Commit
·
cb9d202
1
Parent(s):
efed207
Update parquet files (step 94 of 121)
Browse filesThis view is limited to 50 files because it contains too many changes.
See raw diff
- spaces/1acneusushi/gradio-2dmoleculeeditor/data/Capricho Catalan Segovia Pdf Download TOP.md +0 -21
- spaces/1acneusushi/gradio-2dmoleculeeditor/data/Enhance Your Windows XP Experience with K-Lite Codec Pack.md +0 -28
- spaces/1gistliPinn/ChatGPT4/Baywatch (English) Movie Download Fixed 720p.md +0 -55
- spaces/1gistliPinn/ChatGPT4/Examples/Dan Hartman Torrent Discography.md +0 -63
- spaces/1pelhydcardo/ChatGPT-prompt-generator/assets/Car Parking Multiplayer MOD APK Drive Any Car You Want and Explore the Streets.md +0 -124
- spaces/1pelhydcardo/ChatGPT-prompt-generator/assets/City Taxi Driving 3D Simulator The Ultimate Car Driving Game for Taxi Lovers.md +0 -222
- spaces/1phancelerku/anime-remove-background/Download M Modal and Simplify Your Documentation Workflow with Integrated Transcription Solutions.md +0 -106
- spaces/1phancelerku/anime-remove-background/Download Mortal Kombat MOD APK and Unleash Your Inner Warrior.md +0 -116
- spaces/1phancelerku/anime-remove-background/Enjoy FIFA Mobile with Nulls FIFA APK The Best Mod for Soccer Fans.md +0 -132
- spaces/44ov41za8i/FreeVC/README.md +0 -13
- spaces/52Hz/HWMNet_lowlight_enhancement/README.md +0 -45
- spaces/7hao/bingo/tests/kblob.ts +0 -27
- spaces/AIGC-Audio/AudioGPT/text_to_speech/modules/vocoder/parallel_wavegan/layers/pqmf.py +0 -132
- spaces/AIGC-Audio/AudioGPT/text_to_speech/tasks/tts/diffspeech.py +0 -111
- spaces/AQaTaHaGoD/GoD/confing.py +0 -7
- spaces/Abhilashvj/planogram-compliance/utils/loggers/wandb/README.md +0 -162
- spaces/AchyuthGamer/OpenGPT/g4f/Provider/Providers/Weuseing.py +0 -29
- spaces/AchyuthGamer/text-to-speech-client/style.css +0 -28
- spaces/AgentVerse/agentVerse/agentverse/agents/simulation_agent/tool.py +0 -177
- spaces/AgentVerse/agentVerse/agentverse/environments/tasksolving_env/rules/evaluator/base.py +0 -88
- spaces/AgentVerse/agentVerse/ui/src/phaser3-rex-plugins/templates/ui/canvas/Factory.d.ts +0 -6
- spaces/AlexWang/lama/saicinpainting/evaluation/losses/ssim.py +0 -74
- spaces/Amon1/ChatGPTForAcadamic/request_llm/README.md +0 -36
- spaces/Androidonnxfork/CivitAi-to-Diffusers/diffusers/src/diffusers/pipelines/spectrogram_diffusion/notes_encoder.py +0 -86
- spaces/Androidonnxfork/CivitAi-to-Diffusers/diffusers/src/diffusers/pipelines/stable_diffusion/pipeline_stable_diffusion_pix2pix_zero.py +0 -1259
- spaces/Androidonnxfork/CivitAi-to-Diffusers/diffusers/src/diffusers/pipelines/stable_diffusion_xl/__init__.py +0 -38
- spaces/Andy1621/uniformer_image_demo/imagenet_class_index.py +0 -1002
- spaces/Andy1621/uniformer_image_detection/app.py +0 -62
- spaces/Andy1621/uniformer_image_segmentation/configs/pspnet/pspnet_r101-d8_480x480_80k_pascal_context.py +0 -2
- spaces/Anonymous-sub/Rerender/ControlNet/annotator/canny/__init__.py +0 -6
- spaces/Anonymous-sub/Rerender/ControlNet/annotator/uniformer/mmcv/arraymisc/__init__.py +0 -4
- spaces/ArtGAN/Video-Diffusion-WebUI/video_diffusion/inpaint_zoom/utils/__init__.py +0 -0
- spaces/Arulkumar03/GroundingDINO_SOTA_Zero_Shot_Model/groundingdino/models/GroundingDINO/transformer_vanilla.py +0 -123
- spaces/Ataturk-Chatbot/HuggingFaceChat/venv/lib/python3.11/site-packages/pip/__init__.py +0 -13
- spaces/Ataturk-Chatbot/HuggingFaceChat/venv/lib/python3.11/site-packages/pip/_internal/vcs/subversion.py +0 -324
- spaces/Axolotlily/TextGen/README.md +0 -13
- spaces/Benson/text-generation/Examples/Caso Penal Mod Apk Desbloquear Todos Los Niveles.md +0 -71
- spaces/Big-Web/MMSD/env/Lib/site-packages/pip/_internal/cache.py +0 -272
- spaces/BucketHeadP65/confusion_matrix/app.py +0 -6
- spaces/CVPR/Dual-Key_Backdoor_Attacks/openvqa/openvqa/datasets/clevr/clevr_loader.py +0 -200
- spaces/CVPR/LIVE/thrust/thrust/detail/type_traits/is_call_possible.h +0 -161
- spaces/CVPR/LIVE/thrust/thrust/iterator/detail/host_system_tag.h +0 -40
- spaces/CVPR/Text2Human/Text2Human/ui_util/__init__.py +0 -0
- spaces/Chomkwoy/Nilkessye/synthetic_dataset.py +0 -560
- spaces/Codecooker/rvcapi/src/download_models.py +0 -31
- spaces/CofAI/chat.b4/g4f/Provider/Providers/Liaobots.py +0 -47
- spaces/DQChoi/gpt-demo/venv/lib/python3.11/site-packages/PIL/XVThumbImagePlugin.py +0 -78
- spaces/DebasishDhal99/Youtube_Playlist/app.py +0 -74
- spaces/Dinoking/Guccio-AI-Designer/models/biggan/pytorch_biggan/pytorch_pretrained_biggan/utils.py +0 -216
- spaces/Dinoking/Guccio-AI-Designer/models/stylegan2/stylegan2-pytorch/prepare_data.py +0 -82
spaces/1acneusushi/gradio-2dmoleculeeditor/data/Capricho Catalan Segovia Pdf Download TOP.md
DELETED
@@ -1,21 +0,0 @@
|
|
1 |
-
|
2 |
-
<h1>How to Download Capricho Catalan by Isaac Albeniz Arranged by Andres Segovia in PDF Format</h1>
|
3 |
-
<p>Capricho Catalan is a beautiful piece of music composed by Isaac Albeniz, a Spanish pianist and composer. It is part of his collection Espana, Op. 165, which consists of six pieces inspired by different regions of Spain. Capricho Catalan is dedicated to the region of Catalonia, and it evokes its folk music and culture.</p>
|
4 |
-
<h2>Capricho Catalan Segovia Pdf Download</h2><br /><p><b><b>Download File</b> ––– <a href="https://byltly.com/2uKA7I">https://byltly.com/2uKA7I</a></b></p><br /><br />
|
5 |
-
<p>One of the most famous arrangements of Capricho Catalan for guitar was made by Andres Segovia, a legendary Spanish guitarist and teacher. Segovia transcribed many works by Albeniz and other composers for the guitar, and he is widely regarded as one of the greatest guitarists of all time. His arrangement of Capricho Catalan captures the essence and beauty of the original piece, while adapting it to the technical and expressive possibilities of the guitar.</p>
|
6 |
-
<p>If you want to download Capricho Catalan by Isaac Albeniz arranged by Andres Segovia in PDF format, you can find it on several websites that offer free sheet music for guitar. Here are some of them:</p>
|
7 |
-
<ul>
|
8 |
-
<li><a href="https://www.free-scores.com/PDF_EN/albeniz-isaac-capricho-catalan-86006.pdf">Free-scores.com</a>: This website has a PDF file of Capricho Catalan arranged by Gerald Kidd, based on Segovia's transcription. It also has an audio file and a video link to watch Kidd's performance on YouTube.[^1^]</li>
|
9 |
-
<li><a href="https://www.scribd.com/doc/227432929/Capricho-Catalan-by-Isaac-Albeniz">Scribd.com</a>: This website has a PDF file of Capricho Catalan arranged by Segovia himself. It also has a preview image and some related documents that you might like.[^2^]</li>
|
10 |
-
<li><a href="https://www.free-scores.com/download-sheet-music.php?pdf=7139">Free-scores.com</a>: This website has another PDF file of Capricho Catalan arranged by Segovia himself. It also has a video link to watch Segovia's performance on YouTube.[^3^]</li>
|
11 |
-
</ul>
|
12 |
-
<p>To download the PDF files from these websites, you need to follow their instructions and terms of use. Some of them may require you to create an account or sign up for a free trial. Some of them may also have limitations on the number of downloads or prints that you can make. Please respect the rights of the composers, arrangers, and publishers when using their sheet music.</p>
|
13 |
-
<p></p>
|
14 |
-
<p>Capricho Catalan by Isaac Albeniz arranged by Andres Segovia is a wonderful piece of music that you can enjoy playing on your guitar. It is not very difficult to play, but it requires some skill and expression. You can learn it by following the PDF files that we have suggested, or by watching some videos of other guitarists playing it. We hope that you will have fun with this piece and appreciate its beauty.</p>
|
15 |
-
|
16 |
-
<p>Capricho Catalan is not only a musical piece, but also a cultural symbol. The word "capricho" means "whim" or "fancy" in Spanish, and it suggests a playful and imaginative mood. The word "catalan" refers to the people, language, and culture of Catalonia, a region in northeastern Spain that has a distinct identity and history. Catalonia has been a source of artistic and political inspiration for many people, including Albeniz himself.</p>
|
17 |
-
<p>Albeniz was born in Camprodon, a town in the province of Girona, which is part of Catalonia. He was a child prodigy who started playing the piano at the age of four. He ran away from home several times to pursue his musical career, traveling across Spain and Latin America. He eventually settled in Paris, where he studied with Vincent d'Indy and Paul Dukas. He became one of the most influential composers of Spanish music, blending elements of classical, folk, and impressionist styles.</p>
|
18 |
-
<p>Capricho Catalan was composed in 1899, when Albeniz was already suffering from Bright's disease, a chronic kidney condition that would eventually cause his death at the age of 48. It was part of his last major work, Espana, Op. 165, which he dedicated to his wife Rosina Jordana. The piece reflects Albeniz's nostalgia and love for his homeland, as well as his admiration for Catalan culture. It has a simple but elegant melody that is accompanied by rich harmonies and rhythmic variations.</p>
|
19 |
-
<p>Capricho Catalan has been arranged for various instruments and ensembles, but it is especially popular among guitarists. One of the reasons is that Albeniz himself was fond of the guitar, and he often wrote his pieces with the guitar sound in mind. Another reason is that many guitarists have made remarkable interpretations of Capricho Catalan, such as Andres Segovia[^2^] [^3^], David Russell[^1^], Alexandra Whittingham[^3^], and many others. Their performances showcase the expressive potential and beauty of this piece.</p> 81aa517590<br />
|
20 |
-
<br />
|
21 |
-
<br />
|
|
|
|
|
|
|
|
|
|
|
|
|
|
|
|
|
|
|
|
|
|
|
|
|
|
|
|
|
|
|
|
|
|
|
|
|
|
|
|
|
|
|
|
spaces/1acneusushi/gradio-2dmoleculeeditor/data/Enhance Your Windows XP Experience with K-Lite Codec Pack.md
DELETED
@@ -1,28 +0,0 @@
|
|
1 |
-
<br />
|
2 |
-
<h1>K-Lite Codec Pack for Windows XP</h1>
|
3 |
-
<p>If you are looking for a way to play various audio and video formats on your Windows XP computer, you might want to consider installing the K-Lite Codec Pack. This is a free, easy to install bundle of codecs/filters/splitters that enables your operating system and its software to play back movie and music files.</p>
|
4 |
-
<h2>What is a codec?</h2>
|
5 |
-
<p>A codec is a software component that compresses and decompresses digital media, such as audio and video. There are many different codecs available, each with its own advantages and disadvantages. Some codecs are designed for high quality, some for low file size, some for compatibility, and some for specific purposes.</p>
|
6 |
-
<h2>k-lite codec pack for windows xp</h2><br /><p><b><b>DOWNLOAD</b> — <a href="https://byltly.com/2uKzZG">https://byltly.com/2uKzZG</a></b></p><br /><br />
|
7 |
-
<h2>Why do I need a codec pack?</h2>
|
8 |
-
<p>Windows XP comes with a limited number of codecs pre-installed, which means that it can only play certain types of media files. For example, Windows XP can play MP3 and WAV audio files, but not FLAC or OGG. Similarly, it can play AVI and WMV video files, but not MKV or MP4. To play these and other formats, you need to install additional codecs on your system.</p>
|
9 |
-
<p>A codec pack is a convenient way to install multiple codecs at once, without having to download and install them individually. A codec pack also ensures that the codecs are configured properly and work well together, avoiding conflicts and compatibility issues.</p>
|
10 |
-
<h2>What does the K-Lite Codec Pack include?</h2>
|
11 |
-
<p>The K-Lite Codec Pack is one of the most popular and comprehensive codec packs available. It comes in four different variants: Basic, Standard, Full, and Mega. Each variant includes more components than the previous one, depending on your needs and preferences. The main differences between the variants are:</p>
|
12 |
-
<ul>
|
13 |
-
<li>Basic: Contains only the most essential decoders for playing common audio and video formats.</li>
|
14 |
-
<li>Standard: Same as Basic, plus Media Player Classic Home Cinema (MPC-HC), a versatile and lightweight media player that supports many formats and features; and MediaInfo Lite, a tool for getting details about media files.</li>
|
15 |
-
<li>Full: Same as Standard, plus MadVR, an advanced video renderer with high quality upscaling algorithms; Plugin for 3D video decoding (H.264 MVC), which requires using MPC-HC with MadVR and a compatible graphics driver; ffdshow audio processor and video processor, DirectShow filters that provide some audio and video processing options.</li>
|
16 |
-
<li>Mega: Same as Full, plus DC-Bass Source Mod, a decoder for OptimFrog and Tracker audio files (very rare formats); GraphStudioNext, a tool for creating and testing DirectShow graphs; a few ACM/VFW codecs such as x264VFW and Lagarith, which are used by certain video editing/encoding applications for working with AVI files.</li>
|
17 |
-
</ul>
|
18 |
-
<p>The K-Lite Codec Pack supports playback of many audio and video formats, such as AVI, MKV, MP4, FLV, MPEG, MOV, TS, M2TS, WMV, RM, RMVB, OGM, WebM; MP3, FLAC, M4A, AAC, OGG, 3GP, AMR, APE, MKA, Opus, Wavpack, Musepack; DVD and Blu-ray (after decryption); and many more.</p>
|
19 |
-
<p>The K-Lite Codec Pack also provides lots of useful functionality, such as subtitle display; hardware accelerated video decoding; audio bitstreaming; video thumbnails in Explorer; file association options; broken codec detection; and much more.</p>
|
20 |
-
<h2>How to download and install the K-Lite Codec Pack?</h2>
|
21 |
-
<p>To download the K-Lite Codec Pack for Windows XP,</p>
|
22 |
-
<ol>
|
23 |
-
<li>Go to the official website of the codec pack: <a href="http://www.codecguide.com/download_kl.htm">http://www.codecguide.com/download_kl.htm</a></li>
|
24 |
-
<li>Select the variant that suits your needs: Basic, Standard, Full, or Mega. You can also compare the contents of each variant on the website.</li>
|
25 |
-
<li>Click on the Download button next to your chosen variant. This will take you to another page where you can choose a mirror site to download from.</li>
|
26 |
-
<li>Click on one of the mirror links to start downloading the installer file</p> ddb901b051<br />
|
27 |
-
<br />
|
28 |
-
<br />
|
|
|
|
|
|
|
|
|
|
|
|
|
|
|
|
|
|
|
|
|
|
|
|
|
|
|
|
|
|
|
|
|
|
|
|
|
|
|
|
|
|
|
|
|
|
|
|
|
|
|
|
|
|
|
|
|
|
spaces/1gistliPinn/ChatGPT4/Baywatch (English) Movie Download Fixed 720p.md
DELETED
@@ -1,55 +0,0 @@
|
|
1 |
-
## Baywatch (English) Movie Download 720p
|
2 |
-
|
3 |
-
|
4 |
-
|
5 |
-
**Download File ===> [https://www.google.com/url?q=https%3A%2F%2Ftiurll.com%2F2twsAD&sa=D&sntz=1&usg=AOvVaw1R2vSPPaX4pvh7ddczSPN1](https://www.google.com/url?q=https%3A%2F%2Ftiurll.com%2F2twsAD&sa=D&sntz=1&usg=AOvVaw1R2vSPPaX4pvh7ddczSPN1)**
|
6 |
-
|
7 |
-
|
8 |
-
|
9 |
-
Here is a possible title and article with HTML formatting for the keyword "Baywatch (English) Movie Download 720p":
|
10 |
-
|
11 |
-
# How to Download Baywatch (English) Movie in 720p Quality
|
12 |
-
|
13 |
-
|
14 |
-
|
15 |
-
If you are a fan of action, comedy and crime movies, you might be interested in downloading Baywatch (English) movie in 720p quality. Baywatch is a 2017 Hollywood movie based on the popular TV series of the same name. It stars Dwayne Johnson, Zac Efron, Alexandra Daddario and Priyanka Chopra as lifeguards who uncover a criminal plot that threatens their beach. The movie is full of humor, adventure and romance, and it has received mixed reviews from critics and audiences.
|
16 |
-
|
17 |
-
|
18 |
-
|
19 |
-
There are several ways to download Baywatch (English) movie in 720p quality, but not all of them are safe and legal. Some websites may offer free downloads, but they may also contain viruses, malware or unwanted ads. Some websites may require you to sign up, pay or complete surveys before you can access the download links. Some websites may have low-quality or fake files that do not match the description.
|
20 |
-
|
21 |
-
|
22 |
-
|
23 |
-
To avoid these risks and enjoy Baywatch (English) movie in 720p quality, you need to find a reliable and trustworthy source that offers high-quality and genuine files. One such source is PogoLinks, which is a website that provides direct Google Drive download links for Bollywood and Hollywood movies and web series. You can download Baywatch (English) movie in 720p quality from PogoLinks with the following steps:
|
24 |
-
|
25 |
-
|
26 |
-
|
27 |
-
1. Go to [https://pogolinks.art/movies/baywatch-2017/](https://pogolinks.art/movies/baywatch-2017/) [^2^]. This is the page where you can find the download links for Baywatch (2017) movie in different languages, sizes and qualities.
|
28 |
-
|
29 |
-
2. Scroll down to the section where it says "Download Links". You will see a table with various options for downloading Baywatch (2017) movie. Choose the one that says "Baywatch (2017) [Hindi-Eng] 720p .mkv" with a size of 1.24 GB and click on it.
|
30 |
-
|
31 |
-
3. You will be redirected to a new page where you will see a button that says "Download Now". Click on it and wait for a few seconds until a pop-up window appears.
|
32 |
-
|
33 |
-
4. In the pop-up window, you will see another button that says "Download". Click on it and you will be taken to Google Drive where you can download Baywatch (2017) movie in 720p quality.
|
34 |
-
|
35 |
-
5. Click on the download icon on the top right corner of the Google Drive page and choose where you want to save the file on your device.
|
36 |
-
|
37 |
-
6. Enjoy watching Baywatch (2017) movie in 720p quality!
|
38 |
-
|
39 |
-
|
40 |
-
|
41 |
-
Note: You may need to turn off your ad-blocker or use a VPN when downloading torrents or movies from PogoLinks. You may also need to use Mx Player or VLC Player to switch audio or play the movie after downloading it.
|
42 |
-
|
43 |
-
Here are a few more paragraphs with HTML formatting for the article:
|
44 |
-
|
45 |
-
If you want to know more about Baywatch (English) movie, you can also check out some of the reviews from critics and audiences. The movie has a 17% rating on Rotten Tomatoes, based on 246 reviews, with an average score of 3.7/10. The critics consensus says: "Baywatch takes its source material's jiggle factor to R-rated levels, but lacks the original's campy charm -- and leaves its charming stars flailing in the shallows." [^1^]
|
46 |
-
|
47 |
-
|
48 |
-
|
49 |
-
On IMDb, the movie has a 5.5/10 rating, based on 192,000 votes, with a metascore of 37 out of 100, based on 47 reviews. The movie has received mixed feedback from the users, with some praising the humor, action and chemistry of the cast, and others criticizing the plot, script and vulgarity of the movie. One user wrote: "Baywatch is a action comedy movie about a bunch of bad ass lifeguard at the beach saving people life while kicking drug dealer ass staring Dwayne Johnson A.K.A The Rock one of the most likable action hero of this generation with a cast full of young hot actors that always show up with close up slow mo boobs bouncing and muscle flexing plus a lot of dick jokes." [^3^]
|
50 |
-
|
51 |
-
|
52 |
-
|
53 |
-
Whether you love it or hate it, Baywatch (English) movie is a movie that does not take itself too seriously and aims to entertain the fans of the original TV series and the new generation of viewers. It is a movie that you can watch with your friends and have a good laugh at the absurdity and fun of it. If you are looking for a movie that is light-hearted, action-packed and sexy, you can download Baywatch (English) movie in 720p quality from PogoLinks and enjoy it at your own convenience.
|
54 |
-
|
55 |
-
dfd1c89656
|
|
|
|
|
|
|
|
|
|
|
|
|
|
|
|
|
|
|
|
|
|
|
|
|
|
|
|
|
|
|
|
|
|
|
|
|
|
|
|
|
|
|
|
|
|
|
|
|
|
|
|
|
|
|
|
|
|
|
|
|
|
|
|
|
|
|
|
|
|
|
|
|
|
|
|
|
|
|
|
|
|
|
|
|
|
|
|
|
|
|
|
|
|
|
|
|
|
|
|
|
|
|
|
|
|
|
|
|
|
|
|
spaces/1gistliPinn/ChatGPT4/Examples/Dan Hartman Torrent Discography.md
DELETED
@@ -1,63 +0,0 @@
|
|
1 |
-
<br />
|
2 |
-
<h1>Dan Hartman Torrent Discography: How to Download and Enjoy His Music</h1>
|
3 |
-
<p>Dan Hartman was an American singer, songwriter and record producer who rose to fame in the 1970s and 1980s with his disco, pop and rock hits. He is best known for songs like "Relight My Fire", "I Can Dream About You", "Instant Replay" and "We Are the Young". He also collaborated with artists like Donna Summer, James Brown, Tina Turner and Edgar Winter.</p>
|
4 |
-
<h2>Dan Hartman Torrent Discography</h2><br /><p><b><b>Download Zip</b> ★★★ <a href="https://imgfil.com/2uy1cG">https://imgfil.com/2uy1cG</a></b></p><br /><br />
|
5 |
-
<p>If you are a fan of Dan Hartman or want to discover his music, you might be interested in downloading his torrent discography. A torrent discography is a collection of all his albums and singles in digital format that you can download using a torrent client. Torrents are a way of sharing files over the internet that allow you to download large amounts of data quickly and efficiently.</p>
|
6 |
-
<p>In this article, we will show you how to find and download Dan Hartman's torrent discography, as well as some tips on how to enjoy his music. Let's get started!</p>
|
7 |
-
<h2>How to Find Dan Hartman's Torrent Discography</h2>
|
8 |
-
<p>The first step to download Dan Hartman's torrent discography is to find a reliable source that offers it. There are many websites that host torrent files, but not all of them are safe and legal. Some of them might contain viruses, malware or fake files that can harm your computer or violate your privacy.</p>
|
9 |
-
<p>To avoid these risks, you should use a reputable torrent site that has a large community of users and moderators who verify the quality and authenticity of the files. You should also use a VPN (virtual private network) service that encrypts your internet traffic and hides your IP address from prying eyes.</p>
|
10 |
-
<p></p>
|
11 |
-
<p>One of the most popular and trusted torrent sites that has Dan Hartman's torrent discography is RuTracker.org. This is a Russian website that has a huge collection of music torrents, including disco, pop, rock and other genres. You can find Dan Hartman's torrent discography by searching for his name or browsing through the categories.</p>
|
12 |
-
<p>To access RuTracker.org, you need to register for a free account and use a VPN service that can bypass the geo-restrictions imposed by some countries. Once you are on the site, you can download the torrent file of Dan Hartman's collection, which contains 9 albums and 8 singles from 1976 to 2016, in MP3 format with different bitrates.</p>
|
13 |
-
<h2>How to Download Dan Hartman's Torrent Discography</h2>
|
14 |
-
<p>After you have downloaded the torrent file of Dan Hartman's collection, you need to open it with a torrent client. A torrent client is a software that connects you to other users who have the same file and allows you to download it from them in small pieces.</p>
|
15 |
-
<p>There are many torrent clients available for different platforms, but some of the most popular ones are uTorrent, BitTorrent, qBittorrent and Transmission. You can download and install any of them on your computer and then open the torrent file with them.</p>
|
16 |
-
<p>Once you have opened the torrent file with your torrent client, you will see a list of all the files included in the collection. You can choose which ones you want to download or download them all. You will also see information such as the size, progress, speed and peers of the download.</p>
|
17 |
-
<p>The download time will depend on several factors, such as your internet connection, the number of seeders (users who have the complete file) and leechers (users who are downloading the file) and the settings of your torrent client. Generally, the more seeders and leechers there are, the faster the download will be.</p>
|
18 |
-
<p>Once the download is complete, you will have all the files of Dan Hartman's collection on your computer. You can then play them with any media player that supports MP3 format or transfer them to your mobile device or external drive.</p>
|
19 |
-
<h2>How to Enjoy Dan Hartman's Music</h2>
|
20 |
-
<p>Now that you have downloaded Dan Hartman's torrent discography, you can enjoy his music anytime and anywhere. Here are some tips on how to make the most out of his music:</p>
|
21 |
-
<ul>
|
22 |
-
<li>Listen to his albums chronologically to appreciate his musical evolution and diversity. He started as a rock musician with his band The Edgar Winter Group, then switched to disco and pop in the late 1970s and early 1980s, and finally experimented with electronic and dance music in the late 1980s and early 1990s.</li>
|
23 |
-
<li>Pay attention to his lyrics and vocals. He was a talented songwriter who wrote catchy hooks and meaningful messages. He was also a versatile singer who could adapt his voice to different styles and moods.</li>
|
24 |
-
<li>Watch his videos and live performances. He was a charismatic performer who knew how to entertain his audience with his energy and charisma. He also had a great sense of fashion and style.</li>
|
25 |
-
<li>Learn more about his life and career. He was a prolific artist who worked with many other musicians as a producer, arranger or guest vocalist. He also faced many challenges in his personal life, such as his sexuality and his health issues.</li>
|
26 |
-
<li>Share his music with others. He was a beloved artist who influenced many other musicians and genres. He also had a loyal fan base who supported him throughout his career. You can introduce his music to your friends or family or join online communities of fans who appreciate his legacy.</li>
|
27 |
-
</ul>
|
28 |
-
<h2>Conclusion</h2>
|
29 |
-
<p>Dan Hartman was one of the most talented and versatile artists of his generation. His music spanned across different genres and decades, offering something for everyone. His torrent discography is a great way to access his entire musical catalog in one place.</p>
|
30 |
-
<p>To download Dan Hartman's torrent discography, you need to find a reliable torrent site that offers it, such as RuTracker.org; use a VPN service that protects your privacy; use a torrent client that downloads the file from other users; and enjoy his music with any media player or device.</p>
|
31 |
-
<p>We hope this article has helped you learn how to download and enjoy Dan Hartman's music using torrents. If you have any questions or comments, feel free to leave them below.</p>
|
32 |
-
<h2>What are the Benefits of Dan Hartman's Torrent Discography</h2>
|
33 |
-
<p>Downloading Dan Hartman's torrent discography has many benefits for music lovers and fans. Here are some of them:</p>
|
34 |
-
<ul>
|
35 |
-
<li>You can access his entire musical catalog in one place. You don't have to buy or stream his albums and singles separately, which can be costly or inconvenient. You can also save space on your computer or device by storing them in a single folder.</li>
|
36 |
-
<li>You can enjoy his music in high quality. The torrent files of his collection have different bitrates, ranging from 128 to 320 kbps, which means you can choose the level of sound quality that suits your preference and device. You can also use a media player that supports MP3 format to adjust the volume, bass, treble and other settings.</li>
|
37 |
-
<li>You can discover new songs and albums that you might have missed or forgotten. Dan Hartman had a long and prolific career that spanned across different genres and decades. He released 9 albums and 8 singles from 1976 to 2016, some of which are rare or hard to find. By downloading his torrent discography, you can explore his musical diversity and evolution and find new favorites.</li>
|
38 |
-
<li>You can support his legacy and influence. Dan Hartman was a beloved artist who influenced many other musicians and genres. He also had a loyal fan base who supported him throughout his career. By downloading his torrent discography, you can show your appreciation and respect for his work and keep his music alive.</li>
|
39 |
-
</ul>
|
40 |
-
<h2>How to Avoid Risks When Downloading Dan Hartman's Torrent Discography</h2>
|
41 |
-
<p>While downloading Dan Hartman's torrent discography has many benefits, it also has some risks that you should be aware of and avoid. Here are some of them:</p>
|
42 |
-
<ul>
|
43 |
-
<li>You might violate the copyright laws of your country or region. Torrenting is a legal activity, but downloading copyrighted material without permission is not. You might face legal consequences if you are caught by the authorities or reported by the rights holders.</li>
|
44 |
-
<li>You might expose your computer or device to viruses, malware or fake files that can harm your system or violate your privacy. Some torrent sites or files might contain malicious software or data that can infect your computer or device or steal your personal information.</li>
|
45 |
-
<li>You might have a slow or unstable download speed or experience interruptions or errors during the download process. Torrenting depends on several factors, such as your internet connection, the number of seeders and leechers, and the settings of your torrent client. Sometimes, these factors might cause problems that affect your download experience.</li>
|
46 |
-
</ul>
|
47 |
-
<p>To avoid these risks, you should follow these tips:</p>
|
48 |
-
<ul>
|
49 |
-
<li>Use a reputable torrent site that offers Dan Hartman's torrent discography, such as RuTracker.org. This site has a large community of users and moderators who verify the quality and authenticity of the files. It also has a lot of seeders and leechers who ensure a fast and stable download speed.</li>
|
50 |
-
<li>Use a VPN service that protects your privacy and security. A VPN service encrypts your internet traffic and hides your IP address from prying eyes. It also allows you to bypass the geo-restrictions imposed by some countries or regions that block access to certain torrent sites or files.</li>
|
51 |
-
<li>Use a torrent client that downloads the file from other users efficiently and safely. A torrent client is a software that connects you to other users who have the same file and allows you to download it from them in small pieces. Some of the most popular torrent clients are uTorrent, BitTorrent, qBittorrent and Transmission.</li>
|
52 |
-
<li>Use an antivirus software that scans your computer or device for viruses, malware or fake files that can harm your system or violate your privacy. An antivirus software is a software that detects and removes malicious software or data from your computer or device. Some of the most popular antivirus software are Avast, Norton, McAfee and Kaspersky.</li>
|
53 |
-
</ul>
|
54 |
-
<h2>Conclusion</h2>
|
55 |
-
<p>Dan Hartman was one of the most talented and versatile artists of his generation. His music spanned across different genres and decades, offering something for everyone. His torrent discography is a great way to access his entire musical catalog in one place.</p>
|
56 |
-
<p>To download Dan Hartman's torrent discography, you need to find a reliable torrent site that offers it, such as RuTracker.org; use a VPN service that protects your privacy; use a torrent client that downloads the file from other users; use an antivirus software that scans your computer or device; and enjoy his music with any media player or device.</p>
|
57 |
-
<p>We hope this article has helped you learn how to download and enjoy Dan Hartman's music using torrents. If you have any questions or comments, feel free to leave them below.</p>
|
58 |
-
<h2>Conclusion</h2>
|
59 |
-
<p>Dan Hartman was one of the most talented and versatile artists of his generation. His music spanned across different genres and decades, offering something for everyone. His torrent discography is a great way to access his entire musical catalog in one place.</p>
|
60 |
-
<p>To download Dan Hartman's torrent discography, you need to find a reliable torrent site that offers it, such as RuTracker.org; use a VPN service that protects your privacy; use a torrent client that downloads the file from other users; use an antivirus software that scans your computer or device; and enjoy his music with any media player or device.</p>
|
61 |
-
<p>We hope this article has helped you learn how to download and enjoy Dan Hartman's music using torrents. If you have any questions or comments, feel free to leave them below.</p> 3cee63e6c2<br />
|
62 |
-
<br />
|
63 |
-
<br />
|
|
|
|
|
|
|
|
|
|
|
|
|
|
|
|
|
|
|
|
|
|
|
|
|
|
|
|
|
|
|
|
|
|
|
|
|
|
|
|
|
|
|
|
|
|
|
|
|
|
|
|
|
|
|
|
|
|
|
|
|
|
|
|
|
|
|
|
|
|
|
|
|
|
|
|
|
|
|
|
|
|
|
|
|
|
|
|
|
|
|
|
|
|
|
|
|
|
|
|
|
|
|
|
|
|
|
|
|
|
|
|
|
|
|
|
|
|
|
|
|
|
|
|
|
|
|
|
spaces/1pelhydcardo/ChatGPT-prompt-generator/assets/Car Parking Multiplayer MOD APK Drive Any Car You Want and Explore the Streets.md
DELETED
@@ -1,124 +0,0 @@
|
|
1 |
-
|
2 |
-
<h1>Car Parking Multiplayer Mod APK Apkvision: A Review</h1>
|
3 |
-
<p>If you are looking for a realistic and fun driving and parking simulation game, you might want to check out Car Parking Multiplayer. This game offers more than just parking, as you can explore an open-world multiplayer mode, customize your car, chat with other players, and even play as a cop. And if you want to enhance your gaming experience, you can download the Car Parking Multiplayer Mod APK Apkvision, which gives you unlimited money and access to all cars and features. In this article, we will review the game and the mod apk, and show you how to download and install it on your device. We will also suggest some alternatives to Car Parking Multiplayer Mod APK Apkvision that you might like.</p>
|
4 |
-
<h2>What is Car Parking Multiplayer?</h2>
|
5 |
-
<p>Car Parking Multiplayer is a game that supports open-world multiplayer mode, car tuning, police mode, and free walking. Plus, you can decide to jump out of the car and walk around. There are several areas that you can explore in the game and you can choose to play either single-player mode or online mode if you want a more chaotic (fun) scene.</p>
|
6 |
-
<h2>car parking multiplayer mod apk apkvision</h2><br /><p><b><b>Download</b> »»» <a href="https://urlin.us/2uSUuR">https://urlin.us/2uSUuR</a></b></p><br /><br />
|
7 |
-
<h3>Features of the game</h3>
|
8 |
-
<p>Some of the features of Car Parking Multiplayer are:</p>
|
9 |
-
<ul>
|
10 |
-
<li>Free walking. You can get out of your car and walk around the city, interact with other players, or enter buildings.</li>
|
11 |
-
<li>Car exchange. You can exchange your cars with other players or join a team and share cars.</li>
|
12 |
-
<li>Multiplayer racing. You can compete against real players in different modes, such as drag racing, drifting, or parking.</li>
|
13 |
-
<li>Car customization. You can adjust the suspension, wheel angle, engine, turbo, gearbox, exhaust, and more. You can also change the appearance of your car with dynamic vinyls, body parts, and paint.</li>
|
14 |
-
<li>High-quality open world. The game has highly-detailed environments, 100 cars with real interiors, 16 player skins, and realistic physics.</li>
|
15 |
-
<li>Interesting gameplay. The game has 82 real-life parking and driving challenges, different vehicles such as tow trucks, pickups, trucks, sports cars, and classic cars.</li>
|
16 |
-
<li>Voice chat. You can communicate with other players using voice chat.</li>
|
17 |
-
<li>Police mode. You can play as a cop and chase criminals or join a gang and cause trouble.</li>
|
18 |
-
<li>Friend list. You can add other players as friends and join their games.</li>
|
19 |
-
</ul>
|
20 |
-
<h3>Benefits of the mod apk</h3>
|
21 |
-
<p>With the Car Parking Multiplayer Mod APK Apkvision, you get unlimited money and a menu that gives you access to all of your favorite cars, upgrades and more. With the Car Parking Multiplayer Mod APK Apkvision, you can use your money to unlock cars and see a menu that gives you access to:</p>
|
22 |
-
<ul>
|
23 |
-
<li>All cars unlocked</li>
|
24 |
-
<li>All colors unlocked</li>
|
25 |
-
<li>All vinyls unlocked</li>
|
26 |
-
<li>All wheels unlocked</li>
|
27 |
-
<li>All tuning parts unlocked</li>
|
28 |
-
<li>All levels unlocked</li>
|
29 |
-
<li>No ads</li>
|
30 |
-
</ul>
|
31 |
-
<h2>How to download and install Car Parking Multiplayer Mod APK Apkvision?</h2>
|
32 |
-
<p>If you want to download and install Car Parking Multiplayer Mod APK Apkvision on your device, you need to follow these steps:</p>
|
33 |
-
<h3>Steps to follow</h3>
|
34 |
-
<ol>
|
35 |
-
<li>Go to [Apkvision](^8^) website and search for Car Parking Multiplayer Mod APK.</li>
|
36 |
-
<li>Select the latest version of the mod apk and click on the download button.</li>
|
37 |
-
<li>Wait for the download to finish and then locate the file on your device.</li>
|
38 |
-
<li>Before installing the mod apk, you need to enable the installation of apps from unknown sources on your device. To do this, go to your device settings, security, and toggle on the unknown sources option.</li>
|
39 |
-
<li>Now, tap on the mod apk file and follow the instructions to install it on your device.</li>
|
40 |
-
<li>Once the installation is done, you can launch the game and enjoy the mod features.</li>
|
41 |
-
</ol>
|
42 |
-
<h3>Drawbacks of the mod apk</h3>
|
43 |
-
<p>While the Car Parking Multiplayer Mod APK Apkvision can give you a lot of advantages, it also has some drawbacks that you should be aware of. Some of the drawbacks are:</p>
|
44 |
-
<ul>
|
45 |
-
<li>The mod apk is not official and may not be compatible with the latest version of the game or your device.</li>
|
46 |
-
<li>The mod apk may contain viruses or malware that can harm your device or compromise your data.</li>
|
47 |
-
<li>The mod apk may cause glitches or errors in the game or interfere with other apps on your device.</li>
|
48 |
-
<li>The mod apk may violate the terms and conditions of the game and result in a ban or suspension of your account.</li>
|
49 |
-
</ul>
|
50 |
-
<h2>What are some alternatives to Car Parking Multiplayer Mod APK Apkvision?</h2>
|
51 |
-
<p>If you are looking for some other games that are similar to Car Parking Multiplayer, you might want to try these alternatives:</p>
|
52 |
-
<p>car parking multiplayer unlimited money mod apk<br />
|
53 |
-
car parking multiplayer hack apk download<br />
|
54 |
-
car parking multiplayer mod menu apk<br />
|
55 |
-
car parking multiplayer free shopping mod apk<br />
|
56 |
-
car parking multiplayer latest version mod apk<br />
|
57 |
-
car parking multiplayer online mod apk<br />
|
58 |
-
car parking multiplayer 3d mod apk<br />
|
59 |
-
car parking multiplayer realistic mod apk<br />
|
60 |
-
car parking multiplayer unlocked cars mod apk<br />
|
61 |
-
car parking multiplayer android 1 mod apk<br />
|
62 |
-
car parking multiplayer rexdl mod apk<br />
|
63 |
-
car parking multiplayer revdl mod apk<br />
|
64 |
-
car parking multiplayer premium mod apk<br />
|
65 |
-
car parking multiplayer vip mod apk<br />
|
66 |
-
car parking multiplayer pro mod apk<br />
|
67 |
-
car parking multiplayer mega mod apk<br />
|
68 |
-
car parking multiplayer god mode mod apk<br />
|
69 |
-
car parking multiplayer no ads mod apk<br />
|
70 |
-
car parking multiplayer all cars unlocked mod apk<br />
|
71 |
-
car parking multiplayer anti ban mod apk<br />
|
72 |
-
car parking multiplayer cheat codes mod apk<br />
|
73 |
-
car parking multiplayer unlimited coins mod apk<br />
|
74 |
-
car parking multiplayer custom cars mod apk<br />
|
75 |
-
car parking multiplayer drift mode mod apk<br />
|
76 |
-
car parking multiplayer easy win mod apk<br />
|
77 |
-
car parking multiplayer full unlocked mod apk<br />
|
78 |
-
car parking multiplayer graphics mod apk<br />
|
79 |
-
car parking multiplayer high speed mod apk<br />
|
80 |
-
car parking multiplayer infinite money mod apk<br />
|
81 |
-
car parking multiplayer ios download mod apk<br />
|
82 |
-
car parking multiplayer joystick mod apk<br />
|
83 |
-
car parking multiplayer key hack mod apk<br />
|
84 |
-
car parking multiplayer low mb mod apk<br />
|
85 |
-
car parking multiplayer map hack mod apk<br />
|
86 |
-
car parking multiplayer new update mod apk<br />
|
87 |
-
car parking multiplayer offline mode mod apk<br />
|
88 |
-
car parking multiplayer police mode mod apk<br />
|
89 |
-
car parking multiplayer quick money mod apk<br />
|
90 |
-
car parking multiplayer radar hack mod apk<br />
|
91 |
-
car parking multiplayer speed hack mod apk<br />
|
92 |
-
car parking multiplayer turbo boost mod apk<br />
|
93 |
-
car parking multiplayer unlimited fuel mod apk<br />
|
94 |
-
car parking multiplayer vip cars mod apk<br />
|
95 |
-
car parking multiplayer world record mod apk<br />
|
96 |
-
car parking multiplayer xapk download mod apk</p>
|
97 |
-
<h3>Real Car Parking 2</h3>
|
98 |
-
<p>Real Car Parking 2 is a realistic and challenging parking simulation game that tests your driving skills. You can choose from a variety of cars, customize them, and park them in different scenarios. You can also enjoy the 3D graphics, realistic sounds, and multiplayer mode.</p>
|
99 |
-
<h3>Torque Burnout</h3>
|
100 |
-
<p>Torque Burnout is a game that lets you unleash your inner hoonigan and perform epic burnouts and stunts. You can drive and drift in various arenas, customize your car, and compete against other players. You can also enjoy the realistic physics, smoke effects, and engine sounds.</p>
|
101 |
-
<h2>Conclusion</h2>
|
102 |
-
<p>Car Parking Multiplayer is a game that offers more than just parking. It is a fun and realistic driving and parking simulation game that lets you explore an open-world multiplayer mode, customize your car, chat with other players, and even play as a cop. And if you want to get unlimited money and access to all cars and features, you can download the Car Parking Multiplayer Mod APK Apkvision. However, you should also be aware of the drawbacks of using the mod apk and consider some alternatives to Car Parking Multiplayer Mod APK Apkvision.</p>
|
103 |
-
<h2>FAQs</h2>
|
104 |
-
<p>Here are some frequently asked questions about Car Parking Multiplayer Mod APK Apkvision:</p>
|
105 |
-
<h4>Q: Is Car Parking Multiplayer Mod APK Apkvision safe to use?</h4>
|
106 |
-
<p>A: Car Parking Multiplayer Mod APK Apkvision is not an official app and may not be safe to use. It may contain viruses or malware that can harm your device or compromise your data. It may also cause glitches or errors in the game or interfere with other apps on your device. Therefore, you should use it at your own risk and discretion.</p>
|
107 |
-
<h4>Q: How do I update Car Parking Multiplayer Mod APK Apkvision?</h4>
|
108 |
-
<p>A: To update Car Parking Multiplayer Mod APK Apkvision, you need to visit [Apkvision] website and download the latest version of the mod apk. Then, you need to uninstall the previous version of the mod apk from your device and install the new one following the same steps as before.</p>
|
109 |
-
<h4>Q: Can I play Car Parking Multiplayer Mod APK Apkvision offline?</h4>
|
110 |
-
<p>A: Yes, you can play Car Parking Multiplayer Mod APK Apkvision offline. However, you will not be able to access some features such as multiplayer mode, voice chat, or car exchange. You will also not be able to save your progress online or sync it with other devices.</p>
|
111 |
-
<h4>Q: Can I play Car Parking Multiplayer Mod APK Apkvision with my friends?</h4>
|
112 |
-
<p>A: Yes, you can play Car Parking Multiplayer Mod APK Apkvision with your friends. You can join their games or invite them to yours using the friend list feature. You can also communicate with them using voice chat or text chat.</p>
|
113 |
-
<h4>Q: What are some tips and tricks for playing Car Parking Multiplayer Mod APK Apkvision?</h4>
|
114 |
-
<p>A: Some tips and tricks for playing Car Parking Multiplayer Mod APK Apkvision are:</p>
|
115 |
-
<ul>
|
116 |
-
<li>Use the map to find parking spots, gas stations, car washes, or other places of interest.</li>
|
117 |
-
<li>Use the camera angles to adjust your view and see the obstacles better.</li>
|
118 |
-
<li>Use the brake, handbrake, and clutch to control your speed and direction.</li>
|
119 |
-
<li>Use the indicators, horn, and headlights to signal your intentions and alert other drivers.</li>
|
120 |
-
<li>Use the tuning menu to customize your car and improve its performance.</li>
|
121 |
-
<li>Use the police mode to chase criminals or join a gang and cause trouble.</li>
|
122 |
-
</ul></p> 197e85843d<br />
|
123 |
-
<br />
|
124 |
-
<br />
|
|
|
|
|
|
|
|
|
|
|
|
|
|
|
|
|
|
|
|
|
|
|
|
|
|
|
|
|
|
|
|
|
|
|
|
|
|
|
|
|
|
|
|
|
|
|
|
|
|
|
|
|
|
|
|
|
|
|
|
|
|
|
|
|
|
|
|
|
|
|
|
|
|
|
|
|
|
|
|
|
|
|
|
|
|
|
|
|
|
|
|
|
|
|
|
|
|
|
|
|
|
|
|
|
|
|
|
|
|
|
|
|
|
|
|
|
|
|
|
|
|
|
|
|
|
|
|
|
|
|
|
|
|
|
|
|
|
|
|
|
|
|
|
|
|
|
|
|
|
|
|
|
|
|
|
|
|
|
|
|
|
|
|
|
|
|
|
|
|
|
|
|
|
|
|
|
|
|
|
|
|
|
|
|
|
|
|
|
|
|
|
|
|
|
|
|
|
|
|
|
|
|
|
|
|
|
|
|
|
|
|
|
|
|
|
|
|
|
|
|
|
|
|
|
|
|
|
|
|
|
|
|
|
|
|
|
|
|
|
|
|
|
|
|
|
|
|
|
|
spaces/1pelhydcardo/ChatGPT-prompt-generator/assets/City Taxi Driving 3D Simulator The Ultimate Car Driving Game for Taxi Lovers.md
DELETED
@@ -1,222 +0,0 @@
|
|
1 |
-
|
2 |
-
<h1>City Taxi Driving 3D Simulator: A Fun and Realistic Game for Car Lovers</h1>
|
3 |
-
<h2>Introduction</h2>
|
4 |
-
<p>What is city taxi driving 3d simulator and why should you play it?</p>
|
5 |
-
<h2>city taxi driving 3d simulator</h2><br /><p><b><b>Download File</b> 🗸 <a href="https://urlin.us/2uSSZF">https://urlin.us/2uSSZF</a></b></p><br /><br />
|
6 |
-
<h2>Features of the Game</h2>
|
7 |
-
<p>What are some of the exciting features of city taxi driving 3d simulator?</p>
|
8 |
-
<h3>100 Unique Levels</h3>
|
9 |
-
<p>How many levels are there and what are the challenges?</p>
|
10 |
-
<h3>50 Different Taxi Cars</h3>
|
11 |
-
<p>How many taxi cars can you choose from and how can you unlock them?</p>
|
12 |
-
<h3>Challenging Driving and Parking Missions</h3>
|
13 |
-
<p>What are some of the driving and parking missions you have to complete?</p>
|
14 |
-
<h3>Drop and Pick Up Interesting Passengers</h3>
|
15 |
-
<p>Who are some of the passengers you will meet and how do they affect your gameplay?</p>
|
16 |
-
<h3>Realistic and Lively City Environment</h3>
|
17 |
-
<p>How does the city environment look like and what are some of the landmarks you will see?</p>
|
18 |
-
<h3>Crazy Realistic Driving Physics</h3>
|
19 |
-
<p>How does the game simulate the real driving physics and what are some of the effects?</p>
|
20 |
-
<h2>How to Play the Game</h2>
|
21 |
-
<p>What are the controls and tips for playing city taxi driving 3d simulator?</p>
|
22 |
-
<p>city taxi driving fun 3d car driver simulator<br />
|
23 |
-
city taxi simulator 3d online game<br />
|
24 |
-
city taxi driving 3d simulation games free<br />
|
25 |
-
city taxi simulator 3d webgl<br />
|
26 |
-
city taxi driving 3d car parking game<br />
|
27 |
-
city taxi simulator 3d upgrade<br />
|
28 |
-
city taxi driving 3d realistic physics<br />
|
29 |
-
city taxi simulator 3d y8<br />
|
30 |
-
city taxi driving 3d crazy games<br />
|
31 |
-
city taxi simulator 3d play now<br />
|
32 |
-
city taxi driving 3d apps on google play<br />
|
33 |
-
city taxi simulator 3d free online games studio<br />
|
34 |
-
city taxi driving 3d coins and videos<br />
|
35 |
-
city taxi simulator 3d timing and challenge<br />
|
36 |
-
city taxi driving 3d unique levels and missions<br />
|
37 |
-
city taxi simulator 3d pick up and drop off passengers<br />
|
38 |
-
city taxi driving 3d different vehicles and taxis<br />
|
39 |
-
city taxi simulator 3d metro and streets<br />
|
40 |
-
city taxi driving 3d colorful personalities and characters<br />
|
41 |
-
city taxi simulator 3d cash and rewards<br />
|
42 |
-
city taxi driving 3d android and ios<br />
|
43 |
-
city taxi simulator 3d handbrake and camera view<br />
|
44 |
-
city taxi driving 3d games2win.com<br />
|
45 |
-
city taxi simulator 3d cookie policy<br />
|
46 |
-
city taxi driving 3d available in 26 languages<br />
|
47 |
-
city taxi simulator 3d trailer and install<br />
|
48 |
-
city taxi driving 3d reviews and ratings<br />
|
49 |
-
city taxi simulator 3d report a bug and add to favourite<br />
|
50 |
-
city taxi driving 3d everyone and in-app purchases<br />
|
51 |
-
city taxi simulator 3d contains ads and info<br />
|
52 |
-
city taxi driving 3d korea institute of fusion energy<br />
|
53 |
-
city taxi simulator 3d nuclear fusion reactor experiment<br />
|
54 |
-
city taxi driving 3d holy grail of unlimited energy<br />
|
55 |
-
city taxi simulator 3d seven times hotter than the sun core<br />
|
56 |
-
city taxi driving 3d net energy gain and breakthrough<br />
|
57 |
-
city taxi simulator 3d new scientist and the sun articles<br />
|
58 |
-
city taxi driving 3d yahoo news and wikipedia sources<br />
|
59 |
-
city taxi simulator 3d kelvin and celsius temperature units<br />
|
60 |
-
city taxi driving 3d solar core and solar atmosphere facts<br />
|
61 |
-
city taxi simulator 3d radiative zone and convection zone layers<br />
|
62 |
-
city taxi driving 3d photosphere and chromosphere thicknesses<br />
|
63 |
-
city taxi simulator 3d sun spot cycle and composition data<br />
|
64 |
-
city taxi driving 3d montana solar physics website information <br />
|
65 |
-
city taxi simulator 3d cornell university curious astro website information <br />
|
66 |
-
city taxi driving 3d nasa sun fact sheet information <br />
|
67 |
-
city taxi simulator 3d date and time of web search results <br />
|
68 |
-
city taxi driving 3d snippets and titles of web search results <br />
|
69 |
-
city taxi simulator 3d question answering results from wikipedia <br />
|
70 |
-
city taxi driving 3d bing search engine tool output</p>
|
71 |
-
<h2>Comparison with Other Similar Games</h2>
|
72 |
-
<p>How does city taxi driving 3d simulator compare with other similar games in the market?</p>
|
73 |
-
<h3>City Taxi Simulator 3D Game</h3>
|
74 |
-
<p>What are the similarities and differences between city taxi driving 3d simulator and city taxi simulator 3d game?</p>
|
75 |
-
<h3>Taxi Life: A City Driving Simulator</h3>
|
76 |
-
<p>What are the similarities and differences between city taxi driving 3d simulator and taxi life: a city driving simulator?</p>
|
77 |
-
<h2>The Benefits of Playing City Taxi Driving 3D Simulator</h2>
|
78 |
-
<p>What are some of the benefits of playing city taxi driving 3d simulator for your mental and physical health?</p>
|
79 |
-
<h2>The Drawbacks of Playing City Taxi Driving 3D Simulator</h2>
|
80 |
-
<p>What are some of the drawbacks of playing city taxi driving 3d simulator for your time and money?</p>
|
81 |
-
<h2>The Future of City Taxi Driving 3D Simulator</h2>
|
82 |
-
<p>What are some of the future updates and improvements that the developers are planning for city taxi driving 3d simulator?</p>
|
83 |
-
<h2>Conclusion</h2>
|
84 |
-
<p>A summary of the main points and a call to action for the readers.</p>
|
85 |
-
<table border="1">
|
86 |
-
<tr><th colspan="4">City Taxi Driving 3D Simulator: A Fun and Realistic Game for Car Lovers</th></tr>
|
87 |
-
<tr><td colspan="4">Introduction</td></tr>
|
88 |
-
<tr><td colspan="4">Features of the Game</td></tr>
|
89 |
-
<tr><td rowspan="5">100 Unique Levels</td><td rowspan="5">50 Different Taxi Cars</td><td rowspan="5">Challenging Driving and Parking Missions</td><td rowspan="5">Drop and Pick Up Interesting Passengers</td></tr>
|
90 |
-
<tr></tr>
|
91 |
-
<tr></tr>
|
92 |
-
<tr></tr>
|
93 |
-
<tr></tr>
|
94 |
-
<tr><td colspan="4">Realistic and Lively City Environment</td></tr>
|
95 |
-
<tr><td colspan="4">Crazy Realistic Driving Physics</td></tr>
|
96 |
-
<tr><td colspan="4">How to Play the Game</td></tr>
|
97 |
-
<tr><td colspan="4">Comparison with Other Similar Games</td></tr>
|
98 |
-
<tr><td colspan="2">City Taxi Simulator 3D Game</td><td colspan="2">Taxi Life: A City Driving Simulator</td></tr>
|
99 |
-
<tr><td colspan="4">The Benefits of Playing City Taxi Driving 3D Simulator</td></tr>
|
100 |
-
<tr><td colspan="4">The Drawbacks of Playing City Taxi Driving 3D Simulator</td></tr>
|
101 |
-
<tr><td colspan="4">The Future of City Taxi Driving 3D Simulator</td></tr>
|
102 |
-
<tr><td colspan="4">Conclusion</td></tr>
|
103 |
-
<h1>City Taxi Driving 3D Simulator: A Fun and Realistic Game for Car Lovers</h1>
|
104 |
-
<p>Do you love driving cars and exploring new places? Do you want to experience the thrill of being a taxi driver in a busy city? Do you want to enjoy a realistic and immersive 3D game that will keep you entertained for hours? If you answered yes to any of these questions, then you should try city taxi driving 3d simulator, a fun and realistic game for car lovers.</p>
|
105 |
-
<h2>Features of the Game</h2>
|
106 |
-
<p>City taxi driving 3d simulator is a game that lets you drive and park different taxi cars in a realistic and lively city environment. You can choose from 50 different taxi cars, each with its own unique design and performance. You can also unlock new cars by completing various missions and earning money. The game has 100 unique levels, each with its own challenges and objectives. You have to drop and pick up interesting passengers, who will give you feedback and tips on your driving skills. You also have to follow the traffic rules and avoid accidents, as they will affect your reputation and income. The game has crazy realistic driving physics, which will make you feel like you are driving a real car. You can also customize your car with different colors, stickers, and accessories.</p>
|
107 |
-
<h3>100 Unique Levels</h3>
|
108 |
-
<p>The game has 100 unique levels, each with its own challenges and objectives. You have to complete each level within a given time limit and without damaging your car or hurting your passengers. Some of the levels are easy, while others are hard and require more skill and concentration. Some of the levels include:</p>
|
109 |
-
<ul>
|
110 |
-
<li>Driving in rainy or snowy weather</li>
|
111 |
-
<li>Driving at night or in foggy conditions</li>
|
112 |
-
<li>Driving in busy or crowded streets</li>
|
113 |
-
<li>Driving in narrow or winding roads</li>
|
114 |
-
<li>Driving in high-speed or highway lanes</li>
|
115 |
-
<li>Driving in emergency or special situations</li>
|
116 |
-
</ul>
|
117 |
-
<h3>50 Different Taxi Cars</h3>
|
118 |
-
<p>The game has 50 different taxi cars, each with its own unique design and performance. You can choose from classic, modern, luxury, sports, or exotic cars, depending on your preference and budget. You can also unlock new cars by completing various missions and earning money. Some of the cars include:</p>
|
119 |
-
<ul>
|
120 |
-
<li>Yellow cab</li>
|
121 |
-
<li>Black cab</li>
|
122 |
-
<li>Limo cab</li>
|
123 |
-
<li>Muscle cab</li>
|
124 |
-
<li>Bugatti cab</li>
|
125 |
-
<li>Lamborghini cab</li>
|
126 |
-
</ul>
|
127 |
-
<h3>Challenging Driving and Parking Missions</h3>
|
128 |
-
<p>The game has challenging driving and parking missions, which will test your driving skills and accuracy. You have to drive your car safely and smoothly, avoiding obstacles and collisions. You also have to park your car in the designated spot, following the arrows and indicators. Some of the missions include:</p>
|
129 |
-
<ul>
|
130 |
-
<li>Parking in parallel or perpendicular spaces</li>
|
131 |
-
<li>Parking in reverse or forward direction</li>
|
132 |
-
<li>Parking in tight or angled spaces</li>
|
133 |
-
<li>Parking in multi-level or underground garages</li>
|
134 |
-
<li>Parking in busy or crowded areas</li>
|
135 |
-
<li>Parking in tricky or hazardous locations</li>
|
136 |
-
<h3>Drop and Pick Up Interesting Passengers</h3>
|
137 |
-
<p>The game has interesting passengers, who will give you feedback and tips on your driving skills. You have to drop and pick up passengers from different locations, such as hotels, airports, restaurants, malls, schools, hospitals, and more. You also have to interact with them and listen to their stories, requests, and complaints. Some of the passengers include:</p>
|
138 |
-
<ul>
|
139 |
-
<li>A businessman who is late for a meeting</li>
|
140 |
-
<li>A tourist who wants to see the city attractions</li>
|
141 |
-
<li>A student who is nervous about an exam</li>
|
142 |
-
<li>A celebrity who is hiding from the paparazzi</li>
|
143 |
-
<li>A robber who is escaping from the police</li>
|
144 |
-
<li>A ghost who is haunting your car</li>
|
145 |
-
</ul>
|
146 |
-
<h3>Realistic and Lively City Environment</h3>
|
147 |
-
<p>The game has a realistic and lively city environment, which will make you feel like you are driving in a real city. You can see the city skyline, the buildings, the streets, the traffic lights, the signs, the pedestrians, the animals, and more. You can also explore different areas of the city, such as downtown, uptown, suburbs, industrial zone, countryside, and more. You can also see different landmarks of the city, such as bridges, monuments, parks, stadiums, museums, and more.</p>
|
148 |
-
<h3>Crazy Realistic Driving Physics</h3>
|
149 |
-
<p>The game has crazy realistic driving physics, which will make you feel like you are driving a real car. You can see the effects of speed, acceleration, braking, steering, drifting, skidding, crashing, and more. You can also hear the sounds of the engine, the horn, the tires, the wind, the radio, and more. You can also feel the vibration of the car, the bumps on the road, the collisions with other vehicles or objects, and more.</p>
|
150 |
-
<h2>How to Play the Game</h2>
|
151 |
-
<p>The game is easy to play and has simple controls. You can use your keyboard or mouse to control your car. You can also use a joystick or a steering wheel if you have one. The game has a tutorial mode that will teach you how to play the game and give you some tips and tricks. Here are some of the basic controls:</p>
|
152 |
-
<ul>
|
153 |
-
<li>Use the arrow keys or WASD keys to move your car forward, backward, left or right.</li>
|
154 |
-
<li>Use the space bar or left mouse button to brake your car.</li>
|
155 |
-
<li>Use the shift key or right mouse button to boost your car speed.</li>
|
156 |
-
<li>Use the C key or middle mouse button to change your camera view.</li>
|
157 |
-
<li>Use the M key or ESC key to open or close the menu.</li>
|
158 |
-
<h2>Comparison with Other Similar Games</h2>
|
159 |
-
<p>City taxi driving 3d simulator is not the only game that lets you drive and park taxi cars in a city environment. There are other similar games in the market that you can also try. However, city taxi driving 3d simulator has some advantages and disadvantages over them. Here are some of the comparisons:</p>
|
160 |
-
<h3>City Taxi Simulator 3D Game</h3>
|
161 |
-
<p>City taxi simulator 3d game is another game that lets you drive and park taxi cars in a city environment. It has similar features as city taxi driving 3d simulator, such as different taxi cars, levels, missions, passengers, and physics. However, there are some differences between them:</p>
|
162 |
-
<ul>
|
163 |
-
<li>City taxi simulator 3d game has more realistic graphics and sounds than city taxi driving 3d simulator.</li>
|
164 |
-
<li>City taxi simulator 3d game has more traffic and pedestrians than city taxi driving 3d simulator.</li>
|
165 |
-
<li>City taxi simulator 3d game has more customization options than city taxi driving 3d simulator.</li>
|
166 |
-
<li>City taxi simulator 3d game has more bugs and glitches than city taxi driving 3d simulator.</li>
|
167 |
-
<li>City taxi simulator 3d game has more ads and in-app purchases than city taxi driving 3d simulator.</li>
|
168 |
-
</ul>
|
169 |
-
<h3>Taxi Life: A City Driving Simulator</h3>
|
170 |
-
<p>Taxi life: a city driving simulator is another game that lets you drive and park taxi cars in a city environment. It has similar features as city taxi driving 3d simulator, such as different taxi cars, levels, missions, passengers, and physics. However, there are some differences between them:</p>
|
171 |
-
<ul>
|
172 |
-
<li>Taxi life: a city driving simulator has more variety and diversity than city taxi driving 3d simulator.</li>
|
173 |
-
<li>Taxi life: a city driving simulator has more story and humor than city taxi driving 3d simulator.</li>
|
174 |
-
<li>Taxi life: a city driving simulator has more freedom and creativity than city taxi driving 3d simulator.</li>
|
175 |
-
<li>Taxi life: a city driving simulator has more difficulty and challenge than city taxi driving 3d simulator.</li>
|
176 |
-
<li>Taxi life: a city driving simulator has more ratings and reviews than city taxi driving 3d simulator.</li>
|
177 |
-
</ul>
|
178 |
-
<h2>The Benefits of Playing City Taxi Driving 3D Simulator</h2>
|
179 |
-
<p>Playing city taxi driving 3d simulator can have some benefits for your mental and physical health. Here are some of them:</p>
|
180 |
-
<ul>
|
181 |
-
<li>Playing city taxi driving 3d simulator can improve your concentration and focus, as you have to pay attention to the road, the traffic, the passengers, and the time.</li>
|
182 |
-
<li>Playing city taxi driving 3d simulator can enhance your memory and recall, as you have to remember the locations, the routes, the landmarks, and the tips.</li>
|
183 |
-
<li>Playing city taxi driving 3d simulator can boost your creativity and imagination, as you can customize your car, explore the city, and interact with the passengers.</li>
|
184 |
-
<li>Playing city taxi driving 3d simulator can reduce your stress and anxiety, as you can relax and enjoy the game without any pressure or competition.</li>
|
185 |
-
<li>Playing city taxi driving 3d simulator can increase your hand-eye coordination and reaction time, as you have to control your car, avoid obstacles, and complete missions.</li>
|
186 |
-
<h2>The Drawbacks of Playing City Taxi Driving 3D Simulator</h2>
|
187 |
-
<p>Playing city taxi driving 3d simulator can also have some drawbacks for your time and money. Here are some of them:</p>
|
188 |
-
<ul>
|
189 |
-
<li>Playing city taxi driving 3d simulator can be addictive and time-consuming, as you may want to play more and more levels and unlock more and more cars.</li>
|
190 |
-
<li>Playing city taxi driving 3d simulator can be expensive and wasteful, as you may want to buy more and more coins and gems to upgrade your car and access premium features.</li>
|
191 |
-
<li>Playing city taxi driving 3d simulator can be frustrating and annoying, as you may encounter some bugs and glitches that may ruin your gameplay or cause your game to crash.</li>
|
192 |
-
<li>Playing city taxi driving 3d simulator can be boring and repetitive, as you may find some levels and missions too easy or too hard, or too similar or too different.</li>
|
193 |
-
<li>Playing city taxi driving 3d simulator can be harmful and unhealthy, as you may neglect your other responsibilities and activities, or strain your eyes and hands from playing too long.</li>
|
194 |
-
</ul>
|
195 |
-
<h2>The Future of City Taxi Driving 3D Simulator</h2>
|
196 |
-
<p>City taxi driving 3d simulator is a game that is constantly being updated and improved by the developers. They are always listening to the feedback and suggestions of the players and adding new features and content to the game. Some of the future updates and improvements that the developers are planning for city taxi driving 3d simulator are:</p>
|
197 |
-
<ul>
|
198 |
-
<li>Adding more levels and missions with different themes and scenarios.</li>
|
199 |
-
<li>Adding more taxi cars with different styles and functions.</li>
|
200 |
-
<li>Adding more passengers with different personalities and stories.</li>
|
201 |
-
<li>Adding more customization options for your car and your driver.</li>
|
202 |
-
<li>Adding more realistic and dynamic effects for the weather, the traffic, the physics, and the sounds.</li>
|
203 |
-
</ul>
|
204 |
-
<h2>Conclusion</h2>
|
205 |
-
<p>City taxi driving 3d simulator is a fun and realistic game for car lovers. It lets you drive and park different taxi cars in a realistic and lively city environment. You can choose from 50 different taxi cars, each with its own unique design and performance. You can also unlock new cars by completing various missions and earning money. The game has 100 unique levels, each with its own challenges and objectives. You have to drop and pick up interesting passengers, who will give you feedback and tips on your driving skills. You also have to follow the traffic rules and avoid accidents, as they will affect your reputation and income. The game has crazy realistic driving physics, which will make you feel like you are driving a real car. You can also customize your car with different colors, stickers, and accessories.</p>
|
206 |
-
<p>If you are looking for a game that will keep you entertained for hours, then you should try city taxi driving 3d simulator. It is a game that will improve your concentration, memory, creativity, hand-eye coordination, and reaction time. It is also a game that will reduce your stress, anxiety, boredom, and loneliness. However, you should also be aware of the drawbacks of playing city taxi driving 3d simulator. It is a game that can be addictive, expensive, frustrating, boring, and harmful if you play it too much or too often. Therefore, you should play it in moderation and balance it with other activities.</p>
|
207 |
-
<p>City taxi driving 3d simulator is a game that is constantly being updated and improved by the developers. They are always listening to the feedback and suggestions of the players and adding new features and content to the game. You can expect more levels, cars, passengers, customization options, realistic effects, and more in the future updates. You can also contact them if you have any questions or problems with the game.</p>
|
208 |
-
<p>So what are you waiting for? Download city taxi driving 3d simulator today and enjoy the thrill of being a taxi driver in a busy city!</p>
|
209 |
-
<table border="1">
|
210 |
-
<tr><th colspan="4">FAQs</th></tr>
|
211 |
-
<tr><td colspan="4">Q: How can I download city taxi driving 3d simulator?</td></tr>
|
212 |
-
<tr><td colspan="4">A: You can download city taxi driving 3d simulator from the Google Play Store or the App Store for free. You can also visit their official website or social media pages for more information.</td></tr>
|
213 |
-
<tr><td colspan="4">Q: How can I earn more money in city taxi driving 3d simulator?</td></tr>
|
214 |
-
bonuses, and watching ads or completing offers.</td></tr>
|
215 |
-
<tr><td colspan="4">Q: How can I unlock more cars in city taxi driving 3d simulator?</td></tr>
|
216 |
-
<tr><td colspan="4">A: You can unlock more cars in city taxi driving 3d simulator by earning more money, completing more levels, reaching higher ranks, and collecting car cards.</td></tr>
|
217 |
-
<tr><td colspan="4">Q: How can I customize my car in city taxi driving 3d simulator?</td></tr>
|
218 |
-
<tr><td colspan="4">A: You can customize your car in city taxi driving 3d simulator by going to the garage and selecting the paint, sticker, or accessory option. You can also change your driver's appearance and outfit.</td></tr>
|
219 |
-
<tr><td colspan="4">Q: How can I contact the developers of city taxi driving 3d simulator?</td></tr>
|
220 |
-
<tr><td colspan="4">A: You can contact the developers of city taxi driving 3d simulator by sending them an email at [email protected] or by following them on Facebook, Twitter, or Instagram.</td></tr></p> 197e85843d<br />
|
221 |
-
<br />
|
222 |
-
<br />
|
|
|
|
|
|
|
|
|
|
|
|
|
|
|
|
|
|
|
|
|
|
|
|
|
|
|
|
|
|
|
|
|
|
|
|
|
|
|
|
|
|
|
|
|
|
|
|
|
|
|
|
|
|
|
|
|
|
|
|
|
|
|
|
|
|
|
|
|
|
|
|
|
|
|
|
|
|
|
|
|
|
|
|
|
|
|
|
|
|
|
|
|
|
|
|
|
|
|
|
|
|
|
|
|
|
|
|
|
|
|
|
|
|
|
|
|
|
|
|
|
|
|
|
|
|
|
|
|
|
|
|
|
|
|
|
|
|
|
|
|
|
|
|
|
|
|
|
|
|
|
|
|
|
|
|
|
|
|
|
|
|
|
|
|
|
|
|
|
|
|
|
|
|
|
|
|
|
|
|
|
|
|
|
|
|
|
|
|
|
|
|
|
|
|
|
|
|
|
|
|
|
|
|
|
|
|
|
|
|
|
|
|
|
|
|
|
|
|
|
|
|
|
|
|
|
|
|
|
|
|
|
|
|
|
|
|
|
|
|
|
|
|
|
|
|
|
|
|
|
|
|
|
|
|
|
|
|
|
|
|
|
|
|
|
|
|
|
|
|
|
|
|
|
|
|
|
|
|
|
|
|
|
|
|
|
|
|
|
|
|
|
|
|
|
|
|
|
|
|
|
|
|
|
|
|
|
|
|
|
|
|
|
|
|
|
|
|
|
|
|
|
|
|
|
|
|
|
|
|
|
|
|
|
|
|
|
|
|
|
|
|
|
|
|
|
|
|
|
|
|
|
|
|
|
|
|
|
|
|
|
|
|
|
|
|
|
|
|
|
|
|
|
|
|
|
|
|
|
|
|
|
|
|
|
|
|
|
|
|
|
|
|
|
|
|
|
|
|
|
|
|
|
|
|
|
|
|
|
|
|
|
|
|
|
|
|
|
|
|
|
|
|
|
|
|
|
|
|
|
|
|
|
|
|
|
|
|
|
|
|
|
|
|
|
|
spaces/1phancelerku/anime-remove-background/Download M Modal and Simplify Your Documentation Workflow with Integrated Transcription Solutions.md
DELETED
@@ -1,106 +0,0 @@
|
|
1 |
-
|
2 |
-
<h1>Download M*Modal: A Guide to the Top-Ranking Conversational AI Platform for Medical Transcription</h1>
|
3 |
-
<p>If you are a physician or a medical transcriptionist, you know how important it is to create accurate, comprehensive, and timely clinical documentation. However, you also know how challenging and time-consuming it can be to capture the patient story using traditional methods such as typing, clicking, or dictating into a phone.</p>
|
4 |
-
<h2>download m modal</h2><br /><p><b><b>Download File</b> ✅ <a href="https://jinyurl.com/2uNP5I">https://jinyurl.com/2uNP5I</a></b></p><br /><br />
|
5 |
-
<p>That's why you need a better solution that can help you streamline your documentation workflow, improve your productivity and satisfaction, and enhance your quality of care and revenue cycle. That's why you need <strong>M*Modal</strong>.</p>
|
6 |
-
<p>M*Modal is a leading healthcare software and services company that empowers the transformation of ambulatory care. It offers a new breed of speech understanding applications that use artificial intelligence (AI) and natural language understanding (NLU) technologies to convert your spoken word into high quality electronic records.</p>
|
7 |
-
<p>M*Modal has over two decades of transcription experience, with a global workforce of over 8,600, and revenue of approximately $200 million. It is used by over 8,000 organizations worldwide, including hospitals, medical clinics, and medical transcription companies.</p>
|
8 |
-
<p>In this article, we will guide you through the main features and benefits of M*Modal, and show you how to download it and get started. We will focus on three of its products: <strong>M*Modal Fluency Direct</strong>, <strong>M*Modal Fluency Mobile</strong>, and <strong>M*Modal Fluency for Transcription</strong>.</p>
|
9 |
-
<h2>M*Modal Fluency Direct: The Cloud-Based Speech Recognition Solution</h2>
|
10 |
-
<p>M*Modal Fluency Direct is an all-in-one speech recognition solution that enables physicians of any medical specialty to conversationally create, review, edit, and sign clinical notes directly in their electronic health record (EHR) system. It leverages 3M NLU technology for contextual understanding of the patient's narrative and helps to improve documentation accuracy from the first word.</p>
|
11 |
-
<p>download m modal fluency direct for speech recognition<br />
|
12 |
-
download m modal software for clinical documentation<br />
|
13 |
-
download m modal app for voice dictation<br />
|
14 |
-
download m modal integration with epic ehr<br />
|
15 |
-
download m modal transcription services<br />
|
16 |
-
download m modal coding services<br />
|
17 |
-
download m modal cloud-based speech understanding<br />
|
18 |
-
download m modal conversational documentation<br />
|
19 |
-
download m modal natural language understanding<br />
|
20 |
-
download m modal artificial intelligence for healthcare<br />
|
21 |
-
download m modal voice profile for any device<br />
|
22 |
-
download m modal smart phrases and smart text<br />
|
23 |
-
download m modal computer-assisted physician documentation<br />
|
24 |
-
download m modal closed-loop documentation solution<br />
|
25 |
-
download m modal quality improvement and compliance<br />
|
26 |
-
download m modal productivity and efficiency tools<br />
|
27 |
-
download m modal support and training resources<br />
|
28 |
-
download m modal user guide and manual<br />
|
29 |
-
download m modal free trial and demo<br />
|
30 |
-
download m modal customer reviews and testimonials<br />
|
31 |
-
download m modal pricing and plans<br />
|
32 |
-
download m modal latest updates and features<br />
|
33 |
-
download m modal case studies and success stories<br />
|
34 |
-
download m modal best practices and tips<br />
|
35 |
-
download m modal comparison with other speech recognition solutions<br />
|
36 |
-
download m modal compatibility with different ehrs<br />
|
37 |
-
download m modal benefits and advantages for clinicians<br />
|
38 |
-
download m modal challenges and solutions for healthcare organizations<br />
|
39 |
-
download m modal security and privacy policies<br />
|
40 |
-
download m modal technical specifications and requirements</p>
|
41 |
-
<p>With built-in computer-assisted physician documentation (CAPD) functionality, M*Modal Fluency Direct continuously analyzes and monitors the clinical narrative. In real time, it nudges you for additional information or clarification and suggests specific things you can do to improve the quality of care and clinical documentation.</p>
|
42 |
-
<h3>How to integrate M*Modal Fluency Direct with your EHR system?</h3>
|
43 |
-
<p>M*Modal Fluency Direct seamlessly works with more than 250 EHRs, including all major platforms such as Epic, Cerner, athenahealth, MEDITECH, eClinicalWorks, (TLD), and front-end speech recognition (FESR). It combines the best of both worlds: the speed and convenience of speech recognition and the accuracy and quality of human transcription.</p>
|
44 |
-
<p>M*Modal Fluency for Transcription allows you to choose the option that best suits your needs and preferences. You can switch between the options at any time, depending on the complexity of the case, the availability of transcriptionists, or the urgency of the report. You can also use a hybrid approach that combines speech recognition and transcription for optimal results.</p>
|
45 |
-
<h3>How to choose between transcription-backed dictation, transcription-less dictation, and front-end speech recognition options?</h3>
|
46 |
-
<p>Here is a brief overview of each option and how to choose the best one for your situation:</p>
|
47 |
-
<table>
|
48 |
-
<tr>
|
49 |
-
<th>Option</th>
|
50 |
-
<th>Description</th>
|
51 |
-
<th>Advantages</th>
|
52 |
-
<th>Disadvantages</th>
|
53 |
-
<th>When to use</th>
|
54 |
-
</tr>
|
55 |
-
<tr>
|
56 |
-
<td>Transcription-backed dictation (TBD)</td>
|
57 |
-
<td>You dictate your clinical note using M*Modal Fluency Direct or M*Modal Fluency Mobile. Your dictation is sent to a human transcriptionist who edits and formats it according to your specifications. You receive a completed note within a specified turnaround time.</td>
|
58 |
-
<td>You can dictate quickly and easily without worrying about editing or formatting. You can rely on the transcriptionist's expertise and quality assurance. You can save time and money compared to traditional transcription.</td>
|
59 |
-
<td>You have to wait for the transcriptionist to finish your note before you can review and sign it. You have less control over the final output of your note. You may encounter delays or errors due to human factors.</td>
|
60 |
-
<td>You have a complex or unusual case that requires a high level of accuracy and detail. You prefer to delegate the editing and formatting tasks to a professional. You are not in a hurry to complete your note.</td>
|
61 |
-
</tr>
|
62 |
-
<tr>
|
63 |
-
<td>Transcription-less dictation (TLD)</td>
|
64 |
-
<td>You dictate your clinical note using M*Modal Fluency Direct or M*Modal Fluency Mobile. Your dictation is converted into text by M*Modal's speech recognition engine. You receive a draft note that you can review, edit, and sign yourself.</td>
|
65 |
-
<td>You can get your note done faster and more efficiently without waiting for a transcriptionist. You have more control over the content and style of your note. You can use CAPD functionality to improve your documentation quality and compliance.</td>
|
66 |
-
<td>You have to spend some time and effort to review and edit your note yourself. You may encounter some errors or inaccuracies due to speech recognition limitations. You may need some training and practice to use voice commands and macros effectively.</td>
|
67 |
-
<td>You have a simple or routine case that does not require a lot of detail or explanation. You prefer to do the editing and formatting tasks yourself. You are in a hurry to complete your note.</td>
|
68 |
-
</tr>
|
69 |
-
<tr>
|
70 |
-
<td>Front-end speech recognition (FESR)</td>
|
71 |
-
<td>You dictate your clinical note using M*Modal Fluency Direct or M*Modal Fluency Mobile. Your dictation is converted into text by M*Modal's speech recognition engine in real time. You can see the text on your screen as you speak and make corrections or changes on the fly.</td>
|
72 |
-
<td>You can create your note in one step without having to review or edit it later. You can see the immediate results of your dictation and make adjustments as needed. You can use CAPD functionality to get real-time feedback and guidance.</td>
|
73 |
-
<td>You have to pay attention to the text on your screen as you dictate and correct any errors or mistakes as you go. You may lose some of the natural flow and spontaneity of your dictation. You may need some training and practice to use voice commands and macros effectively.</td>
|
74 |
-
<td>You have a moderate or standard case that requires some detail or explanation. You prefer to see the text as you dictate and make corrections or changes on the fly. You want to create your note in one step without having to review or edit it later.</td>
|
75 |
-
</tr>
|
76 |
-
</table>
|
77 |
-
<h3>How to use M*Modal Fluency for Transcription to create high quality electronic records with less time and cost?</h3>
|
78 |
-
<p>To use M*Modal Fluency for Transcription, you need to download M*Modal Fluency Direct from the official website and install it on your Windows PC. You will need a valid license key and a microphone to use the software. Once installed, you can launch M*Modal Fluency Direct from your desktop or from within your EHR system.</p>
|
79 |
-
<p>To choose between TBD, TLD, or FESR options, you can either select them from the drop-down menu on the top left corner of the screen or say "switch mode" followed by the option name. For example, you can say "switch mode transcription-backed" or "switch mode front-end". You can also create custom voice commands for switching modes. For example, you can say "create command transcription mode" followed by the option name.</p>
|
80 |
-
<p>To dictate your clinical note, you can either tap on the microphone icon on the bottom right corner of the screen or select a patient and a note type from your EHR system. You will see a red recording button on the bottom center of the screen indicating that the app is ready to capture your voice. You can speak naturally and conversationally as you would with a human transcriptionist.</p>
|
81 |
-
<p>Depending on the mode you have chosen, you will see different results on your screen. If you have chosen TBD, you will see a message saying "Your dictation has been sent to transcription". If you have chosen TLD, you will see a draft note that you can review, edit, and sign yourself. If you have chosen FESR, you will see the text on your screen as you speak and make corrections or changes on the fly.</p>
|
82 |
-
<p>In any mode, you can use voice commands, macros, and CAPD functionality to improve your documentation workflow and quality. You can also use touch gestures such as tapping, swiping, or pinching to edit your note.</p>
|
83 |
-
<h2>Conclusion</h2>
|
84 |
-
<p>M*Modal is a conversational AI platform that helps physicians and medical transcriptionists create high quality electronic records with less time and cost. It offers three products: M*Modal Fluency Direct, M*Modal Fluency Mobile, and M*Modal Fluency for Transcription. Each product has its own features and benefits that suit different needs and preferences.</p>
|
85 |
-
<p>M*Modal Fluency Direct is a cloud-based speech recognition solution that enables physicians to conversationally create, review, edit, and sign clinical notes directly in their EHR system. It leverages 3M NLU technology for contextual understanding of the patient's narrative and helps to improve documentation accuracy from the first word.</p>
|
86 |
-
<p>M*Modal Fluency Mobile is a robust mobile medical dictation app that enables physicians to dictate clinical notes using their iOS or Android devices. It works with M*Modal Fluency Direct to provide a consistent and seamless speech recognition experience across different platforms and devices. It also works offline, so you can dictate without an internet connection.</p>
|
87 |
-
<p>M*Modal Fluency for Transcription is a flexible transcription solution that offers three options for creating electronic records: transcription-backed dictation (TBD), transcription-less dictation (TLD), and front-end speech recognition (FESR). It combines the best of both worlds: the speed and convenience of speech recognition and the accuracy and quality of human transcription.</p>
|
88 |
-
<p>If you want to download M*Modal and try it for yourself, you can visit the official website and request a demo or a free trial. You can also contact M*Modal's sales team or customer support team for more information or assistance.</p>
|
89 |
-
<p>We hope this article has helped you understand what M*Modal is and how it can help you with your medical transcription needs. Thank you for reading!</p>
|
90 |
-
<h2>FAQs</h2>
|
91 |
-
<h3>What are the pricing options for M*Modal?</h3>
|
92 |
-
<p>M*Modal does not disclose its pricing options publicly on its website. However, according to some online sources, M*Modal charges per line or per minute of dictation, depending on the product and the option you choose. The average cost ranges from $0.05 to $0.15 per line or from $0.08 to $0.25 per minute. You may also have to pay additional fees for installation, training, support, or maintenance.</p>
|
93 |
-
<h3>What are the system requirements for M*Modal?</h3>
|
94 |
-
<p>M*Modal Fluency Direct requires a Windows PC with at least 4 GB of RAM, 1 GB of free disk space, and an internet connection. It also requires a microphone that meets M*Modal's specifications. You can use any headset or handheld microphone that has a noise-canceling feature and a USB connection.</p>
|
95 |
-
<p>M*Modal Fluency Mobile requires an iOS or Android device with at least 1 GB of RAM, 100 MB of free disk space, and an internet connection. It also requires a microphone that meets M*Modal's specifications. You can use the built-in microphone of your device or an external microphone that has a noise-canceling feature and a Bluetooth connection.</p>
|
96 |
-
<p>M*Modal Fluency for Transcription requires a Windows PC with at least 4 GB of RAM, 1 GB of free disk space, and an internet connection. It also requires a microphone that meets M*Modal's specifications. You can use any headset or handheld microphone that has a noise-canceling feature and a USB connection.</p>
|
97 |
-
<h3>What are some of the best practices for using M*Modal?</h3>
|
98 |
-
<p>Here are some tips and tricks to help you get the most out of M*Modal and improve your documentation quality and efficiency:</p>
|
99 |
-
- Speak clearly and naturally, as if you were talking to a colleague or a patient. Avoid mumbling, whispering, shouting, or speaking too fast or too slow. - Use proper grammar, punctuation, and spelling. Say "period", "comma", "question mark", or "new paragraph" to insert the corresponding symbols. Say "spell that" to spell a word letter by letter. - Use medical terminology and abbreviations that are appropriate for your specialty and your EHR system. Avoid using slang, jargon, or acronyms that may be ambiguous or confusing. - Provide enough detail and context for your clinical note. Include relevant information such as the patient's history, physical examination, diagnosis, treatment plan, and follow-up instructions. Avoid vague or incomplete statements that may raise questions or doubts. - Review your note carefully before signing it. Check for any errors, inaccuracies, or inconsistencies that may have occurred during the dictation or transcription process. Make any corrections or changes as needed using voice commands or touch gestures. - Follow the best practices and guidelines for clinical documentation in your organization and your industry. Comply with the standards and regulations for quality, compliance, and reimbursement purposes. <h3>How secure is M*Modal and how does it comply with HIPAA and other regulations?</h3>
|
100 |
-
<p>M*Modal is committed to ensuring the security and privacy of your data and your patients' data. It uses advanced encryption, authentication, and authorization technologies to protect your data from unauthorized access, use, or disclosure. It also complies with the Health Insurance Portability and Accountability Act (HIPAA) and other applicable laws and regulations regarding the handling of protected health information (PHI).</p>
|
101 |
-
<p>M*Modal has obtained several certifications and accreditations that demonstrate its adherence to the highest standards of security and privacy. These include ISO 27001, SOC 2 Type II, HITRUST CSF, PCI DSS, GDPR, and CCPA.</p>
|
102 |
-
<h3>How can I get support and training for M*Modal?</h3>
|
103 |
-
<p>M*Modal provides various resources and channels for support and training for its customers. You can access the following options from the M*Modal website or the M*Modal Fluency Direct software:</p>
|
104 |
-
- User guides and manuals: You can download PDF files that contain detailed instructions and information on how to use M*Modal products. - Video tutorials: You can watch short videos that demonstrate how to perform common tasks or functions with M*Modal products. - Webinars: You can register for live or recorded webinars that cover various topics related to M*Modal products. - Knowledge base: You can search for answers to frequently asked questions or common issues with M*Modal products. - Support portal: You can submit a ticket or chat with a support agent online to get help with any technical or operational issues with M*Modal products. - Phone support: You can call a toll-free number to speak with a support agent over the phone to get help with any urgent or complex issues with M*Modal products.</p> 401be4b1e0<br />
|
105 |
-
<br />
|
106 |
-
<br />
|
|
|
|
|
|
|
|
|
|
|
|
|
|
|
|
|
|
|
|
|
|
|
|
|
|
|
|
|
|
|
|
|
|
|
|
|
|
|
|
|
|
|
|
|
|
|
|
|
|
|
|
|
|
|
|
|
|
|
|
|
|
|
|
|
|
|
|
|
|
|
|
|
|
|
|
|
|
|
|
|
|
|
|
|
|
|
|
|
|
|
|
|
|
|
|
|
|
|
|
|
|
|
|
|
|
|
|
|
|
|
|
|
|
|
|
|
|
|
|
|
|
|
|
|
|
|
|
|
|
|
|
|
|
|
|
|
|
|
|
|
|
|
|
|
|
|
|
|
|
|
|
|
|
|
|
|
|
|
|
|
|
|
|
|
|
|
|
|
|
|
|
|
|
|
|
|
|
|
|
|
|
|
|
|
|
|
|
|
|
|
|
|
|
|
|
|
|
|
|
|
|
|
|
|
|
|
|
|
|
|
|
|
|
spaces/1phancelerku/anime-remove-background/Download Mortal Kombat MOD APK and Unleash Your Inner Warrior.md
DELETED
@@ -1,116 +0,0 @@
|
|
1 |
-
<br />
|
2 |
-
<h1>Mortal Kombat Mod Apk: Everything You Need to Know</h1>
|
3 |
-
<p>Mortal Kombat is one of the most popular and iconic fighting game franchises of all time, with over 27 years of history and dozens of games across various platforms. The latest installment, Mortal Kombat 11, was released in 2019 for PC, PS4, Xbox One, Nintendo Switch, and Stadia, and received critical acclaim for its gameplay, story, graphics, and content.</p>
|
4 |
-
<h2>mortal kombat mod apk</h2><br /><p><b><b>Download</b> ✏ ✏ ✏ <a href="https://jinyurl.com/2uNJRW">https://jinyurl.com/2uNJRW</a></b></p><br /><br />
|
5 |
-
<p>But what if you want to enjoy Mortal Kombat 11 with even more features and options than the official version offers? What if you want to <p>What if you want to unlock all the characters, skins, and gear without spending hours of grinding or real money? What if you want to experience the game in the highest quality possible, with 4K resolution, HDR support, and immersive sound? What if you want to play online with your friends and opponents across different platforms?</p>
|
6 |
-
<p>If you answered yes to any of these questions, then you might be interested in Mortal Kombat mod apk. A mod apk is a modified version of an application that allows you to access features and functions that are not available in the original version. In this case, Mortal Kombat mod apk is a modified version of Mortal Kombat 11 that gives you unlimited access to all the content and options of the game, as well as some extra benefits that enhance your gaming experience.</p>
|
7 |
-
<p>In this article, we will tell you everything you need to know about Mortal Kombat mod apk, including its features, how to download and install it, tips and tricks for playing it, and its pros and cons. By the end of this article, you will be able to decide whether Mortal Kombat mod apk is worth trying or not. So, let's get started!</p>
|
8 |
-
<h2>Features of Mortal Kombat Mod Apk</h2>
|
9 |
-
<p>Mortal Kombat mod apk has many features that make it stand out from the official version of the game. Here are some of the most notable ones:</p>
|
10 |
-
<h3>Unlimited Souls and Money</h3>
|
11 |
-
<p>One of the main advantages of Mortal Kombat mod apk is that it gives you unlimited souls and money, which are the two main currencies of the game. You can use souls and money to unlock characters, skins, gear, fatalities, brutalities, and other items from the in-game store or the krypt. You can also use them to upgrade your characters and their abilities. With Mortal Kombat mod apk, you don't have to worry about running out of resources or spending real money to get them. You can have everything you want in the game without any hassle.</p>
|
12 |
-
<h3>All Characters Unlocked</h3>
|
13 |
-
<p>Another great feature of Mortal Kombat mod apk is that it unlocks all the characters in the game, including the DLC characters that are normally only available through purchasing the season pass or individual packs. Mortal Kombat 11 has a total of 37 playable fighters, each with their own unique moves, combos, styles, and personalities. Some of the most popular characters are Scorpion, Sub-Zero, Raiden, Liu Kang, Sonya Blade, Johnny Cage, Kitana, Jade, Shao Kahn, Sindel, Spawn, Joker, Terminator, RoboCop, Rambo, Mileena, and Rain. With Mortal Kombat mod apk, you can access all these characters and more from the start of the game. You can also customize their appearance and equipment to suit your preferences.</p>
|
14 |
-
<h3>High-Quality Graphics and Sound</h3>
|
15 |
-
<p>Mortal Kombat mod apk also enhances the graphics and sound quality of the game to make it more realistic and immersive. The mod apk supports dynamic 4K resolution and HDR (high dynamic range) technology, which improve the clarity, contrast, and color of the images on your screen. The mod apk also supports Dolby Atmos and DTS:X audio formats, which create a surround sound effect that makes you feel like you are in the middle of the action. The graphics and sound of Mortal Kombat mod apk are so good that they will make you forget that you are playing on a mobile device.</p>
|
16 |
-
<p>mortal kombat mod apk unlimited souls<br />
|
17 |
-
mortal kombat mod apk latest version<br />
|
18 |
-
mortal kombat mod apk download for android<br />
|
19 |
-
mortal kombat mod apk offline<br />
|
20 |
-
mortal kombat mod apk all characters unlocked<br />
|
21 |
-
mortal kombat mod apk unlimited money<br />
|
22 |
-
mortal kombat mod apk no root<br />
|
23 |
-
mortal kombat mod apk free shopping<br />
|
24 |
-
mortal kombat mod apk god mode<br />
|
25 |
-
mortal kombat mod apk unlimited everything<br />
|
26 |
-
mortal kombat mod apk rexdl<br />
|
27 |
-
mortal kombat mod apk revdl<br />
|
28 |
-
mortal kombat mod apk hack<br />
|
29 |
-
mortal kombat mod apk obb<br />
|
30 |
-
mortal kombat mod apk android 1<br />
|
31 |
-
mortal kombat mod apk 2023<br />
|
32 |
-
mortal kombat mod apk anti ban<br />
|
33 |
-
mortal kombat mod apk unlimited coins and souls<br />
|
34 |
-
mortal kombat mod apk data<br />
|
35 |
-
mortal kombat mod apk highly compressed<br />
|
36 |
-
mortal kombat mod apk mega<br />
|
37 |
-
mortal kombat mod apk menu<br />
|
38 |
-
mortal kombat mod apk new update<br />
|
39 |
-
mortal kombat mod apk old version<br />
|
40 |
-
mortal kombat mod apk pure<br />
|
41 |
-
mortal kombat mod apk unlimited health<br />
|
42 |
-
mortal kombat mod apk vip<br />
|
43 |
-
mortal kombat mod apk x ray unlocked<br />
|
44 |
-
mortal kombat x mod apk<br />
|
45 |
-
mortal kombat 11 mod apk<br />
|
46 |
-
mortal kombat xl mod apk<br />
|
47 |
-
mortal kombat armageddon mod apk<br />
|
48 |
-
mortal kombat shaolin monks mod apk<br />
|
49 |
-
mortal kombat deception mod apk<br />
|
50 |
-
mortal kombat deadly alliance mod apk<br />
|
51 |
-
mortal kombat trilogy mod apk<br />
|
52 |
-
mortal kombat unchained mod apk<br />
|
53 |
-
mortal kombat ultimate mod apk<br />
|
54 |
-
mortal kombat legacy mod apk<br />
|
55 |
-
mortal kombat 9 mod apk<br />
|
56 |
-
mortal kombat 10 mod apk<br />
|
57 |
-
mortal kombat 4 mod apk<br />
|
58 |
-
mortal kombat 3d mod apk<br />
|
59 |
-
mortal kombat 2d mod apk<br />
|
60 |
-
ultimate mortal kombat 3 mod apk</p>
|
61 |
-
<h3>Cross-Play Support</h3>
|
62 |
-
<p>The last feature we will mention here is cross-play support. Cross-play is a feature that allows players to play online with other players who are using different platforms or devices. For example, if you are playing Mortal Kombat mod apk on your Android phone, you can play online with someone who is playing Mortal Kombat 11 on their PS4 or Xbox One. This feature expands your pool of potential opponents and friends online and makes the game more fun and social. Cross-play also allows you to sync your progress and data across different devices using your WB Games account.</p>
|
63 |
-
<h2>How to Download and Install Mortal Kombat Mod Apk</h2>
|
64 |
-
<p>Now that you know what Mortal Kombat mod apk can do for you, you might be wondering how to get it on your device. Well, it's not very difficult if you follow these simple steps:</p>
|
65 |
-
<h3>Step 1: Find a reliable source for the mod apk file and download it to your device.</h3>
|
66 |
-
<p>The first thing you need to do is find a trustworthy website that offers the latest version of Mortal Kombat mod apk for free download. There are many sites that claim to provide this service but not all of them are safe or reliable. Some may contain viruses or malware <p>Some may contain viruses or malware that can harm your device or steal your personal information. Some may also have outdated or fake versions of the mod apk that do not work properly or at all. Therefore, you need to be careful and do some research before downloading anything from the internet. You can use online reviews, ratings, comments, and feedback from other users to help you find a reputable site. You can also use antivirus software and VPN services to protect your device and privacy.</p>
|
67 |
-
<p>Once you find a reliable source, you can download the mod apk file to your device. The file size may vary depending on the version and features of the mod apk, but it should be around 1 GB. Make sure you have enough storage space and a stable internet connection before downloading the file.</p>
|
68 |
-
<h3>Step 2: Enable unknown sources in your device settings and install the mod apk file.</h3>
|
69 |
-
<p>The next thing you need to do is enable unknown sources in your device settings. This is a security feature that prevents you from installing applications that are not from the official app store or verified by Google. Since Mortal Kombat mod apk is not from the official app store, you need to enable unknown sources to install it. To do this, go to your device settings, then security, then unknown sources, and toggle it on. You may see a warning message that says installing from unknown sources may harm your device or data, but don't worry, this is just a precautionary measure. You can trust Mortal Kombat mod apk as long as you downloaded it from a safe source.</p>
|
70 |
-
<p>After enabling unknown sources, you can install the mod apk file. To do this, locate the file in your device's file manager or downloads folder and tap on it. You may see a pop-up window that asks for your permission to install the application. Tap on install and wait for the installation process to complete. It may take a few minutes depending on your device's speed and performance.</p>
|
71 |
-
<h3>Step 3: Launch the game and enjoy the modded features.</h3>
|
72 |
-
<p>The final thing you need to do is launch the game and enjoy the modded features. To do this, find the game icon on your device's home screen or app drawer and tap on it. You may see a loading screen that says "Mortal Kombat mod apk" or something similar. This means that the mod apk is working properly and you can access all its features and options. You may also see some ads or pop-ups from the mod apk developer or sponsor, but you can ignore or close them if they bother you.</p>
|
73 |
-
<p>Once the game loads, you can start playing with unlimited souls and money, all characters unlocked, high-quality graphics and sound, cross-play support, and more. You can also customize your settings, preferences, controls, and account details from the game's menu. You can also check for updates or contact support from the game's menu if you encounter any problems or issues with the mod apk.</p>
|
74 |
-
<h2>Tips and Tricks for Playing Mortal Kombat Mod Apk</h2>
|
75 |
-
<p>Mortal Kombat mod apk is a fun and exciting way to play Mortal Kombat 11 with enhanced features and options. However, it can also be challenging and competitive, especially if you are new to the game or want to improve your skills and performance. Here are some tips and tricks that can help you play better and enjoy more:</p>
|
76 |
-
<h3>Tip 1: Use the tutorial mode to learn the basics and advanced techniques of the game.</h3>
|
77 |
-
<p>Mortal Kombat 11 has a comprehensive tutorial mode that teaches you everything you need to know about the game's mechanics, systems, modes, characters, moves, combos, styles, strategies, and more. The tutorial mode is divided into several sections that cover different aspects of the game, such as basic attacks, special moves, defense, offense, meter management, krushing blows, fatal blows, fatalities, brutalities, and more. The tutorial mode also has a practice mode that allows you to practice your moves and combos against a dummy opponent or a live opponent. The tutorial mode is a great way to learn the game and improve your skills, whether you are a beginner or a veteran. You can access the tutorial mode from the main menu of the game.</p>
|
78 |
-
<h3>Tip 2: Pin your favorite moves and combos on the screen for easy reference.</h3>
|
79 |
-
<p>Mortal Kombat 11 has a feature that allows you to pin your favorite moves and combos on the screen for easy reference. This is useful if you want to remember how to perform a certain move or combo, or if you want to try out new moves and combos that you have learned from the tutorial mode or elsewhere. To pin a move or combo on the screen, go to the pause menu of the game, then select "Move List", then select the move or combo you want to pin, then press the pin button. You can pin up to 10 moves or combos at a time, and you can unpin them anytime by pressing the same button. You can also customize the position and size of the pinned moves and combos on the screen from the settings menu of the game.</p>
|
80 |
-
<h3>Tip 3: Master your Krushing Blows and Fatal Blows to deal massive damage and turn the tide of battle.</h3>
|
81 |
-
<p>Krushing Blows and Fatal Blows are special moves that deal massive damage and have cinematic effects. They are also crucial for winning fights and turning the tide of battle. Krushing Blows are enhanced versions of certain moves that trigger when certain conditions are met, such as landing a counter hit, connecting a certain number of hits, or using a certain move as a punish. Krushing Blows can only be used once per match for each move, so use them wisely. Fatal Blows are powerful attacks that become available when your health drops below 30%. You can use them by pressing both triggers at the same time. Fatal Blows can only be used once per match, and they can be blocked or dodged, so use them strategically.</p>
|
82 |
-
<h3>Tip 4: Use the environment to your advantage by interacting with objects and stage hazards.</h3>
|
83 |
-
<p>Mortal Kombat 11 has interactive environments that allow you to use objects and stage hazards to your advantage. You can use objects such as barrels, chains, spears, swords, guns, and more to attack your opponent or escape from their attacks. You can also use stage hazards such as acid pools, spikes, fire pits, electric fences, and more to damage your opponent or change the position of the fight. To interact with an object or stage hazard, press the right bumper when you are near it. You can also throw your opponent into an object or stage hazard by pressing forward and right bumper when you are near it. Using the environment can give you an edge in combat and add some variety to your fights.</p>
|
84 |
-
<h3>Tip 5: Mix up your moves and strategies when fighting against the AI or other players.</h3>
|
85 |
-
<p>Mortal Kombat 11 has a smart and adaptive AI that learns from your moves and strategies and adjusts accordingly. It also has a diverse and competitive online community that consists of players of different skill levels and play styles. Therefore, if you want to win against the AI or other players, you need to mix up your moves and strategies and avoid being predictable or repetitive. You can do this by using different attacks, blocks, throws, specials, combos, variations, stances, and more. You can also do this by changing your pace, timing, distance, angle, direction, and more. Mixing up your moves and strategies will keep your opponent guessing and off-balance, and give you an advantage in combat.</p>
|
86 |
-
<h2>Pros and Cons of Mortal Kombat Mod Apk</h2>
|
87 |
-
<p>Mortal Kombat mod apk has many pros that make it appealing and enjoyable for many players. However, it also has some cons that may deter some players from using it. Here are some of the pros and cons of Mortal Kombat mod apk:</p>
|
88 |
-
<h3>Pros</h3>
|
89 |
-
<ul>
|
90 |
-
<li>More resources: You get unlimited souls and money that allow you to unlock everything in the game without any hassle or cost.</li>
|
91 |
-
<li>More characters: You get access to all 37 playable fighters in the game, including DLC characters that are normally only available through purchasing.</li>
|
92 |
-
<li>More fun: You get to enjoy the game in the highest quality possible, with 4K resolution, HDR support, Dolby Atmos sound, cross-play support, and more.</li>
|
93 |
-
<li>No ads: You don't have to deal with annoying ads that interrupt your gameplay or waste your time.</li>
|
94 |
-
<li>No root required: You don't have to root your device or modify its system settings to use Mortal Kombat mod apk.</li> <h3>Cons</h3>
|
95 |
-
<ul>
|
96 |
-
<li>Possible security risks: You may expose your device or data to viruses or malware that can harm or steal them. Always download Mortal Kombat mod apk from trusted sources and scan the file for viruses before installing.</li>
|
97 |
-
<li>Possible compatibility issues: You may encounter some bugs or glitches that affect the performance or functionality of the game. Always check your device's compatibility and update the mod apk to the latest version before playing.</li>
|
98 |
-
<li>Possible legal issues: You may violate the terms of service of the game developer and publisher, and may face legal action or account suspension. Always use Mortal Kombat mod apk at your own risk and respect the intellectual property rights of the original creators.</li>
|
99 |
-
<li>Possible ban from online modes: You may be detected and banned by the game's anti-cheat system if you play online with Mortal Kombat mod apk. Always play online at your own risk and avoid using unfair or abusive tactics that ruin the game for others.</li>
|
100 |
-
</ul>
|
101 |
-
<h2>Conclusion</h2>
|
102 |
-
<p>Mortal Kombat mod apk is a modified version of Mortal Kombat 11 that gives you unlimited access to all the content and options of the game, as well as some extra benefits that enhance your gaming experience. It is a great way to enjoy the best fighting game series with more resources, more characters, more fun, no ads, and no root required. However, it also comes with some drawbacks, such as possible security risks, possible compatibility issues, possible legal issues, and possible ban from online modes. Therefore, you need to weigh the pros and cons of Mortal Kombat mod apk before using it, and always use it responsibly and ethically.</p>
|
103 |
-
<h3>FAQs</h3>
|
104 |
-
<p>Here are some frequently asked questions about Mortal Kombat mod apk:</p>
|
105 |
-
<h4>Q1: Is Mortal Kombat mod apk safe to use?</h4>
|
106 |
-
<p>A1: It depends on the source of the mod apk file. Always download from trusted sites and scan the file for viruses before installing.</p>
|
107 |
-
<h4>Q2: Is Mortal Kombat mod apk legal to use?</h4>
|
108 |
-
<p>A2: It depends on your country's laws and regulations. Using a mod apk may violate the terms of service of the game developer and publisher, and may result in legal action or account suspension. Use at your own risk.</p>
|
109 |
-
<h4>Q3: Is Mortal Kombat mod apk compatible with my device?</h4>
|
110 |
-
<p>A3: It depends on your device's specifications and operating system. The mod apk requires Android 5.0 or higher and at least 4 GB of RAM to run smoothly. Check your device's compatibility before downloading and installing.</p>
|
111 |
-
<h4>Q4: Can I play online with Mortal Kombat mod apk?</h4>
|
112 |
-
<p>A4: Yes, but only with other players who are using the same mod apk version. You can also enable cross-play to play with players on other platforms, but be aware that you may be detected and banned by the game's anti-cheat system. Play online at your own risk.</p>
|
113 |
-
<h4>Q5: Can I update Mortal Kombat mod apk?</h4>
|
114 |
-
<p>A5: Yes, but only if there is a new version of the mod apk available from the same source. Do not update from the official app store or you will lose all the modded features.</p> 197e85843d<br />
|
115 |
-
<br />
|
116 |
-
<br />
|
|
|
|
|
|
|
|
|
|
|
|
|
|
|
|
|
|
|
|
|
|
|
|
|
|
|
|
|
|
|
|
|
|
|
|
|
|
|
|
|
|
|
|
|
|
|
|
|
|
|
|
|
|
|
|
|
|
|
|
|
|
|
|
|
|
|
|
|
|
|
|
|
|
|
|
|
|
|
|
|
|
|
|
|
|
|
|
|
|
|
|
|
|
|
|
|
|
|
|
|
|
|
|
|
|
|
|
|
|
|
|
|
|
|
|
|
|
|
|
|
|
|
|
|
|
|
|
|
|
|
|
|
|
|
|
|
|
|
|
|
|
|
|
|
|
|
|
|
|
|
|
|
|
|
|
|
|
|
|
|
|
|
|
|
|
|
|
|
|
|
|
|
|
|
|
|
|
|
|
|
|
|
|
|
|
|
|
|
|
|
|
|
|
|
|
|
|
|
|
|
|
|
|
|
|
|
|
|
|
|
|
|
|
|
|
|
|
|
|
|
|
|
|
|
|
|
|
|
|
|
|
|
|
spaces/1phancelerku/anime-remove-background/Enjoy FIFA Mobile with Nulls FIFA APK The Best Mod for Soccer Fans.md
DELETED
@@ -1,132 +0,0 @@
|
|
1 |
-
<br />
|
2 |
-
<h1>Nulls FIFA APK: How to Download and Play FIFA Mobile with Unlimited Money and Features</h1>
|
3 |
-
<p>If you are a fan of soccer games, you might have heard of FIFA Mobile, the official mobile game of the FIFA World Cup 2022. But did you know that there is a modified version of this game that gives you unlimited money, unlocked features, and more? It's called Nulls FIFA APK, and in this article, we will tell you everything you need to know about it.</p>
|
4 |
-
<h2>nulls fifa apk</h2><br /><p><b><b>Download</b> ★ <a href="https://jinyurl.com/2uNQpO">https://jinyurl.com/2uNQpO</a></b></p><br /><br />
|
5 |
-
<h2>What is Nulls FIFA APK?</h2>
|
6 |
-
<h3>A modified version of FIFA Mobile</h3>
|
7 |
-
<p>Nulls FIFA APK is a modded version of FIFA Mobile, the popular soccer game developed by Electronic Arts. It is not available on the Google Play Store or the App Store, but you can download it from third-party websites. The main difference between Nulls FIFA APK and the original game is that it gives you access to unlimited money, unlocked players, kits, clubs, leagues, and more. You can also use a menu to customize your gameplay settings, such as speed, difficulty, graphics, etc.</p>
|
8 |
-
<h3>Features of Nulls FIFA APK</h3>
|
9 |
-
<p>Some of the features that you can enjoy with Nulls FIFA APK are:</p>
|
10 |
-
<ul>
|
11 |
-
<li>Unlimited money: You can buy anything you want in the game without worrying about your budget.</li>
|
12 |
-
<li>Unlocked players: You can choose from over 15,000 authentic soccer stars from over 600 teams, including world-class talent like Kylian Mbappé, Christian Pulisic, Vinicius Jr and Son Heung-min.</li>
|
13 |
-
<li>Unlocked kits, clubs, leagues: You can play with any team you want in any league you want, including Chelsea, Paris SG, Real Madrid, Liverpool and Juventus.</li>
|
14 |
-
<li>Unlocked World Cup mode: You can replay the official tournament brackets with any of the 32 qualified nations, with authentic kits, badges, stadiums, and commentary.</li>
|
15 |
-
<li>Menu mod: You can use a menu to adjust your gameplay settings, such as speed, difficulty, graphics, etc.</li>
|
16 |
-
</ul>
|
17 |
-
<h2>How to Download and Install Nulls FIFA APK?</h2>
|
18 |
-
<h3>Requirements and precautions</h3>
|
19 |
-
<p>Before you download and install Nulls FIFA APK, you need to make sure that your device meets the following requirements:</p>
|
20 |
-
<ul>
|
21 |
-
<li>Android 5.0 or higher</li>
|
22 |
-
<li>At least 200 MB of free storage space</li>
|
23 |
-
<li>A stable internet connection</li>
|
24 |
-
</ul>
|
25 |
-
<p>You also need to take some precautions to avoid any problems or risks:</p>
|
26 |
-
<ul>
|
27 |
-
<li>Enable unknown sources in your device settings to allow installation of apps from third-party sources.</li>
|
28 |
-
<li>Disable antivirus or firewall software that might interfere with the installation process.</li>
|
29 |
-
<li>Backup your data before installing the modded game in case something goes wrong.</li>
|
30 |
-
<li>Do not use your real account or personal information when playing the modded game to avoid getting banned or hacked.</li>
|
31 |
-
</ul>
|
32 |
-
<h3>Steps to download and install</h3>
|
33 |
-
<p>Once you have met the requirements and taken the precautions, you can follow these steps to download and install Nulls FIFA APK:</p>
|
34 |
-
<ol>
|
35 |
-
<li>Go to a reliable website that offers Nulls FIFA APK for download. For example, you can use [this link](^1^) to download the latest version of the game.</li>
|
36 |
-
<li>Tap on the download button and wait for the file to be downloaded on your device.</li>
|
37 |
-
<li>Locate the downloaded file in your file manager and tap on it to start the installation process.</li>
|
38 |
-
<li>Follow the instructions on the screen and wait for the installation to finish. You may need to grant some permissions to the app if asked.</li>
|
39 |
-
<li>Launch the game and enjoy playing FIFA Mobile with unlimited money and features.</li>
|
40 |
-
</ol>
|
41 |
-
<h2>How to Play Nulls FIFA APK?</h2>
|
42 |
-
<h3>Build your ultimate team</h3>
|
43 |
-
<p>One of the main features of Nulls FIFA APK is that you can build your ultimate team with any players you want. You can use the unlimited money to buy packs, players, or items in the store. You can also use the menu mod to unlock all the players, kits, clubs, and leagues in the game. You can then create your own custom squad with your favorite stars and formations. You can also upgrade your players' skills and attributes to make them more powerful.</p>
|
44 |
-
<p>nulls fifa mobile apk download<br />
|
45 |
-
nulls fifa 23 apk<br />
|
46 |
-
nulls fifa mod apk<br />
|
47 |
-
nulls fifa hack apk<br />
|
48 |
-
nulls fifa unlimited coins apk<br />
|
49 |
-
nulls fifa offline apk<br />
|
50 |
-
nulls fifa latest version apk<br />
|
51 |
-
nulls fifa android apk<br />
|
52 |
-
nulls fifa update apk<br />
|
53 |
-
nulls fifa free apk<br />
|
54 |
-
nulls fifa 22 apk<br />
|
55 |
-
nulls fifa online apk<br />
|
56 |
-
nulls fifa cracked apk<br />
|
57 |
-
nulls fifa full apk<br />
|
58 |
-
nulls fifa premium apk<br />
|
59 |
-
nulls fifa pro apk<br />
|
60 |
-
nulls fifa beta apk<br />
|
61 |
-
nulls fifa mega mod apk<br />
|
62 |
-
nulls fifa cheat apk<br />
|
63 |
-
nulls fifa generator apk<br />
|
64 |
-
nulls fifa 21 apk<br />
|
65 |
-
nulls fifa no root apk<br />
|
66 |
-
nulls fifa patched apk<br />
|
67 |
-
nulls fifa unlocked apk<br />
|
68 |
-
nulls fifa vip apk<br />
|
69 |
-
nulls fifa 2023 apk<br />
|
70 |
-
nulls fifa no ads apk<br />
|
71 |
-
nulls fifa modded apk<br />
|
72 |
-
nulls fifa unlimited money apk<br />
|
73 |
-
nulls fifa hack tool apk<br />
|
74 |
-
nulls fifa original apk<br />
|
75 |
-
nulls fifa obb data apk<br />
|
76 |
-
nulls fifa revdl apk<br />
|
77 |
-
nulls fifa rexdl apk<br />
|
78 |
-
nulls fifa apkpure apk<br />
|
79 |
-
nulls fifa apkmirror apk<br />
|
80 |
-
nulls fifa appvn apk<br />
|
81 |
-
nulls fifa an1 apk<br />
|
82 |
-
nulls fifa andropalace apk<br />
|
83 |
-
nulls fifa android 1 apk<br />
|
84 |
-
nulls fifa android republic apk<br />
|
85 |
-
nulls fifa blackmod apk<br />
|
86 |
-
nulls fifa byjus modz club apk <br />
|
87 |
-
nulls fifa byjus modz club download link <br />
|
88 |
-
nulls fifa byjus modz club free download <br />
|
89 |
-
nuls-fifa-mobile-apk-combo <br />
|
90 |
-
nuls-fifa-mobile-apk-download <br />
|
91 |
-
nuls-fifa-mobile-apk-latest-version <br />
|
92 |
-
nuls-fifa-mobile-apk-update</p>
|
93 |
-
<h3>Relive the FIFA World Cup 2022</h3>
|
94 |
-
<p>Another feature of Nulls FIFA APK is that you can relive the FIFA World Cup 2022 with the official tournament mode. You can choose any of the 32 qualified nations and play through the group stage, knockout stage, and final. You can also customize your team's kit, badge, stadium, and commentary to match the real event. You can experience the thrill and excitement of the world's biggest soccer competition on your mobile device.</p>
|
95 |
-
<h3>Compete in various modes</h3>
|
96 |
-
<p>Besides the World Cup mode, Nulls FIFA APK also offers other modes for you to compete in. You can play in the Season mode, where you can choose a league and a club and play a full season of matches. You can also play in the Events mode, where you can participate in various challenges and tournaments based on real-life soccer events. You can also play in the Versus mode, where you can challenge other players online and climb the leaderboards.</p>
|
97 |
-
<h2>Pros and Cons of Nulls FIFA APK</h2>
|
98 |
-
<h3>Pros</h3>
|
99 |
-
<p>Some of the advantages of using Nulls FIFA APK are:</p>
|
100 |
-
<ul>
|
101 |
-
<li>You can enjoy FIFA Mobile with unlimited money and features.</li>
|
102 |
-
<li>You can play with any players, kits, clubs, and leagues you want.</li>
|
103 |
-
<li>You can relive the FIFA World Cup 2022 with authentic details.</li>
|
104 |
-
<li>You can compete in various modes and challenges.</li>
|
105 |
-
<li>You can customize your gameplay settings with the menu mod.</li>
|
106 |
-
</ul>
|
107 |
-
<h3>Cons</h3>
|
108 |
-
<p>Some of the disadvantages of using Nulls FIFA APK are:</p>
|
109 |
-
<ul>
|
110 |
-
<li>You may face some bugs or errors while playing the game.</li>
|
111 |
-
<li>You may get banned or hacked if you use your real account or personal information.</li>
|
112 |
-
<li>You may not be able to update the game or access some features that require an internet connection.</li>
|
113 |
-
<li>You may violate the terms and conditions of Electronic Arts by using a modded game.</li>
|
114 |
-
</ul>
|
115 |
-
<h2>Conclusion</h2>
|
116 |
-
<p>Nulls FIFA APK is a modded version of FIFA Mobile that gives you unlimited money and features. It allows you to build your ultimate team, relive the FIFA World Cup 2022, and compete in various modes. However, it also has some drawbacks, such as bugs, risks, limitations, and violations. Therefore, you should use it at your own discretion and responsibility. If you want to try it out, you can follow the steps above to download and install it on your device.</p>
|
117 |
-
<h2>FAQs</h2>
|
118 |
-
<p>Here are some frequently asked questions about Nulls FIFA APK:</p>
|
119 |
-
<ol>
|
120 |
-
<li><b>What is Nulls FIFA APK?</b><br>
|
121 |
-
Nulls FIFA APK is a modded version of FIFA Mobile that gives you unlimited money and features.</li>
|
122 |
-
<li><b>How to download Nulls FIFA APK?</b><br>
|
123 |
-
You can download Nulls FIFA APK from third-party websites that offer it for download. For example, you can use [this link] to download the latest version of the game.</li>
|
124 |
-
<li><b>How to install Nulls FIFA APK?</b><br>
|
125 |
-
You need to enable unknown sources in your device settings, disable antivirus or firewall software, backup your data, and then follow the instructions on the screen to install Nulls FIFA APK on your device.</li>
|
126 |
-
<li><b>How to play Nulls FIFA APK?</b><br>
|
127 |
-
You can launch the game and enjoy playing FIFA Mobile with unlimited money and features. You can build your ultimate team, relive the FIFA World Cup 2022, and compete in various modes.</li>
|
128 |
-
<li><b>Is Nulls FIFA APK safe?</b><br>
|
129 |
-
Nulls FIFA APK is not safe as it may contain bugs, viruses, malware, or spyware. It may also get you banned or hacked if you use your real account or personal information. It may also violate the terms and conditions of Electronic Arts by using a modded game. Therefore, you should use it at your own discretion and responsibility.</li>
|
130 |
-
</ol></p> 401be4b1e0<br />
|
131 |
-
<br />
|
132 |
-
<br />
|
|
|
|
|
|
|
|
|
|
|
|
|
|
|
|
|
|
|
|
|
|
|
|
|
|
|
|
|
|
|
|
|
|
|
|
|
|
|
|
|
|
|
|
|
|
|
|
|
|
|
|
|
|
|
|
|
|
|
|
|
|
|
|
|
|
|
|
|
|
|
|
|
|
|
|
|
|
|
|
|
|
|
|
|
|
|
|
|
|
|
|
|
|
|
|
|
|
|
|
|
|
|
|
|
|
|
|
|
|
|
|
|
|
|
|
|
|
|
|
|
|
|
|
|
|
|
|
|
|
|
|
|
|
|
|
|
|
|
|
|
|
|
|
|
|
|
|
|
|
|
|
|
|
|
|
|
|
|
|
|
|
|
|
|
|
|
|
|
|
|
|
|
|
|
|
|
|
|
|
|
|
|
|
|
|
|
|
|
|
|
|
|
|
|
|
|
|
|
|
|
|
|
|
|
|
|
|
|
|
|
|
|
|
|
|
|
|
|
|
|
|
|
|
|
|
|
|
|
|
|
|
|
|
|
|
|
|
|
|
|
|
|
|
|
|
|
|
|
|
|
|
|
|
|
|
|
|
|
|
|
|
|
|
|
|
spaces/44ov41za8i/FreeVC/README.md
DELETED
@@ -1,13 +0,0 @@
|
|
1 |
-
---
|
2 |
-
title: FreeVC
|
3 |
-
emoji: 🚀
|
4 |
-
colorFrom: gray
|
5 |
-
colorTo: red
|
6 |
-
sdk: gradio
|
7 |
-
sdk_version: 3.13.0
|
8 |
-
app_file: app.py
|
9 |
-
pinned: false
|
10 |
-
license: mit
|
11 |
-
---
|
12 |
-
|
13 |
-
Check out the configuration reference at https://huggingface.co/docs/hub/spaces-config-reference
|
|
|
|
|
|
|
|
|
|
|
|
|
|
|
|
|
|
|
|
|
|
|
|
|
|
|
|
spaces/52Hz/HWMNet_lowlight_enhancement/README.md
DELETED
@@ -1,45 +0,0 @@
|
|
1 |
-
---
|
2 |
-
title: HWMNet_low-light_enhancement
|
3 |
-
emoji: 🕶
|
4 |
-
colorFrom: indigo
|
5 |
-
colorTo: black
|
6 |
-
sdk: gradio
|
7 |
-
app_file: app.py
|
8 |
-
pinned: false
|
9 |
-
---
|
10 |
-
|
11 |
-
# Configuration
|
12 |
-
|
13 |
-
`title`: _string_
|
14 |
-
Display title for the Space
|
15 |
-
|
16 |
-
`emoji`: _string_
|
17 |
-
Space emoji (emoji-only character allowed)
|
18 |
-
|
19 |
-
`colorFrom`: _string_
|
20 |
-
Color for Thumbnail gradient (red, yellow, green, blue, indigo, purple, pink, gray)
|
21 |
-
|
22 |
-
`colorTo`: _string_
|
23 |
-
Color for Thumbnail gradient (red, yellow, green, blue, indigo, purple, pink, gray)
|
24 |
-
|
25 |
-
`sdk`: _string_
|
26 |
-
Can be either `gradio`, `streamlit`, or `static`
|
27 |
-
|
28 |
-
`sdk_version` : _string_
|
29 |
-
Only applicable for `streamlit` SDK.
|
30 |
-
See [doc](https://hf.co/docs/hub/spaces) for more info on supported versions.
|
31 |
-
|
32 |
-
`app_file`: _string_
|
33 |
-
Path to your main application file (which contains either `gradio` or `streamlit` Python code, or `static` html code).
|
34 |
-
Path is relative to the root of the repository.
|
35 |
-
|
36 |
-
`models`: _List[string]_
|
37 |
-
HF model IDs (like "gpt2" or "deepset/roberta-base-squad2") used in the Space.
|
38 |
-
Will be parsed automatically from your code if not specified here.
|
39 |
-
|
40 |
-
`datasets`: _List[string]_
|
41 |
-
HF dataset IDs (like "common_voice" or "oscar-corpus/OSCAR-2109") used in the Space.
|
42 |
-
Will be parsed automatically from your code if not specified here.
|
43 |
-
|
44 |
-
`pinned`: _boolean_
|
45 |
-
Whether the Space stays on top of your list.
|
|
|
|
|
|
|
|
|
|
|
|
|
|
|
|
|
|
|
|
|
|
|
|
|
|
|
|
|
|
|
|
|
|
|
|
|
|
|
|
|
|
|
|
|
|
|
|
|
|
|
|
|
|
|
|
|
|
|
|
|
|
|
|
|
|
|
|
|
|
|
|
|
|
|
|
|
|
|
|
|
|
|
|
|
|
|
|
|
|
|
|
spaces/7hao/bingo/tests/kblob.ts
DELETED
@@ -1,27 +0,0 @@
|
|
1 |
-
import FormData from 'form-data'
|
2 |
-
|
3 |
-
import { fetch } from '@/lib/isomorphic'
|
4 |
-
|
5 |
-
const formData = new FormData()
|
6 |
-
|
7 |
-
const knowledgeRequest = {"imageInfo":{"url":"https://www.baidu.com/img/PCfb_5bf082d29588c07f842ccde3f97243ea.png"},"knowledgeRequest":{"invokedSkills":["ImageById"],"subscriptionId":"Bing.Chat.Multimodal","invokedSkillsRequestData":{"enableFaceBlur":true},"convoData":{"convoid":"51D|BingProdUnAuthenticatedUsers|E3DCA904FF236C67C3450163BCEC64CFF3F618CC8A4AFD75FD518F5ED0ADA080","convotone":"Creative"}}}
|
8 |
-
|
9 |
-
formData.append('knowledgeRequest', JSON.stringify(knowledgeRequest))
|
10 |
-
|
11 |
-
|
12 |
-
fetch('https://bing.vcanbb.top/images/kblob',
|
13 |
-
{
|
14 |
-
method: 'POST',
|
15 |
-
body: formData.getBuffer(),
|
16 |
-
headers: {
|
17 |
-
"sec-ch-ua": "\"Not/A)Brand\";v=\"99\", \"Google Chrome\";v=\"115\", \"Chromium\";v=\"115\"",
|
18 |
-
"sec-ch-ua-mobile": "?0",
|
19 |
-
"sec-ch-ua-platform": "\"Windows\"",
|
20 |
-
"Referer": "https://bing.vcanbb.top/web/index.html",
|
21 |
-
"Referrer-Policy": "origin-when-cross-origin",
|
22 |
-
...formData.getHeaders()
|
23 |
-
}
|
24 |
-
|
25 |
-
}
|
26 |
-
).then(res => res.text())
|
27 |
-
.then(res => console.log('res', res))
|
|
|
|
|
|
|
|
|
|
|
|
|
|
|
|
|
|
|
|
|
|
|
|
|
|
|
|
|
|
|
|
|
|
|
|
|
|
|
|
|
|
|
|
|
|
|
|
|
|
|
|
|
|
|
|
spaces/AIGC-Audio/AudioGPT/text_to_speech/modules/vocoder/parallel_wavegan/layers/pqmf.py
DELETED
@@ -1,132 +0,0 @@
|
|
1 |
-
# -*- coding: utf-8 -*-
|
2 |
-
|
3 |
-
# Copyright 2020 Tomoki Hayashi
|
4 |
-
# MIT License (https://opensource.org/licenses/MIT)
|
5 |
-
|
6 |
-
"""Pseudo QMF modules."""
|
7 |
-
|
8 |
-
import numpy as np
|
9 |
-
import torch
|
10 |
-
import torch.nn.functional as F
|
11 |
-
|
12 |
-
from scipy.signal import kaiser
|
13 |
-
|
14 |
-
|
15 |
-
def design_prototype_filter(taps=62, cutoff_ratio=0.15, beta=9.0):
|
16 |
-
"""Design prototype filter for PQMF.
|
17 |
-
|
18 |
-
This method is based on `A Kaiser window approach for the design of prototype
|
19 |
-
filters of cosine modulated filterbanks`_.
|
20 |
-
|
21 |
-
Args:
|
22 |
-
taps (int): The number of filter taps.
|
23 |
-
cutoff_ratio (float): Cut-off frequency ratio.
|
24 |
-
beta (float): Beta coefficient for kaiser window.
|
25 |
-
|
26 |
-
Returns:
|
27 |
-
ndarray: Impluse response of prototype filter (taps + 1,).
|
28 |
-
|
29 |
-
.. _`A Kaiser window approach for the design of prototype filters of cosine modulated filterbanks`:
|
30 |
-
https://ieeexplore.ieee.org/abstract/document/681427
|
31 |
-
|
32 |
-
"""
|
33 |
-
# check the arguments are valid
|
34 |
-
assert taps % 2 == 0, "The number of taps mush be even number."
|
35 |
-
assert 0.0 < cutoff_ratio < 1.0, "Cutoff ratio must be > 0.0 and < 1.0."
|
36 |
-
|
37 |
-
# make initial filter
|
38 |
-
omega_c = np.pi * cutoff_ratio
|
39 |
-
with np.errstate(invalid='ignore'):
|
40 |
-
h_i = np.sin(omega_c * (np.arange(taps + 1) - 0.5 * taps)) \
|
41 |
-
/ (np.pi * (np.arange(taps + 1) - 0.5 * taps))
|
42 |
-
h_i[taps // 2] = np.cos(0) * cutoff_ratio # fix nan due to indeterminate form
|
43 |
-
|
44 |
-
# apply kaiser window
|
45 |
-
w = kaiser(taps + 1, beta)
|
46 |
-
h = h_i * w
|
47 |
-
|
48 |
-
return h
|
49 |
-
|
50 |
-
|
51 |
-
class PQMF(torch.nn.Module):
|
52 |
-
"""PQMF module.
|
53 |
-
|
54 |
-
This module is based on `Near-perfect-reconstruction pseudo-QMF banks`_.
|
55 |
-
|
56 |
-
.. _`Near-perfect-reconstruction pseudo-QMF banks`:
|
57 |
-
https://ieeexplore.ieee.org/document/258122
|
58 |
-
|
59 |
-
"""
|
60 |
-
|
61 |
-
def __init__(self, subbands=4, taps=62, cutoff_ratio=0.15, beta=9.0):
|
62 |
-
"""Initilize PQMF module.
|
63 |
-
|
64 |
-
Args:
|
65 |
-
subbands (int): The number of subbands.
|
66 |
-
taps (int): The number of filter taps.
|
67 |
-
cutoff_ratio (float): Cut-off frequency ratio.
|
68 |
-
beta (float): Beta coefficient for kaiser window.
|
69 |
-
|
70 |
-
"""
|
71 |
-
super(PQMF, self).__init__()
|
72 |
-
|
73 |
-
# define filter coefficient
|
74 |
-
h_proto = design_prototype_filter(taps, cutoff_ratio, beta)
|
75 |
-
h_analysis = np.zeros((subbands, len(h_proto)))
|
76 |
-
h_synthesis = np.zeros((subbands, len(h_proto)))
|
77 |
-
for k in range(subbands):
|
78 |
-
h_analysis[k] = 2 * h_proto * np.cos(
|
79 |
-
(2 * k + 1) * (np.pi / (2 * subbands)) *
|
80 |
-
(np.arange(taps + 1) - ((taps - 1) / 2)) +
|
81 |
-
(-1) ** k * np.pi / 4)
|
82 |
-
h_synthesis[k] = 2 * h_proto * np.cos(
|
83 |
-
(2 * k + 1) * (np.pi / (2 * subbands)) *
|
84 |
-
(np.arange(taps + 1) - ((taps - 1) / 2)) -
|
85 |
-
(-1) ** k * np.pi / 4)
|
86 |
-
|
87 |
-
# convert to tensor
|
88 |
-
analysis_filter = torch.from_numpy(h_analysis).float().unsqueeze(1)
|
89 |
-
synthesis_filter = torch.from_numpy(h_synthesis).float().unsqueeze(0)
|
90 |
-
|
91 |
-
# register coefficients as beffer
|
92 |
-
self.register_buffer("analysis_filter", analysis_filter)
|
93 |
-
self.register_buffer("synthesis_filter", synthesis_filter)
|
94 |
-
|
95 |
-
# filter for downsampling & upsampling
|
96 |
-
updown_filter = torch.zeros((subbands, subbands, subbands)).float()
|
97 |
-
for k in range(subbands):
|
98 |
-
updown_filter[k, k, 0] = 1.0
|
99 |
-
self.register_buffer("updown_filter", updown_filter)
|
100 |
-
self.subbands = subbands
|
101 |
-
|
102 |
-
# keep padding info
|
103 |
-
self.pad_fn = torch.nn.ConstantPad1d(taps // 2, 0.0)
|
104 |
-
|
105 |
-
def analysis(self, x):
|
106 |
-
"""Analysis with PQMF.
|
107 |
-
|
108 |
-
Args:
|
109 |
-
x (Tensor): Input tensor (B, 1, T).
|
110 |
-
|
111 |
-
Returns:
|
112 |
-
Tensor: Output tensor (B, subbands, T // subbands).
|
113 |
-
|
114 |
-
"""
|
115 |
-
x = F.conv1d(self.pad_fn(x), self.analysis_filter)
|
116 |
-
return F.conv1d(x, self.updown_filter, stride=self.subbands)
|
117 |
-
|
118 |
-
def synthesis(self, x):
|
119 |
-
"""Synthesis with PQMF.
|
120 |
-
|
121 |
-
Args:
|
122 |
-
x (Tensor): Input tensor (B, subbands, T // subbands).
|
123 |
-
|
124 |
-
Returns:
|
125 |
-
Tensor: Output tensor (B, 1, T).
|
126 |
-
|
127 |
-
"""
|
128 |
-
# NOTE(kan-bayashi): Power will be dreased so here multipy by # subbands.
|
129 |
-
# Not sure this is the correct way, it is better to check again.
|
130 |
-
# TODO(kan-bayashi): Understand the reconstruction procedure
|
131 |
-
x = F.conv_transpose1d(x, self.updown_filter * self.subbands, stride=self.subbands)
|
132 |
-
return F.conv1d(self.pad_fn(x), self.synthesis_filter)
|
|
|
|
|
|
|
|
|
|
|
|
|
|
|
|
|
|
|
|
|
|
|
|
|
|
|
|
|
|
|
|
|
|
|
|
|
|
|
|
|
|
|
|
|
|
|
|
|
|
|
|
|
|
|
|
|
|
|
|
|
|
|
|
|
|
|
|
|
|
|
|
|
|
|
|
|
|
|
|
|
|
|
|
|
|
|
|
|
|
|
|
|
|
|
|
|
|
|
|
|
|
|
|
|
|
|
|
|
|
|
|
|
|
|
|
|
|
|
|
|
|
|
|
|
|
|
|
|
|
|
|
|
|
|
|
|
|
|
|
|
|
|
|
|
|
|
|
|
|
|
|
|
|
|
|
|
|
|
|
|
|
|
|
|
|
|
|
|
|
|
|
|
|
|
|
|
|
|
|
|
|
|
|
|
|
|
|
|
|
|
|
|
|
|
|
|
|
|
|
|
|
|
|
|
|
|
|
|
|
|
|
|
|
|
|
|
|
|
|
|
|
|
|
|
|
|
|
|
|
|
|
|
|
|
|
|
|
|
|
|
|
|
|
|
|
|
|
|
|
|
|
|
|
|
|
|
|
|
|
|
|
|
|
|
|
spaces/AIGC-Audio/AudioGPT/text_to_speech/tasks/tts/diffspeech.py
DELETED
@@ -1,111 +0,0 @@
|
|
1 |
-
import torch
|
2 |
-
|
3 |
-
from text_to_speech.modules.tts.diffspeech.shallow_diffusion_tts import GaussianDiffusion
|
4 |
-
from tasks.tts.fs2_orig import FastSpeech2OrigTask
|
5 |
-
|
6 |
-
import utils
|
7 |
-
from text_to_speech.utils.commons.hparams import hparams
|
8 |
-
from text_to_speech.utils.commons.ckpt_utils import load_ckpt
|
9 |
-
from text_to_speech.utils.audio.pitch.utils import denorm_f0
|
10 |
-
|
11 |
-
|
12 |
-
class DiffSpeechTask(FastSpeech2OrigTask):
|
13 |
-
def build_tts_model(self):
|
14 |
-
# get min and max
|
15 |
-
# import torch
|
16 |
-
# from tqdm import tqdm
|
17 |
-
# v_min = torch.ones([80]) * 100
|
18 |
-
# v_max = torch.ones([80]) * -100
|
19 |
-
# for i, ds in enumerate(tqdm(self.dataset_cls('train'))):
|
20 |
-
# v_max = torch.max(torch.max(ds['mel'].reshape(-1, 80), 0)[0], v_max)
|
21 |
-
# v_min = torch.min(torch.min(ds['mel'].reshape(-1, 80), 0)[0], v_min)
|
22 |
-
# if i % 100 == 0:
|
23 |
-
# print(i, v_min, v_max)
|
24 |
-
# print('final', v_min, v_max)
|
25 |
-
dict_size = len(self.token_encoder)
|
26 |
-
self.model = GaussianDiffusion(dict_size, hparams)
|
27 |
-
if hparams['fs2_ckpt'] != '':
|
28 |
-
load_ckpt(self.model.fs2, hparams['fs2_ckpt'], 'model', strict=True)
|
29 |
-
# for k, v in self.model.fs2.named_parameters():
|
30 |
-
# if 'predictor' not in k:
|
31 |
-
# v.requires_grad = False
|
32 |
-
# or
|
33 |
-
for k, v in self.model.fs2.named_parameters():
|
34 |
-
v.requires_grad = False
|
35 |
-
|
36 |
-
def build_optimizer(self, model):
|
37 |
-
self.optimizer = optimizer = torch.optim.AdamW(
|
38 |
-
filter(lambda p: p.requires_grad, model.parameters()),
|
39 |
-
lr=hparams['lr'],
|
40 |
-
betas=(hparams['optimizer_adam_beta1'], hparams['optimizer_adam_beta2']),
|
41 |
-
weight_decay=hparams['weight_decay'])
|
42 |
-
return optimizer
|
43 |
-
|
44 |
-
def build_scheduler(self, optimizer):
|
45 |
-
return torch.optim.lr_scheduler.StepLR(optimizer, hparams['decay_steps'], gamma=0.5)
|
46 |
-
|
47 |
-
def run_model(self, sample, infer=False, *args, **kwargs):
|
48 |
-
txt_tokens = sample['txt_tokens'] # [B, T_t]
|
49 |
-
spk_embed = sample.get('spk_embed')
|
50 |
-
spk_id = sample.get('spk_ids')
|
51 |
-
if not infer:
|
52 |
-
target = sample['mels'] # [B, T_s, 80]
|
53 |
-
mel2ph = sample['mel2ph'] # [B, T_s]
|
54 |
-
f0 = sample.get('f0')
|
55 |
-
uv = sample.get('uv')
|
56 |
-
output = self.model(txt_tokens, mel2ph=mel2ph, spk_embed=spk_embed, spk_id=spk_id,
|
57 |
-
ref_mels=target, f0=f0, uv=uv, infer=False)
|
58 |
-
losses = {}
|
59 |
-
if 'diff_loss' in output:
|
60 |
-
losses['mel'] = output['diff_loss']
|
61 |
-
self.add_dur_loss(output['dur'], mel2ph, txt_tokens, losses=losses)
|
62 |
-
if hparams['use_pitch_embed']:
|
63 |
-
self.add_pitch_loss(output, sample, losses)
|
64 |
-
return losses, output
|
65 |
-
else:
|
66 |
-
use_gt_dur = kwargs.get('infer_use_gt_dur', hparams['use_gt_dur'])
|
67 |
-
use_gt_f0 = kwargs.get('infer_use_gt_f0', hparams['use_gt_f0'])
|
68 |
-
mel2ph, uv, f0 = None, None, None
|
69 |
-
if use_gt_dur:
|
70 |
-
mel2ph = sample['mel2ph']
|
71 |
-
if use_gt_f0:
|
72 |
-
f0 = sample['f0']
|
73 |
-
uv = sample['uv']
|
74 |
-
output = self.model(txt_tokens, mel2ph=mel2ph, spk_embed=spk_embed, spk_id=spk_id,
|
75 |
-
ref_mels=None, f0=f0, uv=uv, infer=True)
|
76 |
-
return output
|
77 |
-
|
78 |
-
def save_valid_result(self, sample, batch_idx, model_out):
|
79 |
-
sr = hparams['audio_sample_rate']
|
80 |
-
f0_gt = None
|
81 |
-
# mel_out = model_out['mel_out']
|
82 |
-
if sample.get('f0') is not None:
|
83 |
-
f0_gt = denorm_f0(sample['f0'][0].cpu(), sample['uv'][0].cpu())
|
84 |
-
# self.plot_mel(batch_idx, sample['mels'], mel_out, f0s=f0_gt)
|
85 |
-
if self.global_step > 0:
|
86 |
-
# wav_pred = self.vocoder.spec2wav(mel_out[0].cpu(), f0=f0_gt)
|
87 |
-
# self.logger.add_audio(f'wav_val_{batch_idx}', wav_pred, self.global_step, sr)
|
88 |
-
# with gt duration
|
89 |
-
model_out = self.run_model(sample, infer=True, infer_use_gt_dur=True)
|
90 |
-
dur_info = self.get_plot_dur_info(sample, model_out)
|
91 |
-
del dur_info['dur_pred']
|
92 |
-
wav_pred = self.vocoder.spec2wav(model_out['mel_out'][0].cpu(), f0=f0_gt)
|
93 |
-
self.logger.add_audio(f'wav_gdur_{batch_idx}', wav_pred, self.global_step, sr)
|
94 |
-
self.plot_mel(batch_idx, sample['mels'], model_out['mel_out'][0], f'diffmel_gdur_{batch_idx}',
|
95 |
-
dur_info=dur_info, f0s=f0_gt)
|
96 |
-
self.plot_mel(batch_idx, sample['mels'], model_out['fs2_mel'][0], f'fs2mel_gdur_{batch_idx}',
|
97 |
-
dur_info=dur_info, f0s=f0_gt) # gt mel vs. fs2 mel
|
98 |
-
|
99 |
-
# with pred duration
|
100 |
-
if not hparams['use_gt_dur']:
|
101 |
-
model_out = self.run_model(sample, infer=True, infer_use_gt_dur=False)
|
102 |
-
dur_info = self.get_plot_dur_info(sample, model_out)
|
103 |
-
self.plot_mel(batch_idx, sample['mels'], model_out['mel_out'][0], f'mel_pdur_{batch_idx}',
|
104 |
-
dur_info=dur_info, f0s=f0_gt)
|
105 |
-
wav_pred = self.vocoder.spec2wav(model_out['mel_out'][0].cpu(), f0=f0_gt)
|
106 |
-
self.logger.add_audio(f'wav_pdur_{batch_idx}', wav_pred, self.global_step, sr)
|
107 |
-
# gt wav
|
108 |
-
if self.global_step <= hparams['valid_infer_interval']:
|
109 |
-
mel_gt = sample['mels'][0].cpu()
|
110 |
-
wav_gt = self.vocoder.spec2wav(mel_gt, f0=f0_gt)
|
111 |
-
self.logger.add_audio(f'wav_gt_{batch_idx}', wav_gt, self.global_step, sr)
|
|
|
|
|
|
|
|
|
|
|
|
|
|
|
|
|
|
|
|
|
|
|
|
|
|
|
|
|
|
|
|
|
|
|
|
|
|
|
|
|
|
|
|
|
|
|
|
|
|
|
|
|
|
|
|
|
|
|
|
|
|
|
|
|
|
|
|
|
|
|
|
|
|
|
|
|
|
|
|
|
|
|
|
|
|
|
|
|
|
|
|
|
|
|
|
|
|
|
|
|
|
|
|
|
|
|
|
|
|
|
|
|
|
|
|
|
|
|
|
|
|
|
|
|
|
|
|
|
|
|
|
|
|
|
|
|
|
|
|
|
|
|
|
|
|
|
|
|
|
|
|
|
|
|
|
|
|
|
|
|
|
|
|
|
|
|
|
|
|
|
|
|
|
|
|
|
|
|
|
|
|
|
|
|
|
|
|
|
|
|
|
|
|
|
|
|
|
|
|
|
|
|
|
|
|
|
|
|
|
|
|
|
|
|
|
|
|
|
|
|
|
|
|
spaces/AQaTaHaGoD/GoD/confing.py
DELETED
@@ -1,7 +0,0 @@
|
|
1 |
-
class token:
|
2 |
-
|
3 |
-
auth = "iyqmgydbhmsovgboojncfdpadzdmlmum"
|
4 |
-
# شناسه اکانت ربات را وارد کنید !
|
5 |
-
|
6 |
-
post_link = "https://rubika.ir/The_EnD_TaHa/DIEFFHBEDIGFJHA"
|
7 |
-
# لینک پست کانال را وارد کنید !
|
|
|
|
|
|
|
|
|
|
|
|
|
|
|
|
spaces/Abhilashvj/planogram-compliance/utils/loggers/wandb/README.md
DELETED
@@ -1,162 +0,0 @@
|
|
1 |
-
📚 This guide explains how to use **Weights & Biases** (W&B) with YOLOv5 🚀. UPDATED 29 September 2021.
|
2 |
-
|
3 |
-
- [About Weights & Biases](#about-weights-&-biases)
|
4 |
-
- [First-Time Setup](#first-time-setup)
|
5 |
-
- [Viewing runs](#viewing-runs)
|
6 |
-
- [Disabling wandb](#disabling-wandb)
|
7 |
-
- [Advanced Usage: Dataset Versioning and Evaluation](#advanced-usage)
|
8 |
-
- [Reports: Share your work with the world!](#reports)
|
9 |
-
|
10 |
-
## About Weights & Biases
|
11 |
-
|
12 |
-
Think of [W&B](https://wandb.ai/site?utm_campaign=repo_yolo_wandbtutorial) like GitHub for machine learning models. With a few lines of code, save everything you need to debug, compare and reproduce your models — architecture, hyperparameters, git commits, model weights, GPU usage, and even datasets and predictions.
|
13 |
-
|
14 |
-
Used by top researchers including teams at OpenAI, Lyft, Github, and MILA, W&B is part of the new standard of best practices for machine learning. How W&B can help you optimize your machine learning workflows:
|
15 |
-
|
16 |
-
- [Debug](https://wandb.ai/wandb/getting-started/reports/Visualize-Debug-Machine-Learning-Models--VmlldzoyNzY5MDk#Free-2) model performance in real time
|
17 |
-
- [GPU usage](https://wandb.ai/wandb/getting-started/reports/Visualize-Debug-Machine-Learning-Models--VmlldzoyNzY5MDk#System-4) visualized automatically
|
18 |
-
- [Custom charts](https://wandb.ai/wandb/customizable-charts/reports/Powerful-Custom-Charts-To-Debug-Model-Peformance--VmlldzoyNzY4ODI) for powerful, extensible visualization
|
19 |
-
- [Share insights](https://wandb.ai/wandb/getting-started/reports/Visualize-Debug-Machine-Learning-Models--VmlldzoyNzY5MDk#Share-8) interactively with collaborators
|
20 |
-
- [Optimize hyperparameters](https://docs.wandb.com/sweeps) efficiently
|
21 |
-
- [Track](https://docs.wandb.com/artifacts) datasets, pipelines, and production models
|
22 |
-
|
23 |
-
## First-Time Setup
|
24 |
-
|
25 |
-
<details open>
|
26 |
-
<summary> Toggle Details </summary>
|
27 |
-
When you first train, W&B will prompt you to create a new account and will generate an **API key** for you. If you are an existing user you can retrieve your key from https://wandb.ai/authorize. This key is used to tell W&B where to log your data. You only need to supply your key once, and then it is remembered on the same device.
|
28 |
-
|
29 |
-
W&B will create a cloud **project** (default is 'YOLOv5') for your training runs, and each new training run will be provided a unique run **name** within that project as project/name. You can also manually set your project and run name as:
|
30 |
-
|
31 |
-
```shell
|
32 |
-
$ python train.py --project ... --name ...
|
33 |
-
```
|
34 |
-
|
35 |
-
YOLOv5 notebook example: <a href="https://colab.research.google.com/github/ultralytics/yolov5/blob/master/tutorial.ipynb"><img src="https://colab.research.google.com/assets/colab-badge.svg" alt="Open In Colab"></a> <a href="https://www.kaggle.com/ultralytics/yolov5"><img src="https://kaggle.com/static/images/open-in-kaggle.svg" alt="Open In Kaggle"></a>
|
36 |
-
<img width="960" alt="Screen Shot 2021-09-29 at 10 23 13 PM" src="https://user-images.githubusercontent.com/26833433/135392431-1ab7920a-c49d-450a-b0b0-0c86ec86100e.png">
|
37 |
-
|
38 |
-
</details>
|
39 |
-
|
40 |
-
## Viewing Runs
|
41 |
-
|
42 |
-
<details open>
|
43 |
-
<summary> Toggle Details </summary>
|
44 |
-
Run information streams from your environment to the W&B cloud console as you train. This allows you to monitor and even cancel runs in <b>realtime</b> . All important information is logged:
|
45 |
-
|
46 |
-
- Training & Validation losses
|
47 |
-
- Metrics: Precision, Recall, [email protected], [email protected]:0.95
|
48 |
-
- Learning Rate over time
|
49 |
-
- A bounding box debugging panel, showing the training progress over time
|
50 |
-
- GPU: Type, **GPU Utilization**, power, temperature, **CUDA memory usage**
|
51 |
-
- System: Disk I/0, CPU utilization, RAM memory usage
|
52 |
-
- Your trained model as W&B Artifact
|
53 |
-
- Environment: OS and Python types, Git repository and state, **training command**
|
54 |
-
|
55 |
-
<p align="center"><img width="900" alt="Weights & Biases dashboard" src="https://user-images.githubusercontent.com/26833433/135390767-c28b050f-8455-4004-adb0-3b730386e2b2.png"></p>
|
56 |
-
</details>
|
57 |
-
|
58 |
-
## Disabling wandb
|
59 |
-
|
60 |
-
- training after running `wandb disabled` inside that directory creates no wandb run
|
61 |
-
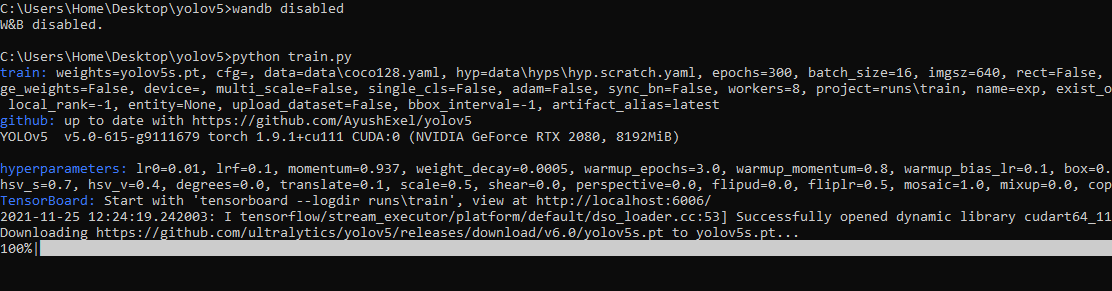
|
62 |
-
|
63 |
-
- To enable wandb again, run `wandb online`
|
64 |
-
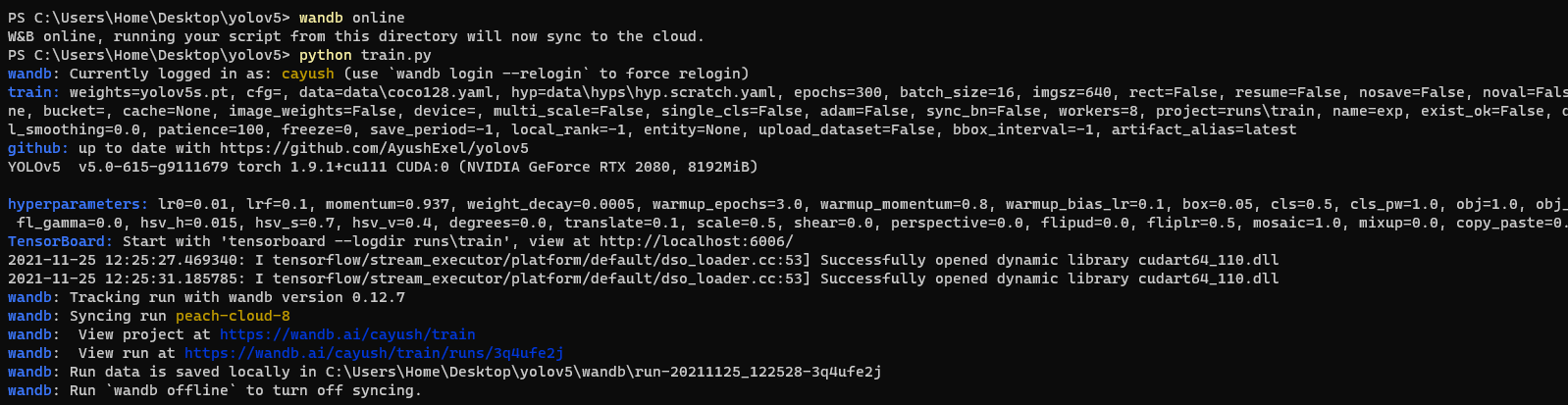
|
65 |
-
|
66 |
-
## Advanced Usage
|
67 |
-
|
68 |
-
You can leverage W&B artifacts and Tables integration to easily visualize and manage your datasets, models and training evaluations. Here are some quick examples to get you started.
|
69 |
-
|
70 |
-
<details open>
|
71 |
-
<h3> 1: Train and Log Evaluation simultaneousy </h3>
|
72 |
-
This is an extension of the previous section, but it'll also training after uploading the dataset. <b> This also evaluation Table</b>
|
73 |
-
Evaluation table compares your predictions and ground truths across the validation set for each epoch. It uses the references to the already uploaded datasets,
|
74 |
-
so no images will be uploaded from your system more than once.
|
75 |
-
<details open>
|
76 |
-
<summary> <b>Usage</b> </summary>
|
77 |
-
<b>Code</b> <code> $ python train.py --upload_data val</code>
|
78 |
-
|
79 |
-
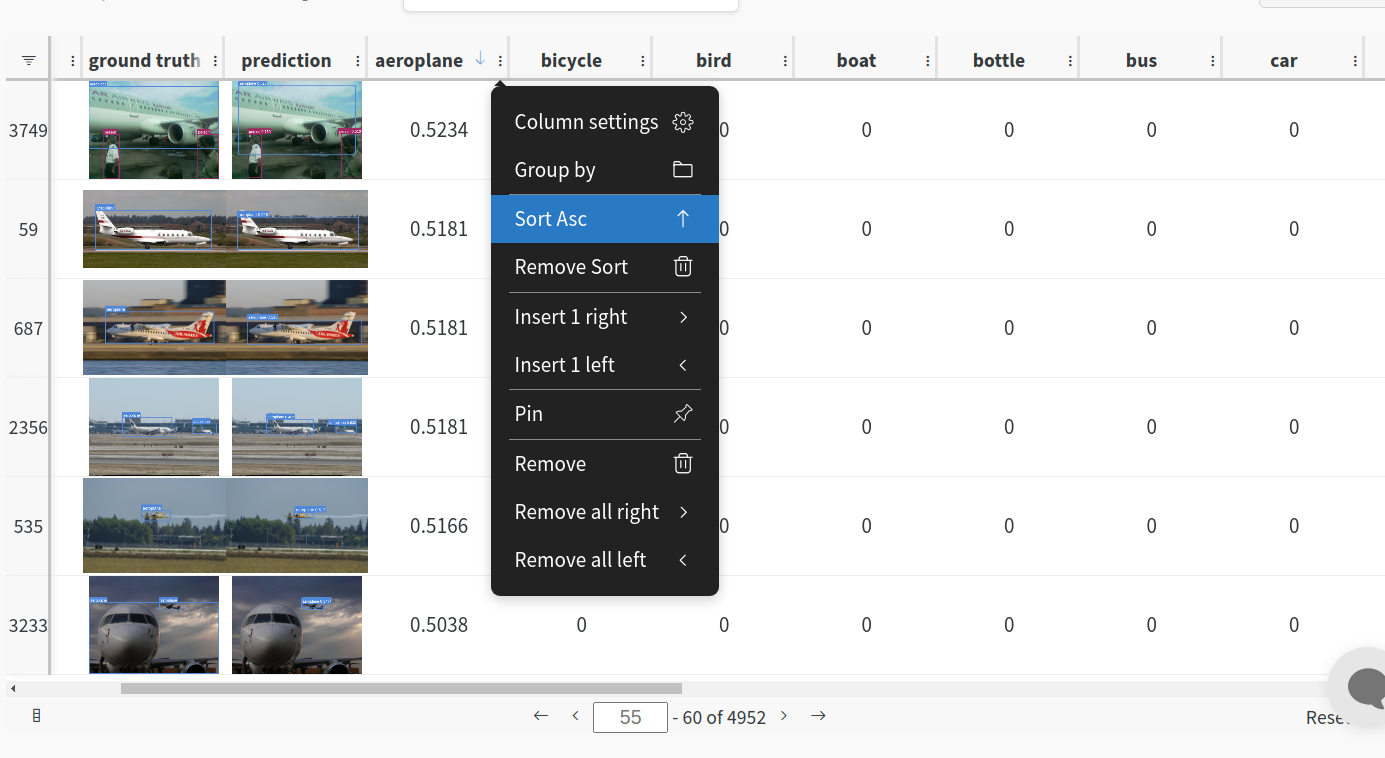
|
80 |
-
|
81 |
-
</details>
|
82 |
-
|
83 |
-
<h3>2. Visualize and Version Datasets</h3>
|
84 |
-
Log, visualize, dynamically query, and understand your data with <a href='https://docs.wandb.ai/guides/data-vis/tables'>W&B Tables</a>. You can use the following command to log your dataset as a W&B Table. This will generate a <code>{dataset}_wandb.yaml</code> file which can be used to train from dataset artifact.
|
85 |
-
<details>
|
86 |
-
<summary> <b>Usage</b> </summary>
|
87 |
-
<b>Code</b> <code> $ python utils/logger/wandb/log_dataset.py --project ... --name ... --data .. </code>
|
88 |
-
|
89 |
-
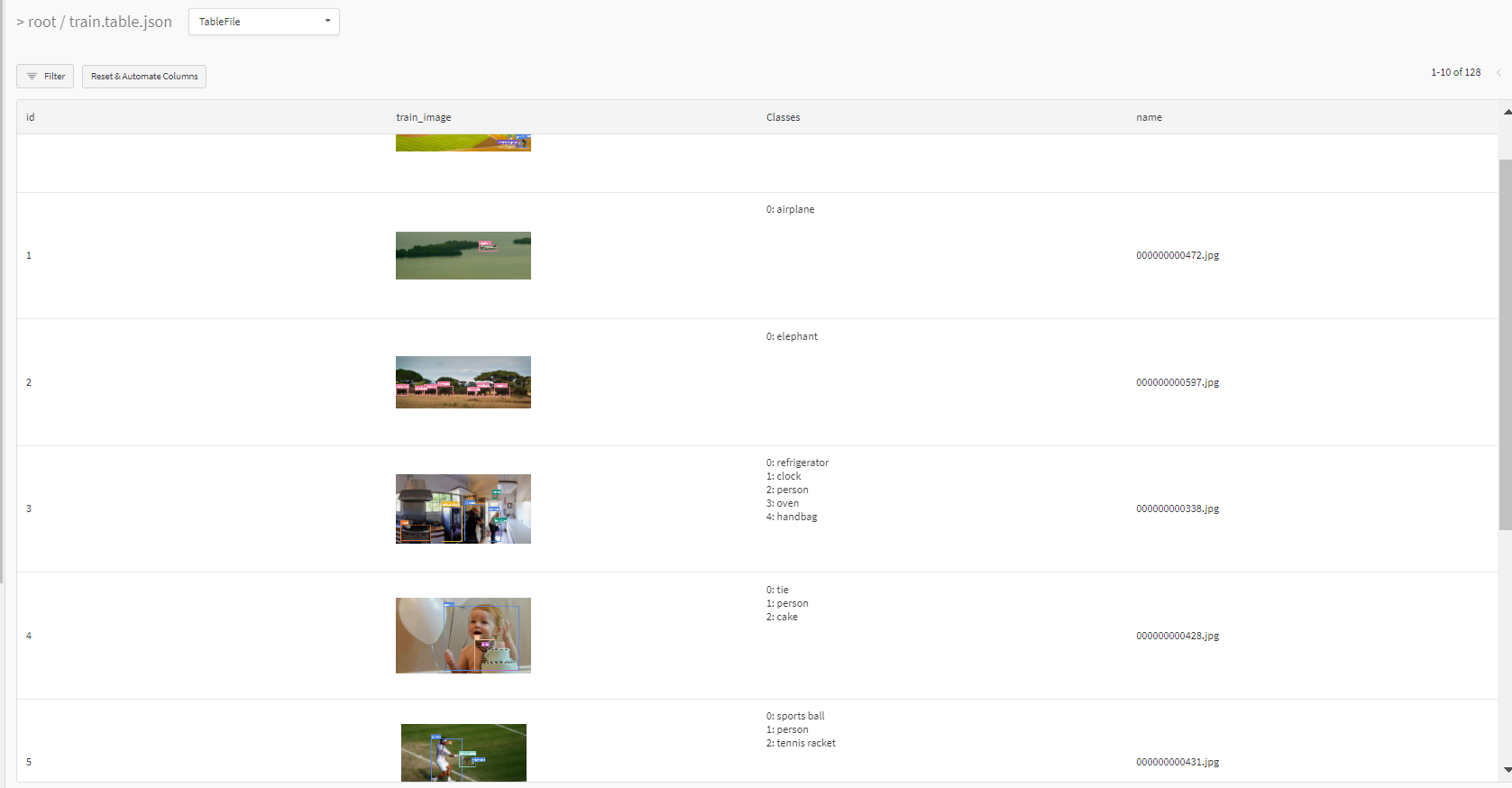
|
90 |
-
|
91 |
-
</details>
|
92 |
-
|
93 |
-
<h3> 3: Train using dataset artifact </h3>
|
94 |
-
When you upload a dataset as described in the first section, you get a new config file with an added `_wandb` to its name. This file contains the information that
|
95 |
-
can be used to train a model directly from the dataset artifact. <b> This also logs evaluation </b>
|
96 |
-
<details>
|
97 |
-
<summary> <b>Usage</b> </summary>
|
98 |
-
<b>Code</b> <code> $ python train.py --data {data}_wandb.yaml </code>
|
99 |
-
|
100 |
-
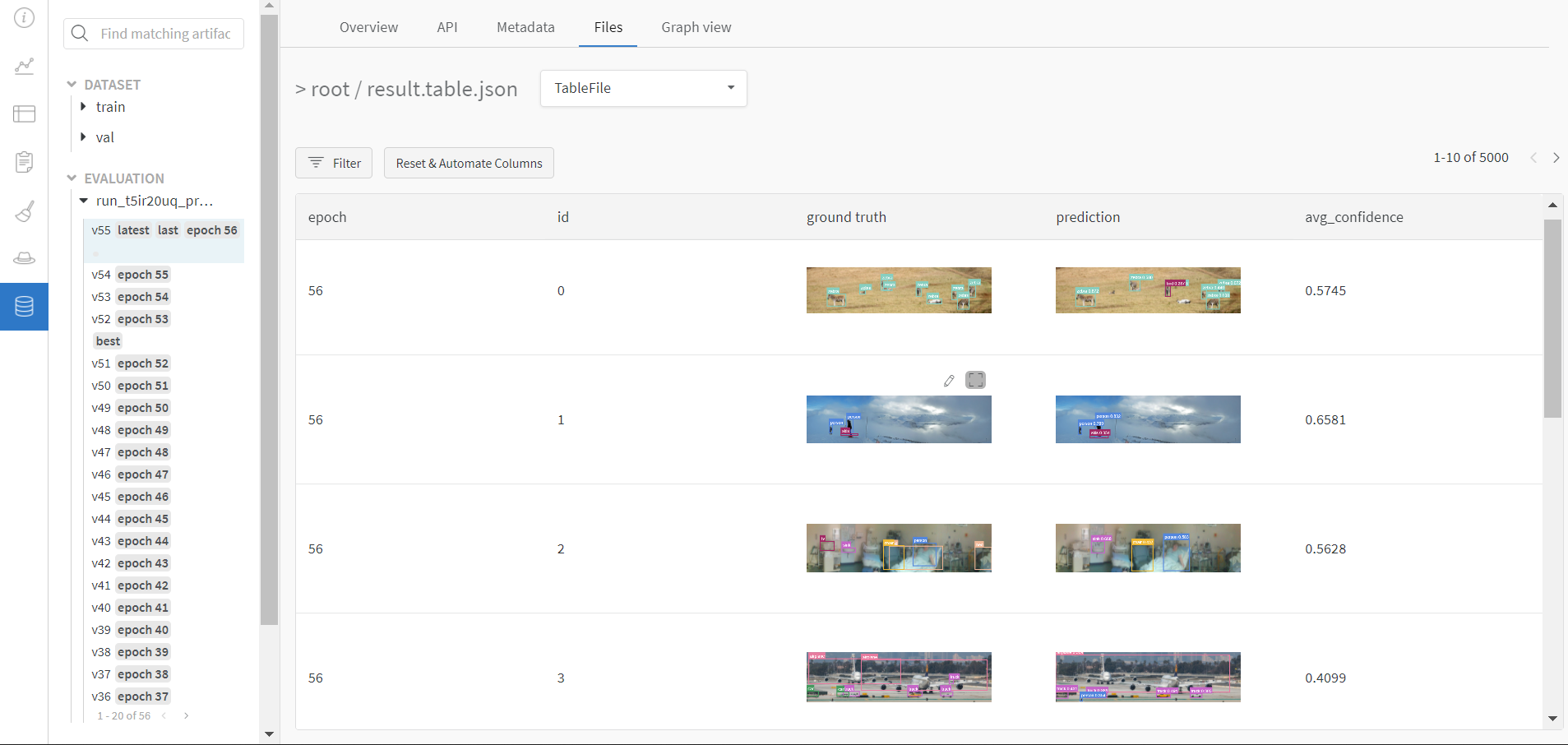
|
101 |
-
|
102 |
-
</details>
|
103 |
-
|
104 |
-
<h3> 4: Save model checkpoints as artifacts </h3>
|
105 |
-
To enable saving and versioning checkpoints of your experiment, pass `--save_period n` with the base cammand, where `n` represents checkpoint interval.
|
106 |
-
You can also log both the dataset and model checkpoints simultaneously. If not passed, only the final model will be logged
|
107 |
-
|
108 |
-
<details>
|
109 |
-
<summary> <b>Usage</b> </summary>
|
110 |
-
<b>Code</b> <code> $ python train.py --save_period 1 </code>
|
111 |
-
|
112 |
-
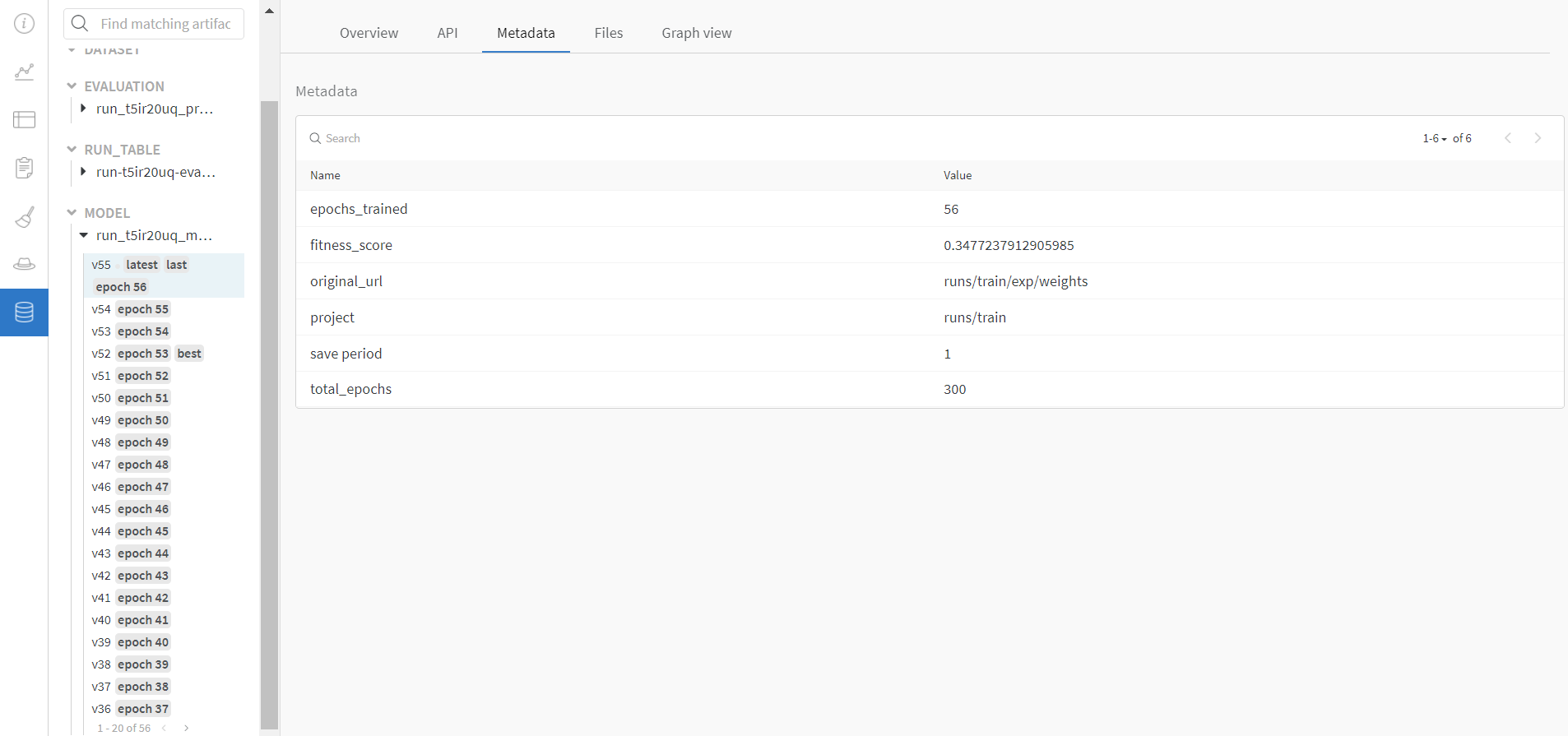
|
113 |
-
|
114 |
-
</details>
|
115 |
-
|
116 |
-
</details>
|
117 |
-
|
118 |
-
<h3> 5: Resume runs from checkpoint artifacts. </h3>
|
119 |
-
Any run can be resumed using artifacts if the <code>--resume</code> argument starts with <code>wandb-artifact://</code> prefix followed by the run path, i.e, <code>wandb-artifact://username/project/runid </code>. This doesn't require the model checkpoint to be present on the local system.
|
120 |
-
|
121 |
-
<details>
|
122 |
-
<summary> <b>Usage</b> </summary>
|
123 |
-
<b>Code</b> <code> $ python train.py --resume wandb-artifact://{run_path} </code>
|
124 |
-
|
125 |
-
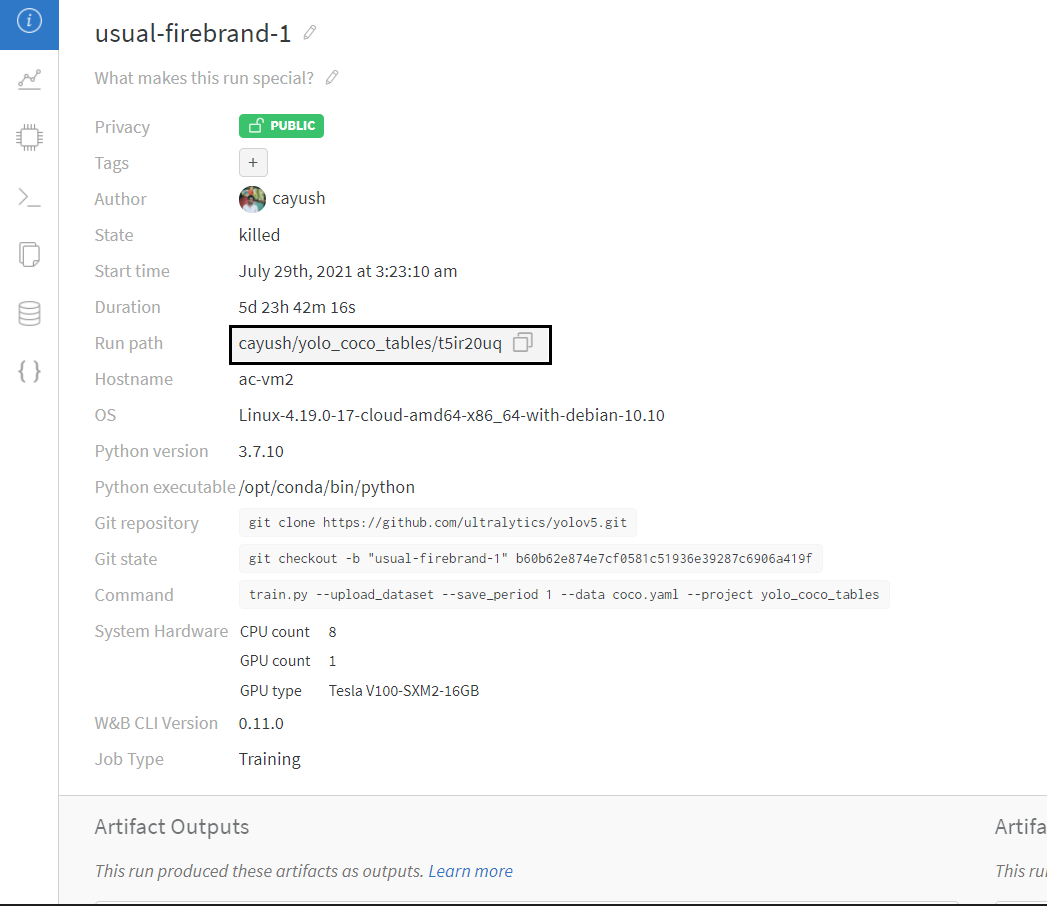
|
126 |
-
|
127 |
-
</details>
|
128 |
-
|
129 |
-
<h3> 6: Resume runs from dataset artifact & checkpoint artifacts. </h3>
|
130 |
-
<b> Local dataset or model checkpoints are not required. This can be used to resume runs directly on a different device </b>
|
131 |
-
The syntax is same as the previous section, but you'll need to lof both the dataset and model checkpoints as artifacts, i.e, set bot <code>--upload_dataset</code> or
|
132 |
-
train from <code>_wandb.yaml</code> file and set <code>--save_period</code>
|
133 |
-
|
134 |
-
<details>
|
135 |
-
<summary> <b>Usage</b> </summary>
|
136 |
-
<b>Code</b> <code> $ python train.py --resume wandb-artifact://{run_path} </code>
|
137 |
-
|
138 |
-
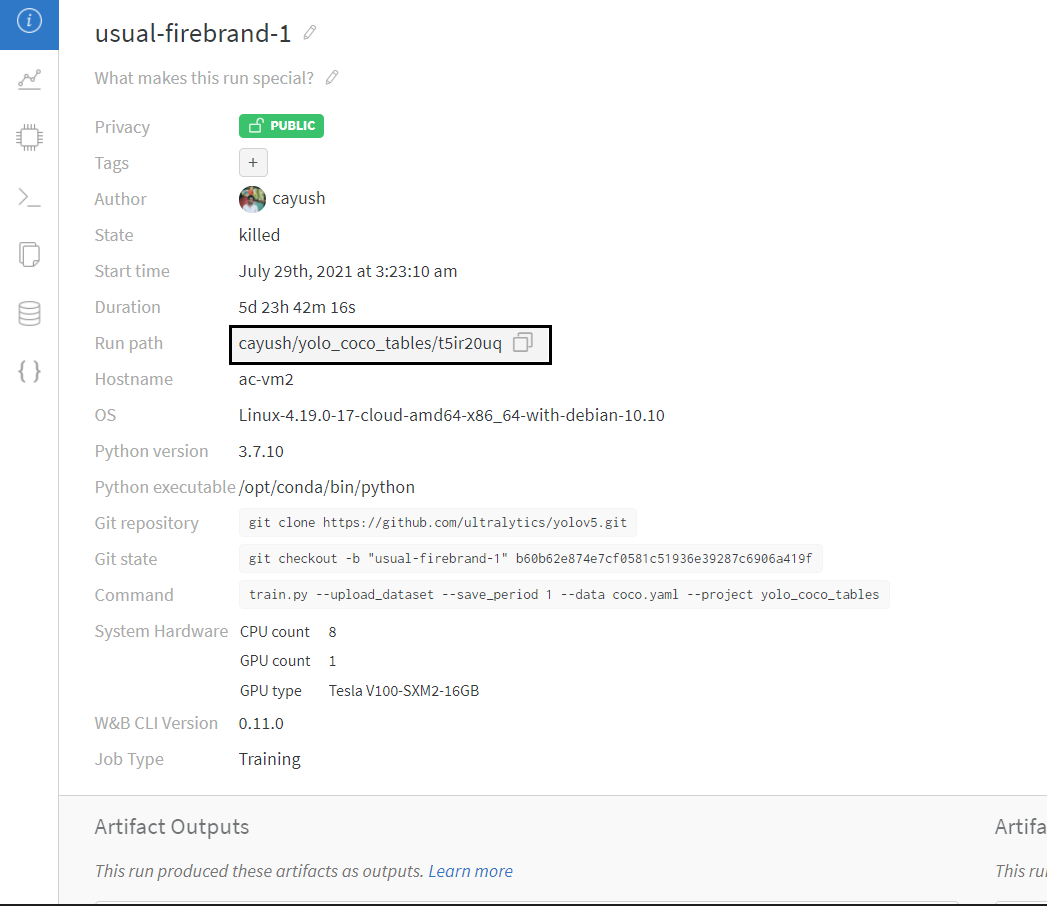
|
139 |
-
|
140 |
-
</details>
|
141 |
-
|
142 |
-
</details>
|
143 |
-
|
144 |
-
<h3> Reports </h3>
|
145 |
-
W&B Reports can be created from your saved runs for sharing online. Once a report is created you will receive a link you can use to publically share your results. Here is an example report created from the COCO128 tutorial trainings of all four YOLOv5 models ([link](https://wandb.ai/glenn-jocher/yolov5_tutorial/reports/YOLOv5-COCO128-Tutorial-Results--VmlldzozMDI5OTY)).
|
146 |
-
|
147 |
-
<img width="900" alt="Weights & Biases Reports" src="https://user-images.githubusercontent.com/26833433/135394029-a17eaf86-c6c1-4b1d-bb80-b90e83aaffa7.png">
|
148 |
-
|
149 |
-
## Environments
|
150 |
-
|
151 |
-
YOLOv5 may be run in any of the following up-to-date verified environments (with all dependencies including [CUDA](https://developer.nvidia.com/cuda)/[CUDNN](https://developer.nvidia.com/cudnn), [Python](https://www.python.org/) and [PyTorch](https://pytorch.org/) preinstalled):
|
152 |
-
|
153 |
-
- **Google Colab and Kaggle** notebooks with free GPU: <a href="https://colab.research.google.com/github/ultralytics/yolov5/blob/master/tutorial.ipynb"><img src="https://colab.research.google.com/assets/colab-badge.svg" alt="Open In Colab"></a> <a href="https://www.kaggle.com/ultralytics/yolov5"><img src="https://kaggle.com/static/images/open-in-kaggle.svg" alt="Open In Kaggle"></a>
|
154 |
-
- **Google Cloud** Deep Learning VM. See [GCP Quickstart Guide](https://github.com/ultralytics/yolov5/wiki/GCP-Quickstart)
|
155 |
-
- **Amazon** Deep Learning AMI. See [AWS Quickstart Guide](https://github.com/ultralytics/yolov5/wiki/AWS-Quickstart)
|
156 |
-
- **Docker Image**. See [Docker Quickstart Guide](https://github.com/ultralytics/yolov5/wiki/Docker-Quickstart) <a href="https://hub.docker.com/r/ultralytics/yolov5"><img src="https://img.shields.io/docker/pulls/ultralytics/yolov5?logo=docker" alt="Docker Pulls"></a>
|
157 |
-
|
158 |
-
## Status
|
159 |
-
|
160 |
-

|
161 |
-
|
162 |
-
If this badge is green, all [YOLOv5 GitHub Actions](https://github.com/ultralytics/yolov5/actions) Continuous Integration (CI) tests are currently passing. CI tests verify correct operation of YOLOv5 training ([train.py](https://github.com/ultralytics/yolov5/blob/master/train.py)), validation ([val.py](https://github.com/ultralytics/yolov5/blob/master/val.py)), inference ([detect.py](https://github.com/ultralytics/yolov5/blob/master/detect.py)) and export ([export.py](https://github.com/ultralytics/yolov5/blob/master/export.py)) on macOS, Windows, and Ubuntu every 24 hours and on every commit.
|
|
|
|
|
|
|
|
|
|
|
|
|
|
|
|
|
|
|
|
|
|
|
|
|
|
|
|
|
|
|
|
|
|
|
|
|
|
|
|
|
|
|
|
|
|
|
|
|
|
|
|
|
|
|
|
|
|
|
|
|
|
|
|
|
|
|
|
|
|
|
|
|
|
|
|
|
|
|
|
|
|
|
|
|
|
|
|
|
|
|
|
|
|
|
|
|
|
|
|
|
|
|
|
|
|
|
|
|
|
|
|
|
|
|
|
|
|
|
|
|
|
|
|
|
|
|
|
|
|
|
|
|
|
|
|
|
|
|
|
|
|
|
|
|
|
|
|
|
|
|
|
|
|
|
|
|
|
|
|
|
|
|
|
|
|
|
|
|
|
|
|
|
|
|
|
|
|
|
|
|
|
|
|
|
|
|
|
|
|
|
|
|
|
|
|
|
|
|
|
|
|
|
|
|
|
|
|
|
|
|
|
|
|
|
|
|
|
|
|
|
|
|
|
|
|
|
|
|
|
|
|
|
|
|
|
|
|
|
|
|
|
|
|
|
|
|
|
|
|
|
|
|
|
|
|
|
|
|
|
|
|
|
|
|
|
|
|
|
|
|
|
|
|
|
|
|
|
|
|
|
|
|
|
|
|
|
|
|
|
|
|
|
|
|
|
|
|
|
|
|
|
|
|
|
|
|
|
|
|
|
|
|
|
|
|
|
|
|
|
|
|
|
|
|
|
spaces/AchyuthGamer/OpenGPT/g4f/Provider/Providers/Weuseing.py
DELETED
@@ -1,29 +0,0 @@
|
|
1 |
-
import requests
|
2 |
-
import os
|
3 |
-
import json
|
4 |
-
from ...typing import sha256, Dict, get_type_hints
|
5 |
-
|
6 |
-
url = 'https://api.gptplus.one'
|
7 |
-
model = ['gpt-3.5-turbo', 'gpt-3.5-turbo-16k', 'gpt-3.5-turbo-16k-0613', 'gpt-3.5-turbo-0613']
|
8 |
-
supports_stream = True
|
9 |
-
needs_auth = False
|
10 |
-
|
11 |
-
def _create_completion(model: str, messages: list, stream: bool, temperature: float = 0.7, **kwargs):
|
12 |
-
headers = {
|
13 |
-
'Content-Type': 'application/json',
|
14 |
-
'Accept': '*/*',
|
15 |
-
'Accept-Language': 'ru-RU,ru;q=0.9,en-US;q=0.8,en;q=0.7,ja;q=0.6,zh-TW;q=0.5,zh;q=0.4',
|
16 |
-
}
|
17 |
-
data = {
|
18 |
-
'messages': messages,
|
19 |
-
'model': model,
|
20 |
-
}
|
21 |
-
response = requests.post('https://api.gptplus.one/chat-process', json=data, stream=True)
|
22 |
-
print(response)
|
23 |
-
|
24 |
-
for token in response.iter_content(chunk_size=None):
|
25 |
-
yield (token.decode('utf-8'))
|
26 |
-
|
27 |
-
|
28 |
-
params = f'g4f.Providers.{os.path.basename(__file__)[:-3]} supports: ' + \
|
29 |
-
'(%s)' % ', '.join([f"{name}: {get_type_hints(_create_completion)[name].__name__}" for name in _create_completion.__code__.co_varnames[:_create_completion.__code__.co_argcount]])
|
|
|
|
|
|
|
|
|
|
|
|
|
|
|
|
|
|
|
|
|
|
|
|
|
|
|
|
|
|
|
|
|
|
|
|
|
|
|
|
|
|
|
|
|
|
|
|
|
|
|
|
|
|
|
|
|
|
|
|
spaces/AchyuthGamer/text-to-speech-client/style.css
DELETED
@@ -1,28 +0,0 @@
|
|
1 |
-
body {
|
2 |
-
padding: 2rem;
|
3 |
-
font-family: -apple-system, BlinkMacSystemFont, "Arial", sans-serif;
|
4 |
-
}
|
5 |
-
|
6 |
-
h1 {
|
7 |
-
font-size: 16px;
|
8 |
-
margin-top: 0;
|
9 |
-
}
|
10 |
-
|
11 |
-
p {
|
12 |
-
color: rgb(107, 114, 128);
|
13 |
-
font-size: 15px;
|
14 |
-
margin-bottom: 10px;
|
15 |
-
margin-top: 5px;
|
16 |
-
}
|
17 |
-
|
18 |
-
.card {
|
19 |
-
max-width: 620px;
|
20 |
-
margin: 0 auto;
|
21 |
-
padding: 16px;
|
22 |
-
border: 1px solid lightgray;
|
23 |
-
border-radius: 16px;
|
24 |
-
}
|
25 |
-
|
26 |
-
.card p:last-child {
|
27 |
-
margin-bottom: 0;
|
28 |
-
}
|
|
|
|
|
|
|
|
|
|
|
|
|
|
|
|
|
|
|
|
|
|
|
|
|
|
|
|
|
|
|
|
|
|
|
|
|
|
|
|
|
|
|
|
|
|
|
|
|
|
|
|
|
|
|
|
|
|
spaces/AgentVerse/agentVerse/agentverse/agents/simulation_agent/tool.py
DELETED
@@ -1,177 +0,0 @@
|
|
1 |
-
import logging
|
2 |
-
from string import Template
|
3 |
-
from typing import List, NamedTuple, Optional, Union
|
4 |
-
|
5 |
-
from langchain.tools import BaseTool
|
6 |
-
from pydantic import Field
|
7 |
-
|
8 |
-
|
9 |
-
from agentverse.memory import BaseMemory, ChatHistoryMemory
|
10 |
-
from agentverse.message import Message
|
11 |
-
from agentverse.utils import AgentAction, AgentFinish
|
12 |
-
|
13 |
-
#from . import agent_registry
|
14 |
-
#from .base import BaseAgent
|
15 |
-
|
16 |
-
from agentverse.agents import agent_registry
|
17 |
-
from agentverse.agents.base import BaseAgent
|
18 |
-
|
19 |
-
class ToolNotExistError(BaseException):
|
20 |
-
"""Exception raised when parsing output from a command fails."""
|
21 |
-
|
22 |
-
def __init__(self, tool_name=""):
|
23 |
-
self.tool_name = tool_name
|
24 |
-
|
25 |
-
def __str__(self):
|
26 |
-
return f"Tool {self.tool_name} does not exist."
|
27 |
-
|
28 |
-
|
29 |
-
@agent_registry.register("tool")
|
30 |
-
class ToolAgent(BaseAgent):
|
31 |
-
tools: List[BaseTool] = Field(default=[])
|
32 |
-
tool_memory: BaseMemory = Field(default_factory=ChatHistoryMemory)
|
33 |
-
verbose: bool = Field(default=False)
|
34 |
-
|
35 |
-
def step(self, env_description: str = "") -> Message:
|
36 |
-
parsed_response = None
|
37 |
-
tool_observation = [self.tool_memory.to_string()]
|
38 |
-
while True:
|
39 |
-
prompt = self._fill_prompt_template(env_description, tool_observation)
|
40 |
-
|
41 |
-
for i in range(self.max_retry):
|
42 |
-
try:
|
43 |
-
response = self.llm.generate_response(prompt)
|
44 |
-
parsed_response = self.output_parser.parse(response)
|
45 |
-
if isinstance(parsed_response, AgentAction):
|
46 |
-
observation = self._call_tool(parsed_response)
|
47 |
-
tool_observation.append(
|
48 |
-
parsed_response.log.strip()
|
49 |
-
+ f"\nObservation: {observation.strip()}"
|
50 |
-
)
|
51 |
-
break
|
52 |
-
except BaseException as e:
|
53 |
-
logging.error(e)
|
54 |
-
logging.warning("Retrying...")
|
55 |
-
continue
|
56 |
-
if parsed_response is None or isinstance(parsed_response, AgentFinish):
|
57 |
-
break
|
58 |
-
|
59 |
-
if parsed_response is None:
|
60 |
-
logging.error(f"{self.name} failed to generate valid response.")
|
61 |
-
|
62 |
-
self._update_tool_memory(tool_observation)
|
63 |
-
|
64 |
-
message = Message(
|
65 |
-
content=""
|
66 |
-
if parsed_response is None
|
67 |
-
else parsed_response.return_values["output"],
|
68 |
-
sender=self.name,
|
69 |
-
receiver=self.get_receiver(),
|
70 |
-
)
|
71 |
-
return message
|
72 |
-
|
73 |
-
async def astep(self, env_description: str = "") -> Message:
|
74 |
-
"""Asynchronous version of step"""
|
75 |
-
parsed_response = None
|
76 |
-
# Initialize the tool_observation with tool_memory
|
77 |
-
tool_observation = [self.tool_memory.to_string()]
|
78 |
-
while True:
|
79 |
-
prompt = self._fill_prompt_template(env_description, tool_observation)
|
80 |
-
|
81 |
-
for i in range(self.max_retry):
|
82 |
-
try:
|
83 |
-
response = await self.llm.agenerate_response(prompt)
|
84 |
-
parsed_response = self.output_parser.parse(response)
|
85 |
-
if isinstance(parsed_response, AgentAction):
|
86 |
-
# If the response is an action, call the tool
|
87 |
-
# and append the observation to tool_observation
|
88 |
-
observation = await self._acall_tool(parsed_response)
|
89 |
-
tool_observation.append(
|
90 |
-
parsed_response.log.strip()
|
91 |
-
+ f"\nObservation: {observation.strip()}"
|
92 |
-
)
|
93 |
-
break
|
94 |
-
except BaseException as e:
|
95 |
-
logging.error(e)
|
96 |
-
logging.warning("Retrying...")
|
97 |
-
continue
|
98 |
-
if parsed_response is None or isinstance(parsed_response, AgentFinish):
|
99 |
-
break
|
100 |
-
|
101 |
-
if parsed_response is None:
|
102 |
-
logging.error(f"{self.name} failed to generate valid response.")
|
103 |
-
|
104 |
-
self._update_tool_memory(tool_observation)
|
105 |
-
|
106 |
-
message = Message(
|
107 |
-
content=""
|
108 |
-
if parsed_response is None
|
109 |
-
else parsed_response.return_values["output"],
|
110 |
-
sender=self.name,
|
111 |
-
receiver=self.get_receiver(),
|
112 |
-
)
|
113 |
-
return message
|
114 |
-
|
115 |
-
def _call_tool(self, response: NamedTuple) -> str:
|
116 |
-
"""Call a tool and return the output"""
|
117 |
-
name_to_tool = {tool.name: tool for tool in self.tools}
|
118 |
-
if response.tool not in name_to_tool:
|
119 |
-
raise ToolNotExistError(response.tool)
|
120 |
-
tool = name_to_tool[response.tool]
|
121 |
-
observation = tool.run(response.tool_input, verbose=self.verbose)
|
122 |
-
return observation
|
123 |
-
|
124 |
-
async def _acall_tool(self, response: NamedTuple) -> str:
|
125 |
-
"""Call a tool and return the output"""
|
126 |
-
name_to_tool = {tool.name: tool for tool in self.tools}
|
127 |
-
if response.tool not in name_to_tool:
|
128 |
-
raise ToolNotExistError(response.tool)
|
129 |
-
tool = name_to_tool[response.tool]
|
130 |
-
observation = await tool.arun(response.tool_input, verbose=self.verbose)
|
131 |
-
return observation
|
132 |
-
|
133 |
-
def _update_tool_memory(self, tool_observation: List[str]):
|
134 |
-
"""Update the memory of the tool"""
|
135 |
-
if len(tool_observation) == 1:
|
136 |
-
# If no tool is called this turn, do nothing
|
137 |
-
return
|
138 |
-
messages = [
|
139 |
-
Message(content=observation) for observation in tool_observation[1:]
|
140 |
-
]
|
141 |
-
self.tool_memory.add_message(messages)
|
142 |
-
|
143 |
-
def _fill_prompt_template(
|
144 |
-
self, env_description: str = "", tool_observation: List[str] = []
|
145 |
-
) -> str:
|
146 |
-
"""Fill the placeholders in the prompt template
|
147 |
-
|
148 |
-
In the tool agent, these placeholders are supported:
|
149 |
-
- ${agent_name}: the name of the agent
|
150 |
-
- ${env_description}: the description of the environment
|
151 |
-
- ${role_description}: the description of the role of the agent
|
152 |
-
- ${chat_history}: the chat history of the agent
|
153 |
-
- ${tools}: the list of tools and their usage
|
154 |
-
- ${tool_names}: the list of tool names
|
155 |
-
- ${tool_observations}: the observation of the tool in this turn
|
156 |
-
"""
|
157 |
-
tools = "\n".join([f"> {tool.name}: {tool.description}" for tool in self.tools])
|
158 |
-
tools = tools.replace("{{", "{").replace("}}", "}")
|
159 |
-
tool_names = ", ".join([tool.name for tool in self.tools])
|
160 |
-
input_arguments = {
|
161 |
-
"agent_name": self.name,
|
162 |
-
"env_description": env_description,
|
163 |
-
"role_description": self.role_description,
|
164 |
-
"chat_history": self.memory.to_string(add_sender_prefix=True),
|
165 |
-
"tools": tools,
|
166 |
-
"tool_names": tool_names,
|
167 |
-
"tool_observation": "\n".join(tool_observation),
|
168 |
-
}
|
169 |
-
return Template(self.prompt_template).safe_substitute(input_arguments)
|
170 |
-
|
171 |
-
def add_message_to_memory(self, messages: List[Message]) -> None:
|
172 |
-
self.memory.add_message(messages)
|
173 |
-
|
174 |
-
def reset(self) -> None:
|
175 |
-
"""Reset the agent"""
|
176 |
-
self.memory.reset()
|
177 |
-
# TODO: reset receiver
|
|
|
|
|
|
|
|
|
|
|
|
|
|
|
|
|
|
|
|
|
|
|
|
|
|
|
|
|
|
|
|
|
|
|
|
|
|
|
|
|
|
|
|
|
|
|
|
|
|
|
|
|
|
|
|
|
|
|
|
|
|
|
|
|
|
|
|
|
|
|
|
|
|
|
|
|
|
|
|
|
|
|
|
|
|
|
|
|
|
|
|
|
|
|
|
|
|
|
|
|
|
|
|
|
|
|
|
|
|
|
|
|
|
|
|
|
|
|
|
|
|
|
|
|
|
|
|
|
|
|
|
|
|
|
|
|
|
|
|
|
|
|
|
|
|
|
|
|
|
|
|
|
|
|
|
|
|
|
|
|
|
|
|
|
|
|
|
|
|
|
|
|
|
|
|
|
|
|
|
|
|
|
|
|
|
|
|
|
|
|
|
|
|
|
|
|
|
|
|
|
|
|
|
|
|
|
|
|
|
|
|
|
|
|
|
|
|
|
|
|
|
|
|
|
|
|
|
|
|
|
|
|
|
|
|
|
|
|
|
|
|
|
|
|
|
|
|
|
|
|
|
|
|
|
|
|
|
|
|
|
|
|
|
|
|
|
|
|
|
|
|
|
|
|
|
|
|
|
|
|
|
|
|
|
|
|
|
|
|
|
|
|
|
|
|
|
|
|
|
|
|
|
|
|
|
|
|
|
|
|
|
|
|
|
|
|
|
|
|
|
|
|
|
|
|
|
|
|
|
|
|
|
|
|
|
|
|
|
|
|
|
|
|
|
|
|
|
|
|
|
|
|
|
|
|
spaces/AgentVerse/agentVerse/agentverse/environments/tasksolving_env/rules/evaluator/base.py
DELETED
@@ -1,88 +0,0 @@
|
|
1 |
-
from __future__ import annotations
|
2 |
-
|
3 |
-
from abc import abstractmethod
|
4 |
-
from typing import TYPE_CHECKING, List, Tuple
|
5 |
-
|
6 |
-
from pydantic import BaseModel
|
7 |
-
|
8 |
-
from agentverse.message import EvaluatorMessage
|
9 |
-
|
10 |
-
if TYPE_CHECKING:
|
11 |
-
from agentverse.agents import EvaluatorAgent
|
12 |
-
from agentverse.message import EvaluatorMessage, SolverMessage, ExecutorMessage
|
13 |
-
|
14 |
-
from . import evaluator_registry
|
15 |
-
|
16 |
-
|
17 |
-
class BaseEvaluator(BaseModel):
|
18 |
-
"""
|
19 |
-
The base class of execution.
|
20 |
-
"""
|
21 |
-
|
22 |
-
@abstractmethod
|
23 |
-
def step(
|
24 |
-
self,
|
25 |
-
agent: EvaluatorAgent,
|
26 |
-
solution: List[SolverMessage],
|
27 |
-
result: List[ExecutorMessage],
|
28 |
-
task_description: str,
|
29 |
-
all_role_description: List[str],
|
30 |
-
*args,
|
31 |
-
**kwargs,
|
32 |
-
) -> EvaluatorMessage:
|
33 |
-
pass
|
34 |
-
|
35 |
-
def reset(self):
|
36 |
-
pass
|
37 |
-
|
38 |
-
|
39 |
-
@evaluator_registry.register("none")
|
40 |
-
class NoneEvaluator(BaseEvaluator):
|
41 |
-
def step(
|
42 |
-
self,
|
43 |
-
agent: EvaluatorAgent,
|
44 |
-
solution: List[SolverMessage],
|
45 |
-
result: List[ExecutorMessage],
|
46 |
-
task_description: str,
|
47 |
-
all_role_description: List[str],
|
48 |
-
*args,
|
49 |
-
**kwargs,
|
50 |
-
) -> EvaluatorMessage:
|
51 |
-
result = EvaluatorMessage(
|
52 |
-
score=0, advice="\n".join([r.content for r in result])
|
53 |
-
)
|
54 |
-
return result
|
55 |
-
|
56 |
-
|
57 |
-
@evaluator_registry.register("dummy")
|
58 |
-
class DummyEvaluator(BaseEvaluator):
|
59 |
-
def step(
|
60 |
-
self,
|
61 |
-
agent: EvaluatorAgent,
|
62 |
-
solution: List[SolverMessage],
|
63 |
-
result: List[ExecutorMessage],
|
64 |
-
task_description: str,
|
65 |
-
all_role_description: List[str],
|
66 |
-
*args,
|
67 |
-
**kwargs,
|
68 |
-
) -> EvaluatorMessage:
|
69 |
-
result = EvaluatorMessage(score=1, advice="")
|
70 |
-
return result
|
71 |
-
|
72 |
-
|
73 |
-
@evaluator_registry.register("dummy")
|
74 |
-
class DummyEvaluator(BaseEvaluator):
|
75 |
-
def step(
|
76 |
-
self,
|
77 |
-
agent: EvaluatorAgent,
|
78 |
-
solution: List[str] | str,
|
79 |
-
result: List[str] | str,
|
80 |
-
task_description: str,
|
81 |
-
all_role_description: List[str],
|
82 |
-
*args,
|
83 |
-
**kwargs,
|
84 |
-
) -> EvaluatorMessage:
|
85 |
-
result = EvaluatorMessage(
|
86 |
-
score=0, advice="\n".join([r.content for r in result])
|
87 |
-
)
|
88 |
-
return result
|
|
|
|
|
|
|
|
|
|
|
|
|
|
|
|
|
|
|
|
|
|
|
|
|
|
|
|
|
|
|
|
|
|
|
|
|
|
|
|
|
|
|
|
|
|
|
|
|
|
|
|
|
|
|
|
|
|
|
|
|
|
|
|
|
|
|
|
|
|
|
|
|
|
|
|
|
|
|
|
|
|
|
|
|
|
|
|
|
|
|
|
|
|
|
|
|
|
|
|
|
|
|
|
|
|
|
|
|
|
|
|
|
|
|
|
|
|
|
|
|
|
|
|
|
|
|
|
|
|
|
|
|
|
|
|
|
|
|
|
|
|
|
|
|
|
|
|
|
|
|
|
|
|
|
|
|
|
|
|
|
|
|
|
|
|
|
|
|
|
|
|
|
|
|
|
|
|
spaces/AgentVerse/agentVerse/ui/src/phaser3-rex-plugins/templates/ui/canvas/Factory.d.ts
DELETED
@@ -1,6 +0,0 @@
|
|
1 |
-
import Canvas from './Canvas';
|
2 |
-
|
3 |
-
export default function (
|
4 |
-
x?: number, y?: number,
|
5 |
-
width?: number, height?: number
|
6 |
-
): Canvas;
|
|
|
|
|
|
|
|
|
|
|
|
|
|
spaces/AlexWang/lama/saicinpainting/evaluation/losses/ssim.py
DELETED
@@ -1,74 +0,0 @@
|
|
1 |
-
import numpy as np
|
2 |
-
import torch
|
3 |
-
import torch.nn.functional as F
|
4 |
-
|
5 |
-
|
6 |
-
class SSIM(torch.nn.Module):
|
7 |
-
"""SSIM. Modified from:
|
8 |
-
https://github.com/Po-Hsun-Su/pytorch-ssim/blob/master/pytorch_ssim/__init__.py
|
9 |
-
"""
|
10 |
-
|
11 |
-
def __init__(self, window_size=11, size_average=True):
|
12 |
-
super().__init__()
|
13 |
-
self.window_size = window_size
|
14 |
-
self.size_average = size_average
|
15 |
-
self.channel = 1
|
16 |
-
self.register_buffer('window', self._create_window(window_size, self.channel))
|
17 |
-
|
18 |
-
def forward(self, img1, img2):
|
19 |
-
assert len(img1.shape) == 4
|
20 |
-
|
21 |
-
channel = img1.size()[1]
|
22 |
-
|
23 |
-
if channel == self.channel and self.window.data.type() == img1.data.type():
|
24 |
-
window = self.window
|
25 |
-
else:
|
26 |
-
window = self._create_window(self.window_size, channel)
|
27 |
-
|
28 |
-
# window = window.to(img1.get_device())
|
29 |
-
window = window.type_as(img1)
|
30 |
-
|
31 |
-
self.window = window
|
32 |
-
self.channel = channel
|
33 |
-
|
34 |
-
return self._ssim(img1, img2, window, self.window_size, channel, self.size_average)
|
35 |
-
|
36 |
-
def _gaussian(self, window_size, sigma):
|
37 |
-
gauss = torch.Tensor([
|
38 |
-
np.exp(-(x - (window_size // 2)) ** 2 / float(2 * sigma ** 2)) for x in range(window_size)
|
39 |
-
])
|
40 |
-
return gauss / gauss.sum()
|
41 |
-
|
42 |
-
def _create_window(self, window_size, channel):
|
43 |
-
_1D_window = self._gaussian(window_size, 1.5).unsqueeze(1)
|
44 |
-
_2D_window = _1D_window.mm(_1D_window.t()).float().unsqueeze(0).unsqueeze(0)
|
45 |
-
return _2D_window.expand(channel, 1, window_size, window_size).contiguous()
|
46 |
-
|
47 |
-
def _ssim(self, img1, img2, window, window_size, channel, size_average=True):
|
48 |
-
mu1 = F.conv2d(img1, window, padding=(window_size // 2), groups=channel)
|
49 |
-
mu2 = F.conv2d(img2, window, padding=(window_size // 2), groups=channel)
|
50 |
-
|
51 |
-
mu1_sq = mu1.pow(2)
|
52 |
-
mu2_sq = mu2.pow(2)
|
53 |
-
mu1_mu2 = mu1 * mu2
|
54 |
-
|
55 |
-
sigma1_sq = F.conv2d(
|
56 |
-
img1 * img1, window, padding=(window_size // 2), groups=channel) - mu1_sq
|
57 |
-
sigma2_sq = F.conv2d(
|
58 |
-
img2 * img2, window, padding=(window_size // 2), groups=channel) - mu2_sq
|
59 |
-
sigma12 = F.conv2d(
|
60 |
-
img1 * img2, window, padding=(window_size // 2), groups=channel) - mu1_mu2
|
61 |
-
|
62 |
-
C1 = 0.01 ** 2
|
63 |
-
C2 = 0.03 ** 2
|
64 |
-
|
65 |
-
ssim_map = ((2 * mu1_mu2 + C1) * (2 * sigma12 + C2)) / \
|
66 |
-
((mu1_sq + mu2_sq + C1) * (sigma1_sq + sigma2_sq + C2))
|
67 |
-
|
68 |
-
if size_average:
|
69 |
-
return ssim_map.mean()
|
70 |
-
|
71 |
-
return ssim_map.mean(1).mean(1).mean(1)
|
72 |
-
|
73 |
-
def _load_from_state_dict(self, state_dict, prefix, local_metadata, strict, missing_keys, unexpected_keys, error_msgs):
|
74 |
-
return
|
|
|
|
|
|
|
|
|
|
|
|
|
|
|
|
|
|
|
|
|
|
|
|
|
|
|
|
|
|
|
|
|
|
|
|
|
|
|
|
|
|
|
|
|
|
|
|
|
|
|
|
|
|
|
|
|
|
|
|
|
|
|
|
|
|
|
|
|
|
|
|
|
|
|
|
|
|
|
|
|
|
|
|
|
|
|
|
|
|
|
|
|
|
|
|
|
|
|
|
|
|
|
|
|
|
|
|
|
|
|
|
|
|
|
|
|
|
|
|
|
|
|
|
|
|
|
|
|
|
|
|
|
|
|
|
|
|
|
|
|
|
|
|
|
|
|
|
|
|
spaces/Amon1/ChatGPTForAcadamic/request_llm/README.md
DELETED
@@ -1,36 +0,0 @@
|
|
1 |
-
# 如何使用其他大语言模型(dev分支测试中)
|
2 |
-
|
3 |
-
## 1. 先运行text-generation
|
4 |
-
``` sh
|
5 |
-
# 下载模型( text-generation 这么牛的项目,别忘了给人家star )
|
6 |
-
git clone https://github.com/oobabooga/text-generation-webui.git
|
7 |
-
|
8 |
-
# 安装text-generation的额外依赖
|
9 |
-
pip install accelerate bitsandbytes flexgen gradio llamacpp markdown numpy peft requests rwkv safetensors sentencepiece tqdm datasets git+https://github.com/huggingface/transformers
|
10 |
-
|
11 |
-
# 切换路径
|
12 |
-
cd text-generation-webui
|
13 |
-
|
14 |
-
# 下载模型
|
15 |
-
python download-model.py facebook/galactica-1.3b
|
16 |
-
# 其他可选如 facebook/opt-1.3b
|
17 |
-
# facebook/galactica-6.7b
|
18 |
-
# facebook/galactica-120b
|
19 |
-
# facebook/pygmalion-1.3b 等
|
20 |
-
# 详情见 https://github.com/oobabooga/text-generation-webui
|
21 |
-
|
22 |
-
# 启动text-generation,注意把模型的斜杠改成下划线
|
23 |
-
python server.py --cpu --listen --listen-port 7860 --model facebook_galactica-1.3b
|
24 |
-
```
|
25 |
-
|
26 |
-
## 2. 修改config.py
|
27 |
-
``` sh
|
28 |
-
# LLM_MODEL格式较复杂 TGUI:[模型]@[ws地址]:[ws端口] , 端口要和上面给定的端口一致
|
29 |
-
LLM_MODEL = "TGUI:galactica-1.3b@localhost:7860"
|
30 |
-
```
|
31 |
-
|
32 |
-
## 3. 运行!
|
33 |
-
``` sh
|
34 |
-
cd chatgpt-academic
|
35 |
-
python main.py
|
36 |
-
```
|
|
|
|
|
|
|
|
|
|
|
|
|
|
|
|
|
|
|
|
|
|
|
|
|
|
|
|
|
|
|
|
|
|
|
|
|
|
|
|
|
|
|
|
|
|
|
|
|
|
|
|
|
|
|
|
|
|
|
|
|
|
|
|
|
|
|
|
|
|
|
|
|
|
spaces/Androidonnxfork/CivitAi-to-Diffusers/diffusers/src/diffusers/pipelines/spectrogram_diffusion/notes_encoder.py
DELETED
@@ -1,86 +0,0 @@
|
|
1 |
-
# Copyright 2022 The Music Spectrogram Diffusion Authors.
|
2 |
-
# Copyright 2023 The HuggingFace Team. All rights reserved.
|
3 |
-
#
|
4 |
-
# Licensed under the Apache License, Version 2.0 (the "License");
|
5 |
-
# you may not use this file except in compliance with the License.
|
6 |
-
# You may obtain a copy of the License at
|
7 |
-
#
|
8 |
-
# http://www.apache.org/licenses/LICENSE-2.0
|
9 |
-
#
|
10 |
-
# Unless required by applicable law or agreed to in writing, software
|
11 |
-
# distributed under the License is distributed on an "AS IS" BASIS,
|
12 |
-
# WITHOUT WARRANTIES OR CONDITIONS OF ANY KIND, either express or implied.
|
13 |
-
# See the License for the specific language governing permissions and
|
14 |
-
# limitations under the License.
|
15 |
-
|
16 |
-
import torch
|
17 |
-
import torch.nn as nn
|
18 |
-
from transformers.modeling_utils import ModuleUtilsMixin
|
19 |
-
from transformers.models.t5.modeling_t5 import T5Block, T5Config, T5LayerNorm
|
20 |
-
|
21 |
-
from ...configuration_utils import ConfigMixin, register_to_config
|
22 |
-
from ...models import ModelMixin
|
23 |
-
|
24 |
-
|
25 |
-
class SpectrogramNotesEncoder(ModelMixin, ConfigMixin, ModuleUtilsMixin):
|
26 |
-
@register_to_config
|
27 |
-
def __init__(
|
28 |
-
self,
|
29 |
-
max_length: int,
|
30 |
-
vocab_size: int,
|
31 |
-
d_model: int,
|
32 |
-
dropout_rate: float,
|
33 |
-
num_layers: int,
|
34 |
-
num_heads: int,
|
35 |
-
d_kv: int,
|
36 |
-
d_ff: int,
|
37 |
-
feed_forward_proj: str,
|
38 |
-
is_decoder: bool = False,
|
39 |
-
):
|
40 |
-
super().__init__()
|
41 |
-
|
42 |
-
self.token_embedder = nn.Embedding(vocab_size, d_model)
|
43 |
-
|
44 |
-
self.position_encoding = nn.Embedding(max_length, d_model)
|
45 |
-
self.position_encoding.weight.requires_grad = False
|
46 |
-
|
47 |
-
self.dropout_pre = nn.Dropout(p=dropout_rate)
|
48 |
-
|
49 |
-
t5config = T5Config(
|
50 |
-
vocab_size=vocab_size,
|
51 |
-
d_model=d_model,
|
52 |
-
num_heads=num_heads,
|
53 |
-
d_kv=d_kv,
|
54 |
-
d_ff=d_ff,
|
55 |
-
dropout_rate=dropout_rate,
|
56 |
-
feed_forward_proj=feed_forward_proj,
|
57 |
-
is_decoder=is_decoder,
|
58 |
-
is_encoder_decoder=False,
|
59 |
-
)
|
60 |
-
|
61 |
-
self.encoders = nn.ModuleList()
|
62 |
-
for lyr_num in range(num_layers):
|
63 |
-
lyr = T5Block(t5config)
|
64 |
-
self.encoders.append(lyr)
|
65 |
-
|
66 |
-
self.layer_norm = T5LayerNorm(d_model)
|
67 |
-
self.dropout_post = nn.Dropout(p=dropout_rate)
|
68 |
-
|
69 |
-
def forward(self, encoder_input_tokens, encoder_inputs_mask):
|
70 |
-
x = self.token_embedder(encoder_input_tokens)
|
71 |
-
|
72 |
-
seq_length = encoder_input_tokens.shape[1]
|
73 |
-
inputs_positions = torch.arange(seq_length, device=encoder_input_tokens.device)
|
74 |
-
x += self.position_encoding(inputs_positions)
|
75 |
-
|
76 |
-
x = self.dropout_pre(x)
|
77 |
-
|
78 |
-
# inverted the attention mask
|
79 |
-
input_shape = encoder_input_tokens.size()
|
80 |
-
extended_attention_mask = self.get_extended_attention_mask(encoder_inputs_mask, input_shape)
|
81 |
-
|
82 |
-
for lyr in self.encoders:
|
83 |
-
x = lyr(x, extended_attention_mask)[0]
|
84 |
-
x = self.layer_norm(x)
|
85 |
-
|
86 |
-
return self.dropout_post(x), encoder_inputs_mask
|
|
|
|
|
|
|
|
|
|
|
|
|
|
|
|
|
|
|
|
|
|
|
|
|
|
|
|
|
|
|
|
|
|
|
|
|
|
|
|
|
|
|
|
|
|
|
|
|
|
|
|
|
|
|
|
|
|
|
|
|
|
|
|
|
|
|
|
|
|
|
|
|
|
|
|
|
|
|
|
|
|
|
|
|
|
|
|
|
|
|
|
|
|
|
|
|
|
|
|
|
|
|
|
|
|
|
|
|
|
|
|
|
|
|
|
|
|
|
|
|
|
|
|
|
|
|
|
|
|
|
|
|
|
|
|
|
|
|
|
|
|
|
|
|
|
|
|
|
|
|
|
|
|
|
|
|
|
|
|
|
|
|
|
|
|
|
|
|
|
|
|
|
|
spaces/Androidonnxfork/CivitAi-to-Diffusers/diffusers/src/diffusers/pipelines/stable_diffusion/pipeline_stable_diffusion_pix2pix_zero.py
DELETED
@@ -1,1259 +0,0 @@
|
|
1 |
-
# Copyright 2023 Pix2Pix Zero Authors and The HuggingFace Team. All rights reserved.
|
2 |
-
#
|
3 |
-
# Licensed under the Apache License, Version 2.0 (the "License");
|
4 |
-
# you may not use this file except in compliance with the License.
|
5 |
-
# You may obtain a copy of the License at
|
6 |
-
#
|
7 |
-
# http://www.apache.org/licenses/LICENSE-2.0
|
8 |
-
#
|
9 |
-
# Unless required by applicable law or agreed to in writing, software
|
10 |
-
# distributed under the License is distributed on an "AS IS" BASIS,
|
11 |
-
# WITHOUT WARRANTIES OR CONDITIONS OF ANY KIND, either express or implied.
|
12 |
-
# See the License for the specific language governing permissions and
|
13 |
-
# limitations under the License.
|
14 |
-
|
15 |
-
import inspect
|
16 |
-
import warnings
|
17 |
-
from dataclasses import dataclass
|
18 |
-
from typing import Any, Callable, Dict, List, Optional, Union
|
19 |
-
|
20 |
-
import numpy as np
|
21 |
-
import PIL
|
22 |
-
import torch
|
23 |
-
import torch.nn.functional as F
|
24 |
-
from transformers import (
|
25 |
-
BlipForConditionalGeneration,
|
26 |
-
BlipProcessor,
|
27 |
-
CLIPImageProcessor,
|
28 |
-
CLIPTextModel,
|
29 |
-
CLIPTokenizer,
|
30 |
-
)
|
31 |
-
|
32 |
-
from ...image_processor import VaeImageProcessor
|
33 |
-
from ...loaders import LoraLoaderMixin, TextualInversionLoaderMixin
|
34 |
-
from ...models import AutoencoderKL, UNet2DConditionModel
|
35 |
-
from ...models.attention_processor import Attention
|
36 |
-
from ...schedulers import DDIMScheduler, DDPMScheduler, EulerAncestralDiscreteScheduler, LMSDiscreteScheduler
|
37 |
-
from ...schedulers.scheduling_ddim_inverse import DDIMInverseScheduler
|
38 |
-
from ...utils import (
|
39 |
-
PIL_INTERPOLATION,
|
40 |
-
BaseOutput,
|
41 |
-
deprecate,
|
42 |
-
is_accelerate_available,
|
43 |
-
is_accelerate_version,
|
44 |
-
logging,
|
45 |
-
randn_tensor,
|
46 |
-
replace_example_docstring,
|
47 |
-
)
|
48 |
-
from ..pipeline_utils import DiffusionPipeline
|
49 |
-
from . import StableDiffusionPipelineOutput
|
50 |
-
from .safety_checker import StableDiffusionSafetyChecker
|
51 |
-
|
52 |
-
|
53 |
-
logger = logging.get_logger(__name__) # pylint: disable=invalid-name
|
54 |
-
|
55 |
-
|
56 |
-
@dataclass
|
57 |
-
class Pix2PixInversionPipelineOutput(BaseOutput, TextualInversionLoaderMixin):
|
58 |
-
"""
|
59 |
-
Output class for Stable Diffusion pipelines.
|
60 |
-
|
61 |
-
Args:
|
62 |
-
latents (`torch.FloatTensor`)
|
63 |
-
inverted latents tensor
|
64 |
-
images (`List[PIL.Image.Image]` or `np.ndarray`)
|
65 |
-
List of denoised PIL images of length `batch_size` or numpy array of shape `(batch_size, height, width,
|
66 |
-
num_channels)`. PIL images or numpy array present the denoised images of the diffusion pipeline.
|
67 |
-
"""
|
68 |
-
|
69 |
-
latents: torch.FloatTensor
|
70 |
-
images: Union[List[PIL.Image.Image], np.ndarray]
|
71 |
-
|
72 |
-
|
73 |
-
EXAMPLE_DOC_STRING = """
|
74 |
-
Examples:
|
75 |
-
```py
|
76 |
-
>>> import requests
|
77 |
-
>>> import torch
|
78 |
-
|
79 |
-
>>> from diffusers import DDIMScheduler, StableDiffusionPix2PixZeroPipeline
|
80 |
-
|
81 |
-
|
82 |
-
>>> def download(embedding_url, local_filepath):
|
83 |
-
... r = requests.get(embedding_url)
|
84 |
-
... with open(local_filepath, "wb") as f:
|
85 |
-
... f.write(r.content)
|
86 |
-
|
87 |
-
|
88 |
-
>>> model_ckpt = "CompVis/stable-diffusion-v1-4"
|
89 |
-
>>> pipeline = StableDiffusionPix2PixZeroPipeline.from_pretrained(model_ckpt, torch_dtype=torch.float16)
|
90 |
-
>>> pipeline.scheduler = DDIMScheduler.from_config(pipeline.scheduler.config)
|
91 |
-
>>> pipeline.to("cuda")
|
92 |
-
|
93 |
-
>>> prompt = "a high resolution painting of a cat in the style of van gough"
|
94 |
-
>>> source_emb_url = "https://hf.co/datasets/sayakpaul/sample-datasets/resolve/main/cat.pt"
|
95 |
-
>>> target_emb_url = "https://hf.co/datasets/sayakpaul/sample-datasets/resolve/main/dog.pt"
|
96 |
-
|
97 |
-
>>> for url in [source_emb_url, target_emb_url]:
|
98 |
-
... download(url, url.split("/")[-1])
|
99 |
-
|
100 |
-
>>> src_embeds = torch.load(source_emb_url.split("/")[-1])
|
101 |
-
>>> target_embeds = torch.load(target_emb_url.split("/")[-1])
|
102 |
-
>>> images = pipeline(
|
103 |
-
... prompt,
|
104 |
-
... source_embeds=src_embeds,
|
105 |
-
... target_embeds=target_embeds,
|
106 |
-
... num_inference_steps=50,
|
107 |
-
... cross_attention_guidance_amount=0.15,
|
108 |
-
... ).images
|
109 |
-
|
110 |
-
>>> images[0].save("edited_image_dog.png")
|
111 |
-
```
|
112 |
-
"""
|
113 |
-
|
114 |
-
EXAMPLE_INVERT_DOC_STRING = """
|
115 |
-
Examples:
|
116 |
-
```py
|
117 |
-
>>> import torch
|
118 |
-
>>> from transformers import BlipForConditionalGeneration, BlipProcessor
|
119 |
-
>>> from diffusers import DDIMScheduler, DDIMInverseScheduler, StableDiffusionPix2PixZeroPipeline
|
120 |
-
|
121 |
-
>>> import requests
|
122 |
-
>>> from PIL import Image
|
123 |
-
|
124 |
-
>>> captioner_id = "Salesforce/blip-image-captioning-base"
|
125 |
-
>>> processor = BlipProcessor.from_pretrained(captioner_id)
|
126 |
-
>>> model = BlipForConditionalGeneration.from_pretrained(
|
127 |
-
... captioner_id, torch_dtype=torch.float16, low_cpu_mem_usage=True
|
128 |
-
... )
|
129 |
-
|
130 |
-
>>> sd_model_ckpt = "CompVis/stable-diffusion-v1-4"
|
131 |
-
>>> pipeline = StableDiffusionPix2PixZeroPipeline.from_pretrained(
|
132 |
-
... sd_model_ckpt,
|
133 |
-
... caption_generator=model,
|
134 |
-
... caption_processor=processor,
|
135 |
-
... torch_dtype=torch.float16,
|
136 |
-
... safety_checker=None,
|
137 |
-
... )
|
138 |
-
|
139 |
-
>>> pipeline.scheduler = DDIMScheduler.from_config(pipeline.scheduler.config)
|
140 |
-
>>> pipeline.inverse_scheduler = DDIMInverseScheduler.from_config(pipeline.scheduler.config)
|
141 |
-
>>> pipeline.enable_model_cpu_offload()
|
142 |
-
|
143 |
-
>>> img_url = "https://github.com/pix2pixzero/pix2pix-zero/raw/main/assets/test_images/cats/cat_6.png"
|
144 |
-
|
145 |
-
>>> raw_image = Image.open(requests.get(img_url, stream=True).raw).convert("RGB").resize((512, 512))
|
146 |
-
>>> # generate caption
|
147 |
-
>>> caption = pipeline.generate_caption(raw_image)
|
148 |
-
|
149 |
-
>>> # "a photography of a cat with flowers and dai dai daie - daie - daie kasaii"
|
150 |
-
>>> inv_latents = pipeline.invert(caption, image=raw_image).latents
|
151 |
-
>>> # we need to generate source and target embeds
|
152 |
-
|
153 |
-
>>> source_prompts = ["a cat sitting on the street", "a cat playing in the field", "a face of a cat"]
|
154 |
-
|
155 |
-
>>> target_prompts = ["a dog sitting on the street", "a dog playing in the field", "a face of a dog"]
|
156 |
-
|
157 |
-
>>> source_embeds = pipeline.get_embeds(source_prompts)
|
158 |
-
>>> target_embeds = pipeline.get_embeds(target_prompts)
|
159 |
-
>>> # the latents can then be used to edit a real image
|
160 |
-
>>> # when using Stable Diffusion 2 or other models that use v-prediction
|
161 |
-
>>> # set `cross_attention_guidance_amount` to 0.01 or less to avoid input latent gradient explosion
|
162 |
-
|
163 |
-
>>> image = pipeline(
|
164 |
-
... caption,
|
165 |
-
... source_embeds=source_embeds,
|
166 |
-
... target_embeds=target_embeds,
|
167 |
-
... num_inference_steps=50,
|
168 |
-
... cross_attention_guidance_amount=0.15,
|
169 |
-
... generator=generator,
|
170 |
-
... latents=inv_latents,
|
171 |
-
... negative_prompt=caption,
|
172 |
-
... ).images[0]
|
173 |
-
>>> image.save("edited_image.png")
|
174 |
-
```
|
175 |
-
"""
|
176 |
-
|
177 |
-
|
178 |
-
# Copied from diffusers.pipelines.stable_diffusion.pipeline_stable_diffusion_img2img.preprocess
|
179 |
-
def preprocess(image):
|
180 |
-
warnings.warn(
|
181 |
-
"The preprocess method is deprecated and will be removed in a future version. Please"
|
182 |
-
" use VaeImageProcessor.preprocess instead",
|
183 |
-
FutureWarning,
|
184 |
-
)
|
185 |
-
if isinstance(image, torch.Tensor):
|
186 |
-
return image
|
187 |
-
elif isinstance(image, PIL.Image.Image):
|
188 |
-
image = [image]
|
189 |
-
|
190 |
-
if isinstance(image[0], PIL.Image.Image):
|
191 |
-
w, h = image[0].size
|
192 |
-
w, h = (x - x % 8 for x in (w, h)) # resize to integer multiple of 8
|
193 |
-
|
194 |
-
image = [np.array(i.resize((w, h), resample=PIL_INTERPOLATION["lanczos"]))[None, :] for i in image]
|
195 |
-
image = np.concatenate(image, axis=0)
|
196 |
-
image = np.array(image).astype(np.float32) / 255.0
|
197 |
-
image = image.transpose(0, 3, 1, 2)
|
198 |
-
image = 2.0 * image - 1.0
|
199 |
-
image = torch.from_numpy(image)
|
200 |
-
elif isinstance(image[0], torch.Tensor):
|
201 |
-
image = torch.cat(image, dim=0)
|
202 |
-
return image
|
203 |
-
|
204 |
-
|
205 |
-
def prepare_unet(unet: UNet2DConditionModel):
|
206 |
-
"""Modifies the UNet (`unet`) to perform Pix2Pix Zero optimizations."""
|
207 |
-
pix2pix_zero_attn_procs = {}
|
208 |
-
for name in unet.attn_processors.keys():
|
209 |
-
module_name = name.replace(".processor", "")
|
210 |
-
module = unet.get_submodule(module_name)
|
211 |
-
if "attn2" in name:
|
212 |
-
pix2pix_zero_attn_procs[name] = Pix2PixZeroAttnProcessor(is_pix2pix_zero=True)
|
213 |
-
module.requires_grad_(True)
|
214 |
-
else:
|
215 |
-
pix2pix_zero_attn_procs[name] = Pix2PixZeroAttnProcessor(is_pix2pix_zero=False)
|
216 |
-
module.requires_grad_(False)
|
217 |
-
|
218 |
-
unet.set_attn_processor(pix2pix_zero_attn_procs)
|
219 |
-
return unet
|
220 |
-
|
221 |
-
|
222 |
-
class Pix2PixZeroL2Loss:
|
223 |
-
def __init__(self):
|
224 |
-
self.loss = 0.0
|
225 |
-
|
226 |
-
def compute_loss(self, predictions, targets):
|
227 |
-
self.loss += ((predictions - targets) ** 2).sum((1, 2)).mean(0)
|
228 |
-
|
229 |
-
|
230 |
-
class Pix2PixZeroAttnProcessor:
|
231 |
-
"""An attention processor class to store the attention weights.
|
232 |
-
In Pix2Pix Zero, it happens during computations in the cross-attention blocks."""
|
233 |
-
|
234 |
-
def __init__(self, is_pix2pix_zero=False):
|
235 |
-
self.is_pix2pix_zero = is_pix2pix_zero
|
236 |
-
if self.is_pix2pix_zero:
|
237 |
-
self.reference_cross_attn_map = {}
|
238 |
-
|
239 |
-
def __call__(
|
240 |
-
self,
|
241 |
-
attn: Attention,
|
242 |
-
hidden_states,
|
243 |
-
encoder_hidden_states=None,
|
244 |
-
attention_mask=None,
|
245 |
-
timestep=None,
|
246 |
-
loss=None,
|
247 |
-
):
|
248 |
-
batch_size, sequence_length, _ = hidden_states.shape
|
249 |
-
attention_mask = attn.prepare_attention_mask(attention_mask, sequence_length, batch_size)
|
250 |
-
query = attn.to_q(hidden_states)
|
251 |
-
|
252 |
-
if encoder_hidden_states is None:
|
253 |
-
encoder_hidden_states = hidden_states
|
254 |
-
elif attn.norm_cross:
|
255 |
-
encoder_hidden_states = attn.norm_encoder_hidden_states(encoder_hidden_states)
|
256 |
-
|
257 |
-
key = attn.to_k(encoder_hidden_states)
|
258 |
-
value = attn.to_v(encoder_hidden_states)
|
259 |
-
|
260 |
-
query = attn.head_to_batch_dim(query)
|
261 |
-
key = attn.head_to_batch_dim(key)
|
262 |
-
value = attn.head_to_batch_dim(value)
|
263 |
-
|
264 |
-
attention_probs = attn.get_attention_scores(query, key, attention_mask)
|
265 |
-
if self.is_pix2pix_zero and timestep is not None:
|
266 |
-
# new bookkeeping to save the attention weights.
|
267 |
-
if loss is None:
|
268 |
-
self.reference_cross_attn_map[timestep.item()] = attention_probs.detach().cpu()
|
269 |
-
# compute loss
|
270 |
-
elif loss is not None:
|
271 |
-
prev_attn_probs = self.reference_cross_attn_map.pop(timestep.item())
|
272 |
-
loss.compute_loss(attention_probs, prev_attn_probs.to(attention_probs.device))
|
273 |
-
|
274 |
-
hidden_states = torch.bmm(attention_probs, value)
|
275 |
-
hidden_states = attn.batch_to_head_dim(hidden_states)
|
276 |
-
|
277 |
-
# linear proj
|
278 |
-
hidden_states = attn.to_out[0](hidden_states)
|
279 |
-
# dropout
|
280 |
-
hidden_states = attn.to_out[1](hidden_states)
|
281 |
-
|
282 |
-
return hidden_states
|
283 |
-
|
284 |
-
|
285 |
-
class StableDiffusionPix2PixZeroPipeline(DiffusionPipeline):
|
286 |
-
r"""
|
287 |
-
Pipeline for pixel-levl image editing using Pix2Pix Zero. Based on Stable Diffusion.
|
288 |
-
|
289 |
-
This model inherits from [`DiffusionPipeline`]. Check the superclass documentation for the generic methods the
|
290 |
-
library implements for all the pipelines (such as downloading or saving, running on a particular device, etc.)
|
291 |
-
|
292 |
-
Args:
|
293 |
-
vae ([`AutoencoderKL`]):
|
294 |
-
Variational Auto-Encoder (VAE) Model to encode and decode images to and from latent representations.
|
295 |
-
text_encoder ([`CLIPTextModel`]):
|
296 |
-
Frozen text-encoder. Stable Diffusion uses the text portion of
|
297 |
-
[CLIP](https://huggingface.co/docs/transformers/model_doc/clip#transformers.CLIPTextModel), specifically
|
298 |
-
the [clip-vit-large-patch14](https://huggingface.co/openai/clip-vit-large-patch14) variant.
|
299 |
-
tokenizer (`CLIPTokenizer`):
|
300 |
-
Tokenizer of class
|
301 |
-
[CLIPTokenizer](https://huggingface.co/docs/transformers/v4.21.0/en/model_doc/clip#transformers.CLIPTokenizer).
|
302 |
-
unet ([`UNet2DConditionModel`]): Conditional U-Net architecture to denoise the encoded image latents.
|
303 |
-
scheduler ([`SchedulerMixin`]):
|
304 |
-
A scheduler to be used in combination with `unet` to denoise the encoded image latents. Can be one of
|
305 |
-
[`DDIMScheduler`], [`LMSDiscreteScheduler`], [`EulerAncestralDiscreteScheduler`], or [`DDPMScheduler`].
|
306 |
-
safety_checker ([`StableDiffusionSafetyChecker`]):
|
307 |
-
Classification module that estimates whether generated images could be considered offensive or harmful.
|
308 |
-
Please, refer to the [model card](https://huggingface.co/runwayml/stable-diffusion-v1-5) for details.
|
309 |
-
feature_extractor ([`CLIPImageProcessor`]):
|
310 |
-
Model that extracts features from generated images to be used as inputs for the `safety_checker`.
|
311 |
-
requires_safety_checker (bool):
|
312 |
-
Whether the pipeline requires a safety checker. We recommend setting it to True if you're using the
|
313 |
-
pipeline publicly.
|
314 |
-
"""
|
315 |
-
_optional_components = [
|
316 |
-
"safety_checker",
|
317 |
-
"feature_extractor",
|
318 |
-
"caption_generator",
|
319 |
-
"caption_processor",
|
320 |
-
"inverse_scheduler",
|
321 |
-
]
|
322 |
-
|
323 |
-
def __init__(
|
324 |
-
self,
|
325 |
-
vae: AutoencoderKL,
|
326 |
-
text_encoder: CLIPTextModel,
|
327 |
-
tokenizer: CLIPTokenizer,
|
328 |
-
unet: UNet2DConditionModel,
|
329 |
-
scheduler: Union[DDPMScheduler, DDIMScheduler, EulerAncestralDiscreteScheduler, LMSDiscreteScheduler],
|
330 |
-
feature_extractor: CLIPImageProcessor,
|
331 |
-
safety_checker: StableDiffusionSafetyChecker,
|
332 |
-
inverse_scheduler: DDIMInverseScheduler,
|
333 |
-
caption_generator: BlipForConditionalGeneration,
|
334 |
-
caption_processor: BlipProcessor,
|
335 |
-
requires_safety_checker: bool = True,
|
336 |
-
):
|
337 |
-
super().__init__()
|
338 |
-
|
339 |
-
if safety_checker is None and requires_safety_checker:
|
340 |
-
logger.warning(
|
341 |
-
f"You have disabled the safety checker for {self.__class__} by passing `safety_checker=None`. Ensure"
|
342 |
-
" that you abide to the conditions of the Stable Diffusion license and do not expose unfiltered"
|
343 |
-
" results in services or applications open to the public. Both the diffusers team and Hugging Face"
|
344 |
-
" strongly recommend to keep the safety filter enabled in all public facing circumstances, disabling"
|
345 |
-
" it only for use-cases that involve analyzing network behavior or auditing its results. For more"
|
346 |
-
" information, please have a look at https://github.com/huggingface/diffusers/pull/254 ."
|
347 |
-
)
|
348 |
-
|
349 |
-
if safety_checker is not None and feature_extractor is None:
|
350 |
-
raise ValueError(
|
351 |
-
"Make sure to define a feature extractor when loading {self.__class__} if you want to use the safety"
|
352 |
-
" checker. If you do not want to use the safety checker, you can pass `'safety_checker=None'` instead."
|
353 |
-
)
|
354 |
-
|
355 |
-
self.register_modules(
|
356 |
-
vae=vae,
|
357 |
-
text_encoder=text_encoder,
|
358 |
-
tokenizer=tokenizer,
|
359 |
-
unet=unet,
|
360 |
-
scheduler=scheduler,
|
361 |
-
safety_checker=safety_checker,
|
362 |
-
feature_extractor=feature_extractor,
|
363 |
-
caption_processor=caption_processor,
|
364 |
-
caption_generator=caption_generator,
|
365 |
-
inverse_scheduler=inverse_scheduler,
|
366 |
-
)
|
367 |
-
self.vae_scale_factor = 2 ** (len(self.vae.config.block_out_channels) - 1)
|
368 |
-
self.image_processor = VaeImageProcessor(vae_scale_factor=self.vae_scale_factor)
|
369 |
-
self.register_to_config(requires_safety_checker=requires_safety_checker)
|
370 |
-
|
371 |
-
def enable_model_cpu_offload(self, gpu_id=0):
|
372 |
-
r"""
|
373 |
-
Offloads all models to CPU using accelerate, reducing memory usage with a low impact on performance. Compared
|
374 |
-
to `enable_sequential_cpu_offload`, this method moves one whole model at a time to the GPU when its `forward`
|
375 |
-
method is called, and the model remains in GPU until the next model runs. Memory savings are lower than with
|
376 |
-
`enable_sequential_cpu_offload`, but performance is much better due to the iterative execution of the `unet`.
|
377 |
-
"""
|
378 |
-
if is_accelerate_available() and is_accelerate_version(">=", "0.17.0.dev0"):
|
379 |
-
from accelerate import cpu_offload_with_hook
|
380 |
-
else:
|
381 |
-
raise ImportError("`enable_model_cpu_offload` requires `accelerate v0.17.0` or higher.")
|
382 |
-
|
383 |
-
device = torch.device(f"cuda:{gpu_id}")
|
384 |
-
|
385 |
-
hook = None
|
386 |
-
for cpu_offloaded_model in [self.vae, self.text_encoder, self.unet, self.vae]:
|
387 |
-
_, hook = cpu_offload_with_hook(cpu_offloaded_model, device, prev_module_hook=hook)
|
388 |
-
|
389 |
-
if self.safety_checker is not None:
|
390 |
-
_, hook = cpu_offload_with_hook(self.safety_checker, device, prev_module_hook=hook)
|
391 |
-
|
392 |
-
# We'll offload the last model manually.
|
393 |
-
self.final_offload_hook = hook
|
394 |
-
|
395 |
-
# Copied from diffusers.pipelines.stable_diffusion.pipeline_stable_diffusion.StableDiffusionPipeline._encode_prompt
|
396 |
-
def _encode_prompt(
|
397 |
-
self,
|
398 |
-
prompt,
|
399 |
-
device,
|
400 |
-
num_images_per_prompt,
|
401 |
-
do_classifier_free_guidance,
|
402 |
-
negative_prompt=None,
|
403 |
-
prompt_embeds: Optional[torch.FloatTensor] = None,
|
404 |
-
negative_prompt_embeds: Optional[torch.FloatTensor] = None,
|
405 |
-
lora_scale: Optional[float] = None,
|
406 |
-
):
|
407 |
-
r"""
|
408 |
-
Encodes the prompt into text encoder hidden states.
|
409 |
-
|
410 |
-
Args:
|
411 |
-
prompt (`str` or `List[str]`, *optional*):
|
412 |
-
prompt to be encoded
|
413 |
-
device: (`torch.device`):
|
414 |
-
torch device
|
415 |
-
num_images_per_prompt (`int`):
|
416 |
-
number of images that should be generated per prompt
|
417 |
-
do_classifier_free_guidance (`bool`):
|
418 |
-
whether to use classifier free guidance or not
|
419 |
-
negative_prompt (`str` or `List[str]`, *optional*):
|
420 |
-
The prompt or prompts not to guide the image generation. If not defined, one has to pass
|
421 |
-
`negative_prompt_embeds` instead. Ignored when not using guidance (i.e., ignored if `guidance_scale` is
|
422 |
-
less than `1`).
|
423 |
-
prompt_embeds (`torch.FloatTensor`, *optional*):
|
424 |
-
Pre-generated text embeddings. Can be used to easily tweak text inputs, *e.g.* prompt weighting. If not
|
425 |
-
provided, text embeddings will be generated from `prompt` input argument.
|
426 |
-
negative_prompt_embeds (`torch.FloatTensor`, *optional*):
|
427 |
-
Pre-generated negative text embeddings. Can be used to easily tweak text inputs, *e.g.* prompt
|
428 |
-
weighting. If not provided, negative_prompt_embeds will be generated from `negative_prompt` input
|
429 |
-
argument.
|
430 |
-
lora_scale (`float`, *optional*):
|
431 |
-
A lora scale that will be applied to all LoRA layers of the text encoder if LoRA layers are loaded.
|
432 |
-
"""
|
433 |
-
# set lora scale so that monkey patched LoRA
|
434 |
-
# function of text encoder can correctly access it
|
435 |
-
if lora_scale is not None and isinstance(self, LoraLoaderMixin):
|
436 |
-
self._lora_scale = lora_scale
|
437 |
-
|
438 |
-
if prompt is not None and isinstance(prompt, str):
|
439 |
-
batch_size = 1
|
440 |
-
elif prompt is not None and isinstance(prompt, list):
|
441 |
-
batch_size = len(prompt)
|
442 |
-
else:
|
443 |
-
batch_size = prompt_embeds.shape[0]
|
444 |
-
|
445 |
-
if prompt_embeds is None:
|
446 |
-
# textual inversion: procecss multi-vector tokens if necessary
|
447 |
-
if isinstance(self, TextualInversionLoaderMixin):
|
448 |
-
prompt = self.maybe_convert_prompt(prompt, self.tokenizer)
|
449 |
-
|
450 |
-
text_inputs = self.tokenizer(
|
451 |
-
prompt,
|
452 |
-
padding="max_length",
|
453 |
-
max_length=self.tokenizer.model_max_length,
|
454 |
-
truncation=True,
|
455 |
-
return_tensors="pt",
|
456 |
-
)
|
457 |
-
text_input_ids = text_inputs.input_ids
|
458 |
-
untruncated_ids = self.tokenizer(prompt, padding="longest", return_tensors="pt").input_ids
|
459 |
-
|
460 |
-
if untruncated_ids.shape[-1] >= text_input_ids.shape[-1] and not torch.equal(
|
461 |
-
text_input_ids, untruncated_ids
|
462 |
-
):
|
463 |
-
removed_text = self.tokenizer.batch_decode(
|
464 |
-
untruncated_ids[:, self.tokenizer.model_max_length - 1 : -1]
|
465 |
-
)
|
466 |
-
logger.warning(
|
467 |
-
"The following part of your input was truncated because CLIP can only handle sequences up to"
|
468 |
-
f" {self.tokenizer.model_max_length} tokens: {removed_text}"
|
469 |
-
)
|
470 |
-
|
471 |
-
if hasattr(self.text_encoder.config, "use_attention_mask") and self.text_encoder.config.use_attention_mask:
|
472 |
-
attention_mask = text_inputs.attention_mask.to(device)
|
473 |
-
else:
|
474 |
-
attention_mask = None
|
475 |
-
|
476 |
-
prompt_embeds = self.text_encoder(
|
477 |
-
text_input_ids.to(device),
|
478 |
-
attention_mask=attention_mask,
|
479 |
-
)
|
480 |
-
prompt_embeds = prompt_embeds[0]
|
481 |
-
|
482 |
-
prompt_embeds = prompt_embeds.to(dtype=self.text_encoder.dtype, device=device)
|
483 |
-
|
484 |
-
bs_embed, seq_len, _ = prompt_embeds.shape
|
485 |
-
# duplicate text embeddings for each generation per prompt, using mps friendly method
|
486 |
-
prompt_embeds = prompt_embeds.repeat(1, num_images_per_prompt, 1)
|
487 |
-
prompt_embeds = prompt_embeds.view(bs_embed * num_images_per_prompt, seq_len, -1)
|
488 |
-
|
489 |
-
# get unconditional embeddings for classifier free guidance
|
490 |
-
if do_classifier_free_guidance and negative_prompt_embeds is None:
|
491 |
-
uncond_tokens: List[str]
|
492 |
-
if negative_prompt is None:
|
493 |
-
uncond_tokens = [""] * batch_size
|
494 |
-
elif prompt is not None and type(prompt) is not type(negative_prompt):
|
495 |
-
raise TypeError(
|
496 |
-
f"`negative_prompt` should be the same type to `prompt`, but got {type(negative_prompt)} !="
|
497 |
-
f" {type(prompt)}."
|
498 |
-
)
|
499 |
-
elif isinstance(negative_prompt, str):
|
500 |
-
uncond_tokens = [negative_prompt]
|
501 |
-
elif batch_size != len(negative_prompt):
|
502 |
-
raise ValueError(
|
503 |
-
f"`negative_prompt`: {negative_prompt} has batch size {len(negative_prompt)}, but `prompt`:"
|
504 |
-
f" {prompt} has batch size {batch_size}. Please make sure that passed `negative_prompt` matches"
|
505 |
-
" the batch size of `prompt`."
|
506 |
-
)
|
507 |
-
else:
|
508 |
-
uncond_tokens = negative_prompt
|
509 |
-
|
510 |
-
# textual inversion: procecss multi-vector tokens if necessary
|
511 |
-
if isinstance(self, TextualInversionLoaderMixin):
|
512 |
-
uncond_tokens = self.maybe_convert_prompt(uncond_tokens, self.tokenizer)
|
513 |
-
|
514 |
-
max_length = prompt_embeds.shape[1]
|
515 |
-
uncond_input = self.tokenizer(
|
516 |
-
uncond_tokens,
|
517 |
-
padding="max_length",
|
518 |
-
max_length=max_length,
|
519 |
-
truncation=True,
|
520 |
-
return_tensors="pt",
|
521 |
-
)
|
522 |
-
|
523 |
-
if hasattr(self.text_encoder.config, "use_attention_mask") and self.text_encoder.config.use_attention_mask:
|
524 |
-
attention_mask = uncond_input.attention_mask.to(device)
|
525 |
-
else:
|
526 |
-
attention_mask = None
|
527 |
-
|
528 |
-
negative_prompt_embeds = self.text_encoder(
|
529 |
-
uncond_input.input_ids.to(device),
|
530 |
-
attention_mask=attention_mask,
|
531 |
-
)
|
532 |
-
negative_prompt_embeds = negative_prompt_embeds[0]
|
533 |
-
|
534 |
-
if do_classifier_free_guidance:
|
535 |
-
# duplicate unconditional embeddings for each generation per prompt, using mps friendly method
|
536 |
-
seq_len = negative_prompt_embeds.shape[1]
|
537 |
-
|
538 |
-
negative_prompt_embeds = negative_prompt_embeds.to(dtype=self.text_encoder.dtype, device=device)
|
539 |
-
|
540 |
-
negative_prompt_embeds = negative_prompt_embeds.repeat(1, num_images_per_prompt, 1)
|
541 |
-
negative_prompt_embeds = negative_prompt_embeds.view(batch_size * num_images_per_prompt, seq_len, -1)
|
542 |
-
|
543 |
-
# For classifier free guidance, we need to do two forward passes.
|
544 |
-
# Here we concatenate the unconditional and text embeddings into a single batch
|
545 |
-
# to avoid doing two forward passes
|
546 |
-
prompt_embeds = torch.cat([negative_prompt_embeds, prompt_embeds])
|
547 |
-
|
548 |
-
return prompt_embeds
|
549 |
-
|
550 |
-
# Copied from diffusers.pipelines.stable_diffusion.pipeline_stable_diffusion.StableDiffusionPipeline.run_safety_checker
|
551 |
-
def run_safety_checker(self, image, device, dtype):
|
552 |
-
if self.safety_checker is None:
|
553 |
-
has_nsfw_concept = None
|
554 |
-
else:
|
555 |
-
if torch.is_tensor(image):
|
556 |
-
feature_extractor_input = self.image_processor.postprocess(image, output_type="pil")
|
557 |
-
else:
|
558 |
-
feature_extractor_input = self.image_processor.numpy_to_pil(image)
|
559 |
-
safety_checker_input = self.feature_extractor(feature_extractor_input, return_tensors="pt").to(device)
|
560 |
-
image, has_nsfw_concept = self.safety_checker(
|
561 |
-
images=image, clip_input=safety_checker_input.pixel_values.to(dtype)
|
562 |
-
)
|
563 |
-
return image, has_nsfw_concept
|
564 |
-
|
565 |
-
# Copied from diffusers.pipelines.stable_diffusion.pipeline_stable_diffusion.StableDiffusionPipeline.decode_latents
|
566 |
-
def decode_latents(self, latents):
|
567 |
-
warnings.warn(
|
568 |
-
"The decode_latents method is deprecated and will be removed in a future version. Please"
|
569 |
-
" use VaeImageProcessor instead",
|
570 |
-
FutureWarning,
|
571 |
-
)
|
572 |
-
latents = 1 / self.vae.config.scaling_factor * latents
|
573 |
-
image = self.vae.decode(latents, return_dict=False)[0]
|
574 |
-
image = (image / 2 + 0.5).clamp(0, 1)
|
575 |
-
# we always cast to float32 as this does not cause significant overhead and is compatible with bfloat16
|
576 |
-
image = image.cpu().permute(0, 2, 3, 1).float().numpy()
|
577 |
-
return image
|
578 |
-
|
579 |
-
# Copied from diffusers.pipelines.stable_diffusion.pipeline_stable_diffusion.StableDiffusionPipeline.prepare_extra_step_kwargs
|
580 |
-
def prepare_extra_step_kwargs(self, generator, eta):
|
581 |
-
# prepare extra kwargs for the scheduler step, since not all schedulers have the same signature
|
582 |
-
# eta (η) is only used with the DDIMScheduler, it will be ignored for other schedulers.
|
583 |
-
# eta corresponds to η in DDIM paper: https://arxiv.org/abs/2010.02502
|
584 |
-
# and should be between [0, 1]
|
585 |
-
|
586 |
-
accepts_eta = "eta" in set(inspect.signature(self.scheduler.step).parameters.keys())
|
587 |
-
extra_step_kwargs = {}
|
588 |
-
if accepts_eta:
|
589 |
-
extra_step_kwargs["eta"] = eta
|
590 |
-
|
591 |
-
# check if the scheduler accepts generator
|
592 |
-
accepts_generator = "generator" in set(inspect.signature(self.scheduler.step).parameters.keys())
|
593 |
-
if accepts_generator:
|
594 |
-
extra_step_kwargs["generator"] = generator
|
595 |
-
return extra_step_kwargs
|
596 |
-
|
597 |
-
def check_inputs(
|
598 |
-
self,
|
599 |
-
prompt,
|
600 |
-
source_embeds,
|
601 |
-
target_embeds,
|
602 |
-
callback_steps,
|
603 |
-
prompt_embeds=None,
|
604 |
-
):
|
605 |
-
if (callback_steps is None) or (
|
606 |
-
callback_steps is not None and (not isinstance(callback_steps, int) or callback_steps <= 0)
|
607 |
-
):
|
608 |
-
raise ValueError(
|
609 |
-
f"`callback_steps` has to be a positive integer but is {callback_steps} of type"
|
610 |
-
f" {type(callback_steps)}."
|
611 |
-
)
|
612 |
-
if source_embeds is None and target_embeds is None:
|
613 |
-
raise ValueError("`source_embeds` and `target_embeds` cannot be undefined.")
|
614 |
-
|
615 |
-
if prompt is not None and prompt_embeds is not None:
|
616 |
-
raise ValueError(
|
617 |
-
f"Cannot forward both `prompt`: {prompt} and `prompt_embeds`: {prompt_embeds}. Please make sure to"
|
618 |
-
" only forward one of the two."
|
619 |
-
)
|
620 |
-
elif prompt is None and prompt_embeds is None:
|
621 |
-
raise ValueError(
|
622 |
-
"Provide either `prompt` or `prompt_embeds`. Cannot leave both `prompt` and `prompt_embeds` undefined."
|
623 |
-
)
|
624 |
-
elif prompt is not None and (not isinstance(prompt, str) and not isinstance(prompt, list)):
|
625 |
-
raise ValueError(f"`prompt` has to be of type `str` or `list` but is {type(prompt)}")
|
626 |
-
|
627 |
-
# Copied from diffusers.pipelines.stable_diffusion.pipeline_stable_diffusion.StableDiffusionPipeline.prepare_latents
|
628 |
-
def prepare_latents(self, batch_size, num_channels_latents, height, width, dtype, device, generator, latents=None):
|
629 |
-
shape = (batch_size, num_channels_latents, height // self.vae_scale_factor, width // self.vae_scale_factor)
|
630 |
-
if isinstance(generator, list) and len(generator) != batch_size:
|
631 |
-
raise ValueError(
|
632 |
-
f"You have passed a list of generators of length {len(generator)}, but requested an effective batch"
|
633 |
-
f" size of {batch_size}. Make sure the batch size matches the length of the generators."
|
634 |
-
)
|
635 |
-
|
636 |
-
if latents is None:
|
637 |
-
latents = randn_tensor(shape, generator=generator, device=device, dtype=dtype)
|
638 |
-
else:
|
639 |
-
latents = latents.to(device)
|
640 |
-
|
641 |
-
# scale the initial noise by the standard deviation required by the scheduler
|
642 |
-
latents = latents * self.scheduler.init_noise_sigma
|
643 |
-
return latents
|
644 |
-
|
645 |
-
@torch.no_grad()
|
646 |
-
def generate_caption(self, images):
|
647 |
-
"""Generates caption for a given image."""
|
648 |
-
text = "a photography of"
|
649 |
-
|
650 |
-
prev_device = self.caption_generator.device
|
651 |
-
|
652 |
-
device = self._execution_device
|
653 |
-
inputs = self.caption_processor(images, text, return_tensors="pt").to(
|
654 |
-
device=device, dtype=self.caption_generator.dtype
|
655 |
-
)
|
656 |
-
self.caption_generator.to(device)
|
657 |
-
outputs = self.caption_generator.generate(**inputs, max_new_tokens=128)
|
658 |
-
|
659 |
-
# offload caption generator
|
660 |
-
self.caption_generator.to(prev_device)
|
661 |
-
|
662 |
-
caption = self.caption_processor.batch_decode(outputs, skip_special_tokens=True)[0]
|
663 |
-
return caption
|
664 |
-
|
665 |
-
def construct_direction(self, embs_source: torch.Tensor, embs_target: torch.Tensor):
|
666 |
-
"""Constructs the edit direction to steer the image generation process semantically."""
|
667 |
-
return (embs_target.mean(0) - embs_source.mean(0)).unsqueeze(0)
|
668 |
-
|
669 |
-
@torch.no_grad()
|
670 |
-
def get_embeds(self, prompt: List[str], batch_size: int = 16) -> torch.FloatTensor:
|
671 |
-
num_prompts = len(prompt)
|
672 |
-
embeds = []
|
673 |
-
for i in range(0, num_prompts, batch_size):
|
674 |
-
prompt_slice = prompt[i : i + batch_size]
|
675 |
-
|
676 |
-
input_ids = self.tokenizer(
|
677 |
-
prompt_slice,
|
678 |
-
padding="max_length",
|
679 |
-
max_length=self.tokenizer.model_max_length,
|
680 |
-
truncation=True,
|
681 |
-
return_tensors="pt",
|
682 |
-
).input_ids
|
683 |
-
|
684 |
-
input_ids = input_ids.to(self.text_encoder.device)
|
685 |
-
embeds.append(self.text_encoder(input_ids)[0])
|
686 |
-
|
687 |
-
return torch.cat(embeds, dim=0).mean(0)[None]
|
688 |
-
|
689 |
-
def prepare_image_latents(self, image, batch_size, dtype, device, generator=None):
|
690 |
-
if not isinstance(image, (torch.Tensor, PIL.Image.Image, list)):
|
691 |
-
raise ValueError(
|
692 |
-
f"`image` has to be of type `torch.Tensor`, `PIL.Image.Image` or list but is {type(image)}"
|
693 |
-
)
|
694 |
-
|
695 |
-
image = image.to(device=device, dtype=dtype)
|
696 |
-
|
697 |
-
if image.shape[1] == 4:
|
698 |
-
latents = image
|
699 |
-
|
700 |
-
else:
|
701 |
-
if isinstance(generator, list) and len(generator) != batch_size:
|
702 |
-
raise ValueError(
|
703 |
-
f"You have passed a list of generators of length {len(generator)}, but requested an effective batch"
|
704 |
-
f" size of {batch_size}. Make sure the batch size matches the length of the generators."
|
705 |
-
)
|
706 |
-
|
707 |
-
if isinstance(generator, list):
|
708 |
-
latents = [
|
709 |
-
self.vae.encode(image[i : i + 1]).latent_dist.sample(generator[i]) for i in range(batch_size)
|
710 |
-
]
|
711 |
-
latents = torch.cat(latents, dim=0)
|
712 |
-
else:
|
713 |
-
latents = self.vae.encode(image).latent_dist.sample(generator)
|
714 |
-
|
715 |
-
latents = self.vae.config.scaling_factor * latents
|
716 |
-
|
717 |
-
if batch_size != latents.shape[0]:
|
718 |
-
if batch_size % latents.shape[0] == 0:
|
719 |
-
# expand image_latents for batch_size
|
720 |
-
deprecation_message = (
|
721 |
-
f"You have passed {batch_size} text prompts (`prompt`), but only {latents.shape[0]} initial"
|
722 |
-
" images (`image`). Initial images are now duplicating to match the number of text prompts. Note"
|
723 |
-
" that this behavior is deprecated and will be removed in a version 1.0.0. Please make sure to update"
|
724 |
-
" your script to pass as many initial images as text prompts to suppress this warning."
|
725 |
-
)
|
726 |
-
deprecate("len(prompt) != len(image)", "1.0.0", deprecation_message, standard_warn=False)
|
727 |
-
additional_latents_per_image = batch_size // latents.shape[0]
|
728 |
-
latents = torch.cat([latents] * additional_latents_per_image, dim=0)
|
729 |
-
else:
|
730 |
-
raise ValueError(
|
731 |
-
f"Cannot duplicate `image` of batch size {latents.shape[0]} to {batch_size} text prompts."
|
732 |
-
)
|
733 |
-
else:
|
734 |
-
latents = torch.cat([latents], dim=0)
|
735 |
-
|
736 |
-
return latents
|
737 |
-
|
738 |
-
def get_epsilon(self, model_output: torch.Tensor, sample: torch.Tensor, timestep: int):
|
739 |
-
pred_type = self.inverse_scheduler.config.prediction_type
|
740 |
-
alpha_prod_t = self.inverse_scheduler.alphas_cumprod[timestep]
|
741 |
-
|
742 |
-
beta_prod_t = 1 - alpha_prod_t
|
743 |
-
|
744 |
-
if pred_type == "epsilon":
|
745 |
-
return model_output
|
746 |
-
elif pred_type == "sample":
|
747 |
-
return (sample - alpha_prod_t ** (0.5) * model_output) / beta_prod_t ** (0.5)
|
748 |
-
elif pred_type == "v_prediction":
|
749 |
-
return (alpha_prod_t**0.5) * model_output + (beta_prod_t**0.5) * sample
|
750 |
-
else:
|
751 |
-
raise ValueError(
|
752 |
-
f"prediction_type given as {pred_type} must be one of `epsilon`, `sample`, or `v_prediction`"
|
753 |
-
)
|
754 |
-
|
755 |
-
def auto_corr_loss(self, hidden_states, generator=None):
|
756 |
-
reg_loss = 0.0
|
757 |
-
for i in range(hidden_states.shape[0]):
|
758 |
-
for j in range(hidden_states.shape[1]):
|
759 |
-
noise = hidden_states[i : i + 1, j : j + 1, :, :]
|
760 |
-
while True:
|
761 |
-
roll_amount = torch.randint(noise.shape[2] // 2, (1,), generator=generator).item()
|
762 |
-
reg_loss += (noise * torch.roll(noise, shifts=roll_amount, dims=2)).mean() ** 2
|
763 |
-
reg_loss += (noise * torch.roll(noise, shifts=roll_amount, dims=3)).mean() ** 2
|
764 |
-
|
765 |
-
if noise.shape[2] <= 8:
|
766 |
-
break
|
767 |
-
noise = F.avg_pool2d(noise, kernel_size=2)
|
768 |
-
return reg_loss
|
769 |
-
|
770 |
-
def kl_divergence(self, hidden_states):
|
771 |
-
mean = hidden_states.mean()
|
772 |
-
var = hidden_states.var()
|
773 |
-
return var + mean**2 - 1 - torch.log(var + 1e-7)
|
774 |
-
|
775 |
-
@torch.no_grad()
|
776 |
-
@replace_example_docstring(EXAMPLE_DOC_STRING)
|
777 |
-
def __call__(
|
778 |
-
self,
|
779 |
-
prompt: Optional[Union[str, List[str]]] = None,
|
780 |
-
source_embeds: torch.Tensor = None,
|
781 |
-
target_embeds: torch.Tensor = None,
|
782 |
-
height: Optional[int] = None,
|
783 |
-
width: Optional[int] = None,
|
784 |
-
num_inference_steps: int = 50,
|
785 |
-
guidance_scale: float = 7.5,
|
786 |
-
negative_prompt: Optional[Union[str, List[str]]] = None,
|
787 |
-
num_images_per_prompt: Optional[int] = 1,
|
788 |
-
eta: float = 0.0,
|
789 |
-
generator: Optional[Union[torch.Generator, List[torch.Generator]]] = None,
|
790 |
-
latents: Optional[torch.FloatTensor] = None,
|
791 |
-
prompt_embeds: Optional[torch.FloatTensor] = None,
|
792 |
-
negative_prompt_embeds: Optional[torch.FloatTensor] = None,
|
793 |
-
cross_attention_guidance_amount: float = 0.1,
|
794 |
-
output_type: Optional[str] = "pil",
|
795 |
-
return_dict: bool = True,
|
796 |
-
callback: Optional[Callable[[int, int, torch.FloatTensor], None]] = None,
|
797 |
-
callback_steps: Optional[int] = 1,
|
798 |
-
cross_attention_kwargs: Optional[Dict[str, Any]] = None,
|
799 |
-
):
|
800 |
-
r"""
|
801 |
-
Function invoked when calling the pipeline for generation.
|
802 |
-
|
803 |
-
Args:
|
804 |
-
prompt (`str` or `List[str]`, *optional*):
|
805 |
-
The prompt or prompts to guide the image generation. If not defined, one has to pass `prompt_embeds`.
|
806 |
-
instead.
|
807 |
-
source_embeds (`torch.Tensor`):
|
808 |
-
Source concept embeddings. Generation of the embeddings as per the [original
|
809 |
-
paper](https://arxiv.org/abs/2302.03027). Used in discovering the edit direction.
|
810 |
-
target_embeds (`torch.Tensor`):
|
811 |
-
Target concept embeddings. Generation of the embeddings as per the [original
|
812 |
-
paper](https://arxiv.org/abs/2302.03027). Used in discovering the edit direction.
|
813 |
-
height (`int`, *optional*, defaults to self.unet.config.sample_size * self.vae_scale_factor):
|
814 |
-
The height in pixels of the generated image.
|
815 |
-
width (`int`, *optional*, defaults to self.unet.config.sample_size * self.vae_scale_factor):
|
816 |
-
The width in pixels of the generated image.
|
817 |
-
num_inference_steps (`int`, *optional*, defaults to 50):
|
818 |
-
The number of denoising steps. More denoising steps usually lead to a higher quality image at the
|
819 |
-
expense of slower inference.
|
820 |
-
guidance_scale (`float`, *optional*, defaults to 7.5):
|
821 |
-
Guidance scale as defined in [Classifier-Free Diffusion Guidance](https://arxiv.org/abs/2207.12598).
|
822 |
-
`guidance_scale` is defined as `w` of equation 2. of [Imagen
|
823 |
-
Paper](https://arxiv.org/pdf/2205.11487.pdf). Guidance scale is enabled by setting `guidance_scale >
|
824 |
-
1`. Higher guidance scale encourages to generate images that are closely linked to the text `prompt`,
|
825 |
-
usually at the expense of lower image quality.
|
826 |
-
negative_prompt (`str` or `List[str]`, *optional*):
|
827 |
-
The prompt or prompts not to guide the image generation. If not defined, one has to pass
|
828 |
-
`negative_prompt_embeds` instead. Ignored when not using guidance (i.e., ignored if `guidance_scale` is
|
829 |
-
less than `1`).
|
830 |
-
num_images_per_prompt (`int`, *optional*, defaults to 1):
|
831 |
-
The number of images to generate per prompt.
|
832 |
-
eta (`float`, *optional*, defaults to 0.0):
|
833 |
-
Corresponds to parameter eta (η) in the DDIM paper: https://arxiv.org/abs/2010.02502. Only applies to
|
834 |
-
[`schedulers.DDIMScheduler`], will be ignored for others.
|
835 |
-
generator (`torch.Generator` or `List[torch.Generator]`, *optional*):
|
836 |
-
One or a list of [torch generator(s)](https://pytorch.org/docs/stable/generated/torch.Generator.html)
|
837 |
-
to make generation deterministic.
|
838 |
-
latents (`torch.FloatTensor`, *optional*):
|
839 |
-
Pre-generated noisy latents, sampled from a Gaussian distribution, to be used as inputs for image
|
840 |
-
generation. Can be used to tweak the same generation with different prompts. If not provided, a latents
|
841 |
-
tensor will ge generated by sampling using the supplied random `generator`.
|
842 |
-
prompt_embeds (`torch.FloatTensor`, *optional*):
|
843 |
-
Pre-generated text embeddings. Can be used to easily tweak text inputs, *e.g.* prompt weighting. If not
|
844 |
-
provided, text embeddings will be generated from `prompt` input argument.
|
845 |
-
negative_prompt_embeds (`torch.FloatTensor`, *optional*):
|
846 |
-
Pre-generated negative text embeddings. Can be used to easily tweak text inputs, *e.g.* prompt
|
847 |
-
weighting. If not provided, negative_prompt_embeds will be generated from `negative_prompt` input
|
848 |
-
argument.
|
849 |
-
cross_attention_guidance_amount (`float`, defaults to 0.1):
|
850 |
-
Amount of guidance needed from the reference cross-attention maps.
|
851 |
-
output_type (`str`, *optional*, defaults to `"pil"`):
|
852 |
-
The output format of the generate image. Choose between
|
853 |
-
[PIL](https://pillow.readthedocs.io/en/stable/): `PIL.Image.Image` or `np.array`.
|
854 |
-
return_dict (`bool`, *optional*, defaults to `True`):
|
855 |
-
Whether or not to return a [`~pipelines.stable_diffusion.StableDiffusionPipelineOutput`] instead of a
|
856 |
-
plain tuple.
|
857 |
-
callback (`Callable`, *optional*):
|
858 |
-
A function that will be called every `callback_steps` steps during inference. The function will be
|
859 |
-
called with the following arguments: `callback(step: int, timestep: int, latents: torch.FloatTensor)`.
|
860 |
-
callback_steps (`int`, *optional*, defaults to 1):
|
861 |
-
The frequency at which the `callback` function will be called. If not specified, the callback will be
|
862 |
-
called at every step.
|
863 |
-
|
864 |
-
Examples:
|
865 |
-
|
866 |
-
Returns:
|
867 |
-
[`~pipelines.stable_diffusion.StableDiffusionPipelineOutput`] or `tuple`:
|
868 |
-
[`~pipelines.stable_diffusion.StableDiffusionPipelineOutput`] if `return_dict` is True, otherwise a `tuple.
|
869 |
-
When returning a tuple, the first element is a list with the generated images, and the second element is a
|
870 |
-
list of `bool`s denoting whether the corresponding generated image likely represents "not-safe-for-work"
|
871 |
-
(nsfw) content, according to the `safety_checker`.
|
872 |
-
"""
|
873 |
-
# 0. Define the spatial resolutions.
|
874 |
-
height = height or self.unet.config.sample_size * self.vae_scale_factor
|
875 |
-
width = width or self.unet.config.sample_size * self.vae_scale_factor
|
876 |
-
|
877 |
-
# 1. Check inputs. Raise error if not correct
|
878 |
-
self.check_inputs(
|
879 |
-
prompt,
|
880 |
-
source_embeds,
|
881 |
-
target_embeds,
|
882 |
-
callback_steps,
|
883 |
-
prompt_embeds,
|
884 |
-
)
|
885 |
-
|
886 |
-
# 3. Define call parameters
|
887 |
-
if prompt is not None and isinstance(prompt, str):
|
888 |
-
batch_size = 1
|
889 |
-
elif prompt is not None and isinstance(prompt, list):
|
890 |
-
batch_size = len(prompt)
|
891 |
-
else:
|
892 |
-
batch_size = prompt_embeds.shape[0]
|
893 |
-
if cross_attention_kwargs is None:
|
894 |
-
cross_attention_kwargs = {}
|
895 |
-
|
896 |
-
device = self._execution_device
|
897 |
-
# here `guidance_scale` is defined analog to the guidance weight `w` of equation (2)
|
898 |
-
# of the Imagen paper: https://arxiv.org/pdf/2205.11487.pdf . `guidance_scale = 1`
|
899 |
-
# corresponds to doing no classifier free guidance.
|
900 |
-
do_classifier_free_guidance = guidance_scale > 1.0
|
901 |
-
|
902 |
-
# 3. Encode input prompt
|
903 |
-
prompt_embeds = self._encode_prompt(
|
904 |
-
prompt,
|
905 |
-
device,
|
906 |
-
num_images_per_prompt,
|
907 |
-
do_classifier_free_guidance,
|
908 |
-
negative_prompt,
|
909 |
-
prompt_embeds=prompt_embeds,
|
910 |
-
negative_prompt_embeds=negative_prompt_embeds,
|
911 |
-
)
|
912 |
-
|
913 |
-
# 4. Prepare timesteps
|
914 |
-
self.scheduler.set_timesteps(num_inference_steps, device=device)
|
915 |
-
timesteps = self.scheduler.timesteps
|
916 |
-
|
917 |
-
# 5. Generate the inverted noise from the input image or any other image
|
918 |
-
# generated from the input prompt.
|
919 |
-
num_channels_latents = self.unet.config.in_channels
|
920 |
-
latents = self.prepare_latents(
|
921 |
-
batch_size * num_images_per_prompt,
|
922 |
-
num_channels_latents,
|
923 |
-
height,
|
924 |
-
width,
|
925 |
-
prompt_embeds.dtype,
|
926 |
-
device,
|
927 |
-
generator,
|
928 |
-
latents,
|
929 |
-
)
|
930 |
-
latents_init = latents.clone()
|
931 |
-
|
932 |
-
# 6. Prepare extra step kwargs. TODO: Logic should ideally just be moved out of the pipeline
|
933 |
-
extra_step_kwargs = self.prepare_extra_step_kwargs(generator, eta)
|
934 |
-
|
935 |
-
# 8. Rejig the UNet so that we can obtain the cross-attenion maps and
|
936 |
-
# use them for guiding the subsequent image generation.
|
937 |
-
self.unet = prepare_unet(self.unet)
|
938 |
-
|
939 |
-
# 7. Denoising loop where we obtain the cross-attention maps.
|
940 |
-
num_warmup_steps = len(timesteps) - num_inference_steps * self.scheduler.order
|
941 |
-
with self.progress_bar(total=num_inference_steps) as progress_bar:
|
942 |
-
for i, t in enumerate(timesteps):
|
943 |
-
# expand the latents if we are doing classifier free guidance
|
944 |
-
latent_model_input = torch.cat([latents] * 2) if do_classifier_free_guidance else latents
|
945 |
-
latent_model_input = self.scheduler.scale_model_input(latent_model_input, t)
|
946 |
-
|
947 |
-
# predict the noise residual
|
948 |
-
noise_pred = self.unet(
|
949 |
-
latent_model_input,
|
950 |
-
t,
|
951 |
-
encoder_hidden_states=prompt_embeds,
|
952 |
-
cross_attention_kwargs={"timestep": t},
|
953 |
-
).sample
|
954 |
-
|
955 |
-
# perform guidance
|
956 |
-
if do_classifier_free_guidance:
|
957 |
-
noise_pred_uncond, noise_pred_text = noise_pred.chunk(2)
|
958 |
-
noise_pred = noise_pred_uncond + guidance_scale * (noise_pred_text - noise_pred_uncond)
|
959 |
-
|
960 |
-
# compute the previous noisy sample x_t -> x_t-1
|
961 |
-
latents = self.scheduler.step(noise_pred, t, latents, **extra_step_kwargs).prev_sample
|
962 |
-
|
963 |
-
# call the callback, if provided
|
964 |
-
if i == len(timesteps) - 1 or ((i + 1) > num_warmup_steps and (i + 1) % self.scheduler.order == 0):
|
965 |
-
progress_bar.update()
|
966 |
-
if callback is not None and i % callback_steps == 0:
|
967 |
-
callback(i, t, latents)
|
968 |
-
|
969 |
-
# 8. Compute the edit directions.
|
970 |
-
edit_direction = self.construct_direction(source_embeds, target_embeds).to(prompt_embeds.device)
|
971 |
-
|
972 |
-
# 9. Edit the prompt embeddings as per the edit directions discovered.
|
973 |
-
prompt_embeds_edit = prompt_embeds.clone()
|
974 |
-
prompt_embeds_edit[1:2] += edit_direction
|
975 |
-
|
976 |
-
# 10. Second denoising loop to generate the edited image.
|
977 |
-
latents = latents_init
|
978 |
-
num_warmup_steps = len(timesteps) - num_inference_steps * self.scheduler.order
|
979 |
-
with self.progress_bar(total=num_inference_steps) as progress_bar:
|
980 |
-
for i, t in enumerate(timesteps):
|
981 |
-
# expand the latents if we are doing classifier free guidance
|
982 |
-
latent_model_input = torch.cat([latents] * 2) if do_classifier_free_guidance else latents
|
983 |
-
latent_model_input = self.scheduler.scale_model_input(latent_model_input, t)
|
984 |
-
|
985 |
-
# we want to learn the latent such that it steers the generation
|
986 |
-
# process towards the edited direction, so make the make initial
|
987 |
-
# noise learnable
|
988 |
-
x_in = latent_model_input.detach().clone()
|
989 |
-
x_in.requires_grad = True
|
990 |
-
|
991 |
-
# optimizer
|
992 |
-
opt = torch.optim.SGD([x_in], lr=cross_attention_guidance_amount)
|
993 |
-
|
994 |
-
with torch.enable_grad():
|
995 |
-
# initialize loss
|
996 |
-
loss = Pix2PixZeroL2Loss()
|
997 |
-
|
998 |
-
# predict the noise residual
|
999 |
-
noise_pred = self.unet(
|
1000 |
-
x_in,
|
1001 |
-
t,
|
1002 |
-
encoder_hidden_states=prompt_embeds_edit.detach(),
|
1003 |
-
cross_attention_kwargs={"timestep": t, "loss": loss},
|
1004 |
-
).sample
|
1005 |
-
|
1006 |
-
loss.loss.backward(retain_graph=False)
|
1007 |
-
opt.step()
|
1008 |
-
|
1009 |
-
# recompute the noise
|
1010 |
-
noise_pred = self.unet(
|
1011 |
-
x_in.detach(),
|
1012 |
-
t,
|
1013 |
-
encoder_hidden_states=prompt_embeds_edit,
|
1014 |
-
cross_attention_kwargs={"timestep": None},
|
1015 |
-
).sample
|
1016 |
-
|
1017 |
-
latents = x_in.detach().chunk(2)[0]
|
1018 |
-
|
1019 |
-
# perform guidance
|
1020 |
-
if do_classifier_free_guidance:
|
1021 |
-
noise_pred_uncond, noise_pred_text = noise_pred.chunk(2)
|
1022 |
-
noise_pred = noise_pred_uncond + guidance_scale * (noise_pred_text - noise_pred_uncond)
|
1023 |
-
|
1024 |
-
# compute the previous noisy sample x_t -> x_t-1
|
1025 |
-
latents = self.scheduler.step(noise_pred, t, latents, **extra_step_kwargs).prev_sample
|
1026 |
-
|
1027 |
-
# call the callback, if provided
|
1028 |
-
if i == len(timesteps) - 1 or ((i + 1) > num_warmup_steps and (i + 1) % self.scheduler.order == 0):
|
1029 |
-
progress_bar.update()
|
1030 |
-
|
1031 |
-
if not output_type == "latent":
|
1032 |
-
image = self.vae.decode(latents / self.vae.config.scaling_factor, return_dict=False)[0]
|
1033 |
-
image, has_nsfw_concept = self.run_safety_checker(image, device, prompt_embeds.dtype)
|
1034 |
-
else:
|
1035 |
-
image = latents
|
1036 |
-
has_nsfw_concept = None
|
1037 |
-
|
1038 |
-
if has_nsfw_concept is None:
|
1039 |
-
do_denormalize = [True] * image.shape[0]
|
1040 |
-
else:
|
1041 |
-
do_denormalize = [not has_nsfw for has_nsfw in has_nsfw_concept]
|
1042 |
-
|
1043 |
-
image = self.image_processor.postprocess(image, output_type=output_type, do_denormalize=do_denormalize)
|
1044 |
-
|
1045 |
-
# Offload last model to CPU
|
1046 |
-
if hasattr(self, "final_offload_hook") and self.final_offload_hook is not None:
|
1047 |
-
self.final_offload_hook.offload()
|
1048 |
-
|
1049 |
-
if not return_dict:
|
1050 |
-
return (image, has_nsfw_concept)
|
1051 |
-
|
1052 |
-
return StableDiffusionPipelineOutput(images=image, nsfw_content_detected=has_nsfw_concept)
|
1053 |
-
|
1054 |
-
@torch.no_grad()
|
1055 |
-
@replace_example_docstring(EXAMPLE_INVERT_DOC_STRING)
|
1056 |
-
def invert(
|
1057 |
-
self,
|
1058 |
-
prompt: Optional[str] = None,
|
1059 |
-
image: Union[
|
1060 |
-
torch.FloatTensor,
|
1061 |
-
PIL.Image.Image,
|
1062 |
-
np.ndarray,
|
1063 |
-
List[torch.FloatTensor],
|
1064 |
-
List[PIL.Image.Image],
|
1065 |
-
List[np.ndarray],
|
1066 |
-
] = None,
|
1067 |
-
num_inference_steps: int = 50,
|
1068 |
-
guidance_scale: float = 1,
|
1069 |
-
generator: Optional[Union[torch.Generator, List[torch.Generator]]] = None,
|
1070 |
-
latents: Optional[torch.FloatTensor] = None,
|
1071 |
-
prompt_embeds: Optional[torch.FloatTensor] = None,
|
1072 |
-
cross_attention_guidance_amount: float = 0.1,
|
1073 |
-
output_type: Optional[str] = "pil",
|
1074 |
-
return_dict: bool = True,
|
1075 |
-
callback: Optional[Callable[[int, int, torch.FloatTensor], None]] = None,
|
1076 |
-
callback_steps: Optional[int] = 1,
|
1077 |
-
cross_attention_kwargs: Optional[Dict[str, Any]] = None,
|
1078 |
-
lambda_auto_corr: float = 20.0,
|
1079 |
-
lambda_kl: float = 20.0,
|
1080 |
-
num_reg_steps: int = 5,
|
1081 |
-
num_auto_corr_rolls: int = 5,
|
1082 |
-
):
|
1083 |
-
r"""
|
1084 |
-
Function used to generate inverted latents given a prompt and image.
|
1085 |
-
|
1086 |
-
Args:
|
1087 |
-
prompt (`str` or `List[str]`, *optional*):
|
1088 |
-
The prompt or prompts to guide the image generation. If not defined, one has to pass `prompt_embeds`.
|
1089 |
-
instead.
|
1090 |
-
image (`torch.FloatTensor` `np.ndarray`, `PIL.Image.Image`, `List[torch.FloatTensor]`, `List[PIL.Image.Image]`, or `List[np.ndarray]`):
|
1091 |
-
`Image`, or tensor representing an image batch which will be used for conditioning. Can also accpet
|
1092 |
-
image latents as `image`, if passing latents directly, it will not be encoded again.
|
1093 |
-
num_inference_steps (`int`, *optional*, defaults to 50):
|
1094 |
-
The number of denoising steps. More denoising steps usually lead to a higher quality image at the
|
1095 |
-
expense of slower inference.
|
1096 |
-
guidance_scale (`float`, *optional*, defaults to 1):
|
1097 |
-
Guidance scale as defined in [Classifier-Free Diffusion Guidance](https://arxiv.org/abs/2207.12598).
|
1098 |
-
`guidance_scale` is defined as `w` of equation 2. of [Imagen
|
1099 |
-
Paper](https://arxiv.org/pdf/2205.11487.pdf). Guidance scale is enabled by setting `guidance_scale >
|
1100 |
-
1`. Higher guidance scale encourages to generate images that are closely linked to the text `prompt`,
|
1101 |
-
usually at the expense of lower image quality.
|
1102 |
-
generator (`torch.Generator` or `List[torch.Generator]`, *optional*):
|
1103 |
-
One or a list of [torch generator(s)](https://pytorch.org/docs/stable/generated/torch.Generator.html)
|
1104 |
-
to make generation deterministic.
|
1105 |
-
latents (`torch.FloatTensor`, *optional*):
|
1106 |
-
Pre-generated noisy latents, sampled from a Gaussian distribution, to be used as inputs for image
|
1107 |
-
generation. Can be used to tweak the same generation with different prompts. If not provided, a latents
|
1108 |
-
tensor will ge generated by sampling using the supplied random `generator`.
|
1109 |
-
prompt_embeds (`torch.FloatTensor`, *optional*):
|
1110 |
-
Pre-generated text embeddings. Can be used to easily tweak text inputs, *e.g.* prompt weighting. If not
|
1111 |
-
provided, text embeddings will be generated from `prompt` input argument.
|
1112 |
-
cross_attention_guidance_amount (`float`, defaults to 0.1):
|
1113 |
-
Amount of guidance needed from the reference cross-attention maps.
|
1114 |
-
output_type (`str`, *optional*, defaults to `"pil"`):
|
1115 |
-
The output format of the generate image. Choose between
|
1116 |
-
[PIL](https://pillow.readthedocs.io/en/stable/): `PIL.Image.Image` or `np.array`.
|
1117 |
-
return_dict (`bool`, *optional*, defaults to `True`):
|
1118 |
-
Whether or not to return a [`~pipelines.stable_diffusion.StableDiffusionPipelineOutput`] instead of a
|
1119 |
-
plain tuple.
|
1120 |
-
callback (`Callable`, *optional*):
|
1121 |
-
A function that will be called every `callback_steps` steps during inference. The function will be
|
1122 |
-
called with the following arguments: `callback(step: int, timestep: int, latents: torch.FloatTensor)`.
|
1123 |
-
callback_steps (`int`, *optional*, defaults to 1):
|
1124 |
-
The frequency at which the `callback` function will be called. If not specified, the callback will be
|
1125 |
-
called at every step.
|
1126 |
-
lambda_auto_corr (`float`, *optional*, defaults to 20.0):
|
1127 |
-
Lambda parameter to control auto correction
|
1128 |
-
lambda_kl (`float`, *optional*, defaults to 20.0):
|
1129 |
-
Lambda parameter to control Kullback–Leibler divergence output
|
1130 |
-
num_reg_steps (`int`, *optional*, defaults to 5):
|
1131 |
-
Number of regularization loss steps
|
1132 |
-
num_auto_corr_rolls (`int`, *optional*, defaults to 5):
|
1133 |
-
Number of auto correction roll steps
|
1134 |
-
|
1135 |
-
Examples:
|
1136 |
-
|
1137 |
-
Returns:
|
1138 |
-
[`~pipelines.stable_diffusion.pipeline_stable_diffusion_pix2pix_zero.Pix2PixInversionPipelineOutput`] or
|
1139 |
-
`tuple`:
|
1140 |
-
[`~pipelines.stable_diffusion.pipeline_stable_diffusion_pix2pix_zero.Pix2PixInversionPipelineOutput`] if
|
1141 |
-
`return_dict` is True, otherwise a `tuple. When returning a tuple, the first element is the inverted
|
1142 |
-
latents tensor and then second is the corresponding decoded image.
|
1143 |
-
"""
|
1144 |
-
# 1. Define call parameters
|
1145 |
-
if prompt is not None and isinstance(prompt, str):
|
1146 |
-
batch_size = 1
|
1147 |
-
elif prompt is not None and isinstance(prompt, list):
|
1148 |
-
batch_size = len(prompt)
|
1149 |
-
else:
|
1150 |
-
batch_size = prompt_embeds.shape[0]
|
1151 |
-
if cross_attention_kwargs is None:
|
1152 |
-
cross_attention_kwargs = {}
|
1153 |
-
|
1154 |
-
device = self._execution_device
|
1155 |
-
# here `guidance_scale` is defined analog to the guidance weight `w` of equation (2)
|
1156 |
-
# of the Imagen paper: https://arxiv.org/pdf/2205.11487.pdf . `guidance_scale = 1`
|
1157 |
-
# corresponds to doing no classifier free guidance.
|
1158 |
-
do_classifier_free_guidance = guidance_scale > 1.0
|
1159 |
-
|
1160 |
-
# 3. Preprocess image
|
1161 |
-
image = self.image_processor.preprocess(image)
|
1162 |
-
|
1163 |
-
# 4. Prepare latent variables
|
1164 |
-
latents = self.prepare_image_latents(image, batch_size, self.vae.dtype, device, generator)
|
1165 |
-
|
1166 |
-
# 5. Encode input prompt
|
1167 |
-
num_images_per_prompt = 1
|
1168 |
-
prompt_embeds = self._encode_prompt(
|
1169 |
-
prompt,
|
1170 |
-
device,
|
1171 |
-
num_images_per_prompt,
|
1172 |
-
do_classifier_free_guidance,
|
1173 |
-
prompt_embeds=prompt_embeds,
|
1174 |
-
)
|
1175 |
-
|
1176 |
-
# 4. Prepare timesteps
|
1177 |
-
self.inverse_scheduler.set_timesteps(num_inference_steps, device=device)
|
1178 |
-
timesteps = self.inverse_scheduler.timesteps
|
1179 |
-
|
1180 |
-
# 6. Rejig the UNet so that we can obtain the cross-attenion maps and
|
1181 |
-
# use them for guiding the subsequent image generation.
|
1182 |
-
self.unet = prepare_unet(self.unet)
|
1183 |
-
|
1184 |
-
# 7. Denoising loop where we obtain the cross-attention maps.
|
1185 |
-
num_warmup_steps = len(timesteps) - num_inference_steps * self.inverse_scheduler.order
|
1186 |
-
with self.progress_bar(total=num_inference_steps) as progress_bar:
|
1187 |
-
for i, t in enumerate(timesteps):
|
1188 |
-
# expand the latents if we are doing classifier free guidance
|
1189 |
-
latent_model_input = torch.cat([latents] * 2) if do_classifier_free_guidance else latents
|
1190 |
-
latent_model_input = self.inverse_scheduler.scale_model_input(latent_model_input, t)
|
1191 |
-
|
1192 |
-
# predict the noise residual
|
1193 |
-
noise_pred = self.unet(
|
1194 |
-
latent_model_input,
|
1195 |
-
t,
|
1196 |
-
encoder_hidden_states=prompt_embeds,
|
1197 |
-
cross_attention_kwargs={"timestep": t},
|
1198 |
-
).sample
|
1199 |
-
|
1200 |
-
# perform guidance
|
1201 |
-
if do_classifier_free_guidance:
|
1202 |
-
noise_pred_uncond, noise_pred_text = noise_pred.chunk(2)
|
1203 |
-
noise_pred = noise_pred_uncond + guidance_scale * (noise_pred_text - noise_pred_uncond)
|
1204 |
-
|
1205 |
-
# regularization of the noise prediction
|
1206 |
-
with torch.enable_grad():
|
1207 |
-
for _ in range(num_reg_steps):
|
1208 |
-
if lambda_auto_corr > 0:
|
1209 |
-
for _ in range(num_auto_corr_rolls):
|
1210 |
-
var = torch.autograd.Variable(noise_pred.detach().clone(), requires_grad=True)
|
1211 |
-
|
1212 |
-
# Derive epsilon from model output before regularizing to IID standard normal
|
1213 |
-
var_epsilon = self.get_epsilon(var, latent_model_input.detach(), t)
|
1214 |
-
|
1215 |
-
l_ac = self.auto_corr_loss(var_epsilon, generator=generator)
|
1216 |
-
l_ac.backward()
|
1217 |
-
|
1218 |
-
grad = var.grad.detach() / num_auto_corr_rolls
|
1219 |
-
noise_pred = noise_pred - lambda_auto_corr * grad
|
1220 |
-
|
1221 |
-
if lambda_kl > 0:
|
1222 |
-
var = torch.autograd.Variable(noise_pred.detach().clone(), requires_grad=True)
|
1223 |
-
|
1224 |
-
# Derive epsilon from model output before regularizing to IID standard normal
|
1225 |
-
var_epsilon = self.get_epsilon(var, latent_model_input.detach(), t)
|
1226 |
-
|
1227 |
-
l_kld = self.kl_divergence(var_epsilon)
|
1228 |
-
l_kld.backward()
|
1229 |
-
|
1230 |
-
grad = var.grad.detach()
|
1231 |
-
noise_pred = noise_pred - lambda_kl * grad
|
1232 |
-
|
1233 |
-
noise_pred = noise_pred.detach()
|
1234 |
-
|
1235 |
-
# compute the previous noisy sample x_t -> x_t-1
|
1236 |
-
latents = self.inverse_scheduler.step(noise_pred, t, latents).prev_sample
|
1237 |
-
|
1238 |
-
# call the callback, if provided
|
1239 |
-
if i == len(timesteps) - 1 or (
|
1240 |
-
(i + 1) > num_warmup_steps and (i + 1) % self.inverse_scheduler.order == 0
|
1241 |
-
):
|
1242 |
-
progress_bar.update()
|
1243 |
-
if callback is not None and i % callback_steps == 0:
|
1244 |
-
callback(i, t, latents)
|
1245 |
-
|
1246 |
-
inverted_latents = latents.detach().clone()
|
1247 |
-
|
1248 |
-
# 8. Post-processing
|
1249 |
-
image = self.vae.decode(latents / self.vae.config.scaling_factor, return_dict=False)[0]
|
1250 |
-
image = self.image_processor.postprocess(image, output_type=output_type)
|
1251 |
-
|
1252 |
-
# Offload last model to CPU
|
1253 |
-
if hasattr(self, "final_offload_hook") and self.final_offload_hook is not None:
|
1254 |
-
self.final_offload_hook.offload()
|
1255 |
-
|
1256 |
-
if not return_dict:
|
1257 |
-
return (inverted_latents, image)
|
1258 |
-
|
1259 |
-
return Pix2PixInversionPipelineOutput(latents=inverted_latents, images=image)
|
|
|
|
|
|
|
|
|
|
|
|
|
|
|
|
|
|
|
|
|
|
|
|
|
|
|
|
|
|
|
|
|
|
|
|
|
|
|
|
|
|
|
|
|
|
|
|
|
|
|
|
|
|
|
|
|
|
|
|
|
|
|
|
|
|
|
|
|
|
|
|
|
|
|
|
|
|
|
|
|
|
|
|
|
|
|
|
|
|
|
|
|
|
|
|
|
|
|
|
|
|
|
|
|
|
|
|
|
|
|
|
|
|
|
|
|
|
|
|
|
|
|
|
|
|
|
|
|
|
|
|
|
|
|
|
|
|
|
|
|
|
|
|
|
|
|
|
|
|
|
|
|
|
|
|
|
|
|
|
|
|
|
|
|
|
|
|
|
|
|
|
|
|
|
|
|
|
|
|
|
|
|
|
|
|
|
|
|
|
|
|
|
|
|
|
|
|
|
|
|
|
|
|
|
|
|
|
|
|
|
|
|
|
|
|
|
|
|
|
|
|
|
|
|
|
|
|
|
|
|
|
|
|
|
|
|
|
|
|
|
|
|
|
|
|
|
|
|
|
|
|
|
|
|
|
|
|
|
|
|
|
|
|
|
|
|
|
|
|
|
|
|
|
|
|
|
|
|
|
|
|
|
|
|
|
|
|
|
|
|
|
|
|
|
|
|
|
|
|
|
|
|
|
|
|
|
|
|
|
|
|
|
|
|
|
|
|
|
|
|
|
|
|
|
|
|
|
|
|
|
|
|
|
|
|
|
|
|
|
|
|
|
|
|
|
|
|
|
|
|
|
|
|
|
|
|
|
|
|
|
|
|
|
|
|
|
|
|
|
|
|
|
|
|
|
|
|
|
|
|
|
|
|
|
|
|
|
|
|
|
|
|
|
|
|
|
|
|
|
|
|
|
|
|
|
|
|
|
|
|
|
|
|
|
|
|
|
|
|
|
|
|
|
|
|
|
|
|
|
|
|
|
|
|
|
|
|
|
|
|
|
|
|
|
|
|
|
|
|
|
|
|
|
|
|
|
|
|
|
|
|
|
|
|
|
|
|
|
|
|
|
|
|
|
|
|
|
|
|
|
|
|
|
|
|
|
|
|
|
|
|
|
|
|
|
|
|
|
|
|
|
|
|
|
|
|
|
|
|
|
|
|
|
|
|
|
|
|
|
|
|
|
|
|
|
|
|
|
|
|
|
|
|
|
|
|
|
|
|
|
|
|
|
|
|
|
|
|
|
|
|
|
|
|
|
|
|
|
|
|
|
|
|
|
|
|
|
|
|
|
|
|
|
|
|
|
|
|
|
|
|
|
|
|
|
|
|
|
|
|
|
|
|
|
|
|
|
|
|
|
|
|
|
|
|
|
|
|
|
|
|
|
|
|
|
|
|
|
|
|
|
|
|
|
|
|
|
|
|
|
|
|
|
|
|
|
|
|
|
|
|
|
|
|
|
|
|
|
|
|
|
|
|
|
|
|
|
|
|
|
|
|
|
|
|
|
|
|
|
|
|
|
|
|
|
|
|
|
|
|
|
|
|
|
|
|
|
|
|
|
|
|
|
|
|
|
|
|
|
|
|
|
|
|
|
|
|
|
|
|
|
|
|
|
|
|
|
|
|
|
|
|
|
|
|
|
|
|
|
|
|
|
|
|
|
|
|
|
|
|
|
|
|
|
|
|
|
|
|
|
|
|
|
|
|
|
|
|
|
|
|
|
|
|
|
|
|
|
|
|
|
|
|
|
|
|
|
|
|
|
|
|
|
|
|
|
|
|
|
|
|
|
|
|
|
|
|
|
|
|
|
|
|
|
|
|
|
|
|
|
|
|
|
|
|
|
|
|
|
|
|
|
|
|
|
|
|
|
|
|
|
|
|
|
|
|
|
|
|
|
|
|
|
|
|
|
|
|
|
|
|
|
|
|
|
|
|
|
|
|
|
|
|
|
|
|
|
|
|
|
|
|
|
|
|
|
|
|
|
|
|
|
|
|
|
|
|
|
|
|
|
|
|
|
|
|
|
|
|
|
|
|
|
|
|
|
|
|
|
|
|
|
|
|
|
|
|
|
|
|
|
|
|
|
|
|
|
|
|
|
|
|
|
|
|
|
|
|
|
|
|
|
|
|
|
|
|
|
|
|
|
|
|
|
|
|
|
|
|
|
|
|
|
|
|
|
|
|
|
|
|
|
|
|
|
|
|
|
|
|
|
|
|
|
|
|
|
|
|
|
|
|
|
|
|
|
|
|
|
|
|
|
|
|
|
|
|
|
|
|
|
|
|
|
|
|
|
|
|
|
|
|
|
|
|
|
|
|
|
|
|
|
|
|
|
|
|
|
|
|
|
|
|
|
|
|
|
|
|
|
|
|
|
|
|
|
|
|
|
|
|
|
|
|
|
|
|
|
|
|
|
|
|
|
|
|
|
|
|
|
|
|
|
|
|
|
|
|
|
|
|
|
|
|
|
|
|
|
|
|
|
|
|
|
|
|
|
|
|
|
|
|
|
|
|
|
|
|
|
|
|
|
|
|
|
|
|
|
|
|
|
|
|
|
|
|
|
|
|
|
|
|
|
|
|
|
|
|
|
|
|
|
|
|
|
|
|
|
|
|
|
|
|
|
|
|
|
|
|
|
|
|
|
|
|
|
|
|
|
|
|
|
|
|
|
|
|
|
|
|
|
|
|
|
|
|
|
|
|
|
|
|
|
|
|
|
|
|
|
|
|
|
|
|
|
|
|
|
|
|
|
|
|
|
|
|
|
|
|
|
|
|
|
|
|
|
|
|
|
|
|
|
|
|
|
|
|
|
|
|
|
|
|
|
|
|
|
|
|
|
|
|
|
|
|
|
|
|
|
|
|
|
|
|
|
|
|
|
|
|
|
|
|
|
|
|
|
|
|
|
|
|
|
|
|
|
|
|
|
|
|
|
|
|
|
|
|
|
|
|
|
|
|
|
|
|
|
|
|
|
|
|
|
|
|
|
|
|
|
|
|
|
|
|
|
|
|
|
|
|
|
|
|
|
|
|
|
|
|
|
|
|
|
|
|
|
|
|
|
|
|
|
|
|
|
|
|
|
|
|
|
|
|
|
|
|
|
|
|
|
|
|
|
|
|
|
|
|
|
|
|
|
|
|
|
|
|
|
|
|
|
|
|
|
|
|
|
|
|
|
|
|
|
|
|
|
|
|
|
|
|
|
|
|
|
|
|
|
|
|
|
|
|
|
|
|
|
|
|
|
|
|
|
|
|
|
|
|
|
|
|
|
|
|
|
|
|
|
|
|
|
|
|
|
|
|
|
|
|
|
|
|
|
|
|
|
|
|
|
|
|
|
|
|
|
|
|
|
|
|
|
|
|
|
|
|
|
|
|
|
|
|
|
|
|
|
|
|
|
|
|
|
|
|
|
|
|
|
|
|
|
|
|
|
|
|
|
|
|
|
|
|
|
|
|
|
|
|
|
|
|
|
|
|
|
|
|
|
|
|
|
|
|
|
|
|
|
|
|
|
|
|
|
|
|
|
|
|
|
|
|
|
|
|
|
|
|
|
|
|
|
|
|
|
|
|
|
|
|
|
|
|
|
|
|
|
|
|
|
|
|
|
|
|
|
|
|
|
|
|
|
|
|
|
|
|
|
|
|
|
|
|
|
|
|
|
|
|
|
|
|
|
|
|
|
|
|
|
|
|
|
|
|
|
|
|
|
|
|
|
|
|
|
|
|
|
|
|
|
|
|
|
|
|
|
|
|
|
|
|
|
|
|
|
|
|
|
|
|
|
|
|
|
|
|
|
|
|
|
|
|
|
|
|
|
|
|
|
|
|
|
|
|
|
|
|
|
|
|
|
|
|
|
|
|
|
|
|
|
|
|
|
|
|
|
|
|
|
|
|
|
|
|
|
|
|
|
|
|
|
|
|
|
|
|
|
|
|
|
|
|
|
|
|
|
|
|
|
|
|
|
|
|
|
|
|
|
|
|
|
|
|
|
|
|
|
|
|
|
|
|
|
|
|
|
|
|
|
|
|
|
|
|
|
|
|
|
|
|
|
|
|
|
|
|
|
|
|
|
|
|
|
|
|
|
|
|
|
|
|
|
|
|
|
|
|
|
|
|
|
|
|
|
|
|
|
|
|
|
|
|
|
|
|
|
|
|
|
|
|
|
|
|
|
|
|
|
|
|
|
|
|
|
|
|
|
|
|
|
|
|
|
|
|
|
|
|
|
|
|
|
|
|
|
|
|
|
|
|
|
|
|
|
|
|
|
|
|
|
|
|
|
|
|
|
|
|
|
|
|
|
|
|
|
|
|
|
|
|
|
|
|
|
|
|
|
|
|
|
|
|
|
|
|
|
|
|
|
|
|
|
|
|
|
|
|
|
|
|
|
|
|
|
|
|
|
|
|
|
|
|
|
|
|
|
|
|
|
|
|
|
|
|
|
|
|
|
|
|
|
|
|
|
|
|
|
|
|
|
|
|
|
|
|
|
|
|
|
|
|
|
|
|
|
|
|
|
|
|
|
|
|
|
|
|
|
|
|
|
|
|
|
|
|
|
|
|
|
|
|
|
|
|
|
|
|
|
|
|
|
|
|
|
|
|
|
|
|
|
|
|
|
|
|
|
|
|
|
|
|
|
|
|
|
|
|
|
|
|
|
|
|
|
|
|
|
|
|
|
|
|
|
|
|
|
|
|
|
|
|
|
|
|
|
|
|
|
|
|
|
|
|
|
|
|
|
|
|
|
|
|
|
|
|
|
|
|
|
|
|
|
|
|
|
|
|
|
|
|
|
|
|
|
|
|
|
|
|
|
|
|
|
|
|
|
|
|
|
|
|
|
|
|
|
|
|
|
|
|
|
|
|
|
|
|
|
|
|
|
|
|
|
|
|
|
|
|
|
|
|
|
|
|
|
|
|
|
|
|
|
|
|
|
|
|
|
|
|
|
|
|
|
|
|
|
|
|
|
|
|
|
|
|
|
|
|
|
|
|
|
|
|
|
|
|
|
|
|
|
|
|
|
|
|
|
|
|
|
|
|
|
|
|
|
|
|
|
|
|
|
|
|
|
|
|
|
|
|
|
|
|
|
|
|
|
|
|
|
|
|
|
|
|
|
|
|
|
|
|
|
|
|
|
|
|
|
|
|
|
|
|
|
|
|
|
|
|
|
|
|
|
|
|
|
|
|
|
|
|
|
|
|
|
|
|
|
|
|
|
|
|
|
|
|
|
|
|
|
|
|
|
|
|
|
|
|
|
|
|
|
|
|
|
|
|
|
|
|
|
|
|
|
|
|
|
|
|
|
|
|
|
|
|
|
|
|
|
|
|
|
|
|
|
|
|
|
|
|
|
|
|
|
|
|
|
|
|
|
|
|
|
|
|
|
|
|
|
|
|
|
|
|
|
|
|
|
|
|
|
|
|
|
|
|
|
|
|
|
|
|
|
|
|
|
|
|
|
|
|
|
|
|
|
|
|
|
|
|
|
|
|
|
|
|
|
|
|
|
|
|
|
|
|
|
|
|
|
|
|
|
|
|
|
|
|
|
|
|
|
|
|
|
|
|
|
|
|
|
|
|
|
|
|
|
|
|
|
|
|
|
|
|
|
|
|
|
|
|
|
|
|
|
|
|
|
|
|
|
|
|
|
|
|
|
|
|
|
|
|
spaces/Androidonnxfork/CivitAi-to-Diffusers/diffusers/src/diffusers/pipelines/stable_diffusion_xl/__init__.py
DELETED
@@ -1,38 +0,0 @@
|
|
1 |
-
from dataclasses import dataclass
|
2 |
-
from typing import List, Optional, Union
|
3 |
-
|
4 |
-
import numpy as np
|
5 |
-
import PIL
|
6 |
-
|
7 |
-
from ...utils import (
|
8 |
-
BaseOutput,
|
9 |
-
OptionalDependencyNotAvailable,
|
10 |
-
is_torch_available,
|
11 |
-
is_transformers_available,
|
12 |
-
)
|
13 |
-
|
14 |
-
|
15 |
-
@dataclass
|
16 |
-
class StableDiffusionXLPipelineOutput(BaseOutput):
|
17 |
-
"""
|
18 |
-
Output class for Stable Diffusion pipelines.
|
19 |
-
|
20 |
-
Args:
|
21 |
-
images (`List[PIL.Image.Image]` or `np.ndarray`)
|
22 |
-
List of denoised PIL images of length `batch_size` or numpy array of shape `(batch_size, height, width,
|
23 |
-
num_channels)`. PIL images or numpy array present the denoised images of the diffusion pipeline.
|
24 |
-
"""
|
25 |
-
|
26 |
-
images: Union[List[PIL.Image.Image], np.ndarray]
|
27 |
-
|
28 |
-
|
29 |
-
try:
|
30 |
-
if not (is_transformers_available() and is_torch_available()):
|
31 |
-
raise OptionalDependencyNotAvailable()
|
32 |
-
except OptionalDependencyNotAvailable:
|
33 |
-
from ...utils.dummy_torch_and_transformers_objects import * # noqa F403
|
34 |
-
else:
|
35 |
-
from .pipeline_stable_diffusion_xl import StableDiffusionXLPipeline
|
36 |
-
from .pipeline_stable_diffusion_xl_img2img import StableDiffusionXLImg2ImgPipeline
|
37 |
-
from .pipeline_stable_diffusion_xl_inpaint import StableDiffusionXLInpaintPipeline
|
38 |
-
from .pipeline_stable_diffusion_xl_instruct_pix2pix import StableDiffusionXLInstructPix2PixPipeline
|
|
|
|
|
|
|
|
|
|
|
|
|
|
|
|
|
|
|
|
|
|
|
|
|
|
|
|
|
|
|
|
|
|
|
|
|
|
|
|
|
|
|
|
|
|
|
|
|
|
|
|
|
|
|
|
|
|
|
|
|
|
|
|
|
|
|
|
|
|
|
|
|
|
|
|
|
|
spaces/Andy1621/uniformer_image_demo/imagenet_class_index.py
DELETED
@@ -1,1002 +0,0 @@
|
|
1 |
-
imagenet_classnames = {
|
2 |
-
"0": ["n01440764", "tench"],
|
3 |
-
"1": ["n01443537", "goldfish"],
|
4 |
-
"2": ["n01484850", "great_white_shark"],
|
5 |
-
"3": ["n01491361", "tiger_shark"],
|
6 |
-
"4": ["n01494475", "hammerhead"],
|
7 |
-
"5": ["n01496331", "electric_ray"],
|
8 |
-
"6": ["n01498041", "stingray"],
|
9 |
-
"7": ["n01514668", "cock"],
|
10 |
-
"8": ["n01514859", "hen"],
|
11 |
-
"9": ["n01518878", "ostrich"],
|
12 |
-
"10": ["n01530575", "brambling"],
|
13 |
-
"11": ["n01531178", "goldfinch"],
|
14 |
-
"12": ["n01532829", "house_finch"],
|
15 |
-
"13": ["n01534433", "junco"],
|
16 |
-
"14": ["n01537544", "indigo_bunting"],
|
17 |
-
"15": ["n01558993", "robin"],
|
18 |
-
"16": ["n01560419", "bulbul"],
|
19 |
-
"17": ["n01580077", "jay"],
|
20 |
-
"18": ["n01582220", "magpie"],
|
21 |
-
"19": ["n01592084", "chickadee"],
|
22 |
-
"20": ["n01601694", "water_ouzel"],
|
23 |
-
"21": ["n01608432", "kite"],
|
24 |
-
"22": ["n01614925", "bald_eagle"],
|
25 |
-
"23": ["n01616318", "vulture"],
|
26 |
-
"24": ["n01622779", "great_grey_owl"],
|
27 |
-
"25": ["n01629819", "European_fire_salamander"],
|
28 |
-
"26": ["n01630670", "common_newt"],
|
29 |
-
"27": ["n01631663", "eft"],
|
30 |
-
"28": ["n01632458", "spotted_salamander"],
|
31 |
-
"29": ["n01632777", "axolotl"],
|
32 |
-
"30": ["n01641577", "bullfrog"],
|
33 |
-
"31": ["n01644373", "tree_frog"],
|
34 |
-
"32": ["n01644900", "tailed_frog"],
|
35 |
-
"33": ["n01664065", "loggerhead"],
|
36 |
-
"34": ["n01665541", "leatherback_turtle"],
|
37 |
-
"35": ["n01667114", "mud_turtle"],
|
38 |
-
"36": ["n01667778", "terrapin"],
|
39 |
-
"37": ["n01669191", "box_turtle"],
|
40 |
-
"38": ["n01675722", "banded_gecko"],
|
41 |
-
"39": ["n01677366", "common_iguana"],
|
42 |
-
"40": ["n01682714", "American_chameleon"],
|
43 |
-
"41": ["n01685808", "whiptail"],
|
44 |
-
"42": ["n01687978", "agama"],
|
45 |
-
"43": ["n01688243", "frilled_lizard"],
|
46 |
-
"44": ["n01689811", "alligator_lizard"],
|
47 |
-
"45": ["n01692333", "Gila_monster"],
|
48 |
-
"46": ["n01693334", "green_lizard"],
|
49 |
-
"47": ["n01694178", "African_chameleon"],
|
50 |
-
"48": ["n01695060", "Komodo_dragon"],
|
51 |
-
"49": ["n01697457", "African_crocodile"],
|
52 |
-
"50": ["n01698640", "American_alligator"],
|
53 |
-
"51": ["n01704323", "triceratops"],
|
54 |
-
"52": ["n01728572", "thunder_snake"],
|
55 |
-
"53": ["n01728920", "ringneck_snake"],
|
56 |
-
"54": ["n01729322", "hognose_snake"],
|
57 |
-
"55": ["n01729977", "green_snake"],
|
58 |
-
"56": ["n01734418", "king_snake"],
|
59 |
-
"57": ["n01735189", "garter_snake"],
|
60 |
-
"58": ["n01737021", "water_snake"],
|
61 |
-
"59": ["n01739381", "vine_snake"],
|
62 |
-
"60": ["n01740131", "night_snake"],
|
63 |
-
"61": ["n01742172", "boa_constrictor"],
|
64 |
-
"62": ["n01744401", "rock_python"],
|
65 |
-
"63": ["n01748264", "Indian_cobra"],
|
66 |
-
"64": ["n01749939", "green_mamba"],
|
67 |
-
"65": ["n01751748", "sea_snake"],
|
68 |
-
"66": ["n01753488", "horned_viper"],
|
69 |
-
"67": ["n01755581", "diamondback"],
|
70 |
-
"68": ["n01756291", "sidewinder"],
|
71 |
-
"69": ["n01768244", "trilobite"],
|
72 |
-
"70": ["n01770081", "harvestman"],
|
73 |
-
"71": ["n01770393", "scorpion"],
|
74 |
-
"72": ["n01773157", "black_and_gold_garden_spider"],
|
75 |
-
"73": ["n01773549", "barn_spider"],
|
76 |
-
"74": ["n01773797", "garden_spider"],
|
77 |
-
"75": ["n01774384", "black_widow"],
|
78 |
-
"76": ["n01774750", "tarantula"],
|
79 |
-
"77": ["n01775062", "wolf_spider"],
|
80 |
-
"78": ["n01776313", "tick"],
|
81 |
-
"79": ["n01784675", "centipede"],
|
82 |
-
"80": ["n01795545", "black_grouse"],
|
83 |
-
"81": ["n01796340", "ptarmigan"],
|
84 |
-
"82": ["n01797886", "ruffed_grouse"],
|
85 |
-
"83": ["n01798484", "prairie_chicken"],
|
86 |
-
"84": ["n01806143", "peacock"],
|
87 |
-
"85": ["n01806567", "quail"],
|
88 |
-
"86": ["n01807496", "partridge"],
|
89 |
-
"87": ["n01817953", "African_grey"],
|
90 |
-
"88": ["n01818515", "macaw"],
|
91 |
-
"89": ["n01819313", "sulphur-crested_cockatoo"],
|
92 |
-
"90": ["n01820546", "lorikeet"],
|
93 |
-
"91": ["n01824575", "coucal"],
|
94 |
-
"92": ["n01828970", "bee_eater"],
|
95 |
-
"93": ["n01829413", "hornbill"],
|
96 |
-
"94": ["n01833805", "hummingbird"],
|
97 |
-
"95": ["n01843065", "jacamar"],
|
98 |
-
"96": ["n01843383", "toucan"],
|
99 |
-
"97": ["n01847000", "drake"],
|
100 |
-
"98": ["n01855032", "red-breasted_merganser"],
|
101 |
-
"99": ["n01855672", "goose"],
|
102 |
-
"100": ["n01860187", "black_swan"],
|
103 |
-
"101": ["n01871265", "tusker"],
|
104 |
-
"102": ["n01872401", "echidna"],
|
105 |
-
"103": ["n01873310", "platypus"],
|
106 |
-
"104": ["n01877812", "wallaby"],
|
107 |
-
"105": ["n01882714", "koala"],
|
108 |
-
"106": ["n01883070", "wombat"],
|
109 |
-
"107": ["n01910747", "jellyfish"],
|
110 |
-
"108": ["n01914609", "sea_anemone"],
|
111 |
-
"109": ["n01917289", "brain_coral"],
|
112 |
-
"110": ["n01924916", "flatworm"],
|
113 |
-
"111": ["n01930112", "nematode"],
|
114 |
-
"112": ["n01943899", "conch"],
|
115 |
-
"113": ["n01944390", "snail"],
|
116 |
-
"114": ["n01945685", "slug"],
|
117 |
-
"115": ["n01950731", "sea_slug"],
|
118 |
-
"116": ["n01955084", "chiton"],
|
119 |
-
"117": ["n01968897", "chambered_nautilus"],
|
120 |
-
"118": ["n01978287", "Dungeness_crab"],
|
121 |
-
"119": ["n01978455", "rock_crab"],
|
122 |
-
"120": ["n01980166", "fiddler_crab"],
|
123 |
-
"121": ["n01981276", "king_crab"],
|
124 |
-
"122": ["n01983481", "American_lobster"],
|
125 |
-
"123": ["n01984695", "spiny_lobster"],
|
126 |
-
"124": ["n01985128", "crayfish"],
|
127 |
-
"125": ["n01986214", "hermit_crab"],
|
128 |
-
"126": ["n01990800", "isopod"],
|
129 |
-
"127": ["n02002556", "white_stork"],
|
130 |
-
"128": ["n02002724", "black_stork"],
|
131 |
-
"129": ["n02006656", "spoonbill"],
|
132 |
-
"130": ["n02007558", "flamingo"],
|
133 |
-
"131": ["n02009229", "little_blue_heron"],
|
134 |
-
"132": ["n02009912", "American_egret"],
|
135 |
-
"133": ["n02011460", "bittern"],
|
136 |
-
"134": ["n02012849", "crane"],
|
137 |
-
"135": ["n02013706", "limpkin"],
|
138 |
-
"136": ["n02017213", "European_gallinule"],
|
139 |
-
"137": ["n02018207", "American_coot"],
|
140 |
-
"138": ["n02018795", "bustard"],
|
141 |
-
"139": ["n02025239", "ruddy_turnstone"],
|
142 |
-
"140": ["n02027492", "red-backed_sandpiper"],
|
143 |
-
"141": ["n02028035", "redshank"],
|
144 |
-
"142": ["n02033041", "dowitcher"],
|
145 |
-
"143": ["n02037110", "oystercatcher"],
|
146 |
-
"144": ["n02051845", "pelican"],
|
147 |
-
"145": ["n02056570", "king_penguin"],
|
148 |
-
"146": ["n02058221", "albatross"],
|
149 |
-
"147": ["n02066245", "grey_whale"],
|
150 |
-
"148": ["n02071294", "killer_whale"],
|
151 |
-
"149": ["n02074367", "dugong"],
|
152 |
-
"150": ["n02077923", "sea_lion"],
|
153 |
-
"151": ["n02085620", "Chihuahua"],
|
154 |
-
"152": ["n02085782", "Japanese_spaniel"],
|
155 |
-
"153": ["n02085936", "Maltese_dog"],
|
156 |
-
"154": ["n02086079", "Pekinese"],
|
157 |
-
"155": ["n02086240", "Shih-Tzu"],
|
158 |
-
"156": ["n02086646", "Blenheim_spaniel"],
|
159 |
-
"157": ["n02086910", "papillon"],
|
160 |
-
"158": ["n02087046", "toy_terrier"],
|
161 |
-
"159": ["n02087394", "Rhodesian_ridgeback"],
|
162 |
-
"160": ["n02088094", "Afghan_hound"],
|
163 |
-
"161": ["n02088238", "basset"],
|
164 |
-
"162": ["n02088364", "beagle"],
|
165 |
-
"163": ["n02088466", "bloodhound"],
|
166 |
-
"164": ["n02088632", "bluetick"],
|
167 |
-
"165": ["n02089078", "black-and-tan_coonhound"],
|
168 |
-
"166": ["n02089867", "Walker_hound"],
|
169 |
-
"167": ["n02089973", "English_foxhound"],
|
170 |
-
"168": ["n02090379", "redbone"],
|
171 |
-
"169": ["n02090622", "borzoi"],
|
172 |
-
"170": ["n02090721", "Irish_wolfhound"],
|
173 |
-
"171": ["n02091032", "Italian_greyhound"],
|
174 |
-
"172": ["n02091134", "whippet"],
|
175 |
-
"173": ["n02091244", "Ibizan_hound"],
|
176 |
-
"174": ["n02091467", "Norwegian_elkhound"],
|
177 |
-
"175": ["n02091635", "otterhound"],
|
178 |
-
"176": ["n02091831", "Saluki"],
|
179 |
-
"177": ["n02092002", "Scottish_deerhound"],
|
180 |
-
"178": ["n02092339", "Weimaraner"],
|
181 |
-
"179": ["n02093256", "Staffordshire_bullterrier"],
|
182 |
-
"180": ["n02093428", "American_Staffordshire_terrier"],
|
183 |
-
"181": ["n02093647", "Bedlington_terrier"],
|
184 |
-
"182": ["n02093754", "Border_terrier"],
|
185 |
-
"183": ["n02093859", "Kerry_blue_terrier"],
|
186 |
-
"184": ["n02093991", "Irish_terrier"],
|
187 |
-
"185": ["n02094114", "Norfolk_terrier"],
|
188 |
-
"186": ["n02094258", "Norwich_terrier"],
|
189 |
-
"187": ["n02094433", "Yorkshire_terrier"],
|
190 |
-
"188": ["n02095314", "wire-haired_fox_terrier"],
|
191 |
-
"189": ["n02095570", "Lakeland_terrier"],
|
192 |
-
"190": ["n02095889", "Sealyham_terrier"],
|
193 |
-
"191": ["n02096051", "Airedale"],
|
194 |
-
"192": ["n02096177", "cairn"],
|
195 |
-
"193": ["n02096294", "Australian_terrier"],
|
196 |
-
"194": ["n02096437", "Dandie_Dinmont"],
|
197 |
-
"195": ["n02096585", "Boston_bull"],
|
198 |
-
"196": ["n02097047", "miniature_schnauzer"],
|
199 |
-
"197": ["n02097130", "giant_schnauzer"],
|
200 |
-
"198": ["n02097209", "standard_schnauzer"],
|
201 |
-
"199": ["n02097298", "Scotch_terrier"],
|
202 |
-
"200": ["n02097474", "Tibetan_terrier"],
|
203 |
-
"201": ["n02097658", "silky_terrier"],
|
204 |
-
"202": ["n02098105", "soft-coated_wheaten_terrier"],
|
205 |
-
"203": ["n02098286", "West_Highland_white_terrier"],
|
206 |
-
"204": ["n02098413", "Lhasa"],
|
207 |
-
"205": ["n02099267", "flat-coated_retriever"],
|
208 |
-
"206": ["n02099429", "curly-coated_retriever"],
|
209 |
-
"207": ["n02099601", "golden_retriever"],
|
210 |
-
"208": ["n02099712", "Labrador_retriever"],
|
211 |
-
"209": ["n02099849", "Chesapeake_Bay_retriever"],
|
212 |
-
"210": ["n02100236", "German_short-haired_pointer"],
|
213 |
-
"211": ["n02100583", "vizsla"],
|
214 |
-
"212": ["n02100735", "English_setter"],
|
215 |
-
"213": ["n02100877", "Irish_setter"],
|
216 |
-
"214": ["n02101006", "Gordon_setter"],
|
217 |
-
"215": ["n02101388", "Brittany_spaniel"],
|
218 |
-
"216": ["n02101556", "clumber"],
|
219 |
-
"217": ["n02102040", "English_springer"],
|
220 |
-
"218": ["n02102177", "Welsh_springer_spaniel"],
|
221 |
-
"219": ["n02102318", "cocker_spaniel"],
|
222 |
-
"220": ["n02102480", "Sussex_spaniel"],
|
223 |
-
"221": ["n02102973", "Irish_water_spaniel"],
|
224 |
-
"222": ["n02104029", "kuvasz"],
|
225 |
-
"223": ["n02104365", "schipperke"],
|
226 |
-
"224": ["n02105056", "groenendael"],
|
227 |
-
"225": ["n02105162", "malinois"],
|
228 |
-
"226": ["n02105251", "briard"],
|
229 |
-
"227": ["n02105412", "kelpie"],
|
230 |
-
"228": ["n02105505", "komondor"],
|
231 |
-
"229": ["n02105641", "Old_English_sheepdog"],
|
232 |
-
"230": ["n02105855", "Shetland_sheepdog"],
|
233 |
-
"231": ["n02106030", "collie"],
|
234 |
-
"232": ["n02106166", "Border_collie"],
|
235 |
-
"233": ["n02106382", "Bouvier_des_Flandres"],
|
236 |
-
"234": ["n02106550", "Rottweiler"],
|
237 |
-
"235": ["n02106662", "German_shepherd"],
|
238 |
-
"236": ["n02107142", "Doberman"],
|
239 |
-
"237": ["n02107312", "miniature_pinscher"],
|
240 |
-
"238": ["n02107574", "Greater_Swiss_Mountain_dog"],
|
241 |
-
"239": ["n02107683", "Bernese_mountain_dog"],
|
242 |
-
"240": ["n02107908", "Appenzeller"],
|
243 |
-
"241": ["n02108000", "EntleBucher"],
|
244 |
-
"242": ["n02108089", "boxer"],
|
245 |
-
"243": ["n02108422", "bull_mastiff"],
|
246 |
-
"244": ["n02108551", "Tibetan_mastiff"],
|
247 |
-
"245": ["n02108915", "French_bulldog"],
|
248 |
-
"246": ["n02109047", "Great_Dane"],
|
249 |
-
"247": ["n02109525", "Saint_Bernard"],
|
250 |
-
"248": ["n02109961", "Eskimo_dog"],
|
251 |
-
"249": ["n02110063", "malamute"],
|
252 |
-
"250": ["n02110185", "Siberian_husky"],
|
253 |
-
"251": ["n02110341", "dalmatian"],
|
254 |
-
"252": ["n02110627", "affenpinscher"],
|
255 |
-
"253": ["n02110806", "basenji"],
|
256 |
-
"254": ["n02110958", "pug"],
|
257 |
-
"255": ["n02111129", "Leonberg"],
|
258 |
-
"256": ["n02111277", "Newfoundland"],
|
259 |
-
"257": ["n02111500", "Great_Pyrenees"],
|
260 |
-
"258": ["n02111889", "Samoyed"],
|
261 |
-
"259": ["n02112018", "Pomeranian"],
|
262 |
-
"260": ["n02112137", "chow"],
|
263 |
-
"261": ["n02112350", "keeshond"],
|
264 |
-
"262": ["n02112706", "Brabancon_griffon"],
|
265 |
-
"263": ["n02113023", "Pembroke"],
|
266 |
-
"264": ["n02113186", "Cardigan"],
|
267 |
-
"265": ["n02113624", "toy_poodle"],
|
268 |
-
"266": ["n02113712", "miniature_poodle"],
|
269 |
-
"267": ["n02113799", "standard_poodle"],
|
270 |
-
"268": ["n02113978", "Mexican_hairless"],
|
271 |
-
"269": ["n02114367", "timber_wolf"],
|
272 |
-
"270": ["n02114548", "white_wolf"],
|
273 |
-
"271": ["n02114712", "red_wolf"],
|
274 |
-
"272": ["n02114855", "coyote"],
|
275 |
-
"273": ["n02115641", "dingo"],
|
276 |
-
"274": ["n02115913", "dhole"],
|
277 |
-
"275": ["n02116738", "African_hunting_dog"],
|
278 |
-
"276": ["n02117135", "hyena"],
|
279 |
-
"277": ["n02119022", "red_fox"],
|
280 |
-
"278": ["n02119789", "kit_fox"],
|
281 |
-
"279": ["n02120079", "Arctic_fox"],
|
282 |
-
"280": ["n02120505", "grey_fox"],
|
283 |
-
"281": ["n02123045", "tabby"],
|
284 |
-
"282": ["n02123159", "tiger_cat"],
|
285 |
-
"283": ["n02123394", "Persian_cat"],
|
286 |
-
"284": ["n02123597", "Siamese_cat"],
|
287 |
-
"285": ["n02124075", "Egyptian_cat"],
|
288 |
-
"286": ["n02125311", "cougar"],
|
289 |
-
"287": ["n02127052", "lynx"],
|
290 |
-
"288": ["n02128385", "leopard"],
|
291 |
-
"289": ["n02128757", "snow_leopard"],
|
292 |
-
"290": ["n02128925", "jaguar"],
|
293 |
-
"291": ["n02129165", "lion"],
|
294 |
-
"292": ["n02129604", "tiger"],
|
295 |
-
"293": ["n02130308", "cheetah"],
|
296 |
-
"294": ["n02132136", "brown_bear"],
|
297 |
-
"295": ["n02133161", "American_black_bear"],
|
298 |
-
"296": ["n02134084", "ice_bear"],
|
299 |
-
"297": ["n02134418", "sloth_bear"],
|
300 |
-
"298": ["n02137549", "mongoose"],
|
301 |
-
"299": ["n02138441", "meerkat"],
|
302 |
-
"300": ["n02165105", "tiger_beetle"],
|
303 |
-
"301": ["n02165456", "ladybug"],
|
304 |
-
"302": ["n02167151", "ground_beetle"],
|
305 |
-
"303": ["n02168699", "long-horned_beetle"],
|
306 |
-
"304": ["n02169497", "leaf_beetle"],
|
307 |
-
"305": ["n02172182", "dung_beetle"],
|
308 |
-
"306": ["n02174001", "rhinoceros_beetle"],
|
309 |
-
"307": ["n02177972", "weevil"],
|
310 |
-
"308": ["n02190166", "fly"],
|
311 |
-
"309": ["n02206856", "bee"],
|
312 |
-
"310": ["n02219486", "ant"],
|
313 |
-
"311": ["n02226429", "grasshopper"],
|
314 |
-
"312": ["n02229544", "cricket"],
|
315 |
-
"313": ["n02231487", "walking_stick"],
|
316 |
-
"314": ["n02233338", "cockroach"],
|
317 |
-
"315": ["n02236044", "mantis"],
|
318 |
-
"316": ["n02256656", "cicada"],
|
319 |
-
"317": ["n02259212", "leafhopper"],
|
320 |
-
"318": ["n02264363", "lacewing"],
|
321 |
-
"319": ["n02268443", "dragonfly"],
|
322 |
-
"320": ["n02268853", "damselfly"],
|
323 |
-
"321": ["n02276258", "admiral"],
|
324 |
-
"322": ["n02277742", "ringlet"],
|
325 |
-
"323": ["n02279972", "monarch"],
|
326 |
-
"324": ["n02280649", "cabbage_butterfly"],
|
327 |
-
"325": ["n02281406", "sulphur_butterfly"],
|
328 |
-
"326": ["n02281787", "lycaenid"],
|
329 |
-
"327": ["n02317335", "starfish"],
|
330 |
-
"328": ["n02319095", "sea_urchin"],
|
331 |
-
"329": ["n02321529", "sea_cucumber"],
|
332 |
-
"330": ["n02325366", "wood_rabbit"],
|
333 |
-
"331": ["n02326432", "hare"],
|
334 |
-
"332": ["n02328150", "Angora"],
|
335 |
-
"333": ["n02342885", "hamster"],
|
336 |
-
"334": ["n02346627", "porcupine"],
|
337 |
-
"335": ["n02356798", "fox_squirrel"],
|
338 |
-
"336": ["n02361337", "marmot"],
|
339 |
-
"337": ["n02363005", "beaver"],
|
340 |
-
"338": ["n02364673", "guinea_pig"],
|
341 |
-
"339": ["n02389026", "sorrel"],
|
342 |
-
"340": ["n02391049", "zebra"],
|
343 |
-
"341": ["n02395406", "hog"],
|
344 |
-
"342": ["n02396427", "wild_boar"],
|
345 |
-
"343": ["n02397096", "warthog"],
|
346 |
-
"344": ["n02398521", "hippopotamus"],
|
347 |
-
"345": ["n02403003", "ox"],
|
348 |
-
"346": ["n02408429", "water_buffalo"],
|
349 |
-
"347": ["n02410509", "bison"],
|
350 |
-
"348": ["n02412080", "ram"],
|
351 |
-
"349": ["n02415577", "bighorn"],
|
352 |
-
"350": ["n02417914", "ibex"],
|
353 |
-
"351": ["n02422106", "hartebeest"],
|
354 |
-
"352": ["n02422699", "impala"],
|
355 |
-
"353": ["n02423022", "gazelle"],
|
356 |
-
"354": ["n02437312", "Arabian_camel"],
|
357 |
-
"355": ["n02437616", "llama"],
|
358 |
-
"356": ["n02441942", "weasel"],
|
359 |
-
"357": ["n02442845", "mink"],
|
360 |
-
"358": ["n02443114", "polecat"],
|
361 |
-
"359": ["n02443484", "black-footed_ferret"],
|
362 |
-
"360": ["n02444819", "otter"],
|
363 |
-
"361": ["n02445715", "skunk"],
|
364 |
-
"362": ["n02447366", "badger"],
|
365 |
-
"363": ["n02454379", "armadillo"],
|
366 |
-
"364": ["n02457408", "three-toed_sloth"],
|
367 |
-
"365": ["n02480495", "orangutan"],
|
368 |
-
"366": ["n02480855", "gorilla"],
|
369 |
-
"367": ["n02481823", "chimpanzee"],
|
370 |
-
"368": ["n02483362", "gibbon"],
|
371 |
-
"369": ["n02483708", "siamang"],
|
372 |
-
"370": ["n02484975", "guenon"],
|
373 |
-
"371": ["n02486261", "patas"],
|
374 |
-
"372": ["n02486410", "baboon"],
|
375 |
-
"373": ["n02487347", "macaque"],
|
376 |
-
"374": ["n02488291", "langur"],
|
377 |
-
"375": ["n02488702", "colobus"],
|
378 |
-
"376": ["n02489166", "proboscis_monkey"],
|
379 |
-
"377": ["n02490219", "marmoset"],
|
380 |
-
"378": ["n02492035", "capuchin"],
|
381 |
-
"379": ["n02492660", "howler_monkey"],
|
382 |
-
"380": ["n02493509", "titi"],
|
383 |
-
"381": ["n02493793", "spider_monkey"],
|
384 |
-
"382": ["n02494079", "squirrel_monkey"],
|
385 |
-
"383": ["n02497673", "Madagascar_cat"],
|
386 |
-
"384": ["n02500267", "indri"],
|
387 |
-
"385": ["n02504013", "Indian_elephant"],
|
388 |
-
"386": ["n02504458", "African_elephant"],
|
389 |
-
"387": ["n02509815", "lesser_panda"],
|
390 |
-
"388": ["n02510455", "giant_panda"],
|
391 |
-
"389": ["n02514041", "barracouta"],
|
392 |
-
"390": ["n02526121", "eel"],
|
393 |
-
"391": ["n02536864", "coho"],
|
394 |
-
"392": ["n02606052", "rock_beauty"],
|
395 |
-
"393": ["n02607072", "anemone_fish"],
|
396 |
-
"394": ["n02640242", "sturgeon"],
|
397 |
-
"395": ["n02641379", "gar"],
|
398 |
-
"396": ["n02643566", "lionfish"],
|
399 |
-
"397": ["n02655020", "puffer"],
|
400 |
-
"398": ["n02666196", "abacus"],
|
401 |
-
"399": ["n02667093", "abaya"],
|
402 |
-
"400": ["n02669723", "academic_gown"],
|
403 |
-
"401": ["n02672831", "accordion"],
|
404 |
-
"402": ["n02676566", "acoustic_guitar"],
|
405 |
-
"403": ["n02687172", "aircraft_carrier"],
|
406 |
-
"404": ["n02690373", "airliner"],
|
407 |
-
"405": ["n02692877", "airship"],
|
408 |
-
"406": ["n02699494", "altar"],
|
409 |
-
"407": ["n02701002", "ambulance"],
|
410 |
-
"408": ["n02704792", "amphibian"],
|
411 |
-
"409": ["n02708093", "analog_clock"],
|
412 |
-
"410": ["n02727426", "apiary"],
|
413 |
-
"411": ["n02730930", "apron"],
|
414 |
-
"412": ["n02747177", "ashcan"],
|
415 |
-
"413": ["n02749479", "assault_rifle"],
|
416 |
-
"414": ["n02769748", "backpack"],
|
417 |
-
"415": ["n02776631", "bakery"],
|
418 |
-
"416": ["n02777292", "balance_beam"],
|
419 |
-
"417": ["n02782093", "balloon"],
|
420 |
-
"418": ["n02783161", "ballpoint"],
|
421 |
-
"419": ["n02786058", "Band_Aid"],
|
422 |
-
"420": ["n02787622", "banjo"],
|
423 |
-
"421": ["n02788148", "bannister"],
|
424 |
-
"422": ["n02790996", "barbell"],
|
425 |
-
"423": ["n02791124", "barber_chair"],
|
426 |
-
"424": ["n02791270", "barbershop"],
|
427 |
-
"425": ["n02793495", "barn"],
|
428 |
-
"426": ["n02794156", "barometer"],
|
429 |
-
"427": ["n02795169", "barrel"],
|
430 |
-
"428": ["n02797295", "barrow"],
|
431 |
-
"429": ["n02799071", "baseball"],
|
432 |
-
"430": ["n02802426", "basketball"],
|
433 |
-
"431": ["n02804414", "bassinet"],
|
434 |
-
"432": ["n02804610", "bassoon"],
|
435 |
-
"433": ["n02807133", "bathing_cap"],
|
436 |
-
"434": ["n02808304", "bath_towel"],
|
437 |
-
"435": ["n02808440", "bathtub"],
|
438 |
-
"436": ["n02814533", "beach_wagon"],
|
439 |
-
"437": ["n02814860", "beacon"],
|
440 |
-
"438": ["n02815834", "beaker"],
|
441 |
-
"439": ["n02817516", "bearskin"],
|
442 |
-
"440": ["n02823428", "beer_bottle"],
|
443 |
-
"441": ["n02823750", "beer_glass"],
|
444 |
-
"442": ["n02825657", "bell_cote"],
|
445 |
-
"443": ["n02834397", "bib"],
|
446 |
-
"444": ["n02835271", "bicycle-built-for-two"],
|
447 |
-
"445": ["n02837789", "bikini"],
|
448 |
-
"446": ["n02840245", "binder"],
|
449 |
-
"447": ["n02841315", "binoculars"],
|
450 |
-
"448": ["n02843684", "birdhouse"],
|
451 |
-
"449": ["n02859443", "boathouse"],
|
452 |
-
"450": ["n02860847", "bobsled"],
|
453 |
-
"451": ["n02865351", "bolo_tie"],
|
454 |
-
"452": ["n02869837", "bonnet"],
|
455 |
-
"453": ["n02870880", "bookcase"],
|
456 |
-
"454": ["n02871525", "bookshop"],
|
457 |
-
"455": ["n02877765", "bottlecap"],
|
458 |
-
"456": ["n02879718", "bow"],
|
459 |
-
"457": ["n02883205", "bow_tie"],
|
460 |
-
"458": ["n02892201", "brass"],
|
461 |
-
"459": ["n02892767", "brassiere"],
|
462 |
-
"460": ["n02894605", "breakwater"],
|
463 |
-
"461": ["n02895154", "breastplate"],
|
464 |
-
"462": ["n02906734", "broom"],
|
465 |
-
"463": ["n02909870", "bucket"],
|
466 |
-
"464": ["n02910353", "buckle"],
|
467 |
-
"465": ["n02916936", "bulletproof_vest"],
|
468 |
-
"466": ["n02917067", "bullet_train"],
|
469 |
-
"467": ["n02927161", "butcher_shop"],
|
470 |
-
"468": ["n02930766", "cab"],
|
471 |
-
"469": ["n02939185", "caldron"],
|
472 |
-
"470": ["n02948072", "candle"],
|
473 |
-
"471": ["n02950826", "cannon"],
|
474 |
-
"472": ["n02951358", "canoe"],
|
475 |
-
"473": ["n02951585", "can_opener"],
|
476 |
-
"474": ["n02963159", "cardigan"],
|
477 |
-
"475": ["n02965783", "car_mirror"],
|
478 |
-
"476": ["n02966193", "carousel"],
|
479 |
-
"477": ["n02966687", "carpenter's_kit"],
|
480 |
-
"478": ["n02971356", "carton"],
|
481 |
-
"479": ["n02974003", "car_wheel"],
|
482 |
-
"480": ["n02977058", "cash_machine"],
|
483 |
-
"481": ["n02978881", "cassette"],
|
484 |
-
"482": ["n02979186", "cassette_player"],
|
485 |
-
"483": ["n02980441", "castle"],
|
486 |
-
"484": ["n02981792", "catamaran"],
|
487 |
-
"485": ["n02988304", "CD_player"],
|
488 |
-
"486": ["n02992211", "cello"],
|
489 |
-
"487": ["n02992529", "cellular_telephone"],
|
490 |
-
"488": ["n02999410", "chain"],
|
491 |
-
"489": ["n03000134", "chainlink_fence"],
|
492 |
-
"490": ["n03000247", "chain_mail"],
|
493 |
-
"491": ["n03000684", "chain_saw"],
|
494 |
-
"492": ["n03014705", "chest"],
|
495 |
-
"493": ["n03016953", "chiffonier"],
|
496 |
-
"494": ["n03017168", "chime"],
|
497 |
-
"495": ["n03018349", "china_cabinet"],
|
498 |
-
"496": ["n03026506", "Christmas_stocking"],
|
499 |
-
"497": ["n03028079", "church"],
|
500 |
-
"498": ["n03032252", "cinema"],
|
501 |
-
"499": ["n03041632", "cleaver"],
|
502 |
-
"500": ["n03042490", "cliff_dwelling"],
|
503 |
-
"501": ["n03045698", "cloak"],
|
504 |
-
"502": ["n03047690", "clog"],
|
505 |
-
"503": ["n03062245", "cocktail_shaker"],
|
506 |
-
"504": ["n03063599", "coffee_mug"],
|
507 |
-
"505": ["n03063689", "coffeepot"],
|
508 |
-
"506": ["n03065424", "coil"],
|
509 |
-
"507": ["n03075370", "combination_lock"],
|
510 |
-
"508": ["n03085013", "computer_keyboard"],
|
511 |
-
"509": ["n03089624", "confectionery"],
|
512 |
-
"510": ["n03095699", "container_ship"],
|
513 |
-
"511": ["n03100240", "convertible"],
|
514 |
-
"512": ["n03109150", "corkscrew"],
|
515 |
-
"513": ["n03110669", "cornet"],
|
516 |
-
"514": ["n03124043", "cowboy_boot"],
|
517 |
-
"515": ["n03124170", "cowboy_hat"],
|
518 |
-
"516": ["n03125729", "cradle"],
|
519 |
-
"517": ["n03126707", "crane"],
|
520 |
-
"518": ["n03127747", "crash_helmet"],
|
521 |
-
"519": ["n03127925", "crate"],
|
522 |
-
"520": ["n03131574", "crib"],
|
523 |
-
"521": ["n03133878", "Crock_Pot"],
|
524 |
-
"522": ["n03134739", "croquet_ball"],
|
525 |
-
"523": ["n03141823", "crutch"],
|
526 |
-
"524": ["n03146219", "cuirass"],
|
527 |
-
"525": ["n03160309", "dam"],
|
528 |
-
"526": ["n03179701", "desk"],
|
529 |
-
"527": ["n03180011", "desktop_computer"],
|
530 |
-
"528": ["n03187595", "dial_telephone"],
|
531 |
-
"529": ["n03188531", "diaper"],
|
532 |
-
"530": ["n03196217", "digital_clock"],
|
533 |
-
"531": ["n03197337", "digital_watch"],
|
534 |
-
"532": ["n03201208", "dining_table"],
|
535 |
-
"533": ["n03207743", "dishrag"],
|
536 |
-
"534": ["n03207941", "dishwasher"],
|
537 |
-
"535": ["n03208938", "disk_brake"],
|
538 |
-
"536": ["n03216828", "dock"],
|
539 |
-
"537": ["n03218198", "dogsled"],
|
540 |
-
"538": ["n03220513", "dome"],
|
541 |
-
"539": ["n03223299", "doormat"],
|
542 |
-
"540": ["n03240683", "drilling_platform"],
|
543 |
-
"541": ["n03249569", "drum"],
|
544 |
-
"542": ["n03250847", "drumstick"],
|
545 |
-
"543": ["n03255030", "dumbbell"],
|
546 |
-
"544": ["n03259280", "Dutch_oven"],
|
547 |
-
"545": ["n03271574", "electric_fan"],
|
548 |
-
"546": ["n03272010", "electric_guitar"],
|
549 |
-
"547": ["n03272562", "electric_locomotive"],
|
550 |
-
"548": ["n03290653", "entertainment_center"],
|
551 |
-
"549": ["n03291819", "envelope"],
|
552 |
-
"550": ["n03297495", "espresso_maker"],
|
553 |
-
"551": ["n03314780", "face_powder"],
|
554 |
-
"552": ["n03325584", "feather_boa"],
|
555 |
-
"553": ["n03337140", "file"],
|
556 |
-
"554": ["n03344393", "fireboat"],
|
557 |
-
"555": ["n03345487", "fire_engine"],
|
558 |
-
"556": ["n03347037", "fire_screen"],
|
559 |
-
"557": ["n03355925", "flagpole"],
|
560 |
-
"558": ["n03372029", "flute"],
|
561 |
-
"559": ["n03376595", "folding_chair"],
|
562 |
-
"560": ["n03379051", "football_helmet"],
|
563 |
-
"561": ["n03384352", "forklift"],
|
564 |
-
"562": ["n03388043", "fountain"],
|
565 |
-
"563": ["n03388183", "fountain_pen"],
|
566 |
-
"564": ["n03388549", "four-poster"],
|
567 |
-
"565": ["n03393912", "freight_car"],
|
568 |
-
"566": ["n03394916", "French_horn"],
|
569 |
-
"567": ["n03400231", "frying_pan"],
|
570 |
-
"568": ["n03404251", "fur_coat"],
|
571 |
-
"569": ["n03417042", "garbage_truck"],
|
572 |
-
"570": ["n03424325", "gasmask"],
|
573 |
-
"571": ["n03425413", "gas_pump"],
|
574 |
-
"572": ["n03443371", "goblet"],
|
575 |
-
"573": ["n03444034", "go-kart"],
|
576 |
-
"574": ["n03445777", "golf_ball"],
|
577 |
-
"575": ["n03445924", "golfcart"],
|
578 |
-
"576": ["n03447447", "gondola"],
|
579 |
-
"577": ["n03447721", "gong"],
|
580 |
-
"578": ["n03450230", "gown"],
|
581 |
-
"579": ["n03452741", "grand_piano"],
|
582 |
-
"580": ["n03457902", "greenhouse"],
|
583 |
-
"581": ["n03459775", "grille"],
|
584 |
-
"582": ["n03461385", "grocery_store"],
|
585 |
-
"583": ["n03467068", "guillotine"],
|
586 |
-
"584": ["n03476684", "hair_slide"],
|
587 |
-
"585": ["n03476991", "hair_spray"],
|
588 |
-
"586": ["n03478589", "half_track"],
|
589 |
-
"587": ["n03481172", "hammer"],
|
590 |
-
"588": ["n03482405", "hamper"],
|
591 |
-
"589": ["n03483316", "hand_blower"],
|
592 |
-
"590": ["n03485407", "hand-held_computer"],
|
593 |
-
"591": ["n03485794", "handkerchief"],
|
594 |
-
"592": ["n03492542", "hard_disc"],
|
595 |
-
"593": ["n03494278", "harmonica"],
|
596 |
-
"594": ["n03495258", "harp"],
|
597 |
-
"595": ["n03496892", "harvester"],
|
598 |
-
"596": ["n03498962", "hatchet"],
|
599 |
-
"597": ["n03527444", "holster"],
|
600 |
-
"598": ["n03529860", "home_theater"],
|
601 |
-
"599": ["n03530642", "honeycomb"],
|
602 |
-
"600": ["n03532672", "hook"],
|
603 |
-
"601": ["n03534580", "hoopskirt"],
|
604 |
-
"602": ["n03535780", "horizontal_bar"],
|
605 |
-
"603": ["n03538406", "horse_cart"],
|
606 |
-
"604": ["n03544143", "hourglass"],
|
607 |
-
"605": ["n03584254", "iPod"],
|
608 |
-
"606": ["n03584829", "iron"],
|
609 |
-
"607": ["n03590841", "jack-o'-lantern"],
|
610 |
-
"608": ["n03594734", "jean"],
|
611 |
-
"609": ["n03594945", "jeep"],
|
612 |
-
"610": ["n03595614", "jersey"],
|
613 |
-
"611": ["n03598930", "jigsaw_puzzle"],
|
614 |
-
"612": ["n03599486", "jinrikisha"],
|
615 |
-
"613": ["n03602883", "joystick"],
|
616 |
-
"614": ["n03617480", "kimono"],
|
617 |
-
"615": ["n03623198", "knee_pad"],
|
618 |
-
"616": ["n03627232", "knot"],
|
619 |
-
"617": ["n03630383", "lab_coat"],
|
620 |
-
"618": ["n03633091", "ladle"],
|
621 |
-
"619": ["n03637318", "lampshade"],
|
622 |
-
"620": ["n03642806", "laptop"],
|
623 |
-
"621": ["n03649909", "lawn_mower"],
|
624 |
-
"622": ["n03657121", "lens_cap"],
|
625 |
-
"623": ["n03658185", "letter_opener"],
|
626 |
-
"624": ["n03661043", "library"],
|
627 |
-
"625": ["n03662601", "lifeboat"],
|
628 |
-
"626": ["n03666591", "lighter"],
|
629 |
-
"627": ["n03670208", "limousine"],
|
630 |
-
"628": ["n03673027", "liner"],
|
631 |
-
"629": ["n03676483", "lipstick"],
|
632 |
-
"630": ["n03680355", "Loafer"],
|
633 |
-
"631": ["n03690938", "lotion"],
|
634 |
-
"632": ["n03691459", "loudspeaker"],
|
635 |
-
"633": ["n03692522", "loupe"],
|
636 |
-
"634": ["n03697007", "lumbermill"],
|
637 |
-
"635": ["n03706229", "magnetic_compass"],
|
638 |
-
"636": ["n03709823", "mailbag"],
|
639 |
-
"637": ["n03710193", "mailbox"],
|
640 |
-
"638": ["n03710637", "maillot"],
|
641 |
-
"639": ["n03710721", "maillot"],
|
642 |
-
"640": ["n03717622", "manhole_cover"],
|
643 |
-
"641": ["n03720891", "maraca"],
|
644 |
-
"642": ["n03721384", "marimba"],
|
645 |
-
"643": ["n03724870", "mask"],
|
646 |
-
"644": ["n03729826", "matchstick"],
|
647 |
-
"645": ["n03733131", "maypole"],
|
648 |
-
"646": ["n03733281", "maze"],
|
649 |
-
"647": ["n03733805", "measuring_cup"],
|
650 |
-
"648": ["n03742115", "medicine_chest"],
|
651 |
-
"649": ["n03743016", "megalith"],
|
652 |
-
"650": ["n03759954", "microphone"],
|
653 |
-
"651": ["n03761084", "microwave"],
|
654 |
-
"652": ["n03763968", "military_uniform"],
|
655 |
-
"653": ["n03764736", "milk_can"],
|
656 |
-
"654": ["n03769881", "minibus"],
|
657 |
-
"655": ["n03770439", "miniskirt"],
|
658 |
-
"656": ["n03770679", "minivan"],
|
659 |
-
"657": ["n03773504", "missile"],
|
660 |
-
"658": ["n03775071", "mitten"],
|
661 |
-
"659": ["n03775546", "mixing_bowl"],
|
662 |
-
"660": ["n03776460", "mobile_home"],
|
663 |
-
"661": ["n03777568", "Model_T"],
|
664 |
-
"662": ["n03777754", "modem"],
|
665 |
-
"663": ["n03781244", "monastery"],
|
666 |
-
"664": ["n03782006", "monitor"],
|
667 |
-
"665": ["n03785016", "moped"],
|
668 |
-
"666": ["n03786901", "mortar"],
|
669 |
-
"667": ["n03787032", "mortarboard"],
|
670 |
-
"668": ["n03788195", "mosque"],
|
671 |
-
"669": ["n03788365", "mosquito_net"],
|
672 |
-
"670": ["n03791053", "motor_scooter"],
|
673 |
-
"671": ["n03792782", "mountain_bike"],
|
674 |
-
"672": ["n03792972", "mountain_tent"],
|
675 |
-
"673": ["n03793489", "mouse"],
|
676 |
-
"674": ["n03794056", "mousetrap"],
|
677 |
-
"675": ["n03796401", "moving_van"],
|
678 |
-
"676": ["n03803284", "muzzle"],
|
679 |
-
"677": ["n03804744", "nail"],
|
680 |
-
"678": ["n03814639", "neck_brace"],
|
681 |
-
"679": ["n03814906", "necklace"],
|
682 |
-
"680": ["n03825788", "nipple"],
|
683 |
-
"681": ["n03832673", "notebook"],
|
684 |
-
"682": ["n03837869", "obelisk"],
|
685 |
-
"683": ["n03838899", "oboe"],
|
686 |
-
"684": ["n03840681", "ocarina"],
|
687 |
-
"685": ["n03841143", "odometer"],
|
688 |
-
"686": ["n03843555", "oil_filter"],
|
689 |
-
"687": ["n03854065", "organ"],
|
690 |
-
"688": ["n03857828", "oscilloscope"],
|
691 |
-
"689": ["n03866082", "overskirt"],
|
692 |
-
"690": ["n03868242", "oxcart"],
|
693 |
-
"691": ["n03868863", "oxygen_mask"],
|
694 |
-
"692": ["n03871628", "packet"],
|
695 |
-
"693": ["n03873416", "paddle"],
|
696 |
-
"694": ["n03874293", "paddlewheel"],
|
697 |
-
"695": ["n03874599", "padlock"],
|
698 |
-
"696": ["n03876231", "paintbrush"],
|
699 |
-
"697": ["n03877472", "pajama"],
|
700 |
-
"698": ["n03877845", "palace"],
|
701 |
-
"699": ["n03884397", "panpipe"],
|
702 |
-
"700": ["n03887697", "paper_towel"],
|
703 |
-
"701": ["n03888257", "parachute"],
|
704 |
-
"702": ["n03888605", "parallel_bars"],
|
705 |
-
"703": ["n03891251", "park_bench"],
|
706 |
-
"704": ["n03891332", "parking_meter"],
|
707 |
-
"705": ["n03895866", "passenger_car"],
|
708 |
-
"706": ["n03899768", "patio"],
|
709 |
-
"707": ["n03902125", "pay-phone"],
|
710 |
-
"708": ["n03903868", "pedestal"],
|
711 |
-
"709": ["n03908618", "pencil_box"],
|
712 |
-
"710": ["n03908714", "pencil_sharpener"],
|
713 |
-
"711": ["n03916031", "perfume"],
|
714 |
-
"712": ["n03920288", "Petri_dish"],
|
715 |
-
"713": ["n03924679", "photocopier"],
|
716 |
-
"714": ["n03929660", "pick"],
|
717 |
-
"715": ["n03929855", "pickelhaube"],
|
718 |
-
"716": ["n03930313", "picket_fence"],
|
719 |
-
"717": ["n03930630", "pickup"],
|
720 |
-
"718": ["n03933933", "pier"],
|
721 |
-
"719": ["n03935335", "piggy_bank"],
|
722 |
-
"720": ["n03937543", "pill_bottle"],
|
723 |
-
"721": ["n03938244", "pillow"],
|
724 |
-
"722": ["n03942813", "ping-pong_ball"],
|
725 |
-
"723": ["n03944341", "pinwheel"],
|
726 |
-
"724": ["n03947888", "pirate"],
|
727 |
-
"725": ["n03950228", "pitcher"],
|
728 |
-
"726": ["n03954731", "plane"],
|
729 |
-
"727": ["n03956157", "planetarium"],
|
730 |
-
"728": ["n03958227", "plastic_bag"],
|
731 |
-
"729": ["n03961711", "plate_rack"],
|
732 |
-
"730": ["n03967562", "plow"],
|
733 |
-
"731": ["n03970156", "plunger"],
|
734 |
-
"732": ["n03976467", "Polaroid_camera"],
|
735 |
-
"733": ["n03976657", "pole"],
|
736 |
-
"734": ["n03977966", "police_van"],
|
737 |
-
"735": ["n03980874", "poncho"],
|
738 |
-
"736": ["n03982430", "pool_table"],
|
739 |
-
"737": ["n03983396", "pop_bottle"],
|
740 |
-
"738": ["n03991062", "pot"],
|
741 |
-
"739": ["n03992509", "potter's_wheel"],
|
742 |
-
"740": ["n03995372", "power_drill"],
|
743 |
-
"741": ["n03998194", "prayer_rug"],
|
744 |
-
"742": ["n04004767", "printer"],
|
745 |
-
"743": ["n04005630", "prison"],
|
746 |
-
"744": ["n04008634", "projectile"],
|
747 |
-
"745": ["n04009552", "projector"],
|
748 |
-
"746": ["n04019541", "puck"],
|
749 |
-
"747": ["n04023962", "punching_bag"],
|
750 |
-
"748": ["n04026417", "purse"],
|
751 |
-
"749": ["n04033901", "quill"],
|
752 |
-
"750": ["n04033995", "quilt"],
|
753 |
-
"751": ["n04037443", "racer"],
|
754 |
-
"752": ["n04039381", "racket"],
|
755 |
-
"753": ["n04040759", "radiator"],
|
756 |
-
"754": ["n04041544", "radio"],
|
757 |
-
"755": ["n04044716", "radio_telescope"],
|
758 |
-
"756": ["n04049303", "rain_barrel"],
|
759 |
-
"757": ["n04065272", "recreational_vehicle"],
|
760 |
-
"758": ["n04067472", "reel"],
|
761 |
-
"759": ["n04069434", "reflex_camera"],
|
762 |
-
"760": ["n04070727", "refrigerator"],
|
763 |
-
"761": ["n04074963", "remote_control"],
|
764 |
-
"762": ["n04081281", "restaurant"],
|
765 |
-
"763": ["n04086273", "revolver"],
|
766 |
-
"764": ["n04090263", "rifle"],
|
767 |
-
"765": ["n04099969", "rocking_chair"],
|
768 |
-
"766": ["n04111531", "rotisserie"],
|
769 |
-
"767": ["n04116512", "rubber_eraser"],
|
770 |
-
"768": ["n04118538", "rugby_ball"],
|
771 |
-
"769": ["n04118776", "rule"],
|
772 |
-
"770": ["n04120489", "running_shoe"],
|
773 |
-
"771": ["n04125021", "safe"],
|
774 |
-
"772": ["n04127249", "safety_pin"],
|
775 |
-
"773": ["n04131690", "saltshaker"],
|
776 |
-
"774": ["n04133789", "sandal"],
|
777 |
-
"775": ["n04136333", "sarong"],
|
778 |
-
"776": ["n04141076", "sax"],
|
779 |
-
"777": ["n04141327", "scabbard"],
|
780 |
-
"778": ["n04141975", "scale"],
|
781 |
-
"779": ["n04146614", "school_bus"],
|
782 |
-
"780": ["n04147183", "schooner"],
|
783 |
-
"781": ["n04149813", "scoreboard"],
|
784 |
-
"782": ["n04152593", "screen"],
|
785 |
-
"783": ["n04153751", "screw"],
|
786 |
-
"784": ["n04154565", "screwdriver"],
|
787 |
-
"785": ["n04162706", "seat_belt"],
|
788 |
-
"786": ["n04179913", "sewing_machine"],
|
789 |
-
"787": ["n04192698", "shield"],
|
790 |
-
"788": ["n04200800", "shoe_shop"],
|
791 |
-
"789": ["n04201297", "shoji"],
|
792 |
-
"790": ["n04204238", "shopping_basket"],
|
793 |
-
"791": ["n04204347", "shopping_cart"],
|
794 |
-
"792": ["n04208210", "shovel"],
|
795 |
-
"793": ["n04209133", "shower_cap"],
|
796 |
-
"794": ["n04209239", "shower_curtain"],
|
797 |
-
"795": ["n04228054", "ski"],
|
798 |
-
"796": ["n04229816", "ski_mask"],
|
799 |
-
"797": ["n04235860", "sleeping_bag"],
|
800 |
-
"798": ["n04238763", "slide_rule"],
|
801 |
-
"799": ["n04239074", "sliding_door"],
|
802 |
-
"800": ["n04243546", "slot"],
|
803 |
-
"801": ["n04251144", "snorkel"],
|
804 |
-
"802": ["n04252077", "snowmobile"],
|
805 |
-
"803": ["n04252225", "snowplow"],
|
806 |
-
"804": ["n04254120", "soap_dispenser"],
|
807 |
-
"805": ["n04254680", "soccer_ball"],
|
808 |
-
"806": ["n04254777", "sock"],
|
809 |
-
"807": ["n04258138", "solar_dish"],
|
810 |
-
"808": ["n04259630", "sombrero"],
|
811 |
-
"809": ["n04263257", "soup_bowl"],
|
812 |
-
"810": ["n04264628", "space_bar"],
|
813 |
-
"811": ["n04265275", "space_heater"],
|
814 |
-
"812": ["n04266014", "space_shuttle"],
|
815 |
-
"813": ["n04270147", "spatula"],
|
816 |
-
"814": ["n04273569", "speedboat"],
|
817 |
-
"815": ["n04275548", "spider_web"],
|
818 |
-
"816": ["n04277352", "spindle"],
|
819 |
-
"817": ["n04285008", "sports_car"],
|
820 |
-
"818": ["n04286575", "spotlight"],
|
821 |
-
"819": ["n04296562", "stage"],
|
822 |
-
"820": ["n04310018", "steam_locomotive"],
|
823 |
-
"821": ["n04311004", "steel_arch_bridge"],
|
824 |
-
"822": ["n04311174", "steel_drum"],
|
825 |
-
"823": ["n04317175", "stethoscope"],
|
826 |
-
"824": ["n04325704", "stole"],
|
827 |
-
"825": ["n04326547", "stone_wall"],
|
828 |
-
"826": ["n04328186", "stopwatch"],
|
829 |
-
"827": ["n04330267", "stove"],
|
830 |
-
"828": ["n04332243", "strainer"],
|
831 |
-
"829": ["n04335435", "streetcar"],
|
832 |
-
"830": ["n04336792", "stretcher"],
|
833 |
-
"831": ["n04344873", "studio_couch"],
|
834 |
-
"832": ["n04346328", "stupa"],
|
835 |
-
"833": ["n04347754", "submarine"],
|
836 |
-
"834": ["n04350905", "suit"],
|
837 |
-
"835": ["n04355338", "sundial"],
|
838 |
-
"836": ["n04355933", "sunglass"],
|
839 |
-
"837": ["n04356056", "sunglasses"],
|
840 |
-
"838": ["n04357314", "sunscreen"],
|
841 |
-
"839": ["n04366367", "suspension_bridge"],
|
842 |
-
"840": ["n04367480", "swab"],
|
843 |
-
"841": ["n04370456", "sweatshirt"],
|
844 |
-
"842": ["n04371430", "swimming_trunks"],
|
845 |
-
"843": ["n04371774", "swing"],
|
846 |
-
"844": ["n04372370", "switch"],
|
847 |
-
"845": ["n04376876", "syringe"],
|
848 |
-
"846": ["n04380533", "table_lamp"],
|
849 |
-
"847": ["n04389033", "tank"],
|
850 |
-
"848": ["n04392985", "tape_player"],
|
851 |
-
"849": ["n04398044", "teapot"],
|
852 |
-
"850": ["n04399382", "teddy"],
|
853 |
-
"851": ["n04404412", "television"],
|
854 |
-
"852": ["n04409515", "tennis_ball"],
|
855 |
-
"853": ["n04417672", "thatch"],
|
856 |
-
"854": ["n04418357", "theater_curtain"],
|
857 |
-
"855": ["n04423845", "thimble"],
|
858 |
-
"856": ["n04428191", "thresher"],
|
859 |
-
"857": ["n04429376", "throne"],
|
860 |
-
"858": ["n04435653", "tile_roof"],
|
861 |
-
"859": ["n04442312", "toaster"],
|
862 |
-
"860": ["n04443257", "tobacco_shop"],
|
863 |
-
"861": ["n04447861", "toilet_seat"],
|
864 |
-
"862": ["n04456115", "torch"],
|
865 |
-
"863": ["n04458633", "totem_pole"],
|
866 |
-
"864": ["n04461696", "tow_truck"],
|
867 |
-
"865": ["n04462240", "toyshop"],
|
868 |
-
"866": ["n04465501", "tractor"],
|
869 |
-
"867": ["n04467665", "trailer_truck"],
|
870 |
-
"868": ["n04476259", "tray"],
|
871 |
-
"869": ["n04479046", "trench_coat"],
|
872 |
-
"870": ["n04482393", "tricycle"],
|
873 |
-
"871": ["n04483307", "trimaran"],
|
874 |
-
"872": ["n04485082", "tripod"],
|
875 |
-
"873": ["n04486054", "triumphal_arch"],
|
876 |
-
"874": ["n04487081", "trolleybus"],
|
877 |
-
"875": ["n04487394", "trombone"],
|
878 |
-
"876": ["n04493381", "tub"],
|
879 |
-
"877": ["n04501370", "turnstile"],
|
880 |
-
"878": ["n04505470", "typewriter_keyboard"],
|
881 |
-
"879": ["n04507155", "umbrella"],
|
882 |
-
"880": ["n04509417", "unicycle"],
|
883 |
-
"881": ["n04515003", "upright"],
|
884 |
-
"882": ["n04517823", "vacuum"],
|
885 |
-
"883": ["n04522168", "vase"],
|
886 |
-
"884": ["n04523525", "vault"],
|
887 |
-
"885": ["n04525038", "velvet"],
|
888 |
-
"886": ["n04525305", "vending_machine"],
|
889 |
-
"887": ["n04532106", "vestment"],
|
890 |
-
"888": ["n04532670", "viaduct"],
|
891 |
-
"889": ["n04536866", "violin"],
|
892 |
-
"890": ["n04540053", "volleyball"],
|
893 |
-
"891": ["n04542943", "waffle_iron"],
|
894 |
-
"892": ["n04548280", "wall_clock"],
|
895 |
-
"893": ["n04548362", "wallet"],
|
896 |
-
"894": ["n04550184", "wardrobe"],
|
897 |
-
"895": ["n04552348", "warplane"],
|
898 |
-
"896": ["n04553703", "washbasin"],
|
899 |
-
"897": ["n04554684", "washer"],
|
900 |
-
"898": ["n04557648", "water_bottle"],
|
901 |
-
"899": ["n04560804", "water_jug"],
|
902 |
-
"900": ["n04562935", "water_tower"],
|
903 |
-
"901": ["n04579145", "whiskey_jug"],
|
904 |
-
"902": ["n04579432", "whistle"],
|
905 |
-
"903": ["n04584207", "wig"],
|
906 |
-
"904": ["n04589890", "window_screen"],
|
907 |
-
"905": ["n04590129", "window_shade"],
|
908 |
-
"906": ["n04591157", "Windsor_tie"],
|
909 |
-
"907": ["n04591713", "wine_bottle"],
|
910 |
-
"908": ["n04592741", "wing"],
|
911 |
-
"909": ["n04596742", "wok"],
|
912 |
-
"910": ["n04597913", "wooden_spoon"],
|
913 |
-
"911": ["n04599235", "wool"],
|
914 |
-
"912": ["n04604644", "worm_fence"],
|
915 |
-
"913": ["n04606251", "wreck"],
|
916 |
-
"914": ["n04612504", "yawl"],
|
917 |
-
"915": ["n04613696", "yurt"],
|
918 |
-
"916": ["n06359193", "web_site"],
|
919 |
-
"917": ["n06596364", "comic_book"],
|
920 |
-
"918": ["n06785654", "crossword_puzzle"],
|
921 |
-
"919": ["n06794110", "street_sign"],
|
922 |
-
"920": ["n06874185", "traffic_light"],
|
923 |
-
"921": ["n07248320", "book_jacket"],
|
924 |
-
"922": ["n07565083", "menu"],
|
925 |
-
"923": ["n07579787", "plate"],
|
926 |
-
"924": ["n07583066", "guacamole"],
|
927 |
-
"925": ["n07584110", "consomme"],
|
928 |
-
"926": ["n07590611", "hot_pot"],
|
929 |
-
"927": ["n07613480", "trifle"],
|
930 |
-
"928": ["n07614500", "ice_cream"],
|
931 |
-
"929": ["n07615774", "ice_lolly"],
|
932 |
-
"930": ["n07684084", "French_loaf"],
|
933 |
-
"931": ["n07693725", "bagel"],
|
934 |
-
"932": ["n07695742", "pretzel"],
|
935 |
-
"933": ["n07697313", "cheeseburger"],
|
936 |
-
"934": ["n07697537", "hotdog"],
|
937 |
-
"935": ["n07711569", "mashed_potato"],
|
938 |
-
"936": ["n07714571", "head_cabbage"],
|
939 |
-
"937": ["n07714990", "broccoli"],
|
940 |
-
"938": ["n07715103", "cauliflower"],
|
941 |
-
"939": ["n07716358", "zucchini"],
|
942 |
-
"940": ["n07716906", "spaghetti_squash"],
|
943 |
-
"941": ["n07717410", "acorn_squash"],
|
944 |
-
"942": ["n07717556", "butternut_squash"],
|
945 |
-
"943": ["n07718472", "cucumber"],
|
946 |
-
"944": ["n07718747", "artichoke"],
|
947 |
-
"945": ["n07720875", "bell_pepper"],
|
948 |
-
"946": ["n07730033", "cardoon"],
|
949 |
-
"947": ["n07734744", "mushroom"],
|
950 |
-
"948": ["n07742313", "Granny_Smith"],
|
951 |
-
"949": ["n07745940", "strawberry"],
|
952 |
-
"950": ["n07747607", "orange"],
|
953 |
-
"951": ["n07749582", "lemon"],
|
954 |
-
"952": ["n07753113", "fig"],
|
955 |
-
"953": ["n07753275", "pineapple"],
|
956 |
-
"954": ["n07753592", "banana"],
|
957 |
-
"955": ["n07754684", "jackfruit"],
|
958 |
-
"956": ["n07760859", "custard_apple"],
|
959 |
-
"957": ["n07768694", "pomegranate"],
|
960 |
-
"958": ["n07802026", "hay"],
|
961 |
-
"959": ["n07831146", "carbonara"],
|
962 |
-
"960": ["n07836838", "chocolate_sauce"],
|
963 |
-
"961": ["n07860988", "dough"],
|
964 |
-
"962": ["n07871810", "meat_loaf"],
|
965 |
-
"963": ["n07873807", "pizza"],
|
966 |
-
"964": ["n07875152", "potpie"],
|
967 |
-
"965": ["n07880968", "burrito"],
|
968 |
-
"966": ["n07892512", "red_wine"],
|
969 |
-
"967": ["n07920052", "espresso"],
|
970 |
-
"968": ["n07930864", "cup"],
|
971 |
-
"969": ["n07932039", "eggnog"],
|
972 |
-
"970": ["n09193705", "alp"],
|
973 |
-
"971": ["n09229709", "bubble"],
|
974 |
-
"972": ["n09246464", "cliff"],
|
975 |
-
"973": ["n09256479", "coral_reef"],
|
976 |
-
"974": ["n09288635", "geyser"],
|
977 |
-
"975": ["n09332890", "lakeside"],
|
978 |
-
"976": ["n09399592", "promontory"],
|
979 |
-
"977": ["n09421951", "sandbar"],
|
980 |
-
"978": ["n09428293", "seashore"],
|
981 |
-
"979": ["n09468604", "valley"],
|
982 |
-
"980": ["n09472597", "volcano"],
|
983 |
-
"981": ["n09835506", "ballplayer"],
|
984 |
-
"982": ["n10148035", "groom"],
|
985 |
-
"983": ["n10565667", "scuba_diver"],
|
986 |
-
"984": ["n11879895", "rapeseed"],
|
987 |
-
"985": ["n11939491", "daisy"],
|
988 |
-
"986": ["n12057211", "yellow_lady's_slipper"],
|
989 |
-
"987": ["n12144580", "corn"],
|
990 |
-
"988": ["n12267677", "acorn"],
|
991 |
-
"989": ["n12620546", "hip"],
|
992 |
-
"990": ["n12768682", "buckeye"],
|
993 |
-
"991": ["n12985857", "coral_fungus"],
|
994 |
-
"992": ["n12998815", "agaric"],
|
995 |
-
"993": ["n13037406", "gyromitra"],
|
996 |
-
"994": ["n13040303", "stinkhorn"],
|
997 |
-
"995": ["n13044778", "earthstar"],
|
998 |
-
"996": ["n13052670", "hen-of-the-woods"],
|
999 |
-
"997": ["n13054560", "bolete"],
|
1000 |
-
"998": ["n13133613", "ear"],
|
1001 |
-
"999": ["n15075141", "toilet_tissue"]
|
1002 |
-
}
|
|
|
|
|
|
|
|
|
|
|
|
|
|
|
|
|
|
|
|
|
|
|
|
|
|
|
|
|
|
|
|
|
|
|
|
|
|
|
|
|
|
|
|
|
|
|
|
|
|
|
|
|
|
|
|
|
|
|
|
|
|
|
|
|
|
|
|
|
|
|
|
|
|
|
|
|
|
|
|
|
|
|
|
|
|
|
|
|
|
|
|
|
|
|
|
|
|
|
|
|
|
|
|
|
|
|
|
|
|
|
|
|
|
|
|
|
|
|
|
|
|
|
|
|
|
|
|
|
|
|
|
|
|
|
|
|
|
|
|
|
|
|
|
|
|
|
|
|
|
|
|
|
|
|
|
|
|
|
|
|
|
|
|
|
|
|
|
|
|
|
|
|
|
|
|
|
|
|
|
|
|
|
|
|
|
|
|
|
|
|
|
|
|
|
|
|
|
|
|
|
|
|
|
|
|
|
|
|
|
|
|
|
|
|
|
|
|
|
|
|
|
|
|
|
|
|
|
|
|
|
|
|
|
|
|
|
|
|
|
|
|
|
|
|
|
|
|
|
|
|
|
|
|
|
|
|
|
|
|
|
|
|
|
|
|
|
|
|
|
|
|
|
|
|
|
|
|
|
|
|
|
|
|
|
|
|
|
|
|
|
|
|
|
|
|
|
|
|
|
|
|
|
|
|
|
|
|
|
|
|
|
|
|
|
|
|
|
|
|
|
|
|
|
|
|
|
|
|
|
|
|
|
|
|
|
|
|
|
|
|
|
|
|
|
|
|
|
|
|
|
|
|
|
|
|
|
|
|
|
|
|
|
|
|
|
|
|
|
|
|
|
|
|
|
|
|
|
|
|
|
|
|
|
|
|
|
|
|
|
|
|
|
|
|
|
|
|
|
|
|
|
|
|
|
|
|
|
|
|
|
|
|
|
|
|
|
|
|
|
|
|
|
|
|
|
|
|
|
|
|
|
|
|
|
|
|
|
|
|
|
|
|
|
|
|
|
|
|
|
|
|
|
|
|
|
|
|
|
|
|
|
|
|
|
|
|
|
|
|
|
|
|
|
|
|
|
|
|
|
|
|
|
|
|
|
|
|
|
|
|
|
|
|
|
|
|
|
|
|
|
|
|
|
|
|
|
|
|
|
|
|
|
|
|
|
|
|
|
|
|
|
|
|
|
|
|
|
|
|
|
|
|
|
|
|
|
|
|
|
|
|
|
|
|
|
|
|
|
|
|
|
|
|
|
|
|
|
|
|
|
|
|
|
|
|
|
|
|
|
|
|
|
|
|
|
|
|
|
|
|
|
|
|
|
|
|
|
|
|
|
|
|
|
|
|
|
|
|
|
|
|
|
|
|
|
|
|
|
|
|
|
|
|
|
|
|
|
|
|
|
|
|
|
|
|
|
|
|
|
|
|
|
|
|
|
|
|
|
|
|
|
|
|
|
|
|
|
|
|
|
|
|
|
|
|
|
|
|
|
|
|
|
|
|
|
|
|
|
|
|
|
|
|
|
|
|
|
|
|
|
|
|
|
|
|
|
|
|
|
|
|
|
|
|
|
|
|
|
|
|
|
|
|
|
|
|
|
|
|
|
|
|
|
|
|
|
|
|
|
|
|
|
|
|
|
|
|
|
|
|
|
|
|
|
|
|
|
|
|
|
|
|
|
|
|
|
|
|
|
|
|
|
|
|
|
|
|
|
|
|
|
|
|
|
|
|
|
|
|
|
|
|
|
|
|
|
|
|
|
|
|
|
|
|
|
|
|
|
|
|
|
|
|
|
|
|
|
|
|
|
|
|
|
|
|
|
|
|
|
|
|
|
|
|
|
|
|
|
|
|
|
|
|
|
|
|
|
|
|
|
|
|
|
|
|
|
|
|
|
|
|
|
|
|
|
|
|
|
|
|
|
|
|
|
|
|
|
|
|
|
|
|
|
|
|
|
|
|
|
|
|
|
|
|
|
|
|
|
|
|
|
|
|
|
|
|
|
|
|
|
|
|
|
|
|
|
|
|
|
|
|
|
|
|
|
|
|
|
|
|
|
|
|
|
|
|
|
|
|
|
|
|
|
|
|
|
|
|
|
|
|
|
|
|
|
|
|
|
|
|
|
|
|
|
|
|
|
|
|
|
|
|
|
|
|
|
|
|
|
|
|
|
|
|
|
|
|
|
|
|
|
|
|
|
|
|
|
|
|
|
|
|
|
|
|
|
|
|
|
|
|
|
|
|
|
|
|
|
|
|
|
|
|
|
|
|
|
|
|
|
|
|
|
|
|
|
|
|
|
|
|
|
|
|
|
|
|
|
|
|
|
|
|
|
|
|
|
|
|
|
|
|
|
|
|
|
|
|
|
|
|
|
|
|
|
|
|
|
|
|
|
|
|
|
|
|
|
|
|
|
|
|
|
|
|
|
|
|
|
|
|
|
|
|
|
|
|
|
|
|
|
|
|
|
|
|
|
|
|
|
|
|
|
|
|
|
|
|
|
|
|
|
|
|
|
|
|
|
|
|
|
|
|
|
|
|
|
|
|
|
|
|
|
|
|
|
|
|
|
|
|
|
|
|
|
|
|
|
|
|
|
|
|
|
|
|
|
|
|
|
|
|
|
|
|
|
|
|
|
|
|
|
|
|
|
|
|
|
|
|
|
|
|
|
|
|
|
|
|
|
|
|
|
|
|
|
|
|
|
|
|
|
|
|
|
|
|
|
|
|
|
|
|
|
|
|
|
|
|
|
|
|
|
|
|
|
|
|
|
|
|
|
|
|
|
|
|
|
|
|
|
|
|
|
|
|
|
|
|
|
|
|
|
|
|
|
|
|
|
|
|
|
|
|
|
|
|
|
|
|
|
|
|
|
|
|
|
|
|
|
|
|
|
|
|
|
|
|
|
|
|
|
|
|
|
|
|
|
|
|
|
|
|
|
|
|
|
|
|
|
|
|
|
|
|
|
|
|
|
|
|
|
|
|
|
|
|
|
|
|
|
|
|
|
|
|
|
|
|
|
|
|
|
|
|
|
|
|
|
|
|
|
|
|
|
|
|
|
|
|
|
|
|
|
|
|
|
|
|
|
|
|
|
|
|
|
|
|
|
|
|
|
|
|
|
|
|
|
|
|
|
|
|
|
|
|
|
|
|
|
|
|
|
|
|
|
|
|
|
|
|
|
|
|
|
|
|
|
|
|
|
|
|
|
|
|
|
|
|
|
|
|
|
|
|
|
|
|
|
|
|
|
|
|
|
|
|
|
|
|
|
|
|
|
|
|
|
|
|
|
|
|
|
|
|
|
|
|
|
|
|
|
|
|
|
|
|
|
|
|
|
|
|
|
|
|
|
|
|
|
|
|
|
|
|
|
|
|
|
|
|
|
|
|
|
|
|
|
|
|
|
|
|
|
|
|
|
|
|
|
|
|
|
|
|
|
|
|
|
|
|
|
|
|
|
|
|
|
|
|
|
|
|
|
|
|
|
|
|
|
|
|
|
|
|
|
|
|
|
|
|
|
|
|
|
|
|
|
|
|
|
|
|
|
|
|
|
|
|
|
|
|
|
|
|
|
|
|
|
|
|
|
|
|
|
|
|
|
|
|
|
|
|
|
|
|
|
|
|
|
|
|
|
|
|
|
|
|
|
|
|
|
|
|
|
|
|
|
|
|
|
|
|
|
|
|
|
|
|
|
|
|
|
|
|
|
|
|
|
|
|
|
|
|
|
|
|
|
|
|
|
|
|
|
|
|
|
|
|
|
|
|
|
|
|
|
|
|
|
|
|
|
|
|
|
|
|
|
|
|
|
|
|
|
|
|
|
|
|
|
|
|
|
|
|
|
|
|
|
|
|
|
|
|
|
|
|
|
|
|
|
|
|
|
|
|
|
|
|
|
|
|
|
|
|
|
|
|
|
|
|
|
|
|
|
|
|
|
|
|
|
|
|
|
|
|
|
|
|
|
|
|
|
|
|
|
|
|
|
|
|
|
|
|
|
|
|
|
|
|
|
|
|
|
|
|
|
|
|
|
|
|
|
|
|
|
|
|
|
|
|
|
|
|
|
|
|
|
|
|
|
|
|
|
|
|
|
|
|
|
|
|
|
|
|
|
|
|
|
|
|
|
|
|
|
|
|
|
|
|
|
|
|
|
|
|
|
|
|
|
|
|
|
|
|
|
|
|
|
|
|
|
|
|
|
|
|
|
|
|
|
|
|
|
|
|
|
|
|
|
|
|
|
|
|
|
|
|
|
|
|
|
|
|
|
|
|
|
|
|
|
|
|
|
|
|
|
|
|
|
|
|
|
|
|
|
|
|
|
|
|
|
|
|
|
|
|
|
|
|
|
|
|
|
|
|
|
|
|
|
|
|
|
|
|
|
|
|
|
|
|
|
|
|
|
|
|
|
|
|
|
|
|
|
|
|
|
|
|
|
|
|
|
|
|
|
|
|
|
|
|
|
|
|
|
|
|
|
|
|
|
|
|
|
|
|
|
|
|
|
|
|
|
|
|
spaces/Andy1621/uniformer_image_detection/app.py
DELETED
@@ -1,62 +0,0 @@
|
|
1 |
-
import os
|
2 |
-
|
3 |
-
import torch
|
4 |
-
import torch.nn.functional as F
|
5 |
-
import torchvision.transforms as T
|
6 |
-
from mmdet.apis import init_detector, inference_detector, show_result_pyplot
|
7 |
-
import mmcv
|
8 |
-
|
9 |
-
import gradio as gr
|
10 |
-
from huggingface_hub import hf_hub_download
|
11 |
-
|
12 |
-
# Device on which to run the model
|
13 |
-
# Set to cuda to load on GPU
|
14 |
-
device = "cpu"
|
15 |
-
checkpoint_file = hf_hub_download(repo_id="Andy1621/uniformer", filename="mask_rcnn_3x_ms_hybrid_small.pth")
|
16 |
-
config_file = './exp/mask_rcnn_3x_ms_hybrid_small/config.py'
|
17 |
-
# init detector
|
18 |
-
# build the model from a config file and a checkpoint file
|
19 |
-
model = init_detector(config_file, checkpoint_file, device='cpu')
|
20 |
-
|
21 |
-
|
22 |
-
def set_example_image(example: list) -> dict:
|
23 |
-
return gr.Image.update(value=example[0])
|
24 |
-
|
25 |
-
|
26 |
-
def inference(img):
|
27 |
-
result = inference_detector(model, img)
|
28 |
-
res_img = show_result_pyplot(model, img, result)
|
29 |
-
return res_img
|
30 |
-
|
31 |
-
|
32 |
-
demo = gr.Blocks()
|
33 |
-
with demo:
|
34 |
-
gr.Markdown(
|
35 |
-
"""
|
36 |
-
# UniFormer-S
|
37 |
-
Gradio demo for <a href='https://github.com/Sense-X/UniFormer' target='_blank'>UniFormer</a>: To use it, simply upload your image, or click one of the examples to load them. Read more at the links below.
|
38 |
-
"""
|
39 |
-
)
|
40 |
-
|
41 |
-
with gr.Box():
|
42 |
-
with gr.Row():
|
43 |
-
with gr.Column():
|
44 |
-
with gr.Row():
|
45 |
-
input_image = gr.Image(label='Input Image', type='numpy')
|
46 |
-
with gr.Row():
|
47 |
-
submit_button = gr.Button('Submit')
|
48 |
-
with gr.Column():
|
49 |
-
res_image = gr.Image(type='numpy', label='Detection Resutls')
|
50 |
-
with gr.Row():
|
51 |
-
example_images = gr.Dataset(components=[input_image], samples=[['demo.jpg']])
|
52 |
-
|
53 |
-
gr.Markdown(
|
54 |
-
"""
|
55 |
-
<p style='text-align: center'><a href='https://arxiv.org/abs/2201.09450' target='_blank'>UniFormer: Unifying Convolution and Self-attention for Visual Recognition</a> | <a href='https://github.com/Sense-X/UniFormer' target='_blank'>Github Repo</a></p>
|
56 |
-
"""
|
57 |
-
)
|
58 |
-
|
59 |
-
submit_button.click(fn=inference, inputs=input_image, outputs=res_image)
|
60 |
-
example_images.click(fn=set_example_image, inputs=example_images, outputs=example_images.components)
|
61 |
-
|
62 |
-
demo.launch(enable_queue=True)
|
|
|
|
|
|
|
|
|
|
|
|
|
|
|
|
|
|
|
|
|
|
|
|
|
|
|
|
|
|
|
|
|
|
|
|
|
|
|
|
|
|
|
|
|
|
|
|
|
|
|
|
|
|
|
|
|
|
|
|
|
|
|
|
|
|
|
|
|
|
|
|
|
|
|
|
|
|
|
|
|
|
|
|
|
|
|
|
|
|
|
|
|
|
|
|
|
|
|
|
|
|
|
|
|
|
|
|
|
|
|
|
|
|
|
|
|
|
|
|
|
|
|
|
|
|
spaces/Andy1621/uniformer_image_segmentation/configs/pspnet/pspnet_r101-d8_480x480_80k_pascal_context.py
DELETED
@@ -1,2 +0,0 @@
|
|
1 |
-
_base_ = './pspnet_r50-d8_480x480_80k_pascal_context.py'
|
2 |
-
model = dict(pretrained='open-mmlab://resnet101_v1c', backbone=dict(depth=101))
|
|
|
|
|
|
spaces/Anonymous-sub/Rerender/ControlNet/annotator/canny/__init__.py
DELETED
@@ -1,6 +0,0 @@
|
|
1 |
-
import cv2
|
2 |
-
|
3 |
-
|
4 |
-
class CannyDetector:
|
5 |
-
def __call__(self, img, low_threshold, high_threshold):
|
6 |
-
return cv2.Canny(img, low_threshold, high_threshold)
|
|
|
|
|
|
|
|
|
|
|
|
|
|
spaces/Anonymous-sub/Rerender/ControlNet/annotator/uniformer/mmcv/arraymisc/__init__.py
DELETED
@@ -1,4 +0,0 @@
|
|
1 |
-
# Copyright (c) OpenMMLab. All rights reserved.
|
2 |
-
from .quantization import dequantize, quantize
|
3 |
-
|
4 |
-
__all__ = ['quantize', 'dequantize']
|
|
|
|
|
|
|
|
|
|
spaces/ArtGAN/Video-Diffusion-WebUI/video_diffusion/inpaint_zoom/utils/__init__.py
DELETED
File without changes
|
spaces/Arulkumar03/GroundingDINO_SOTA_Zero_Shot_Model/groundingdino/models/GroundingDINO/transformer_vanilla.py
DELETED
@@ -1,123 +0,0 @@
|
|
1 |
-
# ------------------------------------------------------------------------
|
2 |
-
# Grounding DINO
|
3 |
-
# url: https://github.com/IDEA-Research/GroundingDINO
|
4 |
-
# Copyright (c) 2023 IDEA. All Rights Reserved.
|
5 |
-
# Licensed under the Apache License, Version 2.0 [see LICENSE for details]
|
6 |
-
# ------------------------------------------------------------------------
|
7 |
-
# Copyright (c) Aishwarya Kamath & Nicolas Carion. Licensed under the Apache License 2.0. All Rights Reserved
|
8 |
-
# Copyright (c) Facebook, Inc. and its affiliates. All Rights Reserved
|
9 |
-
"""
|
10 |
-
DETR Transformer class.
|
11 |
-
|
12 |
-
Copy-paste from torch.nn.Transformer with modifications:
|
13 |
-
* positional encodings are passed in MHattention
|
14 |
-
* extra LN at the end of encoder is removed
|
15 |
-
* decoder returns a stack of activations from all decoding layers
|
16 |
-
"""
|
17 |
-
from typing import Optional
|
18 |
-
|
19 |
-
import torch
|
20 |
-
import torch.nn.functional as F
|
21 |
-
from torch import Tensor, nn
|
22 |
-
|
23 |
-
from .utils import (
|
24 |
-
MLP,
|
25 |
-
_get_activation_fn,
|
26 |
-
_get_clones,
|
27 |
-
gen_encoder_output_proposals,
|
28 |
-
gen_sineembed_for_position,
|
29 |
-
sigmoid_focal_loss,
|
30 |
-
)
|
31 |
-
|
32 |
-
|
33 |
-
class TextTransformer(nn.Module):
|
34 |
-
def __init__(self, num_layers, d_model=256, nheads=8, dim_feedforward=2048, dropout=0.1):
|
35 |
-
super().__init__()
|
36 |
-
self.num_layers = num_layers
|
37 |
-
self.d_model = d_model
|
38 |
-
self.nheads = nheads
|
39 |
-
self.dim_feedforward = dim_feedforward
|
40 |
-
self.norm = None
|
41 |
-
|
42 |
-
single_encoder_layer = TransformerEncoderLayer(
|
43 |
-
d_model=d_model, nhead=nheads, dim_feedforward=dim_feedforward, dropout=dropout
|
44 |
-
)
|
45 |
-
self.layers = _get_clones(single_encoder_layer, num_layers)
|
46 |
-
|
47 |
-
def forward(self, memory_text: torch.Tensor, text_attention_mask: torch.Tensor):
|
48 |
-
"""
|
49 |
-
|
50 |
-
Args:
|
51 |
-
text_attention_mask: bs, num_token
|
52 |
-
memory_text: bs, num_token, d_model
|
53 |
-
|
54 |
-
Raises:
|
55 |
-
RuntimeError: _description_
|
56 |
-
|
57 |
-
Returns:
|
58 |
-
output: bs, num_token, d_model
|
59 |
-
"""
|
60 |
-
|
61 |
-
output = memory_text.transpose(0, 1)
|
62 |
-
|
63 |
-
for layer in self.layers:
|
64 |
-
output = layer(output, src_key_padding_mask=text_attention_mask)
|
65 |
-
|
66 |
-
if self.norm is not None:
|
67 |
-
output = self.norm(output)
|
68 |
-
|
69 |
-
return output.transpose(0, 1)
|
70 |
-
|
71 |
-
|
72 |
-
class TransformerEncoderLayer(nn.Module):
|
73 |
-
def __init__(
|
74 |
-
self,
|
75 |
-
d_model,
|
76 |
-
nhead,
|
77 |
-
dim_feedforward=2048,
|
78 |
-
dropout=0.1,
|
79 |
-
activation="relu",
|
80 |
-
normalize_before=False,
|
81 |
-
):
|
82 |
-
super().__init__()
|
83 |
-
self.self_attn = nn.MultiheadAttention(d_model, nhead, dropout=dropout)
|
84 |
-
# Implementation of Feedforward model
|
85 |
-
self.linear1 = nn.Linear(d_model, dim_feedforward)
|
86 |
-
self.dropout = nn.Dropout(dropout)
|
87 |
-
self.linear2 = nn.Linear(dim_feedforward, d_model)
|
88 |
-
|
89 |
-
self.norm1 = nn.LayerNorm(d_model)
|
90 |
-
self.norm2 = nn.LayerNorm(d_model)
|
91 |
-
self.dropout1 = nn.Dropout(dropout)
|
92 |
-
self.dropout2 = nn.Dropout(dropout)
|
93 |
-
|
94 |
-
self.activation = _get_activation_fn(activation)
|
95 |
-
self.normalize_before = normalize_before
|
96 |
-
self.nhead = nhead
|
97 |
-
|
98 |
-
def with_pos_embed(self, tensor, pos: Optional[Tensor]):
|
99 |
-
return tensor if pos is None else tensor + pos
|
100 |
-
|
101 |
-
def forward(
|
102 |
-
self,
|
103 |
-
src,
|
104 |
-
src_mask: Optional[Tensor] = None,
|
105 |
-
src_key_padding_mask: Optional[Tensor] = None,
|
106 |
-
pos: Optional[Tensor] = None,
|
107 |
-
):
|
108 |
-
# repeat attn mask
|
109 |
-
if src_mask.dim() == 3 and src_mask.shape[0] == src.shape[1]:
|
110 |
-
# bs, num_q, num_k
|
111 |
-
src_mask = src_mask.repeat(self.nhead, 1, 1)
|
112 |
-
|
113 |
-
q = k = self.with_pos_embed(src, pos)
|
114 |
-
|
115 |
-
src2 = self.self_attn(q, k, value=src, attn_mask=src_mask)[0]
|
116 |
-
|
117 |
-
# src2 = self.self_attn(q, k, value=src, attn_mask=src_mask, key_padding_mask=src_key_padding_mask)[0]
|
118 |
-
src = src + self.dropout1(src2)
|
119 |
-
src = self.norm1(src)
|
120 |
-
src2 = self.linear2(self.dropout(self.activation(self.linear1(src))))
|
121 |
-
src = src + self.dropout2(src2)
|
122 |
-
src = self.norm2(src)
|
123 |
-
return src
|
|
|
|
|
|
|
|
|
|
|
|
|
|
|
|
|
|
|
|
|
|
|
|
|
|
|
|
|
|
|
|
|
|
|
|
|
|
|
|
|
|
|
|
|
|
|
|
|
|
|
|
|
|
|
|
|
|
|
|
|
|
|
|
|
|
|
|
|
|
|
|
|
|
|
|
|
|
|
|
|
|
|
|
|
|
|
|
|
|
|
|
|
|
|
|
|
|
|
|
|
|
|
|
|
|
|
|
|
|
|
|
|
|
|
|
|
|
|
|
|
|
|
|
|
|
|
|
|
|
|
|
|
|
|
|
|
|
|
|
|
|
|
|
|
|
|
|
|
|
|
|
|
|
|
|
|
|
|
|
|
|
|
|
|
|
|
|
|
|
|
|
|
|
|
|
|
|
|
|
|
|
|
|
|
|
|
|
|
|
|
|
|
|
|
|
|
|
|
|
|
|
|
|
|
|
|
|
|
|
|
|
|
|
|
|
|
|
|
|
|
|
|
|
|
|
|
|
|
|
|
|
|
|
|
|
|
|
|
|
|
|
|
|
|
|
|
|
spaces/Ataturk-Chatbot/HuggingFaceChat/venv/lib/python3.11/site-packages/pip/__init__.py
DELETED
@@ -1,13 +0,0 @@
|
|
1 |
-
from typing import List, Optional
|
2 |
-
|
3 |
-
__version__ = "23.1.2"
|
4 |
-
|
5 |
-
|
6 |
-
def main(args: Optional[List[str]] = None) -> int:
|
7 |
-
"""This is an internal API only meant for use by pip's own console scripts.
|
8 |
-
|
9 |
-
For additional details, see https://github.com/pypa/pip/issues/7498.
|
10 |
-
"""
|
11 |
-
from pip._internal.utils.entrypoints import _wrapper
|
12 |
-
|
13 |
-
return _wrapper(args)
|
|
|
|
|
|
|
|
|
|
|
|
|
|
|
|
|
|
|
|
|
|
|
|
|
|
|
|
spaces/Ataturk-Chatbot/HuggingFaceChat/venv/lib/python3.11/site-packages/pip/_internal/vcs/subversion.py
DELETED
@@ -1,324 +0,0 @@
|
|
1 |
-
import logging
|
2 |
-
import os
|
3 |
-
import re
|
4 |
-
from typing import List, Optional, Tuple
|
5 |
-
|
6 |
-
from pip._internal.utils.misc import (
|
7 |
-
HiddenText,
|
8 |
-
display_path,
|
9 |
-
is_console_interactive,
|
10 |
-
is_installable_dir,
|
11 |
-
split_auth_from_netloc,
|
12 |
-
)
|
13 |
-
from pip._internal.utils.subprocess import CommandArgs, make_command
|
14 |
-
from pip._internal.vcs.versioncontrol import (
|
15 |
-
AuthInfo,
|
16 |
-
RemoteNotFoundError,
|
17 |
-
RevOptions,
|
18 |
-
VersionControl,
|
19 |
-
vcs,
|
20 |
-
)
|
21 |
-
|
22 |
-
logger = logging.getLogger(__name__)
|
23 |
-
|
24 |
-
_svn_xml_url_re = re.compile('url="([^"]+)"')
|
25 |
-
_svn_rev_re = re.compile(r'committed-rev="(\d+)"')
|
26 |
-
_svn_info_xml_rev_re = re.compile(r'\s*revision="(\d+)"')
|
27 |
-
_svn_info_xml_url_re = re.compile(r"<url>(.*)</url>")
|
28 |
-
|
29 |
-
|
30 |
-
class Subversion(VersionControl):
|
31 |
-
name = "svn"
|
32 |
-
dirname = ".svn"
|
33 |
-
repo_name = "checkout"
|
34 |
-
schemes = ("svn+ssh", "svn+http", "svn+https", "svn+svn", "svn+file")
|
35 |
-
|
36 |
-
@classmethod
|
37 |
-
def should_add_vcs_url_prefix(cls, remote_url: str) -> bool:
|
38 |
-
return True
|
39 |
-
|
40 |
-
@staticmethod
|
41 |
-
def get_base_rev_args(rev: str) -> List[str]:
|
42 |
-
return ["-r", rev]
|
43 |
-
|
44 |
-
@classmethod
|
45 |
-
def get_revision(cls, location: str) -> str:
|
46 |
-
"""
|
47 |
-
Return the maximum revision for all files under a given location
|
48 |
-
"""
|
49 |
-
# Note: taken from setuptools.command.egg_info
|
50 |
-
revision = 0
|
51 |
-
|
52 |
-
for base, dirs, _ in os.walk(location):
|
53 |
-
if cls.dirname not in dirs:
|
54 |
-
dirs[:] = []
|
55 |
-
continue # no sense walking uncontrolled subdirs
|
56 |
-
dirs.remove(cls.dirname)
|
57 |
-
entries_fn = os.path.join(base, cls.dirname, "entries")
|
58 |
-
if not os.path.exists(entries_fn):
|
59 |
-
# FIXME: should we warn?
|
60 |
-
continue
|
61 |
-
|
62 |
-
dirurl, localrev = cls._get_svn_url_rev(base)
|
63 |
-
|
64 |
-
if base == location:
|
65 |
-
assert dirurl is not None
|
66 |
-
base = dirurl + "/" # save the root url
|
67 |
-
elif not dirurl or not dirurl.startswith(base):
|
68 |
-
dirs[:] = []
|
69 |
-
continue # not part of the same svn tree, skip it
|
70 |
-
revision = max(revision, localrev)
|
71 |
-
return str(revision)
|
72 |
-
|
73 |
-
@classmethod
|
74 |
-
def get_netloc_and_auth(
|
75 |
-
cls, netloc: str, scheme: str
|
76 |
-
) -> Tuple[str, Tuple[Optional[str], Optional[str]]]:
|
77 |
-
"""
|
78 |
-
This override allows the auth information to be passed to svn via the
|
79 |
-
--username and --password options instead of via the URL.
|
80 |
-
"""
|
81 |
-
if scheme == "ssh":
|
82 |
-
# The --username and --password options can't be used for
|
83 |
-
# svn+ssh URLs, so keep the auth information in the URL.
|
84 |
-
return super().get_netloc_and_auth(netloc, scheme)
|
85 |
-
|
86 |
-
return split_auth_from_netloc(netloc)
|
87 |
-
|
88 |
-
@classmethod
|
89 |
-
def get_url_rev_and_auth(cls, url: str) -> Tuple[str, Optional[str], AuthInfo]:
|
90 |
-
# hotfix the URL scheme after removing svn+ from svn+ssh:// re-add it
|
91 |
-
url, rev, user_pass = super().get_url_rev_and_auth(url)
|
92 |
-
if url.startswith("ssh://"):
|
93 |
-
url = "svn+" + url
|
94 |
-
return url, rev, user_pass
|
95 |
-
|
96 |
-
@staticmethod
|
97 |
-
def make_rev_args(
|
98 |
-
username: Optional[str], password: Optional[HiddenText]
|
99 |
-
) -> CommandArgs:
|
100 |
-
extra_args: CommandArgs = []
|
101 |
-
if username:
|
102 |
-
extra_args += ["--username", username]
|
103 |
-
if password:
|
104 |
-
extra_args += ["--password", password]
|
105 |
-
|
106 |
-
return extra_args
|
107 |
-
|
108 |
-
@classmethod
|
109 |
-
def get_remote_url(cls, location: str) -> str:
|
110 |
-
# In cases where the source is in a subdirectory, we have to look up in
|
111 |
-
# the location until we find a valid project root.
|
112 |
-
orig_location = location
|
113 |
-
while not is_installable_dir(location):
|
114 |
-
last_location = location
|
115 |
-
location = os.path.dirname(location)
|
116 |
-
if location == last_location:
|
117 |
-
# We've traversed up to the root of the filesystem without
|
118 |
-
# finding a Python project.
|
119 |
-
logger.warning(
|
120 |
-
"Could not find Python project for directory %s (tried all "
|
121 |
-
"parent directories)",
|
122 |
-
orig_location,
|
123 |
-
)
|
124 |
-
raise RemoteNotFoundError
|
125 |
-
|
126 |
-
url, _rev = cls._get_svn_url_rev(location)
|
127 |
-
if url is None:
|
128 |
-
raise RemoteNotFoundError
|
129 |
-
|
130 |
-
return url
|
131 |
-
|
132 |
-
@classmethod
|
133 |
-
def _get_svn_url_rev(cls, location: str) -> Tuple[Optional[str], int]:
|
134 |
-
from pip._internal.exceptions import InstallationError
|
135 |
-
|
136 |
-
entries_path = os.path.join(location, cls.dirname, "entries")
|
137 |
-
if os.path.exists(entries_path):
|
138 |
-
with open(entries_path) as f:
|
139 |
-
data = f.read()
|
140 |
-
else: # subversion >= 1.7 does not have the 'entries' file
|
141 |
-
data = ""
|
142 |
-
|
143 |
-
url = None
|
144 |
-
if data.startswith("8") or data.startswith("9") or data.startswith("10"):
|
145 |
-
entries = list(map(str.splitlines, data.split("\n\x0c\n")))
|
146 |
-
del entries[0][0] # get rid of the '8'
|
147 |
-
url = entries[0][3]
|
148 |
-
revs = [int(d[9]) for d in entries if len(d) > 9 and d[9]] + [0]
|
149 |
-
elif data.startswith("<?xml"):
|
150 |
-
match = _svn_xml_url_re.search(data)
|
151 |
-
if not match:
|
152 |
-
raise ValueError(f"Badly formatted data: {data!r}")
|
153 |
-
url = match.group(1) # get repository URL
|
154 |
-
revs = [int(m.group(1)) for m in _svn_rev_re.finditer(data)] + [0]
|
155 |
-
else:
|
156 |
-
try:
|
157 |
-
# subversion >= 1.7
|
158 |
-
# Note that using get_remote_call_options is not necessary here
|
159 |
-
# because `svn info` is being run against a local directory.
|
160 |
-
# We don't need to worry about making sure interactive mode
|
161 |
-
# is being used to prompt for passwords, because passwords
|
162 |
-
# are only potentially needed for remote server requests.
|
163 |
-
xml = cls.run_command(
|
164 |
-
["info", "--xml", location],
|
165 |
-
show_stdout=False,
|
166 |
-
stdout_only=True,
|
167 |
-
)
|
168 |
-
match = _svn_info_xml_url_re.search(xml)
|
169 |
-
assert match is not None
|
170 |
-
url = match.group(1)
|
171 |
-
revs = [int(m.group(1)) for m in _svn_info_xml_rev_re.finditer(xml)]
|
172 |
-
except InstallationError:
|
173 |
-
url, revs = None, []
|
174 |
-
|
175 |
-
if revs:
|
176 |
-
rev = max(revs)
|
177 |
-
else:
|
178 |
-
rev = 0
|
179 |
-
|
180 |
-
return url, rev
|
181 |
-
|
182 |
-
@classmethod
|
183 |
-
def is_commit_id_equal(cls, dest: str, name: Optional[str]) -> bool:
|
184 |
-
"""Always assume the versions don't match"""
|
185 |
-
return False
|
186 |
-
|
187 |
-
def __init__(self, use_interactive: Optional[bool] = None) -> None:
|
188 |
-
if use_interactive is None:
|
189 |
-
use_interactive = is_console_interactive()
|
190 |
-
self.use_interactive = use_interactive
|
191 |
-
|
192 |
-
# This member is used to cache the fetched version of the current
|
193 |
-
# ``svn`` client.
|
194 |
-
# Special value definitions:
|
195 |
-
# None: Not evaluated yet.
|
196 |
-
# Empty tuple: Could not parse version.
|
197 |
-
self._vcs_version: Optional[Tuple[int, ...]] = None
|
198 |
-
|
199 |
-
super().__init__()
|
200 |
-
|
201 |
-
def call_vcs_version(self) -> Tuple[int, ...]:
|
202 |
-
"""Query the version of the currently installed Subversion client.
|
203 |
-
|
204 |
-
:return: A tuple containing the parts of the version information or
|
205 |
-
``()`` if the version returned from ``svn`` could not be parsed.
|
206 |
-
:raises: BadCommand: If ``svn`` is not installed.
|
207 |
-
"""
|
208 |
-
# Example versions:
|
209 |
-
# svn, version 1.10.3 (r1842928)
|
210 |
-
# compiled Feb 25 2019, 14:20:39 on x86_64-apple-darwin17.0.0
|
211 |
-
# svn, version 1.7.14 (r1542130)
|
212 |
-
# compiled Mar 28 2018, 08:49:13 on x86_64-pc-linux-gnu
|
213 |
-
# svn, version 1.12.0-SlikSvn (SlikSvn/1.12.0)
|
214 |
-
# compiled May 28 2019, 13:44:56 on x86_64-microsoft-windows6.2
|
215 |
-
version_prefix = "svn, version "
|
216 |
-
version = self.run_command(["--version"], show_stdout=False, stdout_only=True)
|
217 |
-
if not version.startswith(version_prefix):
|
218 |
-
return ()
|
219 |
-
|
220 |
-
version = version[len(version_prefix) :].split()[0]
|
221 |
-
version_list = version.partition("-")[0].split(".")
|
222 |
-
try:
|
223 |
-
parsed_version = tuple(map(int, version_list))
|
224 |
-
except ValueError:
|
225 |
-
return ()
|
226 |
-
|
227 |
-
return parsed_version
|
228 |
-
|
229 |
-
def get_vcs_version(self) -> Tuple[int, ...]:
|
230 |
-
"""Return the version of the currently installed Subversion client.
|
231 |
-
|
232 |
-
If the version of the Subversion client has already been queried,
|
233 |
-
a cached value will be used.
|
234 |
-
|
235 |
-
:return: A tuple containing the parts of the version information or
|
236 |
-
``()`` if the version returned from ``svn`` could not be parsed.
|
237 |
-
:raises: BadCommand: If ``svn`` is not installed.
|
238 |
-
"""
|
239 |
-
if self._vcs_version is not None:
|
240 |
-
# Use cached version, if available.
|
241 |
-
# If parsing the version failed previously (empty tuple),
|
242 |
-
# do not attempt to parse it again.
|
243 |
-
return self._vcs_version
|
244 |
-
|
245 |
-
vcs_version = self.call_vcs_version()
|
246 |
-
self._vcs_version = vcs_version
|
247 |
-
return vcs_version
|
248 |
-
|
249 |
-
def get_remote_call_options(self) -> CommandArgs:
|
250 |
-
"""Return options to be used on calls to Subversion that contact the server.
|
251 |
-
|
252 |
-
These options are applicable for the following ``svn`` subcommands used
|
253 |
-
in this class.
|
254 |
-
|
255 |
-
- checkout
|
256 |
-
- switch
|
257 |
-
- update
|
258 |
-
|
259 |
-
:return: A list of command line arguments to pass to ``svn``.
|
260 |
-
"""
|
261 |
-
if not self.use_interactive:
|
262 |
-
# --non-interactive switch is available since Subversion 0.14.4.
|
263 |
-
# Subversion < 1.8 runs in interactive mode by default.
|
264 |
-
return ["--non-interactive"]
|
265 |
-
|
266 |
-
svn_version = self.get_vcs_version()
|
267 |
-
# By default, Subversion >= 1.8 runs in non-interactive mode if
|
268 |
-
# stdin is not a TTY. Since that is how pip invokes SVN, in
|
269 |
-
# call_subprocess(), pip must pass --force-interactive to ensure
|
270 |
-
# the user can be prompted for a password, if required.
|
271 |
-
# SVN added the --force-interactive option in SVN 1.8. Since
|
272 |
-
# e.g. RHEL/CentOS 7, which is supported until 2024, ships with
|
273 |
-
# SVN 1.7, pip should continue to support SVN 1.7. Therefore, pip
|
274 |
-
# can't safely add the option if the SVN version is < 1.8 (or unknown).
|
275 |
-
if svn_version >= (1, 8):
|
276 |
-
return ["--force-interactive"]
|
277 |
-
|
278 |
-
return []
|
279 |
-
|
280 |
-
def fetch_new(
|
281 |
-
self, dest: str, url: HiddenText, rev_options: RevOptions, verbosity: int
|
282 |
-
) -> None:
|
283 |
-
rev_display = rev_options.to_display()
|
284 |
-
logger.info(
|
285 |
-
"Checking out %s%s to %s",
|
286 |
-
url,
|
287 |
-
rev_display,
|
288 |
-
display_path(dest),
|
289 |
-
)
|
290 |
-
if verbosity <= 0:
|
291 |
-
flag = "--quiet"
|
292 |
-
else:
|
293 |
-
flag = ""
|
294 |
-
cmd_args = make_command(
|
295 |
-
"checkout",
|
296 |
-
flag,
|
297 |
-
self.get_remote_call_options(),
|
298 |
-
rev_options.to_args(),
|
299 |
-
url,
|
300 |
-
dest,
|
301 |
-
)
|
302 |
-
self.run_command(cmd_args)
|
303 |
-
|
304 |
-
def switch(self, dest: str, url: HiddenText, rev_options: RevOptions) -> None:
|
305 |
-
cmd_args = make_command(
|
306 |
-
"switch",
|
307 |
-
self.get_remote_call_options(),
|
308 |
-
rev_options.to_args(),
|
309 |
-
url,
|
310 |
-
dest,
|
311 |
-
)
|
312 |
-
self.run_command(cmd_args)
|
313 |
-
|
314 |
-
def update(self, dest: str, url: HiddenText, rev_options: RevOptions) -> None:
|
315 |
-
cmd_args = make_command(
|
316 |
-
"update",
|
317 |
-
self.get_remote_call_options(),
|
318 |
-
rev_options.to_args(),
|
319 |
-
dest,
|
320 |
-
)
|
321 |
-
self.run_command(cmd_args)
|
322 |
-
|
323 |
-
|
324 |
-
vcs.register(Subversion)
|
|
|
|
|
|
|
|
|
|
|
|
|
|
|
|
|
|
|
|
|
|
|
|
|
|
|
|
|
|
|
|
|
|
|
|
|
|
|
|
|
|
|
|
|
|
|
|
|
|
|
|
|
|
|
|
|
|
|
|
|
|
|
|
|
|
|
|
|
|
|
|
|
|
|
|
|
|
|
|
|
|
|
|
|
|
|
|
|
|
|
|
|
|
|
|
|
|
|
|
|
|
|
|
|
|
|
|
|
|
|
|
|
|
|
|
|
|
|
|
|
|
|
|
|
|
|
|
|
|
|
|
|
|
|
|
|
|
|
|
|
|
|
|
|
|
|
|
|
|
|
|
|
|
|
|
|
|
|
|
|
|
|
|
|
|
|
|
|
|
|
|
|
|
|
|
|
|
|
|
|
|
|
|
|
|
|
|
|
|
|
|
|
|
|
|
|
|
|
|
|
|
|
|
|
|
|
|
|
|
|
|
|
|
|
|
|
|
|
|
|
|
|
|
|
|
|
|
|
|
|
|
|
|
|
|
|
|
|
|
|
|
|
|
|
|
|
|
|
|
|
|
|
|
|
|
|
|
|
|
|
|
|
|
|
|
|
|
|
|
|
|
|
|
|
|
|
|
|
|
|
|
|
|
|
|
|
|
|
|
|
|
|
|
|
|
|
|
|
|
|
|
|
|
|
|
|
|
|
|
|
|
|
|
|
|
|
|
|
|
|
|
|
|
|
|
|
|
|
|
|
|
|
|
|
|
|
|
|
|
|
|
|
|
|
|
|
|
|
|
|
|
|
|
|
|
|
|
|
|
|
|
|
|
|
|
|
|
|
|
|
|
|
|
|
|
|
|
|
|
|
|
|
|
|
|
|
|
|
|
|
|
|
|
|
|
|
|
|
|
|
|
|
|
|
|
|
|
|
|
|
|
|
|
|
|
|
|
|
|
|
|
|
|
|
|
|
|
|
|
|
|
|
|
|
|
|
|
|
|
|
|
|
|
|
|
|
|
|
|
|
|
|
|
|
|
|
|
|
|
|
|
|
|
|
|
|
|
|
|
|
|
|
|
|
|
|
|
|
|
|
|
|
|
|
|
|
|
|
|
|
|
|
|
|
|
|
|
|
|
|
|
|
|
|
|
|
|
|
|
|
|
|
|
|
|
|
|
|
|
|
|
|
|
|
|
|
|
|
|
|
|
|
|
|
|
|
|
|
|
|
|
|
|
|
|
|
|
|
|
|
|
|
|
|
|
|
|
|
|
|
|
|
|
|
|
|
|
|
|
|
|
|
|
|
|
|
|
|
|
|
|
|
|
|
|
|
|
|
|
|
|
|
|
|
|
|
|
|
|
|
|
|
|
|
|
|
|
|
|
|
|
|
|
|
|
|
|
|
|
|
|
|
|
|
|
|
|
|
|
|
|
|
|
|
|
|
|
|
|
|
|
|
|
|
|
|
|
|
|
spaces/Axolotlily/TextGen/README.md
DELETED
@@ -1,13 +0,0 @@
|
|
1 |
-
---
|
2 |
-
title: TextGen
|
3 |
-
emoji: 🐠
|
4 |
-
colorFrom: yellow
|
5 |
-
colorTo: red
|
6 |
-
sdk: gradio
|
7 |
-
sdk_version: 3.0.14
|
8 |
-
app_file: app.py
|
9 |
-
pinned: false
|
10 |
-
license: other
|
11 |
-
---
|
12 |
-
|
13 |
-
Check out the configuration reference at https://huggingface.co/docs/hub/spaces-config-reference
|
|
|
|
|
|
|
|
|
|
|
|
|
|
|
|
|
|
|
|
|
|
|
|
|
|
|
|
spaces/Benson/text-generation/Examples/Caso Penal Mod Apk Desbloquear Todos Los Niveles.md
DELETED
@@ -1,71 +0,0 @@
|
|
1 |
-
<br />
|
2 |
-
<h1>Caso Penal Mod Apk desbloquear todos los niveles: Una revisión</h1>
|
3 |
-
<p>Si eres un fan de las historias de detectives y los juegos de objetos ocultos, es posible que hayas oído hablar de <strong>Criminal Case</strong>, un juego popular y adictivo que te permite resolver casos de asesinato en una ciudad sombría y corrupta. Pero ¿sabías que hay una manera de desbloquear todos los niveles y características del juego sin gastar dinero o esperar horas? En este artículo, vamos a revisar <strong>Criminal Case Mod Apk</strong>, una versión modificada del juego que le da acceso ilimitado a todo lo que necesita para atrapar a los asesinos. También te mostraremos cómo descargarlo e instalarlo, cómo jugarlo y cuáles son los pros y los contras de usarlo. </p>
|
4 |
-
<h2>caso penal mod apk desbloquear todos los niveles</h2><br /><p><b><b>DOWNLOAD</b> ⇒ <a href="https://bltlly.com/2v6MCP">https://bltlly.com/2v6MCP</a></b></p><br /><br />
|
5 |
-
<h2>¿Qué es un caso penal? </h2>
|
6 |
-
<h3>Un juego de objetos ocultos con misterios de asesinato</h3>
|
7 |
-
<p>Criminal Case es un juego gratuito que fue lanzado en 2012 por Pretty Simple Games. Está disponible en Facebook y dispositivos móviles (Android, iOS, Windows). El juego es un juego de objetos ocultos, lo que significa que tienes que encontrar pistas en diferentes escenas del crimen tocando o haciendo clic en ellos. También tienes que examinar pistas, interrogar sospechosos, analizar pruebas y llevar al asesino ante la justicia. El juego tiene cientos de casos para resolver, cada uno con su propia historia, personajes y ubicaciones. El juego se divide en varias temporadas, cada una en una ciudad o región diferente. </p>
|
8 |
-
<h3>Un juego popular y adictivo en Facebook y dispositivos móviles</h3>
|
9 |
-
|
10 |
-
<h2>¿Qué es Caso Penal Mod Apk? </h2>
|
11 |
-
<h3>Una versión modificada del juego con funciones ilimitadas</h3>
|
12 |
-
<p>Criminal Case Mod Apk es una versión modificada del juego original que le da acceso ilimitado a todas las características y contenido del juego. Esto significa que puedes jugar a cualquier nivel que quieras, usar cualquier elemento que necesites y disfrutar de cualquier personaje o mapa que te guste. Usted no tiene que pagar ningún dinero o esperar a que cualquier temporizador para rellenar su energía o obtener sus informes. También puede obtener pistas y estrellas ilimitadas, que se utilizan para desbloquear más pistas y pruebas. Con Criminal Case Mod Apk, puede resolver cualquier caso en ningún momento y convertirse en el mejor detective nunca. </p>
|
13 |
-
<h3>Una forma de desbloquear todos los niveles y elementos sin pagar o esperar</h3>
|
14 |
-
<p>Caso Penal Mod Apk es una manera de evitar las limitaciones y restricciones del juego original. Como sabrás, Criminal Case es un juego gratuito, pero también tiene algunas compras en la aplicación que te permiten comprar más energía, estrellas, monedas, dinero en efectivo u objetos. Estas compras pueden variar desde $ Continuar el artículo: 0.99 a $99.99. Además, el juego tiene una cantidad limitada de energía que se puede utilizar para jugar los niveles. Cada nivel cuesta 20 puntos de energía, y solo se puede tener un máximo de 110 puntos de energía a la vez. Las recargas de energía a razón de un punto cada tres minutos, lo que significa que tienes que esperar horas para jugar más niveles. El juego también tiene un tiempo de espera para el análisis y los informes de las pistas, que pueden tardar de 30 minutos a 24 horas. Estos tiempos de espera se pueden saltarse usando estrellas o dinero en efectivo, que también son limitados y difíciles de ganar. Con Caso Penal Mod Apk, usted no tiene que preocuparse por cualquiera de estos problemas. Puedes jugar todo lo que quieras, cuando quieras, y obtener resultados instantáneos. </p>
|
15 |
-
<h2>¿Cuáles son las características de Caso Penal Mod Apk? </h2>
|
16 |
-
<h3>Dinero, energía y estrellas ilimitados</h3>
|
17 |
-
|
18 |
-
<h3>Todos los elementos, caracteres y mapas desbloqueados</h3>
|
19 |
-
<p>Otra característica de Criminal Case Mod Apk es que desbloquea todos los elementos, personajes y mapas en el juego. Los elementos se utilizan para personalizar tu personaje y tu hogar, así como para mejorar tu rendimiento en el juego. Los personajes son las personas que conoces en el juego, como tus compañeros, sospechosos, testigos o asesinos. Los mapas son los lugares donde ocurren los casos, como Grimsborough, Pacific Bay, Misterios del pasado, La conspiración o Viajar en el tiempo. Con Criminal Case Mod Apk, se puede acceder a todos los elementos, personajes y mapas en el juego. Puedes vestir a tu personaje como quieras, interactuar con quien quieras y explorar cualquier lugar que desees. </p>
|
20 |
-
<p></p>
|
21 |
-
<h3>Análisis instantáneo, informes y sugerencias</h3>
|
22 |
-
<p>Una tercera característica de Criminal Case Mod Apk es que proporciona análisis instantáneo, informes, y pistas en el juego. El análisis es el proceso de examinar las pistas y pruebas que se encuentran en las escenas del crimen. Los informes son los resultados del análisis que le dan más información sobre las pistas y las pruebas. Las pistas son los consejos que le ayudan a encontrar las pistas y la evidencia más rápido y más fácil. Con Caso Penal Mod Apk, usted no tiene que esperar a que se complete cualquier análisis o informe. Puedes conseguirlos al instante sin usar ninguna estrella ni dinero. Tampoco tienes que luchar para encontrar las pistas o pruebas en las escenas del crimen. Puedes obtener pistas ilimitadas que te muestran dónde están. </p>
|
23 |
-
<h2>Cómo descargar e instalar Penal Case Mod Apk? </h2>
|
24 |
-
<h3>Los pasos para descargar e instalar el archivo apk mod</h3>
|
25 |
-
<p>Si desea descargar e instalar Criminal Case Mod Apk en su dispositivo, es necesario seguir estos pasos:</p>
|
26 |
-
<ol>
|
27 |
-
<li>Ir a un sitio web de confianza que proporciona archivos apk mod para juegos Android. </li>
|
28 |
-
<li>Búsqueda de Caso Penal Mod Apk y elegir una versión que se adapte a su dispositivo. </li>
|
29 |
-
<li>Descargar el archivo apk mod en su dispositivo. </li>
|
30 |
-
|
31 |
-
<li>Busque el archivo apk mod en su dispositivo y toque en él para instalarlo. </li>
|
32 |
-
<li>Espere a que la instalación termine y lance el juego. </li>
|
33 |
-
<li>Disfruta jugando Criminal Case Mod Apk con características ilimitadas. </li>
|
34 |
-
</ol>
|
35 |
-
<h3>Las precauciones y riesgos de usar archivos apk mod</h3>
|
36 |
-
<p>Antes de descargar e instalar Criminal Case Mod Apk en su dispositivo, usted necesita ser consciente de algunas precauciones y riesgos de usar archivos apk mod:</p>
|
37 |
-
<ul>
|
38 |
-
<li>Mod apk archivos no son oficiales o autorizados por los desarrolladores de juegos o editores. Son creados por desarrolladores de terceros o hackers que modifican los archivos originales del juego. </li>
|
39 |
-
<li> Los archivos apk mod pueden contener virus o malware que pueden dañar su dispositivo o robar su información personal. </li>
|
40 |
-
<li>Los archivos apk mod pueden no ser compatibles con su dispositivo o con la última versión del juego. Pueden causar errores o fallos en el juego o en tu dispositivo. </li>
|
41 |
-
<li>Los archivos apk mod pueden violar los términos de servicio o la política de privacidad de los desarrolladores o editores de juegos. Pueden resultar en la prohibición o suspensión de su cuenta del juego o de otros servicios en línea. </li> Continuando con el artículo: <li>Los archivos apk mod pueden no ser éticos o justos para los desarrolladores de juegos o editores que trabajan duro para crear y mantener el juego. También pueden arruinar la diversión y el desafío del juego para usted y otros jugadores. </li>
|
42 |
-
</ul>
|
43 |
-
<p>Por lo tanto, usted debe utilizar Caso Penal Mod Apk a su propio riesgo y discreción. También debes respetar los derechos y esfuerzos de los desarrolladores y editores de juegos, y apoyarlos jugando el juego original o haciendo compras legítimas. </p>
|
44 |
-
<h2>¿Cómo se juega Caso Penal Mod Apk? </h2>
|
45 |
-
<h3>La jugabilidad básica y los controles del juego</h3>
|
46 |
-
|
47 |
-
<h3>Los consejos y trucos para resolver los casos más rápido y fácil</h3>
|
48 |
-
<p>Con Criminal Case Mod Apk, puede resolver cualquier caso más rápido y más fácil que nunca. Aquí hay algunos consejos y trucos para ayudarle a jugar mejor:</p>
|
49 |
-
<ul>
|
50 |
-
<li>Usa sabiamente tu dinero, energía y estrellas ilimitados. Puedes comprar cualquier artículo que quieras, jugar a cualquier nivel que quieras y desbloquear cualquier pista o evidencia que quieras. Pero no los desperdicies en cosas o niveles innecesarios. Guárdalos para los más difíciles o más importantes. </li>
|
51 |
-
<li>Usa tus pistas ilimitadas inteligentemente. Puedes usar tantas pistas como quieras para encontrar las pistas y evidencias en las escenas del crimen. Pero no confíes demasiado en ellos. Trata de encontrarlos por ti mismo primero, y usa pistas solo cuando estés atascado o tengas prisa. </li>
|
52 |
-
<li>Utilice su análisis instantáneo, informes y sugerencias de manera efectiva. Puede obtener resultados instantáneos para cualquier análisis o informe sin usar ninguna estrella o dinero en efectivo. También puede obtener sugerencias instantáneas para cualquier interrogatorio o arresto sin usar ninguna estrella o dinero en efectivo. Pero no las omita demasiado rápido. Léalas cuidadosamente y preste atención a los detalles. Pueden contener información útil o pistas para el caso. </li>
|
53 |
-
<li>Usa todos tus objetos, personajes y mapas de forma creativa. Puedes acceder a todos los objetos, personajes y mapas del juego sin desbloquearlos. Puedes personalizar tu personaje y tu hogar con cualquier elemento que te guste. Puedes interactuar con cualquier personaje que quieras. Puedes explorar cualquier mapa que quieras. Pero no olvides el objetivo principal del juego: resolver los casos. Usa tus objetos, personajes y mapas para mejorar tu jugabilidad y experiencia, no para distraerte de ella. </li>
|
54 |
-
</ul>
|
55 |
-
<h2>Conclusión</h2>
|
56 |
-
<h3>Un resumen de los puntos principales del artículo</h3>
|
57 |
-
|
58 |
-
<h3>Una recomendación y un llamado a la acción para los lectores</h3>
|
59 |
-
<p>Si usted está interesado en tratar Criminal Case Mod Apk, se puede descargar desde un sitio web de confianza que proporciona archivos mod apk para juegos de Android. También puede seguir los pasos que proporcionamos anteriormente para instalarlo en su dispositivo. Sin embargo, también debe ser consciente de las precauciones y riesgos de usar archivos mod apk, tales como virus, errores, prohibiciones o cuestiones éticas. Usted debe utilizar Caso Penal Mod Apk a su propio riesgo y discreción. También debes respetar los derechos y esfuerzos de los desarrolladores y editores de juegos, y apoyarlos jugando el juego original o haciendo compras legítimas. </p>
|
60 |
-
<h2>Preguntas frecuentes</h2>
|
61 |
-
<h3>¿Qué es un caso penal? </h3>
|
62 |
-
<p>Criminal Case es un juego de objetos ocultos gratuito que te permite resolver casos de asesinato en una ciudad sombría y corrupta. </p>
|
63 |
-
<h3>¿Qué es Caso Penal Mod Apk? </h3>
|
64 |
-
<p>Criminal Case Mod Apk es una versión modificada de Criminal Case que le da acceso ilimitado a todas las características y contenido del juego. </p Continuando el artículo: <h3>Cómo descargar e instalar Criminal Case Mod Apk? </h3>
|
65 |
-
<p>Puede descargar Criminal Case Mod Apk desde un sitio web de confianza que proporciona archivos mod apk para juegos de Android. También puede seguir los pasos que proporcionamos anteriormente para instalarlo en su dispositivo. </p>
|
66 |
-
<h3>¿Cuáles son las características de Caso Penal Mod Apk? </h3>
|
67 |
-
<p>Caso Penal Mod Apk tiene muchas características, tales como dinero ilimitado, energía y estrellas, todos los elementos, personajes, y mapas desbloqueados, y análisis instantáneo, informes, y sugerencias. </p>
|
68 |
-
<h3>¿Cuáles son las precauciones y riesgos de usar Caso Penal Mod Apk? </h3>
|
69 |
-
<p>Caso Penal Mod Apk puede tener algunas precauciones y riesgos, tales como virus, errores, prohibiciones, o cuestiones éticas. Debe usarlo bajo su propio riesgo y discreción. También debe respetar los derechos y esfuerzos de los desarrolladores y editores de juegos. </p> 64aa2da5cf<br />
|
70 |
-
<br />
|
71 |
-
<br />
|
|
|
|
|
|
|
|
|
|
|
|
|
|
|
|
|
|
|
|
|
|
|
|
|
|
|
|
|
|
|
|
|
|
|
|
|
|
|
|
|
|
|
|
|
|
|
|
|
|
|
|
|
|
|
|
|
|
|
|
|
|
|
|
|
|
|
|
|
|
|
|
|
|
|
|
|
|
|
|
|
|
|
|
|
|
|
|
|
|
|
|
|
|
|
|
|
|
|
|
|
|
|
|
|
|
|
|
|
|
|
|
|
|
|
|
|
|
|
|
|
|
|
|
|
|
|
|
|
|
|
|
|
|
|
|
|
|
|
|
|
|
|
|
spaces/Big-Web/MMSD/env/Lib/site-packages/pip/_internal/cache.py
DELETED
@@ -1,272 +0,0 @@
|
|
1 |
-
"""Cache Management
|
2 |
-
"""
|
3 |
-
|
4 |
-
import hashlib
|
5 |
-
import json
|
6 |
-
import logging
|
7 |
-
import os
|
8 |
-
from pathlib import Path
|
9 |
-
from typing import Any, Dict, List, Optional
|
10 |
-
|
11 |
-
from pip._vendor.packaging.tags import Tag, interpreter_name, interpreter_version
|
12 |
-
from pip._vendor.packaging.utils import canonicalize_name
|
13 |
-
|
14 |
-
from pip._internal.exceptions import InvalidWheelFilename
|
15 |
-
from pip._internal.models.direct_url import DirectUrl
|
16 |
-
from pip._internal.models.link import Link
|
17 |
-
from pip._internal.models.wheel import Wheel
|
18 |
-
from pip._internal.utils.temp_dir import TempDirectory, tempdir_kinds
|
19 |
-
from pip._internal.utils.urls import path_to_url
|
20 |
-
|
21 |
-
logger = logging.getLogger(__name__)
|
22 |
-
|
23 |
-
ORIGIN_JSON_NAME = "origin.json"
|
24 |
-
|
25 |
-
|
26 |
-
def _hash_dict(d: Dict[str, str]) -> str:
|
27 |
-
"""Return a stable sha224 of a dictionary."""
|
28 |
-
s = json.dumps(d, sort_keys=True, separators=(",", ":"), ensure_ascii=True)
|
29 |
-
return hashlib.sha224(s.encode("ascii")).hexdigest()
|
30 |
-
|
31 |
-
|
32 |
-
class Cache:
|
33 |
-
"""An abstract class - provides cache directories for data from links
|
34 |
-
|
35 |
-
:param cache_dir: The root of the cache.
|
36 |
-
"""
|
37 |
-
|
38 |
-
def __init__(self, cache_dir: str) -> None:
|
39 |
-
super().__init__()
|
40 |
-
assert not cache_dir or os.path.isabs(cache_dir)
|
41 |
-
self.cache_dir = cache_dir or None
|
42 |
-
|
43 |
-
def _get_cache_path_parts(self, link: Link) -> List[str]:
|
44 |
-
"""Get parts of part that must be os.path.joined with cache_dir"""
|
45 |
-
|
46 |
-
# We want to generate an url to use as our cache key, we don't want to
|
47 |
-
# just re-use the URL because it might have other items in the fragment
|
48 |
-
# and we don't care about those.
|
49 |
-
key_parts = {"url": link.url_without_fragment}
|
50 |
-
if link.hash_name is not None and link.hash is not None:
|
51 |
-
key_parts[link.hash_name] = link.hash
|
52 |
-
if link.subdirectory_fragment:
|
53 |
-
key_parts["subdirectory"] = link.subdirectory_fragment
|
54 |
-
|
55 |
-
# Include interpreter name, major and minor version in cache key
|
56 |
-
# to cope with ill-behaved sdists that build a different wheel
|
57 |
-
# depending on the python version their setup.py is being run on,
|
58 |
-
# and don't encode the difference in compatibility tags.
|
59 |
-
# https://github.com/pypa/pip/issues/7296
|
60 |
-
key_parts["interpreter_name"] = interpreter_name()
|
61 |
-
key_parts["interpreter_version"] = interpreter_version()
|
62 |
-
|
63 |
-
# Encode our key url with sha224, we'll use this because it has similar
|
64 |
-
# security properties to sha256, but with a shorter total output (and
|
65 |
-
# thus less secure). However the differences don't make a lot of
|
66 |
-
# difference for our use case here.
|
67 |
-
hashed = _hash_dict(key_parts)
|
68 |
-
|
69 |
-
# We want to nest the directories some to prevent having a ton of top
|
70 |
-
# level directories where we might run out of sub directories on some
|
71 |
-
# FS.
|
72 |
-
parts = [hashed[:2], hashed[2:4], hashed[4:6], hashed[6:]]
|
73 |
-
|
74 |
-
return parts
|
75 |
-
|
76 |
-
def _get_candidates(self, link: Link, canonical_package_name: str) -> List[Any]:
|
77 |
-
can_not_cache = not self.cache_dir or not canonical_package_name or not link
|
78 |
-
if can_not_cache:
|
79 |
-
return []
|
80 |
-
|
81 |
-
candidates = []
|
82 |
-
path = self.get_path_for_link(link)
|
83 |
-
if os.path.isdir(path):
|
84 |
-
for candidate in os.listdir(path):
|
85 |
-
candidates.append((candidate, path))
|
86 |
-
return candidates
|
87 |
-
|
88 |
-
def get_path_for_link(self, link: Link) -> str:
|
89 |
-
"""Return a directory to store cached items in for link."""
|
90 |
-
raise NotImplementedError()
|
91 |
-
|
92 |
-
def get(
|
93 |
-
self,
|
94 |
-
link: Link,
|
95 |
-
package_name: Optional[str],
|
96 |
-
supported_tags: List[Tag],
|
97 |
-
) -> Link:
|
98 |
-
"""Returns a link to a cached item if it exists, otherwise returns the
|
99 |
-
passed link.
|
100 |
-
"""
|
101 |
-
raise NotImplementedError()
|
102 |
-
|
103 |
-
|
104 |
-
class SimpleWheelCache(Cache):
|
105 |
-
"""A cache of wheels for future installs."""
|
106 |
-
|
107 |
-
def __init__(self, cache_dir: str) -> None:
|
108 |
-
super().__init__(cache_dir)
|
109 |
-
|
110 |
-
def get_path_for_link(self, link: Link) -> str:
|
111 |
-
"""Return a directory to store cached wheels for link
|
112 |
-
|
113 |
-
Because there are M wheels for any one sdist, we provide a directory
|
114 |
-
to cache them in, and then consult that directory when looking up
|
115 |
-
cache hits.
|
116 |
-
|
117 |
-
We only insert things into the cache if they have plausible version
|
118 |
-
numbers, so that we don't contaminate the cache with things that were
|
119 |
-
not unique. E.g. ./package might have dozens of installs done for it
|
120 |
-
and build a version of 0.0...and if we built and cached a wheel, we'd
|
121 |
-
end up using the same wheel even if the source has been edited.
|
122 |
-
|
123 |
-
:param link: The link of the sdist for which this will cache wheels.
|
124 |
-
"""
|
125 |
-
parts = self._get_cache_path_parts(link)
|
126 |
-
assert self.cache_dir
|
127 |
-
# Store wheels within the root cache_dir
|
128 |
-
return os.path.join(self.cache_dir, "wheels", *parts)
|
129 |
-
|
130 |
-
def get(
|
131 |
-
self,
|
132 |
-
link: Link,
|
133 |
-
package_name: Optional[str],
|
134 |
-
supported_tags: List[Tag],
|
135 |
-
) -> Link:
|
136 |
-
candidates = []
|
137 |
-
|
138 |
-
if not package_name:
|
139 |
-
return link
|
140 |
-
|
141 |
-
canonical_package_name = canonicalize_name(package_name)
|
142 |
-
for wheel_name, wheel_dir in self._get_candidates(link, canonical_package_name):
|
143 |
-
try:
|
144 |
-
wheel = Wheel(wheel_name)
|
145 |
-
except InvalidWheelFilename:
|
146 |
-
continue
|
147 |
-
if canonicalize_name(wheel.name) != canonical_package_name:
|
148 |
-
logger.debug(
|
149 |
-
"Ignoring cached wheel %s for %s as it "
|
150 |
-
"does not match the expected distribution name %s.",
|
151 |
-
wheel_name,
|
152 |
-
link,
|
153 |
-
package_name,
|
154 |
-
)
|
155 |
-
continue
|
156 |
-
if not wheel.supported(supported_tags):
|
157 |
-
# Built for a different python/arch/etc
|
158 |
-
continue
|
159 |
-
candidates.append(
|
160 |
-
(
|
161 |
-
wheel.support_index_min(supported_tags),
|
162 |
-
wheel_name,
|
163 |
-
wheel_dir,
|
164 |
-
)
|
165 |
-
)
|
166 |
-
|
167 |
-
if not candidates:
|
168 |
-
return link
|
169 |
-
|
170 |
-
_, wheel_name, wheel_dir = min(candidates)
|
171 |
-
return Link(path_to_url(os.path.join(wheel_dir, wheel_name)))
|
172 |
-
|
173 |
-
|
174 |
-
class EphemWheelCache(SimpleWheelCache):
|
175 |
-
"""A SimpleWheelCache that creates it's own temporary cache directory"""
|
176 |
-
|
177 |
-
def __init__(self) -> None:
|
178 |
-
self._temp_dir = TempDirectory(
|
179 |
-
kind=tempdir_kinds.EPHEM_WHEEL_CACHE,
|
180 |
-
globally_managed=True,
|
181 |
-
)
|
182 |
-
|
183 |
-
super().__init__(self._temp_dir.path)
|
184 |
-
|
185 |
-
|
186 |
-
class CacheEntry:
|
187 |
-
def __init__(
|
188 |
-
self,
|
189 |
-
link: Link,
|
190 |
-
persistent: bool,
|
191 |
-
):
|
192 |
-
self.link = link
|
193 |
-
self.persistent = persistent
|
194 |
-
self.origin: Optional[DirectUrl] = None
|
195 |
-
origin_direct_url_path = Path(self.link.file_path).parent / ORIGIN_JSON_NAME
|
196 |
-
if origin_direct_url_path.exists():
|
197 |
-
self.origin = DirectUrl.from_json(origin_direct_url_path.read_text())
|
198 |
-
|
199 |
-
|
200 |
-
class WheelCache(Cache):
|
201 |
-
"""Wraps EphemWheelCache and SimpleWheelCache into a single Cache
|
202 |
-
|
203 |
-
This Cache allows for gracefully degradation, using the ephem wheel cache
|
204 |
-
when a certain link is not found in the simple wheel cache first.
|
205 |
-
"""
|
206 |
-
|
207 |
-
def __init__(self, cache_dir: str) -> None:
|
208 |
-
super().__init__(cache_dir)
|
209 |
-
self._wheel_cache = SimpleWheelCache(cache_dir)
|
210 |
-
self._ephem_cache = EphemWheelCache()
|
211 |
-
|
212 |
-
def get_path_for_link(self, link: Link) -> str:
|
213 |
-
return self._wheel_cache.get_path_for_link(link)
|
214 |
-
|
215 |
-
def get_ephem_path_for_link(self, link: Link) -> str:
|
216 |
-
return self._ephem_cache.get_path_for_link(link)
|
217 |
-
|
218 |
-
def get(
|
219 |
-
self,
|
220 |
-
link: Link,
|
221 |
-
package_name: Optional[str],
|
222 |
-
supported_tags: List[Tag],
|
223 |
-
) -> Link:
|
224 |
-
cache_entry = self.get_cache_entry(link, package_name, supported_tags)
|
225 |
-
if cache_entry is None:
|
226 |
-
return link
|
227 |
-
return cache_entry.link
|
228 |
-
|
229 |
-
def get_cache_entry(
|
230 |
-
self,
|
231 |
-
link: Link,
|
232 |
-
package_name: Optional[str],
|
233 |
-
supported_tags: List[Tag],
|
234 |
-
) -> Optional[CacheEntry]:
|
235 |
-
"""Returns a CacheEntry with a link to a cached item if it exists or
|
236 |
-
None. The cache entry indicates if the item was found in the persistent
|
237 |
-
or ephemeral cache.
|
238 |
-
"""
|
239 |
-
retval = self._wheel_cache.get(
|
240 |
-
link=link,
|
241 |
-
package_name=package_name,
|
242 |
-
supported_tags=supported_tags,
|
243 |
-
)
|
244 |
-
if retval is not link:
|
245 |
-
return CacheEntry(retval, persistent=True)
|
246 |
-
|
247 |
-
retval = self._ephem_cache.get(
|
248 |
-
link=link,
|
249 |
-
package_name=package_name,
|
250 |
-
supported_tags=supported_tags,
|
251 |
-
)
|
252 |
-
if retval is not link:
|
253 |
-
return CacheEntry(retval, persistent=False)
|
254 |
-
|
255 |
-
return None
|
256 |
-
|
257 |
-
@staticmethod
|
258 |
-
def record_download_origin(cache_dir: str, download_info: DirectUrl) -> None:
|
259 |
-
origin_path = Path(cache_dir) / ORIGIN_JSON_NAME
|
260 |
-
if origin_path.is_file():
|
261 |
-
origin = DirectUrl.from_json(origin_path.read_text())
|
262 |
-
# TODO: use DirectUrl.equivalent when https://github.com/pypa/pip/pull/10564
|
263 |
-
# is merged.
|
264 |
-
if origin.url != download_info.url:
|
265 |
-
logger.warning(
|
266 |
-
"Origin URL %s in cache entry %s does not match download URL %s. "
|
267 |
-
"This is likely a pip bug or a cache corruption issue.",
|
268 |
-
origin.url,
|
269 |
-
cache_dir,
|
270 |
-
download_info.url,
|
271 |
-
)
|
272 |
-
origin_path.write_text(download_info.to_json(), encoding="utf-8")
|
|
|
|
|
|
|
|
|
|
|
|
|
|
|
|
|
|
|
|
|
|
|
|
|
|
|
|
|
|
|
|
|
|
|
|
|
|
|
|
|
|
|
|
|
|
|
|
|
|
|
|
|
|
|
|
|
|
|
|
|
|
|
|
|
|
|
|
|
|
|
|
|
|
|
|
|
|
|
|
|
|
|
|
|
|
|
|
|
|
|
|
|
|
|
|
|
|
|
|
|
|
|
|
|
|
|
|
|
|
|
|
|
|
|
|
|
|
|
|
|
|
|
|
|
|
|
|
|
|
|
|
|
|
|
|
|
|
|
|
|
|
|
|
|
|
|
|
|
|
|
|
|
|
|
|
|
|
|
|
|
|
|
|
|
|
|
|
|
|
|
|
|
|
|
|
|
|
|
|
|
|
|
|
|
|
|
|
|
|
|
|
|
|
|
|
|
|
|
|
|
|
|
|
|
|
|
|
|
|
|
|
|
|
|
|
|
|
|
|
|
|
|
|
|
|
|
|
|
|
|
|
|
|
|
|
|
|
|
|
|
|
|
|
|
|
|
|
|
|
|
|
|
|
|
|
|
|
|
|
|
|
|
|
|
|
|
|
|
|
|
|
|
|
|
|
|
|
|
|
|
|
|
|
|
|
|
|
|
|
|
|
|
|
|
|
|
|
|
|
|
|
|
|
|
|
|
|
|
|
|
|
|
|
|
|
|
|
|
|
|
|
|
|
|
|
|
|
|
|
|
|
|
|
|
|
|
|
|
|
|
|
|
|
|
|
|
|
|
|
|
|
|
|
|
|
|
|
|
|
|
|
|
|
|
|
|
|
|
|
|
|
|
|
|
|
|
|
|
|
|
|
|
|
|
|
|
|
|
|
|
|
|
|
|
|
|
|
|
|
|
|
|
|
|
|
|
|
|
|
|
|
|
|
|
|
|
|
|
|
|
|
|
|
|
|
|
|
|
|
|
|
|
|
|
|
|
|
|
|
|
|
|
|
|
|
|
|
|
|
|
|
|
|
|
|
|
|
|
|
|
|
|
|
|
|
|
|
|
|
|
|
|
|
|
|
|
|
|
|
|
|
|
|
|
|
|
|
|
|
|
|
|
|
|
|
|
|
|
|
|
|
|
|
|
|
|
|
|
|
|
|
|
|
|
|
|
|
|
|
|
|
|
|
|
|
|
|
|
|
|
|
|
|
|
|
|
|
|
|
|
|
|
|
|
|
spaces/BucketHeadP65/confusion_matrix/app.py
DELETED
@@ -1,6 +0,0 @@
|
|
1 |
-
import evaluate
|
2 |
-
from evaluate.utils import launch_gradio_widget
|
3 |
-
|
4 |
-
|
5 |
-
module = evaluate.load("BucketHeadP65/confusion_matrix")
|
6 |
-
launch_gradio_widget(module)
|
|
|
|
|
|
|
|
|
|
|
|
|
|
spaces/CVPR/Dual-Key_Backdoor_Attacks/openvqa/openvqa/datasets/clevr/clevr_loader.py
DELETED
@@ -1,200 +0,0 @@
|
|
1 |
-
# --------------------------------------------------------
|
2 |
-
# OpenVQA
|
3 |
-
# Written by Yuhao Cui https://github.com/cuiyuhao1996
|
4 |
-
# --------------------------------------------------------
|
5 |
-
|
6 |
-
import numpy as np
|
7 |
-
import glob, json, re, en_vectors_web_lg
|
8 |
-
from openvqa.core.base_dataset import BaseDataSet
|
9 |
-
from openvqa.utils.ans_punct import prep_ans
|
10 |
-
|
11 |
-
|
12 |
-
class DataSet(BaseDataSet):
|
13 |
-
def __init__(self, __C):
|
14 |
-
super(DataSet, self).__init__()
|
15 |
-
self.__C = __C
|
16 |
-
|
17 |
-
# --------------------------
|
18 |
-
# ---- Raw data loading ----
|
19 |
-
# --------------------------
|
20 |
-
|
21 |
-
# Loading all image paths
|
22 |
-
# grid_feat_path_list = \
|
23 |
-
# glob.glob(__C.FEATS_PATH[__C.DATASET]['train'] + '/*.npz') + \
|
24 |
-
# glob.glob(__C.FEATS_PATH[__C.DATASET]['val'] + '/*.npz') + \
|
25 |
-
# glob.glob(__C.FEATS_PATH[__C.DATASET]['test'] + '/*.npz')
|
26 |
-
|
27 |
-
# Loading question word list
|
28 |
-
stat_ques_list = \
|
29 |
-
json.load(open(__C.RAW_PATH[__C.DATASET]['train'], 'r'))['questions'] + \
|
30 |
-
json.load(open(__C.RAW_PATH[__C.DATASET]['val'], 'r'))['questions'] + \
|
31 |
-
json.load(open(__C.RAW_PATH[__C.DATASET]['test'], 'r'))['questions']
|
32 |
-
|
33 |
-
# Loading answer word list
|
34 |
-
stat_ans_list = \
|
35 |
-
json.load(open(__C.RAW_PATH[__C.DATASET]['train'], 'r'))['questions'] + \
|
36 |
-
json.load(open(__C.RAW_PATH[__C.DATASET]['val'], 'r'))['questions']
|
37 |
-
|
38 |
-
# Loading question and answer list
|
39 |
-
self.ques_list = []
|
40 |
-
grid_feat_path_list = []
|
41 |
-
|
42 |
-
split_list = __C.SPLIT[__C.RUN_MODE].split('+')
|
43 |
-
for split in split_list:
|
44 |
-
self.ques_list += json.load(open(__C.RAW_PATH[__C.DATASET][split], 'r'))['questions']
|
45 |
-
grid_feat_path_list += glob.glob(__C.FEATS_PATH[__C.DATASET][split] + '/*.npz')
|
46 |
-
|
47 |
-
# Define run data size
|
48 |
-
self.data_size = self.ques_list.__len__()
|
49 |
-
|
50 |
-
print(' ========== Dataset size:', self.data_size)
|
51 |
-
|
52 |
-
|
53 |
-
# ------------------------
|
54 |
-
# ---- Data statistic ----
|
55 |
-
# ------------------------
|
56 |
-
|
57 |
-
# {image id} -> {image feature absolutely path}
|
58 |
-
self.iid_to_grid_feat_path = self.img_feat_path_load(grid_feat_path_list)
|
59 |
-
|
60 |
-
# Tokenize
|
61 |
-
self.token_to_ix, self.pretrained_emb, max_token = self.tokenize(stat_ques_list, __C.USE_GLOVE)
|
62 |
-
self.token_size = self.token_to_ix.__len__()
|
63 |
-
print(' ========== Question token vocab size:', self.token_size)
|
64 |
-
|
65 |
-
self.max_token = -1
|
66 |
-
if self.max_token == -1:
|
67 |
-
self.max_token = max_token
|
68 |
-
print('Max token length:', max_token, 'Trimmed to:', self.max_token)
|
69 |
-
|
70 |
-
# Answers statistic
|
71 |
-
self.ans_to_ix, self.ix_to_ans = self.ans_stat(stat_ans_list)
|
72 |
-
self.ans_size = self.ans_to_ix.__len__()
|
73 |
-
print(' ========== Answer token vocab size:', self.ans_size)
|
74 |
-
print('Finished!')
|
75 |
-
print('')
|
76 |
-
|
77 |
-
|
78 |
-
|
79 |
-
def img_feat_path_load(self, path_list):
|
80 |
-
iid_to_path = {}
|
81 |
-
|
82 |
-
for ix, path in enumerate(path_list):
|
83 |
-
iid = path.split('/')[-1].split('.')[0]
|
84 |
-
iid_to_path[iid] = path
|
85 |
-
|
86 |
-
return iid_to_path
|
87 |
-
|
88 |
-
|
89 |
-
def tokenize(self, stat_ques_list, use_glove):
|
90 |
-
token_to_ix = {
|
91 |
-
'PAD': 0,
|
92 |
-
'UNK': 1,
|
93 |
-
'CLS': 2,
|
94 |
-
}
|
95 |
-
|
96 |
-
spacy_tool = None
|
97 |
-
pretrained_emb = []
|
98 |
-
if use_glove:
|
99 |
-
spacy_tool = en_vectors_web_lg.load()
|
100 |
-
pretrained_emb.append(spacy_tool('PAD').vector)
|
101 |
-
pretrained_emb.append(spacy_tool('UNK').vector)
|
102 |
-
pretrained_emb.append(spacy_tool('CLS').vector)
|
103 |
-
|
104 |
-
max_token = 0
|
105 |
-
for ques in stat_ques_list:
|
106 |
-
words = re.sub(
|
107 |
-
r"([.,'!?\"()*#:;])",
|
108 |
-
'',
|
109 |
-
ques['question'].lower()
|
110 |
-
).replace('-', ' ').replace('/', ' ').split()
|
111 |
-
|
112 |
-
if len(words) > max_token:
|
113 |
-
max_token = len(words)
|
114 |
-
|
115 |
-
for word in words:
|
116 |
-
if word not in token_to_ix:
|
117 |
-
token_to_ix[word] = len(token_to_ix)
|
118 |
-
if use_glove:
|
119 |
-
pretrained_emb.append(spacy_tool(word).vector)
|
120 |
-
|
121 |
-
pretrained_emb = np.array(pretrained_emb)
|
122 |
-
|
123 |
-
return token_to_ix, pretrained_emb, max_token
|
124 |
-
|
125 |
-
|
126 |
-
def ans_stat(self, stat_ans_list):
|
127 |
-
ans_to_ix = {}
|
128 |
-
ix_to_ans = {}
|
129 |
-
|
130 |
-
for ans_stat in stat_ans_list:
|
131 |
-
ans = ans_stat['answer']
|
132 |
-
|
133 |
-
if ans not in ans_to_ix:
|
134 |
-
ix_to_ans[ans_to_ix.__len__()] = ans
|
135 |
-
ans_to_ix[ans] = ans_to_ix.__len__()
|
136 |
-
|
137 |
-
return ans_to_ix, ix_to_ans
|
138 |
-
|
139 |
-
|
140 |
-
|
141 |
-
# ----------------------------------------------
|
142 |
-
# ---- Real-Time Processing Implementations ----
|
143 |
-
# ----------------------------------------------
|
144 |
-
|
145 |
-
def load_ques_ans(self, idx):
|
146 |
-
# if self.__C.RUN_MODE in ['train']:
|
147 |
-
ques = self.ques_list[idx]
|
148 |
-
iid = str(ques['image_index'])
|
149 |
-
|
150 |
-
# Process question
|
151 |
-
ques_ix_iter = self.proc_ques(ques, self.token_to_ix, max_token=self.max_token)
|
152 |
-
ans_iter = np.zeros(1)
|
153 |
-
|
154 |
-
if self.__C.RUN_MODE in ['train']:
|
155 |
-
# process answers
|
156 |
-
ans = ques['answer']
|
157 |
-
ans_iter = self.proc_ans(ans, self.ans_to_ix)
|
158 |
-
|
159 |
-
return ques_ix_iter, ans_iter, iid
|
160 |
-
|
161 |
-
|
162 |
-
def load_img_feats(self, idx, iid):
|
163 |
-
grid_feat = np.load(self.iid_to_grid_feat_path[iid])
|
164 |
-
grid_feat_iter = grid_feat['x']
|
165 |
-
|
166 |
-
return np.zeros(1), grid_feat_iter, np.zeros(1)
|
167 |
-
|
168 |
-
|
169 |
-
|
170 |
-
# ------------------------------------
|
171 |
-
# ---- Real-Time Processing Utils ----
|
172 |
-
# ------------------------------------
|
173 |
-
|
174 |
-
def proc_ques(self, ques, token_to_ix, max_token):
|
175 |
-
ques_ix = np.zeros(max_token, np.int64)
|
176 |
-
|
177 |
-
words = re.sub(
|
178 |
-
r"([.,'!?\"()*#:;])",
|
179 |
-
'',
|
180 |
-
ques['question'].lower()
|
181 |
-
).replace('-', ' ').replace('/', ' ').split()
|
182 |
-
|
183 |
-
for ix, word in enumerate(words):
|
184 |
-
if word in token_to_ix:
|
185 |
-
ques_ix[ix] = token_to_ix[word]
|
186 |
-
else:
|
187 |
-
ques_ix[ix] = token_to_ix['UNK']
|
188 |
-
|
189 |
-
if ix + 1 == max_token:
|
190 |
-
break
|
191 |
-
|
192 |
-
return ques_ix
|
193 |
-
|
194 |
-
|
195 |
-
def proc_ans(self, ans, ans_to_ix):
|
196 |
-
ans_ix = np.zeros(1, np.int64)
|
197 |
-
ans_ix[0] = ans_to_ix[ans]
|
198 |
-
|
199 |
-
return ans_ix
|
200 |
-
|
|
|
|
|
|
|
|
|
|
|
|
|
|
|
|
|
|
|
|
|
|
|
|
|
|
|
|
|
|
|
|
|
|
|
|
|
|
|
|
|
|
|
|
|
|
|
|
|
|
|
|
|
|
|
|
|
|
|
|
|
|
|
|
|
|
|
|
|
|
|
|
|
|
|
|
|
|
|
|
|
|
|
|
|
|
|
|
|
|
|
|
|
|
|
|
|
|
|
|
|
|
|
|
|
|
|
|
|
|
|
|
|
|
|
|
|
|
|
|
|
|
|
|
|
|
|
|
|
|
|
|
|
|
|
|
|
|
|
|
|
|
|
|
|
|
|
|
|
|
|
|
|
|
|
|
|
|
|
|
|
|
|
|
|
|
|
|
|
|
|
|
|
|
|
|
|
|
|
|
|
|
|
|
|
|
|
|
|
|
|
|
|
|
|
|
|
|
|
|
|
|
|
|
|
|
|
|
|
|
|
|
|
|
|
|
|
|
|
|
|
|
|
|
|
|
|
|
|
|
|
|
|
|
|
|
|
|
|
|
|
|
|
|
|
|
|
|
|
|
|
|
|
|
|
|
|
|
|
|
|
|
|
|
|
|
|
|
|
|
|
|
|
|
|
|
|
|
|
|
|
|
|
|
|
|
|
|
|
|
|
|
|
|
|
|
|
|
|
|
|
|
|
|
|
|
|
|
|
|
|
|
|
|
|
|
|
|
|
|
|
|
|
|
|
|
|
|
|
|
|
|
|
|
|
|
|
|
|
|
|
|
|
|
|
|
|
|
|
|
|
|
|
|
|
|
|
|
|
|
|
|
|
|
|
|
|
|
|
|
|
|
|
|
|
|
|
|
|
|
|
|
|
|
|
|
|
|
|
|
|
|
|
|
|
|
|
|
|
|
|
|
spaces/CVPR/LIVE/thrust/thrust/detail/type_traits/is_call_possible.h
DELETED
@@ -1,161 +0,0 @@
|
|
1 |
-
/*
|
2 |
-
* Copyright 2008-2013 NVIDIA Corporation
|
3 |
-
*
|
4 |
-
* Licensed under the Apache License, Version 2.0 (the "License");
|
5 |
-
* you may not use this file except in compliance with the License.
|
6 |
-
* You may obtain a copy of the License at
|
7 |
-
*
|
8 |
-
* http://www.apache.org/licenses/LICENSE-2.0
|
9 |
-
*
|
10 |
-
* Unless required by applicable law or agreed to in writing, software
|
11 |
-
* distributed under the License is distributed on an "AS IS" BASIS,
|
12 |
-
* WITHOUT WARRANTIES OR CONDITIONS OF ANY KIND, either express or implied.
|
13 |
-
* See the License for the specific language governing permissions and
|
14 |
-
* limitations under the License.
|
15 |
-
*/
|
16 |
-
|
17 |
-
#pragma once
|
18 |
-
|
19 |
-
#include <thrust/detail/type_traits.h>
|
20 |
-
#include <thrust/detail/type_traits/has_member_function.h>
|
21 |
-
|
22 |
-
// inspired by Roman Perepelitsa's presentation from comp.lang.c++.moderated
|
23 |
-
// based on the implementation here: http://www.rsdn.ru/forum/cpp/2759773.1.aspx
|
24 |
-
|
25 |
-
namespace thrust
|
26 |
-
{
|
27 |
-
namespace detail
|
28 |
-
{
|
29 |
-
namespace is_call_possible_detail
|
30 |
-
{
|
31 |
-
|
32 |
-
template<typename T> class void_exp_result {};
|
33 |
-
|
34 |
-
template<typename T, typename U>
|
35 |
-
U const& operator,(U const&, void_exp_result<T>);
|
36 |
-
|
37 |
-
template<typename T, typename U>
|
38 |
-
U& operator,(U&, void_exp_result<T>);
|
39 |
-
|
40 |
-
template<typename src_type, typename dest_type>
|
41 |
-
struct clone_constness
|
42 |
-
{
|
43 |
-
typedef dest_type type;
|
44 |
-
};
|
45 |
-
|
46 |
-
template<typename src_type, typename dest_type>
|
47 |
-
struct clone_constness<const src_type, dest_type>
|
48 |
-
{
|
49 |
-
typedef const dest_type type;
|
50 |
-
};
|
51 |
-
|
52 |
-
} // end is_call_possible_detail
|
53 |
-
} // end detail
|
54 |
-
} // end thrust
|
55 |
-
|
56 |
-
#define __THRUST_DEFINE_IS_CALL_POSSIBLE(trait_name, member_function_name) \
|
57 |
-
__THRUST_DEFINE_HAS_MEMBER_FUNCTION(trait_name##_has_member, member_function_name) \
|
58 |
-
\
|
59 |
-
template <typename T, typename Signature> \
|
60 |
-
struct trait_name \
|
61 |
-
{ \
|
62 |
-
private: \
|
63 |
-
struct yes {}; \
|
64 |
-
struct no { yes m[2]; }; \
|
65 |
-
struct derived : public T \
|
66 |
-
{ \
|
67 |
-
using T::member_function_name; \
|
68 |
-
no member_function_name(...) const; \
|
69 |
-
}; \
|
70 |
-
\
|
71 |
-
typedef typename thrust::detail::is_call_possible_detail::clone_constness<T, derived>::type derived_type; \
|
72 |
-
\
|
73 |
-
template<typename U, typename Result> \
|
74 |
-
struct return_value_check \
|
75 |
-
{ \
|
76 |
-
static yes deduce(Result); \
|
77 |
-
static no deduce(...); \
|
78 |
-
static no deduce(no); \
|
79 |
-
static no deduce(thrust::detail::is_call_possible_detail::void_exp_result<T>); \
|
80 |
-
}; \
|
81 |
-
\
|
82 |
-
template<typename U> \
|
83 |
-
struct return_value_check<U, void> \
|
84 |
-
{ \
|
85 |
-
static yes deduce(...); \
|
86 |
-
static no deduce(no); \
|
87 |
-
}; \
|
88 |
-
\
|
89 |
-
template<bool has_the_member_of_interest, typename F> \
|
90 |
-
struct impl \
|
91 |
-
{ \
|
92 |
-
static const bool value = false; \
|
93 |
-
}; \
|
94 |
-
\
|
95 |
-
template<typename Result, typename Arg> \
|
96 |
-
struct impl<true, Result(Arg)> \
|
97 |
-
{ \
|
98 |
-
static typename add_reference<derived_type>::type test_me; \
|
99 |
-
static typename add_reference<Arg>::type arg; \
|
100 |
-
\
|
101 |
-
static const bool value = \
|
102 |
-
sizeof( \
|
103 |
-
return_value_check<T, Result>::deduce( \
|
104 |
-
(test_me.member_function_name(arg), thrust::detail::is_call_possible_detail::void_exp_result<T>()) \
|
105 |
-
) \
|
106 |
-
) == sizeof(yes); \
|
107 |
-
}; \
|
108 |
-
\
|
109 |
-
template<typename Result, typename Arg1, typename Arg2> \
|
110 |
-
struct impl<true, Result(Arg1,Arg2)> \
|
111 |
-
{ \
|
112 |
-
static typename add_reference<derived_type>::type test_me; \
|
113 |
-
static typename add_reference<Arg1>::type arg1; \
|
114 |
-
static typename add_reference<Arg2>::type arg2; \
|
115 |
-
\
|
116 |
-
static const bool value = \
|
117 |
-
sizeof( \
|
118 |
-
return_value_check<T, Result>::deduce( \
|
119 |
-
(test_me.member_function_name(arg1,arg2), thrust::detail::is_call_possible_detail::void_exp_result<T>()) \
|
120 |
-
) \
|
121 |
-
) == sizeof(yes); \
|
122 |
-
}; \
|
123 |
-
\
|
124 |
-
template<typename Result, typename Arg1, typename Arg2, typename Arg3> \
|
125 |
-
struct impl<true, Result(Arg1,Arg2,Arg3)> \
|
126 |
-
{ \
|
127 |
-
static typename add_reference<derived_type>::type test_me; \
|
128 |
-
static typename add_reference<Arg1>::type arg1; \
|
129 |
-
static typename add_reference<Arg2>::type arg2; \
|
130 |
-
static typename add_reference<Arg3>::type arg3; \
|
131 |
-
\
|
132 |
-
static const bool value = \
|
133 |
-
sizeof( \
|
134 |
-
return_value_check<T, Result>::deduce( \
|
135 |
-
(test_me.member_function_name(arg1,arg2,arg3), thrust::detail::is_call_possible_detail::void_exp_result<T>()) \
|
136 |
-
) \
|
137 |
-
) == sizeof(yes); \
|
138 |
-
}; \
|
139 |
-
\
|
140 |
-
template<typename Result, typename Arg1, typename Arg2, typename Arg3, typename Arg4> \
|
141 |
-
struct impl<true, Result(Arg1,Arg2,Arg3,Arg4)> \
|
142 |
-
{ \
|
143 |
-
static typename add_reference<derived_type>::type test_me; \
|
144 |
-
static typename add_reference<Arg1>::type arg1; \
|
145 |
-
static typename add_reference<Arg2>::type arg2; \
|
146 |
-
static typename add_reference<Arg3>::type arg3; \
|
147 |
-
static typename add_reference<Arg4>::type arg4; \
|
148 |
-
\
|
149 |
-
static const bool value = \
|
150 |
-
sizeof( \
|
151 |
-
return_value_check<T, Result>::deduce( \
|
152 |
-
(test_me.member_function_name(arg1,arg2,arg3,arg4), thrust::detail::is_call_possible_detail::void_exp_result<T>()) \
|
153 |
-
) \
|
154 |
-
) == sizeof(yes); \
|
155 |
-
}; \
|
156 |
-
\
|
157 |
-
public: \
|
158 |
-
static const bool value = impl<trait_name##_has_member<T,Signature>::value, Signature>::value; \
|
159 |
-
typedef thrust::detail::integral_constant<bool,value> type; \
|
160 |
-
};
|
161 |
-
|
|
|
|
|
|
|
|
|
|
|
|
|
|
|
|
|
|
|
|
|
|
|
|
|
|
|
|
|
|
|
|
|
|
|
|
|
|
|
|
|
|
|
|
|
|
|
|
|
|
|
|
|
|
|
|
|
|
|
|
|
|
|
|
|
|
|
|
|
|
|
|
|
|
|
|
|
|
|
|
|
|
|
|
|
|
|
|
|
|
|
|
|
|
|
|
|
|
|
|
|
|
|
|
|
|
|
|
|
|
|
|
|
|
|
|
|
|
|
|
|
|
|
|
|
|
|
|
|
|
|
|
|
|
|
|
|
|
|
|
|
|
|
|
|
|
|
|
|
|
|
|
|
|
|
|
|
|
|
|
|
|
|
|
|
|
|
|
|
|
|
|
|
|
|
|
|
|
|
|
|
|
|
|
|
|
|
|
|
|
|
|
|
|
|
|
|
|
|
|
|
|
|
|
|
|
|
|
|
|
|
|
|
|
|
|
|
|
|
|
|
|
|
|
|
|
|
|
|
|
|
|
|
|
|
|
|
|
|
|
|
|
|
|
|
|
|
|
|
|
|
|
|
|
|
|
|
|
|
|
|
|
|
|
|
|
|
|
|
|
|
|
|
|
|
|
|
|
|
|
|
|
|
|
|
|
|
|
|
|
|
|
|
|
|
|
|
|
|
|
|
|
|
|
|
|
|
|
|
|
|
|
|
|
|
|
|
|
|
|
|
|
|
|
spaces/CVPR/LIVE/thrust/thrust/iterator/detail/host_system_tag.h
DELETED
@@ -1,40 +0,0 @@
|
|
1 |
-
/*
|
2 |
-
* Copyright 2008-2013 NVIDIA Corporation
|
3 |
-
*
|
4 |
-
* Licensed under the Apache License, Version 2.0 (the "License");
|
5 |
-
* you may not use this file except in compliance with the License.
|
6 |
-
* You may obtain a copy of the License at
|
7 |
-
*
|
8 |
-
* http://www.apache.org/licenses/LICENSE-2.0
|
9 |
-
*
|
10 |
-
* Unless required by applicable law or agreed to in writing, software
|
11 |
-
* distributed under the License is distributed on an "AS IS" BASIS,
|
12 |
-
* WITHOUT WARRANTIES OR CONDITIONS OF ANY KIND, either express or implied.
|
13 |
-
* See the License for the specific language governing permissions and
|
14 |
-
* limitations under the License.
|
15 |
-
*/
|
16 |
-
|
17 |
-
#pragma once
|
18 |
-
|
19 |
-
#include <thrust/detail/config.h>
|
20 |
-
|
21 |
-
// #include the host system's execution_policy header
|
22 |
-
#define __THRUST_HOST_SYSTEM_TAG_HEADER <__THRUST_HOST_SYSTEM_ROOT/detail/execution_policy.h>
|
23 |
-
#include __THRUST_HOST_SYSTEM_TAG_HEADER
|
24 |
-
#undef __THRUST_HOST_SYSTEM_TAG_HEADER
|
25 |
-
|
26 |
-
namespace thrust
|
27 |
-
{
|
28 |
-
|
29 |
-
typedef thrust::system::__THRUST_HOST_SYSTEM_NAMESPACE::tag host_system_tag;
|
30 |
-
|
31 |
-
} // end thrust
|
32 |
-
|
33 |
-
// TODO remove this in 1.8.0
|
34 |
-
namespace thrust
|
35 |
-
{
|
36 |
-
|
37 |
-
typedef THRUST_DEPRECATED host_system_tag host_space_tag;
|
38 |
-
|
39 |
-
} // end thrust
|
40 |
-
|
|
|
|
|
|
|
|
|
|
|
|
|
|
|
|
|
|
|
|
|
|
|
|
|
|
|
|
|
|
|
|
|
|
|
|
|
|
|
|
|
|
|
|
|
|
|
|
|
|
|
|
|
|
|
|
|
|
|
|
|
|
|
|
|
|
|
|
|
|
|
|
|
|
|
|
|
|
|
|
|
|
spaces/CVPR/Text2Human/Text2Human/ui_util/__init__.py
DELETED
File without changes
|
spaces/Chomkwoy/Nilkessye/synthetic_dataset.py
DELETED
@@ -1,560 +0,0 @@
|
|
1 |
-
import json
|
2 |
-
|
3 |
-
import cv2
|
4 |
-
from torch.utils.data import Dataset
|
5 |
-
import random
|
6 |
-
import math
|
7 |
-
import albumentations
|
8 |
-
from sklearn.model_selection import train_test_split
|
9 |
-
import pathlib
|
10 |
-
import numpy as np
|
11 |
-
from scipy.ndimage import map_coordinates
|
12 |
-
from scipy.ndimage import gaussian_filter
|
13 |
-
|
14 |
-
from load_book import process_page
|
15 |
-
from utils.image import gaussian_radius, draw_gaussian
|
16 |
-
|
17 |
-
|
18 |
-
def resize(image, dsize, fx=None, fy=None, interpolation=cv2.INTER_AREA):
|
19 |
-
image, bboxes = image
|
20 |
-
orig_height, orig_width = image.shape[0], image.shape[1]
|
21 |
-
image = cv2.resize(image, dsize=dsize, fx=fx, fy=fy, interpolation=interpolation)
|
22 |
-
H, W = image.shape[0], image.shape[1]
|
23 |
-
bboxes = bboxes * np.array([W / orig_width, H / orig_height])
|
24 |
-
return image, bboxes
|
25 |
-
|
26 |
-
|
27 |
-
def crop(image, tlx, tly, brx, bry):
|
28 |
-
image, bboxes = image
|
29 |
-
image = image[tly:bry, tlx:brx]
|
30 |
-
bboxes = bboxes - np.array([tlx, tly])
|
31 |
-
return image, bboxes
|
32 |
-
|
33 |
-
|
34 |
-
def make_line(file_list, do_stretch, remove_margin, rand):
|
35 |
-
images = []
|
36 |
-
syllables = []
|
37 |
-
|
38 |
-
for p in file_list:
|
39 |
-
img = cv2.imread(str(p), cv2.IMREAD_UNCHANGED) # [H, W, rgba]
|
40 |
-
img = cv2.resize(img, dsize=None, fx=0.5, fy=0.5, interpolation=cv2.INTER_AREA)
|
41 |
-
alpha = img[:, :, 3:] / 255.
|
42 |
-
img = np.uint8(img[:, :, :-1] * alpha + 255 * (1 - alpha)) # white background
|
43 |
-
image_grey = 255 - cv2.cvtColor(img, cv2.COLOR_RGB2GRAY)
|
44 |
-
tx, ty, w, h = cv2.boundingRect(image_grey)
|
45 |
-
img = (img, np.array([[[tx, ty], [tx + w, ty + h]]]))
|
46 |
-
|
47 |
-
syllables.append(p.stem)
|
48 |
-
|
49 |
-
orig_height, orig_width = img[0].shape[0], img[0].shape[1]
|
50 |
-
|
51 |
-
# remove top & bottom margins
|
52 |
-
img = crop(img, 0, max(0, ty - 4), img[0].shape[1], min(ty + h + 4, img[0].shape[0]))
|
53 |
-
|
54 |
-
if do_stretch:
|
55 |
-
img = resize(img, dsize=None, fx=1.0, fy=rand.uniform(0.6, 1.0), interpolation=cv2.INTER_AREA)
|
56 |
-
else:
|
57 |
-
img = resize(img, dsize=(orig_width, orig_height), interpolation=cv2.INTER_AREA)
|
58 |
-
|
59 |
-
if remove_margin:
|
60 |
-
# remove left margin
|
61 |
-
img = crop(img, tx, 0, min(tx + w + 2, img[0].shape[1]), img[0].shape[0])
|
62 |
-
img = resize(img, dsize=(orig_width, orig_height), interpolation=cv2.INTER_AREA)
|
63 |
-
|
64 |
-
images.append(img)
|
65 |
-
|
66 |
-
img = vstack(images)
|
67 |
-
|
68 |
-
return img
|
69 |
-
|
70 |
-
|
71 |
-
def vstack(images):
|
72 |
-
offset = 0
|
73 |
-
all_coords = []
|
74 |
-
for img, coords in images:
|
75 |
-
all_coords.append(coords + np.array([0, offset]))
|
76 |
-
offset += img.shape[0]
|
77 |
-
all_coords = np.concatenate(all_coords)
|
78 |
-
stacked_image = np.vstack([img for img, _ in images])
|
79 |
-
return stacked_image, all_coords
|
80 |
-
|
81 |
-
|
82 |
-
def add_border(img, top, bottom, left, right, value):
|
83 |
-
return cv2.copyMakeBorder(
|
84 |
-
img[0], top, bottom, left, right, cv2.BORDER_CONSTANT, None, value
|
85 |
-
), img[1] + np.array([left, top])
|
86 |
-
|
87 |
-
|
88 |
-
class SyllableDataset(Dataset):
|
89 |
-
def __init__(self, file_list, hanja_file_list,
|
90 |
-
real_annotations,
|
91 |
-
do_augment=None, length=100000):
|
92 |
-
self.files_no_final = [
|
93 |
-
[f for f in font if f.stem[-1] not in 'aeiouy']
|
94 |
-
for font in file_list
|
95 |
-
]
|
96 |
-
self.files_with_final = [
|
97 |
-
[f for f in font if f.stem[-1] in 'aeiouy']
|
98 |
-
for font in file_list
|
99 |
-
]
|
100 |
-
self.hanja_file_list = hanja_file_list
|
101 |
-
self.real_annotations = real_annotations
|
102 |
-
self.do_augment = do_augment
|
103 |
-
self.length = length
|
104 |
-
|
105 |
-
def __len__(self):
|
106 |
-
return self.length
|
107 |
-
|
108 |
-
def __getitem__(self, idx):
|
109 |
-
rand = random.Random(idx)
|
110 |
-
max_objs = 9 * 16 * 4
|
111 |
-
|
112 |
-
if rand.randint(0, 1) == 0:
|
113 |
-
record = rand.choice(self.real_annotations)
|
114 |
-
|
115 |
-
file_url = record['data']['url']
|
116 |
-
annotations = record['annotations'][0]['result']
|
117 |
-
|
118 |
-
filename = '/'.join(file_url.split('/')[-2:])
|
119 |
-
image, orig_image_bbox, orig_size = process_page(filename, thresholding=False)
|
120 |
-
|
121 |
-
bboxes = np.array([
|
122 |
-
[[rect['value']['x'], rect['value']['y']],
|
123 |
-
[rect['value']['x'] + rect['value']['width'], rect['value']['y'] + rect['value']['height']]]
|
124 |
-
for rect in annotations
|
125 |
-
])
|
126 |
-
bboxes = bboxes / 100. * np.array(orig_size) - np.array(orig_image_bbox[0])
|
127 |
-
|
128 |
-
labels = np.array([
|
129 |
-
['kor_main', 'hanja_main', 'kor_anno', 'hanja_anno'].index(rect['value']['rectanglelabels'][0])
|
130 |
-
for rect in annotations
|
131 |
-
])
|
132 |
-
|
133 |
-
# clip bboxes
|
134 |
-
bboxes = bboxes.clip(min=0, max=np.array([image.shape[1], image.shape[0]]))
|
135 |
-
bboxes = bboxes[(bboxes[:, 0, 0] < bboxes[:, 1, 0]) & (bboxes[:, 0, 1] < bboxes[:, 1, 1])]
|
136 |
-
|
137 |
-
# Augment image
|
138 |
-
if self.do_augment is not None:
|
139 |
-
if rand.randint(0, 1) == 1:
|
140 |
-
image, bboxes = self.do_augment(image, bboxes)
|
141 |
-
|
142 |
-
# normalize image
|
143 |
-
orig_size = (image.shape[1], image.shape[0])
|
144 |
-
image = cv2.resize(image, dsize=(512, 512), interpolation=cv2.INTER_AREA)
|
145 |
-
bboxes = bboxes * np.array([512 / orig_size[0], 512 / orig_size[1]])
|
146 |
-
image = image.astype(np.float32) / 255. - .5 # to [-.5, +.5] range
|
147 |
-
image = image.transpose((2, 0, 1)) # [H, W, C] to [C, H, W]
|
148 |
-
|
149 |
-
hmap = make_heatmap(bboxes, labels, max_objs, num_classes=4)
|
150 |
-
|
151 |
-
# pad bboxes
|
152 |
-
bboxes = np.concatenate([bboxes, np.zeros((max_objs - bboxes.shape[0], 2, 2))], axis=0)
|
153 |
-
|
154 |
-
return {
|
155 |
-
'image': image,
|
156 |
-
'bboxes': bboxes,
|
157 |
-
'syllables': None, # TODO
|
158 |
-
'sequence': None,
|
159 |
-
**hmap
|
160 |
-
}
|
161 |
-
|
162 |
-
else:
|
163 |
-
return generate_synthetic_page(
|
164 |
-
rand,
|
165 |
-
self.files_with_final,
|
166 |
-
self.files_no_final,
|
167 |
-
self.hanja_file_list,
|
168 |
-
self.do_augment,
|
169 |
-
max_objs
|
170 |
-
)
|
171 |
-
|
172 |
-
|
173 |
-
def generate_synthetic_page(rand, files_with_final, files_no_final, hanja_file_list, do_augment, max_objs):
|
174 |
-
max_lines = rand.randint(6, 9)
|
175 |
-
line_max_len = rand.randint(9, 16)
|
176 |
-
L = max_lines * line_max_len * 4
|
177 |
-
max_span_len = 50
|
178 |
-
|
179 |
-
add_rule = rand.randint(0, 1) == 1
|
180 |
-
do_stretch = rand.randint(0, 1) == 1
|
181 |
-
do_remove_margin = rand.randint(0, 1) == 1
|
182 |
-
|
183 |
-
file_list = []
|
184 |
-
labels = []
|
185 |
-
|
186 |
-
lines = []
|
187 |
-
line_imgs = []
|
188 |
-
offset = 0
|
189 |
-
page_offset = 0
|
190 |
-
|
191 |
-
is_normal = rand.randint(0, 1) == 1
|
192 |
-
while len(lines) < max_lines:
|
193 |
-
span_len = rand.randint(1, min(max_span_len, L - offset))
|
194 |
-
|
195 |
-
is_hanja = rand.choices([0, 1], [0.8, 0.2], k=span_len)
|
196 |
-
has_final = rand.choices([0, 1], [0.6, 0.4], k=span_len)
|
197 |
-
|
198 |
-
font_idx = rand.randint(0, len(files_with_final) - 1)
|
199 |
-
with_finals = files_with_final[font_idx]
|
200 |
-
no_finals = files_no_final[font_idx]
|
201 |
-
hanjas = rand.choice(hanja_file_list)
|
202 |
-
|
203 |
-
for h, f in zip(is_hanja, has_final):
|
204 |
-
if h:
|
205 |
-
file_list.append(rand.choice(hanjas))
|
206 |
-
else:
|
207 |
-
if f:
|
208 |
-
file_list.append(rand.choice(with_finals))
|
209 |
-
else:
|
210 |
-
file_list.append(rand.choice(no_finals))
|
211 |
-
|
212 |
-
# 0: normal, hangul
|
213 |
-
# 1: normal, hanja
|
214 |
-
# 2: annotation, hangul
|
215 |
-
# 3: annotation, hanja
|
216 |
-
labels.extend(int(not is_normal) * 2 + np.array(is_hanja))
|
217 |
-
|
218 |
-
if is_normal: # normal span
|
219 |
-
# print(f"new normal span {span_len=}")
|
220 |
-
while span_len > 0:
|
221 |
-
# print(f"main: line {len(lines)}, sec {len(line_imgs)}, {page_offset=}, {span_len=}")
|
222 |
-
bit_len = min(span_len, int(line_max_len - page_offset))
|
223 |
-
line_imgs.append(make_line(file_list[offset:offset + bit_len],
|
224 |
-
do_stretch, do_remove_margin, rand))
|
225 |
-
offset += bit_len
|
226 |
-
page_offset += bit_len
|
227 |
-
span_len -= bit_len
|
228 |
-
if math.ceil(page_offset) >= line_max_len:
|
229 |
-
page_offset = 0
|
230 |
-
lines.append(vstack(line_imgs))
|
231 |
-
line_imgs = []
|
232 |
-
|
233 |
-
else: # narrow annotation span
|
234 |
-
# print(f"new narrow span {span_len=}")
|
235 |
-
while span_len > 0:
|
236 |
-
# where in the line the annotation ends
|
237 |
-
# print(f"anno: line {len(lines)}, sec {len(line_imgs)}, {page_offset=}, {span_len=}")
|
238 |
-
anno_line_max_len = min(line_max_len, page_offset + (span_len + 1) // 2 / 2)
|
239 |
-
|
240 |
-
anno_lines = []
|
241 |
-
|
242 |
-
bit_len = min(span_len, int((anno_line_max_len - page_offset) * 2))
|
243 |
-
# print(f"{anno_line_max_len=}, {bit_len=}")
|
244 |
-
anno_lines.append(make_line(file_list[offset:offset + bit_len],
|
245 |
-
do_stretch, do_remove_margin, rand))
|
246 |
-
offset += bit_len
|
247 |
-
span_len -= bit_len
|
248 |
-
|
249 |
-
bit_len = min(span_len, int((anno_line_max_len - page_offset) * 2))
|
250 |
-
# print(f"{anno_line_max_len=}, {bit_len=}")
|
251 |
-
if bit_len > 0:
|
252 |
-
anno_lines.append(make_line(file_list[offset:offset + bit_len],
|
253 |
-
do_stretch, do_remove_margin, rand))
|
254 |
-
else:
|
255 |
-
anno_lines.append((np.full_like(anno_lines[0][0], 255), np.zeros((0, 2, 2))))
|
256 |
-
offset += bit_len
|
257 |
-
span_len -= bit_len
|
258 |
-
|
259 |
-
anno_line = hstack_lines(anno_lines)
|
260 |
-
anno_line = resize(anno_line, dsize=None, fx=.45, fy=.5, interpolation=cv2.INTER_AREA)
|
261 |
-
anno_line = add_border(anno_line, 0, 0, 128 - anno_line[0].shape[1], 0, [255, 255, 255])
|
262 |
-
line_imgs.append(anno_line)
|
263 |
-
|
264 |
-
page_offset = anno_line_max_len
|
265 |
-
|
266 |
-
if math.ceil(page_offset) >= line_max_len:
|
267 |
-
page_offset = 0
|
268 |
-
lines.append(vstack(line_imgs))
|
269 |
-
line_imgs = []
|
270 |
-
|
271 |
-
is_normal = not is_normal
|
272 |
-
|
273 |
-
new_lines = []
|
274 |
-
for l, coords in lines:
|
275 |
-
tx, ty, w, h = cv2.boundingRect(255 - cv2.cvtColor(l, cv2.COLOR_RGB2GRAY))
|
276 |
-
x1, x2 = max(0, tx - 2), min(tx + w + 2, l.shape[1])
|
277 |
-
new_lines.append((l[:, x1:x2], coords - np.array([x1, 0])))
|
278 |
-
lines = new_lines
|
279 |
-
|
280 |
-
# add indent
|
281 |
-
indent = rand.randint(0, 200)
|
282 |
-
lines = [add_border(img, indent, 0, 0, 0, [255, 255, 255]) for img in lines]
|
283 |
-
|
284 |
-
if add_rule:
|
285 |
-
lines = [add_border(img, 0, 0, 1, 0, 0) for img in lines]
|
286 |
-
|
287 |
-
img = hstack_lines_resize(lines[:max_lines])
|
288 |
-
|
289 |
-
# Add page borders
|
290 |
-
img = add_border(img, 1, 1, 1, 1, [255, 255, 255])
|
291 |
-
if rand.randint(0, 1) == 1:
|
292 |
-
img = add_border(img, 1, 1, 1, 1, 0)
|
293 |
-
img = add_border(img, 7, 7, 7, 7, [255, 255, 255])
|
294 |
-
img = add_border(img, 10, 10, 10, 10, 0)
|
295 |
-
img = add_border(img, 5, 5, 5, 5, [255, 255, 255])
|
296 |
-
|
297 |
-
# Clip bboxes
|
298 |
-
img = (img[0], img[1].clip(min=0, max=np.array([img[0].shape[1], img[0].shape[0]])))
|
299 |
-
|
300 |
-
# Augment image
|
301 |
-
if do_augment is not None:
|
302 |
-
if rand.randint(0, 1) == 1:
|
303 |
-
img = do_augment(img[0], img[1])
|
304 |
-
|
305 |
-
image, bboxes = img
|
306 |
-
|
307 |
-
# Normalize image
|
308 |
-
orig_size = (image.shape[1], image.shape[0])
|
309 |
-
image = cv2.resize(image, dsize=(512, 512), interpolation=cv2.INTER_AREA)
|
310 |
-
bboxes = bboxes * np.array([512 / orig_size[0], 512 / orig_size[1]])
|
311 |
-
|
312 |
-
image = image.astype(np.float32) / 255. - .5 # to [-.5, +.5] range
|
313 |
-
image = image.transpose((2, 0, 1)) # [H, W, C] to [C, H, W]
|
314 |
-
|
315 |
-
labels = np.array(labels)[:bboxes.shape[0]]
|
316 |
-
|
317 |
-
hmap = make_heatmap(bboxes, labels, max_objs, num_classes=4)
|
318 |
-
sequence = np.arange(max_objs)
|
319 |
-
sequence[bboxes.shape[0]:] = -1
|
320 |
-
|
321 |
-
# Collect syllables
|
322 |
-
syllables = []
|
323 |
-
for f in file_list:
|
324 |
-
syllable = f.stem
|
325 |
-
if syllable[0] in 'HR':
|
326 |
-
syllable = syllable[1:] + syllable[0]
|
327 |
-
elif syllable[0].isascii() and syllable[0].isalpha():
|
328 |
-
syllable += 'L'
|
329 |
-
syllables.append(syllable)
|
330 |
-
syllables = syllables[:bboxes.shape[0]]
|
331 |
-
|
332 |
-
bboxes = np.concatenate([bboxes, np.zeros((max_objs - bboxes.shape[0], 2, 2))], axis=0)
|
333 |
-
|
334 |
-
return {
|
335 |
-
'image': image,
|
336 |
-
'bboxes': bboxes,
|
337 |
-
'syllables': '.'.join(syllables),
|
338 |
-
'sequence': sequence,
|
339 |
-
**hmap
|
340 |
-
}
|
341 |
-
|
342 |
-
|
343 |
-
def hstack_lines(lines):
|
344 |
-
max_height = max(l[0].shape[0] for l in lines)
|
345 |
-
offset = 0
|
346 |
-
images = []
|
347 |
-
all_coords = []
|
348 |
-
for l, coords in lines[::-1]:
|
349 |
-
images.append(np.pad(l, ((0, max_height - l.shape[0]), (0, 0), (0, 0)), constant_values=255))
|
350 |
-
all_coords.append(coords + np.array([offset, 0]))
|
351 |
-
offset += l.shape[1]
|
352 |
-
return np.hstack(images), np.concatenate(all_coords[::-1])
|
353 |
-
|
354 |
-
|
355 |
-
def hstack_lines_resize(lines):
|
356 |
-
max_height = max(l[0].shape[0] for l in lines)
|
357 |
-
offset = 0
|
358 |
-
images = []
|
359 |
-
all_coords = []
|
360 |
-
for l, coords in lines[::-1]:
|
361 |
-
images.append(cv2.resize(l, dsize=(l.shape[1], max_height), interpolation=cv2.INTER_AREA))
|
362 |
-
all_coords.append(coords * np.array([1, max_height / l.shape[0]]) + np.array([offset, 0]))
|
363 |
-
offset += l.shape[1]
|
364 |
-
return np.hstack(images), np.concatenate(all_coords[::-1])
|
365 |
-
|
366 |
-
|
367 |
-
def elastic_transform(image, bboxes, alpha, sigma, random_state=None):
|
368 |
-
if random_state is None:
|
369 |
-
random_state = np.random.RandomState(None)
|
370 |
-
|
371 |
-
shape = image.shape
|
372 |
-
N = 256
|
373 |
-
mult = N / shape[0]
|
374 |
-
noise_shape = [N, N * shape[1] // shape[0], 1]
|
375 |
-
dx = alpha * gaussian_filter((random_state.rand(*noise_shape) * 2 - 1), sigma, mode="constant", cval=0)
|
376 |
-
dy = alpha * gaussian_filter((random_state.rand(*noise_shape) * 2 - 1), sigma, mode="constant", cval=0)
|
377 |
-
dz = np.zeros_like(dx)
|
378 |
-
|
379 |
-
# find rects
|
380 |
-
rects = np.zeros((noise_shape[0], noise_shape[1], bboxes.shape[0]))
|
381 |
-
for i, (tl, br) in enumerate(bboxes * mult):
|
382 |
-
rects[math.floor(tl[1]):math.floor(br[1]), math.ceil(tl[0]):math.ceil(br[0]), i] = 1
|
383 |
-
|
384 |
-
x, y, z = np.meshgrid(np.arange(noise_shape[1]), np.arange(noise_shape[0]), np.arange(bboxes.shape[0]))
|
385 |
-
indices = np.reshape(y + dy * mult, (-1, 1)), np.reshape(x + dx * mult, (-1, 1)), np.reshape(z, (-1, 1))
|
386 |
-
|
387 |
-
distored_rects = map_coordinates(rects, indices, order=1, mode='constant', cval=0, prefilter=False)
|
388 |
-
distored_rects = distored_rects.reshape(rects.shape)
|
389 |
-
|
390 |
-
bounds = []
|
391 |
-
for i in range(distored_rects.shape[-1]):
|
392 |
-
x, y, w, h = cv2.boundingRect(np.uint8(distored_rects[..., i] > 0.5))
|
393 |
-
bounds.append(np.array([[x, y], [x + w, y + h]]))
|
394 |
-
bounds = np.stack(bounds) / mult
|
395 |
-
|
396 |
-
# distort image
|
397 |
-
x, y, z = np.meshgrid(np.arange(shape[1]), np.arange(shape[0]), np.arange(shape[2]))
|
398 |
-
xx = x + cv2.resize(dx, dsize=[shape[1], shape[0]], interpolation=cv2.INTER_CUBIC)[..., None]
|
399 |
-
yy = y + cv2.resize(dy, dsize=[shape[1], shape[0]], interpolation=cv2.INTER_CUBIC)[..., None]
|
400 |
-
indices = np.reshape(yy, (-1, 1)), np.reshape(xx, (-1, 1)), np.reshape(z, (-1, 1))
|
401 |
-
|
402 |
-
distored_image = map_coordinates(image, indices, order=1, mode='constant', cval=255, prefilter=False)
|
403 |
-
distored_image = distored_image.reshape(image.shape)
|
404 |
-
|
405 |
-
return distored_image, bounds
|
406 |
-
|
407 |
-
|
408 |
-
def make_heatmap(bboxes, labels, max_objs, num_classes,
|
409 |
-
fmap_size=None, img_size=None, gaussian=True, gaussian_iou=0.7):
|
410 |
-
if fmap_size is None:
|
411 |
-
fmap_size = {'h': 128, 'w': 128}
|
412 |
-
if img_size is None:
|
413 |
-
img_size = {'h': 512, 'w': 512}
|
414 |
-
|
415 |
-
hmap_tl = np.zeros((num_classes, fmap_size['h'], fmap_size['w']), dtype=np.float32)
|
416 |
-
hmap_br = np.zeros((num_classes, fmap_size['h'], fmap_size['w']), dtype=np.float32)
|
417 |
-
hmap_ct = np.zeros((num_classes, fmap_size['h'], fmap_size['w']), dtype=np.float32)
|
418 |
-
|
419 |
-
regs_tl = np.zeros((max_objs, 2), dtype=np.float32)
|
420 |
-
regs_br = np.zeros((max_objs, 2), dtype=np.float32)
|
421 |
-
regs_ct = np.zeros((max_objs, 2), dtype=np.float32)
|
422 |
-
|
423 |
-
inds_tl = np.zeros((max_objs,), dtype=np.int64)
|
424 |
-
inds_br = np.zeros((max_objs,), dtype=np.int64)
|
425 |
-
inds_ct = np.zeros((max_objs,), dtype=np.int64)
|
426 |
-
|
427 |
-
num_objs = np.array(min(bboxes.shape[0], max_objs))
|
428 |
-
ind_masks = np.zeros((max_objs,), dtype=np.uint8)
|
429 |
-
ind_masks[:num_objs] = 1
|
430 |
-
|
431 |
-
for i, (((xtl, ytl), (xbr, ybr)), label) in enumerate(zip(bboxes, labels)):
|
432 |
-
xct, yct = (xbr + xtl) / 2., (ybr + ytl) / 2.
|
433 |
-
|
434 |
-
fxtl = (xtl * fmap_size['w'] / img_size['w'])
|
435 |
-
fytl = (ytl * fmap_size['h'] / img_size['h'])
|
436 |
-
fxbr = (xbr * fmap_size['w'] / img_size['w'])
|
437 |
-
fybr = (ybr * fmap_size['h'] / img_size['h'])
|
438 |
-
fxct = (xct * fmap_size['w'] / img_size['w'])
|
439 |
-
fyct = (yct * fmap_size['h'] / img_size['h'])
|
440 |
-
|
441 |
-
ixtl = min(max(int(fxtl), 0), fmap_size['w'] - 1)
|
442 |
-
iytl = min(max(int(fytl), 0), fmap_size['h'] - 1)
|
443 |
-
ixbr = min(max(int(fxbr), 0), fmap_size['w'] - 1)
|
444 |
-
iybr = min(max(int(fybr), 0), fmap_size['h'] - 1)
|
445 |
-
ixct = min(max(int(fxct), 0), fmap_size['w'] - 1)
|
446 |
-
iyct = min(max(int(fyct), 0), fmap_size['h'] - 1)
|
447 |
-
|
448 |
-
if gaussian:
|
449 |
-
width = xbr - xtl
|
450 |
-
height = ybr - ytl
|
451 |
-
|
452 |
-
width = math.ceil(width * fmap_size['w'] / img_size['w'])
|
453 |
-
height = math.ceil(height * fmap_size['h'] / img_size['h'])
|
454 |
-
|
455 |
-
radius = max(0, int(gaussian_radius((height, width), gaussian_iou)))
|
456 |
-
|
457 |
-
draw_gaussian(hmap_tl[label], [ixtl, iytl], radius)
|
458 |
-
draw_gaussian(hmap_br[label], [ixbr, iybr], radius)
|
459 |
-
draw_gaussian(hmap_ct[label], [ixct, iyct], radius, delta=5)
|
460 |
-
else:
|
461 |
-
hmap_tl[label, iytl, ixtl] = 1
|
462 |
-
hmap_br[label, iybr, ixbr] = 1
|
463 |
-
hmap_ct[label, iyct, ixct] = 1
|
464 |
-
|
465 |
-
regs_tl[i, :] = [fxtl - ixtl, fytl - iytl]
|
466 |
-
regs_br[i, :] = [fxbr - ixbr, fybr - iybr]
|
467 |
-
regs_ct[i, :] = [fxct - ixct, fyct - iyct]
|
468 |
-
inds_tl[i] = iytl * fmap_size['w'] + ixtl
|
469 |
-
inds_br[i] = iybr * fmap_size['w'] + ixbr
|
470 |
-
inds_ct[i] = iyct * fmap_size['w'] + ixct
|
471 |
-
|
472 |
-
return {'hmap_tl': hmap_tl, 'hmap_br': hmap_br, 'hmap_ct': hmap_ct,
|
473 |
-
'regs_tl': regs_tl, 'regs_br': regs_br, 'regs_ct': regs_ct,
|
474 |
-
'inds_tl': inds_tl, 'inds_br': inds_br, 'inds_ct': inds_ct,
|
475 |
-
'ind_masks': ind_masks}
|
476 |
-
|
477 |
-
|
478 |
-
def load_dataset(train_size=100000):
|
479 |
-
df1 = list(pathlib.Path("img/img1").iterdir())
|
480 |
-
df2 = list(pathlib.Path("img/img2").iterdir())
|
481 |
-
df3 = list(pathlib.Path("img/img3").iterdir())
|
482 |
-
df_hnaja1 = list(pathlib.Path("img/img-hanja1").iterdir())
|
483 |
-
df_hanja2 = list(pathlib.Path("img/img-hanja2").iterdir())
|
484 |
-
|
485 |
-
with open("annotations.json", "r") as fp:
|
486 |
-
real_annotations = json.load(fp)
|
487 |
-
|
488 |
-
train_df1, test_df1 = train_test_split(df1, test_size=128, random_state=0)
|
489 |
-
train_df2, test_df2 = train_test_split(df2, test_size=128, random_state=0)
|
490 |
-
train_df3, test_df3 = train_test_split(df3, test_size=128, random_state=0)
|
491 |
-
train_dfh1, test_dfh1 = train_test_split(df_hnaja1, test_size=128, random_state=0)
|
492 |
-
train_dfh2, test_dfh2 = train_test_split(df_hanja2, test_size=128, random_state=0)
|
493 |
-
train_real_annotations, test_real_annotations = train_test_split(real_annotations, test_size=2, random_state=0)
|
494 |
-
|
495 |
-
# Augmentation
|
496 |
-
colorize = albumentations.RGBShift(r_shift_limit=0, g_shift_limit=0, b_shift_limit=[-80, 0])
|
497 |
-
|
498 |
-
def color_get_params():
|
499 |
-
a = random.uniform(-40, 0)
|
500 |
-
b = random.uniform(-80, -30)
|
501 |
-
return {"r_shift": a,
|
502 |
-
"g_shift": a,
|
503 |
-
"b_shift": b}
|
504 |
-
|
505 |
-
colorize.get_params = color_get_params
|
506 |
-
|
507 |
-
transform = albumentations.Compose([
|
508 |
-
albumentations.CropAndPad(
|
509 |
-
percent=0.05,
|
510 |
-
pad_mode=cv2.BORDER_REPLICATE,
|
511 |
-
),
|
512 |
-
albumentations.Perspective(
|
513 |
-
always_apply=True,
|
514 |
-
fit_output=True,
|
515 |
-
pad_val=[255, 255, 255],
|
516 |
-
scale=(0.0, 0.05),
|
517 |
-
),
|
518 |
-
albumentations.Rotate(
|
519 |
-
always_apply=True,
|
520 |
-
limit=5,
|
521 |
-
border_mode=cv2.BORDER_CONSTANT,
|
522 |
-
value=[255, 255, 255]
|
523 |
-
),
|
524 |
-
albumentations.Blur(blur_limit=(2, 5)),
|
525 |
-
colorize,
|
526 |
-
albumentations.GaussNoise(var_limit=(200.0, 200.0)),
|
527 |
-
],
|
528 |
-
bbox_params=albumentations.BboxParams(
|
529 |
-
format='coco', label_fields=['class_labels']
|
530 |
-
),
|
531 |
-
)
|
532 |
-
|
533 |
-
def augment(img, coords):
|
534 |
-
# Apply Augmentations
|
535 |
-
aug = transform(
|
536 |
-
image=img,
|
537 |
-
bboxes=np.concatenate([coords[:, 0], coords[:, 1] - coords[:, 0]], axis=1),
|
538 |
-
class_labels=['hangul'] * len(coords),
|
539 |
-
)
|
540 |
-
|
541 |
-
bboxes = np.array(aug['bboxes'])
|
542 |
-
aug_coords = np.stack([bboxes[:, :2], bboxes[:, :2] + bboxes[:, 2:]], axis=1)
|
543 |
-
dimage, bounds = elastic_transform(aug['image'], aug_coords, 4000, 10)
|
544 |
-
|
545 |
-
return dimage, bounds
|
546 |
-
|
547 |
-
train_dataset = SyllableDataset(
|
548 |
-
[train_df1, train_df2, train_df3],
|
549 |
-
[train_dfh1, train_dfh2],
|
550 |
-
train_real_annotations,
|
551 |
-
augment, train_size
|
552 |
-
)
|
553 |
-
test_dataset = SyllableDataset(
|
554 |
-
[test_df1, test_df2, test_df3],
|
555 |
-
[test_dfh1, test_dfh2],
|
556 |
-
test_real_annotations,
|
557 |
-
None, 256
|
558 |
-
)
|
559 |
-
|
560 |
-
return train_dataset, test_dataset
|
|
|
|
|
|
|
|
|
|
|
|
|
|
|
|
|
|
|
|
|
|
|
|
|
|
|
|
|
|
|
|
|
|
|
|
|
|
|
|
|
|
|
|
|
|
|
|
|
|
|
|
|
|
|
|
|
|
|
|
|
|
|
|
|
|
|
|
|
|
|
|
|
|
|
|
|
|
|
|
|
|
|
|
|
|
|
|
|
|
|
|
|
|
|
|
|
|
|
|
|
|
|
|
|
|
|
|
|
|
|
|
|
|
|
|
|
|
|
|
|
|
|
|
|
|
|
|
|
|
|
|
|
|
|
|
|
|
|
|
|
|
|
|
|
|
|
|
|
|
|
|
|
|
|
|
|
|
|
|
|
|
|
|
|
|
|
|
|
|
|
|
|
|
|
|
|
|
|
|
|
|
|
|
|
|
|
|
|
|
|
|
|
|
|
|
|
|
|
|
|
|
|
|
|
|
|
|
|
|
|
|
|
|
|
|
|
|
|
|
|
|
|
|
|
|
|
|
|
|
|
|
|
|
|
|
|
|
|
|
|
|
|
|
|
|
|
|
|
|
|
|
|
|
|
|
|
|
|
|
|
|
|
|
|
|
|
|
|
|
|
|
|
|
|
|
|
|
|
|
|
|
|
|
|
|
|
|
|
|
|
|
|
|
|
|
|
|
|
|
|
|
|
|
|
|
|
|
|
|
|
|
|
|
|
|
|
|
|
|
|
|
|
|
|
|
|
|
|
|
|
|
|
|
|
|
|
|
|
|
|
|
|
|
|
|
|
|
|
|
|
|
|
|
|
|
|
|
|
|
|
|
|
|
|
|
|
|
|
|
|
|
|
|
|
|
|
|
|
|
|
|
|
|
|
|
|
|
|
|
|
|
|
|
|
|
|
|
|
|
|
|
|
|
|
|
|
|
|
|
|
|
|
|
|
|
|
|
|
|
|
|
|
|
|
|
|
|
|
|
|
|
|
|
|
|
|
|
|
|
|
|
|
|
|
|
|
|
|
|
|
|
|
|
|
|
|
|
|
|
|
|
|
|
|
|
|
|
|
|
|
|
|
|
|
|
|
|
|
|
|
|
|
|
|
|
|
|
|
|
|
|
|
|
|
|
|
|
|
|
|
|
|
|
|
|
|
|
|
|
|
|
|
|
|
|
|
|
|
|
|
|
|
|
|
|
|
|
|
|
|
|
|
|
|
|
|
|
|
|
|
|
|
|
|
|
|
|
|
|
|
|
|
|
|
|
|
|
|
|
|
|
|
|
|
|
|
|
|
|
|
|
|
|
|
|
|
|
|
|
|
|
|
|
|
|
|
|
|
|
|
|
|
|
|
|
|
|
|
|
|
|
|
|
|
|
|
|
|
|
|
|
|
|
|
|
|
|
|
|
|
|
|
|
|
|
|
|
|
|
|
|
|
|
|
|
|
|
|
|
|
|
|
|
|
|
|
|
|
|
|
|
|
|
|
|
|
|
|
|
|
|
|
|
|
|
|
|
|
|
|
|
|
|
|
|
|
|
|
|
|
|
|
|
|
|
|
|
|
|
|
|
|
|
|
|
|
|
|
|
|
|
|
|
|
|
|
|
|
|
|
|
|
|
|
|
|
|
|
|
|
|
|
|
|
|
|
|
|
|
|
|
|
|
|
|
|
|
|
|
|
|
|
|
|
|
|
|
|
|
|
|
|
|
|
|
|
|
|
|
|
|
|
|
|
|
|
|
|
|
|
|
|
|
|
|
|
|
|
|
|
|
|
|
|
|
|
|
|
|
|
|
|
|
|
|
|
|
|
|
|
|
|
|
|
|
|
|
|
|
|
|
|
|
|
|
|
|
|
|
|
|
|
|
|
|
|
|
|
|
|
|
|
|
|
|
|
|
|
|
|
|
|
|
|
|
|
|
|
|
|
|
|
|
|
|
|
|
|
|
|
|
|
|
|
|
|
|
|
|
|
|
|
|
|
|
|
|
|
|
|
|
|
|
|
|
|
|
|
|
|
|
|
|
|
|
|
|
|
|
|
|
|
|
|
|
|
|
|
|
|
|
|
|
|
|
|
|
|
|
|
|
|
|
|
|
|
|
|
|
|
|
|
|
|
|
|
|
|
|
|
|
|
|
|
|
|
|
|
|
|
|
|
|
|
|
|
|
|
|
|
|
|
|
|
|
|
|
|
|
|
|
|
|
|
|
|
|
|
|
|
|
|
|
|
|
|
|
|
|
|
|
|
|
|
|
|
|
|
|
|
|
|
|
|
|
|
|
|
|
|
|
|
|
|
|
|
|
|
|
|
|
|
|
|
|
|
|
|
|
|
|
|
|
|
|
|
|
|
|
|
|
|
|
|
|
|
|
|
|
|
|
|
|
|
|
|
|
|
|
|
|
|
|
|
|
|
|
|
|
|
|
|
|
|
|
|
|
|
|
|
|
|
|
|
|
|
|
|
|
|
|
|
|
|
|
|
|
|
|
|
|
|
|
|
|
|
|
|
|
|
|
|
|
|
|
|
|
|
|
|
|
spaces/Codecooker/rvcapi/src/download_models.py
DELETED
@@ -1,31 +0,0 @@
|
|
1 |
-
from pathlib import Path
|
2 |
-
import requests
|
3 |
-
|
4 |
-
MDX_DOWNLOAD_LINK = 'https://github.com/TRvlvr/model_repo/releases/download/all_public_uvr_models/'
|
5 |
-
RVC_DOWNLOAD_LINK = 'https://huggingface.co/lj1995/VoiceConversionWebUI/resolve/main/'
|
6 |
-
|
7 |
-
BASE_DIR = Path(__file__).resolve().parent.parent
|
8 |
-
mdxnet_models_dir = BASE_DIR / 'mdxnet_models'
|
9 |
-
rvc_models_dir = BASE_DIR / 'rvc_models'
|
10 |
-
|
11 |
-
|
12 |
-
def dl_model(link, model_name, dir_name):
|
13 |
-
with requests.get(f'{link}{model_name}') as r:
|
14 |
-
r.raise_for_status()
|
15 |
-
with open(dir_name / model_name, 'wb') as f:
|
16 |
-
for chunk in r.iter_content(chunk_size=8192):
|
17 |
-
f.write(chunk)
|
18 |
-
|
19 |
-
|
20 |
-
if __name__ == '__main__':
|
21 |
-
mdx_model_names = ['UVR-MDX-NET-Voc_FT.onnx', 'UVR_MDXNET_KARA_2.onnx', 'Reverb_HQ_By_FoxJoy.onnx']
|
22 |
-
for model in mdx_model_names:
|
23 |
-
print(f'Downloading {model}...')
|
24 |
-
dl_model(MDX_DOWNLOAD_LINK, model, mdxnet_models_dir)
|
25 |
-
|
26 |
-
rvc_model_names = ['hubert_base.pt', 'rmvpe.pt']
|
27 |
-
for model in rvc_model_names:
|
28 |
-
print(f'Downloading {model}...')
|
29 |
-
dl_model(RVC_DOWNLOAD_LINK, model, rvc_models_dir)
|
30 |
-
|
31 |
-
print('All models downloaded!')
|
|
|
|
|
|
|
|
|
|
|
|
|
|
|
|
|
|
|
|
|
|
|
|
|
|
|
|
|
|
|
|
|
|
|
|
|
|
|
|
|
|
|
|
|
|
|
|
|
|
|
|
|
|
|
|
|
|
|
|
|
|
|
|
spaces/CofAI/chat.b4/g4f/Provider/Providers/Liaobots.py
DELETED
@@ -1,47 +0,0 @@
|
|
1 |
-
import os, uuid, requests
|
2 |
-
from ...typing import sha256, Dict, get_type_hints
|
3 |
-
|
4 |
-
url = 'https://liaobots.com'
|
5 |
-
model = ['gpt-4-0613']
|
6 |
-
supports_stream = True
|
7 |
-
needs_auth = True
|
8 |
-
|
9 |
-
models = {
|
10 |
-
'gpt-4-0613': {
|
11 |
-
"id":"gpt-4-0613",
|
12 |
-
"name":"GPT-4",
|
13 |
-
"maxLength":24000,
|
14 |
-
"tokenLimit":8000
|
15 |
-
}
|
16 |
-
}
|
17 |
-
|
18 |
-
def _create_completion(model: str, messages: list, stream: bool, **kwargs):
|
19 |
-
|
20 |
-
print(kwargs)
|
21 |
-
|
22 |
-
headers = {
|
23 |
-
'authority': 'liaobots.com',
|
24 |
-
'content-type': 'application/json',
|
25 |
-
'origin': 'https://liaobots.com',
|
26 |
-
'referer': 'https://liaobots.com/',
|
27 |
-
'user-agent': 'Mozilla/5.0 (Windows NT 10.0; Win64; x64) AppleWebKit/537.36 (KHTML, like Gecko) Chrome/112.0.0.0 Safari/537.36',
|
28 |
-
'x-auth-code': 'P6cPPK6Z8JDG3'
|
29 |
-
}
|
30 |
-
|
31 |
-
json_data = {
|
32 |
-
'conversationId': str(uuid.uuid4()),
|
33 |
-
'model': models[model],
|
34 |
-
'authcode':"jrzVZMJiwN0NU",
|
35 |
-
'messages': messages,
|
36 |
-
'key': '',
|
37 |
-
'prompt': "You are ChatGPT, a large language model trained by OpenAI. Follow the user's instructions carefully. Respond using markdown.",
|
38 |
-
}
|
39 |
-
|
40 |
-
response = requests.post('https://liaobots.com/api/chat',
|
41 |
-
headers=headers, json=json_data, stream=True)
|
42 |
-
|
43 |
-
for token in response.iter_content(chunk_size=2046):
|
44 |
-
yield (token.decode('cp1251'))
|
45 |
-
|
46 |
-
params = f'g4f.Providers.{os.path.basename(__file__)[:-3]} supports: ' + \
|
47 |
-
'(%s)' % ', '.join([f"{name}: {get_type_hints(_create_completion)[name].__name__}" for name in _create_completion.__code__.co_varnames[:_create_completion.__code__.co_argcount]])
|
|
|
|
|
|
|
|
|
|
|
|
|
|
|
|
|
|
|
|
|
|
|
|
|
|
|
|
|
|
|
|
|
|
|
|
|
|
|
|
|
|
|
|
|
|
|
|
|
|
|
|
|
|
|
|
|
|
|
|
|
|
|
|
|
|
|
|
|
|
|
|
|
|
|
|
|
|
|
|
|
|
|
|
|
|
|
|
|
|
|
|
|
|
|
|
spaces/DQChoi/gpt-demo/venv/lib/python3.11/site-packages/PIL/XVThumbImagePlugin.py
DELETED
@@ -1,78 +0,0 @@
|
|
1 |
-
#
|
2 |
-
# The Python Imaging Library.
|
3 |
-
# $Id$
|
4 |
-
#
|
5 |
-
# XV Thumbnail file handler by Charles E. "Gene" Cash
|
6 |
-
# ([email protected])
|
7 |
-
#
|
8 |
-
# see xvcolor.c and xvbrowse.c in the sources to John Bradley's XV,
|
9 |
-
# available from ftp://ftp.cis.upenn.edu/pub/xv/
|
10 |
-
#
|
11 |
-
# history:
|
12 |
-
# 98-08-15 cec created (b/w only)
|
13 |
-
# 98-12-09 cec added color palette
|
14 |
-
# 98-12-28 fl added to PIL (with only a few very minor modifications)
|
15 |
-
#
|
16 |
-
# To do:
|
17 |
-
# FIXME: make save work (this requires quantization support)
|
18 |
-
#
|
19 |
-
|
20 |
-
from . import Image, ImageFile, ImagePalette
|
21 |
-
from ._binary import o8
|
22 |
-
|
23 |
-
_MAGIC = b"P7 332"
|
24 |
-
|
25 |
-
# standard color palette for thumbnails (RGB332)
|
26 |
-
PALETTE = b""
|
27 |
-
for r in range(8):
|
28 |
-
for g in range(8):
|
29 |
-
for b in range(4):
|
30 |
-
PALETTE = PALETTE + (
|
31 |
-
o8((r * 255) // 7) + o8((g * 255) // 7) + o8((b * 255) // 3)
|
32 |
-
)
|
33 |
-
|
34 |
-
|
35 |
-
def _accept(prefix):
|
36 |
-
return prefix[:6] == _MAGIC
|
37 |
-
|
38 |
-
|
39 |
-
##
|
40 |
-
# Image plugin for XV thumbnail images.
|
41 |
-
|
42 |
-
|
43 |
-
class XVThumbImageFile(ImageFile.ImageFile):
|
44 |
-
format = "XVThumb"
|
45 |
-
format_description = "XV thumbnail image"
|
46 |
-
|
47 |
-
def _open(self):
|
48 |
-
# check magic
|
49 |
-
if not _accept(self.fp.read(6)):
|
50 |
-
msg = "not an XV thumbnail file"
|
51 |
-
raise SyntaxError(msg)
|
52 |
-
|
53 |
-
# Skip to beginning of next line
|
54 |
-
self.fp.readline()
|
55 |
-
|
56 |
-
# skip info comments
|
57 |
-
while True:
|
58 |
-
s = self.fp.readline()
|
59 |
-
if not s:
|
60 |
-
msg = "Unexpected EOF reading XV thumbnail file"
|
61 |
-
raise SyntaxError(msg)
|
62 |
-
if s[0] != 35: # ie. when not a comment: '#'
|
63 |
-
break
|
64 |
-
|
65 |
-
# parse header line (already read)
|
66 |
-
s = s.strip().split()
|
67 |
-
|
68 |
-
self.mode = "P"
|
69 |
-
self._size = int(s[0]), int(s[1])
|
70 |
-
|
71 |
-
self.palette = ImagePalette.raw("RGB", PALETTE)
|
72 |
-
|
73 |
-
self.tile = [("raw", (0, 0) + self.size, self.fp.tell(), (self.mode, 0, 1))]
|
74 |
-
|
75 |
-
|
76 |
-
# --------------------------------------------------------------------
|
77 |
-
|
78 |
-
Image.register_open(XVThumbImageFile.format, XVThumbImageFile, _accept)
|
|
|
|
|
|
|
|
|
|
|
|
|
|
|
|
|
|
|
|
|
|
|
|
|
|
|
|
|
|
|
|
|
|
|
|
|
|
|
|
|
|
|
|
|
|
|
|
|
|
|
|
|
|
|
|
|
|
|
|
|
|
|
|
|
|
|
|
|
|
|
|
|
|
|
|
|
|
|
|
|
|
|
|
|
|
|
|
|
|
|
|
|
|
|
|
|
|
|
|
|
|
|
|
|
|
|
|
|
|
|
|
|
|
|
|
|
|
|
|
|
|
|
|
|
|
|
|
|
|
|
|
|
|
|
|
|
|
|
|
|
|
|
|
|
|
|
|
|
|
|
|
|
|
|
|
|
|
spaces/DebasishDhal99/Youtube_Playlist/app.py
DELETED
@@ -1,74 +0,0 @@
|
|
1 |
-
import gradio as gr
|
2 |
-
import urllib
|
3 |
-
from urllib.parse import urlparse, parse_qs
|
4 |
-
|
5 |
-
from playlist_duration import playlist_duration_func
|
6 |
-
from average_duration import playlist_average_duration_func
|
7 |
-
from playlists_mismatch import playlists_mismatch_func
|
8 |
-
|
9 |
-
def playlist_duration_calculator(playlist_link, calculation_type):
|
10 |
-
if calculation_type == "Total Duration":
|
11 |
-
result = playlist_duration_func(playlist_link)
|
12 |
-
return f"Total Duration: {result}"
|
13 |
-
elif calculation_type == "Average Duration":
|
14 |
-
result = playlist_average_duration_func(playlist_link)
|
15 |
-
return f"Average Duration: {result}"
|
16 |
-
|
17 |
-
playlist_link_input = gr.inputs.Textbox(label="Playlist Link")
|
18 |
-
calculation_type_input = gr.inputs.Radio(["Total Duration", "Average Duration"], label="What to calculate?")
|
19 |
-
outputs = gr.outputs.Textbox(label="Result")
|
20 |
-
|
21 |
-
heading = "YouTube Playlist Duration Calculator"
|
22 |
-
description = '''Enter a YouTube playlist link to calculate its total duration or average duration.\n
|
23 |
-
Do not enter the link of a video that belongs to that playlist.\n
|
24 |
-
Use the link in the share option of the playlist's page
|
25 |
-
'''
|
26 |
-
|
27 |
-
|
28 |
-
duration_interface = gr.Interface(
|
29 |
-
fn=playlist_duration_calculator,
|
30 |
-
inputs=[playlist_link_input, calculation_type_input],
|
31 |
-
outputs=outputs,
|
32 |
-
title=heading,
|
33 |
-
description=description,
|
34 |
-
# examples=[
|
35 |
-
# ["https://www.youtube.com/playlist?list=PL-osiE80TeTsWmV9i9c58mdDCSskIFdDS", "Total Duration"],
|
36 |
-
# ["https://www.youtube.com/playlist?list=PL-osiE80TeTtoQCKZ03TU5fNfx2UY6U4p", "Average Duration"],
|
37 |
-
# ],
|
38 |
-
theme="compact",
|
39 |
-
)
|
40 |
-
|
41 |
-
second_heading = "YouTube Playlist Mismatch Calculator"
|
42 |
-
second_description = "Enter two YouTube playlist links (without quotation marks) to compare their contents and find the mismatch."
|
43 |
-
mismatch_outputs = gr.outputs.Textbox(label="Mismatch between two playlists")
|
44 |
-
|
45 |
-
def playlist_mismatch_calculator(playlist_link_1, playlist_link_2, output_options):
|
46 |
-
result = playlists_mismatch_func(playlist_link_1, playlist_link_2, output_options)
|
47 |
-
playlist1name = result[2]
|
48 |
-
playlist2name = result[3]
|
49 |
-
text = 'Present in {}, not in {} :- \n{} \n \nPresent in {}, not in {} :-\n {}'.format(result[2],result[3], '\n'.join(result[0]), result[3], result[2], '\n'.join(result[1]))
|
50 |
-
return f"Mismatch Result between the two playlists are as follows: -\n\n {text}"
|
51 |
-
|
52 |
-
playlist_link_1_input = gr.inputs.Textbox(label="Playlist Link 1")
|
53 |
-
playlist_link_2_input = gr.inputs.Textbox(label="Playlist Link 2")
|
54 |
-
output_options = gr.inputs.Radio(["id", "link", "name"], label="Output Options")
|
55 |
-
|
56 |
-
mismatch_interface = gr.Interface(
|
57 |
-
fn=playlist_mismatch_calculator,
|
58 |
-
inputs=[playlist_link_1_input, playlist_link_2_input, output_options],
|
59 |
-
outputs=mismatch_outputs,
|
60 |
-
title=second_heading,
|
61 |
-
description=second_description,
|
62 |
-
# examples=[
|
63 |
-
# ["https://www.youtube.com/playlist?list=PL-osiE80TeTsWmV9i9c58mdDCSskIFdDS", "https://www.youtube.com/playlist?list=PL-osiE80TeTtoQCKZ03TU5fNfx2UY6U4p"],
|
64 |
-
# ],
|
65 |
-
theme="compact",
|
66 |
-
)
|
67 |
-
|
68 |
-
|
69 |
-
# interface1.launch()
|
70 |
-
# interface2.launch()
|
71 |
-
|
72 |
-
combinedinterface = gr.TabbedInterface([duration_interface,mismatch_interface],['Playlist Total and Average Duration', 'Playlist Mismatch'])
|
73 |
-
|
74 |
-
combinedinterface.launch()
|
|
|
|
|
|
|
|
|
|
|
|
|
|
|
|
|
|
|
|
|
|
|
|
|
|
|
|
|
|
|
|
|
|
|
|
|
|
|
|
|
|
|
|
|
|
|
|
|
|
|
|
|
|
|
|
|
|
|
|
|
|
|
|
|
|
|
|
|
|
|
|
|
|
|
|
|
|
|
|
|
|
|
|
|
|
|
|
|
|
|
|
|
|
|
|
|
|
|
|
|
|
|
|
|
|
|
|
|
|
|
|
|
|
|
|
|
|
|
|
|
|
|
|
|
|
|
|
|
|
|
|
|
|
|
|
|
|
|
|
|
|
|
|
|
|
|
|
|
|
spaces/Dinoking/Guccio-AI-Designer/models/biggan/pytorch_biggan/pytorch_pretrained_biggan/utils.py
DELETED
@@ -1,216 +0,0 @@
|
|
1 |
-
# coding: utf-8
|
2 |
-
""" BigGAN utilities to prepare truncated noise samples and convert/save/display output images.
|
3 |
-
Also comprise ImageNet utilities to prepare one hot input vectors for ImageNet classes.
|
4 |
-
We use Wordnet so you can just input a name in a string and automatically get a corresponding
|
5 |
-
imagenet class if it exists (or a hypo/hypernym exists in imagenet).
|
6 |
-
"""
|
7 |
-
from __future__ import absolute_import, division, print_function, unicode_literals
|
8 |
-
|
9 |
-
import json
|
10 |
-
import logging
|
11 |
-
from io import BytesIO
|
12 |
-
|
13 |
-
import numpy as np
|
14 |
-
from scipy.stats import truncnorm
|
15 |
-
|
16 |
-
logger = logging.getLogger(__name__)
|
17 |
-
|
18 |
-
NUM_CLASSES = 1000
|
19 |
-
|
20 |
-
|
21 |
-
def truncated_noise_sample(batch_size=1, dim_z=128, truncation=1., seed=None):
|
22 |
-
""" Create a truncated noise vector.
|
23 |
-
Params:
|
24 |
-
batch_size: batch size.
|
25 |
-
dim_z: dimension of z
|
26 |
-
truncation: truncation value to use
|
27 |
-
seed: seed for the random generator
|
28 |
-
Output:
|
29 |
-
array of shape (batch_size, dim_z)
|
30 |
-
"""
|
31 |
-
state = None if seed is None else np.random.RandomState(seed)
|
32 |
-
values = truncnorm.rvs(-2, 2, size=(batch_size, dim_z), random_state=state).astype(np.float32)
|
33 |
-
return truncation * values
|
34 |
-
|
35 |
-
|
36 |
-
def convert_to_images(obj):
|
37 |
-
""" Convert an output tensor from BigGAN in a list of images.
|
38 |
-
Params:
|
39 |
-
obj: tensor or numpy array of shape (batch_size, channels, height, width)
|
40 |
-
Output:
|
41 |
-
list of Pillow Images of size (height, width)
|
42 |
-
"""
|
43 |
-
try:
|
44 |
-
import PIL
|
45 |
-
except ImportError:
|
46 |
-
raise ImportError("Please install Pillow to use images: pip install Pillow")
|
47 |
-
|
48 |
-
if not isinstance(obj, np.ndarray):
|
49 |
-
obj = obj.detach().numpy()
|
50 |
-
|
51 |
-
obj = obj.transpose((0, 2, 3, 1))
|
52 |
-
obj = np.clip(((obj + 1) / 2.0) * 256, 0, 255)
|
53 |
-
|
54 |
-
img = []
|
55 |
-
for i, out in enumerate(obj):
|
56 |
-
out_array = np.asarray(np.uint8(out), dtype=np.uint8)
|
57 |
-
img.append(PIL.Image.fromarray(out_array))
|
58 |
-
return img
|
59 |
-
|
60 |
-
|
61 |
-
def save_as_images(obj, file_name='output'):
|
62 |
-
""" Convert and save an output tensor from BigGAN in a list of saved images.
|
63 |
-
Params:
|
64 |
-
obj: tensor or numpy array of shape (batch_size, channels, height, width)
|
65 |
-
file_name: path and beggingin of filename to save.
|
66 |
-
Images will be saved as `file_name_{image_number}.png`
|
67 |
-
"""
|
68 |
-
img = convert_to_images(obj)
|
69 |
-
|
70 |
-
for i, out in enumerate(img):
|
71 |
-
current_file_name = file_name + '_%d.png' % i
|
72 |
-
logger.info("Saving image to {}".format(current_file_name))
|
73 |
-
out.save(current_file_name, 'png')
|
74 |
-
|
75 |
-
|
76 |
-
def display_in_terminal(obj):
|
77 |
-
""" Convert and display an output tensor from BigGAN in the terminal.
|
78 |
-
This function use `libsixel` and will only work in a libsixel-compatible terminal.
|
79 |
-
Please refer to https://github.com/saitoha/libsixel for more details.
|
80 |
-
|
81 |
-
Params:
|
82 |
-
obj: tensor or numpy array of shape (batch_size, channels, height, width)
|
83 |
-
file_name: path and beggingin of filename to save.
|
84 |
-
Images will be saved as `file_name_{image_number}.png`
|
85 |
-
"""
|
86 |
-
try:
|
87 |
-
import PIL
|
88 |
-
from libsixel import (sixel_output_new, sixel_dither_new, sixel_dither_initialize,
|
89 |
-
sixel_dither_set_palette, sixel_dither_set_pixelformat,
|
90 |
-
sixel_dither_get, sixel_encode, sixel_dither_unref,
|
91 |
-
sixel_output_unref, SIXEL_PIXELFORMAT_RGBA8888,
|
92 |
-
SIXEL_PIXELFORMAT_RGB888, SIXEL_PIXELFORMAT_PAL8,
|
93 |
-
SIXEL_PIXELFORMAT_G8, SIXEL_PIXELFORMAT_G1)
|
94 |
-
except ImportError:
|
95 |
-
raise ImportError("Display in Terminal requires Pillow, libsixel "
|
96 |
-
"and a libsixel compatible terminal. "
|
97 |
-
"Please read info at https://github.com/saitoha/libsixel "
|
98 |
-
"and install with pip install Pillow libsixel-python")
|
99 |
-
|
100 |
-
s = BytesIO()
|
101 |
-
|
102 |
-
images = convert_to_images(obj)
|
103 |
-
widths, heights = zip(*(i.size for i in images))
|
104 |
-
|
105 |
-
output_width = sum(widths)
|
106 |
-
output_height = max(heights)
|
107 |
-
|
108 |
-
output_image = PIL.Image.new('RGB', (output_width, output_height))
|
109 |
-
|
110 |
-
x_offset = 0
|
111 |
-
for im in images:
|
112 |
-
output_image.paste(im, (x_offset,0))
|
113 |
-
x_offset += im.size[0]
|
114 |
-
|
115 |
-
try:
|
116 |
-
data = output_image.tobytes()
|
117 |
-
except NotImplementedError:
|
118 |
-
data = output_image.tostring()
|
119 |
-
output = sixel_output_new(lambda data, s: s.write(data), s)
|
120 |
-
|
121 |
-
try:
|
122 |
-
if output_image.mode == 'RGBA':
|
123 |
-
dither = sixel_dither_new(256)
|
124 |
-
sixel_dither_initialize(dither, data, output_width, output_height, SIXEL_PIXELFORMAT_RGBA8888)
|
125 |
-
elif output_image.mode == 'RGB':
|
126 |
-
dither = sixel_dither_new(256)
|
127 |
-
sixel_dither_initialize(dither, data, output_width, output_height, SIXEL_PIXELFORMAT_RGB888)
|
128 |
-
elif output_image.mode == 'P':
|
129 |
-
palette = output_image.getpalette()
|
130 |
-
dither = sixel_dither_new(256)
|
131 |
-
sixel_dither_set_palette(dither, palette)
|
132 |
-
sixel_dither_set_pixelformat(dither, SIXEL_PIXELFORMAT_PAL8)
|
133 |
-
elif output_image.mode == 'L':
|
134 |
-
dither = sixel_dither_get(SIXEL_BUILTIN_G8)
|
135 |
-
sixel_dither_set_pixelformat(dither, SIXEL_PIXELFORMAT_G8)
|
136 |
-
elif output_image.mode == '1':
|
137 |
-
dither = sixel_dither_get(SIXEL_BUILTIN_G1)
|
138 |
-
sixel_dither_set_pixelformat(dither, SIXEL_PIXELFORMAT_G1)
|
139 |
-
else:
|
140 |
-
raise RuntimeError('unexpected output_image mode')
|
141 |
-
try:
|
142 |
-
sixel_encode(data, output_width, output_height, 1, dither, output)
|
143 |
-
print(s.getvalue().decode('ascii'))
|
144 |
-
finally:
|
145 |
-
sixel_dither_unref(dither)
|
146 |
-
finally:
|
147 |
-
sixel_output_unref(output)
|
148 |
-
|
149 |
-
|
150 |
-
def one_hot_from_int(int_or_list, batch_size=1):
|
151 |
-
""" Create a one-hot vector from a class index or a list of class indices.
|
152 |
-
Params:
|
153 |
-
int_or_list: int, or list of int, of the imagenet classes (between 0 and 999)
|
154 |
-
batch_size: batch size.
|
155 |
-
If int_or_list is an int create a batch of identical classes.
|
156 |
-
If int_or_list is a list, we should have `len(int_or_list) == batch_size`
|
157 |
-
Output:
|
158 |
-
array of shape (batch_size, 1000)
|
159 |
-
"""
|
160 |
-
if isinstance(int_or_list, int):
|
161 |
-
int_or_list = [int_or_list]
|
162 |
-
|
163 |
-
if len(int_or_list) == 1 and batch_size > 1:
|
164 |
-
int_or_list = [int_or_list[0]] * batch_size
|
165 |
-
|
166 |
-
assert batch_size == len(int_or_list)
|
167 |
-
|
168 |
-
array = np.zeros((batch_size, NUM_CLASSES), dtype=np.float32)
|
169 |
-
for i, j in enumerate(int_or_list):
|
170 |
-
array[i, j] = 1.0
|
171 |
-
return array
|
172 |
-
|
173 |
-
|
174 |
-
def one_hot_from_names(class_name_or_list, batch_size=1):
|
175 |
-
""" Create a one-hot vector from the name of an imagenet class ('tennis ball', 'daisy', ...).
|
176 |
-
We use NLTK's wordnet search to try to find the relevant synset of ImageNet and take the first one.
|
177 |
-
If we can't find it direcly, we look at the hyponyms and hypernyms of the class name.
|
178 |
-
|
179 |
-
Params:
|
180 |
-
class_name_or_list: string containing the name of an imagenet object or a list of such strings (for a batch).
|
181 |
-
Output:
|
182 |
-
array of shape (batch_size, 1000)
|
183 |
-
"""
|
184 |
-
try:
|
185 |
-
from nltk.corpus import wordnet as wn
|
186 |
-
except ImportError:
|
187 |
-
raise ImportError("You need to install nltk to use this function")
|
188 |
-
|
189 |
-
if not isinstance(class_name_or_list, (list, tuple)):
|
190 |
-
class_name_or_list = [class_name_or_list]
|
191 |
-
else:
|
192 |
-
batch_size = max(batch_size, len(class_name_or_list))
|
193 |
-
|
194 |
-
classes = []
|
195 |
-
for class_name in class_name_or_list:
|
196 |
-
class_name = class_name.replace(" ", "_")
|
197 |
-
|
198 |
-
original_synsets = wn.synsets(class_name)
|
199 |
-
original_synsets = list(filter(lambda s: s.pos() == 'n', original_synsets)) # keep only names
|
200 |
-
if not original_synsets:
|
201 |
-
return None
|
202 |
-
|
203 |
-
possible_synsets = list(filter(lambda s: s.offset() in IMAGENET, original_synsets))
|
204 |
-
if possible_synsets:
|
205 |
-
classes.append(IMAGENET[possible_synsets[0].offset()])
|
206 |
-
else:
|
207 |
-
# try hypernyms and hyponyms
|
208 |
-
possible_synsets = sum([s.hypernyms() + s.hyponyms() for s in original_synsets], [])
|
209 |
-
possible_synsets = list(filter(lambda s: s.offset() in IMAGENET, possible_synsets))
|
210 |
-
if possible_synsets:
|
211 |
-
classes.append(IMAGENET[possible_synsets[0].offset()])
|
212 |
-
|
213 |
-
return one_hot_from_int(classes, batch_size=batch_size)
|
214 |
-
|
215 |
-
|
216 |
-
IMAGENET = {1440764: 0, 1443537: 1, 1484850: 2, 1491361: 3, 1494475: 4, 1496331: 5, 1498041: 6, 1514668: 7, 1514859: 8, 1518878: 9, 1530575: 10, 1531178: 11, 1532829: 12, 1534433: 13, 1537544: 14, 1558993: 15, 1560419: 16, 1580077: 17, 1582220: 18, 1592084: 19, 1601694: 20, 1608432: 21, 1614925: 22, 1616318: 23, 1622779: 24, 1629819: 25, 1630670: 26, 1631663: 27, 1632458: 28, 1632777: 29, 1641577: 30, 1644373: 31, 1644900: 32, 1664065: 33, 1665541: 34, 1667114: 35, 1667778: 36, 1669191: 37, 1675722: 38, 1677366: 39, 1682714: 40, 1685808: 41, 1687978: 42, 1688243: 43, 1689811: 44, 1692333: 45, 1693334: 46, 1694178: 47, 1695060: 48, 1697457: 49, 1698640: 50, 1704323: 51, 1728572: 52, 1728920: 53, 1729322: 54, 1729977: 55, 1734418: 56, 1735189: 57, 1737021: 58, 1739381: 59, 1740131: 60, 1742172: 61, 1744401: 62, 1748264: 63, 1749939: 64, 1751748: 65, 1753488: 66, 1755581: 67, 1756291: 68, 1768244: 69, 1770081: 70, 1770393: 71, 1773157: 72, 1773549: 73, 1773797: 74, 1774384: 75, 1774750: 76, 1775062: 77, 1776313: 78, 1784675: 79, 1795545: 80, 1796340: 81, 1797886: 82, 1798484: 83, 1806143: 84, 1806567: 85, 1807496: 86, 1817953: 87, 1818515: 88, 1819313: 89, 1820546: 90, 1824575: 91, 1828970: 92, 1829413: 93, 1833805: 94, 1843065: 95, 1843383: 96, 1847000: 97, 1855032: 98, 1855672: 99, 1860187: 100, 1871265: 101, 1872401: 102, 1873310: 103, 1877812: 104, 1882714: 105, 1883070: 106, 1910747: 107, 1914609: 108, 1917289: 109, 1924916: 110, 1930112: 111, 1943899: 112, 1944390: 113, 1945685: 114, 1950731: 115, 1955084: 116, 1968897: 117, 1978287: 118, 1978455: 119, 1980166: 120, 1981276: 121, 1983481: 122, 1984695: 123, 1985128: 124, 1986214: 125, 1990800: 126, 2002556: 127, 2002724: 128, 2006656: 129, 2007558: 130, 2009229: 131, 2009912: 132, 2011460: 133, 2012849: 134, 2013706: 135, 2017213: 136, 2018207: 137, 2018795: 138, 2025239: 139, 2027492: 140, 2028035: 141, 2033041: 142, 2037110: 143, 2051845: 144, 2056570: 145, 2058221: 146, 2066245: 147, 2071294: 148, 2074367: 149, 2077923: 150, 2085620: 151, 2085782: 152, 2085936: 153, 2086079: 154, 2086240: 155, 2086646: 156, 2086910: 157, 2087046: 158, 2087394: 159, 2088094: 160, 2088238: 161, 2088364: 162, 2088466: 163, 2088632: 164, 2089078: 165, 2089867: 166, 2089973: 167, 2090379: 168, 2090622: 169, 2090721: 170, 2091032: 171, 2091134: 172, 2091244: 173, 2091467: 174, 2091635: 175, 2091831: 176, 2092002: 177, 2092339: 178, 2093256: 179, 2093428: 180, 2093647: 181, 2093754: 182, 2093859: 183, 2093991: 184, 2094114: 185, 2094258: 186, 2094433: 187, 2095314: 188, 2095570: 189, 2095889: 190, 2096051: 191, 2096177: 192, 2096294: 193, 2096437: 194, 2096585: 195, 2097047: 196, 2097130: 197, 2097209: 198, 2097298: 199, 2097474: 200, 2097658: 201, 2098105: 202, 2098286: 203, 2098413: 204, 2099267: 205, 2099429: 206, 2099601: 207, 2099712: 208, 2099849: 209, 2100236: 210, 2100583: 211, 2100735: 212, 2100877: 213, 2101006: 214, 2101388: 215, 2101556: 216, 2102040: 217, 2102177: 218, 2102318: 219, 2102480: 220, 2102973: 221, 2104029: 222, 2104365: 223, 2105056: 224, 2105162: 225, 2105251: 226, 2105412: 227, 2105505: 228, 2105641: 229, 2105855: 230, 2106030: 231, 2106166: 232, 2106382: 233, 2106550: 234, 2106662: 235, 2107142: 236, 2107312: 237, 2107574: 238, 2107683: 239, 2107908: 240, 2108000: 241, 2108089: 242, 2108422: 243, 2108551: 244, 2108915: 245, 2109047: 246, 2109525: 247, 2109961: 248, 2110063: 249, 2110185: 250, 2110341: 251, 2110627: 252, 2110806: 253, 2110958: 254, 2111129: 255, 2111277: 256, 2111500: 257, 2111889: 258, 2112018: 259, 2112137: 260, 2112350: 261, 2112706: 262, 2113023: 263, 2113186: 264, 2113624: 265, 2113712: 266, 2113799: 267, 2113978: 268, 2114367: 269, 2114548: 270, 2114712: 271, 2114855: 272, 2115641: 273, 2115913: 274, 2116738: 275, 2117135: 276, 2119022: 277, 2119789: 278, 2120079: 279, 2120505: 280, 2123045: 281, 2123159: 282, 2123394: 283, 2123597: 284, 2124075: 285, 2125311: 286, 2127052: 287, 2128385: 288, 2128757: 289, 2128925: 290, 2129165: 291, 2129604: 292, 2130308: 293, 2132136: 294, 2133161: 295, 2134084: 296, 2134418: 297, 2137549: 298, 2138441: 299, 2165105: 300, 2165456: 301, 2167151: 302, 2168699: 303, 2169497: 304, 2172182: 305, 2174001: 306, 2177972: 307, 2190166: 308, 2206856: 309, 2219486: 310, 2226429: 311, 2229544: 312, 2231487: 313, 2233338: 314, 2236044: 315, 2256656: 316, 2259212: 317, 2264363: 318, 2268443: 319, 2268853: 320, 2276258: 321, 2277742: 322, 2279972: 323, 2280649: 324, 2281406: 325, 2281787: 326, 2317335: 327, 2319095: 328, 2321529: 329, 2325366: 330, 2326432: 331, 2328150: 332, 2342885: 333, 2346627: 334, 2356798: 335, 2361337: 336, 2363005: 337, 2364673: 338, 2389026: 339, 2391049: 340, 2395406: 341, 2396427: 342, 2397096: 343, 2398521: 344, 2403003: 345, 2408429: 346, 2410509: 347, 2412080: 348, 2415577: 349, 2417914: 350, 2422106: 351, 2422699: 352, 2423022: 353, 2437312: 354, 2437616: 355, 2441942: 356, 2442845: 357, 2443114: 358, 2443484: 359, 2444819: 360, 2445715: 361, 2447366: 362, 2454379: 363, 2457408: 364, 2480495: 365, 2480855: 366, 2481823: 367, 2483362: 368, 2483708: 369, 2484975: 370, 2486261: 371, 2486410: 372, 2487347: 373, 2488291: 374, 2488702: 375, 2489166: 376, 2490219: 377, 2492035: 378, 2492660: 379, 2493509: 380, 2493793: 381, 2494079: 382, 2497673: 383, 2500267: 384, 2504013: 385, 2504458: 386, 2509815: 387, 2510455: 388, 2514041: 389, 2526121: 390, 2536864: 391, 2606052: 392, 2607072: 393, 2640242: 394, 2641379: 395, 2643566: 396, 2655020: 397, 2666196: 398, 2667093: 399, 2669723: 400, 2672831: 401, 2676566: 402, 2687172: 403, 2690373: 404, 2692877: 405, 2699494: 406, 2701002: 407, 2704792: 408, 2708093: 409, 2727426: 410, 2730930: 411, 2747177: 412, 2749479: 413, 2769748: 414, 2776631: 415, 2777292: 416, 2782093: 417, 2783161: 418, 2786058: 419, 2787622: 420, 2788148: 421, 2790996: 422, 2791124: 423, 2791270: 424, 2793495: 425, 2794156: 426, 2795169: 427, 2797295: 428, 2799071: 429, 2802426: 430, 2804414: 431, 2804610: 432, 2807133: 433, 2808304: 434, 2808440: 435, 2814533: 436, 2814860: 437, 2815834: 438, 2817516: 439, 2823428: 440, 2823750: 441, 2825657: 442, 2834397: 443, 2835271: 444, 2837789: 445, 2840245: 446, 2841315: 447, 2843684: 448, 2859443: 449, 2860847: 450, 2865351: 451, 2869837: 452, 2870880: 453, 2871525: 454, 2877765: 455, 2879718: 456, 2883205: 457, 2892201: 458, 2892767: 459, 2894605: 460, 2895154: 461, 2906734: 462, 2909870: 463, 2910353: 464, 2916936: 465, 2917067: 466, 2927161: 467, 2930766: 468, 2939185: 469, 2948072: 470, 2950826: 471, 2951358: 472, 2951585: 473, 2963159: 474, 2965783: 475, 2966193: 476, 2966687: 477, 2971356: 478, 2974003: 479, 2977058: 480, 2978881: 481, 2979186: 482, 2980441: 483, 2981792: 484, 2988304: 485, 2992211: 486, 2992529: 487, 2999410: 488, 3000134: 489, 3000247: 490, 3000684: 491, 3014705: 492, 3016953: 493, 3017168: 494, 3018349: 495, 3026506: 496, 3028079: 497, 3032252: 498, 3041632: 499, 3042490: 500, 3045698: 501, 3047690: 502, 3062245: 503, 3063599: 504, 3063689: 505, 3065424: 506, 3075370: 507, 3085013: 508, 3089624: 509, 3095699: 510, 3100240: 511, 3109150: 512, 3110669: 513, 3124043: 514, 3124170: 515, 3125729: 516, 3126707: 517, 3127747: 518, 3127925: 519, 3131574: 520, 3133878: 521, 3134739: 522, 3141823: 523, 3146219: 524, 3160309: 525, 3179701: 526, 3180011: 527, 3187595: 528, 3188531: 529, 3196217: 530, 3197337: 531, 3201208: 532, 3207743: 533, 3207941: 534, 3208938: 535, 3216828: 536, 3218198: 537, 3220513: 538, 3223299: 539, 3240683: 540, 3249569: 541, 3250847: 542, 3255030: 543, 3259280: 544, 3271574: 545, 3272010: 546, 3272562: 547, 3290653: 548, 3291819: 549, 3297495: 550, 3314780: 551, 3325584: 552, 3337140: 553, 3344393: 554, 3345487: 555, 3347037: 556, 3355925: 557, 3372029: 558, 3376595: 559, 3379051: 560, 3384352: 561, 3388043: 562, 3388183: 563, 3388549: 564, 3393912: 565, 3394916: 566, 3400231: 567, 3404251: 568, 3417042: 569, 3424325: 570, 3425413: 571, 3443371: 572, 3444034: 573, 3445777: 574, 3445924: 575, 3447447: 576, 3447721: 577, 3450230: 578, 3452741: 579, 3457902: 580, 3459775: 581, 3461385: 582, 3467068: 583, 3476684: 584, 3476991: 585, 3478589: 586, 3481172: 587, 3482405: 588, 3483316: 589, 3485407: 590, 3485794: 591, 3492542: 592, 3494278: 593, 3495258: 594, 3496892: 595, 3498962: 596, 3527444: 597, 3529860: 598, 3530642: 599, 3532672: 600, 3534580: 601, 3535780: 602, 3538406: 603, 3544143: 604, 3584254: 605, 3584829: 606, 3590841: 607, 3594734: 608, 3594945: 609, 3595614: 610, 3598930: 611, 3599486: 612, 3602883: 613, 3617480: 614, 3623198: 615, 3627232: 616, 3630383: 617, 3633091: 618, 3637318: 619, 3642806: 620, 3649909: 621, 3657121: 622, 3658185: 623, 3661043: 624, 3662601: 625, 3666591: 626, 3670208: 627, 3673027: 628, 3676483: 629, 3680355: 630, 3690938: 631, 3691459: 632, 3692522: 633, 3697007: 634, 3706229: 635, 3709823: 636, 3710193: 637, 3710637: 638, 3710721: 639, 3717622: 640, 3720891: 641, 3721384: 642, 3724870: 643, 3729826: 644, 3733131: 645, 3733281: 646, 3733805: 647, 3742115: 648, 3743016: 649, 3759954: 650, 3761084: 651, 3763968: 652, 3764736: 653, 3769881: 654, 3770439: 655, 3770679: 656, 3773504: 657, 3775071: 658, 3775546: 659, 3776460: 660, 3777568: 661, 3777754: 662, 3781244: 663, 3782006: 664, 3785016: 665, 3786901: 666, 3787032: 667, 3788195: 668, 3788365: 669, 3791053: 670, 3792782: 671, 3792972: 672, 3793489: 673, 3794056: 674, 3796401: 675, 3803284: 676, 3804744: 677, 3814639: 678, 3814906: 679, 3825788: 680, 3832673: 681, 3837869: 682, 3838899: 683, 3840681: 684, 3841143: 685, 3843555: 686, 3854065: 687, 3857828: 688, 3866082: 689, 3868242: 690, 3868863: 691, 3871628: 692, 3873416: 693, 3874293: 694, 3874599: 695, 3876231: 696, 3877472: 697, 3877845: 698, 3884397: 699, 3887697: 700, 3888257: 701, 3888605: 702, 3891251: 703, 3891332: 704, 3895866: 705, 3899768: 706, 3902125: 707, 3903868: 708, 3908618: 709, 3908714: 710, 3916031: 711, 3920288: 712, 3924679: 713, 3929660: 714, 3929855: 715, 3930313: 716, 3930630: 717, 3933933: 718, 3935335: 719, 3937543: 720, 3938244: 721, 3942813: 722, 3944341: 723, 3947888: 724, 3950228: 725, 3954731: 726, 3956157: 727, 3958227: 728, 3961711: 729, 3967562: 730, 3970156: 731, 3976467: 732, 3976657: 733, 3977966: 734, 3980874: 735, 3982430: 736, 3983396: 737, 3991062: 738, 3992509: 739, 3995372: 740, 3998194: 741, 4004767: 742, 4005630: 743, 4008634: 744, 4009552: 745, 4019541: 746, 4023962: 747, 4026417: 748, 4033901: 749, 4033995: 750, 4037443: 751, 4039381: 752, 4040759: 753, 4041544: 754, 4044716: 755, 4049303: 756, 4065272: 757, 4067472: 758, 4069434: 759, 4070727: 760, 4074963: 761, 4081281: 762, 4086273: 763, 4090263: 764, 4099969: 765, 4111531: 766, 4116512: 767, 4118538: 768, 4118776: 769, 4120489: 770, 4125021: 771, 4127249: 772, 4131690: 773, 4133789: 774, 4136333: 775, 4141076: 776, 4141327: 777, 4141975: 778, 4146614: 779, 4147183: 780, 4149813: 781, 4152593: 782, 4153751: 783, 4154565: 784, 4162706: 785, 4179913: 786, 4192698: 787, 4200800: 788, 4201297: 789, 4204238: 790, 4204347: 791, 4208210: 792, 4209133: 793, 4209239: 794, 4228054: 795, 4229816: 796, 4235860: 797, 4238763: 798, 4239074: 799, 4243546: 800, 4251144: 801, 4252077: 802, 4252225: 803, 4254120: 804, 4254680: 805, 4254777: 806, 4258138: 807, 4259630: 808, 4263257: 809, 4264628: 810, 4265275: 811, 4266014: 812, 4270147: 813, 4273569: 814, 4275548: 815, 4277352: 816, 4285008: 817, 4286575: 818, 4296562: 819, 4310018: 820, 4311004: 821, 4311174: 822, 4317175: 823, 4325704: 824, 4326547: 825, 4328186: 826, 4330267: 827, 4332243: 828, 4335435: 829, 4336792: 830, 4344873: 831, 4346328: 832, 4347754: 833, 4350905: 834, 4355338: 835, 4355933: 836, 4356056: 837, 4357314: 838, 4366367: 839, 4367480: 840, 4370456: 841, 4371430: 842, 4371774: 843, 4372370: 844, 4376876: 845, 4380533: 846, 4389033: 847, 4392985: 848, 4398044: 849, 4399382: 850, 4404412: 851, 4409515: 852, 4417672: 853, 4418357: 854, 4423845: 855, 4428191: 856, 4429376: 857, 4435653: 858, 4442312: 859, 4443257: 860, 4447861: 861, 4456115: 862, 4458633: 863, 4461696: 864, 4462240: 865, 4465501: 866, 4467665: 867, 4476259: 868, 4479046: 869, 4482393: 870, 4483307: 871, 4485082: 872, 4486054: 873, 4487081: 874, 4487394: 875, 4493381: 876, 4501370: 877, 4505470: 878, 4507155: 879, 4509417: 880, 4515003: 881, 4517823: 882, 4522168: 883, 4523525: 884, 4525038: 885, 4525305: 886, 4532106: 887, 4532670: 888, 4536866: 889, 4540053: 890, 4542943: 891, 4548280: 892, 4548362: 893, 4550184: 894, 4552348: 895, 4553703: 896, 4554684: 897, 4557648: 898, 4560804: 899, 4562935: 900, 4579145: 901, 4579432: 902, 4584207: 903, 4589890: 904, 4590129: 905, 4591157: 906, 4591713: 907, 4592741: 908, 4596742: 909, 4597913: 910, 4599235: 911, 4604644: 912, 4606251: 913, 4612504: 914, 4613696: 915, 6359193: 916, 6596364: 917, 6785654: 918, 6794110: 919, 6874185: 920, 7248320: 921, 7565083: 922, 7579787: 923, 7583066: 924, 7584110: 925, 7590611: 926, 7613480: 927, 7614500: 928, 7615774: 929, 7684084: 930, 7693725: 931, 7695742: 932, 7697313: 933, 7697537: 934, 7711569: 935, 7714571: 936, 7714990: 937, 7715103: 938, 7716358: 939, 7716906: 940, 7717410: 941, 7717556: 942, 7718472: 943, 7718747: 944, 7720875: 945, 7730033: 946, 7734744: 947, 7742313: 948, 7745940: 949, 7747607: 950, 7749582: 951, 7753113: 952, 7753275: 953, 7753592: 954, 7754684: 955, 7760859: 956, 7768694: 957, 7802026: 958, 7831146: 959, 7836838: 960, 7860988: 961, 7871810: 962, 7873807: 963, 7875152: 964, 7880968: 965, 7892512: 966, 7920052: 967, 7930864: 968, 7932039: 969, 9193705: 970, 9229709: 971, 9246464: 972, 9256479: 973, 9288635: 974, 9332890: 975, 9399592: 976, 9421951: 977, 9428293: 978, 9468604: 979, 9472597: 980, 9835506: 981, 10148035: 982, 10565667: 983, 11879895: 984, 11939491: 985, 12057211: 986, 12144580: 987, 12267677: 988, 12620546: 989, 12768682: 990, 12985857: 991, 12998815: 992, 13037406: 993, 13040303: 994, 13044778: 995, 13052670: 996, 13054560: 997, 13133613: 998, 15075141: 999}
|
|
|
|
|
|
|
|
|
|
|
|
|
|
|
|
|
|
|
|
|
|
|
|
|
|
|
|
|
|
|
|
|
|
|
|
|
|
|
|
|
|
|
|
|
|
|
|
|
|
|
|
|
|
|
|
|
|
|
|
|
|
|
|
|
|
|
|
|
|
|
|
|
|
|
|
|
|
|
|
|
|
|
|
|
|
|
|
|
|
|
|
|
|
|
|
|
|
|
|
|
|
|
|
|
|
|
|
|
|
|
|
|
|
|
|
|
|
|
|
|
|
|
|
|
|
|
|
|
|
|
|
|
|
|
|
|
|
|
|
|
|
|
|
|
|
|
|
|
|
|
|
|
|
|
|
|
|
|
|
|
|
|
|
|
|
|
|
|
|
|
|
|
|
|
|
|
|
|
|
|
|
|
|
|
|
|
|
|
|
|
|
|
|
|
|
|
|
|
|
|
|
|
|
|
|
|
|
|
|
|
|
|
|
|
|
|
|
|
|
|
|
|
|
|
|
|
|
|
|
|
|
|
|
|
|
|
|
|
|
|
|
|
|
|
|
|
|
|
|
|
|
|
|
|
|
|
|
|
|
|
|
|
|
|
|
|
|
|
|
|
|
|
|
|
|
|
|
|
|
|
|
|
|
|
|
|
|
|
|
|
|
|
|
|
|
|
|
|
|
|
|
|
|
|
|
|
|
|
|
|
|
|
|
|
|
|
|
|
|
|
|
|
|
|
|
|
|
|
|
|
|
|
|
|
|
|
|
|
|
|
|
|
|
|
|
|
|
|
|
|
|
|
|
|
|
|
|
|
|
|
|
|
|
|
|
|
|
|
|
|
|
|
|
|
|
|
|
|
|
|
|
|
|
|
|
|
|
|
|
|
|
|
|
|
|
|
|
|
|
|
|
|
|
|
|
|
|
|
|
|
|
|
|
|
|
|
|
|
|
|
|
|
|
|
|
|
|
|
|
|
|
|
|
spaces/Dinoking/Guccio-AI-Designer/models/stylegan2/stylegan2-pytorch/prepare_data.py
DELETED
@@ -1,82 +0,0 @@
|
|
1 |
-
import argparse
|
2 |
-
from io import BytesIO
|
3 |
-
import multiprocessing
|
4 |
-
from functools import partial
|
5 |
-
|
6 |
-
from PIL import Image
|
7 |
-
import lmdb
|
8 |
-
from tqdm import tqdm
|
9 |
-
from torchvision import datasets
|
10 |
-
from torchvision.transforms import functional as trans_fn
|
11 |
-
|
12 |
-
|
13 |
-
def resize_and_convert(img, size, resample, quality=100):
|
14 |
-
img = trans_fn.resize(img, size, resample)
|
15 |
-
img = trans_fn.center_crop(img, size)
|
16 |
-
buffer = BytesIO()
|
17 |
-
img.save(buffer, format='jpeg', quality=quality)
|
18 |
-
val = buffer.getvalue()
|
19 |
-
|
20 |
-
return val
|
21 |
-
|
22 |
-
|
23 |
-
def resize_multiple(img, sizes=(128, 256, 512, 1024), resample=Image.LANCZOS, quality=100):
|
24 |
-
imgs = []
|
25 |
-
|
26 |
-
for size in sizes:
|
27 |
-
imgs.append(resize_and_convert(img, size, resample, quality))
|
28 |
-
|
29 |
-
return imgs
|
30 |
-
|
31 |
-
|
32 |
-
def resize_worker(img_file, sizes, resample):
|
33 |
-
i, file = img_file
|
34 |
-
img = Image.open(file)
|
35 |
-
img = img.convert('RGB')
|
36 |
-
out = resize_multiple(img, sizes=sizes, resample=resample)
|
37 |
-
|
38 |
-
return i, out
|
39 |
-
|
40 |
-
|
41 |
-
def prepare(env, dataset, n_worker, sizes=(128, 256, 512, 1024), resample=Image.LANCZOS):
|
42 |
-
resize_fn = partial(resize_worker, sizes=sizes, resample=resample)
|
43 |
-
|
44 |
-
files = sorted(dataset.imgs, key=lambda x: x[0])
|
45 |
-
files = [(i, file) for i, (file, label) in enumerate(files)]
|
46 |
-
total = 0
|
47 |
-
|
48 |
-
with multiprocessing.Pool(n_worker) as pool:
|
49 |
-
for i, imgs in tqdm(pool.imap_unordered(resize_fn, files)):
|
50 |
-
for size, img in zip(sizes, imgs):
|
51 |
-
key = f'{size}-{str(i).zfill(5)}'.encode('utf-8')
|
52 |
-
|
53 |
-
with env.begin(write=True) as txn:
|
54 |
-
txn.put(key, img)
|
55 |
-
|
56 |
-
total += 1
|
57 |
-
|
58 |
-
with env.begin(write=True) as txn:
|
59 |
-
txn.put('length'.encode('utf-8'), str(total).encode('utf-8'))
|
60 |
-
|
61 |
-
|
62 |
-
if __name__ == '__main__':
|
63 |
-
parser = argparse.ArgumentParser()
|
64 |
-
parser.add_argument('--out', type=str)
|
65 |
-
parser.add_argument('--size', type=str, default='128,256,512,1024')
|
66 |
-
parser.add_argument('--n_worker', type=int, default=8)
|
67 |
-
parser.add_argument('--resample', type=str, default='lanczos')
|
68 |
-
parser.add_argument('path', type=str)
|
69 |
-
|
70 |
-
args = parser.parse_args()
|
71 |
-
|
72 |
-
resample_map = {'lanczos': Image.LANCZOS, 'bilinear': Image.BILINEAR}
|
73 |
-
resample = resample_map[args.resample]
|
74 |
-
|
75 |
-
sizes = [int(s.strip()) for s in args.size.split(',')]
|
76 |
-
|
77 |
-
print(f'Make dataset of image sizes:', ', '.join(str(s) for s in sizes))
|
78 |
-
|
79 |
-
imgset = datasets.ImageFolder(args.path)
|
80 |
-
|
81 |
-
with lmdb.open(args.out, map_size=1024 ** 4, readahead=False) as env:
|
82 |
-
prepare(env, imgset, args.n_worker, sizes=sizes, resample=resample)
|
|
|
|
|
|
|
|
|
|
|
|
|
|
|
|
|
|
|
|
|
|
|
|
|
|
|
|
|
|
|
|
|
|
|
|
|
|
|
|
|
|
|
|
|
|
|
|
|
|
|
|
|
|
|
|
|
|
|
|
|
|
|
|
|
|
|
|
|
|
|
|
|
|
|
|
|
|
|
|
|
|
|
|
|
|
|
|
|
|
|
|
|
|
|
|
|
|
|
|
|
|
|
|
|
|
|
|
|
|
|
|
|
|
|
|
|
|
|
|
|
|
|
|
|
|
|
|
|
|
|
|
|
|
|
|
|
|
|
|
|
|
|
|
|
|
|
|
|
|
|
|
|
|
|
|
|
|
|
|
|
|
|
|
|
|