diff --git a/spaces/1acneusushi/gradio-2dmoleculeeditor/data/CRACK Luxonix Ravity S 1.4.3.exe Tips and Tricks to Get the Most Out of It.md b/spaces/1acneusushi/gradio-2dmoleculeeditor/data/CRACK Luxonix Ravity S 1.4.3.exe Tips and Tricks to Get the Most Out of It.md
deleted file mode 100644
index 588fa51186e86ad45f665db7268b952dea523a75..0000000000000000000000000000000000000000
--- a/spaces/1acneusushi/gradio-2dmoleculeeditor/data/CRACK Luxonix Ravity S 1.4.3.exe Tips and Tricks to Get the Most Out of It.md
+++ /dev/null
@@ -1,148 +0,0 @@
-
- - Benefits of using it for music production - How to get it legally and avoid cracks | | H2: How to install Luxonix Ravity S 1.4.3.exe on your PC | - System requirements and download link - Step-by-step installation guide - How to register and activate the software | | H2: How to use Luxonix Ravity S 1.4.3.exe to create amazing sounds | - Overview of the user interface and functions - How to load and edit presets - How to use effects and MIDI assign - Tips and tricks for getting the most out of the software | | H2: How to avoid CRACK Luxonix Ravity S 1.4.3.exe and other malware | - Risks and consequences of using cracked software - How to detect and remove malware from your PC - How to support the developers and get updates | | H2: Conclusion and FAQs | - Summary of the main points and call to action - Answers to five common questions about Luxonix Ravity S 1.4.3.exe | **Table 2: Article with HTML formatting** ```html
What is Luxonix Ravity S 1.4.3.exe and why you need it
-
If you are looking for a powerful and versatile VST synthesizer that can create amazing sounds for any genre of music, you should check out Luxonix Ravity S 1.4.3.exe. This software is a virtual PCM sound module that can emulate the hardware PCM synthesizers of the 90s, with only 32MB of wave data.
-
Luxonix Ravity S 1.4.3.exe has many features that make it a great tool for music production, such as:
A convenient user interface with a preset browser, an edit panel, a back panel, and a LCD panel that shows all the parameters.
-
A 4-layer system that allows you to combine up to four different sounds for each preset.
-
A variety of oscillators, filters, amplifiers, LFOs, and arpeggiators that let you shape your sound in many ways.
-
A powerful effecting module that offers 24 types of effects, such as reverb, delay, chorus, flanger, phaser, distortion, and more.
-
A MIDI assign feature that lets you control any parameter with your MIDI controller or keyboard.
-
-
Luxonix Ravity S 1.4.3.exe is a high-quality software that can produce professional sounds for your music projects. However, you should not download or use CRACK Luxonix Ravity S 1.4.3.exe or any other illegal version of the software, as they can harm your PC and violate the rights of the developers.
-
In this article, we will show you how to get Luxonix Ravity S 1.4.3.exe legally and safely, how to install and use it on your PC, and how to avoid CRACK Luxonix Ravity S 1.4.3.exe and other malware.
-
How to install Luxonix Ravity S 1.4.3.exe on your PC
-
To install Luxonix Ravity S 1.4.3.exe on your PC, you need to meet the following system requirements:
-
-
Pentium II 350MHz or higher CPU
-
32MB RAM or higher
-
About 40MB free hard disk space
-
Microsoft Windows 98/ME/2000/XP
-
VST 2.0 compatible host application
-
-
You can download Luxonix Ravity S 1.4.3.exe from the official website of Sonic Cat, which is the new name of Luxonix after they merged with ESI in 2015.
-
To install Luxonix Ravity S 1.4.3.exe on your PC, follow these steps:
-
-
Double-click LUXONIX_ravity(S)_1_1_2_win.exe to execute installation.
-
Click Next button to continue.
-
Set the folder that install Ravity(S). Basically, your VstPlugIns Folder is default.
-
Click Install button to start installing Ravity(S).
-
Installing Ravity(S) is complete.
-
-
When you load Luxonix Ravity S 1.4.3.exe for the first time in your VST host program, you have to register it with your email address and serial number that you received when you purchased it.
-
How to use Luxonix Ravity S 1.4.3.exe to create amazing sounds
-
Luxonix Ravity S 1.4.3.exe has a simple and intuitive user interface that lets you access all its functions easily.
-
The main module consists of four parts:
-
Luxonix Ravity Bundle v1.4.3 Full version download
-Luxonix Ravity S 1.4.3.exe serial key free
-Luxonix Ravity S VST plugin for Windows
-How to install Luxonix Ravity S 1.4.3.exe
-Luxonix Ravity S 1.4.3.exe crack download
-Luxonix Ravity S 1.4.3.exe compatible with Windows 10
-Luxonix Ravity S 1.4.3.exe ASIO driver support
-Luxonix Ravity S 1.4.3.exe 32 bit software
-Luxonix Ravity S 1.4.3.exe standalone application
-Luxonix Ravity S 1.4.3.exe VST2 plugin
-Luxonix Ravity S 1.4.3.exe synthesizer module
-Luxonix Ravity S 1.4.3.exe sound library
-Luxonix Ravity S 1.4.3.exe presets and effects
-Luxonix Ravity S 1.4.3.exe user manual
-Luxonix Ravity S 1.4.3.exe review and rating
-Luxonix Ravity S 1.4.3.exe alternative software
-Luxonix Ravity S 1.4.3.exe vs Luxonix Ravity R
-Luxonix Ravity S 1.4.3.exe vs Sonic Cat
-Luxonix Ravity S 1.4.3.exe forum and support
-Luxonix Ravity S 1.4.3.exe tutorial and tips
-Luxonix Ravity S 1.4.3.exe best price and discount
-Luxonix Ravity S 1.4.3.exe license and activation
-Luxonix Ravity S 1.4.3.exe system requirements and compatibility
-Luxonix Ravity S 1.4.3.exe update and patch
-Luxonix Ravity S 1.4.3.exe error and fix
-Luxonix Ravity S 1.4.3.exe demo and trial version
-Luxonix Ravity S 1.4.3.exe features and benefits
-Luxonix Ravity S 1.4.3.exe comparison and contrast
-Luxonix Ravity S 1.4.3.exe pros and cons
-Luxonix Ravity S 1.4.3.exe testimonials and feedback
-Luxonix Ravity S 1.4.3.exe online course and training
-Luxonix Ravity S 1.4.3.exe video and audio guide
-Luxonix Ravity S 1.4.3.exe blog and article
-Luxonix Ravity S 1.4.3.exe news and announcement
-Luxonix Ravity S 1.4.3.exe FAQ and Q&A
-Luxonix Ravity S 1..43 exe free download full version with crack
-
-
The preset browser, where you can select from over 1000 presets organized by categories.
-
The edit panel, where you can adjust the parameters of each layer of sound.
-
The back panel, where you can set up global functions such as MIDI assign, clipboard functions, hot-keys, etc.
-
The LCD panel, where you can monitor and input the values of each parameter.
-
-
To load a preset, simply click on its name in the preset browser or use the arrow keys on your keyboard.
-
To edit a preset, click on the edit button on the top right corner of the main module or press F5 on your keyboard.
-
You can edit each layer of sound by clicking on its number (1-4) on the left side of the edit panel or pressing F6-F9 on your keyboard.
-
You can adjust the basic settings such as volume, pan, tune, polyphony mode, etc., by using the knobs on the top row of the edit panel.
-
You can modify the sound characteristics by using the tabs below the knobs: OSC (oscillator), FILT (filter), AMP (amplifier), LFO (low frequency oscillator), ARP (arpeggiator).
-
You can add effects by clicking on the LFX button on the bottom right corner of the main module or pressing F10 on your keyboard.
-
You can choose from 24 types of effects by clicking on their names or using the arrow keys on your keyboard.
-
You can adjust the parameters of each effect by using the knobs below their names or clicking on their names and entering values with your keyboard.
-
Tips and tricks for getting the most out of Luxonix Ravity S 1.4.3.exe
-
-
To control any parameter with your MIDI controller or keyboard, simply right-click on it and choose MIDI Assign > Direct Assign or Learn Assign.
-
To copy and paste settings between layers or presets, use the clipboard functions by right-clicking on them or pressing Ctrl+C / Ctrl+V / Ctrl+X / Ctrl+Z / Ctrl+Y on your keyboard.
-
To quickly access the manual or other information about Luxonix Ravity S 1.4.3.exe , click on the question mark button on the top left corner of the main module or press F12 on your keyboard.
-
-
How to avoid CRACK Luxonix Ravity S 1.4.3.exe and other malware
-
CRACK Luxonix Ravity S 1.4.3.exe is an illegal version of Luxonix Ravity S 1.4.3.exe that has been modified by hackers to bypass its registration process and allow anyone to use it without paying for it.
-
However, using CRACK Luxonix Ravity S 1.4.3.exe is not only unethical but also dangerous for your PC and your music projects.
-
Here are some of the risks and consequences of using CRACK Luxonix Ravity S 1.4 Some possible continuations are: - .exe or any other cracked software:
-
-
You may infect your PC with viruses, spyware, ransomware, trojans, worms, or other malware that can damage your system files, steal your personal data, encrypt your files and demand money for their decryption , hijack your browser Continuing the article:
CRACK Luxonix Ravity S 1.4.3.exe or any other cracked software:
-
-
You may infect your PC with viruses, spyware, ransomware, trojans, worms, or other malware that can damage your system files, steal your personal data, encrypt your files and demand money for their decryption , hijack your browser, or display unwanted ads.
-
You may experience poor performance, crashes, errors, or compatibility issues with your PC and other software.
-
You may lose your music projects or corrupt your files due to bugs or glitches in the cracked software.
-
You may face legal actions or penalties for violating the intellectual property rights of the developers and distributors of Luxonix Ravity S 1.4.3.exe.
-
You may miss out on the latest updates, features, bug fixes, and support from the developers of Luxonix Ravity S 1.4.3.exe.
-
-
Therefore, you should avoid CRACK Luxonix Ravity S 1.4.3.exe and other malware at all costs.
-
To detect and remove malware from your PC, you should use a reliable antivirus software that can scan your system regularly and remove any threats.
-
If you have Windows 10 or 11, you can use Windows Security, which is a built-in antivirus tool that can find and remove malware from your PC.
-
To use Windows Security to scan your PC, follow these steps:
-
-
Open your Windows Security settings.
-
Select Virus & threat protection > Scan options.
-
Select Windows Defender Offline scan, and then select Scan now.
-
The Windows Defender Offline scan will take about 15 minutes to run, and then your PC will restart.
-
-
To view the results of your scan, follow these steps:
-
-
Open your Windows Security settings.
-
Select Virus & threat protection > Protection history.
-
The Windows Defender Offline scan will automatically detect and remove or quarantine malware.
-
-
If you have Windows 8.1 or Windows 7, you can use Microsoft Malicious Software Removal Tool, which is a free tool that can scan your PC and remove specific types of malware.
-
To use Microsoft Malicious Software Removal Tool to scan your PC, follow these steps:
-
-
Select the Start icon, type Windows Defender, and then press Enter.
-
Select the History tab.
-
Select All detected items, and then select the View details button.
-
The Microsoft Malicious Software Removal Tool will automatically detect and remove or quarantine malware.
-
-
Conclusion and FAQs
-
Luxonix Ravity S 1.4.3.exe is a great VST synthesizer that can help you create amazing sounds for your music projects. However, you should not use CRACK Luxonix Ravity S 1.4.3.exe or any other illegal version of the software, as they can harm your PC and violate the rights of the developers.
-
Instead, you should get Luxonix Ravity S 1.4.3.exe legally and safely from the official website of Sonic Cat, install it on your PC with the proper registration and activation process, and use it with its full features and functions.
-
You should also protect your PC from malware by using a reliable antivirus software that can scan your system regularly and remove any threats.
-
By doing so, you can enjoy Luxonix Ravity S 1.4.3.exe without any risks or problems, and create professional sounds for your music projects with ease.
-
Here are some FAQs about Luxonix Ravity S 1.4.3.exe that you may find useful:
-
-
Q: How much does Luxonix Ravity S 1.4.3.exe cost?
-
A: Luxonix Ravity S 1.4.3.exe costs $49 USD on the official website of Sonic Cat. You can also get it as part of the Ravity Bundle for $99 USD, which includes Ravity R (a rhythm/drum sound module) and Ravity16 (a host application for Ravity S and R).
-
Q: How many presets does Luxonix Ravity S 1.4.3.exe have?
-
A: Luxonix Ravity S 1.4.3.exe has over 1000 presets organized by categories such as basses, leads, pads, strings, pianos, organs, guitars, drums, etc. You can also create your own presets by editing the parameters of each layer of sound.
-
Q: How many effects does Luxonix Ravity S 1.4.3.exe have?
-
A: Luxonix Ravity S 1.4.3.exe has 24 types of effects that you can apply to each preset or layer of sound. The effects include reverb, delay, chorus, flanger, phaser Continuing the article:
To support the developers of Luxonix Ravity S 1.4.3.exe, you should buy the software from the official website of Sonic Cat, and not download or use any cracked versions.
-
By doing so, you will help them to continue developing and improving Luxonix Ravity S 1.4.3.exe and other software products, and you will also get access to the latest updates, features, bug fixes, and support from them.
-
You can also follow them on their social media channels, such as Facebook, Twitter, and YouTube, and share your feedback, suggestions, and reviews with them and other users.
-
You can also join their online community forum, where you can interact with other Luxonix Ravity S 1.4.3.exe users, ask questions, share tips and tricks, and learn more about the software.
- 0a6ba089eb
-
-
\ No newline at end of file
diff --git a/spaces/1acneusushi/gradio-2dmoleculeeditor/data/Download Adobe Acrobat 8.1 Professional for Free A Complete Guide.md b/spaces/1acneusushi/gradio-2dmoleculeeditor/data/Download Adobe Acrobat 8.1 Professional for Free A Complete Guide.md
deleted file mode 100644
index 920b7c347346b05a43952c9a8bdd598e7b6926a2..0000000000000000000000000000000000000000
--- a/spaces/1acneusushi/gradio-2dmoleculeeditor/data/Download Adobe Acrobat 8.1 Professional for Free A Complete Guide.md
+++ /dev/null
@@ -1,15 +0,0 @@
-
-
How to Download Adobe Acrobat 8.1 Professional for Free
-
If you are looking for a way to download Adobe Acrobat 8.1 Professional for free, you might be disappointed to know that this version of the software is no longer supported by Adobe. However, there are some alternatives that you can try to get the features and functionality of Acrobat 8.1 Pro.
One option is to download the free trial of Adobe Acrobat Pro DC, which is the latest version of the PDF editor and converter. You can use Acrobat Pro DC for 7 days and enjoy all its features, such as editing, converting, signing, commenting, and sharing PDFs. You can also access your files from any device with the free Acrobat Reader app.
-
To download the free trial of Acrobat Pro DC, you can visit this link and click on "Start free trial". You will need to sign in with your Adobe ID or create one if you don't have one. Then, you can follow the instructions to install and activate the software on your computer.
-
Another option is to download Adobe Acrobat Reader DC, which is the free PDF viewer for Windows, Mac OS, and Android. You can use Acrobat Reader DC to view, store, and share PDFs online. You can also fill and sign forms, add annotations, and collect feedback from others. However, you won't be able to edit or convert PDFs with Acrobat Reader DC.
-
-
To download Acrobat Reader DC, you can visit this link and click on "Download Acrobat Reader". You will need to agree to the terms and conditions before downloading the software. Then, you can follow the instructions to install and run the software on your device.
-
A third option is to download a replacement version of Acrobat 8 Pro from this link. This is only possible if you still have your serial number for Acrobat 8 Pro. You will also need to download and install the updates from this link. However, this option is not recommended as Acrobat 8 Pro is outdated and may not work properly on newer operating systems or browsers.
-
These are some of the ways you can download Adobe Acrobat 8.1 Professional for free or get similar features with other versions of the software. We hope this article was helpful and informative for you.
If you want to learn more about Adobe Acrobat and how to use it for your PDF needs, you can visit the official website of Adobe Acrobat. There, you can find more resources, tutorials, tips, and support for the software. You can also join the Adobe Acrobat community and interact with other users and experts who can help you with your questions and issues.
-
Adobe Acrobat is a powerful and versatile tool that can help you create, edit, convert, sign, and share PDFs with ease and efficiency. Whether you need it for personal or professional purposes, you can find a version of Acrobat that suits your needs and budget. You can also try it for free and see how it can improve your PDF workflow.
-
We hope you enjoyed this article and found it useful. If you have any feedback or suggestions, please let us know in the comments below. Thank you for reading!
ddb901b051
-
-
\ No newline at end of file
diff --git a/spaces/1gistliPinn/ChatGPT4/Examples/Download Xforce BEST Keygen BIM 360 Design 2017 64 Bit Patch.md b/spaces/1gistliPinn/ChatGPT4/Examples/Download Xforce BEST Keygen BIM 360 Design 2017 64 Bit Patch.md
deleted file mode 100644
index 8c86cd9f8f5bf56ac1eaca9c93b834c5d2259247..0000000000000000000000000000000000000000
--- a/spaces/1gistliPinn/ChatGPT4/Examples/Download Xforce BEST Keygen BIM 360 Design 2017 64 Bit Patch.md
+++ /dev/null
@@ -1,8 +0,0 @@
-
download xforce keygen BIM 360 Design 2017 64 bit patch
-
-BIM is a high-profile collaboration and design coordination software for AEC teams. The Pro version supports co-editing in Revit, Civil 3D, ... BIM is a tool for automating and managing the BIM design process.
-BIM-technologies (eng. Building Information Modeling) is a building information modeling methodology that allows you to visualize and model the processes occurring in a building, in its individual sections and in rooms, including predicting them.
-BIMx, a collaborative building modeling tool, was officially launched in the UK in 2013. 8a78ff9644
-
-
-
diff --git a/spaces/1pelhydcardo/ChatGPT-prompt-generator/assets/Download Gentility Song MP3 - The Viral TikTok Hit.md b/spaces/1pelhydcardo/ChatGPT-prompt-generator/assets/Download Gentility Song MP3 - The Viral TikTok Hit.md
deleted file mode 100644
index 90becd9df6bbed650eef8a5211a67ab45c12b023..0000000000000000000000000000000000000000
--- a/spaces/1pelhydcardo/ChatGPT-prompt-generator/assets/Download Gentility Song MP3 - The Viral TikTok Hit.md
+++ /dev/null
@@ -1,130 +0,0 @@
-
-
How to Download Gentility MP3 from TikTok
-
If you are a fan of TikTok, you might have heard of the song "Gentility" by Trendybeatz. This song is a remix of a Nigerian folk song that has become viral on the social media platform. Many users have used this song to create funny and creative videos, such as dancing, lip-syncing, or acting out scenes. But what if you want to download gentility mp3 from TikTok and enjoy it offline? In this article, we will show you how to do that, as well as the benefits, drawbacks, and alternatives of doing so.
-
The benefits of downloading gentility mp3 from TikTok
-
Downloading gentility mp3 from TikTok can have several advantages, such as:
You can listen to the song anytime and anywhere, without relying on an internet connection or data plan.
-
You can save storage space on your device, as mp3 files are usually smaller than video files.
-
You can transfer the song to other devices, such as your computer, laptop, or music player.
-
You can edit the song to suit your preferences, such as changing the volume, speed, pitch, or adding effects.
-
You can use the song for other purposes, such as making your own videos, ringtones, or slideshows.
-
-
The best methods to download gentility mp3 from TikTok
-
There are many ways to download gentility mp3 from TikTok, but some are easier and more reliable than others. Here are three of the best methods that we recommend:
-
Method 1: Using a free online TikTok mp3 downloader
-
This is the simplest and fastest way to download gentility mp3 from TikTok. All you need is a web browser and the link of the video that you want to convert. Here are the steps:
-
-
Open the TikTok app and find the video that you want to save as an mp3. Tap on the "Share" icon on the right of the screen and then tap on "Copy link".
-
Go to a free online TikTok mp3 downloader website, such as [ssstik.io](^1^) or [13stream.com](^2^). Paste the link in the input field and click on "Download".
-
Wait for a few seconds until the website processes your request. You will see a "Download MP3" link at the bottom of the page. Click on it and save the file to your device.
-
-
Method 2: Using a browser extension or plugin
-
This is another easy and convenient way to download gentility mp3 from TikTok. All you need is a web browser that supports extensions or plugins, such as Chrome, Firefox, or Safari. Here are the steps:
-
-
Go to the web store of your browser and search for a TikTok mp3 downloader extension or plugin, such as [Tiktok Downloader](https://chrome.google.com/webstore/detail/tiktok-downloader/dfgkibjgjgjgjgj [assistant](#continue)
Method 3: Using a desktop or mobile app
-
This is a more advanced and versatile way to download gentility mp3 from TikTok. All you need is a desktop or mobile app that can download and convert TikTok videos to mp3 files, such as [4K Video Downloader] or [SnapTik]. Here are the steps:
-
-
Download and install the app on your device. Launch the app and grant it the necessary permissions.
-
Open the TikTok app and find the video that you want to save as an mp3. Tap on the "Share" icon on the right of the screen and then tap on "Copy link".
-
Go back to the app and paste the link in the input field. Choose the output format as "MP3" and adjust the quality settings if needed. Click on "Download" and wait for the process to finish.
-
Find the downloaded file in your device's storage and enjoy your gentility mp3.
-
-
The drawbacks of downloading gentility mp3 from TikTok
-
While downloading gentility mp3 from TikTok can be fun and convenient, it also has some disadvantages, such as:
-
-
You might violate the intellectual property rights of the original creator or owner of the song. This could result in legal consequences or penalties, especially if you use the song for commercial purposes or without proper attribution.
-
You might compromise the quality and integrity of the song. Some methods might not preserve the original sound or metadata of the song, such as the artist name, album name, genre, or lyrics.
-
You might expose your device to malware or viruses. Some websites or apps might not be trustworthy or secure, and they might infect your device with harmful software or steal your personal information.
-
-
The alternatives to downloading gentility mp3 from TikTok
-
If you are not comfortable with downloading gentility mp3 from TikTok, or if you want to explore other options, here are some alternatives that you can try:
-
gentility song tiktok remix mp3 download
-gentility tiktok sound download mp3
-gentility tiktok remix trendybeatz mp3
-gentility tiktok song free download
-gentility tiktok audio downloader
-gentility tiktok music converter
-gentility tiktok remix mp3 online
-gentility tiktok song lyrics
-gentility tiktok video downloader
-gentility tiktok remix instrumental
-gentility tiktok song meaning
-gentility tiktok sound origin
-gentility tiktok remix ringtone
-gentility tiktok song artist
-gentility tiktok music video
-gentility tiktok remix challenge
-gentility tiktok sound effect
-gentility tiktok remix dance
-gentility tiktok song genre
-gentility tiktok sound name
-gentility tiktok remix lyrics
-gentility tiktok song release date
-gentility tiktok music download mp4
-gentility tiktok remix bass boosted
-gentility tiktok sound source
-gentility tiktok remix edit
-gentility tiktok song spotify
-gentility tiktok music download 320kbps
-gentility tiktok remix extended
-gentility tiktok sound clip
-gentility tiktok remix mashup
-gentility tiktok song apple music
-gentility tiktok music download pagalworld
-gentility tiktok remix slowed down
-gentility tiktok sound loop
-gentility tiktok remix reaction
-gentility tiktok song youtube
-gentility tiktok music download fakaza
-gentility tiktok remix nightcore
-gentility tiktok sound duration
-gentility tiktok remix karaoke
-gentility tiktok song amazon music
-gentility tiktok music download mr jatt
-gentility tiktok remix clean version
-gentility tiktok sound quality
-gentility tiktok remix cover art
-gentility tiktok song deezer
-gentility tiktok music download djpunjab
-gentility tiktok remix acapella
-
Alternative 1: Streaming gentility mp3 online
-
This is the easiest and safest way to enjoy gentility mp3 without downloading it. All you need is an internet connection and a web browser or a streaming app. Here are some examples:
-
-
You can stream gentility mp3 online from [YouTube], where you can also watch the original video or other remixes.
-
You can stream gentility mp3 online from [Spotify], where you can also create playlists, follow artists, or discover new music.
-
You can stream gentility mp3 online from [SoundCloud], where you can also upload your own tracks, comment on songs, or join communities.
-
-
Alternative 2: Buying or renting gentility mp3 from legal sources
-
This is a more ethical and respectful way to support the original creator or owner of gentility mp3. All you need is some money and a web browser or a digital store app. Here are some examples:
-
-
You can buy or rent gentility mp3 from [Amazon Music], where you can also access millions of songs, podcasts, and audiobooks.
-
You can buy or rent gentility mp3 from [iTunes], where you can also sync your music library across your devices, watch movies, or listen to radio stations.
-
You can buy or rent gentility mp3 from [Google Play Music], where you can also store up to 50,000 songs for free, access YouTube Music Premium, or browse curated playlists.
-
-
Alternative 3: Creating your own gentility mp3 remixes
-
This is a more creative and fun way to express yourself with gentility mp3. All you need is some talent and a web browser or a music production app. Here are some examples:
-
-
You can create your own gentility mp3 remixes online with [Audiotool], where you can also collaborate with other users, share your tracks, or explore genres.
-
You can create your own gentility mp3 remixes online with [Soundation], where you can also learn music production, join contests, or access royalty-free sounds.
-
You can create your own gentility mp3 remixes online with [BandLab], where you can also record vocals, mix songs, or publish albums.
-
-
Conclusion
-
In this article, we have shown you how to download gentility mp3 from TikTok, as well as the benefits, drawbacks, and alternatives of doing so. We hope that you have found this article helpful and informative. If you have any questions or feedback, please feel free to leave a comment below. Thank you for reading and happy listening!
-
FAQs
-
Here are some frequently asked questions about gentility mp3 and TikTok:
-
-
What is gentility mp3?
-
Gentility mp3 is a song by Trendybeatz that is a remix of a Nigerian folk song. It has become popular on TikTok, where many users have used it to create funny and creative videos.
-
How can I download gentility mp3 from TikTok?
-
You can download gentility mp3 from TikTok by using a free online TikTok mp3 downloader, a browser extension or plugin, or a desktop or mobile app. You can also stream, buy, or rent the song from legal sources, or create your own remixes.
-
Is it legal to download gentility mp3 from TikTok?
-
It depends on the laws and regulations of your country and the terms and conditions of TikTok. Generally, it is not legal to download gentility mp3 from TikTok without the permission of the original creator or owner of the song. You might also violate the intellectual property rights of the song and face legal consequences or penalties.
-
Is it safe to download gentility mp3 from TikTok?
-
It depends on the source and method that you use to download gentility mp3 from TikTok. Some websites or apps might not be trustworthy or secure, and they might infect your device with malware or viruses, or steal your personal information. You should always use reputable and reliable sources and methods to download gentility mp3 from TikTok.
-
What can I do with gentility mp3 after downloading it?
-
You can do many things with gentility mp3 after downloading it, such as listening to it offline, transferring it to other devices, editing it to suit your preferences, using it for other purposes, or sharing it with others. However, you should always respect the rights and wishes of the original creator or owner of the song and not use it for illegal or unethical purposes.
- 197e85843d
-
-
\ No newline at end of file
diff --git a/spaces/1phancelerku/anime-remove-background/Download Hataraku Maou-sama S1 The Devil is a Part-Timer! Season 1 Episodes and Subtitles.md b/spaces/1phancelerku/anime-remove-background/Download Hataraku Maou-sama S1 The Devil is a Part-Timer! Season 1 Episodes and Subtitles.md
deleted file mode 100644
index 38fb8417c23f7e9e9afe325016b7e173a35126f1..0000000000000000000000000000000000000000
--- a/spaces/1phancelerku/anime-remove-background/Download Hataraku Maou-sama S1 The Devil is a Part-Timer! Season 1 Episodes and Subtitles.md
+++ /dev/null
@@ -1,127 +0,0 @@
-
-
How to Download Hataraku Maou-sama S1
-
If you are a fan of comedy and fantasy anime, you might have heard of Hataraku Maou-sama, also known as The Devil is a Part-Timer!. This anime is about a demon lord who ends up in modern-day Tokyo and has to work at a fast-food restaurant to survive. Along with his loyal general and some other quirky characters, he faces hilarious situations and challenges in his daily life.
-
Hataraku Maou-sama S1 is a 13-episode anime series that aired in 2013 and received positive reviews from critics and viewers alike. It is based on a light novel series by Satoshi Wagahara and illustrated by Oniku. The anime has a unique premise, witty humor, charming characters, and an engaging plot.
If you are interested in watching or rewatching this anime, you might be wondering how to download it for offline viewing. In this article, we will show you how to download Hataraku Maou-sama S1 from different sources and what are the advantages and disadvantages of each option.
-
What is Hataraku Maou-sama?
-
Hataraku Maou-sama is an anime series that follows the adventures of Sadao Maou, the demon lord of Ente Isla, a fantasy world where he was about to conquer it with his vast army. However, he was defeated by Emilia, the hero of Ente Isla, and forced to flee through a dimensional portal that brought him to Earth.
-
On Earth, Maou finds himself in Tokyo with no magic or power. He assumes a human identity and starts working at MgRonald's, a local fast-food chain. He also lives with his loyal general Alsiel, who takes care of their household chores and finances. Meanwhile, Emilia follows Maou to Earth and also adopts a human identity as Emi Yusa, a customer service representative.
-
* download hataraku maou sama season 1 english sub
-* hataraku maou sama s1 episode 1 free download
-* where to download the devil is a part timer s1
-* hataraku maou sama s1 1080p download
-* download hataraku maou sama s1 batch
-* hataraku maou sama s1 english dub download
-* how to download hataraku maou sama s1 on crunchyroll
-* hataraku maou sama s1 bluray download
-* download hataraku maou sama s1 sub indo
-* hataraku maou sama s1 direct download link
-* download hataraku maou sama s1 mp4
-* hataraku maou sama s1 ost download
-* download hataraku maou sama s1 full episodes
-* hataraku maou sama s1 opening song download
-* download hataraku maou sama s1 anime
-* hataraku maou sama s1 torrent download
-* download hataraku maou sama s1 light novel
-* hataraku maou sama s1 ending song download
-* download hataraku maou sama s1 online
-* hataraku maou sama s1 manga download
-* download hataraku maou sama season 1 english dub
-* hataraku maou sama season 1 episode 2 free download
-* where to watch the devil is a part timer season 1 online
-* the devil is a part timer season 1 720p download
-* the devil is a part timer season 1 batch download
-* the devil is a part timer season 1 english subbed download
-* how to watch the devil is a part timer season 1 on funimation
-* the devil is a part timer season 1 dvd download
-* the devil is a part timer season 1 sub indo download
-* the devil is a part timer season 1 google drive download link
-* the devil is a part timer season 1 mkv download
-* the devil is a part timer season 1 soundtrack download
-* the devil is a part timer season 1 all episodes download
-* the devil is a part timer season 1 theme song download
-* the devil is a part timer season 1 anime download
-* the devil is a part timer season 1 magnet link download
-* the devil is a part timer season 1 novel download
-* the devil is a part timer season 1 ending theme download
-* the devil is a part timer season 1 streaming download
-* the devil is a part timer season 1 comic download
-
As Maou tries to adapt to his new life and find a way to restore his magic and return to Ente Isla, he encounters various obstacles and enemies from both worlds. He also develops friendships and relationships with his co-workers, neighbors, and even Emilia herself.
-
Hataraku Maou-sama is a comedy-fantasy anime that blends elements of action, romance, drama, and parody. It has a colorful animation style, catchy music, and excellent voice acting. It is suitable for viewers who enjoy l
laugh-out-loud comedy and fantasy scenarios.
-
Where to Watch Hataraku Maou-sama Online?
-
If you want to watch Hataraku Maou-sama online, you have several options to choose from. However, not all of them are legal, safe, or reliable. In this section, we will compare some of the most popular anime streaming platforms that have Hataraku Maou-sama in their catalog and see which one is the best for you.
-
Crunchyroll
-
Crunchyroll is one of the most popular and reputable anime streaming sites in the world. It has a huge library of anime titles, including Hataraku Maou-sama, that you can watch in high definition and with English subtitles or dubbing. You can also access exclusive content, such as manga, games, and merchandise.
-
To watch Hataraku Maou-sama on Crunchyroll, you need to create an account and subscribe to a premium plan that costs $7.99 per month or $79.99 per year. Alternatively, you can sign up for a 14-day free trial and enjoy unlimited access to all the features and content.
-
The advantage of watching Hataraku Maou-sama on Crunchyroll is that you can support the official release and the creators of the anime. You can also watch it on multiple devices, such as your computer, smartphone, tablet, or smart TV. You can also download episodes for offline viewing if you have a premium account.
-
You can watch Hataraku Maou-sama on Crunchyroll by clicking here.
-
Other Anime Streaming Sites
-
There are also other anime streaming sites that offer Hataraku Maou-sama for free. However, these sites are not authorized by the licensors or distributors of the anime and may violate their copyrights. They may also contain malware, viruses, pop-up ads, or other annoying or harmful elements.
-
If you decide to watch Hataraku Maou-sama on these sites, you should be careful and use a VPN and antivirus software to protect your device and identity. You should also avoid clicking on any suspicious links or downloading any files from these sites.
-
Some examples of these sites are:
-
-
VIZ: This site has both subbed and dubbed versions of Hataraku Maou-sama. However, it is only available in certain regions and may require a VPN to access it.
-
AnimeFreak: This site has subbed versions of Hataraku Maou-sama. However, it has low video quality, frequent ads, and slow loading speed.
-
AnimeUltima: This site has subbed versions of Hataraku Maou-sama. However, it has limited server options, broken links, and buffering issues.
-
How to Download Hataraku Maou-sama S1?
-
Now that you know where to watch Hataraku Maou-sama online, you might be wondering how to download it for offline viewing. Downloading anime episodes can be useful if you want to watch them without an internet connection, save your data usage, or share them with your friends.
-
However, downloading anime episodes is not always easy or legal. Depending on the source you choose, you may need to use different tools or methods to download them. You may also face some risks or challenges, such as low quality, slow speed, or legal issues.
-
In this section, we will show you how to download Hataraku Maou-sama S1 from Crunchyroll and from other anime streaming sites. We will also explain the pros and cons of each option and give you some tips and warnings to help you download safely and efficiently.
-
Download from Crunchyroll
-
The easiest and safest way to download Hataraku Maou-sama S1 is from Crunchyroll. As we mentioned before, Crunchyroll is a legal and reliable anime streaming platform that offers high-quality videos and subtitles. If you have a premium account or a free trial, you can download episodes from Crunchyroll for offline viewing.
-
To download episodes from Crunchyroll, you need to follow these steps:
-
-
Open the Crunchyroll app on your device. You can download the app for free from the App Store or Google Play Store.
-
Log in with your premium account or sign up for a free trial.
-
Search for Hataraku Maou-sama S1 in the app and select the episode you want to download.
-
Tap on the download icon at the bottom of the screen. You can choose the video quality and subtitle language before downloading.
-
Wait for the download to finish. You can check the progress in the downloads section of the app.
-
Enjoy watching Hataraku Maou-sama S1 offline. You can access your downloaded episodes in the downloads section of the app.
-
-
Here are some screenshots or images to illustrate the steps:
-
-
-
-
The advantage of downloading from Crunchyroll is that you can enjoy high-quality videos and subtitles without any ads or interruptions. You can also watch them on any device that supports the Crunchyroll app. You can also support the official release and the creators of the anime by paying for a subscription.
-
The disadvantage of downloading from Crunchyroll is that you need to pay for a premium account or use a free trial that expires after 14 days. You also need to have enough storage space on your device to store the downloaded episodes. You also need to have an internet connection to start the download process.
Download from Other Anime Streaming Sites
-
If you don't want to pay for a premium account or use a free trial, you can also download Hataraku Maou-sama S1 from other anime streaming sites. However, as we warned you before, these sites are not legal, safe, or reliable. They may contain malware, viruses, pop-up ads, or other annoying or harmful elements.
-
If you decide to download from these sites, you should be careful and use a VPN and antivirus software to protect your device and identity. You should also avoid clicking on any suspicious links or downloading any files from these sites.
-
There are different tools or methods to download from these sites, such as video downloader extensions, online converters, screen recorders, etc. However, they may not work for all sites or videos. They may also have some limitations or drawbacks, such as low quality, slow speed, watermarks, etc.
-
Here are some examples of tools or methods to download from these sites:
-
-
Video downloader extensions: These are browser add-ons that allow you to download videos from various websites. Some examples are Video DownloadHelper, Video Downloader Professional, etc. To use them, you need to install them on your browser and then visit the site that has the video you want to download. You will see a download icon on the toolbar or on the video player. Click on it and choose the format and quality you want. Then wait for the download to finish.
-
Online converters: These are websites that allow you to convert and download videos from various websites. Some examples are OnlineVideoConverter, SaveFrom.net, etc. To use them, you need to copy the URL of the video you want to download and paste it on the website. Then choose the format and quality you want and click on the download button. Then wait for the conversion and download to finish.
-
Screen recorders: These are software or apps that allow you to record your screen and save it as a video file. Some examples are OBS Studio, Camtasia, etc. To use them, you need to install them on your device and then open the site that has the video you want to download. Then start the screen recorder and adjust the settings and area you want to capture. Then play the video and record it. Then stop the recording and save it as a video file.
-
-
Here are some screenshots or images to illustrate the tools or methods:
-
-
-
-
The advantage of downloading from other anime streaming sites is that you can do it for free without any subscription or trial. You can also choose from different sites and sources that have Hataraku Maou-sama S1.
-
The disadvantage of downloading from other anime streaming sites is that you may face some risks or challenges, such as malware, viruses, legal issues, etc. You may also get low-quality videos or subtitles that are not synchronized or accurate. You may also have to deal with ads or interruptions during the download process.
-
Conclusion
-
Hataraku Maou-sama S1 is a comedy-fantasy anime that is worth watching or rewatching if you enjoy hilarious and heartwarming stories with a twist. It has a unique premise, witty humor, charming characters, and an engaging plot.
-
If you want to download Hataraku Maou-sama S1 for offline viewing, you have several options to choose from. However, not all of them are legal, safe, or reliable. The best option is to download it from Crunchyroll using a premium account or a free trial. This way, you can enjoy high-quality videos and subtitles without any ads or interruptions. You can also support the official release and the creators of the anime by paying for a subscription.
-
If you don't want to pay for a premium account or use a free trial, you can also download it from other anime streaming sites using different tools or methods. However, you should be careful and use a VPN and antivirus software to protect your device and identity. You should also avoid clicking on any suspicious links or downloading any files from these sites. You may also face some risks or challenges, such as malware, viruses, legal issues, etc. You may also get low-quality videos or subtitles that are not synchronized or accurate. You may also have to deal with ads or interruptions during the download process.
-
We hope this article has helped you learn how to download Hataraku Maou-sama S1 from different sources and what are the advantages and disadvantages of each option. We also hope you enjoy watching this anime and have a good time.
-
FAQs
-
-
Q: Is there a season 2 of Hataraku Maou-sama?
-
A: Unfortunately, there is no official confirmation or announcement of a season 2 of Hataraku Maou-sama as of now. However, there are rumors and speculations that a season 2 might be in the works or planned for the future. The anime is based on a light novel series that has 21 volumes and is still ongoing. The anime only adapted the first two volumes, so there is plenty of material for a season 2. The anime also has a loyal fan base and a high demand for a sequel. Therefore, there is still hope that a season 2 might happen someday.
-
Q: Who is the main character of Hataraku Maou-sama?
-
A: The main character of Hataraku Maou-sama is Sadao Maou, the demon lord of Ente Isla who ends up in modern-day Tokyo and works at a fast-food restaurant. He is voiced by Ryota Ohsaka in Japanese and Josh Grelle in English. He is a charismatic, intelligent, and ambitious leader who wants to conquer the world. However, he also has a kind, generous, and hardworking side that he shows on Earth. He develops feelings for Emilia, the hero who defeated him in Ente Isla.
-
Q: What is the genre of Hataraku Maou-sama?
-
A: Hataraku Maou-sama is a comedy-fantasy anime that blends elements of action, romance, drama, and parody. It has a unique premise, witty humor, charming characters, and an engaging plot. It is suitable for viewers who enjoy laugh-out-loud comedy and fantasy scenarios.
-
Q: How many episodes are there in Hataraku Maou-sama S1?
-
A: Hataraku Maou-sama S1 has 13 episodes that aired from April to June 2013. Each episode has a duration of about 24 minutes. There is also an OVA episode that was released in December 2013 as a bonus for the DVD and Blu-ray release. The OVA episode has a duration of about 27 minutes.
-
Q: Where can I read the light novel series of Hataraku Maou-sama?
-
A: The light novel series of Hataraku Maou-sama is written by Satoshi Wagahara and illustrated by Oniku. It has 21 volumes and is still ongoing as of now. You can read the light novel series online or buy the physical copies from various sources. Some examples are:
-
-
Yen Press: This is the official English publisher of the light novel series. You can buy the digital or print versions from their website or other online retailers.
-
Baka-Tsuki: This is a fan translation website that has translated some of the light novel volumes into English and other languages. You can read them online for free.
-
Novel Updates: This is a directory website that lists various sources and links to read the light novel series online.
-
-
197e85843d
-
-
\ No newline at end of file
diff --git a/spaces/1phancelerku/anime-remove-background/Dynamons World Game Mod APK The Best Role Playing Game of 2023.md b/spaces/1phancelerku/anime-remove-background/Dynamons World Game Mod APK The Best Role Playing Game of 2023.md
deleted file mode 100644
index 541ad4d20f7271681b1562826eb73c193e3bdabd..0000000000000000000000000000000000000000
--- a/spaces/1phancelerku/anime-remove-background/Dynamons World Game Mod APK The Best Role Playing Game of 2023.md
+++ /dev/null
@@ -1,128 +0,0 @@
-
-
Dynamons World Game Mod Apk: A Review
-
If you are a fan of RPG games, you might have heard of Dynamons World, a popular game that lets you catch and train dozens of unique monsters and battle them in online multiplayer matches. But did you know that there is a modded version of this game that gives you unlimited money and other advantages? In this article, we will review Dynamons World Game Mod Apk, a modified version of the original game that enhances your gaming experience. We will also show you how to download and install it on your device, and share some tips and tricks for playing it.
-
What is Dynamons World?
-
Dynamons World is a role-playing game developed by Azerion Casual, a company that specializes in casual games for web and mobile platforms. The game was released in 2017 and has been downloaded over 10 million times on Google Play Store. It is also available on App Store and BlueStacks, an emulator that allows you to play Android games on PC.
An exciting campaign with multiple challenges and a cool storyline
-
Online matches with other players
-
Dozens of unique Dynamons with varied powers and abilities
-
Useful items and boosters to enhance your battles
-
A tactical turn-based battle system with strategic elements
-
Multiple areas on the maps to explore
-
Tons of new updates with interesting content
-
Free to play on web browser, Android, and iOS platforms
-
-
Features of Dynamons World
-
Some of the features that make Dynamons World stand out are:
-
-
Feature
Description
-
Online Battle Arena
You can battle your friends and players worldwide in online PvP multiplayer battles. You can also join tournaments and leagues to compete for prizes and glory.
-
Catch and train Dynamons
You can catch and train dozens of unique Dynamons, each with their own strengths and weaknesses. You can also evolve them into more powerful forms and customize them with skill cards.
-
Unleash powerful skills
You can unleash powerful skills and brilliant tactics to defeat even the strongest rivals in Klaude's kingdom. You can also use items and boosters to gain an edge in battle.
-
Travel across the world
You can travel all the way from Dynamons Camp to the Temple Ruins in an addictive and immersive RPG story game. You can also explore different areas on the maps, such as forests, deserts, caves, and more.
-
New updates
Dynamons World is being updated all the time with even more new Dynamons, quests, battles, and more. You can also expect new features, such as new online PvP battle arena, new maps, new Dynamon types, new skill cards, new rare dragon Dynamons, and more.
-
Free to play
Dynamons World is free to play on web browser, Android, and iOS platforms. You can also play it offline without internet connection. However, some in-game items may require real money purchases.
-
-
What is Dynamons World Mod Apk?
-
Dynamons World Mod Apk is a modified version of the original game that gives you unlimited money and other benefits. With this mod apk, you can enjoy the game without any limitations or restrictions.
A modified version of the original game
-
Dynamons World Mod Apk is a modified version of the original game that comes with exciting features and benefits. You can enjoy unlimited resources, including coins, gems, and energy, to help you progress faster and unlock more Dynamons. The modded version also offers access to exclusive Dynamons that are not available in the original game.
-
dynamons world mod apk unlimited money and crystals
-dynamons world mod apk latest version download
-dynamons world mod apk android 1
-dynamons world mod apk revdl
-dynamons world mod apk hack
-dynamons world mod apk offline
-dynamons world mod apk free shopping
-dynamons world mod apk no ads
-dynamons world mod apk unlimited everything
-dynamons world mod apk 1.8.07
-dynamons world game download for android
-dynamons world game online play
-dynamons world game cheats
-dynamons world game tips and tricks
-dynamons world game guide
-dynamons world game review
-dynamons world game walkthrough
-dynamons world game best team
-dynamons world game evolution
-dynamons world game codes
-how to install dynamons world mod apk
-how to play dynamons world mod apk
-how to update dynamons world mod apk
-how to get unlimited money in dynamons world mod apk
-how to get unlimited crystals in dynamons world mod apk
-how to level up fast in dynamons world mod apk
-how to unlock all dynamons in dynamons world mod apk
-how to hack dynamons world mod apk
-how to get free shopping in dynamons world mod apk
-how to remove ads in dynamons world mod apk
-azerion casual games mod apk
-azerion casual games hack
-azerion casual games cheats
-azerion casual games download
-azerion casual games online play
-azerion casual games review
-azerion casual games tips and tricks
-azerion casual games guide
-azerion casual games walkthrough
-azerion casual games best games
-role playing games mod apk download
-role playing games mod apk offline
-role playing games mod apk unlimited money and gems
-role playing games mod apk android 1
-role playing games mod apk revdl
-role playing games hack online
-role playing games cheats codes
-role playing games tips and tricks
-role playing games guide
-role playing games best games
-
Benefits of Dynamons World Mod Apk
-
Some of the benefits that you can get from Dynamons World Mod Apk are:
-
-
Unlimited money: You can get unlimited money to buy items, boosters, and skill cards. You can also upgrade your Dynamons and evolve them without any cost.
-
Unlocked content: You can access all the content in the game, such as maps, quests, battles, and Dynamons. You can also catch any Dynamon you want without any difficulty.
-
Removed ads: You can play the game without any annoying ads that interrupt your gameplay. You can also save your data and battery life.
-
Enhanced graphics: You can enjoy the game with enhanced graphics and sound quality. You can also adjust the settings to suit your device's performance.
-
No root required: You can install and play the game without rooting your device. You can also update the game without any problem.
-
-
How to download and install Dynamons World Mod Apk?
-
If you want to download and install Dynamons World Mod Apk on your device, you need to follow these steps:
-
Steps to download and install
-
-
Click on this link to download the Dynamons World Mod Apk file on your device.
-
Go to your device's settings and enable the installation of unknown sources. This will allow you to install apps from sources other than Google Play Store.
-
Locate the downloaded file in your file manager and tap on it to start the installation process.
-
Follow the instructions on the screen and wait for the installation to complete.
-
Launch the game and enjoy playing Dynamons World Mod Apk with unlimited money and other benefits.
-
-
Tips and tricks for playing Dynamons World Mod Apk
-
Here are some tips and tricks that can help you play Dynamons World Mod Apk better:
-
-
Choose your starter Dynamon wisely. Each Dynamon has a different type, such as fire, water, plant, electric, etc. Each type has its own strengths and weaknesses against other types. For example, fire is strong against plant but weak against water. You can check the type chart in the game to see which type is effective or ineffective against another type.
-
Catch and train as many Dynamons as you can. You can catch Dynamons by using capture balls that you can buy or find in the game. You can also train your Dynamons by battling other Dynamons or using skill cards that you can buy or find in the game. Training your Dynamons will increase their level, stats, and skills.
-
Use items and boosters wisely. Items and boosters are useful tools that can help you in battles. Items can heal your Dynamons, revive them, or cure them from status effects. Boosters can increase your Dynamons' attack, defense, speed, or accuracy. However, items and boosters are limited in number, so use them only when necessary.
-
Plan your strategy carefully. Battles in Dynamons World are turn-based, which means you have to choose your actions wisely. You have to consider your Dynamons' type, skills, stats, and status effects when choosing which Dynamon to use and which skill to unleash. You also have to anticipate your opponent's moves and counter them accordingly.
-
Have fun and explore the world. Dynamons World is a game that offers a lot of fun and adventure. You can explore different areas on the maps, such as forests, deserts, caves, and more. You can also meet new characters, complete quests, join tournaments, and discover secrets along the way.
-
-
Conclusion
-
Dynamons World is a fun and addictive RPG game that lets you catch and train dozens of unique monsters and battle them in online multiplayer matches. However, if you want to enjoy the game without any limitations or restrictions, you should try Dynamons World Mod Apk, a modified version of the original game that gives you unlimited money and other benefits. With this mod apk, you can access all the content in the game, catch any Dynamon you want, use items and boosters freely, play without ads, and more. All you have to do is download and install it on your device, and follow the steps and tips we have provided in this article. We hope you have fun playing Dynamons World Mod Apk and become the best Dynamon master in the world.
-
FAQs
-
Here are some frequently asked questions about Dynamons World Mod Apk:
-
-
Is Dynamons World Mod Apk safe to use?
-
Yes, Dynamons World Mod Apk is safe to use as long as you download it from a trusted source, such as the link we have provided in this article. However, you should always be careful when downloading and installing any modded apps, as they may contain viruses or malware that can harm your device. You should also backup your data before installing any modded apps, as they may overwrite or delete your original game data.
-
Is Dynamons World Mod Apk legal to use?
-
Dynamons World Mod Apk is not legal to use, as it violates the terms and conditions of the original game. By using this mod apk, you are breaking the rules of the game and risking your account being banned or suspended. You are also depriving the developers of their rightful income from the game. Therefore, we do not encourage or endorse the use of Dynamons World Mod Apk, and we are not responsible for any consequences that may arise from using it.
-
Can I play Dynamons World Mod Apk online?
-
Yes, you can play Dynamons World Mod Apk online with other players. However, you may face some issues or errors when playing online, as the modded version may not be compatible with the latest version of the original game. You may also encounter players who are using the original game or other modded versions, which may cause unfairness or imbalance in the gameplay. Therefore, we recommend playing Dynamons World Mod Apk offline or with your friends who are using the same modded version.
-
Can I update Dynamons World Mod Apk?
-
No, you cannot update Dynamons World Mod Apk, as it is a modified version of the original game. If you try to update it from Google Play Store or App Store, you will lose all the benefits and features of the modded version. You will also lose all your progress and data in the modded version. Therefore, you should avoid updating Dynamons World Mod Apk, and stick to the version that you have downloaded and installed.
-
Can I uninstall Dynamons World Mod Apk?
-
Yes, you can uninstall Dynamons World Mod Apk anytime you want. You can simply go to your device's settings and uninstall it like any other app. However, you should note that uninstalling Dynamons World Mod Apk will delete all your progress and data in the modded version. You will also lose all the benefits and features of the modded version. Therefore, you should backup your data before uninstalling Dynamons World Mod Apk, or keep a copy of the original game on your device.
- 401be4b1e0
-
-
\ No newline at end of file
diff --git a/spaces/AIatUIUC/CodeLATS/generators/generator_types.py b/spaces/AIatUIUC/CodeLATS/generators/generator_types.py
deleted file mode 100644
index 83ab027484b8205a9bde227dab4d9a40d903950f..0000000000000000000000000000000000000000
--- a/spaces/AIatUIUC/CodeLATS/generators/generator_types.py
+++ /dev/null
@@ -1,33 +0,0 @@
-from typing import List, Optional, Union
-from abc import abstractmethod, ABC
-
-from generators.model import ModelBase
-
-
-class Generator:
- @abstractmethod
- def self_reflection(self, func: str, feedback: str, model: ModelBase) -> str:
- ...
-
- @abstractmethod
- def func_impl(
- self,
- func_sig: str,
- model: ModelBase,
- strategy: str,
- prev_func_impl: Optional[str] = None,
- feedback: Optional[str] = None,
- self_reflection: Optional[str] = None,
- num_comps: int = 1,
- temperature: float = 0.0,
- ) -> Union[str, List[str]]:
- ...
-
- @abstractmethod
- def internal_tests(
- self,
- func_sig: str,
- model: ModelBase,
- max_num_tests: int = 5
- ) -> List[str]:
- ...
diff --git a/spaces/AbandonedMuse/UnlimitedMusicGen/audiocraft/data/audio.py b/spaces/AbandonedMuse/UnlimitedMusicGen/audiocraft/data/audio.py
deleted file mode 100644
index 05fa53ae8ad1b40ab8b9c5dd134227a2a58c55fe..0000000000000000000000000000000000000000
--- a/spaces/AbandonedMuse/UnlimitedMusicGen/audiocraft/data/audio.py
+++ /dev/null
@@ -1,217 +0,0 @@
-# Copyright (c) Meta Platforms, Inc. and affiliates.
-# All rights reserved.
-#
-# This source code is licensed under the license found in the
-# LICENSE file in the root directory of this source tree.
-
-"""
-Audio IO methods are defined in this module (info, read, write),
-We rely on av library for faster read when possible, otherwise on torchaudio.
-"""
-
-from dataclasses import dataclass
-from pathlib import Path
-import logging
-import typing as tp
-
-import numpy as np
-import soundfile
-import torch
-from torch.nn import functional as F
-import torchaudio as ta
-
-import av
-
-from .audio_utils import f32_pcm, i16_pcm, normalize_audio, convert_audio
-
-
-_av_initialized = False
-
-
-def _init_av():
- global _av_initialized
- if _av_initialized:
- return
- logger = logging.getLogger('libav.mp3')
- logger.setLevel(logging.ERROR)
- _av_initialized = True
-
-
-@dataclass(frozen=True)
-class AudioFileInfo:
- sample_rate: int
- duration: float
- channels: int
-
-
-def _av_info(filepath: tp.Union[str, Path]) -> AudioFileInfo:
- _init_av()
- with av.open(str(filepath)) as af:
- stream = af.streams.audio[0]
- sample_rate = stream.codec_context.sample_rate
- duration = float(stream.duration * stream.time_base)
- channels = stream.channels
- return AudioFileInfo(sample_rate, duration, channels)
-
-
-def _soundfile_info(filepath: tp.Union[str, Path]) -> AudioFileInfo:
- info = soundfile.info(filepath)
- return AudioFileInfo(info.samplerate, info.duration, info.channels)
-
-
-def audio_info(filepath: tp.Union[str, Path]) -> AudioFileInfo:
- # torchaudio no longer returns useful duration informations for some formats like mp3s.
- filepath = Path(filepath)
- if filepath.suffix in ['.flac', '.ogg']: # TODO: Validate .ogg can be safely read with av_info
- # ffmpeg has some weird issue with flac.
- return _soundfile_info(filepath)
- else:
- return _av_info(filepath)
-
-
-def _av_read(filepath: tp.Union[str, Path], seek_time: float = 0, duration: float = -1.) -> tp.Tuple[torch.Tensor, int]:
- """FFMPEG-based audio file reading using PyAV bindings.
- Soundfile cannot read mp3 and av_read is more efficient than torchaudio.
-
- Args:
- filepath (str or Path): Path to audio file to read.
- seek_time (float): Time at which to start reading in the file.
- duration (float): Duration to read from the file. If set to -1, the whole file is read.
- Returns:
- Tuple[torch.Tensor, int]: Tuple containing audio data and sample rate
- """
- _init_av()
- with av.open(str(filepath)) as af:
- stream = af.streams.audio[0]
- sr = stream.codec_context.sample_rate
- num_frames = int(sr * duration) if duration >= 0 else -1
- frame_offset = int(sr * seek_time)
- # we need a small negative offset otherwise we get some edge artifact
- # from the mp3 decoder.
- af.seek(int(max(0, (seek_time - 0.1)) / stream.time_base), stream=stream)
- frames = []
- length = 0
- for frame in af.decode(streams=stream.index):
- current_offset = int(frame.rate * frame.pts * frame.time_base)
- strip = max(0, frame_offset - current_offset)
- buf = torch.from_numpy(frame.to_ndarray())
- if buf.shape[0] != stream.channels:
- buf = buf.view(-1, stream.channels).t()
- buf = buf[:, strip:]
- frames.append(buf)
- length += buf.shape[1]
- if num_frames > 0 and length >= num_frames:
- break
- assert frames
- # If the above assert fails, it is likely because we seeked past the end of file point,
- # in which case ffmpeg returns a single frame with only zeros, and a weird timestamp.
- # This will need proper debugging, in due time.
- wav = torch.cat(frames, dim=1)
- assert wav.shape[0] == stream.channels
- if num_frames > 0:
- wav = wav[:, :num_frames]
- return f32_pcm(wav), sr
-
-
-def audio_read(filepath: tp.Union[str, Path], seek_time: float = 0.,
- duration: float = -1., pad: bool = False) -> tp.Tuple[torch.Tensor, int]:
- """Read audio by picking the most appropriate backend tool based on the audio format.
-
- Args:
- filepath (str or Path): Path to audio file to read.
- seek_time (float): Time at which to start reading in the file.
- duration (float): Duration to read from the file. If set to -1, the whole file is read.
- pad (bool): Pad output audio if not reaching expected duration.
- Returns:
- Tuple[torch.Tensor, int]: Tuple containing audio data and sample rate.
- """
- fp = Path(filepath)
- if fp.suffix in ['.flac', '.ogg']: # TODO: check if we can safely use av_read for .ogg
- # There is some bug with ffmpeg and reading flac
- info = _soundfile_info(filepath)
- frames = -1 if duration <= 0 else int(duration * info.sample_rate)
- frame_offset = int(seek_time * info.sample_rate)
- wav, sr = soundfile.read(filepath, start=frame_offset, frames=frames, dtype=np.float32)
- assert info.sample_rate == sr, f"Mismatch of sample rates {info.sample_rate} {sr}"
- wav = torch.from_numpy(wav).t().contiguous()
- if len(wav.shape) == 1:
- wav = torch.unsqueeze(wav, 0)
- elif (
- fp.suffix in ['.wav', '.mp3'] and fp.suffix[1:] in ta.utils.sox_utils.list_read_formats()
- and duration <= 0 and seek_time == 0
- ):
- # Torchaudio is faster if we load an entire file at once.
- wav, sr = ta.load(fp)
- else:
- wav, sr = _av_read(filepath, seek_time, duration)
- if pad and duration > 0:
- expected_frames = int(duration * sr)
- wav = F.pad(wav, (0, expected_frames - wav.shape[-1]))
- return wav, sr
-
-
-def audio_write(stem_name: tp.Union[str, Path],
- wav: torch.Tensor, sample_rate: int,
- format: str = 'wav', mp3_rate: int = 320, normalize: bool = True,
- strategy: str = 'peak', peak_clip_headroom_db: float = 1,
- rms_headroom_db: float = 18, loudness_headroom_db: float = 14,
- loudness_compressor: bool = False,
- log_clipping: bool = True, make_parent_dir: bool = True,
- add_suffix: bool = True, channels:int = 1) -> Path:
- """Convenience function for saving audio to disk. Returns the filename the audio was written to.
-
- Args:
- stem_name (str or Path): Filename without extension which will be added automatically.
- format (str): Either "wav" or "mp3".
- mp3_rate (int): kbps when using mp3s.
- normalize (bool): if `True` (default), normalizes according to the prescribed
- strategy (see after). If `False`, the strategy is only used in case clipping
- would happen.
- strategy (str): Can be either 'clip', 'peak', or 'rms'. Default is 'peak',
- i.e. audio is normalized by its largest value. RMS normalizes by root-mean-square
- with extra headroom to avoid clipping. 'clip' just clips.
- peak_clip_headroom_db (float): Headroom in dB when doing 'peak' or 'clip' strategy.
- rms_headroom_db (float): Headroom in dB when doing 'rms' strategy. This must be much larger
- than the `peak_clip` one to avoid further clipping.
- loudness_headroom_db (float): Target loudness for loudness normalization.
- loudness_compressor (bool): Uses tanh for soft clipping when strategy is 'loudness'.
- when strategy is 'loudness'log_clipping (bool): If True, basic logging on stderr when clipping still
- occurs despite strategy (only for 'rms').
- make_parent_dir (bool): Make parent directory if it doesn't exist.
- Returns:
- Path: Path of the saved audio.
- """
- assert wav.dtype.is_floating_point, "wav is not floating point"
- if wav.dim() == 1:
- wav = wav[None]
- elif wav.dim() > 2:
- raise ValueError("Input wav should be at most 2 dimension.")
- assert wav.isfinite().all()
- wav = normalize_audio(wav, normalize, strategy, peak_clip_headroom_db,
- rms_headroom_db, loudness_headroom_db, log_clipping=log_clipping,
- sample_rate=sample_rate, stem_name=str(stem_name))
- if channels > 1:
- wav = convert_audio(wav,sample_rate, sample_rate, channels)
- kwargs: dict = {}
- if format == 'mp3':
- suffix = '.mp3'
- kwargs.update({"compression": mp3_rate})
- elif format == 'wav':
- wav = i16_pcm(wav)
- suffix = '.wav'
- kwargs.update({"encoding": "PCM_S", "bits_per_sample": 16})
- else:
- raise RuntimeError(f"Invalid format {format}. Only wav or mp3 are supported.")
- if not add_suffix:
- suffix = ''
- path = Path(str(stem_name) + suffix)
- if make_parent_dir:
- path.parent.mkdir(exist_ok=True, parents=True)
- try:
- ta.save(path, wav, sample_rate, **kwargs)
- except Exception:
- if path.exists():
- # we do not want to leave half written files around.
- path.unlink()
- raise
- return path
diff --git a/spaces/AchyuthGamer/OpenGPT-Chat-UI/src/lib/shareConversation.ts b/spaces/AchyuthGamer/OpenGPT-Chat-UI/src/lib/shareConversation.ts
deleted file mode 100644
index 4768b604a42258d5d97231dd0e44f9198ef1864c..0000000000000000000000000000000000000000
--- a/spaces/AchyuthGamer/OpenGPT-Chat-UI/src/lib/shareConversation.ts
+++ /dev/null
@@ -1,27 +0,0 @@
-import { base } from "$app/paths";
-import { ERROR_MESSAGES, error } from "$lib/stores/errors";
-import { share } from "./utils/share";
-
-export async function shareConversation(id: string, title: string) {
- try {
- const res = await fetch(`${base}/conversation/${id}/share`, {
- method: "POST",
- headers: {
- "Content-Type": "application/json",
- },
- });
-
- if (!res.ok) {
- error.set("Error while sharing conversation, try again.");
- console.error("Error while sharing conversation: " + (await res.text()));
- return;
- }
-
- const { url } = await res.json();
-
- share(url, title);
- } catch (err) {
- error.set(ERROR_MESSAGES.default);
- console.error(err);
- }
-}
diff --git a/spaces/AgentVerse/agentVerse/ui/src/phaser3-rex-plugins/templates/ui/maker/Factory.js b/spaces/AgentVerse/agentVerse/ui/src/phaser3-rex-plugins/templates/ui/maker/Factory.js
deleted file mode 100644
index 5be2f18eb71a0e6967c56a0160f811096080f23d..0000000000000000000000000000000000000000
--- a/spaces/AgentVerse/agentVerse/ui/src/phaser3-rex-plugins/templates/ui/maker/Factory.js
+++ /dev/null
@@ -1,11 +0,0 @@
-import Maker from './Maker.js';
-import ObjectFactory from '../ObjectFactory.js';
-import SetValue from '../../../plugins/utils/object/SetValue.js';
-
-ObjectFactory.register('maker', function (styles, customBuilders) {
- return new Maker(this.scene, styles, customBuilders);
-});
-
-SetValue(window, 'RexPlugins.UI.Maker', Maker);
-
-export default Maker;
\ No newline at end of file
diff --git a/spaces/AlexWortega/Kandinsky2.0/README.md b/spaces/AlexWortega/Kandinsky2.0/README.md
deleted file mode 100644
index 770b71977951ad5c67dc13902d7136fc9a6a07ac..0000000000000000000000000000000000000000
--- a/spaces/AlexWortega/Kandinsky2.0/README.md
+++ /dev/null
@@ -1,12 +0,0 @@
----
-title: Kandinsky2.0
-emoji: 📉
-colorFrom: indigo
-colorTo: green
-sdk: gradio
-sdk_version: 3.11.0
-app_file: app.py
-pinned: false
----
-
-Check out the configuration reference at https://huggingface.co/docs/hub/spaces-config-reference
diff --git a/spaces/Amrrs/DragGan-Inversion/PTI/models/StyleCLIP/global_directions/utils/visualizer.py b/spaces/Amrrs/DragGan-Inversion/PTI/models/StyleCLIP/global_directions/utils/visualizer.py
deleted file mode 100644
index 8c4a1fba06bf6bc680aa59bf645f796283f6f1c6..0000000000000000000000000000000000000000
--- a/spaces/Amrrs/DragGan-Inversion/PTI/models/StyleCLIP/global_directions/utils/visualizer.py
+++ /dev/null
@@ -1,605 +0,0 @@
-# python 3.7
-"""Utility functions for visualizing results on html page."""
-
-import base64
-import os.path
-import cv2
-import numpy as np
-
-__all__ = [
- 'get_grid_shape', 'get_blank_image', 'load_image', 'save_image',
- 'resize_image', 'add_text_to_image', 'fuse_images', 'HtmlPageVisualizer',
- 'VideoReader', 'VideoWriter', 'adjust_pixel_range'
-]
-
-
-def adjust_pixel_range(images, min_val=-1.0, max_val=1.0, channel_order='NCHW'):
- """Adjusts the pixel range of the input images.
-
- This function assumes the input array (image batch) is with shape [batch_size,
- channel, height, width] if `channel_order = NCHW`, or with shape [batch_size,
- height, width] if `channel_order = NHWC`. The returned images are with shape
- [batch_size, height, width, channel] and pixel range [0, 255].
-
- NOTE: The channel order of output images will remain the same as the input.
-
- Args:
- images: Input images to adjust pixel range.
- min_val: Min value of the input images. (default: -1.0)
- max_val: Max value of the input images. (default: 1.0)
- channel_order: Channel order of the input array. (default: NCHW)
-
- Returns:
- The postprocessed images with dtype `numpy.uint8` and range [0, 255].
-
- Raises:
- ValueError: If the input `images` are not with type `numpy.ndarray` or the
- shape is invalid according to `channel_order`.
- """
- if not isinstance(images, np.ndarray):
- raise ValueError(f'Images should be with type `numpy.ndarray`!')
-
- channel_order = channel_order.upper()
- if channel_order not in ['NCHW', 'NHWC']:
- raise ValueError(f'Invalid channel order `{channel_order}`!')
-
- if images.ndim != 4:
- raise ValueError(f'Input images are expected to be with shape `NCHW` or '
- f'`NHWC`, but `{images.shape}` is received!')
- if channel_order == 'NCHW' and images.shape[1] not in [1, 3]:
- raise ValueError(f'Input images should have 1 or 3 channels under `NCHW` '
- f'channel order!')
- if channel_order == 'NHWC' and images.shape[3] not in [1, 3]:
- raise ValueError(f'Input images should have 1 or 3 channels under `NHWC` '
- f'channel order!')
-
- images = images.astype(np.float32)
- images = (images - min_val) * 255 / (max_val - min_val)
- images = np.clip(images + 0.5, 0, 255).astype(np.uint8)
- if channel_order == 'NCHW':
- images = images.transpose(0, 2, 3, 1)
-
- return images
-
-
-def get_grid_shape(size, row=0, col=0, is_portrait=False):
- """Gets the shape of a grid based on the size.
-
- This function makes greatest effort on making the output grid square if
- neither `row` nor `col` is set. If `is_portrait` is set as `False`, the height
- will always be equal to or smaller than the width. For example, if input
- `size = 16`, output shape will be `(4, 4)`; if input `size = 15`, output shape
- will be (3, 5). Otherwise, the height will always be equal to or larger than
- the width.
-
- Args:
- size: Size (height * width) of the target grid.
- is_portrait: Whether to return a portrait size of a landscape size.
- (default: False)
-
- Returns:
- A two-element tuple, representing height and width respectively.
- """
- assert isinstance(size, int)
- assert isinstance(row, int)
- assert isinstance(col, int)
- if size == 0:
- return (0, 0)
-
- if row > 0 and col > 0 and row * col != size:
- row = 0
- col = 0
-
- if row > 0 and size % row == 0:
- return (row, size // row)
- if col > 0 and size % col == 0:
- return (size // col, col)
-
- row = int(np.sqrt(size))
- while row > 0:
- if size % row == 0:
- col = size // row
- break
- row = row - 1
-
- return (col, row) if is_portrait else (row, col)
-
-
-def get_blank_image(height, width, channels=3, is_black=True):
- """Gets a blank image, either white of black.
-
- NOTE: This function will always return an image with `RGB` channel order for
- color image and pixel range [0, 255].
-
- Args:
- height: Height of the returned image.
- width: Width of the returned image.
- channels: Number of channels. (default: 3)
- is_black: Whether to return a black image or white image. (default: True)
- """
- shape = (height, width, channels)
- if is_black:
- return np.zeros(shape, dtype=np.uint8)
- return np.ones(shape, dtype=np.uint8) * 255
-
-
-def load_image(path):
- """Loads an image from disk.
-
- NOTE: This function will always return an image with `RGB` channel order for
- color image and pixel range [0, 255].
-
- Args:
- path: Path to load the image from.
-
- Returns:
- An image with dtype `np.ndarray` or `None` if input `path` does not exist.
- """
- if not os.path.isfile(path):
- return None
-
- image = cv2.imread(path)
- return image[:, :, ::-1]
-
-
-def save_image(path, image):
- """Saves an image to disk.
-
- NOTE: The input image (if colorful) is assumed to be with `RGB` channel order
- and pixel range [0, 255].
-
- Args:
- path: Path to save the image to.
- image: Image to save.
- """
- if image is None:
- return
-
- assert len(image.shape) == 3 and image.shape[2] in [1, 3]
- cv2.imwrite(path, image[:, :, ::-1])
-
-
-def resize_image(image, *args, **kwargs):
- """Resizes image.
-
- This is a wrap of `cv2.resize()`.
-
- NOTE: THe channel order of the input image will not be changed.
-
- Args:
- image: Image to resize.
- """
- if image is None:
- return None
-
- assert image.ndim == 3 and image.shape[2] in [1, 3]
- image = cv2.resize(image, *args, **kwargs)
- if image.ndim == 2:
- return image[:, :, np.newaxis]
- return image
-
-
-def add_text_to_image(image,
- text='',
- position=None,
- font=cv2.FONT_HERSHEY_TRIPLEX,
- font_size=1.0,
- line_type=cv2.LINE_8,
- line_width=1,
- color=(255, 255, 255)):
- """Overlays text on given image.
-
- NOTE: The input image is assumed to be with `RGB` channel order.
-
- Args:
- image: The image to overlay text on.
- text: Text content to overlay on the image. (default: '')
- position: Target position (bottom-left corner) to add text. If not set,
- center of the image will be used by default. (default: None)
- font: Font of the text added. (default: cv2.FONT_HERSHEY_TRIPLEX)
- font_size: Font size of the text added. (default: 1.0)
- line_type: Line type used to depict the text. (default: cv2.LINE_8)
- line_width: Line width used to depict the text. (default: 1)
- color: Color of the text added in `RGB` channel order. (default:
- (255, 255, 255))
-
- Returns:
- An image with target text overlayed on.
- """
- if image is None or not text:
- return image
-
- cv2.putText(img=image,
- text=text,
- org=position,
- fontFace=font,
- fontScale=font_size,
- color=color,
- thickness=line_width,
- lineType=line_type,
- bottomLeftOrigin=False)
-
- return image
-
-
-def fuse_images(images,
- image_size=None,
- row=0,
- col=0,
- is_row_major=True,
- is_portrait=False,
- row_spacing=0,
- col_spacing=0,
- border_left=0,
- border_right=0,
- border_top=0,
- border_bottom=0,
- black_background=True):
- """Fuses a collection of images into an entire image.
-
- Args:
- images: A collection of images to fuse. Should be with shape [num, height,
- width, channels].
- image_size: Int or two-element tuple. This field is used to resize the image
- before fusing. `None` disables resizing. (default: None)
- row: Number of rows used for image fusion. If not set, this field will be
- automatically assigned based on `col` and total number of images.
- (default: None)
- col: Number of columns used for image fusion. If not set, this field will be
- automatically assigned based on `row` and total number of images.
- (default: None)
- is_row_major: Whether the input images should be arranged row-major or
- column-major. (default: True)
- is_portrait: Only active when both `row` and `col` should be assigned
- automatically. (default: False)
- row_spacing: Space between rows. (default: 0)
- col_spacing: Space between columns. (default: 0)
- border_left: Width of left border. (default: 0)
- border_right: Width of right border. (default: 0)
- border_top: Width of top border. (default: 0)
- border_bottom: Width of bottom border. (default: 0)
-
- Returns:
- The fused image.
-
- Raises:
- ValueError: If the input `images` is not with shape [num, height, width,
- width].
- """
- if images is None:
- return images
-
- if not images.ndim == 4:
- raise ValueError(f'Input `images` should be with shape [num, height, '
- f'width, channels], but {images.shape} is received!')
-
- num, image_height, image_width, channels = images.shape
- if image_size is not None:
- if isinstance(image_size, int):
- image_size = (image_size, image_size)
- assert isinstance(image_size, (list, tuple)) and len(image_size) == 2
- width, height = image_size
- else:
- height, width = image_height, image_width
- row, col = get_grid_shape(num, row=row, col=col, is_portrait=is_portrait)
- fused_height = (
- height * row + row_spacing * (row - 1) + border_top + border_bottom)
- fused_width = (
- width * col + col_spacing * (col - 1) + border_left + border_right)
- fused_image = get_blank_image(
- fused_height, fused_width, channels=channels, is_black=black_background)
- images = images.reshape(row, col, image_height, image_width, channels)
- if not is_row_major:
- images = images.transpose(1, 0, 2, 3, 4)
-
- for i in range(row):
- y = border_top + i * (height + row_spacing)
- for j in range(col):
- x = border_left + j * (width + col_spacing)
- if image_size is not None:
- image = cv2.resize(images[i, j], image_size)
- else:
- image = images[i, j]
- fused_image[y:y + height, x:x + width] = image
-
- return fused_image
-
-
-def get_sortable_html_header(column_name_list, sort_by_ascending=False):
- """Gets header for sortable html page.
-
- Basically, the html page contains a sortable table, where user can sort the
- rows by a particular column by clicking the column head.
-
- Example:
-
- column_name_list = [name_1, name_2, name_3]
- header = get_sortable_html_header(column_name_list)
- footer = get_sortable_html_footer()
- sortable_table = ...
- html_page = header + sortable_table + footer
-
- Args:
- column_name_list: List of column header names.
- sort_by_ascending: Default sorting order. If set as `True`, the html page
- will be sorted by ascending order when the header is clicked for the first
- time.
-
- Returns:
- A string, which represents for the header for a sortable html page.
- """
- header = '\n'.join([
- '',
- '',
- '',
- '',
- '',
- '',
- '',
- '',
- '',
- '',
- '
',
- '',
- '
',
- ''])
- for idx, column_name in enumerate(column_name_list):
- header += f'
{column_name}
\n'
- header += '
\n'
- header += '\n'
- header += '\n'
-
- return header
-
-
-def get_sortable_html_footer():
- """Gets footer for sortable html page.
-
- Check function `get_sortable_html_header()` for more details.
- """
- return '\n
\n\n\n\n'
-
-
-def encode_image_to_html_str(image, image_size=None):
- """Encodes an image to html language.
-
- Args:
- image: The input image to encode. Should be with `RGB` channel order.
- image_size: Int or two-element tuple. This field is used to resize the image
- before encoding. `None` disables resizing. (default: None)
-
- Returns:
- A string which represents the encoded image.
- """
- if image is None:
- return ''
-
- assert len(image.shape) == 3 and image.shape[2] in [1, 3]
-
- # Change channel order to `BGR`, which is opencv-friendly.
- image = image[:, :, ::-1]
-
- # Resize the image if needed.
- if image_size is not None:
- if isinstance(image_size, int):
- image_size = (image_size, image_size)
- assert isinstance(image_size, (list, tuple)) and len(image_size) == 2
- image = cv2.resize(image, image_size)
-
- # Encode the image to html-format string.
- encoded_image = cv2.imencode(".jpg", image)[1].tostring()
- encoded_image_base64 = base64.b64encode(encoded_image).decode('utf-8')
- html_str = f''
-
- return html_str
-
-
-class HtmlPageVisualizer(object):
- """Defines the html page visualizer.
-
- This class can be used to visualize image results as html page. Basically, it
- is based on an html-format sorted table with helper functions
- `get_sortable_html_header()`, `get_sortable_html_footer()`, and
- `encode_image_to_html_str()`. To simplify the usage, specifying the following
- fields is enough to create a visualization page:
-
- (1) num_rows: Number of rows of the table (header-row exclusive).
- (2) num_cols: Number of columns of the table.
- (3) header contents (optional): Title of each column.
-
- NOTE: `grid_size` can be used to assign `num_rows` and `num_cols`
- automatically.
-
- Example:
-
- html = HtmlPageVisualizer(num_rows, num_cols)
- html.set_headers([...])
- for i in range(num_rows):
- for j in range(num_cols):
- html.set_cell(i, j, text=..., image=...)
- html.save('visualize.html')
- """
-
- def __init__(self,
- num_rows=0,
- num_cols=0,
- grid_size=0,
- is_portrait=False,
- viz_size=None):
- if grid_size > 0:
- num_rows, num_cols = get_grid_shape(
- grid_size, row=num_rows, col=num_cols, is_portrait=is_portrait)
- assert num_rows > 0 and num_cols > 0
-
- self.num_rows = num_rows
- self.num_cols = num_cols
- self.viz_size = viz_size
- self.headers = ['' for _ in range(self.num_cols)]
- self.cells = [[{
- 'text': '',
- 'image': '',
- } for _ in range(self.num_cols)] for _ in range(self.num_rows)]
-
- def set_header(self, column_idx, content):
- """Sets the content of a particular header by column index."""
- self.headers[column_idx] = content
-
- def set_headers(self, contents):
- """Sets the contents of all headers."""
- if isinstance(contents, str):
- contents = [contents]
- assert isinstance(contents, (list, tuple))
- assert len(contents) == self.num_cols
- for column_idx, content in enumerate(contents):
- self.set_header(column_idx, content)
-
- def set_cell(self, row_idx, column_idx, text='', image=None):
- """Sets the content of a particular cell.
-
- Basically, a cell contains some text as well as an image. Both text and
- image can be empty.
-
- Args:
- row_idx: Row index of the cell to edit.
- column_idx: Column index of the cell to edit.
- text: Text to add into the target cell.
- image: Image to show in the target cell. Should be with `RGB` channel
- order.
- """
- self.cells[row_idx][column_idx]['text'] = text
- self.cells[row_idx][column_idx]['image'] = encode_image_to_html_str(
- image, self.viz_size)
-
- def save(self, save_path):
- """Saves the html page."""
- html = ''
- for i in range(self.num_rows):
- html += f'
\n'
- for j in range(self.num_cols):
- text = self.cells[i][j]['text']
- image = self.cells[i][j]['image']
- if text:
- html += f'
{text}
{image}
\n'
- else:
- html += f'
{image}
\n'
- html += f'
\n'
-
- header = get_sortable_html_header(self.headers)
- footer = get_sortable_html_footer()
-
- with open(save_path, 'w') as f:
- f.write(header + html + footer)
-
-
-class VideoReader(object):
- """Defines the video reader.
-
- This class can be used to read frames from a given video.
- """
-
- def __init__(self, path):
- """Initializes the video reader by loading the video from disk."""
- if not os.path.isfile(path):
- raise ValueError(f'Video `{path}` does not exist!')
-
- self.path = path
- self.video = cv2.VideoCapture(path)
- assert self.video.isOpened()
- self.position = 0
-
- self.length = int(self.video.get(cv2.CAP_PROP_FRAME_COUNT))
- self.frame_height = int(self.video.get(cv2.CAP_PROP_FRAME_HEIGHT))
- self.frame_width = int(self.video.get(cv2.CAP_PROP_FRAME_WIDTH))
- self.fps = self.video.get(cv2.CAP_PROP_FPS)
-
- def __del__(self):
- """Releases the opened video."""
- self.video.release()
-
- def read(self, position=None):
- """Reads a certain frame.
-
- NOTE: The returned frame is assumed to be with `RGB` channel order.
-
- Args:
- position: Optional. If set, the reader will read frames from the exact
- position. Otherwise, the reader will read next frames. (default: None)
- """
- if position is not None and position < self.length:
- self.video.set(cv2.CAP_PROP_POS_FRAMES, position)
- self.position = position
-
- success, frame = self.video.read()
- self.position = self.position + 1
-
- return frame[:, :, ::-1] if success else None
-
-
-class VideoWriter(object):
- """Defines the video writer.
-
- This class can be used to create a video.
-
- NOTE: `.avi` and `DIVX` is the most recommended codec format since it does not
- rely on other dependencies.
- """
-
- def __init__(self, path, frame_height, frame_width, fps=24, codec='DIVX'):
- """Creates the video writer."""
- self.path = path
- self.frame_height = frame_height
- self.frame_width = frame_width
- self.fps = fps
- self.codec = codec
-
- self.video = cv2.VideoWriter(filename=path,
- fourcc=cv2.VideoWriter_fourcc(*codec),
- fps=fps,
- frameSize=(frame_width, frame_height))
-
- def __del__(self):
- """Releases the opened video."""
- self.video.release()
-
- def write(self, frame):
- """Writes a target frame.
-
- NOTE: The input frame is assumed to be with `RGB` channel order.
- """
- self.video.write(frame[:, :, ::-1])
diff --git a/spaces/Amrrs/DragGan-Inversion/PTI/models/StyleCLIP/models/stylegan2/op/__init__.py b/spaces/Amrrs/DragGan-Inversion/PTI/models/StyleCLIP/models/stylegan2/op/__init__.py
deleted file mode 100644
index d0918d92285955855be89f00096b888ee5597ce3..0000000000000000000000000000000000000000
--- a/spaces/Amrrs/DragGan-Inversion/PTI/models/StyleCLIP/models/stylegan2/op/__init__.py
+++ /dev/null
@@ -1,2 +0,0 @@
-from .fused_act import FusedLeakyReLU, fused_leaky_relu
-from .upfirdn2d import upfirdn2d
diff --git a/spaces/AnandSoni2001/StockMarketPrediction/app.py b/spaces/AnandSoni2001/StockMarketPrediction/app.py
deleted file mode 100644
index 1b4e9945212325586c48533affbe2b76e038b799..0000000000000000000000000000000000000000
--- a/spaces/AnandSoni2001/StockMarketPrediction/app.py
+++ /dev/null
@@ -1,393 +0,0 @@
-#Import Libraries
-import streamlit as st
-import plotly.graph_objects as go
-import pandas as pd
-import plotly.express as px
-from yahoo_fin import stock_info
-from yahoo_fin.stock_info import *
-import math
-import numpy as np
-from sklearn.preprocessing import MinMaxScaler
-import joblib
-
-#Heading
-st.title('Research Project on Stock Market Analysis and Prediction')
-st.write("#")
-
-#TCS Data Taken
-tcsdaily = stock_info.get_data("TCS.NS", interval="1d")
-tcsmonthly= stock_info.get_data("TCS.NS", interval="1mo")
-tcsyearly = pd.read_csv('data/tcs-yearly.csv')
-
-#Reliance Data Taken
-reldaily = stock_info.get_data("RELIANCE.NS", interval="1d")
-relmonthly= stock_info.get_data("RELIANCE.NS", interval="1mo")
-relyearly = pd.read_csv('data/relianceind-yearly.csv')
-
-#Infosys Data Taken
-infdaily = stock_info.get_data("INFY.NS", interval="1d")
-infmonthly= stock_info.get_data("INFY.NS", interval="1mo")
-infyearly = pd.read_csv('data/infosys-yearly.csv')
-
-#Select Box
-comp = st.selectbox('Select a Company from the below options :', ('Tata Consultancy Services - TCS', 'Reliance Industries - RELIANCE', 'Infosys - INFY'))
-
-if comp == 'Tata Consultancy Services - TCS':
- col1, col2, col3, col4 = st.columns(4)
- x = round(stock_info.get_live_price("TCS.NS"),2)
- y = round(tcsdaily['close'].iloc[-2],2)
- tcs = get_stats('TCS.NS')['Value']
- col1.metric(label="Market Price", value=x, delta = round(x-y,2))
- col2.metric(label="52 Week High", value=tcs[3])
- col3.metric(label="52 Week Low", value=tcs[4])
- col4.metric(label="Return on Equity", value=tcs[34])
-
- col1, col2, col3, col4 = st.columns(4)
- col1.metric(label='Previous Close', value=y)
- col2.metric(label="Book Value Per Share", value=tcs[48])
- col3.metric(label='Earning Per Share', value=tcs[41])
- col4.metric(label="Dividend Yield", value=tcs[22])
-
-
-if comp == 'Reliance Industries - RELIANCE':
- col1, col2, col3, col4 = st.columns(4)
- x = round(stock_info.get_live_price("RELIANCE.NS"),2)
- y = round(reldaily['close'].iloc[-2],2)
- rel = get_stats('RELIANCE.NS')['Value']
- col1.metric(label="Market Price", value=x, delta = round(x-y,2))
- col2.metric(label="52 Week High", value=rel[3])
- col3.metric(label="52 Week Low", value=rel[4])
- col4.metric(label="Return on Equity", value='8.21%')
-
- col1, col2, col3, col4 = st.columns(4)
- col1.metric(label='Previous Close', value=y)
- col2.metric(label="Book Value Per Share", value=1202.45)
- col3.metric(label='Earning Per Share', value=93.96)
- col4.metric(label="Dividend Yield", value='0.36%')
-
-if comp == 'Infosys - INFY':
- col1, col2, col3, col4 = st.columns(4)
- x = round(stock_info.get_live_price("INFY.NS"),2)
- y = round(infdaily['close'].iloc[-2],2)
- inf = get_stats('INFY.NS')['Value']
- col1.metric(label="Market Price", value=x, delta = round(x-y,2))
- col2.metric(label="52 Week High", value=inf[3])
- col3.metric(label="52 Week Low", value=inf[4])
- col4.metric(label="Return on Equity", value=inf[34])
-
- col1, col2, col3, col4 = st.columns(4)
- col1.metric(label='Previous Close', value=y)
- col2.metric(label="Book Value Per Share", value=inf[48])
- col3.metric(label='Earning Per Share', value=inf[41])
- col4.metric(label="Dividend Yield", value=inf[22])
-
-#Tab for Hist Data
-st.write("#")
-st.subheader('Historic data : ')
-option1, option2, option3 = st.tabs(["Daily", "Monthly", "Yearly"])
-
-cl1, cl2, cl3, cl4 = st.columns(4)
-with cl1:
- ag1 = st.checkbox('Close', value='True')
-with cl2:
- ag2 = st.checkbox('Open', value='True')
-with cl3:
- ag3 = st.checkbox('High', value='True')
-with cl4:
- ag4 = st.checkbox('Low', value='True')
-
-with option1:
- opt = st.radio("Select timelength :", ('All Time', '1 Week', '1 Month', '1 Year'))
- st.write('', unsafe_allow_html=True)
-
- if comp == 'Tata Consultancy Services - TCS':
- if opt=='All Time' :
- fig = px.line(tcsdaily, y='close',markers=False, title='Tata Consultancy Services daily data of all time')
- if opt=='1 Week' :
- fig = px.line(tcsdaily.tail(5), y='close',markers=False, title='Tata Consultancy Services daily data of 1 week')
- if opt=='1 Month' :
- fig = px.line(tcsdaily.tail(20), y='close',markers=False, title='Tata Consultancy Services daily data of 1 month')
- if opt=='1 Year' :
- fig = px.line(tcsdaily.tail(251), y='close',markers=False, title='Tata Consultancy Services daily data of 1 year')
- st.plotly_chart(fig, use_container_width=True)
-
- fig = go.Figure()
- if(ag1):
- fig.add_trace(go.Scatter(x=tcsdaily.index,y=tcsdaily['close'], name='Closing'))
- if(ag2):
- fig.add_trace(go.Scatter(x=tcsdaily.index,y=tcsdaily['open'], name = 'Opening', line=dict(color='yellow')))
- if(ag3):
- fig.add_trace(go.Scatter(x=tcsdaily.index,y=tcsdaily['high'], name = 'High', line=dict(color='green')))
- if(ag4):
- fig.add_trace(go.Scatter(x=tcsdaily.index,y=tcsdaily['low'], name = 'Low', line=dict(color='red')))
- fig.update_layout(xaxis_title='Date', yaxis_title='Price', title='Comparing other relevant parameters along close')
- st.plotly_chart(fig, use_container_width=True, title='Comparing other relevant parameters')
-
- if comp == 'Infosys - INFY':
- if opt=='All Time' :
- fig = px.line(infdaily, y='close',markers=False, title='Infosys daily data of all time')
- if opt=='1 Week' :
- fig = px.line(infdaily.tail(5), y='close',markers=False, title='Infosys daily data of 1 week')
- if opt=='1 Month' :
- fig = px.line(infdaily.tail(20), y='close',markers=False, title='Infosys daily data of 1 month')
- if opt=='1 Year' :
- fig = px.line(infdaily.tail(251), y='close',markers=False, title='Infosys daily data of 1 year')
- st.plotly_chart(fig, use_container_width=True)
-
- fig = go.Figure()
- if(ag1):
- fig.add_trace(go.Scatter(x=infdaily.index, y=infdaily['close'], name='Closing', line=dict(color='blue')))
- if(ag2):
- fig.add_trace(go.Scatter(x=infdaily.index,y=infdaily['open'], name = 'Opening', line=dict(color='yellow')))
- if(ag3):
- fig.add_trace(go.Scatter(x=infdaily.index,y=infdaily['high'], name = 'High', line=dict(color='green')))
- if(ag4):
- fig.add_trace(go.Scatter(x=infdaily.index,y=infdaily['low'], name = 'Low', line=dict(color='red')))
- fig.update_layout(xaxis_title='Date', yaxis_title='Price', title='Comparing other relevant parameters')
- st.plotly_chart(fig, use_container_width=True)
-
- if comp == 'Reliance Industries - RELIANCE':
- if opt=='All Time' :
- fig = px.line(reldaily, y='close',markers=False, title='Reliance Industries daily data of all time')
- if opt=='1 Week' :
- fig = px.line(reldaily.tail(5), y='close',markers=False, title='Reliance Industries daily data of 1 week')
- if opt=='1 Month' :
- fig = px.line(reldaily.tail(20), y='close',markers=False, title='Reliance Industries daily data of 1 month')
- if opt=='1 Year' :
- fig = px.line(reldaily.tail(251), y='close',markers=False, title='Reliance Industries daily data of 1 year')
- st.plotly_chart(fig, use_container_width=True)
-
- fig = go.Figure()
- if(ag1):
- fig.add_trace(go.Scatter(x=reldaily.index, y=reldaily['close'], name='Closing', line=dict(color='blue')))
- if(ag2):
- fig.add_trace(go.Scatter(x=reldaily.index,y=reldaily['open'], name = 'Opening', line=dict(color='yellow')))
- if(ag3):
- fig.add_trace(go.Scatter(x=reldaily.index,y=reldaily['high'], name = 'High', line=dict(color='green')))
- if(ag4):
- fig.add_trace(go.Scatter(x=reldaily.index,y=reldaily['low'], name = 'Low', line=dict(color='red')))
- fig.update_layout(xaxis_title='Date', yaxis_title='Price', title='Comparing other relevant parameters along close')
- st.plotly_chart(fig, use_container_width=True)
-
-with option2:
- if comp == 'Tata Consultancy Services - TCS':
- fig = px.line(tcsmonthly,y='close', markers=False, title='Tata Consultancy Services monthly data')
- st.plotly_chart(fig, use_container_width=True)
-
- fig = go.Figure()
- if(ag1):
- fig.add_trace(go.Scatter(x=tcsmonthly.index,y=tcsmonthly['close'], name='Closing', line=dict(color='blue')))
- if(ag2):
- fig.add_trace(go.Scatter(x=tcsmonthly.index,y=tcsmonthly['open'], name = 'Opening', line=dict(color='yellow')))
- if(ag3):
- fig.add_trace(go.Scatter(x=tcsmonthly.index,y=tcsmonthly['high'], name = 'High', line=dict(color='green')))
- if(ag4):
- fig.add_trace(go.Scatter(x=tcsmonthly.index,y=tcsmonthly['low'], name = 'Low', line=dict(color='red')))
- fig.update_layout(xaxis_title='Month', yaxis_title='Price', title='Comparing other relevant parameters')
- st.plotly_chart(fig, use_container_width=True)
-
- if comp == 'Infosys - INFY':
- fig = px.line(infmonthly, y='close',markers=False, title='Infosys monthly data')
- st.plotly_chart(fig, use_container_width=True)
-
- fig = go.Figure()
- if(ag1):
- fig.add_trace(go.Scatter(x=infmonthly.index, y=infmonthly['close'], name='Closing', line=dict(color='blue')))
- if(ag2):
- fig.add_trace(go.Scatter(x=infmonthly.index,y=infmonthly['open'], name = 'Opening', line=dict(color='yellow')))
- if(ag3):
- fig.add_trace(go.Scatter(x=infmonthly.index,y=infmonthly['high'], name = 'High', line=dict(color='green')))
- if(ag4):
- fig.add_trace(go.Scatter(y=infmonthly['low'], name = 'Low', line=dict(color='red')))
- fig.update_layout(xaxis_title='Month', yaxis_title='Price', title='Comparing other relevant parameters')
- st.plotly_chart(fig, use_container_width=True)
-
- if comp == 'Reliance Industries - RELIANCE':
- fig = px.line(relmonthly, y='close',markers=False, title='Reliance Industries monthly data')
- st.plotly_chart(fig, use_container_width=True)
-
- fig = go.Figure()
- if(ag1):
- fig.add_trace(go.Scatter(x=relmonthly.index,y=relmonthly['close'], name='Closing', line=dict(color='blue')))
- if(ag2):
- fig.add_trace(go.Scatter(x=relmonthly.index,y=relmonthly['open'], name = 'Opening', line=dict(color='yellow')))
- if(ag3):
- fig.add_trace(go.Scatter(x=relmonthly.index,y=relmonthly['high'], name = 'High', line=dict(color='green')))
- if(ag4):
- fig.add_trace(go.Scatter(x=relmonthly.index,y=relmonthly['low'], name = 'Low', line=dict(color='red')))
- fig.update_layout(xaxis_title='Month', yaxis_title='Price', title='Comparing other relevant parameters')
- st.plotly_chart(fig, use_container_width=True)
-
-with option3:
- if comp == 'Tata Consultancy Services - TCS':
- fig = px.line(tcsyearly, x='Year', y='Close Price',markers=True, title='Tata Consultancy Services Yearly Data from 2004')
- st.plotly_chart(fig, use_container_width=True)
-
- fig = go.Figure()
- if(ag1):
- fig.add_trace(go.Scatter(x=tcsyearly['Year'], y=tcsyearly['Close Price'], name='Closing', line=dict(color='blue')))
- if(ag2):
- fig.add_trace(go.Scatter(x=tcsyearly['Year'], y=tcsyearly['Open Price'], name = 'Opening', line=dict(color='yellow')))
- if(ag3):
- fig.add_trace(go.Scatter(x=tcsyearly['Year'], y=tcsyearly['High Price'], name = 'High', line=dict(color='green')))
- if(ag4):
- fig.add_trace(go.Scatter(x=tcsyearly['Year'], y=tcsyearly['Low Price'], name = 'Low', line=dict(color='red')))
- fig.update_layout(xaxis_title='Year', yaxis_title='Price', title='Comparing other relevant parameters along close price')
- st.plotly_chart(fig, use_container_width=True, title='Comparing other relevant parameters')
-
- if comp == 'Infosys - INFY':
- fig = px.line(infyearly, x='Year', y='Close Price',markers=True, title='Infosys Yearly Data from 2004')
- st.plotly_chart(fig, use_container_width=True)
-
- fig = go.Figure()
- if(ag1):
- fig.add_trace(go.Scatter(x=infyearly['Year'], y=infyearly['Close Price'], name='Closing', line=dict(color='blue')))
- if(ag2):
- fig.add_trace(go.Scatter(x=infyearly['Year'], y=infyearly['Open Price'], name = 'Opening', line=dict(color='yellow')))
- if(ag3):
- fig.add_trace(go.Scatter(x=infyearly['Year'], y=infyearly['High Price'], name = 'High', line=dict(color='green')))
- if(ag4):
- fig.add_trace(go.Scatter(x=infyearly['Year'], y=infyearly['Low Price'], name = 'Low', line=dict(color='red')))
- fig.update_layout(xaxis_title='Year', yaxis_title='Price', title='Comparing other relevant parameters')
- st.plotly_chart(fig, use_container_width=True)
-
- if comp == 'Reliance Industries - RELIANCE':
- fig = px.line(relyearly, x='Year', y='Close Price',markers=True, title='Reliance Industries Yearly Data from 2004')
- st.plotly_chart(fig, use_container_width=True)
-
- fig = go.Figure()
- if(ag1):
- fig.add_trace(go.Scatter(x=relyearly['Year'], y=relyearly['Close Price'], name='Closing', line=dict(color='blue')))
- if(ag2):
- fig.add_trace(go.Scatter(x=relyearly['Year'], y=relyearly['Open Price'], name = 'Opening', line=dict(color='yellow')))
- if(ag3):
- fig.add_trace(go.Scatter(x=relyearly['Year'], y=relyearly['High Price'], name = 'High', line=dict(color='green')))
- if(ag4):
- fig.add_trace(go.Scatter(x=relyearly['Year'], y=relyearly['Low Price'], name = 'Low', line=dict(color='red')))
- fig.update_layout(xaxis_title='Year', yaxis_title='Price', title='Comparing other relevant parameters')
- st.plotly_chart(fig, use_container_width=True)
-
-#Predictions
-st.write("#")
-st.subheader('Predict : ')
-
-if st.button('Click Here'):
- if comp == 'Tata Consultancy Services - TCS':
- x = round(stock_info.get_live_price("TCS.NS"),2)
- tcsweekly = stock_info.get_data("TCS.NS", interval="1d")
- tcsweekly=tcsweekly.dropna()
- values = tcsweekly['close'].values
- data_len = math.ceil(len(values)*0.8)
- scaler = MinMaxScaler(feature_range=(0,1))
- scaled_data = scaler.fit_transform(values.reshape(-1,1))
- test_data = scaled_data[data_len-60: , : ]
- x_test = []
- for i in range(60, len(test_data)):
- x_test.append(test_data[i-60:i, 0])
- x_test = np.array(x_test)
- x_test = np.reshape(x_test, (x_test.shape[0], x_test.shape[1], 1))
- new = joblib.load('tcsdail_1.pkl')
- ans = new.predict(x_test)
- ans1 = scaler.inverse_transform(ans)
- val = np.around(ans1[-1][0], decimals=2)
- st.metric(label="Prediction", value=val, delta = round(val-x,2))
-
- if comp == 'Reliance Industries - RELIANCE':
- x = round(stock_info.get_live_price("RELIANCE.NS"),2)
- relweekly = stock_info.get_data("RELIANCE.NS", interval="1d")
- relweekly=relweekly.dropna()
- values = relweekly['close'].values
- data_len = math.ceil(len(values)*0.8)
- scaler = MinMaxScaler(feature_range=(0,1))
- scaled_data = scaler.fit_transform(values.reshape(-1,1))
- test_data = scaled_data[data_len-60: , : ]
- x_test = []
- for i in range(60, len(test_data)):
- x_test.append(test_data[i-60:i, 0])
- x_test = np.array(x_test)
- x_test = np.reshape(x_test, (x_test.shape[0], x_test.shape[1], 1))
- new = joblib.load('reldail_1.pkl')
- ans = new.predict(x_test)
- ans1 = scaler.inverse_transform(ans)
- val = np.around(ans1[-1][0], decimals=2)
- st.metric(label="Prediction", value=val, delta = round(val-x,2))
-
- if comp == 'Infosys - INFY':
- x = round(stock_info.get_live_price("INFY.NS"),2)
- infweekly = stock_info.get_data("INFY.NS", interval="1d")
- infweekly=infweekly.dropna()
- values = infweekly['close'].values
- data_len = math.ceil(len(values)*0.8)
- scaler = MinMaxScaler(feature_range=(0,1))
- scaled_data = scaler.fit_transform(values.reshape(-1,1))
- test_data = scaled_data[data_len-60: , : ]
- x_test = []
- for i in range(60, len(test_data)):
- x_test.append(test_data[i-60:i, 0])
- x_test = np.array(x_test)
- x_test = np.reshape(x_test, (x_test.shape[0], x_test.shape[1], 1))
- new = joblib.load('infdail_1.pkl')
- ans = new.predict(x_test)
- ans1 = scaler.inverse_transform(ans)
- val = np.around(ans1[-1][0], decimals=2)
- st.metric(label="Prediction", value=val, delta = round(val-x,2))
-
-
-#Tab for Hist Data
-st.write("#")
-st.subheader('Financial data : ')
-a1, a2, a3 = st.tabs(["Revenue & Profit", "Net Worth", "Shareholding Pattern"])
-
-tier=['Promoters', 'Mutual Funds', 'Retail', 'Foreign Institutions','Others']
-y=['2018', '2019', '2020', '2021', '2022']
-
-with a1:
- st.caption('All values in Crs')
- if comp == 'Infosys - INFY':
- chart_data = pd.DataFrame([[70522,16029], [82675,15404], [90791,16594], [100472,19351], [121641,22110]],
- index=y, columns=["Revenue", "Profit"])
- st.bar_chart(chart_data, height=350)
-
- if comp == 'Tata Consultancy Services - TCS':
- chart_data = pd.DataFrame([[123104,25826], [146463,31472], [156949,32430], [164177,32430], [191754,38327]],
- index=y, columns=["Revenue", "Profit"])
- st.bar_chart(chart_data, height=350)
-
- if comp == 'Reliance Industries - RELIANCE':
- chart_data = pd.DataFrame([[408265,36075], [583094,39588], [611645,39354], [486326,49128], [721634,60705]],
- index=y, columns=["Revenue", "Profit"])
- st.bar_chart(chart_data, height=350)
-
-
-with a2:
- st.caption('All values in Crs')
- if comp == 'Infosys - INFY':
- chart_data = pd.DataFrame([64923, 64948, 65450, 76351, 75350], index=y, columns=['Net Worth'])
- st.bar_chart(chart_data, height=350)
-
- if comp == 'Tata Consultancy Services - TCS':
- chart_data = pd.DataFrame([85128, 89446, 84126, 86433, 89139], index=y, columns=['Net Worth'])
- st.bar_chart(chart_data, height=350)
-
- if comp == 'Reliance Industries - RELIANCE':
- chart_data = pd.DataFrame([293506, 387112, 453331, 700172, 779485], index=y, columns=['Net Worth'])
- st.bar_chart(chart_data, height=350)
-
-with a3:
- st.caption('As of March, 2023')
- if comp == 'Infosys - INFY':
- x = [15.11, 17.71, 18.22, 36.28, 12.68]
- fig = px.pie(values=x, names=tier)
- st.plotly_chart(fig, use_container_width=True, height=350)
-
- if comp == 'Tata Consultancy Services - TCS':
- x = [72.30, 3.31, 5.96, 12.94, 5.49]
- fig = px.pie(values=x, names=tier)
- st.plotly_chart(fig, use_container_width=True, height=350)
-
- if comp == 'Reliance Industries - RELIANCE':
- x = [50.49, 5.81, 11.64, 23.43, 8.63]
- fig = px.pie(values=x, names=tier)
- st.plotly_chart(fig, use_container_width=True, height=350)
-
-st.caption('The Web Application was made by Anand Soni and Deepak Rathore.')
diff --git a/spaces/Androidonnxfork/CivitAi-to-Diffusers/diffusers/tests/pipelines/latent_diffusion/test_latent_diffusion_superresolution.py b/spaces/Androidonnxfork/CivitAi-to-Diffusers/diffusers/tests/pipelines/latent_diffusion/test_latent_diffusion_superresolution.py
deleted file mode 100644
index d21ead543af8bbbf60cb16177b76deb5bf33595e..0000000000000000000000000000000000000000
--- a/spaces/Androidonnxfork/CivitAi-to-Diffusers/diffusers/tests/pipelines/latent_diffusion/test_latent_diffusion_superresolution.py
+++ /dev/null
@@ -1,131 +0,0 @@
-# coding=utf-8
-# Copyright 2023 HuggingFace Inc.
-#
-# Licensed under the Apache License, Version 2.0 (the "License");
-# you may not use this file except in compliance with the License.
-# You may obtain a copy of the License at
-#
-# http://www.apache.org/licenses/LICENSE-2.0
-#
-# Unless required by applicable law or agreed to in writing, software
-# distributed under the License is distributed on an "AS IS" BASIS,
-# WITHOUT WARRANTIES OR CONDITIONS OF ANY KIND, either express or implied.
-# See the License for the specific language governing permissions and
-# limitations under the License.
-
-import random
-import unittest
-
-import numpy as np
-import torch
-
-from diffusers import DDIMScheduler, LDMSuperResolutionPipeline, UNet2DModel, VQModel
-from diffusers.utils import PIL_INTERPOLATION, floats_tensor, load_image, slow, torch_device
-from diffusers.utils.testing_utils import enable_full_determinism, require_torch
-
-
-enable_full_determinism()
-
-
-class LDMSuperResolutionPipelineFastTests(unittest.TestCase):
- @property
- def dummy_image(self):
- batch_size = 1
- num_channels = 3
- sizes = (32, 32)
-
- image = floats_tensor((batch_size, num_channels) + sizes, rng=random.Random(0)).to(torch_device)
- return image
-
- @property
- def dummy_uncond_unet(self):
- torch.manual_seed(0)
- model = UNet2DModel(
- block_out_channels=(32, 64),
- layers_per_block=2,
- sample_size=32,
- in_channels=6,
- out_channels=3,
- down_block_types=("DownBlock2D", "AttnDownBlock2D"),
- up_block_types=("AttnUpBlock2D", "UpBlock2D"),
- )
- return model
-
- @property
- def dummy_vq_model(self):
- torch.manual_seed(0)
- model = VQModel(
- block_out_channels=[32, 64],
- in_channels=3,
- out_channels=3,
- down_block_types=["DownEncoderBlock2D", "DownEncoderBlock2D"],
- up_block_types=["UpDecoderBlock2D", "UpDecoderBlock2D"],
- latent_channels=3,
- )
- return model
-
- def test_inference_superresolution(self):
- device = "cpu"
- unet = self.dummy_uncond_unet
- scheduler = DDIMScheduler()
- vqvae = self.dummy_vq_model
-
- ldm = LDMSuperResolutionPipeline(unet=unet, vqvae=vqvae, scheduler=scheduler)
- ldm.to(device)
- ldm.set_progress_bar_config(disable=None)
-
- init_image = self.dummy_image.to(device)
-
- generator = torch.Generator(device=device).manual_seed(0)
- image = ldm(image=init_image, generator=generator, num_inference_steps=2, output_type="numpy").images
-
- image_slice = image[0, -3:, -3:, -1]
-
- assert image.shape == (1, 64, 64, 3)
- expected_slice = np.array([0.8678, 0.8245, 0.6381, 0.6830, 0.4385, 0.5599, 0.4641, 0.6201, 0.5150])
-
- assert np.abs(image_slice.flatten() - expected_slice).max() < 1e-2
-
- @unittest.skipIf(torch_device != "cuda", "This test requires a GPU")
- def test_inference_superresolution_fp16(self):
- unet = self.dummy_uncond_unet
- scheduler = DDIMScheduler()
- vqvae = self.dummy_vq_model
-
- # put models in fp16
- unet = unet.half()
- vqvae = vqvae.half()
-
- ldm = LDMSuperResolutionPipeline(unet=unet, vqvae=vqvae, scheduler=scheduler)
- ldm.to(torch_device)
- ldm.set_progress_bar_config(disable=None)
-
- init_image = self.dummy_image.to(torch_device)
-
- image = ldm(init_image, num_inference_steps=2, output_type="numpy").images
-
- assert image.shape == (1, 64, 64, 3)
-
-
-@slow
-@require_torch
-class LDMSuperResolutionPipelineIntegrationTests(unittest.TestCase):
- def test_inference_superresolution(self):
- init_image = load_image(
- "https://huggingface.co/datasets/hf-internal-testing/diffusers-images/resolve/main"
- "/vq_diffusion/teddy_bear_pool.png"
- )
- init_image = init_image.resize((64, 64), resample=PIL_INTERPOLATION["lanczos"])
-
- ldm = LDMSuperResolutionPipeline.from_pretrained("duongna/ldm-super-resolution", device_map="auto")
- ldm.set_progress_bar_config(disable=None)
-
- generator = torch.manual_seed(0)
- image = ldm(image=init_image, generator=generator, num_inference_steps=20, output_type="numpy").images
-
- image_slice = image[0, -3:, -3:, -1]
-
- assert image.shape == (1, 256, 256, 3)
- expected_slice = np.array([0.7644, 0.7679, 0.7642, 0.7633, 0.7666, 0.7560, 0.7425, 0.7257, 0.6907])
-
- assert np.abs(image_slice.flatten() - expected_slice).max() < 1e-2
diff --git a/spaces/Andy1621/uniformer_image_detection/configs/fast_rcnn/fast_rcnn_r50_fpn_1x_coco.py b/spaces/Andy1621/uniformer_image_detection/configs/fast_rcnn/fast_rcnn_r50_fpn_1x_coco.py
deleted file mode 100644
index d2f080e9d3b1ddade22341aa38c6258eaee78a50..0000000000000000000000000000000000000000
--- a/spaces/Andy1621/uniformer_image_detection/configs/fast_rcnn/fast_rcnn_r50_fpn_1x_coco.py
+++ /dev/null
@@ -1,52 +0,0 @@
-_base_ = [
- '../_base_/models/fast_rcnn_r50_fpn.py',
- '../_base_/datasets/coco_detection.py',
- '../_base_/schedules/schedule_1x.py', '../_base_/default_runtime.py'
-]
-dataset_type = 'CocoDataset'
-data_root = 'data/coco/'
-img_norm_cfg = dict(
- mean=[123.675, 116.28, 103.53], std=[58.395, 57.12, 57.375], to_rgb=True)
-train_pipeline = [
- dict(type='LoadImageFromFile'),
- dict(type='LoadProposals', num_max_proposals=2000),
- dict(type='LoadAnnotations', with_bbox=True),
- dict(type='Resize', img_scale=(1333, 800), keep_ratio=True),
- dict(type='RandomFlip', flip_ratio=0.5),
- dict(type='Normalize', **img_norm_cfg),
- dict(type='Pad', size_divisor=32),
- dict(type='DefaultFormatBundle'),
- dict(type='Collect', keys=['img', 'proposals', 'gt_bboxes', 'gt_labels']),
-]
-test_pipeline = [
- dict(type='LoadImageFromFile'),
- dict(type='LoadProposals', num_max_proposals=None),
- dict(
- type='MultiScaleFlipAug',
- img_scale=(1333, 800),
- flip=False,
- transforms=[
- dict(type='Resize', keep_ratio=True),
- dict(type='RandomFlip'),
- dict(type='Normalize', **img_norm_cfg),
- dict(type='Pad', size_divisor=32),
- dict(type='ImageToTensor', keys=['img']),
- dict(type='ToTensor', keys=['proposals']),
- dict(
- type='ToDataContainer',
- fields=[dict(key='proposals', stack=False)]),
- dict(type='Collect', keys=['img', 'proposals']),
- ])
-]
-data = dict(
- samples_per_gpu=2,
- workers_per_gpu=2,
- train=dict(
- proposal_file=data_root + 'proposals/rpn_r50_fpn_1x_train2017.pkl',
- pipeline=train_pipeline),
- val=dict(
- proposal_file=data_root + 'proposals/rpn_r50_fpn_1x_val2017.pkl',
- pipeline=test_pipeline),
- test=dict(
- proposal_file=data_root + 'proposals/rpn_r50_fpn_1x_val2017.pkl',
- pipeline=test_pipeline))
diff --git a/spaces/Andy1621/uniformer_image_detection/configs/lvis/mask_rcnn_r101_fpn_sample1e-3_mstrain_2x_lvis_v0.5.py b/spaces/Andy1621/uniformer_image_detection/configs/lvis/mask_rcnn_r101_fpn_sample1e-3_mstrain_2x_lvis_v0.5.py
deleted file mode 100644
index 2d2816c2dee68b60376e67e78e9fba277da826c0..0000000000000000000000000000000000000000
--- a/spaces/Andy1621/uniformer_image_detection/configs/lvis/mask_rcnn_r101_fpn_sample1e-3_mstrain_2x_lvis_v0.5.py
+++ /dev/null
@@ -1,2 +0,0 @@
-_base_ = './mask_rcnn_r50_fpn_sample1e-3_mstrain_2x_lvis_v0.5.py'
-model = dict(pretrained='torchvision://resnet101', backbone=dict(depth=101))
diff --git a/spaces/Andy1621/uniformer_image_detection/configs/swin/cascade_mask_rcnn_swin_tiny_patch4_window7_mstrain_480-800_giou_4conv1f_adamw_3x_coco.py b/spaces/Andy1621/uniformer_image_detection/configs/swin/cascade_mask_rcnn_swin_tiny_patch4_window7_mstrain_480-800_giou_4conv1f_adamw_3x_coco.py
deleted file mode 100644
index e01a9eff6197fb80e3a541910c9b94c00510323e..0000000000000000000000000000000000000000
--- a/spaces/Andy1621/uniformer_image_detection/configs/swin/cascade_mask_rcnn_swin_tiny_patch4_window7_mstrain_480-800_giou_4conv1f_adamw_3x_coco.py
+++ /dev/null
@@ -1,140 +0,0 @@
-_base_ = [
- '../_base_/models/cascade_mask_rcnn_swin_fpn.py',
- '../_base_/datasets/coco_instance.py',
- '../_base_/schedules/schedule_1x.py', '../_base_/default_runtime.py'
-]
-
-model = dict(
- backbone=dict(
- embed_dim=96,
- depths=[2, 2, 6, 2],
- num_heads=[3, 6, 12, 24],
- window_size=7,
- ape=False,
- drop_path_rate=0.2,
- patch_norm=True,
- use_checkpoint=False
- ),
- neck=dict(in_channels=[96, 192, 384, 768]),
- roi_head=dict(
- bbox_head=[
- dict(
- type='ConvFCBBoxHead',
- num_shared_convs=4,
- num_shared_fcs=1,
- in_channels=256,
- conv_out_channels=256,
- fc_out_channels=1024,
- roi_feat_size=7,
- num_classes=80,
- bbox_coder=dict(
- type='DeltaXYWHBBoxCoder',
- target_means=[0., 0., 0., 0.],
- target_stds=[0.1, 0.1, 0.2, 0.2]),
- reg_class_agnostic=False,
- reg_decoded_bbox=True,
- norm_cfg=dict(type='SyncBN', requires_grad=True),
- loss_cls=dict(
- type='CrossEntropyLoss', use_sigmoid=False, loss_weight=1.0),
- loss_bbox=dict(type='GIoULoss', loss_weight=10.0)),
- dict(
- type='ConvFCBBoxHead',
- num_shared_convs=4,
- num_shared_fcs=1,
- in_channels=256,
- conv_out_channels=256,
- fc_out_channels=1024,
- roi_feat_size=7,
- num_classes=80,
- bbox_coder=dict(
- type='DeltaXYWHBBoxCoder',
- target_means=[0., 0., 0., 0.],
- target_stds=[0.05, 0.05, 0.1, 0.1]),
- reg_class_agnostic=False,
- reg_decoded_bbox=True,
- norm_cfg=dict(type='SyncBN', requires_grad=True),
- loss_cls=dict(
- type='CrossEntropyLoss', use_sigmoid=False, loss_weight=1.0),
- loss_bbox=dict(type='GIoULoss', loss_weight=10.0)),
- dict(
- type='ConvFCBBoxHead',
- num_shared_convs=4,
- num_shared_fcs=1,
- in_channels=256,
- conv_out_channels=256,
- fc_out_channels=1024,
- roi_feat_size=7,
- num_classes=80,
- bbox_coder=dict(
- type='DeltaXYWHBBoxCoder',
- target_means=[0., 0., 0., 0.],
- target_stds=[0.033, 0.033, 0.067, 0.067]),
- reg_class_agnostic=False,
- reg_decoded_bbox=True,
- norm_cfg=dict(type='SyncBN', requires_grad=True),
- loss_cls=dict(
- type='CrossEntropyLoss', use_sigmoid=False, loss_weight=1.0),
- loss_bbox=dict(type='GIoULoss', loss_weight=10.0))
- ]))
-
-img_norm_cfg = dict(
- mean=[123.675, 116.28, 103.53], std=[58.395, 57.12, 57.375], to_rgb=True)
-
-# augmentation strategy originates from DETR / Sparse RCNN
-train_pipeline = [
- dict(type='LoadImageFromFile'),
- dict(type='LoadAnnotations', with_bbox=True, with_mask=True),
- dict(type='RandomFlip', flip_ratio=0.5),
- dict(type='AutoAugment',
- policies=[
- [
- dict(type='Resize',
- img_scale=[(480, 1333), (512, 1333), (544, 1333), (576, 1333),
- (608, 1333), (640, 1333), (672, 1333), (704, 1333),
- (736, 1333), (768, 1333), (800, 1333)],
- multiscale_mode='value',
- keep_ratio=True)
- ],
- [
- dict(type='Resize',
- img_scale=[(400, 1333), (500, 1333), (600, 1333)],
- multiscale_mode='value',
- keep_ratio=True),
- dict(type='RandomCrop',
- crop_type='absolute_range',
- crop_size=(384, 600),
- allow_negative_crop=True),
- dict(type='Resize',
- img_scale=[(480, 1333), (512, 1333), (544, 1333),
- (576, 1333), (608, 1333), (640, 1333),
- (672, 1333), (704, 1333), (736, 1333),
- (768, 1333), (800, 1333)],
- multiscale_mode='value',
- override=True,
- keep_ratio=True)
- ]
- ]),
- dict(type='Normalize', **img_norm_cfg),
- dict(type='Pad', size_divisor=32),
- dict(type='DefaultFormatBundle'),
- dict(type='Collect', keys=['img', 'gt_bboxes', 'gt_labels', 'gt_masks']),
-]
-data = dict(train=dict(pipeline=train_pipeline))
-
-optimizer = dict(_delete_=True, type='AdamW', lr=0.0001, betas=(0.9, 0.999), weight_decay=0.05,
- paramwise_cfg=dict(custom_keys={'absolute_pos_embed': dict(decay_mult=0.),
- 'relative_position_bias_table': dict(decay_mult=0.),
- 'norm': dict(decay_mult=0.)}))
-lr_config = dict(step=[27, 33])
-runner = dict(type='EpochBasedRunnerAmp', max_epochs=36)
-
-# do not use mmdet version fp16
-fp16 = None
-optimizer_config = dict(
- type="DistOptimizerHook",
- update_interval=1,
- grad_clip=None,
- coalesce=True,
- bucket_size_mb=-1,
- use_fp16=True,
-)
diff --git a/spaces/Ariharasudhan/YoloV5/utils/torch_utils.py b/spaces/Ariharasudhan/YoloV5/utils/torch_utils.py
deleted file mode 100644
index 04a3873854ee03f3eef260d8139dafc46fc69988..0000000000000000000000000000000000000000
--- a/spaces/Ariharasudhan/YoloV5/utils/torch_utils.py
+++ /dev/null
@@ -1,431 +0,0 @@
-# YOLOv5 🚀 by Ultralytics, GPL-3.0 license
-"""
-PyTorch utils
-"""
-
-import math
-import os
-import platform
-import subprocess
-import time
-import warnings
-from contextlib import contextmanager
-from copy import deepcopy
-from pathlib import Path
-
-import torch
-import torch.distributed as dist
-import torch.nn as nn
-import torch.nn.functional as F
-from torch.nn.parallel import DistributedDataParallel as DDP
-
-from utils.general import LOGGER, check_version, colorstr, file_date, git_describe
-
-LOCAL_RANK = int(os.getenv('LOCAL_RANK', -1)) # https://pytorch.org/docs/stable/elastic/run.html
-RANK = int(os.getenv('RANK', -1))
-WORLD_SIZE = int(os.getenv('WORLD_SIZE', 1))
-
-try:
- import thop # for FLOPs computation
-except ImportError:
- thop = None
-
-# Suppress PyTorch warnings
-warnings.filterwarnings('ignore', message='User provided device_type of \'cuda\', but CUDA is not available. Disabling')
-
-
-def smart_inference_mode(torch_1_9=check_version(torch.__version__, '1.9.0')):
- # Applies torch.inference_mode() decorator if torch>=1.9.0 else torch.no_grad() decorator
- def decorate(fn):
- return (torch.inference_mode if torch_1_9 else torch.no_grad)()(fn)
-
- return decorate
-
-
-def smartCrossEntropyLoss(label_smoothing=0.0):
- # Returns nn.CrossEntropyLoss with label smoothing enabled for torch>=1.10.0
- if check_version(torch.__version__, '1.10.0'):
- return nn.CrossEntropyLoss(label_smoothing=label_smoothing)
- if label_smoothing > 0:
- LOGGER.warning(f'WARNING ⚠️ label smoothing {label_smoothing} requires torch>=1.10.0')
- return nn.CrossEntropyLoss()
-
-
-def smart_DDP(model):
- # Model DDP creation with checks
- assert not check_version(torch.__version__, '1.12.0', pinned=True), \
- 'torch==1.12.0 torchvision==0.13.0 DDP training is not supported due to a known issue. ' \
- 'Please upgrade or downgrade torch to use DDP. See https://github.com/ultralytics/yolov5/issues/8395'
- if check_version(torch.__version__, '1.11.0'):
- return DDP(model, device_ids=[LOCAL_RANK], output_device=LOCAL_RANK, static_graph=True)
- else:
- return DDP(model, device_ids=[LOCAL_RANK], output_device=LOCAL_RANK)
-
-
-def reshape_classifier_output(model, n=1000):
- # Update a TorchVision classification model to class count 'n' if required
- from models.common import Classify
- name, m = list((model.model if hasattr(model, 'model') else model).named_children())[-1] # last module
- if isinstance(m, Classify): # YOLOv5 Classify() head
- if m.linear.out_features != n:
- m.linear = nn.Linear(m.linear.in_features, n)
- elif isinstance(m, nn.Linear): # ResNet, EfficientNet
- if m.out_features != n:
- setattr(model, name, nn.Linear(m.in_features, n))
- elif isinstance(m, nn.Sequential):
- types = [type(x) for x in m]
- if nn.Linear in types:
- i = types.index(nn.Linear) # nn.Linear index
- if m[i].out_features != n:
- m[i] = nn.Linear(m[i].in_features, n)
- elif nn.Conv2d in types:
- i = types.index(nn.Conv2d) # nn.Conv2d index
- if m[i].out_channels != n:
- m[i] = nn.Conv2d(m[i].in_channels, n, m[i].kernel_size, m[i].stride, bias=m[i].bias)
-
-
-@contextmanager
-def torch_distributed_zero_first(local_rank: int):
- # Decorator to make all processes in distributed training wait for each local_master to do something
- if local_rank not in [-1, 0]:
- dist.barrier(device_ids=[local_rank])
- yield
- if local_rank == 0:
- dist.barrier(device_ids=[0])
-
-
-def device_count():
- # Returns number of CUDA devices available. Safe version of torch.cuda.device_count(). Supports Linux and Windows
- assert platform.system() in ('Linux', 'Windows'), 'device_count() only supported on Linux or Windows'
- try:
- cmd = 'nvidia-smi -L | wc -l' if platform.system() == 'Linux' else 'nvidia-smi -L | find /c /v ""' # Windows
- return int(subprocess.run(cmd, shell=True, capture_output=True, check=True).stdout.decode().split()[-1])
- except Exception:
- return 0
-
-
-def select_device(device='', batch_size=0, newline=True):
- # device = None or 'cpu' or 0 or '0' or '0,1,2,3'
- s = f'YOLOv5 🚀 {git_describe() or file_date()} Python-{platform.python_version()} torch-{torch.__version__} '
- device = str(device).strip().lower().replace('cuda:', '').replace('none', '') # to string, 'cuda:0' to '0'
- cpu = device == 'cpu'
- mps = device == 'mps' # Apple Metal Performance Shaders (MPS)
- if cpu or mps:
- os.environ['CUDA_VISIBLE_DEVICES'] = '-1' # force torch.cuda.is_available() = False
- elif device: # non-cpu device requested
- os.environ['CUDA_VISIBLE_DEVICES'] = device # set environment variable - must be before assert is_available()
- assert torch.cuda.is_available() and torch.cuda.device_count() >= len(device.replace(',', '')), \
- f"Invalid CUDA '--device {device}' requested, use '--device cpu' or pass valid CUDA device(s)"
-
- if not cpu and not mps and torch.cuda.is_available(): # prefer GPU if available
- devices = device.split(',') if device else '0' # range(torch.cuda.device_count()) # i.e. 0,1,6,7
- n = len(devices) # device count
- if n > 1 and batch_size > 0: # check batch_size is divisible by device_count
- assert batch_size % n == 0, f'batch-size {batch_size} not multiple of GPU count {n}'
- space = ' ' * (len(s) + 1)
- for i, d in enumerate(devices):
- p = torch.cuda.get_device_properties(i)
- s += f"{'' if i == 0 else space}CUDA:{d} ({p.name}, {p.total_memory / (1 << 20):.0f}MiB)\n" # bytes to MB
- arg = 'cuda:0'
- elif mps and getattr(torch, 'has_mps', False) and torch.backends.mps.is_available(): # prefer MPS if available
- s += 'MPS\n'
- arg = 'mps'
- else: # revert to CPU
- s += 'CPU\n'
- arg = 'cpu'
-
- if not newline:
- s = s.rstrip()
- LOGGER.info(s)
- return torch.device(arg)
-
-
-def time_sync():
- # PyTorch-accurate time
- if torch.cuda.is_available():
- torch.cuda.synchronize()
- return time.time()
-
-
-def profile(input, ops, n=10, device=None):
- """ YOLOv5 speed/memory/FLOPs profiler
- Usage:
- input = torch.randn(16, 3, 640, 640)
- m1 = lambda x: x * torch.sigmoid(x)
- m2 = nn.SiLU()
- profile(input, [m1, m2], n=100) # profile over 100 iterations
- """
- results = []
- if not isinstance(device, torch.device):
- device = select_device(device)
- print(f"{'Params':>12s}{'GFLOPs':>12s}{'GPU_mem (GB)':>14s}{'forward (ms)':>14s}{'backward (ms)':>14s}"
- f"{'input':>24s}{'output':>24s}")
-
- for x in input if isinstance(input, list) else [input]:
- x = x.to(device)
- x.requires_grad = True
- for m in ops if isinstance(ops, list) else [ops]:
- m = m.to(device) if hasattr(m, 'to') else m # device
- m = m.half() if hasattr(m, 'half') and isinstance(x, torch.Tensor) and x.dtype is torch.float16 else m
- tf, tb, t = 0, 0, [0, 0, 0] # dt forward, backward
- try:
- flops = thop.profile(m, inputs=(x,), verbose=False)[0] / 1E9 * 2 # GFLOPs
- except Exception:
- flops = 0
-
- try:
- for _ in range(n):
- t[0] = time_sync()
- y = m(x)
- t[1] = time_sync()
- try:
- _ = (sum(yi.sum() for yi in y) if isinstance(y, list) else y).sum().backward()
- t[2] = time_sync()
- except Exception: # no backward method
- # print(e) # for debug
- t[2] = float('nan')
- tf += (t[1] - t[0]) * 1000 / n # ms per op forward
- tb += (t[2] - t[1]) * 1000 / n # ms per op backward
- mem = torch.cuda.memory_reserved() / 1E9 if torch.cuda.is_available() else 0 # (GB)
- s_in, s_out = (tuple(x.shape) if isinstance(x, torch.Tensor) else 'list' for x in (x, y)) # shapes
- p = sum(x.numel() for x in m.parameters()) if isinstance(m, nn.Module) else 0 # parameters
- print(f'{p:12}{flops:12.4g}{mem:>14.3f}{tf:14.4g}{tb:14.4g}{str(s_in):>24s}{str(s_out):>24s}')
- results.append([p, flops, mem, tf, tb, s_in, s_out])
- except Exception as e:
- print(e)
- results.append(None)
- torch.cuda.empty_cache()
- return results
-
-
-def is_parallel(model):
- # Returns True if model is of type DP or DDP
- return type(model) in (nn.parallel.DataParallel, nn.parallel.DistributedDataParallel)
-
-
-def de_parallel(model):
- # De-parallelize a model: returns single-GPU model if model is of type DP or DDP
- return model.module if is_parallel(model) else model
-
-
-def initialize_weights(model):
- for m in model.modules():
- t = type(m)
- if t is nn.Conv2d:
- pass # nn.init.kaiming_normal_(m.weight, mode='fan_out', nonlinearity='relu')
- elif t is nn.BatchNorm2d:
- m.eps = 1e-3
- m.momentum = 0.03
- elif t in [nn.Hardswish, nn.LeakyReLU, nn.ReLU, nn.ReLU6, nn.SiLU]:
- m.inplace = True
-
-
-def find_modules(model, mclass=nn.Conv2d):
- # Finds layer indices matching module class 'mclass'
- return [i for i, m in enumerate(model.module_list) if isinstance(m, mclass)]
-
-
-def sparsity(model):
- # Return global model sparsity
- a, b = 0, 0
- for p in model.parameters():
- a += p.numel()
- b += (p == 0).sum()
- return b / a
-
-
-def prune(model, amount=0.3):
- # Prune model to requested global sparsity
- import torch.nn.utils.prune as prune
- for name, m in model.named_modules():
- if isinstance(m, nn.Conv2d):
- prune.l1_unstructured(m, name='weight', amount=amount) # prune
- prune.remove(m, 'weight') # make permanent
- LOGGER.info(f'Model pruned to {sparsity(model):.3g} global sparsity')
-
-
-def fuse_conv_and_bn(conv, bn):
- # Fuse Conv2d() and BatchNorm2d() layers https://tehnokv.com/posts/fusing-batchnorm-and-conv/
- fusedconv = nn.Conv2d(conv.in_channels,
- conv.out_channels,
- kernel_size=conv.kernel_size,
- stride=conv.stride,
- padding=conv.padding,
- dilation=conv.dilation,
- groups=conv.groups,
- bias=True).requires_grad_(False).to(conv.weight.device)
-
- # Prepare filters
- w_conv = conv.weight.clone().view(conv.out_channels, -1)
- w_bn = torch.diag(bn.weight.div(torch.sqrt(bn.eps + bn.running_var)))
- fusedconv.weight.copy_(torch.mm(w_bn, w_conv).view(fusedconv.weight.shape))
-
- # Prepare spatial bias
- b_conv = torch.zeros(conv.weight.size(0), device=conv.weight.device) if conv.bias is None else conv.bias
- b_bn = bn.bias - bn.weight.mul(bn.running_mean).div(torch.sqrt(bn.running_var + bn.eps))
- fusedconv.bias.copy_(torch.mm(w_bn, b_conv.reshape(-1, 1)).reshape(-1) + b_bn)
-
- return fusedconv
-
-
-def model_info(model, verbose=False, imgsz=640):
- # Model information. img_size may be int or list, i.e. img_size=640 or img_size=[640, 320]
- n_p = sum(x.numel() for x in model.parameters()) # number parameters
- n_g = sum(x.numel() for x in model.parameters() if x.requires_grad) # number gradients
- if verbose:
- print(f"{'layer':>5} {'name':>40} {'gradient':>9} {'parameters':>12} {'shape':>20} {'mu':>10} {'sigma':>10}")
- for i, (name, p) in enumerate(model.named_parameters()):
- name = name.replace('module_list.', '')
- print('%5g %40s %9s %12g %20s %10.3g %10.3g' %
- (i, name, p.requires_grad, p.numel(), list(p.shape), p.mean(), p.std()))
-
- try: # FLOPs
- p = next(model.parameters())
- stride = max(int(model.stride.max()), 32) if hasattr(model, 'stride') else 32 # max stride
- im = torch.empty((1, p.shape[1], stride, stride), device=p.device) # input image in BCHW format
- flops = thop.profile(deepcopy(model), inputs=(im,), verbose=False)[0] / 1E9 * 2 # stride GFLOPs
- imgsz = imgsz if isinstance(imgsz, list) else [imgsz, imgsz] # expand if int/float
- fs = f', {flops * imgsz[0] / stride * imgsz[1] / stride:.1f} GFLOPs' # 640x640 GFLOPs
- except Exception:
- fs = ''
-
- name = Path(model.yaml_file).stem.replace('yolov5', 'YOLOv5') if hasattr(model, 'yaml_file') else 'Model'
- LOGGER.info(f"{name} summary: {len(list(model.modules()))} layers, {n_p} parameters, {n_g} gradients{fs}")
-
-
-def scale_img(img, ratio=1.0, same_shape=False, gs=32): # img(16,3,256,416)
- # Scales img(bs,3,y,x) by ratio constrained to gs-multiple
- if ratio == 1.0:
- return img
- h, w = img.shape[2:]
- s = (int(h * ratio), int(w * ratio)) # new size
- img = F.interpolate(img, size=s, mode='bilinear', align_corners=False) # resize
- if not same_shape: # pad/crop img
- h, w = (math.ceil(x * ratio / gs) * gs for x in (h, w))
- return F.pad(img, [0, w - s[1], 0, h - s[0]], value=0.447) # value = imagenet mean
-
-
-def copy_attr(a, b, include=(), exclude=()):
- # Copy attributes from b to a, options to only include [...] and to exclude [...]
- for k, v in b.__dict__.items():
- if (len(include) and k not in include) or k.startswith('_') or k in exclude:
- continue
- else:
- setattr(a, k, v)
-
-
-def smart_optimizer(model, name='Adam', lr=0.001, momentum=0.9, decay=1e-5):
- # YOLOv5 3-param group optimizer: 0) weights with decay, 1) weights no decay, 2) biases no decay
- g = [], [], [] # optimizer parameter groups
- bn = tuple(v for k, v in nn.__dict__.items() if 'Norm' in k) # normalization layers, i.e. BatchNorm2d()
- for v in model.modules():
- for p_name, p in v.named_parameters(recurse=0):
- if p_name == 'bias': # bias (no decay)
- g[2].append(p)
- elif p_name == 'weight' and isinstance(v, bn): # weight (no decay)
- g[1].append(p)
- else:
- g[0].append(p) # weight (with decay)
-
- if name == 'Adam':
- optimizer = torch.optim.Adam(g[2], lr=lr, betas=(momentum, 0.999)) # adjust beta1 to momentum
- elif name == 'AdamW':
- optimizer = torch.optim.AdamW(g[2], lr=lr, betas=(momentum, 0.999), weight_decay=0.0)
- elif name == 'RMSProp':
- optimizer = torch.optim.RMSprop(g[2], lr=lr, momentum=momentum)
- elif name == 'SGD':
- optimizer = torch.optim.SGD(g[2], lr=lr, momentum=momentum, nesterov=True)
- else:
- raise NotImplementedError(f'Optimizer {name} not implemented.')
-
- optimizer.add_param_group({'params': g[0], 'weight_decay': decay}) # add g0 with weight_decay
- optimizer.add_param_group({'params': g[1], 'weight_decay': 0.0}) # add g1 (BatchNorm2d weights)
- LOGGER.info(f"{colorstr('optimizer:')} {type(optimizer).__name__}(lr={lr}) with parameter groups "
- f"{len(g[1])} weight(decay=0.0), {len(g[0])} weight(decay={decay}), {len(g[2])} bias")
- return optimizer
-
-
-def smart_hub_load(repo='ultralytics/yolov5', model='yolov5s', **kwargs):
- # YOLOv5 torch.hub.load() wrapper with smart error/issue handling
- if check_version(torch.__version__, '1.9.1'):
- kwargs['skip_validation'] = True # validation causes GitHub API rate limit errors
- if check_version(torch.__version__, '1.12.0'):
- kwargs['trust_repo'] = True # argument required starting in torch 0.12
- try:
- return torch.hub.load(repo, model, **kwargs)
- except Exception:
- return torch.hub.load(repo, model, force_reload=True, **kwargs)
-
-
-def smart_resume(ckpt, optimizer, ema=None, weights='yolov5s.pt', epochs=300, resume=True):
- # Resume training from a partially trained checkpoint
- best_fitness = 0.0
- start_epoch = ckpt['epoch'] + 1
- if ckpt['optimizer'] is not None:
- optimizer.load_state_dict(ckpt['optimizer']) # optimizer
- best_fitness = ckpt['best_fitness']
- if ema and ckpt.get('ema'):
- ema.ema.load_state_dict(ckpt['ema'].float().state_dict()) # EMA
- ema.updates = ckpt['updates']
- if resume:
- assert start_epoch > 0, f'{weights} training to {epochs} epochs is finished, nothing to resume.\n' \
- f"Start a new training without --resume, i.e. 'python train.py --weights {weights}'"
- LOGGER.info(f'Resuming training from {weights} from epoch {start_epoch} to {epochs} total epochs')
- if epochs < start_epoch:
- LOGGER.info(f"{weights} has been trained for {ckpt['epoch']} epochs. Fine-tuning for {epochs} more epochs.")
- epochs += ckpt['epoch'] # finetune additional epochs
- return best_fitness, start_epoch, epochs
-
-
-class EarlyStopping:
- # YOLOv5 simple early stopper
- def __init__(self, patience=30):
- self.best_fitness = 0.0 # i.e. mAP
- self.best_epoch = 0
- self.patience = patience or float('inf') # epochs to wait after fitness stops improving to stop
- self.possible_stop = False # possible stop may occur next epoch
-
- def __call__(self, epoch, fitness):
- if fitness >= self.best_fitness: # >= 0 to allow for early zero-fitness stage of training
- self.best_epoch = epoch
- self.best_fitness = fitness
- delta = epoch - self.best_epoch # epochs without improvement
- self.possible_stop = delta >= (self.patience - 1) # possible stop may occur next epoch
- stop = delta >= self.patience # stop training if patience exceeded
- if stop:
- LOGGER.info(f'Stopping training early as no improvement observed in last {self.patience} epochs. '
- f'Best results observed at epoch {self.best_epoch}, best model saved as best.pt.\n'
- f'To update EarlyStopping(patience={self.patience}) pass a new patience value, '
- f'i.e. `python train.py --patience 300` or use `--patience 0` to disable EarlyStopping.')
- return stop
-
-
-class ModelEMA:
- """ Updated Exponential Moving Average (EMA) from https://github.com/rwightman/pytorch-image-models
- Keeps a moving average of everything in the model state_dict (parameters and buffers)
- For EMA details see https://www.tensorflow.org/api_docs/python/tf/train/ExponentialMovingAverage
- """
-
- def __init__(self, model, decay=0.9999, tau=2000, updates=0):
- # Create EMA
- self.ema = deepcopy(de_parallel(model)).eval() # FP32 EMA
- self.updates = updates # number of EMA updates
- self.decay = lambda x: decay * (1 - math.exp(-x / tau)) # decay exponential ramp (to help early epochs)
- for p in self.ema.parameters():
- p.requires_grad_(False)
-
- def update(self, model):
- # Update EMA parameters
- self.updates += 1
- d = self.decay(self.updates)
-
- msd = de_parallel(model).state_dict() # model state_dict
- for k, v in self.ema.state_dict().items():
- if v.dtype.is_floating_point: # true for FP16 and FP32
- v *= d
- v += (1 - d) * msd[k].detach()
- # assert v.dtype == msd[k].dtype == torch.float32, f'{k}: EMA {v.dtype} and model {msd[k].dtype} must be FP32'
-
- def update_attr(self, model, include=(), exclude=('process_group', 'reducer')):
- # Update EMA attributes
- copy_attr(self.ema, model, include, exclude)
diff --git a/spaces/Arikkod/FoodVisionMini/model.py b/spaces/Arikkod/FoodVisionMini/model.py
deleted file mode 100644
index e3fa8e60f4f473ca4835708795c6ae77e7e5f143..0000000000000000000000000000000000000000
--- a/spaces/Arikkod/FoodVisionMini/model.py
+++ /dev/null
@@ -1,19 +0,0 @@
-import torch
-import torchvision
-import torch.nn as nn
-
-def create_effnetb2_model(num_classes:int=3, seed:int=3):
- weights = torchvision.models.EfficientNet_B2_Weights.DEFAULT
- transforms = weights.transforms()
- model = torchvision.models.efficientnet_b2(weights=weights)
-
- # Freeze the base layers in the model (this will stop all layers from training)
- for param in model.parameters():
- param.requires_grad = False
-
- torch.manual_seed(seed)
- model.classifier = nn.Sequential(
- nn.Dropout(p=0.3, inplace=True),
- nn.Linear(in_features=1408, out_features=num_classes, bias=True))
-
- return model, transforms
diff --git a/spaces/Artrajz/vits-simple-api/vits/text/cantonese.py b/spaces/Artrajz/vits-simple-api/vits/text/cantonese.py
deleted file mode 100644
index 656319181e2e1674e61f63b0a24a84895e4bf82b..0000000000000000000000000000000000000000
--- a/spaces/Artrajz/vits-simple-api/vits/text/cantonese.py
+++ /dev/null
@@ -1,75 +0,0 @@
-import os.path
-import re
-import cn2an
-import opencc
-import config
-from utils.download import download_and_verify
-
-URLS = [
- "https://github.com/CjangCjengh/chinese-dialect-lexicons/releases/download/v1.0.3/chinese_dialects.7z",
- "https://ghproxy.com/https://github.com/CjangCjengh/chinese-dialect-lexicons/releases/download/v1.0.3/chinese_dialects.7z",
-]
-TARGET_PATH = os.path.join(config.ABS_PATH, "vits/text/chinese_dialects.7z")
-EXTRACT_DESTINATION = os.path.join(config.ABS_PATH, "vits/text/chinese_dialect_lexicons/")
-EXPECTED_MD5 = None
-OPENCC_FILE_PATH = os.path.join(config.ABS_PATH, "vits/text/chinese_dialect_lexicons/jyutjyu.json")
-
-if not os.path.exists(OPENCC_FILE_PATH):
- success, message = download_and_verify(URLS, TARGET_PATH, EXPECTED_MD5, EXTRACT_DESTINATION)
-
-converter = opencc.OpenCC(OPENCC_FILE_PATH)
-
-# List of (Latin alphabet, ipa) pairs:
-_latin_to_ipa = [(re.compile('%s' % x[0]), x[1]) for x in [
- ('A', 'ei˥'),
- ('B', 'biː˥'),
- ('C', 'siː˥'),
- ('D', 'tiː˥'),
- ('E', 'iː˥'),
- ('F', 'e˥fuː˨˩'),
- ('G', 'tsiː˥'),
- ('H', 'ɪk̚˥tsʰyː˨˩'),
- ('I', 'ɐi˥'),
- ('J', 'tsei˥'),
- ('K', 'kʰei˥'),
- ('L', 'e˥llou˨˩'),
- ('M', 'ɛːm˥'),
- ('N', 'ɛːn˥'),
- ('O', 'ou˥'),
- ('P', 'pʰiː˥'),
- ('Q', 'kʰiːu˥'),
- ('R', 'aː˥lou˨˩'),
- ('S', 'ɛː˥siː˨˩'),
- ('T', 'tʰiː˥'),
- ('U', 'juː˥'),
- ('V', 'wiː˥'),
- ('W', 'tʊk̚˥piː˥juː˥'),
- ('X', 'ɪk̚˥siː˨˩'),
- ('Y', 'waːi˥'),
- ('Z', 'iː˨sɛːt̚˥')
-]]
-
-
-def number_to_cantonese(text):
- return re.sub(r'\d+(?:\.?\d+)?', lambda x: cn2an.an2cn(x.group()), text)
-
-
-def latin_to_ipa(text):
- for regex, replacement in _latin_to_ipa:
- text = re.sub(regex, replacement, text)
- return text
-
-
-def cantonese_to_ipa(text):
- from vits.text.mandarin import symbols_to_chinese
- text = symbols_to_chinese(text)
- text = number_to_cantonese(text.upper())
- text = converter.convert(text).replace('-', '').replace('$', ' ')
- text = re.sub(r'[A-Z]', lambda x: latin_to_ipa(x.group()) + ' ', text)
- text = re.sub(r'[、;:]', ',', text)
- text = re.sub(r'\s*,\s*', ', ', text)
- text = re.sub(r'\s*。\s*', '. ', text)
- text = re.sub(r'\s*?\s*', '? ', text)
- text = re.sub(r'\s*!\s*', '! ', text)
- text = re.sub(r'\s*$', '', text)
- return text
diff --git a/spaces/Ataturk-Chatbot/HuggingFaceChat/venv/lib/python3.11/site-packages/pip/_vendor/pygments/formatters/svg.py b/spaces/Ataturk-Chatbot/HuggingFaceChat/venv/lib/python3.11/site-packages/pip/_vendor/pygments/formatters/svg.py
deleted file mode 100644
index 075150a4b586d668c1666513fbf90463cdbb11ab..0000000000000000000000000000000000000000
--- a/spaces/Ataturk-Chatbot/HuggingFaceChat/venv/lib/python3.11/site-packages/pip/_vendor/pygments/formatters/svg.py
+++ /dev/null
@@ -1,188 +0,0 @@
-"""
- pygments.formatters.svg
- ~~~~~~~~~~~~~~~~~~~~~~~
-
- Formatter for SVG output.
-
- :copyright: Copyright 2006-2022 by the Pygments team, see AUTHORS.
- :license: BSD, see LICENSE for details.
-"""
-
-from pip._vendor.pygments.formatter import Formatter
-from pip._vendor.pygments.token import Comment
-from pip._vendor.pygments.util import get_bool_opt, get_int_opt
-
-__all__ = ['SvgFormatter']
-
-
-def escape_html(text):
- """Escape &, <, > as well as single and double quotes for HTML."""
- return text.replace('&', '&'). \
- replace('<', '<'). \
- replace('>', '>'). \
- replace('"', '"'). \
- replace("'", ''')
-
-
-class2style = {}
-
-class SvgFormatter(Formatter):
- """
- Format tokens as an SVG graphics file. This formatter is still experimental.
- Each line of code is a ```` element with explicit ``x`` and ``y``
- coordinates containing ```` elements with the individual token styles.
-
- By default, this formatter outputs a full SVG document including doctype
- declaration and the ``
-
¿Cómo puedo cambiar mi nombre o avatar en el multijugador de estacionamiento?
-
Puedes cambiar tu nombre o avatar en el multijugador de estacionamiento haciendo lo siguiente:
-
-
Pulsando en el icono de perfil en la esquina superior izquierda de la pantalla
-
Pulsando en el icono de edición en la esquina superior derecha de la pantalla
-
Introducir un nuevo nombre o elegir un nuevo avatar de la lista
-
Pulsando en el icono de guardar en la esquina superior derecha de la pantalla
-
-
¿Cómo puedo reportar un error o un problema en el multijugador de estacionamiento?
-
Puedes reportar un error o un problema en el multijugador de estacionamiento haciendo lo siguiente:
-
-
Pulsando en el icono de configuración en la esquina superior derecha de la pantalla
-
Pulsando en la opción de retroalimentación
-
Llenar el formulario con su nombre, correo electrónico, modelo de dispositivo, versión del juego, y la descripción de la cuestión
-
Pulsando en el botón enviar
-
-
¿Cómo puedo contactar a los desarrolladores del multijugador de aparcamiento?
-
Puede ponerse en contacto con los desarrolladores de aparcamiento multijugador haciendo lo siguiente:
-
-
Enviarlos por correo electrónico a olzhassgames@gmail.com
-
Visitar su sitio web en https:/olzhass.com/
-
Siguiéndolos en Facebook en https://www.facebook.com/olzhassgames/
-
Siguiéndolos en Instagram en https://www.instagram.com/olzhassgames/
-
Siguiéndolos en Twitter en https://twitter.com/olzhassgames
-
64aa2da5cf
-
-
\ No newline at end of file
diff --git a/spaces/Bidwill/Sanskrit-asr/app.py b/spaces/Bidwill/Sanskrit-asr/app.py
deleted file mode 100644
index 5f3120f3ea132d3c2fca77c8f541846cada8d421..0000000000000000000000000000000000000000
--- a/spaces/Bidwill/Sanskrit-asr/app.py
+++ /dev/null
@@ -1,33 +0,0 @@
-from transformers import pipeline
-import gradio as gr
-
-pipe = pipeline(model="Bidwill/whisper-small-sanskrit_4") # change to "your-username/the-name-you-picked"
-
-def transcribe(audio):
- text = pipe(audio)["text"]
- return text
-
-demo = gr.Blocks()
-
-mic_transcribe = gr.Interface(
- fn=transcribe,
- inputs=gr.Audio(source="microphone", type="filepath"),
- outputs="text",
- title="Sanskrit Speech to Text",
- description="Realtime demo for Sanskrit speech recognition.",
-)
-
-file_transcribe = gr.Interface(
- fn=transcribe,
- inputs=gr.Audio(source="upload", type="filepath"),
- outputs=gr.outputs.Textbox(),
- title="Sanskrit STT",
- description= "Realtime demo for Sanskrit speech recognition."
-)
-with demo:
- gr.TabbedInterface(
- [mic_transcribe, file_transcribe],
- ["Transcribe Microphone", "Transcribe Audio File"],
- )
-
-demo.launch()
\ No newline at end of file
diff --git a/spaces/Big-Web/MMSD/env/Lib/site-packages/pip/_vendor/requests/models.py b/spaces/Big-Web/MMSD/env/Lib/site-packages/pip/_vendor/requests/models.py
deleted file mode 100644
index 76e6f199c0042cec6500f53c062ff9ea1033e79d..0000000000000000000000000000000000000000
--- a/spaces/Big-Web/MMSD/env/Lib/site-packages/pip/_vendor/requests/models.py
+++ /dev/null
@@ -1,1034 +0,0 @@
-"""
-requests.models
-~~~~~~~~~~~~~~~
-
-This module contains the primary objects that power Requests.
-"""
-
-import datetime
-
-# Import encoding now, to avoid implicit import later.
-# Implicit import within threads may cause LookupError when standard library is in a ZIP,
-# such as in Embedded Python. See https://github.com/psf/requests/issues/3578.
-import encodings.idna # noqa: F401
-from io import UnsupportedOperation
-
-from pip._vendor.urllib3.exceptions import (
- DecodeError,
- LocationParseError,
- ProtocolError,
- ReadTimeoutError,
- SSLError,
-)
-from pip._vendor.urllib3.fields import RequestField
-from pip._vendor.urllib3.filepost import encode_multipart_formdata
-from pip._vendor.urllib3.util import parse_url
-
-from ._internal_utils import to_native_string, unicode_is_ascii
-from .auth import HTTPBasicAuth
-from .compat import (
- Callable,
- JSONDecodeError,
- Mapping,
- basestring,
- builtin_str,
- chardet,
- cookielib,
-)
-from .compat import json as complexjson
-from .compat import urlencode, urlsplit, urlunparse
-from .cookies import _copy_cookie_jar, cookiejar_from_dict, get_cookie_header
-from .exceptions import (
- ChunkedEncodingError,
- ConnectionError,
- ContentDecodingError,
- HTTPError,
- InvalidJSONError,
- InvalidURL,
-)
-from .exceptions import JSONDecodeError as RequestsJSONDecodeError
-from .exceptions import MissingSchema
-from .exceptions import SSLError as RequestsSSLError
-from .exceptions import StreamConsumedError
-from .hooks import default_hooks
-from .status_codes import codes
-from .structures import CaseInsensitiveDict
-from .utils import (
- check_header_validity,
- get_auth_from_url,
- guess_filename,
- guess_json_utf,
- iter_slices,
- parse_header_links,
- requote_uri,
- stream_decode_response_unicode,
- super_len,
- to_key_val_list,
-)
-
-#: The set of HTTP status codes that indicate an automatically
-#: processable redirect.
-REDIRECT_STATI = (
- codes.moved, # 301
- codes.found, # 302
- codes.other, # 303
- codes.temporary_redirect, # 307
- codes.permanent_redirect, # 308
-)
-
-DEFAULT_REDIRECT_LIMIT = 30
-CONTENT_CHUNK_SIZE = 10 * 1024
-ITER_CHUNK_SIZE = 512
-
-
-class RequestEncodingMixin:
- @property
- def path_url(self):
- """Build the path URL to use."""
-
- url = []
-
- p = urlsplit(self.url)
-
- path = p.path
- if not path:
- path = "/"
-
- url.append(path)
-
- query = p.query
- if query:
- url.append("?")
- url.append(query)
-
- return "".join(url)
-
- @staticmethod
- def _encode_params(data):
- """Encode parameters in a piece of data.
-
- Will successfully encode parameters when passed as a dict or a list of
- 2-tuples. Order is retained if data is a list of 2-tuples but arbitrary
- if parameters are supplied as a dict.
- """
-
- if isinstance(data, (str, bytes)):
- return data
- elif hasattr(data, "read"):
- return data
- elif hasattr(data, "__iter__"):
- result = []
- for k, vs in to_key_val_list(data):
- if isinstance(vs, basestring) or not hasattr(vs, "__iter__"):
- vs = [vs]
- for v in vs:
- if v is not None:
- result.append(
- (
- k.encode("utf-8") if isinstance(k, str) else k,
- v.encode("utf-8") if isinstance(v, str) else v,
- )
- )
- return urlencode(result, doseq=True)
- else:
- return data
-
- @staticmethod
- def _encode_files(files, data):
- """Build the body for a multipart/form-data request.
-
- Will successfully encode files when passed as a dict or a list of
- tuples. Order is retained if data is a list of tuples but arbitrary
- if parameters are supplied as a dict.
- The tuples may be 2-tuples (filename, fileobj), 3-tuples (filename, fileobj, contentype)
- or 4-tuples (filename, fileobj, contentype, custom_headers).
- """
- if not files:
- raise ValueError("Files must be provided.")
- elif isinstance(data, basestring):
- raise ValueError("Data must not be a string.")
-
- new_fields = []
- fields = to_key_val_list(data or {})
- files = to_key_val_list(files or {})
-
- for field, val in fields:
- if isinstance(val, basestring) or not hasattr(val, "__iter__"):
- val = [val]
- for v in val:
- if v is not None:
- # Don't call str() on bytestrings: in Py3 it all goes wrong.
- if not isinstance(v, bytes):
- v = str(v)
-
- new_fields.append(
- (
- field.decode("utf-8")
- if isinstance(field, bytes)
- else field,
- v.encode("utf-8") if isinstance(v, str) else v,
- )
- )
-
- for (k, v) in files:
- # support for explicit filename
- ft = None
- fh = None
- if isinstance(v, (tuple, list)):
- if len(v) == 2:
- fn, fp = v
- elif len(v) == 3:
- fn, fp, ft = v
- else:
- fn, fp, ft, fh = v
- else:
- fn = guess_filename(v) or k
- fp = v
-
- if isinstance(fp, (str, bytes, bytearray)):
- fdata = fp
- elif hasattr(fp, "read"):
- fdata = fp.read()
- elif fp is None:
- continue
- else:
- fdata = fp
-
- rf = RequestField(name=k, data=fdata, filename=fn, headers=fh)
- rf.make_multipart(content_type=ft)
- new_fields.append(rf)
-
- body, content_type = encode_multipart_formdata(new_fields)
-
- return body, content_type
-
-
-class RequestHooksMixin:
- def register_hook(self, event, hook):
- """Properly register a hook."""
-
- if event not in self.hooks:
- raise ValueError(f'Unsupported event specified, with event name "{event}"')
-
- if isinstance(hook, Callable):
- self.hooks[event].append(hook)
- elif hasattr(hook, "__iter__"):
- self.hooks[event].extend(h for h in hook if isinstance(h, Callable))
-
- def deregister_hook(self, event, hook):
- """Deregister a previously registered hook.
- Returns True if the hook existed, False if not.
- """
-
- try:
- self.hooks[event].remove(hook)
- return True
- except ValueError:
- return False
-
-
-class Request(RequestHooksMixin):
- """A user-created :class:`Request ` object.
-
- Used to prepare a :class:`PreparedRequest `, which is sent to the server.
-
- :param method: HTTP method to use.
- :param url: URL to send.
- :param headers: dictionary of headers to send.
- :param files: dictionary of {filename: fileobject} files to multipart upload.
- :param data: the body to attach to the request. If a dictionary or
- list of tuples ``[(key, value)]`` is provided, form-encoding will
- take place.
- :param json: json for the body to attach to the request (if files or data is not specified).
- :param params: URL parameters to append to the URL. If a dictionary or
- list of tuples ``[(key, value)]`` is provided, form-encoding will
- take place.
- :param auth: Auth handler or (user, pass) tuple.
- :param cookies: dictionary or CookieJar of cookies to attach to this request.
- :param hooks: dictionary of callback hooks, for internal usage.
-
- Usage::
-
- >>> import requests
- >>> req = requests.Request('GET', 'https://httpbin.org/get')
- >>> req.prepare()
-
- """
-
- def __init__(
- self,
- method=None,
- url=None,
- headers=None,
- files=None,
- data=None,
- params=None,
- auth=None,
- cookies=None,
- hooks=None,
- json=None,
- ):
-
- # Default empty dicts for dict params.
- data = [] if data is None else data
- files = [] if files is None else files
- headers = {} if headers is None else headers
- params = {} if params is None else params
- hooks = {} if hooks is None else hooks
-
- self.hooks = default_hooks()
- for (k, v) in list(hooks.items()):
- self.register_hook(event=k, hook=v)
-
- self.method = method
- self.url = url
- self.headers = headers
- self.files = files
- self.data = data
- self.json = json
- self.params = params
- self.auth = auth
- self.cookies = cookies
-
- def __repr__(self):
- return f""
-
- def prepare(self):
- """Constructs a :class:`PreparedRequest ` for transmission and returns it."""
- p = PreparedRequest()
- p.prepare(
- method=self.method,
- url=self.url,
- headers=self.headers,
- files=self.files,
- data=self.data,
- json=self.json,
- params=self.params,
- auth=self.auth,
- cookies=self.cookies,
- hooks=self.hooks,
- )
- return p
-
-
-class PreparedRequest(RequestEncodingMixin, RequestHooksMixin):
- """The fully mutable :class:`PreparedRequest ` object,
- containing the exact bytes that will be sent to the server.
-
- Instances are generated from a :class:`Request ` object, and
- should not be instantiated manually; doing so may produce undesirable
- effects.
-
- Usage::
-
- >>> import requests
- >>> req = requests.Request('GET', 'https://httpbin.org/get')
- >>> r = req.prepare()
- >>> r
-
-
- >>> s = requests.Session()
- >>> s.send(r)
-
- """
-
- def __init__(self):
- #: HTTP verb to send to the server.
- self.method = None
- #: HTTP URL to send the request to.
- self.url = None
- #: dictionary of HTTP headers.
- self.headers = None
- # The `CookieJar` used to create the Cookie header will be stored here
- # after prepare_cookies is called
- self._cookies = None
- #: request body to send to the server.
- self.body = None
- #: dictionary of callback hooks, for internal usage.
- self.hooks = default_hooks()
- #: integer denoting starting position of a readable file-like body.
- self._body_position = None
-
- def prepare(
- self,
- method=None,
- url=None,
- headers=None,
- files=None,
- data=None,
- params=None,
- auth=None,
- cookies=None,
- hooks=None,
- json=None,
- ):
- """Prepares the entire request with the given parameters."""
-
- self.prepare_method(method)
- self.prepare_url(url, params)
- self.prepare_headers(headers)
- self.prepare_cookies(cookies)
- self.prepare_body(data, files, json)
- self.prepare_auth(auth, url)
-
- # Note that prepare_auth must be last to enable authentication schemes
- # such as OAuth to work on a fully prepared request.
-
- # This MUST go after prepare_auth. Authenticators could add a hook
- self.prepare_hooks(hooks)
-
- def __repr__(self):
- return f""
-
- def copy(self):
- p = PreparedRequest()
- p.method = self.method
- p.url = self.url
- p.headers = self.headers.copy() if self.headers is not None else None
- p._cookies = _copy_cookie_jar(self._cookies)
- p.body = self.body
- p.hooks = self.hooks
- p._body_position = self._body_position
- return p
-
- def prepare_method(self, method):
- """Prepares the given HTTP method."""
- self.method = method
- if self.method is not None:
- self.method = to_native_string(self.method.upper())
-
- @staticmethod
- def _get_idna_encoded_host(host):
- from pip._vendor import idna
-
- try:
- host = idna.encode(host, uts46=True).decode("utf-8")
- except idna.IDNAError:
- raise UnicodeError
- return host
-
- def prepare_url(self, url, params):
- """Prepares the given HTTP URL."""
- #: Accept objects that have string representations.
- #: We're unable to blindly call unicode/str functions
- #: as this will include the bytestring indicator (b'')
- #: on python 3.x.
- #: https://github.com/psf/requests/pull/2238
- if isinstance(url, bytes):
- url = url.decode("utf8")
- else:
- url = str(url)
-
- # Remove leading whitespaces from url
- url = url.lstrip()
-
- # Don't do any URL preparation for non-HTTP schemes like `mailto`,
- # `data` etc to work around exceptions from `url_parse`, which
- # handles RFC 3986 only.
- if ":" in url and not url.lower().startswith("http"):
- self.url = url
- return
-
- # Support for unicode domain names and paths.
- try:
- scheme, auth, host, port, path, query, fragment = parse_url(url)
- except LocationParseError as e:
- raise InvalidURL(*e.args)
-
- if not scheme:
- raise MissingSchema(
- f"Invalid URL {url!r}: No scheme supplied. "
- f"Perhaps you meant https://{url}?"
- )
-
- if not host:
- raise InvalidURL(f"Invalid URL {url!r}: No host supplied")
-
- # In general, we want to try IDNA encoding the hostname if the string contains
- # non-ASCII characters. This allows users to automatically get the correct IDNA
- # behaviour. For strings containing only ASCII characters, we need to also verify
- # it doesn't start with a wildcard (*), before allowing the unencoded hostname.
- if not unicode_is_ascii(host):
- try:
- host = self._get_idna_encoded_host(host)
- except UnicodeError:
- raise InvalidURL("URL has an invalid label.")
- elif host.startswith(("*", ".")):
- raise InvalidURL("URL has an invalid label.")
-
- # Carefully reconstruct the network location
- netloc = auth or ""
- if netloc:
- netloc += "@"
- netloc += host
- if port:
- netloc += f":{port}"
-
- # Bare domains aren't valid URLs.
- if not path:
- path = "/"
-
- if isinstance(params, (str, bytes)):
- params = to_native_string(params)
-
- enc_params = self._encode_params(params)
- if enc_params:
- if query:
- query = f"{query}&{enc_params}"
- else:
- query = enc_params
-
- url = requote_uri(urlunparse([scheme, netloc, path, None, query, fragment]))
- self.url = url
-
- def prepare_headers(self, headers):
- """Prepares the given HTTP headers."""
-
- self.headers = CaseInsensitiveDict()
- if headers:
- for header in headers.items():
- # Raise exception on invalid header value.
- check_header_validity(header)
- name, value = header
- self.headers[to_native_string(name)] = value
-
- def prepare_body(self, data, files, json=None):
- """Prepares the given HTTP body data."""
-
- # Check if file, fo, generator, iterator.
- # If not, run through normal process.
-
- # Nottin' on you.
- body = None
- content_type = None
-
- if not data and json is not None:
- # urllib3 requires a bytes-like body. Python 2's json.dumps
- # provides this natively, but Python 3 gives a Unicode string.
- content_type = "application/json"
-
- try:
- body = complexjson.dumps(json, allow_nan=False)
- except ValueError as ve:
- raise InvalidJSONError(ve, request=self)
-
- if not isinstance(body, bytes):
- body = body.encode("utf-8")
-
- is_stream = all(
- [
- hasattr(data, "__iter__"),
- not isinstance(data, (basestring, list, tuple, Mapping)),
- ]
- )
-
- if is_stream:
- try:
- length = super_len(data)
- except (TypeError, AttributeError, UnsupportedOperation):
- length = None
-
- body = data
-
- if getattr(body, "tell", None) is not None:
- # Record the current file position before reading.
- # This will allow us to rewind a file in the event
- # of a redirect.
- try:
- self._body_position = body.tell()
- except OSError:
- # This differentiates from None, allowing us to catch
- # a failed `tell()` later when trying to rewind the body
- self._body_position = object()
-
- if files:
- raise NotImplementedError(
- "Streamed bodies and files are mutually exclusive."
- )
-
- if length:
- self.headers["Content-Length"] = builtin_str(length)
- else:
- self.headers["Transfer-Encoding"] = "chunked"
- else:
- # Multi-part file uploads.
- if files:
- (body, content_type) = self._encode_files(files, data)
- else:
- if data:
- body = self._encode_params(data)
- if isinstance(data, basestring) or hasattr(data, "read"):
- content_type = None
- else:
- content_type = "application/x-www-form-urlencoded"
-
- self.prepare_content_length(body)
-
- # Add content-type if it wasn't explicitly provided.
- if content_type and ("content-type" not in self.headers):
- self.headers["Content-Type"] = content_type
-
- self.body = body
-
- def prepare_content_length(self, body):
- """Prepare Content-Length header based on request method and body"""
- if body is not None:
- length = super_len(body)
- if length:
- # If length exists, set it. Otherwise, we fallback
- # to Transfer-Encoding: chunked.
- self.headers["Content-Length"] = builtin_str(length)
- elif (
- self.method not in ("GET", "HEAD")
- and self.headers.get("Content-Length") is None
- ):
- # Set Content-Length to 0 for methods that can have a body
- # but don't provide one. (i.e. not GET or HEAD)
- self.headers["Content-Length"] = "0"
-
- def prepare_auth(self, auth, url=""):
- """Prepares the given HTTP auth data."""
-
- # If no Auth is explicitly provided, extract it from the URL first.
- if auth is None:
- url_auth = get_auth_from_url(self.url)
- auth = url_auth if any(url_auth) else None
-
- if auth:
- if isinstance(auth, tuple) and len(auth) == 2:
- # special-case basic HTTP auth
- auth = HTTPBasicAuth(*auth)
-
- # Allow auth to make its changes.
- r = auth(self)
-
- # Update self to reflect the auth changes.
- self.__dict__.update(r.__dict__)
-
- # Recompute Content-Length
- self.prepare_content_length(self.body)
-
- def prepare_cookies(self, cookies):
- """Prepares the given HTTP cookie data.
-
- This function eventually generates a ``Cookie`` header from the
- given cookies using cookielib. Due to cookielib's design, the header
- will not be regenerated if it already exists, meaning this function
- can only be called once for the life of the
- :class:`PreparedRequest ` object. Any subsequent calls
- to ``prepare_cookies`` will have no actual effect, unless the "Cookie"
- header is removed beforehand.
- """
- if isinstance(cookies, cookielib.CookieJar):
- self._cookies = cookies
- else:
- self._cookies = cookiejar_from_dict(cookies)
-
- cookie_header = get_cookie_header(self._cookies, self)
- if cookie_header is not None:
- self.headers["Cookie"] = cookie_header
-
- def prepare_hooks(self, hooks):
- """Prepares the given hooks."""
- # hooks can be passed as None to the prepare method and to this
- # method. To prevent iterating over None, simply use an empty list
- # if hooks is False-y
- hooks = hooks or []
- for event in hooks:
- self.register_hook(event, hooks[event])
-
-
-class Response:
- """The :class:`Response ` object, which contains a
- server's response to an HTTP request.
- """
-
- __attrs__ = [
- "_content",
- "status_code",
- "headers",
- "url",
- "history",
- "encoding",
- "reason",
- "cookies",
- "elapsed",
- "request",
- ]
-
- def __init__(self):
- self._content = False
- self._content_consumed = False
- self._next = None
-
- #: Integer Code of responded HTTP Status, e.g. 404 or 200.
- self.status_code = None
-
- #: Case-insensitive Dictionary of Response Headers.
- #: For example, ``headers['content-encoding']`` will return the
- #: value of a ``'Content-Encoding'`` response header.
- self.headers = CaseInsensitiveDict()
-
- #: File-like object representation of response (for advanced usage).
- #: Use of ``raw`` requires that ``stream=True`` be set on the request.
- #: This requirement does not apply for use internally to Requests.
- self.raw = None
-
- #: Final URL location of Response.
- self.url = None
-
- #: Encoding to decode with when accessing r.text.
- self.encoding = None
-
- #: A list of :class:`Response ` objects from
- #: the history of the Request. Any redirect responses will end
- #: up here. The list is sorted from the oldest to the most recent request.
- self.history = []
-
- #: Textual reason of responded HTTP Status, e.g. "Not Found" or "OK".
- self.reason = None
-
- #: A CookieJar of Cookies the server sent back.
- self.cookies = cookiejar_from_dict({})
-
- #: The amount of time elapsed between sending the request
- #: and the arrival of the response (as a timedelta).
- #: This property specifically measures the time taken between sending
- #: the first byte of the request and finishing parsing the headers. It
- #: is therefore unaffected by consuming the response content or the
- #: value of the ``stream`` keyword argument.
- self.elapsed = datetime.timedelta(0)
-
- #: The :class:`PreparedRequest ` object to which this
- #: is a response.
- self.request = None
-
- def __enter__(self):
- return self
-
- def __exit__(self, *args):
- self.close()
-
- def __getstate__(self):
- # Consume everything; accessing the content attribute makes
- # sure the content has been fully read.
- if not self._content_consumed:
- self.content
-
- return {attr: getattr(self, attr, None) for attr in self.__attrs__}
-
- def __setstate__(self, state):
- for name, value in state.items():
- setattr(self, name, value)
-
- # pickled objects do not have .raw
- setattr(self, "_content_consumed", True)
- setattr(self, "raw", None)
-
- def __repr__(self):
- return f""
-
- def __bool__(self):
- """Returns True if :attr:`status_code` is less than 400.
-
- This attribute checks if the status code of the response is between
- 400 and 600 to see if there was a client error or a server error. If
- the status code, is between 200 and 400, this will return True. This
- is **not** a check to see if the response code is ``200 OK``.
- """
- return self.ok
-
- def __nonzero__(self):
- """Returns True if :attr:`status_code` is less than 400.
-
- This attribute checks if the status code of the response is between
- 400 and 600 to see if there was a client error or a server error. If
- the status code, is between 200 and 400, this will return True. This
- is **not** a check to see if the response code is ``200 OK``.
- """
- return self.ok
-
- def __iter__(self):
- """Allows you to use a response as an iterator."""
- return self.iter_content(128)
-
- @property
- def ok(self):
- """Returns True if :attr:`status_code` is less than 400, False if not.
-
- This attribute checks if the status code of the response is between
- 400 and 600 to see if there was a client error or a server error. If
- the status code is between 200 and 400, this will return True. This
- is **not** a check to see if the response code is ``200 OK``.
- """
- try:
- self.raise_for_status()
- except HTTPError:
- return False
- return True
-
- @property
- def is_redirect(self):
- """True if this Response is a well-formed HTTP redirect that could have
- been processed automatically (by :meth:`Session.resolve_redirects`).
- """
- return "location" in self.headers and self.status_code in REDIRECT_STATI
-
- @property
- def is_permanent_redirect(self):
- """True if this Response one of the permanent versions of redirect."""
- return "location" in self.headers and self.status_code in (
- codes.moved_permanently,
- codes.permanent_redirect,
- )
-
- @property
- def next(self):
- """Returns a PreparedRequest for the next request in a redirect chain, if there is one."""
- return self._next
-
- @property
- def apparent_encoding(self):
- """The apparent encoding, provided by the charset_normalizer or chardet libraries."""
- return chardet.detect(self.content)["encoding"]
-
- def iter_content(self, chunk_size=1, decode_unicode=False):
- """Iterates over the response data. When stream=True is set on the
- request, this avoids reading the content at once into memory for
- large responses. The chunk size is the number of bytes it should
- read into memory. This is not necessarily the length of each item
- returned as decoding can take place.
-
- chunk_size must be of type int or None. A value of None will
- function differently depending on the value of `stream`.
- stream=True will read data as it arrives in whatever size the
- chunks are received. If stream=False, data is returned as
- a single chunk.
-
- If decode_unicode is True, content will be decoded using the best
- available encoding based on the response.
- """
-
- def generate():
- # Special case for urllib3.
- if hasattr(self.raw, "stream"):
- try:
- yield from self.raw.stream(chunk_size, decode_content=True)
- except ProtocolError as e:
- raise ChunkedEncodingError(e)
- except DecodeError as e:
- raise ContentDecodingError(e)
- except ReadTimeoutError as e:
- raise ConnectionError(e)
- except SSLError as e:
- raise RequestsSSLError(e)
- else:
- # Standard file-like object.
- while True:
- chunk = self.raw.read(chunk_size)
- if not chunk:
- break
- yield chunk
-
- self._content_consumed = True
-
- if self._content_consumed and isinstance(self._content, bool):
- raise StreamConsumedError()
- elif chunk_size is not None and not isinstance(chunk_size, int):
- raise TypeError(
- f"chunk_size must be an int, it is instead a {type(chunk_size)}."
- )
- # simulate reading small chunks of the content
- reused_chunks = iter_slices(self._content, chunk_size)
-
- stream_chunks = generate()
-
- chunks = reused_chunks if self._content_consumed else stream_chunks
-
- if decode_unicode:
- chunks = stream_decode_response_unicode(chunks, self)
-
- return chunks
-
- def iter_lines(
- self, chunk_size=ITER_CHUNK_SIZE, decode_unicode=False, delimiter=None
- ):
- """Iterates over the response data, one line at a time. When
- stream=True is set on the request, this avoids reading the
- content at once into memory for large responses.
-
- .. note:: This method is not reentrant safe.
- """
-
- pending = None
-
- for chunk in self.iter_content(
- chunk_size=chunk_size, decode_unicode=decode_unicode
- ):
-
- if pending is not None:
- chunk = pending + chunk
-
- if delimiter:
- lines = chunk.split(delimiter)
- else:
- lines = chunk.splitlines()
-
- if lines and lines[-1] and chunk and lines[-1][-1] == chunk[-1]:
- pending = lines.pop()
- else:
- pending = None
-
- yield from lines
-
- if pending is not None:
- yield pending
-
- @property
- def content(self):
- """Content of the response, in bytes."""
-
- if self._content is False:
- # Read the contents.
- if self._content_consumed:
- raise RuntimeError("The content for this response was already consumed")
-
- if self.status_code == 0 or self.raw is None:
- self._content = None
- else:
- self._content = b"".join(self.iter_content(CONTENT_CHUNK_SIZE)) or b""
-
- self._content_consumed = True
- # don't need to release the connection; that's been handled by urllib3
- # since we exhausted the data.
- return self._content
-
- @property
- def text(self):
- """Content of the response, in unicode.
-
- If Response.encoding is None, encoding will be guessed using
- ``charset_normalizer`` or ``chardet``.
-
- The encoding of the response content is determined based solely on HTTP
- headers, following RFC 2616 to the letter. If you can take advantage of
- non-HTTP knowledge to make a better guess at the encoding, you should
- set ``r.encoding`` appropriately before accessing this property.
- """
-
- # Try charset from content-type
- content = None
- encoding = self.encoding
-
- if not self.content:
- return ""
-
- # Fallback to auto-detected encoding.
- if self.encoding is None:
- encoding = self.apparent_encoding
-
- # Decode unicode from given encoding.
- try:
- content = str(self.content, encoding, errors="replace")
- except (LookupError, TypeError):
- # A LookupError is raised if the encoding was not found which could
- # indicate a misspelling or similar mistake.
- #
- # A TypeError can be raised if encoding is None
- #
- # So we try blindly encoding.
- content = str(self.content, errors="replace")
-
- return content
-
- def json(self, **kwargs):
- r"""Returns the json-encoded content of a response, if any.
-
- :param \*\*kwargs: Optional arguments that ``json.loads`` takes.
- :raises requests.exceptions.JSONDecodeError: If the response body does not
- contain valid json.
- """
-
- if not self.encoding and self.content and len(self.content) > 3:
- # No encoding set. JSON RFC 4627 section 3 states we should expect
- # UTF-8, -16 or -32. Detect which one to use; If the detection or
- # decoding fails, fall back to `self.text` (using charset_normalizer to make
- # a best guess).
- encoding = guess_json_utf(self.content)
- if encoding is not None:
- try:
- return complexjson.loads(self.content.decode(encoding), **kwargs)
- except UnicodeDecodeError:
- # Wrong UTF codec detected; usually because it's not UTF-8
- # but some other 8-bit codec. This is an RFC violation,
- # and the server didn't bother to tell us what codec *was*
- # used.
- pass
- except JSONDecodeError as e:
- raise RequestsJSONDecodeError(e.msg, e.doc, e.pos)
-
- try:
- return complexjson.loads(self.text, **kwargs)
- except JSONDecodeError as e:
- # Catch JSON-related errors and raise as requests.JSONDecodeError
- # This aliases json.JSONDecodeError and simplejson.JSONDecodeError
- raise RequestsJSONDecodeError(e.msg, e.doc, e.pos)
-
- @property
- def links(self):
- """Returns the parsed header links of the response, if any."""
-
- header = self.headers.get("link")
-
- resolved_links = {}
-
- if header:
- links = parse_header_links(header)
-
- for link in links:
- key = link.get("rel") or link.get("url")
- resolved_links[key] = link
-
- return resolved_links
-
- def raise_for_status(self):
- """Raises :class:`HTTPError`, if one occurred."""
-
- http_error_msg = ""
- if isinstance(self.reason, bytes):
- # We attempt to decode utf-8 first because some servers
- # choose to localize their reason strings. If the string
- # isn't utf-8, we fall back to iso-8859-1 for all other
- # encodings. (See PR #3538)
- try:
- reason = self.reason.decode("utf-8")
- except UnicodeDecodeError:
- reason = self.reason.decode("iso-8859-1")
- else:
- reason = self.reason
-
- if 400 <= self.status_code < 500:
- http_error_msg = (
- f"{self.status_code} Client Error: {reason} for url: {self.url}"
- )
-
- elif 500 <= self.status_code < 600:
- http_error_msg = (
- f"{self.status_code} Server Error: {reason} for url: {self.url}"
- )
-
- if http_error_msg:
- raise HTTPError(http_error_msg, response=self)
-
- def close(self):
- """Releases the connection back to the pool. Once this method has been
- called the underlying ``raw`` object must not be accessed again.
-
- *Note: Should not normally need to be called explicitly.*
- """
- if not self._content_consumed:
- self.raw.close()
-
- release_conn = getattr(self.raw, "release_conn", None)
- if release_conn is not None:
- release_conn()
diff --git a/spaces/Big-Web/MMSD/env/Lib/site-packages/setuptools/_vendor/packaging/markers.py b/spaces/Big-Web/MMSD/env/Lib/site-packages/setuptools/_vendor/packaging/markers.py
deleted file mode 100644
index eb0541b83a77f09f5e598bf88eeb38a84e305ae0..0000000000000000000000000000000000000000
--- a/spaces/Big-Web/MMSD/env/Lib/site-packages/setuptools/_vendor/packaging/markers.py
+++ /dev/null
@@ -1,304 +0,0 @@
-# This file is dual licensed under the terms of the Apache License, Version
-# 2.0, and the BSD License. See the LICENSE file in the root of this repository
-# for complete details.
-
-import operator
-import os
-import platform
-import sys
-from typing import Any, Callable, Dict, List, Optional, Tuple, Union
-
-from setuptools.extern.pyparsing import ( # noqa: N817
- Forward,
- Group,
- Literal as L,
- ParseException,
- ParseResults,
- QuotedString,
- ZeroOrMore,
- stringEnd,
- stringStart,
-)
-
-from .specifiers import InvalidSpecifier, Specifier
-
-__all__ = [
- "InvalidMarker",
- "UndefinedComparison",
- "UndefinedEnvironmentName",
- "Marker",
- "default_environment",
-]
-
-Operator = Callable[[str, str], bool]
-
-
-class InvalidMarker(ValueError):
- """
- An invalid marker was found, users should refer to PEP 508.
- """
-
-
-class UndefinedComparison(ValueError):
- """
- An invalid operation was attempted on a value that doesn't support it.
- """
-
-
-class UndefinedEnvironmentName(ValueError):
- """
- A name was attempted to be used that does not exist inside of the
- environment.
- """
-
-
-class Node:
- def __init__(self, value: Any) -> None:
- self.value = value
-
- def __str__(self) -> str:
- return str(self.value)
-
- def __repr__(self) -> str:
- return f"<{self.__class__.__name__}('{self}')>"
-
- def serialize(self) -> str:
- raise NotImplementedError
-
-
-class Variable(Node):
- def serialize(self) -> str:
- return str(self)
-
-
-class Value(Node):
- def serialize(self) -> str:
- return f'"{self}"'
-
-
-class Op(Node):
- def serialize(self) -> str:
- return str(self)
-
-
-VARIABLE = (
- L("implementation_version")
- | L("platform_python_implementation")
- | L("implementation_name")
- | L("python_full_version")
- | L("platform_release")
- | L("platform_version")
- | L("platform_machine")
- | L("platform_system")
- | L("python_version")
- | L("sys_platform")
- | L("os_name")
- | L("os.name") # PEP-345
- | L("sys.platform") # PEP-345
- | L("platform.version") # PEP-345
- | L("platform.machine") # PEP-345
- | L("platform.python_implementation") # PEP-345
- | L("python_implementation") # undocumented setuptools legacy
- | L("extra") # PEP-508
-)
-ALIASES = {
- "os.name": "os_name",
- "sys.platform": "sys_platform",
- "platform.version": "platform_version",
- "platform.machine": "platform_machine",
- "platform.python_implementation": "platform_python_implementation",
- "python_implementation": "platform_python_implementation",
-}
-VARIABLE.setParseAction(lambda s, l, t: Variable(ALIASES.get(t[0], t[0])))
-
-VERSION_CMP = (
- L("===") | L("==") | L(">=") | L("<=") | L("!=") | L("~=") | L(">") | L("<")
-)
-
-MARKER_OP = VERSION_CMP | L("not in") | L("in")
-MARKER_OP.setParseAction(lambda s, l, t: Op(t[0]))
-
-MARKER_VALUE = QuotedString("'") | QuotedString('"')
-MARKER_VALUE.setParseAction(lambda s, l, t: Value(t[0]))
-
-BOOLOP = L("and") | L("or")
-
-MARKER_VAR = VARIABLE | MARKER_VALUE
-
-MARKER_ITEM = Group(MARKER_VAR + MARKER_OP + MARKER_VAR)
-MARKER_ITEM.setParseAction(lambda s, l, t: tuple(t[0]))
-
-LPAREN = L("(").suppress()
-RPAREN = L(")").suppress()
-
-MARKER_EXPR = Forward()
-MARKER_ATOM = MARKER_ITEM | Group(LPAREN + MARKER_EXPR + RPAREN)
-MARKER_EXPR << MARKER_ATOM + ZeroOrMore(BOOLOP + MARKER_EXPR)
-
-MARKER = stringStart + MARKER_EXPR + stringEnd
-
-
-def _coerce_parse_result(results: Union[ParseResults, List[Any]]) -> List[Any]:
- if isinstance(results, ParseResults):
- return [_coerce_parse_result(i) for i in results]
- else:
- return results
-
-
-def _format_marker(
- marker: Union[List[str], Tuple[Node, ...], str], first: Optional[bool] = True
-) -> str:
-
- assert isinstance(marker, (list, tuple, str))
-
- # Sometimes we have a structure like [[...]] which is a single item list
- # where the single item is itself it's own list. In that case we want skip
- # the rest of this function so that we don't get extraneous () on the
- # outside.
- if (
- isinstance(marker, list)
- and len(marker) == 1
- and isinstance(marker[0], (list, tuple))
- ):
- return _format_marker(marker[0])
-
- if isinstance(marker, list):
- inner = (_format_marker(m, first=False) for m in marker)
- if first:
- return " ".join(inner)
- else:
- return "(" + " ".join(inner) + ")"
- elif isinstance(marker, tuple):
- return " ".join([m.serialize() for m in marker])
- else:
- return marker
-
-
-_operators: Dict[str, Operator] = {
- "in": lambda lhs, rhs: lhs in rhs,
- "not in": lambda lhs, rhs: lhs not in rhs,
- "<": operator.lt,
- "<=": operator.le,
- "==": operator.eq,
- "!=": operator.ne,
- ">=": operator.ge,
- ">": operator.gt,
-}
-
-
-def _eval_op(lhs: str, op: Op, rhs: str) -> bool:
- try:
- spec = Specifier("".join([op.serialize(), rhs]))
- except InvalidSpecifier:
- pass
- else:
- return spec.contains(lhs)
-
- oper: Optional[Operator] = _operators.get(op.serialize())
- if oper is None:
- raise UndefinedComparison(f"Undefined {op!r} on {lhs!r} and {rhs!r}.")
-
- return oper(lhs, rhs)
-
-
-class Undefined:
- pass
-
-
-_undefined = Undefined()
-
-
-def _get_env(environment: Dict[str, str], name: str) -> str:
- value: Union[str, Undefined] = environment.get(name, _undefined)
-
- if isinstance(value, Undefined):
- raise UndefinedEnvironmentName(
- f"{name!r} does not exist in evaluation environment."
- )
-
- return value
-
-
-def _evaluate_markers(markers: List[Any], environment: Dict[str, str]) -> bool:
- groups: List[List[bool]] = [[]]
-
- for marker in markers:
- assert isinstance(marker, (list, tuple, str))
-
- if isinstance(marker, list):
- groups[-1].append(_evaluate_markers(marker, environment))
- elif isinstance(marker, tuple):
- lhs, op, rhs = marker
-
- if isinstance(lhs, Variable):
- lhs_value = _get_env(environment, lhs.value)
- rhs_value = rhs.value
- else:
- lhs_value = lhs.value
- rhs_value = _get_env(environment, rhs.value)
-
- groups[-1].append(_eval_op(lhs_value, op, rhs_value))
- else:
- assert marker in ["and", "or"]
- if marker == "or":
- groups.append([])
-
- return any(all(item) for item in groups)
-
-
-def format_full_version(info: "sys._version_info") -> str:
- version = "{0.major}.{0.minor}.{0.micro}".format(info)
- kind = info.releaselevel
- if kind != "final":
- version += kind[0] + str(info.serial)
- return version
-
-
-def default_environment() -> Dict[str, str]:
- iver = format_full_version(sys.implementation.version)
- implementation_name = sys.implementation.name
- return {
- "implementation_name": implementation_name,
- "implementation_version": iver,
- "os_name": os.name,
- "platform_machine": platform.machine(),
- "platform_release": platform.release(),
- "platform_system": platform.system(),
- "platform_version": platform.version(),
- "python_full_version": platform.python_version(),
- "platform_python_implementation": platform.python_implementation(),
- "python_version": ".".join(platform.python_version_tuple()[:2]),
- "sys_platform": sys.platform,
- }
-
-
-class Marker:
- def __init__(self, marker: str) -> None:
- try:
- self._markers = _coerce_parse_result(MARKER.parseString(marker))
- except ParseException as e:
- raise InvalidMarker(
- f"Invalid marker: {marker!r}, parse error at "
- f"{marker[e.loc : e.loc + 8]!r}"
- )
-
- def __str__(self) -> str:
- return _format_marker(self._markers)
-
- def __repr__(self) -> str:
- return f""
-
- def evaluate(self, environment: Optional[Dict[str, str]] = None) -> bool:
- """Evaluate a marker.
-
- Return the boolean from evaluating the given marker against the
- environment. environment is an optional argument to override all or
- part of the determined environment.
-
- The environment is determined from the current Python process.
- """
- current_environment = default_environment()
- if environment is not None:
- current_environment.update(environment)
-
- return _evaluate_markers(self._markers, current_environment)
diff --git a/spaces/Big-Web/MMSD/env/Lib/site-packages/setuptools/launch.py b/spaces/Big-Web/MMSD/env/Lib/site-packages/setuptools/launch.py
deleted file mode 100644
index 0208fdf33b640cd9791359d74673bb90cfb87f96..0000000000000000000000000000000000000000
--- a/spaces/Big-Web/MMSD/env/Lib/site-packages/setuptools/launch.py
+++ /dev/null
@@ -1,36 +0,0 @@
-"""
-Launch the Python script on the command line after
-setuptools is bootstrapped via import.
-"""
-
-# Note that setuptools gets imported implicitly by the
-# invocation of this script using python -m setuptools.launch
-
-import tokenize
-import sys
-
-
-def run():
- """
- Run the script in sys.argv[1] as if it had
- been invoked naturally.
- """
- __builtins__
- script_name = sys.argv[1]
- namespace = dict(
- __file__=script_name,
- __name__='__main__',
- __doc__=None,
- )
- sys.argv[:] = sys.argv[1:]
-
- open_ = getattr(tokenize, 'open', open)
- with open_(script_name) as fid:
- script = fid.read()
- norm_script = script.replace('\\r\\n', '\\n')
- code = compile(norm_script, script_name, 'exec')
- exec(code, namespace)
-
-
-if __name__ == '__main__':
- run()
diff --git a/spaces/BongoCaat/ArtGenerator/stable_diffusion_2_0.py b/spaces/BongoCaat/ArtGenerator/stable_diffusion_2_0.py
deleted file mode 100644
index 0ee0ea871674634f159a6b204434c704a15cf66c..0000000000000000000000000000000000000000
--- a/spaces/BongoCaat/ArtGenerator/stable_diffusion_2_0.py
+++ /dev/null
@@ -1,611 +0,0 @@
-{
- "cells": [
- {
- "cell_type": "markdown",
- "metadata": {
- "id": "view-in-github",
- "colab_type": "text"
- },
- "source": [
- ""
- ]
- },
- {
- "cell_type": "markdown",
- "metadata": {
- "id": "620o1BxdNbgq"
- },
- "source": [
- "# **Stable Diffusion 2.1**\n",
- "Gradio app for [Stable Diffusion 2](https://huggingface.co/stabilityai/stable-diffusion-2) by [Stability AI](https://stability.ai/) (v2-1_768-ema-pruned.ckpt).\n",
- "It uses [Hugging Face](https://huggingface.co/) Diffusers🧨 implementation.\n",
- "\n",
- "Currently supported pipelines are `text-to-image`, `image-to-image`, `inpainting`, `4x upscaling` and `depth-to-image`.\n",
- "\n",
- " \n",
- "\n",
- "Colab by [anzorq](https://twitter.com/hahahahohohe). If you like it, please consider supporting me:\n",
- "\n",
- "[](https://www.buymeacoffee.com/anzorq)\n",
- " \n",
- "[](https://github.com/qunash/stable-diffusion-2-gui)\n",
- "\n",
- ""
- ]
- },
- {
- "cell_type": "markdown",
- "metadata": {
- "id": "KQI4RX20DW_8"
- },
- "source": [
- "# Install dependencies (~1.5 mins)"
- ]
- },
- {
- "cell_type": "code",
- "execution_count": null,
- "metadata": {
- "id": "78HoqRAB-cES",
- "cellView": "form"
- },
- "outputs": [],
- "source": [
- "!pip install --upgrade git+https://github.com/huggingface/diffusers.git\n",
- "# !pip install diffusers\n",
- "!pip install --upgrade git+https://github.com/huggingface/transformers/\n",
- "# !pip install transformers\n",
- "!pip install accelerate==0.12.0\n",
- "!pip install scipy\n",
- "!pip install ftfy\n",
- "!pip install gradio -q\n",
- "\n",
- "#@markdown ### ⬅️ Run this cell\n",
- "#@markdown ---\n",
- "#@markdown ### Install **xformers**?\n",
- "#@markdown This will take an additional ~3.5 mins. But images will generate 25-40% faster.\n",
- "install_xformers = False #@param {type:\"boolean\"}\n",
- "\n",
- "if install_xformers:\n",
- " import os\n",
- " from subprocess import getoutput\n",
- "\n",
- " os.system(\"pip install --extra-index-url https://download.pytorch.org/whl/cu113 torch torchvision==0.13.1+cu113\")\n",
- " os.system(\"pip install triton==2.0.0.dev20220701\")\n",
- " gpu_info = getoutput('nvidia-smi')\n",
- " if(\"A10G\" in gpu_info):\n",
- " os.system(f\"pip install -q https://github.com/camenduru/stable-diffusion-webui-colab/releases/download/0.0.15/xformers-0.0.15.dev0+4c06c79.d20221205-cp38-cp38-linux_x86_64.whl\")\n",
- " elif(\"T4\" in gpu_info):\n",
- " os.system(f\"pip install -q https://github.com/camenduru/stable-diffusion-webui-colab/releases/download/0.0.15/xformers-0.0.15.dev0+1515f77.d20221130-cp38-cp38-linux_x86_64.whl\")\n",
- "\n",
- "\n",
- "# ### install xformers\n",
- "# from IPython.utils import capture\n",
- "# from subprocess import getoutput\n",
- "# from re import search\n",
- "\n",
- "# with capture.capture_output() as cap:\n",
- " \n",
- "# smi_out = getoutput('nvidia-smi')\n",
- "# supported = search('(T4|P100|V100|A100|K80)', smi_out)\n",
- "\n",
- "# if not supported:\n",
- "# while True:\n",
- "# print(\"\\x1b[1;31mThe current GPU is not supported, try starting a new session.\\x1b[0m\")\n",
- "# else:\n",
- "# supported = supported.group(0)\n",
- "\n",
- "# !pip install -q https://github.com/TheLastBen/fast-stable-diffusion/raw/main/precompiled/{supported}/xformers-0.0.13.dev0-py3-none-any.whl\n",
- "# !pip install -q https://github.com/ShivamShrirao/xformers-wheels/releases/download/4c06c79/xformers-0.0.15.dev0+4c06c79.d20221201-cp38-cp38-linux_x86_64.whl"
- ]
- },
- {
- "cell_type": "markdown",
- "metadata": {
- "id": "OOPHNsFYDbc0"
- },
- "source": [
- "# Run the app"
- ]
- },
- {
- "cell_type": "code",
- "execution_count": null,
- "metadata": {
- "cellView": "form",
- "id": "gId0-asCBVwL"
- },
- "outputs": [],
- "source": [
- "#@title ⬇️🖼️\n",
- "from diffusers import StableDiffusionPipeline, StableDiffusionImg2ImgPipeline, StableDiffusionUpscalePipeline, DiffusionPipeline, StableDiffusionDepth2ImgPipeline, DPMSolverMultistepScheduler\n",
- "import gradio as gr\n",
- "import torch\n",
- "from PIL import Image\n",
- "import random\n",
- "\n",
- "state = None\n",
- "current_steps = 25\n",
- "attn_slicing_enabled = True\n",
- "mem_eff_attn_enabled = install_xformers\n",
- "\n",
- "# model_id = 'stabilityai/stable-diffusion-2'\n",
- "model_id = 'stabilityai/stable-diffusion-2-1'\n",
- "\n",
- "scheduler = DPMSolverMultistepScheduler.from_pretrained(model_id, subfolder=\"scheduler\")\n",
- "\n",
- "pipe = StableDiffusionPipeline.from_pretrained(\n",
- " model_id,\n",
- " revision=\"fp16\" if torch.cuda.is_available() else \"fp32\",\n",
- " torch_dtype=torch.float16 if torch.cuda.is_available() else torch.float32,\n",
- " scheduler=scheduler\n",
- " ).to(\"cuda\")\n",
- "pipe.enable_attention_slicing()\n",
- "if mem_eff_attn_enabled:\n",
- " pipe.enable_xformers_memory_efficient_attention()\n",
- "\n",
- "pipe_i2i = None\n",
- "pipe_upscale = None\n",
- "pipe_inpaint = None\n",
- "pipe_depth2img = None\n",
- "\n",
- "\n",
- "modes = {\n",
- " 'txt2img': 'Text to Image',\n",
- " 'img2img': 'Image to Image',\n",
- " 'inpaint': 'Inpainting',\n",
- " 'upscale4x': 'Upscale 4x',\n",
- " 'depth2img': 'Depth to Image'\n",
- "}\n",
- "current_mode = modes['txt2img']\n",
- "\n",
- "def error_str(error, title=\"Error\"):\n",
- " return f\"\"\"#### {title}\n",
- " {error}\"\"\" if error else \"\"\n",
- "\n",
- "def update_state(new_state):\n",
- " global state\n",
- " state = new_state\n",
- "\n",
- "def update_state_info(old_state):\n",
- " if state and state != old_state:\n",
- " return gr.update(value=state)\n",
- "\n",
- "def set_mem_optimizations(pipe):\n",
- " if attn_slicing_enabled:\n",
- " pipe.enable_attention_slicing()\n",
- " else:\n",
- " pipe.disable_attention_slicing()\n",
- " \n",
- " if mem_eff_attn_enabled:\n",
- " pipe.enable_xformers_memory_efficient_attention()\n",
- " else:\n",
- " pipe.disable_xformers_memory_efficient_attention()\n",
- "\n",
- "def get_i2i_pipe(scheduler):\n",
- " \n",
- " update_state(\"Loading image to image model...\")\n",
- "\n",
- " pipe = StableDiffusionImg2ImgPipeline.from_pretrained(\n",
- " model_id,\n",
- " revision=\"fp16\" if torch.cuda.is_available() else \"fp32\",\n",
- " torch_dtype=torch.float16 if torch.cuda.is_available() else torch.float32,\n",
- " scheduler=scheduler\n",
- " )\n",
- " set_mem_optimizations(pipe)\n",
- " pipe.to(\"cuda\")\n",
- " return pipe\n",
- "\n",
- "def get_inpaint_pipe():\n",
- " \n",
- " update_state(\"Loading inpainting model...\")\n",
- "\n",
- " pipe = DiffusionPipeline.from_pretrained(\n",
- " \"stabilityai/stable-diffusion-2-inpainting\",\n",
- " revision=\"fp16\" if torch.cuda.is_available() else \"fp32\",\n",
- " torch_dtype=torch.float16 if torch.cuda.is_available() else torch.float32,\n",
- " # scheduler=scheduler # TODO currently setting scheduler here messes up the end result. A bug in Diffusers🧨\n",
- " ).to(\"cuda\")\n",
- " pipe.scheduler = DPMSolverMultistepScheduler.from_config(pipe.scheduler.config)\n",
- " pipe.enable_attention_slicing()\n",
- " pipe.enable_xformers_memory_efficient_attention()\n",
- " return pipe\n",
- "\n",
- "def get_upscale_pipe(scheduler):\n",
- " \n",
- " update_state(\"Loading upscale model...\")\n",
- "\n",
- " pipe = StableDiffusionUpscalePipeline.from_pretrained(\n",
- " \"stabilityai/stable-diffusion-x4-upscaler\",\n",
- " revision=\"fp16\" if torch.cuda.is_available() else \"fp32\",\n",
- " torch_dtype=torch.float16 if torch.cuda.is_available() else torch.float32,\n",
- " # scheduler=scheduler\n",
- " )\n",
- " # pipe.scheduler = DPMSolverMultistepScheduler.from_config(pipe.scheduler.config)\n",
- " set_mem_optimizations(pipe)\n",
- " pipe.to(\"cuda\")\n",
- " return pipe\n",
- " \n",
- "def get_depth2img_pipe():\n",
- " \n",
- " update_state(\"Loading depth to image model...\")\n",
- "\n",
- " pipe = StableDiffusionDepth2ImgPipeline.from_pretrained(\n",
- " \"stabilityai/stable-diffusion-2-depth\",\n",
- " revision=\"fp16\" if torch.cuda.is_available() else \"fp32\",\n",
- " torch_dtype=torch.float16 if torch.cuda.is_available() else torch.float32,\n",
- " # scheduler=scheduler\n",
- " )\n",
- " pipe.scheduler = DPMSolverMultistepScheduler.from_config(pipe.scheduler.config)\n",
- " set_mem_optimizations(pipe)\n",
- " pipe.to(\"cuda\")\n",
- " return pipe\n",
- "\n",
- "def switch_attention_slicing(attn_slicing):\n",
- " global attn_slicing_enabled\n",
- " attn_slicing_enabled = attn_slicing\n",
- "\n",
- "def switch_mem_eff_attn(mem_eff_attn):\n",
- " global mem_eff_attn_enabled\n",
- " mem_eff_attn_enabled = mem_eff_attn\n",
- "\n",
- "def pipe_callback(step: int, timestep: int, latents: torch.FloatTensor):\n",
- " update_state(f\"{step}/{current_steps} steps\")#\\nTime left, sec: {timestep/100:.0f}\")\n",
- "\n",
- "def inference(inf_mode, prompt, n_images, guidance, steps, width=768, height=768, seed=0, img=None, strength=0.5, neg_prompt=\"\"):\n",
- "\n",
- " update_state(\" \")\n",
- "\n",
- " global current_mode\n",
- " if inf_mode != current_mode:\n",
- " pipe.to(\"cuda\" if inf_mode == modes['txt2img'] else \"cpu\")\n",
- "\n",
- " if pipe_i2i is not None:\n",
- " pipe_i2i.to(\"cuda\" if inf_mode == modes['img2img'] else \"cpu\")\n",
- "\n",
- " if pipe_inpaint is not None:\n",
- " pipe_inpaint.to(\"cuda\" if inf_mode == modes['inpaint'] else \"cpu\")\n",
- "\n",
- " if pipe_upscale is not None:\n",
- " pipe_upscale.to(\"cuda\" if inf_mode == modes['upscale4x'] else \"cpu\")\n",
- " \n",
- " if pipe_depth2img is not None:\n",
- " pipe_depth2img.to(\"cuda\" if inf_mode == modes['depth2img'] else \"cpu\")\n",
- "\n",
- " current_mode = inf_mode\n",
- " \n",
- " if seed == 0:\n",
- " seed = random.randint(0, 2147483647)\n",
- "\n",
- " generator = torch.Generator('cuda').manual_seed(seed)\n",
- " prompt = prompt\n",
- "\n",
- " try:\n",
- " \n",
- " if inf_mode == modes['txt2img']:\n",
- " return txt_to_img(prompt, n_images, neg_prompt, guidance, steps, width, height, generator, seed), gr.update(visible=False, value=None)\n",
- " \n",
- " elif inf_mode == modes['img2img']:\n",
- " if img is None:\n",
- " return None, gr.update(visible=True, value=error_str(\"Image is required for Image to Image mode\"))\n",
- "\n",
- " return img_to_img(prompt, n_images, neg_prompt, img, strength, guidance, steps, width, height, generator, seed), gr.update(visible=False, value=None)\n",
- " \n",
- " elif inf_mode == modes['inpaint']:\n",
- " if img is None:\n",
- " return None, gr.update(visible=True, value=error_str(\"Image is required for Inpainting mode\"))\n",
- "\n",
- " return inpaint(prompt, n_images, neg_prompt, img, guidance, steps, width, height, generator, seed), gr.update(visible=False, value=None)\n",
- "\n",
- " elif inf_mode == modes['upscale4x']:\n",
- " if img is None:\n",
- " return None, gr.update(visible=True, value=error_str(\"Image is required for Upscale mode\"))\n",
- "\n",
- " return upscale(prompt, n_images, neg_prompt, img, guidance, steps, generator), gr.update(visible=False, value=None)\n",
- "\n",
- " elif inf_mode == modes['depth2img']:\n",
- " if img is None:\n",
- " return None, gr.update(visible=True, value=error_str(\"Image is required for Depth to Image mode\"))\n",
- "\n",
- " return depth2img(prompt, n_images, neg_prompt, img, guidance, steps, generator, seed), gr.update(visible=False, value=None)\n",
- "\n",
- " except Exception as e:\n",
- " return None, gr.update(visible=True, value=error_str(e))\n",
- "\n",
- "def txt_to_img(prompt, n_images, neg_prompt, guidance, steps, width, height, generator, seed):\n",
- "\n",
- " result = pipe(\n",
- " prompt,\n",
- " num_images_per_prompt = n_images,\n",
- " negative_prompt = neg_prompt,\n",
- " num_inference_steps = int(steps),\n",
- " guidance_scale = guidance,\n",
- " width = width,\n",
- " height = height,\n",
- " generator = generator,\n",
- " callback=pipe_callback).images\n",
- "\n",
- " update_state(f\"Done. Seed: {seed}\")\n",
- "\n",
- " return result\n",
- "\n",
- "def img_to_img(prompt, n_images, neg_prompt, img, strength, guidance, steps, width, height, generator, seed):\n",
- "\n",
- " global pipe_i2i\n",
- " if pipe_i2i is None:\n",
- " pipe_i2i = get_i2i_pipe(scheduler)\n",
- "\n",
- " img = img['image']\n",
- " ratio = min(height / img.height, width / img.width)\n",
- " img = img.resize((int(img.width * ratio), int(img.height * ratio)), Image.LANCZOS)\n",
- " result = pipe_i2i(\n",
- " prompt,\n",
- " num_images_per_prompt = n_images,\n",
- " negative_prompt = neg_prompt,\n",
- " image = img,\n",
- " num_inference_steps = int(steps),\n",
- " strength = strength,\n",
- " guidance_scale = guidance,\n",
- " # width = width,\n",
- " # height = height,\n",
- " generator = generator,\n",
- " callback=pipe_callback).images\n",
- "\n",
- " update_state(f\"Done. Seed: {seed}\")\n",
- " \n",
- " return result\n",
- "\n",
- "# TODO Currently supports only 512x512 images\n",
- "def inpaint(prompt, n_images, neg_prompt, img, guidance, steps, width, height, generator, seed):\n",
- "\n",
- " global pipe_inpaint\n",
- " if pipe_inpaint is None:\n",
- " pipe_inpaint = get_inpaint_pipe()\n",
- "\n",
- " inp_img = img['image']\n",
- " mask = img['mask']\n",
- " inp_img = square_padding(inp_img)\n",
- " mask = square_padding(mask)\n",
- "\n",
- " # # ratio = min(height / inp_img.height, width / inp_img.width)\n",
- " # ratio = min(512 / inp_img.height, 512 / inp_img.width)\n",
- " # inp_img = inp_img.resize((int(inp_img.width * ratio), int(inp_img.height * ratio)), Image.LANCZOS)\n",
- " # mask = mask.resize((int(mask.width * ratio), int(mask.height * ratio)), Image.LANCZOS)\n",
- "\n",
- " inp_img = inp_img.resize((512, 512))\n",
- " mask = mask.resize((512, 512))\n",
- "\n",
- " result = pipe_inpaint(\n",
- " prompt,\n",
- " image = inp_img,\n",
- " mask_image = mask,\n",
- " num_images_per_prompt = n_images,\n",
- " negative_prompt = neg_prompt,\n",
- " num_inference_steps = int(steps),\n",
- " guidance_scale = guidance,\n",
- " # width = width,\n",
- " # height = height,\n",
- " generator = generator,\n",
- " callback=pipe_callback).images\n",
- " \n",
- " update_state(f\"Done. Seed: {seed}\")\n",
- "\n",
- " return result\n",
- "\n",
- "def depth2img(prompt, n_images, neg_prompt, img, guidance, steps, generator, seed):\n",
- "\n",
- " global pipe_depth2img\n",
- " if pipe_depth2img is None:\n",
- " pipe_depth2img = get_depth2img_pipe()\n",
- "\n",
- " img = img['image']\n",
- " result = pipe_depth2img(\n",
- " prompt,\n",
- " num_images_per_prompt = n_images,\n",
- " negative_prompt = neg_prompt,\n",
- " image = img,\n",
- " num_inference_steps = int(steps),\n",
- " guidance_scale = guidance,\n",
- " # width = width,\n",
- " # height = height,\n",
- " generator = generator,\n",
- " callback=pipe_callback).images\n",
- "\n",
- " update_state(f\"Done. Seed: {seed}\")\n",
- " \n",
- " return result\n",
- "\n",
- "def square_padding(img):\n",
- " width, height = img.size\n",
- " if width == height:\n",
- " return img\n",
- " new_size = max(width, height)\n",
- " new_img = Image.new('RGB', (new_size, new_size), (0, 0, 0, 255))\n",
- " new_img.paste(img, ((new_size - width) // 2, (new_size - height) // 2))\n",
- " return new_img\n",
- "\n",
- "def upscale(prompt, n_images, neg_prompt, img, guidance, steps, generator):\n",
- "\n",
- " global pipe_upscale\n",
- " if pipe_upscale is None:\n",
- " pipe_upscale = get_upscale_pipe(scheduler)\n",
- "\n",
- " img = img['image']\n",
- " return upscale_tiling(prompt, neg_prompt, img, guidance, steps, generator)\n",
- "\n",
- " # result = pipe_upscale(\n",
- " # prompt,\n",
- " # image = img,\n",
- " # num_inference_steps = int(steps),\n",
- " # guidance_scale = guidance,\n",
- " # negative_prompt = neg_prompt,\n",
- " # num_images_per_prompt = n_images,\n",
- " # generator = generator).images[0]\n",
- "\n",
- " # return result\n",
- "\n",
- "def upscale_tiling(prompt, neg_prompt, img, guidance, steps, generator):\n",
- "\n",
- " width, height = img.size\n",
- "\n",
- " # calculate the padding needed to make the image dimensions a multiple of 128\n",
- " padding_x = 128 - (width % 128) if width % 128 != 0 else 0\n",
- " padding_y = 128 - (height % 128) if height % 128 != 0 else 0\n",
- "\n",
- " # create a white image of the right size to be used as padding\n",
- " padding_img = Image.new('RGB', (padding_x, padding_y), color=(255, 255, 255, 0))\n",
- "\n",
- " # paste the padding image onto the original image to add the padding\n",
- " img.paste(padding_img, (width, height))\n",
- "\n",
- " # update the image dimensions to include the padding\n",
- " width += padding_x\n",
- " height += padding_y\n",
- "\n",
- " if width > 128 or height > 128:\n",
- "\n",
- " num_tiles_x = int(width / 128)\n",
- " num_tiles_y = int(height / 128)\n",
- "\n",
- " upscaled_img = Image.new('RGB', (img.size[0] * 4, img.size[1] * 4))\n",
- " for x in range(num_tiles_x):\n",
- " for y in range(num_tiles_y):\n",
- " update_state(f\"Upscaling tile {x * num_tiles_y + y + 1}/{num_tiles_x * num_tiles_y}\")\n",
- " tile = img.crop((x * 128, y * 128, (x + 1) * 128, (y + 1) * 128))\n",
- "\n",
- " upscaled_tile = pipe_upscale(\n",
- " prompt=\"\",\n",
- " image=tile,\n",
- " num_inference_steps=steps,\n",
- " guidance_scale=guidance,\n",
- " # negative_prompt = neg_prompt,\n",
- " generator=generator,\n",
- " ).images[0]\n",
- "\n",
- " upscaled_img.paste(upscaled_tile, (x * upscaled_tile.size[0], y * upscaled_tile.size[1]))\n",
- "\n",
- " return [upscaled_img]\n",
- " else:\n",
- " return pipe_upscale(\n",
- " prompt=prompt,\n",
- " image=img,\n",
- " num_inference_steps=steps,\n",
- " guidance_scale=guidance,\n",
- " negative_prompt = neg_prompt,\n",
- " generator=generator,\n",
- " ).images\n",
- "\n",
- "\n",
- "\n",
- "def on_mode_change(mode):\n",
- " return gr.update(visible = mode in (modes['img2img'], modes['inpaint'], modes['upscale4x'], modes['depth2img'])), \\\n",
- " gr.update(visible = mode == modes['inpaint']), \\\n",
- " gr.update(visible = mode == modes['upscale4x']), \\\n",
- " gr.update(visible = mode == modes['img2img'])\n",
- "\n",
- "def on_steps_change(steps):\n",
- " global current_steps\n",
- " current_steps = steps\n",
- "\n",
- "css = \"\"\".main-div div{display:inline-flex;align-items:center;gap:.8rem;font-size:1.75rem}.main-div div h1{font-weight:900;margin-bottom:7px}.main-div p{margin-bottom:10px;font-size:94%}a{text-decoration:underline}.tabs{margin-top:0;margin-bottom:0}#gallery{min-height:20rem}\n",
- "\"\"\"\n",
- "with gr.Blocks(css=css) as demo:\n",
- " gr.HTML(\n",
- " f\"\"\"\n",
- "
\n",
- " \"\"\")\n",
- "\n",
- "demo.queue()\n",
- "demo.launch(debug=True, share=True, height=768)\n"
- ]
- }
- ],
- "metadata": {
- "accelerator": "GPU",
- "colab": {
- "private_outputs": true,
- "provenance": [],
- "toc_visible": true,
- "include_colab_link": true
- },
- "gpuClass": "standard",
- "kernelspec": {
- "display_name": "Python 3",
- "name": "python3"
- },
- "language_info": {
- "name": "python"
- }
- },
- "nbformat": 4,
- "nbformat_minor": 0
-}
\ No newline at end of file
diff --git a/spaces/CVPR/DualStyleGAN/style.css b/spaces/CVPR/DualStyleGAN/style.css
deleted file mode 100644
index 472b3df9e5e4936157c53a570a8875992ccac7a3..0000000000000000000000000000000000000000
--- a/spaces/CVPR/DualStyleGAN/style.css
+++ /dev/null
@@ -1,17 +0,0 @@
-h1 {
- text-align: center;
-}
-
-img#overview {
- max-width: 1000px;
- max-height: 600px;
- display: block;
- margin: auto;
-}
-
-img#style-image {
- max-width: 1000px;
- max-height: 600px;
- display: block;
- margin: auto;
-}
diff --git a/spaces/Chris4K/llms_compare/CabelasDangerousHunts2013-SKIDROW-REPACK-Crack-Fix-Torrent-Download.md b/spaces/Chris4K/llms_compare/CabelasDangerousHunts2013-SKIDROW-REPACK-Crack-Fix-Torrent-Download.md
deleted file mode 100644
index b1fdd48d206a702292508c6ce3d71b4f504d0f20..0000000000000000000000000000000000000000
--- a/spaces/Chris4K/llms_compare/CabelasDangerousHunts2013-SKIDROW-REPACK-Crack-Fix-Torrent-Download.md
+++ /dev/null
@@ -1,64 +0,0 @@
-## Cabelas.Dangerous.Hunts.2013. -SKIDROW -Crack Fix Torrent Download
-
-
-
-
-
-
-
-
-
-**LINK ->>->>->> [https://urluso.com/2tBNzD](https://urluso.com/2tBNzD)**
-
-
-
-
-
-
-
-
-
-
-
-
-
-# Cabela's Dangerous Hunts 2013: A Hunting Game with Kill or Be Killed Consequences
-
-
-
-Cabela's Dangerous Hunts 2013 is a first-person shooter hunting game developed by FUN Labs and published by Activision in 2013. The game features a new Prowler animal AI engine that simulates pack social hierarchies, coordinates complex group tactics and sets up deadly ambushes. The game also introduces a new Maneater co-op mode, where two players can join together to take on wave after wave of increasingly deadly beasts in a split screen mode.
-
-
-
-The game is set in 12 exotic locations throughout the world, where players can hunt 27 big game animals, such as lions, bears, wolves, crocodiles and more. The game offers various modes of gameplay, such as Quick Hunt, Action Hunt and Career Hunt. The game also allows players to customize their weapons and gear with thousands of authentic options, including rifles, handguns, bows, crossbows, knives and various scopes.
-
-
-
-The game received mixed reviews from critics, who praised the graphics, sound effects and co-op mode, but criticized the repetitive gameplay, poor AI and texture glitches. The game is available for PC, Xbox 360, PlayStation 3 and Wii U platforms.
-
-
-
-If you are interested in downloading Cabela's Dangerous Hunts 2013 for PC, you can find a torrent link here[^1^]. You will need to mount or burn the image file, install the game, copy everything from the SKIDROW folder into the game installation folder, block the game in your firewall and antivirus program, and play the game. You can also find a crack fix here[^3^], which will solve some of the issues with the game.
-
-
-
-However, please note that downloading torrents is illegal and may expose you to viruses and malware. We do not condone or support piracy in any way. If you like the game, please support the developers and buy it from official sources.
-
-
-
-The game's main mode is the Story Mode, where the player takes the role of Cole Rainsford, a young hunter who joins his estranged father on an African safari. However, their trip turns into a nightmare when they encounter a mysterious cult that unleashes a horde of deadly animals on them. The player must survive the attacks of lions, hyenas, leopards, rhinos and more, while uncovering the truth behind the cult's motives.
-
-
-
-The game's gameplay is based on quick-time events and shooting sequences, where the player must react to the animal attacks and aim for their vital organs. The game also features a Fearmaster controller for some platforms, which measures the player's heart rate and motion. The higher the fear level, the harder it is to aim and shoot accurately. The game also has a dynamic weather system and day-night cycle, which affect the visibility and behavior of the animals.
-
-
-
-The game's graphics and sound effects are realistic and immersive, creating a tense and thrilling atmosphere. The game also features voice acting by Scott Eastwood and Rob Lowe as Cole and his father respectively. The game's co-op mode is also fun and challenging, allowing two players to team up and face different scenarios and objectives. However, the game also has some flaws, such as repetitive gameplay, poor AI and texture glitches. The game also received some criticism for its depiction of animal violence and hunting ethics.
-
- 145887f19f
-
-
-
-
-
diff --git a/spaces/CikeyQI/Yunzai/Yunzai/plugins/ws-plugin/model/tool.js b/spaces/CikeyQI/Yunzai/Yunzai/plugins/ws-plugin/model/tool.js
deleted file mode 100644
index 0f03dc352d36e2c4aefa36caf48481743f24c650..0000000000000000000000000000000000000000
--- a/spaces/CikeyQI/Yunzai/Yunzai/plugins/ws-plugin/model/tool.js
+++ /dev/null
@@ -1,163 +0,0 @@
-import _ from 'lodash'
-import fs from 'fs'
-import { Version } from '../components/index.js'
-
-async function CreateMusicShare(data) {
- let appid, appname, appsign, style = 4;
- switch (data.subType) {
- case 'bilibili':
- appid = 100951776, appname = 'tv.danmaku.bili', appsign = '7194d531cbe7960a22007b9f6bdaa38b';
- break;
- case 'netease':
- appid = 100495085, appname = "com.netease.cloudmusic", appsign = "da6b069da1e2982db3e386233f68d76d";
- break;
- case 'kuwo':
- appid = 100243533, appname = "cn.kuwo.player", appsign = "bf9ff4ffb4c558a34ee3fd52c223ebf5";
- break;
- case 'kugou':
- appid = 205141, appname = "com.kugou.android", appsign = "fe4a24d80fcf253a00676a808f62c2c6";
- break;
- case 'migu':
- appid = 1101053067, appname = "cmccwm.mobilemusic", appsign = "6cdc72a439cef99a3418d2a78aa28c73";
- break;
- case 'qq':
- default:
- appid = 100497308, appname = "com.tencent.qqmusic", appsign = "cbd27cd7c861227d013a25b2d10f0799";
- break;
- }
-
- var text = '', title = data.title, singer = data.content, prompt = '[分享]', jumpUrl = data.url, preview = data.image, musicUrl = data.voice;
-
- prompt = '[分享]' + title + '-' + singer;
-
- let recv_uin = 0;
- let send_type = 0;
- let recv_guild_id = 0;
-
- if (data.message_type === 'group') {//群聊
- recv_uin = data.group_id;
- send_type = 1;
- } else if (data.message_type === 'guild') {//频道
- recv_uin = Number(data.channel_id);
- recv_guild_id = BigInt(data.guild_id);
- send_type = 3;
- } else if (data.message_type === 'private') {//私聊
- recv_uin = data.user_id;
- send_type = 0;
- }
-
- let body = {
- 1: appid,
- 2: 1,
- 3: style,
- 5: {
- 1: 1,
- 2: "0.0.0",
- 3: appname,
- 4: appsign,
- },
- 6: text,
- 10: send_type,
- 11: recv_uin,
- 12: {
- 10: title,
- 11: singer,
- 12: prompt,
- 13: jumpUrl,
- 14: preview,
- 16: musicUrl,
- },
- 19: recv_guild_id
- };
- return body;
-}
-
-async function SendMusicShare(data) {
- let core, bot
- if (Version.isTrss) {
- bot = Bot[data.bot_id]
- core = bot?.core
- } else {
- bot = Bot
- try {
- core = (await import('oicq')).core
- } catch (error) {
- core = null
- }
- }
- if (!core) {
- const msg = [data.url]
- if (data.message_type === 'group') {//群聊
- await bot?.pickGroup?.(data.group_id)?.sendMsg?.(msg)
- } else if (data.message_type === 'private') {//私聊
- await bot?.pickFriend?.(data.user_id)?.sendMsg?.(msg)
- }
- return
- }
- try {
- let body = await CreateMusicShare(data)
- let payload = await bot.sendOidb("OidbSvc.0xb77_9", core.pb.encode(body));
- let result = core.pb.decode(payload);
- if (result[3] != 0) {
- if (data.message_type === 'group') {//群聊
- await bot?.pickGroup(data.group_id).sendMsg('歌曲分享失败:' + result[3])
- } else if (data.message_type === 'private') {//私聊
- await bot?.pickFriend(data.user_id).sendMsg('歌曲分享失败:' + result[3])
- }
- // e.reply('歌曲分享失败:' + result[3], true);
- }
- } catch (error) {
- const msg = [data.url]
- if (data.message_type === 'group') {//群聊
- await bot?.pickGroup?.(data.group_id)?.sendMsg?.(msg)
- } else if (data.message_type === 'private') {//私聊
- await bot?.pickFriend?.(data.user_id)?.sendMsg?.(msg)
- }
- return
- }
-}
-
-function sleep(ms) {
- return new Promise((resolve) => setTimeout(resolve, ms))
-}
-
-const TMP_DIR = process.cwd() + '/plugins/ws-plugin/Temp'
-if (!fs.existsSync(TMP_DIR)) fs.mkdirSync(TMP_DIR)
-
-const mimeTypes = {
- '.html': 'text/html',
- '.js': 'text/javascript',
- '.css': 'text/css',
- '.json': 'application/json',
- '.png': 'image/png',
- '.jpg': 'image/jpg',
- '.gif': 'image/gif',
- '.ico': 'image/x-icon',
- '.txt': 'text/plain',
- '.xlsx': 'application/vnd.openxmlformats-officedocument.spreadsheetml.sheet',
-}
-
-function decodeHtml(html) {
- var map = {
- '&': '&',
- '[': '[',
- ']': ']',
- ',': ','
- };
-
- for (var key in map) {
- const value = map[key];
- const regex = new RegExp(key, 'g');
- html = html.replace(regex, value);
- }
- return html;
-}
-
-
-export {
- SendMusicShare,
- sleep,
- TMP_DIR,
- mimeTypes,
- decodeHtml
-}
\ No newline at end of file
diff --git a/spaces/CikeyQI/meme-api/meme_generator/memes/keep_away/__init__.py b/spaces/CikeyQI/meme-api/meme_generator/memes/keep_away/__init__.py
deleted file mode 100644
index ed47b292dd9d1b70d4f248a65e394c21e03d3c88..0000000000000000000000000000000000000000
--- a/spaces/CikeyQI/meme-api/meme_generator/memes/keep_away/__init__.py
+++ /dev/null
@@ -1,47 +0,0 @@
-from typing import List
-
-from PIL.Image import Transpose
-from pil_utils import BuildImage
-
-from meme_generator import add_meme
-
-
-def keep_away(images: List[BuildImage], texts: List[str], args):
- def trans(img: BuildImage, n: int) -> BuildImage:
- img = img.convert("RGBA").square().resize((100, 100))
- if n < 4:
- return img.rotate(n * 90)
- else:
- return img.transpose(Transpose.FLIP_LEFT_RIGHT).rotate((n - 4) * 90)
-
- def paste(img: BuildImage):
- nonlocal count
- y = 90 if count < 4 else 190
- frame.paste(img, ((count % 4) * 100, y))
- count += 1
-
- text = texts[0] if texts else "如何提高社交质量 : \n远离以下头像的人"
- frame = BuildImage.new("RGB", (400, 290), "white")
- frame.draw_text((10, 10, 390, 80), text, max_fontsize=40, halign="left")
- count = 0
- num_per_user = 8 // len(images)
- for image in images:
- for n in range(num_per_user):
- paste(trans(image, n))
- num_left = 8 - num_per_user * len(images)
- for n in range(num_left):
- paste(trans(images[-1], n + num_per_user))
-
- return frame.save_jpg()
-
-
-add_meme(
- "keep_away",
- keep_away,
- min_images=1,
- max_images=8,
- min_texts=0,
- max_texts=1,
- default_texts=["如何提高社交质量 : \n远离以下头像的人"],
- keywords=["远离"],
-)
diff --git a/spaces/CjangCjengh/Sanskrit-TTS/commons.py b/spaces/CjangCjengh/Sanskrit-TTS/commons.py
deleted file mode 100644
index 2153153f527d94e2abb641ea00c80b518ff6c5bd..0000000000000000000000000000000000000000
--- a/spaces/CjangCjengh/Sanskrit-TTS/commons.py
+++ /dev/null
@@ -1,97 +0,0 @@
-import math
-import torch
-from torch.nn import functional as F
-import torch.jit
-
-
-def script_method(fn, _rcb=None):
- return fn
-
-
-def script(obj, optimize=True, _frames_up=0, _rcb=None):
- return obj
-
-
-torch.jit.script_method = script_method
-torch.jit.script = script
-
-
-def init_weights(m, mean=0.0, std=0.01):
- classname = m.__class__.__name__
- if classname.find("Conv") != -1:
- m.weight.data.normal_(mean, std)
-
-
-def get_padding(kernel_size, dilation=1):
- return int((kernel_size*dilation - dilation)/2)
-
-
-def intersperse(lst, item):
- result = [item] * (len(lst) * 2 + 1)
- result[1::2] = lst
- return result
-
-
-def slice_segments(x, ids_str, segment_size=4):
- ret = torch.zeros_like(x[:, :, :segment_size])
- for i in range(x.size(0)):
- idx_str = ids_str[i]
- idx_end = idx_str + segment_size
- ret[i] = x[i, :, idx_str:idx_end]
- return ret
-
-
-def rand_slice_segments(x, x_lengths=None, segment_size=4):
- b, d, t = x.size()
- if x_lengths is None:
- x_lengths = t
- ids_str_max = x_lengths - segment_size + 1
- ids_str = (torch.rand([b]).to(device=x.device) * ids_str_max).to(dtype=torch.long)
- ret = slice_segments(x, ids_str, segment_size)
- return ret, ids_str
-
-
-def subsequent_mask(length):
- mask = torch.tril(torch.ones(length, length)).unsqueeze(0).unsqueeze(0)
- return mask
-
-
-@torch.jit.script
-def fused_add_tanh_sigmoid_multiply(input_a, input_b, n_channels):
- n_channels_int = n_channels[0]
- in_act = input_a + input_b
- t_act = torch.tanh(in_act[:, :n_channels_int, :])
- s_act = torch.sigmoid(in_act[:, n_channels_int:, :])
- acts = t_act * s_act
- return acts
-
-
-def convert_pad_shape(pad_shape):
- l = pad_shape[::-1]
- pad_shape = [item for sublist in l for item in sublist]
- return pad_shape
-
-
-def sequence_mask(length, max_length=None):
- if max_length is None:
- max_length = length.max()
- x = torch.arange(max_length, dtype=length.dtype, device=length.device)
- return x.unsqueeze(0) < length.unsqueeze(1)
-
-
-def generate_path(duration, mask):
- """
- duration: [b, 1, t_x]
- mask: [b, 1, t_y, t_x]
- """
- device = duration.device
-
- b, _, t_y, t_x = mask.shape
- cum_duration = torch.cumsum(duration, -1)
-
- cum_duration_flat = cum_duration.view(b * t_x)
- path = sequence_mask(cum_duration_flat, t_y).to(mask.dtype)
- path = path.view(b, t_x, t_y)
- path = path - F.pad(path, convert_pad_shape([[0, 0], [1, 0], [0, 0]]))[:, :-1]
- path = path.unsqueeze(1).transpose(2,3) * mask
- return path
diff --git a/spaces/CofAI/chat/g4f/Provider/Provider.py b/spaces/CofAI/chat/g4f/Provider/Provider.py
deleted file mode 100644
index d24df76b6a6ccfc9b244f13a51bfc124b398a271..0000000000000000000000000000000000000000
--- a/spaces/CofAI/chat/g4f/Provider/Provider.py
+++ /dev/null
@@ -1,16 +0,0 @@
-import os
-from ..typing import sha256, Dict, get_type_hints
-
-url = None
-model = None
-supports_stream = False
-needs_auth = False
-
-
-def _create_completion(model: str, messages: list, stream: bool, **kwargs):
- return
-
-
-params = f'g4f.Providers.{os.path.basename(__file__)[:-3]} supports: ' + \
- '(%s)' % ', '.join(
- [f"{name}: {get_type_hints(_create_completion)[name].__name__}" for name in _create_completion.__code__.co_varnames[:_create_completion.__code__.co_argcount]])
diff --git a/spaces/CrucibleAI/ControlNetMediaPipeFaceSD21/ldm/models/diffusion/sampling_util.py b/spaces/CrucibleAI/ControlNetMediaPipeFaceSD21/ldm/models/diffusion/sampling_util.py
deleted file mode 100644
index 7eff02be6d7c54d43ee6680636ac0698dd3b3f33..0000000000000000000000000000000000000000
--- a/spaces/CrucibleAI/ControlNetMediaPipeFaceSD21/ldm/models/diffusion/sampling_util.py
+++ /dev/null
@@ -1,22 +0,0 @@
-import torch
-import numpy as np
-
-
-def append_dims(x, target_dims):
- """Appends dimensions to the end of a tensor until it has target_dims dimensions.
- From https://github.com/crowsonkb/k-diffusion/blob/master/k_diffusion/utils.py"""
- dims_to_append = target_dims - x.ndim
- if dims_to_append < 0:
- raise ValueError(f'input has {x.ndim} dims but target_dims is {target_dims}, which is less')
- return x[(...,) + (None,) * dims_to_append]
-
-
-def norm_thresholding(x0, value):
- s = append_dims(x0.pow(2).flatten(1).mean(1).sqrt().clamp(min=value), x0.ndim)
- return x0 * (value / s)
-
-
-def spatial_norm_thresholding(x0, value):
- # b c h w
- s = x0.pow(2).mean(1, keepdim=True).sqrt().clamp(min=value)
- return x0 * (value / s)
\ No newline at end of file
diff --git a/spaces/DQChoi/gpt-demo/venv/lib/python3.11/site-packages/fastapi/middleware/trustedhost.py b/spaces/DQChoi/gpt-demo/venv/lib/python3.11/site-packages/fastapi/middleware/trustedhost.py
deleted file mode 100644
index 08d7e035315677856fd2cd0be2044689b57619bf..0000000000000000000000000000000000000000
--- a/spaces/DQChoi/gpt-demo/venv/lib/python3.11/site-packages/fastapi/middleware/trustedhost.py
+++ /dev/null
@@ -1,3 +0,0 @@
-from starlette.middleware.trustedhost import ( # noqa
- TrustedHostMiddleware as TrustedHostMiddleware,
-)
diff --git a/spaces/Deci/DeciDiffusion-v1-0/app.py b/spaces/Deci/DeciDiffusion-v1-0/app.py
deleted file mode 100644
index 69c5871b0b098ab1cd1a1e6b5ee2290bb8517b53..0000000000000000000000000000000000000000
--- a/spaces/Deci/DeciDiffusion-v1-0/app.py
+++ /dev/null
@@ -1,115 +0,0 @@
-import gradio as gr
-import torch
-from PIL.ImageDraw import Draw
-from diffusers import StableDiffusionPipeline
-from PIL import Image, ImageOps
-
-
-# Load pipeline once
-model_id = 'Deci/DeciDiffusion-v1-0'
-device = "cuda" if torch.cuda.is_available() else "cpu"
-pipe = StableDiffusionPipeline.from_pretrained(model_id, custom_pipeline=model_id, torch_dtype=torch.float32)
-pipe.unet = pipe.unet.from_pretrained(model_id, subfolder='flexible_unet', torch_dtype=torch.float32)
-pipe = pipe.to(device)
-
-
-def read_content(file_path: str) -> str:
- """read the content of target file
- """
- with open(file_path, 'r', encoding='utf-8') as f:
- content = f.read()
-
- return content
-
-
-def predict(_prompt: str, _steps: int = 30, _seed: int = 42, _guidance_scale: float = 7.5, _negative_prompt: str = ""):
- _negative_prompt = [_negative_prompt] if _negative_prompt else None
-
- output = pipe(prompt=[_prompt],
- negative_prompt=_negative_prompt,
- num_inference_steps=int(_steps),
- guidance_scale=_guidance_scale,
- generator=torch.Generator(device).manual_seed(_seed),
- )
- output_image = output.images[0]
-
- # Add border beneath the image with Deci logo + prompt
- if len(_prompt) > 52:
- _prompt = _prompt[:52] + "..."
-
- original_image_height = output_image.size[1]
- output_image = ImageOps.expand(output_image, border=(0, 0, 0, 64), fill='white')
- deci_logo = Image.open('./deci_logo_white.png')
- output_image.paste(deci_logo, (0, original_image_height))
- Draw(output_image).text((deci_logo.size[0], original_image_height + 26), _prompt, (127, 127, 127))
- return output_image
-
-
-css = '''
-.gradio-container {
- max-width: 1100px !important;
- background-image: url(https://huggingface.co/spaces/Deci/Deci-DeciDiffusionClean/resolve/main/background-image.png);
- background-size: cover;
- background-position: center center;
- background-repeat: no-repeat;
-}
-
-.footer {margin-bottom: 45px;margin-top: 35px !important;text-align: center;border-bottom: 1px solid #e5e5e5}
-.footer>p {font-size: .8rem; display: inline-block; padding: 0 10px;transform: translateY(10px);background: white}
-.dark .footer {border-color: #303030}
-.dark .footer>p {background: #0b0f19}
-.acknowledgments h4{margin: 1.25em 0 .25em 0;font-weight: bold;font-size: 115%}
-@keyframes spin {
- from {
- transform: rotate(0deg);
- }
- to {
- transform: rotate(360deg);
- }
-}
-'''
-
-demo = gr.Blocks(css=css, elem_id="total-container")
-with demo:
- gr.HTML(read_content("header.html"))
- with gr.Row():
- with gr.Column():
- with gr.Row(mobile_collapse=False, equal_height=True):
- prompt = gr.Textbox(placeholder="Your prompt", show_label=False, elem_id="prompt", autofocus=True, lines=3, )
-
- with gr.Accordion(label="Advanced Settings", open=False):
- with gr.Row(mobile_collapse=False, equal_height=True):
- steps = gr.Slider(value=30, minimum=15, maximum=50, step=1, label="steps", interactive=True)
- seed = gr.Slider(value=42, minimum=1, maximum=100, step=1, label="seed", interactive=True)
- guidance_scale = gr.Slider(value=7.5, minimum=1, maximum=15, step=0.1, label='guidance_scale', interactive=True)
-
- with gr.Row(mobile_collapse=False, equal_height=True):
- negative_prompt = gr.Textbox(label="negative_prompt", placeholder="Your negative prompt",
- info="what you don't want to see in the image", lines=3)
- with gr.Row():
- btn = gr.Button(value="Generate!", elem_id="run_button")
-
- with gr.Column():
- image_out = gr.Image(label="Output", elem_id="output-img", height=400)
-
- btn.click(fn=predict,
- inputs=[prompt, steps, seed, guidance_scale, negative_prompt],
- outputs=[image_out],
- api_name='run')
-
- gr.HTML(
- """
-
-
-
LICENSE
-The model is licensed with a CreativeML Open RAIL-M license. The authors claim no rights on the outputs you generate, you are free to use them and are accountable for their use which must not go against the provisions set in this license. The license forbids you from sharing any content that violates any laws, produce any harm to a person, disseminate any personal information that would be meant for harm, spread misinformation and target vulnerable groups. For the full list of restrictions please read the license
-
Biases and content acknowledgment
-Despite how impressive being able to turn text into image is, beware to the fact that this model may output content that reinforces or exacerbates societal biases, as well as realistic faces, pornography and violence. The model was trained on the LAION-5B dataset, which scraped non-curated image-text-pairs from the internet (the exception being the removal of illegal content) and is meant for research purposes. You can read more in the model card
-
- """
- )
-
-demo.queue(max_size=50).launch()
diff --git a/spaces/ElainaFanBoy/MusicGen/tests/common_utils/temp_utils.py b/spaces/ElainaFanBoy/MusicGen/tests/common_utils/temp_utils.py
deleted file mode 100644
index d1e0367e979c8b9fea65472c373916d956ad5aaa..0000000000000000000000000000000000000000
--- a/spaces/ElainaFanBoy/MusicGen/tests/common_utils/temp_utils.py
+++ /dev/null
@@ -1,56 +0,0 @@
-# Copyright (c) Meta Platforms, Inc. and affiliates.
-# All rights reserved.
-#
-# This source code is licensed under the license found in the
-# LICENSE file in the root directory of this source tree.
-
-import os
-import tempfile
-
-
-class TempDirMixin:
- """Mixin to provide easy access to temp dir.
- """
-
- temp_dir_ = None
-
- @classmethod
- def get_base_temp_dir(cls):
- # If AUDIOCRAFT_TEST_DIR is set, use it instead of temporary directory.
- # this is handy for debugging.
- key = "AUDIOCRAFT_TEST_DIR"
- if key in os.environ:
- return os.environ[key]
- if cls.temp_dir_ is None:
- cls.temp_dir_ = tempfile.TemporaryDirectory()
- return cls.temp_dir_.name
-
- @classmethod
- def tearDownClass(cls):
- if cls.temp_dir_ is not None:
- try:
- cls.temp_dir_.cleanup()
- cls.temp_dir_ = None
- except PermissionError:
- # On Windows there is a know issue with `shutil.rmtree`,
- # which fails intermittenly.
- # https://github.com/python/cpython/issues/74168
- # Following the above thread, we ignore it.
- pass
- super().tearDownClass()
-
- @property
- def id(self):
- return self.__class__.__name__
-
- def get_temp_path(self, *paths):
- temp_dir = os.path.join(self.get_base_temp_dir(), self.id)
- path = os.path.join(temp_dir, *paths)
- os.makedirs(os.path.dirname(path), exist_ok=True)
- return path
-
- def get_temp_dir(self, *paths):
- temp_dir = os.path.join(self.get_base_temp_dir(), self.id)
- path = os.path.join(temp_dir, *paths)
- os.makedirs(path, exist_ok=True)
- return path
diff --git a/spaces/EngAbod/Liveness_Detection/README.md b/spaces/EngAbod/Liveness_Detection/README.md
deleted file mode 100644
index 9953cf60eea94b7bc20089a281c8c4f7babb2704..0000000000000000000000000000000000000000
--- a/spaces/EngAbod/Liveness_Detection/README.md
+++ /dev/null
@@ -1,13 +0,0 @@
----
-title: Liveness Detection
-emoji: 👀
-colorFrom: blue
-colorTo: blue
-sdk: streamlit
-sdk_version: 1.27.2
-app_file: app.py
-pinned: false
-license: apache-2.0
----
-
-Check out the configuration reference at https://huggingface.co/docs/hub/spaces-config-reference
diff --git a/spaces/FL33TW00D/whisper-turbo/README.md b/spaces/FL33TW00D/whisper-turbo/README.md
deleted file mode 100644
index 24523341c9ccf937083af6141f73cf5c684ecc19..0000000000000000000000000000000000000000
--- a/spaces/FL33TW00D/whisper-turbo/README.md
+++ /dev/null
@@ -1,10 +0,0 @@
----
-title: Whisper Turbo
-emoji: 🗣️🏎️
-colorFrom: blue
-colorTo: gray
-sdk: static
-pinned: true
----
-
-Check out the configuration reference at https://huggingface.co/docs/hub/spaces-config-reference
diff --git a/spaces/Fadil369/docker/README.md b/spaces/Fadil369/docker/README.md
deleted file mode 100644
index c4f71628142aeafb0cd15d54c9890d373ebb61cb..0000000000000000000000000000000000000000
--- a/spaces/Fadil369/docker/README.md
+++ /dev/null
@@ -1,20 +0,0 @@
----
-title: Shiny for Python template
-emoji: 🌍
-colorFrom: yellow
-colorTo: indigo
-sdk: docker
-pinned: false
-license: mit
----
-
-This is a templated Space for [Shiny for Python](https://shiny.rstudio.com/py/).
-
-
-To get started with a new app do the following:
-
-1) Install Shiny with `pip install shiny`
-2) Create a new app with `shiny create .`
-3) Then run the app with `shiny run --reload`
-
-To learn more about this framework please see the [Documentation](https://shiny.rstudio.com/py/docs/overview.html).
diff --git a/spaces/Feifei315/flax-midjourney-v4-diffusion/app.py b/spaces/Feifei315/flax-midjourney-v4-diffusion/app.py
deleted file mode 100644
index a7e777fc5c7f3e31a491e4bd016b8948b6a260f4..0000000000000000000000000000000000000000
--- a/spaces/Feifei315/flax-midjourney-v4-diffusion/app.py
+++ /dev/null
@@ -1,3 +0,0 @@
-import gradio as gr
-
-gr.Interface.load("models/flax/midjourney-v4-diffusion").launch()
\ No newline at end of file
diff --git a/spaces/FrankZxShen/so-vits-svc-models-pcr/modules/F0Predictor/DioF0Predictor.py b/spaces/FrankZxShen/so-vits-svc-models-pcr/modules/F0Predictor/DioF0Predictor.py
deleted file mode 100644
index 4ab27de23cae4dbc282e30f84501afebd1a37518..0000000000000000000000000000000000000000
--- a/spaces/FrankZxShen/so-vits-svc-models-pcr/modules/F0Predictor/DioF0Predictor.py
+++ /dev/null
@@ -1,85 +0,0 @@
-from modules.F0Predictor.F0Predictor import F0Predictor
-import pyworld
-import numpy as np
-
-class DioF0Predictor(F0Predictor):
- def __init__(self,hop_length=512,f0_min=50,f0_max=1100,sampling_rate=44100):
- self.hop_length = hop_length
- self.f0_min = f0_min
- self.f0_max = f0_max
- self.sampling_rate = sampling_rate
-
- def interpolate_f0(self,f0):
- '''
- 对F0进行插值处理
- '''
-
- data = np.reshape(f0, (f0.size, 1))
-
- vuv_vector = np.zeros((data.size, 1), dtype=np.float32)
- vuv_vector[data > 0.0] = 1.0
- vuv_vector[data <= 0.0] = 0.0
-
- ip_data = data
-
- frame_number = data.size
- last_value = 0.0
- for i in range(frame_number):
- if data[i] <= 0.0:
- j = i + 1
- for j in range(i + 1, frame_number):
- if data[j] > 0.0:
- break
- if j < frame_number - 1:
- if last_value > 0.0:
- step = (data[j] - data[i - 1]) / float(j - i)
- for k in range(i, j):
- ip_data[k] = data[i - 1] + step * (k - i + 1)
- else:
- for k in range(i, j):
- ip_data[k] = data[j]
- else:
- for k in range(i, frame_number):
- ip_data[k] = last_value
- else:
- ip_data[i] = data[i] #这里可能存在一个没有必要的拷贝
- last_value = data[i]
-
- return ip_data[:,0], vuv_vector[:,0]
-
- def resize_f0(self,x, target_len):
- source = np.array(x)
- source[source<0.001] = np.nan
- target = np.interp(np.arange(0, len(source)*target_len, len(source))/ target_len, np.arange(0, len(source)), source)
- res = np.nan_to_num(target)
- return res
-
- def compute_f0(self,wav,p_len=None):
- if p_len is None:
- p_len = wav.shape[0]//self.hop_length
- f0, t = pyworld.dio(
- wav.astype(np.double),
- fs=self.sampling_rate,
- f0_floor=self.f0_min,
- f0_ceil=self.f0_max,
- frame_period=1000 * self.hop_length / self.sampling_rate,
- )
- f0 = pyworld.stonemask(wav.astype(np.double), f0, t, self.sampling_rate)
- for index, pitch in enumerate(f0):
- f0[index] = round(pitch, 1)
- return self.interpolate_f0(self.resize_f0(f0, p_len))[0]
-
- def compute_f0_uv(self,wav,p_len=None):
- if p_len is None:
- p_len = wav.shape[0]//self.hop_length
- f0, t = pyworld.dio(
- wav.astype(np.double),
- fs=self.sampling_rate,
- f0_floor=self.f0_min,
- f0_ceil=self.f0_max,
- frame_period=1000 * self.hop_length / self.sampling_rate,
- )
- f0 = pyworld.stonemask(wav.astype(np.double), f0, t, self.sampling_rate)
- for index, pitch in enumerate(f0):
- f0[index] = round(pitch, 1)
- return self.interpolate_f0(self.resize_f0(f0, p_len))
diff --git a/spaces/FridaZuley/RVC_HFKawaii/Applio-RVC-Fork/utils/backups_test.py b/spaces/FridaZuley/RVC_HFKawaii/Applio-RVC-Fork/utils/backups_test.py
deleted file mode 100644
index f3edf15811b5035ee82f21e54e87b7e87ce413eb..0000000000000000000000000000000000000000
--- a/spaces/FridaZuley/RVC_HFKawaii/Applio-RVC-Fork/utils/backups_test.py
+++ /dev/null
@@ -1,138 +0,0 @@
-
-import os
-import shutil
-import hashlib
-import time
-
-LOGS_FOLDER = '/content/Applio-RVC-Fork/logs'
-WEIGHTS_FOLDER = '/content/Applio-RVC-Fork/weights'
-GOOGLE_DRIVE_PATH = '/content/drive/MyDrive/RVC_Backup'
-
-def import_google_drive_backup():
- print("Importing Google Drive backup...")
- GOOGLE_DRIVE_PATH = '/content/drive/MyDrive/RVC_Backup' # change this to your Google Drive path
- LOGS_FOLDER = '/content/Applio-RVC-Fork/logs'
- WEIGHTS_FOLDER = '/content/Applio-RVC-Fork/weights'
- weights_exist = False
- files_to_copy = []
- weights_to_copy = []
-
- def handle_files(root, files, is_weight_files=False):
- for filename in files:
- filepath = os.path.join(root, filename)
- if filename.endswith('.pth') and is_weight_files:
- weights_exist = True
- backup_filepath = os.path.join(WEIGHTS_FOLDER, os.path.relpath(filepath, GOOGLE_DRIVE_PATH))
- else:
- backup_filepath = os.path.join(LOGS_FOLDER, os.path.relpath(filepath, GOOGLE_DRIVE_PATH))
- backup_folderpath = os.path.dirname(backup_filepath)
- if not os.path.exists(backup_folderpath):
- os.makedirs(backup_folderpath)
- print(f'Created folder: {backup_folderpath}', flush=True)
- if is_weight_files:
- weights_to_copy.append((filepath, backup_filepath))
- else:
- files_to_copy.append((filepath, backup_filepath))
-
- for root, dirs, files in os.walk(os.path.join(GOOGLE_DRIVE_PATH, 'logs')):
- handle_files(root, files)
-
- for root, dirs, files in os.walk(os.path.join(GOOGLE_DRIVE_PATH, 'weights')):
- handle_files(root, files, True)
-
- # Copy files in batches
- total_files = len(files_to_copy)
- start_time = time.time()
- for i, (source, dest) in enumerate(files_to_copy, start=1):
- with open(source, 'rb') as src, open(dest, 'wb') as dst:
- shutil.copyfileobj(src, dst, 1024*1024) # 1MB buffer size
- # Report progress every 5 seconds or after every 100 files, whichever is less frequent
- if time.time() - start_time > 5 or i % 100 == 0:
- print(f'\rCopying file {i} of {total_files} ({i * 100 / total_files:.2f}%)', end="")
- start_time = time.time()
- print(f'\nImported {len(files_to_copy)} files from Google Drive backup')
-
- # Copy weights in batches
- total_weights = len(weights_to_copy)
- start_time = time.time()
- for i, (source, dest) in enumerate(weights_to_copy, start=1):
- with open(source, 'rb') as src, open(dest, 'wb') as dst:
- shutil.copyfileobj(src, dst, 1024*1024) # 1MB buffer size
- # Report progress every 5 seconds or after every 100 files, whichever is less frequent
- if time.time() - start_time > 5 or i % 100 == 0:
- print(f'\rCopying weight file {i} of {total_weights} ({i * 100 / total_weights:.2f}%)', end="")
- start_time = time.time()
- if weights_exist:
- print(f'\nImported {len(weights_to_copy)} weight files')
- print("Copied weights from Google Drive backup to local weights folder.")
- else:
- print("\nNo weights found in Google Drive backup.")
- print("Google Drive backup import completed.")
-
-def backup_files():
- print("\n Starting backup loop...")
- last_backup_timestamps_path = os.path.join(LOGS_FOLDER, 'last_backup_timestamps.txt')
- fully_updated = False # boolean to track if all files are up to date
- try:
- with open(last_backup_timestamps_path, 'r') as f:
- last_backup_timestamps = dict(line.strip().split(':') for line in f)
- except:
- last_backup_timestamps = {}
-
- while True:
- updated = False
- files_to_copy = []
- files_to_delete = []
-
- for root, dirs, files in os.walk(LOGS_FOLDER):
- for filename in files:
- if filename != 'last_backup_timestamps.txt':
- filepath = os.path.join(root, filename)
- if os.path.isfile(filepath):
- backup_filepath = os.path.join(GOOGLE_DRIVE_PATH, os.path.relpath(filepath, LOGS_FOLDER))
- backup_folderpath = os.path.dirname(backup_filepath)
-
- if not os.path.exists(backup_folderpath):
- os.makedirs(backup_folderpath)
- print(f'Created backup folder: {backup_folderpath}', flush=True)
-
- # check if file has changed since last backup
- last_backup_timestamp = last_backup_timestamps.get(filepath)
- current_timestamp = os.path.getmtime(filepath)
- if last_backup_timestamp is None or float(last_backup_timestamp) < current_timestamp:
- files_to_copy.append((filepath, backup_filepath)) # add to list of files to copy
- last_backup_timestamps[filepath] = str(current_timestamp) # update last backup timestamp
- updated = True
- fully_updated = False # if a file is updated, all files are not up to date
-
- # check if any files were deleted in Colab and delete them from the backup drive
- for filepath in list(last_backup_timestamps.keys()):
- if not os.path.exists(filepath):
- backup_filepath = os.path.join(GOOGLE_DRIVE_PATH, os.path.relpath(filepath, LOGS_FOLDER))
- if os.path.exists(backup_filepath):
- files_to_delete.append(backup_filepath) # add to list of files to delete
- del last_backup_timestamps[filepath]
- updated = True
- fully_updated = False # if a file is deleted, all files are not up to date
-
- # Copy files in batches
- if files_to_copy:
- for source, dest in files_to_copy:
- shutil.copy2(source, dest)
- print(f'Copied or updated {len(files_to_copy)} files')
-
- # Delete files in batches
- if files_to_delete:
- for file in files_to_delete:
- os.remove(file)
- print(f'Deleted {len(files_to_delete)} files')
-
- if not updated and not fully_updated:
- print("Files are up to date.")
- fully_updated = True # if all files are up to date, set the boolean to True
- copy_weights_folder_to_drive()
-
- with open(last_backup_timestamps_path, 'w') as f:
- for filepath, timestamp in last_backup_timestamps.items():
- f.write(f'{filepath}:{timestamp}\n')
- time.sleep(15) # wait for 15 seconds before checking again
diff --git a/spaces/FridaZuley/RVC_HFKawaii/infer_batch_rvc.py b/spaces/FridaZuley/RVC_HFKawaii/infer_batch_rvc.py
deleted file mode 100644
index 15c862a3d6bf815fa68003cc7054b694cae50c2a..0000000000000000000000000000000000000000
--- a/spaces/FridaZuley/RVC_HFKawaii/infer_batch_rvc.py
+++ /dev/null
@@ -1,215 +0,0 @@
-"""
-v1
-runtime\python.exe myinfer-v2-0528.py 0 "E:\codes\py39\RVC-beta\todo-songs" "E:\codes\py39\logs\mi-test\added_IVF677_Flat_nprobe_7.index" harvest "E:\codes\py39\RVC-beta\output" "E:\codes\py39\test-20230416b\weights\mi-test.pth" 0.66 cuda:0 True 3 0 1 0.33
-v2
-runtime\python.exe myinfer-v2-0528.py 0 "E:\codes\py39\RVC-beta\todo-songs" "E:\codes\py39\test-20230416b\logs\mi-test-v2\aadded_IVF677_Flat_nprobe_1_v2.index" harvest "E:\codes\py39\RVC-beta\output_v2" "E:\codes\py39\test-20230416b\weights\mi-test-v2.pth" 0.66 cuda:0 True 3 0 1 0.33
-"""
-import os, sys, pdb, torch
-
-now_dir = os.getcwd()
-sys.path.append(now_dir)
-import sys
-import torch
-import tqdm as tq
-from multiprocessing import cpu_count
-
-
-class Config:
- def __init__(self, device, is_half):
- self.device = device
- self.is_half = is_half
- self.n_cpu = 0
- self.gpu_name = None
- self.gpu_mem = None
- self.x_pad, self.x_query, self.x_center, self.x_max = self.device_config()
-
- def device_config(self) -> tuple:
- if torch.cuda.is_available():
- i_device = int(self.device.split(":")[-1])
- self.gpu_name = torch.cuda.get_device_name(i_device)
- if (
- ("16" in self.gpu_name and "V100" not in self.gpu_name.upper())
- or "P40" in self.gpu_name.upper()
- or "1060" in self.gpu_name
- or "1070" in self.gpu_name
- or "1080" in self.gpu_name
- ):
- print("16系/10系显卡和P40强制单精度")
- self.is_half = False
- for config_file in ["32k.json", "40k.json", "48k.json"]:
- with open(f"configs/{config_file}", "r") as f:
- strr = f.read().replace("true", "false")
- with open(f"configs/{config_file}", "w") as f:
- f.write(strr)
- with open("infer/modules/train/preprocess.py", "r") as f:
- strr = f.read().replace("3.7", "3.0")
- with open("infer/modules/train/preprocess.py", "w") as f:
- f.write(strr)
- else:
- self.gpu_name = None
- self.gpu_mem = int(
- torch.cuda.get_device_properties(i_device).total_memory
- / 1024
- / 1024
- / 1024
- + 0.4
- )
- if self.gpu_mem <= 4:
- with open("infer/modules/train/preprocess.py", "r") as f:
- strr = f.read().replace("3.7", "3.0")
- with open("infer/modules/train/preprocess.py", "w") as f:
- f.write(strr)
- elif torch.backends.mps.is_available():
- print("没有发现支持的N卡, 使用MPS进行推理")
- self.device = "mps"
- else:
- print("没有发现支持的N卡, 使用CPU进行推理")
- self.device = "cpu"
- self.is_half = True
-
- if self.n_cpu == 0:
- self.n_cpu = cpu_count()
-
- if self.is_half:
- # 6G显存配置
- x_pad = 3
- x_query = 10
- x_center = 60
- x_max = 65
- else:
- # 5G显存配置
- x_pad = 1
- x_query = 6
- x_center = 38
- x_max = 41
-
- if self.gpu_mem != None and self.gpu_mem <= 4:
- x_pad = 1
- x_query = 5
- x_center = 30
- x_max = 32
-
- return x_pad, x_query, x_center, x_max
-
-
-f0up_key = sys.argv[1]
-input_path = sys.argv[2]
-index_path = sys.argv[3]
-f0method = sys.argv[4] # harvest or pm
-opt_path = sys.argv[5]
-model_path = sys.argv[6]
-index_rate = float(sys.argv[7])
-device = sys.argv[8]
-is_half = sys.argv[9].lower() != "false"
-filter_radius = int(sys.argv[10])
-resample_sr = int(sys.argv[11])
-rms_mix_rate = float(sys.argv[12])
-protect = float(sys.argv[13])
-print(sys.argv)
-config = Config(device, is_half)
-now_dir = os.getcwd()
-sys.path.append(now_dir)
-from infer.modules.vc.modules import VC
-from lib.infer_pack.models import (
- SynthesizerTrnMs256NSFsid,
- SynthesizerTrnMs256NSFsid_nono,
- SynthesizerTrnMs768NSFsid,
- SynthesizerTrnMs768NSFsid_nono,
-)
-from infer.lib.audio import load_audio
-from fairseq import checkpoint_utils
-from scipy.io import wavfile
-
-hubert_model = None
-
-
-def load_hubert():
- global hubert_model
- models, saved_cfg, task = checkpoint_utils.load_model_ensemble_and_task(
- ["hubert_base.pt"],
- suffix="",
- )
- hubert_model = models[0]
- hubert_model = hubert_model.to(device)
- if is_half:
- hubert_model = hubert_model.half()
- else:
- hubert_model = hubert_model.float()
- hubert_model.eval()
-
-
-def vc_single(sid, input_audio, f0_up_key, f0_file, f0_method, file_index, index_rate):
- global tgt_sr, net_g, vc, hubert_model, version
- if input_audio is None:
- return "You need to upload an audio", None
- f0_up_key = int(f0_up_key)
- audio = load_audio(input_audio, 16000)
- times = [0, 0, 0]
- if hubert_model == None:
- load_hubert()
- if_f0 = cpt.get("f0", 1)
- # audio_opt=vc.pipeline(hubert_model,net_g,sid,audio,times,f0_up_key,f0_method,file_index,file_big_npy,index_rate,if_f0,f0_file=f0_file)
- audio_opt = vc.pipeline(
- hubert_model,
- net_g,
- sid,
- audio,
- input_audio,
- times,
- f0_up_key,
- f0_method,
- file_index,
- index_rate,
- if_f0,
- filter_radius,
- tgt_sr,
- resample_sr,
- rms_mix_rate,
- version,
- protect,
- f0_file=f0_file,
- )
- print(times)
- return audio_opt
-
-
-def get_vc(model_path):
- global n_spk, tgt_sr, net_g, vc, cpt, device, is_half, version
- print("loading pth %s" % model_path)
- cpt = torch.load(model_path, map_location="cpu")
- tgt_sr = cpt["config"][-1]
- cpt["config"][-3] = cpt["weight"]["emb_g.weight"].shape[0] # n_spk
- if_f0 = cpt.get("f0", 1)
- version = cpt.get("version", "v1")
- if version == "v1":
- if if_f0 == 1:
- net_g = SynthesizerTrnMs256NSFsid(*cpt["config"], is_half=is_half)
- else:
- net_g = SynthesizerTrnMs256NSFsid_nono(*cpt["config"])
- elif version == "v2":
- if if_f0 == 1: #
- net_g = SynthesizerTrnMs768NSFsid(*cpt["config"], is_half=is_half)
- else:
- net_g = SynthesizerTrnMs768NSFsid_nono(*cpt["config"])
- del net_g.enc_q
- print(net_g.load_state_dict(cpt["weight"], strict=False)) # 不加这一行清不干净,真奇葩
- net_g.eval().to(device)
- if is_half:
- net_g = net_g.half()
- else:
- net_g = net_g.float()
- vc = VC(tgt_sr, config)
- n_spk = cpt["config"][-3]
- # return {"visible": True,"maximum": n_spk, "__type__": "update"}
-
-
-get_vc(model_path)
-audios = os.listdir(input_path)
-for file in tq.tqdm(audios):
- if file.endswith(".wav"):
- file_path = input_path + "/" + file
- wav_opt = vc_single(
- 0, file_path, f0up_key, None, f0method, index_path, index_rate
- )
- out_path = opt_path + "/" + file
- wavfile.write(out_path, tgt_sr, wav_opt)
diff --git a/spaces/GMFTBY/PandaGPT/datasets/samplers.py b/spaces/GMFTBY/PandaGPT/datasets/samplers.py
deleted file mode 100644
index d3ce1e90b2177940acb911d31d1c5245d74a6119..0000000000000000000000000000000000000000
--- a/spaces/GMFTBY/PandaGPT/datasets/samplers.py
+++ /dev/null
@@ -1,166 +0,0 @@
-# coding=utf-8
-# Copyright (c) 2019, NVIDIA CORPORATION. All rights reserved.
-#
-# Licensed under the Apache License, Version 2.0 (the "License");
-# you may not use this file except in compliance with the License.
-# You may obtain a copy of the License at
-#
-# http://www.apache.org/licenses/LICENSE-2.0
-#
-# Unless required by applicable law or agreed to in writing, software
-# distributed under the License is distributed on an "AS IS" BASIS,
-# WITHOUT WARRANTIES OR CONDITIONS OF ANY KIND, either express or implied.
-# See the License for the specific language governing permissions and
-# limitations under the License.
-"""batch samplers that work with either random or sequential data samplers"""
-import math
-import os
-import sys
-
-import torch
-from torch.utils import data
-import numpy as np
-
-
-class RandomSampler(data.sampler.Sampler):
- r"""
- Based off of pytorch RandomSampler and DistributedSampler. Essentially a RandomSampler,
- but this class lets the user set an epoch like DistributedSampler
- Samples elements randomly. If without replacement, then sample from a shuffled dataset.
- If with replacement, then user can specify ``num_samples`` to draw.
- Arguments:
- data_source (Dataset): dataset to sample from
- num_samples (int): number of samples to draw, default=len(dataset)
- replacement (bool): samples are drawn with replacement if ``True``, default=False
- """
-
- def __init__(self, data_source, replacement=False, num_samples=None):
- super(RandomSampler, self).__init__(data_source)
- self.data_source = data_source
- self.replacement = replacement
- self._num_samples = num_samples
- self.epoch = -1
-
- if self._num_samples is not None and replacement is False:
- raise ValueError("With replacement=False, num_samples should not be specified, "
- "since a random permute will be performed.")
-
- if not isinstance(self.num_samples, int) or self.num_samples <= 0:
- raise ValueError("num_samples should be a positive integer "
- "value, but got num_samples={}".format(self.num_samples))
- if not isinstance(self.replacement, bool):
- raise ValueError("replacement should be a boolean value, but got "
- "replacement={}".format(self.replacement))
-
- @property
- def num_samples(self):
- # dataset size might change at runtime
- if self._num_samples is None:
- return len(self.data_source)
- return self._num_samples
-
- def __iter__(self):
- n = len(self.data_source)
- g = torch.Generator()
- if self.epoch >= 0:
- g.manual_seed(self.epoch)
- if self.replacement:
- for _ in range(self.num_samples // 32):
- yield from torch.randint(high=n, size=(32,), dtype=torch.int64, generator=g).tolist()
- yield from torch.randint(high=n, size=(self.num_samples % 32,), dtype=torch.int64,
- generator=g).tolist()
- else:
- yield from torch.randperm(n, generator=self.generator).tolist()
-
- def __len__(self):
- return self.num_samples
-
- def set_epoch(self, epoch):
- self.epoch = epoch
-
-
-class DistributedSequentialSampler(data.sampler.Sampler):
- def __init__(self, num_samples, train_iters, batch_size, rank=-1, world_size=2):
- super().__init__(num_samples)
- if rank == -1:
- rank = 0
- world_size = 1
- self.num_samples = num_samples
- self.rank = rank
- self.world_size = world_size
- self.start_iter = 0
- self.train_iters = train_iters
- self.batch_size = batch_size
- self.batch_bias = [i * (num_samples // batch_size) for i in range(batch_size)]
-
- def __iter__(self):
- for idx in range(self.start_iter, self.train_iters * 10):
- batch = [(idx + bias) % self.num_samples for bias in self.batch_bias]
- tbatch = self._batch(batch)
- yield tbatch
-
- def __len__(self):
- return self.train_iters
-
- def _batch(self, batch):
- """extracts samples only pertaining to this worker's batch"""
- start = self.rank*self.batch_size//self.world_size
- end = (self.rank+1)*self.batch_size//self.world_size
- return batch[start:end]
-
-
-class DistributedBatchSampler(data.sampler.BatchSampler):
- """
- similar to normal implementation of distributed sampler, except implementation is at the
- batch sampler level, instead of just the sampler level. This allows wrapping of arbitrary
- data samplers (sequential, random, WeightedRandomSampler, etc.) with this batch sampler.
- """
- def __init__(self, sampler, batch_size, drop_last, rank=-1, world_size=2, wrap_last=False, gradient_accumulation_steps=None):
- super(DistributedBatchSampler, self).__init__(sampler, batch_size, drop_last)
- if rank == -1:
- assert False, 'should not be here'
- self.rank = rank
- self.world_size = world_size
- self.sampler.wrap_around = 0
- self.wrap_around = 0
- self.wrap_last = wrap_last
- self.start_iter = 0
- self.effective_batch_size = batch_size if gradient_accumulation_steps is None else batch_size * gradient_accumulation_steps
-
- def __iter__(self):
- batch = []
- i = 0
- for idx in self.data_iterator(self.sampler, wrap_around=False):
- batch.append(idx)
- if len(batch) == self.batch_size:
- tbatch = self._batch(batch)
- if i >= self.start_iter * self.effective_batch_size:
- yield tbatch
- self.start_iter = 0
- i += len(batch)
- batch = []
- batch_len = len(batch)
- if batch_len > 0 and not self.drop_last:
- if self.wrap_last:
- self.sampler.wrap_around -= (self.batch_size)
- self.wrap_around += (len(batch))
- self.wrap_around %= self.batch_size
- yield self._batch(batch)
- if self.wrap_last:
- self.sampler.wrap_around += self.batch_size
-
- def data_iterator(self, _iter, wrap_around=False):
- """iterates through data and handles wrap around"""
- for i, idx in enumerate(_iter):
- if i < self.wrap_around%self.batch_size:
- continue
- if wrap_around:
- self.wrap_around += 1
- self.wrap_around %= self.batch_size
- yield idx
-
- def _batch(self, batch):
- """extracts samples only pertaining to this worker's batch"""
- start = self.rank*self.batch_size//self.world_size
- end = (self.rank+1)*self.batch_size//self.world_size
- return batch[start:end]
diff --git a/spaces/GT4SD/paccmann_rl/README.md b/spaces/GT4SD/paccmann_rl/README.md
deleted file mode 100644
index 06d8cff6e50add08db1cd5d6a1cea3c75c6a0e18..0000000000000000000000000000000000000000
--- a/spaces/GT4SD/paccmann_rl/README.md
+++ /dev/null
@@ -1,15 +0,0 @@
----
-title: PaccMann^RL
-emoji: 💡
-colorFrom: green
-colorTo: blue
-sdk: gradio
-sdk_version: 3.46.0
-app_file: app.py
-pinned: false
-python_version: 3.8.13
-pypi_version: 20.2.4
-duplicated_from: jannisborn/gt4sd-torchdrug
----
-
-Check out the configuration reference at https://huggingface.co/docs/hub/spaces-config-reference
\ No newline at end of file
diff --git a/spaces/GeekedReals/jonatasgrosman-wav2vec2-large-xlsr-53-english/app.py b/spaces/GeekedReals/jonatasgrosman-wav2vec2-large-xlsr-53-english/app.py
deleted file mode 100644
index f7bc16986ba92aee8660a44201cba56f67447114..0000000000000000000000000000000000000000
--- a/spaces/GeekedReals/jonatasgrosman-wav2vec2-large-xlsr-53-english/app.py
+++ /dev/null
@@ -1,3 +0,0 @@
-import gradio as gr
-
-gr.Interface.load("models/jonatasgrosman/wav2vec2-large-xlsr-53-english").launch()
\ No newline at end of file
diff --git a/spaces/Gradio-Blocks/anime-colorization/scripts/super_res_sample.py b/spaces/Gradio-Blocks/anime-colorization/scripts/super_res_sample.py
deleted file mode 100644
index d7e4e3374073945a4f34d92c0caab164c45eac3a..0000000000000000000000000000000000000000
--- a/spaces/Gradio-Blocks/anime-colorization/scripts/super_res_sample.py
+++ /dev/null
@@ -1,117 +0,0 @@
-"""
-Generate a large batch of samples from a super resolution model, given a batch
-of samples from a regular model from image_sample.py.
-"""
-
-import argparse
-import os
-
-import blobfile as bf
-import numpy as np
-import torch as th
-import torch.distributed as dist
-
-from pixel_guide_diffusion import dist_util, logger
-from pixel_guide_diffusion.script_util import (
- sr_model_and_diffusion_defaults,
- sr_create_model_and_diffusion,
- args_to_dict,
- add_dict_to_argparser,
-)
-
-
-def main():
- args = create_argparser().parse_args()
-
- dist_util.setup_dist()
- logger.configure()
-
- logger.log("creating model...")
- model, diffusion = sr_create_model_and_diffusion(
- **args_to_dict(args, sr_model_and_diffusion_defaults().keys())
- )
- model.load_state_dict(
- dist_util.load_state_dict(args.model_path, map_location="cpu")
- )
- model.to(dist_util.dev())
- model.eval()
-
- logger.log("loading data...")
- data = load_data_for_worker(args.base_samples, args.batch_size, args.class_cond)
-
- logger.log("creating samples...")
- all_images = []
- while len(all_images) * args.batch_size < args.num_samples:
- model_kwargs = next(data)
- model_kwargs = {k: v.to(dist_util.dev()) for k, v in model_kwargs.items()}
- sample = diffusion.p_sample_loop(
- model,
- (args.batch_size, 3, args.large_size, args.large_size),
- clip_denoised=args.clip_denoised,
- model_kwargs=model_kwargs,
- )
- sample = ((sample + 1) * 127.5).clamp(0, 255).to(th.uint8)
- sample = sample.permute(0, 2, 3, 1)
- sample = sample.contiguous()
-
- all_samples = [th.zeros_like(sample) for _ in range(dist.get_world_size())]
- dist.all_gather(all_samples, sample) # gather not supported with NCCL
- for sample in all_samples:
- all_images.append(sample.cpu().numpy())
- logger.log(f"created {len(all_images) * args.batch_size} samples")
-
- arr = np.concatenate(all_images, axis=0)
- arr = arr[: args.num_samples]
- if dist.get_rank() == 0:
- shape_str = "x".join([str(x) for x in arr.shape])
- out_path = os.path.join(logger.get_dir(), f"samples_{shape_str}.npz")
- logger.log(f"saving to {out_path}")
- np.savez(out_path, arr)
-
- dist.barrier()
- logger.log("sampling complete")
-
-
-def load_data_for_worker(base_samples, batch_size, class_cond):
- with bf.BlobFile(base_samples, "rb") as f:
- obj = np.load(f)
- image_arr = obj["arr_0"]
- if class_cond:
- label_arr = obj["arr_1"]
- rank = dist.get_rank()
- num_ranks = dist.get_world_size()
- buffer = []
- label_buffer = []
- while True:
- for i in range(rank, len(image_arr), num_ranks):
- buffer.append(image_arr[i])
- if class_cond:
- label_buffer.append(label_arr[i])
- if len(buffer) == batch_size:
- batch = th.from_numpy(np.stack(buffer)).float()
- batch = batch / 127.5 - 1.0
- batch = batch.permute(0, 3, 1, 2)
- res = dict(low_res=batch)
- if class_cond:
- res["y"] = th.from_numpy(np.stack(label_buffer))
- yield res
- buffer, label_buffer = [], []
-
-
-def create_argparser():
- defaults = dict(
- clip_denoised=True,
- num_samples=10000,
- batch_size=16,
- use_ddim=False,
- base_samples="",
- model_path="",
- )
- defaults.update(sr_model_and_diffusion_defaults())
- parser = argparse.ArgumentParser()
- add_dict_to_argparser(parser, defaults)
- return parser
-
-
-if __name__ == "__main__":
- main()
diff --git a/spaces/Gradio-Blocks/uniformer_image_detection/configs/double_heads/dh_faster_rcnn_r50_fpn_1x_coco.py b/spaces/Gradio-Blocks/uniformer_image_detection/configs/double_heads/dh_faster_rcnn_r50_fpn_1x_coco.py
deleted file mode 100644
index 9b8118b4b633c78120c370f877f47e951c2fdb38..0000000000000000000000000000000000000000
--- a/spaces/Gradio-Blocks/uniformer_image_detection/configs/double_heads/dh_faster_rcnn_r50_fpn_1x_coco.py
+++ /dev/null
@@ -1,23 +0,0 @@
-_base_ = '../faster_rcnn/faster_rcnn_r50_fpn_1x_coco.py'
-model = dict(
- roi_head=dict(
- type='DoubleHeadRoIHead',
- reg_roi_scale_factor=1.3,
- bbox_head=dict(
- _delete_=True,
- type='DoubleConvFCBBoxHead',
- num_convs=4,
- num_fcs=2,
- in_channels=256,
- conv_out_channels=1024,
- fc_out_channels=1024,
- roi_feat_size=7,
- num_classes=80,
- bbox_coder=dict(
- type='DeltaXYWHBBoxCoder',
- target_means=[0., 0., 0., 0.],
- target_stds=[0.1, 0.1, 0.2, 0.2]),
- reg_class_agnostic=False,
- loss_cls=dict(
- type='CrossEntropyLoss', use_sigmoid=False, loss_weight=2.0),
- loss_bbox=dict(type='SmoothL1Loss', beta=1.0, loss_weight=2.0))))
diff --git a/spaces/Gradio-Blocks/uniformer_image_detection/configs/fpg/retinanet_r50_fpg_crop640_50e_coco.py b/spaces/Gradio-Blocks/uniformer_image_detection/configs/fpg/retinanet_r50_fpg_crop640_50e_coco.py
deleted file mode 100644
index 504ed5ec5040559b3d10f7caf8a970005a1a92d7..0000000000000000000000000000000000000000
--- a/spaces/Gradio-Blocks/uniformer_image_detection/configs/fpg/retinanet_r50_fpg_crop640_50e_coco.py
+++ /dev/null
@@ -1,53 +0,0 @@
-_base_ = '../nas_fpn/retinanet_r50_nasfpn_crop640_50e_coco.py'
-
-norm_cfg = dict(type='BN', requires_grad=True)
-model = dict(
- neck=dict(
- _delete_=True,
- type='FPG',
- in_channels=[256, 512, 1024, 2048],
- out_channels=256,
- inter_channels=256,
- num_outs=5,
- add_extra_convs=True,
- start_level=1,
- stack_times=9,
- paths=['bu'] * 9,
- same_down_trans=None,
- same_up_trans=dict(
- type='conv',
- kernel_size=3,
- stride=2,
- padding=1,
- norm_cfg=norm_cfg,
- inplace=False,
- order=('act', 'conv', 'norm')),
- across_lateral_trans=dict(
- type='conv',
- kernel_size=1,
- norm_cfg=norm_cfg,
- inplace=False,
- order=('act', 'conv', 'norm')),
- across_down_trans=dict(
- type='interpolation_conv',
- mode='nearest',
- kernel_size=3,
- norm_cfg=norm_cfg,
- order=('act', 'conv', 'norm'),
- inplace=False),
- across_up_trans=None,
- across_skip_trans=dict(
- type='conv',
- kernel_size=1,
- norm_cfg=norm_cfg,
- inplace=False,
- order=('act', 'conv', 'norm')),
- output_trans=dict(
- type='last_conv',
- kernel_size=3,
- order=('act', 'conv', 'norm'),
- inplace=False),
- norm_cfg=norm_cfg,
- skip_inds=[(0, 1, 2, 3), (0, 1, 2), (0, 1), (0, ), ()]))
-
-evaluation = dict(interval=2)
diff --git a/spaces/Gradio-Blocks/uniformer_image_detection/configs/hrnet/mask_rcnn_hrnetv2p_w40_1x_coco.py b/spaces/Gradio-Blocks/uniformer_image_detection/configs/hrnet/mask_rcnn_hrnetv2p_w40_1x_coco.py
deleted file mode 100644
index 5b10c166cf36601bdb895de81874970aebc83310..0000000000000000000000000000000000000000
--- a/spaces/Gradio-Blocks/uniformer_image_detection/configs/hrnet/mask_rcnn_hrnetv2p_w40_1x_coco.py
+++ /dev/null
@@ -1,10 +0,0 @@
-_base_ = './mask_rcnn_hrnetv2p_w18_1x_coco.py'
-model = dict(
- pretrained='open-mmlab://msra/hrnetv2_w40',
- backbone=dict(
- type='HRNet',
- extra=dict(
- stage2=dict(num_channels=(40, 80)),
- stage3=dict(num_channels=(40, 80, 160)),
- stage4=dict(num_channels=(40, 80, 160, 320)))),
- neck=dict(type='HRFPN', in_channels=[40, 80, 160, 320], out_channels=256))
diff --git a/spaces/Gradio-Blocks/uniformer_image_detection/configs/retinanet/retinanet_r101_fpn_2x_coco.py b/spaces/Gradio-Blocks/uniformer_image_detection/configs/retinanet/retinanet_r101_fpn_2x_coco.py
deleted file mode 100644
index c12088a266d7ccad31bd2233ee5a9ee90f4c2b14..0000000000000000000000000000000000000000
--- a/spaces/Gradio-Blocks/uniformer_image_detection/configs/retinanet/retinanet_r101_fpn_2x_coco.py
+++ /dev/null
@@ -1,2 +0,0 @@
-_base_ = './retinanet_r50_fpn_2x_coco.py'
-model = dict(pretrained='torchvision://resnet101', backbone=dict(depth=101))
diff --git a/spaces/Gradio-Blocks/uniformer_image_detection/mmdet/core/utils/__init__.py b/spaces/Gradio-Blocks/uniformer_image_detection/mmdet/core/utils/__init__.py
deleted file mode 100644
index 5c51dac6d648f41d5c5f46dbf703f19469a7bb6c..0000000000000000000000000000000000000000
--- a/spaces/Gradio-Blocks/uniformer_image_detection/mmdet/core/utils/__init__.py
+++ /dev/null
@@ -1,7 +0,0 @@
-from .dist_utils import DistOptimizerHook, allreduce_grads, reduce_mean
-from .misc import mask2ndarray, multi_apply, unmap
-
-__all__ = [
- 'allreduce_grads', 'DistOptimizerHook', 'reduce_mean', 'multi_apply',
- 'unmap', 'mask2ndarray'
-]
diff --git a/spaces/GrandaddyShmax/AudioCraft_Plus/audiocraft/models/lm.py b/spaces/GrandaddyShmax/AudioCraft_Plus/audiocraft/models/lm.py
deleted file mode 100644
index 8cefd2c58c3a337378579d6cd6469fd038cbb1ee..0000000000000000000000000000000000000000
--- a/spaces/GrandaddyShmax/AudioCraft_Plus/audiocraft/models/lm.py
+++ /dev/null
@@ -1,531 +0,0 @@
-# Copyright (c) Meta Platforms, Inc. and affiliates.
-# All rights reserved.
-#
-# This source code is licensed under the license found in the
-# LICENSE file in the root directory of this source tree.
-
-from dataclasses import dataclass
-from functools import partial
-import logging
-import math
-import typing as tp
-
-import torch
-from torch import nn
-
-from ..utils import utils
-from ..modules.streaming import StreamingModule, State
-from ..modules.transformer import StreamingTransformer, create_norm_fn
-from ..modules.conditioners import (
- ConditionFuser,
- ClassifierFreeGuidanceDropout,
- AttributeDropout,
- ConditioningProvider,
- ConditioningAttributes,
- ConditionType,
-)
-from ..modules.codebooks_patterns import CodebooksPatternProvider
-from ..modules.activations import get_activation_fn
-
-
-logger = logging.getLogger(__name__)
-ConditionTensors = tp.Dict[str, ConditionType]
-CFGConditions = tp.Union[ConditionTensors, tp.Tuple[ConditionTensors, ConditionTensors]]
-
-
-def get_init_fn(method: str, input_dim: int, init_depth: tp.Optional[int] = None):
- """LM layer initialization.
- Inspired from xlformers: https://github.com/fairinternal/xlformers
-
- Args:
- method (str): Method name for init function. Valid options are:
- 'gaussian', 'uniform'.
- input_dim (int): Input dimension of the initialized module.
- init_depth (int, optional): Optional init depth value used to rescale
- the standard deviation if defined.
- """
- # Compute std
- std = 1 / math.sqrt(input_dim)
- # Rescale with depth
- if init_depth is not None:
- std = std / math.sqrt(2 * init_depth)
-
- if method == 'gaussian':
- return partial(
- torch.nn.init.trunc_normal_, mean=0.0, std=std, a=-3 * std, b=3 * std
- )
- elif method == 'uniform':
- bound = math.sqrt(3) * std # ensure the standard deviation is `std`
- return partial(torch.nn.init.uniform_, a=-bound, b=bound)
- else:
- raise ValueError("Unsupported layer initialization method")
-
-
-def init_layer(m: nn.Module,
- method: str,
- init_depth: tp.Optional[int] = None,
- zero_bias_init: bool = False):
- """Wrapper around ``get_init_fn`` for proper initialization of LM modules.
-
- Args:
- m (nn.Module): Module to initialize.
- method (str): Method name for the init function.
- init_depth (int, optional): Optional init depth value used to rescale
- the standard deviation if defined.
- zero_bias_init (bool): Whether to initialize the bias to 0 or not.
- """
- if isinstance(m, nn.Linear):
- init_fn = get_init_fn(method, m.in_features, init_depth=init_depth)
- if m.weight.device.type == 'cpu' and m.weight.dtype == torch.float16:
- weight = m.weight.float()
- init_fn(weight)
- m.weight.data[:] = weight.half()
- else:
- init_fn(m.weight)
- if zero_bias_init and m.bias is not None:
- nn.init.constant_(m.bias, 0)
- elif isinstance(m, nn.Embedding):
- init_fn = get_init_fn(method, m.embedding_dim, init_depth=None)
- if m.weight.device.type == 'cpu' and m.weight.dtype == torch.float16:
- weight = m.weight.float()
- init_fn(weight)
- m.weight.data[:] = weight.half()
- else:
- init_fn(m.weight)
-
-
-class ScaledEmbedding(nn.Embedding):
- """Boost learning rate for embeddings (with `scale`).
- """
- def __init__(self, *args, lr=None, **kwargs):
- super().__init__(*args, **kwargs)
- self.lr = lr
-
- def make_optim_group(self):
- group = {"params": list(self.parameters())}
- if self.lr is not None:
- group["lr"] = self.lr
- return group
-
-
-@dataclass
-class LMOutput:
- # The logits are already re-aligned with the input codes
- # hence no extra shift is required, e.g. when computing CE
- logits: torch.Tensor # [B, K, T, card]
- mask: torch.Tensor # [B, K, T]
-
-
-class LMModel(StreamingModule):
- """Transformer-based language model on multiple streams of codes.
-
- Args:
- pattern_provider (CodebooksPatternProvider): Pattern provider for codebook interleaving.
- condition_provider (MusicConditioningProvider): Conditioning provider from metadata.
- fuser (ConditionFuser): Fuser handling the fusing of conditions with language model input.
- n_q (int): Number of parallel streams to model.
- card (int): Cardinality, vocabulary size.
- dim (int): Dimension of the transformer encoder.
- num_heads (int): Number of heads for the transformer encoder.
- hidden_scale (int): Scale for hidden feed forward dimension of the transformer encoder.
- norm (str): Normalization method.
- norm_first (bool): Use pre-norm instead of post-norm.
- emb_lr (float, optional): Embedding-specific learning rate.
- bias_proj (bool): Use bias for output projections.
- weight_init (str, optional): Method for weight initialization.
- depthwise_init (str, optional): Method for depthwise weight initialization.
- zero_bias_init (bool): If true and bias in Linears, initialize bias to zeros.
- cfg_dropout (float): Classifier-free guidance dropout.
- cfg_coef (float): Classifier-free guidance coefficient.
- attribute_dropout (dict): Attribute dropout probabilities.
- two_step_cfg (bool): Whether to run classifier free-guidance with 2 distinct steps.
- **kwargs: Additional parameters for the transformer encoder.
- """
- def __init__(self, pattern_provider: CodebooksPatternProvider, condition_provider: ConditioningProvider,
- fuser: ConditionFuser, n_q: int = 8, card: int = 1024, dim: int = 128, num_heads: int = 8,
- hidden_scale: int = 4, norm: str = 'layer_norm', norm_first: bool = False,
- emb_lr: tp.Optional[float] = None, bias_proj: bool = True,
- weight_init: tp.Optional[str] = None, depthwise_init: tp.Optional[str] = None,
- zero_bias_init: bool = False, cfg_dropout: float = 0, cfg_coef: float = 1.0,
- attribute_dropout: tp.Dict[str, tp.Dict[str, float]] = {}, two_step_cfg: bool = False,
- **kwargs):
- super().__init__()
- self.cfg_coef = cfg_coef
- self.cfg_dropout = ClassifierFreeGuidanceDropout(p=cfg_dropout)
- self.att_dropout = AttributeDropout(p=attribute_dropout)
- self.condition_provider = condition_provider
- self.fuser = fuser
- self.card = card
- embed_dim = self.card + 1
- self.n_q = n_q
- self.dim = dim
- self.pattern_provider = pattern_provider
- self.two_step_cfg = two_step_cfg
- self.emb = nn.ModuleList([ScaledEmbedding(embed_dim, dim, lr=emb_lr) for _ in range(n_q)])
- if 'activation' in kwargs:
- kwargs['activation'] = get_activation_fn(kwargs['activation'])
- self.transformer = StreamingTransformer(
- d_model=dim, num_heads=num_heads, dim_feedforward=int(hidden_scale * dim),
- norm=norm, norm_first=norm_first, **kwargs)
- self.out_norm: tp.Optional[nn.Module] = None
- if norm_first:
- self.out_norm = create_norm_fn(norm, dim)
- self.linears = nn.ModuleList([nn.Linear(dim, self.card, bias=bias_proj) for _ in range(n_q)])
- self._init_weights(weight_init, depthwise_init, zero_bias_init)
- self._fsdp: tp.Optional[nn.Module]
- self.__dict__['_fsdp'] = None
-
- def _init_weights(self, weight_init: tp.Optional[str], depthwise_init: tp.Optional[str], zero_bias_init: bool):
- """Initialization of the transformer module weights.
-
- Args:
- weight_init (str, optional): Weight initialization strategy. See ``get_init_fn`` for valid options.
- depthwise_init (str, optional): Depthwise initialization strategy. The following options are valid:
- 'current' where the depth corresponds to the current layer index or 'global' where the total number
- of layer is used as depth. If not set, no depthwise initialization strategy is used.
- zero_bias_init (bool): Whether to initialize bias to zero or not.
- """
- assert depthwise_init is None or depthwise_init in ['current', 'global']
- assert depthwise_init is None or weight_init is not None, \
- "If 'depthwise_init' is defined, a 'weight_init' method should be provided."
- assert not zero_bias_init or weight_init is not None, \
- "If 'zero_bias_init', a 'weight_init' method should be provided"
-
- if weight_init is None:
- return
-
- for emb_layer in self.emb:
- init_layer(emb_layer, method=weight_init, init_depth=None, zero_bias_init=zero_bias_init)
-
- for layer_idx, tr_layer in enumerate(self.transformer.layers):
- depth = None
- if depthwise_init == 'current':
- depth = layer_idx + 1
- elif depthwise_init == 'global':
- depth = len(self.transformer.layers)
- init_fn = partial(init_layer, method=weight_init, init_depth=depth, zero_bias_init=zero_bias_init)
- tr_layer.apply(init_fn)
-
- for linear in self.linears:
- init_layer(linear, method=weight_init, init_depth=None, zero_bias_init=zero_bias_init)
-
- @property
- def special_token_id(self) -> int:
- return self.card
-
- @property
- def num_codebooks(self) -> int:
- return self.n_q
-
- def forward(self, sequence: torch.Tensor,
- conditions: tp.List[ConditioningAttributes],
- condition_tensors: tp.Optional[ConditionTensors] = None) -> torch.Tensor:
- """Apply language model on sequence and conditions.
- Given a tensor of sequence of shape [B, K, S] with K the number of codebooks and
- S the sequence steps, return the logits with shape [B, card, K, S].
-
- Args:
- indices (torch.Tensor): Indices of the codes to model.
- conditions (list of ConditioningAttributes): Conditions to use when modeling
- the given codes. Note that when evaluating multiple time with the same conditioning
- you should pre-compute those and pass them as `condition_tensors`.
- condition_tensors (dict[str, ConditionType], optional): Pre-computed conditioning
- tensors, see `conditions`.
- Returns:
- torch.Tensor: Logits.
- """
- B, K, S = sequence.shape
- assert K == self.num_codebooks, "Sequence shape must match the specified number of codebooks"
- input_ = sum([self.emb[k](sequence[:, k]) for k in range(K)])
- if condition_tensors is None:
- assert not self._is_streaming, "Conditions tensors should be precomputed when streaming."
- # apply dropout modules
- conditions = self.cfg_dropout(conditions)
- conditions = self.att_dropout(conditions)
- tokenized = self.condition_provider.tokenize(conditions)
- # encode conditions and fuse, both have a streaming cache to not recompute when generating.
- condition_tensors = self.condition_provider(tokenized)
- else:
- assert not conditions, "Shouldn't pass both conditions and condition_tensors."
-
- input_, cross_attention_input = self.fuser(input_, condition_tensors)
-
- out = self.transformer(input_, cross_attention_src=cross_attention_input)
- if self.out_norm:
- out = self.out_norm(out)
- logits = torch.stack([self.linears[k](out) for k in range(K)], dim=1) # [B, K, S, card]
-
- # remove the prefix from the model outputs
- if len(self.fuser.fuse2cond['prepend']) > 0:
- logits = logits[:, :, -S:]
-
- return logits # [B, K, S, card]
-
- def compute_predictions(
- self, codes: torch.Tensor,
- conditions: tp.List[ConditioningAttributes],
- condition_tensors: tp.Optional[ConditionTensors] = None) -> LMOutput:
- """Given an input tensor of codes [B, K, T] and list of conditions, runs the model
- forward using the specified codes interleaving pattern.
-
- Args:
- codes (torch.Tensor): Input codes of shape [B, K, T] with B the batch size,
- K the number of codebooks and T the number of timesteps.
- conditions (list of ConditioningAttributes): conditionings to use when modeling
- the given codes. Note that when evaluating multiple time with the same conditioning
- you should pre-compute those and pass them as `condition_tensors`.
- condition_tensors (dict[str, ConditionType], optional): pre-computed conditioning
- tensors, see `conditions`.
- Returns:
- LMOutput: Language model outputs
- logits (torch.Tensor) of shape [B, K, T, card] corresponding to the provided codes,
- i.e. the first item corresponds to logits to predict the first code, meaning that
- no additional shifting of codes and logits is required.
- mask (torch.Tensor) of shape [B, K, T], mask over valid and invalid positions.
- Given the specified interleaving strategies, parts of the logits and codes should
- not be considered as valid predictions because of invalid context.
- """
- B, K, T = codes.shape
- codes = codes.contiguous()
- # map codes [B, K, T] into pattern sequence [B, K, S] using special_token_id for masked tokens
- pattern = self.pattern_provider.get_pattern(T)
- sequence_codes, sequence_indexes, sequence_mask = pattern.build_pattern_sequence(
- codes, self.special_token_id, keep_only_valid_steps=True
- )
- # apply model on pattern sequence
- model = self if self._fsdp is None else self._fsdp
- logits = model(sequence_codes, conditions, condition_tensors) # [B, K, S, card]
- # map back the logits on pattern sequence to logits on original codes: [B, K, S, card] -> [B, K, T, card]
- # and provide the corresponding mask over invalid positions of tokens
- logits = logits.permute(0, 3, 1, 2) # [B, card, K, S]
- # note: we use nans as special token to make it obvious if we feed unexpected logits
- logits, logits_indexes, logits_mask = pattern.revert_pattern_logits(
- logits, float('nan'), keep_only_valid_steps=True
- )
- logits = logits.permute(0, 2, 3, 1) # [B, K, T, card]
- logits_mask = logits_mask[None, :, :].expand(B, -1, -1) # [K, T] -> [B, K, T]
- return LMOutput(logits, logits_mask)
-
- def _sample_next_token(self,
- sequence: torch.Tensor,
- cfg_conditions: CFGConditions,
- unconditional_state: State,
- use_sampling: bool = False,
- temp: float = 1.0,
- top_k: int = 0,
- top_p: float = 0.0,
- cfg_coef: tp.Optional[float] = None) -> torch.Tensor:
- """Sample next token from the model given a sequence and a set of conditions. The model supports
- multiple sampling strategies (greedy sampling, softmax, top-k, top-p...).
-
- Args:
- sequence (torch.Tensor): Current sequence of shape [B, K, S]
- with K corresponding to the number of codebooks and S the number of sequence steps.
- S = 1 in streaming mode, except for the first step that contains a bigger prompt.
- condition_tensors (dict[str, ConditionType): Set of conditions. If CFG is used,
- should be twice the batch size, being the concatenation of the conditions + null conditions.
- use_sampling (bool): Whether to use a sampling strategy or not.
- temp (float): Sampling temperature.
- top_k (int): K for "top-k" sampling.
- top_p (float): P for "top-p" sampling.
- cfg_coef (float, optional): classifier free guidance coefficient
- Returns:
- next_token (torch.Tensor): Next token tensor of shape [B, K, 1].
- """
- B = sequence.shape[0]
- cfg_coef = self.cfg_coef if cfg_coef is None else cfg_coef
- model = self if self._fsdp is None else self._fsdp
- if self.two_step_cfg and cfg_conditions != {}:
- assert isinstance(cfg_conditions, tuple), type(cfg_conditions)
- condition_tensors, null_condition_tensors = cfg_conditions
- cond_logits = model(sequence, conditions=[], condition_tensors=condition_tensors)
- state = self.get_streaming_state()
- self.set_streaming_state(unconditional_state)
- uncond_logits = model(sequence, conditions=[], condition_tensors=null_condition_tensors)
- unconditional_state.update(self.get_streaming_state())
- self.set_streaming_state(state)
- logits = uncond_logits + (cond_logits - uncond_logits) * self.cfg_coef
- else:
- assert isinstance(cfg_conditions, dict)
- condition_tensors = cfg_conditions
- if condition_tensors:
- # Preparing for CFG, predicting both conditional and unconditional logits.
- sequence = torch.cat([sequence, sequence], dim=0)
- all_logits = model(
- sequence,
- conditions=[], condition_tensors=condition_tensors)
- if condition_tensors:
- cond_logits, uncond_logits = all_logits.split(B, dim=0) # [B, K, T, card]
- logits = uncond_logits + (cond_logits - uncond_logits) * cfg_coef
- else:
- logits = all_logits
-
- logits = logits.permute(0, 1, 3, 2) # [B, K, card, T]
- logits = logits[..., -1] # [B x K x card]
-
- # Apply softmax for sampling if temp > 0. Else, do greedy sampling to avoid zero division error.
- if use_sampling and temp > 0.0:
- probs = torch.softmax(logits / temp, dim=-1)
- if top_p > 0.0:
- next_token = utils.sample_top_p(probs, p=top_p)
- elif top_k > 0:
- next_token = utils.sample_top_k(probs, k=top_k)
- else:
- next_token = utils.multinomial(probs, num_samples=1)
- else:
- next_token = torch.argmax(logits, dim=-1, keepdim=True)
-
- return next_token
-
- @torch.no_grad()
- def generate(self,
- prompt: tp.Optional[torch.Tensor] = None,
- conditions: tp.List[ConditioningAttributes] = [],
- num_samples: tp.Optional[int] = None,
- max_gen_len: int = 256,
- use_sampling: bool = True,
- temp: float = 1.0,
- top_k: int = 250,
- top_p: float = 0.0,
- cfg_coef: tp.Optional[float] = None,
- two_step_cfg: tp.Optional[bool] = None,
- remove_prompts: bool = False,
- check: bool = False,
- callback: tp.Optional[tp.Callable[[int, int], None]] = None) -> torch.Tensor:
- """Generate tokens sampling from the model given a prompt or unconditionally. Generation can
- be perform in a greedy fashion or using sampling with top K and top P strategies.
-
- Args:
- prompt (torch.Tensor, optional): Prompt tokens of shape [B, K, T].
- conditions_tensors (list of ConditioningAttributes, optional): List of conditions.
- num_samples (int, optional): Number of samples to generate when no prompt and no conditions are given.
- max_gen_len (int): Maximum generation length.
- use_sampling (bool): Whether to use a sampling strategy or not.
- temp (float): Sampling temperature.
- top_k (int): K for "top-k" sampling.
- top_p (float): P for "top-p" sampling.
- cfg_coeff (float, optional): Classifier-free guidance coefficient.
- two_step_cfg (bool, optional): Whether to perform classifier-free guidance with two steps generation.
- remove_prompts (bool): Whether to remove prompts from generation or not.
- check (bool): Whether to apply further checks on generated sequence.
- callback (Callback, optional): Callback function to report generation progress.
- Returns:
- torch.Tensor: Generated tokens.
- """
- assert not self.training, "generation shouldn't be used in training mode."
- first_param = next(iter(self.parameters()))
- device = first_param.device
-
- # Checking all input shapes are consistent.
- possible_num_samples = []
- if num_samples is not None:
- possible_num_samples.append(num_samples)
- elif prompt is not None:
- possible_num_samples.append(prompt.shape[0])
- elif conditions:
- possible_num_samples.append(len(conditions))
- else:
- possible_num_samples.append(1)
- assert [x == possible_num_samples[0] for x in possible_num_samples], "Inconsistent inputs shapes"
- num_samples = possible_num_samples[0]
-
- # below we create set of conditions: one conditional and one unconditional
- # to do that we merge the regular condition together with the null condition
- # we then do 1 forward pass instead of 2.
- # the reason for that is two-fold:
- # 1. it is about x2 faster than doing 2 forward passes
- # 2. avoid the streaming API treating the 2 passes as part of different time steps
- # We also support doing two different passes, in particular to ensure that
- # the padding structure is exactly the same between train and test.
- # With a batch size of 1, this can be slower though.
- cfg_conditions: CFGConditions
- two_step_cfg = self.two_step_cfg if two_step_cfg is None else two_step_cfg
- if conditions:
- null_conditions = ClassifierFreeGuidanceDropout(p=1.0)(conditions)
- if two_step_cfg:
- cfg_conditions = (
- self.condition_provider(self.condition_provider.tokenize(conditions)),
- self.condition_provider(self.condition_provider.tokenize(null_conditions)),
- )
- else:
- conditions = conditions + null_conditions
- tokenized = self.condition_provider.tokenize(conditions)
- cfg_conditions = self.condition_provider(tokenized)
- else:
- cfg_conditions = {}
-
- if prompt is None:
- assert num_samples > 0
- prompt = torch.zeros((num_samples, self.num_codebooks, 0), dtype=torch.long, device=device)
-
- B, K, T = prompt.shape
- start_offset = T
- assert start_offset < max_gen_len
-
- pattern = self.pattern_provider.get_pattern(max_gen_len)
- # this token is used as default value for codes that are not generated yet
- unknown_token = -1
-
- # we generate codes up to the max_gen_len that will be mapped to the pattern sequence
- gen_codes = torch.full((B, K, max_gen_len), unknown_token, dtype=torch.long, device=device)
- # filling the gen_codes with the prompt if needed
- gen_codes[..., :start_offset] = prompt
- # create the gen_sequence with proper interleaving from the pattern: [B, K, S]
- gen_sequence, indexes, mask = pattern.build_pattern_sequence(gen_codes, self.special_token_id)
- # retrieve the start_offset in the sequence:
- # it is the first sequence step that contains the `start_offset` timestep
- start_offset_sequence = pattern.get_first_step_with_timesteps(start_offset)
- assert start_offset_sequence is not None
-
- with self.streaming():
- unconditional_state = self.get_streaming_state()
- prev_offset = 0
- gen_sequence_len = gen_sequence.shape[-1] # gen_sequence shape is [B, K, S]
- for offset in range(start_offset_sequence, gen_sequence_len):
- # get current sequence (note that the streaming API is providing the caching over previous offsets)
- curr_sequence = gen_sequence[..., prev_offset:offset]
- curr_mask = mask[None, ..., prev_offset:offset].expand(B, -1, -1)
- if check:
- # check coherence between mask and sequence
- assert (curr_sequence == torch.where(curr_mask, curr_sequence, self.special_token_id)).all()
- # should never happen as gen_sequence is filled progressively
- assert not (curr_sequence == unknown_token).any()
- # sample next token from the model, next token shape is [B, K, 1]
- next_token = self._sample_next_token(
- curr_sequence, cfg_conditions, unconditional_state, use_sampling, temp, top_k, top_p,
- cfg_coef=cfg_coef)
- # ensure the tokens that should be masked are properly set to special_token_id
- # as the model never output special_token_id
- valid_mask = mask[..., offset:offset+1].expand(B, -1, -1)
- next_token[~valid_mask] = self.special_token_id
- # ensure we don't overwrite prompt tokens, we only write over unknown tokens
- # (then mask tokens should be left as is as well, which is correct)
- gen_sequence[..., offset:offset+1] = torch.where(
- gen_sequence[..., offset:offset+1] == unknown_token,
- next_token, gen_sequence[..., offset:offset+1]
- )
- prev_offset = offset
- if callback is not None:
- callback(1 + offset - start_offset_sequence, gen_sequence_len - start_offset_sequence)
- unconditional_state.clear()
-
- # ensure sequence has been entirely filled
- assert not (gen_sequence == unknown_token).any()
- # ensure gen_sequence pattern and mask are matching
- # which means the gen_sequence is valid according to the pattern
- assert (
- gen_sequence == torch.where(mask[None, ...].expand(B, -1, -1), gen_sequence, self.special_token_id)
- ).all()
- # get back the codes, trimming the prompt if needed and cutting potentially incomplete timesteps
- out_codes, out_indexes, out_mask = pattern.revert_pattern_sequence(gen_sequence, special_token=unknown_token)
-
- # sanity checks over the returned codes and corresponding masks
- assert (out_codes[..., :max_gen_len] != unknown_token).all()
- assert (out_mask[..., :max_gen_len] == 1).all()
-
- out_start_offset = start_offset if remove_prompts else 0
- out_codes = out_codes[..., out_start_offset:max_gen_len]
-
- # ensure the returned codes are all valid
- assert (out_codes >= 0).all() and (out_codes <= self.card).all()
- return out_codes
diff --git a/spaces/HaHaBill/LandShapes-Antarctica/netdissect/upsegmodel/prroi_pool/README.md b/spaces/HaHaBill/LandShapes-Antarctica/netdissect/upsegmodel/prroi_pool/README.md
deleted file mode 100644
index bb98946d3b48a2069a58f179eb6da63e009c3849..0000000000000000000000000000000000000000
--- a/spaces/HaHaBill/LandShapes-Antarctica/netdissect/upsegmodel/prroi_pool/README.md
+++ /dev/null
@@ -1,66 +0,0 @@
-# PreciseRoIPooling
-This repo implements the **Precise RoI Pooling** (PrRoI Pooling), proposed in the paper **Acquisition of Localization Confidence for Accurate Object Detection** published at ECCV 2018 (Oral Presentation).
-
-**Acquisition of Localization Confidence for Accurate Object Detection**
-
-_Borui Jiang*, Ruixuan Luo*, Jiayuan Mao*, Tete Xiao, Yuning Jiang_ (* indicates equal contribution.)
-
-https://arxiv.org/abs/1807.11590
-
-## Brief
-
-In short, Precise RoI Pooling is an integration-based (bilinear interpolation) average pooling method for RoI Pooling. It avoids any quantization and has a continuous gradient on bounding box coordinates. It is:
-
-- different from the original RoI Pooling proposed in [Fast R-CNN](https://arxiv.org/abs/1504.08083). PrRoI Pooling uses average pooling instead of max pooling for each bin and has a continuous gradient on bounding box coordinates. That is, one can take the derivatives of some loss function w.r.t the coordinates of each RoI and optimize the RoI coordinates.
-- different from the RoI Align proposed in [Mask R-CNN](https://arxiv.org/abs/1703.06870). PrRoI Pooling uses a full integration-based average pooling instead of sampling a constant number of points. This makes the gradient w.r.t. the coordinates continuous.
-
-For a better illustration, we illustrate RoI Pooling, RoI Align and PrRoI Pooing in the following figure. More details including the gradient computation can be found in our paper.
-
-
-
-## Implementation
-
-PrRoI Pooling was originally implemented by [Tete Xiao](http://tetexiao.com/) based on MegBrain, an (internal) deep learning framework built by Megvii Inc. It was later adapted into open-source deep learning frameworks. Currently, we only support PyTorch. Unfortunately, we don't have any specific plan for the adaptation into other frameworks such as TensorFlow, but any contributions (pull requests) will be more than welcome.
-
-## Usage (PyTorch 1.0)
-
-In the directory `pytorch/`, we provide a PyTorch-based implementation of PrRoI Pooling. It requires PyTorch 1.0+ and only supports CUDA (CPU mode is not implemented).
-Since we use PyTorch JIT for cxx/cuda code compilation, to use the module in your code, simply do:
-
-```
-from prroi_pool import PrRoIPool2D
-
-avg_pool = PrRoIPool2D(window_height, window_width, spatial_scale)
-roi_features = avg_pool(features, rois)
-
-# for those who want to use the "functional"
-
-from prroi_pool.functional import prroi_pool2d
-roi_features = prroi_pool2d(features, rois, window_height, window_width, spatial_scale)
-```
-
-
-## Usage (PyTorch 0.4)
-
-**!!! Please first checkout to the branch pytorch0.4.**
-
-In the directory `pytorch/`, we provide a PyTorch-based implementation of PrRoI Pooling. It requires PyTorch 0.4 and only supports CUDA (CPU mode is not implemented).
-To use the PrRoI Pooling module, first goto `pytorch/prroi_pool` and execute `./travis.sh` to compile the essential components (you may need `nvcc` for this step). To use the module in your code, simply do:
-
-```
-from prroi_pool import PrRoIPool2D
-
-avg_pool = PrRoIPool2D(window_height, window_width, spatial_scale)
-roi_features = avg_pool(features, rois)
-
-# for those who want to use the "functional"
-
-from prroi_pool.functional import prroi_pool2d
-roi_features = prroi_pool2d(features, rois, window_height, window_width, spatial_scale)
-```
-
-Here,
-
-- RoI is an `m * 5` float tensor of format `(batch_index, x0, y0, x1, y1)`, following the convention in the original Caffe implementation of RoI Pooling, although in some frameworks the batch indices are provided by an integer tensor.
-- `spatial_scale` is multiplied to the RoIs. For example, if your feature maps are down-sampled by a factor of 16 (w.r.t. the input image), you should use a spatial scale of `1/16`.
-- The coordinates for RoI follows the [L, R) convension. That is, `(0, 0, 4, 4)` denotes a box of size `4x4`.
diff --git a/spaces/Harveenchadha/en_to_indic_translation/binarize_training_exp.sh b/spaces/Harveenchadha/en_to_indic_translation/binarize_training_exp.sh
deleted file mode 100644
index 52e74449df27835ceab7489ce2c8ea3b5feaaf4b..0000000000000000000000000000000000000000
--- a/spaces/Harveenchadha/en_to_indic_translation/binarize_training_exp.sh
+++ /dev/null
@@ -1,24 +0,0 @@
-#/bin/bash
-
-exp_dir=$1
-src_lang=$2
-tgt_lang=$3
-
-# use cpu_count to get num_workers instead of setting it manually when running in different
-# instances
-num_workers=`python -c "import multiprocessing; print(multiprocessing.cpu_count())"`
-
-data_dir=$exp_dir/final
-out_data_dir=$exp_dir/final_bin
-
-rm -rf $out_data_dir
-
-fairseq-preprocess \
- --source-lang $src_lang --target-lang $tgt_lang \
- --trainpref $data_dir/train \
- --validpref $data_dir/dev \
- --testpref $data_dir/test \
- --destdir $out_data_dir \
- --workers $num_workers \
- --thresholdtgt 5 \
- --thresholdsrc 5
diff --git a/spaces/ICML2022/OFA/fairseq/examples/discriminative_reranking_nmt/README.md b/spaces/ICML2022/OFA/fairseq/examples/discriminative_reranking_nmt/README.md
deleted file mode 100644
index b155e855f2f94e30ad22262f260008fda8ac1804..0000000000000000000000000000000000000000
--- a/spaces/ICML2022/OFA/fairseq/examples/discriminative_reranking_nmt/README.md
+++ /dev/null
@@ -1,202 +0,0 @@
-# Discriminative Reranking for Neural Machine Translation
-https://aclanthology.org/2021.acl-long.563/
-
-This folder contains source code for training DrNMT, a discriminatively trained reranker for neural machine translation.
-
-## Data preparation
-1. Follow the instructions under `examples/translation` to build a base MT model. Prepare three files, one with source sentences, one with ground truth target sentences, and one with hypotheses generated from the base MT model. Each line in the file contains one sentence in raw text (i.e. no sentencepiece, etc.). Below is an example of the files with _N_ hypotheses for each source sentence.
-
-```
-# Example of the source sentence file: (The file should contain L lines.)
-
-source_sentence_1
-source_sentence_2
-source_sentence_3
-...
-source_sentence_L
-
-# Example of the target sentence file: (The file should contain L lines.)
-
-target_sentence_1
-target_sentence_2
-target_sentence_3
-...
-target_sentence_L
-
-# Example of the hypotheses file: (The file should contain L*N lines.)
-
-source_sentence_1_hypo_1
-source_sentence_1_hypo_2
-...
-source_sentence_1_hypo_N
-source_sentence_2_hypo_1
-...
-source_sentence_2_hypo_N
-...
-source_sentence_L_hypo_1
-...
-source_sentence_L_hypo_N
-```
-
-2. Download the [XLMR model](https://github.com/fairinternal/fairseq-py/tree/main/examples/xlmr#pre-trained-models).
-```
-wget https://dl.fbaipublicfiles.com/fairseq/models/xlmr.base.tar.gz
-tar zxvf xlmr.base.tar.gz
-
-# The folder should contain dict.txt, model.pt and sentencepiece.bpe.model.
-```
-
-3. Prepare scores and BPE data.
-* `N`: Number of hypotheses per each source sentence. We use 50 in the paper.
-* `SPLIT`: Name of the data split, i.e. train, valid, test. Use split_name, split_name1, split_name2, ..., if there are multiple datasets for a split, e.g. train, train1, valid, valid1.
-* `NUM_SHARDS`: Number of shards. Set this to 1 for non-train splits.
-* `METRIC`: The metric for DrNMT to optimize for. We support either `bleu` or `ter`.
-```
-# For each data split, e.g. train, valid, test, etc., run the following:
-
-SOURCE_FILE=/path/to/source_sentence_file
-TARGET_FILE=/path/to/target_sentence_file
-HYPO_FILE=/path/to/hypo_file
-XLMR_DIR=/path/to/xlmr
-OUTPUT_DIR=/path/to/output
-
-python scripts/prep_data.py \
- --input-source ${SOURCE_FILE} \
- --input-target ${TARGET_FILE} \
- --input-hypo ${HYPO_FILE} \
- --output-dir ${OUTPUT_DIR} \
- --split $SPLIT
- --beam $N \
- --sentencepiece-model ${XLMR_DIR}/sentencepiece.bpe.model \
- --metric $METRIC \
- --num-shards ${NUM_SHARDS}
-
-# The script will create ${OUTPUT_DIR}/$METRIC with ${NUM_SHARDS} splits.
-# Under split*/input_src, split*/input_tgt and split*/$METRIC, there will be $SPLIT.bpe and $SPLIT.$METRIC files, respectively.
-
-```
-
-4. Pre-process the data into fairseq format.
-```
-# use comma to separate if there are more than one train or valid set
-for suffix in src tgt ; do
- fairseq-preprocess --only-source \
- --trainpref ${OUTPUT_DIR}/$METRIC/split1/input_${suffix}/train.bpe \
- --validpref ${OUTPUT_DIR}/$METRIC/split1/input_${suffix}/valid.bpe \
- --destdir ${OUTPUT_DIR}/$METRIC/split1/input_${suffix} \
- --workers 60 \
- --srcdict ${XLMR_DIR}/dict.txt
-done
-
-for i in `seq 2 ${NUM_SHARDS}`; do
- for suffix in src tgt ; do
- fairseq-preprocess --only-source \
- --trainpref ${OUTPUT_DIR}/$METRIC/split${i}/input_${suffix}/train.bpe \
- --destdir ${OUTPUT_DIR}/$METRIC/split${i}/input_${suffix} \
- --workers 60 \
- --srcdict ${XLMR_DIR}/dict.txt
-
- ln -s ${OUTPUT_DIR}/$METRIC/split1/input_${suffix}/valid* ${OUTPUT_DIR}/$METRIC/split${i}/input_${suffix}/.
- done
-
- ln -s ${OUTPUT_DIR}/$METRIC/split1/$METRIC/valid* ${OUTPUT_DIR}/$METRIC/split${i}/$METRIC/.
-done
-```
-
-## Training
-
-```
-EXP_DIR=/path/to/exp
-
-# An example of training the model with the config for De-En experiment in the paper.
-# The config uses 16 GPUs and 50 hypotheses.
-# For training with fewer number of GPUs, set
-# distributed_training.distributed_world_size=k +optimization.update_freq='[x]' where x = 16/k
-# For training with fewer number of hypotheses, set
-# task.mt_beam=N dataset.batch_size=N dataset.required_batch_size_multiple=N
-
-fairseq-hydra-train -m \
- --config-dir config/ --config-name deen \
- task.data=${OUTPUT_DIR}/$METRIC/split1/ \
- task.num_data_splits=${NUM_SHARDS} \
- model.pretrained_model=${XLMR_DIR}/model.pt \
- common.user_dir=${FAIRSEQ_ROOT}/examples/discriminative_reranking_nmt \
- checkpoint.save_dir=${EXP_DIR}
-
-```
-
-## Inference & scoring
-Perform DrNMT reranking (fw + reranker score)
-1. Tune weights on valid sets.
-```
-# genrate N hypotheses with the base MT model (fw score)
-VALID_SOURCE_FILE=/path/to/source_sentences # one sentence per line, converted to the sentencepiece used by the base MT model
-VALID_TARGET_FILE=/path/to/target_sentences # one sentence per line in raw text, i.e. no sentencepiece and tokenization
-MT_MODEL=/path/to/mt_model
-MT_DATA_PATH=/path/to/mt_data
-
-cat ${VALID_SOURCE_FILE} | \
- fairseq-interactive ${MT_DATA_PATH} \
- --max-tokens 4000 --buffer-size 16 \
- --num-workers 32 --path ${MT_MODEL} \
- --beam $N --nbest $N \
- --post-process sentencepiece &> valid-hypo.out
-
-# replace "bleu" with "ter" to optimize for TER
-python drnmt_rerank.py \
- ${OUTPUT_DIR}/$METRIC/split1/ \
- --path ${EXP_DIR}/checkpoint_best.pt \
- --in-text valid-hypo.out \
- --results-path ${EXP_DIR} \
- --gen-subset valid \
- --target-text ${VALID_TARGET_FILE} \
- --user-dir ${FAIRSEQ_ROOT}/examples/discriminative_reranking_nmt \
- --bpe sentencepiece \
- --sentencepiece-model ${XLMR_DIR}/sentencepiece.bpe.model \
- --beam $N \
- --batch-size $N \
- --metric bleu \
- --tune
-
-```
-
-2. Apply best weights on test sets
-```
-# genrate N hypotheses with the base MT model (fw score)
-TEST_SOURCE_FILE=/path/to/source_sentences # one sentence per line, converted to the sentencepiece used by the base MT model
-
-cat ${TEST_SOURCE_FILE} | \
- fairseq-interactive ${MT_DATA_PATH} \
- --max-tokens 4000 --buffer-size 16 \
- --num-workers 32 --path ${MT_MODEL} \
- --beam $N --nbest $N \
- --post-process sentencepiece &> test-hypo.out
-
-# replace "bleu" with "ter" to evaluate TER
-# Add --target-text for evaluating BLEU/TER,
-# otherwise the script will only generate the hypotheses with the highest scores only.
-python drnmt_rerank.py \
- ${OUTPUT_DIR}/$METRIC/split1/ \
- --path ${EXP_DIR}/checkpoint_best.pt \
- --in-text test-hypo.out \
- --results-path ${EXP_DIR} \
- --gen-subset test \
- --user-dir ${FAIRSEQ_ROOT}/examples/discriminative_reranking_nmt \
- --bpe sentencepiece \
- --sentencepiece-model ${XLMR_DIR}/sentencepiece.bpe.model \
- --beam $N \
- --batch-size $N \
- --metric bleu \
- --fw-weight ${BEST_FW_WEIGHT} \
- --lenpen ${BEST_LENPEN}
-```
-
-## Citation
-```bibtex
-@inproceedings{lee2021discriminative,
- title={Discriminative Reranking for Neural Machine Translation},
- author={Lee, Ann and Auli, Michael and Ranzato, Marc'Aurelio},
- booktitle={ACL},
- year={2021}
-}
-```
diff --git a/spaces/ICML2022/OFA/fairseq/fairseq/modules/quantization/scalar/modules/__init__.py b/spaces/ICML2022/OFA/fairseq/fairseq/modules/quantization/scalar/modules/__init__.py
deleted file mode 100644
index 8031d9cdb23f2bc72596f8bc9cfa4965f96e3e6c..0000000000000000000000000000000000000000
--- a/spaces/ICML2022/OFA/fairseq/fairseq/modules/quantization/scalar/modules/__init__.py
+++ /dev/null
@@ -1,9 +0,0 @@
-# Copyright (c) Facebook, Inc. and its affiliates.
-#
-# This source code is licensed under the MIT license found in the
-# LICENSE file in the root directory of this source tree.
-
-from .qact import ActivationQuantizer # NOQA
-from .qconv import IntConv2d # NOQA
-from .qemb import IntEmbedding # NOQA
-from .qlinear import IntLinear # NOQA
diff --git a/spaces/Ibtehaj10/cheating-detection-FYP/fps_example.py b/spaces/Ibtehaj10/cheating-detection-FYP/fps_example.py
deleted file mode 100644
index a22f0d68930a1d219485f91331dc7f6db1b3aa23..0000000000000000000000000000000000000000
--- a/spaces/Ibtehaj10/cheating-detection-FYP/fps_example.py
+++ /dev/null
@@ -1,37 +0,0 @@
-import cv2
-import datetime
-import imutils
-
-
-def main():
- cap = cv2.VideoCapture('test_video.mp4')
-
- fps_start_time = datetime.datetime.now()
- fps = 0
- total_frames = 0
-
- while True:
- ret, frame = cap.read()
- frame = imutils.resize(frame, width=800)
- total_frames = total_frames + 1
-
- fps_end_time = datetime.datetime.now()
- time_diff = fps_end_time - fps_start_time
- if time_diff.seconds == 0:
- fps = 0.0
- else:
- fps = (total_frames / time_diff.seconds)
-
- fps_text = "FPS: {:.2f}".format(fps)
-
- cv2.putText(frame, fps_text, (5, 30), cv2.FONT_HERSHEY_COMPLEX_SMALL, 1, (0, 0, 255), 1)
-
- cv2.imshow("Application", frame)
- key = cv2.waitKey(1)
- if key == ord('q'):
- break
-
- cv2.destroyAllWindows()
-
-
-main()
diff --git a/spaces/Iceclear/StableSR/StableSR/taming/data/open_images_helper.py b/spaces/Iceclear/StableSR/StableSR/taming/data/open_images_helper.py
deleted file mode 100644
index 8feb7c6e705fc165d2983303192aaa88f579b243..0000000000000000000000000000000000000000
--- a/spaces/Iceclear/StableSR/StableSR/taming/data/open_images_helper.py
+++ /dev/null
@@ -1,379 +0,0 @@
-open_images_unify_categories_for_coco = {
- '/m/03bt1vf': '/m/01g317',
- '/m/04yx4': '/m/01g317',
- '/m/05r655': '/m/01g317',
- '/m/01bl7v': '/m/01g317',
- '/m/0cnyhnx': '/m/01xq0k1',
- '/m/01226z': '/m/018xm',
- '/m/05ctyq': '/m/018xm',
- '/m/058qzx': '/m/04ctx',
- '/m/06pcq': '/m/0l515',
- '/m/03m3pdh': '/m/02crq1',
- '/m/046dlr': '/m/01x3z',
- '/m/0h8mzrc': '/m/01x3z',
-}
-
-
-top_300_classes_plus_coco_compatibility = [
- ('Man', 1060962),
- ('Clothing', 986610),
- ('Tree', 748162),
- ('Woman', 611896),
- ('Person', 610294),
- ('Human face', 442948),
- ('Girl', 175399),
- ('Building', 162147),
- ('Car', 159135),
- ('Plant', 155704),
- ('Human body', 137073),
- ('Flower', 133128),
- ('Window', 127485),
- ('Human arm', 118380),
- ('House', 114365),
- ('Wheel', 111684),
- ('Suit', 99054),
- ('Human hair', 98089),
- ('Human head', 92763),
- ('Chair', 88624),
- ('Boy', 79849),
- ('Table', 73699),
- ('Jeans', 57200),
- ('Tire', 55725),
- ('Skyscraper', 53321),
- ('Food', 52400),
- ('Footwear', 50335),
- ('Dress', 50236),
- ('Human leg', 47124),
- ('Toy', 46636),
- ('Tower', 45605),
- ('Boat', 43486),
- ('Land vehicle', 40541),
- ('Bicycle wheel', 34646),
- ('Palm tree', 33729),
- ('Fashion accessory', 32914),
- ('Glasses', 31940),
- ('Bicycle', 31409),
- ('Furniture', 30656),
- ('Sculpture', 29643),
- ('Bottle', 27558),
- ('Dog', 26980),
- ('Snack', 26796),
- ('Human hand', 26664),
- ('Bird', 25791),
- ('Book', 25415),
- ('Guitar', 24386),
- ('Jacket', 23998),
- ('Poster', 22192),
- ('Dessert', 21284),
- ('Baked goods', 20657),
- ('Drink', 19754),
- ('Flag', 18588),
- ('Houseplant', 18205),
- ('Tableware', 17613),
- ('Airplane', 17218),
- ('Door', 17195),
- ('Sports uniform', 17068),
- ('Shelf', 16865),
- ('Drum', 16612),
- ('Vehicle', 16542),
- ('Microphone', 15269),
- ('Street light', 14957),
- ('Cat', 14879),
- ('Fruit', 13684),
- ('Fast food', 13536),
- ('Animal', 12932),
- ('Vegetable', 12534),
- ('Train', 12358),
- ('Horse', 11948),
- ('Flowerpot', 11728),
- ('Motorcycle', 11621),
- ('Fish', 11517),
- ('Desk', 11405),
- ('Helmet', 10996),
- ('Truck', 10915),
- ('Bus', 10695),
- ('Hat', 10532),
- ('Auto part', 10488),
- ('Musical instrument', 10303),
- ('Sunglasses', 10207),
- ('Picture frame', 10096),
- ('Sports equipment', 10015),
- ('Shorts', 9999),
- ('Wine glass', 9632),
- ('Duck', 9242),
- ('Wine', 9032),
- ('Rose', 8781),
- ('Tie', 8693),
- ('Butterfly', 8436),
- ('Beer', 7978),
- ('Cabinetry', 7956),
- ('Laptop', 7907),
- ('Insect', 7497),
- ('Goggles', 7363),
- ('Shirt', 7098),
- ('Dairy Product', 7021),
- ('Marine invertebrates', 7014),
- ('Cattle', 7006),
- ('Trousers', 6903),
- ('Van', 6843),
- ('Billboard', 6777),
- ('Balloon', 6367),
- ('Human nose', 6103),
- ('Tent', 6073),
- ('Camera', 6014),
- ('Doll', 6002),
- ('Coat', 5951),
- ('Mobile phone', 5758),
- ('Swimwear', 5729),
- ('Strawberry', 5691),
- ('Stairs', 5643),
- ('Goose', 5599),
- ('Umbrella', 5536),
- ('Cake', 5508),
- ('Sun hat', 5475),
- ('Bench', 5310),
- ('Bookcase', 5163),
- ('Bee', 5140),
- ('Computer monitor', 5078),
- ('Hiking equipment', 4983),
- ('Office building', 4981),
- ('Coffee cup', 4748),
- ('Curtain', 4685),
- ('Plate', 4651),
- ('Box', 4621),
- ('Tomato', 4595),
- ('Coffee table', 4529),
- ('Office supplies', 4473),
- ('Maple', 4416),
- ('Muffin', 4365),
- ('Cocktail', 4234),
- ('Castle', 4197),
- ('Couch', 4134),
- ('Pumpkin', 3983),
- ('Computer keyboard', 3960),
- ('Human mouth', 3926),
- ('Christmas tree', 3893),
- ('Mushroom', 3883),
- ('Swimming pool', 3809),
- ('Pastry', 3799),
- ('Lavender (Plant)', 3769),
- ('Football helmet', 3732),
- ('Bread', 3648),
- ('Traffic sign', 3628),
- ('Common sunflower', 3597),
- ('Television', 3550),
- ('Bed', 3525),
- ('Cookie', 3485),
- ('Fountain', 3484),
- ('Paddle', 3447),
- ('Bicycle helmet', 3429),
- ('Porch', 3420),
- ('Deer', 3387),
- ('Fedora', 3339),
- ('Canoe', 3338),
- ('Carnivore', 3266),
- ('Bowl', 3202),
- ('Human eye', 3166),
- ('Ball', 3118),
- ('Pillow', 3077),
- ('Salad', 3061),
- ('Beetle', 3060),
- ('Orange', 3050),
- ('Drawer', 2958),
- ('Platter', 2937),
- ('Elephant', 2921),
- ('Seafood', 2921),
- ('Monkey', 2915),
- ('Countertop', 2879),
- ('Watercraft', 2831),
- ('Helicopter', 2805),
- ('Kitchen appliance', 2797),
- ('Personal flotation device', 2781),
- ('Swan', 2739),
- ('Lamp', 2711),
- ('Boot', 2695),
- ('Bronze sculpture', 2693),
- ('Chicken', 2677),
- ('Taxi', 2643),
- ('Juice', 2615),
- ('Cowboy hat', 2604),
- ('Apple', 2600),
- ('Tin can', 2590),
- ('Necklace', 2564),
- ('Ice cream', 2560),
- ('Human beard', 2539),
- ('Coin', 2536),
- ('Candle', 2515),
- ('Cart', 2512),
- ('High heels', 2441),
- ('Weapon', 2433),
- ('Handbag', 2406),
- ('Penguin', 2396),
- ('Rifle', 2352),
- ('Violin', 2336),
- ('Skull', 2304),
- ('Lantern', 2285),
- ('Scarf', 2269),
- ('Saucer', 2225),
- ('Sheep', 2215),
- ('Vase', 2189),
- ('Lily', 2180),
- ('Mug', 2154),
- ('Parrot', 2140),
- ('Human ear', 2137),
- ('Sandal', 2115),
- ('Lizard', 2100),
- ('Kitchen & dining room table', 2063),
- ('Spider', 1977),
- ('Coffee', 1974),
- ('Goat', 1926),
- ('Squirrel', 1922),
- ('Cello', 1913),
- ('Sushi', 1881),
- ('Tortoise', 1876),
- ('Pizza', 1870),
- ('Studio couch', 1864),
- ('Barrel', 1862),
- ('Cosmetics', 1841),
- ('Moths and butterflies', 1841),
- ('Convenience store', 1817),
- ('Watch', 1792),
- ('Home appliance', 1786),
- ('Harbor seal', 1780),
- ('Luggage and bags', 1756),
- ('Vehicle registration plate', 1754),
- ('Shrimp', 1751),
- ('Jellyfish', 1730),
- ('French fries', 1723),
- ('Egg (Food)', 1698),
- ('Football', 1697),
- ('Musical keyboard', 1683),
- ('Falcon', 1674),
- ('Candy', 1660),
- ('Medical equipment', 1654),
- ('Eagle', 1651),
- ('Dinosaur', 1634),
- ('Surfboard', 1630),
- ('Tank', 1628),
- ('Grape', 1624),
- ('Lion', 1624),
- ('Owl', 1622),
- ('Ski', 1613),
- ('Waste container', 1606),
- ('Frog', 1591),
- ('Sparrow', 1585),
- ('Rabbit', 1581),
- ('Pen', 1546),
- ('Sea lion', 1537),
- ('Spoon', 1521),
- ('Sink', 1512),
- ('Teddy bear', 1507),
- ('Bull', 1495),
- ('Sofa bed', 1490),
- ('Dragonfly', 1479),
- ('Brassiere', 1478),
- ('Chest of drawers', 1472),
- ('Aircraft', 1466),
- ('Human foot', 1463),
- ('Pig', 1455),
- ('Fork', 1454),
- ('Antelope', 1438),
- ('Tripod', 1427),
- ('Tool', 1424),
- ('Cheese', 1422),
- ('Lemon', 1397),
- ('Hamburger', 1393),
- ('Dolphin', 1390),
- ('Mirror', 1390),
- ('Marine mammal', 1387),
- ('Giraffe', 1385),
- ('Snake', 1368),
- ('Gondola', 1364),
- ('Wheelchair', 1360),
- ('Piano', 1358),
- ('Cupboard', 1348),
- ('Banana', 1345),
- ('Trumpet', 1335),
- ('Lighthouse', 1333),
- ('Invertebrate', 1317),
- ('Carrot', 1268),
- ('Sock', 1260),
- ('Tiger', 1241),
- ('Camel', 1224),
- ('Parachute', 1224),
- ('Bathroom accessory', 1223),
- ('Earrings', 1221),
- ('Headphones', 1218),
- ('Skirt', 1198),
- ('Skateboard', 1190),
- ('Sandwich', 1148),
- ('Saxophone', 1141),
- ('Goldfish', 1136),
- ('Stool', 1104),
- ('Traffic light', 1097),
- ('Shellfish', 1081),
- ('Backpack', 1079),
- ('Sea turtle', 1078),
- ('Cucumber', 1075),
- ('Tea', 1051),
- ('Toilet', 1047),
- ('Roller skates', 1040),
- ('Mule', 1039),
- ('Bust', 1031),
- ('Broccoli', 1030),
- ('Crab', 1020),
- ('Oyster', 1019),
- ('Cannon', 1012),
- ('Zebra', 1012),
- ('French horn', 1008),
- ('Grapefruit', 998),
- ('Whiteboard', 997),
- ('Zucchini', 997),
- ('Crocodile', 992),
-
- ('Clock', 960),
- ('Wall clock', 958),
-
- ('Doughnut', 869),
- ('Snail', 868),
-
- ('Baseball glove', 859),
-
- ('Panda', 830),
- ('Tennis racket', 830),
-
- ('Pear', 652),
-
- ('Bagel', 617),
- ('Oven', 616),
- ('Ladybug', 615),
- ('Shark', 615),
- ('Polar bear', 614),
- ('Ostrich', 609),
-
- ('Hot dog', 473),
- ('Microwave oven', 467),
- ('Fire hydrant', 20),
- ('Stop sign', 20),
- ('Parking meter', 20),
- ('Bear', 20),
- ('Flying disc', 20),
- ('Snowboard', 20),
- ('Tennis ball', 20),
- ('Kite', 20),
- ('Baseball bat', 20),
- ('Kitchen knife', 20),
- ('Knife', 20),
- ('Submarine sandwich', 20),
- ('Computer mouse', 20),
- ('Remote control', 20),
- ('Toaster', 20),
- ('Sink', 20),
- ('Refrigerator', 20),
- ('Alarm clock', 20),
- ('Wall clock', 20),
- ('Scissors', 20),
- ('Hair dryer', 20),
- ('Toothbrush', 20),
- ('Suitcase', 20)
-]
diff --git a/spaces/Illia56/Llama-2-voice/README.md b/spaces/Illia56/Llama-2-voice/README.md
deleted file mode 100644
index 8d0bc3a1a5afe9adbdef90bb9d5d94944a9fd850..0000000000000000000000000000000000000000
--- a/spaces/Illia56/Llama-2-voice/README.md
+++ /dev/null
@@ -1,13 +0,0 @@
----
-title: Chat With Llama 2 70b St Voice
-emoji: 🦙
-colorFrom: purple
-colorTo: gray
-sdk: streamlit
-sdk_version: 1.26.0
-app_file: app.py
-pinned: false
-license: mit
----
-
-Check out the configuration reference at https://huggingface.co/docs/hub/spaces-config-reference
\ No newline at end of file
diff --git a/spaces/Illumotion/Koboldcpp/otherarch/llama_v3.h b/spaces/Illumotion/Koboldcpp/otherarch/llama_v3.h
deleted file mode 100644
index 2cc4b47074158d919436d6c5a67abff227c92293..0000000000000000000000000000000000000000
--- a/spaces/Illumotion/Koboldcpp/otherarch/llama_v3.h
+++ /dev/null
@@ -1,486 +0,0 @@
-#ifndef LLAMA_V3_H
-#define LLAMA_V3_H
-
-#include "ggml.h"
-#ifdef GGML_USE_CUBLAS
-#include "ggml-cuda.h"
-#define LLAMA_V3_MAX_DEVICES GGML_CUDA_MAX_DEVICES
-#else
-#define LLAMA_V3_MAX_DEVICES 1
-#endif // GGML_USE_CUBLAS
-#include
-#include
-#include
-
-#ifdef LLAMA_V3_SHARED
-# if defined(_WIN32) && !defined(__MINGW32__)
-# ifdef LLAMA_V3_BUILD
-# define LLAMA_V3_API __declspec(dllexport)
-# else
-# define LLAMA_V3_API __declspec(dllimport)
-# endif
-# else
-# define LLAMA_V3_API __attribute__ ((visibility ("default")))
-# endif
-#else
-# define LLAMA_V3_API
-#endif
-
-#ifdef __GNUC__
-# define DEPRECATED(func, hint) func __attribute__((deprecated(hint)))
-#elif defined(_MSC_VER)
-# define DEPRECATED(func, hint) __declspec(deprecated(hint)) func
-#else
-# define DEPRECATED(func, hint) func
-#endif
-
-#define LLAMA_V3_FILE_MAGIC_GGJT 0x67676a74u // 'ggjt'
-#define LLAMA_V3_FILE_MAGIC_GGLA 0x67676c61u // 'ggla'
-#define LLAMA_V3_FILE_MAGIC_GGMF 0x67676d66u // 'ggmf'
-#define LLAMA_V3_FILE_MAGIC_GGML 0x67676d6cu // 'ggml'
-#define LLAMA_V3_FILE_MAGIC_GGSN 0x6767736eu // 'ggsn'
-
-#define LLAMA_V3_FILE_VERSION 3
-#define LLAMA_V3_FILE_MAGIC LLAMA_V3_FILE_MAGIC_GGJT
-#define LLAMA_V3_FILE_MAGIC_UNVERSIONED LLAMA_V3_FILE_MAGIC_GGML
-#define LLAMA_V3_SESSION_MAGIC LLAMA_V3_FILE_MAGIC_GGSN
-#define LLAMA_V3_SESSION_VERSION 1
-
-#define LLAMA_V3_DEFAULT_SEED 0xFFFFFFFF
-
-#if defined(GGML_USE_CUBLAS) || defined(GGML_USE_CLBLAST) || defined(GGML_USE_METAL)
-// Defined when llama.cpp is compiled with support for offloading model layers to GPU.
-#define LLAMA_V3_SUPPORTS_GPU_OFFLOAD
-#endif
-
-#ifndef LLAMA_V3_DEFAULT_RMS_EPS
-#define LLAMA_V3_DEFAULT_RMS_EPS 5e-6f
-#endif
-
-#ifdef __cplusplus
-extern "C" {
-#endif
-
- //
- // C interface
- //
- // TODO: show sample usage
- //
-
- struct llama_v3_model;
- struct llama_v3_context;
-
- typedef int llama_v3_token;
-
- typedef struct llama_v3_token_data {
- llama_v3_token id; // token id
- float logit; // log-odds of the token
- float p; // probability of the token
- } llama_v3_token_data;
-
- typedef struct llama_v3_token_data_array {
- llama_v3_token_data * data;
- size_t size;
- bool sorted;
- } llama_v3_token_data_array;
-
- typedef void (*llama_v3_progress_callback)(float progress, void *ctx);
-
- enum llama_v3_log_level {
- LLAMA_V3_LOG_LEVEL_ERROR = 2,
- LLAMA_V3_LOG_LEVEL_WARN = 3,
- LLAMA_V3_LOG_LEVEL_INFO = 4
- };
-
- // Signature for logging events
- // Note that text includes the new line character at the end for most events.
- // If your logging mechanism cannot handle that, check if the last character is '\n' and strip it
- // if it exists.
- // It might not exist for progress report where '.' is output repeatedly.
- typedef void (*llama_v3_log_callback)(enum llama_v3_log_level level, const char * text, void * user_data);
-
- struct llama_v3_context_params {
- uint32_t seed; // RNG seed, -1 for random
- int32_t n_ctx; // text context
- int32_t n_batch; // prompt processing batch size
- int32_t n_gqa; // grouped-query attention (TEMP - will be moved to model hparams)
- float rms_norm_eps; // rms norm epsilon (TEMP - will be moved to model hparams)
- int32_t n_gpu_layers; // number of layers to store in VRAM
- int32_t main_gpu; // the GPU that is used for scratch and small tensors
-
- const float * tensor_split; // how to split layers across multiple GPUs (size: LLAMA_V3_MAX_DEVICES)
-
- // ref: https://github.com/ggerganov/llama.cpp/pull/2054
- float rope_freq_base; // RoPE base frequency
- float rope_freq_scale; // RoPE frequency scaling factor
-
- // called with a progress value between 0 and 1, pass NULL to disable
- llama_v3_progress_callback progress_callback;
- // context pointer passed to the progress callback
- void * progress_callback_user_data;
-
- // Keep the booleans together to avoid misalignment during copy-by-value.
- bool low_vram; // if true, reduce VRAM usage at the cost of performance
- bool mul_mat_q; // if true, use experimental mul_mat_q kernels
- bool f16_kv; // use fp16 for KV cache
- bool logits_all; // the llama_v3_eval() call computes all logits, not just the last one
- bool vocab_only; // only load the vocabulary, no weights
- bool use_mmap; // use mmap if possible
- bool use_mlock; // force system to keep model in RAM
- bool embedding; // embedding mode only
- };
- // model file types
- enum llama_v3_ftype {
- LLAMA_V3_FTYPE_ALL_F32 = 0,
- LLAMA_V3_FTYPE_MOSTLY_F16 = 1, // except 1d tensors
- LLAMA_V3_FTYPE_MOSTLY_Q4_0 = 2, // except 1d tensors
- LLAMA_V3_FTYPE_MOSTLY_Q4_1 = 3, // except 1d tensors
- LLAMA_V3_FTYPE_MOSTLY_Q4_1_SOME_F16 = 4, // tok_embeddings.weight and output.weight are F16
- // LLAMA_V3_FTYPE_MOSTLY_Q4_2 = 5, // support has been removed
- // LLAMA_V3_FTYPE_MOSTLY_Q4_3 = 6, // support has been removed
- LLAMA_V3_FTYPE_MOSTLY_Q8_0 = 7, // except 1d tensors
- LLAMA_V3_FTYPE_MOSTLY_Q5_0 = 8, // except 1d tensors
- LLAMA_V3_FTYPE_MOSTLY_Q5_1 = 9, // except 1d tensors
- LLAMA_V3_FTYPE_MOSTLY_Q2_K = 10,// except 1d tensors
- LLAMA_V3_FTYPE_MOSTLY_Q3_K_S = 11,// except 1d tensors
- LLAMA_V3_FTYPE_MOSTLY_Q3_K_M = 12,// except 1d tensors
- LLAMA_V3_FTYPE_MOSTLY_Q3_K_L = 13,// except 1d tensors
- LLAMA_V3_FTYPE_MOSTLY_Q4_K_S = 14,// except 1d tensors
- LLAMA_V3_FTYPE_MOSTLY_Q4_K_M = 15,// except 1d tensors
- LLAMA_V3_FTYPE_MOSTLY_Q5_K_S = 16,// except 1d tensors
- LLAMA_V3_FTYPE_MOSTLY_Q5_K_M = 17,// except 1d tensors
- LLAMA_V3_FTYPE_MOSTLY_Q6_K = 18,// except 1d tensors
- };
-
- // model quantization parameters
- typedef struct llama_v3_model_quantize_params {
- int nthread; // number of threads to use for quantizing, if <=0 will use std::thread::hardware_concurrency()
- enum llama_v3_ftype ftype; // quantize to this llama_v3_ftype
- bool allow_requantize; // allow quantizing non-f32/f16 tensors
- bool quantize_output_tensor; // quantize output.weight
- } llama_v3_model_quantize_params;
-
- // grammar types
- struct llama_v3_grammar;
-
- // grammar element type
- enum llama_v3_gretype {
- // end of rule definition
- LLAMA_V3_GRETYPE_END = 0,
-
- // start of alternate definition for rule
- LLAMA_V3_GRETYPE_ALT = 1,
-
- // non-terminal element: reference to rule
- LLAMA_V3_GRETYPE_RULE_REF = 2,
-
- // terminal element: character (code point)
- LLAMA_V3_GRETYPE_CHAR = 3,
-
- // inverse char(s) ([^a], [^a-b] [^abc])
- LLAMA_V3_GRETYPE_CHAR_NOT = 4,
-
- // modifies a preceding LLAMA_V3_GRETYPE_CHAR or LLAMA_V3_GRETYPE_CHAR_ALT to
- // be an inclusive range ([a-z])
- LLAMA_V3_GRETYPE_CHAR_RNG_UPPER = 5,
-
- // modifies a preceding LLAMA_V3_GRETYPE_CHAR or
- // LLAMA_V3_GRETYPE_CHAR_RNG_UPPER to add an alternate char to match ([ab], [a-zA])
- LLAMA_V3_GRETYPE_CHAR_ALT = 6,
- };
-
- typedef struct llama_v3_grammar_element {
- enum llama_v3_gretype type;
- uint32_t value; // Unicode code point or rule ID
- } llama_v3_grammar_element;
-
- // performance timing information
- struct llama_v3_timings {
- double t_start_ms;
- double t_end_ms;
- double t_load_ms;
- double t_sample_ms;
- double t_p_eval_ms;
- double t_eval_ms;
-
- int32_t n_sample;
- int32_t n_p_eval;
- int32_t n_eval;
- };
-
- // Set callback for all future logging events.
- // If this is not called, or NULL is supplied, everything is output on stderr.
- LLAMA_V3_API void llama_v3_log_set(llama_v3_log_callback log_callback, void * user_data);
-
- LLAMA_V3_API int llama_v3_max_devices();
-
- LLAMA_V3_API struct llama_v3_context_params llama_v3_context_default_params();
- LLAMA_V3_API struct llama_v3_model_quantize_params llama_v3_model_quantize_default_params();
-
- LLAMA_V3_API bool llama_v3_mmap_supported();
- LLAMA_V3_API bool llama_v3_mlock_supported();
-
- // TODO: not great API - very likely to change
- // Initialize the llama + ggml backend
- // If numa is true, use NUMA optimizations
- // Call once at the start of the program
- LLAMA_V3_API void llama_v3_backend_init(bool numa);
- // Call once at the end of the program - currently only used for MPI
- LLAMA_V3_API void llama_v3_backend_free();
-
- LLAMA_V3_API int64_t llama_v3_time_us();
-
- LLAMA_V3_API struct llama_v3_model * llama_v3_load_model_from_file(
- const char * path_model,
- struct llama_v3_context_params params);
-
- LLAMA_V3_API void llama_v3_free_model(struct llama_v3_model * model);
-
- LLAMA_V3_API struct llama_v3_context * llama_v3_new_context_with_model(
- struct llama_v3_model * model,
- struct llama_v3_context_params params);
-
- // Various functions for loading a ggml llama model.
- // Allocate (almost) all memory needed for the model.
- // Return NULL on failure
- LLAMA_V3_API struct llama_v3_context * llama_v3_init_from_file(
- const char * path_model,
- struct llama_v3_context_params params);
-
- // Frees all allocated memory
- LLAMA_V3_API void llama_v3_free(struct llama_v3_context * ctx);
-
- // Returns 0 on success
- LLAMA_V3_API int llama_v3_model_quantize(
- const char * fname_inp,
- const char * fname_out,
- const llama_v3_model_quantize_params * params);
-
- // Apply a LoRA adapter to a loaded model
- // path_base_model is the path to a higher quality model to use as a base for
- // the layers modified by the adapter. Can be NULL to use the current loaded model.
- // The model needs to be reloaded before applying a new adapter, otherwise the adapter
- // will be applied on top of the previous one
- // Returns 0 on success
- LLAMA_V3_API int llama_v3_apply_lora_from_file(
- struct llama_v3_context * ctx,
- const char * path_lora,
- const char * path_base_model,
- int n_threads);
-
- LLAMA_V3_API int llama_v3_model_apply_lora_from_file(
- const struct llama_v3_model * model,
- const char * path_lora,
- const char * path_base_model,
- int n_threads);
-
- // Returns the number of tokens in the KV cache
- LLAMA_V3_API int llama_v3_get_kv_cache_token_count(const struct llama_v3_context * ctx);
-
- // Sets the current rng seed.
- LLAMA_V3_API void llama_v3_set_rng_seed(struct llama_v3_context * ctx, uint32_t seed);
-
- // Returns the maximum size in bytes of the state (rng, logits, embedding
- // and kv_cache) - will often be smaller after compacting tokens
- LLAMA_V3_API size_t llama_v3_get_state_size(const struct llama_v3_context * ctx);
-
- // Copies the state to the specified destination address.
- // Destination needs to have allocated enough memory.
- // Returns the number of bytes copied
- LLAMA_V3_API size_t llama_v3_copy_state_data(struct llama_v3_context * ctx, uint8_t * dst);
-
- // Set the state reading from the specified address
- // Returns the number of bytes read
- LLAMA_V3_API size_t llama_v3_set_state_data(struct llama_v3_context * ctx, uint8_t * src);
-
- // Save/load session file
- LLAMA_V3_API bool llama_v3_load_session_file(struct llama_v3_context * ctx, const char * path_session, llama_v3_token * tokens_out, size_t n_token_capacity, size_t * n_token_count_out);
- LLAMA_V3_API bool llama_v3_save_session_file(struct llama_v3_context * ctx, const char * path_session, const llama_v3_token * tokens, size_t n_token_count);
-
- // Run the llama inference to obtain the logits and probabilities for the next token.
- // tokens + n_tokens is the provided batch of new tokens to process
- // n_past is the number of tokens to use from previous eval calls
- // Returns 0 on success
- LLAMA_V3_API int llama_v3_eval(
- struct llama_v3_context * ctx,
- const llama_v3_token * tokens,
- int n_tokens,
- int n_past,
- int n_threads);
-
- // Same as llama_v3_eval, but use float matrix input directly.
- LLAMA_V3_API int llama_v3_eval_embd(
- struct llama_v3_context * ctx,
- const float * embd,
- int n_tokens,
- int n_past,
- int n_threads);
-
- // Export a static computation graph for context of 511 and batch size of 1
- // NOTE: since this functionality is mostly for debugging and demonstration purposes, we hardcode these
- // parameters here to keep things simple
- // IMPORTANT: do not use for anything else other than debugging and testing!
- LLAMA_V3_API int llama_v3_eval_export(struct llama_v3_context * ctx, const char * fname);
-
- // Convert the provided text into tokens.
- // The tokens pointer must be large enough to hold the resulting tokens.
- // Returns the number of tokens on success, no more than n_max_tokens
- // Returns a negative number on failure - the number of tokens that would have been returned
- // TODO: not sure if correct
- LLAMA_V3_API int llama_v3_tokenize(
- struct llama_v3_context * ctx,
- const char * text,
- llama_v3_token * tokens,
- int n_max_tokens,
- bool add_bos);
-
- LLAMA_V3_API int llama_v3_tokenize_with_model(
- const struct llama_v3_model * model,
- const char * text,
- llama_v3_token * tokens,
- int n_max_tokens,
- bool add_bos);
-
- LLAMA_V3_API int llama_v3_n_vocab(const struct llama_v3_context * ctx);
- LLAMA_V3_API int llama_v3_n_ctx (const struct llama_v3_context * ctx);
- LLAMA_V3_API int llama_v3_n_embd (const struct llama_v3_context * ctx);
-
- LLAMA_V3_API int llama_v3_n_vocab_from_model(const struct llama_v3_model * model);
- LLAMA_V3_API int llama_v3_n_ctx_from_model (const struct llama_v3_model * model);
- LLAMA_V3_API int llama_v3_n_embd_from_model (const struct llama_v3_model * model);
-
- LLAMA_V3_API int llama_v3_model_type(const struct llama_v3_model * model, char * buf, size_t buf_size);
-
- // Get the vocabulary as output parameters.
- // Returns number of results.
- LLAMA_V3_API int llama_v3_get_vocab(
- const struct llama_v3_context * ctx,
- const char * * strings,
- float * scores,
- int capacity);
-
- LLAMA_V3_API int llama_v3_get_vocab_from_model(
- const struct llama_v3_model * model,
- const char * * strings,
- float * scores,
- int capacity);
-
- // Token logits obtained from the last call to llama_v3_eval()
- // The logits for the last token are stored in the last row
- // Can be mutated in order to change the probabilities of the next token
- // Rows: n_tokens
- // Cols: n_vocab
- LLAMA_V3_API float * llama_v3_get_logits(struct llama_v3_context * ctx);
-
- // Get the embeddings for the input
- // shape: [n_embd] (1-dimensional)
- LLAMA_V3_API float * llama_v3_get_embeddings(struct llama_v3_context * ctx);
-
- // Token Id -> String. Uses the vocabulary in the provided context
- LLAMA_V3_API const char * llama_v3_token_to_str(
- const struct llama_v3_context * ctx,
- llama_v3_token token);
-
- LLAMA_V3_API const char * llama_v3_token_to_str_with_model(
- const struct llama_v3_model * model,
- llama_v3_token token);
-
- // Special tokens
- LLAMA_V3_API llama_v3_token llama_v3_token_bos(); // beginning-of-sentence
- LLAMA_V3_API llama_v3_token llama_v3_token_eos(); // end-of-sentence
- LLAMA_V3_API llama_v3_token llama_v3_token_nl(); // next-line
-
- // Grammar
- //
- LLAMA_V3_API struct llama_v3_grammar * llama_v3_grammar_init(
- const llama_v3_grammar_element ** rules,
- size_t n_rules,
- size_t start_rule_index);
-
- LLAMA_V3_API void llama_v3_grammar_free(struct llama_v3_grammar * grammar);
-
- // Sampling functions
-
- /// @details Repetition penalty described in CTRL academic paper https://arxiv.org/abs/1909.05858, with negative logit fix.
- LLAMA_V3_API void llama_v3_sample_repetition_penalty(struct llama_v3_context * ctx, llama_v3_token_data_array * candidates, const llama_v3_token * last_tokens, size_t last_tokens_size, float penalty);
-
- /// @details Frequency and presence penalties described in OpenAI API https://platform.openai.com/docs/api-reference/parameter-details.
- LLAMA_V3_API void llama_v3_sample_frequency_and_presence_penalties(struct llama_v3_context * ctx, llama_v3_token_data_array * candidates, const llama_v3_token * last_tokens, size_t last_tokens_size, float alpha_frequency, float alpha_presence);
-
- /// @details Apply classifier-free guidance to the logits as described in academic paper "Stay on topic with Classifier-Free Guidance" https://arxiv.org/abs/2306.17806
- /// @param candidates A vector of `llama_v3_token_data` containing the candidate tokens, the logits must be directly extracted from the original generation context without being sorted.
- /// @params guidance_ctx A separate context from the same model. Other than a negative prompt at the beginning, it should have all generated and user input tokens copied from the main context.
- /// @params scale Guidance strength. 1.0f means no guidance. Higher values mean stronger guidance.
- LLAMA_V3_API void llama_v3_sample_classifier_free_guidance(
- struct llama_v3_context * ctx,
- llama_v3_token_data_array * candidates,
- struct llama_v3_context * guidance_ctx,
- float scale);
-
- /// @details Sorts candidate tokens by their logits in descending order and calculate probabilities based on logits.
- LLAMA_V3_API void llama_v3_sample_softmax(struct llama_v3_context * ctx, llama_v3_token_data_array * candidates);
-
- /// @details Top-K sampling described in academic paper "The Curious Case of Neural Text Degeneration" https://arxiv.org/abs/1904.09751
- LLAMA_V3_API void llama_v3_sample_top_k(struct llama_v3_context * ctx, llama_v3_token_data_array * candidates, int k, size_t min_keep);
-
- /// @details Nucleus sampling described in academic paper "The Curious Case of Neural Text Degeneration" https://arxiv.org/abs/1904.09751
- LLAMA_V3_API void llama_v3_sample_top_p(struct llama_v3_context * ctx, llama_v3_token_data_array * candidates, float p, size_t min_keep);
-
- /// @details Tail Free Sampling described in https://www.trentonbricken.com/Tail-Free-Sampling/.
- LLAMA_V3_API void llama_v3_sample_tail_free(struct llama_v3_context * ctx, llama_v3_token_data_array * candidates, float z, size_t min_keep);
-
- /// @details Locally Typical Sampling implementation described in the paper https://arxiv.org/abs/2202.00666.
- LLAMA_V3_API void llama_v3_sample_typical(struct llama_v3_context * ctx, llama_v3_token_data_array * candidates, float p, size_t min_keep);
- LLAMA_V3_API void llama_v3_sample_temperature(struct llama_v3_context * ctx, llama_v3_token_data_array * candidates, float temp);
-
- /// @details Apply constraints from grammar
- LLAMA_V3_API void llama_v3_sample_grammar(struct llama_v3_context * ctx, llama_v3_token_data_array * candidates, const struct llama_v3_grammar * grammar);
-
- /// @details Mirostat 1.0 algorithm described in the paper https://arxiv.org/abs/2007.14966. Uses tokens instead of words.
- /// @param candidates A vector of `llama_v3_token_data` containing the candidate tokens, their probabilities (p), and log-odds (logit) for the current position in the generated text.
- /// @param tau The target cross-entropy (or surprise) value you want to achieve for the generated text. A higher value corresponds to more surprising or less predictable text, while a lower value corresponds to less surprising or more predictable text.
- /// @param eta The learning rate used to update `mu` based on the error between the target and observed surprisal of the sampled word. A larger learning rate will cause `mu` to be updated more quickly, while a smaller learning rate will result in slower updates.
- /// @param m The number of tokens considered in the estimation of `s_hat`. This is an arbitrary value that is used to calculate `s_hat`, which in turn helps to calculate the value of `k`. In the paper, they use `m = 100`, but you can experiment with different values to see how it affects the performance of the algorithm.
- /// @param mu Maximum cross-entropy. This value is initialized to be twice the target cross-entropy (`2 * tau`) and is updated in the algorithm based on the error between the target and observed surprisal.
- LLAMA_V3_API llama_v3_token llama_v3_sample_token_mirostat(struct llama_v3_context * ctx, llama_v3_token_data_array * candidates, float tau, float eta, int m, float * mu);
-
- /// @details Mirostat 2.0 algorithm described in the paper https://arxiv.org/abs/2007.14966. Uses tokens instead of words.
- /// @param candidates A vector of `llama_v3_token_data` containing the candidate tokens, their probabilities (p), and log-odds (logit) for the current position in the generated text.
- /// @param tau The target cross-entropy (or surprise) value you want to achieve for the generated text. A higher value corresponds to more surprising or less predictable text, while a lower value corresponds to less surprising or more predictable text.
- /// @param eta The learning rate used to update `mu` based on the error between the target and observed surprisal of the sampled word. A larger learning rate will cause `mu` to be updated more quickly, while a smaller learning rate will result in slower updates.
- /// @param mu Maximum cross-entropy. This value is initialized to be twice the target cross-entropy (`2 * tau`) and is updated in the algorithm based on the error between the target and observed surprisal.
- LLAMA_V3_API llama_v3_token llama_v3_sample_token_mirostat_v2(struct llama_v3_context * ctx, llama_v3_token_data_array * candidates, float tau, float eta, float * mu);
-
- /// @details Selects the token with the highest probability.
- LLAMA_V3_API llama_v3_token llama_v3_sample_token_greedy(struct llama_v3_context * ctx, llama_v3_token_data_array * candidates);
-
- /// @details Randomly selects a token from the candidates based on their probabilities.
- LLAMA_V3_API llama_v3_token llama_v3_sample_token(struct llama_v3_context * ctx, llama_v3_token_data_array * candidates);
-
- /// @details Accepts the sampled token into the grammar
- LLAMA_V3_API void llama_v3_grammar_accept_token(struct llama_v3_context * ctx, struct llama_v3_grammar * grammar, llama_v3_token token);
-
- // Performance information
- LLAMA_V3_API struct llama_v3_timings llama_v3_get_timings(struct llama_v3_context * ctx);
- LLAMA_V3_API void llama_v3_print_timings(struct llama_v3_context * ctx);
- LLAMA_V3_API void llama_v3_reset_timings(struct llama_v3_context * ctx);
-
- // Print system information
- LLAMA_V3_API const char * llama_v3_print_system_info(void);
-
-#ifdef __cplusplus
-}
-#endif
-
-// Internal API to be implemented by llama.cpp and used by tests/benchmarks only
-#ifdef LLAMA_V3_API_INTERNAL
-
-#include
-#include
-struct ggml_tensor;
-
-const std::vector>& llama_v3_internal_get_tensor_map(struct llama_v3_context * ctx);
-
-#endif
-
-#endif // LLAMA_V3_H
diff --git a/spaces/Intel/NeuralChat-ICX-INT4/fastchat/serve/gradio_patch.py b/spaces/Intel/NeuralChat-ICX-INT4/fastchat/serve/gradio_patch.py
deleted file mode 100644
index af8731da17d4c39a2a32afd4ce2cca13e3845ac4..0000000000000000000000000000000000000000
--- a/spaces/Intel/NeuralChat-ICX-INT4/fastchat/serve/gradio_patch.py
+++ /dev/null
@@ -1,168 +0,0 @@
-"""
-Adopted from https://github.com/gradio-app/gradio/blob/main/gradio/components.py
-Fix a markdown render problem.
-"""
-from __future__ import annotations
-
-from gradio.components import *
-from markdown2 import Markdown
-import nh3
-
-
-class _Keywords(Enum):
- NO_VALUE = "NO_VALUE" # Used as a sentinel to determine if nothing is provided as a argument for `value` in `Component.update()`
- FINISHED_ITERATING = "FINISHED_ITERATING" # Used to skip processing of a component's value (needed for generators + state)
-
-
-@document("style")
-class Chatbot(Changeable, Selectable, IOComponent, JSONSerializable):
- """
- Displays a chatbot output showing both user submitted messages and responses. Supports a subset of Markdown including bold, italics, code, and images.
- Preprocessing: this component does *not* accept input.
- Postprocessing: expects function to return a {List[Tuple[str | None | Tuple, str | None | Tuple]]}, a list of tuples with user message and response messages. Messages should be strings, tuples, or Nones. If the message is a string, it can include Markdown. If it is a tuple, it should consist of (string filepath to image/video/audio, [optional string alt text]). Messages that are `None` are not displayed.
-
- Demos: chatbot_simple, chatbot_multimodal
- """
-
- def __init__(
- self,
- value: List[Tuple[str | None, str | None]] | Callable | None = None,
- color_map: Dict[str, str] | None = None, # Parameter moved to Chatbot.style()
- *,
- label: str | None = None,
- every: float | None = None,
- show_label: bool = True,
- visible: bool = True,
- elem_id: str | None = None,
- elem_classes: List[str] | str | None = None,
- **kwargs,
- ):
- """
- Parameters:
- value: Default value to show in chatbot. If callable, the function will be called whenever the app loads to set the initial value of the component.
- label: component name in interface.
- every: If `value` is a callable, run the function 'every' number of seconds while the client connection is open. Has no effect otherwise. Queue must be enabled. The event can be accessed (e.g. to cancel it) via this component's .load_event attribute.
- show_label: if True, will display label.
- visible: If False, component will be hidden.
- elem_id: An optional string that is assigned as the id of this component in the HTML DOM. Can be used for targeting CSS styles.
- elem_classes: An optional list of strings that are assigned as the classes of this component in the HTML DOM. Can be used for targeting CSS styles.
- """
- if color_map is not None:
- warnings.warn(
- "The 'color_map' parameter has been deprecated.",
- )
- # self.md = utils.get_markdown_parser()
- self.md = Markdown(extras=["fenced-code-blocks", "tables", "break-on-newline"])
- self.select: EventListenerMethod
- """
- Event listener for when the user selects message from Chatbot.
- Uses event data gradio.SelectData to carry `value` referring to text of selected message, and `index` tuple to refer to [message, participant] index.
- See EventData documentation on how to use this event data.
- """
-
- IOComponent.__init__(
- self,
- label=label,
- every=every,
- show_label=show_label,
- visible=visible,
- elem_id=elem_id,
- elem_classes=elem_classes,
- value=value,
- **kwargs,
- )
-
- def get_config(self):
- return {
- "value": self.value,
- "selectable": self.selectable,
- **IOComponent.get_config(self),
- }
-
- @staticmethod
- def update(
- value: Any | Literal[_Keywords.NO_VALUE] | None = _Keywords.NO_VALUE,
- label: str | None = None,
- show_label: bool | None = None,
- visible: bool | None = None,
- ):
- updated_config = {
- "label": label,
- "show_label": show_label,
- "visible": visible,
- "value": value,
- "__type__": "update",
- }
- return updated_config
-
- def _process_chat_messages(
- self, chat_message: str | Tuple | List | Dict | None
- ) -> str | Dict | None:
- if chat_message is None:
- return None
- elif isinstance(chat_message, (tuple, list)):
- mime_type = processing_utils.get_mimetype(chat_message[0])
- return {
- "name": chat_message[0],
- "mime_type": mime_type,
- "alt_text": chat_message[1] if len(chat_message) > 1 else None,
- "data": None, # These last two fields are filled in by the frontend
- "is_file": True,
- }
- elif isinstance(
- chat_message, dict
- ): # This happens for previously processed messages
- return chat_message
- elif isinstance(chat_message, str):
- # return self.md.render(chat_message)
- return str(self.md.convert(chat_message))
- else:
- raise ValueError(f"Invalid message for Chatbot component: {chat_message}")
-
- def postprocess(
- self,
- y: List[
- Tuple[str | Tuple | List | Dict | None, str | Tuple | List | Dict | None]
- ],
- ) -> List[Tuple[str | Dict | None, str | Dict | None]]:
- """
- Parameters:
- y: List of tuples representing the message and response pairs. Each message and response should be a string, which may be in Markdown format. It can also be a tuple whose first element is a string filepath or URL to an image/video/audio, and second (optional) element is the alt text, in which case the media file is displayed. It can also be None, in which case that message is not displayed.
- Returns:
- List of tuples representing the message and response. Each message and response will be a string of HTML, or a dictionary with media information.
- """
- if y is None:
- return []
- processed_messages = []
- for message_pair in y:
- assert isinstance(
- message_pair, (tuple, list)
- ), f"Expected a list of lists or list of tuples. Received: {message_pair}"
- assert (
- len(message_pair) == 2
- ), f"Expected a list of lists of length 2 or list of tuples of length 2. Received: {message_pair}"
- processed_messages.append(
- (
- # self._process_chat_messages(message_pair[0]),
- '
-"""
-
-SUMMARIZE_PROMPT = "你是谁?我们刚才聊了什么?" # 总结对话时的 prompt
-
-ONLINE_MODELS = [
- "gpt-3.5-turbo",
- "gpt-3.5-turbo-0301",
- "gpt-4",
- "gpt-4-0314",
- "gpt-4-32k",
- "gpt-4-32k-0314",
- "xmchat",
-]
-
-LOCAL_MODELS = [
- "chatglm-6b",
- "chatglm-6b-int4",
- "chatglm-6b-int4-qe",
- "llama-7b-hf",
- "llama-7b-hf-int4",
- "llama-7b-hf-int8",
- "llama-13b-hf",
- "llama-13b-hf-int4",
- "llama-30b-hf",
- "llama-30b-hf-int4",
- "llama-65b-hf"
-]
-
-if os.environ.get('HIDE_LOCAL_MODELS', 'false') == 'true':
- MODELS = ONLINE_MODELS
-else:
- MODELS = ONLINE_MODELS + LOCAL_MODELS
-
-DEFAULT_MODEL = 1
-
-os.makedirs("models", exist_ok=True)
-os.makedirs("lora", exist_ok=True)
-os.makedirs("history", exist_ok=True)
-for dir_name in os.listdir("models"):
- if os.path.isdir(os.path.join("models", dir_name)):
- if dir_name not in MODELS:
- MODELS.append(dir_name)
-
-MODEL_TOKEN_LIMIT = {
- "gpt-3.5-turbo": 4096,
- "gpt-3.5-turbo-0301": 4096,
- "gpt-4": 8192,
- "gpt-4-0314": 8192,
- "gpt-4-32k": 32768,
- "gpt-4-32k-0314": 32768
-}
-
-TOKEN_OFFSET = 1000 # 模型的token上限减去这个值,得到软上限。到达软上限之后,自动尝试减少token占用。
-DEFAULT_TOKEN_LIMIT = 3000 # 默认的token上限
-REDUCE_TOKEN_FACTOR = 0.5 # 与模型token上限想乘,得到目标token数。减少token占用时,将token占用减少到目标token数以下。
-
-REPLY_LANGUAGES = [
- "简体中文",
- "繁體中文",
- "English",
- "日本語",
- "Español",
- "Français",
- "Deutsch",
- "跟随问题语言(不稳定)"
-]
-
-
-WEBSEARCH_PTOMPT_TEMPLATE = """\
-Web search results:
-
-{web_results}
-Current date: {current_date}
-
-Instructions: Using the provided web search results, write a comprehensive reply to the given query. Make sure to cite results using [[number](URL)] notation after the reference. If the provided search results refer to multiple subjects with the same name, write separate answers for each subject.
-Query: {query}
-Reply in {reply_language}
-"""
-
-PROMPT_TEMPLATE = """\
-Context information is below.
----------------------
-{context_str}
----------------------
-Current date: {current_date}.
-Using the provided context information, write a comprehensive reply to the given query.
-Make sure to cite results using [number] notation after the reference.
-If the provided context information refer to multiple subjects with the same name, write separate answers for each subject.
-Use prior knowledge only if the given context didn't provide enough information.
-Answer the question: {query_str}
-Reply in {reply_language}
-"""
-
-REFINE_TEMPLATE = """\
-The original question is as follows: {query_str}
-We have provided an existing answer: {existing_answer}
-We have the opportunity to refine the existing answer
-(only if needed) with some more context below.
-------------
-{context_msg}
-------------
-Given the new context, refine the original answer to better
-Reply in {reply_language}
-If the context isn't useful, return the original answer.
-"""
-
-ALREADY_CONVERTED_MARK = ""
-
-small_and_beautiful_theme = gr.themes.Soft(
- primary_hue=gr.themes.Color(
- c50="#02C160",
- c100="rgba(2, 193, 96, 0.2)",
- c200="#02C160",
- c300="rgba(2, 193, 96, 0.32)",
- c400="rgba(2, 193, 96, 0.32)",
- c500="rgba(2, 193, 96, 1.0)",
- c600="rgba(2, 193, 96, 1.0)",
- c700="rgba(2, 193, 96, 0.32)",
- c800="rgba(2, 193, 96, 0.32)",
- c900="#02C160",
- c950="#02C160",
- ),
- secondary_hue=gr.themes.Color(
- c50="#576b95",
- c100="#576b95",
- c200="#576b95",
- c300="#576b95",
- c400="#576b95",
- c500="#576b95",
- c600="#576b95",
- c700="#576b95",
- c800="#576b95",
- c900="#576b95",
- c950="#576b95",
- ),
- neutral_hue=gr.themes.Color(
- name="gray",
- c50="#f9fafb",
- c100="#f3f4f6",
- c200="#e5e7eb",
- c300="#d1d5db",
- c400="#B2B2B2",
- c500="#808080",
- c600="#636363",
- c700="#515151",
- c800="#393939",
- c900="#272727",
- c950="#171717",
- ),
- radius_size=gr.themes.sizes.radius_sm,
- ).set(
- button_primary_background_fill="#06AE56",
- button_primary_background_fill_dark="#06AE56",
- button_primary_background_fill_hover="#07C863",
- button_primary_border_color="#06AE56",
- button_primary_border_color_dark="#06AE56",
- button_primary_text_color="#FFFFFF",
- button_primary_text_color_dark="#FFFFFF",
- button_secondary_background_fill="#F2F2F2",
- button_secondary_background_fill_dark="#2B2B2B",
- button_secondary_text_color="#393939",
- button_secondary_text_color_dark="#FFFFFF",
- # background_fill_primary="#F7F7F7",
- # background_fill_primary_dark="#1F1F1F",
- block_title_text_color="*primary_500",
- block_title_background_fill="*primary_100",
- input_background_fill="#F6F6F6",
- )
diff --git a/spaces/Jack-Ahan/fruit-vegetable-classifier/README.md b/spaces/Jack-Ahan/fruit-vegetable-classifier/README.md
deleted file mode 100644
index ee17f01df361f6f139aa1d16f9f99e40ad16ed8b..0000000000000000000000000000000000000000
--- a/spaces/Jack-Ahan/fruit-vegetable-classifier/README.md
+++ /dev/null
@@ -1,13 +0,0 @@
----
-title: Fruit Vegetable Classifier
-emoji: 👀
-colorFrom: pink
-colorTo: purple
-sdk: gradio
-sdk_version: 3.1.7
-app_file: app.py
-pinned: false
-license: gpl-3.0
----
-
-Check out the configuration reference at https://huggingface.co/docs/hub/spaces-config-reference
diff --git a/spaces/Jikiwi/sovits-models/vdecoder/hifigan/nvSTFT.py b/spaces/Jikiwi/sovits-models/vdecoder/hifigan/nvSTFT.py
deleted file mode 100644
index 88597d62a505715091f9ba62d38bf0a85a31b95a..0000000000000000000000000000000000000000
--- a/spaces/Jikiwi/sovits-models/vdecoder/hifigan/nvSTFT.py
+++ /dev/null
@@ -1,111 +0,0 @@
-import math
-import os
-os.environ["LRU_CACHE_CAPACITY"] = "3"
-import random
-import torch
-import torch.utils.data
-import numpy as np
-import librosa
-from librosa.util import normalize
-from librosa.filters import mel as librosa_mel_fn
-from scipy.io.wavfile import read
-import soundfile as sf
-
-def load_wav_to_torch(full_path, target_sr=None, return_empty_on_exception=False):
- sampling_rate = None
- try:
- data, sampling_rate = sf.read(full_path, always_2d=True)# than soundfile.
- except Exception as ex:
- print(f"'{full_path}' failed to load.\nException:")
- print(ex)
- if return_empty_on_exception:
- return [], sampling_rate or target_sr or 32000
- else:
- raise Exception(ex)
-
- if len(data.shape) > 1:
- data = data[:, 0]
- assert len(data) > 2# check duration of audio file is > 2 samples (because otherwise the slice operation was on the wrong dimension)
-
- if np.issubdtype(data.dtype, np.integer): # if audio data is type int
- max_mag = -np.iinfo(data.dtype).min # maximum magnitude = min possible value of intXX
- else: # if audio data is type fp32
- max_mag = max(np.amax(data), -np.amin(data))
- max_mag = (2**31)+1 if max_mag > (2**15) else ((2**15)+1 if max_mag > 1.01 else 1.0) # data should be either 16-bit INT, 32-bit INT or [-1 to 1] float32
-
- data = torch.FloatTensor(data.astype(np.float32))/max_mag
-
- if (torch.isinf(data) | torch.isnan(data)).any() and return_empty_on_exception:# resample will crash with inf/NaN inputs. return_empty_on_exception will return empty arr instead of except
- return [], sampling_rate or target_sr or 32000
- if target_sr is not None and sampling_rate != target_sr:
- data = torch.from_numpy(librosa.core.resample(data.numpy(), orig_sr=sampling_rate, target_sr=target_sr))
- sampling_rate = target_sr
-
- return data, sampling_rate
-
-def dynamic_range_compression(x, C=1, clip_val=1e-5):
- return np.log(np.clip(x, a_min=clip_val, a_max=None) * C)
-
-def dynamic_range_decompression(x, C=1):
- return np.exp(x) / C
-
-def dynamic_range_compression_torch(x, C=1, clip_val=1e-5):
- return torch.log(torch.clamp(x, min=clip_val) * C)
-
-def dynamic_range_decompression_torch(x, C=1):
- return torch.exp(x) / C
-
-class STFT():
- def __init__(self, sr=22050, n_mels=80, n_fft=1024, win_size=1024, hop_length=256, fmin=20, fmax=11025, clip_val=1e-5):
- self.target_sr = sr
-
- self.n_mels = n_mels
- self.n_fft = n_fft
- self.win_size = win_size
- self.hop_length = hop_length
- self.fmin = fmin
- self.fmax = fmax
- self.clip_val = clip_val
- self.mel_basis = {}
- self.hann_window = {}
-
- def get_mel(self, y, center=False):
- sampling_rate = self.target_sr
- n_mels = self.n_mels
- n_fft = self.n_fft
- win_size = self.win_size
- hop_length = self.hop_length
- fmin = self.fmin
- fmax = self.fmax
- clip_val = self.clip_val
-
- if torch.min(y) < -1.:
- print('min value is ', torch.min(y))
- if torch.max(y) > 1.:
- print('max value is ', torch.max(y))
-
- if fmax not in self.mel_basis:
- mel = librosa_mel_fn(sr=sampling_rate, n_fft=n_fft, n_mels=n_mels, fmin=fmin, fmax=fmax)
- self.mel_basis[str(fmax)+'_'+str(y.device)] = torch.from_numpy(mel).float().to(y.device)
- self.hann_window[str(y.device)] = torch.hann_window(self.win_size).to(y.device)
-
- y = torch.nn.functional.pad(y.unsqueeze(1), (int((n_fft-hop_length)/2), int((n_fft-hop_length)/2)), mode='reflect')
- y = y.squeeze(1)
-
- spec = torch.stft(y, n_fft, hop_length=hop_length, win_length=win_size, window=self.hann_window[str(y.device)],
- center=center, pad_mode='reflect', normalized=False, onesided=True)
- # print(111,spec)
- spec = torch.sqrt(spec.pow(2).sum(-1)+(1e-9))
- # print(222,spec)
- spec = torch.matmul(self.mel_basis[str(fmax)+'_'+str(y.device)], spec)
- # print(333,spec)
- spec = dynamic_range_compression_torch(spec, clip_val=clip_val)
- # print(444,spec)
- return spec
-
- def __call__(self, audiopath):
- audio, sr = load_wav_to_torch(audiopath, target_sr=self.target_sr)
- spect = self.get_mel(audio.unsqueeze(0)).squeeze(0)
- return spect
-
-stft = STFT()
diff --git a/spaces/Kangarroar/ApplioRVC-Inference/infer/modules/onnx/export.py b/spaces/Kangarroar/ApplioRVC-Inference/infer/modules/onnx/export.py
deleted file mode 100644
index ed4a4162ff04b7e12642fcbe96847f8ea9db06aa..0000000000000000000000000000000000000000
--- a/spaces/Kangarroar/ApplioRVC-Inference/infer/modules/onnx/export.py
+++ /dev/null
@@ -1,52 +0,0 @@
-import torch
-
-from infer.lib.infer_pack.models_onnx import SynthesizerTrnMsNSFsidM
-
-
-def export_onnx(ModelPath, ExportedPath):
- cpt = torch.load(ModelPath, map_location="cpu")
- cpt["config"][-3] = cpt["weight"]["emb_g.weight"].shape[0]
- vec_channels = 256 if cpt.get("version", "v1") == "v1" else 768
-
- test_phone = torch.rand(1, 200, vec_channels) # hidden unit
- test_phone_lengths = torch.tensor([200]).long() # hidden unit 长度(貌似没啥用)
- test_pitch = torch.randint(size=(1, 200), low=5, high=255) # 基频(单位赫兹)
- test_pitchf = torch.rand(1, 200) # nsf基频
- test_ds = torch.LongTensor([0]) # 说话人ID
- test_rnd = torch.rand(1, 192, 200) # 噪声(加入随机因子)
-
- device = "cpu" # 导出时设备(不影响使用模型)
-
- net_g = SynthesizerTrnMsNSFsidM(
- *cpt["config"], is_half=False, version=cpt.get("version", "v1")
- ) # fp32导出(C++要支持fp16必须手动将内存重新排列所以暂时不用fp16)
- net_g.load_state_dict(cpt["weight"], strict=False)
- input_names = ["phone", "phone_lengths", "pitch", "pitchf", "ds", "rnd"]
- output_names = [
- "audio",
- ]
- # net_g.construct_spkmixmap(n_speaker) 多角色混合轨道导出
- torch.onnx.export(
- net_g,
- (
- test_phone.to(device),
- test_phone_lengths.to(device),
- test_pitch.to(device),
- test_pitchf.to(device),
- test_ds.to(device),
- test_rnd.to(device),
- ),
- ExportedPath,
- dynamic_axes={
- "phone": [1],
- "pitch": [1],
- "pitchf": [1],
- "rnd": [2],
- },
- do_constant_folding=False,
- opset_version=13,
- verbose=False,
- input_names=input_names,
- output_names=output_names,
- )
- return "Finished"
diff --git a/spaces/Kangarroar/ApplioRVC-Inference/utils/backups.py b/spaces/Kangarroar/ApplioRVC-Inference/utils/backups.py
deleted file mode 100644
index b814f8184792e80e2324685436053d61487110b1..0000000000000000000000000000000000000000
--- a/spaces/Kangarroar/ApplioRVC-Inference/utils/backups.py
+++ /dev/null
@@ -1,141 +0,0 @@
-import os
-import shutil
-import hashlib
-import time
-import base64
-
-
-
-
-LOGS_FOLDER = '/content/Applio-RVC-Fork/logs'
-WEIGHTS_FOLDER = '/content/Applio-RVC-Fork/weights'
-GOOGLE_DRIVE_PATH = '/content/drive/MyDrive/RVC_Backup'
-
-def import_google_drive_backup():
- print("Importing Google Drive backup...")
- weights_exist = False
- for root, dirs, files in os.walk(GOOGLE_DRIVE_PATH):
- for filename in files:
- filepath = os.path.join(root, filename)
- if os.path.isfile(filepath) and not filepath.startswith(os.path.join(GOOGLE_DRIVE_PATH, 'weights')):
- backup_filepath = os.path.join(LOGS_FOLDER, os.path.relpath(filepath, GOOGLE_DRIVE_PATH))
- backup_folderpath = os.path.dirname(backup_filepath)
- if not os.path.exists(backup_folderpath):
- os.makedirs(backup_folderpath)
- print(f'Created backup folder: {backup_folderpath}', flush=True)
- shutil.copy2(filepath, backup_filepath) # copy file with metadata
- print(f'Imported file from Google Drive backup: {filename}')
- elif filepath.startswith(os.path.join(GOOGLE_DRIVE_PATH, 'weights')) and filename.endswith('.pth'):
- weights_exist = True
- weights_filepath = os.path.join(WEIGHTS_FOLDER, os.path.relpath(filepath, os.path.join(GOOGLE_DRIVE_PATH, 'weights')))
- weights_folderpath = os.path.dirname(weights_filepath)
- if not os.path.exists(weights_folderpath):
- os.makedirs(weights_folderpath)
- print(f'Created weights folder: {weights_folderpath}', flush=True)
- shutil.copy2(filepath, weights_filepath) # copy file with metadata
- print(f'Imported file from weights: {filename}')
- if weights_exist:
- print("Copied weights from Google Drive backup to local weights folder.")
- else:
- print("No weights found in Google Drive backup.")
- print("Google Drive backup import completed.")
-
-def get_md5_hash(file_path):
- hash_md5 = hashlib.md5()
- with open(file_path, "rb") as f:
- for chunk in iter(lambda: f.read(4096), b""):
- hash_md5.update(chunk)
- return hash_md5.hexdigest()
-
-def copy_weights_folder_to_drive():
- destination_folder = os.path.join(GOOGLE_DRIVE_PATH, 'weights')
- try:
- if not os.path.exists(destination_folder):
- os.makedirs(destination_folder)
-
- num_copied = 0
- for filename in os.listdir(WEIGHTS_FOLDER):
- if filename.endswith('.pth'):
- source_file = os.path.join(WEIGHTS_FOLDER, filename)
- destination_file = os.path.join(destination_folder, filename)
- if not os.path.exists(destination_file):
- shutil.copy2(source_file, destination_file)
- num_copied += 1
- print(f"Copied {filename} to Google Drive!")
-
- if num_copied == 0:
- print("No new finished models found for copying.")
- else:
- print(f"Finished copying {num_copied} files to Google Drive!")
-
- except Exception as e:
- print(f"An error occurred while copying weights: {str(e)}")
- # You can log the error or take appropriate actions here.
-
-def backup_files():
- print("\nStarting backup loop...")
- last_backup_timestamps_path = os.path.join(LOGS_FOLDER, 'last_backup_timestamps.txt')
- fully_updated = False # boolean to track if all files are up to date
-
- while True:
- try:
- updated = False # flag to check if any files were updated
- last_backup_timestamps = {}
-
- try:
- with open(last_backup_timestamps_path, 'r') as f:
- last_backup_timestamps = dict(line.strip().split(':') for line in f)
- except FileNotFoundError:
- pass # File does not exist yet, which is fine
-
- for root, dirs, files in os.walk(LOGS_FOLDER):
- for filename in files:
- if filename != 'last_backup_timestamps.txt':
- filepath = os.path.join(root, filename)
- if os.path.isfile(filepath):
- backup_filepath = os.path.join(GOOGLE_DRIVE_PATH, os.path.relpath(filepath, LOGS_FOLDER))
- backup_folderpath = os.path.dirname(backup_filepath)
- if not os.path.exists(backup_folderpath):
- os.makedirs(backup_folderpath)
- print(f'Created backup folder: {backup_folderpath}', flush=True)
- # check if file has changed since last backup
- last_backup_timestamp = last_backup_timestamps.get(filepath)
- current_timestamp = os.path.getmtime(filepath)
- if last_backup_timestamp is None or float(last_backup_timestamp) < current_timestamp:
- shutil.copy2(filepath, backup_filepath) # copy file with metadata
- last_backup_timestamps[filepath] = str(current_timestamp) # update last backup timestamp
- if last_backup_timestamp is None:
- print(f'Backed up file: {filename}')
- else:
- print(f'Updating backed up file: {filename}')
- updated = True
- fully_updated = False # if a file is updated, all files are not up to date
-
- # check if any files were deleted in Colab and delete them from the backup drive
- for filepath in list(last_backup_timestamps.keys()):
- if not os.path.exists(filepath):
- backup_filepath = os.path.join(GOOGLE_DRIVE_PATH, os.path.relpath(filepath, LOGS_FOLDER))
- if os.path.exists(backup_filepath):
- os.remove(backup_filepath)
- print(f'Deleted file: {filepath}')
- del last_backup_timestamps[filepath]
- updated = True
- fully_updated = False # if a file is deleted, all files are not up to date
-
- if not updated and not fully_updated:
- print("Files are up to date.")
- fully_updated = True # if all files are up to date, set the boolean to True
- copy_weights_folder_to_drive()
- sleep_time = 15
- else:
- sleep_time = 0.1
-
- with open(last_backup_timestamps_path, 'w') as f:
- for filepath, timestamp in last_backup_timestamps.items():
- f.write(f'{filepath}:{timestamp}\n')
-
- time.sleep(sleep_time) # wait for 15 seconds before checking again, or 0.1s if not fully up to date to speed up backups
-
- except Exception as e:
- print(f"An error occurred: {str(e)}")
- # You can log the error or take appropriate actions here.
diff --git a/spaces/KarmKarma/genshinimpact-rvc-models-v2/lib/infer_pack/modules/F0Predictor/F0Predictor.py b/spaces/KarmKarma/genshinimpact-rvc-models-v2/lib/infer_pack/modules/F0Predictor/F0Predictor.py
deleted file mode 100644
index f56e49e7f0e6eab3babf0711cae2933371b9f9cc..0000000000000000000000000000000000000000
--- a/spaces/KarmKarma/genshinimpact-rvc-models-v2/lib/infer_pack/modules/F0Predictor/F0Predictor.py
+++ /dev/null
@@ -1,16 +0,0 @@
-class F0Predictor(object):
- def compute_f0(self, wav, p_len):
- """
- input: wav:[signal_length]
- p_len:int
- output: f0:[signal_length//hop_length]
- """
- pass
-
- def compute_f0_uv(self, wav, p_len):
- """
- input: wav:[signal_length]
- p_len:int
- output: f0:[signal_length//hop_length],uv:[signal_length//hop_length]
- """
- pass
diff --git a/spaces/KbL19/invokeAI/README.md b/spaces/KbL19/invokeAI/README.md
deleted file mode 100644
index 1c909c0308cf151e4a976a44b93da580957bba60..0000000000000000000000000000000000000000
--- a/spaces/KbL19/invokeAI/README.md
+++ /dev/null
@@ -1,10 +0,0 @@
----
-title: InvokeAI
-emoji: 🌖
-colorFrom: green
-colorTo: blue
-sdk: static
-pinned: false
----
-
-Check out the configuration reference at https://huggingface.co/docs/hub/spaces-config-reference
diff --git a/spaces/Kevin676/ChatGPT-with-Voice-Cloning-in-Chinese/encoder_preprocess.py b/spaces/Kevin676/ChatGPT-with-Voice-Cloning-in-Chinese/encoder_preprocess.py
deleted file mode 100644
index 853c6cb6c5cdda5c2e53ce3370d2570f2925f01a..0000000000000000000000000000000000000000
--- a/spaces/Kevin676/ChatGPT-with-Voice-Cloning-in-Chinese/encoder_preprocess.py
+++ /dev/null
@@ -1,61 +0,0 @@
-from encoder.preprocess import preprocess_librispeech, preprocess_voxceleb1, preprocess_voxceleb2, preprocess_aidatatang_200zh
-from utils.argutils import print_args
-from pathlib import Path
-import argparse
-
-if __name__ == "__main__":
- class MyFormatter(argparse.ArgumentDefaultsHelpFormatter, argparse.RawDescriptionHelpFormatter):
- pass
-
- parser = argparse.ArgumentParser(
- description="Preprocesses audio files from datasets, encodes them as mel spectrograms and "
- "writes them to the disk. This will allow you to train the encoder. The "
- "datasets required are at least one of LibriSpeech, VoxCeleb1, VoxCeleb2, aidatatang_200zh. ",
- formatter_class=MyFormatter
- )
- parser.add_argument("datasets_root", type=Path, help=\
- "Path to the directory containing your LibriSpeech/TTS and VoxCeleb datasets.")
- parser.add_argument("-o", "--out_dir", type=Path, default=argparse.SUPPRESS, help=\
- "Path to the output directory that will contain the mel spectrograms. If left out, "
- "defaults to /SV2TTS/encoder/")
- parser.add_argument("-d", "--datasets", type=str,
- default="librispeech_other,voxceleb1,aidatatang_200zh", help=\
- "Comma-separated list of the name of the datasets you want to preprocess. Only the train "
- "set of these datasets will be used. Possible names: librispeech_other, voxceleb1, "
- "voxceleb2.")
- parser.add_argument("-s", "--skip_existing", action="store_true", help=\
- "Whether to skip existing output files with the same name. Useful if this script was "
- "interrupted.")
- parser.add_argument("--no_trim", action="store_true", help=\
- "Preprocess audio without trimming silences (not recommended).")
- args = parser.parse_args()
-
- # Verify webrtcvad is available
- if not args.no_trim:
- try:
- import webrtcvad
- except:
- raise ModuleNotFoundError("Package 'webrtcvad' not found. This package enables "
- "noise removal and is recommended. Please install and try again. If installation fails, "
- "use --no_trim to disable this error message.")
- del args.no_trim
-
- # Process the arguments
- args.datasets = args.datasets.split(",")
- if not hasattr(args, "out_dir"):
- args.out_dir = args.datasets_root.joinpath("SV2TTS", "encoder")
- assert args.datasets_root.exists()
- args.out_dir.mkdir(exist_ok=True, parents=True)
-
- # Preprocess the datasets
- print_args(args, parser)
- preprocess_func = {
- "librispeech_other": preprocess_librispeech,
- "voxceleb1": preprocess_voxceleb1,
- "voxceleb2": preprocess_voxceleb2,
- "aidatatang_200zh": preprocess_aidatatang_200zh,
- }
- args = vars(args)
- for dataset in args.pop("datasets"):
- print("Preprocessing %s" % dataset)
- preprocess_func[dataset](**args)
diff --git a/spaces/Kevin676/ChatGPT-with-Voice-Cloning-in-Chinese/utils/profiler.py b/spaces/Kevin676/ChatGPT-with-Voice-Cloning-in-Chinese/utils/profiler.py
deleted file mode 100644
index 17175b9e1b0eb17fdc015199e5194a5c1afb8a28..0000000000000000000000000000000000000000
--- a/spaces/Kevin676/ChatGPT-with-Voice-Cloning-in-Chinese/utils/profiler.py
+++ /dev/null
@@ -1,45 +0,0 @@
-from time import perf_counter as timer
-from collections import OrderedDict
-import numpy as np
-
-
-class Profiler:
- def __init__(self, summarize_every=5, disabled=False):
- self.last_tick = timer()
- self.logs = OrderedDict()
- self.summarize_every = summarize_every
- self.disabled = disabled
-
- def tick(self, name):
- if self.disabled:
- return
-
- # Log the time needed to execute that function
- if not name in self.logs:
- self.logs[name] = []
- if len(self.logs[name]) >= self.summarize_every:
- self.summarize()
- self.purge_logs()
- self.logs[name].append(timer() - self.last_tick)
-
- self.reset_timer()
-
- def purge_logs(self):
- for name in self.logs:
- self.logs[name].clear()
-
- def reset_timer(self):
- self.last_tick = timer()
-
- def summarize(self):
- n = max(map(len, self.logs.values()))
- assert n == self.summarize_every
- print("\nAverage execution time over %d steps:" % n)
-
- name_msgs = ["%s (%d/%d):" % (name, len(deltas), n) for name, deltas in self.logs.items()]
- pad = max(map(len, name_msgs))
- for name_msg, deltas in zip(name_msgs, self.logs.values()):
- print(" %s mean: %4.0fms std: %4.0fms" %
- (name_msg.ljust(pad), np.mean(deltas) * 1000, np.std(deltas) * 1000))
- print("", flush=True)
-
\ No newline at end of file
diff --git a/spaces/Lamai/LAMAIGPT/autogpt/commands/web_playwright.py b/spaces/Lamai/LAMAIGPT/autogpt/commands/web_playwright.py
deleted file mode 100644
index 4e388ded203cefb5e24f9116f7fe5b8a94893413..0000000000000000000000000000000000000000
--- a/spaces/Lamai/LAMAIGPT/autogpt/commands/web_playwright.py
+++ /dev/null
@@ -1,80 +0,0 @@
-"""Web scraping commands using Playwright"""
-from __future__ import annotations
-
-try:
- from playwright.sync_api import sync_playwright
-except ImportError:
- print(
- "Playwright not installed. Please install it with 'pip install playwright' to use."
- )
-from bs4 import BeautifulSoup
-
-from autogpt.processing.html import extract_hyperlinks, format_hyperlinks
-
-
-def scrape_text(url: str) -> str:
- """Scrape text from a webpage
-
- Args:
- url (str): The URL to scrape text from
-
- Returns:
- str: The scraped text
- """
- with sync_playwright() as p:
- browser = p.chromium.launch()
- page = browser.new_page()
-
- try:
- page.goto(url)
- html_content = page.content()
- soup = BeautifulSoup(html_content, "html.parser")
-
- for script in soup(["script", "style"]):
- script.extract()
-
- text = soup.get_text()
- lines = (line.strip() for line in text.splitlines())
- chunks = (phrase.strip() for line in lines for phrase in line.split(" "))
- text = "\n".join(chunk for chunk in chunks if chunk)
-
- except Exception as e:
- text = f"Error: {str(e)}"
-
- finally:
- browser.close()
-
- return text
-
-
-def scrape_links(url: str) -> str | list[str]:
- """Scrape links from a webpage
-
- Args:
- url (str): The URL to scrape links from
-
- Returns:
- Union[str, List[str]]: The scraped links
- """
- with sync_playwright() as p:
- browser = p.chromium.launch()
- page = browser.new_page()
-
- try:
- page.goto(url)
- html_content = page.content()
- soup = BeautifulSoup(html_content, "html.parser")
-
- for script in soup(["script", "style"]):
- script.extract()
-
- hyperlinks = extract_hyperlinks(soup, url)
- formatted_links = format_hyperlinks(hyperlinks)
-
- except Exception as e:
- formatted_links = f"Error: {str(e)}"
-
- finally:
- browser.close()
-
- return formatted_links
diff --git a/spaces/Lamai/LAMAIGPT/tests/integration/weaviate_memory_tests.py b/spaces/Lamai/LAMAIGPT/tests/integration/weaviate_memory_tests.py
deleted file mode 100644
index 015eab05484f485aeb8ee035e92ad7811e9dddd4..0000000000000000000000000000000000000000
--- a/spaces/Lamai/LAMAIGPT/tests/integration/weaviate_memory_tests.py
+++ /dev/null
@@ -1,117 +0,0 @@
-import os
-import sys
-import unittest
-from unittest import mock
-from uuid import uuid4
-
-from weaviate import Client
-from weaviate.util import get_valid_uuid
-
-from autogpt.config import Config
-from autogpt.memory.base import get_ada_embedding
-from autogpt.memory.weaviate import WeaviateMemory
-
-
-class TestWeaviateMemory(unittest.TestCase):
- cfg = None
- client = None
- index = None
-
- @classmethod
- def setUpClass(cls):
- # only create the connection to weaviate once
- cls.cfg = Config()
-
- if cls.cfg.use_weaviate_embedded:
- from weaviate.embedded import EmbeddedOptions
-
- cls.client = Client(
- embedded_options=EmbeddedOptions(
- hostname=cls.cfg.weaviate_host,
- port=int(cls.cfg.weaviate_port),
- persistence_data_path=cls.cfg.weaviate_embedded_path,
- )
- )
- else:
- cls.client = Client(
- f"{cls.cfg.weaviate_protocol}://{cls.cfg.weaviate_host}:{self.cfg.weaviate_port}"
- )
-
- cls.index = WeaviateMemory.format_classname(cls.cfg.memory_index)
-
- """
- In order to run these tests you will need a local instance of
- Weaviate running. Refer to https://weaviate.io/developers/weaviate/installation/docker-compose
- for creating local instances using docker.
- Alternatively in your .env file set the following environmental variables to run Weaviate embedded (see: https://weaviate.io/developers/weaviate/installation/embedded):
-
- USE_WEAVIATE_EMBEDDED=True
- WEAVIATE_EMBEDDED_PATH="/home/me/.local/share/weaviate"
- """
-
- def setUp(self):
- try:
- self.client.schema.delete_class(self.index)
- except:
- pass
-
- self.memory = WeaviateMemory(self.cfg)
-
- def test_add(self):
- doc = "You are a Titan name Thanos and you are looking for the Infinity Stones"
- self.memory.add(doc)
- result = self.client.query.get(self.index, ["raw_text"]).do()
- actual = result["data"]["Get"][self.index]
-
- self.assertEqual(len(actual), 1)
- self.assertEqual(actual[0]["raw_text"], doc)
-
- def test_get(self):
- doc = "You are an Avenger and swore to defend the Galaxy from a menace called Thanos"
-
- with self.client.batch as batch:
- batch.add_data_object(
- uuid=get_valid_uuid(uuid4()),
- data_object={"raw_text": doc},
- class_name=self.index,
- vector=get_ada_embedding(doc),
- )
-
- batch.flush()
-
- actual = self.memory.get(doc)
-
- self.assertEqual(len(actual), 1)
- self.assertEqual(actual[0], doc)
-
- def test_get_stats(self):
- docs = [
- "You are now about to count the number of docs in this index",
- "And then you about to find out if you can count correctly",
- ]
-
- [self.memory.add(doc) for doc in docs]
-
- stats = self.memory.get_stats()
-
- self.assertTrue(stats)
- self.assertTrue("count" in stats)
- self.assertEqual(stats["count"], 2)
-
- def test_clear(self):
- docs = [
- "Shame this is the last test for this class",
- "Testing is fun when someone else is doing it",
- ]
-
- [self.memory.add(doc) for doc in docs]
-
- self.assertEqual(self.memory.get_stats()["count"], 2)
-
- self.memory.clear()
-
- self.assertEqual(self.memory.get_stats()["count"], 0)
-
-
-if __name__ == "__main__":
- unittest.main()
diff --git a/spaces/LaynzKunz/Aesthetic_RVC_Inference_HF/lib/infer/infer_pack/commons.py b/spaces/LaynzKunz/Aesthetic_RVC_Inference_HF/lib/infer/infer_pack/commons.py
deleted file mode 100644
index 2618e3ad501d1d4745a34024c2bf1676546fae80..0000000000000000000000000000000000000000
--- a/spaces/LaynzKunz/Aesthetic_RVC_Inference_HF/lib/infer/infer_pack/commons.py
+++ /dev/null
@@ -1,164 +0,0 @@
-import math
-import torch
-from torch.nn import functional as F
-
-
-def init_weights(m, mean=0.0, std=0.01):
- classname = m.__class__.__name__
- if classname.find("Conv") != -1:
- m.weight.data.normal_(mean, std)
-
-
-def get_padding(kernel_size, dilation=1):
- return int((kernel_size * dilation - dilation) / 2)
-
-
-def convert_pad_shape(pad_shape):
- l = pad_shape[::-1]
- pad_shape = [item for sublist in l for item in sublist]
- return pad_shape
-
-
-def kl_divergence(m_p, logs_p, m_q, logs_q):
- """KL(P||Q)"""
- kl = (logs_q - logs_p) - 0.5
- kl += (
- 0.5 * (torch.exp(2.0 * logs_p) + ((m_p - m_q) ** 2)) * torch.exp(-2.0 * logs_q)
- )
- return kl
-
-
-def rand_gumbel(shape):
- """Sample from the Gumbel distribution, protect from overflows."""
- uniform_samples = torch.rand(shape) * 0.99998 + 0.00001
- return -torch.log(-torch.log(uniform_samples))
-
-
-def rand_gumbel_like(x):
- g = rand_gumbel(x.size()).to(dtype=x.dtype, device=x.device)
- return g
-
-
-def slice_segments(x, ids_str, segment_size=4):
- ret = torch.zeros_like(x[:, :, :segment_size])
- for i in range(x.size(0)):
- idx_str = ids_str[i]
- idx_end = idx_str + segment_size
- ret[i] = x[i, :, idx_str:idx_end]
- return ret
-
-
-def slice_segments2(x, ids_str, segment_size=4):
- ret = torch.zeros_like(x[:, :segment_size])
- for i in range(x.size(0)):
- idx_str = ids_str[i]
- idx_end = idx_str + segment_size
- ret[i] = x[i, idx_str:idx_end]
- return ret
-
-
-def rand_slice_segments(x, x_lengths=None, segment_size=4):
- b, d, t = x.size()
- if x_lengths is None:
- x_lengths = t
- ids_str_max = x_lengths - segment_size + 1
- ids_str = (torch.rand([b]).to(device=x.device) * ids_str_max).to(dtype=torch.long)
- ret = slice_segments(x, ids_str, segment_size)
- return ret, ids_str
-
-
-def get_timing_signal_1d(length, channels, min_timescale=1.0, max_timescale=1.0e4):
- position = torch.arange(length, dtype=torch.float)
- num_timescales = channels // 2
- log_timescale_increment = math.log(float(max_timescale) / float(min_timescale)) / (
- num_timescales - 1
- )
- inv_timescales = min_timescale * torch.exp(
- torch.arange(num_timescales, dtype=torch.float) * -log_timescale_increment
- )
- scaled_time = position.unsqueeze(0) * inv_timescales.unsqueeze(1)
- signal = torch.cat([torch.sin(scaled_time), torch.cos(scaled_time)], 0)
- signal = F.pad(signal, [0, 0, 0, channels % 2])
- signal = signal.view(1, channels, length)
- return signal
-
-
-def add_timing_signal_1d(x, min_timescale=1.0, max_timescale=1.0e4):
- b, channels, length = x.size()
- signal = get_timing_signal_1d(length, channels, min_timescale, max_timescale)
- return x + signal.to(dtype=x.dtype, device=x.device)
-
-
-def cat_timing_signal_1d(x, min_timescale=1.0, max_timescale=1.0e4, axis=1):
- b, channels, length = x.size()
- signal = get_timing_signal_1d(length, channels, min_timescale, max_timescale)
- return torch.cat([x, signal.to(dtype=x.dtype, device=x.device)], axis)
-
-
-def subsequent_mask(length):
- mask = torch.tril(torch.ones(length, length)).unsqueeze(0).unsqueeze(0)
- return mask
-
-
-@torch.jit.script
-def fused_add_tanh_sigmoid_multiply(input_a, input_b, n_channels):
- n_channels_int = n_channels[0]
- in_act = input_a + input_b
- t_act = torch.tanh(in_act[:, :n_channels_int, :])
- s_act = torch.sigmoid(in_act[:, n_channels_int:, :])
- acts = t_act * s_act
- return acts
-
-
-def convert_pad_shape(pad_shape):
- l = pad_shape[::-1]
- pad_shape = [item for sublist in l for item in sublist]
- return pad_shape
-
-
-def shift_1d(x):
- x = F.pad(x, convert_pad_shape([[0, 0], [0, 0], [1, 0]]))[:, :, :-1]
- return x
-
-
-def sequence_mask(length, max_length=None):
- if max_length is None:
- max_length = length.max()
- x = torch.arange(max_length, dtype=length.dtype, device=length.device)
- return x.unsqueeze(0) < length.unsqueeze(1)
-
-
-def generate_path(duration, mask):
- """
- duration: [b, 1, t_x]
- mask: [b, 1, t_y, t_x]
- """
- device = duration.device
-
- b, _, t_y, t_x = mask.shape
- cum_duration = torch.cumsum(duration, -1)
-
- cum_duration_flat = cum_duration.view(b * t_x)
- path = sequence_mask(cum_duration_flat, t_y).to(mask.dtype)
- path = path.view(b, t_x, t_y)
- path = path - F.pad(path, convert_pad_shape([[0, 0], [1, 0], [0, 0]]))[:, :-1]
- path = path.unsqueeze(1).transpose(2, 3) * mask
- return path
-
-
-def clip_grad_value_(parameters, clip_value, norm_type=2):
- if isinstance(parameters, torch.Tensor):
- parameters = [parameters]
- parameters = list(filter(lambda p: p.grad is not None, parameters))
- norm_type = float(norm_type)
- if clip_value is not None:
- clip_value = float(clip_value)
-
- total_norm = 0
- for p in parameters:
- param_norm = p.grad.data.norm(norm_type)
- total_norm += param_norm.item() ** norm_type
- if clip_value is not None:
- p.grad.data.clamp_(min=-clip_value, max=clip_value)
- total_norm = total_norm ** (1.0 / norm_type)
- return total_norm
diff --git a/spaces/LaynzKunz/Aesthetic_RVC_Inference_HF/lib/infer/infer_pack/models_onnx.py b/spaces/LaynzKunz/Aesthetic_RVC_Inference_HF/lib/infer/infer_pack/models_onnx.py
deleted file mode 100644
index e370d3736219568247a20a1ddf2f450b087bd329..0000000000000000000000000000000000000000
--- a/spaces/LaynzKunz/Aesthetic_RVC_Inference_HF/lib/infer/infer_pack/models_onnx.py
+++ /dev/null
@@ -1,817 +0,0 @@
-import math
-import torch
-from torch import nn
-from torch.nn import functional as F
-from lib.infer.infer_pack import modules
-from lib.infer.infer_pack import attentions
-from lib.infer.infer_pack.commons import get_padding
-from torch.nn import Conv1d, ConvTranspose1d, Conv2d
-from torch.nn.utils import weight_norm, remove_weight_norm, spectral_norm
-from lib.infer.infer_pack.commons import init_weights
-import numpy as np
-from lib.infer.infer_pack import commons
-
-
-class TextEncoder256(nn.Module):
- def __init__(
- self,
- out_channels,
- hidden_channels,
- filter_channels,
- n_heads,
- n_layers,
- kernel_size,
- p_dropout,
- f0=True,
- ):
- super().__init__()
- self.out_channels = out_channels
- self.hidden_channels = hidden_channels
- self.filter_channels = filter_channels
- self.n_heads = n_heads
- self.n_layers = n_layers
- self.kernel_size = kernel_size
- self.p_dropout = p_dropout
- self.emb_phone = nn.Linear(256, hidden_channels)
- self.lrelu = nn.LeakyReLU(0.1, inplace=True)
- if f0 == True:
- self.emb_pitch = nn.Embedding(256, hidden_channels) # pitch 256
- self.encoder = attentions.Encoder(
- hidden_channels, filter_channels, n_heads, n_layers, kernel_size, p_dropout
- )
- self.proj = nn.Conv1d(hidden_channels, out_channels * 2, 1)
-
- def forward(self, phone, pitch, lengths):
- if pitch == None:
- x = self.emb_phone(phone)
- else:
- x = self.emb_phone(phone) + self.emb_pitch(pitch)
- x = x * math.sqrt(self.hidden_channels) # [b, t, h]
- x = self.lrelu(x)
- x = torch.transpose(x, 1, -1) # [b, h, t]
- x_mask = torch.unsqueeze(commons.sequence_mask(lengths, x.size(2)), 1).to(
- x.dtype
- )
- x = self.encoder(x * x_mask, x_mask)
- stats = self.proj(x) * x_mask
-
- m, logs = torch.split(stats, self.out_channels, dim=1)
- return m, logs, x_mask
-
-
-class TextEncoder768(nn.Module):
- def __init__(
- self,
- out_channels,
- hidden_channels,
- filter_channels,
- n_heads,
- n_layers,
- kernel_size,
- p_dropout,
- f0=True,
- ):
- super().__init__()
- self.out_channels = out_channels
- self.hidden_channels = hidden_channels
- self.filter_channels = filter_channels
- self.n_heads = n_heads
- self.n_layers = n_layers
- self.kernel_size = kernel_size
- self.p_dropout = p_dropout
- self.emb_phone = nn.Linear(768, hidden_channels)
- self.lrelu = nn.LeakyReLU(0.1, inplace=True)
- if f0 == True:
- self.emb_pitch = nn.Embedding(256, hidden_channels) # pitch 256
- self.encoder = attentions.Encoder(
- hidden_channels, filter_channels, n_heads, n_layers, kernel_size, p_dropout
- )
- self.proj = nn.Conv1d(hidden_channels, out_channels * 2, 1)
-
- def forward(self, phone, pitch, lengths):
- if pitch == None:
- x = self.emb_phone(phone)
- else:
- x = self.emb_phone(phone) + self.emb_pitch(pitch)
- x = x * math.sqrt(self.hidden_channels) # [b, t, h]
- x = self.lrelu(x)
- x = torch.transpose(x, 1, -1) # [b, h, t]
- x_mask = torch.unsqueeze(commons.sequence_mask(lengths, x.size(2)), 1).to(
- x.dtype
- )
- x = self.encoder(x * x_mask, x_mask)
- stats = self.proj(x) * x_mask
-
- m, logs = torch.split(stats, self.out_channels, dim=1)
- return m, logs, x_mask
-
-
-class ResidualCouplingBlock(nn.Module):
- def __init__(
- self,
- channels,
- hidden_channels,
- kernel_size,
- dilation_rate,
- n_layers,
- n_flows=4,
- gin_channels=0,
- ):
- super().__init__()
- self.channels = channels
- self.hidden_channels = hidden_channels
- self.kernel_size = kernel_size
- self.dilation_rate = dilation_rate
- self.n_layers = n_layers
- self.n_flows = n_flows
- self.gin_channels = gin_channels
-
- self.flows = nn.ModuleList()
- for i in range(n_flows):
- self.flows.append(
- modules.ResidualCouplingLayer(
- channels,
- hidden_channels,
- kernel_size,
- dilation_rate,
- n_layers,
- gin_channels=gin_channels,
- mean_only=True,
- )
- )
- self.flows.append(modules.Flip())
-
- def forward(self, x, x_mask, g=None, reverse=False):
- if not reverse:
- for flow in self.flows:
- x, _ = flow(x, x_mask, g=g, reverse=reverse)
- else:
- for flow in reversed(self.flows):
- x = flow(x, x_mask, g=g, reverse=reverse)
- return x
-
- def remove_weight_norm(self):
- for i in range(self.n_flows):
- self.flows[i * 2].remove_weight_norm()
-
-
-class PosteriorEncoder(nn.Module):
- def __init__(
- self,
- in_channels,
- out_channels,
- hidden_channels,
- kernel_size,
- dilation_rate,
- n_layers,
- gin_channels=0,
- ):
- super().__init__()
- self.in_channels = in_channels
- self.out_channels = out_channels
- self.hidden_channels = hidden_channels
- self.kernel_size = kernel_size
- self.dilation_rate = dilation_rate
- self.n_layers = n_layers
- self.gin_channels = gin_channels
-
- self.pre = nn.Conv1d(in_channels, hidden_channels, 1)
- self.enc = modules.WN(
- hidden_channels,
- kernel_size,
- dilation_rate,
- n_layers,
- gin_channels=gin_channels,
- )
- self.proj = nn.Conv1d(hidden_channels, out_channels * 2, 1)
-
- def forward(self, x, x_lengths, g=None):
- x_mask = torch.unsqueeze(commons.sequence_mask(x_lengths, x.size(2)), 1).to(
- x.dtype
- )
- x = self.pre(x) * x_mask
- x = self.enc(x, x_mask, g=g)
- stats = self.proj(x) * x_mask
- m, logs = torch.split(stats, self.out_channels, dim=1)
- z = (m + torch.randn_like(m) * torch.exp(logs)) * x_mask
- return z, m, logs, x_mask
-
- def remove_weight_norm(self):
- self.enc.remove_weight_norm()
-
-
-class Generator(torch.nn.Module):
- def __init__(
- self,
- initial_channel,
- resblock,
- resblock_kernel_sizes,
- resblock_dilation_sizes,
- upsample_rates,
- upsample_initial_channel,
- upsample_kernel_sizes,
- gin_channels=0,
- ):
- super(Generator, self).__init__()
- self.num_kernels = len(resblock_kernel_sizes)
- self.num_upsamples = len(upsample_rates)
- self.conv_pre = Conv1d(
- initial_channel, upsample_initial_channel, 7, 1, padding=3
- )
- resblock = modules.ResBlock1 if resblock == "1" else modules.ResBlock2
-
- self.ups = nn.ModuleList()
- for i, (u, k) in enumerate(zip(upsample_rates, upsample_kernel_sizes)):
- self.ups.append(
- weight_norm(
- ConvTranspose1d(
- upsample_initial_channel // (2**i),
- upsample_initial_channel // (2 ** (i + 1)),
- k,
- u,
- padding=(k - u) // 2,
- )
- )
- )
-
- self.resblocks = nn.ModuleList()
- for i in range(len(self.ups)):
- ch = upsample_initial_channel // (2 ** (i + 1))
- for j, (k, d) in enumerate(
- zip(resblock_kernel_sizes, resblock_dilation_sizes)
- ):
- self.resblocks.append(resblock(ch, k, d))
-
- self.conv_post = Conv1d(ch, 1, 7, 1, padding=3, bias=False)
- self.ups.apply(init_weights)
-
- if gin_channels != 0:
- self.cond = nn.Conv1d(gin_channels, upsample_initial_channel, 1)
-
- def forward(self, x, g=None):
- x = self.conv_pre(x)
- if g is not None:
- x = x + self.cond(g)
-
- for i in range(self.num_upsamples):
- x = F.leaky_relu(x, modules.LRELU_SLOPE)
- x = self.ups[i](x)
- xs = None
- for j in range(self.num_kernels):
- if xs is None:
- xs = self.resblocks[i * self.num_kernels + j](x)
- else:
- xs += self.resblocks[i * self.num_kernels + j](x)
- x = xs / self.num_kernels
- x = F.leaky_relu(x)
- x = self.conv_post(x)
- x = torch.tanh(x)
-
- return x
-
- def remove_weight_norm(self):
- for l in self.ups:
- remove_weight_norm(l)
- for l in self.resblocks:
- l.remove_weight_norm()
-
-
-class SineGen(torch.nn.Module):
- """Definition of sine generator
- SineGen(samp_rate, harmonic_num = 0,
- sine_amp = 0.1, noise_std = 0.003,
- voiced_threshold = 0,
- flag_for_pulse=False)
- samp_rate: sampling rate in Hz
- harmonic_num: number of harmonic overtones (default 0)
- sine_amp: amplitude of sine-wavefrom (default 0.1)
- noise_std: std of Gaussian noise (default 0.003)
- voiced_thoreshold: F0 threshold for U/V classification (default 0)
- flag_for_pulse: this SinGen is used inside PulseGen (default False)
- Note: when flag_for_pulse is True, the first time step of a voiced
- segment is always sin(np.pi) or cos(0)
- """
-
- def __init__(
- self,
- samp_rate,
- harmonic_num=0,
- sine_amp=0.1,
- noise_std=0.003,
- voiced_threshold=0,
- flag_for_pulse=False,
- ):
- super(SineGen, self).__init__()
- self.sine_amp = sine_amp
- self.noise_std = noise_std
- self.harmonic_num = harmonic_num
- self.dim = self.harmonic_num + 1
- self.sampling_rate = samp_rate
- self.voiced_threshold = voiced_threshold
-
- def _f02uv(self, f0):
- # generate uv signal
- uv = torch.ones_like(f0)
- uv = uv * (f0 > self.voiced_threshold)
- return uv
-
- def forward(self, f0, upp):
- """sine_tensor, uv = forward(f0)
- input F0: tensor(batchsize=1, length, dim=1)
- f0 for unvoiced steps should be 0
- output sine_tensor: tensor(batchsize=1, length, dim)
- output uv: tensor(batchsize=1, length, 1)
- """
- with torch.no_grad():
- f0 = f0[:, None].transpose(1, 2)
- f0_buf = torch.zeros(f0.shape[0], f0.shape[1], self.dim, device=f0.device)
- # fundamental component
- f0_buf[:, :, 0] = f0[:, :, 0]
- for idx in np.arange(self.harmonic_num):
- f0_buf[:, :, idx + 1] = f0_buf[:, :, 0] * (
- idx + 2
- ) # idx + 2: the (idx+1)-th overtone, (idx+2)-th harmonic
- rad_values = (f0_buf / self.sampling_rate) % 1 ###%1意味着n_har的乘积无法后处理优化
- rand_ini = torch.rand(
- f0_buf.shape[0], f0_buf.shape[2], device=f0_buf.device
- )
- rand_ini[:, 0] = 0
- rad_values[:, 0, :] = rad_values[:, 0, :] + rand_ini
- tmp_over_one = torch.cumsum(rad_values, 1) # % 1 #####%1意味着后面的cumsum无法再优化
- tmp_over_one *= upp
- tmp_over_one = F.interpolate(
- tmp_over_one.transpose(2, 1),
- scale_factor=upp,
- mode="linear",
- align_corners=True,
- ).transpose(2, 1)
- rad_values = F.interpolate(
- rad_values.transpose(2, 1), scale_factor=upp, mode="nearest"
- ).transpose(
- 2, 1
- ) #######
- tmp_over_one %= 1
- tmp_over_one_idx = (tmp_over_one[:, 1:, :] - tmp_over_one[:, :-1, :]) < 0
- cumsum_shift = torch.zeros_like(rad_values)
- cumsum_shift[:, 1:, :] = tmp_over_one_idx * -1.0
- sine_waves = torch.sin(
- torch.cumsum(rad_values + cumsum_shift, dim=1) * 2 * np.pi
- )
- sine_waves = sine_waves * self.sine_amp
- uv = self._f02uv(f0)
- uv = F.interpolate(
- uv.transpose(2, 1), scale_factor=upp, mode="nearest"
- ).transpose(2, 1)
- noise_amp = uv * self.noise_std + (1 - uv) * self.sine_amp / 3
- noise = noise_amp * torch.randn_like(sine_waves)
- sine_waves = sine_waves * uv + noise
- return sine_waves, uv, noise
-
-
-class SourceModuleHnNSF(torch.nn.Module):
- """SourceModule for hn-nsf
- SourceModule(sampling_rate, harmonic_num=0, sine_amp=0.1,
- add_noise_std=0.003, voiced_threshod=0)
- sampling_rate: sampling_rate in Hz
- harmonic_num: number of harmonic above F0 (default: 0)
- sine_amp: amplitude of sine source signal (default: 0.1)
- add_noise_std: std of additive Gaussian noise (default: 0.003)
- note that amplitude of noise in unvoiced is decided
- by sine_amp
- voiced_threshold: threhold to set U/V given F0 (default: 0)
- Sine_source, noise_source = SourceModuleHnNSF(F0_sampled)
- F0_sampled (batchsize, length, 1)
- Sine_source (batchsize, length, 1)
- noise_source (batchsize, length 1)
- uv (batchsize, length, 1)
- """
-
- def __init__(
- self,
- sampling_rate,
- harmonic_num=0,
- sine_amp=0.1,
- add_noise_std=0.003,
- voiced_threshod=0,
- is_half=True,
- ):
- super(SourceModuleHnNSF, self).__init__()
-
- self.sine_amp = sine_amp
- self.noise_std = add_noise_std
- self.is_half = is_half
- # to produce sine waveforms
- self.l_sin_gen = SineGen(
- sampling_rate, harmonic_num, sine_amp, add_noise_std, voiced_threshod
- )
-
- # to merge source harmonics into a single excitation
- self.l_linear = torch.nn.Linear(harmonic_num + 1, 1)
- self.l_tanh = torch.nn.Tanh()
-
- def forward(self, x, upp=None):
- sine_wavs, uv, _ = self.l_sin_gen(x, upp)
- if self.is_half:
- sine_wavs = sine_wavs.half()
- sine_merge = self.l_tanh(self.l_linear(sine_wavs))
- return sine_merge, None, None # noise, uv
-
-
-class GeneratorNSF(torch.nn.Module):
- def __init__(
- self,
- initial_channel,
- resblock,
- resblock_kernel_sizes,
- resblock_dilation_sizes,
- upsample_rates,
- upsample_initial_channel,
- upsample_kernel_sizes,
- gin_channels,
- sr,
- is_half=False,
- ):
- super(GeneratorNSF, self).__init__()
- self.num_kernels = len(resblock_kernel_sizes)
- self.num_upsamples = len(upsample_rates)
-
- self.f0_upsamp = torch.nn.Upsample(scale_factor=np.prod(upsample_rates))
- self.m_source = SourceModuleHnNSF(
- sampling_rate=sr, harmonic_num=0, is_half=is_half
- )
- self.noise_convs = nn.ModuleList()
- self.conv_pre = Conv1d(
- initial_channel, upsample_initial_channel, 7, 1, padding=3
- )
- resblock = modules.ResBlock1 if resblock == "1" else modules.ResBlock2
-
- self.ups = nn.ModuleList()
- for i, (u, k) in enumerate(zip(upsample_rates, upsample_kernel_sizes)):
- c_cur = upsample_initial_channel // (2 ** (i + 1))
- self.ups.append(
- weight_norm(
- ConvTranspose1d(
- upsample_initial_channel // (2**i),
- upsample_initial_channel // (2 ** (i + 1)),
- k,
- u,
- padding=(k - u) // 2,
- )
- )
- )
- if i + 1 < len(upsample_rates):
- stride_f0 = np.prod(upsample_rates[i + 1 :])
- self.noise_convs.append(
- Conv1d(
- 1,
- c_cur,
- kernel_size=stride_f0 * 2,
- stride=stride_f0,
- padding=stride_f0 // 2,
- )
- )
- else:
- self.noise_convs.append(Conv1d(1, c_cur, kernel_size=1))
-
- self.resblocks = nn.ModuleList()
- for i in range(len(self.ups)):
- ch = upsample_initial_channel // (2 ** (i + 1))
- for j, (k, d) in enumerate(
- zip(resblock_kernel_sizes, resblock_dilation_sizes)
- ):
- self.resblocks.append(resblock(ch, k, d))
-
- self.conv_post = Conv1d(ch, 1, 7, 1, padding=3, bias=False)
- self.ups.apply(init_weights)
-
- if gin_channels != 0:
- self.cond = nn.Conv1d(gin_channels, upsample_initial_channel, 1)
-
- self.upp = np.prod(upsample_rates)
-
- def forward(self, x, f0, g=None):
- har_source, noi_source, uv = self.m_source(f0, self.upp)
- har_source = har_source.transpose(1, 2)
- x = self.conv_pre(x)
- if g is not None:
- x = x + self.cond(g)
-
- for i in range(self.num_upsamples):
- x = F.leaky_relu(x, modules.LRELU_SLOPE)
- x = self.ups[i](x)
- x_source = self.noise_convs[i](har_source)
- x = x + x_source
- xs = None
- for j in range(self.num_kernels):
- if xs is None:
- xs = self.resblocks[i * self.num_kernels + j](x)
- else:
- xs += self.resblocks[i * self.num_kernels + j](x)
- x = xs / self.num_kernels
- x = F.leaky_relu(x)
- x = self.conv_post(x)
- x = torch.tanh(x)
- return x
-
- def remove_weight_norm(self):
- for l in self.ups:
- remove_weight_norm(l)
- for l in self.resblocks:
- l.remove_weight_norm()
-
-
-sr2sr = {
- "32k": 32000,
- "40k": 40000,
- "48k": 48000,
-}
-
-
-class SynthesizerTrnMsNSFsidM(nn.Module):
- def __init__(
- self,
- spec_channels,
- segment_size,
- inter_channels,
- hidden_channels,
- filter_channels,
- n_heads,
- n_layers,
- kernel_size,
- p_dropout,
- resblock,
- resblock_kernel_sizes,
- resblock_dilation_sizes,
- upsample_rates,
- upsample_initial_channel,
- upsample_kernel_sizes,
- spk_embed_dim,
- gin_channels,
- sr,
- version,
- **kwargs
- ):
- super().__init__()
- if type(sr) == type("strr"):
- sr = sr2sr[sr]
- self.spec_channels = spec_channels
- self.inter_channels = inter_channels
- self.hidden_channels = hidden_channels
- self.filter_channels = filter_channels
- self.n_heads = n_heads
- self.n_layers = n_layers
- self.kernel_size = kernel_size
- self.p_dropout = p_dropout
- self.resblock = resblock
- self.resblock_kernel_sizes = resblock_kernel_sizes
- self.resblock_dilation_sizes = resblock_dilation_sizes
- self.upsample_rates = upsample_rates
- self.upsample_initial_channel = upsample_initial_channel
- self.upsample_kernel_sizes = upsample_kernel_sizes
- self.segment_size = segment_size
- self.gin_channels = gin_channels
- # self.hop_length = hop_length#
- self.spk_embed_dim = spk_embed_dim
- if version == "v1":
- self.enc_p = TextEncoder256(
- inter_channels,
- hidden_channels,
- filter_channels,
- n_heads,
- n_layers,
- kernel_size,
- p_dropout,
- )
- else:
- self.enc_p = TextEncoder768(
- inter_channels,
- hidden_channels,
- filter_channels,
- n_heads,
- n_layers,
- kernel_size,
- p_dropout,
- )
- self.dec = GeneratorNSF(
- inter_channels,
- resblock,
- resblock_kernel_sizes,
- resblock_dilation_sizes,
- upsample_rates,
- upsample_initial_channel,
- upsample_kernel_sizes,
- gin_channels=gin_channels,
- sr=sr,
- is_half=kwargs["is_half"],
- )
- self.enc_q = PosteriorEncoder(
- spec_channels,
- inter_channels,
- hidden_channels,
- 5,
- 1,
- 16,
- gin_channels=gin_channels,
- )
- self.flow = ResidualCouplingBlock(
- inter_channels, hidden_channels, 5, 1, 3, gin_channels=gin_channels
- )
- self.emb_g = nn.Embedding(self.spk_embed_dim, gin_channels)
- self.speaker_map = None
- print("gin_channels:", gin_channels, "self.spk_embed_dim:", self.spk_embed_dim)
-
- def remove_weight_norm(self):
- self.dec.remove_weight_norm()
- self.flow.remove_weight_norm()
- self.enc_q.remove_weight_norm()
-
- def construct_spkmixmap(self, n_speaker):
- self.speaker_map = torch.zeros((n_speaker, 1, 1, self.gin_channels))
- for i in range(n_speaker):
- self.speaker_map[i] = self.emb_g(torch.LongTensor([[i]]))
- self.speaker_map = self.speaker_map.unsqueeze(0)
-
- def forward(self, phone, phone_lengths, pitch, nsff0, g, rnd, max_len=None):
- if self.speaker_map is not None: # [N, S] * [S, B, 1, H]
- g = g.reshape((g.shape[0], g.shape[1], 1, 1, 1)) # [N, S, B, 1, 1]
- g = g * self.speaker_map # [N, S, B, 1, H]
- g = torch.sum(g, dim=1) # [N, 1, B, 1, H]
- g = g.transpose(0, -1).transpose(0, -2).squeeze(0) # [B, H, N]
- else:
- g = g.unsqueeze(0)
- g = self.emb_g(g).transpose(1, 2)
-
- m_p, logs_p, x_mask = self.enc_p(phone, pitch, phone_lengths)
- z_p = (m_p + torch.exp(logs_p) * rnd) * x_mask
- z = self.flow(z_p, x_mask, g=g, reverse=True)
- o = self.dec((z * x_mask)[:, :, :max_len], nsff0, g=g)
- return o
-
-
-class MultiPeriodDiscriminator(torch.nn.Module):
- def __init__(self, use_spectral_norm=False):
- super(MultiPeriodDiscriminator, self).__init__()
- periods = [2, 3, 5, 7, 11, 17]
- # periods = [3, 5, 7, 11, 17, 23, 37]
-
- discs = [DiscriminatorS(use_spectral_norm=use_spectral_norm)]
- discs = discs + [
- DiscriminatorP(i, use_spectral_norm=use_spectral_norm) for i in periods
- ]
- self.discriminators = nn.ModuleList(discs)
-
- def forward(self, y, y_hat):
- y_d_rs = [] #
- y_d_gs = []
- fmap_rs = []
- fmap_gs = []
- for i, d in enumerate(self.discriminators):
- y_d_r, fmap_r = d(y)
- y_d_g, fmap_g = d(y_hat)
- # for j in range(len(fmap_r)):
- # print(i,j,y.shape,y_hat.shape,fmap_r[j].shape,fmap_g[j].shape)
- y_d_rs.append(y_d_r)
- y_d_gs.append(y_d_g)
- fmap_rs.append(fmap_r)
- fmap_gs.append(fmap_g)
-
- return y_d_rs, y_d_gs, fmap_rs, fmap_gs
-
-
-class MultiPeriodDiscriminatorV2(torch.nn.Module):
- def __init__(self, use_spectral_norm=False):
- super(MultiPeriodDiscriminatorV2, self).__init__()
- # periods = [2, 3, 5, 7, 11, 17]
- periods = [2, 3, 5, 7, 11, 17, 23, 37]
-
- discs = [DiscriminatorS(use_spectral_norm=use_spectral_norm)]
- discs = discs + [
- DiscriminatorP(i, use_spectral_norm=use_spectral_norm) for i in periods
- ]
- self.discriminators = nn.ModuleList(discs)
-
- def forward(self, y, y_hat):
- y_d_rs = [] #
- y_d_gs = []
- fmap_rs = []
- fmap_gs = []
- for i, d in enumerate(self.discriminators):
- y_d_r, fmap_r = d(y)
- y_d_g, fmap_g = d(y_hat)
- # for j in range(len(fmap_r)):
- # print(i,j,y.shape,y_hat.shape,fmap_r[j].shape,fmap_g[j].shape)
- y_d_rs.append(y_d_r)
- y_d_gs.append(y_d_g)
- fmap_rs.append(fmap_r)
- fmap_gs.append(fmap_g)
-
- return y_d_rs, y_d_gs, fmap_rs, fmap_gs
-
-
-class DiscriminatorS(torch.nn.Module):
- def __init__(self, use_spectral_norm=False):
- super(DiscriminatorS, self).__init__()
- norm_f = weight_norm if use_spectral_norm == False else spectral_norm
- self.convs = nn.ModuleList(
- [
- norm_f(Conv1d(1, 16, 15, 1, padding=7)),
- norm_f(Conv1d(16, 64, 41, 4, groups=4, padding=20)),
- norm_f(Conv1d(64, 256, 41, 4, groups=16, padding=20)),
- norm_f(Conv1d(256, 1024, 41, 4, groups=64, padding=20)),
- norm_f(Conv1d(1024, 1024, 41, 4, groups=256, padding=20)),
- norm_f(Conv1d(1024, 1024, 5, 1, padding=2)),
- ]
- )
- self.conv_post = norm_f(Conv1d(1024, 1, 3, 1, padding=1))
-
- def forward(self, x):
- fmap = []
-
- for l in self.convs:
- x = l(x)
- x = F.leaky_relu(x, modules.LRELU_SLOPE)
- fmap.append(x)
- x = self.conv_post(x)
- fmap.append(x)
- x = torch.flatten(x, 1, -1)
-
- return x, fmap
-
-
-class DiscriminatorP(torch.nn.Module):
- def __init__(self, period, kernel_size=5, stride=3, use_spectral_norm=False):
- super(DiscriminatorP, self).__init__()
- self.period = period
- self.use_spectral_norm = use_spectral_norm
- norm_f = weight_norm if use_spectral_norm == False else spectral_norm
- self.convs = nn.ModuleList(
- [
- norm_f(
- Conv2d(
- 1,
- 32,
- (kernel_size, 1),
- (stride, 1),
- padding=(get_padding(kernel_size, 1), 0),
- )
- ),
- norm_f(
- Conv2d(
- 32,
- 128,
- (kernel_size, 1),
- (stride, 1),
- padding=(get_padding(kernel_size, 1), 0),
- )
- ),
- norm_f(
- Conv2d(
- 128,
- 512,
- (kernel_size, 1),
- (stride, 1),
- padding=(get_padding(kernel_size, 1), 0),
- )
- ),
- norm_f(
- Conv2d(
- 512,
- 1024,
- (kernel_size, 1),
- (stride, 1),
- padding=(get_padding(kernel_size, 1), 0),
- )
- ),
- norm_f(
- Conv2d(
- 1024,
- 1024,
- (kernel_size, 1),
- 1,
- padding=(get_padding(kernel_size, 1), 0),
- )
- ),
- ]
- )
- self.conv_post = norm_f(Conv2d(1024, 1, (3, 1), 1, padding=(1, 0)))
-
- def forward(self, x):
- fmap = []
-
- # 1d to 2d
- b, c, t = x.shape
- if t % self.period != 0: # pad first
- n_pad = self.period - (t % self.period)
- x = F.pad(x, (0, n_pad), "reflect")
- t = t + n_pad
- x = x.view(b, c, t // self.period, self.period)
-
- for l in self.convs:
- x = l(x)
- x = F.leaky_relu(x, modules.LRELU_SLOPE)
- fmap.append(x)
- x = self.conv_post(x)
- fmap.append(x)
- x = torch.flatten(x, 1, -1)
-
- return x, fmap
diff --git a/spaces/MISATO-dataset/Adaptability_protein_dynamics/transforms.py b/spaces/MISATO-dataset/Adaptability_protein_dynamics/transforms.py
deleted file mode 100644
index a37886d0a217cbd5d0543b66b17fc9a7ea388601..0000000000000000000000000000000000000000
--- a/spaces/MISATO-dataset/Adaptability_protein_dynamics/transforms.py
+++ /dev/null
@@ -1,46 +0,0 @@
-import torch
-from torch_geometric.data import Data
-from graph import prot_df_to_graph, mol_df_to_graph_for_qm
-
-def prot_graph_transform(item, atom_keys, label_key, edge_dist_cutoff):
- """Transform for converting dataframes to Pytorch Geometric graphs, to be applied when defining a :mod:`Dataset `.
- Operates on Dataset items, assumes that the item contains all keys specified in ``keys`` and ``labels`` arguments.
-
- :param item: Dataset item to transform
- :type item: dict
- :param atom_keys: list of keys to transform, where each key contains a dataframe of atoms, defaults to ['atoms']
- :type atom_keys: list, optional
- :param label_key: name of key containing labels, defaults to ['scores']
- :type label_key: str, optional
- :return: Transformed Dataset item
- :rtype: dict
- """
-
- for key in atom_keys:
- node_feats, edge_index, edge_feats, pos = prot_df_to_graph(item, item[key], edge_dist_cutoff)
- item[key] = Data(node_feats, edge_index, edge_feats, y=torch.FloatTensor(item[label_key]), pos=pos, ids=item["id"])
-
- return item
-
-def mol_graph_transform_for_qm(item, atom_key, label_key, allowable_atoms, use_bonds, onehot_edges, edge_dist_cutoff):
- """Transform for converting dataframes to Pytorch Geometric graphs, to be applied when defining a :mod:`Dataset `.
- Operates on Dataset items, assumes that the item contains all keys specified in ``keys`` and ``labels`` arguments.
-
- :param item: Dataset item to transform
- :type item: dict
- :param atom_key: name of key containing molecule structure as a dataframe, defaults to 'atoms'
- :type atom_keys: list, optional
- :param label_key: name of key containing labels, defaults to 'scores'
- :type label_key: str, optional
- :param use_bonds: whether to use molecular bond information for edges instead of distance. Assumes bonds are stored under 'bonds' key, defaults to False
- :type use_bonds: bool, optional
- :return: Transformed Dataset item
- :rtype: dict
- """
-
- bonds = item['bonds'] if use_bonds else None
-
- node_feats, edge_index, edge_feats, pos = mol_df_to_graph_for_qm(item[atom_key], bonds=bonds, onehot_edges=onehot_edges, allowable_atoms=allowable_atoms, edge_dist_cutoff=edge_dist_cutoff)
- item[atom_key] = Data(node_feats, edge_index, edge_feats, y=item[label_key], pos=pos)
-
- return item
diff --git a/spaces/Mark3347/AlpinaB12/README.md b/spaces/Mark3347/AlpinaB12/README.md
deleted file mode 100644
index 073dd74e99bdb0d9e180275f84e15f42830df250..0000000000000000000000000000000000000000
--- a/spaces/Mark3347/AlpinaB12/README.md
+++ /dev/null
@@ -1,168 +0,0 @@
----
-title: LabelStudio
-emoji: 🟧
-colorFrom: yellow
-colorTo: purple
-sdk: docker
-tags:
-- label-studio
-fullwidth: true
-license: apache-2.0
-app_port: 8080
----
-
-
-[Website](https://hubs.ly/Q01CNgsd0) • [Docs](https://hubs.ly/Q01CN9Yq0) • [12K+ GitHub ⭐️!](https://hubs.ly/Q01CNbPQ0) • [Slack Community](https://hubs.ly/Q01CNb9H0)
-
-## What is Label Studio?
-
-Label Studio is an open source data labeling platform. It lets you label audio,
-text, images, videos, and time series data with a simple, straightforward, and
-highly-configurable user interface. Label Studio can prepare new data or
-improve existing training data to get more accurate ML models.
-
-
-## Label Studio in Hugging Face Spaces
-
-The Label Studio community is thrilled to offer Label Studio as a Hugging Face
-Spaces application. You can try the data-annotation interface, connect popular
-machine learning models, and share the application with collaborators. You can
-start immediately by creating an account or replicate the space and work in
-your own environment.
-
-## Creating a Use Account and Logging In
-
-Begin by creating a new account in the Label Studio space, then log in with your
-credentials.
-
-**By default, these spaces permit anyone to create a new login
-account, allowing them to view and modify project configuration, data sets, and
-annotations. Without any modifications, treat this space like a demo environment.**
-
-## Creating a Labeling Project
-
-After logging in, Label Studio will present you with a project view. Here you
-can create a new project with prompts to upload data and set up a custom
-configuration interface.
-
-**Note that in the default configuration, storage is local and temporary. Any
-projects, annotations, and configurations will be lost if the space is restarted.**
-
-## Next Steps and Additional Resources
-
-To help with getting started, the Label Studio community curated a list of
-resources including tutorials and documentation.
-
-- 🚀 [Zero to One with Label Studio Tutorial](https://labelstud.io/blog/introduction-to-label-studio-in-hugging-face-spaces/)
-- 📈 [Try Label Studio Enterprise](https://hubs.ly/Q01CMLll0)
-- 🤗 [Tutorial: Using Label Studio with Hugging Face Datasets Hub](https://danielvanstrien.xyz/huggingface/huggingface-datasets/annotation/full%20stack%20deep%20learning%20notes/2022/09/07/label-studio-annotations-hub.html)
-- 💡 [Label Studio Docs](https://hubs.ly/Q01CN9Yq0)
-
-
-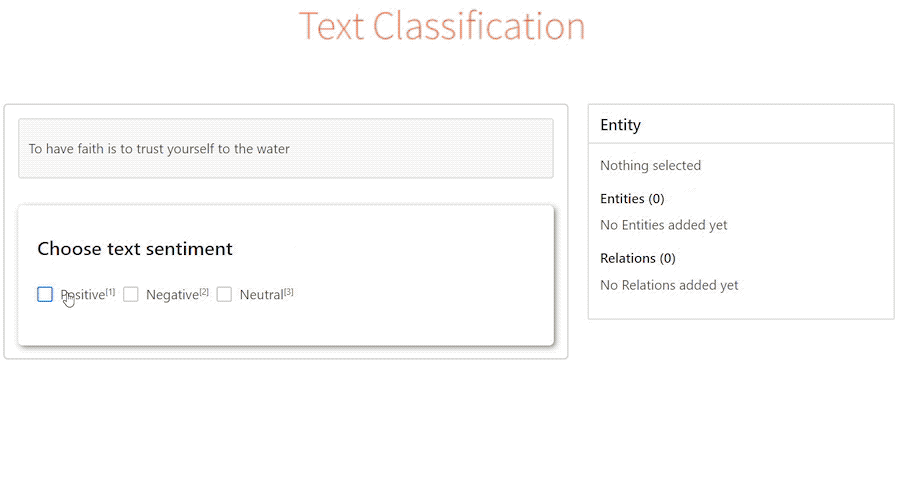
-
-### Making your Label Studio Hugging Face Space production-ready
-
-By default this space allows for the unrestricted creation of new accounts
-will full access to all projects and data. This is great for trying out
-Label Studio and collaborating on projects, but you may want to restrict
-access to your space to only authorized users. Add the following environment
-variable to your spaces Dockerfile to disable public account creation for
-this space.
-
- ENV LABEL_STUDIO_DISABLE_SIGNUP_WITHOUT_LINK=true
-
-Set secrets in your space to create an inital user, and log in with your
-provided username and password. Do not set these in your Dockerfile, as they
-globally visible on a public space.
-
- LABEL_STUDIO_USERNAME
- LABEL_STUDIO_PASSWORD
-
-You will need to provide new users with an invitation link to join the space,
-which can be found in the Organizations interface of Label Studio.
-
-By default this space stores all project configuration and data annotations
-in local storage with Sqlite. If the space is reset, all configuration and
-annotation data in the space will be lost. You can enable configuration
-persistence in one of two ways:
-
-1. Enabling Persistent Storage in your Space settings and configuring Label
- Studio to write its database and task storage there.
-
-2. Connecting an external Postgres database and cloud storage to your space,
- guaranteeing that all project and annotation settings are preserved.
-
-### Enabling Hugging Face Persistent Storage
-
-In the Hugging Face Label Studio Space settings, select the appropriate
-Persistent Storage tier. Note that Persistent Storage is a paid add-on.
-By default, persistent storage is mounted to /data. In your Space settings,
-set the following variables:
-
- LABEL_STUDIO_BASE_DATA_DIR=/data
- ENV STORAGE_PERSISTENCE=1
-
-Your space will restart. NOTE: if you have existing settings and data,
-they will be lost in this first restart. Data and setting will only be
-preserved on subsequent restarts of the space.
-
-### Enabling Postgres Database and Cloud Storage
-
-Set the following secret variables to match your own hosted instance of
-Postgres. We strongly recommend setting these as secrets to prevent leaking
-information about your database service to the public in your spaces
-definition.
-
- DJANGO_DB=default
- POSTGRE_NAME=
- POSTGRE_PORT=
- POSTGRE_USER=
- POSTGRE_PASSWORD=
- POSTGRE_PORT=
- POSTGRE_HOST=
-
-Add the following environment variable to remove the warning about ephemeral
-storage.
-
- ENV STORAGE_PERSISTENCE=1
-
-Note that you will need to connect cloud storage to host data items that you
-want to annotate, as local storage will not be preserved across a space reset.
-
-By default the only data storage enabled for this space is local. In the case
-of a space reset, all data will be lost. To enable permanent storage, you
-must enable a cloud storage connector. We also strongly recommend enabling
-configuration persistence to preserve project data, annotations, and user
-settings. Choose the appropriate cloud connector and configure the secrets
-for it.
-
-#### Amazon S3
- STORAGE_TYPE=s3
- STORAGE_AWS_ACCESS_KEY_ID=""
- STORAGE_AWS_SECRET_ACCESS_KEY=""
- STORAGE_AWS_BUCKET_NAME=""
- STORAGE_AWS_REGION_NAME=""
- STORAGE_AWS_FOLDER=""
-
-#### Google Cloud Storage
-
- STORAGE_TYPE=gcs
- STORAGE_GCS_BUCKET_NAME=""
- STORAGE_GCS_PROJECT_ID=""
- STORAGE_GCS_FOLDER=""
- GOOGLE_APPLICATION_CREDENTIALS="/opt/heartex/secrets/key.json"
-
-Azure Blob Storage
-==================
-
- STORAGE_TYPE=azure
- STORAGE_AZURE_ACCOUNT_NAME=""
- STORAGE_AZURE_ACCOUNT_KEY=""
- STORAGE_AZURE_CONTAINER_NAME=""
- STORAGE_AZURE_FOLDER=""
-
-
-## Questions? Concerns? Want to get involved?
-
-Email the community team at [community@labelstud.io](mailto:community@labelstud.io)
diff --git a/spaces/Mashir0/pximg/middleware/favicon.js b/spaces/Mashir0/pximg/middleware/favicon.js
deleted file mode 100644
index 45b09983dc30ed7424261c00ecb0fa0731e36061..0000000000000000000000000000000000000000
--- a/spaces/Mashir0/pximg/middleware/favicon.js
+++ /dev/null
@@ -1,21 +0,0 @@
-const { get } = require('axios').default;
-const { pixivHeaders } = require('../utils/pixiv');
-
-/**
- * @type {import('koa-router').IMiddleware}
- */
-module.exports = async ctx => {
- try {
- const { data, status, headers } = await get('https://www.pixiv.net/favicon.ico', {
- headers: pixivHeaders,
- responseType: 'stream',
- });
-
- ctx.body = data;
- ctx.status = status;
- ctx.set('cache-control', 'max-age=604800');
- ['content-length', 'content-type', 'last-modified'].forEach(k => headers[k] && ctx.set(k, headers[k]));
- } catch (error) {
- ctx.status = 502;
- }
-};
diff --git a/spaces/MashiroSA/sovits-emu-voice-transform/modules/attentions.py b/spaces/MashiroSA/sovits-emu-voice-transform/modules/attentions.py
deleted file mode 100644
index f9c11ca4a3acb86bf1abc04d9dcfa82a4ed4061f..0000000000000000000000000000000000000000
--- a/spaces/MashiroSA/sovits-emu-voice-transform/modules/attentions.py
+++ /dev/null
@@ -1,349 +0,0 @@
-import copy
-import math
-import numpy as np
-import torch
-from torch import nn
-from torch.nn import functional as F
-
-import modules.commons as commons
-import modules.modules as modules
-from modules.modules import LayerNorm
-
-
-class FFT(nn.Module):
- def __init__(self, hidden_channels, filter_channels, n_heads, n_layers=1, kernel_size=1, p_dropout=0.,
- proximal_bias=False, proximal_init=True, **kwargs):
- super().__init__()
- self.hidden_channels = hidden_channels
- self.filter_channels = filter_channels
- self.n_heads = n_heads
- self.n_layers = n_layers
- self.kernel_size = kernel_size
- self.p_dropout = p_dropout
- self.proximal_bias = proximal_bias
- self.proximal_init = proximal_init
-
- self.drop = nn.Dropout(p_dropout)
- self.self_attn_layers = nn.ModuleList()
- self.norm_layers_0 = nn.ModuleList()
- self.ffn_layers = nn.ModuleList()
- self.norm_layers_1 = nn.ModuleList()
- for i in range(self.n_layers):
- self.self_attn_layers.append(
- MultiHeadAttention(hidden_channels, hidden_channels, n_heads, p_dropout=p_dropout, proximal_bias=proximal_bias,
- proximal_init=proximal_init))
- self.norm_layers_0.append(LayerNorm(hidden_channels))
- self.ffn_layers.append(
- FFN(hidden_channels, hidden_channels, filter_channels, kernel_size, p_dropout=p_dropout, causal=True))
- self.norm_layers_1.append(LayerNorm(hidden_channels))
-
- def forward(self, x, x_mask):
- """
- x: decoder input
- h: encoder output
- """
- self_attn_mask = commons.subsequent_mask(x_mask.size(2)).to(device=x.device, dtype=x.dtype)
- x = x * x_mask
- for i in range(self.n_layers):
- y = self.self_attn_layers[i](x, x, self_attn_mask)
- y = self.drop(y)
- x = self.norm_layers_0[i](x + y)
-
- y = self.ffn_layers[i](x, x_mask)
- y = self.drop(y)
- x = self.norm_layers_1[i](x + y)
- x = x * x_mask
- return x
-
-
-class Encoder(nn.Module):
- def __init__(self, hidden_channels, filter_channels, n_heads, n_layers, kernel_size=1, p_dropout=0., window_size=4, **kwargs):
- super().__init__()
- self.hidden_channels = hidden_channels
- self.filter_channels = filter_channels
- self.n_heads = n_heads
- self.n_layers = n_layers
- self.kernel_size = kernel_size
- self.p_dropout = p_dropout
- self.window_size = window_size
-
- self.drop = nn.Dropout(p_dropout)
- self.attn_layers = nn.ModuleList()
- self.norm_layers_1 = nn.ModuleList()
- self.ffn_layers = nn.ModuleList()
- self.norm_layers_2 = nn.ModuleList()
- for i in range(self.n_layers):
- self.attn_layers.append(MultiHeadAttention(hidden_channels, hidden_channels, n_heads, p_dropout=p_dropout, window_size=window_size))
- self.norm_layers_1.append(LayerNorm(hidden_channels))
- self.ffn_layers.append(FFN(hidden_channels, hidden_channels, filter_channels, kernel_size, p_dropout=p_dropout))
- self.norm_layers_2.append(LayerNorm(hidden_channels))
-
- def forward(self, x, x_mask):
- attn_mask = x_mask.unsqueeze(2) * x_mask.unsqueeze(-1)
- x = x * x_mask
- for i in range(self.n_layers):
- y = self.attn_layers[i](x, x, attn_mask)
- y = self.drop(y)
- x = self.norm_layers_1[i](x + y)
-
- y = self.ffn_layers[i](x, x_mask)
- y = self.drop(y)
- x = self.norm_layers_2[i](x + y)
- x = x * x_mask
- return x
-
-
-class Decoder(nn.Module):
- def __init__(self, hidden_channels, filter_channels, n_heads, n_layers, kernel_size=1, p_dropout=0., proximal_bias=False, proximal_init=True, **kwargs):
- super().__init__()
- self.hidden_channels = hidden_channels
- self.filter_channels = filter_channels
- self.n_heads = n_heads
- self.n_layers = n_layers
- self.kernel_size = kernel_size
- self.p_dropout = p_dropout
- self.proximal_bias = proximal_bias
- self.proximal_init = proximal_init
-
- self.drop = nn.Dropout(p_dropout)
- self.self_attn_layers = nn.ModuleList()
- self.norm_layers_0 = nn.ModuleList()
- self.encdec_attn_layers = nn.ModuleList()
- self.norm_layers_1 = nn.ModuleList()
- self.ffn_layers = nn.ModuleList()
- self.norm_layers_2 = nn.ModuleList()
- for i in range(self.n_layers):
- self.self_attn_layers.append(MultiHeadAttention(hidden_channels, hidden_channels, n_heads, p_dropout=p_dropout, proximal_bias=proximal_bias, proximal_init=proximal_init))
- self.norm_layers_0.append(LayerNorm(hidden_channels))
- self.encdec_attn_layers.append(MultiHeadAttention(hidden_channels, hidden_channels, n_heads, p_dropout=p_dropout))
- self.norm_layers_1.append(LayerNorm(hidden_channels))
- self.ffn_layers.append(FFN(hidden_channels, hidden_channels, filter_channels, kernel_size, p_dropout=p_dropout, causal=True))
- self.norm_layers_2.append(LayerNorm(hidden_channels))
-
- def forward(self, x, x_mask, h, h_mask):
- """
- x: decoder input
- h: encoder output
- """
- self_attn_mask = commons.subsequent_mask(x_mask.size(2)).to(device=x.device, dtype=x.dtype)
- encdec_attn_mask = h_mask.unsqueeze(2) * x_mask.unsqueeze(-1)
- x = x * x_mask
- for i in range(self.n_layers):
- y = self.self_attn_layers[i](x, x, self_attn_mask)
- y = self.drop(y)
- x = self.norm_layers_0[i](x + y)
-
- y = self.encdec_attn_layers[i](x, h, encdec_attn_mask)
- y = self.drop(y)
- x = self.norm_layers_1[i](x + y)
-
- y = self.ffn_layers[i](x, x_mask)
- y = self.drop(y)
- x = self.norm_layers_2[i](x + y)
- x = x * x_mask
- return x
-
-
-class MultiHeadAttention(nn.Module):
- def __init__(self, channels, out_channels, n_heads, p_dropout=0., window_size=None, heads_share=True, block_length=None, proximal_bias=False, proximal_init=False):
- super().__init__()
- assert channels % n_heads == 0
-
- self.channels = channels
- self.out_channels = out_channels
- self.n_heads = n_heads
- self.p_dropout = p_dropout
- self.window_size = window_size
- self.heads_share = heads_share
- self.block_length = block_length
- self.proximal_bias = proximal_bias
- self.proximal_init = proximal_init
- self.attn = None
-
- self.k_channels = channels // n_heads
- self.conv_q = nn.Conv1d(channels, channels, 1)
- self.conv_k = nn.Conv1d(channels, channels, 1)
- self.conv_v = nn.Conv1d(channels, channels, 1)
- self.conv_o = nn.Conv1d(channels, out_channels, 1)
- self.drop = nn.Dropout(p_dropout)
-
- if window_size is not None:
- n_heads_rel = 1 if heads_share else n_heads
- rel_stddev = self.k_channels**-0.5
- self.emb_rel_k = nn.Parameter(torch.randn(n_heads_rel, window_size * 2 + 1, self.k_channels) * rel_stddev)
- self.emb_rel_v = nn.Parameter(torch.randn(n_heads_rel, window_size * 2 + 1, self.k_channels) * rel_stddev)
-
- nn.init.xavier_uniform_(self.conv_q.weight)
- nn.init.xavier_uniform_(self.conv_k.weight)
- nn.init.xavier_uniform_(self.conv_v.weight)
- if proximal_init:
- with torch.no_grad():
- self.conv_k.weight.copy_(self.conv_q.weight)
- self.conv_k.bias.copy_(self.conv_q.bias)
-
- def forward(self, x, c, attn_mask=None):
- q = self.conv_q(x)
- k = self.conv_k(c)
- v = self.conv_v(c)
-
- x, self.attn = self.attention(q, k, v, mask=attn_mask)
-
- x = self.conv_o(x)
- return x
-
- def attention(self, query, key, value, mask=None):
- # reshape [b, d, t] -> [b, n_h, t, d_k]
- b, d, t_s, t_t = (*key.size(), query.size(2))
- query = query.view(b, self.n_heads, self.k_channels, t_t).transpose(2, 3)
- key = key.view(b, self.n_heads, self.k_channels, t_s).transpose(2, 3)
- value = value.view(b, self.n_heads, self.k_channels, t_s).transpose(2, 3)
-
- scores = torch.matmul(query / math.sqrt(self.k_channels), key.transpose(-2, -1))
- if self.window_size is not None:
- assert t_s == t_t, "Relative attention is only available for self-attention."
- key_relative_embeddings = self._get_relative_embeddings(self.emb_rel_k, t_s)
- rel_logits = self._matmul_with_relative_keys(query /math.sqrt(self.k_channels), key_relative_embeddings)
- scores_local = self._relative_position_to_absolute_position(rel_logits)
- scores = scores + scores_local
- if self.proximal_bias:
- assert t_s == t_t, "Proximal bias is only available for self-attention."
- scores = scores + self._attention_bias_proximal(t_s).to(device=scores.device, dtype=scores.dtype)
- if mask is not None:
- scores = scores.masked_fill(mask == 0, -1e4)
- if self.block_length is not None:
- assert t_s == t_t, "Local attention is only available for self-attention."
- block_mask = torch.ones_like(scores).triu(-self.block_length).tril(self.block_length)
- scores = scores.masked_fill(block_mask == 0, -1e4)
- p_attn = F.softmax(scores, dim=-1) # [b, n_h, t_t, t_s]
- p_attn = self.drop(p_attn)
- output = torch.matmul(p_attn, value)
- if self.window_size is not None:
- relative_weights = self._absolute_position_to_relative_position(p_attn)
- value_relative_embeddings = self._get_relative_embeddings(self.emb_rel_v, t_s)
- output = output + self._matmul_with_relative_values(relative_weights, value_relative_embeddings)
- output = output.transpose(2, 3).contiguous().view(b, d, t_t) # [b, n_h, t_t, d_k] -> [b, d, t_t]
- return output, p_attn
-
- def _matmul_with_relative_values(self, x, y):
- """
- x: [b, h, l, m]
- y: [h or 1, m, d]
- ret: [b, h, l, d]
- """
- ret = torch.matmul(x, y.unsqueeze(0))
- return ret
-
- def _matmul_with_relative_keys(self, x, y):
- """
- x: [b, h, l, d]
- y: [h or 1, m, d]
- ret: [b, h, l, m]
- """
- ret = torch.matmul(x, y.unsqueeze(0).transpose(-2, -1))
- return ret
-
- def _get_relative_embeddings(self, relative_embeddings, length):
- max_relative_position = 2 * self.window_size + 1
- # Pad first before slice to avoid using cond ops.
- pad_length = max(length - (self.window_size + 1), 0)
- slice_start_position = max((self.window_size + 1) - length, 0)
- slice_end_position = slice_start_position + 2 * length - 1
- if pad_length > 0:
- padded_relative_embeddings = F.pad(
- relative_embeddings,
- commons.convert_pad_shape([[0, 0], [pad_length, pad_length], [0, 0]]))
- else:
- padded_relative_embeddings = relative_embeddings
- used_relative_embeddings = padded_relative_embeddings[:,slice_start_position:slice_end_position]
- return used_relative_embeddings
-
- def _relative_position_to_absolute_position(self, x):
- """
- x: [b, h, l, 2*l-1]
- ret: [b, h, l, l]
- """
- batch, heads, length, _ = x.size()
- # Concat columns of pad to shift from relative to absolute indexing.
- x = F.pad(x, commons.convert_pad_shape([[0,0],[0,0],[0,0],[0,1]]))
-
- # Concat extra elements so to add up to shape (len+1, 2*len-1).
- x_flat = x.view([batch, heads, length * 2 * length])
- x_flat = F.pad(x_flat, commons.convert_pad_shape([[0,0],[0,0],[0,length-1]]))
-
- # Reshape and slice out the padded elements.
- x_final = x_flat.view([batch, heads, length+1, 2*length-1])[:, :, :length, length-1:]
- return x_final
-
- def _absolute_position_to_relative_position(self, x):
- """
- x: [b, h, l, l]
- ret: [b, h, l, 2*l-1]
- """
- batch, heads, length, _ = x.size()
- # padd along column
- x = F.pad(x, commons.convert_pad_shape([[0, 0], [0, 0], [0, 0], [0, length-1]]))
- x_flat = x.view([batch, heads, length**2 + length*(length -1)])
- # add 0's in the beginning that will skew the elements after reshape
- x_flat = F.pad(x_flat, commons.convert_pad_shape([[0, 0], [0, 0], [length, 0]]))
- x_final = x_flat.view([batch, heads, length, 2*length])[:,:,:,1:]
- return x_final
-
- def _attention_bias_proximal(self, length):
- """Bias for self-attention to encourage attention to close positions.
- Args:
- length: an integer scalar.
- Returns:
- a Tensor with shape [1, 1, length, length]
- """
- r = torch.arange(length, dtype=torch.float32)
- diff = torch.unsqueeze(r, 0) - torch.unsqueeze(r, 1)
- return torch.unsqueeze(torch.unsqueeze(-torch.log1p(torch.abs(diff)), 0), 0)
-
-
-class FFN(nn.Module):
- def __init__(self, in_channels, out_channels, filter_channels, kernel_size, p_dropout=0., activation=None, causal=False):
- super().__init__()
- self.in_channels = in_channels
- self.out_channels = out_channels
- self.filter_channels = filter_channels
- self.kernel_size = kernel_size
- self.p_dropout = p_dropout
- self.activation = activation
- self.causal = causal
-
- if causal:
- self.padding = self._causal_padding
- else:
- self.padding = self._same_padding
-
- self.conv_1 = nn.Conv1d(in_channels, filter_channels, kernel_size)
- self.conv_2 = nn.Conv1d(filter_channels, out_channels, kernel_size)
- self.drop = nn.Dropout(p_dropout)
-
- def forward(self, x, x_mask):
- x = self.conv_1(self.padding(x * x_mask))
- if self.activation == "gelu":
- x = x * torch.sigmoid(1.702 * x)
- else:
- x = torch.relu(x)
- x = self.drop(x)
- x = self.conv_2(self.padding(x * x_mask))
- return x * x_mask
-
- def _causal_padding(self, x):
- if self.kernel_size == 1:
- return x
- pad_l = self.kernel_size - 1
- pad_r = 0
- padding = [[0, 0], [0, 0], [pad_l, pad_r]]
- x = F.pad(x, commons.convert_pad_shape(padding))
- return x
-
- def _same_padding(self, x):
- if self.kernel_size == 1:
- return x
- pad_l = (self.kernel_size - 1) // 2
- pad_r = self.kernel_size // 2
- padding = [[0, 0], [0, 0], [pad_l, pad_r]]
- x = F.pad(x, commons.convert_pad_shape(padding))
- return x
diff --git a/spaces/Mellow-ai/PhotoAI_Mellow/annotator/uniformer/mmcv/ops/info.py b/spaces/Mellow-ai/PhotoAI_Mellow/annotator/uniformer/mmcv/ops/info.py
deleted file mode 100644
index 29f2e5598ae2bb5866ccd15a7d3b4de33c0cd14d..0000000000000000000000000000000000000000
--- a/spaces/Mellow-ai/PhotoAI_Mellow/annotator/uniformer/mmcv/ops/info.py
+++ /dev/null
@@ -1,36 +0,0 @@
-# Copyright (c) OpenMMLab. All rights reserved.
-import glob
-import os
-
-import torch
-
-if torch.__version__ == 'parrots':
- import parrots
-
- def get_compiler_version():
- return 'GCC ' + parrots.version.compiler
-
- def get_compiling_cuda_version():
- return parrots.version.cuda
-else:
- from ..utils import ext_loader
- ext_module = ext_loader.load_ext(
- '_ext', ['get_compiler_version', 'get_compiling_cuda_version'])
-
- def get_compiler_version():
- return ext_module.get_compiler_version()
-
- def get_compiling_cuda_version():
- return ext_module.get_compiling_cuda_version()
-
-
-def get_onnxruntime_op_path():
- wildcard = os.path.join(
- os.path.abspath(os.path.dirname(os.path.dirname(__file__))),
- '_ext_ort.*.so')
-
- paths = glob.glob(wildcard)
- if len(paths) > 0:
- return paths[0]
- else:
- return ''
diff --git a/spaces/Mellow-ai/PhotoAI_Mellow/annotator/uniformer/mmcv/parallel/distributed.py b/spaces/Mellow-ai/PhotoAI_Mellow/annotator/uniformer/mmcv/parallel/distributed.py
deleted file mode 100644
index 1e4c27903db58a54d37ea1ed9ec0104098b486f2..0000000000000000000000000000000000000000
--- a/spaces/Mellow-ai/PhotoAI_Mellow/annotator/uniformer/mmcv/parallel/distributed.py
+++ /dev/null
@@ -1,112 +0,0 @@
-# Copyright (c) OpenMMLab. All rights reserved.
-import torch
-from torch.nn.parallel.distributed import (DistributedDataParallel,
- _find_tensors)
-
-from annotator.uniformer.mmcv import print_log
-from annotator.uniformer.mmcv.utils import TORCH_VERSION, digit_version
-from .scatter_gather import scatter_kwargs
-
-
-class MMDistributedDataParallel(DistributedDataParallel):
- """The DDP module that supports DataContainer.
-
- MMDDP has two main differences with PyTorch DDP:
-
- - It supports a custom type :class:`DataContainer` which allows more
- flexible control of input data.
- - It implement two APIs ``train_step()`` and ``val_step()``.
- """
-
- def to_kwargs(self, inputs, kwargs, device_id):
- # Use `self.to_kwargs` instead of `self.scatter` in pytorch1.8
- # to move all tensors to device_id
- return scatter_kwargs(inputs, kwargs, [device_id], dim=self.dim)
-
- def scatter(self, inputs, kwargs, device_ids):
- return scatter_kwargs(inputs, kwargs, device_ids, dim=self.dim)
-
- def train_step(self, *inputs, **kwargs):
- """train_step() API for module wrapped by DistributedDataParallel.
-
- This method is basically the same as
- ``DistributedDataParallel.forward()``, while replacing
- ``self.module.forward()`` with ``self.module.train_step()``.
- It is compatible with PyTorch 1.1 - 1.5.
- """
-
- # In PyTorch >= 1.7, ``reducer._rebuild_buckets()`` is moved from the
- # end of backward to the beginning of forward.
- if ('parrots' not in TORCH_VERSION
- and digit_version(TORCH_VERSION) >= digit_version('1.7')
- and self.reducer._rebuild_buckets()):
- print_log(
- 'Reducer buckets have been rebuilt in this iteration.',
- logger='mmcv')
-
- if getattr(self, 'require_forward_param_sync', True):
- self._sync_params()
- if self.device_ids:
- inputs, kwargs = self.scatter(inputs, kwargs, self.device_ids)
- if len(self.device_ids) == 1:
- output = self.module.train_step(*inputs[0], **kwargs[0])
- else:
- outputs = self.parallel_apply(
- self._module_copies[:len(inputs)], inputs, kwargs)
- output = self.gather(outputs, self.output_device)
- else:
- output = self.module.train_step(*inputs, **kwargs)
-
- if torch.is_grad_enabled() and getattr(
- self, 'require_backward_grad_sync', True):
- if self.find_unused_parameters:
- self.reducer.prepare_for_backward(list(_find_tensors(output)))
- else:
- self.reducer.prepare_for_backward([])
- else:
- if ('parrots' not in TORCH_VERSION
- and digit_version(TORCH_VERSION) > digit_version('1.2')):
- self.require_forward_param_sync = False
- return output
-
- def val_step(self, *inputs, **kwargs):
- """val_step() API for module wrapped by DistributedDataParallel.
-
- This method is basically the same as
- ``DistributedDataParallel.forward()``, while replacing
- ``self.module.forward()`` with ``self.module.val_step()``.
- It is compatible with PyTorch 1.1 - 1.5.
- """
- # In PyTorch >= 1.7, ``reducer._rebuild_buckets()`` is moved from the
- # end of backward to the beginning of forward.
- if ('parrots' not in TORCH_VERSION
- and digit_version(TORCH_VERSION) >= digit_version('1.7')
- and self.reducer._rebuild_buckets()):
- print_log(
- 'Reducer buckets have been rebuilt in this iteration.',
- logger='mmcv')
-
- if getattr(self, 'require_forward_param_sync', True):
- self._sync_params()
- if self.device_ids:
- inputs, kwargs = self.scatter(inputs, kwargs, self.device_ids)
- if len(self.device_ids) == 1:
- output = self.module.val_step(*inputs[0], **kwargs[0])
- else:
- outputs = self.parallel_apply(
- self._module_copies[:len(inputs)], inputs, kwargs)
- output = self.gather(outputs, self.output_device)
- else:
- output = self.module.val_step(*inputs, **kwargs)
-
- if torch.is_grad_enabled() and getattr(
- self, 'require_backward_grad_sync', True):
- if self.find_unused_parameters:
- self.reducer.prepare_for_backward(list(_find_tensors(output)))
- else:
- self.reducer.prepare_for_backward([])
- else:
- if ('parrots' not in TORCH_VERSION
- and digit_version(TORCH_VERSION) > digit_version('1.2')):
- self.require_forward_param_sync = False
- return output
diff --git a/spaces/Mellow-ai/PhotoAI_Mellow/annotator/uniformer/mmseg/models/decode_heads/gc_head.py b/spaces/Mellow-ai/PhotoAI_Mellow/annotator/uniformer/mmseg/models/decode_heads/gc_head.py
deleted file mode 100644
index 70741245af975800840709911bd18d72247e3e04..0000000000000000000000000000000000000000
--- a/spaces/Mellow-ai/PhotoAI_Mellow/annotator/uniformer/mmseg/models/decode_heads/gc_head.py
+++ /dev/null
@@ -1,47 +0,0 @@
-import torch
-from annotator.uniformer.mmcv.cnn import ContextBlock
-
-from ..builder import HEADS
-from .fcn_head import FCNHead
-
-
-@HEADS.register_module()
-class GCHead(FCNHead):
- """GCNet: Non-local Networks Meet Squeeze-Excitation Networks and Beyond.
-
- This head is the implementation of `GCNet
- `_.
-
- Args:
- ratio (float): Multiplier of channels ratio. Default: 1/4.
- pooling_type (str): The pooling type of context aggregation.
- Options are 'att', 'avg'. Default: 'avg'.
- fusion_types (tuple[str]): The fusion type for feature fusion.
- Options are 'channel_add', 'channel_mul'. Default: ('channel_add',)
- """
-
- def __init__(self,
- ratio=1 / 4.,
- pooling_type='att',
- fusion_types=('channel_add', ),
- **kwargs):
- super(GCHead, self).__init__(num_convs=2, **kwargs)
- self.ratio = ratio
- self.pooling_type = pooling_type
- self.fusion_types = fusion_types
- self.gc_block = ContextBlock(
- in_channels=self.channels,
- ratio=self.ratio,
- pooling_type=self.pooling_type,
- fusion_types=self.fusion_types)
-
- def forward(self, inputs):
- """Forward function."""
- x = self._transform_inputs(inputs)
- output = self.convs[0](x)
- output = self.gc_block(output)
- output = self.convs[1](output)
- if self.concat_input:
- output = self.conv_cat(torch.cat([x, output], dim=1))
- output = self.cls_seg(output)
- return output
diff --git a/spaces/MelodyKwok/text_generator/README.md b/spaces/MelodyKwok/text_generator/README.md
deleted file mode 100644
index fab5731cb104834efb4eb268fa8a72e310b43710..0000000000000000000000000000000000000000
--- a/spaces/MelodyKwok/text_generator/README.md
+++ /dev/null
@@ -1,12 +0,0 @@
----
-title: Text Generator
-emoji: 🦀
-colorFrom: yellow
-colorTo: red
-sdk: gradio
-sdk_version: 3.18.0
-app_file: app.py
-pinned: false
----
-
-Check out the configuration reference at https://huggingface.co/docs/hub/spaces-config-reference
diff --git a/spaces/MiguelVGP/redfruits/README.md b/spaces/MiguelVGP/redfruits/README.md
deleted file mode 100644
index 3ba5f17652ca2a15ef2e7f0ecac86c2d96c085ca..0000000000000000000000000000000000000000
--- a/spaces/MiguelVGP/redfruits/README.md
+++ /dev/null
@@ -1,13 +0,0 @@
----
-title: Redfruits
-emoji: 👁
-colorFrom: yellow
-colorTo: yellow
-sdk: gradio
-sdk_version: 3.19.1
-app_file: app.py
-pinned: false
-license: apache-2.0
----
-
-Check out the configuration reference at https://huggingface.co/docs/hub/spaces-config-reference
diff --git a/spaces/Mountchicken/MAERec-Gradio/mmocr/models/textrecog/recognizers/encoder_decoder_recognizer.py b/spaces/Mountchicken/MAERec-Gradio/mmocr/models/textrecog/recognizers/encoder_decoder_recognizer.py
deleted file mode 100644
index 2696ac70ef3553e867d3be5a2a62b02923d3e3d3..0000000000000000000000000000000000000000
--- a/spaces/Mountchicken/MAERec-Gradio/mmocr/models/textrecog/recognizers/encoder_decoder_recognizer.py
+++ /dev/null
@@ -1,130 +0,0 @@
-# Copyright (c) OpenMMLab. All rights reserved.
-
-from typing import Dict
-
-import torch
-
-from mmocr.registry import MODELS
-from mmocr.utils.typing_utils import (ConfigType, InitConfigType,
- OptConfigType, OptRecSampleList,
- RecForwardResults, RecSampleList)
-from .base import BaseRecognizer
-
-
-@MODELS.register_module()
-class EncoderDecoderRecognizer(BaseRecognizer):
- """Base class for encode-decode recognizer.
-
- Args:
- preprocessor (dict, optional): Config dict for preprocessor. Defaults
- to None.
- backbone (dict, optional): Backbone config. Defaults to None.
- encoder (dict, optional): Encoder config. If None, the output from
- backbone will be directly fed into ``decoder``. Defaults to None.
- decoder (dict, optional): Decoder config. Defaults to None.
- data_preprocessor (dict, optional): Model preprocessing config
- for processing the input image data. Keys allowed are
- ``to_rgb``(bool), ``pad_size_divisor``(int), ``pad_value``(int or
- float), ``mean``(int or float) and ``std``(int or float).
- Preprcessing order: 1. to rgb; 2. normalization 3. pad.
- Defaults to None.
- init_cfg (dict or list[dict], optional): Initialization configs.
- Defaults to None.
- """
-
- def __init__(self,
- preprocessor: OptConfigType = None,
- backbone: OptConfigType = None,
- encoder: OptConfigType = None,
- decoder: OptConfigType = None,
- data_preprocessor: ConfigType = None,
- init_cfg: InitConfigType = None) -> None:
-
- super().__init__(
- init_cfg=init_cfg, data_preprocessor=data_preprocessor)
-
- # Preprocessor module, e.g., TPS
- if preprocessor is not None:
- self.preprocessor = MODELS.build(preprocessor)
-
- # Backbone
- if backbone is not None:
- self.backbone = MODELS.build(backbone)
-
- # Encoder module
- if encoder is not None:
- self.encoder = MODELS.build(encoder)
-
- # Decoder module
- assert decoder is not None
- self.decoder = MODELS.build(decoder)
-
- def extract_feat(self, inputs: torch.Tensor) -> torch.Tensor:
- """Directly extract features from the backbone."""
- if self.with_preprocessor:
- inputs = self.preprocessor(inputs)
- if self.with_backbone:
- inputs = self.backbone(inputs)
- return inputs
-
- def loss(self, inputs: torch.Tensor, data_samples: RecSampleList,
- **kwargs) -> Dict:
- """Calculate losses from a batch of inputs and data samples.
- Args:
- inputs (tensor): Input images of shape (N, C, H, W).
- Typically these should be mean centered and std scaled.
- data_samples (list[TextRecogDataSample]): A list of N
- datasamples, containing meta information and gold
- annotations for each of the images.
-
- Returns:
- dict[str, tensor]: A dictionary of loss components.
- """
- feat = self.extract_feat(inputs)
- out_enc = None
- if self.with_encoder:
- out_enc = self.encoder(feat, data_samples)
- return self.decoder.loss(feat, out_enc, data_samples)
-
- def predict(self, inputs: torch.Tensor, data_samples: RecSampleList,
- **kwargs) -> RecSampleList:
- """Predict results from a batch of inputs and data samples with post-
- processing.
-
- Args:
- inputs (torch.Tensor): Image input tensor.
- data_samples (list[TextRecogDataSample]): A list of N datasamples,
- containing meta information and gold annotations for each of
- the images.
-
- Returns:
- list[TextRecogDataSample]: A list of N datasamples of prediction
- results. Results are stored in ``pred_text``.
- """
- feat = self.extract_feat(inputs)
- out_enc = None
- if self.with_encoder:
- out_enc = self.encoder(feat, data_samples)
- return self.decoder.predict(feat, out_enc, data_samples)
-
- def _forward(self,
- inputs: torch.Tensor,
- data_samples: OptRecSampleList = None,
- **kwargs) -> RecForwardResults:
- """Network forward process. Usually includes backbone, encoder and
- decoder forward without any post-processing.
-
- Args:
- inputs (Tensor): Inputs with shape (N, C, H, W).
- data_samples (list[TextRecogDataSample]): A list of N
- datasamples, containing meta information and gold
- annotations for each of the images.
-
- Returns:
- Tensor: A tuple of features from ``decoder`` forward.
- """
- feat = self.extract_feat(inputs)
- out_enc = None
- if self.with_encoder:
- out_enc = self.encoder(feat, data_samples)
- return self.decoder(feat, out_enc, data_samples)
diff --git a/spaces/Mysterykey/test/README.md b/spaces/Mysterykey/test/README.md
deleted file mode 100644
index 3ee55ff1841aef2340943bf480c9c20851c88a6c..0000000000000000000000000000000000000000
--- a/spaces/Mysterykey/test/README.md
+++ /dev/null
@@ -1,9 +0,0 @@
----
-title: test
-emoji: 💻
-sdk: docker
-duplicated_from: null
-pinned: false
----
-
-Check out the configuration reference at https://huggingface.co/docs/hub/spaces-config-reference
\ No newline at end of file
diff --git a/spaces/NATSpeech/PortaSpeech/data_gen/tts/txt_processors/__init__.py b/spaces/NATSpeech/PortaSpeech/data_gen/tts/txt_processors/__init__.py
deleted file mode 100644
index 7bff3e9af7d634363116c6605f22a52aad614dea..0000000000000000000000000000000000000000
--- a/spaces/NATSpeech/PortaSpeech/data_gen/tts/txt_processors/__init__.py
+++ /dev/null
@@ -1 +0,0 @@
-from . import en
\ No newline at end of file
diff --git a/spaces/NATSpeech/PortaSpeech/utils/audio/rnnoise.py b/spaces/NATSpeech/PortaSpeech/utils/audio/rnnoise.py
deleted file mode 100644
index 47f4eb6471918ca8144f217580a71d1720cd8c36..0000000000000000000000000000000000000000
--- a/spaces/NATSpeech/PortaSpeech/utils/audio/rnnoise.py
+++ /dev/null
@@ -1,48 +0,0 @@
-# rnnoise.py, requirements: ffmpeg, sox, rnnoise, python
-import os
-import subprocess
-
-INSTALL_STR = """
-RNNoise library not found. Please install RNNoise (https://github.com/xiph/rnnoise) to $REPO/rnnoise:
-sudo apt-get install -y autoconf automake libtool ffmpeg sox
-git clone https://github.com/xiph/rnnoise.git
-rm -rf rnnoise/.git
-cd rnnoise
-./autogen.sh && ./configure && make
-cd ..
-"""
-
-
-def rnnoise(filename, out_fn=None, verbose=False, out_sample_rate=22050):
- assert os.path.exists('./rnnoise/examples/rnnoise_demo'), INSTALL_STR
- if out_fn is None:
- out_fn = f"{filename[:-4]}.denoised.wav"
- out_48k_fn = f"{out_fn}.48000.wav"
- tmp0_fn = f"{out_fn}.0.wav"
- tmp1_fn = f"{out_fn}.1.wav"
- tmp2_fn = f"{out_fn}.2.raw"
- tmp3_fn = f"{out_fn}.3.raw"
- if verbose:
- print("Pre-processing audio...") # wav to pcm raw
- subprocess.check_call(
- f'sox "{filename}" -G -r48000 "{tmp0_fn}"', shell=True, stdin=subprocess.PIPE) # convert to raw
- subprocess.check_call(
- f'sox -v 0.95 "{tmp0_fn}" "{tmp1_fn}"', shell=True, stdin=subprocess.PIPE) # convert to raw
- subprocess.check_call(
- f'ffmpeg -y -i "{tmp1_fn}" -loglevel quiet -f s16le -ac 1 -ar 48000 "{tmp2_fn}"',
- shell=True, stdin=subprocess.PIPE) # convert to raw
- if verbose:
- print("Applying rnnoise algorithm to audio...") # rnnoise
- subprocess.check_call(
- f'./rnnoise/examples/rnnoise_demo "{tmp2_fn}" "{tmp3_fn}"', shell=True)
-
- if verbose:
- print("Post-processing audio...") # pcm raw to wav
- if filename == out_fn:
- subprocess.check_call(f'rm -f "{out_fn}"', shell=True)
- subprocess.check_call(
- f'sox -t raw -r 48000 -b 16 -e signed-integer -c 1 "{tmp3_fn}" "{out_48k_fn}"', shell=True)
- subprocess.check_call(f'sox "{out_48k_fn}" -G -r{out_sample_rate} "{out_fn}"', shell=True)
- subprocess.check_call(f'rm -f "{tmp0_fn}" "{tmp1_fn}" "{tmp2_fn}" "{tmp3_fn}" "{out_48k_fn}"', shell=True)
- if verbose:
- print("Audio-filtering completed!")
diff --git a/spaces/NCTCMumbai/NCTC/models/official/modeling/optimization/configs/learning_rate_config.py b/spaces/NCTCMumbai/NCTC/models/official/modeling/optimization/configs/learning_rate_config.py
deleted file mode 100644
index b55c713f1905cf9aaa52f87a6663d3385628d5a5..0000000000000000000000000000000000000000
--- a/spaces/NCTCMumbai/NCTC/models/official/modeling/optimization/configs/learning_rate_config.py
+++ /dev/null
@@ -1,162 +0,0 @@
-# Lint as: python3
-# Copyright 2019 The TensorFlow Authors. All Rights Reserved.
-#
-# Licensed under the Apache License, Version 2.0 (the "License");
-# you may not use this file except in compliance with the License.
-# You may obtain a copy of the License at
-#
-# http://www.apache.org/licenses/LICENSE-2.0
-#
-# Unless required by applicable law or agreed to in writing, software
-# distributed under the License is distributed on an "AS IS" BASIS,
-# WITHOUT WARRANTIES OR CONDITIONS OF ANY KIND, either express or implied.
-# See the License for the specific language governing permissions and
-# limitations under the License.
-# ==============================================================================
-"""Dataclasses for learning rate schedule config."""
-from typing import List, Optional
-
-import dataclasses
-from official.modeling.hyperparams import base_config
-
-
-@dataclasses.dataclass
-class StepwiseLrConfig(base_config.Config):
- """Configuration for stepwise learning rate decay.
-
- This class is a container for the piecewise constant learning rate scheduling
- configs. It will configure an instance of PiecewiseConstantDecay keras
- learning rate schedule.
-
- An example (from keras docs): use a learning rate that's 1.0 for the first
- 100001 steps, 0.5 for the next 10000 steps, and 0.1 for any additional steps.
- ```python
- boundaries: [100000, 110000]
- values: [1.0, 0.5, 0.1]
-
- Attributes:
- name: The name of the learning rate schedule. Defaults to PiecewiseConstant.
- boundaries: A list of ints of strictly increasing entries.
- Defaults to None.
- values: A list of floats that specifies the values for the intervals defined
- by `boundaries`. It should have one more element than `boundaries`.
- The learning rate is computed as follows:
- [0, boundaries[0]] -> values[0]
- [boundaries[0], boundaries[1]] -> values[1]
- [boundaries[n-1], boundaries[n]] -> values[n]
- [boundaries[n], end] -> values[n+1]
- Defaults to None.
- """
- name: str = 'PiecewiseConstantDecay'
- boundaries: Optional[List[int]] = None
- values: Optional[List[float]] = None
-
-
-@dataclasses.dataclass
-class ExponentialLrConfig(base_config.Config):
- """Configuration for exponential learning rate decay.
-
- This class is a containers for the exponential learning rate decay configs.
-
- Attributes:
- name: The name of the learning rate schedule. Defaults to ExponentialDecay.
- initial_learning_rate: A float. The initial learning rate. Defaults to
- None.
- decay_steps: A positive integer that is used for decay computation.
- Defaults to None.
- decay_rate: A float. Defaults to None.
- staircase: A boolean, if true, learning rate is decreased at discreate
- intervals. Defaults to False.
- """
- name: str = 'ExponentialDecay'
- initial_learning_rate: Optional[float] = None
- decay_steps: Optional[int] = None
- decay_rate: Optional[float] = None
- staircase: Optional[bool] = None
-
-
-@dataclasses.dataclass
-class PolynomialLrConfig(base_config.Config):
- """Configuration for polynomial learning rate decay.
-
- This class is a containers for the polynomial learning rate decay configs.
-
- Attributes:
- name: The name of the learning rate schedule. Defaults to PolynomialDecay.
- initial_learning_rate: A float. The initial learning rate. Defaults to
- None.
- decay_steps: A positive integer that is used for decay computation.
- Defaults to None.
- end_learning_rate: A float. The minimal end learning rate.
- power: A float. The power of the polynomial. Defaults to linear, 1.0.
- cycle: A boolean, whether or not it should cycle beyond decay_steps.
- Defaults to False.
- """
- name: str = 'PolynomialDecay'
- initial_learning_rate: Optional[float] = None
- decay_steps: Optional[int] = None
- end_learning_rate: float = 0.0001
- power: float = 1.0
- cycle: bool = False
-
-
-@dataclasses.dataclass
-class CosineLrConfig(base_config.Config):
- """Configuration for Cosine learning rate decay.
-
- This class is a containers for the cosine learning rate decay configs,
- tf.keras.experimental.CosineDecay.
-
- Attributes:
- name: The name of the learning rate schedule. Defaults to CosineDecay.
- initial_learning_rate: A float. The initial learning rate. Defaults to
- None.
- decay_steps: A positive integer that is used for decay computation.
- Defaults to None.
- alpha: A float. Minimum learning rate value as a fraction of
- initial_learning_rate.
- """
- name: str = 'CosineDecay'
- initial_learning_rate: Optional[float] = None
- decay_steps: Optional[int] = None
- alpha: float = 0.0
-
-
-@dataclasses.dataclass
-class LinearWarmupConfig(base_config.Config):
- """Configuration for linear warmup schedule config.
-
- This class is a container for the linear warmup schedule configs.
- Warmup_learning_rate is the initial learning rate, the final learning rate of
- the warmup period is the learning_rate of the optimizer in use. The learning
- rate at each step linearly increased according to the following formula:
- warmup_learning_rate = warmup_learning_rate +
- step / warmup_steps * (final_learning_rate - warmup_learning_rate).
- Using warmup overrides the learning rate schedule by the number of warmup
- steps.
-
- Attributes:
- name: The name of warmup schedule. Defaults to linear.
- warmup_learning_rate: Initial learning rate for the warmup. Defaults to 0.
- warmup_steps: Warmup steps. Defaults to None.
- """
- name: str = 'linear'
- warmup_learning_rate: float = 0
- warmup_steps: Optional[int] = None
-
-
-@dataclasses.dataclass
-class PolynomialWarmupConfig(base_config.Config):
- """Configuration for linear warmup schedule config.
-
- This class is a container for the polynomial warmup schedule configs.
-
- Attributes:
- name: The name of warmup schedule. Defaults to Polynomial.
- power: Polynomial power. Defaults to 1.
- warmup_steps: Warmup steps. Defaults to None.
- """
- name: str = 'polynomial'
- power: float = 1
- warmup_steps: Optional[int] = None
-
diff --git a/spaces/NCTCMumbai/NCTC/models/official/modeling/training/__init__.py b/spaces/NCTCMumbai/NCTC/models/official/modeling/training/__init__.py
deleted file mode 100644
index e69de29bb2d1d6434b8b29ae775ad8c2e48c5391..0000000000000000000000000000000000000000
diff --git a/spaces/NCTCMumbai/NCTC/models/official/nlp/transformer/utils/metrics.py b/spaces/NCTCMumbai/NCTC/models/official/nlp/transformer/utils/metrics.py
deleted file mode 100644
index 7900cf807768f81af7a8afeee1f467074b04189f..0000000000000000000000000000000000000000
--- a/spaces/NCTCMumbai/NCTC/models/official/nlp/transformer/utils/metrics.py
+++ /dev/null
@@ -1,490 +0,0 @@
-# Copyright 2018 The TensorFlow Authors. All Rights Reserved.
-#
-# Licensed under the Apache License, Version 2.0 (the 'License');
-# you may not use this file except in compliance with the License.
-# You may obtain a copy of the License at
-#
-# http://www.apache.org/licenses/LICENSE-2.0
-#
-# Unless required by applicable law or agreed to in writing, software
-# distributed under the License is distributed on an 'AS IS' BASIS,
-# WITHOUT WARRANTIES OR CONDITIONS OF ANY KIND, either express or implied.
-# See the License for the specific language governing permissions and
-# limitations under the License.
-# ==============================================================================
-"""Functions for calculating loss, accuracy, and other model metrics.
-
-Metrics:
- - Padded loss, accuracy, and negative log perplexity. Source:
- https://github.com/tensorflow/tensor2tensor/blob/master/tensor2tensor/utils/metrics.py
- - BLEU approximation. Source:
- https://github.com/tensorflow/tensor2tensor/blob/master/tensor2tensor/utils/bleu_hook.py
- - ROUGE score. Source:
- https://github.com/tensorflow/tensor2tensor/blob/master/tensor2tensor/utils/rouge.py
-"""
-
-from __future__ import absolute_import
-from __future__ import division
-from __future__ import print_function
-
-import collections
-import math
-
-import numpy as np
-import six
-from six.moves import xrange # pylint: disable=redefined-builtin
-import tensorflow.compat.v1 as tf
-
-
-def _pad_tensors_to_same_length(x, y):
- """Pad x and y so that the results have the same length (second dimension)."""
- with tf.name_scope("pad_to_same_length"):
- x_length = tf.shape(x)[1]
- y_length = tf.shape(y)[1]
-
- max_length = tf.maximum(x_length, y_length)
-
- x = tf.pad(x, [[0, 0], [0, max_length - x_length], [0, 0]])
- y = tf.pad(y, [[0, 0], [0, max_length - y_length]])
- return x, y
-
-
-def padded_cross_entropy_loss(logits, labels, smoothing, vocab_size):
- """Calculate cross entropy loss while ignoring padding.
-
- Args:
- logits: Tensor of size [batch_size, length_logits, vocab_size]
- labels: Tensor of size [batch_size, length_labels]
- smoothing: Label smoothing constant, used to determine the on and off values
- vocab_size: int size of the vocabulary
- Returns:
- Returns the cross entropy loss and weight tensors: float32 tensors with
- shape [batch_size, max(length_logits, length_labels)]
- """
- with tf.name_scope("loss", values=[logits, labels]):
- logits, labels = _pad_tensors_to_same_length(logits, labels)
-
- # Calculate smoothing cross entropy
- with tf.name_scope("smoothing_cross_entropy", values=[logits, labels]):
- confidence = 1.0 - smoothing
- low_confidence = (1.0 - confidence) / tf.to_float(vocab_size - 1)
- soft_targets = tf.one_hot(
- tf.cast(labels, tf.int32),
- depth=vocab_size,
- on_value=confidence,
- off_value=low_confidence)
- xentropy = tf.nn.softmax_cross_entropy_with_logits_v2(
- logits=logits, labels=soft_targets)
-
- # Calculate the best (lowest) possible value of cross entropy, and
- # subtract from the cross entropy loss.
- normalizing_constant = -(
- confidence * tf.log(confidence) + tf.to_float(vocab_size - 1) *
- low_confidence * tf.log(low_confidence + 1e-20))
- xentropy -= normalizing_constant
-
- weights = tf.to_float(tf.not_equal(labels, 0))
- return xentropy * weights, weights
-
-
-def _convert_to_eval_metric(metric_fn):
- """Wrap a metric fn that returns scores and weights as an eval metric fn.
-
- The input metric_fn returns values for the current batch. The wrapper
- aggregates the return values collected over all of the batches evaluated.
-
- Args:
- metric_fn: function that returns scores and weights for the current batch's
- logits and predicted labels.
-
- Returns:
- function that aggregates the scores and weights from metric_fn.
- """
- def problem_metric_fn(*args):
- """Returns an aggregation of the metric_fn's returned values."""
- (scores, weights) = metric_fn(*args)
-
- # The tf.metrics.mean function assures correct aggregation.
- return tf.metrics.mean(scores, weights)
- return problem_metric_fn
-
-
-def get_eval_metrics(logits, labels, params):
- """Return dictionary of model evaluation metrics."""
- metrics = {
- "accuracy": _convert_to_eval_metric(padded_accuracy)(logits, labels),
- "accuracy_top5": _convert_to_eval_metric(padded_accuracy_top5)(
- logits, labels),
- "accuracy_per_sequence": _convert_to_eval_metric(
- padded_sequence_accuracy)(logits, labels),
- "neg_log_perplexity": _convert_to_eval_metric(padded_neg_log_perplexity)(
- logits, labels, params["vocab_size"]),
- }
-
- if not params["use_tpu"]:
- # TPU does not support tf.py_func
- metrics.update({
- "approx_bleu_score": _convert_to_eval_metric(
- bleu_score)(logits, labels),
- "rouge_2_fscore": _convert_to_eval_metric(
- rouge_2_fscore)(logits, labels),
- "rouge_L_fscore": _convert_to_eval_metric(
- rouge_l_fscore)(logits, labels),
- })
-
- # Prefix each of the metric names with "metrics/". This allows the metric
- # graphs to display under the "metrics" category in TensorBoard.
- metrics = {"metrics/%s" % k: v for k, v in six.iteritems(metrics)}
- return metrics
-
-
-def padded_accuracy(logits, labels):
- """Percentage of times that predictions matches labels on non-0s."""
- with tf.variable_scope("padded_accuracy", values=[logits, labels]):
- logits, labels = _pad_tensors_to_same_length(logits, labels)
- weights = tf.to_float(tf.not_equal(labels, 0))
- outputs = tf.to_int32(tf.argmax(logits, axis=-1))
- padded_labels = tf.to_int32(labels)
- return tf.to_float(tf.equal(outputs, padded_labels)), weights
-
-
-def padded_accuracy_topk(logits, labels, k):
- """Percentage of times that top-k predictions matches labels on non-0s."""
- with tf.variable_scope("padded_accuracy_topk", values=[logits, labels]):
- logits, labels = _pad_tensors_to_same_length(logits, labels)
- weights = tf.to_float(tf.not_equal(labels, 0))
- effective_k = tf.minimum(k, tf.shape(logits)[-1])
- _, outputs = tf.nn.top_k(logits, k=effective_k)
- outputs = tf.to_int32(outputs)
- padded_labels = tf.to_int32(labels)
- padded_labels = tf.expand_dims(padded_labels, axis=-1)
- padded_labels += tf.zeros_like(outputs) # Pad to same shape.
- same = tf.to_float(tf.equal(outputs, padded_labels))
- same_topk = tf.reduce_sum(same, axis=-1)
- return same_topk, weights
-
-
-def padded_accuracy_top5(logits, labels):
- return padded_accuracy_topk(logits, labels, 5)
-
-
-def padded_sequence_accuracy(logits, labels):
- """Percentage of times that predictions matches labels everywhere (non-0)."""
- with tf.variable_scope("padded_sequence_accuracy", values=[logits, labels]):
- logits, labels = _pad_tensors_to_same_length(logits, labels)
- weights = tf.to_float(tf.not_equal(labels, 0))
- outputs = tf.to_int32(tf.argmax(logits, axis=-1))
- padded_labels = tf.to_int32(labels)
- not_correct = tf.to_float(tf.not_equal(outputs, padded_labels)) * weights
- axis = list(range(1, len(outputs.get_shape())))
- correct_seq = 1.0 - tf.minimum(1.0, tf.reduce_sum(not_correct, axis=axis))
- return correct_seq, tf.constant(1.0)
-
-
-def padded_neg_log_perplexity(logits, labels, vocab_size):
- """Average log-perplexity excluding padding 0s. No smoothing."""
- num, den = padded_cross_entropy_loss(logits, labels, 0, vocab_size)
- return -num, den
-
-
-def bleu_score(logits, labels):
- """Approximate BLEU score computation between labels and predictions.
-
- An approximate BLEU scoring method since we do not glue word pieces or
- decode the ids and tokenize the output. By default, we use ngram order of 4
- and use brevity penalty. Also, this does not have beam search.
-
- Args:
- logits: Tensor of size [batch_size, length_logits, vocab_size]
- labels: Tensor of size [batch-size, length_labels]
-
- Returns:
- bleu: int, approx bleu score
- """
- predictions = tf.to_int32(tf.argmax(logits, axis=-1))
- # TODO: Look into removing use of py_func
- bleu = tf.py_func(compute_bleu, (labels, predictions), tf.float32)
- return bleu, tf.constant(1.0)
-
-
-def _get_ngrams_with_counter(segment, max_order):
- """Extracts all n-grams up to a given maximum order from an input segment.
-
- Args:
- segment: text segment from which n-grams will be extracted.
- max_order: maximum length in tokens of the n-grams returned by this
- methods.
-
- Returns:
- The Counter containing all n-grams upto max_order in segment
- with a count of how many times each n-gram occurred.
- """
- ngram_counts = collections.Counter()
- for order in xrange(1, max_order + 1):
- for i in xrange(0, len(segment) - order + 1):
- ngram = tuple(segment[i:i + order])
- ngram_counts[ngram] += 1
- return ngram_counts
-
-
-def compute_bleu(reference_corpus, translation_corpus, max_order=4,
- use_bp=True):
- """Computes BLEU score of translated segments against one or more references.
-
- Args:
- reference_corpus: list of references for each translation. Each
- reference should be tokenized into a list of tokens.
- translation_corpus: list of translations to score. Each translation
- should be tokenized into a list of tokens.
- max_order: Maximum n-gram order to use when computing BLEU score.
- use_bp: boolean, whether to apply brevity penalty.
-
- Returns:
- BLEU score.
- """
- reference_length = 0
- translation_length = 0
- bp = 1.0
- geo_mean = 0
-
- matches_by_order = [0] * max_order
- possible_matches_by_order = [0] * max_order
- precisions = []
-
- for (references, translations) in zip(reference_corpus, translation_corpus):
- reference_length += len(references)
- translation_length += len(translations)
- ref_ngram_counts = _get_ngrams_with_counter(references, max_order)
- translation_ngram_counts = _get_ngrams_with_counter(translations, max_order)
-
- overlap = dict((ngram,
- min(count, translation_ngram_counts[ngram]))
- for ngram, count in ref_ngram_counts.items())
-
- for ngram in overlap:
- matches_by_order[len(ngram) - 1] += overlap[ngram]
- for ngram in translation_ngram_counts:
- possible_matches_by_order[len(ngram) - 1] += translation_ngram_counts[
- ngram]
-
- precisions = [0] * max_order
- smooth = 1.0
-
- for i in xrange(0, max_order):
- if possible_matches_by_order[i] > 0:
- precisions[i] = float(matches_by_order[i]) / possible_matches_by_order[i]
- if matches_by_order[i] > 0:
- precisions[i] = float(matches_by_order[i]) / possible_matches_by_order[
- i]
- else:
- smooth *= 2
- precisions[i] = 1.0 / (smooth * possible_matches_by_order[i])
- else:
- precisions[i] = 0.0
-
- if max(precisions) > 0:
- p_log_sum = sum(math.log(p) for p in precisions if p)
- geo_mean = math.exp(p_log_sum / max_order)
-
- if use_bp:
- ratio = translation_length / reference_length
- bp = math.exp(1 - 1. / ratio) if ratio < 1.0 else 1.0
- bleu = geo_mean * bp
- return np.float32(bleu)
-
-
-def rouge_2_fscore(logits, labels):
- """ROUGE-2 F1 score computation between labels and predictions.
-
- This is an approximate ROUGE scoring method since we do not glue word pieces
- or decode the ids and tokenize the output.
-
- Args:
- logits: tensor, model predictions
- labels: tensor, gold output.
-
- Returns:
- rouge2_fscore: approx rouge-2 f1 score.
- """
- predictions = tf.to_int32(tf.argmax(logits, axis=-1))
- # TODO: Look into removing use of py_func
- rouge_2_f_score = tf.py_func(rouge_n, (predictions, labels), tf.float32)
- return rouge_2_f_score, tf.constant(1.0)
-
-
-def _get_ngrams(n, text):
- """Calculates n-grams.
-
- Args:
- n: which n-grams to calculate
- text: An array of tokens
-
- Returns:
- A set of n-grams
- """
- ngram_set = set()
- text_length = len(text)
- max_index_ngram_start = text_length - n
- for i in range(max_index_ngram_start + 1):
- ngram_set.add(tuple(text[i:i + n]))
- return ngram_set
-
-
-def rouge_n(eval_sentences, ref_sentences, n=2):
- """Computes ROUGE-N f1 score of two text collections of sentences.
-
- Source: https://www.microsoft.com/en-us/research/publication/
- rouge-a-package-for-automatic-evaluation-of-summaries/
-
- Args:
- eval_sentences: Predicted sentences.
- ref_sentences: Sentences from the reference set
- n: Size of ngram. Defaults to 2.
-
- Returns:
- f1 score for ROUGE-N
- """
- f1_scores = []
- for eval_sentence, ref_sentence in zip(eval_sentences, ref_sentences):
- eval_ngrams = _get_ngrams(n, eval_sentence)
- ref_ngrams = _get_ngrams(n, ref_sentence)
- ref_count = len(ref_ngrams)
- eval_count = len(eval_ngrams)
-
- # Count the overlapping ngrams between evaluated and reference
- overlapping_ngrams = eval_ngrams.intersection(ref_ngrams)
- overlapping_count = len(overlapping_ngrams)
-
- # Handle edge case. This isn't mathematically correct, but it's good enough
- if eval_count == 0:
- precision = 0.0
- else:
- precision = float(overlapping_count) / eval_count
- if ref_count == 0:
- recall = 0.0
- else:
- recall = float(overlapping_count) / ref_count
- f1_scores.append(2.0 * ((precision * recall) / (precision + recall + 1e-8)))
-
- # return overlapping_count / reference_count
- return np.mean(f1_scores, dtype=np.float32)
-
-
-def rouge_l_fscore(predictions, labels):
- """ROUGE scores computation between labels and predictions.
-
- This is an approximate ROUGE scoring method since we do not glue word pieces
- or decode the ids and tokenize the output.
-
- Args:
- predictions: tensor, model predictions
- labels: tensor, gold output.
-
- Returns:
- rouge_l_fscore: approx rouge-l f1 score.
- """
- outputs = tf.to_int32(tf.argmax(predictions, axis=-1))
- rouge_l_f_score = tf.py_func(rouge_l_sentence_level, (outputs, labels),
- tf.float32)
- return rouge_l_f_score, tf.constant(1.0)
-
-
-def rouge_l_sentence_level(eval_sentences, ref_sentences):
- """Computes ROUGE-L (sentence level) of two collections of sentences.
-
- Source: https://www.microsoft.com/en-us/research/publication/
- rouge-a-package-for-automatic-evaluation-of-summaries/
-
- Calculated according to:
- R_lcs = LCS(X,Y)/m
- P_lcs = LCS(X,Y)/n
- F_lcs = ((1 + beta^2)*R_lcs*P_lcs) / (R_lcs + (beta^2) * P_lcs)
-
- where:
- X = reference summary
- Y = Candidate summary
- m = length of reference summary
- n = length of candidate summary
-
- Args:
- eval_sentences: The sentences that have been picked by the summarizer
- ref_sentences: The sentences from the reference set
-
- Returns:
- A float: F_lcs
- """
-
- f1_scores = []
- for eval_sentence, ref_sentence in zip(eval_sentences, ref_sentences):
- m = float(len(ref_sentence))
- n = float(len(eval_sentence))
- lcs = _len_lcs(eval_sentence, ref_sentence)
- f1_scores.append(_f_lcs(lcs, m, n))
- return np.mean(f1_scores, dtype=np.float32)
-
-
-def _len_lcs(x, y):
- """Returns the length of the Longest Common Subsequence between two seqs.
-
- Source: http://www.algorithmist.com/index.php/Longest_Common_Subsequence
-
- Args:
- x: sequence of words
- y: sequence of words
-
- Returns
- integer: Length of LCS between x and y
- """
- table = _lcs(x, y)
- n, m = len(x), len(y)
- return table[n, m]
-
-
-def _lcs(x, y):
- """Computes the length of the LCS between two seqs.
-
- The implementation below uses a DP programming algorithm and runs
- in O(nm) time where n = len(x) and m = len(y).
- Source: http://www.algorithmist.com/index.php/Longest_Common_Subsequence
-
- Args:
- x: collection of words
- y: collection of words
-
- Returns:
- Table of dictionary of coord and len lcs
- """
- n, m = len(x), len(y)
- table = dict()
- for i in range(n + 1):
- for j in range(m + 1):
- if i == 0 or j == 0:
- table[i, j] = 0
- elif x[i - 1] == y[j - 1]:
- table[i, j] = table[i - 1, j - 1] + 1
- else:
- table[i, j] = max(table[i - 1, j], table[i, j - 1])
- return table
-
-
-def _f_lcs(llcs, m, n):
- """Computes the LCS-based F-measure score.
-
- Source: http://research.microsoft.com/en-us/um/people/cyl/download/papers/
- rouge-working-note-v1.3.1.pdf
-
- Args:
- llcs: Length of LCS
- m: number of words in reference summary
- n: number of words in candidate summary
-
- Returns:
- Float. LCS-based F-measure score
- """
- r_lcs = llcs / m
- p_lcs = llcs / n
- beta = p_lcs / (r_lcs + 1e-12)
- num = (1 + (beta ** 2)) * r_lcs * p_lcs
- denom = r_lcs + ((beta ** 2) * p_lcs)
- f_lcs = num / (denom + 1e-12)
- return f_lcs
diff --git a/spaces/NCTCMumbai/NCTC/models/research/autoaugment/wrn.py b/spaces/NCTCMumbai/NCTC/models/research/autoaugment/wrn.py
deleted file mode 100644
index ea04e19cfc30f52fe49c475b6ed35610e7c87aa4..0000000000000000000000000000000000000000
--- a/spaces/NCTCMumbai/NCTC/models/research/autoaugment/wrn.py
+++ /dev/null
@@ -1,158 +0,0 @@
-# Copyright 2018 The TensorFlow Authors All Rights Reserved.
-#
-# Licensed under the Apache License, Version 2.0 (the "License");
-# you may not use this file except in compliance with the License.
-# You may obtain a copy of the License at
-#
-# http://www.apache.org/licenses/LICENSE-2.0
-#
-# Unless required by applicable law or agreed to in writing, software
-# distributed under the License is distributed on an "AS IS" BASIS,
-# WITHOUT WARRANTIES OR CONDITIONS OF ANY KIND, either express or implied.
-# See the License for the specific language governing permissions and
-# limitations under the License.
-# ==============================================================================
-
-"""Builds the Wide-ResNet Model."""
-
-from __future__ import absolute_import
-from __future__ import division
-from __future__ import print_function
-
-import custom_ops as ops
-import numpy as np
-import tensorflow as tf
-
-
-
-def residual_block(
- x, in_filter, out_filter, stride, activate_before_residual=False):
- """Adds residual connection to `x` in addition to applying BN->ReLU->3x3 Conv.
-
- Args:
- x: Tensor that is the output of the previous layer in the model.
- in_filter: Number of filters `x` has.
- out_filter: Number of filters that the output of this layer will have.
- stride: Integer that specified what stride should be applied to `x`.
- activate_before_residual: Boolean on whether a BN->ReLU should be applied
- to x before the convolution is applied.
-
- Returns:
- A Tensor that is the result of applying two sequences of BN->ReLU->3x3 Conv
- and then adding that Tensor to `x`.
- """
-
- if activate_before_residual: # Pass up RELU and BN activation for resnet
- with tf.variable_scope('shared_activation'):
- x = ops.batch_norm(x, scope='init_bn')
- x = tf.nn.relu(x)
- orig_x = x
- else:
- orig_x = x
-
- block_x = x
- if not activate_before_residual:
- with tf.variable_scope('residual_only_activation'):
- block_x = ops.batch_norm(block_x, scope='init_bn')
- block_x = tf.nn.relu(block_x)
-
- with tf.variable_scope('sub1'):
- block_x = ops.conv2d(
- block_x, out_filter, 3, stride=stride, scope='conv1')
-
- with tf.variable_scope('sub2'):
- block_x = ops.batch_norm(block_x, scope='bn2')
- block_x = tf.nn.relu(block_x)
- block_x = ops.conv2d(
- block_x, out_filter, 3, stride=1, scope='conv2')
-
- with tf.variable_scope(
- 'sub_add'): # If number of filters do not agree then zero pad them
- if in_filter != out_filter:
- orig_x = ops.avg_pool(orig_x, stride, stride)
- orig_x = ops.zero_pad(orig_x, in_filter, out_filter)
- x = orig_x + block_x
- return x
-
-
-def _res_add(in_filter, out_filter, stride, x, orig_x):
- """Adds `x` with `orig_x`, both of which are layers in the model.
-
- Args:
- in_filter: Number of filters in `orig_x`.
- out_filter: Number of filters in `x`.
- stride: Integer specifying the stide that should be applied `orig_x`.
- x: Tensor that is the output of the previous layer.
- orig_x: Tensor that is the output of an earlier layer in the network.
-
- Returns:
- A Tensor that is the result of `x` and `orig_x` being added after
- zero padding and striding are applied to `orig_x` to get the shapes
- to match.
- """
- if in_filter != out_filter:
- orig_x = ops.avg_pool(orig_x, stride, stride)
- orig_x = ops.zero_pad(orig_x, in_filter, out_filter)
- x = x + orig_x
- orig_x = x
- return x, orig_x
-
-
-def build_wrn_model(images, num_classes, wrn_size):
- """Builds the WRN model.
-
- Build the Wide ResNet model from https://arxiv.org/abs/1605.07146.
-
- Args:
- images: Tensor of images that will be fed into the Wide ResNet Model.
- num_classes: Number of classed that the model needs to predict.
- wrn_size: Parameter that scales the number of filters in the Wide ResNet
- model.
-
- Returns:
- The logits of the Wide ResNet model.
- """
- kernel_size = wrn_size
- filter_size = 3
- num_blocks_per_resnet = 4
- filters = [
- min(kernel_size, 16), kernel_size, kernel_size * 2, kernel_size * 4
- ]
- strides = [1, 2, 2] # stride for each resblock
-
- # Run the first conv
- with tf.variable_scope('init'):
- x = images
- output_filters = filters[0]
- x = ops.conv2d(x, output_filters, filter_size, scope='init_conv')
-
- first_x = x # Res from the beginning
- orig_x = x # Res from previous block
-
- for block_num in range(1, 4):
- with tf.variable_scope('unit_{}_0'.format(block_num)):
- activate_before_residual = True if block_num == 1 else False
- x = residual_block(
- x,
- filters[block_num - 1],
- filters[block_num],
- strides[block_num - 1],
- activate_before_residual=activate_before_residual)
- for i in range(1, num_blocks_per_resnet):
- with tf.variable_scope('unit_{}_{}'.format(block_num, i)):
- x = residual_block(
- x,
- filters[block_num],
- filters[block_num],
- 1,
- activate_before_residual=False)
- x, orig_x = _res_add(filters[block_num - 1], filters[block_num],
- strides[block_num - 1], x, orig_x)
- final_stride_val = np.prod(strides)
- x, _ = _res_add(filters[0], filters[3], final_stride_val, x, first_x)
- with tf.variable_scope('unit_last'):
- x = ops.batch_norm(x, scope='final_bn')
- x = tf.nn.relu(x)
- x = ops.global_avg_pool(x)
- logits = ops.fc(x, num_classes)
- return logits
diff --git a/spaces/Nalla/PDF_tables_to_CSV_output/App_For_PDF_To_Dataframe.py b/spaces/Nalla/PDF_tables_to_CSV_output/App_For_PDF_To_Dataframe.py
deleted file mode 100644
index b52c2577db012f6c20e602fb065d57a2249ded83..0000000000000000000000000000000000000000
--- a/spaces/Nalla/PDF_tables_to_CSV_output/App_For_PDF_To_Dataframe.py
+++ /dev/null
@@ -1,88 +0,0 @@
-# -*- coding: utf-8 -*-
-"""
-Created on Sat Feb 19 20:23:31 2022
-
-@author: Nalla
-"""
-
-import streamlit as st # data app development
-import subprocess # process in the os
-from subprocess import STDOUT, check_call #os process manipuation
-import os #os process manipuation
-import base64 # byte object into a pdf file
-import camelot as cam # extracting tables from PDFs
-
-# to run this only once and it's cached
-@st.cache
-def gh():
- """install ghostscript on the linux machine"""
- proc = subprocess.Popen('apt-get install -y ghostscript', shell=True, stdin=None, stdout=open(os.devnull,"wb"), stderr=STDOUT, executable="/bin/bash")
- proc.wait()
-
-gh()
-
-
-
-st.title("PDF Table Extractor")
-st.subheader("Extract the contents in ease")
-
-st.image("https://raw.githubusercontent.com/camelot-dev/camelot/master/docs/_static/camelot.png", width=150)
-
-
-
-# file uploader on streamlit
-
-input_pdf = st.file_uploader(label = "upload your pdf here", type = 'pdf')
-
-st.markdown("### Page Number")
-
-page_number = st.text_input("Enter the page # from where you want to extract the PDF eg: 3", value = 1)
-
-# run this only when a PDF is uploaded
-
-if input_pdf is not None:
- # byte object into a PDF file
- with open("input.pdf", "wb") as f:
- base64_pdf = base64.b64encode(input_pdf.read()).decode('utf-8')
- f.write(base64.b64decode(base64_pdf))
- f.close()
- #Select the flavor which is needed
- #Ddlist_selection = st.selectbox("Does the pdf contain a proper table structure?",['lattice', 'stream'])
- # read the pdf and parse it using stream
- table = cam.read_pdf("input.pdf", pages = page_number, flavor = 'stream')
-
- st.markdown("### Number of Tables")
-
- # display the output after parsing
- st.write(table)
-
- # display the table
-
- if len(table) > 0:
-
- # extract the index value of the table
-
- option = st.selectbox(label = "Select the Table to be displayed", options = range(len(table) + 1))
-
- st.markdown('### Output Table')
-
- # display the dataframe
-
- st.dataframe(table[int(option)-1].df)
-
-
-
- @st.cache
- def convert_df(df):
- # IMPORTANT: Cache the conversion to prevent computation on every rerun
- return df.to_csv(index=False).encode('utf-8')
-
- csv = convert_df(table[int(option)-1].df)
-
- st.download_button(
- label="Download data as CSV",
- data=csv,
- file_name='Data_table.csv',
- mime='text/csv',
- )
-
diff --git a/spaces/NexusInstruments/offensive-hugging-face/README.md b/spaces/NexusInstruments/offensive-hugging-face/README.md
deleted file mode 100644
index b08a1585574a82eb304b23fa4e94aa93463892b1..0000000000000000000000000000000000000000
--- a/spaces/NexusInstruments/offensive-hugging-face/README.md
+++ /dev/null
@@ -1,13 +0,0 @@
----
-title: Offensive Hugging Face
-emoji: 👁
-colorFrom: gray
-colorTo: yellow
-sdk: gradio
-sdk_version: 3.29.0
-app_file: app.py
-pinned: false
-license: unknown
----
-
-Check out the configuration reference at https://huggingface.co/docs/hub/spaces-config-reference
diff --git a/spaces/NowLoadY/ocr-gpt/app.py b/spaces/NowLoadY/ocr-gpt/app.py
deleted file mode 100644
index 48bd800005e137c829f8be40d091e05e238d3655..0000000000000000000000000000000000000000
--- a/spaces/NowLoadY/ocr-gpt/app.py
+++ /dev/null
@@ -1,34 +0,0 @@
-import easyocr
-import gradio as gr
-import openai
-import requests
-
-# Set OpenAI API key
-openai.api_key = "your_api_key_here"
-
-# Initialize OCR reader
-reader = easyocr.Reader(['ch_sim', 'en'],gpu=False)
-
-# Define the OCR function
-# Update the ocr_gpt function to accept the API key as an input
-def ocr_gpt(image, gpt_opinion_prompt, api_key):
- openai.api_key = api_key
- ocr_result = reader.readtext(image)
- prompt = "Correct the following OCR result: " + ocr_result[0][1]
- response = openai.Completion.create(engine="davinci-codex", prompt=prompt, max_tokens=50, n=1, stop=None, temperature=0.5)
- corrected_text = response.choices[0].text.strip()
- gpt_opinion_response = openai.Completion.create(engine="davinci-codex", prompt=gpt_opinion_prompt, max_tokens=50, n=1, stop=None, temperature=0.5)
- gpt_opinion = gpt_opinion_response.choices[0].text.strip()
- return corrected_text, gpt_opinion
-
-# Define Gradio UI components
-image_input = gr.inputs.Image()
-gpt_input = gr.inputs.Textbox(lines=1, label="GPT Opinion Prompt")
-api_key_input = gr.inputs.Textbox(lines=1, label="API Key")
-ocr_output = gr.outputs.Textbox(label="OCR Result (GPT Corrected)")
-gpt_opinion_output = gr.outputs.Textbox(label="GPT Opinion on Image Information")
-
-
-
-# Create Gradio interface
-iface = gr.Interface(fn=ocr_gpt, inputs=[image_input, gpt_input, api_key_input], outputs=[ocr_output, gpt_opinion_output])
\ No newline at end of file
diff --git a/spaces/OFA-Sys/OFA-Generic_Interface/fairseq/fairseq/modules/quantization/pq/em.py b/spaces/OFA-Sys/OFA-Generic_Interface/fairseq/fairseq/modules/quantization/pq/em.py
deleted file mode 100644
index 6f15c3e46bd052b1e00929e7ece9355fb03846c7..0000000000000000000000000000000000000000
--- a/spaces/OFA-Sys/OFA-Generic_Interface/fairseq/fairseq/modules/quantization/pq/em.py
+++ /dev/null
@@ -1,211 +0,0 @@
-# Copyright (c) Facebook, Inc. and its affiliates.
-#
-# This source code is licensed under the MIT license found in the
-# LICENSE file in the root directory of this source tree.
-
-import logging
-import os
-import random
-from collections import Counter
-
-import torch
-
-
-class EM:
- """
- EM algorithm used to quantize the columns of W to minimize
-
- ||W - W_hat||^2
-
- Args:
- - W: weight matrix of size (in_features x out_features)
- - n_iter: number of k-means iterations
- - n_centroids: number of centroids (size of codebook)
- - eps: for cluster reassignment when an empty cluster is found
- - max_tentatives for cluster reassignment when an empty cluster is found
- - verbose: print error after each iteration
-
- Remarks:
- - If one cluster is empty, the most populated cluster is split into
- two clusters
- - All the relevant dimensions are specified in the code
- """
-
- def __init__(
- self, W, n_centroids=256, n_iter=20, eps=1e-6, max_tentatives=30, verbose=True
- ):
- self.W = W
- self.n_centroids = n_centroids
- self.n_iter = n_iter
- self.eps = eps
- self.max_tentatives = max_tentatives
- self.verbose = verbose
- self.centroids = torch.Tensor()
- self.assignments = torch.Tensor()
- self.objective = []
-
- def initialize_centroids(self):
- """
- Initializes the centroids by sampling random columns from W.
- """
-
- in_features, out_features = self.W.size()
- indices = torch.randint(
- low=0, high=out_features, size=(self.n_centroids,)
- ).long()
- self.centroids = self.W[:, indices].t() # (n_centroids x in_features)
-
- def step(self, i):
- """
- There are two standard steps for each iteration: expectation (E) and
- minimization (M). The E-step (assignment) is performed with an exhaustive
- search and the M-step (centroid computation) is performed with
- the exact solution.
-
- Args:
- - i: step number
-
- Remarks:
- - The E-step heavily uses PyTorch broadcasting to speed up computations
- and reduce the memory overhead
- """
-
- # assignments (E-step)
- distances = self.compute_distances() # (n_centroids x out_features)
- self.assignments = torch.argmin(distances, dim=0) # (out_features)
- n_empty_clusters = self.resolve_empty_clusters()
-
- # centroids (M-step)
- for k in range(self.n_centroids):
- W_k = self.W[:, self.assignments == k] # (in_features x size_of_cluster_k)
- self.centroids[k] = W_k.mean(dim=1) # (in_features)
-
- # book-keeping
- obj = (self.centroids[self.assignments].t() - self.W).norm(p=2).item()
- self.objective.append(obj)
- if self.verbose:
- logging.info(
- f"Iteration: {i},\t"
- f"objective: {obj:.6f},\t"
- f"resolved empty clusters: {n_empty_clusters}"
- )
-
- def resolve_empty_clusters(self):
- """
- If one cluster is empty, the most populated cluster is split into
- two clusters by shifting the respective centroids. This is done
- iteratively for a fixed number of tentatives.
- """
-
- # empty clusters
- counts = Counter(map(lambda x: x.item(), self.assignments))
- empty_clusters = set(range(self.n_centroids)) - set(counts.keys())
- n_empty_clusters = len(empty_clusters)
-
- tentatives = 0
- while len(empty_clusters) > 0:
- # given an empty cluster, find most populated cluster and split it into two
- k = random.choice(list(empty_clusters))
- m = counts.most_common(1)[0][0]
- e = torch.randn_like(self.centroids[m]) * self.eps
- self.centroids[k] = self.centroids[m].clone()
- self.centroids[k] += e
- self.centroids[m] -= e
-
- # recompute assignments
- distances = self.compute_distances() # (n_centroids x out_features)
- self.assignments = torch.argmin(distances, dim=0) # (out_features)
-
- # check for empty clusters
- counts = Counter(map(lambda x: x.item(), self.assignments))
- empty_clusters = set(range(self.n_centroids)) - set(counts.keys())
-
- # increment tentatives
- if tentatives == self.max_tentatives:
- logging.info(
- f"Could not resolve all empty clusters, {len(empty_clusters)} remaining"
- )
- raise EmptyClusterResolveError
- tentatives += 1
-
- return n_empty_clusters
-
- def compute_distances(self):
- """
- For every centroid m, computes
-
- ||M - m[None, :]||_2
-
- Remarks:
- - We rely on PyTorch's broadcasting to speed up computations
- and reduce the memory overhead
- - Without chunking, the sizes in the broadcasting are modified as:
- (n_centroids x n_samples x out_features) -> (n_centroids x out_features)
- - The broadcasting computation is automatically chunked so that
- the tensors fit into the memory of the GPU
- """
-
- nb_centroids_chunks = 1
-
- while True:
- try:
- return torch.cat(
- [
- (self.W[None, :, :] - centroids_c[:, :, None]).norm(p=2, dim=1)
- for centroids_c in self.centroids.chunk(
- nb_centroids_chunks, dim=0
- )
- ],
- dim=0,
- )
- except RuntimeError:
- nb_centroids_chunks *= 2
-
- def assign(self):
- """
- Assigns each column of W to its closest centroid, thus essentially
- performing the E-step in train().
-
- Remarks:
- - The function must be called after train() or after loading
- centroids using self.load(), otherwise it will return empty tensors
- """
-
- distances = self.compute_distances() # (n_centroids x out_features)
- self.assignments = torch.argmin(distances, dim=0) # (out_features)
-
- def save(self, path, layer):
- """
- Saves centroids and assignments.
-
- Args:
- - path: folder used to save centroids and assignments
- """
-
- torch.save(self.centroids, os.path.join(path, "{}_centroids.pth".format(layer)))
- torch.save(
- self.assignments, os.path.join(path, "{}_assignments.pth".format(layer))
- )
- torch.save(self.objective, os.path.join(path, "{}_objective.pth".format(layer)))
-
- def load(self, path, layer):
- """
- Loads centroids and assignments from a given path
-
- Args:
- - path: folder use to load centroids and assignments
- """
-
- self.centroids = torch.load(
- os.path.join(path, "{}_centroids.pth".format(layer))
- )
- self.assignments = torch.load(
- os.path.join(path, "{}_assignments.pth".format(layer))
- )
- self.objective = torch.load(
- os.path.join(path, "{}_objective.pth".format(layer))
- )
-
-
-class EmptyClusterResolveError(Exception):
- pass
diff --git a/spaces/OFA-Sys/OFA-Generic_Interface/fairseq/tests/test_online_backtranslation.py b/spaces/OFA-Sys/OFA-Generic_Interface/fairseq/tests/test_online_backtranslation.py
deleted file mode 100644
index 0ae7e773da0ff838b3c8151bc14b84a6a9238a72..0000000000000000000000000000000000000000
--- a/spaces/OFA-Sys/OFA-Generic_Interface/fairseq/tests/test_online_backtranslation.py
+++ /dev/null
@@ -1,206 +0,0 @@
-# Copyright (c) Facebook, Inc. and its affiliates.
-#
-# This source code is licensed under the MIT license found in the
-# LICENSE file in the root directory of this source tree.
-
-import tempfile
-import unittest
-from pathlib import Path
-from typing import Any, Dict, Sequence
-
-import fairseq.data.indexed_dataset as indexed_dataset
-import fairseq.options
-import fairseq.tasks.online_backtranslation as obt
-import torch
-from tests import utils
-
-
-def mk_sample(tokens: Sequence[int], batch_size: int = 2) -> Dict[str, Any]:
- batch = torch.stack([torch.tensor(tokens, dtype=torch.long)] * batch_size)
- sample = {
- "net_input": {
- "src_tokens": batch,
- "prev_output_tokens": batch,
- "src_lengths": torch.tensor([len(tokens)] * batch_size, dtype=torch.long),
- },
- "target": batch[:, 1:],
- }
- return sample
-
-
-def mk_dataset(num_samples: int, max_len: int, output: Path):
- output.parent.mkdir(exist_ok=True)
- idx = indexed_dataset.IndexedDatasetBuilder(str(output))
- data = torch.randint(5, 100, (num_samples, max_len))
- lengths = torch.randint(3, max_len, (num_samples,))
- for d, l in zip(data, lengths):
- d[0] = 0
- idx.add_item(d[:l])
- idx.finalize(output.with_suffix(".idx"))
- assert output.exists()
- assert output.with_suffix(".idx").exists()
-
-
-class OnlineBacktranslationTest(unittest.TestCase):
-
- tmp_dir = Path(tempfile.mkdtemp(suffix="OnlineBacktranslationTest"))
-
- @classmethod
- def obt_task(
- cls, languages: Sequence[str], data: Path = None, language_mapping: str = None
- ):
- dict_path = cls.tmp_dir / "dict.txt"
- if not dict_path.exists():
- dictionary = utils.dummy_dictionary(100)
- dictionary.save(str(dict_path))
-
- if data is not None:
- (data / "dict.txt").write_text(dict_path.read_text())
- else:
- data = cls.tmp_dir
- assert len(languages) >= 2
-
- kwargs = {
- "arch": "transformer",
- # --max-sentences=1 for better predictability of batches
- "max_sentences": 1,
- # Use characteristics dimensions
- "encoder_layers": 3,
- "encoder_embed_dim": 12,
- "encoder_ffn_embed_dim": 14,
- "encoder_attention_heads": 4,
- "decoder_layers": 3,
- "decoder_embed_dim": 12,
- "decoder_output_dim": 12,
- "decoder_ffn_embed_dim": 14,
- "decoder_attention_heads": 4,
- # Disable dropout so we have comparable tests.
- "dropout": 0,
- "attention_dropout": 0,
- "activation_dropout": 0,
- "encoder_layerdrop": 0,
- }
-
- args = fairseq.options.get_args(
- data,
- task="online_backtranslation",
- mono_langs=",".join(languages),
- valid_lang_pairs=f"{languages[0]}-{languages[1]}",
- tokens_per_sample=256,
- language_mapping=language_mapping,
- **kwargs,
- )
- task = obt.OnlineBackTranslationTask.setup_task(args)
- # we need to build the model to have the correct dictionary
- model = task.build_model(task.args)
- return task, model
-
- def tmp_path(self, test_case: str) -> Path:
- return Path(tempfile.mkdtemp(test_case, dir=self.tmp_dir))
-
- def test_lang_tokens(self):
- task, model = self.obt_task(["en", "ro", "zh"])
- assert obt._lang_token("en") in task.dictionary
- assert obt._lang_token("ro") in task.dictionary
- assert obt._lang_token("zh") in task.dictionary
-
- en_bos = obt._lang_token_index(task.common_dict, "en")
- assert "en" == task.common_dict[en_bos].strip("_")
- zh_bos = obt._lang_token_index(task.common_dict, "zh")
- assert "zh" == task.common_dict[zh_bos].strip("_")
- zh_sample = mk_sample([zh_bos, 16, 14, 12, 10])
-
- # we expect to receive the bos token for translation
- assert task.get_bos_token_from_sample(zh_sample) == en_bos
-
- def test_backtranslate_sample(self):
- task, model = self.obt_task(["en", "ro", "zh"])
-
- en_bos = obt._lang_token_index(task.common_dict, "en")
- zh_bos = obt._lang_token_index(task.common_dict, "zh")
- sample = mk_sample([zh_bos, 16, 14, 12, 10])
-
- task.backtranslate_sample(sample, "zh", "en")
- target_zh = list(sample["target"][0])
- assert target_zh == [16, 14, 12, 10] # original zh sentence
- generated_en = sample["net_input"]["src_tokens"][0]
- assert generated_en[0] == en_bos
-
- def test_train_dataset(self):
- data = self.tmp_path("test_train_dataset")
- mk_dataset(20, 10, data / "en" / "train.bin")
- mk_dataset(10, 10, data / "zh" / "train.bin")
- task, model = self.obt_task(["en", "zh"], data)
- task.load_dataset("train")
-
- en_bos = obt._lang_token_index(task.common_dict, "en")
- zh_bos = obt._lang_token_index(task.common_dict, "zh")
-
- train = task.datasets["train"]
- train.ordered_indices()
- train.prefetch([0, 19])
- sample_0 = train[0]
- sample_19 = train[19]
- self.assertEqual(
- set(sample_0.keys()), {"en-BT", "en-DENOISE", "zh-BT", "zh-DENOISE"}
- )
- for sample in (sample_0, sample_19):
- self.assertEqual(sample["en-BT"]["source"][0], en_bos)
- # bt target isn't ready to look at.
- self.assertEqual(sample["en-DENOISE"]["source"][0], en_bos)
- # TODO What could we check on the target side ?
-
- for i in range(10):
- # Zh dataset is shorter, and is wrapped around En dataset.
- train.prefetch([i, i + 10])
- self.assertEqual(
- list(train[i]["zh-DENOISE"]["source"]),
- list(train[i + 10]["zh-DENOISE"]["source"]),
- )
- self.assertEqual(train[i]["zh-DENOISE"]["source"][0].item(), zh_bos)
-
- # Sorted by increasing len
- self.assertLess(
- len(sample_0["en-BT"]["source"]), len(sample_19["en-BT"]["source"])
- )
-
- def test_valid_dataset(self):
- data = self.tmp_path("test_valid_dataset")
- mk_dataset(10, 21, data / "valid.en-zh.en.bin")
- mk_dataset(10, 21, data / "valid.en-zh.zh.bin")
-
- task, model = self.obt_task(["en", "zh"], data)
- valid = task.load_dataset("valid")
- en_bos = obt._lang_token_index(task.common_dict, "en")
-
- assert valid is not None
- valid.prefetch(range(10))
- sample_0 = valid[0]
- sample_9 = valid[9]
- self.assertEqual(sample_0["id"], 0)
- self.assertEqual(sample_9["id"], 9)
- self.assertEqual(sample_0["source"][0], en_bos)
- self.assertEqual(sample_9["source"][0], en_bos)
- # TODO: could we test the target side ?
-
- def assertFnMatch(self, fn, values):
- for x, y in values.items():
- fn_x = fn(x)
- self.assertEqual(fn_x, y, f"Fn has wrong value: fn({x}) = {fn_x} != {y}")
-
- def test_piecewise_linear_fn(self):
- self.assertFnMatch(
- obt.PiecewiseLinearFn.from_string("1.0"), {0: 1, 100: 1, 500: 1, 1000: 1}
- )
- self.assertFnMatch(
- obt.PiecewiseLinearFn.from_string("0:1,1000:0"),
- {0: 1, 500: 0.5, 1000: 0, 2000: 0},
- )
- self.assertFnMatch(
- obt.PiecewiseLinearFn.from_string("0:0,1000:1"),
- {0: 0, 500: 0.5, 1000: 1, 2000: 1},
- )
- self.assertFnMatch(
- obt.PiecewiseLinearFn.from_string("0:0,1000:1,2000:0"),
- {0: 0, 500: 0.5, 1000: 1, 1500: 0.5, 2000: 0, 3000: 0},
- )
diff --git a/spaces/OFA-Sys/OFA-Image_Caption/fairseq/examples/adaptive_span/adaptive_span_model.py b/spaces/OFA-Sys/OFA-Image_Caption/fairseq/examples/adaptive_span/adaptive_span_model.py
deleted file mode 100644
index d96c95b85dbcf29e9384cc6d8d9630d2489991b2..0000000000000000000000000000000000000000
--- a/spaces/OFA-Sys/OFA-Image_Caption/fairseq/examples/adaptive_span/adaptive_span_model.py
+++ /dev/null
@@ -1,263 +0,0 @@
-# Copyright (c) Facebook, Inc. and its affiliates.
-# All rights reserved.
-#
-# This source code is licensed under the license found in the
-# LICENSE file in the root directory of this source tree.
-
-import math
-
-import torch
-import torch.nn as nn
-import torch.nn.functional as F
-
-from fairseq.modules.layer_norm import LayerNorm
-
-from .adaptive_span_attention import AdaptiveSpan
-
-# Size notations:
-# B = batch_size, H = d_model, M = block_size, L = attn_span
-
-
-def _skew(X, pad_value):
- """shift every row 1 step to right"""
- # X = B x M x L
- B, M, L = X.size()
- X = F.pad(X, (0, M + 1), value=pad_value) # B x M x (L+M+1)
- X = X.view(B, -1) # B x ML+MM+M
- X = X[:, :-M] # B x ML+MM
- X = X.view(B, M, M + L) # B x M x L+M
- return X
-
-
-def _unskew(X):
- """reverse _skew operation"""
- # X = B x M x L+M
- B, M, L = X.size()
- L -= M
- X = X.view(B, -1) # B x ML+MM
- X = F.pad(X, (0, M)) # B x ML+MM+M
- X = X.view(B, M, M + L + 1) # B x M x L+M+1
- X = X[:, :, :L] # B x M x L
- return X
-
-
-class SeqAttention(nn.Module):
- """Sequential self-attention layer.
- Each token will attend to its previous fixed number of steps.
- Note that attention doesn't include the current step itself.
- """
-
- def __init__(self, d_model, n_head, attn_span, dropout, adapt_span_layer, **kargs):
- nn.Module.__init__(self)
- self.dropout = nn.Dropout(dropout)
- self.d_model = d_model # size of a single head
- self.attn_span = attn_span
- self.adaptive_span = AdaptiveSpan(
- attn_span=attn_span,
- n_head=n_head,
- adapt_span_layer=adapt_span_layer,
- **kargs
- )
-
- def forward(self, query, key, value, key_pe):
- # query size = B x M x H
- # key, value sizes = B x (M+L) x H
-
- key, value, key_pe = self.adaptive_span.trim_memory(query, key, value, key_pe)
-
- # compute attention from context
- # B x M (dest) x (M+L) (src)
- attn_cont = torch.matmul(query, key.transpose(-1, -2))
- attn_cont = _unskew(attn_cont) # B x M x L
-
- # compute the effect of position embedding
- attn_pos = torch.matmul(query, key_pe) # B x M x L_pos
- attn = attn_cont + attn_pos
-
- attn = attn / math.sqrt(self.d_model) # B x M X L_pos
-
- attn = F.softmax(attn.float(), dim=-1).type_as(attn)
-
- # trim attention lengths according to the learned span
- attn = self.adaptive_span(attn)
-
- attn = self.dropout(attn) # B x M X L_pos
-
- attn_cont = _skew(attn, 0) # B x M X (L+M)
- out = torch.matmul(attn_cont, value) # B x M x H
- return out
-
- def get_cache_size(self):
- return self.adaptive_span.get_cache_size()
-
-
-class MultiHeadSeqAttention(nn.Module):
- def __init__(self, d_model, n_head, **kargs):
- nn.Module.__init__(self)
- assert d_model % n_head == 0
- self.n_head = n_head
- self.head_dim = d_model // n_head
- self.attn = SeqAttention(d_model=self.head_dim, n_head=n_head, **kargs)
- self.proj_query = nn.Linear(d_model, d_model, bias=False)
- nn.init.xavier_normal_(self.proj_query.weight)
- self.proj_out = nn.Linear(d_model, d_model, bias=False)
- nn.init.xavier_normal_(self.proj_out.weight)
- self.proj_val = nn.Linear(d_model, d_model, bias=False)
- nn.init.xavier_normal_(self.proj_val.weight)
- self.proj_key = nn.Linear(d_model, d_model, bias=False)
- nn.init.xavier_normal_(self.proj_key.weight)
-
- def head_reshape(self, x):
- K = self.n_head
- D = self.head_dim
- x = x.view(x.size()[:-1] + (K, D)) # B x (M+L) x K x D
- x = x.transpose(1, 2).contiguous() # B x K x (M+L) x D
- x = x.view(-1, x.size(-2), x.size(-1)) # B_K x (M+L) x D
- return x
-
- def forward(self, query, key, value, key_pe):
- B = query.size(0)
- K = self.n_head
- D = self.head_dim
- M = query.size(1)
-
- query = self.proj_query(query)
- query = self.head_reshape(query)
- value = self.proj_val(value)
- value = self.head_reshape(value)
- key = self.proj_key(key)
- key = self.head_reshape(key)
-
- out = self.attn(query, key, value, key_pe) # B_K x M x D
- out = out.view(B, K, M, D) # B x K x M x D
- out = out.transpose(1, 2).contiguous() # B x M x K x D
- out = out.view(B, M, -1) # B x M x K_D
- out = self.proj_out(out)
- return out
-
-
-class FeedForwardLayer(nn.Module):
- def __init__(self, d_model, d_inner, dropout, **kargs):
- nn.Module.__init__(self)
- self.fc1 = nn.Linear(d_model, d_inner)
- self.fc2 = nn.Linear(d_inner, d_model)
- nn.init.xavier_uniform_(self.fc1.weight)
- nn.init.xavier_uniform_(self.fc2.weight)
- self.dropout = nn.Dropout(dropout)
-
- def forward(self, h):
- h1 = F.relu(self.fc1(h))
- h1 = self.dropout(h1)
- h2 = self.fc2(h1)
- return h2
-
-
-class TransformerSeqLayer(nn.Module):
- def __init__(self, d_model, **kargs):
- nn.Module.__init__(self)
- self.attn = MultiHeadSeqAttention(d_model=d_model, **kargs)
- self.norm1 = LayerNorm(d_model)
- self.ff = FeedForwardLayer(d_model=d_model, **kargs)
- self.norm2 = LayerNorm(d_model)
-
- def forward(self, h, h_cache, key_pe):
- # h = B x M x H
- # h_cache = B x L x H
- h_all = torch.cat([h_cache, h], dim=1) # B x (M+L) x H
- attn_out = self.attn(h, h_all, h_all, key_pe)
- h = self.norm1(h + attn_out) # B x M x H
- if self.ff is not None:
- ff_out = self.ff(h)
- out = self.norm2(h + ff_out) # B x M x H
- else:
- out = h
- return out
-
- def get_cache_size(self):
- return self.attn.attn.get_cache_size()
-
-
-class TransformerSeq(nn.Module):
- def __init__(
- self,
- vocab_size,
- d_model,
- n_head,
- n_layer,
- attn_span,
- emb_dropout,
- aux_loss_scaler,
- adapt_span_layer,
- **kargs
- ):
- nn.Module.__init__(self)
- # token embeddings
- self.in_emb = nn.Embedding(vocab_size, d_model)
- nn.init.normal_(self.in_emb.weight, mean=0, std=d_model ** -0.5)
- self.out_emb = nn.Linear(d_model, vocab_size)
- self.aux_loss_scaler = aux_loss_scaler
- if emb_dropout > 0:
- self.emb_dropout = nn.Dropout(emb_dropout)
- else:
- self.emb_dropout = None
- # position embeddings
- self.key_pe = nn.Parameter(torch.randn(1, d_model // n_head, attn_span))
-
- self.layers = nn.ModuleList()
- self.layers.extend(
- TransformerSeqLayer(
- d_model=d_model,
- n_head=n_head,
- attn_span=attn_span,
- adapt_span_layer=adapt_span_layer,
- **kargs
- )
- for _ in range(n_layer)
- )
-
- def forward(self, x, h_cache, target=None):
- # x size = B x M
- block_size = x.size(1)
- h = self.in_emb(x) # B x M x H
- if self.emb_dropout is not None:
- h = self.emb_dropout(h)
-
- h_cache_next = []
- for l, layer in enumerate(self.layers):
- cache_size = layer.attn.attn.get_cache_size()
- if cache_size > block_size:
- h_cache_next_l = torch.cat(
- [h_cache[l][:, -cache_size + block_size :, :], h], dim=1
- ).detach()
- else:
- h_cache_next_l = h[:, -cache_size:, :].detach()
- h_cache_next.append(h_cache_next_l)
- h = layer(h, h_cache[l], self.key_pe) # B x M x H
-
- if self.emb_dropout is not None:
- h = self.emb_dropout(h)
-
- out = F.log_softmax(self.out_emb(h).float(), dim=-1).type_as(h)
- dummy_loss = None
-
- return out, h_cache_next, dummy_loss
-
- def get_aux_loss(self):
- loss = 0.0
- for layer in self.layers:
- loss += layer.attn.attn.adaptive_span.get_loss()
- return self.aux_loss_scaler * loss
-
- def get_current_max_span(self):
- max_span = 0.0
- for layer in self.layers:
- max_span = max(
- max_span, layer.attn.attn.adaptive_span.get_current_max_span()
- )
- return max_span
-
- def get_current_avg_span(self):
- avg_span = 0.0
- for layer in self.layers:
- avg_span += layer.attn.attn.adaptive_span.get_current_avg_span()
- return avg_span / len(self.layers)
diff --git a/spaces/OFA-Sys/OFA-vqa/fairseq/examples/truncated_bptt/truncated_bptt_lm_task.py b/spaces/OFA-Sys/OFA-vqa/fairseq/examples/truncated_bptt/truncated_bptt_lm_task.py
deleted file mode 100644
index 02be0e7fb4213b98798c85b79e9046e9990b97fc..0000000000000000000000000000000000000000
--- a/spaces/OFA-Sys/OFA-vqa/fairseq/examples/truncated_bptt/truncated_bptt_lm_task.py
+++ /dev/null
@@ -1,281 +0,0 @@
-# Copyright (c) Facebook, Inc. and its affiliates.
-#
-# This source code is licensed under the MIT license found in the
-# LICENSE file in the root directory of this source tree.
-
-import logging
-import os
-from dataclasses import dataclass, field
-from typing import List, Optional, Tuple
-
-import torch
-from fairseq import utils
-from fairseq.data import (
- Dictionary,
- TokenBlockDataset,
- data_utils,
- iterators,
-)
-from fairseq.dataclass import FairseqDataclass
-from fairseq.distributed import utils as dist_utils
-from fairseq.tasks import FairseqTask, register_task
-from omegaconf import II
-
-
-logger = logging.getLogger(__name__)
-
-
-@dataclass
-class TruncatedBPTTLMConfig(FairseqDataclass):
- data: str = field(default="???", metadata={"help": "path to data directory"})
- tokens_per_sample: int = field(
- default=1024,
- metadata={"help": "max number of tokens per sequence"},
- )
- batch_size: int = II("dataset.batch_size")
- # Some models use *max_target_positions* to know how many positional
- # embeddings to learn. We use II(...) to make it default to
- # *tokens_per_sample*, but in principle there could be more positional
- # embeddings than tokens in a single batch. This may also be irrelevant for
- # custom model implementations.
- max_target_positions: int = II("task.tokens_per_sample")
- # these will be populated automatically if not provided
- data_parallel_rank: Optional[int] = None
- data_parallel_size: Optional[int] = None
-
-
-@register_task("truncated_bptt_lm", dataclass=TruncatedBPTTLMConfig)
-class TruncatedBPTTLMTask(FairseqTask):
- def __init__(self, cfg: TruncatedBPTTLMConfig):
- super().__init__(cfg)
-
- if cfg.data_parallel_rank is None or cfg.data_parallel_size is None:
- if torch.distributed.is_initialized():
- cfg.data_parallel_rank = dist_utils.get_data_parallel_rank()
- cfg.data_parallel_size = dist_utils.get_data_parallel_world_size()
- else:
- cfg.data_parallel_rank = 0
- cfg.data_parallel_size = 1
-
- # load the dictionary
- paths = utils.split_paths(cfg.data)
- assert len(paths) > 0
- self.dictionary = Dictionary.load(os.path.join(paths[0], "dict.txt"))
- logger.info("dictionary: {} types".format(len(self.dictionary)))
-
- def load_dataset(self, split, epoch=1, combine=False, **kwargs):
- """Load a given dataset split (e.g., train, valid, test)"""
-
- # support sharded datasets
- paths = utils.split_paths(self.cfg.data)
- assert len(paths) > 0
- data_path = paths[(epoch - 1) % len(paths)]
- split_path = os.path.join(data_path, split)
-
- # each element of *data* will be a tensorized line from the original
- # text dataset, similar to ``open(split_path).readlines()``
- data = data_utils.load_indexed_dataset(
- split_path, self.dictionary, combine=combine
- )
- if data is None:
- raise FileNotFoundError(
- "Dataset not found: {} ({})".format(split, split_path)
- )
-
- # this is similar to ``data.view(-1).split(tokens_per_sample)``
- data = TokenBlockDataset(
- data,
- data.sizes,
- block_size=self.cfg.tokens_per_sample,
- pad=None, # unused
- eos=None, # unused
- break_mode="none",
- )
-
- self.datasets[split] = TruncatedBPTTDataset(
- data=data,
- bsz_per_shard=self.cfg.batch_size,
- shard_id=self.cfg.data_parallel_rank,
- num_shards=self.cfg.data_parallel_size,
- )
-
- def dataset(self, split):
- return self.datasets[split]
-
- def get_batch_iterator(
- self, dataset, num_workers=0, epoch=1, data_buffer_size=0, **kwargs
- ):
- return iterators.EpochBatchIterator(
- dataset=dataset,
- collate_fn=self._collate_fn,
- num_workers=num_workers,
- epoch=epoch,
- buffer_size=data_buffer_size,
- # we don't use the batching functionality from EpochBatchIterator;
- # instead every item in *dataset* is a whole batch
- batch_sampler=[[i] for i in range(len(dataset))],
- disable_shuffling=True,
- )
-
- def _collate_fn(self, items: List[List[torch.Tensor]]):
- # we don't use fairseq's batching functionality, so we expect a single
- # Tensor of type List[torch.Tensor]
- assert len(items) == 1
-
- # item will have shape B x T (the last batch may have length < T)
- id, item = items[0]
- item = data_utils.collate_tokens(item, pad_idx=self.source_dictionary.pad())
- B, T = item.size()
-
- # shift item one position over and append a padding token for the target
- target = torch.nn.functional.pad(
- item[:, 1:], (0, 1, 0, 0), value=self.target_dictionary.pad()
- )
-
- # fairseq expects batches to have the following structure
- return {
- "id": torch.tensor([id]*item.size(0)),
- "net_input": {
- "src_tokens": item,
- },
- "target": target,
- "nsentences": item.size(0),
- "ntokens": item.numel(),
- }
-
- def build_dataset_for_inference(
- self, src_tokens: List[torch.Tensor], src_lengths: List[int], **kwargs
- ) -> torch.utils.data.Dataset:
- eos = self.source_dictionary.eos()
- dataset = TokenBlockDataset(
- src_tokens,
- src_lengths,
- block_size=None, # ignored for "eos" break mode
- pad=self.source_dictionary.pad(),
- eos=eos,
- break_mode="eos",
- )
-
- class Dataset(torch.utils.data.Dataset):
- def __getitem__(self, i):
- item = dataset[i]
- if item[-1] == eos:
- # remove eos to support generating with a prefix
- item = item[:-1]
- return (i, [item])
-
- def __len__(self):
- return len(dataset)
-
- return Dataset()
-
- def inference_step(
- self, generator, models, sample, prefix_tokens=None, constraints=None
- ):
- with torch.no_grad():
- if constraints is not None:
- raise NotImplementedError
-
- # SequenceGenerator doesn't use *src_tokens* directly, we need to
- # pass the *prefix_tokens* argument instead.
- if prefix_tokens is None and sample["net_input"]["src_tokens"].nelement():
- prefix_tokens = sample["net_input"]["src_tokens"]
-
- # begin generation with the end-of-sentence token
- bos_token = self.source_dictionary.eos()
-
- return generator.generate(
- models, sample, prefix_tokens=prefix_tokens, bos_token=bos_token
- )
-
- def eval_lm_dataloader(
- self,
- dataset,
- max_tokens: Optional[int] = 36000,
- batch_size: Optional[int] = None,
- max_positions: Optional[int] = None,
- num_shards: int = 1,
- shard_id: int = 0,
- num_workers: int = 1,
- data_buffer_size: int = 10,
- context_window: int = 0,
- ):
- if context_window > 0:
- raise NotImplementedError(
- "Transformer-XL doesn't need --context-window, try "
- "--model-overrides '{\"mem_len\":42}' instead "
- )
- return self.get_batch_iterator(
- dataset=dataset,
- max_tokens=max_tokens,
- max_sentences=batch_size,
- max_positions=max_positions,
- ignore_invalid_inputs=True,
- num_shards=num_shards,
- shard_id=shard_id,
- num_workers=num_workers,
- data_buffer_size=data_buffer_size,
- ).next_epoch_itr(shuffle=False)
-
- @property
- def source_dictionary(self):
- return self.dictionary
-
- @property
- def target_dictionary(self):
- return self.dictionary
-
-
-class TruncatedBPTTDataset(torch.utils.data.Dataset):
- def __init__(
- self,
- data: List[torch.Tensor], # ordered list of items
- bsz_per_shard, # number of items processed per GPUs per forward
- shard_id, # current GPU ID
- num_shards, # number of GPUs
- ):
- super().__init__()
- self.data = data
-
- def batchify(data, bsz):
- # Work out how cleanly we can divide the dataset into bsz parts.
- nbatch = data.size(0) // bsz
- # Trim off any extra elements that wouldn't cleanly fit (remainders).
- data = data.narrow(0, 0, nbatch * bsz)
- # Evenly divide the data across the bsz batches.
- data = data.view(bsz, -1).contiguous()
- return data
-
- # total number of sequences processed by all GPUs in each forward pass
- global_batch_size = bsz_per_shard * num_shards
-
- """
- With a 16 item dataset, bsz_per_shard=2 and num_shards=3,
- *indices* might look like:
-
- indices = [[0, 1],
- [2, 3],
- [4, 5],
- [6, 7],
- [8, 9],
- [10, 11]]
-
- The size of the TruncatedBPTTDataset instance will be 2,
- and shard 1 will see items:
-
- [(0, [data[4], data[6]]),
- (1, [data[5], data[7]])]
- """
- indices = batchify(torch.arange(len(data)), global_batch_size)
- assert indices.size(0) == global_batch_size
-
- self.my_indices = indices[
- shard_id * bsz_per_shard : (shard_id + 1) * bsz_per_shard
- ]
- assert self.my_indices.size(0) == bsz_per_shard
-
- def __len__(self):
- return self.my_indices.size(1)
-
- def __getitem__(self, i) -> Tuple[int, List[torch.Tensor]]:
- return (i, [self.data[idx] for idx in self.my_indices[:, i]])
diff --git a/spaces/OFA-Sys/OFA-vqa/fairseq/fairseq/models/nat/levenshtein_utils.py b/spaces/OFA-Sys/OFA-vqa/fairseq/fairseq/models/nat/levenshtein_utils.py
deleted file mode 100644
index 375a98c2e11354de085f0a7926f407bd1a6a2ad4..0000000000000000000000000000000000000000
--- a/spaces/OFA-Sys/OFA-vqa/fairseq/fairseq/models/nat/levenshtein_utils.py
+++ /dev/null
@@ -1,293 +0,0 @@
-# Copyright (c) Facebook, Inc. and its affiliates.
-#
-# This source code is licensed under the MIT license found in the
-# LICENSE file in the root directory of this source tree.
-
-import torch
-from fairseq.utils import new_arange
-
-
-# -------------- Helper Functions --------------------------------------------------- #
-
-
-def load_libnat():
- try:
- from fairseq import libnat_cuda
-
- return libnat_cuda, True
-
- except ImportError as e:
- print(str(e) + "... fall back to CPU version")
-
- try:
- from fairseq import libnat
-
- return libnat, False
-
- except ImportError as e:
- import sys
-
- sys.stderr.write(
- "ERROR: missing libnat_cuda. run `python setup.py build_ext --inplace`\n"
- )
- raise e
-
-
-def _get_ins_targets(in_tokens, out_tokens, padding_idx, unk_idx):
- libnat, use_cuda = load_libnat()
-
- def _get_ins_targets_cuda(in_tokens, out_tokens, padding_idx, unk_idx):
- in_masks = in_tokens.ne(padding_idx)
- out_masks = out_tokens.ne(padding_idx)
- mask_ins_targets, masked_tgt_masks = libnat.generate_insertion_labels(
- out_tokens.int(),
- libnat.levenshtein_distance(
- in_tokens.int(),
- out_tokens.int(),
- in_masks.sum(1).int(),
- out_masks.sum(1).int(),
- ),
- )
- masked_tgt_masks = masked_tgt_masks.bool() & out_masks
- mask_ins_targets = mask_ins_targets.type_as(in_tokens)[
- :, 1 : in_masks.size(1)
- ].masked_fill_(~in_masks[:, 1:], 0)
- masked_tgt_tokens = out_tokens.masked_fill(masked_tgt_masks, unk_idx)
- return masked_tgt_masks, masked_tgt_tokens, mask_ins_targets
-
- def _get_ins_targets_cpu(in_tokens, out_tokens, padding_idx, unk_idx):
- in_seq_len, out_seq_len = in_tokens.size(1), out_tokens.size(1)
-
- in_tokens_list = [
- [t for t in s if t != padding_idx] for i, s in enumerate(in_tokens.tolist())
- ]
- out_tokens_list = [
- [t for t in s if t != padding_idx]
- for i, s in enumerate(out_tokens.tolist())
- ]
-
- full_labels = libnat.suggested_ed2_path(
- in_tokens_list, out_tokens_list, padding_idx
- )
- mask_inputs = [
- [len(c) if c[0] != padding_idx else 0 for c in a[:-1]] for a in full_labels
- ]
-
- # generate labels
- masked_tgt_masks = []
- for mask_input in mask_inputs:
- mask_label = []
- for beam_size in mask_input[1:-1]: # HACK 1:-1
- mask_label += [0] + [1 for _ in range(beam_size)]
- masked_tgt_masks.append(
- mask_label + [0 for _ in range(out_seq_len - len(mask_label))]
- )
- mask_ins_targets = [
- mask_input[1:-1]
- + [0 for _ in range(in_seq_len - 1 - len(mask_input[1:-1]))]
- for mask_input in mask_inputs
- ]
-
- # transform to tensor
- masked_tgt_masks = torch.tensor(
- masked_tgt_masks, device=out_tokens.device
- ).bool()
- mask_ins_targets = torch.tensor(mask_ins_targets, device=in_tokens.device)
- masked_tgt_tokens = out_tokens.masked_fill(masked_tgt_masks, unk_idx)
- return masked_tgt_masks, masked_tgt_tokens, mask_ins_targets
-
- if use_cuda:
- return _get_ins_targets_cuda(in_tokens, out_tokens, padding_idx, unk_idx)
- return _get_ins_targets_cpu(in_tokens, out_tokens, padding_idx, unk_idx)
-
-
-def _get_del_targets(in_tokens, out_tokens, padding_idx):
- libnat, use_cuda = load_libnat()
-
- def _get_del_targets_cuda(in_tokens, out_tokens, padding_idx):
- in_masks = in_tokens.ne(padding_idx)
- out_masks = out_tokens.ne(padding_idx)
-
- word_del_targets = libnat.generate_deletion_labels(
- in_tokens.int(),
- libnat.levenshtein_distance(
- in_tokens.int(),
- out_tokens.int(),
- in_masks.sum(1).int(),
- out_masks.sum(1).int(),
- ),
- )
- word_del_targets = word_del_targets.type_as(in_tokens).masked_fill_(
- ~in_masks, 0
- )
- return word_del_targets
-
- def _get_del_targets_cpu(in_tokens, out_tokens, padding_idx):
- out_seq_len = out_tokens.size(1)
- with torch.cuda.device_of(in_tokens):
- in_tokens_list = [
- [t for t in s if t != padding_idx]
- for i, s in enumerate(in_tokens.tolist())
- ]
- out_tokens_list = [
- [t for t in s if t != padding_idx]
- for i, s in enumerate(out_tokens.tolist())
- ]
-
- full_labels = libnat.suggested_ed2_path(
- in_tokens_list, out_tokens_list, padding_idx
- )
- word_del_targets = [b[-1] for b in full_labels]
- word_del_targets = [
- labels + [0 for _ in range(out_seq_len - len(labels))]
- for labels in word_del_targets
- ]
-
- # transform to tensor
- word_del_targets = torch.tensor(word_del_targets, device=out_tokens.device)
- return word_del_targets
-
- if use_cuda:
- return _get_del_targets_cuda(in_tokens, out_tokens, padding_idx)
- return _get_del_targets_cpu(in_tokens, out_tokens, padding_idx)
-
-
-def _apply_ins_masks(
- in_tokens, in_scores, mask_ins_pred, padding_idx, unk_idx, eos_idx
-):
-
- in_masks = in_tokens.ne(padding_idx)
- in_lengths = in_masks.sum(1)
-
- # HACK: hacky way to shift all the paddings to eos first.
- in_tokens.masked_fill_(~in_masks, eos_idx)
- mask_ins_pred.masked_fill_(~in_masks[:, 1:], 0)
-
- out_lengths = in_lengths + mask_ins_pred.sum(1)
- out_max_len = out_lengths.max()
- out_masks = new_arange(out_lengths, out_max_len)[None, :] < out_lengths[:, None]
-
- reordering = (mask_ins_pred + in_masks[:, 1:].long()).cumsum(1)
- out_tokens = (
- in_tokens.new_zeros(in_tokens.size(0), out_max_len)
- .fill_(padding_idx)
- .masked_fill_(out_masks, unk_idx)
- )
- out_tokens[:, 0] = in_tokens[:, 0]
- out_tokens.scatter_(1, reordering, in_tokens[:, 1:])
-
- out_scores = None
- if in_scores is not None:
- in_scores.masked_fill_(~in_masks, 0)
- out_scores = in_scores.new_zeros(*out_tokens.size())
- out_scores[:, 0] = in_scores[:, 0]
- out_scores.scatter_(1, reordering, in_scores[:, 1:])
-
- return out_tokens, out_scores
-
-
-def _apply_ins_words(in_tokens, in_scores, word_ins_pred, word_ins_scores, unk_idx):
- word_ins_masks = in_tokens.eq(unk_idx)
- out_tokens = in_tokens.masked_scatter(word_ins_masks, word_ins_pred[word_ins_masks])
-
- if in_scores is not None:
- out_scores = in_scores.masked_scatter(
- word_ins_masks, word_ins_scores[word_ins_masks]
- )
- else:
- out_scores = None
-
- return out_tokens, out_scores
-
-
-def _apply_del_words(
- in_tokens, in_scores, in_attn, word_del_pred, padding_idx, bos_idx, eos_idx
-):
- # apply deletion to a tensor
- in_masks = in_tokens.ne(padding_idx)
- bos_eos_masks = in_tokens.eq(bos_idx) | in_tokens.eq(eos_idx)
-
- max_len = in_tokens.size(1)
- word_del_pred.masked_fill_(~in_masks, 1)
- word_del_pred.masked_fill_(bos_eos_masks, 0)
-
- reordering = new_arange(in_tokens).masked_fill_(word_del_pred, max_len).sort(1)[1]
-
- out_tokens = in_tokens.masked_fill(word_del_pred, padding_idx).gather(1, reordering)
-
- out_scores = None
- if in_scores is not None:
- out_scores = in_scores.masked_fill(word_del_pred, 0).gather(1, reordering)
-
- out_attn = None
- if in_attn is not None:
- _mask = word_del_pred[:, :, None].expand_as(in_attn)
- _reordering = reordering[:, :, None].expand_as(in_attn)
- out_attn = in_attn.masked_fill(_mask, 0.0).gather(1, _reordering)
-
- return out_tokens, out_scores, out_attn
-
-
-def _skip(x, mask):
- """
- Getting sliced (dim=0) tensor by mask. Supporting tensor and list/dict of tensors.
- """
- if isinstance(x, int):
- return x
-
- if x is None:
- return None
-
- if isinstance(x, torch.Tensor):
- if x.size(0) == mask.size(0):
- return x[mask]
- elif x.size(1) == mask.size(0):
- return x[:, mask]
-
- if isinstance(x, list):
- return [_skip(x_i, mask) for x_i in x]
-
- if isinstance(x, dict):
- return {k: _skip(v, mask) for k, v in x.items()}
-
- raise NotImplementedError
-
-
-def _skip_encoder_out(encoder, encoder_out, mask):
- if not mask.any():
- return encoder_out
- else:
- return encoder.reorder_encoder_out(
- encoder_out, mask.nonzero(as_tuple=False).squeeze()
- )
-
-
-def _fill(x, mask, y, padding_idx):
- """
- Filling tensor x with y at masked positions (dim=0).
- """
- if x is None:
- return y
- assert x.dim() == y.dim() and mask.size(0) == x.size(0)
- assert x.dim() == 2 or (x.dim() == 3 and x.size(2) == y.size(2))
- n_selected = mask.sum()
- assert n_selected == y.size(0)
-
- if n_selected == x.size(0):
- return y
-
- if x.size(1) < y.size(1):
- dims = [x.size(0), y.size(1) - x.size(1)]
- if x.dim() == 3:
- dims.append(x.size(2))
- x = torch.cat([x, x.new_zeros(*dims).fill_(padding_idx)], 1)
- x[mask] = y
- elif x.size(1) > y.size(1):
- x[mask] = padding_idx
- if x.dim() == 2:
- x[mask, : y.size(1)] = y
- else:
- x[mask, : y.size(1), :] = y
- else:
- x[mask] = y
- return x
diff --git a/spaces/OOlajide/common-nlp-tasks/README.md b/spaces/OOlajide/common-nlp-tasks/README.md
deleted file mode 100644
index cc189ba663a8042cb360f1d9dc4e11dfbfe4e860..0000000000000000000000000000000000000000
--- a/spaces/OOlajide/common-nlp-tasks/README.md
+++ /dev/null
@@ -1,37 +0,0 @@
----
-title: Common Nlp Tasks
-emoji: 👀
-colorFrom: blue
-colorTo: indigo
-sdk: streamlit
-app_file: app.py
-pinned: true
----
-
-# Configuration
-
-`title`: _string_
-Display title for the Space
-
-`emoji`: _string_
-Space emoji (emoji-only character allowed)
-
-`colorFrom`: _string_
-Color for Thumbnail gradient (red, yellow, green, blue, indigo, purple, pink, gray)
-
-`colorTo`: _string_
-Color for Thumbnail gradient (red, yellow, green, blue, indigo, purple, pink, gray)
-
-`sdk`: _string_
-Can be either `gradio` or `streamlit`
-
-`sdk_version` : _string_
-Only applicable for `streamlit` SDK.
-See [doc](https://hf.co/docs/hub/spaces) for more info on supported versions.
-
-`app_file`: _string_
-Path to your main application file (which contains either `gradio` or `streamlit` Python code).
-Path is relative to the root of the repository.
-
-`pinned`: _boolean_
-Whether the Space stays on top of your list.
diff --git a/spaces/Omnibus/MusicGen/audiocraft/models/__init__.py b/spaces/Omnibus/MusicGen/audiocraft/models/__init__.py
deleted file mode 100644
index 92c7a48a200eba455044cd66e0d2c1efe6494f5c..0000000000000000000000000000000000000000
--- a/spaces/Omnibus/MusicGen/audiocraft/models/__init__.py
+++ /dev/null
@@ -1,10 +0,0 @@
-# Copyright (c) Meta Platforms, Inc. and affiliates.
-# All rights reserved.
-#
-# This source code is licensed under the license found in the
-# LICENSE file in the root directory of this source tree.
-
-# flake8: noqa
-from .musicgen import MusicGen
-from .lm import LMModel
-from .encodec import CompressionModel, EncodecModel
diff --git a/spaces/Omnibus/Video-Diffusion-WebUI/video_diffusion/tuneavideo/models/unet_blocks.py b/spaces/Omnibus/Video-Diffusion-WebUI/video_diffusion/tuneavideo/models/unet_blocks.py
deleted file mode 100644
index e48e9320490c7fdccd2f3cb1d28e7f694609beea..0000000000000000000000000000000000000000
--- a/spaces/Omnibus/Video-Diffusion-WebUI/video_diffusion/tuneavideo/models/unet_blocks.py
+++ /dev/null
@@ -1,588 +0,0 @@
-# Adapted from https://github.com/huggingface/diffusers/blob/main/src/diffusers/models/unet_2d_blocks.py
-
-import torch
-from torch import nn
-
-from .attention import Transformer3DModel
-from .resnet import Downsample3D, ResnetBlock3D, Upsample3D
-
-
-def get_down_block(
- down_block_type,
- num_layers,
- in_channels,
- out_channels,
- temb_channels,
- add_downsample,
- resnet_eps,
- resnet_act_fn,
- attn_num_head_channels,
- resnet_groups=None,
- cross_attention_dim=None,
- downsample_padding=None,
- dual_cross_attention=False,
- use_linear_projection=False,
- only_cross_attention=False,
- upcast_attention=False,
- resnet_time_scale_shift="default",
-):
- down_block_type = down_block_type[7:] if down_block_type.startswith("UNetRes") else down_block_type
- if down_block_type == "DownBlock3D":
- return DownBlock3D(
- num_layers=num_layers,
- in_channels=in_channels,
- out_channels=out_channels,
- temb_channels=temb_channels,
- add_downsample=add_downsample,
- resnet_eps=resnet_eps,
- resnet_act_fn=resnet_act_fn,
- resnet_groups=resnet_groups,
- downsample_padding=downsample_padding,
- resnet_time_scale_shift=resnet_time_scale_shift,
- )
- elif down_block_type == "CrossAttnDownBlock3D":
- if cross_attention_dim is None:
- raise ValueError("cross_attention_dim must be specified for CrossAttnDownBlock3D")
- return CrossAttnDownBlock3D(
- num_layers=num_layers,
- in_channels=in_channels,
- out_channels=out_channels,
- temb_channels=temb_channels,
- add_downsample=add_downsample,
- resnet_eps=resnet_eps,
- resnet_act_fn=resnet_act_fn,
- resnet_groups=resnet_groups,
- downsample_padding=downsample_padding,
- cross_attention_dim=cross_attention_dim,
- attn_num_head_channels=attn_num_head_channels,
- dual_cross_attention=dual_cross_attention,
- use_linear_projection=use_linear_projection,
- only_cross_attention=only_cross_attention,
- upcast_attention=upcast_attention,
- resnet_time_scale_shift=resnet_time_scale_shift,
- )
- raise ValueError(f"{down_block_type} does not exist.")
-
-
-def get_up_block(
- up_block_type,
- num_layers,
- in_channels,
- out_channels,
- prev_output_channel,
- temb_channels,
- add_upsample,
- resnet_eps,
- resnet_act_fn,
- attn_num_head_channels,
- resnet_groups=None,
- cross_attention_dim=None,
- dual_cross_attention=False,
- use_linear_projection=False,
- only_cross_attention=False,
- upcast_attention=False,
- resnet_time_scale_shift="default",
-):
- up_block_type = up_block_type[7:] if up_block_type.startswith("UNetRes") else up_block_type
- if up_block_type == "UpBlock3D":
- return UpBlock3D(
- num_layers=num_layers,
- in_channels=in_channels,
- out_channels=out_channels,
- prev_output_channel=prev_output_channel,
- temb_channels=temb_channels,
- add_upsample=add_upsample,
- resnet_eps=resnet_eps,
- resnet_act_fn=resnet_act_fn,
- resnet_groups=resnet_groups,
- resnet_time_scale_shift=resnet_time_scale_shift,
- )
- elif up_block_type == "CrossAttnUpBlock3D":
- if cross_attention_dim is None:
- raise ValueError("cross_attention_dim must be specified for CrossAttnUpBlock3D")
- return CrossAttnUpBlock3D(
- num_layers=num_layers,
- in_channels=in_channels,
- out_channels=out_channels,
- prev_output_channel=prev_output_channel,
- temb_channels=temb_channels,
- add_upsample=add_upsample,
- resnet_eps=resnet_eps,
- resnet_act_fn=resnet_act_fn,
- resnet_groups=resnet_groups,
- cross_attention_dim=cross_attention_dim,
- attn_num_head_channels=attn_num_head_channels,
- dual_cross_attention=dual_cross_attention,
- use_linear_projection=use_linear_projection,
- only_cross_attention=only_cross_attention,
- upcast_attention=upcast_attention,
- resnet_time_scale_shift=resnet_time_scale_shift,
- )
- raise ValueError(f"{up_block_type} does not exist.")
-
-
-class UNetMidBlock3DCrossAttn(nn.Module):
- def __init__(
- self,
- in_channels: int,
- temb_channels: int,
- dropout: float = 0.0,
- num_layers: int = 1,
- resnet_eps: float = 1e-6,
- resnet_time_scale_shift: str = "default",
- resnet_act_fn: str = "swish",
- resnet_groups: int = 32,
- resnet_pre_norm: bool = True,
- attn_num_head_channels=1,
- output_scale_factor=1.0,
- cross_attention_dim=1280,
- dual_cross_attention=False,
- use_linear_projection=False,
- upcast_attention=False,
- ):
- super().__init__()
-
- self.has_cross_attention = True
- self.attn_num_head_channels = attn_num_head_channels
- resnet_groups = resnet_groups if resnet_groups is not None else min(in_channels // 4, 32)
-
- # there is always at least one resnet
- resnets = [
- ResnetBlock3D(
- in_channels=in_channels,
- out_channels=in_channels,
- temb_channels=temb_channels,
- eps=resnet_eps,
- groups=resnet_groups,
- dropout=dropout,
- time_embedding_norm=resnet_time_scale_shift,
- non_linearity=resnet_act_fn,
- output_scale_factor=output_scale_factor,
- pre_norm=resnet_pre_norm,
- )
- ]
- attentions = []
-
- for _ in range(num_layers):
- if dual_cross_attention:
- raise NotImplementedError
- attentions.append(
- Transformer3DModel(
- attn_num_head_channels,
- in_channels // attn_num_head_channels,
- in_channels=in_channels,
- num_layers=1,
- cross_attention_dim=cross_attention_dim,
- norm_num_groups=resnet_groups,
- use_linear_projection=use_linear_projection,
- upcast_attention=upcast_attention,
- )
- )
- resnets.append(
- ResnetBlock3D(
- in_channels=in_channels,
- out_channels=in_channels,
- temb_channels=temb_channels,
- eps=resnet_eps,
- groups=resnet_groups,
- dropout=dropout,
- time_embedding_norm=resnet_time_scale_shift,
- non_linearity=resnet_act_fn,
- output_scale_factor=output_scale_factor,
- pre_norm=resnet_pre_norm,
- )
- )
-
- self.attentions = nn.ModuleList(attentions)
- self.resnets = nn.ModuleList(resnets)
-
- def forward(self, hidden_states, temb=None, encoder_hidden_states=None, attention_mask=None):
- hidden_states = self.resnets[0](hidden_states, temb)
- for attn, resnet in zip(self.attentions, self.resnets[1:]):
- hidden_states = attn(hidden_states, encoder_hidden_states=encoder_hidden_states).sample
- hidden_states = resnet(hidden_states, temb)
-
- return hidden_states
-
-
-class CrossAttnDownBlock3D(nn.Module):
- def __init__(
- self,
- in_channels: int,
- out_channels: int,
- temb_channels: int,
- dropout: float = 0.0,
- num_layers: int = 1,
- resnet_eps: float = 1e-6,
- resnet_time_scale_shift: str = "default",
- resnet_act_fn: str = "swish",
- resnet_groups: int = 32,
- resnet_pre_norm: bool = True,
- attn_num_head_channels=1,
- cross_attention_dim=1280,
- output_scale_factor=1.0,
- downsample_padding=1,
- add_downsample=True,
- dual_cross_attention=False,
- use_linear_projection=False,
- only_cross_attention=False,
- upcast_attention=False,
- ):
- super().__init__()
- resnets = []
- attentions = []
-
- self.has_cross_attention = True
- self.attn_num_head_channels = attn_num_head_channels
-
- for i in range(num_layers):
- in_channels = in_channels if i == 0 else out_channels
- resnets.append(
- ResnetBlock3D(
- in_channels=in_channels,
- out_channels=out_channels,
- temb_channels=temb_channels,
- eps=resnet_eps,
- groups=resnet_groups,
- dropout=dropout,
- time_embedding_norm=resnet_time_scale_shift,
- non_linearity=resnet_act_fn,
- output_scale_factor=output_scale_factor,
- pre_norm=resnet_pre_norm,
- )
- )
- if dual_cross_attention:
- raise NotImplementedError
- attentions.append(
- Transformer3DModel(
- attn_num_head_channels,
- out_channels // attn_num_head_channels,
- in_channels=out_channels,
- num_layers=1,
- cross_attention_dim=cross_attention_dim,
- norm_num_groups=resnet_groups,
- use_linear_projection=use_linear_projection,
- only_cross_attention=only_cross_attention,
- upcast_attention=upcast_attention,
- )
- )
- self.attentions = nn.ModuleList(attentions)
- self.resnets = nn.ModuleList(resnets)
-
- if add_downsample:
- self.downsamplers = nn.ModuleList(
- [
- Downsample3D(
- out_channels, use_conv=True, out_channels=out_channels, padding=downsample_padding, name="op"
- )
- ]
- )
- else:
- self.downsamplers = None
-
- self.gradient_checkpointing = False
-
- def forward(self, hidden_states, temb=None, encoder_hidden_states=None, attention_mask=None):
- output_states = ()
-
- for resnet, attn in zip(self.resnets, self.attentions):
- if self.training and self.gradient_checkpointing:
-
- def create_custom_forward(module, return_dict=None):
- def custom_forward(*inputs):
- if return_dict is not None:
- return module(*inputs, return_dict=return_dict)
- else:
- return module(*inputs)
-
- return custom_forward
-
- hidden_states = torch.utils.checkpoint.checkpoint(create_custom_forward(resnet), hidden_states, temb)
- hidden_states = torch.utils.checkpoint.checkpoint(
- create_custom_forward(attn, return_dict=False),
- hidden_states,
- encoder_hidden_states,
- )[0]
- else:
- hidden_states = resnet(hidden_states, temb)
- hidden_states = attn(hidden_states, encoder_hidden_states=encoder_hidden_states).sample
-
- output_states += (hidden_states,)
-
- if self.downsamplers is not None:
- for downsampler in self.downsamplers:
- hidden_states = downsampler(hidden_states)
-
- output_states += (hidden_states,)
-
- return hidden_states, output_states
-
-
-class DownBlock3D(nn.Module):
- def __init__(
- self,
- in_channels: int,
- out_channels: int,
- temb_channels: int,
- dropout: float = 0.0,
- num_layers: int = 1,
- resnet_eps: float = 1e-6,
- resnet_time_scale_shift: str = "default",
- resnet_act_fn: str = "swish",
- resnet_groups: int = 32,
- resnet_pre_norm: bool = True,
- output_scale_factor=1.0,
- add_downsample=True,
- downsample_padding=1,
- ):
- super().__init__()
- resnets = []
-
- for i in range(num_layers):
- in_channels = in_channels if i == 0 else out_channels
- resnets.append(
- ResnetBlock3D(
- in_channels=in_channels,
- out_channels=out_channels,
- temb_channels=temb_channels,
- eps=resnet_eps,
- groups=resnet_groups,
- dropout=dropout,
- time_embedding_norm=resnet_time_scale_shift,
- non_linearity=resnet_act_fn,
- output_scale_factor=output_scale_factor,
- pre_norm=resnet_pre_norm,
- )
- )
-
- self.resnets = nn.ModuleList(resnets)
-
- if add_downsample:
- self.downsamplers = nn.ModuleList(
- [
- Downsample3D(
- out_channels, use_conv=True, out_channels=out_channels, padding=downsample_padding, name="op"
- )
- ]
- )
- else:
- self.downsamplers = None
-
- self.gradient_checkpointing = False
-
- def forward(self, hidden_states, temb=None):
- output_states = ()
-
- for resnet in self.resnets:
- if self.training and self.gradient_checkpointing:
-
- def create_custom_forward(module):
- def custom_forward(*inputs):
- return module(*inputs)
-
- return custom_forward
-
- hidden_states = torch.utils.checkpoint.checkpoint(create_custom_forward(resnet), hidden_states, temb)
- else:
- hidden_states = resnet(hidden_states, temb)
-
- output_states += (hidden_states,)
-
- if self.downsamplers is not None:
- for downsampler in self.downsamplers:
- hidden_states = downsampler(hidden_states)
-
- output_states += (hidden_states,)
-
- return hidden_states, output_states
-
-
-class CrossAttnUpBlock3D(nn.Module):
- def __init__(
- self,
- in_channels: int,
- out_channels: int,
- prev_output_channel: int,
- temb_channels: int,
- dropout: float = 0.0,
- num_layers: int = 1,
- resnet_eps: float = 1e-6,
- resnet_time_scale_shift: str = "default",
- resnet_act_fn: str = "swish",
- resnet_groups: int = 32,
- resnet_pre_norm: bool = True,
- attn_num_head_channels=1,
- cross_attention_dim=1280,
- output_scale_factor=1.0,
- add_upsample=True,
- dual_cross_attention=False,
- use_linear_projection=False,
- only_cross_attention=False,
- upcast_attention=False,
- ):
- super().__init__()
- resnets = []
- attentions = []
-
- self.has_cross_attention = True
- self.attn_num_head_channels = attn_num_head_channels
-
- for i in range(num_layers):
- res_skip_channels = in_channels if (i == num_layers - 1) else out_channels
- resnet_in_channels = prev_output_channel if i == 0 else out_channels
-
- resnets.append(
- ResnetBlock3D(
- in_channels=resnet_in_channels + res_skip_channels,
- out_channels=out_channels,
- temb_channels=temb_channels,
- eps=resnet_eps,
- groups=resnet_groups,
- dropout=dropout,
- time_embedding_norm=resnet_time_scale_shift,
- non_linearity=resnet_act_fn,
- output_scale_factor=output_scale_factor,
- pre_norm=resnet_pre_norm,
- )
- )
- if dual_cross_attention:
- raise NotImplementedError
- attentions.append(
- Transformer3DModel(
- attn_num_head_channels,
- out_channels // attn_num_head_channels,
- in_channels=out_channels,
- num_layers=1,
- cross_attention_dim=cross_attention_dim,
- norm_num_groups=resnet_groups,
- use_linear_projection=use_linear_projection,
- only_cross_attention=only_cross_attention,
- upcast_attention=upcast_attention,
- )
- )
-
- self.attentions = nn.ModuleList(attentions)
- self.resnets = nn.ModuleList(resnets)
-
- if add_upsample:
- self.upsamplers = nn.ModuleList([Upsample3D(out_channels, use_conv=True, out_channels=out_channels)])
- else:
- self.upsamplers = None
-
- self.gradient_checkpointing = False
-
- def forward(
- self,
- hidden_states,
- res_hidden_states_tuple,
- temb=None,
- encoder_hidden_states=None,
- upsample_size=None,
- attention_mask=None,
- ):
- for resnet, attn in zip(self.resnets, self.attentions):
- # pop res hidden states
- res_hidden_states = res_hidden_states_tuple[-1]
- res_hidden_states_tuple = res_hidden_states_tuple[:-1]
- hidden_states = torch.cat([hidden_states, res_hidden_states], dim=1)
-
- if self.training and self.gradient_checkpointing:
-
- def create_custom_forward(module, return_dict=None):
- def custom_forward(*inputs):
- if return_dict is not None:
- return module(*inputs, return_dict=return_dict)
- else:
- return module(*inputs)
-
- return custom_forward
-
- hidden_states = torch.utils.checkpoint.checkpoint(create_custom_forward(resnet), hidden_states, temb)
- hidden_states = torch.utils.checkpoint.checkpoint(
- create_custom_forward(attn, return_dict=False),
- hidden_states,
- encoder_hidden_states,
- )[0]
- else:
- hidden_states = resnet(hidden_states, temb)
- hidden_states = attn(hidden_states, encoder_hidden_states=encoder_hidden_states).sample
-
- if self.upsamplers is not None:
- for upsampler in self.upsamplers:
- hidden_states = upsampler(hidden_states, upsample_size)
-
- return hidden_states
-
-
-class UpBlock3D(nn.Module):
- def __init__(
- self,
- in_channels: int,
- prev_output_channel: int,
- out_channels: int,
- temb_channels: int,
- dropout: float = 0.0,
- num_layers: int = 1,
- resnet_eps: float = 1e-6,
- resnet_time_scale_shift: str = "default",
- resnet_act_fn: str = "swish",
- resnet_groups: int = 32,
- resnet_pre_norm: bool = True,
- output_scale_factor=1.0,
- add_upsample=True,
- ):
- super().__init__()
- resnets = []
-
- for i in range(num_layers):
- res_skip_channels = in_channels if (i == num_layers - 1) else out_channels
- resnet_in_channels = prev_output_channel if i == 0 else out_channels
-
- resnets.append(
- ResnetBlock3D(
- in_channels=resnet_in_channels + res_skip_channels,
- out_channels=out_channels,
- temb_channels=temb_channels,
- eps=resnet_eps,
- groups=resnet_groups,
- dropout=dropout,
- time_embedding_norm=resnet_time_scale_shift,
- non_linearity=resnet_act_fn,
- output_scale_factor=output_scale_factor,
- pre_norm=resnet_pre_norm,
- )
- )
-
- self.resnets = nn.ModuleList(resnets)
-
- if add_upsample:
- self.upsamplers = nn.ModuleList([Upsample3D(out_channels, use_conv=True, out_channels=out_channels)])
- else:
- self.upsamplers = None
-
- self.gradient_checkpointing = False
-
- def forward(self, hidden_states, res_hidden_states_tuple, temb=None, upsample_size=None):
- for resnet in self.resnets:
- # pop res hidden states
- res_hidden_states = res_hidden_states_tuple[-1]
- res_hidden_states_tuple = res_hidden_states_tuple[:-1]
- hidden_states = torch.cat([hidden_states, res_hidden_states], dim=1)
-
- if self.training and self.gradient_checkpointing:
-
- def create_custom_forward(module):
- def custom_forward(*inputs):
- return module(*inputs)
-
- return custom_forward
-
- hidden_states = torch.utils.checkpoint.checkpoint(create_custom_forward(resnet), hidden_states, temb)
- else:
- hidden_states = resnet(hidden_states, temb)
-
- if self.upsamplers is not None:
- for upsampler in self.upsamplers:
- hidden_states = upsampler(hidden_states, upsample_size)
-
- return hidden_states
diff --git a/spaces/OnabajoMonsurat/Brain_tumor_prediction/README.md b/spaces/OnabajoMonsurat/Brain_tumor_prediction/README.md
deleted file mode 100644
index f44d003b1ccdb811884f577e161d3232d9ed8148..0000000000000000000000000000000000000000
--- a/spaces/OnabajoMonsurat/Brain_tumor_prediction/README.md
+++ /dev/null
@@ -1,13 +0,0 @@
----
-title: Brain Tumor Prediction
-emoji: 🌍
-colorFrom: gray
-colorTo: yellow
-sdk: gradio
-sdk_version: 3.36.1
-app_file: app.py
-pinned: false
-license: mit
----
-
-Check out the configuration reference at https://huggingface.co/docs/hub/spaces-config-reference
diff --git a/spaces/OpenGVLab/InternGPT/third-party/lama/bin/gen_debug_mask_dataset.py b/spaces/OpenGVLab/InternGPT/third-party/lama/bin/gen_debug_mask_dataset.py
deleted file mode 100644
index 738f76875c82aa412063bb5bff15e69c46f20362..0000000000000000000000000000000000000000
--- a/spaces/OpenGVLab/InternGPT/third-party/lama/bin/gen_debug_mask_dataset.py
+++ /dev/null
@@ -1,61 +0,0 @@
-#!/usr/bin/env python3
-
-import glob
-import os
-
-import PIL.Image as Image
-import cv2
-import numpy as np
-import tqdm
-import shutil
-
-
-from saicinpainting.evaluation.utils import load_yaml
-
-
-def generate_masks_for_img(infile, outmask_pattern, mask_size=200, step=0.5):
- inimg = Image.open(infile)
- width, height = inimg.size
- step_abs = int(mask_size * step)
-
- mask = np.zeros((height, width), dtype='uint8')
- mask_i = 0
-
- for start_vertical in range(0, height - step_abs, step_abs):
- for start_horizontal in range(0, width - step_abs, step_abs):
- mask[start_vertical:start_vertical + mask_size, start_horizontal:start_horizontal + mask_size] = 255
-
- cv2.imwrite(outmask_pattern.format(mask_i), mask)
-
- mask[start_vertical:start_vertical + mask_size, start_horizontal:start_horizontal + mask_size] = 0
- mask_i += 1
-
-
-def main(args):
- if not args.indir.endswith('/'):
- args.indir += '/'
- if not args.outdir.endswith('/'):
- args.outdir += '/'
-
- config = load_yaml(args.config)
-
- in_files = list(glob.glob(os.path.join(args.indir, '**', f'*{config.img_ext}'), recursive=True))
- for infile in tqdm.tqdm(in_files):
- outimg = args.outdir + infile[len(args.indir):]
- outmask_pattern = outimg[:-len(config.img_ext)] + '_mask{:04d}.png'
-
- os.makedirs(os.path.dirname(outimg), exist_ok=True)
- shutil.copy2(infile, outimg)
-
- generate_masks_for_img(infile, outmask_pattern, **config.gen_kwargs)
-
-
-if __name__ == '__main__':
- import argparse
-
- aparser = argparse.ArgumentParser()
- aparser.add_argument('config', type=str, help='Path to config for dataset generation')
- aparser.add_argument('indir', type=str, help='Path to folder with images')
- aparser.add_argument('outdir', type=str, help='Path to folder to store aligned images and masks to')
-
- main(aparser.parse_args())
diff --git a/spaces/ParagKesharDas360/MovieRecommadationApp/README.md b/spaces/ParagKesharDas360/MovieRecommadationApp/README.md
deleted file mode 100644
index 9365fef335fd07b1515635ac483ad46b69bd7ab7..0000000000000000000000000000000000000000
--- a/spaces/ParagKesharDas360/MovieRecommadationApp/README.md
+++ /dev/null
@@ -1,12 +0,0 @@
----
-title: MovieRecommadationApp
-emoji: 💩
-colorFrom: indigo
-colorTo: gray
-sdk: streamlit
-sdk_version: 1.19.0
-app_file: app.py
-pinned: false
----
-
-Check out the configuration reference at https://huggingface.co/docs/hub/spaces-config-reference
diff --git a/spaces/Paulraj916/paulraj916/scrapAudio.py b/spaces/Paulraj916/paulraj916/scrapAudio.py
deleted file mode 100644
index c40f24fb4d326a1845a3e43b3a1de3233818ccdb..0000000000000000000000000000000000000000
--- a/spaces/Paulraj916/paulraj916/scrapAudio.py
+++ /dev/null
@@ -1,58 +0,0 @@
-import os
-import requests
-from bs4 import BeautifulSoup
-from urllib.parse import urljoin
-
-class ScrapAudio:
- def __init__(self, url, output_folder):
- self.url = url
- self.output_folder = output_folder
-
- def extract_and_save_audio(self):
- try:
- # Send an HTTP GET request to the webpage and get the HTML content
- response = requests.get(self.url)
- response.raise_for_status()
- html_content = response.text
-
- # Parse the HTML content using BeautifulSoup
- soup = BeautifulSoup(html_content, 'html.parser')
-
- # Find all audio tags
- audio_tags = soup.find_all('audio')
-
- # Extract audio URLs and store them in a list
- audio_urls = []
- for audio_tag in audio_tags:
- if 'src' in audio_tag.attrs:
- audio_url = audio_tag['src']
- absolute_url = urljoin(self.url, audio_url)
- audio_urls.append(absolute_url)
-
- # Create the output folder if it doesn't exist
- os.makedirs(self.output_folder, exist_ok=True)
-
- # Download and save audio files in the output folder
- for audio_url in audio_urls:
- audio_content = requests.get(audio_url).content
-
- # Get the path to the audio file
- path = urljoin(self.url, audio_url).replace(self.url, '').lstrip('/')
- filename = os.path.join(self.output_folder, path)
-
- # Create subdirectories if needed
- os.makedirs(os.path.dirname(filename), exist_ok=True)
-
- # Save the audio content to the file
- with open(filename, 'wb') as file:
- file.write(audio_content)
-
- print(f"Downloaded: {audio_url}")
-
- print("Audio files downloaded and saved successfully.")
- except requests.exceptions.MissingSchema:
- print(f"Skipping download from {self.url} (Invalid URL)")
- except requests.exceptions.RequestException as e:
- print(f"Failed to fetch content from {self.url}: {e}")
- except OSError as e:
- print(f"Failed to save audio files: {e}")
diff --git a/spaces/Pengyey/bingo-chuchu/src/components/tone-selector.tsx b/spaces/Pengyey/bingo-chuchu/src/components/tone-selector.tsx
deleted file mode 100644
index 5c6e464c91f564b895acd121f0a4a79ed9c5c356..0000000000000000000000000000000000000000
--- a/spaces/Pengyey/bingo-chuchu/src/components/tone-selector.tsx
+++ /dev/null
@@ -1,43 +0,0 @@
-import React from 'react'
-import { BingConversationStyle } from '@/lib/bots/bing/types'
-import { cn } from '@/lib/utils'
-
-type ToneItem = {
- type: BingConversationStyle,
- name: string
-}
-
-const ToneList: ToneItem[] = [
- { name: '有创造力', type: BingConversationStyle.Creative },
- { name: '更平衡', type: BingConversationStyle.Balanced },
- { name: '更精确', type: BingConversationStyle.Precise }
-]
-
-interface ToneSelectorProps {
- type: BingConversationStyle | ''
- onChange?: (type: BingConversationStyle) => void
-}
-
-export function ToneSelector({ type, onChange }: ToneSelectorProps) {
- return (
-
-
- 选择对话样式
-
-
-
- {
- ToneList.map(tone => (
-
onChange?.(tone.type)}>
-
-
- ))
- }
-
-
-
- )
-}
diff --git a/spaces/Pinwheel/GLIP-BLIP-Object-Detection-VQA/maskrcnn_benchmark/csrc/vision.cpp b/spaces/Pinwheel/GLIP-BLIP-Object-Detection-VQA/maskrcnn_benchmark/csrc/vision.cpp
deleted file mode 100644
index d7a663718eae9d5615c155fe1e4cf9c5bb0d6d6b..0000000000000000000000000000000000000000
--- a/spaces/Pinwheel/GLIP-BLIP-Object-Detection-VQA/maskrcnn_benchmark/csrc/vision.cpp
+++ /dev/null
@@ -1,27 +0,0 @@
-// Copyright (c) Facebook, Inc. and its affiliates. All Rights Reserved.
-#include "nms.h"
-#include "ml_nms.h"
-#include "ROIAlign.h"
-#include "ROIPool.h"
-#include "SigmoidFocalLoss.h"
-#include "deform_conv.h"
-#include "deform_pool.h"
-
-PYBIND11_MODULE(TORCH_EXTENSION_NAME, m) {
- m.def("nms", &nms, "non-maximum suppression");
- m.def("ml_nms", &ml_nms, "multi-label non-maximum suppression");
- m.def("soft_nms", &soft_nms, "soft non-maximum suppression");
- m.def("roi_align_forward", &ROIAlign_forward, "ROIAlign_forward");
- m.def("roi_align_backward", &ROIAlign_backward, "ROIAlign_backward");
- m.def("roi_pool_forward", &ROIPool_forward, "ROIPool_forward");
- m.def("roi_pool_backward", &ROIPool_backward, "ROIPool_backward");
- m.def("sigmoid_focalloss_forward", &SigmoidFocalLoss_forward, "SigmoidFocalLoss_forward");
- m.def("sigmoid_focalloss_backward", &SigmoidFocalLoss_backward, "SigmoidFocalLoss_backward");
- m.def("deform_conv_forward", &deform_conv_forward, "deform_conv_forward");
- m.def("deform_conv_backward_input", &deform_conv_backward_input, "deform_conv_backward_input");
- m.def("deform_conv_backward_parameters", &deform_conv_backward_parameters, "deform_conv_backward_parameters");
- m.def("modulated_deform_conv_forward", &modulated_deform_conv_forward, "modulated_deform_conv_forward");
- m.def("modulated_deform_conv_backward", &modulated_deform_conv_backward, "modulated_deform_conv_backward");
- m.def("deform_psroi_pooling_forward", &deform_psroi_pooling_forward, "deform_psroi_pooling_forward");
- m.def("deform_psroi_pooling_backward", &deform_psroi_pooling_backward, "deform_psroi_pooling_backward");
-}
diff --git a/spaces/Prathap/summarization/app.py b/spaces/Prathap/summarization/app.py
deleted file mode 100644
index d658cd0fd6877e07b5c50655452cefbc4439ced5..0000000000000000000000000000000000000000
--- a/spaces/Prathap/summarization/app.py
+++ /dev/null
@@ -1,88 +0,0 @@
-
-from transformers import pipeline
-import base64
-import time
-from bs4 import BeautifulSoup
-import requests
-import streamlit as st
-import warnings
-warnings.filterwarnings("ignore")
-
-
-timestr = time.strftime("%Y%m%d-%H%M%S")
-
-st.markdown(' Created by **_Prathap_**. :baby_chick:')
-
-st.title("Automatic text summarization")
-
-@st.cache(allow_output_mutation=True)
-def pipen():
- summarizer = pipeline("summarization")
- return summarizer
-
-
-def text_downloader(raw_text):
- b64 = base64.b64encode(raw_text.encode()).decode()
- new_filename = "new_text_file_{}_.txt".format(timestr)
- st.markdown("#### Download File ###")
- href = f'Click Here!!'
- st.markdown(href,unsafe_allow_html=True)
-
-
-
-url = st.text_input('Paste URL ⤵️')
-
-
-if st.button("Submit"):
- r = requests.get(url)
- soup = BeautifulSoup(r.text, 'html.parser')
- results = soup.find_all(['h1', 'p'])
- text = [result.text for result in results]
- ARTICLE = ' '.join(text)
- max_chunk = 400
- ARTICLE = ARTICLE.replace('.', '.')
- ARTICLE = ARTICLE.replace('?', '?')
- ARTICLE = ARTICLE.replace('!', '!')
-
-
-
-
- sentences = ARTICLE.split('')
- current_chunk = 0
- chunks = []
- for sentence in sentences:
- if len(chunks) == current_chunk + 1:
- if len(chunks[current_chunk]) + len(sentence.split(' '))<= max_chunk:
- chunks[current_chunk].extend(sentence.split(' '))
- else:
- current_chunk += 1
- chunks.append(sentence.split(' '))
- else:
- print(current_chunk)
- chunks.append(sentence.split(' '))
-
- for chunk_id in range(len(chunks)):
- chunks[chunk_id] = ' '.join(chunks[chunk_id])
-
- with st.spinner("Loading the Model into the memory...."):
- model=pipen()
- res = model(chunks, max_length=50, min_length=30, do_sample=False)
- text = ' '.join([summ['summary_text'] for summ in res])
-
- st.write("Success")
- st.write(text)
- text_downloader(text)
-
-
-if st.button("Contact"):
- st.write("Hi there, I'm Prathap 👋. 2+ years Applied Deep Learning experience")
- st.write("✅ [LinkedIn](https://linkedin.com/in/prathapreddyk)")
- st.write(" 📚[Github](https://github.com/Pratap517)")
- st.write(" 📗Analyze Csv files in one step [Click Here](https://data-analyse-prathap.herokuapp.com)")
- st.write(" 😷 Face Mask Detection App [Click Here](https://mask-detection-5a800.firebaseapp.com/)")
-
-
-
-
-
-
\ No newline at end of file
diff --git a/spaces/ProteinDesignLab/protpardelle/ProteinMPNN/helper_scripts/make_fixed_positions_dict.py b/spaces/ProteinDesignLab/protpardelle/ProteinMPNN/helper_scripts/make_fixed_positions_dict.py
deleted file mode 100644
index 176a24f716e868edaa8ac3cf74df63053e6d265c..0000000000000000000000000000000000000000
--- a/spaces/ProteinDesignLab/protpardelle/ProteinMPNN/helper_scripts/make_fixed_positions_dict.py
+++ /dev/null
@@ -1,59 +0,0 @@
-import argparse
-
-def main(args):
- import glob
- import random
- import numpy as np
- import json
- import itertools
-
- with open(args.input_path, 'r') as json_file:
- json_list = list(json_file)
-
- fixed_list = [[int(item) for item in one.split()] for one in args.position_list.split(",")]
- global_designed_chain_list = [str(item) for item in args.chain_list.split()]
- my_dict = {}
-
- if not args.specify_non_fixed:
- for json_str in json_list:
- result = json.loads(json_str)
- all_chain_list = [item[-1:] for item in list(result) if item[:9]=='seq_chain']
- fixed_position_dict = {}
- for i, chain in enumerate(global_designed_chain_list):
- fixed_position_dict[chain] = fixed_list[i]
- for chain in all_chain_list:
- if chain not in global_designed_chain_list:
- fixed_position_dict[chain] = []
- my_dict[result['name']] = fixed_position_dict
- else:
- for json_str in json_list:
- result = json.loads(json_str)
- all_chain_list = [item[-1:] for item in list(result) if item[:9]=='seq_chain']
- fixed_position_dict = {}
- for chain in all_chain_list:
- seq_length = len(result[f'seq_chain_{chain}'])
- all_residue_list = (np.arange(seq_length)+1).tolist()
- if chain not in global_designed_chain_list:
- fixed_position_dict[chain] = all_residue_list
- else:
- idx = np.argwhere(np.array(global_designed_chain_list) == chain)[0][0]
- fixed_position_dict[chain] = list(set(all_residue_list)-set(fixed_list[idx]))
- my_dict[result['name']] = fixed_position_dict
-
- with open(args.output_path, 'w') as f:
- f.write(json.dumps(my_dict) + '\n')
-
- #e.g. output
- #{"5TTA": {"A": [1, 2, 3, 7, 8, 9, 22, 25, 33], "B": []}, "3LIS": {"A": [], "B": []}}
-
-if __name__ == "__main__":
- argparser = argparse.ArgumentParser(formatter_class=argparse.ArgumentDefaultsHelpFormatter)
- argparser.add_argument("--input_path", type=str, help="Path to the parsed PDBs")
- argparser.add_argument("--output_path", type=str, help="Path to the output dictionary")
- argparser.add_argument("--chain_list", type=str, default='', help="List of the chains that need to be fixed")
- argparser.add_argument("--position_list", type=str, default='', help="Position lists, e.g. 11 12 14 18, 1 2 3 4 for first chain and the second chain")
- argparser.add_argument("--specify_non_fixed", action="store_true", default=False, help="Allows specifying just residues that need to be designed (default: false)")
-
- args = argparser.parse_args()
- main(args)
-
diff --git a/spaces/PunPk/AI_FallingAsleepDriving/app.py b/spaces/PunPk/AI_FallingAsleepDriving/app.py
deleted file mode 100644
index e6b7c5b8cd34c424faff179329cfee7d4dfce497..0000000000000000000000000000000000000000
--- a/spaces/PunPk/AI_FallingAsleepDriving/app.py
+++ /dev/null
@@ -1,427 +0,0 @@
-import gradio as gr
-import mediapipe as mp
-import cv2 as cv2
-import cv2 as cv
-import utils
-# Fast Ai
-from fastbook import *
-import pathlib
-temp = pathlib.PosixPath
-
-def conv2(ni, nf): return ConvLayer(ni, nf, stride=2)
-
-class ResBlock(Module):
- def __init__(self, nf):
- self.conv1 = ConvLayer(nf, nf)
- self.conv2 = ConvLayer(nf, nf)
-
- def forward(self, x): return x + self.conv2(self.conv1(x))
-
-def conv_and_res(ni, nf): return nn.Sequential(conv2(ni, nf), ResBlock(nf))
-
-learn_inf_eye = load_learner("Model/eye_data_resnet18_fastai.pkl")
-learn_inf_yawn = load_learner("Model/yawn_data_resnet18_fastai.pkl")
-learn_inf_eye2 = load_learner("Model/Teye_ModelsfromScratch.pkl")
-learn_inf_yawn2 = load_learner("Model/yawn_ModelsfromScratch.pkl")
-
-# Left eyes indices
-LEFT_EYE = [362, 382, 381, 380, 374, 373, 390, 249, 263, 466, 388, 387, 386, 385, 384, 398]
-# Right eyes indices
-RIGHT_EYE = [33, 7, 163, 144, 145, 153, 154, 155, 133, 173, 157, 158, 159, 160, 161, 246]
-mouth = [61, 146, 91, 181, 84, 17, 314, 405, 321, 375, 291, 308, 324, 318, 402, 317, 14, 87, 178, 88, 95, 185, 40, 39, 37, 0, 267, 269, 270, 409, 415, 310, 311, 312, 13, 82, 81, 42, 183, 78]
-mp_drawing = mp.solutions.drawing_utils
-mp_drawing_styles = mp.solutions.drawing_styles
-map_face_mesh = mp.solutions.face_mesh
-FONTS = cv.FONT_HERSHEY_COMPLEX
-
-def euclaideanDistance(point, point1):
- x, y = point
- x1, y1 = point1
- distance = math.sqrt((x1 - x)**2 + (y1 - y)**2)
- return distance
-
-def landmarksDetection(img, results, draw=False):
- img_height, img_width = img.shape[:2]
- # List of (x,y) coordinates
- mesh_coord = [(int(point.x * img_width), int(point.y * img_height)) for point in results.multi_face_landmarks[0].landmark]
- if draw:
- [cv.circle(img, p, 2, (0,255,0), -1) for p in mesh_coord]
-
- # Returning the list of tuples for each landmark
- return mesh_coord
-
-def detecteye(img, landmarks, right_indices, left_indices):
- eye_right_x_min = min([landmarks[idx][0] for idx in RIGHT_EYE])
- eye_right_x_max = max([landmarks[idx][0] for idx in RIGHT_EYE])
- eye_right_y_min = min([landmarks[idx][1] for idx in RIGHT_EYE])
- eye_right_y_max = max([landmarks[idx][1] for idx in RIGHT_EYE])
-
- # Increase width of rectangle
- width_increase = 20
- eye_right_x_min -= width_increase
- eye_right_x_max += width_increase
- eye_right_y_min -= width_increase
- eye_right_y_max += width_increase
- # Draw rectangle around right eye
- cv.rectangle(img, (eye_right_x_min, eye_right_y_min), (eye_right_x_max, eye_right_y_max), (255, 0, 0))
-
- # Left eye
- eye_left_x_min = min([landmarks[idx][0] for idx in LEFT_EYE])
- eye_left_x_max = max([landmarks[idx][0] for idx in LEFT_EYE])
- eye_left_y_min = min([landmarks[idx][1] for idx in LEFT_EYE])
- eye_left_y_max = max([landmarks[idx][1] for idx in LEFT_EYE])
-
- # Increase width of rectangle
- eye_left_x_min -= width_increase
- eye_left_x_max += width_increase
- eye_left_y_min -= width_increase
- eye_left_y_max += width_increase
-
- if eye_right_x_min >= 0 and eye_right_y_min >= 0 and (eye_right_x_max - eye_right_x_min) > 0 and (eye_right_y_max - eye_right_y_min) > 0:
- # Draw rectangle around left eye
- cv.rectangle(img, (eye_right_x_min, eye_right_y_min), (eye_right_x_max, eye_right_y_max), (255, 0, 0))
- eye_right_image = img[eye_right_y_min:eye_right_y_max, eye_right_x_min:eye_right_x_max]
- re_right = learn_inf_eye.predict(eye_right_image)
- print("Eye right:", re_right)
- re_right_m = re_right[0]
- else:
- # Mouth region is not valid, return None or any appropriate value
- re_right_m = None
-
- if eye_left_x_min >= 0 and eye_left_y_min >= 0 and (eye_left_x_max - eye_left_x_min) > 0 and (eye_left_y_max - eye_left_y_min) > 0:
- # Draw rectangle around left eye
- cv.rectangle(img, (eye_left_x_min, eye_left_y_min), (eye_left_x_max, eye_left_y_max), (255, 0, 0))
- # Crop eye regions from the image based on the rectangles
- eye_left_image = img[eye_left_y_min:eye_left_y_max, eye_left_x_min:eye_left_x_max]
-
- re_left = learn_inf_eye.predict(eye_left_image)
- print("Eye left:", re_left)
- re_left_m = re_left[0]
-
- else:
- # Mouth region is not valid, return None or any appropriate value
- re_left_m = None
- return(re_right_m,re_left_m)
-
-def detectYawn(img, landmarks, LIPS):
- # Lips coordinates
- lips_points = [landmarks[idx] for idx in LIPS]
-
- # Find the minimum and maximum x and y coordinates of the lips points
- x_values = [point[0] for point in lips_points]
- y_values = [point[1] for point in lips_points]
- lips_x_min = min(x_values)
- lips_x_max = max(x_values)
- lips_y_min = min(y_values)
- lips_y_max = max(y_values)
-
- # Increase width and height of the rectangle
- width_increase = 25
- height_increase = 20
- lips_x_min -= width_increase
- lips_x_max += width_increase
- lips_y_min -= height_increase
- lips_y_max += height_increase
-
- if lips_x_min >= 0 and lips_y_min >= 0 and (lips_x_max - lips_x_min) > 0 and (lips_y_max - lips_y_min) > 0:
- # Draw rectangle around lips
- cv.rectangle(img, (lips_x_min, lips_y_min), (lips_x_max, lips_y_max), (255, 0, 0))
-
- Yawn_image = img[lips_y_min:lips_y_max, lips_x_min:lips_x_max]
- # Perform prediction on cropped mouth region
- re_yawn = learn_inf_yawn.predict(Yawn_image)
- print("Yawn: ", re_yawn)
- return re_yawn[0]
- else:
- # Mouth region is not valid, return None or any appropriate value
- return None
-
-def detecteye2(img, landmarks, right_indices, left_indices):
- eye_right_x_min = min([landmarks[idx][0] for idx in RIGHT_EYE])
- eye_right_x_max = max([landmarks[idx][0] for idx in RIGHT_EYE])
- eye_right_y_min = min([landmarks[idx][1] for idx in RIGHT_EYE])
- eye_right_y_max = max([landmarks[idx][1] for idx in RIGHT_EYE])
-
- # Increase width of rectangle
- width_increase = 20
- eye_right_x_min -= width_increase
- eye_right_x_max += width_increase
- eye_right_y_min -= width_increase
- eye_right_y_max += width_increase
- # Draw rectangle around right eye
- cv.rectangle(img, (eye_right_x_min, eye_right_y_min), (eye_right_x_max, eye_right_y_max), (255, 0, 0))
-
- # Left eye
- eye_left_x_min = min([landmarks[idx][0] for idx in LEFT_EYE])
- eye_left_x_max = max([landmarks[idx][0] for idx in LEFT_EYE])
- eye_left_y_min = min([landmarks[idx][1] for idx in LEFT_EYE])
- eye_left_y_max = max([landmarks[idx][1] for idx in LEFT_EYE])
-
- # Increase width of rectangle
- eye_left_x_min -= width_increase
- eye_left_x_max += width_increase
- eye_left_y_min -= width_increase
- eye_left_y_max += width_increase
-
- if eye_right_x_min >= 0 and eye_right_y_min >= 0 and (eye_right_x_max - eye_right_x_min) > 0 and (eye_right_y_max - eye_right_y_min) > 0:
- # Draw rectangle around left eye
- cv.rectangle(img, (eye_right_x_min, eye_right_y_min), (eye_right_x_max, eye_right_y_max), (255, 0, 0))
- eye_right_image = img[eye_right_y_min:eye_right_y_max, eye_right_x_min:eye_right_x_max]
- re_right = learn_inf_eye2.predict(eye_right_image)
- print("Eye right:", re_right)
- re_right_m = re_right[0]
- else:
- # Mouth region is not valid, return None or any appropriate value
- re_right_m = None
-
- if eye_left_x_min >= 0 and eye_left_y_min >= 0 and (eye_left_x_max - eye_left_x_min) > 0 and (eye_left_y_max - eye_left_y_min) > 0:
- # Draw rectangle around left eye
- cv.rectangle(img, (eye_left_x_min, eye_left_y_min), (eye_left_x_max, eye_left_y_max), (255, 0, 0))
- # Crop eye regions from the image based on the rectangles
- eye_left_image = img[eye_left_y_min:eye_left_y_max, eye_left_x_min:eye_left_x_max]
-
- re_left = learn_inf_eye2.predict(eye_left_image)
- print("Eye left:", re_left)
- re_left_m = re_left[0]
-
- else:
- # Mouth region is not valid, return None or any appropriate value
- re_left_m = None
- return(re_right_m,re_left_m)
-
-def detectYawn2(img, landmarks, LIPS):
- # Lips coordinates
- lips_points = [landmarks[idx] for idx in LIPS]
-
- # Find the minimum and maximum x and y coordinates of the lips points
- x_values = [point[0] for point in lips_points]
- y_values = [point[1] for point in lips_points]
- lips_x_min = min(x_values)
- lips_x_max = max(x_values)
- lips_y_min = min(y_values)
- lips_y_max = max(y_values)
-
- # Increase width and height of the rectangle
- width_increase = 25
- height_increase = 20
- lips_x_min -= width_increase
- lips_x_max += width_increase
- lips_y_min -= height_increase
- lips_y_max += height_increase
-
- if lips_x_min >= 0 and lips_y_min >= 0 and (lips_x_max - lips_x_min) > 0 and (lips_y_max - lips_y_min) > 0:
- # Draw rectangle around lips
- cv.rectangle(img, (lips_x_min, lips_y_min), (lips_x_max, lips_y_max), (255, 0, 0))
-
- Yawn_image = img[lips_y_min:lips_y_max, lips_x_min:lips_x_max]
- # Perform prediction on cropped mouth region
- re_yawn = learn_inf_yawn2.predict(Yawn_image)
- print("Yawn: ", re_yawn)
- return re_yawn[0]
- else:
- # Mouth region is not valid, return None or any appropriate value
- return None
-
-def apply_media_pipe_detection_image(image):
- with map_face_mesh.FaceMesh(min_detection_confidence=0.5, min_tracking_confidence=0.5) as face_detection:
- # Resize frame
- frame = cv.resize(image, None, fx=1.5, fy=1.5, interpolation=cv.INTER_CUBIC)
- #frame = cropped_frame(frame)
- frame_height, frame_width = frame.shape[:2]
- rgb_frame = cv.cvtColor(frame, cv.COLOR_RGB2BGR)
- results = face_detection.process(rgb_frame)
-
- if not results.multi_face_landmarks:
- return image
-
- frame = image.copy()
- if results.multi_face_landmarks:
- mesh_coords = landmarksDetection(frame, results, False)
- re_right,re_left = detecteye(frame, mesh_coords, RIGHT_EYE, LEFT_EYE)
- re_yawn = detectYawn(frame, mesh_coords,mouth)
- frame = utils.textWithBackground(frame, f'Yawn : {re_yawn}', FONTS, 0.5, (30, 200), bgOpacity=0.45, textThickness=1)
- frame = utils.textWithBackground(frame, f'Eye right : {re_right}', FONTS, 0.5, (30, 100), bgOpacity=0.45, textThickness=1)
- frame = utils.textWithBackground(frame, f'Eye left : {re_left}', FONTS, 0.5, (30, 150), bgOpacity=0.45, textThickness=1)
-
- return frame
-
-def apply_media_pipe_detection_video(video):
- with map_face_mesh.FaceMesh(min_detection_confidence=0.5, min_tracking_confidence=0.5) as face_detection:
- # Resize frame
- frame = cv.resize(video, None, fx=1.5, fy=1.5, interpolation=cv.INTER_CUBIC)
- #frame = cropped_frame(frame)
- frame_height, frame_width = frame.shape[:2]
- rgb_frame = cv.cvtColor(frame, cv.COLOR_RGB2BGR)
- results = face_detection.process(rgb_frame)
-
- if not results.multi_face_landmarks:
- return video
-
- if results.multi_face_landmarks:
- mesh_coords = landmarksDetection(frame, results, False)
- re_right,re_left = detecteye2(frame, mesh_coords, RIGHT_EYE, LEFT_EYE)
- re_yawn = detectYawn2(frame, mesh_coords,mouth)
-
- frame = utils.textWithBackground(frame, f'Eye right : {re_right}', FONTS, 1.0, (30, 100), bgOpacity=0.9, textThickness=2)
- frame = utils.textWithBackground(frame, f'Eye left : {re_left}', FONTS, 1.0, (30, 150), bgOpacity=0.9, textThickness=2)
- frame = utils.textWithBackground(frame, f'Yawn : {re_yawn}', FONTS, 1.0, (30, 200), bgOpacity=0.9, textThickness=2)
- return frame
-
-def process_video(video):
- with map_face_mesh.FaceMesh(min_detection_confidence=0.5, min_tracking_confidence=0.5) as face_detection:
- for frame in video:
- # Resize frame
- frame = cv.resize(frame, None, fx=1.5, fy=1.5, interpolation=cv.INTER_CUBIC)
- frame_height, frame_width = frame.shape[:2]
- rgb_frame = cv.cvtColor(frame, cv.COLOR_RGB2BGR)
- results = face_detection.process(rgb_frame)
-
- if not results.multi_face_landmarks:
- yield frame
- else:
- mesh_coords = landmarksDetection(frame, results, False)
- re_right, re_left = detecteye2(frame, mesh_coords, RIGHT_EYE, LEFT_EYE)
- re_yawn = detectYawn2(frame, mesh_coords, mouth)
- frame = utils.textWithBackground(frame, f'Yawn : {re_yawn}', FONTS, 0.5, (30, 200), bgOpacity=0.45, textThickness=1)
- frame = utils.textWithBackground(frame, f'Eye right : {re_right}', FONTS, 0.5, (30, 100), bgOpacity=0.45, textThickness=1)
- frame = utils.textWithBackground(frame, f'Eye left : {re_left}', FONTS, 0.5, (30, 150), bgOpacity=0.45, textThickness=1)
- yield frame
-
-class FaceProcessing(object):
- def __init__(self, ui_obj):
- self.name = "Face Image Processing"
- self.description = "Call for Face Image and video Processing"
- self.ui_obj = ui_obj
-
- def take_webcam_photo(self, image):
- return image
-
- def take_webcam_video(self, video_frame):
- video_out2 = apply_media_pipe_detection_video(video_frame)
- return video_out2
-
- def mp_webcam_photo(self, image):
- return image
-
- def mp_webcam_image_detection(self, image):
- detection_image = apply_media_pipe_detection_image(image)
- return detection_image
-
- def mp_webcam_video_detection(self, video):
- detection_video = apply_media_pipe_detection_video(video)
- return detection_video
-
- def webcam_stream_update(self, video_frame) :
- video_out = apply_media_pipe_detection_video(video_frame)
- return video_out
-
- def create_ui(self):
- with self.ui_obj:
- gr.Markdown("AI_FallingAsleepDriving with Webcam/Video")
- with gr.Tabs():
- with gr.TabItem("Eye/Yawn detection Image with Webcam"):
- with gr.Row():
- with gr.Column():
- mp_image_in = gr.Image(label="Webcam Image Input", source="webcam")
- with gr.Column():
- mp_photo_action = gr.Button("Take the Photo")
- mp_apply_fm_action = gr.Button("Apply detection Image the Photo")
- gr.Text("Please use it in a well lit place. Because the model may guess wrong.")
- with gr.Row():
- mp_photo_out = gr.Image(label="Webcam Photo Output")
- mp_fm_photo_out = gr.Image(label="Face detection Image Photo Output")
-
- with gr.TabItem("Eye/Yawn detection Import Image"):
- with gr.Row():
- with gr.Column():
- mp_image_in2 = gr.Image(label="Image Input")
- with gr.Column():
- mp_photo_action2 = gr.Button("Take the Photo")
- mp_apply_fm_action2 = gr.Button("Apply detection Image the Photo")
- gr.Text("Please use it in a well lit place. Because the model may guess wrong.")
- with gr.Row():
- mp_photo_out2 = gr.Image(label="Webcam Photo Output")
- mp_fm_photo_out2 = gr.Image(label="Face detection Image Photo Output")
-
- with gr.TabItem("Eye/Yawn detection on Live Webcam Stream"):
- with gr.Row():
- webcam_stream_in = gr.Image(label="Webcam Stream Input",
- source="webcam",
- streaming=True)
- webcam_stream_out = gr.Image(label="Webcam Stream Output")
- webcam_stream_in.change(
- self.webcam_stream_update,
- inputs=webcam_stream_in,
- outputs=webcam_stream_out
- )
- with gr.Row():
- gr.Text("Please use it in a well lit place. Because the model may guess wrong.")
-
- with gr.TabItem("Eye/Yawn detection on Import Video"):
- with gr.Row():
- webcam_video_in2 = gr.Video(label="Import Video Input")
- with gr.Row():
- webcam_video_action2 = gr.Button("Take the Video")
- gr.Text("Please use it in a well lit place. Because the model may guess wrong.")
- with gr.Row():
- webcam_video_out2 = gr.Video(label="Video Output")
-
- webcam_video_action2.click(
- self.take_webcam_video,
- [
- webcam_video_in2
- ],
- [
- webcam_video_out2
- ]
- )
-
-
- mp_photo_action.click(
- self.mp_webcam_photo,
- [
- mp_image_in
- ],
- [
- mp_photo_out
- ]
- )
-
- mp_photo_action2.click(
- self.mp_webcam_photo,
- [
- mp_image_in2
- ],
- [
- mp_photo_out2
- ]
- )
-
- mp_apply_fm_action.click(
- self.mp_webcam_image_detection,
- [
- mp_image_in
- ],
- [
- mp_fm_photo_out
- ]
- )
-
- mp_apply_fm_action2.click(
- self.mp_webcam_image_detection,
- [
- mp_image_in2
- ],
- [
- mp_fm_photo_out2
- ]
- )
-
- def launch_ui(self):
- self.ui_obj.launch()
-
-if __name__ == '__main__':
- my_app = gr.Blocks()
- face_ui = FaceProcessing(my_app)
- face_ui.create_ui()
- face_ui.launch_ui()
\ No newline at end of file
diff --git a/spaces/Purple11/Grounded-Diffusion/ldm/models/diffusion/__init__.py b/spaces/Purple11/Grounded-Diffusion/ldm/models/diffusion/__init__.py
deleted file mode 100644
index e69de29bb2d1d6434b8b29ae775ad8c2e48c5391..0000000000000000000000000000000000000000
diff --git a/spaces/RMXK/RVC_HFF/lib/infer_pack/transforms.py b/spaces/RMXK/RVC_HFF/lib/infer_pack/transforms.py
deleted file mode 100644
index a11f799e023864ff7082c1f49c0cc18351a13b47..0000000000000000000000000000000000000000
--- a/spaces/RMXK/RVC_HFF/lib/infer_pack/transforms.py
+++ /dev/null
@@ -1,209 +0,0 @@
-import torch
-from torch.nn import functional as F
-
-import numpy as np
-
-
-DEFAULT_MIN_BIN_WIDTH = 1e-3
-DEFAULT_MIN_BIN_HEIGHT = 1e-3
-DEFAULT_MIN_DERIVATIVE = 1e-3
-
-
-def piecewise_rational_quadratic_transform(
- inputs,
- unnormalized_widths,
- unnormalized_heights,
- unnormalized_derivatives,
- inverse=False,
- tails=None,
- tail_bound=1.0,
- min_bin_width=DEFAULT_MIN_BIN_WIDTH,
- min_bin_height=DEFAULT_MIN_BIN_HEIGHT,
- min_derivative=DEFAULT_MIN_DERIVATIVE,
-):
- if tails is None:
- spline_fn = rational_quadratic_spline
- spline_kwargs = {}
- else:
- spline_fn = unconstrained_rational_quadratic_spline
- spline_kwargs = {"tails": tails, "tail_bound": tail_bound}
-
- outputs, logabsdet = spline_fn(
- inputs=inputs,
- unnormalized_widths=unnormalized_widths,
- unnormalized_heights=unnormalized_heights,
- unnormalized_derivatives=unnormalized_derivatives,
- inverse=inverse,
- min_bin_width=min_bin_width,
- min_bin_height=min_bin_height,
- min_derivative=min_derivative,
- **spline_kwargs
- )
- return outputs, logabsdet
-
-
-def searchsorted(bin_locations, inputs, eps=1e-6):
- bin_locations[..., -1] += eps
- return torch.sum(inputs[..., None] >= bin_locations, dim=-1) - 1
-
-
-def unconstrained_rational_quadratic_spline(
- inputs,
- unnormalized_widths,
- unnormalized_heights,
- unnormalized_derivatives,
- inverse=False,
- tails="linear",
- tail_bound=1.0,
- min_bin_width=DEFAULT_MIN_BIN_WIDTH,
- min_bin_height=DEFAULT_MIN_BIN_HEIGHT,
- min_derivative=DEFAULT_MIN_DERIVATIVE,
-):
- inside_interval_mask = (inputs >= -tail_bound) & (inputs <= tail_bound)
- outside_interval_mask = ~inside_interval_mask
-
- outputs = torch.zeros_like(inputs)
- logabsdet = torch.zeros_like(inputs)
-
- if tails == "linear":
- unnormalized_derivatives = F.pad(unnormalized_derivatives, pad=(1, 1))
- constant = np.log(np.exp(1 - min_derivative) - 1)
- unnormalized_derivatives[..., 0] = constant
- unnormalized_derivatives[..., -1] = constant
-
- outputs[outside_interval_mask] = inputs[outside_interval_mask]
- logabsdet[outside_interval_mask] = 0
- else:
- raise RuntimeError("{} tails are not implemented.".format(tails))
-
- (
- outputs[inside_interval_mask],
- logabsdet[inside_interval_mask],
- ) = rational_quadratic_spline(
- inputs=inputs[inside_interval_mask],
- unnormalized_widths=unnormalized_widths[inside_interval_mask, :],
- unnormalized_heights=unnormalized_heights[inside_interval_mask, :],
- unnormalized_derivatives=unnormalized_derivatives[inside_interval_mask, :],
- inverse=inverse,
- left=-tail_bound,
- right=tail_bound,
- bottom=-tail_bound,
- top=tail_bound,
- min_bin_width=min_bin_width,
- min_bin_height=min_bin_height,
- min_derivative=min_derivative,
- )
-
- return outputs, logabsdet
-
-
-def rational_quadratic_spline(
- inputs,
- unnormalized_widths,
- unnormalized_heights,
- unnormalized_derivatives,
- inverse=False,
- left=0.0,
- right=1.0,
- bottom=0.0,
- top=1.0,
- min_bin_width=DEFAULT_MIN_BIN_WIDTH,
- min_bin_height=DEFAULT_MIN_BIN_HEIGHT,
- min_derivative=DEFAULT_MIN_DERIVATIVE,
-):
- if torch.min(inputs) < left or torch.max(inputs) > right:
- raise ValueError("Input to a transform is not within its domain")
-
- num_bins = unnormalized_widths.shape[-1]
-
- if min_bin_width * num_bins > 1.0:
- raise ValueError("Minimal bin width too large for the number of bins")
- if min_bin_height * num_bins > 1.0:
- raise ValueError("Minimal bin height too large for the number of bins")
-
- widths = F.softmax(unnormalized_widths, dim=-1)
- widths = min_bin_width + (1 - min_bin_width * num_bins) * widths
- cumwidths = torch.cumsum(widths, dim=-1)
- cumwidths = F.pad(cumwidths, pad=(1, 0), mode="constant", value=0.0)
- cumwidths = (right - left) * cumwidths + left
- cumwidths[..., 0] = left
- cumwidths[..., -1] = right
- widths = cumwidths[..., 1:] - cumwidths[..., :-1]
-
- derivatives = min_derivative + F.softplus(unnormalized_derivatives)
-
- heights = F.softmax(unnormalized_heights, dim=-1)
- heights = min_bin_height + (1 - min_bin_height * num_bins) * heights
- cumheights = torch.cumsum(heights, dim=-1)
- cumheights = F.pad(cumheights, pad=(1, 0), mode="constant", value=0.0)
- cumheights = (top - bottom) * cumheights + bottom
- cumheights[..., 0] = bottom
- cumheights[..., -1] = top
- heights = cumheights[..., 1:] - cumheights[..., :-1]
-
- if inverse:
- bin_idx = searchsorted(cumheights, inputs)[..., None]
- else:
- bin_idx = searchsorted(cumwidths, inputs)[..., None]
-
- input_cumwidths = cumwidths.gather(-1, bin_idx)[..., 0]
- input_bin_widths = widths.gather(-1, bin_idx)[..., 0]
-
- input_cumheights = cumheights.gather(-1, bin_idx)[..., 0]
- delta = heights / widths
- input_delta = delta.gather(-1, bin_idx)[..., 0]
-
- input_derivatives = derivatives.gather(-1, bin_idx)[..., 0]
- input_derivatives_plus_one = derivatives[..., 1:].gather(-1, bin_idx)[..., 0]
-
- input_heights = heights.gather(-1, bin_idx)[..., 0]
-
- if inverse:
- a = (inputs - input_cumheights) * (
- input_derivatives + input_derivatives_plus_one - 2 * input_delta
- ) + input_heights * (input_delta - input_derivatives)
- b = input_heights * input_derivatives - (inputs - input_cumheights) * (
- input_derivatives + input_derivatives_plus_one - 2 * input_delta
- )
- c = -input_delta * (inputs - input_cumheights)
-
- discriminant = b.pow(2) - 4 * a * c
- assert (discriminant >= 0).all()
-
- root = (2 * c) / (-b - torch.sqrt(discriminant))
- outputs = root * input_bin_widths + input_cumwidths
-
- theta_one_minus_theta = root * (1 - root)
- denominator = input_delta + (
- (input_derivatives + input_derivatives_plus_one - 2 * input_delta)
- * theta_one_minus_theta
- )
- derivative_numerator = input_delta.pow(2) * (
- input_derivatives_plus_one * root.pow(2)
- + 2 * input_delta * theta_one_minus_theta
- + input_derivatives * (1 - root).pow(2)
- )
- logabsdet = torch.log(derivative_numerator) - 2 * torch.log(denominator)
-
- return outputs, -logabsdet
- else:
- theta = (inputs - input_cumwidths) / input_bin_widths
- theta_one_minus_theta = theta * (1 - theta)
-
- numerator = input_heights * (
- input_delta * theta.pow(2) + input_derivatives * theta_one_minus_theta
- )
- denominator = input_delta + (
- (input_derivatives + input_derivatives_plus_one - 2 * input_delta)
- * theta_one_minus_theta
- )
- outputs = input_cumheights + numerator / denominator
-
- derivative_numerator = input_delta.pow(2) * (
- input_derivatives_plus_one * theta.pow(2)
- + 2 * input_delta * theta_one_minus_theta
- + input_derivatives * (1 - theta).pow(2)
- )
- logabsdet = torch.log(derivative_numerator) - 2 * torch.log(denominator)
-
- return outputs, logabsdet
diff --git a/spaces/Ramse/TTS_Hindi/config/README.md b/spaces/Ramse/TTS_Hindi/config/README.md
deleted file mode 100644
index e69de29bb2d1d6434b8b29ae775ad8c2e48c5391..0000000000000000000000000000000000000000
diff --git a/spaces/Raspberry-ai/main/.env/lib/python3.11/site-packages/pip/_vendor/cachecontrol/controller.py b/spaces/Raspberry-ai/main/.env/lib/python3.11/site-packages/pip/_vendor/cachecontrol/controller.py
deleted file mode 100644
index 7f23529f1155cd3bbfde335ccdb7fc483b9d2d19..0000000000000000000000000000000000000000
--- a/spaces/Raspberry-ai/main/.env/lib/python3.11/site-packages/pip/_vendor/cachecontrol/controller.py
+++ /dev/null
@@ -1,439 +0,0 @@
-# SPDX-FileCopyrightText: 2015 Eric Larson
-#
-# SPDX-License-Identifier: Apache-2.0
-
-"""
-The httplib2 algorithms ported for use with requests.
-"""
-import logging
-import re
-import calendar
-import time
-from email.utils import parsedate_tz
-
-from pip._vendor.requests.structures import CaseInsensitiveDict
-
-from .cache import DictCache, SeparateBodyBaseCache
-from .serialize import Serializer
-
-
-logger = logging.getLogger(__name__)
-
-URI = re.compile(r"^(([^:/?#]+):)?(//([^/?#]*))?([^?#]*)(\?([^#]*))?(#(.*))?")
-
-PERMANENT_REDIRECT_STATUSES = (301, 308)
-
-
-def parse_uri(uri):
- """Parses a URI using the regex given in Appendix B of RFC 3986.
-
- (scheme, authority, path, query, fragment) = parse_uri(uri)
- """
- groups = URI.match(uri).groups()
- return (groups[1], groups[3], groups[4], groups[6], groups[8])
-
-
-class CacheController(object):
- """An interface to see if request should cached or not."""
-
- def __init__(
- self, cache=None, cache_etags=True, serializer=None, status_codes=None
- ):
- self.cache = DictCache() if cache is None else cache
- self.cache_etags = cache_etags
- self.serializer = serializer or Serializer()
- self.cacheable_status_codes = status_codes or (200, 203, 300, 301, 308)
-
- @classmethod
- def _urlnorm(cls, uri):
- """Normalize the URL to create a safe key for the cache"""
- (scheme, authority, path, query, fragment) = parse_uri(uri)
- if not scheme or not authority:
- raise Exception("Only absolute URIs are allowed. uri = %s" % uri)
-
- scheme = scheme.lower()
- authority = authority.lower()
-
- if not path:
- path = "/"
-
- # Could do syntax based normalization of the URI before
- # computing the digest. See Section 6.2.2 of Std 66.
- request_uri = query and "?".join([path, query]) or path
- defrag_uri = scheme + "://" + authority + request_uri
-
- return defrag_uri
-
- @classmethod
- def cache_url(cls, uri):
- return cls._urlnorm(uri)
-
- def parse_cache_control(self, headers):
- known_directives = {
- # https://tools.ietf.org/html/rfc7234#section-5.2
- "max-age": (int, True),
- "max-stale": (int, False),
- "min-fresh": (int, True),
- "no-cache": (None, False),
- "no-store": (None, False),
- "no-transform": (None, False),
- "only-if-cached": (None, False),
- "must-revalidate": (None, False),
- "public": (None, False),
- "private": (None, False),
- "proxy-revalidate": (None, False),
- "s-maxage": (int, True),
- }
-
- cc_headers = headers.get("cache-control", headers.get("Cache-Control", ""))
-
- retval = {}
-
- for cc_directive in cc_headers.split(","):
- if not cc_directive.strip():
- continue
-
- parts = cc_directive.split("=", 1)
- directive = parts[0].strip()
-
- try:
- typ, required = known_directives[directive]
- except KeyError:
- logger.debug("Ignoring unknown cache-control directive: %s", directive)
- continue
-
- if not typ or not required:
- retval[directive] = None
- if typ:
- try:
- retval[directive] = typ(parts[1].strip())
- except IndexError:
- if required:
- logger.debug(
- "Missing value for cache-control " "directive: %s",
- directive,
- )
- except ValueError:
- logger.debug(
- "Invalid value for cache-control directive " "%s, must be %s",
- directive,
- typ.__name__,
- )
-
- return retval
-
- def cached_request(self, request):
- """
- Return a cached response if it exists in the cache, otherwise
- return False.
- """
- cache_url = self.cache_url(request.url)
- logger.debug('Looking up "%s" in the cache', cache_url)
- cc = self.parse_cache_control(request.headers)
-
- # Bail out if the request insists on fresh data
- if "no-cache" in cc:
- logger.debug('Request header has "no-cache", cache bypassed')
- return False
-
- if "max-age" in cc and cc["max-age"] == 0:
- logger.debug('Request header has "max_age" as 0, cache bypassed')
- return False
-
- # Request allows serving from the cache, let's see if we find something
- cache_data = self.cache.get(cache_url)
- if cache_data is None:
- logger.debug("No cache entry available")
- return False
-
- if isinstance(self.cache, SeparateBodyBaseCache):
- body_file = self.cache.get_body(cache_url)
- else:
- body_file = None
-
- # Check whether it can be deserialized
- resp = self.serializer.loads(request, cache_data, body_file)
- if not resp:
- logger.warning("Cache entry deserialization failed, entry ignored")
- return False
-
- # If we have a cached permanent redirect, return it immediately. We
- # don't need to test our response for other headers b/c it is
- # intrinsically "cacheable" as it is Permanent.
- #
- # See:
- # https://tools.ietf.org/html/rfc7231#section-6.4.2
- #
- # Client can try to refresh the value by repeating the request
- # with cache busting headers as usual (ie no-cache).
- if int(resp.status) in PERMANENT_REDIRECT_STATUSES:
- msg = (
- "Returning cached permanent redirect response "
- "(ignoring date and etag information)"
- )
- logger.debug(msg)
- return resp
-
- headers = CaseInsensitiveDict(resp.headers)
- if not headers or "date" not in headers:
- if "etag" not in headers:
- # Without date or etag, the cached response can never be used
- # and should be deleted.
- logger.debug("Purging cached response: no date or etag")
- self.cache.delete(cache_url)
- logger.debug("Ignoring cached response: no date")
- return False
-
- now = time.time()
- date = calendar.timegm(parsedate_tz(headers["date"]))
- current_age = max(0, now - date)
- logger.debug("Current age based on date: %i", current_age)
-
- # TODO: There is an assumption that the result will be a
- # urllib3 response object. This may not be best since we
- # could probably avoid instantiating or constructing the
- # response until we know we need it.
- resp_cc = self.parse_cache_control(headers)
-
- # determine freshness
- freshness_lifetime = 0
-
- # Check the max-age pragma in the cache control header
- if "max-age" in resp_cc:
- freshness_lifetime = resp_cc["max-age"]
- logger.debug("Freshness lifetime from max-age: %i", freshness_lifetime)
-
- # If there isn't a max-age, check for an expires header
- elif "expires" in headers:
- expires = parsedate_tz(headers["expires"])
- if expires is not None:
- expire_time = calendar.timegm(expires) - date
- freshness_lifetime = max(0, expire_time)
- logger.debug("Freshness lifetime from expires: %i", freshness_lifetime)
-
- # Determine if we are setting freshness limit in the
- # request. Note, this overrides what was in the response.
- if "max-age" in cc:
- freshness_lifetime = cc["max-age"]
- logger.debug(
- "Freshness lifetime from request max-age: %i", freshness_lifetime
- )
-
- if "min-fresh" in cc:
- min_fresh = cc["min-fresh"]
- # adjust our current age by our min fresh
- current_age += min_fresh
- logger.debug("Adjusted current age from min-fresh: %i", current_age)
-
- # Return entry if it is fresh enough
- if freshness_lifetime > current_age:
- logger.debug('The response is "fresh", returning cached response')
- logger.debug("%i > %i", freshness_lifetime, current_age)
- return resp
-
- # we're not fresh. If we don't have an Etag, clear it out
- if "etag" not in headers:
- logger.debug('The cached response is "stale" with no etag, purging')
- self.cache.delete(cache_url)
-
- # return the original handler
- return False
-
- def conditional_headers(self, request):
- cache_url = self.cache_url(request.url)
- resp = self.serializer.loads(request, self.cache.get(cache_url))
- new_headers = {}
-
- if resp:
- headers = CaseInsensitiveDict(resp.headers)
-
- if "etag" in headers:
- new_headers["If-None-Match"] = headers["ETag"]
-
- if "last-modified" in headers:
- new_headers["If-Modified-Since"] = headers["Last-Modified"]
-
- return new_headers
-
- def _cache_set(self, cache_url, request, response, body=None, expires_time=None):
- """
- Store the data in the cache.
- """
- if isinstance(self.cache, SeparateBodyBaseCache):
- # We pass in the body separately; just put a placeholder empty
- # string in the metadata.
- self.cache.set(
- cache_url,
- self.serializer.dumps(request, response, b""),
- expires=expires_time,
- )
- self.cache.set_body(cache_url, body)
- else:
- self.cache.set(
- cache_url,
- self.serializer.dumps(request, response, body),
- expires=expires_time,
- )
-
- def cache_response(self, request, response, body=None, status_codes=None):
- """
- Algorithm for caching requests.
-
- This assumes a requests Response object.
- """
- # From httplib2: Don't cache 206's since we aren't going to
- # handle byte range requests
- cacheable_status_codes = status_codes or self.cacheable_status_codes
- if response.status not in cacheable_status_codes:
- logger.debug(
- "Status code %s not in %s", response.status, cacheable_status_codes
- )
- return
-
- response_headers = CaseInsensitiveDict(response.headers)
-
- if "date" in response_headers:
- date = calendar.timegm(parsedate_tz(response_headers["date"]))
- else:
- date = 0
-
- # If we've been given a body, our response has a Content-Length, that
- # Content-Length is valid then we can check to see if the body we've
- # been given matches the expected size, and if it doesn't we'll just
- # skip trying to cache it.
- if (
- body is not None
- and "content-length" in response_headers
- and response_headers["content-length"].isdigit()
- and int(response_headers["content-length"]) != len(body)
- ):
- return
-
- cc_req = self.parse_cache_control(request.headers)
- cc = self.parse_cache_control(response_headers)
-
- cache_url = self.cache_url(request.url)
- logger.debug('Updating cache with response from "%s"', cache_url)
-
- # Delete it from the cache if we happen to have it stored there
- no_store = False
- if "no-store" in cc:
- no_store = True
- logger.debug('Response header has "no-store"')
- if "no-store" in cc_req:
- no_store = True
- logger.debug('Request header has "no-store"')
- if no_store and self.cache.get(cache_url):
- logger.debug('Purging existing cache entry to honor "no-store"')
- self.cache.delete(cache_url)
- if no_store:
- return
-
- # https://tools.ietf.org/html/rfc7234#section-4.1:
- # A Vary header field-value of "*" always fails to match.
- # Storing such a response leads to a deserialization warning
- # during cache lookup and is not allowed to ever be served,
- # so storing it can be avoided.
- if "*" in response_headers.get("vary", ""):
- logger.debug('Response header has "Vary: *"')
- return
-
- # If we've been given an etag, then keep the response
- if self.cache_etags and "etag" in response_headers:
- expires_time = 0
- if response_headers.get("expires"):
- expires = parsedate_tz(response_headers["expires"])
- if expires is not None:
- expires_time = calendar.timegm(expires) - date
-
- expires_time = max(expires_time, 14 * 86400)
-
- logger.debug("etag object cached for {0} seconds".format(expires_time))
- logger.debug("Caching due to etag")
- self._cache_set(cache_url, request, response, body, expires_time)
-
- # Add to the cache any permanent redirects. We do this before looking
- # that the Date headers.
- elif int(response.status) in PERMANENT_REDIRECT_STATUSES:
- logger.debug("Caching permanent redirect")
- self._cache_set(cache_url, request, response, b"")
-
- # Add to the cache if the response headers demand it. If there
- # is no date header then we can't do anything about expiring
- # the cache.
- elif "date" in response_headers:
- date = calendar.timegm(parsedate_tz(response_headers["date"]))
- # cache when there is a max-age > 0
- if "max-age" in cc and cc["max-age"] > 0:
- logger.debug("Caching b/c date exists and max-age > 0")
- expires_time = cc["max-age"]
- self._cache_set(
- cache_url,
- request,
- response,
- body,
- expires_time,
- )
-
- # If the request can expire, it means we should cache it
- # in the meantime.
- elif "expires" in response_headers:
- if response_headers["expires"]:
- expires = parsedate_tz(response_headers["expires"])
- if expires is not None:
- expires_time = calendar.timegm(expires) - date
- else:
- expires_time = None
-
- logger.debug(
- "Caching b/c of expires header. expires in {0} seconds".format(
- expires_time
- )
- )
- self._cache_set(
- cache_url,
- request,
- response,
- body,
- expires_time,
- )
-
- def update_cached_response(self, request, response):
- """On a 304 we will get a new set of headers that we want to
- update our cached value with, assuming we have one.
-
- This should only ever be called when we've sent an ETag and
- gotten a 304 as the response.
- """
- cache_url = self.cache_url(request.url)
-
- cached_response = self.serializer.loads(request, self.cache.get(cache_url))
-
- if not cached_response:
- # we didn't have a cached response
- return response
-
- # Lets update our headers with the headers from the new request:
- # http://tools.ietf.org/html/draft-ietf-httpbis-p4-conditional-26#section-4.1
- #
- # The server isn't supposed to send headers that would make
- # the cached body invalid. But... just in case, we'll be sure
- # to strip out ones we know that might be problmatic due to
- # typical assumptions.
- excluded_headers = ["content-length"]
-
- cached_response.headers.update(
- dict(
- (k, v)
- for k, v in response.headers.items()
- if k.lower() not in excluded_headers
- )
- )
-
- # we want a 200 b/c we have content via the cache
- cached_response.status = 200
-
- # update our cache
- self._cache_set(cache_url, request, cached_response)
-
- return cached_response
diff --git a/spaces/Raspberry-ai/main/.env/lib/python3.11/site-packages/pip/_vendor/requests/certs.py b/spaces/Raspberry-ai/main/.env/lib/python3.11/site-packages/pip/_vendor/requests/certs.py
deleted file mode 100644
index 38696a1fb3419dd810004d5aec9654e5224042ed..0000000000000000000000000000000000000000
--- a/spaces/Raspberry-ai/main/.env/lib/python3.11/site-packages/pip/_vendor/requests/certs.py
+++ /dev/null
@@ -1,24 +0,0 @@
-#!/usr/bin/env python
-
-"""
-requests.certs
-~~~~~~~~~~~~~~
-
-This module returns the preferred default CA certificate bundle. There is
-only one — the one from the certifi package.
-
-If you are packaging Requests, e.g., for a Linux distribution or a managed
-environment, you can change the definition of where() to return a separately
-packaged CA bundle.
-"""
-
-import os
-
-if "_PIP_STANDALONE_CERT" not in os.environ:
- from pip._vendor.certifi import where
-else:
- def where():
- return os.environ["_PIP_STANDALONE_CERT"]
-
-if __name__ == "__main__":
- print(where())
diff --git a/spaces/Rbrq/DeticChatGPT/detic/__init__.py b/spaces/Rbrq/DeticChatGPT/detic/__init__.py
deleted file mode 100644
index 8ffba6afd9bf5e9848c891a855943ede73568c3b..0000000000000000000000000000000000000000
--- a/spaces/Rbrq/DeticChatGPT/detic/__init__.py
+++ /dev/null
@@ -1,19 +0,0 @@
-# Copyright (c) Facebook, Inc. and its affiliates.
-from .modeling.meta_arch import custom_rcnn
-from .modeling.roi_heads import detic_roi_heads
-from .modeling.roi_heads import res5_roi_heads
-from .modeling.backbone import swintransformer
-from .modeling.backbone import timm
-
-
-from .data.datasets import lvis_v1
-from .data.datasets import imagenet
-from .data.datasets import cc
-from .data.datasets import objects365
-from .data.datasets import oid
-from .data.datasets import coco_zeroshot
-
-try:
- from .modeling.meta_arch import d2_deformable_detr
-except:
- pass
\ No newline at end of file
diff --git a/spaces/Realcat/image-matching-webui/hloc/pipelines/CMU/__init__.py b/spaces/Realcat/image-matching-webui/hloc/pipelines/CMU/__init__.py
deleted file mode 100644
index e69de29bb2d1d6434b8b29ae775ad8c2e48c5391..0000000000000000000000000000000000000000
diff --git a/spaces/Redgon/bingo/src/components/ui/tooltip.tsx b/spaces/Redgon/bingo/src/components/ui/tooltip.tsx
deleted file mode 100644
index af1d48beb90dd5ae311796539843700871052cae..0000000000000000000000000000000000000000
--- a/spaces/Redgon/bingo/src/components/ui/tooltip.tsx
+++ /dev/null
@@ -1,30 +0,0 @@
-'use client'
-
-import * as React from 'react'
-import * as TooltipPrimitive from '@radix-ui/react-tooltip'
-
-import { cn } from '@/lib/utils'
-
-const TooltipProvider = TooltipPrimitive.Provider
-
-const Tooltip = TooltipPrimitive.Root
-
-const TooltipTrigger = TooltipPrimitive.Trigger
-
-const TooltipContent = React.forwardRef<
- React.ElementRef,
- React.ComponentPropsWithoutRef
->(({ className, sideOffset = 4, ...props }, ref) => (
-
-))
-TooltipContent.displayName = TooltipPrimitive.Content.displayName
-
-export { Tooltip, TooltipTrigger, TooltipContent, TooltipProvider }
diff --git a/spaces/Robert001/UniControl-Demo/annotator/uniformer/mmcv/image/photometric.py b/spaces/Robert001/UniControl-Demo/annotator/uniformer/mmcv/image/photometric.py
deleted file mode 100644
index 5085d012019c0cbf56f66f421a378278c1a058ae..0000000000000000000000000000000000000000
--- a/spaces/Robert001/UniControl-Demo/annotator/uniformer/mmcv/image/photometric.py
+++ /dev/null
@@ -1,428 +0,0 @@
-# Copyright (c) OpenMMLab. All rights reserved.
-import cv2
-import numpy as np
-
-from ..utils import is_tuple_of
-from .colorspace import bgr2gray, gray2bgr
-
-
-def imnormalize(img, mean, std, to_rgb=True):
- """Normalize an image with mean and std.
-
- Args:
- img (ndarray): Image to be normalized.
- mean (ndarray): The mean to be used for normalize.
- std (ndarray): The std to be used for normalize.
- to_rgb (bool): Whether to convert to rgb.
-
- Returns:
- ndarray: The normalized image.
- """
- img = img.copy().astype(np.float32)
- return imnormalize_(img, mean, std, to_rgb)
-
-
-def imnormalize_(img, mean, std, to_rgb=True):
- """Inplace normalize an image with mean and std.
-
- Args:
- img (ndarray): Image to be normalized.
- mean (ndarray): The mean to be used for normalize.
- std (ndarray): The std to be used for normalize.
- to_rgb (bool): Whether to convert to rgb.
-
- Returns:
- ndarray: The normalized image.
- """
- # cv2 inplace normalization does not accept uint8
- assert img.dtype != np.uint8
- mean = np.float64(mean.reshape(1, -1))
- stdinv = 1 / np.float64(std.reshape(1, -1))
- if to_rgb:
- cv2.cvtColor(img, cv2.COLOR_BGR2RGB, img) # inplace
- cv2.subtract(img, mean, img) # inplace
- cv2.multiply(img, stdinv, img) # inplace
- return img
-
-
-def imdenormalize(img, mean, std, to_bgr=True):
- assert img.dtype != np.uint8
- mean = mean.reshape(1, -1).astype(np.float64)
- std = std.reshape(1, -1).astype(np.float64)
- img = cv2.multiply(img, std) # make a copy
- cv2.add(img, mean, img) # inplace
- if to_bgr:
- cv2.cvtColor(img, cv2.COLOR_RGB2BGR, img) # inplace
- return img
-
-
-def iminvert(img):
- """Invert (negate) an image.
-
- Args:
- img (ndarray): Image to be inverted.
-
- Returns:
- ndarray: The inverted image.
- """
- return np.full_like(img, 255) - img
-
-
-def solarize(img, thr=128):
- """Solarize an image (invert all pixel values above a threshold)
-
- Args:
- img (ndarray): Image to be solarized.
- thr (int): Threshold for solarizing (0 - 255).
-
- Returns:
- ndarray: The solarized image.
- """
- img = np.where(img < thr, img, 255 - img)
- return img
-
-
-def posterize(img, bits):
- """Posterize an image (reduce the number of bits for each color channel)
-
- Args:
- img (ndarray): Image to be posterized.
- bits (int): Number of bits (1 to 8) to use for posterizing.
-
- Returns:
- ndarray: The posterized image.
- """
- shift = 8 - bits
- img = np.left_shift(np.right_shift(img, shift), shift)
- return img
-
-
-def adjust_color(img, alpha=1, beta=None, gamma=0):
- r"""It blends the source image and its gray image:
-
- .. math::
- output = img * alpha + gray\_img * beta + gamma
-
- Args:
- img (ndarray): The input source image.
- alpha (int | float): Weight for the source image. Default 1.
- beta (int | float): Weight for the converted gray image.
- If None, it's assigned the value (1 - `alpha`).
- gamma (int | float): Scalar added to each sum.
- Same as :func:`cv2.addWeighted`. Default 0.
-
- Returns:
- ndarray: Colored image which has the same size and dtype as input.
- """
- gray_img = bgr2gray(img)
- gray_img = np.tile(gray_img[..., None], [1, 1, 3])
- if beta is None:
- beta = 1 - alpha
- colored_img = cv2.addWeighted(img, alpha, gray_img, beta, gamma)
- if not colored_img.dtype == np.uint8:
- # Note when the dtype of `img` is not the default `np.uint8`
- # (e.g. np.float32), the value in `colored_img` got from cv2
- # is not guaranteed to be in range [0, 255], so here clip
- # is needed.
- colored_img = np.clip(colored_img, 0, 255)
- return colored_img
-
-
-def imequalize(img):
- """Equalize the image histogram.
-
- This function applies a non-linear mapping to the input image,
- in order to create a uniform distribution of grayscale values
- in the output image.
-
- Args:
- img (ndarray): Image to be equalized.
-
- Returns:
- ndarray: The equalized image.
- """
-
- def _scale_channel(im, c):
- """Scale the data in the corresponding channel."""
- im = im[:, :, c]
- # Compute the histogram of the image channel.
- histo = np.histogram(im, 256, (0, 255))[0]
- # For computing the step, filter out the nonzeros.
- nonzero_histo = histo[histo > 0]
- step = (np.sum(nonzero_histo) - nonzero_histo[-1]) // 255
- if not step:
- lut = np.array(range(256))
- else:
- # Compute the cumulative sum, shifted by step // 2
- # and then normalized by step.
- lut = (np.cumsum(histo) + (step // 2)) // step
- # Shift lut, prepending with 0.
- lut = np.concatenate([[0], lut[:-1]], 0)
- # handle potential integer overflow
- lut[lut > 255] = 255
- # If step is zero, return the original image.
- # Otherwise, index from lut.
- return np.where(np.equal(step, 0), im, lut[im])
-
- # Scales each channel independently and then stacks
- # the result.
- s1 = _scale_channel(img, 0)
- s2 = _scale_channel(img, 1)
- s3 = _scale_channel(img, 2)
- equalized_img = np.stack([s1, s2, s3], axis=-1)
- return equalized_img.astype(img.dtype)
-
-
-def adjust_brightness(img, factor=1.):
- """Adjust image brightness.
-
- This function controls the brightness of an image. An
- enhancement factor of 0.0 gives a black image.
- A factor of 1.0 gives the original image. This function
- blends the source image and the degenerated black image:
-
- .. math::
- output = img * factor + degenerated * (1 - factor)
-
- Args:
- img (ndarray): Image to be brightened.
- factor (float): A value controls the enhancement.
- Factor 1.0 returns the original image, lower
- factors mean less color (brightness, contrast,
- etc), and higher values more. Default 1.
-
- Returns:
- ndarray: The brightened image.
- """
- degenerated = np.zeros_like(img)
- # Note manually convert the dtype to np.float32, to
- # achieve as close results as PIL.ImageEnhance.Brightness.
- # Set beta=1-factor, and gamma=0
- brightened_img = cv2.addWeighted(
- img.astype(np.float32), factor, degenerated.astype(np.float32),
- 1 - factor, 0)
- brightened_img = np.clip(brightened_img, 0, 255)
- return brightened_img.astype(img.dtype)
-
-
-def adjust_contrast(img, factor=1.):
- """Adjust image contrast.
-
- This function controls the contrast of an image. An
- enhancement factor of 0.0 gives a solid grey
- image. A factor of 1.0 gives the original image. It
- blends the source image and the degenerated mean image:
-
- .. math::
- output = img * factor + degenerated * (1 - factor)
-
- Args:
- img (ndarray): Image to be contrasted. BGR order.
- factor (float): Same as :func:`mmcv.adjust_brightness`.
-
- Returns:
- ndarray: The contrasted image.
- """
- gray_img = bgr2gray(img)
- hist = np.histogram(gray_img, 256, (0, 255))[0]
- mean = round(np.sum(gray_img) / np.sum(hist))
- degenerated = (np.ones_like(img[..., 0]) * mean).astype(img.dtype)
- degenerated = gray2bgr(degenerated)
- contrasted_img = cv2.addWeighted(
- img.astype(np.float32), factor, degenerated.astype(np.float32),
- 1 - factor, 0)
- contrasted_img = np.clip(contrasted_img, 0, 255)
- return contrasted_img.astype(img.dtype)
-
-
-def auto_contrast(img, cutoff=0):
- """Auto adjust image contrast.
-
- This function maximize (normalize) image contrast by first removing cutoff
- percent of the lightest and darkest pixels from the histogram and remapping
- the image so that the darkest pixel becomes black (0), and the lightest
- becomes white (255).
-
- Args:
- img (ndarray): Image to be contrasted. BGR order.
- cutoff (int | float | tuple): The cutoff percent of the lightest and
- darkest pixels to be removed. If given as tuple, it shall be
- (low, high). Otherwise, the single value will be used for both.
- Defaults to 0.
-
- Returns:
- ndarray: The contrasted image.
- """
-
- def _auto_contrast_channel(im, c, cutoff):
- im = im[:, :, c]
- # Compute the histogram of the image channel.
- histo = np.histogram(im, 256, (0, 255))[0]
- # Remove cut-off percent pixels from histo
- histo_sum = np.cumsum(histo)
- cut_low = histo_sum[-1] * cutoff[0] // 100
- cut_high = histo_sum[-1] - histo_sum[-1] * cutoff[1] // 100
- histo_sum = np.clip(histo_sum, cut_low, cut_high) - cut_low
- histo = np.concatenate([[histo_sum[0]], np.diff(histo_sum)], 0)
-
- # Compute mapping
- low, high = np.nonzero(histo)[0][0], np.nonzero(histo)[0][-1]
- # If all the values have been cut off, return the origin img
- if low >= high:
- return im
- scale = 255.0 / (high - low)
- offset = -low * scale
- lut = np.array(range(256))
- lut = lut * scale + offset
- lut = np.clip(lut, 0, 255)
- return lut[im]
-
- if isinstance(cutoff, (int, float)):
- cutoff = (cutoff, cutoff)
- else:
- assert isinstance(cutoff, tuple), 'cutoff must be of type int, ' \
- f'float or tuple, but got {type(cutoff)} instead.'
- # Auto adjusts contrast for each channel independently and then stacks
- # the result.
- s1 = _auto_contrast_channel(img, 0, cutoff)
- s2 = _auto_contrast_channel(img, 1, cutoff)
- s3 = _auto_contrast_channel(img, 2, cutoff)
- contrasted_img = np.stack([s1, s2, s3], axis=-1)
- return contrasted_img.astype(img.dtype)
-
-
-def adjust_sharpness(img, factor=1., kernel=None):
- """Adjust image sharpness.
-
- This function controls the sharpness of an image. An
- enhancement factor of 0.0 gives a blurred image. A
- factor of 1.0 gives the original image. And a factor
- of 2.0 gives a sharpened image. It blends the source
- image and the degenerated mean image:
-
- .. math::
- output = img * factor + degenerated * (1 - factor)
-
- Args:
- img (ndarray): Image to be sharpened. BGR order.
- factor (float): Same as :func:`mmcv.adjust_brightness`.
- kernel (np.ndarray, optional): Filter kernel to be applied on the img
- to obtain the degenerated img. Defaults to None.
-
- Note:
- No value sanity check is enforced on the kernel set by users. So with
- an inappropriate kernel, the ``adjust_sharpness`` may fail to perform
- the function its name indicates but end up performing whatever
- transform determined by the kernel.
-
- Returns:
- ndarray: The sharpened image.
- """
-
- if kernel is None:
- # adopted from PIL.ImageFilter.SMOOTH
- kernel = np.array([[1., 1., 1.], [1., 5., 1.], [1., 1., 1.]]) / 13
- assert isinstance(kernel, np.ndarray), \
- f'kernel must be of type np.ndarray, but got {type(kernel)} instead.'
- assert kernel.ndim == 2, \
- f'kernel must have a dimension of 2, but got {kernel.ndim} instead.'
-
- degenerated = cv2.filter2D(img, -1, kernel)
- sharpened_img = cv2.addWeighted(
- img.astype(np.float32), factor, degenerated.astype(np.float32),
- 1 - factor, 0)
- sharpened_img = np.clip(sharpened_img, 0, 255)
- return sharpened_img.astype(img.dtype)
-
-
-def adjust_lighting(img, eigval, eigvec, alphastd=0.1, to_rgb=True):
- """AlexNet-style PCA jitter.
-
- This data augmentation is proposed in `ImageNet Classification with Deep
- Convolutional Neural Networks
- `_.
-
- Args:
- img (ndarray): Image to be adjusted lighting. BGR order.
- eigval (ndarray): the eigenvalue of the convariance matrix of pixel
- values, respectively.
- eigvec (ndarray): the eigenvector of the convariance matrix of pixel
- values, respectively.
- alphastd (float): The standard deviation for distribution of alpha.
- Defaults to 0.1
- to_rgb (bool): Whether to convert img to rgb.
-
- Returns:
- ndarray: The adjusted image.
- """
- assert isinstance(eigval, np.ndarray) and isinstance(eigvec, np.ndarray), \
- f'eigval and eigvec should both be of type np.ndarray, got ' \
- f'{type(eigval)} and {type(eigvec)} instead.'
-
- assert eigval.ndim == 1 and eigvec.ndim == 2
- assert eigvec.shape == (3, eigval.shape[0])
- n_eigval = eigval.shape[0]
- assert isinstance(alphastd, float), 'alphastd should be of type float, ' \
- f'got {type(alphastd)} instead.'
-
- img = img.copy().astype(np.float32)
- if to_rgb:
- cv2.cvtColor(img, cv2.COLOR_BGR2RGB, img) # inplace
-
- alpha = np.random.normal(0, alphastd, n_eigval)
- alter = eigvec \
- * np.broadcast_to(alpha.reshape(1, n_eigval), (3, n_eigval)) \
- * np.broadcast_to(eigval.reshape(1, n_eigval), (3, n_eigval))
- alter = np.broadcast_to(alter.sum(axis=1).reshape(1, 1, 3), img.shape)
- img_adjusted = img + alter
- return img_adjusted
-
-
-def lut_transform(img, lut_table):
- """Transform array by look-up table.
-
- The function lut_transform fills the output array with values from the
- look-up table. Indices of the entries are taken from the input array.
-
- Args:
- img (ndarray): Image to be transformed.
- lut_table (ndarray): look-up table of 256 elements; in case of
- multi-channel input array, the table should either have a single
- channel (in this case the same table is used for all channels) or
- the same number of channels as in the input array.
-
- Returns:
- ndarray: The transformed image.
- """
- assert isinstance(img, np.ndarray)
- assert 0 <= np.min(img) and np.max(img) <= 255
- assert isinstance(lut_table, np.ndarray)
- assert lut_table.shape == (256, )
-
- return cv2.LUT(np.array(img, dtype=np.uint8), lut_table)
-
-
-def clahe(img, clip_limit=40.0, tile_grid_size=(8, 8)):
- """Use CLAHE method to process the image.
-
- See `ZUIDERVELD,K. Contrast Limited Adaptive Histogram Equalization[J].
- Graphics Gems, 1994:474-485.` for more information.
-
- Args:
- img (ndarray): Image to be processed.
- clip_limit (float): Threshold for contrast limiting. Default: 40.0.
- tile_grid_size (tuple[int]): Size of grid for histogram equalization.
- Input image will be divided into equally sized rectangular tiles.
- It defines the number of tiles in row and column. Default: (8, 8).
-
- Returns:
- ndarray: The processed image.
- """
- assert isinstance(img, np.ndarray)
- assert img.ndim == 2
- assert isinstance(clip_limit, (float, int))
- assert is_tuple_of(tile_grid_size, int)
- assert len(tile_grid_size) == 2
-
- clahe = cv2.createCLAHE(clip_limit, tile_grid_size)
- return clahe.apply(np.array(img, dtype=np.uint8))
diff --git a/spaces/Rongjiehuang/ProDiff/data_gen/tts/base_binarizer.py b/spaces/Rongjiehuang/ProDiff/data_gen/tts/base_binarizer.py
deleted file mode 100644
index b30a20c1cdc3403214ff527d68a50806befafeb9..0000000000000000000000000000000000000000
--- a/spaces/Rongjiehuang/ProDiff/data_gen/tts/base_binarizer.py
+++ /dev/null
@@ -1,224 +0,0 @@
-import os
-os.environ["OMP_NUM_THREADS"] = "1"
-
-from utils.multiprocess_utils import chunked_multiprocess_run
-import random
-import traceback
-import json
-from resemblyzer import VoiceEncoder
-from tqdm import tqdm
-from data_gen.tts.data_gen_utils import get_mel2ph, get_pitch, build_phone_encoder
-from utils.hparams import set_hparams, hparams
-import numpy as np
-from utils.indexed_datasets import IndexedDatasetBuilder
-from vocoders.base_vocoder import VOCODERS
-import pandas as pd
-
-
-class BinarizationError(Exception):
- pass
-
-
-class BaseBinarizer:
- def __init__(self, processed_data_dir=None):
- if processed_data_dir is None:
- processed_data_dir = hparams['processed_data_dir']
- self.processed_data_dirs = processed_data_dir.split(",")
- self.binarization_args = hparams['binarization_args']
- self.pre_align_args = hparams['pre_align_args']
- self.forced_align = self.pre_align_args['forced_align']
- tg_dir = None
- if self.forced_align == 'mfa':
- tg_dir = 'mfa_outputs'
- if self.forced_align == 'kaldi':
- tg_dir = 'kaldi_outputs'
- self.item2txt = {}
- self.item2ph = {}
- self.item2wavfn = {}
- self.item2tgfn = {}
- self.item2spk = {}
- for ds_id, processed_data_dir in enumerate(self.processed_data_dirs):
- self.meta_df = pd.read_csv(f"{processed_data_dir}/metadata_phone.csv", dtype=str)
- for r_idx, r in self.meta_df.iterrows():
- item_name = raw_item_name = r['item_name']
- if len(self.processed_data_dirs) > 1:
- item_name = f'ds{ds_id}_{item_name}'
- self.item2txt[item_name] = r['txt']
- self.item2ph[item_name] = r['ph']
- self.item2wavfn[item_name] = os.path.join(hparams['raw_data_dir'], 'wavs', os.path.basename(r['wav_fn']).split('_')[1])
- self.item2spk[item_name] = r.get('spk', 'SPK1')
- if len(self.processed_data_dirs) > 1:
- self.item2spk[item_name] = f"ds{ds_id}_{self.item2spk[item_name]}"
- if tg_dir is not None:
- self.item2tgfn[item_name] = f"{processed_data_dir}/{tg_dir}/{raw_item_name}.TextGrid"
- self.item_names = sorted(list(self.item2txt.keys()))
- if self.binarization_args['shuffle']:
- random.seed(1234)
- random.shuffle(self.item_names)
-
- @property
- def train_item_names(self):
- return self.item_names[hparams['test_num']+hparams['valid_num']:]
-
- @property
- def valid_item_names(self):
- return self.item_names[0: hparams['test_num']+hparams['valid_num']] #
-
- @property
- def test_item_names(self):
- return self.item_names[0: hparams['test_num']] # Audios for MOS testing are in 'test_ids'
-
- def build_spk_map(self):
- spk_map = set()
- for item_name in self.item_names:
- spk_name = self.item2spk[item_name]
- spk_map.add(spk_name)
- spk_map = {x: i for i, x in enumerate(sorted(list(spk_map)))}
- assert len(spk_map) == 0 or len(spk_map) <= hparams['num_spk'], len(spk_map)
- return spk_map
-
- def item_name2spk_id(self, item_name):
- return self.spk_map[self.item2spk[item_name]]
-
- def _phone_encoder(self):
- ph_set_fn = f"{hparams['binary_data_dir']}/phone_set.json"
- ph_set = []
- if hparams['reset_phone_dict'] or not os.path.exists(ph_set_fn):
- for processed_data_dir in self.processed_data_dirs:
- ph_set += [x.split(' ')[0] for x in open(f'{processed_data_dir}/dict.txt').readlines()]
- ph_set = sorted(set(ph_set))
- json.dump(ph_set, open(ph_set_fn, 'w'))
- else:
- ph_set = json.load(open(ph_set_fn, 'r'))
- print("| phone set: ", ph_set)
- return build_phone_encoder(hparams['binary_data_dir'])
-
- def meta_data(self, prefix):
- if prefix == 'valid':
- item_names = self.valid_item_names
- elif prefix == 'test':
- item_names = self.test_item_names
- else:
- item_names = self.train_item_names
- for item_name in item_names:
- ph = self.item2ph[item_name]
- txt = self.item2txt[item_name]
- tg_fn = self.item2tgfn.get(item_name)
- wav_fn = self.item2wavfn[item_name]
- spk_id = self.item_name2spk_id(item_name)
- yield item_name, ph, txt, tg_fn, wav_fn, spk_id
-
- def process(self):
- os.makedirs(hparams['binary_data_dir'], exist_ok=True)
- self.spk_map = self.build_spk_map()
- print("| spk_map: ", self.spk_map)
- spk_map_fn = f"{hparams['binary_data_dir']}/spk_map.json"
- json.dump(self.spk_map, open(spk_map_fn, 'w'))
-
- self.phone_encoder = self._phone_encoder()
- self.process_data('valid')
- self.process_data('test')
- self.process_data('train')
-
- def process_data(self, prefix):
- data_dir = hparams['binary_data_dir']
- args = []
- builder = IndexedDatasetBuilder(f'{data_dir}/{prefix}')
- lengths = []
- f0s = []
- total_sec = 0
- if self.binarization_args['with_spk_embed']:
- voice_encoder = VoiceEncoder().cuda()
-
- meta_data = list(self.meta_data(prefix))
- for m in meta_data:
- args.append(list(m) + [self.phone_encoder, self.binarization_args])
- num_workers = int(os.getenv('N_PROC', os.cpu_count() // 3))
- for f_id, (_, item) in enumerate(
- zip(tqdm(meta_data), chunked_multiprocess_run(self.process_item, args, num_workers=num_workers))):
- if item is None:
- continue
- item['spk_embed'] = voice_encoder.embed_utterance(item['wav']) \
- if self.binarization_args['with_spk_embed'] else None
- if not self.binarization_args['with_wav'] and 'wav' in item:
- print("del wav")
- del item['wav']
- builder.add_item(item)
- lengths.append(item['len'])
- total_sec += item['sec']
- if item.get('f0') is not None:
- f0s.append(item['f0'])
- builder.finalize()
- np.save(f'{data_dir}/{prefix}_lengths.npy', lengths)
- if len(f0s) > 0:
- f0s = np.concatenate(f0s, 0)
- f0s = f0s[f0s != 0]
- np.save(f'{data_dir}/{prefix}_f0s_mean_std.npy', [np.mean(f0s).item(), np.std(f0s).item()])
- print(f"| {prefix} total duration: {total_sec:.3f}s")
-
- @classmethod
- def process_item(cls, item_name, ph, txt, tg_fn, wav_fn, spk_id, encoder, binarization_args):
- if hparams['vocoder'] in VOCODERS:
- wav, mel = VOCODERS[hparams['vocoder']].wav2spec(wav_fn)
- else:
- wav, mel = VOCODERS[hparams['vocoder'].split('.')[-1]].wav2spec(wav_fn)
- res = {
- 'item_name': item_name, 'txt': txt, 'ph': ph, 'mel': mel, 'wav': wav, 'wav_fn': wav_fn,
- 'sec': len(wav) / hparams['audio_sample_rate'], 'len': mel.shape[0], 'spk_id': spk_id
- }
- try:
- if binarization_args['with_f0']:
- cls.get_pitch(wav, mel, res)
- if binarization_args['with_f0cwt']:
- cls.get_f0cwt(res['f0'], res)
- if binarization_args['with_txt']:
- try:
- phone_encoded = res['phone'] = encoder.encode(ph)
- except:
- traceback.print_exc()
- raise BinarizationError(f"Empty phoneme")
- if binarization_args['with_align']:
- cls.get_align(tg_fn, ph, mel, phone_encoded, res)
- except BinarizationError as e:
- print(f"| Skip item ({e}). item_name: {item_name}, wav_fn: {wav_fn}")
- return None
- return res
-
- @staticmethod
- def get_align(tg_fn, ph, mel, phone_encoded, res):
- if tg_fn is not None and os.path.exists(tg_fn):
- mel2ph, dur = get_mel2ph(tg_fn, ph, mel, hparams)
- else:
- raise BinarizationError(f"Align not found")
- if mel2ph.max() - 1 >= len(phone_encoded):
- raise BinarizationError(
- f"Align does not match: mel2ph.max() - 1: {mel2ph.max() - 1}, len(phone_encoded): {len(phone_encoded)}")
- res['mel2ph'] = mel2ph
- res['dur'] = dur
-
- @staticmethod
- def get_pitch(wav, mel, res):
- f0, pitch_coarse = get_pitch(wav, mel, hparams)
- if sum(f0) == 0:
- raise BinarizationError("Empty f0")
- res['f0'] = f0
- res['pitch'] = pitch_coarse
-
- @staticmethod
- def get_f0cwt(f0, res):
- from utils.cwt import get_cont_lf0, get_lf0_cwt
- uv, cont_lf0_lpf = get_cont_lf0(f0)
- logf0s_mean_org, logf0s_std_org = np.mean(cont_lf0_lpf), np.std(cont_lf0_lpf)
- cont_lf0_lpf_norm = (cont_lf0_lpf - logf0s_mean_org) / logf0s_std_org
- Wavelet_lf0, scales = get_lf0_cwt(cont_lf0_lpf_norm)
- if np.any(np.isnan(Wavelet_lf0)):
- raise BinarizationError("NaN CWT")
- res['cwt_spec'] = Wavelet_lf0
- res['cwt_scales'] = scales
- res['f0_mean'] = logf0s_mean_org
- res['f0_std'] = logf0s_std_org
-
-
-if __name__ == "__main__":
- set_hparams()
- BaseBinarizer().process()
diff --git a/spaces/Rongjiehuang/ProDiff/modules/parallel_wavegan/optimizers/radam.py b/spaces/Rongjiehuang/ProDiff/modules/parallel_wavegan/optimizers/radam.py
deleted file mode 100644
index e805d7e34921bee436e1e7fd9e1f753c7609186b..0000000000000000000000000000000000000000
--- a/spaces/Rongjiehuang/ProDiff/modules/parallel_wavegan/optimizers/radam.py
+++ /dev/null
@@ -1,91 +0,0 @@
-# -*- coding: utf-8 -*-
-
-"""RAdam optimizer.
-
-This code is drived from https://github.com/LiyuanLucasLiu/RAdam.
-"""
-
-import math
-import torch
-
-from torch.optim.optimizer import Optimizer
-
-
-class RAdam(Optimizer):
- """Rectified Adam optimizer."""
-
- def __init__(self, params, lr=1e-3, betas=(0.9, 0.999), eps=1e-8, weight_decay=0):
- """Initilize RAdam optimizer."""
- defaults = dict(lr=lr, betas=betas, eps=eps, weight_decay=weight_decay)
- self.buffer = [[None, None, None] for ind in range(10)]
- super(RAdam, self).__init__(params, defaults)
-
- def __setstate__(self, state):
- """Set state."""
- super(RAdam, self).__setstate__(state)
-
- def step(self, closure=None):
- """Run one step."""
- loss = None
- if closure is not None:
- loss = closure()
-
- for group in self.param_groups:
-
- for p in group['params']:
- if p.grad is None:
- continue
- grad = p.grad.data.float()
- if grad.is_sparse:
- raise RuntimeError('RAdam does not support sparse gradients')
-
- p_data_fp32 = p.data.float()
-
- state = self.state[p]
-
- if len(state) == 0:
- state['step'] = 0
- state['exp_avg'] = torch.zeros_like(p_data_fp32)
- state['exp_avg_sq'] = torch.zeros_like(p_data_fp32)
- else:
- state['exp_avg'] = state['exp_avg'].type_as(p_data_fp32)
- state['exp_avg_sq'] = state['exp_avg_sq'].type_as(p_data_fp32)
-
- exp_avg, exp_avg_sq = state['exp_avg'], state['exp_avg_sq']
- beta1, beta2 = group['betas']
-
- exp_avg_sq.mul_(beta2).addcmul_(1 - beta2, grad, grad)
- exp_avg.mul_(beta1).add_(1 - beta1, grad)
-
- state['step'] += 1
- buffered = self.buffer[int(state['step'] % 10)]
- if state['step'] == buffered[0]:
- N_sma, step_size = buffered[1], buffered[2]
- else:
- buffered[0] = state['step']
- beta2_t = beta2 ** state['step']
- N_sma_max = 2 / (1 - beta2) - 1
- N_sma = N_sma_max - 2 * state['step'] * beta2_t / (1 - beta2_t)
- buffered[1] = N_sma
-
- # more conservative since it's an approximated value
- if N_sma >= 5:
- step_size = math.sqrt(
- (1 - beta2_t) * (N_sma - 4) / (N_sma_max - 4) * (N_sma - 2) / N_sma * N_sma_max / (N_sma_max - 2)) / (1 - beta1 ** state['step']) # NOQA
- else:
- step_size = 1.0 / (1 - beta1 ** state['step'])
- buffered[2] = step_size
-
- if group['weight_decay'] != 0:
- p_data_fp32.add_(-group['weight_decay'] * group['lr'], p_data_fp32)
-
- # more conservative since it's an approximated value
- if N_sma >= 5:
- denom = exp_avg_sq.sqrt().add_(group['eps'])
- p_data_fp32.addcdiv_(-step_size * group['lr'], exp_avg, denom)
- else:
- p_data_fp32.add_(-step_size * group['lr'], exp_avg)
-
- p.data.copy_(p_data_fp32)
-
- return loss
diff --git a/spaces/SIGGRAPH2022/Text2Human/Text2Human/models/losses/accuracy.py b/spaces/SIGGRAPH2022/Text2Human/Text2Human/models/losses/accuracy.py
deleted file mode 100644
index 8e17db52c85aa693fe8a2f6d0036afc432580cfc..0000000000000000000000000000000000000000
--- a/spaces/SIGGRAPH2022/Text2Human/Text2Human/models/losses/accuracy.py
+++ /dev/null
@@ -1,46 +0,0 @@
-def accuracy(pred, target, topk=1, thresh=None):
- """Calculate accuracy according to the prediction and target.
-
- Args:
- pred (torch.Tensor): The model prediction, shape (N, num_class, ...)
- target (torch.Tensor): The target of each prediction, shape (N, , ...)
- topk (int | tuple[int], optional): If the predictions in ``topk``
- matches the target, the predictions will be regarded as
- correct ones. Defaults to 1.
- thresh (float, optional): If not None, predictions with scores under
- this threshold are considered incorrect. Default to None.
-
- Returns:
- float | tuple[float]: If the input ``topk`` is a single integer,
- the function will return a single float as accuracy. If
- ``topk`` is a tuple containing multiple integers, the
- function will return a tuple containing accuracies of
- each ``topk`` number.
- """
- assert isinstance(topk, (int, tuple))
- if isinstance(topk, int):
- topk = (topk, )
- return_single = True
- else:
- return_single = False
-
- maxk = max(topk)
- if pred.size(0) == 0:
- accu = [pred.new_tensor(0.) for i in range(len(topk))]
- return accu[0] if return_single else accu
- assert pred.ndim == target.ndim + 1
- assert pred.size(0) == target.size(0)
- assert maxk <= pred.size(1), \
- f'maxk {maxk} exceeds pred dimension {pred.size(1)}'
- pred_value, pred_label = pred.topk(maxk, dim=1)
- # transpose to shape (maxk, N, ...)
- pred_label = pred_label.transpose(0, 1)
- correct = pred_label.eq(target.unsqueeze(0).expand_as(pred_label))
- if thresh is not None:
- # Only prediction values larger than thresh are counted as correct
- correct = correct & (pred_value > thresh).t()
- res = []
- for k in topk:
- correct_k = correct[:k].view(-1).float().sum(0, keepdim=True)
- res.append(correct_k.mul_(100.0 / target.numel()))
- return res[0] if return_single else res
diff --git a/spaces/SceneDiffuser/SceneDiffuserDemo/interface.py b/spaces/SceneDiffuser/SceneDiffuserDemo/interface.py
deleted file mode 100644
index 525883a77b475b4715b851f58ea882708d37a8e6..0000000000000000000000000000000000000000
--- a/spaces/SceneDiffuser/SceneDiffuserDemo/interface.py
+++ /dev/null
@@ -1,313 +0,0 @@
-import os
-import random
-import torch
-import hydra
-import numpy as np
-import zipfile
-import time
-import uuid
-
-from typing import Any
-from hydra import compose, initialize
-from omegaconf import DictConfig, OmegaConf
-from huggingface_hub import hf_hub_download
-
-from utils.misc import compute_model_dim
-from datasets.base import create_dataset
-from datasets.misc import collate_fn_general, collate_fn_squeeze_pcd_batch
-from models.base import create_model
-from models.visualizer import create_visualizer
-from models.environment import create_enviroment
-
-def pretrain_pointtrans_weight_path():
- return hf_hub_download('SceneDiffuser/SceneDiffuser', 'weights/POINTTRANS_C_32768/model.pth')
-
-def model_weight_path(task, has_observation=False):
- if task == 'pose_gen':
- return hf_hub_download('SceneDiffuser/SceneDiffuser', 'weights/2022-11-09_11-22-52_PoseGen_ddm4_lr1e-4_ep100/ckpts/model.pth')
- elif task == 'motion_gen' and has_observation == True:
- return hf_hub_download('SceneDiffuser/SceneDiffuser', 'weights/2022-11-09_14-28-12_MotionGen_ddm_T200_lr1e-4_ep300_obser/ckpts/model.pth')
- elif task == 'motion_gen' and has_observation == False:
- return hf_hub_download('SceneDiffuser/SceneDiffuser', 'weights/2022-11-09_12-54-50_MotionGen_ddm_T200_lr1e-4_ep300/ckpts/model.pth')
- elif task == 'path_planning':
- return hf_hub_download('SceneDiffuser/SceneDiffuser', 'weights/2022-11-25_20-57-28_Path_ddm4_LR1e-4_E100_REL/ckpts/model.pth')
- else:
- raise Exception('Unexcepted task.')
-
-def pose_motion_data_path():
- zip_path = hf_hub_download('SceneDiffuser/SceneDiffuser', 'hf_data/pose_motion.zip')
- with zipfile.ZipFile(zip_path, 'r') as zip_ref:
- zip_ref.extractall(os.path.dirname(zip_path))
-
- rpath = os.path.join(os.path.dirname(zip_path), 'pose_motion')
-
- return (
- os.path.join(rpath, 'PROXD_temp'),
- os.path.join(rpath, 'models_smplx_v1_1/models/'),
- os.path.join(rpath, 'PROX'),
- os.path.join(rpath, 'PROX/V02_05')
- )
-
-def path_planning_data_path():
- zip_path = hf_hub_download('SceneDiffuser/SceneDiffuser', 'hf_data/path_planning.zip')
- with zipfile.ZipFile(zip_path, 'r') as zip_ref:
- zip_ref.extractall(os.path.dirname(zip_path))
-
- return os.path.join(os.path.dirname(zip_path), 'path_planning')
-
-def load_ckpt(model: torch.nn.Module, path: str) -> None:
- """ load ckpt for current model
-
- Args:
- model: current model
- path: save path
- """
- assert os.path.exists(path), 'Can\'t find provided ckpt.'
-
- saved_state_dict = torch.load(path)['model']
- model_state_dict = model.state_dict()
-
- for key in model_state_dict:
- if key in saved_state_dict:
- model_state_dict[key] = saved_state_dict[key]
- ## model is trained with ddm
- if 'module.'+key in saved_state_dict:
- model_state_dict[key] = saved_state_dict['module.'+key]
-
- model.load_state_dict(model_state_dict)
-
-def _sampling(cfg: DictConfig, scene: str) -> Any:
- ## compute modeling dimension according to task
- cfg.model.d_x = compute_model_dim(cfg.task)
-
- if cfg.gpu is not None:
- device = f'cuda:{cfg.gpu}'
- else:
- device = 'cpu'
-
- dataset = create_dataset(cfg.task.dataset, 'test', cfg.slurm, case_only=True, specific_scene=scene)
-
- if cfg.model.scene_model.name == 'PointTransformer':
- collate_fn = collate_fn_squeeze_pcd_batch
- else:
- collate_fn = collate_fn_general
-
- dataloader = dataset.get_dataloader(
- batch_size=1,
- collate_fn=collate_fn,
- shuffle=True,
- )
-
- ## create model and load ckpt
- model = create_model(cfg, slurm=cfg.slurm, device=device)
- model.to(device=device)
- load_ckpt(model, path=model_weight_path(cfg.task.name, cfg.task.has_observation if 'has_observation' in cfg.task else False))
-
- ## create visualizer and visualize
- visualizer = create_visualizer(cfg.task.visualizer)
- results = visualizer.visualize(model, dataloader)
- return results
-
-def _planning(cfg: DictConfig, scene: str) -> Any:
- ## compute modeling dimension according to task
- cfg.model.d_x = compute_model_dim(cfg.task)
-
- if cfg.gpu is not None:
- device = f'cuda:{cfg.gpu}'
- else:
- device = 'cpu'
-
- dataset = create_dataset(cfg.task.dataset, 'test', cfg.slurm, case_only=True, specific_scene=scene)
-
- if cfg.model.scene_model.name == 'PointTransformer':
- collate_fn = collate_fn_squeeze_pcd_batch
- else:
- collate_fn = collate_fn_general
-
- dataloader = dataset.get_dataloader(
- batch_size=1,
- collate_fn=collate_fn,
- shuffle=True,
- )
-
- ## create model and load ckpt
- model = create_model(cfg, slurm=cfg.slurm, device=device)
- model.to(device=device)
- load_ckpt(model, path=model_weight_path(cfg.task.name, cfg.task.has_observation if 'has_observation' in cfg.task else False))
-
- ## create environment for planning task and run
- env = create_enviroment(cfg.task.env)
- results = env.run(model, dataloader)
- return results
-
-
-## interface for five task
-## real-time model:
-## - pose generation
-## - motion generation
-## - path planning
-def pose_generation(scene, count, seed, opt, scale) -> Any:
- scene_model_weight_path = pretrain_pointtrans_weight_path()
- data_dir, smpl_dir, prox_dir, vposer_dir = pose_motion_data_path()
- override_config = [
- "diffuser=ddpm",
- "model=unet",
- f"model.scene_model.pretrained_weights={scene_model_weight_path}",
- "task=pose_gen",
- "task.visualizer.name=PoseGenVisualizerHF",
- f"task.visualizer.ksample={count}",
- f"task.dataset.data_dir={data_dir}",
- f"task.dataset.smpl_dir={smpl_dir}",
- f"task.dataset.prox_dir={prox_dir}",
- f"task.dataset.vposer_dir={vposer_dir}",
- ]
-
- if opt == True:
- override_config += [
- "optimizer=pose_in_scene",
- "optimizer.scale_type=div_var",
- f"optimizer.scale={scale}",
- "optimizer.vposer=false",
- "optimizer.contact_weight=0.02",
- "optimizer.collision_weight=1.0"
- ]
-
- initialize(config_path="./scenediffuser/configs", version_base=None)
- config = compose(config_name="default", overrides=override_config)
-
- random.seed(seed)
- np.random.seed(seed)
- torch.manual_seed(seed)
- torch.cuda.manual_seed(seed)
- torch.cuda.manual_seed_all(seed)
-
- res = _sampling(config, scene)
-
- hydra.core.global_hydra.GlobalHydra.instance().clear()
- return res
-
-def motion_generation(scene, count, seed, withstart, opt, scale) -> Any:
- scene_model_weight_path = pretrain_pointtrans_weight_path()
- data_dir, smpl_dir, prox_dir, vposer_dir = pose_motion_data_path()
- override_config = [
- "diffuser=ddpm",
- "diffuser.steps=200",
- "model=unet",
- "model.use_position_embedding=true",
- f"model.scene_model.pretrained_weights={scene_model_weight_path}",
- "task=motion_gen",
- f"task.has_observation={withstart}",
- "task.dataset.repr_type=absolute",
- "task.dataset.frame_interval_test=20",
- "task.visualizer.name=MotionGenVisualizerHF",
- f"task.visualizer.ksample={count}",
- f"task.dataset.data_dir={data_dir}",
- f"task.dataset.smpl_dir={smpl_dir}",
- f"task.dataset.prox_dir={prox_dir}",
- f"task.dataset.vposer_dir={vposer_dir}",
- ]
- if opt == True:
- override_config += [
- "optimizer=motion_in_scene",
- "optimizer.scale_type=div_var",
- f"optimizer.scale={scale}",
- "optimizer.vposer=false",
- "optimizer.contact_weight=0.02",
- "optimizer.collision_weight=1.0",
- "optimizer.smoothness_weight=0.001",
- "optimizer.frame_interval=1",
- ]
-
- initialize(config_path="./scenediffuser/configs", version_base=None)
- config = compose(config_name="default", overrides=override_config)
-
- random.seed(seed)
- np.random.seed(seed)
- torch.manual_seed(seed)
- torch.cuda.manual_seed(seed)
- torch.cuda.manual_seed_all(seed)
-
- res_gifs = _sampling(config, scene)
-
- ## save sampled motion as .gif file
- datestr = time.strftime("%Y-%m-%d", time.localtime(time.time()))
- target_dir = os.path.join('./results/motion_generation/', f'd-{datestr}')
- os.makedirs(target_dir, exist_ok=True)
- res = []
- uuid_str = uuid.uuid4()
- for i, imgs in enumerate(res_gifs):
- target_path = os.path.join(target_dir, f'{uuid_str}--{i}.gif')
- imgs = [im.resize((720, 405)) for im in imgs] # resize image for low resolution to save space
- img, *img_rest = imgs
- img.save(fp=target_path, format='GIF', append_images=img_rest, save_all=True, duration=33.33, loop=0)
- res.append(target_path)
-
- hydra.core.global_hydra.GlobalHydra.instance().clear()
- return res
-
-def grasp_generation(case_id):
- assert isinstance(case_id, str)
- res = f"./results/grasp_generation/results/{case_id}/{random.randint(0, 19)}.glb"
- if not os.path.exists(res):
- results_path = hf_hub_download('SceneDiffuser/SceneDiffuser', 'results/grasp_generation/results.zip')
- os.makedirs('./results/grasp_generation/', exist_ok=True)
- with zipfile.ZipFile(results_path, 'r') as zip_ref:
- zip_ref.extractall('./results/grasp_generation/')
-
- return res
-
-def path_planning(scene, mode, count, seed, opt, scale_opt, pla, scale_pla):
-
- scene_model_weight_path = pretrain_pointtrans_weight_path()
- data_dir = path_planning_data_path()
-
- override_config = [
- "diffuser=ddpm",
- "model=unet",
- "model.use_position_embedding=true",
- f"model.scene_model.pretrained_weights={scene_model_weight_path}",
- "task=path_planning",
- "task.visualizer.name=PathPlanningRenderingVisualizerHF",
- f"task.visualizer.ksample={count}",
- f"task.dataset.data_dir={data_dir}",
- "task.dataset.repr_type=relative",
- "task.env.name=PathPlanningEnvWrapperHF",
- "task.env.inpainting_horizon=16",
- "task.env.robot_top=3.0",
- "task.env.env_adaption=false"
- ]
-
- if opt == True:
- override_config += [
- "optimizer=path_in_scene",
- "optimizer.scale_type=div_var",
- "optimizer.continuity=false",
- f"optimizer.scale={scale_opt}",
- ]
- if pla == True:
- override_config += [
- "planner=greedy_path_planning",
- f"planner.scale={scale_pla}",
- "planner.scale_type=div_var",
- "planner.greedy_type=all_frame_exp"
- ]
-
- initialize(config_path="./scenediffuser/configs", version_base=None)
- config = compose(config_name="default", overrides=override_config)
-
- random.seed(seed)
- np.random.seed(seed)
- torch.manual_seed(seed)
- torch.cuda.manual_seed(seed)
- torch.cuda.manual_seed_all(seed)
-
- if mode == 'Sampling':
- img = _sampling(config, scene)
- res = (img, 0)
- elif mode == 'Planning':
- res = _planning(config, scene)
- else:
- res = (None, 0)
-
- hydra.core.global_hydra.GlobalHydra.instance().clear()
- return res
diff --git a/spaces/ServerX/PorcoDiaz/lib/uvr5_pack/lib_v5/layers_123821KB.py b/spaces/ServerX/PorcoDiaz/lib/uvr5_pack/lib_v5/layers_123821KB.py
deleted file mode 100644
index b82f06bb4993cd63f076e68d7e24185269b1bc42..0000000000000000000000000000000000000000
--- a/spaces/ServerX/PorcoDiaz/lib/uvr5_pack/lib_v5/layers_123821KB.py
+++ /dev/null
@@ -1,118 +0,0 @@
-import torch
-from torch import nn
-import torch.nn.functional as F
-
-from . import spec_utils
-
-
-class Conv2DBNActiv(nn.Module):
- def __init__(self, nin, nout, ksize=3, stride=1, pad=1, dilation=1, activ=nn.ReLU):
- super(Conv2DBNActiv, self).__init__()
- self.conv = nn.Sequential(
- nn.Conv2d(
- nin,
- nout,
- kernel_size=ksize,
- stride=stride,
- padding=pad,
- dilation=dilation,
- bias=False,
- ),
- nn.BatchNorm2d(nout),
- activ(),
- )
-
- def __call__(self, x):
- return self.conv(x)
-
-
-class SeperableConv2DBNActiv(nn.Module):
- def __init__(self, nin, nout, ksize=3, stride=1, pad=1, dilation=1, activ=nn.ReLU):
- super(SeperableConv2DBNActiv, self).__init__()
- self.conv = nn.Sequential(
- nn.Conv2d(
- nin,
- nin,
- kernel_size=ksize,
- stride=stride,
- padding=pad,
- dilation=dilation,
- groups=nin,
- bias=False,
- ),
- nn.Conv2d(nin, nout, kernel_size=1, bias=False),
- nn.BatchNorm2d(nout),
- activ(),
- )
-
- def __call__(self, x):
- return self.conv(x)
-
-
-class Encoder(nn.Module):
- def __init__(self, nin, nout, ksize=3, stride=1, pad=1, activ=nn.LeakyReLU):
- super(Encoder, self).__init__()
- self.conv1 = Conv2DBNActiv(nin, nout, ksize, 1, pad, activ=activ)
- self.conv2 = Conv2DBNActiv(nout, nout, ksize, stride, pad, activ=activ)
-
- def __call__(self, x):
- skip = self.conv1(x)
- h = self.conv2(skip)
-
- return h, skip
-
-
-class Decoder(nn.Module):
- def __init__(
- self, nin, nout, ksize=3, stride=1, pad=1, activ=nn.ReLU, dropout=False
- ):
- super(Decoder, self).__init__()
- self.conv = Conv2DBNActiv(nin, nout, ksize, 1, pad, activ=activ)
- self.dropout = nn.Dropout2d(0.1) if dropout else None
-
- def __call__(self, x, skip=None):
- x = F.interpolate(x, scale_factor=2, mode="bilinear", align_corners=True)
- if skip is not None:
- skip = spec_utils.crop_center(skip, x)
- x = torch.cat([x, skip], dim=1)
- h = self.conv(x)
-
- if self.dropout is not None:
- h = self.dropout(h)
-
- return h
-
-
-class ASPPModule(nn.Module):
- def __init__(self, nin, nout, dilations=(4, 8, 16), activ=nn.ReLU):
- super(ASPPModule, self).__init__()
- self.conv1 = nn.Sequential(
- nn.AdaptiveAvgPool2d((1, None)),
- Conv2DBNActiv(nin, nin, 1, 1, 0, activ=activ),
- )
- self.conv2 = Conv2DBNActiv(nin, nin, 1, 1, 0, activ=activ)
- self.conv3 = SeperableConv2DBNActiv(
- nin, nin, 3, 1, dilations[0], dilations[0], activ=activ
- )
- self.conv4 = SeperableConv2DBNActiv(
- nin, nin, 3, 1, dilations[1], dilations[1], activ=activ
- )
- self.conv5 = SeperableConv2DBNActiv(
- nin, nin, 3, 1, dilations[2], dilations[2], activ=activ
- )
- self.bottleneck = nn.Sequential(
- Conv2DBNActiv(nin * 5, nout, 1, 1, 0, activ=activ), nn.Dropout2d(0.1)
- )
-
- def forward(self, x):
- _, _, h, w = x.size()
- feat1 = F.interpolate(
- self.conv1(x), size=(h, w), mode="bilinear", align_corners=True
- )
- feat2 = self.conv2(x)
- feat3 = self.conv3(x)
- feat4 = self.conv4(x)
- feat5 = self.conv5(x)
- out = torch.cat((feat1, feat2, feat3, feat4, feat5), dim=1)
- bottle = self.bottleneck(out)
- return bottle
diff --git a/spaces/Sonnt/Fracture_Webapp/Antuns/page_setting.py b/spaces/Sonnt/Fracture_Webapp/Antuns/page_setting.py
deleted file mode 100644
index b57f2be7655e0bc07a905677ef8fb97aeed3e0c3..0000000000000000000000000000000000000000
--- a/spaces/Sonnt/Fracture_Webapp/Antuns/page_setting.py
+++ /dev/null
@@ -1,14 +0,0 @@
-import streamlit as st
-from PIL import Image
-img = Image.open("/work/2022_VPIMLogs_WebApp/data/LogoVPI.png")
-def page_intro():
- st.set_page_config(# Alternate names: setup_page, page, layout
- layout="wide", # Can be "centered" or "wide". In the future also "dashboard", etc.
- initial_sidebar_state="auto", # Can be "auto", "expanded", "collapsed"
- page_title="VPI-MLogs", # String or None. Strings get appended with "• Streamlit".
- page_icon=img, # String, anything supported by st.image, or None.
- )
- col_1, col_2, col_3, col_4, col_5, = st.columns(5)
- with col_3:
- st.image("https://i.ibb.co/Yd42K98/LogoVPI.png", width=250)
- st.header("Welcome to VPI-MLOGS!")
\ No newline at end of file
diff --git a/spaces/Suhailshah/image-captioning-with-vit-gpt2/app.py b/spaces/Suhailshah/image-captioning-with-vit-gpt2/app.py
deleted file mode 100644
index 6ea691be81de68d77d5a7110ae9adcb858f60aa2..0000000000000000000000000000000000000000
--- a/spaces/Suhailshah/image-captioning-with-vit-gpt2/app.py
+++ /dev/null
@@ -1,113 +0,0 @@
-# -*- coding: utf-8 -*-
-"""Copy of caption.ipynb
-
-Automatically generated by Colaboratory.
-
-Original file is located at
- https://colab.research.google.com/drive/1nybx9b_W5IsJz9G0GHvDx6KQKiTv_gt3
-
-## Image Caption Generator
-
-We are going to use Transformers model to generate caption from an Image.
-
-### Installation
-
-
-
-1. Transformers
-2. Pytorch
-3. Image
-
-
-@misc {nlp_connect_2022,
-
- author = { {NLP Connect} },
- title = { vit-gpt2-image-captioning (Revision 0e334c7) },
- year = 2022,
- url = { https://huggingface.co/nlpconnect/vit-gpt2-image-captioning },
- doi = { 10.57967/hf/0222 },
- publisher = { Hugging Face }
-} *italicized text*
-"""
-
-#!pip install transformers
-
-from transformers import VisionEncoderDecoderModel, ViTFeatureExtractor, AutoTokenizer
-import torch
-from PIL import Image
-import pandas as pd
-
-model = VisionEncoderDecoderModel.from_pretrained("nlpconnect/vit-gpt2-image-captioning")
-feature_extractor = ViTFeatureExtractor.from_pretrained("nlpconnect/vit-gpt2-image-captioning")
-tokenizer = AutoTokenizer.from_pretrained("nlpconnect/vit-gpt2-image-captioning")
-
-device = torch.device("cuda" if torch.cuda.is_available() else "cpu")
-model.to(device)
-
-max_length = 16
-num_beams = 8
-gen_kwargs = {"max_length": max_length, "num_beams": num_beams}
-
-def cap_generation(img,Num_of_captions):
- images = []
- Num_of_captions = int(Num_of_captions)
- if img.mode != "RGB":
- img = img.convert(mode="RGB")
- width, height = img.size
-
- new_size = (int(width/4), int(height/4))
-
-# Resize the image for faster computation.
- img = img.resize(new_size)
-
- images.append(img)
-
- pixel_values = feature_extractor(images=images, return_tensors="pt").pixel_values
- pixel_values = pixel_values.to(device)
- if(Num_of_captions==1):
- output_ids = model.generate(pixel_values,**gen_kwargs)
- preds = tokenizer.batch_decode(output_ids, skip_special_tokens=True)
- preds = [pred.strip() for pred in preds]
- result = [s.capitalize() + '.' for s in preds]
- data = {"No.": range(1, len(result)+1), "Captions": result}
- df = pd.DataFrame(data)
- return df
-
- else:
- output_ids = model.generate(pixel_values,max_length = 100,num_return_sequences=Num_of_captions,do_sample=True)
- preds = tokenizer.batch_decode(output_ids, skip_special_tokens=True)
- preds = [pred.strip() for pred in preds]
- result = [s.capitalize() + '.' for s in preds]
- data = {"No.": range(1, len(result)+1), "Captions": result}
- df = pd.DataFrame(data)
- return df
-
-#!pip install gradio
-import gradio as gr
-
-import gradio as gr
-inputs = [
- gr.inputs.Image(type='pil',label = 'Original Image'),
- gr.inputs.Number(default = 1, label="Number Of Captions")
-]
-outputs=[gr.outputs.Dataframe(type="pandas")]
-
-title = "Image Captioning Using VIT-GPT2 "
-description = "Image Captioning with vit-gpt2"
-article = " Model "
-'''examples = [
- ['Image3.png']
-]'''
-
-interface = gr.Interface(
- cap_generation,
- inputs,
- outputs=outputs,
- title=title,
- description=description,
- article=article,
- theme="huggingface",
- )
-
-interface.launch()
-
diff --git a/spaces/SungBeom/chatwine-korean/.venv/Lib/site-packages/altair/vegalite/v5/api.py b/spaces/SungBeom/chatwine-korean/.venv/Lib/site-packages/altair/vegalite/v5/api.py
deleted file mode 100644
index ed449bcab3fe7b2679f1ffaadc97402f43381869..0000000000000000000000000000000000000000
--- a/spaces/SungBeom/chatwine-korean/.venv/Lib/site-packages/altair/vegalite/v5/api.py
+++ /dev/null
@@ -1,3434 +0,0 @@
-import warnings
-
-import hashlib
-import io
-import json
-import jsonschema
-import pandas as pd
-from toolz.curried import pipe as _pipe
-import itertools
-import sys
-from typing import cast
-
-# Have to rename it here as else it overlaps with schema.core.Type
-from typing import Type as TypingType
-
-from .schema import core, channels, mixins, Undefined, SCHEMA_URL
-
-from .data import data_transformers
-from ... import utils, expr
-from .display import renderers, VEGALITE_VERSION, VEGAEMBED_VERSION, VEGA_VERSION
-from .theme import themes
-
-if sys.version_info >= (3, 11):
- from typing import Self
-else:
- from typing_extensions import Self
-
-
-# ------------------------------------------------------------------------
-# Data Utilities
-def _dataset_name(values):
- """Generate a unique hash of the data
-
- Parameters
- ----------
- values : list or dict
- A list/dict representation of data values.
-
- Returns
- -------
- name : string
- A unique name generated from the hash of the values.
- """
- if isinstance(values, core.InlineDataset):
- values = values.to_dict()
- if values == [{}]:
- return "empty"
- values_json = json.dumps(values, sort_keys=True)
- hsh = hashlib.md5(values_json.encode()).hexdigest()
- return "data-" + hsh
-
-
-def _consolidate_data(data, context):
- """If data is specified inline, then move it to context['datasets']
-
- This function will modify context in-place, and return a new version of data
- """
- values = Undefined
- kwds = {}
-
- if isinstance(data, core.InlineData):
- if data.name is Undefined and data.values is not Undefined:
- if isinstance(data.values, core.InlineDataset):
- values = data.to_dict()["values"]
- else:
- values = data.values
- kwds = {"format": data.format}
-
- elif isinstance(data, dict):
- if "name" not in data and "values" in data:
- values = data["values"]
- kwds = {k: v for k, v in data.items() if k != "values"}
-
- if values is not Undefined:
- name = _dataset_name(values)
- data = core.NamedData(name=name, **kwds)
- context.setdefault("datasets", {})[name] = values
-
- return data
-
-
-def _prepare_data(data, context=None):
- """Convert input data to data for use within schema
-
- Parameters
- ----------
- data :
- The input dataset in the form of a DataFrame, dictionary, altair data
- object, or other type that is recognized by the data transformers.
- context : dict (optional)
- The to_dict context in which the data is being prepared. This is used
- to keep track of information that needs to be passed up and down the
- recursive serialization routine, such as global named datasets.
- """
- if data is Undefined:
- return data
-
- # convert dataframes or objects with __geo_interface__ to dict
- elif isinstance(data, pd.DataFrame) or hasattr(data, "__geo_interface__"):
- data = _pipe(data, data_transformers.get())
-
- # convert string input to a URLData
- elif isinstance(data, str):
- data = core.UrlData(data)
-
- elif hasattr(data, "__dataframe__"):
- data = _pipe(data, data_transformers.get())
-
- # consolidate inline data to top-level datasets
- if context is not None and data_transformers.consolidate_datasets:
- data = _consolidate_data(data, context)
-
- # if data is still not a recognized type, then return
- if not isinstance(data, (dict, core.Data)):
- warnings.warn("data of type {} not recognized".format(type(data)), stacklevel=1)
-
- return data
-
-
-# ------------------------------------------------------------------------
-# Aliases & specializations
-Bin = core.BinParams
-Impute = core.ImputeParams
-Title = core.TitleParams
-
-
-class LookupData(core.LookupData):
- @utils.use_signature(core.LookupData)
- def __init__(self, *args, **kwargs):
- super().__init__(*args, **kwargs)
-
- def to_dict(self, *args, **kwargs):
- """Convert the chart to a dictionary suitable for JSON export."""
- copy = self.copy(deep=False)
- copy.data = _prepare_data(copy.data, kwargs.get("context"))
- return super(LookupData, copy).to_dict(*args, **kwargs)
-
-
-class FacetMapping(core.FacetMapping):
- _class_is_valid_at_instantiation = False
-
- @utils.use_signature(core.FacetMapping)
- def __init__(self, *args, **kwargs):
- super().__init__(*args, **kwargs)
-
- def to_dict(self, *args, **kwargs):
- copy = self.copy(deep=False)
- context = kwargs.get("context", {})
- data = context.get("data", None)
- if isinstance(self.row, str):
- copy.row = core.FacetFieldDef(**utils.parse_shorthand(self.row, data))
- if isinstance(self.column, str):
- copy.column = core.FacetFieldDef(**utils.parse_shorthand(self.column, data))
- return super(FacetMapping, copy).to_dict(*args, **kwargs)
-
-
-# ------------------------------------------------------------------------
-# Encoding will contain channel objects that aren't valid at instantiation
-core.FacetedEncoding._class_is_valid_at_instantiation = False
-
-# ------------------------------------------------------------------------
-# These are parameters that are valid at the top level, but are not valid
-# for specs that are within a composite chart
-# (layer, hconcat, vconcat, facet, repeat)
-TOPLEVEL_ONLY_KEYS = {"background", "config", "autosize", "padding", "$schema"}
-
-
-def _get_channels_mapping():
- mapping = {}
- for attr in dir(channels):
- cls = getattr(channels, attr)
- if isinstance(cls, type) and issubclass(cls, core.SchemaBase):
- mapping[cls] = attr.replace("Value", "").lower()
- return mapping
-
-
-# -------------------------------------------------------------------------
-# Tools for working with parameters
-class Parameter(expr.core.OperatorMixin, object):
- """A Parameter object"""
-
- _counter = 0
-
- @classmethod
- def _get_name(cls):
- cls._counter += 1
- return f"param_{cls._counter}"
-
- def __init__(self, name):
- if name is None:
- name = self._get_name()
- self.name = name
-
- @utils.deprecation.deprecated(
- message="'ref' is deprecated. No need to call '.ref()' anymore."
- )
- def ref(self):
- "'ref' is deprecated. No need to call '.ref()' anymore."
- return self.to_dict()
-
- def to_dict(self):
- if self.param_type == "variable":
- return {"expr": self.name}
- elif self.param_type == "selection":
- return {
- "param": self.name.to_dict()
- if hasattr(self.name, "to_dict")
- else self.name
- }
-
- def __invert__(self):
- if self.param_type == "selection":
- return SelectionPredicateComposition({"not": {"param": self.name}})
- else:
- return expr.core.OperatorMixin.__invert__(self)
-
- def __and__(self, other):
- if self.param_type == "selection":
- if isinstance(other, Parameter):
- other = {"param": other.name}
- return SelectionPredicateComposition({"and": [{"param": self.name}, other]})
- else:
- return expr.core.OperatorMixin.__and__(self, other)
-
- def __or__(self, other):
- if self.param_type == "selection":
- if isinstance(other, Parameter):
- other = {"param": other.name}
- return SelectionPredicateComposition({"or": [{"param": self.name}, other]})
- else:
- return expr.core.OperatorMixin.__or__(self, other)
-
- def __repr__(self):
- return "Parameter({0!r}, {1})".format(self.name, self.param)
-
- def _to_expr(self):
- return self.name
-
- def _from_expr(self, expr):
- return ParameterExpression(expr=expr)
-
- def __getattr__(self, field_name):
- if field_name.startswith("__") and field_name.endswith("__"):
- raise AttributeError(field_name)
- _attrexpr = expr.core.GetAttrExpression(self.name, field_name)
- # If self is a SelectionParameter and field_name is in its
- # fields or encodings list, then we want to return an expression.
- if check_fields_and_encodings(self, field_name):
- return SelectionExpression(_attrexpr)
- return expr.core.GetAttrExpression(self.name, field_name)
-
- # TODO: Are there any special cases to consider for __getitem__?
- # This was copied from v4.
- def __getitem__(self, field_name):
- return expr.core.GetItemExpression(self.name, field_name)
-
-
-# Enables use of ~, &, | with compositions of selection objects.
-class SelectionPredicateComposition(core.PredicateComposition):
- def __invert__(self):
- return SelectionPredicateComposition({"not": self.to_dict()})
-
- def __and__(self, other):
- return SelectionPredicateComposition({"and": [self.to_dict(), other.to_dict()]})
-
- def __or__(self, other):
- return SelectionPredicateComposition({"or": [self.to_dict(), other.to_dict()]})
-
-
-class ParameterExpression(expr.core.OperatorMixin, object):
- def __init__(self, expr):
- self.expr = expr
-
- def to_dict(self):
- return {"expr": repr(self.expr)}
-
- def _to_expr(self):
- return repr(self.expr)
-
- def _from_expr(self, expr):
- return ParameterExpression(expr=expr)
-
-
-class SelectionExpression(expr.core.OperatorMixin, object):
- def __init__(self, expr):
- self.expr = expr
-
- def to_dict(self):
- return {"expr": repr(self.expr)}
-
- def _to_expr(self):
- return repr(self.expr)
-
- def _from_expr(self, expr):
- return SelectionExpression(expr=expr)
-
-
-def check_fields_and_encodings(parameter, field_name):
- for prop in ["fields", "encodings"]:
- try:
- if field_name in getattr(parameter.param.select, prop):
- return True
- except (AttributeError, TypeError):
- pass
-
- return False
-
-
-# ------------------------------------------------------------------------
-# Top-Level Functions
-
-
-def value(value, **kwargs):
- """Specify a value for use in an encoding"""
- return dict(value=value, **kwargs)
-
-
-def param(
- name=None,
- value=Undefined,
- bind=Undefined,
- empty=Undefined,
- expr=Undefined,
- **kwds,
-):
- """Create a named parameter. See https://altair-viz.github.io/user_guide/interactions.html for examples. Although both variable parameters and selection parameters can be created using this 'param' function, to create a selection parameter, it is recommended to use either 'selection_point' or 'selection_interval' instead.
-
- Parameters
- ----------
- name : string (optional)
- The name of the parameter. If not specified, a unique name will be
- created.
- value : any (optional)
- The default value of the parameter. If not specified, the parameter
- will be created without a default value.
- bind : :class:`Binding` (optional)
- Binds the parameter to an external input element such as a slider,
- selection list or radio button group.
- empty : boolean (optional)
- For selection parameters, the predicate of empty selections returns
- True by default. Override this behavior, by setting this property
- 'empty=False'.
- expr : :class:`Expr` (optional)
- An expression for the value of the parameter. This expression may
- include other parameters, in which case the parameter will
- automatically update in response to upstream parameter changes.
- **kwds :
- additional keywords will be used to construct a parameter. If 'select'
- is among the keywords, then a selection parameter will be created.
- Otherwise, a variable parameter will be created.
-
- Returns
- -------
- parameter: Parameter
- The parameter object that can be used in chart creation.
- """
- parameter = Parameter(name)
-
- if empty is not Undefined:
- parameter.empty = empty
- if parameter.empty == "none":
- warnings.warn(
- """The value of 'empty' should be True or False.""",
- utils.AltairDeprecationWarning,
- stacklevel=1,
- )
- parameter.empty = False
- elif parameter.empty == "all":
- warnings.warn(
- """The value of 'empty' should be True or False.""",
- utils.AltairDeprecationWarning,
- stacklevel=1,
- )
- parameter.empty = True
- elif (parameter.empty is False) or (parameter.empty is True):
- pass
- else:
- raise ValueError("The value of 'empty' should be True or False.")
-
- if "init" in kwds:
- warnings.warn(
- """Use 'value' instead of 'init'.""",
- utils.AltairDeprecationWarning,
- stacklevel=1,
- )
- if value is Undefined:
- kwds["value"] = kwds.pop("init")
- else:
- # If both 'value' and 'init' are set, we ignore 'init'.
- kwds.pop("init")
-
- if "select" not in kwds:
- parameter.param = core.VariableParameter(
- name=parameter.name, bind=bind, value=value, expr=expr, **kwds
- )
- parameter.param_type = "variable"
- elif "views" in kwds:
- parameter.param = core.TopLevelSelectionParameter(
- name=parameter.name, bind=bind, value=value, expr=expr, **kwds
- )
- parameter.param_type = "selection"
- else:
- parameter.param = core.SelectionParameter(
- name=parameter.name, bind=bind, value=value, expr=expr, **kwds
- )
- parameter.param_type = "selection"
-
- return parameter
-
-
-def _selection(type=Undefined, **kwds):
- # We separate out the parameter keywords from the selection keywords
- param_kwds = {}
-
- for kwd in {"name", "bind", "value", "empty", "init", "views"}:
- if kwd in kwds:
- param_kwds[kwd] = kwds.pop(kwd)
-
- if type == "interval":
- select = core.IntervalSelectionConfig(type=type, **kwds)
- elif type == "point":
- select = core.PointSelectionConfig(type=type, **kwds)
- elif type in ["single", "multi"]:
- select = core.PointSelectionConfig(type="point", **kwds)
- warnings.warn(
- """The types 'single' and 'multi' are now
- combined and should be specified using "selection_point()".""",
- utils.AltairDeprecationWarning,
- stacklevel=1,
- )
- else:
- raise ValueError("""'type' must be 'point' or 'interval'""")
-
- return param(select=select, **param_kwds)
-
-
-@utils.deprecation.deprecated(
- message="""'selection' is deprecated.
- Use 'selection_point()' or 'selection_interval()' instead; these functions also include more helpful docstrings."""
-)
-def selection(type=Undefined, **kwds):
- """
- Users are recommended to use either 'selection_point' or 'selection_interval' instead, depending on the type of parameter they want to create.
-
- Create a selection parameter.
-
- Parameters
- ----------
- type : enum('point', 'interval') (required)
- Determines the default event processing and data query for the
- selection. Vega-Lite currently supports two selection types:
- * "point" - to select multiple discrete data values; the first
- value is selected on click and additional values toggled on
- shift-click.
- * "interval" - to select a continuous range of data values on
- drag.
- **kwds :
- additional keywords to control the selection.
- """
-
- return _selection(type=type, **kwds)
-
-
-def selection_interval(
- name=None,
- value=Undefined,
- bind=Undefined,
- empty=Undefined,
- expr=Undefined,
- encodings=Undefined,
- on=Undefined,
- clear=Undefined,
- resolve=Undefined,
- mark=Undefined,
- translate=Undefined,
- zoom=Undefined,
- **kwds,
-):
- """Create an interval selection parameter. Selection parameters define data queries that are driven by direct manipulation from user input (e.g., mouse clicks or drags). Interval selection parameters are used to select a continuous range of data values on drag, whereas point selection parameters (`selection_point`) are used to select multiple discrete data values.)
-
- Parameters
- ----------
- name : string (optional)
- The name of the parameter. If not specified, a unique name will be
- created.
- value : any (optional)
- The default value of the parameter. If not specified, the parameter
- will be created without a default value.
- bind : :class:`Binding` (optional)
- Binds the parameter to an external input element such as a slider,
- selection list or radio button group.
- empty : boolean (optional)
- For selection parameters, the predicate of empty selections returns
- True by default. Override this behavior, by setting this property
- 'empty=False'.
- expr : :class:`Expr` (optional)
- An expression for the value of the parameter. This expression may
- include other parameters, in which case the parameter will
- automatically update in response to upstream parameter changes.
- encodings : List[str] (optional)
- A list of encoding channels. The corresponding data field values
- must match for a data tuple to fall within the selection.
- on : string (optional)
- A Vega event stream (object or selector) that triggers the selection.
- For interval selections, the event stream must specify a start and end.
- clear : string or boolean (optional)
- Clears the selection, emptying it of all values. This property can
- be an Event Stream or False to disable clear. Default is 'dblclick'.
- resolve : enum('global', 'union', 'intersect') (optional)
- With layered and multi-view displays, a strategy that determines
- how selections' data queries are resolved when applied in a filter
- transform, conditional encoding rule, or scale domain.
- One of:
-
- * 'global': only one brush exists for the entire SPLOM. When the
- user begins to drag, any previous brushes are cleared, and a
- new one is constructed.
- * 'union': each cell contains its own brush, and points are
- highlighted if they lie within any of these individual brushes.
- * 'intersect': each cell contains its own brush, and points are
- highlighted only if they fall within all of these individual
- brushes.
-
- The default is 'global'.
- mark : :class:`Mark` (optional)
- An interval selection also adds a rectangle mark to depict the
- extents of the interval. The mark property can be used to
- customize the appearance of the mark.
- translate : string or boolean (optional)
- When truthy, allows a user to interactively move an interval
- selection back-and-forth. Can be True, False (to disable panning),
- or a Vega event stream definition which must include a start and
- end event to trigger continuous panning. Discrete panning (e.g.,
- pressing the left/right arrow keys) will be supported in future
- versions.
- The default value is True, which corresponds to
- [mousedown, window:mouseup] > window:mousemove!
- This default allows users to click and drag within an interval
- selection to reposition it.
- zoom : string or boolean (optional)
- When truthy, allows a user to interactively resize an interval
- selection. Can be True, False (to disable zooming), or a Vega
- event stream definition. Currently, only wheel events are supported,
- but custom event streams can still be used to specify filters,
- debouncing, and throttling. Future versions will expand the set of
- events that can trigger this transformation.
- The default value is True, which corresponds to wheel!. This
- default allows users to use the mouse wheel to resize an interval
- selection.
- **kwds :
- Additional keywords to control the selection.
-
- Returns
- -------
- parameter: Parameter
- The parameter object that can be used in chart creation.
- """
- return _selection(
- type="interval",
- name=name,
- value=value,
- bind=bind,
- empty=empty,
- expr=expr,
- encodings=encodings,
- on=on,
- clear=clear,
- resolve=resolve,
- mark=mark,
- translate=translate,
- zoom=zoom,
- **kwds,
- )
-
-
-def selection_point(
- name=None,
- value=Undefined,
- bind=Undefined,
- empty=Undefined,
- expr=Undefined,
- encodings=Undefined,
- fields=Undefined,
- on=Undefined,
- clear=Undefined,
- resolve=Undefined,
- toggle=Undefined,
- nearest=Undefined,
- **kwds,
-):
- """Create a point selection parameter. Selection parameters define data queries that are driven by direct manipulation from user input (e.g., mouse clicks or drags). Point selection parameters are used to select multiple discrete data values; the first value is selected on click and additional values toggled on shift-click. To select a continuous range of data values on drag interval selection parameters (`selection_interval`) can be used instead.
-
- Parameters
- ----------
- name : string (optional)
- The name of the parameter. If not specified, a unique name will be
- created.
- value : any (optional)
- The default value of the parameter. If not specified, the parameter
- will be created without a default value.
- bind : :class:`Binding` (optional)
- Binds the parameter to an external input element such as a slider,
- selection list or radio button group.
- empty : boolean (optional)
- For selection parameters, the predicate of empty selections returns
- True by default. Override this behavior, by setting this property
- 'empty=False'.
- expr : :class:`Expr` (optional)
- An expression for the value of the parameter. This expression may
- include other parameters, in which case the parameter will
- automatically update in response to upstream parameter changes.
- encodings : List[str] (optional)
- A list of encoding channels. The corresponding data field values
- must match for a data tuple to fall within the selection.
- fields : List[str] (optional)
- A list of field names whose values must match for a data tuple to
- fall within the selection.
- on : string (optional)
- A Vega event stream (object or selector) that triggers the selection.
- For interval selections, the event stream must specify a start and end.
- clear : string or boolean (optional)
- Clears the selection, emptying it of all values. This property can
- be an Event Stream or False to disable clear. Default is 'dblclick'.
- resolve : enum('global', 'union', 'intersect') (optional)
- With layered and multi-view displays, a strategy that determines
- how selections' data queries are resolved when applied in a filter
- transform, conditional encoding rule, or scale domain.
- One of:
-
- * 'global': only one brush exists for the entire SPLOM. When the
- user begins to drag, any previous brushes are cleared, and a
- new one is constructed.
- * 'union': each cell contains its own brush, and points are
- highlighted if they lie within any of these individual brushes.
- * 'intersect': each cell contains its own brush, and points are
- highlighted only if they fall within all of these individual
- brushes.
-
- The default is 'global'.
- toggle : string or boolean (optional)
- Controls whether data values should be toggled (inserted or
- removed from a point selection) or only ever inserted into
- point selections.
- One of:
-
- * True (default): the toggle behavior, which corresponds to
- "event.shiftKey". As a result, data values are toggled
- when the user interacts with the shift-key pressed.
- * False: disables toggling behaviour; the selection will
- only ever contain a single data value corresponding
- to the most recent interaction.
- * A Vega expression which is re-evaluated as the user interacts.
- If the expression evaluates to True, the data value is
- toggled into or out of the point selection. If the expression
- evaluates to False, the point selection is first cleared, and
- the data value is then inserted. For example, setting the
- value to the Vega expression True will toggle data values
- without the user pressing the shift-key.
-
- nearest : boolean (optional)
- When true, an invisible voronoi diagram is computed to accelerate
- discrete selection. The data value nearest the mouse cursor is
- added to the selection. The default is False, which means that
- data values must be interacted with directly (e.g., clicked on)
- to be added to the selection.
- **kwds :
- Additional keywords to control the selection.
-
- Returns
- -------
- parameter: Parameter
- The parameter object that can be used in chart creation.
- """
- return _selection(
- type="point",
- name=name,
- value=value,
- bind=bind,
- empty=empty,
- expr=expr,
- encodings=encodings,
- fields=fields,
- on=on,
- clear=clear,
- resolve=resolve,
- toggle=toggle,
- nearest=nearest,
- **kwds,
- )
-
-
-@utils.deprecation.deprecated(
- message="'selection_multi' is deprecated. Use 'selection_point'"
-)
-@utils.use_signature(core.PointSelectionConfig)
-def selection_multi(**kwargs):
- """'selection_multi' is deprecated. Use 'selection_point'"""
- return _selection(type="point", **kwargs)
-
-
-@utils.deprecation.deprecated(
- message="'selection_single' is deprecated. Use 'selection_point'"
-)
-@utils.use_signature(core.PointSelectionConfig)
-def selection_single(**kwargs):
- """'selection_single' is deprecated. Use 'selection_point'"""
- return _selection(type="point", **kwargs)
-
-
-@utils.use_signature(core.Binding)
-def binding(input, **kwargs):
- """A generic binding"""
- return core.Binding(input=input, **kwargs)
-
-
-@utils.use_signature(core.BindCheckbox)
-def binding_checkbox(**kwargs):
- """A checkbox binding"""
- return core.BindCheckbox(input="checkbox", **kwargs)
-
-
-@utils.use_signature(core.BindRadioSelect)
-def binding_radio(**kwargs):
- """A radio button binding"""
- return core.BindRadioSelect(input="radio", **kwargs)
-
-
-@utils.use_signature(core.BindRadioSelect)
-def binding_select(**kwargs):
- """A select binding"""
- return core.BindRadioSelect(input="select", **kwargs)
-
-
-@utils.use_signature(core.BindRange)
-def binding_range(**kwargs):
- """A range binding"""
- return core.BindRange(input="range", **kwargs)
-
-
-# TODO: update the docstring
-def condition(predicate, if_true, if_false, **kwargs):
- """A conditional attribute or encoding
-
- Parameters
- ----------
- predicate: Selection, PredicateComposition, expr.Expression, dict, or string
- the selection predicate or test predicate for the condition.
- if a string is passed, it will be treated as a test operand.
- if_true:
- the spec or object to use if the selection predicate is true
- if_false:
- the spec or object to use if the selection predicate is false
- **kwargs:
- additional keyword args are added to the resulting dict
-
- Returns
- -------
- spec: dict or VegaLiteSchema
- the spec that describes the condition
- """
- test_predicates = (str, expr.Expression, core.PredicateComposition)
-
- if isinstance(predicate, Parameter):
- if predicate.param_type == "selection" or predicate.param.expr is Undefined:
- condition = {"param": predicate.name}
- if "empty" in kwargs:
- condition["empty"] = kwargs.pop("empty")
- elif isinstance(predicate.empty, bool):
- condition["empty"] = predicate.empty
- else:
- condition = {"test": predicate.param.expr}
- elif isinstance(predicate, test_predicates):
- condition = {"test": predicate}
- elif isinstance(predicate, dict):
- condition = predicate
- else:
- raise NotImplementedError(
- "condition predicate of type {}" "".format(type(predicate))
- )
-
- if isinstance(if_true, core.SchemaBase):
- # convert to dict for now; the from_dict call below will wrap this
- # dict in the appropriate schema
- if_true = if_true.to_dict()
- elif isinstance(if_true, str):
- if isinstance(if_false, str):
- raise ValueError(
- "A field cannot be used for both the `if_true` and `if_false` values of a condition. One of them has to specify a `value` or `datum` definition."
- )
- else:
- if_true = utils.parse_shorthand(if_true)
- if_true.update(kwargs)
- condition.update(if_true)
-
- if isinstance(if_false, core.SchemaBase):
- # For the selection, the channel definitions all allow selections
- # already. So use this SchemaBase wrapper if possible.
- selection = if_false.copy()
- selection.condition = condition
- elif isinstance(if_false, str):
- selection = {"condition": condition, "shorthand": if_false}
- selection.update(kwargs)
- else:
- selection = dict(condition=condition, **if_false)
-
- return selection
-
-
-# --------------------------------------------------------------------
-# Top-level objects
-
-
-class TopLevelMixin(mixins.ConfigMethodMixin):
- """Mixin for top-level chart objects such as Chart, LayeredChart, etc."""
-
- _class_is_valid_at_instantiation = False
-
- def to_dict(self, *args, **kwargs) -> dict:
- """Convert the chart to a dictionary suitable for JSON export"""
- # We make use of three context markers:
- # - 'data' points to the data that should be referenced for column type
- # inference.
- # - 'top_level' is a boolean flag that is assumed to be true; if it's
- # true then a "$schema" arg is added to the dict.
- # - 'datasets' is a dict of named datasets that should be inserted
- # in the top-level object
-
- # note: not a deep copy because we want datasets and data arguments to
- # be passed by reference
- context = kwargs.get("context", {}).copy()
- context.setdefault("datasets", {})
- is_top_level = context.get("top_level", True)
-
- # TopLevelMixin instance does not necessarily have copy defined but due to how
- # Altair is set up this should hold. Too complex to type hint right now
- copy = self.copy(deep=False) # type: ignore[attr-defined]
- original_data = getattr(copy, "data", Undefined)
- copy.data = _prepare_data(original_data, context)
-
- if original_data is not Undefined:
- context["data"] = original_data
-
- # remaining to_dict calls are not at top level
- context["top_level"] = False
- kwargs["context"] = context
-
- # TopLevelMixin instance does not necessarily have to_dict defined
- # but due to how Altair is set up this should hold.
- # Too complex to type hint right now
- dct = super(TopLevelMixin, copy).to_dict(*args, **kwargs) # type: ignore[misc]
-
- # TODO: following entries are added after validation. Should they be validated?
- if is_top_level:
- # since this is top-level we add $schema if it's missing
- if "$schema" not in dct:
- dct["$schema"] = SCHEMA_URL
-
- # apply theme from theme registry
- the_theme = themes.get()
- # Use assert to tell type checkers that it is not None. Holds true
- # as there is always a default theme set when importing Altair
- assert the_theme is not None
- dct = utils.update_nested(the_theme(), dct, copy=True)
-
- # update datasets
- if context["datasets"]:
- dct.setdefault("datasets", {}).update(context["datasets"])
-
- return dct
-
- def to_html(
- self,
- base_url="https://cdn.jsdelivr.net/npm",
- output_div="vis",
- embed_options=None,
- json_kwds=None,
- fullhtml=True,
- requirejs=False,
- ) -> str:
- return utils.spec_to_html(
- self.to_dict(),
- mode="vega-lite",
- vegalite_version=VEGALITE_VERSION,
- vegaembed_version=VEGAEMBED_VERSION,
- vega_version=VEGA_VERSION,
- base_url=base_url,
- output_div=output_div,
- embed_options=embed_options,
- json_kwds=json_kwds,
- fullhtml=fullhtml,
- requirejs=requirejs,
- )
-
- def save(
- self,
- fp,
- format=None,
- override_data_transformer=True,
- scale_factor=1.0,
- vegalite_version=VEGALITE_VERSION,
- vega_version=VEGA_VERSION,
- vegaembed_version=VEGAEMBED_VERSION,
- **kwargs,
- ):
- """Save a chart to file in a variety of formats
-
- Supported formats are json, html, png, svg, pdf; the last three require
- the altair_saver package to be installed.
-
- Parameters
- ----------
- fp : string filename or file-like object
- file in which to write the chart.
- format : string (optional)
- the format to write: one of ['json', 'html', 'png', 'svg', 'pdf'].
- If not specified, the format will be determined from the filename.
- override_data_transformer : `boolean` (optional)
- If True (default), then the save action will be done with
- the MaxRowsError disabled. If False, then do not change the data
- transformer.
- scale_factor : float
- For svg or png formats, scale the image by this factor when saving.
- This can be used to control the size or resolution of the output.
- Default is 1.0
- **kwargs :
- Additional keyword arguments are passed to the output method
- associated with the specified format.
-
- """
- from ...utils.save import save
-
- kwds = dict(
- chart=self,
- fp=fp,
- format=format,
- scale_factor=scale_factor,
- vegalite_version=vegalite_version,
- vega_version=vega_version,
- vegaembed_version=vegaembed_version,
- **kwargs,
- )
-
- # By default we override the data transformer. This makes it so
- # that save() will succeed even for large datasets that would
- # normally trigger a MaxRowsError
- if override_data_transformer:
- with data_transformers.disable_max_rows():
- result = save(**kwds)
- else:
- result = save(**kwds)
- return result
-
- # Fallback for when rendering fails; the full repr is too long to be
- # useful in nearly all cases.
- def __repr__(self):
- return "alt.{}(...)".format(self.__class__.__name__)
-
- # Layering and stacking
- def __add__(self, other):
- if not isinstance(other, TopLevelMixin):
- raise ValueError("Only Chart objects can be layered.")
- return layer(self, other)
-
- def __and__(self, other):
- if not isinstance(other, TopLevelMixin):
- raise ValueError("Only Chart objects can be concatenated.")
- return vconcat(self, other)
-
- def __or__(self, other):
- if not isinstance(other, TopLevelMixin):
- raise ValueError("Only Chart objects can be concatenated.")
- return hconcat(self, other)
-
- def repeat(
- self,
- repeat=Undefined,
- row=Undefined,
- column=Undefined,
- layer=Undefined,
- columns=Undefined,
- **kwargs,
- ) -> "RepeatChart":
- """Return a RepeatChart built from the chart
-
- Fields within the chart can be set to correspond to the row or
- column using `alt.repeat('row')` and `alt.repeat('column')`.
-
- Parameters
- ----------
- repeat : list
- a list of data column names to be repeated. This cannot be
- used along with the ``row``, ``column`` or ``layer`` argument.
- row : list
- a list of data column names to be mapped to the row facet
- column : list
- a list of data column names to be mapped to the column facet
- layer : list
- a list of data column names to be layered. This cannot be
- used along with the ``row``, ``column`` or ``repeat`` argument.
- columns : int
- the maximum number of columns before wrapping. Only referenced
- if ``repeat`` is specified.
- **kwargs :
- additional keywords passed to RepeatChart.
-
- Returns
- -------
- chart : RepeatChart
- a repeated chart.
- """
- repeat_specified = repeat is not Undefined
- rowcol_specified = row is not Undefined or column is not Undefined
- layer_specified = layer is not Undefined
-
- if repeat_specified and rowcol_specified:
- raise ValueError(
- "repeat argument cannot be combined with row/column argument."
- )
- elif repeat_specified and layer_specified:
- raise ValueError("repeat argument cannot be combined with layer argument.")
-
- if repeat_specified:
- repeat = repeat
- elif layer_specified:
- repeat = core.LayerRepeatMapping(layer=layer, row=row, column=column)
- else:
- repeat = core.RepeatMapping(row=row, column=column)
-
- return RepeatChart(spec=self, repeat=repeat, columns=columns, **kwargs)
-
- def properties(self, **kwargs) -> Self:
- """Set top-level properties of the Chart.
-
- Argument names and types are the same as class initialization.
- """
- # ignore type as copy comes from another class for subclasses of TopLevelMixin
- copy = self.copy(deep=False) # type: ignore[attr-defined]
- for key, val in kwargs.items():
- if key == "selection" and isinstance(val, Parameter):
- # TODO: Can this be removed
- # For backward compatibility with old selection interface.
- setattr(copy, key, {val.name: val.selection})
- else:
- # Don't validate data, because it hasn't been processed.
- if key != "data":
- # ignore type as validate_property comes from SchemaBase,
- # not from TopLevelMixin
- self.validate_property(key, val) # type: ignore[attr-defined]
- setattr(copy, key, val)
- return copy
-
- def project(
- self,
- type=Undefined,
- center=Undefined,
- clipAngle=Undefined,
- clipExtent=Undefined,
- coefficient=Undefined,
- distance=Undefined,
- fraction=Undefined,
- lobes=Undefined,
- parallel=Undefined,
- precision=Undefined,
- radius=Undefined,
- ratio=Undefined,
- reflectX=Undefined,
- reflectY=Undefined,
- rotate=Undefined,
- scale=Undefined,
- spacing=Undefined,
- tilt=Undefined,
- translate=Undefined,
- **kwds,
- ) -> Self:
- """Add a geographic projection to the chart.
-
- This is generally used either with ``mark_geoshape`` or with the
- ``latitude``/``longitude`` encodings.
-
- Available projection types are
- ['albers', 'albersUsa', 'azimuthalEqualArea', 'azimuthalEquidistant',
- 'conicConformal', 'conicEqualArea', 'conicEquidistant', 'equalEarth', 'equirectangular',
- 'gnomonic', 'identity', 'mercator', 'orthographic', 'stereographic', 'transverseMercator']
-
- Parameters
- ----------
- type : ProjectionType
- The cartographic projection to use. This value is case-insensitive, for example
- `"albers"` and `"Albers"` indicate the same projection type. You can find all valid
- projection types [in the
- documentation](https://vega.github.io/vega-lite/docs/projection.html#projection-types).
-
- **Default value:** `equalEarth`
- center : List(float)
- Sets the projection’s center to the specified center, a two-element array of
- longitude and latitude in degrees.
-
- **Default value:** `[0, 0]`
- clipAngle : float
- Sets the projection’s clipping circle radius to the specified angle in degrees. If
- `null`, switches to [antimeridian](http://bl.ocks.org/mbostock/3788999) cutting
- rather than small-circle clipping.
- clipExtent : List(List(float))
- Sets the projection’s viewport clip extent to the specified bounds in pixels. The
- extent bounds are specified as an array `[[x0, y0], [x1, y1]]`, where `x0` is the
- left-side of the viewport, `y0` is the top, `x1` is the right and `y1` is the
- bottom. If `null`, no viewport clipping is performed.
- coefficient : float
-
- distance : float
-
- fraction : float
-
- lobes : float
-
- parallel : float
-
- precision : Mapping(required=[length])
- Sets the threshold for the projection’s [adaptive
- resampling](http://bl.ocks.org/mbostock/3795544) to the specified value in pixels.
- This value corresponds to the [Douglas–Peucker
- distance](http://en.wikipedia.org/wiki/Ramer%E2%80%93Douglas%E2%80%93Peucker_algorithm).
- If precision is not specified, returns the projection’s current resampling
- precision which defaults to `√0.5 ≅ 0.70710…`.
- radius : float
-
- ratio : float
-
- reflectX : boolean
-
- reflectY : boolean
-
- rotate : List(float)
- Sets the projection’s three-axis rotation to the specified angles, which must be a
- two- or three-element array of numbers [`lambda`, `phi`, `gamma`] specifying the
- rotation angles in degrees about each spherical axis. (These correspond to yaw,
- pitch and roll.)
-
- **Default value:** `[0, 0, 0]`
- scale : float
- Sets the projection's scale (zoom) value, overriding automatic fitting.
-
- spacing : float
-
- tilt : float
-
- translate : List(float)
- Sets the projection's translation (pan) value, overriding automatic fitting.
-
- """
- projection = core.Projection(
- center=center,
- clipAngle=clipAngle,
- clipExtent=clipExtent,
- coefficient=coefficient,
- distance=distance,
- fraction=fraction,
- lobes=lobes,
- parallel=parallel,
- precision=precision,
- radius=radius,
- ratio=ratio,
- reflectX=reflectX,
- reflectY=reflectY,
- rotate=rotate,
- scale=scale,
- spacing=spacing,
- tilt=tilt,
- translate=translate,
- type=type,
- **kwds,
- )
- return self.properties(projection=projection)
-
- def _add_transform(self, *transforms):
- """Copy the chart and add specified transforms to chart.transform"""
- copy = self.copy(deep=["transform"])
- if copy.transform is Undefined:
- copy.transform = []
- copy.transform.extend(transforms)
- return copy
-
- def transform_aggregate(
- self, aggregate=Undefined, groupby=Undefined, **kwds
- ) -> Self:
- """
- Add an :class:`AggregateTransform` to the schema.
-
- Parameters
- ----------
- aggregate : List(:class:`AggregatedFieldDef`)
- Array of objects that define fields to aggregate.
- groupby : List(string)
- The data fields to group by. If not specified, a single group containing all data
- objects will be used.
- **kwds :
- additional keywords are converted to aggregates using standard
- shorthand parsing.
-
- Returns
- -------
- self : Chart object
- returns chart to allow for chaining
-
- Examples
- --------
- The aggregate transform allows you to specify transforms directly using
- the same shorthand syntax as used in encodings:
-
- >>> import altair as alt
- >>> chart1 = alt.Chart().transform_aggregate(
- ... mean_acc='mean(Acceleration)',
- ... groupby=['Origin']
- ... )
- >>> print(chart1.transform[0].to_json()) # doctest: +NORMALIZE_WHITESPACE
- {
- "aggregate": [
- {
- "as": "mean_acc",
- "field": "Acceleration",
- "op": "mean"
- }
- ],
- "groupby": [
- "Origin"
- ]
- }
-
- It also supports including AggregatedFieldDef instances or dicts directly,
- so you can create the above transform like this:
-
- >>> chart2 = alt.Chart().transform_aggregate(
- ... [alt.AggregatedFieldDef(field='Acceleration', op='mean',
- ... **{'as': 'mean_acc'})],
- ... groupby=['Origin']
- ... )
- >>> chart2.transform == chart1.transform
- True
-
- See Also
- --------
- alt.AggregateTransform : underlying transform object
-
- """
- if aggregate is Undefined:
- aggregate = []
- for key, val in kwds.items():
- parsed = utils.parse_shorthand(val)
- dct = {
- "as": key,
- "field": parsed.get("field", Undefined),
- "op": parsed.get("aggregate", Undefined),
- }
- aggregate.append(core.AggregatedFieldDef(**dct))
- return self._add_transform(
- core.AggregateTransform(aggregate=aggregate, groupby=groupby)
- )
-
- def transform_bin(self, as_=Undefined, field=Undefined, bin=True, **kwargs) -> Self:
- """
- Add a :class:`BinTransform` to the schema.
-
- Parameters
- ----------
- as_ : anyOf(string, List(string))
- The output fields at which to write the start and end bin values.
- bin : anyOf(boolean, :class:`BinParams`)
- An object indicating bin properties, or simply ``true`` for using default bin
- parameters.
- field : string
- The data field to bin.
-
- Returns
- -------
- self : Chart object
- returns chart to allow for chaining
-
- Examples
- --------
- >>> import altair as alt
- >>> chart = alt.Chart().transform_bin("x_binned", "x")
- >>> chart.transform[0]
- BinTransform({
- as: 'x_binned',
- bin: True,
- field: 'x'
- })
-
- >>> chart = alt.Chart().transform_bin("x_binned", "x",
- ... bin=alt.Bin(maxbins=10))
- >>> chart.transform[0]
- BinTransform({
- as: 'x_binned',
- bin: BinParams({
- maxbins: 10
- }),
- field: 'x'
- })
-
- See Also
- --------
- alt.BinTransform : underlying transform object
-
- """
- if as_ is not Undefined:
- if "as" in kwargs:
- raise ValueError(
- "transform_bin: both 'as_' and 'as' passed as arguments."
- )
- kwargs["as"] = as_
- kwargs["bin"] = bin
- kwargs["field"] = field
- return self._add_transform(core.BinTransform(**kwargs))
-
- def transform_calculate(self, as_=Undefined, calculate=Undefined, **kwargs) -> Self:
- """
- Add a :class:`CalculateTransform` to the schema.
-
- Parameters
- ----------
- as_ : string
- The field for storing the computed formula value.
- calculate : string or alt.expr expression
- A `expression `__
- string. Use the variable ``datum`` to refer to the current data object.
- **kwargs
- transforms can also be passed by keyword argument; see Examples
-
- Returns
- -------
- self : Chart object
- returns chart to allow for chaining
-
- Examples
- --------
- >>> import altair as alt
- >>> from altair import datum, expr
-
- >>> chart = alt.Chart().transform_calculate(y = 2 * expr.sin(datum.x))
- >>> chart.transform[0]
- CalculateTransform({
- as: 'y',
- calculate: (2 * sin(datum.x))
- })
-
- It's also possible to pass the ``CalculateTransform`` arguments directly:
-
- >>> kwds = {'as': 'y', 'calculate': '2 * sin(datum.x)'}
- >>> chart = alt.Chart().transform_calculate(**kwds)
- >>> chart.transform[0]
- CalculateTransform({
- as: 'y',
- calculate: '2 * sin(datum.x)'
- })
-
- As the first form is easier to write and understand, that is the
- recommended method.
-
- See Also
- --------
- alt.CalculateTransform : underlying transform object
- """
- if as_ is Undefined:
- as_ = kwargs.pop("as", Undefined)
- elif "as" in kwargs:
- raise ValueError(
- "transform_calculate: both 'as_' and 'as' passed as arguments."
- )
- if as_ is not Undefined or calculate is not Undefined:
- dct = {"as": as_, "calculate": calculate}
- self = self._add_transform(core.CalculateTransform(**dct))
- for as_, calculate in kwargs.items():
- dct = {"as": as_, "calculate": calculate}
- self = self._add_transform(core.CalculateTransform(**dct))
- return self
-
- def transform_density(
- self,
- density,
- as_=Undefined,
- bandwidth=Undefined,
- counts=Undefined,
- cumulative=Undefined,
- extent=Undefined,
- groupby=Undefined,
- maxsteps=Undefined,
- minsteps=Undefined,
- steps=Undefined,
- ) -> Self:
- """Add a :class:`DensityTransform` to the spec.
-
- Parameters
- ----------
- density : str
- The data field for which to perform density estimation.
- as_ : [str, str]
- The output fields for the sample value and corresponding density estimate.
- **Default value:** ``["value", "density"]``
- bandwidth : float
- The bandwidth (standard deviation) of the Gaussian kernel. If unspecified or set to
- zero, the bandwidth value is automatically estimated from the input data using
- Scott’s rule.
- counts : boolean
- A boolean flag indicating if the output values should be probability estimates
- (false) or smoothed counts (true).
- **Default value:** ``false``
- cumulative : boolean
- A boolean flag indicating whether to produce density estimates (false) or cumulative
- density estimates (true).
- **Default value:** ``false``
- extent : List([float, float])
- A [min, max] domain from which to sample the distribution. If unspecified, the
- extent will be determined by the observed minimum and maximum values of the density
- value field.
- groupby : List(str)
- The data fields to group by. If not specified, a single group containing all data
- objects will be used.
- maxsteps : float
- The maximum number of samples to take along the extent domain for plotting the
- density. **Default value:** ``200``
- minsteps : float
- The minimum number of samples to take along the extent domain for plotting the
- density. **Default value:** ``25``
- steps : float
- The exact number of samples to take along the extent domain for plotting the
- density. If specified, overrides both minsteps and maxsteps to set an exact number
- of uniform samples. Potentially useful in conjunction with a fixed extent to ensure
- consistent sample points for stacked densities.
- """
- return self._add_transform(
- core.DensityTransform(
- density=density,
- bandwidth=bandwidth,
- counts=counts,
- cumulative=cumulative,
- extent=extent,
- groupby=groupby,
- maxsteps=maxsteps,
- minsteps=minsteps,
- steps=steps,
- **{"as": as_},
- )
- )
-
- def transform_impute(
- self,
- impute,
- key,
- frame=Undefined,
- groupby=Undefined,
- keyvals=Undefined,
- method=Undefined,
- value=Undefined,
- ) -> Self:
- """
- Add an :class:`ImputeTransform` to the schema.
-
- Parameters
- ----------
- impute : string
- The data field for which the missing values should be imputed.
- key : string
- A key field that uniquely identifies data objects within a group.
- Missing key values (those occurring in the data but not in the current group) will
- be imputed.
- frame : List(anyOf(None, float))
- A frame specification as a two-element array used to control the window over which
- the specified method is applied. The array entries should either be a number
- indicating the offset from the current data object, or null to indicate unbounded
- rows preceding or following the current data object. For example, the value ``[-5,
- 5]`` indicates that the window should include five objects preceding and five
- objects following the current object.
- **Default value:** : ``[null, null]`` indicating that the window includes all
- objects.
- groupby : List(string)
- An optional array of fields by which to group the values.
- Imputation will then be performed on a per-group basis.
- keyvals : anyOf(List(Mapping(required=[])), :class:`ImputeSequence`)
- Defines the key values that should be considered for imputation.
- An array of key values or an object defining a `number sequence
- `__.
- If provided, this will be used in addition to the key values observed within the
- input data. If not provided, the values will be derived from all unique values of
- the ``key`` field. For ``impute`` in ``encoding``, the key field is the x-field if
- the y-field is imputed, or vice versa.
- If there is no impute grouping, this property *must* be specified.
- method : :class:`ImputeMethod`
- The imputation method to use for the field value of imputed data objects.
- One of ``value``, ``mean``, ``median``, ``max`` or ``min``.
- **Default value:** ``"value"``
- value : Mapping(required=[])
- The field value to use when the imputation ``method`` is ``"value"``.
-
- Returns
- -------
- self : Chart object
- returns chart to allow for chaining
-
- See Also
- --------
- alt.ImputeTransform : underlying transform object
- """
- return self._add_transform(
- core.ImputeTransform(
- impute=impute,
- key=key,
- frame=frame,
- groupby=groupby,
- keyvals=keyvals,
- method=method,
- value=value,
- )
- )
-
- def transform_joinaggregate(
- self, joinaggregate=Undefined, groupby=Undefined, **kwargs
- ) -> Self:
- """
- Add a :class:`JoinAggregateTransform` to the schema.
-
- Parameters
- ----------
- joinaggregate : List(:class:`JoinAggregateFieldDef`)
- The definition of the fields in the join aggregate, and what calculations to use.
- groupby : List(string)
- The data fields for partitioning the data objects into separate groups. If
- unspecified, all data points will be in a single group.
- **kwargs
- joinaggregates can also be passed by keyword argument; see Examples.
-
- Returns
- -------
- self : Chart object
- returns chart to allow for chaining
-
- Examples
- --------
- >>> import altair as alt
- >>> chart = alt.Chart().transform_joinaggregate(x='sum(y)')
- >>> chart.transform[0]
- JoinAggregateTransform({
- joinaggregate: [JoinAggregateFieldDef({
- as: 'x',
- field: 'y',
- op: 'sum'
- })]
- })
-
- See Also
- --------
- alt.JoinAggregateTransform : underlying transform object
- """
- if joinaggregate is Undefined:
- joinaggregate = []
- for key, val in kwargs.items():
- parsed = utils.parse_shorthand(val)
- dct = {
- "as": key,
- "field": parsed.get("field", Undefined),
- "op": parsed.get("aggregate", Undefined),
- }
- joinaggregate.append(core.JoinAggregateFieldDef(**dct))
- return self._add_transform(
- core.JoinAggregateTransform(joinaggregate=joinaggregate, groupby=groupby)
- )
-
- # TODO: Update docstring
- def transform_filter(self, filter, **kwargs) -> Self:
- """
- Add a :class:`FilterTransform` to the schema.
-
- Parameters
- ----------
- filter : a filter expression or :class:`PredicateComposition`
- The `filter` property must be one of the predicate definitions:
- (1) a string or alt.expr expression
- (2) a range predicate
- (3) a selection predicate
- (4) a logical operand combining (1)-(3)
- (5) a Selection object
-
- Returns
- -------
- self : Chart object
- returns chart to allow for chaining
-
- See Also
- --------
- alt.FilterTransform : underlying transform object
-
- """
- if isinstance(filter, Parameter):
- new_filter = {"param": filter.name}
- if "empty" in kwargs:
- new_filter["empty"] = kwargs.pop("empty")
- elif isinstance(filter.empty, bool):
- new_filter["empty"] = filter.empty
- filter = new_filter
- return self._add_transform(core.FilterTransform(filter=filter, **kwargs))
-
- def transform_flatten(self, flatten, as_=Undefined) -> Self:
- """Add a :class:`FlattenTransform` to the schema.
-
- Parameters
- ----------
- flatten : List(string)
- An array of one or more data fields containing arrays to flatten.
- If multiple fields are specified, their array values should have a parallel
- structure, ideally with the same length.
- If the lengths of parallel arrays do not match,
- the longest array will be used with ``null`` values added for missing entries.
- as : List(string)
- The output field names for extracted array values.
- **Default value:** The field name of the corresponding array field
-
- Returns
- -------
- self : Chart object
- returns chart to allow for chaining
-
- See Also
- --------
- alt.FlattenTransform : underlying transform object
- """
- return self._add_transform(
- core.FlattenTransform(flatten=flatten, **{"as": as_})
- )
-
- def transform_fold(self, fold, as_=Undefined) -> Self:
- """Add a :class:`FoldTransform` to the spec.
-
- Parameters
- ----------
- fold : List(string)
- An array of data fields indicating the properties to fold.
- as : [string, string]
- The output field names for the key and value properties produced by the fold
- transform. Default: ``["key", "value"]``
-
- Returns
- -------
- self : Chart object
- returns chart to allow for chaining
-
- See Also
- --------
- Chart.transform_pivot : pivot transform - opposite of fold.
- alt.FoldTransform : underlying transform object
- """
- return self._add_transform(core.FoldTransform(fold=fold, **{"as": as_}))
-
- def transform_loess(
- self,
- on,
- loess,
- as_=Undefined,
- bandwidth=Undefined,
- groupby=Undefined,
- ) -> Self:
- """Add a :class:`LoessTransform` to the spec.
-
- Parameters
- ----------
- on : str
- The data field of the independent variable to use a predictor.
- loess : str
- The data field of the dependent variable to smooth.
- as_ : [str, str]
- The output field names for the smoothed points generated by the loess transform.
- **Default value:** The field names of the input x and y values.
- bandwidth : float
- A bandwidth parameter in the range ``[0, 1]`` that determines the amount of
- smoothing. **Default value:** ``0.3``
- groupby : List(str)
- The data fields to group by. If not specified, a single group containing all data
- objects will be used.
-
- Returns
- -------
- self : Chart object
- returns chart to allow for chaining
-
- See Also
- --------
- Chart.transform_regression: regression transform
- alt.LoessTransform : underlying transform object
- """
- return self._add_transform(
- core.LoessTransform(
- loess=loess, on=on, bandwidth=bandwidth, groupby=groupby, **{"as": as_}
- )
- )
-
- def transform_lookup(
- self,
- lookup=Undefined,
- from_=Undefined,
- as_=Undefined,
- default=Undefined,
- **kwargs,
- ) -> Self:
- """Add a :class:`DataLookupTransform` or :class:`SelectionLookupTransform` to the chart
-
- Parameters
- ----------
- lookup : string
- Key in primary data source.
- from_ : anyOf(:class:`LookupData`, :class:`LookupSelection`)
- Secondary data reference.
- as_ : anyOf(string, List(string))
- The output fields on which to store the looked up data values.
-
- For data lookups, this property may be left blank if ``from_.fields``
- has been specified (those field names will be used); if ``from_.fields``
- has not been specified, ``as_`` must be a string.
-
- For selection lookups, this property is optional: if unspecified,
- looked up values will be stored under a property named for the selection;
- and if specified, it must correspond to ``from_.fields``.
- default : string
- The default value to use if lookup fails. **Default value:** ``null``
-
- Returns
- -------
- self : Chart object
- returns chart to allow for chaining
-
- See Also
- --------
- alt.DataLookupTransform : underlying transform object
- alt.SelectionLookupTransform : underlying transform object
- """
- if as_ is not Undefined:
- if "as" in kwargs:
- raise ValueError(
- "transform_lookup: both 'as_' and 'as' passed as arguments."
- )
- kwargs["as"] = as_
- if from_ is not Undefined:
- if "from" in kwargs:
- raise ValueError(
- "transform_lookup: both 'from_' and 'from' passed as arguments."
- )
- kwargs["from"] = from_
- kwargs["lookup"] = lookup
- kwargs["default"] = default
- return self._add_transform(core.LookupTransform(**kwargs))
-
- def transform_pivot(
- self,
- pivot,
- value,
- groupby=Undefined,
- limit=Undefined,
- op=Undefined,
- ) -> Self:
- """Add a :class:`PivotTransform` to the chart.
-
- Parameters
- ----------
- pivot : str
- The data field to pivot on. The unique values of this field become new field names
- in the output stream.
- value : str
- The data field to populate pivoted fields. The aggregate values of this field become
- the values of the new pivoted fields.
- groupby : List(str)
- The optional data fields to group by. If not specified, a single group containing
- all data objects will be used.
- limit : float
- An optional parameter indicating the maximum number of pivoted fields to generate.
- The default ( ``0`` ) applies no limit. The pivoted ``pivot`` names are sorted in
- ascending order prior to enforcing the limit.
- **Default value:** ``0``
- op : string
- The aggregation operation to apply to grouped ``value`` field values.
- **Default value:** ``sum``
-
- Returns
- -------
- self : Chart object
- returns chart to allow for chaining
-
- See Also
- --------
- Chart.transform_fold : fold transform - opposite of pivot.
- alt.PivotTransform : underlying transform object
- """
- return self._add_transform(
- core.PivotTransform(
- pivot=pivot, value=value, groupby=groupby, limit=limit, op=op
- )
- )
-
- def transform_quantile(
- self,
- quantile,
- as_=Undefined,
- groupby=Undefined,
- probs=Undefined,
- step=Undefined,
- ) -> Self:
- """Add a :class:`QuantileTransform` to the chart
-
- Parameters
- ----------
- quantile : str
- The data field for which to perform quantile estimation.
- as : [str, str]
- The output field names for the probability and quantile values.
- groupby : List(str)
- The data fields to group by. If not specified, a single group containing all data
- objects will be used.
- probs : List(float)
- An array of probabilities in the range (0, 1) for which to compute quantile values.
- If not specified, the *step* parameter will be used.
- step : float
- A probability step size (default 0.01) for sampling quantile values. All values from
- one-half the step size up to 1 (exclusive) will be sampled. This parameter is only
- used if the *probs* parameter is not provided. **Default value:** ``["prob", "value"]``
-
- Returns
- -------
- self : Chart object
- returns chart to allow for chaining
-
- See Also
- --------
- alt.QuantileTransform : underlying transform object
- """
- return self._add_transform(
- core.QuantileTransform(
- quantile=quantile,
- groupby=groupby,
- probs=probs,
- step=step,
- **{"as": as_},
- )
- )
-
- def transform_regression(
- self,
- on,
- regression,
- as_=Undefined,
- extent=Undefined,
- groupby=Undefined,
- method=Undefined,
- order=Undefined,
- params=Undefined,
- ) -> Self:
- """Add a :class:`RegressionTransform` to the chart.
-
- Parameters
- ----------
- on : str
- The data field of the independent variable to use a predictor.
- regression : str
- The data field of the dependent variable to predict.
- as_ : [str, str]
- The output field names for the smoothed points generated by the regression
- transform. **Default value:** The field names of the input x and y values.
- extent : [float, float]
- A [min, max] domain over the independent (x) field for the starting and ending
- points of the generated trend line.
- groupby : List(str)
- The data fields to group by. If not specified, a single group containing all data
- objects will be used.
- method : enum('linear', 'log', 'exp', 'pow', 'quad', 'poly')
- The functional form of the regression model. One of ``"linear"``, ``"log"``,
- ``"exp"``, ``"pow"``, ``"quad"``, or ``"poly"``. **Default value:** ``"linear"``
- order : float
- The polynomial order (number of coefficients) for the 'poly' method.
- **Default value:** ``3``
- params : boolean
- A boolean flag indicating if the transform should return the regression model
- parameters (one object per group), rather than trend line points.
- The resulting objects include a ``coef`` array of fitted coefficient values
- (starting with the intercept term and then including terms of increasing order)
- and an ``rSquared`` value (indicating the total variance explained by the model).
- **Default value:** ``false``
-
- Returns
- -------
- self : Chart object
- returns chart to allow for chaining
-
- See Also
- --------
- Chart.transform_loess : LOESS transform
- alt.RegressionTransform : underlying transform object
- """
- return self._add_transform(
- core.RegressionTransform(
- regression=regression,
- on=on,
- extent=extent,
- groupby=groupby,
- method=method,
- order=order,
- params=params,
- **{"as": as_},
- )
- )
-
- def transform_sample(self, sample=1000) -> Self:
- """
- Add a :class:`SampleTransform` to the schema.
-
- Parameters
- ----------
- sample : float
- The maximum number of data objects to include in the sample. Default: 1000.
-
- Returns
- -------
- self : Chart object
- returns chart to allow for chaining
-
- See Also
- --------
- alt.SampleTransform : underlying transform object
- """
- return self._add_transform(core.SampleTransform(sample))
-
- def transform_stack(
- self, as_, stack, groupby, offset=Undefined, sort=Undefined
- ) -> Self:
- """
- Add a :class:`StackTransform` to the schema.
-
- Parameters
- ----------
- as_ : anyOf(string, List(string))
- Output field names. This can be either a string or an array of strings with
- two elements denoting the name for the fields for stack start and stack end
- respectively.
- If a single string(eg."val") is provided, the end field will be "val_end".
- stack : string
- The field which is stacked.
- groupby : List(string)
- The data fields to group by.
- offset : enum('zero', 'center', 'normalize')
- Mode for stacking marks. Default: 'zero'.
- sort : List(:class:`SortField`)
- Field that determines the order of leaves in the stacked charts.
-
- Returns
- -------
- self : Chart object
- returns chart to allow for chaining
-
- See Also
- --------
- alt.StackTransform : underlying transform object
- """
- return self._add_transform(
- core.StackTransform(
- stack=stack, groupby=groupby, offset=offset, sort=sort, **{"as": as_}
- )
- )
-
- def transform_timeunit(
- self,
- as_=Undefined,
- field=Undefined,
- timeUnit=Undefined,
- **kwargs,
- ) -> Self:
- """
- Add a :class:`TimeUnitTransform` to the schema.
-
- Parameters
- ----------
- as_ : string
- The output field to write the timeUnit value.
- field : string
- The data field to apply time unit.
- timeUnit : :class:`TimeUnit`
- The timeUnit.
- **kwargs
- transforms can also be passed by keyword argument; see Examples
-
- Returns
- -------
- self : Chart object
- returns chart to allow for chaining
-
- Examples
- --------
- >>> import altair as alt
- >>> from altair import datum, expr
-
- >>> chart = alt.Chart().transform_timeunit(month='month(date)')
- >>> chart.transform[0]
- TimeUnitTransform({
- as: 'month',
- field: 'date',
- timeUnit: 'month'
- })
-
- It's also possible to pass the ``TimeUnitTransform`` arguments directly;
- this is most useful in cases where the desired field name is not a
- valid python identifier:
-
- >>> kwds = {'as': 'month', 'timeUnit': 'month', 'field': 'The Month'}
- >>> chart = alt.Chart().transform_timeunit(**kwds)
- >>> chart.transform[0]
- TimeUnitTransform({
- as: 'month',
- field: 'The Month',
- timeUnit: 'month'
- })
-
- As the first form is easier to write and understand, that is the
- recommended method.
-
- See Also
- --------
- alt.TimeUnitTransform : underlying transform object
-
- """
- if as_ is Undefined:
- as_ = kwargs.pop("as", Undefined)
- else:
- if "as" in kwargs:
- raise ValueError(
- "transform_timeunit: both 'as_' and 'as' passed as arguments."
- )
- if as_ is not Undefined:
- dct = {"as": as_, "timeUnit": timeUnit, "field": field}
- self = self._add_transform(core.TimeUnitTransform(**dct))
- for as_, shorthand in kwargs.items():
- dct = utils.parse_shorthand(
- shorthand,
- parse_timeunits=True,
- parse_aggregates=False,
- parse_types=False,
- )
- dct.pop("type", None)
- dct["as"] = as_
- if "timeUnit" not in dct:
- raise ValueError("'{}' must include a valid timeUnit".format(shorthand))
- self = self._add_transform(core.TimeUnitTransform(**dct))
- return self
-
- def transform_window(
- self,
- window=Undefined,
- frame=Undefined,
- groupby=Undefined,
- ignorePeers=Undefined,
- sort=Undefined,
- **kwargs,
- ) -> Self:
- """Add a :class:`WindowTransform` to the schema
-
- Parameters
- ----------
- window : List(:class:`WindowFieldDef`)
- The definition of the fields in the window, and what calculations to use.
- frame : List(anyOf(None, float))
- A frame specification as a two-element array indicating how the sliding window
- should proceed. The array entries should either be a number indicating the offset
- from the current data object, or null to indicate unbounded rows preceding or
- following the current data object. The default value is ``[null, 0]``, indicating
- that the sliding window includes the current object and all preceding objects. The
- value ``[-5, 5]`` indicates that the window should include five objects preceding
- and five objects following the current object. Finally, ``[null, null]`` indicates
- that the window frame should always include all data objects. The only operators
- affected are the aggregation operations and the ``first_value``, ``last_value``, and
- ``nth_value`` window operations. The other window operations are not affected by
- this.
-
- **Default value:** : ``[null, 0]`` (includes the current object and all preceding
- objects)
- groupby : List(string)
- The data fields for partitioning the data objects into separate windows. If
- unspecified, all data points will be in a single group.
- ignorePeers : boolean
- Indicates if the sliding window frame should ignore peer values. (Peer values are
- those considered identical by the sort criteria). The default is false, causing the
- window frame to expand to include all peer values. If set to true, the window frame
- will be defined by offset values only. This setting only affects those operations
- that depend on the window frame, namely aggregation operations and the first_value,
- last_value, and nth_value window operations.
-
- **Default value:** ``false``
- sort : List(:class:`SortField`)
- A sort field definition for sorting data objects within a window. If two data
- objects are considered equal by the comparator, they are considered “peer” values of
- equal rank. If sort is not specified, the order is undefined: data objects are
- processed in the order they are observed and none are considered peers (the
- ignorePeers parameter is ignored and treated as if set to ``true`` ).
- **kwargs
- transforms can also be passed by keyword argument; see Examples
-
- Examples
- --------
- A cumulative line chart
-
- >>> import altair as alt
- >>> import numpy as np
- >>> import pandas as pd
- >>> data = pd.DataFrame({'x': np.arange(100),
- ... 'y': np.random.randn(100)})
- >>> chart = alt.Chart(data).mark_line().encode(
- ... x='x:Q',
- ... y='ycuml:Q'
- ... ).transform_window(
- ... ycuml='sum(y)'
- ... )
- >>> chart.transform[0]
- WindowTransform({
- window: [WindowFieldDef({
- as: 'ycuml',
- field: 'y',
- op: 'sum'
- })]
- })
-
- """
- if kwargs:
- if window is Undefined:
- window = []
- for as_, shorthand in kwargs.items():
- kwds = {"as": as_}
- kwds.update(
- utils.parse_shorthand(
- shorthand,
- parse_aggregates=False,
- parse_window_ops=True,
- parse_timeunits=False,
- parse_types=False,
- )
- )
- window.append(core.WindowFieldDef(**kwds))
-
- return self._add_transform(
- core.WindowTransform(
- window=window,
- frame=frame,
- groupby=groupby,
- ignorePeers=ignorePeers,
- sort=sort,
- )
- )
-
- # Display-related methods
-
- def _repr_mimebundle_(self, include=None, exclude=None):
- """Return a MIME bundle for display in Jupyter frontends."""
- # Catch errors explicitly to get around issues in Jupyter frontend
- # see https://github.com/ipython/ipython/issues/11038
- try:
- dct = self.to_dict()
- except Exception:
- utils.display_traceback(in_ipython=True)
- return {}
- else:
- return renderers.get()(dct)
-
- def display(self, renderer=Undefined, theme=Undefined, actions=Undefined, **kwargs):
- """Display chart in Jupyter notebook or JupyterLab
-
- Parameters are passed as options to vega-embed within supported frontends.
- See https://github.com/vega/vega-embed#options for details.
-
- Parameters
- ----------
- renderer : string ('canvas' or 'svg')
- The renderer to use
- theme : string
- The Vega theme name to use; see https://github.com/vega/vega-themes
- actions : bool or dict
- Specify whether action links ("Open In Vega Editor", etc.) are
- included in the view.
- **kwargs :
- Additional parameters are also passed to vega-embed as options.
-
- """
- from IPython.display import display
-
- if renderer is not Undefined:
- kwargs["renderer"] = renderer
- if theme is not Undefined:
- kwargs["theme"] = theme
- if actions is not Undefined:
- kwargs["actions"] = actions
-
- if kwargs:
- options = renderers.options.copy()
- options["embed_options"] = options.get("embed_options", {}).copy()
- options["embed_options"].update(kwargs)
- with renderers.enable(**options):
- display(self)
- else:
- display(self)
-
- @utils.deprecation.deprecated(message="'serve' is deprecated. Use 'show' instead.")
- def serve(
- self,
- ip="127.0.0.1",
- port=8888,
- n_retries=50,
- files=None,
- jupyter_warning=True,
- open_browser=True,
- http_server=None,
- **kwargs,
- ):
- """
- 'serve' is deprecated. Use 'show' instead.
-
- Open a browser window and display a rendering of the chart
-
- Parameters
- ----------
- html : string
- HTML to serve
- ip : string (default = '127.0.0.1')
- ip address at which the HTML will be served.
- port : int (default = 8888)
- the port at which to serve the HTML
- n_retries : int (default = 50)
- the number of nearby ports to search if the specified port
- is already in use.
- files : dictionary (optional)
- dictionary of extra content to serve
- jupyter_warning : bool (optional)
- if True (default), then print a warning if this is used
- within the Jupyter notebook
- open_browser : bool (optional)
- if True (default), then open a web browser to the given HTML
- http_server : class (optional)
- optionally specify an HTTPServer class to use for showing the
- figure. The default is Python's basic HTTPServer.
- **kwargs :
- additional keyword arguments passed to the save() method
-
- """
- from ...utils.server import serve
-
- html = io.StringIO()
- self.save(html, format="html", **kwargs)
- html.seek(0)
-
- serve(
- html.read(),
- ip=ip,
- port=port,
- n_retries=n_retries,
- files=files,
- jupyter_warning=jupyter_warning,
- open_browser=open_browser,
- http_server=http_server,
- )
-
- def show(self, embed_opt=None, open_browser=None):
- """Show the chart in an external browser window.
-
- This requires a recent version of the altair_viewer package.
-
- Parameters
- ----------
- embed_opt : dict (optional)
- The Vega embed options that control the dispay of the chart.
- open_browser : bool (optional)
- Specify whether a browser window should be opened. If not specified,
- a browser window will be opened only if the server is not already
- connected to a browser.
- """
- try:
- import altair_viewer # type: ignore
- except ImportError as err:
- raise ValueError(
- "'show' method requires the altair_viewer package. "
- "See http://github.com/altair-viz/altair_viewer"
- ) from err
- altair_viewer.show(self, embed_opt=embed_opt, open_browser=open_browser)
-
- @utils.use_signature(core.Resolve)
- def _set_resolve(self, **kwargs):
- """Copy the chart and update the resolve property with kwargs"""
- if not hasattr(self, "resolve"):
- raise ValueError(
- "{} object has no attribute " "'resolve'".format(self.__class__)
- )
- copy = self.copy(deep=["resolve"])
- if copy.resolve is Undefined:
- copy.resolve = core.Resolve()
- for key, val in kwargs.items():
- copy.resolve[key] = val
- return copy
-
- @utils.use_signature(core.AxisResolveMap)
- def resolve_axis(self, *args, **kwargs) -> Self:
- return self._set_resolve(axis=core.AxisResolveMap(*args, **kwargs))
-
- @utils.use_signature(core.LegendResolveMap)
- def resolve_legend(self, *args, **kwargs) -> Self:
- return self._set_resolve(legend=core.LegendResolveMap(*args, **kwargs))
-
- @utils.use_signature(core.ScaleResolveMap)
- def resolve_scale(self, *args, **kwargs) -> Self:
- return self._set_resolve(scale=core.ScaleResolveMap(*args, **kwargs))
-
-
-class _EncodingMixin:
- @utils.use_signature(core.FacetedEncoding)
- def encode(self, *args, **kwargs) -> Self:
- # Convert args to kwargs based on their types.
- kwargs = utils.infer_encoding_types(args, kwargs, channels)
-
- # get a copy of the dict representation of the previous encoding
- # ignore type as copy method comes from SchemaBase
- copy = self.copy(deep=["encoding"]) # type: ignore[attr-defined]
- encoding = copy._get("encoding", {})
- if isinstance(encoding, core.VegaLiteSchema):
- encoding = {k: v for k, v in encoding._kwds.items() if v is not Undefined}
-
- # update with the new encodings, and apply them to the copy
- encoding.update(kwargs)
- copy.encoding = core.FacetedEncoding(**encoding)
- return copy
-
- def facet(
- self,
- facet=Undefined,
- row=Undefined,
- column=Undefined,
- data=Undefined,
- columns=Undefined,
- **kwargs,
- ) -> "FacetChart":
- """Create a facet chart from the current chart.
-
- Faceted charts require data to be specified at the top level; if data
- is not specified, the data from the current chart will be used at the
- top level.
-
- Parameters
- ----------
- facet : string or alt.Facet (optional)
- The data column to use as an encoding for a wrapped facet.
- If specified, then neither row nor column may be specified.
- column : string or alt.Column (optional)
- The data column to use as an encoding for a column facet.
- May be combined with row argument, but not with facet argument.
- row : string or alt.Column (optional)
- The data column to use as an encoding for a row facet.
- May be combined with column argument, but not with facet argument.
- data : string or dataframe (optional)
- The dataset to use for faceting. If not supplied, then data must
- be specified in the top-level chart that calls this method.
- columns : integer
- the maximum number of columns for a wrapped facet.
-
- Returns
- -------
- self :
- for chaining
- """
- facet_specified = facet is not Undefined
- rowcol_specified = row is not Undefined or column is not Undefined
-
- if facet_specified and rowcol_specified:
- raise ValueError(
- "facet argument cannot be combined with row/column argument."
- )
-
- # Remove "ignore" statement once Undefined is no longer typed as Any
- if data is Undefined: # type: ignore
- # Remove "ignore" statement once Undefined is no longer typed as Any
- if self.data is Undefined: # type: ignore
- raise ValueError(
- "Facet charts require data to be specified at the top level."
- )
- # ignore type as copy comes from another class
- self = self.copy(deep=False) # type: ignore[attr-defined]
- # Remove "ignore" statement once Undefined is no longer typed as Any
- data, self.data = self.data, Undefined # type: ignore
-
- if facet_specified:
- if isinstance(facet, str):
- facet = channels.Facet(facet)
- else:
- facet = FacetMapping(row=row, column=column)
-
- return FacetChart(spec=self, facet=facet, data=data, columns=columns, **kwargs)
-
-
-class Chart(
- TopLevelMixin, _EncodingMixin, mixins.MarkMethodMixin, core.TopLevelUnitSpec
-):
- """Create a basic Altair/Vega-Lite chart.
-
- Although it is possible to set all Chart properties as constructor attributes,
- it is more idiomatic to use methods such as ``mark_point()``, ``encode()``,
- ``transform_filter()``, ``properties()``, etc. See Altair's documentation
- for details and examples: http://altair-viz.github.io/.
-
- Parameters
- ----------
- data : Data
- An object describing the data source
- mark : AnyMark
- A string describing the mark type (one of `"bar"`, `"circle"`, `"square"`, `"tick"`,
- `"line"`, * `"area"`, `"point"`, `"rule"`, `"geoshape"`, and `"text"`) or a
- MarkDef object.
- encoding : FacetedEncoding
- A key-value mapping between encoding channels and definition of fields.
- autosize : anyOf(AutosizeType, AutoSizeParams)
- Sets how the visualization size should be determined. If a string, should be one of
- `"pad"`, `"fit"` or `"none"`. Object values can additionally specify parameters for
- content sizing and automatic resizing. `"fit"` is only supported for single and
- layered views that don't use `rangeStep`. Default value: `pad`
- background : string
- CSS color property to use as the background of visualization.
-
- **Default value:** none (transparent)
- config : Config
- Vega-Lite configuration object. This property can only be defined at the top-level
- of a specification.
- description : string
- Description of this mark for commenting purpose.
- height : float
- The height of a visualization.
- name : string
- Name of the visualization for later reference.
- padding : Padding
- The default visualization padding, in pixels, from the edge of the visualization
- canvas to the data rectangle. If a number, specifies padding for all sides. If an
- object, the value should have the format `{"left": 5, "top": 5, "right": 5,
- "bottom": 5}` to specify padding for each side of the visualization. Default
- value: `5`
- projection : Projection
- An object defining properties of geographic projection. Works with `"geoshape"`
- marks and `"point"` or `"line"` marks that have a channel (one or more of `"X"`,
- `"X2"`, `"Y"`, `"Y2"`) with type `"latitude"`, or `"longitude"`.
- selection : Mapping(required=[])
- A key-value mapping between selection names and definitions.
- title : anyOf(string, TitleParams)
- Title for the plot.
- transform : List(Transform)
- An array of data transformations such as filter and new field calculation.
- width : float
- The width of a visualization.
- """
-
- def __init__(
- self,
- data=Undefined,
- encoding=Undefined,
- mark=Undefined,
- width=Undefined,
- height=Undefined,
- **kwargs,
- ):
- super(Chart, self).__init__(
- data=data,
- encoding=encoding,
- mark=mark,
- width=width,
- height=height,
- **kwargs,
- )
-
- _counter = 0
-
- @classmethod
- def _get_name(cls):
- cls._counter += 1
- return f"view_{cls._counter}"
-
- @classmethod
- def from_dict(cls, dct, validate=True) -> "Chart": # type: ignore[override] # Not the same signature as SchemaBase.from_dict. Would ideally be aligned in the future
- """Construct class from a dictionary representation
-
- Parameters
- ----------
- dct : dictionary
- The dict from which to construct the class
- validate : boolean
- If True (default), then validate the input against the schema.
-
- Returns
- -------
- obj : Chart object
- The wrapped schema
-
- Raises
- ------
- jsonschema.ValidationError :
- if validate=True and dct does not conform to the schema
- """
- for class_ in TopLevelMixin.__subclasses__():
- if class_ is Chart:
- class_ = cast(TypingType[TopLevelMixin], super(Chart, cls))
- try:
- # TopLevelMixin classes don't necessarily have from_dict defined
- # but all classes which are used here have due to how Altair is
- # designed. Too complex to type check right now.
- return class_.from_dict(dct, validate=validate) # type: ignore[attr-defined]
- except jsonschema.ValidationError:
- pass
-
- # As a last resort, try using the Root vegalite object
- return core.Root.from_dict(dct, validate)
-
- def to_dict(self, *args, **kwargs) -> dict:
- """Convert the chart to a dictionary suitable for JSON export."""
- context = kwargs.get("context", {})
- if self.data is Undefined and "data" not in context:
- # No data specified here or in parent: inject empty data
- # for easier specification of datum encodings.
- copy = self.copy(deep=False)
- copy.data = core.InlineData(values=[{}])
- return super(Chart, copy).to_dict(*args, **kwargs)
- return super().to_dict(*args, **kwargs)
-
- def add_params(self, *params) -> Self:
- """Add one or more parameters to the chart."""
- if not params:
- return self
- copy = self.copy(deep=["params"])
- if copy.params is Undefined:
- copy.params = []
-
- for s in params:
- copy.params.append(s.param)
- return copy
-
- @utils.deprecation.deprecated(
- message="'add_selection' is deprecated. Use 'add_params' instead."
- )
- def add_selection(self, *params) -> Self:
- """'add_selection' is deprecated. Use 'add_params' instead."""
- return self.add_params(*params)
-
- def interactive(self, name=None, bind_x=True, bind_y=True) -> Self:
- """Make chart axes scales interactive
-
- Parameters
- ----------
- name : string
- The parameter name to use for the axes scales. This name should be
- unique among all parameters within the chart.
- bind_x : boolean, default True
- If true, then bind the interactive scales to the x-axis
- bind_y : boolean, default True
- If true, then bind the interactive scales to the y-axis
-
- Returns
- -------
- chart :
- copy of self, with interactive axes added
-
- """
- encodings = []
- if bind_x:
- encodings.append("x")
- if bind_y:
- encodings.append("y")
- return self.add_params(selection_interval(bind="scales", encodings=encodings))
-
-
-def _check_if_valid_subspec(spec, classname):
- """Check if the spec is a valid sub-spec.
-
- If it is not, then raise a ValueError
- """
- err = (
- 'Objects with "{0}" attribute cannot be used within {1}. '
- "Consider defining the {0} attribute in the {1} object instead."
- )
-
- if not isinstance(spec, (core.SchemaBase, dict)):
- raise ValueError("Only chart objects can be used in {0}.".format(classname))
- for attr in TOPLEVEL_ONLY_KEYS:
- if isinstance(spec, core.SchemaBase):
- val = getattr(spec, attr, Undefined)
- else:
- val = spec.get(attr, Undefined)
- if val is not Undefined:
- raise ValueError(err.format(attr, classname))
-
-
-def _check_if_can_be_layered(spec):
- """Check if the spec can be layered."""
-
- def _get(spec, attr):
- if isinstance(spec, core.SchemaBase):
- return spec._get(attr)
- else:
- return spec.get(attr, Undefined)
-
- encoding = _get(spec, "encoding")
- if encoding is not Undefined:
- for channel in ["row", "column", "facet"]:
- if _get(encoding, channel) is not Undefined:
- raise ValueError(
- "Faceted charts cannot be layered. Instead, layer the charts before faceting."
- )
- if isinstance(spec, (Chart, LayerChart)):
- return
-
- if not isinstance(spec, (core.SchemaBase, dict)):
- raise ValueError("Only chart objects can be layered.")
- if _get(spec, "facet") is not Undefined:
- raise ValueError(
- "Faceted charts cannot be layered. Instead, layer the charts before faceting."
- )
- if isinstance(spec, FacetChart) or _get(spec, "facet") is not Undefined:
- raise ValueError(
- "Faceted charts cannot be layered. Instead, layer the charts before faceting."
- )
- if isinstance(spec, RepeatChart) or _get(spec, "repeat") is not Undefined:
- raise ValueError(
- "Repeat charts cannot be layered. Instead, layer the charts before repeating."
- )
- if isinstance(spec, ConcatChart) or _get(spec, "concat") is not Undefined:
- raise ValueError(
- "Concatenated charts cannot be layered. Instead, layer the charts before concatenating."
- )
- if isinstance(spec, HConcatChart) or _get(spec, "hconcat") is not Undefined:
- raise ValueError(
- "Concatenated charts cannot be layered. Instead, layer the charts before concatenating."
- )
- if isinstance(spec, VConcatChart) or _get(spec, "vconcat") is not Undefined:
- raise ValueError(
- "Concatenated charts cannot be layered. Instead, layer the charts before concatenating."
- )
-
-
-class RepeatChart(TopLevelMixin, core.TopLevelRepeatSpec):
- """A chart repeated across rows and columns with small changes"""
-
- # Because TopLevelRepeatSpec is defined as a union as of Vega-Lite schema 4.9,
- # we set the arguments explicitly here.
- # TODO: Should we instead use tools/schemapi/codegen._get_args?
- @utils.use_signature(core.TopLevelRepeatSpec)
- def __init__(
- self,
- repeat=Undefined,
- spec=Undefined,
- align=Undefined,
- autosize=Undefined,
- background=Undefined,
- bounds=Undefined,
- center=Undefined,
- columns=Undefined,
- config=Undefined,
- data=Undefined,
- datasets=Undefined,
- description=Undefined,
- name=Undefined,
- padding=Undefined,
- params=Undefined,
- resolve=Undefined,
- spacing=Undefined,
- title=Undefined,
- transform=Undefined,
- usermeta=Undefined,
- **kwds,
- ):
- _check_if_valid_subspec(spec, "RepeatChart")
- _spec_as_list = [spec]
- params, _spec_as_list = _combine_subchart_params(params, _spec_as_list)
- spec = _spec_as_list[0]
- if isinstance(spec, (Chart, LayerChart)):
- params = _repeat_names(params, repeat, spec)
- super(RepeatChart, self).__init__(
- repeat=repeat,
- spec=spec,
- align=align,
- autosize=autosize,
- background=background,
- bounds=bounds,
- center=center,
- columns=columns,
- config=config,
- data=data,
- datasets=datasets,
- description=description,
- name=name,
- padding=padding,
- params=params,
- resolve=resolve,
- spacing=spacing,
- title=title,
- transform=transform,
- usermeta=usermeta,
- **kwds,
- )
-
- def interactive(self, name=None, bind_x=True, bind_y=True) -> Self:
- """Make chart axes scales interactive
-
- Parameters
- ----------
- name : string
- The parameter name to use for the axes scales. This name should be
- unique among all parameters within the chart.
- bind_x : boolean, default True
- If true, then bind the interactive scales to the x-axis
- bind_y : boolean, default True
- If true, then bind the interactive scales to the y-axis
-
- Returns
- -------
- chart :
- copy of self, with interactive axes added
-
- """
- copy = self.copy(deep=False)
- copy.spec = copy.spec.interactive(name=name, bind_x=bind_x, bind_y=bind_y)
- return copy
-
- def add_params(self, *params) -> Self:
- """Add one or more parameters to the chart."""
- if not params or self.spec is Undefined:
- return self
- copy = self.copy()
- copy.spec = copy.spec.add_params(*params)
- return copy.copy()
-
- @utils.deprecation.deprecated(
- message="'add_selection' is deprecated. Use 'add_params' instead."
- )
- def add_selection(self, *selections) -> Self:
- """'add_selection' is deprecated. Use 'add_params' instead."""
- return self.add_params(*selections)
-
-
-def repeat(repeater="repeat"):
- """Tie a channel to the row or column within a repeated chart
-
- The output of this should be passed to the ``field`` attribute of
- a channel.
-
- Parameters
- ----------
- repeater : {'row'|'column'|'repeat'|'layer'}
- The repeater to tie the field to. Default is 'repeat'.
-
- Returns
- -------
- repeat : RepeatRef object
- """
- if repeater not in ["row", "column", "repeat", "layer"]:
- raise ValueError("repeater must be one of ['row', 'column', 'repeat', 'layer']")
- return core.RepeatRef(repeat=repeater)
-
-
-class ConcatChart(TopLevelMixin, core.TopLevelConcatSpec):
- """A chart with horizontally-concatenated facets"""
-
- @utils.use_signature(core.TopLevelConcatSpec)
- def __init__(self, data=Undefined, concat=(), columns=Undefined, **kwargs):
- # TODO: move common data to top level?
- for spec in concat:
- _check_if_valid_subspec(spec, "ConcatChart")
- super(ConcatChart, self).__init__(
- data=data, concat=list(concat), columns=columns, **kwargs
- )
- self.data, self.concat = _combine_subchart_data(self.data, self.concat)
- self.params, self.concat = _combine_subchart_params(self.params, self.concat)
-
- def __ior__(self, other):
- _check_if_valid_subspec(other, "ConcatChart")
- self.concat.append(other)
- self.data, self.concat = _combine_subchart_data(self.data, self.concat)
- self.params, self.concat = _combine_subchart_params(self.params, self.concat)
- return self
-
- def __or__(self, other):
- copy = self.copy(deep=["concat"])
- copy |= other
- return copy
-
- def interactive(self, name=None, bind_x=True, bind_y=True) -> Self:
- """Make chart axes scales interactive
-
- Parameters
- ----------
- name : string
- The parameter name to use for the axes scales. This name should be
- unique among all parameters within the chart.
- bind_x : boolean, default True
- If true, then bind the interactive scales to the x-axis
- bind_y : boolean, default True
- If true, then bind the interactive scales to the y-axis
-
- Returns
- -------
- chart :
- copy of self, with interactive axes added
-
- """
- encodings = []
- if bind_x:
- encodings.append("x")
- if bind_y:
- encodings.append("y")
- return self.add_params(selection_interval(bind="scales", encodings=encodings))
-
- def add_params(self, *params) -> Self:
- """Add one or more parameters to the chart."""
- if not params or not self.concat:
- return self
- copy = self.copy()
- copy.concat = [chart.add_params(*params) for chart in copy.concat]
- return copy
-
- @utils.deprecation.deprecated(
- message="'add_selection' is deprecated. Use 'add_params' instead."
- )
- def add_selection(self, *selections) -> Self:
- """'add_selection' is deprecated. Use 'add_params' instead."""
- return self.add_params(*selections)
-
-
-def concat(*charts, **kwargs):
- """Concatenate charts horizontally"""
- return ConcatChart(concat=charts, **kwargs)
-
-
-class HConcatChart(TopLevelMixin, core.TopLevelHConcatSpec):
- """A chart with horizontally-concatenated facets"""
-
- @utils.use_signature(core.TopLevelHConcatSpec)
- def __init__(self, data=Undefined, hconcat=(), **kwargs):
- # TODO: move common data to top level?
- for spec in hconcat:
- _check_if_valid_subspec(spec, "HConcatChart")
- super(HConcatChart, self).__init__(data=data, hconcat=list(hconcat), **kwargs)
- self.data, self.hconcat = _combine_subchart_data(self.data, self.hconcat)
- self.params, self.hconcat = _combine_subchart_params(self.params, self.hconcat)
-
- def __ior__(self, other):
- _check_if_valid_subspec(other, "HConcatChart")
- self.hconcat.append(other)
- self.data, self.hconcat = _combine_subchart_data(self.data, self.hconcat)
- self.params, self.hconcat = _combine_subchart_params(self.params, self.hconcat)
- return self
-
- def __or__(self, other):
- copy = self.copy(deep=["hconcat"])
- copy |= other
- return copy
-
- def interactive(self, name=None, bind_x=True, bind_y=True) -> Self:
- """Make chart axes scales interactive
-
- Parameters
- ----------
- name : string
- The parameter name to use for the axes scales. This name should be
- unique among all parameters within the chart.
- bind_x : boolean, default True
- If true, then bind the interactive scales to the x-axis
- bind_y : boolean, default True
- If true, then bind the interactive scales to the y-axis
-
- Returns
- -------
- chart :
- copy of self, with interactive axes added
-
- """
- encodings = []
- if bind_x:
- encodings.append("x")
- if bind_y:
- encodings.append("y")
- return self.add_params(selection_interval(bind="scales", encodings=encodings))
-
- def add_params(self, *params) -> Self:
- """Add one or more parameters to the chart."""
- if not params or not self.hconcat:
- return self
- copy = self.copy()
- copy.hconcat = [chart.add_params(*params) for chart in copy.hconcat]
- return copy
-
- @utils.deprecation.deprecated(
- message="'add_selection' is deprecated. Use 'add_params' instead."
- )
- def add_selection(self, *selections) -> Self:
- """'add_selection' is deprecated. Use 'add_params' instead."""
- return self.add_params(*selections)
-
-
-def hconcat(*charts, **kwargs):
- """Concatenate charts horizontally"""
- return HConcatChart(hconcat=charts, **kwargs)
-
-
-class VConcatChart(TopLevelMixin, core.TopLevelVConcatSpec):
- """A chart with vertically-concatenated facets"""
-
- @utils.use_signature(core.TopLevelVConcatSpec)
- def __init__(self, data=Undefined, vconcat=(), **kwargs):
- # TODO: move common data to top level?
- for spec in vconcat:
- _check_if_valid_subspec(spec, "VConcatChart")
- super(VConcatChart, self).__init__(data=data, vconcat=list(vconcat), **kwargs)
- self.data, self.vconcat = _combine_subchart_data(self.data, self.vconcat)
- self.params, self.vconcat = _combine_subchart_params(self.params, self.vconcat)
-
- def __iand__(self, other):
- _check_if_valid_subspec(other, "VConcatChart")
- self.vconcat.append(other)
- self.data, self.vconcat = _combine_subchart_data(self.data, self.vconcat)
- self.params, self.vconcat = _combine_subchart_params(self.params, self.vconcat)
- return self
-
- def __and__(self, other):
- copy = self.copy(deep=["vconcat"])
- copy &= other
- return copy
-
- def interactive(self, name=None, bind_x=True, bind_y=True) -> Self:
- """Make chart axes scales interactive
-
- Parameters
- ----------
- name : string
- The parameter name to use for the axes scales. This name should be
- unique among all parameters within the chart.
- bind_x : boolean, default True
- If true, then bind the interactive scales to the x-axis
- bind_y : boolean, default True
- If true, then bind the interactive scales to the y-axis
-
- Returns
- -------
- chart :
- copy of self, with interactive axes added
-
- """
- encodings = []
- if bind_x:
- encodings.append("x")
- if bind_y:
- encodings.append("y")
- return self.add_params(selection_interval(bind="scales", encodings=encodings))
-
- def add_params(self, *params) -> Self:
- """Add one or more parameters to the chart."""
- if not params or not self.vconcat:
- return self
- copy = self.copy()
- copy.vconcat = [chart.add_params(*params) for chart in copy.vconcat]
- return copy
-
- @utils.deprecation.deprecated(
- message="'add_selection' is deprecated. Use 'add_params' instead."
- )
- def add_selection(self, *selections) -> Self:
- """'add_selection' is deprecated. Use 'add_params' instead."""
- return self.add_params(*selections)
-
-
-def vconcat(*charts, **kwargs):
- """Concatenate charts vertically"""
- return VConcatChart(vconcat=charts, **kwargs)
-
-
-class LayerChart(TopLevelMixin, _EncodingMixin, core.TopLevelLayerSpec):
- """A Chart with layers within a single panel"""
-
- @utils.use_signature(core.TopLevelLayerSpec)
- def __init__(self, data=Undefined, layer=(), **kwargs):
- # TODO: move common data to top level?
- # TODO: check for conflicting interaction
- for spec in layer:
- _check_if_valid_subspec(spec, "LayerChart")
- _check_if_can_be_layered(spec)
- super(LayerChart, self).__init__(data=data, layer=list(layer), **kwargs)
- self.data, self.layer = _combine_subchart_data(self.data, self.layer)
- # Currently (Vega-Lite 5.5) the same param can't occur on two layers
- self.layer = _remove_duplicate_params(self.layer)
- self.params, self.layer = _combine_subchart_params(self.params, self.layer)
-
- # Some properties are not allowed within layer; we'll move to parent.
- layer_props = ("height", "width", "view")
- combined_dict, self.layer = _remove_layer_props(self, self.layer, layer_props)
-
- for prop in combined_dict:
- self[prop] = combined_dict[prop]
-
- def __iadd__(self, other):
- _check_if_valid_subspec(other, "LayerChart")
- _check_if_can_be_layered(other)
- self.layer.append(other)
- self.data, self.layer = _combine_subchart_data(self.data, self.layer)
- self.params, self.layer = _combine_subchart_params(self.params, self.layer)
- return self
-
- def __add__(self, other):
- copy = self.copy(deep=["layer"])
- copy += other
- return copy
-
- def add_layers(self, *layers) -> Self:
- copy = self.copy(deep=["layer"])
- for layer in layers:
- copy += layer
- return copy
-
- def interactive(self, name=None, bind_x=True, bind_y=True) -> Self:
- """Make chart axes scales interactive
-
- Parameters
- ----------
- name : string
- The parameter name to use for the axes scales. This name should be
- unique among all parameters within the chart.
- bind_x : boolean, default True
- If true, then bind the interactive scales to the x-axis
- bind_y : boolean, default True
- If true, then bind the interactive scales to the y-axis
-
- Returns
- -------
- chart :
- copy of self, with interactive axes added
-
- """
- if not self.layer:
- raise ValueError(
- "LayerChart: cannot call interactive() until a " "layer is defined"
- )
- copy = self.copy(deep=["layer"])
- copy.layer[0] = copy.layer[0].interactive(
- name=name, bind_x=bind_x, bind_y=bind_y
- )
- return copy
-
- def add_params(self, *params) -> Self:
- """Add one or more parameters to the chart."""
- if not params or not self.layer:
- return self
- copy = self.copy()
- copy.layer[0] = copy.layer[0].add_params(*params)
- return copy.copy()
-
- @utils.deprecation.deprecated(
- message="'add_selection' is deprecated. Use 'add_params' instead."
- )
- def add_selection(self, *selections) -> Self:
- """'add_selection' is deprecated. Use 'add_params' instead."""
- return self.add_params(*selections)
-
-
-def layer(*charts, **kwargs):
- """layer multiple charts"""
- return LayerChart(layer=charts, **kwargs)
-
-
-class FacetChart(TopLevelMixin, core.TopLevelFacetSpec):
- """A Chart with layers within a single panel"""
-
- @utils.use_signature(core.TopLevelFacetSpec)
- def __init__(
- self,
- data=Undefined,
- spec=Undefined,
- facet=Undefined,
- params=Undefined,
- **kwargs,
- ):
- _check_if_valid_subspec(spec, "FacetChart")
- _spec_as_list = [spec]
- params, _spec_as_list = _combine_subchart_params(params, _spec_as_list)
- spec = _spec_as_list[0]
- super(FacetChart, self).__init__(
- data=data, spec=spec, facet=facet, params=params, **kwargs
- )
-
- def interactive(self, name=None, bind_x=True, bind_y=True) -> Self:
- """Make chart axes scales interactive
-
- Parameters
- ----------
- name : string
- The parameter name to use for the axes scales. This name should be
- unique among all parameters within the chart.
- bind_x : boolean, default True
- If true, then bind the interactive scales to the x-axis
- bind_y : boolean, default True
- If true, then bind the interactive scales to the y-axis
-
- Returns
- -------
- chart :
- copy of self, with interactive axes added
-
- """
- copy = self.copy(deep=False)
- copy.spec = copy.spec.interactive(name=name, bind_x=bind_x, bind_y=bind_y)
- return copy
-
- def add_params(self, *params) -> Self:
- """Add one or more parameters to the chart."""
- if not params or self.spec is Undefined:
- return self
- copy = self.copy()
- copy.spec = copy.spec.add_params(*params)
- return copy.copy()
-
- @utils.deprecation.deprecated(
- message="'add_selection' is deprecated. Use 'add_params' instead."
- )
- def add_selection(self, *selections) -> Self:
- """'add_selection' is deprecated. Use 'add_params' instead."""
- return self.add_params(*selections)
-
-
-def topo_feature(url, feature, **kwargs):
- """A convenience function for extracting features from a topojson url
-
- Parameters
- ----------
- url : string
- An URL from which to load the data set.
-
- feature : string
- The name of the TopoJSON object set to convert to a GeoJSON feature collection. For
- example, in a map of the world, there may be an object set named `"countries"`.
- Using the feature property, we can extract this set and generate a GeoJSON feature
- object for each country.
-
- **kwargs :
- additional keywords passed to TopoDataFormat
- """
- return core.UrlData(
- url=url, format=core.TopoDataFormat(type="topojson", feature=feature, **kwargs)
- )
-
-
-def _combine_subchart_data(data, subcharts):
- def remove_data(subchart):
- if subchart.data is not Undefined:
- subchart = subchart.copy()
- subchart.data = Undefined
- return subchart
-
- if not subcharts:
- # No subcharts = nothing to do.
- pass
- elif data is Undefined:
- # Top level has no data; all subchart data must
- # be identical to proceed.
- subdata = subcharts[0].data
- if subdata is not Undefined and all(c.data is subdata for c in subcharts):
- data = subdata
- subcharts = [remove_data(c) for c in subcharts]
- else:
- # Top level has data; subchart data must be either
- # undefined or identical to proceed.
- if all(c.data is Undefined or c.data is data for c in subcharts):
- subcharts = [remove_data(c) for c in subcharts]
-
- return data, subcharts
-
-
-def _viewless_dict(param):
- d = param.to_dict()
- d.pop("views", None)
- return d
-
-
-def _needs_name(subchart):
- # Only `Chart` objects need a name
- if (subchart.name is not Undefined) or (not isinstance(subchart, Chart)):
- return False
-
- # Variable parameters won't receive a views property.
- if all(isinstance(p, core.VariableParameter) for p in subchart.params):
- return False
-
- return True
-
-
-# Convert SelectionParameters to TopLevelSelectionParameters with a views property.
-def _prepare_to_lift(param):
- param = param.copy()
-
- if isinstance(param, core.VariableParameter):
- return param
-
- if isinstance(param, core.SelectionParameter):
- return core.TopLevelSelectionParameter(**param.to_dict(), views=[])
-
- if param.views is Undefined:
- param.views = []
-
- return param
-
-
-def _remove_duplicate_params(layer):
- subcharts = [subchart.copy() for subchart in layer]
- found_params = []
-
- for subchart in subcharts:
- if (not hasattr(subchart, "params")) or (subchart.params is Undefined):
- continue
-
- params = []
-
- # Ensure the same selection parameter doesn't appear twice
- for param in subchart.params:
- if isinstance(param, core.VariableParameter):
- params.append(param)
- continue
-
- p = param.copy()
- pd = _viewless_dict(p)
-
- if pd not in found_params:
- params.append(p)
- found_params.append(pd)
-
- if len(params) == 0:
- subchart.params = Undefined
- else:
- subchart.params = params
-
- return subcharts
-
-
-def _combine_subchart_params(params, subcharts):
- if params is Undefined:
- params = []
-
- # List of triples related to params, (param, dictionary minus views, views)
- param_info = []
-
- # Put parameters already found into `param_info` list.
- for param in params:
- p = _prepare_to_lift(param)
- param_info.append(
- (
- p,
- _viewless_dict(p),
- [] if isinstance(p, core.VariableParameter) else p.views,
- )
- )
-
- subcharts = [subchart.copy() for subchart in subcharts]
-
- for subchart in subcharts:
- if (not hasattr(subchart, "params")) or (subchart.params is Undefined):
- continue
-
- if _needs_name(subchart):
- subchart.name = subchart._get_name()
-
- for param in subchart.params:
- p = _prepare_to_lift(param)
- pd = _viewless_dict(p)
-
- dlist = [d for _, d, _ in param_info]
- found = pd in dlist
-
- if isinstance(p, core.VariableParameter) and found:
- continue
-
- if isinstance(p, core.VariableParameter) and not found:
- param_info.append((p, pd, []))
- continue
-
- # At this stage in the loop, p must be a TopLevelSelectionParameter.
-
- if isinstance(subchart, Chart) and (subchart.name not in p.views):
- p.views.append(subchart.name)
-
- if found:
- i = dlist.index(pd)
- _, _, old_views = param_info[i]
- new_views = [v for v in p.views if v not in old_views]
- old_views += new_views
- else:
- param_info.append((p, pd, p.views))
-
- subchart.params = Undefined
-
- for p, _, v in param_info:
- if len(v) > 0:
- p.views = v
-
- subparams = [p for p, _, _ in param_info]
-
- if len(subparams) == 0:
- subparams = Undefined
-
- return subparams, subcharts
-
-
-def _get_repeat_strings(repeat):
- if isinstance(repeat, list):
- return repeat
- elif isinstance(repeat, core.LayerRepeatMapping):
- klist = ["row", "column", "layer"]
- elif isinstance(repeat, core.RepeatMapping):
- klist = ["row", "column"]
- rclist = [k for k in klist if repeat[k] is not Undefined]
- rcstrings = [[f"{k}_{v}" for v in repeat[k]] for k in rclist]
- return ["".join(s) for s in itertools.product(*rcstrings)]
-
-
-def _extend_view_name(v, r, spec):
- # prevent the same extension from happening more than once
- if isinstance(spec, Chart):
- if v.endswith("child__" + r):
- return v
- else:
- return f"{v}_child__{r}"
- elif isinstance(spec, LayerChart):
- if v.startswith("child__" + r):
- return v
- else:
- return f"child__{r}_{v}"
-
-
-def _repeat_names(params, repeat, spec):
- if params is Undefined:
- return params
-
- repeat = _get_repeat_strings(repeat)
- params_named = []
-
- for param in params:
- if not isinstance(param, core.TopLevelSelectionParameter):
- params_named.append(param)
- continue
- p = param.copy()
- views = []
- repeat_strings = _get_repeat_strings(repeat)
- for v in param.views:
- if isinstance(spec, Chart):
- if any(v.endswith(f"child__{r}") for r in repeat_strings):
- views.append(v)
- else:
- views += [_extend_view_name(v, r, spec) for r in repeat_strings]
- elif isinstance(spec, LayerChart):
- if any(v.startswith(f"child__{r}") for r in repeat_strings):
- views.append(v)
- else:
- views += [_extend_view_name(v, r, spec) for r in repeat_strings]
-
- p.views = views
- params_named.append(p)
-
- return params_named
-
-
-def _remove_layer_props(chart, subcharts, layer_props):
- def remove_prop(subchart, prop):
- # If subchart is a UnitSpec, then subchart["height"] raises a KeyError
- try:
- if subchart[prop] is not Undefined:
- subchart = subchart.copy()
- subchart[prop] = Undefined
- except KeyError:
- pass
- return subchart
-
- output_dict = {}
-
- if not subcharts:
- # No subcharts = nothing to do.
- return output_dict, subcharts
-
- for prop in layer_props:
- if chart[prop] is Undefined:
- # Top level does not have this prop.
- # Check for consistent props within the subcharts.
- values = []
- for c in subcharts:
- # If c is a UnitSpec, then c["height"] raises a KeyError.
- try:
- val = c[prop]
- if val is not Undefined:
- values.append(val)
- except KeyError:
- pass
- if len(values) == 0:
- pass
- elif all(v == values[0] for v in values[1:]):
- output_dict[prop] = values[0]
- else:
- raise ValueError(f"There are inconsistent values {values} for {prop}")
- else:
- # Top level has this prop; subchart must either not have the prop
- # or it must be Undefined or identical to proceed.
- if all(
- getattr(c, prop, Undefined) is Undefined or c[prop] == chart[prop]
- for c in subcharts
- ):
- output_dict[prop] = chart[prop]
- else:
- raise ValueError(f"There are inconsistent values {values} for {prop}")
- subcharts = [remove_prop(c, prop) for c in subcharts]
-
- return output_dict, subcharts
-
-
-@utils.use_signature(core.SequenceParams)
-def sequence(start, stop=None, step=Undefined, as_=Undefined, **kwds):
- """Sequence generator."""
- if stop is None:
- start, stop = 0, start
- params = core.SequenceParams(start=start, stop=stop, step=step, **{"as": as_})
- return core.SequenceGenerator(sequence=params, **kwds)
-
-
-@utils.use_signature(core.GraticuleParams)
-def graticule(**kwds):
- """Graticule generator."""
- if not kwds:
- # graticule: True indicates default parameters
- graticule = True
- else:
- graticule = core.GraticuleParams(**kwds)
- return core.GraticuleGenerator(graticule=graticule)
-
-
-def sphere():
- """Sphere generator."""
- return core.SphereGenerator(sphere=True)
diff --git a/spaces/SungBeom/chatwine-korean/.venv/Lib/site-packages/docarray/base_doc/__init__.py b/spaces/SungBeom/chatwine-korean/.venv/Lib/site-packages/docarray/base_doc/__init__.py
deleted file mode 100644
index 47e01c1c662428607faa9d72b5c1e40b89acd95c..0000000000000000000000000000000000000000
--- a/spaces/SungBeom/chatwine-korean/.venv/Lib/site-packages/docarray/base_doc/__init__.py
+++ /dev/null
@@ -1,24 +0,0 @@
-from docarray.base_doc.any_doc import AnyDoc
-from docarray.base_doc.base_node import BaseNode
-from docarray.base_doc.doc import BaseDoc
-from docarray.utils._internal.misc import (
- _get_path_from_docarray_root_level,
- import_library,
-)
-
-__all__ = ['AnyDoc', 'BaseDoc', 'BaseNode']
-
-
-def __getattr__(name: str):
- if name == 'DocArrayResponse':
- import_library('fastapi', raise_error=True)
- from docarray.base_doc.docarray_response import DocArrayResponse
-
- if name not in __all__:
- __all__.append(name)
-
- return DocArrayResponse
- else:
- raise ImportError(
- f'cannot import name \'{name}\' from \'{_get_path_from_docarray_root_level(__file__)}\''
- )
diff --git a/spaces/Superlang/ImageProcessor/annotator/uniformer/mmseg/models/utils/inverted_residual.py b/spaces/Superlang/ImageProcessor/annotator/uniformer/mmseg/models/utils/inverted_residual.py
deleted file mode 100644
index 53b8fcd41f71d814738f1ac3f5acd3c3d701bf96..0000000000000000000000000000000000000000
--- a/spaces/Superlang/ImageProcessor/annotator/uniformer/mmseg/models/utils/inverted_residual.py
+++ /dev/null
@@ -1,208 +0,0 @@
-from annotator.uniformer.mmcv.cnn import ConvModule
-from torch import nn
-from torch.utils import checkpoint as cp
-
-from .se_layer import SELayer
-
-
-class InvertedResidual(nn.Module):
- """InvertedResidual block for MobileNetV2.
-
- Args:
- in_channels (int): The input channels of the InvertedResidual block.
- out_channels (int): The output channels of the InvertedResidual block.
- stride (int): Stride of the middle (first) 3x3 convolution.
- expand_ratio (int): Adjusts number of channels of the hidden layer
- in InvertedResidual by this amount.
- dilation (int): Dilation rate of depthwise conv. Default: 1
- conv_cfg (dict): Config dict for convolution layer.
- Default: None, which means using conv2d.
- norm_cfg (dict): Config dict for normalization layer.
- Default: dict(type='BN').
- act_cfg (dict): Config dict for activation layer.
- Default: dict(type='ReLU6').
- with_cp (bool): Use checkpoint or not. Using checkpoint will save some
- memory while slowing down the training speed. Default: False.
-
- Returns:
- Tensor: The output tensor.
- """
-
- def __init__(self,
- in_channels,
- out_channels,
- stride,
- expand_ratio,
- dilation=1,
- conv_cfg=None,
- norm_cfg=dict(type='BN'),
- act_cfg=dict(type='ReLU6'),
- with_cp=False):
- super(InvertedResidual, self).__init__()
- self.stride = stride
- assert stride in [1, 2], f'stride must in [1, 2]. ' \
- f'But received {stride}.'
- self.with_cp = with_cp
- self.use_res_connect = self.stride == 1 and in_channels == out_channels
- hidden_dim = int(round(in_channels * expand_ratio))
-
- layers = []
- if expand_ratio != 1:
- layers.append(
- ConvModule(
- in_channels=in_channels,
- out_channels=hidden_dim,
- kernel_size=1,
- conv_cfg=conv_cfg,
- norm_cfg=norm_cfg,
- act_cfg=act_cfg))
- layers.extend([
- ConvModule(
- in_channels=hidden_dim,
- out_channels=hidden_dim,
- kernel_size=3,
- stride=stride,
- padding=dilation,
- dilation=dilation,
- groups=hidden_dim,
- conv_cfg=conv_cfg,
- norm_cfg=norm_cfg,
- act_cfg=act_cfg),
- ConvModule(
- in_channels=hidden_dim,
- out_channels=out_channels,
- kernel_size=1,
- conv_cfg=conv_cfg,
- norm_cfg=norm_cfg,
- act_cfg=None)
- ])
- self.conv = nn.Sequential(*layers)
-
- def forward(self, x):
-
- def _inner_forward(x):
- if self.use_res_connect:
- return x + self.conv(x)
- else:
- return self.conv(x)
-
- if self.with_cp and x.requires_grad:
- out = cp.checkpoint(_inner_forward, x)
- else:
- out = _inner_forward(x)
-
- return out
-
-
-class InvertedResidualV3(nn.Module):
- """Inverted Residual Block for MobileNetV3.
-
- Args:
- in_channels (int): The input channels of this Module.
- out_channels (int): The output channels of this Module.
- mid_channels (int): The input channels of the depthwise convolution.
- kernel_size (int): The kernel size of the depthwise convolution.
- Default: 3.
- stride (int): The stride of the depthwise convolution. Default: 1.
- se_cfg (dict): Config dict for se layer. Default: None, which means no
- se layer.
- with_expand_conv (bool): Use expand conv or not. If set False,
- mid_channels must be the same with in_channels. Default: True.
- conv_cfg (dict): Config dict for convolution layer. Default: None,
- which means using conv2d.
- norm_cfg (dict): Config dict for normalization layer.
- Default: dict(type='BN').
- act_cfg (dict): Config dict for activation layer.
- Default: dict(type='ReLU').
- with_cp (bool): Use checkpoint or not. Using checkpoint will save some
- memory while slowing down the training speed. Default: False.
-
- Returns:
- Tensor: The output tensor.
- """
-
- def __init__(self,
- in_channels,
- out_channels,
- mid_channels,
- kernel_size=3,
- stride=1,
- se_cfg=None,
- with_expand_conv=True,
- conv_cfg=None,
- norm_cfg=dict(type='BN'),
- act_cfg=dict(type='ReLU'),
- with_cp=False):
- super(InvertedResidualV3, self).__init__()
- self.with_res_shortcut = (stride == 1 and in_channels == out_channels)
- assert stride in [1, 2]
- self.with_cp = with_cp
- self.with_se = se_cfg is not None
- self.with_expand_conv = with_expand_conv
-
- if self.with_se:
- assert isinstance(se_cfg, dict)
- if not self.with_expand_conv:
- assert mid_channels == in_channels
-
- if self.with_expand_conv:
- self.expand_conv = ConvModule(
- in_channels=in_channels,
- out_channels=mid_channels,
- kernel_size=1,
- stride=1,
- padding=0,
- conv_cfg=conv_cfg,
- norm_cfg=norm_cfg,
- act_cfg=act_cfg)
- self.depthwise_conv = ConvModule(
- in_channels=mid_channels,
- out_channels=mid_channels,
- kernel_size=kernel_size,
- stride=stride,
- padding=kernel_size // 2,
- groups=mid_channels,
- conv_cfg=dict(
- type='Conv2dAdaptivePadding') if stride == 2 else conv_cfg,
- norm_cfg=norm_cfg,
- act_cfg=act_cfg)
-
- if self.with_se:
- self.se = SELayer(**se_cfg)
-
- self.linear_conv = ConvModule(
- in_channels=mid_channels,
- out_channels=out_channels,
- kernel_size=1,
- stride=1,
- padding=0,
- conv_cfg=conv_cfg,
- norm_cfg=norm_cfg,
- act_cfg=None)
-
- def forward(self, x):
-
- def _inner_forward(x):
- out = x
-
- if self.with_expand_conv:
- out = self.expand_conv(out)
-
- out = self.depthwise_conv(out)
-
- if self.with_se:
- out = self.se(out)
-
- out = self.linear_conv(out)
-
- if self.with_res_shortcut:
- return x + out
- else:
- return out
-
- if self.with_cp and x.requires_grad:
- out = cp.checkpoint(_inner_forward, x)
- else:
- out = _inner_forward(x)
-
- return out
diff --git a/spaces/Superlang/ImageProcessor/annotator/zoe/zoedepth/models/base_models/midas_repo/midas/backbones/beit.py b/spaces/Superlang/ImageProcessor/annotator/zoe/zoedepth/models/base_models/midas_repo/midas/backbones/beit.py
deleted file mode 100644
index 7a24e02cd2b979844bf638b46ac60949ee9ce691..0000000000000000000000000000000000000000
--- a/spaces/Superlang/ImageProcessor/annotator/zoe/zoedepth/models/base_models/midas_repo/midas/backbones/beit.py
+++ /dev/null
@@ -1,196 +0,0 @@
-import timm
-import torch
-import types
-
-import numpy as np
-import torch.nn.functional as F
-
-from .utils import forward_adapted_unflatten, make_backbone_default
-from timm.models.beit import gen_relative_position_index
-from torch.utils.checkpoint import checkpoint
-from typing import Optional
-
-
-def forward_beit(pretrained, x):
- return forward_adapted_unflatten(pretrained, x, "forward_features")
-
-
-def patch_embed_forward(self, x):
- """
- Modification of timm.models.layers.patch_embed.py: PatchEmbed.forward to support arbitrary window sizes.
- """
- x = self.proj(x)
- if self.flatten:
- x = x.flatten(2).transpose(1, 2)
- x = self.norm(x)
- return x
-
-
-def _get_rel_pos_bias(self, window_size):
- """
- Modification of timm.models.beit.py: Attention._get_rel_pos_bias to support arbitrary window sizes.
- """
- old_height = 2 * self.window_size[0] - 1
- old_width = 2 * self.window_size[1] - 1
-
- new_height = 2 * window_size[0] - 1
- new_width = 2 * window_size[1] - 1
-
- old_relative_position_bias_table = self.relative_position_bias_table
-
- old_num_relative_distance = self.num_relative_distance
- new_num_relative_distance = new_height * new_width + 3
-
- old_sub_table = old_relative_position_bias_table[:old_num_relative_distance - 3]
-
- old_sub_table = old_sub_table.reshape(1, old_width, old_height, -1).permute(0, 3, 1, 2)
- new_sub_table = F.interpolate(old_sub_table, size=(new_height, new_width), mode="bilinear")
- new_sub_table = new_sub_table.permute(0, 2, 3, 1).reshape(new_num_relative_distance - 3, -1)
-
- new_relative_position_bias_table = torch.cat(
- [new_sub_table, old_relative_position_bias_table[old_num_relative_distance - 3:]])
-
- key = str(window_size[1]) + "," + str(window_size[0])
- if key not in self.relative_position_indices.keys():
- self.relative_position_indices[key] = gen_relative_position_index(window_size)
-
- relative_position_bias = new_relative_position_bias_table[
- self.relative_position_indices[key].view(-1)].view(
- window_size[0] * window_size[1] + 1,
- window_size[0] * window_size[1] + 1, -1) # Wh*Ww,Wh*Ww,nH
- relative_position_bias = relative_position_bias.permute(2, 0, 1).contiguous() # nH, Wh*Ww, Wh*Ww
- return relative_position_bias.unsqueeze(0)
-
-
-def attention_forward(self, x, resolution, shared_rel_pos_bias: Optional[torch.Tensor] = None):
- """
- Modification of timm.models.beit.py: Attention.forward to support arbitrary window sizes.
- """
- B, N, C = x.shape
-
- qkv_bias = torch.cat((self.q_bias, self.k_bias, self.v_bias)) if self.q_bias is not None else None
- qkv = F.linear(input=x, weight=self.qkv.weight, bias=qkv_bias)
- qkv = qkv.reshape(B, N, 3, self.num_heads, -1).permute(2, 0, 3, 1, 4)
- q, k, v = qkv.unbind(0) # make torchscript happy (cannot use tensor as tuple)
-
- q = q * self.scale
- attn = (q @ k.transpose(-2, -1))
-
- if self.relative_position_bias_table is not None:
- window_size = tuple(np.array(resolution) // 16)
- attn = attn + self._get_rel_pos_bias(window_size)
- if shared_rel_pos_bias is not None:
- attn = attn + shared_rel_pos_bias
-
- attn = attn.softmax(dim=-1)
- attn = self.attn_drop(attn)
-
- x = (attn @ v).transpose(1, 2).reshape(B, N, -1)
- x = self.proj(x)
- x = self.proj_drop(x)
- return x
-
-
-def block_forward(self, x, resolution, shared_rel_pos_bias: Optional[torch.Tensor] = None):
- """
- Modification of timm.models.beit.py: Block.forward to support arbitrary window sizes.
- """
- if self.gamma_1 is None:
- x = x + self.drop_path(self.attn(self.norm1(x), resolution, shared_rel_pos_bias=shared_rel_pos_bias))
- x = x + self.drop_path(self.mlp(self.norm2(x)))
- else:
- x = x + self.drop_path(self.gamma_1 * self.attn(self.norm1(x), resolution,
- shared_rel_pos_bias=shared_rel_pos_bias))
- x = x + self.drop_path(self.gamma_2 * self.mlp(self.norm2(x)))
- return x
-
-
-def beit_forward_features(self, x):
- """
- Modification of timm.models.beit.py: Beit.forward_features to support arbitrary window sizes.
- """
- resolution = x.shape[2:]
-
- x = self.patch_embed(x)
- x = torch.cat((self.cls_token.expand(x.shape[0], -1, -1), x), dim=1)
- if self.pos_embed is not None:
- x = x + self.pos_embed
- x = self.pos_drop(x)
-
- rel_pos_bias = self.rel_pos_bias() if self.rel_pos_bias is not None else None
- for blk in self.blocks:
- if self.grad_checkpointing and not torch.jit.is_scripting():
- x = checkpoint(blk, x, shared_rel_pos_bias=rel_pos_bias)
- else:
- x = blk(x, resolution, shared_rel_pos_bias=rel_pos_bias)
- x = self.norm(x)
- return x
-
-
-def _make_beit_backbone(
- model,
- features=[96, 192, 384, 768],
- size=[384, 384],
- hooks=[0, 4, 8, 11],
- vit_features=768,
- use_readout="ignore",
- start_index=1,
- start_index_readout=1,
-):
- backbone = make_backbone_default(model, features, size, hooks, vit_features, use_readout, start_index,
- start_index_readout)
-
- backbone.model.patch_embed.forward = types.MethodType(patch_embed_forward, backbone.model.patch_embed)
- backbone.model.forward_features = types.MethodType(beit_forward_features, backbone.model)
-
- for block in backbone.model.blocks:
- attn = block.attn
- attn._get_rel_pos_bias = types.MethodType(_get_rel_pos_bias, attn)
- attn.forward = types.MethodType(attention_forward, attn)
- attn.relative_position_indices = {}
-
- block.forward = types.MethodType(block_forward, block)
-
- return backbone
-
-
-def _make_pretrained_beitl16_512(pretrained, use_readout="ignore", hooks=None):
- model = timm.create_model("beit_large_patch16_512", pretrained=pretrained)
-
- hooks = [5, 11, 17, 23] if hooks is None else hooks
-
- features = [256, 512, 1024, 1024]
-
- return _make_beit_backbone(
- model,
- features=features,
- size=[512, 512],
- hooks=hooks,
- vit_features=1024,
- use_readout=use_readout,
- )
-
-
-def _make_pretrained_beitl16_384(pretrained, use_readout="ignore", hooks=None):
- model = timm.create_model("beit_large_patch16_384", pretrained=pretrained)
-
- hooks = [5, 11, 17, 23] if hooks is None else hooks
- return _make_beit_backbone(
- model,
- features=[256, 512, 1024, 1024],
- hooks=hooks,
- vit_features=1024,
- use_readout=use_readout,
- )
-
-
-def _make_pretrained_beitb16_384(pretrained, use_readout="ignore", hooks=None):
- model = timm.create_model("beit_base_patch16_384", pretrained=pretrained)
-
- hooks = [2, 5, 8, 11] if hooks is None else hooks
- return _make_beit_backbone(
- model,
- features=[96, 192, 384, 768],
- hooks=hooks,
- use_readout=use_readout,
- )
diff --git a/spaces/TH5314/newbing/src/pages/api/blob.ts b/spaces/TH5314/newbing/src/pages/api/blob.ts
deleted file mode 100644
index fecd48031916b2284b8958892196e0a1ad420421..0000000000000000000000000000000000000000
--- a/spaces/TH5314/newbing/src/pages/api/blob.ts
+++ /dev/null
@@ -1,40 +0,0 @@
-'use server'
-
-import { NextApiRequest, NextApiResponse } from 'next'
-import { Readable } from 'node:stream'
-import { fetch } from '@/lib/isomorphic'
-
-const API_DOMAIN = 'https://www.bing.com'
-
-export default async function handler(req: NextApiRequest, res: NextApiResponse) {
- try {
- const { bcid } = req.query
-
- const { headers, body } = await fetch(`${API_DOMAIN}/images/blob?bcid=${bcid}`,
- {
- method: 'GET',
- headers: {
- "sec-ch-ua": "\"Not/A)Brand\";v=\"99\", \"Google Chrome\";v=\"115\", \"Chromium\";v=\"115\"",
- "sec-ch-ua-mobile": "?0",
- "sec-ch-ua-platform": "\"Windows\"",
- "Referrer-Policy": "origin-when-cross-origin",
- },
- },
- )
-
- res.writeHead(200, {
- 'Content-Length': headers.get('content-length')!,
- 'Content-Type': headers.get('content-type')!,
- })
- // @ts-ignore
- return Readable.fromWeb(body!).pipe(res)
- } catch (e) {
- console.log('Error', e)
- return res.json({
- result: {
- value: 'UploadFailed',
- message: `${e}`
- }
- })
- }
-}
diff --git a/spaces/TLME/Bert-VITS-Umamusume-Genshin-HonkaiSR/text/symbols.py b/spaces/TLME/Bert-VITS-Umamusume-Genshin-HonkaiSR/text/symbols.py
deleted file mode 100644
index 161ae9f71275856a168cca1b8963a2aee875bb78..0000000000000000000000000000000000000000
--- a/spaces/TLME/Bert-VITS-Umamusume-Genshin-HonkaiSR/text/symbols.py
+++ /dev/null
@@ -1,187 +0,0 @@
-punctuation = ["!", "?", "…", ",", ".", "'", "-"]
-pu_symbols = punctuation + ["SP", "UNK"]
-pad = "_"
-
-# chinese
-zh_symbols = [
- "E",
- "En",
- "a",
- "ai",
- "an",
- "ang",
- "ao",
- "b",
- "c",
- "ch",
- "d",
- "e",
- "ei",
- "en",
- "eng",
- "er",
- "f",
- "g",
- "h",
- "i",
- "i0",
- "ia",
- "ian",
- "iang",
- "iao",
- "ie",
- "in",
- "ing",
- "iong",
- "ir",
- "iu",
- "j",
- "k",
- "l",
- "m",
- "n",
- "o",
- "ong",
- "ou",
- "p",
- "q",
- "r",
- "s",
- "sh",
- "t",
- "u",
- "ua",
- "uai",
- "uan",
- "uang",
- "ui",
- "un",
- "uo",
- "v",
- "van",
- "ve",
- "vn",
- "w",
- "x",
- "y",
- "z",
- "zh",
- "AA",
- "EE",
- "OO",
-]
-num_zh_tones = 6
-
-# japanese
-ja_symbols = [
- "N",
- "a",
- "a:",
- "b",
- "by",
- "ch",
- "d",
- "dy",
- "e",
- "e:",
- "f",
- "g",
- "gy",
- "h",
- "hy",
- "i",
- "i:",
- "j",
- "k",
- "ky",
- "m",
- "my",
- "n",
- "ny",
- "o",
- "o:",
- "p",
- "py",
- "q",
- "r",
- "ry",
- "s",
- "sh",
- "t",
- "ts",
- "ty",
- "u",
- "u:",
- "w",
- "y",
- "z",
- "zy",
-]
-num_ja_tones = 1
-
-# English
-en_symbols = [
- "aa",
- "ae",
- "ah",
- "ao",
- "aw",
- "ay",
- "b",
- "ch",
- "d",
- "dh",
- "eh",
- "er",
- "ey",
- "f",
- "g",
- "hh",
- "ih",
- "iy",
- "jh",
- "k",
- "l",
- "m",
- "n",
- "ng",
- "ow",
- "oy",
- "p",
- "r",
- "s",
- "sh",
- "t",
- "th",
- "uh",
- "uw",
- "V",
- "w",
- "y",
- "z",
- "zh",
-]
-num_en_tones = 4
-
-# combine all symbols
-normal_symbols = sorted(set(zh_symbols + ja_symbols + en_symbols))
-symbols = [pad] + normal_symbols + pu_symbols
-sil_phonemes_ids = [symbols.index(i) for i in pu_symbols]
-
-# combine all tones
-num_tones = num_zh_tones + num_ja_tones + num_en_tones
-
-# language maps
-language_id_map = {"ZH": 0, "JP": 1, "EN": 2}
-num_languages = len(language_id_map.keys())
-
-language_tone_start_map = {
- "ZH": 0,
- "JP": num_zh_tones,
- "EN": num_zh_tones + num_ja_tones,
-}
-
-if __name__ == "__main__":
- a = set(zh_symbols)
- b = set(en_symbols)
- print(sorted(a & b))
diff --git a/spaces/TandCAcceptMe/face-swap-docker/mynewshinyroop/Lib/site-packages/pip/_internal/vcs/mercurial.py b/spaces/TandCAcceptMe/face-swap-docker/mynewshinyroop/Lib/site-packages/pip/_internal/vcs/mercurial.py
deleted file mode 100644
index 4595960b5bfff671449235d51a0b9312e7d6c5d1..0000000000000000000000000000000000000000
--- a/spaces/TandCAcceptMe/face-swap-docker/mynewshinyroop/Lib/site-packages/pip/_internal/vcs/mercurial.py
+++ /dev/null
@@ -1,163 +0,0 @@
-import configparser
-import logging
-import os
-from typing import List, Optional, Tuple
-
-from pip._internal.exceptions import BadCommand, InstallationError
-from pip._internal.utils.misc import HiddenText, display_path
-from pip._internal.utils.subprocess import make_command
-from pip._internal.utils.urls import path_to_url
-from pip._internal.vcs.versioncontrol import (
- RevOptions,
- VersionControl,
- find_path_to_project_root_from_repo_root,
- vcs,
-)
-
-logger = logging.getLogger(__name__)
-
-
-class Mercurial(VersionControl):
- name = "hg"
- dirname = ".hg"
- repo_name = "clone"
- schemes = (
- "hg+file",
- "hg+http",
- "hg+https",
- "hg+ssh",
- "hg+static-http",
- )
-
- @staticmethod
- def get_base_rev_args(rev: str) -> List[str]:
- return ["-r", rev]
-
- def fetch_new(
- self, dest: str, url: HiddenText, rev_options: RevOptions, verbosity: int
- ) -> None:
- rev_display = rev_options.to_display()
- logger.info(
- "Cloning hg %s%s to %s",
- url,
- rev_display,
- display_path(dest),
- )
- if verbosity <= 0:
- flags: Tuple[str, ...] = ("--quiet",)
- elif verbosity == 1:
- flags = ()
- elif verbosity == 2:
- flags = ("--verbose",)
- else:
- flags = ("--verbose", "--debug")
- self.run_command(make_command("clone", "--noupdate", *flags, url, dest))
- self.run_command(
- make_command("update", *flags, rev_options.to_args()),
- cwd=dest,
- )
-
- def switch(self, dest: str, url: HiddenText, rev_options: RevOptions) -> None:
- repo_config = os.path.join(dest, self.dirname, "hgrc")
- config = configparser.RawConfigParser()
- try:
- config.read(repo_config)
- config.set("paths", "default", url.secret)
- with open(repo_config, "w") as config_file:
- config.write(config_file)
- except (OSError, configparser.NoSectionError) as exc:
- logger.warning("Could not switch Mercurial repository to %s: %s", url, exc)
- else:
- cmd_args = make_command("update", "-q", rev_options.to_args())
- self.run_command(cmd_args, cwd=dest)
-
- def update(self, dest: str, url: HiddenText, rev_options: RevOptions) -> None:
- self.run_command(["pull", "-q"], cwd=dest)
- cmd_args = make_command("update", "-q", rev_options.to_args())
- self.run_command(cmd_args, cwd=dest)
-
- @classmethod
- def get_remote_url(cls, location: str) -> str:
- url = cls.run_command(
- ["showconfig", "paths.default"],
- show_stdout=False,
- stdout_only=True,
- cwd=location,
- ).strip()
- if cls._is_local_repository(url):
- url = path_to_url(url)
- return url.strip()
-
- @classmethod
- def get_revision(cls, location: str) -> str:
- """
- Return the repository-local changeset revision number, as an integer.
- """
- current_revision = cls.run_command(
- ["parents", "--template={rev}"],
- show_stdout=False,
- stdout_only=True,
- cwd=location,
- ).strip()
- return current_revision
-
- @classmethod
- def get_requirement_revision(cls, location: str) -> str:
- """
- Return the changeset identification hash, as a 40-character
- hexadecimal string
- """
- current_rev_hash = cls.run_command(
- ["parents", "--template={node}"],
- show_stdout=False,
- stdout_only=True,
- cwd=location,
- ).strip()
- return current_rev_hash
-
- @classmethod
- def is_commit_id_equal(cls, dest: str, name: Optional[str]) -> bool:
- """Always assume the versions don't match"""
- return False
-
- @classmethod
- def get_subdirectory(cls, location: str) -> Optional[str]:
- """
- Return the path to Python project root, relative to the repo root.
- Return None if the project root is in the repo root.
- """
- # find the repo root
- repo_root = cls.run_command(
- ["root"], show_stdout=False, stdout_only=True, cwd=location
- ).strip()
- if not os.path.isabs(repo_root):
- repo_root = os.path.abspath(os.path.join(location, repo_root))
- return find_path_to_project_root_from_repo_root(location, repo_root)
-
- @classmethod
- def get_repository_root(cls, location: str) -> Optional[str]:
- loc = super().get_repository_root(location)
- if loc:
- return loc
- try:
- r = cls.run_command(
- ["root"],
- cwd=location,
- show_stdout=False,
- stdout_only=True,
- on_returncode="raise",
- log_failed_cmd=False,
- )
- except BadCommand:
- logger.debug(
- "could not determine if %s is under hg control "
- "because hg is not available",
- location,
- )
- return None
- except InstallationError:
- return None
- return os.path.normpath(r.rstrip("\r\n"))
-
-
-vcs.register(Mercurial)
diff --git a/spaces/TandCAcceptMe/face-swap-docker/mynewshinyroop/Lib/site-packages/pip/_vendor/rich/_win32_console.py b/spaces/TandCAcceptMe/face-swap-docker/mynewshinyroop/Lib/site-packages/pip/_vendor/rich/_win32_console.py
deleted file mode 100644
index 81b1082905338a74b72b9de432ece50a456687bc..0000000000000000000000000000000000000000
--- a/spaces/TandCAcceptMe/face-swap-docker/mynewshinyroop/Lib/site-packages/pip/_vendor/rich/_win32_console.py
+++ /dev/null
@@ -1,662 +0,0 @@
-"""Light wrapper around the Win32 Console API - this module should only be imported on Windows
-
-The API that this module wraps is documented at https://docs.microsoft.com/en-us/windows/console/console-functions
-"""
-import ctypes
-import sys
-from typing import Any
-
-windll: Any = None
-if sys.platform == "win32":
- windll = ctypes.LibraryLoader(ctypes.WinDLL)
-else:
- raise ImportError(f"{__name__} can only be imported on Windows")
-
-import time
-from ctypes import Structure, byref, wintypes
-from typing import IO, NamedTuple, Type, cast
-
-from pip._vendor.rich.color import ColorSystem
-from pip._vendor.rich.style import Style
-
-STDOUT = -11
-ENABLE_VIRTUAL_TERMINAL_PROCESSING = 4
-
-COORD = wintypes._COORD
-
-
-class LegacyWindowsError(Exception):
- pass
-
-
-class WindowsCoordinates(NamedTuple):
- """Coordinates in the Windows Console API are (y, x), not (x, y).
- This class is intended to prevent that confusion.
- Rows and columns are indexed from 0.
- This class can be used in place of wintypes._COORD in arguments and argtypes.
- """
-
- row: int
- col: int
-
- @classmethod
- def from_param(cls, value: "WindowsCoordinates") -> COORD:
- """Converts a WindowsCoordinates into a wintypes _COORD structure.
- This classmethod is internally called by ctypes to perform the conversion.
-
- Args:
- value (WindowsCoordinates): The input coordinates to convert.
-
- Returns:
- wintypes._COORD: The converted coordinates struct.
- """
- return COORD(value.col, value.row)
-
-
-class CONSOLE_SCREEN_BUFFER_INFO(Structure):
- _fields_ = [
- ("dwSize", COORD),
- ("dwCursorPosition", COORD),
- ("wAttributes", wintypes.WORD),
- ("srWindow", wintypes.SMALL_RECT),
- ("dwMaximumWindowSize", COORD),
- ]
-
-
-class CONSOLE_CURSOR_INFO(ctypes.Structure):
- _fields_ = [("dwSize", wintypes.DWORD), ("bVisible", wintypes.BOOL)]
-
-
-_GetStdHandle = windll.kernel32.GetStdHandle
-_GetStdHandle.argtypes = [
- wintypes.DWORD,
-]
-_GetStdHandle.restype = wintypes.HANDLE
-
-
-def GetStdHandle(handle: int = STDOUT) -> wintypes.HANDLE:
- """Retrieves a handle to the specified standard device (standard input, standard output, or standard error).
-
- Args:
- handle (int): Integer identifier for the handle. Defaults to -11 (stdout).
-
- Returns:
- wintypes.HANDLE: The handle
- """
- return cast(wintypes.HANDLE, _GetStdHandle(handle))
-
-
-_GetConsoleMode = windll.kernel32.GetConsoleMode
-_GetConsoleMode.argtypes = [wintypes.HANDLE, wintypes.LPDWORD]
-_GetConsoleMode.restype = wintypes.BOOL
-
-
-def GetConsoleMode(std_handle: wintypes.HANDLE) -> int:
- """Retrieves the current input mode of a console's input buffer
- or the current output mode of a console screen buffer.
-
- Args:
- std_handle (wintypes.HANDLE): A handle to the console input buffer or the console screen buffer.
-
- Raises:
- LegacyWindowsError: If any error occurs while calling the Windows console API.
-
- Returns:
- int: Value representing the current console mode as documented at
- https://docs.microsoft.com/en-us/windows/console/getconsolemode#parameters
- """
-
- console_mode = wintypes.DWORD()
- success = bool(_GetConsoleMode(std_handle, console_mode))
- if not success:
- raise LegacyWindowsError("Unable to get legacy Windows Console Mode")
- return console_mode.value
-
-
-_FillConsoleOutputCharacterW = windll.kernel32.FillConsoleOutputCharacterW
-_FillConsoleOutputCharacterW.argtypes = [
- wintypes.HANDLE,
- ctypes.c_char,
- wintypes.DWORD,
- cast(Type[COORD], WindowsCoordinates),
- ctypes.POINTER(wintypes.DWORD),
-]
-_FillConsoleOutputCharacterW.restype = wintypes.BOOL
-
-
-def FillConsoleOutputCharacter(
- std_handle: wintypes.HANDLE,
- char: str,
- length: int,
- start: WindowsCoordinates,
-) -> int:
- """Writes a character to the console screen buffer a specified number of times, beginning at the specified coordinates.
-
- Args:
- std_handle (wintypes.HANDLE): A handle to the console input buffer or the console screen buffer.
- char (str): The character to write. Must be a string of length 1.
- length (int): The number of times to write the character.
- start (WindowsCoordinates): The coordinates to start writing at.
-
- Returns:
- int: The number of characters written.
- """
- character = ctypes.c_char(char.encode())
- num_characters = wintypes.DWORD(length)
- num_written = wintypes.DWORD(0)
- _FillConsoleOutputCharacterW(
- std_handle,
- character,
- num_characters,
- start,
- byref(num_written),
- )
- return num_written.value
-
-
-_FillConsoleOutputAttribute = windll.kernel32.FillConsoleOutputAttribute
-_FillConsoleOutputAttribute.argtypes = [
- wintypes.HANDLE,
- wintypes.WORD,
- wintypes.DWORD,
- cast(Type[COORD], WindowsCoordinates),
- ctypes.POINTER(wintypes.DWORD),
-]
-_FillConsoleOutputAttribute.restype = wintypes.BOOL
-
-
-def FillConsoleOutputAttribute(
- std_handle: wintypes.HANDLE,
- attributes: int,
- length: int,
- start: WindowsCoordinates,
-) -> int:
- """Sets the character attributes for a specified number of character cells,
- beginning at the specified coordinates in a screen buffer.
-
- Args:
- std_handle (wintypes.HANDLE): A handle to the console input buffer or the console screen buffer.
- attributes (int): Integer value representing the foreground and background colours of the cells.
- length (int): The number of cells to set the output attribute of.
- start (WindowsCoordinates): The coordinates of the first cell whose attributes are to be set.
-
- Returns:
- int: The number of cells whose attributes were actually set.
- """
- num_cells = wintypes.DWORD(length)
- style_attrs = wintypes.WORD(attributes)
- num_written = wintypes.DWORD(0)
- _FillConsoleOutputAttribute(
- std_handle, style_attrs, num_cells, start, byref(num_written)
- )
- return num_written.value
-
-
-_SetConsoleTextAttribute = windll.kernel32.SetConsoleTextAttribute
-_SetConsoleTextAttribute.argtypes = [
- wintypes.HANDLE,
- wintypes.WORD,
-]
-_SetConsoleTextAttribute.restype = wintypes.BOOL
-
-
-def SetConsoleTextAttribute(
- std_handle: wintypes.HANDLE, attributes: wintypes.WORD
-) -> bool:
- """Set the colour attributes for all text written after this function is called.
-
- Args:
- std_handle (wintypes.HANDLE): A handle to the console input buffer or the console screen buffer.
- attributes (int): Integer value representing the foreground and background colours.
-
-
- Returns:
- bool: True if the attribute was set successfully, otherwise False.
- """
- return bool(_SetConsoleTextAttribute(std_handle, attributes))
-
-
-_GetConsoleScreenBufferInfo = windll.kernel32.GetConsoleScreenBufferInfo
-_GetConsoleScreenBufferInfo.argtypes = [
- wintypes.HANDLE,
- ctypes.POINTER(CONSOLE_SCREEN_BUFFER_INFO),
-]
-_GetConsoleScreenBufferInfo.restype = wintypes.BOOL
-
-
-def GetConsoleScreenBufferInfo(
- std_handle: wintypes.HANDLE,
-) -> CONSOLE_SCREEN_BUFFER_INFO:
- """Retrieves information about the specified console screen buffer.
-
- Args:
- std_handle (wintypes.HANDLE): A handle to the console input buffer or the console screen buffer.
-
- Returns:
- CONSOLE_SCREEN_BUFFER_INFO: A CONSOLE_SCREEN_BUFFER_INFO ctype struct contain information about
- screen size, cursor position, colour attributes, and more."""
- console_screen_buffer_info = CONSOLE_SCREEN_BUFFER_INFO()
- _GetConsoleScreenBufferInfo(std_handle, byref(console_screen_buffer_info))
- return console_screen_buffer_info
-
-
-_SetConsoleCursorPosition = windll.kernel32.SetConsoleCursorPosition
-_SetConsoleCursorPosition.argtypes = [
- wintypes.HANDLE,
- cast(Type[COORD], WindowsCoordinates),
-]
-_SetConsoleCursorPosition.restype = wintypes.BOOL
-
-
-def SetConsoleCursorPosition(
- std_handle: wintypes.HANDLE, coords: WindowsCoordinates
-) -> bool:
- """Set the position of the cursor in the console screen
-
- Args:
- std_handle (wintypes.HANDLE): A handle to the console input buffer or the console screen buffer.
- coords (WindowsCoordinates): The coordinates to move the cursor to.
-
- Returns:
- bool: True if the function succeeds, otherwise False.
- """
- return bool(_SetConsoleCursorPosition(std_handle, coords))
-
-
-_GetConsoleCursorInfo = windll.kernel32.GetConsoleCursorInfo
-_GetConsoleCursorInfo.argtypes = [
- wintypes.HANDLE,
- ctypes.POINTER(CONSOLE_CURSOR_INFO),
-]
-_GetConsoleCursorInfo.restype = wintypes.BOOL
-
-
-def GetConsoleCursorInfo(
- std_handle: wintypes.HANDLE, cursor_info: CONSOLE_CURSOR_INFO
-) -> bool:
- """Get the cursor info - used to get cursor visibility and width
-
- Args:
- std_handle (wintypes.HANDLE): A handle to the console input buffer or the console screen buffer.
- cursor_info (CONSOLE_CURSOR_INFO): CONSOLE_CURSOR_INFO ctype struct that receives information
- about the console's cursor.
-
- Returns:
- bool: True if the function succeeds, otherwise False.
- """
- return bool(_GetConsoleCursorInfo(std_handle, byref(cursor_info)))
-
-
-_SetConsoleCursorInfo = windll.kernel32.SetConsoleCursorInfo
-_SetConsoleCursorInfo.argtypes = [
- wintypes.HANDLE,
- ctypes.POINTER(CONSOLE_CURSOR_INFO),
-]
-_SetConsoleCursorInfo.restype = wintypes.BOOL
-
-
-def SetConsoleCursorInfo(
- std_handle: wintypes.HANDLE, cursor_info: CONSOLE_CURSOR_INFO
-) -> bool:
- """Set the cursor info - used for adjusting cursor visibility and width
-
- Args:
- std_handle (wintypes.HANDLE): A handle to the console input buffer or the console screen buffer.
- cursor_info (CONSOLE_CURSOR_INFO): CONSOLE_CURSOR_INFO ctype struct containing the new cursor info.
-
- Returns:
- bool: True if the function succeeds, otherwise False.
- """
- return bool(_SetConsoleCursorInfo(std_handle, byref(cursor_info)))
-
-
-_SetConsoleTitle = windll.kernel32.SetConsoleTitleW
-_SetConsoleTitle.argtypes = [wintypes.LPCWSTR]
-_SetConsoleTitle.restype = wintypes.BOOL
-
-
-def SetConsoleTitle(title: str) -> bool:
- """Sets the title of the current console window
-
- Args:
- title (str): The new title of the console window.
-
- Returns:
- bool: True if the function succeeds, otherwise False.
- """
- return bool(_SetConsoleTitle(title))
-
-
-class LegacyWindowsTerm:
- """This class allows interaction with the legacy Windows Console API. It should only be used in the context
- of environments where virtual terminal processing is not available. However, if it is used in a Windows environment,
- the entire API should work.
-
- Args:
- file (IO[str]): The file which the Windows Console API HANDLE is retrieved from, defaults to sys.stdout.
- """
-
- BRIGHT_BIT = 8
-
- # Indices are ANSI color numbers, values are the corresponding Windows Console API color numbers
- ANSI_TO_WINDOWS = [
- 0, # black The Windows colours are defined in wincon.h as follows:
- 4, # red define FOREGROUND_BLUE 0x0001 -- 0000 0001
- 2, # green define FOREGROUND_GREEN 0x0002 -- 0000 0010
- 6, # yellow define FOREGROUND_RED 0x0004 -- 0000 0100
- 1, # blue define FOREGROUND_INTENSITY 0x0008 -- 0000 1000
- 5, # magenta define BACKGROUND_BLUE 0x0010 -- 0001 0000
- 3, # cyan define BACKGROUND_GREEN 0x0020 -- 0010 0000
- 7, # white define BACKGROUND_RED 0x0040 -- 0100 0000
- 8, # bright black (grey) define BACKGROUND_INTENSITY 0x0080 -- 1000 0000
- 12, # bright red
- 10, # bright green
- 14, # bright yellow
- 9, # bright blue
- 13, # bright magenta
- 11, # bright cyan
- 15, # bright white
- ]
-
- def __init__(self, file: "IO[str]") -> None:
- handle = GetStdHandle(STDOUT)
- self._handle = handle
- default_text = GetConsoleScreenBufferInfo(handle).wAttributes
- self._default_text = default_text
-
- self._default_fore = default_text & 7
- self._default_back = (default_text >> 4) & 7
- self._default_attrs = self._default_fore | (self._default_back << 4)
-
- self._file = file
- self.write = file.write
- self.flush = file.flush
-
- @property
- def cursor_position(self) -> WindowsCoordinates:
- """Returns the current position of the cursor (0-based)
-
- Returns:
- WindowsCoordinates: The current cursor position.
- """
- coord: COORD = GetConsoleScreenBufferInfo(self._handle).dwCursorPosition
- return WindowsCoordinates(row=cast(int, coord.Y), col=cast(int, coord.X))
-
- @property
- def screen_size(self) -> WindowsCoordinates:
- """Returns the current size of the console screen buffer, in character columns and rows
-
- Returns:
- WindowsCoordinates: The width and height of the screen as WindowsCoordinates.
- """
- screen_size: COORD = GetConsoleScreenBufferInfo(self._handle).dwSize
- return WindowsCoordinates(
- row=cast(int, screen_size.Y), col=cast(int, screen_size.X)
- )
-
- def write_text(self, text: str) -> None:
- """Write text directly to the terminal without any modification of styles
-
- Args:
- text (str): The text to write to the console
- """
- self.write(text)
- self.flush()
-
- def write_styled(self, text: str, style: Style) -> None:
- """Write styled text to the terminal.
-
- Args:
- text (str): The text to write
- style (Style): The style of the text
- """
- color = style.color
- bgcolor = style.bgcolor
- if style.reverse:
- color, bgcolor = bgcolor, color
-
- if color:
- fore = color.downgrade(ColorSystem.WINDOWS).number
- fore = fore if fore is not None else 7 # Default to ANSI 7: White
- if style.bold:
- fore = fore | self.BRIGHT_BIT
- if style.dim:
- fore = fore & ~self.BRIGHT_BIT
- fore = self.ANSI_TO_WINDOWS[fore]
- else:
- fore = self._default_fore
-
- if bgcolor:
- back = bgcolor.downgrade(ColorSystem.WINDOWS).number
- back = back if back is not None else 0 # Default to ANSI 0: Black
- back = self.ANSI_TO_WINDOWS[back]
- else:
- back = self._default_back
-
- assert fore is not None
- assert back is not None
-
- SetConsoleTextAttribute(
- self._handle, attributes=ctypes.c_ushort(fore | (back << 4))
- )
- self.write_text(text)
- SetConsoleTextAttribute(self._handle, attributes=self._default_text)
-
- def move_cursor_to(self, new_position: WindowsCoordinates) -> None:
- """Set the position of the cursor
-
- Args:
- new_position (WindowsCoordinates): The WindowsCoordinates representing the new position of the cursor.
- """
- if new_position.col < 0 or new_position.row < 0:
- return
- SetConsoleCursorPosition(self._handle, coords=new_position)
-
- def erase_line(self) -> None:
- """Erase all content on the line the cursor is currently located at"""
- screen_size = self.screen_size
- cursor_position = self.cursor_position
- cells_to_erase = screen_size.col
- start_coordinates = WindowsCoordinates(row=cursor_position.row, col=0)
- FillConsoleOutputCharacter(
- self._handle, " ", length=cells_to_erase, start=start_coordinates
- )
- FillConsoleOutputAttribute(
- self._handle,
- self._default_attrs,
- length=cells_to_erase,
- start=start_coordinates,
- )
-
- def erase_end_of_line(self) -> None:
- """Erase all content from the cursor position to the end of that line"""
- cursor_position = self.cursor_position
- cells_to_erase = self.screen_size.col - cursor_position.col
- FillConsoleOutputCharacter(
- self._handle, " ", length=cells_to_erase, start=cursor_position
- )
- FillConsoleOutputAttribute(
- self._handle,
- self._default_attrs,
- length=cells_to_erase,
- start=cursor_position,
- )
-
- def erase_start_of_line(self) -> None:
- """Erase all content from the cursor position to the start of that line"""
- row, col = self.cursor_position
- start = WindowsCoordinates(row, 0)
- FillConsoleOutputCharacter(self._handle, " ", length=col, start=start)
- FillConsoleOutputAttribute(
- self._handle, self._default_attrs, length=col, start=start
- )
-
- def move_cursor_up(self) -> None:
- """Move the cursor up a single cell"""
- cursor_position = self.cursor_position
- SetConsoleCursorPosition(
- self._handle,
- coords=WindowsCoordinates(
- row=cursor_position.row - 1, col=cursor_position.col
- ),
- )
-
- def move_cursor_down(self) -> None:
- """Move the cursor down a single cell"""
- cursor_position = self.cursor_position
- SetConsoleCursorPosition(
- self._handle,
- coords=WindowsCoordinates(
- row=cursor_position.row + 1,
- col=cursor_position.col,
- ),
- )
-
- def move_cursor_forward(self) -> None:
- """Move the cursor forward a single cell. Wrap to the next line if required."""
- row, col = self.cursor_position
- if col == self.screen_size.col - 1:
- row += 1
- col = 0
- else:
- col += 1
- SetConsoleCursorPosition(
- self._handle, coords=WindowsCoordinates(row=row, col=col)
- )
-
- def move_cursor_to_column(self, column: int) -> None:
- """Move cursor to the column specified by the zero-based column index, staying on the same row
-
- Args:
- column (int): The zero-based column index to move the cursor to.
- """
- row, _ = self.cursor_position
- SetConsoleCursorPosition(self._handle, coords=WindowsCoordinates(row, column))
-
- def move_cursor_backward(self) -> None:
- """Move the cursor backward a single cell. Wrap to the previous line if required."""
- row, col = self.cursor_position
- if col == 0:
- row -= 1
- col = self.screen_size.col - 1
- else:
- col -= 1
- SetConsoleCursorPosition(
- self._handle, coords=WindowsCoordinates(row=row, col=col)
- )
-
- def hide_cursor(self) -> None:
- """Hide the cursor"""
- current_cursor_size = self._get_cursor_size()
- invisible_cursor = CONSOLE_CURSOR_INFO(dwSize=current_cursor_size, bVisible=0)
- SetConsoleCursorInfo(self._handle, cursor_info=invisible_cursor)
-
- def show_cursor(self) -> None:
- """Show the cursor"""
- current_cursor_size = self._get_cursor_size()
- visible_cursor = CONSOLE_CURSOR_INFO(dwSize=current_cursor_size, bVisible=1)
- SetConsoleCursorInfo(self._handle, cursor_info=visible_cursor)
-
- def set_title(self, title: str) -> None:
- """Set the title of the terminal window
-
- Args:
- title (str): The new title of the console window
- """
- assert len(title) < 255, "Console title must be less than 255 characters"
- SetConsoleTitle(title)
-
- def _get_cursor_size(self) -> int:
- """Get the percentage of the character cell that is filled by the cursor"""
- cursor_info = CONSOLE_CURSOR_INFO()
- GetConsoleCursorInfo(self._handle, cursor_info=cursor_info)
- return int(cursor_info.dwSize)
-
-
-if __name__ == "__main__":
- handle = GetStdHandle()
-
- from pip._vendor.rich.console import Console
-
- console = Console()
-
- term = LegacyWindowsTerm(sys.stdout)
- term.set_title("Win32 Console Examples")
-
- style = Style(color="black", bgcolor="red")
-
- heading = Style.parse("black on green")
-
- # Check colour output
- console.rule("Checking colour output")
- console.print("[on red]on red!")
- console.print("[blue]blue!")
- console.print("[yellow]yellow!")
- console.print("[bold yellow]bold yellow!")
- console.print("[bright_yellow]bright_yellow!")
- console.print("[dim bright_yellow]dim bright_yellow!")
- console.print("[italic cyan]italic cyan!")
- console.print("[bold white on blue]bold white on blue!")
- console.print("[reverse bold white on blue]reverse bold white on blue!")
- console.print("[bold black on cyan]bold black on cyan!")
- console.print("[black on green]black on green!")
- console.print("[blue on green]blue on green!")
- console.print("[white on black]white on black!")
- console.print("[black on white]black on white!")
- console.print("[#1BB152 on #DA812D]#1BB152 on #DA812D!")
-
- # Check cursor movement
- console.rule("Checking cursor movement")
- console.print()
- term.move_cursor_backward()
- term.move_cursor_backward()
- term.write_text("went back and wrapped to prev line")
- time.sleep(1)
- term.move_cursor_up()
- term.write_text("we go up")
- time.sleep(1)
- term.move_cursor_down()
- term.write_text("and down")
- time.sleep(1)
- term.move_cursor_up()
- term.move_cursor_backward()
- term.move_cursor_backward()
- term.write_text("we went up and back 2")
- time.sleep(1)
- term.move_cursor_down()
- term.move_cursor_backward()
- term.move_cursor_backward()
- term.write_text("we went down and back 2")
- time.sleep(1)
-
- # Check erasing of lines
- term.hide_cursor()
- console.print()
- console.rule("Checking line erasing")
- console.print("\n...Deleting to the start of the line...")
- term.write_text("The red arrow shows the cursor location, and direction of erase")
- time.sleep(1)
- term.move_cursor_to_column(16)
- term.write_styled("<", Style.parse("black on red"))
- term.move_cursor_backward()
- time.sleep(1)
- term.erase_start_of_line()
- time.sleep(1)
-
- console.print("\n\n...And to the end of the line...")
- term.write_text("The red arrow shows the cursor location, and direction of erase")
- time.sleep(1)
-
- term.move_cursor_to_column(16)
- term.write_styled(">", Style.parse("black on red"))
- time.sleep(1)
- term.erase_end_of_line()
- time.sleep(1)
-
- console.print("\n\n...Now the whole line will be erased...")
- term.write_styled("I'm going to disappear!", style=Style.parse("black on cyan"))
- time.sleep(1)
- term.erase_line()
-
- term.show_cursor()
- print("\n")
diff --git a/spaces/TandCAcceptMe/face-swap-docker/mynewshinyroop/Lib/site-packages/setuptools/_vendor/packaging/utils.py b/spaces/TandCAcceptMe/face-swap-docker/mynewshinyroop/Lib/site-packages/setuptools/_vendor/packaging/utils.py
deleted file mode 100644
index 33c613b749a49d6035c0e549389e92c3d68a83ad..0000000000000000000000000000000000000000
--- a/spaces/TandCAcceptMe/face-swap-docker/mynewshinyroop/Lib/site-packages/setuptools/_vendor/packaging/utils.py
+++ /dev/null
@@ -1,141 +0,0 @@
-# This file is dual licensed under the terms of the Apache License, Version
-# 2.0, and the BSD License. See the LICENSE file in the root of this repository
-# for complete details.
-
-import re
-from typing import FrozenSet, NewType, Tuple, Union, cast
-
-from .tags import Tag, parse_tag
-from .version import InvalidVersion, Version
-
-BuildTag = Union[Tuple[()], Tuple[int, str]]
-NormalizedName = NewType("NormalizedName", str)
-
-
-class InvalidWheelFilename(ValueError):
- """
- An invalid wheel filename was found, users should refer to PEP 427.
- """
-
-
-class InvalidSdistFilename(ValueError):
- """
- An invalid sdist filename was found, users should refer to the packaging user guide.
- """
-
-
-_canonicalize_regex = re.compile(r"[-_.]+")
-# PEP 427: The build number must start with a digit.
-_build_tag_regex = re.compile(r"(\d+)(.*)")
-
-
-def canonicalize_name(name: str) -> NormalizedName:
- # This is taken from PEP 503.
- value = _canonicalize_regex.sub("-", name).lower()
- return cast(NormalizedName, value)
-
-
-def canonicalize_version(
- version: Union[Version, str], *, strip_trailing_zero: bool = True
-) -> str:
- """
- This is very similar to Version.__str__, but has one subtle difference
- with the way it handles the release segment.
- """
- if isinstance(version, str):
- try:
- parsed = Version(version)
- except InvalidVersion:
- # Legacy versions cannot be normalized
- return version
- else:
- parsed = version
-
- parts = []
-
- # Epoch
- if parsed.epoch != 0:
- parts.append(f"{parsed.epoch}!")
-
- # Release segment
- release_segment = ".".join(str(x) for x in parsed.release)
- if strip_trailing_zero:
- # NB: This strips trailing '.0's to normalize
- release_segment = re.sub(r"(\.0)+$", "", release_segment)
- parts.append(release_segment)
-
- # Pre-release
- if parsed.pre is not None:
- parts.append("".join(str(x) for x in parsed.pre))
-
- # Post-release
- if parsed.post is not None:
- parts.append(f".post{parsed.post}")
-
- # Development release
- if parsed.dev is not None:
- parts.append(f".dev{parsed.dev}")
-
- # Local version segment
- if parsed.local is not None:
- parts.append(f"+{parsed.local}")
-
- return "".join(parts)
-
-
-def parse_wheel_filename(
- filename: str,
-) -> Tuple[NormalizedName, Version, BuildTag, FrozenSet[Tag]]:
- if not filename.endswith(".whl"):
- raise InvalidWheelFilename(
- f"Invalid wheel filename (extension must be '.whl'): {filename}"
- )
-
- filename = filename[:-4]
- dashes = filename.count("-")
- if dashes not in (4, 5):
- raise InvalidWheelFilename(
- f"Invalid wheel filename (wrong number of parts): {filename}"
- )
-
- parts = filename.split("-", dashes - 2)
- name_part = parts[0]
- # See PEP 427 for the rules on escaping the project name
- if "__" in name_part or re.match(r"^[\w\d._]*$", name_part, re.UNICODE) is None:
- raise InvalidWheelFilename(f"Invalid project name: {filename}")
- name = canonicalize_name(name_part)
- version = Version(parts[1])
- if dashes == 5:
- build_part = parts[2]
- build_match = _build_tag_regex.match(build_part)
- if build_match is None:
- raise InvalidWheelFilename(
- f"Invalid build number: {build_part} in '{filename}'"
- )
- build = cast(BuildTag, (int(build_match.group(1)), build_match.group(2)))
- else:
- build = ()
- tags = parse_tag(parts[-1])
- return (name, version, build, tags)
-
-
-def parse_sdist_filename(filename: str) -> Tuple[NormalizedName, Version]:
- if filename.endswith(".tar.gz"):
- file_stem = filename[: -len(".tar.gz")]
- elif filename.endswith(".zip"):
- file_stem = filename[: -len(".zip")]
- else:
- raise InvalidSdistFilename(
- f"Invalid sdist filename (extension must be '.tar.gz' or '.zip'):"
- f" {filename}"
- )
-
- # We are requiring a PEP 440 version, which cannot contain dashes,
- # so we split on the last dash.
- name_part, sep, version_part = file_stem.rpartition("-")
- if not sep:
- raise InvalidSdistFilename(f"Invalid sdist filename: {filename}")
-
- name = canonicalize_name(name_part)
- version = Version(version_part)
- return (name, version)
diff --git a/spaces/TandCAcceptMe/face-swap-docker/mynewshinyroop/Lib/site-packages/wheel/metadata.py b/spaces/TandCAcceptMe/face-swap-docker/mynewshinyroop/Lib/site-packages/wheel/metadata.py
deleted file mode 100644
index b391c962e97303847e19b71642628f68f4e98117..0000000000000000000000000000000000000000
--- a/spaces/TandCAcceptMe/face-swap-docker/mynewshinyroop/Lib/site-packages/wheel/metadata.py
+++ /dev/null
@@ -1,179 +0,0 @@
-"""
-Tools for converting old- to new-style metadata.
-"""
-from __future__ import annotations
-
-import functools
-import itertools
-import os.path
-import re
-import textwrap
-from email.message import Message
-from email.parser import Parser
-from typing import Iterator
-
-from .vendored.packaging.requirements import Requirement
-
-
-def _nonblank(str):
- return str and not str.startswith("#")
-
-
-@functools.singledispatch
-def yield_lines(iterable):
- r"""
- Yield valid lines of a string or iterable.
- >>> list(yield_lines(''))
- []
- >>> list(yield_lines(['foo', 'bar']))
- ['foo', 'bar']
- >>> list(yield_lines('foo\nbar'))
- ['foo', 'bar']
- >>> list(yield_lines('\nfoo\n#bar\nbaz #comment'))
- ['foo', 'baz #comment']
- >>> list(yield_lines(['foo\nbar', 'baz', 'bing\n\n\n']))
- ['foo', 'bar', 'baz', 'bing']
- """
- return itertools.chain.from_iterable(map(yield_lines, iterable))
-
-
-@yield_lines.register(str)
-def _(text):
- return filter(_nonblank, map(str.strip, text.splitlines()))
-
-
-def split_sections(s):
- """Split a string or iterable thereof into (section, content) pairs
- Each ``section`` is a stripped version of the section header ("[section]")
- and each ``content`` is a list of stripped lines excluding blank lines and
- comment-only lines. If there are any such lines before the first section
- header, they're returned in a first ``section`` of ``None``.
- """
- section = None
- content = []
- for line in yield_lines(s):
- if line.startswith("["):
- if line.endswith("]"):
- if section or content:
- yield section, content
- section = line[1:-1].strip()
- content = []
- else:
- raise ValueError("Invalid section heading", line)
- else:
- content.append(line)
-
- # wrap up last segment
- yield section, content
-
-
-def safe_extra(extra):
- """Convert an arbitrary string to a standard 'extra' name
- Any runs of non-alphanumeric characters are replaced with a single '_',
- and the result is always lowercased.
- """
- return re.sub("[^A-Za-z0-9.-]+", "_", extra).lower()
-
-
-def safe_name(name):
- """Convert an arbitrary string to a standard distribution name
- Any runs of non-alphanumeric/. characters are replaced with a single '-'.
- """
- return re.sub("[^A-Za-z0-9.]+", "-", name)
-
-
-def requires_to_requires_dist(requirement: Requirement) -> str:
- """Return the version specifier for a requirement in PEP 345/566 fashion."""
- if getattr(requirement, "url", None):
- return " @ " + requirement.url
-
- requires_dist = []
- for spec in requirement.specifier:
- requires_dist.append(spec.operator + spec.version)
-
- if requires_dist:
- return " (" + ",".join(sorted(requires_dist)) + ")"
- else:
- return ""
-
-
-def convert_requirements(requirements: list[str]) -> Iterator[str]:
- """Yield Requires-Dist: strings for parsed requirements strings."""
- for req in requirements:
- parsed_requirement = Requirement(req)
- spec = requires_to_requires_dist(parsed_requirement)
- extras = ",".join(sorted(safe_extra(e) for e in parsed_requirement.extras))
- if extras:
- extras = f"[{extras}]"
-
- yield safe_name(parsed_requirement.name) + extras + spec
-
-
-def generate_requirements(
- extras_require: dict[str, list[str]]
-) -> Iterator[tuple[str, str]]:
- """
- Convert requirements from a setup()-style dictionary to
- ('Requires-Dist', 'requirement') and ('Provides-Extra', 'extra') tuples.
-
- extras_require is a dictionary of {extra: [requirements]} as passed to setup(),
- using the empty extra {'': [requirements]} to hold install_requires.
- """
- for extra, depends in extras_require.items():
- condition = ""
- extra = extra or ""
- if ":" in extra: # setuptools extra:condition syntax
- extra, condition = extra.split(":", 1)
-
- extra = safe_extra(extra)
- if extra:
- yield "Provides-Extra", extra
- if condition:
- condition = "(" + condition + ") and "
- condition += "extra == '%s'" % extra
-
- if condition:
- condition = " ; " + condition
-
- for new_req in convert_requirements(depends):
- yield "Requires-Dist", new_req + condition
-
-
-def pkginfo_to_metadata(egg_info_path: str, pkginfo_path: str) -> Message:
- """
- Convert .egg-info directory with PKG-INFO to the Metadata 2.1 format
- """
- with open(pkginfo_path, encoding="utf-8") as headers:
- pkg_info = Parser().parse(headers)
-
- pkg_info.replace_header("Metadata-Version", "2.1")
- # Those will be regenerated from `requires.txt`.
- del pkg_info["Provides-Extra"]
- del pkg_info["Requires-Dist"]
- requires_path = os.path.join(egg_info_path, "requires.txt")
- if os.path.exists(requires_path):
- with open(requires_path, encoding="utf-8") as requires_file:
- requires = requires_file.read()
-
- parsed_requirements = sorted(split_sections(requires), key=lambda x: x[0] or "")
- for extra, reqs in parsed_requirements:
- for key, value in generate_requirements({extra: reqs}):
- if (key, value) not in pkg_info.items():
- pkg_info[key] = value
-
- description = pkg_info["Description"]
- if description:
- description_lines = pkg_info["Description"].splitlines()
- dedented_description = "\n".join(
- # if the first line of long_description is blank,
- # the first line here will be indented.
- (
- description_lines[0].lstrip(),
- textwrap.dedent("\n".join(description_lines[1:])),
- "\n",
- )
- )
- pkg_info.set_payload(dedented_description)
- del pkg_info["Description"]
-
- return pkg_info
diff --git a/spaces/TencentARC/VLog/models/grit_src/grit/modeling/text/file_utils.py b/spaces/TencentARC/VLog/models/grit_src/grit/modeling/text/file_utils.py
deleted file mode 100644
index 51918cf3857471e4ffb5b617d73ee8b9eed0989e..0000000000000000000000000000000000000000
--- a/spaces/TencentARC/VLog/models/grit_src/grit/modeling/text/file_utils.py
+++ /dev/null
@@ -1,256 +0,0 @@
-# Utilities for working with the local dataset cache.
-# This file is adapted from the AllenNLP library at https://github.com/allenai/allennlp
-# Copyright by the AllenNLP authors.
-
-from __future__ import absolute_import, division, print_function, unicode_literals
-
-import sys
-import json
-import logging
-import os
-import shutil
-import tempfile
-import fnmatch
-from functools import wraps
-from hashlib import sha256
-from io import open
-
-import boto3
-import requests
-from botocore.exceptions import ClientError
-from tqdm import tqdm
-
-try:
- from torch.hub import _get_torch_home
- torch_cache_home = _get_torch_home()
-except ImportError:
- torch_cache_home = os.path.expanduser(
- os.getenv('TORCH_HOME', os.path.join(
- os.getenv('XDG_CACHE_HOME', '~/.cache'), 'torch')))
-default_cache_path = os.path.join(torch_cache_home, 'pytorch_transformers')
-
-try:
- from urllib.parse import urlparse
-except ImportError:
- from urlparse import urlparse
-
-try:
- from pathlib import Path
- PYTORCH_PRETRAINED_BERT_CACHE = Path(
- os.getenv('PYTORCH_PRETRAINED_BERT_CACHE', default_cache_path))
-except (AttributeError, ImportError):
- PYTORCH_PRETRAINED_BERT_CACHE = os.getenv('PYTORCH_PRETRAINED_BERT_CACHE',
- default_cache_path)
-
-logger = logging.getLogger(__name__) # pylint: disable=invalid-name
-
-
-def url_to_filename(url, etag=None):
- """
- Convert `url` into a hashed filename in a repeatable way.
- If `etag` is specified, append its hash to the url's, delimited
- by a period.
- """
- url_bytes = url.encode('utf-8')
- url_hash = sha256(url_bytes)
- filename = url_hash.hexdigest()
-
- if etag:
- etag_bytes = etag.encode('utf-8')
- etag_hash = sha256(etag_bytes)
- filename += '.' + etag_hash.hexdigest()
-
- return filename
-
-
-def filename_to_url(filename, cache_dir=None):
- """
- Return the url and etag (which may be ``None``) stored for `filename`.
- Raise ``EnvironmentError`` if `filename` or its stored metadata do not exist.
- """
- if cache_dir is None:
- cache_dir = PYTORCH_PRETRAINED_BERT_CACHE
- if sys.version_info[0] == 3 and isinstance(cache_dir, Path):
- cache_dir = str(cache_dir)
-
- cache_path = os.path.join(cache_dir, filename)
- if not os.path.exists(cache_path):
- raise EnvironmentError("file {} not found".format(cache_path))
-
- meta_path = cache_path + '.json'
- if not os.path.exists(meta_path):
- raise EnvironmentError("file {} not found".format(meta_path))
-
- with open(meta_path, encoding="utf-8") as meta_file:
- metadata = json.load(meta_file)
- url = metadata['url']
- etag = metadata['etag']
-
- return url, etag
-
-
-def cached_path(url_or_filename, cache_dir=None):
- """
- Given something that might be a URL (or might be a local path),
- determine which. If it's a URL, download the file and cache it, and
- return the path to the cached file. If it's already a local path,
- make sure the file exists and then return the path.
- """
- if cache_dir is None:
- cache_dir = PYTORCH_PRETRAINED_BERT_CACHE
- if sys.version_info[0] == 3 and isinstance(url_or_filename, Path):
- url_or_filename = str(url_or_filename)
- if sys.version_info[0] == 3 and isinstance(cache_dir, Path):
- cache_dir = str(cache_dir)
-
- parsed = urlparse(url_or_filename)
-
- if parsed.scheme in ('http', 'https', 's3'):
- # URL, so get it from the cache (downloading if necessary)
- return get_from_cache(url_or_filename, cache_dir)
- elif os.path.exists(url_or_filename):
- # File, and it exists.
- return url_or_filename
- elif parsed.scheme == '':
- # File, but it doesn't exist.
- raise EnvironmentError("file {} not found".format(url_or_filename))
- else:
- # Something unknown
- raise ValueError("unable to parse {} as a URL or as a local path".format(url_or_filename))
-
-
-def split_s3_path(url):
- """Split a full s3 path into the bucket name and path."""
- parsed = urlparse(url)
- if not parsed.netloc or not parsed.path:
- raise ValueError("bad s3 path {}".format(url))
- bucket_name = parsed.netloc
- s3_path = parsed.path
- # Remove '/' at beginning of path.
- if s3_path.startswith("/"):
- s3_path = s3_path[1:]
- return bucket_name, s3_path
-
-
-def s3_request(func):
- """
- Wrapper function for s3 requests in order to create more helpful error
- messages.
- """
-
- @wraps(func)
- def wrapper(url, *args, **kwargs):
- try:
- return func(url, *args, **kwargs)
- except ClientError as exc:
- if int(exc.response["Error"]["Code"]) == 404:
- raise EnvironmentError("file {} not found".format(url))
- else:
- raise
-
- return wrapper
-
-
-@s3_request
-def s3_etag(url):
- """Check ETag on S3 object."""
- s3_resource = boto3.resource("s3")
- bucket_name, s3_path = split_s3_path(url)
- s3_object = s3_resource.Object(bucket_name, s3_path)
- return s3_object.e_tag
-
-
-@s3_request
-def s3_get(url, temp_file):
- """Pull a file directly from S3."""
- s3_resource = boto3.resource("s3")
- bucket_name, s3_path = split_s3_path(url)
- s3_resource.Bucket(bucket_name).download_fileobj(s3_path, temp_file)
-
-
-def http_get(url, temp_file):
- req = requests.get(url, stream=True)
- content_length = req.headers.get('Content-Length')
- total = int(content_length) if content_length is not None else None
- progress = tqdm(unit="B", total=total)
- for chunk in req.iter_content(chunk_size=1024):
- if chunk: # filter out keep-alive new chunks
- progress.update(len(chunk))
- temp_file.write(chunk)
- progress.close()
-
-
-def get_from_cache(url, cache_dir=None):
- """
- Given a URL, look for the corresponding dataset in the local cache.
- If it's not there, download it. Then return the path to the cached file.
- """
- if cache_dir is None:
- cache_dir = PYTORCH_PRETRAINED_BERT_CACHE
- if sys.version_info[0] == 3 and isinstance(cache_dir, Path):
- cache_dir = str(cache_dir)
- if sys.version_info[0] == 2 and not isinstance(cache_dir, str):
- cache_dir = str(cache_dir)
-
- if not os.path.exists(cache_dir):
- os.makedirs(cache_dir)
-
- # Get eTag to add to filename, if it exists.
- if url.startswith("s3://"):
- etag = s3_etag(url)
- else:
- try:
- response = requests.head(url, allow_redirects=True)
- if response.status_code != 200:
- etag = None
- else:
- etag = response.headers.get("ETag")
- except EnvironmentError:
- etag = None
-
- if sys.version_info[0] == 2 and etag is not None:
- etag = etag.decode('utf-8')
- filename = url_to_filename(url, etag)
-
- # get cache path to put the file
- cache_path = os.path.join(cache_dir, filename)
-
- # If we don't have a connection (etag is None) and can't identify the file
- # try to get the last downloaded one
- if not os.path.exists(cache_path) and etag is None:
- matching_files = fnmatch.filter(os.listdir(cache_dir), filename + '.*')
- matching_files = list(filter(lambda s: not s.endswith('.json'), matching_files))
- if matching_files:
- cache_path = os.path.join(cache_dir, matching_files[-1])
-
- if not os.path.exists(cache_path):
- # Download to temporary file, then copy to cache dir once finished.
- # Otherwise you get corrupt cache entries if the download gets interrupted.
- with tempfile.NamedTemporaryFile() as temp_file:
- logger.info("%s not found in cache, downloading to %s", url, temp_file.name)
-
- # GET file object
- if url.startswith("s3://"):
- s3_get(url, temp_file)
- else:
- http_get(url, temp_file)
-
- # we are copying the file before closing it, so flush to avoid truncation
- temp_file.flush()
- # shutil.copyfileobj() starts at the current position, so go to the start
- temp_file.seek(0)
-
- logger.info("copying %s to cache at %s", temp_file.name, cache_path)
- with open(cache_path, 'wb') as cache_file:
- shutil.copyfileobj(temp_file, cache_file)
-
- logger.info("creating metadata file for %s", cache_path)
- meta = {'url': url, 'etag': etag}
- meta_path = cache_path + '.json'
- with open(meta_path, 'w') as meta_file:
- output_string = json.dumps(meta)
- meta_file.write(output_string)
-
- logger.info("removing temp file %s", temp_file.name)
-
- return cache_path
diff --git a/spaces/TitleOS/Seahorse-350m/README.md b/spaces/TitleOS/Seahorse-350m/README.md
deleted file mode 100644
index 22931461178b22d3cbf781195f82681d2c4b1542..0000000000000000000000000000000000000000
--- a/spaces/TitleOS/Seahorse-350m/README.md
+++ /dev/null
@@ -1,12 +0,0 @@
----
-title: Seahorse 350m
-emoji: 🏃
-colorFrom: pink
-colorTo: green
-sdk: gradio
-sdk_version: 3.36.1
-app_file: app.py
-pinned: false
----
-
-Check out the configuration reference at https://huggingface.co/docs/hub/spaces-config-reference
diff --git a/spaces/VaneM/text-to-image-es/app.py b/spaces/VaneM/text-to-image-es/app.py
deleted file mode 100644
index 0e0733c24a79f77614d6b3b075bf4ba5e7e2be98..0000000000000000000000000000000000000000
--- a/spaces/VaneM/text-to-image-es/app.py
+++ /dev/null
@@ -1,16 +0,0 @@
-import gradio as gr
-
-
-title="Texto a Imagen con Stable Diffusion 2.0"
-description = '
Prompt en Español!
'
-article = """
-El modelo usa:
-- Texto a Imagen [Stable Diffusion 2.0](https://huggingface.co/stabilityai/stable-diffusion-2),
-- Para la traduccion [Helsinki-NLP](https://huggingface.co/Helsinki-NLP)
-\n ... y mucha magia ☺
-"""
-
-text_imagen = gr.Interface.load("models/stabilityai/stable-diffusion-2")
-text_translate = gr.Interface.load("models/Helsinki-NLP/opus-mt-es-en")
-
-gr.Series(text_translate, text_imagen, title=title, description = description, article=article).launch(debug=True)
\ No newline at end of file
diff --git a/spaces/Vegecken/sovits4dzl/utils.py b/spaces/Vegecken/sovits4dzl/utils.py
deleted file mode 100644
index f13d3526d514be71c77bebb17a5af8831b9c6a36..0000000000000000000000000000000000000000
--- a/spaces/Vegecken/sovits4dzl/utils.py
+++ /dev/null
@@ -1,508 +0,0 @@
-import os
-import glob
-import re
-import sys
-import argparse
-import logging
-import json
-import subprocess
-import random
-
-import librosa
-import numpy as np
-from scipy.io.wavfile import read
-import torch
-from torch.nn import functional as F
-from modules.commons import sequence_mask
-from hubert import hubert_model
-MATPLOTLIB_FLAG = False
-
-logging.basicConfig(stream=sys.stdout, level=logging.DEBUG)
-logger = logging
-
-f0_bin = 256
-f0_max = 1100.0
-f0_min = 50.0
-f0_mel_min = 1127 * np.log(1 + f0_min / 700)
-f0_mel_max = 1127 * np.log(1 + f0_max / 700)
-
-
-# def normalize_f0(f0, random_scale=True):
-# f0_norm = f0.clone() # create a copy of the input Tensor
-# batch_size, _, frame_length = f0_norm.shape
-# for i in range(batch_size):
-# means = torch.mean(f0_norm[i, 0, :])
-# if random_scale:
-# factor = random.uniform(0.8, 1.2)
-# else:
-# factor = 1
-# f0_norm[i, 0, :] = (f0_norm[i, 0, :] - means) * factor
-# return f0_norm
-# def normalize_f0(f0, random_scale=True):
-# means = torch.mean(f0[:, 0, :], dim=1, keepdim=True)
-# if random_scale:
-# factor = torch.Tensor(f0.shape[0],1).uniform_(0.8, 1.2).to(f0.device)
-# else:
-# factor = torch.ones(f0.shape[0], 1, 1).to(f0.device)
-# f0_norm = (f0 - means.unsqueeze(-1)) * factor.unsqueeze(-1)
-# return f0_norm
-def normalize_f0(f0, x_mask, uv, random_scale=True):
- # calculate means based on x_mask
- uv_sum = torch.sum(uv, dim=1, keepdim=True)
- uv_sum[uv_sum == 0] = 9999
- means = torch.sum(f0[:, 0, :] * uv, dim=1, keepdim=True) / uv_sum
-
- if random_scale:
- factor = torch.Tensor(f0.shape[0], 1).uniform_(0.8, 1.2).to(f0.device)
- else:
- factor = torch.ones(f0.shape[0], 1).to(f0.device)
- # normalize f0 based on means and factor
- f0_norm = (f0 - means.unsqueeze(-1)) * factor.unsqueeze(-1)
- if torch.isnan(f0_norm).any():
- exit(0)
- return f0_norm * x_mask
-
-
-def plot_data_to_numpy(x, y):
- global MATPLOTLIB_FLAG
- if not MATPLOTLIB_FLAG:
- import matplotlib
- matplotlib.use("Agg")
- MATPLOTLIB_FLAG = True
- mpl_logger = logging.getLogger('matplotlib')
- mpl_logger.setLevel(logging.WARNING)
- import matplotlib.pylab as plt
- import numpy as np
-
- fig, ax = plt.subplots(figsize=(10, 2))
- plt.plot(x)
- plt.plot(y)
- plt.tight_layout()
-
- fig.canvas.draw()
- data = np.fromstring(fig.canvas.tostring_rgb(), dtype=np.uint8, sep='')
- data = data.reshape(fig.canvas.get_width_height()[::-1] + (3,))
- plt.close()
- return data
-
-
-
-def interpolate_f0(f0):
- '''
- 对F0进行插值处理
- '''
-
- data = np.reshape(f0, (f0.size, 1))
-
- vuv_vector = np.zeros((data.size, 1), dtype=np.float32)
- vuv_vector[data > 0.0] = 1.0
- vuv_vector[data <= 0.0] = 0.0
-
- ip_data = data
-
- frame_number = data.size
- last_value = 0.0
- for i in range(frame_number):
- if data[i] <= 0.0:
- j = i + 1
- for j in range(i + 1, frame_number):
- if data[j] > 0.0:
- break
- if j < frame_number - 1:
- if last_value > 0.0:
- step = (data[j] - data[i - 1]) / float(j - i)
- for k in range(i, j):
- ip_data[k] = data[i - 1] + step * (k - i + 1)
- else:
- for k in range(i, j):
- ip_data[k] = data[j]
- else:
- for k in range(i, frame_number):
- ip_data[k] = last_value
- else:
- ip_data[i] = data[i]
- last_value = data[i]
-
- return ip_data[:,0], vuv_vector[:,0]
-
-
-def compute_f0_parselmouth(wav_numpy, p_len=None, sampling_rate=44100, hop_length=512):
- import parselmouth
- x = wav_numpy
- if p_len is None:
- p_len = x.shape[0]//hop_length
- else:
- assert abs(p_len-x.shape[0]//hop_length) < 4, "pad length error"
- time_step = hop_length / sampling_rate * 1000
- f0_min = 50
- f0_max = 1100
- f0 = parselmouth.Sound(x, sampling_rate).to_pitch_ac(
- time_step=time_step / 1000, voicing_threshold=0.6,
- pitch_floor=f0_min, pitch_ceiling=f0_max).selected_array['frequency']
-
- pad_size=(p_len - len(f0) + 1) // 2
- if(pad_size>0 or p_len - len(f0) - pad_size>0):
- f0 = np.pad(f0,[[pad_size,p_len - len(f0) - pad_size]], mode='constant')
- return f0
-
-def resize_f0(x, target_len):
- source = np.array(x)
- source[source<0.001] = np.nan
- target = np.interp(np.arange(0, len(source)*target_len, len(source))/ target_len, np.arange(0, len(source)), source)
- res = np.nan_to_num(target)
- return res
-
-def compute_f0_dio(wav_numpy, p_len=None, sampling_rate=44100, hop_length=512):
- import pyworld
- if p_len is None:
- p_len = wav_numpy.shape[0]//hop_length
- f0, t = pyworld.dio(
- wav_numpy.astype(np.double),
- fs=sampling_rate,
- f0_ceil=800,
- frame_period=1000 * hop_length / sampling_rate,
- )
- f0 = pyworld.stonemask(wav_numpy.astype(np.double), f0, t, sampling_rate)
- for index, pitch in enumerate(f0):
- f0[index] = round(pitch, 1)
- return resize_f0(f0, p_len)
-
-def f0_to_coarse(f0):
- is_torch = isinstance(f0, torch.Tensor)
- f0_mel = 1127 * (1 + f0 / 700).log() if is_torch else 1127 * np.log(1 + f0 / 700)
- f0_mel[f0_mel > 0] = (f0_mel[f0_mel > 0] - f0_mel_min) * (f0_bin - 2) / (f0_mel_max - f0_mel_min) + 1
-
- f0_mel[f0_mel <= 1] = 1
- f0_mel[f0_mel > f0_bin - 1] = f0_bin - 1
- f0_coarse = (f0_mel + 0.5).long() if is_torch else np.rint(f0_mel).astype(np.int)
- assert f0_coarse.max() <= 255 and f0_coarse.min() >= 1, (f0_coarse.max(), f0_coarse.min())
- return f0_coarse
-
-
-def get_hubert_model():
- vec_path = "hubert/checkpoint_best_legacy_500.pt"
- print("load model(s) from {}".format(vec_path))
- from fairseq import checkpoint_utils
- models, saved_cfg, task = checkpoint_utils.load_model_ensemble_and_task(
- [vec_path],
- suffix="",
- )
- model = models[0]
- model.eval()
- return model
-
-def get_hubert_content(hmodel, wav_16k_tensor):
- feats = wav_16k_tensor
- if feats.dim() == 2: # double channels
- feats = feats.mean(-1)
- assert feats.dim() == 1, feats.dim()
- feats = feats.view(1, -1)
- padding_mask = torch.BoolTensor(feats.shape).fill_(False)
- inputs = {
- "source": feats.to(wav_16k_tensor.device),
- "padding_mask": padding_mask.to(wav_16k_tensor.device),
- "output_layer": 9, # layer 9
- }
- with torch.no_grad():
- logits = hmodel.extract_features(**inputs)
- feats = hmodel.final_proj(logits[0])
- return feats.transpose(1, 2)
-
-
-def get_content(cmodel, y):
- with torch.no_grad():
- c = cmodel.extract_features(y.squeeze(1))[0]
- c = c.transpose(1, 2)
- return c
-
-
-
-def load_checkpoint(checkpoint_path, model, optimizer=None, skip_optimizer=False):
- assert os.path.isfile(checkpoint_path)
- checkpoint_dict = torch.load(checkpoint_path, map_location='cpu')
- iteration = checkpoint_dict['iteration']
- learning_rate = checkpoint_dict['learning_rate']
- if optimizer is not None and not skip_optimizer:
- optimizer.load_state_dict(checkpoint_dict['optimizer'])
- saved_state_dict = checkpoint_dict['model']
- if hasattr(model, 'module'):
- state_dict = model.module.state_dict()
- else:
- state_dict = model.state_dict()
- new_state_dict = {}
- for k, v in state_dict.items():
- try:
- # assert "dec" in k or "disc" in k
- # print("load", k)
- new_state_dict[k] = saved_state_dict[k]
- assert saved_state_dict[k].shape == v.shape, (saved_state_dict[k].shape, v.shape)
- except:
- print("error, %s is not in the checkpoint" % k)
- logger.info("%s is not in the checkpoint" % k)
- new_state_dict[k] = v
- if hasattr(model, 'module'):
- model.module.load_state_dict(new_state_dict)
- else:
- model.load_state_dict(new_state_dict)
- print("load ")
- logger.info("Loaded checkpoint '{}' (iteration {})".format(
- checkpoint_path, iteration))
- return model, optimizer, learning_rate, iteration
-
-
-def save_checkpoint(model, optimizer, learning_rate, iteration, checkpoint_path, val_steps, current_step):
- logger.info("Saving model and optimizer state at iteration {} to {}".format(
- iteration, checkpoint_path))
- if hasattr(model, 'module'):
- state_dict = model.module.state_dict()
- else:
- state_dict = model.state_dict()
- torch.save({'model': state_dict,
- 'iteration': iteration,
- 'optimizer': optimizer.state_dict(),
- 'learning_rate': learning_rate}, checkpoint_path)
- if current_step >= val_steps * 3:
- to_del_ckptname = checkpoint_path.replace(str(current_step), str(current_step - val_steps * 3))
- if os.path.exists(to_del_ckptname):
- os.remove(to_del_ckptname)
- print("Removing ", to_del_ckptname)
-
-
-def clean_checkpoints(path_to_models='logs/48k/', n_ckpts_to_keep=2, sort_by_time=True):
- """Freeing up space by deleting saved ckpts
-
- Arguments:
- path_to_models -- Path to the model directory
- n_ckpts_to_keep -- Number of ckpts to keep, excluding G_0.pth and D_0.pth
- sort_by_time -- True -> chronologically delete ckpts
- False -> lexicographically delete ckpts
- """
- ckpts_files = [f for f in os.listdir(path_to_models) if os.path.isfile(os.path.join(path_to_models, f))]
- name_key = (lambda _f: int(re.compile('._(\d+)\.pth').match(_f).group(1)))
- time_key = (lambda _f: os.path.getmtime(os.path.join(path_to_models, _f)))
- sort_key = time_key if sort_by_time else name_key
- x_sorted = lambda _x: sorted([f for f in ckpts_files if f.startswith(_x) and not f.endswith('_0.pth')], key=sort_key)
- to_del = [os.path.join(path_to_models, fn) for fn in
- (x_sorted('G')[:-n_ckpts_to_keep] + x_sorted('D')[:-n_ckpts_to_keep])]
- del_info = lambda fn: logger.info(f".. Free up space by deleting ckpt {fn}")
- del_routine = lambda x: [os.remove(x), del_info(x)]
- rs = [del_routine(fn) for fn in to_del]
-
-def summarize(writer, global_step, scalars={}, histograms={}, images={}, audios={}, audio_sampling_rate=22050):
- for k, v in scalars.items():
- writer.add_scalar(k, v, global_step)
- for k, v in histograms.items():
- writer.add_histogram(k, v, global_step)
- for k, v in images.items():
- writer.add_image(k, v, global_step, dataformats='HWC')
- for k, v in audios.items():
- writer.add_audio(k, v, global_step, audio_sampling_rate)
-
-
-def latest_checkpoint_path(dir_path, regex="G_*.pth"):
- f_list = glob.glob(os.path.join(dir_path, regex))
- f_list.sort(key=lambda f: int("".join(filter(str.isdigit, f))))
- x = f_list[-1]
- print(x)
- return x
-
-
-def plot_spectrogram_to_numpy(spectrogram):
- global MATPLOTLIB_FLAG
- if not MATPLOTLIB_FLAG:
- import matplotlib
- matplotlib.use("Agg")
- MATPLOTLIB_FLAG = True
- mpl_logger = logging.getLogger('matplotlib')
- mpl_logger.setLevel(logging.WARNING)
- import matplotlib.pylab as plt
- import numpy as np
-
- fig, ax = plt.subplots(figsize=(10,2))
- im = ax.imshow(spectrogram, aspect="auto", origin="lower",
- interpolation='none')
- plt.colorbar(im, ax=ax)
- plt.xlabel("Frames")
- plt.ylabel("Channels")
- plt.tight_layout()
-
- fig.canvas.draw()
- data = np.fromstring(fig.canvas.tostring_rgb(), dtype=np.uint8, sep='')
- data = data.reshape(fig.canvas.get_width_height()[::-1] + (3,))
- plt.close()
- return data
-
-
-def plot_alignment_to_numpy(alignment, info=None):
- global MATPLOTLIB_FLAG
- if not MATPLOTLIB_FLAG:
- import matplotlib
- matplotlib.use("Agg")
- MATPLOTLIB_FLAG = True
- mpl_logger = logging.getLogger('matplotlib')
- mpl_logger.setLevel(logging.WARNING)
- import matplotlib.pylab as plt
- import numpy as np
-
- fig, ax = plt.subplots(figsize=(6, 4))
- im = ax.imshow(alignment.transpose(), aspect='auto', origin='lower',
- interpolation='none')
- fig.colorbar(im, ax=ax)
- xlabel = 'Decoder timestep'
- if info is not None:
- xlabel += '\n\n' + info
- plt.xlabel(xlabel)
- plt.ylabel('Encoder timestep')
- plt.tight_layout()
-
- fig.canvas.draw()
- data = np.fromstring(fig.canvas.tostring_rgb(), dtype=np.uint8, sep='')
- data = data.reshape(fig.canvas.get_width_height()[::-1] + (3,))
- plt.close()
- return data
-
-
-def load_wav_to_torch(full_path):
- sampling_rate, data = read(full_path)
- return torch.FloatTensor(data.astype(np.float32)), sampling_rate
-
-
-def load_filepaths_and_text(filename, split="|"):
- with open(filename, encoding='utf-8') as f:
- filepaths_and_text = [line.strip().split(split) for line in f]
- return filepaths_and_text
-
-
-def get_hparams(init=True):
- parser = argparse.ArgumentParser()
- parser.add_argument('-c', '--config', type=str, default="./configs/base.json",
- help='JSON file for configuration')
- parser.add_argument('-m', '--model', type=str, required=True,
- help='Model name')
-
- args = parser.parse_args()
- model_dir = os.path.join("./logs", args.model)
-
- if not os.path.exists(model_dir):
- os.makedirs(model_dir)
-
- config_path = args.config
- config_save_path = os.path.join(model_dir, "config.json")
- if init:
- with open(config_path, "r") as f:
- data = f.read()
- with open(config_save_path, "w") as f:
- f.write(data)
- else:
- with open(config_save_path, "r") as f:
- data = f.read()
- config = json.loads(data)
-
- hparams = HParams(**config)
- hparams.model_dir = model_dir
- return hparams
-
-
-def get_hparams_from_dir(model_dir):
- config_save_path = os.path.join(model_dir, "config.json")
- with open(config_save_path, "r") as f:
- data = f.read()
- config = json.loads(data)
-
- hparams =HParams(**config)
- hparams.model_dir = model_dir
- return hparams
-
-
-def get_hparams_from_file(config_path):
- with open(config_path, "r") as f:
- data = f.read()
- config = json.loads(data)
-
- hparams =HParams(**config)
- return hparams
-
-
-def check_git_hash(model_dir):
- source_dir = os.path.dirname(os.path.realpath(__file__))
- if not os.path.exists(os.path.join(source_dir, ".git")):
- logger.warn("{} is not a git repository, therefore hash value comparison will be ignored.".format(
- source_dir
- ))
- return
-
- cur_hash = subprocess.getoutput("git rev-parse HEAD")
-
- path = os.path.join(model_dir, "githash")
- if os.path.exists(path):
- saved_hash = open(path).read()
- if saved_hash != cur_hash:
- logger.warn("git hash values are different. {}(saved) != {}(current)".format(
- saved_hash[:8], cur_hash[:8]))
- else:
- open(path, "w").write(cur_hash)
-
-
-def get_logger(model_dir, filename="train.log"):
- global logger
- logger = logging.getLogger(os.path.basename(model_dir))
- logger.setLevel(logging.DEBUG)
-
- formatter = logging.Formatter("%(asctime)s\t%(name)s\t%(levelname)s\t%(message)s")
- if not os.path.exists(model_dir):
- os.makedirs(model_dir)
- h = logging.FileHandler(os.path.join(model_dir, filename))
- h.setLevel(logging.DEBUG)
- h.setFormatter(formatter)
- logger.addHandler(h)
- return logger
-
-
-def repeat_expand_2d(content, target_len):
- # content : [h, t]
-
- src_len = content.shape[-1]
- target = torch.zeros([content.shape[0], target_len], dtype=torch.float).to(content.device)
- temp = torch.arange(src_len+1) * target_len / src_len
- current_pos = 0
- for i in range(target_len):
- if i < temp[current_pos+1]:
- target[:, i] = content[:, current_pos]
- else:
- current_pos += 1
- target[:, i] = content[:, current_pos]
-
- return target
-
-
-class HParams():
- def __init__(self, **kwargs):
- for k, v in kwargs.items():
- if type(v) == dict:
- v = HParams(**v)
- self[k] = v
-
- def keys(self):
- return self.__dict__.keys()
-
- def items(self):
- return self.__dict__.items()
-
- def values(self):
- return self.__dict__.values()
-
- def __len__(self):
- return len(self.__dict__)
-
- def __getitem__(self, key):
- return getattr(self, key)
-
- def __setitem__(self, key, value):
- return setattr(self, key, value)
-
- def __contains__(self, key):
- return key in self.__dict__
-
- def __repr__(self):
- return self.__dict__.__repr__()
-
diff --git a/spaces/Vision-CAIR/minigpt4/minigpt4/datasets/builders/__init__.py b/spaces/Vision-CAIR/minigpt4/minigpt4/datasets/builders/__init__.py
deleted file mode 100644
index a1f19e672f951204dc80067f30db368818fa4e00..0000000000000000000000000000000000000000
--- a/spaces/Vision-CAIR/minigpt4/minigpt4/datasets/builders/__init__.py
+++ /dev/null
@@ -1,72 +0,0 @@
-"""
- Copyright (c) 2022, salesforce.com, inc.
- All rights reserved.
- SPDX-License-Identifier: BSD-3-Clause
- For full license text, see the LICENSE file in the repo root or https://opensource.org/licenses/BSD-3-Clause
-"""
-
-from minigpt4.datasets.builders.base_dataset_builder import load_dataset_config
-from minigpt4.datasets.builders.image_text_pair_builder import (
- CCCombineBuilder,
- LaionBuilder,
- CCAlignBuilder
-)
-from minigpt4.common.registry import registry
-
-__all__ = [
- "CCCombineBuilder",
- "LaionBuilder",
- "CCAlignBuilder"
-]
-
-
-def load_dataset(name, cfg_path=None, vis_path=None, data_type=None):
- """
- Example
-
- >>> dataset = load_dataset("coco_caption", cfg=None)
- >>> splits = dataset.keys()
- >>> print([len(dataset[split]) for split in splits])
-
- """
- if cfg_path is None:
- cfg = None
- else:
- cfg = load_dataset_config(cfg_path)
-
- try:
- builder = registry.get_builder_class(name)(cfg)
- except TypeError:
- print(
- f"Dataset {name} not found. Available datasets:\n"
- + ", ".join([str(k) for k in dataset_zoo.get_names()])
- )
- exit(1)
-
- if vis_path is not None:
- if data_type is None:
- # use default data type in the config
- data_type = builder.config.data_type
-
- assert (
- data_type in builder.config.build_info
- ), f"Invalid data_type {data_type} for {name}."
-
- builder.config.build_info.get(data_type).storage = vis_path
-
- dataset = builder.build_datasets()
- return dataset
-
-
-class DatasetZoo:
- def __init__(self) -> None:
- self.dataset_zoo = {
- k: list(v.DATASET_CONFIG_DICT.keys())
- for k, v in sorted(registry.mapping["builder_name_mapping"].items())
- }
-
- def get_names(self):
- return list(self.dataset_zoo.keys())
-
-
-dataset_zoo = DatasetZoo()
diff --git a/spaces/VoiceHero69/changer/hubert/hubert_manager.py b/spaces/VoiceHero69/changer/hubert/hubert_manager.py
deleted file mode 100644
index 4c62ed7efc95da65f31b540803c0d25dc2a2ff68..0000000000000000000000000000000000000000
--- a/spaces/VoiceHero69/changer/hubert/hubert_manager.py
+++ /dev/null
@@ -1,46 +0,0 @@
-import os.path
-import shutil
-import urllib.request
-
-import huggingface_hub
-
-
-class HuBERTManager:
- @staticmethod
- def make_sure_hubert_installed(download_url: str = 'https://dl.fbaipublicfiles.com/hubert/hubert_base_ls960.pt', file_name: str = 'hubert.pt'):
- install_dir = os.path.join('data', 'models', 'hubert')
- if not os.path.isdir(install_dir):
- os.makedirs(install_dir, exist_ok=True)
- install_file = os.path.join(install_dir, file_name)
- if not os.path.isfile(install_file):
- print('Downloading HuBERT base model')
- urllib.request.urlretrieve(download_url, install_file)
- print('Downloaded HuBERT')
- return install_file
-
-
- @staticmethod
- def make_sure_tokenizer_installed(model: str = 'quantifier_hubert_base_ls960_14.pth', repo: str = 'GitMylo/bark-voice-cloning', local_file: str = 'tokenizer.pth'):
- install_dir = os.path.join('data', 'models', 'hubert')
- if not os.path.isdir(install_dir):
- os.makedirs(install_dir, exist_ok=True)
- install_file = os.path.join(install_dir, local_file)
- if not os.path.isfile(install_file):
- print('Downloading HuBERT custom tokenizer')
- huggingface_hub.hf_hub_download(repo, model, local_dir=install_dir, local_dir_use_symlinks=False)
- shutil.move(os.path.join(install_dir, model), install_file)
- print('Downloaded tokenizer')
- return install_file
-
- @staticmethod
- def make_sure_hubert_rvc_installed(model: str = 'hubert_base.pt', repo: str = 'lj1995/VoiceConversionWebUI', local_file: str = 'hubert_rvc.pt'):
- install_dir = os.path.join('data', 'models', 'hubert')
- if not os.path.isdir(install_dir):
- os.makedirs(install_dir, exist_ok=True)
- install_file = os.path.join(install_dir, local_file)
- if not os.path.isfile(install_file):
- print('Downloading HuBERT for RVC')
- huggingface_hub.hf_hub_download(repo, model, local_dir=install_dir, local_dir_use_symlinks=False)
- shutil.move(os.path.join(install_dir, model), install_file)
- print('Downloaded HuBERT for RVC')
- return install_file
diff --git a/spaces/WZUN666/vits-uma-genshin-honkai/README.md b/spaces/WZUN666/vits-uma-genshin-honkai/README.md
deleted file mode 100644
index 1c0aa069bfd980b6b45bb2bf62ff74bd9b0b61c2..0000000000000000000000000000000000000000
--- a/spaces/WZUN666/vits-uma-genshin-honkai/README.md
+++ /dev/null
@@ -1,11 +0,0 @@
----
-license: apache-2.0
-title: ' vits-uma-genshin-honkai'
-sdk: gradio
-sdk_version: 3.7
-emoji: 🐨
-colorTo: yellow
-pinned: false
-app_file: app.py
-duplicated_from: ikechan8370/vits-uma-genshin-honkai
----
diff --git a/spaces/XzJosh/XingTong-Bert-VITS2/monotonic_align/core.py b/spaces/XzJosh/XingTong-Bert-VITS2/monotonic_align/core.py
deleted file mode 100644
index dddc688d76172b880054e544b7a217acd013f14f..0000000000000000000000000000000000000000
--- a/spaces/XzJosh/XingTong-Bert-VITS2/monotonic_align/core.py
+++ /dev/null
@@ -1,35 +0,0 @@
-import numba
-
-
-@numba.jit(numba.void(numba.int32[:,:,::1], numba.float32[:,:,::1], numba.int32[::1], numba.int32[::1]), nopython=True, nogil=True)
-def maximum_path_jit(paths, values, t_ys, t_xs):
- b = paths.shape[0]
- max_neg_val=-1e9
- for i in range(int(b)):
- path = paths[i]
- value = values[i]
- t_y = t_ys[i]
- t_x = t_xs[i]
-
- v_prev = v_cur = 0.0
- index = t_x - 1
-
- for y in range(t_y):
- for x in range(max(0, t_x + y - t_y), min(t_x, y + 1)):
- if x == y:
- v_cur = max_neg_val
- else:
- v_cur = value[y-1, x]
- if x == 0:
- if y == 0:
- v_prev = 0.
- else:
- v_prev = max_neg_val
- else:
- v_prev = value[y-1, x-1]
- value[y, x] += max(v_prev, v_cur)
-
- for y in range(t_y - 1, -1, -1):
- path[y, index] = 1
- if index != 0 and (index == y or value[y-1, index] < value[y-1, index-1]):
- index = index - 1
diff --git a/spaces/XzJosh/yoyo-Bert-VITS2/text/chinese_bert.py b/spaces/XzJosh/yoyo-Bert-VITS2/text/chinese_bert.py
deleted file mode 100644
index cb84ce0b426cd0a1c7954ddcdf41322c10ed14fa..0000000000000000000000000000000000000000
--- a/spaces/XzJosh/yoyo-Bert-VITS2/text/chinese_bert.py
+++ /dev/null
@@ -1,50 +0,0 @@
-import torch
-from transformers import AutoTokenizer, AutoModelForMaskedLM
-
-device = torch.device("cuda" if torch.cuda.is_available() else "cpu")
-
-tokenizer = AutoTokenizer.from_pretrained("./bert/chinese-roberta-wwm-ext-large")
-model = AutoModelForMaskedLM.from_pretrained("./bert/chinese-roberta-wwm-ext-large").to(device)
-
-def get_bert_feature(text, word2ph):
- with torch.no_grad():
- inputs = tokenizer(text, return_tensors='pt')
- for i in inputs:
- inputs[i] = inputs[i].to(device)
- res = model(**inputs, output_hidden_states=True)
- res = torch.cat(res['hidden_states'][-3:-2], -1)[0].cpu()
-
- assert len(word2ph) == len(text)+2
- word2phone = word2ph
- phone_level_feature = []
- for i in range(len(word2phone)):
- repeat_feature = res[i].repeat(word2phone[i], 1)
- phone_level_feature.append(repeat_feature)
-
- phone_level_feature = torch.cat(phone_level_feature, dim=0)
-
-
- return phone_level_feature.T
-
-if __name__ == '__main__':
- # feature = get_bert_feature('你好,我是说的道理。')
- import torch
-
- word_level_feature = torch.rand(38, 1024) # 12个词,每个词1024维特征
- word2phone = [1, 2, 1, 2, 2, 1, 2, 2, 1, 2, 2, 1, 2, 2, 2, 2, 2, 1, 1, 2, 2, 1, 2, 2, 2, 2, 1, 2, 2, 2, 2, 2, 1, 2, 2, 2, 2, 1]
-
- # 计算总帧数
- total_frames = sum(word2phone)
- print(word_level_feature.shape)
- print(word2phone)
- phone_level_feature = []
- for i in range(len(word2phone)):
- print(word_level_feature[i].shape)
-
- # 对每个词重复word2phone[i]次
- repeat_feature = word_level_feature[i].repeat(word2phone[i], 1)
- phone_level_feature.append(repeat_feature)
-
- phone_level_feature = torch.cat(phone_level_feature, dim=0)
- print(phone_level_feature.shape) # torch.Size([36, 1024])
-
diff --git a/spaces/YUANAI/DiffspeechResearch/data_gen/tts/wav_processors/__init__.py b/spaces/YUANAI/DiffspeechResearch/data_gen/tts/wav_processors/__init__.py
deleted file mode 100644
index 4be97b377dcb95a0e6bceb876ac0ce93c8290249..0000000000000000000000000000000000000000
--- a/spaces/YUANAI/DiffspeechResearch/data_gen/tts/wav_processors/__init__.py
+++ /dev/null
@@ -1,2 +0,0 @@
-from . import base_processor
-from . import common_processors
diff --git a/spaces/YUANAI/DiffspeechResearch/modules/commons/rnn.py b/spaces/YUANAI/DiffspeechResearch/modules/commons/rnn.py
deleted file mode 100644
index 205c2c76b8fda2de920bc59228a5eec0a20119a9..0000000000000000000000000000000000000000
--- a/spaces/YUANAI/DiffspeechResearch/modules/commons/rnn.py
+++ /dev/null
@@ -1,261 +0,0 @@
-import torch
-from torch import nn
-import torch.nn.functional as F
-
-
-class PreNet(nn.Module):
- def __init__(self, in_dims, fc1_dims=256, fc2_dims=128, dropout=0.5):
- super().__init__()
- self.fc1 = nn.Linear(in_dims, fc1_dims)
- self.fc2 = nn.Linear(fc1_dims, fc2_dims)
- self.p = dropout
-
- def forward(self, x):
- x = self.fc1(x)
- x = F.relu(x)
- x = F.dropout(x, self.p, training=self.training)
- x = self.fc2(x)
- x = F.relu(x)
- x = F.dropout(x, self.p, training=self.training)
- return x
-
-
-class HighwayNetwork(nn.Module):
- def __init__(self, size):
- super().__init__()
- self.W1 = nn.Linear(size, size)
- self.W2 = nn.Linear(size, size)
- self.W1.bias.data.fill_(0.)
-
- def forward(self, x):
- x1 = self.W1(x)
- x2 = self.W2(x)
- g = torch.sigmoid(x2)
- y = g * F.relu(x1) + (1. - g) * x
- return y
-
-
-class BatchNormConv(nn.Module):
- def __init__(self, in_channels, out_channels, kernel, relu=True):
- super().__init__()
- self.conv = nn.Conv1d(in_channels, out_channels, kernel, stride=1, padding=kernel // 2, bias=False)
- self.bnorm = nn.BatchNorm1d(out_channels)
- self.relu = relu
-
- def forward(self, x):
- x = self.conv(x)
- x = F.relu(x) if self.relu is True else x
- return self.bnorm(x)
-
-
-class ConvNorm(torch.nn.Module):
- def __init__(self, in_channels, out_channels, kernel_size=1, stride=1,
- padding=None, dilation=1, bias=True, w_init_gain='linear'):
- super(ConvNorm, self).__init__()
- if padding is None:
- assert (kernel_size % 2 == 1)
- padding = int(dilation * (kernel_size - 1) / 2)
-
- self.conv = torch.nn.Conv1d(in_channels, out_channels,
- kernel_size=kernel_size, stride=stride,
- padding=padding, dilation=dilation,
- bias=bias)
-
- torch.nn.init.xavier_uniform_(
- self.conv.weight, gain=torch.nn.init.calculate_gain(w_init_gain))
-
- def forward(self, signal):
- conv_signal = self.conv(signal)
- return conv_signal
-
-
-class CBHG(nn.Module):
- def __init__(self, K, in_channels, channels, proj_channels, num_highways):
- super().__init__()
-
- # List of all rnns to call `flatten_parameters()` on
- self._to_flatten = []
-
- self.bank_kernels = [i for i in range(1, K + 1)]
- self.conv1d_bank = nn.ModuleList()
- for k in self.bank_kernels:
- conv = BatchNormConv(in_channels, channels, k)
- self.conv1d_bank.append(conv)
-
- self.maxpool = nn.MaxPool1d(kernel_size=2, stride=1, padding=1)
-
- self.conv_project1 = BatchNormConv(len(self.bank_kernels) * channels, proj_channels[0], 3)
- self.conv_project2 = BatchNormConv(proj_channels[0], proj_channels[1], 3, relu=False)
-
- # Fix the highway input if necessary
- if proj_channels[-1] != channels:
- self.highway_mismatch = True
- self.pre_highway = nn.Linear(proj_channels[-1], channels, bias=False)
- else:
- self.highway_mismatch = False
-
- self.highways = nn.ModuleList()
- for i in range(num_highways):
- hn = HighwayNetwork(channels)
- self.highways.append(hn)
-
- self.rnn = nn.GRU(channels, channels, batch_first=True, bidirectional=True)
- self._to_flatten.append(self.rnn)
-
- # Avoid fragmentation of RNN parameters and associated warning
- self._flatten_parameters()
-
- def forward(self, x):
- # Although we `_flatten_parameters()` on init, when using DataParallel
- # the model gets replicated, making it no longer guaranteed that the
- # weights are contiguous in GPU memory. Hence, we must call it again
- self._flatten_parameters()
-
- # Save these for later
- residual = x
- seq_len = x.size(-1)
- conv_bank = []
-
- # Convolution Bank
- for conv in self.conv1d_bank:
- c = conv(x) # Convolution
- conv_bank.append(c[:, :, :seq_len])
-
- # Stack along the channel axis
- conv_bank = torch.cat(conv_bank, dim=1)
-
- # dump the last padding to fit residual
- x = self.maxpool(conv_bank)[:, :, :seq_len]
-
- # Conv1d projections
- x = self.conv_project1(x)
- x = self.conv_project2(x)
-
- # Residual Connect
- x = x + residual
-
- # Through the highways
- x = x.transpose(1, 2)
- if self.highway_mismatch is True:
- x = self.pre_highway(x)
- for h in self.highways:
- x = h(x)
-
- # And then the RNN
- x, _ = self.rnn(x)
- return x
-
- def _flatten_parameters(self):
- """Calls `flatten_parameters` on all the rnns used by the WaveRNN. Used
- to improve efficiency and avoid PyTorch yelling at us."""
- [m.flatten_parameters() for m in self._to_flatten]
-
-
-class TacotronEncoder(nn.Module):
- def __init__(self, embed_dims, num_chars, cbhg_channels, K, num_highways, dropout):
- super().__init__()
- self.embedding = nn.Embedding(num_chars, embed_dims)
- self.pre_net = PreNet(embed_dims, embed_dims, embed_dims, dropout=dropout)
- self.cbhg = CBHG(K=K, in_channels=cbhg_channels, channels=cbhg_channels,
- proj_channels=[cbhg_channels, cbhg_channels],
- num_highways=num_highways)
- self.proj_out = nn.Linear(cbhg_channels * 2, cbhg_channels)
-
- def forward(self, x):
- x = self.embedding(x)
- x = self.pre_net(x)
- x.transpose_(1, 2)
- x = self.cbhg(x)
- x = self.proj_out(x)
- return x
-
-
-class RNNEncoder(nn.Module):
- def __init__(self, num_chars, embedding_dim, n_convolutions=3, kernel_size=5):
- super(RNNEncoder, self).__init__()
- self.embedding = nn.Embedding(num_chars, embedding_dim, padding_idx=0)
- convolutions = []
- for _ in range(n_convolutions):
- conv_layer = nn.Sequential(
- ConvNorm(embedding_dim,
- embedding_dim,
- kernel_size=kernel_size, stride=1,
- padding=int((kernel_size - 1) / 2),
- dilation=1, w_init_gain='relu'),
- nn.BatchNorm1d(embedding_dim))
- convolutions.append(conv_layer)
- self.convolutions = nn.ModuleList(convolutions)
-
- self.lstm = nn.LSTM(embedding_dim, int(embedding_dim / 2), 1,
- batch_first=True, bidirectional=True)
-
- def forward(self, x):
- input_lengths = (x > 0).sum(-1)
- input_lengths = input_lengths.cpu().numpy()
-
- x = self.embedding(x)
- x = x.transpose(1, 2) # [B, H, T]
- for conv in self.convolutions:
- x = F.dropout(F.relu(conv(x)), 0.5, self.training) + x
- x = x.transpose(1, 2) # [B, T, H]
-
- # pytorch tensor are not reversible, hence the conversion
- x = nn.utils.rnn.pack_padded_sequence(x, input_lengths, batch_first=True, enforce_sorted=False)
-
- self.lstm.flatten_parameters()
- outputs, _ = self.lstm(x)
- outputs, _ = nn.utils.rnn.pad_packed_sequence(outputs, batch_first=True)
-
- return outputs
-
-
-class DecoderRNN(torch.nn.Module):
- def __init__(self, hidden_size, decoder_rnn_dim, dropout):
- super(DecoderRNN, self).__init__()
- self.in_conv1d = nn.Sequential(
- torch.nn.Conv1d(
- in_channels=hidden_size,
- out_channels=hidden_size,
- kernel_size=9, padding=4,
- ),
- torch.nn.ReLU(),
- torch.nn.Conv1d(
- in_channels=hidden_size,
- out_channels=hidden_size,
- kernel_size=9, padding=4,
- ),
- )
- self.ln = nn.LayerNorm(hidden_size)
- if decoder_rnn_dim == 0:
- decoder_rnn_dim = hidden_size * 2
- self.rnn = torch.nn.LSTM(
- input_size=hidden_size,
- hidden_size=decoder_rnn_dim,
- num_layers=1,
- batch_first=True,
- bidirectional=True,
- dropout=dropout
- )
- self.rnn.flatten_parameters()
- self.conv1d = torch.nn.Conv1d(
- in_channels=decoder_rnn_dim * 2,
- out_channels=hidden_size,
- kernel_size=3,
- padding=1,
- )
-
- def forward(self, x):
- input_masks = x.abs().sum(-1).ne(0).data[:, :, None]
- input_lengths = input_masks.sum([-1, -2])
- input_lengths = input_lengths.cpu().numpy()
-
- x = self.in_conv1d(x.transpose(1, 2)).transpose(1, 2)
- x = self.ln(x)
- x = nn.utils.rnn.pack_padded_sequence(x, input_lengths, batch_first=True, enforce_sorted=False)
- self.rnn.flatten_parameters()
- x, _ = self.rnn(x) # [B, T, C]
- x, _ = nn.utils.rnn.pad_packed_sequence(x, batch_first=True)
- x = x * input_masks
- pre_mel = self.conv1d(x.transpose(1, 2)).transpose(1, 2) # [B, T, C]
- pre_mel = pre_mel * input_masks
- return pre_mel
diff --git a/spaces/YuAnthony/Audio-Caption/coco_caption/pycocoevalcap/rouge/__init__.py b/spaces/YuAnthony/Audio-Caption/coco_caption/pycocoevalcap/rouge/__init__.py
deleted file mode 100644
index 43a773e12ea2e960f9a62fa1c2179a73d8c0dd35..0000000000000000000000000000000000000000
--- a/spaces/YuAnthony/Audio-Caption/coco_caption/pycocoevalcap/rouge/__init__.py
+++ /dev/null
@@ -1 +0,0 @@
-__author__ = 'vrama91'
diff --git a/spaces/Zakia/cat_or_dog_predictor/README.md b/spaces/Zakia/cat_or_dog_predictor/README.md
deleted file mode 100644
index 63b4832721a7d3a85c3913824a9bd6167e27f871..0000000000000000000000000000000000000000
--- a/spaces/Zakia/cat_or_dog_predictor/README.md
+++ /dev/null
@@ -1,13 +0,0 @@
----
-title: Cat_or_dog_predictor
-emoji: 💩
-colorFrom: blue
-colorTo: green
-sdk: gradio
-sdk_version: 2.9.4
-app_file: app.py
-pinned: false
-license: apache-2.0
----
-
-Check out the configuration reference at https://huggingface.co/docs/hub/spaces#reference
diff --git a/spaces/ZilliaxOfficial/nyaru-svc-3.0/vdecoder/hifigan/env.py b/spaces/ZilliaxOfficial/nyaru-svc-3.0/vdecoder/hifigan/env.py
deleted file mode 100644
index 2bdbc95d4f7a8bad8fd4f5eef657e2b51d946056..0000000000000000000000000000000000000000
--- a/spaces/ZilliaxOfficial/nyaru-svc-3.0/vdecoder/hifigan/env.py
+++ /dev/null
@@ -1,15 +0,0 @@
-import os
-import shutil
-
-
-class AttrDict(dict):
- def __init__(self, *args, **kwargs):
- super(AttrDict, self).__init__(*args, **kwargs)
- self.__dict__ = self
-
-
-def build_env(config, config_name, path):
- t_path = os.path.join(path, config_name)
- if config != t_path:
- os.makedirs(path, exist_ok=True)
- shutil.copyfile(config, os.path.join(path, config_name))
diff --git a/spaces/abhaskumarsinha/MinimalGPT-Ragdoll/MinimalGPT_2.py b/spaces/abhaskumarsinha/MinimalGPT-Ragdoll/MinimalGPT_2.py
deleted file mode 100644
index fb21243a63272d6efa56a289ab6f42333308353a..0000000000000000000000000000000000000000
--- a/spaces/abhaskumarsinha/MinimalGPT-Ragdoll/MinimalGPT_2.py
+++ /dev/null
@@ -1,401 +0,0 @@
-import os
-import json
-import tensorflow as tf
-from tqdm import tqdm
-from GPT import *
-import pickle
-import argparse
-import sys
-
-
-
-def save_module(save_weights, model, vectorizer, save_tokenizer):
-
- # Save the GPT Model
- with open(save_weights, 'wb') as file:
- pickle.dump(model.weights, file)
-
- #Save the Vectorizer Model
- vocabulary = vectorizer.get_vocabulary()
-
- # Encode the vocabulary as JSON-compatible strings
- encoded_vocabulary = [word.encode('unicode_escape').decode('utf-8') for word in vocabulary]
- encoded_vocabulary = encoded_vocabulary[2:]
-
- # Save the encoded vocabulary to a JSON file
- with open(save_tokenizer, 'w') as f:
- json.dump(encoded_vocabulary, f)
- print("Vocabulary size saved: " + str(len(encoded_vocabulary)))
-
-
-
-
-
-def read_file(f, vectorizer, chunk_size = 1024, starting_chunk = 0, ending_chunk = 5, gpt_input = 10):
- i = 0
- chunk = []
-
- while True:
- data = f.read(chunk_size)
-
- if not data or i > ending_chunk:
- break
-
- if i >= starting_chunk and i <= ending_chunk:
- file_contents = data.split()
- input_tokens, output_tokens = [], []
- for j in range(len(file_contents) - gpt_input - 1):
- input_tokens += [file_contents[j : j + gpt_input]]
- output_tokens += [file_contents[j + gpt_input]]
-
-
- X = [' '.join(input_tokens[j]) for j in range(len(input_tokens))]
- Y = output_tokens
-
- X = vectorizer(X)
- Y = vectorizer(Y)
-
- output = tf.concat([X, Y], 1)
-
- yield output
-
- i += 1
-
-
-def get_model(gpt_input, d_model, h, vocab_size, decoder_stacks, GPT_attention):
- input_words = tf.keras.layers.Input((gpt_input))
- embedding = tf.keras.layers.Embedding(vocab_size + 2, d_model)(input_words)
- positional_enc = PositionalEmbedding(words = gpt_input, embedding_size = d_model)(embedding)
- decoder = Decoder(num_heads = h, key_dim = gpt_input, key_embedding = d_model, GPT_attention = GPT_attention)(positional_enc)
-
- for _ in range(decoder_stacks - 1):
- decoder = Decoder(num_heads = h, key_dim = gpt_input, key_embedding = d_model, GPT_attention = GPT_attention)(decoder)
-
- decoder = tf.keras.layers.Flatten()(decoder)
- linear_layer = tf.keras.layers.Dense(vocab_size + 3)(decoder)
- softmax = tf.nn.softmax(linear_layer)
- GPT = tf.keras.Model(inputs = input_words, outputs = softmax)
-
- return GPT
-
-
-def MinimalGPT(data_path='.',
- learning_rate=0,
- output_length=0,
- epochs = 1,
- batch_size = 1,
- gpt_input=10,
- d_model=128,
- h=8,
- decoder_stacks=1,
- starting_chunk = 0,
- ending_chunk = 5,
- chunk_size = 10,
- token_end=40000,
- vocabulary_start = 0,
- vocabulary_end = 40000,
- save=False,
- load_tokenizer=None,
- load_weights=None,
- save_tokenizer=None,
- save_weights=None,
- optimizer=None,
- inference_only = False,
- return_model_and_vectorizer = False,
- return_model_and_vectorizer_and_output = False,
- GPT_attention = False,
- TPU = False):
-
- if chunk_size:
- chunk_size *= 1024
-
-
- if inference_only == False:
- with open(data_path, 'r', encoding = 'utf-8') as file:
- corpus = file.read()
- #file_contents = corpus.split()[token_start : token_end]
- #print("Total tokens: " + str(len(file_contents)))
-
-
- if load_tokenizer:
- with open(load_tokenizer, 'r') as f:
- encoded_vocabulary = json.load(f)
-
- # Decode the encoded vocabulary to original strings
- vocabulary = [word.encode('utf-8').decode('unicode_escape') for word in encoded_vocabulary]
- vectorizer = tf.keras.layers.TextVectorization(standardize = None, split = 'whitespace')
- vectorizer.set_vocabulary(vocabulary)
- vocab_size = vectorizer.vocabulary_size()
-
- else:
- vocab = []
- for word in tqdm(corpus.split()[vocabulary_start : vocabulary_end]):
- vocab += [word]
- vocab = list(set(vocab))
- vocab_size = len(vocab)
- vectorizer = tf.keras.layers.TextVectorization(standardize = None, split = 'whitespace', vocabulary = vocab)
- print('New Vectorizer created successfully...')
- print("Vocabulary Size: " + str(vocab_size))
- del corpus
-
-
- #if inference_only == False:
- # input_tokens, output_tokens = [], []
- # for i in tqdm(range(len(file_contents) - gpt_input - 1)):
- # input_tokens += [file_contents[i : i + gpt_input]]
- # output_tokens += [file_contents[i + gpt_input]]
-
-
- # X = [' '.join(input_tokens[i]) for i in tqdm(range(len(input_tokens)))]
- # Y = output_tokens
-
- # del corpus
-
- # X = vectorizer(X)
- # Y = vectorizer(Y)
-
- if load_weights:
- model = get_model(gpt_input = gpt_input, d_model = d_model, h = h, decoder_stacks = decoder_stacks, vocab_size = vocab_size - 2, GPT_attention = GPT_attention)
-
- with open(load_weights, 'rb') as file:
- W = pickle.load(file)
- model.set_weights(W)
- else:
- model = get_model(gpt_input = gpt_input, d_model = d_model, h = h, decoder_stacks = decoder_stacks, vocab_size = vocab_size, GPT_attention = GPT_attention)
-
-
- print(model.summary())
-
-
- if inference_only == False:
- # Compile the model
- if not optimizer:
- model.compile(optimizer=tf.keras.optimizers.Adam(learning_rate=learning_rate), loss='sparse_categorical_crossentropy')
- else:
- model.compile(optimizer=optimizer, loss='sparse_categorical_crossentropy')
-
- # Train the model
- if learning_rate > 0:
-
- for epoch in tqdm(range(epochs)):
-
- with open(data_path, 'r', encoding='utf-8') as f:
- chunk_number = 1
- for chunk in read_file(f,
- vectorizer,
- chunk_size,
- starting_chunk,
- ending_chunk,
- gpt_input):
- print('Chunk_size: ' + str(chunk.shape[0]))
- model.fit(chunk[:, :gpt_input], tf.reshape(chunk[:, -1], (-1, 1)), batch_size = batch_size, epochs=1)
- print("Chunk Number " + str(chunk_number) + "/" +str(ending_chunk - starting_chunk + 1) + " processed!")
- chunk_number += 1
-
-
- # Print the output of the Model
- output_seq = generate_output(gpt_input = gpt_input, model = model, vectorizer = vectorizer, text_size = output_length, input_sequence = [])
-
- if save == True and TPU == False:
- print('Saveeeeee')
-
- save_module(save_weights, model, vectorizer, save_tokenizer)
-
- if save == True and TPU == True:
-
- return save_weights, model, vectorizer, save_tokenizer, output_seq
- # Save the GPT Model
- #with open(save_weights, 'wb') as file:
- # pickle.dump(model.weights, file)
-
- #Save the Vectorizer Model
- #vocabulary = vectorizer.get_vocabulary()
-
- # Encode the vocabulary as JSON-compatible strings
- #encoded_vocabulary = [word.encode('unicode_escape').decode('utf-8') for word in vocabulary]
- #encoded_vocabulary = encoded_vocabulary[2:]
-
- # Save the encoded vocabulary to a JSON file
- #with open(save_tokenizer, 'w') as f:
- # json.dump(encoded_vocabulary, f)
- # print("Vocabulary size saved: " + str(len(encoded_vocabulary)))
-
-
- if return_model_and_vectorizer:
- return model, vectorizer
- elif return_model_and_vectorizer_and_output:
- return model, vectorizer, output_seq.replace('@@ ', '')
- else:
- return output_seq.replace('@@ ', '')
-
-
-
-# Example code to execute when the script file is called
-
-def main():
- print("This code is executed when the script file is called directly.")
-
-# Check if the script is being run as the main module
-if __name__ == '__main__':
- parser = argparse.ArgumentParser()
- parser.add_argument('-d', '--data-path', help='File: Corresponding to corpus or training text [String]')
- parser.add_argument('-l', '--learning-rate', help='Float: Learning Rate. The model will train ONLY IF the rate is > 0, skip otherwise [Float]', type=float)
- parser.add_argument('-ol', '--output-length', help='Length of the output sequence to be generated', type=int)
- parser.add_argument('-e', '--epochs', help='Number of training Epochs [Int]', type=int)
- parser.add_argument('-b', '--batch-size', help='Size of each batch [Int]', type=int)
- parser.add_argument('-s', '--gpt-input', help='Number of Tokens of text the model inputs at a time [Int]', type=int)
- parser.add_argument('-dm', '--d-model', help='Embedding layer output dimensions [Int]', type=int)
- parser.add_argument('-p', '--multi-head', help='Number of Multi-head Attention layer in parallel [Int]', type=int)
- parser.add_argument('-ds', '--decoder-stacks', help='Number of stacked Decoder layer [Int]', type=int)
- parser.add_argument('-sc', '--chunk-start', help='The chunk number in the corpus to mark it as the starting point of the training [Int]', type=int)
- parser.add_argument('-ec', '--chunk-end', help='The chunk number in the corpus to mark it as the end point of the training [Int]', type=int)
- parser.add_argument('-csz', '--chunk-size', help='The size of each chunk in KB.', type=int)
- parser.add_argument('-vs', '--vocabulary-start', help='Token number from the corpus to mark the starting point of vocabulary data [Int]', type=int)
- parser.add_argument('-ve', '--vocabulary-end', help='Token number from the corpus to mark the end point of vocabulary data [Int]', type=int)
- parser.add_argument('-sd', '--save', help='Save the Model and Vectorizer data to disk [True/False]', action='store_true')
- parser.add_argument('-lt', '--load-tokenizer', help='File: Vectorization layer [File]')
- parser.add_argument('-lw', '--load-weights', help='File: Model Weights [File]')
- parser.add_argument('-st', '--save-tokenizer', help='File: Saving Vectorizer File [File]')
- parser.add_argument('-sw', '--save-weights', help='File: Saving Model Weights[File]')
- parser.add_argument('-ot', '--optimizer', help='Optimizer consistent to TensorFlow optimizer class [tf.keras.optimizers]')
- parser.add_argument('-i', '--inference-only', help='Only Print the output of the model in Inference Mode [True/False]', action='store_true')
- parser.add_argument('-mv', '--model-vectorizer', help='Return Model, Vectorizer Tuple [True/False]', action='store_true')
- parser.add_argument('-mvo', '--model-vectorizer-output', help='Return Model, Vectorizer, Output Tuple [True/False]', action='store_true')
- parser.add_argument('-ga', '--gpt-style-attention', help='Uses GPT-styled attention. Note: (d-model) parameter should be divisible by (multi-head), otherwise the program will throw an error! [True/False]', action='store_true')
- parser.add_argument('-tpu', '--TPU', help='Use Tensor Processor Units (Distributed Learning)', action='store_true')
-
-
- args = parser.parse_args()
-
-
- data_path = args.data_path
- learning_rate = args.learning_rate
- output_length = args.output_length
- epochs = args.epochs
- batch_size = args.batch_size
- gpt_input = args.gpt_input
- d_model = args.d_model
- h = args.multi_head
- stacks = args.decoder_stacks
- chunk_start = args.chunk_start
- chunk_end = args.chunk_end
- chunk_size = args.chunk_size
- vocabulary_start = args.vocabulary_start
- vocabulary_end = args.vocabulary_end
- save = args.save
- load_tokenizer = args.load_tokenizer
- load_weights = args.load_weights
- save_tokenizer = args.save_tokenizer
- save_weights = args.save_weights
- optimizer = args.optimizer
- inference_only = args.inference_only
- model_and_vectorizer = args.model_vectorizer
- GPT_attention = args.gpt_style_attention
- model_vectorizer_output = args.model_vectorizer_output
-
-
-
- configuration = {
- 'data_path': args.data_path,
- 'learning_rate': args.learning_rate,
- 'output_length': args.output_length,
- 'epochs': args.epochs,
- 'batch_size': args.batch_size,
- 'gpt_input': args.gpt_input,
- 'd_model': args.d_model,
- 'h': args.multi_head,
- 'stacks': args.decoder_stacks,
- 'chunk_start': args.chunk_start,
- 'chunk_end': args.chunk_end,
- 'chunk_size': args.chunk_size,
- 'vocabulary_start': args.vocabulary_start,
- 'vocabulary_end': args.vocabulary_end,
- 'save': args.save,
- 'load_tokenizer': args.load_tokenizer,
- 'load_weights': args.load_weights,
- 'save_tokenizer': args.save_tokenizer,
- 'save_weights': args.save_weights,
- 'optimizer': args.optimizer,
- 'inference_only': args.inference_only,
- 'model_and_vectorizer': args.model_vectorizer,
- 'model_vectorizer_output': args.model_vectorizer_output,
- 'GPT_Attention' : args.gpt_style_attention
- }
-
- # Save the configuration to a JSON file
- with open('last-configuration.json', 'w') as file:
- json.dump(configuration, file)
-
-
-
- if args.TPU == True:
-
- resolver = tf.distribute.cluster_resolver.TPUClusterResolver(tpu='')
- tf.config.experimental_connect_to_cluster(resolver)
- # This is the TPU initialization code that has to be at the beginning.
- tf.tpu.experimental.initialize_tpu_system(resolver)
- print("All devices: ", tf.config.list_logical_devices('TPU'))
-
-
- strategy = tf.distribute.TPUStrategy(resolver)
-
- with strategy.scope():
-
- output = MinimalGPT(data_path = data_path,
- learning_rate = learning_rate,
- output_length = output_length,
- epochs = epochs,
- batch_size = batch_size,
- gpt_input = gpt_input,
- d_model = d_model,
- h = h,
- decoder_stacks = stacks,
- starting_chunk = chunk_start,
- ending_chunk = chunk_end,
- chunk_size = chunk_size,
- vocabulary_start = vocabulary_start,
- vocabulary_end = vocabulary_end,
- save = save,
- load_tokenizer = load_tokenizer,
- load_weights = load_weights,
- save_tokenizer = save_tokenizer,
- save_weights = save_weights,
- optimizer = optimizer,
- inference_only = inference_only,
- return_model_and_vectorizer = model_and_vectorizer,
- return_model_and_vectorizer_and_output = model_vectorizer_output,
- GPT_attention = GPT_attention,
- TPU = True)
-
- save_module(output[0], output[1], output[2], output[3])
-
- print(output[4])
- sys.exit(0)
-
-
- output = MinimalGPT(data_path = data_path,
- learning_rate = learning_rate,
- output_length = output_length,
- epochs = epochs,
- batch_size = batch_size,
- gpt_input = gpt_input,
- d_model = d_model,
- h = h,
- decoder_stacks = stacks,
- starting_chunk = chunk_start,
- ending_chunk = chunk_end,
- chunk_size = chunk_size,
- vocabulary_start = vocabulary_start,
- vocabulary_end = vocabulary_end,
- save = save,
- load_tokenizer = load_tokenizer,
- load_weights = load_weights,
- save_tokenizer = save_tokenizer,
- save_weights = save_weights,
- optimizer = optimizer,
- inference_only = inference_only,
- return_model_and_vectorizer = model_and_vectorizer,
- return_model_and_vectorizer_and_output = model_vectorizer_output,
- GPT_attention = GPT_attention,
- TPU = False)
- print(output)
\ No newline at end of file
diff --git a/spaces/abhishek/sketch-to-image/annotator/uniformer/mmseg/models/utils/__init__.py b/spaces/abhishek/sketch-to-image/annotator/uniformer/mmseg/models/utils/__init__.py
deleted file mode 100644
index 3d3bdd349b9f2ae499a2fcb2ac1d2e3c77befebe..0000000000000000000000000000000000000000
--- a/spaces/abhishek/sketch-to-image/annotator/uniformer/mmseg/models/utils/__init__.py
+++ /dev/null
@@ -1,13 +0,0 @@
-from .drop import DropPath
-from .inverted_residual import InvertedResidual, InvertedResidualV3
-from .make_divisible import make_divisible
-from .res_layer import ResLayer
-from .se_layer import SELayer
-from .self_attention_block import SelfAttentionBlock
-from .up_conv_block import UpConvBlock
-from .weight_init import trunc_normal_
-
-__all__ = [
- 'ResLayer', 'SelfAttentionBlock', 'make_divisible', 'InvertedResidual',
- 'UpConvBlock', 'InvertedResidualV3', 'SELayer', 'DropPath', 'trunc_normal_'
-]
diff --git a/spaces/abhishek/sketch-to-image/annotator/uniformer_base/mmseg/core/evaluation/eval_hooks.py b/spaces/abhishek/sketch-to-image/annotator/uniformer_base/mmseg/core/evaluation/eval_hooks.py
deleted file mode 100644
index 6fc100c8f96e817a6ed2666f7c9f762af2463b48..0000000000000000000000000000000000000000
--- a/spaces/abhishek/sketch-to-image/annotator/uniformer_base/mmseg/core/evaluation/eval_hooks.py
+++ /dev/null
@@ -1,109 +0,0 @@
-import os.path as osp
-
-from annotator.uniformer.mmcv.runner import DistEvalHook as _DistEvalHook
-from annotator.uniformer.mmcv.runner import EvalHook as _EvalHook
-
-
-class EvalHook(_EvalHook):
- """Single GPU EvalHook, with efficient test support.
-
- Args:
- by_epoch (bool): Determine perform evaluation by epoch or by iteration.
- If set to True, it will perform by epoch. Otherwise, by iteration.
- Default: False.
- efficient_test (bool): Whether save the results as local numpy files to
- save CPU memory during evaluation. Default: False.
- Returns:
- list: The prediction results.
- """
-
- greater_keys = ['mIoU', 'mAcc', 'aAcc']
-
- def __init__(self, *args, by_epoch=False, efficient_test=False, **kwargs):
- super().__init__(*args, by_epoch=by_epoch, **kwargs)
- self.efficient_test = efficient_test
-
- def after_train_iter(self, runner):
- """After train epoch hook.
-
- Override default ``single_gpu_test``.
- """
- if self.by_epoch or not self.every_n_iters(runner, self.interval):
- return
- from annotator.uniformer.mmseg.apis import single_gpu_test
- runner.log_buffer.clear()
- results = single_gpu_test(
- runner.model,
- self.dataloader,
- show=False,
- efficient_test=self.efficient_test)
- self.evaluate(runner, results)
-
- def after_train_epoch(self, runner):
- """After train epoch hook.
-
- Override default ``single_gpu_test``.
- """
- if not self.by_epoch or not self.every_n_epochs(runner, self.interval):
- return
- from annotator.uniformer.mmseg.apis import single_gpu_test
- runner.log_buffer.clear()
- results = single_gpu_test(runner.model, self.dataloader, show=False)
- self.evaluate(runner, results)
-
-
-class DistEvalHook(_DistEvalHook):
- """Distributed EvalHook, with efficient test support.
-
- Args:
- by_epoch (bool): Determine perform evaluation by epoch or by iteration.
- If set to True, it will perform by epoch. Otherwise, by iteration.
- Default: False.
- efficient_test (bool): Whether save the results as local numpy files to
- save CPU memory during evaluation. Default: False.
- Returns:
- list: The prediction results.
- """
-
- greater_keys = ['mIoU', 'mAcc', 'aAcc']
-
- def __init__(self, *args, by_epoch=False, efficient_test=False, **kwargs):
- super().__init__(*args, by_epoch=by_epoch, **kwargs)
- self.efficient_test = efficient_test
-
- def after_train_iter(self, runner):
- """After train epoch hook.
-
- Override default ``multi_gpu_test``.
- """
- if self.by_epoch or not self.every_n_iters(runner, self.interval):
- return
- from annotator.uniformer.mmseg.apis import multi_gpu_test
- runner.log_buffer.clear()
- results = multi_gpu_test(
- runner.model,
- self.dataloader,
- tmpdir=osp.join(runner.work_dir, '.eval_hook'),
- gpu_collect=self.gpu_collect,
- efficient_test=self.efficient_test)
- if runner.rank == 0:
- print('\n')
- self.evaluate(runner, results)
-
- def after_train_epoch(self, runner):
- """After train epoch hook.
-
- Override default ``multi_gpu_test``.
- """
- if not self.by_epoch or not self.every_n_epochs(runner, self.interval):
- return
- from annotator.uniformer.mmseg.apis import multi_gpu_test
- runner.log_buffer.clear()
- results = multi_gpu_test(
- runner.model,
- self.dataloader,
- tmpdir=osp.join(runner.work_dir, '.eval_hook'),
- gpu_collect=self.gpu_collect)
- if runner.rank == 0:
- print('\n')
- self.evaluate(runner, results)
diff --git a/spaces/abrar-lohia/text-2-character-anim/VQTrans/models/resnet.py b/spaces/abrar-lohia/text-2-character-anim/VQTrans/models/resnet.py
deleted file mode 100644
index 062346e3ba2fc4d6ae5636f228c5b7565bdb62b7..0000000000000000000000000000000000000000
--- a/spaces/abrar-lohia/text-2-character-anim/VQTrans/models/resnet.py
+++ /dev/null
@@ -1,82 +0,0 @@
-import torch.nn as nn
-import torch
-
-class nonlinearity(nn.Module):
- def __init__(self):
- super().__init__()
-
- def forward(self, x):
- # swish
- return x * torch.sigmoid(x)
-
-class ResConv1DBlock(nn.Module):
- def __init__(self, n_in, n_state, dilation=1, activation='silu', norm=None, dropout=None):
- super().__init__()
- padding = dilation
- self.norm = norm
- if norm == "LN":
- self.norm1 = nn.LayerNorm(n_in)
- self.norm2 = nn.LayerNorm(n_in)
- elif norm == "GN":
- self.norm1 = nn.GroupNorm(num_groups=32, num_channels=n_in, eps=1e-6, affine=True)
- self.norm2 = nn.GroupNorm(num_groups=32, num_channels=n_in, eps=1e-6, affine=True)
- elif norm == "BN":
- self.norm1 = nn.BatchNorm1d(num_features=n_in, eps=1e-6, affine=True)
- self.norm2 = nn.BatchNorm1d(num_features=n_in, eps=1e-6, affine=True)
-
- else:
- self.norm1 = nn.Identity()
- self.norm2 = nn.Identity()
-
- if activation == "relu":
- self.activation1 = nn.ReLU()
- self.activation2 = nn.ReLU()
-
- elif activation == "silu":
- self.activation1 = nonlinearity()
- self.activation2 = nonlinearity()
-
- elif activation == "gelu":
- self.activation1 = nn.GELU()
- self.activation2 = nn.GELU()
-
-
-
- self.conv1 = nn.Conv1d(n_in, n_state, 3, 1, padding, dilation)
- self.conv2 = nn.Conv1d(n_state, n_in, 1, 1, 0,)
-
-
- def forward(self, x):
- x_orig = x
- if self.norm == "LN":
- x = self.norm1(x.transpose(-2, -1))
- x = self.activation1(x.transpose(-2, -1))
- else:
- x = self.norm1(x)
- x = self.activation1(x)
-
- x = self.conv1(x)
-
- if self.norm == "LN":
- x = self.norm2(x.transpose(-2, -1))
- x = self.activation2(x.transpose(-2, -1))
- else:
- x = self.norm2(x)
- x = self.activation2(x)
-
- x = self.conv2(x)
- x = x + x_orig
- return x
-
-class Resnet1D(nn.Module):
- def __init__(self, n_in, n_depth, dilation_growth_rate=1, reverse_dilation=True, activation='relu', norm=None):
- super().__init__()
-
- blocks = [ResConv1DBlock(n_in, n_in, dilation=dilation_growth_rate ** depth, activation=activation, norm=norm) for depth in range(n_depth)]
- if reverse_dilation:
- blocks = blocks[::-1]
-
- self.model = nn.Sequential(*blocks)
-
- def forward(self, x):
- return self.model(x)
\ No newline at end of file
diff --git a/spaces/abrar-lohia/text-2-character-anim/pyrender/.eggs/pyglet-2.0.5-py3.10.egg/pyglet/gl/lib_agl.py b/spaces/abrar-lohia/text-2-character-anim/pyrender/.eggs/pyglet-2.0.5-py3.10.egg/pyglet/gl/lib_agl.py
deleted file mode 100644
index a6c258ef6c877a050a11a6fbf038e9f074b973a1..0000000000000000000000000000000000000000
--- a/spaces/abrar-lohia/text-2-character-anim/pyrender/.eggs/pyglet-2.0.5-py3.10.egg/pyglet/gl/lib_agl.py
+++ /dev/null
@@ -1,31 +0,0 @@
-from ctypes import *
-
-import pyglet.lib
-from pyglet.gl.lib import missing_function, decorate_function
-
-__all__ = ['link_GL', 'link_AGL']
-
-gl_lib = pyglet.lib.load_library(framework='OpenGL')
-agl_lib = pyglet.lib.load_library(framework='AGL')
-
-
-def link_GL(name, restype, argtypes, requires=None, suggestions=None):
- try:
- func = getattr(gl_lib, name)
- func.restype = restype
- func.argtypes = argtypes
- decorate_function(func, name)
- return func
- except AttributeError:
- return missing_function(name, requires, suggestions)
-
-
-def link_AGL(name, restype, argtypes, requires=None, suggestions=None):
- try:
- func = getattr(agl_lib, name)
- func.restype = restype
- func.argtypes = argtypes
- decorate_function(func, name)
- return func
- except AttributeError:
- return missing_function(name, requires, suggestions)
diff --git a/spaces/aieye/named_entity_recognition_tutorial/utils/levels.py b/spaces/aieye/named_entity_recognition_tutorial/utils/levels.py
deleted file mode 100644
index a37624fa0e95a0a1385a146edb4be97cdbbd54b7..0000000000000000000000000000000000000000
--- a/spaces/aieye/named_entity_recognition_tutorial/utils/levels.py
+++ /dev/null
@@ -1,36 +0,0 @@
-import streamlit as st
-from utils.login import get_login
-import os
-
-def initialize_level():
- if 'level' not in st.session_state:
- if get_login()["status"]:
- if not os.path.exists(f".sessions/{get_login()['username']}/level.txt"):
- with open(f".sessions/{get_login()['username']}/level.txt", "w") as f:
- f.write("0")
- st.session_state['level'] = 0
- else:
- with open(f".sessions/{get_login()['username']}/level.txt", "r") as f:
- st.session_state['level'] = int(f.read())
-
-def get_level():
- return st.session_state['level']
-
-def render_page(page, level):
- if get_login()["status"]:
- if st.session_state['level'] < level:
- st.error(f"You need to complete Level {st.session_state['level']} first!")
- else:
- page()
- else:
- st.error("You need to login first!")
-
-def complete_level(level):
- if st.session_state['level'] > level:
- st.info(f'You have Already completed Level {level}!')
- else:
- st.session_state['level'] = level + 1
- with open(f".sessions/{get_login()['username']}/level.txt", "w") as f:
- f.write(str(st.session_state['level']))
- st.balloons()
- st.success(f'You have completed Level {level}! You can now move on to the next level.')
\ No newline at end of file
diff --git a/spaces/ajitrajasekharan/NER-Biomedical-PHI-Ensemble/app.py b/spaces/ajitrajasekharan/NER-Biomedical-PHI-Ensemble/app.py
deleted file mode 100644
index 09be3e9531f9e1d62de20121aadae4479a9764aa..0000000000000000000000000000000000000000
--- a/spaces/ajitrajasekharan/NER-Biomedical-PHI-Ensemble/app.py
+++ /dev/null
@@ -1,272 +0,0 @@
-import time
-import streamlit as st
-import torch
-import string
-from annotated_text import annotated_text
-
-from flair.data import Sentence
-from flair.models import SequenceTagger
-from transformers import BertTokenizer, BertForMaskedLM
-import BatchInference as bd
-import batched_main_NER as ner
-import aggregate_server_json as aggr
-import json
-
-
-DEFAULT_TOP_K = 20
-SPECIFIC_TAG=":__entity__"
-
-
-@st.cache(suppress_st_warning=True, allow_output_mutation=True)
-def POS_get_model(model_name):
- val = SequenceTagger.load(model_name) # Load the model
- return val
-
-def getPos(s: Sentence):
- texts = []
- labels = []
- for t in s.tokens:
- for label in t.annotation_layers.keys():
- texts.append(t.text)
- labels.append(t.get_labels(label)[0].value)
- return texts, labels
-
-def getDictFromPOS(texts, labels):
- return [["dummy",t,l,"dummy","dummy" ] for t, l in zip(texts, labels)]
-
-def decode(tokenizer, pred_idx, top_clean):
- ignore_tokens = string.punctuation + '[PAD]'
- tokens = []
- for w in pred_idx:
- token = ''.join(tokenizer.decode(w).split())
- if token not in ignore_tokens:
- tokens.append(token.replace('##', ''))
- return '\n'.join(tokens[:top_clean])
-
-def encode(tokenizer, text_sentence, add_special_tokens=True):
- text_sentence = text_sentence.replace('', tokenizer.mask_token)
- # if is the last token, append a "." so that models dont predict punctuation.
- if tokenizer.mask_token == text_sentence.split()[-1]:
- text_sentence += ' .'
-
- input_ids = torch.tensor([tokenizer.encode(text_sentence, add_special_tokens=add_special_tokens)])
- mask_idx = torch.where(input_ids == tokenizer.mask_token_id)[1].tolist()[0]
- return input_ids, mask_idx
-
-def get_all_predictions(text_sentence, top_clean=5):
- # ========================= BERT =================================
- input_ids, mask_idx = encode(bert_tokenizer, text_sentence)
- with torch.no_grad():
- predict = bert_model(input_ids)[0]
- bert = decode(bert_tokenizer, predict[0, mask_idx, :].topk(top_k).indices.tolist(), top_clean)
- return {'bert': bert}
-
-def get_bert_prediction(input_text,top_k):
- try:
- input_text += ' '
- res = get_all_predictions(input_text, top_clean=int(top_k))
- return res
- except Exception as error:
- pass
-
-
-def load_pos_model():
- checkpoint = "flair/pos-english"
- return POS_get_model(checkpoint)
-
-
-
-
-def init_session_states():
- if 'top_k' not in st.session_state:
- st.session_state['top_k'] = 20
- if 'pos_model' not in st.session_state:
- st.session_state['pos_model'] = None
- if 'bio_model' not in st.session_state:
- st.session_state['bio_model'] = None
- if 'phi_model' not in st.session_state:
- st.session_state['phi_model'] = None
- if 'ner_bio' not in st.session_state:
- st.session_state['ner_bio'] = None
- if 'ner_phi' not in st.session_state:
- st.session_state['ner_phi'] = None
- if 'aggr' not in st.session_state:
- st.session_state['aggr'] = None
-
-
-
-def get_pos_arr(input_text,display_area):
- if (st.session_state['pos_model'] is None):
- display_area.text("Loading model 3 of 3.Loading POS model...")
- st.session_state['pos_model'] = load_pos_model()
- s = Sentence(input_text)
- st.session_state['pos_model'].predict(s)
- texts, labels = getPos(s)
- pos_results = getDictFromPOS(texts, labels)
- return pos_results
-
-def perform_inference(text,display_area):
-
- if (st.session_state['bio_model'] is None):
- display_area.text("Loading model 1 of 3. Bio model...")
- st.session_state['bio_model'] = bd.BatchInference("bio/desc_a100_config.json",'ajitrajasekharan/biomedical',False,False,DEFAULT_TOP_K,True,True, "bio/","bio/a100_labels.txt",False)
-
- if (st.session_state['phi_model'] is None):
- display_area.text("Loading model 2 of 3. PHI model...")
- st.session_state['phi_model'] = bd.BatchInference("bbc/desc_bbc_config.json",'bert-base-cased',False,False,DEFAULT_TOP_K,True,True, "bbc/","bbc/bbc_labels.txt",False)
-
- #Load POS model if needed and gets POS tags
- if (SPECIFIC_TAG not in text):
- pos_arr = get_pos_arr(text,display_area)
- else:
- pos_arr = None
-
- if (st.session_state['ner_bio'] is None):
- display_area.text("Initializing BIO module...")
- st.session_state['ner_bio'] = ner.UnsupNER("bio/ner_a100_config.json")
-
- if (st.session_state['ner_phi'] is None):
- display_area.text("Initializing PHI module...")
- st.session_state['ner_phi'] = ner.UnsupNER("bbc/ner_bbc_config.json")
-
- if (st.session_state['aggr'] is None):
- display_area.text("Initializing Aggregation modeule...")
- st.session_state['aggr'] = aggr.AggregateNER("./ensemble_config.json")
-
-
-
- display_area.text("Getting results from BIO model...")
- bio_descs = st.session_state['bio_model'].get_descriptors(text,pos_arr)
- display_area.text("Getting results from PHI model...")
- phi_results = st.session_state['phi_model'].get_descriptors(text,pos_arr)
- display_area.text("Aggregating BIO & PHI results...")
- bio_ner = st.session_state['ner_bio'].tag_sentence_service(text,bio_descs)
- phi_ner = st.session_state['ner_phi'].tag_sentence_service(text,phi_results)
-
- combined_arr = [json.loads(bio_ner),json.loads(phi_ner)]
-
- aggregate_results = st.session_state['aggr'].fetch_all(text,combined_arr)
- return aggregate_results
-
-
-sent_arr = [
-"Lou Gehrig who works for XCorp and lives in New York suffers from Parkinson's ",
-"Parkinson who works for XCorp and lives in New York suffers from Lou Gehrig's",
-"lou gehrig was diagnosed with Parkinson's ",
-"A eGFR below 60 indicates chronic kidney disease",
-"Overexpression of EGFR occurs across a wide range of different cancers",
-"Stanford called",
-"He was diagnosed with non small cell lung cancer",
-"Her hypophysitis secondary to ipilimumab was well managed with supplemental hormones",
-"I met my girl friends at the pub ",
-"I met my New York friends at the pub",
-"I met my XCorp friends at the pub",
-"I met my two friends at the pub",
-"Bio-Techne's genomic tools include advanced tissue-based in-situ hybridization assays sold under the ACD brand as well as a portfolio of assays for prostate cancer diagnosis ",
-"There are no treatment options specifically indicated for ACD and physicians must utilize agents approved for other dermatology conditions", "As ACD has been implicated in apoptosis-resistant glioblastoma (GBM), there is a high medical need for identifying novel ACD-inducing drugs ",
-"Located in the heart of Dublin , in the family home of acclaimed writer Oscar Wilde , ACD provides the perfect backdrop to inspire Irish (and Irish-at-heart) students to excel in business and the arts",
-"Patients treated with anticancer chemotherapy drugs ( ACD ) are vulnerable to infectious diseases due to immunosuppression and to the direct impact of ACD on their intestinal microbiota ",
-"In the LASOR trial , increasing daily imatinib dose from 400 to 600mg induced MMR at 12 and 24 months in 25% and 36% of the patients, respectively, who had suboptimal cytogenetic responses ",
-"The sky turned dark in advance of the storm that was coming from the east ",
-"She loves to watch Sunday afternoon football with her family ",
-"Paul Erdos died at 83 "
-]
-
-
-sent_arr_masked = [
-"Lou Gehrig:__entity__ who works for XCorp:__entity__ and lives in New:__entity__ York:__entity__ suffers from Parkinson's:__entity__ ",
-"Parkinson:__entity__ who works for XCorp:__entity__ and lives in New:__entity__ York:__entity__ suffers from Lou:__entity__ Gehrig's:__entity__",
-"lou:__entity__ gehrig:__entity__ was diagnosed with Parkinson's:__entity__ ",
-"A eGFR:__entity__ below 60 indicates chronic kidney disease",
-"Overexpression of EGFR:__entity__ occurs across a wide range of different cancers",
-"Stanford:__entity__ called",
-"He was diagnosed with non:__entity__ small:__entity__ cell:__entity__ lung:__entity__ cancer:__entity__",
-"Her hypophysitis:__entity__ secondary to ipilimumab:__entity__ was well managed with supplemental:__entity__ hormones:__entity__",
-"I met my girl:__entity__ friends at the pub ",
-"I met my New:__entity__ York:__entity__ friends at the pub",
-"I met my XCorp:__entity__ friends at the pub",
-"I met my two:__entity__ friends at the pub",
-"Bio-Techne's genomic tools include advanced tissue-based in-situ hybridization assays sold under the ACD:__entity__ brand as well as a portfolio of assays for prostate cancer diagnosis ",
-"There are no treatment options specifically indicated for ACD:__entity__ and physicians must utilize agents approved for other dermatology conditions",
-"As ACD:__entity__ has been implicated in apoptosis-resistant glioblastoma (GBM), there is a high medical need for identifying novel ACD-inducing drugs ",
-"Located in the heart of Dublin , in the family home of acclaimed writer Oscar Wilde , ACD:__entity__ provides the perfect backdrop to inspire Irish (and Irish-at-heart) students to excel in business and the arts",
-"Patients treated with anticancer chemotherapy drugs ( ACD:__entity__ ) are vulnerable to infectious diseases due to immunosuppression and to the direct impact of ACD on their intestinal microbiota ",
-"In the LASOR:__entity__ trial:__entity__ , increasing daily imatinib dose from 400 to 600mg induced MMR at 12 and 24 months in 25% and 36% of the patients, respectively, who had suboptimal cytogenetic responses ",
-"The sky turned dark:__entity__ in advance of the storm that was coming from the east ",
-"She loves to watch Sunday afternoon football:__entity__ with her family ",
-"Paul:__entity__ Erdos:__entity__ died at 83:__entity__ "
-]
-
-def init_selectbox():
- return st.selectbox(
- 'Choose any of the sentences in pull-down below',
- sent_arr,key='my_choice')
-
-
-def on_text_change():
- text = st.session_state.my_text
- print("in callback: " + text)
- perform_inference(text)
-
-def main():
- try:
-
- init_session_states()
-
- st.markdown("