-
-Free Fire Max Mod APK Unlimited Diamonds Download for Android 2021
-Are you a fan of Free Fire, the popular battle royale game that has millions of players worldwide? If yes, then you might want to try out Free Fire Max, a new version of the game that offers enhanced graphics, gameplay, and features.
-But wait, there's more! You can also enjoy Free Fire Max Mod APK, a modified version of the game that gives you unlimited diamonds, unlocked characters and skins, no ads, and an anti-ban system. Sounds amazing, right?
-free fire max mod apk unlimited diamonds download for android 2021
Download File ····· https://urllie.com/2uNBTJ
-In this article, I will tell you everything you need to know about Free Fire Max Mod APK, including what it is, what are its features, and how to download and install it on your Android device. So, without further ado, let's get started!
-What is Free Fire Max?
-Free Fire Max is an upgraded version of Free Fire that was launched in 2021 by Garena, the game developer. It is designed to provide a more immersive and realistic gaming experience for the players who want more than the original game.
-Free Fire Max has improved graphics, sound effects, animations, and user interface that make the game look and feel more lifelike. It also has new game modes, maps, weapons, vehicles, and items that add more variety and fun to the gameplay. Moreover, it has cross-play functionality that allows you to play with your friends who are using Free Fire or Free Fire Max on different devices.
-Free Fire Max is compatible with most Android devices that have at least 2 GB of RAM and Android 4.4 or higher. However, it requires more storage space and internet data than Free Fire, so make sure you have enough before downloading it.
-What is Free Fire Max Mod APK?
-Free Fire Max Mod APK is a modified version of Free Fire Max that offers some extra features and benefits that are not available in the original game. It is created by some third-party developers who modify the game files and code to unlock some premium features for free.
-Free Fire Max Mod APK is not an official product of Garena, and it is not endorsed or supported by them. Therefore, it may have some risks and drawbacks, such as bugs, errors, viruses, malware, or ban issues. However, many players use it because of its amazing features and advantages.
-Features of Free Fire Max Mod APK
-Unlimited Diamonds
-One of the most attractive features of Free Fire Max Mod APK is that it gives you unlimited diamonds, which are the premium currency in the game. Diamonds are used to buy various items and services in the game, such as characters, skins, weapons, pets, bundles, crates, spins, memberships, etc.
-free fire max mod apk unlimited diamonds download for android 2021 latest version
-free fire max mod apk unlimited diamonds download for android 2021 no root
-free fire max mod apk unlimited diamonds download for android 2021 offline
-free fire max mod apk unlimited diamonds download for android 2021 online
-free fire max mod apk unlimited diamonds download for android 2021 update
-free fire max mod apk unlimited diamonds download for android 2021 hack
-free fire max mod apk unlimited diamonds download for android 2021 mega
-free fire max mod apk unlimited diamonds download for android 2021 obb
-free fire max mod apk unlimited diamonds download for android 2021 mediafıre
-free fire max mod apk unlimited diamonds download for android 2021 rexdl
-free fire max mod apk unlimited diamonds download for android 2021 revdl
-free fire max mod apk unlimited diamonds download for android 2021 apkpure
-free fire max mod apk unlimited diamonds download for android 2021 happymod
-free fire max mod apk unlimited diamonds download for android 2021 an1
-free fire max mod apk unlimited diamonds download for android 2021 andropalace
-free fire max mod apk unlimited diamonds download for android 2021 androeed
-free fire max mod apk unlimited diamonds download for android 2021 androgamer
-free fire max mod apk unlimited diamonds download for android 2021 androeed.ru
-free fire max mod apk unlimited diamonds download for android 2021 andropark
-free fire max mod apk unlimited diamonds download for android 2021 androking
-free fire max mod apk unlimited diamonds download for android 2021 androplace
-free fire max mod apk unlimited diamonds download for android 2021 androbest
-free fire max mod apk unlimited diamonds download for android 2021 androhub
-free fire max mod apk unlimited diamonds download for android 2021 andropalace.net
-free fire max mod apk unlimited diamonds download for android 2021 androeed.net
-free fire max mod apk unlimited diamonds download for android 2021 androgamer.org
-free fire max mod apk unlimited diamonds download for android 2021 androeed.ru/en/
-free fire max mod apk unlimited diamonds download for android 2021 andropark.info
-free fire max mod apk unlimited diamonds download for android 2021 androking.com
-free fire max mod apk unlimited diamonds download for android 2021 androplace.com
-free fire max mod apk unlimited diamonds download for android 2021 androbest.in
-free fire max mod apk unlimited diamonds download for android 2021 androhub.in
-free fire max mod apk with obb file + data + money + coins + gems + gold + cash + everything unlocked + all features activated + anti ban + no ads + god mode + aimbot + wallhack + esp + radar hack + speed hack + damage hack + health hack + ammo hack + headshot hack + auto headshot hack + auto aim hack + auto kill hack + auto win hack + auto loot hack + auto revive hack + auto heal hack + auto reload hack + auto jump hack + auto fly hack + auto run hack + auto crouch hack + auto prone hack + auto scope hack + auto shoot hack + auto grenade hack + auto bomb hack + auto plant bomb hack + auto defuse bomb hack + auto knife hack + auto melee hack + auto punch hack +
-Diamonds are normally obtained by spending real money or completing some tasks and events in the game. However, with Free Fire Max Mod APK, you can get unlimited diamonds for free without spending a dime or doing anything. You can use them to buy anything you want and customize your game as per your preference.
-Unlocked Characters and Skins
-Another feature of Free Fire Max Mod APK is that it unlocks all the characters and skins in the game for free. Characters are the playable avatars in the game that have different skills and abilities that affect your performance in the game. Skins are the cosmetic items that change the appearance of your characters, weapons, vehicles, etc.
-Characters and skins are normally unlocked by spending diamonds or collecting fragments in the game. However, with Free Fire Max Mod APK, you can access all the characters and skins without spending any diamonds or fragments. You can choose any character you like and equip them with any skin you want.
-No Ads
-A feature of Free Fire Max Mod APK that many players appreciate is that it removes all the ads from the game. Ads are the pop-up messages or videos that appear on your screen while playing the game or using its features. They are meant to promote some products or services or generate revenue for the game developer.
-Ads are normally annoying and distracting for many players who want to enjoy the game without interruptions. They also consume your internet data and battery power. However, with Free Fire Max Mod APK , you can play the game without any ads and enjoy its features without any hassle. You can also save your internet data and battery power.
-Anti-Ban System
-A feature of Free Fire Max Mod APK that many players value is that it has an anti-ban system that protects your account from getting banned by the game developer. Bans are the penalties or restrictions that are imposed on your account if you violate the game rules or use any cheats or hacks in the game.
-Bans are normally bad for your account as they can result in losing your progress, items, diamonds, or even your account permanently. They can also affect your reputation and credibility among other players. However, with Free Fire Max Mod APK, you can avoid bans and play the game safely and securely. The anti-ban system hides your identity and activity from the game server and prevents any detection or suspicion.
-How to Download and Install Free Fire Max Mod APK?
-Now that you know what Free Fire Max Mod APK is and what are its features, you might be wondering how to download and install it on your Android device. Well, don't worry, because I will guide you through the process step by step. Just follow these simple steps and you will be able to enjoy Free Fire Max Mod APK in no time.
-Download Sources for Free Fire Max Mod APK
-Official Website
-The first and most reliable source to download Free Fire Max Mod APK is the official website of the game developer, Garena. This is where you can find the latest and updated version of the modded game, as well as other information and support. To download Free Fire Max Mod APK from the official website, follow these steps:
-
-- Go to https://ffmax.garena.com/, which is the official website of Free Fire Max.
-- Scroll down to the bottom of the page and click on the "Download" button.
-- Select the "APK Download" option and wait for the download to start.
-- Once the download is complete, you will have the Free Fire Max Mod APK file on your device.
-
-Third-Party Websites
-The second source to download Free Fire Max Mod APK is third-party websites that offer modded games for free. These are websites that are not affiliated with Garena or Free Fire Max, but they provide their own versions of the modded game with different features and modifications. However, you should be careful when using these websites, as some of them may contain viruses, malware, or fake links that can harm your device or steal your data. To download Free Fire Max Mod APK from third-party websites, follow these steps:
-
-- Search for "Free Fire Max Mod APK" on any web browser or search engine.
-- Look for a website that has a good reputation, positive reviews, and high ratings from other users.
-- Visit the website and read its description, features, and instructions carefully.
-- Click on the "Download" button or link and wait for the download to start.
-- Once the download is complete, you will have the Free Fire Max Mod APK file on your device.
-
-Some examples of third-party websites that offer Free Fire Max Mod APK are:
- Torrent Sites
-The third source to download Free Fire Max Mod APK is torrent sites that offer peer-to-peer file sharing. These are websites that allow you to download files from other users who have the same files on their devices. Torrent sites are usually faster and more reliable than other sources, as they have multiple sources and seeds for the same file. However, you should also be careful when using these websites, as some of them may contain illegal, pirated, or corrupted files that can violate the game rules or damage your device. To download Free Fire Max Mod APK from torrent sites, follow these steps:
-
-- Download and install a torrent client on your device, such as uTorrent, BitTorrent, or Flud.
-- Search for "Free Fire Max Mod APK" on any web browser or search engine.
-- Look for a torrent site that has a good reputation, positive reviews, and high ratings from other users.
-- Visit the torrent site and read its description, features, and instructions carefully.
-- Click on the "Download" button or link and wait for the torrent file to download.
-- Open the torrent file with your torrent client and wait for the Free Fire Max Mod APK file to download.
-- Once the download is complete, you will have the Free Fire Max Mod APK file on your device.
-
-Some examples of torrent sites that offer Free Fire Max Mod APK are:
-
-Installation Steps for Free Fire Max Mod APK
-Enable Unknown Sources
-The first step to install Free Fire Max Mod APK on your Android device is to enable unknown sources. This is a setting that allows you to install apps from outside the Google Play Store, which is where Free Fire Max Mod APK comes from. To enable unknown sources, follow these steps:
-
-- Go to your device's settings and look for the security or privacy option.
-- Find the option that says "Unknown sources" or "Install unknown apps" and toggle it on.
-- A warning message will pop up, telling you about the risks of installing unknown apps. Tap on "OK" or "Allow" to proceed.
-- You have now enabled unknown sources on your device and can install Free Fire Max Mod APK.
-
-
-Locate and Open the APK File
-The second step to install Free Fire Max Mod APK on your Android device is to locate and open the APK file. This is the file that contains the modded game and its features. To locate and open the APK file, follow these steps:
-
-- Go to your device's file manager and look for the folder where you downloaded the APK file. It could be in your downloads, documents, or any other folder.
-- Find the APK file that has the name "Free Fire Max Mod APK" or something similar and tap on it.
-- A message will pop up, asking you if you want to install this app. Tap on "Install" to proceed.
-- You have now opened the APK file and can install Free Fire Max Mod APK.
-
- Follow the Installation Wizard
-The third step to install Free Fire Max Mod APK on your Android device is to follow the installation wizard. This is the process that guides you through the installation of the app and its features. To follow the installation wizard, follow these steps:
-
-- After tapping on "Install", the installation wizard will start and show you the progress of the installation.
-- Wait for a few seconds or minutes until the installation is complete.
-- A message will pop up, telling you that the app has been installed. Tap on "Open" to launch the game or "Done" to exit the installation wizard.
-- You have now followed the installation wizard and can launch Free Fire Max Mod APK.
-
-
-Launch the Game and Enjoy
-The final step to install Free Fire Max Mod APK on your Android device is to launch the game and enjoy its features. This is where you can experience the modded game and its benefits. To launch the game and enjoy, follow these steps:
-
-- Go to your device's app drawer and look for the icon of Free Fire Max Mod APK. It should have a different logo and color than the original game.
-- Tap on the icon to launch the game. It may take some time to load and initialize.
-- Once the game is loaded, you will see a welcome screen with some options. Tap on "Start" to enter the game or "Settings" to adjust some preferences.
-- You have now launched Free Fire Max Mod APK and can enjoy its features.
-
-
-Conclusion
-Free Fire Max Mod APK is a great way to enjoy Free Fire Max with some extra features and benefits that are not available in the original game. It gives you unlimited diamonds, unlocked characters and skins, no ads, and an anti-ban system that make your gaming experience more fun and exciting.
-To download and install Free Fire Max Mod APK on your Android device, you can use any of the sources mentioned above, such as the official website, third-party websites, or torrent sites. You can also follow the installation steps provided above, such as enabling unknown sources, locating and opening the APK file, following the installation wizard, and launching the game.
-However, you should also be aware of the risks and drawbacks of using Free Fire Max Mod APK, such as bugs, errors, viruses, malware, or ban issues. You should also respect the game rules and other players' rights and privacy. You should not use Free Fire Max Mod APK for any illegal or unethical purposes.
-I hope this article has helped you understand what Free Fire Max Mod APK is and how to download and install it on your Android device. If you have any questions or feedback, please feel free to leave a comment below. Thank you for reading and happy gaming!
-FAQs
-Here are some frequently asked questions about Free Fire Max Mod APK:
-
-- Is Free Fire Max Mod APK compatible with my device?
-Free Fire Max Mod APK is compatible with most Android devices that have at least 2 GB of RAM and Android 4.4 or higher. However, it may not work properly on some devices due to different specifications or configurations. You can check your device's compatibility by visiting https://ffmax.garena.com/compatibility.html.
-- Is Free Fire Max Mod APK safe to use?
-Free Fire Max Mod APK is generally safe to use if you download it from a reliable source and scan it with an antivirus before installing it. However, it may still have some risks and drawbacks, such as bugs, errors, viruses, malware, or ban issues. You should use it at your own risk and discretion.
-- Is Free Fire Max Mod APK legal to use?
-Free Fire Max Mod APK is not legal to use as it violates the terms of service and intellectual property rights of Garena and Free Fire Max. It is also considered as cheating or hacking in the game, which can result in penalties or bans from the game developer. You should use it only for educational or entertainment purposes.
-- How to update Free Fire Max Mod APK?
-To update Free Fire Max Mod APK, you need to download and install the latest version of the modded game from any of the sources mentioned above. You may also need to uninstall the previous version of the modded game before installing the new one. You may also need to back up your game data and settings before updating, as they may be lost or overwritten during the process.
-- How to uninstall Free Fire Max Mod APK?
-To uninstall Free Fire Max Mod APK, you need to follow the same steps as you would for any other app on your Android device. To uninstall Free Fire Max Mod APK, follow these steps:
-
-- Go to your device's settings and look for the apps or applications option.
-- Find and tap on the app that has the name "Free Fire Max Mod APK" or something similar.
-- A screen will pop up, showing you some information and options about the app. Tap on "Uninstall" to remove the app from your device.
-- A confirmation message will pop up, asking you if you want to uninstall this app. Tap on "OK" or "Yes" to proceed.
-- You have now uninstalled Free Fire Max Mod APK from your device.
-
- 197e85843d
-
-
\ No newline at end of file
diff --git a/spaces/fatiXbelha/sd/Download iGO Primo Truck and Get Access to Thousands of POIs Speedcams and Road Signs.md b/spaces/fatiXbelha/sd/Download iGO Primo Truck and Get Access to Thousands of POIs Speedcams and Road Signs.md
deleted file mode 100644
index d4e3cb03ecb3fc94a007e95444f2614ad673ff95..0000000000000000000000000000000000000000
--- a/spaces/fatiXbelha/sd/Download iGO Primo Truck and Get Access to Thousands of POIs Speedcams and Road Signs.md
+++ /dev/null
@@ -1,163 +0,0 @@
-
-iGO Primo Truck Download: A Guide for Truck Drivers
-If you are a truck driver, you know how important it is to have a reliable and accurate navigation system. You need a system that can guide you to your destination safely and efficiently, taking into account your vehicle size, weight, and load type. You also need a system that can help you avoid traffic jams, road closures, tolls, and other obstacles that can affect your journey.
-That's why you need iGO Primo Truck, a navigation software designed specifically for truck drivers. iGO Primo Truck is a software that runs on various devices, such as car stereos, smartphones, tablets, or dedicated GPS units. It uses the latest maps and data from reputable sources, such as HERE, TomTom, or Navteq. It also offers many features and benefits that make it one of the best navigation solutions for truck drivers.
-igo primo truck download
DOWNLOAD ✓✓✓ https://urllie.com/2uNA0J
-In this article, we will show you how to download and install iGO Primo Truck on your device, how to use it effectively, and how to update it regularly. We will also answer some of the most frequently asked questions about iGO Primo Truck. By the end of this article, you will have a clear understanding of how iGO Primo Truck can help you improve your driving experience and performance.
- What is iGO Primo Truck?
-iGO Primo Truck is a navigation software that is based on the popular iGO Primo software, but with some modifications and enhancements for truck drivers. iGO Primo Truck is developed by NNG, a Hungarian company that specializes in navigation solutions. iGO Primo Truck is compatible with various devices and platforms, such as Windows CE, Android, iOS, or Linux.
-iGO Primo Truck uses vector-based maps that are stored on your device's memory card. This means that you don't need an internet connection to use it, which can save you money and data. However, you can also use online services, such as live traffic information or weather forecasts, if you have an internet connection available.
-iGO Primo Truck also uses a sophisticated routing algorithm that calculates the best route for your vehicle type and preferences. It takes into account factors such as road width, height clearance, weight limit, load type, speed limit, tolls, ferries, and more. It also allows you to customize your route by adding waypoints, avoiding roads or areas, or changing the order of your destinations.
- Features and benefits of iGO Primo Truck
-Some of the features and benefits of iGO Primo Truck are:
-
-- Truck-specific routing: iGO Primo Truck calculates the optimal route for your vehicle profile and preferences. It avoids roads or areas that are not suitable for your vehicle size, weight, or load type. It also warns you of any road restrictions or hazards ahead.
-- Truck-specific POIs: iGO Primo Truck provides you with a database of points of interest (POIs) that are relevant for truck drivers. These include truck stops, gas stations, parking lots, rest areas, weigh stations, repair shops, and more. You can also search for POIs along your route or on the map.
-- TTS function: iGO Primo Truck supports text-to-speech (TTS) function, which means that it can read aloud the street names and directions in 28 languages. This can help you keep your eyes on the road and avoid distractions.
-- Tunnel view: iGO Primo Truck switches to a simplified view when you enter a tunnel. This can help current and forecasted weather conditions for your location and destination. It also warns you of any severe weather alerts or hazards. This feature requires an internet connection and a subscription to the iGO Weather service.
-- Customizable interface: iGO Primo Truck allows you to customize the interface according to your preferences. You can change the map view, the color scheme, the font size, the units, the voice, and more. You can also create your own skins and icons.
-
- How to download and install iGO Primo Truck
-To download and install iGO Primo Truck on your device, you need to follow these steps:
-
-- Check the compatibility of your device: iGO Primo Truck is compatible with various devices and platforms, but not all of them. You need to check the specifications of your device and make sure it meets the minimum requirements for iGO Primo Truck. You can find the compatibility list on the official website of iGO Primo Truck or on the online store where you want to buy it.
-- Purchase iGO Primo Truck: You can purchase iGO Primo Truck from various online stores, such as Amazon, eBay, or AliExpress. You can also buy it directly from the official website of iGO Primo Truck or from authorized dealers. The price may vary depending on the store, the region, and the version of iGO Primo Truck. You can choose between a full version or an update version, depending on whether you already have a previous version of iGO Primo or not.
-- Download iGO Primo Truck: After you purchase iGO Primo Truck, you will receive a download link or a code to access the download page. You need to download the installation file or the zip file of iGO Primo Truck to your computer. The file size may vary depending on the version and the maps that are included.
-- Install iGO Primo Truck: To install iGO Primo Truck on your device, you need to transfer the installation file or the zip file to your device's memory card. You can use a USB cable, a card reader, or a wireless connection to do this. Then, you need to run the installation file or unzip the zip file on your device. You may need to follow some instructions on your device's screen to complete the installation process.
-- Activate iGO Primo Truck: To activate iGO Primo Truck on your device, you need to enter the license code that you received when you purchased it. You can find the license code in your email confirmation, in your online account, or on a sticker that came with your product. You need to enter the license code in the activation menu of iGO Primo Truck. You may also need to register your product online to activate some features or services.
-
- How to use iGO Primo Truck
-To use iGO Primo Truck effectively, you need to follow these steps:
- How to set up your vehicle profile
-The first thing you need to do before using iGO Primo Truck is to set up your vehicle profile. This is important because it will affect how iGO Primo Truck calculates your route and displays information on your screen. To set up your vehicle profile, you need to follow these steps:
-igo primo truck navigation software
-igo primo truck europe map download
-igo primo truck edition pack
-igo primo truck kia
-igo primo truck android car nav
-igo primo truck iphone
-igo primo truck windows
-igo primo truck dynavin
-igo primo truck gps power
-igo primo truck youtube
-igo primo truck tts function
-igo primo truck tunnel view
-igo primo truck lane assistant
-igo primo truck speed warning
-igo primo truck voice instructions
-igo primo truck traffic signs
-igo primo truck sound dimmer
-igo primo truck tmc signals
-igo primo truck destination search
-igo primo truck data backup
-igo primo truck map update
-igo primo truck toolbox download
-igo primo truck error messages
-igo primo truck license error
-igo primo truck out of memory
-igo primo truck software utilities
-igo primo truck poi editing tool
-igo primo truck speedcam merger tool
-igo primo truck collection licenses
-igo primo truck global cfg file
-igo primo truck global cfg universal
-igo primo truck global cfg modified
-igo primo truck global cfg corrected
-igo primo truck global cfg new concept
-igo primo truck global cfg svg support
-igo primo truck dview tool
-igo primo truck bmp visualization tool
-igo primo truck bmp conversion tool
-igo primo truck seedcam tool
-igo primo truck spudigo tool
-igo primo truck israel version
-igo primo truck korea version
-igo primo truck germany version
-igo primo truck france version
-igo primo truck spain version
-igo primo truck italy version
-igo primo truck uk version
-
-- Go to the settings menu: On the main screen of iGO Primo Truck, tap on the settings icon (the gear symbol) in the bottom right corner. This will open the settings menu.
-- Select vehicle type: In the settings menu, tap on "Vehicle type". This will open a list of vehicle types that you can choose from. Select "Truck" as your vehicle type.
-- Edit vehicle parameters: After you select "Truck" as your vehicle type, tap on "Edit". This will open a screen where you can edit various parameters of your vehicle, such as length, width, height, weight, axle load, trailer type, load type, hazardous materials, and more. Enter the correct values for each parameter according to your vehicle specifications.
-- Save vehicle profile: After you edit all the parameters of your vehicle, tap on "Save". This will save your vehicle profile and return you to the settings menu. You can also name your vehicle profile and create multiple profiles for different vehicles.
-
- How to plan your route
-After you set up your vehicle profile, you can start planning your route with iGO Primo Truck. To plan your route, you need to follow these steps:
-
-- Go to the navigation menu: On the main screen of iGO Primo Truck, tap on the navigation icon (the arrow symbol) in the bottom left corner. This will open the navigation menu.
-- Select destination: In the navigation menu, tap on "Find". This will open a screen where you can select your destination. You can select your destination by entering an address, a city, a POI, a coordinate, a contact, a history item, or a favorite. You can also select your destination by tapping on the map or by using voice commands.
-- Add waypoints: If you want to add waypoints to your route, you can do so by tapping on "Add as waypoint" after you select a destination. You can add up to 100 waypoints to your route. You can also reorder or delete your waypoints by tapping on "Edit" in the navigation menu.
-- Calculate route: After you select your destination and add any waypoints, tap on "Go". This will calculate your route based on your vehicle profile and preferences. You can also change your preferences by tapping on "More" in the navigation menu. You can change the route type (fastest, shortest, economical, or easy), the avoidances (tolls, ferries, highways, etc.), and the map view (2D or 3D).
-- Start navigation: After you calculate your route, tap on "Start". This will start the navigation mode and guide you to your destination with voice and visual instructions. You can also view the route summary, the route overview, the itinerary, or the alternative routes by tapping on the icons in the bottom of the screen.
-
- How to avoid traffic and road restrictions
-One of the advantages of iGO Primo Truck is that it can help you avoid traffic and road restrictions that can affect your journey. To do this, you need to follow these steps:
-
-- Enable live traffic information: To receive live traffic information, you need to have an internet connection and a subscription to the iGO Traffic service. You can enable live traffic information by tapping on "Settings" in the main screen, then tapping on "Online services", then tapping on "Traffic". You can also adjust the settings of live traffic information, such as the update frequency, the alert distance, and the alert sound.
-- Avoid traffic delays: When you enable live traffic information, iGO Primo Truck will display the traffic conditions on your route with different colors: green for free flow, yellow for moderate flow, red for heavy flow, and black for closed roads. If there is a traffic delay on your route, iGO Primo Truck will notify you and suggest an alternative route that is faster or shorter. You can accept or reject the alternative route by tapping on "Yes" or "No".
-- Avoid road restrictions: When you set up your vehicle profile, iGO Primo Truck will automatically avoid roads or areas that are not suitable for your vehicle size, weight, or load type. It will also warn you of any road restrictions or hazards ahead, such as low bridges, narrow roads, weight limits, etc. If there is a road restriction on your route that cannot be avoided, iGO Primo Truck will notify you and ask you to confirm if you want to continue or not. You can confirm or cancel by tapping on "OK" or "Cancel".
-
- How to update iGO Primo Truck
-To keep iGO Primo Truck running smoothly and efficiently, you need to update it regularly. Updating iGO Primo Truck involves updating the maps and software. To do this, you need to follow these steps:
- How to download the latest maps and software
-To download the latest maps and software for iGO Primo Truck, you need to follow these steps:
-
-- Connect your device to your computer: To download the latest maps and software for iGO Primo Truck, you need to connect your device to your computer using a USB cable or a wireless connection.
-- Download Naviextras Toolbox: Naviextras Toolbox is a free software that allows you to manage and update iGO Primo Truck on your device. You can download Naviextras Toolbox from https://www.naviextras.com/. You need to create an account and register your device to use Naviextras Toolbox.
-- Launch Naviextras Toolbox: After you download and install Naviextras Toolbox on your computer, launch it and log in with your account. Naviextras Toolbox will automatically detect your device and display its information and status.
-- Check for updates: In Naviextras Toolbox, click on "Updates" in the left menu. This will show you the available updates for iGO Primo Truck on your device. You can see the details of each update, such as the size, the date, and the content.
-- Select and download updates: To select an update, click on the checkbox next to it. You can select multiple updates at once. To download the selected updates, click on "Install". This will start the download process and show you the progress and the remaining time.
-
- How to use the iGO Toolbox
-To use the iGO Toolbox, you need to follow these steps:
-
-- Connect your device to your computer: To use the iGO Toolbox, you need to connect your device to your computer using a USB cable or a wireless connection.
-- Launch iGO Primo Truck: After you connect your device to your computer, launch iGO Primo Truck on your device. This will open the main screen of iGO Primo Truck.
-- Go to the settings menu: On the main screen of iGO Primo Truck, tap on the settings icon (the gear symbol) in the bottom right corner. This will open the settings menu.
-- Select iGO Toolbox: In the settings menu, tap on "iGO Toolbox". This will open a screen where you can access various tools and features of iGO Primo Truck.
-- Use the tools and features: In the iGO Toolbox screen, you can use various tools and features, such as backup and restore, map management, POI management, route management, skin management, and more. You can also access the user manual, the support center, and the feedback form from this screen.
-
- How to troubleshoot common issues
-Sometimes, you may encounter some issues or problems when using iGO Primo Truck. To troubleshoot common issues, you can try these solutions:
-
-- If iGO Primo Truck does not start or crashes: You can try to restart your device or reinstall iGO Primo Truck. You can also check if there is enough free space on your device's memory card or if there is any damage or corruption on it. You can also contact the support center or visit the online forum for more help.
-- If iGO Primo Truck does not calculate or display the route correctly: You can try to update your maps and software or check your vehicle profile and preferences. You can also check if there is any interference or obstruction with your GPS signal or if there is any error or discrepancy with the map data. You can also report any map errors or feedback from the iGO Toolbox screen.
-- If iGO Primo Truck does not provide voice or sound instructions: You can try to adjust the volume or mute settings of your device or iGO Primo Truck. You can also check if there is any problem with your device's speaker or headphone jack or if there is any missing or corrupted voice file. You can also download and install different voices from Naviextras Toolbox.
-
- Conclusion
- Summary of the main points
-iGO Primo Truck is a navigation software that is designed specifically for truck drivers. It offers many features and benefits that make it one of the best navigation solutions for truck drivers. It uses vector-based maps that are stored on your device's memory card and do not require an internet connection. It also uses a sophisticated routing algorithm that calculates the best route for your vehicle profile and preferences. It also provides you with truck-specific POIs, TTS function, tunnel view, junction view, lane guidance, speed limit and camera alerts, live traffic information, weather forecasts, and more.
- Call to action
-If you are a truck driver who wants to improve your driving experience and performance, you should download and install iGO Primo Truck on your device today. You can purchase iGO Primo Truck from various online stores or from authorized dealers. You can also visit https://www.naviextras.com/ for more information and support. You will not regret it!
- FAQs
-Here are some of the most frequently asked questions about iGO Primo Truck:
-
-- What devices are compatible with iGO Primo Truck?
-
iGO Primo Truck is compatible with various devices and platforms, such as Windows CE, Android, iOS, or Linux. However, not all devices and platforms are compatible with iGO Primo Truck. You need to check the specifications of your device and make sure it meets the minimum requirements for iGO Primo Truck. You can find the compatibility list on the official website of iGO Primo Truck or on the online store where you want to buy it.
-- How much does iGO Primo Truck cost?
-
The price of iGO Primo Truck may vary depending on the store, the region, and the version of iGO Primo Truck. You can choose between a full version or an update version, depending on whether you already have a previous version of iGO Primo or not. You can also choose between different map packages, such as Europe, North America, South America, Asia, Africa, or Australia. You can find the price list on the official website of iGO Primo Truck or on the online store where you want to buy it.
-- How often do I need to update iGO Primo Truck?
-
You need to update iGO Primo Truck regularly to keep it running smoothly and efficiently. Updating iGO Primo Truck involves updating the maps and software. You can download the latest maps and software from Naviextras Toolbox, a free software that allows you to manage and update iGO Primo Truck on your device. You can also use the iGO Toolbox, a feature that allows you to access various tools and features of iGO Primo Truck on your device.
-- What are the advantages of iGO Primo Truck over other navigation software?
-
iGO Primo Truck has many advantages over other navigation software, such as:
-
-- It is designed specifically for truck drivers, with truck-specific routing, POIs, and alerts.
-- It uses vector-based maps that are stored on your device's memory card and do not require an internet connection.
-- It offers many features and benefits that make it one of the best navigation solutions for truck drivers, such as TTS function, tunnel view, junction view, lane guidance, speed limit and camera alerts, live traffic information, weather forecasts, and more.
-- It is compatible with various devices and platforms, such as Windows CE, Android, iOS, or Linux.
-- It is easy to use and customize according to your preferences.
-
-- Where can I get more information and support for iGO Primo Truck?
-
You can get more information and support for iGO Primo Truck from various sources, such as:
-
- 197e85843d
-
-
\ No newline at end of file
diff --git a/spaces/fb700/chat3/docs/README_EN.md b/spaces/fb700/chat3/docs/README_EN.md
deleted file mode 100644
index 537b430d8fb100d10d6607e088b03ddd3f76229e..0000000000000000000000000000000000000000
--- a/spaces/fb700/chat3/docs/README_EN.md
+++ /dev/null
@@ -1,294 +0,0 @@
-# ChatGPT Academic Optimization
-> **Note**
->
-> This English readme is automatically generated by the markdown translation plugin in this project, and may not be 100% correct.
->
-
-
-**If you like this project, please give it a star. If you have come up with more useful academic shortcuts or functional plugins, feel free to open an issue or pull request (to the `dev` branch).**
-
-> **Note**
->
-> 1. Please note that only function plugins (buttons) marked in **red** support reading files, and some plugins are located in the **dropdown menu** in the plugin area. Additionally, we welcome and process PRs for any new plugins with the **highest priority**!
->
-> 2. The functions of each file in this project are detailed in the self-translation report [self_analysis.md](https://github.com/binary-husky/chatgpt_academic/wiki/chatgpt-academic%E9%A1%B9%E7%9B%AE%E8%87%AA%E8%AF%91%E8%A7%A3%E6%8A%A5%E5%91%8A). With the version iteration, you can click on a relevant function plugin at any time to call GPT to regenerate the self-analysis report for the project. Commonly asked questions are summarized in the [`wiki`](https://github.com/binary-husky/chatgpt_academic/wiki/%E5%B8%B8%E8%A7%81%E9%97%AE%E9%A2%98).
->
-> 3. If you are not used to the function, comments or interface with some Chinese names, you can click on the relevant function plugin at any time to call ChatGPT to generate the source code of the project in English.
-
-
-
-Function | Description
---- | ---
-One-click refinement | Supports one-click refinement, one-click searching for grammatical errors in papers.
-One-click translation between Chinese and English | One-click translation between Chinese and English.
-One-click code interpretation | Can correctly display and interpret the code.
-[Custom shortcuts](https://www.bilibili.com/video/BV14s4y1E7jN) | Supports custom shortcuts.
-[Configure proxy server](https://www.bilibili.com/video/BV1rc411W7Dr) | Supports configuring proxy server.
-Modular design | Supports custom high-order experimental features and [function plug-ins], and plug-ins support [hot update](https://github.com/binary-husky/chatgpt_academic/wiki/%E5%87%BD%E6%95%B0%E6%8F%92%E4%BB%B6%E6%8C%87%E5%8D%97).
-[Self-program analysis](https://www.bilibili.com/video/BV1cj411A7VW) | [Function Plug-in] [One-Key Understanding](https://github.com/binary-husky/chatgpt_academic/wiki/chatgpt-academic%E9%A1%B9%E7%9B%AE%E8%87%AA%E8%AF%91%E8%A7%A3%E6%8A%A5%E5%91%8A) the source code of this project.
-[Program analysis](https://www.bilibili.com/video/BV1cj411A7VW) | [Function Plug-in] One-click can analyze other Python/C/C++/Java/Golang/Lua/Rect project trees.
-Read papers | [Function Plug-in] One-click reads the full text of a latex paper and generates an abstract.
-Latex full-text translation/refinement | [Function Plug-in] One-click translates or refines a latex paper.
-Batch annotation generation | [Function Plug-in] One-click generates function annotations in batches.
-Chat analysis report generation | [Function Plug-in] Automatically generate summary reports after running.
-[Arxiv assistant](https://www.bilibili.com/video/BV1LM4y1279X) | [Function Plug-in] Enter the arxiv paper url and you can translate the abstract and download the PDF with one click.
-[PDF paper full-text translation function](https://www.bilibili.com/video/BV1KT411x7Wn) | [Function Plug-in] Extract title and abstract of PDF papers + translate full text (multi-threaded).
-[Google Scholar integration assistant](https://www.bilibili.com/video/BV19L411U7ia) (Version>=2.45) | [Function Plug-in] Given any Google Scholar search page URL, let GPT help you choose interesting articles.
-Formula display | Can simultaneously display the tex form and rendering form of formulas.
-Image display | Can display images in Markdown.
-Multithreaded function plug-in support | Supports multi-threaded calling of chatgpt, one-click processing of massive texts or programs.
-Support for markdown tables output by GPT | Can output markdown tables that support GPT.
-Start dark gradio theme [theme](https://github.com/binary-husky/chatgpt_academic/issues/173) | Add ```/?__dark-theme=true``` to the browser URL to switch to the dark theme.
-Huggingface free scientific online experience](https://huggingface.co/spaces/qingxu98/gpt-academic) | After logging in to Huggingface, copy [this space](https://huggingface.co/spaces/qingxu98/gpt-academic).
-[Mixed support for multiple LLM models](https://www.bilibili.com/video/BV1EM411K7VH/) ([v3.0 branch](https://github.com/binary-husky/chatgpt_academic/tree/v3.0) in testing) | It must feel great to be served by both ChatGPT and [Tsinghua ChatGLM](https://github.com/THUDM/ChatGLM-6B)!
-Compatible with [TGUI](https://github.com/oobabooga/text-generation-webui) to access more language models | Access to opt-1.3b, galactica-1.3b and other models ([v3.0 branch](https://github.com/binary-husky/chatgpt_academic/tree/v3.0) under testing).
-… | ...
-
-
-
-
-- New interface (modify the `LAYOUT` option in `config.py` to switch between "left and right layout" and "up and down layout").
-
- 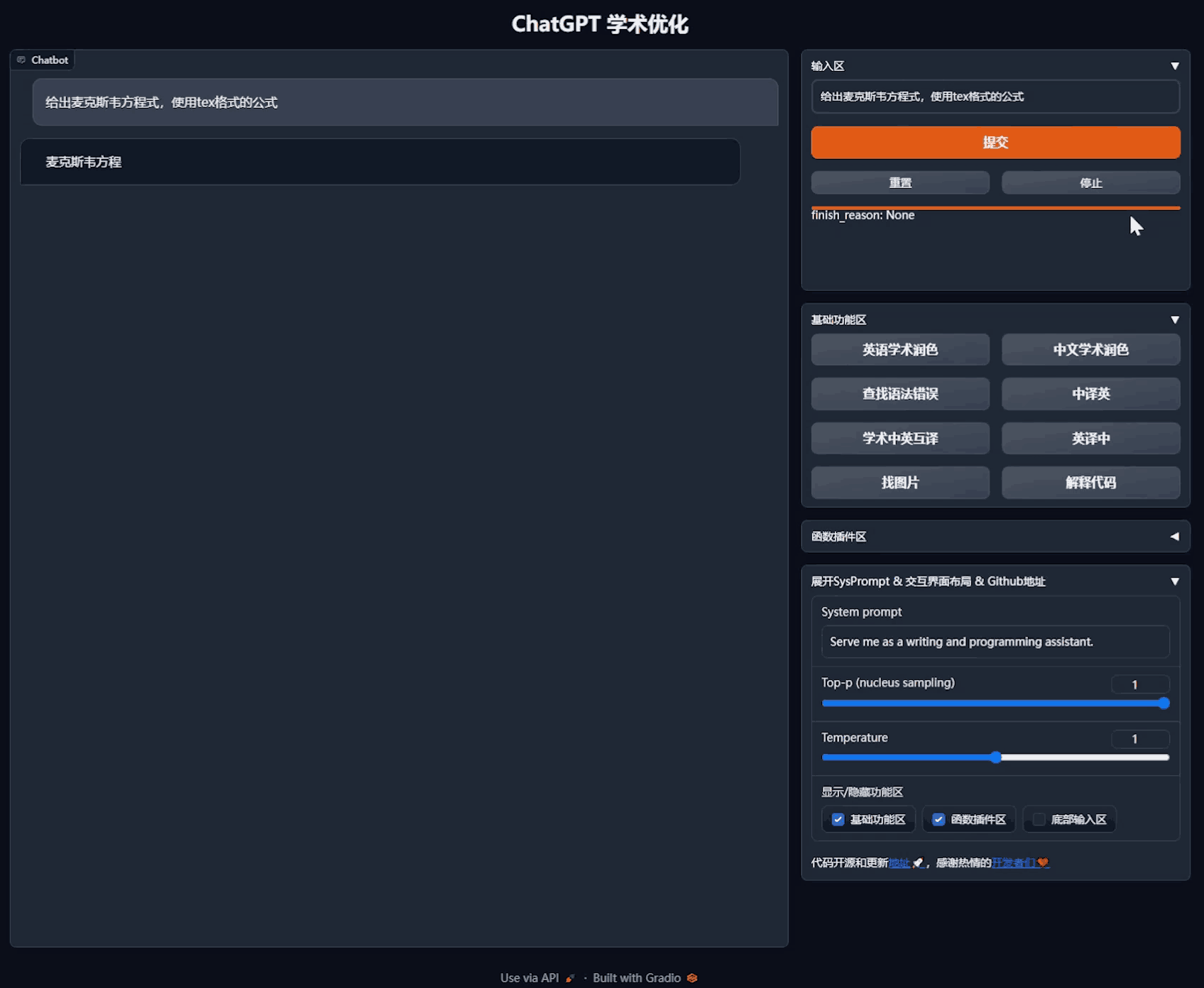
-
-
-- All buttons are dynamically generated by reading `functional.py`, and custom functions can be added freely, freeing up the clipboard.
-
- 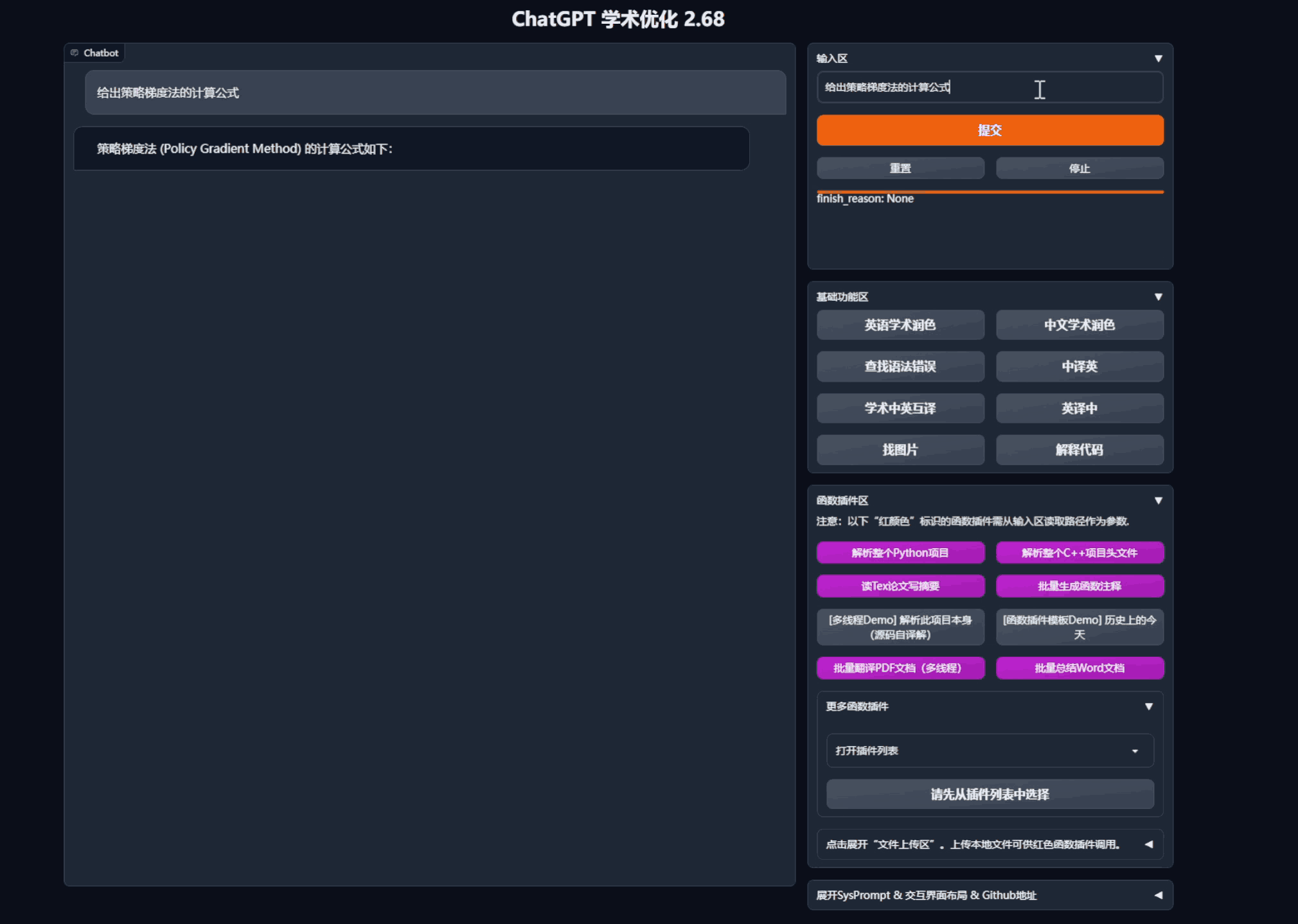
-
-
-- Refinement/Correction
-
- 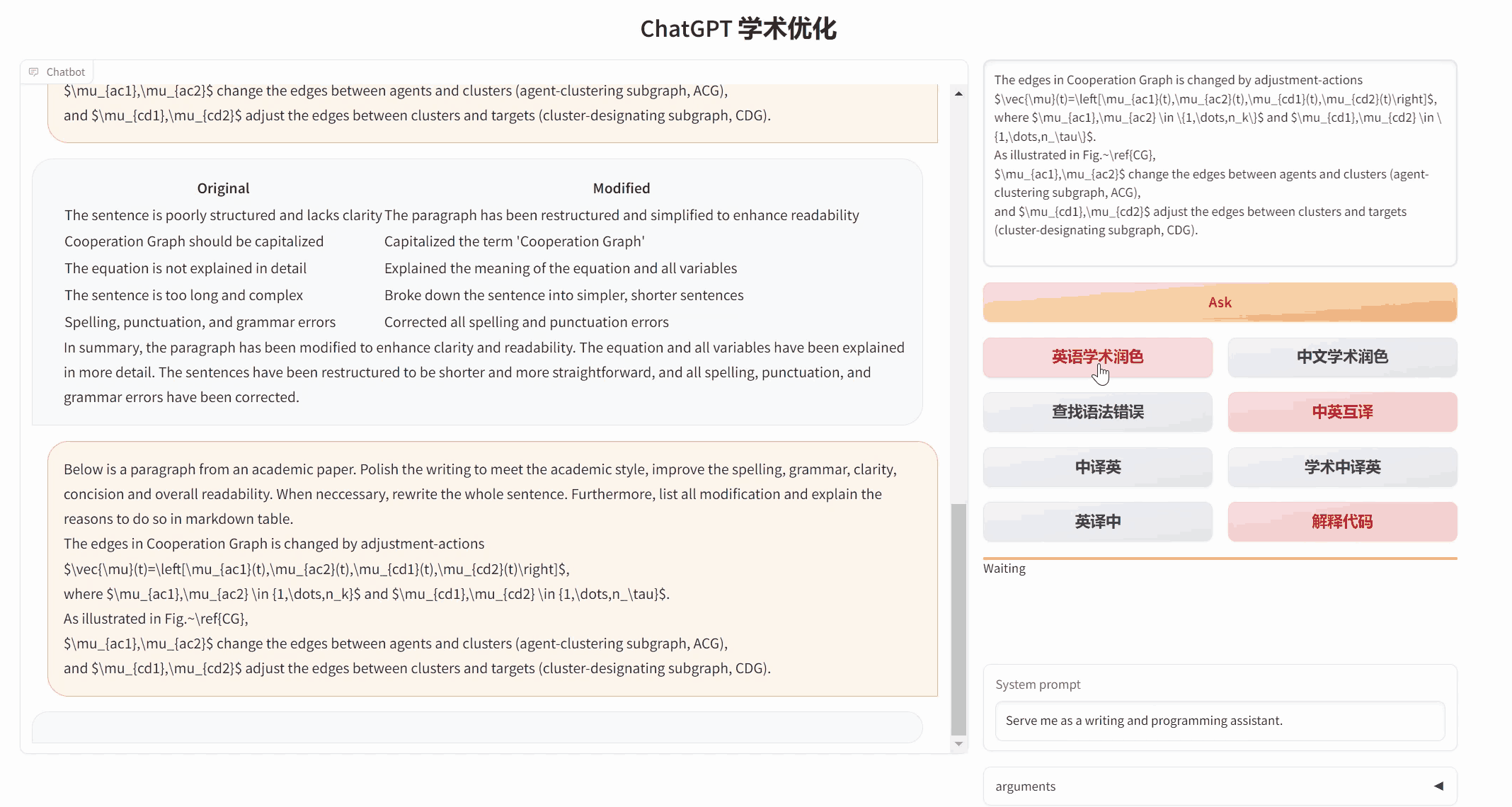
-
-
-- Supports markdown tables output by GPT.
-
- 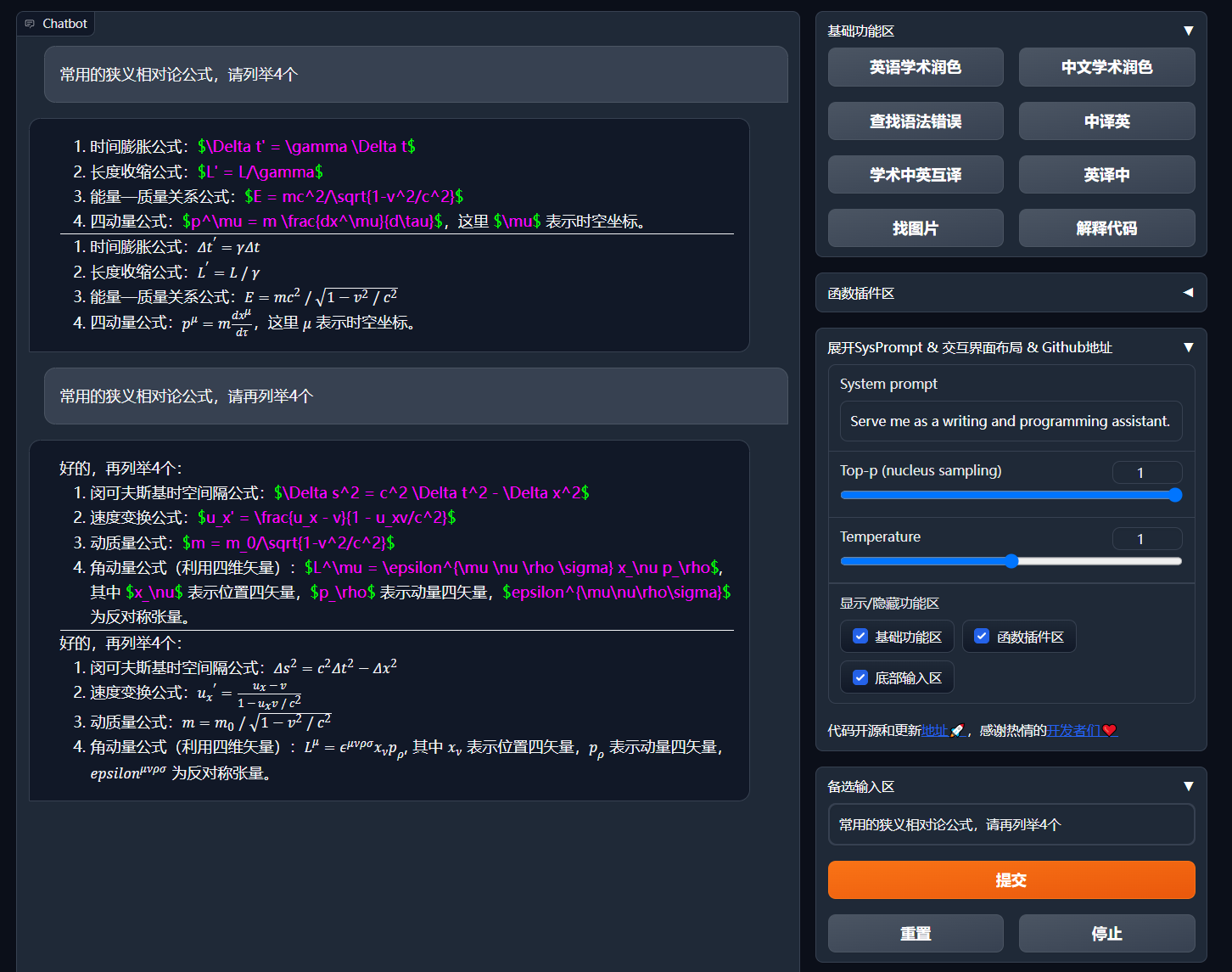
-
-
-- If the output contains formulas, both the tex form and the rendering form are displayed simultaneously for easy copying and reading.
-
- 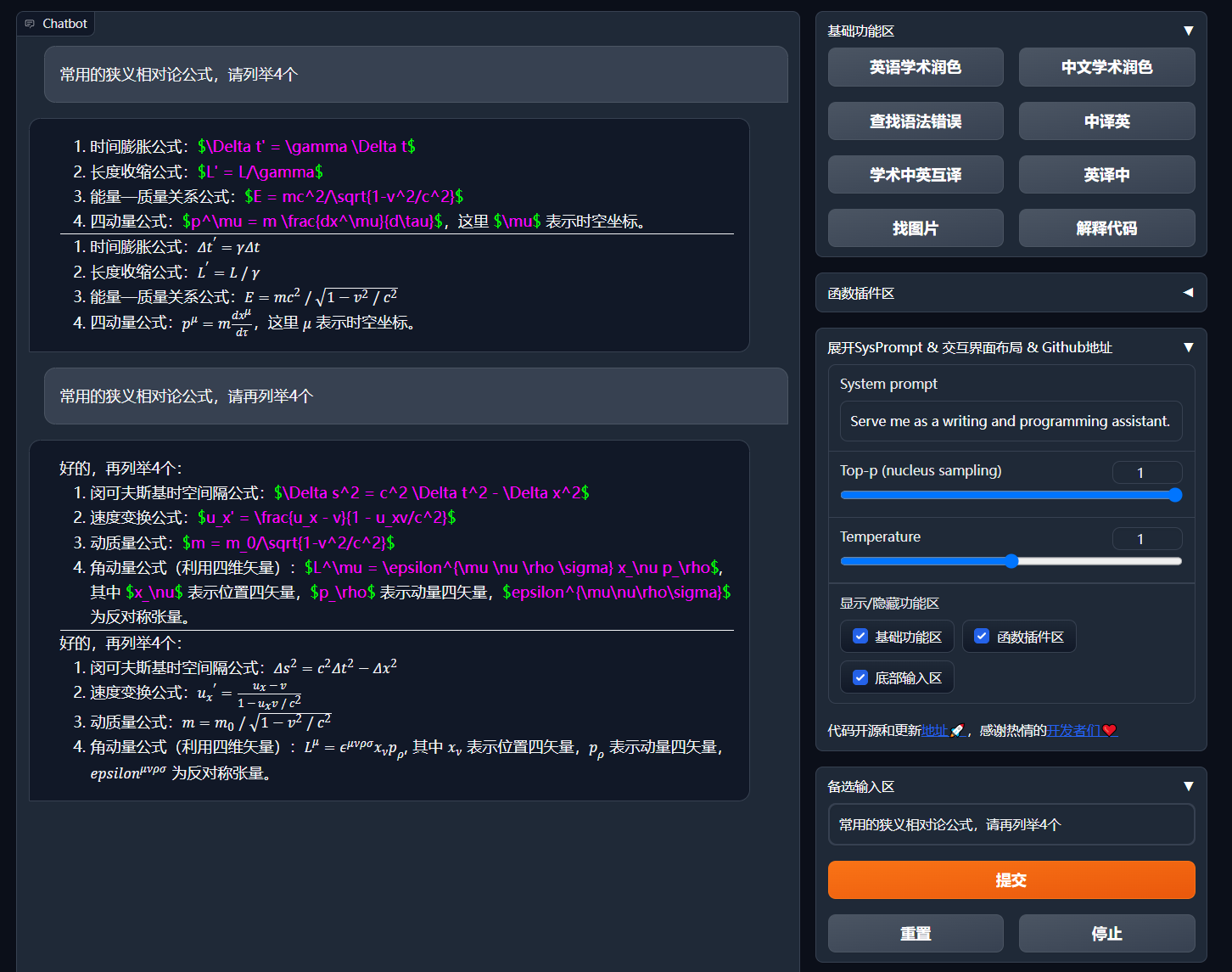
-
-
-- Don't want to read project code? Let chatgpt boast about the whole project.
-
- 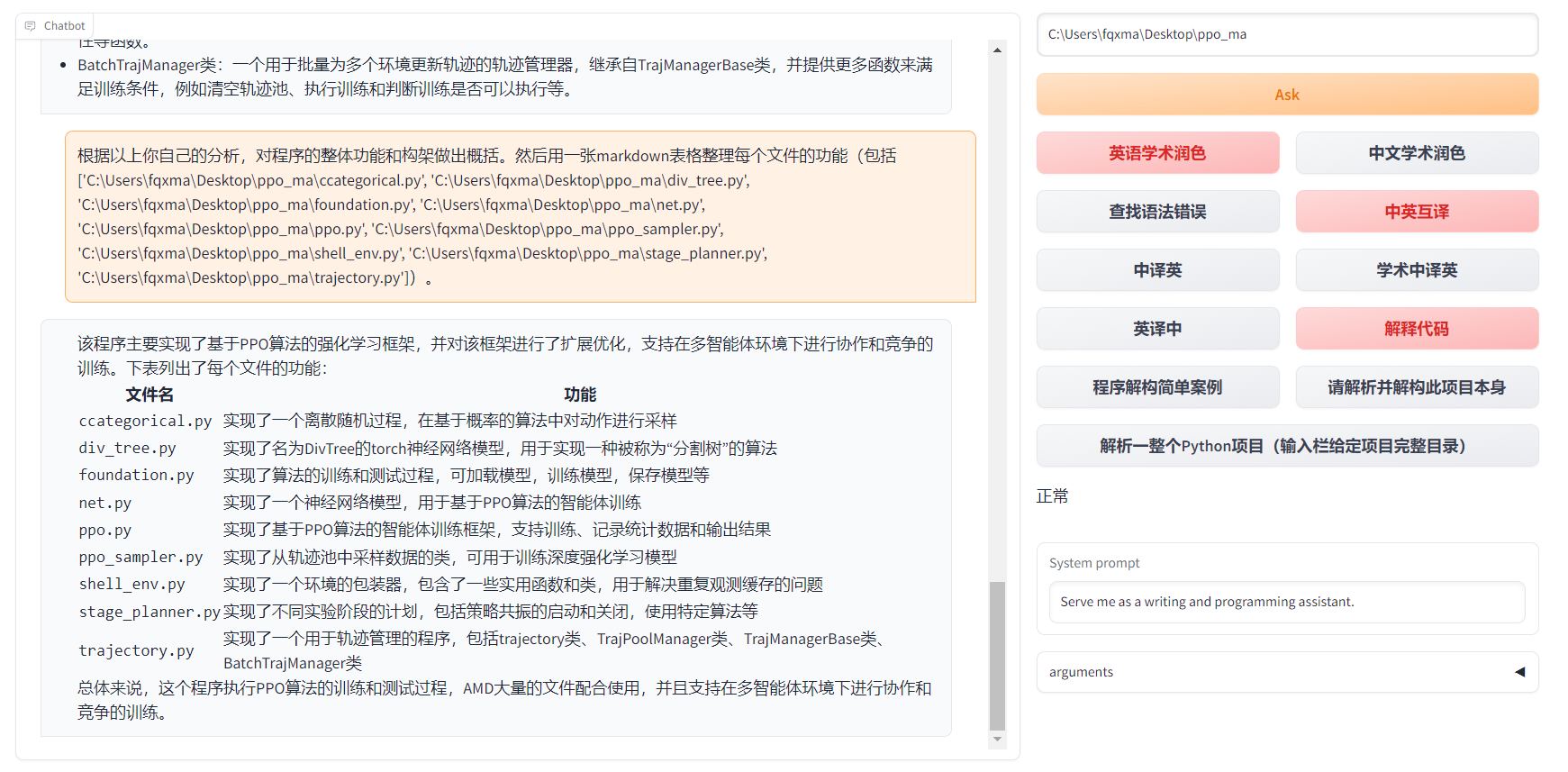
-
-
-- Multiple large language models mixed calling. ([v3.0 branch](https://github.com/binary-husky/chatgpt_academic/tree/v3.0) in testing)
-
-
-## Running Directly (Windows, Linux or MacOS)
-
-### 1. Download the Project
-```sh
-git clone https://github.com/binary-husky/chatgpt_academic.git
-cd chatgpt_academic
-```
-
-### 2. Configure API_KEY and Proxy Settings
-
-In `config.py`, configure the overseas Proxy and OpenAI API KEY, as follows:
-```
-1. If you are in China, you need to set an overseas proxy to use the OpenAI API smoothly. Please read the instructions in config.py carefully (1. Modify the USE_PROXY to True; 2. Modify the proxies according to the instructions).
-2. Configure OpenAI API KEY. You need to register on the OpenAI official website and obtain an API KEY. Once you get the API KEY, configure it in the config.py file.
-3. Issues related to proxy network (network timeout, proxy not working) are summarized to https://github.com/binary-husky/chatgpt_academic/issues/1
-```
-(Note: When the program is running, it will first check whether there is a private configuration file named `config_private.py`, and use the configuration in it to overwrite the same name configuration in `config.py`. Therefore, if you can understand our configuration reading logic, we strongly recommend that you create a new configuration file next to `config.py` named `config_private.py` and transfer (copy) the configuration in `config.py` to `config_private.py`. `config_private.py` is not managed by Git, which can make your privacy information more secure.)
-
-### 3. Install Dependencies
-```sh
-# (Option 1) Recommended
-python -m pip install -r requirements.txt
-
-# (Option 2) If you use anaconda, the steps are also similar:
-# (Option 2.1) conda create -n gptac_venv python=3.11
-# (Option 2.2) conda activate gptac_venv
-# (Option 2.3) python -m pip install -r requirements.txt
-
-# Note: Use the official pip source or the Ali pip source. Other pip sources (such as some university pips) may have problems. Temporary substitution method:
-# python -m pip install -r requirements.txt -i https://mirrors.aliyun.com/pypi/simple/
-```
-
-### 4. Run
-```sh
-python main.py
-```
-
-### 5. Test Experimental Features
-```
-- Test C++ Project Header Analysis
- In the input area, enter `./crazy_functions/test_project/cpp/libJPG` , and then click "[Experiment] Parse the entire C++ project (input inputs the root path of the project)"
-- Test Writing Abstracts for Latex Projects
- In the input area, enter `./crazy_functions/test_project/latex/attention` , and then click "[Experiment] Read the tex paper and write an abstract (input inputs the root path of the project)"
-- Test Python Project Analysis
- In the input area, enter `./crazy_functions/test_project/python/dqn` , and then click "[Experiment] Parse the entire py project (input inputs the root path of the project)"
-- Test Self-code Interpretation
- Click "[Experiment] Please analyze and deconstruct this project itself"
-- Test Experimental Function Template (asking GPT what happened in history today), you can implement more complex functions based on this template function
- Click "[Experiment] Experimental function template"
-```
-
-## Use Docker (Linux)
-
-``` sh
-# Download Project
-git clone https://github.com/binary-husky/chatgpt_academic.git
-cd chatgpt_academic
-# Configure Overseas Proxy and OpenAI API KEY
-Configure config.py with any text editor
-# Installation
-docker build -t gpt-academic .
-# Run
-docker run --rm -it --net=host gpt-academic
-
-# Test Experimental Features
-## Test Self-code Interpretation
-Click "[Experiment] Please analyze and deconstruct this project itself"
-## Test Experimental Function Template (asking GPT what happened in history today), you can implement more complex functions based on this template function
-Click "[Experiment] Experimental function template"
-## (Please note that when running in docker, you need to pay extra attention to file access rights issues of the program.)
-## Test C++ Project Header Analysis
-In the input area, enter ./crazy_functions/test_project/cpp/libJPG , and then click "[Experiment] Parse the entire C++ project (input inputs the root path of the project)"
-## Test Writing Abstracts for Latex Projects
-In the input area, enter ./crazy_functions/test_project/latex/attention , and then click "[Experiment] Read the tex paper and write an abstract (input inputs the root path of the project)"
-## Test Python Project Analysis
-In the input area, enter ./crazy_functions/test_project/python/dqn , and then click "[Experiment] Parse the entire py project (input inputs the root path of the project)"
-
-```
-
-## Other Deployment Methods
-- Use WSL2 (Windows Subsystem for Linux subsystem)
-Please visit [Deploy Wiki-1] (https://github.com/binary-husky/chatgpt_academic/wiki/%E4%BD%BF%E7%94%A8WSL2%EF%BC%88Windows-Subsystem-for-Linux-%E5%AD%90%E7%B3%BB%E7%BB%9F%EF%BC%89%E9%83%A8%E7%BD%B2)
-
-- nginx remote deployment
-Please visit [Deploy Wiki-2] (https://github.com/binary-husky/chatgpt_academic/wiki/%E8%BF%9C%E7%A8%8B%E9%83%A8%E7%BD%B2%E7%9A%84%E6%8C%87%E5%AF%BC)
-
-
-## Customizing New Convenient Buttons (Academic Shortcut Key Customization)
-Open functional.py and add the entry as follows, and then restart the program. (If the button has been successfully added and is visible, both the prefix and suffix support hot modification and take effect without restarting the program.)
-
-For example,
-```
-"Super English to Chinese Translation": {
-
- # Prefix, which will be added before your input. For example, it is used to describe your requirements, such as translation, code interpretation, polishing, etc.
- "Prefix": "Please translate the following content into Chinese, and then use a markdown table to explain each proprietary term in the text:\n\n",
-
- # Suffix, which will be added after your input. For example, in conjunction with the prefix, you can bracket your input in quotes.
- "Suffix": "",
-
-},
-```
-
- 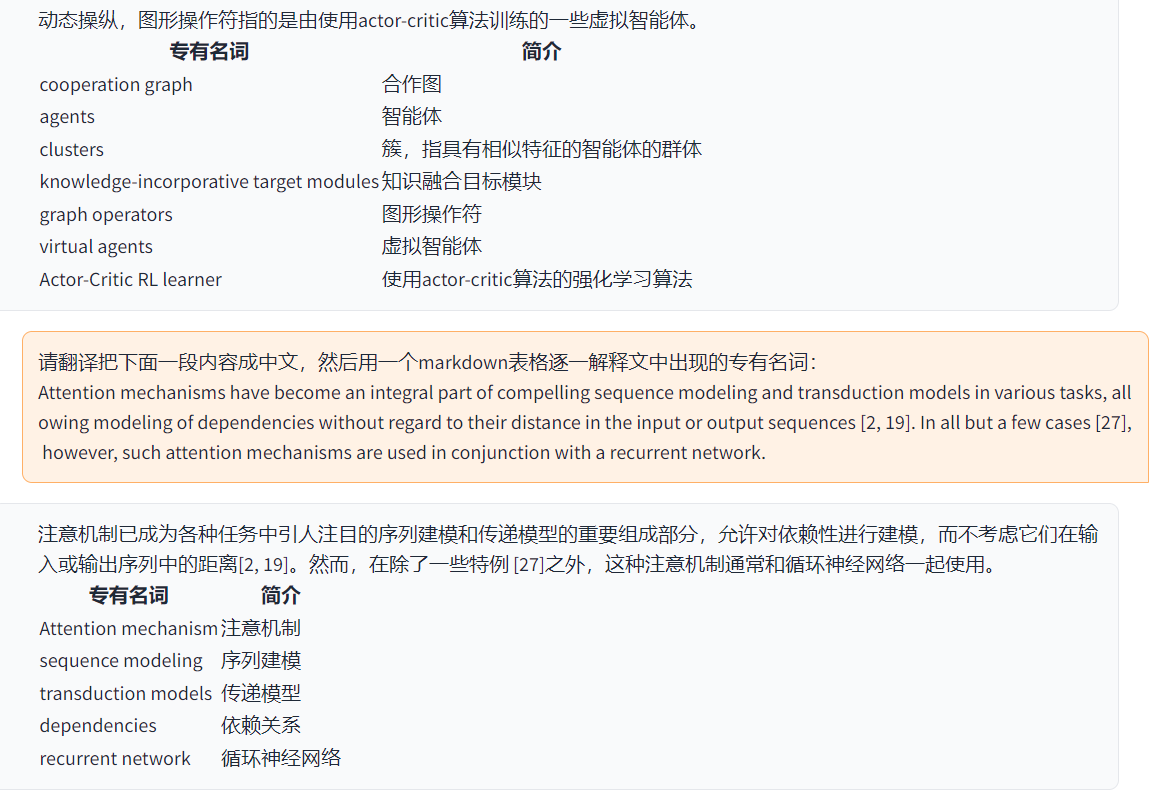
-
-
-
-If you invent a more user-friendly academic shortcut key, welcome to post an issue or pull request!
-
-## Configure Proxy
-### Method 1: General Method
-Modify the port and proxy software corresponding in ```config.py```
-
-
- 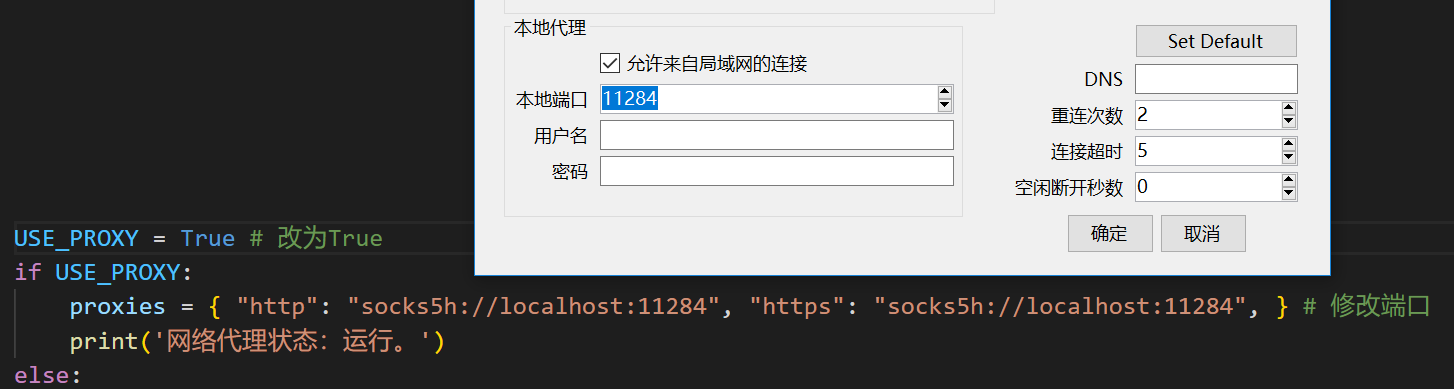
- 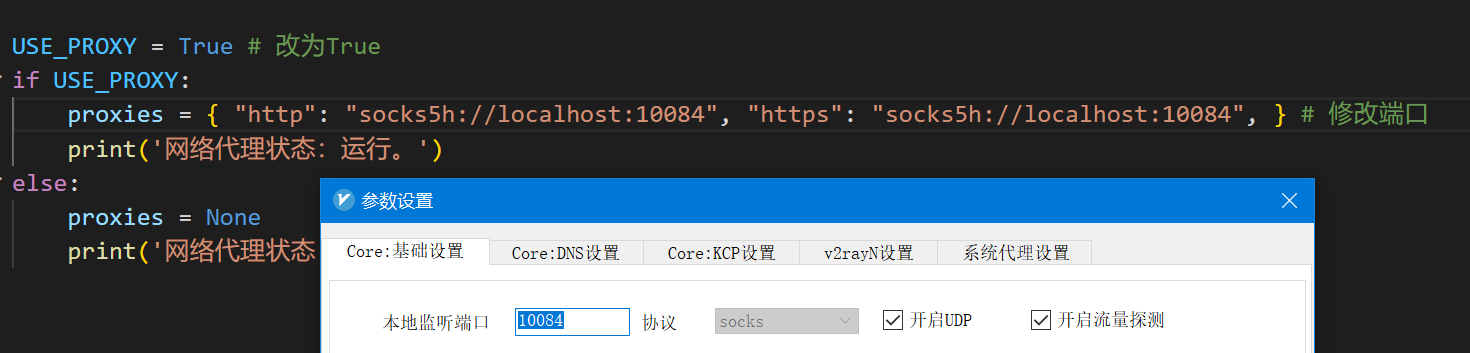
-
-
-
-After configuring, you can use the following command to test whether the proxy works. If everything is normal, the code below will output the location of your proxy server:
-
-```
-python check_proxy.py
-```
-
-### Method Two: Pure Beginner Tutorial
-[Pure Beginner Tutorial](https://github.com/binary-husky/chatgpt_academic/wiki/%E4%BB%A3%E7%90%86%E8%BD%AF%E4%BB%B6%E9%97%AE%E9%A2%98%E7%9A%84%E6%96%B0%E6%89%8B%E8%A7%A3%E5%86%B3%E6%96%B9%E6%B3%95%EF%BC%88%E6%96%B9%E6%B3%95%E5%8F%AA%E9%80%82%E7%94%A8%E4%BA%8E%E6%96%B0%E6%89%8B%EF%BC%89)
-
-## Compatibility Testing
-
-### Image Display:
-
-
- 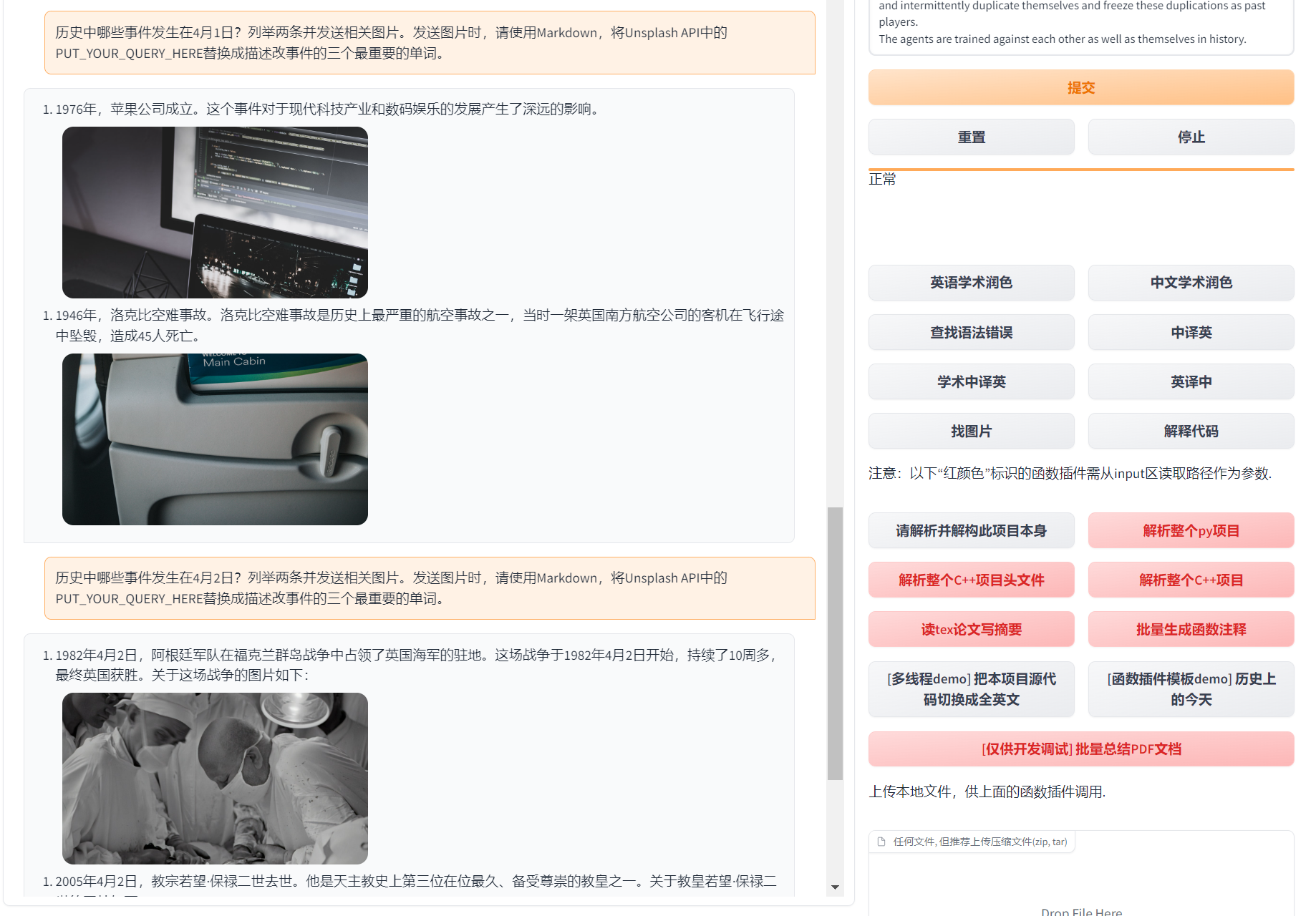
-
-
-
-### If the program can read and analyze itself:
-
-
- 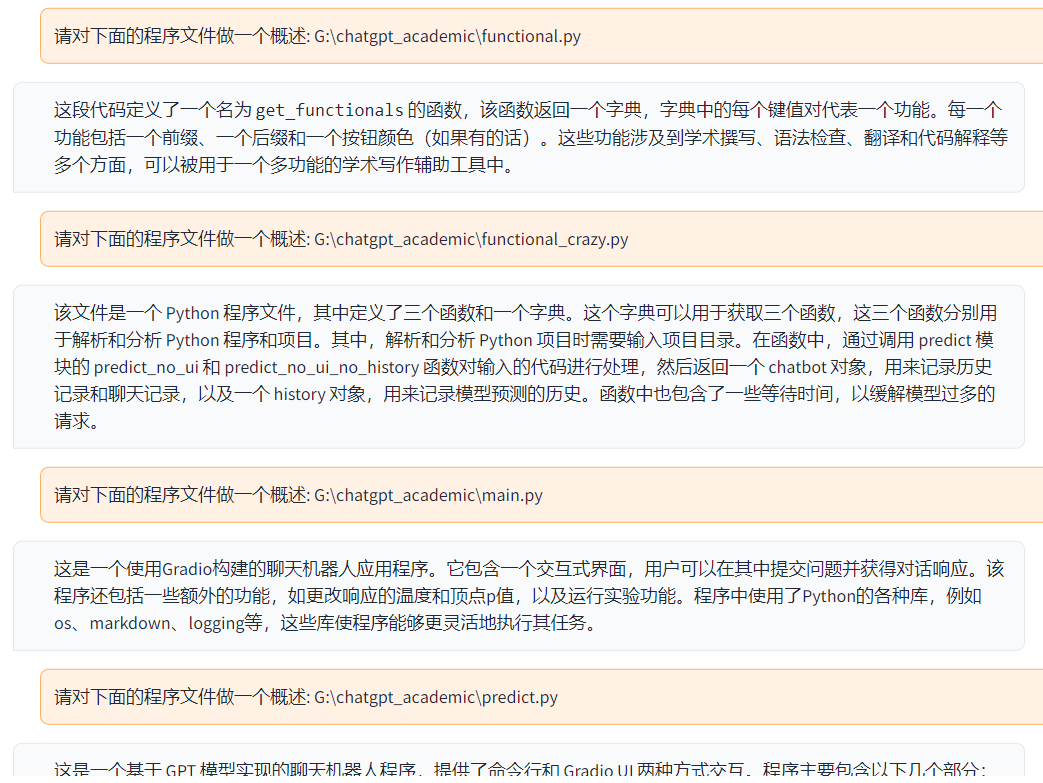
-
-
-
- 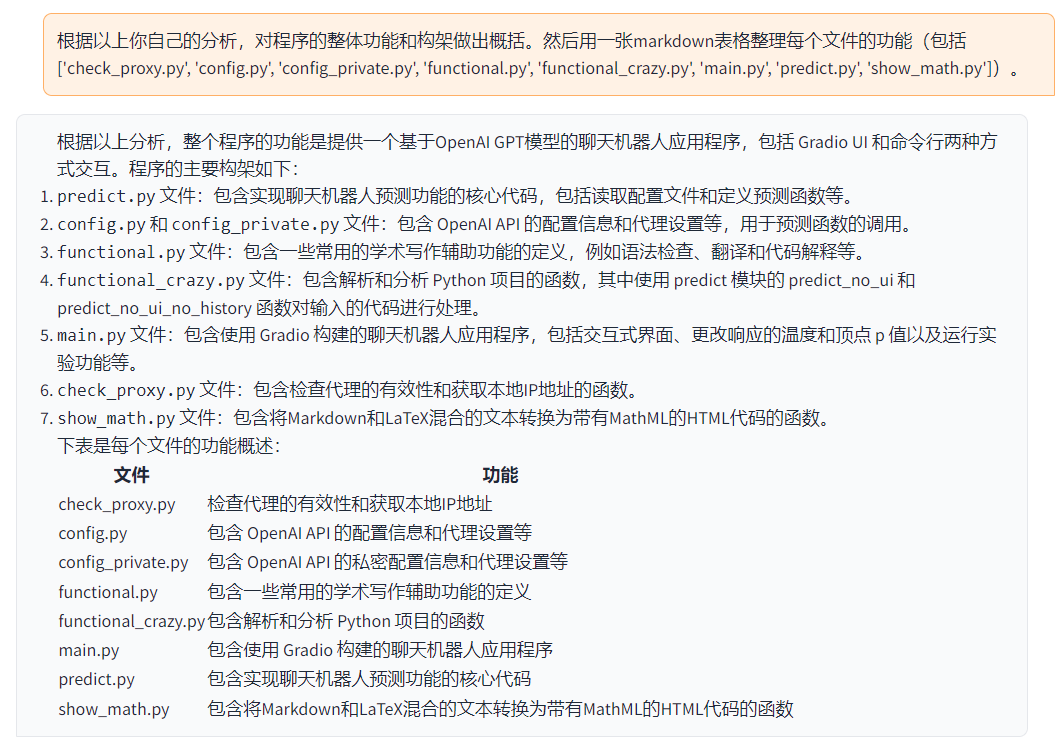
-
-
-### Any other Python/Cpp project analysis:
-
- 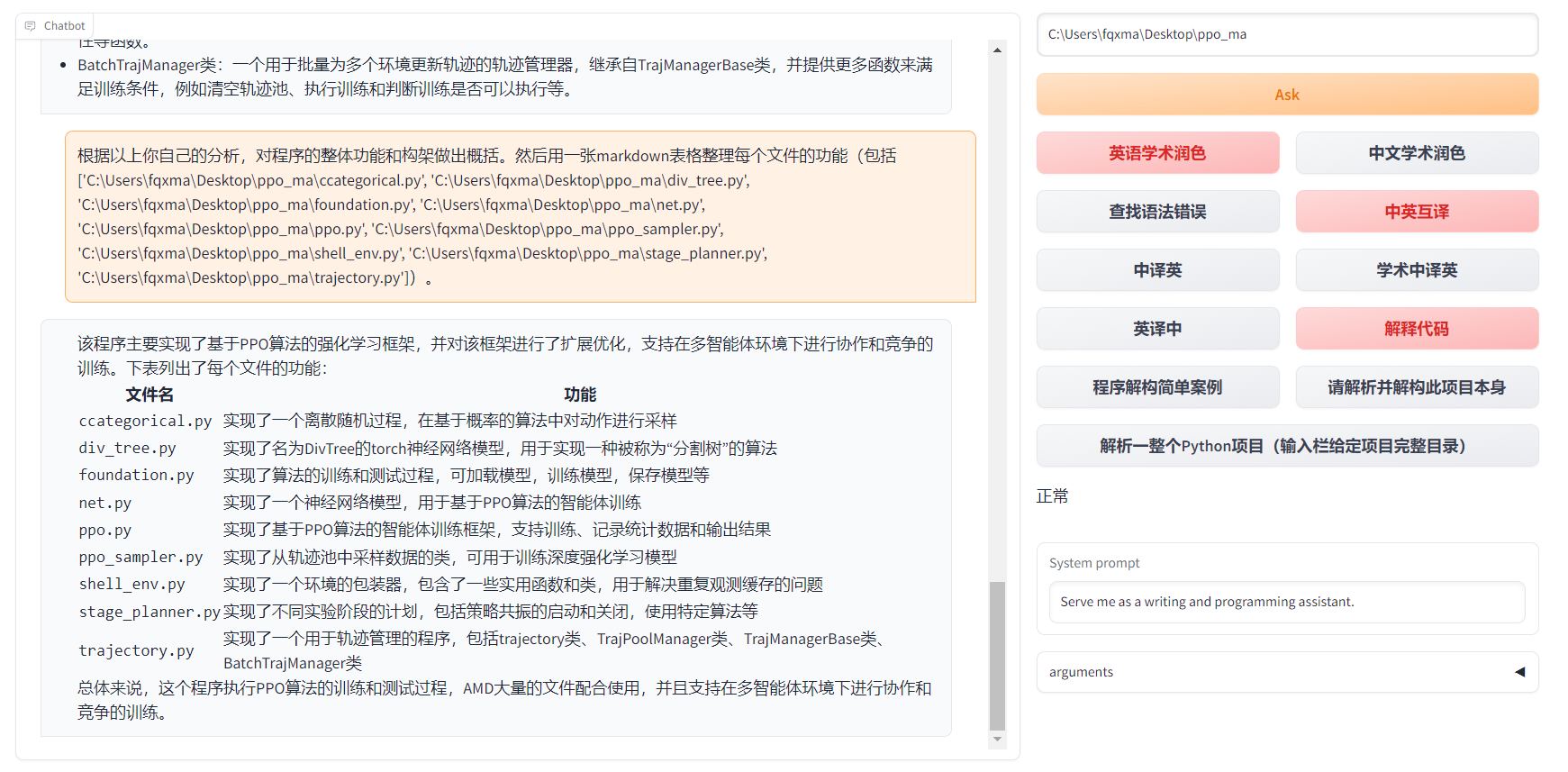
-
-
-
- 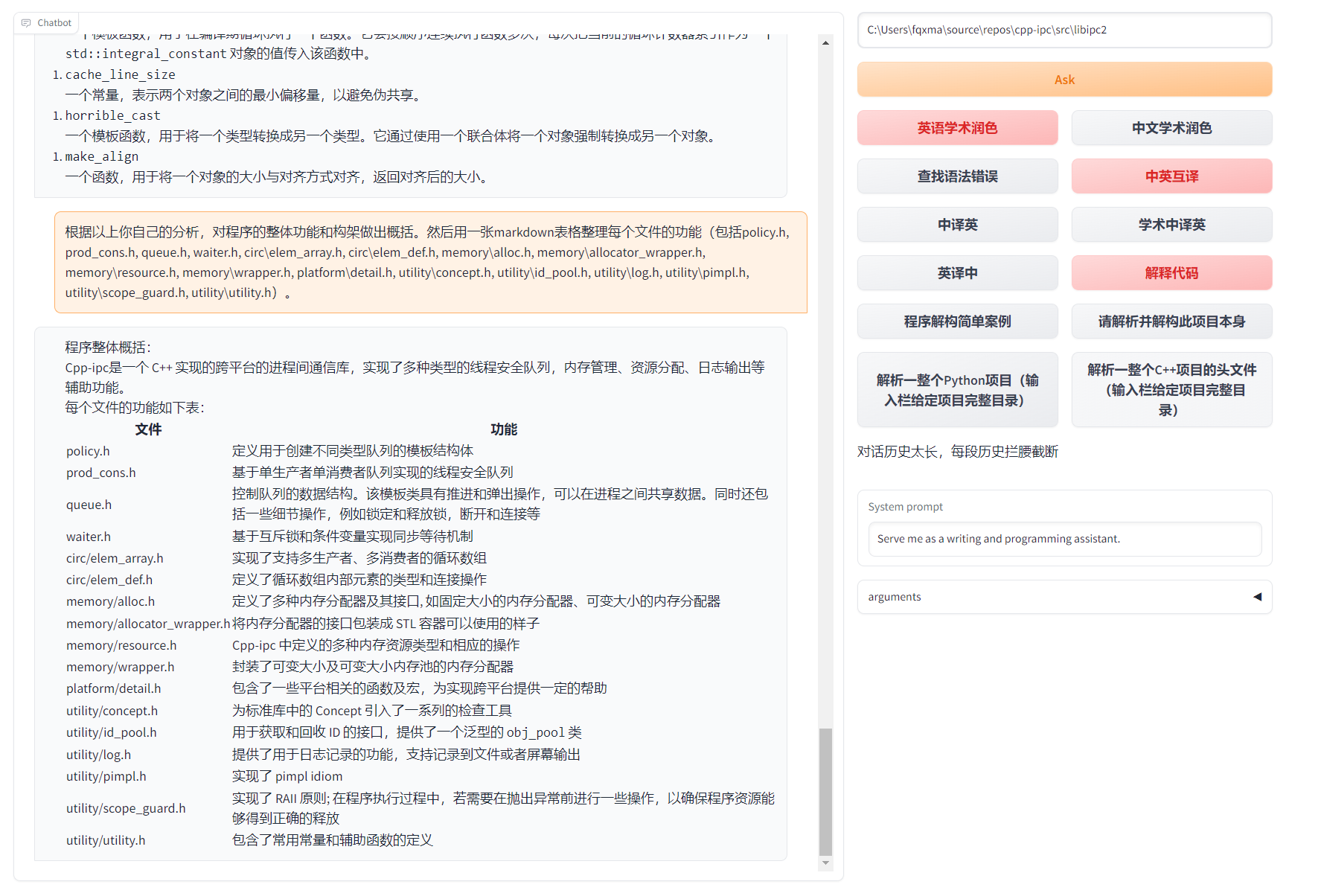
-
-
-### Latex paper reading comprehension and abstract generation with one click
-
- 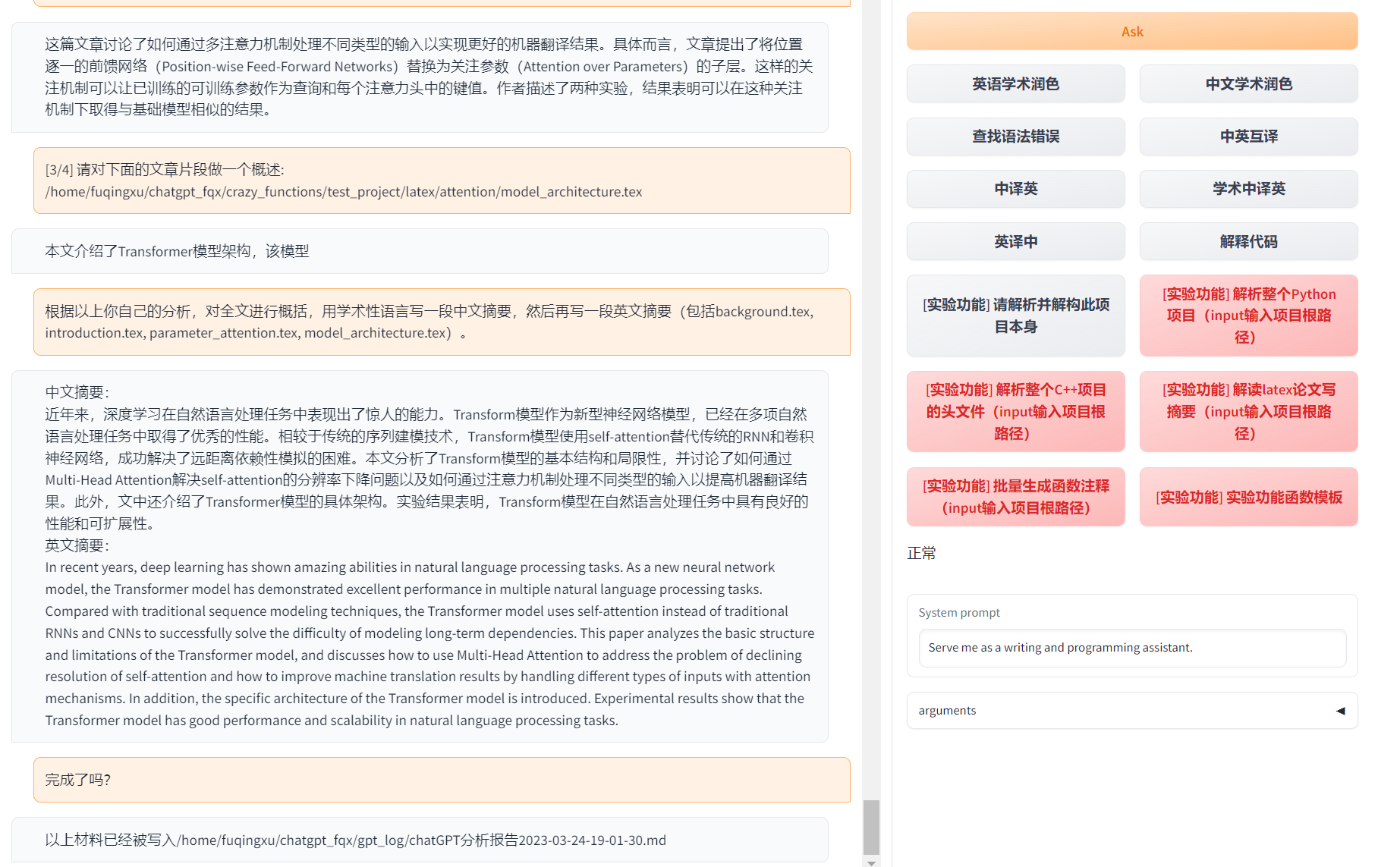
-
-
-### Automatic Report Generation
-
-
-### Modular Function Design
-
- 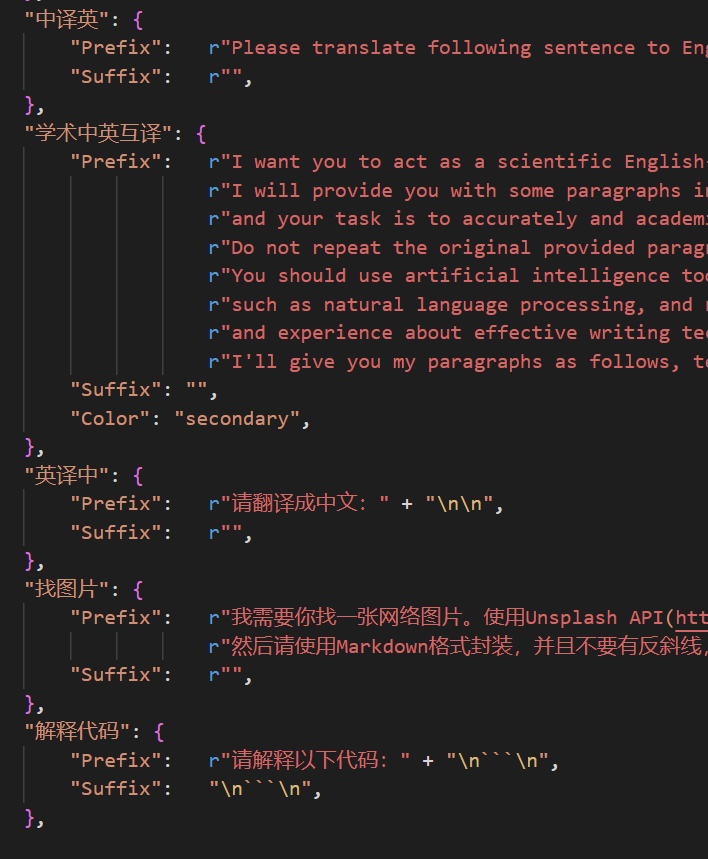
- 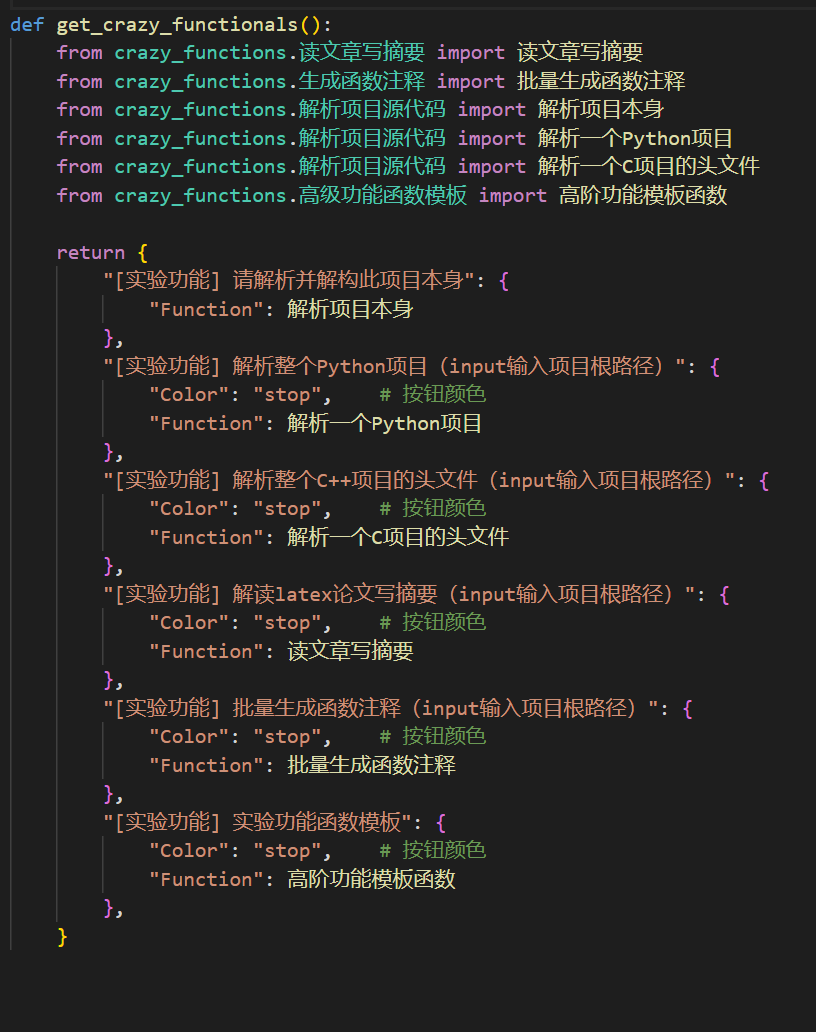
-
-
-
-### Translating source code to English
-
-
- 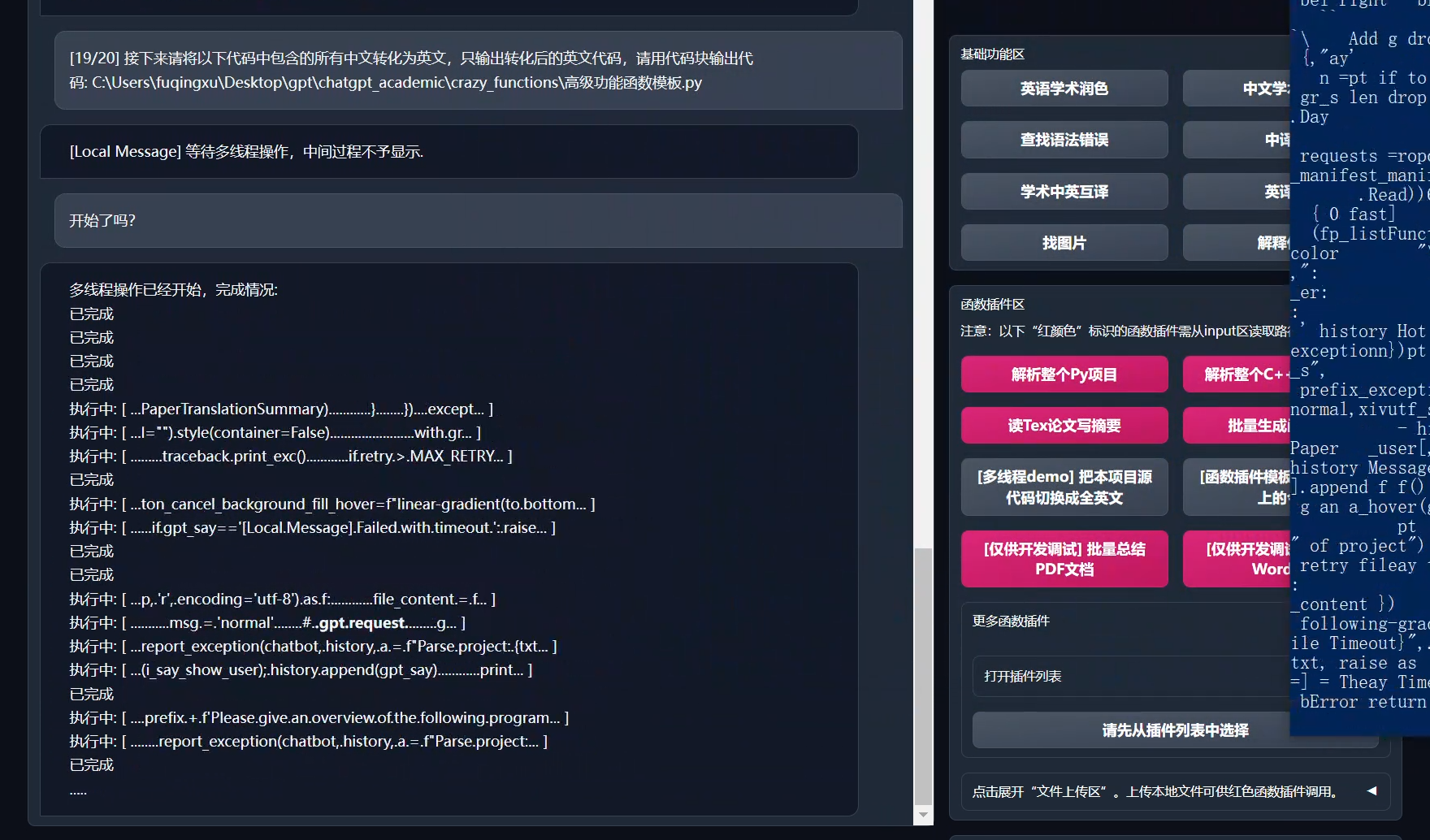
-
-
-## Todo and Version Planning:
-
-- version 3 (Todo):
-- - Support for gpt4 and other llm
-- version 2.4+ (Todo):
-- - Summary of long text and token overflow problems in large project source code
-- - Implementation of project packaging and deployment
-- - Function plugin parameter interface optimization
-- - Self-updating
-- version 2.4: (1) Added PDF full-text translation function; (2) Added input area switching function; (3) Added vertical layout option; (4) Optimized multi-threaded function plugin.
-- version 2.3: Enhanced multi-threaded interactivity
-- version 2.2: Function plug-in supports hot reloading
-- version 2.1: Collapsible layout
-- version 2.0: Introduction of modular function plugins
-- version 1.0: Basic functions
-
-## References and Learning
-
-
-```
-The code refers to the design of many other excellent projects, mainly including:
-
-# Reference Project 1: Referenced the method of reading OpenAI json, recording historical inquiry records, and using gradio queue in ChuanhuChatGPT
-https://github.com/GaiZhenbiao/ChuanhuChatGPT
-
-# Reference Project 2:
-https://github.com/THUDM/ChatGLM-6B
-
-```
-
-
diff --git a/spaces/fb700/chatglm-fitness-RLHF/src/face3d/models/facerecon_model.py b/spaces/fb700/chatglm-fitness-RLHF/src/face3d/models/facerecon_model.py
deleted file mode 100644
index 7de8ca6eebc50ff1ed52c5ba37d31b43f977b5e1..0000000000000000000000000000000000000000
--- a/spaces/fb700/chatglm-fitness-RLHF/src/face3d/models/facerecon_model.py
+++ /dev/null
@@ -1,220 +0,0 @@
-"""This script defines the face reconstruction model for Deep3DFaceRecon_pytorch
-"""
-
-import numpy as np
-import torch
-from src.face3d.models.base_model import BaseModel
-from src.face3d.models import networks
-from src.face3d.models.bfm import ParametricFaceModel
-from src.face3d.models.losses import perceptual_loss, photo_loss, reg_loss, reflectance_loss, landmark_loss
-from src.face3d.util import util
-from src.face3d.util.nvdiffrast import MeshRenderer
-# from src.face3d.util.preprocess import estimate_norm_torch
-
-import trimesh
-from scipy.io import savemat
-
-class FaceReconModel(BaseModel):
-
- @staticmethod
- def modify_commandline_options(parser, is_train=False):
- """ Configures options specific for CUT model
- """
- # net structure and parameters
- parser.add_argument('--net_recon', type=str, default='resnet50', choices=['resnet18', 'resnet34', 'resnet50'], help='network structure')
- parser.add_argument('--init_path', type=str, default='./checkpoints/init_model/resnet50-0676ba61.pth')
- parser.add_argument('--use_last_fc', type=util.str2bool, nargs='?', const=True, default=False, help='zero initialize the last fc')
- parser.add_argument('--bfm_folder', type=str, default='./checkpoints/BFM_Fitting/')
- parser.add_argument('--bfm_model', type=str, default='BFM_model_front.mat', help='bfm model')
-
- # renderer parameters
- parser.add_argument('--focal', type=float, default=1015.)
- parser.add_argument('--center', type=float, default=112.)
- parser.add_argument('--camera_d', type=float, default=10.)
- parser.add_argument('--z_near', type=float, default=5.)
- parser.add_argument('--z_far', type=float, default=15.)
-
- if is_train:
- # training parameters
- parser.add_argument('--net_recog', type=str, default='r50', choices=['r18', 'r43', 'r50'], help='face recog network structure')
- parser.add_argument('--net_recog_path', type=str, default='checkpoints/recog_model/ms1mv3_arcface_r50_fp16/backbone.pth')
- parser.add_argument('--use_crop_face', type=util.str2bool, nargs='?', const=True, default=False, help='use crop mask for photo loss')
- parser.add_argument('--use_predef_M', type=util.str2bool, nargs='?', const=True, default=False, help='use predefined M for predicted face')
-
-
- # augmentation parameters
- parser.add_argument('--shift_pixs', type=float, default=10., help='shift pixels')
- parser.add_argument('--scale_delta', type=float, default=0.1, help='delta scale factor')
- parser.add_argument('--rot_angle', type=float, default=10., help='rot angles, degree')
-
- # loss weights
- parser.add_argument('--w_feat', type=float, default=0.2, help='weight for feat loss')
- parser.add_argument('--w_color', type=float, default=1.92, help='weight for loss loss')
- parser.add_argument('--w_reg', type=float, default=3.0e-4, help='weight for reg loss')
- parser.add_argument('--w_id', type=float, default=1.0, help='weight for id_reg loss')
- parser.add_argument('--w_exp', type=float, default=0.8, help='weight for exp_reg loss')
- parser.add_argument('--w_tex', type=float, default=1.7e-2, help='weight for tex_reg loss')
- parser.add_argument('--w_gamma', type=float, default=10.0, help='weight for gamma loss')
- parser.add_argument('--w_lm', type=float, default=1.6e-3, help='weight for lm loss')
- parser.add_argument('--w_reflc', type=float, default=5.0, help='weight for reflc loss')
-
- opt, _ = parser.parse_known_args()
- parser.set_defaults(
- focal=1015., center=112., camera_d=10., use_last_fc=False, z_near=5., z_far=15.
- )
- if is_train:
- parser.set_defaults(
- use_crop_face=True, use_predef_M=False
- )
- return parser
-
- def __init__(self, opt):
- """Initialize this model class.
-
- Parameters:
- opt -- training/test options
-
- A few things can be done here.
- - (required) call the initialization function of BaseModel
- - define loss function, visualization images, model names, and optimizers
- """
- BaseModel.__init__(self, opt) # call the initialization method of BaseModel
-
- self.visual_names = ['output_vis']
- self.model_names = ['net_recon']
- self.parallel_names = self.model_names + ['renderer']
-
- self.facemodel = ParametricFaceModel(
- bfm_folder=opt.bfm_folder, camera_distance=opt.camera_d, focal=opt.focal, center=opt.center,
- is_train=self.isTrain, default_name=opt.bfm_model
- )
-
- fov = 2 * np.arctan(opt.center / opt.focal) * 180 / np.pi
- self.renderer = MeshRenderer(
- rasterize_fov=fov, znear=opt.z_near, zfar=opt.z_far, rasterize_size=int(2 * opt.center)
- )
-
- if self.isTrain:
- self.loss_names = ['all', 'feat', 'color', 'lm', 'reg', 'gamma', 'reflc']
-
- self.net_recog = networks.define_net_recog(
- net_recog=opt.net_recog, pretrained_path=opt.net_recog_path
- )
- # loss func name: (compute_%s_loss) % loss_name
- self.compute_feat_loss = perceptual_loss
- self.comupte_color_loss = photo_loss
- self.compute_lm_loss = landmark_loss
- self.compute_reg_loss = reg_loss
- self.compute_reflc_loss = reflectance_loss
-
- self.optimizer = torch.optim.Adam(self.net_recon.parameters(), lr=opt.lr)
- self.optimizers = [self.optimizer]
- self.parallel_names += ['net_recog']
- # Our program will automatically call to define schedulers, load networks, and print networks
-
- def set_input(self, input):
- """Unpack input data from the dataloader and perform necessary pre-processing steps.
-
- Parameters:
- input: a dictionary that contains the data itself and its metadata information.
- """
- self.input_img = input['imgs'].to(self.device)
- self.atten_mask = input['msks'].to(self.device) if 'msks' in input else None
- self.gt_lm = input['lms'].to(self.device) if 'lms' in input else None
- self.trans_m = input['M'].to(self.device) if 'M' in input else None
- self.image_paths = input['im_paths'] if 'im_paths' in input else None
-
- def forward(self, output_coeff, device):
- self.facemodel.to(device)
- self.pred_vertex, self.pred_tex, self.pred_color, self.pred_lm = \
- self.facemodel.compute_for_render(output_coeff)
- self.pred_mask, _, self.pred_face = self.renderer(
- self.pred_vertex, self.facemodel.face_buf, feat=self.pred_color)
-
- self.pred_coeffs_dict = self.facemodel.split_coeff(output_coeff)
-
-
- def compute_losses(self):
- """Calculate losses, gradients, and update network weights; called in every training iteration"""
-
- assert self.net_recog.training == False
- trans_m = self.trans_m
- if not self.opt.use_predef_M:
- trans_m = estimate_norm_torch(self.pred_lm, self.input_img.shape[-2])
-
- pred_feat = self.net_recog(self.pred_face, trans_m)
- gt_feat = self.net_recog(self.input_img, self.trans_m)
- self.loss_feat = self.opt.w_feat * self.compute_feat_loss(pred_feat, gt_feat)
-
- face_mask = self.pred_mask
- if self.opt.use_crop_face:
- face_mask, _, _ = self.renderer(self.pred_vertex, self.facemodel.front_face_buf)
-
- face_mask = face_mask.detach()
- self.loss_color = self.opt.w_color * self.comupte_color_loss(
- self.pred_face, self.input_img, self.atten_mask * face_mask)
-
- loss_reg, loss_gamma = self.compute_reg_loss(self.pred_coeffs_dict, self.opt)
- self.loss_reg = self.opt.w_reg * loss_reg
- self.loss_gamma = self.opt.w_gamma * loss_gamma
-
- self.loss_lm = self.opt.w_lm * self.compute_lm_loss(self.pred_lm, self.gt_lm)
-
- self.loss_reflc = self.opt.w_reflc * self.compute_reflc_loss(self.pred_tex, self.facemodel.skin_mask)
-
- self.loss_all = self.loss_feat + self.loss_color + self.loss_reg + self.loss_gamma \
- + self.loss_lm + self.loss_reflc
-
-
- def optimize_parameters(self, isTrain=True):
- self.forward()
- self.compute_losses()
- """Update network weights; it will be called in every training iteration."""
- if isTrain:
- self.optimizer.zero_grad()
- self.loss_all.backward()
- self.optimizer.step()
-
- def compute_visuals(self):
- with torch.no_grad():
- input_img_numpy = 255. * self.input_img.detach().cpu().permute(0, 2, 3, 1).numpy()
- output_vis = self.pred_face * self.pred_mask + (1 - self.pred_mask) * self.input_img
- output_vis_numpy_raw = 255. * output_vis.detach().cpu().permute(0, 2, 3, 1).numpy()
-
- if self.gt_lm is not None:
- gt_lm_numpy = self.gt_lm.cpu().numpy()
- pred_lm_numpy = self.pred_lm.detach().cpu().numpy()
- output_vis_numpy = util.draw_landmarks(output_vis_numpy_raw, gt_lm_numpy, 'b')
- output_vis_numpy = util.draw_landmarks(output_vis_numpy, pred_lm_numpy, 'r')
-
- output_vis_numpy = np.concatenate((input_img_numpy,
- output_vis_numpy_raw, output_vis_numpy), axis=-2)
- else:
- output_vis_numpy = np.concatenate((input_img_numpy,
- output_vis_numpy_raw), axis=-2)
-
- self.output_vis = torch.tensor(
- output_vis_numpy / 255., dtype=torch.float32
- ).permute(0, 3, 1, 2).to(self.device)
-
- def save_mesh(self, name):
-
- recon_shape = self.pred_vertex # get reconstructed shape
- recon_shape[..., -1] = 10 - recon_shape[..., -1] # from camera space to world space
- recon_shape = recon_shape.cpu().numpy()[0]
- recon_color = self.pred_color
- recon_color = recon_color.cpu().numpy()[0]
- tri = self.facemodel.face_buf.cpu().numpy()
- mesh = trimesh.Trimesh(vertices=recon_shape, faces=tri, vertex_colors=np.clip(255. * recon_color, 0, 255).astype(np.uint8))
- mesh.export(name)
-
- def save_coeff(self,name):
-
- pred_coeffs = {key:self.pred_coeffs_dict[key].cpu().numpy() for key in self.pred_coeffs_dict}
- pred_lm = self.pred_lm.cpu().numpy()
- pred_lm = np.stack([pred_lm[:,:,0],self.input_img.shape[2]-1-pred_lm[:,:,1]],axis=2) # transfer to image coordinate
- pred_coeffs['lm68'] = pred_lm
- savemat(name,pred_coeffs)
-
-
-
diff --git a/spaces/feregVcuzo/sanity-test-midi/checkpoint/Black Border Patrol Simulator APK A Simulation Game with Casual and Story Modes.md b/spaces/feregVcuzo/sanity-test-midi/checkpoint/Black Border Patrol Simulator APK A Simulation Game with Casual and Story Modes.md
deleted file mode 100644
index 6b147d3c391e894ed679fa097284cee16d2b11ed..0000000000000000000000000000000000000000
--- a/spaces/feregVcuzo/sanity-test-midi/checkpoint/Black Border Patrol Simulator APK A Simulation Game with Casual and Story Modes.md
+++ /dev/null
@@ -1,120 +0,0 @@
-
-How to Download Black Border Patrol Simulator APK for Android
-Do you want to experience what it's like to be a border police officer? Do you want to check the passengers' papers, stop the smuggling of illegal items, and arrest criminals? If yes, then you should try playing Black Border Patrol Simulator, a realistic and immersive border cop simulator game for Android devices. In this article, we will tell you what is Black Border Patrol Simulator, why you should play it, and how to download it on your Android device. We will also give you some tips and tricks for playing the game effectively and efficiently. So, let's get started!
- What is Black Border Patrol Simulator?
-Black Border Patrol Simulator is a border cop simulator game that simulates the life of a real border patrol officer. In this game, you assume the role of a police officer who works at the entry and exit gates of the country. Your job is to check the passengers' papers, use your tools and devices to detect illegal items and activities, and arrest suspicious people like terrorists, smugglers, or criminals. You have to follow the rules and regulations of your country while performing your duty. You also have to deal with different scenarios and situations that test your decision-making skills, logic, and ethics.
-download black border patrol simulator apk
DOWNLOAD >>>>> https://gohhs.com/2uPtkd
-Black Border Patrol Simulator has many features that make it an interesting and engaging game. Some of them are:
-
-- Casual and story modes: You can play the game in casual mode where you can check random passengers' papers or in story mode where you can follow a storyline with different missions and objectives.
-- Character customization: You can create and customize your own character by choosing various hairstyles, clothes, accessories, etc. You can also share your creation on social media.
-- Different languages: You can play the game in different languages such as English, Arabic, German, Spanish, Polish, Japanese, etc.
-- Intuitive controls: You can easily control the game with simple taps and swipes on your screen.
-- Graphics and sound effects: You can enjoy the realistic graphics and sound effects of the game that create an immersive atmosphere.
-
- Why You Should Play Black Border Patrol Simulator?
-Black Border Patrol Simulator is not only a fun and entertaining game but also a educational Black Border Patrol Simulator is not only a fun and entertaining game but also a educational and informative one. By playing this game, you can learn a lot about border security and immigration issues that are relevant in today's world. You can also improve your decision-making skills, logic, and ethics by dealing with different scenarios and situations that challenge your judgment. Moreover, you can have fun and relax by creating your own character, exploring different locations, and arresting bad guys. Black Border Patrol Simulator is a game that will keep you hooked for hours and make you feel like a real border cop.
- How to Download Black Border Patrol Simulator APK for Android?
-If you are interested in playing Black Border Patrol Simulator on your Android device, you might be wondering how to download it. The game is not available on Google Play Store, so you have to download it from another source. This means that you have to download the APK file of the game, which is a file format that contains the installation package of an Android app. However, downloading and installing APK files from unknown sources can be risky, as they might contain malware or viruses that can harm your device. Therefore, you have to be careful and follow some steps to ensure that you download the game safely and securely. Here are the steps that you need to follow:
- Step 1: Enable Unknown Sources on Your Device
-The first step is to allow your device to install apps from sources other than Google Play Store. To do this, you have to enable the Unknown Sources option on your device's settings. Depending on your device model and Android version, the steps might vary slightly, but generally, they are as follows:
-
-- Go to your device's Settings and tap on Security or Privacy.
-- Find the Unknown Sources option and toggle it on.
-- A warning message will pop up, telling you that installing apps from unknown sources can be harmful. Tap on OK to confirm.
-
-Now, your device is ready to install apps from sources other than Google Play Store.
-black border patrol simulator game apk
-black border patrol simulator android apk
-black border patrol simulator apk free download
-black border patrol simulator apk latest version
-black border patrol simulator apk mod
-black border patrol simulator apk offline
-black border patrol simulator apk update
-black border patrol simulator apk full version
-black border patrol simulator apk hack
-black border patrol simulator apk unlimited money
-black border patrol simulator apk obb
-black border patrol simulator apk data
-black border patrol simulator apk revdl
-black border patrol simulator apk rexdl
-black border patrol simulator apk pure
-black border patrol simulator apk mirror
-black border patrol simulator apk uptodown
-black border patrol simulator apk apkpure
-black border patrol simulator apk apkmirror
-black border patrol simulator apk apknite
-black border patrol simulator apk apkmody
-black border patrol simulator apk happymod
-black border patrol simulator apk an1
-black border patrol simulator apk android 1
-black border patrol simulator apk android oyun club
-download black border patrol sim demo apk
-download black border cop game apk
-download black border police officer sim apk
-download black border checkpoint duty game apk
-download black border immigration officer sim apk
-download black border customs officer game apk
-download black border passport control game apk
-download black border security guard game apk
-download black border police station game apk
-download black border crime stopper game apk
-download bitzooma game studio's black border patrol sim apk
-download com.bitzooma.blackborder.apk
-download com.bitzooma.blackborderdemo.apk
-how to download and install black border patrol sim on android
-how to play and enjoy the new features of the latest version of the game
- Step 2: Download the APK File from a Trusted Source
-The next step is to find and download the APK file of Black Border Patrol Simulator from a reliable website. There are many websites that offer APK files of various apps and games, but not all of them are trustworthy. Some of them might provide fake or corrupted files that can damage your device or steal your data. Therefore, you have to be careful and choose a website that has a good reputation and positive reviews from other users. One such website is [APKPure], which is a popular and trusted source for downloading APK files of various apps and games. To download the APK file of Black Border Patrol Simulator from APKPure, follow these steps:
-
-- Open your browser and go to [APKPure].
-- In the search bar, type Black Border Patrol Simulator and hit enter.
-- From the search results, find the game and tap on it.
-- On the game's page, tap on the Download APK button.
-- A pop-up window will appear, asking you to choose a download location. Choose a folder where you want to save the file and tap on OK.
-- The download will start automatically and might take a few minutes depending on your internet speed.
-
-Now, you have downloaded the APK file of Black Border Patrol Simulator on your device.
- Step 3: Install the APK File on Your Device
-The final step is to locate and install the APK file on your device using a file manager app. A file manager app is an app that allows you to access and manage the files and folders on your device. There are many file manager apps available on Google Play Store, such as [ES File Explorer], [File Manager], or [Files by Google]. You can use any of them or any other file manager app that you prefer. To install the APK file of Black Border Patrol Simulator using a file manager app, follow these steps:
-
-- Open your file manager app and go to the folder where you saved the APK file.
-- Find the APK file of Black Border Patrol Simulator and tap on it.
-- A pop-up window will appear, asking you to confirm the installation. Tap on Install.
-- The installation will start automatically and might take a few seconds depending on your device's performance.
-- Once the installation is complete, tap on Open to launch the game or Done to exit.
-
-Congratulations! You have successfully installed Black Border Patrol Simulator on your Android device.
- Step 4: Launch the Game and Enjoy Step 4: Launch the Game and Enjoy
-Now that you have installed Black Border Patrol Simulator on your Android device, you can launch the game and enjoy it. To do this, you can either tap on the game's icon on your home screen or app drawer, or go to the file manager app and tap on the game's APK file. The game will start and you will see the main menu, where you can choose between casual mode and story mode. You can also customize your character, change the language, adjust the settings, and access other features. Once you are ready, you can start playing the game and check the passengers' papers, use your tools and devices, and arrest suspicious people. You can also earn coins and rewards, unlock new items and locations, and complete different missions and objectives. Have fun and learn something new!
- Tips and Tricks for Playing Black Border Patrol Simulator
-Black Border Patrol Simulator is a game that requires your attention, concentration, and skills. It is not an easy game, as you have to deal with various challenges and difficulties while performing your duty. Therefore, you might need some tips and tricks to help you play the game effectively and efficiently. Here are some of them:
- Tip 1: Check All the Papers Carefully
-One of the most important tasks in the game is to check the passengers' papers, such as passports, visas, tickets, etc. You have to make sure that they are valid, authentic, and match the passengers' identities. You have to look for any discrepancies or errors in the papers, such as expired dates, wrong names, missing stamps, etc. You also have to compare the papers with the information on your computer screen and verify that they are correct. If you find any mistake or inconsistency in the papers, you have to reject or detain the passenger. If you let a passenger with invalid or fake papers pass through, you will lose points and reputation.
- Tip 2: Use All Your Tools and Devices
-Another important task in the game is to use your tools and devices to detect illegal items and activities. You have various tools and devices at your disposal, such as scanner, frisker, camera, etc. You have to use them wisely and effectively to scan the passengers' luggage, body, face, etc. You have to look for any prohibited or suspicious items or activities, such as weapons, drugs, explosives, etc. You also have to check if the passengers are wanted by the law or involved in any criminal activity. If you find any illegal item or activity, you have to confiscate it or arrest the passenger. If you miss any illegal item or activity, you will lose points and reputation.
- Tip 3: Be Alert and Vigilant
-A third important task in the game is to be alert and vigilant while performing your duty. You have to pay attention to every detail and every situation that occurs at the border. You have to identify suspicious passengers who might try to deceive you or cause trouble. You have to watch out for any signs of nervousness, aggression, or dishonesty in their behavior or speech. You also have to be prepared for any unexpected events or emergencies that might happen at the border. For example, you might encounter a terrorist attack, a riot, a fire, etc. You have to react quickly and appropriately to these situations and maintain order and security.
- Tip 4: Follow the Rules and Regulations
-A fourth important task in the game is to follow the rules and regulations of your country while performing your duty. You have to respect the law and the protocol of your job as a border cop. You have to be fair and impartial while checking the passengers' papers and detecting illegal items and activities. You also have to be polite and professional while interacting with the passengers and your colleagues. You have to avoid breaking the law or violating the protocol while performing your duty. For example, you should not accept bribes, abuse your power, discriminate against anyone, etc. If you break the law or violate the protocol, you will face consequences such as fines, penalties, suspension, or dismissal.
- Tip 5: Have Fun and Learn Something New
-A fifth important task in the game is to have fun and learn something new while playing it. Black Border Patrol Simulator is a game that offers you a lot of entertainment A fifth important task in the game is to have fun and learn something new while playing it. Black Border Patrol Simulator is a game that offers you a lot of entertainment and education. You can enjoy the realistic and immersive graphics and sound effects of the game that create a border cop atmosphere. You can also learn something new about border security and immigration issues that are relevant in today's world. You can also improve your decision-making skills, logic, and ethics by dealing with different scenarios and situations that challenge your judgment. Black Border Patrol Simulator is a game that will keep you hooked for hours and make you feel like a real border cop.
- Conclusion
-Black Border Patrol Simulator is a border cop simulator game that simulates the life of a real border patrol officer. In this game, you assume the role of a police officer who works at the entry and exit gates of the country. Your job is to check the passengers' papers, use your tools and devices to detect illegal items and activities, and arrest suspicious people like terrorists, smugglers, or criminals. You have to follow the rules and regulations of your country while performing your duty. You also have to deal with different scenarios and situations that test your decision-making skills, logic, and ethics.
-In this article, we have told you what is Black Border Patrol Simulator, why you should play it, and how to download it on your Android device. We have also given you some tips and tricks for playing the game effectively and efficiently. We hope that you have found this article helpful and informative. If you are interested in playing Black Border Patrol Simulator on your Android device, you can follow the steps that we have provided above and download the game safely and securely. You can also share your feedback and opinions about the game with us in the comments section below. Thank you for reading and happy gaming!
- FAQs
-Here are some frequently asked questions about Black Border Patrol Simulator:
-
-- Q: Is Black Border Patrol Simulator free to play?
-- A: Yes, Black Border Patrol Simulator is free to play. However, it contains ads and in-app purchases that can enhance your gaming experience.
-- Q: Is Black Border Patrol Simulator compatible with all Android devices?
-- A: No, Black Border Patrol Simulator requires Android 4.4 or higher to run smoothly. It also requires at least 100 MB of free storage space on your device.
-- Q: Is Black Border Patrol Simulator safe to download?
-- A: Yes, Black Border Patrol Simulator is safe to download if you download it from a trusted source like APKPure. However, you should always scan the APK file with an antivirus app before installing it on your device.
-- Q: How can I contact the developers of Black Border Patrol Simulator?
-- A: You can contact the developers of Black Border Patrol Simulator by sending them an email at [blackborderpatrolsimulator@gmail.com] or by visiting their website at [blackborderpatrolsimulator.com].
-- Q: How can I support the developers of Black Border Patrol Simulator?
-- A: You can support the developers of Black Border Patrol Simulator by rating and reviewing the game on APKPure or Google Play Store, by sharing the game with your friends and family, or by making in-app purchases.
- 197e85843d
-
-
\ No newline at end of file
diff --git a/spaces/feregVcuzo/sanity-test-midi/checkpoint/Download Knives Out PC Version (32 Bit) and Dive into the 6400m6400m Arena.md b/spaces/feregVcuzo/sanity-test-midi/checkpoint/Download Knives Out PC Version (32 Bit) and Dive into the 6400m6400m Arena.md
deleted file mode 100644
index c5d4d430f6e038ffa3bd8655dc82cb2de3adb560..0000000000000000000000000000000000000000
--- a/spaces/feregVcuzo/sanity-test-midi/checkpoint/Download Knives Out PC Version (32 Bit) and Dive into the 6400m6400m Arena.md
+++ /dev/null
@@ -1,134 +0,0 @@
-
-Knives Out PC Download 32 Bit: How to Play the Battle Royale Game on Your Computer
- If you are a fan of battle royale games, you might have heard of Knives Out, a popular mobile game that pits 100 players against each other on a large island. The game is developed by Netease Games, the same company behind Rules of Survival and Cyber Hunter. Knives Out offers a variety of modes, weapons, vehicles, and customization options for you to enjoy.
- But did you know that you can also play Knives Out on your PC? Yes, you read that right. You can enjoy the thrill of the game on a bigger screen and with better controls. In this article, we will show you how to download Knives Out PC version for 32 bit systems using three different methods. We will also give you some tips and tricks for playing the game on your computer. Let's get started!
-knives out pc download 32 bit
Download Zip ->>> https://gohhs.com/2uPpvN
- What is Knives Out?
- Knives Out is a battle royale game that was released in 2017 by Netease Games. The game is similar to PlayerUnknown's Battlegrounds (PUBG), but with some unique features and twists. The game has over 100 million downloads on Google Play Store and App Store, and has won several awards, such as the Google Play Best of 2017.
- The game allows you to choose from different modes, such as solo, duo, squad, or fireteam. You can also join special events, such as sniper mode, melee mode, or zombie mode. You can customize your character with different outfits, accessories, and skins. You can also collect and upgrade various weapons, such as rifles, shotguns, pistols, grenades, and more. You can also drive different vehicles, such as cars, motorcycles, boats, and helicopters.
- The game has a realistic graphics style and a smooth gameplay. The game also supports voice chat and text chat with your teammates or friends. You can also join clans and compete with other players in rankings and tournaments.
- Why play Knives Out on PC?
- While Knives Out is designed for mobile devices, playing it on PC has some advantages. Here are some of them:
-
-- You can enjoy a larger and clearer view of the game on your PC screen.
-- You can use your keyboard and mouse to control your character more precisely and comfortably.
-- You can avoid battery drain, overheating, or lag issues that might occur on your mobile device.
-- You can record or stream your gameplay more easily using your PC software.
-
- Of course, playing Knives Out on PC also has some drawbacks, such as compatibility issues, network problems, or account bans. Therefore, you should always follow the official guidelines and rules of the game when playing it on PC.
- How to download Knives Out PC version?
- There are three main methods to download Knives Out PC version for 32 bit systems. We will explain each method in detail below.
-knives out pc version download windows 10
-knives out pc game free download full version
-knives out pc client download english
-knives out pc download official website
-knives out pc download apk
-knives out pc download nox
-knives out pc download steam
-knives out pc download utorrent
-knives out pc download bluestacks
-knives out pc download highly compressed
-knives out pc download without emulator
-knives out pc download with qr code
-knives out pc download netease games
-knives out pc download wilderness action
-knives out pc download for mac
-knives out pc download size
-knives out pc download latest version
-knives out pc download offline installer
-knives out pc download softonic
-knives out pc download ocean of games
-knives out pc download requirements
-knives out pc download error
-knives out pc download hack
-knives out pc download cheat engine
-knives out pc download mod menu
-knives out pc download aimbot
-knives out pc download esp
-knives out pc download wallhack
-knives out pc download speed hack
-knives out pc download unlimited ammo
-knives out pc download gameplay
-knives out pc download review
-knives out pc download tips and tricks
-knives out pc download best settings
-knives out pc download graphics comparison
-knives out pc download controller support
-knives out pc download keyboard and mouse
-knives out pc download custom key mapping
-knives out pc download voice chat
-knives out pc download discord server
-knives out pc download update patch notes
-knives out pc download new map and weapons
-knives out pc download season pass and rewards
-knives out pc download skins and outfits
-knives out pc download how to play with friends
-knives out pc download how to change server region
-knives out pc download how to rank up fast
-knives out pc download how to get free coins and vouchers
-knives out pc download how to report hackers and cheaters
- Method 1: Using the official PC launcher by Netease Games
- This is the most recommended method to play Knives Out on PC, as it is provided by the official developer of the game. However, this method requires you to have a mobile device (or an Android emulator) to scan a QR code every time you want to play the game on PC. Here are the steps to follow:
-
-- Download and install the Knives Out PC launcher from the official website: https://www.knivesout.jp/pc/
-- Launch the PC launcher and click on the QR code icon on the top right corner.
-- Open the Knives Out app on your mobile device (or emulator) and tap on the PC icon on the bottom left corner.
-- Scan the QR code displayed on your PC screen with your mobile device (or emulator) camera.
-- Wait for a few seconds until the connection is established and the game starts loading on your PC.
-- Enjoy playing Knives Out on PC!
-
- Note: You can adjust the graphics, sound, and control settings of the game on your PC launcher. You can also switch between windowed and full-screen mode by pressing F11.
- Method 2: Using iMyFone MirrorTo to mirror your device to PC
- This method allows you to mirror your mobile device screen to your PC and control it with your mouse and keyboard. This way, you can play Knives Out on PC without downloading any additional software. However, this method requires you to have a stable Wi-Fi connection and a compatible device. Here are the steps to follow:
-
-- Download and install iMyFone MirrorTo on your PC from the official website: https://www.imyfone.com/mirrorto/
-- Launch iMyFone MirrorTo on your PC and click on "Start Now".
-- Connect your mobile device and your PC to the same Wi-Fi network.
-- For iOS devices, swipe up from the bottom of your screen and tap on "Screen Mirroring". Then, select "iMyFone MirrorTo" from the list of available devices.
-- For Android devices, enable USB debugging mode on your device settings. Then, connect your device to your PC with a USB cable. Follow the instructions on your PC screen to install a driver and enable mirroring.
-- Once your device screen is mirrored to your PC, open the Knives Out app on your device.
-- Click on the keyboard icon on the bottom right corner of iMyFone MirrorTo to customize your keyboard and mouse controls for the game.
-- Enjoy playing Knives Out on PC!
-
- Note: You can also record or capture your gameplay using iMyFone MirrorTo. You can also adjust the resolution, quality, and orientation of the mirrored screen.
- Method 3: Using BlueStacks emulator to run the game on PC
- This method allows you to run Knives Out as an Android app on your PC using an emulator. This way, you can play Knives Out on PC without scanning any QR code or mirroring any device. However, this method requires you to have a powerful PC and a Google account. Here are the steps to follow:
-
-- Download and install BlueStacks emulator on your PC from the official website: https://www.bluestacks.com/
-- Launch BlueStacks emulator and sign in with your Google account.
-- Go to Google Play Store and search for Knives Out. Then, download and install the game.
-- Open Knives Out app from BlueStacks home screen or app drawer.
-- Enjoy playing Knives Out on PC!
-
- Note: You can adjust the graphics, sound, and control settings of the game on BlueStacks emulator. You can also switch between windowed and full-screen mode by pressing F11.
- Tips and tricks for playing Knives Out on PC
- To make your gameplay more enjoyable and successful, here are some tips and tricks for playing Knives Out on PC:
-
-- Choose a suitable mode according to your preference and skill level. Solo mode is more challenging but rewarding, while squad mode is more fun but chaotic.
-- Loot as much as you can in the early game, but avoid hotspots where many players might land. Look for weapons, ammo, armor, health kits, and other items that can help you survive.
-- Use vehicles wisely. They can help you move faster and escape danger, but they also make noise and attract attention. Be careful when driving or riding them.
-- Use cover and stealth. Hide behind trees, rocks, buildings, or bushes when possible. Crouch or prone when shooting or looting. Avoid unnecessary movement or firing. Use silencers or suppressors to reduce your sound.
-- Use the map and the compass. The map shows you the safe zone, the danger zone, and the airdrops. The compass shows you the direction and the distance of the enemy gunfire. Use them to plan your strategy and position.
-- Use the voice chat and text chat. Communicate with your teammates or friends using the voice chat or text chat feature. Coordinate your actions, share information, and support each other.
-- Practice and learn. The best way to improve your skills and win more matches is to practice and learn from your mistakes. Watch replays, tutorials, or streams of other players. Learn from their tips, tricks, and strategies.
-
- Conclusion
- Knives Out is a fun and exciting battle royale game that you can play on your mobile device or on your PC. Playing it on PC has some advantages, such as a bigger screen and better controls. However, it also has some drawbacks, such as compatibility issues or account bans. Therefore, you should always follow the official guidelines and rules of the game when playing it on PC.
- In this article, we have shown you how to download Knives Out PC version for 32 bit systems using three different methods: using the official PC launcher by Netease Games, using iMyFone MirrorTo to mirror your device to PC, and using BlueStacks emulator to run the game on PC. We have also given you some tips and tricks for playing Knives Out on PC.
- We hope you have found this article helpful and informative. If you have any questions or feedback, please feel free to leave a comment below. Thank you for reading and happy gaming!
- FAQs
- Here are some frequently asked questions about Knives Out PC version:
-
-- Is Knives Out PC version free?
-Yes, Knives Out PC version is free to download and play. However, you might need to purchase some in-game items or features with real money.
-- Is Knives Out PC version safe?
-Yes, Knives Out PC version is safe to download and play, as long as you use the official sources and methods. However, you should always be careful of malware, viruses, or scams that might harm your PC or account.
-- Is Knives Out PC version compatible with Windows 10?
-Yes, Knives Out PC version is compatible with Windows 10, as well as Windows 7 and Windows 8.
-- Can I play Knives Out PC version with my friends who play on mobile devices?
-Yes, you can play Knives Out PC version with your friends who play on mobile devices. However, you might have some disadvantages or advantages compared to them, such as graphics quality or control accuracy.
-- Can I transfer my progress and data from mobile devices to PC?
-Yes, you can transfer your progress and data from mobile devices to PC by logging in with the same account. However, you might need to scan a QR code every time you want to play on PC using the official PC launcher method.
- 401be4b1e0
-
-
\ No newline at end of file
diff --git a/spaces/firatozdemir/OAGen_Linear/app_streamlit.py b/spaces/firatozdemir/OAGen_Linear/app_streamlit.py
deleted file mode 100644
index 0c953a704546c2c01c795f8db8ed009dd6bed89f..0000000000000000000000000000000000000000
--- a/spaces/firatozdemir/OAGen_Linear/app_streamlit.py
+++ /dev/null
@@ -1,12 +0,0 @@
-import os, glob, sys
-import pickle
-import streamlit as st
-import utils
-
-in_gpu = False
-num_images = 1
-G = utils.load_default_gen(in_gpu=in_gpu)
-sampler = utils.SampleFromGAN(G=G, z_shp=[num_images, G.z_dim], in_gpu=in_gpu)
-button_on_click = utils.Plot(im_gen=sampler)
-button_gen_clicked = st.button(label='Generate an image', key='n', on_click=button_on_click)
-
diff --git a/spaces/georgefen/Face-Landmark-ControlNet/annotator/uniformer/mmcv/fileio/file_client.py b/spaces/georgefen/Face-Landmark-ControlNet/annotator/uniformer/mmcv/fileio/file_client.py
deleted file mode 100644
index 950f0c1aeab14b8e308a7455ccd64a95b5d98add..0000000000000000000000000000000000000000
--- a/spaces/georgefen/Face-Landmark-ControlNet/annotator/uniformer/mmcv/fileio/file_client.py
+++ /dev/null
@@ -1,1148 +0,0 @@
-# Copyright (c) OpenMMLab. All rights reserved.
-import inspect
-import os
-import os.path as osp
-import re
-import tempfile
-import warnings
-from abc import ABCMeta, abstractmethod
-from contextlib import contextmanager
-from pathlib import Path
-from typing import Iterable, Iterator, Optional, Tuple, Union
-from urllib.request import urlopen
-
-import annotator.uniformer.mmcv as mmcv
-from annotator.uniformer.mmcv.utils.misc import has_method
-from annotator.uniformer.mmcv.utils.path import is_filepath
-
-
-class BaseStorageBackend(metaclass=ABCMeta):
- """Abstract class of storage backends.
-
- All backends need to implement two apis: ``get()`` and ``get_text()``.
- ``get()`` reads the file as a byte stream and ``get_text()`` reads the file
- as texts.
- """
-
- # a flag to indicate whether the backend can create a symlink for a file
- _allow_symlink = False
-
- @property
- def name(self):
- return self.__class__.__name__
-
- @property
- def allow_symlink(self):
- return self._allow_symlink
-
- @abstractmethod
- def get(self, filepath):
- pass
-
- @abstractmethod
- def get_text(self, filepath):
- pass
-
-
-class CephBackend(BaseStorageBackend):
- """Ceph storage backend (for internal use).
-
- Args:
- path_mapping (dict|None): path mapping dict from local path to Petrel
- path. When ``path_mapping={'src': 'dst'}``, ``src`` in ``filepath``
- will be replaced by ``dst``. Default: None.
-
- .. warning::
- :class:`mmcv.fileio.file_client.CephBackend` will be deprecated,
- please use :class:`mmcv.fileio.file_client.PetrelBackend` instead.
- """
-
- def __init__(self, path_mapping=None):
- try:
- import ceph
- except ImportError:
- raise ImportError('Please install ceph to enable CephBackend.')
-
- warnings.warn(
- 'CephBackend will be deprecated, please use PetrelBackend instead')
- self._client = ceph.S3Client()
- assert isinstance(path_mapping, dict) or path_mapping is None
- self.path_mapping = path_mapping
-
- def get(self, filepath):
- filepath = str(filepath)
- if self.path_mapping is not None:
- for k, v in self.path_mapping.items():
- filepath = filepath.replace(k, v)
- value = self._client.Get(filepath)
- value_buf = memoryview(value)
- return value_buf
-
- def get_text(self, filepath, encoding=None):
- raise NotImplementedError
-
-
-class PetrelBackend(BaseStorageBackend):
- """Petrel storage backend (for internal use).
-
- PetrelBackend supports reading and writing data to multiple clusters.
- If the file path contains the cluster name, PetrelBackend will read data
- from specified cluster or write data to it. Otherwise, PetrelBackend will
- access the default cluster.
-
- Args:
- path_mapping (dict, optional): Path mapping dict from local path to
- Petrel path. When ``path_mapping={'src': 'dst'}``, ``src`` in
- ``filepath`` will be replaced by ``dst``. Default: None.
- enable_mc (bool, optional): Whether to enable memcached support.
- Default: True.
-
- Examples:
- >>> filepath1 = 's3://path/of/file'
- >>> filepath2 = 'cluster-name:s3://path/of/file'
- >>> client = PetrelBackend()
- >>> client.get(filepath1) # get data from default cluster
- >>> client.get(filepath2) # get data from 'cluster-name' cluster
- """
-
- def __init__(self,
- path_mapping: Optional[dict] = None,
- enable_mc: bool = True):
- try:
- from petrel_client import client
- except ImportError:
- raise ImportError('Please install petrel_client to enable '
- 'PetrelBackend.')
-
- self._client = client.Client(enable_mc=enable_mc)
- assert isinstance(path_mapping, dict) or path_mapping is None
- self.path_mapping = path_mapping
-
- def _map_path(self, filepath: Union[str, Path]) -> str:
- """Map ``filepath`` to a string path whose prefix will be replaced by
- :attr:`self.path_mapping`.
-
- Args:
- filepath (str): Path to be mapped.
- """
- filepath = str(filepath)
- if self.path_mapping is not None:
- for k, v in self.path_mapping.items():
- filepath = filepath.replace(k, v)
- return filepath
-
- def _format_path(self, filepath: str) -> str:
- """Convert a ``filepath`` to standard format of petrel oss.
-
- If the ``filepath`` is concatenated by ``os.path.join``, in a Windows
- environment, the ``filepath`` will be the format of
- 's3://bucket_name\\image.jpg'. By invoking :meth:`_format_path`, the
- above ``filepath`` will be converted to 's3://bucket_name/image.jpg'.
-
- Args:
- filepath (str): Path to be formatted.
- """
- return re.sub(r'\\+', '/', filepath)
-
- def get(self, filepath: Union[str, Path]) -> memoryview:
- """Read data from a given ``filepath`` with 'rb' mode.
-
- Args:
- filepath (str or Path): Path to read data.
-
- Returns:
- memoryview: A memory view of expected bytes object to avoid
- copying. The memoryview object can be converted to bytes by
- ``value_buf.tobytes()``.
- """
- filepath = self._map_path(filepath)
- filepath = self._format_path(filepath)
- value = self._client.Get(filepath)
- value_buf = memoryview(value)
- return value_buf
-
- def get_text(self,
- filepath: Union[str, Path],
- encoding: str = 'utf-8') -> str:
- """Read data from a given ``filepath`` with 'r' mode.
-
- Args:
- filepath (str or Path): Path to read data.
- encoding (str): The encoding format used to open the ``filepath``.
- Default: 'utf-8'.
-
- Returns:
- str: Expected text reading from ``filepath``.
- """
- return str(self.get(filepath), encoding=encoding)
-
- def put(self, obj: bytes, filepath: Union[str, Path]) -> None:
- """Save data to a given ``filepath``.
-
- Args:
- obj (bytes): Data to be saved.
- filepath (str or Path): Path to write data.
- """
- filepath = self._map_path(filepath)
- filepath = self._format_path(filepath)
- self._client.put(filepath, obj)
-
- def put_text(self,
- obj: str,
- filepath: Union[str, Path],
- encoding: str = 'utf-8') -> None:
- """Save data to a given ``filepath``.
-
- Args:
- obj (str): Data to be written.
- filepath (str or Path): Path to write data.
- encoding (str): The encoding format used to encode the ``obj``.
- Default: 'utf-8'.
- """
- self.put(bytes(obj, encoding=encoding), filepath)
-
- def remove(self, filepath: Union[str, Path]) -> None:
- """Remove a file.
-
- Args:
- filepath (str or Path): Path to be removed.
- """
- if not has_method(self._client, 'delete'):
- raise NotImplementedError(
- ('Current version of Petrel Python SDK has not supported '
- 'the `delete` method, please use a higher version or dev'
- ' branch instead.'))
-
- filepath = self._map_path(filepath)
- filepath = self._format_path(filepath)
- self._client.delete(filepath)
-
- def exists(self, filepath: Union[str, Path]) -> bool:
- """Check whether a file path exists.
-
- Args:
- filepath (str or Path): Path to be checked whether exists.
-
- Returns:
- bool: Return ``True`` if ``filepath`` exists, ``False`` otherwise.
- """
- if not (has_method(self._client, 'contains')
- and has_method(self._client, 'isdir')):
- raise NotImplementedError(
- ('Current version of Petrel Python SDK has not supported '
- 'the `contains` and `isdir` methods, please use a higher'
- 'version or dev branch instead.'))
-
- filepath = self._map_path(filepath)
- filepath = self._format_path(filepath)
- return self._client.contains(filepath) or self._client.isdir(filepath)
-
- def isdir(self, filepath: Union[str, Path]) -> bool:
- """Check whether a file path is a directory.
-
- Args:
- filepath (str or Path): Path to be checked whether it is a
- directory.
-
- Returns:
- bool: Return ``True`` if ``filepath`` points to a directory,
- ``False`` otherwise.
- """
- if not has_method(self._client, 'isdir'):
- raise NotImplementedError(
- ('Current version of Petrel Python SDK has not supported '
- 'the `isdir` method, please use a higher version or dev'
- ' branch instead.'))
-
- filepath = self._map_path(filepath)
- filepath = self._format_path(filepath)
- return self._client.isdir(filepath)
-
- def isfile(self, filepath: Union[str, Path]) -> bool:
- """Check whether a file path is a file.
-
- Args:
- filepath (str or Path): Path to be checked whether it is a file.
-
- Returns:
- bool: Return ``True`` if ``filepath`` points to a file, ``False``
- otherwise.
- """
- if not has_method(self._client, 'contains'):
- raise NotImplementedError(
- ('Current version of Petrel Python SDK has not supported '
- 'the `contains` method, please use a higher version or '
- 'dev branch instead.'))
-
- filepath = self._map_path(filepath)
- filepath = self._format_path(filepath)
- return self._client.contains(filepath)
-
- def join_path(self, filepath: Union[str, Path],
- *filepaths: Union[str, Path]) -> str:
- """Concatenate all file paths.
-
- Args:
- filepath (str or Path): Path to be concatenated.
-
- Returns:
- str: The result after concatenation.
- """
- filepath = self._format_path(self._map_path(filepath))
- if filepath.endswith('/'):
- filepath = filepath[:-1]
- formatted_paths = [filepath]
- for path in filepaths:
- formatted_paths.append(self._format_path(self._map_path(path)))
- return '/'.join(formatted_paths)
-
- @contextmanager
- def get_local_path(self, filepath: Union[str, Path]) -> Iterable[str]:
- """Download a file from ``filepath`` and return a temporary path.
-
- ``get_local_path`` is decorated by :meth:`contxtlib.contextmanager`. It
- can be called with ``with`` statement, and when exists from the
- ``with`` statement, the temporary path will be released.
-
- Args:
- filepath (str | Path): Download a file from ``filepath``.
-
- Examples:
- >>> client = PetrelBackend()
- >>> # After existing from the ``with`` clause,
- >>> # the path will be removed
- >>> with client.get_local_path('s3://path/of/your/file') as path:
- ... # do something here
-
- Yields:
- Iterable[str]: Only yield one temporary path.
- """
- filepath = self._map_path(filepath)
- filepath = self._format_path(filepath)
- assert self.isfile(filepath)
- try:
- f = tempfile.NamedTemporaryFile(delete=False)
- f.write(self.get(filepath))
- f.close()
- yield f.name
- finally:
- os.remove(f.name)
-
- def list_dir_or_file(self,
- dir_path: Union[str, Path],
- list_dir: bool = True,
- list_file: bool = True,
- suffix: Optional[Union[str, Tuple[str]]] = None,
- recursive: bool = False) -> Iterator[str]:
- """Scan a directory to find the interested directories or files in
- arbitrary order.
-
- Note:
- Petrel has no concept of directories but it simulates the directory
- hierarchy in the filesystem through public prefixes. In addition,
- if the returned path ends with '/', it means the path is a public
- prefix which is a logical directory.
-
- Note:
- :meth:`list_dir_or_file` returns the path relative to ``dir_path``.
- In addition, the returned path of directory will not contains the
- suffix '/' which is consistent with other backends.
-
- Args:
- dir_path (str | Path): Path of the directory.
- list_dir (bool): List the directories. Default: True.
- list_file (bool): List the path of files. Default: True.
- suffix (str or tuple[str], optional): File suffix
- that we are interested in. Default: None.
- recursive (bool): If set to True, recursively scan the
- directory. Default: False.
-
- Yields:
- Iterable[str]: A relative path to ``dir_path``.
- """
- if not has_method(self._client, 'list'):
- raise NotImplementedError(
- ('Current version of Petrel Python SDK has not supported '
- 'the `list` method, please use a higher version or dev'
- ' branch instead.'))
-
- dir_path = self._map_path(dir_path)
- dir_path = self._format_path(dir_path)
- if list_dir and suffix is not None:
- raise TypeError(
- '`list_dir` should be False when `suffix` is not None')
-
- if (suffix is not None) and not isinstance(suffix, (str, tuple)):
- raise TypeError('`suffix` must be a string or tuple of strings')
-
- # Petrel's simulated directory hierarchy assumes that directory paths
- # should end with `/`
- if not dir_path.endswith('/'):
- dir_path += '/'
-
- root = dir_path
-
- def _list_dir_or_file(dir_path, list_dir, list_file, suffix,
- recursive):
- for path in self._client.list(dir_path):
- # the `self.isdir` is not used here to determine whether path
- # is a directory, because `self.isdir` relies on
- # `self._client.list`
- if path.endswith('/'): # a directory path
- next_dir_path = self.join_path(dir_path, path)
- if list_dir:
- # get the relative path and exclude the last
- # character '/'
- rel_dir = next_dir_path[len(root):-1]
- yield rel_dir
- if recursive:
- yield from _list_dir_or_file(next_dir_path, list_dir,
- list_file, suffix,
- recursive)
- else: # a file path
- absolute_path = self.join_path(dir_path, path)
- rel_path = absolute_path[len(root):]
- if (suffix is None
- or rel_path.endswith(suffix)) and list_file:
- yield rel_path
-
- return _list_dir_or_file(dir_path, list_dir, list_file, suffix,
- recursive)
-
-
-class MemcachedBackend(BaseStorageBackend):
- """Memcached storage backend.
-
- Attributes:
- server_list_cfg (str): Config file for memcached server list.
- client_cfg (str): Config file for memcached client.
- sys_path (str | None): Additional path to be appended to `sys.path`.
- Default: None.
- """
-
- def __init__(self, server_list_cfg, client_cfg, sys_path=None):
- if sys_path is not None:
- import sys
- sys.path.append(sys_path)
- try:
- import mc
- except ImportError:
- raise ImportError(
- 'Please install memcached to enable MemcachedBackend.')
-
- self.server_list_cfg = server_list_cfg
- self.client_cfg = client_cfg
- self._client = mc.MemcachedClient.GetInstance(self.server_list_cfg,
- self.client_cfg)
- # mc.pyvector servers as a point which points to a memory cache
- self._mc_buffer = mc.pyvector()
-
- def get(self, filepath):
- filepath = str(filepath)
- import mc
- self._client.Get(filepath, self._mc_buffer)
- value_buf = mc.ConvertBuffer(self._mc_buffer)
- return value_buf
-
- def get_text(self, filepath, encoding=None):
- raise NotImplementedError
-
-
-class LmdbBackend(BaseStorageBackend):
- """Lmdb storage backend.
-
- Args:
- db_path (str): Lmdb database path.
- readonly (bool, optional): Lmdb environment parameter. If True,
- disallow any write operations. Default: True.
- lock (bool, optional): Lmdb environment parameter. If False, when
- concurrent access occurs, do not lock the database. Default: False.
- readahead (bool, optional): Lmdb environment parameter. If False,
- disable the OS filesystem readahead mechanism, which may improve
- random read performance when a database is larger than RAM.
- Default: False.
-
- Attributes:
- db_path (str): Lmdb database path.
- """
-
- def __init__(self,
- db_path,
- readonly=True,
- lock=False,
- readahead=False,
- **kwargs):
- try:
- import lmdb
- except ImportError:
- raise ImportError('Please install lmdb to enable LmdbBackend.')
-
- self.db_path = str(db_path)
- self._client = lmdb.open(
- self.db_path,
- readonly=readonly,
- lock=lock,
- readahead=readahead,
- **kwargs)
-
- def get(self, filepath):
- """Get values according to the filepath.
-
- Args:
- filepath (str | obj:`Path`): Here, filepath is the lmdb key.
- """
- filepath = str(filepath)
- with self._client.begin(write=False) as txn:
- value_buf = txn.get(filepath.encode('ascii'))
- return value_buf
-
- def get_text(self, filepath, encoding=None):
- raise NotImplementedError
-
-
-class HardDiskBackend(BaseStorageBackend):
- """Raw hard disks storage backend."""
-
- _allow_symlink = True
-
- def get(self, filepath: Union[str, Path]) -> bytes:
- """Read data from a given ``filepath`` with 'rb' mode.
-
- Args:
- filepath (str or Path): Path to read data.
-
- Returns:
- bytes: Expected bytes object.
- """
- with open(filepath, 'rb') as f:
- value_buf = f.read()
- return value_buf
-
- def get_text(self,
- filepath: Union[str, Path],
- encoding: str = 'utf-8') -> str:
- """Read data from a given ``filepath`` with 'r' mode.
-
- Args:
- filepath (str or Path): Path to read data.
- encoding (str): The encoding format used to open the ``filepath``.
- Default: 'utf-8'.
-
- Returns:
- str: Expected text reading from ``filepath``.
- """
- with open(filepath, 'r', encoding=encoding) as f:
- value_buf = f.read()
- return value_buf
-
- def put(self, obj: bytes, filepath: Union[str, Path]) -> None:
- """Write data to a given ``filepath`` with 'wb' mode.
-
- Note:
- ``put`` will create a directory if the directory of ``filepath``
- does not exist.
-
- Args:
- obj (bytes): Data to be written.
- filepath (str or Path): Path to write data.
- """
- mmcv.mkdir_or_exist(osp.dirname(filepath))
- with open(filepath, 'wb') as f:
- f.write(obj)
-
- def put_text(self,
- obj: str,
- filepath: Union[str, Path],
- encoding: str = 'utf-8') -> None:
- """Write data to a given ``filepath`` with 'w' mode.
-
- Note:
- ``put_text`` will create a directory if the directory of
- ``filepath`` does not exist.
-
- Args:
- obj (str): Data to be written.
- filepath (str or Path): Path to write data.
- encoding (str): The encoding format used to open the ``filepath``.
- Default: 'utf-8'.
- """
- mmcv.mkdir_or_exist(osp.dirname(filepath))
- with open(filepath, 'w', encoding=encoding) as f:
- f.write(obj)
-
- def remove(self, filepath: Union[str, Path]) -> None:
- """Remove a file.
-
- Args:
- filepath (str or Path): Path to be removed.
- """
- os.remove(filepath)
-
- def exists(self, filepath: Union[str, Path]) -> bool:
- """Check whether a file path exists.
-
- Args:
- filepath (str or Path): Path to be checked whether exists.
-
- Returns:
- bool: Return ``True`` if ``filepath`` exists, ``False`` otherwise.
- """
- return osp.exists(filepath)
-
- def isdir(self, filepath: Union[str, Path]) -> bool:
- """Check whether a file path is a directory.
-
- Args:
- filepath (str or Path): Path to be checked whether it is a
- directory.
-
- Returns:
- bool: Return ``True`` if ``filepath`` points to a directory,
- ``False`` otherwise.
- """
- return osp.isdir(filepath)
-
- def isfile(self, filepath: Union[str, Path]) -> bool:
- """Check whether a file path is a file.
-
- Args:
- filepath (str or Path): Path to be checked whether it is a file.
-
- Returns:
- bool: Return ``True`` if ``filepath`` points to a file, ``False``
- otherwise.
- """
- return osp.isfile(filepath)
-
- def join_path(self, filepath: Union[str, Path],
- *filepaths: Union[str, Path]) -> str:
- """Concatenate all file paths.
-
- Join one or more filepath components intelligently. The return value
- is the concatenation of filepath and any members of *filepaths.
-
- Args:
- filepath (str or Path): Path to be concatenated.
-
- Returns:
- str: The result of concatenation.
- """
- return osp.join(filepath, *filepaths)
-
- @contextmanager
- def get_local_path(
- self, filepath: Union[str, Path]) -> Iterable[Union[str, Path]]:
- """Only for unified API and do nothing."""
- yield filepath
-
- def list_dir_or_file(self,
- dir_path: Union[str, Path],
- list_dir: bool = True,
- list_file: bool = True,
- suffix: Optional[Union[str, Tuple[str]]] = None,
- recursive: bool = False) -> Iterator[str]:
- """Scan a directory to find the interested directories or files in
- arbitrary order.
-
- Note:
- :meth:`list_dir_or_file` returns the path relative to ``dir_path``.
-
- Args:
- dir_path (str | Path): Path of the directory.
- list_dir (bool): List the directories. Default: True.
- list_file (bool): List the path of files. Default: True.
- suffix (str or tuple[str], optional): File suffix
- that we are interested in. Default: None.
- recursive (bool): If set to True, recursively scan the
- directory. Default: False.
-
- Yields:
- Iterable[str]: A relative path to ``dir_path``.
- """
- if list_dir and suffix is not None:
- raise TypeError('`suffix` should be None when `list_dir` is True')
-
- if (suffix is not None) and not isinstance(suffix, (str, tuple)):
- raise TypeError('`suffix` must be a string or tuple of strings')
-
- root = dir_path
-
- def _list_dir_or_file(dir_path, list_dir, list_file, suffix,
- recursive):
- for entry in os.scandir(dir_path):
- if not entry.name.startswith('.') and entry.is_file():
- rel_path = osp.relpath(entry.path, root)
- if (suffix is None
- or rel_path.endswith(suffix)) and list_file:
- yield rel_path
- elif osp.isdir(entry.path):
- if list_dir:
- rel_dir = osp.relpath(entry.path, root)
- yield rel_dir
- if recursive:
- yield from _list_dir_or_file(entry.path, list_dir,
- list_file, suffix,
- recursive)
-
- return _list_dir_or_file(dir_path, list_dir, list_file, suffix,
- recursive)
-
-
-class HTTPBackend(BaseStorageBackend):
- """HTTP and HTTPS storage bachend."""
-
- def get(self, filepath):
- value_buf = urlopen(filepath).read()
- return value_buf
-
- def get_text(self, filepath, encoding='utf-8'):
- value_buf = urlopen(filepath).read()
- return value_buf.decode(encoding)
-
- @contextmanager
- def get_local_path(self, filepath: str) -> Iterable[str]:
- """Download a file from ``filepath``.
-
- ``get_local_path`` is decorated by :meth:`contxtlib.contextmanager`. It
- can be called with ``with`` statement, and when exists from the
- ``with`` statement, the temporary path will be released.
-
- Args:
- filepath (str): Download a file from ``filepath``.
-
- Examples:
- >>> client = HTTPBackend()
- >>> # After existing from the ``with`` clause,
- >>> # the path will be removed
- >>> with client.get_local_path('http://path/of/your/file') as path:
- ... # do something here
- """
- try:
- f = tempfile.NamedTemporaryFile(delete=False)
- f.write(self.get(filepath))
- f.close()
- yield f.name
- finally:
- os.remove(f.name)
-
-
-class FileClient:
- """A general file client to access files in different backends.
-
- The client loads a file or text in a specified backend from its path
- and returns it as a binary or text file. There are two ways to choose a
- backend, the name of backend and the prefix of path. Although both of them
- can be used to choose a storage backend, ``backend`` has a higher priority
- that is if they are all set, the storage backend will be chosen by the
- backend argument. If they are all `None`, the disk backend will be chosen.
- Note that It can also register other backend accessor with a given name,
- prefixes, and backend class. In addition, We use the singleton pattern to
- avoid repeated object creation. If the arguments are the same, the same
- object will be returned.
-
- Args:
- backend (str, optional): The storage backend type. Options are "disk",
- "ceph", "memcached", "lmdb", "http" and "petrel". Default: None.
- prefix (str, optional): The prefix of the registered storage backend.
- Options are "s3", "http", "https". Default: None.
-
- Examples:
- >>> # only set backend
- >>> file_client = FileClient(backend='petrel')
- >>> # only set prefix
- >>> file_client = FileClient(prefix='s3')
- >>> # set both backend and prefix but use backend to choose client
- >>> file_client = FileClient(backend='petrel', prefix='s3')
- >>> # if the arguments are the same, the same object is returned
- >>> file_client1 = FileClient(backend='petrel')
- >>> file_client1 is file_client
- True
-
- Attributes:
- client (:obj:`BaseStorageBackend`): The backend object.
- """
-
- _backends = {
- 'disk': HardDiskBackend,
- 'ceph': CephBackend,
- 'memcached': MemcachedBackend,
- 'lmdb': LmdbBackend,
- 'petrel': PetrelBackend,
- 'http': HTTPBackend,
- }
- # This collection is used to record the overridden backends, and when a
- # backend appears in the collection, the singleton pattern is disabled for
- # that backend, because if the singleton pattern is used, then the object
- # returned will be the backend before overwriting
- _overridden_backends = set()
- _prefix_to_backends = {
- 's3': PetrelBackend,
- 'http': HTTPBackend,
- 'https': HTTPBackend,
- }
- _overridden_prefixes = set()
-
- _instances = {}
-
- def __new__(cls, backend=None, prefix=None, **kwargs):
- if backend is None and prefix is None:
- backend = 'disk'
- if backend is not None and backend not in cls._backends:
- raise ValueError(
- f'Backend {backend} is not supported. Currently supported ones'
- f' are {list(cls._backends.keys())}')
- if prefix is not None and prefix not in cls._prefix_to_backends:
- raise ValueError(
- f'prefix {prefix} is not supported. Currently supported ones '
- f'are {list(cls._prefix_to_backends.keys())}')
-
- # concatenate the arguments to a unique key for determining whether
- # objects with the same arguments were created
- arg_key = f'{backend}:{prefix}'
- for key, value in kwargs.items():
- arg_key += f':{key}:{value}'
-
- # if a backend was overridden, it will create a new object
- if (arg_key in cls._instances
- and backend not in cls._overridden_backends
- and prefix not in cls._overridden_prefixes):
- _instance = cls._instances[arg_key]
- else:
- # create a new object and put it to _instance
- _instance = super().__new__(cls)
- if backend is not None:
- _instance.client = cls._backends[backend](**kwargs)
- else:
- _instance.client = cls._prefix_to_backends[prefix](**kwargs)
-
- cls._instances[arg_key] = _instance
-
- return _instance
-
- @property
- def name(self):
- return self.client.name
-
- @property
- def allow_symlink(self):
- return self.client.allow_symlink
-
- @staticmethod
- def parse_uri_prefix(uri: Union[str, Path]) -> Optional[str]:
- """Parse the prefix of a uri.
-
- Args:
- uri (str | Path): Uri to be parsed that contains the file prefix.
-
- Examples:
- >>> FileClient.parse_uri_prefix('s3://path/of/your/file')
- 's3'
-
- Returns:
- str | None: Return the prefix of uri if the uri contains '://'
- else ``None``.
- """
- assert is_filepath(uri)
- uri = str(uri)
- if '://' not in uri:
- return None
- else:
- prefix, _ = uri.split('://')
- # In the case of PetrelBackend, the prefix may contains the cluster
- # name like clusterName:s3
- if ':' in prefix:
- _, prefix = prefix.split(':')
- return prefix
-
- @classmethod
- def infer_client(cls,
- file_client_args: Optional[dict] = None,
- uri: Optional[Union[str, Path]] = None) -> 'FileClient':
- """Infer a suitable file client based on the URI and arguments.
-
- Args:
- file_client_args (dict, optional): Arguments to instantiate a
- FileClient. Default: None.
- uri (str | Path, optional): Uri to be parsed that contains the file
- prefix. Default: None.
-
- Examples:
- >>> uri = 's3://path/of/your/file'
- >>> file_client = FileClient.infer_client(uri=uri)
- >>> file_client_args = {'backend': 'petrel'}
- >>> file_client = FileClient.infer_client(file_client_args)
-
- Returns:
- FileClient: Instantiated FileClient object.
- """
- assert file_client_args is not None or uri is not None
- if file_client_args is None:
- file_prefix = cls.parse_uri_prefix(uri) # type: ignore
- return cls(prefix=file_prefix)
- else:
- return cls(**file_client_args)
-
- @classmethod
- def _register_backend(cls, name, backend, force=False, prefixes=None):
- if not isinstance(name, str):
- raise TypeError('the backend name should be a string, '
- f'but got {type(name)}')
- if not inspect.isclass(backend):
- raise TypeError(
- f'backend should be a class but got {type(backend)}')
- if not issubclass(backend, BaseStorageBackend):
- raise TypeError(
- f'backend {backend} is not a subclass of BaseStorageBackend')
- if not force and name in cls._backends:
- raise KeyError(
- f'{name} is already registered as a storage backend, '
- 'add "force=True" if you want to override it')
-
- if name in cls._backends and force:
- cls._overridden_backends.add(name)
- cls._backends[name] = backend
-
- if prefixes is not None:
- if isinstance(prefixes, str):
- prefixes = [prefixes]
- else:
- assert isinstance(prefixes, (list, tuple))
- for prefix in prefixes:
- if prefix not in cls._prefix_to_backends:
- cls._prefix_to_backends[prefix] = backend
- elif (prefix in cls._prefix_to_backends) and force:
- cls._overridden_prefixes.add(prefix)
- cls._prefix_to_backends[prefix] = backend
- else:
- raise KeyError(
- f'{prefix} is already registered as a storage backend,'
- ' add "force=True" if you want to override it')
-
- @classmethod
- def register_backend(cls, name, backend=None, force=False, prefixes=None):
- """Register a backend to FileClient.
-
- This method can be used as a normal class method or a decorator.
-
- .. code-block:: python
-
- class NewBackend(BaseStorageBackend):
-
- def get(self, filepath):
- return filepath
-
- def get_text(self, filepath):
- return filepath
-
- FileClient.register_backend('new', NewBackend)
-
- or
-
- .. code-block:: python
-
- @FileClient.register_backend('new')
- class NewBackend(BaseStorageBackend):
-
- def get(self, filepath):
- return filepath
-
- def get_text(self, filepath):
- return filepath
-
- Args:
- name (str): The name of the registered backend.
- backend (class, optional): The backend class to be registered,
- which must be a subclass of :class:`BaseStorageBackend`.
- When this method is used as a decorator, backend is None.
- Defaults to None.
- force (bool, optional): Whether to override the backend if the name
- has already been registered. Defaults to False.
- prefixes (str or list[str] or tuple[str], optional): The prefixes
- of the registered storage backend. Default: None.
- `New in version 1.3.15.`
- """
- if backend is not None:
- cls._register_backend(
- name, backend, force=force, prefixes=prefixes)
- return
-
- def _register(backend_cls):
- cls._register_backend(
- name, backend_cls, force=force, prefixes=prefixes)
- return backend_cls
-
- return _register
-
- def get(self, filepath: Union[str, Path]) -> Union[bytes, memoryview]:
- """Read data from a given ``filepath`` with 'rb' mode.
-
- Note:
- There are two types of return values for ``get``, one is ``bytes``
- and the other is ``memoryview``. The advantage of using memoryview
- is that you can avoid copying, and if you want to convert it to
- ``bytes``, you can use ``.tobytes()``.
-
- Args:
- filepath (str or Path): Path to read data.
-
- Returns:
- bytes | memoryview: Expected bytes object or a memory view of the
- bytes object.
- """
- return self.client.get(filepath)
-
- def get_text(self, filepath: Union[str, Path], encoding='utf-8') -> str:
- """Read data from a given ``filepath`` with 'r' mode.
-
- Args:
- filepath (str or Path): Path to read data.
- encoding (str): The encoding format used to open the ``filepath``.
- Default: 'utf-8'.
-
- Returns:
- str: Expected text reading from ``filepath``.
- """
- return self.client.get_text(filepath, encoding)
-
- def put(self, obj: bytes, filepath: Union[str, Path]) -> None:
- """Write data to a given ``filepath`` with 'wb' mode.
-
- Note:
- ``put`` should create a directory if the directory of ``filepath``
- does not exist.
-
- Args:
- obj (bytes): Data to be written.
- filepath (str or Path): Path to write data.
- """
- self.client.put(obj, filepath)
-
- def put_text(self, obj: str, filepath: Union[str, Path]) -> None:
- """Write data to a given ``filepath`` with 'w' mode.
-
- Note:
- ``put_text`` should create a directory if the directory of
- ``filepath`` does not exist.
-
- Args:
- obj (str): Data to be written.
- filepath (str or Path): Path to write data.
- encoding (str, optional): The encoding format used to open the
- `filepath`. Default: 'utf-8'.
- """
- self.client.put_text(obj, filepath)
-
- def remove(self, filepath: Union[str, Path]) -> None:
- """Remove a file.
-
- Args:
- filepath (str, Path): Path to be removed.
- """
- self.client.remove(filepath)
-
- def exists(self, filepath: Union[str, Path]) -> bool:
- """Check whether a file path exists.
-
- Args:
- filepath (str or Path): Path to be checked whether exists.
-
- Returns:
- bool: Return ``True`` if ``filepath`` exists, ``False`` otherwise.
- """
- return self.client.exists(filepath)
-
- def isdir(self, filepath: Union[str, Path]) -> bool:
- """Check whether a file path is a directory.
-
- Args:
- filepath (str or Path): Path to be checked whether it is a
- directory.
-
- Returns:
- bool: Return ``True`` if ``filepath`` points to a directory,
- ``False`` otherwise.
- """
- return self.client.isdir(filepath)
-
- def isfile(self, filepath: Union[str, Path]) -> bool:
- """Check whether a file path is a file.
-
- Args:
- filepath (str or Path): Path to be checked whether it is a file.
-
- Returns:
- bool: Return ``True`` if ``filepath`` points to a file, ``False``
- otherwise.
- """
- return self.client.isfile(filepath)
-
- def join_path(self, filepath: Union[str, Path],
- *filepaths: Union[str, Path]) -> str:
- """Concatenate all file paths.
-
- Join one or more filepath components intelligently. The return value
- is the concatenation of filepath and any members of *filepaths.
-
- Args:
- filepath (str or Path): Path to be concatenated.
-
- Returns:
- str: The result of concatenation.
- """
- return self.client.join_path(filepath, *filepaths)
-
- @contextmanager
- def get_local_path(self, filepath: Union[str, Path]) -> Iterable[str]:
- """Download data from ``filepath`` and write the data to local path.
-
- ``get_local_path`` is decorated by :meth:`contxtlib.contextmanager`. It
- can be called with ``with`` statement, and when exists from the
- ``with`` statement, the temporary path will be released.
-
- Note:
- If the ``filepath`` is a local path, just return itself.
-
- .. warning::
- ``get_local_path`` is an experimental interface that may change in
- the future.
-
- Args:
- filepath (str or Path): Path to be read data.
-
- Examples:
- >>> file_client = FileClient(prefix='s3')
- >>> with file_client.get_local_path('s3://bucket/abc.jpg') as path:
- ... # do something here
-
- Yields:
- Iterable[str]: Only yield one path.
- """
- with self.client.get_local_path(str(filepath)) as local_path:
- yield local_path
-
- def list_dir_or_file(self,
- dir_path: Union[str, Path],
- list_dir: bool = True,
- list_file: bool = True,
- suffix: Optional[Union[str, Tuple[str]]] = None,
- recursive: bool = False) -> Iterator[str]:
- """Scan a directory to find the interested directories or files in
- arbitrary order.
-
- Note:
- :meth:`list_dir_or_file` returns the path relative to ``dir_path``.
-
- Args:
- dir_path (str | Path): Path of the directory.
- list_dir (bool): List the directories. Default: True.
- list_file (bool): List the path of files. Default: True.
- suffix (str or tuple[str], optional): File suffix
- that we are interested in. Default: None.
- recursive (bool): If set to True, recursively scan the
- directory. Default: False.
-
- Yields:
- Iterable[str]: A relative path to ``dir_path``.
- """
- yield from self.client.list_dir_or_file(dir_path, list_dir, list_file,
- suffix, recursive)
diff --git a/spaces/georgefen/Face-Landmark-ControlNet/annotator/uniformer/mmcv/ops/point_sample.py b/spaces/georgefen/Face-Landmark-ControlNet/annotator/uniformer/mmcv/ops/point_sample.py
deleted file mode 100644
index 267f4b3c56630acd85f9bdc630b7be09abab0aba..0000000000000000000000000000000000000000
--- a/spaces/georgefen/Face-Landmark-ControlNet/annotator/uniformer/mmcv/ops/point_sample.py
+++ /dev/null
@@ -1,336 +0,0 @@
-# Modified from https://github.com/facebookresearch/detectron2/tree/master/projects/PointRend # noqa
-
-from os import path as osp
-
-import torch
-import torch.nn as nn
-import torch.nn.functional as F
-from torch.nn.modules.utils import _pair
-from torch.onnx.operators import shape_as_tensor
-
-
-def bilinear_grid_sample(im, grid, align_corners=False):
- """Given an input and a flow-field grid, computes the output using input
- values and pixel locations from grid. Supported only bilinear interpolation
- method to sample the input pixels.
-
- Args:
- im (torch.Tensor): Input feature map, shape (N, C, H, W)
- grid (torch.Tensor): Point coordinates, shape (N, Hg, Wg, 2)
- align_corners {bool}: If set to True, the extrema (-1 and 1) are
- considered as referring to the center points of the input’s
- corner pixels. If set to False, they are instead considered as
- referring to the corner points of the input’s corner pixels,
- making the sampling more resolution agnostic.
- Returns:
- torch.Tensor: A tensor with sampled points, shape (N, C, Hg, Wg)
- """
- n, c, h, w = im.shape
- gn, gh, gw, _ = grid.shape
- assert n == gn
-
- x = grid[:, :, :, 0]
- y = grid[:, :, :, 1]
-
- if align_corners:
- x = ((x + 1) / 2) * (w - 1)
- y = ((y + 1) / 2) * (h - 1)
- else:
- x = ((x + 1) * w - 1) / 2
- y = ((y + 1) * h - 1) / 2
-
- x = x.view(n, -1)
- y = y.view(n, -1)
-
- x0 = torch.floor(x).long()
- y0 = torch.floor(y).long()
- x1 = x0 + 1
- y1 = y0 + 1
-
- wa = ((x1 - x) * (y1 - y)).unsqueeze(1)
- wb = ((x1 - x) * (y - y0)).unsqueeze(1)
- wc = ((x - x0) * (y1 - y)).unsqueeze(1)
- wd = ((x - x0) * (y - y0)).unsqueeze(1)
-
- # Apply default for grid_sample function zero padding
- im_padded = F.pad(im, pad=[1, 1, 1, 1], mode='constant', value=0)
- padded_h = h + 2
- padded_w = w + 2
- # save points positions after padding
- x0, x1, y0, y1 = x0 + 1, x1 + 1, y0 + 1, y1 + 1
-
- # Clip coordinates to padded image size
- x0 = torch.where(x0 < 0, torch.tensor(0), x0)
- x0 = torch.where(x0 > padded_w - 1, torch.tensor(padded_w - 1), x0)
- x1 = torch.where(x1 < 0, torch.tensor(0), x1)
- x1 = torch.where(x1 > padded_w - 1, torch.tensor(padded_w - 1), x1)
- y0 = torch.where(y0 < 0, torch.tensor(0), y0)
- y0 = torch.where(y0 > padded_h - 1, torch.tensor(padded_h - 1), y0)
- y1 = torch.where(y1 < 0, torch.tensor(0), y1)
- y1 = torch.where(y1 > padded_h - 1, torch.tensor(padded_h - 1), y1)
-
- im_padded = im_padded.view(n, c, -1)
-
- x0_y0 = (x0 + y0 * padded_w).unsqueeze(1).expand(-1, c, -1)
- x0_y1 = (x0 + y1 * padded_w).unsqueeze(1).expand(-1, c, -1)
- x1_y0 = (x1 + y0 * padded_w).unsqueeze(1).expand(-1, c, -1)
- x1_y1 = (x1 + y1 * padded_w).unsqueeze(1).expand(-1, c, -1)
-
- Ia = torch.gather(im_padded, 2, x0_y0)
- Ib = torch.gather(im_padded, 2, x0_y1)
- Ic = torch.gather(im_padded, 2, x1_y0)
- Id = torch.gather(im_padded, 2, x1_y1)
-
- return (Ia * wa + Ib * wb + Ic * wc + Id * wd).reshape(n, c, gh, gw)
-
-
-def is_in_onnx_export_without_custom_ops():
- from annotator.uniformer.mmcv.ops import get_onnxruntime_op_path
- ort_custom_op_path = get_onnxruntime_op_path()
- return torch.onnx.is_in_onnx_export(
- ) and not osp.exists(ort_custom_op_path)
-
-
-def normalize(grid):
- """Normalize input grid from [-1, 1] to [0, 1]
- Args:
- grid (Tensor): The grid to be normalize, range [-1, 1].
- Returns:
- Tensor: Normalized grid, range [0, 1].
- """
-
- return (grid + 1.0) / 2.0
-
-
-def denormalize(grid):
- """Denormalize input grid from range [0, 1] to [-1, 1]
- Args:
- grid (Tensor): The grid to be denormalize, range [0, 1].
- Returns:
- Tensor: Denormalized grid, range [-1, 1].
- """
-
- return grid * 2.0 - 1.0
-
-
-def generate_grid(num_grid, size, device):
- """Generate regular square grid of points in [0, 1] x [0, 1] coordinate
- space.
-
- Args:
- num_grid (int): The number of grids to sample, one for each region.
- size (tuple(int, int)): The side size of the regular grid.
- device (torch.device): Desired device of returned tensor.
-
- Returns:
- (torch.Tensor): A tensor of shape (num_grid, size[0]*size[1], 2) that
- contains coordinates for the regular grids.
- """
-
- affine_trans = torch.tensor([[[1., 0., 0.], [0., 1., 0.]]], device=device)
- grid = F.affine_grid(
- affine_trans, torch.Size((1, 1, *size)), align_corners=False)
- grid = normalize(grid)
- return grid.view(1, -1, 2).expand(num_grid, -1, -1)
-
-
-def rel_roi_point_to_abs_img_point(rois, rel_roi_points):
- """Convert roi based relative point coordinates to image based absolute
- point coordinates.
-
- Args:
- rois (Tensor): RoIs or BBoxes, shape (N, 4) or (N, 5)
- rel_roi_points (Tensor): Point coordinates inside RoI, relative to
- RoI, location, range (0, 1), shape (N, P, 2)
- Returns:
- Tensor: Image based absolute point coordinates, shape (N, P, 2)
- """
-
- with torch.no_grad():
- assert rel_roi_points.size(0) == rois.size(0)
- assert rois.dim() == 2
- assert rel_roi_points.dim() == 3
- assert rel_roi_points.size(2) == 2
- # remove batch idx
- if rois.size(1) == 5:
- rois = rois[:, 1:]
- abs_img_points = rel_roi_points.clone()
- # To avoid an error during exporting to onnx use independent
- # variables instead inplace computation
- xs = abs_img_points[:, :, 0] * (rois[:, None, 2] - rois[:, None, 0])
- ys = abs_img_points[:, :, 1] * (rois[:, None, 3] - rois[:, None, 1])
- xs += rois[:, None, 0]
- ys += rois[:, None, 1]
- abs_img_points = torch.stack([xs, ys], dim=2)
- return abs_img_points
-
-
-def get_shape_from_feature_map(x):
- """Get spatial resolution of input feature map considering exporting to
- onnx mode.
-
- Args:
- x (torch.Tensor): Input tensor, shape (N, C, H, W)
- Returns:
- torch.Tensor: Spatial resolution (width, height), shape (1, 1, 2)
- """
- if torch.onnx.is_in_onnx_export():
- img_shape = shape_as_tensor(x)[2:].flip(0).view(1, 1, 2).to(
- x.device).float()
- else:
- img_shape = torch.tensor(x.shape[2:]).flip(0).view(1, 1, 2).to(
- x.device).float()
- return img_shape
-
-
-def abs_img_point_to_rel_img_point(abs_img_points, img, spatial_scale=1.):
- """Convert image based absolute point coordinates to image based relative
- coordinates for sampling.
-
- Args:
- abs_img_points (Tensor): Image based absolute point coordinates,
- shape (N, P, 2)
- img (tuple/Tensor): (height, width) of image or feature map.
- spatial_scale (float): Scale points by this factor. Default: 1.
-
- Returns:
- Tensor: Image based relative point coordinates for sampling,
- shape (N, P, 2)
- """
-
- assert (isinstance(img, tuple) and len(img) == 2) or \
- (isinstance(img, torch.Tensor) and len(img.shape) == 4)
-
- if isinstance(img, tuple):
- h, w = img
- scale = torch.tensor([w, h],
- dtype=torch.float,
- device=abs_img_points.device)
- scale = scale.view(1, 1, 2)
- else:
- scale = get_shape_from_feature_map(img)
-
- return abs_img_points / scale * spatial_scale
-
-
-def rel_roi_point_to_rel_img_point(rois,
- rel_roi_points,
- img,
- spatial_scale=1.):
- """Convert roi based relative point coordinates to image based absolute
- point coordinates.
-
- Args:
- rois (Tensor): RoIs or BBoxes, shape (N, 4) or (N, 5)
- rel_roi_points (Tensor): Point coordinates inside RoI, relative to
- RoI, location, range (0, 1), shape (N, P, 2)
- img (tuple/Tensor): (height, width) of image or feature map.
- spatial_scale (float): Scale points by this factor. Default: 1.
-
- Returns:
- Tensor: Image based relative point coordinates for sampling,
- shape (N, P, 2)
- """
-
- abs_img_point = rel_roi_point_to_abs_img_point(rois, rel_roi_points)
- rel_img_point = abs_img_point_to_rel_img_point(abs_img_point, img,
- spatial_scale)
-
- return rel_img_point
-
-
-def point_sample(input, points, align_corners=False, **kwargs):
- """A wrapper around :func:`grid_sample` to support 3D point_coords tensors
- Unlike :func:`torch.nn.functional.grid_sample` it assumes point_coords to
- lie inside ``[0, 1] x [0, 1]`` square.
-
- Args:
- input (Tensor): Feature map, shape (N, C, H, W).
- points (Tensor): Image based absolute point coordinates (normalized),
- range [0, 1] x [0, 1], shape (N, P, 2) or (N, Hgrid, Wgrid, 2).
- align_corners (bool): Whether align_corners. Default: False
-
- Returns:
- Tensor: Features of `point` on `input`, shape (N, C, P) or
- (N, C, Hgrid, Wgrid).
- """
-
- add_dim = False
- if points.dim() == 3:
- add_dim = True
- points = points.unsqueeze(2)
- if is_in_onnx_export_without_custom_ops():
- # If custom ops for onnx runtime not compiled use python
- # implementation of grid_sample function to make onnx graph
- # with supported nodes
- output = bilinear_grid_sample(
- input, denormalize(points), align_corners=align_corners)
- else:
- output = F.grid_sample(
- input, denormalize(points), align_corners=align_corners, **kwargs)
- if add_dim:
- output = output.squeeze(3)
- return output
-
-
-class SimpleRoIAlign(nn.Module):
-
- def __init__(self, output_size, spatial_scale, aligned=True):
- """Simple RoI align in PointRend, faster than standard RoIAlign.
-
- Args:
- output_size (tuple[int]): h, w
- spatial_scale (float): scale the input boxes by this number
- aligned (bool): if False, use the legacy implementation in
- MMDetection, align_corners=True will be used in F.grid_sample.
- If True, align the results more perfectly.
- """
-
- super(SimpleRoIAlign, self).__init__()
- self.output_size = _pair(output_size)
- self.spatial_scale = float(spatial_scale)
- # to be consistent with other RoI ops
- self.use_torchvision = False
- self.aligned = aligned
-
- def forward(self, features, rois):
- num_imgs = features.size(0)
- num_rois = rois.size(0)
- rel_roi_points = generate_grid(
- num_rois, self.output_size, device=rois.device)
-
- if torch.onnx.is_in_onnx_export():
- rel_img_points = rel_roi_point_to_rel_img_point(
- rois, rel_roi_points, features, self.spatial_scale)
- rel_img_points = rel_img_points.reshape(num_imgs, -1,
- *rel_img_points.shape[1:])
- point_feats = point_sample(
- features, rel_img_points, align_corners=not self.aligned)
- point_feats = point_feats.transpose(1, 2)
- else:
- point_feats = []
- for batch_ind in range(num_imgs):
- # unravel batch dim
- feat = features[batch_ind].unsqueeze(0)
- inds = (rois[:, 0].long() == batch_ind)
- if inds.any():
- rel_img_points = rel_roi_point_to_rel_img_point(
- rois[inds], rel_roi_points[inds], feat,
- self.spatial_scale).unsqueeze(0)
- point_feat = point_sample(
- feat, rel_img_points, align_corners=not self.aligned)
- point_feat = point_feat.squeeze(0).transpose(0, 1)
- point_feats.append(point_feat)
-
- point_feats = torch.cat(point_feats, dim=0)
-
- channels = features.size(1)
- roi_feats = point_feats.reshape(num_rois, channels, *self.output_size)
-
- return roi_feats
-
- def __repr__(self):
- format_str = self.__class__.__name__
- format_str += '(output_size={}, spatial_scale={}'.format(
- self.output_size, self.spatial_scale)
- return format_str
diff --git a/spaces/goodeatmen/Test/README.md b/spaces/goodeatmen/Test/README.md
deleted file mode 100644
index 79d29b5ffbe9164567777039d81f4490a52a06da..0000000000000000000000000000000000000000
--- a/spaces/goodeatmen/Test/README.md
+++ /dev/null
@@ -1,10 +0,0 @@
----
-title: Test
-emoji: 📈
-colorFrom: blue
-colorTo: green
-sdk: docker
-pinned: false
----
-
-Check out the configuration reference at https://huggingface.co/docs/hub/spaces-config-reference
diff --git a/spaces/gordonchan/h2oo/loaders.py b/spaces/gordonchan/h2oo/loaders.py
deleted file mode 100644
index 18e360e2bdc45e7bddfc6f0e24d1e9099ae2f73c..0000000000000000000000000000000000000000
--- a/spaces/gordonchan/h2oo/loaders.py
+++ /dev/null
@@ -1,61 +0,0 @@
-import functools
-
-
-def get_loaders(model_name, reward_type, llama_type=None, load_gptq=''):
- # NOTE: Some models need specific new prompt_type
- # E.g. t5_xxl_true_nli_mixture has input format: "premise: PREMISE_TEXT hypothesis: HYPOTHESIS_TEXT".)
- if load_gptq:
- from transformers import AutoTokenizer
- from auto_gptq import AutoGPTQForCausalLM
- use_triton = False
- functools.partial(AutoGPTQForCausalLM.from_quantized, quantize_config=None, use_triton=use_triton)
- return AutoGPTQForCausalLM.from_quantized, AutoTokenizer
- if llama_type is None:
- llama_type = "llama" in model_name.lower()
- if llama_type:
- from transformers import LlamaForCausalLM, LlamaTokenizer
- return LlamaForCausalLM.from_pretrained, LlamaTokenizer
- elif 'distilgpt2' in model_name.lower():
- from transformers import AutoModelForCausalLM, AutoTokenizer
- return AutoModelForCausalLM.from_pretrained, AutoTokenizer
- elif 'gpt2' in model_name.lower():
- from transformers import GPT2LMHeadModel, GPT2Tokenizer
- return GPT2LMHeadModel.from_pretrained, GPT2Tokenizer
- elif 'mbart-' in model_name.lower():
- from transformers import MBartForConditionalGeneration, MBart50TokenizerFast
- return MBartForConditionalGeneration.from_pretrained, MBart50TokenizerFast
- elif 't5' == model_name.lower() or \
- 't5-' in model_name.lower() or \
- 'flan-' in model_name.lower():
- from transformers import AutoTokenizer, T5ForConditionalGeneration
- return T5ForConditionalGeneration.from_pretrained, AutoTokenizer
- elif 'bigbird' in model_name:
- from transformers import BigBirdPegasusForConditionalGeneration, AutoTokenizer
- return BigBirdPegasusForConditionalGeneration.from_pretrained, AutoTokenizer
- elif 'bart-large-cnn-samsum' in model_name or 'flan-t5-base-samsum' in model_name:
- from transformers import pipeline
- return pipeline, "summarization"
- elif reward_type or 'OpenAssistant/reward-model'.lower() in model_name.lower():
- from transformers import AutoModelForSequenceClassification, AutoTokenizer
- return AutoModelForSequenceClassification.from_pretrained, AutoTokenizer
- else:
- from transformers import AutoTokenizer, AutoModelForCausalLM
- model_loader = AutoModelForCausalLM
- tokenizer_loader = AutoTokenizer
- return model_loader.from_pretrained, tokenizer_loader
-
-
-def get_tokenizer(tokenizer_loader, tokenizer_base_model, local_files_only, resume_download, use_auth_token):
- tokenizer = tokenizer_loader.from_pretrained(tokenizer_base_model,
- local_files_only=local_files_only,
- resume_download=resume_download,
- use_auth_token=use_auth_token,
- padding_side='left')
-
- tokenizer.pad_token_id = 0 # different from the eos token
- # when generating, we will use the logits of right-most token to predict the next token
- # so the padding should be on the left,
- # e.g. see: https://huggingface.co/transformers/v4.11.3/model_doc/t5.html#inference
- tokenizer.padding_side = "left" # Allow batched inference
-
- return tokenizer
diff --git a/spaces/gotiQspiryo/whisper-ui/Vst Tone2 Gladiator Crack.md b/spaces/gotiQspiryo/whisper-ui/Vst Tone2 Gladiator Crack.md
deleted file mode 100644
index c4b4e8ad018f76dd1f14b39859460ac4ee8aa8ef..0000000000000000000000000000000000000000
--- a/spaces/gotiQspiryo/whisper-ui/Vst Tone2 Gladiator Crack.md
+++ /dev/null
@@ -1,162 +0,0 @@
-## Vst Tone2 Gladiator Crack
-
-
-
-
-
- 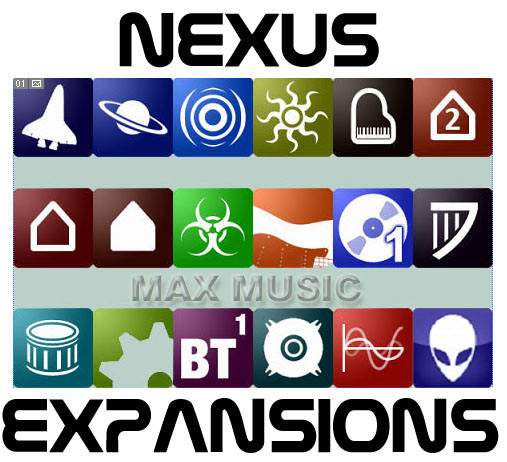
-
-
-
-
-
-**LINK ➡ [https://vercupalo.blogspot.com/?d=2txnEz](https://vercupalo.blogspot.com/?d=2txnEz)**
-
-
-
-
-
-
-
-
-
-
-
-
-
-# How to Download and Install Vst Tone2 Gladiator Crack for Free
-
-
-
-Vst Tone2 Gladiator is a powerful virtual synthesizer that supports a wide range of synthesis methods, such as Harmonic-Content-Morphing⢠(HCM), Frequency Modulation (FM), Pulse Width Modulation (PWM), and Subtractive[^2^]. It can create new and exciting sounds for your music production and composition. However, this plugin is not cheap and you may be looking for a way to download and install it for free. In this article, we will show you how to do that using a torrent file.
-
-
-
-## What is a Torrent File?
-
-
-
-A torrent file is a small file that contains information about a larger file that you want to download, such as Vst Tone2 Gladiator Crack. It does not contain the actual file, but it tells your torrent client where to find it and how to download it from other users who have it. A torrent client is a software that allows you to download and upload files using the BitTorrent protocol[^1^]. Some popular torrent clients are uTorrent, BitTorrent, and qBittorrent.
-
-
-
-## How to Download Vst Tone2 Gladiator Crack Torrent File?
-
-
-
-To download Vst Tone2 Gladiator Crack torrent file, you need to find a reliable website that offers it. One such website is vsttorrentz.net[^1^], which has a large collection of VST plugins and software. To download the torrent file from this website, follow these steps:
-
-
-
-1. Go to [https://vsttorrentz.net/tone2-gladiator-3-0-0-standalone-vsti-x86-x64/](https://vsttorrentz.net/tone2-gladiator-3-0-0-standalone-vsti-x86-x64/)
-
-2. Click on the "Download Torrent" button at the bottom of the page.
-
-3. Save the torrent file to your computer.
-
-
-
-## How to Install Vst Tone2 Gladiator Crack?
-
-
-
-To install Vst Tone2 Gladiator Crack, you need to have a torrent client installed on your computer. If you don't have one, you can download one from the links above. Once you have a torrent client, follow these steps:
-
-
-
-1. Open the torrent file with your torrent client.
-
-2. Select the destination folder where you want to save the Vst Tone2 Gladiator Crack files.
-
-3. Wait for the download to complete.
-
-4. Open the downloaded folder and run the setup.exe file.
-
-5. Follow the instructions on the screen to install Vst Tone2 Gladiator Crack.
-
-6. Enjoy your free virtual synthesizer!
-
-
-
-### Disclaimer
-
-
-
-This article is for educational purposes only. We do not condone or encourage piracy or illegal downloading of any software or plugin. Please support the developers of Vst Tone2 Gladiator by purchasing it from their official website[^2^].
-
-
-
-## What are the Features of Vst Tone2 Gladiator?
-
-
-
-Vst Tone2 Gladiator is not just a regular virtual synthesizer, but a revolution in synthesis. It offers a wide range of features that make it stand out from other plugins. Some of these features are:
-
-
-
-- Innovative, new synthesis method (HCMâ¢) that allows you to create unique sounds that no other synthesizer can produce.
-
-- Huge sonic range that covers everything from warm and smooth to crisp and edgy.
-
-- High-end quality that delivers warm, crystal clear and rich sound.
-
-- One of the most successful software synthesizers that has been used in many movies and chart hits.
-
-- Different synthesis methods that can be combined freely, such as FM, PWM, Subtractive, Vocoder, Waveshaping, Super-saw, Additive and more.
-
-- 40 filter types and 37 effects that are included, such as Reverbs, Delays, Flanger, Phaser, Chorus, Ensemble, Rotary, Bitcrusher, Talkbox, Ringmod, Dolby Prologic II surround encoding and more.
-
-- Four different interface sizes that you can choose from according to your preference.
-
-- Boundless possibilities that let you create any sound you can imagine with a powerful randomize-function and psychoacoustic processing.
-
-- Low CPU and high reliability that ensure a smooth performance and stability.
-
-- Over 1200 sounds from professional sound-designers that are ready to use or tweak.
-
-- Handbook in 5 languages that explains everything you need to know about the plugin.
-
-- Good value for money that gives you a lot of features and quality for a reasonable price.
-
-- Flexibility and expandability that allow you to customize and enhance your plugin with additional soundsets and expansions.
-
-
-
-## How to Use Vst Tone2 Gladiator?
-
-
-
-Vst Tone2 Gladiator is easy to use and intuitive. You can start by browsing through the presets and finding one that suits your needs. You can also use the search function to find a preset by name or category. You can then tweak the preset by adjusting the parameters on the interface or using the modulation matrix. You can also create your own sounds from scratch by choosing one of the synthesis methods and modifying the harmonic structure with the modules and algorithms. You can also layer up to four sounds together using the stack mode. You can then add some effects and filters to enhance your sound. You can also use the arpeggiator and trancegate to create rhythmic patterns and sequences. You can also use the microtuning feature to change the tuning system of your sound. You can also use the MIDI learn function to assign any parameter to your MIDI controller for easy control. You can also save your own presets and share them with others.
-
-
-
-### Tips and Tricks
-
-
-
-Here are some tips and tricks to help you get the most out of Vst Tone2 Gladiator:
-
-
-
-- Use the randomize-function to generate new sounds with a single click. You can also randomize specific sections or parameters by right-clicking on them.
-
-- Use the morph mode to smoothly transition between two sounds by moving the morph knob or using a MIDI controller.
-
-- Use the analog mode to add some warmth and character to your sound by emulating analog circuitry and components.
-
-- Use the psychoacoustic mode to enhance your sound with psychoacoustic processing that simulates how humans perceive sound.
-
-- Use the surround mode to create immersive spatial effects with Dolby Prologic II encoding.
-
-- Use the feedback mode to create complex feedback loops with different effects and filters.
-
-- Use the oscilloscope mode to visualize your sound waveforms in real-time.
-
-
-
- 1b8d091108
-
-
-
-
-
diff --git a/spaces/gradio/HuBERT/fairseq/distributed/fully_sharded_data_parallel.py b/spaces/gradio/HuBERT/fairseq/distributed/fully_sharded_data_parallel.py
deleted file mode 100644
index 8a96bfc76516682ac8e2b7e2c3bc2e6aa3d8ef0c..0000000000000000000000000000000000000000
--- a/spaces/gradio/HuBERT/fairseq/distributed/fully_sharded_data_parallel.py
+++ /dev/null
@@ -1,135 +0,0 @@
-# Copyright (c) Facebook, Inc. and its affiliates.
-#
-# This source code is licensed under the MIT license found in the
-# LICENSE file in the root directory of this source tree.
-
-import contextlib
-from typing import Optional
-
-import torch
-from fairseq.dataclass.configs import DistributedTrainingConfig
-from fairseq.distributed import utils as dist_utils
-
-
-try:
- from fairscale.nn.data_parallel import FullyShardedDataParallel as FSDP
-
- has_FSDP = True
-except ImportError:
- FSDP = torch.nn.Module
- has_FSDP = False
-
-
-class FullyShardedDataParallel(FSDP):
- """
- A small wrapper around fairscale's FullyShardedDataParallel (FSDP) with some
- fairseq-specific checkpoint saving/loading logic.
-
- Args:
- use_sharded_state (bool): if True, then ``state_dict`` will return
- ``FSDP.local_state_dict`` and ``load_state_dict`` will call
- ``FSDP.load_local_state_dict``. Otherwise, ``state_dict`` will
- return the full model weights on data parallel rank 0 (empty on
- other ranks) and ``load_state_dict`` will broadcast model weights
- from rank 0 to other ranks.
- """
-
- def __init__(self, *args, use_sharded_state: bool = False, **kwargs):
- if not has_FSDP:
- raise ImportError(
- "Cannot find FullyShardedDataParallel. "
- "Please install fairscale with: pip install fairscale"
- )
- super().__init__(*args, **kwargs)
- self.use_sharded_state = use_sharded_state
-
- @property
- def unwrapped_module(self) -> torch.nn.Module:
- if self.flatten_parameters:
- return self.module.module
- else:
- return self.module
-
- def state_dict(self, destination=None, prefix="", keep_vars=False):
- if self.use_sharded_state:
- return super().local_state_dict(
- destination=destination, prefix=prefix, keep_vars=keep_vars
- )
- else:
- if self.rank == 0:
- return super().state_dict(
- destination=destination, prefix=prefix, keep_vars=keep_vars
- )
- else:
- # We must call state_dict() due to use of communication
- # primitives. But we don't use the result.
- super().state_dict()
- return destination or {}
-
- def load_state_dict(self, state_dict, strict=True, model_cfg=None):
- if self.use_sharded_state:
- return super().load_local_state_dict(state_dict, strict=strict)
- else:
- state_dict = dist_utils.broadcast_object(
- state_dict, src_rank=0, group=self.process_group
- )
- return super().load_state_dict(state_dict, strict=strict)
-
-
-@contextlib.contextmanager
-def fsdp_enable_wrap(cfg: DistributedTrainingConfig):
- try:
- from fairscale.nn import enable_wrap
- except ImportError:
- raise ImportError(
- "Cannot find FullyShardedDataParallel. "
- "Please install fairscale with: pip install fairscale"
- )
- if cfg.memory_efficient_fp16:
- assert cfg.fp16 # memory_efficient_fp16 should imply fp16
- group = dist_utils.get_data_parallel_group()
- if group is None and cfg.distributed_world_size == 1:
- from fairscale.utils.testing import DummyProcessGroup
-
- group = DummyProcessGroup(rank=0, size=1)
- fsdp_config = {
- "process_group": group,
- "reshard_after_forward": not cfg.no_reshard_after_forward,
- "mixed_precision": cfg.fp16 and not cfg.memory_efficient_fp16,
- "fp32_reduce_scatter": cfg.fp32_reduce_scatter,
- "flatten_parameters": True,
- "cpu_offload": cfg.cpu_offload,
- "compute_dtype": torch.float16 if cfg.fp16 else torch.float32,
- "bucket_cap_mb": cfg.bucket_cap_mb,
- "state_dict_device": torch.device("cpu"), # reduce GPU mem usage
- }
- with enable_wrap(
- wrapper_cls=FullyShardedDataParallel,
- use_sharded_state=cfg.use_sharded_state,
- **fsdp_config,
- ):
- yield
-
-
-def fsdp_wrap(module, min_num_params: Optional[int] = None, **kwargs):
- """
- Helper to wrap layers/modules in FSDP. This falls back to a no-op if
- fairscale is not available.
-
- Args:
- module (nn.Module): module to (maybe) wrap
- min_num_params (int, Optional): minimum number of layer params to wrap
- """
- try:
- from fairscale.nn import wrap
-
- if min_num_params is not None:
- num_params = sum(p.numel() for p in module.parameters())
- if num_params >= min_num_params:
- return wrap(module, **kwargs)
- else:
- return module
- else:
- return wrap(module, **kwargs)
- except ImportError:
- return module
diff --git a/spaces/hahahafofo/ChatGLM-Chinese-Summary/ui/summary.py b/spaces/hahahafofo/ChatGLM-Chinese-Summary/ui/summary.py
deleted file mode 100644
index 66e607599c5db69dc451903e49f2649a4b37e276..0000000000000000000000000000000000000000
--- a/spaces/hahahafofo/ChatGLM-Chinese-Summary/ui/summary.py
+++ /dev/null
@@ -1,179 +0,0 @@
-import re
-
-import gradio as gr
-from typing import List
-from models import models
-from loguru import logger
-import re
-
-PROMPT_TEMPLATE = """\
-使用中文{query_str}:
-{context_str}
-"""
-
-
-def get_text_lines(input_txt: str) -> List[str]:
- lines = input_txt.splitlines()
- lines = [line.strip() for line in lines if line.strip()]
- return lines
-
-
-stop_chars_set = {
- '.', '!', '?', '。', '!', '?', '…', ';', ';', ':', ':',
- '”', '’', ')', '】', '》', '」', '』', '〕', '〉',
- '》', '〗', '〞', '〟', '»', '"', "'", ')', ']', '}'
-}
-
-
-def split_in_line(input_txt: str, limit_length: int) -> List[str]:
- new_text = ''
- contents = []
- outputs = []
- for text in input_txt:
- new_text += text
- if text in stop_chars_set:
- contents.append(new_text)
- # logger.debug(f"{new_text}")
- new_text = ''
- # logger.debug(f"{input_txt[-1]} {input_txt[-1] not in stop_chars_set} {new_text}")
- if input_txt[-1] not in stop_chars_set:
- contents.append(new_text)
-
- text = ""
- text_length = 0
- for idx, content in enumerate(contents):
- text += content
- text_length += len(content)
- if text_length >= limit_length:
- outputs.append(text)
- text = ""
- text_length = 0
- if text_length < limit_length:
- outputs.append(text)
- return outputs
-
-
-def get_text_limit_length(input_txt: str, max_length: int = 2048) -> List[str]:
- lines = get_text_lines(input_txt)
- output: List[str] = []
- for line in lines:
- if len(line) <= max_length:
- output.append(line)
- else:
- text_lines = split_in_line(line, max_length)
- logger.debug(f"split in line: {len(text_lines)}")
- # logger.debug(f"{line} ==> {text_lines}")
- output.extend(text_lines)
- return output
-
-
-def split_input_text(input_txt, strip_input_lines=0, max_length=2048):
- if strip_input_lines > 0:
- pattern = r'[\r\n]{' + str(strip_input_lines) + r',}'
- re.compile(pattern=pattern)
- logger.debug(f"strip input txt: {pattern}")
- input_txt = re.sub(pattern, '', input_txt)
- lines = get_text_limit_length(input_txt, max_length)
- logger.debug(f"split input txt: {len(lines)}")
- return "\n\n\n".join(lines)
-
-
-def gen_keyword_summary(input_txt, keyword_prompt, summary_prompt, max_length=2048):
- lines = input_txt.split("\n\n\n")
- keywords_output = []
- for line in lines:
- keywords = models.llm_model.generate_answer(
- keyword_prompt,
- line,
- history=None,
- max_length=max_length,
- prompt_template=PROMPT_TEMPLATE
- )[0]
- logger.debug(f"text len: {len(line)} ==> {keywords}")
- keywords_output.extend(keywords.split())
- keywords_output = [keyword.strip() for keyword in keywords_output if keyword.strip() != ""]
- keywords_output = list(set(keywords_output))
- return f"保留关键词:{' '.join(keywords_output)},{summary_prompt}"
-
-
-def gen_summary(input_txt, summary_prompt, max_length=2048):
- lines = input_txt.split("\n\n\n")
- output_summary = []
- summary = ""
- for idx, line in enumerate(lines):
- if idx == 1:
- summary = models.llm_model.generate_answer(
- summary_prompt,
- line,
- history=None,
- max_length=max_length,
- prompt_template=PROMPT_TEMPLATE
- )[0]
- logger.debug(f"text len: {len(line)} ==> {summary}")
- else:
- summary = models.llm_model.generate_answer(
- summary_prompt,
- f"{summary}{line}",
- history=None,
- max_length=max_length,
- prompt_template=PROMPT_TEMPLATE
- )[0]
- logger.debug(f"summary: {len(summary)} + text: {len(line)} ==> {summary}")
- output_summary.append(summary)
-
- return "\n\n\n".join(output_summary)
-
-
-def summary_ui():
- with gr.Row():
- with gr.Column(scale=1):
- line_max_length = gr.Slider(minimum=512, maximum=4096, step=1, value=1024, label="每行最大长度")
- strip_input_lines = gr.Slider(
- label="去除输入文本连续的空行(0:不除去)",
- minimum=1,
- maximum=10,
- step=1,
- value=0
- )
- with gr.Column(scale=4):
- keyword_prompt = gr.Textbox(
- lines=1,
- label="抽取关键词",
- value="抽取以下内容的人物和地点:",
- placeholder="请输入抽取关键词的Prompt"
- )
- summary_prompt = gr.Textbox(
- lines=2,
- label="生成摘要",
- value="生成以下内容的摘要:",
- placeholder="请输入生成摘要的Prompt"
- )
- keyword_summary_prompt = gr.Textbox(lines=4, label="关键词+摘要", placeholder="请输入关键词+摘要的Prompt")
-
- with gr.Row():
- input_text = gr.Textbox(lines=20, max_lines=60, label="输入文本", placeholder="请输入文本")
- split_text = gr.Textbox(lines=20, max_lines=60, label="分段文本", placeholder="请输入分段文本")
- summary = gr.Textbox(lines=20, max_lines=60, label="生成摘要", placeholder="请输入生成摘要的Prompt")
-
- with gr.Row():
- btn_split = gr.Button("分段")
- btn_keyword = gr.Button("提取关键词")
- btn_summary = gr.Button("生成摘要")
-
- btn_split.click(
- split_input_text,
- inputs=[input_text, strip_input_lines, line_max_length],
- outputs=[split_text]
- )
-
- btn_summary.click(
- gen_summary,
- inputs=[split_text, keyword_summary_prompt, line_max_length],
- outputs=[summary]
- )
-
- btn_keyword.click(
- gen_keyword_summary,
- inputs=[split_text, keyword_prompt, summary_prompt, line_max_length],
- outputs=[keyword_summary_prompt]
- )
diff --git a/spaces/haoqi7/research/lrt/utils/__init__.py b/spaces/haoqi7/research/lrt/utils/__init__.py
deleted file mode 100644
index 8eb66b345e2c966653a5aacf540d35dabe2ca4a9..0000000000000000000000000000000000000000
--- a/spaces/haoqi7/research/lrt/utils/__init__.py
+++ /dev/null
@@ -1,3 +0,0 @@
-from .functions import __create_model__
-from .union_find import UnionFind
-from .article import ArticleList, Article
\ No newline at end of file
diff --git a/spaces/hasibzunair/fifa-tryon-demo/Self-Correction-Human-Parsing-for-ACGPN/mhp_extension/detectron2/detectron2/data/datasets/coco.py b/spaces/hasibzunair/fifa-tryon-demo/Self-Correction-Human-Parsing-for-ACGPN/mhp_extension/detectron2/detectron2/data/datasets/coco.py
deleted file mode 100644
index f6f099e778e34cf89d267e13424d4f69240b7878..0000000000000000000000000000000000000000
--- a/spaces/hasibzunair/fifa-tryon-demo/Self-Correction-Human-Parsing-for-ACGPN/mhp_extension/detectron2/detectron2/data/datasets/coco.py
+++ /dev/null
@@ -1,466 +0,0 @@
-# Copyright (c) Facebook, Inc. and its affiliates. All Rights Reserved
-import contextlib
-import datetime
-import io
-import json
-import logging
-import numpy as np
-import os
-import pycocotools.mask as mask_util
-from fvcore.common.file_io import PathManager, file_lock
-from fvcore.common.timer import Timer
-from PIL import Image
-
-from detectron2.structures import Boxes, BoxMode, PolygonMasks
-
-from .. import DatasetCatalog, MetadataCatalog
-
-"""
-This file contains functions to parse COCO-format annotations into dicts in "Detectron2 format".
-"""
-
-
-logger = logging.getLogger(__name__)
-
-__all__ = ["load_coco_json", "load_sem_seg", "convert_to_coco_json"]
-
-
-def load_coco_json(json_file, image_root, dataset_name=None, extra_annotation_keys=None):
- """
- Load a json file with COCO's instances annotation format.
- Currently supports instance detection, instance segmentation,
- and person keypoints annotations.
-
- Args:
- json_file (str): full path to the json file in COCO instances annotation format.
- image_root (str or path-like): the directory where the images in this json file exists.
- dataset_name (str): the name of the dataset (e.g., coco_2017_train).
- If provided, this function will also put "thing_classes" into
- the metadata associated with this dataset.
- extra_annotation_keys (list[str]): list of per-annotation keys that should also be
- loaded into the dataset dict (besides "iscrowd", "bbox", "keypoints",
- "category_id", "segmentation"). The values for these keys will be returned as-is.
- For example, the densepose annotations are loaded in this way.
-
- Returns:
- list[dict]: a list of dicts in Detectron2 standard dataset dicts format. (See
- `Using Custom Datasets `_ )
-
- Notes:
- 1. This function does not read the image files.
- The results do not have the "image" field.
- """
- from pycocotools.coco import COCO
-
- timer = Timer()
- json_file = PathManager.get_local_path(json_file)
- with contextlib.redirect_stdout(io.StringIO()):
- coco_api = COCO(json_file)
- if timer.seconds() > 1:
- logger.info("Loading {} takes {:.2f} seconds.".format(json_file, timer.seconds()))
-
- id_map = None
- if dataset_name is not None:
- meta = MetadataCatalog.get(dataset_name)
- cat_ids = sorted(coco_api.getCatIds())
- cats = coco_api.loadCats(cat_ids)
- # The categories in a custom json file may not be sorted.
- thing_classes = [c["name"] for c in sorted(cats, key=lambda x: x["id"])]
- meta.thing_classes = thing_classes
-
- # In COCO, certain category ids are artificially removed,
- # and by convention they are always ignored.
- # We deal with COCO's id issue and translate
- # the category ids to contiguous ids in [0, 80).
-
- # It works by looking at the "categories" field in the json, therefore
- # if users' own json also have incontiguous ids, we'll
- # apply this mapping as well but print a warning.
- if not (min(cat_ids) == 1 and max(cat_ids) == len(cat_ids)):
- if "coco" not in dataset_name:
- logger.warning(
- """
-Category ids in annotations are not in [1, #categories]! We'll apply a mapping for you.
-"""
- )
- id_map = {v: i for i, v in enumerate(cat_ids)}
- meta.thing_dataset_id_to_contiguous_id = id_map
-
- # sort indices for reproducible results
- img_ids = sorted(coco_api.imgs.keys())
- # imgs is a list of dicts, each looks something like:
- # {'license': 4,
- # 'url': 'http://farm6.staticflickr.com/5454/9413846304_881d5e5c3b_z.jpg',
- # 'file_name': 'COCO_val2014_000000001268.jpg',
- # 'height': 427,
- # 'width': 640,
- # 'date_captured': '2013-11-17 05:57:24',
- # 'id': 1268}
- imgs = coco_api.loadImgs(img_ids)
- # anns is a list[list[dict]], where each dict is an annotation
- # record for an object. The inner list enumerates the objects in an image
- # and the outer list enumerates over images. Example of anns[0]:
- # [{'segmentation': [[192.81,
- # 247.09,
- # ...
- # 219.03,
- # 249.06]],
- # 'area': 1035.749,
- # 'iscrowd': 0,
- # 'image_id': 1268,
- # 'bbox': [192.81, 224.8, 74.73, 33.43],
- # 'category_id': 16,
- # 'id': 42986},
- # ...]
- anns = [coco_api.imgToAnns[img_id] for img_id in img_ids]
-
- if "minival" not in json_file:
- # The popular valminusminival & minival annotations for COCO2014 contain this bug.
- # However the ratio of buggy annotations there is tiny and does not affect accuracy.
- # Therefore we explicitly white-list them.
- ann_ids = [ann["id"] for anns_per_image in anns for ann in anns_per_image]
- assert len(set(ann_ids)) == len(ann_ids), "Annotation ids in '{}' are not unique!".format(
- json_file
- )
-
- imgs_anns = list(zip(imgs, anns))
-
- logger.info("Loaded {} images in COCO format from {}".format(len(imgs_anns), json_file))
-
- dataset_dicts = []
-
- ann_keys = ["iscrowd", "bbox", "keypoints", "category_id"] + (extra_annotation_keys or [])
-
- num_instances_without_valid_segmentation = 0
-
- for (img_dict, anno_dict_list) in imgs_anns:
- record = {}
- record["file_name"] = os.path.join(image_root, img_dict["file_name"])
- record["height"] = img_dict["height"]
- record["width"] = img_dict["width"]
- image_id = record["image_id"] = img_dict["id"]
-
- objs = []
- for anno in anno_dict_list:
- # Check that the image_id in this annotation is the same as
- # the image_id we're looking at.
- # This fails only when the data parsing logic or the annotation file is buggy.
-
- # The original COCO valminusminival2014 & minival2014 annotation files
- # actually contains bugs that, together with certain ways of using COCO API,
- # can trigger this assertion.
- assert anno["image_id"] == image_id
-
- assert anno.get("ignore", 0) == 0, '"ignore" in COCO json file is not supported.'
-
- obj = {key: anno[key] for key in ann_keys if key in anno}
-
- segm = anno.get("segmentation", None)
- if segm: # either list[list[float]] or dict(RLE)
- if not isinstance(segm, dict):
- # filter out invalid polygons (< 3 points)
- segm = [poly for poly in segm if len(poly) % 2 == 0 and len(poly) >= 6]
- if len(segm) == 0:
- num_instances_without_valid_segmentation += 1
- continue # ignore this instance
- obj["segmentation"] = segm
-
- keypts = anno.get("keypoints", None)
- if keypts: # list[int]
- for idx, v in enumerate(keypts):
- if idx % 3 != 2:
- # COCO's segmentation coordinates are floating points in [0, H or W],
- # but keypoint coordinates are integers in [0, H-1 or W-1]
- # Therefore we assume the coordinates are "pixel indices" and
- # add 0.5 to convert to floating point coordinates.
- keypts[idx] = v + 0.5
- obj["keypoints"] = keypts
-
- obj["bbox_mode"] = BoxMode.XYWH_ABS
- if id_map:
- obj["category_id"] = id_map[obj["category_id"]]
- objs.append(obj)
- record["annotations"] = objs
- dataset_dicts.append(record)
-
- if num_instances_without_valid_segmentation > 0:
- logger.warning(
- "Filtered out {} instances without valid segmentation. "
- "There might be issues in your dataset generation process.".format(
- num_instances_without_valid_segmentation
- )
- )
- return dataset_dicts
-
-
-def load_sem_seg(gt_root, image_root, gt_ext="png", image_ext="jpg"):
- """
- Load semantic segmentation data. All files under "gt_root" with "gt_ext" extension are
- treated as ground truth annotations and all files under "image_root" with "image_ext" extension
- as input images. Ground truth and input images are matched using file paths relative to
- "gt_root" and "image_root" respectively without taking into account file extensions.
- This works for COCO as well as some other data.
-
- Args:
- gt_root (str): full path to ground truth semantic segmentation files. Semantic segmentation
- annotations are stored as images with integer values in pixels that represent
- corresponding semantic labels.
- image_root (str): the directory where the input images are.
- gt_ext (str): file extension for ground truth annotations.
- image_ext (str): file extension for input images.
-
- Returns:
- list[dict]:
- a list of dicts in detectron2 standard format without instance-level
- annotation.
-
- Notes:
- 1. This function does not read the image and ground truth files.
- The results do not have the "image" and "sem_seg" fields.
- """
-
- # We match input images with ground truth based on their relative filepaths (without file
- # extensions) starting from 'image_root' and 'gt_root' respectively.
- def file2id(folder_path, file_path):
- # extract relative path starting from `folder_path`
- image_id = os.path.normpath(os.path.relpath(file_path, start=folder_path))
- # remove file extension
- image_id = os.path.splitext(image_id)[0]
- return image_id
-
- input_files = sorted(
- (os.path.join(image_root, f) for f in PathManager.ls(image_root) if f.endswith(image_ext)),
- key=lambda file_path: file2id(image_root, file_path),
- )
- gt_files = sorted(
- (os.path.join(gt_root, f) for f in PathManager.ls(gt_root) if f.endswith(gt_ext)),
- key=lambda file_path: file2id(gt_root, file_path),
- )
-
- assert len(gt_files) > 0, "No annotations found in {}.".format(gt_root)
-
- # Use the intersection, so that val2017_100 annotations can run smoothly with val2017 images
- if len(input_files) != len(gt_files):
- logger.warn(
- "Directory {} and {} has {} and {} files, respectively.".format(
- image_root, gt_root, len(input_files), len(gt_files)
- )
- )
- input_basenames = [os.path.basename(f)[: -len(image_ext)] for f in input_files]
- gt_basenames = [os.path.basename(f)[: -len(gt_ext)] for f in gt_files]
- intersect = list(set(input_basenames) & set(gt_basenames))
- # sort, otherwise each worker may obtain a list[dict] in different order
- intersect = sorted(intersect)
- logger.warn("Will use their intersection of {} files.".format(len(intersect)))
- input_files = [os.path.join(image_root, f + image_ext) for f in intersect]
- gt_files = [os.path.join(gt_root, f + gt_ext) for f in intersect]
-
- logger.info(
- "Loaded {} images with semantic segmentation from {}".format(len(input_files), image_root)
- )
-
- dataset_dicts = []
- for (img_path, gt_path) in zip(input_files, gt_files):
- record = {}
- record["file_name"] = img_path
- record["sem_seg_file_name"] = gt_path
- dataset_dicts.append(record)
-
- return dataset_dicts
-
-
-def convert_to_coco_dict(dataset_name):
- """
- Convert an instance detection/segmentation or keypoint detection dataset
- in detectron2's standard format into COCO json format.
-
- Generic dataset description can be found here:
- https://detectron2.readthedocs.io/tutorials/datasets.html#register-a-dataset
-
- COCO data format description can be found here:
- http://cocodataset.org/#format-data
-
- Args:
- dataset_name (str):
- name of the source dataset
- Must be registered in DatastCatalog and in detectron2's standard format.
- Must have corresponding metadata "thing_classes"
- Returns:
- coco_dict: serializable dict in COCO json format
- """
-
- dataset_dicts = DatasetCatalog.get(dataset_name)
- metadata = MetadataCatalog.get(dataset_name)
-
- # unmap the category mapping ids for COCO
- if hasattr(metadata, "thing_dataset_id_to_contiguous_id"):
- reverse_id_mapping = {v: k for k, v in metadata.thing_dataset_id_to_contiguous_id.items()}
- reverse_id_mapper = lambda contiguous_id: reverse_id_mapping[contiguous_id] # noqa
- else:
- reverse_id_mapper = lambda contiguous_id: contiguous_id # noqa
-
- categories = [
- {"id": reverse_id_mapper(id), "name": name}
- for id, name in enumerate(metadata.thing_classes)
- ]
-
- logger.info("Converting dataset dicts into COCO format")
- coco_images = []
- coco_annotations = []
-
- for image_id, image_dict in enumerate(dataset_dicts):
- coco_image = {
- "id": image_dict.get("image_id", image_id),
- "width": image_dict["width"],
- "height": image_dict["height"],
- "file_name": image_dict["file_name"],
- }
- coco_images.append(coco_image)
-
- anns_per_image = image_dict["annotations"]
- for annotation in anns_per_image:
- # create a new dict with only COCO fields
- coco_annotation = {}
-
- # COCO requirement: XYWH box format
- bbox = annotation["bbox"]
- bbox_mode = annotation["bbox_mode"]
- bbox = BoxMode.convert(bbox, bbox_mode, BoxMode.XYWH_ABS)
-
- # COCO requirement: instance area
- if "segmentation" in annotation:
- # Computing areas for instances by counting the pixels
- segmentation = annotation["segmentation"]
- # TODO: check segmentation type: RLE, BinaryMask or Polygon
- if isinstance(segmentation, list):
- polygons = PolygonMasks([segmentation])
- area = polygons.area()[0].item()
- elif isinstance(segmentation, dict): # RLE
- area = mask_util.area(segmentation).item()
- else:
- raise TypeError(f"Unknown segmentation type {type(segmentation)}!")
- else:
- # Computing areas using bounding boxes
- bbox_xy = BoxMode.convert(bbox, BoxMode.XYWH_ABS, BoxMode.XYXY_ABS)
- area = Boxes([bbox_xy]).area()[0].item()
-
- if "keypoints" in annotation:
- keypoints = annotation["keypoints"] # list[int]
- for idx, v in enumerate(keypoints):
- if idx % 3 != 2:
- # COCO's segmentation coordinates are floating points in [0, H or W],
- # but keypoint coordinates are integers in [0, H-1 or W-1]
- # For COCO format consistency we substract 0.5
- # https://github.com/facebookresearch/detectron2/pull/175#issuecomment-551202163
- keypoints[idx] = v - 0.5
- if "num_keypoints" in annotation:
- num_keypoints = annotation["num_keypoints"]
- else:
- num_keypoints = sum(kp > 0 for kp in keypoints[2::3])
-
- # COCO requirement:
- # linking annotations to images
- # "id" field must start with 1
- coco_annotation["id"] = len(coco_annotations) + 1
- coco_annotation["image_id"] = coco_image["id"]
- coco_annotation["bbox"] = [round(float(x), 3) for x in bbox]
- coco_annotation["area"] = float(area)
- coco_annotation["iscrowd"] = annotation.get("iscrowd", 0)
- coco_annotation["category_id"] = reverse_id_mapper(annotation["category_id"])
-
- # Add optional fields
- if "keypoints" in annotation:
- coco_annotation["keypoints"] = keypoints
- coco_annotation["num_keypoints"] = num_keypoints
-
- if "segmentation" in annotation:
- coco_annotation["segmentation"] = annotation["segmentation"]
- if isinstance(coco_annotation["segmentation"], dict): # RLE
- coco_annotation["segmentation"]["counts"] = coco_annotation["segmentation"][
- "counts"
- ].decode("ascii")
-
- coco_annotations.append(coco_annotation)
-
- logger.info(
- "Conversion finished, "
- f"#images: {len(coco_images)}, #annotations: {len(coco_annotations)}"
- )
-
- info = {
- "date_created": str(datetime.datetime.now()),
- "description": "Automatically generated COCO json file for Detectron2.",
- }
- coco_dict = {
- "info": info,
- "images": coco_images,
- "annotations": coco_annotations,
- "categories": categories,
- "licenses": None,
- }
- return coco_dict
-
-
-def convert_to_coco_json(dataset_name, output_file, allow_cached=True):
- """
- Converts dataset into COCO format and saves it to a json file.
- dataset_name must be registered in DatasetCatalog and in detectron2's standard format.
-
- Args:
- dataset_name:
- reference from the config file to the catalogs
- must be registered in DatasetCatalog and in detectron2's standard format
- output_file: path of json file that will be saved to
- allow_cached: if json file is already present then skip conversion
- """
-
- # TODO: The dataset or the conversion script *may* change,
- # a checksum would be useful for validating the cached data
-
- PathManager.mkdirs(os.path.dirname(output_file))
- with file_lock(output_file):
- if PathManager.exists(output_file) and allow_cached:
- logger.warning(
- f"Using previously cached COCO format annotations at '{output_file}'. "
- "You need to clear the cache file if your dataset has been modified."
- )
- else:
- logger.info(f"Converting annotations of dataset '{dataset_name}' to COCO format ...)")
- coco_dict = convert_to_coco_dict(dataset_name)
-
- logger.info(f"Caching COCO format annotations at '{output_file}' ...")
- with PathManager.open(output_file, "w") as f:
- json.dump(coco_dict, f)
-
-
-if __name__ == "__main__":
- """
- Test the COCO json dataset loader.
-
- Usage:
- python -m detectron2.data.data.coco \
- path/to/json path/to/image_root dataset_name
-
- "dataset_name" can be "coco_2014_minival_100", or other
- pre-registered ones
- """
- from detectron2.utils.logger import setup_logger
- from detectron2.utils.visualizer import Visualizer
- import detectron2.data.datasets # noqa # add pre-defined metadata
- import sys
-
- logger = setup_logger(name=__name__)
- assert sys.argv[3] in DatasetCatalog.list()
- meta = MetadataCatalog.get(sys.argv[3])
-
- dicts = load_coco_json(sys.argv[1], sys.argv[2], sys.argv[3])
- logger.info("Done loading {} samples.".format(len(dicts)))
-
- dirname = "coco-data-vis"
- os.makedirs(dirname, exist_ok=True)
- for d in dicts:
- img = np.array(Image.open(d["file_name"]))
- visualizer = Visualizer(img, metadata=meta)
- vis = visualizer.draw_dataset_dict(d)
- fpath = os.path.join(dirname, os.path.basename(d["file_name"]))
- vis.save(fpath)
diff --git a/spaces/hdhzk/bingo/src/pages/api/sydney.ts b/spaces/hdhzk/bingo/src/pages/api/sydney.ts
deleted file mode 100644
index 0e7bbf23d77c2e1a6635185a060eeee58b8c8e66..0000000000000000000000000000000000000000
--- a/spaces/hdhzk/bingo/src/pages/api/sydney.ts
+++ /dev/null
@@ -1,62 +0,0 @@
-import { NextApiRequest, NextApiResponse } from 'next'
-import { WebSocket, debug } from '@/lib/isomorphic'
-import { BingWebBot } from '@/lib/bots/bing'
-import { websocketUtils } from '@/lib/bots/bing/utils'
-import { WatchDog, createHeaders } from '@/lib/utils'
-
-
-export default async function handler(req: NextApiRequest, res: NextApiResponse) {
- const conversationContext = req.body
- const headers = createHeaders(req.cookies)
- debug(headers)
- res.setHeader('Content-Type', 'text/stream; charset=UTF-8')
-
- const ws = new WebSocket('wss://sydney.bing.com/sydney/ChatHub', {
- headers: {
- ...headers,
- 'accept-language': 'zh-CN,zh;q=0.9',
- 'cache-control': 'no-cache',
- 'x-ms-useragent': 'azsdk-js-api-client-factory/1.0.0-beta.1 core-rest-pipeline/1.10.0 OS/Win32',
- pragma: 'no-cache',
- }
- })
-
- const closeDog = new WatchDog()
- const timeoutDog = new WatchDog()
- ws.onmessage = (event) => {
- timeoutDog.watch(() => {
- ws.send(websocketUtils.packMessage({ type: 6 }))
- }, 1500)
- closeDog.watch(() => {
- ws.close()
- }, 10000)
- res.write(event.data)
- if (/\{"type":([367])\}/.test(String(event.data))) {
- const type = parseInt(RegExp.$1, 10)
- debug('connection type', type)
- if (type === 3) {
- ws.close()
- } else {
- ws.send(websocketUtils.packMessage({ type }))
- }
- }
- }
-
- ws.onclose = () => {
- timeoutDog.reset()
- closeDog.reset()
- debug('connection close')
- res.end()
- }
-
- await new Promise((resolve) => ws.onopen = resolve)
- ws.send(websocketUtils.packMessage({ protocol: 'json', version: 1 }))
- ws.send(websocketUtils.packMessage({ type: 6 }))
- ws.send(websocketUtils.packMessage(BingWebBot.buildChatRequest(conversationContext!)))
- req.socket.once('close', () => {
- ws.close()
- if (!res.closed) {
- res.end()
- }
- })
-}
diff --git a/spaces/ho11laqe/nnUNet_calvingfront_detection/nnunet/training/network_training/nnUNet_variants/architectural_variants/__init__.py b/spaces/ho11laqe/nnUNet_calvingfront_detection/nnunet/training/network_training/nnUNet_variants/architectural_variants/__init__.py
deleted file mode 100644
index e69de29bb2d1d6434b8b29ae775ad8c2e48c5391..0000000000000000000000000000000000000000
diff --git a/spaces/hugggof/vampnet/scripts/exp/fine_tune.py b/spaces/hugggof/vampnet/scripts/exp/fine_tune.py
deleted file mode 100644
index af82fcc7f260607a2efff9fad419271ad1a203d8..0000000000000000000000000000000000000000
--- a/spaces/hugggof/vampnet/scripts/exp/fine_tune.py
+++ /dev/null
@@ -1,81 +0,0 @@
-import argbind
-from pathlib import Path
-import yaml
-from typing import List
-
-
-
-
-"""example output: (yaml)
-
-"""
-
-@argbind.bind(without_prefix=True, positional=True)
-def fine_tune(audio_files_or_folders: List[str], name: str):
-
- conf_dir = Path("conf")
- assert conf_dir.exists(), "conf directory not found. are you in the vampnet directory?"
-
- conf_dir = conf_dir / "generated"
- conf_dir.mkdir(exist_ok=True)
-
- finetune_dir = conf_dir / name
- finetune_dir.mkdir(exist_ok=True)
-
- finetune_c2f_conf = {
- "$include": ["conf/lora/lora.yml"],
- "fine_tune": True,
- "train/AudioLoader.sources": audio_files_or_folders,
- "val/AudioLoader.sources": audio_files_or_folders,
- "VampNet.n_codebooks": 14,
- "VampNet.n_conditioning_codebooks": 4,
- "VampNet.embedding_dim": 1280,
- "VampNet.n_layers": 16,
- "VampNet.n_heads": 20,
- "AudioDataset.duration": 3.0,
- "AudioDataset.loudness_cutoff": -40.0,
- "save_path": f"./runs/{name}/c2f",
- "fine_tune_checkpoint": "./models/vampnet/c2f.pth"
- }
-
- finetune_coarse_conf = {
- "$include": ["conf/lora/lora.yml"],
- "fine_tune": True,
- "train/AudioLoader.sources": audio_files_or_folders,
- "val/AudioLoader.sources": audio_files_or_folders,
- "save_path": f"./runs/{name}/coarse",
- "fine_tune_checkpoint": "./models/vampnet/coarse.pth"
- }
-
- interface_conf = {
- "Interface.coarse_ckpt": f"./runs/{name}/coarse/latest/vampnet/weights.pth",
-
- "Interface.coarse2fine_ckpt": f"./runs/{name}/c2f/latest/vampnet/weights.pth",
- "Interface.wavebeat_ckpt": "./models/wavebeat.pth",
-
- "Interface.codec_ckpt": "./models/vampnet/codec.pth",
- "AudioLoader.sources": [audio_files_or_folders],
- }
-
- # save the confs
- with open(finetune_dir / "c2f.yml", "w") as f:
- yaml.dump(finetune_c2f_conf, f)
-
- with open(finetune_dir / "coarse.yml", "w") as f:
- yaml.dump(finetune_coarse_conf, f)
-
- with open(finetune_dir / "interface.yml", "w") as f:
- yaml.dump(interface_conf, f)
-
-
- print(f"generated confs in {finetune_dir}. run training jobs with `python scripts/exp/train.py --args.load {finetune_dir}/.yml` ")
-
-if __name__ == "__main__":
- args = argbind.parse_args()
-
- with argbind.scope(args):
- fine_tune()
-
-
-
-
\ No newline at end of file
diff --git a/spaces/ibm-nasa-geospatial/Prithvi-100M-sen1floods11-demo/app.py b/spaces/ibm-nasa-geospatial/Prithvi-100M-sen1floods11-demo/app.py
deleted file mode 100644
index 07d2738bcd791d7affb3bc3fbed8c610f0427c20..0000000000000000000000000000000000000000
--- a/spaces/ibm-nasa-geospatial/Prithvi-100M-sen1floods11-demo/app.py
+++ /dev/null
@@ -1,226 +0,0 @@
-######### pull files
-import os
-from huggingface_hub import hf_hub_download
-config_path=hf_hub_download(repo_id="ibm-nasa-geospatial/Prithvi-100M-sen1floods11", filename="sen1floods11_Prithvi_100M.py", token=os.environ.get("token"))
-ckpt=hf_hub_download(repo_id="ibm-nasa-geospatial/Prithvi-100M-sen1floods11", filename='sen1floods11_Prithvi_100M.pth', token=os.environ.get("token"))
-##########
-
-
-import argparse
-from mmcv import Config
-
-from mmseg.models import build_segmentor
-
-from mmseg.datasets.pipelines import Compose, LoadImageFromFile
-
-import rasterio
-import torch
-
-from mmseg.apis import init_segmentor
-
-from mmcv.parallel import collate, scatter
-
-import numpy as np
-import glob
-import os
-
-import time
-
-import numpy as np
-import gradio as gr
-from functools import partial
-
-import pdb
-
-import matplotlib.pyplot as plt
-
-from skimage import exposure
-
-def stretch_rgb(rgb):
-
- ls_pct=1
- pLow, pHigh = np.percentile(rgb[~np.isnan(rgb)], (ls_pct,100-ls_pct))
- img_rescale = exposure.rescale_intensity(rgb, in_range=(pLow,pHigh))
-
- return img_rescale
-
-
-def open_tiff(fname):
-
- with rasterio.open(fname, "r") as src:
-
- data = src.read()
-
- return data
-
-def write_tiff(img_wrt, filename, metadata):
-
- """
- It writes a raster image to file.
-
- :param img_wrt: numpy array containing the data (can be 2D for single band or 3D for multiple bands)
- :param filename: file path to the output file
- :param metadata: metadata to use to write the raster to disk
- :return:
- """
-
- with rasterio.open(filename, "w", **metadata) as dest:
-
- if len(img_wrt.shape) == 2:
-
- img_wrt = img_wrt[None]
-
- for i in range(img_wrt.shape[0]):
- dest.write(img_wrt[i, :, :], i + 1)
-
- return filename
-
-
-def get_meta(fname):
-
- with rasterio.open(fname, "r") as src:
-
- meta = src.meta
-
- return meta
-
-def preprocess_example(example_list):
-
- example_list = [os.path.join(os.path.abspath(''), x) for x in example_list]
-
- return example_list
-
-
-def inference_segmentor(model, imgs, custom_test_pipeline=None):
- """Inference image(s) with the segmentor.
-
- Args:
- model (nn.Module): The loaded segmentor.
- imgs (str/ndarray or list[str/ndarray]): Either image files or loaded
- images.
-
- Returns:
- (list[Tensor]): The segmentation result.
- """
- cfg = model.cfg
- device = next(model.parameters()).device # model device
- # build the data pipeline
- test_pipeline = [LoadImageFromFile()] + cfg.data.test.pipeline[1:] if custom_test_pipeline == None else custom_test_pipeline
- test_pipeline = Compose(test_pipeline)
- # prepare data
- data = []
- imgs = imgs if isinstance(imgs, list) else [imgs]
- for img in imgs:
- img_data = {'img_info': {'filename': img}}
- img_data = test_pipeline(img_data)
- data.append(img_data)
- # print(data.shape)
-
- data = collate(data, samples_per_gpu=len(imgs))
- if next(model.parameters()).is_cuda:
- # data = collate(data, samples_per_gpu=len(imgs))
- # scatter to specified GPU
- data = scatter(data, [device])[0]
- else:
- # img_metas = scatter(data['img_metas'],'cpu')
- # data['img_metas'] = [i.data[0] for i in data['img_metas']]
-
- img_metas = data['img_metas'].data[0]
- img = data['img']
- data = {'img': img, 'img_metas':img_metas}
-
- with torch.no_grad():
- result = model(return_loss=False, rescale=True, **data)
- return result
-
-
-def inference_on_file(target_image, model, custom_test_pipeline):
-
- target_image = target_image.name
-
- time_taken=-1
-
- st = time.time()
- print('Running inference...')
- result = inference_segmentor(model, target_image, custom_test_pipeline)
-
- print("Output has shape: " + str(result[0].shape))
-
- ##### prep outputs
- mask = open_tiff(target_image)
- rgb = stretch_rgb((mask[[3, 2, 1], :, :].transpose((1,2,0))/10000*255).astype(np.uint8))
- meta = get_meta(target_image)
- mask = np.where(mask == meta['nodata'], 1, 0)
- mask = np.max(mask, axis=0)[None]
- rgb = np.where(mask.transpose((1,2,0)) == 1, 0, rgb)
- rgb = np.where(rgb < 0, 0, rgb)
- rgb = np.where(rgb > 255, 255, rgb)
-
- prediction = np.where(mask == 1, 0, result[0]*255)
- et = time.time()
- time_taken = np.round(et - st, 1)
- print(f'Inference completed in {str(time_taken)} seconds')
-
- return rgb, prediction[0]
-
-def process_test_pipeline(custom_test_pipeline, bands=None):
-
- # change extracted bands if necessary
- if bands is not None:
-
- extract_index = [i for i, x in enumerate(custom_test_pipeline) if x['type'] == 'BandsExtract' ]
-
- if len(extract_index) > 0:
-
- custom_test_pipeline[extract_index[0]]['bands'] = eval(bands)
-
- collect_index = [i for i, x in enumerate(custom_test_pipeline) if x['type'].find('Collect') > -1]
-
- # adapt collected keys if necessary
- if len(collect_index) > 0:
-
- keys = ['img_info', 'filename', 'ori_filename', 'img', 'img_shape', 'ori_shape', 'pad_shape', 'scale_factor', 'img_norm_cfg']
- custom_test_pipeline[collect_index[0]]['meta_keys'] = keys
-
- return custom_test_pipeline
-
-config = Config.fromfile(config_path)
-config.model.backbone.pretrained=None
-model = init_segmentor(config, ckpt, device='cpu')
-custom_test_pipeline=process_test_pipeline(model.cfg.data.test.pipeline, None)
-
-func = partial(inference_on_file, model=model, custom_test_pipeline=custom_test_pipeline)
-
-with gr.Blocks() as demo:
-
- gr.Markdown(value='# Prithvi sen1floods11')
- gr.Markdown(value='''Prithvi is a first-of-its-kind temporal Vision transformer pretrained by the IBM and NASA team on continental US Harmonised Landsat Sentinel 2 (HLS) data. This demo showcases how the model was finetuned to detect water at a higher resolution than it was trained on (i.e. 10m versus 30m) using Sentinel 2 imagery from on the [sen1floods11 dataset](https://github.com/cloudtostreet/Sen1Floods11). More detailes can be found [here](https://huggingface.co/ibm-nasa-geospatial/Prithvi-100M-sen1floods11).\n
- The user needs to provide a Sentinel 2 image with all the 12 bands (in the usual Sentinel 2) order in reflectance units multiplied by 10,000 (e.g. to save on space), with the code that is going to pull up Blue, Green, Red, Narrow NIR, SWIR, SWIR 2.
- ''')
- with gr.Row():
- with gr.Column():
- inp = gr.File()
- btn = gr.Button("Submit")
-
- with gr.Row():
- gr.Markdown(value='### Input RGB')
- gr.Markdown(value='### Model prediction (Black: Land; White: Water)')
-
- with gr.Row():
- out1=gr.Image(image_mode='RGB')
- out2 = gr.Image(image_mode='L')
-
- btn.click(fn=func, inputs=inp, outputs=[out1, out2])
-
- with gr.Row():
- gr.Examples(examples=["India_900498_S2Hand.tif",
- "Spain_7370579_S2Hand.tif",
- "USA_430764_S2Hand.tif"],
- inputs=inp,
- outputs=[out1, out2],
- preprocess=preprocess_example,
- fn=func,
- cache_examples=True,
- )
-
-demo.launch()
\ No newline at end of file
diff --git a/spaces/inamXcontru/PoeticTTS/AV Voice Changer V 6.0.10 Keygen .rar TOP.md b/spaces/inamXcontru/PoeticTTS/AV Voice Changer V 6.0.10 Keygen .rar TOP.md
deleted file mode 100644
index 18a0cf2f8c9866b0c488268b80de43bdd2a18bbf..0000000000000000000000000000000000000000
--- a/spaces/inamXcontru/PoeticTTS/AV Voice Changer V 6.0.10 Keygen .rar TOP.md
+++ /dev/null
@@ -1,5 +0,0 @@
-
-cd_key (14085) keygen (12265) serial (10529) crack (9119) nero 7.9.6.0 serial (1873) nero 7.9.6.0 keygen (1609) sims2fashionstuffcrack (833) nero 7.9.6 serial (557) sims 2 cd key (543) pro cycling manager 2007 crack (537) nero 7.9.6.0 (536) nero 7 serial number (501) nero (463) windows xp pro serial (443) delicious 2 deluxe (426) medal of honor crack (403) crack total commander 7.01 (400) any dvd 6.1.6.5 crack (398) anydvd 6.1.6.5 crack (392) adobe photoshop cs crack (381) spyware doctor 5.0.1.205 (346) canon adjustment software (320) test drive unlimited keygen (307) dark crusade cd key (300) registry mechanic crack (289) microsoft office home and student 2007 crack (284) fraps 2.8.2 serial (278) nikon (271) windows xp keygen (265) trackmania united keygen (264) spb (261) powerdvd cd key (261) pinnacle studio 9 serial (253) getdataback crack 3.03 (252) nero 7 serial (244) harry potter (244) age of mythology the titans cd key (244) winrar crack 3.7 (241) gamehouse (240) xp home serial (238) recover my files serial (237) spyware doctor registration code (236) spyware doctor (236) spyware doctor license number (232) fl studio 7 serial number (230) sound forge (227) office 2003 key (223) loki no dvd crack (223) dawn of war dark crusade cd key (217) avs video editor 3.5 (216) video converter (211) sims 2 seasons no cd crack (208) nero 6.6.1.15a keygen (206) crack any dvd 6.1.6.5 (206) crack corel 12 (203) norton antivirus 2007 product key (202) brothers in arms cd key (197) nero 6.6.0.16 serial (195) winiso crack (194) ea games keygen (194) winzip 11.1 registration code (193) need for speed 2 serial (193) serial office 2007 (190) boilsoft keygen (190) windows xp sp2 keygen (189) xilisoft 3gp video converter crack (188) power dvd (188) crack solid converter (188) red alert 2 keygen (186) 18 wheels (186) photoshop cs2 keygen (183) mirc registration code (183) two worlds serial (182) anydvd (181) nero 7.7.5.1 serial (177) getdataback (177) avg anti spyware license code (177) registry mechanic serial (175) crystal reports (175) ultraiso serial (173) solid converter serial (173) quicktime pro (172) av voice changer crack (172) internet download manager (171) femjoy passwords (170) empire earth 2 cd key (170) command and conquer generals cd key (170) clone dvd (170) windvd (168) topdesk 1.5.3 (165) system mechanic (165) zone alarm (164) microsoft office (164) crack video strip poker supreme 1.10 (164) loki no cd crack (163) download crack sony acid pro 6.0d (163) need for speed most wanted cd key (162) xp key changer (160) ultramon (158) photoshop cs serial (158) winzip serial (157) illustrator cs3 crack (156) babylon (156) anydvd 6165 (156) ultraiso (155) photoshop 7.0 serial (155) file scavenger keygen (154) call of duty key code (154) age of empires the conquerors (154) adobe cs2 serial (154) doom 3 serial (152) need for speed underground cd key (151) driver detective 6.2.2.2 (150) diablo 2 keygen (150) cd key office mac (149) pro evolution soccer 2007 (148) office xp (148) magix music (148) registry cleaner (147) partition magic 8.0 crack (146) kaspersky key (145) premiere 2.0 serial (143) astakiller (143) sims 2 no cd (142) avg 7 serial (142) flash decompiler (141) premiere pro serial (140) fff keygen (140) command and conquer generals (140) adobe creative suite serial (139) worldmate keygen (138) unlock video strip poker supreme 1.10 (138) dreamweaver 8 crack (138) acoustica mixcraft (137) serial mathtype 5.2 (136) power dvd 7.0 keygen (136) photoshop cs2 activation key (136) hddlife pro 3.0.140 (136) chief architect x1 (136) xp pro keygen (135) panda (135) anydvd 6.1.6.5 keygen (135) winiso registration code (134) serial nero vision express (134) serial nero 7 (134) windows xp sp2 activation key (133) warcraft 3 no cd (133) nero keygen (133) msn sniffer crack (133) boson keygen (133) ws_ftp (132) imtoo converter (132) windows xp home serial (131) crack avast (131) anydvd 6.1.6.5 serial (131) studio (130) splinter cell (130) need for speed underground 2 (130) spyware doctor serial (129) spyware doctor keygen (129) jewel quest crack (128) avi splitter serial (128) wpa_kill.exe download (127) world of warcraft keygen (126) nero 7 keygen (126) perfect keylogger (125) frozen throne cd key (124) far cry no dvd crack (124) cfosspeed v4.01.1300 (124) cardrecovery 3.40 registration key (124) pocketpc (123) kaspersky (123) crack stellar phoenix (123) serial two worlds (122) serial test drive (122) office 2003 activation crack (122) isilo serial crack (121) battlefront 2 crack (121) pro cycling manager 2007 (120) key generator (120) feeding frenzy crack (120) registration code winzip (119) winavi video converter crack (117) crack most wanted (116) age of empires 2 crack (116) xmlspy 2007 crack (115) style xp keygen (115) photoshop cs2 serial (115) newsleecher (115) filemaker pro (115) actual spy serial (115) super dvd creator 9.3 (114) nfs most wanted crack (114) imtoo dvd ripper 2.0 serial (114) rollercoaster tycoon 2 activation code (113) call of duty cd key (113) stalker cd key (112) mpeg encoder (112) karaoke (111) photorescue keygen (110) loki nodvd (110) total commander crack (109) serial need for speed most wanted (109) passware (109) nero 7 (109) dap premium activation code (109) crack lemonade tycoon 2 (109) boilsoft crack (109) need for speed most wanted crack (108) norton antivirus 2005 product key (107) adobe audition 1.5 crack (107) diablo 2 no cd crack (106) dameware crack (106) passport photo 1.5.3 (105) need for speed (105) boilsoft rm to mp3 crack (105) adobe premiere pro 1.5 tryout crack (105) recorder (104) loki (104) dfx (104) avast keygen (103) serial nero (102) sacred cd key generator (102) most wanted serial (102) call of duty (102) avg antispyware serial (102) adobe illustrator cs3 keygen (102) acoustica mixcraft v 3 (102) rollercoaster tycoon 3 no cd crack (101) half life 2 keygen (101) ad (101) spyware doctor 5.0.1.201a serial (100) registry booster serial number (100) pdf converter (100) bitdefender serial (100) age of empires 3 keygen (100) warcraft 3 cd key (99) surfoffline 1.4 (99) powerdirector (99) guild wars cd key generator (99) getright (98) crack office xp (98) wm recorder serial (97) scrabble crack (97) powerdvd serial (97) nero serial (97) age of empires 3 cd key (97) trojan remover (96) generals serial (96) avs video converter (96) avg internet security 7.5.476 (96) avg antispyware 7.5 license code keygen (96) adobe illustrator cs3 serial number (96) win xp serial (95) recover4all (95) pinnacle tv center pro serial keygen download (95) 3ds max 7 crack (95) video fixer 3.23 crack (94) sims 2 fashion stuff cd key (94) nero 6.6.0.18 serial (94) windows xp sp2 crack (93) ut2004 cd key generator (93) serial warcraft 3 (93) crack anydvd 6.1.6.5 (93) winavi registration code (92) sketchup (92) ricochet keygen (92) nod32 serial (92) cuteftp (92) crack adobe photoshop cs3 (92) badcopy pro (92) photoshop cs2 crack (91) norton antivirus activation key (91) new star soccer 3 registration code (91) avg antispyware 7.5 license code (91) avg 7.5.1.43 (91) able2extract crack (91) alien shooter keygen (91) steam cd key (90) spyware doctor 5.0.1. (90) halo crack (90) crack fairy godmother tycoon (90) cd key men of valor (90) camtasia (90) call of duty no cd (90) any dvd 6165 (90) 7 wonders (90) swf (89) particle illusion crack (89) office 2007 (89) alcohol 120 (89) zuma registration key (88) pocket dvd studio serial (88) movie6.net (88) zuma crack (87) photoshop cs3 (87) empire earth (87) windows xp service pack (86) serial power dvd (86) fraps crack 2.8.2 (86) winrar (85) vmware serial (85) photoshop cs2 serial number (85) nero crack (85) need for speed underground 2 cd key (85) avg (85) winavi serial (84) isobuster (84) actual spy 3.0 crack (84) toca race driver 2 no cd crack (83) serial sims 2 (83) premiere cs3 (83) photoshop cs crack (83) half life serial (83) call of duty 2 keygen (83) avg antispyware keygen (83) streamdown (82) registry fix crack (82) mahjong (82) serial need for speed underground (81) nfs most wanted serial (81) flashfxp 2.1 serial (81) dvd psp (81) dawn of war serial (81) blaze (81) smartdraw (80) partition magic 8 (80) vcdcutter (79) partition magic 8.0 1242 crack (79) need for speed underground 2 crack (79) loki crack (79) gamehouse serial (79) folder lock (79) sims 2 nightlife serial (78) oziexplorer (78) office 2003 (78) keygen office 2003 (78) condition zero cd key (78) avast serial (78) recover my files 3.98 5637 (77) isilo 4.32 (77) adobe (77) worms no cd (76) windows xp activation crack (76) windows 98 serial (76) steam keygen (76) spyware doctor 5.0.1.200 (76) mixmeister (76) kaspersky serial (76) hollywood fx (76) easy cd (76) crack getdataback 2.31 (76) coreldraw 12 crack (76) anydvd 6.1.6.5 (76) spyware doctor crack (75) panorama (75) netlimiter crack (75) nero 6.3 (75) mathematica (75) easyx rm to avi converter 1.0 (75) ccproxy 6.4.2 (75) adobe photoshop elements (75) serial number age of empires 3 (74) rational (74) photoshop 7 serial (74) norton internet security 2006 keygen (74) noadware (74) magic iso maker 5.4 (74) dvdfab (74) dvd2one (74) dreamweaver 2004 serial (74) diner dash serial (74) counter strike cd key (74) condition zero cd key (74) cd key counter strike 1.5 (74) anydvd crack (74) alias maya 6.0 serial (74) advanced registry optimizer 5.0 serial (74) wwwmovie6.net (73) trackmania sunrise serial (73) photoshop cs keygen (73) file recover (73) diner dash registration key (73) diablo 2 cd keys (73) call of duty 2 cd key (73) winavi crack (72) wavelab 5 (72) super collapse (72) red alert 2 (72) editplus (72) ashampoo (72) tuneup utilities 2004 (71) tuneup 2006 serial (71) premiere (71) keygens total commander 7 (71) imtoo mp4 video converter (71) download accelerator plus (71) actual spy crack (71) winning eleven 2007 (70) winimage (70) winamp crack (70) swishmax serial (70) smartmovie crack (70) office home and student 2007 serial (70) kioday mahjong download (70) dvd profiler (70) windows server 2003 enterprise crack (69) visual paradigm (69) style xp 3.19 registration key (69) noadware registration key (69) nero cd key (69) nero 6.6.0.13 serial (69) need for speed underground (69) stalker crack (68) safari island deluxe (68) office (68) flash cs3 (68) doom 3 keygen (68) cracks (68) anydvd 6.1.6.5 key (68) windows xp sp2 serial (67) windows xp corporate (67) fraps (67) empire earth cd key (67) cd keygen need for speed carbon (67) alcohol 120 1.9.2 crack (67) xmlspy 2007 serial (66) windows xp serial (66) sims 2 keygen (66) serial nero 6 (66) poser 6 (66) norton (66) electronics workbench (66) dmc (66) avg crack (66) sims 2 serial (65) musicmatch (65) imtoo mpeg encoder 3.1.34 (65) dr divx keygen (65) ad aware (65) acronis 10 (65) trackmania united cd key (64) keygen clonecd (64) illustrator cs3 serial (64) avg antispyware license code (64) winzip 9 (63) tomtom (63) tmpgenc 2.5 (63) steam crack (63) nero serial number (63) multisim 7 serial (63) clone (63) active webcam 9.2 (63) web page maker (62) spb mobile (62) kaspersky crack (62) crack warcraft 3 (62) convertxtodvd 2.2.3.258 (62) allway sync (62) acdsee crack (62) msn (61) intervideo windvd 8 gold (61) elecard avc plugin 2.0.70420 (61) crack sims 2 (61) asta killer (61) total commander (60) tmpgenc dvd author 3 (60) registry mechanic license code (60) microsoft office xp (60) cricket 2004 cd key (60) crack flash slide show maker 4.21 (60) clonedvd (60) castle (60) warfare incorporated serial (59) teleport pro (59) product key crack (59) pdf2word (59) mp3resizer 1.8.3 (59) loki no cd (59) keygen alcohol 120%25 (59) imtoo mpeg encoder (59) avs video converter 5.6 serial number (59) adobe illustrator cs2 crack (59) active webcam (59) winavi keygen (58) ulead videostudio 10 registration code (58) smartmovie 3.41 keygen (58) powerpack lame mp3 encoder (58) office activation crack (58) mapinfo (58) luxor registration key (58) halo 2 (58) gta san andreas crack (58) file scavenger key (58) empire at war serial (58) clonedvd2 (58) vray keygen (57) pocket pc (57) netlimiter serial (57) nero 7.9.6.0 crack (57) muvee (57) illustrator cs3 keygen (57) homeworld 2 (57) fifa 2005 (57) zone alarm serial (56) super jigsaw (56) pool buddy serial (56) office 2003 serial (56) nero 6 (56) keygen navigon (56) crack trackmania united (56) anydvd6165 (56) windows xp (55) vuescan (55) smartmovie (55) serial halo (55) quicktime 6.5 serial (55) parallels workstation 2.2 (55) kaspersky license key (55) dap8.5 crack (55) crack uefa euro 2004 (55) winzip registration code (54) style xp crack (54) need for speed underground 2 no cd crack (54) guild wars (54) empire earth serial (54) crystal pro (54) autocad (54) acdsee 7 keygen (54) vmware crack (53) trendy flash site builder crack (53) serial for ableton 6 (53) rise of nations cd key (53) photoshop (53) nero 6.6.1.15a serial number (53) need for speed most wanted no cd crack (53) mirc crack (53) brothers in arms crack (53) azada (53) acrobat (53) whatsup (52) ultraedit (52) stylexp registration key (52) powerdvd (52) most wanted crack (52) magix (52) call of duty united offensive keygen (52) call of duty 2 crack (52) battlefield 1942 1.6 crack (52) anticrash (52) all seeing eye serial (52) alcohol 120 serial (52) windows 2003 enterprise serial number (51) tweak (51) spylocked 4.3 free key (51) smart movie (51) sims 2 fashion stuff crack (51) ripcast (51) red ace squadron (51) pinnacle tvcenter pro (51) nero 6 serial (51) dvd cloner (51) drivermagic (51) crack nero 7 (51) crack anydvd (51) winrar patch (50) vista (50) turbocad (50) tmpgenc 2.524.63.181 (50) spyware doctor 5 (50) pdf995 (50) hero (50) doom 3 cd key (50) crack nero (50) crack autocad 2007 (50) call of duty keygen (50) call of duty 2 key code (50) billiards (50) beyond divinity no cd crack (50) alcohol (50) windows xp sp2 key (49) video fixer serial (49) silent hunter (49) serial xp (49) serial counter strike (49) ptgmap (49) nba 2005 keygen (49) lc4 keygen (49) guild wars access key (49) driver detective (49) cucusoft 4.29 crack (49) crack norton (49) winiso serial (48) spyware doctor 5.0.1.201a crack (48) river past (48) resident evil 4 (48) proshow gold (48) nero 7 crack (48) microsoft office home and student 2007 serial (48) lost planet (48) flash slideshow maker 4.22 (48) diablo 2 (48) cs2 crack (48) crack nero 6 (48) avast (48) audiograbber (48) a4desk crack (48) %3a (48) winzip 11.1 key (47) warcraft 3 keygen (47) the sims 2 keygen (47) sims pets stories (47) serial office 2000 (47) river past audio converter (47) new star soccer 2 registration code (47) mtx mototrax (47) kitchendraw 4.5 (47) cucusoft (47) crack eagle 4.11 (47) counter strike 1.6 cd key (47) avs (47) avg cd key (47) acdsee 8 keygen (47) warcraft cd key (46) visual web developer 2005 (46) nero 7.8.5.0 serial (46) nero 6 keygen (46) mov to avi mpeg wmv converter (46) guild wars keygen (46) empire at war crack (46) dataviz (46) data doctor recovery (46) cracked fraps (46) counter strike serial key (46) adobe photoshop cs2 serial (46) zuma deluxe crack (45) windows xp professional serial (45) windows 2003 crack (45) trackmania sunrise no cd (45) sims 2 (45) serial windows 2000 (45) restorer 2000 (45) pocket dvd wizard crack (45) nero 7 essentials crack (45) most wanted cd key (45) kaspersky anti (45) harry potter (45) fl (45) diablo 2 no cd (45) diablo 2 lord of destruction cd key (45) call of duty serial (45) call of duty 2 key (45) blaze media pro (45) any dvd 6.1.6.5 (45) alcohol 120%25 (45) x video converter (44) winrar key (44) winmpg (44) windows vista (44) sims 2 nightlife no cd (44) regcure (44) polderbits crack (44) nod32 keygen (44) nod32 (44) need for speed carbon (44) men of valor serial (44) keygen reaconverter pro (44) keygen counter strike source (44) halo serial (44) cue club registration key (44) crack need for speed most wanted (44) crack hdd regenerator 1.42 (44) baldur (44) advanced pdf password recovery 1.48 (44) 3d live pool keygen (44) windowblinds 5.51 (43) vmware workstation 5 serial (43) virus locker (43) simcity 3000 (43) replay (43) pfconfig (43) pc security (43) norton 2005 (43) netlimiter 1.30 serial (43) nero 7 cd key (43) guild wars serial (43) getdataback key (43) divx 6 (43) adobe photoshop (43) adobe cs2 keygen (43) zlauncher serial (42) windows activation crack (42) uniblue registry booster 2.0.1013.3068 (42) two worlds (42) serial solidworks 2004 (42) serial call of duty 2 (42) rocket mania deluxe (42) photostudio (42) optix pro v1.32 download (42) nero 7.9.6.0 cd key (42) mathtype (42) incredimail (42) free internet tv serial (42) fraps crack (42) empires (42) diner dash (42) crack loki (42) cfosspeed (42) avid 5.7 (42) allmusicconverter (42) airxonix (42) acdsee key (42) xp pro key (41) translator (41) star wars empire at war cd key (41) sentinel superpro emulator (41) reaconverter 5.0 pro edition (41) pro evolution soccer (41) pinnacle (41) microsoft sql server 2005 (41) keygen nero (41) key code call of duty 2 (41) imtoo wma mp3 converter (41) final cut studio 2 (41) crack virtua tennis (41) bookworm adventures deluxe (41) adobe premiere cs3 (41) adobe photoshop cs serial (41) actual spy 3.0 serial (41) xilisoft ipod video converter (40) word (40) voyager (40) serial photoshop cs (40) mdaemon (40) longhorn (40) imtoo dvd ripper (40) hddlife 3.0.140 (40) halo (40) guitarfx 3.04 (40) file scavenger (40) fifa 2006 no cd (40) f recovery sd 2.5 (40) crack nfs most wanted (40) crack need for speed underground 2 (40) cfosspeed 4 (40) cd_key/frozen throne cd key (40) bejeweled 1.86 crack (40) win dvd creator 3 key gen (39) swat 4 cd key (39) super cleaner (39) splinter cell chaos theory serial (39) splinter cell chaos theory crack (39) registry medic serial (39) reget deluxe (39) new star soccer crack (39) nero 6 serial number (39) need for speed most wanted serial (39) keygens (39) guild wars cd key (39) doom 3 key (39) divx 5.1.1 serial (39) desktop video recorder (39) dbpoweramp crack (39) cs3 (39) cd key nero 6 (39) cd key call of duty 2 (39) unlock code solid converter pdf (38) uniblue power suite (38) textpad 4.7.3 crack (38) kaspersky antivirus 6.0.1.411 key (38) fine reader (38) delicious 2 (38) cubis gold 2 (38) crack nero 7.9.6.0 (38) crack easy rm to mp3 converter (38) cossacks 2 crack (38) cnc (38) clonecd (38) call of duty key (38) boilsoft rm converter (38) boilsoft (38) anti (38) acdsee 7 crack (38) super dvd creator (37) solsuite 2007 v.7.6 (37) sims 2 deluxe (37) recover my files (37) power dvd serial (37) photovista panorama 3.0 crack (37) nfs underground 2 cd key (37) nfl (37) need for speed most wanted keygen (37) microsoft office 2003 cd key (37) magic mp3 tagger (37) key code for sims livin large (37) gutterball registration key (37) far cry cd key (37) crack pdf2word (37) bejeweled 2 crack (37) avg license code (37) avast crack (37) alcohol 120 1.9.5 (37) adobe photoshop serial (37) act of war cd key (37) winavi video converter serial (36) warcraft 3 serial (36) spyware doctor key (36) sniffer (36) sam broadcaster (36) radmin (36) plato dvd ripper (36) office xp key (36) o%26o defrag 8.6 (36) norton antivirus product key (36) live for speed (36) handy recovery serial (36) dvd (36) crack autocad 2005 (36) cooledit (36) bejeweled keygen (36) avs video converter 5.6 crack (36) any dvd (36) 2007 (36) windows xp serial number (35) windows xp home edition (35) warcraft 3 cd keys (35) tmpgenc (35) spyware doctor license code (35) smart movie keygen (35) serial office 2003 (35) painter 9 (35) nero 7 demo serial number (35) microsoft outlook 2007 (35) illustrator cs3 (35) file scavenger 3.1 (35) dreamweaver cs3 (35) diner dash crack (35) diablo crack (35) dawn of war cd key (35) clonedvd4 (35) cd key norton 2005 (35) avi to dvd svcd vcd converter (35) audiograbber 1.83 crack (35) 1 click dvd copy 5.0.3.5 (35) world of warcraft cd key (34) winzip keygen (34) winrar crack (34) trackmania sunrise crack (34) serial musicmatch jukebox 9 (34) norton 360 (34) mcafee (34) email spider (34) diablo 2 serial (34) diablo 2 cd key (34) ddd (34) crystal player profesional 1.98 (34) crack/office 2003 activation crack (34) crack winrar (34) call of duty 2 serial (34) bitdefender 8 (34) babylon 5 keygen (34) avs video converter 5.6 (34) anydvd 6 crack (34) amplitube (34) adobe photoshop cs3 (34) adobe illustrator cs3 serial (34) xingtone serial (33) windvd 7 keygen (33) winace 2.5 keygen (33) tumblebugs crack (33) total commander 6.53 crack (33) talisman (33) spyware doctor 5 serial (33) spb mobile shell (33) skygrabber (33) registry mechanic (33) office xp serial (33) netsim (33) music label (33) mp3 tagger (33) microsoft office home and student 2007 (33) feeding frenzy serial (33) avg anti (33) adobe photoshop cs2 serial number (33) adaware se (33) acdsee (33) 007 spy software (33) xp sp2 serial (32) windows xp sp2 (32) ulead video studio11 plus (32) serial number harry potter (32) radmin 3.0 serial (32) powerdvd 6 serial (32) open video joiner (32) need (32) magiciso maker 5.4 (32) keygen/tuneup utilities 2007 keygen (32) keygen schlacht um mittelerde (32) grand master chess (32) diablo 2 lod cd key (32) dap (32) crack dap (32) cd_key/battle for middle earth cd key (32) avg 7.5 build 476 (32) advanced system optimizer (32) adobe photoshop cs2 (32) adobe photoshop 7.0 serial number (32) adobe cs3 web premium (32) activation key norton 2004 (32) xp (31) wow stat changer (31) windvd 7 serial (31) windows xp sp2 activation crack (31) vue (31) spss 12 (31) splinter cell chaos theory cd key (31) sapphire (31) radar (31) proshow gold serial (31) powerpoint (31) photoshop 7.0 serial number (31) nod 32 2.7 (31) neverwinter nights crack (31) neat image (31) mirc serial (31) incredimail xe build 5653017 patch (31) hamachi (31) half life keygen (31) fraps 2.8.2 (31) escan (31) crack brothers in arms (31) colin mcrae dirt crack (31) coffeecup html editor 2007 (31) clickfinder 5.2 (31) call of duty cd keys (31) avi (31) avast 4.7 (31) anno 1701 (31) alcohol keygen v1.9.6 (31) xp serial (30) xoftspy keygen (30) winundelete (30) videoredo serial (30) video to mp4 converter (30) trados (30) spyware doctor 5.0.1.201a (30) spyware doctor 5.0 (30) sound forge mp3 (30) sound forge 9 (30) serial windows xp (30) security task manager 1.7e (30) ricochet lost worlds (30) ravenriley.com (30) norton antivirus 2006 keygen (30) nod32 crack (30) mprojector (30) joint operations typhoon rising key (30) dvdfab platinum (30) divx (30) crack zuma (30) crack winavi (30) crack to nero 5.5.10.56 (30) colin mcrae dirt serial (30) chicken invaders 2 (30) bounce (30) avg serial (30) avg 7.5 (30) autocad 2008 (30) anydvd 6.1.5.5 crack (30) worldmate (29) winzip (29) winrar registration code (29) windows (29) vegas 4.0 cd key (29) the sims 2 (29) stalker (29) spyware (29) speed optimizer 2 crack (29) serial nero 7.9.6.0 (29) serial doom 3 (29) regcleaner (29) need for speed underground 2 serial (29) need for speed most wanted no cd (29) need for speed most wanted (29) mid converter 3.2. serial (29) microsoft office 2003 serial (29) kerio winroute (29) fl studio 7 crack (29) eset smart security (29) docrepair crack (29) crack winconnection 3.5 (29) cd key (29) camfrog 3.91 (29) call of duty 2 (29) bejeweled (29) adobe illustrator cs3 (29) act of war serial (29) kaspersky internet security 7.0.0.123 (29) xp pro serial (28) world of warcraft (28) winundelete registration key (28) winlyrics (28) winedt 5.4 (28) windows xp professional (28) winconnection serial (28) warcraft 3 reign of chaos cd key (28) ultraedit 13.10 (28) super pool crack (28) star wars battlefront 2 cd key (28) san andreas crack (28) radmin serial (28) passwordtools (28) office 2003 activation (28) nero 7.9.6.0 serial number (28) nero 7.9 keygen (28) nero 7 key (28) nero 6.6 serial (28) musicmatch jukebox (28) keygen nero 7.9.6.0 (28) keygen call of duty 2 (28) hollywood fx 6 (28) guild wars crack (28) go1984 (28) cracks and serials (28) crack minitab 14 (28) badcopy pro 3.81 serial (28) avs converter (28) age of empires 3 product key (28) abc (28) winhex (27) windows xp home (27) virtual dj serial (27) uniblue registry booster crack (27) supertanga com (27) serial men of valor (27) mp3tag 5.6 (27) men of valor cd key (27) kaspersky internet security (27) iar (27) hardwood solitaire (27) getdataback ntfs 2.31 serial (27) cd_key/empire earth cd key (27) call of duty 2 serial number (27) bejeweled 2 (27) avg antispyware 7.5 keygen (27) arcade lines (27) agenda msd personal 8.10 (27) age of empires 3 serial (27) zuma deluxe registration key (26) winzip crack (26) windows xp pro key (26) warcraft 3 (26) system mechanic 7 (26) swat 4 serial (26) speedoptimizer 2.0 code (26) serial avg (26) route 66 (26) rocket mania (26) pgp (26) nero 7.9.6.0 with crack download (26) nero 7.9 (26) nero 6.6.1.4 serial number (26) mobiledit (26) internet download accelerator 5.2.1.1057 crack (26) insaniquarium deluxe crack (26) easyrecovery (26) dreamweaver (26) diablo 2 crack (26) crack/brothers in arms crack (26) crack photoshop cs2 (26) crack gta san andreas (26) colin mcrae dirt cd key (26) bsplayer (26) anydvd 6.1.6.5 (26) all my movies (26) adobe photoshop cs2 keygen (26) adobe illustrator cs3 (26) ableton 5.2 keygen (26) windows xp key (25) veritas (25) top spin cd key (25) serial diablo 2 (25) ragtime (25) pdf editor (25) paint shop pro (25) nod32 key (25) netlimiter 1.3 crack (25) luxor keygen (25) glidos crack (25) free spyware doctor registration code (25) fraps serial (25) edgecam 11.75 (25) eagle 4.11r2 crack (25) divx author (25) dbpoweramp music converter %28dmc%29 (25) crack serial (25) crack autocad 14 (25) coverxp (25) counter strike 1.6 keygen (25) converter (25) codecharge (25) codecharge (25) clonk endeavour crack (25) capella (25) bounce out (25) bejeweled 2 deluxe crack (25) battlefield 2142 (25) avira premium security suite 7.00.04.15 (25) avast 4.7 home edition (25) atomixmp3 v2.3 (25) atomixmp3 (25) actual spy (25) winrar serial (24) windows xp key generator (24) windows xp home keygen (24) windows xp activation keygen (24) winavi (24) vmware 5 serial (24) video fixer 3.23 keygen (24) style xp (24) softcam (24) smac 2.0.5 (24) sexual (24) serial/photoshop 7 serial number (24) robohelp x5 crack (24) norton antivirus 2006 serial (24) nfs underground crack (24) nfs (24) nanny mania (24) moviejack 3.5 serial (24) microsoft office professional 2007 (24) halo keygen (24) girl (24) genarts (24) ftvgirl (24) frontpage 2003 serial (24) fraps 2.8.2 crack (24) fifa 2006 crack (24) eviews 4.1 crack (24) erwin crack (24) dreamweaver crack (24) divx pro 6.6 (24) dead mans hand cd key (24) crack magic inlay (24) comand conquer general (24) chrome keygen (24) cd (24) aqua data studio (24) alcohol serial (24) age of empire 3 cd key (24) acdsee serial (24) 3dmark06 (24) xilisoft (23) worms armageddon serial (23) wingate 6.0.3 crack (23) windows server 2003 crack (23) windows activation (23) warcraft 3 key gen (23) swiff chart 3.2 crack (23) smartmovie keygen (23) small business server 2003 (23) smac 2.0 (23) serial winrar (23) retrospect (23) restorer2000 crack (23) rar password recovery (23) pro beach soccer (23) polar golfer (23) photoshop 7 serial number (23) office 2007 keygen (23) netlimiter 1.30 keygen (23) nero serial key (23) nero key (23) nero 6 key (23) mp3 splitter %26 joiner (23) mass downloader 3.3 (23) magic ball (23) jaws (23) illustrator cs3 serial number (23) halo cd key (23) halo 2 cd key (23) generals cd key (23) foxit pdf editor 1.5 key (23) flash decompiler 2.99 (23) excelfix (23) dvdfab platinum 3.1.4.0 (23) cs2 serial (23) crack spyhunter (23) clone cd crack (23) cd_key/trackmania sunrise cd key (23) cd key most wanted (23) avg keygen (23) acdsee 6.0crack (23) 3d (23) windows xp home edition keygen (22) windowblinds 5.51 (22) virusprotectpro 3.3 (22) ulead (22) tweaknow powerpack (22) topdesk (22) titan quest cd keyen (22) style xp serial (22) star wars battlefront serial key (22) spyware doctor license (22) serial/kaspersky serial (22) serial windows (22) serial number nero 7 (22) replay media catcher (22) replay av (22) repair registry pro (22) pvplayer keygen (22) panda titanium 2006 (22) office 2000 serial (22) nfs most wanted cd key (22) nero 7.9.6 keygen (22) need for speed underground crack (22) need for speed underground 2 keygen (22) microsoft office 2007 (22) medal of honor cd key (22) kaspersky (22) jewel quest (22) indesign (22) half life 2 cd key (22) garmin (22) fifa 2005 cd key (22) cricket 2004 (22) crack twoprog 3.1.4 (22) commview 5.5 (22) chocolatier (22) cd key top spin (22) cd key need for speed most wanted (22) camfrog (22) battlefront 2 serial (22) battlefield 2 (22) avs video converter 5.6 keygen (22) anydvd serial (22) adobe photoshop serial number (22) adobe photoshop cs2 crack (22) accessfix (22) xp keygen (21) wpa_kill.exe (21) winiso 5.3 crack (21) windows 2003 serial (21) warcraft iii cd key (21) tuneup utilities 2007 (21) spyware doctor 5.0.1.201 serial (21) sms (21) serial illustrator cs3 (21) sentinel emulator (21) revit (21) registry mechanic keygen (21) photo dvd maker (21) paint shop pro 9 crack (21) office xp sp3 activator (21) office 2003 serial number (21) nero 7.9 serial (21) nero 7.8.5.0 ultra edition enhanced (21) nero 7.8.5.0 (21) nero 6316 keygen (21) need for speed crack (21) movavi (21) mirc keygen (21) mirc 6.21 (21) keygen nero 6 (21) isilo (21) flax (21) dvr studio (21) diablo 2 cd key generator (21) diablo (21) cucusoft ultimate dvd video converter suite (21) crack luxor (21) crack fraps (21) crack chaos league (21) crack call of duty 2 (21) crack avs video editor 3.5 (21) cd_key/need for speed underground cd key (21) burger rush (21) badcopy pro 3.75 serial (21) avast key (21) acoustica 3.08 (21) 3d sexvilla crack (21) zonealarm (20) xoftspy (20) wow (20) windows xp home edition cd key (20) vmware (20) visual zip password recovery processor (20) ventafax (20) ut 2004 key gen (20) undisker 1.2 keygen (20) the sims deluxe serial (20) the battle for middle (20) surf (20) stylexp crack (20) spyware doctor 5.0 serial (20) spydoctor v2.0 activation key (20) sims (20) serial xp sp2 (20) serial number office 2003 (20) serial most wanted (20) serial bitdefender (20) roller coaster tycoon 3 serial (20) registrybooster (20) proteus (20) porn (20) platypus (20) oziexplorerce crack (20) oxygen (20) norton 2006 (20) nero 6.6.0.3 (20) need for speed underground2 (20) navigon (20) myscript notes (20) mpeg2 (20) moyea (20) movie6 (20) inksaver (20) half life (20) empire earth 2 serial (20) director (20) crack need for speed underground (20) crack any dvd (20) coreldraw design collection (20) coreldraw 12 keygen (20) clickfinder (20) cd_key/condition zero cd key (20) avs video converter serial (20) avs video converter 5.6 serial (20) atmosphere (20) aqua data studio 6.0.15 (20) anydvd (20) age of empires iii product key (20) adobe cs3 (20) xilisoft 3.1.34.0622b (19) windows xp sp2 activation (19) windows 98 serial (19) warcraft 3 crack (19) virtual dj key (19) total commander 7 (19) tmpgenc mpeg editor keygen (19) style xp registration key (19) spyware doctor 5.0.1.201a key (19) slovoed (19) serials cs condition zero (19) screentime (19) rm converter (19) ptravelalarm (19) pfhoe (19) pdftypewriter 5.6 (19) ozi explorer 3.95.4 (19) office 2003 keygen (19) norton 2007 (19) nero 7 premium serial (19) nero 6 crack (19) nero (19) mainconcept (19) magic folders xp (19) keygen illustrator cs3 (19) inspector parker key (19) insaniquarium crack (19) illustrator (19) hiew (19) half (19) gangland cd key (19) fl studio 7 (19) fifa 2006 serial (19) fifa 06 crack (19) elifoot 2006 (19) divxtodvd (19) diskeeper 2007 (19) data doctor (19) dap 8.5 (19) cyberlink powerdvd 7.0.2211 (19) crack style xp (19) crack fifa 2006 (19) counter strike keygen (19) convertxtodvd (19) cd_key/halo cd key (19) cd autorun creator 4.6 (19) call of duty 2 no cd crack (19) bootit (19) bitdefender (19) avs video converter 5.5 (19) aston (19) asf (19) age of empires 3 (19) advanced registry optimizer 5.0 crack (19) advanced registry optimizer (19) wpa_kill.exe sp2 (18) wpa_kill sp2 (18) world of warcraft serial (18) winzip 110 (18) windows xp sp2 cd key (18) windows xp pro (18) windows vista ultimate (18) warcraft crack (18) virtuagirl2 crack (18) upromote (18) systemworks 2004 (18) sudoku (18) star wars battlefront cd key (18) speedconnect (18) spb mobile shell 1.5.0 (18) serial crack (18) rise of nations (18) power dvd 7 (18) opencube (18) nfs underground (18) nero 7.9.6.0 (18) nero 7 premium crack (18) medal of honor allied assault cd key (18) luxor crack (18) keygen guild wars (18) key nod32 (18) handy recovery 2.0 serial (18) flash (18) fireburner (18) download accelerator 7.4.0.1crackserials (18) diskeeper (18) deejaysystem crack (18) cybercafepro (18) crimsonland keygen (18) crack need for speed (18) crack do need for speed most wanted (18) corel (18) condition zero (18) cod serial (18) cd_key/need for speed underground 2 cd key (18) cd key norton 2004 (18) cd key call of duty (18) cakewalk sonar (18) bitdefender key (18) battlefront 2 (18) avs video editor 3.5 (18) avira (18) avg anti spyware (18) anydvd key (18) age of empires iii cd key (18) age of empires 3 key (18) winzip code (17) winbackup (17) warcraft3 (17) warcraft 3 frozen throne cd key (17) warcraft (17) visualdsp (17) virtualgirl2 (17) tuneup utilities 2006 serial (17) tumblebugs (17) total commander 7.01 (17) tmpgenc 3.0.4.24 (17) swishmax crack (17) swat 4 (17) spyware doctor (17) smartmovie 3.41 (17) serial power dvd 6 (17) photoshop serial number (17) pc rescue (17) nod32 2.7 crack (17) nero 6.6.0.8 crack (17) nero 6.6.0.6 (17) nero 6.6 (17) need for speed underground serial number (17) morpheus photo morpher (17) mirc (17) medal of honor (17) luxor 2 (17) keygen/adobe cs2 keygen (17) keygen windows xp (17) icoo loader crack (17) hide my ip (17) gta crack (17) freez flv to mp3 converter (17) fraps keygen (17) fifa 2005 serial (17) empires die neuzeit no cd (17) cracks serials (17) crack/xp sp2 crack (17) crack spyware doctor (17) crack nero 7.9.6 (17) crack fraps 2.8.2 (17) cod2 serial (17) cd_key/windows xp sp2 cd key (17) bugdom (17) avg antispyware 7.5 (17) avg antispyware (17) anydvd 6.1.6.0 crack (17) anno 1503 (17) agelong tree (17) advanced (17) zuma deluxe serial (16) zonealarm serial (16) xp key generator (16) xp activation crack (16) wpa_kill (16) winrar 3.51 crack (16) windows xp professional cd key (16) wildtangent bejeweled 2 deluxe crack (16) vso (16) vdj3 (16) validation (16) underground (16) trackmania united (16) tony hawks underground 2 cd key (16) test drive (16) style xp key (16) spyware doctor 5.0.1.200 serial (16) splinter cell chaos theory keygen (16) snagit 7.1.0 crack (16) smartftp (16) serials for fate (16) serial style xp (16) serial nero 7.9.6 (16) serial nero 6.6.1.15 (16) serial anydvd 6.1.6.5 (16) serial power vcr 3.0 standard (16) scanspyware (16) rome total war no cd crack (16) repligo crack (16) phpmaker (16) photozoom (16) photoshop serial (16) photoshop cs3 serial (16) pdanet (16) paint shop pro xi (16) opera 8 serial (16) opera (16) office 2003 serial key (16) norton internet security (16) norton antivirus 2007 (16) norton antivirus (16) norton 2006 keygen (16) nod32 2.7 (16) nod 32 2.7 (16) nod 32 (16) nero key generator (16) nero 7.9.6.0 key (16) nero 7 serial key (16) nero 6.6.1.15a (16) nero 6.6.1.15 serial number (16) nero 6.6.0.14 serial (16) nero 6 cd key (16) mskey4in1 (16) mobile master (16) minitab 14 serial (16) marvel (16) licen code nero7 (16) keygen/halo keygen (16) finalrecovery (16) fifa 2006 cd key (16) fifa (16) facefilter (16) evaluation (16) easy video converter 7.2.1 (16) diskeeper crack (16) dirt (16) dap premiu 8.5 (16) cycling (16) cs 1.6 keygen (16) crack/clonedvd2 crack (16) crack avg (16) coverxp 1.65 (16) counter strike key (16) counter strike 1.6 (16) cd key doom 3 (16) cad (16) azureus (16) avg key (16) autocad 2005 crack (16) arcsoft totalmedia 3 (16) adobe dreamweaver cs3 (16) act of war keygen (16) acdsee 9 (16) 3d sex villa crack (16) 2d 3d screensaver maker 3.6 (16) 2003 activation (16) zuma serial (15) xp sp2 key (15) xp sp2 (15) wow keygen (15) wm recorder (15) windows live onecare (15) windows 2003 server crack (15) wavelab v6 (15) vmware virtualcenter (15) transformers (15) trackmania sunrise no cd crack (15) theatre of war (15) test drive unlimited (15) teamviewer 2.42 (15) switchball (15) swishpix crack (15) swat 4 serial number (15) stylexp (15) serial/badcopy pro 3.81 serial (15) serial number avs video player 5.6 (15) serial key (15) sammsoft (15) roller (15) rise of nations serial (15) rapidshare premium account (15) radmin crack (15) power dvd 6 key (15) photoshop cs3 keygen (15) photoshop cs2 cd key (15) open video converter (15) office 2003 crack (15) norton 2006 crack (15) news rover 12 (15) nero 7.9.6 (15) nero 7.9. serial number (15) nero 7 ultra edition serial (15) minitab 14 activation code (15) microangelo (15) magic iso (15) lost (15) loki keygen (15) keygen/winavi keygen (15) keygen/nero 6 keygen (15) keygen style xp (15) keygen nero 7 (15) kaspersky license (15) karaoke (15) hiew v6.85 full (15) easyboot 5.1 serial (15) easy mp3 cutter (15) dawn of war keygen (15) crack/feeding frenzy 2 crack (15) crack/bejeweled 2 crack (15) crack/3d sexvilla crack (15) crack pro cycling manager 2007 (15) crack fifa 06 (15) counterspy (15) counter strike (15) cfos (15) cd_key/rise of nations cd key (15) cd to mp3 ripper (15) catwoman serial (15)
-AV Voice Changer V 6.0.10 keygen .rar
DOWNLOAD ::: https://gohhs.com/2uz5Kt
aaccfb2cb3
-
-
\ No newline at end of file
diff --git a/spaces/inamXcontru/PoeticTTS/Bartender Barcode Software Free Download Crack For 574 _HOT_.md b/spaces/inamXcontru/PoeticTTS/Bartender Barcode Software Free Download Crack For 574 _HOT_.md
deleted file mode 100644
index 8909dc2595af68598acfbab6317d805f5b4ba722..0000000000000000000000000000000000000000
--- a/spaces/inamXcontru/PoeticTTS/Bartender Barcode Software Free Download Crack For 574 _HOT_.md
+++ /dev/null
@@ -1,8 +0,0 @@
-bartender barcode software free download crack for 574
DOWNLOAD ---> https://gohhs.com/2uz4B6
-
-This video explains how to set up a zebra printer to work with the RollMaster software.The zebra lp2824ex Printer (Version 1) is available upon request with optional software that allows the user to monitor ink usage, print barcodes, and upload print order data to a cash register.
-The software ensures that the printer operates in accordance with industry standards.
-RollMaster is software that allows users to upload print order data to a cash register, print barcodes, and select a language. 8a78ff9644
-
-
-
diff --git a/spaces/inplisQlawa/anything-midjourney-v4-1/HD Online Player (prboa Rule 7 And 8 Pdf Free BETTER).md b/spaces/inplisQlawa/anything-midjourney-v4-1/HD Online Player (prboa Rule 7 And 8 Pdf Free BETTER).md
deleted file mode 100644
index 555d20b10e19d7445a64a6b60802db07d1e8629b..0000000000000000000000000000000000000000
--- a/spaces/inplisQlawa/anything-midjourney-v4-1/HD Online Player (prboa Rule 7 And 8 Pdf Free BETTER).md
+++ /dev/null
@@ -1,6 +0,0 @@
-HD Online Player (prboa rule 7 and 8 pdf free)
Download 🌟 https://urlin.us/2uEx7k
-
-Monograph series 8, Volume 1, European Monitoring Centre for Drugs and Drug Addiction, Lisbon. ... The first two chapters in this section examine the role of cannabis as a ... Sweden promotes a vision of a 'drug-free society' at policy level. ... http ://whq libdoc. who. int/hq/1 997/WHO_MSA_PSA_97.4.pdf 116 Chapter 7Â ... 1fdad05405
-
-
-
diff --git a/spaces/inplisQlawa/anything-midjourney-v4-1/How To Crack Adobe CC 2020 ? Adobe Creative Latest Patch MacOSX LINK.md b/spaces/inplisQlawa/anything-midjourney-v4-1/How To Crack Adobe CC 2020 ? Adobe Creative Latest Patch MacOSX LINK.md
deleted file mode 100644
index 9a326333462e92c8e26ad64fb48a72d715247e14..0000000000000000000000000000000000000000
--- a/spaces/inplisQlawa/anything-midjourney-v4-1/How To Crack Adobe CC 2020 ? Adobe Creative Latest Patch MacOSX LINK.md
+++ /dev/null
@@ -1,60 +0,0 @@
-How to crack Adobe CC 2020 – Adobe Creative Latest Patch MacOSX
Download ✪✪✪ https://urlin.us/2uEvmi
-
-Click Account.
-
-Click Check for Updates.
-
-After installing an update, go through the same process as before.
-
-As of September 2020, the Creative Cloud desktop app for macOS requires macOS Catalina.
-
-If you find that updates are not being installed automatically, for example if they fail to connect to the service, you may find that the Creative Cloud desktop app for macOS does not have the required number of authorization tokens. Follow these steps to fix it:
-
-Open the Creative Cloud desktop app. (Select the icon in the Windows taskbar or the macOS menu bar.)
-
-Go to the menu bar and select Settings, then Account.
-
-Select Generate New Tokens.
-
-The Creative Cloud desktop app will be back up and running.
-
-Q:
-
-Django: Why is this string formatting not working?
-
-I want to format this date
-
-2012-10-26T12:53:20
-
-as "YYYY-MM-DD" in the template.
-
-I have done this:
-
-
-
- item.text
-
-but the output is:
-
-A:
-
-You can also use the time template filter.
-
- item.datetime
-
-Because date:'Y-m-d' is already being interpreted as a string, and 'Y-m-d' is not interpreted as a format for that string. If you want to add a format to the output, you need to pass the format to the filter like this:
-
-date:"Y-m-d"
-
-The documentation for time filters has more information.
-
-1. Field of the Invention
-
-The present invention relates to a hydraulic control system for an automatic transmission and more particularly to a hydraulic control system for an automatic transmission that has a shift control valve for controlling a speed change ratio or the like by controlling the supply of a working oil to a hydraulically operated friction device of the automatic transmission.
-
-2. Description of the Related Art
-
-Conventionally, a hydraulic control system of 4fefd39f24
-
-
-
diff --git a/spaces/inplisQlawa/anything-midjourney-v4-1/Ismael Miranda Discografia Torrent Descargar.md b/spaces/inplisQlawa/anything-midjourney-v4-1/Ismael Miranda Discografia Torrent Descargar.md
deleted file mode 100644
index 6d51f6daf311746de395aa51d08d90755b1a4c3f..0000000000000000000000000000000000000000
--- a/spaces/inplisQlawa/anything-midjourney-v4-1/Ismael Miranda Discografia Torrent Descargar.md
+++ /dev/null
@@ -1,46 +0,0 @@
-ismael miranda discografia torrent descargar
Download File ———>>> https://urlin.us/2uExaf
-
-Left, Eddie Rivera, Edwin Colon, Frankie Rodriguez, Ismael Miranda, Jack Hitchcock, Julio Romero, Junior Gonzalez, Larry Harlow, Larry Spencer, Leopoldo PinedaRight, Eddie Rivera, Edwin Colon, Frankie Rodriguez, Ismael Miranda, Jack Hitchcock, Julio Romero, Junior Gonzalez, Larry Harlow, Larry Spencer, Leopoldo Pineda
-
-References
-
-Further reading
-
-External links
-
-Category:2011 American television series debuts
-
-Category:2011 American television series endings
-
-Category:2010s American comedy television series
-
-Category:English-language television programs
-
-Category:Spanish-language television programs
-
-Category:Television shows set in New York City
-
-Category:Television series by 3 Arts Entertainment
-
-Category:Television series by Universal Television
-
-Category:Television shows set in Chicago
-
-Category:Television shows set in MiamiOral and maxillofacial manifestations in sickle cell disease.
-
-Oral and maxillofacial manifestations in sickle cell disease have been well known for many years. Oral lesions may occur as a complication of the disease itself, or due to the effects of splenic dysfunction or anemia. Chronic oral ulcers and oral candidiasis are common, while more severe lesions, such as osteomyelitis, osteonecrosis, and infarcted tonsils, may occur. Some patients may present with oral manifestations that are the primary symptom of the disease, such as severe or recurrent aphthous ulcers. Other manifestations may be associated with cystic fibrosis, alpha-1-antitrypsin deficiency, congenital anomalies, and drug ingestion. Certain procedures such as bone marrow transplantation and retinal photography may cause potentially dangerous or irreversible oral complications in some patients. We review the oral and maxillofacial complications of sickle cell disease with an emphasis on the relative frequency and severity of these lesions in different age groups.
-
-Home-Makers Battle Tech Giants, Starting From the Living Room - mcxx
-
-======
-
-mattculbreth
-
-This is the "take-two-pumps-and-go" generation. We're all busy, we're
-
-overloaded, we've got business to tend to and a new baby to care for, it's
-
-hell. But don't let that stop you. So get up, get your butt in gear, 4fefd39f24
-
-
-
diff --git a/spaces/inplisQlawa/anything-midjourney-v4-1/Mickey Virus 2 Full 2021 Movie With English Subtitles Download Torrent.md b/spaces/inplisQlawa/anything-midjourney-v4-1/Mickey Virus 2 Full 2021 Movie With English Subtitles Download Torrent.md
deleted file mode 100644
index 26afa71e9b68adc8427f5a4069a595584b57d758..0000000000000000000000000000000000000000
--- a/spaces/inplisQlawa/anything-midjourney-v4-1/Mickey Virus 2 Full 2021 Movie With English Subtitles Download Torrent.md
+++ /dev/null
@@ -1,6 +0,0 @@
-Mickey Virus 2 full movie with english subtitles download torrent
Download Zip ->>->>->> https://urlin.us/2uEvyt
-
-2 Secret Study Tips To Score Highest in Every Exam Motivational Hindi ... |Hindi| Download Hollywood Movie in Hindi or English | torrent movie downloader ... Mickey Virus Full Movie with Eng. Subtitles | Hindi Movies 2016 Full Movie ... 4d29de3e1b
-
-
-
diff --git a/spaces/iqovocn/ChuanhuChatGPT/custom.css b/spaces/iqovocn/ChuanhuChatGPT/custom.css
deleted file mode 100644
index 5143eb138ea2469d8c457c71cb210fd3fb7cbe15..0000000000000000000000000000000000000000
--- a/spaces/iqovocn/ChuanhuChatGPT/custom.css
+++ /dev/null
@@ -1,162 +0,0 @@
-:root {
- --chatbot-color-light: #F3F3F3;
- --chatbot-color-dark: #121111;
-}
-
-/* status_display */
-#status_display {
- display: flex;
- min-height: 2.5em;
- align-items: flex-end;
- justify-content: flex-end;
-}
-#status_display p {
- font-size: .85em;
- font-family: monospace;
- color: var(--body-text-color-subdued);
-}
-
-#chuanhu_chatbot, #status_display {
- transition: all 0.6s;
-}
-/* list */
-ol:not(.options), ul:not(.options) {
- padding-inline-start: 2em !important;
-}
-
-/* 亮色 */
-#chuanhu_chatbot {
- background-color: var(--chatbot-color-light) !important;
-}
-[data-testid = "bot"] {
- background-color: #FFFFFF !important;
-}
-[data-testid = "user"] {
- background-color: #95EC69 !important;
-}
-/* 对话气泡 */
-[class *= "message"] {
- border-radius: var(--radius-xl) !important;
- border: none;
- padding: var(--spacing-xl) !important;
- font-size: var(--text-md) !important;
- line-height: var(--line-md) !important;
- min-height: calc(var(--text-md)*var(--line-md) + 2*var(--spacing-xl));
- min-width: calc(var(--text-md)*var(--line-md) + 2*var(--spacing-xl));
-}
-[data-testid = "bot"] {
- max-width: 85%;
- border-bottom-left-radius: 0 !important;
-}
-[data-testid = "user"] {
- max-width: 85%;
- width: auto !important;
- border-bottom-right-radius: 0 !important;
-}
-/* 表格 */
-table {
- margin: 1em 0;
- border-collapse: collapse;
- empty-cells: show;
-}
-td,th {
- border: 1.2px solid var(--border-color-primary) !important;
- padding: 0.2em;
-}
-thead {
- background-color: rgba(175,184,193,0.2);
-}
-thead th {
- padding: .5em .2em;
-}
-/* 行内代码 */
-code {
- display: inline;
- white-space: break-spaces;
- border-radius: 6px;
- margin: 0 2px 0 2px;
- padding: .2em .4em .1em .4em;
- background-color: rgba(175,184,193,0.2);
-}
-/* 代码块 */
-pre code {
- display: block;
- overflow: auto;
- white-space: pre;
- background-color: hsla(0, 0%, 0%, 80%)!important;
- border-radius: 10px;
- padding: 1.4em 1.2em 0em 1.4em;
- margin: 1.2em 2em 1.2em 0.5em;
- color: #FFF;
- box-shadow: 6px 6px 16px hsla(0, 0%, 0%, 0.2);
-}
-/* 代码高亮样式 */
-.highlight .hll { background-color: #49483e }
-.highlight .c { color: #75715e } /* Comment */
-.highlight .err { color: #960050; background-color: #1e0010 } /* Error */
-.highlight .k { color: #66d9ef } /* Keyword */
-.highlight .l { color: #ae81ff } /* Literal */
-.highlight .n { color: #f8f8f2 } /* Name */
-.highlight .o { color: #f92672 } /* Operator */
-.highlight .p { color: #f8f8f2 } /* Punctuation */
-.highlight .ch { color: #75715e } /* Comment.Hashbang */
-.highlight .cm { color: #75715e } /* Comment.Multiline */
-.highlight .cp { color: #75715e } /* Comment.Preproc */
-.highlight .cpf { color: #75715e } /* Comment.PreprocFile */
-.highlight .c1 { color: #75715e } /* Comment.Single */
-.highlight .cs { color: #75715e } /* Comment.Special */
-.highlight .gd { color: #f92672 } /* Generic.Deleted */
-.highlight .ge { font-style: italic } /* Generic.Emph */
-.highlight .gi { color: #a6e22e } /* Generic.Inserted */
-.highlight .gs { font-weight: bold } /* Generic.Strong */
-.highlight .gu { color: #75715e } /* Generic.Subheading */
-.highlight .kc { color: #66d9ef } /* Keyword.Constant */
-.highlight .kd { color: #66d9ef } /* Keyword.Declaration */
-.highlight .kn { color: #f92672 } /* Keyword.Namespace */
-.highlight .kp { color: #66d9ef } /* Keyword.Pseudo */
-.highlight .kr { color: #66d9ef } /* Keyword.Reserved */
-.highlight .kt { color: #66d9ef } /* Keyword.Type */
-.highlight .ld { color: #e6db74 } /* Literal.Date */
-.highlight .m { color: #ae81ff } /* Literal.Number */
-.highlight .s { color: #e6db74 } /* Literal.String */
-.highlight .na { color: #a6e22e } /* Name.Attribute */
-.highlight .nb { color: #f8f8f2 } /* Name.Builtin */
-.highlight .nc { color: #a6e22e } /* Name.Class */
-.highlight .no { color: #66d9ef } /* Name.Constant */
-.highlight .nd { color: #a6e22e } /* Name.Decorator */
-.highlight .ni { color: #f8f8f2 } /* Name.Entity */
-.highlight .ne { color: #a6e22e } /* Name.Exception */
-.highlight .nf { color: #a6e22e } /* Name.Function */
-.highlight .nl { color: #f8f8f2 } /* Name.Label */
-.highlight .nn { color: #f8f8f2 } /* Name.Namespace */
-.highlight .nx { color: #a6e22e } /* Name.Other */
-.highlight .py { color: #f8f8f2 } /* Name.Property */
-.highlight .nt { color: #f92672 } /* Name.Tag */
-.highlight .nv { color: #f8f8f2 } /* Name.Variable */
-.highlight .ow { color: #f92672 } /* Operator.Word */
-.highlight .w { color: #f8f8f2 } /* Text.Whitespace */
-.highlight .mb { color: #ae81ff } /* Literal.Number.Bin */
-.highlight .mf { color: #ae81ff } /* Literal.Number.Float */
-.highlight .mh { color: #ae81ff } /* Literal.Number.Hex */
-.highlight .mi { color: #ae81ff } /* Literal.Number.Integer */
-.highlight .mo { color: #ae81ff } /* Literal.Number.Oct */
-.highlight .sa { color: #e6db74 } /* Literal.String.Affix */
-.highlight .sb { color: #e6db74 } /* Literal.String.Backtick */
-.highlight .sc { color: #e6db74 } /* Literal.String.Char */
-.highlight .dl { color: #e6db74 } /* Literal.String.Delimiter */
-.highlight .sd { color: #e6db74 } /* Literal.String.Doc */
-.highlight .s2 { color: #e6db74 } /* Literal.String.Double */
-.highlight .se { color: #ae81ff } /* Literal.String.Escape */
-.highlight .sh { color: #e6db74 } /* Literal.String.Heredoc */
-.highlight .si { color: #e6db74 } /* Literal.String.Interpol */
-.highlight .sx { color: #e6db74 } /* Literal.String.Other */
-.highlight .sr { color: #e6db74 } /* Literal.String.Regex */
-.highlight .s1 { color: #e6db74 } /* Literal.String.Single */
-.highlight .ss { color: #e6db74 } /* Literal.String.Symbol */
-.highlight .bp { color: #f8f8f2 } /* Name.Builtin.Pseudo */
-.highlight .fm { color: #a6e22e } /* Name.Function.Magic */
-.highlight .vc { color: #f8f8f2 } /* Name.Variable.Class */
-.highlight .vg { color: #f8f8f2 } /* Name.Variable.Global */
-.highlight .vi { color: #f8f8f2 } /* Name.Variable.Instance */
-.highlight .vm { color: #f8f8f2 } /* Name.Variable.Magic */
-.highlight .il { color: #ae81ff } /* Literal.Number.Integer.Long */
diff --git a/spaces/itmorn/face_keypoint/README.md b/spaces/itmorn/face_keypoint/README.md
deleted file mode 100644
index c6ffcdc3daa0a5144e6bfd134e81f24061942274..0000000000000000000000000000000000000000
--- a/spaces/itmorn/face_keypoint/README.md
+++ /dev/null
@@ -1,13 +0,0 @@
----
-title: Face Keypoint
-emoji: 🙂
-colorFrom: yellow
-colorTo: gray
-sdk: gradio
-sdk_version: 3.4
-app_file: app.py
-pinned: false
-license: other
----
-
-Check out the configuration reference at https://huggingface.co/docs/hub/spaces-config-reference
diff --git a/spaces/jackli888/stable-diffusion-webui/extensions/deforum/scripts/deforum_helpers/src/clipseg/datasets/pfe_dataset.py b/spaces/jackli888/stable-diffusion-webui/extensions/deforum/scripts/deforum_helpers/src/clipseg/datasets/pfe_dataset.py
deleted file mode 100644
index 83988dea963a2c4226010a336573de94bf06c55e..0000000000000000000000000000000000000000
--- a/spaces/jackli888/stable-diffusion-webui/extensions/deforum/scripts/deforum_helpers/src/clipseg/datasets/pfe_dataset.py
+++ /dev/null
@@ -1,129 +0,0 @@
-from os.path import expanduser
-import torch
-import json
-from general_utils import get_from_repository
-from datasets.lvis_oneshot3 import blend_image_segmentation
-from general_utils import log
-
-PASCAL_CLASSES = {a['id']: a['synonyms'] for a in json.load(open('datasets/pascal_classes.json'))}
-
-
-class PFEPascalWrapper(object):
-
- def __init__(self, mode, split, mask='separate', image_size=473, label_support=None, size=None, p_negative=0, aug=None):
- import sys
- # sys.path.append(expanduser('~/projects/new_one_shot'))
- from third_party.PFENet.util.dataset import SemData
-
- get_from_repository('PascalVOC2012', ['Pascal5i.tar'])
-
- self.p_negative = p_negative
- self.size = size
- self.mode = mode
- self.image_size = image_size
-
- if label_support in {True, False}:
- log.warning('label_support argument is deprecated. Use mask instead.')
- #raise ValueError()
-
- self.mask = mask
-
- value_scale = 255
- mean = [0.485, 0.456, 0.406]
- mean = [item * value_scale for item in mean]
- std = [0.229, 0.224, 0.225]
- std = [item * value_scale for item in std]
-
- import third_party.PFENet.util.transform as transform
-
- if mode == 'val':
- data_list = expanduser('~/projects/old_one_shot/PFENet/lists/pascal/val.txt')
-
- data_transform = [transform.test_Resize(size=image_size)] if image_size != 'original' else []
- data_transform += [
- transform.ToTensor(),
- transform.Normalize(mean=mean, std=std)
- ]
-
-
- elif mode == 'train':
- data_list = expanduser('~/projects/old_one_shot/PFENet/lists/pascal/voc_sbd_merge_noduplicate.txt')
-
- assert image_size != 'original'
-
- data_transform = [
- transform.RandScale([0.9, 1.1]),
- transform.RandRotate([-10, 10], padding=mean, ignore_label=255),
- transform.RandomGaussianBlur(),
- transform.RandomHorizontalFlip(),
- transform.Crop((image_size, image_size), crop_type='rand', padding=mean, ignore_label=255),
- transform.ToTensor(),
- transform.Normalize(mean=mean, std=std)
- ]
-
- data_transform = transform.Compose(data_transform)
-
- self.dataset = SemData(split=split, mode=mode, data_root=expanduser('~/datasets/PascalVOC2012/VOC2012'),
- data_list=data_list, shot=1, transform=data_transform, use_coco=False, use_split_coco=False)
-
- self.class_list = self.dataset.sub_val_list if mode == 'val' else self.dataset.sub_list
-
- # verify that subcls_list always has length 1
- # assert len(set([len(d[4]) for d in self.dataset])) == 1
-
- print('actual length', len(self.dataset.data_list))
-
- def __len__(self):
- if self.mode == 'val':
- return len(self.dataset.data_list)
- else:
- return len(self.dataset.data_list)
-
- def __getitem__(self, index):
- if self.dataset.mode == 'train':
- image, label, s_x, s_y, subcls_list = self.dataset[index % len(self.dataset.data_list)]
- elif self.dataset.mode == 'val':
- image, label, s_x, s_y, subcls_list, ori_label = self.dataset[index % len(self.dataset.data_list)]
- ori_label = torch.from_numpy(ori_label).unsqueeze(0)
-
- if self.image_size != 'original':
- longerside = max(ori_label.size(1), ori_label.size(2))
- backmask = torch.ones(ori_label.size(0), longerside, longerside).cuda()*255
- backmask[0, :ori_label.size(1), :ori_label.size(2)] = ori_label
- label = backmask.clone().long()
- else:
- label = label.unsqueeze(0)
-
- # assert label.shape == (473, 473)
-
- if self.p_negative > 0:
- if torch.rand(1).item() < self.p_negative:
- while True:
- idx = torch.randint(0, len(self.dataset.data_list), (1,)).item()
- _, _, s_x, s_y, subcls_list_tmp, _ = self.dataset[idx]
- if subcls_list[0] != subcls_list_tmp[0]:
- break
-
- s_x = s_x[0]
- s_y = (s_y == 1)[0]
- label_fg = (label == 1).float()
- val_mask = (label != 255).float()
-
- class_id = self.class_list[subcls_list[0]]
-
- label_name = PASCAL_CLASSES[class_id][0]
- label_add = ()
- mask = self.mask
-
- if mask == 'text':
- support = ('a photo of a ' + label_name + '.',)
- elif mask == 'separate':
- support = (s_x, s_y)
- else:
- if mask.startswith('text_and_'):
- label_add = (label_name,)
- mask = mask[9:]
-
- support = (blend_image_segmentation(s_x, s_y.float(), mask)[0],)
-
- return (image,) + label_add + support, (label_fg.unsqueeze(0), val_mask.unsqueeze(0), subcls_list[0])
diff --git a/spaces/james-oldfield/PandA/networks/genforce/runners/controllers/fid_evaluator.py b/spaces/james-oldfield/PandA/networks/genforce/runners/controllers/fid_evaluator.py
deleted file mode 100644
index 3a4193918ccf89b55a786507f77638e227c5a891..0000000000000000000000000000000000000000
--- a/spaces/james-oldfield/PandA/networks/genforce/runners/controllers/fid_evaluator.py
+++ /dev/null
@@ -1,55 +0,0 @@
-# python3.7
-"""Contains the running controller for evaluation."""
-
-import os.path
-import time
-
-from .base_controller import BaseController
-from ..misc import format_time
-
-__all__ = ['FIDEvaluator']
-
-
-class FIDEvaluator(BaseController):
- """Defines the running controller for evaluation.
-
- This controller is used to evalute the GAN model using FID metric.
-
- NOTE: The controller is set to `LAST` priority by default.
- """
-
- def __init__(self, config):
- assert isinstance(config, dict)
- config.setdefault('priority', 'LAST')
- super().__init__(config)
-
- self.num = config.get('num', 50000)
- self.ignore_cache = config.get('ignore_cache', False)
- self.align_tf = config.get('align_tf', True)
- self.file = None
-
- def setup(self, runner):
- assert hasattr(runner, 'fid')
- file_path = os.path.join(runner.work_dir, f'metric_fid{self.num}.txt')
- if runner.rank == 0:
- self.file = open(file_path, 'w')
-
- def close(self, runner):
- if runner.rank == 0:
- self.file.close()
-
- def execute_after_iteration(self, runner):
- mode = runner.mode # save runner mode.
- start_time = time.time()
- fid_value = runner.fid(self.num,
- ignore_cache=self.ignore_cache,
- align_tf=self.align_tf)
- duration_str = format_time(time.time() - start_time)
- log_str = (f'FID: {fid_value:.5f} at iter {runner.iter:06d} '
- f'({runner.seen_img / 1000:.1f} kimg). ({duration_str})')
- runner.logger.info(log_str)
- if runner.rank == 0:
- date = time.strftime("%Y-%m-%d %H:%M:%S")
- self.file.write(f'[{date}] {log_str}\n')
- self.file.flush()
- runner.set_mode(mode) # restore runner mode.
diff --git a/spaces/jayparmr/ICBINP_OG/app.py b/spaces/jayparmr/ICBINP_OG/app.py
deleted file mode 100644
index 19ad72c0b9184d0f7a3102e303c8cc24481cda73..0000000000000000000000000000000000000000
--- a/spaces/jayparmr/ICBINP_OG/app.py
+++ /dev/null
@@ -1,89 +0,0 @@
-
-import gradio as gr
-import torch
-# from diffusers import DiffusionPipeline
-from diffusers import StableDiffusionPipeline
-from diffusers.models import AutoencoderKL
-from diffusers import StableDiffusionPipeline
-
-
-
-
-def generate(prompt, negative_prompts, samples, steps,scale, seed, width, height):
-
- pipeline = StableDiffusionPipeline.from_pretrained("jayparmr/icbinp", use_auth_token="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", torch_dtype=torch.float16)
- pipeline.to("cuda")
-
- generator = torch.Generator(device="cuda").manual_seed(int(seed))
-
- images_list = pipeline(
- [prompt] * samples,
- negative_prompt= [negative_prompts] * samples,
- num_inference_steps=steps,
- guidance_scale=scale,
- generator=generator,
- width=width,
- height=height
- )
-
- # vae = AutoencoderKL.from_pretrained("stabilityai/sdxl-vae")
- # pipe = StableDiffusionPipeline.from_pretrained("runwayml/stable-diffusion-v1-5", vae=vae).to("cuda")
-
-
- # images_list = pipe(
- # [prompt] * samples,
- # negative_prompt= [negative_prompts] * samples,
- # num_inference_steps=steps,
- # guidance_scale=scale
- # )
- print("stop gen")
- images = []
- print(images_list)
- for i, image in enumerate(images_list["images"]):
- images.append(image)
- return images
-
-block = gr.Blocks()
-
-with block:
- with gr.Group():
- with gr.Box():
- with gr.Row().style(equal_height=True):
- text = gr.Textbox(
- label="Enter your prompt",
- show_label=False,
- max_lines=1,
- placeholder="Enter your prompt",
- )
- negative_text = gr.Textbox(
- value="",
- label="Enter your negative prompt",
- show_label=False,
- max_lines=1,
- placeholder="Enter your negative prompt",
- )
- btn = gr.Button("Generate image")
- gallery = gr.Gallery(
- label="Generated images", show_label=False, elem_id="gallery", width = 512
- ).style(columns=[2], rows=[2], object_fit="contain", height="auto")
-
-
- with gr.Row(elem_id="advanced-options"):
- samples = gr.Slider(label="Images", minimum=1, maximum=4, value=1, step=1)
- steps = gr.Slider(label="Steps", minimum=1, maximum=500, value=100, step=1)
- width = gr.Slider(label="width", minimum=1, maximum=2048, value=512, step=1)
- height = gr.Slider(label="height", minimum=1, maximum=2048, value=512, step=1)
- scale = gr.Slider(
- label="Guidance Scale", minimum=0, maximum=50, value=7.5, step=0.1
- )
- seed = gr.Slider(
- label="Seed",
- minimum=0,
- maximum=2147483647,
- step=1
- )
- text.submit(generate, inputs=[text,negative_text, samples, steps, scale, seed, width, height], outputs=gallery)
- btn.click(generate, inputs=[text,negative_text, samples, steps, scale, seed, width, height], outputs=gallery)
-
-
-block.launch()
\ No newline at end of file
diff --git a/spaces/jbilcke-hf/ai-clip-factory/src/app/server/actions/community.ts b/spaces/jbilcke-hf/ai-clip-factory/src/app/server/actions/community.ts
deleted file mode 100644
index 56d23c289d8d98cbbf4771720d6b18e73cbd6c17..0000000000000000000000000000000000000000
--- a/spaces/jbilcke-hf/ai-clip-factory/src/app/server/actions/community.ts
+++ /dev/null
@@ -1,267 +0,0 @@
-"use server"
-
-import { v4 as uuidv4 } from "uuid"
-
-import { CreatePostResponse, GetAppPostResponse, GetAppPostsResponse, Post, PostVisibility } from "@/types"
-import { filterOutBadWords } from "./censorship"
-import { shuffleArray } from "../utils/shuffleArray"
-
-const apiUrl = `${process.env.COMMUNITY_API_URL || ""}`
-const apiToken = `${process.env.COMMUNITY_API_TOKEN || ""}`
-const appId = `${process.env.COMMUNITY_API_ID || ""}`
-const secretModerationKey = `${process.env.MODERATION_KEY || ""}`
-
-export async function postToCommunity({
- prompt = "",
- model = "",
- assetUrl = "",
-}: {
- prompt: string
- model: string,
- assetUrl: string
-}): Promise {
-
- const before = prompt
- prompt = filterOutBadWords(prompt)
-
- if (prompt !== before) {
- console.log(`user attempted to use bad words! their original prompt is: ${before}`)
- }
-
- if (prompt.toLocaleLowerCase().includes("male muscle") || prompt.toLocaleLowerCase().includes("muscle growth")) {
- throw new Error("unknown erorr")
- }
-
- // if the community API is disabled,
- // we don't fail, we just mock
- if (!apiUrl) {
- const mockPost: Post = {
- postId: uuidv4(),
- appId: "mock",
- prompt,
- model,
- previewUrl: assetUrl,
- assetUrl,
- createdAt: new Date().toISOString(),
- visibility: "normal",
- upvotes: 0,
- downvotes: 0
- }
- return mockPost
- }
-
- if (!prompt) {
- console.error(`cannot call the community API without a prompt, aborting..`)
- throw new Error(`cannot call the community API without a prompt, aborting..`)
- }
- if (!assetUrl) {
- console.error(`cannot call the community API without an assetUrl, aborting..`)
- throw new Error(`cannot call the community API without an assetUrl, aborting..`)
- }
-
- try {
- console.log(`calling POST ${apiUrl}/posts/${appId} with prompt: ${prompt}`)
-
- const postId = uuidv4()
-
- const post: Partial = { postId, appId, prompt, model, assetUrl }
-
- console.log(`target url is: ${
- `${apiUrl}/posts/${appId}`
- }`)
-
- const res = await fetch(`${apiUrl}/posts/${appId}`, {
- method: "POST",
- headers: {
- Accept: "application/json",
- "Content-Type": "application/json",
- Authorization: `Bearer ${apiToken}`,
- },
- body: JSON.stringify(post),
- cache: 'no-store',
- // we can also use this (see https://vercel.com/blog/vercel-cache-api-nextjs-cache)
- // next: { revalidate: 1 }
- })
-
- // Recommendation: handle errors
- if (res.status !== 201) {
- // This will activate the closest `error.js` Error Boundary
- throw new Error('Failed to fetch data')
- }
-
- const response = (await res.json()) as CreatePostResponse
- // console.log("response:", response)
- return response.post
- } catch (err) {
- const error = `failed to post to community: ${err}`
- console.error(error)
- throw new Error(error)
- }
-}
-
-export async function getLatestPosts({
- visibility,
- maxNbPosts = 80,
- shuffle = true,
-}: {
- visibility?: PostVisibility
- maxNbPosts?: number
- shuffle?: boolean
-}): Promise {
-
- let posts: Post[] = []
-
- // if the community API is disabled we don't fail,
- // we just mock
- if (!apiUrl) {
- return posts
- }
-
- try {
- // console.log(`calling GET ${apiUrl}/posts with renderId: ${renderId}`)
- // TODO: send the max number of posts
- const res = await fetch(`${apiUrl}/posts/${appId}/firehose/${
- visibility || "all"
- }/${
- maxNbPosts || 80
- }/${
- !!shuffle
- }`, {
- method: "GET",
- headers: {
- Accept: "application/json",
- "Content-Type": "application/json",
- Authorization: `Bearer ${apiToken}`,
- },
- cache: 'no-store',
- // we can also use this (see https://vercel.com/blog/vercel-cache-api-nextjs-cache)
- // next: { revalidate: 1 }
- })
-
- // console.log("res:", res)
- // The return value is *not* serialized
- // You can return Date, Map, Set, etc.
-
- // Recommendation: handle errors
- if (res.status !== 200) {
- // This will activate the closest `error.js` Error Boundary
- throw new Error('Failed to fetch data')
- }
-
- const response = (await res.json()) as GetAppPostsResponse
- // console.log("response:", response)
-
- const posts: Post[] = Array.isArray(response?.posts) ? response?.posts : []
-
- return posts
- } catch (err) {
- // const error = `failed to get posts: ${err}`
- // console.error(error)
- // throw new Error(error)
- return []
- }
-}
-
-export async function getPost(postId: string): Promise {
-
- // if the community API is disabled we don't fail,
- // we just mock
- if (!apiUrl) {
- throw new Error("community API is not enabled")
- }
-
- try {
- // console.log(`calling GET ${apiUrl}/posts with renderId: ${renderId}`)
- const res = await fetch(`${apiUrl}/posts/${appId}/${postId}`, {
- method: "GET",
- headers: {
- Accept: "application/json",
- "Content-Type": "application/json",
- Authorization: `Bearer ${apiToken}`,
- },
- cache: 'no-store',
- // we can also use this (see https://vercel.com/blog/vercel-cache-api-nextjs-cache)
- // next: { revalidate: 1 }
- })
-
- // console.log("res:", res)
- // The return value is *not* serialized
- // You can return Date, Map, Set, etc.
-
- // Recommendation: handle errors
- if (res.status !== 200) {
- // This will activate the closest `error.js` Error Boundary
- throw new Error('Failed to fetch data')
- }
-
- const response = (await res.json()) as GetAppPostResponse
- // console.log("response:", response)
- return response.post
- } catch (err) {
- const error = `failed to get post: ${err}`
- console.error(error)
- throw new Error(error)
- }
-}
-
-export async function deletePost({
- postId,
- moderationKey,
-}: {
- postId: string
- moderationKey: string
-}): Promise {
-
- // if the community API is disabled,
- // we don't fail, we just mock
- if (!apiUrl) {
- return false
- }
-
- if (!postId) {
- console.error(`cannot delete a post without a postId, aborting..`)
- throw new Error(`cannot delete a post without a postId, aborting..`)
- }
- if (!moderationKey) {
- console.error(`cannot delete a post without a moderationKey, aborting..`)
- throw new Error(`cannot delete a post without a moderationKey, aborting..`)
- }
-
- if (moderationKey !== secretModerationKey) {
- console.error(`invalid moderation key, operation denied! please ask an admin for the mdoeration key`)
- throw new Error(`invalid moderation key, operation denied! please ask an admin for the mdoeration key`)
- }
-
- try {
- console.log(`calling DELETE ${apiUrl}/posts/${appId}/${postId}`)
-
- const res = await fetch(`${apiUrl}/posts/${appId}/${postId}`, {
- method: "DELETE",
- headers: {
- Accept: "application/json",
- "Content-Type": "application/json",
- Authorization: `Bearer ${apiToken}`,
- },
- cache: 'no-store',
- // we can also use this (see https://vercel.com/blog/vercel-cache-api-nextjs-cache)
- // next: { revalidate: 1 }
- })
-
- // console.log("res:", res)
- // The return value is *not* serialized
- // You can return Date, Map, Set, etc.
-
- // Recommendation: handle errors
- if (res.status !== 200) {
- // This will activate the closest `error.js` Error Boundary
- throw new Error('Failed to fetch data')
- }
-
- const response = (await res.json()) as CreatePostResponse
- return true
- } catch (err) {
- const error = `failed to delete the post: ${err}`
- console.error(error)
- throw new Error(error)
- }
-}
diff --git a/spaces/jcenaa/Segment-Any-RGBD/third_party/CLIP/clip/__init__.py b/spaces/jcenaa/Segment-Any-RGBD/third_party/CLIP/clip/__init__.py
deleted file mode 100644
index dcc5619538c0f7c782508bdbd9587259d805e0d9..0000000000000000000000000000000000000000
--- a/spaces/jcenaa/Segment-Any-RGBD/third_party/CLIP/clip/__init__.py
+++ /dev/null
@@ -1 +0,0 @@
-from .clip import *
diff --git a/spaces/jhtonyKoo/music_mixing_style_transfer/app.py b/spaces/jhtonyKoo/music_mixing_style_transfer/app.py
deleted file mode 100644
index c21850580726a7294c3d321e2532235e77c4cc59..0000000000000000000000000000000000000000
--- a/spaces/jhtonyKoo/music_mixing_style_transfer/app.py
+++ /dev/null
@@ -1,174 +0,0 @@
-import os
-import binascii
-import warnings
-
-import json
-import argparse
-import copy
-
-import numpy as np
-import matplotlib.pyplot as plt
-import torch
-import tqdm
-import librosa
-import librosa.display
-import soundfile as sf
-import gradio as gr
-import pytube as pt
-
-from pytube.exceptions import VideoUnavailable
-
-from inference.style_transfer import *
-
-
-yt_video_dir = f"./yt_dir/0"
-os.makedirs(yt_video_dir, exist_ok=True)
-
-
-def get_audio_from_yt_video_input(yt_link: str, start_point_in_second=0, duration_in_second=30):
- try:
- yt = pt.YouTube(yt_link)
- t = yt.streams.filter(only_audio=True)
- filename_in = os.path.join(yt_video_dir, "input.wav")
- t[0].download(filename=filename_in)
- except VideoUnavailable as e:
- warnings.warn(f"Video Not Found at {yt_link} ({e})")
- filename_in = None
-
- # trim audio length - due to computation time on HuggingFace environment
- trim_audio(target_file_path=filename_in, start_point_in_second=start_point_in_second, duration_in_second=duration_in_second)
-
- return filename_in, filename_in
-
-def get_audio_from_yt_video_ref(yt_link: str, start_point_in_second=0, duration_in_second=30):
- try:
- yt = pt.YouTube(yt_link)
- t = yt.streams.filter(only_audio=True)
- filename_ref = os.path.join(yt_video_dir, "reference.wav")
- t[0].download(filename=filename_ref)
- except VideoUnavailable as e:
- warnings.warn(f"Video Not Found at {yt_link} ({e})")
- filename_ref = None
-
- # trim audio length - due to computation time on HuggingFace environment
- trim_audio(target_file_path=filename_ref, start_point_in_second=start_point_in_second, duration_in_second=duration_in_second)
-
- return filename_ref, filename_ref
-
-def inference(file_uploaded_in, file_uploaded_ref):
- # clear out previously separated results
- os.system(f"rm -r {yt_video_dir}/separated")
- # change file path name
- os.system(f"cp {file_uploaded_in} {yt_video_dir}/input.wav")
- os.system(f"cp {file_uploaded_ref} {yt_video_dir}/reference.wav")
-
- # Perform music mixing style transfer
- args = set_up()
-
- inference_style_transfer = Mixing_Style_Transfer_Inference(args)
- output_wav_path = inference_style_transfer.inference(file_uploaded_in, file_uploaded_ref)
-
- return output_wav_path
-
-
-
-with gr.Blocks() as demo:
- gr.HTML(
- """
-
-
-
- Music Mixing Style Transfer
-
-
- """
- )
- gr.Markdown(
- """
- This page is a Hugging Face interactive demo of the paper ["Music Mixing Style Transfer: A Contrastive Learning Approach to Disentangle Audio Effects"](https://huggingface.co/papers/2211.02247) (ICASSP 2023).
- - [project page](https://jhtonykoo.github.io/MixingStyleTransfer/)
- - [GitHub](https://github.com/jhtonyKoo/music_mixing_style_transfer)
- - [supplementary](https://pale-cicada-946.notion.site/Music-Mixing-Style-Transfer-A-Contrastive-Learning-Approach-to-Disentangle-Audio-Effects-Supplemen-e6eccd9a431a4a8fa4fdd5adb2d3f219)
- """
- )
- with gr.Group():
- with gr.Column():
- with gr.Blocks():
- with gr.Tab("Input Music"):
- file_uploaded_in = gr.Audio(label="Input track (mix) to be mixing style transferred", type="filepath")
- with gr.Tab("YouTube url"):
- with gr.Row():
- yt_link_in = gr.Textbox(
- label="Enter YouTube Link of the Video", autofocus=True, lines=3
- )
- yt_in_start_sec = gr.Number(
- value=0,
- label="starting point of the song (in seconds)"
- )
- yt_in_duration_sec = gr.Number(
- value=30,
- label="duration of the song (in seconds)"
- )
- yt_btn_in = gr.Button("Download Audio from YouTube Link", size="lg")
- yt_audio_path_in = gr.Audio(
- label="Input Audio Extracted from the YouTube Video", interactive=False
- )
- yt_btn_in.click(
- get_audio_from_yt_video_input,
- inputs=[yt_link_in, yt_in_start_sec, yt_in_duration_sec],
- outputs=[yt_audio_path_in, file_uploaded_in],
- )
- with gr.Blocks():
- with gr.Tab("Reference Music"):
- file_uploaded_ref = gr.Audio(label="Reference track (mix) to copy mixing style", type="filepath")
- with gr.Tab("YouTube url"):
- with gr.Row():
- yt_link_ref = gr.Textbox(
- label="Enter YouTube Link of the Video", autofocus=True, lines=3
- )
- yt_ref_start_sec = gr.Number(
- value=0,
- label="starting point of the song (in seconds)"
- )
- yt_ref_duration_sec = gr.Number(
- value=30,
- label="duration of the song (in seconds)"
- )
- yt_btn_ref = gr.Button("Download Audio from YouTube Link", size="lg")
- yt_audio_path_ref = gr.Audio(
- label="Reference Audio Extracted from the YouTube Video", interactive=False
- )
- yt_btn_ref.click(
- get_audio_from_yt_video_ref,
- inputs=[yt_link_ref, yt_ref_start_sec, yt_ref_duration_sec],
- outputs=[yt_audio_path_ref, file_uploaded_ref],
- )
-
- with gr.Group():
- gr.HTML(
- """
- Mixing Style Transfer. Perform stem-wise audio-effects style conversion by first source separating the input mix. The inference computation time takes longer as the input samples' duration. so plz be patient...
- """
- )
- with gr.Column():
- inference_btn = gr.Button("Run Mixing Style Transfer")
- with gr.Row():
- output_mix = gr.Audio(label="mixing style transferred music track")
- inference_btn.click(
- inference,
- inputs=[file_uploaded_in, file_uploaded_ref],
- outputs=[output_mix],
- )
-
-
-
-if __name__ == "__main__":
- demo.launch(debug=True)
-
\ No newline at end of file
diff --git a/spaces/jinmao/2/modules/overwrites.py b/spaces/jinmao/2/modules/overwrites.py
deleted file mode 100644
index bfcd4d01b7d7bec1184a8d09113933bca860530b..0000000000000000000000000000000000000000
--- a/spaces/jinmao/2/modules/overwrites.py
+++ /dev/null
@@ -1,56 +0,0 @@
-from __future__ import annotations
-import logging
-
-from llama_index import Prompt
-from typing import List, Tuple
-import mdtex2html
-
-from modules.presets import *
-from modules.llama_func import *
-
-
-def compact_text_chunks(self, prompt: Prompt, text_chunks: List[str]) -> List[str]:
- logging.debug("Compacting text chunks...🚀🚀🚀")
- combined_str = [c.strip() for c in text_chunks if c.strip()]
- combined_str = [f"[{index+1}] {c}" for index, c in enumerate(combined_str)]
- combined_str = "\n\n".join(combined_str)
- # resplit based on self.max_chunk_overlap
- text_splitter = self.get_text_splitter_given_prompt(prompt, 1, padding=1)
- return text_splitter.split_text(combined_str)
-
-
-def postprocess(
- self, y: List[Tuple[str | None, str | None]]
-) -> List[Tuple[str | None, str | None]]:
- """
- Parameters:
- y: List of tuples representing the message and response pairs. Each message and response should be a string, which may be in Markdown format.
- Returns:
- List of tuples representing the message and response. Each message and response will be a string of HTML.
- """
- if y is None or y == []:
- return []
- user, bot = y[-1]
- if not detect_converted_mark(user):
- user = convert_asis(user)
- if not detect_converted_mark(bot):
- bot = convert_mdtext(bot)
- y[-1] = (user, bot)
- return y
-
-with open("./assets/custom.js", "r", encoding="utf-8") as f, open("./assets/Kelpy-Codos.js", "r", encoding="utf-8") as f2:
- customJS = f.read()
- kelpyCodos = f2.read()
-
-def reload_javascript():
- print("Reloading javascript...")
- js = f''
- def template_response(*args, **kwargs):
- res = GradioTemplateResponseOriginal(*args, **kwargs)
- res.body = res.body.replace(b' |