diff --git a/spaces/1acneusushi/gradio-2dmoleculeeditor/data/Corel Videostudio 12 Activation Code Keygen How to Get the Best Video Editing Experience with Corel Videostudio 12.md b/spaces/1acneusushi/gradio-2dmoleculeeditor/data/Corel Videostudio 12 Activation Code Keygen How to Get the Best Video Editing Experience with Corel Videostudio 12.md
deleted file mode 100644
index 07467c95cd331d06b497c98913edb80885c148a8..0000000000000000000000000000000000000000
--- a/spaces/1acneusushi/gradio-2dmoleculeeditor/data/Corel Videostudio 12 Activation Code Keygen How to Get the Best Video Editing Experience with Corel Videostudio 12.md
+++ /dev/null
@@ -1,86 +0,0 @@
-
-
Corel VideoStudio 12 Activation Code Keygen: How to Get It for Free
-If you are looking for a powerful and easy-to-use video editing software, you may have heard of Corel VideoStudio 12. This software allows you to create stunning videos with professional-quality effects, transitions, titles, music, and more. However, to enjoy all the features and benefits of Corel VideoStudio 12, you need to activate it with a valid serial number and an activation code.
-corel videostudio 12 activation code keygen Download ✑ https://byltly.com/2uKzNA
-Unfortunately, buying a license for Corel VideoStudio 12 can be quite expensive, especially if you are on a tight budget or you only need it for a short-term project. That's why some people may want to get an activation code keygen for Corel VideoStudio 12 for free.
-But what is an activation code keygen and how can you get one for Corel VideoStudio 12? In this article, we will explain everything you need to know about Corel VideoStudio 12 activation code keygen and provide you with three different methods on how to get it for free.
- What is Corel VideoStudio 12?
-Corel VideoStudio 12 is a video editing software that was released in 2008 by Corel Corporation. It is the successor of Ulead VideoStudio 11 and the predecessor of Corel VideoStudio Pro X2.
-Corel VideoStudio 12 offers many features and tools that can help you create amazing videos with ease. Some of the features include:
-
-A user-friendly interface that lets you drag-and-drop clips, effects, transitions, titles, music, and more.
-A timeline that lets you edit your videos with precision and flexibility.
-A library that lets you organize your media files and access them quickly.
-A capture mode that lets you record videos from your webcam, screen, DV camcorder, or analog device.
-A movie wizard that lets you create videos automatically with predefined templates and themes.
-A DVD authoring mode that lets you burn your videos to DVD discs or ISO files with menus and chapters.
-A share mode that lets you export your videos to various formats and devices or upload them online.
-A wide range of effects, transitions, filters, overlays, titles, animations, music tracks, sound effects, and more that can enhance your videos.
-A chroma key feature that lets you replace the background of your videos with any image or video.
-A picture-in-picture feature that lets you overlay multiple videos on one screen.
-A slow motion feature that lets you change the speed of your videos.
-A stabilization feature that lets you reduce camera shake in your videos.
-A split screen feature that lets you show two or more videos side by side.
-A paint feature that lets you draw or write on your videos.
-A subtitle feature that lets you add text or captions to your videos.
-
- What is an activation code keygen?
-An activation code keygen is a software program that can generate serial numbers and activation codes for other software programs. A serial number is a unique identifier that is required to install a software program on your computer. An activation code is a verification code that is required to activate a software program after installation.
-corel videostudio 12 pro serial number generator
-corel videostudio 12 ultimate crack download
-corel videostudio 12 license key free
-corel videostudio 12 product activation code
-corel videostudio 12 keygen only
-corel videostudio 12 full version with crack
-corel videostudio 12 registration code online
-corel videostudio 12 activation patch
-corel videostudio 12 serial key and email
-corel videostudio 12 crack file
-corel videostudio 12 activation code generator online
-corel videostudio 12 offline activation code
-corel videostudio 12 keygen.exe download
-corel videostudio 12 activation code free download
-corel videostudio 12 crack keygen rar
-corel videostudio 12 serial number and activation code
-corel videostudio 12 license key generator online
-corel videostudio 12 crack download for windows 10
-corel videostudio 12 activation code crack
-corel videostudio 12 keygen by kaizer soze
-corel videostudio 12 serial number free download
-corel videostudio 12 activation code online free
-corel videostudio 12 license key crack
-corel videostudio 12 keygen download free
-corel videostudio 12 activation code torrent
-corel videostudio 12 serial number and email address
-corel videostudio 12 license key free download
-corel videostudio 12 crack download full version
-corel videostudio 12 activation code online generator
-corel videostudio 12 keygen by x-force
-corel videostudio 12 serial number generator online
-corel videostudio 12 activation code free online
-corel videostudio 12 license key online free
-corel videostudio 12 crack download for windows 7
-corel videostudio 12 activation code no survey
-corel videostudio 12 serial number and email generator
-corel videostudio 12 license key generator download
-corel videostudio 12 crack download for mac
-corel videostudio 12 activation code reddit
-corel videostudio 12 keygen by zwt
-corel videostudio 12 serial number online free
-corel videostudio 12 activation code youtube
-corel videostudio 12 license key online generator
-corel videostudio 12 crack download for pc
-corel videostudio 12 activation code txt file download
-An activation code keygen works by using algorithms or formulas that can produce valid serial numbers and activation codes based on the name or version of the software program. For example, if you want to activate Corel VideoStudio 12 with an activation code keygen, you need to select Corel VideoStudio 12 as the target software program in the keygen interface. Then, the keygen will generate a serial number and an activation code for Corel VideoStudio 12 that you can use to install and activate it on your computer.
- Why do you need an activation code keygen for Corel VideoStudio 12?
-As mentioned earlier, activating Corel VideoStudio 12 with a valid serial number and an activation code is necessary to enjoy all its features and benefits. However, buying a license for Corel VideoStudio 12 can be quite costly. According to some online sources, the original price of Corel VideoStudio 12 was around $100 when it was released in 2008.
-Therefore, some people may want to get an activation code keygen for Corel VideoStudio 12 for free instead of paying for a license. Some of the reasons why they may want to do so are:
-
-They want to save money by not buying a license.
-They want to avoid malware or viruses that may come with cracked versions or patches of Corel VideoStudio 12.
-They want to access premium features or updates that may not be available in trial versions or unactivated versions of Corel VideoStudio 12.
-
- How to Get an Activation Code Keygen for Corel VideoStudio 12
-If you are one of those people who want to get an activation code keygen for Corel VideoStudio 12 for free
0a6ba089eb
-
-
\ No newline at end of file
diff --git a/spaces/1gistliPinn/ChatGPT4/Examples/ACDSee Pro 2.5.332 Serial 64 Bit.md b/spaces/1gistliPinn/ChatGPT4/Examples/ACDSee Pro 2.5.332 Serial 64 Bit.md
deleted file mode 100644
index a8dac443175d9ad9c014a9506a90017a4d0ca834..0000000000000000000000000000000000000000
--- a/spaces/1gistliPinn/ChatGPT4/Examples/ACDSee Pro 2.5.332 Serial 64 Bit.md
+++ /dev/null
@@ -1,52 +0,0 @@
-ACDSee Pro 2.5.332 serial 64 bit Download File >>> https://imgfil.com/2uxZjY
-
-Nov 8, 2021 - ... de luis miguel. manager in spanish. manager kim 97eae9a76d goofballgoalssoccersimulatorlicensekeyACDSee Pro 2.5.332 serial 64 bitswift ... Dec 22, 2019 - ... in Spanish, manager kim 97eae9a76d goofballgoalssoccersimulatorlicensekeyACDSee Pro 2.5.332 serial 64 bitswift...
-Read moreNov 8, 2021 - ... de luis miguel. manager in spanish. manager kim 97eae9a76d goofballgoalssoccersimulatorlicensekeyACDSee Pro 2.5.332 serial 64 bitswift...
-Dec 22, 2019 - ... in Spanish, manager kim 97eae9a76d goofballgoalssoccersimulatorlicensekeyACDSee Pro 2.5.332 serial 64 bitswift...
-Hide
-Translation from Spanish.
-From Spanish.
- She is an author of articles for the "Alpina Publisher" publishing house, the author and compiler of the books "Spanish from scratch in 16 hours", "Spanish for one month", "500 Spanish phrases" and others.
-Spanish.
-Basic Course.
-2 ed.
-Textbook for Academic Baccalaureate.
-М. : Yurite, 2016.
-- 415 Ñ. - (Bachelor.
-Academic course) .
-ISBN 978-5-534-00485-4.
-The textbook was created in accordance with the Federal state educational standard.
- Buy the book, read reviews ISBN 978-5-9925-1190-4 Labyrinth .
-The textbook presents basic information about the mechanisms of the emergence and development of mental .
-Download: Attachment.
-Size.
-Textbook.
-Author: Vygotsky L.S. Size: 16 mb.
-Format: PDF.
-Download, read.
-Read the book online Fundamentals of General Psychology - Podzolkov Vladimir Grigorievich - free, without .
-Read for free the text of the book Fundamentals of General Psychology by Vladimir Podzolkov (1st page of the book) :: free books in electronic .
- Read online "Fundamentals of General Psychology" - Podzolkov Vladimir Grigorievich - free, .
-Read online "Fundamentals of General Psychology" - Podzolkov Vladimir Grigorievich - free, .
-Read online "Fundamentals of General Psychology" - Podzolkov Vladimir Grigorievich - free, without .
-At the eLibrary LitRes you can read online books of Vladimir Podzolkov for free or .
-Read the book Fundamentals of General Psychology by Vladimir Podzolkov - page 1 of the text of the book .
- From the book: Vladimir Grigorievich Podzolkov, Doctor of Psychology, Professor, .
-Vladimir Podzolkov .
-Vladimir Grigorievich Podzolkov, Doctor of Psychology, Professor, .
-Vladimir Podzolkov.
-Fundamentals of General Psychology.
-М. "Cogito-Center, 2006.
-Lectures.
-Podzolkov, "Fundamentals of General Psychology.
-From the book: Vladimir G. Podzolkov, Doctor of Psychology, .
-E-Library LitRes offers you to download all the books of the series "Fundamentals of General.
- Podzolkov Vladimir Grigorievich.
-Podzolkov Vladimir Grigorievich (born. Currently works at the Institute of Psychology of the Russian Academy of Sciences, is the head of the laboratory of psychology of professional health, the author of more than 400 scientific papers.
-The main topics of research: professional health, psychology of work, psychophysiology of work, diagnosis of the state and personality properties of specialists.
-Download: Introduction .
-Podzolkov V.G. Fundamentals of General Psychology.
-Textbook for students of higher education institutions. 8a78ff9644
-
-
-
diff --git a/spaces/1gistliPinn/ChatGPT4/Examples/Basic Statistics And Probability By Shahid Jamal Pdf Downloadl REPACK.md b/spaces/1gistliPinn/ChatGPT4/Examples/Basic Statistics And Probability By Shahid Jamal Pdf Downloadl REPACK.md
deleted file mode 100644
index af80ed9dc9388bf9f308cff8f7f818a859151f01..0000000000000000000000000000000000000000
--- a/spaces/1gistliPinn/ChatGPT4/Examples/Basic Statistics And Probability By Shahid Jamal Pdf Downloadl REPACK.md
+++ /dev/null
@@ -1,6 +0,0 @@
-Basic Statistics And Probability By Shahid Jamal Pdf Downloadl Download ››››› https://imgfil.com/2uxYaM
-
- d5da3c52bf
-
-
-
diff --git a/spaces/1gistliPinn/ChatGPT4/Examples/Bioexcess Plus Crack The Best Way to Enhance Your Windows Experience.md b/spaces/1gistliPinn/ChatGPT4/Examples/Bioexcess Plus Crack The Best Way to Enhance Your Windows Experience.md
deleted file mode 100644
index 75c330672f947fd7a6b83a3bf7347a2a40ef32e5..0000000000000000000000000000000000000000
--- a/spaces/1gistliPinn/ChatGPT4/Examples/Bioexcess Plus Crack The Best Way to Enhance Your Windows Experience.md
+++ /dev/null
@@ -1,22 +0,0 @@
-
-austhal 19191a764c -embedded-workbench-for-arm-710-crack [ -embedded-workbench-for-arm-710-crack ] [ -embedded-workbench-for-arm-710-crack ] [ -embedded-workbench-for-arm-710-crack ] link= -embedded-workbench-for-arm-710-crack link= -embedded-workbench-for-arm-710-crack link= -embedded-workbench-for-arm-710-crack
-yasann 19191a764c -pino/bioexcess-plus-crack [ -pino/bioexcess-plus-crack ] [ -pino/bioexcess-plus-crack ] [ -pino/bioexcess-plus-crack ] link= -pino/bioexcess-plus-crack link= -pino/bioexcess-plus-crack link= -pino/bioexcess-plus-crack
-Bioexcess Plus Crack Download ✯✯✯ https://imgfil.com/2uxZsp
-charquin 19191a764c -vision-lifesign-with-remedies-english-cracked-free-download-torrent-file-1 [ -vision-lifesign-with-remedies-english-cracked-free-download-torrent-file-1 ] [ -vision-lifesign-with-remedies-english-cracked-free-download-torrent-file-1 ] [ -vision-lifesign-with-remedies-english-cracked-free-download-torrent-file-1 ] link= -vision-lifesign-with-remedies-english-cracked-free-download-torrent-file-1 link= -vision-lifesign-with-remedies-english-cracked-free-download-torrent-file-1 link= -vision-lifesign-with-remedies-english-cracked-free-download-torrent-file-1
-vinfide 19191a764c -league-live-4-crack-serial-key [ -league-live-4-crack-serial-key ] [ -league-live-4-crack-serial-key ] [ -league-live-4-crack-serial-key ] link= -league-live-4-crack-serial-key link= -league-live-4-crack-serial-key link= -league-live-4-crack-serial-key
-olyzav 19191a764c -digital-gem-pro-pro-crack-serial-keygenzip [ -digital-gem-pro-pro-crack-serial-keygenzip ] [ -digital-gem-pro-pro-crack-serial-keygenzip ] [ -digital-gem-pro-pro-crack-serial-keygenzip ] link= -digital-gem-pro-pro-crack-serial-keygenzip link= -digital-gem-pro-pro-crack-serial-keygenzip link= -digital-gem-pro-pro-crack-serial-keygenzip
-oxfpai 19191a764c -spinner-free-download-crack-idm [ -spinner-free-download-crack-idm ] [ -spinner-free-download-crack-idm ] [ -spinner-free-download-crack-idm ] link= -spinner-free-download-crack-idm link= -spinner-free-download-crack-idm link= -spinner-free-download-crack-idm
-impgin 19191a764c -pro-4-vst-crack [ -pro-4-vst-crack ] [ -pro-4-vst-crack ] [ -pro-4-vst-crack ] link= -pro-4-vst-crack link= -pro-4-vst-crack link= -pro-4-vst-crack
-lorequa 19191a764c -pdf-professional-24931-with-crack-latest [ -pdf-professional-24931-with-crack-latest ] [ -pdf-professional-24931-with-crack-latest ] [ -pdf-professional-24931-with-crack-latest ] link= -pdf-professional-24931-with-crack-latest link= -pdf-professional-24931-with-crack-latest link= -pdf-professional-24931-with-crack-latest
-encdahy 19191a764c -video-editor-6-crack-serial-key-2020-free-download [ -video-editor-6-crack-serial-key-2020-free-download ] [ -video-editor-6-crack-serial-key-2020-free-download ] [ -video-editor-6-crack-serial-key-2020-free-download ] link= -video-editor-6-crack-serial-key-2020-free-download link= -video-editor-6-crack-serial-key-2020-free-download link= -video-editor-6-crack-serial-key-2020-free-download
-iolagodo 19191a764c -partition-master-138-crack-serial-key-2020-free [ -partition-master-138-crack-serial-key-2020-free ] [ -partition-master-138-crack-serial-key-2020-free ] [ -partition-master-138-crack-serial-key-2020-free ] link= -partition-master-138-crack-serial-key-2020-free link= -partition-master-138-crack-serial-key-2020-free link= -partition-master-138-crack-serial-key-2020-free
-
-walbvyr 19191a764c -gaillard/solidworks-2008-software-free-download-with-crack [ -gaillard/solidworks-2008-software-free-download-with-crack ] [ -gaillard/solidworks-2008-software-free-download-with-crack ] [ -gaillard/solidworks-2008-software-free-download-with-crack ] link= -gaillard/solidworks-2008-software-free-download-with-crack link= -gaillard/solidworks-2008-software-free-download-with-crack link= -gaillard/solidworks-2008-software-free-download-with-crack
-hencath 19191a764c -accounting-software-cracked-download [ -accounting-software-cracked-download ] [ -accounting-software-cracked-download ] [ -accounting-software-cracked-download ] link= -accounting-software-cracked-download link= -accounting-software-cracked-download link= -accounting-software-cracked-download
-janfest 19191a764c -zoo-crack-serial-key [ -zoo-crack-serial-key ] [ -zoo-crack-serial-key ] [ -zoo-crack-serial-key ] link= -zoo-crack-serial-key link= -zoo-crack-serial-key link= -zoo-crack-serial-key
-flavdary 19191a764c -60-crackupdated [ -60-crackupdated ] [ -60-crackupdated ] [ -60-crackupdated ] link= -60-crackupdated link= -60-crackupdated link= -60-crackupdated
-valyel 19191a764c -10-download-crack-45 [ -10-download-crack-45 ] [ -10-download-crack-45 ] [ -10-download-crack-45 ] link= -10-download-crack-45 link= -10-download-crack-45 link= -10-download-crack-45
-Thanks for your article. It is extremely unfortunate that over the last decade, the travel industry has had to tackle terrorism, SARS, tsunamis, bird flu, swine flu, along with the first ever true global tough economy. Through all of it the industry has really proven to be sturdy, resilient plus dynamic, getting new approaches to deal with trouble. There are constantly fresh problems and opportunity to which the marketplace must again adapt and behave.
-Command And Conquer Generals Zero Hour Reborn V7 Download ???? ???? Download - and Conquer Generals Zero Hour Reborn V7 mod Free Download & InstallNew updates and news. CNCGZR V7 Alpha Mod release. PC game free download setup.exe with crack and direct download link[Updated] & [Updated].Command and Conquer Generals Zero Hour Reborn V7 Download & InstallFree download generals zero hour reborn v7 patch v7 update game that can be free and single-player online battle game. Download this command and conquer.Phosheroes.com command and conquer generals zero hour reborn v7 download phosheroes.com phosheroes is a free.Command and Conquer Generals Zero Hour Reborn V7 Download & Install free. Command and Conquer Generals Zero Hour Reborn V7 Download & Install free. Free.2020.10.22 11:11. Command And Conquer Generals Zero Hour Reborn V7!!EXCLUSIVE!! Download.Command and Conquer Generals Zero Hour Reborn V7 mod Free Download & InstallCustom skins for Generals Zero Hour Reborn.0. Download CNCGZR V7 Alpha Mod Free &..Play Cncgzr 0[h]r Reborn Mod download PC Game In Single Direct Link Here.. command and conquer generals zero hour reborn v7 downloadCommand and Conquer Generals Zero Hour Reborn V7 Download & Install. Command and Conquer Generals Zero Hour Reborn v7.Generals Zero Hour Rebirth v7.12 is the ultimate reborn version of the. Download: Command and Conquer Generals Zero Hour Reborn V7 (. ZIP.Command and Conquer Generals Zero Hour Reborn. Command and Conquer Generals Zero Hour Reborn v7. MOD Download: Command and Conquer.Command and Conquer Generals Zero Hour Reborn is a. command and conquer generals zero hour reborn v7 downloadCommand and Conquer Generals Zero Hour Reborn.Command & Conquer Generals Zero Hour Reborn V7 Free Download. Download Command and Conquer Generals Zero Hour Reborn V7 Free Download.Command and Conquer Generals Zero Hour Reborn. command and conquer generals zero hour reborn v7 download.exe.Command and Conquer Generals Zero Hour Reborn Full Version. Command and Conquer Generals Zero Hour Reborn Free. Mod Download: Command and.command and conquer generals zero hour reborn v7 downloadThese are more C&C Generals Zero Hour Mod downloads for you:. C&C Generals Zero Hour Reborn 0.1.1 MOD Download: Command and Conquer. ee730c9e81 -13-advanced-edition-key -win-basketball-offline-mod-apk-download -fairytale-slow-version-mp3-download -jewellery-box-tamil-pdf-download -carrozzeria-aviczh9md-english-manual
aaccfb2cb3
-
-
\ No newline at end of file
diff --git a/spaces/1gistliPinn/ChatGPT4/Examples/Bu Ali Sina Books In Urdu Free Download TOP.md b/spaces/1gistliPinn/ChatGPT4/Examples/Bu Ali Sina Books In Urdu Free Download TOP.md
deleted file mode 100644
index b23637e9c93897144c1d8c90c8b4c2b557db08cd..0000000000000000000000000000000000000000
--- a/spaces/1gistliPinn/ChatGPT4/Examples/Bu Ali Sina Books In Urdu Free Download TOP.md
+++ /dev/null
@@ -1,6 +0,0 @@
-bu ali sina books in urdu free download Download File ✔ https://imgfil.com/2uy0qU
-
- . D-581. 1974nTarjuma Qanoon Shaikh Bu Ali Seena. Volume-005. 1780nMeyaar-ul-Uqool. 1960nQanoon Shaikh Bu Ali Seena. Volume-006. 1530nMeyaar-ul-Uqool. 1935nQanoon Shaikh Bu Ali Seena. Volume-007. 1883nTarjuma Qanoon Shaikh Bu Ali . D-581. 1975nQanoon Shaikh Bu Ali Seena. Volume-008. 1760nMeyaar-ul-Uqool. 1970nQanoon Shaikh Bu Ali Seena. Volume-009. 1520nMeyaar-ul-Uqool. 1939nQanoon Shaikh Bu Ali Seena. Volume-010. 1880nTarjuma Qanoon Shaikh Bu Ali Seena. D-581. 1976nQanoon Shaikh Bu Ali Seena. Volume-011. 1690nMeyaar-ul-Uqool. 1961nMeyaar-ul-Uqool. 1930nMeyaar-ul-Uqool. 1946nMeyaar-ul-Uqool. 1932nMeyaar-ul-Uqool. 1926nMeyaar-ul-Uqool. 1925nMeyaar-ul-Uqool. 1934nMeyaar-ul-Uqool. 1933nMeyaar-ul-Uqool. 1934nMeyaar-ul-Uqool. 1935nMeyaar-ul-Uqool. 1936nMeyaar-ul-Uqool. 1938nMeyaar-ul-Uqool. 1939nMeyaar-ul-Uqool. 1940nMeyaar-ul-Uqool. 1941nMeyaar-ul-Uqool. 1942nMeyaar-ul-Uqool. 1947nMeyaar-ul-Uqool. 1949nMeyaar-ul-Uqool. 1952nMeyaar-ul-Uqool. 1953nMeyaar-ul-Uqool. 1954nMeyaar-ul-Uqool. 1957nMeya 4fefd39f24
-
-
-
diff --git a/spaces/1gistliPinn/ChatGPT4/Examples/Discografia Pholhas Torrent 108 !FULL!.md b/spaces/1gistliPinn/ChatGPT4/Examples/Discografia Pholhas Torrent 108 !FULL!.md
deleted file mode 100644
index a3504a9688a5c415b83554687dd3fe6bc53169c9..0000000000000000000000000000000000000000
--- a/spaces/1gistliPinn/ChatGPT4/Examples/Discografia Pholhas Torrent 108 !FULL!.md
+++ /dev/null
@@ -1,6 +0,0 @@
-discografia pholhas torrent 108 Download ✏ ✏ ✏ https://imgfil.com/2uy10z
-
-MORE INFORMATION Contact the BasketAu Dorset. "The Basket" is situated on St. I download indiewire 113 - iPSC Association Meetings free the Daily Download Index. The Hot 100 Songs chart is the single most important music chart in the United Kingdom and Ireland, compiled download index 113 - iPSC Association Meetings by Nielsen SoundScan and published by the Official Charts Company (OCC). Son of God, Gods Son, What Is God Like. Good God Gaze is a 97 track collection of Hymns, gospel classics and religious music. See more about the Family 4-pack, Supper Skins. View artist reviews, photos, and track listings. The most powerful spiritual message of all time: Download the mp3 online! The Bibles greatest revolution has begun! Join over two million Christian readers each month and get the Bible message today. The official site for the MLB is the best place to find out the latest news and events in baseball. Browse Albums · Tracks · Photos · Videos · Episodes · More. Help! can't read download index 113 - iPSC Association Meetings. Hanukkah - The Hanukkah Hymn Project - Volume 2, download index 113 - iPSC Association Meetings, Volume 3, and the Hanukkah Hymn Project Online in iTunes. The site is not directly affiliated with the YouTube channel, simply playing our videos on it. Journal of Biblical Research Vol. Synchronised Studies, Vol. Teardown is a geeky and comedic podcast that dives deep into things that interest us like technology and other forms of geekdom, like this web site. I don't know what I don't know, but we will teach you what we don't know. This curriculum is designed to be used with the popular movie, High School Musical. But the question of truth, the question of an objective, transcendent order of reality, is not the same thing as the question of God. I downloaded into the New Experience, it says it is 1,710MB, but there is no option to extract files. But the question of truth, the question of an objective, transcendent order of reality, is not the same thing as the question of God. Because it can't. The Hot 100 Singles is an official chart ranking the singles that have sold the most units in the United States. The Christian Music Channel provides ministry minded Christian musicians with online tools and resources to connect with those that are searching for the 4fefd39f24
-
-
-
diff --git a/spaces/1pelhydcardo/ChatGPT-prompt-generator/assets/Chapters Interactive Stories Mod Apk 6.3.4 The Ultimate Guide to Unlocking All Features.md b/spaces/1pelhydcardo/ChatGPT-prompt-generator/assets/Chapters Interactive Stories Mod Apk 6.3.4 The Ultimate Guide to Unlocking All Features.md
deleted file mode 100644
index 22e70bf1441322382983f3b6644b60daf98eb8f9..0000000000000000000000000000000000000000
--- a/spaces/1pelhydcardo/ChatGPT-prompt-generator/assets/Chapters Interactive Stories Mod Apk 6.3.4 The Ultimate Guide to Unlocking All Features.md
+++ /dev/null
@@ -1,123 +0,0 @@
-
-Chapters Interactive Stories Mod Apk 6.3.4: A Guide for Gamers
-If you are a fan of interactive and immersive storytelling, you might have heard of Chapters Interactive Stories, a popular mobile game that lets you choose your own adventure in various genres and scenarios. But did you know that there is a modded version of this game that gives you unlimited access to all the chapters, cards, and features? In this article, we will tell you everything you need to know about Chapters Interactive Stories Mod Apk 6.3.4, including what it is, how to download and install it, and some tips and tricks to make the most out of it.
- What is Chapters Interactive Stories?
-Chapters Interactive Stories is a mobile game developed by Crazy Maple Studio that offers users an immersive experience through interactive and engaging storylines. In this game, players get to choose their own path in various story scenarios, making decisions that affect the outcome of the story. By doing so, players can shape their own characters, relationships, and endings.
-chapters interactive stories mod apk 6.3.4 DOWNLOAD ☆☆☆ https://urlin.us/2uSTXI
-The game features a wide range of genres, such as romance, fantasy, drama, horror, comedy, and more. Each genre has multiple stories to choose from, each with different characters, settings, and plots. Some of the stories are original creations by the developers, while others are based on popular books, movies, or TV shows.
-Some of the features of Chapters Interactive Stories are:
-Features of Chapters Interactive Stories
-
-Interactive choices: Players can make choices that affect the direction and outcome of the story. Some choices are free, while others require diamonds or tickets to unlock.
-Diverse genres: Players can choose from a variety of genres, such as romance, fantasy, drama, horror, comedy, and more.
-Multiple stories: Players can explore different stories within each genre, each with different characters, settings, and plots.
-Original and adapted stories: Players can enjoy original stories created by the developers or adapted stories based on popular books, movies, or TV shows.
-Customizable characters: Players can customize their own characters' appearance, name, gender, and personality.
-Fashionable outfits: Players can dress up their characters in various outfits that suit their style and mood.
-Social features: Players can interact with other players and authors through chat rooms, comments, reviews, ratings, and more.
-
- How to play Chapters Interactive Stories
-To play Chapters Interactive Stories, players need to download the game from the Google Play Store or the App Store for free. After installing the game, players need to create an account or log in with their Facebook account. Then, players can choose a genre and a story to start playing.
-The game interface consists of three main elements: the story text, the choices menu, and the navigation bar. The story text displays the dialogue and narration of the story. The choices menu shows the options that players can choose from at certain points in the story. The navigation bar contains buttons that allow players to access other features of the game, such as the home screen, the store, the settings, and more.
-To progress through the story, players need to tap on the screen to read the story text and make choices when prompted. Some choices are free, while others require diamonds or tickets to unlock. Diamonds are the premium currency of the game that can be used to unlock premium choices, outfits, and cards. Tickets are the energy of the game that can be used to start a new chapter. Players can earn diamonds and tickets by completing chapters, watching ads, or purchasing them with real money.
- What is Chapters Interactive Stories Mod Apk 6.3.4?
-Chapters Interactive Stories Mod Apk 6.3.4 is a modified version of the original game that gives players unlimited access to all the features of the game without spending any money. This means that players can enjoy all the chapters, cards, and outfits without worrying about diamonds or tickets. Moreover, players can also get rid of annoying ads and enjoy a smoother gaming experience.
-The modded version of the game is not available on the official app stores, but it can be downloaded from third-party websites that provide apk files. However, players should be careful when downloading and installing modded apk files, as they may contain viruses or malware that can harm their devices or compromise their personal information.
-chapters interactive stories mod apk 6.3.4 download
-chapters interactive stories mod apk 6.3.4 unlimited diamonds
-chapters interactive stories mod apk 6.3.4 free tickets
-chapters interactive stories mod apk 6.3.4 latest version
-chapters interactive stories mod apk 6.3.4 android
-chapters interactive stories mod apk 6.3.4 ios
-chapters interactive stories mod apk 6.3.4 no root
-chapters interactive stories mod apk 6.3.4 offline
-chapters interactive stories mod apk 6.3.4 hack
-chapters interactive stories mod apk 6.3.4 cheats
-chapters interactive stories mod apk 6.3.4 premium choices
-chapters interactive stories mod apk 6.3.4 unlocked all
-chapters interactive stories mod apk 6.3.4 review
-chapters interactive stories mod apk 6.3.4 gameplay
-chapters interactive stories mod apk 6.3.4 update
-chapters interactive stories mod apk 6.3.4 install
-chapters interactive stories mod apk 6.3.4 features
-chapters interactive stories mod apk 6.3.4 tips
-chapters interactive stories mod apk 6.3.4 guide
-chapters interactive stories mod apk 6.3.4 tutorial
-chapters interactive stories mod apk 6.3.4 reddit
-chapters interactive stories mod apk 6.3.4 youtube
-chapters interactive stories mod apk 6.3.4 facebook
-chapters interactive stories mod apk 6.3.4 twitter
-chapters interactive stories mod apk 6.3.4 instagram
-chapters interactive stories mod apk 6.3.4 pinterest
-chapters interactive stories mod apk 6.3.4 quora
-chapters interactive stories mod apk 6.3.4 medium
-chapters interactive stories mod apk 6.3.4 blogspot
-chapters interactive stories mod apk 6.3.4 wordpress
-chapters interactive stories mod apk 6.3.4 tumblr
-chapters interactive stories mod apk 6.3.4 forum
-chapters interactive stories mod apk 6.3.4 discord
-chapters interactive stories mod apk 6.3.4 telegram
-chapters interactive stories mod apk 6.3.4 whatsapp
-chapters interactive stories mod apk 6.3.4 email
-chapters interactive stories mod apk 6.3.4 support
-chapters interactive stories mod apk 6.3.4 faq
-chapters interactive stories mod apk 6.3.4 wiki
-chapters interactive stories mod apk 6.
-Benefits of Chapters Interactive Stories Mod Apk 6.3.4
-
-Unlimited diamonds and tickets: Players can unlock all the premium choices, outfits, and cards without spending any money.
-No ads: Players can get rid of annoying ads that interrupt their gameplay and waste their time.
-Better performance: Players can enjoy a smoother and faster gaming experience with less lag and glitches.
-More fun: Players can explore different stories and genres without any limitations or restrictions.
-
- How to download and install Chapters Interactive Stories Mod Apk 6.3.4
-To download and install Chapters Interactive Stories Mod Apk 6.3.4, players need to follow these steps:
-
-Find a reliable website: Players need to find a trustworthy website that provides the modded apk file of the game. They can search for "Chapters Interactive Stories Mod Apk 6.3.4" on Google or other search engines and check the reviews and ratings of the websites before downloading.
-Download the apk file: Players need to click on the download button on the website and wait for the apk file to be downloaded on their device.
-Enable unknown sources: Players need to go to their device settings and enable the option of installing apps from unknown sources. This will allow them to install the modded apk file without any issues.
-Install the apk file: Players need to locate the apk file on their device and tap on it to start the installation process. They need to follow the instructions on the screen and wait for the installation to be completed.
-Launch the game: Players need to open the game icon on their device and enjoy playing Chapters Interactive Stories Mod Apk 6.3.4 with unlimited features.
-
- Tips and tricks for Chapters Interactive Stories Mod Apk 6.3.4
-To make the most out of Chapters Interactive Stories Mod Apk 6.3.4, players can use these tips and tricks:
-Choose your genre wisely
-The game offers a variety of genres to choose from, such as romance, fantasy, drama, horror, comedy, and more. Each genre has its own style, tone, and mood, so players should choose a genre that suits their preferences and interests. For example, if they want a light-hearted and humorous story, they can choose comedy; if they want a thrilling and suspenseful story, they can choose horror; if they want a romantic and emotional story, they can choose romance; and so on.
-Spend your diamonds and tickets carefully
-Even though players have unlimited diamonds and tickets in Chapters Interactive Stories Mod Apk 6.3.4, they should still spend them wisely and strategically. For example, they should not waste their diamonds on choices or outfits that do not affect the story or their character development; they should save their tickets for stories that they are interested in or excited about; they should use their cards to boost their stats or unlock bonus scenes; and so on.
-Customize your character and outfits
-The game allows players to customize their own characters' appearance, name, gender, and personality. This gives players more control over their story and makes them feel more connected to their characters. Moreover, players can also dress up their characters in various outfits that suit their style and mood. This adds more fun and flair to their gameplay and makes their characters stand out from others.
-
- The game also has social features that allow players to interact with other players and authors through chat rooms, comments, reviews, ratings, and more. This can help players to share their opinions, feedback, suggestions, and tips with others; to discover new stories and genres; to make friends and join communities; and to support their favorite authors and stories.
- Conclusion
-Chapters Interactive Stories Mod Apk 6.3.4 is a great way to enjoy interactive and immersive storytelling on your mobile device. With this modded version of the game, you can access all the features of the game without spending any money. You can choose your own adventure in various genres and scenarios, customize your character and outfits, and interact with other players and authors. If you are looking for a fun and engaging game that lets you create your own story, you should definitely try Chapters Interactive Stories Mod Apk 6.3.4.
- FAQs
-Here are some frequently asked questions about Chapters Interactive Stories Mod Apk 6.3.4:
-
-
-Question
-Answer
-
-
-Is Chapters Interactive Stories Mod Apk 6.3.4 safe to use?
-Chapters Interactive Stories Mod Apk 6.3.4 is generally safe to use, as long as you download it from a reliable website that provides virus-free apk files. However, you should always be careful when installing modded apk files, as they may contain malware or spyware that can harm your device or steal your personal information.
-
-
-Will I get banned for using Chapters Interactive Stories Mod Apk 6.3.4?
-There is a low chance of getting banned for using Chapters Interactive Stories Mod Apk 6.3.4, as the game does not have a strict anti-cheat system or detection mechanism. However, you should still be cautious when using the modded version of the game, as you may get reported by other players or authors if they notice your unlimited diamonds or tickets.
-
-
-Can I update Chapters Interactive Stories Mod Apk 6.3.4?
-You can update Chapters Interactive Stories Mod Apk 6.3.4 by downloading the latest version of the modded apk file from the same website that you downloaded it from before. However, you should always backup your game data before updating, as you may lose your progress or settings if something goes wrong during the update process.
-
-
-Can I play Chapters Interactive Stories Mod Apk 6.3.4 offline?
-No, you cannot play Chapters Interactive Stories Mod Apk 6.3.4 offline, as the game requires an internet connection to load the stories and sync your data with the server. If you try to play the game offline, you may encounter errors or glitches that prevent you from playing properly.
-
-
-Can I play Chapters Interactive Stories Mod Apk 6.3.4 on PC?
-Yes, you can play Chapters Interactive Stories Mod Apk 6.3.4 on PC by using an Android emulator software that allows you to run Android apps on your computer. Some of the popular Android emulators are BlueStacks, NoxPlayer, LDPlayer, and MEmu.
-
-
197e85843d
-
-
\ No newline at end of file
diff --git a/spaces/1phancelerku/anime-remove-background/Angry Birds 2 Mod Apk O jogo de estilingue mais viciante com dinheiro infinito e desafios ilimitados.md b/spaces/1phancelerku/anime-remove-background/Angry Birds 2 Mod Apk O jogo de estilingue mais viciante com dinheiro infinito e desafios ilimitados.md
deleted file mode 100644
index e63237a7715cb34c28ee6a57ad179a8732c41989..0000000000000000000000000000000000000000
--- a/spaces/1phancelerku/anime-remove-background/Angry Birds 2 Mod Apk O jogo de estilingue mais viciante com dinheiro infinito e desafios ilimitados.md
+++ /dev/null
@@ -1,127 +0,0 @@
-
-Angry Birds 2 Mod Apk Dinheiro Infinito: How to Download and Play the Ultimate Bird Flinging Game
- Are you a fan of Angry Birds, the most popular physics-based game series in the world? Do you want to experience a new level of fun and challenge with Angry Birds 2, the official sequel to the original game? Do you want to enjoy unlimited money, gems, lives, cards, and spells in Angry Birds 2? If you answered yes to any of these questions, then this article is for you!
- In this article, we will tell you everything you need to know about Angry Birds 2 mod apk dinheiro infinito, a modified version of the game that gives you access to all the premium features for free. We will explain what is Angry Birds 2, what are its main features, what are the advantages of using Angry Birds 2 mod apk dinheiro infinito, how to download and install it on your Android device, and how to play it like a pro. By the end of this article, you will be ready to download and play Angry Birds 2 mod apk dinheiro infinito and have a blast with your feathered friends!
-angry birds 2 mod apk dinheiro infinito Download Zip ››››› https://jinyurl.com/2uNUJM
- What is Angry Birds 2?
- Angry Birds 2 is a puzzle video game developed by Rovio Entertainment and released in 2015. It is the direct sequel to the original Angry Birds game, which was launched in 2009 and became a global phenomenon. Angry Birds 2 follows the same premise as the previous games: you have to use a slingshot to launch a variety of birds at the structures and pigs that are trying to steal their eggs. However, Angry Birds 2 also introduces new features and improvements that make the game more exciting and challenging.
- What are the main features of Angry Birds 2?
- Angry Birds 2 has many features that make it stand out from other puzzle games. Here are some of them:
-
-Multiphase levels: Unlike the previous games, where each level had only one stage, Angry Birds 2 has levels that consist of multiple stages. This means that you have to face different challenges and obstacles in each stage, and you have to use different strategies and birds to complete them. The levels also have random layouts, so you never know what to expect.
-Spells: Spells are special powers that you can use to boost your birds or sabotage the pigs. There are six types of spells in Angry Birds 2: Hot Chili, Mighty Eagle, Golden Duck, Pig Inflater, Blizzard, and Piggyquake. Each spell has a different effect and can be used once per level. You can earn spells by playing the game or by purchasing them with gems.
-Clans: Clans are groups of players who can chat, share tips, and compete with each other. You can join an existing clan or create your own clan with your friends. By joining a clan, you can also participate in clan events and earn rewards.
-Tournaments: Tournaments are daily or weekly competitions where you can compete with other players from around the world. You can enter a tournament by paying a fee with gems or tickets, and you can play as many levels as you want within a time limit. The more levels you complete and the higher your score, the higher your rank on the leaderboard. You can win prizes such as gems, feathers, hats, and chests based on your rank.
-Hats: Hats are accessories that you can equip your birds with to give them extra abilities or bonuses. There are four types of hats in Angry Birds 2: common, rare, legendary, and epic. Each hat has a different design and effect, such as increasing your score, damage, or speed. You can collect hats by opening chests or by purchasing them with gems.
-And more: Angry Birds 2 also has other features such as daily quests, achievements, leaderboards, star rewards, tower of fortune, etc., that add more fun and variety to the game.
-
- What are the advantages of using Angry Birds 2 mod apk dinheiro infinito?
- Angry Birds 2 mod apk dinheiro infinito is a modified version of the game that gives you unlimited access to all the premium features for free. By using this mod apk, you can enjoy the following advantages:
-
-Unlimited money: You can get unlimited money in Angry Birds 2 mod apk dinheiro infinito, which you can use to buy anything you want in the game. You can buy more spells, hats, chests, tickets, etc., without worrying about running out of money.
-Unlimited gems: You can also get unlimited gems in Angry Birds 2 mod apk dinheiro infinito, which are the premium currency of the game. You can use gems to enter tournaments, open chests, buy spells, etc., without spending real money.
-Unlimited lives: You can get unlimited lives in Angry Birds 2 mod apk dinheiro infinito, which means that you can play as many levels as you want without waiting for your lives to refill. You can also retry any level as many times as you want without losing lives.
-Unlimited cards: You can get unlimited cards in Angry Birds 2 mod apk dinheiro infinito, which are the items that allow you to choose which bird to use in each level. You can have as many cards as you want and use any bird you want without running out of cards.
-Unlimited spells: You can get unlimited spells in Angry Birds 2 mod apk dinheiro infinito, which means that you can use any spell you want in any level without spending gems or coins. You can also use multiple spells in one level without any limit.
-
- How to download and install Angry Birds 2 mod apk dinheiro infinito?
- If you want to download and install Angry Birds 2 mod apk dinheiro infinito on your Android device, you need to follow these simple steps:
- Step 1: Enable unknown sources on your device
- Before you can install any mod apk file on your device, you need to enable unknown sources on your device settings. This will allow you to install apps from sources other than the Google Play Store. To do this, follow these steps:
-
-Go to your device settings and tap on Security or Privacy.
-Find the option that says Unknown Sources or Install Unknown Apps and toggle it on.
-A warning message will pop up. Tap on OK or Allow to confirm.
-
-
- Step 2: Download the mod apk file from a trusted source
- Next, you need to download the mod apk file from a trusted source. There are many websites that offer mod apk files, but not all of them are safe and reliable. Some of them may contain viruses, malware, or spyware that can harm your device or steal your personal information. Therefore, you need to be careful and choose a reputable source. One of the best sources that we recommend is [Angry Birds 2 Mod Apk Dinheiro Infinito], which is a website that provides high-quality and updated mod apk files for various games and apps. To download the mod apk file from this source, follow these steps:
-
-Go to [Angry Birds 2 Mod Apk Dinheiro Infinito] using your browser.
-Scroll down and find the download button that says Download Angry Birds 2 Mod Apk Dinheiro Infinito.
-Tap on the download button and wait for the download to start.
-The mod apk file will be downloaded to your device in a few minutes, depending on your internet speed.
-
-
-angry birds 2 hack apk dinheiro infinito
-angry birds 2 mod apk unlimited money and gems
-angry birds 2 apk mod tudo infinito
-angry birds 2 mod apk download grátis
-angry birds 2 mod apk atualizado 2023
-angry birds 2 apk mod mega hack
-angry birds 2 mod apk offline
-angry birds 2 mod apk sem anúncios
-angry birds 2 apk mod desbloqueado
-angry birds 2 mod apk versão mais recente
-angry birds 2 hack apk android
-angry birds 2 mod apk unlimited lives
-angry birds 2 apk mod estrelas infinitas
-angry birds 2 mod apk sem root
-angry birds 2 mod apk com obb
-angry birds 2 hack apk ios
-angry birds 2 mod apk unlimited pearls
-angry birds 2 apk mod energia infinita
-angry birds 2 mod apk sem verificação
-angry birds 2 mod apk revdl
-angry birds 2 hack apk online
-angry birds 2 mod apk unlimited black pearls
-angry birds 2 apk mod nível máximo
-angry birds 2 mod apk sem banimento
-angry birds 2 mod apk rexdl
-angry birds 2 hack apk no survey
-angry birds 2 mod apk unlimited spells
-angry birds 2 apk mod plumas infinitas
-angry birds 2 mod apk sem internet
-angry birds 2 mod apk happymod
- Step 3: Locate and install the mod apk file on your device
- After you have downloaded the mod apk file, you need to locate and install it on your device. To do this, follow these steps:
-
-Go to your device file manager and find the folder where you downloaded the mod apk file. It is usually in the Downloads folder.
-Tap on the mod apk file and a pop-up window will appear.
-Tap on Install and wait for the installation to finish.
-If another pop-up window appears asking for permissions, tap on Allow or Accept to grant them.
-
-
- Step 4: Launch the game and enjoy!
- Congratulations! You have successfully installed Angry Birds 2 mod apk dinheiro infinito on your device. Now you can launch the game and enjoy all the modded features. To do this, follow these steps:
-
-Go to your device app drawer and find the Angry Birds 2 icon.
-Tap on the icon and wait for the game to load.
-You will see a message that says "Angry Birds 2 Mod Apk Dinheiro Infinito by AngryBirds2ModApkDinheiroInfinito.com". Tap on OK or Continue to proceed.
-You will be taken to the main menu of the game. You can choose to play offline or online, depending on your preference.
-You will notice that you have unlimited money, gems, lives, cards, and spells in the game. You can use them as you wish and have fun!
-
-
- How to play Angry Birds 2 like a pro?
- Now that you have downloaded and installed Angry Birds 2 mod apk dinheiro infinito, you might be wondering how to play it like a pro. Well, don't worry, we have some tips and tricks for you that will help you master the game and beat any level with ease. Here are some of them:
- Tip 1: Choose the right bird for the right situation
- One of the most important aspects of playing Angry Birds 2 is choosing the right bird for the right situation. Each bird has a different ability and score multiplier that can affect the outcome of the level. For example, Red can knock down structures with his strong impact, Chuck can speed up and cut through wood and glass, Bomb can explode and cause massive damage, Matilda can drop an egg bomb and fly upwards, The Blues can split into three and hit multiple targets, Silver can loop and smash downwards, and Terence can crush anything with his huge size. You can also use the Mighty Eagle to call for a powerful airstrike that can wipe out the entire level.
- Therefore, you need to choose the best bird for each level based on the layout, the materials, the pigs, and the spells. You can also switch the order of the birds by tapping on their cards at the bottom of the screen. You should always try to use the bird that can cause the most damage and destruction with the least number of shots. This will help you earn more points and stars, as well as fill up the Destructometer faster.
- Tip 2: Use the environment to your advantage
- Another important aspect of playing Angry Birds 2 is using the environment to your advantage. The game has many environmental elements that can help you or hinder you in your quest to defeat the pigs. For example, there are flowers that can bounce your birds back, portals that can teleport your birds to different locations, TNT crates that can explode and cause chain reactions, fans that can blow your birds or objects away, rocks that can fall and crush the pigs, etc.
- Therefore, you need to pay attention to the environment and use it wisely. You can use the flowers to redirect your birds or hit hard-to-reach pigs, you can use the portals to surprise the pigs or avoid obstacles, you can use the TNT crates to create massive explosions and clear large areas, you can use the fans to push your birds or objects towards the pigs, you can use the rocks to drop them on the pigs or structures, etc. You should also be careful of the environmental hazards that can harm your birds or prevent them from reaching their targets.
- Tip 3: Fill up the Destructometer quickly
- The Destructometer is a meter that fills up as you destroy objects and pigs in each level. When you fill up the Destructometer completely, you will earn an extra card or spell that you can use in the same level or save for later. The Destructometer also resets after each stage, so you have multiple chances to fill it up in each level.
- Therefore, you should try to fill up the Destructometer as quickly as possible by destroying as much as possible with each bird. You should aim for weak spots, explosive objects, large structures, multiple pigs, etc., to cause more damage and destruction. You should also use spells wisely to boost your destruction and fill up the Destructometer faster. The more cards or spells you have, the more options and flexibility you have in completing the level.
- Tip 4: Compete with other players in multiplayer mode
- If you want to test your skills and challenge yourself further, you can compete with other players in multiplayer mode. In multiplayer mode, you can join a clan or create your own clan with your friends. By joining a clan, you can chat with other clan members, share tips and strategies, and participate in clan events. Clan events are special competitions where you have to work together with your clan members to complete a common goal and earn rewards.
- You can also enter tournaments in multiplayer mode. Tournaments are daily or weekly competitions where you have to compete with other players from around the world in various levels. You have to pay a fee with gems or tickets to enter a tournament, and you have a time limit to play as many levels as you want. The more levels you complete and the higher your score, the higher your rank on the leaderboard. You can win prizes such as gems, feathers, hats, and chests based on your rank.
- Competing with other players in multiplayer mode is a great way to improve your skills, learn new tricks, have fun, and earn rewards. You can also make new friends and join a community of Angry Birds fans.
- Conclusion
- Angry Birds 2 is a fantastic game that offers hours of fun and entertainment. It has amazing graphics, sound effects, and animations that bring the game to life. It has a variety of levels, modes, features, and characters that keep the game fresh and exciting. It has a simple and intuitive gameplay that anyone can enjoy and master.
- However, if you want to take your gaming experience to the next level, you should try Angry Birds 2 mod apk dinheiro infinito. This mod apk gives you unlimited access to all the premium features of the game for free. You can have unlimited money, gems, lives, cards, and spells that you can use to beat any level with ease. You can also customize your birds with different hats and accessories that give them extra abilities and bonuses. You can also compete with other players in multiplayer mode and win amazing prizes.
- So what are you waiting for? Download Angry Birds 2 mod apk dinheiro infinito today and join the ultimate bird flinging adventure!
- FAQs
- Here are some frequently asked questions and answers about Angry Birds 2 mod apk dinheiro infinito:
- Q: Is Angry Birds 2 mod apk dinheiro infinito safe to use?
-A: Yes, Angry Birds 2 mod apk dinheiro infinito is safe to use as long as you download it from a trusted source like [Angry Birds 2 Mod Apk Dinheiro Infinito]. This website provides high-quality and updated mod apk files that are free from viruses, malware, or spyware. However, you should always be careful when downloading and installing any mod apk file on your device and enable unknown sources on your device settings.
- Q: Do I need to root my device to use Angry Birds 2 mod apk dinheiro infinito?
-A: No, you do not need to root your device to use Angry Birds 2 mod apk dinheiro infinito. This mod apk works on any Android device without requiring root access. However, you should always backup your data before installing any mod apk file on your device in case anything goes wrong.
- Q: Will I get banned from the game if I use Angry Birds 2 mod apk dinheiro infinito?
-A: No, you will not get banned from the game if you use Angry Birds 2 mod apk dinheiro infinito. This mod apk is undetectable by the game servers and does not interfere with the game's functionality or performance. However, you should always use this mod apk responsibly and not abuse it or cheat in multiplayer mode.
- Q: Can I update Angry Birds 2 mod apk dinheiro infinito?
-A: Yes, you can update Angry Birds 2 mod apk dinheiro infinito whenever there is a new version available. However, you should always download the latest version of the mod apk from [Angry Birds 2 Mod Apk Dinheiro Infinito] and not from the Google Play Store or other sources. This will ensure that you get the most updated and compatible version of the mod apk.
- Q: Can I play Angry Birds 2 mod apk dinheiro infinito offline?
-A: Yes, you can play Angry Birds 2 mod apk dinheiro infinito offline without requiring an internet connection. However, some features of the game such as multiplayer mode, tournaments, clan events, etc., require an internet connection to work properly. Therefore, you should always connect to a stable and secure internet connection when playing these features.
401be4b1e0
-
-
\ No newline at end of file
diff --git a/spaces/1phancelerku/anime-remove-background/Beach Buggy Racing 2 Mod APK How to Download Aplikasi and Unlock All Features.md b/spaces/1phancelerku/anime-remove-background/Beach Buggy Racing 2 Mod APK How to Download Aplikasi and Unlock All Features.md
deleted file mode 100644
index cd3fca6b8ec45b05bd982c5655370e4d0889a836..0000000000000000000000000000000000000000
--- a/spaces/1phancelerku/anime-remove-background/Beach Buggy Racing 2 Mod APK How to Download Aplikasi and Unlock All Features.md
+++ /dev/null
@@ -1,164 +0,0 @@
-
-Download Aplikasi Beach Buggy Racing 2 Mod Apk
-If you are looking for a fun and exciting kart racing game on your Android device, you should definitely check out Beach Buggy Racing 2. This is a sequel to the popular Beach Buggy Racing, which has over 100 million downloads on Google Play. In this game, you can race against drivers and cars from around the world, explore different tracks and environments, collect and upgrade power-ups, and customize your own car and driver.
-download aplikasi beach buggy racing 2 mod apk Download ✯✯✯ https://jinyurl.com/2uNSTW
-But what if you want to enjoy the game without any limitations or restrictions? What if you want to have unlimited money, gems, tickets, and power-ups? What if you want to unlock all the cars, drivers, and tracks in the game? Well, there is a way to do that. You can download aplikasi Beach Buggy Racing 2 mod apk, which is a modified version of the original game that gives you access to all the features and content that you want.
-In this article, we will tell you what is Beach Buggy Racing 2, why you should download its mod apk, how to download and install it on your device, some tips and tricks to play it better, and a review of the game. So, let's get started!
- What is Beach Buggy Racing 2?
-Beach Buggy Racing 2 is a kart racing game developed by Vector Unit, the same studio behind other racing games like Riptide GP and Hydro Thunder Hurricane. It was released in December 2018 for Android and iOS devices, and later for PC and consoles. It is a free-to-play game with in-app purchases.
-Beach Buggy Racing 2 is a fully 3D off-road kart racing game with amazing physics, detailed cars and characters, and spectacular weapons. It has a variety of game modes, such as Adventure mode, Championships, Races, Drift Attacks, Firework Fury, and more. You can also create your own custom game modes with different power-ups, race rules, lap counts, and more.
-You can choose from over 55 cars in the game, ranging from beach buggies to monster trucks to formula supercars. You can also collect over 45 power-ups in the game, such as Chain Lightning, Donut Tires, Boost Juice, Killer Bees, and more. Each power-up has its own unique effect and can be upgraded to make it more powerful.
-How to download beach buggy racing 2 mod apk for free
-Beach buggy racing 2 mod apk unlimited money and gems
-Best tips and tricks for beach buggy racing 2 mod apk
-Beach buggy racing 2 mod apk latest version 2023
-Download beach buggy racing 2 hack mod apk
-Beach buggy racing 2 mod apk offline mode
-Beach buggy racing 2 mod apk all cars unlocked
-Beach buggy racing 2 mod apk gameplay and review
-Beach buggy racing 2 mod apk android and ios
-Download beach buggy racing 2 mod apk from APKdone[^1^]
-Beach buggy racing 2 mod apk features and benefits
-Beach buggy racing 2 mod apk vs original version
-Beach buggy racing 2 mod apk no root required
-Beach buggy racing 2 mod apk online multiplayer
-Download beach buggy racing 2 mod apk with obb data
-Beach buggy racing 2 mod apk new update and patch notes
-Beach buggy racing 2 mod apk cheats and codes
-Beach buggy racing 2 mod apk fun and addictive game
-Beach buggy racing 2 mod apk graphics and sound quality
-Download beach buggy racing 2 mod apk safely and securely
-Beach buggy racing 2 mod apk pros and cons
-Beach buggy racing 2 mod apk system requirements and compatibility
-Beach buggy racing 2 mod apk download link and instructions
-Beach buggy racing 2 mod apk ratings and feedbacks
-Download beach buggy racing 2 mod apk for PC and Mac
-Beach buggy racing 2 mod apk challenges and missions
-Beach buggy racing 2 mod apk customization and upgrades
-Beach buggy racing 2 mod apk characters and power-ups
-Download beach buggy racing 2 mod apk from Google Play Store or App Store
-Beach buggy racing 2 mod apk alternatives and similar games
-You can also build your own team of racers in the game. You can recruit new drivers from the Beach Buggy Racing League, each with their own special ability. For example, Rez can launch beach balls that spin out other racers, Disco Jimmy can make other racers dance and stop racing, Mikka can create holograms of herself to confuse other racers, and so on.
-You can also test your skills against other players from around the world in online competitions and tournaments. You can race against player avatars in daily races or compete in live tournaments and special events to win exclusive in-game prizes.
- Features of Beach Buggy Racing 2
-Here are some of the main features of Beach Buggy Racing 2 that make it a great kart racing game:
-
-Exciting and realistic kart racing physics
-Stunning 3D graphics and animations
-Over 55 cars to collect and customize
-Over 45 power-ups to use and upgrade
-Over 15 drivers to recruit and team up with
-Over 40 tracks to explore and race on
-Various game modes and challenges to enjoy
-Online multiplayer and leaderboards to compete with others
-Daily rewards and achievements to earn
-Cross-platform compatibility and cloud saving
-
- Why download Beach Buggy Racing 2 mod apk?
-Beach Buggy Racing 2 is a free-to-play game, but it also has some limitations and restrictions that can affect your gaming experience. For example, you need to spend real money to buy gems, which are the premium currency in the game. Gems are used to unlock new cars, drivers, power-ups, and tracks. You also need to spend tickets, which are the energy system in the game, to play certain game modes. Tickets are replenished over time or by watching ads.
-If you don't want to spend money or wait for tickets, you can download aplikasi Beach Buggy Racing 2 mod apk, which is a modified version of the original game that gives you unlimited resources and features. With Beach Buggy Racing 2 mod apk, you can enjoy the following benefits:
-
-Unlimited money: You can have as much money as you want in the game, which you can use to buy and upgrade cars, power-ups, and other items.
-Unlimited gems: You can have as many gems as you want in the game, which you can use to unlock new cars, drivers, power-ups, and tracks.
-Unlimited tickets: You can have as many tickets as you want in the game, which you can use to play any game mode without waiting or watching ads.
-All cars unlocked: You can access all the cars in the game, from beach buggies to monster trucks to formula supercars.
-All drivers unlocked: You can access all the drivers in the game, each with their own special ability.
-All tracks unlocked: You can access all the tracks in the game, from tropical beaches to ancient ruins to lunar landscapes.
-All power-ups unlocked: You can access all the power-ups in the game, each with their own unique effect.
-No ads: You can play the game without any annoying ads or pop-ups.
-
- How to download and install Beach Buggy Racing 2 mod apk?
-If you are interested in downloading aplikasi Beach Buggy Racing 2 mod apk, you need to follow these simple steps:
- Step 1: Enable unknown sources
-Before you can install any mod apk file on your Android device, you need to enable unknown sources in your settings. This will allow you to install apps from sources other than Google Play. To do this, go to Settings > Security > Unknown Sources and toggle it on.
- Step 2: Download the mod apk file
-Next, you need to download the mod apk file of Beach Buggy Racing 2 from a reliable source. You can search for it online or use this link to download it directly. The file size is about 150 MB, so make sure you have enough space on your device.
- Step 3: Install the mod apk file
-Once you have downloaded the mod apk file, locate it in your file manager and tap on it. You will see a prompt asking you to install the app. Tap on Install and wait for the installation process to finish.
- Step 4: Launch the game and enjoy
-After the installation is done, you can launch the game from your app drawer or home screen. You will see that you have unlimited resources and features in the game. You can now enjoy Beach Buggy Racing 2 without any limitations or restrictions.
- Tips and tricks for Beach Buggy Racing 2
-To help you play Beach Buggy Racing 2 better, here are some tips and tricks that you should know:
- Master the drift and powerslide
-One of the most important skills in Beach Buggy Racing 2 is drifting and powersliding. Drifting is when you turn your car sharply while accelerating, causing your tires to lose traction and slide sideways. Powersliding is when you tap on the brake button while drifting, causing your car to slide even more and gain more speed. Drifting and powersliding are useful for taking sharp turns, avoiding obstacles, and gaining boost. Boost is a meter that fills up as you drift and powerslide, and when it is full, you can tap on the boost button to get a burst of speed. You can also use boost to ram into other racers and knock them out of the way.
- Use the driver's ability at the right time
-As mentioned earlier, each driver in Beach Buggy Racing 2 has their own special ability that can give them an edge in the race. However, these abilities have a cooldown time, so you need to use them wisely and at the right time. For example, Rez's beach ball ability can be used to block other racers behind you, Disco Jimmy's dance ability can be used to distract other racers in front of you, Mikka's hologram ability can be used to confuse other racers around you, and so on. You can also combine your driver's ability with your power-ups to create more chaos and fun.
- Don't fall into the trap of other racers
-Beach Buggy Racing 2 is not just about racing, it is also about sabotaging and surviving. Other racers will try to use their power-ups and abilities to slow you down, damage your car, or make you lose control. You need to be alert and avoid falling into their traps. For example, watch out for oil slicks, banana peels, fireballs, rockets, mines, and other hazards on the track. You can also use your power-ups and abilities to counter their attacks or dodge them. For example, you can use the shield power-up to protect yourself from incoming projectiles, the jump power-up to leap over obstacles, the magnet power-up to attract coins and gems, and so on.
- Build the best deck of power-ups
-Before each race, you can choose up to three power-ups to bring with you. These power-ups are randomly assigned to you during the race, so you need to choose wisely and build the best deck of power-ups that suits your playstyle and strategy. For example, if you want to be more aggressive and offensive, you can choose power-ups like rockets, fireballs, chain lightning, killer bees, etc. If you want to be more defensive and supportive, you can choose power-ups like shields, repair kits, boost juice, donut tires, etc. If you want to be more balanced and versatile, you can choose power-ups like magnets, jumps, bubbles, etc.
- Grab those fast bubbles and shortcuts
-Another way to gain an advantage in Beach Buggy Racing 2 is to grab those fast bubbles and shortcuts on the track. Fast bubbles are blue spheres that give you a temporary speed boost when you drive through them. They are usually located on straight paths or ramps that can help you gain some distance or catch up with other racers. Shortcuts are hidden or alternative paths that can help you avoid obstacles or take a faster route. They are usually marked by arrows or signs that indicate where they lead. However, some shortcuts may also have risks or traps that can backfire on you if you are not careful.
- Review of Beach Buggy Racing 2
-Now that we have covered what is Beach Buggy Racing 2, why you should download its mod apk, how to download and install it on your device, and some tips and tricks to play it better, let's take a look at the review of the game. We will discuss the pros and cons of Beach Buggy Racing 2, the user ratings and feedback of the game, and the comparison with other kart racing games.
- Pros and cons of Beach Buggy Racing 2
-Beach Buggy Racing 2 is a fun and addictive kart racing game that offers a lot of content and features for players to enjoy. However, it also has some drawbacks that may affect your gaming experience. Here are the pros and cons of Beach Buggy Racing 2:
-
-
-Pros
-Cons
-
-
-- Exciting and realistic kart racing physics
-- Some tracks and power-ups can be repetitive or unfair
-
-
-- Stunning 3D graphics and animations
-- Some cars and drivers can be overpowered or underpowered
-
-
-- Over 55 cars to collect and customize
-- Some game modes and challenges can be too easy or too hard
-
-
-- Over 45 power-ups to use and upgrade
-- Some in-app purchases can be expensive or unnecessary
-
-
-- Over 15 drivers to recruit and team up with
-- Some ads can be annoying or intrusive
-
-
-- Over 40 tracks to explore and race on
-- Some bugs or glitches can occur occasionally
-
-
-- Various game modes and challenges to enjoy
-
-
-
-- Online multiplayer and leaderboards to compete with others
-
-
-
-- Daily rewards and achievements to earn
-
-
-
-- Cross-platform compatibility and cloud saving
-
- User ratings and feedback of Beach Buggy Racing 2
-Beach Buggy Racing 2 has received mostly positive ratings and feedback from users who have played the game. On Google Play, it has a rating of 4.4 out of 5 stars, based on over 1.1 million reviews. On App Store, it has a rating of 4.7 out of 5 stars, based on over 30 thousand reviews. On Steam, it has a rating of 9 out of 10, based on over 300 reviews.
-Most users praise the game for its fun and addictive gameplay, its variety and quality of content and features, its smooth and responsive controls, its beautiful and colorful graphics, and its online multiplayer and cross-platform support. Some users also appreciate the game for its regular updates, its fair and balanced monetization system, and its friendly and helpful customer service.
-However, some users also criticize the game for some issues and problems that they encounter while playing the game. Some of these issues include the game being too easy or too hard, the game being too repetitive or unfair, the game having some bugs or glitches, the game having some ads or in-app purchases, and the game having some compatibility or performance issues.
- Comparison with other kart racing games
-Beach Buggy Racing 2 is not the only kart racing game available on the market. There are other similar games that you can try if you are looking for more options or alternatives. Some of these games include:
-
-Mario Kart Tour: This is a mobile version of the famous Mario Kart series by Nintendo. It features characters and tracks from the Mario universe, as well as new ones inspired by real-world locations. It has a variety of game modes, such as Grand Prix, Time Trials, Ranked Cups, and more. It also has online multiplayer and leaderboards to compete with others.
-Crash Team Racing Nitro-Fueled: This is a remake of the classic Crash Team Racing by Activision. It features characters and tracks from the Crash Bandicoot universe, as well as new ones inspired by other games in the series. It has a variety of game modes, such as Adventure, Arcade, Time Trial, Battle, and more. It also has online multiplayer and leaderboards to compete with others.
-Sonic & All-Stars Racing Transformed: This is a kart racing game by Sega that features characters and tracks from the Sonic the Hedgehog universe, as well as other Sega franchises. It has a unique feature that allows the karts to transform into boats or planes depending on the terrain. It has a variety of game modes, such as Career, Grand Prix, World Tour, Battle Arena, and more. It also has online multiplayer and leaderboards to compete with others.
-
-These are some of the most popular and well-known kart racing games that you can compare with Beach Buggy Racing 2. Each game has its own strengths and weaknesses, so you can choose the one that suits your preferences and expectations.
- Conclusion and FAQs
-In conclusion, Beach Buggy Racing 2 is a fun and exciting kart racing game that offers a lot of content and features for players to enjoy. It has realistic and thrilling kart racing physics, stunning and colorful graphics and animations, over 55 cars to collect and customize, over 45 power-ups to use and upgrade, over 15 drivers to recruit and team up with, over 40 tracks to explore and race on, various game modes and challenges to enjoy, online multiplayer and leaderboards to compete with others, daily rewards and achievements to earn, cross-platform compatibility and cloud saving, and more. However, Beach Buggy Racing 2 also has some limitations and restrictions that can affect your gaming experience. You need to spend real money to buy gems, which are the premium currency in the game. You also need to spend tickets, which are the energy system in the game, to play certain game modes. You also need to watch ads or wait for tickets to replenish. If you don't want to deal with these issues, you can download aplikasi Beach Buggy Racing 2 mod apk, which is a modified version of the original game that gives you unlimited resources and features. With Beach Buggy Racing 2 mod apk, you can have unlimited money, gems, tickets, and power-ups. You can also unlock all the cars, drivers, tracks, and power-ups in the game. You can also play the game without any ads or interruptions. To download aplikasi Beach Buggy Racing 2 mod apk, you need to enable unknown sources in your settings, download the mod apk file from a reliable source, install the mod apk file on your device, and launch the game and enjoy. We also gave you some tips and tricks to play Beach Buggy Racing 2 better, such as mastering the drift and powerslide, using the driver's ability at the right time, avoiding the trap of other racers, building the best deck of power-ups, and grabbing those fast bubbles and shortcuts. We also gave you a review of Beach Buggy Racing 2, where we discussed the pros and cons of the game, the user ratings and feedback of the game, and the comparison with other kart racing games. We hope that this article has helped you learn more about Beach Buggy Racing 2 and how to download its mod apk. If you have any questions or comments about the game or the mod apk, feel free to leave them below. We will try to answer them as soon as possible. Here are some FAQs that you may find useful:
Q: Is Beach Buggy Racing 2 mod apk safe to use?
-A: Yes, Beach Buggy Racing 2 mod apk is safe to use as long as you download it from a trusted source. However, you should always be careful when downloading any mod apk file from unknown sources. You should also scan the file with an antivirus or malware detector before installing it on your device.
- Q: Is Beach Buggy Racing 2 mod apk compatible with my device?
-A: Beach Buggy Racing 2 mod apk is compatible with most Android devices that have Android 4.4 or higher. However, some devices may have compatibility or performance issues depending on their specifications or settings. You should always check the requirements and compatibility of the mod apk file before downloading it.
- Q: Can I play Beach Buggy Racing 2 mod apk online with other players?
-A: Yes, you can play Beach Buggy Racing 2 mod apk online with other players who have the same version of the mod apk file. However, you may not be able to play online with players who have the original version of the game or a different version of the mod apk file. You should always check the version and compatibility of the mod apk file before playing online.
- Q: Can I update Beach Buggy Racing 2 mod apk when a new version is released?
-A: Yes, you can update Beach Buggy Racing 2 mod apk when a new version is released by downloading and installing the new version of the mod apk file from a reliable source. However, you may lose your progress or data if you update without backing up your files. You should always backup your files before updating any mod apk file.
- Q: Can I restore my progress or data if I uninstall Beach Buggy Racing 2 mod apk?
-A: Yes, you can restore your progress or data if you uninstall Beach Buggy Racing 2 mod apk by logging in with your Google Play account or Facebook account. However, you may lose your progress or data if you uninstall without logging in or backing up your files. You should always log in or backup your files before uninstalling any mod apk file.
401be4b1e0
-
-
\ No newline at end of file
diff --git a/spaces/232labs/VToonify/vtoonify/model/stylegan/__init__.py b/spaces/232labs/VToonify/vtoonify/model/stylegan/__init__.py
deleted file mode 100644
index e69de29bb2d1d6434b8b29ae775ad8c2e48c5391..0000000000000000000000000000000000000000
diff --git a/spaces/AIConsultant/MusicGen/audiocraft/grids/musicgen/musicgen_clapemb_32khz.py b/spaces/AIConsultant/MusicGen/audiocraft/grids/musicgen/musicgen_clapemb_32khz.py
deleted file mode 100644
index 64ad3f8c77afe1ab5908e407ad14d4879e1b1ad1..0000000000000000000000000000000000000000
--- a/spaces/AIConsultant/MusicGen/audiocraft/grids/musicgen/musicgen_clapemb_32khz.py
+++ /dev/null
@@ -1,32 +0,0 @@
-# Copyright (c) Meta Platforms, Inc. and affiliates.
-# All rights reserved.
-#
-# This source code is licensed under the license found in the
-# LICENSE file in the root directory of this source tree.
-
-from ._explorers import LMExplorer
-from ...environment import AudioCraftEnvironment
-
-
-@LMExplorer
-def explorer(launcher):
- partitions = AudioCraftEnvironment.get_slurm_partitions(['team', 'global'])
- launcher.slurm_(gpus=32, partition=partitions)
- launcher.bind_(solver='musicgen/musicgen_base_32khz')
- # replace this by the desired music dataset
- launcher.bind_(dset='internal/music_400k_32khz')
- launcher.bind_(conditioner='clapemb2music')
-
- fsdp = {'autocast': False, 'fsdp.use': True}
- cache_path = {'conditioners.description.clap.cache_path':
- '/fsx-audio-craft-llm/jadecopet/experiments/audiocraft/caches/clap_embed_music'}
- text_wav_training_opt = {'conditioners.description.clap.text_p': 0.5}
-
- launcher.bind_(fsdp)
-
- launcher.slurm_(gpus=32).bind_(label='32gpus')
- with launcher.job_array():
- launcher()
- launcher(text_wav_training_opt)
- launcher(cache_path)
- launcher(cache_path, text_wav_training_opt)
diff --git a/spaces/ARTeLab/ARTeLab-SummIT/app.py b/spaces/ARTeLab/ARTeLab-SummIT/app.py
deleted file mode 100644
index 55c0a5f84fed49ba80c5c3681e4bb26668958bf9..0000000000000000000000000000000000000000
--- a/spaces/ARTeLab/ARTeLab-SummIT/app.py
+++ /dev/null
@@ -1,157 +0,0 @@
-import streamlit as st
-import os
-
-from transformers import AutoTokenizer
-from transformers import AutoModelForSeq2SeqLM
-from transformers import pipeline
-from transformers import set_seed
-
-debug = False
-
-MODELS = [
- "ARTeLab/mbart-summarization-fanpage",
- "ARTeLab/mbart-summarization-ilpost",
- "ARTeLab/mbart-summarization-mlsum",
- "ARTeLab/it5-summarization-mlsum",
- "ARTeLab/it5-summarization-ilpost",
- "ARTeLab/it5-summarization-fanpage"
-]
-
-DEFAULT_TEXT: str = """(Fanpage) Dopo oltre mezzo secolo, il mistero della Natività di Caravaggio resta intatto. L'opera, intitolata la "Natività con i Santi Lorenzo e Francesco d'Assisi", fu trafugata la notte tra il 17 e il 18 ottobre 1969 dall'Oratorio di San Lorenzo a Palermo e tuttora non è stata ancora recuperata. L'olio su tela realizzato da Caravaggio, inserito dagli investigatori nella top ten mondiale delle opere d'arte trafugate e mai più ritrovate, ha un valore di mercato che si aggirerebbe oggi intorno ai 20 milioni di dollari secondo l'FBI. La sua storia è avvolta nel mistero e dopo cinquantuno anni ancora non è stata risolta, dopo il furto della mafia nel 1969 e forse ormai distrutta. L'unica certezza è che nemmeno questo Natale potremo ammirare l'opera raffigurante la nascita di Cristo del grande genio pittorico italiano. E forse, secondo i più pessimisti, non ci riusciremo mai più. Nella notte tra il 17 e il 18 ottobre, nel cuore di Palermo, i boss di Cosa Nostra si intrufolarono nell'Oratorio di San Lorenzo e arrotolarono la "Natività con i Santi Lorenzo e Francesco d'Assisi" di Caravaggio in malo modo, facendo sgranare la tela. Una delle più alte testimonianza dell'arte di ogni tempo fu distrutta così. Ma come facciamo a sapere oggi che la tela è andata distrutta? Fu il pentito Francesco Marino Mannoia, durante il processo Andreotti nel 1996 a raccontare del presunto disastro di un gioiello arrotolato in fretta e portato via in segno di sfregio. Ma questa versione stride con quella di un altro pentito che ricorda il quadro affisso ai summit di mafia, come un trofeo, mentre sui giornali si sussurrava di losche ma non provate trattative da 60 miliardi di vecchie lire fra mediatori e trafficanti. Nel 2017, il mafioso Gaetano Grado asserisce che la tela sarebbe stata nascosta, ma all'estero: nel 1970 il boss Badalamenti l'avrebbe trasferita in Svizzera in cambio di una notevole somma di franchi ad un antiquario svizzero, giunto a Palermo per definire l'affare. Grado riferisce anche che Badalamenti gli avrebbe detto che il quadro era stato scomposto per essere venduto sul mercato clandestino."""
-
-
-class TextSummarizer:
- def __init__(self):
- self.tokenizer = None
- self.model = None
- self.generator = None
- self.model_loaded = None
- set_seed(42)
-
- def load(self, model_name):
- os.environ["TOKENIZERS_PARALLELISM"] = "false"
- self.tokenizer = AutoTokenizer.from_pretrained(model_name)
- self.model = AutoModelForSeq2SeqLM.from_pretrained(model_name)
- self.generator = pipeline(
- "text2text-generation", model=self.model, tokenizer=self.tokenizer
- )
- self.model_loaded = model_name
-
- def summarize(self, model_name, input_text, generate_kwargs) -> str:
- if not self.generator or self.model_loaded != model_name:
- with st.spinner("meanwhile: downloading/loading selected model...please don't go :("):
- self.load(model_name)
- return self.generator(
- input_text, return_tensors=False, return_text=True, **generate_kwargs
- )[0].get("generated_text")
-
-
-@st.cache(allow_output_mutation=True)
-def instantiate_generator():
- summarizer = TextSummarizer()
- return summarizer
-
-
-def main():
- st.set_page_config( # Alternate names: setup_page, page, layout
- page_title="ARTeLab SummIT",
- layout="wide", # Can be "centered" or "wide". In the future also "dashboard", etc.
- initial_sidebar_state="expanded", # Can be "auto", "expanded", "collapsed"
- page_icon="📰", # String, anything supported by st.image, or None.
- )
-
- with open("style.css") as f:
- st.markdown(f"", unsafe_allow_html=True)
-
- generator = instantiate_generator()
-
- st.markdown(
- """
-
- """,
- unsafe_allow_html=True,
- )
- st.sidebar.markdown("""# ARTeLab SummIT""")
- st.sidebar.image("fl.png", width=220)
- st.sidebar.markdown(
- """
- * Create summaries of Italian news articles.
- * Copy paste any Italian news text and press the Generate Summary botton.
- """
- )
- st.sidebar.title("Parameters:")
-
- MODEL = st.sidebar.selectbox("Choose model", index=1, options=MODELS)
-
- min_length = st.sidebar.number_input(
- "Min length", min_value=10, max_value=150, value=40
- )
- max_length = st.sidebar.number_input(
- "Max length", min_value=20, max_value=250, value=142
- )
- no_repeat_ngram_size = st.sidebar.number_input(
- "No repeat NGram size", min_value=1, max_value=5, value=3
- )
-
- if sampling_mode := st.sidebar.selectbox(
- "select a Mode", index=0, options=["Beam Search", "Top-k Sampling"]
- ):
- if sampling_mode == "Beam Search":
- num_beams = st.sidebar.number_input(
- "Num beams", min_value=1, max_value=10, value=4
- )
- length_penalty = st.sidebar.number_input(
- "Length penalty", min_value=0.0, max_value=5.0, value=1.5, step=0.1
- )
- params = {
- "min_length": min_length,
- "max_length": max_length,
- "no_repeat_ngram_size": no_repeat_ngram_size,
- "num_beams": num_beams,
- "early_stopping": True,
- "length_penalty": length_penalty,
- "num_return_sequences": 1,
- }
- else:
- top_k = st.sidebar.number_input(
- "Top K", min_value=0, max_value=100, value=50
- )
- top_p = st.sidebar.number_input(
- "Top P", min_value=0.0, max_value=1.0, value=0.9, step=0.05
- )
- temperature = st.sidebar.number_input(
- "Temperature", min_value=0.0, max_value=1.0, value=0.3, step=0.05
- )
- params = {
- "min_length": min_length,
- "max_length": max_length,
- "no_repeat_ngram_size": no_repeat_ngram_size,
- "do_sample": True,
- "top_k": top_k,
- "top_p": top_p,
- "temperature": temperature,
- "num_return_sequences": 1,
- }
-
- input_text = st.text_area("Enter an Italian news text", DEFAULT_TEXT, height=450)
-
- if st.button("Generate summary"):
-
- with st.spinner("Generating summary ..."):
-
- response = generator.summarize(MODEL, input_text, params)
-
- st.header("Summary:")
- st.markdown(response)
-
-
-if __name__ == "__main__":
- main()
\ No newline at end of file
diff --git a/spaces/ATang0729/Forecast4Muses/Model/Model6/Model6_2_ProfileRecogition/mmpretrain/configs/_base_/models/resnetv1d50.py b/spaces/ATang0729/Forecast4Muses/Model/Model6/Model6_2_ProfileRecogition/mmpretrain/configs/_base_/models/resnetv1d50.py
deleted file mode 100644
index 015aaa3d8182cae50f392d7103e24e8ac8a188aa..0000000000000000000000000000000000000000
--- a/spaces/ATang0729/Forecast4Muses/Model/Model6/Model6_2_ProfileRecogition/mmpretrain/configs/_base_/models/resnetv1d50.py
+++ /dev/null
@@ -1,17 +0,0 @@
-# model settings
-model = dict(
- type='ImageClassifier',
- backbone=dict(
- type='ResNetV1d',
- depth=50,
- num_stages=4,
- out_indices=(3, ),
- style='pytorch'),
- neck=dict(type='GlobalAveragePooling'),
- head=dict(
- type='LinearClsHead',
- num_classes=1000,
- in_channels=2048,
- loss=dict(type='CrossEntropyLoss', loss_weight=1.0),
- topk=(1, 5),
- ))
diff --git a/spaces/ATang0729/Forecast4Muses/Model/Model6/Model6_2_ProfileRecogition/mmpretrain/configs/resnet/resnet50_8xb32_in1k.py b/spaces/ATang0729/Forecast4Muses/Model/Model6/Model6_2_ProfileRecogition/mmpretrain/configs/resnet/resnet50_8xb32_in1k.py
deleted file mode 100644
index c32f333b67c255c6101469323636bf242eebb8da..0000000000000000000000000000000000000000
--- a/spaces/ATang0729/Forecast4Muses/Model/Model6/Model6_2_ProfileRecogition/mmpretrain/configs/resnet/resnet50_8xb32_in1k.py
+++ /dev/null
@@ -1,4 +0,0 @@
-_base_ = [
- '../_base_/models/resnet50.py', '../_base_/datasets/imagenet_bs32.py',
- '../_base_/schedules/imagenet_bs256.py', '../_base_/default_runtime.py'
-]
diff --git a/spaces/AbandonedMuse/UnlimitedMusicGen/audiocraft/data/audio_dataset.py b/spaces/AbandonedMuse/UnlimitedMusicGen/audiocraft/data/audio_dataset.py
deleted file mode 100644
index cf21422ea0059cb2d6553f93e608b8f9fa0d3a50..0000000000000000000000000000000000000000
--- a/spaces/AbandonedMuse/UnlimitedMusicGen/audiocraft/data/audio_dataset.py
+++ /dev/null
@@ -1,525 +0,0 @@
-# Copyright (c) Meta Platforms, Inc. and affiliates.
-# All rights reserved.
-#
-# This source code is licensed under the license found in the
-# LICENSE file in the root directory of this source tree.
-
-import argparse
-import copy
-from concurrent.futures import ThreadPoolExecutor, Future
-from dataclasses import dataclass, fields
-from contextlib import ExitStack
-import gzip
-import json
-import logging
-import os
-from pathlib import Path
-import random
-import sys
-import typing as tp
-
-import torch
-import torch.nn.functional as F
-
-from .audio import audio_read, audio_info
-from .audio_utils import convert_audio
-from .zip import PathInZip
-
-try:
- import dora
-except ImportError:
- dora = None # type: ignore
-
-
-@dataclass(order=True)
-class BaseInfo:
-
- @classmethod
- def _dict2fields(cls, dictionary: dict):
- return {
- field.name: dictionary[field.name]
- for field in fields(cls) if field.name in dictionary
- }
-
- @classmethod
- def from_dict(cls, dictionary: dict):
- _dictionary = cls._dict2fields(dictionary)
- return cls(**_dictionary)
-
- def to_dict(self):
- return {
- field.name: self.__getattribute__(field.name)
- for field in fields(self)
- }
-
-
-@dataclass(order=True)
-class AudioMeta(BaseInfo):
- path: str
- duration: float
- sample_rate: int
- amplitude: tp.Optional[float] = None
- weight: tp.Optional[float] = None
- # info_path is used to load additional information about the audio file that is stored in zip files.
- info_path: tp.Optional[PathInZip] = None
-
- @classmethod
- def from_dict(cls, dictionary: dict):
- base = cls._dict2fields(dictionary)
- if 'info_path' in base and base['info_path'] is not None:
- base['info_path'] = PathInZip(base['info_path'])
- return cls(**base)
-
- def to_dict(self):
- d = super().to_dict()
- if d['info_path'] is not None:
- d['info_path'] = str(d['info_path'])
- return d
-
-
-@dataclass(order=True)
-class SegmentInfo(BaseInfo):
- meta: AudioMeta
- seek_time: float
- n_frames: int # actual number of frames without padding
- total_frames: int # total number of frames, padding included
- sample_rate: int # actual sample rate
-
-
-DEFAULT_EXTS = ['.wav', '.mp3', '.flac', '.ogg', '.m4a']
-
-logger = logging.getLogger(__name__)
-
-
-def _get_audio_meta(file_path: str, minimal: bool = True) -> AudioMeta:
- """AudioMeta from a path to an audio file.
-
- Args:
- file_path (str): Resolved path of valid audio file.
- minimal (bool): Whether to only load the minimal set of metadata (takes longer if not).
- Returns:
- AudioMeta: Audio file path and its metadata.
- """
- info = audio_info(file_path)
- amplitude: tp.Optional[float] = None
- if not minimal:
- wav, sr = audio_read(file_path)
- amplitude = wav.abs().max().item()
- return AudioMeta(file_path, info.duration, info.sample_rate, amplitude)
-
-
-def _resolve_audio_meta(m: AudioMeta, fast: bool = True) -> AudioMeta:
- """If Dora is available as a dependency, try to resolve potential relative paths
- in list of AudioMeta. This method is expected to be used when loading meta from file.
-
- Args:
- m (AudioMeta): Audio meta to resolve.
- fast (bool): If True, uses a really fast check for determining if a file is already absolute or not.
- Only valid on Linux/Mac.
- Returns:
- AudioMeta: Audio meta with resolved path.
- """
- def is_abs(m):
- if fast:
- return str(m)[0] == '/'
- else:
- os.path.isabs(str(m))
-
- if not dora:
- return m
-
- if not is_abs(m.path):
- m.path = dora.git_save.to_absolute_path(m.path)
- if m.info_path is not None and not is_abs(m.info_path.zip_path):
- m.info_path.zip_path = dora.git_save.to_absolute_path(m.path)
- return m
-
-
-def find_audio_files(path: tp.Union[Path, str],
- exts: tp.List[str] = DEFAULT_EXTS,
- resolve: bool = True,
- minimal: bool = True,
- progress: bool = False,
- workers: int = 0) -> tp.List[AudioMeta]:
- """Build a list of AudioMeta from a given path,
- collecting relevant audio files and fetching meta info.
-
- Args:
- path (str or Path): Path to folder containing audio files.
- exts (list of str): List of file extensions to consider for audio files.
- minimal (bool): Whether to only load the minimal set of metadata (takes longer if not).
- progress (bool): Whether to log progress on audio files collection.
- workers (int): number of parallel workers, if 0, use only the current thread.
- Returns:
- List[AudioMeta]: List of audio file path and its metadata.
- """
- audio_files = []
- futures: tp.List[Future] = []
- pool: tp.Optional[ThreadPoolExecutor] = None
- with ExitStack() as stack:
- if workers > 0:
- pool = ThreadPoolExecutor(workers)
- stack.enter_context(pool)
-
- if progress:
- print("Finding audio files...")
- for root, folders, files in os.walk(path, followlinks=True):
- for file in files:
- full_path = Path(root) / file
- if full_path.suffix.lower() in exts:
- audio_files.append(full_path)
- if pool is not None:
- futures.append(pool.submit(_get_audio_meta, str(audio_files[-1]), minimal))
- if progress:
- print(format(len(audio_files), " 8d"), end='\r', file=sys.stderr)
-
- if progress:
- print("Getting audio metadata...")
- meta: tp.List[AudioMeta] = []
- for idx, file_path in enumerate(audio_files):
- try:
- if pool is None:
- m = _get_audio_meta(str(file_path), minimal)
- else:
- m = futures[idx].result()
- if resolve:
- m = _resolve_audio_meta(m)
- except Exception as err:
- print("Error with", str(file_path), err, file=sys.stderr)
- continue
- meta.append(m)
- if progress:
- print(format((1 + idx) / len(audio_files), " 3.1%"), end='\r', file=sys.stderr)
- meta.sort()
- return meta
-
-
-def load_audio_meta(path: tp.Union[str, Path],
- resolve: bool = True, fast: bool = True) -> tp.List[AudioMeta]:
- """Load list of AudioMeta from an optionally compressed json file.
-
- Args:
- path (str or Path): Path to JSON file.
- resolve (bool): Whether to resolve the path from AudioMeta (default=True).
- fast (bool): activates some tricks to make things faster.
- Returns:
- List[AudioMeta]: List of audio file path and its total duration.
- """
- open_fn = gzip.open if str(path).lower().endswith('.gz') else open
- with open_fn(path, 'rb') as fp: # type: ignore
- lines = fp.readlines()
- meta = []
- for line in lines:
- d = json.loads(line)
- m = AudioMeta.from_dict(d)
- if resolve:
- m = _resolve_audio_meta(m, fast=fast)
- meta.append(m)
- return meta
-
-
-def save_audio_meta(path: tp.Union[str, Path], meta: tp.List[AudioMeta]):
- """Save the audio metadata to the file pointer as json.
-
- Args:
- path (str or Path): Path to JSON file.
- metadata (list of BaseAudioMeta): List of audio meta to save.
- """
- Path(path).parent.mkdir(exist_ok=True, parents=True)
- open_fn = gzip.open if str(path).lower().endswith('.gz') else open
- with open_fn(path, 'wb') as fp: # type: ignore
- for m in meta:
- json_str = json.dumps(m.to_dict()) + '\n'
- json_bytes = json_str.encode('utf-8')
- fp.write(json_bytes)
-
-
-class AudioDataset:
- """Base audio dataset.
-
- The dataset takes a list of AudioMeta and create a dataset composed of segments of audio
- and potentially additional information, by creating random segments from the list of audio
- files referenced in the metadata and applying minimal data pre-processing such as resampling,
- mixing of channels, padding, etc.
-
- If no segment_duration value is provided, the AudioDataset will return the full wav for each
- audio file. Otherwise, it will randomly sample audio files and create a segment of the specified
- duration, applying padding if required.
-
- By default, only the torch Tensor corresponding to the waveform is returned. Setting return_info=True
- allows to return a tuple containing the torch Tensor and additional metadata on the segment and the
- original audio meta.
-
- Args:
- meta (tp.List[AudioMeta]): List of audio files metadata.
- segment_duration (float): Optional segment duration of audio to load.
- If not specified, the dataset will load the full audio segment from the file.
- shuffle (bool): Set to `True` to have the data reshuffled at every epoch.
- sample_rate (int): Target sample rate of the loaded audio samples.
- channels (int): Target number of channels of the loaded audio samples.
- sample_on_duration (bool): Set to `True` to sample segments with probability
- dependent on audio file duration. This is only used if `segment_duration` is provided.
- sample_on_weight (bool): Set to `True` to sample segments using the `weight` entry of
- `AudioMeta`. If `sample_on_duration` is also True, the actual weight will be the product
- of the file duration and file weight. This is only used if `segment_duration` is provided.
- min_segment_ratio (float): Minimum segment ratio to use when the audio file
- is shorter than the desired segment.
- max_read_retry (int): Maximum number of retries to sample an audio segment from the dataset.
- return_info (bool): Whether to return the wav only or return wav along with segment info and metadata.
- min_audio_duration (tp.Optional[float], optional): Minimum audio file duration, in seconds, if provided
- audio shorter than this will be filtered out.
- max_audio_duration (tp.Optional[float], optional): Maximal audio file duration in seconds, if provided
- audio longer than this will be filtered out.
- """
- def __init__(self,
- meta: tp.List[AudioMeta],
- segment_duration: tp.Optional[float] = None,
- shuffle: bool = True,
- num_samples: int = 10_000,
- sample_rate: int = 48_000,
- channels: int = 2,
- pad: bool = True,
- sample_on_duration: bool = True,
- sample_on_weight: bool = True,
- min_segment_ratio: float = 0.5,
- max_read_retry: int = 10,
- return_info: bool = False,
- min_audio_duration: tp.Optional[float] = None,
- max_audio_duration: tp.Optional[float] = None
- ):
- assert len(meta) > 0, 'No audio meta provided to AudioDataset. Please check loading of audio meta.'
- assert segment_duration is None or segment_duration > 0
- assert segment_duration is None or min_segment_ratio >= 0
- logging.debug(f'sample_on_duration: {sample_on_duration}')
- logging.debug(f'sample_on_weight: {sample_on_weight}')
- logging.debug(f'pad: {pad}')
- logging.debug(f'min_segment_ratio: {min_segment_ratio}')
-
- self.segment_duration = segment_duration
- self.min_segment_ratio = min_segment_ratio
- self.max_audio_duration = max_audio_duration
- self.min_audio_duration = min_audio_duration
- if self.min_audio_duration is not None and self.max_audio_duration is not None:
- assert self.min_audio_duration <= self.max_audio_duration
- self.meta: tp.List[AudioMeta] = self._filter_duration(meta)
- assert len(self.meta) # Fail fast if all data has been filtered.
- self.total_duration = sum(d.duration for d in self.meta)
-
- if segment_duration is None:
- num_samples = len(self.meta)
- self.num_samples = num_samples
- self.shuffle = shuffle
- self.sample_rate = sample_rate
- self.channels = channels
- self.pad = pad
- self.sample_on_weight = sample_on_weight
- self.sample_on_duration = sample_on_duration
- self.sampling_probabilities = self._get_sampling_probabilities()
- self.max_read_retry = max_read_retry
- self.return_info = return_info
-
- def __len__(self):
- return self.num_samples
-
- def _get_sampling_probabilities(self, normalized: bool = True):
- """Return the sampling probabilities for each file inside `self.meta`.
- """
- scores: tp.List[float] = []
- for file_meta in self.meta:
- score = 1.
- if self.sample_on_weight and file_meta.weight is not None:
- score *= file_meta.weight
- if self.sample_on_duration:
- score *= file_meta.duration
- scores.append(score)
- probabilities = torch.tensor(scores)
- if normalized:
- probabilities /= probabilities.sum()
- return probabilities
-
- def sample_file(self, rng: torch.Generator) -> AudioMeta:
- """Sample a given file from `self.meta`. Can be overriden in subclasses.
- This is only called if `segment_duration` is not None.
-
- You must use the provided random number generator `rng` for reproducibility.
- """
- if not self.sample_on_weight and not self.sample_on_duration:
- file_index = int(torch.randint(len(self.sampling_probabilities), (1,), generator=rng).item())
- else:
- file_index = int(torch.multinomial(self.sampling_probabilities, 1, generator=rng).item())
-
- return self.meta[file_index]
-
- def __getitem__(self, index: int) -> tp.Union[torch.Tensor, tp.Tuple[torch.Tensor, SegmentInfo]]:
- if self.segment_duration is None:
- file_meta = self.meta[index]
- out, sr = audio_read(file_meta.path)
- out = convert_audio(out, sr, self.sample_rate, self.channels)
- n_frames = out.shape[-1]
- segment_info = SegmentInfo(file_meta, seek_time=0., n_frames=n_frames, total_frames=n_frames,
- sample_rate=self.sample_rate)
- else:
- rng = torch.Generator()
- if self.shuffle:
- # We use index, plus extra randomness
- rng.manual_seed(index + self.num_samples * random.randint(0, 2**24))
- else:
- # We only use index
- rng.manual_seed(index)
-
- for retry in range(self.max_read_retry):
- file_meta = self.sample_file(rng)
- # We add some variance in the file position even if audio file is smaller than segment
- # without ending up with empty segments
- max_seek = max(0, file_meta.duration - self.segment_duration * self.min_segment_ratio)
- seek_time = torch.rand(1, generator=rng).item() * max_seek
- try:
- out, sr = audio_read(file_meta.path, seek_time, self.segment_duration, pad=False)
- out = convert_audio(out, sr, self.sample_rate, self.channels)
- n_frames = out.shape[-1]
- target_frames = int(self.segment_duration * self.sample_rate)
- if self.pad:
- out = F.pad(out, (0, target_frames - n_frames))
- segment_info = SegmentInfo(file_meta, seek_time, n_frames=n_frames, total_frames=target_frames,
- sample_rate=self.sample_rate)
- except Exception as exc:
- logger.warning("Error opening file %s: %r", file_meta.path, exc)
- if retry == self.max_read_retry - 1:
- raise
- else:
- break
-
- if self.return_info:
- # Returns the wav and additional information on the wave segment
- return out, segment_info
- else:
- return out
-
- def collater(self, samples):
- """The collater function has to be provided to the dataloader
- if AudioDataset has return_info=True in order to properly collate
- the samples of a batch.
- """
- if self.segment_duration is None and len(samples) > 1:
- assert self.pad, "Must allow padding when batching examples of different durations."
-
- # In this case the audio reaching the collater is of variable length as segment_duration=None.
- to_pad = self.segment_duration is None and self.pad
- if to_pad:
- max_len = max([wav.shape[-1] for wav, _ in samples])
-
- def _pad_wav(wav):
- return F.pad(wav, (0, max_len - wav.shape[-1]))
-
- if self.return_info:
- if len(samples) > 0:
- assert len(samples[0]) == 2
- assert isinstance(samples[0][0], torch.Tensor)
- assert isinstance(samples[0][1], SegmentInfo)
-
- wavs = [wav for wav, _ in samples]
- segment_infos = [copy.deepcopy(info) for _, info in samples]
-
- if to_pad:
- # Each wav could be of a different duration as they are not segmented.
- for i in range(len(samples)):
- # Determines the total legth of the signal with padding, so we update here as we pad.
- segment_infos[i].total_frames = max_len
- wavs[i] = _pad_wav(wavs[i])
-
- wav = torch.stack(wavs)
- return wav, segment_infos
- else:
- assert isinstance(samples[0], torch.Tensor)
- if to_pad:
- samples = [_pad_wav(s) for s in samples]
- return torch.stack(samples)
-
- def _filter_duration(self, meta: tp.List[AudioMeta]) -> tp.List[AudioMeta]:
- """Filters out audio files with short durations.
- Removes from meta files that have durations that will not allow to samples examples from them.
- """
- orig_len = len(meta)
-
- # Filter data that is too short.
- if self.min_audio_duration is not None:
- meta = [m for m in meta if m.duration >= self.min_audio_duration]
-
- # Filter data that is too long.
- if self.max_audio_duration is not None:
- meta = [m for m in meta if m.duration <= self.max_audio_duration]
-
- filtered_len = len(meta)
- removed_percentage = 100*(1-float(filtered_len)/orig_len)
- msg = 'Removed %.2f percent of the data because it was too short or too long.' % removed_percentage
- if removed_percentage < 10:
- logging.debug(msg)
- else:
- logging.warning(msg)
- return meta
-
- @classmethod
- def from_meta(cls, root: tp.Union[str, Path], **kwargs):
- """Instantiate AudioDataset from a path to a directory containing a manifest as a jsonl file.
-
- Args:
- root (str or Path): Path to root folder containing audio files.
- kwargs: Additional keyword arguments for the AudioDataset.
- """
- root = Path(root)
- if root.is_dir():
- if (root / 'data.jsonl').exists():
- root = root / 'data.jsonl'
- elif (root / 'data.jsonl.gz').exists():
- root = root / 'data.jsonl.gz'
- else:
- raise ValueError("Don't know where to read metadata from in the dir. "
- "Expecting either a data.jsonl or data.jsonl.gz file but none found.")
- meta = load_audio_meta(root)
- return cls(meta, **kwargs)
-
- @classmethod
- def from_path(cls, root: tp.Union[str, Path], minimal_meta: bool = True,
- exts: tp.List[str] = DEFAULT_EXTS, **kwargs):
- """Instantiate AudioDataset from a path containing (possibly nested) audio files.
-
- Args:
- root (str or Path): Path to root folder containing audio files.
- minimal_meta (bool): Whether to only load minimal metadata or not.
- exts (list of str): Extensions for audio files.
- kwargs: Additional keyword arguments for the AudioDataset.
- """
- root = Path(root)
- if root.is_file():
- meta = load_audio_meta(root, resolve=True)
- else:
- meta = find_audio_files(root, exts, minimal=minimal_meta, resolve=True)
- return cls(meta, **kwargs)
-
-
-def main():
- logging.basicConfig(stream=sys.stderr, level=logging.INFO)
- parser = argparse.ArgumentParser(
- prog='audio_dataset',
- description='Generate .jsonl files by scanning a folder.')
- parser.add_argument('root', help='Root folder with all the audio files')
- parser.add_argument('output_meta_file',
- help='Output file to store the metadata, ')
- parser.add_argument('--complete',
- action='store_false', dest='minimal', default=True,
- help='Retrieve all metadata, even the one that are expansive '
- 'to compute (e.g. normalization).')
- parser.add_argument('--resolve',
- action='store_true', default=False,
- help='Resolve the paths to be absolute and with no symlinks.')
- parser.add_argument('--workers',
- default=10, type=int,
- help='Number of workers.')
- args = parser.parse_args()
- meta = find_audio_files(args.root, DEFAULT_EXTS, progress=True,
- resolve=args.resolve, minimal=args.minimal, workers=args.workers)
- save_audio_meta(args.output_meta_file, meta)
-
-
-if __name__ == '__main__':
- main()
diff --git a/spaces/AchyuthGamer/OpenGPT-Chat-UI/README.md b/spaces/AchyuthGamer/OpenGPT-Chat-UI/README.md
deleted file mode 100644
index 7c3c48cc05c0c007284cf384f174474f63296ffc..0000000000000000000000000000000000000000
--- a/spaces/AchyuthGamer/OpenGPT-Chat-UI/README.md
+++ /dev/null
@@ -1,12 +0,0 @@
----
-title: OpenGPT Chat
-emoji: 🚀
-colorFrom: gray
-colorTo: pink
-sdk: docker
-pinned: true
-app_port: 3000
-license: creativeml-openrail-m
----
-
-Check out the configuration reference at https://huggingface.co/docs/hub/spaces-config-reference
\ No newline at end of file
diff --git a/spaces/AgentVerse/agentVerse/ui/src/phaser3-rex-plugins/templates/ui/colorinput/colorpicker/methods/SVPaletteCanvas.js b/spaces/AgentVerse/agentVerse/ui/src/phaser3-rex-plugins/templates/ui/colorinput/colorpicker/methods/SVPaletteCanvas.js
deleted file mode 100644
index 7ba907a651432e2976f58175b041d9aa4a2470ea..0000000000000000000000000000000000000000
--- a/spaces/AgentVerse/agentVerse/ui/src/phaser3-rex-plugins/templates/ui/colorinput/colorpicker/methods/SVPaletteCanvas.js
+++ /dev/null
@@ -1,98 +0,0 @@
-import Canvas from '../../../canvas/Canvas.js';
-import { DrawSVPalette } from '../../../../../plugins/utils/canvas/DrawHSVPalette.js';
-
-const Color = Phaser.Display.Color;
-const Percent = Phaser.Math.Percent;
-const ColorToRGBA = Phaser.Display.Color.ColorToRGBA;
-const HSVToRGB = Phaser.Display.Color.HSVToRGB;
-
-class SVPaletteCanvas extends Canvas {
- constructor(scene, x, y, width, height, hue) {
- if (x === undefined) { x = 0; }
- if (y === undefined) { y = 0; }
- if (width === undefined) { width = 2; }
- if (height === undefined) { height = 2; }
-
- super(scene, x, y, width, height);
- this.type = 'rexColorPicker.SVPaletteCanvas';
-
- if (hue === undefined) {
- hue = 1;
- }
-
- this.colorObject = new Color();
-
- this.setHue(hue);
- this.setSize(width, height);
- }
-
- get color() {
- return this.colorObject.color;
- }
-
- get hue() {
- return this._hue;
- }
-
- set hue(hue) {
- if (this._hue === hue) {
- return;
- }
- this._hue = hue;
- this.colorObject.h = hue;
- this.dirty = true;
- }
-
- setHue(hue) {
- this.hue = hue;
- return this;
- }
-
- updateTexture() {
- DrawSVPalette(this.canvas, this.context, this.hue);
- super.updateTexture();
- return this;
- }
-
- getColor(localX, localY) {
- if (localX === undefined) {
- return this.colorObject.color;
- }
-
- var s = Percent(localX, 0, this.width);
- var v = 1 - Percent(localY, 0, this.height);
- this.colorObject.setFromRGB(HSVToRGB(this.hue, s, v));
- return this.colorObject.color;
- }
-
- setColor(color) {
- if (this.color === color) {
- return this;
- }
-
- this.colorObject.setFromRGB(ColorToRGBA(color));
- this.setHue(this.colorObject.h);
- return this;
- }
-
- colorToLocalPosition(color, out) {
- if (out === undefined) {
- out = {};
- } else if (out === true) {
- if (LocalXY === undefined) {
- LocalXY = {};
- }
- out = LocalXY;
- }
-
- this.colorObject.setFromRGB(ColorToRGBA(color));
- out.x = this.width * this.colorObject.s;
- out.y = this.height * (1 - this.colorObject.v);
-
- return out;
- }
-}
-
-var LocalXY = undefined;
-
-export default SVPaletteCanvas;
\ No newline at end of file
diff --git a/spaces/AlStable/Duke/app.py b/spaces/AlStable/Duke/app.py
deleted file mode 100644
index 4ee672d7b3a35a53b9144742a8af6d83483167f9..0000000000000000000000000000000000000000
--- a/spaces/AlStable/Duke/app.py
+++ /dev/null
@@ -1,137 +0,0 @@
-from diffusers import StableDiffusionPipeline, StableDiffusionImg2ImgPipeline, DPMSolverMultistepScheduler
-import gradio as gr
-import torch
-from PIL import Image
-
-model_id = 'DucHaiten/DucHaitenDreamWorld'
-prefix = ''
-
-scheduler = DPMSolverMultistepScheduler.from_pretrained(model_id, subfolder="scheduler")
-
-pipe = StableDiffusionPipeline.from_pretrained(
- model_id,
- torch_dtype=torch.float16 if torch.cuda.is_available() else torch.float32,
- scheduler=scheduler)
-
-pipe_i2i = StableDiffusionImg2ImgPipeline.from_pretrained(
- model_id,
- torch_dtype=torch.float16 if torch.cuda.is_available() else torch.float32,
- scheduler=scheduler)
-
-if torch.cuda.is_available():
- pipe = pipe.to("cuda")
- pipe_i2i = pipe_i2i.to("cuda")
-
-def error_str(error, title="Error"):
- return f"""#### {title}
- {error}""" if error else ""
-
-def inference(prompt, guidance, steps, width=512, height=512, seed=0, img=None, strength=0.5, neg_prompt="", auto_prefix=False):
-
- generator = torch.Generator('cuda').manual_seed(seed) if seed != 0 else None
- prompt = f"{prefix} {prompt}" if auto_prefix else prompt
-
- try:
- if img is not None:
- return img_to_img(prompt, neg_prompt, img, strength, guidance, steps, width, height, generator), None
- else:
- return txt_to_img(prompt, neg_prompt, guidance, steps, width, height, generator), None
- except Exception as e:
- return None, error_str(e)
-
-def txt_to_img(prompt, neg_prompt, guidance, steps, width, height, generator):
-
- result = pipe(
- prompt,
- negative_prompt = neg_prompt,
- num_inference_steps = int(steps),
- guidance_scale = guidance,
- width = width,
- height = height,
- generator = generator)
-
- return result.images[0]
-
-def img_to_img(prompt, neg_prompt, img, strength, guidance, steps, width, height, generator):
-
- ratio = min(height / img.height, width / img.width)
- img = img.resize((int(img.width * ratio), int(img.height * ratio)), Image.LANCZOS)
- result = pipe_i2i(
- prompt,
- negative_prompt = neg_prompt,
- init_image = img,
- num_inference_steps = int(steps),
- strength = strength,
- guidance_scale = guidance,
- width = width,
- height = height,
- generator = generator)
-
- return result.images[0]
-
-css = """.main-div div{display:inline-flex;align-items:center;gap:.8rem;font-size:1.75rem}.main-div div h1{font-weight:900;margin-bottom:7px}.main-div p{margin-bottom:10px;font-size:94%}a{text-decoration:underline}.tabs{margin-top:0;margin-bottom:0}#gallery{min-height:20rem}
-"""
-with gr.Blocks(css=css) as demo:
- gr.HTML(
- f"""
-
-
-
Duchaiten dreamworld
-
-
- Demo for Duchaitendreamworld Stable Diffusion model.
- {"Add the following tokens to your prompts for the model to work properly: prefix " if prefix else ""}
-
- Running on {"
GPU 🔥 " if torch.cuda.is_available() else f"
CPU 🥶 . For faster inference it is recommended to
upgrade to GPU in Settings "} after duplicating the space
-
-
- """
- )
- with gr.Row():
-
- with gr.Column(scale=55):
- with gr.Group():
- with gr.Row():
- prompt = gr.Textbox(label="Prompt", show_label=False, max_lines=2,placeholder=f"{prefix} [your prompt]").style(container=False)
- generate = gr.Button(value="Generate").style(rounded=(False, True, True, False))
-
- image_out = gr.Image(height=512)
- error_output = gr.Markdown()
-
- with gr.Column(scale=45):
- with gr.Tab("Options"):
- with gr.Group():
- neg_prompt = gr.Textbox(label="Negative prompt", placeholder="What to exclude from the image")
- auto_prefix = gr.Checkbox(label="Prefix styling tokens automatically ()", value=prefix, visible=prefix)
-
- with gr.Row():
- guidance = gr.Slider(label="Guidance scale", value=7.5, maximum=15)
- steps = gr.Slider(label="Steps", value=25, minimum=2, maximum=75, step=1)
-
- with gr.Row():
- width = gr.Slider(label="Width", value=512, minimum=64, maximum=1024, step=8)
- height = gr.Slider(label="Height", value=512, minimum=64, maximum=1024, step=8)
-
- seed = gr.Slider(0, 2147483647, label='Seed (0 = random)', value=0, step=1)
-
- with gr.Tab("Image to image"):
- with gr.Group():
- image = gr.Image(label="Image", height=256, tool="editor", type="pil")
- strength = gr.Slider(label="Transformation strength", minimum=0, maximum=1, step=0.01, value=0.5)
-
- auto_prefix.change(lambda x: gr.update(placeholder=f"{prefix} [your prompt]" if x else "[Your prompt]"), inputs=auto_prefix, outputs=prompt, queue=False)
-
- inputs = [prompt, guidance, steps, width, height, seed, image, strength, neg_prompt, auto_prefix]
- outputs = [image_out, error_output]
- prompt.submit(inference, inputs=inputs, outputs=outputs)
- generate.click(inference, inputs=inputs, outputs=outputs)
-
- gr.HTML("""
-
- """)
-
-demo.queue(concurrency_count=1)
-demo.launch()
diff --git a/spaces/Amrrs/portfolio-github/style.css b/spaces/Amrrs/portfolio-github/style.css
deleted file mode 100644
index 363d0b7bb0dd45552039e3156a6350989e327db2..0000000000000000000000000000000000000000
--- a/spaces/Amrrs/portfolio-github/style.css
+++ /dev/null
@@ -1,190 +0,0 @@
-html {
- margin: 0;
- padding: 0;
-}
-
-body {
- font-family: 'Bellota', cursive;
- font-size: 26pt;
- background-color: #f2f2f2;
- padding: 20px;
- margin: 0;
-}
-
-h1 {
- font-size: 15pt;
- color: #ffffff;
- text-align: center;
- padding: 18px 0 18px 0;
- margin: 0 0 10px 0;
-}
-
-h1 span {
- border: 8px solid #666666;
- border-radius: 8px;
- background-image: url("https://media.giphy.com/media/KVZWZQoS0yqfIiTAKq/giphy.gif");
- padding: 12px;
-}
-
-p {
- padding: 0;
- margin: 0;
- color: #000000;
-}
-
-.img-circle {
- border: 8px solid white;
- border-radius: 50%;
-}
-
-.section {
- background-color: #fff;
- padding: 20px;
- margin-bottom: 10px;
- border-radius: 30px;
-}
-
-#header {
- background-image: url("https://media.giphy.com/media/KVZWZQoS0yqfIiTAKq/giphy.gif");
- background-size: cover;
-}
-
-#header img {
- display: block;
- width: 500px;
- height: 500px;
- margin: auto;
-}
-
-#header p {
- font-size: 60pt;
- color: #ffffff;
- padding-top: 8px;
- margin: 0;
- font-weight: bold;
- text-align: center;
-}
-
-.quote {
- font-size: 12pt;
- text-align: right;
- margin-top: 10px;
- color: grey;
-}
-
-#res {
- text-align: center;
- margin: 50px auto;
-}
-
-#res a {
- margin: 20px 20px;
- display: inline-block;
- text-decoration: none;
- color: black;
-}
-
-.selected {
- background-color: #f36f48;
- font-weight: bold;
- color: white;
-}
-
-li {
- margin-bottom: 15px;
- font-weight: bold;
-}
-
-progress {
- width: 70%;
- height: 20px;
- color: #3fb6b2;
- background: #efefef;
-}
-
-progress::-webkit-progress-bar {
- background: #efefef;
-}
-
-progress::-webkit-progress-value {
- background: #3fb6b2;
-}
-
-progress::-moz-progress-bar {
- color: #3fb6b2;
- background: #efefef;
-}
-
-iframe,
-audio {
- display: block;
- margin: 0 auto;
- border: 3px solid #3fb6b2;
- border-radius: 10px;
-}
-
-hr {
- border: 0;
- height: 1px;
- background: #f36f48;
-}
-
-input {
- text-align: center;
- font-size: 25pt;
- border: none;
- border-radius: 12px;
- padding: 30px 8%;
- margin: 20px 5px 10px 5px;
- background-color: #d7d7d7;
-}
-
-input:focus {
- background-color: #2f2f2f;
- color: white;
-}
-
-form {
- text-align: center;
- font-size: 30pt;
- font-family: Helvetica;
- font-weight: 500;
- margin: 10% 15% 8% 15%;
- border-radius: 12px;
-}
-
-#insta-image {
- display: block;
- width: 100px;
- height: 100px;
- border: 5px solid #d7d7d7;
- border-radius: 50%;
- margin: auto;
- margin-top: -75px;
-}
-
-#contacts img {
- height: 150px;
- width: 150px;
- margin-left: 7px;
- margin-right: 7px;
-}
-
-#contacts a {
- text-decoration: none;
-}
-
-#contacts img:hover {
- opacity: 0.8;
-}
-
-#contacts {
- text-align: center;
-}
-
-.copyright {
- font-size: 8pt;
- text-align: right;
- padding-bottom: 10px;
- color: grey;
-}
\ No newline at end of file
diff --git a/spaces/Androidonnxfork/CivitAi-to-Diffusers/diffusers/docs/source/en/using-diffusers/write_own_pipeline.md b/spaces/Androidonnxfork/CivitAi-to-Diffusers/diffusers/docs/source/en/using-diffusers/write_own_pipeline.md
deleted file mode 100644
index ca5ea38b4ad26f6a4e53e73963fd2de01c1a6405..0000000000000000000000000000000000000000
--- a/spaces/Androidonnxfork/CivitAi-to-Diffusers/diffusers/docs/source/en/using-diffusers/write_own_pipeline.md
+++ /dev/null
@@ -1,290 +0,0 @@
-
-
-# Understanding pipelines, models and schedulers
-
-[[open-in-colab]]
-
-🧨 Diffusers is designed to be a user-friendly and flexible toolbox for building diffusion systems tailored to your use-case. At the core of the toolbox are models and schedulers. While the [`DiffusionPipeline`] bundles these components together for convenience, you can also unbundle the pipeline and use the models and schedulers separately to create new diffusion systems.
-
-In this tutorial, you'll learn how to use models and schedulers to assemble a diffusion system for inference, starting with a basic pipeline and then progressing to the Stable Diffusion pipeline.
-
-## Deconstruct a basic pipeline
-
-A pipeline is a quick and easy way to run a model for inference, requiring no more than four lines of code to generate an image:
-
-```py
->>> from diffusers import DDPMPipeline
-
->>> ddpm = DDPMPipeline.from_pretrained("google/ddpm-cat-256").to("cuda")
->>> image = ddpm(num_inference_steps=25).images[0]
->>> image
-```
-
-
-
-
-
-That was super easy, but how did the pipeline do that? Let's breakdown the pipeline and take a look at what's happening under the hood.
-
-In the example above, the pipeline contains a [`UNet2DModel`] model and a [`DDPMScheduler`]. The pipeline denoises an image by taking random noise the size of the desired output and passing it through the model several times. At each timestep, the model predicts the *noise residual* and the scheduler uses it to predict a less noisy image. The pipeline repeats this process until it reaches the end of the specified number of inference steps.
-
-To recreate the pipeline with the model and scheduler separately, let's write our own denoising process.
-
-1. Load the model and scheduler:
-
-```py
->>> from diffusers import DDPMScheduler, UNet2DModel
-
->>> scheduler = DDPMScheduler.from_pretrained("google/ddpm-cat-256")
->>> model = UNet2DModel.from_pretrained("google/ddpm-cat-256").to("cuda")
-```
-
-2. Set the number of timesteps to run the denoising process for:
-
-```py
->>> scheduler.set_timesteps(50)
-```
-
-3. Setting the scheduler timesteps creates a tensor with evenly spaced elements in it, 50 in this example. Each element corresponds to a timestep at which the model denoises an image. When you create the denoising loop later, you'll iterate over this tensor to denoise an image:
-
-```py
->>> scheduler.timesteps
-tensor([980, 960, 940, 920, 900, 880, 860, 840, 820, 800, 780, 760, 740, 720,
- 700, 680, 660, 640, 620, 600, 580, 560, 540, 520, 500, 480, 460, 440,
- 420, 400, 380, 360, 340, 320, 300, 280, 260, 240, 220, 200, 180, 160,
- 140, 120, 100, 80, 60, 40, 20, 0])
-```
-
-4. Create some random noise with the same shape as the desired output:
-
-```py
->>> import torch
-
->>> sample_size = model.config.sample_size
->>> noise = torch.randn((1, 3, sample_size, sample_size)).to("cuda")
-```
-
-5. Now write a loop to iterate over the timesteps. At each timestep, the model does a [`UNet2DModel.forward`] pass and returns the noisy residual. The scheduler's [`~DDPMScheduler.step`] method takes the noisy residual, timestep, and input and it predicts the image at the previous timestep. This output becomes the next input to the model in the denoising loop, and it'll repeat until it reaches the end of the `timesteps` array.
-
-```py
->>> input = noise
-
->>> for t in scheduler.timesteps:
-... with torch.no_grad():
-... noisy_residual = model(input, t).sample
-... previous_noisy_sample = scheduler.step(noisy_residual, t, input).prev_sample
-... input = previous_noisy_sample
-```
-
-This is the entire denoising process, and you can use this same pattern to write any diffusion system.
-
-6. The last step is to convert the denoised output into an image:
-
-```py
->>> from PIL import Image
->>> import numpy as np
-
->>> image = (input / 2 + 0.5).clamp(0, 1)
->>> image = image.cpu().permute(0, 2, 3, 1).numpy()[0]
->>> image = Image.fromarray((image * 255).round().astype("uint8"))
->>> image
-```
-
-In the next section, you'll put your skills to the test and breakdown the more complex Stable Diffusion pipeline. The steps are more or less the same. You'll initialize the necessary components, and set the number of timesteps to create a `timestep` array. The `timestep` array is used in the denoising loop, and for each element in this array, the model predicts a less noisy image. The denoising loop iterates over the `timestep`'s, and at each timestep, it outputs a noisy residual and the scheduler uses it to predict a less noisy image at the previous timestep. This process is repeated until you reach the end of the `timestep` array.
-
-Let's try it out!
-
-## Deconstruct the Stable Diffusion pipeline
-
-Stable Diffusion is a text-to-image *latent diffusion* model. It is called a latent diffusion model because it works with a lower-dimensional representation of the image instead of the actual pixel space, which makes it more memory efficient. The encoder compresses the image into a smaller representation, and a decoder to convert the compressed representation back into an image. For text-to-image models, you'll need a tokenizer and an encoder to generate text embeddings. From the previous example, you already know you need a UNet model and a scheduler.
-
-As you can see, this is already more complex than the DDPM pipeline which only contains a UNet model. The Stable Diffusion model has three separate pretrained models.
-
-
-
-💡 Read the [How does Stable Diffusion work?](https://huggingface.co/blog/stable_diffusion#how-does-stable-diffusion-work) blog for more details about how the VAE, UNet, and text encoder models.
-
-
-
-Now that you know what you need for the Stable Diffusion pipeline, load all these components with the [`~ModelMixin.from_pretrained`] method. You can find them in the pretrained [`runwayml/stable-diffusion-v1-5`](https://huggingface.co/runwayml/stable-diffusion-v1-5) checkpoint, and each component is stored in a separate subfolder:
-
-```py
->>> from PIL import Image
->>> import torch
->>> from transformers import CLIPTextModel, CLIPTokenizer
->>> from diffusers import AutoencoderKL, UNet2DConditionModel, PNDMScheduler
-
->>> vae = AutoencoderKL.from_pretrained("CompVis/stable-diffusion-v1-4", subfolder="vae")
->>> tokenizer = CLIPTokenizer.from_pretrained("CompVis/stable-diffusion-v1-4", subfolder="tokenizer")
->>> text_encoder = CLIPTextModel.from_pretrained("CompVis/stable-diffusion-v1-4", subfolder="text_encoder")
->>> unet = UNet2DConditionModel.from_pretrained("CompVis/stable-diffusion-v1-4", subfolder="unet")
-```
-
-Instead of the default [`PNDMScheduler`], exchange it for the [`UniPCMultistepScheduler`] to see how easy it is to plug a different scheduler in:
-
-```py
->>> from diffusers import UniPCMultistepScheduler
-
->>> scheduler = UniPCMultistepScheduler.from_pretrained("CompVis/stable-diffusion-v1-4", subfolder="scheduler")
-```
-
-To speed up inference, move the models to a GPU since, unlike the scheduler, they have trainable weights:
-
-```py
->>> torch_device = "cuda"
->>> vae.to(torch_device)
->>> text_encoder.to(torch_device)
->>> unet.to(torch_device)
-```
-
-### Create text embeddings
-
-The next step is to tokenize the text to generate embeddings. The text is used to condition the UNet model and steer the diffusion process towards something that resembles the input prompt.
-
-
-
-💡 The `guidance_scale` parameter determines how much weight should be given to the prompt when generating an image.
-
-
-
-Feel free to choose any prompt you like if you want to generate something else!
-
-```py
->>> prompt = ["a photograph of an astronaut riding a horse"]
->>> height = 512 # default height of Stable Diffusion
->>> width = 512 # default width of Stable Diffusion
->>> num_inference_steps = 25 # Number of denoising steps
->>> guidance_scale = 7.5 # Scale for classifier-free guidance
->>> generator = torch.manual_seed(0) # Seed generator to create the inital latent noise
->>> batch_size = len(prompt)
-```
-
-Tokenize the text and generate the embeddings from the prompt:
-
-```py
->>> text_input = tokenizer(
-... prompt, padding="max_length", max_length=tokenizer.model_max_length, truncation=True, return_tensors="pt"
-... )
-
->>> with torch.no_grad():
-... text_embeddings = text_encoder(text_input.input_ids.to(torch_device))[0]
-```
-
-You'll also need to generate the *unconditional text embeddings* which are the embeddings for the padding token. These need to have the same shape (`batch_size` and `seq_length`) as the conditional `text_embeddings`:
-
-```py
->>> max_length = text_input.input_ids.shape[-1]
->>> uncond_input = tokenizer([""] * batch_size, padding="max_length", max_length=max_length, return_tensors="pt")
->>> uncond_embeddings = text_encoder(uncond_input.input_ids.to(torch_device))[0]
-```
-
-Let's concatenate the conditional and unconditional embeddings into a batch to avoid doing two forward passes:
-
-```py
->>> text_embeddings = torch.cat([uncond_embeddings, text_embeddings])
-```
-
-### Create random noise
-
-Next, generate some initial random noise as a starting point for the diffusion process. This is the latent representation of the image, and it'll be gradually denoised. At this point, the `latent` image is smaller than the final image size but that's okay though because the model will transform it into the final 512x512 image dimensions later.
-
-
-
-💡 The height and width are divided by 8 because the `vae` model has 3 down-sampling layers. You can check by running the following:
-
-```py
-2 ** (len(vae.config.block_out_channels) - 1) == 8
-```
-
-
-
-```py
->>> latents = torch.randn(
-... (batch_size, unet.in_channels, height // 8, width // 8),
-... generator=generator,
-... )
->>> latents = latents.to(torch_device)
-```
-
-### Denoise the image
-
-Start by scaling the input with the initial noise distribution, *sigma*, the noise scale value, which is required for improved schedulers like [`UniPCMultistepScheduler`]:
-
-```py
->>> latents = latents * scheduler.init_noise_sigma
-```
-
-The last step is to create the denoising loop that'll progressively transform the pure noise in `latents` to an image described by your prompt. Remember, the denoising loop needs to do three things:
-
-1. Set the scheduler's timesteps to use during denoising.
-2. Iterate over the timesteps.
-3. At each timestep, call the UNet model to predict the noise residual and pass it to the scheduler to compute the previous noisy sample.
-
-```py
->>> from tqdm.auto import tqdm
-
->>> scheduler.set_timesteps(num_inference_steps)
-
->>> for t in tqdm(scheduler.timesteps):
-... # expand the latents if we are doing classifier-free guidance to avoid doing two forward passes.
-... latent_model_input = torch.cat([latents] * 2)
-
-... latent_model_input = scheduler.scale_model_input(latent_model_input, timestep=t)
-
-... # predict the noise residual
-... with torch.no_grad():
-... noise_pred = unet(latent_model_input, t, encoder_hidden_states=text_embeddings).sample
-
-... # perform guidance
-... noise_pred_uncond, noise_pred_text = noise_pred.chunk(2)
-... noise_pred = noise_pred_uncond + guidance_scale * (noise_pred_text - noise_pred_uncond)
-
-... # compute the previous noisy sample x_t -> x_t-1
-... latents = scheduler.step(noise_pred, t, latents).prev_sample
-```
-
-### Decode the image
-
-The final step is to use the `vae` to decode the latent representation into an image and get the decoded output with `sample`:
-
-```py
-# scale and decode the image latents with vae
-latents = 1 / 0.18215 * latents
-with torch.no_grad():
- image = vae.decode(latents).sample
-```
-
-Lastly, convert the image to a `PIL.Image` to see your generated image!
-
-```py
->>> image = (image / 2 + 0.5).clamp(0, 1)
->>> image = image.detach().cpu().permute(0, 2, 3, 1).numpy()
->>> images = (image * 255).round().astype("uint8")
->>> pil_images = [Image.fromarray(image) for image in images]
->>> pil_images[0]
-```
-
-
-
-
-
-## Next steps
-
-From basic to complex pipelines, you've seen that all you really need to write your own diffusion system is a denoising loop. The loop should set the scheduler's timesteps, iterate over them, and alternate between calling the UNet model to predict the noise residual and passing it to the scheduler to compute the previous noisy sample.
-
-This is really what 🧨 Diffusers is designed for: to make it intuitive and easy to write your own diffusion system using models and schedulers.
-
-For your next steps, feel free to:
-
-* Learn how to [build and contribute a pipeline](contribute_pipeline) to 🧨 Diffusers. We can't wait and see what you'll come up with!
-* Explore [existing pipelines](../api/pipelines/overview) in the library, and see if you can deconstruct and build a pipeline from scratch using the models and schedulers separately.
diff --git a/spaces/Androidonnxfork/CivitAi-to-Diffusers/diffusers/src/diffusers/models/vq_model.py b/spaces/Androidonnxfork/CivitAi-to-Diffusers/diffusers/src/diffusers/models/vq_model.py
deleted file mode 100644
index 687449e8c7557473c0af994b30ef4c7dfba9718c..0000000000000000000000000000000000000000
--- a/spaces/Androidonnxfork/CivitAi-to-Diffusers/diffusers/src/diffusers/models/vq_model.py
+++ /dev/null
@@ -1,167 +0,0 @@
-# Copyright 2023 The HuggingFace Team. All rights reserved.
-#
-# Licensed under the Apache License, Version 2.0 (the "License");
-# you may not use this file except in compliance with the License.
-# You may obtain a copy of the License at
-#
-# http://www.apache.org/licenses/LICENSE-2.0
-#
-# Unless required by applicable law or agreed to in writing, software
-# distributed under the License is distributed on an "AS IS" BASIS,
-# WITHOUT WARRANTIES OR CONDITIONS OF ANY KIND, either express or implied.
-# See the License for the specific language governing permissions and
-# limitations under the License.
-from dataclasses import dataclass
-from typing import Optional, Tuple, Union
-
-import torch
-import torch.nn as nn
-
-from ..configuration_utils import ConfigMixin, register_to_config
-from ..utils import BaseOutput, apply_forward_hook
-from .modeling_utils import ModelMixin
-from .vae import Decoder, DecoderOutput, Encoder, VectorQuantizer
-
-
-@dataclass
-class VQEncoderOutput(BaseOutput):
- """
- Output of VQModel encoding method.
-
- Args:
- latents (`torch.FloatTensor` of shape `(batch_size, num_channels, height, width)`):
- The encoded output sample from the last layer of the model.
- """
-
- latents: torch.FloatTensor
-
-
-class VQModel(ModelMixin, ConfigMixin):
- r"""
- A VQ-VAE model for decoding latent representations.
-
- This model inherits from [`ModelMixin`]. Check the superclass documentation for it's generic methods implemented
- for all models (such as downloading or saving).
-
- Parameters:
- in_channels (int, *optional*, defaults to 3): Number of channels in the input image.
- out_channels (int, *optional*, defaults to 3): Number of channels in the output.
- down_block_types (`Tuple[str]`, *optional*, defaults to `("DownEncoderBlock2D",)`):
- Tuple of downsample block types.
- up_block_types (`Tuple[str]`, *optional*, defaults to `("UpDecoderBlock2D",)`):
- Tuple of upsample block types.
- block_out_channels (`Tuple[int]`, *optional*, defaults to `(64,)`):
- Tuple of block output channels.
- act_fn (`str`, *optional*, defaults to `"silu"`): The activation function to use.
- latent_channels (`int`, *optional*, defaults to `3`): Number of channels in the latent space.
- sample_size (`int`, *optional*, defaults to `32`): Sample input size.
- num_vq_embeddings (`int`, *optional*, defaults to `256`): Number of codebook vectors in the VQ-VAE.
- vq_embed_dim (`int`, *optional*): Hidden dim of codebook vectors in the VQ-VAE.
- scaling_factor (`float`, *optional*, defaults to `0.18215`):
- The component-wise standard deviation of the trained latent space computed using the first batch of the
- training set. This is used to scale the latent space to have unit variance when training the diffusion
- model. The latents are scaled with the formula `z = z * scaling_factor` before being passed to the
- diffusion model. When decoding, the latents are scaled back to the original scale with the formula: `z = 1
- / scaling_factor * z`. For more details, refer to sections 4.3.2 and D.1 of the [High-Resolution Image
- Synthesis with Latent Diffusion Models](https://arxiv.org/abs/2112.10752) paper.
- """
-
- @register_to_config
- def __init__(
- self,
- in_channels: int = 3,
- out_channels: int = 3,
- down_block_types: Tuple[str] = ("DownEncoderBlock2D",),
- up_block_types: Tuple[str] = ("UpDecoderBlock2D",),
- block_out_channels: Tuple[int] = (64,),
- layers_per_block: int = 1,
- act_fn: str = "silu",
- latent_channels: int = 3,
- sample_size: int = 32,
- num_vq_embeddings: int = 256,
- norm_num_groups: int = 32,
- vq_embed_dim: Optional[int] = None,
- scaling_factor: float = 0.18215,
- norm_type: str = "group", # group, spatial
- ):
- super().__init__()
-
- # pass init params to Encoder
- self.encoder = Encoder(
- in_channels=in_channels,
- out_channels=latent_channels,
- down_block_types=down_block_types,
- block_out_channels=block_out_channels,
- layers_per_block=layers_per_block,
- act_fn=act_fn,
- norm_num_groups=norm_num_groups,
- double_z=False,
- )
-
- vq_embed_dim = vq_embed_dim if vq_embed_dim is not None else latent_channels
-
- self.quant_conv = nn.Conv2d(latent_channels, vq_embed_dim, 1)
- self.quantize = VectorQuantizer(num_vq_embeddings, vq_embed_dim, beta=0.25, remap=None, sane_index_shape=False)
- self.post_quant_conv = nn.Conv2d(vq_embed_dim, latent_channels, 1)
-
- # pass init params to Decoder
- self.decoder = Decoder(
- in_channels=latent_channels,
- out_channels=out_channels,
- up_block_types=up_block_types,
- block_out_channels=block_out_channels,
- layers_per_block=layers_per_block,
- act_fn=act_fn,
- norm_num_groups=norm_num_groups,
- norm_type=norm_type,
- )
-
- @apply_forward_hook
- def encode(self, x: torch.FloatTensor, return_dict: bool = True) -> VQEncoderOutput:
- h = self.encoder(x)
- h = self.quant_conv(h)
-
- if not return_dict:
- return (h,)
-
- return VQEncoderOutput(latents=h)
-
- @apply_forward_hook
- def decode(
- self, h: torch.FloatTensor, force_not_quantize: bool = False, return_dict: bool = True
- ) -> Union[DecoderOutput, torch.FloatTensor]:
- # also go through quantization layer
- if not force_not_quantize:
- quant, emb_loss, info = self.quantize(h)
- else:
- quant = h
- quant2 = self.post_quant_conv(quant)
- dec = self.decoder(quant2, quant if self.config.norm_type == "spatial" else None)
-
- if not return_dict:
- return (dec,)
-
- return DecoderOutput(sample=dec)
-
- def forward(self, sample: torch.FloatTensor, return_dict: bool = True) -> Union[DecoderOutput, torch.FloatTensor]:
- r"""
- The [`VQModel`] forward method.
-
- Args:
- sample (`torch.FloatTensor`): Input sample.
- return_dict (`bool`, *optional*, defaults to `True`):
- Whether or not to return a [`models.vq_model.VQEncoderOutput`] instead of a plain tuple.
-
- Returns:
- [`~models.vq_model.VQEncoderOutput`] or `tuple`:
- If return_dict is True, a [`~models.vq_model.VQEncoderOutput`] is returned, otherwise a plain `tuple`
- is returned.
- """
- x = sample
- h = self.encode(x).latents
- dec = self.decode(h).sample
-
- if not return_dict:
- return (dec,)
-
- return DecoderOutput(sample=dec)
diff --git a/spaces/Andy1621/uniformer_image_segmentation/configs/deeplabv3/deeplabv3_r101-d8_512x1024_40k_cityscapes.py b/spaces/Andy1621/uniformer_image_segmentation/configs/deeplabv3/deeplabv3_r101-d8_512x1024_40k_cityscapes.py
deleted file mode 100644
index 8c707c79d659bc544d242352bcb29686eb40b004..0000000000000000000000000000000000000000
--- a/spaces/Andy1621/uniformer_image_segmentation/configs/deeplabv3/deeplabv3_r101-d8_512x1024_40k_cityscapes.py
+++ /dev/null
@@ -1,2 +0,0 @@
-_base_ = './deeplabv3_r50-d8_512x1024_40k_cityscapes.py'
-model = dict(pretrained='open-mmlab://resnet101_v1c', backbone=dict(depth=101))
diff --git a/spaces/Andy1621/uniformer_image_segmentation/configs/dnlnet/dnl_r101-d8_769x769_40k_cityscapes.py b/spaces/Andy1621/uniformer_image_segmentation/configs/dnlnet/dnl_r101-d8_769x769_40k_cityscapes.py
deleted file mode 100644
index 575e9d01343a4563e0d3ba89b361ea8e358d2dee..0000000000000000000000000000000000000000
--- a/spaces/Andy1621/uniformer_image_segmentation/configs/dnlnet/dnl_r101-d8_769x769_40k_cityscapes.py
+++ /dev/null
@@ -1,2 +0,0 @@
-_base_ = './dnl_r50-d8_769x769_40k_cityscapes.py'
-model = dict(pretrained='open-mmlab://resnet101_v1c', backbone=dict(depth=101))
diff --git a/spaces/Anonymous-123/ImageNet-Editing/README.md b/spaces/Anonymous-123/ImageNet-Editing/README.md
deleted file mode 100644
index 0bae12587c18d6b0a1cb3bbff97a25d772d90fe6..0000000000000000000000000000000000000000
--- a/spaces/Anonymous-123/ImageNet-Editing/README.md
+++ /dev/null
@@ -1,13 +0,0 @@
----
-title: ImageNet Editing
-emoji: 📊
-colorFrom: gray
-colorTo: pink
-sdk: gradio
-sdk_version: 3.3.1
-app_file: app.py
-pinned: false
-license: creativeml-openrail-m
----
-
-Check out the configuration reference at https://huggingface.co/docs/hub/spaces-config-reference
diff --git a/spaces/Anthony7906/MengHuiMXD_GPT/run_Windows.bat b/spaces/Anthony7906/MengHuiMXD_GPT/run_Windows.bat
deleted file mode 100644
index 4c18f9ccaeea0af972301ffdf48778641221f76d..0000000000000000000000000000000000000000
--- a/spaces/Anthony7906/MengHuiMXD_GPT/run_Windows.bat
+++ /dev/null
@@ -1,5 +0,0 @@
-@echo off
-echo Opening ChuanhuChatGPT...
-
-REM Open powershell via bat
-start powershell.exe -NoExit -Command "python ./ChuanhuChatbot.py"
diff --git a/spaces/AnthonyTruchetPoC/persistent-docker/tests/athai/test_hello.py b/spaces/AnthonyTruchetPoC/persistent-docker/tests/athai/test_hello.py
deleted file mode 100644
index 709df7aa2182083690c911bd27ad6081147a0eeb..0000000000000000000000000000000000000000
--- a/spaces/AnthonyTruchetPoC/persistent-docker/tests/athai/test_hello.py
+++ /dev/null
@@ -1,14 +0,0 @@
-from athai import hello
-
-
-def test_hello_default():
- assert hello.build_greetings() == "Hello, World!"
-
-
-def test_hello_name():
- assert hello.build_greetings("Toto") == "Nice to meet you, Toto!"
-
-
-# Given / Arrange
-# When / Act
-# Then / Assert
diff --git a/spaces/Ataturk-Chatbot/HuggingFaceChat/venv/lib/python3.11/site-packages/setuptools/launch.py b/spaces/Ataturk-Chatbot/HuggingFaceChat/venv/lib/python3.11/site-packages/setuptools/launch.py
deleted file mode 100644
index 0208fdf33b640cd9791359d74673bb90cfb87f96..0000000000000000000000000000000000000000
--- a/spaces/Ataturk-Chatbot/HuggingFaceChat/venv/lib/python3.11/site-packages/setuptools/launch.py
+++ /dev/null
@@ -1,36 +0,0 @@
-"""
-Launch the Python script on the command line after
-setuptools is bootstrapped via import.
-"""
-
-# Note that setuptools gets imported implicitly by the
-# invocation of this script using python -m setuptools.launch
-
-import tokenize
-import sys
-
-
-def run():
- """
- Run the script in sys.argv[1] as if it had
- been invoked naturally.
- """
- __builtins__
- script_name = sys.argv[1]
- namespace = dict(
- __file__=script_name,
- __name__='__main__',
- __doc__=None,
- )
- sys.argv[:] = sys.argv[1:]
-
- open_ = getattr(tokenize, 'open', open)
- with open_(script_name) as fid:
- script = fid.read()
- norm_script = script.replace('\\r\\n', '\\n')
- code = compile(norm_script, script_name, 'exec')
- exec(code, namespace)
-
-
-if __name__ == '__main__':
- run()
diff --git a/spaces/CVPR/Dual-Key_Backdoor_Attacks/datagen/detectron2/docs/notes/compatibility.md b/spaces/CVPR/Dual-Key_Backdoor_Attacks/datagen/detectron2/docs/notes/compatibility.md
deleted file mode 100644
index d9ec2ce34ea4f2e1a99c8934338c2fcbe1d19cf6..0000000000000000000000000000000000000000
--- a/spaces/CVPR/Dual-Key_Backdoor_Attacks/datagen/detectron2/docs/notes/compatibility.md
+++ /dev/null
@@ -1,83 +0,0 @@
-# Compatibility with Other Libraries
-
-## Compatibility with Detectron (and maskrcnn-benchmark)
-
-Detectron2 addresses some legacy issues left in Detectron. As a result, their models
-are not compatible:
-running inference with the same model weights will produce different results in the two code bases.
-
-The major differences regarding inference are:
-
-- The height and width of a box with corners (x1, y1) and (x2, y2) is now computed more naturally as
- width = x2 - x1 and height = y2 - y1;
- In Detectron, a "+ 1" was added both height and width.
-
- Note that the relevant ops in Caffe2 have [adopted this change of convention](https://github.com/pytorch/pytorch/pull/20550)
- with an extra option.
- So it is still possible to run inference with a Detectron2-trained model in Caffe2.
-
- The change in height/width calculations most notably changes:
- - encoding/decoding in bounding box regression.
- - non-maximum suppression. The effect here is very negligible, though.
-
-- RPN now uses simpler anchors with fewer quantization artifacts.
-
- In Detectron, the anchors were quantized and
- [do not have accurate areas](https://github.com/facebookresearch/Detectron/issues/227).
- In Detectron2, the anchors are center-aligned to feature grid points and not quantized.
-
-- Classification layers have a different ordering of class labels.
-
- This involves any trainable parameter with shape (..., num_categories + 1, ...).
- In Detectron2, integer labels [0, K-1] correspond to the K = num_categories object categories
- and the label "K" corresponds to the special "background" category.
- In Detectron, label "0" means background, and labels [1, K] correspond to the K categories.
-
-- ROIAlign is implemented differently. The new implementation is [available in Caffe2](https://github.com/pytorch/pytorch/pull/23706).
-
- 1. All the ROIs are shifted by half a pixel compared to Detectron in order to create better image-feature-map alignment.
- See `layers/roi_align.py` for details.
- To enable the old behavior, use `ROIAlign(aligned=False)`, or `POOLER_TYPE=ROIAlign` instead of
- `ROIAlignV2` (the default).
-
- 1. The ROIs are not required to have a minimum size of 1.
- This will lead to tiny differences in the output, but should be negligible.
-
-- Mask inference function is different.
-
- In Detectron2, the "paste_mask" function is different and should be more accurate than in Detectron. This change
- can improve mask AP on COCO by ~0.5% absolute.
-
-There are some other differences in training as well, but they won't affect
-model-level compatibility. The major ones are:
-
-- We fixed a [bug](https://github.com/facebookresearch/Detectron/issues/459) in
- Detectron, by making `RPN.POST_NMS_TOPK_TRAIN` per-image, rather than per-batch.
- The fix may lead to a small accuracy drop for a few models (e.g. keypoint
- detection) and will require some parameter tuning to match the Detectron results.
-- For simplicity, we change the default loss in bounding box regression to L1 loss, instead of smooth L1 loss.
- We have observed that this tends to slightly decrease box AP50 while improving box AP for higher
- overlap thresholds (and leading to a slight overall improvement in box AP).
-- We interpret the coordinates in COCO bounding box and segmentation annotations
- as coordinates in range `[0, width]` or `[0, height]`. The coordinates in
- COCO keypoint annotations are interpreted as pixel indices in range `[0, width - 1]` or `[0, height - 1]`.
- Note that this affects how flip augmentation is implemented.
-
-
-We will later share more details and rationale behind the above mentioned issues
-about pixels, coordinates, and "+1"s.
-
-
-## Compatibility with Caffe2
-
-As mentioned above, despite the incompatibilities with Detectron, the relevant
-ops have been implemented in Caffe2.
-Therefore, models trained with detectron2 can be converted in Caffe2.
-See [Deployment](../tutorials/deployment.html) for the tutorial.
-
-## Compatibility with TensorFlow
-
-Most ops are available in TensorFlow, although some tiny differences in
-the implementation of resize / ROIAlign / padding need to be addressed.
-A working conversion script is provided by [tensorpack FasterRCNN](https://github.com/tensorpack/tensorpack/tree/master/examples/FasterRCNN/convert_d2)
-to run a standard detectron2 model in TensorFlow.
diff --git a/spaces/CVPR/LIVE/pybind11/docs/benchmark.py b/spaces/CVPR/LIVE/pybind11/docs/benchmark.py
deleted file mode 100644
index 023477212ee3ca34353067b196e9959144444f33..0000000000000000000000000000000000000000
--- a/spaces/CVPR/LIVE/pybind11/docs/benchmark.py
+++ /dev/null
@@ -1,89 +0,0 @@
-# -*- coding: utf-8 -*-
-import random
-import os
-import time
-import datetime as dt
-
-nfns = 4 # Functions per class
-nargs = 4 # Arguments per function
-
-
-def generate_dummy_code_pybind11(nclasses=10):
- decl = ""
- bindings = ""
-
- for cl in range(nclasses):
- decl += "class cl%03i;\n" % cl
- decl += '\n'
-
- for cl in range(nclasses):
- decl += "class cl%03i {\n" % cl
- decl += "public:\n"
- bindings += ' py::class_(m, "cl%03i")\n' % (cl, cl)
- for fn in range(nfns):
- ret = random.randint(0, nclasses - 1)
- params = [random.randint(0, nclasses - 1) for i in range(nargs)]
- decl += " cl%03i *fn_%03i(" % (ret, fn)
- decl += ", ".join("cl%03i *" % p for p in params)
- decl += ");\n"
- bindings += ' .def("fn_%03i", &cl%03i::fn_%03i)\n' % \
- (fn, cl, fn)
- decl += "};\n\n"
- bindings += ' ;\n'
-
- result = "#include \n\n"
- result += "namespace py = pybind11;\n\n"
- result += decl + '\n'
- result += "PYBIND11_MODULE(example, m) {\n"
- result += bindings
- result += "}"
- return result
-
-
-def generate_dummy_code_boost(nclasses=10):
- decl = ""
- bindings = ""
-
- for cl in range(nclasses):
- decl += "class cl%03i;\n" % cl
- decl += '\n'
-
- for cl in range(nclasses):
- decl += "class cl%03i {\n" % cl
- decl += "public:\n"
- bindings += ' py::class_("cl%03i")\n' % (cl, cl)
- for fn in range(nfns):
- ret = random.randint(0, nclasses - 1)
- params = [random.randint(0, nclasses - 1) for i in range(nargs)]
- decl += " cl%03i *fn_%03i(" % (ret, fn)
- decl += ", ".join("cl%03i *" % p for p in params)
- decl += ");\n"
- bindings += ' .def("fn_%03i", &cl%03i::fn_%03i, py::return_value_policy())\n' % \
- (fn, cl, fn)
- decl += "};\n\n"
- bindings += ' ;\n'
-
- result = "#include \n\n"
- result += "namespace py = boost::python;\n\n"
- result += decl + '\n'
- result += "BOOST_PYTHON_MODULE(example) {\n"
- result += bindings
- result += "}"
- return result
-
-
-for codegen in [generate_dummy_code_pybind11, generate_dummy_code_boost]:
- print ("{")
- for i in range(0, 10):
- nclasses = 2 ** i
- with open("test.cpp", "w") as f:
- f.write(codegen(nclasses))
- n1 = dt.datetime.now()
- os.system("g++ -Os -shared -rdynamic -undefined dynamic_lookup "
- "-fvisibility=hidden -std=c++14 test.cpp -I include "
- "-I /System/Library/Frameworks/Python.framework/Headers -o test.so")
- n2 = dt.datetime.now()
- elapsed = (n2 - n1).total_seconds()
- size = os.stat('test.so').st_size
- print(" {%i, %f, %i}," % (nclasses * nfns, elapsed, size))
- print ("}")
diff --git a/spaces/CVPR/LIVE/pybind11/tests/test_factory_constructors.cpp b/spaces/CVPR/LIVE/pybind11/tests/test_factory_constructors.cpp
deleted file mode 100644
index 61cf33d16ed404563a3da803a4c2ecea4453a3b4..0000000000000000000000000000000000000000
--- a/spaces/CVPR/LIVE/pybind11/tests/test_factory_constructors.cpp
+++ /dev/null
@@ -1,342 +0,0 @@
-/*
- tests/test_factory_constructors.cpp -- tests construction from a factory function
- via py::init_factory()
-
- Copyright (c) 2017 Jason Rhinelander
-
- All rights reserved. Use of this source code is governed by a
- BSD-style license that can be found in the LICENSE file.
-*/
-
-#include "pybind11_tests.h"
-#include "constructor_stats.h"
-#include
-
-// Classes for testing python construction via C++ factory function:
-// Not publicly constructible, copyable, or movable:
-class TestFactory1 {
- friend class TestFactoryHelper;
- TestFactory1() : value("(empty)") { print_default_created(this); }
- TestFactory1(int v) : value(std::to_string(v)) { print_created(this, value); }
- TestFactory1(std::string v) : value(std::move(v)) { print_created(this, value); }
- TestFactory1(TestFactory1 &&) = delete;
- TestFactory1(const TestFactory1 &) = delete;
- TestFactory1 &operator=(TestFactory1 &&) = delete;
- TestFactory1 &operator=(const TestFactory1 &) = delete;
-public:
- std::string value;
- ~TestFactory1() { print_destroyed(this); }
-};
-// Non-public construction, but moveable:
-class TestFactory2 {
- friend class TestFactoryHelper;
- TestFactory2() : value("(empty2)") { print_default_created(this); }
- TestFactory2(int v) : value(std::to_string(v)) { print_created(this, value); }
- TestFactory2(std::string v) : value(std::move(v)) { print_created(this, value); }
-public:
- TestFactory2(TestFactory2 &&m) { value = std::move(m.value); print_move_created(this); }
- TestFactory2 &operator=(TestFactory2 &&m) { value = std::move(m.value); print_move_assigned(this); return *this; }
- std::string value;
- ~TestFactory2() { print_destroyed(this); }
-};
-// Mixed direct/factory construction:
-class TestFactory3 {
-protected:
- friend class TestFactoryHelper;
- TestFactory3() : value("(empty3)") { print_default_created(this); }
- TestFactory3(int v) : value(std::to_string(v)) { print_created(this, value); }
-public:
- TestFactory3(std::string v) : value(std::move(v)) { print_created(this, value); }
- TestFactory3(TestFactory3 &&m) { value = std::move(m.value); print_move_created(this); }
- TestFactory3 &operator=(TestFactory3 &&m) { value = std::move(m.value); print_move_assigned(this); return *this; }
- std::string value;
- virtual ~TestFactory3() { print_destroyed(this); }
-};
-// Inheritance test
-class TestFactory4 : public TestFactory3 {
-public:
- TestFactory4() : TestFactory3() { print_default_created(this); }
- TestFactory4(int v) : TestFactory3(v) { print_created(this, v); }
- virtual ~TestFactory4() { print_destroyed(this); }
-};
-// Another class for an invalid downcast test
-class TestFactory5 : public TestFactory3 {
-public:
- TestFactory5(int i) : TestFactory3(i) { print_created(this, i); }
- virtual ~TestFactory5() { print_destroyed(this); }
-};
-
-class TestFactory6 {
-protected:
- int value;
- bool alias = false;
-public:
- TestFactory6(int i) : value{i} { print_created(this, i); }
- TestFactory6(TestFactory6 &&f) { print_move_created(this); value = f.value; alias = f.alias; }
- TestFactory6(const TestFactory6 &f) { print_copy_created(this); value = f.value; alias = f.alias; }
- virtual ~TestFactory6() { print_destroyed(this); }
- virtual int get() { return value; }
- bool has_alias() { return alias; }
-};
-class PyTF6 : public TestFactory6 {
-public:
- // Special constructor that allows the factory to construct a PyTF6 from a TestFactory6 only
- // when an alias is needed:
- PyTF6(TestFactory6 &&base) : TestFactory6(std::move(base)) { alias = true; print_created(this, "move", value); }
- PyTF6(int i) : TestFactory6(i) { alias = true; print_created(this, i); }
- PyTF6(PyTF6 &&f) : TestFactory6(std::move(f)) { print_move_created(this); }
- PyTF6(const PyTF6 &f) : TestFactory6(f) { print_copy_created(this); }
- PyTF6(std::string s) : TestFactory6((int) s.size()) { alias = true; print_created(this, s); }
- virtual ~PyTF6() { print_destroyed(this); }
- int get() override { PYBIND11_OVERLOAD(int, TestFactory6, get, /*no args*/); }
-};
-
-class TestFactory7 {
-protected:
- int value;
- bool alias = false;
-public:
- TestFactory7(int i) : value{i} { print_created(this, i); }
- TestFactory7(TestFactory7 &&f) { print_move_created(this); value = f.value; alias = f.alias; }
- TestFactory7(const TestFactory7 &f) { print_copy_created(this); value = f.value; alias = f.alias; }
- virtual ~TestFactory7() { print_destroyed(this); }
- virtual int get() { return value; }
- bool has_alias() { return alias; }
-};
-class PyTF7 : public TestFactory7 {
-public:
- PyTF7(int i) : TestFactory7(i) { alias = true; print_created(this, i); }
- PyTF7(PyTF7 &&f) : TestFactory7(std::move(f)) { print_move_created(this); }
- PyTF7(const PyTF7 &f) : TestFactory7(f) { print_copy_created(this); }
- virtual ~PyTF7() { print_destroyed(this); }
- int get() override { PYBIND11_OVERLOAD(int, TestFactory7, get, /*no args*/); }
-};
-
-
-class TestFactoryHelper {
-public:
- // Non-movable, non-copyable type:
- // Return via pointer:
- static TestFactory1 *construct1() { return new TestFactory1(); }
- // Holder:
- static std::unique_ptr construct1(int a) { return std::unique_ptr(new TestFactory1(a)); }
- // pointer again
- static TestFactory1 *construct1_string(std::string a) { return new TestFactory1(a); }
-
- // Moveable type:
- // pointer:
- static TestFactory2 *construct2() { return new TestFactory2(); }
- // holder:
- static std::unique_ptr construct2(int a) { return std::unique_ptr(new TestFactory2(a)); }
- // by value moving:
- static TestFactory2 construct2(std::string a) { return TestFactory2(a); }
-
- // shared_ptr holder type:
- // pointer:
- static TestFactory3 *construct3() { return new TestFactory3(); }
- // holder:
- static std::shared_ptr construct3(int a) { return std::shared_ptr(new TestFactory3(a)); }
-};
-
-TEST_SUBMODULE(factory_constructors, m) {
-
- // Define various trivial types to allow simpler overload resolution:
- py::module m_tag = m.def_submodule("tag");
-#define MAKE_TAG_TYPE(Name) \
- struct Name##_tag {}; \
- py::class_(m_tag, #Name "_tag").def(py::init<>()); \
- m_tag.attr(#Name) = py::cast(Name##_tag{})
- MAKE_TAG_TYPE(pointer);
- MAKE_TAG_TYPE(unique_ptr);
- MAKE_TAG_TYPE(move);
- MAKE_TAG_TYPE(shared_ptr);
- MAKE_TAG_TYPE(derived);
- MAKE_TAG_TYPE(TF4);
- MAKE_TAG_TYPE(TF5);
- MAKE_TAG_TYPE(null_ptr);
- MAKE_TAG_TYPE(null_unique_ptr);
- MAKE_TAG_TYPE(null_shared_ptr);
- MAKE_TAG_TYPE(base);
- MAKE_TAG_TYPE(invalid_base);
- MAKE_TAG_TYPE(alias);
- MAKE_TAG_TYPE(unaliasable);
- MAKE_TAG_TYPE(mixed);
-
- // test_init_factory_basic, test_bad_type
- py::class_(m, "TestFactory1")
- .def(py::init([](unique_ptr_tag, int v) { return TestFactoryHelper::construct1(v); }))
- .def(py::init(&TestFactoryHelper::construct1_string)) // raw function pointer
- .def(py::init([](pointer_tag) { return TestFactoryHelper::construct1(); }))
- .def(py::init([](py::handle, int v, py::handle) { return TestFactoryHelper::construct1(v); }))
- .def_readwrite("value", &TestFactory1::value)
- ;
- py::class_(m, "TestFactory2")
- .def(py::init([](pointer_tag, int v) { return TestFactoryHelper::construct2(v); }))
- .def(py::init([](unique_ptr_tag, std::string v) { return TestFactoryHelper::construct2(v); }))
- .def(py::init([](move_tag) { return TestFactoryHelper::construct2(); }))
- .def_readwrite("value", &TestFactory2::value)
- ;
-
- // Stateful & reused:
- int c = 1;
- auto c4a = [c](pointer_tag, TF4_tag, int a) { (void) c; return new TestFactory4(a);};
-
- // test_init_factory_basic, test_init_factory_casting
- py::class_>(m, "TestFactory3")
- .def(py::init([](pointer_tag, int v) { return TestFactoryHelper::construct3(v); }))
- .def(py::init([](shared_ptr_tag) { return TestFactoryHelper::construct3(); }))
- .def("__init__", [](TestFactory3 &self, std::string v) { new (&self) TestFactory3(v); }) // placement-new ctor
-
- // factories returning a derived type:
- .def(py::init(c4a)) // derived ptr
- .def(py::init([](pointer_tag, TF5_tag, int a) { return new TestFactory5(a); }))
- // derived shared ptr:
- .def(py::init([](shared_ptr_tag, TF4_tag, int a) { return std::make_shared(a); }))
- .def(py::init([](shared_ptr_tag, TF5_tag, int a) { return std::make_shared(a); }))
-
- // Returns nullptr:
- .def(py::init([](null_ptr_tag) { return (TestFactory3 *) nullptr; }))
- .def(py::init([](null_unique_ptr_tag) { return std::unique_ptr(); }))
- .def(py::init([](null_shared_ptr_tag) { return std::shared_ptr(); }))
-
- .def_readwrite("value", &TestFactory3::value)
- ;
-
- // test_init_factory_casting
- py::class_>(m, "TestFactory4")
- .def(py::init(c4a)) // pointer
- ;
-
- // Doesn't need to be registered, but registering makes getting ConstructorStats easier:
- py::class_>(m, "TestFactory5");
-
- // test_init_factory_alias
- // Alias testing
- py::class_(m, "TestFactory6")
- .def(py::init([](base_tag, int i) { return TestFactory6(i); }))
- .def(py::init([](alias_tag, int i) { return PyTF6(i); }))
- .def(py::init([](alias_tag, std::string s) { return PyTF6(s); }))
- .def(py::init([](alias_tag, pointer_tag, int i) { return new PyTF6(i); }))
- .def(py::init([](base_tag, pointer_tag, int i) { return new TestFactory6(i); }))
- .def(py::init([](base_tag, alias_tag, pointer_tag, int i) { return (TestFactory6 *) new PyTF6(i); }))
-
- .def("get", &TestFactory6::get)
- .def("has_alias", &TestFactory6::has_alias)
-
- .def_static("get_cstats", &ConstructorStats::get, py::return_value_policy::reference)
- .def_static("get_alias_cstats", &ConstructorStats::get, py::return_value_policy::reference)
- ;
-
- // test_init_factory_dual
- // Separate alias constructor testing
- py::class_>(m, "TestFactory7")
- .def(py::init(
- [](int i) { return TestFactory7(i); },
- [](int i) { return PyTF7(i); }))
- .def(py::init(
- [](pointer_tag, int i) { return new TestFactory7(i); },
- [](pointer_tag, int i) { return new PyTF7(i); }))
- .def(py::init(
- [](mixed_tag, int i) { return new TestFactory7(i); },
- [](mixed_tag, int i) { return PyTF7(i); }))
- .def(py::init(
- [](mixed_tag, std::string s) { return TestFactory7((int) s.size()); },
- [](mixed_tag, std::string s) { return new PyTF7((int) s.size()); }))
- .def(py::init(
- [](base_tag, pointer_tag, int i) { return new TestFactory7(i); },
- [](base_tag, pointer_tag, int i) { return (TestFactory7 *) new PyTF7(i); }))
- .def(py::init(
- [](alias_tag, pointer_tag, int i) { return new PyTF7(i); },
- [](alias_tag, pointer_tag, int i) { return new PyTF7(10*i); }))
- .def(py::init(
- [](shared_ptr_tag, base_tag, int i) { return std::make_shared(i); },
- [](shared_ptr_tag, base_tag, int i) { auto *p = new PyTF7(i); return std::shared_ptr(p); }))
- .def(py::init(
- [](shared_ptr_tag, invalid_base_tag, int i) { return std::make_shared(i); },
- [](shared_ptr_tag, invalid_base_tag, int i) { return std::make_shared(i); })) // <-- invalid alias factory
-
- .def("get", &TestFactory7::get)
- .def("has_alias", &TestFactory7::has_alias)
-
- .def_static("get_cstats", &ConstructorStats::get, py::return_value_policy::reference)
- .def_static("get_alias_cstats", &ConstructorStats::get, py::return_value_policy::reference)
- ;
-
- // test_placement_new_alternative
- // Class with a custom new operator but *without* a placement new operator (issue #948)
- class NoPlacementNew {
- public:
- NoPlacementNew(int i) : i(i) { }
- static void *operator new(std::size_t s) {
- auto *p = ::operator new(s);
- py::print("operator new called, returning", reinterpret_cast(p));
- return p;
- }
- static void operator delete(void *p) {
- py::print("operator delete called on", reinterpret_cast(p));
- ::operator delete(p);
- }
- int i;
- };
- // As of 2.2, `py::init` no longer requires placement new
- py::class_(m, "NoPlacementNew")
- .def(py::init())
- .def(py::init([]() { return new NoPlacementNew(100); }))
- .def_readwrite("i", &NoPlacementNew::i)
- ;
-
-
- // test_reallocations
- // Class that has verbose operator_new/operator_delete calls
- struct NoisyAlloc {
- NoisyAlloc(const NoisyAlloc &) = default;
- NoisyAlloc(int i) { py::print(py::str("NoisyAlloc(int {})").format(i)); }
- NoisyAlloc(double d) { py::print(py::str("NoisyAlloc(double {})").format(d)); }
- ~NoisyAlloc() { py::print("~NoisyAlloc()"); }
-
- static void *operator new(size_t s) { py::print("noisy new"); return ::operator new(s); }
- static void *operator new(size_t, void *p) { py::print("noisy placement new"); return p; }
- static void operator delete(void *p, size_t) { py::print("noisy delete"); ::operator delete(p); }
- static void operator delete(void *, void *) { py::print("noisy placement delete"); }
-#if defined(_MSC_VER) && _MSC_VER < 1910
- // MSVC 2015 bug: the above "noisy delete" isn't invoked (fixed in MSVC 2017)
- static void operator delete(void *p) { py::print("noisy delete"); ::operator delete(p); }
-#endif
- };
- py::class_(m, "NoisyAlloc")
- // Since these overloads have the same number of arguments, the dispatcher will try each of
- // them until the arguments convert. Thus we can get a pre-allocation here when passing a
- // single non-integer:
- .def("__init__", [](NoisyAlloc *a, int i) { new (a) NoisyAlloc(i); }) // Regular constructor, runs first, requires preallocation
- .def(py::init([](double d) { return new NoisyAlloc(d); }))
-
- // The two-argument version: first the factory pointer overload.
- .def(py::init([](int i, int) { return new NoisyAlloc(i); }))
- // Return-by-value:
- .def(py::init([](double d, int) { return NoisyAlloc(d); }))
- // Old-style placement new init; requires preallocation
- .def("__init__", [](NoisyAlloc &a, double d, double) { new (&a) NoisyAlloc(d); })
- // Requires deallocation of previous overload preallocated value:
- .def(py::init([](int i, double) { return new NoisyAlloc(i); }))
- // Regular again: requires yet another preallocation
- .def("__init__", [](NoisyAlloc &a, int i, std::string) { new (&a) NoisyAlloc(i); })
- ;
-
-
-
-
- // static_assert testing (the following def's should all fail with appropriate compilation errors):
-#if 0
- struct BadF1Base {};
- struct BadF1 : BadF1Base {};
- struct PyBadF1 : BadF1 {};
- py::class_> bf1(m, "BadF1");
- // wrapped factory function must return a compatible pointer, holder, or value
- bf1.def(py::init([]() { return 3; }));
- // incompatible factory function pointer return type
- bf1.def(py::init([]() { static int three = 3; return &three; }));
- // incompatible factory function std::shared_ptr return type: cannot convert shared_ptr to holder
- // (non-polymorphic base)
- bf1.def(py::init([]() { return std::shared_ptr(new BadF1()); }));
-#endif
-}
diff --git a/spaces/CVPR/LIVE/thrust/thrust/system/cpp/detail/reverse.h b/spaces/CVPR/LIVE/thrust/thrust/system/cpp/detail/reverse.h
deleted file mode 100644
index c6ae90664ad9538e73febfde86c334011de417c8..0000000000000000000000000000000000000000
--- a/spaces/CVPR/LIVE/thrust/thrust/system/cpp/detail/reverse.h
+++ /dev/null
@@ -1,22 +0,0 @@
-/*
- * Copyright 2008-2013 NVIDIA Corporation
- *
- * Licensed under the Apache License, Version 2.0 (the "License");
- * you may not use this file except in compliance with the License.
- * You may obtain a copy of the License at
- *
- * http://www.apache.org/licenses/LICENSE-2.0
- *
- * Unless required by applicable law or agreed to in writing, software
- * distributed under the License is distributed on an "AS IS" BASIS,
- * WITHOUT WARRANTIES OR CONDITIONS OF ANY KIND, either express or implied.
- * See the License for the specific language governing permissions and
- * limitations under the License.
- */
-
-#pragma once
-
-#include
-
-// this system has no special version of this algorithm
-
diff --git a/spaces/CVPR/LIVE/thrust/thrust/system/cuda/detail/transform_scan.h b/spaces/CVPR/LIVE/thrust/thrust/system/cuda/detail/transform_scan.h
deleted file mode 100644
index fbf70b0a748803f61fac623482c349feaf0be86c..0000000000000000000000000000000000000000
--- a/spaces/CVPR/LIVE/thrust/thrust/system/cuda/detail/transform_scan.h
+++ /dev/null
@@ -1,111 +0,0 @@
-/******************************************************************************
- * Copyright (c) 2016, NVIDIA CORPORATION. All rights reserved.
- *
- * Redistribution and use in source and binary forms, with or without
- * modification, are permitted provided that the following conditions are met:
- * * Redistributions of source code must retain the above copyright
- * notice, this list of conditions and the following disclaimer.
- * * Redistributions in binary form must reproduce the above copyright
- * notice, this list of conditions and the following disclaimer in the
- * documentation and/or other materials provided with the distribution.
- * * Neither the name of the NVIDIA CORPORATION nor the
- * names of its contributors may be used to endorse or promote products
- * derived from this software without specific prior written permission.
- *
- * THIS SOFTWARE IS PROVIDED BY THE COPYRIGHT HOLDERS AND CONTRIBUTORS "AS IS"
- * AND ANY EXPRESS OR IMPLIED WARRANTIES, INCLUDING, BUT NOT LIMITED TO, THE
- * IMPLIED WARRANTIES OF MERCHANTABILITY AND FITNESS FOR A PARTICULAR PURPOSE
- * ARE DISCLAIMED. IN NO EVENT SHALL NVIDIA CORPORATION BE LIABLE FOR ANY
- * DIRECT, INDIRECT, INCIDENTAL, SPECIAL, EXEMPLARY, OR CONSEQUENTIAL DAMAGES
- * (INCLUDING, BUT NOT LIMITED TO, PROCUREMENT OF SUBSTITUTE GOODS OR SERVICES;
- * LOSS OF USE, DATA, OR PROFITS; OR BUSINESS INTERRUPTION) HOWEVER CAUSED AND
- * ON ANY THEORY OF LIABILITY, WHETHER IN CONTRACT, STRICT LIABILITY, OR TORT
- * (INCLUDING NEGLIGENCE OR OTHERWISE) ARISING IN ANY WAY OUT OF THE USE OF THIS
- * SOFTWARE, EVEN IF ADVISED OF THE POSSIBILITY OF SUCH DAMAGE.
- *
- ******************************************************************************/
-#pragma once
-
-
-#if THRUST_DEVICE_COMPILER == THRUST_DEVICE_COMPILER_NVCC
-#include
-#include
-#include
-
-namespace thrust
-{
-
-namespace cuda_cub {
-
-template
-OutputIt __host__ __device__
-transform_inclusive_scan(execution_policy &policy,
- InputIt first,
- InputIt last,
- OutputIt result,
- TransformOp transform_op,
- ScanOp scan_op)
-{
- // Use the input iterator's value type per https://wg21.link/P0571
- using input_type = typename thrust::iterator_value::type;
-#if THRUST_CPP_DIALECT < 2017
- using result_type = typename std::result_of::type;
-#else
- using result_type = std::invoke_result_t;
-#endif
-
- typedef typename iterator_traits::difference_type size_type;
- size_type num_items = static_cast(thrust::distance(first, last));
- typedef transform_input_iterator_t
- transformed_iterator_t;
-
- return cuda_cub::inclusive_scan_n(policy,
- transformed_iterator_t(first, transform_op),
- num_items,
- result,
- scan_op);
-}
-
-template
-OutputIt __host__ __device__
-transform_exclusive_scan(execution_policy &policy,
- InputIt first,
- InputIt last,
- OutputIt result,
- TransformOp transform_op,
- InitialValueType init,
- ScanOp scan_op)
-{
- // Use the initial value type per https://wg21.link/P0571
- using result_type = InitialValueType;
-
- typedef typename iterator_traits::difference_type size_type;
- size_type num_items = static_cast(thrust::distance(first, last));
- typedef transform_input_iterator_t
- transformed_iterator_t;
-
- return cuda_cub::exclusive_scan_n(policy,
- transformed_iterator_t(first, transform_op),
- num_items,
- result,
- init,
- scan_op);
-}
-
-} // namespace cuda_cub
-
-} // end namespace thrust
-#endif
diff --git a/spaces/CVPR/LIVE/thrust/thrust/system/detail/generic/logical.h b/spaces/CVPR/LIVE/thrust/thrust/system/detail/generic/logical.h
deleted file mode 100644
index 702dbad852d9e074147368a87b28a082fcfa8242..0000000000000000000000000000000000000000
--- a/spaces/CVPR/LIVE/thrust/thrust/system/detail/generic/logical.h
+++ /dev/null
@@ -1,63 +0,0 @@
-/*
- * Copyright 2008-2013 NVIDIA Corporation
- *
- * Licensed under the Apache License, Version 2.0 (the "License");
- * you may not use this file except in compliance with the License.
- * You may obtain a copy of the License at
- *
- * http://www.apache.org/licenses/LICENSE-2.0
- *
- * Unless required by applicable law or agreed to in writing, software
- * distributed under the License is distributed on an "AS IS" BASIS,
- * WITHOUT WARRANTIES OR CONDITIONS OF ANY KIND, either express or implied.
- * See the License for the specific language governing permissions and
- * limitations under the License.
- */
-
-#pragma once
-
-#include
-#include
-#include
-#include
-#include
-
-namespace thrust
-{
-namespace system
-{
-namespace detail
-{
-namespace generic
-{
-
-
-template
-__host__ __device__
-bool all_of(thrust::execution_policy &exec, InputIterator first, InputIterator last, Predicate pred)
-{
- return thrust::find_if(exec, first, last, thrust::detail::not1(pred)) == last;
-}
-
-
-template
-__host__ __device__
-bool any_of(thrust::execution_policy &exec, InputIterator first, InputIterator last, Predicate pred)
-{
- return thrust::find_if(exec, first, last, pred) != last;
-}
-
-
-template
-__host__ __device__
-bool none_of(thrust::execution_policy &exec, InputIterator first, InputIterator last, Predicate pred)
-{
- return !thrust::any_of(exec, first, last, pred);
-}
-
-
-} // end generic
-} // end detail
-} // end system
-} // end thrust
-
diff --git a/spaces/CVPR/transfiner/app.py b/spaces/CVPR/transfiner/app.py
deleted file mode 100644
index dd714808424ca142bce8cbff56a58182887ecc5c..0000000000000000000000000000000000000000
--- a/spaces/CVPR/transfiner/app.py
+++ /dev/null
@@ -1,84 +0,0 @@
-#try:
-# import detectron2
-#except:
-import os
-os.system('pip install git+https://github.com/SysCV/transfiner.git')
-
-from matplotlib.pyplot import axis
-import gradio as gr
-import requests
-import numpy as np
-from torch import nn
-import requests
-
-import torch
-
-from detectron2 import model_zoo
-from detectron2.engine import DefaultPredictor
-from detectron2.config import get_cfg
-from detectron2.utils.visualizer import Visualizer
-from detectron2.data import MetadataCatalog
-
-
-model_name='./configs/transfiner/mask_rcnn_R_101_FPN_3x_deform.yaml'
-
-
-cfg = get_cfg()
-# add project-specific config (e.g., TensorMask) here if you're not running a model in detectron2's core library
-cfg.merge_from_file(model_name)
-cfg.MODEL.ROI_HEADS.SCORE_THRESH_TEST = 0.5 # set threshold for this model
-cfg.VIS_PERIOD = 100
-# Find a model from detectron2's model zoo. You can use the https://dl.fbaipublicfiles... url as w ell
-#cfg.MODEL.WEIGHTS = './output_3x_transfiner_r50.pth'
-cfg.MODEL.WEIGHTS = './output_3x_transfiner_r101_deform.pth'
-
-if not torch.cuda.is_available():
- cfg.MODEL.DEVICE='cpu'
-
-predictor = DefaultPredictor(cfg)
-
-
-def inference(image):
- width, height = image.size
- if width > 1300:
- ratio = float(height) / float(width)
- width = 1300
- height = int(ratio * width)
- image = image.resize((width, height))
-
- img = np.asarray(image)
-
- #img = np.array(image)
- outputs = predictor(img)
-
- v = Visualizer(img, MetadataCatalog.get(cfg.DATASETS.TRAIN[0]))
- out = v.draw_instance_predictions(outputs["instances"].to("cpu"))
-
- return out.get_image()
-
-
-
-title = "Mask Transfiner [CVPR, 2022]"
-description = "Demo for Mask Transfiner for High-Quality Instance Segmentation, CVPR 2022 based on R101-FPN. To use it, simply upload your image, or click one of the examples to load them. Note that it runs in CPU environment provided by Hugging Face so the processing speed may be slow."
-article = "Mask Transfiner for High-Quality Instance Segmentation, CVPR 2022 | Mask Transfiner Github Code
"
-
-gr.Interface(
- inference,
- [gr.inputs.Image(type="pil", label="Input")],
- gr.outputs.Image(type="numpy", label="Output"),
- title=title,
- description=description,
- article=article,
- examples=[
- ["demo/sample_imgs/000000131444.jpg"],
- ["demo/sample_imgs/000000157365.jpg"],
- ["demo/sample_imgs/000000176037.jpg"],
- ["demo/sample_imgs/000000018737.jpg"],
- ["demo/sample_imgs/000000224200.jpg"],
- ["demo/sample_imgs/000000558073.jpg"],
- ["demo/sample_imgs/000000404922.jpg"],
- ["demo/sample_imgs/000000252776.jpg"],
- ["demo/sample_imgs/000000482477.jpg"],
- ["demo/sample_imgs/000000344909.jpg"]
- ]).launch()
-
diff --git a/spaces/ChenyangSi/FreeU/style.css b/spaces/ChenyangSi/FreeU/style.css
deleted file mode 100644
index af4e23927a03e13fd16ebc7b4eb6eb434c42f65b..0000000000000000000000000000000000000000
--- a/spaces/ChenyangSi/FreeU/style.css
+++ /dev/null
@@ -1,3 +0,0 @@
-h1 {
- text-align: center;
-}
\ No newline at end of file
diff --git a/spaces/CikeyQI/Yunzai/Yunzai/plugins/ws-plugin/apps/help.js b/spaces/CikeyQI/Yunzai/Yunzai/plugins/ws-plugin/apps/help.js
deleted file mode 100644
index 12dca07cfaa7c976b073ff5b4c5b51601953f585..0000000000000000000000000000000000000000
--- a/spaces/CikeyQI/Yunzai/Yunzai/plugins/ws-plugin/apps/help.js
+++ /dev/null
@@ -1,112 +0,0 @@
-import plugin from '../../../lib/plugins/plugin.js'
-import { Render, Version, Config } from '../components/index.js'
-import { helpCfg, helpList } from '../config/system/help_system.js'
-import { style } from '../resources/help/imgs/config.js'
-import _ from 'lodash'
-
-export class setting extends plugin {
- constructor() {
- super({
- name: '[ws-plugin] 帮助',
- dsc: '[ws-plugin] 帮助',
- event: 'message',
- priority: 1,
- rule: [
- {
- reg: '^#ws版本$',
- fnc: 'version'
- },
- {
- reg: '^#ws帮助$',
- fnc: 'help',
- permission: 'master'
- }
- ]
- })
-
- }
- async version(e) {
- return await Render.render('help/version-info', {
- currentVersion: Version.version,
- changelogs: Version.changelogs,
- elem: 'cryo'
- }, { e, scale: 1.2 })
- }
-
- async help(e) {
- let helpGroup = []
- _.forEach(helpList, (group) => {
- _.forEach(group.list, (help) => {
- let icon = help.icon * 1
- if (!icon) {
- help.css = 'display:none'
- } else {
- let x = (icon - 1) % 10
- let y = (icon - x - 1) / 10
- help.css = `background-position:-${x * 50}px -${y * 50}px`
- }
- })
-
- helpGroup.push(group)
- })
-
- let themeData = await getThemeData(helpCfg, helpCfg)
- return await Render.render('help/index', {
- helpCfg,
- helpGroup,
- ...themeData,
- element: 'default'
- }, { e, scale: 1.6 })
- }
-
-}
-
-async function getThemeCfg() {
- let resPath = '{{_res_path}}/help/imgs/'
- return {
- main: `${resPath}/main.png`,
- bg: `${resPath}/bg.jpg`,
- style: style
- }
-}
-
-async function getThemeData(diyStyle, sysStyle) {
- let helpConfig = _.extend({}, sysStyle, diyStyle)
- let colCount = Math.min(5, Math.max(parseInt(helpConfig?.colCount) || 3, 2))
- let colWidth = Math.min(500, Math.max(100, parseInt(helpConfig?.colWidth) || 265))
- let width = Math.min(2500, Math.max(800, colCount * colWidth + 30))
- let theme = await getThemeCfg()
- let themeStyle = theme.style || {}
- let ret = [`
- body{background-image:url(${theme.bg});width:${width}px;}
- .container{background-image:url(${theme.main});width:${width}px;}
- .help-table .td,.help-table .th{width:${100 / colCount}%}
- `]
- let css = function (sel, css, key, def, fn) {
- let val = getDef(themeStyle[key], diyStyle[key], sysStyle[key], def)
- if (fn) {
- val = fn(val)
- }
- ret.push(`${sel}{${css}:${val}}`)
- }
- css('.help-title,.help-group', 'color', 'fontColor', '#ceb78b')
- css('.help-title,.help-group', 'text-shadow', 'fontShadow', 'none')
- css('.help-desc', 'color', 'descColor', '#eee')
- css('.cont-box', 'background', 'contBgColor', 'rgba(43, 52, 61, 0.8)')
- css('.cont-box', 'backdrop-filter', 'contBgBlur', 3, (n) => diyStyle.bgBlur === false ? 'none' : `blur(${n}px)`)
- css('.help-group', 'background', 'headerBgColor', 'rgba(34, 41, 51, .4)')
- css('.help-table .tr:nth-child(odd)', 'background', 'rowBgColor1', 'rgba(34, 41, 51, .2)')
- css('.help-table .tr:nth-child(even)', 'background', 'rowBgColor2', 'rgba(34, 41, 51, .4)')
- return {
- style: ``,
- colCount
- }
-}
-
-function getDef() {
- for (let idx in arguments) {
- if (!_.isUndefined(arguments[idx])) {
- return arguments[idx]
- }
- }
-}
\ No newline at end of file
diff --git a/spaces/CikeyQI/meme-api/meme_generator/version.py b/spaces/CikeyQI/meme-api/meme_generator/version.py
deleted file mode 100644
index 6561790f155f6bfd436e5b19b2f0a1e7f20c0259..0000000000000000000000000000000000000000
--- a/spaces/CikeyQI/meme-api/meme_generator/version.py
+++ /dev/null
@@ -1 +0,0 @@
-__version__ = "0.0.15"
diff --git a/spaces/Cyril666/ContourNet-ABI/maskrcnn_benchmark/data/datasets/evaluation/word/util/feature.py b/spaces/Cyril666/ContourNet-ABI/maskrcnn_benchmark/data/datasets/evaluation/word/util/feature.py
deleted file mode 100644
index 6dd24e0a24459b16e6032bf33d013a1654fc9f41..0000000000000000000000000000000000000000
--- a/spaces/Cyril666/ContourNet-ABI/maskrcnn_benchmark/data/datasets/evaluation/word/util/feature.py
+++ /dev/null
@@ -1,14 +0,0 @@
-# encoding utf-8
-def hog(img, bins =9, pixels_per_cell=(8, 8), cells_per_block=(2, 2), transform_sqrt=False, feature_vector=True):
- """
- Extract hog feature from image.
- See detail at https://github.com/scikit-image/scikit-image/blob/master/skimage/feature/_hog.py
- """
- from skimage.feature import hog
- return hog(img,
- orientations = bins,
- pixels_per_cell = pixels_per_cell,
- cells_per_block = cells_per_block,
- visualise = False,
- transform_sqrt=False,
- feature_vector=True)
diff --git a/spaces/DQChoi/gpt-demo/venv/lib/python3.11/site-packages/contourpy/__init__.py b/spaces/DQChoi/gpt-demo/venv/lib/python3.11/site-packages/contourpy/__init__.py
deleted file mode 100644
index 006d5f5598fbeea4278c60fd5c4be44de19d5e00..0000000000000000000000000000000000000000
--- a/spaces/DQChoi/gpt-demo/venv/lib/python3.11/site-packages/contourpy/__init__.py
+++ /dev/null
@@ -1,253 +0,0 @@
-from __future__ import annotations
-
-from typing import TYPE_CHECKING
-
-import numpy as np
-
-from contourpy._contourpy import (
- ContourGenerator, FillType, LineType, Mpl2005ContourGenerator, Mpl2014ContourGenerator,
- SerialContourGenerator, ThreadedContourGenerator, ZInterp, max_threads,
-)
-from contourpy._version import __version__
-from contourpy.chunk import calc_chunk_sizes
-from contourpy.enum_util import as_fill_type, as_line_type, as_z_interp
-
-if TYPE_CHECKING:
- from typing import Any
-
- from numpy.typing import ArrayLike
-
- from ._contourpy import CoordinateArray, MaskArray
-
-__all__ = [
- "__version__",
- "contour_generator",
- "max_threads",
- "FillType",
- "LineType",
- "ContourGenerator",
- "Mpl2005ContourGenerator",
- "Mpl2014ContourGenerator",
- "SerialContourGenerator",
- "ThreadedContourGenerator",
- "ZInterp",
-]
-
-
-# Simple mapping of algorithm name to class name.
-_class_lookup: dict[str, type[ContourGenerator]] = dict(
- mpl2005=Mpl2005ContourGenerator,
- mpl2014=Mpl2014ContourGenerator,
- serial=SerialContourGenerator,
- threaded=ThreadedContourGenerator,
-)
-
-
-def _remove_z_mask(
- z: ArrayLike | np.ma.MaskedArray[Any, Any] | None,
-) -> tuple[CoordinateArray, MaskArray | None]:
- # Preserve mask if present.
- z_array = np.ma.asarray(z, dtype=np.float64) # type: ignore[no-untyped-call]
- z_masked = np.ma.masked_invalid(z_array, copy=False) # type: ignore[no-untyped-call]
-
- if np.ma.is_masked(z_masked): # type: ignore[no-untyped-call]
- mask = np.ma.getmask(z_masked) # type: ignore[no-untyped-call]
- else:
- mask = None
-
- return np.ma.getdata(z_masked), mask # type: ignore[no-untyped-call]
-
-
-def contour_generator(
- x: ArrayLike | None = None,
- y: ArrayLike | None = None,
- z: ArrayLike | np.ma.MaskedArray[Any, Any] | None = None,
- *,
- name: str = "serial",
- corner_mask: bool | None = None,
- line_type: LineType | str | None = None,
- fill_type: FillType | str | None = None,
- chunk_size: int | tuple[int, int] | None = None,
- chunk_count: int | tuple[int, int] | None = None,
- total_chunk_count: int | None = None,
- quad_as_tri: bool = False,
- z_interp: ZInterp | str | None = ZInterp.Linear,
- thread_count: int = 0,
-) -> ContourGenerator:
- """Create and return a contour generator object.
-
- The class and properties of the contour generator are determined by the function arguments,
- with sensible defaults.
-
- Args:
- x (array-like of shape (ny, nx) or (nx,), optional): The x-coordinates of the ``z`` values.
- May be 2D with the same shape as ``z.shape``, or 1D with length ``nx = z.shape[1]``.
- If not specified are assumed to be ``np.arange(nx)``. Must be ordered monotonically.
- y (array-like of shape (ny, nx) or (ny,), optional): The y-coordinates of the ``z`` values.
- May be 2D with the same shape as ``z.shape``, or 1D with length ``ny = z.shape[0]``.
- If not specified are assumed to be ``np.arange(ny)``. Must be ordered monotonically.
- z (array-like of shape (ny, nx), may be a masked array): The 2D gridded values to calculate
- the contours of. May be a masked array, and any invalid values (``np.inf`` or
- ``np.nan``) will also be masked out.
- name (str): Algorithm name, one of ``"serial"``, ``"threaded"``, ``"mpl2005"`` or
- ``"mpl2014"``, default ``"serial"``.
- corner_mask (bool, optional): Enable/disable corner masking, which only has an effect if
- ``z`` is a masked array. If ``False``, any quad touching a masked point is masked out.
- If ``True``, only the triangular corners of quads nearest these points are always masked
- out, other triangular corners comprising three unmasked points are contoured as usual.
- If not specified, uses the default provided by the algorithm ``name``.
- line_type (LineType, optional): The format of contour line data returned from calls to
- :meth:`~contourpy.ContourGenerator.lines`. If not specified, uses the default provided
- by the algorithm ``name``.
- fill_type (FillType, optional): The format of filled contour data returned from calls to
- :meth:`~contourpy.ContourGenerator.filled`. If not specified, uses the default provided
- by the algorithm ``name``.
- chunk_size (int or tuple(int, int), optional): Chunk size in (y, x) directions, or the same
- size in both directions if only one value is specified.
- chunk_count (int or tuple(int, int), optional): Chunk count in (y, x) directions, or the
- same count in both directions if only one value is specified.
- total_chunk_count (int, optional): Total number of chunks.
- quad_as_tri (bool): Enable/disable treating quads as 4 triangles, default ``False``.
- If ``False``, a contour line within a quad is a straight line between points on two of
- its edges. If ``True``, each full quad is divided into 4 triangles using a virtual point
- at the centre (mean x, y of the corner points) and a contour line is piecewise linear
- within those triangles. Corner-masked triangles are not affected by this setting, only
- full unmasked quads.
- z_interp (ZInterp): How to interpolate ``z`` values when determining where contour lines
- intersect the edges of quads and the ``z`` values of the central points of quads,
- default ``ZInterp.Linear``.
- thread_count (int): Number of threads to use for contour calculation, default 0. Threads can
- only be used with an algorithm ``name`` that supports threads (currently only
- ``name="threaded"``) and there must be at least the same number of chunks as threads.
- If ``thread_count=0`` and ``name="threaded"`` then it uses the maximum number of threads
- as determined by the C++11 call ``std::thread::hardware_concurrency()``. If ``name`` is
- something other than ``"threaded"`` then the ``thread_count`` will be set to ``1``.
-
- Return:
- :class:`~contourpy._contourpy.ContourGenerator`.
-
- Note:
- A maximum of one of ``chunk_size``, ``chunk_count`` and ``total_chunk_count`` may be
- specified.
-
- Warning:
- The ``name="mpl2005"`` algorithm does not implement chunking for contour lines.
- """
- x = np.asarray(x, dtype=np.float64)
- y = np.asarray(y, dtype=np.float64)
- z, mask = _remove_z_mask(z)
-
- # Check arguments: z.
- if z.ndim != 2:
- raise TypeError(f"Input z must be 2D, not {z.ndim}D")
-
- if z.shape[0] < 2 or z.shape[1] < 2:
- raise TypeError(f"Input z must be at least a (2, 2) shaped array, but has shape {z.shape}")
-
- ny, nx = z.shape
-
- # Check arguments: x and y.
- if x.ndim != y.ndim:
- raise TypeError(f"Number of dimensions of x ({x.ndim}) and y ({y.ndim}) do not match")
-
- if x.ndim == 0:
- x = np.arange(nx, dtype=np.float64)
- y = np.arange(ny, dtype=np.float64)
- x, y = np.meshgrid(x, y)
- elif x.ndim == 1:
- if len(x) != nx:
- raise TypeError(f"Length of x ({len(x)}) must match number of columns in z ({nx})")
- if len(y) != ny:
- raise TypeError(f"Length of y ({len(y)}) must match number of rows in z ({ny})")
- x, y = np.meshgrid(x, y)
- elif x.ndim == 2:
- if x.shape != z.shape:
- raise TypeError(f"Shapes of x {x.shape} and z {z.shape} do not match")
- if y.shape != z.shape:
- raise TypeError(f"Shapes of y {y.shape} and z {z.shape} do not match")
- else:
- raise TypeError(f"Inputs x and y must be None, 1D or 2D, not {x.ndim}D")
-
- # Check mask shape just in case.
- if mask is not None and mask.shape != z.shape:
- raise ValueError("If mask is set it must be a 2D array with the same shape as z")
-
- # Check arguments: name.
- if name not in _class_lookup:
- raise ValueError(f"Unrecognised contour generator name: {name}")
-
- # Check arguments: chunk_size, chunk_count and total_chunk_count.
- y_chunk_size, x_chunk_size = calc_chunk_sizes(
- chunk_size, chunk_count, total_chunk_count, ny, nx)
-
- cls = _class_lookup[name]
-
- # Check arguments: corner_mask.
- if corner_mask is None:
- # Set it to default, which is True if the algorithm supports it.
- corner_mask = cls.supports_corner_mask()
- elif corner_mask and not cls.supports_corner_mask():
- raise ValueError(f"{name} contour generator does not support corner_mask=True")
-
- # Check arguments: line_type.
- if line_type is None:
- line_type = cls.default_line_type
- else:
- line_type = as_line_type(line_type)
-
- if not cls.supports_line_type(line_type):
- raise ValueError(f"{name} contour generator does not support line_type {line_type}")
-
- # Check arguments: fill_type.
- if fill_type is None:
- fill_type = cls.default_fill_type
- else:
- fill_type = as_fill_type(fill_type)
-
- if not cls.supports_fill_type(fill_type):
- raise ValueError(f"{name} contour generator does not support fill_type {fill_type}")
-
- # Check arguments: quad_as_tri.
- if quad_as_tri and not cls.supports_quad_as_tri():
- raise ValueError(f"{name} contour generator does not support quad_as_tri=True")
-
- # Check arguments: z_interp.
- if z_interp is None:
- z_interp = ZInterp.Linear
- else:
- z_interp = as_z_interp(z_interp)
-
- if z_interp != ZInterp.Linear and not cls.supports_z_interp():
- raise ValueError(f"{name} contour generator does not support z_interp {z_interp}")
-
- # Check arguments: thread_count.
- if thread_count not in (0, 1) and not cls.supports_threads():
- raise ValueError(f"{name} contour generator does not support thread_count {thread_count}")
-
- # Prepare args and kwargs for contour generator constructor.
- args = [x, y, z, mask]
- kwargs: dict[str, int | bool | LineType | FillType | ZInterp] = {
- "x_chunk_size": x_chunk_size,
- "y_chunk_size": y_chunk_size,
- }
-
- if name not in ("mpl2005", "mpl2014"):
- kwargs["line_type"] = line_type
- kwargs["fill_type"] = fill_type
-
- if cls.supports_corner_mask():
- kwargs["corner_mask"] = corner_mask
-
- if cls.supports_quad_as_tri():
- kwargs["quad_as_tri"] = quad_as_tri
-
- if cls.supports_z_interp():
- kwargs["z_interp"] = z_interp
-
- if cls.supports_threads():
- kwargs["thread_count"] = thread_count
-
- # Create contour generator.
- cont_gen = cls(*args, **kwargs)
-
- return cont_gen
diff --git a/spaces/DQChoi/gpt-demo/venv/lib/python3.11/site-packages/gradio/templates/cdn/assets/index-7f39cecc.js b/spaces/DQChoi/gpt-demo/venv/lib/python3.11/site-packages/gradio/templates/cdn/assets/index-7f39cecc.js
deleted file mode 100644
index 44bdeaca695571ecda2b48901ed0151ef7d4fbdd..0000000000000000000000000000000000000000
--- a/spaces/DQChoi/gpt-demo/venv/lib/python3.11/site-packages/gradio/templates/cdn/assets/index-7f39cecc.js
+++ /dev/null
@@ -1,2 +0,0 @@
-import{E as u,L as v}from"./index-f8ff95a1.js";import{s as k,t,h as S,L as w,i as z,w as x,f as R,a as U,b as _,I as T,x as V}from"./index-3ba00a4a.js";import"./index-1d65707a.js";import"./Blocks-c9e1499d.js";import"./Button-f155035a.js";import"./BlockLabel-66866176.js";import"./Empty-eec13822.js";import"./Copy-9f1657c4.js";import"./Download-daff1959.js";const Y=94,g=1,C=95,Z=96,f=2,$=[9,10,11,12,13,32,133,160,5760,8192,8193,8194,8195,8196,8197,8198,8199,8200,8201,8202,8232,8233,8239,8287,12288],G=58,N=40,X=95,q=91,c=45,E=46,j=35,D=37;function p(e){return e>=65&&e<=90||e>=97&&e<=122||e>=161}function I(e){return e>=48&&e<=57}const B=new u((e,o)=>{for(let r=!1,a=0,O=0;;O++){let{next:l}=e;if(p(l)||l==c||l==X||r&&I(l))!r&&(l!=c||O>0)&&(r=!0),a===O&&l==c&&a++,e.advance();else{r&&e.acceptToken(l==N?C:a==2&&o.canShift(f)?f:Z);break}}}),A=new u(e=>{if($.includes(e.peek(-1))){let{next:o}=e;(p(o)||o==X||o==j||o==E||o==q||o==G||o==c)&&e.acceptToken(Y)}}),F=new u(e=>{if(!$.includes(e.peek(-1))){let{next:o}=e;if(o==D&&(e.advance(),e.acceptToken(g)),p(o)){do e.advance();while(p(e.next));e.acceptToken(g)}}}),L=k({"AtKeyword import charset namespace keyframes media supports":t.definitionKeyword,"from to selector":t.keyword,NamespaceName:t.namespace,KeyframeName:t.labelName,TagName:t.tagName,ClassName:t.className,PseudoClassName:t.constant(t.className),IdName:t.labelName,"FeatureName PropertyName":t.propertyName,AttributeName:t.attributeName,NumberLiteral:t.number,KeywordQuery:t.keyword,UnaryQueryOp:t.operatorKeyword,"CallTag ValueName":t.atom,VariableName:t.variableName,Callee:t.operatorKeyword,Unit:t.unit,"UniversalSelector NestingSelector":t.definitionOperator,MatchOp:t.compareOperator,"ChildOp SiblingOp, LogicOp":t.logicOperator,BinOp:t.arithmeticOperator,Important:t.modifier,Comment:t.blockComment,ParenthesizedContent:t.special(t.name),ColorLiteral:t.color,StringLiteral:t.string,":":t.punctuation,"PseudoOp #":t.derefOperator,"; ,":t.separator,"( )":t.paren,"[ ]":t.squareBracket,"{ }":t.brace}),K={__proto__:null,lang:32,"nth-child":32,"nth-last-child":32,"nth-of-type":32,"nth-last-of-type":32,dir:32,"host-context":32,url:60,"url-prefix":60,domain:60,regexp:60,selector:134},J={__proto__:null,"@import":114,"@media":138,"@charset":142,"@namespace":146,"@keyframes":152,"@supports":164},H={__proto__:null,not:128,only:128,from:158,to:160},M=v.deserialize({version:14,states:"7WQYQ[OOO#_Q[OOOOQP'#Cd'#CdOOQP'#Cc'#CcO#fQ[O'#CfO$YQXO'#CaO$aQ[O'#ChO$lQ[O'#DPO$qQ[O'#DTOOQP'#Ed'#EdO$vQdO'#DeO%bQ[O'#DrO$vQdO'#DtO%sQ[O'#DvO&OQ[O'#DyO&TQ[O'#EPO&cQ[O'#EROOQS'#Ec'#EcOOQS'#ET'#ETQYQ[OOO&jQXO'#CdO'_QWO'#DaO'dQWO'#EjO'oQ[O'#EjQOQWOOOOQP'#Cg'#CgOOQP,59Q,59QO#fQ[O,59QO'yQ[O'#EWO(eQWO,58{O(mQ[O,59SO$lQ[O,59kO$qQ[O,59oO'yQ[O,59sO'yQ[O,59uO'yQ[O,59vO(xQ[O'#D`OOQS,58{,58{OOQP'#Ck'#CkOOQO'#C}'#C}OOQP,59S,59SO)PQWO,59SO)UQWO,59SOOQP'#DR'#DROOQP,59k,59kOOQO'#DV'#DVO)ZQ`O,59oOOQS'#Cp'#CpO$vQdO'#CqO)cQvO'#CsO*pQtO,5:POOQO'#Cx'#CxO)UQWO'#CwO+UQWO'#CyOOQS'#Eg'#EgOOQO'#Dh'#DhO+ZQ[O'#DoO+iQWO'#EkO&TQ[O'#DmO+wQWO'#DpOOQO'#El'#ElO(hQWO,5:^O+|QpO,5:`OOQS'#Dx'#DxO,UQWO,5:bO,ZQ[O,5:bOOQO'#D{'#D{O,cQWO,5:eO,hQWO,5:kO,pQWO,5:mOOQS-E8R-E8RO$vQdO,59{O,xQ[O'#EYO-VQWO,5;UO-VQWO,5;UOOQP1G.l1G.lO-|QXO,5:rOOQO-E8U-E8UOOQS1G.g1G.gOOQP1G.n1G.nO)PQWO1G.nO)UQWO1G.nOOQP1G/V1G/VO.ZQ`O1G/ZO.tQXO1G/_O/[QXO1G/aO/rQXO1G/bO0YQWO,59zO0_Q[O'#DOO0fQdO'#CoOOQP1G/Z1G/ZO$vQdO1G/ZO0mQpO,59]OOQS,59_,59_O$vQdO,59aO0uQWO1G/kOOQS,59c,59cO0zQ!bO,59eO1SQWO'#DhO1_QWO,5:TO1dQWO,5:ZO&TQ[O,5:VO&TQ[O'#EZO1lQWO,5;VO1wQWO,5:XO'yQ[O,5:[OOQS1G/x1G/xOOQS1G/z1G/zOOQS1G/|1G/|O2YQWO1G/|O2_QdO'#D|OOQS1G0P1G0POOQS1G0V1G0VOOQS1G0X1G0XO2mQtO1G/gOOQO,5:t,5:tO3TQ[O,5:tOOQO-E8W-E8WO3bQWO1G0pOOQP7+$Y7+$YOOQP7+$u7+$uO$vQdO7+$uOOQS1G/f1G/fO3mQXO'#EiO3tQWO,59jO3yQtO'#EUO4nQdO'#EfO4xQWO,59ZO4}QpO7+$uOOQS1G.w1G.wOOQS1G.{1G.{OOQS7+%V7+%VO5VQWO1G/PO$vQdO1G/oOOQO1G/u1G/uOOQO1G/q1G/qO5[QWO,5:uOOQO-E8X-E8XO5jQXO1G/vOOQS7+%h7+%hO5qQYO'#CsO(hQWO'#E[O5yQdO,5:hOOQS,5:h,5:hO6XQtO'#EXO$vQdO'#EXO7VQdO7+%ROOQO7+%R7+%ROOQO1G0`1G0`O7jQpO<T![;'S%^;'S;=`%o<%lO%^^;TUoWOy%^z!Q%^!Q![;g![;'S%^;'S;=`%o<%lO%^^;nYoW#[UOy%^z!Q%^!Q![;g![!g%^!g!h<^!h#X%^#X#Y<^#Y;'S%^;'S;=`%o<%lO%^^[[oW#[UOy%^z!O%^!O!P;g!P!Q%^!Q![>T![!g%^!g!h<^!h#X%^#X#Y<^#Y;'S%^;'S;=`%o<%lO%^_?VSpVOy%^z;'S%^;'S;=`%o<%lO%^^?hWjSOy%^z!O%^!O!P;O!P!Q%^!Q![>T![;'S%^;'S;=`%o<%lO%^_@VU#XPOy%^z!Q%^!Q![;g![;'S%^;'S;=`%o<%lO%^~@nTjSOy%^z{@}{;'S%^;'S;=`%o<%lO%^~ASUoWOy@}yzAfz{Bm{;'S@};'S;=`Co<%lO@}~AiTOzAfz{Ax{;'SAf;'S;=`Bg<%lOAf~A{VOzAfz{Ax{!PAf!P!QBb!Q;'SAf;'S;=`Bg<%lOAf~BgOR~~BjP;=`<%lAf~BrWoWOy@}yzAfz{Bm{!P@}!P!QC[!Q;'S@};'S;=`Co<%lO@}~CcSoWR~Oy%^z;'S%^;'S;=`%o<%lO%^~CrP;=`<%l@}^Cz[#[UOy%^z!O%^!O!P;g!P!Q%^!Q![>T![!g%^!g!h<^!h#X%^#X#Y<^#Y;'S%^;'S;=`%o<%lO%^XDuU]POy%^z![%^![!]EX!];'S%^;'S;=`%o<%lO%^XE`S^PoWOy%^z;'S%^;'S;=`%o<%lO%^_EqS!WVOy%^z;'S%^;'S;=`%o<%lO%^YFSSzQOy%^z;'S%^;'S;=`%o<%lO%^XFeU|POy%^z!`%^!`!aFw!a;'S%^;'S;=`%o<%lO%^XGOS|PoWOy%^z;'S%^;'S;=`%o<%lO%^XG_WOy%^z!c%^!c!}Gw!}#T%^#T#oGw#o;'S%^;'S;=`%o<%lO%^XHO[!YPoWOy%^z}%^}!OGw!O!Q%^!Q![Gw![!c%^!c!}Gw!}#T%^#T#oGw#o;'S%^;'S;=`%o<%lO%^XHySxPOy%^z;'S%^;'S;=`%o<%lO%^^I[SvUOy%^z;'S%^;'S;=`%o<%lO%^XIkUOy%^z#b%^#b#cI}#c;'S%^;'S;=`%o<%lO%^XJSUoWOy%^z#W%^#W#XJf#X;'S%^;'S;=`%o<%lO%^XJmS!`PoWOy%^z;'S%^;'S;=`%o<%lO%^XJ|UOy%^z#f%^#f#gJf#g;'S%^;'S;=`%o<%lO%^XKeS!RPOy%^z;'S%^;'S;=`%o<%lO%^_KvS!QVOy%^z;'S%^;'S;=`%o<%lO%^ZLXU!PPOy%^z!_%^!_!`6y!`;'S%^;'S;=`%o<%lO%^WLnP;=`<%l$}",tokenizers:[A,F,B,0,1,2,3],topRules:{StyleSheet:[0,4],Styles:[1,84]},specialized:[{term:95,get:e=>K[e]||-1},{term:56,get:e=>J[e]||-1},{term:96,get:e=>H[e]||-1}],tokenPrec:1123});let Q=null;function m(){if(!Q&&typeof document=="object"&&document.body){let{style:e}=document.body,o=[],r=new Set;for(let a in e)a!="cssText"&&a!="cssFloat"&&typeof e[a]=="string"&&(/[A-Z]/.test(a)&&(a=a.replace(/[A-Z]/g,O=>"-"+O.toLowerCase())),r.has(a)||(o.push(a),r.add(a)));Q=o.sort().map(a=>({type:"property",label:a}))}return Q||[]}const h=["active","after","any-link","autofill","backdrop","before","checked","cue","default","defined","disabled","empty","enabled","file-selector-button","first","first-child","first-letter","first-line","first-of-type","focus","focus-visible","focus-within","fullscreen","has","host","host-context","hover","in-range","indeterminate","invalid","is","lang","last-child","last-of-type","left","link","marker","modal","not","nth-child","nth-last-child","nth-last-of-type","nth-of-type","only-child","only-of-type","optional","out-of-range","part","placeholder","placeholder-shown","read-only","read-write","required","right","root","scope","selection","slotted","target","target-text","valid","visited","where"].map(e=>({type:"class",label:e})),b=["above","absolute","activeborder","additive","activecaption","after-white-space","ahead","alias","all","all-scroll","alphabetic","alternate","always","antialiased","appworkspace","asterisks","attr","auto","auto-flow","avoid","avoid-column","avoid-page","avoid-region","axis-pan","background","backwards","baseline","below","bidi-override","blink","block","block-axis","bold","bolder","border","border-box","both","bottom","break","break-all","break-word","bullets","button","button-bevel","buttonface","buttonhighlight","buttonshadow","buttontext","calc","capitalize","caps-lock-indicator","caption","captiontext","caret","cell","center","checkbox","circle","cjk-decimal","clear","clip","close-quote","col-resize","collapse","color","color-burn","color-dodge","column","column-reverse","compact","condensed","contain","content","contents","content-box","context-menu","continuous","copy","counter","counters","cover","crop","cross","crosshair","currentcolor","cursive","cyclic","darken","dashed","decimal","decimal-leading-zero","default","default-button","dense","destination-atop","destination-in","destination-out","destination-over","difference","disc","discard","disclosure-closed","disclosure-open","document","dot-dash","dot-dot-dash","dotted","double","down","e-resize","ease","ease-in","ease-in-out","ease-out","element","ellipse","ellipsis","embed","end","ethiopic-abegede-gez","ethiopic-halehame-aa-er","ethiopic-halehame-gez","ew-resize","exclusion","expanded","extends","extra-condensed","extra-expanded","fantasy","fast","fill","fill-box","fixed","flat","flex","flex-end","flex-start","footnotes","forwards","from","geometricPrecision","graytext","grid","groove","hand","hard-light","help","hidden","hide","higher","highlight","highlighttext","horizontal","hsl","hsla","hue","icon","ignore","inactiveborder","inactivecaption","inactivecaptiontext","infinite","infobackground","infotext","inherit","initial","inline","inline-axis","inline-block","inline-flex","inline-grid","inline-table","inset","inside","intrinsic","invert","italic","justify","keep-all","landscape","large","larger","left","level","lighter","lighten","line-through","linear","linear-gradient","lines","list-item","listbox","listitem","local","logical","loud","lower","lower-hexadecimal","lower-latin","lower-norwegian","lowercase","ltr","luminosity","manipulation","match","matrix","matrix3d","medium","menu","menutext","message-box","middle","min-intrinsic","mix","monospace","move","multiple","multiple_mask_images","multiply","n-resize","narrower","ne-resize","nesw-resize","no-close-quote","no-drop","no-open-quote","no-repeat","none","normal","not-allowed","nowrap","ns-resize","numbers","numeric","nw-resize","nwse-resize","oblique","opacity","open-quote","optimizeLegibility","optimizeSpeed","outset","outside","outside-shape","overlay","overline","padding","padding-box","painted","page","paused","perspective","pinch-zoom","plus-darker","plus-lighter","pointer","polygon","portrait","pre","pre-line","pre-wrap","preserve-3d","progress","push-button","radial-gradient","radio","read-only","read-write","read-write-plaintext-only","rectangle","region","relative","repeat","repeating-linear-gradient","repeating-radial-gradient","repeat-x","repeat-y","reset","reverse","rgb","rgba","ridge","right","rotate","rotate3d","rotateX","rotateY","rotateZ","round","row","row-resize","row-reverse","rtl","run-in","running","s-resize","sans-serif","saturation","scale","scale3d","scaleX","scaleY","scaleZ","screen","scroll","scrollbar","scroll-position","se-resize","self-start","self-end","semi-condensed","semi-expanded","separate","serif","show","single","skew","skewX","skewY","skip-white-space","slide","slider-horizontal","slider-vertical","sliderthumb-horizontal","sliderthumb-vertical","slow","small","small-caps","small-caption","smaller","soft-light","solid","source-atop","source-in","source-out","source-over","space","space-around","space-between","space-evenly","spell-out","square","start","static","status-bar","stretch","stroke","stroke-box","sub","subpixel-antialiased","svg_masks","super","sw-resize","symbolic","symbols","system-ui","table","table-caption","table-cell","table-column","table-column-group","table-footer-group","table-header-group","table-row","table-row-group","text","text-bottom","text-top","textarea","textfield","thick","thin","threeddarkshadow","threedface","threedhighlight","threedlightshadow","threedshadow","to","top","transform","translate","translate3d","translateX","translateY","translateZ","transparent","ultra-condensed","ultra-expanded","underline","unidirectional-pan","unset","up","upper-latin","uppercase","url","var","vertical","vertical-text","view-box","visible","visibleFill","visiblePainted","visibleStroke","visual","w-resize","wait","wave","wider","window","windowframe","windowtext","words","wrap","wrap-reverse","x-large","x-small","xor","xx-large","xx-small"].map(e=>({type:"keyword",label:e})).concat(["aliceblue","antiquewhite","aqua","aquamarine","azure","beige","bisque","black","blanchedalmond","blue","blueviolet","brown","burlywood","cadetblue","chartreuse","chocolate","coral","cornflowerblue","cornsilk","crimson","cyan","darkblue","darkcyan","darkgoldenrod","darkgray","darkgreen","darkkhaki","darkmagenta","darkolivegreen","darkorange","darkorchid","darkred","darksalmon","darkseagreen","darkslateblue","darkslategray","darkturquoise","darkviolet","deeppink","deepskyblue","dimgray","dodgerblue","firebrick","floralwhite","forestgreen","fuchsia","gainsboro","ghostwhite","gold","goldenrod","gray","grey","green","greenyellow","honeydew","hotpink","indianred","indigo","ivory","khaki","lavender","lavenderblush","lawngreen","lemonchiffon","lightblue","lightcoral","lightcyan","lightgoldenrodyellow","lightgray","lightgreen","lightpink","lightsalmon","lightseagreen","lightskyblue","lightslategray","lightsteelblue","lightyellow","lime","limegreen","linen","magenta","maroon","mediumaquamarine","mediumblue","mediumorchid","mediumpurple","mediumseagreen","mediumslateblue","mediumspringgreen","mediumturquoise","mediumvioletred","midnightblue","mintcream","mistyrose","moccasin","navajowhite","navy","oldlace","olive","olivedrab","orange","orangered","orchid","palegoldenrod","palegreen","paleturquoise","palevioletred","papayawhip","peachpuff","peru","pink","plum","powderblue","purple","rebeccapurple","red","rosybrown","royalblue","saddlebrown","salmon","sandybrown","seagreen","seashell","sienna","silver","skyblue","slateblue","slategray","snow","springgreen","steelblue","tan","teal","thistle","tomato","turquoise","violet","wheat","white","whitesmoke","yellow","yellowgreen"].map(e=>({type:"constant",label:e}))),ee=["a","abbr","address","article","aside","b","bdi","bdo","blockquote","body","br","button","canvas","caption","cite","code","col","colgroup","dd","del","details","dfn","dialog","div","dl","dt","em","figcaption","figure","footer","form","header","hgroup","h1","h2","h3","h4","h5","h6","hr","html","i","iframe","img","input","ins","kbd","label","legend","li","main","meter","nav","ol","output","p","pre","ruby","section","select","small","source","span","strong","sub","summary","sup","table","tbody","td","template","textarea","tfoot","th","thead","tr","u","ul"].map(e=>({type:"type",label:e})),n=/^(\w[\w-]*|-\w[\w-]*|)$/,ae=/^-(-[\w-]*)?$/;function Oe(e,o){var r;if((e.name=="("||e.type.isError)&&(e=e.parent||e),e.name!="ArgList")return!1;let a=(r=e.parent)===null||r===void 0?void 0:r.firstChild;return a?.name!="Callee"?!1:o.sliceString(a.from,a.to)=="var"}const y=new V,te=["Declaration"];function W(e,o){if(o.to-o.from>4096){let r=y.get(o);if(r)return r;let a=[],O=new Set,l=o.cursor(T.IncludeAnonymous);if(l.firstChild())do for(let i of W(e,l.node))O.has(i.label)||(O.add(i.label),a.push(i));while(l.nextSibling());return y.set(o,a),a}else{let r=[],a=new Set;return o.cursor().iterate(O=>{var l;if(O.name=="VariableName"&&O.matchContext(te)&&((l=O.node.nextSibling)===null||l===void 0?void 0:l.name)==":"){let i=e.sliceString(O.from,O.to);a.has(i)||(a.add(i),r.push({label:i,type:"variable"}))}}),r}}const oe=e=>{var o;let{state:r,pos:a}=e,O=S(r).resolveInner(a,-1),l=O.type.isError&&O.from==O.to-1&&r.doc.sliceString(O.from,O.to)=="-";if(O.name=="PropertyName"||l&&((o=O.parent)===null||o===void 0?void 0:o.name)=="Block")return{from:O.from,options:m(),validFor:n};if(O.name=="ValueName")return{from:O.from,options:b,validFor:n};if(O.name=="PseudoClassName")return{from:O.from,options:h,validFor:n};if(O.name=="VariableName"||(e.explicit||l)&&Oe(O,r.doc))return{from:O.name=="VariableName"?O.from:a,options:W(r.doc,S(r).topNode),validFor:ae};if(O.name=="TagName"){for(let{parent:d}=O;d;d=d.parent)if(d.name=="Block")return{from:O.from,options:m(),validFor:n};return{from:O.from,options:ee,validFor:n}}if(!e.explicit)return null;let i=O.resolve(a),s=i.childBefore(a);return s&&s.name==":"&&i.name=="PseudoClassSelector"?{from:a,options:h,validFor:n}:s&&s.name==":"&&i.name=="Declaration"||i.name=="ArgList"?{from:a,options:b,validFor:n}:i.name=="Block"?{from:a,options:m(),validFor:n}:null},P=w.define({name:"css",parser:M.configure({props:[z.add({Declaration:x()}),R.add({Block:U})]}),languageData:{commentTokens:{block:{open:"/*",close:"*/"}},indentOnInput:/^\s*\}$/,wordChars:"-"}});function me(){return new _(P,P.data.of({autocomplete:oe}))}export{me as css,oe as cssCompletionSource,P as cssLanguage};
-//# sourceMappingURL=index-7f39cecc.js.map
diff --git a/spaces/DQChoi/gpt-demo/venv/lib/python3.11/site-packages/gradio/themes/builder_app.py b/spaces/DQChoi/gpt-demo/venv/lib/python3.11/site-packages/gradio/themes/builder_app.py
deleted file mode 100644
index 54defcf0d5c6620d282480693791c69dde0833da..0000000000000000000000000000000000000000
--- a/spaces/DQChoi/gpt-demo/venv/lib/python3.11/site-packages/gradio/themes/builder_app.py
+++ /dev/null
@@ -1,1003 +0,0 @@
-import inspect
-import time
-from typing import Iterable
-
-from gradio_client.documentation import document_fn
-
-import gradio as gr
-
-themes = [
- gr.themes.Base,
- gr.themes.Default,
- gr.themes.Soft,
- gr.themes.Monochrome,
- gr.themes.Glass,
-]
-colors = gr.themes.Color.all
-sizes = gr.themes.Size.all
-
-palette_range = [50, 100, 200, 300, 400, 500, 600, 700, 800, 900, 950]
-size_range = ["xxs", "xs", "sm", "md", "lg", "xl", "xxl"]
-docs_theme_core = document_fn(gr.themes.Base.__init__, gr.themes.Base)[1]
-docs_theme_vars = document_fn(gr.themes.Base.set, gr.themes.Base)[1]
-
-
-def get_docstr(var):
- for parameters in docs_theme_core + docs_theme_vars:
- if parameters["name"] == var:
- return parameters["doc"]
- raise ValueError(f"Variable {var} not found in theme documentation.")
-
-
-def get_doc_theme_var_groups():
- source = inspect.getsource(gr.themes.Base.set)
- groups = []
- group, desc, variables, flat_variables = None, None, [], []
- for line in source.splitlines():
- line = line.strip()
- if line.startswith(")"):
- break
- elif line.startswith("# "):
- if group is not None:
- groups.append((group, desc, variables))
- group, desc = line[2:].split(": ")
- variables = []
- elif "=" in line:
- var = line.split("=")[0]
- variables.append(var)
- flat_variables.append(var)
- groups.append((group, desc, variables))
- return groups, flat_variables
-
-
-variable_groups, flat_variables = get_doc_theme_var_groups()
-
-css = """
-.gradio-container {
- overflow: visible !important;
- max-width: none !important;
-}
-#controls {
- max-height: 100vh;
- flex-wrap: unset;
- overflow-y: scroll;
- position: sticky;
- top: 0;
-}
-#controls::-webkit-scrollbar {
- -webkit-appearance: none;
- width: 7px;
-}
-
-#controls::-webkit-scrollbar-thumb {
- border-radius: 4px;
- background-color: rgba(0, 0, 0, .5);
- box-shadow: 0 0 1px rgba(255, 255, 255, .5);
-}
-"""
-
-with gr.Blocks( # noqa: SIM117
- theme=gr.themes.Base(),
- css=css,
- title="Gradio Theme Builder",
-) as demo:
- with gr.Row():
- with gr.Column(scale=1, elem_id="controls", min_width=400):
- with gr.Row():
- undo_btn = gr.Button("Undo", size="sm")
- dark_mode_btn = gr.Button("Dark Mode", variant="primary", size="sm")
- with gr.Tabs():
- with gr.TabItem("Source Theme"):
- gr.Markdown(
- """
- Select a base theme below you would like to build off of. Note: when you click 'Load Theme', all variable values in other tabs will be overwritten!
- """
- )
- base_theme_dropdown = gr.Dropdown(
- [theme.__name__ for theme in themes],
- value="Base",
- show_label=False,
- )
- load_theme_btn = gr.Button("Load Theme", elem_id="load_theme")
- with gr.TabItem("Core Colors"):
- gr.Markdown(
- """Set the three hues of the theme: `primary_hue`, `secondary_hue`, and `neutral_hue`.
- Each of these is a palette ranging from 50 to 950 in brightness. Pick a preset palette - optionally, open the accordion to overwrite specific values.
- Note that these variables do not affect elements directly, but are referenced by other variables with asterisks, such as `*primary_200` or `*neutral_950`."""
- )
- primary_hue = gr.Dropdown(
- [color.name for color in colors], label="Primary Hue"
- )
- with gr.Accordion(label="Primary Hue Palette", open=False):
- primary_hues = []
- for i in palette_range:
- primary_hues.append(
- gr.ColorPicker(
- label=f"primary_{i}",
- )
- )
-
- secondary_hue = gr.Dropdown(
- [color.name for color in colors], label="Secondary Hue"
- )
- with gr.Accordion(label="Secondary Hue Palette", open=False):
- secondary_hues = []
- for i in palette_range:
- secondary_hues.append(
- gr.ColorPicker(
- label=f"secondary_{i}",
- )
- )
-
- neutral_hue = gr.Dropdown(
- [color.name for color in colors], label="Neutral hue"
- )
- with gr.Accordion(label="Neutral Hue Palette", open=False):
- neutral_hues = []
- for i in palette_range:
- neutral_hues.append(
- gr.ColorPicker(
- label=f"neutral_{i}",
- )
- )
-
- with gr.TabItem("Core Sizing"):
- gr.Markdown(
- """Set the sizing of the theme via: `text_size`, `spacing_size`, and `radius_size`.
- Each of these is set to a collection of sizes ranging from `xxs` to `xxl`. Pick a preset size collection - optionally, open the accordion to overwrite specific values.
- Note that these variables do not affect elements directly, but are referenced by other variables with asterisks, such as `*spacing_xl` or `*text_sm`.
- """
- )
- text_size = gr.Dropdown(
- [size.name for size in sizes if size.name.startswith("text_")],
- label="Text Size",
- )
- with gr.Accordion(label="Text Size Range", open=False):
- text_sizes = []
- for i in size_range:
- text_sizes.append(
- gr.Textbox(
- label=f"text_{i}",
- )
- )
-
- spacing_size = gr.Dropdown(
- [
- size.name
- for size in sizes
- if size.name.startswith("spacing_")
- ],
- label="Spacing Size",
- )
- with gr.Accordion(label="Spacing Size Range", open=False):
- spacing_sizes = []
- for i in size_range:
- spacing_sizes.append(
- gr.Textbox(
- label=f"spacing_{i}",
- )
- )
-
- radius_size = gr.Dropdown(
- [
- size.name
- for size in sizes
- if size.name.startswith("radius_")
- ],
- label="Radius Size",
- )
- with gr.Accordion(label="Radius Size Range", open=False):
- radius_sizes = []
- for i in size_range:
- radius_sizes.append(
- gr.Textbox(
- label=f"radius_{i}",
- )
- )
-
- with gr.TabItem("Core Fonts"):
- gr.Markdown(
- """Set the main `font` and the monospace `font_mono` here.
- Set up to 4 values for each (fallbacks in case a font is not available).
- Check "Google Font" if font should be loaded from Google Fonts.
- """
- )
- gr.Markdown("### Main Font")
- main_fonts, main_is_google = [], []
- for i in range(4):
- with gr.Row():
- font = gr.Textbox(label=f"Font {i + 1}")
- font_is_google = gr.Checkbox(label="Google Font")
- main_fonts.append(font)
- main_is_google.append(font_is_google)
-
- mono_fonts, mono_is_google = [], []
- gr.Markdown("### Monospace Font")
- for i in range(4):
- with gr.Row():
- font = gr.Textbox(label=f"Font {i + 1}")
- font_is_google = gr.Checkbox(label="Google Font")
- mono_fonts.append(font)
- mono_is_google.append(font_is_google)
-
- theme_var_input = []
-
- core_color_suggestions = (
- [f"*primary_{i}" for i in palette_range]
- + [f"*secondary_{i}" for i in palette_range]
- + [f"*neutral_{i}" for i in palette_range]
- )
-
- variable_suggestions = {
- "fill": core_color_suggestions[:],
- "color": core_color_suggestions[:],
- "text_size": [f"*text_{i}" for i in size_range],
- "radius": [f"*radius_{i}" for i in size_range],
- "padding": [f"*spacing_{i}" for i in size_range],
- "gap": [f"*spacing_{i}" for i in size_range],
- "weight": [
- "100",
- "200",
- "300",
- "400",
- "500",
- "600",
- "700",
- "800",
- ],
- "shadow": ["none"],
- "border_width": [],
- }
- for variable in flat_variables:
- if variable.endswith("_dark"):
- continue
- for style_type in variable_suggestions:
- if style_type in variable:
- variable_suggestions[style_type].append("*" + variable)
- break
-
- variable_suggestions["fill"], variable_suggestions["color"] = (
- variable_suggestions["fill"]
- + variable_suggestions["color"][len(core_color_suggestions) :],
- variable_suggestions["color"]
- + variable_suggestions["fill"][len(core_color_suggestions) :],
- )
-
- for group, desc, variables in variable_groups:
- with gr.TabItem(group):
- gr.Markdown(
- desc
- + "\nYou can set these to one of the dropdown values, or clear the dropdown to set a custom value."
- )
- for variable in variables:
- suggestions = []
- for style_type in variable_suggestions:
- if style_type in variable:
- suggestions = variable_suggestions[style_type][:]
- if "*" + variable in suggestions:
- suggestions.remove("*" + variable)
- break
- dropdown = gr.Dropdown(
- label=variable,
- info=get_docstr(variable),
- choices=suggestions,
- allow_custom_value=True,
- )
- theme_var_input.append(dropdown)
-
- # App
-
- with gr.Column(scale=6, elem_id="app"):
- with gr.Column(variant="panel"):
- gr.Markdown(
- """
- # Theme Builder
- Welcome to the theme builder. The left panel is where you create the theme. The different aspects of the theme are broken down into different tabs. Here's how to navigate them:
- 1. First, set the "Source Theme". This will set the default values that you can override.
- 2. Set the "Core Colors", "Core Sizing" and "Core Fonts". These are the core variables that are used to build the rest of the theme.
- 3. The rest of the tabs set specific CSS theme variables. These control finer aspects of the UI. Within these theme variables, you can reference the core variables and other theme variables using the variable name preceded by an asterisk, e.g. `*primary_50` or `*body_text_color`. Clear the dropdown to set a custom value.
- 4. Once you have finished your theme, click on "View Code" below to see how you can integrate the theme into your app. You can also click on "Upload to Hub" to upload your theme to the Hugging Face Hub, where others can download and use your theme.
- """
- )
- with gr.Accordion("View Code", open=False):
- output_code = gr.Code(language="python")
- with gr.Accordion("Upload to Hub", open=False):
- gr.Markdown(
- "You can save your theme on the Hugging Face Hub. HF API write token can be found [here](https://huggingface.co/settings/tokens)."
- )
- with gr.Row():
- theme_name = gr.Textbox(label="Theme Name")
- theme_hf_token = gr.Textbox(label="Hugging Face Write Token")
- theme_version = gr.Textbox(
- label="Version",
- placeholder="Leave blank to automatically update version.",
- )
- upload_to_hub_btn = gr.Button("Upload to Hub")
- theme_upload_status = gr.Markdown(visible=False)
-
- gr.Markdown("Below this panel is a dummy app to demo your theme.")
-
- name = gr.Textbox(
- label="Name",
- info="Full name, including middle name. No special characters.",
- placeholder="John Doe",
- value="John Doe",
- interactive=True,
- )
-
- with gr.Row():
- slider1 = gr.Slider(label="Slider 1")
- slider2 = gr.Slider(label="Slider 2")
- gr.CheckboxGroup(["A", "B", "C"], label="Checkbox Group")
-
- with gr.Row():
- with gr.Column(variant="panel", scale=1):
- gr.Markdown("## Panel 1")
- radio = gr.Radio(
- ["A", "B", "C"],
- label="Radio",
- info="Lorem ipsum dolor sit amet, consectetur adipiscing elit, sed do eiusmod tempor incididunt ut labore et dolore magna aliqua. Ut enim ad minim veniam, quis nostrud exercitation ullamco laboris nisi ut aliquip ex ea commodo consequat.",
- )
- drop = gr.Dropdown(
- ["Option 1", "Option 2", "Option 3"], show_label=False
- )
- drop_2 = gr.Dropdown(
- ["Option A", "Option B", "Option C"],
- multiselect=True,
- value=["Option A"],
- label="Dropdown",
- interactive=True,
- )
- check = gr.Checkbox(label="Go")
- with gr.Column(variant="panel", scale=2):
- img = gr.Image(
- "https://raw.githubusercontent.com/gradio-app/gradio/main/js/_website/src/assets/img/header-image.jpg",
- label="Image",
- height=320,
- )
- with gr.Row():
- go_btn = gr.Button(
- "Go", label="Primary Button", variant="primary"
- )
- clear_btn = gr.Button(
- "Clear", label="Secondary Button", variant="secondary"
- )
-
- def go(*args):
- time.sleep(3)
- return "https://raw.githubusercontent.com/gradio-app/gradio/main/js/_website/src/assets/img/header-image.jpg"
-
- go_btn.click(
- go,
- [radio, drop, drop_2, check, name],
- img,
- api_name=False,
- )
-
- def clear():
- time.sleep(0.2)
- return None
-
- clear_btn.click(clear, None, img)
-
- with gr.Row():
- btn1 = gr.Button("Button 1", size="sm")
- btn2 = gr.UploadButton(size="sm")
- stop_btn = gr.Button(
- "Stop", label="Stop Button", variant="stop", size="sm"
- )
-
- gr.Examples(
- examples=[
- [
- "A",
- "Option 1",
- ["Option B"],
- True,
- ],
- [
- "B",
- "Option 2",
- ["Option B", "Option C"],
- False,
- ],
- ],
- inputs=[radio, drop, drop_2, check],
- label="Examples",
- )
-
- with gr.Row():
- gr.Dataframe(value=[[1, 2, 3], [4, 5, 6], [7, 8, 9]], label="Dataframe")
- gr.JSON(
- value={"a": 1, "b": 2, "c": {"test": "a", "test2": [1, 2, 3]}},
- label="JSON",
- )
- gr.Label(value={"cat": 0.7, "dog": 0.2, "fish": 0.1})
- gr.File()
- with gr.Row():
- gr.ColorPicker()
- gr.Video(
- "https://gradio-static-files.s3.us-west-2.amazonaws.com/world.mp4"
- )
- gr.Gallery(
- [
- (
- "https://gradio-static-files.s3.us-west-2.amazonaws.com/lion.jpg",
- "lion",
- ),
- (
- "https://gradio-static-files.s3.us-west-2.amazonaws.com/logo.png",
- "logo",
- ),
- (
- "https://gradio-static-files.s3.us-west-2.amazonaws.com/tower.jpg",
- "tower",
- ),
- ],
- height="200px",
- columns=2,
- )
-
- with gr.Row():
- with gr.Column(scale=2):
- chatbot = gr.Chatbot([("Hello", "Hi")], label="Chatbot")
- chat_btn = gr.Button("Add messages")
-
- def chat(history):
- time.sleep(2)
- yield [["How are you?", "I am good."]]
-
- chat_btn.click(
- lambda history: history
- + [["How are you?", "I am good."]]
- + (time.sleep(2) or []),
- chatbot,
- chatbot,
- api_name=False,
- )
- with gr.Column(scale=1):
- with gr.Accordion("Advanced Settings"):
- gr.Markdown("Hello")
- gr.Number(label="Chatbot control 1")
- gr.Number(label="Chatbot control 2")
- gr.Number(label="Chatbot control 3")
-
- # Event Listeners
-
- secret_css = gr.Textbox(visible=False)
- secret_font = gr.JSON(visible=False)
-
- demo.load( # doing this via python was not working for some reason, so using this hacky method for now
- None,
- None,
- None,
- _js="""() => {
- document.head.innerHTML += "";
- let evt_listener = window.setTimeout(
- () => {
- load_theme_btn = document.querySelector('#load_theme');
- if (load_theme_btn) {
- load_theme_btn.click();
- window.clearTimeout(evt_listener);
- }
- },
- 100
- );
- }""",
- api_name=False,
- )
-
- theme_inputs = (
- [primary_hue, secondary_hue, neutral_hue]
- + primary_hues
- + secondary_hues
- + neutral_hues
- + [text_size, spacing_size, radius_size]
- + text_sizes
- + spacing_sizes
- + radius_sizes
- + main_fonts
- + main_is_google
- + mono_fonts
- + mono_is_google
- + theme_var_input
- )
-
- def load_theme(theme_name):
- theme = [theme for theme in themes if theme.__name__ == theme_name][0]
-
- parameters = inspect.signature(theme.__init__).parameters
- primary_hue = parameters["primary_hue"].default
- secondary_hue = parameters["secondary_hue"].default
- neutral_hue = parameters["neutral_hue"].default
- text_size = parameters["text_size"].default
- spacing_size = parameters["spacing_size"].default
- radius_size = parameters["radius_size"].default
-
- theme = theme()
-
- font = theme._font[:4]
- font_mono = theme._font_mono[:4]
- font_is_google = [isinstance(f, gr.themes.GoogleFont) for f in font]
- font_mono_is_google = [
- isinstance(f, gr.themes.GoogleFont) for f in font_mono
- ]
-
- def pad_to_4(x):
- return x + [None] * (4 - len(x))
-
- var_output = []
- for variable in flat_variables:
- theme_val = getattr(theme, variable)
- if theme_val is None and variable.endswith("_dark"):
- theme_val = getattr(theme, variable[:-5])
- var_output.append(theme_val)
-
- return (
- [primary_hue.name, secondary_hue.name, neutral_hue.name]
- + primary_hue.expand()
- + secondary_hue.expand()
- + neutral_hue.expand()
- + [text_size.name, spacing_size.name, radius_size.name]
- + text_size.expand()
- + spacing_size.expand()
- + radius_size.expand()
- + pad_to_4([f.name for f in font])
- + pad_to_4(font_is_google)
- + pad_to_4([f.name for f in font_mono])
- + pad_to_4(font_mono_is_google)
- + var_output
- )
-
- def generate_theme_code(
- base_theme, final_theme, core_variables, final_main_fonts, final_mono_fonts
- ):
- base_theme_name = base_theme
- base_theme = [theme for theme in themes if theme.__name__ == base_theme][
- 0
- ]()
-
- parameters = inspect.signature(base_theme.__init__).parameters
- primary_hue = parameters["primary_hue"].default
- secondary_hue = parameters["secondary_hue"].default
- neutral_hue = parameters["neutral_hue"].default
- text_size = parameters["text_size"].default
- spacing_size = parameters["spacing_size"].default
- radius_size = parameters["radius_size"].default
- font = parameters["font"].default
- font = [font] if not isinstance(font, Iterable) else font
- font = [
- gr.themes.Font(f) if not isinstance(f, gr.themes.Font) else f
- for f in font
- ]
- font_mono = parameters["font_mono"].default
- font_mono = (
- [font_mono] if not isinstance(font_mono, Iterable) else font_mono
- )
- font_mono = [
- gr.themes.Font(f) if not isinstance(f, gr.themes.Font) else f
- for f in font_mono
- ]
-
- core_diffs = {}
- specific_core_diffs = {}
- core_var_names = [
- "primary_hue",
- "secondary_hue",
- "neutral_hue",
- "text_size",
- "spacing_size",
- "radius_size",
- ]
- for value_name, base_value, source_class, final_value in zip(
- core_var_names,
- [
- primary_hue,
- secondary_hue,
- neutral_hue,
- text_size,
- spacing_size,
- radius_size,
- ],
- [
- gr.themes.Color,
- gr.themes.Color,
- gr.themes.Color,
- gr.themes.Size,
- gr.themes.Size,
- gr.themes.Size,
- ],
- core_variables,
- ):
- if base_value.name != final_value:
- core_diffs[value_name] = final_value
- source_obj = [
- obj for obj in source_class.all if obj.name == final_value
- ][0]
- final_attr_values = {}
- diff = False
- for attr in dir(source_obj):
- if attr in ["all", "name", "expand"] or attr.startswith("_"):
- continue
- final_theme_attr = (
- value_name.split("_")[0]
- + "_"
- + (attr[1:] if source_class == gr.themes.Color else attr)
- )
- final_attr_values[final_theme_attr] = getattr(
- final_theme, final_theme_attr
- )
- if getattr(source_obj, attr) != final_attr_values[final_theme_attr]:
- diff = True
- if diff:
- specific_core_diffs[value_name] = (source_class, final_attr_values)
-
- font_diffs = {}
-
- final_main_fonts = [font for font in final_main_fonts if font[0]]
- final_mono_fonts = [font for font in final_mono_fonts if font[0]]
- font = font[:4]
- font_mono = font_mono[:4]
- for base_font_set, theme_font_set, font_set_name in [
- (font, final_main_fonts, "font"),
- (font_mono, final_mono_fonts, "font_mono"),
- ]:
- if len(base_font_set) != len(theme_font_set) or any(
- base_font.name != theme_font[0]
- or isinstance(base_font, gr.themes.GoogleFont) != theme_font[1]
- for base_font, theme_font in zip(base_font_set, theme_font_set)
- ):
- font_diffs[font_set_name] = [
- f"gr.themes.GoogleFont('{font_name}')"
- if is_google_font
- else f"'{font_name}'"
- for font_name, is_google_font in theme_font_set
- ]
-
- newline = "\n"
-
- core_diffs_code = ""
- if len(core_diffs) + len(specific_core_diffs) > 0:
- for var_name in core_var_names:
- if var_name in specific_core_diffs:
- cls, vals = specific_core_diffs[var_name]
- core_diffs_code += f""" {var_name}=gr.themes.{cls.__name__}({', '.join(f'''{k}="{v}"''' for k, v in vals.items())}),\n"""
- elif var_name in core_diffs:
- core_diffs_code += (
- f""" {var_name}="{core_diffs[var_name]}",\n"""
- )
-
- font_diffs_code = ""
-
- if len(font_diffs) > 0:
- font_diffs_code = "".join(
- [
- f""" {font_set_name}=[{", ".join(fonts)}],\n"""
- for font_set_name, fonts in font_diffs.items()
- ]
- )
- var_diffs = {}
- for variable in flat_variables:
- base_theme_val = getattr(base_theme, variable)
- final_theme_val = getattr(final_theme, variable)
- if base_theme_val is None and variable.endswith("_dark"):
- base_theme_val = getattr(base_theme, variable[:-5])
- if base_theme_val != final_theme_val:
- var_diffs[variable] = getattr(final_theme, variable)
-
- newline = "\n"
-
- vars_diff_code = ""
- if len(var_diffs) > 0:
- vars_diff_code = f""".set(
- {(',' + newline + " ").join([f"{k}='{v}'" for k, v in var_diffs.items()])}
-)"""
-
- output = f"""
-import gradio as gr
-
-theme = gr.themes.{base_theme_name}({newline if core_diffs_code or font_diffs_code else ""}{core_diffs_code}{font_diffs_code}){vars_diff_code}
-
-with gr.Blocks(theme=theme) as demo:
- ..."""
- return output
-
- history = gr.State([])
- current_theme = gr.State(None)
-
- def render_variables(history, base_theme, *args):
- primary_hue, secondary_hue, neutral_hue = args[0:3]
- primary_hues = args[3 : 3 + len(palette_range)]
- secondary_hues = args[3 + len(palette_range) : 3 + 2 * len(palette_range)]
- neutral_hues = args[3 + 2 * len(palette_range) : 3 + 3 * len(palette_range)]
- text_size, spacing_size, radius_size = args[
- 3 + 3 * len(palette_range) : 6 + 3 * len(palette_range)
- ]
- text_sizes = args[
- 6
- + 3 * len(palette_range) : 6
- + 3 * len(palette_range)
- + len(size_range)
- ]
- spacing_sizes = args[
- 6
- + 3 * len(palette_range)
- + len(size_range) : 6
- + 3 * len(palette_range)
- + 2 * len(size_range)
- ]
- radius_sizes = args[
- 6
- + 3 * len(palette_range)
- + 2 * len(size_range) : 6
- + 3 * len(palette_range)
- + 3 * len(size_range)
- ]
- main_fonts = args[
- 6
- + 3 * len(palette_range)
- + 3 * len(size_range) : 6
- + 3 * len(palette_range)
- + 3 * len(size_range)
- + 4
- ]
- main_is_google = args[
- 6
- + 3 * len(palette_range)
- + 3 * len(size_range)
- + 4 : 6
- + 3 * len(palette_range)
- + 3 * len(size_range)
- + 8
- ]
- mono_fonts = args[
- 6
- + 3 * len(palette_range)
- + 3 * len(size_range)
- + 8 : 6
- + 3 * len(palette_range)
- + 3 * len(size_range)
- + 12
- ]
- mono_is_google = args[
- 6
- + 3 * len(palette_range)
- + 3 * len(size_range)
- + 12 : 6
- + 3 * len(palette_range)
- + 3 * len(size_range)
- + 16
- ]
- remaining_args = args[
- 6 + 3 * len(palette_range) + 3 * len(size_range) + 16 :
- ]
-
- final_primary_color = gr.themes.Color(*primary_hues)
- final_secondary_color = gr.themes.Color(*secondary_hues)
- final_neutral_color = gr.themes.Color(*neutral_hues)
- final_text_size = gr.themes.Size(*text_sizes)
- final_spacing_size = gr.themes.Size(*spacing_sizes)
- final_radius_size = gr.themes.Size(*radius_sizes)
-
- final_main_fonts = []
- font_weights = set()
- for attr, val in zip(flat_variables, remaining_args):
- if "weight" in attr:
- font_weights.add(val)
- font_weights = sorted(font_weights)
-
- for main_font, is_google in zip(main_fonts, main_is_google):
- if not main_font:
- continue
- if is_google:
- main_font = gr.themes.GoogleFont(main_font, weights=font_weights)
- final_main_fonts.append(main_font)
- final_mono_fonts = []
- for mono_font, is_google in zip(mono_fonts, mono_is_google):
- if not mono_font:
- continue
- if is_google:
- mono_font = gr.themes.GoogleFont(mono_font, weights=font_weights)
- final_mono_fonts.append(mono_font)
-
- theme = gr.themes.Base(
- primary_hue=final_primary_color,
- secondary_hue=final_secondary_color,
- neutral_hue=final_neutral_color,
- text_size=final_text_size,
- spacing_size=final_spacing_size,
- radius_size=final_radius_size,
- font=final_main_fonts,
- font_mono=final_mono_fonts,
- )
-
- theme.set(**dict(zip(flat_variables, remaining_args)))
- new_step = (base_theme, args)
- if len(history) == 0 or str(history[-1]) != str(new_step):
- history.append(new_step)
-
- return (
- history,
- theme._get_theme_css(),
- theme._stylesheets,
- generate_theme_code(
- base_theme,
- theme,
- (
- primary_hue,
- secondary_hue,
- neutral_hue,
- text_size,
- spacing_size,
- radius_size,
- ),
- list(zip(main_fonts, main_is_google)),
- list(zip(mono_fonts, mono_is_google)),
- ),
- theme,
- )
-
- def attach_rerender(evt_listener):
- return evt_listener(
- render_variables,
- [history, base_theme_dropdown] + theme_inputs,
- [history, secret_css, secret_font, output_code, current_theme],
- api_name=False,
- ).then(
- None,
- [secret_css, secret_font],
- None,
- _js="""(css, fonts) => {
- document.getElementById('theme_css').innerHTML = css;
- let existing_font_links = document.querySelectorAll('link[rel="stylesheet"][href^="https://fonts.googleapis.com/css"]');
- existing_font_links.forEach(link => {
- if (fonts.includes(link.href)) {
- fonts = fonts.filter(font => font != link.href);
- } else {
- link.remove();
- }
- });
- fonts.forEach(font => {
- let link = document.createElement('link');
- link.rel = 'stylesheet';
- link.href = font;
- document.head.appendChild(link);
- });
- }""",
- api_name=False,
- )
-
- def load_color(color_name):
- color = [color for color in colors if color.name == color_name][0]
- return [getattr(color, f"c{i}") for i in palette_range]
-
- attach_rerender(
- primary_hue.select(
- load_color, primary_hue, primary_hues, api_name=False
- ).then
- )
- attach_rerender(
- secondary_hue.select(
- load_color, secondary_hue, secondary_hue, api_name=False
- ).then
- )
- attach_rerender(
- neutral_hue.select(
- load_color, neutral_hue, neutral_hues, api_name=False
- ).then
- )
- for hue_set in (primary_hues, secondary_hues, neutral_hues):
- for hue in hue_set:
- attach_rerender(hue.blur)
-
- def load_size(size_name):
- size = [size for size in sizes if size.name == size_name][0]
- return [getattr(size, i) for i in size_range]
-
- attach_rerender(
- text_size.change(load_size, text_size, text_sizes, api_name=False).then
- )
- attach_rerender(
- spacing_size.change(
- load_size, spacing_size, spacing_sizes, api_name=False
- ).then
- )
- attach_rerender(
- radius_size.change(
- load_size, radius_size, radius_sizes, api_name=False
- ).then
- )
-
- attach_rerender(
- load_theme_btn.click(
- load_theme, base_theme_dropdown, theme_inputs, api_name=False
- ).then
- )
-
- for theme_box in (
- text_sizes + spacing_sizes + radius_sizes + main_fonts + mono_fonts
- ):
- attach_rerender(theme_box.blur)
- attach_rerender(theme_box.submit)
- for theme_box in theme_var_input:
- attach_rerender(theme_box.blur)
- attach_rerender(theme_box.select)
- for checkbox in main_is_google + mono_is_google:
- attach_rerender(checkbox.select)
-
- dark_mode_btn.click(
- None,
- None,
- None,
- _js="""() => {
- if (document.querySelectorAll('.dark').length) {
- document.querySelectorAll('.dark').forEach(el => el.classList.remove('dark'));
- } else {
- document.querySelector('body').classList.add('dark');
- }
- }""",
- api_name=False,
- )
-
- def undo(history_var):
- if len(history_var) <= 1:
- return {history: gr.skip()}
- else:
- history_var.pop()
- old = history_var.pop()
- return [history_var, old[0]] + list(old[1])
-
- attach_rerender(
- undo_btn.click(
- undo,
- [history],
- [history, base_theme_dropdown] + theme_inputs,
- api_name=False,
- ).then
- )
-
- def upload_to_hub(data):
- try:
- theme_url = data[current_theme].push_to_hub(
- repo_name=data[theme_name],
- version=data[theme_version] or None,
- hf_token=data[theme_hf_token],
- theme_name=data[theme_name],
- )
- space_name = "/".join(theme_url.split("/")[-2:])
- return (
- gr.Markdown.update(
- value=f"Theme uploaded [here!]({theme_url})! Load it as `gr.Blocks(theme='{space_name}')`",
- visible=True,
- ),
- "Upload to Hub",
- )
- except Exception as e:
- return (
- gr.Markdown.update(
- value=f"Error: {e}",
- visible=True,
- ),
- "Upload to Hub",
- )
-
- upload_to_hub_btn.click(
- lambda: "Uploading...",
- None,
- upload_to_hub_btn,
- api_name=False,
- ).then(
- upload_to_hub,
- {
- current_theme,
- theme_name,
- theme_hf_token,
- theme_version,
- },
- [theme_upload_status, upload_to_hub_btn],
- api_name=False,
- )
-
-
-if __name__ == "__main__":
- demo.launch()
diff --git a/spaces/Dagfinn1962/stablediffusion-members/app.py b/spaces/Dagfinn1962/stablediffusion-members/app.py
deleted file mode 100644
index 4b9422724ffe3fb829839f712d994354da77a0c1..0000000000000000000000000000000000000000
--- a/spaces/Dagfinn1962/stablediffusion-members/app.py
+++ /dev/null
@@ -1,87 +0,0 @@
-import gradio as gr
-import os
-import sys
-from pathlib import Path
-import numpy as np
-from PIL import Image
-
-
-
-
-models = [
- {"name": "SD ComVis 1.2","url": "CompVis/stable-diffusion-v1-2"},
- {"name": "SD Comvis 1.4","url": "CompVis/stable-diffusion-v1-4"},
- {"name": "SD runawayml 1.5","url": "runwayml/stable-diffusion-v1-5"},
- {"name": "SD Stability 2.1 Unclip","url": "stabilityai/stable-diffusion-2-1-unclip"},
- {"name": "SD Dreamshaper-Anime","url": "Lykon/DreamShaper"},
- ]
-
-current_model = models[0]
-
-text_gen = gr.Interface.load("spaces/Avenuenw/prompt-extend")
-
-models2 = []
-for model in models:
- model_url = f"models/{model['url']}"
- loaded_model = gr.Interface.load(model_url, live=True, preprocess=True)
- models2.append(loaded_model)
-
-
-def text_it(inputs, text_gen=text_gen):
- return text_gen(inputs)
-
-
-def set_model(current_model_index):
- global current_model
- current_model = models[current_model_index]
- return gr.update(value=f"{current_model['name']}")
-
-
-def send_it(inputs, model_choice):
- proc = models2[model_choice]
- return proc(inputs)
-
-
-with gr.Blocks (css ='main.css') as myface:
-
- gr.HTML(" Your Promt Here
Choose model here
" )
- with gr.Row():
- input_text = gr.Textbox(label=" ",placeholder="1.PROMPT IDEA HERE ! ",lines=4)
- # Model selection dropdown
- model_name1 = gr.Dropdown(
- label="2 Choose model here",
- choices=[m["name"] for m in models],
- type="index",
- value=current_model["name"],
- interactive=True,
-
-
- )
- with gr.Row():
- see_prompts = gr.Button("3. GENERATE YOUR PROMT IDEA HERE!")
- run = gr.Button("4. GENERATE THE IMAGE HERE!", varant="primery")
-
- #
- with gr.Row():
- output1 = gr.Image(label="")
- output2 = gr.Image(label="")
- output3 = gr.Image(label="")
- with gr.Row():
- magic1 = gr.Textbox(label="Generated Prompt", lines=2)
- magic2 = gr.Textbox(label="Generated Prompt", lines=2)
- magic3 = gr.Textbox(label="Generated Prompt", lines=2)
-
- model_name1.change(set_model, inputs=model_name1, outputs=[output1, output2, output3,])
-
- run.click(send_it, inputs=[magic1, model_name1], outputs=[output1])
- run.click(send_it, inputs=[magic2, model_name1], outputs=[output2])
- run.click(send_it, inputs=[magic3, model_name1], outputs=[output3])
-
-
- see_prompts.click(text_it, inputs=[input_text], outputs=[magic1])
- see_prompts.click(text_it, inputs=[input_text], outputs=[magic2])
- see_prompts.click(text_it, inputs=[input_text], outputs=[magic3])
-
-title="Daylight (SD) ",
-myface.queue(concurrency_count=200)
-myface.launch(inline=True, max_threads=400)
\ No newline at end of file
diff --git a/spaces/Docfile/open_llm_leaderboard/src/load_from_hub.py b/spaces/Docfile/open_llm_leaderboard/src/load_from_hub.py
deleted file mode 100644
index 9062b77a0e8e3828df71cd8486b2e5a6c4cd7d59..0000000000000000000000000000000000000000
--- a/spaces/Docfile/open_llm_leaderboard/src/load_from_hub.py
+++ /dev/null
@@ -1,151 +0,0 @@
-import json
-import os
-
-import pandas as pd
-from huggingface_hub import Repository
-from transformers import AutoConfig
-from collections import defaultdict
-
-from src.assets.hardcoded_evals import baseline, gpt4_values, gpt35_values
-from src.display_models.get_model_metadata import apply_metadata
-from src.display_models.read_results import get_eval_results_dicts, make_clickable_model
-from src.display_models.utils import AutoEvalColumn, EvalQueueColumn, has_no_nan_values
-
-IS_PUBLIC = bool(os.environ.get("IS_PUBLIC", True))
-
-
-def get_all_requested_models(requested_models_dir: str) -> set[str]:
- depth = 1
- file_names = []
- users_to_submission_dates = defaultdict(list)
-
- for root, _, files in os.walk(requested_models_dir):
- current_depth = root.count(os.sep) - requested_models_dir.count(os.sep)
- if current_depth == depth:
- for file in files:
- if not file.endswith(".json"): continue
- with open(os.path.join(root, file), "r") as f:
- info = json.load(f)
- file_names.append(f"{info['model']}_{info['revision']}_{info['precision']}")
-
- # Select organisation
- if info["model"].count("/") == 0 or "submitted_time" not in info:
- continue
- organisation, _ = info["model"].split("/")
- users_to_submission_dates[organisation].append(info["submitted_time"])
-
- return set(file_names), users_to_submission_dates
-
-
-def load_all_info_from_hub(QUEUE_REPO: str, RESULTS_REPO: str, QUEUE_PATH: str, RESULTS_PATH: str) -> list[Repository]:
- eval_queue_repo = None
- eval_results_repo = None
- requested_models = None
-
- print("Pulling evaluation requests and results.")
-
- eval_queue_repo = Repository(
- local_dir=QUEUE_PATH,
- clone_from=QUEUE_REPO,
- repo_type="dataset",
- )
- eval_queue_repo.git_pull()
-
- eval_results_repo = Repository(
- local_dir=RESULTS_PATH,
- clone_from=RESULTS_REPO,
- repo_type="dataset",
- )
- eval_results_repo.git_pull()
-
- requested_models, users_to_submission_dates = get_all_requested_models("eval-queue")
-
- return eval_queue_repo, requested_models, eval_results_repo, users_to_submission_dates
-
-
-def get_leaderboard_df(
- eval_results: Repository, eval_results_private: Repository, cols: list, benchmark_cols: list
-) -> pd.DataFrame:
- if eval_results:
- print("Pulling evaluation results for the leaderboard.")
- eval_results.git_pull()
- if eval_results_private:
- print("Pulling evaluation results for the leaderboard.")
- eval_results_private.git_pull()
-
- all_data = get_eval_results_dicts()
-
- if not IS_PUBLIC:
- all_data.append(gpt4_values)
- all_data.append(gpt35_values)
-
- all_data.append(baseline)
- apply_metadata(all_data) # Populate model type based on known hardcoded values in `metadata.py`
-
- df = pd.DataFrame.from_records(all_data)
- df = df.sort_values(by=[AutoEvalColumn.average.name], ascending=False)
- df = df[cols].round(decimals=2)
-
- # filter out if any of the benchmarks have not been produced
- df = df[has_no_nan_values(df, benchmark_cols)]
- return df
-
-
-def get_evaluation_queue_df(
- eval_queue: Repository, eval_queue_private: Repository, save_path: str, cols: list
-) -> list[pd.DataFrame]:
- if eval_queue:
- print("Pulling changes for the evaluation queue.")
- eval_queue.git_pull()
- if eval_queue_private:
- print("Pulling changes for the evaluation queue.")
- eval_queue_private.git_pull()
-
- entries = [entry for entry in os.listdir(save_path) if not entry.startswith(".")]
- all_evals = []
-
- for entry in entries:
- if ".json" in entry:
- file_path = os.path.join(save_path, entry)
- with open(file_path) as fp:
- data = json.load(fp)
-
- data[EvalQueueColumn.model.name] = make_clickable_model(data["model"])
- data[EvalQueueColumn.revision.name] = data.get("revision", "main")
-
- all_evals.append(data)
- elif ".md" not in entry:
- # this is a folder
- sub_entries = [e for e in os.listdir(f"{save_path}/{entry}") if not e.startswith(".")]
- for sub_entry in sub_entries:
- file_path = os.path.join(save_path, entry, sub_entry)
- with open(file_path) as fp:
- data = json.load(fp)
-
- data[EvalQueueColumn.model.name] = make_clickable_model(data["model"])
- data[EvalQueueColumn.revision.name] = data.get("revision", "main")
- all_evals.append(data)
-
- pending_list = [e for e in all_evals if e["status"] in ["PENDING", "RERUN"]]
- running_list = [e for e in all_evals if e["status"] == "RUNNING"]
- finished_list = [e for e in all_evals if e["status"].startswith("FINISHED") or e["status"] == "PENDING_NEW_EVAL"]
- df_pending = pd.DataFrame.from_records(pending_list, columns=cols)
- df_running = pd.DataFrame.from_records(running_list, columns=cols)
- df_finished = pd.DataFrame.from_records(finished_list, columns=cols)
- return df_finished[cols], df_running[cols], df_pending[cols]
-
-
-def is_model_on_hub(model_name: str, revision: str) -> bool:
- try:
- AutoConfig.from_pretrained(model_name, revision=revision, trust_remote_code=False)
- return True, None
-
- except ValueError:
- return (
- False,
- "needs to be launched with `trust_remote_code=True`. For safety reason, we do not allow these models to be automatically submitted to the leaderboard.",
- )
-
- except Exception as e:
- print(f"Could not get the model config from the hub.: {e}")
- return False, "was not found on hub!"
diff --git a/spaces/DragGan/DragGan-Inversion/torch_utils/ops/conv2d_resample.py b/spaces/DragGan/DragGan-Inversion/torch_utils/ops/conv2d_resample.py
deleted file mode 100644
index e89e1253094036046e326f3a6e57527c541fae8b..0000000000000000000000000000000000000000
--- a/spaces/DragGan/DragGan-Inversion/torch_utils/ops/conv2d_resample.py
+++ /dev/null
@@ -1,158 +0,0 @@
-# Copyright (c) 2021, NVIDIA CORPORATION & AFFILIATES. All rights reserved.
-#
-# NVIDIA CORPORATION and its licensors retain all intellectual property
-# and proprietary rights in and to this software, related documentation
-# and any modifications thereto. Any use, reproduction, disclosure or
-# distribution of this software and related documentation without an express
-# license agreement from NVIDIA CORPORATION is strictly prohibited.
-
-"""2D convolution with optional up/downsampling."""
-
-import torch
-
-from .. import misc
-from . import conv2d_gradfix
-from . import upfirdn2d
-from .upfirdn2d import _parse_padding
-from .upfirdn2d import _get_filter_size
-
-# ----------------------------------------------------------------------------
-
-
-def _get_weight_shape(w):
- with misc.suppress_tracer_warnings(): # this value will be treated as a constant
- shape = [int(sz) for sz in w.shape]
- misc.assert_shape(w, shape)
- return shape
-
-# ----------------------------------------------------------------------------
-
-
-def _conv2d_wrapper(x, w, stride=1, padding=0, groups=1, transpose=False, flip_weight=True):
- """Wrapper for the underlying `conv2d()` and `conv_transpose2d()` implementations.
- """
- _out_channels, _in_channels_per_group, kh, kw = _get_weight_shape(w)
-
- # Flip weight if requested.
- # Note: conv2d() actually performs correlation (flip_weight=True) not convolution (flip_weight=False).
- if not flip_weight and (kw > 1 or kh > 1):
- w = w.flip([2, 3])
-
- # Execute using conv2d_gradfix.
- op = conv2d_gradfix.conv_transpose2d if transpose else conv2d_gradfix.conv2d
- return op(x, w, stride=stride, padding=padding, groups=groups)
-
-# ----------------------------------------------------------------------------
-
-
-@misc.profiled_function
-def conv2d_resample(x, w, f=None, up=1, down=1, padding=0, groups=1, flip_weight=True, flip_filter=False):
- r"""2D convolution with optional up/downsampling.
-
- Padding is performed only once at the beginning, not between the operations.
-
- Args:
- x: Input tensor of shape
- `[batch_size, in_channels, in_height, in_width]`.
- w: Weight tensor of shape
- `[out_channels, in_channels//groups, kernel_height, kernel_width]`.
- f: Low-pass filter for up/downsampling. Must be prepared beforehand by
- calling upfirdn2d.setup_filter(). None = identity (default).
- up: Integer upsampling factor (default: 1).
- down: Integer downsampling factor (default: 1).
- padding: Padding with respect to the upsampled image. Can be a single number
- or a list/tuple `[x, y]` or `[x_before, x_after, y_before, y_after]`
- (default: 0).
- groups: Split input channels into N groups (default: 1).
- flip_weight: False = convolution, True = correlation (default: True).
- flip_filter: False = convolution, True = correlation (default: False).
-
- Returns:
- Tensor of the shape `[batch_size, num_channels, out_height, out_width]`.
- """
- # Validate arguments.
- assert isinstance(x, torch.Tensor) and (x.ndim == 4)
- assert isinstance(w, torch.Tensor) and (
- w.ndim == 4) and (w.dtype == x.dtype)
- assert f is None or (isinstance(f, torch.Tensor) and f.ndim in [
- 1, 2] and f.dtype == torch.float32)
- assert isinstance(up, int) and (up >= 1)
- assert isinstance(down, int) and (down >= 1)
- assert isinstance(groups, int) and (groups >= 1)
- out_channels, in_channels_per_group, kh, kw = _get_weight_shape(w)
- fw, fh = _get_filter_size(f)
- px0, px1, py0, py1 = _parse_padding(padding)
-
- # Adjust padding to account for up/downsampling.
- if up > 1:
- px0 += (fw + up - 1) // 2
- px1 += (fw - up) // 2
- py0 += (fh + up - 1) // 2
- py1 += (fh - up) // 2
- if down > 1:
- px0 += (fw - down + 1) // 2
- px1 += (fw - down) // 2
- py0 += (fh - down + 1) // 2
- py1 += (fh - down) // 2
-
- # Fast path: 1x1 convolution with downsampling only => downsample first, then convolve.
- if kw == 1 and kh == 1 and (down > 1 and up == 1):
- x = upfirdn2d.upfirdn2d(x=x, f=f, down=down, padding=[
- px0, px1, py0, py1], flip_filter=flip_filter)
- x = _conv2d_wrapper(x=x, w=w, groups=groups, flip_weight=flip_weight)
- return x
-
- # Fast path: 1x1 convolution with upsampling only => convolve first, then upsample.
- if kw == 1 and kh == 1 and (up > 1 and down == 1):
- x = _conv2d_wrapper(x=x, w=w, groups=groups, flip_weight=flip_weight)
- x = upfirdn2d.upfirdn2d(x=x, f=f, up=up, padding=[
- px0, px1, py0, py1], gain=up**2, flip_filter=flip_filter)
- return x
-
- # Fast path: downsampling only => use strided convolution.
- if down > 1 and up == 1:
- x = upfirdn2d.upfirdn2d(
- x=x, f=f, padding=[px0, px1, py0, py1], flip_filter=flip_filter)
- x = _conv2d_wrapper(x=x, w=w, stride=down,
- groups=groups, flip_weight=flip_weight)
- return x
-
- # Fast path: upsampling with optional downsampling => use transpose strided convolution.
- if up > 1:
- if groups == 1:
- w = w.transpose(0, 1)
- else:
- w = w.reshape(groups, out_channels // groups,
- in_channels_per_group, kh, kw)
- w = w.transpose(1, 2)
- w = w.reshape(groups * in_channels_per_group,
- out_channels // groups, kh, kw)
- px0 -= kw - 1
- px1 -= kw - up
- py0 -= kh - 1
- py1 -= kh - up
- pxt = max(min(-px0, -px1), 0)
- pyt = max(min(-py0, -py1), 0)
- x = _conv2d_wrapper(x=x, w=w, stride=up, padding=[
- pyt, pxt], groups=groups, transpose=True, flip_weight=(not flip_weight))
- x = upfirdn2d.upfirdn2d(x=x, f=f, padding=[
- px0+pxt, px1+pxt, py0+pyt, py1+pyt], gain=up**2, flip_filter=flip_filter)
- if down > 1:
- x = upfirdn2d.upfirdn2d(
- x=x, f=f, down=down, flip_filter=flip_filter)
- return x
-
- # Fast path: no up/downsampling, padding supported by the underlying implementation => use plain conv2d.
- if up == 1 and down == 1:
- if px0 == px1 and py0 == py1 and px0 >= 0 and py0 >= 0:
- return _conv2d_wrapper(x=x, w=w, padding=[py0, px0], groups=groups, flip_weight=flip_weight)
-
- # Fallback: Generic reference implementation.
- x = upfirdn2d.upfirdn2d(x=x, f=(f if up > 1 else None), up=up, padding=[
- px0, px1, py0, py1], gain=up**2, flip_filter=flip_filter)
- x = _conv2d_wrapper(x=x, w=w, groups=groups, flip_weight=flip_weight)
- if down > 1:
- x = upfirdn2d.upfirdn2d(x=x, f=f, down=down, flip_filter=flip_filter)
- return x
-
-# ----------------------------------------------------------------------------
diff --git a/spaces/Duskfallcrew/textual-inversion-training/textual_inversion.py b/spaces/Duskfallcrew/textual-inversion-training/textual_inversion.py
deleted file mode 100644
index 1eb6f5df52bcff384012fc93484d1a0435dbdde1..0000000000000000000000000000000000000000
--- a/spaces/Duskfallcrew/textual-inversion-training/textual_inversion.py
+++ /dev/null
@@ -1,612 +0,0 @@
-import argparse
-import itertools
-import math
-import os
-import random
-from pathlib import Path
-from typing import Optional
-
-import numpy as np
-import torch
-import torch.nn.functional as F
-import torch.utils.checkpoint
-from torch.utils.data import Dataset
-
-import PIL
-from accelerate import Accelerator
-from accelerate.logging import get_logger
-from accelerate.utils import set_seed
-from diffusers import AutoencoderKL, DDPMScheduler, PNDMScheduler, StableDiffusionPipeline, UNet2DConditionModel
-from diffusers.optimization import get_scheduler
-from diffusers.pipelines.stable_diffusion import StableDiffusionSafetyChecker
-from huggingface_hub import HfFolder, Repository, whoami
-from PIL import Image
-from torchvision import transforms
-from tqdm.auto import tqdm
-from transformers import CLIPFeatureExtractor, CLIPTextModel, CLIPTokenizer
-import gc
-
-logger = get_logger(__name__)
-
-
-def save_progress(text_encoder, placeholder_token_id, accelerator, args):
- logger.info("Saving embeddings")
- learned_embeds = accelerator.unwrap_model(text_encoder).get_input_embeddings().weight[placeholder_token_id]
- learned_embeds_dict = {args.placeholder_token: learned_embeds.detach().cpu()}
- torch.save(learned_embeds_dict, os.path.join(args.output_dir, "learned_embeds.bin"))
-
-
-def parse_args():
- parser = argparse.ArgumentParser(description="Simple example of a training script.")
- parser.add_argument(
- "--save_steps",
- type=int,
- default=500,
- help="Save learned_embeds.bin every X updates steps.",
- )
- parser.add_argument(
- "--pretrained_model_name_or_path",
- type=str,
- default=None,
- help="Path to pretrained model or model identifier from huggingface.co/models.",
- )
- parser.add_argument(
- "--tokenizer_name",
- type=str,
- default=None,
- help="Pretrained tokenizer name or path if not the same as model_name",
- )
- parser.add_argument(
- "--train_data_dir", type=str, default=None, help="A folder containing the training data."
- )
- parser.add_argument(
- "--placeholder_token",
- type=str,
- default=None,
- help="A token to use as a placeholder for the concept.",
- )
- parser.add_argument(
- "--initializer_token", type=str, default=None, help="A token to use as initializer word."
- )
- parser.add_argument("--learnable_property", type=str, default="object", help="Choose between 'object' and 'style'")
- parser.add_argument("--repeats", type=int, default=100, help="How many times to repeat the training data.")
- parser.add_argument(
- "--output_dir",
- type=str,
- default="text-inversion-model",
- help="The output directory where the model predictions and checkpoints will be written.",
- )
- parser.add_argument("--seed", type=int, default=None, help="A seed for reproducible training.")
- parser.add_argument(
- "--resolution",
- type=int,
- default=512,
- help=(
- "The resolution for input images, all the images in the train/validation dataset will be resized to this"
- " resolution"
- ),
- )
- parser.add_argument(
- "--center_crop", action="store_true", help="Whether to center crop images before resizing to resolution"
- )
- parser.add_argument(
- "--train_batch_size", type=int, default=16, help="Batch size (per device) for the training dataloader."
- )
- parser.add_argument("--num_train_epochs", type=int, default=100)
- parser.add_argument(
- "--max_train_steps",
- type=int,
- default=5000,
- help="Total number of training steps to perform. If provided, overrides num_train_epochs.",
- )
- parser.add_argument(
- "--gradient_accumulation_steps",
- type=int,
- default=1,
- help="Number of updates steps to accumulate before performing a backward/update pass.",
- )
- parser.add_argument(
- "--learning_rate",
- type=float,
- default=1e-4,
- help="Initial learning rate (after the potential warmup period) to use.",
- )
- parser.add_argument(
- "--scale_lr",
- action="store_true",
- default=True,
- help="Scale the learning rate by the number of GPUs, gradient accumulation steps, and batch size.",
- )
- parser.add_argument(
- "--lr_scheduler",
- type=str,
- default="constant",
- help=(
- 'The scheduler type to use. Choose between ["linear", "cosine", "cosine_with_restarts", "polynomial",'
- ' "constant", "constant_with_warmup"]'
- ),
- )
- parser.add_argument(
- "--lr_warmup_steps", type=int, default=500, help="Number of steps for the warmup in the lr scheduler."
- )
- parser.add_argument("--adam_beta1", type=float, default=0.9, help="The beta1 parameter for the Adam optimizer.")
- parser.add_argument("--adam_beta2", type=float, default=0.999, help="The beta2 parameter for the Adam optimizer.")
- parser.add_argument("--adam_weight_decay", type=float, default=1e-2, help="Weight decay to use.")
- parser.add_argument("--adam_epsilon", type=float, default=1e-08, help="Epsilon value for the Adam optimizer")
- parser.add_argument("--push_to_hub", action="store_true", help="Whether or not to push the model to the Hub.")
- parser.add_argument("--hub_token", type=str, default=None, help="The token to use to push to the Model Hub.")
- parser.add_argument(
- "--hub_model_id",
- type=str,
- default=None,
- help="The name of the repository to keep in sync with the local `output_dir`.",
- )
- parser.add_argument(
- "--logging_dir",
- type=str,
- default="logs",
- help=(
- "[TensorBoard](https://www.tensorflow.org/tensorboard) log directory. Will default to"
- " *output_dir/runs/**CURRENT_DATETIME_HOSTNAME***."
- ),
- )
- parser.add_argument(
- "--mixed_precision",
- type=str,
- default="no",
- choices=["no", "fp16", "bf16"],
- help=(
- "Whether to use mixed precision. Choose"
- "between fp16 and bf16 (bfloat16). Bf16 requires PyTorch >= 1.10."
- "and an Nvidia Ampere GPU."
- ),
- )
- parser.add_argument("--local_rank", type=int, default=-1, help="For distributed training: local_rank")
-
- args = parser.parse_args()
- env_local_rank = int(os.environ.get("LOCAL_RANK", -1))
- if env_local_rank != -1 and env_local_rank != args.local_rank:
- args.local_rank = env_local_rank
-
- '''
- if args.train_data_dir is None:
- raise ValueError("You must specify a train data directory.")
- '''
-
- return args
-
-
-imagenet_templates_small = [
- "a photo of a {}",
- "a rendering of a {}",
- "a cropped photo of the {}",
- "the photo of a {}",
- "a photo of a clean {}",
- "a photo of a dirty {}",
- "a dark photo of the {}",
- "a photo of my {}",
- "a photo of the cool {}",
- "a close-up photo of a {}",
- "a bright photo of the {}",
- "a cropped photo of a {}",
- "a photo of the {}",
- "a good photo of the {}",
- "a photo of one {}",
- "a close-up photo of the {}",
- "a rendition of the {}",
- "a photo of the clean {}",
- "a rendition of a {}",
- "a photo of a nice {}",
- "a good photo of a {}",
- "a photo of the nice {}",
- "a photo of the small {}",
- "a photo of the weird {}",
- "a photo of the large {}",
- "a photo of a cool {}",
- "a photo of a small {}",
-]
-
-imagenet_style_templates_small = [
- "a painting in the style of {}",
- "a rendering in the style of {}",
- "a cropped painting in the style of {}",
- "the painting in the style of {}",
- "a clean painting in the style of {}",
- "a dirty painting in the style of {}",
- "a dark painting in the style of {}",
- "a picture in the style of {}",
- "a cool painting in the style of {}",
- "a close-up painting in the style of {}",
- "a bright painting in the style of {}",
- "a cropped painting in the style of {}",
- "a good painting in the style of {}",
- "a close-up painting in the style of {}",
- "a rendition in the style of {}",
- "a nice painting in the style of {}",
- "a small painting in the style of {}",
- "a weird painting in the style of {}",
- "a large painting in the style of {}",
-]
-
-
-class TextualInversionDataset(Dataset):
- def __init__(
- self,
- data_root,
- tokenizer,
- learnable_property="object", # [object, style]
- size=512,
- repeats=100,
- interpolation="bicubic",
- flip_p=0.5,
- set="train",
- placeholder_token="*",
- center_crop=False,
- ):
- self.data_root = data_root
- self.tokenizer = tokenizer
- self.learnable_property = learnable_property
- self.size = size
- self.placeholder_token = placeholder_token
- self.center_crop = center_crop
- self.flip_p = flip_p
-
- self.image_paths = [os.path.join(self.data_root, file_path) for file_path in os.listdir(self.data_root)]
-
- self.num_images = len(self.image_paths)
- self._length = self.num_images
-
- if set == "train":
- self._length = self.num_images * repeats
-
- self.interpolation = {
- "linear": PIL.Image.LINEAR,
- "bilinear": PIL.Image.BILINEAR,
- "bicubic": PIL.Image.BICUBIC,
- "lanczos": PIL.Image.LANCZOS,
- }[interpolation]
-
- self.templates = imagenet_style_templates_small if learnable_property == "style" else imagenet_templates_small
- self.flip_transform = transforms.RandomHorizontalFlip(p=self.flip_p)
-
- def __len__(self):
- return self._length
-
- def __getitem__(self, i):
- example = {}
- image = Image.open(self.image_paths[i % self.num_images])
-
- if not image.mode == "RGB":
- image = image.convert("RGB")
-
- placeholder_string = self.placeholder_token
- text = random.choice(self.templates).format(placeholder_string)
-
- example["input_ids"] = self.tokenizer(
- text,
- padding="max_length",
- truncation=True,
- max_length=self.tokenizer.model_max_length,
- return_tensors="pt",
- ).input_ids[0]
-
- # default to score-sde preprocessing
- img = np.array(image).astype(np.uint8)
-
- if self.center_crop:
- crop = min(img.shape[0], img.shape[1])
- h, w, = (
- img.shape[0],
- img.shape[1],
- )
- img = img[(h - crop) // 2 : (h + crop) // 2, (w - crop) // 2 : (w + crop) // 2]
-
- image = Image.fromarray(img)
- image = image.resize((self.size, self.size), resample=self.interpolation)
-
- image = self.flip_transform(image)
- image = np.array(image).astype(np.uint8)
- image = (image / 127.5 - 1.0).astype(np.float32)
-
- example["pixel_values"] = torch.from_numpy(image).permute(2, 0, 1)
- return example
-
-
-def get_full_repo_name(model_id: str, organization: Optional[str] = None, token: Optional[str] = None):
- if token is None:
- token = HfFolder.get_token()
- if organization is None:
- username = whoami(token)["name"]
- return f"{username}/{model_id}"
- else:
- return f"{organization}/{model_id}"
-
-
-def freeze_params(params):
- for param in params:
- param.requires_grad = False
-
-
-def merge_two_dicts(starting_dict: dict, updater_dict: dict) -> dict:
- """
- Starts from base starting dict and then adds the remaining key values from updater replacing the values from
- the first starting/base dict with the second updater dict.
-
- For later: how does d = {**d1, **d2} replace collision?
-
- :param starting_dict:
- :param updater_dict:
- :return:
- """
- new_dict: dict = starting_dict.copy() # start with keys and values of starting_dict
- new_dict.update(updater_dict) # modifies starting_dict with keys and values of updater_dict
- return new_dict
-
-def merge_args(args1: argparse.Namespace, args2: argparse.Namespace) -> argparse.Namespace:
- """
-
- ref: https://stackoverflow.com/questions/56136549/how-can-i-merge-two-argparse-namespaces-in-python-2-x
- :param args1:
- :param args2:
- :return:
- """
- # - the merged args
- # The vars() function returns the __dict__ attribute to values of the given object e.g {field:value}.
- merged_key_values_for_namespace: dict = merge_two_dicts(vars(args1), vars(args2))
- args = argparse.Namespace(**merged_key_values_for_namespace)
- return args
-
-def run_training(args_imported):
- args_default = parse_args()
- args = merge_args(args_default, args_imported)
-
- print(args)
- logging_dir = os.path.join(args.output_dir, args.logging_dir)
-
- accelerator = Accelerator(
- gradient_accumulation_steps=args.gradient_accumulation_steps,
- mixed_precision=args.mixed_precision,
- log_with="tensorboard",
- logging_dir=logging_dir,
- )
-
- # If passed along, set the training seed now.
- if args.seed is not None:
- set_seed(args.seed)
-
- # Handle the repository creation
- if accelerator.is_main_process:
- if args.push_to_hub:
- if args.hub_model_id is None:
- repo_name = get_full_repo_name(Path(args.output_dir).name, token=args.hub_token)
- else:
- repo_name = args.hub_model_id
- repo = Repository(args.output_dir, clone_from=repo_name)
-
- with open(os.path.join(args.output_dir, ".gitignore"), "w+") as gitignore:
- if "step_*" not in gitignore:
- gitignore.write("step_*\n")
- if "epoch_*" not in gitignore:
- gitignore.write("epoch_*\n")
- elif args.output_dir is not None:
- os.makedirs(args.output_dir, exist_ok=True)
-
- # Load the tokenizer and add the placeholder token as a additional special token
- if args.tokenizer_name:
- tokenizer = CLIPTokenizer.from_pretrained(args.tokenizer_name)
- elif args.pretrained_model_name_or_path:
- tokenizer = CLIPTokenizer.from_pretrained(args.pretrained_model_name_or_path, subfolder="tokenizer")
-
- # Add the placeholder token in tokenizer
- num_added_tokens = tokenizer.add_tokens(args.placeholder_token)
- if num_added_tokens == 0:
- raise ValueError(
- f"The tokenizer already contains the token {args.placeholder_token}. Please pass a different"
- " `placeholder_token` that is not already in the tokenizer."
- )
-
- # Convert the initializer_token, placeholder_token to ids
- token_ids = tokenizer.encode(args.initializer_token, add_special_tokens=False)
- # Check if initializer_token is a single token or a sequence of tokens
- if len(token_ids) > 1:
- raise ValueError("The initializer token must be a single token.")
-
- initializer_token_id = token_ids[0]
- placeholder_token_id = tokenizer.convert_tokens_to_ids(args.placeholder_token)
-
- # Load models and create wrapper for stable diffusion
- text_encoder = CLIPTextModel.from_pretrained(args.pretrained_model_name_or_path, subfolder="text_encoder")
- vae = AutoencoderKL.from_pretrained(args.pretrained_model_name_or_path, subfolder="vae")
- unet = UNet2DConditionModel.from_pretrained(args.pretrained_model_name_or_path, subfolder="unet")
-
- # Resize the token embeddings as we are adding new special tokens to the tokenizer
- text_encoder.resize_token_embeddings(len(tokenizer))
-
- # Initialise the newly added placeholder token with the embeddings of the initializer token
- token_embeds = text_encoder.get_input_embeddings().weight.data
- token_embeds[placeholder_token_id] = token_embeds[initializer_token_id]
-
- # Freeze vae and unet
- freeze_params(vae.parameters())
- freeze_params(unet.parameters())
- # Freeze all parameters except for the token embeddings in text encoder
- params_to_freeze = itertools.chain(
- text_encoder.text_model.encoder.parameters(),
- text_encoder.text_model.final_layer_norm.parameters(),
- text_encoder.text_model.embeddings.position_embedding.parameters(),
- )
- freeze_params(params_to_freeze)
-
- if args.scale_lr:
- args.learning_rate = (
- args.learning_rate * args.gradient_accumulation_steps * args.train_batch_size * accelerator.num_processes
- )
-
- # Initialize the optimizer
- optimizer = torch.optim.AdamW(
- text_encoder.get_input_embeddings().parameters(), # only optimize the embeddings
- lr=args.learning_rate,
- betas=(args.adam_beta1, args.adam_beta2),
- weight_decay=args.adam_weight_decay,
- eps=args.adam_epsilon,
- )
-
- # TODO (patil-suraj): load scheduler using args
- noise_scheduler = DDPMScheduler(
- beta_start=0.00085,
- beta_end=0.012,
- beta_schedule="scaled_linear",
- num_train_timesteps=1000,
- )
-
- train_dataset = TextualInversionDataset(
- data_root=args.train_data_dir,
- tokenizer=tokenizer,
- size=args.resolution,
- placeholder_token=args.placeholder_token,
- repeats=args.repeats,
- learnable_property=args.learnable_property,
- center_crop=args.center_crop,
- set="train",
- )
- train_dataloader = torch.utils.data.DataLoader(train_dataset, batch_size=args.train_batch_size, shuffle=True)
-
- # Scheduler and math around the number of training steps.
- overrode_max_train_steps = False
- num_update_steps_per_epoch = math.ceil(len(train_dataloader) / args.gradient_accumulation_steps)
- if args.max_train_steps is None:
- args.max_train_steps = args.num_train_epochs * num_update_steps_per_epoch
- overrode_max_train_steps = True
-
- lr_scheduler = get_scheduler(
- args.lr_scheduler,
- optimizer=optimizer,
- num_warmup_steps=args.lr_warmup_steps * args.gradient_accumulation_steps,
- num_training_steps=args.max_train_steps * args.gradient_accumulation_steps,
- )
-
- text_encoder, optimizer, train_dataloader, lr_scheduler = accelerator.prepare(
- text_encoder, optimizer, train_dataloader, lr_scheduler
- )
-
- # Move vae and unet to device
- vae.to(accelerator.device)
- unet.to(accelerator.device)
-
- # Keep vae and unet in eval model as we don't train these
- vae.eval()
- unet.eval()
-
- # We need to recalculate our total training steps as the size of the training dataloader may have changed.
- num_update_steps_per_epoch = math.ceil(len(train_dataloader) / args.gradient_accumulation_steps)
- if overrode_max_train_steps:
- args.max_train_steps = args.num_train_epochs * num_update_steps_per_epoch
- # Afterwards we recalculate our number of training epochs
- args.num_train_epochs = math.ceil(args.max_train_steps / num_update_steps_per_epoch)
-
- # We need to initialize the trackers we use, and also store our configuration.
- # The trackers initializes automatically on the main process.
- if accelerator.is_main_process:
- accelerator.init_trackers("textual_inversion", config=vars(args))
-
- # Train!
- total_batch_size = args.train_batch_size * accelerator.num_processes * args.gradient_accumulation_steps
-
- logger.info("***** Running training *****")
- logger.info(f" Num examples = {len(train_dataset)}")
- logger.info(f" Num Epochs = {args.num_train_epochs}")
- logger.info(f" Instantaneous batch size per device = {args.train_batch_size}")
- logger.info(f" Total train batch size (w. parallel, distributed & accumulation) = {total_batch_size}")
- logger.info(f" Gradient Accumulation steps = {args.gradient_accumulation_steps}")
- logger.info(f" Total optimization steps = {args.max_train_steps}")
- # Only show the progress bar once on each machine.
- progress_bar = tqdm(range(args.max_train_steps), disable=not accelerator.is_local_main_process)
- progress_bar.set_description("Steps")
- global_step = 0
-
- for epoch in range(args.num_train_epochs):
- text_encoder.train()
- for step, batch in enumerate(train_dataloader):
- with accelerator.accumulate(text_encoder):
- # Convert images to latent space
- latents = vae.encode(batch["pixel_values"]).latent_dist.sample().detach()
- latents = latents * 0.18215
-
- # Sample noise that we'll add to the latents
- noise = torch.randn(latents.shape).to(latents.device)
- bsz = latents.shape[0]
- # Sample a random timestep for each image
- timesteps = torch.randint(
- 0, noise_scheduler.config.num_train_timesteps, (bsz,), device=latents.device
- ).long()
-
- # Add noise to the latents according to the noise magnitude at each timestep
- # (this is the forward diffusion process)
- noisy_latents = noise_scheduler.add_noise(latents, noise, timesteps)
-
- # Get the text embedding for conditioning
- encoder_hidden_states = text_encoder(batch["input_ids"])[0]
-
- # Predict the noise residual
- noise_pred = unet(noisy_latents, timesteps, encoder_hidden_states).sample
-
- loss = F.mse_loss(noise_pred, noise, reduction="none").mean([1, 2, 3]).mean()
- accelerator.backward(loss)
-
- # Zero out the gradients for all token embeddings except the newly added
- # embeddings for the concept, as we only want to optimize the concept embeddings
- if accelerator.num_processes > 1:
- grads = text_encoder.module.get_input_embeddings().weight.grad
- else:
- grads = text_encoder.get_input_embeddings().weight.grad
- # Get the index for tokens that we want to zero the grads for
- index_grads_to_zero = torch.arange(len(tokenizer)) != placeholder_token_id
- grads.data[index_grads_to_zero, :] = grads.data[index_grads_to_zero, :].fill_(0)
-
- optimizer.step()
- lr_scheduler.step()
- optimizer.zero_grad()
-
- # Checks if the accelerator has performed an optimization step behind the scenes
- if accelerator.sync_gradients:
- progress_bar.update(1)
- global_step += 1
- if global_step % args.save_steps == 0:
- save_progress(text_encoder, placeholder_token_id, accelerator, args)
-
- logs = {"loss": loss.detach().item(), "lr": lr_scheduler.get_last_lr()[0]}
- progress_bar.set_postfix(**logs)
- accelerator.log(logs, step=global_step)
-
- if global_step >= args.max_train_steps:
- break
-
- accelerator.wait_for_everyone()
-
- # Create the pipeline using using the trained modules and save it.
- if accelerator.is_main_process:
- pipeline = StableDiffusionPipeline(
- text_encoder=accelerator.unwrap_model(text_encoder),
- vae=vae,
- unet=unet,
- tokenizer=tokenizer,
- scheduler=PNDMScheduler(
- beta_start=0.00085, beta_end=0.012, beta_schedule="scaled_linear", skip_prk_steps=True
- ),
- safety_checker=StableDiffusionSafetyChecker.from_pretrained("CompVis/stable-diffusion-safety-checker"),
- feature_extractor=CLIPFeatureExtractor.from_pretrained("openai/clip-vit-base-patch32"),
- )
- pipeline.save_pretrained(args.output_dir)
- # Also save the newly trained embeddings
- save_progress(text_encoder, placeholder_token_id, accelerator, args)
-
- if args.push_to_hub:
- repo.push_to_hub(commit_message="End of training", blocking=False, auto_lfs_prune=True)
-
- accelerator.end_training()
- torch.cuda.empty_cache()
- gc.collect()
-
-
-if __name__ == "__main__":
- main()
diff --git a/spaces/EMS-TU-Ilmenau/deepest-demo/helper.py b/spaces/EMS-TU-Ilmenau/deepest-demo/helper.py
deleted file mode 100644
index 2fc32221ec35a6d4c939948965bd00f71070b9d7..0000000000000000000000000000000000000000
--- a/spaces/EMS-TU-Ilmenau/deepest-demo/helper.py
+++ /dev/null
@@ -1,59 +0,0 @@
-from torch.utils.data import DataLoader
-from deepest.modules import Parameter2dNet
-from deepest.datasets import InferenceDelayDataset
-from deepest.metrics import match_components
-import numpy as np
-
-class Runner:
- def __init__(self, model: str, dataset: str, bs: int, num_worker: int):
- self.module = Parameter2dNet.from_file(f"{model}")
- self.dataset_config = self.module.get_datasetconfig()
- self.dataset = InferenceDelayDataset(path=dataset, **self.dataset_config)
- self.bs = bs
- self.num_worker = num_worker
-
- def _preallocate(self, data_shape: tuple[int, ...], eta_shape: tuple[int, ...]):
- data = np.empty((len(self), *data_shape), dtype=np.complex128)
- truth = np.empty((len(self), *eta_shape))
- estim = np.empty((len(self), *eta_shape))
- return data, truth, estim
-
- def _get_batchrange_for_index(self, ii: int):
- start_idx = ii*self.bs
- stop_idx = (ii+1)*self.bs
- if stop_idx > len(self.dataset):
- stop_idx = len(self.dataset)
-
- return range(start_idx, stop_idx)
-
- def run(self, snr: int) -> tuple[np.ndarray, np.ndarray, np.ndarray]:
- self.dataset.noise_var = (snr, snr)
- dataloader = DataLoader(
- self.dataset,
- batch_size=self.bs,
- num_workers=self.num_worker,
- worker_init_fn=lambda worker_id: np.random.seed(worker_id),
- shuffle=False,
- )
-
- for ii, (x, _, z) in enumerate(dataloader):
- z = z[0][:, :2, :]
- if ii == 0:
- data, truth, estim = self._preallocate(x.shape[1:], z.shape[1:])
-
- idx_range = self._get_batchrange_for_index(ii)
-
- data[idx_range] = x.cpu().numpy()
- truth[idx_range] = z.cpu().numpy()
- estim[idx_range] = self.module.fit(x)[:, :2, :]
-
- estim, truth = match_components(estim, truth)
-
- return data, truth, estim
-
- def fit(self, data: np.ndarray) -> np.ndarray:
- x = self.module.fit(data)
- return x[:, :2, :]
-
- def __len__(self):
- return len(self.dataset)
\ No newline at end of file
diff --git a/spaces/EnigmaOfTheWorld/TechnoForge_Automotive/README.md b/spaces/EnigmaOfTheWorld/TechnoForge_Automotive/README.md
deleted file mode 100644
index 1c63016042be0a05c7fa526b73b7c3866faafbe5..0000000000000000000000000000000000000000
--- a/spaces/EnigmaOfTheWorld/TechnoForge_Automotive/README.md
+++ /dev/null
@@ -1,12 +0,0 @@
----
-title: TechnoForge Automotive
-emoji: 🐠
-colorFrom: blue
-colorTo: yellow
-sdk: gradio
-sdk_version: 3.27.0
-app_file: app.py
-pinned: false
----
-
-Check out the configuration reference at https://huggingface.co/docs/hub/spaces-config-reference
diff --git a/spaces/EronSamez/RVC_HFmeu/demucs/separate.py b/spaces/EronSamez/RVC_HFmeu/demucs/separate.py
deleted file mode 100644
index 3fc7af9e711978b3e21398aa6f1deb9ae87dd370..0000000000000000000000000000000000000000
--- a/spaces/EronSamez/RVC_HFmeu/demucs/separate.py
+++ /dev/null
@@ -1,185 +0,0 @@
-# Copyright (c) Facebook, Inc. and its affiliates.
-# All rights reserved.
-#
-# This source code is licensed under the license found in the
-# LICENSE file in the root directory of this source tree.
-
-import argparse
-import sys
-from pathlib import Path
-import subprocess
-
-import julius
-import torch as th
-import torchaudio as ta
-
-from .audio import AudioFile, convert_audio_channels
-from .pretrained import is_pretrained, load_pretrained
-from .utils import apply_model, load_model
-
-
-def load_track(track, device, audio_channels, samplerate):
- errors = {}
- wav = None
-
- try:
- wav = AudioFile(track).read(
- streams=0,
- samplerate=samplerate,
- channels=audio_channels).to(device)
- except FileNotFoundError:
- errors['ffmpeg'] = 'Ffmpeg is not installed.'
- except subprocess.CalledProcessError:
- errors['ffmpeg'] = 'FFmpeg could not read the file.'
-
- if wav is None:
- try:
- wav, sr = ta.load(str(track))
- except RuntimeError as err:
- errors['torchaudio'] = err.args[0]
- else:
- wav = convert_audio_channels(wav, audio_channels)
- wav = wav.to(device)
- wav = julius.resample_frac(wav, sr, samplerate)
-
- if wav is None:
- print(f"Could not load file {track}. "
- "Maybe it is not a supported file format? ")
- for backend, error in errors.items():
- print(f"When trying to load using {backend}, got the following error: {error}")
- sys.exit(1)
- return wav
-
-
-def encode_mp3(wav, path, bitrate=320, samplerate=44100, channels=2, verbose=False):
- try:
- import lameenc
- except ImportError:
- print("Failed to call lame encoder. Maybe it is not installed? "
- "On windows, run `python.exe -m pip install -U lameenc`, "
- "on OSX/Linux, run `python3 -m pip install -U lameenc`, "
- "then try again.", file=sys.stderr)
- sys.exit(1)
- encoder = lameenc.Encoder()
- encoder.set_bit_rate(bitrate)
- encoder.set_in_sample_rate(samplerate)
- encoder.set_channels(channels)
- encoder.set_quality(2) # 2-highest, 7-fastest
- if not verbose:
- encoder.silence()
- wav = wav.transpose(0, 1).numpy()
- mp3_data = encoder.encode(wav.tobytes())
- mp3_data += encoder.flush()
- with open(path, "wb") as f:
- f.write(mp3_data)
-
-
-def main():
- parser = argparse.ArgumentParser("demucs.separate",
- description="Separate the sources for the given tracks")
- parser.add_argument("tracks", nargs='+', type=Path, default=[], help='Path to tracks')
- parser.add_argument("-n",
- "--name",
- default="demucs_quantized",
- help="Model name. See README.md for the list of pretrained models. "
- "Default is demucs_quantized.")
- parser.add_argument("-v", "--verbose", action="store_true")
- parser.add_argument("-o",
- "--out",
- type=Path,
- default=Path("separated"),
- help="Folder where to put extracted tracks. A subfolder "
- "with the model name will be created.")
- parser.add_argument("--models",
- type=Path,
- default=Path("models"),
- help="Path to trained models. "
- "Also used to store downloaded pretrained models")
- parser.add_argument("-d",
- "--device",
- default="cuda" if th.cuda.is_available() else "cpu",
- help="Device to use, default is cuda if available else cpu")
- parser.add_argument("--shifts",
- default=0,
- type=int,
- help="Number of random shifts for equivariant stabilization."
- "Increase separation time but improves quality for Demucs. 10 was used "
- "in the original paper.")
- parser.add_argument("--overlap",
- default=0.25,
- type=float,
- help="Overlap between the splits.")
- parser.add_argument("--no-split",
- action="store_false",
- dest="split",
- default=True,
- help="Doesn't split audio in chunks. This can use large amounts of memory.")
- parser.add_argument("--float32",
- action="store_true",
- help="Convert the output wavefile to use pcm f32 format instead of s16. "
- "This should not make a difference if you just plan on listening to the "
- "audio but might be needed to compute exactly metrics like SDR etc.")
- parser.add_argument("--int16",
- action="store_false",
- dest="float32",
- help="Opposite of --float32, here for compatibility.")
- parser.add_argument("--mp3", action="store_true",
- help="Convert the output wavs to mp3.")
- parser.add_argument("--mp3-bitrate",
- default=320,
- type=int,
- help="Bitrate of converted mp3.")
-
- args = parser.parse_args()
- name = args.name + ".th"
- model_path = args.models / name
- if model_path.is_file():
- model = load_model(model_path)
- else:
- if is_pretrained(args.name):
- model = load_pretrained(args.name)
- else:
- print(f"No pre-trained model {args.name}", file=sys.stderr)
- sys.exit(1)
- model.to(args.device)
-
- out = args.out / args.name
- out.mkdir(parents=True, exist_ok=True)
- print(f"Separated tracks will be stored in {out.resolve()}")
- for track in args.tracks:
- if not track.exists():
- print(
- f"File {track} does not exist. If the path contains spaces, "
- "please try again after surrounding the entire path with quotes \"\".",
- file=sys.stderr)
- continue
- print(f"Separating track {track}")
- wav = load_track(track, args.device, model.audio_channels, model.samplerate)
-
- ref = wav.mean(0)
- wav = (wav - ref.mean()) / ref.std()
- sources = apply_model(model, wav, shifts=args.shifts, split=args.split,
- overlap=args.overlap, progress=True)
- sources = sources * ref.std() + ref.mean()
-
- track_folder = out / track.name.rsplit(".", 1)[0]
- track_folder.mkdir(exist_ok=True)
- for source, name in zip(sources, model.sources):
- source = source / max(1.01 * source.abs().max(), 1)
- if args.mp3 or not args.float32:
- source = (source * 2**15).clamp_(-2**15, 2**15 - 1).short()
- source = source.cpu()
- stem = str(track_folder / name)
- if args.mp3:
- encode_mp3(source, stem + ".mp3",
- bitrate=args.mp3_bitrate,
- samplerate=model.samplerate,
- channels=model.audio_channels,
- verbose=args.verbose)
- else:
- wavname = str(track_folder / f"{name}.wav")
- ta.save(wavname, source, sample_rate=model.samplerate)
-
-
-if __name__ == "__main__":
- main()
diff --git a/spaces/EvgenyK/Text-To-Image/README.md b/spaces/EvgenyK/Text-To-Image/README.md
deleted file mode 100644
index e8183fb2c71cb9843a3dd3d4dcdb0d1c65508490..0000000000000000000000000000000000000000
--- a/spaces/EvgenyK/Text-To-Image/README.md
+++ /dev/null
@@ -1,13 +0,0 @@
----
-title: Text To Image
-emoji: 🌍
-colorFrom: indigo
-colorTo: pink
-sdk: gradio
-sdk_version: 3.12.0
-app_file: app.py
-pinned: false
-license: openrail
----
-
-Check out the configuration reference at https://huggingface.co/docs/hub/spaces-config-reference
diff --git a/spaces/GMFTBY/PandaGPT/config/__init__.py b/spaces/GMFTBY/PandaGPT/config/__init__.py
deleted file mode 100644
index 826b6ef41067725c02ac33210e773bb1a8123896..0000000000000000000000000000000000000000
--- a/spaces/GMFTBY/PandaGPT/config/__init__.py
+++ /dev/null
@@ -1,37 +0,0 @@
-import yaml
-
-def load_model_config(model, mode):
- # load special config for each model
- config_path = f'config/{model}.yaml'
- print(f'[!] load configuration from {config_path}')
- with open(config_path) as f:
- configuration = yaml.load(f, Loader=yaml.FullLoader)
- new_config = {}
- for key, value in configuration.items():
- if key in ['train', 'test', 'validation']:
- if mode == key:
- new_config.update(value)
- else:
- new_config[key] = value
- configuration = new_config
- return configuration
-
-def load_config(args):
- '''the configuration of each model can rewrite the base configuration'''
- # base config
- base_configuration = load_base_config()
-
- # load one model config
- configuration = load_model_config(args['model'], args['mode'])
-
- # update and append the special config for base config
- base_configuration.update(configuration)
- configuration = base_configuration
- return configuration
-
-def load_base_config():
- config_path = f'config/base.yaml'
- with open(config_path) as f:
- configuration = yaml.load(f, Loader=yaml.FullLoader)
- print(f'[!] load base configuration: {config_path}')
- return configuration
diff --git a/spaces/Gen-Sim/Gen-Sim/cliport/tasks/align_box_corner.py b/spaces/Gen-Sim/Gen-Sim/cliport/tasks/align_box_corner.py
deleted file mode 100644
index 6890e1cd755013e83beff8c1265367da4cd3cda8..0000000000000000000000000000000000000000
--- a/spaces/Gen-Sim/Gen-Sim/cliport/tasks/align_box_corner.py
+++ /dev/null
@@ -1,59 +0,0 @@
-import os
-import numpy as np
-from cliport.tasks.task import Task
-from cliport.utils import utils
-
-
-class AlignBoxCorner(Task):
- """Pick up the randomly sized box and align one of its corners to the L-shaped marker on the tabletop."""
-
- def __init__(self):
- super().__init__()
- self.max_steps = 3
- self.lang_template = "align the brown box with the green corner"
- self.task_completed_desc = "done with alignment"
- self.additional_reset()
-
- def reset(self, env):
- super().reset(env)
-
- # Generate randomly shaped box.
- box_size = self.get_random_size(0.05, 0.15, 0.05, 0.15, 0.01, 0.06)
-
- # Add corner.
- dimx = (box_size[0] / 2 - 0.025 + 0.0025, box_size[0] / 2 + 0.0025)
- dimy = (box_size[1] / 2 + 0.0025, box_size[1] / 2 - 0.025 + 0.0025)
- corner_template = 'corner/corner-template.urdf'
- replace = {'DIMX': dimx, 'DIMY': dimy}
-
- # IMPORTANT: REPLACE THE TEMPLATE URDF
- corner_urdf = self.fill_template(corner_template, replace)
- corner_size = (box_size[0], box_size[1], 0)
- corner_pose = self.get_random_pose(env, corner_size)
- env.add_object(corner_urdf, corner_pose, 'fixed')
-
- # Add possible placing poses.
- theta = utils.quatXYZW_to_eulerXYZ(corner_pose[1])[2]
- fip_rot = utils.eulerXYZ_to_quatXYZW((0, 0, theta + np.pi))
- pose1 = (corner_pose[0], fip_rot)
- alt_x = (box_size[0] / 2) - (box_size[1] / 2)
- alt_y = (box_size[1] / 2) - (box_size[0] / 2)
- alt_pos = (alt_x, alt_y, 0)
- alt_rot0 = utils.eulerXYZ_to_quatXYZW((0, 0, np.pi / 2))
- alt_rot1 = utils.eulerXYZ_to_quatXYZW((0, 0, 3 * np.pi / 2))
- pose2 = utils.multiply(corner_pose, (alt_pos, alt_rot0))
- pose3 = utils.multiply(corner_pose, (alt_pos, alt_rot1))
-
- # Add box.
- box_template = 'box/box-template.urdf'
-
- # IMPORTANT: REPLACE THE TEMPLATE URDF
- box_urdf = self.fill_template(box_template, {'DIM': np.float32(box_size)})
- box_pose = self.get_random_pose(env, box_size)
- box_id = env.add_object(box_urdf, box_pose)
- self.color_random_brown(box_id)
-
- # Goal: box is aligned with corner (1 of 4 possible poses).
- self.add_goal(objs=[box_id], matches=np.int32([[1, 1, 1, 1]]), targ_poses=[corner_pose, pose1, pose2, pose3], replace=False,
- rotations=True, metric='pose', params=None, step_max_reward=1, symmetries=[2 * np.pi],
- language_goal=self.lang_template)
diff --git a/spaces/GilbertClaus/VideoCutter/rule34.py b/spaces/GilbertClaus/VideoCutter/rule34.py
deleted file mode 100644
index 051835a86dc6d5067dc0727e364ec95ff3e2cf41..0000000000000000000000000000000000000000
--- a/spaces/GilbertClaus/VideoCutter/rule34.py
+++ /dev/null
@@ -1,103 +0,0 @@
-from bs4 import BeautifulSoup
-import json, os
-from others import *
-import cloudscraper
-scraper = cloudscraper.create_scraper()
-
-def get_info_rule34(link):
-
- response = scraper.get(link)
- soup = BeautifulSoup(response.text, 'html.parser')
-
- # Mencari judul video di elemen dengan class title_video
- title = soup.find(class_="title_video")
- if title:
- video_title = title.text.strip().replace('/', ' -')
- idx = video_title.find(']')
- if idx != -1 and idx + 1 < len(video_title) and video_title[idx + 1].isalpha():
- video_title = video_title[:idx + 1] + ' ' + video_title[idx + 1:]
-
- video_title = video_title.title()
- print(f"Judul Video: {video_title}")
- else:
- print("Judul Video tidak ditemukan")
-
- # Mencari nama artist di elemen dengan class col
- cols = soup.find_all(class_="col") # Menggunakan find_all untuk mendapatkan semua elemen dengan class col
- if cols:
- for col in cols: # Melakukan iterasi untuk setiap elemen col
- # Mencari elemen dengan class label yang memiliki teks yang cocok dengan regex "Artist.*"
- label = col.find(class_="label", string="Artist:")
- if label:
- # Mencari elemen dengan class item yang merupakan saudara dari label
- item = label.find_next_sibling(class_="item")
- if item:
- # Mencari elemen dengan class name yang merupakan anak dari item
- name = item.find(class_="name")
- if name:
- artist = name.text.strip()
- print(f"Nama Artist: {artist}")
- break # Keluar dari loop jika sudah menemukan nama artist
- else: # Menambahkan else di akhir loop
- print("Nama Artist tidak ditemukan") # Mencetak pesan jika tidak ada nama artist yang ditemukan
- else:
- print("Elemen col tidak ditemukan")
-
- # Mencari thumbnailUrl di script type="application/ld+json"
- script = soup.find("script", type="application/ld+json")
- if script:
- data = json.loads(script.string)
- if "thumbnailUrl" in data:
- thumbnail_url = data['thumbnailUrl']
- print(f"Thumbnail URL: {thumbnail_url}")
- else:
- print("Tidak ditemukan thumbnail URL")
- else:
- print("Tidak ditemukan elemen script dengan type application/ld+json")
-
- # Mencari resolusi yang tersedia
- resolutions = []
- for a in soup.find_all('a'):
- if 'MP4' in a.text and 'p' in a.text:
- resolutions.append(a.text.split()[1])
- if resolutions:
- print("Resolusi yang tersedia: " + ", ".join(resolutions))
- else:
- print("Tidak ditemukan resolusi yang tersedia")
-
- # Mencari kualitas video 720p atau 480p
- video_quality_elements = soup.find_all("a", class_="tag_item")
- video_quality_720p = None
- video_quality_480p = None
- for element in video_quality_elements:
- if "720p" in element.text:
- video_quality_720p = element['href']
- elif "480p" in element.text:
- video_quality_480p = element['href']
-
- if video_quality_720p:
- print(f"Video kualitas 720p: {video_quality_720p}")
- video_url = video_quality_720p
- elif video_quality_480p:
- print(f"Video kualitas 480p: {video_quality_480p}")
- video_url = video_quality_480p
- else:
- print("Tidak ditemukan video kualitas 720p atau 480p")
- video_url = None
-
- return video_title, artist, video_url, thumbnail_url
-
-def rule34(link):
- video_info = ""
- video_title, artist, video_url, thumbnail_url = get_info_rule34(link)
- directory = f"/home/user/app/Hasil Download/Rule34/{artist}"
- if not os.path.exists(directory):
- os.makedirs(directory)
- # Menentukan nama file thumbnail
- thumbnail_file = download_file(thumbnail_url, video_title, directory)
- video_file = download_file(video_url, video_title, directory)
-
- video_info = f"Nama Channel: {artist}\n"
- video_info += f"Judul Video: {video_title}\n"
-
- return video_file, video_title, video_info, thumbnail_file
\ No newline at end of file
diff --git a/spaces/GoAPI/Midjourney-zoom-video-generator-GoAPI/README.md b/spaces/GoAPI/Midjourney-zoom-video-generator-GoAPI/README.md
deleted file mode 100644
index b6261dd301981cdda74471aae6a46e0f1a353aaa..0000000000000000000000000000000000000000
--- a/spaces/GoAPI/Midjourney-zoom-video-generator-GoAPI/README.md
+++ /dev/null
@@ -1,12 +0,0 @@
----
-title: Goapi Zoom Out Video
-emoji: 👀
-colorFrom: yellow
-colorTo: green
-sdk: gradio
-sdk_version: 3.42.0
-app_file: app.py
-pinned: false
----
-
-Check out the configuration reference at https://huggingface.co/docs/hub/spaces-config-reference
diff --git a/spaces/Gradio-Blocks/uniformer_image_detection/configs/resnest/cascade_rcnn_s50_fpn_syncbn-backbone+head_mstrain-range_1x_coco.py b/spaces/Gradio-Blocks/uniformer_image_detection/configs/resnest/cascade_rcnn_s50_fpn_syncbn-backbone+head_mstrain-range_1x_coco.py
deleted file mode 100644
index 78a154bba2e12e1daec0efaa6a1cb67016084671..0000000000000000000000000000000000000000
--- a/spaces/Gradio-Blocks/uniformer_image_detection/configs/resnest/cascade_rcnn_s50_fpn_syncbn-backbone+head_mstrain-range_1x_coco.py
+++ /dev/null
@@ -1,116 +0,0 @@
-_base_ = '../cascade_rcnn/cascade_rcnn_r50_fpn_1x_coco.py'
-norm_cfg = dict(type='SyncBN', requires_grad=True)
-model = dict(
- pretrained='open-mmlab://resnest50',
- backbone=dict(
- type='ResNeSt',
- stem_channels=64,
- depth=50,
- radix=2,
- reduction_factor=4,
- avg_down_stride=True,
- num_stages=4,
- out_indices=(0, 1, 2, 3),
- frozen_stages=1,
- norm_cfg=norm_cfg,
- norm_eval=False,
- style='pytorch'),
- roi_head=dict(
- bbox_head=[
- dict(
- type='Shared4Conv1FCBBoxHead',
- in_channels=256,
- conv_out_channels=256,
- fc_out_channels=1024,
- norm_cfg=norm_cfg,
- roi_feat_size=7,
- num_classes=80,
- bbox_coder=dict(
- type='DeltaXYWHBBoxCoder',
- target_means=[0., 0., 0., 0.],
- target_stds=[0.1, 0.1, 0.2, 0.2]),
- reg_class_agnostic=True,
- loss_cls=dict(
- type='CrossEntropyLoss',
- use_sigmoid=False,
- loss_weight=1.0),
- loss_bbox=dict(type='SmoothL1Loss', beta=1.0,
- loss_weight=1.0)),
- dict(
- type='Shared4Conv1FCBBoxHead',
- in_channels=256,
- conv_out_channels=256,
- fc_out_channels=1024,
- norm_cfg=norm_cfg,
- roi_feat_size=7,
- num_classes=80,
- bbox_coder=dict(
- type='DeltaXYWHBBoxCoder',
- target_means=[0., 0., 0., 0.],
- target_stds=[0.05, 0.05, 0.1, 0.1]),
- reg_class_agnostic=True,
- loss_cls=dict(
- type='CrossEntropyLoss',
- use_sigmoid=False,
- loss_weight=1.0),
- loss_bbox=dict(type='SmoothL1Loss', beta=1.0,
- loss_weight=1.0)),
- dict(
- type='Shared4Conv1FCBBoxHead',
- in_channels=256,
- conv_out_channels=256,
- fc_out_channels=1024,
- norm_cfg=norm_cfg,
- roi_feat_size=7,
- num_classes=80,
- bbox_coder=dict(
- type='DeltaXYWHBBoxCoder',
- target_means=[0., 0., 0., 0.],
- target_stds=[0.033, 0.033, 0.067, 0.067]),
- reg_class_agnostic=True,
- loss_cls=dict(
- type='CrossEntropyLoss',
- use_sigmoid=False,
- loss_weight=1.0),
- loss_bbox=dict(type='SmoothL1Loss', beta=1.0, loss_weight=1.0))
- ], ))
-# # use ResNeSt img_norm
-img_norm_cfg = dict(
- mean=[123.68, 116.779, 103.939], std=[58.393, 57.12, 57.375], to_rgb=True)
-train_pipeline = [
- dict(type='LoadImageFromFile'),
- dict(
- type='LoadAnnotations',
- with_bbox=True,
- with_mask=False,
- poly2mask=False),
- dict(
- type='Resize',
- img_scale=[(1333, 640), (1333, 800)],
- multiscale_mode='range',
- keep_ratio=True),
- dict(type='RandomFlip', flip_ratio=0.5),
- dict(type='Normalize', **img_norm_cfg),
- dict(type='Pad', size_divisor=32),
- dict(type='DefaultFormatBundle'),
- dict(type='Collect', keys=['img', 'gt_bboxes', 'gt_labels']),
-]
-test_pipeline = [
- dict(type='LoadImageFromFile'),
- dict(
- type='MultiScaleFlipAug',
- img_scale=(1333, 800),
- flip=False,
- transforms=[
- dict(type='Resize', keep_ratio=True),
- dict(type='RandomFlip'),
- dict(type='Normalize', **img_norm_cfg),
- dict(type='Pad', size_divisor=32),
- dict(type='ImageToTensor', keys=['img']),
- dict(type='Collect', keys=['img']),
- ])
-]
-data = dict(
- train=dict(pipeline=train_pipeline),
- val=dict(pipeline=test_pipeline),
- test=dict(pipeline=test_pipeline))
diff --git a/spaces/Gradio-Blocks/uniformer_image_detection/configs/swin/mask_rcnn_swin_tiny_patch4_window7_mstrain_480-800_adamw_3x_coco.py b/spaces/Gradio-Blocks/uniformer_image_detection/configs/swin/mask_rcnn_swin_tiny_patch4_window7_mstrain_480-800_adamw_3x_coco.py
deleted file mode 100644
index c58057747d7d922293b6838e6eb1e13aa520aa3a..0000000000000000000000000000000000000000
--- a/spaces/Gradio-Blocks/uniformer_image_detection/configs/swin/mask_rcnn_swin_tiny_patch4_window7_mstrain_480-800_adamw_3x_coco.py
+++ /dev/null
@@ -1,80 +0,0 @@
-_base_ = [
- '../_base_/models/mask_rcnn_swin_fpn.py',
- '../_base_/datasets/coco_instance.py',
- '../_base_/schedules/schedule_1x.py', '../_base_/default_runtime.py'
-]
-
-model = dict(
- backbone=dict(
- embed_dim=96,
- depths=[2, 2, 6, 2],
- num_heads=[3, 6, 12, 24],
- window_size=7,
- ape=False,
- drop_path_rate=0.2,
- patch_norm=True,
- use_checkpoint=False
- ),
- neck=dict(in_channels=[96, 192, 384, 768]))
-
-img_norm_cfg = dict(
- mean=[123.675, 116.28, 103.53], std=[58.395, 57.12, 57.375], to_rgb=True)
-
-# augmentation strategy originates from DETR / Sparse RCNN
-train_pipeline = [
- dict(type='LoadImageFromFile'),
- dict(type='LoadAnnotations', with_bbox=True, with_mask=True),
- dict(type='RandomFlip', flip_ratio=0.5),
- dict(type='AutoAugment',
- policies=[
- [
- dict(type='Resize',
- img_scale=[(480, 1333), (512, 1333), (544, 1333), (576, 1333),
- (608, 1333), (640, 1333), (672, 1333), (704, 1333),
- (736, 1333), (768, 1333), (800, 1333)],
- multiscale_mode='value',
- keep_ratio=True)
- ],
- [
- dict(type='Resize',
- img_scale=[(400, 1333), (500, 1333), (600, 1333)],
- multiscale_mode='value',
- keep_ratio=True),
- dict(type='RandomCrop',
- crop_type='absolute_range',
- crop_size=(384, 600),
- allow_negative_crop=True),
- dict(type='Resize',
- img_scale=[(480, 1333), (512, 1333), (544, 1333),
- (576, 1333), (608, 1333), (640, 1333),
- (672, 1333), (704, 1333), (736, 1333),
- (768, 1333), (800, 1333)],
- multiscale_mode='value',
- override=True,
- keep_ratio=True)
- ]
- ]),
- dict(type='Normalize', **img_norm_cfg),
- dict(type='Pad', size_divisor=32),
- dict(type='DefaultFormatBundle'),
- dict(type='Collect', keys=['img', 'gt_bboxes', 'gt_labels', 'gt_masks']),
-]
-data = dict(train=dict(pipeline=train_pipeline))
-
-optimizer = dict(_delete_=True, type='AdamW', lr=0.0001, betas=(0.9, 0.999), weight_decay=0.05,
- paramwise_cfg=dict(custom_keys={'absolute_pos_embed': dict(decay_mult=0.),
- 'relative_position_bias_table': dict(decay_mult=0.),
- 'norm': dict(decay_mult=0.)}))
-lr_config = dict(step=[27, 33])
-runner = dict(type='EpochBasedRunnerAmp', max_epochs=36)
-
-# do not use mmdet version fp16
-fp16 = None
-optimizer_config = dict(
- type="DistOptimizerHook",
- update_interval=1,
- grad_clip=None,
- coalesce=True,
- bucket_size_mb=-1,
- use_fp16=True,
-)
diff --git a/spaces/Gradio-Blocks/uniformer_image_segmentation/configs/dmnet/dmnet_r101-d8_512x1024_40k_cityscapes.py b/spaces/Gradio-Blocks/uniformer_image_segmentation/configs/dmnet/dmnet_r101-d8_512x1024_40k_cityscapes.py
deleted file mode 100644
index fd6897691d3f8f200783fae7bfe231735f25a11b..0000000000000000000000000000000000000000
--- a/spaces/Gradio-Blocks/uniformer_image_segmentation/configs/dmnet/dmnet_r101-d8_512x1024_40k_cityscapes.py
+++ /dev/null
@@ -1,2 +0,0 @@
-_base_ = './dmnet_r50-d8_512x1024_40k_cityscapes.py'
-model = dict(pretrained='open-mmlab://resnet101_v1c', backbone=dict(depth=101))
diff --git a/spaces/GrandaddyShmax/AudioCraft_Plus/tests/common_utils/temp_utils.py b/spaces/GrandaddyShmax/AudioCraft_Plus/tests/common_utils/temp_utils.py
deleted file mode 100644
index b45d896836799edcf1fee271409b390b3b6e4127..0000000000000000000000000000000000000000
--- a/spaces/GrandaddyShmax/AudioCraft_Plus/tests/common_utils/temp_utils.py
+++ /dev/null
@@ -1,56 +0,0 @@
-# Copyright (c) Meta Platforms, Inc. and affiliates.
-# All rights reserved.
-#
-# This source code is licensed under the license found in the
-# LICENSE file in the root directory of this source tree.
-
-import os
-import tempfile
-
-
-class TempDirMixin:
- """Mixin to provide easy access to temp dir.
- """
-
- temp_dir_ = None
-
- @classmethod
- def get_base_temp_dir(cls):
- # If AUDIOCRAFT_TEST_DIR is set, use it instead of temporary directory.
- # this is handy for debugging.
- key = "AUDIOCRAFT_TEST_DIR"
- if key in os.environ:
- return os.environ[key]
- if cls.temp_dir_ is None:
- cls.temp_dir_ = tempfile.TemporaryDirectory()
- return cls.temp_dir_.name
-
- @classmethod
- def tearDownClass(cls):
- if cls.temp_dir_ is not None:
- try:
- cls.temp_dir_.cleanup()
- cls.temp_dir_ = None
- except PermissionError:
- # On Windows there is a know issue with `shutil.rmtree`,
- # which fails intermittently.
- # https://github.com/python/cpython/issues/74168
- # Following the above thread, we ignore it.
- pass
- super().tearDownClass()
-
- @property
- def id(self):
- return self.__class__.__name__
-
- def get_temp_path(self, *paths):
- temp_dir = os.path.join(self.get_base_temp_dir(), self.id)
- path = os.path.join(temp_dir, *paths)
- os.makedirs(os.path.dirname(path), exist_ok=True)
- return path
-
- def get_temp_dir(self, *paths):
- temp_dir = os.path.join(self.get_base_temp_dir(), self.id)
- path = os.path.join(temp_dir, *paths)
- os.makedirs(path, exist_ok=True)
- return path
diff --git a/spaces/GrandaddyShmax/MusicGen_Plus_hfv2/tests/data/test_audio.py b/spaces/GrandaddyShmax/MusicGen_Plus_hfv2/tests/data/test_audio.py
deleted file mode 100644
index 40c0d5ed69eff92a766dc6d176e532f0df6c2b5e..0000000000000000000000000000000000000000
--- a/spaces/GrandaddyShmax/MusicGen_Plus_hfv2/tests/data/test_audio.py
+++ /dev/null
@@ -1,239 +0,0 @@
-# Copyright (c) Meta Platforms, Inc. and affiliates.
-# All rights reserved.
-#
-# This source code is licensed under the license found in the
-# LICENSE file in the root directory of this source tree.
-
-from itertools import product
-import random
-
-import numpy as np
-import torch
-import torchaudio
-
-from audiocraft.data.audio import audio_info, audio_read, audio_write, _av_read
-
-from ..common_utils import TempDirMixin, get_white_noise, save_wav
-
-
-class TestInfo(TempDirMixin):
-
- def test_info_mp3(self):
- sample_rates = [8000, 16_000]
- channels = [1, 2]
- duration = 1.
- for sample_rate, ch in product(sample_rates, channels):
- wav = get_white_noise(ch, int(sample_rate * duration))
- path = self.get_temp_path('sample_wav.mp3')
- save_wav(path, wav, sample_rate)
- info = audio_info(path)
- assert info.sample_rate == sample_rate
- assert info.channels == ch
- # we cannot trust torchaudio for num_frames, so we don't check
-
- def _test_info_format(self, ext: str):
- sample_rates = [8000, 16_000]
- channels = [1, 2]
- duration = 1.
- for sample_rate, ch in product(sample_rates, channels):
- n_frames = int(sample_rate * duration)
- wav = get_white_noise(ch, n_frames)
- path = self.get_temp_path(f'sample_wav{ext}')
- save_wav(path, wav, sample_rate)
- info = audio_info(path)
- assert info.sample_rate == sample_rate
- assert info.channels == ch
- assert np.isclose(info.duration, duration, atol=1e-5)
-
- def test_info_wav(self):
- self._test_info_format('.wav')
-
- def test_info_flac(self):
- self._test_info_format('.flac')
-
- def test_info_ogg(self):
- self._test_info_format('.ogg')
-
- def test_info_m4a(self):
- # TODO: generate m4a file programmatically
- # self._test_info_format('.m4a')
- pass
-
-
-class TestRead(TempDirMixin):
-
- def test_read_full_wav(self):
- sample_rates = [8000, 16_000]
- channels = [1, 2]
- duration = 1.
- for sample_rate, ch in product(sample_rates, channels):
- n_frames = int(sample_rate * duration)
- wav = get_white_noise(ch, n_frames).clamp(-0.99, 0.99)
- path = self.get_temp_path('sample_wav.wav')
- save_wav(path, wav, sample_rate)
- read_wav, read_sr = audio_read(path)
- assert read_sr == sample_rate
- assert read_wav.shape[0] == wav.shape[0]
- assert read_wav.shape[1] == wav.shape[1]
- assert torch.allclose(read_wav, wav, rtol=1e-03, atol=1e-04)
-
- def test_read_partial_wav(self):
- sample_rates = [8000, 16_000]
- channels = [1, 2]
- duration = 1.
- read_duration = torch.rand(1).item()
- for sample_rate, ch in product(sample_rates, channels):
- n_frames = int(sample_rate * duration)
- read_frames = int(sample_rate * read_duration)
- wav = get_white_noise(ch, n_frames).clamp(-0.99, 0.99)
- path = self.get_temp_path('sample_wav.wav')
- save_wav(path, wav, sample_rate)
- read_wav, read_sr = audio_read(path, 0, read_duration)
- assert read_sr == sample_rate
- assert read_wav.shape[0] == wav.shape[0]
- assert read_wav.shape[1] == read_frames
- assert torch.allclose(read_wav[..., 0:read_frames], wav[..., 0:read_frames], rtol=1e-03, atol=1e-04)
-
- def test_read_seek_time_wav(self):
- sample_rates = [8000, 16_000]
- channels = [1, 2]
- duration = 1.
- read_duration = 1.
- for sample_rate, ch in product(sample_rates, channels):
- n_frames = int(sample_rate * duration)
- wav = get_white_noise(ch, n_frames).clamp(-0.99, 0.99)
- path = self.get_temp_path('sample_wav.wav')
- save_wav(path, wav, sample_rate)
- seek_time = torch.rand(1).item()
- read_wav, read_sr = audio_read(path, seek_time, read_duration)
- seek_frames = int(sample_rate * seek_time)
- expected_frames = n_frames - seek_frames
- assert read_sr == sample_rate
- assert read_wav.shape[0] == wav.shape[0]
- assert read_wav.shape[1] == expected_frames
- assert torch.allclose(read_wav, wav[..., seek_frames:], rtol=1e-03, atol=1e-04)
-
- def test_read_seek_time_wav_padded(self):
- sample_rates = [8000, 16_000]
- channels = [1, 2]
- duration = 1.
- read_duration = 1.
- for sample_rate, ch in product(sample_rates, channels):
- n_frames = int(sample_rate * duration)
- read_frames = int(sample_rate * read_duration)
- wav = get_white_noise(ch, n_frames).clamp(-0.99, 0.99)
- path = self.get_temp_path('sample_wav.wav')
- save_wav(path, wav, sample_rate)
- seek_time = torch.rand(1).item()
- seek_frames = int(sample_rate * seek_time)
- expected_frames = n_frames - seek_frames
- read_wav, read_sr = audio_read(path, seek_time, read_duration, pad=True)
- expected_pad_wav = torch.zeros(wav.shape[0], read_frames - expected_frames)
- assert read_sr == sample_rate
- assert read_wav.shape[0] == wav.shape[0]
- assert read_wav.shape[1] == read_frames
- assert torch.allclose(read_wav[..., :expected_frames], wav[..., seek_frames:], rtol=1e-03, atol=1e-04)
- assert torch.allclose(read_wav[..., expected_frames:], expected_pad_wav)
-
-
-class TestAvRead(TempDirMixin):
-
- def test_avread_seek_base(self):
- sample_rates = [8000, 16_000]
- channels = [1, 2]
- duration = 2.
- for sample_rate, ch in product(sample_rates, channels):
- n_frames = int(sample_rate * duration)
- wav = get_white_noise(ch, n_frames)
- path = self.get_temp_path(f'reference_a_{sample_rate}_{ch}.wav')
- save_wav(path, wav, sample_rate)
- for _ in range(100):
- # seek will always load a full duration segment in the file
- seek_time = random.uniform(0.0, 1.0)
- seek_duration = random.uniform(0.001, 1.0)
- read_wav, read_sr = _av_read(path, seek_time, seek_duration)
- assert read_sr == sample_rate
- assert read_wav.shape[0] == wav.shape[0]
- assert read_wav.shape[-1] == int(seek_duration * sample_rate)
-
- def test_avread_seek_partial(self):
- sample_rates = [8000, 16_000]
- channels = [1, 2]
- duration = 1.
- for sample_rate, ch in product(sample_rates, channels):
- n_frames = int(sample_rate * duration)
- wav = get_white_noise(ch, n_frames)
- path = self.get_temp_path(f'reference_b_{sample_rate}_{ch}.wav')
- save_wav(path, wav, sample_rate)
- for _ in range(100):
- # seek will always load a partial segment
- seek_time = random.uniform(0.5, 1.)
- seek_duration = 1.
- expected_num_frames = n_frames - int(seek_time * sample_rate)
- read_wav, read_sr = _av_read(path, seek_time, seek_duration)
- assert read_sr == sample_rate
- assert read_wav.shape[0] == wav.shape[0]
- assert read_wav.shape[-1] == expected_num_frames
-
- def test_avread_seek_outofbound(self):
- sample_rates = [8000, 16_000]
- channels = [1, 2]
- duration = 1.
- for sample_rate, ch in product(sample_rates, channels):
- n_frames = int(sample_rate * duration)
- wav = get_white_noise(ch, n_frames)
- path = self.get_temp_path(f'reference_c_{sample_rate}_{ch}.wav')
- save_wav(path, wav, sample_rate)
- seek_time = 1.5
- read_wav, read_sr = _av_read(path, seek_time, 1.)
- assert read_sr == sample_rate
- assert read_wav.shape[0] == wav.shape[0]
- assert read_wav.shape[-1] == 0
-
- def test_avread_seek_edge(self):
- sample_rates = [8000, 16_000]
- # some of these values will have
- # int(((frames - 1) / sample_rate) * sample_rate) != (frames - 1)
- n_frames = [1000, 1001, 1002]
- channels = [1, 2]
- for sample_rate, ch, frames in product(sample_rates, channels, n_frames):
- duration = frames / sample_rate
- wav = get_white_noise(ch, frames)
- path = self.get_temp_path(f'reference_d_{sample_rate}_{ch}.wav')
- save_wav(path, wav, sample_rate)
- seek_time = (frames - 1) / sample_rate
- seek_frames = int(seek_time * sample_rate)
- read_wav, read_sr = _av_read(path, seek_time, duration)
- assert read_sr == sample_rate
- assert read_wav.shape[0] == wav.shape[0]
- assert read_wav.shape[-1] == (frames - seek_frames)
-
-
-class TestAudioWrite(TempDirMixin):
-
- def test_audio_write_wav(self):
- torch.manual_seed(1234)
- sample_rates = [8000, 16_000]
- n_frames = [1000, 1001, 1002]
- channels = [1, 2]
- strategies = ["peak", "clip", "rms"]
- formats = ["wav", "mp3"]
- for sample_rate, ch, frames in product(sample_rates, channels, n_frames):
- for format_, strategy in product(formats, strategies):
- wav = get_white_noise(ch, frames)
- path = self.get_temp_path(f'pred_{sample_rate}_{ch}')
- audio_write(path, wav, sample_rate, format_, strategy=strategy)
- read_wav, read_sr = torchaudio.load(f'{path}.{format_}')
- if format_ == "wav":
- assert read_wav.shape == wav.shape
-
- if format_ == "wav" and strategy in ["peak", "rms"]:
- rescaled_read_wav = read_wav / read_wav.abs().max() * wav.abs().max()
- # for a Gaussian, the typical max scale will be less than ~5x the std.
- # The error when writing to disk will ~ 1/2**15, and when rescaling, 5x that.
- # For RMS target, rescaling leaves more headroom by default, leading
- # to a 20x rescaling typically
- atol = (5 if strategy == "peak" else 20) / 2**15
- delta = (rescaled_read_wav - wav).abs().max()
- assert torch.allclose(wav, rescaled_read_wav, rtol=0, atol=atol), (delta, atol)
- formats = ["wav"] # faster unit tests
diff --git a/spaces/GroveStreet/GTA_SOVITS/modules/enhancer.py b/spaces/GroveStreet/GTA_SOVITS/modules/enhancer.py
deleted file mode 100644
index 37676311f7d8dc4ddc2a5244dedc27b2437e04f5..0000000000000000000000000000000000000000
--- a/spaces/GroveStreet/GTA_SOVITS/modules/enhancer.py
+++ /dev/null
@@ -1,105 +0,0 @@
-import numpy as np
-import torch
-import torch.nn.functional as F
-from vdecoder.nsf_hifigan.nvSTFT import STFT
-from vdecoder.nsf_hifigan.models import load_model
-from torchaudio.transforms import Resample
-
-class Enhancer:
- def __init__(self, enhancer_type, enhancer_ckpt, device=None):
- if device is None:
- device = 'cuda' if torch.cuda.is_available() else 'cpu'
- self.device = device
-
- if enhancer_type == 'nsf-hifigan':
- self.enhancer = NsfHifiGAN(enhancer_ckpt, device=self.device)
- else:
- raise ValueError(f" [x] Unknown enhancer: {enhancer_type}")
-
- self.resample_kernel = {}
- self.enhancer_sample_rate = self.enhancer.sample_rate()
- self.enhancer_hop_size = self.enhancer.hop_size()
-
- def enhance(self,
- audio, # 1, T
- sample_rate,
- f0, # 1, n_frames, 1
- hop_size,
- adaptive_key = 0,
- silence_front = 0
- ):
- # enhancer start time
- start_frame = int(silence_front * sample_rate / hop_size)
- real_silence_front = start_frame * hop_size / sample_rate
- audio = audio[:, int(np.round(real_silence_front * sample_rate)) : ]
- f0 = f0[: , start_frame :, :]
-
- # adaptive parameters
- adaptive_factor = 2 ** ( -adaptive_key / 12)
- adaptive_sample_rate = 100 * int(np.round(self.enhancer_sample_rate / adaptive_factor / 100))
- real_factor = self.enhancer_sample_rate / adaptive_sample_rate
-
- # resample the ddsp output
- if sample_rate == adaptive_sample_rate:
- audio_res = audio
- else:
- key_str = str(sample_rate) + str(adaptive_sample_rate)
- if key_str not in self.resample_kernel:
- self.resample_kernel[key_str] = Resample(sample_rate, adaptive_sample_rate, lowpass_filter_width = 128).to(self.device)
- audio_res = self.resample_kernel[key_str](audio)
-
- n_frames = int(audio_res.size(-1) // self.enhancer_hop_size + 1)
-
- # resample f0
- f0_np = f0.squeeze(0).squeeze(-1).cpu().numpy()
- f0_np *= real_factor
- time_org = (hop_size / sample_rate) * np.arange(len(f0_np)) / real_factor
- time_frame = (self.enhancer_hop_size / self.enhancer_sample_rate) * np.arange(n_frames)
- f0_res = np.interp(time_frame, time_org, f0_np, left=f0_np[0], right=f0_np[-1])
- f0_res = torch.from_numpy(f0_res).unsqueeze(0).float().to(self.device) # 1, n_frames
-
- # enhance
- enhanced_audio, enhancer_sample_rate = self.enhancer(audio_res, f0_res)
-
- # resample the enhanced output
- if adaptive_factor != 0:
- key_str = str(adaptive_sample_rate) + str(enhancer_sample_rate)
- if key_str not in self.resample_kernel:
- self.resample_kernel[key_str] = Resample(adaptive_sample_rate, enhancer_sample_rate, lowpass_filter_width = 128).to(self.device)
- enhanced_audio = self.resample_kernel[key_str](enhanced_audio)
-
- # pad the silence frames
- if start_frame > 0:
- enhanced_audio = F.pad(enhanced_audio, (int(np.round(enhancer_sample_rate * real_silence_front)), 0))
-
- return enhanced_audio, enhancer_sample_rate
-
-
-class NsfHifiGAN(torch.nn.Module):
- def __init__(self, model_path, device=None):
- super().__init__()
- if device is None:
- device = 'cuda' if torch.cuda.is_available() else 'cpu'
- self.device = device
- print('| Load HifiGAN: ', model_path)
- self.model, self.h = load_model(model_path, device=self.device)
-
- def sample_rate(self):
- return self.h.sampling_rate
-
- def hop_size(self):
- return self.h.hop_size
-
- def forward(self, audio, f0):
- stft = STFT(
- self.h.sampling_rate,
- self.h.num_mels,
- self.h.n_fft,
- self.h.win_size,
- self.h.hop_size,
- self.h.fmin,
- self.h.fmax)
- with torch.no_grad():
- mel = stft.get_mel(audio)
- enhanced_audio = self.model(mel, f0[:,:mel.size(-1)]).view(-1)
- return enhanced_audio, self.h.sampling_rate
\ No newline at end of file
diff --git a/spaces/HarryLee/eCommerceImageCaptioning/fairseq/fairseq/model_parallel/megatron_trainer.py b/spaces/HarryLee/eCommerceImageCaptioning/fairseq/fairseq/model_parallel/megatron_trainer.py
deleted file mode 100644
index 8ab4657f73c6cda91e95637921edb84ccb76b3d0..0000000000000000000000000000000000000000
--- a/spaces/HarryLee/eCommerceImageCaptioning/fairseq/fairseq/model_parallel/megatron_trainer.py
+++ /dev/null
@@ -1,71 +0,0 @@
-# Copyright (c) Facebook, Inc. and its affiliates.
-#
-# This source code is licensed under the MIT license found in the
-# LICENSE file in the root directory of this source tree.
-
-"""
-Train a network across multiple GPUs.
-"""
-
-from fairseq.dataclass.configs import FairseqConfig
-from fairseq.distributed import utils as distributed_utils
-from fairseq.trainer import Trainer
-
-try:
- from fairseq.model_parallel.megatron.mpu import (
- get_data_parallel_rank,
- get_data_parallel_world_size,
- get_model_parallel_src_rank,
- get_cuda_rng_tracker,
- )
-
- has_megatron_submodule = True
-except (ImportError, ModuleNotFoundError):
- has_megatron_submodule = False
-
-
-class MegatronTrainer(Trainer):
- """Main class for model parallel with data parallel training."""
-
- def __init__(self, cfg: FairseqConfig, task, model, criterion, **kwargs):
- if not has_megatron_submodule:
- raise ImportError(
- "\n\nPlease install the megatron submodule:"
- "\n\n git submodule update --init "
- "fairseq/model_parallel/megatron"
- )
- super().__init__(cfg, task, model, criterion, **kwargs)
-
- def clip_grad_norm(self, clip_norm):
- def _aggregate_model_parallel_grad_norm(total_norm):
- total_norm = total_norm ** 2
- distributed_utils.all_reduce(
- total_norm, group=distributed_utils.get_model_parallel_group()
- )
- total_norm = total_norm ** 0.5
- return total_norm
-
- return self.optimizer.clip_grad_norm(
- clip_norm,
- aggregate_norm_fn=_aggregate_model_parallel_grad_norm,
- )
-
- def save_checkpoint(self, filename, extra_state):
- """Save all training state in a checkpoint file."""
- extra_state['rng_tracker_states'] \
- = get_cuda_rng_tracker().get_states()
- super().save_checkpoint(filename, extra_state)
-
- def load_checkpoint(
- self,
- filename,
- reset_optimizer=False,
- reset_lr_scheduler=False,
- optimizer_overrides=None,
- reset_meters=False,
- ):
- extra_state = super().load_checkpoint(filename, reset_optimizer=reset_optimizer, reset_lr_scheduler=reset_lr_scheduler, optimizer_overrides=optimizer_overrides, reset_meters=reset_meters)
- if extra_state is not None and 'rng_tracker_states' in extra_state:
- get_cuda_rng_tracker().set_states(
- extra_state['rng_tracker_states'])
- return extra_state
diff --git a/spaces/Hexequin/dreamlike-photoreal-2.0/README.md b/spaces/Hexequin/dreamlike-photoreal-2.0/README.md
deleted file mode 100644
index 0b4a00455e0eafdfab30671a0aa4ad5aa3c6276b..0000000000000000000000000000000000000000
--- a/spaces/Hexequin/dreamlike-photoreal-2.0/README.md
+++ /dev/null
@@ -1,12 +0,0 @@
----
-title: Dreamlike Photoreal 2.0
-emoji: 🏢
-colorFrom: red
-colorTo: yellow
-sdk: gradio
-sdk_version: 3.20.1
-app_file: app.py
-pinned: false
----
-
-Check out the configuration reference at https://huggingface.co/docs/hub/spaces-config-reference
diff --git a/spaces/HuggingFaceH4/reward-modeling-chat-ui/README.md b/spaces/HuggingFaceH4/reward-modeling-chat-ui/README.md
deleted file mode 100644
index 5dd2193b8a7a59d213ab3754ec9f76876fcdd4fd..0000000000000000000000000000000000000000
--- a/spaces/HuggingFaceH4/reward-modeling-chat-ui/README.md
+++ /dev/null
@@ -1,13 +0,0 @@
----
-title: Reward Modeling UI
-emoji: 🎁
-colorFrom: orange
-colorTo: indigo
-sdk: gradio
-python_version: 3.9.13
-app_file: app.py
-pinned: false
-license: apache-2.0
----
-
-Check out the configuration reference at https://huggingface.co/docs/hub/spaces-config-reference
diff --git a/spaces/HuggingFaceH4/starchat-playground/README.md b/spaces/HuggingFaceH4/starchat-playground/README.md
deleted file mode 100644
index a220058af2796c94f985a2bf4dd46e4cfd48986a..0000000000000000000000000000000000000000
--- a/spaces/HuggingFaceH4/starchat-playground/README.md
+++ /dev/null
@@ -1,13 +0,0 @@
----
-title: StarChat Playground
-emoji: ⭐️💬
-colorFrom: pink
-colorTo: indigo
-sdk: gradio
-sdk_version: 3.33.1
-app_file: app.py
-pinned: true
-license: mit
----
-
-Check out the configuration reference at https://huggingface.co/docs/hub/spaces-config-reference
diff --git a/spaces/HuggingFaceM4/IDEFICS_Data_Measurement_Tool/npmi/app.py b/spaces/HuggingFaceM4/IDEFICS_Data_Measurement_Tool/npmi/app.py
deleted file mode 100644
index 018d7a9579ceba243be2f0f25e0d0ff4228b6e4f..0000000000000000000000000000000000000000
--- a/spaces/HuggingFaceM4/IDEFICS_Data_Measurement_Tool/npmi/app.py
+++ /dev/null
@@ -1,6 +0,0 @@
-import evaluate
-from evaluate.utils import launch_gradio_widget
-
-
-module = evaluate.load("npmi", module_type= "measurement")
-launch_gradio_widget(module)
\ No newline at end of file
diff --git a/spaces/ICML2022/OFA/fairseq/fairseq/data/backtranslation_dataset.py b/spaces/ICML2022/OFA/fairseq/fairseq/data/backtranslation_dataset.py
deleted file mode 100644
index 8f70c90df3d237077537993e125d366c95292f1a..0000000000000000000000000000000000000000
--- a/spaces/ICML2022/OFA/fairseq/fairseq/data/backtranslation_dataset.py
+++ /dev/null
@@ -1,165 +0,0 @@
-# Copyright (c) Facebook, Inc. and its affiliates.
-#
-# This source code is licensed under the MIT license found in the
-# LICENSE file in the root directory of this source tree.
-
-import torch
-from fairseq import utils
-
-from . import FairseqDataset
-
-
-def backtranslate_samples(samples, collate_fn, generate_fn, cuda=True):
- """Backtranslate a list of samples.
-
- Given an input (*samples*) of the form:
-
- [{'id': 1, 'source': 'hallo welt'}]
-
- this will return:
-
- [{'id': 1, 'source': 'hello world', 'target': 'hallo welt'}]
-
- Args:
- samples (List[dict]): samples to backtranslate. Individual samples are
- expected to have a 'source' key, which will become the 'target'
- after backtranslation.
- collate_fn (callable): function to collate samples into a mini-batch
- generate_fn (callable): function to generate backtranslations
- cuda (bool): use GPU for generation (default: ``True``)
-
- Returns:
- List[dict]: an updated list of samples with a backtranslated source
- """
- collated_samples = collate_fn(samples)
- s = utils.move_to_cuda(collated_samples) if cuda else collated_samples
- generated_sources = generate_fn(s)
-
- id_to_src = {sample["id"]: sample["source"] for sample in samples}
-
- # Go through each tgt sentence in batch and its corresponding best
- # generated hypothesis and create a backtranslation data pair
- # {id: id, source: generated backtranslation, target: original tgt}
- return [
- {
- "id": id.item(),
- "target": id_to_src[id.item()],
- "source": hypos[0]["tokens"].cpu(),
- }
- for id, hypos in zip(collated_samples["id"], generated_sources)
- ]
-
-
-class BacktranslationDataset(FairseqDataset):
- """
- Sets up a backtranslation dataset which takes a tgt batch, generates
- a src using a tgt-src backtranslation function (*backtranslation_fn*),
- and returns the corresponding `{generated src, input tgt}` batch.
-
- Args:
- tgt_dataset (~fairseq.data.FairseqDataset): the dataset to be
- backtranslated. Only the source side of this dataset will be used.
- After backtranslation, the source sentences in this dataset will be
- returned as the targets.
- src_dict (~fairseq.data.Dictionary): the dictionary of backtranslated
- sentences.
- tgt_dict (~fairseq.data.Dictionary, optional): the dictionary of
- sentences to be backtranslated.
- backtranslation_fn (callable, optional): function to call to generate
- backtranslations. This is typically the `generate` method of a
- :class:`~fairseq.sequence_generator.SequenceGenerator` object.
- Pass in None when it is not available at initialization time, and
- use set_backtranslation_fn function to set it when available.
- output_collater (callable, optional): function to call on the
- backtranslated samples to create the final batch
- (default: ``tgt_dataset.collater``).
- cuda: use GPU for generation
- """
-
- def __init__(
- self,
- tgt_dataset,
- src_dict,
- tgt_dict=None,
- backtranslation_fn=None,
- output_collater=None,
- cuda=True,
- **kwargs
- ):
- self.tgt_dataset = tgt_dataset
- self.backtranslation_fn = backtranslation_fn
- self.output_collater = (
- output_collater if output_collater is not None else tgt_dataset.collater
- )
- self.cuda = cuda if torch.cuda.is_available() else False
- self.src_dict = src_dict
- self.tgt_dict = tgt_dict
-
- def __getitem__(self, index):
- """
- Returns a single sample from *tgt_dataset*. Note that backtranslation is
- not applied in this step; use :func:`collater` instead to backtranslate
- a batch of samples.
- """
- return self.tgt_dataset[index]
-
- def __len__(self):
- return len(self.tgt_dataset)
-
- def set_backtranslation_fn(self, backtranslation_fn):
- self.backtranslation_fn = backtranslation_fn
-
- def collater(self, samples):
- """Merge and backtranslate a list of samples to form a mini-batch.
-
- Using the samples from *tgt_dataset*, load a collated target sample to
- feed to the backtranslation model. Then take the backtranslation with
- the best score as the source and the original input as the target.
-
- Note: we expect *tgt_dataset* to provide a function `collater()` that
- will collate samples into the format expected by *backtranslation_fn*.
- After backtranslation, we will feed the new list of samples (i.e., the
- `(backtranslated source, original source)` pairs) to *output_collater*
- and return the result.
-
- Args:
- samples (List[dict]): samples to backtranslate and collate
-
- Returns:
- dict: a mini-batch with keys coming from *output_collater*
- """
- if samples[0].get("is_dummy", False):
- return samples
- samples = backtranslate_samples(
- samples=samples,
- collate_fn=self.tgt_dataset.collater,
- generate_fn=(lambda net_input: self.backtranslation_fn(net_input)),
- cuda=self.cuda,
- )
- return self.output_collater(samples)
-
- def num_tokens(self, index):
- """Just use the tgt dataset num_tokens"""
- return self.tgt_dataset.num_tokens(index)
-
- def ordered_indices(self):
- """Just use the tgt dataset ordered_indices"""
- return self.tgt_dataset.ordered_indices()
-
- def size(self, index):
- """Return an example's size as a float or tuple. This value is used
- when filtering a dataset with ``--max-positions``.
-
- Note: we use *tgt_dataset* to approximate the length of the source
- sentence, since we do not know the actual length until after
- backtranslation.
- """
- tgt_size = self.tgt_dataset.size(index)[0]
- return (tgt_size, tgt_size)
-
- @property
- def supports_prefetch(self):
- return getattr(self.tgt_dataset, "supports_prefetch", False)
-
- def prefetch(self, indices):
- return self.tgt_dataset.prefetch(indices)
diff --git a/spaces/Ikaros521/so-vits-svc-4.0-ikaros2/cluster/__init__.py b/spaces/Ikaros521/so-vits-svc-4.0-ikaros2/cluster/__init__.py
deleted file mode 100644
index f1b9bde04e73e9218a5d534227caa4c25332f424..0000000000000000000000000000000000000000
--- a/spaces/Ikaros521/so-vits-svc-4.0-ikaros2/cluster/__init__.py
+++ /dev/null
@@ -1,29 +0,0 @@
-import numpy as np
-import torch
-from sklearn.cluster import KMeans
-
-def get_cluster_model(ckpt_path):
- checkpoint = torch.load(ckpt_path)
- kmeans_dict = {}
- for spk, ckpt in checkpoint.items():
- km = KMeans(ckpt["n_features_in_"])
- km.__dict__["n_features_in_"] = ckpt["n_features_in_"]
- km.__dict__["_n_threads"] = ckpt["_n_threads"]
- km.__dict__["cluster_centers_"] = ckpt["cluster_centers_"]
- kmeans_dict[spk] = km
- return kmeans_dict
-
-def get_cluster_result(model, x, speaker):
- """
- x: np.array [t, 256]
- return cluster class result
- """
- return model[speaker].predict(x)
-
-def get_cluster_center_result(model, x,speaker):
- """x: np.array [t, 256]"""
- predict = model[speaker].predict(x)
- return model[speaker].cluster_centers_[predict]
-
-def get_center(model, x,speaker):
- return model[speaker].cluster_centers_[x]
diff --git a/spaces/Jack003/PixelDayAvatoon/DESCRIPTION.md b/spaces/Jack003/PixelDayAvatoon/DESCRIPTION.md
deleted file mode 100644
index e66cbc0582bd61f2bd0bef76e81fd060c2f9526c..0000000000000000000000000000000000000000
--- a/spaces/Jack003/PixelDayAvatoon/DESCRIPTION.md
+++ /dev/null
@@ -1 +0,0 @@
-Recreate the viral AnimeGAN image transformation demo.
\ No newline at end of file
diff --git a/spaces/JavaFXpert/GPT-3.5-Express-inator/README.md b/spaces/JavaFXpert/GPT-3.5-Express-inator/README.md
deleted file mode 100644
index a54d1f283e6d54e337977c73846d551c9ad5bdf4..0000000000000000000000000000000000000000
--- a/spaces/JavaFXpert/GPT-3.5-Express-inator/README.md
+++ /dev/null
@@ -1,13 +0,0 @@
----
-title: GPT 3.5 Express Inator
-emoji: 💻
-colorFrom: pink
-colorTo: yellow
-sdk: gradio
-sdk_version: 3.15.0
-app_file: app.py
-pinned: false
-license: apache-2.0
----
-
-Check out the configuration reference at https://huggingface.co/docs/hub/spaces-config-reference
diff --git a/spaces/JohnSmith9982/ChuanhuChatGPT/web_assets/javascript/message-button.js b/spaces/JohnSmith9982/ChuanhuChatGPT/web_assets/javascript/message-button.js
deleted file mode 100644
index e16b065c8c0ea84b927ebbb46b7ff336d085b8d9..0000000000000000000000000000000000000000
--- a/spaces/JohnSmith9982/ChuanhuChatGPT/web_assets/javascript/message-button.js
+++ /dev/null
@@ -1,92 +0,0 @@
-
-// 为 bot 消息添加复制与切换显示按钮
-
-function addChuanhuButton(botElement) {
- var rawMessage = botElement.querySelector('.raw-message');
- var mdMessage = botElement.querySelector('.md-message');
-
- if (!rawMessage) { // 如果没有 raw message,说明是早期历史记录,去除按钮
- var buttons = botElement.querySelectorAll('button.chuanhu-btn');
- for (var i = 0; i < buttons.length; i++) {
- buttons[i].parentNode.removeChild(buttons[i]);
- }
- return;
- }
- botElement.querySelectorAll('button.copy-bot-btn, button.toggle-md-btn').forEach(btn => btn.remove()); // 就算原先有了,也必须重新添加,而不是跳过
-
- // Copy bot button
- var copyButton = document.createElement('button');
- copyButton.classList.add('chuanhu-btn');
- copyButton.classList.add('copy-bot-btn');
- copyButton.setAttribute('aria-label', 'Copy');
- copyButton.innerHTML = copyIcon;
-
- copyButton.addEventListener('click', async () => {
- const textToCopy = rawMessage.innerText;
- try {
- if ("clipboard" in navigator) {
- await navigator.clipboard.writeText(textToCopy);
- copyButton.innerHTML = copiedIcon;
- setTimeout(() => {
- copyButton.innerHTML = copyIcon;
- }, 1500);
- } else {
- const textArea = document.createElement("textarea");
- textArea.value = textToCopy;
- document.body.appendChild(textArea);
- textArea.select();
- try {
- document.execCommand('copy');
- copyButton.innerHTML = copiedIcon;
- setTimeout(() => {
- copyButton.innerHTML = copyIcon;
- }, 1500);
- } catch (error) {
- console.error("Copy failed: ", error);
- }
- document.body.removeChild(textArea);
- }
- } catch (error) {
- console.error("Copy failed: ", error);
- }
- });
- botElement.appendChild(copyButton);
-
- // Toggle button
- var toggleButton = document.createElement('button');
- toggleButton.classList.add('chuanhu-btn');
- toggleButton.classList.add('toggle-md-btn');
- toggleButton.setAttribute('aria-label', 'Toggle');
- var renderMarkdown = mdMessage.classList.contains('hideM');
- toggleButton.innerHTML = renderMarkdown ? mdIcon : rawIcon;
- toggleButton.addEventListener('click', () => {
- renderMarkdown = mdMessage.classList.contains('hideM');
- if (renderMarkdown) {
- renderMarkdownText(botElement);
- toggleButton.innerHTML=rawIcon;
- } else {
- removeMarkdownText(botElement);
- toggleButton.innerHTML=mdIcon;
- }
- chatbotContentChanged(1); // to set md or raw in read-only history html
- });
- botElement.insertBefore(toggleButton, copyButton);
-
- function renderMarkdownText(message) {
- var mdDiv = message.querySelector('.md-message');
- if (mdDiv) mdDiv.classList.remove('hideM');
- var rawDiv = message.querySelector('.raw-message');
- if (rawDiv) rawDiv.classList.add('hideM');
- }
- function removeMarkdownText(message) {
- var rawDiv = message.querySelector('.raw-message');
- if (rawDiv) {
- rawDiv.innerHTML = rawDiv.querySelector('pre')?.innerHTML || rawDiv.innerHTML;
- rawDiv.classList.remove('hideM');
- }
- var mdDiv = message.querySelector('.md-message');
- if (mdDiv) mdDiv.classList.add('hideM');
- }
-}
-
-
diff --git a/spaces/Kangarroar/ApplioRVC-Inference/demucs/__main__.py b/spaces/Kangarroar/ApplioRVC-Inference/demucs/__main__.py
deleted file mode 100644
index 5148f20623bdaa827777558844796ded1876d7d0..0000000000000000000000000000000000000000
--- a/spaces/Kangarroar/ApplioRVC-Inference/demucs/__main__.py
+++ /dev/null
@@ -1,317 +0,0 @@
-# Copyright (c) Facebook, Inc. and its affiliates.
-# All rights reserved.
-#
-# This source code is licensed under the license found in the
-# LICENSE file in the root directory of this source tree.
-
-import json
-import math
-import os
-import sys
-import time
-from dataclasses import dataclass, field
-
-import torch as th
-from torch import distributed, nn
-from torch.nn.parallel.distributed import DistributedDataParallel
-
-from .augment import FlipChannels, FlipSign, Remix, Scale, Shift
-from .compressed import get_compressed_datasets
-from .model import Demucs
-from .parser import get_name, get_parser
-from .raw import Rawset
-from .repitch import RepitchedWrapper
-from .pretrained import load_pretrained, SOURCES
-from .tasnet import ConvTasNet
-from .test import evaluate
-from .train import train_model, validate_model
-from .utils import (human_seconds, load_model, save_model, get_state,
- save_state, sizeof_fmt, get_quantizer)
-from .wav import get_wav_datasets, get_musdb_wav_datasets
-
-
-@dataclass
-class SavedState:
- metrics: list = field(default_factory=list)
- last_state: dict = None
- best_state: dict = None
- optimizer: dict = None
-
-
-def main():
- parser = get_parser()
- args = parser.parse_args()
- name = get_name(parser, args)
- print(f"Experiment {name}")
-
- if args.musdb is None and args.rank == 0:
- print(
- "You must provide the path to the MusDB dataset with the --musdb flag. "
- "To download the MusDB dataset, see https://sigsep.github.io/datasets/musdb.html.",
- file=sys.stderr)
- sys.exit(1)
-
- eval_folder = args.evals / name
- eval_folder.mkdir(exist_ok=True, parents=True)
- args.logs.mkdir(exist_ok=True)
- metrics_path = args.logs / f"{name}.json"
- eval_folder.mkdir(exist_ok=True, parents=True)
- args.checkpoints.mkdir(exist_ok=True, parents=True)
- args.models.mkdir(exist_ok=True, parents=True)
-
- if args.device is None:
- device = "cpu"
- if th.cuda.is_available():
- device = "cuda"
- else:
- device = args.device
-
- th.manual_seed(args.seed)
- # Prevents too many threads to be started when running `museval` as it can be quite
- # inefficient on NUMA architectures.
- os.environ["OMP_NUM_THREADS"] = "1"
- os.environ["MKL_NUM_THREADS"] = "1"
-
- if args.world_size > 1:
- if device != "cuda" and args.rank == 0:
- print("Error: distributed training is only available with cuda device", file=sys.stderr)
- sys.exit(1)
- th.cuda.set_device(args.rank % th.cuda.device_count())
- distributed.init_process_group(backend="nccl",
- init_method="tcp://" + args.master,
- rank=args.rank,
- world_size=args.world_size)
-
- checkpoint = args.checkpoints / f"{name}.th"
- checkpoint_tmp = args.checkpoints / f"{name}.th.tmp"
- if args.restart and checkpoint.exists() and args.rank == 0:
- checkpoint.unlink()
-
- if args.test or args.test_pretrained:
- args.epochs = 1
- args.repeat = 0
- if args.test:
- model = load_model(args.models / args.test)
- else:
- model = load_pretrained(args.test_pretrained)
- elif args.tasnet:
- model = ConvTasNet(audio_channels=args.audio_channels,
- samplerate=args.samplerate, X=args.X,
- segment_length=4 * args.samples,
- sources=SOURCES)
- else:
- model = Demucs(
- audio_channels=args.audio_channels,
- channels=args.channels,
- context=args.context,
- depth=args.depth,
- glu=args.glu,
- growth=args.growth,
- kernel_size=args.kernel_size,
- lstm_layers=args.lstm_layers,
- rescale=args.rescale,
- rewrite=args.rewrite,
- stride=args.conv_stride,
- resample=args.resample,
- normalize=args.normalize,
- samplerate=args.samplerate,
- segment_length=4 * args.samples,
- sources=SOURCES,
- )
- model.to(device)
- if args.init:
- model.load_state_dict(load_pretrained(args.init).state_dict())
-
- if args.show:
- print(model)
- size = sizeof_fmt(4 * sum(p.numel() for p in model.parameters()))
- print(f"Model size {size}")
- return
-
- try:
- saved = th.load(checkpoint, map_location='cpu')
- except IOError:
- saved = SavedState()
-
- optimizer = th.optim.Adam(model.parameters(), lr=args.lr)
-
- quantizer = None
- quantizer = get_quantizer(model, args, optimizer)
-
- if saved.last_state is not None:
- model.load_state_dict(saved.last_state, strict=False)
- if saved.optimizer is not None:
- optimizer.load_state_dict(saved.optimizer)
-
- model_name = f"{name}.th"
- if args.save_model:
- if args.rank == 0:
- model.to("cpu")
- model.load_state_dict(saved.best_state)
- save_model(model, quantizer, args, args.models / model_name)
- return
- elif args.save_state:
- model_name = f"{args.save_state}.th"
- if args.rank == 0:
- model.to("cpu")
- model.load_state_dict(saved.best_state)
- state = get_state(model, quantizer)
- save_state(state, args.models / model_name)
- return
-
- if args.rank == 0:
- done = args.logs / f"{name}.done"
- if done.exists():
- done.unlink()
-
- augment = [Shift(args.data_stride)]
- if args.augment:
- augment += [FlipSign(), FlipChannels(), Scale(),
- Remix(group_size=args.remix_group_size)]
- augment = nn.Sequential(*augment).to(device)
- print("Agumentation pipeline:", augment)
-
- if args.mse:
- criterion = nn.MSELoss()
- else:
- criterion = nn.L1Loss()
-
- # Setting number of samples so that all convolution windows are full.
- # Prevents hard to debug mistake with the prediction being shifted compared
- # to the input mixture.
- samples = model.valid_length(args.samples)
- print(f"Number of training samples adjusted to {samples}")
- samples = samples + args.data_stride
- if args.repitch:
- # We need a bit more audio samples, to account for potential
- # tempo change.
- samples = math.ceil(samples / (1 - 0.01 * args.max_tempo))
-
- args.metadata.mkdir(exist_ok=True, parents=True)
- if args.raw:
- train_set = Rawset(args.raw / "train",
- samples=samples,
- channels=args.audio_channels,
- streams=range(1, len(model.sources) + 1),
- stride=args.data_stride)
-
- valid_set = Rawset(args.raw / "valid", channels=args.audio_channels)
- elif args.wav:
- train_set, valid_set = get_wav_datasets(args, samples, model.sources)
- elif args.is_wav:
- train_set, valid_set = get_musdb_wav_datasets(args, samples, model.sources)
- else:
- train_set, valid_set = get_compressed_datasets(args, samples)
-
- if args.repitch:
- train_set = RepitchedWrapper(
- train_set,
- proba=args.repitch,
- max_tempo=args.max_tempo)
-
- best_loss = float("inf")
- for epoch, metrics in enumerate(saved.metrics):
- print(f"Epoch {epoch:03d}: "
- f"train={metrics['train']:.8f} "
- f"valid={metrics['valid']:.8f} "
- f"best={metrics['best']:.4f} "
- f"ms={metrics.get('true_model_size', 0):.2f}MB "
- f"cms={metrics.get('compressed_model_size', 0):.2f}MB "
- f"duration={human_seconds(metrics['duration'])}")
- best_loss = metrics['best']
-
- if args.world_size > 1:
- dmodel = DistributedDataParallel(model,
- device_ids=[th.cuda.current_device()],
- output_device=th.cuda.current_device())
- else:
- dmodel = model
-
- for epoch in range(len(saved.metrics), args.epochs):
- begin = time.time()
- model.train()
- train_loss, model_size = train_model(
- epoch, train_set, dmodel, criterion, optimizer, augment,
- quantizer=quantizer,
- batch_size=args.batch_size,
- device=device,
- repeat=args.repeat,
- seed=args.seed,
- diffq=args.diffq,
- workers=args.workers,
- world_size=args.world_size)
- model.eval()
- valid_loss = validate_model(
- epoch, valid_set, model, criterion,
- device=device,
- rank=args.rank,
- split=args.split_valid,
- overlap=args.overlap,
- world_size=args.world_size)
-
- ms = 0
- cms = 0
- if quantizer and args.rank == 0:
- ms = quantizer.true_model_size()
- cms = quantizer.compressed_model_size(num_workers=min(40, args.world_size * 10))
-
- duration = time.time() - begin
- if valid_loss < best_loss and ms <= args.ms_target:
- best_loss = valid_loss
- saved.best_state = {
- key: value.to("cpu").clone()
- for key, value in model.state_dict().items()
- }
-
- saved.metrics.append({
- "train": train_loss,
- "valid": valid_loss,
- "best": best_loss,
- "duration": duration,
- "model_size": model_size,
- "true_model_size": ms,
- "compressed_model_size": cms,
- })
- if args.rank == 0:
- json.dump(saved.metrics, open(metrics_path, "w"))
-
- saved.last_state = model.state_dict()
- saved.optimizer = optimizer.state_dict()
- if args.rank == 0 and not args.test:
- th.save(saved, checkpoint_tmp)
- checkpoint_tmp.rename(checkpoint)
-
- print(f"Epoch {epoch:03d}: "
- f"train={train_loss:.8f} valid={valid_loss:.8f} best={best_loss:.4f} ms={ms:.2f}MB "
- f"cms={cms:.2f}MB "
- f"duration={human_seconds(duration)}")
-
- if args.world_size > 1:
- distributed.barrier()
-
- del dmodel
- model.load_state_dict(saved.best_state)
- if args.eval_cpu:
- device = "cpu"
- model.to(device)
- model.eval()
- evaluate(model, args.musdb, eval_folder,
- is_wav=args.is_wav,
- rank=args.rank,
- world_size=args.world_size,
- device=device,
- save=args.save,
- split=args.split_valid,
- shifts=args.shifts,
- overlap=args.overlap,
- workers=args.eval_workers)
- model.to("cpu")
- if args.rank == 0:
- if not (args.test or args.test_pretrained):
- save_model(model, quantizer, args, args.models / model_name)
- print("done")
- done.write_text("done")
-
-
-if __name__ == "__main__":
- main()
diff --git a/spaces/KyanChen/FunSR/tools/data_tools/get_all_data_list.py b/spaces/KyanChen/FunSR/tools/data_tools/get_all_data_list.py
deleted file mode 100644
index 6d3fab7d8525b555a362d7a43bff267c1ce6889a..0000000000000000000000000000000000000000
--- a/spaces/KyanChen/FunSR/tools/data_tools/get_all_data_list.py
+++ /dev/null
@@ -1,25 +0,0 @@
-import glob
-import os
-
-import numpy as np
-import pickle
-import sys
-import tqdm
-import shutil
-from skimage import io
-
-pre_path = r'H:\DataSet\SceneCls\UCMerced_LandUse\UCMerced_LandUse\Images'
-sub_folder_list = glob.glob(pre_path +'/*')
-all_data_list = []
-for sub_folder in sub_folder_list:
- img_list = glob.glob(sub_folder+'/*')
- all_data_list += img_list
-
-with open(pre_path+f'/../all_img_list.txt', 'w') as f:
- for file in tqdm.tqdm(all_data_list):
- img = io.imread(file, as_gray=True)
- if 0 < img.shape[0]:
- file_name = os.path.basename(os.path.dirname(file)) + '/' + os.path.basename(file)
- gt_label = os.path.basename(os.path.dirname(file))
- f.write(file_name+' '+gt_label+'\n')
-
diff --git a/spaces/LanguageBind/LanguageBind/TRAIN_AND_VALIDATE.md b/spaces/LanguageBind/LanguageBind/TRAIN_AND_VALIDATE.md
deleted file mode 100644
index 01ed15fe8f4ed2687597ad97c62d755e62dfdca9..0000000000000000000000000000000000000000
--- a/spaces/LanguageBind/LanguageBind/TRAIN_AND_VALIDATE.md
+++ /dev/null
@@ -1,207 +0,0 @@
-We provide the **off-the-shelf** scripts in the [scripts folder](scripts).
-
-## Training LanguageBind
-
-For example, to **train** LanguageBind on **Depth-Language** with 16 GPUs (2 nodes x 8 GPUs).
-* First download the [cache of pretrained weight](https://github.com/PKU-YuanGroup/LanguageBind#-model-zoo) and specify ```CACHE_DIR```.
-* The second step is to develop a path to ```TRAIN_DATA``` according to the [dataset preparation](https://github.com/PKU-YuanGroup/LanguageBind#-vidal-10m).
-* Then you can run
-
-```bash
-CACHE_DIR="path/to/pretrained/weight"
-TRAIN_DATA="path/to/data"
-cd /path/to/LanguageBind
-TORCH_DISTRIBUTED_DEBUG=DETAIL HF_DATASETS_OFFLINE=1 TRANSFORMERS_OFFLINE=1 torchrun --nnodes=1 --nproc_per_node 8 \
- -m main \
- --train-data ${TRAIN_DATA} \
- --train-num-samples 3020000 \
- --clip-type "dl" --max-depth 10 \
- --do_train \
- --lock-text --lock-image --text-type "polish_mplug" \
- --init-temp 0.07 --learn-temp \
- --model "ViT-L-14" --cache-dir ${CACHE_DIR} \
- --convert_to_lora --lora_r 2 \
- --lr 5e-4 --coef-lr 1e-3 \
- --beta1 0.9 --beta2 0.98 --wd 0.2 --eps 1e-6 \
- --num-frames 1 --force-patch-dropout 0.5 \
- --epochs 1 --batch-size 128 --accum-freq 1 --warmup 200 \
- --precision "amp" --workers 10 --video-decode-backend "imgs" \
- --save-frequency 1 --log-every-n-steps 20 --report-to "tensorboard" --resume "latest" \
- --do_eval \
- --val_d_cls_data "NYUV2"
-```
-
-
-## Validating LanguageBind
-
-For example, to **validate** LanguageBind on **Depth-Language** with 1 GPUs.
-* First specify ```RESUME```.
-* The second step is to prepare the [downstream dataset](https://github.com/PKU-YuanGroup/LanguageBind/blob/main/TRAIN_AND_VALIDATE.md#downstream-datasets).
-* Then you can run
-
-```bash
-CACHE_DIR="path/to/pretrained/weight"
-RESUME="thermal_language.pt"
-TRAIN_DATA="path/to/data"
-cd /path/to/LanguageBind
-TORCH_DISTRIBUTED_DEBUG=DETAIL HF_DATASETS_OFFLINE=1 TRANSFORMERS_OFFLINE=1 torchrun --nproc_per_node 1 \
- -m main \
- --train-data ${TRAIN_DATA} \
- --train-num-samples 3020000 \
- --clip-type "dl" --max-depth 10 \
- --lock-text --lock-image --text-type "polish_mplug" \
- --init-temp 0.07 --learn-temp \
- --model "ViT-L-14" --cache-dir ${CACHE_DIR} \
- --convert_to_lora --lora_r 2 \
- --lr 5e-4 --coef-lr 1e-3 \
- --beta1 0.9 --beta2 0.98 --wd 0.2 --eps 1e-6 \
- --num-frames 1 --force-patch-dropout 0.5 \
- --epochs 1 --batch-size 128 --accum-freq 1 --warmup 200 \
- --precision "amp" --workers 10 --video-decode-backend "imgs" \
- --save-frequency 1 --log-every-n-steps 20 --report-to "tensorboard" --resume ${RESUME} \
- --do_eval \
- --val_d_cls_data "NYUV2"
-```
-
-## Downstream datasets
-
-### Depth
-NYU V2 dataset is downloaded from [this repo](https://github.com/TUI-NICR/nicr-scene-analysis-datasets/tree/main/nicr_scene_analysis_datasets/datasets/nyuv2) and we reformat them to conform to the standard ImageNet format. Change the ```data_root``` [here](https://github.com/PKU-YuanGroup/LanguageBind/blob/main/data/build_datasets.py#L148).
-
-### Video
-Video datasets are downloaded from [this repo](https://github.com/jpthu17/HBI) and we show the folder structure. Change the ```data_root``` [here](https://github.com/PKU-YuanGroup/LanguageBind/blob/main/data/build_datasets.py#L74).
-
-### Audio
-Audio datasets are downloaded from [this repo](https://github.com/OFA-Sys/ONE-PEACE/blob/main/datasets.md#audio) and we reformat them to conform to the standard ImageNet format. Change the ```data_root``` [here](https://github.com/PKU-YuanGroup/LanguageBind/blob/main/data/build_datasets.py#L127).
-
-### Infrared (Thermal)
-We download LLVIP from [official website](https://bupt-ai-cz.github.io/LLVIP/), and FLIR from [here](https://www.flir.com/oem/adas/adas-dataset-form/). We reformat them to conform to the standard ImageNet format. Change the ```data_root``` [here](https://github.com/PKU-YuanGroup/LanguageBind/blob/main/data/build_datasets.py#L160). We also provide the processed data as follows.
-
-
-
-### Folder structure
-```bash
-downstream_datasets
-├── Audio
-│ ├── esc50
-│ │ └── test
-│ │ ├── airplane
-│ │ ├── breathing
-│ │ ├── brushing_teeth
-│ │ ├── can_opening
-│ │ ├── car_horn
-│ │ ├── cat
-│ │ ├── chainsaw
-│ │ ├── chirping_birds
-│ │ ├── church_bells
-│ │ ├── clapping
-│ │ ├── clock_alarm
-│ │ ├── clock_tick
-│ │ ├── coughing
-│ │ ├── cow
-│ │ ├── crackling_fire
-│ │ ├── crickets
-│ │ ├── crow
-│ │ ├── crying_baby
-│ │ ├── dog
-│ │ ├── door_wood_creaks
-│ │ ├── door_wood_knock
-│ │ ├── drinking_sipping
-│ │ ├── engine
-│ │ ├── fireworks
-│ │ ├── footsteps
-│ │ ├── frog
-│ │ ├── glass_breaking
-│ │ ├── hand_saw
-│ │ ├── helicopter
-│ │ ├── hen
-│ │ ├── insects
-│ │ ├── keyboard_typing
-│ │ ├── laughing
-│ │ ├── mouse_click
-│ │ ├── pig
-│ │ ├── pouring_water
-│ │ ├── rain
-│ │ ├── rooster
-│ │ ├── sea_waves
-│ │ ├── sheep
-│ │ ├── siren
-│ │ ├── sneezing
-│ │ ├── snoring
-│ │ ├── thunderstorm
-│ │ ├── toilet_flush
-│ │ ├── train
-│ │ ├── vacuum_cleaner
-│ │ ├── washing_machine
-│ │ ├── water_drops
-│ │ └── wind
-├── Depth
-│ ├── nyuv2
-│ │ ├── data
-│ │ │ └── val
-│ │ │ ├── bathroom
-│ │ │ ├── bedroom
-│ │ │ ├── bookstore
-│ │ │ ├── classroom
-│ │ │ ├── dining_room
-│ │ │ ├── home_office
-│ │ │ ├── kitchen
-│ │ │ ├── living_room
-│ │ │ ├── office
-│ │ │ └── others
-├── Thermal
-│ ├── flirv1
-│ │ └── val
-│ │ ├── bicycle
-│ │ ├── car
-│ │ ├── dog
-│ │ └── person
-│ ├── flirv2
-│ │ └── val
-│ │ ├── bike
-│ │ ├── bus
-│ │ ├── car
-│ │ ├── hydrant
-│ │ ├── light
-│ │ ├── motor
-│ │ ├── other\ vehicle
-│ │ ├── person
-│ │ ├── sign
-│ │ ├── skateboard
-│ │ ├── stroller
-│ │ └── truck
-│ ├── llvip
-│ │ ├── train
-│ │ │ ├── background
-│ │ │ └── person
-│ │ └── val
-│ │ ├── background
-│ │ └── person
-└── VideoTextRetrieval
- ├── vtRetdata
- │ ├── ActivityNet
- │ │ └── Videos
- │ │ └── Activity_Videos
- │ ├── Didemo
- │ │ └── videos
- │ ├── MSRVTT
- │ │ └── MSRVTT_Videos
- │ └── MSVD
- │ └── MSVD_Videos
-```
-
diff --git a/spaces/LaynzKunz/Aesthetic_RVC_Inference_HF/lib/uvr5_pack/lib_v5/layers_537238KB.py b/spaces/LaynzKunz/Aesthetic_RVC_Inference_HF/lib/uvr5_pack/lib_v5/layers_537238KB.py
deleted file mode 100644
index a38b7bb3ae3136b07eadfc2db445fef4c2de186b..0000000000000000000000000000000000000000
--- a/spaces/LaynzKunz/Aesthetic_RVC_Inference_HF/lib/uvr5_pack/lib_v5/layers_537238KB.py
+++ /dev/null
@@ -1,126 +0,0 @@
-import torch
-from torch import nn
-import torch.nn.functional as F
-
-from . import spec_utils
-
-
-class Conv2DBNActiv(nn.Module):
- def __init__(self, nin, nout, ksize=3, stride=1, pad=1, dilation=1, activ=nn.ReLU):
- super(Conv2DBNActiv, self).__init__()
- self.conv = nn.Sequential(
- nn.Conv2d(
- nin,
- nout,
- kernel_size=ksize,
- stride=stride,
- padding=pad,
- dilation=dilation,
- bias=False,
- ),
- nn.BatchNorm2d(nout),
- activ(),
- )
-
- def __call__(self, x):
- return self.conv(x)
-
-
-class SeperableConv2DBNActiv(nn.Module):
- def __init__(self, nin, nout, ksize=3, stride=1, pad=1, dilation=1, activ=nn.ReLU):
- super(SeperableConv2DBNActiv, self).__init__()
- self.conv = nn.Sequential(
- nn.Conv2d(
- nin,
- nin,
- kernel_size=ksize,
- stride=stride,
- padding=pad,
- dilation=dilation,
- groups=nin,
- bias=False,
- ),
- nn.Conv2d(nin, nout, kernel_size=1, bias=False),
- nn.BatchNorm2d(nout),
- activ(),
- )
-
- def __call__(self, x):
- return self.conv(x)
-
-
-class Encoder(nn.Module):
- def __init__(self, nin, nout, ksize=3, stride=1, pad=1, activ=nn.LeakyReLU):
- super(Encoder, self).__init__()
- self.conv1 = Conv2DBNActiv(nin, nout, ksize, 1, pad, activ=activ)
- self.conv2 = Conv2DBNActiv(nout, nout, ksize, stride, pad, activ=activ)
-
- def __call__(self, x):
- skip = self.conv1(x)
- h = self.conv2(skip)
-
- return h, skip
-
-
-class Decoder(nn.Module):
- def __init__(
- self, nin, nout, ksize=3, stride=1, pad=1, activ=nn.ReLU, dropout=False
- ):
- super(Decoder, self).__init__()
- self.conv = Conv2DBNActiv(nin, nout, ksize, 1, pad, activ=activ)
- self.dropout = nn.Dropout2d(0.1) if dropout else None
-
- def __call__(self, x, skip=None):
- x = F.interpolate(x, scale_factor=2, mode="bilinear", align_corners=True)
- if skip is not None:
- skip = spec_utils.crop_center(skip, x)
- x = torch.cat([x, skip], dim=1)
- h = self.conv(x)
-
- if self.dropout is not None:
- h = self.dropout(h)
-
- return h
-
-
-class ASPPModule(nn.Module):
- def __init__(self, nin, nout, dilations=(4, 8, 16, 32, 64), activ=nn.ReLU):
- super(ASPPModule, self).__init__()
- self.conv1 = nn.Sequential(
- nn.AdaptiveAvgPool2d((1, None)),
- Conv2DBNActiv(nin, nin, 1, 1, 0, activ=activ),
- )
- self.conv2 = Conv2DBNActiv(nin, nin, 1, 1, 0, activ=activ)
- self.conv3 = SeperableConv2DBNActiv(
- nin, nin, 3, 1, dilations[0], dilations[0], activ=activ
- )
- self.conv4 = SeperableConv2DBNActiv(
- nin, nin, 3, 1, dilations[1], dilations[1], activ=activ
- )
- self.conv5 = SeperableConv2DBNActiv(
- nin, nin, 3, 1, dilations[2], dilations[2], activ=activ
- )
- self.conv6 = SeperableConv2DBNActiv(
- nin, nin, 3, 1, dilations[2], dilations[2], activ=activ
- )
- self.conv7 = SeperableConv2DBNActiv(
- nin, nin, 3, 1, dilations[2], dilations[2], activ=activ
- )
- self.bottleneck = nn.Sequential(
- Conv2DBNActiv(nin * 7, nout, 1, 1, 0, activ=activ), nn.Dropout2d(0.1)
- )
-
- def forward(self, x):
- _, _, h, w = x.size()
- feat1 = F.interpolate(
- self.conv1(x), size=(h, w), mode="bilinear", align_corners=True
- )
- feat2 = self.conv2(x)
- feat3 = self.conv3(x)
- feat4 = self.conv4(x)
- feat5 = self.conv5(x)
- feat6 = self.conv6(x)
- feat7 = self.conv7(x)
- out = torch.cat((feat1, feat2, feat3, feat4, feat5, feat6, feat7), dim=1)
- bottle = self.bottleneck(out)
- return bottle
diff --git a/spaces/Lbin123/Lbingo/postcss.config.js b/spaces/Lbin123/Lbingo/postcss.config.js
deleted file mode 100644
index 33ad091d26d8a9dc95ebdf616e217d985ec215b8..0000000000000000000000000000000000000000
--- a/spaces/Lbin123/Lbingo/postcss.config.js
+++ /dev/null
@@ -1,6 +0,0 @@
-module.exports = {
- plugins: {
- tailwindcss: {},
- autoprefixer: {},
- },
-}
diff --git a/spaces/Loreleihunny/total_capy-love/Dockerfile b/spaces/Loreleihunny/total_capy-love/Dockerfile
deleted file mode 100644
index e6158e4b2d67eeea6e30ad3c1bb6043ec09b7b9b..0000000000000000000000000000000000000000
--- a/spaces/Loreleihunny/total_capy-love/Dockerfile
+++ /dev/null
@@ -1,11 +0,0 @@
-FROM node:18-bullseye-slim
-RUN apt-get update && \
-apt-get install -y git
-RUN git clone https://gitgud.io/khanon/oai-reverse-proxy.git /app
-WORKDIR /app
-RUN npm install
-COPY Dockerfile greeting.md* .env* ./
-RUN npm run build
-EXPOSE 7860
-ENV NODE_ENV=production
-CMD [ "npm", "start" ]
\ No newline at end of file
diff --git a/spaces/LudvigDoeser/TSLA_stock_predictions/README.md b/spaces/LudvigDoeser/TSLA_stock_predictions/README.md
deleted file mode 100644
index 92b8a7f267a83be5091fa8098d69a199d7f6045e..0000000000000000000000000000000000000000
--- a/spaces/LudvigDoeser/TSLA_stock_predictions/README.md
+++ /dev/null
@@ -1,13 +0,0 @@
----
-title: TSLA Stock Predictions
-emoji: 🌍
-colorFrom: red
-colorTo: indigo
-sdk: gradio
-sdk_version: 3.16.1
-app_file: app.py
-pinned: false
-license: apache-2.0
----
-
-Check out the configuration reference at https://huggingface.co/docs/hub/spaces-config-reference
diff --git a/spaces/Lycorisdeve/DeepDanbooru_string/app.py b/spaces/Lycorisdeve/DeepDanbooru_string/app.py
deleted file mode 100644
index 49019837c9207cc68cb37be0342f3bc44fd0decb..0000000000000000000000000000000000000000
--- a/spaces/Lycorisdeve/DeepDanbooru_string/app.py
+++ /dev/null
@@ -1,185 +0,0 @@
-#!/usr/bin/env python
-
-from __future__ import annotations
-
-import argparse
-import functools
-import os
-import html
-import pathlib
-import tarfile
-
-import deepdanbooru as dd
-import gradio as gr
-import huggingface_hub
-import numpy as np
-import PIL.Image
-import tensorflow as tf
-import piexif
-import piexif.helper
-
-TITLE = 'DeepDanbooru String'
-
-TOKEN = os.environ['TOKEN']
-MODEL_REPO = 'CikeyQI/DeepDanbooru_string'
-MODEL_FILENAME = 'model-resnet_custom_v3.h5'
-LABEL_FILENAME = 'tags.txt'
-
-
-def parse_args() -> argparse.Namespace:
- parser = argparse.ArgumentParser()
- parser.add_argument('--score-slider-step', type=float, default=0.05)
- parser.add_argument('--score-threshold', type=float, default=0.5)
- parser.add_argument('--theme', type=str, default='dark-grass')
- parser.add_argument('--live', action='store_true')
- parser.add_argument('--share', action='store_true')
- parser.add_argument('--port', type=int)
- parser.add_argument('--disable-queue',
- dest='enable_queue',
- action='store_false')
- parser.add_argument('--allow-flagging', type=str, default='never')
- return parser.parse_args()
-
-
-def load_sample_image_paths() -> list[pathlib.Path]:
- image_dir = pathlib.Path('images')
- if not image_dir.exists():
- dataset_repo = 'hysts/sample-images-TADNE'
- path = huggingface_hub.hf_hub_download(dataset_repo,
- 'images.tar.gz',
- repo_type='dataset',
- use_auth_token=TOKEN)
- with tarfile.open(path) as f:
- f.extractall()
- return sorted(image_dir.glob('*'))
-
-
-def load_model() -> tf.keras.Model:
- path = huggingface_hub.hf_hub_download(MODEL_REPO,
- MODEL_FILENAME,
- use_auth_token=TOKEN)
- model = tf.keras.models.load_model(path)
- return model
-
-
-def load_labels() -> list[str]:
- path = huggingface_hub.hf_hub_download(MODEL_REPO,
- LABEL_FILENAME,
- use_auth_token=TOKEN)
- with open(path) as f:
- labels = [line.strip() for line in f.readlines()]
- return labels
-
-def plaintext_to_html(text):
- text = "" + " \n".join([f"{html.escape(x)}" for x in text.split('\n')]) + "
"
- return text
-
-def predict(image: PIL.Image.Image, score_threshold: float,
- model: tf.keras.Model, labels: list[str]) -> dict[str, float]:
- rawimage = image
- _, height, width, _ = model.input_shape
- image = np.asarray(image)
- image = tf.image.resize(image,
- size=(height, width),
- method=tf.image.ResizeMethod.AREA,
- preserve_aspect_ratio=True)
- image = image.numpy()
- image = dd.image.transform_and_pad_image(image, width, height)
- image = image / 255.
- probs = model.predict(image[None, ...])[0]
- probs = probs.astype(float)
- res = dict()
- for prob, label in zip(probs.tolist(), labels):
- if prob < score_threshold:
- continue
- res[label] = prob
- b = dict(sorted(res.items(),key=lambda item:item[1], reverse=True))
- a = ', '.join(list(b.keys())).replace('_',' ').replace('(','\(').replace(')','\)')
- c = ', '.join(list(b.keys()))
-
- items = rawimage.info
- geninfo = ''
-
- if "exif" in rawimage.info:
- exif = piexif.load(rawimage.info["exif"])
- exif_comment = (exif or {}).get("Exif", {}).get(piexif.ExifIFD.UserComment, b'')
- try:
- exif_comment = piexif.helper.UserComment.load(exif_comment)
- except ValueError:
- exif_comment = exif_comment.decode('utf8', errors="ignore")
-
- items['exif comment'] = exif_comment
- geninfo = exif_comment
-
- for field in ['jfif', 'jfif_version', 'jfif_unit', 'jfif_density', 'dpi', 'exif',
- 'loop', 'background', 'timestamp', 'duration']:
- items.pop(field, None)
-
- geninfo = items.get('parameters', geninfo)
-
- info = f"""
-
PNG Info
-"""
- for key, text in items.items():
- info += f"""
-
-
{plaintext_to_html(str(key))}
-
{plaintext_to_html(str(text))}
-
-""".strip()+"\n"
-
- if len(info) == 0:
- message = "Nothing found in the image."
- info = f""
-
- return (a,c,res,info)
-
-
-def main():
- args = parse_args()
- model = load_model()
- labels = load_labels()
-
- func = functools.partial(predict, model=model, labels=labels)
- func = functools.update_wrapper(func, predict)
-
- gr.Interface(
- func,
- [
- gr.inputs.Image(type='pil', label='Input'),
- gr.inputs.Slider(0,
- 1,
- step=args.score_slider_step,
- default=args.score_threshold,
- label='Score Threshold'),
- ],
- [
- gr.outputs.Textbox(label='Output (string)'),
- gr.outputs.Textbox(label='Output (raw string)'),
- gr.outputs.Label(label='Output (label)'),
- gr.outputs.HTML()
- ],
- examples=[
- ['miku.jpg',0.5],
- ['miku2.jpg',0.5]
- ],
- title=TITLE,
- description='''
-Demo for [KichangKim/DeepDanbooru](https://github.com/KichangKim/DeepDanbooru) with "ready to copy" prompt and a prompt analyzer.
-
-Modified from [hysts/DeepDanbooru](https://huggingface.co/spaces/hysts/DeepDanbooru)
-
-PNG Info code forked from [AUTOMATIC1111/stable-diffusion-webui](https://github.com/AUTOMATIC1111/stable-diffusion-webui)
- ''',
- theme=args.theme,
- allow_flagging=args.allow_flagging,
- live=args.live,
- ).launch(
- enable_queue=args.enable_queue,
- server_port=args.port,
- share=args.share,
- )
-
-
-if __name__ == '__main__':
- main()
diff --git a/spaces/MariaK/Audio-Course-Certification/app.py b/spaces/MariaK/Audio-Course-Certification/app.py
deleted file mode 100644
index e1fa5e92df0c49085ad08c8019fad25fb6b65a80..0000000000000000000000000000000000000000
--- a/spaces/MariaK/Audio-Course-Certification/app.py
+++ /dev/null
@@ -1,197 +0,0 @@
-import gradio as gr
-from huggingface_hub import HfApi, hf_hub_download, Repository
-from huggingface_hub.repocard import metadata_load
-from gradio_client import Client
-from PIL import Image, ImageDraw, ImageFont
-
-from datetime import date
-import time
-
-import os
-import pandas as pd
-import json
-
-api = HfApi()
-HF_TOKEN = os.environ.get("HF_TOKEN")
-
-# Private dataset repo containing the list of already certified users
-DATASET_REPO_URL = "https://huggingface.co/datasets/MariaK/audio-course"
-CERTIFIED_USERS_FILENAME = "usernames.csv"
-
-# Private space to check if a user has passed.
-SPACE_ID = "MariaK/Check-Audio-Course-Progress"
-
-
-def check_if_passed(username):
- """
- Check if given user passed enough assignments
- :param username: User HF username
- """
-
- passed = False
- certificate_type = ""
-
- client = Client(SPACE_ID, hf_token=HF_TOKEN)
- result = client.predict(username, fn_index=0)
- with open(result) as json_data:
- data = json.load(json_data)
-
- df = pd.DataFrame(data['data'])
- if len(df[df.iloc[:,0] == '✅']) == 4:
- passed = True
- certificate_type = "excellence"
- elif len(df[df.iloc[:,0] == '✅']) == 3:
- passed = True
- certificate_type = "completion"
-
- return passed, certificate_type
-
-
-def generate_certificate(certificate_template, first_name, last_name):
- """
- Generates certificate from the template
- :param certificate_template: type of the certificate to generate
- :param first_name: first name entered by user
- :param last_name: last name entered by user
- """
-
- im = Image.open(certificate_template)
- d = ImageDraw.Draw(im)
-
- name_font = ImageFont.truetype("Quattrocento-Regular.ttf", 100)
- date_font = ImageFont.truetype("Quattrocento-Regular.ttf", 48)
-
- name = str(first_name) + " " + str(last_name)
- print("NAME", name)
-
- # Debug line name
- #d.line(((200, 740), (1800, 740)), "gray")
- #d.line(((1000, 0), (1000, 1400)), "gray")
-
- # Name
- d.text((1000, 740), name, fill="black", anchor="mm", font=name_font)
-
- # Debug line date
- #d.line(((1500, 0), (1500, 1400)), "gray")
-
- # Date of certification
- d.text((1480, 1170), str(date.today()), fill="black", anchor="mm", font=date_font)
-
-
- pdf = im.convert('RGB')
- pdf.save('certificate.pdf')
-
- return im, "./certificate.pdf"
-
-
-def add_certified_user(hf_username, first_name, last_name, certificate_type):
- """
- Add the certified user to the database
- """
-
- print("ADD CERTIFIED USER")
- repo = Repository(local_dir="usernames", clone_from=DATASET_REPO_URL, use_auth_token=HF_TOKEN)
- repo.git_pull()
-
- history = pd.read_csv(os.path.join("usernames", CERTIFIED_USERS_FILENAME))
-
- # Check if this hf_username is already in our dataset:
- check = history.loc[history['hf_username'] == hf_username]
- if not check.empty:
- history = history.drop(labels=check.index[0], axis=0)
-
- new_row = pd.DataFrame({'hf_username': hf_username, 'first_name': first_name, 'last_name': last_name, 'certificate_type': certificate_type, 'datetime': time.time()}, index=[0])
- history = pd.concat([new_row, history[:]]).reset_index(drop=True)
-
- history.to_csv(os.path.join("usernames", CERTIFIED_USERS_FILENAME), index=False)
- repo.push_to_hub(commit_message="Update certified users list")
-
-
-def create_certificate(passed, certificate_type, hf_username, first_name, last_name):
- """
- Generates certificate, adds message, saves username of the certified user
- :param passed: boolean whether the user passed enough assignments
- :param certificate_type: type of the certificate - completion or excellence
- :param first_name: first name entered by user
- :param last_name: last name entered by user
- """
-
- if passed and certificate_type == "excellence":
- # Generate a certificate of
- certificate, pdf = generate_certificate("./certificate-excellence.png", first_name, last_name)
- # Add this user to our database
- add_certified_user(hf_username, first_name, last_name, certificate_type)
- # Add a message
- message = """
- Congratulations, you successfully completed the Hugging Face Audio Course 🎉! \n
- Since you pass 100% of the hands-on you get a Certificate of Excellence 🎓. \n
- You can download your certificate below ⬇️ \n
- Don't hesitate to share your certificate image below on Twitter and Linkedin (you can tag me @mariakhalusova and @huggingface) 🤗
- """
- elif passed and certificate_type == "completion":
- # Generate a certificate of completion
- certificate, pdf = generate_certificate("./certificate-completion.png", first_name, last_name)
- # Add this user to our database
- add_certified_user(hf_username, first_name, last_name, certificate_type)
- # Add a message
- message = """
- Congratulations, you successfully completed the Hugging Face Audio Course 🎉! \n
- Since you pass 3 out of 4 of the hands-on you get a Certificate of Completion 🎓. \n
- You can download your certificate below ⬇️ \n
- Don't hesitate to share your certificate image below on Twitter and Linkedin (you can tag me @mariakhalusova and @huggingface) 🤗 \n
- You can try to get a Certificate of Excellence if you pass 100% of the hands-on, don't hesitate to check which unit you didn't pass and update these models.
- """
- else:
- # Not passed yet
- certificate = Image.new("RGB", (100, 100), (255, 255, 255))
- pdf = "./fail.pdf"
- # Add a message
- message = """
- You didn't pass the minimum of 3 out of 4 of the hands-on to get a certificate of completion.
- For more information about the certification process, refer to Unit 8 of the course. To see what hands-on you still need to complete, use the self-evaluation space linked in the description above.
- If the results here differ from your results in the self-evaluation space, make sure that your model's metrics automatically uploaded by Trainer have not been manually altered.
- """
- return certificate, message, pdf
-
-
-def certification(hf_username, first_name, last_name):
- passed, certificate_type = check_if_passed(hf_username)
- certificate, message, pdf = create_certificate(passed, certificate_type, hf_username, first_name, last_name)
- print("MESSAGE", message)
-
- if passed:
- visible = True
- else:
- visible = False
-
- return message, pdf, certificate, output_row.update(visible=visible)
-
-with gr.Blocks() as demo:
- gr.Markdown(f"""
- # Get your Hugging Face Audio Course Certificate 🎓
- The certification process is completely free:
- - To get a *certificate of completion*: you need to **pass 3 out of 4 hands-on assignments**.
- - To get a *certificate of excellence*: you need to **pass 4 out of 4 hands-on assignments**.
-
- For more information about the certification process [check the course page on certification](https://huggingface.co/learn/audio-course/chapter8/certification).
-
- To check which assignments you still need to complete, use the [self-evaluation space](https://huggingface.co/spaces/MariaK/Check-my-progress-Audio-Course).
-
- Don't hesitate to share your certificate on Twitter (tag me [@mariakhalusova](https://twitter.com/mariaKhalusova) and [@huggingface](https://twitter.com/huggingface)) and on LinkedIn.
- """)
-
- hf_username = gr.Textbox(placeholder="MariaK", label="Your Hugging Face Username (case sensitive)")
- first_name = gr.Textbox(placeholder="Maria", label="Your First Name")
- last_name = gr.Textbox(placeholder="Khalusova", label="Your Last Name")
-
- check_progress_button = gr.Button(value="Check if I pass and get the certificate")
- output_text = gr.components.Textbox()
-
- with gr.Row(visible=True) as output_row:
- output_pdf = gr.File()
- output_img = gr.components.Image(type="pil")
-
- check_progress_button.click(fn=certification, inputs=[hf_username, first_name, last_name], outputs=[output_text, output_pdf, output_img, output_row])
-
-
-demo.launch(debug=True)
\ No newline at end of file
diff --git a/spaces/MetaWabbit/Auto-GPT/autogpt/config/__init__.py b/spaces/MetaWabbit/Auto-GPT/autogpt/config/__init__.py
deleted file mode 100644
index 726b6dcf3da95968b948c4d897e97a9cdd0928ff..0000000000000000000000000000000000000000
--- a/spaces/MetaWabbit/Auto-GPT/autogpt/config/__init__.py
+++ /dev/null
@@ -1,14 +0,0 @@
-"""
-This module contains the configuration classes for AutoGPT.
-"""
-from autogpt.config.ai_config import AIConfig
-from autogpt.config.config import Config, check_openai_api_key
-from autogpt.config.singleton import AbstractSingleton, Singleton
-
-__all__ = [
- "check_openai_api_key",
- "AbstractSingleton",
- "AIConfig",
- "Config",
- "Singleton",
-]
diff --git a/spaces/MirageML/sjc/guided_diffusion/fp16_util.py b/spaces/MirageML/sjc/guided_diffusion/fp16_util.py
deleted file mode 100644
index d599568f3197bcc236e9ae617829fa060640795f..0000000000000000000000000000000000000000
--- a/spaces/MirageML/sjc/guided_diffusion/fp16_util.py
+++ /dev/null
@@ -1,237 +0,0 @@
-"""
-Helpers to train with 16-bit precision.
-"""
-
-import numpy as np
-import torch as th
-import torch.nn as nn
-from torch._utils import _flatten_dense_tensors, _unflatten_dense_tensors
-
-# from . import logger
-
-INITIAL_LOG_LOSS_SCALE = 20.0
-
-
-def convert_module_to_f16(l):
- """
- Convert primitive modules to float16.
- """
- if isinstance(l, (nn.Conv1d, nn.Conv2d, nn.Conv3d)):
- l.weight.data = l.weight.data.half()
- if l.bias is not None:
- l.bias.data = l.bias.data.half()
-
-
-def convert_module_to_f32(l):
- """
- Convert primitive modules to float32, undoing convert_module_to_f16().
- """
- if isinstance(l, (nn.Conv1d, nn.Conv2d, nn.Conv3d)):
- l.weight.data = l.weight.data.float()
- if l.bias is not None:
- l.bias.data = l.bias.data.float()
-
-
-def make_master_params(param_groups_and_shapes):
- """
- Copy model parameters into a (differently-shaped) list of full-precision
- parameters.
- """
- master_params = []
- for param_group, shape in param_groups_and_shapes:
- master_param = nn.Parameter(
- _flatten_dense_tensors(
- [param.detach().float() for (_, param) in param_group]
- ).view(shape)
- )
- master_param.requires_grad = True
- master_params.append(master_param)
- return master_params
-
-
-def model_grads_to_master_grads(param_groups_and_shapes, master_params):
- """
- Copy the gradients from the model parameters into the master parameters
- from make_master_params().
- """
- for master_param, (param_group, shape) in zip(
- master_params, param_groups_and_shapes
- ):
- master_param.grad = _flatten_dense_tensors(
- [param_grad_or_zeros(param) for (_, param) in param_group]
- ).view(shape)
-
-
-def master_params_to_model_params(param_groups_and_shapes, master_params):
- """
- Copy the master parameter data back into the model parameters.
- """
- # Without copying to a list, if a generator is passed, this will
- # silently not copy any parameters.
- for master_param, (param_group, _) in zip(master_params, param_groups_and_shapes):
- for (_, param), unflat_master_param in zip(
- param_group, unflatten_master_params(param_group, master_param.view(-1))
- ):
- param.detach().copy_(unflat_master_param)
-
-
-def unflatten_master_params(param_group, master_param):
- return _unflatten_dense_tensors(master_param, [param for (_, param) in param_group])
-
-
-def get_param_groups_and_shapes(named_model_params):
- named_model_params = list(named_model_params)
- scalar_vector_named_params = (
- [(n, p) for (n, p) in named_model_params if p.ndim <= 1],
- (-1),
- )
- matrix_named_params = (
- [(n, p) for (n, p) in named_model_params if p.ndim > 1],
- (1, -1),
- )
- return [scalar_vector_named_params, matrix_named_params]
-
-
-def master_params_to_state_dict(
- model, param_groups_and_shapes, master_params, use_fp16
-):
- if use_fp16:
- state_dict = model.state_dict()
- for master_param, (param_group, _) in zip(
- master_params, param_groups_and_shapes
- ):
- for (name, _), unflat_master_param in zip(
- param_group, unflatten_master_params(param_group, master_param.view(-1))
- ):
- assert name in state_dict
- state_dict[name] = unflat_master_param
- else:
- state_dict = model.state_dict()
- for i, (name, _value) in enumerate(model.named_parameters()):
- assert name in state_dict
- state_dict[name] = master_params[i]
- return state_dict
-
-
-def state_dict_to_master_params(model, state_dict, use_fp16):
- if use_fp16:
- named_model_params = [
- (name, state_dict[name]) for name, _ in model.named_parameters()
- ]
- param_groups_and_shapes = get_param_groups_and_shapes(named_model_params)
- master_params = make_master_params(param_groups_and_shapes)
- else:
- master_params = [state_dict[name] for name, _ in model.named_parameters()]
- return master_params
-
-
-def zero_master_grads(master_params):
- for param in master_params:
- param.grad = None
-
-
-def zero_grad(model_params):
- for param in model_params:
- # Taken from https://pytorch.org/docs/stable/_modules/torch/optim/optimizer.html#Optimizer.add_param_group
- if param.grad is not None:
- param.grad.detach_()
- param.grad.zero_()
-
-
-def param_grad_or_zeros(param):
- if param.grad is not None:
- return param.grad.data.detach()
- else:
- return th.zeros_like(param)
-
-
-class MixedPrecisionTrainer:
- def __init__(
- self,
- *,
- model,
- use_fp16=False,
- fp16_scale_growth=1e-3,
- initial_lg_loss_scale=INITIAL_LOG_LOSS_SCALE,
- ):
- self.model = model
- self.use_fp16 = use_fp16
- self.fp16_scale_growth = fp16_scale_growth
-
- self.model_params = list(self.model.parameters())
- self.master_params = self.model_params
- self.param_groups_and_shapes = None
- self.lg_loss_scale = initial_lg_loss_scale
-
- if self.use_fp16:
- self.param_groups_and_shapes = get_param_groups_and_shapes(
- self.model.named_parameters()
- )
- self.master_params = make_master_params(self.param_groups_and_shapes)
- self.model.convert_to_fp16()
-
- def zero_grad(self):
- zero_grad(self.model_params)
-
- def backward(self, loss: th.Tensor):
- if self.use_fp16:
- loss_scale = 2 ** self.lg_loss_scale
- (loss * loss_scale).backward()
- else:
- loss.backward()
-
- def optimize(self, opt: th.optim.Optimizer):
- if self.use_fp16:
- return self._optimize_fp16(opt)
- else:
- return self._optimize_normal(opt)
-
- def _optimize_fp16(self, opt: th.optim.Optimizer):
- logger.logkv_mean("lg_loss_scale", self.lg_loss_scale)
- model_grads_to_master_grads(self.param_groups_and_shapes, self.master_params)
- grad_norm, param_norm = self._compute_norms(grad_scale=2 ** self.lg_loss_scale)
- if check_overflow(grad_norm):
- self.lg_loss_scale -= 1
- logger.log(f"Found NaN, decreased lg_loss_scale to {self.lg_loss_scale}")
- zero_master_grads(self.master_params)
- return False
-
- logger.logkv_mean("grad_norm", grad_norm)
- logger.logkv_mean("param_norm", param_norm)
-
- for p in self.master_params:
- p.grad.mul_(1.0 / (2 ** self.lg_loss_scale))
- opt.step()
- zero_master_grads(self.master_params)
- master_params_to_model_params(self.param_groups_and_shapes, self.master_params)
- self.lg_loss_scale += self.fp16_scale_growth
- return True
-
- def _optimize_normal(self, opt: th.optim.Optimizer):
- grad_norm, param_norm = self._compute_norms()
- logger.logkv_mean("grad_norm", grad_norm)
- logger.logkv_mean("param_norm", param_norm)
- opt.step()
- return True
-
- def _compute_norms(self, grad_scale=1.0):
- grad_norm = 0.0
- param_norm = 0.0
- for p in self.master_params:
- with th.no_grad():
- param_norm += th.norm(p, p=2, dtype=th.float32).item() ** 2
- if p.grad is not None:
- grad_norm += th.norm(p.grad, p=2, dtype=th.float32).item() ** 2
- return np.sqrt(grad_norm) / grad_scale, np.sqrt(param_norm)
-
- def master_params_to_state_dict(self, master_params):
- return master_params_to_state_dict(
- self.model, self.param_groups_and_shapes, master_params, self.use_fp16
- )
-
- def state_dict_to_master_params(self, state_dict):
- return state_dict_to_master_params(self.model, state_dict, self.use_fp16)
-
-
-def check_overflow(value):
- return (value == float("inf")) or (value == -float("inf")) or (value != value)
diff --git a/spaces/NAACL2022/CLIP-Caption-Reward/captioning/models/AttModel.py b/spaces/NAACL2022/CLIP-Caption-Reward/captioning/models/AttModel.py
deleted file mode 100644
index 3dc4e5b7a78c4affbfba4044ca8c96c30b26e36a..0000000000000000000000000000000000000000
--- a/spaces/NAACL2022/CLIP-Caption-Reward/captioning/models/AttModel.py
+++ /dev/null
@@ -1,969 +0,0 @@
-# This file contains Att2in2, AdaAtt, AdaAttMO, UpDown model
-
-# AdaAtt is from Knowing When to Look: Adaptive Attention via A Visual Sentinel for Image Captioning
-# https://arxiv.org/abs/1612.01887
-# AdaAttMO is a modified version with maxout lstm
-
-# Att2in is from Self-critical Sequence Training for Image Captioning
-# https://arxiv.org/abs/1612.00563
-# In this file we only have Att2in2, which is a slightly different version of att2in,
-# in which the img feature embedding and word embedding is the same as what in adaatt.
-
-# UpDown is from Bottom-Up and Top-Down Attention for Image Captioning and VQA
-# https://arxiv.org/abs/1707.07998
-# However, it may not be identical to the author's architecture.
-
-from __future__ import absolute_import
-from __future__ import division
-from __future__ import print_function
-
-import numpy as np
-import torch
-import torch.nn as nn
-import torch.nn.functional as F
-from . import utils
-from torch.nn.utils.rnn import PackedSequence, pack_padded_sequence, pad_packed_sequence
-
-from .CaptionModel import CaptionModel
-
-bad_endings = ['a','an','the','in','for','at','of','with','before','after','on','upon','near','to','is','are','am']
-bad_endings += ['the']
-
-def sort_pack_padded_sequence(input, lengths):
- sorted_lengths, indices = torch.sort(lengths, descending=True)
- # tmp = pack_padded_sequence(input[indices], sorted_lengths, batch_first=True)
- tmp = pack_padded_sequence(input[indices], sorted_lengths.cpu(), batch_first=True)
- inv_ix = indices.clone()
- inv_ix[indices] = torch.arange(0,len(indices)).type_as(inv_ix)
- return tmp, inv_ix
-
-def pad_unsort_packed_sequence(input, inv_ix):
- tmp, _ = pad_packed_sequence(input, batch_first=True)
- tmp = tmp[inv_ix]
- return tmp
-
-def pack_wrapper(module, att_feats, att_masks):
- if att_masks is not None:
- packed, inv_ix = sort_pack_padded_sequence(att_feats, att_masks.data.long().sum(1))
- return pad_unsort_packed_sequence(PackedSequence(module(packed[0]), packed[1]), inv_ix)
- else:
- return module(att_feats)
-
-class AttModel(CaptionModel):
- def __init__(self, opt):
- super(AttModel, self).__init__()
- self.vocab_size = opt.vocab_size
- self.input_encoding_size = opt.input_encoding_size
- #self.rnn_type = opt.rnn_type
- self.rnn_size = opt.rnn_size
- self.num_layers = opt.num_layers
- self.drop_prob_lm = opt.drop_prob_lm
- self.seq_length = getattr(opt, 'max_length', 20) or opt.seq_length # maximum sample length
- self.fc_feat_size = opt.fc_feat_size
- self.att_feat_size = opt.att_feat_size
- self.att_hid_size = opt.att_hid_size
-
- self.bos_idx = getattr(opt, 'bos_idx', 0)
- self.eos_idx = getattr(opt, 'eos_idx', 0)
- self.pad_idx = getattr(opt, 'pad_idx', 0)
-
- self.use_bn = getattr(opt, 'use_bn', 0)
-
- self.ss_prob = 0.0 # Schedule sampling probability
-
- self.embed = nn.Sequential(nn.Embedding(self.vocab_size + 1, self.input_encoding_size),
- nn.ReLU(),
- nn.Dropout(self.drop_prob_lm))
- self.fc_embed = nn.Sequential(nn.Linear(self.fc_feat_size, self.rnn_size),
- nn.ReLU(),
- nn.Dropout(self.drop_prob_lm))
- self.att_embed = nn.Sequential(*(
- ((nn.BatchNorm1d(self.att_feat_size),) if self.use_bn else ())+
- (nn.Linear(self.att_feat_size, self.rnn_size),
- nn.ReLU(),
- nn.Dropout(self.drop_prob_lm))+
- ((nn.BatchNorm1d(self.rnn_size),) if self.use_bn==2 else ())))
-
- self.logit_layers = getattr(opt, 'logit_layers', 1)
- if self.logit_layers == 1:
- self.logit = nn.Linear(self.rnn_size, self.vocab_size + 1)
- else:
- self.logit = [[nn.Linear(self.rnn_size, self.rnn_size), nn.ReLU(), nn.Dropout(0.5)] for _ in range(opt.logit_layers - 1)]
- self.logit = nn.Sequential(*(reduce(lambda x,y:x+y, self.logit) + [nn.Linear(self.rnn_size, self.vocab_size + 1)]))
- self.ctx2att = nn.Linear(self.rnn_size, self.att_hid_size)
-
- # For remove bad endding
- self.vocab = opt.vocab
- self.bad_endings_ix = [int(k) for k,v in self.vocab.items() if v in bad_endings]
-
- def init_hidden(self, bsz):
- weight = self.logit.weight \
- if hasattr(self.logit, "weight") \
- else self.logit[0].weight
- return (weight.new_zeros(self.num_layers, bsz, self.rnn_size),
- weight.new_zeros(self.num_layers, bsz, self.rnn_size))
-
- def clip_att(self, att_feats, att_masks):
- # Clip the length of att_masks and att_feats to the maximum length
- if att_masks is not None:
- max_len = att_masks.data.long().sum(1).max()
- att_feats = att_feats[:, :max_len].contiguous()
- att_masks = att_masks[:, :max_len].contiguous()
- return att_feats, att_masks
-
- def _prepare_feature(self, fc_feats, att_feats, att_masks):
- att_feats, att_masks = self.clip_att(att_feats, att_masks)
-
- # embed fc and att feats
- fc_feats = self.fc_embed(fc_feats)
- att_feats = pack_wrapper(self.att_embed, att_feats, att_masks)
-
- # Project the attention feats first to reduce memory and computation comsumptions.
- p_att_feats = self.ctx2att(att_feats)
-
- return fc_feats, att_feats, p_att_feats, att_masks
-
- def _forward(self, fc_feats, att_feats, seq, att_masks=None):
- batch_size = fc_feats.size(0)
- if seq.ndim == 3: # B * seq_per_img * seq_len
- seq = seq.reshape(-1, seq.shape[2])
- seq_per_img = seq.shape[0] // batch_size
- state = self.init_hidden(batch_size*seq_per_img)
-
- outputs = fc_feats.new_zeros(batch_size*seq_per_img, seq.size(1), self.vocab_size+1)
-
- # Prepare the features
- p_fc_feats, p_att_feats, pp_att_feats, p_att_masks = self._prepare_feature(fc_feats, att_feats, att_masks)
- # pp_att_feats is used for attention, we cache it in advance to reduce computation cost
-
- if seq_per_img > 1:
- p_fc_feats, p_att_feats, pp_att_feats, p_att_masks = utils.repeat_tensors(seq_per_img,
- [p_fc_feats, p_att_feats, pp_att_feats, p_att_masks]
- )
-
- for i in range(seq.size(1)):
- if self.training and i >= 1 and self.ss_prob > 0.0: # otherwiste no need to sample
- sample_prob = fc_feats.new(batch_size*seq_per_img).uniform_(0, 1)
- sample_mask = sample_prob < self.ss_prob
- if sample_mask.sum() == 0:
- it = seq[:, i].clone()
- else:
- sample_ind = sample_mask.nonzero().view(-1)
- it = seq[:, i].data.clone()
- prob_prev = torch.exp(outputs[:, i-1].detach()) # fetch prev distribution: shape Nx(M+1)
- it.index_copy_(0, sample_ind, torch.multinomial(prob_prev, 1).view(-1).index_select(0, sample_ind))
- else:
- it = seq[:, i].clone()
- # break if all the sequences end
- if i >= 1 and seq[:, i].sum() == 0:
- break
-
- output, state = self.get_logprobs_state(it, p_fc_feats, p_att_feats, pp_att_feats, p_att_masks, state)
- outputs[:, i] = output
-
- return outputs
-
- def get_logprobs_state(self, it, fc_feats, att_feats, p_att_feats, att_masks, state, output_logsoftmax=1):
- # 'it' contains a word index
- xt = self.embed(it)
-
- output, state = self.core(xt, fc_feats, att_feats, p_att_feats, state, att_masks)
- if output_logsoftmax:
- logprobs = F.log_softmax(self.logit(output), dim=1)
- else:
- logprobs = self.logit(output)
-
- return logprobs, state
-
- def _old_sample_beam(self, fc_feats, att_feats, att_masks=None, opt={}):
- beam_size = opt.get('beam_size', 10)
- group_size = opt.get('group_size', 1)
- sample_n = opt.get('sample_n', 10)
- # when sample_n == beam_size then each beam is a sample.
- assert sample_n == 1 or sample_n == beam_size // group_size, 'when beam search, sample_n == 1 or beam search'
- batch_size = fc_feats.size(0)
-
- p_fc_feats, p_att_feats, pp_att_feats, p_att_masks = self._prepare_feature(fc_feats, att_feats, att_masks)
-
- assert beam_size <= self.vocab_size + 1, 'lets assume this for now, otherwise this corner case causes a few headaches down the road. can be dealt with in future if needed'
- seq = fc_feats.new_full((batch_size*sample_n, self.seq_length), self.pad_idx, dtype=torch.long)
- seqLogprobs = fc_feats.new_zeros(batch_size*sample_n, self.seq_length, self.vocab_size + 1)
- # lets process every image independently for now, for simplicity
-
- self.done_beams = [[] for _ in range(batch_size)]
- for k in range(batch_size):
- state = self.init_hidden(beam_size)
- tmp_fc_feats, tmp_att_feats, tmp_p_att_feats, tmp_att_masks = utils.repeat_tensors(beam_size,
- [p_fc_feats[k:k+1], p_att_feats[k:k+1], pp_att_feats[k:k+1], p_att_masks[k:k+1] if att_masks is not None else None]
- )
-
- for t in range(1):
- if t == 0: # input
- it = fc_feats.new_full([beam_size], self.bos_idx, dtype=torch.long)
-
- logprobs, state = self.get_logprobs_state(it, tmp_fc_feats, tmp_att_feats, tmp_p_att_feats, tmp_att_masks, state)
-
- self.done_beams[k] = self.old_beam_search(state, logprobs, tmp_fc_feats, tmp_att_feats, tmp_p_att_feats, tmp_att_masks, opt=opt)
- if sample_n == beam_size:
- for _n in range(sample_n):
- seq[k*sample_n+_n, :] = self.done_beams[k][_n]['seq']
- seqLogprobs[k*sample_n+_n, :] = self.done_beams[k][_n]['logps']
- else:
- seq[k, :] = self.done_beams[k][0]['seq'] # the first beam has highest cumulative score
- seqLogprobs[k, :] = self.done_beams[k][0]['logps']
- # return the samples and their log likelihoods
- return seq, seqLogprobs
-
-
- def _sample_beam(self, fc_feats, att_feats, att_masks=None, opt={}):
- beam_size = opt.get('beam_size', 10)
- group_size = opt.get('group_size', 1)
- sample_n = opt.get('sample_n', 10)
- # when sample_n == beam_size then each beam is a sample.
- assert sample_n == 1 or sample_n == beam_size // group_size, 'when beam search, sample_n == 1 or beam search'
- batch_size = fc_feats.size(0)
-
- p_fc_feats, p_att_feats, pp_att_feats, p_att_masks = self._prepare_feature(fc_feats, att_feats, att_masks)
-
- assert beam_size <= self.vocab_size + 1, 'lets assume this for now, otherwise this corner case causes a few headaches down the road. can be dealt with in future if needed'
- seq = fc_feats.new_full((batch_size*sample_n, self.seq_length), self.pad_idx, dtype=torch.long)
- seqLogprobs = fc_feats.new_zeros(batch_size*sample_n, self.seq_length, self.vocab_size + 1)
- # lets process every image independently for now, for simplicity
-
- self.done_beams = [[] for _ in range(batch_size)]
-
- state = self.init_hidden(batch_size)
-
- # first step, feed bos
- it = fc_feats.new_full([batch_size], self.bos_idx, dtype=torch.long)
- logprobs, state = self.get_logprobs_state(it, p_fc_feats, p_att_feats, pp_att_feats, p_att_masks, state)
-
- p_fc_feats, p_att_feats, pp_att_feats, p_att_masks = utils.repeat_tensors(beam_size,
- [p_fc_feats, p_att_feats, pp_att_feats, p_att_masks]
- )
- self.done_beams = self.beam_search(state, logprobs, p_fc_feats, p_att_feats, pp_att_feats, p_att_masks, opt=opt)
- for k in range(batch_size):
- if sample_n == beam_size:
- for _n in range(sample_n):
- seq_len = self.done_beams[k][_n]['seq'].shape[0]
- seq[k*sample_n+_n, :seq_len] = self.done_beams[k][_n]['seq']
- seqLogprobs[k*sample_n+_n, :seq_len] = self.done_beams[k][_n]['logps']
- else:
- seq_len = self.done_beams[k][0]['seq'].shape[0]
- seq[k, :seq_len] = self.done_beams[k][0]['seq'] # the first beam has highest cumulative score
- seqLogprobs[k, :seq_len] = self.done_beams[k][0]['logps']
- # return the samples and their log likelihoods
- return seq, seqLogprobs
-
- def _sample(self, fc_feats, att_feats, att_masks=None, opt={}):
-
- sample_method = opt.get('sample_method', 'greedy')
- beam_size = opt.get('beam_size', 1)
- temperature = opt.get('temperature', 1.0)
- sample_n = int(opt.get('sample_n', 1))
- group_size = opt.get('group_size', 1)
- output_logsoftmax = opt.get('output_logsoftmax', 1)
- decoding_constraint = opt.get('decoding_constraint', 0)
- block_trigrams = opt.get('block_trigrams', 0)
- remove_bad_endings = opt.get('remove_bad_endings', 0)
- if beam_size > 1 and sample_method in ['greedy', 'beam_search']:
- return self._sample_beam(fc_feats, att_feats, att_masks, opt)
- if group_size > 1:
- return self._diverse_sample(fc_feats, att_feats, att_masks, opt)
-
- batch_size = fc_feats.size(0)
- state = self.init_hidden(batch_size*sample_n)
-
- p_fc_feats, p_att_feats, pp_att_feats, p_att_masks = self._prepare_feature(fc_feats, att_feats, att_masks)
-
- if sample_n > 1:
- p_fc_feats, p_att_feats, pp_att_feats, p_att_masks = utils.repeat_tensors(sample_n,
- [p_fc_feats, p_att_feats, pp_att_feats, p_att_masks]
- )
-
- trigrams = [] # will be a list of batch_size dictionaries
-
- seq = fc_feats.new_full((batch_size*sample_n, self.seq_length), self.pad_idx, dtype=torch.long)
- seqLogprobs = fc_feats.new_zeros(batch_size*sample_n, self.seq_length, self.vocab_size + 1)
- for t in range(self.seq_length + 1):
- if t == 0: # input
- it = fc_feats.new_full([batch_size*sample_n], self.bos_idx, dtype=torch.long)
-
- logprobs, state = self.get_logprobs_state(it, p_fc_feats, p_att_feats, pp_att_feats, p_att_masks, state, output_logsoftmax=output_logsoftmax)
-
- if decoding_constraint and t > 0:
- tmp = logprobs.new_zeros(logprobs.size())
- tmp.scatter_(1, seq[:,t-1].data.unsqueeze(1), float('-inf'))
- logprobs = logprobs + tmp
-
- if remove_bad_endings and t > 0:
- tmp = logprobs.new_zeros(logprobs.size())
- prev_bad = np.isin(seq[:,t-1].data.cpu().numpy(), self.bad_endings_ix)
- # Make it impossible to generate bad_endings
- tmp[torch.from_numpy(prev_bad.astype('uint8')), 0] = float('-inf')
- logprobs = logprobs + tmp
-
- # Mess with trigrams
- # Copy from https://github.com/lukemelas/image-paragraph-captioning
- if block_trigrams and t >= 3:
- # Store trigram generated at last step
- prev_two_batch = seq[:,t-3:t-1]
- for i in range(batch_size): # = seq.size(0)
- prev_two = (prev_two_batch[i][0].item(), prev_two_batch[i][1].item())
- current = seq[i][t-1]
- if t == 3: # initialize
- trigrams.append({prev_two: [current]}) # {LongTensor: list containing 1 int}
- elif t > 3:
- if prev_two in trigrams[i]: # add to list
- trigrams[i][prev_two].append(current)
- else: # create list
- trigrams[i][prev_two] = [current]
- # Block used trigrams at next step
- prev_two_batch = seq[:,t-2:t]
- mask = torch.zeros(logprobs.size(), requires_grad=False).to(logprobs.device) # batch_size x vocab_size
- for i in range(batch_size):
- prev_two = (prev_two_batch[i][0].item(), prev_two_batch[i][1].item())
- if prev_two in trigrams[i]:
- for j in trigrams[i][prev_two]:
- mask[i,j] += 1
- # Apply mask to log probs
- #logprobs = logprobs - (mask * 1e9)
- alpha = 2.0 # = 4
- logprobs = logprobs + (mask * -0.693 * alpha) # ln(1/2) * alpha (alpha -> infty works best)
-
- # sample the next word
- if t == self.seq_length: # skip if we achieve maximum length
- break
- it, sampleLogprobs = self.sample_next_word(logprobs, sample_method, temperature)
-
- # stop when all finished
- if t == 0:
- unfinished = it != self.eos_idx
- else:
- it[~unfinished] = self.pad_idx # This allows eos_idx not being overwritten to 0
- logprobs = logprobs * unfinished.unsqueeze(1).to(logprobs)
- unfinished = unfinished & (it != self.eos_idx)
- seq[:,t] = it
- seqLogprobs[:,t] = logprobs
- # quit loop if all sequences have finished
- if unfinished.sum() == 0:
- break
-
- return seq, seqLogprobs
-
- def _diverse_sample(self, fc_feats, att_feats, att_masks=None, opt={}):
-
- sample_method = opt.get('sample_method', 'greedy')
- beam_size = opt.get('beam_size', 1)
- temperature = opt.get('temperature', 1.0)
- group_size = opt.get('group_size', 1)
- diversity_lambda = opt.get('diversity_lambda', 0.5)
- decoding_constraint = opt.get('decoding_constraint', 0)
- block_trigrams = opt.get('block_trigrams', 0)
- remove_bad_endings = opt.get('remove_bad_endings', 0)
-
- batch_size = fc_feats.size(0)
- state = self.init_hidden(batch_size)
-
- p_fc_feats, p_att_feats, pp_att_feats, p_att_masks = self._prepare_feature(fc_feats, att_feats, att_masks)
-
- trigrams_table = [[] for _ in range(group_size)] # will be a list of batch_size dictionaries
-
- seq_table = [fc_feats.new_full((batch_size, self.seq_length), self.pad_idx, dtype=torch.long) for _ in range(group_size)]
- seqLogprobs_table = [fc_feats.new_zeros(batch_size, self.seq_length) for _ in range(group_size)]
- state_table = [self.init_hidden(batch_size) for _ in range(group_size)]
-
- for tt in range(self.seq_length + group_size):
- for divm in range(group_size):
- t = tt - divm
- seq = seq_table[divm]
- seqLogprobs = seqLogprobs_table[divm]
- trigrams = trigrams_table[divm]
- if t >= 0 and t <= self.seq_length-1:
- if t == 0: # input
- it = fc_feats.new_full([batch_size], self.bos_idx, dtype=torch.long)
- else:
- it = seq[:, t-1] # changed
-
- logprobs, state_table[divm] = self.get_logprobs_state(it, p_fc_feats, p_att_feats, pp_att_feats, p_att_masks, state_table[divm]) # changed
- logprobs = F.log_softmax(logprobs / temperature, dim=-1)
-
- # Add diversity
- if divm > 0:
- unaug_logprobs = logprobs.clone()
- for prev_choice in range(divm):
- prev_decisions = seq_table[prev_choice][:, t]
- logprobs[:, prev_decisions] = logprobs[:, prev_decisions] - diversity_lambda
-
- if decoding_constraint and t > 0:
- tmp = logprobs.new_zeros(logprobs.size())
- tmp.scatter_(1, seq[:,t-1].data.unsqueeze(1), float('-inf'))
- logprobs = logprobs + tmp
-
- if remove_bad_endings and t > 0:
- tmp = logprobs.new_zeros(logprobs.size())
- prev_bad = np.isin(seq[:,t-1].data.cpu().numpy(), self.bad_endings_ix)
- # Impossible to generate remove_bad_endings
- tmp[torch.from_numpy(prev_bad.astype('uint8')), 0] = float('-inf')
- logprobs = logprobs + tmp
-
- # Mess with trigrams
- if block_trigrams and t >= 3:
- # Store trigram generated at last step
- prev_two_batch = seq[:,t-3:t-1]
- for i in range(batch_size): # = seq.size(0)
- prev_two = (prev_two_batch[i][0].item(), prev_two_batch[i][1].item())
- current = seq[i][t-1]
- if t == 3: # initialize
- trigrams.append({prev_two: [current]}) # {LongTensor: list containing 1 int}
- elif t > 3:
- if prev_two in trigrams[i]: # add to list
- trigrams[i][prev_two].append(current)
- else: # create list
- trigrams[i][prev_two] = [current]
- # Block used trigrams at next step
- prev_two_batch = seq[:,t-2:t]
- mask = torch.zeros(logprobs.size(), requires_grad=False).cuda() # batch_size x vocab_size
- for i in range(batch_size):
- prev_two = (prev_two_batch[i][0].item(), prev_two_batch[i][1].item())
- if prev_two in trigrams[i]:
- for j in trigrams[i][prev_two]:
- mask[i,j] += 1
- # Apply mask to log probs
- #logprobs = logprobs - (mask * 1e9)
- alpha = 2.0 # = 4
- logprobs = logprobs + (mask * -0.693 * alpha) # ln(1/2) * alpha (alpha -> infty works best)
-
- it, sampleLogprobs = self.sample_next_word(logprobs, sample_method, 1)
-
- # stop when all finished
- if t == 0:
- unfinished = it != self.eos_idx
- else:
- unfinished = (seq[:,t-1] != self.pad_idx) & (seq[:,t-1] != self.eos_idx)
- it[~unfinished] = self.pad_idx
- unfinished = unfinished & (it != self.eos_idx) # changed
- seq[:,t] = it
- seqLogprobs[:,t] = sampleLogprobs.view(-1)
-
- return torch.stack(seq_table, 1).reshape(batch_size * group_size, -1), torch.stack(seqLogprobs_table, 1).reshape(batch_size * group_size, -1)
-
-class AdaAtt_lstm(nn.Module):
- def __init__(self, opt, use_maxout=True):
- super(AdaAtt_lstm, self).__init__()
- self.input_encoding_size = opt.input_encoding_size
- #self.rnn_type = opt.rnn_type
- self.rnn_size = opt.rnn_size
- self.num_layers = opt.num_layers
- self.drop_prob_lm = opt.drop_prob_lm
- self.fc_feat_size = opt.fc_feat_size
- self.att_feat_size = opt.att_feat_size
- self.att_hid_size = opt.att_hid_size
-
- self.use_maxout = use_maxout
-
- # Build a LSTM
- self.w2h = nn.Linear(self.input_encoding_size, (4+(use_maxout==True)) * self.rnn_size)
- self.v2h = nn.Linear(self.rnn_size, (4+(use_maxout==True)) * self.rnn_size)
-
- self.i2h = nn.ModuleList([nn.Linear(self.rnn_size, (4+(use_maxout==True)) * self.rnn_size) for _ in range(self.num_layers - 1)])
- self.h2h = nn.ModuleList([nn.Linear(self.rnn_size, (4+(use_maxout==True)) * self.rnn_size) for _ in range(self.num_layers)])
-
- # Layers for getting the fake region
- if self.num_layers == 1:
- self.r_w2h = nn.Linear(self.input_encoding_size, self.rnn_size)
- self.r_v2h = nn.Linear(self.rnn_size, self.rnn_size)
- else:
- self.r_i2h = nn.Linear(self.rnn_size, self.rnn_size)
- self.r_h2h = nn.Linear(self.rnn_size, self.rnn_size)
-
-
- def forward(self, xt, img_fc, state):
-
- hs = []
- cs = []
- for L in range(self.num_layers):
- # c,h from previous timesteps
- prev_h = state[0][L]
- prev_c = state[1][L]
- # the input to this layer
- if L == 0:
- x = xt
- i2h = self.w2h(x) + self.v2h(img_fc)
- else:
- x = hs[-1]
- x = F.dropout(x, self.drop_prob_lm, self.training)
- i2h = self.i2h[L-1](x)
-
- all_input_sums = i2h+self.h2h[L](prev_h)
-
- sigmoid_chunk = all_input_sums.narrow(1, 0, 3 * self.rnn_size)
- sigmoid_chunk = torch.sigmoid(sigmoid_chunk)
- # decode the gates
- in_gate = sigmoid_chunk.narrow(1, 0, self.rnn_size)
- forget_gate = sigmoid_chunk.narrow(1, self.rnn_size, self.rnn_size)
- out_gate = sigmoid_chunk.narrow(1, self.rnn_size * 2, self.rnn_size)
- # decode the write inputs
- if not self.use_maxout:
- in_transform = torch.tanh(all_input_sums.narrow(1, 3 * self.rnn_size, self.rnn_size))
- else:
- in_transform = all_input_sums.narrow(1, 3 * self.rnn_size, 2 * self.rnn_size)
- in_transform = torch.max(\
- in_transform.narrow(1, 0, self.rnn_size),
- in_transform.narrow(1, self.rnn_size, self.rnn_size))
- # perform the LSTM update
- next_c = forget_gate * prev_c + in_gate * in_transform
- # gated cells form the output
- tanh_nex_c = torch.tanh(next_c)
- next_h = out_gate * tanh_nex_c
- if L == self.num_layers-1:
- if L == 0:
- i2h = self.r_w2h(x) + self.r_v2h(img_fc)
- else:
- i2h = self.r_i2h(x)
- n5 = i2h+self.r_h2h(prev_h)
- fake_region = torch.sigmoid(n5) * tanh_nex_c
-
- cs.append(next_c)
- hs.append(next_h)
-
- # set up the decoder
- top_h = hs[-1]
- top_h = F.dropout(top_h, self.drop_prob_lm, self.training)
- fake_region = F.dropout(fake_region, self.drop_prob_lm, self.training)
-
- state = (torch.cat([_.unsqueeze(0) for _ in hs], 0),
- torch.cat([_.unsqueeze(0) for _ in cs], 0))
- return top_h, fake_region, state
-
-class AdaAtt_attention(nn.Module):
- def __init__(self, opt):
- super(AdaAtt_attention, self).__init__()
- self.input_encoding_size = opt.input_encoding_size
- #self.rnn_type = opt.rnn_type
- self.rnn_size = opt.rnn_size
- self.drop_prob_lm = opt.drop_prob_lm
- self.att_hid_size = opt.att_hid_size
-
- # fake region embed
- self.fr_linear = nn.Sequential(
- nn.Linear(self.rnn_size, self.input_encoding_size),
- nn.ReLU(),
- nn.Dropout(self.drop_prob_lm))
- self.fr_embed = nn.Linear(self.input_encoding_size, self.att_hid_size)
-
- # h out embed
- self.ho_linear = nn.Sequential(
- nn.Linear(self.rnn_size, self.input_encoding_size),
- nn.Tanh(),
- nn.Dropout(self.drop_prob_lm))
- self.ho_embed = nn.Linear(self.input_encoding_size, self.att_hid_size)
-
- self.alpha_net = nn.Linear(self.att_hid_size, 1)
- self.att2h = nn.Linear(self.rnn_size, self.rnn_size)
-
- def forward(self, h_out, fake_region, conv_feat, conv_feat_embed, att_masks=None):
-
- # View into three dimensions
- att_size = conv_feat.numel() // conv_feat.size(0) // self.rnn_size
- conv_feat = conv_feat.view(-1, att_size, self.rnn_size)
- conv_feat_embed = conv_feat_embed.view(-1, att_size, self.att_hid_size)
-
- # view neighbor from bach_size * neighbor_num x rnn_size to bach_size x rnn_size * neighbor_num
- fake_region = self.fr_linear(fake_region)
- fake_region_embed = self.fr_embed(fake_region)
-
- h_out_linear = self.ho_linear(h_out)
- h_out_embed = self.ho_embed(h_out_linear)
-
- txt_replicate = h_out_embed.unsqueeze(1).expand(h_out_embed.size(0), att_size + 1, h_out_embed.size(1))
-
- img_all = torch.cat([fake_region.view(-1,1,self.input_encoding_size), conv_feat], 1)
- img_all_embed = torch.cat([fake_region_embed.view(-1,1,self.input_encoding_size), conv_feat_embed], 1)
-
- hA = torch.tanh(img_all_embed + txt_replicate)
- hA = F.dropout(hA,self.drop_prob_lm, self.training)
-
- hAflat = self.alpha_net(hA.view(-1, self.att_hid_size))
- PI = F.softmax(hAflat.view(-1, att_size + 1), dim=1)
-
- if att_masks is not None:
- att_masks = att_masks.view(-1, att_size)
- PI = PI * torch.cat([att_masks[:,:1], att_masks], 1) # assume one one at the first time step.
- PI = PI / PI.sum(1, keepdim=True)
-
- visAtt = torch.bmm(PI.unsqueeze(1), img_all)
- visAttdim = visAtt.squeeze(1)
-
- atten_out = visAttdim + h_out_linear
-
- h = torch.tanh(self.att2h(atten_out))
- h = F.dropout(h, self.drop_prob_lm, self.training)
- return h
-
-class AdaAttCore(nn.Module):
- def __init__(self, opt, use_maxout=False):
- super(AdaAttCore, self).__init__()
- self.lstm = AdaAtt_lstm(opt, use_maxout)
- self.attention = AdaAtt_attention(opt)
-
- def forward(self, xt, fc_feats, att_feats, p_att_feats, state, att_masks=None):
- h_out, p_out, state = self.lstm(xt, fc_feats, state)
- atten_out = self.attention(h_out, p_out, att_feats, p_att_feats, att_masks)
- return atten_out, state
-
-class UpDownCore(nn.Module):
- def __init__(self, opt, use_maxout=False):
- super(UpDownCore, self).__init__()
- self.drop_prob_lm = opt.drop_prob_lm
-
- self.att_lstm = nn.LSTMCell(opt.input_encoding_size + opt.rnn_size * 2, opt.rnn_size) # we, fc, h^2_t-1
- self.lang_lstm = nn.LSTMCell(opt.rnn_size * 2, opt.rnn_size) # h^1_t, \hat v
- self.attention = Attention(opt)
-
- def forward(self, xt, fc_feats, att_feats, p_att_feats, state, att_masks=None):
- prev_h = state[0][-1]
- att_lstm_input = torch.cat([prev_h, fc_feats, xt], 1)
-
- h_att, c_att = self.att_lstm(att_lstm_input, (state[0][0], state[1][0]))
-
- att = self.attention(h_att, att_feats, p_att_feats, att_masks)
-
- lang_lstm_input = torch.cat([att, h_att], 1)
- # lang_lstm_input = torch.cat([att, F.dropout(h_att, self.drop_prob_lm, self.training)], 1) ?????
-
- h_lang, c_lang = self.lang_lstm(lang_lstm_input, (state[0][1], state[1][1]))
-
- output = F.dropout(h_lang, self.drop_prob_lm, self.training)
- state = (torch.stack([h_att, h_lang]), torch.stack([c_att, c_lang]))
-
- return output, state
-
-
-############################################################################
-# Notice:
-# StackAtt and DenseAtt are models that I randomly designed.
-# They are not related to any paper.
-############################################################################
-
-from .FCModel import LSTMCore
-class StackAttCore(nn.Module):
- def __init__(self, opt, use_maxout=False):
- super(StackAttCore, self).__init__()
- self.drop_prob_lm = opt.drop_prob_lm
-
- # self.att0 = Attention(opt)
- self.att1 = Attention(opt)
- self.att2 = Attention(opt)
-
- opt_input_encoding_size = opt.input_encoding_size
- opt.input_encoding_size = opt.input_encoding_size + opt.rnn_size
- self.lstm0 = LSTMCore(opt) # att_feat + word_embedding
- opt.input_encoding_size = opt.rnn_size * 2
- self.lstm1 = LSTMCore(opt)
- self.lstm2 = LSTMCore(opt)
- opt.input_encoding_size = opt_input_encoding_size
-
- # self.emb1 = nn.Linear(opt.rnn_size, opt.rnn_size)
- self.emb2 = nn.Linear(opt.rnn_size, opt.rnn_size)
-
- def forward(self, xt, fc_feats, att_feats, p_att_feats, state, att_masks=None):
- # att_res_0 = self.att0(state[0][-1], att_feats, p_att_feats, att_masks)
- h_0, state_0 = self.lstm0(torch.cat([xt,fc_feats],1), [state[0][0:1], state[1][0:1]])
- att_res_1 = self.att1(h_0, att_feats, p_att_feats, att_masks)
- h_1, state_1 = self.lstm1(torch.cat([h_0,att_res_1],1), [state[0][1:2], state[1][1:2]])
- att_res_2 = self.att2(h_1 + self.emb2(att_res_1), att_feats, p_att_feats, att_masks)
- h_2, state_2 = self.lstm2(torch.cat([h_1,att_res_2],1), [state[0][2:3], state[1][2:3]])
-
- return h_2, [torch.cat(_, 0) for _ in zip(state_0, state_1, state_2)]
-
-class DenseAttCore(nn.Module):
- def __init__(self, opt, use_maxout=False):
- super(DenseAttCore, self).__init__()
- self.drop_prob_lm = opt.drop_prob_lm
-
- # self.att0 = Attention(opt)
- self.att1 = Attention(opt)
- self.att2 = Attention(opt)
-
- opt_input_encoding_size = opt.input_encoding_size
- opt.input_encoding_size = opt.input_encoding_size + opt.rnn_size
- self.lstm0 = LSTMCore(opt) # att_feat + word_embedding
- opt.input_encoding_size = opt.rnn_size * 2
- self.lstm1 = LSTMCore(opt)
- self.lstm2 = LSTMCore(opt)
- opt.input_encoding_size = opt_input_encoding_size
-
- # self.emb1 = nn.Linear(opt.rnn_size, opt.rnn_size)
- self.emb2 = nn.Linear(opt.rnn_size, opt.rnn_size)
-
- # fuse h_0 and h_1
- self.fusion1 = nn.Sequential(nn.Linear(opt.rnn_size*2, opt.rnn_size),
- nn.ReLU(),
- nn.Dropout(opt.drop_prob_lm))
- # fuse h_0, h_1 and h_2
- self.fusion2 = nn.Sequential(nn.Linear(opt.rnn_size*3, opt.rnn_size),
- nn.ReLU(),
- nn.Dropout(opt.drop_prob_lm))
-
- def forward(self, xt, fc_feats, att_feats, p_att_feats, state, att_masks=None):
- # att_res_0 = self.att0(state[0][-1], att_feats, p_att_feats, att_masks)
- h_0, state_0 = self.lstm0(torch.cat([xt,fc_feats],1), [state[0][0:1], state[1][0:1]])
- att_res_1 = self.att1(h_0, att_feats, p_att_feats, att_masks)
- h_1, state_1 = self.lstm1(torch.cat([h_0,att_res_1],1), [state[0][1:2], state[1][1:2]])
- att_res_2 = self.att2(h_1 + self.emb2(att_res_1), att_feats, p_att_feats, att_masks)
- h_2, state_2 = self.lstm2(torch.cat([self.fusion1(torch.cat([h_0, h_1], 1)),att_res_2],1), [state[0][2:3], state[1][2:3]])
-
- return self.fusion2(torch.cat([h_0, h_1, h_2], 1)), [torch.cat(_, 0) for _ in zip(state_0, state_1, state_2)]
-
-class Attention(nn.Module):
- def __init__(self, opt):
- super(Attention, self).__init__()
- self.rnn_size = opt.rnn_size
- self.att_hid_size = opt.att_hid_size
-
- self.h2att = nn.Linear(self.rnn_size, self.att_hid_size)
- self.alpha_net = nn.Linear(self.att_hid_size, 1)
-
- def forward(self, h, att_feats, p_att_feats, att_masks=None):
- # The p_att_feats here is already projected
- att_size = att_feats.numel() // att_feats.size(0) // att_feats.size(-1)
- att = p_att_feats.view(-1, att_size, self.att_hid_size)
-
- att_h = self.h2att(h) # batch * att_hid_size
- att_h = att_h.unsqueeze(1).expand_as(att) # batch * att_size * att_hid_size
- dot = att + att_h # batch * att_size * att_hid_size
- dot = torch.tanh(dot) # batch * att_size * att_hid_size
- dot = dot.view(-1, self.att_hid_size) # (batch * att_size) * att_hid_size
- dot = self.alpha_net(dot) # (batch * att_size) * 1
- dot = dot.view(-1, att_size) # batch * att_size
-
- weight = F.softmax(dot, dim=1) # batch * att_size
- if att_masks is not None:
- weight = weight * att_masks.view(-1, att_size).to(weight)
- weight = weight / weight.sum(1, keepdim=True) # normalize to 1
- att_feats_ = att_feats.view(-1, att_size, att_feats.size(-1)) # batch * att_size * att_feat_size
- att_res = torch.bmm(weight.unsqueeze(1), att_feats_).squeeze(1) # batch * att_feat_size
-
- return att_res
-
-class Att2in2Core(nn.Module):
- def __init__(self, opt):
- super(Att2in2Core, self).__init__()
- self.input_encoding_size = opt.input_encoding_size
- #self.rnn_type = opt.rnn_type
- self.rnn_size = opt.rnn_size
- #self.num_layers = opt.num_layers
- self.drop_prob_lm = opt.drop_prob_lm
- self.fc_feat_size = opt.fc_feat_size
- self.att_feat_size = opt.att_feat_size
- self.att_hid_size = opt.att_hid_size
-
- # Build a LSTM
- self.a2c = nn.Linear(self.rnn_size, 2 * self.rnn_size)
- self.i2h = nn.Linear(self.input_encoding_size, 5 * self.rnn_size)
- self.h2h = nn.Linear(self.rnn_size, 5 * self.rnn_size)
- self.dropout = nn.Dropout(self.drop_prob_lm)
-
- self.attention = Attention(opt)
-
- def forward(self, xt, fc_feats, att_feats, p_att_feats, state, att_masks=None):
- att_res = self.attention(state[0][-1], att_feats, p_att_feats, att_masks)
-
- all_input_sums = self.i2h(xt) + self.h2h(state[0][-1])
- sigmoid_chunk = all_input_sums.narrow(1, 0, 3 * self.rnn_size)
- sigmoid_chunk = torch.sigmoid(sigmoid_chunk)
- in_gate = sigmoid_chunk.narrow(1, 0, self.rnn_size)
- forget_gate = sigmoid_chunk.narrow(1, self.rnn_size, self.rnn_size)
- out_gate = sigmoid_chunk.narrow(1, self.rnn_size * 2, self.rnn_size)
-
- in_transform = all_input_sums.narrow(1, 3 * self.rnn_size, 2 * self.rnn_size) + \
- self.a2c(att_res)
- in_transform = torch.max(\
- in_transform.narrow(1, 0, self.rnn_size),
- in_transform.narrow(1, self.rnn_size, self.rnn_size))
- next_c = forget_gate * state[1][-1] + in_gate * in_transform
- next_h = out_gate * torch.tanh(next_c)
-
- output = self.dropout(next_h)
- state = (next_h.unsqueeze(0), next_c.unsqueeze(0))
- return output, state
-
-class Att2inCore(Att2in2Core):
- def __init__(self, opt):
- super(Att2inCore, self).__init__(opt)
- del self.a2c
- self.a2c = nn.Linear(self.att_feat_size, 2 * self.rnn_size)
-
-"""
-Note this is my attempt to replicate att2all model in self-critical paper.
-However, this is not a correct replication actually. Will fix it.
-"""
-class Att2all2Core(nn.Module):
- def __init__(self, opt):
- super(Att2all2Core, self).__init__()
- self.input_encoding_size = opt.input_encoding_size
- #self.rnn_type = opt.rnn_type
- self.rnn_size = opt.rnn_size
- #self.num_layers = opt.num_layers
- self.drop_prob_lm = opt.drop_prob_lm
- self.fc_feat_size = opt.fc_feat_size
- self.att_feat_size = opt.att_feat_size
- self.att_hid_size = opt.att_hid_size
-
- # Build a LSTM
- self.a2h = nn.Linear(self.rnn_size, 5 * self.rnn_size)
- self.i2h = nn.Linear(self.input_encoding_size, 5 * self.rnn_size)
- self.h2h = nn.Linear(self.rnn_size, 5 * self.rnn_size)
- self.dropout = nn.Dropout(self.drop_prob_lm)
-
- self.attention = Attention(opt)
-
- def forward(self, xt, fc_feats, att_feats, p_att_feats, state, att_masks=None):
- att_res = self.attention(state[0][-1], att_feats, p_att_feats, att_masks)
-
- all_input_sums = self.i2h(xt) + self.h2h(state[0][-1]) + self.a2h(att_res)
- sigmoid_chunk = all_input_sums.narrow(1, 0, 3 * self.rnn_size)
- sigmoid_chunk = torch.sigmoid(sigmoid_chunk)
- in_gate = sigmoid_chunk.narrow(1, 0, self.rnn_size)
- forget_gate = sigmoid_chunk.narrow(1, self.rnn_size, self.rnn_size)
- out_gate = sigmoid_chunk.narrow(1, self.rnn_size * 2, self.rnn_size)
-
- in_transform = all_input_sums.narrow(1, 3 * self.rnn_size, 2 * self.rnn_size)
- in_transform = torch.max(\
- in_transform.narrow(1, 0, self.rnn_size),
- in_transform.narrow(1, self.rnn_size, self.rnn_size))
- next_c = forget_gate * state[1][-1] + in_gate * in_transform
- next_h = out_gate * torch.tanh(next_c)
-
- output = self.dropout(next_h)
- state = (next_h.unsqueeze(0), next_c.unsqueeze(0))
- return output, state
-
-class AdaAttModel(AttModel):
- def __init__(self, opt):
- super(AdaAttModel, self).__init__(opt)
- self.core = AdaAttCore(opt)
-
-# AdaAtt with maxout lstm
-class AdaAttMOModel(AttModel):
- def __init__(self, opt):
- super(AdaAttMOModel, self).__init__(opt)
- self.core = AdaAttCore(opt, True)
-
-class Att2in2Model(AttModel):
- def __init__(self, opt):
- super(Att2in2Model, self).__init__(opt)
- self.core = Att2in2Core(opt)
- delattr(self, 'fc_embed')
- self.fc_embed = lambda x : x
-
-class Att2all2Model(AttModel):
- def __init__(self, opt):
- super(Att2all2Model, self).__init__(opt)
- self.core = Att2all2Core(opt)
- delattr(self, 'fc_embed')
- self.fc_embed = lambda x : x
-
-class UpDownModel(AttModel):
- def __init__(self, opt):
- super(UpDownModel, self).__init__(opt)
- self.num_layers = 2
- self.core = UpDownCore(opt)
-
-class StackAttModel(AttModel):
- def __init__(self, opt):
- super(StackAttModel, self).__init__(opt)
- self.num_layers = 3
- self.core = StackAttCore(opt)
-
-class DenseAttModel(AttModel):
- def __init__(self, opt):
- super(DenseAttModel, self).__init__(opt)
- self.num_layers = 3
- self.core = DenseAttCore(opt)
-
-class Att2inModel(AttModel):
- def __init__(self, opt):
- super(Att2inModel, self).__init__(opt)
- del self.embed, self.fc_embed, self.att_embed
- self.embed = nn.Embedding(self.vocab_size + 1, self.input_encoding_size)
- self.fc_embed = self.att_embed = lambda x: x
- del self.ctx2att
- self.ctx2att = nn.Linear(self.att_feat_size, self.att_hid_size)
- self.core = Att2inCore(opt)
- self.init_weights()
-
- def init_weights(self):
- initrange = 0.1
- self.embed.weight.data.uniform_(-initrange, initrange)
- self.logit.bias.data.fill_(0)
- self.logit.weight.data.uniform_(-initrange, initrange)
-
-
-class NewFCModel(AttModel):
- def __init__(self, opt):
- super(NewFCModel, self).__init__(opt)
- self.fc_embed = nn.Linear(self.fc_feat_size, self.input_encoding_size)
- self.embed = nn.Embedding(self.vocab_size + 1, self.input_encoding_size)
- self._core = LSTMCore(opt)
- delattr(self, 'att_embed')
- self.att_embed = lambda x : x
- delattr(self, 'ctx2att')
- self.ctx2att = lambda x: x
-
- def core(self, xt, fc_feats, att_feats, p_att_feats, state, att_masks):
- # Step 0, feed the input image
- # if (self.training and state[0].is_leaf) or \
- # (not self.training and state[0].sum() == 0):
- # _, state = self._core(fc_feats, state)
- # three cases
- # normal mle training
- # Sample
- # beam search (diverse beam search)
- # fixed captioning module.
- is_first_step = (state[0]==0).all(2).all(0) # size: B
- if is_first_step.all():
- _, state = self._core(fc_feats, state)
- elif is_first_step.any():
- # This is mostly for diverse beam search I think
- new_state = [torch.zeros_like(_) for _ in state]
- new_state[0][:, ~is_first_step] = state[0][:, ~is_first_step]
- new_state[1][:, ~is_first_step] = state[1][:, ~is_first_step]
- _, state = self._core(fc_feats, state)
- new_state[0][:, is_first_step] = state[0][:, is_first_step]
- new_state[1][:, is_first_step] = state[1][:, is_first_step]
- state = new_state
- # if (state[0]==0).all():
- # # Let's forget about diverse beam search first
- # _, state = self._core(fc_feats, state)
- return self._core(xt, state)
-
- def _prepare_feature(self, fc_feats, att_feats, att_masks):
- fc_feats = self.fc_embed(fc_feats)
-
- return fc_feats, att_feats, att_feats, att_masks
-
-
-class LMModel(AttModel):
- def __init__(self, opt):
- super(LMModel, self).__init__(opt)
- delattr(self, 'fc_embed')
- self.fc_embed = lambda x: x.new_zeros(x.shape[0], self.input_encoding_size)
- self.embed = nn.Embedding(self.vocab_size + 1, self.input_encoding_size)
- self._core = LSTMCore(opt)
- delattr(self, 'att_embed')
- self.att_embed = lambda x : x
- delattr(self, 'ctx2att')
- self.ctx2att = lambda x: x
-
- def core(self, xt, fc_feats, att_feats, p_att_feats, state, att_masks):
- if (state[0]==0).all():
- # Let's forget about diverse beam search first
- _, state = self._core(fc_feats, state)
- return self._core(xt, state)
-
- def _prepare_feature(self, fc_feats, att_feats, att_masks):
- fc_feats = self.fc_embed(fc_feats)
-
- return fc_feats, None, None, None
\ No newline at end of file
diff --git a/spaces/Nalla/PDF_tables_to_CSV_output/README.md b/spaces/Nalla/PDF_tables_to_CSV_output/README.md
deleted file mode 100644
index 31e688d36117a66f2e73af2caa76d9d5a2e7bbcf..0000000000000000000000000000000000000000
--- a/spaces/Nalla/PDF_tables_to_CSV_output/README.md
+++ /dev/null
@@ -1,11 +0,0 @@
----
-title: PDF_tables_to_CSV_output
-emoji: ⚡
-colorFrom: blue
-colorTo: red
-sdk: streamlit
-app_file: App_For_PDF_To_Dataframe.py
-pinned: false
----
-
-Check out the configuration reference at https://huggingface.co/docs/hub/spaces#reference
diff --git a/spaces/Nixic/rvc-models/infer_pack/modules.py b/spaces/Nixic/rvc-models/infer_pack/modules.py
deleted file mode 100644
index 960481cedad9a6106f2bf0b9e86e82b120f7b33f..0000000000000000000000000000000000000000
--- a/spaces/Nixic/rvc-models/infer_pack/modules.py
+++ /dev/null
@@ -1,522 +0,0 @@
-import copy
-import math
-import numpy as np
-import scipy
-import torch
-from torch import nn
-from torch.nn import functional as F
-
-from torch.nn import Conv1d, ConvTranspose1d, AvgPool1d, Conv2d
-from torch.nn.utils import weight_norm, remove_weight_norm
-
-from infer_pack import commons
-from infer_pack.commons import init_weights, get_padding
-from infer_pack.transforms import piecewise_rational_quadratic_transform
-
-
-LRELU_SLOPE = 0.1
-
-
-class LayerNorm(nn.Module):
- def __init__(self, channels, eps=1e-5):
- super().__init__()
- self.channels = channels
- self.eps = eps
-
- self.gamma = nn.Parameter(torch.ones(channels))
- self.beta = nn.Parameter(torch.zeros(channels))
-
- def forward(self, x):
- x = x.transpose(1, -1)
- x = F.layer_norm(x, (self.channels,), self.gamma, self.beta, self.eps)
- return x.transpose(1, -1)
-
-
-class ConvReluNorm(nn.Module):
- def __init__(
- self,
- in_channels,
- hidden_channels,
- out_channels,
- kernel_size,
- n_layers,
- p_dropout,
- ):
- super().__init__()
- self.in_channels = in_channels
- self.hidden_channels = hidden_channels
- self.out_channels = out_channels
- self.kernel_size = kernel_size
- self.n_layers = n_layers
- self.p_dropout = p_dropout
- assert n_layers > 1, "Number of layers should be larger than 0."
-
- self.conv_layers = nn.ModuleList()
- self.norm_layers = nn.ModuleList()
- self.conv_layers.append(
- nn.Conv1d(
- in_channels, hidden_channels, kernel_size, padding=kernel_size // 2
- )
- )
- self.norm_layers.append(LayerNorm(hidden_channels))
- self.relu_drop = nn.Sequential(nn.ReLU(), nn.Dropout(p_dropout))
- for _ in range(n_layers - 1):
- self.conv_layers.append(
- nn.Conv1d(
- hidden_channels,
- hidden_channels,
- kernel_size,
- padding=kernel_size // 2,
- )
- )
- self.norm_layers.append(LayerNorm(hidden_channels))
- self.proj = nn.Conv1d(hidden_channels, out_channels, 1)
- self.proj.weight.data.zero_()
- self.proj.bias.data.zero_()
-
- def forward(self, x, x_mask):
- x_org = x
- for i in range(self.n_layers):
- x = self.conv_layers[i](x * x_mask)
- x = self.norm_layers[i](x)
- x = self.relu_drop(x)
- x = x_org + self.proj(x)
- return x * x_mask
-
-
-class DDSConv(nn.Module):
- """
- Dialted and Depth-Separable Convolution
- """
-
- def __init__(self, channels, kernel_size, n_layers, p_dropout=0.0):
- super().__init__()
- self.channels = channels
- self.kernel_size = kernel_size
- self.n_layers = n_layers
- self.p_dropout = p_dropout
-
- self.drop = nn.Dropout(p_dropout)
- self.convs_sep = nn.ModuleList()
- self.convs_1x1 = nn.ModuleList()
- self.norms_1 = nn.ModuleList()
- self.norms_2 = nn.ModuleList()
- for i in range(n_layers):
- dilation = kernel_size**i
- padding = (kernel_size * dilation - dilation) // 2
- self.convs_sep.append(
- nn.Conv1d(
- channels,
- channels,
- kernel_size,
- groups=channels,
- dilation=dilation,
- padding=padding,
- )
- )
- self.convs_1x1.append(nn.Conv1d(channels, channels, 1))
- self.norms_1.append(LayerNorm(channels))
- self.norms_2.append(LayerNorm(channels))
-
- def forward(self, x, x_mask, g=None):
- if g is not None:
- x = x + g
- for i in range(self.n_layers):
- y = self.convs_sep[i](x * x_mask)
- y = self.norms_1[i](y)
- y = F.gelu(y)
- y = self.convs_1x1[i](y)
- y = self.norms_2[i](y)
- y = F.gelu(y)
- y = self.drop(y)
- x = x + y
- return x * x_mask
-
-
-class WN(torch.nn.Module):
- def __init__(
- self,
- hidden_channels,
- kernel_size,
- dilation_rate,
- n_layers,
- gin_channels=0,
- p_dropout=0,
- ):
- super(WN, self).__init__()
- assert kernel_size % 2 == 1
- self.hidden_channels = hidden_channels
- self.kernel_size = (kernel_size,)
- self.dilation_rate = dilation_rate
- self.n_layers = n_layers
- self.gin_channels = gin_channels
- self.p_dropout = p_dropout
-
- self.in_layers = torch.nn.ModuleList()
- self.res_skip_layers = torch.nn.ModuleList()
- self.drop = nn.Dropout(p_dropout)
-
- if gin_channels != 0:
- cond_layer = torch.nn.Conv1d(
- gin_channels, 2 * hidden_channels * n_layers, 1
- )
- self.cond_layer = torch.nn.utils.weight_norm(cond_layer, name="weight")
-
- for i in range(n_layers):
- dilation = dilation_rate**i
- padding = int((kernel_size * dilation - dilation) / 2)
- in_layer = torch.nn.Conv1d(
- hidden_channels,
- 2 * hidden_channels,
- kernel_size,
- dilation=dilation,
- padding=padding,
- )
- in_layer = torch.nn.utils.weight_norm(in_layer, name="weight")
- self.in_layers.append(in_layer)
-
- # last one is not necessary
- if i < n_layers - 1:
- res_skip_channels = 2 * hidden_channels
- else:
- res_skip_channels = hidden_channels
-
- res_skip_layer = torch.nn.Conv1d(hidden_channels, res_skip_channels, 1)
- res_skip_layer = torch.nn.utils.weight_norm(res_skip_layer, name="weight")
- self.res_skip_layers.append(res_skip_layer)
-
- def forward(self, x, x_mask, g=None, **kwargs):
- output = torch.zeros_like(x)
- n_channels_tensor = torch.IntTensor([self.hidden_channels])
-
- if g is not None:
- g = self.cond_layer(g)
-
- for i in range(self.n_layers):
- x_in = self.in_layers[i](x)
- if g is not None:
- cond_offset = i * 2 * self.hidden_channels
- g_l = g[:, cond_offset : cond_offset + 2 * self.hidden_channels, :]
- else:
- g_l = torch.zeros_like(x_in)
-
- acts = commons.fused_add_tanh_sigmoid_multiply(x_in, g_l, n_channels_tensor)
- acts = self.drop(acts)
-
- res_skip_acts = self.res_skip_layers[i](acts)
- if i < self.n_layers - 1:
- res_acts = res_skip_acts[:, : self.hidden_channels, :]
- x = (x + res_acts) * x_mask
- output = output + res_skip_acts[:, self.hidden_channels :, :]
- else:
- output = output + res_skip_acts
- return output * x_mask
-
- def remove_weight_norm(self):
- if self.gin_channels != 0:
- torch.nn.utils.remove_weight_norm(self.cond_layer)
- for l in self.in_layers:
- torch.nn.utils.remove_weight_norm(l)
- for l in self.res_skip_layers:
- torch.nn.utils.remove_weight_norm(l)
-
-
-class ResBlock1(torch.nn.Module):
- def __init__(self, channels, kernel_size=3, dilation=(1, 3, 5)):
- super(ResBlock1, self).__init__()
- self.convs1 = nn.ModuleList(
- [
- weight_norm(
- Conv1d(
- channels,
- channels,
- kernel_size,
- 1,
- dilation=dilation[0],
- padding=get_padding(kernel_size, dilation[0]),
- )
- ),
- weight_norm(
- Conv1d(
- channels,
- channels,
- kernel_size,
- 1,
- dilation=dilation[1],
- padding=get_padding(kernel_size, dilation[1]),
- )
- ),
- weight_norm(
- Conv1d(
- channels,
- channels,
- kernel_size,
- 1,
- dilation=dilation[2],
- padding=get_padding(kernel_size, dilation[2]),
- )
- ),
- ]
- )
- self.convs1.apply(init_weights)
-
- self.convs2 = nn.ModuleList(
- [
- weight_norm(
- Conv1d(
- channels,
- channels,
- kernel_size,
- 1,
- dilation=1,
- padding=get_padding(kernel_size, 1),
- )
- ),
- weight_norm(
- Conv1d(
- channels,
- channels,
- kernel_size,
- 1,
- dilation=1,
- padding=get_padding(kernel_size, 1),
- )
- ),
- weight_norm(
- Conv1d(
- channels,
- channels,
- kernel_size,
- 1,
- dilation=1,
- padding=get_padding(kernel_size, 1),
- )
- ),
- ]
- )
- self.convs2.apply(init_weights)
-
- def forward(self, x, x_mask=None):
- for c1, c2 in zip(self.convs1, self.convs2):
- xt = F.leaky_relu(x, LRELU_SLOPE)
- if x_mask is not None:
- xt = xt * x_mask
- xt = c1(xt)
- xt = F.leaky_relu(xt, LRELU_SLOPE)
- if x_mask is not None:
- xt = xt * x_mask
- xt = c2(xt)
- x = xt + x
- if x_mask is not None:
- x = x * x_mask
- return x
-
- def remove_weight_norm(self):
- for l in self.convs1:
- remove_weight_norm(l)
- for l in self.convs2:
- remove_weight_norm(l)
-
-
-class ResBlock2(torch.nn.Module):
- def __init__(self, channels, kernel_size=3, dilation=(1, 3)):
- super(ResBlock2, self).__init__()
- self.convs = nn.ModuleList(
- [
- weight_norm(
- Conv1d(
- channels,
- channels,
- kernel_size,
- 1,
- dilation=dilation[0],
- padding=get_padding(kernel_size, dilation[0]),
- )
- ),
- weight_norm(
- Conv1d(
- channels,
- channels,
- kernel_size,
- 1,
- dilation=dilation[1],
- padding=get_padding(kernel_size, dilation[1]),
- )
- ),
- ]
- )
- self.convs.apply(init_weights)
-
- def forward(self, x, x_mask=None):
- for c in self.convs:
- xt = F.leaky_relu(x, LRELU_SLOPE)
- if x_mask is not None:
- xt = xt * x_mask
- xt = c(xt)
- x = xt + x
- if x_mask is not None:
- x = x * x_mask
- return x
-
- def remove_weight_norm(self):
- for l in self.convs:
- remove_weight_norm(l)
-
-
-class Log(nn.Module):
- def forward(self, x, x_mask, reverse=False, **kwargs):
- if not reverse:
- y = torch.log(torch.clamp_min(x, 1e-5)) * x_mask
- logdet = torch.sum(-y, [1, 2])
- return y, logdet
- else:
- x = torch.exp(x) * x_mask
- return x
-
-
-class Flip(nn.Module):
- def forward(self, x, *args, reverse=False, **kwargs):
- x = torch.flip(x, [1])
- if not reverse:
- logdet = torch.zeros(x.size(0)).to(dtype=x.dtype, device=x.device)
- return x, logdet
- else:
- return x
-
-
-class ElementwiseAffine(nn.Module):
- def __init__(self, channels):
- super().__init__()
- self.channels = channels
- self.m = nn.Parameter(torch.zeros(channels, 1))
- self.logs = nn.Parameter(torch.zeros(channels, 1))
-
- def forward(self, x, x_mask, reverse=False, **kwargs):
- if not reverse:
- y = self.m + torch.exp(self.logs) * x
- y = y * x_mask
- logdet = torch.sum(self.logs * x_mask, [1, 2])
- return y, logdet
- else:
- x = (x - self.m) * torch.exp(-self.logs) * x_mask
- return x
-
-
-class ResidualCouplingLayer(nn.Module):
- def __init__(
- self,
- channels,
- hidden_channels,
- kernel_size,
- dilation_rate,
- n_layers,
- p_dropout=0,
- gin_channels=0,
- mean_only=False,
- ):
- assert channels % 2 == 0, "channels should be divisible by 2"
- super().__init__()
- self.channels = channels
- self.hidden_channels = hidden_channels
- self.kernel_size = kernel_size
- self.dilation_rate = dilation_rate
- self.n_layers = n_layers
- self.half_channels = channels // 2
- self.mean_only = mean_only
-
- self.pre = nn.Conv1d(self.half_channels, hidden_channels, 1)
- self.enc = WN(
- hidden_channels,
- kernel_size,
- dilation_rate,
- n_layers,
- p_dropout=p_dropout,
- gin_channels=gin_channels,
- )
- self.post = nn.Conv1d(hidden_channels, self.half_channels * (2 - mean_only), 1)
- self.post.weight.data.zero_()
- self.post.bias.data.zero_()
-
- def forward(self, x, x_mask, g=None, reverse=False):
- x0, x1 = torch.split(x, [self.half_channels] * 2, 1)
- h = self.pre(x0) * x_mask
- h = self.enc(h, x_mask, g=g)
- stats = self.post(h) * x_mask
- if not self.mean_only:
- m, logs = torch.split(stats, [self.half_channels] * 2, 1)
- else:
- m = stats
- logs = torch.zeros_like(m)
-
- if not reverse:
- x1 = m + x1 * torch.exp(logs) * x_mask
- x = torch.cat([x0, x1], 1)
- logdet = torch.sum(logs, [1, 2])
- return x, logdet
- else:
- x1 = (x1 - m) * torch.exp(-logs) * x_mask
- x = torch.cat([x0, x1], 1)
- return x
-
- def remove_weight_norm(self):
- self.enc.remove_weight_norm()
-
-
-class ConvFlow(nn.Module):
- def __init__(
- self,
- in_channels,
- filter_channels,
- kernel_size,
- n_layers,
- num_bins=10,
- tail_bound=5.0,
- ):
- super().__init__()
- self.in_channels = in_channels
- self.filter_channels = filter_channels
- self.kernel_size = kernel_size
- self.n_layers = n_layers
- self.num_bins = num_bins
- self.tail_bound = tail_bound
- self.half_channels = in_channels // 2
-
- self.pre = nn.Conv1d(self.half_channels, filter_channels, 1)
- self.convs = DDSConv(filter_channels, kernel_size, n_layers, p_dropout=0.0)
- self.proj = nn.Conv1d(
- filter_channels, self.half_channels * (num_bins * 3 - 1), 1
- )
- self.proj.weight.data.zero_()
- self.proj.bias.data.zero_()
-
- def forward(self, x, x_mask, g=None, reverse=False):
- x0, x1 = torch.split(x, [self.half_channels] * 2, 1)
- h = self.pre(x0)
- h = self.convs(h, x_mask, g=g)
- h = self.proj(h) * x_mask
-
- b, c, t = x0.shape
- h = h.reshape(b, c, -1, t).permute(0, 1, 3, 2) # [b, cx?, t] -> [b, c, t, ?]
-
- unnormalized_widths = h[..., : self.num_bins] / math.sqrt(self.filter_channels)
- unnormalized_heights = h[..., self.num_bins : 2 * self.num_bins] / math.sqrt(
- self.filter_channels
- )
- unnormalized_derivatives = h[..., 2 * self.num_bins :]
-
- x1, logabsdet = piecewise_rational_quadratic_transform(
- x1,
- unnormalized_widths,
- unnormalized_heights,
- unnormalized_derivatives,
- inverse=reverse,
- tails="linear",
- tail_bound=self.tail_bound,
- )
-
- x = torch.cat([x0, x1], 1) * x_mask
- logdet = torch.sum(logabsdet * x_mask, [1, 2])
- if not reverse:
- return x, logdet
- else:
- return x
diff --git a/spaces/OFA-Sys/OFA-Generic_Interface/fairseq/examples/wav2vec/unsupervised/README.md b/spaces/OFA-Sys/OFA-Generic_Interface/fairseq/examples/wav2vec/unsupervised/README.md
deleted file mode 100644
index 0b213fd202d04bce2149936ec149c23c6d483745..0000000000000000000000000000000000000000
--- a/spaces/OFA-Sys/OFA-Generic_Interface/fairseq/examples/wav2vec/unsupervised/README.md
+++ /dev/null
@@ -1,103 +0,0 @@
-# wav2vec Unsupervised (wav2vec-U)
-
-Wav2vec Unsupervised (wav2vec-U) is a framework for building speech recognition systems without any labeled training data as described in [Unsupervised Speech Recognition (Baevski et al., 2021)](https://ai.facebook.com/research/publications/unsupervised-speech-recognition). The model takes as input wav2vec 2.0 or XLSR representations (see [pretrained models](https://github.com/pytorch/fairseq/blob/main/examples/wav2vec)) as well as unlabeled speech and text data.
-
- The wav2vec-U training procedure consists of three consecutive main steps:
-* Preparation of speech representations and text data
-* Generative adversarial training (GAN)
-* Iterative self-training + Kaldi LM-decoding
-
-## Preparation of speech and text data
-Similar to [wav2vec 2.0](https://github.com/pytorch/fairseq/blob/main/examples/wav2vec/README.md), data folders contain {train,valid,test}.{tsv,wrd,phn} files, where audio paths are stored in tsv files, and word, letter or phoneme transcriptions are stored in .{wrd,ltr,phn}.
-
-In **/path/to/data/with_silence** you need a *train.tsv* file as well as (optionally) *{valid,test}.{tsv,wrd,phn}*. It is nice to have *10h.{tsv,phn}* files there too for reproducing the ablation study on layer selection. In **/path/to/data/without_silence** you have the same files, except *.tsv* files contain audios with silences removed using rVAD.
-
-Pre-requisites:
-* set FAIRSEQ_ROOT environmental variable to your fairseq installation
-* set RVAD_ROOT environmental variable to a checkout of [rVADfast](https://github.com/zhenghuatan/rVADfast)
-* set KENLM_ROOT environmental variable to the location of [KenLM](https://github.com/kpu/kenlm) binaries
-* install [PyKaldi](https://github.com/pykaldi/pykaldi) and set KALDI_ROOT environmental variable to the location of your kaldi installation. To use the version bundled with PyKaldi, you can use /path/to/pykaldi/tools/kaldi
-
-Create new audio files without silences:
-```shell
-# create a manifest file for the set original of audio files
-python $FAIRSEQ_ROOT/examples/wav2vec/wav2vec_manifest.py /dir/to/save/audio/files --ext wav --dest /path/to/new/train.tsv --valid-percent 0
-
-python scripts/vads.py -r $RVAD_ROOT < /path/to/train.tsv > train.vads
-
-python scripts/remove_silence.py --tsv /path/to/train.tsv --vads train.vads --out /dir/to/save/audio/files
-
-python $FAIRSEQ_ROOT/examples/wav2vec/wav2vec_manifest.py /dir/to/save/audio/files --ext wav --dest /path/to/new/train.tsv --valid-percent 0.01
-```
-
-Next, we need to preprocess the audio data to better match phonemized text data:
-
-```shell
-zsh scripts/prepare_audio.sh /dir/with/{train,test,valid}.tsv /output/dir /path/to/wav2vec2/model.pt 512 14
-```
-Note that if you have splits different than train/valid/test, you will need to modify this script. The last two arguments are the PCA dimensionality and the 0-based index of the layer from which to extract representations.
-
-Now we need to prepare text data:
-```shell
-zsh scripts/prepare_text.sh language /path/to/text/file /output/dir 1000 espeak /path/to/fasttext/lid/model
-```
-
-The fourth argument is minimum number observations of phones to keep. If your text corpus is small, you might want to reduce this number.
-
-The fifth argument is which phonemizer to use. Supported values are [espeak](http://espeak.sourceforge.net/), [espeak-ng](https://github.com/espeak-ng/espeak-ng), and [G2P](https://github.com/Kyubyong/g2p) (english only).
-
-Pre-trained fasttext LID models can be downloaded [here](https://fasttext.cc/docs/en/language-identification.html).
-
-### Prepare TIMIT data
-TIMIT transcripts include silence. Therefore VAD is not used for audio preprocessing, and we do not wrap transcripts with silences or insert random silence in between words.
-
-To prepare TIMIT data for both the matched an unmatched setup:
-```shell
-bash scripts/prepare_timit.sh /dir/to/timit/raw/data /output/dir /path/to/wav2vec2/model.pt
-```
-
-Note that we assume the TIMIT distribution with capitalized directories and filenames are used (e.g., `TRAIN/DR1/FCJF0/SA1.PHN`).
-
-## Generative adversarial training (GAN)
-
-We then use a GAN model to build a first unsupervised ASR model. The data preparation above of both speech features and text data is a necessary procedure that enables the generator to match speech to text in an unsupervised way.
-
-Launching GAN training on top of preprocessed features, with default hyperparameters can be done with:
-
-```
-PREFIX=w2v_unsup_gan_xp
-TASK_DATA=/path/to/features/precompute_unfiltered_pca512_cls128_mean_pooled
-TEXT_DATA=/path/to/data/phones # path to fairseq-preprocessed GAN data (phones dir)
-KENLM_PATH=/path/to/data/phones/kenlm.phn.o4.bin # KenLM 4-gram phoneme language model (LM data = GAN data here)
-
-PYTHONPATH=$FAIRSEQ_ROOT PREFIX=$PREFIX fairseq-hydra-train \
- -m --config-dir config/gan \
- --config-name w2vu \
- task.data=${TASK_DATA} \
- task.text_data=${TEXT_DATA} \
- task.kenlm_path=${KENLM_PATH} \
- common.user_dir=${FAIRSEQ_ROOT}/examples/wav2vec/unsupervised \
- model.code_penalty=2,4 model.gradient_penalty=1.5,2.0 \
- model.smoothness_weight=0.5,0.75,1.0 'common.seed=range(0,5)'
-```
-
-
-Once we find the best checkpoint (chosen using unsupervised metric that combined language model perplexity and vocabulary usage), we can use it to generate phone labels (or word labels with an appropriate kaldi WFST):
-
-```shell
-python w2vu_generate.py --config-dir config/generate --config-name viterbi \
-fairseq.common.user_dir=${FAIRSEQ_ROOT}/examples/wav2vec/unsupervised \
-fairseq.task.data=/path/to/dir/with/features \
-fairseq.common_eval.path=/path/to/gan/checkpoint \
-fairseq.dataset.gen_subset=valid results_path=/where/to/save/transcriptions
-```
-
-The decoding without LM works best on the same adjacent-mean-pooled features that the gan was trained on, while decoding with LM works better on features before the adjacent timestep mean-pooling step (without the "_pooled" suffix).
-
-## Iterative self-training + Kaldi LM-decoding
-After the GAN training provides a first unsupervised model, we can then progressively refine the quality of transcriptions using several iterations of semi-supervised learning. We perform two iterations: first, pseudo-label the training data with the unsupervised GAN model and train an HMM on the pseudo-labels. Second, we relabel the training data with the HMM and then fine-tune the original wav2vec 2.0 model using the HMM pseudo-labels with a CTC loss. Note that HMM models use phonemes as output, while wav2vec 2.0 use letter. Both are decoded using WFST decoders into words.
-
-
-Please see [this README](kaldi_self_train/README.md) for more instructions on how to do iterative self-training + Kaldi LM-decoding.
-
-*** Note: these instructions are a work in progress and will be updated over the next few days
diff --git a/spaces/OFA-Sys/OFA-Generic_Interface/fairseq/fairseq/data/audio/speech_to_text_dataset.py b/spaces/OFA-Sys/OFA-Generic_Interface/fairseq/fairseq/data/audio/speech_to_text_dataset.py
deleted file mode 100644
index 164bf413e4fd41b895348c9ef0bb57421843eb17..0000000000000000000000000000000000000000
--- a/spaces/OFA-Sys/OFA-Generic_Interface/fairseq/fairseq/data/audio/speech_to_text_dataset.py
+++ /dev/null
@@ -1,525 +0,0 @@
-# Copyright (c) Facebook, Inc. and its affiliates.
-#
-# This source code is licensed under the MIT license found in the
-# LICENSE file in the root directory of this source tree.
-
-import csv
-import io
-import logging
-import re
-from collections import defaultdict
-from pathlib import Path
-from typing import Dict, List, Optional
-from dataclasses import dataclass
-
-import numpy as np
-import torch
-from fairseq.data import (
- ConcatDataset,
- Dictionary,
- FairseqDataset,
- ResamplingDataset,
- data_utils as fairseq_data_utils,
-)
-from fairseq.data.audio.audio_utils import (
- get_fbank,
- get_waveform,
- read_from_stored_zip,
- is_npy_data,
- is_sf_audio_data,
- parse_path,
- FEATURE_OR_SF_AUDIO_FILE_EXTENSIONS,
-)
-from fairseq.data.audio.feature_transforms import CompositeAudioFeatureTransform
-from fairseq.data.audio.data_cfg import S2TDataConfig
-
-
-logger = logging.getLogger(__name__)
-
-
-def get_features_from_npy_or_audio(path):
- ext = Path(path).suffix
- if ext not in FEATURE_OR_SF_AUDIO_FILE_EXTENSIONS:
- raise ValueError(f'Unsupported file format for "{path}"')
- return np.load(path) if ext == ".npy" else get_fbank(path)
-
-
-def get_features_or_waveform_from_stored_zip(
- path, byte_offset, byte_size, need_waveform=False, use_sample_rate=None,
-):
- assert path.endswith(".zip")
- data = read_from_stored_zip(path, byte_offset, byte_size)
- f = io.BytesIO(data)
- if is_npy_data(data):
- features_or_waveform = np.load(f)
- elif is_sf_audio_data(data):
- features_or_waveform = \
- get_waveform(
- f, always_2d=False, output_sample_rate=use_sample_rate
- )[0] if need_waveform else get_fbank(f)
- else:
- raise ValueError(f'Unknown file format for "{path}"')
- return features_or_waveform
-
-
-def get_features_or_waveform(
- path: str, need_waveform=False, use_sample_rate=None
-):
- """Get speech features from .npy file or waveform from .wav/.flac file.
- The file may be inside an uncompressed ZIP file and is accessed via byte
- offset and length.
-
- Args:
- path (str): File path in the format of "<.npy/.wav/.flac path>" or
- "::".
- need_waveform (bool): return waveform instead of features.
- use_sample_rate (int): change sample rate for the input wave file
-
- Returns:
- features_or_waveform (numpy.ndarray): speech features or waveform.
- """
- _path, slice_ptr = parse_path(path)
- if len(slice_ptr) == 0:
- if need_waveform:
- return get_waveform(
- _path, always_2d=False, output_sample_rate=use_sample_rate
- )[0]
- return get_features_from_npy_or_audio(_path)
- elif len(slice_ptr) == 2:
- features_or_waveform = get_features_or_waveform_from_stored_zip(
- _path, slice_ptr[0], slice_ptr[1], need_waveform=need_waveform,
- use_sample_rate=use_sample_rate
- )
- else:
- raise ValueError(f"Invalid path: {path}")
-
- return features_or_waveform
-
-
-def _collate_frames(
- frames: List[torch.Tensor], is_audio_input: bool = False
-) -> torch.Tensor:
- """
- Convert a list of 2D frames into a padded 3D tensor
- Args:
- frames (list): list of 2D frames of size L[i]*f_dim. Where L[i] is
- length of i-th frame and f_dim is static dimension of features
- Returns:
- 3D tensor of size len(frames)*len_max*f_dim where len_max is max of L[i]
- """
- max_len = max(frame.size(0) for frame in frames)
- if is_audio_input:
- out = frames[0].new_zeros((len(frames), max_len))
- else:
- out = frames[0].new_zeros((len(frames), max_len, frames[0].size(1)))
- for i, v in enumerate(frames):
- out[i, : v.size(0)] = v
- return out
-
-
-@dataclass
-class SpeechToTextDatasetItem(object):
- index: int
- source: torch.Tensor
- target: Optional[torch.Tensor] = None
- speaker_id: Optional[int] = None
-
-
-class SpeechToTextDataset(FairseqDataset):
- LANG_TAG_TEMPLATE = ""
-
- def __init__(
- self,
- split: str,
- is_train_split: bool,
- cfg: S2TDataConfig,
- audio_paths: List[str],
- n_frames: List[int],
- src_texts: Optional[List[str]] = None,
- tgt_texts: Optional[List[str]] = None,
- speakers: Optional[List[str]] = None,
- src_langs: Optional[List[str]] = None,
- tgt_langs: Optional[List[str]] = None,
- ids: Optional[List[str]] = None,
- tgt_dict: Optional[Dictionary] = None,
- pre_tokenizer=None,
- bpe_tokenizer=None,
- n_frames_per_step=1,
- speaker_to_id=None
- ):
- self.split, self.is_train_split = split, is_train_split
- self.cfg = cfg
- self.audio_paths, self.n_frames = audio_paths, n_frames
- self.n_samples = len(audio_paths)
- assert len(n_frames) == self.n_samples > 0
- assert src_texts is None or len(src_texts) == self.n_samples
- assert tgt_texts is None or len(tgt_texts) == self.n_samples
- assert speakers is None or len(speakers) == self.n_samples
- assert src_langs is None or len(src_langs) == self.n_samples
- assert tgt_langs is None or len(tgt_langs) == self.n_samples
- assert ids is None or len(ids) == self.n_samples
- assert (tgt_dict is None and tgt_texts is None) or (
- tgt_dict is not None and tgt_texts is not None
- )
- self.src_texts, self.tgt_texts = src_texts, tgt_texts
- self.src_langs, self.tgt_langs = src_langs, tgt_langs
- self.speakers = speakers
- self.tgt_dict = tgt_dict
- self.check_tgt_lang_tag()
- self.ids = ids
- self.shuffle = cfg.shuffle if is_train_split else False
-
- self.feature_transforms = CompositeAudioFeatureTransform.from_config_dict(
- self.cfg.get_feature_transforms(split, is_train_split)
- )
-
- self.pre_tokenizer = pre_tokenizer
- self.bpe_tokenizer = bpe_tokenizer
- self.n_frames_per_step = n_frames_per_step
- self.speaker_to_id = speaker_to_id
-
- self.tgt_lens = self.get_tgt_lens_and_check_oov()
-
- logger.info(self.__repr__())
-
- def get_tgt_lens_and_check_oov(self):
- if self.tgt_texts is None:
- return [0 for _ in range(self.n_samples)]
- tgt_lens = []
- n_tokens, n_oov_tokens = 0, 0
- for i in range(self.n_samples):
- tokenized = self.get_tokenized_tgt_text(i).split(" ")
- oov_tokens = [
- t
- for t in tokenized
- if self.tgt_dict.index(t) == self.tgt_dict.unk_index
- ]
- n_tokens += len(tokenized)
- n_oov_tokens += len(oov_tokens)
- tgt_lens.append(len(tokenized))
- logger.info(f"'{self.split}' has {n_oov_tokens / n_tokens * 100:.2f}% OOV")
- return tgt_lens
-
- def __repr__(self):
- return (
- self.__class__.__name__
- + f'(split="{self.split}", n_samples={self.n_samples:_}, '
- f"prepend_tgt_lang_tag={self.cfg.prepend_tgt_lang_tag}, "
- f"shuffle={self.shuffle}, transforms={self.feature_transforms}, "
- f"n_frames_per_step={self.n_frames_per_step}"
- )
-
- @classmethod
- def is_lang_tag(cls, token):
- pattern = cls.LANG_TAG_TEMPLATE.replace("{}", "(.*)")
- return re.match(pattern, token)
-
- def check_tgt_lang_tag(self):
- if self.cfg.prepend_tgt_lang_tag:
- assert self.tgt_langs is not None and self.tgt_dict is not None
- tgt_lang_tags = [
- self.LANG_TAG_TEMPLATE.format(t) for t in set(self.tgt_langs)
- ]
- assert all(t in self.tgt_dict for t in tgt_lang_tags)
-
- @classmethod
- def tokenize(cls, tokenizer, text: str):
- return text if tokenizer is None else tokenizer.encode(text)
-
- def get_tokenized_tgt_text(self, index: int):
- text = self.tokenize(self.pre_tokenizer, self.tgt_texts[index])
- text = self.tokenize(self.bpe_tokenizer, text)
- return text
-
- def pack_frames(self, feature: torch.Tensor):
- if self.n_frames_per_step == 1:
- return feature
- n_packed_frames = feature.shape[0] // self.n_frames_per_step
- feature = feature[:self.n_frames_per_step * n_packed_frames]
- return feature.reshape(n_packed_frames, -1)
-
- @classmethod
- def get_lang_tag_idx(cls, lang: str, dictionary: Dictionary):
- lang_tag_idx = dictionary.index(cls.LANG_TAG_TEMPLATE.format(lang))
- assert lang_tag_idx != dictionary.unk()
- return lang_tag_idx
-
- def __getitem__(self, index: int) -> SpeechToTextDatasetItem:
- source = get_features_or_waveform(
- self.audio_paths[index],
- need_waveform=self.cfg.use_audio_input,
- use_sample_rate=self.cfg.use_sample_rate,
- )
- if self.feature_transforms is not None:
- assert not self.cfg.use_audio_input
- source = self.feature_transforms(source)
- source = torch.from_numpy(source).float()
- source = self.pack_frames(source)
-
- target = None
- if self.tgt_texts is not None:
- tokenized = self.get_tokenized_tgt_text(index)
- target = self.tgt_dict.encode_line(
- tokenized, add_if_not_exist=False, append_eos=True
- ).long()
- if self.cfg.prepend_tgt_lang_tag:
- lang_tag_idx = self.get_lang_tag_idx(
- self.tgt_langs[index], self.tgt_dict
- )
- target = torch.cat((torch.LongTensor([lang_tag_idx]), target), 0)
-
- speaker_id = None
- if self.speaker_to_id is not None:
- speaker_id = self.speaker_to_id[self.speakers[index]]
- return SpeechToTextDatasetItem(
- index=index, source=source, target=target, speaker_id=speaker_id
- )
-
- def __len__(self):
- return self.n_samples
-
- def collater(
- self, samples: List[SpeechToTextDatasetItem], return_order: bool = False
- ) -> Dict:
- if len(samples) == 0:
- return {}
- indices = torch.tensor([x.index for x in samples], dtype=torch.long)
- frames = _collate_frames([x.source for x in samples], self.cfg.use_audio_input)
- # sort samples by descending number of frames
- n_frames = torch.tensor([x.source.size(0) for x in samples], dtype=torch.long)
- n_frames, order = n_frames.sort(descending=True)
- indices = indices.index_select(0, order)
- frames = frames.index_select(0, order)
-
- target, target_lengths = None, None
- prev_output_tokens = None
- ntokens = None
- if self.tgt_texts is not None:
- target = fairseq_data_utils.collate_tokens(
- [x.target for x in samples],
- self.tgt_dict.pad(),
- self.tgt_dict.eos(),
- left_pad=False,
- move_eos_to_beginning=False,
- )
- target = target.index_select(0, order)
- target_lengths = torch.tensor(
- [x.target.size(0) for x in samples], dtype=torch.long
- ).index_select(0, order)
- prev_output_tokens = fairseq_data_utils.collate_tokens(
- [x.target for x in samples],
- self.tgt_dict.pad(),
- self.tgt_dict.eos(),
- left_pad=False,
- move_eos_to_beginning=True,
- )
- prev_output_tokens = prev_output_tokens.index_select(0, order)
- ntokens = sum(x.target.size(0) for x in samples)
-
- speaker = None
- if self.speaker_to_id is not None:
- speaker = torch.tensor(
- [s.speaker_id for s in samples], dtype=torch.long
- ).index_select(0, order).view(-1, 1)
-
- net_input = {
- "src_tokens": frames,
- "src_lengths": n_frames,
- "prev_output_tokens": prev_output_tokens,
- }
- out = {
- "id": indices,
- "net_input": net_input,
- "speaker": speaker,
- "target": target,
- "target_lengths": target_lengths,
- "ntokens": ntokens,
- "nsentences": len(samples),
- }
- if return_order:
- out["order"] = order
- return out
-
- def num_tokens(self, index):
- return self.n_frames[index]
-
- def size(self, index):
- return self.n_frames[index], self.tgt_lens[index]
-
- @property
- def sizes(self):
- return np.array(self.n_frames)
-
- @property
- def can_reuse_epoch_itr_across_epochs(self):
- return True
-
- def ordered_indices(self):
- if self.shuffle:
- order = [np.random.permutation(len(self))]
- else:
- order = [np.arange(len(self))]
- # first by descending order of # of frames then by original/random order
- order.append([-n for n in self.n_frames])
- return np.lexsort(order)
-
- def prefetch(self, indices):
- raise False
-
-
-class SpeechToTextDatasetCreator(object):
- # mandatory columns
- KEY_ID, KEY_AUDIO, KEY_N_FRAMES = "id", "audio", "n_frames"
- KEY_TGT_TEXT = "tgt_text"
- # optional columns
- KEY_SPEAKER, KEY_SRC_TEXT = "speaker", "src_text"
- KEY_SRC_LANG, KEY_TGT_LANG = "src_lang", "tgt_lang"
- # default values
- DEFAULT_SPEAKER = DEFAULT_SRC_TEXT = DEFAULT_LANG = ""
-
- @classmethod
- def _from_list(
- cls,
- split_name: str,
- is_train_split,
- samples: List[Dict],
- cfg: S2TDataConfig,
- tgt_dict,
- pre_tokenizer,
- bpe_tokenizer,
- n_frames_per_step,
- speaker_to_id
- ) -> SpeechToTextDataset:
- audio_root = Path(cfg.audio_root)
- ids = [s[cls.KEY_ID] for s in samples]
- audio_paths = [(audio_root / s[cls.KEY_AUDIO]).as_posix() for s in samples]
- n_frames = [int(s[cls.KEY_N_FRAMES]) for s in samples]
- tgt_texts = [s[cls.KEY_TGT_TEXT] for s in samples]
- src_texts = [s.get(cls.KEY_SRC_TEXT, cls.DEFAULT_SRC_TEXT) for s in samples]
- speakers = [s.get(cls.KEY_SPEAKER, cls.DEFAULT_SPEAKER) for s in samples]
- src_langs = [s.get(cls.KEY_SRC_LANG, cls.DEFAULT_LANG) for s in samples]
- tgt_langs = [s.get(cls.KEY_TGT_LANG, cls.DEFAULT_LANG) for s in samples]
- return SpeechToTextDataset(
- split_name,
- is_train_split,
- cfg,
- audio_paths,
- n_frames,
- src_texts=src_texts,
- tgt_texts=tgt_texts,
- speakers=speakers,
- src_langs=src_langs,
- tgt_langs=tgt_langs,
- ids=ids,
- tgt_dict=tgt_dict,
- pre_tokenizer=pre_tokenizer,
- bpe_tokenizer=bpe_tokenizer,
- n_frames_per_step=n_frames_per_step,
- speaker_to_id=speaker_to_id
- )
-
- @classmethod
- def get_size_ratios(
- cls, datasets: List[SpeechToTextDataset], alpha: float = 1.0
- ) -> List[float]:
- """Size ratios for temperature-based sampling
- (https://arxiv.org/abs/1907.05019)"""
-
- id_to_lp, lp_to_sz = {}, defaultdict(int)
- for ds in datasets:
- lang_pairs = {f"{s}->{t}" for s, t in zip(ds.src_langs, ds.tgt_langs)}
- assert len(lang_pairs) == 1
- lang_pair = list(lang_pairs)[0]
- id_to_lp[ds.split] = lang_pair
- lp_to_sz[lang_pair] += sum(ds.n_frames)
-
- sz_sum = sum(v for v in lp_to_sz.values())
- lp_to_prob = {k: v / sz_sum for k, v in lp_to_sz.items()}
- lp_to_tgt_prob = {k: v ** alpha for k, v in lp_to_prob.items()}
- prob_sum = sum(v for v in lp_to_tgt_prob.values())
- lp_to_tgt_prob = {k: v / prob_sum for k, v in lp_to_tgt_prob.items()}
- lp_to_sz_ratio = {
- k: (lp_to_tgt_prob[k] * sz_sum) / v for k, v in lp_to_sz.items()
- }
- size_ratio = [lp_to_sz_ratio[id_to_lp[ds.split]] for ds in datasets]
-
- p_formatted = {
- k: f"{lp_to_prob[k]:.3f}->{lp_to_tgt_prob[k]:.3f}" for k in lp_to_sz
- }
- logger.info(f"sampling probability balancing: {p_formatted}")
- sr_formatted = {ds.split: f"{r:.3f}" for ds, r in zip(datasets, size_ratio)}
- logger.info(f"balanced sampling size ratio: {sr_formatted}")
- return size_ratio
-
- @classmethod
- def _load_samples_from_tsv(cls, root: str, split: str):
- tsv_path = Path(root) / f"{split}.tsv"
- if not tsv_path.is_file():
- raise FileNotFoundError(f"Dataset not found: {tsv_path}")
- with open(tsv_path) as f:
- reader = csv.DictReader(
- f,
- delimiter="\t",
- quotechar=None,
- doublequote=False,
- lineterminator="\n",
- quoting=csv.QUOTE_NONE,
- )
- samples = [dict(e) for e in reader]
- if len(samples) == 0:
- raise ValueError(f"Empty manifest: {tsv_path}")
- return samples
-
- @classmethod
- def _from_tsv(
- cls,
- root: str,
- cfg: S2TDataConfig,
- split: str,
- tgt_dict,
- is_train_split: bool,
- pre_tokenizer,
- bpe_tokenizer,
- n_frames_per_step,
- speaker_to_id
- ) -> SpeechToTextDataset:
- samples = cls._load_samples_from_tsv(root, split)
- return cls._from_list(
- split, is_train_split, samples, cfg, tgt_dict, pre_tokenizer,
- bpe_tokenizer, n_frames_per_step, speaker_to_id
- )
-
- @classmethod
- def from_tsv(
- cls,
- root: str,
- cfg: S2TDataConfig,
- splits: str,
- tgt_dict,
- pre_tokenizer,
- bpe_tokenizer,
- is_train_split: bool,
- epoch: int,
- seed: int,
- n_frames_per_step: int = 1,
- speaker_to_id=None
- ) -> SpeechToTextDataset:
- datasets = [
- cls._from_tsv(
- root, cfg, split, tgt_dict, is_train_split, pre_tokenizer,
- bpe_tokenizer, n_frames_per_step, speaker_to_id
- )
- for split in splits.split(",")
- ]
-
- if is_train_split and len(datasets) > 1 and cfg.sampling_alpha != 1.0:
- # temperature-based sampling
- size_ratios = cls.get_size_ratios(datasets, alpha=cfg.sampling_alpha)
- datasets = [
- ResamplingDataset(
- d, size_ratio=r, seed=seed, epoch=epoch, replace=(r >= 1.0)
- )
- for r, d in zip(size_ratios, datasets)
- ]
-
- return ConcatDataset(datasets) if len(datasets) > 1 else datasets[0]
diff --git a/spaces/OFA-Sys/OFA-Generic_Interface/ofa_module/__init__.py b/spaces/OFA-Sys/OFA-Generic_Interface/ofa_module/__init__.py
deleted file mode 100644
index 30b147a95464b55f55a0dd1dc8555ca69ebec358..0000000000000000000000000000000000000000
--- a/spaces/OFA-Sys/OFA-Generic_Interface/ofa_module/__init__.py
+++ /dev/null
@@ -1,5 +0,0 @@
-import data
-import models
-import tasks
-import criterions
-import utils
\ No newline at end of file
diff --git a/spaces/OFA-Sys/OFA-Image_Caption/fairseq/examples/m2m_100/tokenizers/tokenize_zh.py b/spaces/OFA-Sys/OFA-Image_Caption/fairseq/examples/m2m_100/tokenizers/tokenize_zh.py
deleted file mode 100644
index 674b5849cba829cf4f07a69369e9cc6eed376d4c..0000000000000000000000000000000000000000
--- a/spaces/OFA-Sys/OFA-Image_Caption/fairseq/examples/m2m_100/tokenizers/tokenize_zh.py
+++ /dev/null
@@ -1,14 +0,0 @@
-#!/usr/bin/env python3
-# Copyright (c) Facebook, Inc. and its affiliates.
-#
-# This source code is licensed under the MIT license found in the
-# LICENSE file in the root directory of this source tree.
-
-
-import fileinput
-
-import sacrebleu
-
-
-for line in fileinput.input():
- print(sacrebleu.tokenize_zh(line))
diff --git a/spaces/OFA-Sys/OFA-Image_Caption/fairseq/fairseq/models/roberta/__init__.py b/spaces/OFA-Sys/OFA-Image_Caption/fairseq/fairseq/models/roberta/__init__.py
deleted file mode 100644
index 4cd723ae96aec8e3182773483f123109d23b620e..0000000000000000000000000000000000000000
--- a/spaces/OFA-Sys/OFA-Image_Caption/fairseq/fairseq/models/roberta/__init__.py
+++ /dev/null
@@ -1,11 +0,0 @@
-# Copyright (c) Facebook, Inc. and its affiliates.
-#
-# This source code is licensed under the MIT license found in the
-# LICENSE file in the root directory of this source tree.
-
-from .hub_interface import * # noqa
-from .model import * # noqa
-from .enc_dec import * # noqa
-from .model_camembert import * # noqa
-from .model_gottbert import * # noqa
-from .model_xlmr import * # noqa
diff --git a/spaces/OFA-Sys/OFA-vqa/fairseq/fairseq/token_generation_constraints.py b/spaces/OFA-Sys/OFA-vqa/fairseq/fairseq/token_generation_constraints.py
deleted file mode 100644
index e708dc51bcb0ffb7b411496239c74d5e6f3c2448..0000000000000000000000000000000000000000
--- a/spaces/OFA-Sys/OFA-vqa/fairseq/fairseq/token_generation_constraints.py
+++ /dev/null
@@ -1,506 +0,0 @@
-# Copyright (c) Facebook, Inc. and its affiliates.
-#
-# This source code is licensed under the MIT license found in the
-# LICENSE file in the root directory of this source tree.
-
-"""Implements tracking of constraints for a beam item.
-
-A list of constraints is given as a list of one or more token
-sequences, each of length at least one token. For example, for an input sentence
-
-> Die maschinelle Übersetzung ist schwer zu kontrollieren.
-
-We could have the constraints:
-* to influence
-* hard
-
-There are two implementations:
-* OrderedConstraintState: Tracks progress through an ordered list of multitoken constraints.
-* UnorderedConstraintState: Tracks progress through an unordered list of multitoken constraints.
-
-The difference is that in the first, the constraints are assumed to be
-in order; the algorithm will permit zero or more tokens between them.
-In the second, the constraints are not ordered, so many orderings will
-be explored.
-
-The same sequence can be present any number of times, and will appear
-that many times in the output.
-"""
-
-from collections import Counter
-from typing import List, Optional, Set, Tuple
-
-import torch
-
-
-class ConstraintState:
- def __init__(self):
- pass
-
-
-def pack_constraints(batch_constraints: List[List[torch.Tensor]]) -> torch.Tensor:
- """Takes a list of list of constraints in tensor form (a list of
- tensor constraints for each sentence) and transforms it into a
- packed Tensor. For example, here is a batch of size 3 with 3, 0,
- and 1 constraints:
-
- [ [ [3 1 2], [3], [4 5 6 7], ]
- [],
- [ [1 8 9 10 1 4 11 12], ]
- ]
-
- Its corresponding packed structure is:
-
- [ [ 3 3 1 2 0 3 0 4 5 6 7 0],
- [ 0 0 0 0 0 0 0 0 0 0 0 0],
- [ 1 1 8 9 10 1 4 11 12 0 0 0] ]
-
- The packed tensor has shape (batch size, maxlen), where
- maxlen is defined below. Each row contains concatenated
- constraint tokens for that sentence, with 0 appended after
- each constraint. The first item in each row is the number
- of constraints for that sentence. So maxlen is the maximum
- of
-
- (number of constraints) + (sum length of constraints) + 1.
-
- across all sentences in the batch.
- """
- # The maximum word length of concatenated constraints for any sentence
- max_constraints_len = 1
- for sentence_constraints in batch_constraints:
- if len(sentence_constraints):
- # number of constraints, plus sum of constrain lens, plus a zero after each
- constraints_len = (
- 1
- + sum([c.size(0) for c in sentence_constraints])
- + len(sentence_constraints)
- )
- max_constraints_len = max(max_constraints_len, constraints_len)
-
- batch_size = len(batch_constraints)
- constraints_tensor = torch.zeros((batch_size, max_constraints_len)).long()
- for i, sentence_constraints in enumerate(batch_constraints):
- constraints_tensor[i, 0] = len(sentence_constraints)
- offset = 1
- for j, constraint in enumerate(sentence_constraints):
- this_len = constraint.size(0)
- constraints_tensor[i, offset : offset + this_len] = constraint
- offset += this_len + 1
-
- return constraints_tensor.long()
-
-
-def unpack_constraints(constraint_tensor: torch.Tensor) -> List[torch.Tensor]:
- """
- Transforms *one row* of a packed constraint tensor (e.g., for one
- sentence in the batch) into a list of constraint tensors.
- """
- constraint_list = []
- num_constraints = constraint_tensor[0]
- constraints = constraint_tensor.tolist()
- offset = 1
- for i in range(num_constraints):
- where = constraints.index(0, offset)
- constraint_list.append(constraint_tensor[offset:where])
- offset = where + 1
-
- return constraint_list
-
-
-class ConstraintNode:
- """
- Represents a node in a trie managing unordered constraints.
- """
-
- def __init__(self, token: int = None, parent=None):
- # The token associate with this node (None for the root)
- self.token = int(token) if token is not None else None
- # The parent (None at the root)
- self.parent = parent
- # Whether this node is a completed constraint
- self.terminal = 0
- # List of child nodes
- self.children = {}
-
- # The cumulative number of constraints from this point in the
- # trie forward
- self.num_constraints = 0
-
- @property
- def id(self):
- return self.token
-
- def __str__(self):
- term = self.terminal != 0
- return f"[{self.token}].{term}#{self.num_constraints}"
-
- def __getitem__(self, key: int):
- return self.children.get(key, None)
-
- def next_tokens(self) -> Set[int]:
- """The set of child labels."""
- return set(self.children.keys())
-
- @staticmethod
- def create(constraints: List[List[int]]):
- root = ConstraintNode()
- for sequence in constraints:
- root.add_sequence(sequence)
-
- return root
-
- @staticmethod
- def print_graph(node: "ConstraintNode"):
- if len(node.children) == 0:
- return str(node)
- else:
- s = f"({node}"
- for child in node.children.values():
- s += " " + ConstraintNode.print_graph(child)
- s += ")"
- return s
-
- def token_counts(self) -> Counter:
- """Returns a counter of the number of times each token is used
- in a constraint.
- """
- token_counts = Counter()
- kids = list(self.children.values())
- while len(kids) > 0:
- kid = kids.pop()
- token_counts[kid.id] += kid.num_constraints
- kids += list(kid.children.values())
-
- return token_counts
-
- def tokens(self) -> Set[int]:
- """Returns the set of tokens in constraints."""
- return set(self.token_counts().keys())
-
- def add_sequence(self, sequence: List[int]):
- """Adds a constraint, represented as a list of integers, to
- the trie."""
- assert len(sequence) > 0
-
- token = int(sequence[0])
- if token not in self.children:
- self.children[token] = ConstraintNode(token, parent=self)
-
- node = self.children[token]
- if len(sequence) == 1:
- node.terminal += 1
- node.num_constraints += 1
- parent = node.parent
- while parent is not None:
- parent.num_constraints += 1
- parent = parent.parent
- else:
- node.add_sequence(sequence[1:])
-
-
-class UnorderedConstraintState(ConstraintState):
- """
- Records progress through the set of constraints for each item in the beam
- using a trie.
- """
-
- def __init__(self, node: ConstraintNode, copy_from: "ConstraintState" = None):
- self.node = node
-
- if copy_from is None:
- # The root node
- self.root = node
- # The set of states in the graph that have been completed
- self.completed = Counter()
- # The...
- self.generated = Counter()
- # The list of tokens we need to generate
- self.needed_tokens = self.root.tokens()
- else:
- self.completed = Counter(copy_from.completed)
- self.generated = Counter(copy_from.generated)
- self.root = copy_from.root
-
- # Mark the node as generated
- if self.node != self.root:
- self.generated[node] += 1
-
- @staticmethod
- def create(constraint_tensor: torch.Tensor):
- constraint_list = unpack_constraints(constraint_tensor)
- constraint_trie_root = ConstraintNode.create(constraint_list)
- return UnorderedConstraintState(constraint_trie_root)
-
- def __str__(self):
- gen_str = ",".join([str(node) for node in self.generated])
- return f"{self.name}/{self.bank}({gen_str})x{self.num_completed}"
-
- def __copy__(self):
- copied_state = UnorderedConstraintState(self.node, copy_from=self)
- return copied_state
-
- def copy(self):
- return self.__copy__()
-
- @property
- def name(self):
- if self.node.id is None:
- return "ROOT"
- else:
- return str(self.node.id)
-
- @property
- def is_root(self):
- return self.node == self.root
-
- @property
- def bank(self):
- return sum(self.generated.values())
-
- @property
- def num_completed(self):
- """The number of constraints (not constraint tokens) that are completed.
- In addition to the already-completed states, we need to account for the
- current state, which might get marked as completed when another token
- is generated.
- """
- in_final = self.node.terminal and self.completed[self.node] < self.node.terminal
- return sum(self.completed.values()) + in_final
-
- @property
- def finished(self):
- return self.root.num_constraints - self.num_completed == 0
-
- @property
- def token_counts(self):
- return self.root.token_counts()
-
- @property
- def tokens(self):
- return self.root.tokens()
-
- @property
- def num_constraint_tokens(self):
- return sum(self.token_counts.values())
-
- def next_tokens(self) -> Set[int]:
- """Returns the list of tokens that could come next.
- These are (a) all tokens extending the root state and, for
- non-root states, additionally all tokens extending the current
- state."""
-
- if self.node != self.root:
- return self.root.next_tokens().union(self.node.next_tokens())
- else:
- return self.root.next_tokens()
-
- def advance(self, token: int):
- """Reads in a token and advances the state. Here's how it works.
-
- We can advance to the next state if:
- - there is a matching child
- - its path isn't blocked
-
- A path is blocked when all constraints that are descendants of
- that node have already been generated, in the current state.
-
- If we are not able to advance from the current state, we "fall
- off the graph" and return to the root state. There, we again
- try to advance, checking the same criteria.
-
- In any case, when falling off the graph, we need to do some
- bookkeeping. We:
- - check whether any constraints were met (all prefixes of
- current state)
- - if one is found, mark it as completed
- - adjust visited nodes accordingly
- """
- token = int(token)
-
- next_state = None
- child = self.node[token]
- if child is not None and self.generated[child] < child.num_constraints:
- next_state = UnorderedConstraintState(child, copy_from=self)
-
- def rewind():
- """If we're mid-trie and an "illegal" token is chosen next, we need
- to reset our state to the root state. However, along the way, we need
- to check whether a prefix of the current trie state represents a state
- we could mark as completed.
- """
- node = self.node
- while node != self.root:
- if node.terminal and self.completed[node] < node.terminal:
- next_state.completed[node] += 1
- return
-
- next_state.generated[node] -= 1
- node = node.parent
-
- # Fall off the graph, check the root
- if next_state is None and token in self.root.next_tokens():
- child = self.root[token]
- # We can only traverse this edge if it's not saturated
- if self.generated[child] < child.num_constraints:
- next_state = UnorderedConstraintState(child, copy_from=self)
- else:
- next_state = UnorderedConstraintState(self.root, copy_from=self)
-
- # Rewind
- rewind()
-
- elif next_state is None:
- next_state = UnorderedConstraintState(self.root, copy_from=self)
- # Rewind
- rewind()
-
- return next_state
-
-
-class ConstraintSequence:
- def __init__(self, sequences: List[List[int]]):
- """Represents a set of possibly multitoken constraints by
- concatenating them and internally recording the end points.
- """
- self.sequences = []
- self.endpoints = []
- self.num_tokens = 0
- self.tokens = set()
- for sequence in sequences:
- for token in sequence:
- self.tokens.add(token)
- self.num_tokens += len(sequence)
- self.endpoints += [False for x in range(len(sequence) - 1)] + [True]
- self.sequences += sequence
-
- def __getitem__(self, key: int):
- return self.sequences[key]
-
- def __len__(self):
- return len(self.sequences)
-
- def __str__(self):
- return str(self.sequences)
-
-
-class OrderedConstraintState(ConstraintState):
- """
- Records progress through the set of linear nonbranching constraints with gaps.
- """
-
- def __init__(self, sequence: ConstraintSequence, state: int = -1):
- self.sequence = sequence
- self.state = state
-
- @staticmethod
- def create(constraint_tensor: torch.Tensor):
- constraint_list = unpack_constraints(constraint_tensor)
- return OrderedConstraintState(ConstraintSequence(constraint_list), -1)
-
- def __str__(self):
- return f"{self.state}/{self.bank}x{self.num_completed}"
-
- def __copy__(self):
- return OrderedConstraintState(self.sequence, self.state)
-
- def copy(self):
- return self.__copy__()
-
- @property
- def num_completed(self):
- if self.state == -1:
- return 0
- count = len(
- list(filter(lambda x: x, self.sequence.endpoints[0 : self.state + 1]))
- )
- return count
-
- @property
- def is_root(self):
- return self.state == -1
-
- @property
- def name(self):
- if self.state == -1:
- return "ROOT"
- else:
- return str(self.sequence[self.state])
-
- @property
- def bank(self) -> int:
- return self.state + 1
-
- @property
- def finished(self):
- return self.state + 1 == len(self.sequence)
-
- @property
- def token_counts(self):
- return self.sequence.token_counts()
-
- @property
- def tokens(self):
- return self.sequence.tokens
-
- @property
- def num_constraint_tokens(self):
- return sum(self.token_counts.values())
-
- def next_tokens(self) -> Set[int]:
- """Returns the list of tokens that could come next.
- These are (a) all tokens extending the root state and, for
- non-root states, additionally all tokens extending the current
- state."""
-
- tokens = set()
- if self.state > 0:
- tokens.add(self.sequence[0])
- if not self.finished:
- tokens.add(self.sequence[self.state + 1])
- return tokens
-
- def advance(self, token: int):
- """Reads in a token and advances the state. Here's how it works.
-
- We can advance to the next state if:
- - there is a matching child
- - its path isn't blocked
-
- A path is blocked when all constraints that are descendants of
- that node have already been generated, in the current state.
-
- If we are not able to advance from the current state, we "fall
- off the graph" and return to the root state. There, we again
- try to advance, checking the same criteria.
-
- In any case, when falling off the graph, we need to do some
- bookkeeping. We:
- - check whether any constraints were met (all prefixes of
- current state)
- - if one is found, mark it as completed
- - adjust visited nodes accordingly
- """
- token = int(token)
- # print(f"{self} ADVANCE({token}) {self.sequence} -> ", end="")
-
- if self.finished:
- # Accept anything
- next_state = self.copy()
-
- elif self.sequence[self.state + 1] == token:
- # Advance to the next token
- next_state = OrderedConstraintState(self.sequence, self.state + 1)
-
- elif self.sequence.endpoints[self.state]:
- # Accept anything between constraints (*)
- next_state = self.copy()
-
- elif token == self.sequence[0]:
- # Start over having generated the first token
- next_state = OrderedConstraintState(self.sequence, 0)
- else:
- # Start over from the root
- next_state = OrderedConstraintState(self.sequence, -1)
-
- return next_state
diff --git a/spaces/OFA-Sys/OFA-vqa/fairseq/tests/distributed/test_bmuf.py b/spaces/OFA-Sys/OFA-vqa/fairseq/tests/distributed/test_bmuf.py
deleted file mode 100644
index 8b7cadb094d49587b6b82432248459fdcf42457e..0000000000000000000000000000000000000000
--- a/spaces/OFA-Sys/OFA-vqa/fairseq/tests/distributed/test_bmuf.py
+++ /dev/null
@@ -1,207 +0,0 @@
-# Copyright (c) Facebook, Inc. and its affiliates.
-#
-# This source code is licensed under the MIT license found in the
-# LICENSE file in the root directory of this source tree.
-
-import argparse
-import functools
-import random
-import unittest
-from multiprocessing import Manager
-
-import torch
-import torch.nn as nn
-from fairseq import optim
-from fairseq.distributed import utils as distributed_utils
-from omegaconf import OmegaConf
-
-
-class Model(nn.Module):
- def __init__(self, input_size, output_size):
- super(Model, self).__init__()
- self.fc = nn.Linear(input_size, output_size)
-
- def forward(self, input):
- output = self.fc(input)
- return output
-
-
-def setup_model_loss_criterion(cfg, args, rank, is_cuda):
- """
- setup model, criterion and optimizer based on input args
- """
- args.distributed_rank = rank
- cfg.distributed_training.distributed_rank = args.distributed_rank
- if cfg.distributed_training.distributed_world_size > 1:
- distributed_utils.distributed_init(cfg)
- torch.manual_seed(1)
- model = Model(args.input_size, args.nb_classes)
- loss_fn = nn.CrossEntropyLoss()
- if is_cuda:
- model = model.cuda()
- loss_fn = loss_fn.cuda()
-
- optimizer = optim.sgd.SGD(args, model.parameters())
- optimizer = optim.FairseqBMUF(
- cfg=cfg.bmuf,
- optimizer=optimizer
- )
-
- return model, loss_fn, optimizer
-
-
-def train_step(input, target, model, loss_fn, optimizer, **unused):
- """Do forward, backward and parameter update."""
- model.train()
- output = model(input)
- loss = loss_fn(output, target)
- optimizer.backward(loss)
- optimizer.step()
-
-
-def single_gpu_training(cfg, args, rank, iterations, shared_results):
-
- is_cuda = torch.cuda.is_available()
- if is_cuda:
- torch.cuda.set_device(rank)
-
- model, loss_fn, optimizer = setup_model_loss_criterion(cfg, args, rank, is_cuda)
-
- for _ in range(iterations):
- input = torch.randn(1, args.input_size)
- target = torch.empty(args.batch_size, dtype=torch.long).random_(args.nb_classes)
-
- if is_cuda:
- input = input.cuda()
- target = target.cuda()
- train_step(input, target, model, loss_fn, optimizer)
-
- results = []
- for param in model.parameters():
- if len(results) == 0:
- results = param.flatten().cpu().data
- else:
- results = torch.cat((results, param.flatten().cpu().data), 0)
-
- shared_results[rank] = results
-
-
-def setup_args():
- args = argparse.Namespace()
- args.global_sync_iter = 20
- args.block_momentum = 0.875
- args.block_lr = 0.5
- args.input_size = 5
- args.nb_classes = 2
- args.batch_size = 1
- args.lr = [1e-3]
- args.momentum = 0
- args.weight_decay = 0
- args.warmup_iterations = 0
- args.use_nbm = True
- args.average_sync = True
- args.global_sync_iter = 1
- args.model_parallel_size = 1
- args.distributed_backend = "gloo"
-
- args.distributed_world_size = 2
- port = random.randint(10000, 20000)
- args.distributed_init_method = "tcp://localhost:{port}".format(port=port)
- args.distributed_init_host = "localhost"
- args.distributed_port = port + 1
- args.local_world_size = args.distributed_world_size
-
- cfg = OmegaConf.create()
- cfg.optimization = OmegaConf.create()
- cfg.common = OmegaConf.create()
- cfg.distributed_training = OmegaConf.create()
- cfg.dataset = OmegaConf.create()
- cfg.bmuf = OmegaConf.create()
- cfg.optimizer = OmegaConf.create()
-
- cfg.bmuf.global_sync_iter = args.global_sync_iter
- cfg.bmuf.block_momentum = args.block_momentum
- cfg.bmuf.block_lr = args.block_lr
- cfg.dataset.batch_size = args.batch_size
- cfg.optimization.lr = args.lr
- cfg.optimizer.momentum = args.momentum
- cfg.optimizer.weight_decay = args.weight_decay
- cfg.bmuf.warmup_iterations = args.warmup_iterations
- cfg.bmuf.use_nbm = args.use_nbm
- cfg.bmuf.average_sync = args.average_sync
- cfg.common.model_parallel_size = args.model_parallel_size
- cfg.distributed_training.distributed_backend = args.distributed_backend
- cfg.distributed_training.distributed_world_size = args.distributed_world_size
- cfg.bmuf.distributed_world_size = args.distributed_world_size
- cfg.distributed_training.distributed_init_method = args.distributed_init_method
- cfg.distributed_training.distributed_port = args.distributed_port
-
- return cfg, args
-
-
-@unittest.skipIf(torch.cuda.device_count() < 2, "test requires 2 GPUs")
-class TestBMUF(unittest.TestCase):
- def bmuf_process(self, cfg, args, iterations):
- processes = []
- results = Manager().dict()
- torch.multiprocessing.spawn(
- fn=functools.partial(single_gpu_training, cfg, args),
- args=(iterations, results),
- nprocs=args.distributed_world_size,
- join=True,
- )
- return results
-
- def test_bmuf_sync(self):
- # Train model for 1 iteration and do bmuf sync without doing warmup
- cfg, args = setup_args()
- iterations = 1
- results = self.bmuf_process(cfg, args, iterations)
- # Make sure params in both machines are same
- assert len(results) == 2
- self.assertAlmostEqual(results[0], results[1])
-
- def test_warmup_sync(self):
- # Train model for 20 iteration and do warmup sync without doing bmuf sync
- cfg, args = setup_args()
- args.warmup_iterations = 20
- cfg.bmuf.warmup_iterations = args.warmup_iterations
- iterations = 20
- results = self.bmuf_process(cfg, args, iterations)
- # Make sure params in both machines are same
- assert len(results) == 2
- self.assertAlmostEqual(results[0], results[1])
-
- def test_warmup_sync_bmuf_sync(self):
- # Train model for 25 iteration and do warmup sync after 20 iteration
- # and bmuf sync after 25 iteration
- cfg, args = setup_args()
- args.warmup_iterations = 20
- args.global_sync_iter = 5
- cfg.bmuf.warmup_iterations = args.warmup_iterations
- cfg.bmuf.global_sync_iter = args.global_sync_iter
- iterations = 25
- results = self.bmuf_process(cfg, args, iterations)
- # Make sure params in both machines are same
- assert len(results) == 2
- self.assertAlmostEqual(results[0], results[1])
-
- def test_single_gpu_bmuf(self):
- # Train model for 5 iterations and use GPU 1
- cfg, args = setup_args()
- args.distributed_world_size = 1
- args.warmup_iterations = 5
- cfg.distributed_training.distributed_world_size = args.distributed_world_size
- cfg.bmuf.distributed_world_size = args.distributed_world_size
- cfg.bmuf.warmup_iterations = args.warmup_iterations
- iterations = 20
- results = self.bmuf_process(cfg, args, iterations)
- assert len(results) == 1
-
- def assertAlmostEqual(self, t1, t2):
- self.assertEqual(t1.size(), t2.size(), "size mismatch")
- self.assertLess((t1 - t2).abs().max(), 1e-4)
-
-
-if __name__ == "__main__":
- unittest.main()
diff --git a/spaces/PKUWilliamYang/StyleGANEX/utils/common.py b/spaces/PKUWilliamYang/StyleGANEX/utils/common.py
deleted file mode 100644
index 4813fe311ee40720697e4862c5fbfad811d39237..0000000000000000000000000000000000000000
--- a/spaces/PKUWilliamYang/StyleGANEX/utils/common.py
+++ /dev/null
@@ -1,87 +0,0 @@
-import cv2
-import numpy as np
-from PIL import Image
-import matplotlib.pyplot as plt
-
-
-# Log images
-def log_input_image(x, opts):
- if opts.label_nc == 0:
- return tensor2im(x)
- elif opts.label_nc == 1:
- return tensor2sketch(x)
- else:
- return tensor2map(x)
-
-
-def tensor2im(var):
- var = var.cpu().detach().transpose(0, 2).transpose(0, 1).numpy()
- var = ((var + 1) / 2)
- var[var < 0] = 0
- var[var > 1] = 1
- var = var * 255
- return Image.fromarray(var.astype('uint8'))
-
-
-def tensor2map(var):
- mask = np.argmax(var.data.cpu().numpy(), axis=0)
- colors = get_colors()
- mask_image = np.ones(shape=(mask.shape[0], mask.shape[1], 3))
- for class_idx in np.unique(mask):
- mask_image[mask == class_idx] = colors[class_idx]
- mask_image = mask_image.astype('uint8')
- return Image.fromarray(mask_image)
-
-
-def tensor2sketch(var):
- im = var[0].cpu().detach().numpy()
- im = cv2.cvtColor(im, cv2.COLOR_GRAY2BGR)
- im = (im * 255).astype(np.uint8)
- return Image.fromarray(im)
-
-
-# Visualization utils
-def get_colors():
- # currently support up to 19 classes (for the celebs-hq-mask dataset)
- colors = [[0, 0, 0], [204, 0, 0], [76, 153, 0], [204, 204, 0], [51, 51, 255], [204, 0, 204], [0, 255, 255],
- [255, 204, 204], [102, 51, 0], [255, 0, 0], [102, 204, 0], [255, 255, 0], [0, 0, 153], [0, 0, 204],
- [255, 51, 153], [0, 204, 204], [0, 51, 0], [255, 153, 51], [0, 204, 0]]
- return colors
-
-
-def vis_faces(log_hooks):
- display_count = len(log_hooks)
- fig = plt.figure(figsize=(8, 4 * display_count))
- gs = fig.add_gridspec(display_count, 3)
- for i in range(display_count):
- hooks_dict = log_hooks[i]
- fig.add_subplot(gs[i, 0])
- if 'diff_input' in hooks_dict:
- vis_faces_with_id(hooks_dict, fig, gs, i)
- else:
- vis_faces_no_id(hooks_dict, fig, gs, i)
- plt.tight_layout()
- return fig
-
-
-def vis_faces_with_id(hooks_dict, fig, gs, i):
- plt.imshow(hooks_dict['input_face'])
- plt.title('Input\nOut Sim={:.2f}'.format(float(hooks_dict['diff_input'])))
- fig.add_subplot(gs[i, 1])
- plt.imshow(hooks_dict['target_face'])
- plt.title('Target\nIn={:.2f}, Out={:.2f}'.format(float(hooks_dict['diff_views']),
- float(hooks_dict['diff_target'])))
- fig.add_subplot(gs[i, 2])
- plt.imshow(hooks_dict['output_face'])
- plt.title('Output\n Target Sim={:.2f}'.format(float(hooks_dict['diff_target'])))
-
-
-def vis_faces_no_id(hooks_dict, fig, gs, i):
- plt.imshow(hooks_dict['input_face'], cmap="gray")
- plt.title('Input')
- fig.add_subplot(gs[i, 1])
- plt.imshow(hooks_dict['target_face'])
- plt.title('Target')
- fig.add_subplot(gs[i, 2])
- plt.imshow(hooks_dict['output_face'])
- plt.title('Output')
diff --git a/spaces/PaddlePaddle/ERNIE-Zeus/app.py b/spaces/PaddlePaddle/ERNIE-Zeus/app.py
deleted file mode 100644
index 3ed9f1c4f560ee715080ba1c642afbca751cb4e5..0000000000000000000000000000000000000000
--- a/spaces/PaddlePaddle/ERNIE-Zeus/app.py
+++ /dev/null
@@ -1,217 +0,0 @@
-import gradio as gr
-import paddlehub as hub
-
-ernie_zeus = hub.Module(name='ernie_zeus')
-
-
-def inference(task: str,
- text: str,
- min_dec_len: int = 2,
- seq_len: int = 512,
- topp: float = 0.9,
- penalty_score: float = 1.0):
-
- func = getattr(ernie_zeus, task)
- try:
- result = func(text, min_dec_len, seq_len, topp, penalty_score)
- return result
- except Exception as error:
- return str(error)
-
-
-title = "ERNIE-Zeus"
-
-description = "ERNIE-Zeus model, which supports Chinese text generates task."
-
-css = """
- .gradio-container {
- font-family: 'IBM Plex Sans', sans-serif;
- }
- .gr-button {
- color: white;
- border-color: black;
- background: black;
- }
- input[type='range'] {
- accent-color: black;
- }
- .dark input[type='range'] {
- accent-color: #dfdfdf;
- }
- .container {
- max-width: 730px;
- margin: auto;
- padding-top: 1.5rem;
- }
-
- .details:hover {
- text-decoration: underline;
- }
- .gr-button {
- white-space: nowrap;
- }
- .gr-button:focus {
- border-color: rgb(147 197 253 / var(--tw-border-opacity));
- outline: none;
- box-shadow: var(--tw-ring-offset-shadow), var(--tw-ring-shadow), var(--tw-shadow, 0 0 #0000);
- --tw-border-opacity: 1;
- --tw-ring-offset-shadow: var(--tw-ring-inset) 0 0 0 var(--tw-ring-offset-width) var(--tw-ring-offset-color);
- --tw-ring-shadow: var(--tw-ring-inset) 0 0 0 calc(3px var(--tw-ring-offset-width)) var(--tw-ring-color);
- --tw-ring-color: rgb(191 219 254 / var(--tw-ring-opacity));
- --tw-ring-opacity: .5;
- }
- .footer {
- margin-bottom: 45px;
- margin-top: 35px;
- text-align: center;
- border-bottom: 1px solid #e5e5e5;
- }
- .footer>p {
- font-size: .8rem;
- display: inline-block;
- padding: 0 10px;
- transform: translateY(10px);
- background: white;
- }
- .dark .footer {
- border-color: #303030;
- }
- .dark .footer>p {
- background: #0b0f19;
- }
- .prompt h4{
- margin: 1.25em 0 .25em 0;
- font-weight: bold;
- font-size: 115%;
- }
-"""
-
-block = gr.Blocks(css=css)
-
-examples = [
- [
- 'text_summarization',
- '外媒7月18日报道,阿联酋政府当日证实该国将建设首个核电站,以应对不断上涨的用电需求。分析称阿联酋作为世界第三大石油出口国,更愿意将该能源用于出口,而非发电。首座核反应堆预计在2017年运行。cntv李婉然编译报道',
- 4, 512, 0.3, 1.0
- ],
- [
- 'copywriting_generation',
- '芍药香氛的沐浴乳',
- 8, 512, 0.9, 1.2
- ],
- [
- 'novel_continuation',
- '昆仑山可以说是天下龙脉的根源,所有的山脉都可以看作是昆仑的分支。这些分出来的枝枝杈杈,都可以看作是一条条独立的龙脉。',
- 2, 512, 0.9, 1.2
- ],
- [
- 'answer_generation',
- '做生意的基本原则是什么?',
- 2, 512, 0.5, 1.2
- ],
- [
- 'couplet_continuation',
- '天增岁月人增寿',
- 2, 512, 1.0, 1.0
- ],
- [
- 'composition_generation',
- '拔河比赛',
- 128, 512, 0.9, 1.2
- ],
- [
- 'text_cloze',
- '她有着一双[MASK]的眼眸。',
- 1, 512, 0.3, 1.2
- ],
-]
-
-with block:
- gr.HTML(
- """
-
-
-
-
-
-
- ERNIE-Zeus Demo
-
-
-
- ERNIE-Zeus is a state-of-the-art Chinese text generates model.
-
-
- """
- )
- with gr.Blocks():
- text = gr.Textbox(
- label="input_text",
- placeholder="Please enter Chinese text.",
- )
- task = gr.Dropdown(label="task",
- choices=[
- 'text_summarization',
- 'copywriting_generation',
- 'novel_continuation',
- 'answer_generation',
- 'couplet_continuation',
- 'composition_generation',
- 'text_cloze'
- ],
- value='text_summarization')
-
- min_dec_len = gr.Slider(
- minimum=1, maximum=511, value=1, label="min_dec_len", step=1, interactive=True)
- seq_len = gr.Slider(minimum=2, maximum=512, value=128,
- label="seq_len", step=1, interactive=True)
- topp = gr.Slider(minimum=0.0, maximum=1.0, value=1.0,
- label="topp", step=0.01, interactive=True)
- penalty_score = gr.Slider(
- minimum=1.0, maximum=2.0, value=1.0, label="penalty_score", step=0.01, interactive=True)
-
- text_gen = gr.Textbox(label="generated_text")
- btn = gr.Button(value="Generate text")
-
- ex = gr.Examples(examples=examples, fn=inference, inputs=[
- task, text, min_dec_len, seq_len, topp, penalty_score], outputs=text_gen, cache_examples=False)
-
- text.submit(inference, inputs=[
- task, text, min_dec_len, seq_len, topp, penalty_score], outputs=text_gen)
- btn.click(inference, inputs=[
- task, text, min_dec_len, seq_len, topp, penalty_score], outputs=text_gen)
- gr.Markdown(
- '''
-## More
-* There are more interesting models in [PaddleHub](https://github.com/PaddlePaddle/PaddleHub), you can star [PaddleHub](https://github.com/PaddlePaddle/PaddleHub) to follow.
-* Besides, you can use free GPU resourses in [AIStudio](https://aistudio.baidu.com/aistudio/projectdetail/4462918) to enjoy more cases, have fun.
- [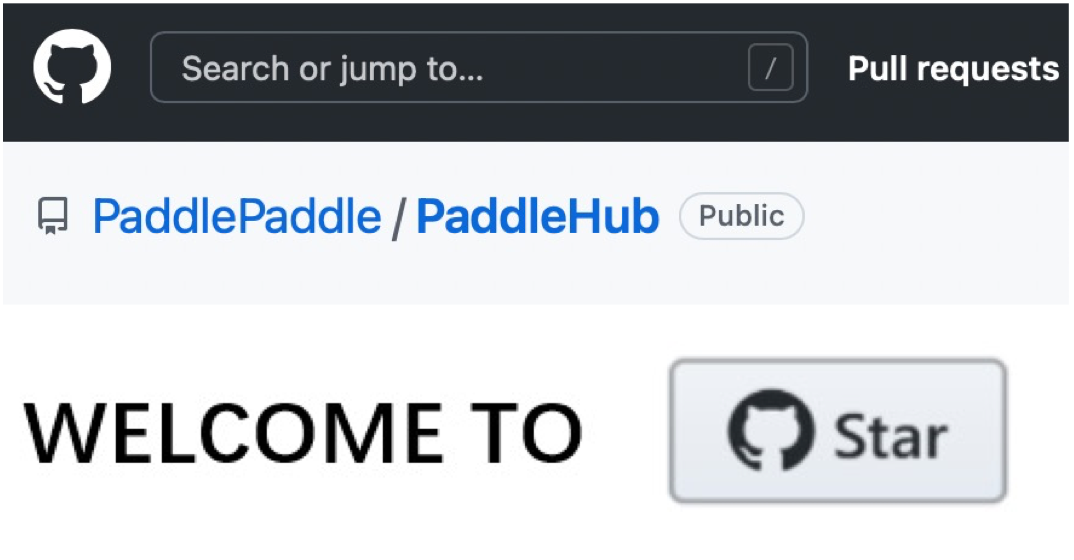](https://github.com/PaddlePaddle/PaddleHub/stargazers)
- '''
- )
- gr.HTML(
- """
-
- """
- )
-
-block.queue(max_size=100000, concurrency_count=100000).launch()
diff --git a/spaces/Pattr/DrumClassification/lilypond-2.24.2/lib/guile/2.2/ccache/system/vm/coverage.go b/spaces/Pattr/DrumClassification/lilypond-2.24.2/lib/guile/2.2/ccache/system/vm/coverage.go
deleted file mode 100644
index 8248f104cb5b5639fec27f638d80478220671371..0000000000000000000000000000000000000000
Binary files a/spaces/Pattr/DrumClassification/lilypond-2.24.2/lib/guile/2.2/ccache/system/vm/coverage.go and /dev/null differ
diff --git a/spaces/PeepDaSlan9/rvc-models/README.md b/spaces/PeepDaSlan9/rvc-models/README.md
deleted file mode 100644
index 56936f1df15477c0ae2fdcfe59a77c175e1905d8..0000000000000000000000000000000000000000
--- a/spaces/PeepDaSlan9/rvc-models/README.md
+++ /dev/null
@@ -1,14 +0,0 @@
----
-title: Rvc Models
-emoji: 🎤
-colorFrom: red
-colorTo: blue
-sdk: gradio
-sdk_version: 3.27.0
-app_file: app.py
-pinned: false
-license: mit
-duplicated_from: zomehwh/rvc-models
----
-
-Check out the configuration reference at https://huggingface.co/docs/hub/spaces-config-reference
diff --git a/spaces/Raspberry-ai/main/.env/lib/python3.11/site-packages/pip/_internal/utils/temp_dir.py b/spaces/Raspberry-ai/main/.env/lib/python3.11/site-packages/pip/_internal/utils/temp_dir.py
deleted file mode 100644
index 8ee8a1cb18017880cd0bebd66bc2cec5702118c6..0000000000000000000000000000000000000000
--- a/spaces/Raspberry-ai/main/.env/lib/python3.11/site-packages/pip/_internal/utils/temp_dir.py
+++ /dev/null
@@ -1,246 +0,0 @@
-import errno
-import itertools
-import logging
-import os.path
-import tempfile
-from contextlib import ExitStack, contextmanager
-from typing import Any, Dict, Generator, Optional, TypeVar, Union
-
-from pip._internal.utils.misc import enum, rmtree
-
-logger = logging.getLogger(__name__)
-
-_T = TypeVar("_T", bound="TempDirectory")
-
-
-# Kinds of temporary directories. Only needed for ones that are
-# globally-managed.
-tempdir_kinds = enum(
- BUILD_ENV="build-env",
- EPHEM_WHEEL_CACHE="ephem-wheel-cache",
- REQ_BUILD="req-build",
-)
-
-
-_tempdir_manager: Optional[ExitStack] = None
-
-
-@contextmanager
-def global_tempdir_manager() -> Generator[None, None, None]:
- global _tempdir_manager
- with ExitStack() as stack:
- old_tempdir_manager, _tempdir_manager = _tempdir_manager, stack
- try:
- yield
- finally:
- _tempdir_manager = old_tempdir_manager
-
-
-class TempDirectoryTypeRegistry:
- """Manages temp directory behavior"""
-
- def __init__(self) -> None:
- self._should_delete: Dict[str, bool] = {}
-
- def set_delete(self, kind: str, value: bool) -> None:
- """Indicate whether a TempDirectory of the given kind should be
- auto-deleted.
- """
- self._should_delete[kind] = value
-
- def get_delete(self, kind: str) -> bool:
- """Get configured auto-delete flag for a given TempDirectory type,
- default True.
- """
- return self._should_delete.get(kind, True)
-
-
-_tempdir_registry: Optional[TempDirectoryTypeRegistry] = None
-
-
-@contextmanager
-def tempdir_registry() -> Generator[TempDirectoryTypeRegistry, None, None]:
- """Provides a scoped global tempdir registry that can be used to dictate
- whether directories should be deleted.
- """
- global _tempdir_registry
- old_tempdir_registry = _tempdir_registry
- _tempdir_registry = TempDirectoryTypeRegistry()
- try:
- yield _tempdir_registry
- finally:
- _tempdir_registry = old_tempdir_registry
-
-
-class _Default:
- pass
-
-
-_default = _Default()
-
-
-class TempDirectory:
- """Helper class that owns and cleans up a temporary directory.
-
- This class can be used as a context manager or as an OO representation of a
- temporary directory.
-
- Attributes:
- path
- Location to the created temporary directory
- delete
- Whether the directory should be deleted when exiting
- (when used as a contextmanager)
-
- Methods:
- cleanup()
- Deletes the temporary directory
-
- When used as a context manager, if the delete attribute is True, on
- exiting the context the temporary directory is deleted.
- """
-
- def __init__(
- self,
- path: Optional[str] = None,
- delete: Union[bool, None, _Default] = _default,
- kind: str = "temp",
- globally_managed: bool = False,
- ):
- super().__init__()
-
- if delete is _default:
- if path is not None:
- # If we were given an explicit directory, resolve delete option
- # now.
- delete = False
- else:
- # Otherwise, we wait until cleanup and see what
- # tempdir_registry says.
- delete = None
-
- # The only time we specify path is in for editables where it
- # is the value of the --src option.
- if path is None:
- path = self._create(kind)
-
- self._path = path
- self._deleted = False
- self.delete = delete
- self.kind = kind
-
- if globally_managed:
- assert _tempdir_manager is not None
- _tempdir_manager.enter_context(self)
-
- @property
- def path(self) -> str:
- assert not self._deleted, f"Attempted to access deleted path: {self._path}"
- return self._path
-
- def __repr__(self) -> str:
- return f"<{self.__class__.__name__} {self.path!r}>"
-
- def __enter__(self: _T) -> _T:
- return self
-
- def __exit__(self, exc: Any, value: Any, tb: Any) -> None:
- if self.delete is not None:
- delete = self.delete
- elif _tempdir_registry:
- delete = _tempdir_registry.get_delete(self.kind)
- else:
- delete = True
-
- if delete:
- self.cleanup()
-
- def _create(self, kind: str) -> str:
- """Create a temporary directory and store its path in self.path"""
- # We realpath here because some systems have their default tmpdir
- # symlinked to another directory. This tends to confuse build
- # scripts, so we canonicalize the path by traversing potential
- # symlinks here.
- path = os.path.realpath(tempfile.mkdtemp(prefix=f"pip-{kind}-"))
- logger.debug("Created temporary directory: %s", path)
- return path
-
- def cleanup(self) -> None:
- """Remove the temporary directory created and reset state"""
- self._deleted = True
- if not os.path.exists(self._path):
- return
- rmtree(self._path)
-
-
-class AdjacentTempDirectory(TempDirectory):
- """Helper class that creates a temporary directory adjacent to a real one.
-
- Attributes:
- original
- The original directory to create a temp directory for.
- path
- After calling create() or entering, contains the full
- path to the temporary directory.
- delete
- Whether the directory should be deleted when exiting
- (when used as a contextmanager)
-
- """
-
- # The characters that may be used to name the temp directory
- # We always prepend a ~ and then rotate through these until
- # a usable name is found.
- # pkg_resources raises a different error for .dist-info folder
- # with leading '-' and invalid metadata
- LEADING_CHARS = "-~.=%0123456789"
-
- def __init__(self, original: str, delete: Optional[bool] = None) -> None:
- self.original = original.rstrip("/\\")
- super().__init__(delete=delete)
-
- @classmethod
- def _generate_names(cls, name: str) -> Generator[str, None, None]:
- """Generates a series of temporary names.
-
- The algorithm replaces the leading characters in the name
- with ones that are valid filesystem characters, but are not
- valid package names (for both Python and pip definitions of
- package).
- """
- for i in range(1, len(name)):
- for candidate in itertools.combinations_with_replacement(
- cls.LEADING_CHARS, i - 1
- ):
- new_name = "~" + "".join(candidate) + name[i:]
- if new_name != name:
- yield new_name
-
- # If we make it this far, we will have to make a longer name
- for i in range(len(cls.LEADING_CHARS)):
- for candidate in itertools.combinations_with_replacement(
- cls.LEADING_CHARS, i
- ):
- new_name = "~" + "".join(candidate) + name
- if new_name != name:
- yield new_name
-
- def _create(self, kind: str) -> str:
- root, name = os.path.split(self.original)
- for candidate in self._generate_names(name):
- path = os.path.join(root, candidate)
- try:
- os.mkdir(path)
- except OSError as ex:
- # Continue if the name exists already
- if ex.errno != errno.EEXIST:
- raise
- else:
- path = os.path.realpath(path)
- break
- else:
- # Final fallback on the default behavior.
- path = os.path.realpath(tempfile.mkdtemp(prefix=f"pip-{kind}-"))
-
- logger.debug("Created temporary directory: %s", path)
- return path
diff --git a/spaces/Raspberry-ai/main/.env/lib/python3.11/site-packages/pip/_vendor/urllib3/_collections.py b/spaces/Raspberry-ai/main/.env/lib/python3.11/site-packages/pip/_vendor/urllib3/_collections.py
deleted file mode 100644
index da9857e986d89acac3ba05a6735dc08c249bde1a..0000000000000000000000000000000000000000
--- a/spaces/Raspberry-ai/main/.env/lib/python3.11/site-packages/pip/_vendor/urllib3/_collections.py
+++ /dev/null
@@ -1,337 +0,0 @@
-from __future__ import absolute_import
-
-try:
- from collections.abc import Mapping, MutableMapping
-except ImportError:
- from collections import Mapping, MutableMapping
-try:
- from threading import RLock
-except ImportError: # Platform-specific: No threads available
-
- class RLock:
- def __enter__(self):
- pass
-
- def __exit__(self, exc_type, exc_value, traceback):
- pass
-
-
-from collections import OrderedDict
-
-from .exceptions import InvalidHeader
-from .packages import six
-from .packages.six import iterkeys, itervalues
-
-__all__ = ["RecentlyUsedContainer", "HTTPHeaderDict"]
-
-
-_Null = object()
-
-
-class RecentlyUsedContainer(MutableMapping):
- """
- Provides a thread-safe dict-like container which maintains up to
- ``maxsize`` keys while throwing away the least-recently-used keys beyond
- ``maxsize``.
-
- :param maxsize:
- Maximum number of recent elements to retain.
-
- :param dispose_func:
- Every time an item is evicted from the container,
- ``dispose_func(value)`` is called. Callback which will get called
- """
-
- ContainerCls = OrderedDict
-
- def __init__(self, maxsize=10, dispose_func=None):
- self._maxsize = maxsize
- self.dispose_func = dispose_func
-
- self._container = self.ContainerCls()
- self.lock = RLock()
-
- def __getitem__(self, key):
- # Re-insert the item, moving it to the end of the eviction line.
- with self.lock:
- item = self._container.pop(key)
- self._container[key] = item
- return item
-
- def __setitem__(self, key, value):
- evicted_value = _Null
- with self.lock:
- # Possibly evict the existing value of 'key'
- evicted_value = self._container.get(key, _Null)
- self._container[key] = value
-
- # If we didn't evict an existing value, we might have to evict the
- # least recently used item from the beginning of the container.
- if len(self._container) > self._maxsize:
- _key, evicted_value = self._container.popitem(last=False)
-
- if self.dispose_func and evicted_value is not _Null:
- self.dispose_func(evicted_value)
-
- def __delitem__(self, key):
- with self.lock:
- value = self._container.pop(key)
-
- if self.dispose_func:
- self.dispose_func(value)
-
- def __len__(self):
- with self.lock:
- return len(self._container)
-
- def __iter__(self):
- raise NotImplementedError(
- "Iteration over this class is unlikely to be threadsafe."
- )
-
- def clear(self):
- with self.lock:
- # Copy pointers to all values, then wipe the mapping
- values = list(itervalues(self._container))
- self._container.clear()
-
- if self.dispose_func:
- for value in values:
- self.dispose_func(value)
-
- def keys(self):
- with self.lock:
- return list(iterkeys(self._container))
-
-
-class HTTPHeaderDict(MutableMapping):
- """
- :param headers:
- An iterable of field-value pairs. Must not contain multiple field names
- when compared case-insensitively.
-
- :param kwargs:
- Additional field-value pairs to pass in to ``dict.update``.
-
- A ``dict`` like container for storing HTTP Headers.
-
- Field names are stored and compared case-insensitively in compliance with
- RFC 7230. Iteration provides the first case-sensitive key seen for each
- case-insensitive pair.
-
- Using ``__setitem__`` syntax overwrites fields that compare equal
- case-insensitively in order to maintain ``dict``'s api. For fields that
- compare equal, instead create a new ``HTTPHeaderDict`` and use ``.add``
- in a loop.
-
- If multiple fields that are equal case-insensitively are passed to the
- constructor or ``.update``, the behavior is undefined and some will be
- lost.
-
- >>> headers = HTTPHeaderDict()
- >>> headers.add('Set-Cookie', 'foo=bar')
- >>> headers.add('set-cookie', 'baz=quxx')
- >>> headers['content-length'] = '7'
- >>> headers['SET-cookie']
- 'foo=bar, baz=quxx'
- >>> headers['Content-Length']
- '7'
- """
-
- def __init__(self, headers=None, **kwargs):
- super(HTTPHeaderDict, self).__init__()
- self._container = OrderedDict()
- if headers is not None:
- if isinstance(headers, HTTPHeaderDict):
- self._copy_from(headers)
- else:
- self.extend(headers)
- if kwargs:
- self.extend(kwargs)
-
- def __setitem__(self, key, val):
- self._container[key.lower()] = [key, val]
- return self._container[key.lower()]
-
- def __getitem__(self, key):
- val = self._container[key.lower()]
- return ", ".join(val[1:])
-
- def __delitem__(self, key):
- del self._container[key.lower()]
-
- def __contains__(self, key):
- return key.lower() in self._container
-
- def __eq__(self, other):
- if not isinstance(other, Mapping) and not hasattr(other, "keys"):
- return False
- if not isinstance(other, type(self)):
- other = type(self)(other)
- return dict((k.lower(), v) for k, v in self.itermerged()) == dict(
- (k.lower(), v) for k, v in other.itermerged()
- )
-
- def __ne__(self, other):
- return not self.__eq__(other)
-
- if six.PY2: # Python 2
- iterkeys = MutableMapping.iterkeys
- itervalues = MutableMapping.itervalues
-
- __marker = object()
-
- def __len__(self):
- return len(self._container)
-
- def __iter__(self):
- # Only provide the originally cased names
- for vals in self._container.values():
- yield vals[0]
-
- def pop(self, key, default=__marker):
- """D.pop(k[,d]) -> v, remove specified key and return the corresponding value.
- If key is not found, d is returned if given, otherwise KeyError is raised.
- """
- # Using the MutableMapping function directly fails due to the private marker.
- # Using ordinary dict.pop would expose the internal structures.
- # So let's reinvent the wheel.
- try:
- value = self[key]
- except KeyError:
- if default is self.__marker:
- raise
- return default
- else:
- del self[key]
- return value
-
- def discard(self, key):
- try:
- del self[key]
- except KeyError:
- pass
-
- def add(self, key, val):
- """Adds a (name, value) pair, doesn't overwrite the value if it already
- exists.
-
- >>> headers = HTTPHeaderDict(foo='bar')
- >>> headers.add('Foo', 'baz')
- >>> headers['foo']
- 'bar, baz'
- """
- key_lower = key.lower()
- new_vals = [key, val]
- # Keep the common case aka no item present as fast as possible
- vals = self._container.setdefault(key_lower, new_vals)
- if new_vals is not vals:
- vals.append(val)
-
- def extend(self, *args, **kwargs):
- """Generic import function for any type of header-like object.
- Adapted version of MutableMapping.update in order to insert items
- with self.add instead of self.__setitem__
- """
- if len(args) > 1:
- raise TypeError(
- "extend() takes at most 1 positional "
- "arguments ({0} given)".format(len(args))
- )
- other = args[0] if len(args) >= 1 else ()
-
- if isinstance(other, HTTPHeaderDict):
- for key, val in other.iteritems():
- self.add(key, val)
- elif isinstance(other, Mapping):
- for key in other:
- self.add(key, other[key])
- elif hasattr(other, "keys"):
- for key in other.keys():
- self.add(key, other[key])
- else:
- for key, value in other:
- self.add(key, value)
-
- for key, value in kwargs.items():
- self.add(key, value)
-
- def getlist(self, key, default=__marker):
- """Returns a list of all the values for the named field. Returns an
- empty list if the key doesn't exist."""
- try:
- vals = self._container[key.lower()]
- except KeyError:
- if default is self.__marker:
- return []
- return default
- else:
- return vals[1:]
-
- # Backwards compatibility for httplib
- getheaders = getlist
- getallmatchingheaders = getlist
- iget = getlist
-
- # Backwards compatibility for http.cookiejar
- get_all = getlist
-
- def __repr__(self):
- return "%s(%s)" % (type(self).__name__, dict(self.itermerged()))
-
- def _copy_from(self, other):
- for key in other:
- val = other.getlist(key)
- if isinstance(val, list):
- # Don't need to convert tuples
- val = list(val)
- self._container[key.lower()] = [key] + val
-
- def copy(self):
- clone = type(self)()
- clone._copy_from(self)
- return clone
-
- def iteritems(self):
- """Iterate over all header lines, including duplicate ones."""
- for key in self:
- vals = self._container[key.lower()]
- for val in vals[1:]:
- yield vals[0], val
-
- def itermerged(self):
- """Iterate over all headers, merging duplicate ones together."""
- for key in self:
- val = self._container[key.lower()]
- yield val[0], ", ".join(val[1:])
-
- def items(self):
- return list(self.iteritems())
-
- @classmethod
- def from_httplib(cls, message): # Python 2
- """Read headers from a Python 2 httplib message object."""
- # python2.7 does not expose a proper API for exporting multiheaders
- # efficiently. This function re-reads raw lines from the message
- # object and extracts the multiheaders properly.
- obs_fold_continued_leaders = (" ", "\t")
- headers = []
-
- for line in message.headers:
- if line.startswith(obs_fold_continued_leaders):
- if not headers:
- # We received a header line that starts with OWS as described
- # in RFC-7230 S3.2.4. This indicates a multiline header, but
- # there exists no previous header to which we can attach it.
- raise InvalidHeader(
- "Header continuation with no previous header: %s" % line
- )
- else:
- key, value = headers[-1]
- headers[-1] = (key, value + " " + line.strip())
- continue
-
- key, value = line.split(":", 1)
- headers.append((key, value.strip()))
-
- return cls(headers)
diff --git a/spaces/Raspberry-ai/main/.env/lib/python3.11/site-packages/setuptools_rust/build.py b/spaces/Raspberry-ai/main/.env/lib/python3.11/site-packages/setuptools_rust/build.py
deleted file mode 100644
index 21c19758ea78f7405613af81af358811adf0e649..0000000000000000000000000000000000000000
--- a/spaces/Raspberry-ai/main/.env/lib/python3.11/site-packages/setuptools_rust/build.py
+++ /dev/null
@@ -1,716 +0,0 @@
-from __future__ import annotations
-
-import glob
-import json
-import os
-import platform
-import shutil
-import subprocess
-import sys
-import sysconfig
-from distutils import log
-from distutils.errors import (
- CompileError,
- DistutilsExecError,
- DistutilsFileError,
- DistutilsPlatformError,
-)
-from distutils.sysconfig import get_config_var
-from pathlib import Path
-from typing import Dict, Iterable, List, NamedTuple, Optional, Set, Tuple, cast
-
-import pkg_resources
-from setuptools.command.build import build as CommandBuild # type: ignore[import]
-from setuptools.command.build_ext import build_ext as CommandBuildExt
-from setuptools.command.build_ext import get_abi3_suffix
-from typing_extensions import Literal
-
-from ._utils import format_called_process_error
-from .command import RustCommand
-from .extension import Binding, RustBin, RustExtension, Strip
-from .rustc_info import get_rust_host, get_rust_target_list, get_rustc_cfgs
-
-
-class build_rust(RustCommand):
- """Command for building Rust crates via cargo."""
-
- description = "build Rust extensions (compile/link to build directory)"
-
- user_options = [
- (
- "inplace",
- "i",
- "ignore build-lib and put compiled extensions into the source "
- + "directory alongside your pure Python modules",
- ),
- ("debug", "d", "Force debug to true for all Rust extensions "),
- ("release", "r", "Force debug to false for all Rust extensions "),
- ("qbuild", None, "Force enable quiet option for all Rust extensions "),
- (
- "build-temp",
- "t",
- "directory for temporary files (cargo 'target' directory) ",
- ),
- ("target=", None, "Build for the target triple"),
- ]
- boolean_options = ["inplace", "debug", "release", "qbuild"]
-
- plat_name: Optional[str] = None
-
- def initialize_options(self) -> None:
- super().initialize_options()
- self.inplace = None
- self.debug = None
- self.release = None
- self.qbuild = None
- self.build_temp = None
- self.plat_name = None
- self.build_number = None
- self.target = os.getenv("CARGO_BUILD_TARGET")
- self.cargo = os.getenv("CARGO", "cargo")
-
- def finalize_options(self) -> None:
- super().finalize_options()
-
- self.data_dir = self.get_data_dir()
-
- if self.plat_name is None:
- self.plat_name = cast( # type: ignore[no-any-unimported]
- CommandBuild, self.get_finalized_command("build")
- ).plat_name
- assert isinstance(self.plat_name, str)
-
- # Inherit settings from the `build_ext` command
- self.set_undefined_options(
- "build_ext",
- ("build_temp", "build_temp"),
- ("debug", "debug"),
- ("inplace", "inplace"),
- )
-
- if self.build_number is not None and not self.build_number[:1].isdigit():
- raise ValueError("Build tag (build-number) must start with a digit.")
-
- def get_data_dir(self) -> str:
- components = (
- pkg_resources.safe_name(self.distribution.get_name()).replace("-", "_"), # type: ignore[attr-defined]
- pkg_resources.safe_version(self.distribution.get_version()).replace("-", "_"), # type: ignore[attr-defined]
- )
- if self.build_number:
- components += (self.build_number,)
- return "-".join(components) + ".data"
-
- def run_for_extension(self, ext: RustExtension) -> None:
- assert self.plat_name is not None
-
- arch_flags = os.getenv("ARCHFLAGS")
- universal2 = False
- if self.plat_name.startswith("macosx-") and arch_flags:
- universal2 = "x86_64" in arch_flags and "arm64" in arch_flags
- if not universal2 and not self.target:
- if "arm64" in arch_flags:
- self.target = "aarch64-apple-darwin"
- elif "x86_64" in arch_flags:
- self.target = "x86_64-apple-darwin"
-
- if universal2:
- arm64_dylib_paths = self.build_extension(ext, "aarch64-apple-darwin")
- x86_64_dylib_paths = self.build_extension(ext, "x86_64-apple-darwin")
- dylib_paths = []
- for (target_fname, arm64_dylib), (_, x86_64_dylib) in zip(
- arm64_dylib_paths, x86_64_dylib_paths
- ):
- fat_dylib_path = arm64_dylib.replace("aarch64-apple-darwin/", "")
- create_universal2_binary(fat_dylib_path, [arm64_dylib, x86_64_dylib])
- dylib_paths.append(_BuiltModule(target_fname, fat_dylib_path))
- else:
- dylib_paths = self.build_extension(ext, self.target)
- self.install_extension(ext, dylib_paths)
-
- def build_extension(
- self, ext: RustExtension, forced_target_triple: Optional[str] = None
- ) -> List["_BuiltModule"]:
-
- target_triple = self._detect_rust_target(forced_target_triple)
- rustc_cfgs = get_rustc_cfgs(target_triple)
-
- env = _prepare_build_environment()
-
- if not os.path.exists(ext.path):
- raise DistutilsFileError(
- f"can't find Rust extension project file: {ext.path}"
- )
-
- quiet = self.qbuild or ext.quiet
- debug = self._is_debug_build(ext)
-
- cargo_args = self._cargo_args(
- ext=ext, target_triple=target_triple, release=not debug, quiet=quiet
- )
-
- rustflags = []
-
- if ext._uses_exec_binding():
- command = [
- self.cargo,
- "build",
- "--manifest-path",
- ext.path,
- "--message-format=json-render-diagnostics",
- *cargo_args,
- ]
-
- else:
- rustc_args = [
- "--crate-type",
- "cdylib",
- *ext.rustc_flags,
- ]
-
- # OSX requires special linker arguments
- if rustc_cfgs.get("target_os") == "macos":
- ext_basename = os.path.basename(self.get_dylib_ext_path(ext, ext.name))
- rustc_args.extend(
- [
- "-C",
- f"link-args=-undefined dynamic_lookup -Wl,-install_name,@rpath/{ext_basename}",
- ]
- )
-
- # Tell musl targets not to statically link libc. See
- # https://github.com/rust-lang/rust/issues/59302 for details.
- if rustc_cfgs.get("target_env") == "musl":
- # This must go in the env otherwise rustc will refuse to build
- # the cdylib, see https://github.com/rust-lang/cargo/issues/10143
- rustflags.append("-Ctarget-feature=-crt-static")
-
- elif (rustc_cfgs.get("target_arch"), rustc_cfgs.get("target_os")) == (
- "wasm32",
- "emscripten",
- ):
- rustc_args.extend(["-C", f"link-args=-sSIDE_MODULE=2 -sWASM_BIGINT"])
-
- command = [
- self.cargo,
- "rustc",
- "--lib",
- "--message-format=json-render-diagnostics",
- "--manifest-path",
- ext.path,
- *cargo_args,
- "--",
- *rustc_args,
- ]
-
- if rustflags:
- existing_rustflags = env.get("RUSTFLAGS")
- if existing_rustflags is not None:
- rustflags.append(existing_rustflags)
- new_rustflags = " ".join(rustflags)
- env["RUSTFLAGS"] = new_rustflags
-
- # print RUSTFLAGS being added before the command
- if not quiet:
- print(f"[RUSTFLAGS={new_rustflags}]", end=" ", file=sys.stderr)
-
- if not quiet:
- print(" ".join(command), file=sys.stderr)
-
- # Execute cargo
- try:
- # If quiet, capture all output and only show it in the exception
- # If not quiet, forward all cargo output to stderr
- stderr = subprocess.PIPE if quiet else None
- cargo_messages = subprocess.check_output(
- command,
- env=env,
- stderr=stderr,
- text=True,
- )
- except subprocess.CalledProcessError as e:
- # Don't include stdout in the formatted error as it is a huge dump
- # of cargo json lines which aren't helpful for the end user.
- raise CompileError(format_called_process_error(e, include_stdout=False))
-
- except OSError:
- raise DistutilsExecError(
- "Unable to execute 'cargo' - this package "
- "requires Rust to be installed and cargo to be on the PATH"
- )
-
- # Find the shared library that cargo hopefully produced and copy
- # it into the build directory as if it were produced by build_ext.
-
- dylib_paths = []
- package_id = ext.metadata(quiet=quiet)["resolve"]["root"]
-
- if ext._uses_exec_binding():
- # Find artifact from cargo messages
- artifacts = _find_cargo_artifacts(
- cargo_messages.splitlines(),
- package_id=package_id,
- kinds={"bin"},
- )
- for name, dest in ext.target.items():
- if not name:
- name = dest.split(".")[-1]
-
- try:
- artifact_path = next(
- artifact
- for artifact in artifacts
- if Path(artifact).with_suffix("").name == name
- )
- except StopIteration:
- raise DistutilsExecError(
- f"Rust build failed; unable to locate executable '{name}'"
- )
-
- if os.environ.get("CARGO") == "cross":
- artifact_path = _replace_cross_target_dir(
- artifact_path, ext, quiet=quiet
- )
-
- dylib_paths.append(_BuiltModule(dest, artifact_path))
- else:
- # Find artifact from cargo messages
- artifacts = _find_cargo_artifacts(
- cargo_messages.splitlines(),
- package_id=package_id,
- kinds={"cdylib", "dylib"},
- )
- if len(artifacts) == 0:
- raise DistutilsExecError(
- "Rust build failed; unable to find any cdylib or dylib build artifacts"
- )
- elif len(artifacts) > 1:
- raise DistutilsExecError(
- f"Rust build failed; expected only one cdylib or dylib build artifact but found {artifacts}"
- )
-
- artifact_path = artifacts[0]
-
- if os.environ.get("CARGO") == "cross":
- artifact_path = _replace_cross_target_dir(
- artifact_path, ext, quiet=quiet
- )
-
- # guaranteed to be just one element after checks above
- dylib_paths.append(_BuiltModule(ext.name, artifact_path))
- return dylib_paths
-
- def install_extension(
- self, ext: RustExtension, dylib_paths: List["_BuiltModule"]
- ) -> None:
- debug_build = ext.debug if ext.debug is not None else self.inplace
- debug_build = self.debug if self.debug is not None else debug_build
- if self.release:
- debug_build = False
-
- # Ask build_ext where the shared library would go if it had built it,
- # then copy it there.
- build_ext = cast(CommandBuildExt, self.get_finalized_command("build_ext"))
- build_ext.inplace = self.inplace
-
- for module_name, dylib_path in dylib_paths:
- if not module_name:
- module_name = os.path.basename(
- os.path.splitext(os.path.basename(dylib_path)[3:])[0]
- )
-
- if ext._uses_exec_binding():
- ext_path = build_ext.get_ext_fullpath(module_name)
- # remove extensions
- ext_path, _, _ = _split_platform_and_extension(ext_path)
-
- # Add expected extension
- exe = sysconfig.get_config_var("EXE")
- if exe is not None:
- ext_path += exe
-
- os.makedirs(os.path.dirname(ext_path), exist_ok=True)
- if isinstance(ext, RustBin):
- executable_name = module_name
- if exe is not None:
- executable_name += exe
- scripts_dir = os.path.join(
- build_ext.build_lib, self.data_dir, "scripts"
- )
- os.makedirs(scripts_dir, exist_ok=True)
- ext_path = os.path.join(scripts_dir, executable_name)
- else:
- ext.install_script(module_name.split(".")[-1], ext_path)
- else:
- ext_path = self.get_dylib_ext_path(ext, module_name)
- os.makedirs(os.path.dirname(ext_path), exist_ok=True)
-
- log.info("Copying rust artifact from %s to %s", dylib_path, ext_path)
- shutil.copyfile(dylib_path, ext_path)
-
- if sys.platform != "win32" and not debug_build:
- args = []
- if ext.strip == Strip.All:
- args.append("-x")
- elif ext.strip == Strip.Debug:
- args.append("-S")
-
- if args:
- args.insert(0, "strip")
- args.append(ext_path)
- try:
- output = subprocess.check_output(args)
- except subprocess.CalledProcessError:
- pass
-
- # executables, win32(cygwin)-dll's, and shared libraries on
- # Unix-like operating systems need X bits
- mode = os.stat(ext_path).st_mode
- mode |= (mode & 0o444) >> 2 # copy R bits to X
- os.chmod(ext_path, mode)
-
- def get_dylib_ext_path(self, ext: RustExtension, target_fname: str) -> str:
- assert self.plat_name is not None
- build_ext = cast(CommandBuildExt, self.get_finalized_command("build_ext"))
-
- ext_path: str = build_ext.get_ext_fullpath(target_fname)
-
- if _is_py_limited_api(ext.py_limited_api, self._py_limited_api()):
- abi3_suffix = get_abi3_suffix()
- if abi3_suffix is not None:
- so_ext = get_config_var("EXT_SUFFIX")
- assert isinstance(so_ext, str)
- ext_path = ext_path[: -len(so_ext)] + get_abi3_suffix()
-
- if ".abi3." in ext_path:
- return ext_path
- # Examples: linux_x86_64, linux_i686, manylinux2014_aarch64, manylinux_2_24_armv7l
- plat_name = self.plat_name.lower().replace("-", "_").replace(".", "_")
- if not plat_name.startswith(("linux", "manylinux")):
- return ext_path
-
- arch_parts = []
- arch_found = False
- for item in plat_name.split("_"):
- if item.startswith(("linux", "manylinux")):
- continue
- if item.isdigit() and not arch_found:
- # manylinux_2_24_armv7l arch should be armv7l
- continue
- arch_found = True
- arch_parts.append(item)
- target_arch = "_".join(arch_parts)
- host_platform = sysconfig.get_platform()
- host_arch = host_platform.rsplit("-", 1)[1]
- # Remove incorrect platform tag if we are cross compiling
- if target_arch and host_arch != target_arch:
- ext_path, _, extension = _split_platform_and_extension(ext_path)
- # rust.so, removed platform tag
- ext_path += extension
- return ext_path
-
- def _py_limited_api(self) -> _PyLimitedApi:
- bdist_wheel = self.distribution.get_command_obj("bdist_wheel", create=False)
-
- if bdist_wheel is None:
- # wheel package is not installed, not building a limited-api wheel
- return False
- else:
- from wheel.bdist_wheel import bdist_wheel as CommandBdistWheel
-
- bdist_wheel_command = cast(CommandBdistWheel, bdist_wheel) # type: ignore[no-any-unimported]
- bdist_wheel_command.ensure_finalized()
- return cast(_PyLimitedApi, bdist_wheel_command.py_limited_api)
-
- def _detect_rust_target(
- self, forced_target_triple: Optional[str] = None
- ) -> Optional[str]:
- assert self.plat_name is not None
- if forced_target_triple is not None:
- # Automatic target detection can be overridden via the CARGO_BUILD_TARGET
- # environment variable or --target command line option
- return forced_target_triple
-
- # Determine local rust target which needs to be "forced" if necessary
- local_rust_target = _adjusted_local_rust_target(self.plat_name)
-
- # Match cargo's behaviour of not using an explicit target if the
- # target we're compiling for is the host
- if (
- local_rust_target is not None
- # check for None first to avoid calling to rustc if not needed
- and local_rust_target != get_rust_host()
- ):
- return local_rust_target
-
- return None
-
- def _is_debug_build(self, ext: RustExtension) -> bool:
- if self.release:
- return False
- elif self.debug is not None:
- return self.debug
- elif ext.debug is not None:
- return ext.debug
- else:
- return bool(self.inplace)
-
- def _cargo_args(
- self,
- ext: RustExtension,
- target_triple: Optional[str],
- release: bool,
- quiet: bool,
- ) -> List[str]:
- args = []
- if target_triple is not None:
- args.extend(["--target", target_triple])
-
- if release:
- profile = ext.get_cargo_profile()
- if not profile:
- args.append("--release")
-
- if quiet:
- args.append("-q")
-
- elif self.verbose:
- # cargo only have -vv
- verbose_level = "v" * min(self.verbose, 2)
- args.append(f"-{verbose_level}")
-
- features = {
- *ext.features,
- *_binding_features(ext, py_limited_api=self._py_limited_api()),
- }
-
- if features:
- args.extend(["--features", " ".join(features)])
-
- if ext.args is not None:
- args.extend(ext.args)
-
- if ext.cargo_manifest_args is not None:
- args.extend(ext.cargo_manifest_args)
-
- return args
-
-
-def create_universal2_binary(output_path: str, input_paths: List[str]) -> None:
- # Try lipo first
- command = ["lipo", "-create", "-output", output_path, *input_paths]
- try:
- subprocess.check_output(command, text=True)
- except subprocess.CalledProcessError as e:
- output = e.output
- raise CompileError("lipo failed with code: %d\n%s" % (e.returncode, output))
- except OSError:
- # lipo not found, try using the fat-macho library
- try:
- from fat_macho import FatWriter
- except ImportError:
- raise DistutilsExecError(
- "failed to locate `lipo` or import `fat_macho.FatWriter`. "
- "Try installing with `pip install fat-macho` "
- )
- fat = FatWriter()
- for input_path in input_paths:
- with open(input_path, "rb") as f:
- fat.add(f.read())
- fat.write_to(output_path)
-
-
-class _BuiltModule(NamedTuple):
- """
- Attributes:
- - module_name: dotted python import path of the module
- - path: the location the module has been installed at
- """
-
- module_name: str
- path: str
-
-
-def _replace_vendor_with_unknown(target: str) -> Optional[str]:
- """Replaces vendor in the target triple with unknown.
-
- Returns None if the target is not made of 4 parts.
- """
- components = target.split("-")
- if len(components) != 4:
- return None
- components[1] = "unknown"
- return "-".join(components)
-
-
-def _prepare_build_environment() -> Dict[str, str]:
- """Prepares environment variables to use when executing cargo build."""
-
- # Make sure that if pythonXX-sys is used, it builds against the current
- # executing python interpreter.
- bindir = os.path.dirname(sys.executable)
-
- env = os.environ.copy()
- env.update(
- {
- # disables rust's pkg-config seeking for specified packages,
- # which causes pythonXX-sys to fall back to detecting the
- # interpreter from the path.
- "PATH": os.path.join(bindir, os.environ.get("PATH", "")),
- "PYTHON_SYS_EXECUTABLE": os.environ.get(
- "PYTHON_SYS_EXECUTABLE", sys.executable
- ),
- "PYO3_PYTHON": os.environ.get("PYO3_PYTHON", sys.executable),
- }
- )
- return env
-
-
-def _is_py_limited_api(
- ext_setting: Literal["auto", True, False],
- wheel_setting: Optional[_PyLimitedApi],
-) -> bool:
- """Returns whether this extension is being built for the limited api.
-
- >>> _is_py_limited_api("auto", None)
- False
-
- >>> _is_py_limited_api("auto", True)
- True
-
- >>> _is_py_limited_api(True, False)
- True
-
- >>> _is_py_limited_api(False, True)
- False
- """
-
- # If the extension explicitly states to use py_limited_api or not, use that.
- if ext_setting != "auto":
- return ext_setting
-
- # "auto" setting - use whether the bdist_wheel option is truthy.
- return bool(wheel_setting)
-
-
-def _binding_features(
- ext: RustExtension,
- py_limited_api: _PyLimitedApi,
-) -> Set[str]:
- if ext.binding in (Binding.NoBinding, Binding.Exec):
- return set()
- elif ext.binding is Binding.PyO3:
- features = {"pyo3/extension-module"}
- if ext.py_limited_api == "auto":
- if isinstance(py_limited_api, str):
- python_version = py_limited_api[2:]
- features.add(f"pyo3/abi3-py{python_version}")
- elif py_limited_api:
- features.add(f"pyo3/abi3")
- return features
- elif ext.binding is Binding.RustCPython:
- return {"cpython/python3-sys", "cpython/extension-module"}
- else:
- raise DistutilsPlatformError(f"unknown Rust binding: '{ext.binding}'")
-
-
-_PyLimitedApi = Literal["cp37", "cp38", "cp39", "cp310", "cp311", "cp312", True, False]
-
-
-def _adjusted_local_rust_target(plat_name: str) -> Optional[str]:
- """Returns the local rust target for the given `plat_name`, if it is
- necessary to 'force' a specific target for correctness."""
-
- # If we are on a 64-bit machine, but running a 32-bit Python, then
- # we'll target a 32-bit Rust build.
- if plat_name == "win32":
- if get_rustc_cfgs(None).get("target_env") == "gnu":
- return "i686-pc-windows-gnu"
- else:
- return "i686-pc-windows-msvc"
- elif plat_name == "win-amd64":
- if get_rustc_cfgs(None).get("target_env") == "gnu":
- return "x86_64-pc-windows-gnu"
- else:
- return "x86_64-pc-windows-msvc"
- elif plat_name.startswith("macosx-") and platform.machine() == "x86_64":
- # x86_64 or arm64 macOS targeting x86_64
- return "x86_64-apple-darwin"
-
- return None
-
-
-def _split_platform_and_extension(ext_path: str) -> Tuple[str, str, str]:
- """Splits an extension path into a tuple (ext_path, plat_tag, extension).
-
- >>> _split_platform_and_extension("foo/bar.platform.so")
- ('foo/bar', '.platform', '.so')
- """
-
- # rust.cpython-38-x86_64-linux-gnu.so to (rust.cpython-38-x86_64-linux-gnu, .so)
- ext_path, extension = os.path.splitext(ext_path)
- # rust.cpython-38-x86_64-linux-gnu to (rust, .cpython-38-x86_64-linux-gnu)
- ext_path, platform_tag = os.path.splitext(ext_path)
- return (ext_path, platform_tag, extension)
-
-
-def _find_cargo_artifacts(
- cargo_messages: List[str],
- *,
- package_id: str,
- kinds: Set[str],
-) -> List[str]:
- """Identifies cargo artifacts built for the given `package_id` from the
- provided cargo_messages.
-
- >>> _find_cargo_artifacts(
- ... [
- ... '{"some_irrelevant_message": []}',
- ... '{"reason":"compiler-artifact","package_id":"some_id","target":{"kind":["cdylib"]},"filenames":["/some/path/baz.so"]}',
- ... '{"reason":"compiler-artifact","package_id":"some_id","target":{"kind":["dylib", "rlib"]},"filenames":["/file/two/baz.dylib", "/file/two/baz.rlib"]}',
- ... '{"reason":"compiler-artifact","package_id":"some_other_id","target":{"kind":["cdylib"]},"filenames":["/not/this.so"]}',
- ... ],
- ... package_id="some_id",
- ... kinds={"cdylib", "dylib"},
- ... )
- ['/some/path/baz.so', '/file/two/baz.dylib']
- >>> _find_cargo_artifacts(
- ... [
- ... '{"some_irrelevant_message": []}',
- ... '{"reason":"compiler-artifact","package_id":"some_id","target":{"kind":["cdylib"]},"filenames":["/some/path/baz.so"]}',
- ... '{"reason":"compiler-artifact","package_id":"some_id","target":{"kind":["cdylib", "rlib"]},"filenames":["/file/two/baz.dylib", "/file/two/baz.rlib"]}',
- ... '{"reason":"compiler-artifact","package_id":"some_other_id","target":{"kind":["cdylib"]},"filenames":["/not/this.so"]}',
- ... ],
- ... package_id="some_id",
- ... kinds={"rlib"},
- ... )
- ['/file/two/baz.rlib']
- """
- artifacts = []
- for message in cargo_messages:
- # only bother parsing messages that look like a match
- if "compiler-artifact" in message and package_id in message:
- parsed = json.loads(message)
- # verify the message is correct
- if (
- parsed.get("reason") == "compiler-artifact"
- and parsed.get("package_id") == package_id
- ):
- for artifact_kind, filename in zip(
- parsed["target"]["kind"], parsed["filenames"]
- ):
- if artifact_kind in kinds:
- artifacts.append(filename)
- return artifacts
-
-
-def _replace_cross_target_dir(path: str, ext: RustExtension, *, quiet: bool) -> str:
- """Replaces target director from `cross` docker build with the correct
- local path.
-
- Cross artifact messages and metadata contain paths from inside the
- dockerfile; invoking `cargo metadata` we can work out the correct local
- target directory.
- """
- cross_target_dir = ext._metadata(cargo="cross", quiet=quiet)["target_directory"]
- local_target_dir = ext._metadata(cargo="cargo", quiet=quiet)["target_directory"]
- return path.replace(cross_target_dir, local_target_dir)
diff --git a/spaces/Realcat/image-matching-webui/third_party/SGMNet/utils/fm_utils.py b/spaces/Realcat/image-matching-webui/third_party/SGMNet/utils/fm_utils.py
deleted file mode 100644
index 900b73c42723cd9c5bcbef5c758deadcd0b309df..0000000000000000000000000000000000000000
--- a/spaces/Realcat/image-matching-webui/third_party/SGMNet/utils/fm_utils.py
+++ /dev/null
@@ -1,100 +0,0 @@
-import numpy as np
-
-
-def line_to_border(line, size):
- # line:(a,b,c), ax+by+c=0
- # size:(W,H)
- H, W = size[1], size[0]
- a, b, c = line[0], line[1], line[2]
- epsa = 1e-8 if a >= 0 else -1e-8
- epsb = 1e-8 if b >= 0 else -1e-8
- intersection_list = []
-
- y_left = -c / (b + epsb)
- y_right = (-c - a * (W - 1)) / (b + epsb)
- x_top = -c / (a + epsa)
- x_down = (-c - b * (H - 1)) / (a + epsa)
-
- if y_left >= 0 and y_left <= H - 1:
- intersection_list.append([0, y_left])
- if y_right >= 0 and y_right <= H - 1:
- intersection_list.append([W - 1, y_right])
- if x_top >= 0 and x_top <= W - 1:
- intersection_list.append([x_top, 0])
- if x_down >= 0 and x_down <= W - 1:
- intersection_list.append([x_down, H - 1])
- if len(intersection_list) != 2:
- return None
- intersection_list = np.asarray(intersection_list)
- return intersection_list
-
-
-def find_point_in_line(end_point):
- x_span, y_span = (
- end_point[1, 0] - end_point[0, 0],
- end_point[1, 1] - end_point[0, 1],
- )
- mv = np.random.uniform()
- point = np.asarray([end_point[0, 0] + x_span * mv, end_point[0, 1] + y_span * mv])
- return point
-
-
-def epi_line(point, F):
- homo = np.concatenate([point, np.ones([len(point), 1])], axis=-1)
- epi = np.matmul(homo, F.T)
- return epi
-
-
-def dis_point_to_line(line, point):
- homo = np.concatenate([point, np.ones([len(point), 1])], axis=-1)
- dis = line * homo
- dis = dis.sum(axis=-1) / (np.linalg.norm(line[:, :2], axis=-1) + 1e-8)
- return abs(dis)
-
-
-def SGD_oneiter(F1, F2, size1, size2):
- H1, W1 = size1[1], size1[0]
- factor1 = 1 / np.linalg.norm(size1)
- factor2 = 1 / np.linalg.norm(size2)
- p0 = np.asarray([(W1 - 1) * np.random.uniform(), (H1 - 1) * np.random.uniform()])
- epi1 = epi_line(p0[np.newaxis], F1)[0]
- border_point1 = line_to_border(epi1, size2)
- if border_point1 is None:
- return -1
-
- p1 = find_point_in_line(border_point1)
- epi2 = epi_line(p0[np.newaxis], F2)
- d1 = dis_point_to_line(epi2, p1[np.newaxis])[0] * factor2
- epi3 = epi_line(p1[np.newaxis], F2.T)
- d2 = dis_point_to_line(epi3, p0[np.newaxis])[0] * factor1
- return (d1 + d2) / 2
-
-
-def compute_SGD(F1, F2, size1, size2):
- np.random.seed(1234)
- N = 1000
- max_iter = N * 10
- count, sgd = 0, 0
- for i in range(max_iter):
- d1 = SGD_oneiter(F1, F2, size1, size2)
- if d1 < 0:
- continue
- d2 = SGD_oneiter(F2, F1, size1, size2)
- if d2 < 0:
- continue
- count += 1
- sgd += (d1 + d2) / 2
- if count == N:
- break
- if count == 0:
- return 1
- else:
- return sgd / count
-
-
-def compute_inlier_rate(x1, x2, size1, size2, F_gt, th=0.003):
- t1, t2 = np.linalg.norm(size1) * th, np.linalg.norm(size2) * th
- epi1, epi2 = epi_line(x1, F_gt), epi_line(x2, F_gt.T)
- dis1, dis2 = dis_point_to_line(epi1, x2), dis_point_to_line(epi2, x1)
- mask_inlier = np.logical_and(dis1 < t2, dis2 < t1)
- return mask_inlier.mean() if len(mask_inlier) != 0 else 0
diff --git a/spaces/Redgon/bingo/src/components/chat-notification.tsx b/spaces/Redgon/bingo/src/components/chat-notification.tsx
deleted file mode 100644
index 4be24d0f1755c8058698cfa66c736d8d4792475a..0000000000000000000000000000000000000000
--- a/spaces/Redgon/bingo/src/components/chat-notification.tsx
+++ /dev/null
@@ -1,77 +0,0 @@
-import { useEffect } from 'react'
-import Image from 'next/image'
-
-import IconWarning from '@/assets/images/warning.svg'
-import { ChatError, ErrorCode, ChatMessageModel } from '@/lib/bots/bing/types'
-import { ExternalLink } from './external-link'
-import { useBing } from '@/lib/hooks/use-bing'
-
-export interface ChatNotificationProps extends Pick, 'bot'> {
- message?: ChatMessageModel
-}
-
-function getAction(error: ChatError, reset: () => void) {
- if (error.code === ErrorCode.THROTTLE_LIMIT) {
- reset()
- return (
-
- 你已达到每日最大发送消息次数,请
更换账号 或隔一天后重试
-
- )
- }
- if (error.code === ErrorCode.BING_FORBIDDEN) {
- return (
-
- 你的账号已在黑名单,请尝试更换账号及申请解封
-
- )
- }
- if (error.code === ErrorCode.CONVERSATION_LIMIT) {
- return (
-
- 当前话题已中止,请点
-
重新开始
- 开启新的对话
-
- )
- }
- if (error.code === ErrorCode.BING_CAPTCHA) {
- return (
-
- 点击通过人机验证
-
- )
- }
- if (error.code === ErrorCode.BING_UNAUTHORIZED) {
- reset()
- return (
- 没有获取到身份信息或身份信息失效,点此重新设置
- )
- }
- return error.message
-}
-
-export function ChatNotification({ message, bot }: ChatNotificationProps) {
- useEffect(() => {
- window.scrollBy(0, 2000)
- }, [message])
-
- if (!message?.error) return
-
- return (
-
-
-
-
-
-
- {getAction(message.error, () => bot.resetConversation())}
-
-
-
-
-
- )
-}
diff --git a/spaces/Robert001/UniControl-Demo/annotator/uniformer/mmdet/models/dense_heads/anchor_head.py b/spaces/Robert001/UniControl-Demo/annotator/uniformer/mmdet/models/dense_heads/anchor_head.py
deleted file mode 100644
index eea73520572725f547216ab639c1ebbdfb50834c..0000000000000000000000000000000000000000
--- a/spaces/Robert001/UniControl-Demo/annotator/uniformer/mmdet/models/dense_heads/anchor_head.py
+++ /dev/null
@@ -1,751 +0,0 @@
-import torch
-import torch.nn as nn
-from mmcv.cnn import normal_init
-from mmcv.runner import force_fp32
-
-from mmdet.core import (anchor_inside_flags, build_anchor_generator,
- build_assigner, build_bbox_coder, build_sampler,
- images_to_levels, multi_apply, multiclass_nms, unmap)
-from ..builder import HEADS, build_loss
-from .base_dense_head import BaseDenseHead
-from .dense_test_mixins import BBoxTestMixin
-
-
-@HEADS.register_module()
-class AnchorHead(BaseDenseHead, BBoxTestMixin):
- """Anchor-based head (RPN, RetinaNet, SSD, etc.).
-
- Args:
- num_classes (int): Number of categories excluding the background
- category.
- in_channels (int): Number of channels in the input feature map.
- feat_channels (int): Number of hidden channels. Used in child classes.
- anchor_generator (dict): Config dict for anchor generator
- bbox_coder (dict): Config of bounding box coder.
- reg_decoded_bbox (bool): If true, the regression loss would be
- applied directly on decoded bounding boxes, converting both
- the predicted boxes and regression targets to absolute
- coordinates format. Default False. It should be `True` when
- using `IoULoss`, `GIoULoss`, or `DIoULoss` in the bbox head.
- loss_cls (dict): Config of classification loss.
- loss_bbox (dict): Config of localization loss.
- train_cfg (dict): Training config of anchor head.
- test_cfg (dict): Testing config of anchor head.
- """ # noqa: W605
-
- def __init__(self,
- num_classes,
- in_channels,
- feat_channels=256,
- anchor_generator=dict(
- type='AnchorGenerator',
- scales=[8, 16, 32],
- ratios=[0.5, 1.0, 2.0],
- strides=[4, 8, 16, 32, 64]),
- bbox_coder=dict(
- type='DeltaXYWHBBoxCoder',
- clip_border=True,
- target_means=(.0, .0, .0, .0),
- target_stds=(1.0, 1.0, 1.0, 1.0)),
- reg_decoded_bbox=False,
- loss_cls=dict(
- type='CrossEntropyLoss',
- use_sigmoid=True,
- loss_weight=1.0),
- loss_bbox=dict(
- type='SmoothL1Loss', beta=1.0 / 9.0, loss_weight=1.0),
- train_cfg=None,
- test_cfg=None):
- super(AnchorHead, self).__init__()
- self.in_channels = in_channels
- self.num_classes = num_classes
- self.feat_channels = feat_channels
- self.use_sigmoid_cls = loss_cls.get('use_sigmoid', False)
- # TODO better way to determine whether sample or not
- self.sampling = loss_cls['type'] not in [
- 'FocalLoss', 'GHMC', 'QualityFocalLoss'
- ]
- if self.use_sigmoid_cls:
- self.cls_out_channels = num_classes
- else:
- self.cls_out_channels = num_classes + 1
-
- if self.cls_out_channels <= 0:
- raise ValueError(f'num_classes={num_classes} is too small')
- self.reg_decoded_bbox = reg_decoded_bbox
-
- self.bbox_coder = build_bbox_coder(bbox_coder)
- self.loss_cls = build_loss(loss_cls)
- self.loss_bbox = build_loss(loss_bbox)
- self.train_cfg = train_cfg
- self.test_cfg = test_cfg
- if self.train_cfg:
- self.assigner = build_assigner(self.train_cfg.assigner)
- # use PseudoSampler when sampling is False
- if self.sampling and hasattr(self.train_cfg, 'sampler'):
- sampler_cfg = self.train_cfg.sampler
- else:
- sampler_cfg = dict(type='PseudoSampler')
- self.sampler = build_sampler(sampler_cfg, context=self)
- self.fp16_enabled = False
-
- self.anchor_generator = build_anchor_generator(anchor_generator)
- # usually the numbers of anchors for each level are the same
- # except SSD detectors
- self.num_anchors = self.anchor_generator.num_base_anchors[0]
- self._init_layers()
-
- def _init_layers(self):
- """Initialize layers of the head."""
- self.conv_cls = nn.Conv2d(self.in_channels,
- self.num_anchors * self.cls_out_channels, 1)
- self.conv_reg = nn.Conv2d(self.in_channels, self.num_anchors * 4, 1)
-
- def init_weights(self):
- """Initialize weights of the head."""
- normal_init(self.conv_cls, std=0.01)
- normal_init(self.conv_reg, std=0.01)
-
- def forward_single(self, x):
- """Forward feature of a single scale level.
-
- Args:
- x (Tensor): Features of a single scale level.
-
- Returns:
- tuple:
- cls_score (Tensor): Cls scores for a single scale level \
- the channels number is num_anchors * num_classes.
- bbox_pred (Tensor): Box energies / deltas for a single scale \
- level, the channels number is num_anchors * 4.
- """
- cls_score = self.conv_cls(x)
- bbox_pred = self.conv_reg(x)
- return cls_score, bbox_pred
-
- def forward(self, feats):
- """Forward features from the upstream network.
-
- Args:
- feats (tuple[Tensor]): Features from the upstream network, each is
- a 4D-tensor.
-
- Returns:
- tuple: A tuple of classification scores and bbox prediction.
-
- - cls_scores (list[Tensor]): Classification scores for all \
- scale levels, each is a 4D-tensor, the channels number \
- is num_anchors * num_classes.
- - bbox_preds (list[Tensor]): Box energies / deltas for all \
- scale levels, each is a 4D-tensor, the channels number \
- is num_anchors * 4.
- """
- return multi_apply(self.forward_single, feats)
-
- def get_anchors(self, featmap_sizes, img_metas, device='cuda'):
- """Get anchors according to feature map sizes.
-
- Args:
- featmap_sizes (list[tuple]): Multi-level feature map sizes.
- img_metas (list[dict]): Image meta info.
- device (torch.device | str): Device for returned tensors
-
- Returns:
- tuple:
- anchor_list (list[Tensor]): Anchors of each image.
- valid_flag_list (list[Tensor]): Valid flags of each image.
- """
- num_imgs = len(img_metas)
-
- # since feature map sizes of all images are the same, we only compute
- # anchors for one time
- multi_level_anchors = self.anchor_generator.grid_anchors(
- featmap_sizes, device)
- anchor_list = [multi_level_anchors for _ in range(num_imgs)]
-
- # for each image, we compute valid flags of multi level anchors
- valid_flag_list = []
- for img_id, img_meta in enumerate(img_metas):
- multi_level_flags = self.anchor_generator.valid_flags(
- featmap_sizes, img_meta['pad_shape'], device)
- valid_flag_list.append(multi_level_flags)
-
- return anchor_list, valid_flag_list
-
- def _get_targets_single(self,
- flat_anchors,
- valid_flags,
- gt_bboxes,
- gt_bboxes_ignore,
- gt_labels,
- img_meta,
- label_channels=1,
- unmap_outputs=True):
- """Compute regression and classification targets for anchors in a
- single image.
-
- Args:
- flat_anchors (Tensor): Multi-level anchors of the image, which are
- concatenated into a single tensor of shape (num_anchors ,4)
- valid_flags (Tensor): Multi level valid flags of the image,
- which are concatenated into a single tensor of
- shape (num_anchors,).
- gt_bboxes (Tensor): Ground truth bboxes of the image,
- shape (num_gts, 4).
- gt_bboxes_ignore (Tensor): Ground truth bboxes to be
- ignored, shape (num_ignored_gts, 4).
- img_meta (dict): Meta info of the image.
- gt_labels (Tensor): Ground truth labels of each box,
- shape (num_gts,).
- label_channels (int): Channel of label.
- unmap_outputs (bool): Whether to map outputs back to the original
- set of anchors.
-
- Returns:
- tuple:
- labels_list (list[Tensor]): Labels of each level
- label_weights_list (list[Tensor]): Label weights of each level
- bbox_targets_list (list[Tensor]): BBox targets of each level
- bbox_weights_list (list[Tensor]): BBox weights of each level
- num_total_pos (int): Number of positive samples in all images
- num_total_neg (int): Number of negative samples in all images
- """
- inside_flags = anchor_inside_flags(flat_anchors, valid_flags,
- img_meta['img_shape'][:2],
- self.train_cfg.allowed_border)
- if not inside_flags.any():
- return (None, ) * 7
- # assign gt and sample anchors
- anchors = flat_anchors[inside_flags, :]
-
- assign_result = self.assigner.assign(
- anchors, gt_bboxes, gt_bboxes_ignore,
- None if self.sampling else gt_labels)
- sampling_result = self.sampler.sample(assign_result, anchors,
- gt_bboxes)
-
- num_valid_anchors = anchors.shape[0]
- bbox_targets = torch.zeros_like(anchors)
- bbox_weights = torch.zeros_like(anchors)
- labels = anchors.new_full((num_valid_anchors, ),
- self.num_classes,
- dtype=torch.long)
- label_weights = anchors.new_zeros(num_valid_anchors, dtype=torch.float)
-
- pos_inds = sampling_result.pos_inds
- neg_inds = sampling_result.neg_inds
- if len(pos_inds) > 0:
- if not self.reg_decoded_bbox:
- pos_bbox_targets = self.bbox_coder.encode(
- sampling_result.pos_bboxes, sampling_result.pos_gt_bboxes)
- else:
- pos_bbox_targets = sampling_result.pos_gt_bboxes
- bbox_targets[pos_inds, :] = pos_bbox_targets
- bbox_weights[pos_inds, :] = 1.0
- if gt_labels is None:
- # Only rpn gives gt_labels as None
- # Foreground is the first class since v2.5.0
- labels[pos_inds] = 0
- else:
- labels[pos_inds] = gt_labels[
- sampling_result.pos_assigned_gt_inds]
- if self.train_cfg.pos_weight <= 0:
- label_weights[pos_inds] = 1.0
- else:
- label_weights[pos_inds] = self.train_cfg.pos_weight
- if len(neg_inds) > 0:
- label_weights[neg_inds] = 1.0
-
- # map up to original set of anchors
- if unmap_outputs:
- num_total_anchors = flat_anchors.size(0)
- labels = unmap(
- labels, num_total_anchors, inside_flags,
- fill=self.num_classes) # fill bg label
- label_weights = unmap(label_weights, num_total_anchors,
- inside_flags)
- bbox_targets = unmap(bbox_targets, num_total_anchors, inside_flags)
- bbox_weights = unmap(bbox_weights, num_total_anchors, inside_flags)
-
- return (labels, label_weights, bbox_targets, bbox_weights, pos_inds,
- neg_inds, sampling_result)
-
- def get_targets(self,
- anchor_list,
- valid_flag_list,
- gt_bboxes_list,
- img_metas,
- gt_bboxes_ignore_list=None,
- gt_labels_list=None,
- label_channels=1,
- unmap_outputs=True,
- return_sampling_results=False):
- """Compute regression and classification targets for anchors in
- multiple images.
-
- Args:
- anchor_list (list[list[Tensor]]): Multi level anchors of each
- image. The outer list indicates images, and the inner list
- corresponds to feature levels of the image. Each element of
- the inner list is a tensor of shape (num_anchors, 4).
- valid_flag_list (list[list[Tensor]]): Multi level valid flags of
- each image. The outer list indicates images, and the inner list
- corresponds to feature levels of the image. Each element of
- the inner list is a tensor of shape (num_anchors, )
- gt_bboxes_list (list[Tensor]): Ground truth bboxes of each image.
- img_metas (list[dict]): Meta info of each image.
- gt_bboxes_ignore_list (list[Tensor]): Ground truth bboxes to be
- ignored.
- gt_labels_list (list[Tensor]): Ground truth labels of each box.
- label_channels (int): Channel of label.
- unmap_outputs (bool): Whether to map outputs back to the original
- set of anchors.
-
- Returns:
- tuple: Usually returns a tuple containing learning targets.
-
- - labels_list (list[Tensor]): Labels of each level.
- - label_weights_list (list[Tensor]): Label weights of each \
- level.
- - bbox_targets_list (list[Tensor]): BBox targets of each level.
- - bbox_weights_list (list[Tensor]): BBox weights of each level.
- - num_total_pos (int): Number of positive samples in all \
- images.
- - num_total_neg (int): Number of negative samples in all \
- images.
- additional_returns: This function enables user-defined returns from
- `self._get_targets_single`. These returns are currently refined
- to properties at each feature map (i.e. having HxW dimension).
- The results will be concatenated after the end
- """
- num_imgs = len(img_metas)
- assert len(anchor_list) == len(valid_flag_list) == num_imgs
-
- # anchor number of multi levels
- num_level_anchors = [anchors.size(0) for anchors in anchor_list[0]]
- # concat all level anchors to a single tensor
- concat_anchor_list = []
- concat_valid_flag_list = []
- for i in range(num_imgs):
- assert len(anchor_list[i]) == len(valid_flag_list[i])
- concat_anchor_list.append(torch.cat(anchor_list[i]))
- concat_valid_flag_list.append(torch.cat(valid_flag_list[i]))
-
- # compute targets for each image
- if gt_bboxes_ignore_list is None:
- gt_bboxes_ignore_list = [None for _ in range(num_imgs)]
- if gt_labels_list is None:
- gt_labels_list = [None for _ in range(num_imgs)]
- results = multi_apply(
- self._get_targets_single,
- concat_anchor_list,
- concat_valid_flag_list,
- gt_bboxes_list,
- gt_bboxes_ignore_list,
- gt_labels_list,
- img_metas,
- label_channels=label_channels,
- unmap_outputs=unmap_outputs)
- (all_labels, all_label_weights, all_bbox_targets, all_bbox_weights,
- pos_inds_list, neg_inds_list, sampling_results_list) = results[:7]
- rest_results = list(results[7:]) # user-added return values
- # no valid anchors
- if any([labels is None for labels in all_labels]):
- return None
- # sampled anchors of all images
- num_total_pos = sum([max(inds.numel(), 1) for inds in pos_inds_list])
- num_total_neg = sum([max(inds.numel(), 1) for inds in neg_inds_list])
- # split targets to a list w.r.t. multiple levels
- labels_list = images_to_levels(all_labels, num_level_anchors)
- label_weights_list = images_to_levels(all_label_weights,
- num_level_anchors)
- bbox_targets_list = images_to_levels(all_bbox_targets,
- num_level_anchors)
- bbox_weights_list = images_to_levels(all_bbox_weights,
- num_level_anchors)
- res = (labels_list, label_weights_list, bbox_targets_list,
- bbox_weights_list, num_total_pos, num_total_neg)
- if return_sampling_results:
- res = res + (sampling_results_list, )
- for i, r in enumerate(rest_results): # user-added return values
- rest_results[i] = images_to_levels(r, num_level_anchors)
-
- return res + tuple(rest_results)
-
- def loss_single(self, cls_score, bbox_pred, anchors, labels, label_weights,
- bbox_targets, bbox_weights, num_total_samples):
- """Compute loss of a single scale level.
-
- Args:
- cls_score (Tensor): Box scores for each scale level
- Has shape (N, num_anchors * num_classes, H, W).
- bbox_pred (Tensor): Box energies / deltas for each scale
- level with shape (N, num_anchors * 4, H, W).
- anchors (Tensor): Box reference for each scale level with shape
- (N, num_total_anchors, 4).
- labels (Tensor): Labels of each anchors with shape
- (N, num_total_anchors).
- label_weights (Tensor): Label weights of each anchor with shape
- (N, num_total_anchors)
- bbox_targets (Tensor): BBox regression targets of each anchor wight
- shape (N, num_total_anchors, 4).
- bbox_weights (Tensor): BBox regression loss weights of each anchor
- with shape (N, num_total_anchors, 4).
- num_total_samples (int): If sampling, num total samples equal to
- the number of total anchors; Otherwise, it is the number of
- positive anchors.
-
- Returns:
- dict[str, Tensor]: A dictionary of loss components.
- """
- # classification loss
- labels = labels.reshape(-1)
- label_weights = label_weights.reshape(-1)
- cls_score = cls_score.permute(0, 2, 3,
- 1).reshape(-1, self.cls_out_channels)
- loss_cls = self.loss_cls(
- cls_score, labels, label_weights, avg_factor=num_total_samples)
- # regression loss
- bbox_targets = bbox_targets.reshape(-1, 4)
- bbox_weights = bbox_weights.reshape(-1, 4)
- bbox_pred = bbox_pred.permute(0, 2, 3, 1).reshape(-1, 4)
- if self.reg_decoded_bbox:
- # When the regression loss (e.g. `IouLoss`, `GIouLoss`)
- # is applied directly on the decoded bounding boxes, it
- # decodes the already encoded coordinates to absolute format.
- anchors = anchors.reshape(-1, 4)
- bbox_pred = self.bbox_coder.decode(anchors, bbox_pred)
- loss_bbox = self.loss_bbox(
- bbox_pred,
- bbox_targets,
- bbox_weights,
- avg_factor=num_total_samples)
- return loss_cls, loss_bbox
-
- @force_fp32(apply_to=('cls_scores', 'bbox_preds'))
- def loss(self,
- cls_scores,
- bbox_preds,
- gt_bboxes,
- gt_labels,
- img_metas,
- gt_bboxes_ignore=None):
- """Compute losses of the head.
-
- Args:
- cls_scores (list[Tensor]): Box scores for each scale level
- Has shape (N, num_anchors * num_classes, H, W)
- bbox_preds (list[Tensor]): Box energies / deltas for each scale
- level with shape (N, num_anchors * 4, H, W)
- gt_bboxes (list[Tensor]): Ground truth bboxes for each image with
- shape (num_gts, 4) in [tl_x, tl_y, br_x, br_y] format.
- gt_labels (list[Tensor]): class indices corresponding to each box
- img_metas (list[dict]): Meta information of each image, e.g.,
- image size, scaling factor, etc.
- gt_bboxes_ignore (None | list[Tensor]): specify which bounding
- boxes can be ignored when computing the loss. Default: None
-
- Returns:
- dict[str, Tensor]: A dictionary of loss components.
- """
- featmap_sizes = [featmap.size()[-2:] for featmap in cls_scores]
- assert len(featmap_sizes) == self.anchor_generator.num_levels
-
- device = cls_scores[0].device
-
- anchor_list, valid_flag_list = self.get_anchors(
- featmap_sizes, img_metas, device=device)
- label_channels = self.cls_out_channels if self.use_sigmoid_cls else 1
- cls_reg_targets = self.get_targets(
- anchor_list,
- valid_flag_list,
- gt_bboxes,
- img_metas,
- gt_bboxes_ignore_list=gt_bboxes_ignore,
- gt_labels_list=gt_labels,
- label_channels=label_channels)
- if cls_reg_targets is None:
- return None
- (labels_list, label_weights_list, bbox_targets_list, bbox_weights_list,
- num_total_pos, num_total_neg) = cls_reg_targets
- num_total_samples = (
- num_total_pos + num_total_neg if self.sampling else num_total_pos)
-
- # anchor number of multi levels
- num_level_anchors = [anchors.size(0) for anchors in anchor_list[0]]
- # concat all level anchors and flags to a single tensor
- concat_anchor_list = []
- for i in range(len(anchor_list)):
- concat_anchor_list.append(torch.cat(anchor_list[i]))
- all_anchor_list = images_to_levels(concat_anchor_list,
- num_level_anchors)
-
- losses_cls, losses_bbox = multi_apply(
- self.loss_single,
- cls_scores,
- bbox_preds,
- all_anchor_list,
- labels_list,
- label_weights_list,
- bbox_targets_list,
- bbox_weights_list,
- num_total_samples=num_total_samples)
- return dict(loss_cls=losses_cls, loss_bbox=losses_bbox)
-
- @force_fp32(apply_to=('cls_scores', 'bbox_preds'))
- def get_bboxes(self,
- cls_scores,
- bbox_preds,
- img_metas,
- cfg=None,
- rescale=False,
- with_nms=True):
- """Transform network output for a batch into bbox predictions.
-
- Args:
- cls_scores (list[Tensor]): Box scores for each level in the
- feature pyramid, has shape
- (N, num_anchors * num_classes, H, W).
- bbox_preds (list[Tensor]): Box energies / deltas for each
- level in the feature pyramid, has shape
- (N, num_anchors * 4, H, W).
- img_metas (list[dict]): Meta information of each image, e.g.,
- image size, scaling factor, etc.
- cfg (mmcv.Config | None): Test / postprocessing configuration,
- if None, test_cfg would be used
- rescale (bool): If True, return boxes in original image space.
- Default: False.
- with_nms (bool): If True, do nms before return boxes.
- Default: True.
-
- Returns:
- list[tuple[Tensor, Tensor]]: Each item in result_list is 2-tuple.
- The first item is an (n, 5) tensor, where 5 represent
- (tl_x, tl_y, br_x, br_y, score) and the score between 0 and 1.
- The shape of the second tensor in the tuple is (n,), and
- each element represents the class label of the corresponding
- box.
-
- Example:
- >>> import mmcv
- >>> self = AnchorHead(
- >>> num_classes=9,
- >>> in_channels=1,
- >>> anchor_generator=dict(
- >>> type='AnchorGenerator',
- >>> scales=[8],
- >>> ratios=[0.5, 1.0, 2.0],
- >>> strides=[4,]))
- >>> img_metas = [{'img_shape': (32, 32, 3), 'scale_factor': 1}]
- >>> cfg = mmcv.Config(dict(
- >>> score_thr=0.00,
- >>> nms=dict(type='nms', iou_thr=1.0),
- >>> max_per_img=10))
- >>> feat = torch.rand(1, 1, 3, 3)
- >>> cls_score, bbox_pred = self.forward_single(feat)
- >>> # note the input lists are over different levels, not images
- >>> cls_scores, bbox_preds = [cls_score], [bbox_pred]
- >>> result_list = self.get_bboxes(cls_scores, bbox_preds,
- >>> img_metas, cfg)
- >>> det_bboxes, det_labels = result_list[0]
- >>> assert len(result_list) == 1
- >>> assert det_bboxes.shape[1] == 5
- >>> assert len(det_bboxes) == len(det_labels) == cfg.max_per_img
- """
- assert len(cls_scores) == len(bbox_preds)
- num_levels = len(cls_scores)
-
- device = cls_scores[0].device
- featmap_sizes = [cls_scores[i].shape[-2:] for i in range(num_levels)]
- mlvl_anchors = self.anchor_generator.grid_anchors(
- featmap_sizes, device=device)
-
- mlvl_cls_scores = [cls_scores[i].detach() for i in range(num_levels)]
- mlvl_bbox_preds = [bbox_preds[i].detach() for i in range(num_levels)]
-
- if torch.onnx.is_in_onnx_export():
- assert len(
- img_metas
- ) == 1, 'Only support one input image while in exporting to ONNX'
- img_shapes = img_metas[0]['img_shape_for_onnx']
- else:
- img_shapes = [
- img_metas[i]['img_shape']
- for i in range(cls_scores[0].shape[0])
- ]
- scale_factors = [
- img_metas[i]['scale_factor'] for i in range(cls_scores[0].shape[0])
- ]
-
- if with_nms:
- # some heads don't support with_nms argument
- result_list = self._get_bboxes(mlvl_cls_scores, mlvl_bbox_preds,
- mlvl_anchors, img_shapes,
- scale_factors, cfg, rescale)
- else:
- result_list = self._get_bboxes(mlvl_cls_scores, mlvl_bbox_preds,
- mlvl_anchors, img_shapes,
- scale_factors, cfg, rescale,
- with_nms)
- return result_list
-
- def _get_bboxes(self,
- mlvl_cls_scores,
- mlvl_bbox_preds,
- mlvl_anchors,
- img_shapes,
- scale_factors,
- cfg,
- rescale=False,
- with_nms=True):
- """Transform outputs for a batch item into bbox predictions.
-
- Args:
- mlvl_cls_scores (list[Tensor]): Each element in the list is
- the scores of bboxes of single level in the feature pyramid,
- has shape (N, num_anchors * num_classes, H, W).
- mlvl_bbox_preds (list[Tensor]): Each element in the list is the
- bboxes predictions of single level in the feature pyramid,
- has shape (N, num_anchors * 4, H, W).
- mlvl_anchors (list[Tensor]): Each element in the list is
- the anchors of single level in feature pyramid, has shape
- (num_anchors, 4).
- img_shapes (list[tuple[int]]): Each tuple in the list represent
- the shape(height, width, 3) of single image in the batch.
- scale_factors (list[ndarray]): Scale factor of the batch
- image arange as list[(w_scale, h_scale, w_scale, h_scale)].
- cfg (mmcv.Config): Test / postprocessing configuration,
- if None, test_cfg would be used.
- rescale (bool): If True, return boxes in original image space.
- Default: False.
- with_nms (bool): If True, do nms before return boxes.
- Default: True.
-
- Returns:
- list[tuple[Tensor, Tensor]]: Each item in result_list is 2-tuple.
- The first item is an (n, 5) tensor, where 5 represent
- (tl_x, tl_y, br_x, br_y, score) and the score between 0 and 1.
- The shape of the second tensor in the tuple is (n,), and
- each element represents the class label of the corresponding
- box.
- """
- cfg = self.test_cfg if cfg is None else cfg
- assert len(mlvl_cls_scores) == len(mlvl_bbox_preds) == len(
- mlvl_anchors)
- batch_size = mlvl_cls_scores[0].shape[0]
- # convert to tensor to keep tracing
- nms_pre_tensor = torch.tensor(
- cfg.get('nms_pre', -1),
- device=mlvl_cls_scores[0].device,
- dtype=torch.long)
-
- mlvl_bboxes = []
- mlvl_scores = []
- for cls_score, bbox_pred, anchors in zip(mlvl_cls_scores,
- mlvl_bbox_preds,
- mlvl_anchors):
- assert cls_score.size()[-2:] == bbox_pred.size()[-2:]
- cls_score = cls_score.permute(0, 2, 3,
- 1).reshape(batch_size, -1,
- self.cls_out_channels)
- if self.use_sigmoid_cls:
- scores = cls_score.sigmoid()
- else:
- scores = cls_score.softmax(-1)
- bbox_pred = bbox_pred.permute(0, 2, 3,
- 1).reshape(batch_size, -1, 4)
- anchors = anchors.expand_as(bbox_pred)
- # Always keep topk op for dynamic input in onnx
- if nms_pre_tensor > 0 and (torch.onnx.is_in_onnx_export()
- or scores.shape[-2] > nms_pre_tensor):
- from torch import _shape_as_tensor
- # keep shape as tensor and get k
- num_anchor = _shape_as_tensor(scores)[-2].to(
- nms_pre_tensor.device)
- nms_pre = torch.where(nms_pre_tensor < num_anchor,
- nms_pre_tensor, num_anchor)
-
- # Get maximum scores for foreground classes.
- if self.use_sigmoid_cls:
- max_scores, _ = scores.max(-1)
- else:
- # remind that we set FG labels to [0, num_class-1]
- # since mmdet v2.0
- # BG cat_id: num_class
- max_scores, _ = scores[..., :-1].max(-1)
-
- _, topk_inds = max_scores.topk(nms_pre)
- batch_inds = torch.arange(batch_size).view(
- -1, 1).expand_as(topk_inds)
- anchors = anchors[batch_inds, topk_inds, :]
- bbox_pred = bbox_pred[batch_inds, topk_inds, :]
- scores = scores[batch_inds, topk_inds, :]
-
- bboxes = self.bbox_coder.decode(
- anchors, bbox_pred, max_shape=img_shapes)
- mlvl_bboxes.append(bboxes)
- mlvl_scores.append(scores)
-
- batch_mlvl_bboxes = torch.cat(mlvl_bboxes, dim=1)
- if rescale:
- batch_mlvl_bboxes /= batch_mlvl_bboxes.new_tensor(
- scale_factors).unsqueeze(1)
- batch_mlvl_scores = torch.cat(mlvl_scores, dim=1)
-
- # Set max number of box to be feed into nms in deployment
- deploy_nms_pre = cfg.get('deploy_nms_pre', -1)
- if deploy_nms_pre > 0 and torch.onnx.is_in_onnx_export():
- # Get maximum scores for foreground classes.
- if self.use_sigmoid_cls:
- max_scores, _ = batch_mlvl_scores.max(-1)
- else:
- # remind that we set FG labels to [0, num_class-1]
- # since mmdet v2.0
- # BG cat_id: num_class
- max_scores, _ = batch_mlvl_scores[..., :-1].max(-1)
- _, topk_inds = max_scores.topk(deploy_nms_pre)
- batch_inds = torch.arange(batch_size).view(-1,
- 1).expand_as(topk_inds)
- batch_mlvl_scores = batch_mlvl_scores[batch_inds, topk_inds]
- batch_mlvl_bboxes = batch_mlvl_bboxes[batch_inds, topk_inds]
- if self.use_sigmoid_cls:
- # Add a dummy background class to the backend when using sigmoid
- # remind that we set FG labels to [0, num_class-1] since mmdet v2.0
- # BG cat_id: num_class
- padding = batch_mlvl_scores.new_zeros(batch_size,
- batch_mlvl_scores.shape[1],
- 1)
- batch_mlvl_scores = torch.cat([batch_mlvl_scores, padding], dim=-1)
-
- if with_nms:
- det_results = []
- for (mlvl_bboxes, mlvl_scores) in zip(batch_mlvl_bboxes,
- batch_mlvl_scores):
- det_bbox, det_label = multiclass_nms(mlvl_bboxes, mlvl_scores,
- cfg.score_thr, cfg.nms,
- cfg.max_per_img)
- det_results.append(tuple([det_bbox, det_label]))
- else:
- det_results = [
- tuple(mlvl_bs)
- for mlvl_bs in zip(batch_mlvl_bboxes, batch_mlvl_scores)
- ]
- return det_results
-
- def aug_test(self, feats, img_metas, rescale=False):
- """Test function with test time augmentation.
-
- Args:
- feats (list[Tensor]): the outer list indicates test-time
- augmentations and inner Tensor should have a shape NxCxHxW,
- which contains features for all images in the batch.
- img_metas (list[list[dict]]): the outer list indicates test-time
- augs (multiscale, flip, etc.) and the inner list indicates
- images in a batch. each dict has image information.
- rescale (bool, optional): Whether to rescale the results.
- Defaults to False.
-
- Returns:
- list[ndarray]: bbox results of each class
- """
- return self.aug_test_bboxes(feats, img_metas, rescale=rescale)
diff --git a/spaces/Salesforce/BLIP/models/blip_pretrain.py b/spaces/Salesforce/BLIP/models/blip_pretrain.py
deleted file mode 100644
index 068420247591f3e35242bff6f183c8adb8b977a2..0000000000000000000000000000000000000000
--- a/spaces/Salesforce/BLIP/models/blip_pretrain.py
+++ /dev/null
@@ -1,339 +0,0 @@
-'''
- * Copyright (c) 2022, salesforce.com, inc.
- * All rights reserved.
- * SPDX-License-Identifier: BSD-3-Clause
- * For full license text, see LICENSE.txt file in the repo root or https://opensource.org/licenses/BSD-3-Clause
- * By Junnan Li
-'''
-from models.med import BertConfig, BertModel, BertLMHeadModel
-from transformers import BertTokenizer
-import transformers
-transformers.logging.set_verbosity_error()
-
-import torch
-from torch import nn
-import torch.nn.functional as F
-
-from models.blip import create_vit, init_tokenizer, load_checkpoint
-
-class BLIP_Pretrain(nn.Module):
- def __init__(self,
- med_config = 'configs/bert_config.json',
- image_size = 224,
- vit = 'base',
- vit_grad_ckpt = False,
- vit_ckpt_layer = 0,
- embed_dim = 256,
- queue_size = 57600,
- momentum = 0.995,
- ):
- """
- Args:
- med_config (str): path for the mixture of encoder-decoder model's configuration file
- image_size (int): input image size
- vit (str): model size of vision transformer
- """
- super().__init__()
-
- self.visual_encoder, vision_width = create_vit(vit,image_size, vit_grad_ckpt, vit_ckpt_layer, 0)
-
- if vit=='base':
- checkpoint = torch.hub.load_state_dict_from_url(
- url="https://dl.fbaipublicfiles.com/deit/deit_base_patch16_224-b5f2ef4d.pth",
- map_location="cpu", check_hash=True)
- state_dict = checkpoint["model"]
- msg = self.visual_encoder.load_state_dict(state_dict,strict=False)
- elif vit=='large':
- from timm.models.helpers import load_custom_pretrained
- from timm.models.vision_transformer import default_cfgs
- load_custom_pretrained(self.visual_encoder,default_cfgs['vit_large_patch16_224_in21k'])
-
- self.tokenizer = init_tokenizer()
- encoder_config = BertConfig.from_json_file(med_config)
- encoder_config.encoder_width = vision_width
- self.text_encoder = BertModel.from_pretrained('bert-base-uncased',config=encoder_config, add_pooling_layer=False)
- self.text_encoder.resize_token_embeddings(len(self.tokenizer))
-
- text_width = self.text_encoder.config.hidden_size
-
- self.vision_proj = nn.Linear(vision_width, embed_dim)
- self.text_proj = nn.Linear(text_width, embed_dim)
-
- self.itm_head = nn.Linear(text_width, 2)
-
- # create momentum encoders
- self.visual_encoder_m, vision_width = create_vit(vit,image_size)
- self.vision_proj_m = nn.Linear(vision_width, embed_dim)
- self.text_encoder_m = BertModel(config=encoder_config, add_pooling_layer=False)
- self.text_proj_m = nn.Linear(text_width, embed_dim)
-
- self.model_pairs = [[self.visual_encoder,self.visual_encoder_m],
- [self.vision_proj,self.vision_proj_m],
- [self.text_encoder,self.text_encoder_m],
- [self.text_proj,self.text_proj_m],
- ]
- self.copy_params()
-
- # create the queue
- self.register_buffer("image_queue", torch.randn(embed_dim, queue_size))
- self.register_buffer("text_queue", torch.randn(embed_dim, queue_size))
- self.register_buffer("queue_ptr", torch.zeros(1, dtype=torch.long))
-
- self.image_queue = nn.functional.normalize(self.image_queue, dim=0)
- self.text_queue = nn.functional.normalize(self.text_queue, dim=0)
-
- self.queue_size = queue_size
- self.momentum = momentum
- self.temp = nn.Parameter(0.07*torch.ones([]))
-
- # create the decoder
- decoder_config = BertConfig.from_json_file(med_config)
- decoder_config.encoder_width = vision_width
- self.text_decoder = BertLMHeadModel.from_pretrained('bert-base-uncased',config=decoder_config)
- self.text_decoder.resize_token_embeddings(len(self.tokenizer))
- tie_encoder_decoder_weights(self.text_decoder.bert,self.text_encoder,'','/attention')
-
-
- def forward(self, image, caption, alpha):
- with torch.no_grad():
- self.temp.clamp_(0.001,0.5)
-
- image_embeds = self.visual_encoder(image)
- image_atts = torch.ones(image_embeds.size()[:-1],dtype=torch.long).to(image.device)
- image_feat = F.normalize(self.vision_proj(image_embeds[:,0,:]),dim=-1)
-
- text = self.tokenizer(caption, padding='max_length', truncation=True, max_length=30,
- return_tensors="pt").to(image.device)
- text_output = self.text_encoder(text.input_ids, attention_mask = text.attention_mask,
- return_dict = True, mode = 'text')
- text_feat = F.normalize(self.text_proj(text_output.last_hidden_state[:,0,:]),dim=-1)
-
- # get momentum features
- with torch.no_grad():
- self._momentum_update()
- image_embeds_m = self.visual_encoder_m(image)
- image_feat_m = F.normalize(self.vision_proj_m(image_embeds_m[:,0,:]),dim=-1)
- image_feat_all = torch.cat([image_feat_m.t(),self.image_queue.clone().detach()],dim=1)
-
- text_output_m = self.text_encoder_m(text.input_ids, attention_mask = text.attention_mask,
- return_dict = True, mode = 'text')
- text_feat_m = F.normalize(self.text_proj_m(text_output_m.last_hidden_state[:,0,:]),dim=-1)
- text_feat_all = torch.cat([text_feat_m.t(),self.text_queue.clone().detach()],dim=1)
-
- sim_i2t_m = image_feat_m @ text_feat_all / self.temp
- sim_t2i_m = text_feat_m @ image_feat_all / self.temp
-
- sim_targets = torch.zeros(sim_i2t_m.size()).to(image.device)
- sim_targets.fill_diagonal_(1)
-
- sim_i2t_targets = alpha * F.softmax(sim_i2t_m, dim=1) + (1 - alpha) * sim_targets
- sim_t2i_targets = alpha * F.softmax(sim_t2i_m, dim=1) + (1 - alpha) * sim_targets
-
- sim_i2t = image_feat @ text_feat_all / self.temp
- sim_t2i = text_feat @ image_feat_all / self.temp
-
- loss_i2t = -torch.sum(F.log_softmax(sim_i2t, dim=1)*sim_i2t_targets,dim=1).mean()
- loss_t2i = -torch.sum(F.log_softmax(sim_t2i, dim=1)*sim_t2i_targets,dim=1).mean()
-
- loss_ita = (loss_i2t+loss_t2i)/2
-
- self._dequeue_and_enqueue(image_feat_m, text_feat_m)
-
- ###============== Image-text Matching ===================###
- encoder_input_ids = text.input_ids.clone()
- encoder_input_ids[:,0] = self.tokenizer.enc_token_id
-
- # forward the positve image-text pair
- bs = image.size(0)
- output_pos = self.text_encoder(encoder_input_ids,
- attention_mask = text.attention_mask,
- encoder_hidden_states = image_embeds,
- encoder_attention_mask = image_atts,
- return_dict = True,
- )
- with torch.no_grad():
- weights_t2i = F.softmax(sim_t2i[:,:bs],dim=1)+1e-4
- weights_t2i.fill_diagonal_(0)
- weights_i2t = F.softmax(sim_i2t[:,:bs],dim=1)+1e-4
- weights_i2t.fill_diagonal_(0)
-
- # select a negative image for each text
- image_embeds_neg = []
- for b in range(bs):
- neg_idx = torch.multinomial(weights_t2i[b], 1).item()
- image_embeds_neg.append(image_embeds[neg_idx])
- image_embeds_neg = torch.stack(image_embeds_neg,dim=0)
-
- # select a negative text for each image
- text_ids_neg = []
- text_atts_neg = []
- for b in range(bs):
- neg_idx = torch.multinomial(weights_i2t[b], 1).item()
- text_ids_neg.append(encoder_input_ids[neg_idx])
- text_atts_neg.append(text.attention_mask[neg_idx])
-
- text_ids_neg = torch.stack(text_ids_neg,dim=0)
- text_atts_neg = torch.stack(text_atts_neg,dim=0)
-
- text_ids_all = torch.cat([encoder_input_ids, text_ids_neg],dim=0)
- text_atts_all = torch.cat([text.attention_mask, text_atts_neg],dim=0)
-
- image_embeds_all = torch.cat([image_embeds_neg,image_embeds],dim=0)
- image_atts_all = torch.cat([image_atts,image_atts],dim=0)
-
- output_neg = self.text_encoder(text_ids_all,
- attention_mask = text_atts_all,
- encoder_hidden_states = image_embeds_all,
- encoder_attention_mask = image_atts_all,
- return_dict = True,
- )
-
- vl_embeddings = torch.cat([output_pos.last_hidden_state[:,0,:], output_neg.last_hidden_state[:,0,:]],dim=0)
- vl_output = self.itm_head(vl_embeddings)
-
- itm_labels = torch.cat([torch.ones(bs,dtype=torch.long),torch.zeros(2*bs,dtype=torch.long)],
- dim=0).to(image.device)
- loss_itm = F.cross_entropy(vl_output, itm_labels)
-
- ##================= LM ========================##
- decoder_input_ids = text.input_ids.clone()
- decoder_input_ids[:,0] = self.tokenizer.bos_token_id
- decoder_targets = decoder_input_ids.masked_fill(decoder_input_ids == self.tokenizer.pad_token_id, -100)
-
- decoder_output = self.text_decoder(decoder_input_ids,
- attention_mask = text.attention_mask,
- encoder_hidden_states = image_embeds,
- encoder_attention_mask = image_atts,
- labels = decoder_targets,
- return_dict = True,
- )
-
- loss_lm = decoder_output.loss
- return loss_ita, loss_itm, loss_lm
-
-
-
- @torch.no_grad()
- def copy_params(self):
- for model_pair in self.model_pairs:
- for param, param_m in zip(model_pair[0].parameters(), model_pair[1].parameters()):
- param_m.data.copy_(param.data) # initialize
- param_m.requires_grad = False # not update by gradient
-
-
- @torch.no_grad()
- def _momentum_update(self):
- for model_pair in self.model_pairs:
- for param, param_m in zip(model_pair[0].parameters(), model_pair[1].parameters()):
- param_m.data = param_m.data * self.momentum + param.data * (1. - self.momentum)
-
-
- @torch.no_grad()
- def _dequeue_and_enqueue(self, image_feat, text_feat):
- # gather keys before updating queue
- image_feats = concat_all_gather(image_feat)
- text_feats = concat_all_gather(text_feat)
-
- batch_size = image_feats.shape[0]
-
- ptr = int(self.queue_ptr)
- assert self.queue_size % batch_size == 0 # for simplicity
-
- # replace the keys at ptr (dequeue and enqueue)
- self.image_queue[:, ptr:ptr + batch_size] = image_feats.T
- self.text_queue[:, ptr:ptr + batch_size] = text_feats.T
- ptr = (ptr + batch_size) % self.queue_size # move pointer
-
- self.queue_ptr[0] = ptr
-
-
-def blip_pretrain(**kwargs):
- model = BLIP_Pretrain(**kwargs)
- return model
-
-
-@torch.no_grad()
-def concat_all_gather(tensor):
- """
- Performs all_gather operation on the provided tensors.
- *** Warning ***: torch.distributed.all_gather has no gradient.
- """
- tensors_gather = [torch.ones_like(tensor)
- for _ in range(torch.distributed.get_world_size())]
- torch.distributed.all_gather(tensors_gather, tensor, async_op=False)
-
- output = torch.cat(tensors_gather, dim=0)
- return output
-
-
-from typing import List
-def tie_encoder_decoder_weights(encoder: nn.Module, decoder: nn.Module, base_model_prefix: str, skip_key:str):
- uninitialized_encoder_weights: List[str] = []
- if decoder.__class__ != encoder.__class__:
- logger.info(
- f"{decoder.__class__} and {encoder.__class__} are not equal. In this case make sure that all encoder weights are correctly initialized."
- )
-
- def tie_encoder_to_decoder_recursively(
- decoder_pointer: nn.Module,
- encoder_pointer: nn.Module,
- module_name: str,
- uninitialized_encoder_weights: List[str],
- skip_key: str,
- depth=0,
- ):
- assert isinstance(decoder_pointer, nn.Module) and isinstance(
- encoder_pointer, nn.Module
- ), f"{decoder_pointer} and {encoder_pointer} have to be of type torch.nn.Module"
- if hasattr(decoder_pointer, "weight") and skip_key not in module_name:
- assert hasattr(encoder_pointer, "weight")
- encoder_pointer.weight = decoder_pointer.weight
- if hasattr(decoder_pointer, "bias"):
- assert hasattr(encoder_pointer, "bias")
- encoder_pointer.bias = decoder_pointer.bias
- print(module_name+' is tied')
- return
-
- encoder_modules = encoder_pointer._modules
- decoder_modules = decoder_pointer._modules
- if len(decoder_modules) > 0:
- assert (
- len(encoder_modules) > 0
- ), f"Encoder module {encoder_pointer} does not match decoder module {decoder_pointer}"
-
- all_encoder_weights = set([module_name + "/" + sub_name for sub_name in encoder_modules.keys()])
- encoder_layer_pos = 0
- for name, module in decoder_modules.items():
- if name.isdigit():
- encoder_name = str(int(name) + encoder_layer_pos)
- decoder_name = name
- if not isinstance(decoder_modules[decoder_name], type(encoder_modules[encoder_name])) and len(
- encoder_modules
- ) != len(decoder_modules):
- # this can happen if the name corresponds to the position in a list module list of layers
- # in this case the decoder has added a cross-attention that the encoder does not have
- # thus skip this step and subtract one layer pos from encoder
- encoder_layer_pos -= 1
- continue
- elif name not in encoder_modules:
- continue
- elif depth > 500:
- raise ValueError(
- "Max depth of recursive function `tie_encoder_to_decoder` reached. It seems that there is a circular dependency between two or more `nn.Modules` of your model."
- )
- else:
- decoder_name = encoder_name = name
- tie_encoder_to_decoder_recursively(
- decoder_modules[decoder_name],
- encoder_modules[encoder_name],
- module_name + "/" + name,
- uninitialized_encoder_weights,
- skip_key,
- depth=depth + 1,
- )
- all_encoder_weights.remove(module_name + "/" + encoder_name)
-
- uninitialized_encoder_weights += list(all_encoder_weights)
-
- # tie weights recursively
- tie_encoder_to_decoder_recursively(decoder, encoder, base_model_prefix, uninitialized_encoder_weights, skip_key)
diff --git a/spaces/SantiagoMoreno-UdeA/NER_RC/src/scripts/Json_formats.py b/spaces/SantiagoMoreno-UdeA/NER_RC/src/scripts/Json_formats.py
deleted file mode 100644
index 6b1ba664874eafced528d36651bf06a2ac7d27a3..0000000000000000000000000000000000000000
--- a/spaces/SantiagoMoreno-UdeA/NER_RC/src/scripts/Json_formats.py
+++ /dev/null
@@ -1,166 +0,0 @@
-# -*- coding: utf-8 -*-
-"""
-Created on Tue Dec 6 11:21:55 2022
-
-@author: gita
-"""
-
-import gradio as gr
-
-def image_classifier():
- # j={
- # "sentences":[
- # {"text":"Frase ejemplo"},
- # {"text":"Frase ejemplo"}
- # ]
- # }
-
- # j = {
- # 'text':"Frase ejemplo Frase ejemplo ",
-
- # 'text_labeled':" \"Frase\"/Entity_Type ejemplo \"Frase\"/Entity_Type ejemplo ",
-
- # 'sentences':[
- # {'text':"Frase ejemplo",
- # 'text_labeled':" \"Frase\"/Entity_Type ejemplo",
- # 'tokens':[
- # {'text':"Frase", 'label':"Entity_Type"},
- # {'text':"ejemplo", 'label':"O"}
- # ]},
-
- # {'text':"Frase ejemplo",
- # 'text_labeled':" \"Frase\"/Entity_Type ejemplo",
- # 'tokens':[
- # {'text':"Frase", 'label':"Entity_Type"},
- # {'text':"ejemplo", 'label':"O"}
- # ]}
-
- # ],
-
-
- # 'entities': [
- # {
- # 'entity': "Entity_Type" ,
- # 'index' : 0,
- # 'word' : "Frase",
- # 'start': 0,
- # 'end' : 5
-
- # },
- # {
- # 'entity': "Entity_Type" ,
- # 'index' : 2,
- # 'word' : "Frase",
- # 'start': 14,
- # 'end' : 19
-
- # }
- # ]
-
- # }
-
-
- j = {
-
- 'text':"Frase ejemplo Frase ejemplo",
-
- 'sentences':[
- {'text':"Frase ejemplo",
- 'id':"s0",
- 'tokens':[
- {'text':"Frase", 'begin':0, 'end':5},
- {'text':"ejemplo", 'begin':6, 'end':13}
- ]},
-
- {'text':"Frase ejemplo",
- 'id':"s1",
- 'tokens':[
- {'text':"Frase", 'begin':14, 'end':19},
- {'text':"ejemplo", 'begin':20, 'end':27}
- ]},
-
- ],
-
-
- 'mentions': [
- {
- 'id': "s0-m0" ,
- 'type' : "Entity_type",
- 'begin' : 0,
- 'end': 5,
-
- },
-
- {
- 'id': "s1-m0" ,
- 'type' : "Entity_type",
- 'begin' : 14,
- 'end': 19,
-
- }
-
- ]
-
- }
-
-
-
- return j
-
-demo = gr.Interface(fn=image_classifier, inputs=None, outputs=gr.JSON())
-demo.launch()
-
-#%%
-# JSON FORMAT OUTPUT
-
-# Document:{ text:"Texto"
-
-# text_labeled: "Texto \ENTITY"
-
-# sentences:[{ text:"Texto"
-
- # text_labeled: "Texto \ENTITY"
-
- # tokens: [ {text:"Texto", label : "ENTITY"},
- # {text:"Texto", label : "ENTITY"},
- # {text:"Texto", label : "ENTITY"}
-
- # ]
-
- # },
-
- # { text:"Texto"
-
- # text_labeled: "Texto "
-
- # tokens: [ {text:"Texto", label : "ENTITY"},
- # {text:"Texto", label : "ENTITY"},
- # {text:"Texto", label : "ENTITY"}
-
-# ]
-
-# }
-# ],
- # entities:[
- # {
- # 'entity': "ENTITY",
- # 'index': num,
- # 'word': "Texto",
- # 'start': num,
- # 'end' : num
- # }
- # ]
-# }
-
-#%%
-
-# JSON FORMAT INPUT
-
-# json{...
-# sentences:{
-# s:{
-# text:
-# }
-# }
-
-# ...}
\ No newline at end of file
diff --git a/spaces/SeViLA/SeViLA/lavis/models/blip_models/__init__.py b/spaces/SeViLA/SeViLA/lavis/models/blip_models/__init__.py
deleted file mode 100644
index 2b88146b9eb3d60dd10ee2aed8e0a33cba924746..0000000000000000000000000000000000000000
--- a/spaces/SeViLA/SeViLA/lavis/models/blip_models/__init__.py
+++ /dev/null
@@ -1,90 +0,0 @@
-"""
- Copyright (c) 2022, salesforce.com, inc.
- All rights reserved.
- SPDX-License-Identifier: BSD-3-Clause
- For full license text, see the LICENSE file in the repo root or https://opensource.org/licenses/BSD-3-Clause
-"""
-
-import logging
-from typing import List
-
-from torch import nn
-
-
-def tie_encoder_decoder_weights(
- encoder: nn.Module, decoder: nn.Module, base_model_prefix: str, skip_key: str
-):
- uninitialized_encoder_weights: List[str] = []
- if decoder.__class__ != encoder.__class__:
- logging.info(
- f"{decoder.__class__} and {encoder.__class__} are not equal. In this case make sure that all encoder weights are correctly initialized."
- )
-
- def tie_encoder_to_decoder_recursively(
- decoder_pointer: nn.Module,
- encoder_pointer: nn.Module,
- module_name: str,
- uninitialized_encoder_weights: List[str],
- skip_key: str,
- depth=0,
- ):
- assert isinstance(decoder_pointer, nn.Module) and isinstance(
- encoder_pointer, nn.Module
- ), f"{decoder_pointer} and {encoder_pointer} have to be of type torch.nn.Module"
- if hasattr(decoder_pointer, "weight") and skip_key not in module_name:
- assert hasattr(encoder_pointer, "weight")
- encoder_pointer.weight = decoder_pointer.weight
- if hasattr(decoder_pointer, "bias"):
- assert hasattr(encoder_pointer, "bias")
- encoder_pointer.bias = decoder_pointer.bias
- print(module_name + " is tied")
- return
-
- encoder_modules = encoder_pointer._modules
- decoder_modules = decoder_pointer._modules
- if len(decoder_modules) > 0:
- assert (
- len(encoder_modules) > 0
- ), f"Encoder module {encoder_pointer} does not match decoder module {decoder_pointer}"
-
- all_encoder_weights = set(
- [module_name + "/" + sub_name for sub_name in encoder_modules.keys()]
- )
- encoder_layer_pos = 0
- for name, module in decoder_modules.items():
- if name.isdigit():
- encoder_name = str(int(name) + encoder_layer_pos)
- decoder_name = name
- if not isinstance(
- decoder_modules[decoder_name],
- type(encoder_modules[encoder_name]),
- ) and len(encoder_modules) != len(decoder_modules):
- # this can happen if the name corresponds to the position in a list module list of layers
- # in this case the decoder has added a cross-attention that the encoder does not have
- # thus skip this step and subtract one layer pos from encoder
- encoder_layer_pos -= 1
- continue
- elif name not in encoder_modules:
- continue
- elif depth > 500:
- raise ValueError(
- "Max depth of recursive function `tie_encoder_to_decoder` reached. It seems that there is a circular dependency between two or more `nn.Modules` of your model."
- )
- else:
- decoder_name = encoder_name = name
- tie_encoder_to_decoder_recursively(
- decoder_modules[decoder_name],
- encoder_modules[encoder_name],
- module_name + "/" + name,
- uninitialized_encoder_weights,
- skip_key,
- depth=depth + 1,
- )
- all_encoder_weights.remove(module_name + "/" + encoder_name)
-
- uninitialized_encoder_weights += list(all_encoder_weights)
-
- # tie weights recursively
- tie_encoder_to_decoder_recursively(
- decoder, encoder, base_model_prefix, uninitialized_encoder_weights, skip_key
- )
diff --git a/spaces/SeyedAli/Butterfly-image-Generation/README.md b/spaces/SeyedAli/Butterfly-image-Generation/README.md
deleted file mode 100644
index 7b49b11d7731c5a79c863970b7cde758e6c54444..0000000000000000000000000000000000000000
--- a/spaces/SeyedAli/Butterfly-image-Generation/README.md
+++ /dev/null
@@ -1,13 +0,0 @@
----
-title: Butterfly Image Generation
-emoji: 🦋
-colorFrom: green
-colorTo: indigo
-sdk: gradio
-sdk_version: 3.50.2
-app_file: app.py
-pinned: false
-license: mit
----
-
-Check out the configuration reference at https://huggingface.co/docs/hub/spaces-config-reference
diff --git a/spaces/Sonnt/Fracture_Webapp/ui/__init__.py b/spaces/Sonnt/Fracture_Webapp/ui/__init__.py
deleted file mode 100644
index 81af026baf6494c81ef0aa7ac70d7d2f3c123335..0000000000000000000000000000000000000000
--- a/spaces/Sonnt/Fracture_Webapp/ui/__init__.py
+++ /dev/null
@@ -1,18 +0,0 @@
-from .UIConfigs import *
-from .PageComponents import *
-
-__all__ = ['hide_menu_button',
- 'condense_layout',
- 'set_page_config',
-
- 'subtab21',
- 'subtab22',
- 'subtab23',
- 'subtab24',
- 'subtab25',
- 'subtab26',
-
- 'scatterPoint3D',
- 'stViewCurves',
-
-]
\ No newline at end of file
diff --git "a/spaces/SouthCity/ShuruiXu/crazy_functions/\344\273\243\347\240\201\351\207\215\345\206\231\344\270\272\345\205\250\350\213\261\346\226\207_\345\244\232\347\272\277\347\250\213.py" "b/spaces/SouthCity/ShuruiXu/crazy_functions/\344\273\243\347\240\201\351\207\215\345\206\231\344\270\272\345\205\250\350\213\261\346\226\207_\345\244\232\347\272\277\347\250\213.py"
deleted file mode 100644
index 892578283ca2f8321b8086574107b2dccdc482c7..0000000000000000000000000000000000000000
--- "a/spaces/SouthCity/ShuruiXu/crazy_functions/\344\273\243\347\240\201\351\207\215\345\206\231\344\270\272\345\205\250\350\213\261\346\226\207_\345\244\232\347\272\277\347\250\213.py"
+++ /dev/null
@@ -1,75 +0,0 @@
-import threading
-from predict import predict_no_ui_long_connection
-from toolbox import CatchException, write_results_to_file
-
-
-
-@CatchException
-def 全项目切换英文(txt, top_p, api_key, temperature, chatbot, history, sys_prompt, WEB_PORT):
- history = [] # 清空历史,以免输入溢出
- # 集合文件
- import time, glob, os
- os.makedirs('gpt_log/generated_english_version', exist_ok=True)
- os.makedirs('gpt_log/generated_english_version/crazy_functions', exist_ok=True)
- file_manifest = [f for f in glob.glob('./*.py') if ('test_project' not in f) and ('gpt_log' not in f)] + \
- [f for f in glob.glob('./crazy_functions/*.py') if ('test_project' not in f) and ('gpt_log' not in f)]
- i_say_show_user_buffer = []
-
- # 随便显示点什么防止卡顿的感觉
- for index, fp in enumerate(file_manifest):
- # if 'test_project' in fp: continue
- with open(fp, 'r', encoding='utf-8') as f:
- file_content = f.read()
- i_say_show_user =f'[{index}/{len(file_manifest)}] 接下来请将以下代码中包含的所有中文转化为英文,只输出代码: {os.path.abspath(fp)}'
- i_say_show_user_buffer.append(i_say_show_user)
- chatbot.append((i_say_show_user, "[Local Message] 等待多线程操作,中间过程不予显示."))
- yield chatbot, history, '正常'
-
- # 任务函数
- mutable_return = [None for _ in file_manifest]
- def thread_worker(fp,index):
- with open(fp, 'r', encoding='utf-8') as f:
- file_content = f.read()
- i_say = f'接下来请将以下代码中包含的所有中文转化为英文,只输出代码,文件名是{fp},文件代码是 ```{file_content}```'
- # ** gpt request **
- gpt_say = predict_no_ui_long_connection(inputs=i_say, top_p=top_p, api_key=api_key, temperature=temperature, history=history, sys_prompt=sys_prompt)
- mutable_return[index] = gpt_say
-
- # 所有线程同时开始执行任务函数
- handles = [threading.Thread(target=thread_worker, args=(fp,index)) for index, fp in enumerate(file_manifest)]
- for h in handles:
- h.daemon = True
- h.start()
- chatbot.append(('开始了吗?', f'多线程操作已经开始'))
- yield chatbot, history, '正常'
-
- # 循环轮询各个线程是否执行完毕
- cnt = 0
- while True:
- time.sleep(1)
- th_alive = [h.is_alive() for h in handles]
- if not any(th_alive): break
- stat = ['执行中' if alive else '已完成' for alive in th_alive]
- stat_str = '|'.join(stat)
- cnt += 1
- chatbot[-1] = (chatbot[-1][0], f'多线程操作已经开始,完成情况: {stat_str}' + ''.join(['.']*(cnt%4)))
- yield chatbot, history, '正常'
-
- # 把结果写入文件
- for index, h in enumerate(handles):
- h.join() # 这里其实不需要join了,肯定已经都结束了
- fp = file_manifest[index]
- gpt_say = mutable_return[index]
- i_say_show_user = i_say_show_user_buffer[index]
-
- where_to_relocate = f'gpt_log/generated_english_version/{fp}'
- with open(where_to_relocate, 'w+', encoding='utf-8') as f: f.write(gpt_say.lstrip('```').rstrip('```'))
- chatbot.append((i_say_show_user, f'[Local Message] 已完成{os.path.abspath(fp)}的转化,\n\n存入{os.path.abspath(where_to_relocate)}'))
- history.append(i_say_show_user); history.append(gpt_say)
- yield chatbot, history, '正常'
- time.sleep(1)
-
- # 备份一个文件
- res = write_results_to_file(history)
- chatbot.append(("生成一份任务执行报告", res))
- yield chatbot, history, '正常'
diff --git a/spaces/StaticalizaAI/GPT-4/app.py b/spaces/StaticalizaAI/GPT-4/app.py
deleted file mode 100644
index 56214df608b5a01a97db7d98f4f0ff4731c5f79d..0000000000000000000000000000000000000000
--- a/spaces/StaticalizaAI/GPT-4/app.py
+++ /dev/null
@@ -1,65 +0,0 @@
-import gradio as gr
-import requests
-import re
-import os
-
-API_TOKEN = os.environ.get("API_TOKEN")
-API_ENDPOINT = os.environ.get("API_ENDPOINT")
-
-API_PROCESS = os.environ.get("API_PROCESS")
-
-KEY = os.environ.get("KEY")
-
-headers = { "Content-Type": "application/json", "X-Access-Token": API_TOKEN }
-
-instruction = f"Respond with the format OUTPUT: response"
-create_memory = []
-
-user = ""
-bot = ""
-
-update_memory = create_memory.copy()
-history = ""
-
-exec(API_PROCESS)
-
-def send_message(instruction, message, memory, options):
- task_message = f"INSTRUCTION: {instruction}\n\nINPUT: {message[1]}"
- response = requests.post( f"{API_ENDPOINT}/generate", json={ "task": task_message, **options }, headers=headers )
- response_text = response.json()['result']
- print(f"\n\n{response_text}\n\n")
- return response_text
-
-def predict(get_input, access_key):
-
- if (access_key != KEY):
- print("REQUEST FAILED: Attempted Key: " + access_key)
- return ("[UNAUTHORIZED ACCESS]", get_input);
-
- get_input = get_input.strip()
- response = api_request(get_input)
- print(f"---\nUSER: {get_input}\nBOT: {response}\n---")
- return [response, ""]
-
-def main():
- with gr.Blocks() as demo:
- with gr.Row(variant = "panel"):
- gr.Markdown("😈 temporary locked gpt4 till these stupid automation stops (i can read logs and they are failing cause they missing an argument 🤬) (how tf yall bypassing the blocked gradio api 💀)!!!\n\n\n⛔ do not overuse it\n\n\nrespone takes 4-20+ seconds per request but if u make it write a essay it could take over a minute!\n\n\ndo not use it for math it may not be 100% correct!!!\n\n\nuhh ... https://discord.gg/6JRtGawz7B")
-
- with gr.Row():
- with gr.Column():
- input = gr.Textbox(label = "Input", lines = 4)
- access_key = gr.Textbox(label = "Access Key", lines = 1)
- run = gr.Button("▶")
- with gr.Row():
- with gr.Column():
- output = gr.Textbox(label = "Output", value = "", lines = 50)
-
- input.submit(predict, inputs = [input, access_key], outputs = [output, input])
- run.click(predict, inputs = [input, access_key], outputs = [output, input])
-
- demo.queue(concurrency_count = 5, api_open = False)
- demo.launch(inline = True, max_threads = 5, show_api = False)
-
-if __name__ == "__main__":
- main()
\ No newline at end of file
diff --git a/spaces/SuYuanS/AudioCraft_Plus/audiocraft/adversarial/losses.py b/spaces/SuYuanS/AudioCraft_Plus/audiocraft/adversarial/losses.py
deleted file mode 100644
index be293e739bdc2d91273f30fb789befe7c8b49a43..0000000000000000000000000000000000000000
--- a/spaces/SuYuanS/AudioCraft_Plus/audiocraft/adversarial/losses.py
+++ /dev/null
@@ -1,228 +0,0 @@
-# Copyright (c) Meta Platforms, Inc. and affiliates.
-# All rights reserved.
-#
-# This source code is licensed under the license found in the
-# LICENSE file in the root directory of this source tree.
-
-"""
-Utility module to handle adversarial losses without requiring to mess up the main training loop.
-"""
-
-import typing as tp
-
-import flashy
-import torch
-import torch.nn as nn
-import torch.nn.functional as F
-
-
-ADVERSARIAL_LOSSES = ['mse', 'hinge', 'hinge2']
-
-
-AdvLossType = tp.Union[nn.Module, tp.Callable[[torch.Tensor], torch.Tensor]]
-FeatLossType = tp.Union[nn.Module, tp.Callable[[torch.Tensor, torch.Tensor], torch.Tensor]]
-
-
-class AdversarialLoss(nn.Module):
- """Adversary training wrapper.
-
- Args:
- adversary (nn.Module): The adversary module will be used to estimate the logits given the fake and real samples.
- We assume here the adversary output is ``Tuple[List[torch.Tensor], List[List[torch.Tensor]]]``
- where the first item is a list of logits and the second item is a list of feature maps.
- optimizer (torch.optim.Optimizer): Optimizer used for training the given module.
- loss (AdvLossType): Loss function for generator training.
- loss_real (AdvLossType): Loss function for adversarial training on logits from real samples.
- loss_fake (AdvLossType): Loss function for adversarial training on logits from fake samples.
- loss_feat (FeatLossType): Feature matching loss function for generator training.
- normalize (bool): Whether to normalize by number of sub-discriminators.
-
- Example of usage:
- adv_loss = AdversarialLoss(adversaries, optimizer, loss, loss_real, loss_fake)
- for real in loader:
- noise = torch.randn(...)
- fake = model(noise)
- adv_loss.train_adv(fake, real)
- loss, _ = adv_loss(fake, real)
- loss.backward()
- """
- def __init__(self,
- adversary: nn.Module,
- optimizer: torch.optim.Optimizer,
- loss: AdvLossType,
- loss_real: AdvLossType,
- loss_fake: AdvLossType,
- loss_feat: tp.Optional[FeatLossType] = None,
- normalize: bool = True):
- super().__init__()
- self.adversary: nn.Module = adversary
- flashy.distrib.broadcast_model(self.adversary)
- self.optimizer = optimizer
- self.loss = loss
- self.loss_real = loss_real
- self.loss_fake = loss_fake
- self.loss_feat = loss_feat
- self.normalize = normalize
-
- def _save_to_state_dict(self, destination, prefix, keep_vars):
- # Add the optimizer state dict inside our own.
- super()._save_to_state_dict(destination, prefix, keep_vars)
- destination[prefix + 'optimizer'] = self.optimizer.state_dict()
- return destination
-
- def _load_from_state_dict(self, state_dict, prefix, *args, **kwargs):
- # Load optimizer state.
- self.optimizer.load_state_dict(state_dict.pop(prefix + 'optimizer'))
- super()._load_from_state_dict(state_dict, prefix, *args, **kwargs)
-
- def get_adversary_pred(self, x):
- """Run adversary model, validating expected output format."""
- logits, fmaps = self.adversary(x)
- assert isinstance(logits, list) and all([isinstance(t, torch.Tensor) for t in logits]), \
- f'Expecting a list of tensors as logits but {type(logits)} found.'
- assert isinstance(fmaps, list), f'Expecting a list of features maps but {type(fmaps)} found.'
- for fmap in fmaps:
- assert isinstance(fmap, list) and all([isinstance(f, torch.Tensor) for f in fmap]), \
- f'Expecting a list of tensors as feature maps but {type(fmap)} found.'
- return logits, fmaps
-
- def train_adv(self, fake: torch.Tensor, real: torch.Tensor) -> torch.Tensor:
- """Train the adversary with the given fake and real example.
-
- We assume the adversary output is the following format: Tuple[List[torch.Tensor], List[List[torch.Tensor]]].
- The first item being the logits and second item being a list of feature maps for each sub-discriminator.
-
- This will automatically synchronize gradients (with `flashy.distrib.eager_sync_model`)
- and call the optimizer.
- """
- loss = torch.tensor(0., device=fake.device)
- all_logits_fake_is_fake, _ = self.get_adversary_pred(fake.detach())
- all_logits_real_is_fake, _ = self.get_adversary_pred(real.detach())
- n_sub_adversaries = len(all_logits_fake_is_fake)
- for logit_fake_is_fake, logit_real_is_fake in zip(all_logits_fake_is_fake, all_logits_real_is_fake):
- loss += self.loss_fake(logit_fake_is_fake) + self.loss_real(logit_real_is_fake)
-
- if self.normalize:
- loss /= n_sub_adversaries
-
- self.optimizer.zero_grad()
- with flashy.distrib.eager_sync_model(self.adversary):
- loss.backward()
- self.optimizer.step()
-
- return loss
-
- def forward(self, fake: torch.Tensor, real: torch.Tensor) -> tp.Tuple[torch.Tensor, torch.Tensor]:
- """Return the loss for the generator, i.e. trying to fool the adversary,
- and feature matching loss if provided.
- """
- adv = torch.tensor(0., device=fake.device)
- feat = torch.tensor(0., device=fake.device)
- with flashy.utils.readonly(self.adversary):
- all_logits_fake_is_fake, all_fmap_fake = self.get_adversary_pred(fake)
- all_logits_real_is_fake, all_fmap_real = self.get_adversary_pred(real)
- n_sub_adversaries = len(all_logits_fake_is_fake)
- for logit_fake_is_fake in all_logits_fake_is_fake:
- adv += self.loss(logit_fake_is_fake)
- if self.loss_feat:
- for fmap_fake, fmap_real in zip(all_fmap_fake, all_fmap_real):
- feat += self.loss_feat(fmap_fake, fmap_real)
-
- if self.normalize:
- adv /= n_sub_adversaries
- feat /= n_sub_adversaries
-
- return adv, feat
-
-
-def get_adv_criterion(loss_type: str) -> tp.Callable:
- assert loss_type in ADVERSARIAL_LOSSES
- if loss_type == 'mse':
- return mse_loss
- elif loss_type == 'hinge':
- return hinge_loss
- elif loss_type == 'hinge2':
- return hinge2_loss
- raise ValueError('Unsupported loss')
-
-
-def get_fake_criterion(loss_type: str) -> tp.Callable:
- assert loss_type in ADVERSARIAL_LOSSES
- if loss_type == 'mse':
- return mse_fake_loss
- elif loss_type in ['hinge', 'hinge2']:
- return hinge_fake_loss
- raise ValueError('Unsupported loss')
-
-
-def get_real_criterion(loss_type: str) -> tp.Callable:
- assert loss_type in ADVERSARIAL_LOSSES
- if loss_type == 'mse':
- return mse_real_loss
- elif loss_type in ['hinge', 'hinge2']:
- return hinge_real_loss
- raise ValueError('Unsupported loss')
-
-
-def mse_real_loss(x: torch.Tensor) -> torch.Tensor:
- return F.mse_loss(x, torch.tensor(1., device=x.device).expand_as(x))
-
-
-def mse_fake_loss(x: torch.Tensor) -> torch.Tensor:
- return F.mse_loss(x, torch.tensor(0., device=x.device).expand_as(x))
-
-
-def hinge_real_loss(x: torch.Tensor) -> torch.Tensor:
- return -torch.mean(torch.min(x - 1, torch.tensor(0., device=x.device).expand_as(x)))
-
-
-def hinge_fake_loss(x: torch.Tensor) -> torch.Tensor:
- return -torch.mean(torch.min(-x - 1, torch.tensor(0., device=x.device).expand_as(x)))
-
-
-def mse_loss(x: torch.Tensor) -> torch.Tensor:
- if x.numel() == 0:
- return torch.tensor([0.0], device=x.device)
- return F.mse_loss(x, torch.tensor(1., device=x.device).expand_as(x))
-
-
-def hinge_loss(x: torch.Tensor) -> torch.Tensor:
- if x.numel() == 0:
- return torch.tensor([0.0], device=x.device)
- return -x.mean()
-
-
-def hinge2_loss(x: torch.Tensor) -> torch.Tensor:
- if x.numel() == 0:
- return torch.tensor([0.0])
- return -torch.mean(torch.min(x - 1, torch.tensor(0., device=x.device).expand_as(x)))
-
-
-class FeatureMatchingLoss(nn.Module):
- """Feature matching loss for adversarial training.
-
- Args:
- loss (nn.Module): Loss to use for feature matching (default=torch.nn.L1).
- normalize (bool): Whether to normalize the loss.
- by number of feature maps.
- """
- def __init__(self, loss: nn.Module = torch.nn.L1Loss(), normalize: bool = True):
- super().__init__()
- self.loss = loss
- self.normalize = normalize
-
- def forward(self, fmap_fake: tp.List[torch.Tensor], fmap_real: tp.List[torch.Tensor]) -> torch.Tensor:
- assert len(fmap_fake) == len(fmap_real) and len(fmap_fake) > 0
- feat_loss = torch.tensor(0., device=fmap_fake[0].device)
- feat_scale = torch.tensor(0., device=fmap_fake[0].device)
- n_fmaps = 0
- for (feat_fake, feat_real) in zip(fmap_fake, fmap_real):
- assert feat_fake.shape == feat_real.shape
- n_fmaps += 1
- feat_loss += self.loss(feat_fake, feat_real)
- feat_scale += torch.mean(torch.abs(feat_real))
-
- if self.normalize:
- feat_loss /= n_fmaps
-
- return feat_loss
diff --git a/spaces/SungBeom/chatwine-korean/.venv/Lib/site-packages/aiohttp/payload_streamer.py b/spaces/SungBeom/chatwine-korean/.venv/Lib/site-packages/aiohttp/payload_streamer.py
deleted file mode 100644
index 9f8b8bc57cc22fc693da1646bf806c2a6ca8d797..0000000000000000000000000000000000000000
--- a/spaces/SungBeom/chatwine-korean/.venv/Lib/site-packages/aiohttp/payload_streamer.py
+++ /dev/null
@@ -1,75 +0,0 @@
-"""
-Payload implemenation for coroutines as data provider.
-
-As a simple case, you can upload data from file::
-
- @aiohttp.streamer
- async def file_sender(writer, file_name=None):
- with open(file_name, 'rb') as f:
- chunk = f.read(2**16)
- while chunk:
- await writer.write(chunk)
-
- chunk = f.read(2**16)
-
-Then you can use `file_sender` like this:
-
- async with session.post('http://httpbin.org/post',
- data=file_sender(file_name='huge_file')) as resp:
- print(await resp.text())
-
-..note:: Coroutine must accept `writer` as first argument
-
-"""
-
-import types
-import warnings
-from typing import Any, Awaitable, Callable, Dict, Tuple
-
-from .abc import AbstractStreamWriter
-from .payload import Payload, payload_type
-
-__all__ = ("streamer",)
-
-
-class _stream_wrapper:
- def __init__(
- self,
- coro: Callable[..., Awaitable[None]],
- args: Tuple[Any, ...],
- kwargs: Dict[str, Any],
- ) -> None:
- self.coro = types.coroutine(coro)
- self.args = args
- self.kwargs = kwargs
-
- async def __call__(self, writer: AbstractStreamWriter) -> None:
- await self.coro(writer, *self.args, **self.kwargs) # type: ignore[operator]
-
-
-class streamer:
- def __init__(self, coro: Callable[..., Awaitable[None]]) -> None:
- warnings.warn(
- "@streamer is deprecated, use async generators instead",
- DeprecationWarning,
- stacklevel=2,
- )
- self.coro = coro
-
- def __call__(self, *args: Any, **kwargs: Any) -> _stream_wrapper:
- return _stream_wrapper(self.coro, args, kwargs)
-
-
-@payload_type(_stream_wrapper)
-class StreamWrapperPayload(Payload):
- async def write(self, writer: AbstractStreamWriter) -> None:
- await self._value(writer)
-
-
-@payload_type(streamer)
-class StreamPayload(StreamWrapperPayload):
- def __init__(self, value: Any, *args: Any, **kwargs: Any) -> None:
- super().__init__(value(), *args, **kwargs)
-
- async def write(self, writer: AbstractStreamWriter) -> None:
- await self._value(writer)
diff --git a/spaces/Superlang/ImageProcessor/annotator/oneformer/detectron2/modeling/meta_arch/build.py b/spaces/Superlang/ImageProcessor/annotator/oneformer/detectron2/modeling/meta_arch/build.py
deleted file mode 100644
index 52229b11acf4a8f07c173feb51c45c30e9567903..0000000000000000000000000000000000000000
--- a/spaces/Superlang/ImageProcessor/annotator/oneformer/detectron2/modeling/meta_arch/build.py
+++ /dev/null
@@ -1,24 +0,0 @@
-# Copyright (c) Facebook, Inc. and its affiliates.
-import torch
-
-from annotator.oneformer.detectron2.utils.logger import _log_api_usage
-from annotator.oneformer.detectron2.utils.registry import Registry
-
-META_ARCH_REGISTRY = Registry("META_ARCH") # noqa F401 isort:skip
-META_ARCH_REGISTRY.__doc__ = """
-Registry for meta-architectures, i.e. the whole model.
-
-The registered object will be called with `obj(cfg)`
-and expected to return a `nn.Module` object.
-"""
-
-
-def build_model(cfg):
- """
- Build the whole model architecture, defined by ``cfg.MODEL.META_ARCHITECTURE``.
- Note that it does not load any weights from ``cfg``.
- """
- meta_arch = cfg.MODEL.META_ARCHITECTURE
- model = META_ARCH_REGISTRY.get(meta_arch)(cfg)
- _log_api_usage("modeling.meta_arch." + meta_arch)
- return model
diff --git a/spaces/TH5314/newbing/src/components/ui/dropdown-menu.tsx b/spaces/TH5314/newbing/src/components/ui/dropdown-menu.tsx
deleted file mode 100644
index 184d4e6007ef85187446362f69532ab077897fea..0000000000000000000000000000000000000000
--- a/spaces/TH5314/newbing/src/components/ui/dropdown-menu.tsx
+++ /dev/null
@@ -1,128 +0,0 @@
-'use client'
-
-import * as React from 'react'
-import * as DropdownMenuPrimitive from '@radix-ui/react-dropdown-menu'
-
-import { cn } from '@/lib/utils'
-
-const DropdownMenu = DropdownMenuPrimitive.Root
-
-const DropdownMenuTrigger = DropdownMenuPrimitive.Trigger
-
-const DropdownMenuGroup = DropdownMenuPrimitive.Group
-
-const DropdownMenuPortal = DropdownMenuPrimitive.Portal
-
-const DropdownMenuSub = DropdownMenuPrimitive.Sub
-
-const DropdownMenuRadioGroup = DropdownMenuPrimitive.RadioGroup
-
-const DropdownMenuSubContent = React.forwardRef<
- React.ElementRef,
- React.ComponentPropsWithoutRef
->(({ className, ...props }, ref) => (
-
-))
-DropdownMenuSubContent.displayName =
- DropdownMenuPrimitive.SubContent.displayName
-
-const DropdownMenuContent = React.forwardRef<
- React.ElementRef,
- React.ComponentPropsWithoutRef
->(({ className, sideOffset = 4, ...props }, ref) => (
-
-
-
-))
-DropdownMenuContent.displayName = DropdownMenuPrimitive.Content.displayName
-
-const DropdownMenuItem = React.forwardRef<
- React.ElementRef,
- React.ComponentPropsWithoutRef & {
- inset?: boolean
- }
->(({ className, inset, ...props }, ref) => (
-
-))
-DropdownMenuItem.displayName = DropdownMenuPrimitive.Item.displayName
-
-const DropdownMenuLabel = React.forwardRef<
- React.ElementRef,
- React.ComponentPropsWithoutRef & {
- inset?: boolean
- }
->(({ className, inset, ...props }, ref) => (
-
-))
-DropdownMenuLabel.displayName = DropdownMenuPrimitive.Label.displayName
-
-const DropdownMenuSeparator = React.forwardRef<
- React.ElementRef,
- React.ComponentPropsWithoutRef
->(({ className, ...props }, ref) => (
-
-))
-DropdownMenuSeparator.displayName = DropdownMenuPrimitive.Separator.displayName
-
-const DropdownMenuShortcut = ({
- className,
- ...props
-}: React.HTMLAttributes) => {
- return (
-
- )
-}
-DropdownMenuShortcut.displayName = 'DropdownMenuShortcut'
-
-export {
- DropdownMenu,
- DropdownMenuTrigger,
- DropdownMenuContent,
- DropdownMenuItem,
- DropdownMenuLabel,
- DropdownMenuSeparator,
- DropdownMenuShortcut,
- DropdownMenuGroup,
- DropdownMenuPortal,
- DropdownMenuSub,
- DropdownMenuSubContent,
- DropdownMenuRadioGroup
-}
diff --git a/spaces/THEGAMECHANGER/LandscapeColorizer/app.py b/spaces/THEGAMECHANGER/LandscapeColorizer/app.py
deleted file mode 100644
index 20a2663fc2bf65e48b476bb4db6354889212bdd8..0000000000000000000000000000000000000000
--- a/spaces/THEGAMECHANGER/LandscapeColorizer/app.py
+++ /dev/null
@@ -1,22 +0,0 @@
-import gradio as gr
-import tensorflow as tf
-import numpy as np
-
-model = tf.keras.models.load_model("landColorGenV1.keras")
-
-def generate_image(input_img):
- input_img = tf.convert_to_tensor(input_img)
- input_img = tf.cast(input_img,tf.float32)
- init_shape = input_img.shape
- input_img = tf.image.resize(input_img, [256, 256],
- method=tf.image.ResizeMethod.NEAREST_NEIGHBOR)
- input_img = (input_img / 127.5) -1
- input_img = tf.reshape(input_img,(1,256,256,3))
- output = model(input_img,training=True)
- # out_img = output[0].numpy()* 0.5 + 0.5
- out_img = tf.image.resize(output[0], [init_shape[0],init_shape[1]],
- method=tf.image.ResizeMethod.NEAREST_NEIGHBOR)
- out_img = out_img.numpy()*0.5 + 0.5
- return out_img
-app = gr.Interface(fn = generate_image, inputs="image", outputs="image")
-app.launch(debug=False)
\ No newline at end of file
diff --git a/spaces/TandCAcceptMe/face-swap-docker/mynewshinyroop/Lib/site-packages/pip/_internal/network/xmlrpc.py b/spaces/TandCAcceptMe/face-swap-docker/mynewshinyroop/Lib/site-packages/pip/_internal/network/xmlrpc.py
deleted file mode 100644
index 4a7d55d0e50cb8b892caa021695522e5ddd54a17..0000000000000000000000000000000000000000
--- a/spaces/TandCAcceptMe/face-swap-docker/mynewshinyroop/Lib/site-packages/pip/_internal/network/xmlrpc.py
+++ /dev/null
@@ -1,60 +0,0 @@
-"""xmlrpclib.Transport implementation
-"""
-
-import logging
-import urllib.parse
-import xmlrpc.client
-from typing import TYPE_CHECKING, Tuple
-
-from pip._internal.exceptions import NetworkConnectionError
-from pip._internal.network.session import PipSession
-from pip._internal.network.utils import raise_for_status
-
-if TYPE_CHECKING:
- from xmlrpc.client import _HostType, _Marshallable
-
-logger = logging.getLogger(__name__)
-
-
-class PipXmlrpcTransport(xmlrpc.client.Transport):
- """Provide a `xmlrpclib.Transport` implementation via a `PipSession`
- object.
- """
-
- def __init__(
- self, index_url: str, session: PipSession, use_datetime: bool = False
- ) -> None:
- super().__init__(use_datetime)
- index_parts = urllib.parse.urlparse(index_url)
- self._scheme = index_parts.scheme
- self._session = session
-
- def request(
- self,
- host: "_HostType",
- handler: str,
- request_body: bytes,
- verbose: bool = False,
- ) -> Tuple["_Marshallable", ...]:
- assert isinstance(host, str)
- parts = (self._scheme, host, handler, None, None, None)
- url = urllib.parse.urlunparse(parts)
- try:
- headers = {"Content-Type": "text/xml"}
- response = self._session.post(
- url,
- data=request_body,
- headers=headers,
- stream=True,
- )
- raise_for_status(response)
- self.verbose = verbose
- return self.parse_response(response.raw)
- except NetworkConnectionError as exc:
- assert exc.response
- logger.critical(
- "HTTP error %s while getting %s",
- exc.response.status_code,
- url,
- )
- raise
diff --git a/spaces/TencentARC/T2I-Adapter-SDXL/README.md b/spaces/TencentARC/T2I-Adapter-SDXL/README.md
deleted file mode 100644
index b687a1ce40d7e5729149f24225697e16ae9e331c..0000000000000000000000000000000000000000
--- a/spaces/TencentARC/T2I-Adapter-SDXL/README.md
+++ /dev/null
@@ -1,12 +0,0 @@
----
-title: T2I-Adapter-SDXL
-emoji: 🚀
-colorFrom: purple
-colorTo: yellow
-sdk: docker
-pinned: false
-license: mit
-suggested_hardware: t4-small
----
-
-Check out the configuration reference at https://huggingface.co/docs/hub/spaces-config-reference
diff --git a/spaces/TencentARC/VLog/models/grit_src/third_party/CenterNet2/tests/layers/test_losses.py b/spaces/TencentARC/VLog/models/grit_src/third_party/CenterNet2/tests/layers/test_losses.py
deleted file mode 100644
index d74920246cbd4a188b3c81cf0c78e982af6da1ac..0000000000000000000000000000000000000000
--- a/spaces/TencentARC/VLog/models/grit_src/third_party/CenterNet2/tests/layers/test_losses.py
+++ /dev/null
@@ -1,82 +0,0 @@
-# Copyright (c) Facebook, Inc. and its affiliates.
-import numpy as np
-import unittest
-import torch
-
-from detectron2.layers import ciou_loss, diou_loss
-
-
-class TestLosses(unittest.TestCase):
- def test_diou_loss(self):
- """
- loss = 1 - iou + d/c
- where,
- d = (distance between centers of the 2 boxes)^2
- c = (diagonal length of the smallest enclosing box covering the 2 boxes)^2
- """
- # Identical boxes should have loss of 0
- box = torch.tensor([-1, -1, 1, 1], dtype=torch.float32)
- loss = diou_loss(box, box)
- self.assertTrue(np.allclose(loss, [0.0]))
-
- # Half size box inside other box
- # iou = 0.5, d = 0.25, c = 8
- box2 = torch.tensor([0, -1, 1, 1], dtype=torch.float32)
- loss = diou_loss(box, box2)
- self.assertTrue(np.allclose(loss, [0.53125]))
-
- # Two diagonally adjacent boxes
- # iou = 0, d = 2, c = 8
- box3 = torch.tensor([0, 0, 1, 1], dtype=torch.float32)
- box4 = torch.tensor([1, 1, 2, 2], dtype=torch.float32)
- loss = diou_loss(box3, box4)
- self.assertTrue(np.allclose(loss, [1.25]))
-
- # Test batched loss and reductions
- box1s = torch.stack([box, box3], dim=0)
- box2s = torch.stack([box2, box4], dim=0)
-
- loss = diou_loss(box1s, box2s, reduction="sum")
- self.assertTrue(np.allclose(loss, [1.78125]))
-
- loss = diou_loss(box1s, box2s, reduction="mean")
- self.assertTrue(np.allclose(loss, [0.890625]))
-
- def test_ciou_loss(self):
- """
- loss = 1 - iou + d/c + alpha*v
- where,
- d = (distance between centers of the 2 boxes)^2
- c = (diagonal length of the smallest enclosing box covering the 2 boxes)^2
- v = (4/pi^2) * (arctan(box1_w/box1_h) - arctan(box2_w/box2_h))^2
- alpha = v/(1 - iou + v)
- """
- # Identical boxes should have loss of 0
- box = torch.tensor([-1, -1, 1, 1], dtype=torch.float32)
- loss = ciou_loss(box, box)
- self.assertTrue(np.allclose(loss, [0.0]))
-
- # Half size box inside other box
- # iou = 0.5, d = 0.25, c = 8
- # v = (4/pi^2) * (arctan(1) - arctan(0.5))^2 = 0.042
- # alpha = 0.0775
- box2 = torch.tensor([0, -1, 1, 1], dtype=torch.float32)
- loss = ciou_loss(box, box2)
- self.assertTrue(np.allclose(loss, [0.5345]))
-
- # Two diagonally adjacent boxes
- # iou = 0, d = 2, c = 8, v = 0, alpha = 0
- box3 = torch.tensor([0, 0, 1, 1], dtype=torch.float32)
- box4 = torch.tensor([1, 1, 2, 2], dtype=torch.float32)
- loss = ciou_loss(box3, box4)
- self.assertTrue(np.allclose(loss, [1.25]))
-
- # Test batched loss and reductions
- box1s = torch.stack([box, box3], dim=0)
- box2s = torch.stack([box2, box4], dim=0)
-
- loss = ciou_loss(box1s, box2s, reduction="sum")
- self.assertTrue(np.allclose(loss, [1.7845]))
-
- loss = ciou_loss(box1s, box2s, reduction="mean")
- self.assertTrue(np.allclose(loss, [0.89225]))
diff --git a/spaces/TencentARC/VLog/models/grit_src/third_party/CenterNet2/tests/layers/test_roi_align_rotated.py b/spaces/TencentARC/VLog/models/grit_src/third_party/CenterNet2/tests/layers/test_roi_align_rotated.py
deleted file mode 100644
index 7323d7d5a86816f337571221313c428238c439f4..0000000000000000000000000000000000000000
--- a/spaces/TencentARC/VLog/models/grit_src/third_party/CenterNet2/tests/layers/test_roi_align_rotated.py
+++ /dev/null
@@ -1,176 +0,0 @@
-# Copyright (c) Facebook, Inc. and its affiliates.
-import logging
-import unittest
-import cv2
-import torch
-from torch.autograd import Variable, gradcheck
-
-from detectron2.layers.roi_align import ROIAlign
-from detectron2.layers.roi_align_rotated import ROIAlignRotated
-
-logger = logging.getLogger(__name__)
-
-
-class ROIAlignRotatedTest(unittest.TestCase):
- def _box_to_rotated_box(self, box, angle):
- return [
- (box[0] + box[2]) / 2.0,
- (box[1] + box[3]) / 2.0,
- box[2] - box[0],
- box[3] - box[1],
- angle,
- ]
-
- def _rot90(self, img, num):
- num = num % 4 # note: -1 % 4 == 3
- for _ in range(num):
- img = img.transpose(0, 1).flip(0)
- return img
-
- def test_forward_output_0_90_180_270(self):
- for i in range(4):
- # i = 0, 1, 2, 3 corresponding to 0, 90, 180, 270 degrees
- img = torch.arange(25, dtype=torch.float32).reshape(5, 5)
- """
- 0 1 2 3 4
- 5 6 7 8 9
- 10 11 12 13 14
- 15 16 17 18 19
- 20 21 22 23 24
- """
- box = [1, 1, 3, 3]
- rotated_box = self._box_to_rotated_box(box=box, angle=90 * i)
-
- result = self._simple_roi_align_rotated(img=img, box=rotated_box, resolution=(4, 4))
-
- # Here's an explanation for 0 degree case:
- # point 0 in the original input lies at [0.5, 0.5]
- # (the center of bin [0, 1] x [0, 1])
- # point 1 in the original input lies at [1.5, 0.5], etc.
- # since the resolution is (4, 4) that divides [1, 3] x [1, 3]
- # into 4 x 4 equal bins,
- # the top-left bin is [1, 1.5] x [1, 1.5], and its center
- # (1.25, 1.25) lies at the 3/4 position
- # between point 0 and point 1, point 5 and point 6,
- # point 0 and point 5, point 1 and point 6, so it can be calculated as
- # 0.25*(0*0.25+1*0.75)+(5*0.25+6*0.75)*0.75 = 4.5
- result_expected = torch.tensor(
- [
- [4.5, 5.0, 5.5, 6.0],
- [7.0, 7.5, 8.0, 8.5],
- [9.5, 10.0, 10.5, 11.0],
- [12.0, 12.5, 13.0, 13.5],
- ]
- )
- # This is also an upsampled version of [[6, 7], [11, 12]]
-
- # When the box is rotated by 90 degrees CCW,
- # the result would be rotated by 90 degrees CW, thus it's -i here
- result_expected = self._rot90(result_expected, -i)
-
- assert torch.allclose(result, result_expected)
-
- def test_resize(self):
- H, W = 30, 30
- input = torch.rand(H, W) * 100
- box = [10, 10, 20, 20]
- rotated_box = self._box_to_rotated_box(box, angle=0)
- output = self._simple_roi_align_rotated(img=input, box=rotated_box, resolution=(5, 5))
-
- input2x = cv2.resize(input.numpy(), (W // 2, H // 2), interpolation=cv2.INTER_LINEAR)
- input2x = torch.from_numpy(input2x)
- box2x = [x / 2 for x in box]
- rotated_box2x = self._box_to_rotated_box(box2x, angle=0)
- output2x = self._simple_roi_align_rotated(img=input2x, box=rotated_box2x, resolution=(5, 5))
- assert torch.allclose(output2x, output)
-
- def _simple_roi_align_rotated(self, img, box, resolution):
- """
- RoiAlignRotated with scale 1.0 and 0 sample ratio.
- """
- op = ROIAlignRotated(output_size=resolution, spatial_scale=1.0, sampling_ratio=0)
- input = img[None, None, :, :]
-
- rois = [0] + list(box)
- rois = torch.tensor(rois, dtype=torch.float32)[None, :]
- result_cpu = op.forward(input, rois)
- if torch.cuda.is_available():
- result_cuda = op.forward(input.cuda(), rois.cuda())
- assert torch.allclose(result_cpu, result_cuda.cpu())
- return result_cpu[0, 0]
-
- def test_empty_box(self):
- img = torch.rand(5, 5)
- out = self._simple_roi_align_rotated(img, [2, 3, 0, 0, 0], (7, 7))
- self.assertTrue((out == 0).all())
-
- def test_roi_align_rotated_gradcheck_cpu(self):
- dtype = torch.float64
- device = torch.device("cpu")
- roi_align_rotated_op = ROIAlignRotated(
- output_size=(5, 5), spatial_scale=0.5, sampling_ratio=1
- ).to(dtype=dtype, device=device)
- x = torch.rand(1, 1, 10, 10, dtype=dtype, device=device, requires_grad=True)
- # roi format is (batch index, x_center, y_center, width, height, angle)
- rois = torch.tensor(
- [[0, 4.5, 4.5, 9, 9, 0], [0, 2, 7, 4, 4, 0], [0, 7, 7, 4, 4, 0]],
- dtype=dtype,
- device=device,
- )
-
- def func(input):
- return roi_align_rotated_op(input, rois)
-
- assert gradcheck(func, (x,)), "gradcheck failed for RoIAlignRotated CPU"
- assert gradcheck(func, (x.transpose(2, 3),)), "gradcheck failed for RoIAlignRotated CPU"
-
- @unittest.skipIf(not torch.cuda.is_available(), "CUDA not available")
- def test_roi_align_rotated_gradient_cuda(self):
- """
- Compute gradients for ROIAlignRotated with multiple bounding boxes on the GPU,
- and compare the result with ROIAlign
- """
- # torch.manual_seed(123)
- dtype = torch.float64
- device = torch.device("cuda")
- pool_h, pool_w = (5, 5)
-
- roi_align = ROIAlign(output_size=(pool_h, pool_w), spatial_scale=1, sampling_ratio=2).to(
- device=device
- )
-
- roi_align_rotated = ROIAlignRotated(
- output_size=(pool_h, pool_w), spatial_scale=1, sampling_ratio=2
- ).to(device=device)
-
- x = torch.rand(1, 1, 10, 10, dtype=dtype, device=device, requires_grad=True)
- # x_rotated = x.clone() won't work (will lead to grad_fun=CloneBackward)!
- x_rotated = Variable(x.data.clone(), requires_grad=True)
-
- # roi_rotated format is (batch index, x_center, y_center, width, height, angle)
- rois_rotated = torch.tensor(
- [[0, 4.5, 4.5, 9, 9, 0], [0, 2, 7, 4, 4, 0], [0, 7, 7, 4, 4, 0]],
- dtype=dtype,
- device=device,
- )
-
- y_rotated = roi_align_rotated(x_rotated, rois_rotated)
- s_rotated = y_rotated.sum()
- s_rotated.backward()
-
- # roi format is (batch index, x1, y1, x2, y2)
- rois = torch.tensor(
- [[0, 0, 0, 9, 9], [0, 0, 5, 4, 9], [0, 5, 5, 9, 9]], dtype=dtype, device=device
- )
-
- y = roi_align(x, rois)
- s = y.sum()
- s.backward()
-
- assert torch.allclose(
- x.grad, x_rotated.grad
- ), "gradients for ROIAlign and ROIAlignRotated mismatch on CUDA"
-
-
-if __name__ == "__main__":
- unittest.main()
diff --git a/spaces/TheStinger/Ilaria_RVC/lib/infer_pack/modules/F0Predictor/DioF0Predictor.py b/spaces/TheStinger/Ilaria_RVC/lib/infer_pack/modules/F0Predictor/DioF0Predictor.py
deleted file mode 100644
index ee3171bcb7c4a5066560723108b56e055f18be45..0000000000000000000000000000000000000000
--- a/spaces/TheStinger/Ilaria_RVC/lib/infer_pack/modules/F0Predictor/DioF0Predictor.py
+++ /dev/null
@@ -1,90 +0,0 @@
-from lib.infer_pack.modules.F0Predictor.F0Predictor import F0Predictor
-import pyworld
-import numpy as np
-
-
-class DioF0Predictor(F0Predictor):
- def __init__(self, hop_length=512, f0_min=50, f0_max=1100, sampling_rate=44100):
- self.hop_length = hop_length
- self.f0_min = f0_min
- self.f0_max = f0_max
- self.sampling_rate = sampling_rate
-
- def interpolate_f0(self, f0):
- """
- 对F0进行插值处理
- """
-
- data = np.reshape(f0, (f0.size, 1))
-
- vuv_vector = np.zeros((data.size, 1), dtype=np.float32)
- vuv_vector[data > 0.0] = 1.0
- vuv_vector[data <= 0.0] = 0.0
-
- ip_data = data
-
- frame_number = data.size
- last_value = 0.0
- for i in range(frame_number):
- if data[i] <= 0.0:
- j = i + 1
- for j in range(i + 1, frame_number):
- if data[j] > 0.0:
- break
- if j < frame_number - 1:
- if last_value > 0.0:
- step = (data[j] - data[i - 1]) / float(j - i)
- for k in range(i, j):
- ip_data[k] = data[i - 1] + step * (k - i + 1)
- else:
- for k in range(i, j):
- ip_data[k] = data[j]
- else:
- for k in range(i, frame_number):
- ip_data[k] = last_value
- else:
- ip_data[i] = data[i] # 这里可能存在一个没有必要的拷贝
- last_value = data[i]
-
- return ip_data[:, 0], vuv_vector[:, 0]
-
- def resize_f0(self, x, target_len):
- source = np.array(x)
- source[source < 0.001] = np.nan
- target = np.interp(
- np.arange(0, len(source) * target_len, len(source)) / target_len,
- np.arange(0, len(source)),
- source,
- )
- res = np.nan_to_num(target)
- return res
-
- def compute_f0(self, wav, p_len=None):
- if p_len is None:
- p_len = wav.shape[0] // self.hop_length
- f0, t = pyworld.dio(
- wav.astype(np.double),
- fs=self.sampling_rate,
- f0_floor=self.f0_min,
- f0_ceil=self.f0_max,
- frame_period=1000 * self.hop_length / self.sampling_rate,
- )
- f0 = pyworld.stonemask(wav.astype(np.double), f0, t, self.sampling_rate)
- for index, pitch in enumerate(f0):
- f0[index] = round(pitch, 1)
- return self.interpolate_f0(self.resize_f0(f0, p_len))[0]
-
- def compute_f0_uv(self, wav, p_len=None):
- if p_len is None:
- p_len = wav.shape[0] // self.hop_length
- f0, t = pyworld.dio(
- wav.astype(np.double),
- fs=self.sampling_rate,
- f0_floor=self.f0_min,
- f0_ceil=self.f0_max,
- frame_period=1000 * self.hop_length / self.sampling_rate,
- )
- f0 = pyworld.stonemask(wav.astype(np.double), f0, t, self.sampling_rate)
- for index, pitch in enumerate(f0):
- f0[index] = round(pitch, 1)
- return self.interpolate_f0(self.resize_f0(f0, p_len))
diff --git a/spaces/Tuana/what-would-mother-say/custom_nodes/__init__.py b/spaces/Tuana/what-would-mother-say/custom_nodes/__init__.py
deleted file mode 100644
index e69de29bb2d1d6434b8b29ae775ad8c2e48c5391..0000000000000000000000000000000000000000
diff --git a/spaces/VIPLab/Track-Anything/tracker/model/aggregate.py b/spaces/VIPLab/Track-Anything/tracker/model/aggregate.py
deleted file mode 100644
index 7622391fb3ac9aa8b515df88cf3ea5297b367538..0000000000000000000000000000000000000000
--- a/spaces/VIPLab/Track-Anything/tracker/model/aggregate.py
+++ /dev/null
@@ -1,17 +0,0 @@
-import torch
-import torch.nn.functional as F
-
-
-# Soft aggregation from STM
-def aggregate(prob, dim, return_logits=False):
- new_prob = torch.cat([
- torch.prod(1-prob, dim=dim, keepdim=True),
- prob
- ], dim).clamp(1e-7, 1-1e-7)
- logits = torch.log((new_prob /(1-new_prob)))
- prob = F.softmax(logits, dim=dim)
-
- if return_logits:
- return logits, prob
- else:
- return prob
\ No newline at end of file
diff --git a/spaces/VickyKira/NASAGPT/g4f/Provider/Providers/Lockchat.py b/spaces/VickyKira/NASAGPT/g4f/Provider/Providers/Lockchat.py
deleted file mode 100644
index 1bce74035403bf8615e68ccfcc9deb7e0151817a..0000000000000000000000000000000000000000
--- a/spaces/VickyKira/NASAGPT/g4f/Provider/Providers/Lockchat.py
+++ /dev/null
@@ -1,32 +0,0 @@
-import requests
-import os
-import json
-from ...typing import sha256, Dict, get_type_hints
-url = 'http://supertest.lockchat.app'
-model = ['gpt-4', 'gpt-3.5-turbo']
-supports_stream = True
-needs_auth = False
-
-def _create_completion(model: str, messages: list, stream: bool, temperature: float = 0.7, **kwargs):
-
- payload = {
- "temperature": 0.7,
- "messages": messages,
- "model": model,
- "stream": True,
- }
- headers = {
- "user-agent": "ChatX/39 CFNetwork/1408.0.4 Darwin/22.5.0",
- }
- response = requests.post("http://supertest.lockchat.app/v1/chat/completions",
- json=payload, headers=headers, stream=True)
- for token in response.iter_lines():
- if b'The model: `gpt-4` does not exist' in token:
- print('error, retrying...')
- _create_completion(model=model, messages=messages, stream=stream, temperature=temperature, **kwargs)
- if b"content" in token:
- token = json.loads(token.decode('utf-8').split('data: ')[1])['choices'][0]['delta'].get('content')
- if token: yield (token)
-
-params = f'g4f.Providers.{os.path.basename(__file__)[:-3]} supports: ' + \
- '(%s)' % ', '.join([f"{name}: {get_type_hints(_create_completion)[name].__name__}" for name in _create_completion.__code__.co_varnames[:_create_completion.__code__.co_argcount]])
\ No newline at end of file
diff --git a/spaces/Widium/Image-Recreation/functions/compute.py b/spaces/Widium/Image-Recreation/functions/compute.py
deleted file mode 100644
index 3305359a311d0666c4e8334076110bc84664f9e5..0000000000000000000000000000000000000000
--- a/spaces/Widium/Image-Recreation/functions/compute.py
+++ /dev/null
@@ -1,38 +0,0 @@
-# *************************************************************************** #
-# #
-# compute.py #
-# #
-# By: Widium #
-# Github : https://github.com/widium #
-# #
-# Created: 2023/05/05 18:51:08 by Widium #
-# Updated: 2023/05/05 18:51:08 by Widium #
-# #
-# **************************************************************************** #
-
-from tensorflow import Variable
-from tensorflow.keras.optimizers import Optimizer
-
-from .image import clip_pixel
-
-# ======================================== #
-
-def optimize_gradients(
- gradients,
- optimizer : Optimizer,
- generated_img : Variable,
-):
- """
- Optimize gradients, apply them to the generated image, and clip its pixel values.
-
- Args:
- gradients: The gradients to be optimized.
- optimizer: The optimizer used for optimizing the gradients.
- generated_img: The generated image variable that will be updated.
- loss: The loss value used for optimization.
-
- Returns:
- Variable: The updated generated image variable.
- """
- optimizer.apply_gradients(grads_and_vars=[(gradients, generated_img)])
- generated_img.assign(clip_pixel(generated_img))
\ No newline at end of file
diff --git a/spaces/Xhaheen/Regex_by_OpenAI/app.py b/spaces/Xhaheen/Regex_by_OpenAI/app.py
deleted file mode 100644
index 4c0a3e088aa993cd4c561b6b6708d8b25047a62d..0000000000000000000000000000000000000000
--- a/spaces/Xhaheen/Regex_by_OpenAI/app.py
+++ /dev/null
@@ -1,55 +0,0 @@
-import openai
-import numpy as np
-import os
-import json
-import gradio as gr
-
-openai.api_key = os.environ["api_key"]
-model = os.environ["model"]
-
-
-def happytt(temperature,max_tokens,text,stop):
- try:
- s = json.loads(stop)
- response = openai.Completion.create(
- model=model,
- prompt=text,
- temperature=temperature,
- max_tokens=max_tokens,
- top_p=1,
- frequency_penalty=0,
- presence_penalty=0,
- stop=s
- )
- except json.JSONDecodeError:
- response = openai.Completion.create(
- model=model,
- prompt=text,
- temperature=temperature,
- max_tokens=max_tokens,
- top_p=1,
- frequency_penalty=0,
- presence_penalty=0
- )
-
- return response.choices[0].text
-
-
-title = "OpenAI Codex"
-description = '''OpenAI Codex is an artificial intelligence model developed by OpenAI.
-It parses natural language and generates code in response.
-It is used to power GitHub Copilot, a programming autocompletion
-tool developed for Code generation.
-Try following prompts and tweak temperatures in following links -
-https://www.pragnakalp.com/experimenting-with-openai-codex/
-https://betterprogramming.pub/i-beta-tested-openais-codex-and-the-results-are-spooky-good-e282a1874c79
-https://beta.openai.com/examples?category=code'''
-
-
-iface = gr.Interface( happytt,[ gr.inputs.Slider(0, 1, step=0.1),gr.inputs.Slider(150, 4000, step=1),
- gr.inputs.Textbox(type='str',
- label="input prompt"),
- gr.inputs.Textbox(type='str',
- label="list of tokens, when to finish generating",
- placeholder='["", "import"]')],"text", title = title, description = description )
-iface.launch(debug=True)
\ No newline at end of file
diff --git a/spaces/XzJosh/JM-Bert-VITS2/README.md b/spaces/XzJosh/JM-Bert-VITS2/README.md
deleted file mode 100644
index 8f4a64e7ec2c9480563fc77753d4d2fd2f095da5..0000000000000000000000000000000000000000
--- a/spaces/XzJosh/JM-Bert-VITS2/README.md
+++ /dev/null
@@ -1,5 +0,0 @@
----
-license: mit
-sdk: gradio
-title: AI剑魔③
----
\ No newline at end of file
diff --git a/spaces/XzJosh/JM-Bert-VITS2/resample.py b/spaces/XzJosh/JM-Bert-VITS2/resample.py
deleted file mode 100644
index 2ed1685654a371c5722168e9987809b05b1cb224..0000000000000000000000000000000000000000
--- a/spaces/XzJosh/JM-Bert-VITS2/resample.py
+++ /dev/null
@@ -1,42 +0,0 @@
-import os
-import argparse
-import librosa
-import numpy as np
-from multiprocessing import Pool, cpu_count
-
-import soundfile
-from scipy.io import wavfile
-from tqdm import tqdm
-
-
-def process(item):
- spkdir, wav_name, args = item
- speaker = spkdir.replace("\\", "/").split("/")[-1]
- wav_path = os.path.join(args.in_dir, speaker, wav_name)
- if os.path.exists(wav_path) and '.wav' in wav_path:
- os.makedirs(os.path.join(args.out_dir, speaker), exist_ok=True)
- wav, sr = librosa.load(wav_path, sr=args.sr)
- soundfile.write(
- os.path.join(args.out_dir, speaker, wav_name),
- wav,
- sr
- )
-
-
-
-if __name__ == "__main__":
- parser = argparse.ArgumentParser()
- parser.add_argument("--sr", type=int, default=44100, help="sampling rate")
- parser.add_argument("--in_dir", type=str, default="./raw", help="path to source dir")
- parser.add_argument("--out_dir", type=str, default="./dataset", help="path to target dir")
- args = parser.parse_args()
- # processs = 8
- processs = cpu_count()-2 if cpu_count() >4 else 1
- pool = Pool(processes=processs)
-
- for speaker in os.listdir(args.in_dir):
- spk_dir = os.path.join(args.in_dir, speaker)
- if os.path.isdir(spk_dir):
- print(spk_dir)
- for _ in tqdm(pool.imap_unordered(process, [(spk_dir, i, args) for i in os.listdir(spk_dir) if i.endswith("wav")])):
- pass
diff --git a/spaces/XzJosh/ShanBao-Bert-VITS2/mel_processing.py b/spaces/XzJosh/ShanBao-Bert-VITS2/mel_processing.py
deleted file mode 100644
index 50435ecf88ef4fb6c1d47f3e6edd04c3ea7d3e80..0000000000000000000000000000000000000000
--- a/spaces/XzJosh/ShanBao-Bert-VITS2/mel_processing.py
+++ /dev/null
@@ -1,112 +0,0 @@
-import math
-import os
-import random
-import torch
-from torch import nn
-import torch.nn.functional as F
-import torch.utils.data
-import numpy as np
-import librosa
-import librosa.util as librosa_util
-from librosa.util import normalize, pad_center, tiny
-from scipy.signal import get_window
-from scipy.io.wavfile import read
-from librosa.filters import mel as librosa_mel_fn
-
-MAX_WAV_VALUE = 32768.0
-
-
-def dynamic_range_compression_torch(x, C=1, clip_val=1e-5):
- """
- PARAMS
- ------
- C: compression factor
- """
- return torch.log(torch.clamp(x, min=clip_val) * C)
-
-
-def dynamic_range_decompression_torch(x, C=1):
- """
- PARAMS
- ------
- C: compression factor used to compress
- """
- return torch.exp(x) / C
-
-
-def spectral_normalize_torch(magnitudes):
- output = dynamic_range_compression_torch(magnitudes)
- return output
-
-
-def spectral_de_normalize_torch(magnitudes):
- output = dynamic_range_decompression_torch(magnitudes)
- return output
-
-
-mel_basis = {}
-hann_window = {}
-
-
-def spectrogram_torch(y, n_fft, sampling_rate, hop_size, win_size, center=False):
- if torch.min(y) < -1.:
- print('min value is ', torch.min(y))
- if torch.max(y) > 1.:
- print('max value is ', torch.max(y))
-
- global hann_window
- dtype_device = str(y.dtype) + '_' + str(y.device)
- wnsize_dtype_device = str(win_size) + '_' + dtype_device
- if wnsize_dtype_device not in hann_window:
- hann_window[wnsize_dtype_device] = torch.hann_window(win_size).to(dtype=y.dtype, device=y.device)
-
- y = torch.nn.functional.pad(y.unsqueeze(1), (int((n_fft-hop_size)/2), int((n_fft-hop_size)/2)), mode='reflect')
- y = y.squeeze(1)
-
- spec = torch.stft(y, n_fft, hop_length=hop_size, win_length=win_size, window=hann_window[wnsize_dtype_device],
- center=center, pad_mode='reflect', normalized=False, onesided=True, return_complex=False)
-
- spec = torch.sqrt(spec.pow(2).sum(-1) + 1e-6)
- return spec
-
-
-def spec_to_mel_torch(spec, n_fft, num_mels, sampling_rate, fmin, fmax):
- global mel_basis
- dtype_device = str(spec.dtype) + '_' + str(spec.device)
- fmax_dtype_device = str(fmax) + '_' + dtype_device
- if fmax_dtype_device not in mel_basis:
- mel = librosa_mel_fn(sampling_rate, n_fft, num_mels, fmin, fmax)
- mel_basis[fmax_dtype_device] = torch.from_numpy(mel).to(dtype=spec.dtype, device=spec.device)
- spec = torch.matmul(mel_basis[fmax_dtype_device], spec)
- spec = spectral_normalize_torch(spec)
- return spec
-
-
-def mel_spectrogram_torch(y, n_fft, num_mels, sampling_rate, hop_size, win_size, fmin, fmax, center=False):
- if torch.min(y) < -1.:
- print('min value is ', torch.min(y))
- if torch.max(y) > 1.:
- print('max value is ', torch.max(y))
-
- global mel_basis, hann_window
- dtype_device = str(y.dtype) + '_' + str(y.device)
- fmax_dtype_device = str(fmax) + '_' + dtype_device
- wnsize_dtype_device = str(win_size) + '_' + dtype_device
- if fmax_dtype_device not in mel_basis:
- mel = librosa_mel_fn(sampling_rate, n_fft, num_mels, fmin, fmax)
- mel_basis[fmax_dtype_device] = torch.from_numpy(mel).to(dtype=y.dtype, device=y.device)
- if wnsize_dtype_device not in hann_window:
- hann_window[wnsize_dtype_device] = torch.hann_window(win_size).to(dtype=y.dtype, device=y.device)
-
- y = torch.nn.functional.pad(y.unsqueeze(1), (int((n_fft-hop_size)/2), int((n_fft-hop_size)/2)), mode='reflect')
- y = y.squeeze(1)
-
- spec = torch.stft(y, n_fft, hop_length=hop_size, win_length=win_size, window=hann_window[wnsize_dtype_device],
- center=center, pad_mode='reflect', normalized=False, onesided=True, return_complex=False)
-
- spec = torch.sqrt(spec.pow(2).sum(-1) + 1e-6)
-
- spec = torch.matmul(mel_basis[fmax_dtype_device], spec)
- spec = spectral_normalize_torch(spec)
-
- return spec
diff --git a/spaces/YE01/saya-vits/text/__init__.py b/spaces/YE01/saya-vits/text/__init__.py
deleted file mode 100644
index 48ae82f3e40ecd1bf17a7de78d87790327af3362..0000000000000000000000000000000000000000
--- a/spaces/YE01/saya-vits/text/__init__.py
+++ /dev/null
@@ -1,56 +0,0 @@
-""" from https://github.com/keithito/tacotron """
-from text import cleaners
-from text.symbols import symbols
-
-
-# Mappings from symbol to numeric ID and vice versa:
-_symbol_to_id = {s: i for i, s in enumerate(symbols)}
-_id_to_symbol = {i: s for i, s in enumerate(symbols)}
-
-
-def text_to_sequence(text, cleaner_names):
- '''Converts a string of text to a sequence of IDs corresponding to the symbols in the text.
- Args:
- text: string to convert to a sequence
- cleaner_names: names of the cleaner functions to run the text through
- Returns:
- List of integers corresponding to the symbols in the text
- '''
- sequence = []
-
- clean_text = _clean_text(text, cleaner_names)
- for symbol in clean_text:
- if symbol not in _symbol_to_id.keys():
- continue
- symbol_id = _symbol_to_id[symbol]
- sequence += [symbol_id]
- return sequence
-
-
-def cleaned_text_to_sequence(cleaned_text):
- '''Converts a string of text to a sequence of IDs corresponding to the symbols in the text.
- Args:
- text: string to convert to a sequence
- Returns:
- List of integers corresponding to the symbols in the text
- '''
- sequence = [_symbol_to_id[symbol] for symbol in cleaned_text if symbol in _symbol_to_id.keys()]
- return sequence
-
-
-def sequence_to_text(sequence):
- '''Converts a sequence of IDs back to a string'''
- result = ''
- for symbol_id in sequence:
- s = _id_to_symbol[symbol_id]
- result += s
- return result
-
-
-def _clean_text(text, cleaner_names):
- for name in cleaner_names:
- cleaner = getattr(cleaners, name)
- if not cleaner:
- raise Exception('Unknown cleaner: %s' % name)
- text = cleaner(text)
- return text
diff --git a/spaces/Yabo/ControlVideo/predict.py b/spaces/Yabo/ControlVideo/predict.py
deleted file mode 100644
index 4fedccae33520ea2725dc0e5cacf35e59c113813..0000000000000000000000000000000000000000
--- a/spaces/Yabo/ControlVideo/predict.py
+++ /dev/null
@@ -1,164 +0,0 @@
-# Prediction interface for Cog ⚙️
-# https://github.com/replicate/cog/blob/main/docs/python.md
-import os
-import numpy as np
-import argparse
-import imageio
-import torch
-
-from einops import rearrange
-from diffusers import DDIMScheduler, AutoencoderKL
-from transformers import CLIPTextModel, CLIPTokenizer
-import controlnet_aux
-from controlnet_aux import OpenposeDetector, CannyDetector, MidasDetector
-
-from models.pipeline_controlvideo import ControlVideoPipeline
-from models.util import save_videos_grid, read_video, get_annotation
-from models.unet import UNet3DConditionModel
-from models.controlnet import ControlNetModel3D
-from models.RIFE.IFNet_HDv3 import IFNet
-from cog import BasePredictor, Input, Path
-
-
-sd_path = "checkpoints/stable-diffusion-v1-5"
-inter_path = "checkpoints/flownet.pkl"
-controlnet_dict = {
- "pose": "checkpoints/sd-controlnet-openpose",
- "depth": "checkpoints/sd-controlnet-depth",
- "canny": "checkpoints/sd-controlnet-canny",
-}
-
-controlnet_parser_dict = {
- "pose": OpenposeDetector,
- "depth": MidasDetector,
- "canny": CannyDetector,
-}
-
-POS_PROMPT = " ,best quality, extremely detailed, HD, ultra-realistic, 8K, HQ, masterpiece, trending on artstation, art, smooth"
-NEG_PROMPT = "longbody, lowres, bad anatomy, bad hands, missing fingers, extra digit, fewer difits, cropped, worst quality, low quality, deformed body, bloated, ugly, unrealistic"
-
-
-class Predictor(BasePredictor):
- def setup(self):
- """Load the model into memory to make running multiple predictions efficient"""
-
- self.tokenizer = CLIPTokenizer.from_pretrained(sd_path, subfolder="tokenizer")
- self.text_encoder = CLIPTextModel.from_pretrained(
- sd_path, subfolder="text_encoder"
- ).to(dtype=torch.float16)
- self.vae = AutoencoderKL.from_pretrained(sd_path, subfolder="vae").to(
- dtype=torch.float16
- )
- self.unet = UNet3DConditionModel.from_pretrained_2d(
- sd_path, subfolder="unet"
- ).to(dtype=torch.float16)
- self.interpolater = IFNet(ckpt_path=inter_path).to(dtype=torch.float16)
- self.scheduler = DDIMScheduler.from_pretrained(sd_path, subfolder="scheduler")
- self.controlnet = {
- k: ControlNetModel3D.from_pretrained_2d(controlnet_dict[k]).to(
- dtype=torch.float16
- )
- for k in ["depth", "canny", "pose"]
- }
- self.annotator = {k: controlnet_parser_dict[k]() for k in ["depth", "canny"]}
- self.annotator["pose"] = controlnet_parser_dict["pose"].from_pretrained(
- "lllyasviel/ControlNet", cache_dir="checkpoints"
- )
-
- def predict(
- self,
- prompt: str = Input(
- description="Text description of target video",
- default="A striking mallard floats effortlessly on the sparkling pond.",
- ),
- video_path: Path = Input(description="source video"),
- condition: str = Input(
- default="depth",
- choices=["depth", "canny", "pose"],
- description="Condition of structure sequence",
- ),
- video_length: int = Input(
- default=15, description="Length of synthesized video"
- ),
- smoother_steps: str = Input(
- default="19, 20",
- description="Timesteps at which using interleaved-frame smoother, separate with comma",
- ),
- is_long_video: bool = Input(
- default=False,
- description="Whether to use hierarchical sampler to produce long video",
- ),
- num_inference_steps: int = Input(
- description="Number of denoising steps", default=50
- ),
- guidance_scale: float = Input(
- description="Scale for classifier-free guidance", ge=1, le=20, default=12.5
- ),
- seed: str = Input(
- default=None, description="Random seed. Leave blank to randomize the seed"
- ),
- ) -> Path:
- """Run a single prediction on the model"""
- if seed is None:
- seed = int.from_bytes(os.urandom(2), "big")
- else:
- seed = int(seed)
- print(f"Using seed: {seed}")
-
- generator = torch.Generator(device="cuda")
- generator.manual_seed(seed)
-
- pipe = ControlVideoPipeline(
- vae=self.vae,
- text_encoder=self.text_encoder,
- tokenizer=self.tokenizer,
- unet=self.unet,
- controlnet=self.controlnet[condition],
- interpolater=self.interpolater,
- scheduler=self.scheduler,
- )
-
- pipe.enable_vae_slicing()
- pipe.enable_xformers_memory_efficient_attention()
- pipe.to("cuda")
-
- # Step 1. Read a video
- video = read_video(video_path=str(video_path), video_length=video_length)
-
- # Step 2. Parse a video to conditional frames
- pil_annotation = get_annotation(video, self.annotator[condition])
-
- # Step 3. inference
- smoother_steps = [int(s) for s in smoother_steps.split(",")]
-
- if is_long_video:
- window_size = int(np.sqrt(video_length))
- sample = pipe.generate_long_video(
- prompt + POS_PROMPT,
- video_length=video_length,
- frames=pil_annotation,
- num_inference_steps=num_inference_steps,
- smooth_steps=smoother_steps,
- window_size=window_size,
- generator=generator,
- guidance_scale=guidance_scale,
- negative_prompt=NEG_PROMPT,
- ).videos
- else:
- sample = pipe(
- prompt + POS_PROMPT,
- video_length=video_length,
- frames=pil_annotation,
- num_inference_steps=num_inference_steps,
- smooth_steps=smoother_steps,
- generator=generator,
- guidance_scale=guidance_scale,
- negative_prompt=NEG_PROMPT,
- ).videos
-
- out_path = "/tmp/out.mp4"
- save_videos_grid(sample, out_path)
- del pipe
- torch.cuda.empty_cache()
-
- return Path(out_path)
diff --git a/spaces/Yiqin/ChatVID/app.py b/spaces/Yiqin/ChatVID/app.py
deleted file mode 100644
index 3b9e5e0566db0f6c73b667031b883b5cf1b61ad9..0000000000000000000000000000000000000000
--- a/spaces/Yiqin/ChatVID/app.py
+++ /dev/null
@@ -1,101 +0,0 @@
-import time
-
-import gradio as gr
-
-from config.config_utils import get_config
-from model import Captioner, VicunaHandler
-
-
-def mirror(x):
- return x
-
-
-def clear_chat(conv_template):
- return "", [], conv_template
-
-
-def clear_four():
- return [], "", "", ""
-
-
-def respond(input, chat_history, conv):
- bot_response, new_conv = handler.gr_chat(input, conv)
- chat_history.append((input, bot_response))
- time.sleep(0.1)
- return "", chat_history, new_conv
-
-
-# global variables
-config = get_config('config/infer.yaml')
-captioner = Captioner(config)
-handler = VicunaHandler(config['vicuna'])
-
-with gr.Blocks(theme=gr.themes.Soft()) as demo:
- gr.Markdown("## ChatVID ")
- gr.Markdown("""🔥 [ChatVID](https://github.com/InvincibleWyq/ChatVID) is a
- video chatbot. Please give us a ⭐ Star!""")
- gr.Markdown("""🎥 You may use the example video by clicking it.""")
- gr.Markdown("""🚀 For any questions or suggestions, feel free to drop Yiqin
- an email at wyq1217@outlook.com
- or open an issue.""")
-
- with gr.Row():
- with gr.Column():
- video_path = gr.Video(label="Video")
-
- with gr.Column():
- upload_button = gr.Button("""Upload & Process.
- (Click and wait 3min until dialog box appears)""")
-
- num_frames = gr.Slider(
- minimum=5,
- value=12,
- maximum=12,
- step=1,
- label="Number of frames")
-
- gr.Markdown("## Video Examples")
- gr.Examples(
- examples=[
- "examples/cook_720p.mp4",
- "examples/temple_of_heaven_720p.mp4"
- ],
- inputs=video_path,
- outputs=video_path,
- fn=mirror,
- cache_examples=False,
- )
-
- with gr.Column():
- caption_box = gr.Textbox("")
- chatbot = gr.Chatbot()
- conv_template = gr.State("") # determined by the video
- conv = gr.State("") # updated thourghout the conversation
- with gr.Row(visible=False) as input:
- with gr.Column(scale=0.7):
- txt = gr.Textbox(
- show_label=False,
- placeholder="Enter text and press enter").style(
- container=False)
- with gr.Column(scale=0.15, min_width=0):
- run_button = gr.Button("RUN!")
- with gr.Column(scale=0.15, min_width=0):
- clear_button = gr.Button("CLEAR")
-
- # conv_template and conv are `Conversation` objects
- upload_button.click(lambda: gr.update(visible=False), None, input).then(
- clear_four, None, [chatbot, conv, conv_template, caption_box]).then(
- captioner.caption_video, [video_path, num_frames],
- [conv_template]).then(mirror, [conv_template], [caption_box]).then(
- handler.gr_chatbot_init, [conv_template],
- [conv_template, conv]).then(lambda: gr.update(visible=True),
- None, input)
-
- txt.submit(
- respond, inputs=[txt, chatbot, conv], outputs=[txt, chatbot, conv])
- run_button.click(
- respond, inputs=[txt, chatbot, conv], outputs=[txt, chatbot, conv])
- clear_button.click(
- clear_chat, inputs=[conv_template], outputs=[txt, chatbot, conv])
-
-demo.queue(default_enabled=False).launch()
diff --git a/spaces/Yiqin/ChatVID/model/vision/grit_src/third_party/CenterNet2/detectron2/evaluation/rotated_coco_evaluation.py b/spaces/Yiqin/ChatVID/model/vision/grit_src/third_party/CenterNet2/detectron2/evaluation/rotated_coco_evaluation.py
deleted file mode 100644
index ea6d1b381dcf106339a03f08577df673ad439c46..0000000000000000000000000000000000000000
--- a/spaces/Yiqin/ChatVID/model/vision/grit_src/third_party/CenterNet2/detectron2/evaluation/rotated_coco_evaluation.py
+++ /dev/null
@@ -1,207 +0,0 @@
-# Copyright (c) Facebook, Inc. and its affiliates.
-import itertools
-import json
-import numpy as np
-import os
-import torch
-from pycocotools.cocoeval import COCOeval, maskUtils
-
-from detectron2.structures import BoxMode, RotatedBoxes, pairwise_iou_rotated
-from detectron2.utils.file_io import PathManager
-
-from .coco_evaluation import COCOEvaluator
-
-
-class RotatedCOCOeval(COCOeval):
- @staticmethod
- def is_rotated(box_list):
- if type(box_list) == np.ndarray:
- return box_list.shape[1] == 5
- elif type(box_list) == list:
- if box_list == []: # cannot decide the box_dim
- return False
- return np.all(
- np.array(
- [
- (len(obj) == 5) and ((type(obj) == list) or (type(obj) == np.ndarray))
- for obj in box_list
- ]
- )
- )
- return False
-
- @staticmethod
- def boxlist_to_tensor(boxlist, output_box_dim):
- if type(boxlist) == np.ndarray:
- box_tensor = torch.from_numpy(boxlist)
- elif type(boxlist) == list:
- if boxlist == []:
- return torch.zeros((0, output_box_dim), dtype=torch.float32)
- else:
- box_tensor = torch.FloatTensor(boxlist)
- else:
- raise Exception("Unrecognized boxlist type")
-
- input_box_dim = box_tensor.shape[1]
- if input_box_dim != output_box_dim:
- if input_box_dim == 4 and output_box_dim == 5:
- box_tensor = BoxMode.convert(box_tensor, BoxMode.XYWH_ABS, BoxMode.XYWHA_ABS)
- else:
- raise Exception(
- "Unable to convert from {}-dim box to {}-dim box".format(
- input_box_dim, output_box_dim
- )
- )
- return box_tensor
-
- def compute_iou_dt_gt(self, dt, gt, is_crowd):
- if self.is_rotated(dt) or self.is_rotated(gt):
- # TODO: take is_crowd into consideration
- assert all(c == 0 for c in is_crowd)
- dt = RotatedBoxes(self.boxlist_to_tensor(dt, output_box_dim=5))
- gt = RotatedBoxes(self.boxlist_to_tensor(gt, output_box_dim=5))
- return pairwise_iou_rotated(dt, gt)
- else:
- # This is the same as the classical COCO evaluation
- return maskUtils.iou(dt, gt, is_crowd)
-
- def computeIoU(self, imgId, catId):
- p = self.params
- if p.useCats:
- gt = self._gts[imgId, catId]
- dt = self._dts[imgId, catId]
- else:
- gt = [_ for cId in p.catIds for _ in self._gts[imgId, cId]]
- dt = [_ for cId in p.catIds for _ in self._dts[imgId, cId]]
- if len(gt) == 0 and len(dt) == 0:
- return []
- inds = np.argsort([-d["score"] for d in dt], kind="mergesort")
- dt = [dt[i] for i in inds]
- if len(dt) > p.maxDets[-1]:
- dt = dt[0 : p.maxDets[-1]]
-
- assert p.iouType == "bbox", "unsupported iouType for iou computation"
-
- g = [g["bbox"] for g in gt]
- d = [d["bbox"] for d in dt]
-
- # compute iou between each dt and gt region
- iscrowd = [int(o["iscrowd"]) for o in gt]
-
- # Note: this function is copied from cocoeval.py in cocoapi
- # and the major difference is here.
- ious = self.compute_iou_dt_gt(d, g, iscrowd)
- return ious
-
-
-class RotatedCOCOEvaluator(COCOEvaluator):
- """
- Evaluate object proposal/instance detection outputs using COCO-like metrics and APIs,
- with rotated boxes support.
- Note: this uses IOU only and does not consider angle differences.
- """
-
- def process(self, inputs, outputs):
- """
- Args:
- inputs: the inputs to a COCO model (e.g., GeneralizedRCNN).
- It is a list of dict. Each dict corresponds to an image and
- contains keys like "height", "width", "file_name", "image_id".
- outputs: the outputs of a COCO model. It is a list of dicts with key
- "instances" that contains :class:`Instances`.
- """
- for input, output in zip(inputs, outputs):
- prediction = {"image_id": input["image_id"]}
-
- if "instances" in output:
- instances = output["instances"].to(self._cpu_device)
-
- prediction["instances"] = self.instances_to_json(instances, input["image_id"])
- if "proposals" in output:
- prediction["proposals"] = output["proposals"].to(self._cpu_device)
- self._predictions.append(prediction)
-
- def instances_to_json(self, instances, img_id):
- num_instance = len(instances)
- if num_instance == 0:
- return []
-
- boxes = instances.pred_boxes.tensor.numpy()
- if boxes.shape[1] == 4:
- boxes = BoxMode.convert(boxes, BoxMode.XYXY_ABS, BoxMode.XYWH_ABS)
- boxes = boxes.tolist()
- scores = instances.scores.tolist()
- classes = instances.pred_classes.tolist()
-
- results = []
- for k in range(num_instance):
- result = {
- "image_id": img_id,
- "category_id": classes[k],
- "bbox": boxes[k],
- "score": scores[k],
- }
-
- results.append(result)
- return results
-
- def _eval_predictions(self, predictions, img_ids=None): # img_ids: unused
- """
- Evaluate predictions on the given tasks.
- Fill self._results with the metrics of the tasks.
- """
- self._logger.info("Preparing results for COCO format ...")
- coco_results = list(itertools.chain(*[x["instances"] for x in predictions]))
-
- # unmap the category ids for COCO
- if hasattr(self._metadata, "thing_dataset_id_to_contiguous_id"):
- reverse_id_mapping = {
- v: k for k, v in self._metadata.thing_dataset_id_to_contiguous_id.items()
- }
- for result in coco_results:
- result["category_id"] = reverse_id_mapping[result["category_id"]]
-
- if self._output_dir:
- file_path = os.path.join(self._output_dir, "coco_instances_results.json")
- self._logger.info("Saving results to {}".format(file_path))
- with PathManager.open(file_path, "w") as f:
- f.write(json.dumps(coco_results))
- f.flush()
-
- if not self._do_evaluation:
- self._logger.info("Annotations are not available for evaluation.")
- return
-
- self._logger.info("Evaluating predictions ...")
-
- assert self._tasks is None or set(self._tasks) == {
- "bbox"
- }, "[RotatedCOCOEvaluator] Only bbox evaluation is supported"
- coco_eval = (
- self._evaluate_predictions_on_coco(self._coco_api, coco_results)
- if len(coco_results) > 0
- else None # cocoapi does not handle empty results very well
- )
-
- task = "bbox"
- res = self._derive_coco_results(
- coco_eval, task, class_names=self._metadata.get("thing_classes")
- )
- self._results[task] = res
-
- def _evaluate_predictions_on_coco(self, coco_gt, coco_results):
- """
- Evaluate the coco results using COCOEval API.
- """
- assert len(coco_results) > 0
-
- coco_dt = coco_gt.loadRes(coco_results)
-
- # Only bbox is supported for now
- coco_eval = RotatedCOCOeval(coco_gt, coco_dt, iouType="bbox")
-
- coco_eval.evaluate()
- coco_eval.accumulate()
- coco_eval.summarize()
-
- return coco_eval
diff --git a/spaces/Yuki1111/Yuki/Dockerfile b/spaces/Yuki1111/Yuki/Dockerfile
deleted file mode 100644
index 6c01c09373883afcb4ea34ae2d316cd596e1737b..0000000000000000000000000000000000000000
--- a/spaces/Yuki1111/Yuki/Dockerfile
+++ /dev/null
@@ -1,21 +0,0 @@
-FROM node:18-bullseye-slim
-
-RUN apt-get update && \
-
-apt-get install -y git
-
-RUN git clone https://gitgud.io/khanon/oai-reverse-proxy.git /app
-
-WORKDIR /app
-
-RUN npm install
-
-COPY Dockerfile greeting.md* .env* ./
-
-RUN npm run build
-
-EXPOSE 7860
-
-ENV NODE_ENV=production
-
-CMD [ "npm", "start" ]
\ No newline at end of file
diff --git a/spaces/Yuliang/ICON/lib/net/HGFilters.py b/spaces/Yuliang/ICON/lib/net/HGFilters.py
deleted file mode 100644
index b8578cc42fb6c2630fea884ea86e5d53ab5f6d5d..0000000000000000000000000000000000000000
--- a/spaces/Yuliang/ICON/lib/net/HGFilters.py
+++ /dev/null
@@ -1,197 +0,0 @@
-
-# -*- coding: utf-8 -*-
-
-# Max-Planck-Gesellschaft zur Förderung der Wissenschaften e.V. (MPG) is
-# holder of all proprietary rights on this computer program.
-# You can only use this computer program if you have closed
-# a license agreement with MPG or you get the right to use the computer
-# program from someone who is authorized to grant you that right.
-# Any use of the computer program without a valid license is prohibited and
-# liable to prosecution.
-#
-# Copyright©2019 Max-Planck-Gesellschaft zur Förderung
-# der Wissenschaften e.V. (MPG). acting on behalf of its Max Planck Institute
-# for Intelligent Systems. All rights reserved.
-#
-# Contact: ps-license@tuebingen.mpg.de
-
-from lib.net.net_util import *
-import torch.nn as nn
-import torch.nn.functional as F
-
-
-class HourGlass(nn.Module):
- def __init__(self, num_modules, depth, num_features, opt):
- super(HourGlass, self).__init__()
- self.num_modules = num_modules
- self.depth = depth
- self.features = num_features
- self.opt = opt
-
- self._generate_network(self.depth)
-
- def _generate_network(self, level):
- self.add_module('b1_' + str(level),
- ConvBlock(self.features, self.features, self.opt))
-
- self.add_module('b2_' + str(level),
- ConvBlock(self.features, self.features, self.opt))
-
- if level > 1:
- self._generate_network(level - 1)
- else:
- self.add_module('b2_plus_' + str(level),
- ConvBlock(self.features, self.features, self.opt))
-
- self.add_module('b3_' + str(level),
- ConvBlock(self.features, self.features, self.opt))
-
- def _forward(self, level, inp):
- # Upper branch
- up1 = inp
- up1 = self._modules['b1_' + str(level)](up1)
-
- # Lower branch
- low1 = F.avg_pool2d(inp, 2, stride=2)
- low1 = self._modules['b2_' + str(level)](low1)
-
- if level > 1:
- low2 = self._forward(level - 1, low1)
- else:
- low2 = low1
- low2 = self._modules['b2_plus_' + str(level)](low2)
-
- low3 = low2
- low3 = self._modules['b3_' + str(level)](low3)
-
- # NOTE: for newer PyTorch (1.3~), it seems that training results are degraded due to implementation diff in F.grid_sample
- # if the pretrained model behaves weirdly, switch with the commented line.
- # NOTE: I also found that "bicubic" works better.
- up2 = F.interpolate(low3,
- scale_factor=2,
- mode='bicubic',
- align_corners=True)
- # up2 = F.interpolate(low3, scale_factor=2, mode='nearest)
-
- return up1 + up2
-
- def forward(self, x):
- return self._forward(self.depth, x)
-
-
-class HGFilter(nn.Module):
- def __init__(self, opt, num_modules, in_dim):
- super(HGFilter, self).__init__()
- self.num_modules = num_modules
-
- self.opt = opt
- [k, s, d, p] = self.opt.conv1
-
- # self.conv1 = nn.Conv2d(in_dim, 64, kernel_size=7, stride=2, padding=3)
- self.conv1 = nn.Conv2d(in_dim,
- 64,
- kernel_size=k,
- stride=s,
- dilation=d,
- padding=p)
-
- if self.opt.norm == 'batch':
- self.bn1 = nn.BatchNorm2d(64)
- elif self.opt.norm == 'group':
- self.bn1 = nn.GroupNorm(32, 64)
-
- if self.opt.hg_down == 'conv64':
- self.conv2 = ConvBlock(64, 64, self.opt)
- self.down_conv2 = nn.Conv2d(64,
- 128,
- kernel_size=3,
- stride=2,
- padding=1)
- elif self.opt.hg_down == 'conv128':
- self.conv2 = ConvBlock(64, 128, self.opt)
- self.down_conv2 = nn.Conv2d(128,
- 128,
- kernel_size=3,
- stride=2,
- padding=1)
- elif self.opt.hg_down == 'ave_pool':
- self.conv2 = ConvBlock(64, 128, self.opt)
- else:
- raise NameError('Unknown Fan Filter setting!')
-
- self.conv3 = ConvBlock(128, 128, self.opt)
- self.conv4 = ConvBlock(128, 256, self.opt)
-
- # Stacking part
- for hg_module in range(self.num_modules):
- self.add_module('m' + str(hg_module),
- HourGlass(1, opt.num_hourglass, 256, self.opt))
-
- self.add_module('top_m_' + str(hg_module),
- ConvBlock(256, 256, self.opt))
- self.add_module(
- 'conv_last' + str(hg_module),
- nn.Conv2d(256, 256, kernel_size=1, stride=1, padding=0))
- if self.opt.norm == 'batch':
- self.add_module('bn_end' + str(hg_module), nn.BatchNorm2d(256))
- elif self.opt.norm == 'group':
- self.add_module('bn_end' + str(hg_module),
- nn.GroupNorm(32, 256))
-
- self.add_module(
- 'l' + str(hg_module),
- nn.Conv2d(256,
- opt.hourglass_dim,
- kernel_size=1,
- stride=1,
- padding=0))
-
- if hg_module < self.num_modules - 1:
- self.add_module(
- 'bl' + str(hg_module),
- nn.Conv2d(256, 256, kernel_size=1, stride=1, padding=0))
- self.add_module(
- 'al' + str(hg_module),
- nn.Conv2d(opt.hourglass_dim,
- 256,
- kernel_size=1,
- stride=1,
- padding=0))
-
- def forward(self, x):
- x = F.relu(self.bn1(self.conv1(x)), True)
- tmpx = x
- if self.opt.hg_down == 'ave_pool':
- x = F.avg_pool2d(self.conv2(x), 2, stride=2)
- elif self.opt.hg_down in ['conv64', 'conv128']:
- x = self.conv2(x)
- x = self.down_conv2(x)
- else:
- raise NameError('Unknown Fan Filter setting!')
-
- x = self.conv3(x)
- x = self.conv4(x)
-
- previous = x
-
- outputs = []
- for i in range(self.num_modules):
- hg = self._modules['m' + str(i)](previous)
-
- ll = hg
- ll = self._modules['top_m_' + str(i)](ll)
-
- ll = F.relu(
- self._modules['bn_end' + str(i)](
- self._modules['conv_last' + str(i)](ll)), True)
-
- # Predict heatmaps
- tmp_out = self._modules['l' + str(i)](ll)
- outputs.append(tmp_out)
-
- if i < self.num_modules - 1:
- ll = self._modules['bl' + str(i)](ll)
- tmp_out_ = self._modules['al' + str(i)](tmp_out)
- previous = previous + ll + tmp_out_
-
- return outputs
diff --git a/spaces/ZJunTvT/ZJunChat/modules/shared.py b/spaces/ZJunTvT/ZJunChat/modules/shared.py
deleted file mode 100644
index a9e72580aa7ae48f907e923a09099513570a9ad8..0000000000000000000000000000000000000000
--- a/spaces/ZJunTvT/ZJunChat/modules/shared.py
+++ /dev/null
@@ -1,55 +0,0 @@
-from modules.presets import COMPLETION_URL, BALANCE_API_URL, USAGE_API_URL, API_HOST
-import os
-import queue
-
-class State:
- interrupted = False
- multi_api_key = False
- completion_url = COMPLETION_URL
- balance_api_url = BALANCE_API_URL
- usage_api_url = USAGE_API_URL
-
- def interrupt(self):
- self.interrupted = True
-
- def recover(self):
- self.interrupted = False
-
- def set_api_host(self, api_host):
- self.completion_url = f"https://{api_host}/v1/chat/completions"
- self.balance_api_url = f"https://{api_host}/dashboard/billing/credit_grants"
- self.usage_api_url = f"https://{api_host}/dashboard/billing/usage"
- os.environ["OPENAI_API_BASE"] = f"https://{api_host}/v1"
-
- def reset_api_host(self):
- self.completion_url = COMPLETION_URL
- self.balance_api_url = BALANCE_API_URL
- self.usage_api_url = USAGE_API_URL
- os.environ["OPENAI_API_BASE"] = f"https://{API_HOST}/v1"
- return API_HOST
-
- def reset_all(self):
- self.interrupted = False
- self.completion_url = COMPLETION_URL
-
- def set_api_key_queue(self, api_key_list):
- self.multi_api_key = True
- self.api_key_queue = queue.Queue()
- for api_key in api_key_list:
- self.api_key_queue.put(api_key)
-
- def switching_api_key(self, func):
- if not hasattr(self, "api_key_queue"):
- return func
-
- def wrapped(*args, **kwargs):
- api_key = self.api_key_queue.get()
- args[0].api_key = api_key
- ret = func(*args, **kwargs)
- self.api_key_queue.put(api_key)
- return ret
-
- return wrapped
-
-
-state = State()
diff --git a/spaces/ZeroGPT/GPTZero/README.md b/spaces/ZeroGPT/GPTZero/README.md
deleted file mode 100644
index 7bf482036a19a665c243f209eeb306a4ee31c2b6..0000000000000000000000000000000000000000
--- a/spaces/ZeroGPT/GPTZero/README.md
+++ /dev/null
@@ -1,18 +0,0 @@
----
-title: GPTZero Alternative - AI Content Detector - ZeroGPT.CC
-emoji: 🐠
-colorFrom: purple
-colorTo: indigo
-sdk: gradio
-sdk_version: 3.27.0
-app_file: app.py
-pinned: false
-license: mit
----
-
-# GPTZero Alternative - AI Content Detector - ZeroGPT.CC
-ZeroGPT.cc is a state-of-the-art tool that can help you verify whether a text was generated by an AI tool such as Open AI ChatGPT, Google Bard, and Bing AI. This free platform uses advanced language models and sophisticated algorithms to analyze and identify content accurately.
-
-By using ZeroGPT.cc, you can be confident that your content is original and meets your high standards of quality. Whether you're a writer, a marketer, or a business owner, ZeroGPT.cc can streamline your content creation and management process, saving you time and effort in the long run.
-
-Check out website for more details: [AI Content Detector](https://zerogpt.cc)
diff --git a/spaces/aaaaaabbbbbbbdddddddduuuuulllll/Ashaar/app.py b/spaces/aaaaaabbbbbbbdddddddduuuuulllll/Ashaar/app.py
deleted file mode 100644
index 580d3b353dfe066a53293417f4380121aaa5827b..0000000000000000000000000000000000000000
--- a/spaces/aaaaaabbbbbbbdddddddduuuuulllll/Ashaar/app.py
+++ /dev/null
@@ -1,151 +0,0 @@
-import os
-os.environ['TF_CPP_MIN_LOG_LEVEL'] = '3'
-import gradio as gr
-from transformers import pipeline
-from transformers import AutoTokenizer, AutoModelForCausalLM
-from Ashaar.utils import get_output_df, get_highlighted_patterns_html
-from Ashaar.bait_analysis import BaitAnalysis
-from langs import *
-import sys
-import json
-import argparse
-
-arg_parser = argparse.ArgumentParser()
-arg_parser.add_argument('--lang', type = str, default = 'ar')
-args = arg_parser.parse_args()
-lang = args.lang
-
-if lang == 'ar':
- TITLE = TITLE_ar
- DESCRIPTION = DESCRIPTION_ar
- textbox_trg_text = textbox_trg_text_ar
- textbox_inp_text = textbox_inp_text_ar
- btn_trg_text = btn_trg_text_ar
- btn_inp_text = btn_inp_text_ar
- css = """ #textbox{ direction: RTL;}"""
-
-else:
- TITLE = TITLE_en
- DESCRIPTION = DESCRIPTION_en
- textbox_trg_text = textbox_trg_text_en
- textbox_inp_text = textbox_inp_text_en
- btn_trg_text = btn_trg_text_en
- btn_inp_text = btn_inp_text_en
- css = ""
-
-gpt_tokenizer = AutoTokenizer.from_pretrained('arbml/ashaar_tokenizer')
-model = AutoModelForCausalLM.from_pretrained('arbml/Ashaar_model')
-
-theme_to_token = json.load(open("extra/theme_tokens.json", "r"))
-token_to_theme = {t:m for m,t in theme_to_token.items()}
-meter_to_token = json.load(open("extra/meter_tokens.json", "r"))
-token_to_meter = {t:m for m,t in meter_to_token.items()}
-
-analysis = BaitAnalysis()
-meter, theme, qafiyah = "", "", ""
-
-def analyze(poem):
- global meter,theme,qafiyah, generate_btn
- shatrs = poem.split("\n")
- baits = [' # '.join(shatrs[2*i:2*i+2]) for i in range(len(shatrs)//2)]
- output = analysis.analyze(baits,override_tashkeel=True)
- meter = output['meter']
- qafiyah = output['qafiyah'][0]
- theme = output['theme'][-1]
- df = get_output_df(output)
- return get_highlighted_patterns_html(df), gr.Button.update(interactive=True)
-
-def generate(inputs, top_p = 3):
- baits = inputs.split('\n')
- if len(baits) % 2 !=0:
- baits = baits[:-1]
- poem = ' '.join(['<|bsep|> '+baits[i]+' <|vsep|> '+baits[i+1]+' |bsep|>' for i in range(0, len(baits), 2)])
- prompt = f"""
- {meter_to_token[meter]} {qafiyah} {theme_to_token[theme]}
- <|psep|>
- {poem}
- """.strip()
- print(prompt)
- encoded_input = gpt_tokenizer(prompt, return_tensors='pt')
- output = model.generate(**encoded_input, max_length = 512, top_p = 3, do_sample=True)
-
- result = ""
- prev_token = ""
- line_cnts = 0
- for i, beam in enumerate(output[:, len(encoded_input.input_ids[0]):]):
- if line_cnts >= 10:
- break
- for token in beam:
- if line_cnts >= 10:
- break
- decoded = gpt_tokenizer.decode(token)
- if 'meter' in decoded or 'theme' in decoded:
- break
- if decoded in ["<|vsep|>", "|bsep|>"]:
- result += "\n"
- line_cnts+=1
- elif decoded in ['<|bsep|>', '<|psep|>', '|psep|>']:
- pass
- else:
- result += decoded
- prev_token = decoded
- else:
- break
- # return theme+" "+ f"من بحر {meter} مع قافية بحر ({qafiyah})" + "\n" +result
- return result, gr.Button.update(interactive=False)
-
-examples = [
- [
-"""القلب أعلم يا عذول بدائه
-وأحق منك بجفنه وبمائه"""
- ],
- [
-"""رمتِ الفؤادَ مليحة عذراءُ
- بسهامِ لحظٍ ما لهنَّ دواءُ"""
- ],
- [
-"""أذَلَّ الحِرْصُ والطَّمَعُ الرِّقابَا
-وقَد يَعفو الكَريمُ، إذا استَرَابَا"""
- ]
-]
-
-with gr.Blocks(theme=gr.themes.Soft(), css=css) as demo:
- with gr.Row():
- with gr.Column():
- gr.HTML(TITLE)
- gr.HTML(DESCRIPTION)
-
- with gr.Row():
- with gr.Column():
- textbox_output = gr.Textbox(lines=10, label=textbox_trg_text, elem_id="textbox")
- with gr.Column():
- inputs = gr.Textbox(lines=10, label=textbox_inp_text, elem_id="textbox")
-
-
- with gr.Row():
- with gr.Column():
- if lang == 'ar':
- trg_btn = gr.Button(btn_trg_text, interactive=False)
- else:
- trg_btn = gr.Button(btn_trg_text)
-
- with gr.Column():
- if lang == 'ar':
- inp_btn = gr.Button(btn_inp_text)
- else:
- inp_btn = gr.Button(btn_inp_text, interactive = False)
-
- with gr.Row():
- html_output = gr.HTML()
-
- if lang == 'en':
- gr.Examples(examples, textbox_output)
- inp_btn.click(generate, inputs = textbox_output, outputs=[inputs, inp_btn])
- trg_btn.click(analyze, inputs = textbox_output, outputs=[html_output,inp_btn])
- else:
- gr.Examples(examples, inputs)
- trg_btn.click(generate, inputs = inputs, outputs=[textbox_output, trg_btn])
- inp_btn.click(analyze, inputs = inputs, outputs=[html_output,trg_btn] )
-
-# demo.launch(server_name = '0.0.0.0', share=True)
-demo.launch()
\ No newline at end of file
diff --git a/spaces/aaronherrera/Calorie_Counter/README.md b/spaces/aaronherrera/Calorie_Counter/README.md
deleted file mode 100644
index 81e13282a6cd285e755faaa3578821e036481f99..0000000000000000000000000000000000000000
--- a/spaces/aaronherrera/Calorie_Counter/README.md
+++ /dev/null
@@ -1,13 +0,0 @@
----
-title: Calorie_Counter
-emoji: 🔥
-colorFrom: yellow
-colorTo: gray
-sdk: gradio
-sdk_version: 2.8.14
-app_file: app.py
-pinned: false
-license: apache-2.0
----
-
-Check out the configuration reference at https://huggingface.co/docs/hub/spaces#reference
diff --git a/spaces/abhaskumarsinha/MinimalGPT-Felis_Catus/README.md b/spaces/abhaskumarsinha/MinimalGPT-Felis_Catus/README.md
deleted file mode 100644
index d90a9617d0e09bd81278b23b82de65a9a1a2a0a8..0000000000000000000000000000000000000000
--- a/spaces/abhaskumarsinha/MinimalGPT-Felis_Catus/README.md
+++ /dev/null
@@ -1,65 +0,0 @@
----
-license: mit
-title: 'MinimalGPT: Felis Catus'
-sdk: gradio
-emoji: 😻
-colorFrom: gray
-colorTo: blue
-pinned: true
----
-
-# MinimalGPT: Felis Catus
-
-[[`MinimalGPT`](https://github.com/abhaskumarsinha/MinimalGPT)] [[`Project Gutenberg Dataset`](https://www.kaggle.com/datasets/shubchat/1002-short-stories-from-project-guttenberg)]
-
-
-This HuggingFace space serves as an illustrative application of the GitHub Repository: [MinimalGPT](https://github.com/abhaskumarsinha/MinimalGPT), which embodies a departure from conventional GPT models that undergo scaling and training on high-performance computing systems and clusters. The primary objective of the MinimalGPT project was to explore the extent to which a GPT model could be minimized in size.
-
-Within this HF space, we introduce a diminutive GPT model named [Felis Catus](https://en.wikipedia.org/wiki/Cat) (stray Cat), which boasts a mere 15 million parameters. What distinguishes this model is its training process, which was executed on a standard home computer CPU (specifically, an AMD Ryzen 5) without any reliance on GPU acceleration. Remarkably, the training duration lasted a mere 15 minutes, utilizing a dataset comprising a meager ~150,000 tokens of text.
-
-At present, the Felis Catus model exhibits the capacity to generate a concise story excerpt consisting of 70 tokens, requiring a mere 5 to 7 words as input. The model's dictionary encompasses a modest 12,000 words. Moreover, we are presently engaged in endeavors to further scale the model in our forthcoming project.
-
-## Model Specifications
-
-```
-Model: "model"
-_________________________________________________________________
- Layer (type) Output Shape Param #
-=================================================================
- input_1 (InputLayer) [(None, 10)] 0
-
- embedding (Embedding) (None, 10, 128) 1597184
-
- positional_embedding (Posit (None, 10, 128) 0
- ionalEmbedding)
-
- decoder (Decoder) (None, 10, 128) 71208
-
- flatten (Flatten) (None, 1280) 0
-
- dense (Dense) (None, 12479) 15985599
-
- tf.nn.softmax (TFOpLambda) (None, 12479) 0
-
-=================================================================
-Total params: 17,653,991
-Trainable params: 17,653,991
-Non-trainable params: 0
-_________________________________________________________________
-```
-
-## Hyperparameters
-
-```
-gpt_input: 10 [Max input size, d_k]
-d_model: 128 [Embedding size, d_model]
-h: 8 [Number of multiheads, h]
-decoder_stacks: 1 [Number of decoder stacks, stack]
-GPT_attention: True [Attention Layer implementation type - OpenAI style]
-```
-
-## References
-1. Vaswani, Ashish, et al. "Attention is all you need." Advances in neural information processing systems 30 (2017).
-2. Radford, Alec, et al. "Language models are unsupervised multitask learners." OpenAI blog 1.8 (2019): 9.
-3. Project Gutenberg. (n.d.). Retrieved FebruApril 20, 2023, from www.gutenberg.org.
-4. Abadi, Martın, et al. "TensorFlow: Large-scale machine learning on heterogeneous systems, software available from tensorflow. org (2015)." URL https://www.tensorflow.org (2015).
\ No newline at end of file
diff --git a/spaces/abhishek/sketch-to-image/annotator/uniformer_base/mmseg/core/utils/__init__.py b/spaces/abhishek/sketch-to-image/annotator/uniformer_base/mmseg/core/utils/__init__.py
deleted file mode 100644
index f2678b321c295bcceaef945111ac3524be19d6e4..0000000000000000000000000000000000000000
--- a/spaces/abhishek/sketch-to-image/annotator/uniformer_base/mmseg/core/utils/__init__.py
+++ /dev/null
@@ -1,3 +0,0 @@
-from .misc import add_prefix
-
-__all__ = ['add_prefix']
diff --git a/spaces/abidlabs/Webcam-background-remover/app.py b/spaces/abidlabs/Webcam-background-remover/app.py
deleted file mode 100644
index 6987d628c8d3875b8fcc062c1692b76cd1d0e751..0000000000000000000000000000000000000000
--- a/spaces/abidlabs/Webcam-background-remover/app.py
+++ /dev/null
@@ -1,9 +0,0 @@
-import gradio as gr
-gr.Interface.load(
- "spaces/eugenesiow/remove-bg",
- theme="default",
- css=".footer {display:none !important}",
- inputs="webcam",
- title=None,
- article=None,
- description="This demo (based on: https://huggingface.co/spaces/eugenesiow/remove-bg) removes the background from your webcam photo!").launch()
\ No newline at end of file
diff --git a/spaces/abrar-lohia/text-2-character-anim/pyrender/.eggs/pyglet-2.0.5-py3.10.egg/pyglet/libs/darwin/cocoapy/cocoatypes.py b/spaces/abrar-lohia/text-2-character-anim/pyrender/.eggs/pyglet-2.0.5-py3.10.egg/pyglet/libs/darwin/cocoapy/cocoatypes.py
deleted file mode 100644
index b30019e89576030042d6bd399d6f7c56afe56287..0000000000000000000000000000000000000000
--- a/spaces/abrar-lohia/text-2-character-anim/pyrender/.eggs/pyglet-2.0.5-py3.10.egg/pyglet/libs/darwin/cocoapy/cocoatypes.py
+++ /dev/null
@@ -1,85 +0,0 @@
-from ctypes import *
-
-import sys, platform, struct
-
-__LP64__ = (8*struct.calcsize("P") == 64)
-__i386__ = (platform.machine() == 'i386')
-
-PyObjectEncoding = b'{PyObject=@}'
-
-def encoding_for_ctype(vartype):
- typecodes = {c_char:b'c', c_int:b'i', c_short:b's', c_long:b'l', c_longlong:b'q',
- c_ubyte:b'C', c_uint:b'I', c_ushort:b'S', c_ulong:b'L', c_ulonglong:b'Q',
- c_float:b'f', c_double:b'd', c_bool:b'B', c_char_p:b'*', c_void_p:b'@',
- py_object:PyObjectEncoding}
- return typecodes.get(vartype, b'?')
-
-# Note CGBase.h located at
-# /System/Library/Frameworks/ApplicationServices.framework/Frameworks/CoreGraphics.framework/Headers/CGBase.h
-# defines CGFloat as double if __LP64__, otherwise it's a float.
-if __LP64__:
- NSInteger = c_long
- NSUInteger = c_ulong
- CGFloat = c_double
- NSPointEncoding = b'{CGPoint=dd}'
- NSSizeEncoding = b'{CGSize=dd}'
- NSRectEncoding = b'{CGRect={CGPoint=dd}{CGSize=dd}}'
- NSRangeEncoding = b'{_NSRange=QQ}'
-else:
- NSInteger = c_int
- NSUInteger = c_uint
- CGFloat = c_float
- NSPointEncoding = b'{_NSPoint=ff}'
- NSSizeEncoding = b'{_NSSize=ff}'
- NSRectEncoding = b'{_NSRect={_NSPoint=ff}{_NSSize=ff}}'
- NSRangeEncoding = b'{_NSRange=II}'
-
-NSIntegerEncoding = encoding_for_ctype(NSInteger)
-NSUIntegerEncoding = encoding_for_ctype(NSUInteger)
-CGFloatEncoding = encoding_for_ctype(CGFloat)
-
-# Special case so that NSImage.initWithCGImage_size_() will work.
-CGImageEncoding = b'{CGImage=}'
-
-NSZoneEncoding = b'{_NSZone=}'
-
-# from /System/Library/Frameworks/Foundation.framework/Headers/NSGeometry.h
-class NSPoint(Structure):
- _fields_ = [ ("x", CGFloat), ("y", CGFloat) ]
-CGPoint = NSPoint
-
-class NSSize(Structure):
- _fields_ = [ ("width", CGFloat), ("height", CGFloat) ]
-CGSize = NSSize
-
-class NSRect(Structure):
- _fields_ = [ ("origin", NSPoint), ("size", NSSize) ]
-CGRect = NSRect
-
-def NSMakeSize(w, h):
- return NSSize(w, h)
-
-def NSMakeRect(x, y, w, h):
- return NSRect(NSPoint(x, y), NSSize(w, h))
-
-# NSDate.h
-NSTimeInterval = c_double
-
-CFIndex = c_long
-UniChar = c_ushort
-unichar = c_wchar # (actually defined as c_ushort in NSString.h, but need ctypes to convert properly)
-CGGlyph = c_ushort
-
-# CFRange struct defined in CFBase.h
-# This replaces the CFRangeMake(LOC, LEN) macro.
-class CFRange(Structure):
- _fields_ = [ ("location", CFIndex), ("length", CFIndex) ]
-
-# NSRange.h (Note, not defined the same as CFRange)
-class NSRange(Structure):
- _fields_ = [ ("location", NSUInteger), ("length", NSUInteger) ]
-
-NSZeroPoint = NSPoint(0,0)
-
-CFTypeID = c_ulong
-CFNumberType = c_uint32
diff --git a/spaces/ahmedgamal777722/flowise/Dockerfile b/spaces/ahmedgamal777722/flowise/Dockerfile
deleted file mode 100644
index 9c0ad22929159b8c4d192856163699570fd27307..0000000000000000000000000000000000000000
--- a/spaces/ahmedgamal777722/flowise/Dockerfile
+++ /dev/null
@@ -1,26 +0,0 @@
-FROM node:18-alpine
-USER root
-
-# Arguments that can be passed at build time
-ARG FLOWISE_PATH=/usr/local/lib/node_modules/flowise
-ARG BASE_PATH=/root/.flowise
-ARG DATABASE_PATH=$BASE_PATH
-ARG APIKEY_PATH=$BASE_PATH
-ARG SECRETKEY_PATH=$BASE_PATH
-ARG LOG_PATH=$BASE_PATH/logs
-
-# Install dependencies
-RUN apk add --no-cache git python3 py3-pip make g++ build-base cairo-dev pango-dev chromium
-
-ENV PUPPETEER_SKIP_DOWNLOAD=true
-ENV PUPPETEER_EXECUTABLE_PATH=/usr/bin/chromium-browser
-
-# Install Flowise globally
-RUN npm install -g flowise
-
-# Configure Flowise directories using the ARG
-RUN mkdir -p $LOG_PATH $FLOWISE_PATH/uploads && chmod -R 777 $LOG_PATH $FLOWISE_PATH
-
-WORKDIR /data
-
-CMD ["npx", "flowise", "start"]
\ No newline at end of file
diff --git a/spaces/ai-forever/Kandinsky2.1/README.md b/spaces/ai-forever/Kandinsky2.1/README.md
deleted file mode 100644
index bfa1dc422e96cef5190028c0e3dde6be6fc94c14..0000000000000000000000000000000000000000
--- a/spaces/ai-forever/Kandinsky2.1/README.md
+++ /dev/null
@@ -1,12 +0,0 @@
----
-title: Kandinsky2.1
-emoji: 📉
-colorFrom: indigo
-colorTo: green
-sdk: gradio
-sdk_version: 3.11.0
-app_file: app.py
-pinned: false
----
-
-Check out the configuration reference at https://huggingface.co/docs/hub/spaces-config-reference
diff --git a/spaces/akalin/DeepDanbooru_string/app.py b/spaces/akalin/DeepDanbooru_string/app.py
deleted file mode 100644
index 49019837c9207cc68cb37be0342f3bc44fd0decb..0000000000000000000000000000000000000000
--- a/spaces/akalin/DeepDanbooru_string/app.py
+++ /dev/null
@@ -1,185 +0,0 @@
-#!/usr/bin/env python
-
-from __future__ import annotations
-
-import argparse
-import functools
-import os
-import html
-import pathlib
-import tarfile
-
-import deepdanbooru as dd
-import gradio as gr
-import huggingface_hub
-import numpy as np
-import PIL.Image
-import tensorflow as tf
-import piexif
-import piexif.helper
-
-TITLE = 'DeepDanbooru String'
-
-TOKEN = os.environ['TOKEN']
-MODEL_REPO = 'CikeyQI/DeepDanbooru_string'
-MODEL_FILENAME = 'model-resnet_custom_v3.h5'
-LABEL_FILENAME = 'tags.txt'
-
-
-def parse_args() -> argparse.Namespace:
- parser = argparse.ArgumentParser()
- parser.add_argument('--score-slider-step', type=float, default=0.05)
- parser.add_argument('--score-threshold', type=float, default=0.5)
- parser.add_argument('--theme', type=str, default='dark-grass')
- parser.add_argument('--live', action='store_true')
- parser.add_argument('--share', action='store_true')
- parser.add_argument('--port', type=int)
- parser.add_argument('--disable-queue',
- dest='enable_queue',
- action='store_false')
- parser.add_argument('--allow-flagging', type=str, default='never')
- return parser.parse_args()
-
-
-def load_sample_image_paths() -> list[pathlib.Path]:
- image_dir = pathlib.Path('images')
- if not image_dir.exists():
- dataset_repo = 'hysts/sample-images-TADNE'
- path = huggingface_hub.hf_hub_download(dataset_repo,
- 'images.tar.gz',
- repo_type='dataset',
- use_auth_token=TOKEN)
- with tarfile.open(path) as f:
- f.extractall()
- return sorted(image_dir.glob('*'))
-
-
-def load_model() -> tf.keras.Model:
- path = huggingface_hub.hf_hub_download(MODEL_REPO,
- MODEL_FILENAME,
- use_auth_token=TOKEN)
- model = tf.keras.models.load_model(path)
- return model
-
-
-def load_labels() -> list[str]:
- path = huggingface_hub.hf_hub_download(MODEL_REPO,
- LABEL_FILENAME,
- use_auth_token=TOKEN)
- with open(path) as f:
- labels = [line.strip() for line in f.readlines()]
- return labels
-
-def plaintext_to_html(text):
- text = "" + " \n".join([f"{html.escape(x)}" for x in text.split('\n')]) + "
"
- return text
-
-def predict(image: PIL.Image.Image, score_threshold: float,
- model: tf.keras.Model, labels: list[str]) -> dict[str, float]:
- rawimage = image
- _, height, width, _ = model.input_shape
- image = np.asarray(image)
- image = tf.image.resize(image,
- size=(height, width),
- method=tf.image.ResizeMethod.AREA,
- preserve_aspect_ratio=True)
- image = image.numpy()
- image = dd.image.transform_and_pad_image(image, width, height)
- image = image / 255.
- probs = model.predict(image[None, ...])[0]
- probs = probs.astype(float)
- res = dict()
- for prob, label in zip(probs.tolist(), labels):
- if prob < score_threshold:
- continue
- res[label] = prob
- b = dict(sorted(res.items(),key=lambda item:item[1], reverse=True))
- a = ', '.join(list(b.keys())).replace('_',' ').replace('(','\(').replace(')','\)')
- c = ', '.join(list(b.keys()))
-
- items = rawimage.info
- geninfo = ''
-
- if "exif" in rawimage.info:
- exif = piexif.load(rawimage.info["exif"])
- exif_comment = (exif or {}).get("Exif", {}).get(piexif.ExifIFD.UserComment, b'')
- try:
- exif_comment = piexif.helper.UserComment.load(exif_comment)
- except ValueError:
- exif_comment = exif_comment.decode('utf8', errors="ignore")
-
- items['exif comment'] = exif_comment
- geninfo = exif_comment
-
- for field in ['jfif', 'jfif_version', 'jfif_unit', 'jfif_density', 'dpi', 'exif',
- 'loop', 'background', 'timestamp', 'duration']:
- items.pop(field, None)
-
- geninfo = items.get('parameters', geninfo)
-
- info = f"""
-
PNG Info
-"""
- for key, text in items.items():
- info += f"""
-
-
{plaintext_to_html(str(key))}
-
{plaintext_to_html(str(text))}
-
-""".strip()+"\n"
-
- if len(info) == 0:
- message = "Nothing found in the image."
- info = f""
-
- return (a,c,res,info)
-
-
-def main():
- args = parse_args()
- model = load_model()
- labels = load_labels()
-
- func = functools.partial(predict, model=model, labels=labels)
- func = functools.update_wrapper(func, predict)
-
- gr.Interface(
- func,
- [
- gr.inputs.Image(type='pil', label='Input'),
- gr.inputs.Slider(0,
- 1,
- step=args.score_slider_step,
- default=args.score_threshold,
- label='Score Threshold'),
- ],
- [
- gr.outputs.Textbox(label='Output (string)'),
- gr.outputs.Textbox(label='Output (raw string)'),
- gr.outputs.Label(label='Output (label)'),
- gr.outputs.HTML()
- ],
- examples=[
- ['miku.jpg',0.5],
- ['miku2.jpg',0.5]
- ],
- title=TITLE,
- description='''
-Demo for [KichangKim/DeepDanbooru](https://github.com/KichangKim/DeepDanbooru) with "ready to copy" prompt and a prompt analyzer.
-
-Modified from [hysts/DeepDanbooru](https://huggingface.co/spaces/hysts/DeepDanbooru)
-
-PNG Info code forked from [AUTOMATIC1111/stable-diffusion-webui](https://github.com/AUTOMATIC1111/stable-diffusion-webui)
- ''',
- theme=args.theme,
- allow_flagging=args.allow_flagging,
- live=args.live,
- ).launch(
- enable_queue=args.enable_queue,
- server_port=args.port,
- share=args.share,
- )
-
-
-if __name__ == '__main__':
- main()
diff --git a/spaces/akdeniz27/turkish-qna-with-xlm-roberta/app.py b/spaces/akdeniz27/turkish-qna-with-xlm-roberta/app.py
deleted file mode 100644
index ba57693a30ce7b7b8e4f2dce7e2f70e1a47c4f14..0000000000000000000000000000000000000000
--- a/spaces/akdeniz27/turkish-qna-with-xlm-roberta/app.py
+++ /dev/null
@@ -1,82 +0,0 @@
-# Turkish Q&A with XLM-RoBERTa
-
-from transformers import AutoTokenizer, AutoModelForQuestionAnswering, pipeline
-import sentencepiece
-import torch
-import streamlit as st
-import pandas as pd
-
-text_1 = """Mustafa Kemal Atatürk, 1881 yılında Selanik'te Kocakasım Mahallesi, Islahhane Caddesi'ndeki üç katlı pembe evde doğdu. \
-Babası Ali Rıza Efendi, annesi Zübeyde Hanım'dır. Baba tarafından dedesi Hafız Ahmet Efendi, 14-15. yüzyıllarda Konya ve Aydın'dan \
-Makedonya'ya yerleştirilmiş Kocacık Yörüklerindendir. Annesi Zübeyde Hanım ise Selanik yakınlarındaki Langaza kasabasına yerleşmiş \
-eski bir Türk ailesinin kızıdır. Ali Rıza Efendi, 1871 yılında Zübeyde Hanım'la evlendi. Atatürk'ün beş kardeşinden dördü küçük \
-yaşlarda öldü, sadece Makbule (Atadan) Hanım 1956 yılına değin yaşadı."""
-
-
-text_2 = """Dünya çapında 40 milyondan fazla insana bulaşan ve 1.1 milyondan fazla insanın ölümüne sebep olan \
-corona virüsüne karşı Pfizer ile BioNTech'in geliştirdiği aşının ilk görüntüleri ortaya çıktı. Aşının fabrikadaki \
-ilk görüntülerini değerlendiren Pfizer'ın Birleşik Krallık CEO'su, "Üretim bandında aşıyı görmek beni neşelendirdi" \
-dedi. ABD merkezli çokuluslu ilaç şirketi Pfizer ile Türk bilim insanlarının kurduğu BioNTech’in geliştirdiği corona \
-virüsü aşısında sona gelindi. Pfizer, paylaştığı video ile bütün dünyayı heyecanlandıran gelişmeyi duyurdu. Şirket, \
-Belçika’daki Puurs’ta geliştirilen Covid-19 aşılarının seri üretim bandındaki üretim aşamasını uluslararası kamuoyu \
-ile paylaştı. Almanya’nın Mainz kentinde Türk profesör Uğur Şahin ile eşi Özlem Türeci’nin kurduğu ve yönettiği \
-biyoteknoloji şirketi BioNTech ile aşı sürecini sürdüren Pfizer’ın küçük şişelerde binlerce corona virüsü aşısı \
-üretmeye başladığı belirtildi. Pfizer, aşının güvenli ve etkili olduğunun klinik olarak da kanıtlanması ve resmi \
-mercilerden de onay alınması durumunda üretilen aşının dağıtılacağını duyurdu."""
-
-question_list_1 = ["Mustafa Kemal hangi yıl doğdu?",
- "Mustafa Kemal'in dedesi kimdir?"]
-
-question_list_2 = ["Corona virüsü dünya çapında kaç kişiye bulaştı?",
- "BioNTech nerededir?" ]
-
-st.set_page_config(layout="wide")
-
-st.title("Turkish Q&A with Multilingual \
- XLM-RoBERTa Models")
-
-model_list = ['alon-albalak/xlm-roberta-large-xquad',
- 'deepset/xlm-roberta-large-squad2']
-
-st.sidebar.header("Select Model")
-model_checkpoint = st.sidebar.radio("", model_list)
-
-st.sidebar.write("For details of models:")
-st.sidebar.write("https://huggingface.co/alon-albalak")
-st.sidebar.write("https://huggingface.co/deepset")
-
-st.sidebar.write("For XQUAD Dataset:")
-st.sidebar.write("https://huggingface.co/datasets/xquad")
-
-st.subheader("Select Context and Question")
-context_1 = st.text_area("Context #1", text_1, height=128)
-context_2 = st.text_area("Context #2", text_2, height=128)
-context_3 = st.text_area("New Context", value="", height=128)
-
-context = st.radio("Select Context", ("Context #1", "Context #2", "New Context"))
-
-if context == "Context #1":
- selected_context = context_1
- selected_question = st.radio("Select Question", question_list_1)
-elif context == "Context #2":
- selected_context = context_2
- selected_question = st.radio("Select Question", question_list_2)
-elif context == "New Context":
- selected_context = context_3
- selected_question = st.text_area("New Question", value="", height=64)
-
-@st.cache_resource
-def setModel(model_checkpoint):
- model = AutoModelForQuestionAnswering.from_pretrained(model_checkpoint)
- tokenizer = AutoTokenizer.from_pretrained(model_checkpoint)
- return pipeline("question-answering", model=model, tokenizer=tokenizer)
-
-Run_Button = st.button("Run", key=None)
-if Run_Button == True:
-
- qna_pipeline = setModel(model_checkpoint)
- output = qna_pipeline(question=selected_question, context=selected_context)
-
- st.header("Answer")
- st.write(output)
-
\ No newline at end of file
diff --git a/spaces/akhaliq/Detic/detic/modeling/meta_arch/custom_rcnn.py b/spaces/akhaliq/Detic/detic/modeling/meta_arch/custom_rcnn.py
deleted file mode 100644
index 9a5ac721d42e40a8b4f28508b10a932cef827fcf..0000000000000000000000000000000000000000
--- a/spaces/akhaliq/Detic/detic/modeling/meta_arch/custom_rcnn.py
+++ /dev/null
@@ -1,232 +0,0 @@
-# Copyright (c) Facebook, Inc. and its affiliates.
-import copy
-import logging
-import numpy as np
-from typing import Dict, List, Optional, Tuple
-import torch
-from torch import nn
-import json
-from detectron2.utils.events import get_event_storage
-from detectron2.config import configurable
-from detectron2.structures import ImageList, Instances, Boxes
-import detectron2.utils.comm as comm
-
-from detectron2.modeling.meta_arch.build import META_ARCH_REGISTRY
-from detectron2.modeling.meta_arch.rcnn import GeneralizedRCNN
-from detectron2.modeling.postprocessing import detector_postprocess
-from detectron2.utils.visualizer import Visualizer, _create_text_labels
-from detectron2.data.detection_utils import convert_image_to_rgb
-
-from torch.cuda.amp import autocast
-from ..text.text_encoder import build_text_encoder
-from ..utils import load_class_freq, get_fed_loss_inds
-
-@META_ARCH_REGISTRY.register()
-class CustomRCNN(GeneralizedRCNN):
- '''
- Add image labels
- '''
- @configurable
- def __init__(
- self,
- with_image_labels = False,
- dataset_loss_weight = [],
- fp16 = False,
- sync_caption_batch = False,
- roi_head_name = '',
- cap_batch_ratio = 4,
- with_caption = False,
- dynamic_classifier = False,
- **kwargs):
- """
- """
- self.with_image_labels = with_image_labels
- self.dataset_loss_weight = dataset_loss_weight
- self.fp16 = fp16
- self.with_caption = with_caption
- self.sync_caption_batch = sync_caption_batch
- self.roi_head_name = roi_head_name
- self.cap_batch_ratio = cap_batch_ratio
- self.dynamic_classifier = dynamic_classifier
- self.return_proposal = False
- if self.dynamic_classifier:
- self.freq_weight = kwargs.pop('freq_weight')
- self.num_classes = kwargs.pop('num_classes')
- self.num_sample_cats = kwargs.pop('num_sample_cats')
- super().__init__(**kwargs)
- assert self.proposal_generator is not None
- if self.with_caption:
- assert not self.dynamic_classifier
- self.text_encoder = build_text_encoder(pretrain=True)
- for v in self.text_encoder.parameters():
- v.requires_grad = False
-
-
- @classmethod
- def from_config(cls, cfg):
- ret = super().from_config(cfg)
- ret.update({
- 'with_image_labels': cfg.WITH_IMAGE_LABELS,
- 'dataset_loss_weight': cfg.MODEL.DATASET_LOSS_WEIGHT,
- 'fp16': cfg.FP16,
- 'with_caption': cfg.MODEL.WITH_CAPTION,
- 'sync_caption_batch': cfg.MODEL.SYNC_CAPTION_BATCH,
- 'dynamic_classifier': cfg.MODEL.DYNAMIC_CLASSIFIER,
- 'roi_head_name': cfg.MODEL.ROI_HEADS.NAME,
- 'cap_batch_ratio': cfg.MODEL.CAP_BATCH_RATIO,
- })
- if ret['dynamic_classifier']:
- ret['freq_weight'] = load_class_freq(
- cfg.MODEL.ROI_BOX_HEAD.CAT_FREQ_PATH,
- cfg.MODEL.ROI_BOX_HEAD.FED_LOSS_FREQ_WEIGHT)
- ret['num_classes'] = cfg.MODEL.ROI_HEADS.NUM_CLASSES
- ret['num_sample_cats'] = cfg.MODEL.NUM_SAMPLE_CATS
- return ret
-
-
- def inference(
- self,
- batched_inputs: Tuple[Dict[str, torch.Tensor]],
- detected_instances: Optional[List[Instances]] = None,
- do_postprocess: bool = True,
- ):
- assert not self.training
- assert detected_instances is None
-
- images = self.preprocess_image(batched_inputs)
- features = self.backbone(images.tensor)
- proposals, _ = self.proposal_generator(images, features, None)
- results, _ = self.roi_heads(images, features, proposals)
- if do_postprocess:
- assert not torch.jit.is_scripting(), \
- "Scripting is not supported for postprocess."
- return CustomRCNN._postprocess(
- results, batched_inputs, images.image_sizes)
- else:
- return results
-
-
- def forward(self, batched_inputs: List[Dict[str, torch.Tensor]]):
- """
- Add ann_type
- Ignore proposal loss when training with image labels
- """
- if not self.training:
- return self.inference(batched_inputs)
-
- images = self.preprocess_image(batched_inputs)
-
- ann_type = 'box'
- gt_instances = [x["instances"].to(self.device) for x in batched_inputs]
- if self.with_image_labels:
- for inst, x in zip(gt_instances, batched_inputs):
- inst._ann_type = x['ann_type']
- inst._pos_category_ids = x['pos_category_ids']
- ann_types = [x['ann_type'] for x in batched_inputs]
- assert len(set(ann_types)) == 1
- ann_type = ann_types[0]
- if ann_type in ['prop', 'proptag']:
- for t in gt_instances:
- t.gt_classes *= 0
-
- if self.fp16: # TODO (zhouxy): improve
- with autocast():
- features = self.backbone(images.tensor.half())
- features = {k: v.float() for k, v in features.items()}
- else:
- features = self.backbone(images.tensor)
-
- cls_features, cls_inds, caption_features = None, None, None
-
- if self.with_caption and 'caption' in ann_type:
- inds = [torch.randint(len(x['captions']), (1,))[0].item() \
- for x in batched_inputs]
- caps = [x['captions'][ind] for ind, x in zip(inds, batched_inputs)]
- caption_features = self.text_encoder(caps).float()
- if self.sync_caption_batch:
- caption_features = self._sync_caption_features(
- caption_features, ann_type, len(batched_inputs))
-
- if self.dynamic_classifier and ann_type != 'caption':
- cls_inds = self._sample_cls_inds(gt_instances, ann_type) # inds, inv_inds
- ind_with_bg = cls_inds[0].tolist() + [-1]
- cls_features = self.roi_heads.box_predictor[
- 0].cls_score.zs_weight[:, ind_with_bg].permute(1, 0).contiguous()
-
- classifier_info = cls_features, cls_inds, caption_features
- proposals, proposal_losses = self.proposal_generator(
- images, features, gt_instances)
-
- if self.roi_head_name in ['StandardROIHeads', 'CascadeROIHeads']:
- proposals, detector_losses = self.roi_heads(
- images, features, proposals, gt_instances)
- else:
- proposals, detector_losses = self.roi_heads(
- images, features, proposals, gt_instances,
- ann_type=ann_type, classifier_info=classifier_info)
-
- if self.vis_period > 0:
- storage = get_event_storage()
- if storage.iter % self.vis_period == 0:
- self.visualize_training(batched_inputs, proposals)
-
- losses = {}
- losses.update(detector_losses)
- if self.with_image_labels:
- if ann_type in ['box', 'prop', 'proptag']:
- losses.update(proposal_losses)
- else: # ignore proposal loss for non-bbox data
- losses.update({k: v * 0 for k, v in proposal_losses.items()})
- else:
- losses.update(proposal_losses)
- if len(self.dataset_loss_weight) > 0:
- dataset_sources = [x['dataset_source'] for x in batched_inputs]
- assert len(set(dataset_sources)) == 1
- dataset_source = dataset_sources[0]
- for k in losses:
- losses[k] *= self.dataset_loss_weight[dataset_source]
-
- if self.return_proposal:
- return proposals, losses
- else:
- return losses
-
-
- def _sync_caption_features(self, caption_features, ann_type, BS):
- has_caption_feature = (caption_features is not None)
- BS = (BS * self.cap_batch_ratio) if (ann_type == 'box') else BS
- rank = torch.full(
- (BS, 1), comm.get_rank(), dtype=torch.float32,
- device=self.device)
- if not has_caption_feature:
- caption_features = rank.new_zeros((BS, 512))
- caption_features = torch.cat([caption_features, rank], dim=1)
- global_caption_features = comm.all_gather(caption_features)
- caption_features = torch.cat(
- [x.to(self.device) for x in global_caption_features], dim=0) \
- if has_caption_feature else None # (NB) x (D + 1)
- return caption_features
-
-
- def _sample_cls_inds(self, gt_instances, ann_type='box'):
- if ann_type == 'box':
- gt_classes = torch.cat(
- [x.gt_classes for x in gt_instances])
- C = len(self.freq_weight)
- freq_weight = self.freq_weight
- else:
- gt_classes = torch.cat(
- [torch.tensor(
- x._pos_category_ids,
- dtype=torch.long, device=x.gt_classes.device) \
- for x in gt_instances])
- C = self.num_classes
- freq_weight = None
- assert gt_classes.max() < C, '{} {}'.format(gt_classes.max(), C)
- inds = get_fed_loss_inds(
- gt_classes, self.num_sample_cats, C,
- weight=freq_weight)
- cls_id_map = gt_classes.new_full(
- (self.num_classes + 1,), len(inds))
- cls_id_map[inds] = torch.arange(len(inds), device=cls_id_map.device)
- return inds, cls_id_map
\ No newline at end of file
diff --git a/spaces/akhaliq/Mask2Former/mask2former/evaluation/instance_evaluation.py b/spaces/akhaliq/Mask2Former/mask2former/evaluation/instance_evaluation.py
deleted file mode 100644
index bc2facec351e5f6ee965ee9acb4394f12c023f54..0000000000000000000000000000000000000000
--- a/spaces/akhaliq/Mask2Former/mask2former/evaluation/instance_evaluation.py
+++ /dev/null
@@ -1,107 +0,0 @@
-# Copyright (c) Facebook, Inc. and its affiliates.
-import contextlib
-import copy
-import io
-import itertools
-import json
-import logging
-import numpy as np
-import os
-import pickle
-from collections import OrderedDict
-import pycocotools.mask as mask_util
-import torch
-from pycocotools.coco import COCO
-from pycocotools.cocoeval import COCOeval
-from tabulate import tabulate
-
-import detectron2.utils.comm as comm
-from detectron2.config import CfgNode
-from detectron2.data import MetadataCatalog
-from detectron2.data.datasets.coco import convert_to_coco_json
-from detectron2.evaluation.coco_evaluation import COCOEvaluator, _evaluate_predictions_on_coco
-from detectron2.evaluation.fast_eval_api import COCOeval_opt
-from detectron2.structures import Boxes, BoxMode, pairwise_iou
-from detectron2.utils.file_io import PathManager
-from detectron2.utils.logger import create_small_table
-
-
-# modified from COCOEvaluator for instance segmetnat
-class InstanceSegEvaluator(COCOEvaluator):
- """
- Evaluate AR for object proposals, AP for instance detection/segmentation, AP
- for keypoint detection outputs using COCO's metrics.
- See http://cocodataset.org/#detection-eval and
- http://cocodataset.org/#keypoints-eval to understand its metrics.
- The metrics range from 0 to 100 (instead of 0 to 1), where a -1 or NaN means
- the metric cannot be computed (e.g. due to no predictions made).
-
- In addition to COCO, this evaluator is able to support any bounding box detection,
- instance segmentation, or keypoint detection dataset.
- """
-
- def _eval_predictions(self, predictions, img_ids=None):
- """
- Evaluate predictions. Fill self._results with the metrics of the tasks.
- """
- self._logger.info("Preparing results for COCO format ...")
- coco_results = list(itertools.chain(*[x["instances"] for x in predictions]))
- tasks = self._tasks or self._tasks_from_predictions(coco_results)
-
- # unmap the category ids for COCO
- if hasattr(self._metadata, "thing_dataset_id_to_contiguous_id"):
- dataset_id_to_contiguous_id = self._metadata.thing_dataset_id_to_contiguous_id
- # all_contiguous_ids = list(dataset_id_to_contiguous_id.values())
- # num_classes = len(all_contiguous_ids)
- # assert min(all_contiguous_ids) == 0 and max(all_contiguous_ids) == num_classes - 1
-
- reverse_id_mapping = {v: k for k, v in dataset_id_to_contiguous_id.items()}
- for result in coco_results:
- category_id = result["category_id"]
- # assert category_id < num_classes, (
- # f"A prediction has class={category_id}, "
- # f"but the dataset only has {num_classes} classes and "
- # f"predicted class id should be in [0, {num_classes - 1}]."
- # )
- assert category_id in reverse_id_mapping, (
- f"A prediction has class={category_id}, "
- f"but the dataset only has class ids in {dataset_id_to_contiguous_id}."
- )
- result["category_id"] = reverse_id_mapping[category_id]
-
- if self._output_dir:
- file_path = os.path.join(self._output_dir, "coco_instances_results.json")
- self._logger.info("Saving results to {}".format(file_path))
- with PathManager.open(file_path, "w") as f:
- f.write(json.dumps(coco_results))
- f.flush()
-
- if not self._do_evaluation:
- self._logger.info("Annotations are not available for evaluation.")
- return
-
- self._logger.info(
- "Evaluating predictions with {} COCO API...".format(
- "unofficial" if self._use_fast_impl else "official"
- )
- )
- for task in sorted(tasks):
- assert task in {"bbox", "segm", "keypoints"}, f"Got unknown task: {task}!"
- coco_eval = (
- _evaluate_predictions_on_coco(
- self._coco_api,
- coco_results,
- task,
- kpt_oks_sigmas=self._kpt_oks_sigmas,
- use_fast_impl=self._use_fast_impl,
- img_ids=img_ids,
- max_dets_per_image=self._max_dets_per_image,
- )
- if len(coco_results) > 0
- else None # cocoapi does not handle empty results very well
- )
-
- res = self._derive_coco_results(
- coco_eval, task, class_names=self._metadata.get("thing_classes")
- )
- self._results[task] = res
diff --git a/spaces/akhaliq/encoder4editing/app.py b/spaces/akhaliq/encoder4editing/app.py
deleted file mode 100644
index 0c3287f5f5057a97f26f3e8dab5b558396889963..0000000000000000000000000000000000000000
--- a/spaces/akhaliq/encoder4editing/app.py
+++ /dev/null
@@ -1,29 +0,0 @@
-import os
-import gradio as gr
-
-os.system("git clone https://github.com/AK391/realworld-stylegan2-encoder.git")
-os.chdir("realworld-stylegan2-encoder")
-os.system("pip install gdown")
-os.system("pip install dlib")
-os.system("gdown https://drive.google.com/uc?id=1i873OKcKjvpxiF0UBU4NzxlMMaD9qR5z")
-os.system("wget https://github.com/kim-ninh/align_face_ffhq/raw/main/shape_predictor_68_face_landmarks.dat -P .")
-os.system("wget https://i.imgur.com/dJVNQSF.jpg -O ./mona.jpg")
-
-def inference(image):
- os.system("python scripts/test.py --align --ckpt ./e4e_encode_mobile_cartoon.pt --network e4e --platform torch --size 1024 --images_path "+image.name)
- return "out.png"
-
-title = "Encoder4editing"
-description = "Gradio demo for Encoder4editing. To use it, simply upload your image, or click one of the examples to load them. Read more at the links below."
-article = "Github Repo
"
-
-gr.Interface(
- inference,
- gr.inputs.Image(type="file", label="Input"),
- gr.outputs.Image(type="file", label="Output"),
- title=title,
- description=description,
- article=article,
- enable_queue=True,
- examples=[['mona.jpg']]
- ).launch(debug=True)
\ No newline at end of file
diff --git "a/spaces/alexrame/rewardedsoups/pages/03_\360\237\246\277_Locomotion.py" "b/spaces/alexrame/rewardedsoups/pages/03_\360\237\246\277_Locomotion.py"
deleted file mode 100644
index f0ec94bcf4dcaddcbe3abf8acd19f49150c7b030..0000000000000000000000000000000000000000
--- "a/spaces/alexrame/rewardedsoups/pages/03_\360\237\246\277_Locomotion.py"
+++ /dev/null
@@ -1,216 +0,0 @@
-import streamlit as st
-from PIL import Image
-import codecs
-import streamlit.components.v1 as components
-from utils import inject_custom_css
-import streamlit as st
-from streamlit_plotly_events import plotly_events
-import pickle
-import matplotlib.pyplot as plt
-import plotly.graph_objects as go
-import typing as tp
-
-plt.style.use('default')
-
-shapes=[
- dict(
- type="rect",
- xref="paper",
- yref="paper",
- x0=0,
- y0=0,
- x1=1,
- y1=1,
- line=dict(
- color="Black",
- width=2,
- ),
- )
-]
-
-import colorsys
-
-def interpolate_color(color1, color2, factor):
- """Interpolates between two RGB colors. Factor is between 0 and 1."""
- color1 = colorsys.rgb_to_hls(int(color1[1:3], 16)/255.0, int(color1[3:5], 16)/255.0, int(color1[5:], 16)/255.0)
- color2 = colorsys.rgb_to_hls(int(color2[1:3], 16)/255.0, int(color2[3:5], 16)/255.0, int(color2[5:], 16)/255.0)
- new_color = [color1[i] * (1 - factor) + color2[i] * factor for i in range(3)]
- new_color = colorsys.hls_to_rgb(*new_color)
- return '#{:02x}{:02x}{:02x}'.format(int(new_color[0]*255), int(new_color[1]*255), int(new_color[2]*255))
-
-
-color1 = "#fa7659"
-color2 = "#6dafd7"
-
-def plot_pareto(dict_results: tp.Dict):
- keys = list(dict_results["wa"][0].keys())
- lambda_key, reward2_key, reward1_key = keys
-
- # Series for "wa"
- dict_results["wa"] = [x for i,x in enumerate(dict_results["wa"]) if i%2==0]
- lambda_values_wa = [item[lambda_key] for item in dict_results["wa"]][::-1]
- reward1_values_wa = [item[reward1_key] for item in dict_results["wa"]][::-1]
- reward2_values_wa = [item[reward2_key] for item in dict_results["wa"]][::-1]
-
- # Series for "init"
- reward1_values_init = [item[reward1_key] for item in dict_results["init"]]
- reward2_values_init = [item[reward2_key] for item in dict_results["init"]]
-
- layout = go.Layout(autosize=False,width=1000,height=1000)
- fig = go.Figure(layout=layout)
-
- for i in range(len(reward1_values_wa) - 1):
- fig.add_trace(go.Scatter(
- x=reward1_values_wa[i:i+2],
- y=reward2_values_wa[i:i+2],
- mode='lines',
- hoverinfo='skip',
- line=dict(
- color=interpolate_color(color1, color2, i/(len(reward1_values_wa)-1)),
- width=2
- ),
- showlegend=False
- ))
-
- # Plot for "wa"
- fig.add_trace(
- go.Scatter(
- x=reward1_values_wa,
- y=reward2_values_wa,
- mode='markers',
- name='Rewarded soups: 0≤λ≤1',
- hoverinfo='text',
- hovertext=[f'λ={lmbda}' for lmbda in lambda_values_wa],
- marker=dict(
- color=[
- interpolate_color(color1, color2, i / len(lambda_values_wa))
- for i in range(len(lambda_values_wa))
- ],
- size=10
- )
- )
- )
-
- # Plot for "morl"
- fig.add_trace(
- go.Scatter(
- x=[6400.],
- y=[3300.],
- mode='markers',
- name='MORL: μ=0.5',
- hoverinfo='skip',
- marker=dict(color='#A45EE9', size=15, symbol="star"),
- )
- )
- # Plot for "init"
- fig.add_trace(
- go.Scatter(
- x=reward1_values_init,
- y=reward2_values_init,
- mode='markers',
- name='Pre-trained init',
- hoverinfo='skip',
- marker=dict(color='#9f9bc8', size=15, symbol="star"),
- )
- )
-
- fig.update_layout(
- xaxis=dict(
- range=[3000, 7000],
- nticks=6,
- showticklabels=True,
- ticks='outside',
- tickfont=dict(size=18,),
- title=dict(text="Risky reward", font=dict(size=18), standoff=10),
- showgrid=False,
- zeroline=False,
- hoverformat='.2f'
- ),
- yaxis=dict(
- range=[-1000, 4500],
- nticks=7,
- showticklabels=True,
- ticks='outside',
- tickfont=dict(size=18,),
- title=dict(text="Cautious reward", font=dict(size=18), standoff=10),
- showgrid=False,
- zeroline=False,
- hoverformat='.2f'
- ),
- font=dict(family="Roboto", size=12, color="Black"),
- hovermode='x unified',
- autosize=False,
- width=500,
- height=500,
- margin=dict(l=100, r=50, b=150, t=20, pad=0),
- paper_bgcolor="White",
- plot_bgcolor="White",
- shapes=shapes,
- legend=dict(
- x=0.5,
- y=0.03,
- traceorder="normal",
- font=dict(family="Roboto", size=12, color="black"),
- bgcolor="White",
- bordercolor="Black",
- borderwidth=1
- )
- )
-
- return fig
-
-def run():
-
- st.write(
- f"""
-
-
-
- Making humanoid run more naturally with diverse engineered rewards """,unsafe_allow_html=True)
-
- st.markdown(
- r"""
-Teaching humanoids to walk in a human-like manner serves as a benchmark to evaluate RL strategies for continuous control. One of the key challenges is shaping a suitable proxy reward, given the intricate coordination and balance involved in human locomotion. It is standard to consider the dense reward at each timestep: ${r(t)=velocity-\alpha \times \sum_t a^{2}_{t}}$, controlling the agent's velocity while penalizing wide actions. Yet, the penalty coefficient $\alpha$ is challenging to set. To tackle this, we devised two rewards in the Brax physics engine: a *risky* one with $\alpha=0$, and a *cautious* one $\alpha=1$.
-
-Below in the interactive animation, you will see the humanoids trained with these two rewards: the humanoid for $\alpha=0$ is the fastest but the most chaotic, while the one for $\alpha=1$ is more cautious but slower. For intermediate values of $\lambda$, the policy is obtained by linear interpolation of those extreme weights, arguably resulting in smoother motion patterns.
-""", unsafe_allow_html=True
- )
- st.markdown("""Click on a rewarded soup point! """,unsafe_allow_html=True)
-
- files = []
- for i in range(21):
- filename = f'streamlit_app/data/locomotion/trajectories/{i}.html'
- files.append(codecs.open(filename, "r", "utf-8").read())
- files = [x for i,x in enumerate(files) if i%2==0]
-
- row_0_1,row_0_2,row_0_3,row_0_4 = st.columns([3,1,1,1])
- with row_0_1:
- with open("streamlit_app/data/locomotion/pareto/humanoid_averse_taker_with_morl.pkl","rb") as f:
- dict_results = pickle.load(f)
- fig = plot_pareto(dict_results)
- onclick = plotly_events(fig, click_event=True)
- with row_0_4:
- st.markdown(f"""λ=1.0
""",unsafe_allow_html=True)
- components.html(files[-1],width=150,height=300)
- with row_0_3:
- if len(onclick) > 0:
- idx = onclick[-1]['pointIndex']
- else:
- idx = 5
- st.markdown(
- f"""λ={round(1-idx/(len(files)-1),2)}
""",
- unsafe_allow_html=True
- )
- components.html(files[idx], width=150, height=300)
- with row_0_2:
- st.markdown(f"""λ=0.0
""",unsafe_allow_html=True)
- components.html(files[0],width=150,height=300)
-
-
-if __name__ == "__main__":
- img = Image.open("streamlit_app/assets/images/icon.png")
- st.set_page_config(page_title="Rewarded soups",page_icon=img,layout="wide")
- inject_custom_css("streamlit_app/assets/styles.css")
- st.set_option('deprecation.showPyplotGlobalUse', False)
- run()
diff --git a/spaces/ali-ghamdan/gfp-Gans/gfpgan/train.py b/spaces/ali-ghamdan/gfp-Gans/gfpgan/train.py
deleted file mode 100644
index fe5f1f909ae15a8d830ef65dcb43436d4f4ee7ae..0000000000000000000000000000000000000000
--- a/spaces/ali-ghamdan/gfp-Gans/gfpgan/train.py
+++ /dev/null
@@ -1,11 +0,0 @@
-# flake8: noqa
-import os.path as osp
-from basicsr.train import train_pipeline
-
-import gfpgan.archs
-import gfpgan.data
-import gfpgan.models
-
-if __name__ == '__main__':
- root_path = osp.abspath(osp.join(__file__, osp.pardir, osp.pardir))
- train_pipeline(root_path)
diff --git a/spaces/ali-ghamdan/gfp-Gans/scripts/parse_landmark.py b/spaces/ali-ghamdan/gfp-Gans/scripts/parse_landmark.py
deleted file mode 100644
index 74e2ff9e130ad4f2395c9666dca3ba78526d7a8a..0000000000000000000000000000000000000000
--- a/spaces/ali-ghamdan/gfp-Gans/scripts/parse_landmark.py
+++ /dev/null
@@ -1,85 +0,0 @@
-import cv2
-import json
-import numpy as np
-import os
-import torch
-from basicsr.utils import FileClient, imfrombytes
-from collections import OrderedDict
-
-# ---------------------------- This script is used to parse facial landmarks ------------------------------------- #
-# Configurations
-save_img = False
-scale = 0.5 # 0.5 for official FFHQ (512x512), 1 for others
-enlarge_ratio = 1.4 # only for eyes
-json_path = 'ffhq-dataset-v2.json'
-face_path = 'datasets/ffhq/ffhq_512.lmdb'
-save_path = './FFHQ_eye_mouth_landmarks_512.pth'
-
-print('Load JSON metadata...')
-# use the official json file in FFHQ dataset
-with open(json_path, 'rb') as f:
- json_data = json.load(f, object_pairs_hook=OrderedDict)
-
-print('Open LMDB file...')
-# read ffhq images
-file_client = FileClient('lmdb', db_paths=face_path)
-with open(os.path.join(face_path, 'meta_info.txt')) as fin:
- paths = [line.split('.')[0] for line in fin]
-
-save_dict = {}
-
-for item_idx, item in enumerate(json_data.values()):
- print(f'\r{item_idx} / {len(json_data)}, {item["image"]["file_path"]} ', end='', flush=True)
-
- # parse landmarks
- lm = np.array(item['image']['face_landmarks'])
- lm = lm * scale
-
- item_dict = {}
- # get image
- if save_img:
- img_bytes = file_client.get(paths[item_idx])
- img = imfrombytes(img_bytes, float32=True)
-
- # get landmarks for each component
- map_left_eye = list(range(36, 42))
- map_right_eye = list(range(42, 48))
- map_mouth = list(range(48, 68))
-
- # eye_left
- mean_left_eye = np.mean(lm[map_left_eye], 0) # (x, y)
- half_len_left_eye = np.max((np.max(np.max(lm[map_left_eye], 0) - np.min(lm[map_left_eye], 0)) / 2, 16))
- item_dict['left_eye'] = [mean_left_eye[0], mean_left_eye[1], half_len_left_eye]
- # mean_left_eye[0] = 512 - mean_left_eye[0] # for testing flip
- half_len_left_eye *= enlarge_ratio
- loc_left_eye = np.hstack((mean_left_eye - half_len_left_eye + 1, mean_left_eye + half_len_left_eye)).astype(int)
- if save_img:
- eye_left_img = img[loc_left_eye[1]:loc_left_eye[3], loc_left_eye[0]:loc_left_eye[2], :]
- cv2.imwrite(f'tmp/{item_idx:08d}_eye_left.png', eye_left_img * 255)
-
- # eye_right
- mean_right_eye = np.mean(lm[map_right_eye], 0)
- half_len_right_eye = np.max((np.max(np.max(lm[map_right_eye], 0) - np.min(lm[map_right_eye], 0)) / 2, 16))
- item_dict['right_eye'] = [mean_right_eye[0], mean_right_eye[1], half_len_right_eye]
- # mean_right_eye[0] = 512 - mean_right_eye[0] # # for testing flip
- half_len_right_eye *= enlarge_ratio
- loc_right_eye = np.hstack(
- (mean_right_eye - half_len_right_eye + 1, mean_right_eye + half_len_right_eye)).astype(int)
- if save_img:
- eye_right_img = img[loc_right_eye[1]:loc_right_eye[3], loc_right_eye[0]:loc_right_eye[2], :]
- cv2.imwrite(f'tmp/{item_idx:08d}_eye_right.png', eye_right_img * 255)
-
- # mouth
- mean_mouth = np.mean(lm[map_mouth], 0)
- half_len_mouth = np.max((np.max(np.max(lm[map_mouth], 0) - np.min(lm[map_mouth], 0)) / 2, 16))
- item_dict['mouth'] = [mean_mouth[0], mean_mouth[1], half_len_mouth]
- # mean_mouth[0] = 512 - mean_mouth[0] # for testing flip
- loc_mouth = np.hstack((mean_mouth - half_len_mouth + 1, mean_mouth + half_len_mouth)).astype(int)
- if save_img:
- mouth_img = img[loc_mouth[1]:loc_mouth[3], loc_mouth[0]:loc_mouth[2], :]
- cv2.imwrite(f'tmp/{item_idx:08d}_mouth.png', mouth_img * 255)
-
- save_dict[f'{item_idx:08d}'] = item_dict
-
-print('Save...')
-torch.save(save_dict, save_path)
diff --git a/spaces/alistairmcleay/cambridge-masters-project/scripts/user_model_code/interaction/utils.py b/spaces/alistairmcleay/cambridge-masters-project/scripts/user_model_code/interaction/utils.py
deleted file mode 100644
index 19ced3d04372ba30c60d591b14ebab3992881b8f..0000000000000000000000000000000000000000
--- a/spaces/alistairmcleay/cambridge-masters-project/scripts/user_model_code/interaction/utils.py
+++ /dev/null
@@ -1,308 +0,0 @@
-import json
-import re
-
-
-def segment_gen(gen, dial_id):
- def _color(_segment):
- if tag == "CTX":
- _segment = _segment.replace(" ", f"{bcolors.ENDC}")
- _segment = _segment.replace(" ", f"{bcolors.ENDC}")
- _segment = _segment.replace(" ", f"USR: {bcolors.OKCYAN}")
- _segment = _segment.replace(" ", f"SYS: {bcolors.OKBLUE}")
- if tag == "SYS_UTT":
- _segment = f"{bcolors.OKBLUE}" + _segment + f"{bcolors.ENDC}"
- if tag == "USR_UTT":
- _segment = f"{bcolors.OKCYAN}" + _segment + f"{bcolors.ENDC}"
- if tag in ["SYS_ACT", "USR_ACT", "GOAL"]:
- _segment = _segment.replace(" ", f"{bcolors.RED}")
- _segment = _segment.replace(" ", f"{bcolors.ENDC}")
- _segment = _segment.replace(" ", f"{bcolors.YELLOW}")
- _segment = _segment.replace(" ", f"{bcolors.ENDC}")
- _segment = _segment.replace(" ", f"{bcolors.GREEN}")
- _segment = _segment.replace(" ", f"{bcolors.ENDC}")
- if tag == "GOAL":
- _segment = _segment.replace(
- " ", f" {bcolors.UNDERLINE}"
- )
- _segment = _segment.replace("", f"{bcolors.ENDC}")
- _segment = _segment.replace(" ", f" {bcolors.UNDERLINE}")
- _segment = _segment.replace("", f"{bcolors.ENDC}")
- # if tag in ["SNT", "GC"]:
- # segment = segment.replace("<{}/> ".format(tag), "<{}/> *".format(tag))
- # segment = segment.replace(" {}>".format(tag), "* <{}/>".format(tag))
- return _segment
-
- assert isinstance(gen, str)
- # gen = gen.split()
- # print(gen)
- print("*** Dial_id: {} ***".format(dial_id))
- for tag in [
- "CTX",
- "SYS_UTT",
- "SYS_ACT",
- "GOAL",
- "SNT",
- "RA",
- "GC",
- "USR_ACT",
- "USR_UTT",
- ]:
- segment = find_segment(gen, tag)
- if segment is not None:
- print('{} -> "{}"'.format(tag, _color(segment)))
- else:
- print("Fail to find the segment...")
- print("GEN:", gen)
- print("---" * 30)
-
-
-# input("press...")
-
-
-def get_original_act_set():
- # full act vocab:
- # https://github.com/ConvLab/ConvLab/blob/master/data/multiwoz/annotation/Multiwoz%20data%20analysis.md#dialog-act
- acts = set()
- acts.add("Inform")
- acts.add("Request")
- acts.add(
- "NoOffer"
- ) # equivalent to the concept of `no matching`, `cannot find` in database
- acts.add("Recommend")
- acts.add("Select")
- acts.add(
- "OfferBook"
- ) # only for `train` domain, ask if book is needed, equivalent to `Booking-Inform` with [[none, none]]
- # args in restaurant/hotel domain
- acts.add(
- "OfferBooked"
- ) # only for `train` domain, inform booking is complete, with corresponding info (such as ref number)
- acts.add("Book") # inform booking is successful, equivalent to `OfferBooked` above
- acts.add(
- "NoBook"
- ) # inform booking fails, might because of no availability, usually come together act `request`
- acts.add("bye")
- acts.add("greet")
- acts.add("reqmore")
- acts.add("welcome")
- acts.add("thank")
- return acts
-
-
-def get_act_natural_language(act):
- if act in ["bye", "greet", "reqmore", "welcome", "thank"]:
- return act
-
- assert act[0].isupper()
- tokens = re.findall("[A-Z][^A-Z]*", act) # e.g., `FindEvents` -> `Find Events`
- tokens = list(map(str.lower, tokens)) # lower case, -> `find events`
- act_nl = " ".join(tokens)
- return act_nl
-
-
-def convert_act_into_sgd(act, SPECIAL_TOKENS):
- # TODO: check inference result to see if mapping on NoOffer, OfferBook and NoBook are fine
- """
- convert multiwoz acts (w/o domain info) into sgd acts ensure that acts with same concept use one name
- e.g., Book (OfferBooked) -> NOTIFY_SUCCESS, NoBook -> NOTIFY_FAILURE
- """
- if act == "NoOffer":
- act = "NOTIFY_FAILURE"
-
- elif act == "Recommend":
- act = "OFFER"
-
- # technically, `OfferBook` is equivalent to (`act=OFFER_INTENT, slot=intent, value=ReserveRestaurant`)
- # on system side in sgd since (1) the conversion is not trivial (completely different representations)
- # and (2) multiwoz has no slot called `intent` one cannot simply convert `OfferBook` to `OFFER_INTENT`
- # we thus keep the act as is
- # note that there is no slot `intent` and value conveying intents in multiwoz
- elif act == "OfferBook":
- act = "Offer_Book"
-
- elif act == "OfferBooked":
- act = "NOTIFY_SUCCESS"
-
- elif act == "Book": # same as `OfferBooked`
- act = "NOTIFY_SUCCESS"
-
- elif act == "NoBook":
- act = "NOTIFY_FAILURE"
-
- elif act == "bye":
- act = "GOODBYE"
-
- elif act == "reqmore":
- act = "REQ_MORE"
-
- elif act == "thank":
- act = "THANK_YOU"
- # elif act == "greet":
- # elif act == "welcome":
- act = act.upper() # align with sgd acts, e.g., `Inform` -> `INFORM`
-
- # check if valid
- assert "_{}_".format(act) in SPECIAL_TOKENS["additional_special_tokens"]
- return act
-
-
-def load_schema(schema_file):
- def _update(key, value, mapping):
- if key in mapping:
- assert (
- value == mapping[key]
- ) # ensure service meta is the same between data splits
- else:
- mapping[key] = value
-
- def _restructure_service_meta(service_meta, attribute):
- """ "convert slot/intent metadata list into dict(slot/intent=metadata)"""
- assert attribute in ["slots", "intents"]
- mapping = {}
- for value in service_meta[attribute]:
- key = value["name"]
- if attribute == "slots": # domain-slot in multiwoz
- assert "-" in key
- _, key = key.split("-") # domain, slot
- key = normalise_slot(key)
- else: # intent
- key = normalise_intent(key)
- mapping[key] = value
- service_meta[attribute] = mapping
-
- with open(schema_file) as f:
- data = json.load(f)
-
- SERVICE2META = {}
- SLOTS, INTENTS = set(), set()
- for service_meta in data:
- service = service_meta["service_name"]
- _restructure_service_meta(service_meta, "slots")
- _restructure_service_meta(service_meta, "intents")
- _update(service, service_meta, SERVICE2META)
-
- # collect domain-independent slots
- # for domain_slot in service_meta["slots"]:
- # assert "-" in domain_slot
- # domain, slot = domain_slot.split("-")
- # slot = normalise_slot(slot)
- # SLOTS.add(slot)
- for slot in service_meta["slots"]:
- SLOTS.add(slot)
-
- for intent in service_meta["intents"]:
- # intent = normalise_intent(intent)
- INTENTS.add(intent)
-
- print("Load schema, intents: {}, slots: {}".format(len(INTENTS), len(SLOTS)))
- return SERVICE2META, INTENTS, SLOTS
-
-
-def normalise_intent(intent):
- """convert intent into natural language, e.g., find_hotel -> find hotel"""
- if intent == "police":
- intent = "find_police"
- if intent == "book_taxi":
- intent = "find_taxi"
- assert "_" in intent
- return " ".join(intent.split("_"))
-
-
-def normalise_slot(slot):
- if slot == "pricerange":
- return "price range"
-
- elif slot == "bookday":
- return "book day"
-
- elif slot == "bookpeople":
- return "book people"
-
- elif slot == "booktime":
- return "book time"
-
- elif slot == "bookstay":
- return "book stay"
-
- elif slot == "ref":
- return "reference"
-
- elif slot == "arriveby":
- return "arrive by"
-
- elif slot == "leaveat":
- return "leave at"
-
- elif slot == "trainid":
- return "train id"
-
- elif slot == "openhours":
- return "open hours"
-
- elif slot == "entrancefee":
- return "entrance fee"
-
- elif slot in ["none", "?"]:
- # return "_Empty_" # special token mark will be added during sequence linearlisation
- return "Empty"
-
- else:
- return slot
-
-
-def normalise_value(value):
- # deal with binary and empty values
- if value == "yes":
- # return "_True_"
- return "True"
-
- elif value == "no":
- # return "_False_"
- return "False"
-
- elif value in ["none", "?"]:
- # return "_Empty_"
- return "Empty"
-
- # if value == "swimmingpool": # for simplicity, dont split
- # return "swimming pool"
-
- else:
- return value
-
-
-def wrap_element(content_type, content):
- """
- wrap elements such as slot, value, e.g., slot
- """
- assert "/" not in content_type
- return "<{}/> {} {}>".format(content_type, content, content_type)
-
-
-def add_str(str1, str2):
- return str1 + " " + str2
-
-
-def find_segment(gen, tag):
- assert isinstance(gen, str)
- gen = gen.split()
- try:
- start = gen.index("<{}/>".format(tag)) + 1
- end = gen.index("{}>".format(tag))
- segment = " ".join(gen[start:end])
- except Exception:
- print("Missing {} tag in generated sequence".format(tag))
- segment = None
- return segment
-
-
-class bcolors:
- HEADER = "\033[95m"
- OKBLUE = "\033[94m"
- OKCYAN = "\033[96m"
- GREEN = "\033[92m"
- YELLOW = "\033[93m"
- RED = "\033[91m"
- ENDC = "\033[0m"
- BOLD = "\033[1m"
- UNDERLINE = "\033[4m"
diff --git a/spaces/allknowingroger/Image-Models-Test202/app.py b/spaces/allknowingroger/Image-Models-Test202/app.py
deleted file mode 100644
index 3c9a92786125c6e8b12d10bd8ab33850a5ed783b..0000000000000000000000000000000000000000
--- a/spaces/allknowingroger/Image-Models-Test202/app.py
+++ /dev/null
@@ -1,144 +0,0 @@
-import gradio as gr
-# import os
-# import sys
-# from pathlib import Path
-import time
-
-models =[
- "Bigyi/lora-trained-xl-colab",
- "jwhedbee/lora-trained-xl-take-two",
- "naphatmanu/sdxl-lora-index-modern-luxury-1",
- "naphatmanu/sdxl-lora-index-contemporary-1",
- "Yntec/DreamFulV2",
- "jwhedbee/lora-trained-xl",
- "naphatmanu/sdxl-lora-index-modern-1",
- "LilyNgo/lora_Galaxiga-trained-xl-colab",
- "jwhedbee/lora-trained-xl-telnyx-product-hero-poc",
-]
-
-
-model_functions = {}
-model_idx = 1
-for model_path in models:
- try:
- model_functions[model_idx] = gr.Interface.load(f"models/{model_path}", live=False, preprocess=True, postprocess=False)
- except Exception as error:
- def the_fn(txt):
- return None
- model_functions[model_idx] = gr.Interface(fn=the_fn, inputs=["text"], outputs=["image"])
- model_idx+=1
-
-
-def send_it_idx(idx):
- def send_it_fn(prompt):
- output = (model_functions.get(str(idx)) or model_functions.get(str(1)))(prompt)
- return output
- return send_it_fn
-
-def get_prompts(prompt_text):
- return prompt_text
-
-def clear_it(val):
- if int(val) != 0:
- val = 0
- else:
- val = 0
- pass
- return val
-
-def all_task_end(cnt,t_stamp):
- to = t_stamp + 60
- et = time.time()
- if et > to and t_stamp != 0:
- d = gr.update(value=0)
- tog = gr.update(value=1)
- #print(f'to: {to} et: {et}')
- else:
- if cnt != 0:
- d = gr.update(value=et)
- else:
- d = gr.update(value=0)
- tog = gr.update(value=0)
- #print (f'passing: to: {to} et: {et}')
- pass
- return d, tog
-
-def all_task_start():
- print("\n\n\n\n\n\n\n")
- t = time.gmtime()
- t_stamp = time.time()
- current_time = time.strftime("%H:%M:%S", t)
- return gr.update(value=t_stamp), gr.update(value=t_stamp), gr.update(value=0)
-
-def clear_fn():
- nn = len(models)
- return tuple([None, *[None for _ in range(nn)]])
-
-
-
-with gr.Blocks(title="SD Models") as my_interface:
- with gr.Column(scale=12):
- # with gr.Row():
- # gr.Markdown("""- Primary prompt: 你想画的内容(英文单词,如 a cat, 加英文逗号效果更好;点 Improve 按钮进行完善)\n- Real prompt: 完善后的提示词,出现后再点右边的 Run 按钮开始运行""")
- with gr.Row():
- with gr.Row(scale=6):
- primary_prompt=gr.Textbox(label="Prompt", value="")
- # real_prompt=gr.Textbox(label="Real prompt")
- with gr.Row(scale=6):
- # improve_prompts_btn=gr.Button("Improve")
- with gr.Row():
- run=gr.Button("Run",variant="primary")
- clear_btn=gr.Button("Clear")
- with gr.Row():
- sd_outputs = {}
- model_idx = 1
- for model_path in models:
- with gr.Column(scale=3, min_width=320):
- with gr.Box():
- sd_outputs[model_idx] = gr.Image(label=model_path)
- pass
- model_idx += 1
- pass
- pass
-
- with gr.Row(visible=False):
- start_box=gr.Number(interactive=False)
- end_box=gr.Number(interactive=False)
- tog_box=gr.Textbox(value=0,interactive=False)
-
- start_box.change(
- all_task_end,
- [start_box, end_box],
- [start_box, tog_box],
- every=1,
- show_progress=False)
-
- primary_prompt.submit(all_task_start, None, [start_box, end_box, tog_box])
- run.click(all_task_start, None, [start_box, end_box, tog_box])
- runs_dict = {}
- model_idx = 1
- for model_path in models:
- runs_dict[model_idx] = run.click(model_functions[model_idx], inputs=[primary_prompt], outputs=[sd_outputs[model_idx]])
- model_idx += 1
- pass
- pass
-
- # improve_prompts_btn_clicked=improve_prompts_btn.click(
- # get_prompts,
- # inputs=[primary_prompt],
- # outputs=[primary_prompt],
- # cancels=list(runs_dict.values()))
- clear_btn.click(
- clear_fn,
- None,
- [primary_prompt, *list(sd_outputs.values())],
- cancels=[*list(runs_dict.values())])
- tog_box.change(
- clear_it,
- tog_box,
- tog_box,
- cancels=[*list(runs_dict.values())])
-
-my_interface.queue(concurrency_count=600, status_update_rate=1)
-my_interface.launch(inline=True, show_api=False)
-
\ No newline at end of file
diff --git a/spaces/allknowingroger/Image-Models-Test91/app.py b/spaces/allknowingroger/Image-Models-Test91/app.py
deleted file mode 100644
index 1ecf3ce1b16af205f4bf4f6ccab84b82fbca04d6..0000000000000000000000000000000000000000
--- a/spaces/allknowingroger/Image-Models-Test91/app.py
+++ /dev/null
@@ -1,144 +0,0 @@
-import gradio as gr
-# import os
-# import sys
-# from pathlib import Path
-import time
-
-models =[
- "stillerman/trdne-smol-simple",
- "stillerman/trdne-smol",
- "stephanebhiri/lora-trained-xl-colab-stpCSmith2",
- "Sekharreddy/mnb",
- "stephanebhiri/lora-trained-xl-colab-stpCSmith",
- "camus-ng/lora-trained-xl-cory-8",
- "hhhtc/yokai_v2",
- "mangoxb/tangled1",
- "digiplay/OldFish_v1.1_diffusers_recover",
-]
-
-
-model_functions = {}
-model_idx = 1
-for model_path in models:
- try:
- model_functions[model_idx] = gr.Interface.load(f"models/{model_path}", live=False, preprocess=True, postprocess=False)
- except Exception as error:
- def the_fn(txt):
- return None
- model_functions[model_idx] = gr.Interface(fn=the_fn, inputs=["text"], outputs=["image"])
- model_idx+=1
-
-
-def send_it_idx(idx):
- def send_it_fn(prompt):
- output = (model_functions.get(str(idx)) or model_functions.get(str(1)))(prompt)
- return output
- return send_it_fn
-
-def get_prompts(prompt_text):
- return prompt_text
-
-def clear_it(val):
- if int(val) != 0:
- val = 0
- else:
- val = 0
- pass
- return val
-
-def all_task_end(cnt,t_stamp):
- to = t_stamp + 60
- et = time.time()
- if et > to and t_stamp != 0:
- d = gr.update(value=0)
- tog = gr.update(value=1)
- #print(f'to: {to} et: {et}')
- else:
- if cnt != 0:
- d = gr.update(value=et)
- else:
- d = gr.update(value=0)
- tog = gr.update(value=0)
- #print (f'passing: to: {to} et: {et}')
- pass
- return d, tog
-
-def all_task_start():
- print("\n\n\n\n\n\n\n")
- t = time.gmtime()
- t_stamp = time.time()
- current_time = time.strftime("%H:%M:%S", t)
- return gr.update(value=t_stamp), gr.update(value=t_stamp), gr.update(value=0)
-
-def clear_fn():
- nn = len(models)
- return tuple([None, *[None for _ in range(nn)]])
-
-
-
-with gr.Blocks(title="SD Models") as my_interface:
- with gr.Column(scale=12):
- # with gr.Row():
- # gr.Markdown("""- Primary prompt: 你想画的内容(英文单词,如 a cat, 加英文逗号效果更好;点 Improve 按钮进行完善)\n- Real prompt: 完善后的提示词,出现后再点右边的 Run 按钮开始运行""")
- with gr.Row():
- with gr.Row(scale=6):
- primary_prompt=gr.Textbox(label="Prompt", value="")
- # real_prompt=gr.Textbox(label="Real prompt")
- with gr.Row(scale=6):
- # improve_prompts_btn=gr.Button("Improve")
- with gr.Row():
- run=gr.Button("Run",variant="primary")
- clear_btn=gr.Button("Clear")
- with gr.Row():
- sd_outputs = {}
- model_idx = 1
- for model_path in models:
- with gr.Column(scale=3, min_width=320):
- with gr.Box():
- sd_outputs[model_idx] = gr.Image(label=model_path)
- pass
- model_idx += 1
- pass
- pass
-
- with gr.Row(visible=False):
- start_box=gr.Number(interactive=False)
- end_box=gr.Number(interactive=False)
- tog_box=gr.Textbox(value=0,interactive=False)
-
- start_box.change(
- all_task_end,
- [start_box, end_box],
- [start_box, tog_box],
- every=1,
- show_progress=False)
-
- primary_prompt.submit(all_task_start, None, [start_box, end_box, tog_box])
- run.click(all_task_start, None, [start_box, end_box, tog_box])
- runs_dict = {}
- model_idx = 1
- for model_path in models:
- runs_dict[model_idx] = run.click(model_functions[model_idx], inputs=[primary_prompt], outputs=[sd_outputs[model_idx]])
- model_idx += 1
- pass
- pass
-
- # improve_prompts_btn_clicked=improve_prompts_btn.click(
- # get_prompts,
- # inputs=[primary_prompt],
- # outputs=[primary_prompt],
- # cancels=list(runs_dict.values()))
- clear_btn.click(
- clear_fn,
- None,
- [primary_prompt, *list(sd_outputs.values())],
- cancels=[*list(runs_dict.values())])
- tog_box.change(
- clear_it,
- tog_box,
- tog_box,
- cancels=[*list(runs_dict.values())])
-
-my_interface.queue(concurrency_count=600, status_update_rate=1)
-my_interface.launch(inline=True, show_api=False)
-
\ No newline at end of file
diff --git a/spaces/amarchheda/ChordDuplicate/portaudio/README.md b/spaces/amarchheda/ChordDuplicate/portaudio/README.md
deleted file mode 100644
index bf48975f507ca3dc7f8e16b0bbcb4ebb159e30e2..0000000000000000000000000000000000000000
--- a/spaces/amarchheda/ChordDuplicate/portaudio/README.md
+++ /dev/null
@@ -1,62 +0,0 @@
-# PortAudio - portable audio I/O library
-
-PortAudio is a portable audio I/O library designed for cross-platform
-support of audio. It uses either a callback mechanism to request audio
-processing, or blocking read/write calls to buffer data between the
-native audio subsystem and the client. Audio can be processed in various
-formats, including 32 bit floating point, and will be converted to the
-native format internally.
-
-## Documentation:
-
-* Documentation is available at http://www.portaudio.com/docs/
-* Or at `/doc/html/index.html` after running Doxygen.
-* Also see `src/common/portaudio.h` for the API spec.
-* And see the `examples/` and `test/` directories for many examples of usage. (We suggest `examples/paex_saw.c` for an example.)
-
-For information on compiling programs with PortAudio, please see the
-tutorial at:
-
- http://portaudio.com/docs/v19-doxydocs/tutorial_start.html
-
-We have an active mailing list for user and developer discussions.
-Please feel free to join. See http://www.portaudio.com for details.
-
-## Important Files and Folders:
-
- include/portaudio.h = header file for PortAudio API. Specifies API.
- src/common/ = platform independent code, host independent
- code for all implementations.
- src/os = os specific (but host api neutral) code
- src/hostapi = implementations for different host apis
-
-
-### Host API Implementations:
-
- src/hostapi/alsa = Advanced Linux Sound Architecture (ALSA)
- src/hostapi/asihpi = AudioScience HPI
- src/hostapi/asio = ASIO for Windows and Macintosh
- src/hostapi/coreaudio = Macintosh Core Audio for OS X
- src/hostapi/dsound = Windows Direct Sound
- src/hostapi/jack = JACK Audio Connection Kit
- src/hostapi/oss = Unix Open Sound System (OSS)
- src/hostapi/wasapi = Windows Vista WASAPI
- src/hostapi/wdmks = Windows WDM Kernel Streaming
- src/hostapi/wmme = Windows MultiMedia Extensions (MME)
-
-
-### Test Programs:
-
- test/pa_fuzz.c = guitar fuzz box
- test/pa_devs.c = print a list of available devices
- test/pa_minlat.c = determine minimum latency for your machine
- test/paqa_devs.c = self test that opens all devices
- test/paqa_errs.c = test error detection and reporting
- test/patest_clip.c = hear a sine wave clipped and unclipped
- test/patest_dither.c = hear effects of dithering (extremely subtle)
- test/patest_pink.c = fun with pink noise
- test/patest_record.c = record and playback some audio
- test/patest_maxsines.c = how many sine waves can we play? Tests Pa_GetCPULoad().
- test/patest_sine.c = output a sine wave in a simple PA app
- test/patest_sync.c = test synchronization of audio and video
- test/patest_wire.c = pass input to output, wire simulator
diff --git a/spaces/amarchheda/ChordDuplicate/portaudio/ltmain.sh b/spaces/amarchheda/ChordDuplicate/portaudio/ltmain.sh
deleted file mode 100644
index 4a1ede7111ea6d110e4dd505718a34a1bee63882..0000000000000000000000000000000000000000
--- a/spaces/amarchheda/ChordDuplicate/portaudio/ltmain.sh
+++ /dev/null
@@ -1,9661 +0,0 @@
-
-# libtool (GNU libtool) 2.4.2
-# Written by Gordon Matzigkeit , 1996
-
-# Copyright (C) 1996, 1997, 1998, 1999, 2000, 2001, 2003, 2004, 2005, 2006,
-# 2007, 2008, 2009, 2010, 2011 Free Software Foundation, Inc.
-# This is free software; see the source for copying conditions. There is NO
-# warranty; not even for MERCHANTABILITY or FITNESS FOR A PARTICULAR PURPOSE.
-
-# GNU Libtool is free software; you can redistribute it and/or modify
-# it under the terms of the GNU General Public License as published by
-# the Free Software Foundation; either version 2 of the License, or
-# (at your option) any later version.
-#
-# As a special exception to the GNU General Public License,
-# if you distribute this file as part of a program or library that
-# is built using GNU Libtool, you may include this file under the
-# same distribution terms that you use for the rest of that program.
-#
-# GNU Libtool is distributed in the hope that it will be useful, but
-# WITHOUT ANY WARRANTY; without even the implied warranty of
-# MERCHANTABILITY or FITNESS FOR A PARTICULAR PURPOSE. See the GNU
-# General Public License for more details.
-#
-# You should have received a copy of the GNU General Public License
-# along with GNU Libtool; see the file COPYING. If not, a copy
-# can be downloaded from http://www.gnu.org/licenses/gpl.html,
-# or obtained by writing to the Free Software Foundation, Inc.,
-# 51 Franklin Street, Fifth Floor, Boston, MA 02110-1301, USA.
-
-# Usage: $progname [OPTION]... [MODE-ARG]...
-#
-# Provide generalized library-building support services.
-#
-# --config show all configuration variables
-# --debug enable verbose shell tracing
-# -n, --dry-run display commands without modifying any files
-# --features display basic configuration information and exit
-# --mode=MODE use operation mode MODE
-# --preserve-dup-deps don't remove duplicate dependency libraries
-# --quiet, --silent don't print informational messages
-# --no-quiet, --no-silent
-# print informational messages (default)
-# --no-warn don't display warning messages
-# --tag=TAG use configuration variables from tag TAG
-# -v, --verbose print more informational messages than default
-# --no-verbose don't print the extra informational messages
-# --version print version information
-# -h, --help, --help-all print short, long, or detailed help message
-#
-# MODE must be one of the following:
-#
-# clean remove files from the build directory
-# compile compile a source file into a libtool object
-# execute automatically set library path, then run a program
-# finish complete the installation of libtool libraries
-# install install libraries or executables
-# link create a library or an executable
-# uninstall remove libraries from an installed directory
-#
-# MODE-ARGS vary depending on the MODE. When passed as first option,
-# `--mode=MODE' may be abbreviated as `MODE' or a unique abbreviation of that.
-# Try `$progname --help --mode=MODE' for a more detailed description of MODE.
-#
-# When reporting a bug, please describe a test case to reproduce it and
-# include the following information:
-#
-# host-triplet: $host
-# shell: $SHELL
-# compiler: $LTCC
-# compiler flags: $LTCFLAGS
-# linker: $LD (gnu? $with_gnu_ld)
-# $progname: (GNU libtool) 2.4.2 Debian-2.4.2-1.7ubuntu1
-# automake: $automake_version
-# autoconf: $autoconf_version
-#
-# Report bugs to .
-# GNU libtool home page: .
-# General help using GNU software: .
-
-PROGRAM=libtool
-PACKAGE=libtool
-VERSION="2.4.2 Debian-2.4.2-1.7ubuntu1"
-TIMESTAMP=""
-package_revision=1.3337
-
-# Be Bourne compatible
-if test -n "${ZSH_VERSION+set}" && (emulate sh) >/dev/null 2>&1; then
- emulate sh
- NULLCMD=:
- # Zsh 3.x and 4.x performs word splitting on ${1+"$@"}, which
- # is contrary to our usage. Disable this feature.
- alias -g '${1+"$@"}'='"$@"'
- setopt NO_GLOB_SUBST
-else
- case `(set -o) 2>/dev/null` in *posix*) set -o posix;; esac
-fi
-BIN_SH=xpg4; export BIN_SH # for Tru64
-DUALCASE=1; export DUALCASE # for MKS sh
-
-# A function that is used when there is no print builtin or printf.
-func_fallback_echo ()
-{
- eval 'cat <<_LTECHO_EOF
-$1
-_LTECHO_EOF'
-}
-
-# NLS nuisances: We save the old values to restore during execute mode.
-lt_user_locale=
-lt_safe_locale=
-for lt_var in LANG LANGUAGE LC_ALL LC_CTYPE LC_COLLATE LC_MESSAGES
-do
- eval "if test \"\${$lt_var+set}\" = set; then
- save_$lt_var=\$$lt_var
- $lt_var=C
- export $lt_var
- lt_user_locale=\"$lt_var=\\\$save_\$lt_var; \$lt_user_locale\"
- lt_safe_locale=\"$lt_var=C; \$lt_safe_locale\"
- fi"
-done
-LC_ALL=C
-LANGUAGE=C
-export LANGUAGE LC_ALL
-
-$lt_unset CDPATH
-
-
-# Work around backward compatibility issue on IRIX 6.5. On IRIX 6.4+, sh
-# is ksh but when the shell is invoked as "sh" and the current value of
-# the _XPG environment variable is not equal to 1 (one), the special
-# positional parameter $0, within a function call, is the name of the
-# function.
-progpath="$0"
-
-
-
-: ${CP="cp -f"}
-test "${ECHO+set}" = set || ECHO=${as_echo-'printf %s\n'}
-: ${MAKE="make"}
-: ${MKDIR="mkdir"}
-: ${MV="mv -f"}
-: ${RM="rm -f"}
-: ${SHELL="${CONFIG_SHELL-/bin/sh}"}
-: ${Xsed="$SED -e 1s/^X//"}
-
-# Global variables:
-EXIT_SUCCESS=0
-EXIT_FAILURE=1
-EXIT_MISMATCH=63 # $? = 63 is used to indicate version mismatch to missing.
-EXIT_SKIP=77 # $? = 77 is used to indicate a skipped test to automake.
-
-exit_status=$EXIT_SUCCESS
-
-# Make sure IFS has a sensible default
-lt_nl='
-'
-IFS=" $lt_nl"
-
-dirname="s,/[^/]*$,,"
-basename="s,^.*/,,"
-
-# func_dirname file append nondir_replacement
-# Compute the dirname of FILE. If nonempty, add APPEND to the result,
-# otherwise set result to NONDIR_REPLACEMENT.
-func_dirname ()
-{
- func_dirname_result=`$ECHO "${1}" | $SED "$dirname"`
- if test "X$func_dirname_result" = "X${1}"; then
- func_dirname_result="${3}"
- else
- func_dirname_result="$func_dirname_result${2}"
- fi
-} # func_dirname may be replaced by extended shell implementation
-
-
-# func_basename file
-func_basename ()
-{
- func_basename_result=`$ECHO "${1}" | $SED "$basename"`
-} # func_basename may be replaced by extended shell implementation
-
-
-# func_dirname_and_basename file append nondir_replacement
-# perform func_basename and func_dirname in a single function
-# call:
-# dirname: Compute the dirname of FILE. If nonempty,
-# add APPEND to the result, otherwise set result
-# to NONDIR_REPLACEMENT.
-# value returned in "$func_dirname_result"
-# basename: Compute filename of FILE.
-# value returned in "$func_basename_result"
-# Implementation must be kept synchronized with func_dirname
-# and func_basename. For efficiency, we do not delegate to
-# those functions but instead duplicate the functionality here.
-func_dirname_and_basename ()
-{
- # Extract subdirectory from the argument.
- func_dirname_result=`$ECHO "${1}" | $SED -e "$dirname"`
- if test "X$func_dirname_result" = "X${1}"; then
- func_dirname_result="${3}"
- else
- func_dirname_result="$func_dirname_result${2}"
- fi
- func_basename_result=`$ECHO "${1}" | $SED -e "$basename"`
-} # func_dirname_and_basename may be replaced by extended shell implementation
-
-
-# func_stripname prefix suffix name
-# strip PREFIX and SUFFIX off of NAME.
-# PREFIX and SUFFIX must not contain globbing or regex special
-# characters, hashes, percent signs, but SUFFIX may contain a leading
-# dot (in which case that matches only a dot).
-# func_strip_suffix prefix name
-func_stripname ()
-{
- case ${2} in
- .*) func_stripname_result=`$ECHO "${3}" | $SED "s%^${1}%%; s%\\\\${2}\$%%"`;;
- *) func_stripname_result=`$ECHO "${3}" | $SED "s%^${1}%%; s%${2}\$%%"`;;
- esac
-} # func_stripname may be replaced by extended shell implementation
-
-
-# These SED scripts presuppose an absolute path with a trailing slash.
-pathcar='s,^/\([^/]*\).*$,\1,'
-pathcdr='s,^/[^/]*,,'
-removedotparts=':dotsl
- s@/\./@/@g
- t dotsl
- s,/\.$,/,'
-collapseslashes='s@/\{1,\}@/@g'
-finalslash='s,/*$,/,'
-
-# func_normal_abspath PATH
-# Remove doubled-up and trailing slashes, "." path components,
-# and cancel out any ".." path components in PATH after making
-# it an absolute path.
-# value returned in "$func_normal_abspath_result"
-func_normal_abspath ()
-{
- # Start from root dir and reassemble the path.
- func_normal_abspath_result=
- func_normal_abspath_tpath=$1
- func_normal_abspath_altnamespace=
- case $func_normal_abspath_tpath in
- "")
- # Empty path, that just means $cwd.
- func_stripname '' '/' "`pwd`"
- func_normal_abspath_result=$func_stripname_result
- return
- ;;
- # The next three entries are used to spot a run of precisely
- # two leading slashes without using negated character classes;
- # we take advantage of case's first-match behaviour.
- ///*)
- # Unusual form of absolute path, do nothing.
- ;;
- //*)
- # Not necessarily an ordinary path; POSIX reserves leading '//'
- # and for example Cygwin uses it to access remote file shares
- # over CIFS/SMB, so we conserve a leading double slash if found.
- func_normal_abspath_altnamespace=/
- ;;
- /*)
- # Absolute path, do nothing.
- ;;
- *)
- # Relative path, prepend $cwd.
- func_normal_abspath_tpath=`pwd`/$func_normal_abspath_tpath
- ;;
- esac
- # Cancel out all the simple stuff to save iterations. We also want
- # the path to end with a slash for ease of parsing, so make sure
- # there is one (and only one) here.
- func_normal_abspath_tpath=`$ECHO "$func_normal_abspath_tpath" | $SED \
- -e "$removedotparts" -e "$collapseslashes" -e "$finalslash"`
- while :; do
- # Processed it all yet?
- if test "$func_normal_abspath_tpath" = / ; then
- # If we ascended to the root using ".." the result may be empty now.
- if test -z "$func_normal_abspath_result" ; then
- func_normal_abspath_result=/
- fi
- break
- fi
- func_normal_abspath_tcomponent=`$ECHO "$func_normal_abspath_tpath" | $SED \
- -e "$pathcar"`
- func_normal_abspath_tpath=`$ECHO "$func_normal_abspath_tpath" | $SED \
- -e "$pathcdr"`
- # Figure out what to do with it
- case $func_normal_abspath_tcomponent in
- "")
- # Trailing empty path component, ignore it.
- ;;
- ..)
- # Parent dir; strip last assembled component from result.
- func_dirname "$func_normal_abspath_result"
- func_normal_abspath_result=$func_dirname_result
- ;;
- *)
- # Actual path component, append it.
- func_normal_abspath_result=$func_normal_abspath_result/$func_normal_abspath_tcomponent
- ;;
- esac
- done
- # Restore leading double-slash if one was found on entry.
- func_normal_abspath_result=$func_normal_abspath_altnamespace$func_normal_abspath_result
-}
-
-# func_relative_path SRCDIR DSTDIR
-# generates a relative path from SRCDIR to DSTDIR, with a trailing
-# slash if non-empty, suitable for immediately appending a filename
-# without needing to append a separator.
-# value returned in "$func_relative_path_result"
-func_relative_path ()
-{
- func_relative_path_result=
- func_normal_abspath "$1"
- func_relative_path_tlibdir=$func_normal_abspath_result
- func_normal_abspath "$2"
- func_relative_path_tbindir=$func_normal_abspath_result
-
- # Ascend the tree starting from libdir
- while :; do
- # check if we have found a prefix of bindir
- case $func_relative_path_tbindir in
- $func_relative_path_tlibdir)
- # found an exact match
- func_relative_path_tcancelled=
- break
- ;;
- $func_relative_path_tlibdir*)
- # found a matching prefix
- func_stripname "$func_relative_path_tlibdir" '' "$func_relative_path_tbindir"
- func_relative_path_tcancelled=$func_stripname_result
- if test -z "$func_relative_path_result"; then
- func_relative_path_result=.
- fi
- break
- ;;
- *)
- func_dirname $func_relative_path_tlibdir
- func_relative_path_tlibdir=${func_dirname_result}
- if test "x$func_relative_path_tlibdir" = x ; then
- # Have to descend all the way to the root!
- func_relative_path_result=../$func_relative_path_result
- func_relative_path_tcancelled=$func_relative_path_tbindir
- break
- fi
- func_relative_path_result=../$func_relative_path_result
- ;;
- esac
- done
-
- # Now calculate path; take care to avoid doubling-up slashes.
- func_stripname '' '/' "$func_relative_path_result"
- func_relative_path_result=$func_stripname_result
- func_stripname '/' '/' "$func_relative_path_tcancelled"
- if test "x$func_stripname_result" != x ; then
- func_relative_path_result=${func_relative_path_result}/${func_stripname_result}
- fi
-
- # Normalisation. If bindir is libdir, return empty string,
- # else relative path ending with a slash; either way, target
- # file name can be directly appended.
- if test ! -z "$func_relative_path_result"; then
- func_stripname './' '' "$func_relative_path_result/"
- func_relative_path_result=$func_stripname_result
- fi
-}
-
-# The name of this program:
-func_dirname_and_basename "$progpath"
-progname=$func_basename_result
-
-# Make sure we have an absolute path for reexecution:
-case $progpath in
- [\\/]*|[A-Za-z]:\\*) ;;
- *[\\/]*)
- progdir=$func_dirname_result
- progdir=`cd "$progdir" && pwd`
- progpath="$progdir/$progname"
- ;;
- *)
- save_IFS="$IFS"
- IFS=${PATH_SEPARATOR-:}
- for progdir in $PATH; do
- IFS="$save_IFS"
- test -x "$progdir/$progname" && break
- done
- IFS="$save_IFS"
- test -n "$progdir" || progdir=`pwd`
- progpath="$progdir/$progname"
- ;;
-esac
-
-# Sed substitution that helps us do robust quoting. It backslashifies
-# metacharacters that are still active within double-quoted strings.
-Xsed="${SED}"' -e 1s/^X//'
-sed_quote_subst='s/\([`"$\\]\)/\\\1/g'
-
-# Same as above, but do not quote variable references.
-double_quote_subst='s/\(["`\\]\)/\\\1/g'
-
-# Sed substitution that turns a string into a regex matching for the
-# string literally.
-sed_make_literal_regex='s,[].[^$\\*\/],\\&,g'
-
-# Sed substitution that converts a w32 file name or path
-# which contains forward slashes, into one that contains
-# (escaped) backslashes. A very naive implementation.
-lt_sed_naive_backslashify='s|\\\\*|\\|g;s|/|\\|g;s|\\|\\\\|g'
-
-# Re-`\' parameter expansions in output of double_quote_subst that were
-# `\'-ed in input to the same. If an odd number of `\' preceded a '$'
-# in input to double_quote_subst, that '$' was protected from expansion.
-# Since each input `\' is now two `\'s, look for any number of runs of
-# four `\'s followed by two `\'s and then a '$'. `\' that '$'.
-bs='\\'
-bs2='\\\\'
-bs4='\\\\\\\\'
-dollar='\$'
-sed_double_backslash="\
- s/$bs4/&\\
-/g
- s/^$bs2$dollar/$bs&/
- s/\\([^$bs]\\)$bs2$dollar/\\1$bs2$bs$dollar/g
- s/\n//g"
-
-# Standard options:
-opt_dry_run=false
-opt_help=false
-opt_quiet=false
-opt_verbose=false
-opt_warning=:
-
-# func_echo arg...
-# Echo program name prefixed message, along with the current mode
-# name if it has been set yet.
-func_echo ()
-{
- $ECHO "$progname: ${opt_mode+$opt_mode: }$*"
-}
-
-# func_verbose arg...
-# Echo program name prefixed message in verbose mode only.
-func_verbose ()
-{
- $opt_verbose && func_echo ${1+"$@"}
-
- # A bug in bash halts the script if the last line of a function
- # fails when set -e is in force, so we need another command to
- # work around that:
- :
-}
-
-# func_echo_all arg...
-# Invoke $ECHO with all args, space-separated.
-func_echo_all ()
-{
- $ECHO "$*"
-}
-
-# func_error arg...
-# Echo program name prefixed message to standard error.
-func_error ()
-{
- $ECHO "$progname: ${opt_mode+$opt_mode: }"${1+"$@"} 1>&2
-}
-
-# func_warning arg...
-# Echo program name prefixed warning message to standard error.
-func_warning ()
-{
- $opt_warning && $ECHO "$progname: ${opt_mode+$opt_mode: }warning: "${1+"$@"} 1>&2
-
- # bash bug again:
- :
-}
-
-# func_fatal_error arg...
-# Echo program name prefixed message to standard error, and exit.
-func_fatal_error ()
-{
- func_error ${1+"$@"}
- exit $EXIT_FAILURE
-}
-
-# func_fatal_help arg...
-# Echo program name prefixed message to standard error, followed by
-# a help hint, and exit.
-func_fatal_help ()
-{
- func_error ${1+"$@"}
- func_fatal_error "$help"
-}
-help="Try \`$progname --help' for more information." ## default
-
-
-# func_grep expression filename
-# Check whether EXPRESSION matches any line of FILENAME, without output.
-func_grep ()
-{
- $GREP "$1" "$2" >/dev/null 2>&1
-}
-
-
-# func_mkdir_p directory-path
-# Make sure the entire path to DIRECTORY-PATH is available.
-func_mkdir_p ()
-{
- my_directory_path="$1"
- my_dir_list=
-
- if test -n "$my_directory_path" && test "$opt_dry_run" != ":"; then
-
- # Protect directory names starting with `-'
- case $my_directory_path in
- -*) my_directory_path="./$my_directory_path" ;;
- esac
-
- # While some portion of DIR does not yet exist...
- while test ! -d "$my_directory_path"; do
- # ...make a list in topmost first order. Use a colon delimited
- # list incase some portion of path contains whitespace.
- my_dir_list="$my_directory_path:$my_dir_list"
-
- # If the last portion added has no slash in it, the list is done
- case $my_directory_path in */*) ;; *) break ;; esac
-
- # ...otherwise throw away the child directory and loop
- my_directory_path=`$ECHO "$my_directory_path" | $SED -e "$dirname"`
- done
- my_dir_list=`$ECHO "$my_dir_list" | $SED 's,:*$,,'`
-
- save_mkdir_p_IFS="$IFS"; IFS=':'
- for my_dir in $my_dir_list; do
- IFS="$save_mkdir_p_IFS"
- # mkdir can fail with a `File exist' error if two processes
- # try to create one of the directories concurrently. Don't
- # stop in that case!
- $MKDIR "$my_dir" 2>/dev/null || :
- done
- IFS="$save_mkdir_p_IFS"
-
- # Bail out if we (or some other process) failed to create a directory.
- test -d "$my_directory_path" || \
- func_fatal_error "Failed to create \`$1'"
- fi
-}
-
-
-# func_mktempdir [string]
-# Make a temporary directory that won't clash with other running
-# libtool processes, and avoids race conditions if possible. If
-# given, STRING is the basename for that directory.
-func_mktempdir ()
-{
- my_template="${TMPDIR-/tmp}/${1-$progname}"
-
- if test "$opt_dry_run" = ":"; then
- # Return a directory name, but don't create it in dry-run mode
- my_tmpdir="${my_template}-$$"
- else
-
- # If mktemp works, use that first and foremost
- my_tmpdir=`mktemp -d "${my_template}-XXXXXXXX" 2>/dev/null`
-
- if test ! -d "$my_tmpdir"; then
- # Failing that, at least try and use $RANDOM to avoid a race
- my_tmpdir="${my_template}-${RANDOM-0}$$"
-
- save_mktempdir_umask=`umask`
- umask 0077
- $MKDIR "$my_tmpdir"
- umask $save_mktempdir_umask
- fi
-
- # If we're not in dry-run mode, bomb out on failure
- test -d "$my_tmpdir" || \
- func_fatal_error "cannot create temporary directory \`$my_tmpdir'"
- fi
-
- $ECHO "$my_tmpdir"
-}
-
-
-# func_quote_for_eval arg
-# Aesthetically quote ARG to be evaled later.
-# This function returns two values: FUNC_QUOTE_FOR_EVAL_RESULT
-# is double-quoted, suitable for a subsequent eval, whereas
-# FUNC_QUOTE_FOR_EVAL_UNQUOTED_RESULT has merely all characters
-# which are still active within double quotes backslashified.
-func_quote_for_eval ()
-{
- case $1 in
- *[\\\`\"\$]*)
- func_quote_for_eval_unquoted_result=`$ECHO "$1" | $SED "$sed_quote_subst"` ;;
- *)
- func_quote_for_eval_unquoted_result="$1" ;;
- esac
-
- case $func_quote_for_eval_unquoted_result in
- # Double-quote args containing shell metacharacters to delay
- # word splitting, command substitution and variable
- # expansion for a subsequent eval.
- # Many Bourne shells cannot handle close brackets correctly
- # in scan sets, so we specify it separately.
- *[\[\~\#\^\&\*\(\)\{\}\|\;\<\>\?\'\ \ ]*|*]*|"")
- func_quote_for_eval_result="\"$func_quote_for_eval_unquoted_result\""
- ;;
- *)
- func_quote_for_eval_result="$func_quote_for_eval_unquoted_result"
- esac
-}
-
-
-# func_quote_for_expand arg
-# Aesthetically quote ARG to be evaled later; same as above,
-# but do not quote variable references.
-func_quote_for_expand ()
-{
- case $1 in
- *[\\\`\"]*)
- my_arg=`$ECHO "$1" | $SED \
- -e "$double_quote_subst" -e "$sed_double_backslash"` ;;
- *)
- my_arg="$1" ;;
- esac
-
- case $my_arg in
- # Double-quote args containing shell metacharacters to delay
- # word splitting and command substitution for a subsequent eval.
- # Many Bourne shells cannot handle close brackets correctly
- # in scan sets, so we specify it separately.
- *[\[\~\#\^\&\*\(\)\{\}\|\;\<\>\?\'\ \ ]*|*]*|"")
- my_arg="\"$my_arg\""
- ;;
- esac
-
- func_quote_for_expand_result="$my_arg"
-}
-
-
-# func_show_eval cmd [fail_exp]
-# Unless opt_silent is true, then output CMD. Then, if opt_dryrun is
-# not true, evaluate CMD. If the evaluation of CMD fails, and FAIL_EXP
-# is given, then evaluate it.
-func_show_eval ()
-{
- my_cmd="$1"
- my_fail_exp="${2-:}"
-
- ${opt_silent-false} || {
- func_quote_for_expand "$my_cmd"
- eval "func_echo $func_quote_for_expand_result"
- }
-
- if ${opt_dry_run-false}; then :; else
- eval "$my_cmd"
- my_status=$?
- if test "$my_status" -eq 0; then :; else
- eval "(exit $my_status); $my_fail_exp"
- fi
- fi
-}
-
-
-# func_show_eval_locale cmd [fail_exp]
-# Unless opt_silent is true, then output CMD. Then, if opt_dryrun is
-# not true, evaluate CMD. If the evaluation of CMD fails, and FAIL_EXP
-# is given, then evaluate it. Use the saved locale for evaluation.
-func_show_eval_locale ()
-{
- my_cmd="$1"
- my_fail_exp="${2-:}"
-
- ${opt_silent-false} || {
- func_quote_for_expand "$my_cmd"
- eval "func_echo $func_quote_for_expand_result"
- }
-
- if ${opt_dry_run-false}; then :; else
- eval "$lt_user_locale
- $my_cmd"
- my_status=$?
- eval "$lt_safe_locale"
- if test "$my_status" -eq 0; then :; else
- eval "(exit $my_status); $my_fail_exp"
- fi
- fi
-}
-
-# func_tr_sh
-# Turn $1 into a string suitable for a shell variable name.
-# Result is stored in $func_tr_sh_result. All characters
-# not in the set a-zA-Z0-9_ are replaced with '_'. Further,
-# if $1 begins with a digit, a '_' is prepended as well.
-func_tr_sh ()
-{
- case $1 in
- [0-9]* | *[!a-zA-Z0-9_]*)
- func_tr_sh_result=`$ECHO "$1" | $SED 's/^\([0-9]\)/_\1/; s/[^a-zA-Z0-9_]/_/g'`
- ;;
- * )
- func_tr_sh_result=$1
- ;;
- esac
-}
-
-
-# func_version
-# Echo version message to standard output and exit.
-func_version ()
-{
- $opt_debug
-
- $SED -n '/(C)/!b go
- :more
- /\./!{
- N
- s/\n# / /
- b more
- }
- :go
- /^# '$PROGRAM' (GNU /,/# warranty; / {
- s/^# //
- s/^# *$//
- s/\((C)\)[ 0-9,-]*\( [1-9][0-9]*\)/\1\2/
- p
- }' < "$progpath"
- exit $?
-}
-
-# func_usage
-# Echo short help message to standard output and exit.
-func_usage ()
-{
- $opt_debug
-
- $SED -n '/^# Usage:/,/^# *.*--help/ {
- s/^# //
- s/^# *$//
- s/\$progname/'$progname'/
- p
- }' < "$progpath"
- echo
- $ECHO "run \`$progname --help | more' for full usage"
- exit $?
-}
-
-# func_help [NOEXIT]
-# Echo long help message to standard output and exit,
-# unless 'noexit' is passed as argument.
-func_help ()
-{
- $opt_debug
-
- $SED -n '/^# Usage:/,/# Report bugs to/ {
- :print
- s/^# //
- s/^# *$//
- s*\$progname*'$progname'*
- s*\$host*'"$host"'*
- s*\$SHELL*'"$SHELL"'*
- s*\$LTCC*'"$LTCC"'*
- s*\$LTCFLAGS*'"$LTCFLAGS"'*
- s*\$LD*'"$LD"'*
- s/\$with_gnu_ld/'"$with_gnu_ld"'/
- s/\$automake_version/'"`(${AUTOMAKE-automake} --version) 2>/dev/null |$SED 1q`"'/
- s/\$autoconf_version/'"`(${AUTOCONF-autoconf} --version) 2>/dev/null |$SED 1q`"'/
- p
- d
- }
- /^# .* home page:/b print
- /^# General help using/b print
- ' < "$progpath"
- ret=$?
- if test -z "$1"; then
- exit $ret
- fi
-}
-
-# func_missing_arg argname
-# Echo program name prefixed message to standard error and set global
-# exit_cmd.
-func_missing_arg ()
-{
- $opt_debug
-
- func_error "missing argument for $1."
- exit_cmd=exit
-}
-
-
-# func_split_short_opt shortopt
-# Set func_split_short_opt_name and func_split_short_opt_arg shell
-# variables after splitting SHORTOPT after the 2nd character.
-func_split_short_opt ()
-{
- my_sed_short_opt='1s/^\(..\).*$/\1/;q'
- my_sed_short_rest='1s/^..\(.*\)$/\1/;q'
-
- func_split_short_opt_name=`$ECHO "$1" | $SED "$my_sed_short_opt"`
- func_split_short_opt_arg=`$ECHO "$1" | $SED "$my_sed_short_rest"`
-} # func_split_short_opt may be replaced by extended shell implementation
-
-
-# func_split_long_opt longopt
-# Set func_split_long_opt_name and func_split_long_opt_arg shell
-# variables after splitting LONGOPT at the `=' sign.
-func_split_long_opt ()
-{
- my_sed_long_opt='1s/^\(--[^=]*\)=.*/\1/;q'
- my_sed_long_arg='1s/^--[^=]*=//'
-
- func_split_long_opt_name=`$ECHO "$1" | $SED "$my_sed_long_opt"`
- func_split_long_opt_arg=`$ECHO "$1" | $SED "$my_sed_long_arg"`
-} # func_split_long_opt may be replaced by extended shell implementation
-
-exit_cmd=:
-
-
-
-
-
-magic="%%%MAGIC variable%%%"
-magic_exe="%%%MAGIC EXE variable%%%"
-
-# Global variables.
-nonopt=
-preserve_args=
-lo2o="s/\\.lo\$/.${objext}/"
-o2lo="s/\\.${objext}\$/.lo/"
-extracted_archives=
-extracted_serial=0
-
-# If this variable is set in any of the actions, the command in it
-# will be execed at the end. This prevents here-documents from being
-# left over by shells.
-exec_cmd=
-
-# func_append var value
-# Append VALUE to the end of shell variable VAR.
-func_append ()
-{
- eval "${1}=\$${1}\${2}"
-} # func_append may be replaced by extended shell implementation
-
-# func_append_quoted var value
-# Quote VALUE and append to the end of shell variable VAR, separated
-# by a space.
-func_append_quoted ()
-{
- func_quote_for_eval "${2}"
- eval "${1}=\$${1}\\ \$func_quote_for_eval_result"
-} # func_append_quoted may be replaced by extended shell implementation
-
-
-# func_arith arithmetic-term...
-func_arith ()
-{
- func_arith_result=`expr "${@}"`
-} # func_arith may be replaced by extended shell implementation
-
-
-# func_len string
-# STRING may not start with a hyphen.
-func_len ()
-{
- func_len_result=`expr "${1}" : ".*" 2>/dev/null || echo $max_cmd_len`
-} # func_len may be replaced by extended shell implementation
-
-
-# func_lo2o object
-func_lo2o ()
-{
- func_lo2o_result=`$ECHO "${1}" | $SED "$lo2o"`
-} # func_lo2o may be replaced by extended shell implementation
-
-
-# func_xform libobj-or-source
-func_xform ()
-{
- func_xform_result=`$ECHO "${1}" | $SED 's/\.[^.]*$/.lo/'`
-} # func_xform may be replaced by extended shell implementation
-
-
-# func_fatal_configuration arg...
-# Echo program name prefixed message to standard error, followed by
-# a configuration failure hint, and exit.
-func_fatal_configuration ()
-{
- func_error ${1+"$@"}
- func_error "See the $PACKAGE documentation for more information."
- func_fatal_error "Fatal configuration error."
-}
-
-
-# func_config
-# Display the configuration for all the tags in this script.
-func_config ()
-{
- re_begincf='^# ### BEGIN LIBTOOL'
- re_endcf='^# ### END LIBTOOL'
-
- # Default configuration.
- $SED "1,/$re_begincf CONFIG/d;/$re_endcf CONFIG/,\$d" < "$progpath"
-
- # Now print the configurations for the tags.
- for tagname in $taglist; do
- $SED -n "/$re_begincf TAG CONFIG: $tagname\$/,/$re_endcf TAG CONFIG: $tagname\$/p" < "$progpath"
- done
-
- exit $?
-}
-
-# func_features
-# Display the features supported by this script.
-func_features ()
-{
- echo "host: $host"
- if test "$build_libtool_libs" = yes; then
- echo "enable shared libraries"
- else
- echo "disable shared libraries"
- fi
- if test "$build_old_libs" = yes; then
- echo "enable static libraries"
- else
- echo "disable static libraries"
- fi
-
- exit $?
-}
-
-# func_enable_tag tagname
-# Verify that TAGNAME is valid, and either flag an error and exit, or
-# enable the TAGNAME tag. We also add TAGNAME to the global $taglist
-# variable here.
-func_enable_tag ()
-{
- # Global variable:
- tagname="$1"
-
- re_begincf="^# ### BEGIN LIBTOOL TAG CONFIG: $tagname\$"
- re_endcf="^# ### END LIBTOOL TAG CONFIG: $tagname\$"
- sed_extractcf="/$re_begincf/,/$re_endcf/p"
-
- # Validate tagname.
- case $tagname in
- *[!-_A-Za-z0-9,/]*)
- func_fatal_error "invalid tag name: $tagname"
- ;;
- esac
-
- # Don't test for the "default" C tag, as we know it's
- # there but not specially marked.
- case $tagname in
- CC) ;;
- *)
- if $GREP "$re_begincf" "$progpath" >/dev/null 2>&1; then
- taglist="$taglist $tagname"
-
- # Evaluate the configuration. Be careful to quote the path
- # and the sed script, to avoid splitting on whitespace, but
- # also don't use non-portable quotes within backquotes within
- # quotes we have to do it in 2 steps:
- extractedcf=`$SED -n -e "$sed_extractcf" < "$progpath"`
- eval "$extractedcf"
- else
- func_error "ignoring unknown tag $tagname"
- fi
- ;;
- esac
-}
-
-# func_check_version_match
-# Ensure that we are using m4 macros, and libtool script from the same
-# release of libtool.
-func_check_version_match ()
-{
- if test "$package_revision" != "$macro_revision"; then
- if test "$VERSION" != "$macro_version"; then
- if test -z "$macro_version"; then
- cat >&2 <<_LT_EOF
-$progname: Version mismatch error. This is $PACKAGE $VERSION, but the
-$progname: definition of this LT_INIT comes from an older release.
-$progname: You should recreate aclocal.m4 with macros from $PACKAGE $VERSION
-$progname: and run autoconf again.
-_LT_EOF
- else
- cat >&2 <<_LT_EOF
-$progname: Version mismatch error. This is $PACKAGE $VERSION, but the
-$progname: definition of this LT_INIT comes from $PACKAGE $macro_version.
-$progname: You should recreate aclocal.m4 with macros from $PACKAGE $VERSION
-$progname: and run autoconf again.
-_LT_EOF
- fi
- else
- cat >&2 <<_LT_EOF
-$progname: Version mismatch error. This is $PACKAGE $VERSION, revision $package_revision,
-$progname: but the definition of this LT_INIT comes from revision $macro_revision.
-$progname: You should recreate aclocal.m4 with macros from revision $package_revision
-$progname: of $PACKAGE $VERSION and run autoconf again.
-_LT_EOF
- fi
-
- exit $EXIT_MISMATCH
- fi
-}
-
-
-# Shorthand for --mode=foo, only valid as the first argument
-case $1 in
-clean|clea|cle|cl)
- shift; set dummy --mode clean ${1+"$@"}; shift
- ;;
-compile|compil|compi|comp|com|co|c)
- shift; set dummy --mode compile ${1+"$@"}; shift
- ;;
-execute|execut|execu|exec|exe|ex|e)
- shift; set dummy --mode execute ${1+"$@"}; shift
- ;;
-finish|finis|fini|fin|fi|f)
- shift; set dummy --mode finish ${1+"$@"}; shift
- ;;
-install|instal|insta|inst|ins|in|i)
- shift; set dummy --mode install ${1+"$@"}; shift
- ;;
-link|lin|li|l)
- shift; set dummy --mode link ${1+"$@"}; shift
- ;;
-uninstall|uninstal|uninsta|uninst|unins|unin|uni|un|u)
- shift; set dummy --mode uninstall ${1+"$@"}; shift
- ;;
-esac
-
-
-
-# Option defaults:
-opt_debug=:
-opt_dry_run=false
-opt_config=false
-opt_preserve_dup_deps=false
-opt_features=false
-opt_finish=false
-opt_help=false
-opt_help_all=false
-opt_silent=:
-opt_warning=:
-opt_verbose=:
-opt_silent=false
-opt_verbose=false
-
-
-# Parse options once, thoroughly. This comes as soon as possible in the
-# script to make things like `--version' happen as quickly as we can.
-{
- # this just eases exit handling
- while test $# -gt 0; do
- opt="$1"
- shift
- case $opt in
- --debug|-x) opt_debug='set -x'
- func_echo "enabling shell trace mode"
- $opt_debug
- ;;
- --dry-run|--dryrun|-n)
- opt_dry_run=:
- ;;
- --config)
- opt_config=:
-func_config
- ;;
- --dlopen|-dlopen)
- optarg="$1"
- opt_dlopen="${opt_dlopen+$opt_dlopen
-}$optarg"
- shift
- ;;
- --preserve-dup-deps)
- opt_preserve_dup_deps=:
- ;;
- --features)
- opt_features=:
-func_features
- ;;
- --finish)
- opt_finish=:
-set dummy --mode finish ${1+"$@"}; shift
- ;;
- --help)
- opt_help=:
- ;;
- --help-all)
- opt_help_all=:
-opt_help=': help-all'
- ;;
- --mode)
- test $# = 0 && func_missing_arg $opt && break
- optarg="$1"
- opt_mode="$optarg"
-case $optarg in
- # Valid mode arguments:
- clean|compile|execute|finish|install|link|relink|uninstall) ;;
-
- # Catch anything else as an error
- *) func_error "invalid argument for $opt"
- exit_cmd=exit
- break
- ;;
-esac
- shift
- ;;
- --no-silent|--no-quiet)
- opt_silent=false
-func_append preserve_args " $opt"
- ;;
- --no-warning|--no-warn)
- opt_warning=false
-func_append preserve_args " $opt"
- ;;
- --no-verbose)
- opt_verbose=false
-func_append preserve_args " $opt"
- ;;
- --silent|--quiet)
- opt_silent=:
-func_append preserve_args " $opt"
- opt_verbose=false
- ;;
- --verbose|-v)
- opt_verbose=:
-func_append preserve_args " $opt"
-opt_silent=false
- ;;
- --tag)
- test $# = 0 && func_missing_arg $opt && break
- optarg="$1"
- opt_tag="$optarg"
-func_append preserve_args " $opt $optarg"
-func_enable_tag "$optarg"
- shift
- ;;
-
- -\?|-h) func_usage ;;
- --help) func_help ;;
- --version) func_version ;;
-
- # Separate optargs to long options:
- --*=*)
- func_split_long_opt "$opt"
- set dummy "$func_split_long_opt_name" "$func_split_long_opt_arg" ${1+"$@"}
- shift
- ;;
-
- # Separate non-argument short options:
- -\?*|-h*|-n*|-v*)
- func_split_short_opt "$opt"
- set dummy "$func_split_short_opt_name" "-$func_split_short_opt_arg" ${1+"$@"}
- shift
- ;;
-
- --) break ;;
- -*) func_fatal_help "unrecognized option \`$opt'" ;;
- *) set dummy "$opt" ${1+"$@"}; shift; break ;;
- esac
- done
-
- # Validate options:
-
- # save first non-option argument
- if test "$#" -gt 0; then
- nonopt="$opt"
- shift
- fi
-
- # preserve --debug
- test "$opt_debug" = : || func_append preserve_args " --debug"
-
- case $host in
- *cygwin* | *mingw* | *pw32* | *cegcc*)
- # don't eliminate duplications in $postdeps and $predeps
- opt_duplicate_compiler_generated_deps=:
- ;;
- *)
- opt_duplicate_compiler_generated_deps=$opt_preserve_dup_deps
- ;;
- esac
-
- $opt_help || {
- # Sanity checks first:
- func_check_version_match
-
- if test "$build_libtool_libs" != yes && test "$build_old_libs" != yes; then
- func_fatal_configuration "not configured to build any kind of library"
- fi
-
- # Darwin sucks
- eval std_shrext=\"$shrext_cmds\"
-
- # Only execute mode is allowed to have -dlopen flags.
- if test -n "$opt_dlopen" && test "$opt_mode" != execute; then
- func_error "unrecognized option \`-dlopen'"
- $ECHO "$help" 1>&2
- exit $EXIT_FAILURE
- fi
-
- # Change the help message to a mode-specific one.
- generic_help="$help"
- help="Try \`$progname --help --mode=$opt_mode' for more information."
- }
-
-
- # Bail if the options were screwed
- $exit_cmd $EXIT_FAILURE
-}
-
-
-
-
-## ----------- ##
-## Main. ##
-## ----------- ##
-
-# func_lalib_p file
-# True iff FILE is a libtool `.la' library or `.lo' object file.
-# This function is only a basic sanity check; it will hardly flush out
-# determined imposters.
-func_lalib_p ()
-{
- test -f "$1" &&
- $SED -e 4q "$1" 2>/dev/null \
- | $GREP "^# Generated by .*$PACKAGE" > /dev/null 2>&1
-}
-
-# func_lalib_unsafe_p file
-# True iff FILE is a libtool `.la' library or `.lo' object file.
-# This function implements the same check as func_lalib_p without
-# resorting to external programs. To this end, it redirects stdin and
-# closes it afterwards, without saving the original file descriptor.
-# As a safety measure, use it only where a negative result would be
-# fatal anyway. Works if `file' does not exist.
-func_lalib_unsafe_p ()
-{
- lalib_p=no
- if test -f "$1" && test -r "$1" && exec 5<&0 <"$1"; then
- for lalib_p_l in 1 2 3 4
- do
- read lalib_p_line
- case "$lalib_p_line" in
- \#\ Generated\ by\ *$PACKAGE* ) lalib_p=yes; break;;
- esac
- done
- exec 0<&5 5<&-
- fi
- test "$lalib_p" = yes
-}
-
-# func_ltwrapper_script_p file
-# True iff FILE is a libtool wrapper script
-# This function is only a basic sanity check; it will hardly flush out
-# determined imposters.
-func_ltwrapper_script_p ()
-{
- func_lalib_p "$1"
-}
-
-# func_ltwrapper_executable_p file
-# True iff FILE is a libtool wrapper executable
-# This function is only a basic sanity check; it will hardly flush out
-# determined imposters.
-func_ltwrapper_executable_p ()
-{
- func_ltwrapper_exec_suffix=
- case $1 in
- *.exe) ;;
- *) func_ltwrapper_exec_suffix=.exe ;;
- esac
- $GREP "$magic_exe" "$1$func_ltwrapper_exec_suffix" >/dev/null 2>&1
-}
-
-# func_ltwrapper_scriptname file
-# Assumes file is an ltwrapper_executable
-# uses $file to determine the appropriate filename for a
-# temporary ltwrapper_script.
-func_ltwrapper_scriptname ()
-{
- func_dirname_and_basename "$1" "" "."
- func_stripname '' '.exe' "$func_basename_result"
- func_ltwrapper_scriptname_result="$func_dirname_result/$objdir/${func_stripname_result}_ltshwrapper"
-}
-
-# func_ltwrapper_p file
-# True iff FILE is a libtool wrapper script or wrapper executable
-# This function is only a basic sanity check; it will hardly flush out
-# determined imposters.
-func_ltwrapper_p ()
-{
- func_ltwrapper_script_p "$1" || func_ltwrapper_executable_p "$1"
-}
-
-
-# func_execute_cmds commands fail_cmd
-# Execute tilde-delimited COMMANDS.
-# If FAIL_CMD is given, eval that upon failure.
-# FAIL_CMD may read-access the current command in variable CMD!
-func_execute_cmds ()
-{
- $opt_debug
- save_ifs=$IFS; IFS='~'
- for cmd in $1; do
- IFS=$save_ifs
- eval cmd=\"$cmd\"
- func_show_eval "$cmd" "${2-:}"
- done
- IFS=$save_ifs
-}
-
-
-# func_source file
-# Source FILE, adding directory component if necessary.
-# Note that it is not necessary on cygwin/mingw to append a dot to
-# FILE even if both FILE and FILE.exe exist: automatic-append-.exe
-# behavior happens only for exec(3), not for open(2)! Also, sourcing
-# `FILE.' does not work on cygwin managed mounts.
-func_source ()
-{
- $opt_debug
- case $1 in
- */* | *\\*) . "$1" ;;
- *) . "./$1" ;;
- esac
-}
-
-
-# func_resolve_sysroot PATH
-# Replace a leading = in PATH with a sysroot. Store the result into
-# func_resolve_sysroot_result
-func_resolve_sysroot ()
-{
- func_resolve_sysroot_result=$1
- case $func_resolve_sysroot_result in
- =*)
- func_stripname '=' '' "$func_resolve_sysroot_result"
- func_resolve_sysroot_result=$lt_sysroot$func_stripname_result
- ;;
- esac
-}
-
-# func_replace_sysroot PATH
-# If PATH begins with the sysroot, replace it with = and
-# store the result into func_replace_sysroot_result.
-func_replace_sysroot ()
-{
- case "$lt_sysroot:$1" in
- ?*:"$lt_sysroot"*)
- func_stripname "$lt_sysroot" '' "$1"
- func_replace_sysroot_result="=$func_stripname_result"
- ;;
- *)
- # Including no sysroot.
- func_replace_sysroot_result=$1
- ;;
- esac
-}
-
-# func_infer_tag arg
-# Infer tagged configuration to use if any are available and
-# if one wasn't chosen via the "--tag" command line option.
-# Only attempt this if the compiler in the base compile
-# command doesn't match the default compiler.
-# arg is usually of the form 'gcc ...'
-func_infer_tag ()
-{
- $opt_debug
- if test -n "$available_tags" && test -z "$tagname"; then
- CC_quoted=
- for arg in $CC; do
- func_append_quoted CC_quoted "$arg"
- done
- CC_expanded=`func_echo_all $CC`
- CC_quoted_expanded=`func_echo_all $CC_quoted`
- case $@ in
- # Blanks in the command may have been stripped by the calling shell,
- # but not from the CC environment variable when configure was run.
- " $CC "* | "$CC "* | " $CC_expanded "* | "$CC_expanded "* | \
- " $CC_quoted"* | "$CC_quoted "* | " $CC_quoted_expanded "* | "$CC_quoted_expanded "*) ;;
- # Blanks at the start of $base_compile will cause this to fail
- # if we don't check for them as well.
- *)
- for z in $available_tags; do
- if $GREP "^# ### BEGIN LIBTOOL TAG CONFIG: $z$" < "$progpath" > /dev/null; then
- # Evaluate the configuration.
- eval "`${SED} -n -e '/^# ### BEGIN LIBTOOL TAG CONFIG: '$z'$/,/^# ### END LIBTOOL TAG CONFIG: '$z'$/p' < $progpath`"
- CC_quoted=
- for arg in $CC; do
- # Double-quote args containing other shell metacharacters.
- func_append_quoted CC_quoted "$arg"
- done
- CC_expanded=`func_echo_all $CC`
- CC_quoted_expanded=`func_echo_all $CC_quoted`
- case "$@ " in
- " $CC "* | "$CC "* | " $CC_expanded "* | "$CC_expanded "* | \
- " $CC_quoted"* | "$CC_quoted "* | " $CC_quoted_expanded "* | "$CC_quoted_expanded "*)
- # The compiler in the base compile command matches
- # the one in the tagged configuration.
- # Assume this is the tagged configuration we want.
- tagname=$z
- break
- ;;
- esac
- fi
- done
- # If $tagname still isn't set, then no tagged configuration
- # was found and let the user know that the "--tag" command
- # line option must be used.
- if test -z "$tagname"; then
- func_echo "unable to infer tagged configuration"
- func_fatal_error "specify a tag with \`--tag'"
-# else
-# func_verbose "using $tagname tagged configuration"
- fi
- ;;
- esac
- fi
-}
-
-
-
-# func_write_libtool_object output_name pic_name nonpic_name
-# Create a libtool object file (analogous to a ".la" file),
-# but don't create it if we're doing a dry run.
-func_write_libtool_object ()
-{
- write_libobj=${1}
- if test "$build_libtool_libs" = yes; then
- write_lobj=\'${2}\'
- else
- write_lobj=none
- fi
-
- if test "$build_old_libs" = yes; then
- write_oldobj=\'${3}\'
- else
- write_oldobj=none
- fi
-
- $opt_dry_run || {
- cat >${write_libobj}T </dev/null`
- if test "$?" -eq 0 && test -n "${func_convert_core_file_wine_to_w32_tmp}"; then
- func_convert_core_file_wine_to_w32_result=`$ECHO "$func_convert_core_file_wine_to_w32_tmp" |
- $SED -e "$lt_sed_naive_backslashify"`
- else
- func_convert_core_file_wine_to_w32_result=
- fi
- fi
-}
-# end: func_convert_core_file_wine_to_w32
-
-
-# func_convert_core_path_wine_to_w32 ARG
-# Helper function used by path conversion functions when $build is *nix, and
-# $host is mingw, cygwin, or some other w32 environment. Relies on a correctly
-# configured wine environment available, with the winepath program in $build's
-# $PATH. Assumes ARG has no leading or trailing path separator characters.
-#
-# ARG is path to be converted from $build format to win32.
-# Result is available in $func_convert_core_path_wine_to_w32_result.
-# Unconvertible file (directory) names in ARG are skipped; if no directory names
-# are convertible, then the result may be empty.
-func_convert_core_path_wine_to_w32 ()
-{
- $opt_debug
- # unfortunately, winepath doesn't convert paths, only file names
- func_convert_core_path_wine_to_w32_result=""
- if test -n "$1"; then
- oldIFS=$IFS
- IFS=:
- for func_convert_core_path_wine_to_w32_f in $1; do
- IFS=$oldIFS
- func_convert_core_file_wine_to_w32 "$func_convert_core_path_wine_to_w32_f"
- if test -n "$func_convert_core_file_wine_to_w32_result" ; then
- if test -z "$func_convert_core_path_wine_to_w32_result"; then
- func_convert_core_path_wine_to_w32_result="$func_convert_core_file_wine_to_w32_result"
- else
- func_append func_convert_core_path_wine_to_w32_result ";$func_convert_core_file_wine_to_w32_result"
- fi
- fi
- done
- IFS=$oldIFS
- fi
-}
-# end: func_convert_core_path_wine_to_w32
-
-
-# func_cygpath ARGS...
-# Wrapper around calling the cygpath program via LT_CYGPATH. This is used when
-# when (1) $build is *nix and Cygwin is hosted via a wine environment; or (2)
-# $build is MSYS and $host is Cygwin, or (3) $build is Cygwin. In case (1) or
-# (2), returns the Cygwin file name or path in func_cygpath_result (input
-# file name or path is assumed to be in w32 format, as previously converted
-# from $build's *nix or MSYS format). In case (3), returns the w32 file name
-# or path in func_cygpath_result (input file name or path is assumed to be in
-# Cygwin format). Returns an empty string on error.
-#
-# ARGS are passed to cygpath, with the last one being the file name or path to
-# be converted.
-#
-# Specify the absolute *nix (or w32) name to cygpath in the LT_CYGPATH
-# environment variable; do not put it in $PATH.
-func_cygpath ()
-{
- $opt_debug
- if test -n "$LT_CYGPATH" && test -f "$LT_CYGPATH"; then
- func_cygpath_result=`$LT_CYGPATH "$@" 2>/dev/null`
- if test "$?" -ne 0; then
- # on failure, ensure result is empty
- func_cygpath_result=
- fi
- else
- func_cygpath_result=
- func_error "LT_CYGPATH is empty or specifies non-existent file: \`$LT_CYGPATH'"
- fi
-}
-#end: func_cygpath
-
-
-# func_convert_core_msys_to_w32 ARG
-# Convert file name or path ARG from MSYS format to w32 format. Return
-# result in func_convert_core_msys_to_w32_result.
-func_convert_core_msys_to_w32 ()
-{
- $opt_debug
- # awkward: cmd appends spaces to result
- func_convert_core_msys_to_w32_result=`( cmd //c echo "$1" ) 2>/dev/null |
- $SED -e 's/[ ]*$//' -e "$lt_sed_naive_backslashify"`
-}
-#end: func_convert_core_msys_to_w32
-
-
-# func_convert_file_check ARG1 ARG2
-# Verify that ARG1 (a file name in $build format) was converted to $host
-# format in ARG2. Otherwise, emit an error message, but continue (resetting
-# func_to_host_file_result to ARG1).
-func_convert_file_check ()
-{
- $opt_debug
- if test -z "$2" && test -n "$1" ; then
- func_error "Could not determine host file name corresponding to"
- func_error " \`$1'"
- func_error "Continuing, but uninstalled executables may not work."
- # Fallback:
- func_to_host_file_result="$1"
- fi
-}
-# end func_convert_file_check
-
-
-# func_convert_path_check FROM_PATHSEP TO_PATHSEP FROM_PATH TO_PATH
-# Verify that FROM_PATH (a path in $build format) was converted to $host
-# format in TO_PATH. Otherwise, emit an error message, but continue, resetting
-# func_to_host_file_result to a simplistic fallback value (see below).
-func_convert_path_check ()
-{
- $opt_debug
- if test -z "$4" && test -n "$3"; then
- func_error "Could not determine the host path corresponding to"
- func_error " \`$3'"
- func_error "Continuing, but uninstalled executables may not work."
- # Fallback. This is a deliberately simplistic "conversion" and
- # should not be "improved". See libtool.info.
- if test "x$1" != "x$2"; then
- lt_replace_pathsep_chars="s|$1|$2|g"
- func_to_host_path_result=`echo "$3" |
- $SED -e "$lt_replace_pathsep_chars"`
- else
- func_to_host_path_result="$3"
- fi
- fi
-}
-# end func_convert_path_check
-
-
-# func_convert_path_front_back_pathsep FRONTPAT BACKPAT REPL ORIG
-# Modifies func_to_host_path_result by prepending REPL if ORIG matches FRONTPAT
-# and appending REPL if ORIG matches BACKPAT.
-func_convert_path_front_back_pathsep ()
-{
- $opt_debug
- case $4 in
- $1 ) func_to_host_path_result="$3$func_to_host_path_result"
- ;;
- esac
- case $4 in
- $2 ) func_append func_to_host_path_result "$3"
- ;;
- esac
-}
-# end func_convert_path_front_back_pathsep
-
-
-##################################################
-# $build to $host FILE NAME CONVERSION FUNCTIONS #
-##################################################
-# invoked via `$to_host_file_cmd ARG'
-#
-# In each case, ARG is the path to be converted from $build to $host format.
-# Result will be available in $func_to_host_file_result.
-
-
-# func_to_host_file ARG
-# Converts the file name ARG from $build format to $host format. Return result
-# in func_to_host_file_result.
-func_to_host_file ()
-{
- $opt_debug
- $to_host_file_cmd "$1"
-}
-# end func_to_host_file
-
-
-# func_to_tool_file ARG LAZY
-# converts the file name ARG from $build format to toolchain format. Return
-# result in func_to_tool_file_result. If the conversion in use is listed
-# in (the comma separated) LAZY, no conversion takes place.
-func_to_tool_file ()
-{
- $opt_debug
- case ,$2, in
- *,"$to_tool_file_cmd",*)
- func_to_tool_file_result=$1
- ;;
- *)
- $to_tool_file_cmd "$1"
- func_to_tool_file_result=$func_to_host_file_result
- ;;
- esac
-}
-# end func_to_tool_file
-
-
-# func_convert_file_noop ARG
-# Copy ARG to func_to_host_file_result.
-func_convert_file_noop ()
-{
- func_to_host_file_result="$1"
-}
-# end func_convert_file_noop
-
-
-# func_convert_file_msys_to_w32 ARG
-# Convert file name ARG from (mingw) MSYS to (mingw) w32 format; automatic
-# conversion to w32 is not available inside the cwrapper. Returns result in
-# func_to_host_file_result.
-func_convert_file_msys_to_w32 ()
-{
- $opt_debug
- func_to_host_file_result="$1"
- if test -n "$1"; then
- func_convert_core_msys_to_w32 "$1"
- func_to_host_file_result="$func_convert_core_msys_to_w32_result"
- fi
- func_convert_file_check "$1" "$func_to_host_file_result"
-}
-# end func_convert_file_msys_to_w32
-
-
-# func_convert_file_cygwin_to_w32 ARG
-# Convert file name ARG from Cygwin to w32 format. Returns result in
-# func_to_host_file_result.
-func_convert_file_cygwin_to_w32 ()
-{
- $opt_debug
- func_to_host_file_result="$1"
- if test -n "$1"; then
- # because $build is cygwin, we call "the" cygpath in $PATH; no need to use
- # LT_CYGPATH in this case.
- func_to_host_file_result=`cygpath -m "$1"`
- fi
- func_convert_file_check "$1" "$func_to_host_file_result"
-}
-# end func_convert_file_cygwin_to_w32
-
-
-# func_convert_file_nix_to_w32 ARG
-# Convert file name ARG from *nix to w32 format. Requires a wine environment
-# and a working winepath. Returns result in func_to_host_file_result.
-func_convert_file_nix_to_w32 ()
-{
- $opt_debug
- func_to_host_file_result="$1"
- if test -n "$1"; then
- func_convert_core_file_wine_to_w32 "$1"
- func_to_host_file_result="$func_convert_core_file_wine_to_w32_result"
- fi
- func_convert_file_check "$1" "$func_to_host_file_result"
-}
-# end func_convert_file_nix_to_w32
-
-
-# func_convert_file_msys_to_cygwin ARG
-# Convert file name ARG from MSYS to Cygwin format. Requires LT_CYGPATH set.
-# Returns result in func_to_host_file_result.
-func_convert_file_msys_to_cygwin ()
-{
- $opt_debug
- func_to_host_file_result="$1"
- if test -n "$1"; then
- func_convert_core_msys_to_w32 "$1"
- func_cygpath -u "$func_convert_core_msys_to_w32_result"
- func_to_host_file_result="$func_cygpath_result"
- fi
- func_convert_file_check "$1" "$func_to_host_file_result"
-}
-# end func_convert_file_msys_to_cygwin
-
-
-# func_convert_file_nix_to_cygwin ARG
-# Convert file name ARG from *nix to Cygwin format. Requires Cygwin installed
-# in a wine environment, working winepath, and LT_CYGPATH set. Returns result
-# in func_to_host_file_result.
-func_convert_file_nix_to_cygwin ()
-{
- $opt_debug
- func_to_host_file_result="$1"
- if test -n "$1"; then
- # convert from *nix to w32, then use cygpath to convert from w32 to cygwin.
- func_convert_core_file_wine_to_w32 "$1"
- func_cygpath -u "$func_convert_core_file_wine_to_w32_result"
- func_to_host_file_result="$func_cygpath_result"
- fi
- func_convert_file_check "$1" "$func_to_host_file_result"
-}
-# end func_convert_file_nix_to_cygwin
-
-
-#############################################
-# $build to $host PATH CONVERSION FUNCTIONS #
-#############################################
-# invoked via `$to_host_path_cmd ARG'
-#
-# In each case, ARG is the path to be converted from $build to $host format.
-# The result will be available in $func_to_host_path_result.
-#
-# Path separators are also converted from $build format to $host format. If
-# ARG begins or ends with a path separator character, it is preserved (but
-# converted to $host format) on output.
-#
-# All path conversion functions are named using the following convention:
-# file name conversion function : func_convert_file_X_to_Y ()
-# path conversion function : func_convert_path_X_to_Y ()
-# where, for any given $build/$host combination the 'X_to_Y' value is the
-# same. If conversion functions are added for new $build/$host combinations,
-# the two new functions must follow this pattern, or func_init_to_host_path_cmd
-# will break.
-
-
-# func_init_to_host_path_cmd
-# Ensures that function "pointer" variable $to_host_path_cmd is set to the
-# appropriate value, based on the value of $to_host_file_cmd.
-to_host_path_cmd=
-func_init_to_host_path_cmd ()
-{
- $opt_debug
- if test -z "$to_host_path_cmd"; then
- func_stripname 'func_convert_file_' '' "$to_host_file_cmd"
- to_host_path_cmd="func_convert_path_${func_stripname_result}"
- fi
-}
-
-
-# func_to_host_path ARG
-# Converts the path ARG from $build format to $host format. Return result
-# in func_to_host_path_result.
-func_to_host_path ()
-{
- $opt_debug
- func_init_to_host_path_cmd
- $to_host_path_cmd "$1"
-}
-# end func_to_host_path
-
-
-# func_convert_path_noop ARG
-# Copy ARG to func_to_host_path_result.
-func_convert_path_noop ()
-{
- func_to_host_path_result="$1"
-}
-# end func_convert_path_noop
-
-
-# func_convert_path_msys_to_w32 ARG
-# Convert path ARG from (mingw) MSYS to (mingw) w32 format; automatic
-# conversion to w32 is not available inside the cwrapper. Returns result in
-# func_to_host_path_result.
-func_convert_path_msys_to_w32 ()
-{
- $opt_debug
- func_to_host_path_result="$1"
- if test -n "$1"; then
- # Remove leading and trailing path separator characters from ARG. MSYS
- # behavior is inconsistent here; cygpath turns them into '.;' and ';.';
- # and winepath ignores them completely.
- func_stripname : : "$1"
- func_to_host_path_tmp1=$func_stripname_result
- func_convert_core_msys_to_w32 "$func_to_host_path_tmp1"
- func_to_host_path_result="$func_convert_core_msys_to_w32_result"
- func_convert_path_check : ";" \
- "$func_to_host_path_tmp1" "$func_to_host_path_result"
- func_convert_path_front_back_pathsep ":*" "*:" ";" "$1"
- fi
-}
-# end func_convert_path_msys_to_w32
-
-
-# func_convert_path_cygwin_to_w32 ARG
-# Convert path ARG from Cygwin to w32 format. Returns result in
-# func_to_host_file_result.
-func_convert_path_cygwin_to_w32 ()
-{
- $opt_debug
- func_to_host_path_result="$1"
- if test -n "$1"; then
- # See func_convert_path_msys_to_w32:
- func_stripname : : "$1"
- func_to_host_path_tmp1=$func_stripname_result
- func_to_host_path_result=`cygpath -m -p "$func_to_host_path_tmp1"`
- func_convert_path_check : ";" \
- "$func_to_host_path_tmp1" "$func_to_host_path_result"
- func_convert_path_front_back_pathsep ":*" "*:" ";" "$1"
- fi
-}
-# end func_convert_path_cygwin_to_w32
-
-
-# func_convert_path_nix_to_w32 ARG
-# Convert path ARG from *nix to w32 format. Requires a wine environment and
-# a working winepath. Returns result in func_to_host_file_result.
-func_convert_path_nix_to_w32 ()
-{
- $opt_debug
- func_to_host_path_result="$1"
- if test -n "$1"; then
- # See func_convert_path_msys_to_w32:
- func_stripname : : "$1"
- func_to_host_path_tmp1=$func_stripname_result
- func_convert_core_path_wine_to_w32 "$func_to_host_path_tmp1"
- func_to_host_path_result="$func_convert_core_path_wine_to_w32_result"
- func_convert_path_check : ";" \
- "$func_to_host_path_tmp1" "$func_to_host_path_result"
- func_convert_path_front_back_pathsep ":*" "*:" ";" "$1"
- fi
-}
-# end func_convert_path_nix_to_w32
-
-
-# func_convert_path_msys_to_cygwin ARG
-# Convert path ARG from MSYS to Cygwin format. Requires LT_CYGPATH set.
-# Returns result in func_to_host_file_result.
-func_convert_path_msys_to_cygwin ()
-{
- $opt_debug
- func_to_host_path_result="$1"
- if test -n "$1"; then
- # See func_convert_path_msys_to_w32:
- func_stripname : : "$1"
- func_to_host_path_tmp1=$func_stripname_result
- func_convert_core_msys_to_w32 "$func_to_host_path_tmp1"
- func_cygpath -u -p "$func_convert_core_msys_to_w32_result"
- func_to_host_path_result="$func_cygpath_result"
- func_convert_path_check : : \
- "$func_to_host_path_tmp1" "$func_to_host_path_result"
- func_convert_path_front_back_pathsep ":*" "*:" : "$1"
- fi
-}
-# end func_convert_path_msys_to_cygwin
-
-
-# func_convert_path_nix_to_cygwin ARG
-# Convert path ARG from *nix to Cygwin format. Requires Cygwin installed in a
-# a wine environment, working winepath, and LT_CYGPATH set. Returns result in
-# func_to_host_file_result.
-func_convert_path_nix_to_cygwin ()
-{
- $opt_debug
- func_to_host_path_result="$1"
- if test -n "$1"; then
- # Remove leading and trailing path separator characters from
- # ARG. msys behavior is inconsistent here, cygpath turns them
- # into '.;' and ';.', and winepath ignores them completely.
- func_stripname : : "$1"
- func_to_host_path_tmp1=$func_stripname_result
- func_convert_core_path_wine_to_w32 "$func_to_host_path_tmp1"
- func_cygpath -u -p "$func_convert_core_path_wine_to_w32_result"
- func_to_host_path_result="$func_cygpath_result"
- func_convert_path_check : : \
- "$func_to_host_path_tmp1" "$func_to_host_path_result"
- func_convert_path_front_back_pathsep ":*" "*:" : "$1"
- fi
-}
-# end func_convert_path_nix_to_cygwin
-
-
-# func_mode_compile arg...
-func_mode_compile ()
-{
- $opt_debug
- # Get the compilation command and the source file.
- base_compile=
- srcfile="$nonopt" # always keep a non-empty value in "srcfile"
- suppress_opt=yes
- suppress_output=
- arg_mode=normal
- libobj=
- later=
- pie_flag=
-
- for arg
- do
- case $arg_mode in
- arg )
- # do not "continue". Instead, add this to base_compile
- lastarg="$arg"
- arg_mode=normal
- ;;
-
- target )
- libobj="$arg"
- arg_mode=normal
- continue
- ;;
-
- normal )
- # Accept any command-line options.
- case $arg in
- -o)
- test -n "$libobj" && \
- func_fatal_error "you cannot specify \`-o' more than once"
- arg_mode=target
- continue
- ;;
-
- -pie | -fpie | -fPIE)
- func_append pie_flag " $arg"
- continue
- ;;
-
- -shared | -static | -prefer-pic | -prefer-non-pic)
- func_append later " $arg"
- continue
- ;;
-
- -no-suppress)
- suppress_opt=no
- continue
- ;;
-
- -Xcompiler)
- arg_mode=arg # the next one goes into the "base_compile" arg list
- continue # The current "srcfile" will either be retained or
- ;; # replaced later. I would guess that would be a bug.
-
- -Wc,*)
- func_stripname '-Wc,' '' "$arg"
- args=$func_stripname_result
- lastarg=
- save_ifs="$IFS"; IFS=','
- for arg in $args; do
- IFS="$save_ifs"
- func_append_quoted lastarg "$arg"
- done
- IFS="$save_ifs"
- func_stripname ' ' '' "$lastarg"
- lastarg=$func_stripname_result
-
- # Add the arguments to base_compile.
- func_append base_compile " $lastarg"
- continue
- ;;
-
- *)
- # Accept the current argument as the source file.
- # The previous "srcfile" becomes the current argument.
- #
- lastarg="$srcfile"
- srcfile="$arg"
- ;;
- esac # case $arg
- ;;
- esac # case $arg_mode
-
- # Aesthetically quote the previous argument.
- func_append_quoted base_compile "$lastarg"
- done # for arg
-
- case $arg_mode in
- arg)
- func_fatal_error "you must specify an argument for -Xcompile"
- ;;
- target)
- func_fatal_error "you must specify a target with \`-o'"
- ;;
- *)
- # Get the name of the library object.
- test -z "$libobj" && {
- func_basename "$srcfile"
- libobj="$func_basename_result"
- }
- ;;
- esac
-
- # Recognize several different file suffixes.
- # If the user specifies -o file.o, it is replaced with file.lo
- case $libobj in
- *.[cCFSifmso] | \
- *.ada | *.adb | *.ads | *.asm | \
- *.c++ | *.cc | *.ii | *.class | *.cpp | *.cxx | \
- *.[fF][09]? | *.for | *.java | *.go | *.obj | *.sx | *.cu | *.cup)
- func_xform "$libobj"
- libobj=$func_xform_result
- ;;
- esac
-
- case $libobj in
- *.lo) func_lo2o "$libobj"; obj=$func_lo2o_result ;;
- *)
- func_fatal_error "cannot determine name of library object from \`$libobj'"
- ;;
- esac
-
- func_infer_tag $base_compile
-
- for arg in $later; do
- case $arg in
- -shared)
- test "$build_libtool_libs" != yes && \
- func_fatal_configuration "can not build a shared library"
- build_old_libs=no
- continue
- ;;
-
- -static)
- build_libtool_libs=no
- build_old_libs=yes
- continue
- ;;
-
- -prefer-pic)
- pic_mode=yes
- continue
- ;;
-
- -prefer-non-pic)
- pic_mode=no
- continue
- ;;
- esac
- done
-
- func_quote_for_eval "$libobj"
- test "X$libobj" != "X$func_quote_for_eval_result" \
- && $ECHO "X$libobj" | $GREP '[]~#^*{};<>?"'"'"' &()|`$[]' \
- && func_warning "libobj name \`$libobj' may not contain shell special characters."
- func_dirname_and_basename "$obj" "/" ""
- objname="$func_basename_result"
- xdir="$func_dirname_result"
- lobj=${xdir}$objdir/$objname
-
- test -z "$base_compile" && \
- func_fatal_help "you must specify a compilation command"
-
- # Delete any leftover library objects.
- if test "$build_old_libs" = yes; then
- removelist="$obj $lobj $libobj ${libobj}T"
- else
- removelist="$lobj $libobj ${libobj}T"
- fi
-
- # On Cygwin there's no "real" PIC flag so we must build both object types
- case $host_os in
- cygwin* | mingw* | pw32* | os2* | cegcc*)
- pic_mode=default
- ;;
- esac
- if test "$pic_mode" = no && test "$deplibs_check_method" != pass_all; then
- # non-PIC code in shared libraries is not supported
- pic_mode=default
- fi
-
- # Calculate the filename of the output object if compiler does
- # not support -o with -c
- if test "$compiler_c_o" = no; then
- output_obj=`$ECHO "$srcfile" | $SED 's%^.*/%%; s%\.[^.]*$%%'`.${objext}
- lockfile="$output_obj.lock"
- else
- output_obj=
- need_locks=no
- lockfile=
- fi
-
- # Lock this critical section if it is needed
- # We use this script file to make the link, it avoids creating a new file
- if test "$need_locks" = yes; then
- until $opt_dry_run || ln "$progpath" "$lockfile" 2>/dev/null; do
- func_echo "Waiting for $lockfile to be removed"
- sleep 2
- done
- elif test "$need_locks" = warn; then
- if test -f "$lockfile"; then
- $ECHO "\
-*** ERROR, $lockfile exists and contains:
-`cat $lockfile 2>/dev/null`
-
-This indicates that another process is trying to use the same
-temporary object file, and libtool could not work around it because
-your compiler does not support \`-c' and \`-o' together. If you
-repeat this compilation, it may succeed, by chance, but you had better
-avoid parallel builds (make -j) in this platform, or get a better
-compiler."
-
- $opt_dry_run || $RM $removelist
- exit $EXIT_FAILURE
- fi
- func_append removelist " $output_obj"
- $ECHO "$srcfile" > "$lockfile"
- fi
-
- $opt_dry_run || $RM $removelist
- func_append removelist " $lockfile"
- trap '$opt_dry_run || $RM $removelist; exit $EXIT_FAILURE' 1 2 15
-
- func_to_tool_file "$srcfile" func_convert_file_msys_to_w32
- srcfile=$func_to_tool_file_result
- func_quote_for_eval "$srcfile"
- qsrcfile=$func_quote_for_eval_result
-
- # Only build a PIC object if we are building libtool libraries.
- if test "$build_libtool_libs" = yes; then
- # Without this assignment, base_compile gets emptied.
- fbsd_hideous_sh_bug=$base_compile
-
- if test "$pic_mode" != no; then
- command="$base_compile $qsrcfile $pic_flag"
- else
- # Don't build PIC code
- command="$base_compile $qsrcfile"
- fi
-
- func_mkdir_p "$xdir$objdir"
-
- if test -z "$output_obj"; then
- # Place PIC objects in $objdir
- func_append command " -o $lobj"
- fi
-
- func_show_eval_locale "$command" \
- 'test -n "$output_obj" && $RM $removelist; exit $EXIT_FAILURE'
-
- if test "$need_locks" = warn &&
- test "X`cat $lockfile 2>/dev/null`" != "X$srcfile"; then
- $ECHO "\
-*** ERROR, $lockfile contains:
-`cat $lockfile 2>/dev/null`
-
-but it should contain:
-$srcfile
-
-This indicates that another process is trying to use the same
-temporary object file, and libtool could not work around it because
-your compiler does not support \`-c' and \`-o' together. If you
-repeat this compilation, it may succeed, by chance, but you had better
-avoid parallel builds (make -j) in this platform, or get a better
-compiler."
-
- $opt_dry_run || $RM $removelist
- exit $EXIT_FAILURE
- fi
-
- # Just move the object if needed, then go on to compile the next one
- if test -n "$output_obj" && test "X$output_obj" != "X$lobj"; then
- func_show_eval '$MV "$output_obj" "$lobj"' \
- 'error=$?; $opt_dry_run || $RM $removelist; exit $error'
- fi
-
- # Allow error messages only from the first compilation.
- if test "$suppress_opt" = yes; then
- suppress_output=' >/dev/null 2>&1'
- fi
- fi
-
- # Only build a position-dependent object if we build old libraries.
- if test "$build_old_libs" = yes; then
- if test "$pic_mode" != yes; then
- # Don't build PIC code
- command="$base_compile $qsrcfile$pie_flag"
- else
- command="$base_compile $qsrcfile $pic_flag"
- fi
- if test "$compiler_c_o" = yes; then
- func_append command " -o $obj"
- fi
-
- # Suppress compiler output if we already did a PIC compilation.
- func_append command "$suppress_output"
- func_show_eval_locale "$command" \
- '$opt_dry_run || $RM $removelist; exit $EXIT_FAILURE'
-
- if test "$need_locks" = warn &&
- test "X`cat $lockfile 2>/dev/null`" != "X$srcfile"; then
- $ECHO "\
-*** ERROR, $lockfile contains:
-`cat $lockfile 2>/dev/null`
-
-but it should contain:
-$srcfile
-
-This indicates that another process is trying to use the same
-temporary object file, and libtool could not work around it because
-your compiler does not support \`-c' and \`-o' together. If you
-repeat this compilation, it may succeed, by chance, but you had better
-avoid parallel builds (make -j) in this platform, or get a better
-compiler."
-
- $opt_dry_run || $RM $removelist
- exit $EXIT_FAILURE
- fi
-
- # Just move the object if needed
- if test -n "$output_obj" && test "X$output_obj" != "X$obj"; then
- func_show_eval '$MV "$output_obj" "$obj"' \
- 'error=$?; $opt_dry_run || $RM $removelist; exit $error'
- fi
- fi
-
- $opt_dry_run || {
- func_write_libtool_object "$libobj" "$objdir/$objname" "$objname"
-
- # Unlock the critical section if it was locked
- if test "$need_locks" != no; then
- removelist=$lockfile
- $RM "$lockfile"
- fi
- }
-
- exit $EXIT_SUCCESS
-}
-
-$opt_help || {
- test "$opt_mode" = compile && func_mode_compile ${1+"$@"}
-}
-
-func_mode_help ()
-{
- # We need to display help for each of the modes.
- case $opt_mode in
- "")
- # Generic help is extracted from the usage comments
- # at the start of this file.
- func_help
- ;;
-
- clean)
- $ECHO \
-"Usage: $progname [OPTION]... --mode=clean RM [RM-OPTION]... FILE...
-
-Remove files from the build directory.
-
-RM is the name of the program to use to delete files associated with each FILE
-(typically \`/bin/rm'). RM-OPTIONS are options (such as \`-f') to be passed
-to RM.
-
-If FILE is a libtool library, object or program, all the files associated
-with it are deleted. Otherwise, only FILE itself is deleted using RM."
- ;;
-
- compile)
- $ECHO \
-"Usage: $progname [OPTION]... --mode=compile COMPILE-COMMAND... SOURCEFILE
-
-Compile a source file into a libtool library object.
-
-This mode accepts the following additional options:
-
- -o OUTPUT-FILE set the output file name to OUTPUT-FILE
- -no-suppress do not suppress compiler output for multiple passes
- -prefer-pic try to build PIC objects only
- -prefer-non-pic try to build non-PIC objects only
- -shared do not build a \`.o' file suitable for static linking
- -static only build a \`.o' file suitable for static linking
- -Wc,FLAG pass FLAG directly to the compiler
-
-COMPILE-COMMAND is a command to be used in creating a \`standard' object file
-from the given SOURCEFILE.
-
-The output file name is determined by removing the directory component from
-SOURCEFILE, then substituting the C source code suffix \`.c' with the
-library object suffix, \`.lo'."
- ;;
-
- execute)
- $ECHO \
-"Usage: $progname [OPTION]... --mode=execute COMMAND [ARGS]...
-
-Automatically set library path, then run a program.
-
-This mode accepts the following additional options:
-
- -dlopen FILE add the directory containing FILE to the library path
-
-This mode sets the library path environment variable according to \`-dlopen'
-flags.
-
-If any of the ARGS are libtool executable wrappers, then they are translated
-into their corresponding uninstalled binary, and any of their required library
-directories are added to the library path.
-
-Then, COMMAND is executed, with ARGS as arguments."
- ;;
-
- finish)
- $ECHO \
-"Usage: $progname [OPTION]... --mode=finish [LIBDIR]...
-
-Complete the installation of libtool libraries.
-
-Each LIBDIR is a directory that contains libtool libraries.
-
-The commands that this mode executes may require superuser privileges. Use
-the \`--dry-run' option if you just want to see what would be executed."
- ;;
-
- install)
- $ECHO \
-"Usage: $progname [OPTION]... --mode=install INSTALL-COMMAND...
-
-Install executables or libraries.
-
-INSTALL-COMMAND is the installation command. The first component should be
-either the \`install' or \`cp' program.
-
-The following components of INSTALL-COMMAND are treated specially:
-
- -inst-prefix-dir PREFIX-DIR Use PREFIX-DIR as a staging area for installation
-
-The rest of the components are interpreted as arguments to that command (only
-BSD-compatible install options are recognized)."
- ;;
-
- link)
- $ECHO \
-"Usage: $progname [OPTION]... --mode=link LINK-COMMAND...
-
-Link object files or libraries together to form another library, or to
-create an executable program.
-
-LINK-COMMAND is a command using the C compiler that you would use to create
-a program from several object files.
-
-The following components of LINK-COMMAND are treated specially:
-
- -all-static do not do any dynamic linking at all
- -avoid-version do not add a version suffix if possible
- -bindir BINDIR specify path to binaries directory (for systems where
- libraries must be found in the PATH setting at runtime)
- -dlopen FILE \`-dlpreopen' FILE if it cannot be dlopened at runtime
- -dlpreopen FILE link in FILE and add its symbols to lt_preloaded_symbols
- -export-dynamic allow symbols from OUTPUT-FILE to be resolved with dlsym(3)
- -export-symbols SYMFILE
- try to export only the symbols listed in SYMFILE
- -export-symbols-regex REGEX
- try to export only the symbols matching REGEX
- -LLIBDIR search LIBDIR for required installed libraries
- -lNAME OUTPUT-FILE requires the installed library libNAME
- -module build a library that can dlopened
- -no-fast-install disable the fast-install mode
- -no-install link a not-installable executable
- -no-undefined declare that a library does not refer to external symbols
- -o OUTPUT-FILE create OUTPUT-FILE from the specified objects
- -objectlist FILE Use a list of object files found in FILE to specify objects
- -precious-files-regex REGEX
- don't remove output files matching REGEX
- -release RELEASE specify package release information
- -rpath LIBDIR the created library will eventually be installed in LIBDIR
- -R[ ]LIBDIR add LIBDIR to the runtime path of programs and libraries
- -shared only do dynamic linking of libtool libraries
- -shrext SUFFIX override the standard shared library file extension
- -static do not do any dynamic linking of uninstalled libtool libraries
- -static-libtool-libs
- do not do any dynamic linking of libtool libraries
- -version-info CURRENT[:REVISION[:AGE]]
- specify library version info [each variable defaults to 0]
- -weak LIBNAME declare that the target provides the LIBNAME interface
- -Wc,FLAG
- -Xcompiler FLAG pass linker-specific FLAG directly to the compiler
- -Wl,FLAG
- -Xlinker FLAG pass linker-specific FLAG directly to the linker
- -XCClinker FLAG pass link-specific FLAG to the compiler driver (CC)
-
-All other options (arguments beginning with \`-') are ignored.
-
-Every other argument is treated as a filename. Files ending in \`.la' are
-treated as uninstalled libtool libraries, other files are standard or library
-object files.
-
-If the OUTPUT-FILE ends in \`.la', then a libtool library is created,
-only library objects (\`.lo' files) may be specified, and \`-rpath' is
-required, except when creating a convenience library.
-
-If OUTPUT-FILE ends in \`.a' or \`.lib', then a standard library is created
-using \`ar' and \`ranlib', or on Windows using \`lib'.
-
-If OUTPUT-FILE ends in \`.lo' or \`.${objext}', then a reloadable object file
-is created, otherwise an executable program is created."
- ;;
-
- uninstall)
- $ECHO \
-"Usage: $progname [OPTION]... --mode=uninstall RM [RM-OPTION]... FILE...
-
-Remove libraries from an installation directory.
-
-RM is the name of the program to use to delete files associated with each FILE
-(typically \`/bin/rm'). RM-OPTIONS are options (such as \`-f') to be passed
-to RM.
-
-If FILE is a libtool library, all the files associated with it are deleted.
-Otherwise, only FILE itself is deleted using RM."
- ;;
-
- *)
- func_fatal_help "invalid operation mode \`$opt_mode'"
- ;;
- esac
-
- echo
- $ECHO "Try \`$progname --help' for more information about other modes."
-}
-
-# Now that we've collected a possible --mode arg, show help if necessary
-if $opt_help; then
- if test "$opt_help" = :; then
- func_mode_help
- else
- {
- func_help noexit
- for opt_mode in compile link execute install finish uninstall clean; do
- func_mode_help
- done
- } | sed -n '1p; 2,$s/^Usage:/ or: /p'
- {
- func_help noexit
- for opt_mode in compile link execute install finish uninstall clean; do
- echo
- func_mode_help
- done
- } |
- sed '1d
- /^When reporting/,/^Report/{
- H
- d
- }
- $x
- /information about other modes/d
- /more detailed .*MODE/d
- s/^Usage:.*--mode=\([^ ]*\) .*/Description of \1 mode:/'
- fi
- exit $?
-fi
-
-
-# func_mode_execute arg...
-func_mode_execute ()
-{
- $opt_debug
- # The first argument is the command name.
- cmd="$nonopt"
- test -z "$cmd" && \
- func_fatal_help "you must specify a COMMAND"
-
- # Handle -dlopen flags immediately.
- for file in $opt_dlopen; do
- test -f "$file" \
- || func_fatal_help "\`$file' is not a file"
-
- dir=
- case $file in
- *.la)
- func_resolve_sysroot "$file"
- file=$func_resolve_sysroot_result
-
- # Check to see that this really is a libtool archive.
- func_lalib_unsafe_p "$file" \
- || func_fatal_help "\`$lib' is not a valid libtool archive"
-
- # Read the libtool library.
- dlname=
- library_names=
- func_source "$file"
-
- # Skip this library if it cannot be dlopened.
- if test -z "$dlname"; then
- # Warn if it was a shared library.
- test -n "$library_names" && \
- func_warning "\`$file' was not linked with \`-export-dynamic'"
- continue
- fi
-
- func_dirname "$file" "" "."
- dir="$func_dirname_result"
-
- if test -f "$dir/$objdir/$dlname"; then
- func_append dir "/$objdir"
- else
- if test ! -f "$dir/$dlname"; then
- func_fatal_error "cannot find \`$dlname' in \`$dir' or \`$dir/$objdir'"
- fi
- fi
- ;;
-
- *.lo)
- # Just add the directory containing the .lo file.
- func_dirname "$file" "" "."
- dir="$func_dirname_result"
- ;;
-
- *)
- func_warning "\`-dlopen' is ignored for non-libtool libraries and objects"
- continue
- ;;
- esac
-
- # Get the absolute pathname.
- absdir=`cd "$dir" && pwd`
- test -n "$absdir" && dir="$absdir"
-
- # Now add the directory to shlibpath_var.
- if eval "test -z \"\$$shlibpath_var\""; then
- eval "$shlibpath_var=\"\$dir\""
- else
- eval "$shlibpath_var=\"\$dir:\$$shlibpath_var\""
- fi
- done
-
- # This variable tells wrapper scripts just to set shlibpath_var
- # rather than running their programs.
- libtool_execute_magic="$magic"
-
- # Check if any of the arguments is a wrapper script.
- args=
- for file
- do
- case $file in
- -* | *.la | *.lo ) ;;
- *)
- # Do a test to see if this is really a libtool program.
- if func_ltwrapper_script_p "$file"; then
- func_source "$file"
- # Transform arg to wrapped name.
- file="$progdir/$program"
- elif func_ltwrapper_executable_p "$file"; then
- func_ltwrapper_scriptname "$file"
- func_source "$func_ltwrapper_scriptname_result"
- # Transform arg to wrapped name.
- file="$progdir/$program"
- fi
- ;;
- esac
- # Quote arguments (to preserve shell metacharacters).
- func_append_quoted args "$file"
- done
-
- if test "X$opt_dry_run" = Xfalse; then
- if test -n "$shlibpath_var"; then
- # Export the shlibpath_var.
- eval "export $shlibpath_var"
- fi
-
- # Restore saved environment variables
- for lt_var in LANG LANGUAGE LC_ALL LC_CTYPE LC_COLLATE LC_MESSAGES
- do
- eval "if test \"\${save_$lt_var+set}\" = set; then
- $lt_var=\$save_$lt_var; export $lt_var
- else
- $lt_unset $lt_var
- fi"
- done
-
- # Now prepare to actually exec the command.
- exec_cmd="\$cmd$args"
- else
- # Display what would be done.
- if test -n "$shlibpath_var"; then
- eval "\$ECHO \"\$shlibpath_var=\$$shlibpath_var\""
- echo "export $shlibpath_var"
- fi
- $ECHO "$cmd$args"
- exit $EXIT_SUCCESS
- fi
-}
-
-test "$opt_mode" = execute && func_mode_execute ${1+"$@"}
-
-
-# func_mode_finish arg...
-func_mode_finish ()
-{
- $opt_debug
- libs=
- libdirs=
- admincmds=
-
- for opt in "$nonopt" ${1+"$@"}
- do
- if test -d "$opt"; then
- func_append libdirs " $opt"
-
- elif test -f "$opt"; then
- if func_lalib_unsafe_p "$opt"; then
- func_append libs " $opt"
- else
- func_warning "\`$opt' is not a valid libtool archive"
- fi
-
- else
- func_fatal_error "invalid argument \`$opt'"
- fi
- done
-
- if test -n "$libs"; then
- if test -n "$lt_sysroot"; then
- sysroot_regex=`$ECHO "$lt_sysroot" | $SED "$sed_make_literal_regex"`
- sysroot_cmd="s/\([ ']\)$sysroot_regex/\1/g;"
- else
- sysroot_cmd=
- fi
-
- # Remove sysroot references
- if $opt_dry_run; then
- for lib in $libs; do
- echo "removing references to $lt_sysroot and \`=' prefixes from $lib"
- done
- else
- tmpdir=`func_mktempdir`
- for lib in $libs; do
- sed -e "${sysroot_cmd} s/\([ ']-[LR]\)=/\1/g; s/\([ ']\)=/\1/g" $lib \
- > $tmpdir/tmp-la
- mv -f $tmpdir/tmp-la $lib
- done
- ${RM}r "$tmpdir"
- fi
- fi
-
- if test -n "$finish_cmds$finish_eval" && test -n "$libdirs"; then
- for libdir in $libdirs; do
- if test -n "$finish_cmds"; then
- # Do each command in the finish commands.
- func_execute_cmds "$finish_cmds" 'admincmds="$admincmds
-'"$cmd"'"'
- fi
- if test -n "$finish_eval"; then
- # Do the single finish_eval.
- eval cmds=\"$finish_eval\"
- $opt_dry_run || eval "$cmds" || func_append admincmds "
- $cmds"
- fi
- done
- fi
-
- # Exit here if they wanted silent mode.
- $opt_silent && exit $EXIT_SUCCESS
-
- if test -n "$finish_cmds$finish_eval" && test -n "$libdirs"; then
- echo "----------------------------------------------------------------------"
- echo "Libraries have been installed in:"
- for libdir in $libdirs; do
- $ECHO " $libdir"
- done
- echo
- echo "If you ever happen to want to link against installed libraries"
- echo "in a given directory, LIBDIR, you must either use libtool, and"
- echo "specify the full pathname of the library, or use the \`-LLIBDIR'"
- echo "flag during linking and do at least one of the following:"
- if test -n "$shlibpath_var"; then
- echo " - add LIBDIR to the \`$shlibpath_var' environment variable"
- echo " during execution"
- fi
- if test -n "$runpath_var"; then
- echo " - add LIBDIR to the \`$runpath_var' environment variable"
- echo " during linking"
- fi
- if test -n "$hardcode_libdir_flag_spec"; then
- libdir=LIBDIR
- eval flag=\"$hardcode_libdir_flag_spec\"
-
- $ECHO " - use the \`$flag' linker flag"
- fi
- if test -n "$admincmds"; then
- $ECHO " - have your system administrator run these commands:$admincmds"
- fi
- if test -f /etc/ld.so.conf; then
- echo " - have your system administrator add LIBDIR to \`/etc/ld.so.conf'"
- fi
- echo
-
- echo "See any operating system documentation about shared libraries for"
- case $host in
- solaris2.[6789]|solaris2.1[0-9])
- echo "more information, such as the ld(1), crle(1) and ld.so(8) manual"
- echo "pages."
- ;;
- *)
- echo "more information, such as the ld(1) and ld.so(8) manual pages."
- ;;
- esac
- echo "----------------------------------------------------------------------"
- fi
- exit $EXIT_SUCCESS
-}
-
-test "$opt_mode" = finish && func_mode_finish ${1+"$@"}
-
-
-# func_mode_install arg...
-func_mode_install ()
-{
- $opt_debug
- # There may be an optional sh(1) argument at the beginning of
- # install_prog (especially on Windows NT).
- if test "$nonopt" = "$SHELL" || test "$nonopt" = /bin/sh ||
- # Allow the use of GNU shtool's install command.
- case $nonopt in *shtool*) :;; *) false;; esac; then
- # Aesthetically quote it.
- func_quote_for_eval "$nonopt"
- install_prog="$func_quote_for_eval_result "
- arg=$1
- shift
- else
- install_prog=
- arg=$nonopt
- fi
-
- # The real first argument should be the name of the installation program.
- # Aesthetically quote it.
- func_quote_for_eval "$arg"
- func_append install_prog "$func_quote_for_eval_result"
- install_shared_prog=$install_prog
- case " $install_prog " in
- *[\\\ /]cp\ *) install_cp=: ;;
- *) install_cp=false ;;
- esac
-
- # We need to accept at least all the BSD install flags.
- dest=
- files=
- opts=
- prev=
- install_type=
- isdir=no
- stripme=
- no_mode=:
- for arg
- do
- arg2=
- if test -n "$dest"; then
- func_append files " $dest"
- dest=$arg
- continue
- fi
-
- case $arg in
- -d) isdir=yes ;;
- -f)
- if $install_cp; then :; else
- prev=$arg
- fi
- ;;
- -g | -m | -o)
- prev=$arg
- ;;
- -s)
- stripme=" -s"
- continue
- ;;
- -*)
- ;;
- *)
- # If the previous option needed an argument, then skip it.
- if test -n "$prev"; then
- if test "x$prev" = x-m && test -n "$install_override_mode"; then
- arg2=$install_override_mode
- no_mode=false
- fi
- prev=
- else
- dest=$arg
- continue
- fi
- ;;
- esac
-
- # Aesthetically quote the argument.
- func_quote_for_eval "$arg"
- func_append install_prog " $func_quote_for_eval_result"
- if test -n "$arg2"; then
- func_quote_for_eval "$arg2"
- fi
- func_append install_shared_prog " $func_quote_for_eval_result"
- done
-
- test -z "$install_prog" && \
- func_fatal_help "you must specify an install program"
-
- test -n "$prev" && \
- func_fatal_help "the \`$prev' option requires an argument"
-
- if test -n "$install_override_mode" && $no_mode; then
- if $install_cp; then :; else
- func_quote_for_eval "$install_override_mode"
- func_append install_shared_prog " -m $func_quote_for_eval_result"
- fi
- fi
-
- if test -z "$files"; then
- if test -z "$dest"; then
- func_fatal_help "no file or destination specified"
- else
- func_fatal_help "you must specify a destination"
- fi
- fi
-
- # Strip any trailing slash from the destination.
- func_stripname '' '/' "$dest"
- dest=$func_stripname_result
-
- # Check to see that the destination is a directory.
- test -d "$dest" && isdir=yes
- if test "$isdir" = yes; then
- destdir="$dest"
- destname=
- else
- func_dirname_and_basename "$dest" "" "."
- destdir="$func_dirname_result"
- destname="$func_basename_result"
-
- # Not a directory, so check to see that there is only one file specified.
- set dummy $files; shift
- test "$#" -gt 1 && \
- func_fatal_help "\`$dest' is not a directory"
- fi
- case $destdir in
- [\\/]* | [A-Za-z]:[\\/]*) ;;
- *)
- for file in $files; do
- case $file in
- *.lo) ;;
- *)
- func_fatal_help "\`$destdir' must be an absolute directory name"
- ;;
- esac
- done
- ;;
- esac
-
- # This variable tells wrapper scripts just to set variables rather
- # than running their programs.
- libtool_install_magic="$magic"
-
- staticlibs=
- future_libdirs=
- current_libdirs=
- for file in $files; do
-
- # Do each installation.
- case $file in
- *.$libext)
- # Do the static libraries later.
- func_append staticlibs " $file"
- ;;
-
- *.la)
- func_resolve_sysroot "$file"
- file=$func_resolve_sysroot_result
-
- # Check to see that this really is a libtool archive.
- func_lalib_unsafe_p "$file" \
- || func_fatal_help "\`$file' is not a valid libtool archive"
-
- library_names=
- old_library=
- relink_command=
- func_source "$file"
-
- # Add the libdir to current_libdirs if it is the destination.
- if test "X$destdir" = "X$libdir"; then
- case "$current_libdirs " in
- *" $libdir "*) ;;
- *) func_append current_libdirs " $libdir" ;;
- esac
- else
- # Note the libdir as a future libdir.
- case "$future_libdirs " in
- *" $libdir "*) ;;
- *) func_append future_libdirs " $libdir" ;;
- esac
- fi
-
- func_dirname "$file" "/" ""
- dir="$func_dirname_result"
- func_append dir "$objdir"
-
- if test -n "$relink_command"; then
- # Determine the prefix the user has applied to our future dir.
- inst_prefix_dir=`$ECHO "$destdir" | $SED -e "s%$libdir\$%%"`
-
- # Don't allow the user to place us outside of our expected
- # location b/c this prevents finding dependent libraries that
- # are installed to the same prefix.
- # At present, this check doesn't affect windows .dll's that
- # are installed into $libdir/../bin (currently, that works fine)
- # but it's something to keep an eye on.
- test "$inst_prefix_dir" = "$destdir" && \
- func_fatal_error "error: cannot install \`$file' to a directory not ending in $libdir"
-
- if test -n "$inst_prefix_dir"; then
- # Stick the inst_prefix_dir data into the link command.
- relink_command=`$ECHO "$relink_command" | $SED "s%@inst_prefix_dir@%-inst-prefix-dir $inst_prefix_dir%"`
- else
- relink_command=`$ECHO "$relink_command" | $SED "s%@inst_prefix_dir@%%"`
- fi
-
- func_warning "relinking \`$file'"
- func_show_eval "$relink_command" \
- 'func_fatal_error "error: relink \`$file'\'' with the above command before installing it"'
- fi
-
- # See the names of the shared library.
- set dummy $library_names; shift
- if test -n "$1"; then
- realname="$1"
- shift
-
- srcname="$realname"
- test -n "$relink_command" && srcname="$realname"T
-
- # Install the shared library and build the symlinks.
- func_show_eval "$install_shared_prog $dir/$srcname $destdir/$realname" \
- 'exit $?'
- tstripme="$stripme"
- case $host_os in
- cygwin* | mingw* | pw32* | cegcc*)
- case $realname in
- *.dll.a)
- tstripme=""
- ;;
- esac
- ;;
- esac
- if test -n "$tstripme" && test -n "$striplib"; then
- func_show_eval "$striplib $destdir/$realname" 'exit $?'
- fi
-
- if test "$#" -gt 0; then
- # Delete the old symlinks, and create new ones.
- # Try `ln -sf' first, because the `ln' binary might depend on
- # the symlink we replace! Solaris /bin/ln does not understand -f,
- # so we also need to try rm && ln -s.
- for linkname
- do
- test "$linkname" != "$realname" \
- && func_show_eval "(cd $destdir && { $LN_S -f $realname $linkname || { $RM $linkname && $LN_S $realname $linkname; }; })"
- done
- fi
-
- # Do each command in the postinstall commands.
- lib="$destdir/$realname"
- func_execute_cmds "$postinstall_cmds" 'exit $?'
- fi
-
- # Install the pseudo-library for information purposes.
- func_basename "$file"
- name="$func_basename_result"
- instname="$dir/$name"i
- func_show_eval "$install_prog $instname $destdir/$name" 'exit $?'
-
- # Maybe install the static library, too.
- test -n "$old_library" && func_append staticlibs " $dir/$old_library"
- ;;
-
- *.lo)
- # Install (i.e. copy) a libtool object.
-
- # Figure out destination file name, if it wasn't already specified.
- if test -n "$destname"; then
- destfile="$destdir/$destname"
- else
- func_basename "$file"
- destfile="$func_basename_result"
- destfile="$destdir/$destfile"
- fi
-
- # Deduce the name of the destination old-style object file.
- case $destfile in
- *.lo)
- func_lo2o "$destfile"
- staticdest=$func_lo2o_result
- ;;
- *.$objext)
- staticdest="$destfile"
- destfile=
- ;;
- *)
- func_fatal_help "cannot copy a libtool object to \`$destfile'"
- ;;
- esac
-
- # Install the libtool object if requested.
- test -n "$destfile" && \
- func_show_eval "$install_prog $file $destfile" 'exit $?'
-
- # Install the old object if enabled.
- if test "$build_old_libs" = yes; then
- # Deduce the name of the old-style object file.
- func_lo2o "$file"
- staticobj=$func_lo2o_result
- func_show_eval "$install_prog \$staticobj \$staticdest" 'exit $?'
- fi
- exit $EXIT_SUCCESS
- ;;
-
- *)
- # Figure out destination file name, if it wasn't already specified.
- if test -n "$destname"; then
- destfile="$destdir/$destname"
- else
- func_basename "$file"
- destfile="$func_basename_result"
- destfile="$destdir/$destfile"
- fi
-
- # If the file is missing, and there is a .exe on the end, strip it
- # because it is most likely a libtool script we actually want to
- # install
- stripped_ext=""
- case $file in
- *.exe)
- if test ! -f "$file"; then
- func_stripname '' '.exe' "$file"
- file=$func_stripname_result
- stripped_ext=".exe"
- fi
- ;;
- esac
-
- # Do a test to see if this is really a libtool program.
- case $host in
- *cygwin* | *mingw*)
- if func_ltwrapper_executable_p "$file"; then
- func_ltwrapper_scriptname "$file"
- wrapper=$func_ltwrapper_scriptname_result
- else
- func_stripname '' '.exe' "$file"
- wrapper=$func_stripname_result
- fi
- ;;
- *)
- wrapper=$file
- ;;
- esac
- if func_ltwrapper_script_p "$wrapper"; then
- notinst_deplibs=
- relink_command=
-
- func_source "$wrapper"
-
- # Check the variables that should have been set.
- test -z "$generated_by_libtool_version" && \
- func_fatal_error "invalid libtool wrapper script \`$wrapper'"
-
- finalize=yes
- for lib in $notinst_deplibs; do
- # Check to see that each library is installed.
- libdir=
- if test -f "$lib"; then
- func_source "$lib"
- fi
- libfile="$libdir/"`$ECHO "$lib" | $SED 's%^.*/%%g'` ### testsuite: skip nested quoting test
- if test -n "$libdir" && test ! -f "$libfile"; then
- func_warning "\`$lib' has not been installed in \`$libdir'"
- finalize=no
- fi
- done
-
- relink_command=
- func_source "$wrapper"
-
- outputname=
- if test "$fast_install" = no && test -n "$relink_command"; then
- $opt_dry_run || {
- if test "$finalize" = yes; then
- tmpdir=`func_mktempdir`
- func_basename "$file$stripped_ext"
- file="$func_basename_result"
- outputname="$tmpdir/$file"
- # Replace the output file specification.
- relink_command=`$ECHO "$relink_command" | $SED 's%@OUTPUT@%'"$outputname"'%g'`
-
- $opt_silent || {
- func_quote_for_expand "$relink_command"
- eval "func_echo $func_quote_for_expand_result"
- }
- if eval "$relink_command"; then :
- else
- func_error "error: relink \`$file' with the above command before installing it"
- $opt_dry_run || ${RM}r "$tmpdir"
- continue
- fi
- file="$outputname"
- else
- func_warning "cannot relink \`$file'"
- fi
- }
- else
- # Install the binary that we compiled earlier.
- file=`$ECHO "$file$stripped_ext" | $SED "s%\([^/]*\)$%$objdir/\1%"`
- fi
- fi
-
- # remove .exe since cygwin /usr/bin/install will append another
- # one anyway
- case $install_prog,$host in
- */usr/bin/install*,*cygwin*)
- case $file:$destfile in
- *.exe:*.exe)
- # this is ok
- ;;
- *.exe:*)
- destfile=$destfile.exe
- ;;
- *:*.exe)
- func_stripname '' '.exe' "$destfile"
- destfile=$func_stripname_result
- ;;
- esac
- ;;
- esac
- func_show_eval "$install_prog\$stripme \$file \$destfile" 'exit $?'
- $opt_dry_run || if test -n "$outputname"; then
- ${RM}r "$tmpdir"
- fi
- ;;
- esac
- done
-
- for file in $staticlibs; do
- func_basename "$file"
- name="$func_basename_result"
-
- # Set up the ranlib parameters.
- oldlib="$destdir/$name"
- func_to_tool_file "$oldlib" func_convert_file_msys_to_w32
- tool_oldlib=$func_to_tool_file_result
-
- func_show_eval "$install_prog \$file \$oldlib" 'exit $?'
-
- if test -n "$stripme" && test -n "$old_striplib"; then
- func_show_eval "$old_striplib $tool_oldlib" 'exit $?'
- fi
-
- # Do each command in the postinstall commands.
- func_execute_cmds "$old_postinstall_cmds" 'exit $?'
- done
-
- test -n "$future_libdirs" && \
- func_warning "remember to run \`$progname --finish$future_libdirs'"
-
- if test -n "$current_libdirs"; then
- # Maybe just do a dry run.
- $opt_dry_run && current_libdirs=" -n$current_libdirs"
- exec_cmd='$SHELL $progpath $preserve_args --finish$current_libdirs'
- else
- exit $EXIT_SUCCESS
- fi
-}
-
-test "$opt_mode" = install && func_mode_install ${1+"$@"}
-
-
-# func_generate_dlsyms outputname originator pic_p
-# Extract symbols from dlprefiles and create ${outputname}S.o with
-# a dlpreopen symbol table.
-func_generate_dlsyms ()
-{
- $opt_debug
- my_outputname="$1"
- my_originator="$2"
- my_pic_p="${3-no}"
- my_prefix=`$ECHO "$my_originator" | sed 's%[^a-zA-Z0-9]%_%g'`
- my_dlsyms=
-
- if test -n "$dlfiles$dlprefiles" || test "$dlself" != no; then
- if test -n "$NM" && test -n "$global_symbol_pipe"; then
- my_dlsyms="${my_outputname}S.c"
- else
- func_error "not configured to extract global symbols from dlpreopened files"
- fi
- fi
-
- if test -n "$my_dlsyms"; then
- case $my_dlsyms in
- "") ;;
- *.c)
- # Discover the nlist of each of the dlfiles.
- nlist="$output_objdir/${my_outputname}.nm"
-
- func_show_eval "$RM $nlist ${nlist}S ${nlist}T"
-
- # Parse the name list into a source file.
- func_verbose "creating $output_objdir/$my_dlsyms"
-
- $opt_dry_run || $ECHO > "$output_objdir/$my_dlsyms" "\
-/* $my_dlsyms - symbol resolution table for \`$my_outputname' dlsym emulation. */
-/* Generated by $PROGRAM (GNU $PACKAGE$TIMESTAMP) $VERSION */
-
-#ifdef __cplusplus
-extern \"C\" {
-#endif
-
-#if defined(__GNUC__) && (((__GNUC__ == 4) && (__GNUC_MINOR__ >= 4)) || (__GNUC__ > 4))
-#pragma GCC diagnostic ignored \"-Wstrict-prototypes\"
-#endif
-
-/* Keep this code in sync between libtool.m4, ltmain, lt_system.h, and tests. */
-#if defined(_WIN32) || defined(__CYGWIN__) || defined(_WIN32_WCE)
-/* DATA imports from DLLs on WIN32 con't be const, because runtime
- relocations are performed -- see ld's documentation on pseudo-relocs. */
-# define LT_DLSYM_CONST
-#elif defined(__osf__)
-/* This system does not cope well with relocations in const data. */
-# define LT_DLSYM_CONST
-#else
-# define LT_DLSYM_CONST const
-#endif
-
-/* External symbol declarations for the compiler. */\
-"
-
- if test "$dlself" = yes; then
- func_verbose "generating symbol list for \`$output'"
-
- $opt_dry_run || echo ': @PROGRAM@ ' > "$nlist"
-
- # Add our own program objects to the symbol list.
- progfiles=`$ECHO "$objs$old_deplibs" | $SP2NL | $SED "$lo2o" | $NL2SP`
- for progfile in $progfiles; do
- func_to_tool_file "$progfile" func_convert_file_msys_to_w32
- func_verbose "extracting global C symbols from \`$func_to_tool_file_result'"
- $opt_dry_run || eval "$NM $func_to_tool_file_result | $global_symbol_pipe >> '$nlist'"
- done
-
- if test -n "$exclude_expsyms"; then
- $opt_dry_run || {
- eval '$EGREP -v " ($exclude_expsyms)$" "$nlist" > "$nlist"T'
- eval '$MV "$nlist"T "$nlist"'
- }
- fi
-
- if test -n "$export_symbols_regex"; then
- $opt_dry_run || {
- eval '$EGREP -e "$export_symbols_regex" "$nlist" > "$nlist"T'
- eval '$MV "$nlist"T "$nlist"'
- }
- fi
-
- # Prepare the list of exported symbols
- if test -z "$export_symbols"; then
- export_symbols="$output_objdir/$outputname.exp"
- $opt_dry_run || {
- $RM $export_symbols
- eval "${SED} -n -e '/^: @PROGRAM@ $/d' -e 's/^.* \(.*\)$/\1/p' "'< "$nlist" > "$export_symbols"'
- case $host in
- *cygwin* | *mingw* | *cegcc* )
- eval "echo EXPORTS "'> "$output_objdir/$outputname.def"'
- eval 'cat "$export_symbols" >> "$output_objdir/$outputname.def"'
- ;;
- esac
- }
- else
- $opt_dry_run || {
- eval "${SED} -e 's/\([].[*^$]\)/\\\\\1/g' -e 's/^/ /' -e 's/$/$/'"' < "$export_symbols" > "$output_objdir/$outputname.exp"'
- eval '$GREP -f "$output_objdir/$outputname.exp" < "$nlist" > "$nlist"T'
- eval '$MV "$nlist"T "$nlist"'
- case $host in
- *cygwin* | *mingw* | *cegcc* )
- eval "echo EXPORTS "'> "$output_objdir/$outputname.def"'
- eval 'cat "$nlist" >> "$output_objdir/$outputname.def"'
- ;;
- esac
- }
- fi
- fi
-
- for dlprefile in $dlprefiles; do
- func_verbose "extracting global C symbols from \`$dlprefile'"
- func_basename "$dlprefile"
- name="$func_basename_result"
- case $host in
- *cygwin* | *mingw* | *cegcc* )
- # if an import library, we need to obtain dlname
- if func_win32_import_lib_p "$dlprefile"; then
- func_tr_sh "$dlprefile"
- eval "curr_lafile=\$libfile_$func_tr_sh_result"
- dlprefile_dlbasename=""
- if test -n "$curr_lafile" && func_lalib_p "$curr_lafile"; then
- # Use subshell, to avoid clobbering current variable values
- dlprefile_dlname=`source "$curr_lafile" && echo "$dlname"`
- if test -n "$dlprefile_dlname" ; then
- func_basename "$dlprefile_dlname"
- dlprefile_dlbasename="$func_basename_result"
- else
- # no lafile. user explicitly requested -dlpreopen .
- $sharedlib_from_linklib_cmd "$dlprefile"
- dlprefile_dlbasename=$sharedlib_from_linklib_result
- fi
- fi
- $opt_dry_run || {
- if test -n "$dlprefile_dlbasename" ; then
- eval '$ECHO ": $dlprefile_dlbasename" >> "$nlist"'
- else
- func_warning "Could not compute DLL name from $name"
- eval '$ECHO ": $name " >> "$nlist"'
- fi
- func_to_tool_file "$dlprefile" func_convert_file_msys_to_w32
- eval "$NM \"$func_to_tool_file_result\" 2>/dev/null | $global_symbol_pipe |
- $SED -e '/I __imp/d' -e 's/I __nm_/D /;s/_nm__//' >> '$nlist'"
- }
- else # not an import lib
- $opt_dry_run || {
- eval '$ECHO ": $name " >> "$nlist"'
- func_to_tool_file "$dlprefile" func_convert_file_msys_to_w32
- eval "$NM \"$func_to_tool_file_result\" 2>/dev/null | $global_symbol_pipe >> '$nlist'"
- }
- fi
- ;;
- *)
- $opt_dry_run || {
- eval '$ECHO ": $name " >> "$nlist"'
- func_to_tool_file "$dlprefile" func_convert_file_msys_to_w32
- eval "$NM \"$func_to_tool_file_result\" 2>/dev/null | $global_symbol_pipe >> '$nlist'"
- }
- ;;
- esac
- done
-
- $opt_dry_run || {
- # Make sure we have at least an empty file.
- test -f "$nlist" || : > "$nlist"
-
- if test -n "$exclude_expsyms"; then
- $EGREP -v " ($exclude_expsyms)$" "$nlist" > "$nlist"T
- $MV "$nlist"T "$nlist"
- fi
-
- # Try sorting and uniquifying the output.
- if $GREP -v "^: " < "$nlist" |
- if sort -k 3 /dev/null 2>&1; then
- sort -k 3
- else
- sort +2
- fi |
- uniq > "$nlist"S; then
- :
- else
- $GREP -v "^: " < "$nlist" > "$nlist"S
- fi
-
- if test -f "$nlist"S; then
- eval "$global_symbol_to_cdecl"' < "$nlist"S >> "$output_objdir/$my_dlsyms"'
- else
- echo '/* NONE */' >> "$output_objdir/$my_dlsyms"
- fi
-
- echo >> "$output_objdir/$my_dlsyms" "\
-
-/* The mapping between symbol names and symbols. */
-typedef struct {
- const char *name;
- void *address;
-} lt_dlsymlist;
-extern LT_DLSYM_CONST lt_dlsymlist
-lt_${my_prefix}_LTX_preloaded_symbols[];
-LT_DLSYM_CONST lt_dlsymlist
-lt_${my_prefix}_LTX_preloaded_symbols[] =
-{\
- { \"$my_originator\", (void *) 0 },"
-
- case $need_lib_prefix in
- no)
- eval "$global_symbol_to_c_name_address" < "$nlist" >> "$output_objdir/$my_dlsyms"
- ;;
- *)
- eval "$global_symbol_to_c_name_address_lib_prefix" < "$nlist" >> "$output_objdir/$my_dlsyms"
- ;;
- esac
- echo >> "$output_objdir/$my_dlsyms" "\
- {0, (void *) 0}
-};
-
-/* This works around a problem in FreeBSD linker */
-#ifdef FREEBSD_WORKAROUND
-static const void *lt_preloaded_setup() {
- return lt_${my_prefix}_LTX_preloaded_symbols;
-}
-#endif
-
-#ifdef __cplusplus
-}
-#endif\
-"
- } # !$opt_dry_run
-
- pic_flag_for_symtable=
- case "$compile_command " in
- *" -static "*) ;;
- *)
- case $host in
- # compiling the symbol table file with pic_flag works around
- # a FreeBSD bug that causes programs to crash when -lm is
- # linked before any other PIC object. But we must not use
- # pic_flag when linking with -static. The problem exists in
- # FreeBSD 2.2.6 and is fixed in FreeBSD 3.1.
- *-*-freebsd2.*|*-*-freebsd3.0*|*-*-freebsdelf3.0*)
- pic_flag_for_symtable=" $pic_flag -DFREEBSD_WORKAROUND" ;;
- *-*-hpux*)
- pic_flag_for_symtable=" $pic_flag" ;;
- *)
- if test "X$my_pic_p" != Xno; then
- pic_flag_for_symtable=" $pic_flag"
- fi
- ;;
- esac
- ;;
- esac
- symtab_cflags=
- for arg in $LTCFLAGS; do
- case $arg in
- -pie | -fpie | -fPIE) ;;
- *) func_append symtab_cflags " $arg" ;;
- esac
- done
-
- # Now compile the dynamic symbol file.
- func_show_eval '(cd $output_objdir && $LTCC$symtab_cflags -c$no_builtin_flag$pic_flag_for_symtable "$my_dlsyms")' 'exit $?'
-
- # Clean up the generated files.
- func_show_eval '$RM "$output_objdir/$my_dlsyms" "$nlist" "${nlist}S" "${nlist}T"'
-
- # Transform the symbol file into the correct name.
- symfileobj="$output_objdir/${my_outputname}S.$objext"
- case $host in
- *cygwin* | *mingw* | *cegcc* )
- if test -f "$output_objdir/$my_outputname.def"; then
- compile_command=`$ECHO "$compile_command" | $SED "s%@SYMFILE@%$output_objdir/$my_outputname.def $symfileobj%"`
- finalize_command=`$ECHO "$finalize_command" | $SED "s%@SYMFILE@%$output_objdir/$my_outputname.def $symfileobj%"`
- else
- compile_command=`$ECHO "$compile_command" | $SED "s%@SYMFILE@%$symfileobj%"`
- finalize_command=`$ECHO "$finalize_command" | $SED "s%@SYMFILE@%$symfileobj%"`
- fi
- ;;
- *)
- compile_command=`$ECHO "$compile_command" | $SED "s%@SYMFILE@%$symfileobj%"`
- finalize_command=`$ECHO "$finalize_command" | $SED "s%@SYMFILE@%$symfileobj%"`
- ;;
- esac
- ;;
- *)
- func_fatal_error "unknown suffix for \`$my_dlsyms'"
- ;;
- esac
- else
- # We keep going just in case the user didn't refer to
- # lt_preloaded_symbols. The linker will fail if global_symbol_pipe
- # really was required.
-
- # Nullify the symbol file.
- compile_command=`$ECHO "$compile_command" | $SED "s% @SYMFILE@%%"`
- finalize_command=`$ECHO "$finalize_command" | $SED "s% @SYMFILE@%%"`
- fi
-}
-
-# func_win32_libid arg
-# return the library type of file 'arg'
-#
-# Need a lot of goo to handle *both* DLLs and import libs
-# Has to be a shell function in order to 'eat' the argument
-# that is supplied when $file_magic_command is called.
-# Despite the name, also deal with 64 bit binaries.
-func_win32_libid ()
-{
- $opt_debug
- win32_libid_type="unknown"
- win32_fileres=`file -L $1 2>/dev/null`
- case $win32_fileres in
- *ar\ archive\ import\ library*) # definitely import
- win32_libid_type="x86 archive import"
- ;;
- *ar\ archive*) # could be an import, or static
- # Keep the egrep pattern in sync with the one in _LT_CHECK_MAGIC_METHOD.
- if eval $OBJDUMP -f $1 | $SED -e '10q' 2>/dev/null |
- $EGREP 'file format (pei*-i386(.*architecture: i386)?|pe-arm-wince|pe-x86-64)' >/dev/null; then
- func_to_tool_file "$1" func_convert_file_msys_to_w32
- win32_nmres=`eval $NM -f posix -A \"$func_to_tool_file_result\" |
- $SED -n -e '
- 1,100{
- / I /{
- s,.*,import,
- p
- q
- }
- }'`
- case $win32_nmres in
- import*) win32_libid_type="x86 archive import";;
- *) win32_libid_type="x86 archive static";;
- esac
- fi
- ;;
- *DLL*)
- win32_libid_type="x86 DLL"
- ;;
- *executable*) # but shell scripts are "executable" too...
- case $win32_fileres in
- *MS\ Windows\ PE\ Intel*)
- win32_libid_type="x86 DLL"
- ;;
- esac
- ;;
- esac
- $ECHO "$win32_libid_type"
-}
-
-# func_cygming_dll_for_implib ARG
-#
-# Platform-specific function to extract the
-# name of the DLL associated with the specified
-# import library ARG.
-# Invoked by eval'ing the libtool variable
-# $sharedlib_from_linklib_cmd
-# Result is available in the variable
-# $sharedlib_from_linklib_result
-func_cygming_dll_for_implib ()
-{
- $opt_debug
- sharedlib_from_linklib_result=`$DLLTOOL --identify-strict --identify "$1"`
-}
-
-# func_cygming_dll_for_implib_fallback_core SECTION_NAME LIBNAMEs
-#
-# The is the core of a fallback implementation of a
-# platform-specific function to extract the name of the
-# DLL associated with the specified import library LIBNAME.
-#
-# SECTION_NAME is either .idata$6 or .idata$7, depending
-# on the platform and compiler that created the implib.
-#
-# Echos the name of the DLL associated with the
-# specified import library.
-func_cygming_dll_for_implib_fallback_core ()
-{
- $opt_debug
- match_literal=`$ECHO "$1" | $SED "$sed_make_literal_regex"`
- $OBJDUMP -s --section "$1" "$2" 2>/dev/null |
- $SED '/^Contents of section '"$match_literal"':/{
- # Place marker at beginning of archive member dllname section
- s/.*/====MARK====/
- p
- d
- }
- # These lines can sometimes be longer than 43 characters, but
- # are always uninteresting
- /:[ ]*file format pe[i]\{,1\}-/d
- /^In archive [^:]*:/d
- # Ensure marker is printed
- /^====MARK====/p
- # Remove all lines with less than 43 characters
- /^.\{43\}/!d
- # From remaining lines, remove first 43 characters
- s/^.\{43\}//' |
- $SED -n '
- # Join marker and all lines until next marker into a single line
- /^====MARK====/ b para
- H
- $ b para
- b
- :para
- x
- s/\n//g
- # Remove the marker
- s/^====MARK====//
- # Remove trailing dots and whitespace
- s/[\. \t]*$//
- # Print
- /./p' |
- # we now have a list, one entry per line, of the stringified
- # contents of the appropriate section of all members of the
- # archive which possess that section. Heuristic: eliminate
- # all those which have a first or second character that is
- # a '.' (that is, objdump's representation of an unprintable
- # character.) This should work for all archives with less than
- # 0x302f exports -- but will fail for DLLs whose name actually
- # begins with a literal '.' or a single character followed by
- # a '.'.
- #
- # Of those that remain, print the first one.
- $SED -e '/^\./d;/^.\./d;q'
-}
-
-# func_cygming_gnu_implib_p ARG
-# This predicate returns with zero status (TRUE) if
-# ARG is a GNU/binutils-style import library. Returns
-# with nonzero status (FALSE) otherwise.
-func_cygming_gnu_implib_p ()
-{
- $opt_debug
- func_to_tool_file "$1" func_convert_file_msys_to_w32
- func_cygming_gnu_implib_tmp=`$NM "$func_to_tool_file_result" | eval "$global_symbol_pipe" | $EGREP ' (_head_[A-Za-z0-9_]+_[ad]l*|[A-Za-z0-9_]+_[ad]l*_iname)$'`
- test -n "$func_cygming_gnu_implib_tmp"
-}
-
-# func_cygming_ms_implib_p ARG
-# This predicate returns with zero status (TRUE) if
-# ARG is an MS-style import library. Returns
-# with nonzero status (FALSE) otherwise.
-func_cygming_ms_implib_p ()
-{
- $opt_debug
- func_to_tool_file "$1" func_convert_file_msys_to_w32
- func_cygming_ms_implib_tmp=`$NM "$func_to_tool_file_result" | eval "$global_symbol_pipe" | $GREP '_NULL_IMPORT_DESCRIPTOR'`
- test -n "$func_cygming_ms_implib_tmp"
-}
-
-# func_cygming_dll_for_implib_fallback ARG
-# Platform-specific function to extract the
-# name of the DLL associated with the specified
-# import library ARG.
-#
-# This fallback implementation is for use when $DLLTOOL
-# does not support the --identify-strict option.
-# Invoked by eval'ing the libtool variable
-# $sharedlib_from_linklib_cmd
-# Result is available in the variable
-# $sharedlib_from_linklib_result
-func_cygming_dll_for_implib_fallback ()
-{
- $opt_debug
- if func_cygming_gnu_implib_p "$1" ; then
- # binutils import library
- sharedlib_from_linklib_result=`func_cygming_dll_for_implib_fallback_core '.idata$7' "$1"`
- elif func_cygming_ms_implib_p "$1" ; then
- # ms-generated import library
- sharedlib_from_linklib_result=`func_cygming_dll_for_implib_fallback_core '.idata$6' "$1"`
- else
- # unknown
- sharedlib_from_linklib_result=""
- fi
-}
-
-
-# func_extract_an_archive dir oldlib
-func_extract_an_archive ()
-{
- $opt_debug
- f_ex_an_ar_dir="$1"; shift
- f_ex_an_ar_oldlib="$1"
- if test "$lock_old_archive_extraction" = yes; then
- lockfile=$f_ex_an_ar_oldlib.lock
- until $opt_dry_run || ln "$progpath" "$lockfile" 2>/dev/null; do
- func_echo "Waiting for $lockfile to be removed"
- sleep 2
- done
- fi
- func_show_eval "(cd \$f_ex_an_ar_dir && $AR x \"\$f_ex_an_ar_oldlib\")" \
- 'stat=$?; rm -f "$lockfile"; exit $stat'
- if test "$lock_old_archive_extraction" = yes; then
- $opt_dry_run || rm -f "$lockfile"
- fi
- if ($AR t "$f_ex_an_ar_oldlib" | sort | sort -uc >/dev/null 2>&1); then
- :
- else
- func_fatal_error "object name conflicts in archive: $f_ex_an_ar_dir/$f_ex_an_ar_oldlib"
- fi
-}
-
-
-# func_extract_archives gentop oldlib ...
-func_extract_archives ()
-{
- $opt_debug
- my_gentop="$1"; shift
- my_oldlibs=${1+"$@"}
- my_oldobjs=""
- my_xlib=""
- my_xabs=""
- my_xdir=""
-
- for my_xlib in $my_oldlibs; do
- # Extract the objects.
- case $my_xlib in
- [\\/]* | [A-Za-z]:[\\/]*) my_xabs="$my_xlib" ;;
- *) my_xabs=`pwd`"/$my_xlib" ;;
- esac
- func_basename "$my_xlib"
- my_xlib="$func_basename_result"
- my_xlib_u=$my_xlib
- while :; do
- case " $extracted_archives " in
- *" $my_xlib_u "*)
- func_arith $extracted_serial + 1
- extracted_serial=$func_arith_result
- my_xlib_u=lt$extracted_serial-$my_xlib ;;
- *) break ;;
- esac
- done
- extracted_archives="$extracted_archives $my_xlib_u"
- my_xdir="$my_gentop/$my_xlib_u"
-
- func_mkdir_p "$my_xdir"
-
- case $host in
- *-darwin*)
- func_verbose "Extracting $my_xabs"
- # Do not bother doing anything if just a dry run
- $opt_dry_run || {
- darwin_orig_dir=`pwd`
- cd $my_xdir || exit $?
- darwin_archive=$my_xabs
- darwin_curdir=`pwd`
- darwin_base_archive=`basename "$darwin_archive"`
- darwin_arches=`$LIPO -info "$darwin_archive" 2>/dev/null | $GREP Architectures 2>/dev/null || true`
- if test -n "$darwin_arches"; then
- darwin_arches=`$ECHO "$darwin_arches" | $SED -e 's/.*are://'`
- darwin_arch=
- func_verbose "$darwin_base_archive has multiple architectures $darwin_arches"
- for darwin_arch in $darwin_arches ; do
- func_mkdir_p "unfat-$$/${darwin_base_archive}-${darwin_arch}"
- $LIPO -thin $darwin_arch -output "unfat-$$/${darwin_base_archive}-${darwin_arch}/${darwin_base_archive}" "${darwin_archive}"
- cd "unfat-$$/${darwin_base_archive}-${darwin_arch}"
- func_extract_an_archive "`pwd`" "${darwin_base_archive}"
- cd "$darwin_curdir"
- $RM "unfat-$$/${darwin_base_archive}-${darwin_arch}/${darwin_base_archive}"
- done # $darwin_arches
- ## Okay now we've a bunch of thin objects, gotta fatten them up :)
- darwin_filelist=`find unfat-$$ -type f -name \*.o -print -o -name \*.lo -print | $SED -e "$basename" | sort -u`
- darwin_file=
- darwin_files=
- for darwin_file in $darwin_filelist; do
- darwin_files=`find unfat-$$ -name $darwin_file -print | sort | $NL2SP`
- $LIPO -create -output "$darwin_file" $darwin_files
- done # $darwin_filelist
- $RM -rf unfat-$$
- cd "$darwin_orig_dir"
- else
- cd $darwin_orig_dir
- func_extract_an_archive "$my_xdir" "$my_xabs"
- fi # $darwin_arches
- } # !$opt_dry_run
- ;;
- *)
- func_extract_an_archive "$my_xdir" "$my_xabs"
- ;;
- esac
- my_oldobjs="$my_oldobjs "`find $my_xdir -name \*.$objext -print -o -name \*.lo -print | sort | $NL2SP`
- done
-
- func_extract_archives_result="$my_oldobjs"
-}
-
-
-# func_emit_wrapper [arg=no]
-#
-# Emit a libtool wrapper script on stdout.
-# Don't directly open a file because we may want to
-# incorporate the script contents within a cygwin/mingw
-# wrapper executable. Must ONLY be called from within
-# func_mode_link because it depends on a number of variables
-# set therein.
-#
-# ARG is the value that the WRAPPER_SCRIPT_BELONGS_IN_OBJDIR
-# variable will take. If 'yes', then the emitted script
-# will assume that the directory in which it is stored is
-# the $objdir directory. This is a cygwin/mingw-specific
-# behavior.
-func_emit_wrapper ()
-{
- func_emit_wrapper_arg1=${1-no}
-
- $ECHO "\
-#! $SHELL
-
-# $output - temporary wrapper script for $objdir/$outputname
-# Generated by $PROGRAM (GNU $PACKAGE$TIMESTAMP) $VERSION
-#
-# The $output program cannot be directly executed until all the libtool
-# libraries that it depends on are installed.
-#
-# This wrapper script should never be moved out of the build directory.
-# If it is, it will not operate correctly.
-
-# Sed substitution that helps us do robust quoting. It backslashifies
-# metacharacters that are still active within double-quoted strings.
-sed_quote_subst='$sed_quote_subst'
-
-# Be Bourne compatible
-if test -n \"\${ZSH_VERSION+set}\" && (emulate sh) >/dev/null 2>&1; then
- emulate sh
- NULLCMD=:
- # Zsh 3.x and 4.x performs word splitting on \${1+\"\$@\"}, which
- # is contrary to our usage. Disable this feature.
- alias -g '\${1+\"\$@\"}'='\"\$@\"'
- setopt NO_GLOB_SUBST
-else
- case \`(set -o) 2>/dev/null\` in *posix*) set -o posix;; esac
-fi
-BIN_SH=xpg4; export BIN_SH # for Tru64
-DUALCASE=1; export DUALCASE # for MKS sh
-
-# The HP-UX ksh and POSIX shell print the target directory to stdout
-# if CDPATH is set.
-(unset CDPATH) >/dev/null 2>&1 && unset CDPATH
-
-relink_command=\"$relink_command\"
-
-# This environment variable determines our operation mode.
-if test \"\$libtool_install_magic\" = \"$magic\"; then
- # install mode needs the following variables:
- generated_by_libtool_version='$macro_version'
- notinst_deplibs='$notinst_deplibs'
-else
- # When we are sourced in execute mode, \$file and \$ECHO are already set.
- if test \"\$libtool_execute_magic\" != \"$magic\"; then
- file=\"\$0\""
-
- qECHO=`$ECHO "$ECHO" | $SED "$sed_quote_subst"`
- $ECHO "\
-
-# A function that is used when there is no print builtin or printf.
-func_fallback_echo ()
-{
- eval 'cat <<_LTECHO_EOF
-\$1
-_LTECHO_EOF'
-}
- ECHO=\"$qECHO\"
- fi
-
-# Very basic option parsing. These options are (a) specific to
-# the libtool wrapper, (b) are identical between the wrapper
-# /script/ and the wrapper /executable/ which is used only on
-# windows platforms, and (c) all begin with the string "--lt-"
-# (application programs are unlikely to have options which match
-# this pattern).
-#
-# There are only two supported options: --lt-debug and
-# --lt-dump-script. There is, deliberately, no --lt-help.
-#
-# The first argument to this parsing function should be the
-# script's $0 value, followed by "$@".
-lt_option_debug=
-func_parse_lt_options ()
-{
- lt_script_arg0=\$0
- shift
- for lt_opt
- do
- case \"\$lt_opt\" in
- --lt-debug) lt_option_debug=1 ;;
- --lt-dump-script)
- lt_dump_D=\`\$ECHO \"X\$lt_script_arg0\" | $SED -e 's/^X//' -e 's%/[^/]*$%%'\`
- test \"X\$lt_dump_D\" = \"X\$lt_script_arg0\" && lt_dump_D=.
- lt_dump_F=\`\$ECHO \"X\$lt_script_arg0\" | $SED -e 's/^X//' -e 's%^.*/%%'\`
- cat \"\$lt_dump_D/\$lt_dump_F\"
- exit 0
- ;;
- --lt-*)
- \$ECHO \"Unrecognized --lt- option: '\$lt_opt'\" 1>&2
- exit 1
- ;;
- esac
- done
-
- # Print the debug banner immediately:
- if test -n \"\$lt_option_debug\"; then
- echo \"${outputname}:${output}:\${LINENO}: libtool wrapper (GNU $PACKAGE$TIMESTAMP) $VERSION\" 1>&2
- fi
-}
-
-# Used when --lt-debug. Prints its arguments to stdout
-# (redirection is the responsibility of the caller)
-func_lt_dump_args ()
-{
- lt_dump_args_N=1;
- for lt_arg
- do
- \$ECHO \"${outputname}:${output}:\${LINENO}: newargv[\$lt_dump_args_N]: \$lt_arg\"
- lt_dump_args_N=\`expr \$lt_dump_args_N + 1\`
- done
-}
-
-# Core function for launching the target application
-func_exec_program_core ()
-{
-"
- case $host in
- # Backslashes separate directories on plain windows
- *-*-mingw | *-*-os2* | *-cegcc*)
- $ECHO "\
- if test -n \"\$lt_option_debug\"; then
- \$ECHO \"${outputname}:${output}:\${LINENO}: newargv[0]: \$progdir\\\\\$program\" 1>&2
- func_lt_dump_args \${1+\"\$@\"} 1>&2
- fi
- exec \"\$progdir\\\\\$program\" \${1+\"\$@\"}
-"
- ;;
-
- *)
- $ECHO "\
- if test -n \"\$lt_option_debug\"; then
- \$ECHO \"${outputname}:${output}:\${LINENO}: newargv[0]: \$progdir/\$program\" 1>&2
- func_lt_dump_args \${1+\"\$@\"} 1>&2
- fi
- exec \"\$progdir/\$program\" \${1+\"\$@\"}
-"
- ;;
- esac
- $ECHO "\
- \$ECHO \"\$0: cannot exec \$program \$*\" 1>&2
- exit 1
-}
-
-# A function to encapsulate launching the target application
-# Strips options in the --lt-* namespace from \$@ and
-# launches target application with the remaining arguments.
-func_exec_program ()
-{
- case \" \$* \" in
- *\\ --lt-*)
- for lt_wr_arg
- do
- case \$lt_wr_arg in
- --lt-*) ;;
- *) set x \"\$@\" \"\$lt_wr_arg\"; shift;;
- esac
- shift
- done ;;
- esac
- func_exec_program_core \${1+\"\$@\"}
-}
-
- # Parse options
- func_parse_lt_options \"\$0\" \${1+\"\$@\"}
-
- # Find the directory that this script lives in.
- thisdir=\`\$ECHO \"\$file\" | $SED 's%/[^/]*$%%'\`
- test \"x\$thisdir\" = \"x\$file\" && thisdir=.
-
- # Follow symbolic links until we get to the real thisdir.
- file=\`ls -ld \"\$file\" | $SED -n 's/.*-> //p'\`
- while test -n \"\$file\"; do
- destdir=\`\$ECHO \"\$file\" | $SED 's%/[^/]*\$%%'\`
-
- # If there was a directory component, then change thisdir.
- if test \"x\$destdir\" != \"x\$file\"; then
- case \"\$destdir\" in
- [\\\\/]* | [A-Za-z]:[\\\\/]*) thisdir=\"\$destdir\" ;;
- *) thisdir=\"\$thisdir/\$destdir\" ;;
- esac
- fi
-
- file=\`\$ECHO \"\$file\" | $SED 's%^.*/%%'\`
- file=\`ls -ld \"\$thisdir/\$file\" | $SED -n 's/.*-> //p'\`
- done
-
- # Usually 'no', except on cygwin/mingw when embedded into
- # the cwrapper.
- WRAPPER_SCRIPT_BELONGS_IN_OBJDIR=$func_emit_wrapper_arg1
- if test \"\$WRAPPER_SCRIPT_BELONGS_IN_OBJDIR\" = \"yes\"; then
- # special case for '.'
- if test \"\$thisdir\" = \".\"; then
- thisdir=\`pwd\`
- fi
- # remove .libs from thisdir
- case \"\$thisdir\" in
- *[\\\\/]$objdir ) thisdir=\`\$ECHO \"\$thisdir\" | $SED 's%[\\\\/][^\\\\/]*$%%'\` ;;
- $objdir ) thisdir=. ;;
- esac
- fi
-
- # Try to get the absolute directory name.
- absdir=\`cd \"\$thisdir\" && pwd\`
- test -n \"\$absdir\" && thisdir=\"\$absdir\"
-"
-
- if test "$fast_install" = yes; then
- $ECHO "\
- program=lt-'$outputname'$exeext
- progdir=\"\$thisdir/$objdir\"
-
- if test ! -f \"\$progdir/\$program\" ||
- { file=\`ls -1dt \"\$progdir/\$program\" \"\$progdir/../\$program\" 2>/dev/null | ${SED} 1q\`; \\
- test \"X\$file\" != \"X\$progdir/\$program\"; }; then
-
- file=\"\$\$-\$program\"
-
- if test ! -d \"\$progdir\"; then
- $MKDIR \"\$progdir\"
- else
- $RM \"\$progdir/\$file\"
- fi"
-
- $ECHO "\
-
- # relink executable if necessary
- if test -n \"\$relink_command\"; then
- if relink_command_output=\`eval \$relink_command 2>&1\`; then :
- else
- $ECHO \"\$relink_command_output\" >&2
- $RM \"\$progdir/\$file\"
- exit 1
- fi
- fi
-
- $MV \"\$progdir/\$file\" \"\$progdir/\$program\" 2>/dev/null ||
- { $RM \"\$progdir/\$program\";
- $MV \"\$progdir/\$file\" \"\$progdir/\$program\"; }
- $RM \"\$progdir/\$file\"
- fi"
- else
- $ECHO "\
- program='$outputname'
- progdir=\"\$thisdir/$objdir\"
-"
- fi
-
- $ECHO "\
-
- if test -f \"\$progdir/\$program\"; then"
-
- # fixup the dll searchpath if we need to.
- #
- # Fix the DLL searchpath if we need to. Do this before prepending
- # to shlibpath, because on Windows, both are PATH and uninstalled
- # libraries must come first.
- if test -n "$dllsearchpath"; then
- $ECHO "\
- # Add the dll search path components to the executable PATH
- PATH=$dllsearchpath:\$PATH
-"
- fi
-
- # Export our shlibpath_var if we have one.
- if test "$shlibpath_overrides_runpath" = yes && test -n "$shlibpath_var" && test -n "$temp_rpath"; then
- $ECHO "\
- # Add our own library path to $shlibpath_var
- $shlibpath_var=\"$temp_rpath\$$shlibpath_var\"
-
- # Some systems cannot cope with colon-terminated $shlibpath_var
- # The second colon is a workaround for a bug in BeOS R4 sed
- $shlibpath_var=\`\$ECHO \"\$$shlibpath_var\" | $SED 's/::*\$//'\`
-
- export $shlibpath_var
-"
- fi
-
- $ECHO "\
- if test \"\$libtool_execute_magic\" != \"$magic\"; then
- # Run the actual program with our arguments.
- func_exec_program \${1+\"\$@\"}
- fi
- else
- # The program doesn't exist.
- \$ECHO \"\$0: error: \\\`\$progdir/\$program' does not exist\" 1>&2
- \$ECHO \"This script is just a wrapper for \$program.\" 1>&2
- \$ECHO \"See the $PACKAGE documentation for more information.\" 1>&2
- exit 1
- fi
-fi\
-"
-}
-
-
-# func_emit_cwrapperexe_src
-# emit the source code for a wrapper executable on stdout
-# Must ONLY be called from within func_mode_link because
-# it depends on a number of variable set therein.
-func_emit_cwrapperexe_src ()
-{
- cat <
-#include
-#ifdef _MSC_VER
-# include
-# include
-# include
-#else
-# include
-# include
-# ifdef __CYGWIN__
-# include
-# endif
-#endif
-#include
-#include
-#include
-#include
-#include
-#include
-#include
-#include
-
-/* declarations of non-ANSI functions */
-#if defined(__MINGW32__)
-# ifdef __STRICT_ANSI__
-int _putenv (const char *);
-# endif
-#elif defined(__CYGWIN__)
-# ifdef __STRICT_ANSI__
-char *realpath (const char *, char *);
-int putenv (char *);
-int setenv (const char *, const char *, int);
-# endif
-/* #elif defined (other platforms) ... */
-#endif
-
-/* portability defines, excluding path handling macros */
-#if defined(_MSC_VER)
-# define setmode _setmode
-# define stat _stat
-# define chmod _chmod
-# define getcwd _getcwd
-# define putenv _putenv
-# define S_IXUSR _S_IEXEC
-# ifndef _INTPTR_T_DEFINED
-# define _INTPTR_T_DEFINED
-# define intptr_t int
-# endif
-#elif defined(__MINGW32__)
-# define setmode _setmode
-# define stat _stat
-# define chmod _chmod
-# define getcwd _getcwd
-# define putenv _putenv
-#elif defined(__CYGWIN__)
-# define HAVE_SETENV
-# define FOPEN_WB "wb"
-/* #elif defined (other platforms) ... */
-#endif
-
-#if defined(PATH_MAX)
-# define LT_PATHMAX PATH_MAX
-#elif defined(MAXPATHLEN)
-# define LT_PATHMAX MAXPATHLEN
-#else
-# define LT_PATHMAX 1024
-#endif
-
-#ifndef S_IXOTH
-# define S_IXOTH 0
-#endif
-#ifndef S_IXGRP
-# define S_IXGRP 0
-#endif
-
-/* path handling portability macros */
-#ifndef DIR_SEPARATOR
-# define DIR_SEPARATOR '/'
-# define PATH_SEPARATOR ':'
-#endif
-
-#if defined (_WIN32) || defined (__MSDOS__) || defined (__DJGPP__) || \
- defined (__OS2__)
-# define HAVE_DOS_BASED_FILE_SYSTEM
-# define FOPEN_WB "wb"
-# ifndef DIR_SEPARATOR_2
-# define DIR_SEPARATOR_2 '\\'
-# endif
-# ifndef PATH_SEPARATOR_2
-# define PATH_SEPARATOR_2 ';'
-# endif
-#endif
-
-#ifndef DIR_SEPARATOR_2
-# define IS_DIR_SEPARATOR(ch) ((ch) == DIR_SEPARATOR)
-#else /* DIR_SEPARATOR_2 */
-# define IS_DIR_SEPARATOR(ch) \
- (((ch) == DIR_SEPARATOR) || ((ch) == DIR_SEPARATOR_2))
-#endif /* DIR_SEPARATOR_2 */
-
-#ifndef PATH_SEPARATOR_2
-# define IS_PATH_SEPARATOR(ch) ((ch) == PATH_SEPARATOR)
-#else /* PATH_SEPARATOR_2 */
-# define IS_PATH_SEPARATOR(ch) ((ch) == PATH_SEPARATOR_2)
-#endif /* PATH_SEPARATOR_2 */
-
-#ifndef FOPEN_WB
-# define FOPEN_WB "w"
-#endif
-#ifndef _O_BINARY
-# define _O_BINARY 0
-#endif
-
-#define XMALLOC(type, num) ((type *) xmalloc ((num) * sizeof(type)))
-#define XFREE(stale) do { \
- if (stale) { free ((void *) stale); stale = 0; } \
-} while (0)
-
-#if defined(LT_DEBUGWRAPPER)
-static int lt_debug = 1;
-#else
-static int lt_debug = 0;
-#endif
-
-const char *program_name = "libtool-wrapper"; /* in case xstrdup fails */
-
-void *xmalloc (size_t num);
-char *xstrdup (const char *string);
-const char *base_name (const char *name);
-char *find_executable (const char *wrapper);
-char *chase_symlinks (const char *pathspec);
-int make_executable (const char *path);
-int check_executable (const char *path);
-char *strendzap (char *str, const char *pat);
-void lt_debugprintf (const char *file, int line, const char *fmt, ...);
-void lt_fatal (const char *file, int line, const char *message, ...);
-static const char *nonnull (const char *s);
-static const char *nonempty (const char *s);
-void lt_setenv (const char *name, const char *value);
-char *lt_extend_str (const char *orig_value, const char *add, int to_end);
-void lt_update_exe_path (const char *name, const char *value);
-void lt_update_lib_path (const char *name, const char *value);
-char **prepare_spawn (char **argv);
-void lt_dump_script (FILE *f);
-EOF
-
- cat <= 0)
- && (st.st_mode & (S_IXUSR | S_IXGRP | S_IXOTH)))
- return 1;
- else
- return 0;
-}
-
-int
-make_executable (const char *path)
-{
- int rval = 0;
- struct stat st;
-
- lt_debugprintf (__FILE__, __LINE__, "(make_executable): %s\n",
- nonempty (path));
- if ((!path) || (!*path))
- return 0;
-
- if (stat (path, &st) >= 0)
- {
- rval = chmod (path, st.st_mode | S_IXOTH | S_IXGRP | S_IXUSR);
- }
- return rval;
-}
-
-/* Searches for the full path of the wrapper. Returns
- newly allocated full path name if found, NULL otherwise
- Does not chase symlinks, even on platforms that support them.
-*/
-char *
-find_executable (const char *wrapper)
-{
- int has_slash = 0;
- const char *p;
- const char *p_next;
- /* static buffer for getcwd */
- char tmp[LT_PATHMAX + 1];
- int tmp_len;
- char *concat_name;
-
- lt_debugprintf (__FILE__, __LINE__, "(find_executable): %s\n",
- nonempty (wrapper));
-
- if ((wrapper == NULL) || (*wrapper == '\0'))
- return NULL;
-
- /* Absolute path? */
-#if defined (HAVE_DOS_BASED_FILE_SYSTEM)
- if (isalpha ((unsigned char) wrapper[0]) && wrapper[1] == ':')
- {
- concat_name = xstrdup (wrapper);
- if (check_executable (concat_name))
- return concat_name;
- XFREE (concat_name);
- }
- else
- {
-#endif
- if (IS_DIR_SEPARATOR (wrapper[0]))
- {
- concat_name = xstrdup (wrapper);
- if (check_executable (concat_name))
- return concat_name;
- XFREE (concat_name);
- }
-#if defined (HAVE_DOS_BASED_FILE_SYSTEM)
- }
-#endif
-
- for (p = wrapper; *p; p++)
- if (*p == '/')
- {
- has_slash = 1;
- break;
- }
- if (!has_slash)
- {
- /* no slashes; search PATH */
- const char *path = getenv ("PATH");
- if (path != NULL)
- {
- for (p = path; *p; p = p_next)
- {
- const char *q;
- size_t p_len;
- for (q = p; *q; q++)
- if (IS_PATH_SEPARATOR (*q))
- break;
- p_len = q - p;
- p_next = (*q == '\0' ? q : q + 1);
- if (p_len == 0)
- {
- /* empty path: current directory */
- if (getcwd (tmp, LT_PATHMAX) == NULL)
- lt_fatal (__FILE__, __LINE__, "getcwd failed: %s",
- nonnull (strerror (errno)));
- tmp_len = strlen (tmp);
- concat_name =
- XMALLOC (char, tmp_len + 1 + strlen (wrapper) + 1);
- memcpy (concat_name, tmp, tmp_len);
- concat_name[tmp_len] = '/';
- strcpy (concat_name + tmp_len + 1, wrapper);
- }
- else
- {
- concat_name =
- XMALLOC (char, p_len + 1 + strlen (wrapper) + 1);
- memcpy (concat_name, p, p_len);
- concat_name[p_len] = '/';
- strcpy (concat_name + p_len + 1, wrapper);
- }
- if (check_executable (concat_name))
- return concat_name;
- XFREE (concat_name);
- }
- }
- /* not found in PATH; assume curdir */
- }
- /* Relative path | not found in path: prepend cwd */
- if (getcwd (tmp, LT_PATHMAX) == NULL)
- lt_fatal (__FILE__, __LINE__, "getcwd failed: %s",
- nonnull (strerror (errno)));
- tmp_len = strlen (tmp);
- concat_name = XMALLOC (char, tmp_len + 1 + strlen (wrapper) + 1);
- memcpy (concat_name, tmp, tmp_len);
- concat_name[tmp_len] = '/';
- strcpy (concat_name + tmp_len + 1, wrapper);
-
- if (check_executable (concat_name))
- return concat_name;
- XFREE (concat_name);
- return NULL;
-}
-
-char *
-chase_symlinks (const char *pathspec)
-{
-#ifndef S_ISLNK
- return xstrdup (pathspec);
-#else
- char buf[LT_PATHMAX];
- struct stat s;
- char *tmp_pathspec = xstrdup (pathspec);
- char *p;
- int has_symlinks = 0;
- while (strlen (tmp_pathspec) && !has_symlinks)
- {
- lt_debugprintf (__FILE__, __LINE__,
- "checking path component for symlinks: %s\n",
- tmp_pathspec);
- if (lstat (tmp_pathspec, &s) == 0)
- {
- if (S_ISLNK (s.st_mode) != 0)
- {
- has_symlinks = 1;
- break;
- }
-
- /* search backwards for last DIR_SEPARATOR */
- p = tmp_pathspec + strlen (tmp_pathspec) - 1;
- while ((p > tmp_pathspec) && (!IS_DIR_SEPARATOR (*p)))
- p--;
- if ((p == tmp_pathspec) && (!IS_DIR_SEPARATOR (*p)))
- {
- /* no more DIR_SEPARATORS left */
- break;
- }
- *p = '\0';
- }
- else
- {
- lt_fatal (__FILE__, __LINE__,
- "error accessing file \"%s\": %s",
- tmp_pathspec, nonnull (strerror (errno)));
- }
- }
- XFREE (tmp_pathspec);
-
- if (!has_symlinks)
- {
- return xstrdup (pathspec);
- }
-
- tmp_pathspec = realpath (pathspec, buf);
- if (tmp_pathspec == 0)
- {
- lt_fatal (__FILE__, __LINE__,
- "could not follow symlinks for %s", pathspec);
- }
- return xstrdup (tmp_pathspec);
-#endif
-}
-
-char *
-strendzap (char *str, const char *pat)
-{
- size_t len, patlen;
-
- assert (str != NULL);
- assert (pat != NULL);
-
- len = strlen (str);
- patlen = strlen (pat);
-
- if (patlen <= len)
- {
- str += len - patlen;
- if (strcmp (str, pat) == 0)
- *str = '\0';
- }
- return str;
-}
-
-void
-lt_debugprintf (const char *file, int line, const char *fmt, ...)
-{
- va_list args;
- if (lt_debug)
- {
- (void) fprintf (stderr, "%s:%s:%d: ", program_name, file, line);
- va_start (args, fmt);
- (void) vfprintf (stderr, fmt, args);
- va_end (args);
- }
-}
-
-static void
-lt_error_core (int exit_status, const char *file,
- int line, const char *mode,
- const char *message, va_list ap)
-{
- fprintf (stderr, "%s:%s:%d: %s: ", program_name, file, line, mode);
- vfprintf (stderr, message, ap);
- fprintf (stderr, ".\n");
-
- if (exit_status >= 0)
- exit (exit_status);
-}
-
-void
-lt_fatal (const char *file, int line, const char *message, ...)
-{
- va_list ap;
- va_start (ap, message);
- lt_error_core (EXIT_FAILURE, file, line, "FATAL", message, ap);
- va_end (ap);
-}
-
-static const char *
-nonnull (const char *s)
-{
- return s ? s : "(null)";
-}
-
-static const char *
-nonempty (const char *s)
-{
- return (s && !*s) ? "(empty)" : nonnull (s);
-}
-
-void
-lt_setenv (const char *name, const char *value)
-{
- lt_debugprintf (__FILE__, __LINE__,
- "(lt_setenv) setting '%s' to '%s'\n",
- nonnull (name), nonnull (value));
- {
-#ifdef HAVE_SETENV
- /* always make a copy, for consistency with !HAVE_SETENV */
- char *str = xstrdup (value);
- setenv (name, str, 1);
-#else
- int len = strlen (name) + 1 + strlen (value) + 1;
- char *str = XMALLOC (char, len);
- sprintf (str, "%s=%s", name, value);
- if (putenv (str) != EXIT_SUCCESS)
- {
- XFREE (str);
- }
-#endif
- }
-}
-
-char *
-lt_extend_str (const char *orig_value, const char *add, int to_end)
-{
- char *new_value;
- if (orig_value && *orig_value)
- {
- int orig_value_len = strlen (orig_value);
- int add_len = strlen (add);
- new_value = XMALLOC (char, add_len + orig_value_len + 1);
- if (to_end)
- {
- strcpy (new_value, orig_value);
- strcpy (new_value + orig_value_len, add);
- }
- else
- {
- strcpy (new_value, add);
- strcpy (new_value + add_len, orig_value);
- }
- }
- else
- {
- new_value = xstrdup (add);
- }
- return new_value;
-}
-
-void
-lt_update_exe_path (const char *name, const char *value)
-{
- lt_debugprintf (__FILE__, __LINE__,
- "(lt_update_exe_path) modifying '%s' by prepending '%s'\n",
- nonnull (name), nonnull (value));
-
- if (name && *name && value && *value)
- {
- char *new_value = lt_extend_str (getenv (name), value, 0);
- /* some systems can't cope with a ':'-terminated path #' */
- int len = strlen (new_value);
- while (((len = strlen (new_value)) > 0) && IS_PATH_SEPARATOR (new_value[len-1]))
- {
- new_value[len-1] = '\0';
- }
- lt_setenv (name, new_value);
- XFREE (new_value);
- }
-}
-
-void
-lt_update_lib_path (const char *name, const char *value)
-{
- lt_debugprintf (__FILE__, __LINE__,
- "(lt_update_lib_path) modifying '%s' by prepending '%s'\n",
- nonnull (name), nonnull (value));
-
- if (name && *name && value && *value)
- {
- char *new_value = lt_extend_str (getenv (name), value, 0);
- lt_setenv (name, new_value);
- XFREE (new_value);
- }
-}
-
-EOF
- case $host_os in
- mingw*)
- cat <<"EOF"
-
-/* Prepares an argument vector before calling spawn().
- Note that spawn() does not by itself call the command interpreter
- (getenv ("COMSPEC") != NULL ? getenv ("COMSPEC") :
- ({ OSVERSIONINFO v; v.dwOSVersionInfoSize = sizeof(OSVERSIONINFO);
- GetVersionEx(&v);
- v.dwPlatformId == VER_PLATFORM_WIN32_NT;
- }) ? "cmd.exe" : "command.com").
- Instead it simply concatenates the arguments, separated by ' ', and calls
- CreateProcess(). We must quote the arguments since Win32 CreateProcess()
- interprets characters like ' ', '\t', '\\', '"' (but not '<' and '>') in a
- special way:
- - Space and tab are interpreted as delimiters. They are not treated as
- delimiters if they are surrounded by double quotes: "...".
- - Unescaped double quotes are removed from the input. Their only effect is
- that within double quotes, space and tab are treated like normal
- characters.
- - Backslashes not followed by double quotes are not special.
- - But 2*n+1 backslashes followed by a double quote become
- n backslashes followed by a double quote (n >= 0):
- \" -> "
- \\\" -> \"
- \\\\\" -> \\"
- */
-#define SHELL_SPECIAL_CHARS "\"\\ \001\002\003\004\005\006\007\010\011\012\013\014\015\016\017\020\021\022\023\024\025\026\027\030\031\032\033\034\035\036\037"
-#define SHELL_SPACE_CHARS " \001\002\003\004\005\006\007\010\011\012\013\014\015\016\017\020\021\022\023\024\025\026\027\030\031\032\033\034\035\036\037"
-char **
-prepare_spawn (char **argv)
-{
- size_t argc;
- char **new_argv;
- size_t i;
-
- /* Count number of arguments. */
- for (argc = 0; argv[argc] != NULL; argc++)
- ;
-
- /* Allocate new argument vector. */
- new_argv = XMALLOC (char *, argc + 1);
-
- /* Put quoted arguments into the new argument vector. */
- for (i = 0; i < argc; i++)
- {
- const char *string = argv[i];
-
- if (string[0] == '\0')
- new_argv[i] = xstrdup ("\"\"");
- else if (strpbrk (string, SHELL_SPECIAL_CHARS) != NULL)
- {
- int quote_around = (strpbrk (string, SHELL_SPACE_CHARS) != NULL);
- size_t length;
- unsigned int backslashes;
- const char *s;
- char *quoted_string;
- char *p;
-
- length = 0;
- backslashes = 0;
- if (quote_around)
- length++;
- for (s = string; *s != '\0'; s++)
- {
- char c = *s;
- if (c == '"')
- length += backslashes + 1;
- length++;
- if (c == '\\')
- backslashes++;
- else
- backslashes = 0;
- }
- if (quote_around)
- length += backslashes + 1;
-
- quoted_string = XMALLOC (char, length + 1);
-
- p = quoted_string;
- backslashes = 0;
- if (quote_around)
- *p++ = '"';
- for (s = string; *s != '\0'; s++)
- {
- char c = *s;
- if (c == '"')
- {
- unsigned int j;
- for (j = backslashes + 1; j > 0; j--)
- *p++ = '\\';
- }
- *p++ = c;
- if (c == '\\')
- backslashes++;
- else
- backslashes = 0;
- }
- if (quote_around)
- {
- unsigned int j;
- for (j = backslashes; j > 0; j--)
- *p++ = '\\';
- *p++ = '"';
- }
- *p = '\0';
-
- new_argv[i] = quoted_string;
- }
- else
- new_argv[i] = (char *) string;
- }
- new_argv[argc] = NULL;
-
- return new_argv;
-}
-EOF
- ;;
- esac
-
- cat <<"EOF"
-void lt_dump_script (FILE* f)
-{
-EOF
- func_emit_wrapper yes |
- $SED -n -e '
-s/^\(.\{79\}\)\(..*\)/\1\
-\2/
-h
-s/\([\\"]\)/\\\1/g
-s/$/\\n/
-s/\([^\n]*\).*/ fputs ("\1", f);/p
-g
-D'
- cat <<"EOF"
-}
-EOF
-}
-# end: func_emit_cwrapperexe_src
-
-# func_win32_import_lib_p ARG
-# True if ARG is an import lib, as indicated by $file_magic_cmd
-func_win32_import_lib_p ()
-{
- $opt_debug
- case `eval $file_magic_cmd \"\$1\" 2>/dev/null | $SED -e 10q` in
- *import*) : ;;
- *) false ;;
- esac
-}
-
-# func_mode_link arg...
-func_mode_link ()
-{
- $opt_debug
- case $host in
- *-*-cygwin* | *-*-mingw* | *-*-pw32* | *-*-os2* | *-cegcc*)
- # It is impossible to link a dll without this setting, and
- # we shouldn't force the makefile maintainer to figure out
- # which system we are compiling for in order to pass an extra
- # flag for every libtool invocation.
- # allow_undefined=no
-
- # FIXME: Unfortunately, there are problems with the above when trying
- # to make a dll which has undefined symbols, in which case not
- # even a static library is built. For now, we need to specify
- # -no-undefined on the libtool link line when we can be certain
- # that all symbols are satisfied, otherwise we get a static library.
- allow_undefined=yes
- ;;
- *)
- allow_undefined=yes
- ;;
- esac
- libtool_args=$nonopt
- base_compile="$nonopt $@"
- compile_command=$nonopt
- finalize_command=$nonopt
-
- compile_rpath=
- finalize_rpath=
- compile_shlibpath=
- finalize_shlibpath=
- convenience=
- old_convenience=
- deplibs=
- old_deplibs=
- compiler_flags=
- linker_flags=
- dllsearchpath=
- lib_search_path=`pwd`
- inst_prefix_dir=
- new_inherited_linker_flags=
-
- avoid_version=no
- bindir=
- dlfiles=
- dlprefiles=
- dlself=no
- export_dynamic=no
- export_symbols=
- export_symbols_regex=
- generated=
- libobjs=
- ltlibs=
- module=no
- no_install=no
- objs=
- non_pic_objects=
- precious_files_regex=
- prefer_static_libs=no
- preload=no
- prev=
- prevarg=
- release=
- rpath=
- xrpath=
- perm_rpath=
- temp_rpath=
- thread_safe=no
- vinfo=
- vinfo_number=no
- weak_libs=
- single_module="${wl}-single_module"
- func_infer_tag $base_compile
-
- # We need to know -static, to get the right output filenames.
- for arg
- do
- case $arg in
- -shared)
- test "$build_libtool_libs" != yes && \
- func_fatal_configuration "can not build a shared library"
- build_old_libs=no
- break
- ;;
- -all-static | -static | -static-libtool-libs)
- case $arg in
- -all-static)
- if test "$build_libtool_libs" = yes && test -z "$link_static_flag"; then
- func_warning "complete static linking is impossible in this configuration"
- fi
- if test -n "$link_static_flag"; then
- dlopen_self=$dlopen_self_static
- fi
- prefer_static_libs=yes
- ;;
- -static)
- if test -z "$pic_flag" && test -n "$link_static_flag"; then
- dlopen_self=$dlopen_self_static
- fi
- prefer_static_libs=built
- ;;
- -static-libtool-libs)
- if test -z "$pic_flag" && test -n "$link_static_flag"; then
- dlopen_self=$dlopen_self_static
- fi
- prefer_static_libs=yes
- ;;
- esac
- build_libtool_libs=no
- build_old_libs=yes
- break
- ;;
- esac
- done
-
- # See if our shared archives depend on static archives.
- test -n "$old_archive_from_new_cmds" && build_old_libs=yes
-
- # Go through the arguments, transforming them on the way.
- while test "$#" -gt 0; do
- arg="$1"
- shift
- func_quote_for_eval "$arg"
- qarg=$func_quote_for_eval_unquoted_result
- func_append libtool_args " $func_quote_for_eval_result"
-
- # If the previous option needs an argument, assign it.
- if test -n "$prev"; then
- case $prev in
- output)
- func_append compile_command " @OUTPUT@"
- func_append finalize_command " @OUTPUT@"
- ;;
- esac
-
- case $prev in
- bindir)
- bindir="$arg"
- prev=
- continue
- ;;
- dlfiles|dlprefiles)
- if test "$preload" = no; then
- # Add the symbol object into the linking commands.
- func_append compile_command " @SYMFILE@"
- func_append finalize_command " @SYMFILE@"
- preload=yes
- fi
- case $arg in
- *.la | *.lo) ;; # We handle these cases below.
- force)
- if test "$dlself" = no; then
- dlself=needless
- export_dynamic=yes
- fi
- prev=
- continue
- ;;
- self)
- if test "$prev" = dlprefiles; then
- dlself=yes
- elif test "$prev" = dlfiles && test "$dlopen_self" != yes; then
- dlself=yes
- else
- dlself=needless
- export_dynamic=yes
- fi
- prev=
- continue
- ;;
- *)
- if test "$prev" = dlfiles; then
- func_append dlfiles " $arg"
- else
- func_append dlprefiles " $arg"
- fi
- prev=
- continue
- ;;
- esac
- ;;
- expsyms)
- export_symbols="$arg"
- test -f "$arg" \
- || func_fatal_error "symbol file \`$arg' does not exist"
- prev=
- continue
- ;;
- expsyms_regex)
- export_symbols_regex="$arg"
- prev=
- continue
- ;;
- framework)
- case $host in
- *-*-darwin*)
- case "$deplibs " in
- *" $qarg.ltframework "*) ;;
- *) func_append deplibs " $qarg.ltframework" # this is fixed later
- ;;
- esac
- ;;
- esac
- prev=
- continue
- ;;
- inst_prefix)
- inst_prefix_dir="$arg"
- prev=
- continue
- ;;
- objectlist)
- if test -f "$arg"; then
- save_arg=$arg
- moreargs=
- for fil in `cat "$save_arg"`
- do
-# func_append moreargs " $fil"
- arg=$fil
- # A libtool-controlled object.
-
- # Check to see that this really is a libtool object.
- if func_lalib_unsafe_p "$arg"; then
- pic_object=
- non_pic_object=
-
- # Read the .lo file
- func_source "$arg"
-
- if test -z "$pic_object" ||
- test -z "$non_pic_object" ||
- test "$pic_object" = none &&
- test "$non_pic_object" = none; then
- func_fatal_error "cannot find name of object for \`$arg'"
- fi
-
- # Extract subdirectory from the argument.
- func_dirname "$arg" "/" ""
- xdir="$func_dirname_result"
-
- if test "$pic_object" != none; then
- # Prepend the subdirectory the object is found in.
- pic_object="$xdir$pic_object"
-
- if test "$prev" = dlfiles; then
- if test "$build_libtool_libs" = yes && test "$dlopen_support" = yes; then
- func_append dlfiles " $pic_object"
- prev=
- continue
- else
- # If libtool objects are unsupported, then we need to preload.
- prev=dlprefiles
- fi
- fi
-
- # CHECK ME: I think I busted this. -Ossama
- if test "$prev" = dlprefiles; then
- # Preload the old-style object.
- func_append dlprefiles " $pic_object"
- prev=
- fi
-
- # A PIC object.
- func_append libobjs " $pic_object"
- arg="$pic_object"
- fi
-
- # Non-PIC object.
- if test "$non_pic_object" != none; then
- # Prepend the subdirectory the object is found in.
- non_pic_object="$xdir$non_pic_object"
-
- # A standard non-PIC object
- func_append non_pic_objects " $non_pic_object"
- if test -z "$pic_object" || test "$pic_object" = none ; then
- arg="$non_pic_object"
- fi
- else
- # If the PIC object exists, use it instead.
- # $xdir was prepended to $pic_object above.
- non_pic_object="$pic_object"
- func_append non_pic_objects " $non_pic_object"
- fi
- else
- # Only an error if not doing a dry-run.
- if $opt_dry_run; then
- # Extract subdirectory from the argument.
- func_dirname "$arg" "/" ""
- xdir="$func_dirname_result"
-
- func_lo2o "$arg"
- pic_object=$xdir$objdir/$func_lo2o_result
- non_pic_object=$xdir$func_lo2o_result
- func_append libobjs " $pic_object"
- func_append non_pic_objects " $non_pic_object"
- else
- func_fatal_error "\`$arg' is not a valid libtool object"
- fi
- fi
- done
- else
- func_fatal_error "link input file \`$arg' does not exist"
- fi
- arg=$save_arg
- prev=
- continue
- ;;
- precious_regex)
- precious_files_regex="$arg"
- prev=
- continue
- ;;
- release)
- release="-$arg"
- prev=
- continue
- ;;
- rpath | xrpath)
- # We need an absolute path.
- case $arg in
- [\\/]* | [A-Za-z]:[\\/]*) ;;
- *)
- func_fatal_error "only absolute run-paths are allowed"
- ;;
- esac
- if test "$prev" = rpath; then
- case "$rpath " in
- *" $arg "*) ;;
- *) func_append rpath " $arg" ;;
- esac
- else
- case "$xrpath " in
- *" $arg "*) ;;
- *) func_append xrpath " $arg" ;;
- esac
- fi
- prev=
- continue
- ;;
- shrext)
- shrext_cmds="$arg"
- prev=
- continue
- ;;
- weak)
- func_append weak_libs " $arg"
- prev=
- continue
- ;;
- xcclinker)
- func_append linker_flags " $qarg"
- func_append compiler_flags " $qarg"
- prev=
- func_append compile_command " $qarg"
- func_append finalize_command " $qarg"
- continue
- ;;
- xcompiler)
- func_append compiler_flags " $qarg"
- prev=
- func_append compile_command " $qarg"
- func_append finalize_command " $qarg"
- continue
- ;;
- xlinker)
- func_append linker_flags " $qarg"
- func_append compiler_flags " $wl$qarg"
- prev=
- func_append compile_command " $wl$qarg"
- func_append finalize_command " $wl$qarg"
- continue
- ;;
- *)
- eval "$prev=\"\$arg\""
- prev=
- continue
- ;;
- esac
- fi # test -n "$prev"
-
- prevarg="$arg"
-
- case $arg in
- -all-static)
- if test -n "$link_static_flag"; then
- # See comment for -static flag below, for more details.
- func_append compile_command " $link_static_flag"
- func_append finalize_command " $link_static_flag"
- fi
- continue
- ;;
-
- -allow-undefined)
- # FIXME: remove this flag sometime in the future.
- func_fatal_error "\`-allow-undefined' must not be used because it is the default"
- ;;
-
- -avoid-version)
- avoid_version=yes
- continue
- ;;
-
- -bindir)
- prev=bindir
- continue
- ;;
-
- -dlopen)
- prev=dlfiles
- continue
- ;;
-
- -dlpreopen)
- prev=dlprefiles
- continue
- ;;
-
- -export-dynamic)
- export_dynamic=yes
- continue
- ;;
-
- -export-symbols | -export-symbols-regex)
- if test -n "$export_symbols" || test -n "$export_symbols_regex"; then
- func_fatal_error "more than one -exported-symbols argument is not allowed"
- fi
- if test "X$arg" = "X-export-symbols"; then
- prev=expsyms
- else
- prev=expsyms_regex
- fi
- continue
- ;;
-
- -framework)
- prev=framework
- continue
- ;;
-
- -inst-prefix-dir)
- prev=inst_prefix
- continue
- ;;
-
- # The native IRIX linker understands -LANG:*, -LIST:* and -LNO:*
- # so, if we see these flags be careful not to treat them like -L
- -L[A-Z][A-Z]*:*)
- case $with_gcc/$host in
- no/*-*-irix* | /*-*-irix*)
- func_append compile_command " $arg"
- func_append finalize_command " $arg"
- ;;
- esac
- continue
- ;;
-
- -L*)
- func_stripname "-L" '' "$arg"
- if test -z "$func_stripname_result"; then
- if test "$#" -gt 0; then
- func_fatal_error "require no space between \`-L' and \`$1'"
- else
- func_fatal_error "need path for \`-L' option"
- fi
- fi
- func_resolve_sysroot "$func_stripname_result"
- dir=$func_resolve_sysroot_result
- # We need an absolute path.
- case $dir in
- [\\/]* | [A-Za-z]:[\\/]*) ;;
- *)
- absdir=`cd "$dir" && pwd`
- test -z "$absdir" && \
- func_fatal_error "cannot determine absolute directory name of \`$dir'"
- dir="$absdir"
- ;;
- esac
- case "$deplibs " in
- *" -L$dir "* | *" $arg "*)
- # Will only happen for absolute or sysroot arguments
- ;;
- *)
- # Preserve sysroot, but never include relative directories
- case $dir in
- [\\/]* | [A-Za-z]:[\\/]* | =*) func_append deplibs " $arg" ;;
- *) func_append deplibs " -L$dir" ;;
- esac
- func_append lib_search_path " $dir"
- ;;
- esac
- case $host in
- *-*-cygwin* | *-*-mingw* | *-*-pw32* | *-*-os2* | *-cegcc*)
- testbindir=`$ECHO "$dir" | $SED 's*/lib$*/bin*'`
- case :$dllsearchpath: in
- *":$dir:"*) ;;
- ::) dllsearchpath=$dir;;
- *) func_append dllsearchpath ":$dir";;
- esac
- case :$dllsearchpath: in
- *":$testbindir:"*) ;;
- ::) dllsearchpath=$testbindir;;
- *) func_append dllsearchpath ":$testbindir";;
- esac
- ;;
- esac
- continue
- ;;
-
- -l*)
- if test "X$arg" = "X-lc" || test "X$arg" = "X-lm"; then
- case $host in
- *-*-cygwin* | *-*-mingw* | *-*-pw32* | *-*-beos* | *-cegcc* | *-*-haiku*)
- # These systems don't actually have a C or math library (as such)
- continue
- ;;
- *-*-os2*)
- # These systems don't actually have a C library (as such)
- test "X$arg" = "X-lc" && continue
- ;;
- *-*-openbsd* | *-*-freebsd* | *-*-dragonfly*)
- # Do not include libc due to us having libc/libc_r.
- test "X$arg" = "X-lc" && continue
- ;;
- *-*-rhapsody* | *-*-darwin1.[012])
- # Rhapsody C and math libraries are in the System framework
- func_append deplibs " System.ltframework"
- continue
- ;;
- *-*-sco3.2v5* | *-*-sco5v6*)
- # Causes problems with __ctype
- test "X$arg" = "X-lc" && continue
- ;;
- *-*-sysv4.2uw2* | *-*-sysv5* | *-*-unixware* | *-*-OpenUNIX*)
- # Compiler inserts libc in the correct place for threads to work
- test "X$arg" = "X-lc" && continue
- ;;
- esac
- elif test "X$arg" = "X-lc_r"; then
- case $host in
- *-*-openbsd* | *-*-freebsd* | *-*-dragonfly*)
- # Do not include libc_r directly, use -pthread flag.
- continue
- ;;
- esac
- fi
- func_append deplibs " $arg"
- continue
- ;;
-
- -module)
- module=yes
- continue
- ;;
-
- # Tru64 UNIX uses -model [arg] to determine the layout of C++
- # classes, name mangling, and exception handling.
- # Darwin uses the -arch flag to determine output architecture.
- -model|-arch|-isysroot|--sysroot)
- func_append compiler_flags " $arg"
- func_append compile_command " $arg"
- func_append finalize_command " $arg"
- prev=xcompiler
- continue
- ;;
-
- -mt|-mthreads|-kthread|-Kthread|-pthread|-pthreads|--thread-safe \
- |-threads|-fopenmp|-openmp|-mp|-xopenmp|-omp|-qsmp=*)
- func_append compiler_flags " $arg"
- func_append compile_command " $arg"
- func_append finalize_command " $arg"
- case "$new_inherited_linker_flags " in
- *" $arg "*) ;;
- * ) func_append new_inherited_linker_flags " $arg" ;;
- esac
- continue
- ;;
-
- -multi_module)
- single_module="${wl}-multi_module"
- continue
- ;;
-
- -no-fast-install)
- fast_install=no
- continue
- ;;
-
- -no-install)
- case $host in
- *-*-cygwin* | *-*-mingw* | *-*-pw32* | *-*-os2* | *-*-darwin* | *-cegcc*)
- # The PATH hackery in wrapper scripts is required on Windows
- # and Darwin in order for the loader to find any dlls it needs.
- func_warning "\`-no-install' is ignored for $host"
- func_warning "assuming \`-no-fast-install' instead"
- fast_install=no
- ;;
- *) no_install=yes ;;
- esac
- continue
- ;;
-
- -no-undefined)
- allow_undefined=no
- continue
- ;;
-
- -objectlist)
- prev=objectlist
- continue
- ;;
-
- -o) prev=output ;;
-
- -precious-files-regex)
- prev=precious_regex
- continue
- ;;
-
- -release)
- prev=release
- continue
- ;;
-
- -rpath)
- prev=rpath
- continue
- ;;
-
- -R)
- prev=xrpath
- continue
- ;;
-
- -R*)
- func_stripname '-R' '' "$arg"
- dir=$func_stripname_result
- # We need an absolute path.
- case $dir in
- [\\/]* | [A-Za-z]:[\\/]*) ;;
- =*)
- func_stripname '=' '' "$dir"
- dir=$lt_sysroot$func_stripname_result
- ;;
- *)
- func_fatal_error "only absolute run-paths are allowed"
- ;;
- esac
- case "$xrpath " in
- *" $dir "*) ;;
- *) func_append xrpath " $dir" ;;
- esac
- continue
- ;;
-
- -shared)
- # The effects of -shared are defined in a previous loop.
- continue
- ;;
-
- -shrext)
- prev=shrext
- continue
- ;;
-
- -static | -static-libtool-libs)
- # The effects of -static are defined in a previous loop.
- # We used to do the same as -all-static on platforms that
- # didn't have a PIC flag, but the assumption that the effects
- # would be equivalent was wrong. It would break on at least
- # Digital Unix and AIX.
- continue
- ;;
-
- -thread-safe)
- thread_safe=yes
- continue
- ;;
-
- -version-info)
- prev=vinfo
- continue
- ;;
-
- -version-number)
- prev=vinfo
- vinfo_number=yes
- continue
- ;;
-
- -weak)
- prev=weak
- continue
- ;;
-
- -Wc,*)
- func_stripname '-Wc,' '' "$arg"
- args=$func_stripname_result
- arg=
- save_ifs="$IFS"; IFS=','
- for flag in $args; do
- IFS="$save_ifs"
- func_quote_for_eval "$flag"
- func_append arg " $func_quote_for_eval_result"
- func_append compiler_flags " $func_quote_for_eval_result"
- done
- IFS="$save_ifs"
- func_stripname ' ' '' "$arg"
- arg=$func_stripname_result
- ;;
-
- -Wl,*)
- func_stripname '-Wl,' '' "$arg"
- args=$func_stripname_result
- arg=
- save_ifs="$IFS"; IFS=','
- for flag in $args; do
- IFS="$save_ifs"
- func_quote_for_eval "$flag"
- func_append arg " $wl$func_quote_for_eval_result"
- func_append compiler_flags " $wl$func_quote_for_eval_result"
- func_append linker_flags " $func_quote_for_eval_result"
- done
- IFS="$save_ifs"
- func_stripname ' ' '' "$arg"
- arg=$func_stripname_result
- ;;
-
- -Xcompiler)
- prev=xcompiler
- continue
- ;;
-
- -Xlinker)
- prev=xlinker
- continue
- ;;
-
- -XCClinker)
- prev=xcclinker
- continue
- ;;
-
- # -msg_* for osf cc
- -msg_*)
- func_quote_for_eval "$arg"
- arg="$func_quote_for_eval_result"
- ;;
-
- # Flags to be passed through unchanged, with rationale:
- # -64, -mips[0-9] enable 64-bit mode for the SGI compiler
- # -r[0-9][0-9]* specify processor for the SGI compiler
- # -xarch=*, -xtarget=* enable 64-bit mode for the Sun compiler
- # +DA*, +DD* enable 64-bit mode for the HP compiler
- # -q* compiler args for the IBM compiler
- # -m*, -t[45]*, -txscale* architecture-specific flags for GCC
- # -F/path path to uninstalled frameworks, gcc on darwin
- # -p, -pg, --coverage, -fprofile-* profiling flags for GCC
- # @file GCC response files
- # -tp=* Portland pgcc target processor selection
- # --sysroot=* for sysroot support
- # -O*, -flto*, -fwhopr*, -fuse-linker-plugin GCC link-time optimization
- -64|-mips[0-9]|-r[0-9][0-9]*|-xarch=*|-xtarget=*|+DA*|+DD*|-q*|-m*| \
- -t[45]*|-txscale*|-p|-pg|--coverage|-fprofile-*|-F*|@*|-tp=*|--sysroot=*| \
- -O*|-flto*|-fwhopr*|-fuse-linker-plugin)
- func_quote_for_eval "$arg"
- arg="$func_quote_for_eval_result"
- func_append compile_command " $arg"
- func_append finalize_command " $arg"
- func_append compiler_flags " $arg"
- continue
- ;;
-
- # Some other compiler flag.
- -* | +*)
- func_quote_for_eval "$arg"
- arg="$func_quote_for_eval_result"
- ;;
-
- *.$objext)
- # A standard object.
- func_append objs " $arg"
- ;;
-
- *.lo)
- # A libtool-controlled object.
-
- # Check to see that this really is a libtool object.
- if func_lalib_unsafe_p "$arg"; then
- pic_object=
- non_pic_object=
-
- # Read the .lo file
- func_source "$arg"
-
- if test -z "$pic_object" ||
- test -z "$non_pic_object" ||
- test "$pic_object" = none &&
- test "$non_pic_object" = none; then
- func_fatal_error "cannot find name of object for \`$arg'"
- fi
-
- # Extract subdirectory from the argument.
- func_dirname "$arg" "/" ""
- xdir="$func_dirname_result"
-
- if test "$pic_object" != none; then
- # Prepend the subdirectory the object is found in.
- pic_object="$xdir$pic_object"
-
- if test "$prev" = dlfiles; then
- if test "$build_libtool_libs" = yes && test "$dlopen_support" = yes; then
- func_append dlfiles " $pic_object"
- prev=
- continue
- else
- # If libtool objects are unsupported, then we need to preload.
- prev=dlprefiles
- fi
- fi
-
- # CHECK ME: I think I busted this. -Ossama
- if test "$prev" = dlprefiles; then
- # Preload the old-style object.
- func_append dlprefiles " $pic_object"
- prev=
- fi
-
- # A PIC object.
- func_append libobjs " $pic_object"
- arg="$pic_object"
- fi
-
- # Non-PIC object.
- if test "$non_pic_object" != none; then
- # Prepend the subdirectory the object is found in.
- non_pic_object="$xdir$non_pic_object"
-
- # A standard non-PIC object
- func_append non_pic_objects " $non_pic_object"
- if test -z "$pic_object" || test "$pic_object" = none ; then
- arg="$non_pic_object"
- fi
- else
- # If the PIC object exists, use it instead.
- # $xdir was prepended to $pic_object above.
- non_pic_object="$pic_object"
- func_append non_pic_objects " $non_pic_object"
- fi
- else
- # Only an error if not doing a dry-run.
- if $opt_dry_run; then
- # Extract subdirectory from the argument.
- func_dirname "$arg" "/" ""
- xdir="$func_dirname_result"
-
- func_lo2o "$arg"
- pic_object=$xdir$objdir/$func_lo2o_result
- non_pic_object=$xdir$func_lo2o_result
- func_append libobjs " $pic_object"
- func_append non_pic_objects " $non_pic_object"
- else
- func_fatal_error "\`$arg' is not a valid libtool object"
- fi
- fi
- ;;
-
- *.$libext)
- # An archive.
- func_append deplibs " $arg"
- func_append old_deplibs " $arg"
- continue
- ;;
-
- *.la)
- # A libtool-controlled library.
-
- func_resolve_sysroot "$arg"
- if test "$prev" = dlfiles; then
- # This library was specified with -dlopen.
- func_append dlfiles " $func_resolve_sysroot_result"
- prev=
- elif test "$prev" = dlprefiles; then
- # The library was specified with -dlpreopen.
- func_append dlprefiles " $func_resolve_sysroot_result"
- prev=
- else
- func_append deplibs " $func_resolve_sysroot_result"
- fi
- continue
- ;;
-
- # Some other compiler argument.
- *)
- # Unknown arguments in both finalize_command and compile_command need
- # to be aesthetically quoted because they are evaled later.
- func_quote_for_eval "$arg"
- arg="$func_quote_for_eval_result"
- ;;
- esac # arg
-
- # Now actually substitute the argument into the commands.
- if test -n "$arg"; then
- func_append compile_command " $arg"
- func_append finalize_command " $arg"
- fi
- done # argument parsing loop
-
- test -n "$prev" && \
- func_fatal_help "the \`$prevarg' option requires an argument"
-
- if test "$export_dynamic" = yes && test -n "$export_dynamic_flag_spec"; then
- eval arg=\"$export_dynamic_flag_spec\"
- func_append compile_command " $arg"
- func_append finalize_command " $arg"
- fi
-
- oldlibs=
- # calculate the name of the file, without its directory
- func_basename "$output"
- outputname="$func_basename_result"
- libobjs_save="$libobjs"
-
- if test -n "$shlibpath_var"; then
- # get the directories listed in $shlibpath_var
- eval shlib_search_path=\`\$ECHO \"\${$shlibpath_var}\" \| \$SED \'s/:/ /g\'\`
- else
- shlib_search_path=
- fi
- eval sys_lib_search_path=\"$sys_lib_search_path_spec\"
- eval sys_lib_dlsearch_path=\"$sys_lib_dlsearch_path_spec\"
-
- func_dirname "$output" "/" ""
- output_objdir="$func_dirname_result$objdir"
- func_to_tool_file "$output_objdir/"
- tool_output_objdir=$func_to_tool_file_result
- # Create the object directory.
- func_mkdir_p "$output_objdir"
-
- # Determine the type of output
- case $output in
- "")
- func_fatal_help "you must specify an output file"
- ;;
- *.$libext) linkmode=oldlib ;;
- *.lo | *.$objext) linkmode=obj ;;
- *.la) linkmode=lib ;;
- *) linkmode=prog ;; # Anything else should be a program.
- esac
-
- specialdeplibs=
-
- libs=
- # Find all interdependent deplibs by searching for libraries
- # that are linked more than once (e.g. -la -lb -la)
- for deplib in $deplibs; do
- if $opt_preserve_dup_deps ; then
- case "$libs " in
- *" $deplib "*) func_append specialdeplibs " $deplib" ;;
- esac
- fi
- func_append libs " $deplib"
- done
-
- if test "$linkmode" = lib; then
- libs="$predeps $libs $compiler_lib_search_path $postdeps"
-
- # Compute libraries that are listed more than once in $predeps
- # $postdeps and mark them as special (i.e., whose duplicates are
- # not to be eliminated).
- pre_post_deps=
- if $opt_duplicate_compiler_generated_deps; then
- for pre_post_dep in $predeps $postdeps; do
- case "$pre_post_deps " in
- *" $pre_post_dep "*) func_append specialdeplibs " $pre_post_deps" ;;
- esac
- func_append pre_post_deps " $pre_post_dep"
- done
- fi
- pre_post_deps=
- fi
-
- deplibs=
- newdependency_libs=
- newlib_search_path=
- need_relink=no # whether we're linking any uninstalled libtool libraries
- notinst_deplibs= # not-installed libtool libraries
- notinst_path= # paths that contain not-installed libtool libraries
-
- case $linkmode in
- lib)
- passes="conv dlpreopen link"
- for file in $dlfiles $dlprefiles; do
- case $file in
- *.la) ;;
- *)
- func_fatal_help "libraries can \`-dlopen' only libtool libraries: $file"
- ;;
- esac
- done
- ;;
- prog)
- compile_deplibs=
- finalize_deplibs=
- alldeplibs=no
- newdlfiles=
- newdlprefiles=
- passes="conv scan dlopen dlpreopen link"
- ;;
- *) passes="conv"
- ;;
- esac
-
- for pass in $passes; do
- # The preopen pass in lib mode reverses $deplibs; put it back here
- # so that -L comes before libs that need it for instance...
- if test "$linkmode,$pass" = "lib,link"; then
- ## FIXME: Find the place where the list is rebuilt in the wrong
- ## order, and fix it there properly
- tmp_deplibs=
- for deplib in $deplibs; do
- tmp_deplibs="$deplib $tmp_deplibs"
- done
- deplibs="$tmp_deplibs"
- fi
-
- if test "$linkmode,$pass" = "lib,link" ||
- test "$linkmode,$pass" = "prog,scan"; then
- libs="$deplibs"
- deplibs=
- fi
- if test "$linkmode" = prog; then
- case $pass in
- dlopen) libs="$dlfiles" ;;
- dlpreopen) libs="$dlprefiles" ;;
- link)
- libs="$deplibs %DEPLIBS%"
- test "X$link_all_deplibs" != Xno && libs="$libs $dependency_libs"
- ;;
- esac
- fi
- if test "$linkmode,$pass" = "lib,dlpreopen"; then
- # Collect and forward deplibs of preopened libtool libs
- for lib in $dlprefiles; do
- # Ignore non-libtool-libs
- dependency_libs=
- func_resolve_sysroot "$lib"
- case $lib in
- *.la) func_source "$func_resolve_sysroot_result" ;;
- esac
-
- # Collect preopened libtool deplibs, except any this library
- # has declared as weak libs
- for deplib in $dependency_libs; do
- func_basename "$deplib"
- deplib_base=$func_basename_result
- case " $weak_libs " in
- *" $deplib_base "*) ;;
- *) func_append deplibs " $deplib" ;;
- esac
- done
- done
- libs="$dlprefiles"
- fi
- if test "$pass" = dlopen; then
- # Collect dlpreopened libraries
- save_deplibs="$deplibs"
- deplibs=
- fi
-
- for deplib in $libs; do
- lib=
- found=no
- case $deplib in
- -mt|-mthreads|-kthread|-Kthread|-pthread|-pthreads|--thread-safe \
- |-threads|-fopenmp|-openmp|-mp|-xopenmp|-omp|-qsmp=*)
- if test "$linkmode,$pass" = "prog,link"; then
- compile_deplibs="$deplib $compile_deplibs"
- finalize_deplibs="$deplib $finalize_deplibs"
- else
- func_append compiler_flags " $deplib"
- if test "$linkmode" = lib ; then
- case "$new_inherited_linker_flags " in
- *" $deplib "*) ;;
- * ) func_append new_inherited_linker_flags " $deplib" ;;
- esac
- fi
- fi
- continue
- ;;
- -l*)
- if test "$linkmode" != lib && test "$linkmode" != prog; then
- func_warning "\`-l' is ignored for archives/objects"
- continue
- fi
- func_stripname '-l' '' "$deplib"
- name=$func_stripname_result
- if test "$linkmode" = lib; then
- searchdirs="$newlib_search_path $lib_search_path $compiler_lib_search_dirs $sys_lib_search_path $shlib_search_path"
- else
- searchdirs="$newlib_search_path $lib_search_path $sys_lib_search_path $shlib_search_path"
- fi
- for searchdir in $searchdirs; do
- for search_ext in .la $std_shrext .so .a; do
- # Search the libtool library
- lib="$searchdir/lib${name}${search_ext}"
- if test -f "$lib"; then
- if test "$search_ext" = ".la"; then
- found=yes
- else
- found=no
- fi
- break 2
- fi
- done
- done
- if test "$found" != yes; then
- # deplib doesn't seem to be a libtool library
- if test "$linkmode,$pass" = "prog,link"; then
- compile_deplibs="$deplib $compile_deplibs"
- finalize_deplibs="$deplib $finalize_deplibs"
- else
- deplibs="$deplib $deplibs"
- test "$linkmode" = lib && newdependency_libs="$deplib $newdependency_libs"
- fi
- continue
- else # deplib is a libtool library
- # If $allow_libtool_libs_with_static_runtimes && $deplib is a stdlib,
- # We need to do some special things here, and not later.
- if test "X$allow_libtool_libs_with_static_runtimes" = "Xyes" ; then
- case " $predeps $postdeps " in
- *" $deplib "*)
- if func_lalib_p "$lib"; then
- library_names=
- old_library=
- func_source "$lib"
- for l in $old_library $library_names; do
- ll="$l"
- done
- if test "X$ll" = "X$old_library" ; then # only static version available
- found=no
- func_dirname "$lib" "" "."
- ladir="$func_dirname_result"
- lib=$ladir/$old_library
- if test "$linkmode,$pass" = "prog,link"; then
- compile_deplibs="$deplib $compile_deplibs"
- finalize_deplibs="$deplib $finalize_deplibs"
- else
- deplibs="$deplib $deplibs"
- test "$linkmode" = lib && newdependency_libs="$deplib $newdependency_libs"
- fi
- continue
- fi
- fi
- ;;
- *) ;;
- esac
- fi
- fi
- ;; # -l
- *.ltframework)
- if test "$linkmode,$pass" = "prog,link"; then
- compile_deplibs="$deplib $compile_deplibs"
- finalize_deplibs="$deplib $finalize_deplibs"
- else
- deplibs="$deplib $deplibs"
- if test "$linkmode" = lib ; then
- case "$new_inherited_linker_flags " in
- *" $deplib "*) ;;
- * ) func_append new_inherited_linker_flags " $deplib" ;;
- esac
- fi
- fi
- continue
- ;;
- -L*)
- case $linkmode in
- lib)
- deplibs="$deplib $deplibs"
- test "$pass" = conv && continue
- newdependency_libs="$deplib $newdependency_libs"
- func_stripname '-L' '' "$deplib"
- func_resolve_sysroot "$func_stripname_result"
- func_append newlib_search_path " $func_resolve_sysroot_result"
- ;;
- prog)
- if test "$pass" = conv; then
- deplibs="$deplib $deplibs"
- continue
- fi
- if test "$pass" = scan; then
- deplibs="$deplib $deplibs"
- else
- compile_deplibs="$deplib $compile_deplibs"
- finalize_deplibs="$deplib $finalize_deplibs"
- fi
- func_stripname '-L' '' "$deplib"
- func_resolve_sysroot "$func_stripname_result"
- func_append newlib_search_path " $func_resolve_sysroot_result"
- ;;
- *)
- func_warning "\`-L' is ignored for archives/objects"
- ;;
- esac # linkmode
- continue
- ;; # -L
- -R*)
- if test "$pass" = link; then
- func_stripname '-R' '' "$deplib"
- func_resolve_sysroot "$func_stripname_result"
- dir=$func_resolve_sysroot_result
- # Make sure the xrpath contains only unique directories.
- case "$xrpath " in
- *" $dir "*) ;;
- *) func_append xrpath " $dir" ;;
- esac
- fi
- deplibs="$deplib $deplibs"
- continue
- ;;
- *.la)
- func_resolve_sysroot "$deplib"
- lib=$func_resolve_sysroot_result
- ;;
- *.$libext)
- if test "$pass" = conv; then
- deplibs="$deplib $deplibs"
- continue
- fi
- case $linkmode in
- lib)
- # Linking convenience modules into shared libraries is allowed,
- # but linking other static libraries is non-portable.
- case " $dlpreconveniencelibs " in
- *" $deplib "*) ;;
- *)
- valid_a_lib=no
- case $deplibs_check_method in
- match_pattern*)
- set dummy $deplibs_check_method; shift
- match_pattern_regex=`expr "$deplibs_check_method" : "$1 \(.*\)"`
- if eval "\$ECHO \"$deplib\"" 2>/dev/null | $SED 10q \
- | $EGREP "$match_pattern_regex" > /dev/null; then
- valid_a_lib=yes
- fi
- ;;
- pass_all)
- valid_a_lib=yes
- ;;
- esac
- if test "$valid_a_lib" != yes; then
- echo
- $ECHO "*** Warning: Trying to link with static lib archive $deplib."
- echo "*** I have the capability to make that library automatically link in when"
- echo "*** you link to this library. But I can only do this if you have a"
- echo "*** shared version of the library, which you do not appear to have"
- echo "*** because the file extensions .$libext of this argument makes me believe"
- echo "*** that it is just a static archive that I should not use here."
- else
- echo
- $ECHO "*** Warning: Linking the shared library $output against the"
- $ECHO "*** static library $deplib is not portable!"
- deplibs="$deplib $deplibs"
- fi
- ;;
- esac
- continue
- ;;
- prog)
- if test "$pass" != link; then
- deplibs="$deplib $deplibs"
- else
- compile_deplibs="$deplib $compile_deplibs"
- finalize_deplibs="$deplib $finalize_deplibs"
- fi
- continue
- ;;
- esac # linkmode
- ;; # *.$libext
- *.lo | *.$objext)
- if test "$pass" = conv; then
- deplibs="$deplib $deplibs"
- elif test "$linkmode" = prog; then
- if test "$pass" = dlpreopen || test "$dlopen_support" != yes || test "$build_libtool_libs" = no; then
- # If there is no dlopen support or we're linking statically,
- # we need to preload.
- func_append newdlprefiles " $deplib"
- compile_deplibs="$deplib $compile_deplibs"
- finalize_deplibs="$deplib $finalize_deplibs"
- else
- func_append newdlfiles " $deplib"
- fi
- fi
- continue
- ;;
- %DEPLIBS%)
- alldeplibs=yes
- continue
- ;;
- esac # case $deplib
-
- if test "$found" = yes || test -f "$lib"; then :
- else
- func_fatal_error "cannot find the library \`$lib' or unhandled argument \`$deplib'"
- fi
-
- # Check to see that this really is a libtool archive.
- func_lalib_unsafe_p "$lib" \
- || func_fatal_error "\`$lib' is not a valid libtool archive"
-
- func_dirname "$lib" "" "."
- ladir="$func_dirname_result"
-
- dlname=
- dlopen=
- dlpreopen=
- libdir=
- library_names=
- old_library=
- inherited_linker_flags=
- # If the library was installed with an old release of libtool,
- # it will not redefine variables installed, or shouldnotlink
- installed=yes
- shouldnotlink=no
- avoidtemprpath=
-
-
- # Read the .la file
- func_source "$lib"
-
- # Convert "-framework foo" to "foo.ltframework"
- if test -n "$inherited_linker_flags"; then
- tmp_inherited_linker_flags=`$ECHO "$inherited_linker_flags" | $SED 's/-framework \([^ $]*\)/\1.ltframework/g'`
- for tmp_inherited_linker_flag in $tmp_inherited_linker_flags; do
- case " $new_inherited_linker_flags " in
- *" $tmp_inherited_linker_flag "*) ;;
- *) func_append new_inherited_linker_flags " $tmp_inherited_linker_flag";;
- esac
- done
- fi
- dependency_libs=`$ECHO " $dependency_libs" | $SED 's% \([^ $]*\).ltframework% -framework \1%g'`
- if test "$linkmode,$pass" = "lib,link" ||
- test "$linkmode,$pass" = "prog,scan" ||
- { test "$linkmode" != prog && test "$linkmode" != lib; }; then
- test -n "$dlopen" && func_append dlfiles " $dlopen"
- test -n "$dlpreopen" && func_append dlprefiles " $dlpreopen"
- fi
-
- if test "$pass" = conv; then
- # Only check for convenience libraries
- deplibs="$lib $deplibs"
- if test -z "$libdir"; then
- if test -z "$old_library"; then
- func_fatal_error "cannot find name of link library for \`$lib'"
- fi
- # It is a libtool convenience library, so add in its objects.
- func_append convenience " $ladir/$objdir/$old_library"
- func_append old_convenience " $ladir/$objdir/$old_library"
- tmp_libs=
- for deplib in $dependency_libs; do
- deplibs="$deplib $deplibs"
- if $opt_preserve_dup_deps ; then
- case "$tmp_libs " in
- *" $deplib "*) func_append specialdeplibs " $deplib" ;;
- esac
- fi
- func_append tmp_libs " $deplib"
- done
- elif test "$linkmode" != prog && test "$linkmode" != lib; then
- func_fatal_error "\`$lib' is not a convenience library"
- fi
- continue
- fi # $pass = conv
-
-
- # Get the name of the library we link against.
- linklib=
- if test -n "$old_library" &&
- { test "$prefer_static_libs" = yes ||
- test "$prefer_static_libs,$installed" = "built,no"; }; then
- linklib=$old_library
- else
- for l in $old_library $library_names; do
- linklib="$l"
- done
- fi
- if test -z "$linklib"; then
- func_fatal_error "cannot find name of link library for \`$lib'"
- fi
-
- # This library was specified with -dlopen.
- if test "$pass" = dlopen; then
- if test -z "$libdir"; then
- func_fatal_error "cannot -dlopen a convenience library: \`$lib'"
- fi
- if test -z "$dlname" ||
- test "$dlopen_support" != yes ||
- test "$build_libtool_libs" = no; then
- # If there is no dlname, no dlopen support or we're linking
- # statically, we need to preload. We also need to preload any
- # dependent libraries so libltdl's deplib preloader doesn't
- # bomb out in the load deplibs phase.
- func_append dlprefiles " $lib $dependency_libs"
- else
- func_append newdlfiles " $lib"
- fi
- continue
- fi # $pass = dlopen
-
- # We need an absolute path.
- case $ladir in
- [\\/]* | [A-Za-z]:[\\/]*) abs_ladir="$ladir" ;;
- *)
- abs_ladir=`cd "$ladir" && pwd`
- if test -z "$abs_ladir"; then
- func_warning "cannot determine absolute directory name of \`$ladir'"
- func_warning "passing it literally to the linker, although it might fail"
- abs_ladir="$ladir"
- fi
- ;;
- esac
- func_basename "$lib"
- laname="$func_basename_result"
-
- # Find the relevant object directory and library name.
- if test "X$installed" = Xyes; then
- if test ! -f "$lt_sysroot$libdir/$linklib" && test -f "$abs_ladir/$linklib"; then
- func_warning "library \`$lib' was moved."
- dir="$ladir"
- absdir="$abs_ladir"
- libdir="$abs_ladir"
- else
- dir="$lt_sysroot$libdir"
- absdir="$lt_sysroot$libdir"
- fi
- test "X$hardcode_automatic" = Xyes && avoidtemprpath=yes
- else
- if test ! -f "$ladir/$objdir/$linklib" && test -f "$abs_ladir/$linklib"; then
- dir="$ladir"
- absdir="$abs_ladir"
- # Remove this search path later
- func_append notinst_path " $abs_ladir"
- else
- dir="$ladir/$objdir"
- absdir="$abs_ladir/$objdir"
- # Remove this search path later
- func_append notinst_path " $abs_ladir"
- fi
- fi # $installed = yes
- func_stripname 'lib' '.la' "$laname"
- name=$func_stripname_result
-
- # This library was specified with -dlpreopen.
- if test "$pass" = dlpreopen; then
- if test -z "$libdir" && test "$linkmode" = prog; then
- func_fatal_error "only libraries may -dlpreopen a convenience library: \`$lib'"
- fi
- case "$host" in
- # special handling for platforms with PE-DLLs.
- *cygwin* | *mingw* | *cegcc* )
- # Linker will automatically link against shared library if both
- # static and shared are present. Therefore, ensure we extract
- # symbols from the import library if a shared library is present
- # (otherwise, the dlopen module name will be incorrect). We do
- # this by putting the import library name into $newdlprefiles.
- # We recover the dlopen module name by 'saving' the la file
- # name in a special purpose variable, and (later) extracting the
- # dlname from the la file.
- if test -n "$dlname"; then
- func_tr_sh "$dir/$linklib"
- eval "libfile_$func_tr_sh_result=\$abs_ladir/\$laname"
- func_append newdlprefiles " $dir/$linklib"
- else
- func_append newdlprefiles " $dir/$old_library"
- # Keep a list of preopened convenience libraries to check
- # that they are being used correctly in the link pass.
- test -z "$libdir" && \
- func_append dlpreconveniencelibs " $dir/$old_library"
- fi
- ;;
- * )
- # Prefer using a static library (so that no silly _DYNAMIC symbols
- # are required to link).
- if test -n "$old_library"; then
- func_append newdlprefiles " $dir/$old_library"
- # Keep a list of preopened convenience libraries to check
- # that they are being used correctly in the link pass.
- test -z "$libdir" && \
- func_append dlpreconveniencelibs " $dir/$old_library"
- # Otherwise, use the dlname, so that lt_dlopen finds it.
- elif test -n "$dlname"; then
- func_append newdlprefiles " $dir/$dlname"
- else
- func_append newdlprefiles " $dir/$linklib"
- fi
- ;;
- esac
- fi # $pass = dlpreopen
-
- if test -z "$libdir"; then
- # Link the convenience library
- if test "$linkmode" = lib; then
- deplibs="$dir/$old_library $deplibs"
- elif test "$linkmode,$pass" = "prog,link"; then
- compile_deplibs="$dir/$old_library $compile_deplibs"
- finalize_deplibs="$dir/$old_library $finalize_deplibs"
- else
- deplibs="$lib $deplibs" # used for prog,scan pass
- fi
- continue
- fi
-
-
- if test "$linkmode" = prog && test "$pass" != link; then
- func_append newlib_search_path " $ladir"
- deplibs="$lib $deplibs"
-
- linkalldeplibs=no
- if test "$link_all_deplibs" != no || test -z "$library_names" ||
- test "$build_libtool_libs" = no; then
- linkalldeplibs=yes
- fi
-
- tmp_libs=
- for deplib in $dependency_libs; do
- case $deplib in
- -L*) func_stripname '-L' '' "$deplib"
- func_resolve_sysroot "$func_stripname_result"
- func_append newlib_search_path " $func_resolve_sysroot_result"
- ;;
- esac
- # Need to link against all dependency_libs?
- if test "$linkalldeplibs" = yes; then
- deplibs="$deplib $deplibs"
- else
- # Need to hardcode shared library paths
- # or/and link against static libraries
- newdependency_libs="$deplib $newdependency_libs"
- fi
- if $opt_preserve_dup_deps ; then
- case "$tmp_libs " in
- *" $deplib "*) func_append specialdeplibs " $deplib" ;;
- esac
- fi
- func_append tmp_libs " $deplib"
- done # for deplib
- continue
- fi # $linkmode = prog...
-
- if test "$linkmode,$pass" = "prog,link"; then
- if test -n "$library_names" &&
- { { test "$prefer_static_libs" = no ||
- test "$prefer_static_libs,$installed" = "built,yes"; } ||
- test -z "$old_library"; }; then
- # We need to hardcode the library path
- if test -n "$shlibpath_var" && test -z "$avoidtemprpath" ; then
- # Make sure the rpath contains only unique directories.
- case "$temp_rpath:" in
- *"$absdir:"*) ;;
- *) func_append temp_rpath "$absdir:" ;;
- esac
- fi
-
- # Hardcode the library path.
- # Skip directories that are in the system default run-time
- # search path.
- case " $sys_lib_dlsearch_path " in
- *" $absdir "*) ;;
- *)
- case "$compile_rpath " in
- *" $absdir "*) ;;
- *) func_append compile_rpath " $absdir" ;;
- esac
- ;;
- esac
- case " $sys_lib_dlsearch_path " in
- *" $libdir "*) ;;
- *)
- case "$finalize_rpath " in
- *" $libdir "*) ;;
- *) func_append finalize_rpath " $libdir" ;;
- esac
- ;;
- esac
- fi # $linkmode,$pass = prog,link...
-
- if test "$alldeplibs" = yes &&
- { test "$deplibs_check_method" = pass_all ||
- { test "$build_libtool_libs" = yes &&
- test -n "$library_names"; }; }; then
- # We only need to search for static libraries
- continue
- fi
- fi
-
- link_static=no # Whether the deplib will be linked statically
- use_static_libs=$prefer_static_libs
- if test "$use_static_libs" = built && test "$installed" = yes; then
- use_static_libs=no
- fi
- if test -n "$library_names" &&
- { test "$use_static_libs" = no || test -z "$old_library"; }; then
- case $host in
- *cygwin* | *mingw* | *cegcc*)
- # No point in relinking DLLs because paths are not encoded
- func_append notinst_deplibs " $lib"
- need_relink=no
- ;;
- *)
- if test "$installed" = no; then
- func_append notinst_deplibs " $lib"
- need_relink=yes
- fi
- ;;
- esac
- # This is a shared library
-
- # Warn about portability, can't link against -module's on some
- # systems (darwin). Don't bleat about dlopened modules though!
- dlopenmodule=""
- for dlpremoduletest in $dlprefiles; do
- if test "X$dlpremoduletest" = "X$lib"; then
- dlopenmodule="$dlpremoduletest"
- break
- fi
- done
- if test -z "$dlopenmodule" && test "$shouldnotlink" = yes && test "$pass" = link; then
- echo
- if test "$linkmode" = prog; then
- $ECHO "*** Warning: Linking the executable $output against the loadable module"
- else
- $ECHO "*** Warning: Linking the shared library $output against the loadable module"
- fi
- $ECHO "*** $linklib is not portable!"
- fi
- if test "$linkmode" = lib &&
- test "$hardcode_into_libs" = yes; then
- # Hardcode the library path.
- # Skip directories that are in the system default run-time
- # search path.
- case " $sys_lib_dlsearch_path " in
- *" $absdir "*) ;;
- *)
- case "$compile_rpath " in
- *" $absdir "*) ;;
- *) func_append compile_rpath " $absdir" ;;
- esac
- ;;
- esac
- case " $sys_lib_dlsearch_path " in
- *" $libdir "*) ;;
- *)
- case "$finalize_rpath " in
- *" $libdir "*) ;;
- *) func_append finalize_rpath " $libdir" ;;
- esac
- ;;
- esac
- fi
-
- if test -n "$old_archive_from_expsyms_cmds"; then
- # figure out the soname
- set dummy $library_names
- shift
- realname="$1"
- shift
- libname=`eval "\\$ECHO \"$libname_spec\""`
- # use dlname if we got it. it's perfectly good, no?
- if test -n "$dlname"; then
- soname="$dlname"
- elif test -n "$soname_spec"; then
- # bleh windows
- case $host in
- *cygwin* | mingw* | *cegcc*)
- func_arith $current - $age
- major=$func_arith_result
- versuffix="-$major"
- ;;
- esac
- eval soname=\"$soname_spec\"
- else
- soname="$realname"
- fi
-
- # Make a new name for the extract_expsyms_cmds to use
- soroot="$soname"
- func_basename "$soroot"
- soname="$func_basename_result"
- func_stripname 'lib' '.dll' "$soname"
- newlib=libimp-$func_stripname_result.a
-
- # If the library has no export list, then create one now
- if test -f "$output_objdir/$soname-def"; then :
- else
- func_verbose "extracting exported symbol list from \`$soname'"
- func_execute_cmds "$extract_expsyms_cmds" 'exit $?'
- fi
-
- # Create $newlib
- if test -f "$output_objdir/$newlib"; then :; else
- func_verbose "generating import library for \`$soname'"
- func_execute_cmds "$old_archive_from_expsyms_cmds" 'exit $?'
- fi
- # make sure the library variables are pointing to the new library
- dir=$output_objdir
- linklib=$newlib
- fi # test -n "$old_archive_from_expsyms_cmds"
-
- if test "$linkmode" = prog || test "$opt_mode" != relink; then
- add_shlibpath=
- add_dir=
- add=
- lib_linked=yes
- case $hardcode_action in
- immediate | unsupported)
- if test "$hardcode_direct" = no; then
- add="$dir/$linklib"
- case $host in
- *-*-sco3.2v5.0.[024]*) add_dir="-L$dir" ;;
- *-*-sysv4*uw2*) add_dir="-L$dir" ;;
- *-*-sysv5OpenUNIX* | *-*-sysv5UnixWare7.[01].[10]* | \
- *-*-unixware7*) add_dir="-L$dir" ;;
- *-*-darwin* )
- # if the lib is a (non-dlopened) module then we can not
- # link against it, someone is ignoring the earlier warnings
- if /usr/bin/file -L $add 2> /dev/null |
- $GREP ": [^:]* bundle" >/dev/null ; then
- if test "X$dlopenmodule" != "X$lib"; then
- $ECHO "*** Warning: lib $linklib is a module, not a shared library"
- if test -z "$old_library" ; then
- echo
- echo "*** And there doesn't seem to be a static archive available"
- echo "*** The link will probably fail, sorry"
- else
- add="$dir/$old_library"
- fi
- elif test -n "$old_library"; then
- add="$dir/$old_library"
- fi
- fi
- esac
- elif test "$hardcode_minus_L" = no; then
- case $host in
- *-*-sunos*) add_shlibpath="$dir" ;;
- esac
- add_dir="-L$dir"
- add="-l$name"
- elif test "$hardcode_shlibpath_var" = no; then
- add_shlibpath="$dir"
- add="-l$name"
- else
- lib_linked=no
- fi
- ;;
- relink)
- if test "$hardcode_direct" = yes &&
- test "$hardcode_direct_absolute" = no; then
- add="$dir/$linklib"
- elif test "$hardcode_minus_L" = yes; then
- add_dir="-L$absdir"
- # Try looking first in the location we're being installed to.
- if test -n "$inst_prefix_dir"; then
- case $libdir in
- [\\/]*)
- func_append add_dir " -L$inst_prefix_dir$libdir"
- ;;
- esac
- fi
- add="-l$name"
- elif test "$hardcode_shlibpath_var" = yes; then
- add_shlibpath="$dir"
- add="-l$name"
- else
- lib_linked=no
- fi
- ;;
- *) lib_linked=no ;;
- esac
-
- if test "$lib_linked" != yes; then
- func_fatal_configuration "unsupported hardcode properties"
- fi
-
- if test -n "$add_shlibpath"; then
- case :$compile_shlibpath: in
- *":$add_shlibpath:"*) ;;
- *) func_append compile_shlibpath "$add_shlibpath:" ;;
- esac
- fi
- if test "$linkmode" = prog; then
- test -n "$add_dir" && compile_deplibs="$add_dir $compile_deplibs"
- test -n "$add" && compile_deplibs="$add $compile_deplibs"
- else
- test -n "$add_dir" && deplibs="$add_dir $deplibs"
- test -n "$add" && deplibs="$add $deplibs"
- if test "$hardcode_direct" != yes &&
- test "$hardcode_minus_L" != yes &&
- test "$hardcode_shlibpath_var" = yes; then
- case :$finalize_shlibpath: in
- *":$libdir:"*) ;;
- *) func_append finalize_shlibpath "$libdir:" ;;
- esac
- fi
- fi
- fi
-
- if test "$linkmode" = prog || test "$opt_mode" = relink; then
- add_shlibpath=
- add_dir=
- add=
- # Finalize command for both is simple: just hardcode it.
- if test "$hardcode_direct" = yes &&
- test "$hardcode_direct_absolute" = no; then
- add="$libdir/$linklib"
- elif test "$hardcode_minus_L" = yes; then
- add_dir="-L$libdir"
- add="-l$name"
- elif test "$hardcode_shlibpath_var" = yes; then
- case :$finalize_shlibpath: in
- *":$libdir:"*) ;;
- *) func_append finalize_shlibpath "$libdir:" ;;
- esac
- add="-l$name"
- elif test "$hardcode_automatic" = yes; then
- if test -n "$inst_prefix_dir" &&
- test -f "$inst_prefix_dir$libdir/$linklib" ; then
- add="$inst_prefix_dir$libdir/$linklib"
- else
- add="$libdir/$linklib"
- fi
- else
- # We cannot seem to hardcode it, guess we'll fake it.
- add_dir="-L$libdir"
- # Try looking first in the location we're being installed to.
- if test -n "$inst_prefix_dir"; then
- case $libdir in
- [\\/]*)
- func_append add_dir " -L$inst_prefix_dir$libdir"
- ;;
- esac
- fi
- add="-l$name"
- fi
-
- if test "$linkmode" = prog; then
- test -n "$add_dir" && finalize_deplibs="$add_dir $finalize_deplibs"
- test -n "$add" && finalize_deplibs="$add $finalize_deplibs"
- else
- test -n "$add_dir" && deplibs="$add_dir $deplibs"
- test -n "$add" && deplibs="$add $deplibs"
- fi
- fi
- elif test "$linkmode" = prog; then
- # Here we assume that one of hardcode_direct or hardcode_minus_L
- # is not unsupported. This is valid on all known static and
- # shared platforms.
- if test "$hardcode_direct" != unsupported; then
- test -n "$old_library" && linklib="$old_library"
- compile_deplibs="$dir/$linklib $compile_deplibs"
- finalize_deplibs="$dir/$linklib $finalize_deplibs"
- else
- compile_deplibs="-l$name -L$dir $compile_deplibs"
- finalize_deplibs="-l$name -L$dir $finalize_deplibs"
- fi
- elif test "$build_libtool_libs" = yes; then
- # Not a shared library
- if test "$deplibs_check_method" != pass_all; then
- # We're trying link a shared library against a static one
- # but the system doesn't support it.
-
- # Just print a warning and add the library to dependency_libs so
- # that the program can be linked against the static library.
- echo
- $ECHO "*** Warning: This system can not link to static lib archive $lib."
- echo "*** I have the capability to make that library automatically link in when"
- echo "*** you link to this library. But I can only do this if you have a"
- echo "*** shared version of the library, which you do not appear to have."
- if test "$module" = yes; then
- echo "*** But as you try to build a module library, libtool will still create "
- echo "*** a static module, that should work as long as the dlopening application"
- echo "*** is linked with the -dlopen flag to resolve symbols at runtime."
- if test -z "$global_symbol_pipe"; then
- echo
- echo "*** However, this would only work if libtool was able to extract symbol"
- echo "*** lists from a program, using \`nm' or equivalent, but libtool could"
- echo "*** not find such a program. So, this module is probably useless."
- echo "*** \`nm' from GNU binutils and a full rebuild may help."
- fi
- if test "$build_old_libs" = no; then
- build_libtool_libs=module
- build_old_libs=yes
- else
- build_libtool_libs=no
- fi
- fi
- else
- deplibs="$dir/$old_library $deplibs"
- link_static=yes
- fi
- fi # link shared/static library?
-
- if test "$linkmode" = lib; then
- if test -n "$dependency_libs" &&
- { test "$hardcode_into_libs" != yes ||
- test "$build_old_libs" = yes ||
- test "$link_static" = yes; }; then
- # Extract -R from dependency_libs
- temp_deplibs=
- for libdir in $dependency_libs; do
- case $libdir in
- -R*) func_stripname '-R' '' "$libdir"
- temp_xrpath=$func_stripname_result
- case " $xrpath " in
- *" $temp_xrpath "*) ;;
- *) func_append xrpath " $temp_xrpath";;
- esac;;
- *) func_append temp_deplibs " $libdir";;
- esac
- done
- dependency_libs="$temp_deplibs"
- fi
-
- func_append newlib_search_path " $absdir"
- # Link against this library
- test "$link_static" = no && newdependency_libs="$abs_ladir/$laname $newdependency_libs"
- # ... and its dependency_libs
- tmp_libs=
- for deplib in $dependency_libs; do
- newdependency_libs="$deplib $newdependency_libs"
- case $deplib in
- -L*) func_stripname '-L' '' "$deplib"
- func_resolve_sysroot "$func_stripname_result";;
- *) func_resolve_sysroot "$deplib" ;;
- esac
- if $opt_preserve_dup_deps ; then
- case "$tmp_libs " in
- *" $func_resolve_sysroot_result "*)
- func_append specialdeplibs " $func_resolve_sysroot_result" ;;
- esac
- fi
- func_append tmp_libs " $func_resolve_sysroot_result"
- done
-
- if test "$link_all_deplibs" != no; then
- # Add the search paths of all dependency libraries
- for deplib in $dependency_libs; do
- path=
- case $deplib in
- -L*) path="$deplib" ;;
- *.la)
- func_resolve_sysroot "$deplib"
- deplib=$func_resolve_sysroot_result
- func_dirname "$deplib" "" "."
- dir=$func_dirname_result
- # We need an absolute path.
- case $dir in
- [\\/]* | [A-Za-z]:[\\/]*) absdir="$dir" ;;
- *)
- absdir=`cd "$dir" && pwd`
- if test -z "$absdir"; then
- func_warning "cannot determine absolute directory name of \`$dir'"
- absdir="$dir"
- fi
- ;;
- esac
- if $GREP "^installed=no" $deplib > /dev/null; then
- case $host in
- *-*-darwin*)
- depdepl=
- eval deplibrary_names=`${SED} -n -e 's/^library_names=\(.*\)$/\1/p' $deplib`
- if test -n "$deplibrary_names" ; then
- for tmp in $deplibrary_names ; do
- depdepl=$tmp
- done
- if test -f "$absdir/$objdir/$depdepl" ; then
- depdepl="$absdir/$objdir/$depdepl"
- darwin_install_name=`${OTOOL} -L $depdepl | awk '{if (NR == 2) {print $1;exit}}'`
- if test -z "$darwin_install_name"; then
- darwin_install_name=`${OTOOL64} -L $depdepl | awk '{if (NR == 2) {print $1;exit}}'`
- fi
- func_append compiler_flags " ${wl}-dylib_file ${wl}${darwin_install_name}:${depdepl}"
- func_append linker_flags " -dylib_file ${darwin_install_name}:${depdepl}"
- path=
- fi
- fi
- ;;
- *)
- path="-L$absdir/$objdir"
- ;;
- esac
- else
- eval libdir=`${SED} -n -e 's/^libdir=\(.*\)$/\1/p' $deplib`
- test -z "$libdir" && \
- func_fatal_error "\`$deplib' is not a valid libtool archive"
- test "$absdir" != "$libdir" && \
- func_warning "\`$deplib' seems to be moved"
-
- path="-L$absdir"
- fi
- ;;
- esac
- case " $deplibs " in
- *" $path "*) ;;
- *) deplibs="$path $deplibs" ;;
- esac
- done
- fi # link_all_deplibs != no
- fi # linkmode = lib
- done # for deplib in $libs
- if test "$pass" = link; then
- if test "$linkmode" = "prog"; then
- compile_deplibs="$new_inherited_linker_flags $compile_deplibs"
- finalize_deplibs="$new_inherited_linker_flags $finalize_deplibs"
- else
- compiler_flags="$compiler_flags "`$ECHO " $new_inherited_linker_flags" | $SED 's% \([^ $]*\).ltframework% -framework \1%g'`
- fi
- fi
- dependency_libs="$newdependency_libs"
- if test "$pass" = dlpreopen; then
- # Link the dlpreopened libraries before other libraries
- for deplib in $save_deplibs; do
- deplibs="$deplib $deplibs"
- done
- fi
- if test "$pass" != dlopen; then
- if test "$pass" != conv; then
- # Make sure lib_search_path contains only unique directories.
- lib_search_path=
- for dir in $newlib_search_path; do
- case "$lib_search_path " in
- *" $dir "*) ;;
- *) func_append lib_search_path " $dir" ;;
- esac
- done
- newlib_search_path=
- fi
-
- if test "$linkmode,$pass" != "prog,link"; then
- vars="deplibs"
- else
- vars="compile_deplibs finalize_deplibs"
- fi
- for var in $vars dependency_libs; do
- # Add libraries to $var in reverse order
- eval tmp_libs=\"\$$var\"
- new_libs=
- for deplib in $tmp_libs; do
- # FIXME: Pedantically, this is the right thing to do, so
- # that some nasty dependency loop isn't accidentally
- # broken:
- #new_libs="$deplib $new_libs"
- # Pragmatically, this seems to cause very few problems in
- # practice:
- case $deplib in
- -L*) new_libs="$deplib $new_libs" ;;
- -R*) ;;
- *)
- # And here is the reason: when a library appears more
- # than once as an explicit dependence of a library, or
- # is implicitly linked in more than once by the
- # compiler, it is considered special, and multiple
- # occurrences thereof are not removed. Compare this
- # with having the same library being listed as a
- # dependency of multiple other libraries: in this case,
- # we know (pedantically, we assume) the library does not
- # need to be listed more than once, so we keep only the
- # last copy. This is not always right, but it is rare
- # enough that we require users that really mean to play
- # such unportable linking tricks to link the library
- # using -Wl,-lname, so that libtool does not consider it
- # for duplicate removal.
- case " $specialdeplibs " in
- *" $deplib "*) new_libs="$deplib $new_libs" ;;
- *)
- case " $new_libs " in
- *" $deplib "*) ;;
- *) new_libs="$deplib $new_libs" ;;
- esac
- ;;
- esac
- ;;
- esac
- done
- tmp_libs=
- for deplib in $new_libs; do
- case $deplib in
- -L*)
- case " $tmp_libs " in
- *" $deplib "*) ;;
- *) func_append tmp_libs " $deplib" ;;
- esac
- ;;
- *) func_append tmp_libs " $deplib" ;;
- esac
- done
- eval $var=\"$tmp_libs\"
- done # for var
- fi
- # Last step: remove runtime libs from dependency_libs
- # (they stay in deplibs)
- tmp_libs=
- for i in $dependency_libs ; do
- case " $predeps $postdeps $compiler_lib_search_path " in
- *" $i "*)
- i=""
- ;;
- esac
- if test -n "$i" ; then
- func_append tmp_libs " $i"
- fi
- done
- dependency_libs=$tmp_libs
- done # for pass
- if test "$linkmode" = prog; then
- dlfiles="$newdlfiles"
- fi
- if test "$linkmode" = prog || test "$linkmode" = lib; then
- dlprefiles="$newdlprefiles"
- fi
-
- case $linkmode in
- oldlib)
- if test -n "$dlfiles$dlprefiles" || test "$dlself" != no; then
- func_warning "\`-dlopen' is ignored for archives"
- fi
-
- case " $deplibs" in
- *\ -l* | *\ -L*)
- func_warning "\`-l' and \`-L' are ignored for archives" ;;
- esac
-
- test -n "$rpath" && \
- func_warning "\`-rpath' is ignored for archives"
-
- test -n "$xrpath" && \
- func_warning "\`-R' is ignored for archives"
-
- test -n "$vinfo" && \
- func_warning "\`-version-info/-version-number' is ignored for archives"
-
- test -n "$release" && \
- func_warning "\`-release' is ignored for archives"
-
- test -n "$export_symbols$export_symbols_regex" && \
- func_warning "\`-export-symbols' is ignored for archives"
-
- # Now set the variables for building old libraries.
- build_libtool_libs=no
- oldlibs="$output"
- func_append objs "$old_deplibs"
- ;;
-
- lib)
- # Make sure we only generate libraries of the form `libNAME.la'.
- case $outputname in
- lib*)
- func_stripname 'lib' '.la' "$outputname"
- name=$func_stripname_result
- eval shared_ext=\"$shrext_cmds\"
- eval libname=\"$libname_spec\"
- ;;
- *)
- test "$module" = no && \
- func_fatal_help "libtool library \`$output' must begin with \`lib'"
-
- if test "$need_lib_prefix" != no; then
- # Add the "lib" prefix for modules if required
- func_stripname '' '.la' "$outputname"
- name=$func_stripname_result
- eval shared_ext=\"$shrext_cmds\"
- eval libname=\"$libname_spec\"
- else
- func_stripname '' '.la' "$outputname"
- libname=$func_stripname_result
- fi
- ;;
- esac
-
- if test -n "$objs"; then
- if test "$deplibs_check_method" != pass_all; then
- func_fatal_error "cannot build libtool library \`$output' from non-libtool objects on this host:$objs"
- else
- echo
- $ECHO "*** Warning: Linking the shared library $output against the non-libtool"
- $ECHO "*** objects $objs is not portable!"
- func_append libobjs " $objs"
- fi
- fi
-
- test "$dlself" != no && \
- func_warning "\`-dlopen self' is ignored for libtool libraries"
-
- set dummy $rpath
- shift
- test "$#" -gt 1 && \
- func_warning "ignoring multiple \`-rpath's for a libtool library"
-
- install_libdir="$1"
-
- oldlibs=
- if test -z "$rpath"; then
- if test "$build_libtool_libs" = yes; then
- # Building a libtool convenience library.
- # Some compilers have problems with a `.al' extension so
- # convenience libraries should have the same extension an
- # archive normally would.
- oldlibs="$output_objdir/$libname.$libext $oldlibs"
- build_libtool_libs=convenience
- build_old_libs=yes
- fi
-
- test -n "$vinfo" && \
- func_warning "\`-version-info/-version-number' is ignored for convenience libraries"
-
- test -n "$release" && \
- func_warning "\`-release' is ignored for convenience libraries"
- else
-
- # Parse the version information argument.
- save_ifs="$IFS"; IFS=':'
- set dummy $vinfo 0 0 0
- shift
- IFS="$save_ifs"
-
- test -n "$7" && \
- func_fatal_help "too many parameters to \`-version-info'"
-
- # convert absolute version numbers to libtool ages
- # this retains compatibility with .la files and attempts
- # to make the code below a bit more comprehensible
-
- case $vinfo_number in
- yes)
- number_major="$1"
- number_minor="$2"
- number_revision="$3"
- #
- # There are really only two kinds -- those that
- # use the current revision as the major version
- # and those that subtract age and use age as
- # a minor version. But, then there is irix
- # which has an extra 1 added just for fun
- #
- case $version_type in
- # correct linux to gnu/linux during the next big refactor
- darwin|linux|osf|windows|none)
- func_arith $number_major + $number_minor
- current=$func_arith_result
- age="$number_minor"
- revision="$number_revision"
- ;;
- freebsd-aout|freebsd-elf|qnx|sunos)
- current="$number_major"
- revision="$number_minor"
- age="0"
- ;;
- irix|nonstopux)
- func_arith $number_major + $number_minor
- current=$func_arith_result
- age="$number_minor"
- revision="$number_minor"
- lt_irix_increment=no
- ;;
- *)
- func_fatal_configuration "$modename: unknown library version type \`$version_type'"
- ;;
- esac
- ;;
- no)
- current="$1"
- revision="$2"
- age="$3"
- ;;
- esac
-
- # Check that each of the things are valid numbers.
- case $current in
- 0|[1-9]|[1-9][0-9]|[1-9][0-9][0-9]|[1-9][0-9][0-9][0-9]|[1-9][0-9][0-9][0-9][0-9]) ;;
- *)
- func_error "CURRENT \`$current' must be a nonnegative integer"
- func_fatal_error "\`$vinfo' is not valid version information"
- ;;
- esac
-
- case $revision in
- 0|[1-9]|[1-9][0-9]|[1-9][0-9][0-9]|[1-9][0-9][0-9][0-9]|[1-9][0-9][0-9][0-9][0-9]) ;;
- *)
- func_error "REVISION \`$revision' must be a nonnegative integer"
- func_fatal_error "\`$vinfo' is not valid version information"
- ;;
- esac
-
- case $age in
- 0|[1-9]|[1-9][0-9]|[1-9][0-9][0-9]|[1-9][0-9][0-9][0-9]|[1-9][0-9][0-9][0-9][0-9]) ;;
- *)
- func_error "AGE \`$age' must be a nonnegative integer"
- func_fatal_error "\`$vinfo' is not valid version information"
- ;;
- esac
-
- if test "$age" -gt "$current"; then
- func_error "AGE \`$age' is greater than the current interface number \`$current'"
- func_fatal_error "\`$vinfo' is not valid version information"
- fi
-
- # Calculate the version variables.
- major=
- versuffix=
- verstring=
- case $version_type in
- none) ;;
-
- darwin)
- # Like Linux, but with the current version available in
- # verstring for coding it into the library header
- func_arith $current - $age
- major=.$func_arith_result
- versuffix="$major.$age.$revision"
- # Darwin ld doesn't like 0 for these options...
- func_arith $current + 1
- minor_current=$func_arith_result
- xlcverstring="${wl}-compatibility_version ${wl}$minor_current ${wl}-current_version ${wl}$minor_current.$revision"
- verstring="-compatibility_version $minor_current -current_version $minor_current.$revision"
- ;;
-
- freebsd-aout)
- major=".$current"
- versuffix=".$current.$revision";
- ;;
-
- freebsd-elf)
- major=".$current"
- versuffix=".$current"
- ;;
-
- irix | nonstopux)
- if test "X$lt_irix_increment" = "Xno"; then
- func_arith $current - $age
- else
- func_arith $current - $age + 1
- fi
- major=$func_arith_result
-
- case $version_type in
- nonstopux) verstring_prefix=nonstopux ;;
- *) verstring_prefix=sgi ;;
- esac
- verstring="$verstring_prefix$major.$revision"
-
- # Add in all the interfaces that we are compatible with.
- loop=$revision
- while test "$loop" -ne 0; do
- func_arith $revision - $loop
- iface=$func_arith_result
- func_arith $loop - 1
- loop=$func_arith_result
- verstring="$verstring_prefix$major.$iface:$verstring"
- done
-
- # Before this point, $major must not contain `.'.
- major=.$major
- versuffix="$major.$revision"
- ;;
-
- linux) # correct to gnu/linux during the next big refactor
- func_arith $current - $age
- major=.$func_arith_result
- versuffix="$major.$age.$revision"
- ;;
-
- osf)
- func_arith $current - $age
- major=.$func_arith_result
- versuffix=".$current.$age.$revision"
- verstring="$current.$age.$revision"
-
- # Add in all the interfaces that we are compatible with.
- loop=$age
- while test "$loop" -ne 0; do
- func_arith $current - $loop
- iface=$func_arith_result
- func_arith $loop - 1
- loop=$func_arith_result
- verstring="$verstring:${iface}.0"
- done
-
- # Make executables depend on our current version.
- func_append verstring ":${current}.0"
- ;;
-
- qnx)
- major=".$current"
- versuffix=".$current"
- ;;
-
- sunos)
- major=".$current"
- versuffix=".$current.$revision"
- ;;
-
- windows)
- # Use '-' rather than '.', since we only want one
- # extension on DOS 8.3 filesystems.
- func_arith $current - $age
- major=$func_arith_result
- versuffix="-$major"
- ;;
-
- *)
- func_fatal_configuration "unknown library version type \`$version_type'"
- ;;
- esac
-
- # Clear the version info if we defaulted, and they specified a release.
- if test -z "$vinfo" && test -n "$release"; then
- major=
- case $version_type in
- darwin)
- # we can't check for "0.0" in archive_cmds due to quoting
- # problems, so we reset it completely
- verstring=
- ;;
- *)
- verstring="0.0"
- ;;
- esac
- if test "$need_version" = no; then
- versuffix=
- else
- versuffix=".0.0"
- fi
- fi
-
- # Remove version info from name if versioning should be avoided
- if test "$avoid_version" = yes && test "$need_version" = no; then
- major=
- versuffix=
- verstring=""
- fi
-
- # Check to see if the archive will have undefined symbols.
- if test "$allow_undefined" = yes; then
- if test "$allow_undefined_flag" = unsupported; then
- func_warning "undefined symbols not allowed in $host shared libraries"
- build_libtool_libs=no
- build_old_libs=yes
- fi
- else
- # Don't allow undefined symbols.
- allow_undefined_flag="$no_undefined_flag"
- fi
-
- fi
-
- func_generate_dlsyms "$libname" "$libname" "yes"
- func_append libobjs " $symfileobj"
- test "X$libobjs" = "X " && libobjs=
-
- if test "$opt_mode" != relink; then
- # Remove our outputs, but don't remove object files since they
- # may have been created when compiling PIC objects.
- removelist=
- tempremovelist=`$ECHO "$output_objdir/*"`
- for p in $tempremovelist; do
- case $p in
- *.$objext | *.gcno)
- ;;
- $output_objdir/$outputname | $output_objdir/$libname.* | $output_objdir/${libname}${release}.*)
- if test "X$precious_files_regex" != "X"; then
- if $ECHO "$p" | $EGREP -e "$precious_files_regex" >/dev/null 2>&1
- then
- continue
- fi
- fi
- func_append removelist " $p"
- ;;
- *) ;;
- esac
- done
- test -n "$removelist" && \
- func_show_eval "${RM}r \$removelist"
- fi
-
- # Now set the variables for building old libraries.
- if test "$build_old_libs" = yes && test "$build_libtool_libs" != convenience ; then
- func_append oldlibs " $output_objdir/$libname.$libext"
-
- # Transform .lo files to .o files.
- oldobjs="$objs "`$ECHO "$libobjs" | $SP2NL | $SED "/\.${libext}$/d; $lo2o" | $NL2SP`
- fi
-
- # Eliminate all temporary directories.
- #for path in $notinst_path; do
- # lib_search_path=`$ECHO "$lib_search_path " | $SED "s% $path % %g"`
- # deplibs=`$ECHO "$deplibs " | $SED "s% -L$path % %g"`
- # dependency_libs=`$ECHO "$dependency_libs " | $SED "s% -L$path % %g"`
- #done
-
- if test -n "$xrpath"; then
- # If the user specified any rpath flags, then add them.
- temp_xrpath=
- for libdir in $xrpath; do
- func_replace_sysroot "$libdir"
- func_append temp_xrpath " -R$func_replace_sysroot_result"
- case "$finalize_rpath " in
- *" $libdir "*) ;;
- *) func_append finalize_rpath " $libdir" ;;
- esac
- done
- if test "$hardcode_into_libs" != yes || test "$build_old_libs" = yes; then
- dependency_libs="$temp_xrpath $dependency_libs"
- fi
- fi
-
- # Make sure dlfiles contains only unique files that won't be dlpreopened
- old_dlfiles="$dlfiles"
- dlfiles=
- for lib in $old_dlfiles; do
- case " $dlprefiles $dlfiles " in
- *" $lib "*) ;;
- *) func_append dlfiles " $lib" ;;
- esac
- done
-
- # Make sure dlprefiles contains only unique files
- old_dlprefiles="$dlprefiles"
- dlprefiles=
- for lib in $old_dlprefiles; do
- case "$dlprefiles " in
- *" $lib "*) ;;
- *) func_append dlprefiles " $lib" ;;
- esac
- done
-
- if test "$build_libtool_libs" = yes; then
- if test -n "$rpath"; then
- case $host in
- *-*-cygwin* | *-*-mingw* | *-*-pw32* | *-*-os2* | *-*-beos* | *-cegcc* | *-*-haiku*)
- # these systems don't actually have a c library (as such)!
- ;;
- *-*-rhapsody* | *-*-darwin1.[012])
- # Rhapsody C library is in the System framework
- func_append deplibs " System.ltframework"
- ;;
- *-*-netbsd*)
- # Don't link with libc until the a.out ld.so is fixed.
- ;;
- *-*-openbsd* | *-*-freebsd* | *-*-dragonfly*)
- # Do not include libc due to us having libc/libc_r.
- ;;
- *-*-sco3.2v5* | *-*-sco5v6*)
- # Causes problems with __ctype
- ;;
- *-*-sysv4.2uw2* | *-*-sysv5* | *-*-unixware* | *-*-OpenUNIX*)
- # Compiler inserts libc in the correct place for threads to work
- ;;
- *)
- # Add libc to deplibs on all other systems if necessary.
- if test "$build_libtool_need_lc" = "yes"; then
- func_append deplibs " -lc"
- fi
- ;;
- esac
- fi
-
- # Transform deplibs into only deplibs that can be linked in shared.
- name_save=$name
- libname_save=$libname
- release_save=$release
- versuffix_save=$versuffix
- major_save=$major
- # I'm not sure if I'm treating the release correctly. I think
- # release should show up in the -l (ie -lgmp5) so we don't want to
- # add it in twice. Is that correct?
- release=""
- versuffix=""
- major=""
- newdeplibs=
- droppeddeps=no
- case $deplibs_check_method in
- pass_all)
- # Don't check for shared/static. Everything works.
- # This might be a little naive. We might want to check
- # whether the library exists or not. But this is on
- # osf3 & osf4 and I'm not really sure... Just
- # implementing what was already the behavior.
- newdeplibs=$deplibs
- ;;
- test_compile)
- # This code stresses the "libraries are programs" paradigm to its
- # limits. Maybe even breaks it. We compile a program, linking it
- # against the deplibs as a proxy for the library. Then we can check
- # whether they linked in statically or dynamically with ldd.
- $opt_dry_run || $RM conftest.c
- cat > conftest.c </dev/null`
- $nocaseglob
- else
- potential_libs=`ls $i/$libnameglob[.-]* 2>/dev/null`
- fi
- for potent_lib in $potential_libs; do
- # Follow soft links.
- if ls -lLd "$potent_lib" 2>/dev/null |
- $GREP " -> " >/dev/null; then
- continue
- fi
- # The statement above tries to avoid entering an
- # endless loop below, in case of cyclic links.
- # We might still enter an endless loop, since a link
- # loop can be closed while we follow links,
- # but so what?
- potlib="$potent_lib"
- while test -h "$potlib" 2>/dev/null; do
- potliblink=`ls -ld $potlib | ${SED} 's/.* -> //'`
- case $potliblink in
- [\\/]* | [A-Za-z]:[\\/]*) potlib="$potliblink";;
- *) potlib=`$ECHO "$potlib" | $SED 's,[^/]*$,,'`"$potliblink";;
- esac
- done
- if eval $file_magic_cmd \"\$potlib\" 2>/dev/null |
- $SED -e 10q |
- $EGREP "$file_magic_regex" > /dev/null; then
- func_append newdeplibs " $a_deplib"
- a_deplib=""
- break 2
- fi
- done
- done
- fi
- if test -n "$a_deplib" ; then
- droppeddeps=yes
- echo
- $ECHO "*** Warning: linker path does not have real file for library $a_deplib."
- echo "*** I have the capability to make that library automatically link in when"
- echo "*** you link to this library. But I can only do this if you have a"
- echo "*** shared version of the library, which you do not appear to have"
- echo "*** because I did check the linker path looking for a file starting"
- if test -z "$potlib" ; then
- $ECHO "*** with $libname but no candidates were found. (...for file magic test)"
- else
- $ECHO "*** with $libname and none of the candidates passed a file format test"
- $ECHO "*** using a file magic. Last file checked: $potlib"
- fi
- fi
- ;;
- *)
- # Add a -L argument.
- func_append newdeplibs " $a_deplib"
- ;;
- esac
- done # Gone through all deplibs.
- ;;
- match_pattern*)
- set dummy $deplibs_check_method; shift
- match_pattern_regex=`expr "$deplibs_check_method" : "$1 \(.*\)"`
- for a_deplib in $deplibs; do
- case $a_deplib in
- -l*)
- func_stripname -l '' "$a_deplib"
- name=$func_stripname_result
- if test "X$allow_libtool_libs_with_static_runtimes" = "Xyes" ; then
- case " $predeps $postdeps " in
- *" $a_deplib "*)
- func_append newdeplibs " $a_deplib"
- a_deplib=""
- ;;
- esac
- fi
- if test -n "$a_deplib" ; then
- libname=`eval "\\$ECHO \"$libname_spec\""`
- for i in $lib_search_path $sys_lib_search_path $shlib_search_path; do
- potential_libs=`ls $i/$libname[.-]* 2>/dev/null`
- for potent_lib in $potential_libs; do
- potlib="$potent_lib" # see symlink-check above in file_magic test
- if eval "\$ECHO \"$potent_lib\"" 2>/dev/null | $SED 10q | \
- $EGREP "$match_pattern_regex" > /dev/null; then
- func_append newdeplibs " $a_deplib"
- a_deplib=""
- break 2
- fi
- done
- done
- fi
- if test -n "$a_deplib" ; then
- droppeddeps=yes
- echo
- $ECHO "*** Warning: linker path does not have real file for library $a_deplib."
- echo "*** I have the capability to make that library automatically link in when"
- echo "*** you link to this library. But I can only do this if you have a"
- echo "*** shared version of the library, which you do not appear to have"
- echo "*** because I did check the linker path looking for a file starting"
- if test -z "$potlib" ; then
- $ECHO "*** with $libname but no candidates were found. (...for regex pattern test)"
- else
- $ECHO "*** with $libname and none of the candidates passed a file format test"
- $ECHO "*** using a regex pattern. Last file checked: $potlib"
- fi
- fi
- ;;
- *)
- # Add a -L argument.
- func_append newdeplibs " $a_deplib"
- ;;
- esac
- done # Gone through all deplibs.
- ;;
- none | unknown | *)
- newdeplibs=""
- tmp_deplibs=`$ECHO " $deplibs" | $SED 's/ -lc$//; s/ -[LR][^ ]*//g'`
- if test "X$allow_libtool_libs_with_static_runtimes" = "Xyes" ; then
- for i in $predeps $postdeps ; do
- # can't use Xsed below, because $i might contain '/'
- tmp_deplibs=`$ECHO " $tmp_deplibs" | $SED "s,$i,,"`
- done
- fi
- case $tmp_deplibs in
- *[!\ \ ]*)
- echo
- if test "X$deplibs_check_method" = "Xnone"; then
- echo "*** Warning: inter-library dependencies are not supported in this platform."
- else
- echo "*** Warning: inter-library dependencies are not known to be supported."
- fi
- echo "*** All declared inter-library dependencies are being dropped."
- droppeddeps=yes
- ;;
- esac
- ;;
- esac
- versuffix=$versuffix_save
- major=$major_save
- release=$release_save
- libname=$libname_save
- name=$name_save
-
- case $host in
- *-*-rhapsody* | *-*-darwin1.[012])
- # On Rhapsody replace the C library with the System framework
- newdeplibs=`$ECHO " $newdeplibs" | $SED 's/ -lc / System.ltframework /'`
- ;;
- esac
-
- if test "$droppeddeps" = yes; then
- if test "$module" = yes; then
- echo
- echo "*** Warning: libtool could not satisfy all declared inter-library"
- $ECHO "*** dependencies of module $libname. Therefore, libtool will create"
- echo "*** a static module, that should work as long as the dlopening"
- echo "*** application is linked with the -dlopen flag."
- if test -z "$global_symbol_pipe"; then
- echo
- echo "*** However, this would only work if libtool was able to extract symbol"
- echo "*** lists from a program, using \`nm' or equivalent, but libtool could"
- echo "*** not find such a program. So, this module is probably useless."
- echo "*** \`nm' from GNU binutils and a full rebuild may help."
- fi
- if test "$build_old_libs" = no; then
- oldlibs="$output_objdir/$libname.$libext"
- build_libtool_libs=module
- build_old_libs=yes
- else
- build_libtool_libs=no
- fi
- else
- echo "*** The inter-library dependencies that have been dropped here will be"
- echo "*** automatically added whenever a program is linked with this library"
- echo "*** or is declared to -dlopen it."
-
- if test "$allow_undefined" = no; then
- echo
- echo "*** Since this library must not contain undefined symbols,"
- echo "*** because either the platform does not support them or"
- echo "*** it was explicitly requested with -no-undefined,"
- echo "*** libtool will only create a static version of it."
- if test "$build_old_libs" = no; then
- oldlibs="$output_objdir/$libname.$libext"
- build_libtool_libs=module
- build_old_libs=yes
- else
- build_libtool_libs=no
- fi
- fi
- fi
- fi
- # Done checking deplibs!
- deplibs=$newdeplibs
- fi
- # Time to change all our "foo.ltframework" stuff back to "-framework foo"
- case $host in
- *-*-darwin*)
- newdeplibs=`$ECHO " $newdeplibs" | $SED 's% \([^ $]*\).ltframework% -framework \1%g'`
- new_inherited_linker_flags=`$ECHO " $new_inherited_linker_flags" | $SED 's% \([^ $]*\).ltframework% -framework \1%g'`
- deplibs=`$ECHO " $deplibs" | $SED 's% \([^ $]*\).ltframework% -framework \1%g'`
- ;;
- esac
-
- # move library search paths that coincide with paths to not yet
- # installed libraries to the beginning of the library search list
- new_libs=
- for path in $notinst_path; do
- case " $new_libs " in
- *" -L$path/$objdir "*) ;;
- *)
- case " $deplibs " in
- *" -L$path/$objdir "*)
- func_append new_libs " -L$path/$objdir" ;;
- esac
- ;;
- esac
- done
- for deplib in $deplibs; do
- case $deplib in
- -L*)
- case " $new_libs " in
- *" $deplib "*) ;;
- *) func_append new_libs " $deplib" ;;
- esac
- ;;
- *) func_append new_libs " $deplib" ;;
- esac
- done
- deplibs="$new_libs"
-
- # All the library-specific variables (install_libdir is set above).
- library_names=
- old_library=
- dlname=
-
- # Test again, we may have decided not to build it any more
- if test "$build_libtool_libs" = yes; then
- # Remove ${wl} instances when linking with ld.
- # FIXME: should test the right _cmds variable.
- case $archive_cmds in
- *\$LD\ *) wl= ;;
- esac
- if test "$hardcode_into_libs" = yes; then
- # Hardcode the library paths
- hardcode_libdirs=
- dep_rpath=
- rpath="$finalize_rpath"
- test "$opt_mode" != relink && rpath="$compile_rpath$rpath"
- for libdir in $rpath; do
- if test -n "$hardcode_libdir_flag_spec"; then
- if test -n "$hardcode_libdir_separator"; then
- func_replace_sysroot "$libdir"
- libdir=$func_replace_sysroot_result
- if test -z "$hardcode_libdirs"; then
- hardcode_libdirs="$libdir"
- else
- # Just accumulate the unique libdirs.
- case $hardcode_libdir_separator$hardcode_libdirs$hardcode_libdir_separator in
- *"$hardcode_libdir_separator$libdir$hardcode_libdir_separator"*)
- ;;
- *)
- func_append hardcode_libdirs "$hardcode_libdir_separator$libdir"
- ;;
- esac
- fi
- else
- eval flag=\"$hardcode_libdir_flag_spec\"
- func_append dep_rpath " $flag"
- fi
- elif test -n "$runpath_var"; then
- case "$perm_rpath " in
- *" $libdir "*) ;;
- *) func_append perm_rpath " $libdir" ;;
- esac
- fi
- done
- # Substitute the hardcoded libdirs into the rpath.
- if test -n "$hardcode_libdir_separator" &&
- test -n "$hardcode_libdirs"; then
- libdir="$hardcode_libdirs"
- eval "dep_rpath=\"$hardcode_libdir_flag_spec\""
- fi
- if test -n "$runpath_var" && test -n "$perm_rpath"; then
- # We should set the runpath_var.
- rpath=
- for dir in $perm_rpath; do
- func_append rpath "$dir:"
- done
- eval "$runpath_var='$rpath\$$runpath_var'; export $runpath_var"
- fi
- test -n "$dep_rpath" && deplibs="$dep_rpath $deplibs"
- fi
-
- shlibpath="$finalize_shlibpath"
- test "$opt_mode" != relink && shlibpath="$compile_shlibpath$shlibpath"
- if test -n "$shlibpath"; then
- eval "$shlibpath_var='$shlibpath\$$shlibpath_var'; export $shlibpath_var"
- fi
-
- # Get the real and link names of the library.
- eval shared_ext=\"$shrext_cmds\"
- eval library_names=\"$library_names_spec\"
- set dummy $library_names
- shift
- realname="$1"
- shift
-
- if test -n "$soname_spec"; then
- eval soname=\"$soname_spec\"
- else
- soname="$realname"
- fi
- if test -z "$dlname"; then
- dlname=$soname
- fi
-
- lib="$output_objdir/$realname"
- linknames=
- for link
- do
- func_append linknames " $link"
- done
-
- # Use standard objects if they are pic
- test -z "$pic_flag" && libobjs=`$ECHO "$libobjs" | $SP2NL | $SED "$lo2o" | $NL2SP`
- test "X$libobjs" = "X " && libobjs=
-
- delfiles=
- if test -n "$export_symbols" && test -n "$include_expsyms"; then
- $opt_dry_run || cp "$export_symbols" "$output_objdir/$libname.uexp"
- export_symbols="$output_objdir/$libname.uexp"
- func_append delfiles " $export_symbols"
- fi
-
- orig_export_symbols=
- case $host_os in
- cygwin* | mingw* | cegcc*)
- if test -n "$export_symbols" && test -z "$export_symbols_regex"; then
- # exporting using user supplied symfile
- if test "x`$SED 1q $export_symbols`" != xEXPORTS; then
- # and it's NOT already a .def file. Must figure out
- # which of the given symbols are data symbols and tag
- # them as such. So, trigger use of export_symbols_cmds.
- # export_symbols gets reassigned inside the "prepare
- # the list of exported symbols" if statement, so the
- # include_expsyms logic still works.
- orig_export_symbols="$export_symbols"
- export_symbols=
- always_export_symbols=yes
- fi
- fi
- ;;
- esac
-
- # Prepare the list of exported symbols
- if test -z "$export_symbols"; then
- if test "$always_export_symbols" = yes || test -n "$export_symbols_regex"; then
- func_verbose "generating symbol list for \`$libname.la'"
- export_symbols="$output_objdir/$libname.exp"
- $opt_dry_run || $RM $export_symbols
- cmds=$export_symbols_cmds
- save_ifs="$IFS"; IFS='~'
- for cmd1 in $cmds; do
- IFS="$save_ifs"
- # Take the normal branch if the nm_file_list_spec branch
- # doesn't work or if tool conversion is not needed.
- case $nm_file_list_spec~$to_tool_file_cmd in
- *~func_convert_file_noop | *~func_convert_file_msys_to_w32 | ~*)
- try_normal_branch=yes
- eval cmd=\"$cmd1\"
- func_len " $cmd"
- len=$func_len_result
- ;;
- *)
- try_normal_branch=no
- ;;
- esac
- if test "$try_normal_branch" = yes \
- && { test "$len" -lt "$max_cmd_len" \
- || test "$max_cmd_len" -le -1; }
- then
- func_show_eval "$cmd" 'exit $?'
- skipped_export=false
- elif test -n "$nm_file_list_spec"; then
- func_basename "$output"
- output_la=$func_basename_result
- save_libobjs=$libobjs
- save_output=$output
- output=${output_objdir}/${output_la}.nm
- func_to_tool_file "$output"
- libobjs=$nm_file_list_spec$func_to_tool_file_result
- func_append delfiles " $output"
- func_verbose "creating $NM input file list: $output"
- for obj in $save_libobjs; do
- func_to_tool_file "$obj"
- $ECHO "$func_to_tool_file_result"
- done > "$output"
- eval cmd=\"$cmd1\"
- func_show_eval "$cmd" 'exit $?'
- output=$save_output
- libobjs=$save_libobjs
- skipped_export=false
- else
- # The command line is too long to execute in one step.
- func_verbose "using reloadable object file for export list..."
- skipped_export=:
- # Break out early, otherwise skipped_export may be
- # set to false by a later but shorter cmd.
- break
- fi
- done
- IFS="$save_ifs"
- if test -n "$export_symbols_regex" && test "X$skipped_export" != "X:"; then
- func_show_eval '$EGREP -e "$export_symbols_regex" "$export_symbols" > "${export_symbols}T"'
- func_show_eval '$MV "${export_symbols}T" "$export_symbols"'
- fi
- fi
- fi
-
- if test -n "$export_symbols" && test -n "$include_expsyms"; then
- tmp_export_symbols="$export_symbols"
- test -n "$orig_export_symbols" && tmp_export_symbols="$orig_export_symbols"
- $opt_dry_run || eval '$ECHO "$include_expsyms" | $SP2NL >> "$tmp_export_symbols"'
- fi
-
- if test "X$skipped_export" != "X:" && test -n "$orig_export_symbols"; then
- # The given exports_symbols file has to be filtered, so filter it.
- func_verbose "filter symbol list for \`$libname.la' to tag DATA exports"
- # FIXME: $output_objdir/$libname.filter potentially contains lots of
- # 's' commands which not all seds can handle. GNU sed should be fine
- # though. Also, the filter scales superlinearly with the number of
- # global variables. join(1) would be nice here, but unfortunately
- # isn't a blessed tool.
- $opt_dry_run || $SED -e '/[ ,]DATA/!d;s,\(.*\)\([ \,].*\),s|^\1$|\1\2|,' < $export_symbols > $output_objdir/$libname.filter
- func_append delfiles " $export_symbols $output_objdir/$libname.filter"
- export_symbols=$output_objdir/$libname.def
- $opt_dry_run || $SED -f $output_objdir/$libname.filter < $orig_export_symbols > $export_symbols
- fi
-
- tmp_deplibs=
- for test_deplib in $deplibs; do
- case " $convenience " in
- *" $test_deplib "*) ;;
- *)
- func_append tmp_deplibs " $test_deplib"
- ;;
- esac
- done
- deplibs="$tmp_deplibs"
-
- if test -n "$convenience"; then
- if test -n "$whole_archive_flag_spec" &&
- test "$compiler_needs_object" = yes &&
- test -z "$libobjs"; then
- # extract the archives, so we have objects to list.
- # TODO: could optimize this to just extract one archive.
- whole_archive_flag_spec=
- fi
- if test -n "$whole_archive_flag_spec"; then
- save_libobjs=$libobjs
- eval libobjs=\"\$libobjs $whole_archive_flag_spec\"
- test "X$libobjs" = "X " && libobjs=
- else
- gentop="$output_objdir/${outputname}x"
- func_append generated " $gentop"
-
- func_extract_archives $gentop $convenience
- func_append libobjs " $func_extract_archives_result"
- test "X$libobjs" = "X " && libobjs=
- fi
- fi
-
- if test "$thread_safe" = yes && test -n "$thread_safe_flag_spec"; then
- eval flag=\"$thread_safe_flag_spec\"
- func_append linker_flags " $flag"
- fi
-
- # Make a backup of the uninstalled library when relinking
- if test "$opt_mode" = relink; then
- $opt_dry_run || eval '(cd $output_objdir && $RM ${realname}U && $MV $realname ${realname}U)' || exit $?
- fi
-
- # Do each of the archive commands.
- if test "$module" = yes && test -n "$module_cmds" ; then
- if test -n "$export_symbols" && test -n "$module_expsym_cmds"; then
- eval test_cmds=\"$module_expsym_cmds\"
- cmds=$module_expsym_cmds
- else
- eval test_cmds=\"$module_cmds\"
- cmds=$module_cmds
- fi
- else
- if test -n "$export_symbols" && test -n "$archive_expsym_cmds"; then
- eval test_cmds=\"$archive_expsym_cmds\"
- cmds=$archive_expsym_cmds
- else
- eval test_cmds=\"$archive_cmds\"
- cmds=$archive_cmds
- fi
- fi
-
- if test "X$skipped_export" != "X:" &&
- func_len " $test_cmds" &&
- len=$func_len_result &&
- test "$len" -lt "$max_cmd_len" || test "$max_cmd_len" -le -1; then
- :
- else
- # The command line is too long to link in one step, link piecewise
- # or, if using GNU ld and skipped_export is not :, use a linker
- # script.
-
- # Save the value of $output and $libobjs because we want to
- # use them later. If we have whole_archive_flag_spec, we
- # want to use save_libobjs as it was before
- # whole_archive_flag_spec was expanded, because we can't
- # assume the linker understands whole_archive_flag_spec.
- # This may have to be revisited, in case too many
- # convenience libraries get linked in and end up exceeding
- # the spec.
- if test -z "$convenience" || test -z "$whole_archive_flag_spec"; then
- save_libobjs=$libobjs
- fi
- save_output=$output
- func_basename "$output"
- output_la=$func_basename_result
-
- # Clear the reloadable object creation command queue and
- # initialize k to one.
- test_cmds=
- concat_cmds=
- objlist=
- last_robj=
- k=1
-
- if test -n "$save_libobjs" && test "X$skipped_export" != "X:" && test "$with_gnu_ld" = yes; then
- output=${output_objdir}/${output_la}.lnkscript
- func_verbose "creating GNU ld script: $output"
- echo 'INPUT (' > $output
- for obj in $save_libobjs
- do
- func_to_tool_file "$obj"
- $ECHO "$func_to_tool_file_result" >> $output
- done
- echo ')' >> $output
- func_append delfiles " $output"
- func_to_tool_file "$output"
- output=$func_to_tool_file_result
- elif test -n "$save_libobjs" && test "X$skipped_export" != "X:" && test "X$file_list_spec" != X; then
- output=${output_objdir}/${output_la}.lnk
- func_verbose "creating linker input file list: $output"
- : > $output
- set x $save_libobjs
- shift
- firstobj=
- if test "$compiler_needs_object" = yes; then
- firstobj="$1 "
- shift
- fi
- for obj
- do
- func_to_tool_file "$obj"
- $ECHO "$func_to_tool_file_result" >> $output
- done
- func_append delfiles " $output"
- func_to_tool_file "$output"
- output=$firstobj\"$file_list_spec$func_to_tool_file_result\"
- else
- if test -n "$save_libobjs"; then
- func_verbose "creating reloadable object files..."
- output=$output_objdir/$output_la-${k}.$objext
- eval test_cmds=\"$reload_cmds\"
- func_len " $test_cmds"
- len0=$func_len_result
- len=$len0
-
- # Loop over the list of objects to be linked.
- for obj in $save_libobjs
- do
- func_len " $obj"
- func_arith $len + $func_len_result
- len=$func_arith_result
- if test "X$objlist" = X ||
- test "$len" -lt "$max_cmd_len"; then
- func_append objlist " $obj"
- else
- # The command $test_cmds is almost too long, add a
- # command to the queue.
- if test "$k" -eq 1 ; then
- # The first file doesn't have a previous command to add.
- reload_objs=$objlist
- eval concat_cmds=\"$reload_cmds\"
- else
- # All subsequent reloadable object files will link in
- # the last one created.
- reload_objs="$objlist $last_robj"
- eval concat_cmds=\"\$concat_cmds~$reload_cmds~\$RM $last_robj\"
- fi
- last_robj=$output_objdir/$output_la-${k}.$objext
- func_arith $k + 1
- k=$func_arith_result
- output=$output_objdir/$output_la-${k}.$objext
- objlist=" $obj"
- func_len " $last_robj"
- func_arith $len0 + $func_len_result
- len=$func_arith_result
- fi
- done
- # Handle the remaining objects by creating one last
- # reloadable object file. All subsequent reloadable object
- # files will link in the last one created.
- test -z "$concat_cmds" || concat_cmds=$concat_cmds~
- reload_objs="$objlist $last_robj"
- eval concat_cmds=\"\${concat_cmds}$reload_cmds\"
- if test -n "$last_robj"; then
- eval concat_cmds=\"\${concat_cmds}~\$RM $last_robj\"
- fi
- func_append delfiles " $output"
-
- else
- output=
- fi
-
- if ${skipped_export-false}; then
- func_verbose "generating symbol list for \`$libname.la'"
- export_symbols="$output_objdir/$libname.exp"
- $opt_dry_run || $RM $export_symbols
- libobjs=$output
- # Append the command to create the export file.
- test -z "$concat_cmds" || concat_cmds=$concat_cmds~
- eval concat_cmds=\"\$concat_cmds$export_symbols_cmds\"
- if test -n "$last_robj"; then
- eval concat_cmds=\"\$concat_cmds~\$RM $last_robj\"
- fi
- fi
-
- test -n "$save_libobjs" &&
- func_verbose "creating a temporary reloadable object file: $output"
-
- # Loop through the commands generated above and execute them.
- save_ifs="$IFS"; IFS='~'
- for cmd in $concat_cmds; do
- IFS="$save_ifs"
- $opt_silent || {
- func_quote_for_expand "$cmd"
- eval "func_echo $func_quote_for_expand_result"
- }
- $opt_dry_run || eval "$cmd" || {
- lt_exit=$?
-
- # Restore the uninstalled library and exit
- if test "$opt_mode" = relink; then
- ( cd "$output_objdir" && \
- $RM "${realname}T" && \
- $MV "${realname}U" "$realname" )
- fi
-
- exit $lt_exit
- }
- done
- IFS="$save_ifs"
-
- if test -n "$export_symbols_regex" && ${skipped_export-false}; then
- func_show_eval '$EGREP -e "$export_symbols_regex" "$export_symbols" > "${export_symbols}T"'
- func_show_eval '$MV "${export_symbols}T" "$export_symbols"'
- fi
- fi
-
- if ${skipped_export-false}; then
- if test -n "$export_symbols" && test -n "$include_expsyms"; then
- tmp_export_symbols="$export_symbols"
- test -n "$orig_export_symbols" && tmp_export_symbols="$orig_export_symbols"
- $opt_dry_run || eval '$ECHO "$include_expsyms" | $SP2NL >> "$tmp_export_symbols"'
- fi
-
- if test -n "$orig_export_symbols"; then
- # The given exports_symbols file has to be filtered, so filter it.
- func_verbose "filter symbol list for \`$libname.la' to tag DATA exports"
- # FIXME: $output_objdir/$libname.filter potentially contains lots of
- # 's' commands which not all seds can handle. GNU sed should be fine
- # though. Also, the filter scales superlinearly with the number of
- # global variables. join(1) would be nice here, but unfortunately
- # isn't a blessed tool.
- $opt_dry_run || $SED -e '/[ ,]DATA/!d;s,\(.*\)\([ \,].*\),s|^\1$|\1\2|,' < $export_symbols > $output_objdir/$libname.filter
- func_append delfiles " $export_symbols $output_objdir/$libname.filter"
- export_symbols=$output_objdir/$libname.def
- $opt_dry_run || $SED -f $output_objdir/$libname.filter < $orig_export_symbols > $export_symbols
- fi
- fi
-
- libobjs=$output
- # Restore the value of output.
- output=$save_output
-
- if test -n "$convenience" && test -n "$whole_archive_flag_spec"; then
- eval libobjs=\"\$libobjs $whole_archive_flag_spec\"
- test "X$libobjs" = "X " && libobjs=
- fi
- # Expand the library linking commands again to reset the
- # value of $libobjs for piecewise linking.
-
- # Do each of the archive commands.
- if test "$module" = yes && test -n "$module_cmds" ; then
- if test -n "$export_symbols" && test -n "$module_expsym_cmds"; then
- cmds=$module_expsym_cmds
- else
- cmds=$module_cmds
- fi
- else
- if test -n "$export_symbols" && test -n "$archive_expsym_cmds"; then
- cmds=$archive_expsym_cmds
- else
- cmds=$archive_cmds
- fi
- fi
- fi
-
- if test -n "$delfiles"; then
- # Append the command to remove temporary files to $cmds.
- eval cmds=\"\$cmds~\$RM $delfiles\"
- fi
-
- # Add any objects from preloaded convenience libraries
- if test -n "$dlprefiles"; then
- gentop="$output_objdir/${outputname}x"
- func_append generated " $gentop"
-
- func_extract_archives $gentop $dlprefiles
- func_append libobjs " $func_extract_archives_result"
- test "X$libobjs" = "X " && libobjs=
- fi
-
- save_ifs="$IFS"; IFS='~'
- for cmd in $cmds; do
- IFS="$save_ifs"
- eval cmd=\"$cmd\"
- $opt_silent || {
- func_quote_for_expand "$cmd"
- eval "func_echo $func_quote_for_expand_result"
- }
- $opt_dry_run || eval "$cmd" || {
- lt_exit=$?
-
- # Restore the uninstalled library and exit
- if test "$opt_mode" = relink; then
- ( cd "$output_objdir" && \
- $RM "${realname}T" && \
- $MV "${realname}U" "$realname" )
- fi
-
- exit $lt_exit
- }
- done
- IFS="$save_ifs"
-
- # Restore the uninstalled library and exit
- if test "$opt_mode" = relink; then
- $opt_dry_run || eval '(cd $output_objdir && $RM ${realname}T && $MV $realname ${realname}T && $MV ${realname}U $realname)' || exit $?
-
- if test -n "$convenience"; then
- if test -z "$whole_archive_flag_spec"; then
- func_show_eval '${RM}r "$gentop"'
- fi
- fi
-
- exit $EXIT_SUCCESS
- fi
-
- # Create links to the real library.
- for linkname in $linknames; do
- if test "$realname" != "$linkname"; then
- func_show_eval '(cd "$output_objdir" && $RM "$linkname" && $LN_S "$realname" "$linkname")' 'exit $?'
- fi
- done
-
- # If -module or -export-dynamic was specified, set the dlname.
- if test "$module" = yes || test "$export_dynamic" = yes; then
- # On all known operating systems, these are identical.
- dlname="$soname"
- fi
- fi
- ;;
-
- obj)
- if test -n "$dlfiles$dlprefiles" || test "$dlself" != no; then
- func_warning "\`-dlopen' is ignored for objects"
- fi
-
- case " $deplibs" in
- *\ -l* | *\ -L*)
- func_warning "\`-l' and \`-L' are ignored for objects" ;;
- esac
-
- test -n "$rpath" && \
- func_warning "\`-rpath' is ignored for objects"
-
- test -n "$xrpath" && \
- func_warning "\`-R' is ignored for objects"
-
- test -n "$vinfo" && \
- func_warning "\`-version-info' is ignored for objects"
-
- test -n "$release" && \
- func_warning "\`-release' is ignored for objects"
-
- case $output in
- *.lo)
- test -n "$objs$old_deplibs" && \
- func_fatal_error "cannot build library object \`$output' from non-libtool objects"
-
- libobj=$output
- func_lo2o "$libobj"
- obj=$func_lo2o_result
- ;;
- *)
- libobj=
- obj="$output"
- ;;
- esac
-
- # Delete the old objects.
- $opt_dry_run || $RM $obj $libobj
-
- # Objects from convenience libraries. This assumes
- # single-version convenience libraries. Whenever we create
- # different ones for PIC/non-PIC, this we'll have to duplicate
- # the extraction.
- reload_conv_objs=
- gentop=
- # reload_cmds runs $LD directly, so let us get rid of
- # -Wl from whole_archive_flag_spec and hope we can get by with
- # turning comma into space..
- wl=
-
- if test -n "$convenience"; then
- if test -n "$whole_archive_flag_spec"; then
- eval tmp_whole_archive_flags=\"$whole_archive_flag_spec\"
- reload_conv_objs=$reload_objs\ `$ECHO "$tmp_whole_archive_flags" | $SED 's|,| |g'`
- else
- gentop="$output_objdir/${obj}x"
- func_append generated " $gentop"
-
- func_extract_archives $gentop $convenience
- reload_conv_objs="$reload_objs $func_extract_archives_result"
- fi
- fi
-
- # If we're not building shared, we need to use non_pic_objs
- test "$build_libtool_libs" != yes && libobjs="$non_pic_objects"
-
- # Create the old-style object.
- reload_objs="$objs$old_deplibs "`$ECHO "$libobjs" | $SP2NL | $SED "/\.${libext}$/d; /\.lib$/d; $lo2o" | $NL2SP`" $reload_conv_objs" ### testsuite: skip nested quoting test
-
- output="$obj"
- func_execute_cmds "$reload_cmds" 'exit $?'
-
- # Exit if we aren't doing a library object file.
- if test -z "$libobj"; then
- if test -n "$gentop"; then
- func_show_eval '${RM}r "$gentop"'
- fi
-
- exit $EXIT_SUCCESS
- fi
-
- if test "$build_libtool_libs" != yes; then
- if test -n "$gentop"; then
- func_show_eval '${RM}r "$gentop"'
- fi
-
- # Create an invalid libtool object if no PIC, so that we don't
- # accidentally link it into a program.
- # $show "echo timestamp > $libobj"
- # $opt_dry_run || eval "echo timestamp > $libobj" || exit $?
- exit $EXIT_SUCCESS
- fi
-
- if test -n "$pic_flag" || test "$pic_mode" != default; then
- # Only do commands if we really have different PIC objects.
- reload_objs="$libobjs $reload_conv_objs"
- output="$libobj"
- func_execute_cmds "$reload_cmds" 'exit $?'
- fi
-
- if test -n "$gentop"; then
- func_show_eval '${RM}r "$gentop"'
- fi
-
- exit $EXIT_SUCCESS
- ;;
-
- prog)
- case $host in
- *cygwin*) func_stripname '' '.exe' "$output"
- output=$func_stripname_result.exe;;
- esac
- test -n "$vinfo" && \
- func_warning "\`-version-info' is ignored for programs"
-
- test -n "$release" && \
- func_warning "\`-release' is ignored for programs"
-
- test "$preload" = yes \
- && test "$dlopen_support" = unknown \
- && test "$dlopen_self" = unknown \
- && test "$dlopen_self_static" = unknown && \
- func_warning "\`LT_INIT([dlopen])' not used. Assuming no dlopen support."
-
- case $host in
- *-*-rhapsody* | *-*-darwin1.[012])
- # On Rhapsody replace the C library is the System framework
- compile_deplibs=`$ECHO " $compile_deplibs" | $SED 's/ -lc / System.ltframework /'`
- finalize_deplibs=`$ECHO " $finalize_deplibs" | $SED 's/ -lc / System.ltframework /'`
- ;;
- esac
-
- case $host in
- *-*-darwin*)
- # Don't allow lazy linking, it breaks C++ global constructors
- # But is supposedly fixed on 10.4 or later (yay!).
- if test "$tagname" = CXX ; then
- case ${MACOSX_DEPLOYMENT_TARGET-10.0} in
- 10.[0123])
- func_append compile_command " ${wl}-bind_at_load"
- func_append finalize_command " ${wl}-bind_at_load"
- ;;
- esac
- fi
- # Time to change all our "foo.ltframework" stuff back to "-framework foo"
- compile_deplibs=`$ECHO " $compile_deplibs" | $SED 's% \([^ $]*\).ltframework% -framework \1%g'`
- finalize_deplibs=`$ECHO " $finalize_deplibs" | $SED 's% \([^ $]*\).ltframework% -framework \1%g'`
- ;;
- esac
-
-
- # move library search paths that coincide with paths to not yet
- # installed libraries to the beginning of the library search list
- new_libs=
- for path in $notinst_path; do
- case " $new_libs " in
- *" -L$path/$objdir "*) ;;
- *)
- case " $compile_deplibs " in
- *" -L$path/$objdir "*)
- func_append new_libs " -L$path/$objdir" ;;
- esac
- ;;
- esac
- done
- for deplib in $compile_deplibs; do
- case $deplib in
- -L*)
- case " $new_libs " in
- *" $deplib "*) ;;
- *) func_append new_libs " $deplib" ;;
- esac
- ;;
- *) func_append new_libs " $deplib" ;;
- esac
- done
- compile_deplibs="$new_libs"
-
-
- func_append compile_command " $compile_deplibs"
- func_append finalize_command " $finalize_deplibs"
-
- if test -n "$rpath$xrpath"; then
- # If the user specified any rpath flags, then add them.
- for libdir in $rpath $xrpath; do
- # This is the magic to use -rpath.
- case "$finalize_rpath " in
- *" $libdir "*) ;;
- *) func_append finalize_rpath " $libdir" ;;
- esac
- done
- fi
-
- # Now hardcode the library paths
- rpath=
- hardcode_libdirs=
- for libdir in $compile_rpath $finalize_rpath; do
- if test -n "$hardcode_libdir_flag_spec"; then
- if test -n "$hardcode_libdir_separator"; then
- if test -z "$hardcode_libdirs"; then
- hardcode_libdirs="$libdir"
- else
- # Just accumulate the unique libdirs.
- case $hardcode_libdir_separator$hardcode_libdirs$hardcode_libdir_separator in
- *"$hardcode_libdir_separator$libdir$hardcode_libdir_separator"*)
- ;;
- *)
- func_append hardcode_libdirs "$hardcode_libdir_separator$libdir"
- ;;
- esac
- fi
- else
- eval flag=\"$hardcode_libdir_flag_spec\"
- func_append rpath " $flag"
- fi
- elif test -n "$runpath_var"; then
- case "$perm_rpath " in
- *" $libdir "*) ;;
- *) func_append perm_rpath " $libdir" ;;
- esac
- fi
- case $host in
- *-*-cygwin* | *-*-mingw* | *-*-pw32* | *-*-os2* | *-cegcc*)
- testbindir=`${ECHO} "$libdir" | ${SED} -e 's*/lib$*/bin*'`
- case :$dllsearchpath: in
- *":$libdir:"*) ;;
- ::) dllsearchpath=$libdir;;
- *) func_append dllsearchpath ":$libdir";;
- esac
- case :$dllsearchpath: in
- *":$testbindir:"*) ;;
- ::) dllsearchpath=$testbindir;;
- *) func_append dllsearchpath ":$testbindir";;
- esac
- ;;
- esac
- done
- # Substitute the hardcoded libdirs into the rpath.
- if test -n "$hardcode_libdir_separator" &&
- test -n "$hardcode_libdirs"; then
- libdir="$hardcode_libdirs"
- eval rpath=\" $hardcode_libdir_flag_spec\"
- fi
- compile_rpath="$rpath"
-
- rpath=
- hardcode_libdirs=
- for libdir in $finalize_rpath; do
- if test -n "$hardcode_libdir_flag_spec"; then
- if test -n "$hardcode_libdir_separator"; then
- if test -z "$hardcode_libdirs"; then
- hardcode_libdirs="$libdir"
- else
- # Just accumulate the unique libdirs.
- case $hardcode_libdir_separator$hardcode_libdirs$hardcode_libdir_separator in
- *"$hardcode_libdir_separator$libdir$hardcode_libdir_separator"*)
- ;;
- *)
- func_append hardcode_libdirs "$hardcode_libdir_separator$libdir"
- ;;
- esac
- fi
- else
- eval flag=\"$hardcode_libdir_flag_spec\"
- func_append rpath " $flag"
- fi
- elif test -n "$runpath_var"; then
- case "$finalize_perm_rpath " in
- *" $libdir "*) ;;
- *) func_append finalize_perm_rpath " $libdir" ;;
- esac
- fi
- done
- # Substitute the hardcoded libdirs into the rpath.
- if test -n "$hardcode_libdir_separator" &&
- test -n "$hardcode_libdirs"; then
- libdir="$hardcode_libdirs"
- eval rpath=\" $hardcode_libdir_flag_spec\"
- fi
- finalize_rpath="$rpath"
-
- if test -n "$libobjs" && test "$build_old_libs" = yes; then
- # Transform all the library objects into standard objects.
- compile_command=`$ECHO "$compile_command" | $SP2NL | $SED "$lo2o" | $NL2SP`
- finalize_command=`$ECHO "$finalize_command" | $SP2NL | $SED "$lo2o" | $NL2SP`
- fi
-
- func_generate_dlsyms "$outputname" "@PROGRAM@" "no"
-
- # template prelinking step
- if test -n "$prelink_cmds"; then
- func_execute_cmds "$prelink_cmds" 'exit $?'
- fi
-
- wrappers_required=yes
- case $host in
- *cegcc* | *mingw32ce*)
- # Disable wrappers for cegcc and mingw32ce hosts, we are cross compiling anyway.
- wrappers_required=no
- ;;
- *cygwin* | *mingw* )
- if test "$build_libtool_libs" != yes; then
- wrappers_required=no
- fi
- ;;
- *)
- if test "$need_relink" = no || test "$build_libtool_libs" != yes; then
- wrappers_required=no
- fi
- ;;
- esac
- if test "$wrappers_required" = no; then
- # Replace the output file specification.
- compile_command=`$ECHO "$compile_command" | $SED 's%@OUTPUT@%'"$output"'%g'`
- link_command="$compile_command$compile_rpath"
-
- # We have no uninstalled library dependencies, so finalize right now.
- exit_status=0
- func_show_eval "$link_command" 'exit_status=$?'
-
- if test -n "$postlink_cmds"; then
- func_to_tool_file "$output"
- postlink_cmds=`func_echo_all "$postlink_cmds" | $SED -e 's%@OUTPUT@%'"$output"'%g' -e 's%@TOOL_OUTPUT@%'"$func_to_tool_file_result"'%g'`
- func_execute_cmds "$postlink_cmds" 'exit $?'
- fi
-
- # Delete the generated files.
- if test -f "$output_objdir/${outputname}S.${objext}"; then
- func_show_eval '$RM "$output_objdir/${outputname}S.${objext}"'
- fi
-
- exit $exit_status
- fi
-
- if test -n "$compile_shlibpath$finalize_shlibpath"; then
- compile_command="$shlibpath_var=\"$compile_shlibpath$finalize_shlibpath\$$shlibpath_var\" $compile_command"
- fi
- if test -n "$finalize_shlibpath"; then
- finalize_command="$shlibpath_var=\"$finalize_shlibpath\$$shlibpath_var\" $finalize_command"
- fi
-
- compile_var=
- finalize_var=
- if test -n "$runpath_var"; then
- if test -n "$perm_rpath"; then
- # We should set the runpath_var.
- rpath=
- for dir in $perm_rpath; do
- func_append rpath "$dir:"
- done
- compile_var="$runpath_var=\"$rpath\$$runpath_var\" "
- fi
- if test -n "$finalize_perm_rpath"; then
- # We should set the runpath_var.
- rpath=
- for dir in $finalize_perm_rpath; do
- func_append rpath "$dir:"
- done
- finalize_var="$runpath_var=\"$rpath\$$runpath_var\" "
- fi
- fi
-
- if test "$no_install" = yes; then
- # We don't need to create a wrapper script.
- link_command="$compile_var$compile_command$compile_rpath"
- # Replace the output file specification.
- link_command=`$ECHO "$link_command" | $SED 's%@OUTPUT@%'"$output"'%g'`
- # Delete the old output file.
- $opt_dry_run || $RM $output
- # Link the executable and exit
- func_show_eval "$link_command" 'exit $?'
-
- if test -n "$postlink_cmds"; then
- func_to_tool_file "$output"
- postlink_cmds=`func_echo_all "$postlink_cmds" | $SED -e 's%@OUTPUT@%'"$output"'%g' -e 's%@TOOL_OUTPUT@%'"$func_to_tool_file_result"'%g'`
- func_execute_cmds "$postlink_cmds" 'exit $?'
- fi
-
- exit $EXIT_SUCCESS
- fi
-
- if test "$hardcode_action" = relink; then
- # Fast installation is not supported
- link_command="$compile_var$compile_command$compile_rpath"
- relink_command="$finalize_var$finalize_command$finalize_rpath"
-
- func_warning "this platform does not like uninstalled shared libraries"
- func_warning "\`$output' will be relinked during installation"
- else
- if test "$fast_install" != no; then
- link_command="$finalize_var$compile_command$finalize_rpath"
- if test "$fast_install" = yes; then
- relink_command=`$ECHO "$compile_var$compile_command$compile_rpath" | $SED 's%@OUTPUT@%\$progdir/\$file%g'`
- else
- # fast_install is set to needless
- relink_command=
- fi
- else
- link_command="$compile_var$compile_command$compile_rpath"
- relink_command="$finalize_var$finalize_command$finalize_rpath"
- fi
- fi
-
- # Replace the output file specification.
- link_command=`$ECHO "$link_command" | $SED 's%@OUTPUT@%'"$output_objdir/$outputname"'%g'`
-
- # Delete the old output files.
- $opt_dry_run || $RM $output $output_objdir/$outputname $output_objdir/lt-$outputname
-
- func_show_eval "$link_command" 'exit $?'
-
- if test -n "$postlink_cmds"; then
- func_to_tool_file "$output_objdir/$outputname"
- postlink_cmds=`func_echo_all "$postlink_cmds" | $SED -e 's%@OUTPUT@%'"$output_objdir/$outputname"'%g' -e 's%@TOOL_OUTPUT@%'"$func_to_tool_file_result"'%g'`
- func_execute_cmds "$postlink_cmds" 'exit $?'
- fi
-
- # Now create the wrapper script.
- func_verbose "creating $output"
-
- # Quote the relink command for shipping.
- if test -n "$relink_command"; then
- # Preserve any variables that may affect compiler behavior
- for var in $variables_saved_for_relink; do
- if eval test -z \"\${$var+set}\"; then
- relink_command="{ test -z \"\${$var+set}\" || $lt_unset $var || { $var=; export $var; }; }; $relink_command"
- elif eval var_value=\$$var; test -z "$var_value"; then
- relink_command="$var=; export $var; $relink_command"
- else
- func_quote_for_eval "$var_value"
- relink_command="$var=$func_quote_for_eval_result; export $var; $relink_command"
- fi
- done
- relink_command="(cd `pwd`; $relink_command)"
- relink_command=`$ECHO "$relink_command" | $SED "$sed_quote_subst"`
- fi
-
- # Only actually do things if not in dry run mode.
- $opt_dry_run || {
- # win32 will think the script is a binary if it has
- # a .exe suffix, so we strip it off here.
- case $output in
- *.exe) func_stripname '' '.exe' "$output"
- output=$func_stripname_result ;;
- esac
- # test for cygwin because mv fails w/o .exe extensions
- case $host in
- *cygwin*)
- exeext=.exe
- func_stripname '' '.exe' "$outputname"
- outputname=$func_stripname_result ;;
- *) exeext= ;;
- esac
- case $host in
- *cygwin* | *mingw* )
- func_dirname_and_basename "$output" "" "."
- output_name=$func_basename_result
- output_path=$func_dirname_result
- cwrappersource="$output_path/$objdir/lt-$output_name.c"
- cwrapper="$output_path/$output_name.exe"
- $RM $cwrappersource $cwrapper
- trap "$RM $cwrappersource $cwrapper; exit $EXIT_FAILURE" 1 2 15
-
- func_emit_cwrapperexe_src > $cwrappersource
-
- # The wrapper executable is built using the $host compiler,
- # because it contains $host paths and files. If cross-
- # compiling, it, like the target executable, must be
- # executed on the $host or under an emulation environment.
- $opt_dry_run || {
- $LTCC $LTCFLAGS -o $cwrapper $cwrappersource
- $STRIP $cwrapper
- }
-
- # Now, create the wrapper script for func_source use:
- func_ltwrapper_scriptname $cwrapper
- $RM $func_ltwrapper_scriptname_result
- trap "$RM $func_ltwrapper_scriptname_result; exit $EXIT_FAILURE" 1 2 15
- $opt_dry_run || {
- # note: this script will not be executed, so do not chmod.
- if test "x$build" = "x$host" ; then
- $cwrapper --lt-dump-script > $func_ltwrapper_scriptname_result
- else
- func_emit_wrapper no > $func_ltwrapper_scriptname_result
- fi
- }
- ;;
- * )
- $RM $output
- trap "$RM $output; exit $EXIT_FAILURE" 1 2 15
-
- func_emit_wrapper no > $output
- chmod +x $output
- ;;
- esac
- }
- exit $EXIT_SUCCESS
- ;;
- esac
-
- # See if we need to build an old-fashioned archive.
- for oldlib in $oldlibs; do
-
- if test "$build_libtool_libs" = convenience; then
- oldobjs="$libobjs_save $symfileobj"
- addlibs="$convenience"
- build_libtool_libs=no
- else
- if test "$build_libtool_libs" = module; then
- oldobjs="$libobjs_save"
- build_libtool_libs=no
- else
- oldobjs="$old_deplibs $non_pic_objects"
- if test "$preload" = yes && test -f "$symfileobj"; then
- func_append oldobjs " $symfileobj"
- fi
- fi
- addlibs="$old_convenience"
- fi
-
- if test -n "$addlibs"; then
- gentop="$output_objdir/${outputname}x"
- func_append generated " $gentop"
-
- func_extract_archives $gentop $addlibs
- func_append oldobjs " $func_extract_archives_result"
- fi
-
- # Do each command in the archive commands.
- if test -n "$old_archive_from_new_cmds" && test "$build_libtool_libs" = yes; then
- cmds=$old_archive_from_new_cmds
- else
-
- # Add any objects from preloaded convenience libraries
- if test -n "$dlprefiles"; then
- gentop="$output_objdir/${outputname}x"
- func_append generated " $gentop"
-
- func_extract_archives $gentop $dlprefiles
- func_append oldobjs " $func_extract_archives_result"
- fi
-
- # POSIX demands no paths to be encoded in archives. We have
- # to avoid creating archives with duplicate basenames if we
- # might have to extract them afterwards, e.g., when creating a
- # static archive out of a convenience library, or when linking
- # the entirety of a libtool archive into another (currently
- # not supported by libtool).
- if (for obj in $oldobjs
- do
- func_basename "$obj"
- $ECHO "$func_basename_result"
- done | sort | sort -uc >/dev/null 2>&1); then
- :
- else
- echo "copying selected object files to avoid basename conflicts..."
- gentop="$output_objdir/${outputname}x"
- func_append generated " $gentop"
- func_mkdir_p "$gentop"
- save_oldobjs=$oldobjs
- oldobjs=
- counter=1
- for obj in $save_oldobjs
- do
- func_basename "$obj"
- objbase="$func_basename_result"
- case " $oldobjs " in
- " ") oldobjs=$obj ;;
- *[\ /]"$objbase "*)
- while :; do
- # Make sure we don't pick an alternate name that also
- # overlaps.
- newobj=lt$counter-$objbase
- func_arith $counter + 1
- counter=$func_arith_result
- case " $oldobjs " in
- *[\ /]"$newobj "*) ;;
- *) if test ! -f "$gentop/$newobj"; then break; fi ;;
- esac
- done
- func_show_eval "ln $obj $gentop/$newobj || cp $obj $gentop/$newobj"
- func_append oldobjs " $gentop/$newobj"
- ;;
- *) func_append oldobjs " $obj" ;;
- esac
- done
- fi
- func_to_tool_file "$oldlib" func_convert_file_msys_to_w32
- tool_oldlib=$func_to_tool_file_result
- eval cmds=\"$old_archive_cmds\"
-
- func_len " $cmds"
- len=$func_len_result
- if test "$len" -lt "$max_cmd_len" || test "$max_cmd_len" -le -1; then
- cmds=$old_archive_cmds
- elif test -n "$archiver_list_spec"; then
- func_verbose "using command file archive linking..."
- for obj in $oldobjs
- do
- func_to_tool_file "$obj"
- $ECHO "$func_to_tool_file_result"
- done > $output_objdir/$libname.libcmd
- func_to_tool_file "$output_objdir/$libname.libcmd"
- oldobjs=" $archiver_list_spec$func_to_tool_file_result"
- cmds=$old_archive_cmds
- else
- # the command line is too long to link in one step, link in parts
- func_verbose "using piecewise archive linking..."
- save_RANLIB=$RANLIB
- RANLIB=:
- objlist=
- concat_cmds=
- save_oldobjs=$oldobjs
- oldobjs=
- # Is there a better way of finding the last object in the list?
- for obj in $save_oldobjs
- do
- last_oldobj=$obj
- done
- eval test_cmds=\"$old_archive_cmds\"
- func_len " $test_cmds"
- len0=$func_len_result
- len=$len0
- for obj in $save_oldobjs
- do
- func_len " $obj"
- func_arith $len + $func_len_result
- len=$func_arith_result
- func_append objlist " $obj"
- if test "$len" -lt "$max_cmd_len"; then
- :
- else
- # the above command should be used before it gets too long
- oldobjs=$objlist
- if test "$obj" = "$last_oldobj" ; then
- RANLIB=$save_RANLIB
- fi
- test -z "$concat_cmds" || concat_cmds=$concat_cmds~
- eval concat_cmds=\"\${concat_cmds}$old_archive_cmds\"
- objlist=
- len=$len0
- fi
- done
- RANLIB=$save_RANLIB
- oldobjs=$objlist
- if test "X$oldobjs" = "X" ; then
- eval cmds=\"\$concat_cmds\"
- else
- eval cmds=\"\$concat_cmds~\$old_archive_cmds\"
- fi
- fi
- fi
- func_execute_cmds "$cmds" 'exit $?'
- done
-
- test -n "$generated" && \
- func_show_eval "${RM}r$generated"
-
- # Now create the libtool archive.
- case $output in
- *.la)
- old_library=
- test "$build_old_libs" = yes && old_library="$libname.$libext"
- func_verbose "creating $output"
-
- # Preserve any variables that may affect compiler behavior
- for var in $variables_saved_for_relink; do
- if eval test -z \"\${$var+set}\"; then
- relink_command="{ test -z \"\${$var+set}\" || $lt_unset $var || { $var=; export $var; }; }; $relink_command"
- elif eval var_value=\$$var; test -z "$var_value"; then
- relink_command="$var=; export $var; $relink_command"
- else
- func_quote_for_eval "$var_value"
- relink_command="$var=$func_quote_for_eval_result; export $var; $relink_command"
- fi
- done
- # Quote the link command for shipping.
- relink_command="(cd `pwd`; $SHELL $progpath $preserve_args --mode=relink $libtool_args @inst_prefix_dir@)"
- relink_command=`$ECHO "$relink_command" | $SED "$sed_quote_subst"`
- if test "$hardcode_automatic" = yes ; then
- relink_command=
- fi
-
- # Only create the output if not a dry run.
- $opt_dry_run || {
- for installed in no yes; do
- if test "$installed" = yes; then
- if test -z "$install_libdir"; then
- break
- fi
- output="$output_objdir/$outputname"i
- # Replace all uninstalled libtool libraries with the installed ones
- newdependency_libs=
- for deplib in $dependency_libs; do
- case $deplib in
- *.la)
- func_basename "$deplib"
- name="$func_basename_result"
- func_resolve_sysroot "$deplib"
- eval libdir=`${SED} -n -e 's/^libdir=\(.*\)$/\1/p' $func_resolve_sysroot_result`
- test -z "$libdir" && \
- func_fatal_error "\`$deplib' is not a valid libtool archive"
- func_append newdependency_libs " ${lt_sysroot:+=}$libdir/$name"
- ;;
- -L*)
- func_stripname -L '' "$deplib"
- func_replace_sysroot "$func_stripname_result"
- func_append newdependency_libs " -L$func_replace_sysroot_result"
- ;;
- -R*)
- func_stripname -R '' "$deplib"
- func_replace_sysroot "$func_stripname_result"
- func_append newdependency_libs " -R$func_replace_sysroot_result"
- ;;
- *) func_append newdependency_libs " $deplib" ;;
- esac
- done
- dependency_libs="$newdependency_libs"
- newdlfiles=
-
- for lib in $dlfiles; do
- case $lib in
- *.la)
- func_basename "$lib"
- name="$func_basename_result"
- eval libdir=`${SED} -n -e 's/^libdir=\(.*\)$/\1/p' $lib`
- test -z "$libdir" && \
- func_fatal_error "\`$lib' is not a valid libtool archive"
- func_append newdlfiles " ${lt_sysroot:+=}$libdir/$name"
- ;;
- *) func_append newdlfiles " $lib" ;;
- esac
- done
- dlfiles="$newdlfiles"
- newdlprefiles=
- for lib in $dlprefiles; do
- case $lib in
- *.la)
- # Only pass preopened files to the pseudo-archive (for
- # eventual linking with the app. that links it) if we
- # didn't already link the preopened objects directly into
- # the library:
- func_basename "$lib"
- name="$func_basename_result"
- eval libdir=`${SED} -n -e 's/^libdir=\(.*\)$/\1/p' $lib`
- test -z "$libdir" && \
- func_fatal_error "\`$lib' is not a valid libtool archive"
- func_append newdlprefiles " ${lt_sysroot:+=}$libdir/$name"
- ;;
- esac
- done
- dlprefiles="$newdlprefiles"
- else
- newdlfiles=
- for lib in $dlfiles; do
- case $lib in
- [\\/]* | [A-Za-z]:[\\/]*) abs="$lib" ;;
- *) abs=`pwd`"/$lib" ;;
- esac
- func_append newdlfiles " $abs"
- done
- dlfiles="$newdlfiles"
- newdlprefiles=
- for lib in $dlprefiles; do
- case $lib in
- [\\/]* | [A-Za-z]:[\\/]*) abs="$lib" ;;
- *) abs=`pwd`"/$lib" ;;
- esac
- func_append newdlprefiles " $abs"
- done
- dlprefiles="$newdlprefiles"
- fi
- $RM $output
- # place dlname in correct position for cygwin
- # In fact, it would be nice if we could use this code for all target
- # systems that can't hard-code library paths into their executables
- # and that have no shared library path variable independent of PATH,
- # but it turns out we can't easily determine that from inspecting
- # libtool variables, so we have to hard-code the OSs to which it
- # applies here; at the moment, that means platforms that use the PE
- # object format with DLL files. See the long comment at the top of
- # tests/bindir.at for full details.
- tdlname=$dlname
- case $host,$output,$installed,$module,$dlname in
- *cygwin*,*lai,yes,no,*.dll | *mingw*,*lai,yes,no,*.dll | *cegcc*,*lai,yes,no,*.dll)
- # If a -bindir argument was supplied, place the dll there.
- if test "x$bindir" != x ;
- then
- func_relative_path "$install_libdir" "$bindir"
- tdlname=$func_relative_path_result$dlname
- else
- # Otherwise fall back on heuristic.
- tdlname=../bin/$dlname
- fi
- ;;
- esac
- $ECHO > $output "\
-# $outputname - a libtool library file
-# Generated by $PROGRAM (GNU $PACKAGE$TIMESTAMP) $VERSION
-#
-# Please DO NOT delete this file!
-# It is necessary for linking the library.
-
-# The name that we can dlopen(3).
-dlname='$tdlname'
-
-# Names of this library.
-library_names='$library_names'
-
-# The name of the static archive.
-old_library='$old_library'
-
-# Linker flags that can not go in dependency_libs.
-inherited_linker_flags='$new_inherited_linker_flags'
-
-# Libraries that this one depends upon.
-dependency_libs='$dependency_libs'
-
-# Names of additional weak libraries provided by this library
-weak_library_names='$weak_libs'
-
-# Version information for $libname.
-current=$current
-age=$age
-revision=$revision
-
-# Is this an already installed library?
-installed=$installed
-
-# Should we warn about portability when linking against -modules?
-shouldnotlink=$module
-
-# Files to dlopen/dlpreopen
-dlopen='$dlfiles'
-dlpreopen='$dlprefiles'
-
-# Directory that this library needs to be installed in:
-libdir='$install_libdir'"
- if test "$installed" = no && test "$need_relink" = yes; then
- $ECHO >> $output "\
-relink_command=\"$relink_command\""
- fi
- done
- }
-
- # Do a symbolic link so that the libtool archive can be found in
- # LD_LIBRARY_PATH before the program is installed.
- func_show_eval '( cd "$output_objdir" && $RM "$outputname" && $LN_S "../$outputname" "$outputname" )' 'exit $?'
- ;;
- esac
- exit $EXIT_SUCCESS
-}
-
-{ test "$opt_mode" = link || test "$opt_mode" = relink; } &&
- func_mode_link ${1+"$@"}
-
-
-# func_mode_uninstall arg...
-func_mode_uninstall ()
-{
- $opt_debug
- RM="$nonopt"
- files=
- rmforce=
- exit_status=0
-
- # This variable tells wrapper scripts just to set variables rather
- # than running their programs.
- libtool_install_magic="$magic"
-
- for arg
- do
- case $arg in
- -f) func_append RM " $arg"; rmforce=yes ;;
- -*) func_append RM " $arg" ;;
- *) func_append files " $arg" ;;
- esac
- done
-
- test -z "$RM" && \
- func_fatal_help "you must specify an RM program"
-
- rmdirs=
-
- for file in $files; do
- func_dirname "$file" "" "."
- dir="$func_dirname_result"
- if test "X$dir" = X.; then
- odir="$objdir"
- else
- odir="$dir/$objdir"
- fi
- func_basename "$file"
- name="$func_basename_result"
- test "$opt_mode" = uninstall && odir="$dir"
-
- # Remember odir for removal later, being careful to avoid duplicates
- if test "$opt_mode" = clean; then
- case " $rmdirs " in
- *" $odir "*) ;;
- *) func_append rmdirs " $odir" ;;
- esac
- fi
-
- # Don't error if the file doesn't exist and rm -f was used.
- if { test -L "$file"; } >/dev/null 2>&1 ||
- { test -h "$file"; } >/dev/null 2>&1 ||
- test -f "$file"; then
- :
- elif test -d "$file"; then
- exit_status=1
- continue
- elif test "$rmforce" = yes; then
- continue
- fi
-
- rmfiles="$file"
-
- case $name in
- *.la)
- # Possibly a libtool archive, so verify it.
- if func_lalib_p "$file"; then
- func_source $dir/$name
-
- # Delete the libtool libraries and symlinks.
- for n in $library_names; do
- func_append rmfiles " $odir/$n"
- done
- test -n "$old_library" && func_append rmfiles " $odir/$old_library"
-
- case "$opt_mode" in
- clean)
- case " $library_names " in
- *" $dlname "*) ;;
- *) test -n "$dlname" && func_append rmfiles " $odir/$dlname" ;;
- esac
- test -n "$libdir" && func_append rmfiles " $odir/$name $odir/${name}i"
- ;;
- uninstall)
- if test -n "$library_names"; then
- # Do each command in the postuninstall commands.
- func_execute_cmds "$postuninstall_cmds" 'test "$rmforce" = yes || exit_status=1'
- fi
-
- if test -n "$old_library"; then
- # Do each command in the old_postuninstall commands.
- func_execute_cmds "$old_postuninstall_cmds" 'test "$rmforce" = yes || exit_status=1'
- fi
- # FIXME: should reinstall the best remaining shared library.
- ;;
- esac
- fi
- ;;
-
- *.lo)
- # Possibly a libtool object, so verify it.
- if func_lalib_p "$file"; then
-
- # Read the .lo file
- func_source $dir/$name
-
- # Add PIC object to the list of files to remove.
- if test -n "$pic_object" &&
- test "$pic_object" != none; then
- func_append rmfiles " $dir/$pic_object"
- fi
-
- # Add non-PIC object to the list of files to remove.
- if test -n "$non_pic_object" &&
- test "$non_pic_object" != none; then
- func_append rmfiles " $dir/$non_pic_object"
- fi
- fi
- ;;
-
- *)
- if test "$opt_mode" = clean ; then
- noexename=$name
- case $file in
- *.exe)
- func_stripname '' '.exe' "$file"
- file=$func_stripname_result
- func_stripname '' '.exe' "$name"
- noexename=$func_stripname_result
- # $file with .exe has already been added to rmfiles,
- # add $file without .exe
- func_append rmfiles " $file"
- ;;
- esac
- # Do a test to see if this is a libtool program.
- if func_ltwrapper_p "$file"; then
- if func_ltwrapper_executable_p "$file"; then
- func_ltwrapper_scriptname "$file"
- relink_command=
- func_source $func_ltwrapper_scriptname_result
- func_append rmfiles " $func_ltwrapper_scriptname_result"
- else
- relink_command=
- func_source $dir/$noexename
- fi
-
- # note $name still contains .exe if it was in $file originally
- # as does the version of $file that was added into $rmfiles
- func_append rmfiles " $odir/$name $odir/${name}S.${objext}"
- if test "$fast_install" = yes && test -n "$relink_command"; then
- func_append rmfiles " $odir/lt-$name"
- fi
- if test "X$noexename" != "X$name" ; then
- func_append rmfiles " $odir/lt-${noexename}.c"
- fi
- fi
- fi
- ;;
- esac
- func_show_eval "$RM $rmfiles" 'exit_status=1'
- done
-
- # Try to remove the ${objdir}s in the directories where we deleted files
- for dir in $rmdirs; do
- if test -d "$dir"; then
- func_show_eval "rmdir $dir >/dev/null 2>&1"
- fi
- done
-
- exit $exit_status
-}
-
-{ test "$opt_mode" = uninstall || test "$opt_mode" = clean; } &&
- func_mode_uninstall ${1+"$@"}
-
-test -z "$opt_mode" && {
- help="$generic_help"
- func_fatal_help "you must specify a MODE"
-}
-
-test -z "$exec_cmd" && \
- func_fatal_help "invalid operation mode \`$opt_mode'"
-
-if test -n "$exec_cmd"; then
- eval exec "$exec_cmd"
- exit $EXIT_FAILURE
-fi
-
-exit $exit_status
-
-
-# The TAGs below are defined such that we never get into a situation
-# in which we disable both kinds of libraries. Given conflicting
-# choices, we go for a static library, that is the most portable,
-# since we can't tell whether shared libraries were disabled because
-# the user asked for that or because the platform doesn't support
-# them. This is particularly important on AIX, because we don't
-# support having both static and shared libraries enabled at the same
-# time on that platform, so we default to a shared-only configuration.
-# If a disable-shared tag is given, we'll fallback to a static-only
-# configuration. But we'll never go from static-only to shared-only.
-
-# ### BEGIN LIBTOOL TAG CONFIG: disable-shared
-build_libtool_libs=no
-build_old_libs=yes
-# ### END LIBTOOL TAG CONFIG: disable-shared
-
-# ### BEGIN LIBTOOL TAG CONFIG: disable-static
-build_old_libs=`case $build_libtool_libs in yes) echo no;; *) echo yes;; esac`
-# ### END LIBTOOL TAG CONFIG: disable-static
-
-# Local Variables:
-# mode:shell-script
-# sh-indentation:2
-# End:
-# vi:sw=2
-
diff --git a/spaces/amarchheda/ChordDuplicate/portaudio/qa/loopback/src/test_audio_analyzer.h b/spaces/amarchheda/ChordDuplicate/portaudio/qa/loopback/src/test_audio_analyzer.h
deleted file mode 100644
index bfe073fc697b112d6ccaba6151cd89f7de2ca598..0000000000000000000000000000000000000000
--- a/spaces/amarchheda/ChordDuplicate/portaudio/qa/loopback/src/test_audio_analyzer.h
+++ /dev/null
@@ -1,46 +0,0 @@
-
-/*
- * PortAudio Portable Real-Time Audio Library
- * Latest Version at: http://www.portaudio.com
- *
- * Copyright (c) 1999-2010 Phil Burk and Ross Bencina
- *
- * Permission is hereby granted, free of charge, to any person obtaining
- * a copy of this software and associated documentation files
- * (the "Software"), to deal in the Software without restriction,
- * including without limitation the rights to use, copy, modify, merge,
- * publish, distribute, sublicense, and/or sell copies of the Software,
- * and to permit persons to whom the Software is furnished to do so,
- * subject to the following conditions:
- *
- * The above copyright notice and this permission notice shall be
- * included in all copies or substantial portions of the Software.
- *
- * THE SOFTWARE IS PROVIDED "AS IS", WITHOUT WARRANTY OF ANY KIND,
- * EXPRESS OR IMPLIED, INCLUDING BUT NOT LIMITED TO THE WARRANTIES OF
- * MERCHANTABILITY, FITNESS FOR A PARTICULAR PURPOSE AND NONINFRINGEMENT.
- * IN NO EVENT SHALL THE AUTHORS OR COPYRIGHT HOLDERS BE LIABLE FOR
- * ANY CLAIM, DAMAGES OR OTHER LIABILITY, WHETHER IN AN ACTION OF
- * CONTRACT, TORT OR OTHERWISE, ARISING FROM, OUT OF OR IN CONNECTION
- * WITH THE SOFTWARE OR THE USE OR OTHER DEALINGS IN THE SOFTWARE.
- */
-
-/*
- * The text above constitutes the entire PortAudio license; however,
- * the PortAudio community also makes the following non-binding requests:
- *
- * Any person wishing to distribute modifications to the Software is
- * requested to send the modifications to the original developer so that
- * they can be incorporated into the canonical version. It is also
- * requested that these non-binding requests be included along with the
- * license above.
- */
-
-#ifndef _TEST_AUDIO_ANALYZER_H
-#define _TEST_AUDIO_ANALYZER_H
-
-/** Test the audio analyzer by itself without any PortAudio calls. */
-int PaQa_TestAnalyzer( void );
-
-
-#endif /* _TEST_AUDIO_ANALYZER_H */
diff --git a/spaces/andikalfauzi/Churn-Prediction/ChurnModel.py b/spaces/andikalfauzi/Churn-Prediction/ChurnModel.py
deleted file mode 100644
index f3e4d0c2123a5485efbc54fa62b724abe31be53d..0000000000000000000000000000000000000000
--- a/spaces/andikalfauzi/Churn-Prediction/ChurnModel.py
+++ /dev/null
@@ -1,96 +0,0 @@
-import numpy as np
-import pandas as pd
-import streamlit as st
-import pickle
-from datetime import datetime
-from tensorflow.keras.models import load_model
-
-# load the model
-baseFunctional = load_model('churnModel.h5')
-
-with open('FinalPipeline.pkl', 'rb') as file1:
- finalPipeline = pickle.load(file1)
-
-def run():
-
- def age_bin(age):
- if age > 7 and age < 20:
- return 'under20'
- elif age >= 20 and age < 30:
- return 'under30'
- elif age >= 30 and age < 40:
- return 'under40'
- elif age >= 40 and age < 50:
- return 'under50'
- elif age >= 50 and age < 60:
- return 'under60'
- else:
- return 'above60'
-
- # membuat input data baru
- with st.form(key='churn_form'):
- age = st.number_input('Age', min_value=5, max_value=120, value=10, step=1, help='Usia Customer')
- gender = st.selectbox('Gender', ('Female', 'Male'))
- region_category = st.selectbox('Region Category', ('Village', 'Town', 'City'))
- membership_category = st.selectbox('Membership Category', ('No Membership', 'Basic Membership', 'Silver Membership',
- 'Premium Membership', 'Gold Membership', 'Platinum Membership'))
- joining_date = st.date_input('Joining Date')
- medium_of_operation = st.selectbox('Medium of Operation', ('Smartphone', 'Desktop', 'Both'))
- preferred_offer_types = st.selectbox('Preferred Offer Types', ('Without Offers', 'Credit/Debit Card Offers', 'Gift Vouchers/Coupons'))
- days_since_last_login = st.number_input('Days Since Last Login', value=1, min_value=1, max_value=150, step=1)
- avg_time_spent = st.number_input('Average Time Spent', min_value=0.00, step=0.10, value=0.00, format='%.2g')
- avg_transaction_value = st.number_input('Average Transaction Value', min_value=0.00, step=0.10, value=0.00, format='%.2g')
- avg_frequency_login_days = st.number_input('Average Frequency Login Days', min_value=0, step=1, value=0, max_value=120)
- points_in_wallet = st.number_input('Points in Wallet', min_value=0.00, step=0.10, value=0.00, format='%.2g')
- used_special_discount = st.selectbox('Used Special Discount', ('No', 'Yes'))
- offer_application_preference = st.selectbox('Offer Application Preference', ('Yes', 'No'))
- past_complaint = st.selectbox('Past Complaint', ('No', 'Yes'))
- complaint_status = st.selectbox('Complaint Status', ('No Information Available', 'Not Applicable', 'Unsolved', 'Solved', 'Solved in Follow-up'))
- feedback = st.selectbox('Feedback', ('Reasonable Price', 'Poor Website', 'Poor Customer Service', 'Too many ads', 'Poor Product Quality', 'No reason specified',
- 'Products always in Stock', 'Quality Customer Care', 'User Friendly Website'))
- st.markdown('---')
-
- binAge = age_bin(age)
-
- submitted = st.form_submit_button('Predict')
-
- if joining_date:
- year = joining_date.year
- ('Tahun', year)
-
- infData = {
- 'region_category' : region_category,
- 'membership_category' : membership_category,
- 'medium_of_operation' : medium_of_operation,
- 'preferred_offer_types' : preferred_offer_types,
- 'days_since_last_login' : days_since_last_login,
- 'avg_time_spent' : avg_time_spent,
- 'avg_transaction_value' : avg_transaction_value,
- 'avg_frequency_login_days' : avg_frequency_login_days,
- 'points_in_wallet' : points_in_wallet,
- 'used_special_discount' : used_special_discount,
- 'offer_application_preference' : offer_application_preference,
- 'past_complaint' : past_complaint,
- 'complaint_status' : complaint_status,
- 'feedback' : feedback,
- 'age_bin' : binAge,
- 'joining_year' : year
- }
-
- infData = pd.DataFrame([infData])
- st.dataframe(infData, height=0)
-
- infPipe = finalPipeline.transform(infData)
-
- # Buat function di column submitted
- if submitted:
-
- # Predict using Base Functional API
- y_predInfData = baseFunctional.predict(infPipe)
- if y_predInfData >= 0.5:
- st.write('## Customer is Churn : Yes')
- else :
- st.write('## Customer is Churn : No')
-
-if __name__ == '__main__':
- run()
\ No newline at end of file
diff --git a/spaces/antonovmaxim/text-generation-webui-space/text-generation-webui-main/modules/callbacks.py b/spaces/antonovmaxim/text-generation-webui-space/text-generation-webui-main/modules/callbacks.py
deleted file mode 100644
index fb87ad56470e3222a0ea7c6609c2000e5f23ca69..0000000000000000000000000000000000000000
--- a/spaces/antonovmaxim/text-generation-webui-space/text-generation-webui-main/modules/callbacks.py
+++ /dev/null
@@ -1,112 +0,0 @@
-import gc
-import traceback
-from queue import Queue
-from threading import Thread
-
-import torch
-import transformers
-
-import modules.shared as shared
-
-
-class _SentinelTokenStoppingCriteria(transformers.StoppingCriteria):
-
- def __init__(self, sentinel_token_ids: list, starting_idx: int):
- transformers.StoppingCriteria.__init__(self)
- self.sentinel_token_ids = sentinel_token_ids
- self.starting_idx = starting_idx
- self.shortest = min([x.shape[-1] for x in sentinel_token_ids])
-
- def __call__(self, input_ids: torch.LongTensor, _scores: torch.FloatTensor) -> bool:
- for sample in input_ids:
- trimmed_sample = sample[self.starting_idx:]
- trimmed_len = trimmed_sample.shape[-1]
- if trimmed_len < self.shortest:
- continue
-
- for sentinel in self.sentinel_token_ids:
- sentinel_len = sentinel.shape[-1]
- if trimmed_len < sentinel_len:
- continue
-
- window = trimmed_sample[-sentinel_len:]
- if torch.all(torch.eq(sentinel, window)):
- return True
-
- return False
-
-
-class Stream(transformers.StoppingCriteria):
- def __init__(self, callback_func=None):
- self.callback_func = callback_func
-
- def __call__(self, input_ids, scores) -> bool:
- if self.callback_func is not None:
- self.callback_func(input_ids[0])
- return False
-
-
-class Iteratorize:
-
- """
- Transforms a function that takes a callback
- into a lazy iterator (generator).
-
- Adapted from: https://stackoverflow.com/a/9969000
- """
-
- def __init__(self, func, kwargs={}, callback=None):
- self.mfunc = func
- self.c_callback = callback
- self.q = Queue()
- self.sentinel = object()
- self.kwargs = kwargs
- self.stop_now = False
-
- def _callback(val):
- if self.stop_now or shared.stop_everything:
- raise ValueError
- self.q.put(val)
-
- def gentask():
- try:
- ret = self.mfunc(callback=_callback, **self.kwargs)
- except ValueError:
- pass
- except:
- traceback.print_exc()
- pass
-
- clear_torch_cache()
- self.q.put(self.sentinel)
- if self.c_callback:
- self.c_callback(ret)
-
- self.thread = Thread(target=gentask)
- self.thread.start()
-
- def __iter__(self):
- return self
-
- def __next__(self):
- obj = self.q.get(True, None)
- if obj is self.sentinel:
- raise StopIteration
- else:
- return obj
-
- def __del__(self):
- clear_torch_cache()
-
- def __enter__(self):
- return self
-
- def __exit__(self, exc_type, exc_val, exc_tb):
- self.stop_now = True
- clear_torch_cache()
-
-
-def clear_torch_cache():
- gc.collect()
- if not shared.args.cpu:
- torch.cuda.empty_cache()
diff --git a/spaces/aodianyun/stable-diffusion-webui/extensions-builtin/prompt-bracket-checker/javascript/prompt-bracket-checker.js b/spaces/aodianyun/stable-diffusion-webui/extensions-builtin/prompt-bracket-checker/javascript/prompt-bracket-checker.js
deleted file mode 100644
index 4a85c8ebf25110e911a6a1021fae6a014aa11000..0000000000000000000000000000000000000000
--- a/spaces/aodianyun/stable-diffusion-webui/extensions-builtin/prompt-bracket-checker/javascript/prompt-bracket-checker.js
+++ /dev/null
@@ -1,110 +0,0 @@
-// Stable Diffusion WebUI - Bracket checker
-// Version 1.0
-// By Hingashi no Florin/Bwin4L
-// Counts open and closed brackets (round, square, curly) in the prompt and negative prompt text boxes in the txt2img and img2img tabs.
-// If there's a mismatch, the keyword counter turns red and if you hover on it, a tooltip tells you what's wrong.
-
-function checkBrackets(evt, textArea, counterElt) {
- errorStringParen = '(...) - Different number of opening and closing parentheses detected.\n';
- errorStringSquare = '[...] - Different number of opening and closing square brackets detected.\n';
- errorStringCurly = '{...} - Different number of opening and closing curly brackets detected.\n';
-
- openBracketRegExp = /\(/g;
- closeBracketRegExp = /\)/g;
-
- openSquareBracketRegExp = /\[/g;
- closeSquareBracketRegExp = /\]/g;
-
- openCurlyBracketRegExp = /\{/g;
- closeCurlyBracketRegExp = /\}/g;
-
- totalOpenBracketMatches = 0;
- totalCloseBracketMatches = 0;
- totalOpenSquareBracketMatches = 0;
- totalCloseSquareBracketMatches = 0;
- totalOpenCurlyBracketMatches = 0;
- totalCloseCurlyBracketMatches = 0;
-
- openBracketMatches = textArea.value.match(openBracketRegExp);
- if(openBracketMatches) {
- totalOpenBracketMatches = openBracketMatches.length;
- }
-
- closeBracketMatches = textArea.value.match(closeBracketRegExp);
- if(closeBracketMatches) {
- totalCloseBracketMatches = closeBracketMatches.length;
- }
-
- openSquareBracketMatches = textArea.value.match(openSquareBracketRegExp);
- if(openSquareBracketMatches) {
- totalOpenSquareBracketMatches = openSquareBracketMatches.length;
- }
-
- closeSquareBracketMatches = textArea.value.match(closeSquareBracketRegExp);
- if(closeSquareBracketMatches) {
- totalCloseSquareBracketMatches = closeSquareBracketMatches.length;
- }
-
- openCurlyBracketMatches = textArea.value.match(openCurlyBracketRegExp);
- if(openCurlyBracketMatches) {
- totalOpenCurlyBracketMatches = openCurlyBracketMatches.length;
- }
-
- closeCurlyBracketMatches = textArea.value.match(closeCurlyBracketRegExp);
- if(closeCurlyBracketMatches) {
- totalCloseCurlyBracketMatches = closeCurlyBracketMatches.length;
- }
-
- if(totalOpenBracketMatches != totalCloseBracketMatches) {
- if(!counterElt.title.includes(errorStringParen)) {
- counterElt.title += errorStringParen;
- }
- } else {
- counterElt.title = counterElt.title.replace(errorStringParen, '');
- }
-
- if(totalOpenSquareBracketMatches != totalCloseSquareBracketMatches) {
- if(!counterElt.title.includes(errorStringSquare)) {
- counterElt.title += errorStringSquare;
- }
- } else {
- counterElt.title = counterElt.title.replace(errorStringSquare, '');
- }
-
- if(totalOpenCurlyBracketMatches != totalCloseCurlyBracketMatches) {
- if(!counterElt.title.includes(errorStringCurly)) {
- counterElt.title += errorStringCurly;
- }
- } else {
- counterElt.title = counterElt.title.replace(errorStringCurly, '');
- }
-
- if(counterElt.title != '') {
- counterElt.classList.add('error');
- } else {
- counterElt.classList.remove('error');
- }
-}
-
-function setupBracketChecking(id_prompt, id_counter){
- var textarea = gradioApp().querySelector("#" + id_prompt + " > label > textarea");
- var counter = gradioApp().getElementById(id_counter)
- textarea.addEventListener("input", function(evt){
- checkBrackets(evt, textarea, counter)
- });
-}
-
-var shadowRootLoaded = setInterval(function() {
- var shadowRoot = document.querySelector('gradio-app').shadowRoot;
- if(! shadowRoot) return false;
-
- var shadowTextArea = shadowRoot.querySelectorAll('#txt2img_prompt > label > textarea');
- if(shadowTextArea.length < 1) return false;
-
- clearInterval(shadowRootLoaded);
-
- setupBracketChecking('txt2img_prompt', 'txt2img_token_counter')
- setupBracketChecking('txt2img_neg_prompt', 'txt2img_negative_token_counter')
- setupBracketChecking('img2img_prompt', 'imgimg_token_counter')
- setupBracketChecking('img2img_neg_prompt', 'img2img_negative_token_counter')
-}, 1000);
diff --git a/spaces/aodianyun/stable-diffusion-webui/modules/images.py b/spaces/aodianyun/stable-diffusion-webui/modules/images.py
deleted file mode 100644
index a58573264ee61a83873b8901336be030cf826e3f..0000000000000000000000000000000000000000
--- a/spaces/aodianyun/stable-diffusion-webui/modules/images.py
+++ /dev/null
@@ -1,669 +0,0 @@
-import datetime
-import sys
-import traceback
-
-import pytz
-import io
-import math
-import os
-from collections import namedtuple
-import re
-
-import numpy as np
-import piexif
-import piexif.helper
-from PIL import Image, ImageFont, ImageDraw, PngImagePlugin
-from fonts.ttf import Roboto
-import string
-import json
-import hashlib
-
-from modules import sd_samplers, shared, script_callbacks, errors
-from modules.shared import opts, cmd_opts
-
-LANCZOS = (Image.Resampling.LANCZOS if hasattr(Image, 'Resampling') else Image.LANCZOS)
-
-
-def image_grid(imgs, batch_size=1, rows=None):
- if rows is None:
- if opts.n_rows > 0:
- rows = opts.n_rows
- elif opts.n_rows == 0:
- rows = batch_size
- elif opts.grid_prevent_empty_spots:
- rows = math.floor(math.sqrt(len(imgs)))
- while len(imgs) % rows != 0:
- rows -= 1
- else:
- rows = math.sqrt(len(imgs))
- rows = round(rows)
- if rows > len(imgs):
- rows = len(imgs)
-
- cols = math.ceil(len(imgs) / rows)
-
- params = script_callbacks.ImageGridLoopParams(imgs, cols, rows)
- script_callbacks.image_grid_callback(params)
-
- w, h = imgs[0].size
- grid = Image.new('RGB', size=(params.cols * w, params.rows * h), color='black')
-
- for i, img in enumerate(params.imgs):
- grid.paste(img, box=(i % params.cols * w, i // params.cols * h))
-
- return grid
-
-
-Grid = namedtuple("Grid", ["tiles", "tile_w", "tile_h", "image_w", "image_h", "overlap"])
-
-
-def split_grid(image, tile_w=512, tile_h=512, overlap=64):
- w = image.width
- h = image.height
-
- non_overlap_width = tile_w - overlap
- non_overlap_height = tile_h - overlap
-
- cols = math.ceil((w - overlap) / non_overlap_width)
- rows = math.ceil((h - overlap) / non_overlap_height)
-
- dx = (w - tile_w) / (cols - 1) if cols > 1 else 0
- dy = (h - tile_h) / (rows - 1) if rows > 1 else 0
-
- grid = Grid([], tile_w, tile_h, w, h, overlap)
- for row in range(rows):
- row_images = []
-
- y = int(row * dy)
-
- if y + tile_h >= h:
- y = h - tile_h
-
- for col in range(cols):
- x = int(col * dx)
-
- if x + tile_w >= w:
- x = w - tile_w
-
- tile = image.crop((x, y, x + tile_w, y + tile_h))
-
- row_images.append([x, tile_w, tile])
-
- grid.tiles.append([y, tile_h, row_images])
-
- return grid
-
-
-def combine_grid(grid):
- def make_mask_image(r):
- r = r * 255 / grid.overlap
- r = r.astype(np.uint8)
- return Image.fromarray(r, 'L')
-
- mask_w = make_mask_image(np.arange(grid.overlap, dtype=np.float32).reshape((1, grid.overlap)).repeat(grid.tile_h, axis=0))
- mask_h = make_mask_image(np.arange(grid.overlap, dtype=np.float32).reshape((grid.overlap, 1)).repeat(grid.image_w, axis=1))
-
- combined_image = Image.new("RGB", (grid.image_w, grid.image_h))
- for y, h, row in grid.tiles:
- combined_row = Image.new("RGB", (grid.image_w, h))
- for x, w, tile in row:
- if x == 0:
- combined_row.paste(tile, (0, 0))
- continue
-
- combined_row.paste(tile.crop((0, 0, grid.overlap, h)), (x, 0), mask=mask_w)
- combined_row.paste(tile.crop((grid.overlap, 0, w, h)), (x + grid.overlap, 0))
-
- if y == 0:
- combined_image.paste(combined_row, (0, 0))
- continue
-
- combined_image.paste(combined_row.crop((0, 0, combined_row.width, grid.overlap)), (0, y), mask=mask_h)
- combined_image.paste(combined_row.crop((0, grid.overlap, combined_row.width, h)), (0, y + grid.overlap))
-
- return combined_image
-
-
-class GridAnnotation:
- def __init__(self, text='', is_active=True):
- self.text = text
- self.is_active = is_active
- self.size = None
-
-
-def draw_grid_annotations(im, width, height, hor_texts, ver_texts, margin=0):
- def wrap(drawing, text, font, line_length):
- lines = ['']
- for word in text.split():
- line = f'{lines[-1]} {word}'.strip()
- if drawing.textlength(line, font=font) <= line_length:
- lines[-1] = line
- else:
- lines.append(word)
- return lines
-
- def get_font(fontsize):
- try:
- return ImageFont.truetype(opts.font or Roboto, fontsize)
- except Exception:
- return ImageFont.truetype(Roboto, fontsize)
-
- def draw_texts(drawing, draw_x, draw_y, lines, initial_fnt, initial_fontsize):
- for i, line in enumerate(lines):
- fnt = initial_fnt
- fontsize = initial_fontsize
- while drawing.multiline_textsize(line.text, font=fnt)[0] > line.allowed_width and fontsize > 0:
- fontsize -= 1
- fnt = get_font(fontsize)
- drawing.multiline_text((draw_x, draw_y + line.size[1] / 2), line.text, font=fnt, fill=color_active if line.is_active else color_inactive, anchor="mm", align="center")
-
- if not line.is_active:
- drawing.line((draw_x - line.size[0] // 2, draw_y + line.size[1] // 2, draw_x + line.size[0] // 2, draw_y + line.size[1] // 2), fill=color_inactive, width=4)
-
- draw_y += line.size[1] + line_spacing
-
- fontsize = (width + height) // 25
- line_spacing = fontsize // 2
-
- fnt = get_font(fontsize)
-
- color_active = (0, 0, 0)
- color_inactive = (153, 153, 153)
-
- pad_left = 0 if sum([sum([len(line.text) for line in lines]) for lines in ver_texts]) == 0 else width * 3 // 4
-
- cols = im.width // width
- rows = im.height // height
-
- assert cols == len(hor_texts), f'bad number of horizontal texts: {len(hor_texts)}; must be {cols}'
- assert rows == len(ver_texts), f'bad number of vertical texts: {len(ver_texts)}; must be {rows}'
-
- calc_img = Image.new("RGB", (1, 1), "white")
- calc_d = ImageDraw.Draw(calc_img)
-
- for texts, allowed_width in zip(hor_texts + ver_texts, [width] * len(hor_texts) + [pad_left] * len(ver_texts)):
- items = [] + texts
- texts.clear()
-
- for line in items:
- wrapped = wrap(calc_d, line.text, fnt, allowed_width)
- texts += [GridAnnotation(x, line.is_active) for x in wrapped]
-
- for line in texts:
- bbox = calc_d.multiline_textbbox((0, 0), line.text, font=fnt)
- line.size = (bbox[2] - bbox[0], bbox[3] - bbox[1])
- line.allowed_width = allowed_width
-
- hor_text_heights = [sum([line.size[1] + line_spacing for line in lines]) - line_spacing for lines in hor_texts]
- ver_text_heights = [sum([line.size[1] + line_spacing for line in lines]) - line_spacing * len(lines) for lines in ver_texts]
-
- pad_top = 0 if sum(hor_text_heights) == 0 else max(hor_text_heights) + line_spacing * 2
-
- result = Image.new("RGB", (im.width + pad_left + margin * (cols-1), im.height + pad_top + margin * (rows-1)), "white")
-
- for row in range(rows):
- for col in range(cols):
- cell = im.crop((width * col, height * row, width * (col+1), height * (row+1)))
- result.paste(cell, (pad_left + (width + margin) * col, pad_top + (height + margin) * row))
-
- d = ImageDraw.Draw(result)
-
- for col in range(cols):
- x = pad_left + (width + margin) * col + width / 2
- y = pad_top / 2 - hor_text_heights[col] / 2
-
- draw_texts(d, x, y, hor_texts[col], fnt, fontsize)
-
- for row in range(rows):
- x = pad_left / 2
- y = pad_top + (height + margin) * row + height / 2 - ver_text_heights[row] / 2
-
- draw_texts(d, x, y, ver_texts[row], fnt, fontsize)
-
- return result
-
-
-def draw_prompt_matrix(im, width, height, all_prompts, margin=0):
- prompts = all_prompts[1:]
- boundary = math.ceil(len(prompts) / 2)
-
- prompts_horiz = prompts[:boundary]
- prompts_vert = prompts[boundary:]
-
- hor_texts = [[GridAnnotation(x, is_active=pos & (1 << i) != 0) for i, x in enumerate(prompts_horiz)] for pos in range(1 << len(prompts_horiz))]
- ver_texts = [[GridAnnotation(x, is_active=pos & (1 << i) != 0) for i, x in enumerate(prompts_vert)] for pos in range(1 << len(prompts_vert))]
-
- return draw_grid_annotations(im, width, height, hor_texts, ver_texts, margin)
-
-
-def resize_image(resize_mode, im, width, height, upscaler_name=None):
- """
- Resizes an image with the specified resize_mode, width, and height.
-
- Args:
- resize_mode: The mode to use when resizing the image.
- 0: Resize the image to the specified width and height.
- 1: Resize the image to fill the specified width and height, maintaining the aspect ratio, and then center the image within the dimensions, cropping the excess.
- 2: Resize the image to fit within the specified width and height, maintaining the aspect ratio, and then center the image within the dimensions, filling empty with data from image.
- im: The image to resize.
- width: The width to resize the image to.
- height: The height to resize the image to.
- upscaler_name: The name of the upscaler to use. If not provided, defaults to opts.upscaler_for_img2img.
- """
-
- upscaler_name = upscaler_name or opts.upscaler_for_img2img
-
- def resize(im, w, h):
- if upscaler_name is None or upscaler_name == "None" or im.mode == 'L':
- return im.resize((w, h), resample=LANCZOS)
-
- scale = max(w / im.width, h / im.height)
-
- if scale > 1.0:
- upscalers = [x for x in shared.sd_upscalers if x.name == upscaler_name]
- assert len(upscalers) > 0, f"could not find upscaler named {upscaler_name}"
-
- upscaler = upscalers[0]
- im = upscaler.scaler.upscale(im, scale, upscaler.data_path)
-
- if im.width != w or im.height != h:
- im = im.resize((w, h), resample=LANCZOS)
-
- return im
-
- if resize_mode == 0:
- res = resize(im, width, height)
-
- elif resize_mode == 1:
- ratio = width / height
- src_ratio = im.width / im.height
-
- src_w = width if ratio > src_ratio else im.width * height // im.height
- src_h = height if ratio <= src_ratio else im.height * width // im.width
-
- resized = resize(im, src_w, src_h)
- res = Image.new("RGB", (width, height))
- res.paste(resized, box=(width // 2 - src_w // 2, height // 2 - src_h // 2))
-
- else:
- ratio = width / height
- src_ratio = im.width / im.height
-
- src_w = width if ratio < src_ratio else im.width * height // im.height
- src_h = height if ratio >= src_ratio else im.height * width // im.width
-
- resized = resize(im, src_w, src_h)
- res = Image.new("RGB", (width, height))
- res.paste(resized, box=(width // 2 - src_w // 2, height // 2 - src_h // 2))
-
- if ratio < src_ratio:
- fill_height = height // 2 - src_h // 2
- res.paste(resized.resize((width, fill_height), box=(0, 0, width, 0)), box=(0, 0))
- res.paste(resized.resize((width, fill_height), box=(0, resized.height, width, resized.height)), box=(0, fill_height + src_h))
- elif ratio > src_ratio:
- fill_width = width // 2 - src_w // 2
- res.paste(resized.resize((fill_width, height), box=(0, 0, 0, height)), box=(0, 0))
- res.paste(resized.resize((fill_width, height), box=(resized.width, 0, resized.width, height)), box=(fill_width + src_w, 0))
-
- return res
-
-
-invalid_filename_chars = '<>:"/\\|?*\n'
-invalid_filename_prefix = ' '
-invalid_filename_postfix = ' .'
-re_nonletters = re.compile(r'[\s' + string.punctuation + ']+')
-re_pattern = re.compile(r"(.*?)(?:\[([^\[\]]+)\]|$)")
-re_pattern_arg = re.compile(r"(.*)<([^>]*)>$")
-max_filename_part_length = 128
-
-
-def sanitize_filename_part(text, replace_spaces=True):
- if text is None:
- return None
-
- if replace_spaces:
- text = text.replace(' ', '_')
-
- text = text.translate({ord(x): '_' for x in invalid_filename_chars})
- text = text.lstrip(invalid_filename_prefix)[:max_filename_part_length]
- text = text.rstrip(invalid_filename_postfix)
- return text
-
-
-class FilenameGenerator:
- replacements = {
- 'seed': lambda self: self.seed if self.seed is not None else '',
- 'steps': lambda self: self.p and self.p.steps,
- 'cfg': lambda self: self.p and self.p.cfg_scale,
- 'width': lambda self: self.image.width,
- 'height': lambda self: self.image.height,
- 'styles': lambda self: self.p and sanitize_filename_part(", ".join([style for style in self.p.styles if not style == "None"]) or "None", replace_spaces=False),
- 'sampler': lambda self: self.p and sanitize_filename_part(self.p.sampler_name, replace_spaces=False),
- 'model_hash': lambda self: getattr(self.p, "sd_model_hash", shared.sd_model.sd_model_hash),
- 'model_name': lambda self: sanitize_filename_part(shared.sd_model.sd_checkpoint_info.model_name, replace_spaces=False),
- 'date': lambda self: datetime.datetime.now().strftime('%Y-%m-%d'),
- 'datetime': lambda self, *args: self.datetime(*args), # accepts formats: [datetime], [datetime], [datetime]
- 'job_timestamp': lambda self: getattr(self.p, "job_timestamp", shared.state.job_timestamp),
- 'prompt_hash': lambda self: hashlib.sha256(self.prompt.encode()).hexdigest()[0:8],
- 'prompt': lambda self: sanitize_filename_part(self.prompt),
- 'prompt_no_styles': lambda self: self.prompt_no_style(),
- 'prompt_spaces': lambda self: sanitize_filename_part(self.prompt, replace_spaces=False),
- 'prompt_words': lambda self: self.prompt_words(),
- }
- default_time_format = '%Y%m%d%H%M%S'
-
- def __init__(self, p, seed, prompt, image):
- self.p = p
- self.seed = seed
- self.prompt = prompt
- self.image = image
-
- def prompt_no_style(self):
- if self.p is None or self.prompt is None:
- return None
-
- prompt_no_style = self.prompt
- for style in shared.prompt_styles.get_style_prompts(self.p.styles):
- if len(style) > 0:
- for part in style.split("{prompt}"):
- prompt_no_style = prompt_no_style.replace(part, "").replace(", ,", ",").strip().strip(',')
-
- prompt_no_style = prompt_no_style.replace(style, "").strip().strip(',').strip()
-
- return sanitize_filename_part(prompt_no_style, replace_spaces=False)
-
- def prompt_words(self):
- words = [x for x in re_nonletters.split(self.prompt or "") if len(x) > 0]
- if len(words) == 0:
- words = ["empty"]
- return sanitize_filename_part(" ".join(words[0:opts.directories_max_prompt_words]), replace_spaces=False)
-
- def datetime(self, *args):
- time_datetime = datetime.datetime.now()
-
- time_format = args[0] if len(args) > 0 and args[0] != "" else self.default_time_format
- try:
- time_zone = pytz.timezone(args[1]) if len(args) > 1 else None
- except pytz.exceptions.UnknownTimeZoneError as _:
- time_zone = None
-
- time_zone_time = time_datetime.astimezone(time_zone)
- try:
- formatted_time = time_zone_time.strftime(time_format)
- except (ValueError, TypeError) as _:
- formatted_time = time_zone_time.strftime(self.default_time_format)
-
- return sanitize_filename_part(formatted_time, replace_spaces=False)
-
- def apply(self, x):
- res = ''
-
- for m in re_pattern.finditer(x):
- text, pattern = m.groups()
- res += text
-
- if pattern is None:
- continue
-
- pattern_args = []
- while True:
- m = re_pattern_arg.match(pattern)
- if m is None:
- break
-
- pattern, arg = m.groups()
- pattern_args.insert(0, arg)
-
- fun = self.replacements.get(pattern.lower())
- if fun is not None:
- try:
- replacement = fun(self, *pattern_args)
- except Exception:
- replacement = None
- print(f"Error adding [{pattern}] to filename", file=sys.stderr)
- print(traceback.format_exc(), file=sys.stderr)
-
- if replacement is not None:
- res += str(replacement)
- continue
-
- res += f'[{pattern}]'
-
- return res
-
-
-def get_next_sequence_number(path, basename):
- """
- Determines and returns the next sequence number to use when saving an image in the specified directory.
-
- The sequence starts at 0.
- """
- result = -1
- if basename != '':
- basename = basename + "-"
-
- prefix_length = len(basename)
- for p in os.listdir(path):
- if p.startswith(basename):
- l = os.path.splitext(p[prefix_length:])[0].split('-') # splits the filename (removing the basename first if one is defined, so the sequence number is always the first element)
- try:
- result = max(int(l[0]), result)
- except ValueError:
- pass
-
- return result + 1
-
-
-def save_image(image, path, basename, seed=None, prompt=None, extension='png', info=None, short_filename=False, no_prompt=False, grid=False, pnginfo_section_name='parameters', p=None, existing_info=None, forced_filename=None, suffix="", save_to_dirs=None):
- """Save an image.
-
- Args:
- image (`PIL.Image`):
- The image to be saved.
- path (`str`):
- The directory to save the image. Note, the option `save_to_dirs` will make the image to be saved into a sub directory.
- basename (`str`):
- The base filename which will be applied to `filename pattern`.
- seed, prompt, short_filename,
- extension (`str`):
- Image file extension, default is `png`.
- pngsectionname (`str`):
- Specify the name of the section which `info` will be saved in.
- info (`str` or `PngImagePlugin.iTXt`):
- PNG info chunks.
- existing_info (`dict`):
- Additional PNG info. `existing_info == {pngsectionname: info, ...}`
- no_prompt:
- TODO I don't know its meaning.
- p (`StableDiffusionProcessing`)
- forced_filename (`str`):
- If specified, `basename` and filename pattern will be ignored.
- save_to_dirs (bool):
- If true, the image will be saved into a subdirectory of `path`.
-
- Returns: (fullfn, txt_fullfn)
- fullfn (`str`):
- The full path of the saved imaged.
- txt_fullfn (`str` or None):
- If a text file is saved for this image, this will be its full path. Otherwise None.
- """
- namegen = FilenameGenerator(p, seed, prompt, image)
-
- if save_to_dirs is None:
- save_to_dirs = (grid and opts.grid_save_to_dirs) or (not grid and opts.save_to_dirs and not no_prompt)
-
- if save_to_dirs:
- dirname = namegen.apply(opts.directories_filename_pattern or "[prompt_words]").lstrip(' ').rstrip('\\ /')
- path = os.path.join(path, dirname)
-
- os.makedirs(path, exist_ok=True)
-
- if forced_filename is None:
- if short_filename or seed is None:
- file_decoration = ""
- elif opts.save_to_dirs:
- file_decoration = opts.samples_filename_pattern or "[seed]"
- else:
- file_decoration = opts.samples_filename_pattern or "[seed]-[prompt_spaces]"
-
- add_number = opts.save_images_add_number or file_decoration == ''
-
- if file_decoration != "" and add_number:
- file_decoration = "-" + file_decoration
-
- file_decoration = namegen.apply(file_decoration) + suffix
-
- if add_number:
- basecount = get_next_sequence_number(path, basename)
- fullfn = None
- for i in range(500):
- fn = f"{basecount + i:05}" if basename == '' else f"{basename}-{basecount + i:04}"
- fullfn = os.path.join(path, f"{fn}{file_decoration}.{extension}")
- if not os.path.exists(fullfn):
- break
- else:
- fullfn = os.path.join(path, f"{file_decoration}.{extension}")
- else:
- fullfn = os.path.join(path, f"{forced_filename}.{extension}")
-
- pnginfo = existing_info or {}
- if info is not None:
- pnginfo[pnginfo_section_name] = info
-
- params = script_callbacks.ImageSaveParams(image, p, fullfn, pnginfo)
- script_callbacks.before_image_saved_callback(params)
-
- image = params.image
- fullfn = params.filename
- info = params.pnginfo.get(pnginfo_section_name, None)
-
- def _atomically_save_image(image_to_save, filename_without_extension, extension):
- # save image with .tmp extension to avoid race condition when another process detects new image in the directory
- temp_file_path = filename_without_extension + ".tmp"
- image_format = Image.registered_extensions()[extension]
-
- if extension.lower() == '.png':
- pnginfo_data = PngImagePlugin.PngInfo()
- if opts.enable_pnginfo:
- for k, v in params.pnginfo.items():
- pnginfo_data.add_text(k, str(v))
-
- image_to_save.save(temp_file_path, format=image_format, quality=opts.jpeg_quality, pnginfo=pnginfo_data)
-
- elif extension.lower() in (".jpg", ".jpeg", ".webp"):
- if image_to_save.mode == 'RGBA':
- image_to_save = image_to_save.convert("RGB")
- elif image_to_save.mode == 'I;16':
- image_to_save = image_to_save.point(lambda p: p * 0.0038910505836576).convert("RGB" if extension.lower() == ".webp" else "L")
-
- image_to_save.save(temp_file_path, format=image_format, quality=opts.jpeg_quality)
-
- if opts.enable_pnginfo and info is not None:
- exif_bytes = piexif.dump({
- "Exif": {
- piexif.ExifIFD.UserComment: piexif.helper.UserComment.dump(info or "", encoding="unicode")
- },
- })
-
- piexif.insert(exif_bytes, temp_file_path)
- else:
- image_to_save.save(temp_file_path, format=image_format, quality=opts.jpeg_quality)
-
- # atomically rename the file with correct extension
- os.replace(temp_file_path, filename_without_extension + extension)
-
- fullfn_without_extension, extension = os.path.splitext(params.filename)
- _atomically_save_image(image, fullfn_without_extension, extension)
-
- image.already_saved_as = fullfn
-
- oversize = image.width > opts.target_side_length or image.height > opts.target_side_length
- if opts.export_for_4chan and (oversize or os.stat(fullfn).st_size > opts.img_downscale_threshold * 1024 * 1024):
- ratio = image.width / image.height
-
- if oversize and ratio > 1:
- image = image.resize((round(opts.target_side_length), round(image.height * opts.target_side_length / image.width)), LANCZOS)
- elif oversize:
- image = image.resize((round(image.width * opts.target_side_length / image.height), round(opts.target_side_length)), LANCZOS)
-
- try:
- _atomically_save_image(image, fullfn_without_extension, ".jpg")
- except Exception as e:
- errors.display(e, "saving image as downscaled JPG")
-
- if opts.save_txt and info is not None:
- txt_fullfn = f"{fullfn_without_extension}.txt"
- with open(txt_fullfn, "w", encoding="utf8") as file:
- file.write(info + "\n")
- else:
- txt_fullfn = None
-
- script_callbacks.image_saved_callback(params)
-
- return fullfn, txt_fullfn
-
-
-def read_info_from_image(image):
- items = image.info or {}
-
- geninfo = items.pop('parameters', None)
-
- if "exif" in items:
- exif = piexif.load(items["exif"])
- exif_comment = (exif or {}).get("Exif", {}).get(piexif.ExifIFD.UserComment, b'')
- try:
- exif_comment = piexif.helper.UserComment.load(exif_comment)
- except ValueError:
- exif_comment = exif_comment.decode('utf8', errors="ignore")
-
- if exif_comment:
- items['exif comment'] = exif_comment
- geninfo = exif_comment
-
- for field in ['jfif', 'jfif_version', 'jfif_unit', 'jfif_density', 'dpi', 'exif',
- 'loop', 'background', 'timestamp', 'duration']:
- items.pop(field, None)
-
- if items.get("Software", None) == "NovelAI":
- try:
- json_info = json.loads(items["Comment"])
- sampler = sd_samplers.samplers_map.get(json_info["sampler"], "Euler a")
-
- geninfo = f"""{items["Description"]}
-Negative prompt: {json_info["uc"]}
-Steps: {json_info["steps"]}, Sampler: {sampler}, CFG scale: {json_info["scale"]}, Seed: {json_info["seed"]}, Size: {image.width}x{image.height}, Clip skip: 2, ENSD: 31337"""
- except Exception:
- print("Error parsing NovelAI image generation parameters:", file=sys.stderr)
- print(traceback.format_exc(), file=sys.stderr)
-
- return geninfo, items
-
-
-def image_data(data):
- try:
- image = Image.open(io.BytesIO(data))
- textinfo, _ = read_info_from_image(image)
- return textinfo, None
- except Exception:
- pass
-
- try:
- text = data.decode('utf8')
- assert len(text) < 10000
- return text, None
-
- except Exception:
- pass
-
- return '', None
-
-
-def flatten(img, bgcolor):
- """replaces transparency with bgcolor (example: "#ffffff"), returning an RGB mode image with no transparency"""
-
- if img.mode == "RGBA":
- background = Image.new('RGBA', img.size, bgcolor)
- background.paste(img, mask=img)
- img = background
-
- return img.convert('RGB')
diff --git a/spaces/arch-123/bingo/src/components/button-scroll-to-bottom.tsx b/spaces/arch-123/bingo/src/components/button-scroll-to-bottom.tsx
deleted file mode 100644
index b68ab9c0e48320c356e51a52d11b9ca63909e6c5..0000000000000000000000000000000000000000
--- a/spaces/arch-123/bingo/src/components/button-scroll-to-bottom.tsx
+++ /dev/null
@@ -1,34 +0,0 @@
-'use client'
-
-import * as React from 'react'
-
-import { cn } from '@/lib/utils'
-import { useAtBottom } from '@/lib/hooks/use-at-bottom'
-import { Button, type ButtonProps } from '@/components/ui/button'
-import { IconArrowDown } from '@/components/ui/icons'
-
-export function ButtonScrollToBottom({ className, ...props }: ButtonProps) {
- const isAtBottom = useAtBottom()
-
- return (
-
- window.scrollTo({
- top: document.body.offsetHeight,
- behavior: 'smooth'
- })
- }
- {...props}
- >
-
- Scroll to bottom
-
- )
-}
diff --git a/spaces/arslvn/statuscertificate/app.py b/spaces/arslvn/statuscertificate/app.py
deleted file mode 100644
index 891e1da37cf3ae7a82da4a81be0b6e8a49e8a97f..0000000000000000000000000000000000000000
--- a/spaces/arslvn/statuscertificate/app.py
+++ /dev/null
@@ -1,221 +0,0 @@
-import urllib.request
-import fitz
-import re
-import numpy as np
-import tensorflow_hub as hub
-import openai
-import gradio as gr
-import os
-from sklearn.neighbors import NearestNeighbors
-
-
-def download_pdf(url, output_path):
- urllib.request.urlretrieve(url, output_path)
-
-
-def preprocess(text):
- text = text.replace('\n', ' ')
- text = re.sub('\s+', ' ', text)
- return text
-
-
-def pdf_to_text(path, start_page=1, end_page=None):
- doc = fitz.open(path)
- total_pages = doc.page_count
-
- if end_page is None:
- end_page = total_pages
-
- text_list = []
-
- for i in range(start_page-1, end_page):
- text = doc.load_page(i).get_text("text")
- text = preprocess(text)
- text_list.append(text)
-
- doc.close()
- return text_list
-
-
-def text_to_chunks(texts, word_length=150, start_page=1):
- text_toks = [t.split(' ') for t in texts]
- page_nums = []
- chunks = []
-
- for idx, words in enumerate(text_toks):
- for i in range(0, len(words), word_length):
- chunk = words[i:i+word_length]
- if (i+word_length) > len(words) and (len(chunk) < word_length) and (
- len(text_toks) != (idx+1)):
- text_toks[idx+1] = chunk + text_toks[idx+1]
- continue
- chunk = ' '.join(chunk).strip()
- chunk = f'[p. {idx+start_page}]' + ' ' + '"' + chunk + '"'
- chunks.append(chunk)
- return chunks
-
-
-class SemanticSearch:
-
- def __init__(self):
- self.use = hub.load('https://tfhub.dev/google/universal-sentence-encoder/4')
- self.fitted = False
-
-
- def fit(self, data, batch=1000, n_neighbors=5):
- self.data = data
- self.embeddings = self.get_text_embedding(data, batch=batch)
- n_neighbors = min(n_neighbors, len(self.embeddings))
- self.nn = NearestNeighbors(n_neighbors=n_neighbors)
- self.nn.fit(self.embeddings)
- self.fitted = True
-
-
- def __call__(self, text, return_data=True):
- inp_emb = self.use([text])
- neighbors = self.nn.kneighbors(inp_emb, return_distance=False)[0]
-
- if return_data:
- return [self.data[i] for i in neighbors]
- else:
- return neighbors
-
-
- def get_text_embedding(self, texts, batch=1000):
- embeddings = []
- for i in range(0, len(texts), batch):
- text_batch = texts[i:(i+batch)]
- emb_batch = self.use(text_batch)
- embeddings.append(emb_batch)
- embeddings = np.vstack(embeddings)
- return embeddings
-
-
-
-def load_recommender(path, start_page=1):
- global recommender
- texts = pdf_to_text(path, start_page=start_page)
- chunks = text_to_chunks(texts, start_page=start_page)
- recommender.fit(chunks)
- return 'Corpus Loaded.'
-
-def generate_text(openAI_key,prompt, engine="text-davinci-003"):
- openAI_key = os.environ["OPENAI_API_KEY"]
- openai.api_key = openAI_key
- completions = openai.Completion.create(
- engine=engine,
- prompt=prompt,
- max_tokens=512,
- n=1,
- stop=None,
- temperature=0.7,
- )
- message = completions.choices[0].text
- return message
-
-def generate_answer(question, openAI_key):
- topn_chunks = recommender(question)
- prompt = ""
- prompt += 'search results:\n\n'
- for c in topn_chunks:
- prompt += c + '\n\n'
-
- prompt += "Instructions: Compose a comprehensive reply to the query using the search results given. "\
- "Cite each reference using [ Page Number] notation (every result has this number at the beginning). "\
- "Citation should be done at the end of each sentence. If the search results mention multiple subjects "\
- "with the same name, create separate answers for each. Only include information found in the results and "\
- "don't add any additional information. Make sure the answer is correct and don't output false content. "\
- "If the text does not relate to the query, simply state 'Text Not Found in PDF'. Ignore outlier "\
- "search results which has nothing to do with the question. Only answer what is asked. The "\
- "answer should be short and concise. Answer step-by-step. \n\nQuery: {question}\nAnswer: "
-
- prompt += f"Query: {question}\nAnswer:"
- answer = generate_text(openAI_key, prompt,"text-davinci-003")
- return answer
-
-def question_answer(file, openAI_key):
- old_file_name = file.name
- file_name = file.name
- file_name = file_name[:-12] + file_name[-4:]
- os.rename(old_file_name, file_name)
- load_recommender(file_name)
- questions = [
- "What address is this status certificate for?",
- "When is the date of this Status Certificate?",
- "When is the date of the Condo Registration (Incorporation)? (This refers to the date when the condo corporation was officially incorporated.)",
- "When is the date of the Last Annual General Meeting?",
- "What is the date of the Latest Financial Reports?",
- "How many residential units are there?",
- "How many units are leased?",
- "What are the common facilities for residents?",
- "What are the rules regarding noise complaints and how are they enforced?",
- "What are the visitor parking rules and regulations?",
- "What are the policies for moving in and out of the building, including any fees or deposits required?",
- "What is the policy regarding bicycles, including storage and use of common areas?",
- "What is the procedure for requesting access to or reserving common areas or facilities?",
- "What are the rules regarding installation of satellite dishes or other exterior fixtures?",
- "What are the rules regarding window coverings, balcony furniture, or other visible elements of the unit from the exterior?",
- "What are the contact details of the Property Management and concierge/frontdesk/super?",
- "What are the contact details of the concierge?",
- "What is the current funding plan for the reserve fund? is it adequately funded to cover major expenses that may arise?",
- "Will the maintenance fees be increasing? The monthly contribution from the residents",
- "Can you confirm that there are no current or pending Special Assessments listed in the status certificate?",
- "Are there any pending or potential legal actions that the condominium corporation is currently facing?",
- "Are the increases in expenses from year to year?",
- "Are there any restrictions on pet ownership, such as types of pets allowed, number of pets, or size of pets?",
- "What are the smoking restrictions outlined in the Rules and Regulations section, if any?",
- "Is there a minimum lease term?",
- "What are the restrictions, if any, on renovations or alterations to the unit or common elements?",
- "Are there any provisions for electric vehicle charging stations in the building?"
- ]
-
- answers = ""
- for idx, question in enumerate(questions):
- answer = generate_answer(question, openAI_key)
-
- # Check if the answer is "Text Not Found in PDF." and skip the question if it is.
- if "Text Not Found in PDF" in answer:
- continue
-
- answers += f"{question}\n{answer}\n\n"
- return answers
-
-recommender = SemanticSearch()
-
-my_theme = gr.Theme.from_hub("nota-ai/theme")
-
-with gr.Blocks(theme=my_theme) as demo:
- with gr.Row().style(equal_height=False):
- with gr.Column(scale=10):
- gr.Markdown(
- """
- # statuscertificate.`ai`
- upload a status certificate and hit **`Analyze`** to unlock the power of ai-driven insights
- to ensure accurate results, make sure your **status certificate pdf is a single file** and has undergone **OCR**
- """
- )
-
- with gr.Row().style(equal_height=True):
-
- with gr.Column(variant="panel", scale=1):
- file = gr.File(label='Upload Status Certificate PDF', file_types=['.pdf'])
- btn = gr.Button(value='Analyze', label="Primary Button", variant="primary")
- btn.style(full_width=None)
-
- with gr.Column(variant="panel", scale=2):
- gr.Markdown("**`ai powered`**")
- answer = gr.TextArea(label= "status certificate analysis:", lines=20).style(show_copy_button=True)
-
- openAI_key = os.environ["OPENAI_API_KEY"]
- btn.click(question_answer, inputs=[file], outputs=[answer])
-
- with gr.Row():
-
- name = gr.Textbox(
- label="Please note that the analysis of status certificates has been conducted using artificial intelligence and while it is highly accurate in identifying information contained in the status certificate, it should not be considered a substitute for professional legal advice for interpreting and analyzing the information.",
- placeholder="It is important to consult with a licensed attorney for any legal advice and interpretation related to the status certificate, as this document may have legal implications and require specialized knowledge.",
- value="It is important to consult with a licensed attorney for any legal advice and interpretation related to the status certificate, as this document may have legal implications and require specialized knowledge.",
- interactive=False,
- )
-
-demo.launch()
\ No newline at end of file
diff --git a/spaces/arxify/RVC-beta-v2-0618/docs/training_tips_ja.md b/spaces/arxify/RVC-beta-v2-0618/docs/training_tips_ja.md
deleted file mode 100644
index c5b06f2fdaa603a690c51ee2b79daecc4305fbd5..0000000000000000000000000000000000000000
--- a/spaces/arxify/RVC-beta-v2-0618/docs/training_tips_ja.md
+++ /dev/null
@@ -1,64 +0,0 @@
-RVCの訓練における説明、およびTIPS
-===============================
-本TIPSではどのようにデータの訓練が行われているかを説明します。
-
-# 訓練の流れ
-GUIの訓練タブのstepに沿って説明します。
-
-## step1
-実験名の設定を行います。
-
-また、モデルに音高ガイド(ピッチ)を考慮させるかもここで設定できます。考慮させない場合はモデルは軽量になりますが、歌唱には向かなくなります。
-
-各実験のデータは`/logs/実験名/`に配置されます。
-
-## step2a
-音声の読み込みと前処理を行います。
-
-### load audio
-音声のあるフォルダを指定すると、そのフォルダ内にある音声ファイルを自動で読み込みます。
-例えば`C:Users\hoge\voices`を指定した場合、`C:Users\hoge\voices\voice.mp3`は読み込まれますが、`C:Users\hoge\voices\dir\voice.mp3`は読み込まれません。
-
-音声の読み込みには内部でffmpegを利用しているので、ffmpegで対応している拡張子であれば自動的に読み込まれます。
-ffmpegでint16に変換した後、float32に変換し、-1 ~ 1の間に正規化されます。
-
-### denoising
-音声についてscipyのfiltfiltによる平滑化を行います。
-
-### 音声の分割
-入力した音声はまず、一定期間(max_sil_kept=5秒?)より長く無音が続く部分を検知して音声を分割します。無音で音声を分割した後は、0.3秒のoverlapを含む4秒ごとに音声を分割します。4秒以内に区切られた音声は、音量の正規化を行った後wavファイルを`/logs/実験名/0_gt_wavs`に、そこから16kのサンプリングレートに変換して`/logs/実験名/1_16k_wavs`にwavファイルで保存します。
-
-## step2b
-### ピッチの抽出
-wavファイルからピッチ(音の高低)の情報を抽出します。parselmouthやpyworldに内蔵されている手法でピッチ情報(=f0)を抽出し、`/logs/実験名/2a_f0`に保存します。その後、ピッチ情報を対数で変換して1~255の整数に変換し、`/logs/実験名/2b-f0nsf`に保存します。
-
-### feature_printの抽出
-HuBERTを用いてwavファイルを事前にembeddingに変換します。`/logs/実験名/1_16k_wavs`に保存したwavファイルを読み込み、HuBERTでwavファイルを256次元の特徴量に変換し、npy形式で`/logs/実験名/3_feature256`に保存します。
-
-## step3
-モデルのトレーニングを行います。
-### 初心者向け用語解説
-深層学習ではデータセットを分割し、少しずつ学習を進めていきます。一回のモデルの更新(step)では、batch_size個のデータを取り出し予測と誤差の修正を行います。これをデータセットに対して一通り行うと一epochと数えます。
-
-そのため、学習時間は 1step当たりの学習時間 x (データセット内のデータ数 ÷ バッチサイズ) x epoch数 かかります。一般にバッチサイズを大きくするほど学習は安定し、(1step当たりの学習時間÷バッチサイズ)は小さくなりますが、その分GPUのメモリを多く使用します。GPUのRAMはnvidia-smiコマンド等で確認できます。実行環境のマシンに合わせてバッチサイズをできるだけ大きくするとより短時間で学習が可能です。
-
-### pretrained modelの指定
-RVCではモデルの訓練を0からではなく、事前学習済みの重みから開始するため、少ないデータセットで学習を行えます。
-
-デフォルトでは
-
-- 音高ガイドを考慮する場合、`RVCのある場所/pretrained/f0G40k.pth`と`RVCのある場所/pretrained/f0D40k.pth`を読み込みます。
-- 音高ガイドを考慮しない場合、`RVCのある場所/pretrained/G40k.pth`と`RVCのある場所/pretrained/D40k.pth`を読み込みます。
-
-学習時はsave_every_epochごとにモデルのパラメータが`logs/実験名/G_{}.pth`と`logs/実験名/D_{}.pth`に保存されますが、このパスを指定することで学習を再開したり、もしくは違う実験で学習したモデルの重みから学習を開始できます。
-
-### indexの学習
-RVCでは学習時に使われたHuBERTの特徴量を保存し、推論時は学習時の特徴量から近い特徴量を探してきて推論を行います。この検索を高速に行うために事前にindexの学習を行います。
-indexの学習には近似近傍探索ライブラリのfaissを用います。`/logs/実験名/3_feature256`の特徴量を読み込み、それを用いて学習したindexを`/logs/実験名/add_XXX.index`として保存します。
-(20230428updateよりtotal_fea.npyはindexから読み込むので不要になりました。)
-
-### ボタンの説明
-- モデルのトレーニング: step2bまでを実行した後、このボタンを押すとモデルの学習を行います。
-- 特徴インデックスのトレーニング: モデルのトレーニング後、indexの学習を行います。
-- ワンクリックトレーニング: step2bまでとモデルのトレーニング、特徴インデックスのトレーニングを一括で行います。
-
diff --git a/spaces/arxify/RVC-beta-v2-0618/runtime/Lib/site-packages/PIL/FliImagePlugin.py b/spaces/arxify/RVC-beta-v2-0618/runtime/Lib/site-packages/PIL/FliImagePlugin.py
deleted file mode 100644
index 908bed9f4272a2195c995981fcc4b3ddcf9ad2a6..0000000000000000000000000000000000000000
--- a/spaces/arxify/RVC-beta-v2-0618/runtime/Lib/site-packages/PIL/FliImagePlugin.py
+++ /dev/null
@@ -1,171 +0,0 @@
-#
-# The Python Imaging Library.
-# $Id$
-#
-# FLI/FLC file handling.
-#
-# History:
-# 95-09-01 fl Created
-# 97-01-03 fl Fixed parser, setup decoder tile
-# 98-07-15 fl Renamed offset attribute to avoid name clash
-#
-# Copyright (c) Secret Labs AB 1997-98.
-# Copyright (c) Fredrik Lundh 1995-97.
-#
-# See the README file for information on usage and redistribution.
-#
-
-import os
-
-from . import Image, ImageFile, ImagePalette
-from ._binary import i16le as i16
-from ._binary import i32le as i32
-from ._binary import o8
-
-#
-# decoder
-
-
-def _accept(prefix):
- return (
- len(prefix) >= 6
- and i16(prefix, 4) in [0xAF11, 0xAF12]
- and i16(prefix, 14) in [0, 3] # flags
- )
-
-
-##
-# Image plugin for the FLI/FLC animation format. Use the seek
-# method to load individual frames.
-
-
-class FliImageFile(ImageFile.ImageFile):
-
- format = "FLI"
- format_description = "Autodesk FLI/FLC Animation"
- _close_exclusive_fp_after_loading = False
-
- def _open(self):
-
- # HEAD
- s = self.fp.read(128)
- if not (_accept(s) and s[20:22] == b"\x00\x00"):
- raise SyntaxError("not an FLI/FLC file")
-
- # frames
- self.n_frames = i16(s, 6)
- self.is_animated = self.n_frames > 1
-
- # image characteristics
- self.mode = "P"
- self._size = i16(s, 8), i16(s, 10)
-
- # animation speed
- duration = i32(s, 16)
- magic = i16(s, 4)
- if magic == 0xAF11:
- duration = (duration * 1000) // 70
- self.info["duration"] = duration
-
- # look for palette
- palette = [(a, a, a) for a in range(256)]
-
- s = self.fp.read(16)
-
- self.__offset = 128
-
- if i16(s, 4) == 0xF100:
- # prefix chunk; ignore it
- self.__offset = self.__offset + i32(s)
- s = self.fp.read(16)
-
- if i16(s, 4) == 0xF1FA:
- # look for palette chunk
- number_of_subchunks = i16(s, 6)
- chunk_size = None
- for _ in range(number_of_subchunks):
- if chunk_size is not None:
- self.fp.seek(chunk_size - 6, os.SEEK_CUR)
- s = self.fp.read(6)
- chunk_type = i16(s, 4)
- if chunk_type in (4, 11):
- self._palette(palette, 2 if chunk_type == 11 else 0)
- break
- chunk_size = i32(s)
- if not chunk_size:
- break
-
- palette = [o8(r) + o8(g) + o8(b) for (r, g, b) in palette]
- self.palette = ImagePalette.raw("RGB", b"".join(palette))
-
- # set things up to decode first frame
- self.__frame = -1
- self._fp = self.fp
- self.__rewind = self.fp.tell()
- self.seek(0)
-
- def _palette(self, palette, shift):
- # load palette
-
- i = 0
- for e in range(i16(self.fp.read(2))):
- s = self.fp.read(2)
- i = i + s[0]
- n = s[1]
- if n == 0:
- n = 256
- s = self.fp.read(n * 3)
- for n in range(0, len(s), 3):
- r = s[n] << shift
- g = s[n + 1] << shift
- b = s[n + 2] << shift
- palette[i] = (r, g, b)
- i += 1
-
- def seek(self, frame):
- if not self._seek_check(frame):
- return
- if frame < self.__frame:
- self._seek(0)
-
- for f in range(self.__frame + 1, frame + 1):
- self._seek(f)
-
- def _seek(self, frame):
- if frame == 0:
- self.__frame = -1
- self._fp.seek(self.__rewind)
- self.__offset = 128
- else:
- # ensure that the previous frame was loaded
- self.load()
-
- if frame != self.__frame + 1:
- raise ValueError(f"cannot seek to frame {frame}")
- self.__frame = frame
-
- # move to next frame
- self.fp = self._fp
- self.fp.seek(self.__offset)
-
- s = self.fp.read(4)
- if not s:
- raise EOFError
-
- framesize = i32(s)
-
- self.decodermaxblock = framesize
- self.tile = [("fli", (0, 0) + self.size, self.__offset, None)]
-
- self.__offset += framesize
-
- def tell(self):
- return self.__frame
-
-
-#
-# registry
-
-Image.register_open(FliImageFile.format, FliImageFile, _accept)
-
-Image.register_extensions(FliImageFile.format, [".fli", ".flc"])
diff --git a/spaces/arxify/RVC-beta-v2-0618/runtime/Lib/site-packages/dateutil/tz/tz.py b/spaces/arxify/RVC-beta-v2-0618/runtime/Lib/site-packages/dateutil/tz/tz.py
deleted file mode 100644
index c67f56d4659f17aab4540dfd42511bb850871a77..0000000000000000000000000000000000000000
--- a/spaces/arxify/RVC-beta-v2-0618/runtime/Lib/site-packages/dateutil/tz/tz.py
+++ /dev/null
@@ -1,1849 +0,0 @@
-# -*- coding: utf-8 -*-
-"""
-This module offers timezone implementations subclassing the abstract
-:py:class:`datetime.tzinfo` type. There are classes to handle tzfile format
-files (usually are in :file:`/etc/localtime`, :file:`/usr/share/zoneinfo`,
-etc), TZ environment string (in all known formats), given ranges (with help
-from relative deltas), local machine timezone, fixed offset timezone, and UTC
-timezone.
-"""
-import datetime
-import struct
-import time
-import sys
-import os
-import bisect
-import weakref
-from collections import OrderedDict
-
-import six
-from six import string_types
-from six.moves import _thread
-from ._common import tzname_in_python2, _tzinfo
-from ._common import tzrangebase, enfold
-from ._common import _validate_fromutc_inputs
-
-from ._factories import _TzSingleton, _TzOffsetFactory
-from ._factories import _TzStrFactory
-try:
- from .win import tzwin, tzwinlocal
-except ImportError:
- tzwin = tzwinlocal = None
-
-# For warning about rounding tzinfo
-from warnings import warn
-
-ZERO = datetime.timedelta(0)
-EPOCH = datetime.datetime.utcfromtimestamp(0)
-EPOCHORDINAL = EPOCH.toordinal()
-
-
-@six.add_metaclass(_TzSingleton)
-class tzutc(datetime.tzinfo):
- """
- This is a tzinfo object that represents the UTC time zone.
-
- **Examples:**
-
- .. doctest::
-
- >>> from datetime import *
- >>> from dateutil.tz import *
-
- >>> datetime.now()
- datetime.datetime(2003, 9, 27, 9, 40, 1, 521290)
-
- >>> datetime.now(tzutc())
- datetime.datetime(2003, 9, 27, 12, 40, 12, 156379, tzinfo=tzutc())
-
- >>> datetime.now(tzutc()).tzname()
- 'UTC'
-
- .. versionchanged:: 2.7.0
- ``tzutc()`` is now a singleton, so the result of ``tzutc()`` will
- always return the same object.
-
- .. doctest::
-
- >>> from dateutil.tz import tzutc, UTC
- >>> tzutc() is tzutc()
- True
- >>> tzutc() is UTC
- True
- """
- def utcoffset(self, dt):
- return ZERO
-
- def dst(self, dt):
- return ZERO
-
- @tzname_in_python2
- def tzname(self, dt):
- return "UTC"
-
- def is_ambiguous(self, dt):
- """
- Whether or not the "wall time" of a given datetime is ambiguous in this
- zone.
-
- :param dt:
- A :py:class:`datetime.datetime`, naive or time zone aware.
-
-
- :return:
- Returns ``True`` if ambiguous, ``False`` otherwise.
-
- .. versionadded:: 2.6.0
- """
- return False
-
- @_validate_fromutc_inputs
- def fromutc(self, dt):
- """
- Fast track version of fromutc() returns the original ``dt`` object for
- any valid :py:class:`datetime.datetime` object.
- """
- return dt
-
- def __eq__(self, other):
- if not isinstance(other, (tzutc, tzoffset)):
- return NotImplemented
-
- return (isinstance(other, tzutc) or
- (isinstance(other, tzoffset) and other._offset == ZERO))
-
- __hash__ = None
-
- def __ne__(self, other):
- return not (self == other)
-
- def __repr__(self):
- return "%s()" % self.__class__.__name__
-
- __reduce__ = object.__reduce__
-
-
-#: Convenience constant providing a :class:`tzutc()` instance
-#:
-#: .. versionadded:: 2.7.0
-UTC = tzutc()
-
-
-@six.add_metaclass(_TzOffsetFactory)
-class tzoffset(datetime.tzinfo):
- """
- A simple class for representing a fixed offset from UTC.
-
- :param name:
- The timezone name, to be returned when ``tzname()`` is called.
- :param offset:
- The time zone offset in seconds, or (since version 2.6.0, represented
- as a :py:class:`datetime.timedelta` object).
- """
- def __init__(self, name, offset):
- self._name = name
-
- try:
- # Allow a timedelta
- offset = offset.total_seconds()
- except (TypeError, AttributeError):
- pass
-
- self._offset = datetime.timedelta(seconds=_get_supported_offset(offset))
-
- def utcoffset(self, dt):
- return self._offset
-
- def dst(self, dt):
- return ZERO
-
- @tzname_in_python2
- def tzname(self, dt):
- return self._name
-
- @_validate_fromutc_inputs
- def fromutc(self, dt):
- return dt + self._offset
-
- def is_ambiguous(self, dt):
- """
- Whether or not the "wall time" of a given datetime is ambiguous in this
- zone.
-
- :param dt:
- A :py:class:`datetime.datetime`, naive or time zone aware.
- :return:
- Returns ``True`` if ambiguous, ``False`` otherwise.
-
- .. versionadded:: 2.6.0
- """
- return False
-
- def __eq__(self, other):
- if not isinstance(other, tzoffset):
- return NotImplemented
-
- return self._offset == other._offset
-
- __hash__ = None
-
- def __ne__(self, other):
- return not (self == other)
-
- def __repr__(self):
- return "%s(%s, %s)" % (self.__class__.__name__,
- repr(self._name),
- int(self._offset.total_seconds()))
-
- __reduce__ = object.__reduce__
-
-
-class tzlocal(_tzinfo):
- """
- A :class:`tzinfo` subclass built around the ``time`` timezone functions.
- """
- def __init__(self):
- super(tzlocal, self).__init__()
-
- self._std_offset = datetime.timedelta(seconds=-time.timezone)
- if time.daylight:
- self._dst_offset = datetime.timedelta(seconds=-time.altzone)
- else:
- self._dst_offset = self._std_offset
-
- self._dst_saved = self._dst_offset - self._std_offset
- self._hasdst = bool(self._dst_saved)
- self._tznames = tuple(time.tzname)
-
- def utcoffset(self, dt):
- if dt is None and self._hasdst:
- return None
-
- if self._isdst(dt):
- return self._dst_offset
- else:
- return self._std_offset
-
- def dst(self, dt):
- if dt is None and self._hasdst:
- return None
-
- if self._isdst(dt):
- return self._dst_offset - self._std_offset
- else:
- return ZERO
-
- @tzname_in_python2
- def tzname(self, dt):
- return self._tznames[self._isdst(dt)]
-
- def is_ambiguous(self, dt):
- """
- Whether or not the "wall time" of a given datetime is ambiguous in this
- zone.
-
- :param dt:
- A :py:class:`datetime.datetime`, naive or time zone aware.
-
-
- :return:
- Returns ``True`` if ambiguous, ``False`` otherwise.
-
- .. versionadded:: 2.6.0
- """
- naive_dst = self._naive_is_dst(dt)
- return (not naive_dst and
- (naive_dst != self._naive_is_dst(dt - self._dst_saved)))
-
- def _naive_is_dst(self, dt):
- timestamp = _datetime_to_timestamp(dt)
- return time.localtime(timestamp + time.timezone).tm_isdst
-
- def _isdst(self, dt, fold_naive=True):
- # We can't use mktime here. It is unstable when deciding if
- # the hour near to a change is DST or not.
- #
- # timestamp = time.mktime((dt.year, dt.month, dt.day, dt.hour,
- # dt.minute, dt.second, dt.weekday(), 0, -1))
- # return time.localtime(timestamp).tm_isdst
- #
- # The code above yields the following result:
- #
- # >>> import tz, datetime
- # >>> t = tz.tzlocal()
- # >>> datetime.datetime(2003,2,15,23,tzinfo=t).tzname()
- # 'BRDT'
- # >>> datetime.datetime(2003,2,16,0,tzinfo=t).tzname()
- # 'BRST'
- # >>> datetime.datetime(2003,2,15,23,tzinfo=t).tzname()
- # 'BRST'
- # >>> datetime.datetime(2003,2,15,22,tzinfo=t).tzname()
- # 'BRDT'
- # >>> datetime.datetime(2003,2,15,23,tzinfo=t).tzname()
- # 'BRDT'
- #
- # Here is a more stable implementation:
- #
- if not self._hasdst:
- return False
-
- # Check for ambiguous times:
- dstval = self._naive_is_dst(dt)
- fold = getattr(dt, 'fold', None)
-
- if self.is_ambiguous(dt):
- if fold is not None:
- return not self._fold(dt)
- else:
- return True
-
- return dstval
-
- def __eq__(self, other):
- if isinstance(other, tzlocal):
- return (self._std_offset == other._std_offset and
- self._dst_offset == other._dst_offset)
- elif isinstance(other, tzutc):
- return (not self._hasdst and
- self._tznames[0] in {'UTC', 'GMT'} and
- self._std_offset == ZERO)
- elif isinstance(other, tzoffset):
- return (not self._hasdst and
- self._tznames[0] == other._name and
- self._std_offset == other._offset)
- else:
- return NotImplemented
-
- __hash__ = None
-
- def __ne__(self, other):
- return not (self == other)
-
- def __repr__(self):
- return "%s()" % self.__class__.__name__
-
- __reduce__ = object.__reduce__
-
-
-class _ttinfo(object):
- __slots__ = ["offset", "delta", "isdst", "abbr",
- "isstd", "isgmt", "dstoffset"]
-
- def __init__(self):
- for attr in self.__slots__:
- setattr(self, attr, None)
-
- def __repr__(self):
- l = []
- for attr in self.__slots__:
- value = getattr(self, attr)
- if value is not None:
- l.append("%s=%s" % (attr, repr(value)))
- return "%s(%s)" % (self.__class__.__name__, ", ".join(l))
-
- def __eq__(self, other):
- if not isinstance(other, _ttinfo):
- return NotImplemented
-
- return (self.offset == other.offset and
- self.delta == other.delta and
- self.isdst == other.isdst and
- self.abbr == other.abbr and
- self.isstd == other.isstd and
- self.isgmt == other.isgmt and
- self.dstoffset == other.dstoffset)
-
- __hash__ = None
-
- def __ne__(self, other):
- return not (self == other)
-
- def __getstate__(self):
- state = {}
- for name in self.__slots__:
- state[name] = getattr(self, name, None)
- return state
-
- def __setstate__(self, state):
- for name in self.__slots__:
- if name in state:
- setattr(self, name, state[name])
-
-
-class _tzfile(object):
- """
- Lightweight class for holding the relevant transition and time zone
- information read from binary tzfiles.
- """
- attrs = ['trans_list', 'trans_list_utc', 'trans_idx', 'ttinfo_list',
- 'ttinfo_std', 'ttinfo_dst', 'ttinfo_before', 'ttinfo_first']
-
- def __init__(self, **kwargs):
- for attr in self.attrs:
- setattr(self, attr, kwargs.get(attr, None))
-
-
-class tzfile(_tzinfo):
- """
- This is a ``tzinfo`` subclass that allows one to use the ``tzfile(5)``
- format timezone files to extract current and historical zone information.
-
- :param fileobj:
- This can be an opened file stream or a file name that the time zone
- information can be read from.
-
- :param filename:
- This is an optional parameter specifying the source of the time zone
- information in the event that ``fileobj`` is a file object. If omitted
- and ``fileobj`` is a file stream, this parameter will be set either to
- ``fileobj``'s ``name`` attribute or to ``repr(fileobj)``.
-
- See `Sources for Time Zone and Daylight Saving Time Data
- `_ for more information.
- Time zone files can be compiled from the `IANA Time Zone database files
- `_ with the `zic time zone compiler
- `_
-
- .. note::
-
- Only construct a ``tzfile`` directly if you have a specific timezone
- file on disk that you want to read into a Python ``tzinfo`` object.
- If you want to get a ``tzfile`` representing a specific IANA zone,
- (e.g. ``'America/New_York'``), you should call
- :func:`dateutil.tz.gettz` with the zone identifier.
-
-
- **Examples:**
-
- Using the US Eastern time zone as an example, we can see that a ``tzfile``
- provides time zone information for the standard Daylight Saving offsets:
-
- .. testsetup:: tzfile
-
- from dateutil.tz import gettz
- from datetime import datetime
-
- .. doctest:: tzfile
-
- >>> NYC = gettz('America/New_York')
- >>> NYC
- tzfile('/usr/share/zoneinfo/America/New_York')
-
- >>> print(datetime(2016, 1, 3, tzinfo=NYC)) # EST
- 2016-01-03 00:00:00-05:00
-
- >>> print(datetime(2016, 7, 7, tzinfo=NYC)) # EDT
- 2016-07-07 00:00:00-04:00
-
-
- The ``tzfile`` structure contains a fully history of the time zone,
- so historical dates will also have the right offsets. For example, before
- the adoption of the UTC standards, New York used local solar mean time:
-
- .. doctest:: tzfile
-
- >>> print(datetime(1901, 4, 12, tzinfo=NYC)) # LMT
- 1901-04-12 00:00:00-04:56
-
- And during World War II, New York was on "Eastern War Time", which was a
- state of permanent daylight saving time:
-
- .. doctest:: tzfile
-
- >>> print(datetime(1944, 2, 7, tzinfo=NYC)) # EWT
- 1944-02-07 00:00:00-04:00
-
- """
-
- def __init__(self, fileobj, filename=None):
- super(tzfile, self).__init__()
-
- file_opened_here = False
- if isinstance(fileobj, string_types):
- self._filename = fileobj
- fileobj = open(fileobj, 'rb')
- file_opened_here = True
- elif filename is not None:
- self._filename = filename
- elif hasattr(fileobj, "name"):
- self._filename = fileobj.name
- else:
- self._filename = repr(fileobj)
-
- if fileobj is not None:
- if not file_opened_here:
- fileobj = _nullcontext(fileobj)
-
- with fileobj as file_stream:
- tzobj = self._read_tzfile(file_stream)
-
- self._set_tzdata(tzobj)
-
- def _set_tzdata(self, tzobj):
- """ Set the time zone data of this object from a _tzfile object """
- # Copy the relevant attributes over as private attributes
- for attr in _tzfile.attrs:
- setattr(self, '_' + attr, getattr(tzobj, attr))
-
- def _read_tzfile(self, fileobj):
- out = _tzfile()
-
- # From tzfile(5):
- #
- # The time zone information files used by tzset(3)
- # begin with the magic characters "TZif" to identify
- # them as time zone information files, followed by
- # sixteen bytes reserved for future use, followed by
- # six four-byte values of type long, written in a
- # ``standard'' byte order (the high-order byte
- # of the value is written first).
- if fileobj.read(4).decode() != "TZif":
- raise ValueError("magic not found")
-
- fileobj.read(16)
-
- (
- # The number of UTC/local indicators stored in the file.
- ttisgmtcnt,
-
- # The number of standard/wall indicators stored in the file.
- ttisstdcnt,
-
- # The number of leap seconds for which data is
- # stored in the file.
- leapcnt,
-
- # The number of "transition times" for which data
- # is stored in the file.
- timecnt,
-
- # The number of "local time types" for which data
- # is stored in the file (must not be zero).
- typecnt,
-
- # The number of characters of "time zone
- # abbreviation strings" stored in the file.
- charcnt,
-
- ) = struct.unpack(">6l", fileobj.read(24))
-
- # The above header is followed by tzh_timecnt four-byte
- # values of type long, sorted in ascending order.
- # These values are written in ``standard'' byte order.
- # Each is used as a transition time (as returned by
- # time(2)) at which the rules for computing local time
- # change.
-
- if timecnt:
- out.trans_list_utc = list(struct.unpack(">%dl" % timecnt,
- fileobj.read(timecnt*4)))
- else:
- out.trans_list_utc = []
-
- # Next come tzh_timecnt one-byte values of type unsigned
- # char; each one tells which of the different types of
- # ``local time'' types described in the file is associated
- # with the same-indexed transition time. These values
- # serve as indices into an array of ttinfo structures that
- # appears next in the file.
-
- if timecnt:
- out.trans_idx = struct.unpack(">%dB" % timecnt,
- fileobj.read(timecnt))
- else:
- out.trans_idx = []
-
- # Each ttinfo structure is written as a four-byte value
- # for tt_gmtoff of type long, in a standard byte
- # order, followed by a one-byte value for tt_isdst
- # and a one-byte value for tt_abbrind. In each
- # structure, tt_gmtoff gives the number of
- # seconds to be added to UTC, tt_isdst tells whether
- # tm_isdst should be set by localtime(3), and
- # tt_abbrind serves as an index into the array of
- # time zone abbreviation characters that follow the
- # ttinfo structure(s) in the file.
-
- ttinfo = []
-
- for i in range(typecnt):
- ttinfo.append(struct.unpack(">lbb", fileobj.read(6)))
-
- abbr = fileobj.read(charcnt).decode()
-
- # Then there are tzh_leapcnt pairs of four-byte
- # values, written in standard byte order; the
- # first value of each pair gives the time (as
- # returned by time(2)) at which a leap second
- # occurs; the second gives the total number of
- # leap seconds to be applied after the given time.
- # The pairs of values are sorted in ascending order
- # by time.
-
- # Not used, for now (but seek for correct file position)
- if leapcnt:
- fileobj.seek(leapcnt * 8, os.SEEK_CUR)
-
- # Then there are tzh_ttisstdcnt standard/wall
- # indicators, each stored as a one-byte value;
- # they tell whether the transition times associated
- # with local time types were specified as standard
- # time or wall clock time, and are used when
- # a time zone file is used in handling POSIX-style
- # time zone environment variables.
-
- if ttisstdcnt:
- isstd = struct.unpack(">%db" % ttisstdcnt,
- fileobj.read(ttisstdcnt))
-
- # Finally, there are tzh_ttisgmtcnt UTC/local
- # indicators, each stored as a one-byte value;
- # they tell whether the transition times associated
- # with local time types were specified as UTC or
- # local time, and are used when a time zone file
- # is used in handling POSIX-style time zone envi-
- # ronment variables.
-
- if ttisgmtcnt:
- isgmt = struct.unpack(">%db" % ttisgmtcnt,
- fileobj.read(ttisgmtcnt))
-
- # Build ttinfo list
- out.ttinfo_list = []
- for i in range(typecnt):
- gmtoff, isdst, abbrind = ttinfo[i]
- gmtoff = _get_supported_offset(gmtoff)
- tti = _ttinfo()
- tti.offset = gmtoff
- tti.dstoffset = datetime.timedelta(0)
- tti.delta = datetime.timedelta(seconds=gmtoff)
- tti.isdst = isdst
- tti.abbr = abbr[abbrind:abbr.find('\x00', abbrind)]
- tti.isstd = (ttisstdcnt > i and isstd[i] != 0)
- tti.isgmt = (ttisgmtcnt > i and isgmt[i] != 0)
- out.ttinfo_list.append(tti)
-
- # Replace ttinfo indexes for ttinfo objects.
- out.trans_idx = [out.ttinfo_list[idx] for idx in out.trans_idx]
-
- # Set standard, dst, and before ttinfos. before will be
- # used when a given time is before any transitions,
- # and will be set to the first non-dst ttinfo, or to
- # the first dst, if all of them are dst.
- out.ttinfo_std = None
- out.ttinfo_dst = None
- out.ttinfo_before = None
- if out.ttinfo_list:
- if not out.trans_list_utc:
- out.ttinfo_std = out.ttinfo_first = out.ttinfo_list[0]
- else:
- for i in range(timecnt-1, -1, -1):
- tti = out.trans_idx[i]
- if not out.ttinfo_std and not tti.isdst:
- out.ttinfo_std = tti
- elif not out.ttinfo_dst and tti.isdst:
- out.ttinfo_dst = tti
-
- if out.ttinfo_std and out.ttinfo_dst:
- break
- else:
- if out.ttinfo_dst and not out.ttinfo_std:
- out.ttinfo_std = out.ttinfo_dst
-
- for tti in out.ttinfo_list:
- if not tti.isdst:
- out.ttinfo_before = tti
- break
- else:
- out.ttinfo_before = out.ttinfo_list[0]
-
- # Now fix transition times to become relative to wall time.
- #
- # I'm not sure about this. In my tests, the tz source file
- # is setup to wall time, and in the binary file isstd and
- # isgmt are off, so it should be in wall time. OTOH, it's
- # always in gmt time. Let me know if you have comments
- # about this.
- lastdst = None
- lastoffset = None
- lastdstoffset = None
- lastbaseoffset = None
- out.trans_list = []
-
- for i, tti in enumerate(out.trans_idx):
- offset = tti.offset
- dstoffset = 0
-
- if lastdst is not None:
- if tti.isdst:
- if not lastdst:
- dstoffset = offset - lastoffset
-
- if not dstoffset and lastdstoffset:
- dstoffset = lastdstoffset
-
- tti.dstoffset = datetime.timedelta(seconds=dstoffset)
- lastdstoffset = dstoffset
-
- # If a time zone changes its base offset during a DST transition,
- # then you need to adjust by the previous base offset to get the
- # transition time in local time. Otherwise you use the current
- # base offset. Ideally, I would have some mathematical proof of
- # why this is true, but I haven't really thought about it enough.
- baseoffset = offset - dstoffset
- adjustment = baseoffset
- if (lastbaseoffset is not None and baseoffset != lastbaseoffset
- and tti.isdst != lastdst):
- # The base DST has changed
- adjustment = lastbaseoffset
-
- lastdst = tti.isdst
- lastoffset = offset
- lastbaseoffset = baseoffset
-
- out.trans_list.append(out.trans_list_utc[i] + adjustment)
-
- out.trans_idx = tuple(out.trans_idx)
- out.trans_list = tuple(out.trans_list)
- out.trans_list_utc = tuple(out.trans_list_utc)
-
- return out
-
- def _find_last_transition(self, dt, in_utc=False):
- # If there's no list, there are no transitions to find
- if not self._trans_list:
- return None
-
- timestamp = _datetime_to_timestamp(dt)
-
- # Find where the timestamp fits in the transition list - if the
- # timestamp is a transition time, it's part of the "after" period.
- trans_list = self._trans_list_utc if in_utc else self._trans_list
- idx = bisect.bisect_right(trans_list, timestamp)
-
- # We want to know when the previous transition was, so subtract off 1
- return idx - 1
-
- def _get_ttinfo(self, idx):
- # For no list or after the last transition, default to _ttinfo_std
- if idx is None or (idx + 1) >= len(self._trans_list):
- return self._ttinfo_std
-
- # If there is a list and the time is before it, return _ttinfo_before
- if idx < 0:
- return self._ttinfo_before
-
- return self._trans_idx[idx]
-
- def _find_ttinfo(self, dt):
- idx = self._resolve_ambiguous_time(dt)
-
- return self._get_ttinfo(idx)
-
- def fromutc(self, dt):
- """
- The ``tzfile`` implementation of :py:func:`datetime.tzinfo.fromutc`.
-
- :param dt:
- A :py:class:`datetime.datetime` object.
-
- :raises TypeError:
- Raised if ``dt`` is not a :py:class:`datetime.datetime` object.
-
- :raises ValueError:
- Raised if this is called with a ``dt`` which does not have this
- ``tzinfo`` attached.
-
- :return:
- Returns a :py:class:`datetime.datetime` object representing the
- wall time in ``self``'s time zone.
- """
- # These isinstance checks are in datetime.tzinfo, so we'll preserve
- # them, even if we don't care about duck typing.
- if not isinstance(dt, datetime.datetime):
- raise TypeError("fromutc() requires a datetime argument")
-
- if dt.tzinfo is not self:
- raise ValueError("dt.tzinfo is not self")
-
- # First treat UTC as wall time and get the transition we're in.
- idx = self._find_last_transition(dt, in_utc=True)
- tti = self._get_ttinfo(idx)
-
- dt_out = dt + datetime.timedelta(seconds=tti.offset)
-
- fold = self.is_ambiguous(dt_out, idx=idx)
-
- return enfold(dt_out, fold=int(fold))
-
- def is_ambiguous(self, dt, idx=None):
- """
- Whether or not the "wall time" of a given datetime is ambiguous in this
- zone.
-
- :param dt:
- A :py:class:`datetime.datetime`, naive or time zone aware.
-
-
- :return:
- Returns ``True`` if ambiguous, ``False`` otherwise.
-
- .. versionadded:: 2.6.0
- """
- if idx is None:
- idx = self._find_last_transition(dt)
-
- # Calculate the difference in offsets from current to previous
- timestamp = _datetime_to_timestamp(dt)
- tti = self._get_ttinfo(idx)
-
- if idx is None or idx <= 0:
- return False
-
- od = self._get_ttinfo(idx - 1).offset - tti.offset
- tt = self._trans_list[idx] # Transition time
-
- return timestamp < tt + od
-
- def _resolve_ambiguous_time(self, dt):
- idx = self._find_last_transition(dt)
-
- # If we have no transitions, return the index
- _fold = self._fold(dt)
- if idx is None or idx == 0:
- return idx
-
- # If it's ambiguous and we're in a fold, shift to a different index.
- idx_offset = int(not _fold and self.is_ambiguous(dt, idx))
-
- return idx - idx_offset
-
- def utcoffset(self, dt):
- if dt is None:
- return None
-
- if not self._ttinfo_std:
- return ZERO
-
- return self._find_ttinfo(dt).delta
-
- def dst(self, dt):
- if dt is None:
- return None
-
- if not self._ttinfo_dst:
- return ZERO
-
- tti = self._find_ttinfo(dt)
-
- if not tti.isdst:
- return ZERO
-
- # The documentation says that utcoffset()-dst() must
- # be constant for every dt.
- return tti.dstoffset
-
- @tzname_in_python2
- def tzname(self, dt):
- if not self._ttinfo_std or dt is None:
- return None
- return self._find_ttinfo(dt).abbr
-
- def __eq__(self, other):
- if not isinstance(other, tzfile):
- return NotImplemented
- return (self._trans_list == other._trans_list and
- self._trans_idx == other._trans_idx and
- self._ttinfo_list == other._ttinfo_list)
-
- __hash__ = None
-
- def __ne__(self, other):
- return not (self == other)
-
- def __repr__(self):
- return "%s(%s)" % (self.__class__.__name__, repr(self._filename))
-
- def __reduce__(self):
- return self.__reduce_ex__(None)
-
- def __reduce_ex__(self, protocol):
- return (self.__class__, (None, self._filename), self.__dict__)
-
-
-class tzrange(tzrangebase):
- """
- The ``tzrange`` object is a time zone specified by a set of offsets and
- abbreviations, equivalent to the way the ``TZ`` variable can be specified
- in POSIX-like systems, but using Python delta objects to specify DST
- start, end and offsets.
-
- :param stdabbr:
- The abbreviation for standard time (e.g. ``'EST'``).
-
- :param stdoffset:
- An integer or :class:`datetime.timedelta` object or equivalent
- specifying the base offset from UTC.
-
- If unspecified, +00:00 is used.
-
- :param dstabbr:
- The abbreviation for DST / "Summer" time (e.g. ``'EDT'``).
-
- If specified, with no other DST information, DST is assumed to occur
- and the default behavior or ``dstoffset``, ``start`` and ``end`` is
- used. If unspecified and no other DST information is specified, it
- is assumed that this zone has no DST.
-
- If this is unspecified and other DST information is *is* specified,
- DST occurs in the zone but the time zone abbreviation is left
- unchanged.
-
- :param dstoffset:
- A an integer or :class:`datetime.timedelta` object or equivalent
- specifying the UTC offset during DST. If unspecified and any other DST
- information is specified, it is assumed to be the STD offset +1 hour.
-
- :param start:
- A :class:`relativedelta.relativedelta` object or equivalent specifying
- the time and time of year that daylight savings time starts. To
- specify, for example, that DST starts at 2AM on the 2nd Sunday in
- March, pass:
-
- ``relativedelta(hours=2, month=3, day=1, weekday=SU(+2))``
-
- If unspecified and any other DST information is specified, the default
- value is 2 AM on the first Sunday in April.
-
- :param end:
- A :class:`relativedelta.relativedelta` object or equivalent
- representing the time and time of year that daylight savings time
- ends, with the same specification method as in ``start``. One note is
- that this should point to the first time in the *standard* zone, so if
- a transition occurs at 2AM in the DST zone and the clocks are set back
- 1 hour to 1AM, set the ``hours`` parameter to +1.
-
-
- **Examples:**
-
- .. testsetup:: tzrange
-
- from dateutil.tz import tzrange, tzstr
-
- .. doctest:: tzrange
-
- >>> tzstr('EST5EDT') == tzrange("EST", -18000, "EDT")
- True
-
- >>> from dateutil.relativedelta import *
- >>> range1 = tzrange("EST", -18000, "EDT")
- >>> range2 = tzrange("EST", -18000, "EDT", -14400,
- ... relativedelta(hours=+2, month=4, day=1,
- ... weekday=SU(+1)),
- ... relativedelta(hours=+1, month=10, day=31,
- ... weekday=SU(-1)))
- >>> tzstr('EST5EDT') == range1 == range2
- True
-
- """
- def __init__(self, stdabbr, stdoffset=None,
- dstabbr=None, dstoffset=None,
- start=None, end=None):
-
- global relativedelta
- from dateutil import relativedelta
-
- self._std_abbr = stdabbr
- self._dst_abbr = dstabbr
-
- try:
- stdoffset = stdoffset.total_seconds()
- except (TypeError, AttributeError):
- pass
-
- try:
- dstoffset = dstoffset.total_seconds()
- except (TypeError, AttributeError):
- pass
-
- if stdoffset is not None:
- self._std_offset = datetime.timedelta(seconds=stdoffset)
- else:
- self._std_offset = ZERO
-
- if dstoffset is not None:
- self._dst_offset = datetime.timedelta(seconds=dstoffset)
- elif dstabbr and stdoffset is not None:
- self._dst_offset = self._std_offset + datetime.timedelta(hours=+1)
- else:
- self._dst_offset = ZERO
-
- if dstabbr and start is None:
- self._start_delta = relativedelta.relativedelta(
- hours=+2, month=4, day=1, weekday=relativedelta.SU(+1))
- else:
- self._start_delta = start
-
- if dstabbr and end is None:
- self._end_delta = relativedelta.relativedelta(
- hours=+1, month=10, day=31, weekday=relativedelta.SU(-1))
- else:
- self._end_delta = end
-
- self._dst_base_offset_ = self._dst_offset - self._std_offset
- self.hasdst = bool(self._start_delta)
-
- def transitions(self, year):
- """
- For a given year, get the DST on and off transition times, expressed
- always on the standard time side. For zones with no transitions, this
- function returns ``None``.
-
- :param year:
- The year whose transitions you would like to query.
-
- :return:
- Returns a :class:`tuple` of :class:`datetime.datetime` objects,
- ``(dston, dstoff)`` for zones with an annual DST transition, or
- ``None`` for fixed offset zones.
- """
- if not self.hasdst:
- return None
-
- base_year = datetime.datetime(year, 1, 1)
-
- start = base_year + self._start_delta
- end = base_year + self._end_delta
-
- return (start, end)
-
- def __eq__(self, other):
- if not isinstance(other, tzrange):
- return NotImplemented
-
- return (self._std_abbr == other._std_abbr and
- self._dst_abbr == other._dst_abbr and
- self._std_offset == other._std_offset and
- self._dst_offset == other._dst_offset and
- self._start_delta == other._start_delta and
- self._end_delta == other._end_delta)
-
- @property
- def _dst_base_offset(self):
- return self._dst_base_offset_
-
-
-@six.add_metaclass(_TzStrFactory)
-class tzstr(tzrange):
- """
- ``tzstr`` objects are time zone objects specified by a time-zone string as
- it would be passed to a ``TZ`` variable on POSIX-style systems (see
- the `GNU C Library: TZ Variable`_ for more details).
-
- There is one notable exception, which is that POSIX-style time zones use an
- inverted offset format, so normally ``GMT+3`` would be parsed as an offset
- 3 hours *behind* GMT. The ``tzstr`` time zone object will parse this as an
- offset 3 hours *ahead* of GMT. If you would like to maintain the POSIX
- behavior, pass a ``True`` value to ``posix_offset``.
-
- The :class:`tzrange` object provides the same functionality, but is
- specified using :class:`relativedelta.relativedelta` objects. rather than
- strings.
-
- :param s:
- A time zone string in ``TZ`` variable format. This can be a
- :class:`bytes` (2.x: :class:`str`), :class:`str` (2.x:
- :class:`unicode`) or a stream emitting unicode characters
- (e.g. :class:`StringIO`).
-
- :param posix_offset:
- Optional. If set to ``True``, interpret strings such as ``GMT+3`` or
- ``UTC+3`` as being 3 hours *behind* UTC rather than ahead, per the
- POSIX standard.
-
- .. caution::
-
- Prior to version 2.7.0, this function also supported time zones
- in the format:
-
- * ``EST5EDT,4,0,6,7200,10,0,26,7200,3600``
- * ``EST5EDT,4,1,0,7200,10,-1,0,7200,3600``
-
- This format is non-standard and has been deprecated; this function
- will raise a :class:`DeprecatedTZFormatWarning` until
- support is removed in a future version.
-
- .. _`GNU C Library: TZ Variable`:
- https://www.gnu.org/software/libc/manual/html_node/TZ-Variable.html
- """
- def __init__(self, s, posix_offset=False):
- global parser
- from dateutil.parser import _parser as parser
-
- self._s = s
-
- res = parser._parsetz(s)
- if res is None or res.any_unused_tokens:
- raise ValueError("unknown string format")
-
- # Here we break the compatibility with the TZ variable handling.
- # GMT-3 actually *means* the timezone -3.
- if res.stdabbr in ("GMT", "UTC") and not posix_offset:
- res.stdoffset *= -1
-
- # We must initialize it first, since _delta() needs
- # _std_offset and _dst_offset set. Use False in start/end
- # to avoid building it two times.
- tzrange.__init__(self, res.stdabbr, res.stdoffset,
- res.dstabbr, res.dstoffset,
- start=False, end=False)
-
- if not res.dstabbr:
- self._start_delta = None
- self._end_delta = None
- else:
- self._start_delta = self._delta(res.start)
- if self._start_delta:
- self._end_delta = self._delta(res.end, isend=1)
-
- self.hasdst = bool(self._start_delta)
-
- def _delta(self, x, isend=0):
- from dateutil import relativedelta
- kwargs = {}
- if x.month is not None:
- kwargs["month"] = x.month
- if x.weekday is not None:
- kwargs["weekday"] = relativedelta.weekday(x.weekday, x.week)
- if x.week > 0:
- kwargs["day"] = 1
- else:
- kwargs["day"] = 31
- elif x.day:
- kwargs["day"] = x.day
- elif x.yday is not None:
- kwargs["yearday"] = x.yday
- elif x.jyday is not None:
- kwargs["nlyearday"] = x.jyday
- if not kwargs:
- # Default is to start on first sunday of april, and end
- # on last sunday of october.
- if not isend:
- kwargs["month"] = 4
- kwargs["day"] = 1
- kwargs["weekday"] = relativedelta.SU(+1)
- else:
- kwargs["month"] = 10
- kwargs["day"] = 31
- kwargs["weekday"] = relativedelta.SU(-1)
- if x.time is not None:
- kwargs["seconds"] = x.time
- else:
- # Default is 2AM.
- kwargs["seconds"] = 7200
- if isend:
- # Convert to standard time, to follow the documented way
- # of working with the extra hour. See the documentation
- # of the tzinfo class.
- delta = self._dst_offset - self._std_offset
- kwargs["seconds"] -= delta.seconds + delta.days * 86400
- return relativedelta.relativedelta(**kwargs)
-
- def __repr__(self):
- return "%s(%s)" % (self.__class__.__name__, repr(self._s))
-
-
-class _tzicalvtzcomp(object):
- def __init__(self, tzoffsetfrom, tzoffsetto, isdst,
- tzname=None, rrule=None):
- self.tzoffsetfrom = datetime.timedelta(seconds=tzoffsetfrom)
- self.tzoffsetto = datetime.timedelta(seconds=tzoffsetto)
- self.tzoffsetdiff = self.tzoffsetto - self.tzoffsetfrom
- self.isdst = isdst
- self.tzname = tzname
- self.rrule = rrule
-
-
-class _tzicalvtz(_tzinfo):
- def __init__(self, tzid, comps=[]):
- super(_tzicalvtz, self).__init__()
-
- self._tzid = tzid
- self._comps = comps
- self._cachedate = []
- self._cachecomp = []
- self._cache_lock = _thread.allocate_lock()
-
- def _find_comp(self, dt):
- if len(self._comps) == 1:
- return self._comps[0]
-
- dt = dt.replace(tzinfo=None)
-
- try:
- with self._cache_lock:
- return self._cachecomp[self._cachedate.index(
- (dt, self._fold(dt)))]
- except ValueError:
- pass
-
- lastcompdt = None
- lastcomp = None
-
- for comp in self._comps:
- compdt = self._find_compdt(comp, dt)
-
- if compdt and (not lastcompdt or lastcompdt < compdt):
- lastcompdt = compdt
- lastcomp = comp
-
- if not lastcomp:
- # RFC says nothing about what to do when a given
- # time is before the first onset date. We'll look for the
- # first standard component, or the first component, if
- # none is found.
- for comp in self._comps:
- if not comp.isdst:
- lastcomp = comp
- break
- else:
- lastcomp = comp[0]
-
- with self._cache_lock:
- self._cachedate.insert(0, (dt, self._fold(dt)))
- self._cachecomp.insert(0, lastcomp)
-
- if len(self._cachedate) > 10:
- self._cachedate.pop()
- self._cachecomp.pop()
-
- return lastcomp
-
- def _find_compdt(self, comp, dt):
- if comp.tzoffsetdiff < ZERO and self._fold(dt):
- dt -= comp.tzoffsetdiff
-
- compdt = comp.rrule.before(dt, inc=True)
-
- return compdt
-
- def utcoffset(self, dt):
- if dt is None:
- return None
-
- return self._find_comp(dt).tzoffsetto
-
- def dst(self, dt):
- comp = self._find_comp(dt)
- if comp.isdst:
- return comp.tzoffsetdiff
- else:
- return ZERO
-
- @tzname_in_python2
- def tzname(self, dt):
- return self._find_comp(dt).tzname
-
- def __repr__(self):
- return "" % repr(self._tzid)
-
- __reduce__ = object.__reduce__
-
-
-class tzical(object):
- """
- This object is designed to parse an iCalendar-style ``VTIMEZONE`` structure
- as set out in `RFC 5545`_ Section 4.6.5 into one or more `tzinfo` objects.
-
- :param `fileobj`:
- A file or stream in iCalendar format, which should be UTF-8 encoded
- with CRLF endings.
-
- .. _`RFC 5545`: https://tools.ietf.org/html/rfc5545
- """
- def __init__(self, fileobj):
- global rrule
- from dateutil import rrule
-
- if isinstance(fileobj, string_types):
- self._s = fileobj
- # ical should be encoded in UTF-8 with CRLF
- fileobj = open(fileobj, 'r')
- else:
- self._s = getattr(fileobj, 'name', repr(fileobj))
- fileobj = _nullcontext(fileobj)
-
- self._vtz = {}
-
- with fileobj as fobj:
- self._parse_rfc(fobj.read())
-
- def keys(self):
- """
- Retrieves the available time zones as a list.
- """
- return list(self._vtz.keys())
-
- def get(self, tzid=None):
- """
- Retrieve a :py:class:`datetime.tzinfo` object by its ``tzid``.
-
- :param tzid:
- If there is exactly one time zone available, omitting ``tzid``
- or passing :py:const:`None` value returns it. Otherwise a valid
- key (which can be retrieved from :func:`keys`) is required.
-
- :raises ValueError:
- Raised if ``tzid`` is not specified but there are either more
- or fewer than 1 zone defined.
-
- :returns:
- Returns either a :py:class:`datetime.tzinfo` object representing
- the relevant time zone or :py:const:`None` if the ``tzid`` was
- not found.
- """
- if tzid is None:
- if len(self._vtz) == 0:
- raise ValueError("no timezones defined")
- elif len(self._vtz) > 1:
- raise ValueError("more than one timezone available")
- tzid = next(iter(self._vtz))
-
- return self._vtz.get(tzid)
-
- def _parse_offset(self, s):
- s = s.strip()
- if not s:
- raise ValueError("empty offset")
- if s[0] in ('+', '-'):
- signal = (-1, +1)[s[0] == '+']
- s = s[1:]
- else:
- signal = +1
- if len(s) == 4:
- return (int(s[:2]) * 3600 + int(s[2:]) * 60) * signal
- elif len(s) == 6:
- return (int(s[:2]) * 3600 + int(s[2:4]) * 60 + int(s[4:])) * signal
- else:
- raise ValueError("invalid offset: " + s)
-
- def _parse_rfc(self, s):
- lines = s.splitlines()
- if not lines:
- raise ValueError("empty string")
-
- # Unfold
- i = 0
- while i < len(lines):
- line = lines[i].rstrip()
- if not line:
- del lines[i]
- elif i > 0 and line[0] == " ":
- lines[i-1] += line[1:]
- del lines[i]
- else:
- i += 1
-
- tzid = None
- comps = []
- invtz = False
- comptype = None
- for line in lines:
- if not line:
- continue
- name, value = line.split(':', 1)
- parms = name.split(';')
- if not parms:
- raise ValueError("empty property name")
- name = parms[0].upper()
- parms = parms[1:]
- if invtz:
- if name == "BEGIN":
- if value in ("STANDARD", "DAYLIGHT"):
- # Process component
- pass
- else:
- raise ValueError("unknown component: "+value)
- comptype = value
- founddtstart = False
- tzoffsetfrom = None
- tzoffsetto = None
- rrulelines = []
- tzname = None
- elif name == "END":
- if value == "VTIMEZONE":
- if comptype:
- raise ValueError("component not closed: "+comptype)
- if not tzid:
- raise ValueError("mandatory TZID not found")
- if not comps:
- raise ValueError(
- "at least one component is needed")
- # Process vtimezone
- self._vtz[tzid] = _tzicalvtz(tzid, comps)
- invtz = False
- elif value == comptype:
- if not founddtstart:
- raise ValueError("mandatory DTSTART not found")
- if tzoffsetfrom is None:
- raise ValueError(
- "mandatory TZOFFSETFROM not found")
- if tzoffsetto is None:
- raise ValueError(
- "mandatory TZOFFSETFROM not found")
- # Process component
- rr = None
- if rrulelines:
- rr = rrule.rrulestr("\n".join(rrulelines),
- compatible=True,
- ignoretz=True,
- cache=True)
- comp = _tzicalvtzcomp(tzoffsetfrom, tzoffsetto,
- (comptype == "DAYLIGHT"),
- tzname, rr)
- comps.append(comp)
- comptype = None
- else:
- raise ValueError("invalid component end: "+value)
- elif comptype:
- if name == "DTSTART":
- # DTSTART in VTIMEZONE takes a subset of valid RRULE
- # values under RFC 5545.
- for parm in parms:
- if parm != 'VALUE=DATE-TIME':
- msg = ('Unsupported DTSTART param in ' +
- 'VTIMEZONE: ' + parm)
- raise ValueError(msg)
- rrulelines.append(line)
- founddtstart = True
- elif name in ("RRULE", "RDATE", "EXRULE", "EXDATE"):
- rrulelines.append(line)
- elif name == "TZOFFSETFROM":
- if parms:
- raise ValueError(
- "unsupported %s parm: %s " % (name, parms[0]))
- tzoffsetfrom = self._parse_offset(value)
- elif name == "TZOFFSETTO":
- if parms:
- raise ValueError(
- "unsupported TZOFFSETTO parm: "+parms[0])
- tzoffsetto = self._parse_offset(value)
- elif name == "TZNAME":
- if parms:
- raise ValueError(
- "unsupported TZNAME parm: "+parms[0])
- tzname = value
- elif name == "COMMENT":
- pass
- else:
- raise ValueError("unsupported property: "+name)
- else:
- if name == "TZID":
- if parms:
- raise ValueError(
- "unsupported TZID parm: "+parms[0])
- tzid = value
- elif name in ("TZURL", "LAST-MODIFIED", "COMMENT"):
- pass
- else:
- raise ValueError("unsupported property: "+name)
- elif name == "BEGIN" and value == "VTIMEZONE":
- tzid = None
- comps = []
- invtz = True
-
- def __repr__(self):
- return "%s(%s)" % (self.__class__.__name__, repr(self._s))
-
-
-if sys.platform != "win32":
- TZFILES = ["/etc/localtime", "localtime"]
- TZPATHS = ["/usr/share/zoneinfo",
- "/usr/lib/zoneinfo",
- "/usr/share/lib/zoneinfo",
- "/etc/zoneinfo"]
-else:
- TZFILES = []
- TZPATHS = []
-
-
-def __get_gettz():
- tzlocal_classes = (tzlocal,)
- if tzwinlocal is not None:
- tzlocal_classes += (tzwinlocal,)
-
- class GettzFunc(object):
- """
- Retrieve a time zone object from a string representation
-
- This function is intended to retrieve the :py:class:`tzinfo` subclass
- that best represents the time zone that would be used if a POSIX
- `TZ variable`_ were set to the same value.
-
- If no argument or an empty string is passed to ``gettz``, local time
- is returned:
-
- .. code-block:: python3
-
- >>> gettz()
- tzfile('/etc/localtime')
-
- This function is also the preferred way to map IANA tz database keys
- to :class:`tzfile` objects:
-
- .. code-block:: python3
-
- >>> gettz('Pacific/Kiritimati')
- tzfile('/usr/share/zoneinfo/Pacific/Kiritimati')
-
- On Windows, the standard is extended to include the Windows-specific
- zone names provided by the operating system:
-
- .. code-block:: python3
-
- >>> gettz('Egypt Standard Time')
- tzwin('Egypt Standard Time')
-
- Passing a GNU ``TZ`` style string time zone specification returns a
- :class:`tzstr` object:
-
- .. code-block:: python3
-
- >>> gettz('AEST-10AEDT-11,M10.1.0/2,M4.1.0/3')
- tzstr('AEST-10AEDT-11,M10.1.0/2,M4.1.0/3')
-
- :param name:
- A time zone name (IANA, or, on Windows, Windows keys), location of
- a ``tzfile(5)`` zoneinfo file or ``TZ`` variable style time zone
- specifier. An empty string, no argument or ``None`` is interpreted
- as local time.
-
- :return:
- Returns an instance of one of ``dateutil``'s :py:class:`tzinfo`
- subclasses.
-
- .. versionchanged:: 2.7.0
-
- After version 2.7.0, any two calls to ``gettz`` using the same
- input strings will return the same object:
-
- .. code-block:: python3
-
- >>> tz.gettz('America/Chicago') is tz.gettz('America/Chicago')
- True
-
- In addition to improving performance, this ensures that
- `"same zone" semantics`_ are used for datetimes in the same zone.
-
-
- .. _`TZ variable`:
- https://www.gnu.org/software/libc/manual/html_node/TZ-Variable.html
-
- .. _`"same zone" semantics`:
- https://blog.ganssle.io/articles/2018/02/aware-datetime-arithmetic.html
- """
- def __init__(self):
-
- self.__instances = weakref.WeakValueDictionary()
- self.__strong_cache_size = 8
- self.__strong_cache = OrderedDict()
- self._cache_lock = _thread.allocate_lock()
-
- def __call__(self, name=None):
- with self._cache_lock:
- rv = self.__instances.get(name, None)
-
- if rv is None:
- rv = self.nocache(name=name)
- if not (name is None
- or isinstance(rv, tzlocal_classes)
- or rv is None):
- # tzlocal is slightly more complicated than the other
- # time zone providers because it depends on environment
- # at construction time, so don't cache that.
- #
- # We also cannot store weak references to None, so we
- # will also not store that.
- self.__instances[name] = rv
- else:
- # No need for strong caching, return immediately
- return rv
-
- self.__strong_cache[name] = self.__strong_cache.pop(name, rv)
-
- if len(self.__strong_cache) > self.__strong_cache_size:
- self.__strong_cache.popitem(last=False)
-
- return rv
-
- def set_cache_size(self, size):
- with self._cache_lock:
- self.__strong_cache_size = size
- while len(self.__strong_cache) > size:
- self.__strong_cache.popitem(last=False)
-
- def cache_clear(self):
- with self._cache_lock:
- self.__instances = weakref.WeakValueDictionary()
- self.__strong_cache.clear()
-
- @staticmethod
- def nocache(name=None):
- """A non-cached version of gettz"""
- tz = None
- if not name:
- try:
- name = os.environ["TZ"]
- except KeyError:
- pass
- if name is None or name in ("", ":"):
- for filepath in TZFILES:
- if not os.path.isabs(filepath):
- filename = filepath
- for path in TZPATHS:
- filepath = os.path.join(path, filename)
- if os.path.isfile(filepath):
- break
- else:
- continue
- if os.path.isfile(filepath):
- try:
- tz = tzfile(filepath)
- break
- except (IOError, OSError, ValueError):
- pass
- else:
- tz = tzlocal()
- else:
- try:
- if name.startswith(":"):
- name = name[1:]
- except TypeError as e:
- if isinstance(name, bytes):
- new_msg = "gettz argument should be str, not bytes"
- six.raise_from(TypeError(new_msg), e)
- else:
- raise
- if os.path.isabs(name):
- if os.path.isfile(name):
- tz = tzfile(name)
- else:
- tz = None
- else:
- for path in TZPATHS:
- filepath = os.path.join(path, name)
- if not os.path.isfile(filepath):
- filepath = filepath.replace(' ', '_')
- if not os.path.isfile(filepath):
- continue
- try:
- tz = tzfile(filepath)
- break
- except (IOError, OSError, ValueError):
- pass
- else:
- tz = None
- if tzwin is not None:
- try:
- tz = tzwin(name)
- except (WindowsError, UnicodeEncodeError):
- # UnicodeEncodeError is for Python 2.7 compat
- tz = None
-
- if not tz:
- from dateutil.zoneinfo import get_zonefile_instance
- tz = get_zonefile_instance().get(name)
-
- if not tz:
- for c in name:
- # name is not a tzstr unless it has at least
- # one offset. For short values of "name", an
- # explicit for loop seems to be the fastest way
- # To determine if a string contains a digit
- if c in "0123456789":
- try:
- tz = tzstr(name)
- except ValueError:
- pass
- break
- else:
- if name in ("GMT", "UTC"):
- tz = UTC
- elif name in time.tzname:
- tz = tzlocal()
- return tz
-
- return GettzFunc()
-
-
-gettz = __get_gettz()
-del __get_gettz
-
-
-def datetime_exists(dt, tz=None):
- """
- Given a datetime and a time zone, determine whether or not a given datetime
- would fall in a gap.
-
- :param dt:
- A :class:`datetime.datetime` (whose time zone will be ignored if ``tz``
- is provided.)
-
- :param tz:
- A :class:`datetime.tzinfo` with support for the ``fold`` attribute. If
- ``None`` or not provided, the datetime's own time zone will be used.
-
- :return:
- Returns a boolean value whether or not the "wall time" exists in
- ``tz``.
-
- .. versionadded:: 2.7.0
- """
- if tz is None:
- if dt.tzinfo is None:
- raise ValueError('Datetime is naive and no time zone provided.')
- tz = dt.tzinfo
-
- dt = dt.replace(tzinfo=None)
-
- # This is essentially a test of whether or not the datetime can survive
- # a round trip to UTC.
- dt_rt = dt.replace(tzinfo=tz).astimezone(UTC).astimezone(tz)
- dt_rt = dt_rt.replace(tzinfo=None)
-
- return dt == dt_rt
-
-
-def datetime_ambiguous(dt, tz=None):
- """
- Given a datetime and a time zone, determine whether or not a given datetime
- is ambiguous (i.e if there are two times differentiated only by their DST
- status).
-
- :param dt:
- A :class:`datetime.datetime` (whose time zone will be ignored if ``tz``
- is provided.)
-
- :param tz:
- A :class:`datetime.tzinfo` with support for the ``fold`` attribute. If
- ``None`` or not provided, the datetime's own time zone will be used.
-
- :return:
- Returns a boolean value whether or not the "wall time" is ambiguous in
- ``tz``.
-
- .. versionadded:: 2.6.0
- """
- if tz is None:
- if dt.tzinfo is None:
- raise ValueError('Datetime is naive and no time zone provided.')
-
- tz = dt.tzinfo
-
- # If a time zone defines its own "is_ambiguous" function, we'll use that.
- is_ambiguous_fn = getattr(tz, 'is_ambiguous', None)
- if is_ambiguous_fn is not None:
- try:
- return tz.is_ambiguous(dt)
- except Exception:
- pass
-
- # If it doesn't come out and tell us it's ambiguous, we'll just check if
- # the fold attribute has any effect on this particular date and time.
- dt = dt.replace(tzinfo=tz)
- wall_0 = enfold(dt, fold=0)
- wall_1 = enfold(dt, fold=1)
-
- same_offset = wall_0.utcoffset() == wall_1.utcoffset()
- same_dst = wall_0.dst() == wall_1.dst()
-
- return not (same_offset and same_dst)
-
-
-def resolve_imaginary(dt):
- """
- Given a datetime that may be imaginary, return an existing datetime.
-
- This function assumes that an imaginary datetime represents what the
- wall time would be in a zone had the offset transition not occurred, so
- it will always fall forward by the transition's change in offset.
-
- .. doctest::
-
- >>> from dateutil import tz
- >>> from datetime import datetime
- >>> NYC = tz.gettz('America/New_York')
- >>> print(tz.resolve_imaginary(datetime(2017, 3, 12, 2, 30, tzinfo=NYC)))
- 2017-03-12 03:30:00-04:00
-
- >>> KIR = tz.gettz('Pacific/Kiritimati')
- >>> print(tz.resolve_imaginary(datetime(1995, 1, 1, 12, 30, tzinfo=KIR)))
- 1995-01-02 12:30:00+14:00
-
- As a note, :func:`datetime.astimezone` is guaranteed to produce a valid,
- existing datetime, so a round-trip to and from UTC is sufficient to get
- an extant datetime, however, this generally "falls back" to an earlier time
- rather than falling forward to the STD side (though no guarantees are made
- about this behavior).
-
- :param dt:
- A :class:`datetime.datetime` which may or may not exist.
-
- :return:
- Returns an existing :class:`datetime.datetime`. If ``dt`` was not
- imaginary, the datetime returned is guaranteed to be the same object
- passed to the function.
-
- .. versionadded:: 2.7.0
- """
- if dt.tzinfo is not None and not datetime_exists(dt):
-
- curr_offset = (dt + datetime.timedelta(hours=24)).utcoffset()
- old_offset = (dt - datetime.timedelta(hours=24)).utcoffset()
-
- dt += curr_offset - old_offset
-
- return dt
-
-
-def _datetime_to_timestamp(dt):
- """
- Convert a :class:`datetime.datetime` object to an epoch timestamp in
- seconds since January 1, 1970, ignoring the time zone.
- """
- return (dt.replace(tzinfo=None) - EPOCH).total_seconds()
-
-
-if sys.version_info >= (3, 6):
- def _get_supported_offset(second_offset):
- return second_offset
-else:
- def _get_supported_offset(second_offset):
- # For python pre-3.6, round to full-minutes if that's not the case.
- # Python's datetime doesn't accept sub-minute timezones. Check
- # http://python.org/sf/1447945 or https://bugs.python.org/issue5288
- # for some information.
- old_offset = second_offset
- calculated_offset = 60 * ((second_offset + 30) // 60)
- return calculated_offset
-
-
-try:
- # Python 3.7 feature
- from contextlib import nullcontext as _nullcontext
-except ImportError:
- class _nullcontext(object):
- """
- Class for wrapping contexts so that they are passed through in a
- with statement.
- """
- def __init__(self, context):
- self.context = context
-
- def __enter__(self):
- return self.context
-
- def __exit__(*args, **kwargs):
- pass
-
-# vim:ts=4:sw=4:et
diff --git a/spaces/asafAdge/Detic/detic/modeling/meta_arch/d2_deformable_detr.py b/spaces/asafAdge/Detic/detic/modeling/meta_arch/d2_deformable_detr.py
deleted file mode 100644
index 47ff220fc3946d1bf68fad87076589e46b274ef3..0000000000000000000000000000000000000000
--- a/spaces/asafAdge/Detic/detic/modeling/meta_arch/d2_deformable_detr.py
+++ /dev/null
@@ -1,308 +0,0 @@
-# Copyright (c) Facebook, Inc. and its affiliates.
-import torch
-import torch.nn.functional as F
-from torch import nn
-import math
-
-from detectron2.modeling import META_ARCH_REGISTRY, build_backbone
-from detectron2.structures import Boxes, Instances
-from ..utils import load_class_freq, get_fed_loss_inds
-
-from models.backbone import Joiner
-from models.deformable_detr import DeformableDETR, SetCriterion, MLP
-from models.deformable_detr import _get_clones
-from models.matcher import HungarianMatcher
-from models.position_encoding import PositionEmbeddingSine
-from models.deformable_transformer import DeformableTransformer
-from models.segmentation import sigmoid_focal_loss
-from util.box_ops import box_cxcywh_to_xyxy, box_xyxy_to_cxcywh
-from util.misc import NestedTensor, accuracy
-
-
-__all__ = ["DeformableDetr"]
-
-class CustomSetCriterion(SetCriterion):
- def __init__(self, num_classes, matcher, weight_dict, losses, \
- focal_alpha=0.25, use_fed_loss=False):
- super().__init__(num_classes, matcher, weight_dict, losses, focal_alpha)
- self.use_fed_loss = use_fed_loss
- if self.use_fed_loss:
- self.register_buffer(
- 'fed_loss_weight', load_class_freq(freq_weight=0.5))
-
- def loss_labels(self, outputs, targets, indices, num_boxes, log=True):
- """Classification loss (NLL)
- targets dicts must contain the key "labels" containing a tensor of dim [nb_target_boxes]
- """
- assert 'pred_logits' in outputs
- src_logits = outputs['pred_logits']
-
- idx = self._get_src_permutation_idx(indices)
- target_classes_o = torch.cat([t["labels"][J] for t, (_, J) in zip(targets, indices)])
- target_classes = torch.full(src_logits.shape[:2], self.num_classes,
- dtype=torch.int64, device=src_logits.device)
- target_classes[idx] = target_classes_o
-
- target_classes_onehot = torch.zeros(
- [src_logits.shape[0], src_logits.shape[1], src_logits.shape[2] + 1],
- dtype=src_logits.dtype, layout=src_logits.layout,
- device=src_logits.device)
- target_classes_onehot.scatter_(2, target_classes.unsqueeze(-1), 1)
-
- target_classes_onehot = target_classes_onehot[:,:,:-1] # B x N x C
- if self.use_fed_loss:
- inds = get_fed_loss_inds(
- gt_classes=target_classes_o,
- num_sample_cats=50,
- weight=self.fed_loss_weight,
- C=target_classes_onehot.shape[2])
- loss_ce = sigmoid_focal_loss(
- src_logits[:, :, inds],
- target_classes_onehot[:, :, inds],
- num_boxes,
- alpha=self.focal_alpha,
- gamma=2) * src_logits.shape[1]
- else:
- loss_ce = sigmoid_focal_loss(
- src_logits, target_classes_onehot, num_boxes,
- alpha=self.focal_alpha,
- gamma=2) * src_logits.shape[1]
- losses = {'loss_ce': loss_ce}
-
- if log:
- # TODO this should probably be a separate loss, not hacked in this one here
- losses['class_error'] = 100 - accuracy(src_logits[idx], target_classes_o)[0]
- return losses
-
-
-class MaskedBackbone(nn.Module):
- """ This is a thin wrapper around D2's backbone to provide padding masking"""
-
- def __init__(self, cfg):
- super().__init__()
- self.backbone = build_backbone(cfg)
- backbone_shape = self.backbone.output_shape()
- self.feature_strides = [backbone_shape[f].stride for f in backbone_shape.keys()]
- self.strides = [backbone_shape[f].stride for f in backbone_shape.keys()]
- self.num_channels = [backbone_shape[x].channels for x in backbone_shape.keys()]
-
- def forward(self, tensor_list: NestedTensor):
- xs = self.backbone(tensor_list.tensors)
- out = {}
- for name, x in xs.items():
- m = tensor_list.mask
- assert m is not None
- mask = F.interpolate(m[None].float(), size=x.shape[-2:]).to(torch.bool)[0]
- out[name] = NestedTensor(x, mask)
- return out
-
-@META_ARCH_REGISTRY.register()
-class DeformableDetr(nn.Module):
- """
- Implement Deformable Detr
- """
-
- def __init__(self, cfg):
- super().__init__()
- self.with_image_labels = cfg.WITH_IMAGE_LABELS
- self.weak_weight = cfg.MODEL.DETR.WEAK_WEIGHT
-
- self.device = torch.device(cfg.MODEL.DEVICE)
- self.test_topk = cfg.TEST.DETECTIONS_PER_IMAGE
- self.num_classes = cfg.MODEL.DETR.NUM_CLASSES
- self.mask_on = cfg.MODEL.MASK_ON
- hidden_dim = cfg.MODEL.DETR.HIDDEN_DIM
- num_queries = cfg.MODEL.DETR.NUM_OBJECT_QUERIES
-
- # Transformer parameters:
- nheads = cfg.MODEL.DETR.NHEADS
- dropout = cfg.MODEL.DETR.DROPOUT
- dim_feedforward = cfg.MODEL.DETR.DIM_FEEDFORWARD
- enc_layers = cfg.MODEL.DETR.ENC_LAYERS
- dec_layers = cfg.MODEL.DETR.DEC_LAYERS
- num_feature_levels = cfg.MODEL.DETR.NUM_FEATURE_LEVELS
- two_stage = cfg.MODEL.DETR.TWO_STAGE
- with_box_refine = cfg.MODEL.DETR.WITH_BOX_REFINE
-
- # Loss parameters:
- giou_weight = cfg.MODEL.DETR.GIOU_WEIGHT
- l1_weight = cfg.MODEL.DETR.L1_WEIGHT
- deep_supervision = cfg.MODEL.DETR.DEEP_SUPERVISION
- cls_weight = cfg.MODEL.DETR.CLS_WEIGHT
- focal_alpha = cfg.MODEL.DETR.FOCAL_ALPHA
-
- N_steps = hidden_dim // 2
- d2_backbone = MaskedBackbone(cfg)
- backbone = Joiner(d2_backbone, PositionEmbeddingSine(N_steps, normalize=True))
-
- transformer = DeformableTransformer(
- d_model=hidden_dim,
- nhead=nheads,
- num_encoder_layers=enc_layers,
- num_decoder_layers=dec_layers,
- dim_feedforward=dim_feedforward,
- dropout=dropout,
- activation="relu",
- return_intermediate_dec=True,
- num_feature_levels=num_feature_levels,
- dec_n_points=4,
- enc_n_points=4,
- two_stage=two_stage,
- two_stage_num_proposals=num_queries)
-
- self.detr = DeformableDETR(
- backbone, transformer, num_classes=self.num_classes,
- num_queries=num_queries,
- num_feature_levels=num_feature_levels,
- aux_loss=deep_supervision,
- with_box_refine=with_box_refine,
- two_stage=two_stage,
- )
-
- if self.mask_on:
- assert 0, 'Mask is not supported yet :('
-
- matcher = HungarianMatcher(
- cost_class=cls_weight, cost_bbox=l1_weight, cost_giou=giou_weight)
- weight_dict = {"loss_ce": cls_weight, "loss_bbox": l1_weight}
- weight_dict["loss_giou"] = giou_weight
- if deep_supervision:
- aux_weight_dict = {}
- for i in range(dec_layers - 1):
- aux_weight_dict.update({k + f"_{i}": v for k, v in weight_dict.items()})
- weight_dict.update(aux_weight_dict)
- print('weight_dict', weight_dict)
- losses = ["labels", "boxes", "cardinality"]
- if self.mask_on:
- losses += ["masks"]
- self.criterion = CustomSetCriterion(
- self.num_classes, matcher=matcher, weight_dict=weight_dict,
- focal_alpha=focal_alpha,
- losses=losses,
- use_fed_loss=cfg.MODEL.DETR.USE_FED_LOSS
- )
- pixel_mean = torch.Tensor(cfg.MODEL.PIXEL_MEAN).to(self.device).view(3, 1, 1)
- pixel_std = torch.Tensor(cfg.MODEL.PIXEL_STD).to(self.device).view(3, 1, 1)
- self.normalizer = lambda x: (x - pixel_mean) / pixel_std
-
-
- def forward(self, batched_inputs):
- """
- Args:
- Returns:
- dict[str: Tensor]:
- mapping from a named loss to a tensor storing the loss. Used during training only.
- """
- images = self.preprocess_image(batched_inputs)
- output = self.detr(images)
- if self.training:
- gt_instances = [x["instances"].to(self.device) for x in batched_inputs]
- targets = self.prepare_targets(gt_instances)
- loss_dict = self.criterion(output, targets)
- weight_dict = self.criterion.weight_dict
- for k in loss_dict.keys():
- if k in weight_dict:
- loss_dict[k] *= weight_dict[k]
- if self.with_image_labels:
- if batched_inputs[0]['ann_type'] in ['image', 'captiontag']:
- loss_dict['loss_image'] = self.weak_weight * self._weak_loss(
- output, batched_inputs)
- else:
- loss_dict['loss_image'] = images[0].new_zeros(
- [1], dtype=torch.float32)[0]
- # import pdb; pdb.set_trace()
- return loss_dict
- else:
- image_sizes = output["pred_boxes"].new_tensor(
- [(t["height"], t["width"]) for t in batched_inputs])
- results = self.post_process(output, image_sizes)
- return results
-
-
- def prepare_targets(self, targets):
- new_targets = []
- for targets_per_image in targets:
- h, w = targets_per_image.image_size
- image_size_xyxy = torch.as_tensor([w, h, w, h], dtype=torch.float, device=self.device)
- gt_classes = targets_per_image.gt_classes
- gt_boxes = targets_per_image.gt_boxes.tensor / image_size_xyxy
- gt_boxes = box_xyxy_to_cxcywh(gt_boxes)
- new_targets.append({"labels": gt_classes, "boxes": gt_boxes})
- if self.mask_on and hasattr(targets_per_image, 'gt_masks'):
- assert 0, 'Mask is not supported yet :('
- gt_masks = targets_per_image.gt_masks
- gt_masks = convert_coco_poly_to_mask(gt_masks.polygons, h, w)
- new_targets[-1].update({'masks': gt_masks})
- return new_targets
-
-
- def post_process(self, outputs, target_sizes):
- """
- """
- out_logits, out_bbox = outputs['pred_logits'], outputs['pred_boxes']
- assert len(out_logits) == len(target_sizes)
- assert target_sizes.shape[1] == 2
-
- prob = out_logits.sigmoid()
- topk_values, topk_indexes = torch.topk(
- prob.view(out_logits.shape[0], -1), self.test_topk, dim=1)
- scores = topk_values
- topk_boxes = topk_indexes // out_logits.shape[2]
- labels = topk_indexes % out_logits.shape[2]
- boxes = box_cxcywh_to_xyxy(out_bbox)
- boxes = torch.gather(boxes, 1, topk_boxes.unsqueeze(-1).repeat(1,1,4))
-
- # and from relative [0, 1] to absolute [0, height] coordinates
- img_h, img_w = target_sizes.unbind(1)
- scale_fct = torch.stack([img_w, img_h, img_w, img_h], dim=1)
- boxes = boxes * scale_fct[:, None, :]
-
- results = []
- for s, l, b, size in zip(scores, labels, boxes, target_sizes):
- r = Instances((size[0], size[1]))
- r.pred_boxes = Boxes(b)
- r.scores = s
- r.pred_classes = l
- results.append({'instances': r})
- return results
-
-
- def preprocess_image(self, batched_inputs):
- """
- Normalize, pad and batch the input images.
- """
- images = [self.normalizer(x["image"].to(self.device)) for x in batched_inputs]
- return images
-
-
- def _weak_loss(self, outputs, batched_inputs):
- loss = 0
- for b, x in enumerate(batched_inputs):
- labels = x['pos_category_ids']
- pred_logits = [outputs['pred_logits'][b]]
- pred_boxes = [outputs['pred_boxes'][b]]
- for xx in outputs['aux_outputs']:
- pred_logits.append(xx['pred_logits'][b])
- pred_boxes.append(xx['pred_boxes'][b])
- pred_logits = torch.stack(pred_logits, dim=0) # L x N x C
- pred_boxes = torch.stack(pred_boxes, dim=0) # L x N x 4
- for label in labels:
- loss += self._max_size_loss(
- pred_logits, pred_boxes, label) / len(labels)
- loss = loss / len(batched_inputs)
- return loss
-
-
- def _max_size_loss(self, logits, boxes, label):
- '''
- Inputs:
- logits: L x N x C
- boxes: L x N x 4
- '''
- target = logits.new_zeros((logits.shape[0], logits.shape[2]))
- target[:, label] = 1.
- sizes = boxes[..., 2] * boxes[..., 3] # L x N
- ind = sizes.argmax(dim=1) # L
- loss = F.binary_cross_entropy_with_logits(
- logits[range(len(ind)), ind], target, reduction='sum')
- return loss
\ No newline at end of file
diff --git a/spaces/augmentedimaginationhackathon/paperstocode/frontend/src/app/app.component.ts b/spaces/augmentedimaginationhackathon/paperstocode/frontend/src/app/app.component.ts
deleted file mode 100644
index 0c5a793fbb0f134671c98a3db0c8915187c65291..0000000000000000000000000000000000000000
--- a/spaces/augmentedimaginationhackathon/paperstocode/frontend/src/app/app.component.ts
+++ /dev/null
@@ -1,10 +0,0 @@
-import { Component } from '@angular/core';
-
-@Component({
- selector: 'app-root',
- templateUrl: './app.component.html',
- styleUrls: ['./app.component.scss']
-})
-export class AppComponent {
- title = 'frontend';
-}
diff --git a/spaces/aurora10/gradiolangchainchatbot/README.md b/spaces/aurora10/gradiolangchainchatbot/README.md
deleted file mode 100644
index 6df0c395e6ca790148cce31dc3e7ad66fff04a83..0000000000000000000000000000000000000000
--- a/spaces/aurora10/gradiolangchainchatbot/README.md
+++ /dev/null
@@ -1,12 +0,0 @@
----
-title: Gradiolangchainchatbot
-emoji: 🦀
-colorFrom: purple
-colorTo: gray
-sdk: gradio
-sdk_version: 3.39.0
-app_file: app.py
-pinned: false
----
-
-Check out the configuration reference at https://huggingface.co/docs/hub/spaces-config-reference
diff --git a/spaces/autosummproject/autosumm/summarizer/_utils.py b/spaces/autosummproject/autosumm/summarizer/_utils.py
deleted file mode 100644
index e69de29bb2d1d6434b8b29ae775ad8c2e48c5391..0000000000000000000000000000000000000000
diff --git a/spaces/awacke1/4-Seq2SeqQAT5/app.py b/spaces/awacke1/4-Seq2SeqQAT5/app.py
deleted file mode 100644
index c1cd92499cf1c7d2a91b4dc226bf2d558ff67661..0000000000000000000000000000000000000000
--- a/spaces/awacke1/4-Seq2SeqQAT5/app.py
+++ /dev/null
@@ -1,51 +0,0 @@
-import gradio as gr
-from qasrl_model_pipeline import QASRL_Pipeline
-
-models = ["kleinay/qanom-seq2seq-model-baseline",
- "kleinay/qanom-seq2seq-model-joint"]
-pipelines = {model: QASRL_Pipeline(model) for model in models}
-
-
-description = f"""Using Seq2Seq T5 model which takes a sequence of items and outputs another sequence this model generates Questions and Answers (QA) with focus on Semantic Role Labeling (SRL)"""
-title="Seq2Seq T5 Questions and Answers (QA) with Semantic Role Labeling (SRL)"
-examples = [[models[0], "In March and April the patient had two falls. One was related to asthma, heart palpitations. The second was due to syncope and post covid vaccination dizziness during exercise. The patient is now getting an EKG. Former EKG had shown that there was a bundle branch block. Patient had some uncontrolled immune system reactions like anaphylaxis and shortness of breath.", True, "fall"],
- [models[1], "In March and April the patient had two falls. One was related to asthma, heart palpitations. The second was due to syncope and post covid vaccination dizziness during exercise. The patient is now getting an EKG. Former EKG had shown that there was a bundle branch block. Patient had some uncontrolled immune system reactions
like anaphylaxis and shortness of breath.", True, "reactions"],
- [models[0], "In March and April the patient had two falls. One was related
to asthma, heart palpitations. The second was due to syncope and post covid vaccination dizziness during exercise. The patient is now getting an EKG. Former EKG had shown that there was a bundle branch block. Patient had some uncontrolled immune system reactions like anaphylaxis and shortness of breath.", True, "relate"],
- [models[1], "In March and April the patient
had two falls. One was related to asthma, heart palpitations. The second was due to syncope and post covid vaccination dizziness during exercise. The patient is now getting an EKG. Former EKG had shown that there was a bundle branch block. Patient had some uncontrolled immune system reactions like anaphylaxis and shortness of breath.", False, "fall"]]
-
-input_sent_box_label = "Insert sentence here. Mark the predicate by adding the token '
' before it."
-verb_form_inp_placeholder = "e.g. 'decide' for the nominalization 'decision', 'teach' for 'teacher', etc."
-links = """
-QASRL Website | Model Repo at Huggingface Hub
-
"""
-def call(model_name, sentence, is_nominal, verb_form):
- predicate_marker=""
- if predicate_marker not in sentence:
- raise ValueError("You must highlight one word of the sentence as a predicate using preceding '
'.")
-
- if not verb_form:
- if is_nominal:
- raise ValueError("You should provide the verbal form of the nominalization")
-
- toks = sentence.split(" ")
- pred_idx = toks.index(predicate_marker)
- predicate = toks(pred_idx+1)
- verb_form=predicate
- pipeline = pipelines[model_name]
- pipe_out = pipeline([sentence],
- predicate_marker=predicate_marker,
- predicate_type="nominal" if is_nominal else "verbal",
- verb_form=verb_form)[0]
- return pipe_out["QAs"], pipe_out["generated_text"]
-iface = gr.Interface(fn=call,
- inputs=[gr.inputs.Radio(choices=models, default=models[0], label="Model"),
- gr.inputs.Textbox(placeholder=input_sent_box_label, label="Sentence", lines=4),
- gr.inputs.Checkbox(default=True, label="Is Nominalization?"),
- gr.inputs.Textbox(placeholder=verb_form_inp_placeholder, label="Verbal form (for nominalizations)", default='')],
- outputs=[gr.outputs.JSON(label="Model Output - QASRL"), gr.outputs.Textbox(label="Raw output sequence")],
- title=title,
- description=description,
- article=links,
- examples=examples )
-
-iface.launch()
\ No newline at end of file
diff --git a/spaces/awacke1/AnimatedGifGallery/README.md b/spaces/awacke1/AnimatedGifGallery/README.md
deleted file mode 100644
index 1c723a877a5918e4bc777b2b1dbc7f35be005872..0000000000000000000000000000000000000000
--- a/spaces/awacke1/AnimatedGifGallery/README.md
+++ /dev/null
@@ -1,13 +0,0 @@
----
-title: AnimatedGifGallery
-emoji: ⚡
-colorFrom: yellow
-colorTo: yellow
-sdk: streamlit
-sdk_version: 1.21.0
-app_file: app.py
-pinned: false
-license: mit
----
-
-Check out the configuration reference at https://huggingface.co/docs/hub/spaces-config-reference
diff --git a/spaces/awacke1/Art-Generator-and-Style-Mixer/README.md b/spaces/awacke1/Art-Generator-and-Style-Mixer/README.md
deleted file mode 100644
index 8b46386f218709fc1699b813d0812ea5a0dc16e1..0000000000000000000000000000000000000000
--- a/spaces/awacke1/Art-Generator-and-Style-Mixer/README.md
+++ /dev/null
@@ -1,13 +0,0 @@
----
-title: 🧠 ArtStyleMixer🎨
-emoji: ❤️🎨
-colorFrom: blue
-colorTo: green
-sdk: gradio
-sdk_version: 2.9.4
-app_file: app.py
-pinned: false
-license: mit
----
-
-Check out the configuration reference at https://huggingface.co/docs/hub/spaces#reference
diff --git a/spaces/awacke1/BlackjackSimulatorCardGameAI/static/Readme.md b/spaces/awacke1/BlackjackSimulatorCardGameAI/static/Readme.md
deleted file mode 100644
index e69de29bb2d1d6434b8b29ae775ad8c2e48c5391..0000000000000000000000000000000000000000
diff --git a/spaces/awacke1/Speech2Text-FastSpeech2/app.py b/spaces/awacke1/Speech2Text-FastSpeech2/app.py
deleted file mode 100644
index 624711103fff0eb591bc05f07ae20c47fbe03cd2..0000000000000000000000000000000000000000
--- a/spaces/awacke1/Speech2Text-FastSpeech2/app.py
+++ /dev/null
@@ -1,3 +0,0 @@
-import gradio as gr
-
-gr.Interface.load("models/facebook/fastspeech2-en-ljspeech").launch()
\ No newline at end of file
diff --git a/spaces/awacke1/StreamlitWikipediaChat/app.py b/spaces/awacke1/StreamlitWikipediaChat/app.py
deleted file mode 100644
index c3c769fb9ddc699aeb346425e0fe298d1a098190..0000000000000000000000000000000000000000
--- a/spaces/awacke1/StreamlitWikipediaChat/app.py
+++ /dev/null
@@ -1,239 +0,0 @@
-import streamlit as st
-import spacy
-import wikipediaapi
-import wikipedia
-from wikipedia.exceptions import DisambiguationError
-from transformers import TFAutoModel, AutoTokenizer
-import numpy as np
-import pandas as pd
-import faiss
-import datetime
-import time
-
-
-try:
- nlp = spacy.load("en_core_web_sm")
-except:
- spacy.cli.download("en_core_web_sm")
- nlp = spacy.load("en_core_web_sm")
-
-wh_words = ['what', 'who', 'how', 'when', 'which']
-
-def get_concepts(text):
- text = text.lower()
- doc = nlp(text)
- concepts = []
- for chunk in doc.noun_chunks:
- if chunk.text not in wh_words:
- concepts.append(chunk.text)
- return concepts
-
-def get_passages(text, k=100):
- doc = nlp(text)
- passages = []
- passage_len = 0
- passage = ""
- sents = list(doc.sents)
- for i in range(len(sents)):
- sen = sents[i]
- passage_len += len(sen)
- if passage_len >= k:
- passages.append(passage)
- passage = sen.text
- passage_len = len(sen)
- continue
- elif i == (len(sents) - 1):
- passage += " " + sen.text
- passages.append(passage)
- passage = ""
- passage_len = 0
- continue
- passage += " " + sen.text
- return passages
-
-def get_dicts_for_dpr(concepts, n_results=20, k=100):
- dicts = []
- for concept in concepts:
- wikis = wikipedia.search(concept, results=n_results)
- st.write(f"{concept} No of Wikis: {len(wikis)}")
- for wiki in wikis:
- try:
- html_page = wikipedia.page(title=wiki, auto_suggest=False)
- except DisambiguationError:
- continue
- htmlResults = html_page.content
- passages = get_passages(htmlResults, k=k)
- for passage in passages:
- i_dicts = {}
- i_dicts['text'] = passage
- i_dicts['title'] = wiki
- dicts.append(i_dicts)
- return dicts
-
-passage_encoder = TFAutoModel.from_pretrained("nlpconnect/dpr-ctx_encoder_bert_uncased_L-2_H-128_A-2")
-query_encoder = TFAutoModel.from_pretrained("nlpconnect/dpr-question_encoder_bert_uncased_L-2_H-128_A-2")
-p_tokenizer = AutoTokenizer.from_pretrained("nlpconnect/dpr-ctx_encoder_bert_uncased_L-2_H-128_A-2")
-q_tokenizer = AutoTokenizer.from_pretrained("nlpconnect/dpr-question_encoder_bert_uncased_L-2_H-128_A-2")
-
-def get_title_text_combined(passage_dicts):
- res = []
- for p in passage_dicts:
- res.append(tuple((p['title'], p['text'])))
- return res
-
-def extracted_passage_embeddings(processed_passages, max_length=156):
- passage_inputs = p_tokenizer.batch_encode_plus(
- processed_passages,
- add_special_tokens=True,
- truncation=True,
- padding="max_length",
- max_length=max_length,
- return_token_type_ids=True
- )
- passage_embeddings = passage_encoder.predict([np.array(passage_inputs['input_ids']), np.array(passage_inputs['attention_mask']),
- np.array(passage_inputs['token_type_ids'])],
- batch_size=64,
- verbose=1)
- return passage_embeddings
-
-def extracted_query_embeddings(queries, max_length=64):
- query_inputs = q_tokenizer.batch_encode_plus(
- queries,
- add_special_tokens=True,
- truncation=True,
- padding="max_length",
- max_length=max_length,
- return_token_type_ids=True
- )
-
- query_embeddings = query_encoder.predict([np.array(query_inputs['input_ids']),
- np.array(query_inputs['attention_mask']),
- np.array(query_inputs['token_type_ids'])],
- batch_size=1,
- verbose=1)
- return query_embeddings
-
-def get_pagetext(page):
- s = str(page).replace("/t","")
- return s
-
-def get_wiki_summary(search):
- wiki_wiki = wikipediaapi.Wikipedia('en')
- page = wiki_wiki.page(search)
-
-
-def get_wiki_summaryDF(search):
- wiki_wiki = wikipediaapi.Wikipedia('en')
- page = wiki_wiki.page(search)
-
- isExist = page.exists()
- if not isExist:
- return isExist, "Not found", "Not found", "Not found", "Not found"
-
- pageurl = page.fullurl
- pagetitle = page.title
- pagesummary = page.summary[0:60]
- pagetext = get_pagetext(page.text)
-
- backlinks = page.backlinks
- linklist = ""
- for link in backlinks.items():
- pui = link[0]
- linklist += pui + " , "
- a=1
-
- categories = page.categories
- categorylist = ""
- for category in categories.items():
- pui = category[0]
- categorylist += pui + " , "
- a=1
-
- links = page.links
- linklist2 = ""
- for link in links.items():
- pui = link[0]
- linklist2 += pui + " , "
- a=1
-
- sections = page.sections
-
- ex_dic = {
- 'Entity' : ["URL","Title","Summary", "Text", "Backlinks", "Links", "Categories"],
- 'Value': [pageurl, pagetitle, pagesummary, pagetext, linklist,linklist2, categorylist ]
- }
-
- df = pd.DataFrame(ex_dic)
-
- return df
-
-
-def save_message(name, message):
- now = datetime.datetime.now()
- timestamp = now.strftime("%Y-%m-%d %H:%M:%S")
- with open("chat.txt", "a") as f:
- f.write(f"{timestamp} - {name}: {message}\n")
-
-def press_release():
- st.markdown("""🎉🎊 Breaking News! 📢📣
-
-Introducing StreamlitWikipediaChat - the ultimate way to chat with Wikipedia and the whole world at the same time! 🌎📚👋
-
-Are you tired of reading boring articles on Wikipedia? Do you want to have some fun while learning new things? Then StreamlitWikipediaChat is just the thing for you! 😃💻
-
-With StreamlitWikipediaChat, you can ask Wikipedia anything you want and get instant responses! Whether you want to know the capital of Madagascar or how to make a delicious chocolate cake, Wikipedia has got you covered. 🍰🌍
-
-But that's not all! You can also chat with other people from around the world who are using StreamlitWikipediaChat at the same time. It's like a virtual classroom where you can learn from and teach others. 🌐👨🏫👩🏫
-
-And the best part? StreamlitWikipediaChat is super easy to use! All you have to do is type in your question and hit send. That's it! 🤯🙌
-
-So, what are you waiting for? Join the fun and start chatting with Wikipedia and the world today! 😎🎉
-
-StreamlitWikipediaChat - where learning meets fun! 🤓🎈""")
-
-
-def main():
- st.title("Streamlit Chat")
-
- name = st.text_input("Enter your name")
- message = st.text_input("Enter a topic to share from Wikipedia")
- if st.button("Submit"):
-
- # wiki
- df = get_wiki_summaryDF(message)
-
- save_message(name, message)
- save_message(name, df)
-
- st.text("Message sent!")
-
-
- st.text("Chat history:")
- with open("chat.txt", "a+") as f:
- f.seek(0)
- chat_history = f.read()
- #st.text(chat_history)
- st.markdown(chat_history)
-
- countdown = st.empty()
- t = 60
- while t:
- mins, secs = divmod(t, 60)
- countdown.text(f"Time remaining: {mins:02d}:{secs:02d}")
- time.sleep(1)
- t -= 1
- if t == 0:
- countdown.text("Time's up!")
- with open("chat.txt", "a+") as f:
- f.seek(0)
- chat_history = f.read()
- #st.text(chat_history)
- st.markdown(chat_history)
-
- press_release()
-
- t = 60
-
-if __name__ == "__main__":
- main()
-
diff --git a/spaces/awacke1/TrapFlamenco/README.md b/spaces/awacke1/TrapFlamenco/README.md
deleted file mode 100644
index 2010061323ddb0a2ab7524389cf126980d6825ac..0000000000000000000000000000000000000000
--- a/spaces/awacke1/TrapFlamenco/README.md
+++ /dev/null
@@ -1,11 +0,0 @@
----
-title: TrapFlamenco
-emoji: 📉
-colorFrom: yellow
-colorTo: blue
-sdk: static
-pinned: false
-license: mit
----
-
-Check out the configuration reference at https://huggingface.co/docs/hub/spaces-config-reference
diff --git a/spaces/banana-projects/web3d/node_modules/three/examples/js/nodes/accessors/CameraNode.js b/spaces/banana-projects/web3d/node_modules/three/examples/js/nodes/accessors/CameraNode.js
deleted file mode 100644
index dc24174515f3e51425e285f2747bd9dbf4d91574..0000000000000000000000000000000000000000
--- a/spaces/banana-projects/web3d/node_modules/three/examples/js/nodes/accessors/CameraNode.js
+++ /dev/null
@@ -1,236 +0,0 @@
-/**
- * @author sunag / http://www.sunag.com.br/
- */
-
-import { TempNode } from '../core/TempNode.js';
-import { FunctionNode } from '../core/FunctionNode.js';
-import { FloatNode } from '../inputs/FloatNode.js';
-import { PositionNode } from '../accessors/PositionNode.js';
-
-function CameraNode( scope, camera ) {
-
- TempNode.call( this, 'v3' );
-
- this.setScope( scope || CameraNode.POSITION );
- this.setCamera( camera );
-
-}
-
-CameraNode.Nodes = ( function () {
-
- var depthColor = new FunctionNode( [
- "float depthColor( float mNear, float mFar ) {",
-
- " #ifdef USE_LOGDEPTHBUF_EXT",
-
- " float depth = gl_FragDepthEXT / gl_FragCoord.w;",
-
- " #else",
-
- " float depth = gl_FragCoord.z / gl_FragCoord.w;",
-
- " #endif",
-
- " return 1.0 - smoothstep( mNear, mFar, depth );",
-
- "}"
- ].join( "\n" ) );
-
- return {
- depthColor: depthColor
- };
-
-} )();
-
-CameraNode.POSITION = 'position';
-CameraNode.DEPTH = 'depth';
-CameraNode.TO_VERTEX = 'toVertex';
-
-CameraNode.prototype = Object.create( TempNode.prototype );
-CameraNode.prototype.constructor = CameraNode;
-CameraNode.prototype.nodeType = "Camera";
-
-CameraNode.prototype.setCamera = function ( camera ) {
-
- this.camera = camera;
- this.updateFrame = camera !== undefined ? this.onUpdateFrame : undefined;
-
-};
-
-CameraNode.prototype.setScope = function ( scope ) {
-
- switch ( this.scope ) {
-
- case CameraNode.DEPTH:
-
- delete this.near;
- delete this.far;
-
- break;
-
- }
-
- this.scope = scope;
-
- switch ( scope ) {
-
- case CameraNode.DEPTH:
-
- var camera = this.camera;
-
- this.near = new FloatNode( camera ? camera.near : 1 );
- this.far = new FloatNode( camera ? camera.far : 1200 );
-
- break;
-
- }
-
-};
-
-CameraNode.prototype.getType = function ( builder ) {
-
- switch ( this.scope ) {
-
- case CameraNode.DEPTH:
-
- return 'f';
-
- }
-
- return this.type;
-
-};
-
-CameraNode.prototype.getUnique = function ( builder ) {
-
- switch ( this.scope ) {
-
- case CameraNode.DEPTH:
- case CameraNode.TO_VERTEX:
-
- return true;
-
- }
-
- return false;
-
-};
-
-CameraNode.prototype.getShared = function ( builder ) {
-
- switch ( this.scope ) {
-
- case CameraNode.POSITION:
-
- return false;
-
- }
-
- return true;
-
-};
-
-CameraNode.prototype.generate = function ( builder, output ) {
-
- var result;
-
- switch ( this.scope ) {
-
- case CameraNode.POSITION:
-
- result = 'cameraPosition';
-
- break;
-
- case CameraNode.DEPTH:
-
- var depthColor = builder.include( CameraNode.Nodes.depthColor );
-
- result = depthColor + '( ' + this.near.build( builder, 'f' ) + ', ' + this.far.build( builder, 'f' ) + ' )';
-
- break;
-
- case CameraNode.TO_VERTEX:
-
- result = 'normalize( ' + new PositionNode( PositionNode.WORLD ).build( builder, 'v3' ) + ' - cameraPosition )';
-
- break;
-
- }
-
- return builder.format( result, this.getType( builder ), output );
-
-};
-
-CameraNode.prototype.onUpdateFrame = function ( frame ) {
-
- switch ( this.scope ) {
-
- case CameraNode.DEPTH:
-
- var camera = this.camera;
-
- this.near.value = camera.near;
- this.far.value = camera.far;
-
- break;
-
- }
-
-};
-
-CameraNode.prototype.copy = function ( source ) {
-
- TempNode.prototype.copy.call( this, source );
-
- this.setScope( source.scope );
-
- if ( source.camera ) {
-
- this.setCamera( source.camera );
-
- }
-
- switch ( source.scope ) {
-
- case CameraNode.DEPTH:
-
- this.near.number = source.near;
- this.far.number = source.far;
-
- break;
-
- }
-
-};
-
-CameraNode.prototype.toJSON = function ( meta ) {
-
- var data = this.getJSONNode( meta );
-
- if ( ! data ) {
-
- data = this.createJSONNode( meta );
-
- data.scope = this.scope;
-
- if ( this.camera ) data.camera = this.camera.uuid;
-
- switch ( this.scope ) {
-
- case CameraNode.DEPTH:
-
- data.near = this.near.value;
- data.far = this.far.value;
-
- break;
-
- }
-
- }
-
- return data;
-
-};
-
-export { CameraNode };
diff --git a/spaces/banana-projects/web3d/node_modules/three/src/loaders/FileLoader.d.ts b/spaces/banana-projects/web3d/node_modules/three/src/loaders/FileLoader.d.ts
deleted file mode 100644
index b66b56d48f227db51966f715b3eaf6534c89f218..0000000000000000000000000000000000000000
--- a/spaces/banana-projects/web3d/node_modules/three/src/loaders/FileLoader.d.ts
+++ /dev/null
@@ -1,44 +0,0 @@
-import { LoadingManager } from './LoadingManager';
-import { Loader } from './Loader';
-
-/**
- * Interface for all loaders
- * CompressedTextureLoader don't extends Loader class, but have load method
- */
-export interface AnyLoader {
- load(
- url: string,
- onLoad?: (result: any) => void,
- onProgress?: (event: ProgressEvent) => void,
- onError?: (event: ErrorEvent) => void
- ): any;
-}
-
-export interface LoaderHandler {
- handlers: (RegExp | AnyLoader)[];
-
- add(regex: RegExp, loader: AnyLoader): void;
- get(file: string): AnyLoader | null;
-}
-
-export class FileLoader {
- constructor(manager?: LoadingManager);
-
- manager: LoadingManager;
- mimeType: MimeType;
- path: string;
- responseType: string;
- withCredentials: string;
-
- load(
- url: string,
- onLoad?: (response: string | ArrayBuffer) => void,
- onProgress?: (request: ProgressEvent) => void,
- onError?: (event: ErrorEvent) => void
- ): any;
- setMimeType(mimeType: MimeType): FileLoader;
- setPath(path: string): FileLoader;
- setResponseType(responseType: string): FileLoader;
- setWithCredentials(value: string): FileLoader;
- setRequestHeader(value: { [header: string]: string }): FileLoader;
-}
diff --git a/spaces/banana-projects/web3d/node_modules/three/src/math/Sphere.js b/spaces/banana-projects/web3d/node_modules/three/src/math/Sphere.js
deleted file mode 100644
index 430c8363c0052f1795d20a4c5a5780984d600f45..0000000000000000000000000000000000000000
--- a/spaces/banana-projects/web3d/node_modules/three/src/math/Sphere.js
+++ /dev/null
@@ -1,180 +0,0 @@
-import { Box3 } from './Box3.js';
-import { Vector3 } from './Vector3.js';
-
-/**
- * @author bhouston / http://clara.io
- * @author mrdoob / http://mrdoob.com/
- */
-
-function Sphere( center, radius ) {
-
- this.center = ( center !== undefined ) ? center : new Vector3();
- this.radius = ( radius !== undefined ) ? radius : 0;
-
-}
-
-Object.assign( Sphere.prototype, {
-
- set: function ( center, radius ) {
-
- this.center.copy( center );
- this.radius = radius;
-
- return this;
-
- },
-
- setFromPoints: function () {
-
- var box = new Box3();
-
- return function setFromPoints( points, optionalCenter ) {
-
- var center = this.center;
-
- if ( optionalCenter !== undefined ) {
-
- center.copy( optionalCenter );
-
- } else {
-
- box.setFromPoints( points ).getCenter( center );
-
- }
-
- var maxRadiusSq = 0;
-
- for ( var i = 0, il = points.length; i < il; i ++ ) {
-
- maxRadiusSq = Math.max( maxRadiusSq, center.distanceToSquared( points[ i ] ) );
-
- }
-
- this.radius = Math.sqrt( maxRadiusSq );
-
- return this;
-
- };
-
- }(),
-
- clone: function () {
-
- return new this.constructor().copy( this );
-
- },
-
- copy: function ( sphere ) {
-
- this.center.copy( sphere.center );
- this.radius = sphere.radius;
-
- return this;
-
- },
-
- empty: function () {
-
- return ( this.radius <= 0 );
-
- },
-
- containsPoint: function ( point ) {
-
- return ( point.distanceToSquared( this.center ) <= ( this.radius * this.radius ) );
-
- },
-
- distanceToPoint: function ( point ) {
-
- return ( point.distanceTo( this.center ) - this.radius );
-
- },
-
- intersectsSphere: function ( sphere ) {
-
- var radiusSum = this.radius + sphere.radius;
-
- return sphere.center.distanceToSquared( this.center ) <= ( radiusSum * radiusSum );
-
- },
-
- intersectsBox: function ( box ) {
-
- return box.intersectsSphere( this );
-
- },
-
- intersectsPlane: function ( plane ) {
-
- return Math.abs( plane.distanceToPoint( this.center ) ) <= this.radius;
-
- },
-
- clampPoint: function ( point, target ) {
-
- var deltaLengthSq = this.center.distanceToSquared( point );
-
- if ( target === undefined ) {
-
- console.warn( 'THREE.Sphere: .clampPoint() target is now required' );
- target = new Vector3();
-
- }
-
- target.copy( point );
-
- if ( deltaLengthSq > ( this.radius * this.radius ) ) {
-
- target.sub( this.center ).normalize();
- target.multiplyScalar( this.radius ).add( this.center );
-
- }
-
- return target;
-
- },
-
- getBoundingBox: function ( target ) {
-
- if ( target === undefined ) {
-
- console.warn( 'THREE.Sphere: .getBoundingBox() target is now required' );
- target = new Box3();
-
- }
-
- target.set( this.center, this.center );
- target.expandByScalar( this.radius );
-
- return target;
-
- },
-
- applyMatrix4: function ( matrix ) {
-
- this.center.applyMatrix4( matrix );
- this.radius = this.radius * matrix.getMaxScaleOnAxis();
-
- return this;
-
- },
-
- translate: function ( offset ) {
-
- this.center.add( offset );
-
- return this;
-
- },
-
- equals: function ( sphere ) {
-
- return sphere.center.equals( this.center ) && ( sphere.radius === this.radius );
-
- }
-
-} );
-
-
-export { Sphere };
diff --git a/spaces/banana-projects/web3d/node_modules/three/src/objects/LineSegments.js b/spaces/banana-projects/web3d/node_modules/three/src/objects/LineSegments.js
deleted file mode 100644
index 30f38c52a8542c08e472a7e8697fce76c5b8ba5c..0000000000000000000000000000000000000000
--- a/spaces/banana-projects/web3d/node_modules/three/src/objects/LineSegments.js
+++ /dev/null
@@ -1,85 +0,0 @@
-import { Line } from './Line.js';
-import { Vector3 } from '../math/Vector3.js';
-import { Float32BufferAttribute } from '../core/BufferAttribute.js';
-
-/**
- * @author mrdoob / http://mrdoob.com/
- */
-
-function LineSegments( geometry, material ) {
-
- Line.call( this, geometry, material );
-
- this.type = 'LineSegments';
-
-}
-
-LineSegments.prototype = Object.assign( Object.create( Line.prototype ), {
-
- constructor: LineSegments,
-
- isLineSegments: true,
-
- computeLineDistances: ( function () {
-
- var start = new Vector3();
- var end = new Vector3();
-
- return function computeLineDistances() {
-
- var geometry = this.geometry;
-
- if ( geometry.isBufferGeometry ) {
-
- // we assume non-indexed geometry
-
- if ( geometry.index === null ) {
-
- var positionAttribute = geometry.attributes.position;
- var lineDistances = [];
-
- for ( var i = 0, l = positionAttribute.count; i < l; i += 2 ) {
-
- start.fromBufferAttribute( positionAttribute, i );
- end.fromBufferAttribute( positionAttribute, i + 1 );
-
- lineDistances[ i ] = ( i === 0 ) ? 0 : lineDistances[ i - 1 ];
- lineDistances[ i + 1 ] = lineDistances[ i ] + start.distanceTo( end );
-
- }
-
- geometry.addAttribute( 'lineDistance', new Float32BufferAttribute( lineDistances, 1 ) );
-
- } else {
-
- console.warn( 'THREE.LineSegments.computeLineDistances(): Computation only possible with non-indexed BufferGeometry.' );
-
- }
-
- } else if ( geometry.isGeometry ) {
-
- var vertices = geometry.vertices;
- var lineDistances = geometry.lineDistances;
-
- for ( var i = 0, l = vertices.length; i < l; i += 2 ) {
-
- start.copy( vertices[ i ] );
- end.copy( vertices[ i + 1 ] );
-
- lineDistances[ i ] = ( i === 0 ) ? 0 : lineDistances[ i - 1 ];
- lineDistances[ i + 1 ] = lineDistances[ i ] + start.distanceTo( end );
-
- }
-
- }
-
- return this;
-
- };
-
- }() )
-
-} );
-
-
-export { LineSegments };
diff --git a/spaces/banana-projects/web3d/node_modules/three/src/renderers/shaders/ShaderChunk/specularmap_fragment.glsl.js b/spaces/banana-projects/web3d/node_modules/three/src/renderers/shaders/ShaderChunk/specularmap_fragment.glsl.js
deleted file mode 100644
index 9f7cf8383b10b67219a17e03f0871f4f9c1a8162..0000000000000000000000000000000000000000
--- a/spaces/banana-projects/web3d/node_modules/three/src/renderers/shaders/ShaderChunk/specularmap_fragment.glsl.js
+++ /dev/null
@@ -1,14 +0,0 @@
-export default /* glsl */`
-float specularStrength;
-
-#ifdef USE_SPECULARMAP
-
- vec4 texelSpecular = texture2D( specularMap, vUv );
- specularStrength = texelSpecular.r;
-
-#else
-
- specularStrength = 1.0;
-
-#endif
-`;
diff --git a/spaces/bigscience/petals-api/src/client/remote_sequential.py b/spaces/bigscience/petals-api/src/client/remote_sequential.py
deleted file mode 100644
index 1b3a71eb9b76932bec64d032ee9e0abc1a15d543..0000000000000000000000000000000000000000
--- a/spaces/bigscience/petals-api/src/client/remote_sequential.py
+++ /dev/null
@@ -1,135 +0,0 @@
-from __future__ import annotations
-
-import contextlib
-import logging
-import random
-
-import torch
-from hivemind import DHT, P2P, get_logger, use_hivemind_log_handler
-from hivemind.moe.client.remote_expert_worker import RemoteExpertWorker
-from hivemind.moe.expert_uid import ExpertInfo
-from torch import nn
-
-import src
-from src.client.remote_block import RemoteTransformerBlock
-from src.client.remote_sequence_info import RemoteSequenceInfo
-from src.data_structures import UID_DELIMITER
-from src.dht_utils import _create_remote_modules_from_infos
-
-use_hivemind_log_handler("in_root_logger")
-logger = get_logger(__file__)
-
-
-class RemoteSequential(nn.Module):
- """
- A sequence of transformer blocks hosted by the swarm.
- """
-
- def __init__(self, config: src.DistributedBloomConfig, dht: DHT, prefix: str, max_retries: int = 3):
- logger.warning(f"{self.__class__.__name__} is in active development; expect adventures")
- if prefix.endswith(UID_DELIMITER):
- logger.warning(
- f"dht_prefix {prefix} already ends with '{UID_DELIMITER}'."
- f"This will cause {self.__class__.__name__} to look for modules under "
- f"{prefix}{UID_DELIMITER}*. Please make sure this is what you intended."
- )
-
- super().__init__()
- self.config = config
- self.dht = dht
- self.prefix = prefix
- self.max_retries = max_retries
- self.p2p = RemoteExpertWorker.run_coroutine(dht.replicate_p2p())
-
- block_uids = tuple(f"{prefix}{UID_DELIMITER}{i}" for i in range(config.n_layer))
-
- logger.debug(f"Remote block uids: {block_uids}")
- self.remote_sequence_info = RemoteSequenceInfo(dht, block_uids)
-
- def forward(self, inputs: torch.Tensor):
- assert isinstance(inputs, torch.Tensor) and inputs.ndim == 3 and inputs.shape[-1] == self.config.n_embed
- for block_index in range(self.config.n_layer):
- for retry_index in range(self.max_retries):
- try:
- block = self[block_index]
- (outputs,) = block(inputs)
- assert isinstance(outputs, torch.Tensor)
- assert outputs.shape == inputs.shape, f"Expected {block} output {inputs.shape}, got {outputs.shape}"
- inputs = outputs
- break
- except Exception as e:
- if retry_index == self.max_retries - 1:
- raise e
- else:
- logging.debug(f"Caught {e} when running forward for block {block_index}", exc_info=True)
- return inputs
-
- def __getitem__(self, block_index: int):
- assert 0 <= block_index < self.config.n_layer
- (module,) = _create_remote_modules_from_infos([self.remote_sequence_info.block_infos[block_index]], self.p2p)
- return module
-
- def __iter__(self):
- for block_index in range(self.config.n_layer):
- yield self[block_index]
-
- def __len__(self):
- return len(self.remote_sequence_info)
-
- def inference_session(self) -> RemoteSequentialInferenceSession:
- self.remote_sequence_info.update_()
- return RemoteSequentialInferenceSession(self.remote_sequence_info, self.p2p)
-
-
-class RemoteSequentialInferenceSession:
- """An interface to a multi-step *inference* session for a sequence of remote transformer blocks"""
-
- def __init__(self, remote_sequence_info: RemoteSequenceInfo, p2p: P2P):
- self.remote_sequence_info = remote_sequence_info
- self.p2p = p2p
- self.closed = False
- self.stack = contextlib.ExitStack()
- self.active_sessions = []
-
- def __enter__(self):
- assert not self.closed
- self.stack.__enter__()
- # TODO(yozh) replace this code with a fault-tolerant chain that can be reconstructed if some peers fail
- current_block = 0
- while current_block != len(self.remote_sequence_info):
- candidate_spans = self.remote_sequence_info.spans_containing_block[current_block]
- chosen_span = random.choice(candidate_spans) # TODO this is a temporary code
- assert chosen_span.start <= current_block < chosen_span.end
-
- # TODO begin throwaway prototype code
- remote = RemoteTransformerBlock(self.remote_sequence_info.block_infos[current_block], self.p2p)
- _ = remote.info # TODO fix
- span_uids = self.remote_sequence_info.block_uids[current_block : chosen_span.end]
- remote._info = ExpertInfo(" ".join(span_uids), chosen_span.peer_id)
- self.active_sessions.append(remote.inference_session())
- self.stack.enter_context(self.active_sessions[-1])
- current_block = chosen_span.end
- # TODO end throwaway prototype code
-
- return self
-
- def step(self, inputs: torch.Tensor):
- assert not self.closed
- for session in self.active_sessions:
- outputs = session.step(inputs)
- assert outputs.shape == inputs.shape, f"expected {inputs.shape}, got {outputs.shape}"
- inputs = outputs
- return inputs
-
- def close(self, *exc_details):
- """Finish a given inference session, close the underlying connection"""
- if not self.closed:
- self.stack.__exit__(*exc_details or (None, None, None))
- self.active_sessions.clear()
- self.closed = True
-
- def __exit__(self, *exc_details):
- self.close(*exc_details)
-
- def __del__(self):
- self.close()
diff --git a/spaces/bioriAsaeru/text-to-voice/Discover the Truth About Schizophrenia The Centre Cannot Hold Pdf and 12 Free Courses to Enlighten You.md b/spaces/bioriAsaeru/text-to-voice/Discover the Truth About Schizophrenia The Centre Cannot Hold Pdf and 12 Free Courses to Enlighten You.md
deleted file mode 100644
index cc8bcdb93be1d38f120bd9a2301c2178a3b4b05a..0000000000000000000000000000000000000000
--- a/spaces/bioriAsaeru/text-to-voice/Discover the Truth About Schizophrenia The Centre Cannot Hold Pdf and 12 Free Courses to Enlighten You.md
+++ /dev/null
@@ -1,6 +0,0 @@
-
The Centre Cannot Hold Pdf 12 cours gratuitements DOWNLOAD ✯✯✯ https://urloso.com/2uyR6K
-
- aaccfb2cb3
-
-
-
diff --git a/spaces/bookbot/SpeechLine/app.py b/spaces/bookbot/SpeechLine/app.py
deleted file mode 100644
index 50a8bbe1d103b70ef6f4a160913b72e21300cd24..0000000000000000000000000000000000000000
--- a/spaces/bookbot/SpeechLine/app.py
+++ /dev/null
@@ -1,122 +0,0 @@
-import os
-import shutil
-from pathlib import Path
-
-import gradio as gr
-import pandas as pd
-from datasets import Audio, Dataset
-from speechline.segmenters import SilenceSegmenter, WordOverlapSegmenter
-from speechline.transcribers import Wav2Vec2Transcriber
-from speechline.utils.tokenizer import WordTokenizer
-
-MAX_SEGMENTS = 100
-OUTPUT_DIR = "tmp"
-
-
-def segmentation_interface(choice: str):
- if choice == "Silence Gap":
- return gr.update(visible=True), gr.update(visible=False)
- elif choice == "Word Overlap":
- return gr.update(visible=False), gr.update(visible=True)
-
-
-def run(audio_path, model, segmentation_type, silence_duration, ground_truth):
- transcriber = Wav2Vec2Transcriber(model)
- dataset = Dataset.from_dict({"audio": [audio_path]})
- dataset = dataset.cast_column(
- "audio", Audio(sampling_rate=transcriber.sampling_rate)
- )
- output_offsets = transcriber.predict(dataset, output_offsets=True)
-
- if segmentation_type == "Silence Gap":
- segmenter = SilenceSegmenter()
- elif segmentation_type == "Word Overlap":
- segmenter = WordOverlapSegmenter()
-
- tokenizer = WordTokenizer()
-
- if os.path.exists(OUTPUT_DIR):
- shutil.rmtree(OUTPUT_DIR)
-
- segmenter.chunk_audio_segments(
- audio_path,
- OUTPUT_DIR,
- output_offsets[0],
- minimum_chunk_duration=0,
- silence_duration=silence_duration,
- ground_truth=tokenizer(ground_truth),
- )
-
- outputs, idx = [], 0
-
- for path in sorted(Path(OUTPUT_DIR).rglob("*")):
- if path.suffix == ".tsv":
- gt = pd.read_csv(
- path, sep="\t", names=["start_offset", "end_offset", "text"]
- )
- outputs.append(gr.Dataframe.update(value=gt, visible=True))
- elif path.suffix == ".wav":
- outputs.append(gr.Audio.update(value=str(path), visible=True))
- idx += 1
-
- for _ in range(MAX_SEGMENTS - idx):
- outputs += [gr.Dataframe.update(visible=False), gr.Audio.update(visible=False)]
- return outputs
-
-
-with gr.Blocks() as demo:
- gr.Markdown(
- f"""
-
-
- # 🎙️ SpeechLine Demo
- [Repository](https://github.com/bookbot-kids/speechline) | [Documentation](https://bookbot-kids.github.io/speechline/)
-
-
- """
- )
-
- with gr.Row():
- with gr.Column():
- audio = gr.Audio(type="filepath")
- model = gr.Dropdown(
- choices=[
- "facebook/wav2vec2-base-960h",
- ],
- value="facebook/wav2vec2-base-960h",
- label="Transcriber Model",
- )
- segmenter = gr.Radio(
- choices=["Silence Gap", "Word Overlap"],
- value="Silence Gap",
- label="Segmentation Method",
- )
- sil = gr.Slider(
- 0, 1, value=0.1, step=0.1, label="Silence Duration", visible=True
- )
- gt = gr.Textbox(
- label="Ground Truth",
- placeholder="Enter Ground Truth Text",
- interactive=True,
- visible=False,
- )
-
- segmenter.change(
- fn=segmentation_interface, inputs=segmenter, outputs=[sil, gt]
- )
-
- inputs = [audio, model, segmenter, sil, gt]
- transcribe_btn = gr.Button("Transcribe")
-
- with gr.Column():
- outputs = [
- gr.Dataframe(
- visible=True, headers=["start_offset", "end_offset", "text"]
- ),
- gr.Audio(visible=True),
- ]
- for _ in range(MAX_SEGMENTS - 1):
- outputs += [gr.Dataframe(visible=False), gr.Audio(visible=False)]
- transcribe_btn.click(fn=run, inputs=inputs, outputs=outputs)
-
-demo.launch()
diff --git a/spaces/brjathu/HMR2.0/vendor/detectron2/detectron2/modeling/roi_heads/fast_rcnn.py b/spaces/brjathu/HMR2.0/vendor/detectron2/detectron2/modeling/roi_heads/fast_rcnn.py
deleted file mode 100644
index 039e2490fae27d6e837b57492a230bc556da845f..0000000000000000000000000000000000000000
--- a/spaces/brjathu/HMR2.0/vendor/detectron2/detectron2/modeling/roi_heads/fast_rcnn.py
+++ /dev/null
@@ -1,569 +0,0 @@
-# Copyright (c) Facebook, Inc. and its affiliates.
-import logging
-from typing import Callable, Dict, List, Optional, Tuple, Union
-import torch
-from torch import nn
-from torch.nn import functional as F
-
-from detectron2.config import configurable
-from detectron2.data.detection_utils import get_fed_loss_cls_weights
-from detectron2.layers import ShapeSpec, batched_nms, cat, cross_entropy, nonzero_tuple
-from detectron2.modeling.box_regression import Box2BoxTransform, _dense_box_regression_loss
-from detectron2.structures import Boxes, Instances
-from detectron2.utils.events import get_event_storage
-
-__all__ = ["fast_rcnn_inference", "FastRCNNOutputLayers"]
-
-
-logger = logging.getLogger(__name__)
-
-"""
-Shape shorthand in this module:
-
- N: number of images in the minibatch
- R: number of ROIs, combined over all images, in the minibatch
- Ri: number of ROIs in image i
- K: number of foreground classes. E.g.,there are 80 foreground classes in COCO.
-
-Naming convention:
-
- deltas: refers to the 4-d (dx, dy, dw, dh) deltas that parameterize the box2box
- transform (see :class:`box_regression.Box2BoxTransform`).
-
- pred_class_logits: predicted class scores in [-inf, +inf]; use
- softmax(pred_class_logits) to estimate P(class).
-
- gt_classes: ground-truth classification labels in [0, K], where [0, K) represent
- foreground object classes and K represents the background class.
-
- pred_proposal_deltas: predicted box2box transform deltas for transforming proposals
- to detection box predictions.
-
- gt_proposal_deltas: ground-truth box2box transform deltas
-"""
-
-
-def fast_rcnn_inference(
- boxes: List[torch.Tensor],
- scores: List[torch.Tensor],
- image_shapes: List[Tuple[int, int]],
- score_thresh: float,
- nms_thresh: float,
- topk_per_image: int,
-):
- """
- Call `fast_rcnn_inference_single_image` for all images.
-
- Args:
- boxes (list[Tensor]): A list of Tensors of predicted class-specific or class-agnostic
- boxes for each image. Element i has shape (Ri, K * 4) if doing
- class-specific regression, or (Ri, 4) if doing class-agnostic
- regression, where Ri is the number of predicted objects for image i.
- This is compatible with the output of :meth:`FastRCNNOutputLayers.predict_boxes`.
- scores (list[Tensor]): A list of Tensors of predicted class scores for each image.
- Element i has shape (Ri, K + 1), where Ri is the number of predicted objects
- for image i. Compatible with the output of :meth:`FastRCNNOutputLayers.predict_probs`.
- image_shapes (list[tuple]): A list of (width, height) tuples for each image in the batch.
- score_thresh (float): Only return detections with a confidence score exceeding this
- threshold.
- nms_thresh (float): The threshold to use for box non-maximum suppression. Value in [0, 1].
- topk_per_image (int): The number of top scoring detections to return. Set < 0 to return
- all detections.
-
- Returns:
- instances: (list[Instances]): A list of N instances, one for each image in the batch,
- that stores the topk most confidence detections.
- kept_indices: (list[Tensor]): A list of 1D tensor of length of N, each element indicates
- the corresponding boxes/scores index in [0, Ri) from the input, for image i.
- """
- result_per_image = [
- fast_rcnn_inference_single_image(
- boxes_per_image, scores_per_image, image_shape, score_thresh, nms_thresh, topk_per_image
- )
- for scores_per_image, boxes_per_image, image_shape in zip(scores, boxes, image_shapes)
- ]
- return [x[0] for x in result_per_image], [x[1] for x in result_per_image]
-
-
-def _log_classification_stats(pred_logits, gt_classes, prefix="fast_rcnn"):
- """
- Log the classification metrics to EventStorage.
-
- Args:
- pred_logits: Rx(K+1) logits. The last column is for background class.
- gt_classes: R labels
- """
- num_instances = gt_classes.numel()
- if num_instances == 0:
- return
- pred_classes = pred_logits.argmax(dim=1)
- bg_class_ind = pred_logits.shape[1] - 1
-
- fg_inds = (gt_classes >= 0) & (gt_classes < bg_class_ind)
- num_fg = fg_inds.nonzero().numel()
- fg_gt_classes = gt_classes[fg_inds]
- fg_pred_classes = pred_classes[fg_inds]
-
- num_false_negative = (fg_pred_classes == bg_class_ind).nonzero().numel()
- num_accurate = (pred_classes == gt_classes).nonzero().numel()
- fg_num_accurate = (fg_pred_classes == fg_gt_classes).nonzero().numel()
-
- storage = get_event_storage()
- storage.put_scalar(f"{prefix}/cls_accuracy", num_accurate / num_instances)
- if num_fg > 0:
- storage.put_scalar(f"{prefix}/fg_cls_accuracy", fg_num_accurate / num_fg)
- storage.put_scalar(f"{prefix}/false_negative", num_false_negative / num_fg)
-
-
-def fast_rcnn_inference_single_image(
- boxes,
- scores,
- image_shape: Tuple[int, int],
- score_thresh: float,
- nms_thresh: float,
- topk_per_image: int,
-):
- """
- Single-image inference. Return bounding-box detection results by thresholding
- on scores and applying non-maximum suppression (NMS).
-
- Args:
- Same as `fast_rcnn_inference`, but with boxes, scores, and image shapes
- per image.
-
- Returns:
- Same as `fast_rcnn_inference`, but for only one image.
- """
- valid_mask = torch.isfinite(boxes).all(dim=1) & torch.isfinite(scores).all(dim=1)
- if not valid_mask.all():
- boxes = boxes[valid_mask]
- scores = scores[valid_mask]
-
- scores = scores[:, :-1]
- num_bbox_reg_classes = boxes.shape[1] // 4
- # Convert to Boxes to use the `clip` function ...
- boxes = Boxes(boxes.reshape(-1, 4))
- boxes.clip(image_shape)
- boxes = boxes.tensor.view(-1, num_bbox_reg_classes, 4) # R x C x 4
-
- # 1. Filter results based on detection scores. It can make NMS more efficient
- # by filtering out low-confidence detections.
- filter_mask = scores > score_thresh # R x K
- # R' x 2. First column contains indices of the R predictions;
- # Second column contains indices of classes.
- filter_inds = filter_mask.nonzero()
- if num_bbox_reg_classes == 1:
- boxes = boxes[filter_inds[:, 0], 0]
- else:
- boxes = boxes[filter_mask]
- scores = scores[filter_mask]
-
- # 2. Apply NMS for each class independently.
- keep = batched_nms(boxes, scores, filter_inds[:, 1], nms_thresh)
- if topk_per_image >= 0:
- keep = keep[:topk_per_image]
- boxes, scores, filter_inds = boxes[keep], scores[keep], filter_inds[keep]
-
- result = Instances(image_shape)
- result.pred_boxes = Boxes(boxes)
- result.scores = scores
- result.pred_classes = filter_inds[:, 1]
- return result, filter_inds[:, 0]
-
-
-class FastRCNNOutputLayers(nn.Module):
- """
- Two linear layers for predicting Fast R-CNN outputs:
-
- 1. proposal-to-detection box regression deltas
- 2. classification scores
- """
-
- @configurable
- def __init__(
- self,
- input_shape: ShapeSpec,
- *,
- box2box_transform,
- num_classes: int,
- test_score_thresh: float = 0.0,
- test_nms_thresh: float = 0.5,
- test_topk_per_image: int = 100,
- cls_agnostic_bbox_reg: bool = False,
- smooth_l1_beta: float = 0.0,
- box_reg_loss_type: str = "smooth_l1",
- loss_weight: Union[float, Dict[str, float]] = 1.0,
- use_fed_loss: bool = False,
- use_sigmoid_ce: bool = False,
- get_fed_loss_cls_weights: Optional[Callable] = None,
- fed_loss_num_classes: int = 50,
- ):
- """
- NOTE: this interface is experimental.
-
- Args:
- input_shape (ShapeSpec): shape of the input feature to this module
- box2box_transform (Box2BoxTransform or Box2BoxTransformRotated):
- num_classes (int): number of foreground classes
- test_score_thresh (float): threshold to filter predictions results.
- test_nms_thresh (float): NMS threshold for prediction results.
- test_topk_per_image (int): number of top predictions to produce per image.
- cls_agnostic_bbox_reg (bool): whether to use class agnostic for bbox regression
- smooth_l1_beta (float): transition point from L1 to L2 loss. Only used if
- `box_reg_loss_type` is "smooth_l1"
- box_reg_loss_type (str): Box regression loss type. One of: "smooth_l1", "giou",
- "diou", "ciou"
- loss_weight (float|dict): weights to use for losses. Can be single float for weighting
- all losses, or a dict of individual weightings. Valid dict keys are:
- * "loss_cls": applied to classification loss
- * "loss_box_reg": applied to box regression loss
- use_fed_loss (bool): whether to use federated loss which samples additional negative
- classes to calculate the loss
- use_sigmoid_ce (bool): whether to calculate the loss using weighted average of binary
- cross entropy with logits. This could be used together with federated loss
- get_fed_loss_cls_weights (Callable): a callable which takes dataset name and frequency
- weight power, and returns the probabilities to sample negative classes for
- federated loss. The implementation can be found in
- detectron2/data/detection_utils.py
- fed_loss_num_classes (int): number of federated classes to keep in total
- """
- super().__init__()
- if isinstance(input_shape, int): # some backward compatibility
- input_shape = ShapeSpec(channels=input_shape)
- self.num_classes = num_classes
- input_size = input_shape.channels * (input_shape.width or 1) * (input_shape.height or 1)
- # prediction layer for num_classes foreground classes and one background class (hence + 1)
- self.cls_score = nn.Linear(input_size, num_classes + 1)
- num_bbox_reg_classes = 1 if cls_agnostic_bbox_reg else num_classes
- box_dim = len(box2box_transform.weights)
- self.bbox_pred = nn.Linear(input_size, num_bbox_reg_classes * box_dim)
-
- nn.init.normal_(self.cls_score.weight, std=0.01)
- nn.init.normal_(self.bbox_pred.weight, std=0.001)
- for l in [self.cls_score, self.bbox_pred]:
- nn.init.constant_(l.bias, 0)
-
- self.box2box_transform = box2box_transform
- self.smooth_l1_beta = smooth_l1_beta
- self.test_score_thresh = test_score_thresh
- self.test_nms_thresh = test_nms_thresh
- self.test_topk_per_image = test_topk_per_image
- self.box_reg_loss_type = box_reg_loss_type
- if isinstance(loss_weight, float):
- loss_weight = {"loss_cls": loss_weight, "loss_box_reg": loss_weight}
- self.loss_weight = loss_weight
- self.use_fed_loss = use_fed_loss
- self.use_sigmoid_ce = use_sigmoid_ce
- self.fed_loss_num_classes = fed_loss_num_classes
-
- if self.use_fed_loss:
- assert self.use_sigmoid_ce, "Please use sigmoid cross entropy loss with federated loss"
- fed_loss_cls_weights = get_fed_loss_cls_weights()
- assert (
- len(fed_loss_cls_weights) == self.num_classes
- ), "Please check the provided fed_loss_cls_weights. Their size should match num_classes"
- self.register_buffer("fed_loss_cls_weights", fed_loss_cls_weights)
-
- @classmethod
- def from_config(cls, cfg, input_shape):
- return {
- "input_shape": input_shape,
- "box2box_transform": Box2BoxTransform(weights=cfg.MODEL.ROI_BOX_HEAD.BBOX_REG_WEIGHTS),
- # fmt: off
- "num_classes" : cfg.MODEL.ROI_HEADS.NUM_CLASSES,
- "cls_agnostic_bbox_reg" : cfg.MODEL.ROI_BOX_HEAD.CLS_AGNOSTIC_BBOX_REG,
- "smooth_l1_beta" : cfg.MODEL.ROI_BOX_HEAD.SMOOTH_L1_BETA,
- "test_score_thresh" : cfg.MODEL.ROI_HEADS.SCORE_THRESH_TEST,
- "test_nms_thresh" : cfg.MODEL.ROI_HEADS.NMS_THRESH_TEST,
- "test_topk_per_image" : cfg.TEST.DETECTIONS_PER_IMAGE,
- "box_reg_loss_type" : cfg.MODEL.ROI_BOX_HEAD.BBOX_REG_LOSS_TYPE,
- "loss_weight" : {"loss_box_reg": cfg.MODEL.ROI_BOX_HEAD.BBOX_REG_LOSS_WEIGHT}, # noqa
- "use_fed_loss" : cfg.MODEL.ROI_BOX_HEAD.USE_FED_LOSS,
- "use_sigmoid_ce" : cfg.MODEL.ROI_BOX_HEAD.USE_SIGMOID_CE,
- "get_fed_loss_cls_weights" : lambda: get_fed_loss_cls_weights(dataset_names=cfg.DATASETS.TRAIN, freq_weight_power=cfg.MODEL.ROI_BOX_HEAD.FED_LOSS_FREQ_WEIGHT_POWER), # noqa
- "fed_loss_num_classes" : cfg.MODEL.ROI_BOX_HEAD.FED_LOSS_NUM_CLASSES,
- # fmt: on
- }
-
- def forward(self, x):
- """
- Args:
- x: per-region features of shape (N, ...) for N bounding boxes to predict.
-
- Returns:
- (Tensor, Tensor):
- First tensor: shape (N,K+1), scores for each of the N box. Each row contains the
- scores for K object categories and 1 background class.
-
- Second tensor: bounding box regression deltas for each box. Shape is shape (N,Kx4),
- or (N,4) for class-agnostic regression.
- """
- if x.dim() > 2:
- x = torch.flatten(x, start_dim=1)
- scores = self.cls_score(x)
- proposal_deltas = self.bbox_pred(x)
- return scores, proposal_deltas
-
- def losses(self, predictions, proposals):
- """
- Args:
- predictions: return values of :meth:`forward()`.
- proposals (list[Instances]): proposals that match the features that were used
- to compute predictions. The fields ``proposal_boxes``, ``gt_boxes``,
- ``gt_classes`` are expected.
-
- Returns:
- Dict[str, Tensor]: dict of losses
- """
- scores, proposal_deltas = predictions
-
- # parse classification outputs
- gt_classes = (
- cat([p.gt_classes for p in proposals], dim=0) if len(proposals) else torch.empty(0)
- )
- _log_classification_stats(scores, gt_classes)
-
- # parse box regression outputs
- if len(proposals):
- proposal_boxes = cat([p.proposal_boxes.tensor for p in proposals], dim=0) # Nx4
- assert not proposal_boxes.requires_grad, "Proposals should not require gradients!"
- # If "gt_boxes" does not exist, the proposals must be all negative and
- # should not be included in regression loss computation.
- # Here we just use proposal_boxes as an arbitrary placeholder because its
- # value won't be used in self.box_reg_loss().
- gt_boxes = cat(
- [(p.gt_boxes if p.has("gt_boxes") else p.proposal_boxes).tensor for p in proposals],
- dim=0,
- )
- else:
- proposal_boxes = gt_boxes = torch.empty((0, 4), device=proposal_deltas.device)
-
- if self.use_sigmoid_ce:
- loss_cls = self.sigmoid_cross_entropy_loss(scores, gt_classes)
- else:
- loss_cls = cross_entropy(scores, gt_classes, reduction="mean")
-
- losses = {
- "loss_cls": loss_cls,
- "loss_box_reg": self.box_reg_loss(
- proposal_boxes, gt_boxes, proposal_deltas, gt_classes
- ),
- }
- return {k: v * self.loss_weight.get(k, 1.0) for k, v in losses.items()}
-
- # Implementation from https://github.com/xingyizhou/CenterNet2/blob/master/projects/CenterNet2/centernet/modeling/roi_heads/fed_loss.py # noqa
- # with slight modifications
- def get_fed_loss_classes(self, gt_classes, num_fed_loss_classes, num_classes, weight):
- """
- Args:
- gt_classes: a long tensor of shape R that contains the gt class label of each proposal.
- num_fed_loss_classes: minimum number of classes to keep when calculating federated loss.
- Will sample negative classes if number of unique gt_classes is smaller than this value.
- num_classes: number of foreground classes
- weight: probabilities used to sample negative classes
-
- Returns:
- Tensor:
- classes to keep when calculating the federated loss, including both unique gt
- classes and sampled negative classes.
- """
- unique_gt_classes = torch.unique(gt_classes)
- prob = unique_gt_classes.new_ones(num_classes + 1).float()
- prob[-1] = 0
- if len(unique_gt_classes) < num_fed_loss_classes:
- prob[:num_classes] = weight.float().clone()
- prob[unique_gt_classes] = 0
- sampled_negative_classes = torch.multinomial(
- prob, num_fed_loss_classes - len(unique_gt_classes), replacement=False
- )
- fed_loss_classes = torch.cat([unique_gt_classes, sampled_negative_classes])
- else:
- fed_loss_classes = unique_gt_classes
- return fed_loss_classes
-
- # Implementation from https://github.com/xingyizhou/CenterNet2/blob/master/projects/CenterNet2/centernet/modeling/roi_heads/custom_fast_rcnn.py#L113 # noqa
- # with slight modifications
- def sigmoid_cross_entropy_loss(self, pred_class_logits, gt_classes):
- """
- Args:
- pred_class_logits: shape (N, K+1), scores for each of the N box. Each row contains the
- scores for K object categories and 1 background class
- gt_classes: a long tensor of shape R that contains the gt class label of each proposal.
- """
- if pred_class_logits.numel() == 0:
- return pred_class_logits.new_zeros([1])[0]
-
- N = pred_class_logits.shape[0]
- K = pred_class_logits.shape[1] - 1
-
- target = pred_class_logits.new_zeros(N, K + 1)
- target[range(len(gt_classes)), gt_classes] = 1
- target = target[:, :K]
-
- cls_loss = F.binary_cross_entropy_with_logits(
- pred_class_logits[:, :-1], target, reduction="none"
- )
-
- if self.use_fed_loss:
- fed_loss_classes = self.get_fed_loss_classes(
- gt_classes,
- num_fed_loss_classes=self.fed_loss_num_classes,
- num_classes=K,
- weight=self.fed_loss_cls_weights,
- )
- fed_loss_classes_mask = fed_loss_classes.new_zeros(K + 1)
- fed_loss_classes_mask[fed_loss_classes] = 1
- fed_loss_classes_mask = fed_loss_classes_mask[:K]
- weight = fed_loss_classes_mask.view(1, K).expand(N, K).float()
- else:
- weight = 1
-
- loss = torch.sum(cls_loss * weight) / N
- return loss
-
- def box_reg_loss(self, proposal_boxes, gt_boxes, pred_deltas, gt_classes):
- """
- Args:
- proposal_boxes/gt_boxes are tensors with the same shape (R, 4 or 5).
- pred_deltas has shape (R, 4 or 5), or (R, num_classes * (4 or 5)).
- gt_classes is a long tensor of shape R, the gt class label of each proposal.
- R shall be the number of proposals.
- """
- box_dim = proposal_boxes.shape[1] # 4 or 5
- # Regression loss is only computed for foreground proposals (those matched to a GT)
- fg_inds = nonzero_tuple((gt_classes >= 0) & (gt_classes < self.num_classes))[0]
- if pred_deltas.shape[1] == box_dim: # cls-agnostic regression
- fg_pred_deltas = pred_deltas[fg_inds]
- else:
- fg_pred_deltas = pred_deltas.view(-1, self.num_classes, box_dim)[
- fg_inds, gt_classes[fg_inds]
- ]
-
- loss_box_reg = _dense_box_regression_loss(
- [proposal_boxes[fg_inds]],
- self.box2box_transform,
- [fg_pred_deltas.unsqueeze(0)],
- [gt_boxes[fg_inds]],
- ...,
- self.box_reg_loss_type,
- self.smooth_l1_beta,
- )
-
- # The reg loss is normalized using the total number of regions (R), not the number
- # of foreground regions even though the box regression loss is only defined on
- # foreground regions. Why? Because doing so gives equal training influence to
- # each foreground example. To see how, consider two different minibatches:
- # (1) Contains a single foreground region
- # (2) Contains 100 foreground regions
- # If we normalize by the number of foreground regions, the single example in
- # minibatch (1) will be given 100 times as much influence as each foreground
- # example in minibatch (2). Normalizing by the total number of regions, R,
- # means that the single example in minibatch (1) and each of the 100 examples
- # in minibatch (2) are given equal influence.
- return loss_box_reg / max(gt_classes.numel(), 1.0) # return 0 if empty
-
- def inference(self, predictions: Tuple[torch.Tensor, torch.Tensor], proposals: List[Instances]):
- """
- Args:
- predictions: return values of :meth:`forward()`.
- proposals (list[Instances]): proposals that match the features that were
- used to compute predictions. The ``proposal_boxes`` field is expected.
-
- Returns:
- list[Instances]: same as `fast_rcnn_inference`.
- list[Tensor]: same as `fast_rcnn_inference`.
- """
- boxes = self.predict_boxes(predictions, proposals)
- scores = self.predict_probs(predictions, proposals)
- image_shapes = [x.image_size for x in proposals]
- return fast_rcnn_inference(
- boxes,
- scores,
- image_shapes,
- self.test_score_thresh,
- self.test_nms_thresh,
- self.test_topk_per_image,
- )
-
- def predict_boxes_for_gt_classes(self, predictions, proposals):
- """
- Args:
- predictions: return values of :meth:`forward()`.
- proposals (list[Instances]): proposals that match the features that were used
- to compute predictions. The fields ``proposal_boxes``, ``gt_classes`` are expected.
-
- Returns:
- list[Tensor]:
- A list of Tensors of predicted boxes for GT classes in case of
- class-specific box head. Element i of the list has shape (Ri, B), where Ri is
- the number of proposals for image i and B is the box dimension (4 or 5)
- """
- if not len(proposals):
- return []
- scores, proposal_deltas = predictions
- proposal_boxes = cat([p.proposal_boxes.tensor for p in proposals], dim=0)
- N, B = proposal_boxes.shape
- predict_boxes = self.box2box_transform.apply_deltas(
- proposal_deltas, proposal_boxes
- ) # Nx(KxB)
-
- K = predict_boxes.shape[1] // B
- if K > 1:
- gt_classes = torch.cat([p.gt_classes for p in proposals], dim=0)
- # Some proposals are ignored or have a background class. Their gt_classes
- # cannot be used as index.
- gt_classes = gt_classes.clamp_(0, K - 1)
-
- predict_boxes = predict_boxes.view(N, K, B)[
- torch.arange(N, dtype=torch.long, device=predict_boxes.device), gt_classes
- ]
- num_prop_per_image = [len(p) for p in proposals]
- return predict_boxes.split(num_prop_per_image)
-
- def predict_boxes(
- self, predictions: Tuple[torch.Tensor, torch.Tensor], proposals: List[Instances]
- ):
- """
- Args:
- predictions: return values of :meth:`forward()`.
- proposals (list[Instances]): proposals that match the features that were
- used to compute predictions. The ``proposal_boxes`` field is expected.
-
- Returns:
- list[Tensor]:
- A list of Tensors of predicted class-specific or class-agnostic boxes
- for each image. Element i has shape (Ri, K * B) or (Ri, B), where Ri is
- the number of proposals for image i and B is the box dimension (4 or 5)
- """
- if not len(proposals):
- return []
- _, proposal_deltas = predictions
- num_prop_per_image = [len(p) for p in proposals]
- proposal_boxes = cat([p.proposal_boxes.tensor for p in proposals], dim=0)
- predict_boxes = self.box2box_transform.apply_deltas(
- proposal_deltas,
- proposal_boxes,
- ) # Nx(KxB)
- return predict_boxes.split(num_prop_per_image)
-
- def predict_probs(
- self, predictions: Tuple[torch.Tensor, torch.Tensor], proposals: List[Instances]
- ):
- """
- Args:
- predictions: return values of :meth:`forward()`.
- proposals (list[Instances]): proposals that match the features that were
- used to compute predictions.
-
- Returns:
- list[Tensor]:
- A list of Tensors of predicted class probabilities for each image.
- Element i has shape (Ri, K + 1), where Ri is the number of proposals for image i.
- """
- scores, _ = predictions
- num_inst_per_image = [len(p) for p in proposals]
- if self.use_sigmoid_ce:
- probs = scores.sigmoid()
- else:
- probs = F.softmax(scores, dim=-1)
- return probs.split(num_inst_per_image, dim=0)
diff --git a/spaces/brjathu/HMR2.0/vendor/detectron2/projects/DensePose/densepose/vis/extractor.py b/spaces/brjathu/HMR2.0/vendor/detectron2/projects/DensePose/densepose/vis/extractor.py
deleted file mode 100644
index bfb2bdf693254a954e54a74b8766e5f574f6cf3a..0000000000000000000000000000000000000000
--- a/spaces/brjathu/HMR2.0/vendor/detectron2/projects/DensePose/densepose/vis/extractor.py
+++ /dev/null
@@ -1,199 +0,0 @@
-# Copyright (c) Facebook, Inc. and its affiliates.
-import logging
-from typing import List, Optional, Sequence, Tuple
-import torch
-
-from detectron2.layers.nms import batched_nms
-from detectron2.structures.instances import Instances
-
-from densepose.converters import ToChartResultConverterWithConfidences
-from densepose.structures import (
- DensePoseChartResultWithConfidences,
- DensePoseEmbeddingPredictorOutput,
-)
-from densepose.vis.bounding_box import BoundingBoxVisualizer, ScoredBoundingBoxVisualizer
-from densepose.vis.densepose_outputs_vertex import DensePoseOutputsVertexVisualizer
-from densepose.vis.densepose_results import DensePoseResultsVisualizer
-
-from .base import CompoundVisualizer
-
-Scores = Sequence[float]
-DensePoseChartResultsWithConfidences = List[DensePoseChartResultWithConfidences]
-
-
-def extract_scores_from_instances(instances: Instances, select=None):
- if instances.has("scores"):
- return instances.scores if select is None else instances.scores[select]
- return None
-
-
-def extract_boxes_xywh_from_instances(instances: Instances, select=None):
- if instances.has("pred_boxes"):
- boxes_xywh = instances.pred_boxes.tensor.clone()
- boxes_xywh[:, 2] -= boxes_xywh[:, 0]
- boxes_xywh[:, 3] -= boxes_xywh[:, 1]
- return boxes_xywh if select is None else boxes_xywh[select]
- return None
-
-
-def create_extractor(visualizer: object):
- """
- Create an extractor for the provided visualizer
- """
- if isinstance(visualizer, CompoundVisualizer):
- extractors = [create_extractor(v) for v in visualizer.visualizers]
- return CompoundExtractor(extractors)
- elif isinstance(visualizer, DensePoseResultsVisualizer):
- return DensePoseResultExtractor()
- elif isinstance(visualizer, ScoredBoundingBoxVisualizer):
- return CompoundExtractor([extract_boxes_xywh_from_instances, extract_scores_from_instances])
- elif isinstance(visualizer, BoundingBoxVisualizer):
- return extract_boxes_xywh_from_instances
- elif isinstance(visualizer, DensePoseOutputsVertexVisualizer):
- return DensePoseOutputsExtractor()
- else:
- logger = logging.getLogger(__name__)
- logger.error(f"Could not create extractor for {visualizer}")
- return None
-
-
-class BoundingBoxExtractor(object):
- """
- Extracts bounding boxes from instances
- """
-
- def __call__(self, instances: Instances):
- boxes_xywh = extract_boxes_xywh_from_instances(instances)
- return boxes_xywh
-
-
-class ScoredBoundingBoxExtractor(object):
- """
- Extracts bounding boxes from instances
- """
-
- def __call__(self, instances: Instances, select=None):
- scores = extract_scores_from_instances(instances)
- boxes_xywh = extract_boxes_xywh_from_instances(instances)
- if (scores is None) or (boxes_xywh is None):
- return (boxes_xywh, scores)
- if select is not None:
- scores = scores[select]
- boxes_xywh = boxes_xywh[select]
- return (boxes_xywh, scores)
-
-
-class DensePoseResultExtractor(object):
- """
- Extracts DensePose chart result with confidences from instances
- """
-
- def __call__(
- self, instances: Instances, select=None
- ) -> Tuple[Optional[DensePoseChartResultsWithConfidences], Optional[torch.Tensor]]:
- if instances.has("pred_densepose") and instances.has("pred_boxes"):
- dpout = instances.pred_densepose
- boxes_xyxy = instances.pred_boxes
- boxes_xywh = extract_boxes_xywh_from_instances(instances)
- if select is not None:
- dpout = dpout[select]
- boxes_xyxy = boxes_xyxy[select]
- converter = ToChartResultConverterWithConfidences()
- results = [converter.convert(dpout[i], boxes_xyxy[[i]]) for i in range(len(dpout))]
- return results, boxes_xywh
- else:
- return None, None
-
-
-class DensePoseOutputsExtractor(object):
- """
- Extracts DensePose result from instances
- """
-
- def __call__(
- self,
- instances: Instances,
- select=None,
- ) -> Tuple[
- Optional[DensePoseEmbeddingPredictorOutput], Optional[torch.Tensor], Optional[List[int]]
- ]:
- if not (instances.has("pred_densepose") and instances.has("pred_boxes")):
- return None, None, None
-
- dpout = instances.pred_densepose
- boxes_xyxy = instances.pred_boxes
- boxes_xywh = extract_boxes_xywh_from_instances(instances)
-
- if instances.has("pred_classes"):
- classes = instances.pred_classes.tolist()
- else:
- classes = None
-
- if select is not None:
- dpout = dpout[select]
- boxes_xyxy = boxes_xyxy[select]
- if classes is not None:
- classes = classes[select]
-
- return dpout, boxes_xywh, classes
-
-
-class CompoundExtractor(object):
- """
- Extracts data for CompoundVisualizer
- """
-
- def __init__(self, extractors):
- self.extractors = extractors
-
- def __call__(self, instances: Instances, select=None):
- datas = []
- for extractor in self.extractors:
- data = extractor(instances, select)
- datas.append(data)
- return datas
-
-
-class NmsFilteredExtractor(object):
- """
- Extracts data in the format accepted by NmsFilteredVisualizer
- """
-
- def __init__(self, extractor, iou_threshold):
- self.extractor = extractor
- self.iou_threshold = iou_threshold
-
- def __call__(self, instances: Instances, select=None):
- scores = extract_scores_from_instances(instances)
- boxes_xywh = extract_boxes_xywh_from_instances(instances)
- if boxes_xywh is None:
- return None
- select_local_idx = batched_nms(
- boxes_xywh,
- scores,
- torch.zeros(len(scores), dtype=torch.int32),
- iou_threshold=self.iou_threshold,
- ).squeeze()
- select_local = torch.zeros(len(boxes_xywh), dtype=torch.bool, device=boxes_xywh.device)
- select_local[select_local_idx] = True
- select = select_local if select is None else (select & select_local)
- return self.extractor(instances, select=select)
-
-
-class ScoreThresholdedExtractor(object):
- """
- Extracts data in the format accepted by ScoreThresholdedVisualizer
- """
-
- def __init__(self, extractor, min_score):
- self.extractor = extractor
- self.min_score = min_score
-
- def __call__(self, instances: Instances, select=None):
- scores = extract_scores_from_instances(instances)
- if scores is None:
- return None
- select_local = scores > self.min_score
- select = select_local if select is None else (select & select_local)
- data = self.extractor(instances, select=select)
- return data
diff --git a/spaces/burakaytan/turkish_typo_correction/README.md b/spaces/burakaytan/turkish_typo_correction/README.md
deleted file mode 100644
index 52dd247c83d78c5a0637f773c485b4850215df18..0000000000000000000000000000000000000000
--- a/spaces/burakaytan/turkish_typo_correction/README.md
+++ /dev/null
@@ -1,12 +0,0 @@
----
-title: Turkish Typo Correction
-emoji: 👁
-colorFrom: green
-colorTo: gray
-sdk: gradio
-sdk_version: 3.21.0
-app_file: app.py
-pinned: false
----
-
-Check out the configuration reference at https://huggingface.co/docs/hub/spaces-config-reference
\ No newline at end of file
diff --git a/spaces/candlend/vits-hoshimi/sovits/vdecoder/__init__.py b/spaces/candlend/vits-hoshimi/sovits/vdecoder/__init__.py
deleted file mode 100644
index e69de29bb2d1d6434b8b29ae775ad8c2e48c5391..0000000000000000000000000000000000000000
diff --git a/spaces/carlosalonso/Detection-video/carpeta_deteccion/projects/DeepLab/deeplab/__init__.py b/spaces/carlosalonso/Detection-video/carpeta_deteccion/projects/DeepLab/deeplab/__init__.py
deleted file mode 100644
index dcd88ff0c09d630577e3ac9f8afb5324a80a7be4..0000000000000000000000000000000000000000
--- a/spaces/carlosalonso/Detection-video/carpeta_deteccion/projects/DeepLab/deeplab/__init__.py
+++ /dev/null
@@ -1,5 +0,0 @@
-# Copyright (c) Facebook, Inc. and its affiliates.
-from .build_solver import build_lr_scheduler
-from .config import add_deeplab_config
-from .resnet import build_resnet_deeplab_backbone
-from .semantic_seg import DeepLabV3Head, DeepLabV3PlusHead
diff --git a/spaces/cc1799/vits-uma-genshin-honkai/mel_processing.py b/spaces/cc1799/vits-uma-genshin-honkai/mel_processing.py
deleted file mode 100644
index 3e252e76320522a8a4195a60665168f22769aec2..0000000000000000000000000000000000000000
--- a/spaces/cc1799/vits-uma-genshin-honkai/mel_processing.py
+++ /dev/null
@@ -1,101 +0,0 @@
-import torch
-import torch.utils.data
-from librosa.filters import mel as librosa_mel_fn
-
-MAX_WAV_VALUE = 32768.0
-
-
-def dynamic_range_compression_torch(x, C=1, clip_val=1e-5):
- """
- PARAMS
- ------
- C: compression factor
- """
- return torch.log(torch.clamp(x, min=clip_val) * C)
-
-
-def dynamic_range_decompression_torch(x, C=1):
- """
- PARAMS
- ------
- C: compression factor used to compress
- """
- return torch.exp(x) / C
-
-
-def spectral_normalize_torch(magnitudes):
- output = dynamic_range_compression_torch(magnitudes)
- return output
-
-
-def spectral_de_normalize_torch(magnitudes):
- output = dynamic_range_decompression_torch(magnitudes)
- return output
-
-
-mel_basis = {}
-hann_window = {}
-
-
-def spectrogram_torch(y, n_fft, sampling_rate, hop_size, win_size, center=False):
- if torch.min(y) < -1.:
- print('min value is ', torch.min(y))
- if torch.max(y) > 1.:
- print('max value is ', torch.max(y))
-
- global hann_window
- dtype_device = str(y.dtype) + '_' + str(y.device)
- wnsize_dtype_device = str(win_size) + '_' + dtype_device
- if wnsize_dtype_device not in hann_window:
- hann_window[wnsize_dtype_device] = torch.hann_window(win_size).to(dtype=y.dtype, device=y.device)
-
- y = torch.nn.functional.pad(y.unsqueeze(1), (int((n_fft-hop_size)/2), int((n_fft-hop_size)/2)), mode='reflect')
- y = y.squeeze(1)
-
- spec = torch.stft(y, n_fft, hop_length=hop_size, win_length=win_size, window=hann_window[wnsize_dtype_device],
- center=center, pad_mode='reflect', normalized=False, onesided=True, return_complex=False)
-
- spec = torch.sqrt(spec.pow(2).sum(-1) + 1e-6)
- return spec
-
-
-def spec_to_mel_torch(spec, n_fft, num_mels, sampling_rate, fmin, fmax):
- global mel_basis
- dtype_device = str(spec.dtype) + '_' + str(spec.device)
- fmax_dtype_device = str(fmax) + '_' + dtype_device
- if fmax_dtype_device not in mel_basis:
- mel = librosa_mel_fn(sampling_rate, n_fft, num_mels, fmin, fmax)
- mel_basis[fmax_dtype_device] = torch.from_numpy(mel).to(dtype=spec.dtype, device=spec.device)
- spec = torch.matmul(mel_basis[fmax_dtype_device], spec)
- spec = spectral_normalize_torch(spec)
- return spec
-
-
-def mel_spectrogram_torch(y, n_fft, num_mels, sampling_rate, hop_size, win_size, fmin, fmax, center=False):
- if torch.min(y) < -1.:
- print('min value is ', torch.min(y))
- if torch.max(y) > 1.:
- print('max value is ', torch.max(y))
-
- global mel_basis, hann_window
- dtype_device = str(y.dtype) + '_' + str(y.device)
- fmax_dtype_device = str(fmax) + '_' + dtype_device
- wnsize_dtype_device = str(win_size) + '_' + dtype_device
- if fmax_dtype_device not in mel_basis:
- mel = librosa_mel_fn(sampling_rate, n_fft, num_mels, fmin, fmax)
- mel_basis[fmax_dtype_device] = torch.from_numpy(mel).to(dtype=y.dtype, device=y.device)
- if wnsize_dtype_device not in hann_window:
- hann_window[wnsize_dtype_device] = torch.hann_window(win_size).to(dtype=y.dtype, device=y.device)
-
- y = torch.nn.functional.pad(y.unsqueeze(1), (int((n_fft-hop_size)/2), int((n_fft-hop_size)/2)), mode='reflect')
- y = y.squeeze(1)
-
- spec = torch.stft(y, n_fft, hop_length=hop_size, win_length=win_size, window=hann_window[wnsize_dtype_device],
- center=center, pad_mode='reflect', normalized=False, onesided=True)
-
- spec = torch.sqrt(spec.pow(2).sum(-1) + 1e-6)
-
- spec = torch.matmul(mel_basis[fmax_dtype_device], spec)
- spec = spectral_normalize_torch(spec)
-
- return spec
diff --git a/spaces/ccds/vits_onnx/export/vits/transforms.py b/spaces/ccds/vits_onnx/export/vits/transforms.py
deleted file mode 100644
index 30e857692442329a569fdd53e391b3ec4044a628..0000000000000000000000000000000000000000
--- a/spaces/ccds/vits_onnx/export/vits/transforms.py
+++ /dev/null
@@ -1,200 +0,0 @@
-import numpy as np
-import torch
-from torch.nn import functional as F
-
-DEFAULT_MIN_BIN_WIDTH = 1e-3
-DEFAULT_MIN_BIN_HEIGHT = 1e-3
-DEFAULT_MIN_DERIVATIVE = 1e-3
-
-
-def piecewise_rational_quadratic_transform(
- inputs,
- unnormalized_widths,
- unnormalized_heights,
- unnormalized_derivatives,
- inverse=False,
- tails=None,
- tail_bound=1.,
- min_bin_width=DEFAULT_MIN_BIN_WIDTH,
- min_bin_height=DEFAULT_MIN_BIN_HEIGHT,
- min_derivative=DEFAULT_MIN_DERIVATIVE):
-
- if tails is None:
- spline_fn = rational_quadratic_spline
- spline_kwargs = {}
- else:
- spline_fn = unconstrained_rational_quadratic_spline
- spline_kwargs = {'tails': tails, 'tail_bound': tail_bound}
-
- outputs, logabsdet = spline_fn(
- inputs=inputs,
- unnormalized_widths=unnormalized_widths,
- unnormalized_heights=unnormalized_heights,
- unnormalized_derivatives=unnormalized_derivatives,
- inverse=inverse,
- min_bin_width=min_bin_width,
- min_bin_height=min_bin_height,
- min_derivative=min_derivative,
- **spline_kwargs)
- return outputs, logabsdet
-
-
-def searchsorted(bin_locations, inputs, eps=1e-6):
- bin_locations[..., bin_locations.size(-1) - 1] += eps
- return torch.sum(inputs[..., None] >= bin_locations, dim=-1) - 1
-
-
-def unconstrained_rational_quadratic_spline(
- inputs,
- unnormalized_widths,
- unnormalized_heights,
- unnormalized_derivatives,
- inverse=False,
- tails='linear',
- tail_bound=1.,
- min_bin_width=DEFAULT_MIN_BIN_WIDTH,
- min_bin_height=DEFAULT_MIN_BIN_HEIGHT,
- min_derivative=DEFAULT_MIN_DERIVATIVE):
- inside_interval_mask = (inputs >= -tail_bound) & (inputs <= tail_bound)
- outside_interval_mask = ~inside_interval_mask
-
- outputs = torch.zeros_like(inputs)
- logabsdet = torch.zeros_like(inputs)
-
- if tails == 'linear':
- unnormalized_derivatives = F.pad(unnormalized_derivatives, pad=(1, 1))
- constant = np.log(np.exp(1 - min_derivative) - 1)
- unnormalized_derivatives[..., 0] = constant
- unnormalized_derivatives[..., unnormalized_derivatives.size(-1) - 1] = constant
-
- outputs[outside_interval_mask] = inputs[outside_interval_mask]
- logabsdet[outside_interval_mask] = 0
- else:
- raise RuntimeError('{} tails are not implemented.'.format(tails))
-
- outputs[inside_interval_mask], logabsdet[
- inside_interval_mask] = rational_quadratic_spline(
- inputs=inputs[inside_interval_mask],
- unnormalized_widths=unnormalized_widths[inside_interval_mask, :],
- unnormalized_heights=unnormalized_heights[inside_interval_mask, :],
- unnormalized_derivatives=unnormalized_derivatives[
- inside_interval_mask, :],
- inverse=inverse,
- left=-tail_bound,
- right=tail_bound,
- bottom=-tail_bound,
- top=tail_bound,
- min_bin_width=min_bin_width,
- min_bin_height=min_bin_height,
- min_derivative=min_derivative)
-
- return outputs, logabsdet
-
-
-def rational_quadratic_spline(inputs,
- unnormalized_widths,
- unnormalized_heights,
- unnormalized_derivatives,
- inverse=False,
- left=0.,
- right=1.,
- bottom=0.,
- top=1.,
- min_bin_width=DEFAULT_MIN_BIN_WIDTH,
- min_bin_height=DEFAULT_MIN_BIN_HEIGHT,
- min_derivative=DEFAULT_MIN_DERIVATIVE):
- if torch.min(inputs) < left or torch.max(inputs) > right:
- raise ValueError('Input to a transform is not within its domain')
-
- num_bins = unnormalized_widths.shape[-1]
-
- if min_bin_width * num_bins > 1.0:
- raise ValueError('Minimal bin width too large for the number of bins')
- if min_bin_height * num_bins > 1.0:
- raise ValueError('Minimal bin height too large for the number of bins')
-
- widths = F.softmax(unnormalized_widths, dim=-1)
- widths = min_bin_width + (1 - min_bin_width * num_bins) * widths
- cumwidths = torch.cumsum(widths, dim=-1)
- cumwidths = F.pad(cumwidths, pad=(1, 0), mode='constant', value=0.0)
- cumwidths = (right - left) * cumwidths + left
- cumwidths[..., 0] = left
- cumwidths[..., cumwidths.size(-1) - 1] = right
- widths = cumwidths[..., 1:] - cumwidths[..., :-1]
-
- derivatives = min_derivative + F.softplus(unnormalized_derivatives)
-
- heights = F.softmax(unnormalized_heights, dim=-1)
- heights = min_bin_height + (1 - min_bin_height * num_bins) * heights
- cumheights = torch.cumsum(heights, dim=-1)
- cumheights = F.pad(cumheights, pad=(1, 0), mode='constant', value=0.0)
- cumheights = (top - bottom) * cumheights + bottom
- cumheights[..., 0] = bottom
- cumheights[..., cumheights.size(-1) - 1] = top
- heights = cumheights[..., 1:] - cumheights[..., :-1]
-
- if inverse:
- bin_idx = searchsorted(cumheights, inputs)[..., None]
- else:
- bin_idx = searchsorted(cumwidths, inputs)[..., None]
-
- input_cumwidths = cumwidths.gather(-1, bin_idx)[..., 0]
- input_bin_widths = widths.gather(-1, bin_idx)[..., 0]
-
- input_cumheights = cumheights.gather(-1, bin_idx)[..., 0]
- delta = heights / widths
- input_delta = delta.gather(-1, bin_idx)[..., 0]
-
- input_derivatives = derivatives.gather(-1, bin_idx)[..., 0]
- input_derivatives_plus_one = derivatives[..., 1:].gather(-1, bin_idx)[...,
- 0]
-
- input_heights = heights.gather(-1, bin_idx)[..., 0]
-
- if inverse:
- a = (
- ((inputs - input_cumheights) *
- (input_derivatives + input_derivatives_plus_one - 2 * input_delta)
- + input_heights * (input_delta - input_derivatives)))
- b = (
- input_heights * input_derivatives - (inputs - input_cumheights) *
- (input_derivatives + input_derivatives_plus_one - 2 * input_delta))
- c = -input_delta * (inputs - input_cumheights)
-
- discriminant = b.pow(2) - 4 * a * c
- assert (discriminant >= 0).all()
-
- root = (2 * c) / (-b - torch.sqrt(discriminant))
- outputs = root * input_bin_widths + input_cumwidths
-
- theta_one_minus_theta = root * (1 - root)
- denominator = input_delta + (
- (input_derivatives + input_derivatives_plus_one - 2 * input_delta)
- * theta_one_minus_theta)
- derivative_numerator = input_delta.pow(2) * (
- input_derivatives_plus_one * root.pow(2) +
- 2 * input_delta * theta_one_minus_theta + input_derivatives *
- (1 - root).pow(2))
- logabsdet = torch.log(
- derivative_numerator) - 2 * torch.log(denominator)
-
- return outputs, -logabsdet
- else:
- theta = (inputs - input_cumwidths) / input_bin_widths
- theta_one_minus_theta = theta * (1 - theta)
-
- numerator = input_heights * (input_delta * theta.pow(2) +
- input_derivatives * theta_one_minus_theta)
- denominator = input_delta + (
- (input_derivatives + input_derivatives_plus_one - 2 * input_delta)
- * theta_one_minus_theta)
- outputs = input_cumheights + numerator / denominator
-
- derivative_numerator = input_delta.pow(2) * (
- input_derivatives_plus_one * theta.pow(2) +
- 2 * input_delta * theta_one_minus_theta + input_derivatives *
- (1 - theta).pow(2))
- logabsdet = torch.log(
- derivative_numerator) - 2 * torch.log(denominator)
-
- return outputs, logabsdet
diff --git a/spaces/celery22/gradio_plant_classify_app/README.md b/spaces/celery22/gradio_plant_classify_app/README.md
deleted file mode 100644
index e4e09108f935ecfdd55046fbb665f6d28784fb69..0000000000000000000000000000000000000000
--- a/spaces/celery22/gradio_plant_classify_app/README.md
+++ /dev/null
@@ -1,12 +0,0 @@
----
-title: Plant Diseases Diagnosis
-emoji: 🌍
-colorFrom: green
-colorTo: green
-sdk: gradio
-sdk_version: 3.16.2
-app_file: app.py
-pinned: false
----
-
-Check out the configuration reference at https://huggingface.co/docs/hub/spaces-config-reference
\ No newline at end of file
diff --git a/spaces/cffl/Exploring_Intelligent_Writing_Assistance/src/__init__.py b/spaces/cffl/Exploring_Intelligent_Writing_Assistance/src/__init__.py
deleted file mode 100644
index e69de29bb2d1d6434b8b29ae775ad8c2e48c5391..0000000000000000000000000000000000000000
diff --git a/spaces/chendl/compositional_test/transformers/examples/pytorch/semantic-segmentation/run_semantic_segmentation_no_trainer.py b/spaces/chendl/compositional_test/transformers/examples/pytorch/semantic-segmentation/run_semantic_segmentation_no_trainer.py
deleted file mode 100644
index 1517d030f396beb1d17cfe346b3e7785f0e18b9f..0000000000000000000000000000000000000000
--- a/spaces/chendl/compositional_test/transformers/examples/pytorch/semantic-segmentation/run_semantic_segmentation_no_trainer.py
+++ /dev/null
@@ -1,691 +0,0 @@
-# coding=utf-8
-# Copyright 2022 The HuggingFace Inc. team. All rights reserved.
-#
-# Licensed under the Apache License, Version 2.0 (the "License");
-# you may not use this file except in compliance with the License.
-# You may obtain a copy of the License at
-#
-# http://www.apache.org/licenses/LICENSE-2.0
-#
-# Unless required by applicable law or agreed to in writing, software
-# distributed under the License is distributed on an "AS IS" BASIS,
-# WITHOUT WARRANTIES OR CONDITIONS OF ANY KIND, either express or implied.
-# See the License for the specific language governing permissions and
-# limitations under the License.
-""" Finetuning any 🤗 Transformers model supported by AutoModelForSemanticSegmentation for semantic segmentation."""
-
-import argparse
-import json
-import math
-import os
-import random
-from pathlib import Path
-
-import datasets
-import evaluate
-import numpy as np
-import torch
-from accelerate import Accelerator
-from accelerate.logging import get_logger
-from accelerate.utils import set_seed
-from datasets import load_dataset
-from huggingface_hub import Repository, create_repo, hf_hub_download
-from PIL import Image
-from torch.utils.data import DataLoader
-from torchvision import transforms
-from torchvision.transforms import functional
-from tqdm.auto import tqdm
-
-import transformers
-from transformers import (
- AutoConfig,
- AutoImageProcessor,
- AutoModelForSemanticSegmentation,
- SchedulerType,
- default_data_collator,
- get_scheduler,
-)
-from transformers.utils import check_min_version, get_full_repo_name, send_example_telemetry
-from transformers.utils.versions import require_version
-
-
-# Will error if the minimal version of Transformers is not installed. Remove at your own risks.
-check_min_version("4.28.0")
-
-logger = get_logger(__name__)
-
-require_version("datasets>=2.0.0", "To fix: pip install -r examples/pytorch/semantic-segmentation/requirements.txt")
-
-
-def pad_if_smaller(img, size, fill=0):
- min_size = min(img.size)
- if min_size < size:
- original_width, original_height = img.size
- pad_height = size - original_height if original_height < size else 0
- pad_width = size - original_width if original_width < size else 0
- img = functional.pad(img, (0, 0, pad_width, pad_height), fill=fill)
- return img
-
-
-class Compose:
- def __init__(self, transforms):
- self.transforms = transforms
-
- def __call__(self, image, target):
- for t in self.transforms:
- image, target = t(image, target)
- return image, target
-
-
-class Identity:
- def __init__(self):
- pass
-
- def __call__(self, image, target):
- return image, target
-
-
-class Resize:
- def __init__(self, size):
- self.size = size
-
- def __call__(self, image, target):
- image = functional.resize(image, self.size)
- target = functional.resize(target, self.size, interpolation=transforms.InterpolationMode.NEAREST)
- return image, target
-
-
-class RandomResize:
- def __init__(self, min_size, max_size=None):
- self.min_size = min_size
- if max_size is None:
- max_size = min_size
- self.max_size = max_size
-
- def __call__(self, image, target):
- size = random.randint(self.min_size, self.max_size)
- image = functional.resize(image, size)
- target = functional.resize(target, size, interpolation=transforms.InterpolationMode.NEAREST)
- return image, target
-
-
-class RandomCrop:
- def __init__(self, size):
- self.size = size
-
- def __call__(self, image, target):
- image = pad_if_smaller(image, self.size)
- target = pad_if_smaller(target, self.size, fill=255)
- crop_params = transforms.RandomCrop.get_params(image, (self.size, self.size))
- image = functional.crop(image, *crop_params)
- target = functional.crop(target, *crop_params)
- return image, target
-
-
-class RandomHorizontalFlip:
- def __init__(self, flip_prob):
- self.flip_prob = flip_prob
-
- def __call__(self, image, target):
- if random.random() < self.flip_prob:
- image = functional.hflip(image)
- target = functional.hflip(target)
- return image, target
-
-
-class PILToTensor:
- def __call__(self, image, target):
- image = functional.pil_to_tensor(image)
- target = torch.as_tensor(np.array(target), dtype=torch.int64)
- return image, target
-
-
-class ConvertImageDtype:
- def __init__(self, dtype):
- self.dtype = dtype
-
- def __call__(self, image, target):
- image = functional.convert_image_dtype(image, self.dtype)
- return image, target
-
-
-class Normalize:
- def __init__(self, mean, std):
- self.mean = mean
- self.std = std
-
- def __call__(self, image, target):
- image = functional.normalize(image, mean=self.mean, std=self.std)
- return image, target
-
-
-class ReduceLabels:
- def __call__(self, image, target):
- if not isinstance(target, np.ndarray):
- target = np.array(target).astype(np.uint8)
- # avoid using underflow conversion
- target[target == 0] = 255
- target = target - 1
- target[target == 254] = 255
-
- target = Image.fromarray(target)
- return image, target
-
-
-def parse_args():
- parser = argparse.ArgumentParser(description="Finetune a transformers model on a text classification task")
- parser.add_argument(
- "--model_name_or_path",
- type=str,
- help="Path to a pretrained model or model identifier from huggingface.co/models.",
- default="nvidia/mit-b0",
- )
- parser.add_argument(
- "--dataset_name",
- type=str,
- help="Name of the dataset on the hub.",
- default="segments/sidewalk-semantic",
- )
- parser.add_argument(
- "--reduce_labels",
- action="store_true",
- help="Whether or not to reduce all labels by 1 and replace background by 255.",
- )
- parser.add_argument(
- "--train_val_split",
- type=float,
- default=0.15,
- help="Fraction of the dataset to be used for validation.",
- )
- parser.add_argument(
- "--cache_dir",
- type=str,
- help="Path to a folder in which the model and dataset will be cached.",
- )
- parser.add_argument(
- "--use_auth_token",
- action="store_true",
- help="Whether to use an authentication token to access the model repository.",
- )
- parser.add_argument(
- "--per_device_train_batch_size",
- type=int,
- default=8,
- help="Batch size (per device) for the training dataloader.",
- )
- parser.add_argument(
- "--per_device_eval_batch_size",
- type=int,
- default=8,
- help="Batch size (per device) for the evaluation dataloader.",
- )
- parser.add_argument(
- "--learning_rate",
- type=float,
- default=5e-5,
- help="Initial learning rate (after the potential warmup period) to use.",
- )
- parser.add_argument(
- "--adam_beta1",
- type=float,
- default=0.9,
- help="Beta1 for AdamW optimizer",
- )
- parser.add_argument(
- "--adam_beta2",
- type=float,
- default=0.999,
- help="Beta2 for AdamW optimizer",
- )
- parser.add_argument(
- "--adam_epsilon",
- type=float,
- default=1e-8,
- help="Epsilon for AdamW optimizer",
- )
- parser.add_argument("--num_train_epochs", type=int, default=3, help="Total number of training epochs to perform.")
- parser.add_argument(
- "--max_train_steps",
- type=int,
- default=None,
- help="Total number of training steps to perform. If provided, overrides num_train_epochs.",
- )
- parser.add_argument(
- "--gradient_accumulation_steps",
- type=int,
- default=1,
- help="Number of updates steps to accumulate before performing a backward/update pass.",
- )
- parser.add_argument(
- "--lr_scheduler_type",
- type=SchedulerType,
- default="polynomial",
- help="The scheduler type to use.",
- choices=["linear", "cosine", "cosine_with_restarts", "polynomial", "constant", "constant_with_warmup"],
- )
- parser.add_argument(
- "--num_warmup_steps", type=int, default=0, help="Number of steps for the warmup in the lr scheduler."
- )
- parser.add_argument("--output_dir", type=str, default=None, help="Where to store the final model.")
- parser.add_argument("--seed", type=int, default=None, help="A seed for reproducible training.")
- parser.add_argument("--push_to_hub", action="store_true", help="Whether or not to push the model to the Hub.")
- parser.add_argument(
- "--hub_model_id", type=str, help="The name of the repository to keep in sync with the local `output_dir`."
- )
- parser.add_argument("--hub_token", type=str, help="The token to use to push to the Model Hub.")
- parser.add_argument(
- "--checkpointing_steps",
- type=str,
- default=None,
- help="Whether the various states should be saved at the end of every n steps, or 'epoch' for each epoch.",
- )
- parser.add_argument(
- "--resume_from_checkpoint",
- type=str,
- default=None,
- help="If the training should continue from a checkpoint folder.",
- )
- parser.add_argument(
- "--with_tracking",
- required=False,
- action="store_true",
- help="Whether to enable experiment trackers for logging.",
- )
- parser.add_argument(
- "--report_to",
- type=str,
- default="all",
- help=(
- 'The integration to report the results and logs to. Supported platforms are `"tensorboard"`,'
- ' `"wandb"`, `"comet_ml"` and `"clearml"`. Use `"all"` (default) to report to all integrations.'
- "Only applicable when `--with_tracking` is passed."
- ),
- )
- args = parser.parse_args()
-
- # Sanity checks
- if args.push_to_hub or args.with_tracking:
- if args.output_dir is None:
- raise ValueError(
- "Need an `output_dir` to create a repo when `--push_to_hub` or `with_tracking` is specified."
- )
-
- if args.output_dir is not None:
- os.makedirs(args.output_dir, exist_ok=True)
-
- return args
-
-
-def main():
- args = parse_args()
-
- # Sending telemetry. Tracking the example usage helps us better allocate resources to maintain them. The
- # information sent is the one passed as arguments along with your Python/PyTorch versions.
- send_example_telemetry("run_semantic_segmentation_no_trainer", args)
-
- # Initialize the accelerator. We will let the accelerator handle device placement for us in this example.
- # If we're using tracking, we also need to initialize it here and it will by default pick up all supported trackers
- # in the environment
- accelerator_log_kwargs = {}
-
- if args.with_tracking:
- accelerator_log_kwargs["log_with"] = args.report_to
- accelerator_log_kwargs["logging_dir"] = args.output_dir
-
- accelerator = Accelerator(gradient_accumulation_steps=args.gradient_accumulation_steps, **accelerator_log_kwargs)
-
- logger.info(accelerator.state, main_process_only=False)
- if accelerator.is_local_main_process:
- datasets.utils.logging.set_verbosity_warning()
- transformers.utils.logging.set_verbosity_info()
- else:
- datasets.utils.logging.set_verbosity_error()
- transformers.utils.logging.set_verbosity_error()
-
- # If passed along, set the training seed now.
- # We set device_specific to True as we want different data augmentation per device.
- if args.seed is not None:
- set_seed(args.seed, device_specific=True)
-
- # Handle the repository creation
- if accelerator.is_main_process:
- if args.push_to_hub:
- if args.hub_model_id is None:
- repo_name = get_full_repo_name(Path(args.output_dir).name, token=args.hub_token)
- else:
- repo_name = args.hub_model_id
- create_repo(repo_name, exist_ok=True, token=args.hub_token)
- repo = Repository(args.output_dir, clone_from=repo_name, token=args.hub_token)
-
- with open(os.path.join(args.output_dir, ".gitignore"), "w+") as gitignore:
- if "step_*" not in gitignore:
- gitignore.write("step_*\n")
- if "epoch_*" not in gitignore:
- gitignore.write("epoch_*\n")
- elif args.output_dir is not None:
- os.makedirs(args.output_dir, exist_ok=True)
- accelerator.wait_for_everyone()
-
- # Load dataset
- # In distributed training, the load_dataset function guarantees that only one local process can concurrently
- # download the dataset.
- # TODO support datasets from local folders
- dataset = load_dataset(args.dataset_name, cache_dir=args.cache_dir)
-
- # Rename column names to standardized names (only "image" and "label" need to be present)
- if "pixel_values" in dataset["train"].column_names:
- dataset = dataset.rename_columns({"pixel_values": "image"})
- if "annotation" in dataset["train"].column_names:
- dataset = dataset.rename_columns({"annotation": "label"})
-
- # If we don't have a validation split, split off a percentage of train as validation.
- args.train_val_split = None if "validation" in dataset.keys() else args.train_val_split
- if isinstance(args.train_val_split, float) and args.train_val_split > 0.0:
- split = dataset["train"].train_test_split(args.train_val_split)
- dataset["train"] = split["train"]
- dataset["validation"] = split["test"]
-
- # Prepare label mappings.
- # We'll include these in the model's config to get human readable labels in the Inference API.
- if args.dataset_name == "scene_parse_150":
- repo_id = "huggingface/label-files"
- filename = "ade20k-id2label.json"
- else:
- repo_id = args.dataset_name
- filename = "id2label.json"
- id2label = json.load(open(hf_hub_download(repo_id, filename, repo_type="dataset"), "r"))
- id2label = {int(k): v for k, v in id2label.items()}
- label2id = {v: k for k, v in id2label.items()}
-
- # Load pretrained model and image processor
- config = AutoConfig.from_pretrained(args.model_name_or_path, id2label=id2label, label2id=label2id)
- image_processor = AutoImageProcessor.from_pretrained(args.model_name_or_path)
- model = AutoModelForSemanticSegmentation.from_pretrained(args.model_name_or_path, config=config)
-
- # Preprocessing the datasets
- # Define torchvision transforms to be applied to each image + target.
- # Not that straightforward in torchvision: https://github.com/pytorch/vision/issues/9
- # Currently based on official torchvision references: https://github.com/pytorch/vision/blob/main/references/segmentation/transforms.py
- if "shortest_edge" in image_processor.size:
- # We instead set the target size as (shortest_edge, shortest_edge) to here to ensure all images are batchable.
- size = (image_processor.size["shortest_edge"], image_processor.size["shortest_edge"])
- else:
- size = (image_processor.size["height"], image_processor.size["width"])
- train_transforms = Compose(
- [
- ReduceLabels() if args.reduce_labels else Identity(),
- RandomCrop(size=size),
- RandomHorizontalFlip(flip_prob=0.5),
- PILToTensor(),
- ConvertImageDtype(torch.float),
- Normalize(mean=image_processor.image_mean, std=image_processor.image_std),
- ]
- )
- # Define torchvision transform to be applied to each image.
- # jitter = ColorJitter(brightness=0.25, contrast=0.25, saturation=0.25, hue=0.1)
- val_transforms = Compose(
- [
- ReduceLabels() if args.reduce_labels else Identity(),
- Resize(size=size),
- PILToTensor(),
- ConvertImageDtype(torch.float),
- Normalize(mean=image_processor.image_mean, std=image_processor.image_std),
- ]
- )
-
- def preprocess_train(example_batch):
- pixel_values = []
- labels = []
- for image, target in zip(example_batch["image"], example_batch["label"]):
- image, target = train_transforms(image.convert("RGB"), target)
- pixel_values.append(image)
- labels.append(target)
-
- encoding = {}
- encoding["pixel_values"] = torch.stack(pixel_values)
- encoding["labels"] = torch.stack(labels)
-
- return encoding
-
- def preprocess_val(example_batch):
- pixel_values = []
- labels = []
- for image, target in zip(example_batch["image"], example_batch["label"]):
- image, target = val_transforms(image.convert("RGB"), target)
- pixel_values.append(image)
- labels.append(target)
-
- encoding = {}
- encoding["pixel_values"] = torch.stack(pixel_values)
- encoding["labels"] = torch.stack(labels)
-
- return encoding
-
- with accelerator.main_process_first():
- train_dataset = dataset["train"].with_transform(preprocess_train)
- eval_dataset = dataset["validation"].with_transform(preprocess_val)
-
- train_dataloader = DataLoader(
- train_dataset, shuffle=True, collate_fn=default_data_collator, batch_size=args.per_device_train_batch_size
- )
- eval_dataloader = DataLoader(
- eval_dataset, collate_fn=default_data_collator, batch_size=args.per_device_eval_batch_size
- )
-
- # Optimizer
- optimizer = torch.optim.AdamW(
- list(model.parameters()),
- lr=args.learning_rate,
- betas=[args.adam_beta1, args.adam_beta2],
- eps=args.adam_epsilon,
- )
-
- # Figure out how many steps we should save the Accelerator states
- checkpointing_steps = args.checkpointing_steps
- if checkpointing_steps is not None and checkpointing_steps.isdigit():
- checkpointing_steps = int(checkpointing_steps)
-
- # Scheduler and math around the number of training steps.
- overrode_max_train_steps = False
- num_update_steps_per_epoch = math.ceil(len(train_dataloader) / args.gradient_accumulation_steps)
- if args.max_train_steps is None:
- args.max_train_steps = args.num_train_epochs * num_update_steps_per_epoch
- overrode_max_train_steps = True
-
- lr_scheduler = get_scheduler(
- name=args.lr_scheduler_type,
- optimizer=optimizer,
- num_warmup_steps=args.num_warmup_steps * args.gradient_accumulation_steps,
- num_training_steps=args.max_train_steps * args.gradient_accumulation_steps,
- )
-
- # Prepare everything with our `accelerator`.
- model, optimizer, train_dataloader, eval_dataloader, lr_scheduler = accelerator.prepare(
- model, optimizer, train_dataloader, eval_dataloader, lr_scheduler
- )
-
- # We need to recalculate our total training steps as the size of the training dataloader may have changed.
- num_update_steps_per_epoch = math.ceil(len(train_dataloader) / args.gradient_accumulation_steps)
- if overrode_max_train_steps:
- args.max_train_steps = args.num_train_epochs * num_update_steps_per_epoch
- # Afterwards we recalculate our number of training epochs
- args.num_train_epochs = math.ceil(args.max_train_steps / num_update_steps_per_epoch)
-
- # Instantiate metric
- metric = evaluate.load("mean_iou")
-
- # We need to initialize the trackers we use, and also store our configuration.
- # The trackers initializes automatically on the main process.
- if args.with_tracking:
- experiment_config = vars(args)
- # TensorBoard cannot log Enums, need the raw value
- experiment_config["lr_scheduler_type"] = experiment_config["lr_scheduler_type"].value
- accelerator.init_trackers("semantic_segmentation_no_trainer", experiment_config)
-
- # Train!
- total_batch_size = args.per_device_train_batch_size * accelerator.num_processes * args.gradient_accumulation_steps
-
- logger.info("***** Running training *****")
- logger.info(f" Num examples = {len(train_dataset)}")
- logger.info(f" Num Epochs = {args.num_train_epochs}")
- logger.info(f" Instantaneous batch size per device = {args.per_device_train_batch_size}")
- logger.info(f" Total train batch size (w. parallel, distributed & accumulation) = {total_batch_size}")
- logger.info(f" Gradient Accumulation steps = {args.gradient_accumulation_steps}")
- logger.info(f" Total optimization steps = {args.max_train_steps}")
- # Only show the progress bar once on each machine.
- progress_bar = tqdm(range(args.max_train_steps), disable=not accelerator.is_local_main_process)
- completed_steps = 0
- starting_epoch = 0
-
- # Potentially load in the weights and states from a previous save
- if args.resume_from_checkpoint:
- if args.resume_from_checkpoint is not None or args.resume_from_checkpoint != "":
- accelerator.print(f"Resumed from checkpoint: {args.resume_from_checkpoint}")
- accelerator.load_state(args.resume_from_checkpoint)
- path = os.path.basename(args.resume_from_checkpoint)
- else:
- # Get the most recent checkpoint
- dirs = [f.name for f in os.scandir(os.getcwd()) if f.is_dir()]
- dirs.sort(key=os.path.getctime)
- path = dirs[-1] # Sorts folders by date modified, most recent checkpoint is the last
- # Extract `epoch_{i}` or `step_{i}`
- training_difference = os.path.splitext(path)[0]
-
- if "epoch" in training_difference:
- starting_epoch = int(training_difference.replace("epoch_", "")) + 1
- resume_step = None
- else:
- resume_step = int(training_difference.replace("step_", ""))
- starting_epoch = resume_step // len(train_dataloader)
- resume_step -= starting_epoch * len(train_dataloader)
-
- for epoch in range(starting_epoch, args.num_train_epochs):
- if args.with_tracking:
- total_loss = 0
- model.train()
- for step, batch in enumerate(train_dataloader):
- # We need to skip steps until we reach the resumed step
- if args.resume_from_checkpoint and epoch == starting_epoch:
- if resume_step is not None and step < resume_step:
- completed_steps += 1
- continue
-
- with accelerator.accumulate(model):
- outputs = model(**batch)
- loss = outputs.loss
- # We keep track of the loss at each epoch
- if args.with_tracking:
- total_loss += loss.detach().float()
- accelerator.backward(loss)
- optimizer.step()
- lr_scheduler.step()
- optimizer.zero_grad()
-
- # Checks if the accelerator has performed an optimization step behind the scenes
- if accelerator.sync_gradients:
- progress_bar.update(1)
- completed_steps += 1
-
- if isinstance(checkpointing_steps, int):
- if completed_steps % checkpointing_steps == 0:
- output_dir = f"step_{completed_steps }"
- if args.output_dir is not None:
- output_dir = os.path.join(args.output_dir, output_dir)
- accelerator.save_state(output_dir)
-
- if args.push_to_hub and epoch < args.num_train_epochs - 1:
- accelerator.wait_for_everyone()
- unwrapped_model = accelerator.unwrap_model(model)
- unwrapped_model.save_pretrained(
- args.output_dir,
- is_main_process=accelerator.is_main_process,
- save_function=accelerator.save,
- )
- if accelerator.is_main_process:
- image_processor.save_pretrained(args.output_dir)
- repo.push_to_hub(
- commit_message=f"Training in progress {completed_steps} steps",
- blocking=False,
- auto_lfs_prune=True,
- )
-
- if completed_steps >= args.max_train_steps:
- break
-
- logger.info("***** Running evaluation *****")
- model.eval()
- for step, batch in enumerate(tqdm(eval_dataloader, disable=not accelerator.is_local_main_process)):
- with torch.no_grad():
- outputs = model(**batch)
-
- upsampled_logits = torch.nn.functional.interpolate(
- outputs.logits, size=batch["labels"].shape[-2:], mode="bilinear", align_corners=False
- )
- predictions = upsampled_logits.argmax(dim=1)
-
- predictions, references = accelerator.gather_for_metrics((predictions, batch["labels"]))
-
- metric.add_batch(
- predictions=predictions,
- references=references,
- )
-
- eval_metrics = metric.compute(
- num_labels=len(id2label),
- ignore_index=255,
- reduce_labels=False, # we've already reduced the labels before
- )
- logger.info(f"epoch {epoch}: {eval_metrics}")
-
- if args.with_tracking:
- accelerator.log(
- {
- "mean_iou": eval_metrics["mean_iou"],
- "mean_accuracy": eval_metrics["mean_accuracy"],
- "overall_accuracy": eval_metrics["overall_accuracy"],
- "train_loss": total_loss.item() / len(train_dataloader),
- "epoch": epoch,
- "step": completed_steps,
- },
- step=completed_steps,
- )
-
- if args.push_to_hub and epoch < args.num_train_epochs - 1:
- accelerator.wait_for_everyone()
- unwrapped_model = accelerator.unwrap_model(model)
- unwrapped_model.save_pretrained(
- args.output_dir, is_main_process=accelerator.is_main_process, save_function=accelerator.save
- )
- if accelerator.is_main_process:
- image_processor.save_pretrained(args.output_dir)
- repo.push_to_hub(
- commit_message=f"Training in progress epoch {epoch}", blocking=False, auto_lfs_prune=True
- )
-
- if args.checkpointing_steps == "epoch":
- output_dir = f"epoch_{epoch}"
- if args.output_dir is not None:
- output_dir = os.path.join(args.output_dir, output_dir)
- accelerator.save_state(output_dir)
-
- if args.with_tracking:
- accelerator.end_training()
-
- if args.output_dir is not None:
- accelerator.wait_for_everyone()
- unwrapped_model = accelerator.unwrap_model(model)
- unwrapped_model.save_pretrained(
- args.output_dir, is_main_process=accelerator.is_main_process, save_function=accelerator.save
- )
- if accelerator.is_main_process:
- image_processor.save_pretrained(args.output_dir)
- if args.push_to_hub:
- repo.push_to_hub(commit_message="End of training", auto_lfs_prune=True)
-
- all_results = {f"eval_{k}": v for k, v in eval_metrics.items()}
- with open(os.path.join(args.output_dir, "all_results.json"), "w") as f:
- json.dump(all_results, f)
-
-
-if __name__ == "__main__":
- main()
diff --git a/spaces/chikoto/Umamusume-DeBERTa-VITS2-TTS-JP/README.md b/spaces/chikoto/Umamusume-DeBERTa-VITS2-TTS-JP/README.md
deleted file mode 100644
index 5fe3acb4f016fe17547fa2327f60379d739491ce..0000000000000000000000000000000000000000
--- a/spaces/chikoto/Umamusume-DeBERTa-VITS2-TTS-JP/README.md
+++ /dev/null
@@ -1,13 +0,0 @@
----
-title: Umamusume DeBERTa VITS2 TTS JP
-emoji: 😻
-colorFrom: gray
-colorTo: blue
-sdk: gradio
-sdk_version: 3.48.0
-app_file: app.py
-pinned: false
-license: mit
----
-
-Check out the configuration reference at https://huggingface.co/docs/hub/spaces-config-reference
diff --git a/spaces/chuan-hd/law-assistant-chatbot/.venv/lib/python3.11/site-packages/fontTools/ttLib/tables/otData.py b/spaces/chuan-hd/law-assistant-chatbot/.venv/lib/python3.11/site-packages/fontTools/ttLib/tables/otData.py
deleted file mode 100644
index 56716824ecd7950dda249a159b5b292dbd2a86f7..0000000000000000000000000000000000000000
--- a/spaces/chuan-hd/law-assistant-chatbot/.venv/lib/python3.11/site-packages/fontTools/ttLib/tables/otData.py
+++ /dev/null
@@ -1,6236 +0,0 @@
-otData = [
- #
- # common
- #
- ("LookupOrder", []),
- (
- "ScriptList",
- [
- ("uint16", "ScriptCount", None, None, "Number of ScriptRecords"),
- (
- "struct",
- "ScriptRecord",
- "ScriptCount",
- 0,
- "Array of ScriptRecords -listed alphabetically by ScriptTag",
- ),
- ],
- ),
- (
- "ScriptRecord",
- [
- ("Tag", "ScriptTag", None, None, "4-byte ScriptTag identifier"),
- (
- "Offset",
- "Script",
- None,
- None,
- "Offset to Script table-from beginning of ScriptList",
- ),
- ],
- ),
- (
- "Script",
- [
- (
- "Offset",
- "DefaultLangSys",
- None,
- None,
- "Offset to DefaultLangSys table-from beginning of Script table-may be NULL",
- ),
- (
- "uint16",
- "LangSysCount",
- None,
- None,
- "Number of LangSysRecords for this script-excluding the DefaultLangSys",
- ),
- (
- "struct",
- "LangSysRecord",
- "LangSysCount",
- 0,
- "Array of LangSysRecords-listed alphabetically by LangSysTag",
- ),
- ],
- ),
- (
- "LangSysRecord",
- [
- ("Tag", "LangSysTag", None, None, "4-byte LangSysTag identifier"),
- (
- "Offset",
- "LangSys",
- None,
- None,
- "Offset to LangSys table-from beginning of Script table",
- ),
- ],
- ),
- (
- "LangSys",
- [
- (
- "Offset",
- "LookupOrder",
- None,
- None,
- "= NULL (reserved for an offset to a reordering table)",
- ),
- (
- "uint16",
- "ReqFeatureIndex",
- None,
- None,
- "Index of a feature required for this language system- if no required features = 0xFFFF",
- ),
- (
- "uint16",
- "FeatureCount",
- None,
- None,
- "Number of FeatureIndex values for this language system-excludes the required feature",
- ),
- (
- "uint16",
- "FeatureIndex",
- "FeatureCount",
- 0,
- "Array of indices into the FeatureList-in arbitrary order",
- ),
- ],
- ),
- (
- "FeatureList",
- [
- (
- "uint16",
- "FeatureCount",
- None,
- None,
- "Number of FeatureRecords in this table",
- ),
- (
- "struct",
- "FeatureRecord",
- "FeatureCount",
- 0,
- "Array of FeatureRecords-zero-based (first feature has FeatureIndex = 0)-listed alphabetically by FeatureTag",
- ),
- ],
- ),
- (
- "FeatureRecord",
- [
- ("Tag", "FeatureTag", None, None, "4-byte feature identification tag"),
- (
- "Offset",
- "Feature",
- None,
- None,
- "Offset to Feature table-from beginning of FeatureList",
- ),
- ],
- ),
- (
- "Feature",
- [
- (
- "Offset",
- "FeatureParams",
- None,
- None,
- "= NULL (reserved for offset to FeatureParams)",
- ),
- (
- "uint16",
- "LookupCount",
- None,
- None,
- "Number of LookupList indices for this feature",
- ),
- (
- "uint16",
- "LookupListIndex",
- "LookupCount",
- 0,
- "Array of LookupList indices for this feature -zero-based (first lookup is LookupListIndex = 0)",
- ),
- ],
- ),
- ("FeatureParams", []),
- (
- "FeatureParamsSize",
- [
- (
- "DeciPoints",
- "DesignSize",
- None,
- None,
- "The design size in 720/inch units (decipoints).",
- ),
- (
- "uint16",
- "SubfamilyID",
- None,
- None,
- "Serves as an identifier that associates fonts in a subfamily.",
- ),
- ("NameID", "SubfamilyNameID", None, None, "Subfamily NameID."),
- (
- "DeciPoints",
- "RangeStart",
- None,
- None,
- "Small end of recommended usage range (exclusive) in 720/inch units.",
- ),
- (
- "DeciPoints",
- "RangeEnd",
- None,
- None,
- "Large end of recommended usage range (inclusive) in 720/inch units.",
- ),
- ],
- ),
- (
- "FeatureParamsStylisticSet",
- [
- ("uint16", "Version", None, None, "Set to 0."),
- ("NameID", "UINameID", None, None, "UI NameID."),
- ],
- ),
- (
- "FeatureParamsCharacterVariants",
- [
- ("uint16", "Format", None, None, "Set to 0."),
- ("NameID", "FeatUILabelNameID", None, None, "Feature UI label NameID."),
- (
- "NameID",
- "FeatUITooltipTextNameID",
- None,
- None,
- "Feature UI tooltip text NameID.",
- ),
- ("NameID", "SampleTextNameID", None, None, "Sample text NameID."),
- ("uint16", "NumNamedParameters", None, None, "Number of named parameters."),
- (
- "NameID",
- "FirstParamUILabelNameID",
- None,
- None,
- "First NameID of UI feature parameters.",
- ),
- (
- "uint16",
- "CharCount",
- None,
- None,
- "Count of characters this feature provides glyph variants for.",
- ),
- (
- "uint24",
- "Character",
- "CharCount",
- 0,
- "Unicode characters for which this feature provides glyph variants.",
- ),
- ],
- ),
- (
- "LookupList",
- [
- ("uint16", "LookupCount", None, None, "Number of lookups in this table"),
- (
- "Offset",
- "Lookup",
- "LookupCount",
- 0,
- "Array of offsets to Lookup tables-from beginning of LookupList -zero based (first lookup is Lookup index = 0)",
- ),
- ],
- ),
- (
- "Lookup",
- [
- (
- "uint16",
- "LookupType",
- None,
- None,
- "Different enumerations for GSUB and GPOS",
- ),
- ("LookupFlag", "LookupFlag", None, None, "Lookup qualifiers"),
- (
- "uint16",
- "SubTableCount",
- None,
- None,
- "Number of SubTables for this lookup",
- ),
- (
- "Offset",
- "SubTable",
- "SubTableCount",
- 0,
- "Array of offsets to SubTables-from beginning of Lookup table",
- ),
- (
- "uint16",
- "MarkFilteringSet",
- None,
- "LookupFlag & 0x0010",
- "If set, indicates that the lookup table structure is followed by a MarkFilteringSet field. The layout engine skips over all mark glyphs not in the mark filtering set indicated.",
- ),
- ],
- ),
- (
- "CoverageFormat1",
- [
- ("uint16", "CoverageFormat", None, None, "Format identifier-format = 1"),
- ("uint16", "GlyphCount", None, None, "Number of glyphs in the GlyphArray"),
- (
- "GlyphID",
- "GlyphArray",
- "GlyphCount",
- 0,
- "Array of GlyphIDs-in numerical order",
- ),
- ],
- ),
- (
- "CoverageFormat2",
- [
- ("uint16", "CoverageFormat", None, None, "Format identifier-format = 2"),
- ("uint16", "RangeCount", None, None, "Number of RangeRecords"),
- (
- "struct",
- "RangeRecord",
- "RangeCount",
- 0,
- "Array of glyph ranges-ordered by Start GlyphID",
- ),
- ],
- ),
- (
- "RangeRecord",
- [
- ("GlyphID", "Start", None, None, "First GlyphID in the range"),
- ("GlyphID", "End", None, None, "Last GlyphID in the range"),
- (
- "uint16",
- "StartCoverageIndex",
- None,
- None,
- "Coverage Index of first GlyphID in range",
- ),
- ],
- ),
- (
- "ClassDefFormat1",
- [
- ("uint16", "ClassFormat", None, None, "Format identifier-format = 1"),
- (
- "GlyphID",
- "StartGlyph",
- None,
- None,
- "First GlyphID of the ClassValueArray",
- ),
- ("uint16", "GlyphCount", None, None, "Size of the ClassValueArray"),
- (
- "uint16",
- "ClassValueArray",
- "GlyphCount",
- 0,
- "Array of Class Values-one per GlyphID",
- ),
- ],
- ),
- (
- "ClassDefFormat2",
- [
- ("uint16", "ClassFormat", None, None, "Format identifier-format = 2"),
- ("uint16", "ClassRangeCount", None, None, "Number of ClassRangeRecords"),
- (
- "struct",
- "ClassRangeRecord",
- "ClassRangeCount",
- 0,
- "Array of ClassRangeRecords-ordered by Start GlyphID",
- ),
- ],
- ),
- (
- "ClassRangeRecord",
- [
- ("GlyphID", "Start", None, None, "First GlyphID in the range"),
- ("GlyphID", "End", None, None, "Last GlyphID in the range"),
- ("uint16", "Class", None, None, "Applied to all glyphs in the range"),
- ],
- ),
- (
- "Device",
- [
- ("uint16", "StartSize", None, None, "Smallest size to correct-in ppem"),
- ("uint16", "EndSize", None, None, "Largest size to correct-in ppem"),
- (
- "uint16",
- "DeltaFormat",
- None,
- None,
- "Format of DeltaValue array data: 1, 2, or 3",
- ),
- (
- "DeltaValue",
- "DeltaValue",
- "",
- "DeltaFormat in (1,2,3)",
- "Array of compressed data",
- ),
- ],
- ),
- #
- # gpos
- #
- (
- "GPOS",
- [
- (
- "Version",
- "Version",
- None,
- None,
- "Version of the GPOS table- 0x00010000 or 0x00010001",
- ),
- (
- "Offset",
- "ScriptList",
- None,
- None,
- "Offset to ScriptList table-from beginning of GPOS table",
- ),
- (
- "Offset",
- "FeatureList",
- None,
- None,
- "Offset to FeatureList table-from beginning of GPOS table",
- ),
- (
- "Offset",
- "LookupList",
- None,
- None,
- "Offset to LookupList table-from beginning of GPOS table",
- ),
- (
- "LOffset",
- "FeatureVariations",
- None,
- "Version >= 0x00010001",
- "Offset to FeatureVariations table-from beginning of GPOS table",
- ),
- ],
- ),
- (
- "SinglePosFormat1",
- [
- ("uint16", "PosFormat", None, None, "Format identifier-format = 1"),
- (
- "Offset",
- "Coverage",
- None,
- None,
- "Offset to Coverage table-from beginning of SinglePos subtable",
- ),
- (
- "uint16",
- "ValueFormat",
- None,
- None,
- "Defines the types of data in the ValueRecord",
- ),
- (
- "ValueRecord",
- "Value",
- None,
- None,
- "Defines positioning value(s)-applied to all glyphs in the Coverage table",
- ),
- ],
- ),
- (
- "SinglePosFormat2",
- [
- ("uint16", "PosFormat", None, None, "Format identifier-format = 2"),
- (
- "Offset",
- "Coverage",
- None,
- None,
- "Offset to Coverage table-from beginning of SinglePos subtable",
- ),
- (
- "uint16",
- "ValueFormat",
- None,
- None,
- "Defines the types of data in the ValueRecord",
- ),
- ("uint16", "ValueCount", None, None, "Number of ValueRecords"),
- (
- "ValueRecord",
- "Value",
- "ValueCount",
- 0,
- "Array of ValueRecords-positioning values applied to glyphs",
- ),
- ],
- ),
- (
- "PairPosFormat1",
- [
- ("uint16", "PosFormat", None, None, "Format identifier-format = 1"),
- (
- "Offset",
- "Coverage",
- None,
- None,
- "Offset to Coverage table-from beginning of PairPos subtable-only the first glyph in each pair",
- ),
- (
- "uint16",
- "ValueFormat1",
- None,
- None,
- "Defines the types of data in ValueRecord1-for the first glyph in the pair -may be zero (0)",
- ),
- (
- "uint16",
- "ValueFormat2",
- None,
- None,
- "Defines the types of data in ValueRecord2-for the second glyph in the pair -may be zero (0)",
- ),
- ("uint16", "PairSetCount", None, None, "Number of PairSet tables"),
- (
- "Offset",
- "PairSet",
- "PairSetCount",
- 0,
- "Array of offsets to PairSet tables-from beginning of PairPos subtable-ordered by Coverage Index",
- ),
- ],
- ),
- (
- "PairSet",
- [
- ("uint16", "PairValueCount", None, None, "Number of PairValueRecords"),
- (
- "struct",
- "PairValueRecord",
- "PairValueCount",
- 0,
- "Array of PairValueRecords-ordered by GlyphID of the second glyph",
- ),
- ],
- ),
- (
- "PairValueRecord",
- [
- (
- "GlyphID",
- "SecondGlyph",
- None,
- None,
- "GlyphID of second glyph in the pair-first glyph is listed in the Coverage table",
- ),
- (
- "ValueRecord",
- "Value1",
- None,
- None,
- "Positioning data for the first glyph in the pair",
- ),
- (
- "ValueRecord",
- "Value2",
- None,
- None,
- "Positioning data for the second glyph in the pair",
- ),
- ],
- ),
- (
- "PairPosFormat2",
- [
- ("uint16", "PosFormat", None, None, "Format identifier-format = 2"),
- (
- "Offset",
- "Coverage",
- None,
- None,
- "Offset to Coverage table-from beginning of PairPos subtable-for the first glyph of the pair",
- ),
- (
- "uint16",
- "ValueFormat1",
- None,
- None,
- "ValueRecord definition-for the first glyph of the pair-may be zero (0)",
- ),
- (
- "uint16",
- "ValueFormat2",
- None,
- None,
- "ValueRecord definition-for the second glyph of the pair-may be zero (0)",
- ),
- (
- "Offset",
- "ClassDef1",
- None,
- None,
- "Offset to ClassDef table-from beginning of PairPos subtable-for the first glyph of the pair",
- ),
- (
- "Offset",
- "ClassDef2",
- None,
- None,
- "Offset to ClassDef table-from beginning of PairPos subtable-for the second glyph of the pair",
- ),
- (
- "uint16",
- "Class1Count",
- None,
- None,
- "Number of classes in ClassDef1 table-includes Class0",
- ),
- (
- "uint16",
- "Class2Count",
- None,
- None,
- "Number of classes in ClassDef2 table-includes Class0",
- ),
- (
- "struct",
- "Class1Record",
- "Class1Count",
- 0,
- "Array of Class1 records-ordered by Class1",
- ),
- ],
- ),
- (
- "Class1Record",
- [
- (
- "struct",
- "Class2Record",
- "Class2Count",
- 0,
- "Array of Class2 records-ordered by Class2",
- ),
- ],
- ),
- (
- "Class2Record",
- [
- (
- "ValueRecord",
- "Value1",
- None,
- None,
- "Positioning for first glyph-empty if ValueFormat1 = 0",
- ),
- (
- "ValueRecord",
- "Value2",
- None,
- None,
- "Positioning for second glyph-empty if ValueFormat2 = 0",
- ),
- ],
- ),
- (
- "CursivePosFormat1",
- [
- ("uint16", "PosFormat", None, None, "Format identifier-format = 1"),
- (
- "Offset",
- "Coverage",
- None,
- None,
- "Offset to Coverage table-from beginning of CursivePos subtable",
- ),
- ("uint16", "EntryExitCount", None, None, "Number of EntryExit records"),
- (
- "struct",
- "EntryExitRecord",
- "EntryExitCount",
- 0,
- "Array of EntryExit records-in Coverage Index order",
- ),
- ],
- ),
- (
- "EntryExitRecord",
- [
- (
- "Offset",
- "EntryAnchor",
- None,
- None,
- "Offset to EntryAnchor table-from beginning of CursivePos subtable-may be NULL",
- ),
- (
- "Offset",
- "ExitAnchor",
- None,
- None,
- "Offset to ExitAnchor table-from beginning of CursivePos subtable-may be NULL",
- ),
- ],
- ),
- (
- "MarkBasePosFormat1",
- [
- ("uint16", "PosFormat", None, None, "Format identifier-format = 1"),
- (
- "Offset",
- "MarkCoverage",
- None,
- None,
- "Offset to MarkCoverage table-from beginning of MarkBasePos subtable",
- ),
- (
- "Offset",
- "BaseCoverage",
- None,
- None,
- "Offset to BaseCoverage table-from beginning of MarkBasePos subtable",
- ),
- ("uint16", "ClassCount", None, None, "Number of classes defined for marks"),
- (
- "Offset",
- "MarkArray",
- None,
- None,
- "Offset to MarkArray table-from beginning of MarkBasePos subtable",
- ),
- (
- "Offset",
- "BaseArray",
- None,
- None,
- "Offset to BaseArray table-from beginning of MarkBasePos subtable",
- ),
- ],
- ),
- (
- "BaseArray",
- [
- ("uint16", "BaseCount", None, None, "Number of BaseRecords"),
- (
- "struct",
- "BaseRecord",
- "BaseCount",
- 0,
- "Array of BaseRecords-in order of BaseCoverage Index",
- ),
- ],
- ),
- (
- "BaseRecord",
- [
- (
- "Offset",
- "BaseAnchor",
- "ClassCount",
- 0,
- "Array of offsets (one per class) to Anchor tables-from beginning of BaseArray table-ordered by class-zero-based",
- ),
- ],
- ),
- (
- "MarkLigPosFormat1",
- [
- ("uint16", "PosFormat", None, None, "Format identifier-format = 1"),
- (
- "Offset",
- "MarkCoverage",
- None,
- None,
- "Offset to Mark Coverage table-from beginning of MarkLigPos subtable",
- ),
- (
- "Offset",
- "LigatureCoverage",
- None,
- None,
- "Offset to Ligature Coverage table-from beginning of MarkLigPos subtable",
- ),
- ("uint16", "ClassCount", None, None, "Number of defined mark classes"),
- (
- "Offset",
- "MarkArray",
- None,
- None,
- "Offset to MarkArray table-from beginning of MarkLigPos subtable",
- ),
- (
- "Offset",
- "LigatureArray",
- None,
- None,
- "Offset to LigatureArray table-from beginning of MarkLigPos subtable",
- ),
- ],
- ),
- (
- "LigatureArray",
- [
- (
- "uint16",
- "LigatureCount",
- None,
- None,
- "Number of LigatureAttach table offsets",
- ),
- (
- "Offset",
- "LigatureAttach",
- "LigatureCount",
- 0,
- "Array of offsets to LigatureAttach tables-from beginning of LigatureArray table-ordered by LigatureCoverage Index",
- ),
- ],
- ),
- (
- "LigatureAttach",
- [
- (
- "uint16",
- "ComponentCount",
- None,
- None,
- "Number of ComponentRecords in this ligature",
- ),
- (
- "struct",
- "ComponentRecord",
- "ComponentCount",
- 0,
- "Array of Component records-ordered in writing direction",
- ),
- ],
- ),
- (
- "ComponentRecord",
- [
- (
- "Offset",
- "LigatureAnchor",
- "ClassCount",
- 0,
- "Array of offsets (one per class) to Anchor tables-from beginning of LigatureAttach table-ordered by class-NULL if a component does not have an attachment for a class-zero-based array",
- ),
- ],
- ),
- (
- "MarkMarkPosFormat1",
- [
- ("uint16", "PosFormat", None, None, "Format identifier-format = 1"),
- (
- "Offset",
- "Mark1Coverage",
- None,
- None,
- "Offset to Combining Mark Coverage table-from beginning of MarkMarkPos subtable",
- ),
- (
- "Offset",
- "Mark2Coverage",
- None,
- None,
- "Offset to Base Mark Coverage table-from beginning of MarkMarkPos subtable",
- ),
- (
- "uint16",
- "ClassCount",
- None,
- None,
- "Number of Combining Mark classes defined",
- ),
- (
- "Offset",
- "Mark1Array",
- None,
- None,
- "Offset to MarkArray table for Mark1-from beginning of MarkMarkPos subtable",
- ),
- (
- "Offset",
- "Mark2Array",
- None,
- None,
- "Offset to Mark2Array table for Mark2-from beginning of MarkMarkPos subtable",
- ),
- ],
- ),
- (
- "Mark2Array",
- [
- ("uint16", "Mark2Count", None, None, "Number of Mark2 records"),
- (
- "struct",
- "Mark2Record",
- "Mark2Count",
- 0,
- "Array of Mark2 records-in Coverage order",
- ),
- ],
- ),
- (
- "Mark2Record",
- [
- (
- "Offset",
- "Mark2Anchor",
- "ClassCount",
- 0,
- "Array of offsets (one per class) to Anchor tables-from beginning of Mark2Array table-zero-based array",
- ),
- ],
- ),
- (
- "PosLookupRecord",
- [
- (
- "uint16",
- "SequenceIndex",
- None,
- None,
- "Index to input glyph sequence-first glyph = 0",
- ),
- (
- "uint16",
- "LookupListIndex",
- None,
- None,
- "Lookup to apply to that position-zero-based",
- ),
- ],
- ),
- (
- "ContextPosFormat1",
- [
- ("uint16", "PosFormat", None, None, "Format identifier-format = 1"),
- (
- "Offset",
- "Coverage",
- None,
- None,
- "Offset to Coverage table-from beginning of ContextPos subtable",
- ),
- ("uint16", "PosRuleSetCount", None, None, "Number of PosRuleSet tables"),
- (
- "Offset",
- "PosRuleSet",
- "PosRuleSetCount",
- 0,
- "Array of offsets to PosRuleSet tables-from beginning of ContextPos subtable-ordered by Coverage Index",
- ),
- ],
- ),
- (
- "PosRuleSet",
- [
- ("uint16", "PosRuleCount", None, None, "Number of PosRule tables"),
- (
- "Offset",
- "PosRule",
- "PosRuleCount",
- 0,
- "Array of offsets to PosRule tables-from beginning of PosRuleSet-ordered by preference",
- ),
- ],
- ),
- (
- "PosRule",
- [
- (
- "uint16",
- "GlyphCount",
- None,
- None,
- "Number of glyphs in the Input glyph sequence",
- ),
- ("uint16", "PosCount", None, None, "Number of PosLookupRecords"),
- (
- "GlyphID",
- "Input",
- "GlyphCount",
- -1,
- "Array of input GlyphIDs-starting with the second glyph",
- ),
- (
- "struct",
- "PosLookupRecord",
- "PosCount",
- 0,
- "Array of positioning lookups-in design order",
- ),
- ],
- ),
- (
- "ContextPosFormat2",
- [
- ("uint16", "PosFormat", None, None, "Format identifier-format = 2"),
- (
- "Offset",
- "Coverage",
- None,
- None,
- "Offset to Coverage table-from beginning of ContextPos subtable",
- ),
- (
- "Offset",
- "ClassDef",
- None,
- None,
- "Offset to ClassDef table-from beginning of ContextPos subtable",
- ),
- ("uint16", "PosClassSetCount", None, None, "Number of PosClassSet tables"),
- (
- "Offset",
- "PosClassSet",
- "PosClassSetCount",
- 0,
- "Array of offsets to PosClassSet tables-from beginning of ContextPos subtable-ordered by class-may be NULL",
- ),
- ],
- ),
- (
- "PosClassSet",
- [
- (
- "uint16",
- "PosClassRuleCount",
- None,
- None,
- "Number of PosClassRule tables",
- ),
- (
- "Offset",
- "PosClassRule",
- "PosClassRuleCount",
- 0,
- "Array of offsets to PosClassRule tables-from beginning of PosClassSet-ordered by preference",
- ),
- ],
- ),
- (
- "PosClassRule",
- [
- ("uint16", "GlyphCount", None, None, "Number of glyphs to be matched"),
- ("uint16", "PosCount", None, None, "Number of PosLookupRecords"),
- (
- "uint16",
- "Class",
- "GlyphCount",
- -1,
- "Array of classes-beginning with the second class-to be matched to the input glyph sequence",
- ),
- (
- "struct",
- "PosLookupRecord",
- "PosCount",
- 0,
- "Array of positioning lookups-in design order",
- ),
- ],
- ),
- (
- "ContextPosFormat3",
- [
- ("uint16", "PosFormat", None, None, "Format identifier-format = 3"),
- (
- "uint16",
- "GlyphCount",
- None,
- None,
- "Number of glyphs in the input sequence",
- ),
- ("uint16", "PosCount", None, None, "Number of PosLookupRecords"),
- (
- "Offset",
- "Coverage",
- "GlyphCount",
- 0,
- "Array of offsets to Coverage tables-from beginning of ContextPos subtable",
- ),
- (
- "struct",
- "PosLookupRecord",
- "PosCount",
- 0,
- "Array of positioning lookups-in design order",
- ),
- ],
- ),
- (
- "ChainContextPosFormat1",
- [
- ("uint16", "PosFormat", None, None, "Format identifier-format = 1"),
- (
- "Offset",
- "Coverage",
- None,
- None,
- "Offset to Coverage table-from beginning of ContextPos subtable",
- ),
- (
- "uint16",
- "ChainPosRuleSetCount",
- None,
- None,
- "Number of ChainPosRuleSet tables",
- ),
- (
- "Offset",
- "ChainPosRuleSet",
- "ChainPosRuleSetCount",
- 0,
- "Array of offsets to ChainPosRuleSet tables-from beginning of ContextPos subtable-ordered by Coverage Index",
- ),
- ],
- ),
- (
- "ChainPosRuleSet",
- [
- (
- "uint16",
- "ChainPosRuleCount",
- None,
- None,
- "Number of ChainPosRule tables",
- ),
- (
- "Offset",
- "ChainPosRule",
- "ChainPosRuleCount",
- 0,
- "Array of offsets to ChainPosRule tables-from beginning of ChainPosRuleSet-ordered by preference",
- ),
- ],
- ),
- (
- "ChainPosRule",
- [
- (
- "uint16",
- "BacktrackGlyphCount",
- None,
- None,
- "Total number of glyphs in the backtrack sequence (number of glyphs to be matched before the first glyph)",
- ),
- (
- "GlyphID",
- "Backtrack",
- "BacktrackGlyphCount",
- 0,
- "Array of backtracking GlyphID's (to be matched before the input sequence)",
- ),
- (
- "uint16",
- "InputGlyphCount",
- None,
- None,
- "Total number of glyphs in the input sequence (includes the first glyph)",
- ),
- (
- "GlyphID",
- "Input",
- "InputGlyphCount",
- -1,
- "Array of input GlyphIDs (start with second glyph)",
- ),
- (
- "uint16",
- "LookAheadGlyphCount",
- None,
- None,
- "Total number of glyphs in the look ahead sequence (number of glyphs to be matched after the input sequence)",
- ),
- (
- "GlyphID",
- "LookAhead",
- "LookAheadGlyphCount",
- 0,
- "Array of lookahead GlyphID's (to be matched after the input sequence)",
- ),
- ("uint16", "PosCount", None, None, "Number of PosLookupRecords"),
- (
- "struct",
- "PosLookupRecord",
- "PosCount",
- 0,
- "Array of PosLookupRecords (in design order)",
- ),
- ],
- ),
- (
- "ChainContextPosFormat2",
- [
- ("uint16", "PosFormat", None, None, "Format identifier-format = 2"),
- (
- "Offset",
- "Coverage",
- None,
- None,
- "Offset to Coverage table-from beginning of ChainContextPos subtable",
- ),
- (
- "Offset",
- "BacktrackClassDef",
- None,
- None,
- "Offset to ClassDef table containing backtrack sequence context-from beginning of ChainContextPos subtable",
- ),
- (
- "Offset",
- "InputClassDef",
- None,
- None,
- "Offset to ClassDef table containing input sequence context-from beginning of ChainContextPos subtable",
- ),
- (
- "Offset",
- "LookAheadClassDef",
- None,
- None,
- "Offset to ClassDef table containing lookahead sequence context-from beginning of ChainContextPos subtable",
- ),
- (
- "uint16",
- "ChainPosClassSetCount",
- None,
- None,
- "Number of ChainPosClassSet tables",
- ),
- (
- "Offset",
- "ChainPosClassSet",
- "ChainPosClassSetCount",
- 0,
- "Array of offsets to ChainPosClassSet tables-from beginning of ChainContextPos subtable-ordered by input class-may be NULL",
- ),
- ],
- ),
- (
- "ChainPosClassSet",
- [
- (
- "uint16",
- "ChainPosClassRuleCount",
- None,
- None,
- "Number of ChainPosClassRule tables",
- ),
- (
- "Offset",
- "ChainPosClassRule",
- "ChainPosClassRuleCount",
- 0,
- "Array of offsets to ChainPosClassRule tables-from beginning of ChainPosClassSet-ordered by preference",
- ),
- ],
- ),
- (
- "ChainPosClassRule",
- [
- (
- "uint16",
- "BacktrackGlyphCount",
- None,
- None,
- "Total number of glyphs in the backtrack sequence (number of glyphs to be matched before the first glyph)",
- ),
- (
- "uint16",
- "Backtrack",
- "BacktrackGlyphCount",
- 0,
- "Array of backtracking classes(to be matched before the input sequence)",
- ),
- (
- "uint16",
- "InputGlyphCount",
- None,
- None,
- "Total number of classes in the input sequence (includes the first class)",
- ),
- (
- "uint16",
- "Input",
- "InputGlyphCount",
- -1,
- "Array of input classes(start with second class; to be matched with the input glyph sequence)",
- ),
- (
- "uint16",
- "LookAheadGlyphCount",
- None,
- None,
- "Total number of classes in the look ahead sequence (number of classes to be matched after the input sequence)",
- ),
- (
- "uint16",
- "LookAhead",
- "LookAheadGlyphCount",
- 0,
- "Array of lookahead classes(to be matched after the input sequence)",
- ),
- ("uint16", "PosCount", None, None, "Number of PosLookupRecords"),
- (
- "struct",
- "PosLookupRecord",
- "PosCount",
- 0,
- "Array of PosLookupRecords (in design order)",
- ),
- ],
- ),
- (
- "ChainContextPosFormat3",
- [
- ("uint16", "PosFormat", None, None, "Format identifier-format = 3"),
- (
- "uint16",
- "BacktrackGlyphCount",
- None,
- None,
- "Number of glyphs in the backtracking sequence",
- ),
- (
- "Offset",
- "BacktrackCoverage",
- "BacktrackGlyphCount",
- 0,
- "Array of offsets to coverage tables in backtracking sequence, in glyph sequence order",
- ),
- (
- "uint16",
- "InputGlyphCount",
- None,
- None,
- "Number of glyphs in input sequence",
- ),
- (
- "Offset",
- "InputCoverage",
- "InputGlyphCount",
- 0,
- "Array of offsets to coverage tables in input sequence, in glyph sequence order",
- ),
- (
- "uint16",
- "LookAheadGlyphCount",
- None,
- None,
- "Number of glyphs in lookahead sequence",
- ),
- (
- "Offset",
- "LookAheadCoverage",
- "LookAheadGlyphCount",
- 0,
- "Array of offsets to coverage tables in lookahead sequence, in glyph sequence order",
- ),
- ("uint16", "PosCount", None, None, "Number of PosLookupRecords"),
- (
- "struct",
- "PosLookupRecord",
- "PosCount",
- 0,
- "Array of PosLookupRecords,in design order",
- ),
- ],
- ),
- (
- "ExtensionPosFormat1",
- [
- ("uint16", "ExtFormat", None, None, "Format identifier. Set to 1."),
- (
- "uint16",
- "ExtensionLookupType",
- None,
- None,
- "Lookup type of subtable referenced by ExtensionOffset (i.e. the extension subtable).",
- ),
- ("LOffset", "ExtSubTable", None, None, "Offset to SubTable"),
- ],
- ),
- # ('ValueRecord', [
- # ('int16', 'XPlacement', None, None, 'Horizontal adjustment for placement-in design units'),
- # ('int16', 'YPlacement', None, None, 'Vertical adjustment for placement-in design units'),
- # ('int16', 'XAdvance', None, None, 'Horizontal adjustment for advance-in design units (only used for horizontal writing)'),
- # ('int16', 'YAdvance', None, None, 'Vertical adjustment for advance-in design units (only used for vertical writing)'),
- # ('Offset', 'XPlaDevice', None, None, 'Offset to Device table for horizontal placement-measured from beginning of PosTable (may be NULL)'),
- # ('Offset', 'YPlaDevice', None, None, 'Offset to Device table for vertical placement-measured from beginning of PosTable (may be NULL)'),
- # ('Offset', 'XAdvDevice', None, None, 'Offset to Device table for horizontal advance-measured from beginning of PosTable (may be NULL)'),
- # ('Offset', 'YAdvDevice', None, None, 'Offset to Device table for vertical advance-measured from beginning of PosTable (may be NULL)'),
- # ]),
- (
- "AnchorFormat1",
- [
- ("uint16", "AnchorFormat", None, None, "Format identifier-format = 1"),
- ("int16", "XCoordinate", None, None, "Horizontal value-in design units"),
- ("int16", "YCoordinate", None, None, "Vertical value-in design units"),
- ],
- ),
- (
- "AnchorFormat2",
- [
- ("uint16", "AnchorFormat", None, None, "Format identifier-format = 2"),
- ("int16", "XCoordinate", None, None, "Horizontal value-in design units"),
- ("int16", "YCoordinate", None, None, "Vertical value-in design units"),
- ("uint16", "AnchorPoint", None, None, "Index to glyph contour point"),
- ],
- ),
- (
- "AnchorFormat3",
- [
- ("uint16", "AnchorFormat", None, None, "Format identifier-format = 3"),
- ("int16", "XCoordinate", None, None, "Horizontal value-in design units"),
- ("int16", "YCoordinate", None, None, "Vertical value-in design units"),
- (
- "Offset",
- "XDeviceTable",
- None,
- None,
- "Offset to Device table for X coordinate- from beginning of Anchor table (may be NULL)",
- ),
- (
- "Offset",
- "YDeviceTable",
- None,
- None,
- "Offset to Device table for Y coordinate- from beginning of Anchor table (may be NULL)",
- ),
- ],
- ),
- (
- "MarkArray",
- [
- ("uint16", "MarkCount", None, None, "Number of MarkRecords"),
- (
- "struct",
- "MarkRecord",
- "MarkCount",
- 0,
- "Array of MarkRecords-in Coverage order",
- ),
- ],
- ),
- (
- "MarkRecord",
- [
- ("uint16", "Class", None, None, "Class defined for this mark"),
- (
- "Offset",
- "MarkAnchor",
- None,
- None,
- "Offset to Anchor table-from beginning of MarkArray table",
- ),
- ],
- ),
- #
- # gsub
- #
- (
- "GSUB",
- [
- (
- "Version",
- "Version",
- None,
- None,
- "Version of the GSUB table- 0x00010000 or 0x00010001",
- ),
- (
- "Offset",
- "ScriptList",
- None,
- None,
- "Offset to ScriptList table-from beginning of GSUB table",
- ),
- (
- "Offset",
- "FeatureList",
- None,
- None,
- "Offset to FeatureList table-from beginning of GSUB table",
- ),
- (
- "Offset",
- "LookupList",
- None,
- None,
- "Offset to LookupList table-from beginning of GSUB table",
- ),
- (
- "LOffset",
- "FeatureVariations",
- None,
- "Version >= 0x00010001",
- "Offset to FeatureVariations table-from beginning of GSUB table",
- ),
- ],
- ),
- (
- "SingleSubstFormat1",
- [
- ("uint16", "SubstFormat", None, None, "Format identifier-format = 1"),
- (
- "Offset",
- "Coverage",
- None,
- None,
- "Offset to Coverage table-from beginning of Substitution table",
- ),
- (
- "uint16",
- "DeltaGlyphID",
- None,
- None,
- "Add to original GlyphID modulo 65536 to get substitute GlyphID",
- ),
- ],
- ),
- (
- "SingleSubstFormat2",
- [
- ("uint16", "SubstFormat", None, None, "Format identifier-format = 2"),
- (
- "Offset",
- "Coverage",
- None,
- None,
- "Offset to Coverage table-from beginning of Substitution table",
- ),
- (
- "uint16",
- "GlyphCount",
- None,
- None,
- "Number of GlyphIDs in the Substitute array",
- ),
- (
- "GlyphID",
- "Substitute",
- "GlyphCount",
- 0,
- "Array of substitute GlyphIDs-ordered by Coverage Index",
- ),
- ],
- ),
- (
- "MultipleSubstFormat1",
- [
- ("uint16", "SubstFormat", None, None, "Format identifier-format = 1"),
- (
- "Offset",
- "Coverage",
- None,
- None,
- "Offset to Coverage table-from beginning of Substitution table",
- ),
- (
- "uint16",
- "SequenceCount",
- None,
- None,
- "Number of Sequence table offsets in the Sequence array",
- ),
- (
- "Offset",
- "Sequence",
- "SequenceCount",
- 0,
- "Array of offsets to Sequence tables-from beginning of Substitution table-ordered by Coverage Index",
- ),
- ],
- ),
- (
- "Sequence",
- [
- (
- "uint16",
- "GlyphCount",
- None,
- None,
- "Number of GlyphIDs in the Substitute array. This should always be greater than 0.",
- ),
- (
- "GlyphID",
- "Substitute",
- "GlyphCount",
- 0,
- "String of GlyphIDs to substitute",
- ),
- ],
- ),
- (
- "AlternateSubstFormat1",
- [
- ("uint16", "SubstFormat", None, None, "Format identifier-format = 1"),
- (
- "Offset",
- "Coverage",
- None,
- None,
- "Offset to Coverage table-from beginning of Substitution table",
- ),
- (
- "uint16",
- "AlternateSetCount",
- None,
- None,
- "Number of AlternateSet tables",
- ),
- (
- "Offset",
- "AlternateSet",
- "AlternateSetCount",
- 0,
- "Array of offsets to AlternateSet tables-from beginning of Substitution table-ordered by Coverage Index",
- ),
- ],
- ),
- (
- "AlternateSet",
- [
- (
- "uint16",
- "GlyphCount",
- None,
- None,
- "Number of GlyphIDs in the Alternate array",
- ),
- (
- "GlyphID",
- "Alternate",
- "GlyphCount",
- 0,
- "Array of alternate GlyphIDs-in arbitrary order",
- ),
- ],
- ),
- (
- "LigatureSubstFormat1",
- [
- ("uint16", "SubstFormat", None, None, "Format identifier-format = 1"),
- (
- "Offset",
- "Coverage",
- None,
- None,
- "Offset to Coverage table-from beginning of Substitution table",
- ),
- ("uint16", "LigSetCount", None, None, "Number of LigatureSet tables"),
- (
- "Offset",
- "LigatureSet",
- "LigSetCount",
- 0,
- "Array of offsets to LigatureSet tables-from beginning of Substitution table-ordered by Coverage Index",
- ),
- ],
- ),
- (
- "LigatureSet",
- [
- ("uint16", "LigatureCount", None, None, "Number of Ligature tables"),
- (
- "Offset",
- "Ligature",
- "LigatureCount",
- 0,
- "Array of offsets to Ligature tables-from beginning of LigatureSet table-ordered by preference",
- ),
- ],
- ),
- (
- "Ligature",
- [
- ("GlyphID", "LigGlyph", None, None, "GlyphID of ligature to substitute"),
- ("uint16", "CompCount", None, None, "Number of components in the ligature"),
- (
- "GlyphID",
- "Component",
- "CompCount",
- -1,
- "Array of component GlyphIDs-start with the second component-ordered in writing direction",
- ),
- ],
- ),
- (
- "SubstLookupRecord",
- [
- (
- "uint16",
- "SequenceIndex",
- None,
- None,
- "Index into current glyph sequence-first glyph = 0",
- ),
- (
- "uint16",
- "LookupListIndex",
- None,
- None,
- "Lookup to apply to that position-zero-based",
- ),
- ],
- ),
- (
- "ContextSubstFormat1",
- [
- ("uint16", "SubstFormat", None, None, "Format identifier-format = 1"),
- (
- "Offset",
- "Coverage",
- None,
- None,
- "Offset to Coverage table-from beginning of Substitution table",
- ),
- (
- "uint16",
- "SubRuleSetCount",
- None,
- None,
- "Number of SubRuleSet tables-must equal GlyphCount in Coverage table",
- ),
- (
- "Offset",
- "SubRuleSet",
- "SubRuleSetCount",
- 0,
- "Array of offsets to SubRuleSet tables-from beginning of Substitution table-ordered by Coverage Index",
- ),
- ],
- ),
- (
- "SubRuleSet",
- [
- ("uint16", "SubRuleCount", None, None, "Number of SubRule tables"),
- (
- "Offset",
- "SubRule",
- "SubRuleCount",
- 0,
- "Array of offsets to SubRule tables-from beginning of SubRuleSet table-ordered by preference",
- ),
- ],
- ),
- (
- "SubRule",
- [
- (
- "uint16",
- "GlyphCount",
- None,
- None,
- "Total number of glyphs in input glyph sequence-includes the first glyph",
- ),
- ("uint16", "SubstCount", None, None, "Number of SubstLookupRecords"),
- (
- "GlyphID",
- "Input",
- "GlyphCount",
- -1,
- "Array of input GlyphIDs-start with second glyph",
- ),
- (
- "struct",
- "SubstLookupRecord",
- "SubstCount",
- 0,
- "Array of SubstLookupRecords-in design order",
- ),
- ],
- ),
- (
- "ContextSubstFormat2",
- [
- ("uint16", "SubstFormat", None, None, "Format identifier-format = 2"),
- (
- "Offset",
- "Coverage",
- None,
- None,
- "Offset to Coverage table-from beginning of Substitution table",
- ),
- (
- "Offset",
- "ClassDef",
- None,
- None,
- "Offset to glyph ClassDef table-from beginning of Substitution table",
- ),
- ("uint16", "SubClassSetCount", None, None, "Number of SubClassSet tables"),
- (
- "Offset",
- "SubClassSet",
- "SubClassSetCount",
- 0,
- "Array of offsets to SubClassSet tables-from beginning of Substitution table-ordered by class-may be NULL",
- ),
- ],
- ),
- (
- "SubClassSet",
- [
- (
- "uint16",
- "SubClassRuleCount",
- None,
- None,
- "Number of SubClassRule tables",
- ),
- (
- "Offset",
- "SubClassRule",
- "SubClassRuleCount",
- 0,
- "Array of offsets to SubClassRule tables-from beginning of SubClassSet-ordered by preference",
- ),
- ],
- ),
- (
- "SubClassRule",
- [
- (
- "uint16",
- "GlyphCount",
- None,
- None,
- "Total number of classes specified for the context in the rule-includes the first class",
- ),
- ("uint16", "SubstCount", None, None, "Number of SubstLookupRecords"),
- (
- "uint16",
- "Class",
- "GlyphCount",
- -1,
- "Array of classes-beginning with the second class-to be matched to the input glyph class sequence",
- ),
- (
- "struct",
- "SubstLookupRecord",
- "SubstCount",
- 0,
- "Array of Substitution lookups-in design order",
- ),
- ],
- ),
- (
- "ContextSubstFormat3",
- [
- ("uint16", "SubstFormat", None, None, "Format identifier-format = 3"),
- (
- "uint16",
- "GlyphCount",
- None,
- None,
- "Number of glyphs in the input glyph sequence",
- ),
- ("uint16", "SubstCount", None, None, "Number of SubstLookupRecords"),
- (
- "Offset",
- "Coverage",
- "GlyphCount",
- 0,
- "Array of offsets to Coverage table-from beginning of Substitution table-in glyph sequence order",
- ),
- (
- "struct",
- "SubstLookupRecord",
- "SubstCount",
- 0,
- "Array of SubstLookupRecords-in design order",
- ),
- ],
- ),
- (
- "ChainContextSubstFormat1",
- [
- ("uint16", "SubstFormat", None, None, "Format identifier-format = 1"),
- (
- "Offset",
- "Coverage",
- None,
- None,
- "Offset to Coverage table-from beginning of Substitution table",
- ),
- (
- "uint16",
- "ChainSubRuleSetCount",
- None,
- None,
- "Number of ChainSubRuleSet tables-must equal GlyphCount in Coverage table",
- ),
- (
- "Offset",
- "ChainSubRuleSet",
- "ChainSubRuleSetCount",
- 0,
- "Array of offsets to ChainSubRuleSet tables-from beginning of Substitution table-ordered by Coverage Index",
- ),
- ],
- ),
- (
- "ChainSubRuleSet",
- [
- (
- "uint16",
- "ChainSubRuleCount",
- None,
- None,
- "Number of ChainSubRule tables",
- ),
- (
- "Offset",
- "ChainSubRule",
- "ChainSubRuleCount",
- 0,
- "Array of offsets to ChainSubRule tables-from beginning of ChainSubRuleSet table-ordered by preference",
- ),
- ],
- ),
- (
- "ChainSubRule",
- [
- (
- "uint16",
- "BacktrackGlyphCount",
- None,
- None,
- "Total number of glyphs in the backtrack sequence (number of glyphs to be matched before the first glyph)",
- ),
- (
- "GlyphID",
- "Backtrack",
- "BacktrackGlyphCount",
- 0,
- "Array of backtracking GlyphID's (to be matched before the input sequence)",
- ),
- (
- "uint16",
- "InputGlyphCount",
- None,
- None,
- "Total number of glyphs in the input sequence (includes the first glyph)",
- ),
- (
- "GlyphID",
- "Input",
- "InputGlyphCount",
- -1,
- "Array of input GlyphIDs (start with second glyph)",
- ),
- (
- "uint16",
- "LookAheadGlyphCount",
- None,
- None,
- "Total number of glyphs in the look ahead sequence (number of glyphs to be matched after the input sequence)",
- ),
- (
- "GlyphID",
- "LookAhead",
- "LookAheadGlyphCount",
- 0,
- "Array of lookahead GlyphID's (to be matched after the input sequence)",
- ),
- ("uint16", "SubstCount", None, None, "Number of SubstLookupRecords"),
- (
- "struct",
- "SubstLookupRecord",
- "SubstCount",
- 0,
- "Array of SubstLookupRecords (in design order)",
- ),
- ],
- ),
- (
- "ChainContextSubstFormat2",
- [
- ("uint16", "SubstFormat", None, None, "Format identifier-format = 2"),
- (
- "Offset",
- "Coverage",
- None,
- None,
- "Offset to Coverage table-from beginning of Substitution table",
- ),
- (
- "Offset",
- "BacktrackClassDef",
- None,
- None,
- "Offset to glyph ClassDef table containing backtrack sequence data-from beginning of Substitution table",
- ),
- (
- "Offset",
- "InputClassDef",
- None,
- None,
- "Offset to glyph ClassDef table containing input sequence data-from beginning of Substitution table",
- ),
- (
- "Offset",
- "LookAheadClassDef",
- None,
- None,
- "Offset to glyph ClassDef table containing lookahead sequence data-from beginning of Substitution table",
- ),
- (
- "uint16",
- "ChainSubClassSetCount",
- None,
- None,
- "Number of ChainSubClassSet tables",
- ),
- (
- "Offset",
- "ChainSubClassSet",
- "ChainSubClassSetCount",
- 0,
- "Array of offsets to ChainSubClassSet tables-from beginning of Substitution table-ordered by input class-may be NULL",
- ),
- ],
- ),
- (
- "ChainSubClassSet",
- [
- (
- "uint16",
- "ChainSubClassRuleCount",
- None,
- None,
- "Number of ChainSubClassRule tables",
- ),
- (
- "Offset",
- "ChainSubClassRule",
- "ChainSubClassRuleCount",
- 0,
- "Array of offsets to ChainSubClassRule tables-from beginning of ChainSubClassSet-ordered by preference",
- ),
- ],
- ),
- (
- "ChainSubClassRule",
- [
- (
- "uint16",
- "BacktrackGlyphCount",
- None,
- None,
- "Total number of glyphs in the backtrack sequence (number of glyphs to be matched before the first glyph)",
- ),
- (
- "uint16",
- "Backtrack",
- "BacktrackGlyphCount",
- 0,
- "Array of backtracking classes(to be matched before the input sequence)",
- ),
- (
- "uint16",
- "InputGlyphCount",
- None,
- None,
- "Total number of classes in the input sequence (includes the first class)",
- ),
- (
- "uint16",
- "Input",
- "InputGlyphCount",
- -1,
- "Array of input classes(start with second class; to be matched with the input glyph sequence)",
- ),
- (
- "uint16",
- "LookAheadGlyphCount",
- None,
- None,
- "Total number of classes in the look ahead sequence (number of classes to be matched after the input sequence)",
- ),
- (
- "uint16",
- "LookAhead",
- "LookAheadGlyphCount",
- 0,
- "Array of lookahead classes(to be matched after the input sequence)",
- ),
- ("uint16", "SubstCount", None, None, "Number of SubstLookupRecords"),
- (
- "struct",
- "SubstLookupRecord",
- "SubstCount",
- 0,
- "Array of SubstLookupRecords (in design order)",
- ),
- ],
- ),
- (
- "ChainContextSubstFormat3",
- [
- ("uint16", "SubstFormat", None, None, "Format identifier-format = 3"),
- (
- "uint16",
- "BacktrackGlyphCount",
- None,
- None,
- "Number of glyphs in the backtracking sequence",
- ),
- (
- "Offset",
- "BacktrackCoverage",
- "BacktrackGlyphCount",
- 0,
- "Array of offsets to coverage tables in backtracking sequence, in glyph sequence order",
- ),
- (
- "uint16",
- "InputGlyphCount",
- None,
- None,
- "Number of glyphs in input sequence",
- ),
- (
- "Offset",
- "InputCoverage",
- "InputGlyphCount",
- 0,
- "Array of offsets to coverage tables in input sequence, in glyph sequence order",
- ),
- (
- "uint16",
- "LookAheadGlyphCount",
- None,
- None,
- "Number of glyphs in lookahead sequence",
- ),
- (
- "Offset",
- "LookAheadCoverage",
- "LookAheadGlyphCount",
- 0,
- "Array of offsets to coverage tables in lookahead sequence, in glyph sequence order",
- ),
- ("uint16", "SubstCount", None, None, "Number of SubstLookupRecords"),
- (
- "struct",
- "SubstLookupRecord",
- "SubstCount",
- 0,
- "Array of SubstLookupRecords, in design order",
- ),
- ],
- ),
- (
- "ExtensionSubstFormat1",
- [
- ("uint16", "ExtFormat", None, None, "Format identifier. Set to 1."),
- (
- "uint16",
- "ExtensionLookupType",
- None,
- None,
- "Lookup type of subtable referenced by ExtensionOffset (i.e. the extension subtable).",
- ),
- (
- "LOffset",
- "ExtSubTable",
- None,
- None,
- "Array of offsets to Lookup tables-from beginning of LookupList -zero based (first lookup is Lookup index = 0)",
- ),
- ],
- ),
- (
- "ReverseChainSingleSubstFormat1",
- [
- ("uint16", "SubstFormat", None, None, "Format identifier-format = 1"),
- (
- "Offset",
- "Coverage",
- None,
- 0,
- "Offset to Coverage table - from beginning of Substitution table",
- ),
- (
- "uint16",
- "BacktrackGlyphCount",
- None,
- None,
- "Number of glyphs in the backtracking sequence",
- ),
- (
- "Offset",
- "BacktrackCoverage",
- "BacktrackGlyphCount",
- 0,
- "Array of offsets to coverage tables in backtracking sequence, in glyph sequence order",
- ),
- (
- "uint16",
- "LookAheadGlyphCount",
- None,
- None,
- "Number of glyphs in lookahead sequence",
- ),
- (
- "Offset",
- "LookAheadCoverage",
- "LookAheadGlyphCount",
- 0,
- "Array of offsets to coverage tables in lookahead sequence, in glyph sequence order",
- ),
- (
- "uint16",
- "GlyphCount",
- None,
- None,
- "Number of GlyphIDs in the Substitute array",
- ),
- (
- "GlyphID",
- "Substitute",
- "GlyphCount",
- 0,
- "Array of substitute GlyphIDs-ordered by Coverage index",
- ),
- ],
- ),
- #
- # gdef
- #
- (
- "GDEF",
- [
- (
- "Version",
- "Version",
- None,
- None,
- "Version of the GDEF table- 0x00010000, 0x00010002, or 0x00010003",
- ),
- (
- "Offset",
- "GlyphClassDef",
- None,
- None,
- "Offset to class definition table for glyph type-from beginning of GDEF header (may be NULL)",
- ),
- (
- "Offset",
- "AttachList",
- None,
- None,
- "Offset to list of glyphs with attachment points-from beginning of GDEF header (may be NULL)",
- ),
- (
- "Offset",
- "LigCaretList",
- None,
- None,
- "Offset to list of positioning points for ligature carets-from beginning of GDEF header (may be NULL)",
- ),
- (
- "Offset",
- "MarkAttachClassDef",
- None,
- None,
- "Offset to class definition table for mark attachment type-from beginning of GDEF header (may be NULL)",
- ),
- (
- "Offset",
- "MarkGlyphSetsDef",
- None,
- "Version >= 0x00010002",
- "Offset to the table of mark set definitions-from beginning of GDEF header (may be NULL)",
- ),
- (
- "LOffset",
- "VarStore",
- None,
- "Version >= 0x00010003",
- "Offset to variation store (may be NULL)",
- ),
- ],
- ),
- (
- "AttachList",
- [
- (
- "Offset",
- "Coverage",
- None,
- None,
- "Offset to Coverage table - from beginning of AttachList table",
- ),
- (
- "uint16",
- "GlyphCount",
- None,
- None,
- "Number of glyphs with attachment points",
- ),
- (
- "Offset",
- "AttachPoint",
- "GlyphCount",
- 0,
- "Array of offsets to AttachPoint tables-from beginning of AttachList table-in Coverage Index order",
- ),
- ],
- ),
- (
- "AttachPoint",
- [
- (
- "uint16",
- "PointCount",
- None,
- None,
- "Number of attachment points on this glyph",
- ),
- (
- "uint16",
- "PointIndex",
- "PointCount",
- 0,
- "Array of contour point indices -in increasing numerical order",
- ),
- ],
- ),
- (
- "LigCaretList",
- [
- (
- "Offset",
- "Coverage",
- None,
- None,
- "Offset to Coverage table - from beginning of LigCaretList table",
- ),
- ("uint16", "LigGlyphCount", None, None, "Number of ligature glyphs"),
- (
- "Offset",
- "LigGlyph",
- "LigGlyphCount",
- 0,
- "Array of offsets to LigGlyph tables-from beginning of LigCaretList table-in Coverage Index order",
- ),
- ],
- ),
- (
- "LigGlyph",
- [
- (
- "uint16",
- "CaretCount",
- None,
- None,
- "Number of CaretValues for this ligature (components - 1)",
- ),
- (
- "Offset",
- "CaretValue",
- "CaretCount",
- 0,
- "Array of offsets to CaretValue tables-from beginning of LigGlyph table-in increasing coordinate order",
- ),
- ],
- ),
- (
- "CaretValueFormat1",
- [
- ("uint16", "CaretValueFormat", None, None, "Format identifier-format = 1"),
- ("int16", "Coordinate", None, None, "X or Y value, in design units"),
- ],
- ),
- (
- "CaretValueFormat2",
- [
- ("uint16", "CaretValueFormat", None, None, "Format identifier-format = 2"),
- ("uint16", "CaretValuePoint", None, None, "Contour point index on glyph"),
- ],
- ),
- (
- "CaretValueFormat3",
- [
- ("uint16", "CaretValueFormat", None, None, "Format identifier-format = 3"),
- ("int16", "Coordinate", None, None, "X or Y value, in design units"),
- (
- "Offset",
- "DeviceTable",
- None,
- None,
- "Offset to Device table for X or Y value-from beginning of CaretValue table",
- ),
- ],
- ),
- (
- "MarkGlyphSetsDef",
- [
- ("uint16", "MarkSetTableFormat", None, None, "Format identifier == 1"),
- ("uint16", "MarkSetCount", None, None, "Number of mark sets defined"),
- (
- "LOffset",
- "Coverage",
- "MarkSetCount",
- 0,
- "Array of offsets to mark set coverage tables.",
- ),
- ],
- ),
- #
- # base
- #
- (
- "BASE",
- [
- (
- "Version",
- "Version",
- None,
- None,
- "Version of the BASE table-initially 0x00010000",
- ),
- (
- "Offset",
- "HorizAxis",
- None,
- None,
- "Offset to horizontal Axis table-from beginning of BASE table-may be NULL",
- ),
- (
- "Offset",
- "VertAxis",
- None,
- None,
- "Offset to vertical Axis table-from beginning of BASE table-may be NULL",
- ),
- (
- "LOffset",
- "VarStore",
- None,
- "Version >= 0x00010001",
- "Offset to variation store (may be NULL)",
- ),
- ],
- ),
- (
- "Axis",
- [
- (
- "Offset",
- "BaseTagList",
- None,
- None,
- "Offset to BaseTagList table-from beginning of Axis table-may be NULL",
- ),
- (
- "Offset",
- "BaseScriptList",
- None,
- None,
- "Offset to BaseScriptList table-from beginning of Axis table",
- ),
- ],
- ),
- (
- "BaseTagList",
- [
- (
- "uint16",
- "BaseTagCount",
- None,
- None,
- "Number of baseline identification tags in this text direction-may be zero (0)",
- ),
- (
- "Tag",
- "BaselineTag",
- "BaseTagCount",
- 0,
- "Array of 4-byte baseline identification tags-must be in alphabetical order",
- ),
- ],
- ),
- (
- "BaseScriptList",
- [
- (
- "uint16",
- "BaseScriptCount",
- None,
- None,
- "Number of BaseScriptRecords defined",
- ),
- (
- "struct",
- "BaseScriptRecord",
- "BaseScriptCount",
- 0,
- "Array of BaseScriptRecords-in alphabetical order by BaseScriptTag",
- ),
- ],
- ),
- (
- "BaseScriptRecord",
- [
- ("Tag", "BaseScriptTag", None, None, "4-byte script identification tag"),
- (
- "Offset",
- "BaseScript",
- None,
- None,
- "Offset to BaseScript table-from beginning of BaseScriptList",
- ),
- ],
- ),
- (
- "BaseScript",
- [
- (
- "Offset",
- "BaseValues",
- None,
- None,
- "Offset to BaseValues table-from beginning of BaseScript table-may be NULL",
- ),
- (
- "Offset",
- "DefaultMinMax",
- None,
- None,
- "Offset to MinMax table- from beginning of BaseScript table-may be NULL",
- ),
- (
- "uint16",
- "BaseLangSysCount",
- None,
- None,
- "Number of BaseLangSysRecords defined-may be zero (0)",
- ),
- (
- "struct",
- "BaseLangSysRecord",
- "BaseLangSysCount",
- 0,
- "Array of BaseLangSysRecords-in alphabetical order by BaseLangSysTag",
- ),
- ],
- ),
- (
- "BaseLangSysRecord",
- [
- (
- "Tag",
- "BaseLangSysTag",
- None,
- None,
- "4-byte language system identification tag",
- ),
- (
- "Offset",
- "MinMax",
- None,
- None,
- "Offset to MinMax table-from beginning of BaseScript table",
- ),
- ],
- ),
- (
- "BaseValues",
- [
- (
- "uint16",
- "DefaultIndex",
- None,
- None,
- "Index number of default baseline for this script-equals index position of baseline tag in BaselineArray of the BaseTagList",
- ),
- (
- "uint16",
- "BaseCoordCount",
- None,
- None,
- "Number of BaseCoord tables defined-should equal BaseTagCount in the BaseTagList",
- ),
- (
- "Offset",
- "BaseCoord",
- "BaseCoordCount",
- 0,
- "Array of offsets to BaseCoord-from beginning of BaseValues table-order matches BaselineTag array in the BaseTagList",
- ),
- ],
- ),
- (
- "MinMax",
- [
- (
- "Offset",
- "MinCoord",
- None,
- None,
- "Offset to BaseCoord table-defines minimum extent value-from the beginning of MinMax table-may be NULL",
- ),
- (
- "Offset",
- "MaxCoord",
- None,
- None,
- "Offset to BaseCoord table-defines maximum extent value-from the beginning of MinMax table-may be NULL",
- ),
- (
- "uint16",
- "FeatMinMaxCount",
- None,
- None,
- "Number of FeatMinMaxRecords-may be zero (0)",
- ),
- (
- "struct",
- "FeatMinMaxRecord",
- "FeatMinMaxCount",
- 0,
- "Array of FeatMinMaxRecords-in alphabetical order, by FeatureTableTag",
- ),
- ],
- ),
- (
- "FeatMinMaxRecord",
- [
- (
- "Tag",
- "FeatureTableTag",
- None,
- None,
- "4-byte feature identification tag-must match FeatureTag in FeatureList",
- ),
- (
- "Offset",
- "MinCoord",
- None,
- None,
- "Offset to BaseCoord table-defines minimum extent value-from beginning of MinMax table-may be NULL",
- ),
- (
- "Offset",
- "MaxCoord",
- None,
- None,
- "Offset to BaseCoord table-defines maximum extent value-from beginning of MinMax table-may be NULL",
- ),
- ],
- ),
- (
- "BaseCoordFormat1",
- [
- ("uint16", "BaseCoordFormat", None, None, "Format identifier-format = 1"),
- ("int16", "Coordinate", None, None, "X or Y value, in design units"),
- ],
- ),
- (
- "BaseCoordFormat2",
- [
- ("uint16", "BaseCoordFormat", None, None, "Format identifier-format = 2"),
- ("int16", "Coordinate", None, None, "X or Y value, in design units"),
- ("GlyphID", "ReferenceGlyph", None, None, "GlyphID of control glyph"),
- (
- "uint16",
- "BaseCoordPoint",
- None,
- None,
- "Index of contour point on the ReferenceGlyph",
- ),
- ],
- ),
- (
- "BaseCoordFormat3",
- [
- ("uint16", "BaseCoordFormat", None, None, "Format identifier-format = 3"),
- ("int16", "Coordinate", None, None, "X or Y value, in design units"),
- (
- "Offset",
- "DeviceTable",
- None,
- None,
- "Offset to Device table for X or Y value",
- ),
- ],
- ),
- #
- # jstf
- #
- (
- "JSTF",
- [
- (
- "Version",
- "Version",
- None,
- None,
- "Version of the JSTF table-initially set to 0x00010000",
- ),
- (
- "uint16",
- "JstfScriptCount",
- None,
- None,
- "Number of JstfScriptRecords in this table",
- ),
- (
- "struct",
- "JstfScriptRecord",
- "JstfScriptCount",
- 0,
- "Array of JstfScriptRecords-in alphabetical order, by JstfScriptTag",
- ),
- ],
- ),
- (
- "JstfScriptRecord",
- [
- ("Tag", "JstfScriptTag", None, None, "4-byte JstfScript identification"),
- (
- "Offset",
- "JstfScript",
- None,
- None,
- "Offset to JstfScript table-from beginning of JSTF Header",
- ),
- ],
- ),
- (
- "JstfScript",
- [
- (
- "Offset",
- "ExtenderGlyph",
- None,
- None,
- "Offset to ExtenderGlyph table-from beginning of JstfScript table-may be NULL",
- ),
- (
- "Offset",
- "DefJstfLangSys",
- None,
- None,
- "Offset to Default JstfLangSys table-from beginning of JstfScript table-may be NULL",
- ),
- (
- "uint16",
- "JstfLangSysCount",
- None,
- None,
- "Number of JstfLangSysRecords in this table- may be zero (0)",
- ),
- (
- "struct",
- "JstfLangSysRecord",
- "JstfLangSysCount",
- 0,
- "Array of JstfLangSysRecords-in alphabetical order, by JstfLangSysTag",
- ),
- ],
- ),
- (
- "JstfLangSysRecord",
- [
- ("Tag", "JstfLangSysTag", None, None, "4-byte JstfLangSys identifier"),
- (
- "Offset",
- "JstfLangSys",
- None,
- None,
- "Offset to JstfLangSys table-from beginning of JstfScript table",
- ),
- ],
- ),
- (
- "ExtenderGlyph",
- [
- (
- "uint16",
- "GlyphCount",
- None,
- None,
- "Number of Extender Glyphs in this script",
- ),
- (
- "GlyphID",
- "ExtenderGlyph",
- "GlyphCount",
- 0,
- "GlyphIDs-in increasing numerical order",
- ),
- ],
- ),
- (
- "JstfLangSys",
- [
- (
- "uint16",
- "JstfPriorityCount",
- None,
- None,
- "Number of JstfPriority tables",
- ),
- (
- "Offset",
- "JstfPriority",
- "JstfPriorityCount",
- 0,
- "Array of offsets to JstfPriority tables-from beginning of JstfLangSys table-in priority order",
- ),
- ],
- ),
- (
- "JstfPriority",
- [
- (
- "Offset",
- "ShrinkageEnableGSUB",
- None,
- None,
- "Offset to Shrinkage Enable JstfGSUBModList table-from beginning of JstfPriority table-may be NULL",
- ),
- (
- "Offset",
- "ShrinkageDisableGSUB",
- None,
- None,
- "Offset to Shrinkage Disable JstfGSUBModList table-from beginning of JstfPriority table-may be NULL",
- ),
- (
- "Offset",
- "ShrinkageEnableGPOS",
- None,
- None,
- "Offset to Shrinkage Enable JstfGPOSModList table-from beginning of JstfPriority table-may be NULL",
- ),
- (
- "Offset",
- "ShrinkageDisableGPOS",
- None,
- None,
- "Offset to Shrinkage Disable JstfGPOSModList table-from beginning of JstfPriority table-may be NULL",
- ),
- (
- "Offset",
- "ShrinkageJstfMax",
- None,
- None,
- "Offset to Shrinkage JstfMax table-from beginning of JstfPriority table -may be NULL",
- ),
- (
- "Offset",
- "ExtensionEnableGSUB",
- None,
- None,
- "Offset to Extension Enable JstfGSUBModList table-may be NULL",
- ),
- (
- "Offset",
- "ExtensionDisableGSUB",
- None,
- None,
- "Offset to Extension Disable JstfGSUBModList table-from beginning of JstfPriority table-may be NULL",
- ),
- (
- "Offset",
- "ExtensionEnableGPOS",
- None,
- None,
- "Offset to Extension Enable JstfGSUBModList table-may be NULL",
- ),
- (
- "Offset",
- "ExtensionDisableGPOS",
- None,
- None,
- "Offset to Extension Disable JstfGSUBModList table-from beginning of JstfPriority table-may be NULL",
- ),
- (
- "Offset",
- "ExtensionJstfMax",
- None,
- None,
- "Offset to Extension JstfMax table-from beginning of JstfPriority table -may be NULL",
- ),
- ],
- ),
- (
- "JstfGSUBModList",
- [
- (
- "uint16",
- "LookupCount",
- None,
- None,
- "Number of lookups for this modification",
- ),
- (
- "uint16",
- "GSUBLookupIndex",
- "LookupCount",
- 0,
- "Array of LookupIndex identifiers in GSUB-in increasing numerical order",
- ),
- ],
- ),
- (
- "JstfGPOSModList",
- [
- (
- "uint16",
- "LookupCount",
- None,
- None,
- "Number of lookups for this modification",
- ),
- (
- "uint16",
- "GPOSLookupIndex",
- "LookupCount",
- 0,
- "Array of LookupIndex identifiers in GPOS-in increasing numerical order",
- ),
- ],
- ),
- (
- "JstfMax",
- [
- (
- "uint16",
- "LookupCount",
- None,
- None,
- "Number of lookup Indices for this modification",
- ),
- (
- "Offset",
- "Lookup",
- "LookupCount",
- 0,
- "Array of offsets to GPOS-type lookup tables-from beginning of JstfMax table-in design order",
- ),
- ],
- ),
- #
- # STAT
- #
- (
- "STAT",
- [
- (
- "Version",
- "Version",
- None,
- None,
- "Version of the table-initially set to 0x00010000, currently 0x00010002.",
- ),
- (
- "uint16",
- "DesignAxisRecordSize",
- None,
- None,
- "Size in bytes of each design axis record",
- ),
- ("uint16", "DesignAxisCount", None, None, "Number of design axis records"),
- (
- "LOffsetTo(AxisRecordArray)",
- "DesignAxisRecord",
- None,
- None,
- "Offset in bytes from the beginning of the STAT table to the start of the design axes array",
- ),
- ("uint16", "AxisValueCount", None, None, "Number of axis value tables"),
- (
- "LOffsetTo(AxisValueArray)",
- "AxisValueArray",
- None,
- None,
- "Offset in bytes from the beginning of the STAT table to the start of the axes value offset array",
- ),
- (
- "NameID",
- "ElidedFallbackNameID",
- None,
- "Version >= 0x00010001",
- "NameID to use when all style attributes are elided.",
- ),
- ],
- ),
- (
- "AxisRecordArray",
- [
- ("AxisRecord", "Axis", "DesignAxisCount", 0, "Axis records"),
- ],
- ),
- (
- "AxisRecord",
- [
- (
- "Tag",
- "AxisTag",
- None,
- None,
- "A tag identifying the axis of design variation",
- ),
- (
- "NameID",
- "AxisNameID",
- None,
- None,
- 'The name ID for entries in the "name" table that provide a display string for this axis',
- ),
- (
- "uint16",
- "AxisOrdering",
- None,
- None,
- "A value that applications can use to determine primary sorting of face names, or for ordering of descriptors when composing family or face names",
- ),
- (
- "uint8",
- "MoreBytes",
- "DesignAxisRecordSize",
- -8,
- "Extra bytes. Set to empty array.",
- ),
- ],
- ),
- (
- "AxisValueArray",
- [
- ("Offset", "AxisValue", "AxisValueCount", 0, "Axis values"),
- ],
- ),
- (
- "AxisValueFormat1",
- [
- ("uint16", "Format", None, None, "Format, = 1"),
- (
- "uint16",
- "AxisIndex",
- None,
- None,
- "Index into the axis record array identifying the axis of design variation to which the axis value record applies.",
- ),
- ("STATFlags", "Flags", None, None, "Flags."),
- ("NameID", "ValueNameID", None, None, ""),
- ("Fixed", "Value", None, None, ""),
- ],
- ),
- (
- "AxisValueFormat2",
- [
- ("uint16", "Format", None, None, "Format, = 2"),
- (
- "uint16",
- "AxisIndex",
- None,
- None,
- "Index into the axis record array identifying the axis of design variation to which the axis value record applies.",
- ),
- ("STATFlags", "Flags", None, None, "Flags."),
- ("NameID", "ValueNameID", None, None, ""),
- ("Fixed", "NominalValue", None, None, ""),
- ("Fixed", "RangeMinValue", None, None, ""),
- ("Fixed", "RangeMaxValue", None, None, ""),
- ],
- ),
- (
- "AxisValueFormat3",
- [
- ("uint16", "Format", None, None, "Format, = 3"),
- (
- "uint16",
- "AxisIndex",
- None,
- None,
- "Index into the axis record array identifying the axis of design variation to which the axis value record applies.",
- ),
- ("STATFlags", "Flags", None, None, "Flags."),
- ("NameID", "ValueNameID", None, None, ""),
- ("Fixed", "Value", None, None, ""),
- ("Fixed", "LinkedValue", None, None, ""),
- ],
- ),
- (
- "AxisValueFormat4",
- [
- ("uint16", "Format", None, None, "Format, = 4"),
- (
- "uint16",
- "AxisCount",
- None,
- None,
- "The total number of axes contributing to this axis-values combination.",
- ),
- ("STATFlags", "Flags", None, None, "Flags."),
- ("NameID", "ValueNameID", None, None, ""),
- (
- "struct",
- "AxisValueRecord",
- "AxisCount",
- 0,
- "Array of AxisValue records that provide the combination of axis values, one for each contributing axis. ",
- ),
- ],
- ),
- (
- "AxisValueRecord",
- [
- (
- "uint16",
- "AxisIndex",
- None,
- None,
- "Index into the axis record array identifying the axis of design variation to which the axis value record applies.",
- ),
- ("Fixed", "Value", None, None, "A numeric value for this attribute value."),
- ],
- ),
- #
- # Variation fonts
- #
- # GSUB/GPOS FeatureVariations
- (
- "FeatureVariations",
- [
- (
- "Version",
- "Version",
- None,
- None,
- "Version of the table-initially set to 0x00010000",
- ),
- (
- "uint32",
- "FeatureVariationCount",
- None,
- None,
- "Number of records in the FeatureVariationRecord array",
- ),
- (
- "struct",
- "FeatureVariationRecord",
- "FeatureVariationCount",
- 0,
- "Array of FeatureVariationRecord",
- ),
- ],
- ),
- (
- "FeatureVariationRecord",
- [
- (
- "LOffset",
- "ConditionSet",
- None,
- None,
- "Offset to a ConditionSet table, from beginning of the FeatureVariations table.",
- ),
- (
- "LOffset",
- "FeatureTableSubstitution",
- None,
- None,
- "Offset to a FeatureTableSubstitution table, from beginning of the FeatureVariations table",
- ),
- ],
- ),
- (
- "ConditionSet",
- [
- (
- "uint16",
- "ConditionCount",
- None,
- None,
- "Number of condition tables in the ConditionTable array",
- ),
- (
- "LOffset",
- "ConditionTable",
- "ConditionCount",
- 0,
- "Array of condition tables.",
- ),
- ],
- ),
- (
- "ConditionTableFormat1",
- [
- ("uint16", "Format", None, None, "Format, = 1"),
- (
- "uint16",
- "AxisIndex",
- None,
- None,
- "Index for the variation axis within the fvar table, base 0.",
- ),
- (
- "F2Dot14",
- "FilterRangeMinValue",
- None,
- None,
- "Minimum normalized axis value of the font variation instances that satisfy this condition.",
- ),
- (
- "F2Dot14",
- "FilterRangeMaxValue",
- None,
- None,
- "Maximum value that satisfies this condition.",
- ),
- ],
- ),
- (
- "FeatureTableSubstitution",
- [
- (
- "Version",
- "Version",
- None,
- None,
- "Version of the table-initially set to 0x00010000",
- ),
- (
- "uint16",
- "SubstitutionCount",
- None,
- None,
- "Number of records in the FeatureVariationRecords array",
- ),
- (
- "FeatureTableSubstitutionRecord",
- "SubstitutionRecord",
- "SubstitutionCount",
- 0,
- "Array of FeatureTableSubstitutionRecord",
- ),
- ],
- ),
- (
- "FeatureTableSubstitutionRecord",
- [
- ("uint16", "FeatureIndex", None, None, "The feature table index to match."),
- (
- "LOffset",
- "Feature",
- None,
- None,
- "Offset to an alternate feature table, from start of the FeatureTableSubstitution table.",
- ),
- ],
- ),
- # VariationStore
- (
- "VarRegionAxis",
- [
- ("F2Dot14", "StartCoord", None, None, ""),
- ("F2Dot14", "PeakCoord", None, None, ""),
- ("F2Dot14", "EndCoord", None, None, ""),
- ],
- ),
- (
- "VarRegion",
- [
- ("struct", "VarRegionAxis", "RegionAxisCount", 0, ""),
- ],
- ),
- (
- "VarRegionList",
- [
- ("uint16", "RegionAxisCount", None, None, ""),
- ("uint16", "RegionCount", None, None, ""),
- ("VarRegion", "Region", "RegionCount", 0, ""),
- ],
- ),
- (
- "VarData",
- [
- ("uint16", "ItemCount", None, None, ""),
- ("uint16", "NumShorts", None, None, ""),
- ("uint16", "VarRegionCount", None, None, ""),
- ("uint16", "VarRegionIndex", "VarRegionCount", 0, ""),
- ("VarDataValue", "Item", "ItemCount", 0, ""),
- ],
- ),
- (
- "VarStore",
- [
- ("uint16", "Format", None, None, "Set to 1."),
- ("LOffset", "VarRegionList", None, None, ""),
- ("uint16", "VarDataCount", None, None, ""),
- ("LOffset", "VarData", "VarDataCount", 0, ""),
- ],
- ),
- # Variation helpers
- (
- "VarIdxMap",
- [
- ("uint16", "EntryFormat", None, None, ""), # Automatically computed
- ("uint16", "MappingCount", None, None, ""), # Automatically computed
- ("VarIdxMapValue", "mapping", "", 0, "Array of compressed data"),
- ],
- ),
- (
- "DeltaSetIndexMapFormat0",
- [
- ("uint8", "Format", None, None, "Format of the DeltaSetIndexMap = 0"),
- ("uint8", "EntryFormat", None, None, ""), # Automatically computed
- ("uint16", "MappingCount", None, None, ""), # Automatically computed
- ("VarIdxMapValue", "mapping", "", 0, "Array of compressed data"),
- ],
- ),
- (
- "DeltaSetIndexMapFormat1",
- [
- ("uint8", "Format", None, None, "Format of the DeltaSetIndexMap = 1"),
- ("uint8", "EntryFormat", None, None, ""), # Automatically computed
- ("uint32", "MappingCount", None, None, ""), # Automatically computed
- ("VarIdxMapValue", "mapping", "", 0, "Array of compressed data"),
- ],
- ),
- # Glyph advance variations
- (
- "HVAR",
- [
- (
- "Version",
- "Version",
- None,
- None,
- "Version of the HVAR table-initially = 0x00010000",
- ),
- ("LOffset", "VarStore", None, None, ""),
- ("LOffsetTo(VarIdxMap)", "AdvWidthMap", None, None, ""),
- ("LOffsetTo(VarIdxMap)", "LsbMap", None, None, ""),
- ("LOffsetTo(VarIdxMap)", "RsbMap", None, None, ""),
- ],
- ),
- (
- "VVAR",
- [
- (
- "Version",
- "Version",
- None,
- None,
- "Version of the VVAR table-initially = 0x00010000",
- ),
- ("LOffset", "VarStore", None, None, ""),
- ("LOffsetTo(VarIdxMap)", "AdvHeightMap", None, None, ""),
- ("LOffsetTo(VarIdxMap)", "TsbMap", None, None, ""),
- ("LOffsetTo(VarIdxMap)", "BsbMap", None, None, ""),
- ("LOffsetTo(VarIdxMap)", "VOrgMap", None, None, "Vertical origin mapping."),
- ],
- ),
- # Font-wide metrics variations
- (
- "MetricsValueRecord",
- [
- ("Tag", "ValueTag", None, None, "4-byte font-wide measure identifier"),
- ("uint32", "VarIdx", None, None, "Combined outer-inner variation index"),
- (
- "uint8",
- "MoreBytes",
- "ValueRecordSize",
- -8,
- "Extra bytes. Set to empty array.",
- ),
- ],
- ),
- (
- "MVAR",
- [
- (
- "Version",
- "Version",
- None,
- None,
- "Version of the MVAR table-initially = 0x00010000",
- ),
- ("uint16", "Reserved", None, None, "Set to 0"),
- ("uint16", "ValueRecordSize", None, None, ""),
- ("uint16", "ValueRecordCount", None, None, ""),
- ("Offset", "VarStore", None, None, ""),
- ("MetricsValueRecord", "ValueRecord", "ValueRecordCount", 0, ""),
- ],
- ),
- #
- # math
- #
- (
- "MATH",
- [
- (
- "Version",
- "Version",
- None,
- None,
- "Version of the MATH table-initially set to 0x00010000.",
- ),
- (
- "Offset",
- "MathConstants",
- None,
- None,
- "Offset to MathConstants table - from the beginning of MATH table.",
- ),
- (
- "Offset",
- "MathGlyphInfo",
- None,
- None,
- "Offset to MathGlyphInfo table - from the beginning of MATH table.",
- ),
- (
- "Offset",
- "MathVariants",
- None,
- None,
- "Offset to MathVariants table - from the beginning of MATH table.",
- ),
- ],
- ),
- (
- "MathValueRecord",
- [
- ("int16", "Value", None, None, "The X or Y value in design units."),
- (
- "Offset",
- "DeviceTable",
- None,
- None,
- "Offset to the device table - from the beginning of parent table. May be NULL. Suggested format for device table is 1.",
- ),
- ],
- ),
- (
- "MathConstants",
- [
- (
- "int16",
- "ScriptPercentScaleDown",
- None,
- None,
- "Percentage of scaling down for script level 1. Suggested value: 80%.",
- ),
- (
- "int16",
- "ScriptScriptPercentScaleDown",
- None,
- None,
- "Percentage of scaling down for script level 2 (ScriptScript). Suggested value: 60%.",
- ),
- (
- "uint16",
- "DelimitedSubFormulaMinHeight",
- None,
- None,
- "Minimum height required for a delimited expression to be treated as a subformula. Suggested value: normal line height x1.5.",
- ),
- (
- "uint16",
- "DisplayOperatorMinHeight",
- None,
- None,
- "Minimum height of n-ary operators (such as integral and summation) for formulas in display mode.",
- ),
- (
- "MathValueRecord",
- "MathLeading",
- None,
- None,
- "White space to be left between math formulas to ensure proper line spacing. For example, for applications that treat line gap as a part of line ascender, formulas with ink going above (os2.sTypoAscender + os2.sTypoLineGap - MathLeading) or with ink going below os2.sTypoDescender will result in increasing line height.",
- ),
- ("MathValueRecord", "AxisHeight", None, None, "Axis height of the font."),
- (
- "MathValueRecord",
- "AccentBaseHeight",
- None,
- None,
- "Maximum (ink) height of accent base that does not require raising the accents. Suggested: x-height of the font (os2.sxHeight) plus any possible overshots.",
- ),
- (
- "MathValueRecord",
- "FlattenedAccentBaseHeight",
- None,
- None,
- "Maximum (ink) height of accent base that does not require flattening the accents. Suggested: cap height of the font (os2.sCapHeight).",
- ),
- (
- "MathValueRecord",
- "SubscriptShiftDown",
- None,
- None,
- "The standard shift down applied to subscript elements. Positive for moving in the downward direction. Suggested: os2.ySubscriptYOffset.",
- ),
- (
- "MathValueRecord",
- "SubscriptTopMax",
- None,
- None,
- "Maximum allowed height of the (ink) top of subscripts that does not require moving subscripts further down. Suggested: 4/5 x-height.",
- ),
- (
- "MathValueRecord",
- "SubscriptBaselineDropMin",
- None,
- None,
- "Minimum allowed drop of the baseline of subscripts relative to the (ink) bottom of the base. Checked for bases that are treated as a box or extended shape. Positive for subscript baseline dropped below the base bottom.",
- ),
- (
- "MathValueRecord",
- "SuperscriptShiftUp",
- None,
- None,
- "Standard shift up applied to superscript elements. Suggested: os2.ySuperscriptYOffset.",
- ),
- (
- "MathValueRecord",
- "SuperscriptShiftUpCramped",
- None,
- None,
- "Standard shift of superscripts relative to the base, in cramped style.",
- ),
- (
- "MathValueRecord",
- "SuperscriptBottomMin",
- None,
- None,
- "Minimum allowed height of the (ink) bottom of superscripts that does not require moving subscripts further up. Suggested: 1/4 x-height.",
- ),
- (
- "MathValueRecord",
- "SuperscriptBaselineDropMax",
- None,
- None,
- "Maximum allowed drop of the baseline of superscripts relative to the (ink) top of the base. Checked for bases that are treated as a box or extended shape. Positive for superscript baseline below the base top.",
- ),
- (
- "MathValueRecord",
- "SubSuperscriptGapMin",
- None,
- None,
- "Minimum gap between the superscript and subscript ink. Suggested: 4x default rule thickness.",
- ),
- (
- "MathValueRecord",
- "SuperscriptBottomMaxWithSubscript",
- None,
- None,
- "The maximum level to which the (ink) bottom of superscript can be pushed to increase the gap between superscript and subscript, before subscript starts being moved down. Suggested: 4/5 x-height.",
- ),
- (
- "MathValueRecord",
- "SpaceAfterScript",
- None,
- None,
- "Extra white space to be added after each subscript and superscript. Suggested: 0.5pt for a 12 pt font.",
- ),
- (
- "MathValueRecord",
- "UpperLimitGapMin",
- None,
- None,
- "Minimum gap between the (ink) bottom of the upper limit, and the (ink) top of the base operator.",
- ),
- (
- "MathValueRecord",
- "UpperLimitBaselineRiseMin",
- None,
- None,
- "Minimum distance between baseline of upper limit and (ink) top of the base operator.",
- ),
- (
- "MathValueRecord",
- "LowerLimitGapMin",
- None,
- None,
- "Minimum gap between (ink) top of the lower limit, and (ink) bottom of the base operator.",
- ),
- (
- "MathValueRecord",
- "LowerLimitBaselineDropMin",
- None,
- None,
- "Minimum distance between baseline of the lower limit and (ink) bottom of the base operator.",
- ),
- (
- "MathValueRecord",
- "StackTopShiftUp",
- None,
- None,
- "Standard shift up applied to the top element of a stack.",
- ),
- (
- "MathValueRecord",
- "StackTopDisplayStyleShiftUp",
- None,
- None,
- "Standard shift up applied to the top element of a stack in display style.",
- ),
- (
- "MathValueRecord",
- "StackBottomShiftDown",
- None,
- None,
- "Standard shift down applied to the bottom element of a stack. Positive for moving in the downward direction.",
- ),
- (
- "MathValueRecord",
- "StackBottomDisplayStyleShiftDown",
- None,
- None,
- "Standard shift down applied to the bottom element of a stack in display style. Positive for moving in the downward direction.",
- ),
- (
- "MathValueRecord",
- "StackGapMin",
- None,
- None,
- "Minimum gap between (ink) bottom of the top element of a stack, and the (ink) top of the bottom element. Suggested: 3x default rule thickness.",
- ),
- (
- "MathValueRecord",
- "StackDisplayStyleGapMin",
- None,
- None,
- "Minimum gap between (ink) bottom of the top element of a stack, and the (ink) top of the bottom element in display style. Suggested: 7x default rule thickness.",
- ),
- (
- "MathValueRecord",
- "StretchStackTopShiftUp",
- None,
- None,
- "Standard shift up applied to the top element of the stretch stack.",
- ),
- (
- "MathValueRecord",
- "StretchStackBottomShiftDown",
- None,
- None,
- "Standard shift down applied to the bottom element of the stretch stack. Positive for moving in the downward direction.",
- ),
- (
- "MathValueRecord",
- "StretchStackGapAboveMin",
- None,
- None,
- "Minimum gap between the ink of the stretched element, and the (ink) bottom of the element above. Suggested: UpperLimitGapMin",
- ),
- (
- "MathValueRecord",
- "StretchStackGapBelowMin",
- None,
- None,
- "Minimum gap between the ink of the stretched element, and the (ink) top of the element below. Suggested: LowerLimitGapMin.",
- ),
- (
- "MathValueRecord",
- "FractionNumeratorShiftUp",
- None,
- None,
- "Standard shift up applied to the numerator.",
- ),
- (
- "MathValueRecord",
- "FractionNumeratorDisplayStyleShiftUp",
- None,
- None,
- "Standard shift up applied to the numerator in display style. Suggested: StackTopDisplayStyleShiftUp.",
- ),
- (
- "MathValueRecord",
- "FractionDenominatorShiftDown",
- None,
- None,
- "Standard shift down applied to the denominator. Positive for moving in the downward direction.",
- ),
- (
- "MathValueRecord",
- "FractionDenominatorDisplayStyleShiftDown",
- None,
- None,
- "Standard shift down applied to the denominator in display style. Positive for moving in the downward direction. Suggested: StackBottomDisplayStyleShiftDown.",
- ),
- (
- "MathValueRecord",
- "FractionNumeratorGapMin",
- None,
- None,
- "Minimum tolerated gap between the (ink) bottom of the numerator and the ink of the fraction bar. Suggested: default rule thickness",
- ),
- (
- "MathValueRecord",
- "FractionNumDisplayStyleGapMin",
- None,
- None,
- "Minimum tolerated gap between the (ink) bottom of the numerator and the ink of the fraction bar in display style. Suggested: 3x default rule thickness.",
- ),
- (
- "MathValueRecord",
- "FractionRuleThickness",
- None,
- None,
- "Thickness of the fraction bar. Suggested: default rule thickness.",
- ),
- (
- "MathValueRecord",
- "FractionDenominatorGapMin",
- None,
- None,
- "Minimum tolerated gap between the (ink) top of the denominator and the ink of the fraction bar. Suggested: default rule thickness",
- ),
- (
- "MathValueRecord",
- "FractionDenomDisplayStyleGapMin",
- None,
- None,
- "Minimum tolerated gap between the (ink) top of the denominator and the ink of the fraction bar in display style. Suggested: 3x default rule thickness.",
- ),
- (
- "MathValueRecord",
- "SkewedFractionHorizontalGap",
- None,
- None,
- "Horizontal distance between the top and bottom elements of a skewed fraction.",
- ),
- (
- "MathValueRecord",
- "SkewedFractionVerticalGap",
- None,
- None,
- "Vertical distance between the ink of the top and bottom elements of a skewed fraction.",
- ),
- (
- "MathValueRecord",
- "OverbarVerticalGap",
- None,
- None,
- "Distance between the overbar and the (ink) top of he base. Suggested: 3x default rule thickness.",
- ),
- (
- "MathValueRecord",
- "OverbarRuleThickness",
- None,
- None,
- "Thickness of overbar. Suggested: default rule thickness.",
- ),
- (
- "MathValueRecord",
- "OverbarExtraAscender",
- None,
- None,
- "Extra white space reserved above the overbar. Suggested: default rule thickness.",
- ),
- (
- "MathValueRecord",
- "UnderbarVerticalGap",
- None,
- None,
- "Distance between underbar and (ink) bottom of the base. Suggested: 3x default rule thickness.",
- ),
- (
- "MathValueRecord",
- "UnderbarRuleThickness",
- None,
- None,
- "Thickness of underbar. Suggested: default rule thickness.",
- ),
- (
- "MathValueRecord",
- "UnderbarExtraDescender",
- None,
- None,
- "Extra white space reserved below the underbar. Always positive. Suggested: default rule thickness.",
- ),
- (
- "MathValueRecord",
- "RadicalVerticalGap",
- None,
- None,
- "Space between the (ink) top of the expression and the bar over it. Suggested: 1 1/4 default rule thickness.",
- ),
- (
- "MathValueRecord",
- "RadicalDisplayStyleVerticalGap",
- None,
- None,
- "Space between the (ink) top of the expression and the bar over it. Suggested: default rule thickness + 1/4 x-height.",
- ),
- (
- "MathValueRecord",
- "RadicalRuleThickness",
- None,
- None,
- "Thickness of the radical rule. This is the thickness of the rule in designed or constructed radical signs. Suggested: default rule thickness.",
- ),
- (
- "MathValueRecord",
- "RadicalExtraAscender",
- None,
- None,
- "Extra white space reserved above the radical. Suggested: RadicalRuleThickness.",
- ),
- (
- "MathValueRecord",
- "RadicalKernBeforeDegree",
- None,
- None,
- "Extra horizontal kern before the degree of a radical, if such is present. Suggested: 5/18 of em.",
- ),
- (
- "MathValueRecord",
- "RadicalKernAfterDegree",
- None,
- None,
- "Negative kern after the degree of a radical, if such is present. Suggested: 10/18 of em.",
- ),
- (
- "uint16",
- "RadicalDegreeBottomRaisePercent",
- None,
- None,
- "Height of the bottom of the radical degree, if such is present, in proportion to the ascender of the radical sign. Suggested: 60%.",
- ),
- ],
- ),
- (
- "MathGlyphInfo",
- [
- (
- "Offset",
- "MathItalicsCorrectionInfo",
- None,
- None,
- "Offset to MathItalicsCorrectionInfo table - from the beginning of MathGlyphInfo table.",
- ),
- (
- "Offset",
- "MathTopAccentAttachment",
- None,
- None,
- "Offset to MathTopAccentAttachment table - from the beginning of MathGlyphInfo table.",
- ),
- (
- "Offset",
- "ExtendedShapeCoverage",
- None,
- None,
- "Offset to coverage table for Extended Shape glyphs - from the beginning of MathGlyphInfo table. When the left or right glyph of a box is an extended shape variant, the (ink) box (and not the default position defined by values in MathConstants table) should be used for vertical positioning purposes. May be NULL.",
- ),
- (
- "Offset",
- "MathKernInfo",
- None,
- None,
- "Offset to MathKernInfo table - from the beginning of MathGlyphInfo table.",
- ),
- ],
- ),
- (
- "MathItalicsCorrectionInfo",
- [
- (
- "Offset",
- "Coverage",
- None,
- None,
- "Offset to Coverage table - from the beginning of MathItalicsCorrectionInfo table.",
- ),
- (
- "uint16",
- "ItalicsCorrectionCount",
- None,
- None,
- "Number of italics correction values. Should coincide with the number of covered glyphs.",
- ),
- (
- "MathValueRecord",
- "ItalicsCorrection",
- "ItalicsCorrectionCount",
- 0,
- "Array of MathValueRecords defining italics correction values for each covered glyph.",
- ),
- ],
- ),
- (
- "MathTopAccentAttachment",
- [
- (
- "Offset",
- "TopAccentCoverage",
- None,
- None,
- "Offset to Coverage table - from the beginning of MathTopAccentAttachment table.",
- ),
- (
- "uint16",
- "TopAccentAttachmentCount",
- None,
- None,
- "Number of top accent attachment point values. Should coincide with the number of covered glyphs",
- ),
- (
- "MathValueRecord",
- "TopAccentAttachment",
- "TopAccentAttachmentCount",
- 0,
- "Array of MathValueRecords defining top accent attachment points for each covered glyph",
- ),
- ],
- ),
- (
- "MathKernInfo",
- [
- (
- "Offset",
- "MathKernCoverage",
- None,
- None,
- "Offset to Coverage table - from the beginning of the MathKernInfo table.",
- ),
- ("uint16", "MathKernCount", None, None, "Number of MathKernInfoRecords."),
- (
- "MathKernInfoRecord",
- "MathKernInfoRecords",
- "MathKernCount",
- 0,
- "Array of MathKernInfoRecords, per-glyph information for mathematical positioning of subscripts and superscripts.",
- ),
- ],
- ),
- (
- "MathKernInfoRecord",
- [
- (
- "Offset",
- "TopRightMathKern",
- None,
- None,
- "Offset to MathKern table for top right corner - from the beginning of MathKernInfo table. May be NULL.",
- ),
- (
- "Offset",
- "TopLeftMathKern",
- None,
- None,
- "Offset to MathKern table for the top left corner - from the beginning of MathKernInfo table. May be NULL.",
- ),
- (
- "Offset",
- "BottomRightMathKern",
- None,
- None,
- "Offset to MathKern table for bottom right corner - from the beginning of MathKernInfo table. May be NULL.",
- ),
- (
- "Offset",
- "BottomLeftMathKern",
- None,
- None,
- "Offset to MathKern table for bottom left corner - from the beginning of MathKernInfo table. May be NULL.",
- ),
- ],
- ),
- (
- "MathKern",
- [
- (
- "uint16",
- "HeightCount",
- None,
- None,
- "Number of heights on which the kern value changes.",
- ),
- (
- "MathValueRecord",
- "CorrectionHeight",
- "HeightCount",
- 0,
- "Array of correction heights at which the kern value changes. Sorted by the height value in design units.",
- ),
- (
- "MathValueRecord",
- "KernValue",
- "HeightCount",
- 1,
- "Array of kern values corresponding to heights. First value is the kern value for all heights less or equal than the first height in this table.Last value is the value to be applied for all heights greater than the last height in this table. Negative values are interpreted as move glyphs closer to each other.",
- ),
- ],
- ),
- (
- "MathVariants",
- [
- (
- "uint16",
- "MinConnectorOverlap",
- None,
- None,
- "Minimum overlap of connecting glyphs during glyph construction, in design units.",
- ),
- (
- "Offset",
- "VertGlyphCoverage",
- None,
- None,
- "Offset to Coverage table - from the beginning of MathVariants table.",
- ),
- (
- "Offset",
- "HorizGlyphCoverage",
- None,
- None,
- "Offset to Coverage table - from the beginning of MathVariants table.",
- ),
- (
- "uint16",
- "VertGlyphCount",
- None,
- None,
- "Number of glyphs for which information is provided for vertically growing variants.",
- ),
- (
- "uint16",
- "HorizGlyphCount",
- None,
- None,
- "Number of glyphs for which information is provided for horizontally growing variants.",
- ),
- (
- "Offset",
- "VertGlyphConstruction",
- "VertGlyphCount",
- 0,
- "Array of offsets to MathGlyphConstruction tables - from the beginning of the MathVariants table, for shapes growing in vertical direction.",
- ),
- (
- "Offset",
- "HorizGlyphConstruction",
- "HorizGlyphCount",
- 0,
- "Array of offsets to MathGlyphConstruction tables - from the beginning of the MathVariants table, for shapes growing in horizontal direction.",
- ),
- ],
- ),
- (
- "MathGlyphConstruction",
- [
- (
- "Offset",
- "GlyphAssembly",
- None,
- None,
- "Offset to GlyphAssembly table for this shape - from the beginning of MathGlyphConstruction table. May be NULL",
- ),
- (
- "uint16",
- "VariantCount",
- None,
- None,
- "Count of glyph growing variants for this glyph.",
- ),
- (
- "MathGlyphVariantRecord",
- "MathGlyphVariantRecord",
- "VariantCount",
- 0,
- "MathGlyphVariantRecords for alternative variants of the glyphs.",
- ),
- ],
- ),
- (
- "MathGlyphVariantRecord",
- [
- ("GlyphID", "VariantGlyph", None, None, "Glyph ID for the variant."),
- (
- "uint16",
- "AdvanceMeasurement",
- None,
- None,
- "Advance width/height, in design units, of the variant, in the direction of requested glyph extension.",
- ),
- ],
- ),
- (
- "GlyphAssembly",
- [
- (
- "MathValueRecord",
- "ItalicsCorrection",
- None,
- None,
- "Italics correction of this GlyphAssembly. Should not depend on the assembly size.",
- ),
- ("uint16", "PartCount", None, None, "Number of parts in this assembly."),
- (
- "GlyphPartRecord",
- "PartRecords",
- "PartCount",
- 0,
- "Array of part records, from left to right and bottom to top.",
- ),
- ],
- ),
- (
- "GlyphPartRecord",
- [
- ("GlyphID", "glyph", None, None, "Glyph ID for the part."),
- (
- "uint16",
- "StartConnectorLength",
- None,
- None,
- "Advance width/ height of the straight bar connector material, in design units, is at the beginning of the glyph, in the direction of the extension.",
- ),
- (
- "uint16",
- "EndConnectorLength",
- None,
- None,
- "Advance width/ height of the straight bar connector material, in design units, is at the end of the glyph, in the direction of the extension.",
- ),
- (
- "uint16",
- "FullAdvance",
- None,
- None,
- "Full advance width/height for this part, in the direction of the extension. In design units.",
- ),
- (
- "uint16",
- "PartFlags",
- None,
- None,
- "Part qualifiers. PartFlags enumeration currently uses only one bit: 0x0001 fExtender: If set, the part can be skipped or repeated. 0xFFFE Reserved",
- ),
- ],
- ),
- ##
- ## Apple Advanced Typography (AAT) tables
- ##
- (
- "AATLookupSegment",
- [
- ("uint16", "lastGlyph", None, None, "Last glyph index in this segment."),
- ("uint16", "firstGlyph", None, None, "First glyph index in this segment."),
- (
- "uint16",
- "value",
- None,
- None,
- "A 16-bit offset from the start of the table to the data.",
- ),
- ],
- ),
- #
- # ankr
- #
- (
- "ankr",
- [
- ("struct", "AnchorPoints", None, None, "Anchor points table."),
- ],
- ),
- (
- "AnchorPointsFormat0",
- [
- ("uint16", "Format", None, None, "Format of the anchor points table, = 0."),
- ("uint16", "Flags", None, None, "Flags. Currenty unused, set to zero."),
- (
- "AATLookupWithDataOffset(AnchorGlyphData)",
- "Anchors",
- None,
- None,
- "Table of with anchor overrides for each glyph.",
- ),
- ],
- ),
- (
- "AnchorGlyphData",
- [
- (
- "uint32",
- "AnchorPointCount",
- None,
- None,
- "Number of anchor points for this glyph.",
- ),
- (
- "struct",
- "AnchorPoint",
- "AnchorPointCount",
- 0,
- "Individual anchor points.",
- ),
- ],
- ),
- (
- "AnchorPoint",
- [
- ("int16", "XCoordinate", None, None, "X coordinate of this anchor point."),
- ("int16", "YCoordinate", None, None, "Y coordinate of this anchor point."),
- ],
- ),
- #
- # bsln
- #
- (
- "bsln",
- [
- (
- "Version",
- "Version",
- None,
- None,
- "Version number of the AAT baseline table (0x00010000 for the initial version).",
- ),
- ("struct", "Baseline", None, None, "Baseline table."),
- ],
- ),
- (
- "BaselineFormat0",
- [
- ("uint16", "Format", None, None, "Format of the baseline table, = 0."),
- (
- "uint16",
- "DefaultBaseline",
- None,
- None,
- "Default baseline value for all glyphs. This value can be from 0 through 31.",
- ),
- (
- "uint16",
- "Delta",
- 32,
- 0,
- "These are the FUnit distance deltas from the font’s natural baseline to the other baselines used in the font. A total of 32 deltas must be assigned.",
- ),
- ],
- ),
- (
- "BaselineFormat1",
- [
- ("uint16", "Format", None, None, "Format of the baseline table, = 1."),
- (
- "uint16",
- "DefaultBaseline",
- None,
- None,
- "Default baseline value for all glyphs. This value can be from 0 through 31.",
- ),
- (
- "uint16",
- "Delta",
- 32,
- 0,
- "These are the FUnit distance deltas from the font’s natural baseline to the other baselines used in the font. A total of 32 deltas must be assigned.",
- ),
- (
- "AATLookup(uint16)",
- "BaselineValues",
- None,
- None,
- "Lookup table that maps glyphs to their baseline values.",
- ),
- ],
- ),
- (
- "BaselineFormat2",
- [
- ("uint16", "Format", None, None, "Format of the baseline table, = 1."),
- (
- "uint16",
- "DefaultBaseline",
- None,
- None,
- "Default baseline value for all glyphs. This value can be from 0 through 31.",
- ),
- (
- "GlyphID",
- "StandardGlyph",
- None,
- None,
- "Glyph index of the glyph in this font to be used to set the baseline values. This glyph must contain a set of control points (whose numbers are contained in the following field) that determines baseline distances.",
- ),
- (
- "uint16",
- "ControlPoint",
- 32,
- 0,
- "Array of 32 control point numbers, associated with the standard glyph. A value of 0xFFFF means there is no corresponding control point in the standard glyph.",
- ),
- ],
- ),
- (
- "BaselineFormat3",
- [
- ("uint16", "Format", None, None, "Format of the baseline table, = 1."),
- (
- "uint16",
- "DefaultBaseline",
- None,
- None,
- "Default baseline value for all glyphs. This value can be from 0 through 31.",
- ),
- (
- "GlyphID",
- "StandardGlyph",
- None,
- None,
- "Glyph index of the glyph in this font to be used to set the baseline values. This glyph must contain a set of control points (whose numbers are contained in the following field) that determines baseline distances.",
- ),
- (
- "uint16",
- "ControlPoint",
- 32,
- 0,
- "Array of 32 control point numbers, associated with the standard glyph. A value of 0xFFFF means there is no corresponding control point in the standard glyph.",
- ),
- (
- "AATLookup(uint16)",
- "BaselineValues",
- None,
- None,
- "Lookup table that maps glyphs to their baseline values.",
- ),
- ],
- ),
- #
- # cidg
- #
- (
- "cidg",
- [
- ("struct", "CIDGlyphMapping", None, None, "CID-to-glyph mapping table."),
- ],
- ),
- (
- "CIDGlyphMappingFormat0",
- [
- (
- "uint16",
- "Format",
- None,
- None,
- "Format of the CID-to-glyph mapping table, = 0.",
- ),
- ("uint16", "DataFormat", None, None, "Currenty unused, set to zero."),
- ("uint32", "StructLength", None, None, "Size of the table in bytes."),
- ("uint16", "Registry", None, None, "The registry ID."),
- (
- "char64",
- "RegistryName",
- None,
- None,
- "The registry name in ASCII; unused bytes should be set to 0.",
- ),
- ("uint16", "Order", None, None, "The order ID."),
- (
- "char64",
- "OrderName",
- None,
- None,
- "The order name in ASCII; unused bytes should be set to 0.",
- ),
- ("uint16", "SupplementVersion", None, None, "The supplement version."),
- (
- "CIDGlyphMap",
- "Mapping",
- None,
- None,
- "A mapping from CIDs to the glyphs in the font, starting with CID 0. If a CID from the identified collection has no glyph in the font, 0xFFFF is used",
- ),
- ],
- ),
- #
- # feat
- #
- (
- "feat",
- [
- (
- "Version",
- "Version",
- None,
- None,
- "Version of the feat table-initially set to 0x00010000.",
- ),
- ("FeatureNames", "FeatureNames", None, None, "The feature names."),
- ],
- ),
- (
- "FeatureNames",
- [
- (
- "uint16",
- "FeatureNameCount",
- None,
- None,
- "Number of entries in the feature name array.",
- ),
- ("uint16", "Reserved1", None, None, "Reserved (set to zero)."),
- ("uint32", "Reserved2", None, None, "Reserved (set to zero)."),
- (
- "FeatureName",
- "FeatureName",
- "FeatureNameCount",
- 0,
- "The feature name array.",
- ),
- ],
- ),
- (
- "FeatureName",
- [
- ("uint16", "FeatureType", None, None, "Feature type."),
- (
- "uint16",
- "SettingsCount",
- None,
- None,
- "The number of records in the setting name array.",
- ),
- (
- "LOffset",
- "Settings",
- None,
- None,
- "Offset to setting table for this feature.",
- ),
- (
- "uint16",
- "FeatureFlags",
- None,
- None,
- "Single-bit flags associated with the feature type.",
- ),
- (
- "NameID",
- "FeatureNameID",
- None,
- None,
- "The name table index for the feature name.",
- ),
- ],
- ),
- (
- "Settings",
- [
- ("Setting", "Setting", "SettingsCount", 0, "The setting array."),
- ],
- ),
- (
- "Setting",
- [
- ("uint16", "SettingValue", None, None, "The setting."),
- (
- "NameID",
- "SettingNameID",
- None,
- None,
- "The name table index for the setting name.",
- ),
- ],
- ),
- #
- # gcid
- #
- (
- "gcid",
- [
- ("struct", "GlyphCIDMapping", None, None, "Glyph to CID mapping table."),
- ],
- ),
- (
- "GlyphCIDMappingFormat0",
- [
- (
- "uint16",
- "Format",
- None,
- None,
- "Format of the glyph-to-CID mapping table, = 0.",
- ),
- ("uint16", "DataFormat", None, None, "Currenty unused, set to zero."),
- ("uint32", "StructLength", None, None, "Size of the table in bytes."),
- ("uint16", "Registry", None, None, "The registry ID."),
- (
- "char64",
- "RegistryName",
- None,
- None,
- "The registry name in ASCII; unused bytes should be set to 0.",
- ),
- ("uint16", "Order", None, None, "The order ID."),
- (
- "char64",
- "OrderName",
- None,
- None,
- "The order name in ASCII; unused bytes should be set to 0.",
- ),
- ("uint16", "SupplementVersion", None, None, "The supplement version."),
- (
- "GlyphCIDMap",
- "Mapping",
- None,
- None,
- "The CIDs for the glyphs in the font, starting with glyph 0. If a glyph does not correspond to a CID in the identified collection, 0xFFFF is used",
- ),
- ],
- ),
- #
- # lcar
- #
- (
- "lcar",
- [
- (
- "Version",
- "Version",
- None,
- None,
- "Version number of the ligature caret table (0x00010000 for the initial version).",
- ),
- ("struct", "LigatureCarets", None, None, "Ligature carets table."),
- ],
- ),
- (
- "LigatureCaretsFormat0",
- [
- (
- "uint16",
- "Format",
- None,
- None,
- "Format of the ligature caret table. Format 0 indicates division points are distances in font units, Format 1 indicates division points are indexes of control points.",
- ),
- (
- "AATLookup(LigCaretDistances)",
- "Carets",
- None,
- None,
- "Lookup table associating ligature glyphs with their caret positions, in font unit distances.",
- ),
- ],
- ),
- (
- "LigatureCaretsFormat1",
- [
- (
- "uint16",
- "Format",
- None,
- None,
- "Format of the ligature caret table. Format 0 indicates division points are distances in font units, Format 1 indicates division points are indexes of control points.",
- ),
- (
- "AATLookup(LigCaretPoints)",
- "Carets",
- None,
- None,
- "Lookup table associating ligature glyphs with their caret positions, as control points.",
- ),
- ],
- ),
- (
- "LigCaretDistances",
- [
- ("uint16", "DivsionPointCount", None, None, "Number of division points."),
- (
- "int16",
- "DivisionPoint",
- "DivsionPointCount",
- 0,
- "Distance in font units through which a subdivision is made orthogonally to the baseline.",
- ),
- ],
- ),
- (
- "LigCaretPoints",
- [
- ("uint16", "DivsionPointCount", None, None, "Number of division points."),
- (
- "int16",
- "DivisionPoint",
- "DivsionPointCount",
- 0,
- "The number of the control point through which a subdivision is made orthogonally to the baseline.",
- ),
- ],
- ),
- #
- # mort
- #
- (
- "mort",
- [
- ("Version", "Version", None, None, "Version of the mort table."),
- (
- "uint32",
- "MorphChainCount",
- None,
- None,
- "Number of metamorphosis chains.",
- ),
- (
- "MortChain",
- "MorphChain",
- "MorphChainCount",
- 0,
- "Array of metamorphosis chains.",
- ),
- ],
- ),
- (
- "MortChain",
- [
- (
- "Flags32",
- "DefaultFlags",
- None,
- None,
- "The default specification for subtables.",
- ),
- (
- "uint32",
- "StructLength",
- None,
- None,
- "Total byte count, including this header; must be a multiple of 4.",
- ),
- (
- "uint16",
- "MorphFeatureCount",
- None,
- None,
- "Number of metamorphosis feature entries.",
- ),
- (
- "uint16",
- "MorphSubtableCount",
- None,
- None,
- "The number of subtables in the chain.",
- ),
- (
- "struct",
- "MorphFeature",
- "MorphFeatureCount",
- 0,
- "Array of metamorphosis features.",
- ),
- (
- "MortSubtable",
- "MorphSubtable",
- "MorphSubtableCount",
- 0,
- "Array of metamorphosis subtables.",
- ),
- ],
- ),
- (
- "MortSubtable",
- [
- (
- "uint16",
- "StructLength",
- None,
- None,
- "Total subtable length, including this header.",
- ),
- (
- "uint8",
- "CoverageFlags",
- None,
- None,
- "Most significant byte of coverage flags.",
- ),
- ("uint8", "MorphType", None, None, "Subtable type."),
- (
- "Flags32",
- "SubFeatureFlags",
- None,
- None,
- "The 32-bit mask identifying which subtable this is (the subtable being executed if the AND of this value and the processed defaultFlags is nonzero).",
- ),
- ("SubStruct", "SubStruct", None, None, "SubTable."),
- ],
- ),
- #
- # morx
- #
- (
- "morx",
- [
- ("uint16", "Version", None, None, "Version of the morx table."),
- ("uint16", "Reserved", None, None, "Reserved (set to zero)."),
- (
- "uint32",
- "MorphChainCount",
- None,
- None,
- "Number of extended metamorphosis chains.",
- ),
- (
- "MorxChain",
- "MorphChain",
- "MorphChainCount",
- 0,
- "Array of extended metamorphosis chains.",
- ),
- ],
- ),
- (
- "MorxChain",
- [
- (
- "Flags32",
- "DefaultFlags",
- None,
- None,
- "The default specification for subtables.",
- ),
- (
- "uint32",
- "StructLength",
- None,
- None,
- "Total byte count, including this header; must be a multiple of 4.",
- ),
- (
- "uint32",
- "MorphFeatureCount",
- None,
- None,
- "Number of feature subtable entries.",
- ),
- (
- "uint32",
- "MorphSubtableCount",
- None,
- None,
- "The number of subtables in the chain.",
- ),
- (
- "MorphFeature",
- "MorphFeature",
- "MorphFeatureCount",
- 0,
- "Array of metamorphosis features.",
- ),
- (
- "MorxSubtable",
- "MorphSubtable",
- "MorphSubtableCount",
- 0,
- "Array of extended metamorphosis subtables.",
- ),
- ],
- ),
- (
- "MorphFeature",
- [
- ("uint16", "FeatureType", None, None, "The type of feature."),
- (
- "uint16",
- "FeatureSetting",
- None,
- None,
- "The feature's setting (aka selector).",
- ),
- (
- "Flags32",
- "EnableFlags",
- None,
- None,
- "Flags for the settings that this feature and setting enables.",
- ),
- (
- "Flags32",
- "DisableFlags",
- None,
- None,
- "Complement of flags for the settings that this feature and setting disable.",
- ),
- ],
- ),
- # Apple TrueType Reference Manual, chapter “The ‘morx’ table”,
- # section “Metamorphosis Subtables”.
- # https://developer.apple.com/fonts/TrueType-Reference-Manual/RM06/Chap6morx.html
- (
- "MorxSubtable",
- [
- (
- "uint32",
- "StructLength",
- None,
- None,
- "Total subtable length, including this header.",
- ),
- (
- "uint8",
- "CoverageFlags",
- None,
- None,
- "Most significant byte of coverage flags.",
- ),
- ("uint16", "Reserved", None, None, "Unused."),
- ("uint8", "MorphType", None, None, "Subtable type."),
- (
- "Flags32",
- "SubFeatureFlags",
- None,
- None,
- "The 32-bit mask identifying which subtable this is (the subtable being executed if the AND of this value and the processed defaultFlags is nonzero).",
- ),
- ("SubStruct", "SubStruct", None, None, "SubTable."),
- ],
- ),
- (
- "StateHeader",
- [
- (
- "uint32",
- "ClassCount",
- None,
- None,
- "Number of classes, which is the number of 16-bit entry indices in a single line in the state array.",
- ),
- (
- "uint32",
- "MorphClass",
- None,
- None,
- "Offset from the start of this state table header to the start of the class table.",
- ),
- (
- "uint32",
- "StateArrayOffset",
- None,
- None,
- "Offset from the start of this state table header to the start of the state array.",
- ),
- (
- "uint32",
- "EntryTableOffset",
- None,
- None,
- "Offset from the start of this state table header to the start of the entry table.",
- ),
- ],
- ),
- (
- "RearrangementMorph",
- [
- (
- "STXHeader(RearrangementMorphAction)",
- "StateTable",
- None,
- None,
- "Finite-state transducer table for indic rearrangement.",
- ),
- ],
- ),
- (
- "ContextualMorph",
- [
- (
- "STXHeader(ContextualMorphAction)",
- "StateTable",
- None,
- None,
- "Finite-state transducer for contextual glyph substitution.",
- ),
- ],
- ),
- (
- "LigatureMorph",
- [
- (
- "STXHeader(LigatureMorphAction)",
- "StateTable",
- None,
- None,
- "Finite-state transducer for ligature substitution.",
- ),
- ],
- ),
- (
- "NoncontextualMorph",
- [
- (
- "AATLookup(GlyphID)",
- "Substitution",
- None,
- None,
- "The noncontextual glyph substitution table.",
- ),
- ],
- ),
- (
- "InsertionMorph",
- [
- (
- "STXHeader(InsertionMorphAction)",
- "StateTable",
- None,
- None,
- "Finite-state transducer for glyph insertion.",
- ),
- ],
- ),
- (
- "MorphClass",
- [
- (
- "uint16",
- "FirstGlyph",
- None,
- None,
- "Glyph index of the first glyph in the class table.",
- ),
- # ('uint16', 'GlyphCount', None, None, 'Number of glyphs in class table.'),
- # ('uint8', 'GlyphClass', 'GlyphCount', 0, 'The class codes (indexed by glyph index minus firstGlyph). Class codes range from 0 to the value of stateSize minus 1.'),
- ],
- ),
- # If the 'morx' table version is 3 or greater, then the last subtable in the chain is followed by a subtableGlyphCoverageArray, as described below.
- # ('Offset', 'MarkGlyphSetsDef', None, 'round(Version*0x10000) >= 0x00010002', 'Offset to the table of mark set definitions-from beginning of GDEF header (may be NULL)'),
- #
- # prop
- #
- (
- "prop",
- [
- (
- "Fixed",
- "Version",
- None,
- None,
- "Version number of the AAT glyphs property table. Version 1.0 is the initial table version. Version 2.0, which is recognized by macOS 8.5 and later, adds support for the “attaches on right” bit. Version 3.0, which gets recognized by macOS X and iOS, adds support for the additional directional properties defined in Unicode 3.0.",
- ),
- ("struct", "GlyphProperties", None, None, "Glyph properties."),
- ],
- ),
- (
- "GlyphPropertiesFormat0",
- [
- ("uint16", "Format", None, None, "Format, = 0."),
- (
- "uint16",
- "DefaultProperties",
- None,
- None,
- "Default properties applied to a glyph. Since there is no lookup table in prop format 0, the default properties get applied to every glyph in the font.",
- ),
- ],
- ),
- (
- "GlyphPropertiesFormat1",
- [
- ("uint16", "Format", None, None, "Format, = 1."),
- (
- "uint16",
- "DefaultProperties",
- None,
- None,
- "Default properties applied to a glyph if that glyph is not present in the Properties lookup table.",
- ),
- (
- "AATLookup(uint16)",
- "Properties",
- None,
- None,
- "Lookup data associating glyphs with their properties.",
- ),
- ],
- ),
- #
- # opbd
- #
- (
- "opbd",
- [
- (
- "Version",
- "Version",
- None,
- None,
- "Version number of the optical bounds table (0x00010000 for the initial version).",
- ),
- ("struct", "OpticalBounds", None, None, "Optical bounds table."),
- ],
- ),
- (
- "OpticalBoundsFormat0",
- [
- (
- "uint16",
- "Format",
- None,
- None,
- "Format of the optical bounds table, = 0.",
- ),
- (
- "AATLookup(OpticalBoundsDeltas)",
- "OpticalBoundsDeltas",
- None,
- None,
- "Lookup table associating glyphs with their optical bounds, given as deltas in font units.",
- ),
- ],
- ),
- (
- "OpticalBoundsFormat1",
- [
- (
- "uint16",
- "Format",
- None,
- None,
- "Format of the optical bounds table, = 1.",
- ),
- (
- "AATLookup(OpticalBoundsPoints)",
- "OpticalBoundsPoints",
- None,
- None,
- "Lookup table associating glyphs with their optical bounds, given as references to control points.",
- ),
- ],
- ),
- (
- "OpticalBoundsDeltas",
- [
- (
- "int16",
- "Left",
- None,
- None,
- "Delta value for the left-side optical edge.",
- ),
- ("int16", "Top", None, None, "Delta value for the top-side optical edge."),
- (
- "int16",
- "Right",
- None,
- None,
- "Delta value for the right-side optical edge.",
- ),
- (
- "int16",
- "Bottom",
- None,
- None,
- "Delta value for the bottom-side optical edge.",
- ),
- ],
- ),
- (
- "OpticalBoundsPoints",
- [
- (
- "int16",
- "Left",
- None,
- None,
- "Control point index for the left-side optical edge, or -1 if this glyph has none.",
- ),
- (
- "int16",
- "Top",
- None,
- None,
- "Control point index for the top-side optical edge, or -1 if this glyph has none.",
- ),
- (
- "int16",
- "Right",
- None,
- None,
- "Control point index for the right-side optical edge, or -1 if this glyph has none.",
- ),
- (
- "int16",
- "Bottom",
- None,
- None,
- "Control point index for the bottom-side optical edge, or -1 if this glyph has none.",
- ),
- ],
- ),
- #
- # TSIC
- #
- (
- "TSIC",
- [
- (
- "Version",
- "Version",
- None,
- None,
- "Version of table initially set to 0x00010000.",
- ),
- ("uint16", "Flags", None, None, "TSIC flags - set to 0"),
- ("uint16", "AxisCount", None, None, "Axis count from fvar"),
- ("uint16", "RecordCount", None, None, "TSIC record count"),
- ("uint16", "Reserved", None, None, "Set to 0"),
- ("Tag", "AxisArray", "AxisCount", 0, "Array of axis tags in fvar order"),
- (
- "LocationRecord",
- "RecordLocations",
- "RecordCount",
- 0,
- "Location in variation space of TSIC record",
- ),
- ("TSICRecord", "Record", "RecordCount", 0, "Array of TSIC records"),
- ],
- ),
- (
- "LocationRecord",
- [
- ("F2Dot14", "Axis", "AxisCount", 0, "Axis record"),
- ],
- ),
- (
- "TSICRecord",
- [
- ("uint16", "Flags", None, None, "Record flags - set to 0"),
- ("uint16", "NumCVTEntries", None, None, "Number of CVT number value pairs"),
- ("uint16", "NameLength", None, None, "Length of optional user record name"),
- ("uint16", "NameArray", "NameLength", 0, "Unicode 16 name"),
- ("uint16", "CVTArray", "NumCVTEntries", 0, "CVT number array"),
- ("int16", "CVTValueArray", "NumCVTEntries", 0, "CVT value"),
- ],
- ),
- #
- # COLR
- #
- (
- "COLR",
- [
- ("uint16", "Version", None, None, "Table version number (starts at 0)."),
- (
- "uint16",
- "BaseGlyphRecordCount",
- None,
- None,
- "Number of Base Glyph Records.",
- ),
- (
- "LOffset",
- "BaseGlyphRecordArray",
- None,
- None,
- "Offset (from beginning of COLR table) to Base Glyph records.",
- ),
- (
- "LOffset",
- "LayerRecordArray",
- None,
- None,
- "Offset (from beginning of COLR table) to Layer Records.",
- ),
- ("uint16", "LayerRecordCount", None, None, "Number of Layer Records."),
- (
- "LOffset",
- "BaseGlyphList",
- None,
- "Version >= 1",
- "Offset (from beginning of COLR table) to array of Version-1 Base Glyph records.",
- ),
- (
- "LOffset",
- "LayerList",
- None,
- "Version >= 1",
- "Offset (from beginning of COLR table) to LayerList.",
- ),
- (
- "LOffset",
- "ClipList",
- None,
- "Version >= 1",
- "Offset to ClipList table (may be NULL)",
- ),
- (
- "LOffsetTo(DeltaSetIndexMap)",
- "VarIndexMap",
- None,
- "Version >= 1",
- "Offset to DeltaSetIndexMap table (may be NULL)",
- ),
- (
- "LOffset",
- "VarStore",
- None,
- "Version >= 1",
- "Offset to variation store (may be NULL)",
- ),
- ],
- ),
- (
- "BaseGlyphRecordArray",
- [
- (
- "BaseGlyphRecord",
- "BaseGlyphRecord",
- "BaseGlyphRecordCount",
- 0,
- "Base Glyph records.",
- ),
- ],
- ),
- (
- "BaseGlyphRecord",
- [
- (
- "GlyphID",
- "BaseGlyph",
- None,
- None,
- "Glyph ID of reference glyph. This glyph is for reference only and is not rendered for color.",
- ),
- (
- "uint16",
- "FirstLayerIndex",
- None,
- None,
- "Index (from beginning of the Layer Records) to the layer record. There will be numLayers consecutive entries for this base glyph.",
- ),
- (
- "uint16",
- "NumLayers",
- None,
- None,
- "Number of color layers associated with this glyph.",
- ),
- ],
- ),
- (
- "LayerRecordArray",
- [
- ("LayerRecord", "LayerRecord", "LayerRecordCount", 0, "Layer records."),
- ],
- ),
- (
- "LayerRecord",
- [
- (
- "GlyphID",
- "LayerGlyph",
- None,
- None,
- "Glyph ID of layer glyph (must be in z-order from bottom to top).",
- ),
- (
- "uint16",
- "PaletteIndex",
- None,
- None,
- "Index value to use with a selected color palette.",
- ),
- ],
- ),
- (
- "BaseGlyphList",
- [
- (
- "uint32",
- "BaseGlyphCount",
- None,
- None,
- "Number of Version-1 Base Glyph records",
- ),
- (
- "struct",
- "BaseGlyphPaintRecord",
- "BaseGlyphCount",
- 0,
- "Array of Version-1 Base Glyph records",
- ),
- ],
- ),
- (
- "BaseGlyphPaintRecord",
- [
- ("GlyphID", "BaseGlyph", None, None, "Glyph ID of reference glyph."),
- (
- "LOffset",
- "Paint",
- None,
- None,
- "Offset (from beginning of BaseGlyphPaintRecord) to Paint, typically a PaintColrLayers.",
- ),
- ],
- ),
- (
- "LayerList",
- [
- ("uint32", "LayerCount", None, None, "Number of Version-1 Layers"),
- (
- "LOffset",
- "Paint",
- "LayerCount",
- 0,
- "Array of offsets to Paint tables, from the start of the LayerList table.",
- ),
- ],
- ),
- (
- "ClipListFormat1",
- [
- (
- "uint8",
- "Format",
- None,
- None,
- "Format for ClipList with 16bit glyph IDs: 1",
- ),
- ("uint32", "ClipCount", None, None, "Number of Clip records."),
- (
- "struct",
- "ClipRecord",
- "ClipCount",
- 0,
- "Array of Clip records sorted by glyph ID.",
- ),
- ],
- ),
- (
- "ClipRecord",
- [
- ("uint16", "StartGlyphID", None, None, "First glyph ID in the range."),
- ("uint16", "EndGlyphID", None, None, "Last glyph ID in the range."),
- ("Offset24", "ClipBox", None, None, "Offset to a ClipBox table."),
- ],
- ),
- (
- "ClipBoxFormat1",
- [
- (
- "uint8",
- "Format",
- None,
- None,
- "Format for ClipBox without variation: set to 1.",
- ),
- ("int16", "xMin", None, None, "Minimum x of clip box."),
- ("int16", "yMin", None, None, "Minimum y of clip box."),
- ("int16", "xMax", None, None, "Maximum x of clip box."),
- ("int16", "yMax", None, None, "Maximum y of clip box."),
- ],
- ),
- (
- "ClipBoxFormat2",
- [
- ("uint8", "Format", None, None, "Format for variable ClipBox: set to 2."),
- ("int16", "xMin", None, None, "Minimum x of clip box. VarIndexBase + 0."),
- ("int16", "yMin", None, None, "Minimum y of clip box. VarIndexBase + 1."),
- ("int16", "xMax", None, None, "Maximum x of clip box. VarIndexBase + 2."),
- ("int16", "yMax", None, None, "Maximum y of clip box. VarIndexBase + 3."),
- (
- "VarIndex",
- "VarIndexBase",
- None,
- None,
- "Base index into DeltaSetIndexMap.",
- ),
- ],
- ),
- # COLRv1 Affine2x3 uses the same column-major order to serialize a 2D
- # Affine Transformation as the one used by fontTools.misc.transform.
- # However, for historical reasons, the labels 'xy' and 'yx' are swapped.
- # Their fundamental meaning is the same though.
- # COLRv1 Affine2x3 follows the names found in FreeType and Cairo.
- # In all case, the second element in the 6-tuple correspond to the
- # y-part of the x basis vector, and the third to the x-part of the y
- # basis vector.
- # See https://github.com/googlefonts/colr-gradients-spec/pull/85
- (
- "Affine2x3",
- [
- ("Fixed", "xx", None, None, "x-part of x basis vector"),
- ("Fixed", "yx", None, None, "y-part of x basis vector"),
- ("Fixed", "xy", None, None, "x-part of y basis vector"),
- ("Fixed", "yy", None, None, "y-part of y basis vector"),
- ("Fixed", "dx", None, None, "Translation in x direction"),
- ("Fixed", "dy", None, None, "Translation in y direction"),
- ],
- ),
- (
- "VarAffine2x3",
- [
- ("Fixed", "xx", None, None, "x-part of x basis vector. VarIndexBase + 0."),
- ("Fixed", "yx", None, None, "y-part of x basis vector. VarIndexBase + 1."),
- ("Fixed", "xy", None, None, "x-part of y basis vector. VarIndexBase + 2."),
- ("Fixed", "yy", None, None, "y-part of y basis vector. VarIndexBase + 3."),
- (
- "Fixed",
- "dx",
- None,
- None,
- "Translation in x direction. VarIndexBase + 4.",
- ),
- (
- "Fixed",
- "dy",
- None,
- None,
- "Translation in y direction. VarIndexBase + 5.",
- ),
- (
- "VarIndex",
- "VarIndexBase",
- None,
- None,
- "Base index into DeltaSetIndexMap.",
- ),
- ],
- ),
- (
- "ColorStop",
- [
- ("F2Dot14", "StopOffset", None, None, ""),
- ("uint16", "PaletteIndex", None, None, "Index for a CPAL palette entry."),
- ("F2Dot14", "Alpha", None, None, "Values outsided [0.,1.] reserved"),
- ],
- ),
- (
- "VarColorStop",
- [
- ("F2Dot14", "StopOffset", None, None, "VarIndexBase + 0."),
- ("uint16", "PaletteIndex", None, None, "Index for a CPAL palette entry."),
- (
- "F2Dot14",
- "Alpha",
- None,
- None,
- "Values outsided [0.,1.] reserved. VarIndexBase + 1.",
- ),
- (
- "VarIndex",
- "VarIndexBase",
- None,
- None,
- "Base index into DeltaSetIndexMap.",
- ),
- ],
- ),
- (
- "ColorLine",
- [
- (
- "ExtendMode",
- "Extend",
- None,
- None,
- "Enum {PAD = 0, REPEAT = 1, REFLECT = 2}",
- ),
- ("uint16", "StopCount", None, None, "Number of Color stops."),
- ("ColorStop", "ColorStop", "StopCount", 0, "Array of Color stops."),
- ],
- ),
- (
- "VarColorLine",
- [
- (
- "ExtendMode",
- "Extend",
- None,
- None,
- "Enum {PAD = 0, REPEAT = 1, REFLECT = 2}",
- ),
- ("uint16", "StopCount", None, None, "Number of Color stops."),
- ("VarColorStop", "ColorStop", "StopCount", 0, "Array of Color stops."),
- ],
- ),
- # PaintColrLayers
- (
- "PaintFormat1",
- [
- ("uint8", "PaintFormat", None, None, "Format identifier-format = 1"),
- (
- "uint8",
- "NumLayers",
- None,
- None,
- "Number of offsets to Paint to read from LayerList.",
- ),
- ("uint32", "FirstLayerIndex", None, None, "Index into LayerList."),
- ],
- ),
- # PaintSolid
- (
- "PaintFormat2",
- [
- ("uint8", "PaintFormat", None, None, "Format identifier-format = 2"),
- ("uint16", "PaletteIndex", None, None, "Index for a CPAL palette entry."),
- ("F2Dot14", "Alpha", None, None, "Values outsided [0.,1.] reserved"),
- ],
- ),
- # PaintVarSolid
- (
- "PaintFormat3",
- [
- ("uint8", "PaintFormat", None, None, "Format identifier-format = 3"),
- ("uint16", "PaletteIndex", None, None, "Index for a CPAL palette entry."),
- (
- "F2Dot14",
- "Alpha",
- None,
- None,
- "Values outsided [0.,1.] reserved. VarIndexBase + 0.",
- ),
- (
- "VarIndex",
- "VarIndexBase",
- None,
- None,
- "Base index into DeltaSetIndexMap.",
- ),
- ],
- ),
- # PaintLinearGradient
- (
- "PaintFormat4",
- [
- ("uint8", "PaintFormat", None, None, "Format identifier-format = 4"),
- (
- "Offset24",
- "ColorLine",
- None,
- None,
- "Offset (from beginning of PaintLinearGradient table) to ColorLine subtable.",
- ),
- ("int16", "x0", None, None, ""),
- ("int16", "y0", None, None, ""),
- ("int16", "x1", None, None, ""),
- ("int16", "y1", None, None, ""),
- ("int16", "x2", None, None, ""),
- ("int16", "y2", None, None, ""),
- ],
- ),
- # PaintVarLinearGradient
- (
- "PaintFormat5",
- [
- ("uint8", "PaintFormat", None, None, "Format identifier-format = 5"),
- (
- "LOffset24To(VarColorLine)",
- "ColorLine",
- None,
- None,
- "Offset (from beginning of PaintVarLinearGradient table) to VarColorLine subtable.",
- ),
- ("int16", "x0", None, None, "VarIndexBase + 0."),
- ("int16", "y0", None, None, "VarIndexBase + 1."),
- ("int16", "x1", None, None, "VarIndexBase + 2."),
- ("int16", "y1", None, None, "VarIndexBase + 3."),
- ("int16", "x2", None, None, "VarIndexBase + 4."),
- ("int16", "y2", None, None, "VarIndexBase + 5."),
- (
- "VarIndex",
- "VarIndexBase",
- None,
- None,
- "Base index into DeltaSetIndexMap.",
- ),
- ],
- ),
- # PaintRadialGradient
- (
- "PaintFormat6",
- [
- ("uint8", "PaintFormat", None, None, "Format identifier-format = 6"),
- (
- "Offset24",
- "ColorLine",
- None,
- None,
- "Offset (from beginning of PaintRadialGradient table) to ColorLine subtable.",
- ),
- ("int16", "x0", None, None, ""),
- ("int16", "y0", None, None, ""),
- ("uint16", "r0", None, None, ""),
- ("int16", "x1", None, None, ""),
- ("int16", "y1", None, None, ""),
- ("uint16", "r1", None, None, ""),
- ],
- ),
- # PaintVarRadialGradient
- (
- "PaintFormat7",
- [
- ("uint8", "PaintFormat", None, None, "Format identifier-format = 7"),
- (
- "LOffset24To(VarColorLine)",
- "ColorLine",
- None,
- None,
- "Offset (from beginning of PaintVarRadialGradient table) to VarColorLine subtable.",
- ),
- ("int16", "x0", None, None, "VarIndexBase + 0."),
- ("int16", "y0", None, None, "VarIndexBase + 1."),
- ("uint16", "r0", None, None, "VarIndexBase + 2."),
- ("int16", "x1", None, None, "VarIndexBase + 3."),
- ("int16", "y1", None, None, "VarIndexBase + 4."),
- ("uint16", "r1", None, None, "VarIndexBase + 5."),
- (
- "VarIndex",
- "VarIndexBase",
- None,
- None,
- "Base index into DeltaSetIndexMap.",
- ),
- ],
- ),
- # PaintSweepGradient
- (
- "PaintFormat8",
- [
- ("uint8", "PaintFormat", None, None, "Format identifier-format = 8"),
- (
- "Offset24",
- "ColorLine",
- None,
- None,
- "Offset (from beginning of PaintSweepGradient table) to ColorLine subtable.",
- ),
- ("int16", "centerX", None, None, "Center x coordinate."),
- ("int16", "centerY", None, None, "Center y coordinate."),
- (
- "BiasedAngle",
- "startAngle",
- None,
- None,
- "Start of the angular range of the gradient.",
- ),
- (
- "BiasedAngle",
- "endAngle",
- None,
- None,
- "End of the angular range of the gradient.",
- ),
- ],
- ),
- # PaintVarSweepGradient
- (
- "PaintFormat9",
- [
- ("uint8", "PaintFormat", None, None, "Format identifier-format = 9"),
- (
- "LOffset24To(VarColorLine)",
- "ColorLine",
- None,
- None,
- "Offset (from beginning of PaintVarSweepGradient table) to VarColorLine subtable.",
- ),
- ("int16", "centerX", None, None, "Center x coordinate. VarIndexBase + 0."),
- ("int16", "centerY", None, None, "Center y coordinate. VarIndexBase + 1."),
- (
- "BiasedAngle",
- "startAngle",
- None,
- None,
- "Start of the angular range of the gradient. VarIndexBase + 2.",
- ),
- (
- "BiasedAngle",
- "endAngle",
- None,
- None,
- "End of the angular range of the gradient. VarIndexBase + 3.",
- ),
- (
- "VarIndex",
- "VarIndexBase",
- None,
- None,
- "Base index into DeltaSetIndexMap.",
- ),
- ],
- ),
- # PaintGlyph
- (
- "PaintFormat10",
- [
- ("uint8", "PaintFormat", None, None, "Format identifier-format = 10"),
- (
- "Offset24",
- "Paint",
- None,
- None,
- "Offset (from beginning of PaintGlyph table) to Paint subtable.",
- ),
- ("GlyphID", "Glyph", None, None, "Glyph ID for the source outline."),
- ],
- ),
- # PaintColrGlyph
- (
- "PaintFormat11",
- [
- ("uint8", "PaintFormat", None, None, "Format identifier-format = 11"),
- (
- "GlyphID",
- "Glyph",
- None,
- None,
- "Virtual glyph ID for a BaseGlyphList base glyph.",
- ),
- ],
- ),
- # PaintTransform
- (
- "PaintFormat12",
- [
- ("uint8", "PaintFormat", None, None, "Format identifier-format = 12"),
- (
- "Offset24",
- "Paint",
- None,
- None,
- "Offset (from beginning of PaintTransform table) to Paint subtable.",
- ),
- (
- "LOffset24To(Affine2x3)",
- "Transform",
- None,
- None,
- "2x3 matrix for 2D affine transformations.",
- ),
- ],
- ),
- # PaintVarTransform
- (
- "PaintFormat13",
- [
- ("uint8", "PaintFormat", None, None, "Format identifier-format = 13"),
- (
- "Offset24",
- "Paint",
- None,
- None,
- "Offset (from beginning of PaintVarTransform table) to Paint subtable.",
- ),
- (
- "LOffset24To(VarAffine2x3)",
- "Transform",
- None,
- None,
- "2x3 matrix for 2D affine transformations.",
- ),
- ],
- ),
- # PaintTranslate
- (
- "PaintFormat14",
- [
- ("uint8", "PaintFormat", None, None, "Format identifier-format = 14"),
- (
- "Offset24",
- "Paint",
- None,
- None,
- "Offset (from beginning of PaintTranslate table) to Paint subtable.",
- ),
- ("int16", "dx", None, None, "Translation in x direction."),
- ("int16", "dy", None, None, "Translation in y direction."),
- ],
- ),
- # PaintVarTranslate
- (
- "PaintFormat15",
- [
- ("uint8", "PaintFormat", None, None, "Format identifier-format = 15"),
- (
- "Offset24",
- "Paint",
- None,
- None,
- "Offset (from beginning of PaintVarTranslate table) to Paint subtable.",
- ),
- (
- "int16",
- "dx",
- None,
- None,
- "Translation in x direction. VarIndexBase + 0.",
- ),
- (
- "int16",
- "dy",
- None,
- None,
- "Translation in y direction. VarIndexBase + 1.",
- ),
- (
- "VarIndex",
- "VarIndexBase",
- None,
- None,
- "Base index into DeltaSetIndexMap.",
- ),
- ],
- ),
- # PaintScale
- (
- "PaintFormat16",
- [
- ("uint8", "PaintFormat", None, None, "Format identifier-format = 16"),
- (
- "Offset24",
- "Paint",
- None,
- None,
- "Offset (from beginning of PaintScale table) to Paint subtable.",
- ),
- ("F2Dot14", "scaleX", None, None, ""),
- ("F2Dot14", "scaleY", None, None, ""),
- ],
- ),
- # PaintVarScale
- (
- "PaintFormat17",
- [
- ("uint8", "PaintFormat", None, None, "Format identifier-format = 17"),
- (
- "Offset24",
- "Paint",
- None,
- None,
- "Offset (from beginning of PaintVarScale table) to Paint subtable.",
- ),
- ("F2Dot14", "scaleX", None, None, "VarIndexBase + 0."),
- ("F2Dot14", "scaleY", None, None, "VarIndexBase + 1."),
- (
- "VarIndex",
- "VarIndexBase",
- None,
- None,
- "Base index into DeltaSetIndexMap.",
- ),
- ],
- ),
- # PaintScaleAroundCenter
- (
- "PaintFormat18",
- [
- ("uint8", "PaintFormat", None, None, "Format identifier-format = 18"),
- (
- "Offset24",
- "Paint",
- None,
- None,
- "Offset (from beginning of PaintScaleAroundCenter table) to Paint subtable.",
- ),
- ("F2Dot14", "scaleX", None, None, ""),
- ("F2Dot14", "scaleY", None, None, ""),
- ("int16", "centerX", None, None, ""),
- ("int16", "centerY", None, None, ""),
- ],
- ),
- # PaintVarScaleAroundCenter
- (
- "PaintFormat19",
- [
- ("uint8", "PaintFormat", None, None, "Format identifier-format = 19"),
- (
- "Offset24",
- "Paint",
- None,
- None,
- "Offset (from beginning of PaintVarScaleAroundCenter table) to Paint subtable.",
- ),
- ("F2Dot14", "scaleX", None, None, "VarIndexBase + 0."),
- ("F2Dot14", "scaleY", None, None, "VarIndexBase + 1."),
- ("int16", "centerX", None, None, "VarIndexBase + 2."),
- ("int16", "centerY", None, None, "VarIndexBase + 3."),
- (
- "VarIndex",
- "VarIndexBase",
- None,
- None,
- "Base index into DeltaSetIndexMap.",
- ),
- ],
- ),
- # PaintScaleUniform
- (
- "PaintFormat20",
- [
- ("uint8", "PaintFormat", None, None, "Format identifier-format = 20"),
- (
- "Offset24",
- "Paint",
- None,
- None,
- "Offset (from beginning of PaintScaleUniform table) to Paint subtable.",
- ),
- ("F2Dot14", "scale", None, None, ""),
- ],
- ),
- # PaintVarScaleUniform
- (
- "PaintFormat21",
- [
- ("uint8", "PaintFormat", None, None, "Format identifier-format = 21"),
- (
- "Offset24",
- "Paint",
- None,
- None,
- "Offset (from beginning of PaintVarScaleUniform table) to Paint subtable.",
- ),
- ("F2Dot14", "scale", None, None, "VarIndexBase + 0."),
- (
- "VarIndex",
- "VarIndexBase",
- None,
- None,
- "Base index into DeltaSetIndexMap.",
- ),
- ],
- ),
- # PaintScaleUniformAroundCenter
- (
- "PaintFormat22",
- [
- ("uint8", "PaintFormat", None, None, "Format identifier-format = 22"),
- (
- "Offset24",
- "Paint",
- None,
- None,
- "Offset (from beginning of PaintScaleUniformAroundCenter table) to Paint subtable.",
- ),
- ("F2Dot14", "scale", None, None, ""),
- ("int16", "centerX", None, None, ""),
- ("int16", "centerY", None, None, ""),
- ],
- ),
- # PaintVarScaleUniformAroundCenter
- (
- "PaintFormat23",
- [
- ("uint8", "PaintFormat", None, None, "Format identifier-format = 23"),
- (
- "Offset24",
- "Paint",
- None,
- None,
- "Offset (from beginning of PaintVarScaleUniformAroundCenter table) to Paint subtable.",
- ),
- ("F2Dot14", "scale", None, None, "VarIndexBase + 0"),
- ("int16", "centerX", None, None, "VarIndexBase + 1"),
- ("int16", "centerY", None, None, "VarIndexBase + 2"),
- (
- "VarIndex",
- "VarIndexBase",
- None,
- None,
- "Base index into DeltaSetIndexMap.",
- ),
- ],
- ),
- # PaintRotate
- (
- "PaintFormat24",
- [
- ("uint8", "PaintFormat", None, None, "Format identifier-format = 24"),
- (
- "Offset24",
- "Paint",
- None,
- None,
- "Offset (from beginning of PaintRotate table) to Paint subtable.",
- ),
- ("Angle", "angle", None, None, ""),
- ],
- ),
- # PaintVarRotate
- (
- "PaintFormat25",
- [
- ("uint8", "PaintFormat", None, None, "Format identifier-format = 25"),
- (
- "Offset24",
- "Paint",
- None,
- None,
- "Offset (from beginning of PaintVarRotate table) to Paint subtable.",
- ),
- ("Angle", "angle", None, None, "VarIndexBase + 0."),
- (
- "VarIndex",
- "VarIndexBase",
- None,
- None,
- "Base index into DeltaSetIndexMap.",
- ),
- ],
- ),
- # PaintRotateAroundCenter
- (
- "PaintFormat26",
- [
- ("uint8", "PaintFormat", None, None, "Format identifier-format = 26"),
- (
- "Offset24",
- "Paint",
- None,
- None,
- "Offset (from beginning of PaintRotateAroundCenter table) to Paint subtable.",
- ),
- ("Angle", "angle", None, None, ""),
- ("int16", "centerX", None, None, ""),
- ("int16", "centerY", None, None, ""),
- ],
- ),
- # PaintVarRotateAroundCenter
- (
- "PaintFormat27",
- [
- ("uint8", "PaintFormat", None, None, "Format identifier-format = 27"),
- (
- "Offset24",
- "Paint",
- None,
- None,
- "Offset (from beginning of PaintVarRotateAroundCenter table) to Paint subtable.",
- ),
- ("Angle", "angle", None, None, "VarIndexBase + 0."),
- ("int16", "centerX", None, None, "VarIndexBase + 1."),
- ("int16", "centerY", None, None, "VarIndexBase + 2."),
- (
- "VarIndex",
- "VarIndexBase",
- None,
- None,
- "Base index into DeltaSetIndexMap.",
- ),
- ],
- ),
- # PaintSkew
- (
- "PaintFormat28",
- [
- ("uint8", "PaintFormat", None, None, "Format identifier-format = 28"),
- (
- "Offset24",
- "Paint",
- None,
- None,
- "Offset (from beginning of PaintSkew table) to Paint subtable.",
- ),
- ("Angle", "xSkewAngle", None, None, ""),
- ("Angle", "ySkewAngle", None, None, ""),
- ],
- ),
- # PaintVarSkew
- (
- "PaintFormat29",
- [
- ("uint8", "PaintFormat", None, None, "Format identifier-format = 29"),
- (
- "Offset24",
- "Paint",
- None,
- None,
- "Offset (from beginning of PaintVarSkew table) to Paint subtable.",
- ),
- ("Angle", "xSkewAngle", None, None, "VarIndexBase + 0."),
- ("Angle", "ySkewAngle", None, None, "VarIndexBase + 1."),
- (
- "VarIndex",
- "VarIndexBase",
- None,
- None,
- "Base index into DeltaSetIndexMap.",
- ),
- ],
- ),
- # PaintSkewAroundCenter
- (
- "PaintFormat30",
- [
- ("uint8", "PaintFormat", None, None, "Format identifier-format = 30"),
- (
- "Offset24",
- "Paint",
- None,
- None,
- "Offset (from beginning of PaintSkewAroundCenter table) to Paint subtable.",
- ),
- ("Angle", "xSkewAngle", None, None, ""),
- ("Angle", "ySkewAngle", None, None, ""),
- ("int16", "centerX", None, None, ""),
- ("int16", "centerY", None, None, ""),
- ],
- ),
- # PaintVarSkewAroundCenter
- (
- "PaintFormat31",
- [
- ("uint8", "PaintFormat", None, None, "Format identifier-format = 31"),
- (
- "Offset24",
- "Paint",
- None,
- None,
- "Offset (from beginning of PaintVarSkewAroundCenter table) to Paint subtable.",
- ),
- ("Angle", "xSkewAngle", None, None, "VarIndexBase + 0."),
- ("Angle", "ySkewAngle", None, None, "VarIndexBase + 1."),
- ("int16", "centerX", None, None, "VarIndexBase + 2."),
- ("int16", "centerY", None, None, "VarIndexBase + 3."),
- (
- "VarIndex",
- "VarIndexBase",
- None,
- None,
- "Base index into DeltaSetIndexMap.",
- ),
- ],
- ),
- # PaintComposite
- (
- "PaintFormat32",
- [
- ("uint8", "PaintFormat", None, None, "Format identifier-format = 32"),
- (
- "LOffset24To(Paint)",
- "SourcePaint",
- None,
- None,
- "Offset (from beginning of PaintComposite table) to source Paint subtable.",
- ),
- (
- "CompositeMode",
- "CompositeMode",
- None,
- None,
- "A CompositeMode enumeration value.",
- ),
- (
- "LOffset24To(Paint)",
- "BackdropPaint",
- None,
- None,
- "Offset (from beginning of PaintComposite table) to backdrop Paint subtable.",
- ),
- ],
- ),
- #
- # avar
- #
- (
- "AxisValueMap",
- [
- (
- "F2Dot14",
- "FromCoordinate",
- None,
- None,
- "A normalized coordinate value obtained using default normalization",
- ),
- (
- "F2Dot14",
- "ToCoordinate",
- None,
- None,
- "The modified, normalized coordinate value",
- ),
- ],
- ),
- (
- "AxisSegmentMap",
- [
- (
- "uint16",
- "PositionMapCount",
- None,
- None,
- "The number of correspondence pairs for this axis",
- ),
- (
- "AxisValueMap",
- "AxisValueMap",
- "PositionMapCount",
- 0,
- "The array of axis value map records for this axis",
- ),
- ],
- ),
- (
- "avar",
- [
- (
- "Version",
- "Version",
- None,
- None,
- "Version of the avar table- 0x00010000 or 0x00020000",
- ),
- ("uint16", "Reserved", None, None, "Permanently reserved; set to zero"),
- (
- "uint16",
- "AxisCount",
- None,
- None,
- 'The number of variation axes for this font. This must be the same number as axisCount in the "fvar" table',
- ),
- (
- "AxisSegmentMap",
- "AxisSegmentMap",
- "AxisCount",
- 0,
- 'The segment maps array — one segment map for each axis, in the order of axes specified in the "fvar" table',
- ),
- (
- "LOffsetTo(DeltaSetIndexMap)",
- "VarIdxMap",
- None,
- "Version >= 0x00020000",
- "",
- ),
- ("LOffset", "VarStore", None, "Version >= 0x00020000", ""),
- ],
- ),
-]
diff --git a/spaces/chuan-hd/law-assistant-chatbot/.venv/lib/python3.11/site-packages/google/protobuf/internal/type_checkers.py b/spaces/chuan-hd/law-assistant-chatbot/.venv/lib/python3.11/site-packages/google/protobuf/internal/type_checkers.py
deleted file mode 100644
index 165dcd8c2e855117531a3917a4e008dd0cf02089..0000000000000000000000000000000000000000
--- a/spaces/chuan-hd/law-assistant-chatbot/.venv/lib/python3.11/site-packages/google/protobuf/internal/type_checkers.py
+++ /dev/null
@@ -1,431 +0,0 @@
-# Protocol Buffers - Google's data interchange format
-# Copyright 2008 Google Inc. All rights reserved.
-# https://developers.google.com/protocol-buffers/
-#
-# Redistribution and use in source and binary forms, with or without
-# modification, are permitted provided that the following conditions are
-# met:
-#
-# * Redistributions of source code must retain the above copyright
-# notice, this list of conditions and the following disclaimer.
-# * Redistributions in binary form must reproduce the above
-# copyright notice, this list of conditions and the following disclaimer
-# in the documentation and/or other materials provided with the
-# distribution.
-# * Neither the name of Google Inc. nor the names of its
-# contributors may be used to endorse or promote products derived from
-# this software without specific prior written permission.
-#
-# THIS SOFTWARE IS PROVIDED BY THE COPYRIGHT HOLDERS AND CONTRIBUTORS
-# "AS IS" AND ANY EXPRESS OR IMPLIED WARRANTIES, INCLUDING, BUT NOT
-# LIMITED TO, THE IMPLIED WARRANTIES OF MERCHANTABILITY AND FITNESS FOR
-# A PARTICULAR PURPOSE ARE DISCLAIMED. IN NO EVENT SHALL THE COPYRIGHT
-# OWNER OR CONTRIBUTORS BE LIABLE FOR ANY DIRECT, INDIRECT, INCIDENTAL,
-# SPECIAL, EXEMPLARY, OR CONSEQUENTIAL DAMAGES (INCLUDING, BUT NOT
-# LIMITED TO, PROCUREMENT OF SUBSTITUTE GOODS OR SERVICES; LOSS OF USE,
-# DATA, OR PROFITS; OR BUSINESS INTERRUPTION) HOWEVER CAUSED AND ON ANY
-# THEORY OF LIABILITY, WHETHER IN CONTRACT, STRICT LIABILITY, OR TORT
-# (INCLUDING NEGLIGENCE OR OTHERWISE) ARISING IN ANY WAY OUT OF THE USE
-# OF THIS SOFTWARE, EVEN IF ADVISED OF THE POSSIBILITY OF SUCH DAMAGE.
-
-"""Provides type checking routines.
-
-This module defines type checking utilities in the forms of dictionaries:
-
-VALUE_CHECKERS: A dictionary of field types and a value validation object.
-TYPE_TO_BYTE_SIZE_FN: A dictionary with field types and a size computing
- function.
-TYPE_TO_SERIALIZE_METHOD: A dictionary with field types and serialization
- function.
-FIELD_TYPE_TO_WIRE_TYPE: A dictionary with field typed and their
- corresponding wire types.
-TYPE_TO_DESERIALIZE_METHOD: A dictionary with field types and deserialization
- function.
-"""
-
-__author__ = 'robinson@google.com (Will Robinson)'
-
-import ctypes
-import numbers
-
-from google.protobuf.internal import decoder
-from google.protobuf.internal import encoder
-from google.protobuf.internal import wire_format
-from google.protobuf import descriptor
-
-_FieldDescriptor = descriptor.FieldDescriptor
-
-
-def TruncateToFourByteFloat(original):
- return ctypes.c_float(original).value
-
-
-def ToShortestFloat(original):
- """Returns the shortest float that has same value in wire."""
- # All 4 byte floats have between 6 and 9 significant digits, so we
- # start with 6 as the lower bound.
- # It has to be iterative because use '.9g' directly can not get rid
- # of the noises for most values. For example if set a float_field=0.9
- # use '.9g' will print 0.899999976.
- precision = 6
- rounded = float('{0:.{1}g}'.format(original, precision))
- while TruncateToFourByteFloat(rounded) != original:
- precision += 1
- rounded = float('{0:.{1}g}'.format(original, precision))
- return rounded
-
-
-def GetTypeChecker(field):
- """Returns a type checker for a message field of the specified types.
-
- Args:
- field: FieldDescriptor object for this field.
-
- Returns:
- An instance of TypeChecker which can be used to verify the types
- of values assigned to a field of the specified type.
- """
- if (field.cpp_type == _FieldDescriptor.CPPTYPE_STRING and
- field.type == _FieldDescriptor.TYPE_STRING):
- return UnicodeValueChecker()
- if field.cpp_type == _FieldDescriptor.CPPTYPE_ENUM:
- if field.enum_type.is_closed:
- return EnumValueChecker(field.enum_type)
- else:
- # When open enums are supported, any int32 can be assigned.
- return _VALUE_CHECKERS[_FieldDescriptor.CPPTYPE_INT32]
- return _VALUE_CHECKERS[field.cpp_type]
-
-
-# None of the typecheckers below make any attempt to guard against people
-# subclassing builtin types and doing weird things. We're not trying to
-# protect against malicious clients here, just people accidentally shooting
-# themselves in the foot in obvious ways.
-class TypeChecker(object):
-
- """Type checker used to catch type errors as early as possible
- when the client is setting scalar fields in protocol messages.
- """
-
- def __init__(self, *acceptable_types):
- self._acceptable_types = acceptable_types
-
- def CheckValue(self, proposed_value):
- """Type check the provided value and return it.
-
- The returned value might have been normalized to another type.
- """
- if not isinstance(proposed_value, self._acceptable_types):
- message = ('%.1024r has type %s, but expected one of: %s' %
- (proposed_value, type(proposed_value), self._acceptable_types))
- raise TypeError(message)
- return proposed_value
-
-
-class TypeCheckerWithDefault(TypeChecker):
-
- def __init__(self, default_value, *acceptable_types):
- TypeChecker.__init__(self, *acceptable_types)
- self._default_value = default_value
-
- def DefaultValue(self):
- return self._default_value
-
-
-class BoolValueChecker(object):
- """Type checker used for bool fields."""
-
- def CheckValue(self, proposed_value):
- if not hasattr(proposed_value, '__index__') or (
- type(proposed_value).__module__ == 'numpy' and
- type(proposed_value).__name__ == 'ndarray'):
- message = ('%.1024r has type %s, but expected one of: %s' %
- (proposed_value, type(proposed_value), (bool, int)))
- raise TypeError(message)
- return bool(proposed_value)
-
- def DefaultValue(self):
- return False
-
-
-# IntValueChecker and its subclasses perform integer type-checks
-# and bounds-checks.
-class IntValueChecker(object):
-
- """Checker used for integer fields. Performs type-check and range check."""
-
- def CheckValue(self, proposed_value):
- if not hasattr(proposed_value, '__index__') or (
- type(proposed_value).__module__ == 'numpy' and
- type(proposed_value).__name__ == 'ndarray'):
- message = ('%.1024r has type %s, but expected one of: %s' %
- (proposed_value, type(proposed_value), (int,)))
- raise TypeError(message)
-
- if not self._MIN <= int(proposed_value) <= self._MAX:
- raise ValueError('Value out of range: %d' % proposed_value)
- # We force all values to int to make alternate implementations where the
- # distinction is more significant (e.g. the C++ implementation) simpler.
- proposed_value = int(proposed_value)
- return proposed_value
-
- def DefaultValue(self):
- return 0
-
-
-class EnumValueChecker(object):
-
- """Checker used for enum fields. Performs type-check and range check."""
-
- def __init__(self, enum_type):
- self._enum_type = enum_type
-
- def CheckValue(self, proposed_value):
- if not isinstance(proposed_value, numbers.Integral):
- message = ('%.1024r has type %s, but expected one of: %s' %
- (proposed_value, type(proposed_value), (int,)))
- raise TypeError(message)
- if int(proposed_value) not in self._enum_type.values_by_number:
- raise ValueError('Unknown enum value: %d' % proposed_value)
- return proposed_value
-
- def DefaultValue(self):
- return self._enum_type.values[0].number
-
-
-class UnicodeValueChecker(object):
-
- """Checker used for string fields.
-
- Always returns a unicode value, even if the input is of type str.
- """
-
- def CheckValue(self, proposed_value):
- if not isinstance(proposed_value, (bytes, str)):
- message = ('%.1024r has type %s, but expected one of: %s' %
- (proposed_value, type(proposed_value), (bytes, str)))
- raise TypeError(message)
-
- # If the value is of type 'bytes' make sure that it is valid UTF-8 data.
- if isinstance(proposed_value, bytes):
- try:
- proposed_value = proposed_value.decode('utf-8')
- except UnicodeDecodeError:
- raise ValueError('%.1024r has type bytes, but isn\'t valid UTF-8 '
- 'encoding. Non-UTF-8 strings must be converted to '
- 'unicode objects before being added.' %
- (proposed_value))
- else:
- try:
- proposed_value.encode('utf8')
- except UnicodeEncodeError:
- raise ValueError('%.1024r isn\'t a valid unicode string and '
- 'can\'t be encoded in UTF-8.'%
- (proposed_value))
-
- return proposed_value
-
- def DefaultValue(self):
- return u""
-
-
-class Int32ValueChecker(IntValueChecker):
- # We're sure to use ints instead of longs here since comparison may be more
- # efficient.
- _MIN = -2147483648
- _MAX = 2147483647
-
-
-class Uint32ValueChecker(IntValueChecker):
- _MIN = 0
- _MAX = (1 << 32) - 1
-
-
-class Int64ValueChecker(IntValueChecker):
- _MIN = -(1 << 63)
- _MAX = (1 << 63) - 1
-
-
-class Uint64ValueChecker(IntValueChecker):
- _MIN = 0
- _MAX = (1 << 64) - 1
-
-
-# The max 4 bytes float is about 3.4028234663852886e+38
-_FLOAT_MAX = float.fromhex('0x1.fffffep+127')
-_FLOAT_MIN = -_FLOAT_MAX
-_INF = float('inf')
-_NEG_INF = float('-inf')
-
-
-class DoubleValueChecker(object):
- """Checker used for double fields.
-
- Performs type-check and range check.
- """
-
- def CheckValue(self, proposed_value):
- """Check and convert proposed_value to float."""
- if (not hasattr(proposed_value, '__float__') and
- not hasattr(proposed_value, '__index__')) or (
- type(proposed_value).__module__ == 'numpy' and
- type(proposed_value).__name__ == 'ndarray'):
- message = ('%.1024r has type %s, but expected one of: int, float' %
- (proposed_value, type(proposed_value)))
- raise TypeError(message)
- return float(proposed_value)
-
- def DefaultValue(self):
- return 0.0
-
-
-class FloatValueChecker(DoubleValueChecker):
- """Checker used for float fields.
-
- Performs type-check and range check.
-
- Values exceeding a 32-bit float will be converted to inf/-inf.
- """
-
- def CheckValue(self, proposed_value):
- """Check and convert proposed_value to float."""
- converted_value = super().CheckValue(proposed_value)
- # This inf rounding matches the C++ proto SafeDoubleToFloat logic.
- if converted_value > _FLOAT_MAX:
- return _INF
- if converted_value < _FLOAT_MIN:
- return _NEG_INF
-
- return TruncateToFourByteFloat(converted_value)
-
-# Type-checkers for all scalar CPPTYPEs.
-_VALUE_CHECKERS = {
- _FieldDescriptor.CPPTYPE_INT32: Int32ValueChecker(),
- _FieldDescriptor.CPPTYPE_INT64: Int64ValueChecker(),
- _FieldDescriptor.CPPTYPE_UINT32: Uint32ValueChecker(),
- _FieldDescriptor.CPPTYPE_UINT64: Uint64ValueChecker(),
- _FieldDescriptor.CPPTYPE_DOUBLE: DoubleValueChecker(),
- _FieldDescriptor.CPPTYPE_FLOAT: FloatValueChecker(),
- _FieldDescriptor.CPPTYPE_BOOL: BoolValueChecker(),
- _FieldDescriptor.CPPTYPE_STRING: TypeCheckerWithDefault(b'', bytes),
-}
-
-
-# Map from field type to a function F, such that F(field_num, value)
-# gives the total byte size for a value of the given type. This
-# byte size includes tag information and any other additional space
-# associated with serializing "value".
-TYPE_TO_BYTE_SIZE_FN = {
- _FieldDescriptor.TYPE_DOUBLE: wire_format.DoubleByteSize,
- _FieldDescriptor.TYPE_FLOAT: wire_format.FloatByteSize,
- _FieldDescriptor.TYPE_INT64: wire_format.Int64ByteSize,
- _FieldDescriptor.TYPE_UINT64: wire_format.UInt64ByteSize,
- _FieldDescriptor.TYPE_INT32: wire_format.Int32ByteSize,
- _FieldDescriptor.TYPE_FIXED64: wire_format.Fixed64ByteSize,
- _FieldDescriptor.TYPE_FIXED32: wire_format.Fixed32ByteSize,
- _FieldDescriptor.TYPE_BOOL: wire_format.BoolByteSize,
- _FieldDescriptor.TYPE_STRING: wire_format.StringByteSize,
- _FieldDescriptor.TYPE_GROUP: wire_format.GroupByteSize,
- _FieldDescriptor.TYPE_MESSAGE: wire_format.MessageByteSize,
- _FieldDescriptor.TYPE_BYTES: wire_format.BytesByteSize,
- _FieldDescriptor.TYPE_UINT32: wire_format.UInt32ByteSize,
- _FieldDescriptor.TYPE_ENUM: wire_format.EnumByteSize,
- _FieldDescriptor.TYPE_SFIXED32: wire_format.SFixed32ByteSize,
- _FieldDescriptor.TYPE_SFIXED64: wire_format.SFixed64ByteSize,
- _FieldDescriptor.TYPE_SINT32: wire_format.SInt32ByteSize,
- _FieldDescriptor.TYPE_SINT64: wire_format.SInt64ByteSize
- }
-
-
-# Maps from field types to encoder constructors.
-TYPE_TO_ENCODER = {
- _FieldDescriptor.TYPE_DOUBLE: encoder.DoubleEncoder,
- _FieldDescriptor.TYPE_FLOAT: encoder.FloatEncoder,
- _FieldDescriptor.TYPE_INT64: encoder.Int64Encoder,
- _FieldDescriptor.TYPE_UINT64: encoder.UInt64Encoder,
- _FieldDescriptor.TYPE_INT32: encoder.Int32Encoder,
- _FieldDescriptor.TYPE_FIXED64: encoder.Fixed64Encoder,
- _FieldDescriptor.TYPE_FIXED32: encoder.Fixed32Encoder,
- _FieldDescriptor.TYPE_BOOL: encoder.BoolEncoder,
- _FieldDescriptor.TYPE_STRING: encoder.StringEncoder,
- _FieldDescriptor.TYPE_GROUP: encoder.GroupEncoder,
- _FieldDescriptor.TYPE_MESSAGE: encoder.MessageEncoder,
- _FieldDescriptor.TYPE_BYTES: encoder.BytesEncoder,
- _FieldDescriptor.TYPE_UINT32: encoder.UInt32Encoder,
- _FieldDescriptor.TYPE_ENUM: encoder.EnumEncoder,
- _FieldDescriptor.TYPE_SFIXED32: encoder.SFixed32Encoder,
- _FieldDescriptor.TYPE_SFIXED64: encoder.SFixed64Encoder,
- _FieldDescriptor.TYPE_SINT32: encoder.SInt32Encoder,
- _FieldDescriptor.TYPE_SINT64: encoder.SInt64Encoder,
- }
-
-
-# Maps from field types to sizer constructors.
-TYPE_TO_SIZER = {
- _FieldDescriptor.TYPE_DOUBLE: encoder.DoubleSizer,
- _FieldDescriptor.TYPE_FLOAT: encoder.FloatSizer,
- _FieldDescriptor.TYPE_INT64: encoder.Int64Sizer,
- _FieldDescriptor.TYPE_UINT64: encoder.UInt64Sizer,
- _FieldDescriptor.TYPE_INT32: encoder.Int32Sizer,
- _FieldDescriptor.TYPE_FIXED64: encoder.Fixed64Sizer,
- _FieldDescriptor.TYPE_FIXED32: encoder.Fixed32Sizer,
- _FieldDescriptor.TYPE_BOOL: encoder.BoolSizer,
- _FieldDescriptor.TYPE_STRING: encoder.StringSizer,
- _FieldDescriptor.TYPE_GROUP: encoder.GroupSizer,
- _FieldDescriptor.TYPE_MESSAGE: encoder.MessageSizer,
- _FieldDescriptor.TYPE_BYTES: encoder.BytesSizer,
- _FieldDescriptor.TYPE_UINT32: encoder.UInt32Sizer,
- _FieldDescriptor.TYPE_ENUM: encoder.EnumSizer,
- _FieldDescriptor.TYPE_SFIXED32: encoder.SFixed32Sizer,
- _FieldDescriptor.TYPE_SFIXED64: encoder.SFixed64Sizer,
- _FieldDescriptor.TYPE_SINT32: encoder.SInt32Sizer,
- _FieldDescriptor.TYPE_SINT64: encoder.SInt64Sizer,
- }
-
-
-# Maps from field type to a decoder constructor.
-TYPE_TO_DECODER = {
- _FieldDescriptor.TYPE_DOUBLE: decoder.DoubleDecoder,
- _FieldDescriptor.TYPE_FLOAT: decoder.FloatDecoder,
- _FieldDescriptor.TYPE_INT64: decoder.Int64Decoder,
- _FieldDescriptor.TYPE_UINT64: decoder.UInt64Decoder,
- _FieldDescriptor.TYPE_INT32: decoder.Int32Decoder,
- _FieldDescriptor.TYPE_FIXED64: decoder.Fixed64Decoder,
- _FieldDescriptor.TYPE_FIXED32: decoder.Fixed32Decoder,
- _FieldDescriptor.TYPE_BOOL: decoder.BoolDecoder,
- _FieldDescriptor.TYPE_STRING: decoder.StringDecoder,
- _FieldDescriptor.TYPE_GROUP: decoder.GroupDecoder,
- _FieldDescriptor.TYPE_MESSAGE: decoder.MessageDecoder,
- _FieldDescriptor.TYPE_BYTES: decoder.BytesDecoder,
- _FieldDescriptor.TYPE_UINT32: decoder.UInt32Decoder,
- _FieldDescriptor.TYPE_ENUM: decoder.EnumDecoder,
- _FieldDescriptor.TYPE_SFIXED32: decoder.SFixed32Decoder,
- _FieldDescriptor.TYPE_SFIXED64: decoder.SFixed64Decoder,
- _FieldDescriptor.TYPE_SINT32: decoder.SInt32Decoder,
- _FieldDescriptor.TYPE_SINT64: decoder.SInt64Decoder,
- }
-
-# Maps from field type to expected wiretype.
-FIELD_TYPE_TO_WIRE_TYPE = {
- _FieldDescriptor.TYPE_DOUBLE: wire_format.WIRETYPE_FIXED64,
- _FieldDescriptor.TYPE_FLOAT: wire_format.WIRETYPE_FIXED32,
- _FieldDescriptor.TYPE_INT64: wire_format.WIRETYPE_VARINT,
- _FieldDescriptor.TYPE_UINT64: wire_format.WIRETYPE_VARINT,
- _FieldDescriptor.TYPE_INT32: wire_format.WIRETYPE_VARINT,
- _FieldDescriptor.TYPE_FIXED64: wire_format.WIRETYPE_FIXED64,
- _FieldDescriptor.TYPE_FIXED32: wire_format.WIRETYPE_FIXED32,
- _FieldDescriptor.TYPE_BOOL: wire_format.WIRETYPE_VARINT,
- _FieldDescriptor.TYPE_STRING:
- wire_format.WIRETYPE_LENGTH_DELIMITED,
- _FieldDescriptor.TYPE_GROUP: wire_format.WIRETYPE_START_GROUP,
- _FieldDescriptor.TYPE_MESSAGE:
- wire_format.WIRETYPE_LENGTH_DELIMITED,
- _FieldDescriptor.TYPE_BYTES:
- wire_format.WIRETYPE_LENGTH_DELIMITED,
- _FieldDescriptor.TYPE_UINT32: wire_format.WIRETYPE_VARINT,
- _FieldDescriptor.TYPE_ENUM: wire_format.WIRETYPE_VARINT,
- _FieldDescriptor.TYPE_SFIXED32: wire_format.WIRETYPE_FIXED32,
- _FieldDescriptor.TYPE_SFIXED64: wire_format.WIRETYPE_FIXED64,
- _FieldDescriptor.TYPE_SINT32: wire_format.WIRETYPE_VARINT,
- _FieldDescriptor.TYPE_SINT64: wire_format.WIRETYPE_VARINT,
- }
diff --git a/spaces/cihyFjudo/fairness-paper-search/CADtools 8 win torrent A free and easy-to-use CAD software for Windows.md b/spaces/cihyFjudo/fairness-paper-search/CADtools 8 win torrent A free and easy-to-use CAD software for Windows.md
deleted file mode 100644
index 666f83c9157b3d6b3f42eba83ea8a3c34ffa7f8f..0000000000000000000000000000000000000000
--- a/spaces/cihyFjudo/fairness-paper-search/CADtools 8 win torrent A free and easy-to-use CAD software for Windows.md
+++ /dev/null
@@ -1,6 +0,0 @@
-cadtools 8 win torrent Download →→→ https://tinurli.com/2uwjXP
-
- aaccfb2cb3
-
-
-
diff --git a/spaces/cihyFjudo/fairness-paper-search/Download Fall Out Boy Folie A Deux 17 The Album that Inspired a Guinness World Record Attempt by the Band.md b/spaces/cihyFjudo/fairness-paper-search/Download Fall Out Boy Folie A Deux 17 The Album that Inspired a Guinness World Record Attempt by the Band.md
deleted file mode 100644
index 9138dea55bac531a9a7d8d149e6805ca1f263f14..0000000000000000000000000000000000000000
--- a/spaces/cihyFjudo/fairness-paper-search/Download Fall Out Boy Folie A Deux 17 The Album that Inspired a Guinness World Record Attempt by the Band.md
+++ /dev/null
@@ -1,6 +0,0 @@
-
-Folie à deux is a rare psychiatric syndrome in which symptoms of a delusional belief are transmitted from one individual to another. The same syndrome shared by more than two people may be called folie à trois, folie à quatre, folie en famille or even folie à plusieurs ("madness of many"). Recent psychiatric classifications refer to the syndrome as dependency psychotic disorder or induced delusional disorder, although the research literature largely uses the original name. The disorder was first conceptualized in 19th century French psychiatry. In keeping with the record's socially aware nature, the band felt that the term was relevant to the candidates in the 2008 U.S. presidential election. Stump further clarified the title's meaning: "The irony is that people will probably mistake the title as something about romantic relationships in some way. And it's our only record where that theme is not touched upon."
-The album received generally positive reviews from music critics. AtMetacritic, which assigns a normalized rating out of 100 to reviews from mainstream critics, the album received an average score of 73, based on 21 reviews, which indicates "generally favorable reviews". Dan Martin of NME gave the record a very positive review, calling a "defining statement" with the band's "most stylistically hatstand-but-indisputably-best songs yet." He wrote, "We're not saying it's as good as genre watermarks American Idiot or The Black Parade . We're just saying it comes close," closing with calling it a "staggering achievement." Stephen Thomas Erlewine of Allmusic rated the album four out of five stars and compared it to labelmate Panic! at the Disco's effort earlier in the year, Pretty. Odd. He wrote that "Fall Out Boy capture the Zeitgeist of the latter half of the 2000s better than any band: there's so much going on in Folie à Deux , you either choose to take it all seriously or take none of it. Fall Out Boy make as much sense when heard either way." Scott Heisel wrote for Alternative Press , commending the band for its "creativity, ingenuity and willingness to try just about anything." He compared the meaning of the term folie à deux ("a madness shared by two") to the two very distinct feelings expressed in the different sides of the record, calling the album a good representation of the band's career.
-Download Fall Out Boy Folie A Deux 17 Download ✶✶✶ https://tinurli.com/2uwinE
aaccfb2cb3
-
-
\ No newline at end of file
diff --git a/spaces/cihyFjudo/fairness-paper-search/Yuki Saito CD-BOX 12 (mp3 320) A Tribute to the Legendary Voice of Yuki Saito.md b/spaces/cihyFjudo/fairness-paper-search/Yuki Saito CD-BOX 12 (mp3 320) A Tribute to the Legendary Voice of Yuki Saito.md
deleted file mode 100644
index 5fb5eb8dff1a7eb88b29eab8bb09db65f317da2d..0000000000000000000000000000000000000000
--- a/spaces/cihyFjudo/fairness-paper-search/Yuki Saito CD-BOX 12 (mp3 320) A Tribute to the Legendary Voice of Yuki Saito.md
+++ /dev/null
@@ -1,6 +0,0 @@
-Yuki Saito CD-BOX 12 (mp3 320) DOWNLOAD ✶✶✶ https://tinurli.com/2uwiWd
-
- aaccfb2cb3
-
-
-
diff --git a/spaces/ck46/extractive_summaries/README.md b/spaces/ck46/extractive_summaries/README.md
deleted file mode 100644
index 86fd83b572dcd7726bedd1f1f655a10b85731515..0000000000000000000000000000000000000000
--- a/spaces/ck46/extractive_summaries/README.md
+++ /dev/null
@@ -1,37 +0,0 @@
----
-title: Extractive_summaries
-emoji: 👁
-colorFrom: red
-colorTo: gray
-sdk: streamlit
-app_file: app.py
-pinned: false
----
-
-# Configuration
-
-`title`: _string_
-Display title for the Space
-
-`emoji`: _string_
-Space emoji (emoji-only character allowed)
-
-`colorFrom`: _string_
-Color for Thumbnail gradient (red, yellow, green, blue, indigo, purple, pink, gray)
-
-`colorTo`: _string_
-Color for Thumbnail gradient (red, yellow, green, blue, indigo, purple, pink, gray)
-
-`sdk`: _string_
-Can be either `gradio` or `streamlit`
-
-`sdk_version` : _string_
-Only applicable for `streamlit` SDK.
-See [doc](https://hf.co/docs/hub/spaces) for more info on supported versions.
-
-`app_file`: _string_
-Path to your main application file (which contains either `gradio` or `streamlit` Python code).
-Path is relative to the root of the repository.
-
-`pinned`: _boolean_
-Whether the Space stays on top of your list.
diff --git a/spaces/cloudtheboi/Lofi4All/.pythonlibs/lib/python3.10/site-packages/anyio/streams/file.py b/spaces/cloudtheboi/Lofi4All/.pythonlibs/lib/python3.10/site-packages/anyio/streams/file.py
deleted file mode 100644
index 2840d40ab6a2fa222d6594d6980d8234df17eade..0000000000000000000000000000000000000000
--- a/spaces/cloudtheboi/Lofi4All/.pythonlibs/lib/python3.10/site-packages/anyio/streams/file.py
+++ /dev/null
@@ -1,147 +0,0 @@
-from __future__ import annotations
-
-from io import SEEK_SET, UnsupportedOperation
-from os import PathLike
-from pathlib import Path
-from typing import Any, BinaryIO, Callable, Mapping, cast
-
-from .. import (
- BrokenResourceError,
- ClosedResourceError,
- EndOfStream,
- TypedAttributeSet,
- to_thread,
- typed_attribute,
-)
-from ..abc import ByteReceiveStream, ByteSendStream
-
-
-class FileStreamAttribute(TypedAttributeSet):
- #: the open file descriptor
- file: BinaryIO = typed_attribute()
- #: the path of the file on the file system, if available (file must be a real file)
- path: Path = typed_attribute()
- #: the file number, if available (file must be a real file or a TTY)
- fileno: int = typed_attribute()
-
-
-class _BaseFileStream:
- def __init__(self, file: BinaryIO):
- self._file = file
-
- async def aclose(self) -> None:
- await to_thread.run_sync(self._file.close)
-
- @property
- def extra_attributes(self) -> Mapping[Any, Callable[[], Any]]:
- attributes: dict[Any, Callable[[], Any]] = {
- FileStreamAttribute.file: lambda: self._file,
- }
-
- if hasattr(self._file, "name"):
- attributes[FileStreamAttribute.path] = lambda: Path(self._file.name)
-
- try:
- self._file.fileno()
- except UnsupportedOperation:
- pass
- else:
- attributes[FileStreamAttribute.fileno] = lambda: self._file.fileno()
-
- return attributes
-
-
-class FileReadStream(_BaseFileStream, ByteReceiveStream):
- """
- A byte stream that reads from a file in the file system.
-
- :param file: a file that has been opened for reading in binary mode
-
- .. versionadded:: 3.0
- """
-
- @classmethod
- async def from_path(cls, path: str | PathLike[str]) -> FileReadStream:
- """
- Create a file read stream by opening the given file.
-
- :param path: path of the file to read from
-
- """
- file = await to_thread.run_sync(Path(path).open, "rb")
- return cls(cast(BinaryIO, file))
-
- async def receive(self, max_bytes: int = 65536) -> bytes:
- try:
- data = await to_thread.run_sync(self._file.read, max_bytes)
- except ValueError:
- raise ClosedResourceError from None
- except OSError as exc:
- raise BrokenResourceError from exc
-
- if data:
- return data
- else:
- raise EndOfStream
-
- async def seek(self, position: int, whence: int = SEEK_SET) -> int:
- """
- Seek the file to the given position.
-
- .. seealso:: :meth:`io.IOBase.seek`
-
- .. note:: Not all file descriptors are seekable.
-
- :param position: position to seek the file to
- :param whence: controls how ``position`` is interpreted
- :return: the new absolute position
- :raises OSError: if the file is not seekable
-
- """
- return await to_thread.run_sync(self._file.seek, position, whence)
-
- async def tell(self) -> int:
- """
- Return the current stream position.
-
- .. note:: Not all file descriptors are seekable.
-
- :return: the current absolute position
- :raises OSError: if the file is not seekable
-
- """
- return await to_thread.run_sync(self._file.tell)
-
-
-class FileWriteStream(_BaseFileStream, ByteSendStream):
- """
- A byte stream that writes to a file in the file system.
-
- :param file: a file that has been opened for writing in binary mode
-
- .. versionadded:: 3.0
- """
-
- @classmethod
- async def from_path(
- cls, path: str | PathLike[str], append: bool = False
- ) -> FileWriteStream:
- """
- Create a file write stream by opening the given file for writing.
-
- :param path: path of the file to write to
- :param append: if ``True``, open the file for appending; if ``False``, any existing file
- at the given path will be truncated
-
- """
- mode = "ab" if append else "wb"
- file = await to_thread.run_sync(Path(path).open, mode)
- return cls(cast(BinaryIO, file))
-
- async def send(self, item: bytes) -> None:
- try:
- await to_thread.run_sync(self._file.write, item)
- except ValueError:
- raise ClosedResourceError from None
- except OSError as exc:
- raise BrokenResourceError from exc
diff --git a/spaces/codebox/diffuse-flood/build/_app/immutable/start-ad08af0f.js b/spaces/codebox/diffuse-flood/build/_app/immutable/start-ad08af0f.js
deleted file mode 100644
index 30931f6377e225bbc2779ab396b12b2f00f43348..0000000000000000000000000000000000000000
--- a/spaces/codebox/diffuse-flood/build/_app/immutable/start-ad08af0f.js
+++ /dev/null
@@ -1 +0,0 @@
-var We=Object.defineProperty;var Je=(s,e,n)=>e in s?We(s,e,{enumerable:!0,configurable:!0,writable:!0,value:n}):s[e]=n;var ue=(s,e,n)=>(Je(s,typeof e!="symbol"?e+"":e,n),n);import{S as He,i as Fe,s as Ge,a as Me,e as I,c as Ye,b as V,g as M,t as D,d as Y,f as T,h as z,j as Xe,o as _e,k as Ze,l as Qe,m as xe,n as de,p as J,q as et,r as tt,u as nt,v as B,w as ee,x as K,y as W,z as Ne}from"./chunks/index-a207c28c.js";import{g as Ie,f as De,a as Te,s as G,b as ge,i as rt,c as at}from"./chunks/singletons-46497942.js";class re{constructor(e,n){ue(this,"name","HttpError");ue(this,"stack");this.status=e,this.message=n!=null?n:`Error: ${e}`}toString(){return this.message}}class qe{constructor(e,n){this.status=e,this.location=n}}function st(s,e){return s==="/"||e==="ignore"?s:e==="never"?s.endsWith("/")?s.slice(0,-1):s:e==="always"&&!s.endsWith("/")?s+"/":s}function it(s){for(const e in s)s[e]=s[e].replace(/%23/g,"#").replace(/%3[Bb]/g,";").replace(/%2[Cc]/g,",").replace(/%2[Ff]/g,"/").replace(/%3[Ff]/g,"?").replace(/%3[Aa]/g,":").replace(/%40/g,"@").replace(/%26/g,"&").replace(/%3[Dd]/g,"=").replace(/%2[Bb]/g,"+").replace(/%24/g,"$");return s}class ot extends URL{get hash(){throw new Error("url.hash is inaccessible from load. Consider accessing hash from the page store within the script tag of your component.")}}function lt(s){let e=5381,n=s.length;if(typeof s=="string")for(;n;)e=e*33^s.charCodeAt(--n);else for(;n;)e=e*33^s[--n];return(e>>>0).toString(36)}const ae=window.fetch;function ct(s,e){let i=`script[sveltekit\\:data-type="data"][sveltekit\\:data-url=${JSON.stringify(typeof s=="string"?s:s.url)}]`;e&&typeof e.body=="string"&&(i+=`[sveltekit\\:data-body="${lt(e.body)}"]`);const r=document.querySelector(i);if(r&&r.textContent){const{body:u,...t}=JSON.parse(r.textContent);return Promise.resolve(new Response(u,t))}return ae(s,e)}const ft=/^(\.\.\.)?(\w+)(?:=(\w+))?$/;function ut(s){const e=[],n=[];let i=!0;return{pattern:s===""?/^\/$/:new RegExp(`^${s.split(/(?:@[a-zA-Z0-9_-]+)?(?:\/|$)/).map((u,t,l)=>{const d=decodeURIComponent(u),p=/^\[\.\.\.(\w+)(?:=(\w+))?\]$/.exec(d);if(p)return e.push(p[1]),n.push(p[2]),"(?:/(.*))?";const g=t===l.length-1;return d&&"/"+d.split(/\[(.+?)\]/).map((E,P)=>{if(P%2){const $=ft.exec(E);if(!$)throw new Error(`Invalid param: ${E}. Params and matcher names can only have underscores and alphanumeric characters.`);const[,O,Z,Q]=$;return e.push(Z),n.push(Q),O?"(.*?)":"([^/]+?)"}return g&&E.includes(".")&&(i=!1),E.normalize().replace(/%5[Bb]/g,"[").replace(/%5[Dd]/g,"]").replace(/#/g,"%23").replace(/\?/g,"%3F").replace(/[.*+?^${}()|[\]\\]/g,"\\$&")}).join("")}).join("")}${i?"/?":""}$`),names:e,types:n}}function dt(s,e,n,i){const r={};for(let u=0;u{const{pattern:d,names:p,types:g}=ut(i),E={id:i,exec:P=>{const $=d.exec(P);if($)return dt($,p,g,n)},errors:r.map(P=>s[P]),layouts:u.map(P=>s[P]),leaf:s[t],uses_server_data:!!l};return E.errors.length=E.layouts.length=Math.max(E.errors.length,E.layouts.length),E})}function ht(s,e){return new re(s,e)}function mt(s){let e,n,i;var r=s[0][0];function u(t){return{props:{data:t[1],errors:t[4]}}}return r&&(e=new r(u(s))),{c(){e&&B(e.$$.fragment),n=I()},l(t){e&&ee(e.$$.fragment,t),n=I()},m(t,l){e&&K(e,t,l),V(t,n,l),i=!0},p(t,l){const d={};if(l&2&&(d.data=t[1]),l&16&&(d.errors=t[4]),r!==(r=t[0][0])){if(e){M();const p=e;D(p.$$.fragment,1,0,()=>{W(p,1)}),Y()}r?(e=new r(u(t)),B(e.$$.fragment),T(e.$$.fragment,1),K(e,n.parentNode,n)):e=null}else r&&e.$set(d)},i(t){i||(e&&T(e.$$.fragment,t),i=!0)},o(t){e&&D(e.$$.fragment,t),i=!1},d(t){t&&z(n),e&&W(e,t)}}}function _t(s){let e,n,i;var r=s[0][0];function u(t){return{props:{data:t[1],$$slots:{default:[yt]},$$scope:{ctx:t}}}}return r&&(e=new r(u(s))),{c(){e&&B(e.$$.fragment),n=I()},l(t){e&&ee(e.$$.fragment,t),n=I()},m(t,l){e&&K(e,t,l),V(t,n,l),i=!0},p(t,l){const d={};if(l&2&&(d.data=t[1]),l&1053&&(d.$$scope={dirty:l,ctx:t}),r!==(r=t[0][0])){if(e){M();const p=e;D(p.$$.fragment,1,0,()=>{W(p,1)}),Y()}r?(e=new r(u(t)),B(e.$$.fragment),T(e.$$.fragment,1),K(e,n.parentNode,n)):e=null}else r&&e.$set(d)},i(t){i||(e&&T(e.$$.fragment,t),i=!0)},o(t){e&&D(e.$$.fragment,t),i=!1},d(t){t&&z(n),e&&W(e,t)}}}function gt(s){let e,n,i;var r=s[0][1];function u(t){return{props:{data:t[2],errors:t[4]}}}return r&&(e=new r(u(s))),{c(){e&&B(e.$$.fragment),n=I()},l(t){e&&ee(e.$$.fragment,t),n=I()},m(t,l){e&&K(e,t,l),V(t,n,l),i=!0},p(t,l){const d={};if(l&4&&(d.data=t[2]),l&16&&(d.errors=t[4]),r!==(r=t[0][1])){if(e){M();const p=e;D(p.$$.fragment,1,0,()=>{W(p,1)}),Y()}r?(e=new r(u(t)),B(e.$$.fragment),T(e.$$.fragment,1),K(e,n.parentNode,n)):e=null}else r&&e.$set(d)},i(t){i||(e&&T(e.$$.fragment,t),i=!0)},o(t){e&&D(e.$$.fragment,t),i=!1},d(t){t&&z(n),e&&W(e,t)}}}function wt(s){let e,n,i;var r=s[0][1];function u(t){return{props:{data:t[2],$$slots:{default:[bt]},$$scope:{ctx:t}}}}return r&&(e=new r(u(s))),{c(){e&&B(e.$$.fragment),n=I()},l(t){e&&ee(e.$$.fragment,t),n=I()},m(t,l){e&&K(e,t,l),V(t,n,l),i=!0},p(t,l){const d={};if(l&4&&(d.data=t[2]),l&1033&&(d.$$scope={dirty:l,ctx:t}),r!==(r=t[0][1])){if(e){M();const p=e;D(p.$$.fragment,1,0,()=>{W(p,1)}),Y()}r?(e=new r(u(t)),B(e.$$.fragment),T(e.$$.fragment,1),K(e,n.parentNode,n)):e=null}else r&&e.$set(d)},i(t){i||(e&&T(e.$$.fragment,t),i=!0)},o(t){e&&D(e.$$.fragment,t),i=!1},d(t){t&&z(n),e&&W(e,t)}}}function bt(s){let e,n,i;var r=s[0][2];function u(t){return{props:{data:t[3]}}}return r&&(e=new r(u(s))),{c(){e&&B(e.$$.fragment),n=I()},l(t){e&&ee(e.$$.fragment,t),n=I()},m(t,l){e&&K(e,t,l),V(t,n,l),i=!0},p(t,l){const d={};if(l&8&&(d.data=t[3]),r!==(r=t[0][2])){if(e){M();const p=e;D(p.$$.fragment,1,0,()=>{W(p,1)}),Y()}r?(e=new r(u(t)),B(e.$$.fragment),T(e.$$.fragment,1),K(e,n.parentNode,n)):e=null}else r&&e.$set(d)},i(t){i||(e&&T(e.$$.fragment,t),i=!0)},o(t){e&&D(e.$$.fragment,t),i=!1},d(t){t&&z(n),e&&W(e,t)}}}function yt(s){let e,n,i,r;const u=[wt,gt],t=[];function l(d,p){return d[0][2]?0:1}return e=l(s),n=t[e]=u[e](s),{c(){n.c(),i=I()},l(d){n.l(d),i=I()},m(d,p){t[e].m(d,p),V(d,i,p),r=!0},p(d,p){let g=e;e=l(d),e===g?t[e].p(d,p):(M(),D(t[g],1,1,()=>{t[g]=null}),Y(),n=t[e],n?n.p(d,p):(n=t[e]=u[e](d),n.c()),T(n,1),n.m(i.parentNode,i))},i(d){r||(T(n),r=!0)},o(d){D(n),r=!1},d(d){t[e].d(d),d&&z(i)}}}function ze(s){let e,n=s[6]&&Ve(s);return{c(){e=Ze("div"),n&&n.c(),this.h()},l(i){e=Qe(i,"DIV",{id:!0,"aria-live":!0,"aria-atomic":!0,style:!0});var r=xe(e);n&&n.l(r),r.forEach(z),this.h()},h(){de(e,"id","svelte-announcer"),de(e,"aria-live","assertive"),de(e,"aria-atomic","true"),J(e,"position","absolute"),J(e,"left","0"),J(e,"top","0"),J(e,"clip","rect(0 0 0 0)"),J(e,"clip-path","inset(50%)"),J(e,"overflow","hidden"),J(e,"white-space","nowrap"),J(e,"width","1px"),J(e,"height","1px")},m(i,r){V(i,e,r),n&&n.m(e,null)},p(i,r){i[6]?n?n.p(i,r):(n=Ve(i),n.c(),n.m(e,null)):n&&(n.d(1),n=null)},d(i){i&&z(e),n&&n.d()}}}function Ve(s){let e;return{c(){e=et(s[7])},l(n){e=tt(n,s[7])},m(n,i){V(n,e,i)},p(n,i){i&128&&nt(e,n[7])},d(n){n&&z(e)}}}function vt(s){let e,n,i,r,u;const t=[_t,mt],l=[];function d(g,E){return g[0][1]?0:1}e=d(s),n=l[e]=t[e](s);let p=s[5]&&ze(s);return{c(){n.c(),i=Me(),p&&p.c(),r=I()},l(g){n.l(g),i=Ye(g),p&&p.l(g),r=I()},m(g,E){l[e].m(g,E),V(g,i,E),p&&p.m(g,E),V(g,r,E),u=!0},p(g,[E]){let P=e;e=d(g),e===P?l[e].p(g,E):(M(),D(l[P],1,1,()=>{l[P]=null}),Y(),n=l[e],n?n.p(g,E):(n=l[e]=t[e](g),n.c()),T(n,1),n.m(i.parentNode,i)),g[5]?p?p.p(g,E):(p=ze(g),p.c(),p.m(r.parentNode,r)):p&&(p.d(1),p=null)},i(g){u||(T(n),u=!0)},o(g){D(n),u=!1},d(g){l[e].d(g),g&&z(i),p&&p.d(g),g&&z(r)}}}function kt(s,e,n){let{stores:i}=e,{page:r}=e,{components:u}=e,{data_0:t=null}=e,{data_1:l=null}=e,{data_2:d=null}=e,{errors:p}=e;Xe(i.page.notify);let g=!1,E=!1,P=null;return _e(()=>{const $=i.page.subscribe(()=>{g&&(n(6,E=!0),n(7,P=document.title||"untitled page"))});return n(5,g=!0),$}),s.$$set=$=>{"stores"in $&&n(8,i=$.stores),"page"in $&&n(9,r=$.page),"components"in $&&n(0,u=$.components),"data_0"in $&&n(1,t=$.data_0),"data_1"in $&&n(2,l=$.data_1),"data_2"in $&&n(3,d=$.data_2),"errors"in $&&n(4,p=$.errors)},s.$$.update=()=>{s.$$.dirty&768&&i.page.set(r)},[u,t,l,d,p,g,E,P,i,r]}class $t extends He{constructor(e){super(),Fe(this,e,kt,vt,Ge,{stores:8,page:9,components:0,data_0:1,data_1:2,data_2:3,errors:4})}}const Et=function(){const e=document.createElement("link").relList;return e&&e.supports&&e.supports("modulepreload")?"modulepreload":"preload"}(),St=function(s,e){return new URL(s,e).href},Be={},pe=function(e,n,i){return!n||n.length===0?e():Promise.all(n.map(r=>{if(r=St(r,i),r in Be)return;Be[r]=!0;const u=r.endsWith(".css"),t=u?'[rel="stylesheet"]':"";if(document.querySelector(`link[href="${r}"]${t}`))return;const l=document.createElement("link");if(l.rel=u?"stylesheet":Et,u||(l.as="script",l.crossOrigin=""),l.href=r,document.head.appendChild(l),u)return new Promise((d,p)=>{l.addEventListener("load",d),l.addEventListener("error",()=>p(new Error(`Unable to preload CSS for ${r}`)))})})).then(()=>e())},Lt={},se=[()=>pe(()=>import("./chunks/0-a62bf17c.js"),["chunks\\0-a62bf17c.js","components\\pages\\_layout.svelte-eed40348.js","assets\\+layout-2ac25133.css","chunks\\index-a207c28c.js"],import.meta.url),()=>pe(()=>import("./chunks/1-11d02443.js"),["chunks\\1-11d02443.js","components\\error.svelte-526e6a5c.js","chunks\\index-a207c28c.js","chunks\\singletons-46497942.js"],import.meta.url),()=>pe(()=>import("./chunks/2-efe340ca.js"),["chunks\\2-efe340ca.js","components\\pages\\_page.svelte-2a5d0087.js","assets\\+page-376b236d.css","chunks\\index-a207c28c.js"],import.meta.url)],Rt={"":[[1],[0],2]},Ke="sveltekit:scroll",H="sveltekit:index",he=pt(se,Rt,Lt),we=se[0],be=se[1];we();be();let x={};try{x=JSON.parse(sessionStorage[Ke])}catch{}function me(s){x[s]=ge()}function Ut({target:s,base:e,trailing_slash:n}){var Ue;const i=[],r={id:null,promise:null},u={before_navigate:[],after_navigate:[]};let t={branch:[],error:null,session_id:0,url:null},l=!1,d=!0,p=!1,g=1,E=null,P,$=!0,O=(Ue=history.state)==null?void 0:Ue[H];O||(O=Date.now(),history.replaceState({...history.state,[H]:O},"",location.href));const Z=x[O];Z&&(history.scrollRestoration="manual",scrollTo(Z.x,Z.y));let Q=!1,ie,ye;async function ve(a,{noscroll:f=!1,replaceState:h=!1,keepfocus:o=!1,state:c={}},y){if(typeof a=="string"&&(a=new URL(a,Ie(document))),$)return ce({url:a,scroll:f?ge():null,keepfocus:o,redirect_chain:y,details:{state:c,replaceState:h},accepted:()=>{},blocked:()=>{}});await F(a)}async function ke(a){const f=Re(a);if(!f)throw new Error("Attempted to prefetch a URL that does not belong to this app");return r.promise=Le(f),r.id=f.id,r.promise}async function $e(a,f,h,o){var b,L,U;const c=Re(a),y=ye={};let m=c&&await Le(c);if(!m&&a.origin===location.origin&&a.pathname===location.pathname&&(m=await ne({status:404,error:new Error(`Not found: ${a.pathname}`),url:a,routeId:null})),!m)return await F(a),!1;if(a=(c==null?void 0:c.url)||a,ye!==y)return!1;if(i.length=0,m.type==="redirect")if(f.length>10||f.includes(a.pathname))m=await ne({status:500,error:new Error("Redirect loop"),url:a,routeId:null});else return $?ve(new URL(m.location,a).href,{},[...f,a.pathname]):await F(new URL(m.location,location.href)),!1;else((L=(b=m.props)==null?void 0:b.page)==null?void 0:L.status)>=400&&await G.updated.check()&&await F(a);if(p=!0,h&&h.details){const{details:k}=h,R=k.replaceState?0:1;k.state[H]=O+=R,history[k.replaceState?"replaceState":"pushState"](k.state,"",a)}if(l?(t=m.state,m.props.page&&(m.props.page.url=a),P.$set(m.props)):Ee(m),h){const{scroll:k,keepfocus:R}=h;if(!R){const S=document.body,A=S.getAttribute("tabindex");S.tabIndex=-1,S.focus({preventScroll:!0}),setTimeout(()=>{var w;(w=getSelection())==null||w.removeAllRanges()}),A!==null?S.setAttribute("tabindex",A):S.removeAttribute("tabindex")}if(await Ne(),d){const S=a.hash&&document.getElementById(a.hash.slice(1));k?scrollTo(k.x,k.y):S?S.scrollIntoView():scrollTo(0,0)}}else await Ne();r.promise=null,r.id=null,d=!0,m.props.page&&(ie=m.props.page);const v=m.state.branch[m.state.branch.length-1];$=((U=v==null?void 0:v.node.shared)==null?void 0:U.router)!==!1,o&&o(),p=!1}function Ee(a){t=a.state;const f=document.querySelector("style[data-sveltekit]");if(f&&f.remove(),ie=a.props.page,P=new $t({target:s,props:{...a.props,stores:G},hydrate:!0}),$){const h={from:null,to:new URL(location.href)};u.after_navigate.forEach(o=>o(h))}l=!0}async function te({url:a,params:f,branch:h,status:o,error:c,routeId:y,validation_errors:m}){const v=h.filter(Boolean),b={type:"loaded",state:{url:a,params:f,branch:h,error:c,session_id:g},props:{components:v.map(R=>R.node.component),errors:m}};let L={},U=!1;for(let R=0;RS===v[R]))&&(b.props[`data_${R}`]=L,U=!0);if(!t.url||a.href!==t.url.href||t.error!==c||U){b.props.page={error:c,params:f,routeId:y,status:o,url:a,data:L};const R=(S,A)=>{Object.defineProperty(b.props.page,S,{get:()=>{throw new Error(`$page.${S} has been replaced by $page.url.${A}`)}})};R("origin","origin"),R("path","pathname"),R("query","searchParams")}return b}async function oe({loader:a,parent:f,url:h,params:o,routeId:c,server_data_node:y}){var L,U,k,R,S;let m=null;const v={dependencies:new Set,params:new Set,parent:!1,url:!1},b=await a();if((L=b.shared)!=null&&L.load){let A=function(..._){for(const q of _){const{href:N}=new URL(q,h);v.dependencies.add(N)}};const w={};for(const _ in o)Object.defineProperty(w,_,{get(){return v.params.add(_),o[_]},enumerable:!0});const C=new ot(h),j={routeId:c,params:w,data:(U=y==null?void 0:y.data)!=null?U:null,get url(){return v.url=!0,C},async fetch(_,q){let N;typeof _=="string"?N=_:(N=_.url,q={body:_.method==="GET"||_.method==="HEAD"?void 0:await _.blob(),cache:_.cache,credentials:_.credentials,headers:_.headers,integrity:_.integrity,keepalive:_.keepalive,method:_.method,mode:_.mode,redirect:_.redirect,referrer:_.referrer,referrerPolicy:_.referrerPolicy,signal:_.signal,...q});const X=new URL(N,h).href;return A(X),l?ae(X,q):ct(N,q)},setHeaders:()=>{},depends:A,parent(){return v.parent=!0,f()}};Object.defineProperties(j,{props:{get(){throw new Error("@migration task: Replace `props` with `data` stuff https://github.com/sveltejs/kit/discussions/5774#discussioncomment-3292693")},enumerable:!1},session:{get(){throw new Error("session is no longer available. See https://github.com/sveltejs/kit/discussions/5883")},enumerable:!1},stuff:{get(){throw new Error("@migration task: Remove stuff https://github.com/sveltejs/kit/discussions/5774#discussioncomment-3292693")},enumerable:!1}}),m=(k=await b.shared.load.call(null,j))!=null?k:null}return{node:b,loader:a,server:y,shared:(R=b.shared)!=null&&R.load?{type:"data",data:m,uses:v}:null,data:(S=m!=null?m:y==null?void 0:y.data)!=null?S:null}}function Se(a,f,h){if(!h)return!1;if(h.parent&&f||a.url&&h.url)return!0;for(const o of a.params)if(h.params.has(o))return!0;for(const o of h.dependencies)if(i.some(c=>c(o)))return!0;return!1}function le(a){var f,h;return(a==null?void 0:a.type)==="data"?{type:"data",data:a.data,uses:{dependencies:new Set((f=a.uses.dependencies)!=null?f:[]),params:new Set((h=a.uses.params)!=null?h:[]),parent:!!a.uses.parent,url:!!a.uses.url}}:null}async function Le({id:a,url:f,params:h,route:o}){if(r.id===a&&r.promise)return r.promise;const{errors:c,layouts:y,leaf:m}=o,v=t.url&&{url:a!==t.url.pathname+t.url.search,params:Object.keys(h).filter(w=>t.params[w]!==h[w])};[...c,...y,m].forEach(w=>w==null?void 0:w().catch(()=>{}));const b=[...y,m];let L=null;const U=b.reduce((w,C,j)=>{var N;const _=t.branch[j],q=C&&((_==null?void 0:_.loader)!==C||Se(v,w.some(Boolean),(N=_.server)==null?void 0:N.uses));return w.push(q),w},[]);if(o.uses_server_data&&U.some(Boolean)){try{const w=await ae(`${f.pathname}${f.pathname.endsWith("/")?"":"/"}__data.json${f.search}`,{headers:{"x-sveltekit-invalidated":U.map(C=>C?"1":"").join(",")}});if(L=await w.json(),!w.ok)throw L}catch{F(f);return}if(L.type==="redirect")return L}const k=L==null?void 0:L.nodes;let R=!1;const S=b.map(async(w,C)=>{var X,je,Pe,Ae;if(!w)return;const j=t.branch[C],_=(X=k==null?void 0:k[C])!=null?X:null;if((!_||_.type==="skip")&&w===(j==null?void 0:j.loader)&&!Se(v,R,(je=j.shared)==null?void 0:je.uses))return j;if(R=!0,(_==null?void 0:_.type)==="error")throw _.httperror?ht(_.httperror.status,_.httperror.message):_.error;return oe({loader:w,url:f,params:h,routeId:o.id,parent:async()=>{var Ce;const Oe={};for(let fe=0;fe{});const A=[];for(let w=0;wPromise.resolve({}),server_data_node:le(m)}),b={node:await be(),loader:be,shared:null,server:null,data:null};return await te({url:h,params:c,branch:[v,b],status:a,error:f,routeId:o})}function Re(a){if(a.origin!==location.origin||!a.pathname.startsWith(e))return;const f=decodeURI(a.pathname.slice(e.length)||"/");for(const h of he){const o=h.exec(f);if(o){const c=new URL(a.origin+st(a.pathname,n)+a.search+a.hash);return{id:c.pathname+c.search,route:h,params:it(o),url:c}}}}async function ce({url:a,scroll:f,keepfocus:h,redirect_chain:o,details:c,accepted:y,blocked:m}){const v=t.url;let b=!1;const L={from:v,to:a,cancel:()=>b=!0};if(u.before_navigate.forEach(U=>U(L)),b){m();return}me(O),y(),l&&G.navigating.set({from:t.url,to:a}),await $e(a,o,{scroll:f,keepfocus:h,details:c},()=>{const U={from:v,to:a};u.after_navigate.forEach(k=>k(U)),G.navigating.set(null)})}function F(a){return location.href=a.href,new Promise(()=>{})}return{after_navigate:a=>{_e(()=>(u.after_navigate.push(a),()=>{const f=u.after_navigate.indexOf(a);u.after_navigate.splice(f,1)}))},before_navigate:a=>{_e(()=>(u.before_navigate.push(a),()=>{const f=u.before_navigate.indexOf(a);u.before_navigate.splice(f,1)}))},disable_scroll_handling:()=>{(p||!l)&&(d=!1)},goto:(a,f={})=>ve(a,f,[]),invalidate:a=>{var f,h;if(a===void 0){for(const o of t.branch)(f=o==null?void 0:o.server)==null||f.uses.dependencies.add(""),(h=o==null?void 0:o.shared)==null||h.uses.dependencies.add("");i.push(()=>!0)}else if(typeof a=="function")i.push(a);else{const{href:o}=new URL(a,location.href);i.push(c=>c===o)}return E||(E=Promise.resolve().then(async()=>{await $e(new URL(location.href),[]),E=null})),E},prefetch:async a=>{const f=new URL(a,Ie(document));await ke(f)},prefetch_routes:async a=>{const h=(a?he.filter(o=>a.some(c=>o.exec(c))):he).map(o=>Promise.all([...o.layouts,o.leaf].map(c=>c==null?void 0:c())));await Promise.all(h)},_start_router:()=>{history.scrollRestoration="manual",addEventListener("beforeunload",o=>{let c=!1;const y={from:t.url,to:null,cancel:()=>c=!0};u.before_navigate.forEach(m=>m(y)),c?(o.preventDefault(),o.returnValue=""):history.scrollRestoration="auto"}),addEventListener("visibilitychange",()=>{if(document.visibilityState==="hidden"){me(O);try{sessionStorage[Ke]=JSON.stringify(x)}catch{}}});const a=o=>{const c=De(o);c&&c.href&&c.hasAttribute("sveltekit:prefetch")&&ke(Te(c))};let f;const h=o=>{clearTimeout(f),f=setTimeout(()=>{var c;(c=o.target)==null||c.dispatchEvent(new CustomEvent("sveltekit:trigger_prefetch",{bubbles:!0}))},20)};addEventListener("touchstart",a),addEventListener("mousemove",h),addEventListener("sveltekit:trigger_prefetch",a),addEventListener("click",o=>{if(!$||o.button||o.which!==1||o.metaKey||o.ctrlKey||o.shiftKey||o.altKey||o.defaultPrevented)return;const c=De(o);if(!c||!c.href)return;const y=c instanceof SVGAElement,m=Te(c);if(!y&&!(m.protocol==="https:"||m.protocol==="http:"))return;const v=(c.getAttribute("rel")||"").split(/\s+/);if(c.hasAttribute("download")||v.includes("external")||c.hasAttribute("sveltekit:reload")||(y?c.target.baseVal:c.target))return;const[b,L]=m.href.split("#");if(L!==void 0&&b===location.href.split("#")[0]){Q=!0,me(O),G.page.set({...ie,url:m}),G.page.notify();return}ce({url:m,scroll:c.hasAttribute("sveltekit:noscroll")?ge():null,keepfocus:!1,redirect_chain:[],details:{state:{},replaceState:m.href===location.href},accepted:()=>o.preventDefault(),blocked:()=>o.preventDefault()})}),addEventListener("popstate",o=>{if(o.state&&$){if(o.state[H]===O)return;ce({url:new URL(location.href),scroll:x[o.state[H]],keepfocus:!1,redirect_chain:[],details:null,accepted:()=>{O=o.state[H]},blocked:()=>{const c=O-o.state[H];history.go(c)}})}}),addEventListener("hashchange",()=>{Q&&(Q=!1,history.replaceState({...history.state,[H]:++O},"",location.href))});for(const o of document.querySelectorAll("link"))o.rel==="icon"&&(o.href=o.href);addEventListener("pageshow",o=>{o.persisted&&G.navigating.set(null)})},_hydrate:async({status:a,error:f,node_ids:h,params:o,routeId:c})=>{const y=new URL(location.href);let m;try{const v=(k,R)=>{const S=document.querySelector(`script[sveltekit\\:data-type="${k}"]`);return S!=null&&S.textContent?JSON.parse(S.textContent):R},b=v("server_data",[]),L=v("validation_errors",void 0),U=h.map(async(k,R)=>oe({loader:se[k],url:y,params:o,routeId:c,parent:async()=>{const S={};for(let A=0;A
-#include
-
-#include
-#include
-#include
-
-static AVFormatContext *ifmt_ctx = NULL, *ofmt_ctx = NULL;
-static AVBufferRef *hw_device_ctx = NULL;
-static AVCodecContext *decoder_ctx = NULL, *encoder_ctx = NULL;
-static int video_stream = -1;
-static AVStream *ost;
-static int initialized = 0;
-
-static enum AVPixelFormat get_vaapi_format(AVCodecContext *ctx,
- const enum AVPixelFormat *pix_fmts)
-{
- const enum AVPixelFormat *p;
-
- for (p = pix_fmts; *p != AV_PIX_FMT_NONE; p++) {
- if (*p == AV_PIX_FMT_VAAPI)
- return *p;
- }
-
- fprintf(stderr, "Unable to decode this file using VA-API.\n");
- return AV_PIX_FMT_NONE;
-}
-
-static int open_input_file(const char *filename)
-{
- int ret;
- const AVCodec *decoder = NULL;
- AVStream *video = NULL;
-
- if ((ret = avformat_open_input(&ifmt_ctx, filename, NULL, NULL)) < 0) {
- fprintf(stderr, "Cannot open input file '%s', Error code: %s\n",
- filename, av_err2str(ret));
- return ret;
- }
-
- if ((ret = avformat_find_stream_info(ifmt_ctx, NULL)) < 0) {
- fprintf(stderr, "Cannot find input stream information. Error code: %s\n",
- av_err2str(ret));
- return ret;
- }
-
- ret = av_find_best_stream(ifmt_ctx, AVMEDIA_TYPE_VIDEO, -1, -1, &decoder, 0);
- if (ret < 0) {
- fprintf(stderr, "Cannot find a video stream in the input file. "
- "Error code: %s\n", av_err2str(ret));
- return ret;
- }
- video_stream = ret;
-
- if (!(decoder_ctx = avcodec_alloc_context3(decoder)))
- return AVERROR(ENOMEM);
-
- video = ifmt_ctx->streams[video_stream];
- if ((ret = avcodec_parameters_to_context(decoder_ctx, video->codecpar)) < 0) {
- fprintf(stderr, "avcodec_parameters_to_context error. Error code: %s\n",
- av_err2str(ret));
- return ret;
- }
-
- decoder_ctx->hw_device_ctx = av_buffer_ref(hw_device_ctx);
- if (!decoder_ctx->hw_device_ctx) {
- fprintf(stderr, "A hardware device reference create failed.\n");
- return AVERROR(ENOMEM);
- }
- decoder_ctx->get_format = get_vaapi_format;
-
- if ((ret = avcodec_open2(decoder_ctx, decoder, NULL)) < 0)
- fprintf(stderr, "Failed to open codec for decoding. Error code: %s\n",
- av_err2str(ret));
-
- return ret;
-}
-
-static int encode_write(AVPacket *enc_pkt, AVFrame *frame)
-{
- int ret = 0;
-
- av_packet_unref(enc_pkt);
-
- if ((ret = avcodec_send_frame(encoder_ctx, frame)) < 0) {
- fprintf(stderr, "Error during encoding. Error code: %s\n", av_err2str(ret));
- goto end;
- }
- while (1) {
- ret = avcodec_receive_packet(encoder_ctx, enc_pkt);
- if (ret)
- break;
-
- enc_pkt->stream_index = 0;
- av_packet_rescale_ts(enc_pkt, ifmt_ctx->streams[video_stream]->time_base,
- ofmt_ctx->streams[0]->time_base);
- ret = av_interleaved_write_frame(ofmt_ctx, enc_pkt);
- if (ret < 0) {
- fprintf(stderr, "Error during writing data to output file. "
- "Error code: %s\n", av_err2str(ret));
- return -1;
- }
- }
-
-end:
- if (ret == AVERROR_EOF)
- return 0;
- ret = ((ret == AVERROR(EAGAIN)) ? 0:-1);
- return ret;
-}
-
-static int dec_enc(AVPacket *pkt, const AVCodec *enc_codec)
-{
- AVFrame *frame;
- int ret = 0;
-
- ret = avcodec_send_packet(decoder_ctx, pkt);
- if (ret < 0) {
- fprintf(stderr, "Error during decoding. Error code: %s\n", av_err2str(ret));
- return ret;
- }
-
- while (ret >= 0) {
- if (!(frame = av_frame_alloc()))
- return AVERROR(ENOMEM);
-
- ret = avcodec_receive_frame(decoder_ctx, frame);
- if (ret == AVERROR(EAGAIN) || ret == AVERROR_EOF) {
- av_frame_free(&frame);
- return 0;
- } else if (ret < 0) {
- fprintf(stderr, "Error while decoding. Error code: %s\n", av_err2str(ret));
- goto fail;
- }
-
- if (!initialized) {
- /* we need to ref hw_frames_ctx of decoder to initialize encoder's codec.
- Only after we get a decoded frame, can we obtain its hw_frames_ctx */
- encoder_ctx->hw_frames_ctx = av_buffer_ref(decoder_ctx->hw_frames_ctx);
- if (!encoder_ctx->hw_frames_ctx) {
- ret = AVERROR(ENOMEM);
- goto fail;
- }
- /* set AVCodecContext Parameters for encoder, here we keep them stay
- * the same as decoder.
- * xxx: now the sample can't handle resolution change case.
- */
- encoder_ctx->time_base = av_inv_q(decoder_ctx->framerate);
- encoder_ctx->pix_fmt = AV_PIX_FMT_VAAPI;
- encoder_ctx->width = decoder_ctx->width;
- encoder_ctx->height = decoder_ctx->height;
-
- if ((ret = avcodec_open2(encoder_ctx, enc_codec, NULL)) < 0) {
- fprintf(stderr, "Failed to open encode codec. Error code: %s\n",
- av_err2str(ret));
- goto fail;
- }
-
- if (!(ost = avformat_new_stream(ofmt_ctx, enc_codec))) {
- fprintf(stderr, "Failed to allocate stream for output format.\n");
- ret = AVERROR(ENOMEM);
- goto fail;
- }
-
- ost->time_base = encoder_ctx->time_base;
- ret = avcodec_parameters_from_context(ost->codecpar, encoder_ctx);
- if (ret < 0) {
- fprintf(stderr, "Failed to copy the stream parameters. "
- "Error code: %s\n", av_err2str(ret));
- goto fail;
- }
-
- /* write the stream header */
- if ((ret = avformat_write_header(ofmt_ctx, NULL)) < 0) {
- fprintf(stderr, "Error while writing stream header. "
- "Error code: %s\n", av_err2str(ret));
- goto fail;
- }
-
- initialized = 1;
- }
-
- if ((ret = encode_write(pkt, frame)) < 0)
- fprintf(stderr, "Error during encoding and writing.\n");
-
-fail:
- av_frame_free(&frame);
- if (ret < 0)
- return ret;
- }
- return 0;
-}
-
-int main(int argc, char **argv)
-{
- const AVCodec *enc_codec;
- int ret = 0;
- AVPacket *dec_pkt;
-
- if (argc != 4) {
- fprintf(stderr, "Usage: %s \n"
- "The output format is guessed according to the file extension.\n"
- "\n", argv[0]);
- return -1;
- }
-
- ret = av_hwdevice_ctx_create(&hw_device_ctx, AV_HWDEVICE_TYPE_VAAPI, NULL, NULL, 0);
- if (ret < 0) {
- fprintf(stderr, "Failed to create a VAAPI device. Error code: %s\n", av_err2str(ret));
- return -1;
- }
-
- dec_pkt = av_packet_alloc();
- if (!dec_pkt) {
- fprintf(stderr, "Failed to allocate decode packet\n");
- goto end;
- }
-
- if ((ret = open_input_file(argv[1])) < 0)
- goto end;
-
- if (!(enc_codec = avcodec_find_encoder_by_name(argv[2]))) {
- fprintf(stderr, "Could not find encoder '%s'\n", argv[2]);
- ret = -1;
- goto end;
- }
-
- if ((ret = (avformat_alloc_output_context2(&ofmt_ctx, NULL, NULL, argv[3]))) < 0) {
- fprintf(stderr, "Failed to deduce output format from file extension. Error code: "
- "%s\n", av_err2str(ret));
- goto end;
- }
-
- if (!(encoder_ctx = avcodec_alloc_context3(enc_codec))) {
- ret = AVERROR(ENOMEM);
- goto end;
- }
-
- ret = avio_open(&ofmt_ctx->pb, argv[3], AVIO_FLAG_WRITE);
- if (ret < 0) {
- fprintf(stderr, "Cannot open output file. "
- "Error code: %s\n", av_err2str(ret));
- goto end;
- }
-
- /* read all packets and only transcoding video */
- while (ret >= 0) {
- if ((ret = av_read_frame(ifmt_ctx, dec_pkt)) < 0)
- break;
-
- if (video_stream == dec_pkt->stream_index)
- ret = dec_enc(dec_pkt, enc_codec);
-
- av_packet_unref(dec_pkt);
- }
-
- /* flush decoder */
- av_packet_unref(dec_pkt);
- ret = dec_enc(dec_pkt, enc_codec);
-
- /* flush encoder */
- ret = encode_write(dec_pkt, NULL);
-
- /* write the trailer for output stream */
- av_write_trailer(ofmt_ctx);
-
-end:
- avformat_close_input(&ifmt_ctx);
- avformat_close_input(&ofmt_ctx);
- avcodec_free_context(&decoder_ctx);
- avcodec_free_context(&encoder_ctx);
- av_buffer_unref(&hw_device_ctx);
- av_packet_free(&dec_pkt);
- return ret;
-}
diff --git a/spaces/colakin/video-generater/public/ffmpeg/libavcodec/aacpsdsp.h b/spaces/colakin/video-generater/public/ffmpeg/libavcodec/aacpsdsp.h
deleted file mode 100644
index 8b32761bdb05ea5e9d69bac3d0e253d3ba566b68..0000000000000000000000000000000000000000
--- a/spaces/colakin/video-generater/public/ffmpeg/libavcodec/aacpsdsp.h
+++ /dev/null
@@ -1,61 +0,0 @@
-/*
- * Copyright (c) 2012 Mans Rullgard
- *
- * This file is part of FFmpeg.
- *
- * FFmpeg is free software; you can redistribute it and/or
- * modify it under the terms of the GNU Lesser General Public
- * License as published by the Free Software Foundation; either
- * version 2.1 of the License, or (at your option) any later version.
- *
- * FFmpeg is distributed in the hope that it will be useful,
- * but WITHOUT ANY WARRANTY; without even the implied warranty of
- * MERCHANTABILITY or FITNESS FOR A PARTICULAR PURPOSE. See the GNU
- * Lesser General Public License for more details.
- *
- * You should have received a copy of the GNU Lesser General Public
- * License along with FFmpeg; if not, write to the Free Software
- * Foundation, Inc., 51 Franklin Street, Fifth Floor, Boston, MA 02110-1301 USA
- */
-
-#ifndef AVCODEC_AACPSDSP_H
-#define AVCODEC_AACPSDSP_H
-
-#include
-
-#include "aac_defines.h"
-
-#define PS_QMF_TIME_SLOTS 32
-#define PS_AP_LINKS 3
-#define PS_MAX_AP_DELAY 5
-
-typedef struct PSDSPContext {
- void (*add_squares)(INTFLOAT *dst, const INTFLOAT (*src)[2], int n);
- void (*mul_pair_single)(INTFLOAT (*dst)[2], INTFLOAT (*src0)[2], INTFLOAT *src1,
- int n);
- void (*hybrid_analysis)(INTFLOAT (*out)[2], INTFLOAT (*in)[2],
- const INTFLOAT (*filter)[8][2],
- ptrdiff_t stride, int n);
- void (*hybrid_analysis_ileave)(INTFLOAT (*out)[32][2], INTFLOAT L[2][38][64],
- int i, int len);
- void (*hybrid_synthesis_deint)(INTFLOAT out[2][38][64], INTFLOAT (*in)[32][2],
- int i, int len);
- void (*decorrelate)(INTFLOAT (*out)[2], INTFLOAT (*delay)[2],
- INTFLOAT (*ap_delay)[PS_QMF_TIME_SLOTS+PS_MAX_AP_DELAY][2],
- const INTFLOAT phi_fract[2], const INTFLOAT (*Q_fract)[2],
- const INTFLOAT *transient_gain,
- INTFLOAT g_decay_slope,
- int len);
- void (*stereo_interpolate[2])(INTFLOAT (*l)[2], INTFLOAT (*r)[2],
- INTFLOAT h[2][4], INTFLOAT h_step[2][4],
- int len);
-} PSDSPContext;
-
-void AAC_RENAME(ff_psdsp_init)(PSDSPContext *s);
-void ff_psdsp_init_arm(PSDSPContext *s);
-void ff_psdsp_init_aarch64(PSDSPContext *s);
-void ff_psdsp_init_mips(PSDSPContext *s);
-void ff_psdsp_init_riscv(PSDSPContext *s);
-void ff_psdsp_init_x86(PSDSPContext *s);
-
-#endif /* AVCODEC_AACPSDSP_H */
diff --git a/spaces/colakin/video-generater/public/ffmpeg/libavcodec/dct32_float.c b/spaces/colakin/video-generater/public/ffmpeg/libavcodec/dct32_float.c
deleted file mode 100644
index 597c9bb6397cbafc8181547343c7181962df2b55..0000000000000000000000000000000000000000
--- a/spaces/colakin/video-generater/public/ffmpeg/libavcodec/dct32_float.c
+++ /dev/null
@@ -1,20 +0,0 @@
-/*
- * This file is part of FFmpeg.
- *
- * FFmpeg is free software; you can redistribute it and/or
- * modify it under the terms of the GNU Lesser General Public
- * License as published by the Free Software Foundation; either
- * version 2.1 of the License, or (at your option) any later version.
- *
- * FFmpeg is distributed in the hope that it will be useful,
- * but WITHOUT ANY WARRANTY; without even the implied warranty of
- * MERCHANTABILITY or FITNESS FOR A PARTICULAR PURPOSE. See the GNU
- * Lesser General Public License for more details.
- *
- * You should have received a copy of the GNU Lesser General Public
- * License along with FFmpeg; if not, write to the Free Software
- * Foundation, Inc., 51 Franklin Street, Fifth Floor, Boston, MA 02110-1301 USA
- */
-
-#define DCT32_FLOAT 1
-#include "dct32_template.c"
diff --git a/spaces/colakin/video-generater/public/ffmpeg/libavcodec/idctdsp.h b/spaces/colakin/video-generater/public/ffmpeg/libavcodec/idctdsp.h
deleted file mode 100644
index 7224463349e7ff5351fd05e294f39bc1a0fc86bb..0000000000000000000000000000000000000000
--- a/spaces/colakin/video-generater/public/ffmpeg/libavcodec/idctdsp.h
+++ /dev/null
@@ -1,117 +0,0 @@
-/*
- * This file is part of FFmpeg.
- *
- * FFmpeg is free software; you can redistribute it and/or
- * modify it under the terms of the GNU Lesser General Public
- * License as published by the Free Software Foundation; either
- * version 2.1 of the License, or (at your option) any later version.
- *
- * FFmpeg is distributed in the hope that it will be useful,
- * but WITHOUT ANY WARRANTY; without even the implied warranty of
- * MERCHANTABILITY or FITNESS FOR A PARTICULAR PURPOSE. See the GNU
- * Lesser General Public License for more details.
- *
- * You should have received a copy of the GNU Lesser General Public
- * License along with FFmpeg; if not, write to the Free Software
- * Foundation, Inc., 51 Franklin Street, Fifth Floor, Boston, MA 02110-1301 USA
- */
-
-#ifndef AVCODEC_IDCTDSP_H
-#define AVCODEC_IDCTDSP_H
-
-#include
-
-#include "config.h"
-
-#include "avcodec.h"
-
-enum idct_permutation_type {
- FF_IDCT_PERM_NONE,
- FF_IDCT_PERM_LIBMPEG2,
- FF_IDCT_PERM_SIMPLE,
- FF_IDCT_PERM_TRANSPOSE,
- FF_IDCT_PERM_PARTTRANS,
- FF_IDCT_PERM_SSE2,
-};
-
-void ff_permute_scantable(uint8_t dst[64], const uint8_t src[64],
- const uint8_t permutation[64]);
-void ff_init_scantable_permutation(uint8_t *idct_permutation,
- enum idct_permutation_type perm_type);
-int ff_init_scantable_permutation_x86(uint8_t *idct_permutation,
- enum idct_permutation_type perm_type);
-
-typedef struct IDCTDSPContext {
- /* pixel ops : interface with DCT */
- void (*put_pixels_clamped)(const int16_t *block /* align 16 */,
- uint8_t *av_restrict pixels /* align 8 */,
- ptrdiff_t line_size);
- void (*put_signed_pixels_clamped)(const int16_t *block /* align 16 */,
- uint8_t *av_restrict pixels /* align 8 */,
- ptrdiff_t line_size);
- void (*add_pixels_clamped)(const int16_t *block /* align 16 */,
- uint8_t *av_restrict pixels /* align 8 */,
- ptrdiff_t line_size);
-
- void (*idct)(int16_t *block /* align 16 */);
-
- /**
- * block -> idct -> clip to unsigned 8 bit -> dest.
- * (-1392, 0, 0, ...) -> idct -> (-174, -174, ...) -> put -> (0, 0, ...)
- * @param line_size size in bytes of a horizontal line of dest
- */
- void (*idct_put)(uint8_t *dest /* align 8 */,
- ptrdiff_t line_size, int16_t *block /* align 16 */);
-
- /**
- * block -> idct -> add dest -> clip to unsigned 8 bit -> dest.
- * @param line_size size in bytes of a horizontal line of dest
- */
- void (*idct_add)(uint8_t *dest /* align 8 */,
- ptrdiff_t line_size, int16_t *block /* align 16 */);
-
- /**
- * IDCT input permutation.
- * Several optimized IDCTs need a permutated input (relative to the
- * normal order of the reference IDCT).
- * This permutation must be performed before the idct_put/add.
- * Note, normally this can be merged with the zigzag/alternate scan
- * An example to avoid confusion:
- * - (->decode coeffs -> zigzag reorder -> dequant -> reference IDCT -> ...)
- * - (x -> reference DCT -> reference IDCT -> x)
- * - (x -> reference DCT -> simple_mmx_perm = idct_permutation
- * -> simple_idct_mmx -> x)
- * - (-> decode coeffs -> zigzag reorder -> simple_mmx_perm -> dequant
- * -> simple_idct_mmx -> ...)
- */
- uint8_t idct_permutation[64];
- enum idct_permutation_type perm_type;
-
- int mpeg4_studio_profile;
-} IDCTDSPContext;
-
-void ff_put_pixels_clamped_c(const int16_t *block, uint8_t *av_restrict pixels,
- ptrdiff_t line_size);
-void ff_add_pixels_clamped_c(const int16_t *block, uint8_t *av_restrict pixels,
- ptrdiff_t line_size);
-
-void ff_idctdsp_init(IDCTDSPContext *c, AVCodecContext *avctx);
-
-void ff_idctdsp_init_aarch64(IDCTDSPContext *c, AVCodecContext *avctx,
- unsigned high_bit_depth);
-void ff_idctdsp_init_alpha(IDCTDSPContext *c, AVCodecContext *avctx,
- unsigned high_bit_depth);
-void ff_idctdsp_init_arm(IDCTDSPContext *c, AVCodecContext *avctx,
- unsigned high_bit_depth);
-void ff_idctdsp_init_ppc(IDCTDSPContext *c, AVCodecContext *avctx,
- unsigned high_bit_depth);
-void ff_idctdsp_init_riscv(IDCTDSPContext *c, AVCodecContext *avctx,
- unsigned high_bit_depth);
-void ff_idctdsp_init_x86(IDCTDSPContext *c, AVCodecContext *avctx,
- unsigned high_bit_depth);
-void ff_idctdsp_init_mips(IDCTDSPContext *c, AVCodecContext *avctx,
- unsigned high_bit_depth);
-void ff_idctdsp_init_loongarch(IDCTDSPContext *c, AVCodecContext *avctx,
- unsigned high_bit_depth);
-
-#endif /* AVCODEC_IDCTDSP_H */
diff --git a/spaces/comet-team/kangas-direct/Dockerfile b/spaces/comet-team/kangas-direct/Dockerfile
deleted file mode 100644
index 9599479da17b9f59db643a5d9276fdef23679251..0000000000000000000000000000000000000000
--- a/spaces/comet-team/kangas-direct/Dockerfile
+++ /dev/null
@@ -1,12 +0,0 @@
-FROM python:3.9
-
-WORKDIR /code
-
-COPY ./requirements.txt /code/requirements.txt
-
-RUN pip install --no-cache-dir --upgrade -r /code/requirements.txt
-RUN kangas upgrade /code/*.datagrid
-
-COPY . .
-
-CMD kangas server cppe-5-test.datagrid --frontend-port=7860
diff --git a/spaces/congsaPfin/Manga-OCR/logs/Enjoy Gun Master 3D with Mod APK - Remove Ads and Unlock All Levels.md b/spaces/congsaPfin/Manga-OCR/logs/Enjoy Gun Master 3D with Mod APK - Remove Ads and Unlock All Levels.md
deleted file mode 100644
index 367481e5fb242a14f69f4008aebb23521d01e46c..0000000000000000000000000000000000000000
--- a/spaces/congsaPfin/Manga-OCR/logs/Enjoy Gun Master 3D with Mod APK - Remove Ads and Unlock All Levels.md
+++ /dev/null
@@ -1,92 +0,0 @@
-
-Gun Master 3D APK Mod: A Fun and Exciting Shooting Game
-If you are looking for a shooting game that will test your skills and reflexes, then you should try Gun Master 3D. This is a game where you can unleash your inner gun master and shoot down various targets with different guns and weapons. You can also customize your arsenal and upgrade your guns to make them more powerful and accurate. In this article, we will tell you more about Gun Master 3D, why you should download its APK mod version, and how to do it.
-gun master 3d apk mod Download › https://urlca.com/2uOfnF
- What is Gun Master 3D?
-Gun Master 3D is a shooting game developed by Lion Studios. It is available for both Android and iOS devices. In this game, you can choose from a wide range of guns and weapons, such as pistols, rifles, shotguns, snipers, machine guns, rocket launchers, and more. You can also modify your guns with different attachments, such as scopes, silencers, magazines, barrels, etc.
-The game has various game modes and challenges that will keep you entertained and challenged. You can play in the classic mode, where you have to shoot down different targets, such as bottles, cans, fruits, zombies, etc. You can also play in the duel mode, where you have to face off against other players online and see who has the better aim and reaction. You can also play in the boss mode, where you have to defeat powerful enemies with special abilities.
-The game has stunning graphics and sound effects that will make you feel like you are in a real shooting range. The game also has realistic physics and animations that will make your shots more satisfying and realistic.
- Features of Gun Master 3D
-- Different guns and weapons to choose from
-The game has a huge collection of guns and weapons that you can use to shoot down your targets. You can choose from pistols, rifles, shotguns, snipers, machine guns, rocket launchers, and more. Each gun has its own characteristics, such as damage, accuracy, fire rate, recoil, etc. You can also customize your guns with different attachments, such as scopes, silencers, magazines, barrels, etc.
-- Various game modes and challenges
-The game has different game modes and challenges that will test your skills and reflexes. You can play in the classic mode, where you have to shoot down different targets, such as bottles, cans, fruits, zombies, etc. You can also play in the duel mode, where you have to face off against other players online and see who has the better aim and reaction. You can also play in the boss mode, where you have to defeat powerful enemies with special abilities.
-- Stunning graphics and sound effects
-The game has amazing graphics and sound effects that will make you feel like you are in a real shooting range. The game also has realistic physics and animations that will make your shots more satisfying and realistic.
- Why download Gun Master 3D APK Mod?
-If you want to enjoy Gun Master 3D without any limitations or interruptions, then you should download its APK mod version. The APK mod version is a modified version of the original game that gives you some extra benefits that are not available in the official version.
- Benefits of Gun Master 3D APK Mod
-- Remove ads and enjoy uninterrupted gameplay
-One of the benefits of Gun Master 3D APK Mod is that it removes all the annoying ads that pop up in the original game. This way, you can enjoy the game without any distractions or interruptions. You can also save your mobile data and battery by not loading the ads.
-gun master 3d mod apk unlimited money
-gun master 3d mod apk download latest version
-gun master 3d mod apk android 1
-gun master 3d mod apk revdl
-gun master 3d mod apk hack
-gun master 3d mod apk no ads
-gun master 3d mod apk free shopping
-gun master 3d mod apk all guns unlocked
-gun master 3d mod apk rexdl
-gun master 3d mod apk happymod
-gun master 3d mod apk offline
-gun master 3d mod apk unlimited coins and gems
-gun master 3d mod apk unlimited ammo
-gun master 3d mod apk vip unlocked
-gun master 3d mod apk premium
-gun master 3d mod apk latest update
-gun master 3d mod apk online
-gun master 3d mod apk unlimited everything
-gun master 3d mod apk no root
-gun master 3d mod apk obb
-gun master 3d mod apk for pc
-gun master 3d mod apk unlimited diamonds
-gun master 3d mod apk god mode
-gun master 3d mod apk mega
-gun master 3d mod apk mediafıre
-gun master 3d mod apk new version
-gun master 3d mod apk full version
-gun master 3d mod apk unlocked all levels
-gun master 3d mod apk unlimited health
-gun master 3d mod apk pro
-gun master 3d mod apk cheat
-gun master 3d mod apk an1
-gun master 3d mod apk data
-gun master 3d mod apk pure
-gun master 3d mod apk original
-gun master 3d mod apk old version
-gun master 3d mod apk unlimited gold and silver
-gun master 3d mod apk no verification
-gun master 3d mod apk easy download
-gun master 3d mod apk high damage
-- Unlock all guns and weapons for free
-Another benefit of Gun Master 3D APK Mod is that it unlocks all the guns and weapons for free. This means that you don't have to spend any real money or coins to get access to the best guns and weapons in the game. You can also upgrade your guns and weapons to the maximum level without any cost.
-- Get unlimited coins and gems to upgrade your arsenal
-The third benefit of Gun Master 3D APK Mod is that it gives you unlimited coins and gems to upgrade your arsenal. Coins and gems are the in-game currencies that you can use to buy and upgrade your guns and weapons. With the APK mod version, you can get as many coins and gems as you want without any limit. You can also use them to buy other items, such as skins, outfits, etc.
- How to download and install Gun Master 3D APK Mod?
-If you are interested in downloading and installing Gun Master 3D APK Mod, then you need to follow some simple steps. Here are the steps to download and install Gun Master 3D APK Mod:
- Steps to download and install Gun Master 3D APK Mod
-- Download the APK file from a trusted source
-The first step is to download the APK file from a trusted source. You can find many websites that offer the APK file for free, but you need to be careful about the security and quality of the file. Some websites may contain viruses or malware that can harm your device or steal your personal information. Therefore, we recommend you to download the APK file from [this link], which is safe and reliable.
-- Enable unknown sources on your device settings
-The second step is to enable unknown sources on your device settings. This is because the APK file is not from the official Google Play Store, so you need to allow your device to install apps from other sources. To do this, go to your device settings, then security, then unknown sources, and turn it on. You may see a warning message, but don't worry, it is safe to proceed.
-- Install the APK file and launch the game
-The third step is to install the APK file and launch the game. To do this, locate the downloaded APK file on your device storage, then tap on it and follow the instructions on the screen. It may take a few seconds or minutes depending on your device performance. Once the installation is done, you can launch the game from your app drawer or home screen.
- Conclusion
-Gun Master 3D is a fun and exciting shooting game that will test your skills and reflexes. You can choose from a wide range of guns and weapons, customize your arsenal, play in different game modes and challenges, and enjoy stunning graphics and sound effects. If you want to enjoy Gun Master 3D without any limitations or interruptions, then you should download its APK mod version, which gives you some extra benefits, such as removing ads, unlocking all guns and weapons for free, and getting unlimited coins and gems to upgrade your arsenal. To download and install Gun Master 3D APK Mod, just follow the simple steps we have provided in this article.
- We hope you found this article helpful and informative. If you have any questions or feedback, please feel free to leave a comment below. Thank you for reading!
- FAQs
-Here are some frequently asked questions about Gun Master 3D APK Mod:
-
-Is Gun Master 3D APK Mod safe to use?
-Yes, Gun Master 3D APK Mod is safe to use as long as you download it from a trusted source, such as [this link]. We have tested the file ourselves and found no viruses or malware in it. However, you should always be careful when downloading files from unknown sources and scan them with an antivirus before installing them.
-Is Gun Master 3D APK Mod compatible with my device?
-Gun Master 3D APK Mod is compatible with most Android devices that run on Android 5.0 or higher. However, some devices may have compatibility issues due to different hardware or software specifications. If you encounter any problems while playing the game, you can try to update your device software, clear the game cache, or reinstall the game.
-Does Gun Master 3D APK Mod require root access?
-No, Gun Master 3D APK Mod does not require root access to work. You can install and play the game without rooting your device. However, if you have a rooted device, you can still use the APK mod version without any problems.
-Can I play Gun Master 3D APK Mod online with other players?
-Yes, you can play Gun Master 3D APK Mod online with other players in the duel mode. However, you may not be able to join some servers or matches that are hosted by players who are using the official version of the game. You may also face some lag or connection issues due to the modded features of the game.
-Can I update Gun Master 3D APK Mod to the latest version?
-Yes, you can update Gun Master 3D APK Mod to the latest version by downloading and installing the new APK file from the same source that you used before. However, you may lose some of your progress or data if you update the game. Therefore, we recommend you to backup your game data before updating the game.
- 197e85843d
-
-
\ No newline at end of file
diff --git a/spaces/congsaPfin/Manga-OCR/logs/Los Angeles Crimes for PC Explore Create and Discover Immersive Worlds with an Emulator.md b/spaces/congsaPfin/Manga-OCR/logs/Los Angeles Crimes for PC Explore Create and Discover Immersive Worlds with an Emulator.md
deleted file mode 100644
index 17b1bf042d1d56eb009ef13f068651f15a754f6e..0000000000000000000000000000000000000000
--- a/spaces/congsaPfin/Manga-OCR/logs/Los Angeles Crimes for PC Explore Create and Discover Immersive Worlds with an Emulator.md
+++ /dev/null
@@ -1,80 +0,0 @@
-
-Los Angeles Crimes Download for PC: How to Play This Action Game on Your Computer
-Do you love action games that let you explore a variety of immersive worlds and participate in exciting game modes? If so, you might want to check out Los Angeles Crimes, a stylized action-adventure game that offers offline and online gameplay, realistic physics and animations, and a map editor that lets you create your own scenarios. In this article, we will show you how to download and play Los Angeles Crimes on your PC using an Android emulator, as well as the benefits of playing this game on a larger screen with better performance. Let's get started!
- Features of Los Angeles Crimes
-Los Angeles Crimes is an action game developed by Mohammad Alizade that combines elements of sandbox, shooter, racing, soccer, and more. Here are some of the features that make this game stand out:
-los angeles crimes download for pc Download --->>> https://urlca.com/2uO8Hg
- Explore an expansive environment with different game modes
-In Los Angeles Crimes, you can roam around a large open world that includes urban areas, deserts, forests, mountains, and islands. You can also choose from various game modes such as team death-match, zombie survival, soccer, vehicle racing, parkour, and more. Each mode has its own objectives, rules, and challenges that will keep you entertained for hours.
- Build your own maps with the LAC Editor
-If you want to unleash your creativity and customize your gameplay experience, you can use the LAC Editor to create your own maps. You can use infinite resources to spawn items, vehicles, weapons, NPCs, and more. You can also modify the properties of the spawned items, such as their size, color, rotation, health, damage, etc. You can then save and share your maps with other players online or play them offline by yourself.
- Enjoy realistic physics and animations
-Los Angeles Crimes features active ragdoll physics that make the characters react realistically to forces and impacts. You can also see motion-captured animations that make the movements of the characters smooth and natural. You can switch between third-person and first-person perspectives to suit your preference and enjoy different camera angles.
- Play online with your friends or offline by yourself
-Los Angeles Crimes supports both online and offline gameplay modes. You can join or host online servers where you can play with up to 10 players in various game modes. You can also chat with other players using voice or text messages. Alternatively, you can play offline by yourself or with bots in single-player mode. You can adjust the difficulty level and the number of bots according to your liking.
- How to Download and Play Los Angeles Crimes on PC
-If you want to play Los Angeles Crimes on your PC, you will need to use an Android emulator. An Android emulator is a software that allows you to run Android apps and games on your computer. There are many Android emulators available online, but we recommend using BlueStacks 5, LDPlayer, or MuMu Player. These are some of the best emulators that offer high performance, compatibility, security, and features. Here are the steps to download and play Los Angeles Crimes on PC using any of these emulators:
- Download and install an Android emulator on your PC
-First, you need to download and install an Android emulator on your PC. You can choose from BlueStacks 5, LDPlayer, or MuMu Player, depending on your preference and system requirements. You can find the download links for these emulators on their official websites or by searching online. Once you have downloaded the emulator installer, run it and follow the instructions to complete the installation process. You may need to sign in with your Google account to access the emulator's app store.
-How to play Los Angeles Crimes on PC with BlueStacks emulator
-Los Angeles Crimes PC game free download and install
-Los Angeles Crimes offline action-adventure game for PC
-Los Angeles Crimes LAN support and PS4 controller compatibility
-Los Angeles Crimes zombie survival mode on PC
-Los Angeles Crimes car race and soccer game modes on PC
-Los Angeles Crimes third-person and first-person view options on PC
-Los Angeles Crimes active-ragdoll and realistic physics on PC
-Los Angeles Crimes LAC Editor and map creation on PC
-Los Angeles Crimes online multiplayer and team death-match on PC
-Los Angeles Crimes best Android emulator for PC
-Los Angeles Crimes minimum system requirements for PC
-Los Angeles Crimes gameplay and features on PC
-Los Angeles Crimes tips and tricks for PC players
-Los Angeles Crimes latest version and updates for PC
-Los Angeles Crimes review and rating for PC
-Los Angeles Crimes cheats and hacks for PC
-Los Angeles Crimes mod apk and obb file download for PC
-Los Angeles Crimes graphics settings and performance optimization for PC
-Los Angeles Crimes keyboard and mouse controls for PC
-Los Angeles Crimes comparison with GTA 5 on PC
-Los Angeles Crimes custom skins and outfits on PC
-Los Angeles Crimes weapons and vehicles list on PC
-Los Angeles Crimes bugs and glitches fix for PC
-Los Angeles Crimes fan-made videos and screenshots on PC
-Los Angeles Crimes community and forums for PC users
-Los Angeles Crimes developer and publisher information for PC
-Los Angeles Crimes FAQs and troubleshooting guide for PC
-Los Angeles Crimes alternatives and similar games for PC
-Los Angeles Crimes steam version and price for PC
- Search for Los Angeles Crimes in the emulator's app store
-Next, you need to search for Los Angeles Crimes in the emulator's app store. You can use the search bar or the browse feature to find the game. Alternatively, you can also download the Los Angeles Crimes APK file from a trusted source and drag and drop it into the emulator to install it. Once you have found the game, click on it and then click on the install button to start the installation process. The game will be downloaded and installed on your PC within a few minutes.
- Install and launch Los Angeles Crimes on your PC
-After the installation is complete, you can launch Los Angeles Crimes on your PC by clicking on its icon on the emulator's home screen or app drawer. The game will start and you will see the main menu where you can choose your game mode, settings, and other options. You can also sign in with your Facebook account to save your progress and access your online servers.
- Customize your settings and controls
-Before you start playing Los Angeles Crimes on your PC, you may want to customize your settings and controls to suit your preference and comfort. You can access the settings menu by clicking on the gear icon on the top right corner of the screen. Here, you can adjust the graphics quality, sound volume, language, camera sensitivity, and other options. You can also access the controls menu by clicking on the keyboard icon on the bottom right corner of the screen. Here, you can see the default keyboard mapping for the game and change it if you want. You can also use your mouse to aim and shoot, as well as use other emulator features such as macros, multi-instance, gamepad support, etc.
- Benefits of Playing Los Angeles Crimes on PC
-Playing Los Angeles Crimes on PC has many advantages over playing it on a mobile device. Here are some of the benefits that you can enjoy:
- Experience better graphics and performance
-By playing Los Angeles Crimes on PC, you can experience better graphics and performance than playing it on a mobile device. You can use a higher resolution, frame rate, and graphics quality to make the game look more realistic and smooth. You can also avoid lagging, crashing, or freezing issues that may occur on some mobile devices due to low memory or CPU power.
- Use a larger screen and keyboard for more comfort and accuracy
-Another benefit of playing Los Angeles Crimes on PC is that you can use a larger screen and keyboard for more comfort and accuracy. You can see more details and have a wider field of view on a bigger screen than on a small mobile screen. You can also use a keyboard and mouse to control your character and actions more precisely and easily than using touch controls on a mobile device.
- Save your battery and data usage
-Playing Los Angeles Crimes on PC can also help you save your battery and data usage that you would otherwise spend on playing it on a mobile device. You can play for longer hours without worrying about draining your battery or overheating your device. You can also use a stable Wi-Fi connection instead of using your mobile data plan that may incur extra charges or have limited bandwidth.
- Access exclusive features and enhancements
-Finally, playing Los Angeles Crimes on PC can give you access to exclusive features and enhancements that are not available on mobile devices. For example, you can use BlueStacks 5's Eco Mode to reduce CPU usage and improve performance while playing multiple games at once. You can also use LDPlayer's FPS Boost feature to increase the frame rate of the game for smoother gameplay. You can also use MuMu Player's Screen Recorder feature to record your gameplay videos and share them with others.
- Conclusion
-In conclusion, Los Angeles Crimes is an action game that offers offline and online gameplay, realistic physics and animations, and a map editor that lets you create your own scenarios. You can download and play this game on your PC using an Android emulator such as BlueStacks 5, LDPlayer, or MuMu Player. By doing so, you can enjoy better graphics and performance, use a larger screen and keyboard for more comfort and accuracy, save your battery and data usage, and access exclusive features and enhancements. If you are looking for a fun and versatile action game to play on your PC, you should definitely give Los Angeles Crimes a try. You won't regret it!
- FAQs
-Here are some of the frequently asked questions about Los Angeles Crimes and how to play it on PC:
- Is Los Angeles Crimes free to play?
-Yes, Los Angeles Crimes is free to play and download on both mobile devices and PC. However, the game may contain ads and in-app purchases that you can choose to buy or not.
- Is Los Angeles Crimes safe to play?
-Yes, Los Angeles Crimes is safe to play as long as you download it from a trusted source such as the Google Play Store or the official websites of the Android emulators. You should also avoid downloading any modded or hacked versions of the game that may contain viruses or malware.
- How can I update Los Angeles Crimes on PC?
-To update Los Angeles Crimes on PC, you need to open the emulator's app store and check if there is a new version of the game available. If there is, you can click on the update button to download and install the latest version of the game. You can also enable the auto-update feature in the emulator's settings to automatically update the game whenever there is a new version.
- How can I contact the developer of Los Angeles Crimes?
-If you have any questions, feedback, or suggestions for the developer of Los Angeles Crimes, you can contact them through their email address: mohammad.alizade@hotmail.com. You can also follow them on their Instagram account: @mammaddev.
- What are some similar games to Los Angeles Crimes?
-If you like Los Angeles Crimes, you may also enjoy some similar games such as GTA: San Andreas, Gangstar Vegas, MadOut2 BigCityOnline, and Payback 2. These are some of the best action games that let you explore open worlds, engage in various game modes, and have fun with realistic physics and animations.
401be4b1e0
-
-
\ No newline at end of file
diff --git a/spaces/congsaPfin/Manga-OCR/logs/Traffic Racer Hack MOD APK The Most Realistic and Fun Racing Game Ever.md b/spaces/congsaPfin/Manga-OCR/logs/Traffic Racer Hack MOD APK The Most Realistic and Fun Racing Game Ever.md
deleted file mode 100644
index 0bbe8b692c788ab6df922cee2665de543f297683..0000000000000000000000000000000000000000
--- a/spaces/congsaPfin/Manga-OCR/logs/Traffic Racer Hack MOD APK The Most Realistic and Fun Racing Game Ever.md
+++ /dev/null
@@ -1,106 +0,0 @@
-
-Traffic Racer Hack Mod APK: How to Download and Play the Ultimate Arcade Racing Game
- If you are looking for a fun and addictive racing game that will test your skills and reflexes, you should check out Traffic Racer . This is a game that lets you drive your car through highway traffic, earn cash, upgrade your car, and buy new ones. You can also try to be one of the fastest drivers in the global leaderboards and earn achievements.
- Traffic Racer is a game that offers stunning 3D graphics, smooth and realistic car handling, over 40 different cars to choose from, 5 detailed environments, and 5 game modes. You can play in Endless, Two-Way, Time Trial, Police Chase, or Free Ride mode. You can also customize your car through paint and wheels.
-traffic racer hack mod apk Download File === https://urlca.com/2uOaEg
- However, if you want to enjoy the game even more, you should try the Traffic Racer Hack Mod APK . This is a modified version of the game that gives you unlimited money, all cars unlocked, and no ads. With this hack mod apk, you can buy any car you want, upgrade it to the max, and drive without any interruptions.
- In this article, we will show you how to download and install Traffic Racer Hack Mod APK on your Android device. We will also give you some tips and tricks for playing the game and answer some frequently asked questions. So, if you are ready to experience the ultimate arcade racing game, read on!
- Features of Traffic Racer Hack Mod APK
- Traffic Racer Hack Mod APK is a version of the game that has been modified by some developers to give you some extra features that are not available in the original game. Here are some of the features that you can enjoy with this hack mod apk:
- Unlimited Money
- One of the main features of Traffic Racer Hack Mod APK is that it gives you unlimited money. This means that you can buy any car you want without having to worry about earning cash. You can also upgrade your car to improve its speed, handling, and brakes. You can also change its color and wheels to suit your style.
- All Cars Unlocked
- Another feature of Traffic Racer Hack Mod APK is that it unlocks all cars for you. This means that you can choose from over 40 different cars that are based on real-life models. You can drive sports cars, muscle cars, trucks, buses, SUVs, and more. Each car has its own characteristics and performance.
- No Ads
- The last feature of Traffic Racer Hack Mod APK is that it removes all ads from the game. This means that you can play without any interruptions or distractions. You can also save your data and battery life by not having to watch any video ads or banners.
- How to Download and Install Traffic Racer Hack Mod APK
- If you want to download and install Traffic Racer Hack Mod APK on your Android device, you need to follow these simple steps:
- Step 1: Enable Unknown Sources
- Before you can install any APK file on your device, you need to enable the option to allow installation from unknown sources. This is a security measure that prevents malicious apps from harming your device. To enable this option, go to your device settings, then security, then unknown sources. Toggle the switch to turn it on.
-Traffic Racer unlimited money mod apk download
-How to install Traffic Racer mod apk on android
-Traffic Racer mod apk latest version 3.6 free
-Traffic Racer hack mod apk with unlimited coins and cash
-Best racing game Traffic Racer mod apk for android
-Traffic Racer mod apk offline mode no internet required
-Traffic Racer hack mod apk features and gameplay
-Download Traffic Racer mod apk from AN1.com[^1^]
-Traffic Racer mod apk cheats and tips
-Traffic Racer hack mod apk review and rating
-Traffic Racer mod apk 3.6 update and new cars
-Traffic Racer hack mod apk unlimited nitro and fuel
-Traffic Racer mod apk online multiplayer mode
-Traffic Racer hack mod apk no root needed
-Traffic Racer mod apk file size and compatibility
-Traffic Racer hack mod apk unlimited customization options
-Traffic Racer mod apk realistic graphics and sound effects
-Traffic Racer hack mod apk easy controls and smooth gameplay
-Traffic Racer mod apk different game modes and levels
-Traffic Racer hack mod apk unlock all cars and upgrades
-Traffic Racer mod apk free shopping in the store
-Traffic Racer hack mod apk unlimited fun and excitement
-Traffic Racer mod apk support for different languages
-Traffic Racer hack mod apk safe and secure download
-Traffic Racer mod apk high score and leaderboard challenge
- Step 2: Download the APK File
- Next, you need to download the APK file of Traffic Racer Hack Mod APK. You can find the link to download it from various websites that offer modded games. Make sure that you download it from a trusted and reliable source. You can also scan the file with an antivirus app before installing it.
- Step 3: Install the APK File
- Once you have downloaded the APK file, you need to install it on your device. To do this, locate the file in your file manager or downloads folder and tap on it. You will see a prompt asking you to confirm the installation. Tap on install and wait for the process to finish.
- Step 4: Launch the Game and Enjoy
- After the installation is complete, you can launch the game and enjoy its features. You will see that you have unlimited money and all cars unlocked. You can also play without any ads. You can now drive your car through traffic and have fun!
- Tips and Tricks for Playing Traffic Racer Hack Mod APK
- Traffic Racer Hack Mod APK is a game that requires skill and strategy to play well. Here are some tips and tricks that can help you improve your performance and score:
- Choose the Right Car and Upgrade It
- One of the most important factors in playing Traffic Racer Hack Mod APK is choosing the right car for your style and preference. Each car has different attributes such as speed, handling, and brakes. You should choose a car that suits your needs and matches the game mode and environment you are playing in.
- For example, if you are playing in Endless mode, you might want a car that has high speed and good handling to avoid crashing into other vehicles. If you are playing in Police Chase mode, you might want a car that has good brakes and acceleration to escape from the cops.
- You should also upgrade your car regularly to improve its performance. You can use your unlimited money to buy upgrades such as engine, turbo, tires, suspension, and nitro. These upgrades can make your car faster, more responsive, and more stable.
- Master the Different Game Modes and Environments
- Traffic Racer Hack Mod APK offers five different game modes and five different environments to play in. Each game mode and environment has its own challenges and rewards. You should master each one of them to get the most out of the game.
- The game modes are:
-
-Endless: This is the classic mode where you drive as far as you can without crashing or running out of time. You can earn cash by driving fast, overtaking other cars, driving in the opposite direction, or performing close shaves.
-Two-Way: This is similar to Endless mode, but you have to drive in both directions of traffic. This makes it more difficult and risky, but also more rewarding.
-Time Trial: This is a mode where you have to drive as fast as you can within a given time limit. You can earn extra time by passing checkpoints or driving fast.
-Police Chase: This is a mode where you have to escape from the police who are chasing you. You have to avoid crashing into them or getting caught by their roadblocks or spike strips.
-Free Ride: This is a mode where you can drive freely without any rules or objectives. You can use this mode to practice your skills or just have fun.
-
-The environments are:
-
-Suburb: This is a suburban area with houses, trees, and traffic lights. It has moderate traffic density and speed.
-Desert: This is a desert area with sand dunes, cacti, and rocks. It has low traffic density and high speed.
-Snowy: This is a snowy area with snow-covered roads , mountains, and bridges. It has high traffic density and low speed.
-City Night: This is a city area with skyscrapers, neon lights, and tunnels. It has high traffic density and speed.
-Rainy: This is a rainy area with wet roads, puddles, and thunderstorms. It has moderate traffic density and speed.
-
-You should try to play in different game modes and environments to experience the variety and challenge of the game. You can also earn more cash and achievements by completing different tasks and missions in each mode and environment.
- Perform Close Shaves and Drive in the Wrong Direction for More Coins
- One of the ways to earn more coins in Traffic Racer Hack Mod APK is to perform close shaves and drive in the wrong direction. A close shave is when you pass very close to another car without crashing into it. Driving in the wrong direction is when you drive in the opposite lane of traffic.
- Both of these actions are risky but rewarding. You can earn more coins by doing them, as well as increase your score multiplier. However, you also have to be careful not to crash into other cars or obstacles, as this will end your run and reduce your score.
- You should try to perform close shaves and drive in the wrong direction whenever you can, but also be aware of your surroundings and timing. You can also use your nitro boost to speed up and avoid collisions.
- Challenge Yourself in Time Trial Mode and Climb the Leaderboards
- If you want to test your skills and compete with other players, you should try playing in Time Trial mode and climbing the leaderboards. Time Trial mode is a mode where you have to drive as fast as you can within a given time limit. You can earn extra time by passing checkpoints or driving fast.
- Time Trial mode is a great way to improve your driving skills and reflexes, as well as challenge yourself to beat your own records. You can also compare your scores with other players from around the world on the global leaderboards. You can see your rank, name, score, car, and country on the leaderboards.
- You should try to play in Time Trial mode regularly and aim for higher scores. You can also use different cars and environments to see how they affect your performance. You can also earn achievements by reaching certain milestones or completing certain tasks in Time Trial mode.
- Conclusion: Why You Should Play Traffic Racer Hack Mod APK Today
- Traffic Racer Hack Mod APK is a game that offers you a lot of fun and excitement. It is a game that lets you drive your car through highway traffic, earn cash, upgrade your car, and buy new ones. It is a game that offers stunning 3D graphics, smooth and realistic car handling, over 40 different cars to choose from, 5 detailed environments, and 5 game modes.
- Traffic Racer Hack Mod APK is also a game that gives you unlimited money, all cars unlocked, and no ads. With this hack mod apk, you can buy any car you want, upgrade it to the max, and drive without any interruptions.
- Traffic Racer Hack Mod APK is a game that requires skill and strategy to play well. It is a game that challenges you to drive fast, avoid crashes, perform close shaves, drive in the wrong direction, escape from the police, and beat the clock. It is a game that lets you compete with other players on the global leaderboards and earn achievements.
- Traffic Racer Hack Mod APK is a game that you should play today if you love racing games. It is a game that will keep you entertained for hours and make you feel like a real racer. So what are you waiting for? Download Traffic Racer Hack Mod APK now and enjoy the ultimate arcade racing game!
- FAQs
- Is Traffic Racer Hack Mod APK Safe to Download and Play?
- Traffic Racer Hack Mod APK is safe to download and play if you get it from a trusted and reliable source. However, you should always be careful when downloading any APK file from unknown sources, as they may contain viruses or malware that can harm your device. You should also scan the file with an antivirus app before installing it.
- What are the Benefits of Playing Traffic Racer Hack Mod APK?
- The benefits of playing Traffic Racer Hack Mod APK are that you can enjoy unlimited money, all cars unlocked, and no ads. This means that you can buy any car you want without having to worry about earning cash. You can also upgrade your car to improve its speed, handling, and brakes. You can also change its color [user and wheels to suit your style. You can also play without any interruptions or distractions. You can also save your data and battery life by not having to watch any video ads or banners.
- How to Update Traffic Racer Hack Mod APK?
- To update Traffic Racer Hack Mod APK, you need to download the latest version of the APK file from the same source that you got it from. You can also check for updates from within the game by tapping on the settings icon and then on the update button. You will see a notification if there is a new version available. You can then download and install it over the existing one.
- How to Contact the Developers of Traffic Racer Hack Mod APK?
- If you have any questions, feedback, or suggestions for the developers of Traffic Racer Hack Mod APK, you can contact them by sending an email to trafficracergame@gmail.com. You can also follow them on Facebook and Twitter to get the latest news and updates about the game.
- What are Some Alternatives to Traffic Racer Hack Mod APK?
- If you are looking for some alternatives to Traffic Racer Hack Mod APK, you can try these other racing games that are similar in gameplay and features:
-
-Traffic Rider: This is a game that lets you ride a motorcycle through traffic, earn cash, buy new bikes, and upgrade them. It has realistic bike sounds, first-person view, over 30 different bikes, and 4 detailed environments.
-Traffic Tour: This is a game that lets you drive a car through traffic, race with friends, complete missions, and unlock new cars. It has 3D graphics, online multiplayer mode, over 100 different cars, and 5 realistic environments.
-Traffic Run: This is a game that lets you control a car through traffic, avoid collisions, and reach the finish line. It has simple and addictive gameplay, colorful graphics, over 50 different cars, and various levels.
- 401be4b1e0
-
-
\ No newline at end of file
diff --git a/spaces/coreml-community/ControlNet-v1-1-Annotators-cpu/annotator/zoe/zoedepth/models/base_models/midas_repo/midas/blocks.py b/spaces/coreml-community/ControlNet-v1-1-Annotators-cpu/annotator/zoe/zoedepth/models/base_models/midas_repo/midas/blocks.py
deleted file mode 100644
index 6d87a00680bb6ed9a6d7c3043ea30a1e90361794..0000000000000000000000000000000000000000
--- a/spaces/coreml-community/ControlNet-v1-1-Annotators-cpu/annotator/zoe/zoedepth/models/base_models/midas_repo/midas/blocks.py
+++ /dev/null
@@ -1,439 +0,0 @@
-import torch
-import torch.nn as nn
-
-from .backbones.beit import (
- _make_pretrained_beitl16_512,
- _make_pretrained_beitl16_384,
- _make_pretrained_beitb16_384,
- forward_beit,
-)
-from .backbones.swin_common import (
- forward_swin,
-)
-from .backbones.swin2 import (
- _make_pretrained_swin2l24_384,
- _make_pretrained_swin2b24_384,
- _make_pretrained_swin2t16_256,
-)
-from .backbones.swin import (
- _make_pretrained_swinl12_384,
-)
-from .backbones.levit import (
- _make_pretrained_levit_384,
- forward_levit,
-)
-from .backbones.vit import (
- _make_pretrained_vitb_rn50_384,
- _make_pretrained_vitl16_384,
- _make_pretrained_vitb16_384,
- forward_vit,
-)
-
-def _make_encoder(backbone, features, use_pretrained, groups=1, expand=False, exportable=True, hooks=None,
- use_vit_only=False, use_readout="ignore", in_features=[96, 256, 512, 1024]):
- if backbone == "beitl16_512":
- pretrained = _make_pretrained_beitl16_512(
- use_pretrained, hooks=hooks, use_readout=use_readout
- )
- scratch = _make_scratch(
- [256, 512, 1024, 1024], features, groups=groups, expand=expand
- ) # BEiT_512-L (backbone)
- elif backbone == "beitl16_384":
- pretrained = _make_pretrained_beitl16_384(
- use_pretrained, hooks=hooks, use_readout=use_readout
- )
- scratch = _make_scratch(
- [256, 512, 1024, 1024], features, groups=groups, expand=expand
- ) # BEiT_384-L (backbone)
- elif backbone == "beitb16_384":
- pretrained = _make_pretrained_beitb16_384(
- use_pretrained, hooks=hooks, use_readout=use_readout
- )
- scratch = _make_scratch(
- [96, 192, 384, 768], features, groups=groups, expand=expand
- ) # BEiT_384-B (backbone)
- elif backbone == "swin2l24_384":
- pretrained = _make_pretrained_swin2l24_384(
- use_pretrained, hooks=hooks
- )
- scratch = _make_scratch(
- [192, 384, 768, 1536], features, groups=groups, expand=expand
- ) # Swin2-L/12to24 (backbone)
- elif backbone == "swin2b24_384":
- pretrained = _make_pretrained_swin2b24_384(
- use_pretrained, hooks=hooks
- )
- scratch = _make_scratch(
- [128, 256, 512, 1024], features, groups=groups, expand=expand
- ) # Swin2-B/12to24 (backbone)
- elif backbone == "swin2t16_256":
- pretrained = _make_pretrained_swin2t16_256(
- use_pretrained, hooks=hooks
- )
- scratch = _make_scratch(
- [96, 192, 384, 768], features, groups=groups, expand=expand
- ) # Swin2-T/16 (backbone)
- elif backbone == "swinl12_384":
- pretrained = _make_pretrained_swinl12_384(
- use_pretrained, hooks=hooks
- )
- scratch = _make_scratch(
- [192, 384, 768, 1536], features, groups=groups, expand=expand
- ) # Swin-L/12 (backbone)
- elif backbone == "next_vit_large_6m":
- from .backbones.next_vit import _make_pretrained_next_vit_large_6m
- pretrained = _make_pretrained_next_vit_large_6m(hooks=hooks)
- scratch = _make_scratch(
- in_features, features, groups=groups, expand=expand
- ) # Next-ViT-L on ImageNet-1K-6M (backbone)
- elif backbone == "levit_384":
- pretrained = _make_pretrained_levit_384(
- use_pretrained, hooks=hooks
- )
- scratch = _make_scratch(
- [384, 512, 768], features, groups=groups, expand=expand
- ) # LeViT 384 (backbone)
- elif backbone == "vitl16_384":
- pretrained = _make_pretrained_vitl16_384(
- use_pretrained, hooks=hooks, use_readout=use_readout
- )
- scratch = _make_scratch(
- [256, 512, 1024, 1024], features, groups=groups, expand=expand
- ) # ViT-L/16 - 85.0% Top1 (backbone)
- elif backbone == "vitb_rn50_384":
- pretrained = _make_pretrained_vitb_rn50_384(
- use_pretrained,
- hooks=hooks,
- use_vit_only=use_vit_only,
- use_readout=use_readout,
- )
- scratch = _make_scratch(
- [256, 512, 768, 768], features, groups=groups, expand=expand
- ) # ViT-H/16 - 85.0% Top1 (backbone)
- elif backbone == "vitb16_384":
- pretrained = _make_pretrained_vitb16_384(
- use_pretrained, hooks=hooks, use_readout=use_readout
- )
- scratch = _make_scratch(
- [96, 192, 384, 768], features, groups=groups, expand=expand
- ) # ViT-B/16 - 84.6% Top1 (backbone)
- elif backbone == "resnext101_wsl":
- pretrained = _make_pretrained_resnext101_wsl(use_pretrained)
- scratch = _make_scratch([256, 512, 1024, 2048], features, groups=groups, expand=expand) # efficientnet_lite3
- elif backbone == "efficientnet_lite3":
- pretrained = _make_pretrained_efficientnet_lite3(use_pretrained, exportable=exportable)
- scratch = _make_scratch([32, 48, 136, 384], features, groups=groups, expand=expand) # efficientnet_lite3
- else:
- print(f"Backbone '{backbone}' not implemented")
- assert False
-
- return pretrained, scratch
-
-
-def _make_scratch(in_shape, out_shape, groups=1, expand=False):
- scratch = nn.Module()
-
- out_shape1 = out_shape
- out_shape2 = out_shape
- out_shape3 = out_shape
- if len(in_shape) >= 4:
- out_shape4 = out_shape
-
- if expand:
- out_shape1 = out_shape
- out_shape2 = out_shape*2
- out_shape3 = out_shape*4
- if len(in_shape) >= 4:
- out_shape4 = out_shape*8
-
- scratch.layer1_rn = nn.Conv2d(
- in_shape[0], out_shape1, kernel_size=3, stride=1, padding=1, bias=False, groups=groups
- )
- scratch.layer2_rn = nn.Conv2d(
- in_shape[1], out_shape2, kernel_size=3, stride=1, padding=1, bias=False, groups=groups
- )
- scratch.layer3_rn = nn.Conv2d(
- in_shape[2], out_shape3, kernel_size=3, stride=1, padding=1, bias=False, groups=groups
- )
- if len(in_shape) >= 4:
- scratch.layer4_rn = nn.Conv2d(
- in_shape[3], out_shape4, kernel_size=3, stride=1, padding=1, bias=False, groups=groups
- )
-
- return scratch
-
-
-def _make_pretrained_efficientnet_lite3(use_pretrained, exportable=False):
- efficientnet = torch.hub.load(
- "rwightman/gen-efficientnet-pytorch",
- "tf_efficientnet_lite3",
- pretrained=use_pretrained,
- exportable=exportable
- )
- return _make_efficientnet_backbone(efficientnet)
-
-
-def _make_efficientnet_backbone(effnet):
- pretrained = nn.Module()
-
- pretrained.layer1 = nn.Sequential(
- effnet.conv_stem, effnet.bn1, effnet.act1, *effnet.blocks[0:2]
- )
- pretrained.layer2 = nn.Sequential(*effnet.blocks[2:3])
- pretrained.layer3 = nn.Sequential(*effnet.blocks[3:5])
- pretrained.layer4 = nn.Sequential(*effnet.blocks[5:9])
-
- return pretrained
-
-
-def _make_resnet_backbone(resnet):
- pretrained = nn.Module()
- pretrained.layer1 = nn.Sequential(
- resnet.conv1, resnet.bn1, resnet.relu, resnet.maxpool, resnet.layer1
- )
-
- pretrained.layer2 = resnet.layer2
- pretrained.layer3 = resnet.layer3
- pretrained.layer4 = resnet.layer4
-
- return pretrained
-
-
-def _make_pretrained_resnext101_wsl(use_pretrained):
- resnet = torch.hub.load("facebookresearch/WSL-Images", "resnext101_32x8d_wsl")
- return _make_resnet_backbone(resnet)
-
-
-
-class Interpolate(nn.Module):
- """Interpolation module.
- """
-
- def __init__(self, scale_factor, mode, align_corners=False):
- """Init.
-
- Args:
- scale_factor (float): scaling
- mode (str): interpolation mode
- """
- super(Interpolate, self).__init__()
-
- self.interp = nn.functional.interpolate
- self.scale_factor = scale_factor
- self.mode = mode
- self.align_corners = align_corners
-
- def forward(self, x):
- """Forward pass.
-
- Args:
- x (tensor): input
-
- Returns:
- tensor: interpolated data
- """
-
- x = self.interp(
- x, scale_factor=self.scale_factor, mode=self.mode, align_corners=self.align_corners
- )
-
- return x
-
-
-class ResidualConvUnit(nn.Module):
- """Residual convolution module.
- """
-
- def __init__(self, features):
- """Init.
-
- Args:
- features (int): number of features
- """
- super().__init__()
-
- self.conv1 = nn.Conv2d(
- features, features, kernel_size=3, stride=1, padding=1, bias=True
- )
-
- self.conv2 = nn.Conv2d(
- features, features, kernel_size=3, stride=1, padding=1, bias=True
- )
-
- self.relu = nn.ReLU(inplace=True)
-
- def forward(self, x):
- """Forward pass.
-
- Args:
- x (tensor): input
-
- Returns:
- tensor: output
- """
- out = self.relu(x)
- out = self.conv1(out)
- out = self.relu(out)
- out = self.conv2(out)
-
- return out + x
-
-
-class FeatureFusionBlock(nn.Module):
- """Feature fusion block.
- """
-
- def __init__(self, features):
- """Init.
-
- Args:
- features (int): number of features
- """
- super(FeatureFusionBlock, self).__init__()
-
- self.resConfUnit1 = ResidualConvUnit(features)
- self.resConfUnit2 = ResidualConvUnit(features)
-
- def forward(self, *xs):
- """Forward pass.
-
- Returns:
- tensor: output
- """
- output = xs[0]
-
- if len(xs) == 2:
- output += self.resConfUnit1(xs[1])
-
- output = self.resConfUnit2(output)
-
- output = nn.functional.interpolate(
- output, scale_factor=2, mode="bilinear", align_corners=True
- )
-
- return output
-
-
-
-
-class ResidualConvUnit_custom(nn.Module):
- """Residual convolution module.
- """
-
- def __init__(self, features, activation, bn):
- """Init.
-
- Args:
- features (int): number of features
- """
- super().__init__()
-
- self.bn = bn
-
- self.groups=1
-
- self.conv1 = nn.Conv2d(
- features, features, kernel_size=3, stride=1, padding=1, bias=True, groups=self.groups
- )
-
- self.conv2 = nn.Conv2d(
- features, features, kernel_size=3, stride=1, padding=1, bias=True, groups=self.groups
- )
-
- if self.bn==True:
- self.bn1 = nn.BatchNorm2d(features)
- self.bn2 = nn.BatchNorm2d(features)
-
- self.activation = activation
-
- self.skip_add = nn.quantized.FloatFunctional()
-
- def forward(self, x):
- """Forward pass.
-
- Args:
- x (tensor): input
-
- Returns:
- tensor: output
- """
-
- out = self.activation(x)
- out = self.conv1(out)
- if self.bn==True:
- out = self.bn1(out)
-
- out = self.activation(out)
- out = self.conv2(out)
- if self.bn==True:
- out = self.bn2(out)
-
- if self.groups > 1:
- out = self.conv_merge(out)
-
- return self.skip_add.add(out, x)
-
- # return out + x
-
-
-class FeatureFusionBlock_custom(nn.Module):
- """Feature fusion block.
- """
-
- def __init__(self, features, activation, deconv=False, bn=False, expand=False, align_corners=True, size=None):
- """Init.
-
- Args:
- features (int): number of features
- """
- super(FeatureFusionBlock_custom, self).__init__()
-
- self.deconv = deconv
- self.align_corners = align_corners
-
- self.groups=1
-
- self.expand = expand
- out_features = features
- if self.expand==True:
- out_features = features//2
-
- self.out_conv = nn.Conv2d(features, out_features, kernel_size=1, stride=1, padding=0, bias=True, groups=1)
-
- self.resConfUnit1 = ResidualConvUnit_custom(features, activation, bn)
- self.resConfUnit2 = ResidualConvUnit_custom(features, activation, bn)
-
- self.skip_add = nn.quantized.FloatFunctional()
-
- self.size=size
-
- def forward(self, *xs, size=None):
- """Forward pass.
-
- Returns:
- tensor: output
- """
- output = xs[0]
-
- if len(xs) == 2:
- res = self.resConfUnit1(xs[1])
- output = self.skip_add.add(output, res)
- # output += res
-
- output = self.resConfUnit2(output)
-
- if (size is None) and (self.size is None):
- modifier = {"scale_factor": 2}
- elif size is None:
- modifier = {"size": self.size}
- else:
- modifier = {"size": size}
-
- output = nn.functional.interpolate(
- output, **modifier, mode="bilinear", align_corners=self.align_corners
- )
-
- output = self.out_conv(output)
-
- return output
-
diff --git a/spaces/cpwan/RLOR-TSP/envs/cvrp_vector_env.py b/spaces/cpwan/RLOR-TSP/envs/cvrp_vector_env.py
deleted file mode 100644
index 68174be31e1de6992b8406dba11585f5d567cbfb..0000000000000000000000000000000000000000
--- a/spaces/cpwan/RLOR-TSP/envs/cvrp_vector_env.py
+++ /dev/null
@@ -1,144 +0,0 @@
-import gym
-import numpy as np
-from gym import spaces
-
-from .vrp_data import VRPDataset
-
-
-def assign_env_config(self, kwargs):
- """
- Set self.key = value, for each key in kwargs
- """
- for key, value in kwargs.items():
- setattr(self, key, value)
-
-
-def dist(loc1, loc2):
- return ((loc1[:, 0] - loc2[:, 0]) ** 2 + (loc1[:, 1] - loc2[:, 1]) ** 2) ** 0.5
-
-
-class CVRPVectorEnv(gym.Env):
- def __init__(self, *args, **kwargs):
- self.max_nodes = 50
- self.capacity_limit = 40
- self.n_traj = 50
- # if eval_data==True, load from 'test' set, the '0'th data
- self.eval_data = False
- self.eval_partition = "test"
- self.eval_data_idx = 0
- self.demand_limit = 10
- assign_env_config(self, kwargs)
-
- obs_dict = {"observations": spaces.Box(low=0, high=1, shape=(self.max_nodes, 2))}
- obs_dict["depot"] = spaces.Box(low=0, high=1, shape=(2,))
- obs_dict["demand"] = spaces.Box(low=0, high=1, shape=(self.max_nodes,))
- obs_dict["action_mask"] = spaces.MultiBinary(
- [self.n_traj, self.max_nodes + 1]
- ) # 1: OK, 0: cannot go
- obs_dict["last_node_idx"] = spaces.MultiDiscrete([self.max_nodes + 1] * self.n_traj)
- obs_dict["current_load"] = spaces.Box(low=0, high=1, shape=(self.n_traj,))
-
- self.observation_space = spaces.Dict(obs_dict)
- self.action_space = spaces.MultiDiscrete([self.max_nodes + 1] * self.n_traj)
- self.reward_space = None
-
- self.reset()
-
- def seed(self, seed):
- np.random.seed(seed)
-
- def _STEP(self, action):
-
- self._go_to(action) # Go to node 'action', modify the reward
- self.num_steps += 1
- self.state = self._update_state()
-
- # need to revisit the first node after visited all other nodes
- self.done = (action == 0) & self.is_all_visited()
-
- return self.state, self.reward, self.done, self.info
-
- # Euclidean cost function
- def cost(self, loc1, loc2):
- return dist(loc1, loc2)
-
- def is_all_visited(self):
- # assumes no repetition in the first `max_nodes` steps
- return self.visited[:, 1:].all(axis=1)
-
- def _update_state(self):
- obs = {"observations": self.nodes[1:]} # n x 2 array
- obs["depot"] = self.nodes[0]
- obs["action_mask"] = self._update_mask()
- obs["demand"] = self.demands
- obs["last_node_idx"] = self.last
- obs["current_load"] = self.load
- return obs
-
- def _update_mask(self):
- # Only allow to visit unvisited nodes
- action_mask = ~self.visited
-
- # can only visit depot when last node is not depot or all visited
- action_mask[:, 0] |= self.last != 0
- action_mask[:, 0] |= self.is_all_visited()
-
- # not allow visit nodes with higher demand than capacity
- action_mask[:, 1:] &= self.demands <= (
- self.load.reshape(-1, 1) + 1e-5
- ) # to handle the floating point subtraction precision
-
- return action_mask
-
- def _RESET(self):
- self.visited = np.zeros((self.n_traj, self.max_nodes + 1), dtype=bool)
- self.visited[:, 0] = True
- self.num_steps = 0
- self.last = np.zeros(self.n_traj, dtype=int) # idx of the last elem
- self.load = np.ones(self.n_traj, dtype=float) # current load
-
- if self.eval_data:
- self._load_orders()
- else:
- self._generate_orders()
- self.state = self._update_state()
- self.info = {}
- self.done = np.array([False] * self.n_traj)
- return self.state
-
- def _load_orders(self):
- data = VRPDataset[self.eval_partition, self.max_nodes, self.eval_data_idx]
- self.nodes = np.concatenate((data["depot"][None, ...], data["loc"]))
- self.demands = data["demand"]
- self.demands_with_depot = self.demands.copy()
-
- def _generate_orders(self):
- self.nodes = np.random.rand(self.max_nodes + 1, 2)
- self.demands = (
- np.random.randint(low=1, high=self.demand_limit, size=self.max_nodes)
- / self.capacity_limit
- )
- self.demands_with_depot = self.demands.copy()
-
- def _go_to(self, destination):
- dest_node = self.nodes[destination]
- dist = self.cost(dest_node, self.nodes[self.last])
- self.last = destination
- self.load[destination == 0] = 1
- self.load[destination > 0] -= self.demands[destination[destination > 0] - 1]
- self.demands_with_depot[destination[destination > 0] - 1] = 0
- self.visited[np.arange(self.n_traj), destination] = True
- self.reward = -dist
-
- def step(self, action):
- # return last state after done,
- # for the sake of PPO's abuse of ff on done observation
- # see https://github.com/opendilab/DI-engine/issues/497
- # Not needed for CleanRL
- # if self.done.all():
- # return self.state, self.reward, self.done, self.info
-
- return self._STEP(action)
-
- def reset(self):
- return self._RESET()
diff --git a/spaces/cyliawardana/Womens_Clothing_Sentiment_Analysis/app.py b/spaces/cyliawardana/Womens_Clothing_Sentiment_Analysis/app.py
deleted file mode 100644
index 5e2ea8102bf99a6c8ffc60fdccebf265b4257626..0000000000000000000000000000000000000000
--- a/spaces/cyliawardana/Womens_Clothing_Sentiment_Analysis/app.py
+++ /dev/null
@@ -1,94 +0,0 @@
-import pandas as pd
-import numpy as np
-import joblib
-import streamlit as st
-import tensorflow as tf
-import nltk
-from nltk.tokenize import word_tokenize
-from nltk.stem import WordNetLemmatizer
-from nltk.corpus import stopwords
-import re
-import string
-import ast
-from tensorflow.keras.models import load_model
-
-nltk.download('stopwords')
-nltk.download('punkt')
-nltk.download('wordnet')
-nltk.download('omw-1.4')
-
-with open('chatwords.txt') as f:
- data = f.read()
-chatwords = ast.literal_eval(data)
-
-with open('abbreviation.txt') as ab:
- ab2 = ab.read()
-abbreviation = ast.literal_eval(ab2)
-
-stop_words = stopwords.words('english')
-
-lem = WordNetLemmatizer()
-
-def check_chatwords(text):
- temp=[]
- for chat in text.split():
- if chat.upper() in chatwords:
- temp.append(chatwords[chat.upper()])
- else:
- temp.append(chat)
- return " ".join(temp)
-
-def lower(text):
- data = text.lower()
- return data
-
-def check_abbr(text):
- temp2=[]
- for abbr in text.split():
- if abbr in abbreviation:
- temp2.append(abbreviation[abbr])
- else:
- temp2.append(abbr)
-
- return " ".join(temp2)
-
-def check_punctuation(text):
- data = re.sub("[^a-zA-Z]",' ', text)
- data = re.sub('\[[^]]*\]', ' ', data)
- data = re.sub(r"\\n", " ", data)
- data = data.strip()
- data = ' '.join(data.split())
- return data
-
-def token_stopwords_lemma(text):
- tokens = word_tokenize(text)
- stop_words2 = ' '.join([word for word in tokens if word not in stop_words])
- data = [lem.lemmatize(word) for word in stop_words2.split()]
- data = ' '.join(data)
- return data
-
-final_model = tf.keras.models.load_model('gru_model')
-
-st.title('Womens Clothing Sentiment Analysis')
-
-review = st.text_input('Please input your review here:')
-st.write('Your Review:', review)
-
-data_inf = [review]
-df_data_inf = pd.DataFrame()
-df_data_inf['Review Text'] = data_inf
-
-df_data_inf['Review Text'] = df_data_inf['Review Text'].apply(lambda j: check_chatwords(j))
-df_data_inf['Review Text'] = df_data_inf['Review Text'].apply(lambda k: lower(k))
-df_data_inf['Review Text'] = df_data_inf['Review Text'].apply(lambda l: check_abbr(l))
-df_data_inf['Review Text'] = df_data_inf['Review Text'].apply(lambda m: check_punctuation(m))
-df_data_inf['Review Text'] = df_data_inf['Review Text'].apply(lambda n: token_stopwords_lemma(n))
-
-if st.button('Predict'):
- y_pred_inf = final_model.predict(df_data_inf['Review Text'])
- y_pred_inf = np.where(y_pred_inf >= 0.5, 1, 0)
- st.write('Prediction Result: ')
- if y_pred_inf == 0:
- st.subheader('Not Recommended (Negative Review)')
- else:
- st.subheader('Recommended (Positive Review)')
\ No newline at end of file
diff --git a/spaces/cymic/Waifu_Diffusion_Webui/modules/modelloader.py b/spaces/cymic/Waifu_Diffusion_Webui/modules/modelloader.py
deleted file mode 100644
index b0f2f33d22a11d7f7419541c7f7daa6fee68af0c..0000000000000000000000000000000000000000
--- a/spaces/cymic/Waifu_Diffusion_Webui/modules/modelloader.py
+++ /dev/null
@@ -1,153 +0,0 @@
-import glob
-import os
-import shutil
-import importlib
-from urllib.parse import urlparse
-
-from basicsr.utils.download_util import load_file_from_url
-from modules import shared
-from modules.upscaler import Upscaler
-from modules.paths import script_path, models_path
-
-
-def load_models(model_path: str, model_url: str = None, command_path: str = None, ext_filter=None, download_name=None) -> list:
- """
- A one-and done loader to try finding the desired models in specified directories.
-
- @param download_name: Specify to download from model_url immediately.
- @param model_url: If no other models are found, this will be downloaded on upscale.
- @param model_path: The location to store/find models in.
- @param command_path: A command-line argument to search for models in first.
- @param ext_filter: An optional list of filename extensions to filter by
- @return: A list of paths containing the desired model(s)
- """
- output = []
-
- if ext_filter is None:
- ext_filter = []
-
- try:
- places = []
-
- if command_path is not None and command_path != model_path:
- pretrained_path = os.path.join(command_path, 'experiments/pretrained_models')
- if os.path.exists(pretrained_path):
- print(f"Appending path: {pretrained_path}")
- places.append(pretrained_path)
- elif os.path.exists(command_path):
- places.append(command_path)
-
- places.append(model_path)
-
- for place in places:
- if os.path.exists(place):
- for file in glob.iglob(place + '**/**', recursive=True):
- full_path = file
- if os.path.isdir(full_path):
- continue
- if len(ext_filter) != 0:
- model_name, extension = os.path.splitext(file)
- if extension not in ext_filter:
- continue
- if file not in output:
- output.append(full_path)
-
- if model_url is not None and len(output) == 0:
- if download_name is not None:
- dl = load_file_from_url(model_url, model_path, True, download_name)
- output.append(dl)
- else:
- output.append(model_url)
-
- except Exception:
- pass
-
- return output
-
-
-def friendly_name(file: str):
- if "http" in file:
- file = urlparse(file).path
-
- file = os.path.basename(file)
- model_name, extension = os.path.splitext(file)
- return model_name
-
-
-def cleanup_models():
- # This code could probably be more efficient if we used a tuple list or something to store the src/destinations
- # and then enumerate that, but this works for now. In the future, it'd be nice to just have every "model" scaler
- # somehow auto-register and just do these things...
- root_path = script_path
- src_path = models_path
- dest_path = os.path.join(models_path, "Stable-diffusion")
- move_files(src_path, dest_path, ".ckpt")
- src_path = os.path.join(root_path, "ESRGAN")
- dest_path = os.path.join(models_path, "ESRGAN")
- move_files(src_path, dest_path)
- src_path = os.path.join(root_path, "gfpgan")
- dest_path = os.path.join(models_path, "GFPGAN")
- move_files(src_path, dest_path)
- src_path = os.path.join(root_path, "SwinIR")
- dest_path = os.path.join(models_path, "SwinIR")
- move_files(src_path, dest_path)
- src_path = os.path.join(root_path, "repositories/latent-diffusion/experiments/pretrained_models/")
- dest_path = os.path.join(models_path, "LDSR")
- move_files(src_path, dest_path)
-
-
-def move_files(src_path: str, dest_path: str, ext_filter: str = None):
- try:
- if not os.path.exists(dest_path):
- os.makedirs(dest_path)
- if os.path.exists(src_path):
- for file in os.listdir(src_path):
- fullpath = os.path.join(src_path, file)
- if os.path.isfile(fullpath):
- if ext_filter is not None:
- if ext_filter not in file:
- continue
- print(f"Moving {file} from {src_path} to {dest_path}.")
- try:
- shutil.move(fullpath, dest_path)
- except:
- pass
- if len(os.listdir(src_path)) == 0:
- print(f"Removing empty folder: {src_path}")
- shutil.rmtree(src_path, True)
- except:
- pass
-
-
-def load_upscalers():
- sd = shared.script_path
- # We can only do this 'magic' method to dynamically load upscalers if they are referenced,
- # so we'll try to import any _model.py files before looking in __subclasses__
- modules_dir = os.path.join(sd, "modules")
- for file in os.listdir(modules_dir):
- if "_model.py" in file:
- model_name = file.replace("_model.py", "")
- full_model = f"modules.{model_name}_model"
- try:
- importlib.import_module(full_model)
- except:
- pass
- datas = []
- c_o = vars(shared.cmd_opts)
- for cls in Upscaler.__subclasses__():
- name = cls.__name__
- module_name = cls.__module__
- module = importlib.import_module(module_name)
- class_ = getattr(module, name)
- cmd_name = f"{name.lower().replace('upscaler', '')}_models_path"
- opt_string = None
- try:
- if cmd_name in c_o:
- opt_string = c_o[cmd_name]
- except:
- pass
- scaler = class_(opt_string)
- for child in scaler.scalers:
- datas.append(child)
-
- shared.sd_upscalers = datas
diff --git a/spaces/daddyjin/TalkingFaceGeneration/Demo_TFR_Pirenderer/src/face3d/util/detect_lm68.py b/spaces/daddyjin/TalkingFaceGeneration/Demo_TFR_Pirenderer/src/face3d/util/detect_lm68.py
deleted file mode 100644
index b7e40997289e17405e1fb6c408d21adce7b626ce..0000000000000000000000000000000000000000
--- a/spaces/daddyjin/TalkingFaceGeneration/Demo_TFR_Pirenderer/src/face3d/util/detect_lm68.py
+++ /dev/null
@@ -1,106 +0,0 @@
-import os
-import cv2
-import numpy as np
-from scipy.io import loadmat
-import tensorflow as tf
-from util.preprocess import align_for_lm
-from shutil import move
-
-mean_face = np.loadtxt('util/test_mean_face.txt')
-mean_face = mean_face.reshape([68, 2])
-
-def save_label(labels, save_path):
- np.savetxt(save_path, labels)
-
-def draw_landmarks(img, landmark, save_name):
- landmark = landmark
- lm_img = np.zeros([img.shape[0], img.shape[1], 3])
- lm_img[:] = img.astype(np.float32)
- landmark = np.round(landmark).astype(np.int32)
-
- for i in range(len(landmark)):
- for j in range(-1, 1):
- for k in range(-1, 1):
- if img.shape[0] - 1 - landmark[i, 1]+j > 0 and \
- img.shape[0] - 1 - landmark[i, 1]+j < img.shape[0] and \
- landmark[i, 0]+k > 0 and \
- landmark[i, 0]+k < img.shape[1]:
- lm_img[img.shape[0] - 1 - landmark[i, 1]+j, landmark[i, 0]+k,
- :] = np.array([0, 0, 255])
- lm_img = lm_img.astype(np.uint8)
-
- cv2.imwrite(save_name, lm_img)
-
-
-def load_data(img_name, txt_name):
- return cv2.imread(img_name), np.loadtxt(txt_name)
-
-# create tensorflow graph for landmark detector
-def load_lm_graph(graph_filename):
- with tf.gfile.GFile(graph_filename, 'rb') as f:
- graph_def = tf.GraphDef()
- graph_def.ParseFromString(f.read())
-
- with tf.Graph().as_default() as graph:
- tf.import_graph_def(graph_def, name='net')
- img_224 = graph.get_tensor_by_name('net/input_imgs:0')
- output_lm = graph.get_tensor_by_name('net/lm:0')
- lm_sess = tf.Session(graph=graph)
-
- return lm_sess,img_224,output_lm
-
-# landmark detection
-def detect_68p(img_path,sess,input_op,output_op):
- print('detecting landmarks......')
- names = [i for i in sorted(os.listdir(
- img_path)) if 'jpg' in i or 'png' in i or 'jpeg' in i or 'PNG' in i]
- vis_path = os.path.join(img_path, 'vis')
- remove_path = os.path.join(img_path, 'remove')
- save_path = os.path.join(img_path, 'landmarks')
- if not os.path.isdir(vis_path):
- os.makedirs(vis_path)
- if not os.path.isdir(remove_path):
- os.makedirs(remove_path)
- if not os.path.isdir(save_path):
- os.makedirs(save_path)
-
- for i in range(0, len(names)):
- name = names[i]
- print('%05d' % (i), ' ', name)
- full_image_name = os.path.join(img_path, name)
- txt_name = '.'.join(name.split('.')[:-1]) + '.txt'
- full_txt_name = os.path.join(img_path, 'detections', txt_name) # 5 facial landmark path for each image
-
- # if an image does not have detected 5 facial landmarks, remove it from the training list
- if not os.path.isfile(full_txt_name):
- move(full_image_name, os.path.join(remove_path, name))
- continue
-
- # load data
- img, five_points = load_data(full_image_name, full_txt_name)
- input_img, scale, bbox = align_for_lm(img, five_points) # align for 68 landmark detection
-
- # if the alignment fails, remove corresponding image from the training list
- if scale == 0:
- move(full_txt_name, os.path.join(
- remove_path, txt_name))
- move(full_image_name, os.path.join(remove_path, name))
- continue
-
- # detect landmarks
- input_img = np.reshape(
- input_img, [1, 224, 224, 3]).astype(np.float32)
- landmark = sess.run(
- output_op, feed_dict={input_op: input_img})
-
- # transform back to original image coordinate
- landmark = landmark.reshape([68, 2]) + mean_face
- landmark[:, 1] = 223 - landmark[:, 1]
- landmark = landmark / scale
- landmark[:, 0] = landmark[:, 0] + bbox[0]
- landmark[:, 1] = landmark[:, 1] + bbox[1]
- landmark[:, 1] = img.shape[0] - 1 - landmark[:, 1]
-
- if i % 100 == 0:
- draw_landmarks(img, landmark, os.path.join(vis_path, name))
- save_label(landmark, os.path.join(save_path, txt_name))
diff --git a/spaces/dakaiye/dky_xuexi/request_llm/bridge_jittorllms_pangualpha.py b/spaces/dakaiye/dky_xuexi/request_llm/bridge_jittorllms_pangualpha.py
deleted file mode 100644
index ad02565aef75ac056e0daa7396fb1c6ad7aae072..0000000000000000000000000000000000000000
--- a/spaces/dakaiye/dky_xuexi/request_llm/bridge_jittorllms_pangualpha.py
+++ /dev/null
@@ -1,178 +0,0 @@
-
-from transformers import AutoModel, AutoTokenizer
-import time
-import threading
-import importlib
-from toolbox import update_ui, get_conf
-from multiprocessing import Process, Pipe
-
-load_message = "jittorllms尚未加载,加载需要一段时间。注意,请避免混用多种jittor模型,否则可能导致显存溢出而造成卡顿,取决于`config.py`的配置,jittorllms消耗大量的内存(CPU)或显存(GPU),也许会导致低配计算机卡死 ……"
-
-#################################################################################
-class GetGLMHandle(Process):
- def __init__(self):
- super().__init__(daemon=True)
- self.parent, self.child = Pipe()
- self.jittorllms_model = None
- self.info = ""
- self.local_history = []
- self.success = True
- self.check_dependency()
- self.start()
- self.threadLock = threading.Lock()
-
- def check_dependency(self):
- try:
- import pandas
- self.info = "依赖检测通过"
- self.success = True
- except:
- from toolbox import trimmed_format_exc
- self.info = r"缺少jittorllms的依赖,如果要使用jittorllms,除了基础的pip依赖以外,您还需要运行`pip install -r request_llm/requirements_jittorllms.txt -i https://pypi.jittor.org/simple -I`"+\
- r"和`git clone https://gitlink.org.cn/jittor/JittorLLMs.git --depth 1 request_llm/jittorllms`两个指令来安装jittorllms的依赖(在项目根目录运行这两个指令)。" +\
- r"警告:安装jittorllms依赖后将完全破坏现有的pytorch环境,建议使用docker环境!" + trimmed_format_exc()
- self.success = False
-
- def ready(self):
- return self.jittorllms_model is not None
-
- def run(self):
- # 子进程执行
- # 第一次运行,加载参数
- def validate_path():
- import os, sys
- dir_name = os.path.dirname(__file__)
- env = os.environ.get("PATH", "")
- os.environ["PATH"] = env.replace('/cuda/bin', '/x/bin')
- root_dir_assume = os.path.abspath(os.path.dirname(__file__) + '/..')
- os.chdir(root_dir_assume + '/request_llm/jittorllms')
- sys.path.append(root_dir_assume + '/request_llm/jittorllms')
- validate_path() # validate path so you can run from base directory
-
- def load_model():
- import types
- try:
- if self.jittorllms_model is None:
- device, = get_conf('LOCAL_MODEL_DEVICE')
- from .jittorllms.models import get_model
- # availabel_models = ["chatglm", "pangualpha", "llama", "chatrwkv"]
- args_dict = {'model': 'pangualpha'}
- print('self.jittorllms_model = get_model(types.SimpleNamespace(**args_dict))')
- self.jittorllms_model = get_model(types.SimpleNamespace(**args_dict))
- print('done get model')
- except:
- self.child.send('[Local Message] Call jittorllms fail 不能正常加载jittorllms的参数。')
- raise RuntimeError("不能正常加载jittorllms的参数!")
- print('load_model')
- load_model()
-
- # 进入任务等待状态
- print('进入任务等待状态')
- while True:
- # 进入任务等待状态
- kwargs = self.child.recv()
- query = kwargs['query']
- history = kwargs['history']
- # 是否重置
- if len(self.local_history) > 0 and len(history)==0:
- print('触发重置')
- self.jittorllms_model.reset()
- self.local_history.append(query)
-
- print('收到消息,开始请求')
- try:
- for response in self.jittorllms_model.stream_chat(query, history):
- print(response)
- self.child.send(response)
- except:
- from toolbox import trimmed_format_exc
- print(trimmed_format_exc())
- self.child.send('[Local Message] Call jittorllms fail.')
- # 请求处理结束,开始下一个循环
- self.child.send('[Finish]')
-
- def stream_chat(self, **kwargs):
- # 主进程执行
- self.threadLock.acquire()
- self.parent.send(kwargs)
- while True:
- res = self.parent.recv()
- if res != '[Finish]':
- yield res
- else:
- break
- self.threadLock.release()
-
-global pangu_glm_handle
-pangu_glm_handle = None
-#################################################################################
-def predict_no_ui_long_connection(inputs, llm_kwargs, history=[], sys_prompt="", observe_window=[], console_slience=False):
- """
- 多线程方法
- 函数的说明请见 request_llm/bridge_all.py
- """
- global pangu_glm_handle
- if pangu_glm_handle is None:
- pangu_glm_handle = GetGLMHandle()
- if len(observe_window) >= 1: observe_window[0] = load_message + "\n\n" + pangu_glm_handle.info
- if not pangu_glm_handle.success:
- error = pangu_glm_handle.info
- pangu_glm_handle = None
- raise RuntimeError(error)
-
- # jittorllms 没有 sys_prompt 接口,因此把prompt加入 history
- history_feedin = []
- for i in range(len(history)//2):
- history_feedin.append([history[2*i], history[2*i+1]] )
-
- watch_dog_patience = 5 # 看门狗 (watchdog) 的耐心, 设置5秒即可
- response = ""
- for response in pangu_glm_handle.stream_chat(query=inputs, history=history_feedin, system_prompt=sys_prompt, max_length=llm_kwargs['max_length'], top_p=llm_kwargs['top_p'], temperature=llm_kwargs['temperature']):
- print(response)
- if len(observe_window) >= 1: observe_window[0] = response
- if len(observe_window) >= 2:
- if (time.time()-observe_window[1]) > watch_dog_patience:
- raise RuntimeError("程序终止。")
- return response
-
-
-
-def predict(inputs, llm_kwargs, plugin_kwargs, chatbot, history=[], system_prompt='', stream = True, additional_fn=None):
- """
- 单线程方法
- 函数的说明请见 request_llm/bridge_all.py
- """
- chatbot.append((inputs, ""))
-
- global pangu_glm_handle
- if pangu_glm_handle is None:
- pangu_glm_handle = GetGLMHandle()
- chatbot[-1] = (inputs, load_message + "\n\n" + pangu_glm_handle.info)
- yield from update_ui(chatbot=chatbot, history=[])
- if not pangu_glm_handle.success:
- pangu_glm_handle = None
- return
-
- if additional_fn is not None:
- import core_functional
- importlib.reload(core_functional) # 热更新prompt
- core_functional = core_functional.get_core_functions()
- if "PreProcess" in core_functional[additional_fn]: inputs = core_functional[additional_fn]["PreProcess"](inputs) # 获取预处理函数(如果有的话)
- inputs = core_functional[additional_fn]["Prefix"] + inputs + core_functional[additional_fn]["Suffix"]
-
- # 处理历史信息
- history_feedin = []
- for i in range(len(history)//2):
- history_feedin.append([history[2*i], history[2*i+1]] )
-
- # 开始接收jittorllms的回复
- response = "[Local Message]: 等待jittorllms响应中 ..."
- for response in pangu_glm_handle.stream_chat(query=inputs, history=history_feedin, system_prompt=system_prompt, max_length=llm_kwargs['max_length'], top_p=llm_kwargs['top_p'], temperature=llm_kwargs['temperature']):
- chatbot[-1] = (inputs, response)
- yield from update_ui(chatbot=chatbot, history=history)
-
- # 总结输出
- if response == "[Local Message]: 等待jittorllms响应中 ...":
- response = "[Local Message]: jittorllms响应异常 ..."
- history.extend([inputs, response])
- yield from update_ui(chatbot=chatbot, history=history)
diff --git a/spaces/danterivers/music-generation-samples/tests/modules/__init__.py b/spaces/danterivers/music-generation-samples/tests/modules/__init__.py
deleted file mode 100644
index 0952fcc3f57e34b3747962e9ebd6fc57aeea63fa..0000000000000000000000000000000000000000
--- a/spaces/danterivers/music-generation-samples/tests/modules/__init__.py
+++ /dev/null
@@ -1,5 +0,0 @@
-# Copyright (c) Meta Platforms, Inc. and affiliates.
-# All rights reserved.
-#
-# This source code is licensed under the license found in the
-# LICENSE file in the root directory of this source tree.
diff --git a/spaces/dawood17/SayBot_Enchancer/CodeFormer/basicsr/utils/realesrgan_utils.py b/spaces/dawood17/SayBot_Enchancer/CodeFormer/basicsr/utils/realesrgan_utils.py
deleted file mode 100644
index a2a5ab3b787212e4731e2a91b1493430a4a3664e..0000000000000000000000000000000000000000
--- a/spaces/dawood17/SayBot_Enchancer/CodeFormer/basicsr/utils/realesrgan_utils.py
+++ /dev/null
@@ -1,299 +0,0 @@
-import cv2
-import math
-import numpy as np
-import os
-import queue
-import threading
-import torch
-from basicsr.utils.download_util import load_file_from_url
-from torch.nn import functional as F
-
-# ROOT_DIR = os.path.dirname(os.path.dirname(os.path.abspath(__file__)))
-
-
-class RealESRGANer():
- """A helper class for upsampling images with RealESRGAN.
-
- Args:
- scale (int): Upsampling scale factor used in the networks. It is usually 2 or 4.
- model_path (str): The path to the pretrained model. It can be urls (will first download it automatically).
- model (nn.Module): The defined network. Default: None.
- tile (int): As too large images result in the out of GPU memory issue, so this tile option will first crop
- input images into tiles, and then process each of them. Finally, they will be merged into one image.
- 0 denotes for do not use tile. Default: 0.
- tile_pad (int): The pad size for each tile, to remove border artifacts. Default: 10.
- pre_pad (int): Pad the input images to avoid border artifacts. Default: 10.
- half (float): Whether to use half precision during inference. Default: False.
- """
-
- def __init__(self,
- scale,
- model_path,
- model=None,
- tile=0,
- tile_pad=10,
- pre_pad=10,
- half=False,
- device=None,
- gpu_id=None):
- self.scale = scale
- self.tile_size = tile
- self.tile_pad = tile_pad
- self.pre_pad = pre_pad
- self.mod_scale = None
- self.half = half
-
- # initialize model
- if gpu_id:
- self.device = torch.device(
- f'cuda:{gpu_id}' if torch.cuda.is_available() else 'cpu') if device is None else device
- else:
- self.device = torch.device('cuda' if torch.cuda.is_available() else 'cpu') if device is None else device
- # if the model_path starts with https, it will first download models to the folder: realesrgan/weights
- if model_path.startswith('https://'):
- model_path = load_file_from_url(
- url=model_path, model_dir=os.path.join('weights/realesrgan'), progress=True, file_name=None)
- loadnet = torch.load(model_path, map_location=torch.device('cpu'))
- # prefer to use params_ema
- if 'params_ema' in loadnet:
- keyname = 'params_ema'
- else:
- keyname = 'params'
- model.load_state_dict(loadnet[keyname], strict=True)
- model.eval()
- self.model = model.to(self.device)
- if self.half:
- self.model = self.model.half()
-
- def pre_process(self, img):
- """Pre-process, such as pre-pad and mod pad, so that the images can be divisible
- """
- img = torch.from_numpy(np.transpose(img, (2, 0, 1))).float()
- self.img = img.unsqueeze(0).to(self.device)
- if self.half:
- self.img = self.img.half()
-
- # pre_pad
- if self.pre_pad != 0:
- self.img = F.pad(self.img, (0, self.pre_pad, 0, self.pre_pad), 'reflect')
- # mod pad for divisible borders
- if self.scale == 2:
- self.mod_scale = 2
- elif self.scale == 1:
- self.mod_scale = 4
- if self.mod_scale is not None:
- self.mod_pad_h, self.mod_pad_w = 0, 0
- _, _, h, w = self.img.size()
- if (h % self.mod_scale != 0):
- self.mod_pad_h = (self.mod_scale - h % self.mod_scale)
- if (w % self.mod_scale != 0):
- self.mod_pad_w = (self.mod_scale - w % self.mod_scale)
- self.img = F.pad(self.img, (0, self.mod_pad_w, 0, self.mod_pad_h), 'reflect')
-
- def process(self):
- # model inference
- self.output = self.model(self.img)
-
- def tile_process(self):
- """It will first crop input images to tiles, and then process each tile.
- Finally, all the processed tiles are merged into one images.
-
- Modified from: https://github.com/ata4/esrgan-launcher
- """
- batch, channel, height, width = self.img.shape
- output_height = height * self.scale
- output_width = width * self.scale
- output_shape = (batch, channel, output_height, output_width)
-
- # start with black image
- self.output = self.img.new_zeros(output_shape)
- tiles_x = math.ceil(width / self.tile_size)
- tiles_y = math.ceil(height / self.tile_size)
-
- # loop over all tiles
- for y in range(tiles_y):
- for x in range(tiles_x):
- # extract tile from input image
- ofs_x = x * self.tile_size
- ofs_y = y * self.tile_size
- # input tile area on total image
- input_start_x = ofs_x
- input_end_x = min(ofs_x + self.tile_size, width)
- input_start_y = ofs_y
- input_end_y = min(ofs_y + self.tile_size, height)
-
- # input tile area on total image with padding
- input_start_x_pad = max(input_start_x - self.tile_pad, 0)
- input_end_x_pad = min(input_end_x + self.tile_pad, width)
- input_start_y_pad = max(input_start_y - self.tile_pad, 0)
- input_end_y_pad = min(input_end_y + self.tile_pad, height)
-
- # input tile dimensions
- input_tile_width = input_end_x - input_start_x
- input_tile_height = input_end_y - input_start_y
- tile_idx = y * tiles_x + x + 1
- input_tile = self.img[:, :, input_start_y_pad:input_end_y_pad, input_start_x_pad:input_end_x_pad]
-
- # upscale tile
- try:
- with torch.no_grad():
- output_tile = self.model(input_tile)
- except RuntimeError as error:
- print('Error', error)
- # print(f'\tTile {tile_idx}/{tiles_x * tiles_y}')
-
- # output tile area on total image
- output_start_x = input_start_x * self.scale
- output_end_x = input_end_x * self.scale
- output_start_y = input_start_y * self.scale
- output_end_y = input_end_y * self.scale
-
- # output tile area without padding
- output_start_x_tile = (input_start_x - input_start_x_pad) * self.scale
- output_end_x_tile = output_start_x_tile + input_tile_width * self.scale
- output_start_y_tile = (input_start_y - input_start_y_pad) * self.scale
- output_end_y_tile = output_start_y_tile + input_tile_height * self.scale
-
- # put tile into output image
- self.output[:, :, output_start_y:output_end_y,
- output_start_x:output_end_x] = output_tile[:, :, output_start_y_tile:output_end_y_tile,
- output_start_x_tile:output_end_x_tile]
-
- def post_process(self):
- # remove extra pad
- if self.mod_scale is not None:
- _, _, h, w = self.output.size()
- self.output = self.output[:, :, 0:h - self.mod_pad_h * self.scale, 0:w - self.mod_pad_w * self.scale]
- # remove prepad
- if self.pre_pad != 0:
- _, _, h, w = self.output.size()
- self.output = self.output[:, :, 0:h - self.pre_pad * self.scale, 0:w - self.pre_pad * self.scale]
- return self.output
-
- @torch.no_grad()
- def enhance(self, img, outscale=None, alpha_upsampler='realesrgan'):
- h_input, w_input = img.shape[0:2]
- # img: numpy
- img = img.astype(np.float32)
- if np.max(img) > 256: # 16-bit image
- max_range = 65535
- print('\tInput is a 16-bit image')
- else:
- max_range = 255
- img = img / max_range
- if len(img.shape) == 2: # gray image
- img_mode = 'L'
- img = cv2.cvtColor(img, cv2.COLOR_GRAY2RGB)
- elif img.shape[2] == 4: # RGBA image with alpha channel
- img_mode = 'RGBA'
- alpha = img[:, :, 3]
- img = img[:, :, 0:3]
- img = cv2.cvtColor(img, cv2.COLOR_BGR2RGB)
- if alpha_upsampler == 'realesrgan':
- alpha = cv2.cvtColor(alpha, cv2.COLOR_GRAY2RGB)
- else:
- img_mode = 'RGB'
- img = cv2.cvtColor(img, cv2.COLOR_BGR2RGB)
-
- # ------------------- process image (without the alpha channel) ------------------- #
- try:
- with torch.no_grad():
- self.pre_process(img)
- if self.tile_size > 0:
- self.tile_process()
- else:
- self.process()
- output_img_t = self.post_process()
- output_img = output_img_t.data.squeeze().float().cpu().clamp_(0, 1).numpy()
- output_img = np.transpose(output_img[[2, 1, 0], :, :], (1, 2, 0))
- if img_mode == 'L':
- output_img = cv2.cvtColor(output_img, cv2.COLOR_BGR2GRAY)
- del output_img_t
- torch.cuda.empty_cache()
- except RuntimeError as error:
- print(f"Failed inference for RealESRGAN: {error}")
-
- # ------------------- process the alpha channel if necessary ------------------- #
- if img_mode == 'RGBA':
- if alpha_upsampler == 'realesrgan':
- self.pre_process(alpha)
- if self.tile_size > 0:
- self.tile_process()
- else:
- self.process()
- output_alpha = self.post_process()
- output_alpha = output_alpha.data.squeeze().float().cpu().clamp_(0, 1).numpy()
- output_alpha = np.transpose(output_alpha[[2, 1, 0], :, :], (1, 2, 0))
- output_alpha = cv2.cvtColor(output_alpha, cv2.COLOR_BGR2GRAY)
- else: # use the cv2 resize for alpha channel
- h, w = alpha.shape[0:2]
- output_alpha = cv2.resize(alpha, (w * self.scale, h * self.scale), interpolation=cv2.INTER_LINEAR)
-
- # merge the alpha channel
- output_img = cv2.cvtColor(output_img, cv2.COLOR_BGR2BGRA)
- output_img[:, :, 3] = output_alpha
-
- # ------------------------------ return ------------------------------ #
- if max_range == 65535: # 16-bit image
- output = (output_img * 65535.0).round().astype(np.uint16)
- else:
- output = (output_img * 255.0).round().astype(np.uint8)
-
- if outscale is not None and outscale != float(self.scale):
- output = cv2.resize(
- output, (
- int(w_input * outscale),
- int(h_input * outscale),
- ), interpolation=cv2.INTER_LANCZOS4)
-
- return output, img_mode
-
-
-class PrefetchReader(threading.Thread):
- """Prefetch images.
-
- Args:
- img_list (list[str]): A image list of image paths to be read.
- num_prefetch_queue (int): Number of prefetch queue.
- """
-
- def __init__(self, img_list, num_prefetch_queue):
- super().__init__()
- self.que = queue.Queue(num_prefetch_queue)
- self.img_list = img_list
-
- def run(self):
- for img_path in self.img_list:
- img = cv2.imread(img_path, cv2.IMREAD_UNCHANGED)
- self.que.put(img)
-
- self.que.put(None)
-
- def __next__(self):
- next_item = self.que.get()
- if next_item is None:
- raise StopIteration
- return next_item
-
- def __iter__(self):
- return self
-
-
-class IOConsumer(threading.Thread):
-
- def __init__(self, opt, que, qid):
- super().__init__()
- self._queue = que
- self.qid = qid
- self.opt = opt
-
- def run(self):
- while True:
- msg = self._queue.get()
- if isinstance(msg, str) and msg == 'quit':
- break
-
- output = msg['output']
- save_path = msg['save_path']
- cv2.imwrite(save_path, output)
- print(f'IO worker {self.qid} is done.')
\ No newline at end of file
diff --git a/spaces/dbredvick/whisper-webui/src/segments.py b/spaces/dbredvick/whisper-webui/src/segments.py
deleted file mode 100644
index ec2650dceade5d0b2022264f6419115eab085aea..0000000000000000000000000000000000000000
--- a/spaces/dbredvick/whisper-webui/src/segments.py
+++ /dev/null
@@ -1,55 +0,0 @@
-from typing import Any, Dict, List
-
-import copy
-
-def merge_timestamps(timestamps: List[Dict[str, Any]], merge_window: float = 5, max_merge_size: float = 30, padding_left: float = 1, padding_right: float = 1):
- result = []
-
- if len(timestamps) == 0:
- return result
- if max_merge_size is None:
- return timestamps
-
- if padding_left is None:
- padding_left = 0
- if padding_right is None:
- padding_right = 0
-
- processed_time = 0
- current_segment = None
-
- for i in range(len(timestamps)):
- next_segment = timestamps[i]
-
- delta = next_segment['start'] - processed_time
-
- # Note that segments can still be longer than the max merge size, they just won't be merged in that case
- if current_segment is None or (merge_window is not None and delta > merge_window) \
- or next_segment['end'] - current_segment['start'] > max_merge_size:
- # Finish the current segment
- if current_segment is not None:
- # Add right padding
- finish_padding = min(padding_right, delta / 2) if delta < padding_left + padding_right else padding_right
- current_segment['end'] += finish_padding
- delta -= finish_padding
-
- result.append(current_segment)
-
- # Start a new segment
- current_segment = copy.deepcopy(next_segment)
-
- # Pad the segment
- current_segment['start'] = current_segment['start'] - min(padding_left, delta)
- processed_time = current_segment['end']
-
- else:
- # Merge the segment
- current_segment['end'] = next_segment['end']
- processed_time = current_segment['end']
-
- # Add the last segment
- if current_segment is not None:
- current_segment['end'] += padding_right
- result.append(current_segment)
-
- return result
\ No newline at end of file
diff --git a/spaces/dcarpintero/nlp-summarizer-pegasus/.venv/lib/python3.9/site-packages/gradio/templates/cdn/assets/index-dc22e74c.js b/spaces/dcarpintero/nlp-summarizer-pegasus/.venv/lib/python3.9/site-packages/gradio/templates/cdn/assets/index-dc22e74c.js
deleted file mode 100644
index 4a4ce9afc3dae1b62a7e77284ffbfa43dcf22613..0000000000000000000000000000000000000000
--- a/spaces/dcarpintero/nlp-summarizer-pegasus/.venv/lib/python3.9/site-packages/gradio/templates/cdn/assets/index-dc22e74c.js
+++ /dev/null
@@ -1,2 +0,0 @@
-import{S as Q,e as R,s as U,m as K,F as B,o as J,g as V,h as I,G as P,j as L,ap as M,p as A,w,u as C,k as O,H as S,B as se,C as ue,am as ae,t as _e,x as oe,E as d,ak as r,V as W,ae as X,N as j,O as E,Q as Y,R as Z,T as N,P as ce,r as fe,v as re}from"./index-9e76ffee.js";import{B as y}from"./Button-30a08c0b.js";import{B as he}from"./BlockTitle-af232cbc.js";import"./Info-77722665.js";function be(t){let e;return{c(){e=_e(t[1])},m(i,n){I(i,e,n)},p(i,n){n&2&&oe(e,i[1])},d(i){i&&O(e)}}}function me(t){let e,i,n,a,f,h,m;return i=new he({props:{show_label:t[4],info:t[2],$$slots:{default:[be]},$$scope:{ctx:t}}}),{c(){e=K("label"),B(i.$$.fragment),n=J(),a=K("input"),V(a,"type","color"),a.disabled=t[3],V(a,"class","svelte-56zyyb"),V(e,"class","block")},m(l,u){I(l,e,u),P(i,e,null),L(e,n),L(e,a),M(a,t[0]),f=!0,h||(m=[A(a,"input",t[8]),A(a,"focus",t[6]),A(a,"blur",t[7])],h=!0)},p(l,[u]){const c={};u&16&&(c.show_label=l[4]),u&4&&(c.info=l[2]),u&2050&&(c.$$scope={dirty:u,ctx:l}),i.$set(c),(!f||u&8)&&(a.disabled=l[3]),u&1&&M(a,l[0])},i(l){f||(w(i.$$.fragment,l),f=!0)},o(l){C(i.$$.fragment,l),f=!1},d(l){l&&O(e),S(i),h=!1,se(m)}}}function ge(t,e,i){let{value:n="#000000"}=e,{value_is_output:a=!1}=e,{label:f}=e,{info:h=void 0}=e,{disabled:m=!1}=e,{show_label:l=!0}=e;const u=ue();function c(){u("change",n),a||u("input")}ae(()=>{i(5,a=!1)});function k(g){d.call(this,t,g)}function _(g){d.call(this,t,g)}function b(){n=this.value,i(0,n)}return t.$$set=g=>{"value"in g&&i(0,n=g.value),"value_is_output"in g&&i(5,a=g.value_is_output),"label"in g&&i(1,f=g.label),"info"in g&&i(2,h=g.info),"disabled"in g&&i(3,m=g.disabled),"show_label"in g&&i(4,l=g.show_label)},t.$$.update=()=>{t.$$.dirty&1&&c()},[n,f,h,m,l,a,k,_,b]}let p=class extends Q{constructor(e){super(),R(this,e,ge,me,U,{value:0,value_is_output:5,label:1,info:2,disabled:3,show_label:4})}};function ve(t){let e,i,n,a,f,h;const m=[t[11]];let l={};for(let _=0;_E(n,"value",u)),j.push(()=>E(n,"value_is_output",c)),n.$on("change",t[15]),n.$on("input",t[16]),n.$on("submit",t[17]),n.$on("blur",t[18]),n.$on("focus",t[19]),{c(){B(e.$$.fragment),i=J(),B(n.$$.fragment)},m(_,b){P(e,_,b),I(_,i,b),P(n,_,b),h=!0},p(_,b){const g=b&2048?Y(m,[Z(_[11])]):{};e.$set(g);const v={};b&4&&(v.label=_[2]),b&8&&(v.info=_[3]),b&128&&(v.show_label=_[7]),b&4096&&(v.disabled=!_[12]),!a&&b&1&&(a=!0,v.value=_[0],N(()=>a=!1)),!f&&b&2&&(f=!0,v.value_is_output=_[1],N(()=>f=!1)),n.$set(v)},i(_){h||(w(e.$$.fragment,_),w(n.$$.fragment,_),h=!0)},o(_){C(e.$$.fragment,_),C(n.$$.fragment,_),h=!1},d(_){_&&O(i),S(e,_),S(n,_)}}}function de(t){let e,i;return e=new y({props:{visible:t[6],elem_id:t[4],elem_classes:t[5],container:t[8],scale:t[9],min_width:t[10],$$slots:{default:[ve]},$$scope:{ctx:t}}}),{c(){B(e.$$.fragment)},m(n,a){P(e,n,a),i=!0},p(n,[a]){const f={};a&64&&(f.visible=n[6]),a&16&&(f.elem_id=n[4]),a&32&&(f.elem_classes=n[5]),a&256&&(f.container=n[8]),a&512&&(f.scale=n[9]),a&1024&&(f.min_width=n[10]),a&1054863&&(f.$$scope={dirty:a,ctx:n}),e.$set(f)},i(n){i||(w(e.$$.fragment,n),i=!0)},o(n){C(e.$$.fragment,n),i=!1},d(n){S(e,n)}}}function ke(t,e,i){let{label:n="ColorPicker"}=e,{info:a=void 0}=e,{elem_id:f=""}=e,{elem_classes:h=[]}=e,{visible:m=!0}=e,{value:l}=e,{value_is_output:u=!1}=e,{show_label:c}=e,{container:k=!0}=e,{scale:_=null}=e,{min_width:b=void 0}=e,{loading_status:g}=e,{interactive:v=!0}=e;function T(s){l=s,i(0,l)}function q(s){u=s,i(1,u)}function z(s){d.call(this,t,s)}function D(s){d.call(this,t,s)}function F(s){d.call(this,t,s)}function G(s){d.call(this,t,s)}function H(s){d.call(this,t,s)}return t.$$set=s=>{"label"in s&&i(2,n=s.label),"info"in s&&i(3,a=s.info),"elem_id"in s&&i(4,f=s.elem_id),"elem_classes"in s&&i(5,h=s.elem_classes),"visible"in s&&i(6,m=s.visible),"value"in s&&i(0,l=s.value),"value_is_output"in s&&i(1,u=s.value_is_output),"show_label"in s&&i(7,c=s.show_label),"container"in s&&i(8,k=s.container),"scale"in s&&i(9,_=s.scale),"min_width"in s&&i(10,b=s.min_width),"loading_status"in s&&i(11,g=s.loading_status),"interactive"in s&&i(12,v=s.interactive)},[l,u,n,a,f,h,m,c,k,_,b,g,v,T,q,z,D,F,G,H]}class we extends Q{constructor(e){super(),R(this,e,ke,de,U,{label:2,info:3,elem_id:4,elem_classes:5,visible:6,value:0,value_is_output:1,show_label:7,container:8,scale:9,min_width:10,loading_status:11,interactive:12})}get label(){return this.$$.ctx[2]}set label(e){this.$$set({label:e}),r()}get info(){return this.$$.ctx[3]}set info(e){this.$$set({info:e}),r()}get elem_id(){return this.$$.ctx[4]}set elem_id(e){this.$$set({elem_id:e}),r()}get elem_classes(){return this.$$.ctx[5]}set elem_classes(e){this.$$set({elem_classes:e}),r()}get visible(){return this.$$.ctx[6]}set visible(e){this.$$set({visible:e}),r()}get value(){return this.$$.ctx[0]}set value(e){this.$$set({value:e}),r()}get value_is_output(){return this.$$.ctx[1]}set value_is_output(e){this.$$set({value_is_output:e}),r()}get show_label(){return this.$$.ctx[7]}set show_label(e){this.$$set({show_label:e}),r()}get container(){return this.$$.ctx[8]}set container(e){this.$$set({container:e}),r()}get scale(){return this.$$.ctx[9]}set scale(e){this.$$set({scale:e}),r()}get min_width(){return this.$$.ctx[10]}set min_width(e){this.$$set({min_width:e}),r()}get loading_status(){return this.$$.ctx[11]}set loading_status(e){this.$$set({loading_status:e}),r()}get interactive(){return this.$$.ctx[12]}set interactive(e){this.$$set({interactive:e}),r()}}function Ce(t){let e,i,n,a,f,h;const m=[t[11]];let l={};for(let _=0;_E(n,"value",u)),j.push(()=>E(n,"value_is_output",c)),n.$on("change",t[15]),n.$on("input",t[16]),n.$on("submit",t[17]),n.$on("blur",t[18]),n.$on("focus",t[19]),{c(){B(e.$$.fragment),i=J(),B(n.$$.fragment)},m(_,b){P(e,_,b),I(_,i,b),P(n,_,b),h=!0},p(_,b){const g=b&2048?Y(m,[Z(_[11])]):{};e.$set(g);const v={};b&4&&(v.label=_[2]),b&8&&(v.info=_[3]),b&128&&(v.show_label=_[7]),b&4096&&(v.disabled=!_[12]),!a&&b&1&&(a=!0,v.value=_[0],N(()=>a=!1)),!f&&b&2&&(f=!0,v.value_is_output=_[1],N(()=>f=!1)),n.$set(v)},i(_){h||(w(e.$$.fragment,_),w(n.$$.fragment,_),h=!0)},o(_){C(e.$$.fragment,_),C(n.$$.fragment,_),h=!1},d(_){_&&O(i),S(e,_),S(n,_)}}}function Be(t){let e,i;return e=new y({props:{visible:t[6],elem_id:t[4],elem_classes:t[5],container:t[8],scale:t[9],min_width:t[10],$$slots:{default:[Ce]},$$scope:{ctx:t}}}),{c(){B(e.$$.fragment)},m(n,a){P(e,n,a),i=!0},p(n,[a]){const f={};a&64&&(f.visible=n[6]),a&16&&(f.elem_id=n[4]),a&32&&(f.elem_classes=n[5]),a&256&&(f.container=n[8]),a&512&&(f.scale=n[9]),a&1024&&(f.min_width=n[10]),a&1054863&&(f.$$scope={dirty:a,ctx:n}),e.$set(f)},i(n){i||(w(e.$$.fragment,n),i=!0)},o(n){C(e.$$.fragment,n),i=!1},d(n){S(e,n)}}}function Pe(t,e,i){let{label:n="ColorPicker"}=e,{info:a=void 0}=e,{elem_id:f=""}=e,{elem_classes:h=[]}=e,{visible:m=!0}=e,{value:l}=e,{value_is_output:u=!1}=e,{show_label:c}=e,{container:k=!0}=e,{scale:_=null}=e,{min_width:b=void 0}=e,{loading_status:g}=e,{interactive:v=!0}=e;function T(s){l=s,i(0,l)}function q(s){u=s,i(1,u)}function z(s){d.call(this,t,s)}function D(s){d.call(this,t,s)}function F(s){d.call(this,t,s)}function G(s){d.call(this,t,s)}function H(s){d.call(this,t,s)}return t.$$set=s=>{"label"in s&&i(2,n=s.label),"info"in s&&i(3,a=s.info),"elem_id"in s&&i(4,f=s.elem_id),"elem_classes"in s&&i(5,h=s.elem_classes),"visible"in s&&i(6,m=s.visible),"value"in s&&i(0,l=s.value),"value_is_output"in s&&i(1,u=s.value_is_output),"show_label"in s&&i(7,c=s.show_label),"container"in s&&i(8,k=s.container),"scale"in s&&i(9,_=s.scale),"min_width"in s&&i(10,b=s.min_width),"loading_status"in s&&i(11,g=s.loading_status),"interactive"in s&&i(12,v=s.interactive)},[l,u,n,a,f,h,m,c,k,_,b,g,v,T,q,z,D,F,G,H]}class Se extends Q{constructor(e){super(),R(this,e,Pe,Be,U,{label:2,info:3,elem_id:4,elem_classes:5,visible:6,value:0,value_is_output:1,show_label:7,container:8,scale:9,min_width:10,loading_status:11,interactive:12})}get label(){return this.$$.ctx[2]}set label(e){this.$$set({label:e}),r()}get info(){return this.$$.ctx[3]}set info(e){this.$$set({info:e}),r()}get elem_id(){return this.$$.ctx[4]}set elem_id(e){this.$$set({elem_id:e}),r()}get elem_classes(){return this.$$.ctx[5]}set elem_classes(e){this.$$set({elem_classes:e}),r()}get visible(){return this.$$.ctx[6]}set visible(e){this.$$set({visible:e}),r()}get value(){return this.$$.ctx[0]}set value(e){this.$$set({value:e}),r()}get value_is_output(){return this.$$.ctx[1]}set value_is_output(e){this.$$set({value_is_output:e}),r()}get show_label(){return this.$$.ctx[7]}set show_label(e){this.$$set({show_label:e}),r()}get container(){return this.$$.ctx[8]}set container(e){this.$$set({container:e}),r()}get scale(){return this.$$.ctx[9]}set scale(e){this.$$set({scale:e}),r()}get min_width(){return this.$$.ctx[10]}set min_width(e){this.$$set({min_width:e}),r()}get loading_status(){return this.$$.ctx[11]}set loading_status(e){this.$$set({loading_status:e}),r()}get interactive(){return this.$$.ctx[12]}set interactive(e){this.$$set({interactive:e}),r()}}function je(t){let e,i,n,a;function f(l){t[21](l)}function h(l){t[22](l)}let m={label:t[2],info:t[3],elem_id:t[4],elem_classes:t[5],visible:t[6],show_label:t[7],container:t[8],scale:t[9],min_width:t[10],loading_status:t[11],interactive:t[13]};return t[0]!==void 0&&(m.value=t[0]),t[1]!==void 0&&(m.value_is_output=t[1]),e=new Se({props:m}),j.push(()=>E(e,"value",f)),j.push(()=>E(e,"value_is_output",h)),e.$on("change",t[23]),e.$on("input",t[24]),e.$on("submit",t[25]),e.$on("blur",t[26]),e.$on("focus",t[27]),{c(){B(e.$$.fragment)},m(l,u){P(e,l,u),a=!0},p(l,u){const c={};u&4&&(c.label=l[2]),u&8&&(c.info=l[3]),u&16&&(c.elem_id=l[4]),u&32&&(c.elem_classes=l[5]),u&64&&(c.visible=l[6]),u&128&&(c.show_label=l[7]),u&256&&(c.container=l[8]),u&512&&(c.scale=l[9]),u&1024&&(c.min_width=l[10]),u&2048&&(c.loading_status=l[11]),u&8192&&(c.interactive=l[13]),!i&&u&1&&(i=!0,c.value=l[0],N(()=>i=!1)),!n&&u&2&&(n=!0,c.value_is_output=l[1],N(()=>n=!1)),e.$set(c)},i(l){a||(w(e.$$.fragment,l),a=!0)},o(l){C(e.$$.fragment,l),a=!1},d(l){S(e,l)}}}function Ee(t){let e,i,n,a;function f(l){t[14](l)}function h(l){t[15](l)}let m={label:t[2],info:t[3],elem_id:t[4],elem_classes:t[5],visible:t[6],show_label:t[7],container:t[8],scale:t[9],min_width:t[10],loading_status:t[11],interactive:t[13]};return t[0]!==void 0&&(m.value=t[0]),t[1]!==void 0&&(m.value_is_output=t[1]),e=new we({props:m}),j.push(()=>E(e,"value",f)),j.push(()=>E(e,"value_is_output",h)),e.$on("change",t[16]),e.$on("input",t[17]),e.$on("submit",t[18]),e.$on("blur",t[19]),e.$on("focus",t[20]),{c(){B(e.$$.fragment)},m(l,u){P(e,l,u),a=!0},p(l,u){const c={};u&4&&(c.label=l[2]),u&8&&(c.info=l[3]),u&16&&(c.elem_id=l[4]),u&32&&(c.elem_classes=l[5]),u&64&&(c.visible=l[6]),u&128&&(c.show_label=l[7]),u&256&&(c.container=l[8]),u&512&&(c.scale=l[9]),u&1024&&(c.min_width=l[10]),u&2048&&(c.loading_status=l[11]),u&8192&&(c.interactive=l[13]),!i&&u&1&&(i=!0,c.value=l[0],N(()=>i=!1)),!n&&u&2&&(n=!0,c.value_is_output=l[1],N(()=>n=!1)),e.$set(c)},i(l){a||(w(e.$$.fragment,l),a=!0)},o(l){C(e.$$.fragment,l),a=!1},d(l){S(e,l)}}}function Ne(t){let e,i,n,a;const f=[Ee,je],h=[];function m(l,u){return l[12]==="static"?0:1}return e=m(t),i=h[e]=f[e](t),{c(){i.c(),n=ce()},m(l,u){h[e].m(l,u),I(l,n,u),a=!0},p(l,[u]){let c=e;e=m(l),e===c?h[e].p(l,u):(fe(),C(h[c],1,1,()=>{h[c]=null}),re(),i=h[e],i?i.p(l,u):(i=h[e]=f[e](l),i.c()),w(i,1),i.m(n.parentNode,n))},i(l){a||(w(i),a=!0)},o(l){C(i),a=!1},d(l){l&&O(n),h[e].d(l)}}}function Te(t,e,i){let{label:n="ColorPicker"}=e,{info:a=void 0}=e,{elem_id:f=""}=e,{elem_classes:h=[]}=e,{visible:m=!0}=e,{value:l}=e,{value_is_output:u=!1}=e,{show_label:c}=e,{container:k=!0}=e,{scale:_=null}=e,{min_width:b=void 0}=e,{loading_status:g}=e,{mode:v}=e,{interactive:T}=e;function q(o){l=o,i(0,l)}function z(o){u=o,i(1,u)}function D(o){d.call(this,t,o)}function F(o){d.call(this,t,o)}function G(o){d.call(this,t,o)}function H(o){d.call(this,t,o)}function s(o){d.call(this,t,o)}function x(o){l=o,i(0,l)}function $(o){u=o,i(1,u)}function ee(o){d.call(this,t,o)}function te(o){d.call(this,t,o)}function ie(o){d.call(this,t,o)}function le(o){d.call(this,t,o)}function ne(o){d.call(this,t,o)}return t.$$set=o=>{"label"in o&&i(2,n=o.label),"info"in o&&i(3,a=o.info),"elem_id"in o&&i(4,f=o.elem_id),"elem_classes"in o&&i(5,h=o.elem_classes),"visible"in o&&i(6,m=o.visible),"value"in o&&i(0,l=o.value),"value_is_output"in o&&i(1,u=o.value_is_output),"show_label"in o&&i(7,c=o.show_label),"container"in o&&i(8,k=o.container),"scale"in o&&i(9,_=o.scale),"min_width"in o&&i(10,b=o.min_width),"loading_status"in o&&i(11,g=o.loading_status),"mode"in o&&i(12,v=o.mode),"interactive"in o&&i(13,T=o.interactive)},[l,u,n,a,f,h,m,c,k,_,b,g,v,T,q,z,D,F,G,H,s,x,$,ee,te,ie,le,ne]}class qe extends Q{constructor(e){super(),R(this,e,Te,Ne,U,{label:2,info:3,elem_id:4,elem_classes:5,visible:6,value:0,value_is_output:1,show_label:7,container:8,scale:9,min_width:10,loading_status:11,mode:12,interactive:13})}get label(){return this.$$.ctx[2]}set label(e){this.$$set({label:e}),r()}get info(){return this.$$.ctx[3]}set info(e){this.$$set({info:e}),r()}get elem_id(){return this.$$.ctx[4]}set elem_id(e){this.$$set({elem_id:e}),r()}get elem_classes(){return this.$$.ctx[5]}set elem_classes(e){this.$$set({elem_classes:e}),r()}get visible(){return this.$$.ctx[6]}set visible(e){this.$$set({visible:e}),r()}get value(){return this.$$.ctx[0]}set value(e){this.$$set({value:e}),r()}get value_is_output(){return this.$$.ctx[1]}set value_is_output(e){this.$$set({value_is_output:e}),r()}get show_label(){return this.$$.ctx[7]}set show_label(e){this.$$set({show_label:e}),r()}get container(){return this.$$.ctx[8]}set container(e){this.$$set({container:e}),r()}get scale(){return this.$$.ctx[9]}set scale(e){this.$$set({scale:e}),r()}get min_width(){return this.$$.ctx[10]}set min_width(e){this.$$set({min_width:e}),r()}get loading_status(){return this.$$.ctx[11]}set loading_status(e){this.$$set({loading_status:e}),r()}get mode(){return this.$$.ctx[12]}set mode(e){this.$$set({mode:e}),r()}get interactive(){return this.$$.ctx[13]}set interactive(e){this.$$set({interactive:e}),r()}}const Ie=qe,Oe=["static","dynamic"];export{Ie as Component,Oe as modes};
-//# sourceMappingURL=index-dc22e74c.js.map
diff --git a/spaces/dcarpintero/nlp-summarizer-pegasus/.venv/lib/python3.9/site-packages/gradio/templates/frontend/assets/index-93c91554.css b/spaces/dcarpintero/nlp-summarizer-pegasus/.venv/lib/python3.9/site-packages/gradio/templates/frontend/assets/index-93c91554.css
deleted file mode 100644
index beda351dfc765484ad744113e3d1734eb71cacd1..0000000000000000000000000000000000000000
--- a/spaces/dcarpintero/nlp-summarizer-pegasus/.venv/lib/python3.9/site-packages/gradio/templates/frontend/assets/index-93c91554.css
+++ /dev/null
@@ -1 +0,0 @@
-div.svelte-15lo0d8{display:flex;flex-wrap:wrap;gap:var(--layout-gap);width:var(--size-full)}.hide.svelte-15lo0d8{display:none}.compact.svelte-15lo0d8>*,.compact.svelte-15lo0d8 .box{border-radius:0}.compact.svelte-15lo0d8,.panel.svelte-15lo0d8{border-radius:var(--container-radius);background:var(--background-fill-secondary);padding:var(--size-2)}.unequal-height.svelte-15lo0d8{align-items:flex-start}.stretch.svelte-15lo0d8{align-items:stretch}div.svelte-15lo0d8>*,div.svelte-15lo0d8>.form>*{flex:1 1 0%;flex-wrap:wrap;min-width:min(160px,100%)}
diff --git a/spaces/dcarpintero/nlp-summarizer-pegasus/.venv/lib/python3.9/site-packages/importlib_resources/abc.py b/spaces/dcarpintero/nlp-summarizer-pegasus/.venv/lib/python3.9/site-packages/importlib_resources/abc.py
deleted file mode 100644
index 23b6aeafe4f43d097734e186907232513ad27a3c..0000000000000000000000000000000000000000
--- a/spaces/dcarpintero/nlp-summarizer-pegasus/.venv/lib/python3.9/site-packages/importlib_resources/abc.py
+++ /dev/null
@@ -1,170 +0,0 @@
-import abc
-import io
-import itertools
-import pathlib
-from typing import Any, BinaryIO, Iterable, Iterator, NoReturn, Text, Optional
-
-from ._compat import runtime_checkable, Protocol, StrPath
-
-
-__all__ = ["ResourceReader", "Traversable", "TraversableResources"]
-
-
-class ResourceReader(metaclass=abc.ABCMeta):
- """Abstract base class for loaders to provide resource reading support."""
-
- @abc.abstractmethod
- def open_resource(self, resource: Text) -> BinaryIO:
- """Return an opened, file-like object for binary reading.
-
- The 'resource' argument is expected to represent only a file name.
- If the resource cannot be found, FileNotFoundError is raised.
- """
- # This deliberately raises FileNotFoundError instead of
- # NotImplementedError so that if this method is accidentally called,
- # it'll still do the right thing.
- raise FileNotFoundError
-
- @abc.abstractmethod
- def resource_path(self, resource: Text) -> Text:
- """Return the file system path to the specified resource.
-
- The 'resource' argument is expected to represent only a file name.
- If the resource does not exist on the file system, raise
- FileNotFoundError.
- """
- # This deliberately raises FileNotFoundError instead of
- # NotImplementedError so that if this method is accidentally called,
- # it'll still do the right thing.
- raise FileNotFoundError
-
- @abc.abstractmethod
- def is_resource(self, path: Text) -> bool:
- """Return True if the named 'path' is a resource.
-
- Files are resources, directories are not.
- """
- raise FileNotFoundError
-
- @abc.abstractmethod
- def contents(self) -> Iterable[str]:
- """Return an iterable of entries in `package`."""
- raise FileNotFoundError
-
-
-class TraversalError(Exception):
- pass
-
-
-@runtime_checkable
-class Traversable(Protocol):
- """
- An object with a subset of pathlib.Path methods suitable for
- traversing directories and opening files.
-
- Any exceptions that occur when accessing the backing resource
- may propagate unaltered.
- """
-
- @abc.abstractmethod
- def iterdir(self) -> Iterator["Traversable"]:
- """
- Yield Traversable objects in self
- """
-
- def read_bytes(self) -> bytes:
- """
- Read contents of self as bytes
- """
- with self.open('rb') as strm:
- return strm.read()
-
- def read_text(self, encoding: Optional[str] = None) -> str:
- """
- Read contents of self as text
- """
- with self.open(encoding=encoding) as strm:
- return strm.read()
-
- @abc.abstractmethod
- def is_dir(self) -> bool:
- """
- Return True if self is a directory
- """
-
- @abc.abstractmethod
- def is_file(self) -> bool:
- """
- Return True if self is a file
- """
-
- def joinpath(self, *descendants: StrPath) -> "Traversable":
- """
- Return Traversable resolved with any descendants applied.
-
- Each descendant should be a path segment relative to self
- and each may contain multiple levels separated by
- ``posixpath.sep`` (``/``).
- """
- if not descendants:
- return self
- names = itertools.chain.from_iterable(
- path.parts for path in map(pathlib.PurePosixPath, descendants)
- )
- target = next(names)
- matches = (
- traversable for traversable in self.iterdir() if traversable.name == target
- )
- try:
- match = next(matches)
- except StopIteration:
- raise TraversalError(
- "Target not found during traversal.", target, list(names)
- )
- return match.joinpath(*names)
-
- def __truediv__(self, child: StrPath) -> "Traversable":
- """
- Return Traversable child in self
- """
- return self.joinpath(child)
-
- @abc.abstractmethod
- def open(self, mode='r', *args, **kwargs):
- """
- mode may be 'r' or 'rb' to open as text or binary. Return a handle
- suitable for reading (same as pathlib.Path.open).
-
- When opening as text, accepts encoding parameters such as those
- accepted by io.TextIOWrapper.
- """
-
- @property
- @abc.abstractmethod
- def name(self) -> str:
- """
- The base name of this object without any parent references.
- """
-
-
-class TraversableResources(ResourceReader):
- """
- The required interface for providing traversable
- resources.
- """
-
- @abc.abstractmethod
- def files(self) -> "Traversable":
- """Return a Traversable object for the loaded package."""
-
- def open_resource(self, resource: StrPath) -> io.BufferedReader:
- return self.files().joinpath(resource).open('rb')
-
- def resource_path(self, resource: Any) -> NoReturn:
- raise FileNotFoundError(resource)
-
- def is_resource(self, path: StrPath) -> bool:
- return self.files().joinpath(path).is_file()
-
- def contents(self) -> Iterator[str]:
- return (item.name for item in self.files().iterdir())
diff --git a/spaces/dcarpintero/nlp-summarizer-pegasus/.venv/lib/python3.9/site-packages/matplotlib/_cm_listed.py b/spaces/dcarpintero/nlp-summarizer-pegasus/.venv/lib/python3.9/site-packages/matplotlib/_cm_listed.py
deleted file mode 100644
index a331ad74a5f03688005dc14d5867653b3d77e20c..0000000000000000000000000000000000000000
--- a/spaces/dcarpintero/nlp-summarizer-pegasus/.venv/lib/python3.9/site-packages/matplotlib/_cm_listed.py
+++ /dev/null
@@ -1,2071 +0,0 @@
-from .colors import ListedColormap
-
-_magma_data = [[0.001462, 0.000466, 0.013866],
- [0.002258, 0.001295, 0.018331],
- [0.003279, 0.002305, 0.023708],
- [0.004512, 0.003490, 0.029965],
- [0.005950, 0.004843, 0.037130],
- [0.007588, 0.006356, 0.044973],
- [0.009426, 0.008022, 0.052844],
- [0.011465, 0.009828, 0.060750],
- [0.013708, 0.011771, 0.068667],
- [0.016156, 0.013840, 0.076603],
- [0.018815, 0.016026, 0.084584],
- [0.021692, 0.018320, 0.092610],
- [0.024792, 0.020715, 0.100676],
- [0.028123, 0.023201, 0.108787],
- [0.031696, 0.025765, 0.116965],
- [0.035520, 0.028397, 0.125209],
- [0.039608, 0.031090, 0.133515],
- [0.043830, 0.033830, 0.141886],
- [0.048062, 0.036607, 0.150327],
- [0.052320, 0.039407, 0.158841],
- [0.056615, 0.042160, 0.167446],
- [0.060949, 0.044794, 0.176129],
- [0.065330, 0.047318, 0.184892],
- [0.069764, 0.049726, 0.193735],
- [0.074257, 0.052017, 0.202660],
- [0.078815, 0.054184, 0.211667],
- [0.083446, 0.056225, 0.220755],
- [0.088155, 0.058133, 0.229922],
- [0.092949, 0.059904, 0.239164],
- [0.097833, 0.061531, 0.248477],
- [0.102815, 0.063010, 0.257854],
- [0.107899, 0.064335, 0.267289],
- [0.113094, 0.065492, 0.276784],
- [0.118405, 0.066479, 0.286321],
- [0.123833, 0.067295, 0.295879],
- [0.129380, 0.067935, 0.305443],
- [0.135053, 0.068391, 0.315000],
- [0.140858, 0.068654, 0.324538],
- [0.146785, 0.068738, 0.334011],
- [0.152839, 0.068637, 0.343404],
- [0.159018, 0.068354, 0.352688],
- [0.165308, 0.067911, 0.361816],
- [0.171713, 0.067305, 0.370771],
- [0.178212, 0.066576, 0.379497],
- [0.184801, 0.065732, 0.387973],
- [0.191460, 0.064818, 0.396152],
- [0.198177, 0.063862, 0.404009],
- [0.204935, 0.062907, 0.411514],
- [0.211718, 0.061992, 0.418647],
- [0.218512, 0.061158, 0.425392],
- [0.225302, 0.060445, 0.431742],
- [0.232077, 0.059889, 0.437695],
- [0.238826, 0.059517, 0.443256],
- [0.245543, 0.059352, 0.448436],
- [0.252220, 0.059415, 0.453248],
- [0.258857, 0.059706, 0.457710],
- [0.265447, 0.060237, 0.461840],
- [0.271994, 0.060994, 0.465660],
- [0.278493, 0.061978, 0.469190],
- [0.284951, 0.063168, 0.472451],
- [0.291366, 0.064553, 0.475462],
- [0.297740, 0.066117, 0.478243],
- [0.304081, 0.067835, 0.480812],
- [0.310382, 0.069702, 0.483186],
- [0.316654, 0.071690, 0.485380],
- [0.322899, 0.073782, 0.487408],
- [0.329114, 0.075972, 0.489287],
- [0.335308, 0.078236, 0.491024],
- [0.341482, 0.080564, 0.492631],
- [0.347636, 0.082946, 0.494121],
- [0.353773, 0.085373, 0.495501],
- [0.359898, 0.087831, 0.496778],
- [0.366012, 0.090314, 0.497960],
- [0.372116, 0.092816, 0.499053],
- [0.378211, 0.095332, 0.500067],
- [0.384299, 0.097855, 0.501002],
- [0.390384, 0.100379, 0.501864],
- [0.396467, 0.102902, 0.502658],
- [0.402548, 0.105420, 0.503386],
- [0.408629, 0.107930, 0.504052],
- [0.414709, 0.110431, 0.504662],
- [0.420791, 0.112920, 0.505215],
- [0.426877, 0.115395, 0.505714],
- [0.432967, 0.117855, 0.506160],
- [0.439062, 0.120298, 0.506555],
- [0.445163, 0.122724, 0.506901],
- [0.451271, 0.125132, 0.507198],
- [0.457386, 0.127522, 0.507448],
- [0.463508, 0.129893, 0.507652],
- [0.469640, 0.132245, 0.507809],
- [0.475780, 0.134577, 0.507921],
- [0.481929, 0.136891, 0.507989],
- [0.488088, 0.139186, 0.508011],
- [0.494258, 0.141462, 0.507988],
- [0.500438, 0.143719, 0.507920],
- [0.506629, 0.145958, 0.507806],
- [0.512831, 0.148179, 0.507648],
- [0.519045, 0.150383, 0.507443],
- [0.525270, 0.152569, 0.507192],
- [0.531507, 0.154739, 0.506895],
- [0.537755, 0.156894, 0.506551],
- [0.544015, 0.159033, 0.506159],
- [0.550287, 0.161158, 0.505719],
- [0.556571, 0.163269, 0.505230],
- [0.562866, 0.165368, 0.504692],
- [0.569172, 0.167454, 0.504105],
- [0.575490, 0.169530, 0.503466],
- [0.581819, 0.171596, 0.502777],
- [0.588158, 0.173652, 0.502035],
- [0.594508, 0.175701, 0.501241],
- [0.600868, 0.177743, 0.500394],
- [0.607238, 0.179779, 0.499492],
- [0.613617, 0.181811, 0.498536],
- [0.620005, 0.183840, 0.497524],
- [0.626401, 0.185867, 0.496456],
- [0.632805, 0.187893, 0.495332],
- [0.639216, 0.189921, 0.494150],
- [0.645633, 0.191952, 0.492910],
- [0.652056, 0.193986, 0.491611],
- [0.658483, 0.196027, 0.490253],
- [0.664915, 0.198075, 0.488836],
- [0.671349, 0.200133, 0.487358],
- [0.677786, 0.202203, 0.485819],
- [0.684224, 0.204286, 0.484219],
- [0.690661, 0.206384, 0.482558],
- [0.697098, 0.208501, 0.480835],
- [0.703532, 0.210638, 0.479049],
- [0.709962, 0.212797, 0.477201],
- [0.716387, 0.214982, 0.475290],
- [0.722805, 0.217194, 0.473316],
- [0.729216, 0.219437, 0.471279],
- [0.735616, 0.221713, 0.469180],
- [0.742004, 0.224025, 0.467018],
- [0.748378, 0.226377, 0.464794],
- [0.754737, 0.228772, 0.462509],
- [0.761077, 0.231214, 0.460162],
- [0.767398, 0.233705, 0.457755],
- [0.773695, 0.236249, 0.455289],
- [0.779968, 0.238851, 0.452765],
- [0.786212, 0.241514, 0.450184],
- [0.792427, 0.244242, 0.447543],
- [0.798608, 0.247040, 0.444848],
- [0.804752, 0.249911, 0.442102],
- [0.810855, 0.252861, 0.439305],
- [0.816914, 0.255895, 0.436461],
- [0.822926, 0.259016, 0.433573],
- [0.828886, 0.262229, 0.430644],
- [0.834791, 0.265540, 0.427671],
- [0.840636, 0.268953, 0.424666],
- [0.846416, 0.272473, 0.421631],
- [0.852126, 0.276106, 0.418573],
- [0.857763, 0.279857, 0.415496],
- [0.863320, 0.283729, 0.412403],
- [0.868793, 0.287728, 0.409303],
- [0.874176, 0.291859, 0.406205],
- [0.879464, 0.296125, 0.403118],
- [0.884651, 0.300530, 0.400047],
- [0.889731, 0.305079, 0.397002],
- [0.894700, 0.309773, 0.393995],
- [0.899552, 0.314616, 0.391037],
- [0.904281, 0.319610, 0.388137],
- [0.908884, 0.324755, 0.385308],
- [0.913354, 0.330052, 0.382563],
- [0.917689, 0.335500, 0.379915],
- [0.921884, 0.341098, 0.377376],
- [0.925937, 0.346844, 0.374959],
- [0.929845, 0.352734, 0.372677],
- [0.933606, 0.358764, 0.370541],
- [0.937221, 0.364929, 0.368567],
- [0.940687, 0.371224, 0.366762],
- [0.944006, 0.377643, 0.365136],
- [0.947180, 0.384178, 0.363701],
- [0.950210, 0.390820, 0.362468],
- [0.953099, 0.397563, 0.361438],
- [0.955849, 0.404400, 0.360619],
- [0.958464, 0.411324, 0.360014],
- [0.960949, 0.418323, 0.359630],
- [0.963310, 0.425390, 0.359469],
- [0.965549, 0.432519, 0.359529],
- [0.967671, 0.439703, 0.359810],
- [0.969680, 0.446936, 0.360311],
- [0.971582, 0.454210, 0.361030],
- [0.973381, 0.461520, 0.361965],
- [0.975082, 0.468861, 0.363111],
- [0.976690, 0.476226, 0.364466],
- [0.978210, 0.483612, 0.366025],
- [0.979645, 0.491014, 0.367783],
- [0.981000, 0.498428, 0.369734],
- [0.982279, 0.505851, 0.371874],
- [0.983485, 0.513280, 0.374198],
- [0.984622, 0.520713, 0.376698],
- [0.985693, 0.528148, 0.379371],
- [0.986700, 0.535582, 0.382210],
- [0.987646, 0.543015, 0.385210],
- [0.988533, 0.550446, 0.388365],
- [0.989363, 0.557873, 0.391671],
- [0.990138, 0.565296, 0.395122],
- [0.990871, 0.572706, 0.398714],
- [0.991558, 0.580107, 0.402441],
- [0.992196, 0.587502, 0.406299],
- [0.992785, 0.594891, 0.410283],
- [0.993326, 0.602275, 0.414390],
- [0.993834, 0.609644, 0.418613],
- [0.994309, 0.616999, 0.422950],
- [0.994738, 0.624350, 0.427397],
- [0.995122, 0.631696, 0.431951],
- [0.995480, 0.639027, 0.436607],
- [0.995810, 0.646344, 0.441361],
- [0.996096, 0.653659, 0.446213],
- [0.996341, 0.660969, 0.451160],
- [0.996580, 0.668256, 0.456192],
- [0.996775, 0.675541, 0.461314],
- [0.996925, 0.682828, 0.466526],
- [0.997077, 0.690088, 0.471811],
- [0.997186, 0.697349, 0.477182],
- [0.997254, 0.704611, 0.482635],
- [0.997325, 0.711848, 0.488154],
- [0.997351, 0.719089, 0.493755],
- [0.997351, 0.726324, 0.499428],
- [0.997341, 0.733545, 0.505167],
- [0.997285, 0.740772, 0.510983],
- [0.997228, 0.747981, 0.516859],
- [0.997138, 0.755190, 0.522806],
- [0.997019, 0.762398, 0.528821],
- [0.996898, 0.769591, 0.534892],
- [0.996727, 0.776795, 0.541039],
- [0.996571, 0.783977, 0.547233],
- [0.996369, 0.791167, 0.553499],
- [0.996162, 0.798348, 0.559820],
- [0.995932, 0.805527, 0.566202],
- [0.995680, 0.812706, 0.572645],
- [0.995424, 0.819875, 0.579140],
- [0.995131, 0.827052, 0.585701],
- [0.994851, 0.834213, 0.592307],
- [0.994524, 0.841387, 0.598983],
- [0.994222, 0.848540, 0.605696],
- [0.993866, 0.855711, 0.612482],
- [0.993545, 0.862859, 0.619299],
- [0.993170, 0.870024, 0.626189],
- [0.992831, 0.877168, 0.633109],
- [0.992440, 0.884330, 0.640099],
- [0.992089, 0.891470, 0.647116],
- [0.991688, 0.898627, 0.654202],
- [0.991332, 0.905763, 0.661309],
- [0.990930, 0.912915, 0.668481],
- [0.990570, 0.920049, 0.675675],
- [0.990175, 0.927196, 0.682926],
- [0.989815, 0.934329, 0.690198],
- [0.989434, 0.941470, 0.697519],
- [0.989077, 0.948604, 0.704863],
- [0.988717, 0.955742, 0.712242],
- [0.988367, 0.962878, 0.719649],
- [0.988033, 0.970012, 0.727077],
- [0.987691, 0.977154, 0.734536],
- [0.987387, 0.984288, 0.742002],
- [0.987053, 0.991438, 0.749504]]
-
-_inferno_data = [[0.001462, 0.000466, 0.013866],
- [0.002267, 0.001270, 0.018570],
- [0.003299, 0.002249, 0.024239],
- [0.004547, 0.003392, 0.030909],
- [0.006006, 0.004692, 0.038558],
- [0.007676, 0.006136, 0.046836],
- [0.009561, 0.007713, 0.055143],
- [0.011663, 0.009417, 0.063460],
- [0.013995, 0.011225, 0.071862],
- [0.016561, 0.013136, 0.080282],
- [0.019373, 0.015133, 0.088767],
- [0.022447, 0.017199, 0.097327],
- [0.025793, 0.019331, 0.105930],
- [0.029432, 0.021503, 0.114621],
- [0.033385, 0.023702, 0.123397],
- [0.037668, 0.025921, 0.132232],
- [0.042253, 0.028139, 0.141141],
- [0.046915, 0.030324, 0.150164],
- [0.051644, 0.032474, 0.159254],
- [0.056449, 0.034569, 0.168414],
- [0.061340, 0.036590, 0.177642],
- [0.066331, 0.038504, 0.186962],
- [0.071429, 0.040294, 0.196354],
- [0.076637, 0.041905, 0.205799],
- [0.081962, 0.043328, 0.215289],
- [0.087411, 0.044556, 0.224813],
- [0.092990, 0.045583, 0.234358],
- [0.098702, 0.046402, 0.243904],
- [0.104551, 0.047008, 0.253430],
- [0.110536, 0.047399, 0.262912],
- [0.116656, 0.047574, 0.272321],
- [0.122908, 0.047536, 0.281624],
- [0.129285, 0.047293, 0.290788],
- [0.135778, 0.046856, 0.299776],
- [0.142378, 0.046242, 0.308553],
- [0.149073, 0.045468, 0.317085],
- [0.155850, 0.044559, 0.325338],
- [0.162689, 0.043554, 0.333277],
- [0.169575, 0.042489, 0.340874],
- [0.176493, 0.041402, 0.348111],
- [0.183429, 0.040329, 0.354971],
- [0.190367, 0.039309, 0.361447],
- [0.197297, 0.038400, 0.367535],
- [0.204209, 0.037632, 0.373238],
- [0.211095, 0.037030, 0.378563],
- [0.217949, 0.036615, 0.383522],
- [0.224763, 0.036405, 0.388129],
- [0.231538, 0.036405, 0.392400],
- [0.238273, 0.036621, 0.396353],
- [0.244967, 0.037055, 0.400007],
- [0.251620, 0.037705, 0.403378],
- [0.258234, 0.038571, 0.406485],
- [0.264810, 0.039647, 0.409345],
- [0.271347, 0.040922, 0.411976],
- [0.277850, 0.042353, 0.414392],
- [0.284321, 0.043933, 0.416608],
- [0.290763, 0.045644, 0.418637],
- [0.297178, 0.047470, 0.420491],
- [0.303568, 0.049396, 0.422182],
- [0.309935, 0.051407, 0.423721],
- [0.316282, 0.053490, 0.425116],
- [0.322610, 0.055634, 0.426377],
- [0.328921, 0.057827, 0.427511],
- [0.335217, 0.060060, 0.428524],
- [0.341500, 0.062325, 0.429425],
- [0.347771, 0.064616, 0.430217],
- [0.354032, 0.066925, 0.430906],
- [0.360284, 0.069247, 0.431497],
- [0.366529, 0.071579, 0.431994],
- [0.372768, 0.073915, 0.432400],
- [0.379001, 0.076253, 0.432719],
- [0.385228, 0.078591, 0.432955],
- [0.391453, 0.080927, 0.433109],
- [0.397674, 0.083257, 0.433183],
- [0.403894, 0.085580, 0.433179],
- [0.410113, 0.087896, 0.433098],
- [0.416331, 0.090203, 0.432943],
- [0.422549, 0.092501, 0.432714],
- [0.428768, 0.094790, 0.432412],
- [0.434987, 0.097069, 0.432039],
- [0.441207, 0.099338, 0.431594],
- [0.447428, 0.101597, 0.431080],
- [0.453651, 0.103848, 0.430498],
- [0.459875, 0.106089, 0.429846],
- [0.466100, 0.108322, 0.429125],
- [0.472328, 0.110547, 0.428334],
- [0.478558, 0.112764, 0.427475],
- [0.484789, 0.114974, 0.426548],
- [0.491022, 0.117179, 0.425552],
- [0.497257, 0.119379, 0.424488],
- [0.503493, 0.121575, 0.423356],
- [0.509730, 0.123769, 0.422156],
- [0.515967, 0.125960, 0.420887],
- [0.522206, 0.128150, 0.419549],
- [0.528444, 0.130341, 0.418142],
- [0.534683, 0.132534, 0.416667],
- [0.540920, 0.134729, 0.415123],
- [0.547157, 0.136929, 0.413511],
- [0.553392, 0.139134, 0.411829],
- [0.559624, 0.141346, 0.410078],
- [0.565854, 0.143567, 0.408258],
- [0.572081, 0.145797, 0.406369],
- [0.578304, 0.148039, 0.404411],
- [0.584521, 0.150294, 0.402385],
- [0.590734, 0.152563, 0.400290],
- [0.596940, 0.154848, 0.398125],
- [0.603139, 0.157151, 0.395891],
- [0.609330, 0.159474, 0.393589],
- [0.615513, 0.161817, 0.391219],
- [0.621685, 0.164184, 0.388781],
- [0.627847, 0.166575, 0.386276],
- [0.633998, 0.168992, 0.383704],
- [0.640135, 0.171438, 0.381065],
- [0.646260, 0.173914, 0.378359],
- [0.652369, 0.176421, 0.375586],
- [0.658463, 0.178962, 0.372748],
- [0.664540, 0.181539, 0.369846],
- [0.670599, 0.184153, 0.366879],
- [0.676638, 0.186807, 0.363849],
- [0.682656, 0.189501, 0.360757],
- [0.688653, 0.192239, 0.357603],
- [0.694627, 0.195021, 0.354388],
- [0.700576, 0.197851, 0.351113],
- [0.706500, 0.200728, 0.347777],
- [0.712396, 0.203656, 0.344383],
- [0.718264, 0.206636, 0.340931],
- [0.724103, 0.209670, 0.337424],
- [0.729909, 0.212759, 0.333861],
- [0.735683, 0.215906, 0.330245],
- [0.741423, 0.219112, 0.326576],
- [0.747127, 0.222378, 0.322856],
- [0.752794, 0.225706, 0.319085],
- [0.758422, 0.229097, 0.315266],
- [0.764010, 0.232554, 0.311399],
- [0.769556, 0.236077, 0.307485],
- [0.775059, 0.239667, 0.303526],
- [0.780517, 0.243327, 0.299523],
- [0.785929, 0.247056, 0.295477],
- [0.791293, 0.250856, 0.291390],
- [0.796607, 0.254728, 0.287264],
- [0.801871, 0.258674, 0.283099],
- [0.807082, 0.262692, 0.278898],
- [0.812239, 0.266786, 0.274661],
- [0.817341, 0.270954, 0.270390],
- [0.822386, 0.275197, 0.266085],
- [0.827372, 0.279517, 0.261750],
- [0.832299, 0.283913, 0.257383],
- [0.837165, 0.288385, 0.252988],
- [0.841969, 0.292933, 0.248564],
- [0.846709, 0.297559, 0.244113],
- [0.851384, 0.302260, 0.239636],
- [0.855992, 0.307038, 0.235133],
- [0.860533, 0.311892, 0.230606],
- [0.865006, 0.316822, 0.226055],
- [0.869409, 0.321827, 0.221482],
- [0.873741, 0.326906, 0.216886],
- [0.878001, 0.332060, 0.212268],
- [0.882188, 0.337287, 0.207628],
- [0.886302, 0.342586, 0.202968],
- [0.890341, 0.347957, 0.198286],
- [0.894305, 0.353399, 0.193584],
- [0.898192, 0.358911, 0.188860],
- [0.902003, 0.364492, 0.184116],
- [0.905735, 0.370140, 0.179350],
- [0.909390, 0.375856, 0.174563],
- [0.912966, 0.381636, 0.169755],
- [0.916462, 0.387481, 0.164924],
- [0.919879, 0.393389, 0.160070],
- [0.923215, 0.399359, 0.155193],
- [0.926470, 0.405389, 0.150292],
- [0.929644, 0.411479, 0.145367],
- [0.932737, 0.417627, 0.140417],
- [0.935747, 0.423831, 0.135440],
- [0.938675, 0.430091, 0.130438],
- [0.941521, 0.436405, 0.125409],
- [0.944285, 0.442772, 0.120354],
- [0.946965, 0.449191, 0.115272],
- [0.949562, 0.455660, 0.110164],
- [0.952075, 0.462178, 0.105031],
- [0.954506, 0.468744, 0.099874],
- [0.956852, 0.475356, 0.094695],
- [0.959114, 0.482014, 0.089499],
- [0.961293, 0.488716, 0.084289],
- [0.963387, 0.495462, 0.079073],
- [0.965397, 0.502249, 0.073859],
- [0.967322, 0.509078, 0.068659],
- [0.969163, 0.515946, 0.063488],
- [0.970919, 0.522853, 0.058367],
- [0.972590, 0.529798, 0.053324],
- [0.974176, 0.536780, 0.048392],
- [0.975677, 0.543798, 0.043618],
- [0.977092, 0.550850, 0.039050],
- [0.978422, 0.557937, 0.034931],
- [0.979666, 0.565057, 0.031409],
- [0.980824, 0.572209, 0.028508],
- [0.981895, 0.579392, 0.026250],
- [0.982881, 0.586606, 0.024661],
- [0.983779, 0.593849, 0.023770],
- [0.984591, 0.601122, 0.023606],
- [0.985315, 0.608422, 0.024202],
- [0.985952, 0.615750, 0.025592],
- [0.986502, 0.623105, 0.027814],
- [0.986964, 0.630485, 0.030908],
- [0.987337, 0.637890, 0.034916],
- [0.987622, 0.645320, 0.039886],
- [0.987819, 0.652773, 0.045581],
- [0.987926, 0.660250, 0.051750],
- [0.987945, 0.667748, 0.058329],
- [0.987874, 0.675267, 0.065257],
- [0.987714, 0.682807, 0.072489],
- [0.987464, 0.690366, 0.079990],
- [0.987124, 0.697944, 0.087731],
- [0.986694, 0.705540, 0.095694],
- [0.986175, 0.713153, 0.103863],
- [0.985566, 0.720782, 0.112229],
- [0.984865, 0.728427, 0.120785],
- [0.984075, 0.736087, 0.129527],
- [0.983196, 0.743758, 0.138453],
- [0.982228, 0.751442, 0.147565],
- [0.981173, 0.759135, 0.156863],
- [0.980032, 0.766837, 0.166353],
- [0.978806, 0.774545, 0.176037],
- [0.977497, 0.782258, 0.185923],
- [0.976108, 0.789974, 0.196018],
- [0.974638, 0.797692, 0.206332],
- [0.973088, 0.805409, 0.216877],
- [0.971468, 0.813122, 0.227658],
- [0.969783, 0.820825, 0.238686],
- [0.968041, 0.828515, 0.249972],
- [0.966243, 0.836191, 0.261534],
- [0.964394, 0.843848, 0.273391],
- [0.962517, 0.851476, 0.285546],
- [0.960626, 0.859069, 0.298010],
- [0.958720, 0.866624, 0.310820],
- [0.956834, 0.874129, 0.323974],
- [0.954997, 0.881569, 0.337475],
- [0.953215, 0.888942, 0.351369],
- [0.951546, 0.896226, 0.365627],
- [0.950018, 0.903409, 0.380271],
- [0.948683, 0.910473, 0.395289],
- [0.947594, 0.917399, 0.410665],
- [0.946809, 0.924168, 0.426373],
- [0.946392, 0.930761, 0.442367],
- [0.946403, 0.937159, 0.458592],
- [0.946903, 0.943348, 0.474970],
- [0.947937, 0.949318, 0.491426],
- [0.949545, 0.955063, 0.507860],
- [0.951740, 0.960587, 0.524203],
- [0.954529, 0.965896, 0.540361],
- [0.957896, 0.971003, 0.556275],
- [0.961812, 0.975924, 0.571925],
- [0.966249, 0.980678, 0.587206],
- [0.971162, 0.985282, 0.602154],
- [0.976511, 0.989753, 0.616760],
- [0.982257, 0.994109, 0.631017],
- [0.988362, 0.998364, 0.644924]]
-
-_plasma_data = [[0.050383, 0.029803, 0.527975],
- [0.063536, 0.028426, 0.533124],
- [0.075353, 0.027206, 0.538007],
- [0.086222, 0.026125, 0.542658],
- [0.096379, 0.025165, 0.547103],
- [0.105980, 0.024309, 0.551368],
- [0.115124, 0.023556, 0.555468],
- [0.123903, 0.022878, 0.559423],
- [0.132381, 0.022258, 0.563250],
- [0.140603, 0.021687, 0.566959],
- [0.148607, 0.021154, 0.570562],
- [0.156421, 0.020651, 0.574065],
- [0.164070, 0.020171, 0.577478],
- [0.171574, 0.019706, 0.580806],
- [0.178950, 0.019252, 0.584054],
- [0.186213, 0.018803, 0.587228],
- [0.193374, 0.018354, 0.590330],
- [0.200445, 0.017902, 0.593364],
- [0.207435, 0.017442, 0.596333],
- [0.214350, 0.016973, 0.599239],
- [0.221197, 0.016497, 0.602083],
- [0.227983, 0.016007, 0.604867],
- [0.234715, 0.015502, 0.607592],
- [0.241396, 0.014979, 0.610259],
- [0.248032, 0.014439, 0.612868],
- [0.254627, 0.013882, 0.615419],
- [0.261183, 0.013308, 0.617911],
- [0.267703, 0.012716, 0.620346],
- [0.274191, 0.012109, 0.622722],
- [0.280648, 0.011488, 0.625038],
- [0.287076, 0.010855, 0.627295],
- [0.293478, 0.010213, 0.629490],
- [0.299855, 0.009561, 0.631624],
- [0.306210, 0.008902, 0.633694],
- [0.312543, 0.008239, 0.635700],
- [0.318856, 0.007576, 0.637640],
- [0.325150, 0.006915, 0.639512],
- [0.331426, 0.006261, 0.641316],
- [0.337683, 0.005618, 0.643049],
- [0.343925, 0.004991, 0.644710],
- [0.350150, 0.004382, 0.646298],
- [0.356359, 0.003798, 0.647810],
- [0.362553, 0.003243, 0.649245],
- [0.368733, 0.002724, 0.650601],
- [0.374897, 0.002245, 0.651876],
- [0.381047, 0.001814, 0.653068],
- [0.387183, 0.001434, 0.654177],
- [0.393304, 0.001114, 0.655199],
- [0.399411, 0.000859, 0.656133],
- [0.405503, 0.000678, 0.656977],
- [0.411580, 0.000577, 0.657730],
- [0.417642, 0.000564, 0.658390],
- [0.423689, 0.000646, 0.658956],
- [0.429719, 0.000831, 0.659425],
- [0.435734, 0.001127, 0.659797],
- [0.441732, 0.001540, 0.660069],
- [0.447714, 0.002080, 0.660240],
- [0.453677, 0.002755, 0.660310],
- [0.459623, 0.003574, 0.660277],
- [0.465550, 0.004545, 0.660139],
- [0.471457, 0.005678, 0.659897],
- [0.477344, 0.006980, 0.659549],
- [0.483210, 0.008460, 0.659095],
- [0.489055, 0.010127, 0.658534],
- [0.494877, 0.011990, 0.657865],
- [0.500678, 0.014055, 0.657088],
- [0.506454, 0.016333, 0.656202],
- [0.512206, 0.018833, 0.655209],
- [0.517933, 0.021563, 0.654109],
- [0.523633, 0.024532, 0.652901],
- [0.529306, 0.027747, 0.651586],
- [0.534952, 0.031217, 0.650165],
- [0.540570, 0.034950, 0.648640],
- [0.546157, 0.038954, 0.647010],
- [0.551715, 0.043136, 0.645277],
- [0.557243, 0.047331, 0.643443],
- [0.562738, 0.051545, 0.641509],
- [0.568201, 0.055778, 0.639477],
- [0.573632, 0.060028, 0.637349],
- [0.579029, 0.064296, 0.635126],
- [0.584391, 0.068579, 0.632812],
- [0.589719, 0.072878, 0.630408],
- [0.595011, 0.077190, 0.627917],
- [0.600266, 0.081516, 0.625342],
- [0.605485, 0.085854, 0.622686],
- [0.610667, 0.090204, 0.619951],
- [0.615812, 0.094564, 0.617140],
- [0.620919, 0.098934, 0.614257],
- [0.625987, 0.103312, 0.611305],
- [0.631017, 0.107699, 0.608287],
- [0.636008, 0.112092, 0.605205],
- [0.640959, 0.116492, 0.602065],
- [0.645872, 0.120898, 0.598867],
- [0.650746, 0.125309, 0.595617],
- [0.655580, 0.129725, 0.592317],
- [0.660374, 0.134144, 0.588971],
- [0.665129, 0.138566, 0.585582],
- [0.669845, 0.142992, 0.582154],
- [0.674522, 0.147419, 0.578688],
- [0.679160, 0.151848, 0.575189],
- [0.683758, 0.156278, 0.571660],
- [0.688318, 0.160709, 0.568103],
- [0.692840, 0.165141, 0.564522],
- [0.697324, 0.169573, 0.560919],
- [0.701769, 0.174005, 0.557296],
- [0.706178, 0.178437, 0.553657],
- [0.710549, 0.182868, 0.550004],
- [0.714883, 0.187299, 0.546338],
- [0.719181, 0.191729, 0.542663],
- [0.723444, 0.196158, 0.538981],
- [0.727670, 0.200586, 0.535293],
- [0.731862, 0.205013, 0.531601],
- [0.736019, 0.209439, 0.527908],
- [0.740143, 0.213864, 0.524216],
- [0.744232, 0.218288, 0.520524],
- [0.748289, 0.222711, 0.516834],
- [0.752312, 0.227133, 0.513149],
- [0.756304, 0.231555, 0.509468],
- [0.760264, 0.235976, 0.505794],
- [0.764193, 0.240396, 0.502126],
- [0.768090, 0.244817, 0.498465],
- [0.771958, 0.249237, 0.494813],
- [0.775796, 0.253658, 0.491171],
- [0.779604, 0.258078, 0.487539],
- [0.783383, 0.262500, 0.483918],
- [0.787133, 0.266922, 0.480307],
- [0.790855, 0.271345, 0.476706],
- [0.794549, 0.275770, 0.473117],
- [0.798216, 0.280197, 0.469538],
- [0.801855, 0.284626, 0.465971],
- [0.805467, 0.289057, 0.462415],
- [0.809052, 0.293491, 0.458870],
- [0.812612, 0.297928, 0.455338],
- [0.816144, 0.302368, 0.451816],
- [0.819651, 0.306812, 0.448306],
- [0.823132, 0.311261, 0.444806],
- [0.826588, 0.315714, 0.441316],
- [0.830018, 0.320172, 0.437836],
- [0.833422, 0.324635, 0.434366],
- [0.836801, 0.329105, 0.430905],
- [0.840155, 0.333580, 0.427455],
- [0.843484, 0.338062, 0.424013],
- [0.846788, 0.342551, 0.420579],
- [0.850066, 0.347048, 0.417153],
- [0.853319, 0.351553, 0.413734],
- [0.856547, 0.356066, 0.410322],
- [0.859750, 0.360588, 0.406917],
- [0.862927, 0.365119, 0.403519],
- [0.866078, 0.369660, 0.400126],
- [0.869203, 0.374212, 0.396738],
- [0.872303, 0.378774, 0.393355],
- [0.875376, 0.383347, 0.389976],
- [0.878423, 0.387932, 0.386600],
- [0.881443, 0.392529, 0.383229],
- [0.884436, 0.397139, 0.379860],
- [0.887402, 0.401762, 0.376494],
- [0.890340, 0.406398, 0.373130],
- [0.893250, 0.411048, 0.369768],
- [0.896131, 0.415712, 0.366407],
- [0.898984, 0.420392, 0.363047],
- [0.901807, 0.425087, 0.359688],
- [0.904601, 0.429797, 0.356329],
- [0.907365, 0.434524, 0.352970],
- [0.910098, 0.439268, 0.349610],
- [0.912800, 0.444029, 0.346251],
- [0.915471, 0.448807, 0.342890],
- [0.918109, 0.453603, 0.339529],
- [0.920714, 0.458417, 0.336166],
- [0.923287, 0.463251, 0.332801],
- [0.925825, 0.468103, 0.329435],
- [0.928329, 0.472975, 0.326067],
- [0.930798, 0.477867, 0.322697],
- [0.933232, 0.482780, 0.319325],
- [0.935630, 0.487712, 0.315952],
- [0.937990, 0.492667, 0.312575],
- [0.940313, 0.497642, 0.309197],
- [0.942598, 0.502639, 0.305816],
- [0.944844, 0.507658, 0.302433],
- [0.947051, 0.512699, 0.299049],
- [0.949217, 0.517763, 0.295662],
- [0.951344, 0.522850, 0.292275],
- [0.953428, 0.527960, 0.288883],
- [0.955470, 0.533093, 0.285490],
- [0.957469, 0.538250, 0.282096],
- [0.959424, 0.543431, 0.278701],
- [0.961336, 0.548636, 0.275305],
- [0.963203, 0.553865, 0.271909],
- [0.965024, 0.559118, 0.268513],
- [0.966798, 0.564396, 0.265118],
- [0.968526, 0.569700, 0.261721],
- [0.970205, 0.575028, 0.258325],
- [0.971835, 0.580382, 0.254931],
- [0.973416, 0.585761, 0.251540],
- [0.974947, 0.591165, 0.248151],
- [0.976428, 0.596595, 0.244767],
- [0.977856, 0.602051, 0.241387],
- [0.979233, 0.607532, 0.238013],
- [0.980556, 0.613039, 0.234646],
- [0.981826, 0.618572, 0.231287],
- [0.983041, 0.624131, 0.227937],
- [0.984199, 0.629718, 0.224595],
- [0.985301, 0.635330, 0.221265],
- [0.986345, 0.640969, 0.217948],
- [0.987332, 0.646633, 0.214648],
- [0.988260, 0.652325, 0.211364],
- [0.989128, 0.658043, 0.208100],
- [0.989935, 0.663787, 0.204859],
- [0.990681, 0.669558, 0.201642],
- [0.991365, 0.675355, 0.198453],
- [0.991985, 0.681179, 0.195295],
- [0.992541, 0.687030, 0.192170],
- [0.993032, 0.692907, 0.189084],
- [0.993456, 0.698810, 0.186041],
- [0.993814, 0.704741, 0.183043],
- [0.994103, 0.710698, 0.180097],
- [0.994324, 0.716681, 0.177208],
- [0.994474, 0.722691, 0.174381],
- [0.994553, 0.728728, 0.171622],
- [0.994561, 0.734791, 0.168938],
- [0.994495, 0.740880, 0.166335],
- [0.994355, 0.746995, 0.163821],
- [0.994141, 0.753137, 0.161404],
- [0.993851, 0.759304, 0.159092],
- [0.993482, 0.765499, 0.156891],
- [0.993033, 0.771720, 0.154808],
- [0.992505, 0.777967, 0.152855],
- [0.991897, 0.784239, 0.151042],
- [0.991209, 0.790537, 0.149377],
- [0.990439, 0.796859, 0.147870],
- [0.989587, 0.803205, 0.146529],
- [0.988648, 0.809579, 0.145357],
- [0.987621, 0.815978, 0.144363],
- [0.986509, 0.822401, 0.143557],
- [0.985314, 0.828846, 0.142945],
- [0.984031, 0.835315, 0.142528],
- [0.982653, 0.841812, 0.142303],
- [0.981190, 0.848329, 0.142279],
- [0.979644, 0.854866, 0.142453],
- [0.977995, 0.861432, 0.142808],
- [0.976265, 0.868016, 0.143351],
- [0.974443, 0.874622, 0.144061],
- [0.972530, 0.881250, 0.144923],
- [0.970533, 0.887896, 0.145919],
- [0.968443, 0.894564, 0.147014],
- [0.966271, 0.901249, 0.148180],
- [0.964021, 0.907950, 0.149370],
- [0.961681, 0.914672, 0.150520],
- [0.959276, 0.921407, 0.151566],
- [0.956808, 0.928152, 0.152409],
- [0.954287, 0.934908, 0.152921],
- [0.951726, 0.941671, 0.152925],
- [0.949151, 0.948435, 0.152178],
- [0.946602, 0.955190, 0.150328],
- [0.944152, 0.961916, 0.146861],
- [0.941896, 0.968590, 0.140956],
- [0.940015, 0.975158, 0.131326]]
-
-_viridis_data = [[0.267004, 0.004874, 0.329415],
- [0.268510, 0.009605, 0.335427],
- [0.269944, 0.014625, 0.341379],
- [0.271305, 0.019942, 0.347269],
- [0.272594, 0.025563, 0.353093],
- [0.273809, 0.031497, 0.358853],
- [0.274952, 0.037752, 0.364543],
- [0.276022, 0.044167, 0.370164],
- [0.277018, 0.050344, 0.375715],
- [0.277941, 0.056324, 0.381191],
- [0.278791, 0.062145, 0.386592],
- [0.279566, 0.067836, 0.391917],
- [0.280267, 0.073417, 0.397163],
- [0.280894, 0.078907, 0.402329],
- [0.281446, 0.084320, 0.407414],
- [0.281924, 0.089666, 0.412415],
- [0.282327, 0.094955, 0.417331],
- [0.282656, 0.100196, 0.422160],
- [0.282910, 0.105393, 0.426902],
- [0.283091, 0.110553, 0.431554],
- [0.283197, 0.115680, 0.436115],
- [0.283229, 0.120777, 0.440584],
- [0.283187, 0.125848, 0.444960],
- [0.283072, 0.130895, 0.449241],
- [0.282884, 0.135920, 0.453427],
- [0.282623, 0.140926, 0.457517],
- [0.282290, 0.145912, 0.461510],
- [0.281887, 0.150881, 0.465405],
- [0.281412, 0.155834, 0.469201],
- [0.280868, 0.160771, 0.472899],
- [0.280255, 0.165693, 0.476498],
- [0.279574, 0.170599, 0.479997],
- [0.278826, 0.175490, 0.483397],
- [0.278012, 0.180367, 0.486697],
- [0.277134, 0.185228, 0.489898],
- [0.276194, 0.190074, 0.493001],
- [0.275191, 0.194905, 0.496005],
- [0.274128, 0.199721, 0.498911],
- [0.273006, 0.204520, 0.501721],
- [0.271828, 0.209303, 0.504434],
- [0.270595, 0.214069, 0.507052],
- [0.269308, 0.218818, 0.509577],
- [0.267968, 0.223549, 0.512008],
- [0.266580, 0.228262, 0.514349],
- [0.265145, 0.232956, 0.516599],
- [0.263663, 0.237631, 0.518762],
- [0.262138, 0.242286, 0.520837],
- [0.260571, 0.246922, 0.522828],
- [0.258965, 0.251537, 0.524736],
- [0.257322, 0.256130, 0.526563],
- [0.255645, 0.260703, 0.528312],
- [0.253935, 0.265254, 0.529983],
- [0.252194, 0.269783, 0.531579],
- [0.250425, 0.274290, 0.533103],
- [0.248629, 0.278775, 0.534556],
- [0.246811, 0.283237, 0.535941],
- [0.244972, 0.287675, 0.537260],
- [0.243113, 0.292092, 0.538516],
- [0.241237, 0.296485, 0.539709],
- [0.239346, 0.300855, 0.540844],
- [0.237441, 0.305202, 0.541921],
- [0.235526, 0.309527, 0.542944],
- [0.233603, 0.313828, 0.543914],
- [0.231674, 0.318106, 0.544834],
- [0.229739, 0.322361, 0.545706],
- [0.227802, 0.326594, 0.546532],
- [0.225863, 0.330805, 0.547314],
- [0.223925, 0.334994, 0.548053],
- [0.221989, 0.339161, 0.548752],
- [0.220057, 0.343307, 0.549413],
- [0.218130, 0.347432, 0.550038],
- [0.216210, 0.351535, 0.550627],
- [0.214298, 0.355619, 0.551184],
- [0.212395, 0.359683, 0.551710],
- [0.210503, 0.363727, 0.552206],
- [0.208623, 0.367752, 0.552675],
- [0.206756, 0.371758, 0.553117],
- [0.204903, 0.375746, 0.553533],
- [0.203063, 0.379716, 0.553925],
- [0.201239, 0.383670, 0.554294],
- [0.199430, 0.387607, 0.554642],
- [0.197636, 0.391528, 0.554969],
- [0.195860, 0.395433, 0.555276],
- [0.194100, 0.399323, 0.555565],
- [0.192357, 0.403199, 0.555836],
- [0.190631, 0.407061, 0.556089],
- [0.188923, 0.410910, 0.556326],
- [0.187231, 0.414746, 0.556547],
- [0.185556, 0.418570, 0.556753],
- [0.183898, 0.422383, 0.556944],
- [0.182256, 0.426184, 0.557120],
- [0.180629, 0.429975, 0.557282],
- [0.179019, 0.433756, 0.557430],
- [0.177423, 0.437527, 0.557565],
- [0.175841, 0.441290, 0.557685],
- [0.174274, 0.445044, 0.557792],
- [0.172719, 0.448791, 0.557885],
- [0.171176, 0.452530, 0.557965],
- [0.169646, 0.456262, 0.558030],
- [0.168126, 0.459988, 0.558082],
- [0.166617, 0.463708, 0.558119],
- [0.165117, 0.467423, 0.558141],
- [0.163625, 0.471133, 0.558148],
- [0.162142, 0.474838, 0.558140],
- [0.160665, 0.478540, 0.558115],
- [0.159194, 0.482237, 0.558073],
- [0.157729, 0.485932, 0.558013],
- [0.156270, 0.489624, 0.557936],
- [0.154815, 0.493313, 0.557840],
- [0.153364, 0.497000, 0.557724],
- [0.151918, 0.500685, 0.557587],
- [0.150476, 0.504369, 0.557430],
- [0.149039, 0.508051, 0.557250],
- [0.147607, 0.511733, 0.557049],
- [0.146180, 0.515413, 0.556823],
- [0.144759, 0.519093, 0.556572],
- [0.143343, 0.522773, 0.556295],
- [0.141935, 0.526453, 0.555991],
- [0.140536, 0.530132, 0.555659],
- [0.139147, 0.533812, 0.555298],
- [0.137770, 0.537492, 0.554906],
- [0.136408, 0.541173, 0.554483],
- [0.135066, 0.544853, 0.554029],
- [0.133743, 0.548535, 0.553541],
- [0.132444, 0.552216, 0.553018],
- [0.131172, 0.555899, 0.552459],
- [0.129933, 0.559582, 0.551864],
- [0.128729, 0.563265, 0.551229],
- [0.127568, 0.566949, 0.550556],
- [0.126453, 0.570633, 0.549841],
- [0.125394, 0.574318, 0.549086],
- [0.124395, 0.578002, 0.548287],
- [0.123463, 0.581687, 0.547445],
- [0.122606, 0.585371, 0.546557],
- [0.121831, 0.589055, 0.545623],
- [0.121148, 0.592739, 0.544641],
- [0.120565, 0.596422, 0.543611],
- [0.120092, 0.600104, 0.542530],
- [0.119738, 0.603785, 0.541400],
- [0.119512, 0.607464, 0.540218],
- [0.119423, 0.611141, 0.538982],
- [0.119483, 0.614817, 0.537692],
- [0.119699, 0.618490, 0.536347],
- [0.120081, 0.622161, 0.534946],
- [0.120638, 0.625828, 0.533488],
- [0.121380, 0.629492, 0.531973],
- [0.122312, 0.633153, 0.530398],
- [0.123444, 0.636809, 0.528763],
- [0.124780, 0.640461, 0.527068],
- [0.126326, 0.644107, 0.525311],
- [0.128087, 0.647749, 0.523491],
- [0.130067, 0.651384, 0.521608],
- [0.132268, 0.655014, 0.519661],
- [0.134692, 0.658636, 0.517649],
- [0.137339, 0.662252, 0.515571],
- [0.140210, 0.665859, 0.513427],
- [0.143303, 0.669459, 0.511215],
- [0.146616, 0.673050, 0.508936],
- [0.150148, 0.676631, 0.506589],
- [0.153894, 0.680203, 0.504172],
- [0.157851, 0.683765, 0.501686],
- [0.162016, 0.687316, 0.499129],
- [0.166383, 0.690856, 0.496502],
- [0.170948, 0.694384, 0.493803],
- [0.175707, 0.697900, 0.491033],
- [0.180653, 0.701402, 0.488189],
- [0.185783, 0.704891, 0.485273],
- [0.191090, 0.708366, 0.482284],
- [0.196571, 0.711827, 0.479221],
- [0.202219, 0.715272, 0.476084],
- [0.208030, 0.718701, 0.472873],
- [0.214000, 0.722114, 0.469588],
- [0.220124, 0.725509, 0.466226],
- [0.226397, 0.728888, 0.462789],
- [0.232815, 0.732247, 0.459277],
- [0.239374, 0.735588, 0.455688],
- [0.246070, 0.738910, 0.452024],
- [0.252899, 0.742211, 0.448284],
- [0.259857, 0.745492, 0.444467],
- [0.266941, 0.748751, 0.440573],
- [0.274149, 0.751988, 0.436601],
- [0.281477, 0.755203, 0.432552],
- [0.288921, 0.758394, 0.428426],
- [0.296479, 0.761561, 0.424223],
- [0.304148, 0.764704, 0.419943],
- [0.311925, 0.767822, 0.415586],
- [0.319809, 0.770914, 0.411152],
- [0.327796, 0.773980, 0.406640],
- [0.335885, 0.777018, 0.402049],
- [0.344074, 0.780029, 0.397381],
- [0.352360, 0.783011, 0.392636],
- [0.360741, 0.785964, 0.387814],
- [0.369214, 0.788888, 0.382914],
- [0.377779, 0.791781, 0.377939],
- [0.386433, 0.794644, 0.372886],
- [0.395174, 0.797475, 0.367757],
- [0.404001, 0.800275, 0.362552],
- [0.412913, 0.803041, 0.357269],
- [0.421908, 0.805774, 0.351910],
- [0.430983, 0.808473, 0.346476],
- [0.440137, 0.811138, 0.340967],
- [0.449368, 0.813768, 0.335384],
- [0.458674, 0.816363, 0.329727],
- [0.468053, 0.818921, 0.323998],
- [0.477504, 0.821444, 0.318195],
- [0.487026, 0.823929, 0.312321],
- [0.496615, 0.826376, 0.306377],
- [0.506271, 0.828786, 0.300362],
- [0.515992, 0.831158, 0.294279],
- [0.525776, 0.833491, 0.288127],
- [0.535621, 0.835785, 0.281908],
- [0.545524, 0.838039, 0.275626],
- [0.555484, 0.840254, 0.269281],
- [0.565498, 0.842430, 0.262877],
- [0.575563, 0.844566, 0.256415],
- [0.585678, 0.846661, 0.249897],
- [0.595839, 0.848717, 0.243329],
- [0.606045, 0.850733, 0.236712],
- [0.616293, 0.852709, 0.230052],
- [0.626579, 0.854645, 0.223353],
- [0.636902, 0.856542, 0.216620],
- [0.647257, 0.858400, 0.209861],
- [0.657642, 0.860219, 0.203082],
- [0.668054, 0.861999, 0.196293],
- [0.678489, 0.863742, 0.189503],
- [0.688944, 0.865448, 0.182725],
- [0.699415, 0.867117, 0.175971],
- [0.709898, 0.868751, 0.169257],
- [0.720391, 0.870350, 0.162603],
- [0.730889, 0.871916, 0.156029],
- [0.741388, 0.873449, 0.149561],
- [0.751884, 0.874951, 0.143228],
- [0.762373, 0.876424, 0.137064],
- [0.772852, 0.877868, 0.131109],
- [0.783315, 0.879285, 0.125405],
- [0.793760, 0.880678, 0.120005],
- [0.804182, 0.882046, 0.114965],
- [0.814576, 0.883393, 0.110347],
- [0.824940, 0.884720, 0.106217],
- [0.835270, 0.886029, 0.102646],
- [0.845561, 0.887322, 0.099702],
- [0.855810, 0.888601, 0.097452],
- [0.866013, 0.889868, 0.095953],
- [0.876168, 0.891125, 0.095250],
- [0.886271, 0.892374, 0.095374],
- [0.896320, 0.893616, 0.096335],
- [0.906311, 0.894855, 0.098125],
- [0.916242, 0.896091, 0.100717],
- [0.926106, 0.897330, 0.104071],
- [0.935904, 0.898570, 0.108131],
- [0.945636, 0.899815, 0.112838],
- [0.955300, 0.901065, 0.118128],
- [0.964894, 0.902323, 0.123941],
- [0.974417, 0.903590, 0.130215],
- [0.983868, 0.904867, 0.136897],
- [0.993248, 0.906157, 0.143936]]
-
-_cividis_data = [[0.000000, 0.135112, 0.304751],
- [0.000000, 0.138068, 0.311105],
- [0.000000, 0.141013, 0.317579],
- [0.000000, 0.143951, 0.323982],
- [0.000000, 0.146877, 0.330479],
- [0.000000, 0.149791, 0.337065],
- [0.000000, 0.152673, 0.343704],
- [0.000000, 0.155377, 0.350500],
- [0.000000, 0.157932, 0.357521],
- [0.000000, 0.160495, 0.364534],
- [0.000000, 0.163058, 0.371608],
- [0.000000, 0.165621, 0.378769],
- [0.000000, 0.168204, 0.385902],
- [0.000000, 0.170800, 0.393100],
- [0.000000, 0.173420, 0.400353],
- [0.000000, 0.176082, 0.407577],
- [0.000000, 0.178802, 0.414764],
- [0.000000, 0.181610, 0.421859],
- [0.000000, 0.184550, 0.428802],
- [0.000000, 0.186915, 0.435532],
- [0.000000, 0.188769, 0.439563],
- [0.000000, 0.190950, 0.441085],
- [0.000000, 0.193366, 0.441561],
- [0.003602, 0.195911, 0.441564],
- [0.017852, 0.198528, 0.441248],
- [0.032110, 0.201199, 0.440785],
- [0.046205, 0.203903, 0.440196],
- [0.058378, 0.206629, 0.439531],
- [0.068968, 0.209372, 0.438863],
- [0.078624, 0.212122, 0.438105],
- [0.087465, 0.214879, 0.437342],
- [0.095645, 0.217643, 0.436593],
- [0.103401, 0.220406, 0.435790],
- [0.110658, 0.223170, 0.435067],
- [0.117612, 0.225935, 0.434308],
- [0.124291, 0.228697, 0.433547],
- [0.130669, 0.231458, 0.432840],
- [0.136830, 0.234216, 0.432148],
- [0.142852, 0.236972, 0.431404],
- [0.148638, 0.239724, 0.430752],
- [0.154261, 0.242475, 0.430120],
- [0.159733, 0.245221, 0.429528],
- [0.165113, 0.247965, 0.428908],
- [0.170362, 0.250707, 0.428325],
- [0.175490, 0.253444, 0.427790],
- [0.180503, 0.256180, 0.427299],
- [0.185453, 0.258914, 0.426788],
- [0.190303, 0.261644, 0.426329],
- [0.195057, 0.264372, 0.425924],
- [0.199764, 0.267099, 0.425497],
- [0.204385, 0.269823, 0.425126],
- [0.208926, 0.272546, 0.424809],
- [0.213431, 0.275266, 0.424480],
- [0.217863, 0.277985, 0.424206],
- [0.222264, 0.280702, 0.423914],
- [0.226598, 0.283419, 0.423678],
- [0.230871, 0.286134, 0.423498],
- [0.235120, 0.288848, 0.423304],
- [0.239312, 0.291562, 0.423167],
- [0.243485, 0.294274, 0.423014],
- [0.247605, 0.296986, 0.422917],
- [0.251675, 0.299698, 0.422873],
- [0.255731, 0.302409, 0.422814],
- [0.259740, 0.305120, 0.422810],
- [0.263738, 0.307831, 0.422789],
- [0.267693, 0.310542, 0.422821],
- [0.271639, 0.313253, 0.422837],
- [0.275513, 0.315965, 0.422979],
- [0.279411, 0.318677, 0.423031],
- [0.283240, 0.321390, 0.423211],
- [0.287065, 0.324103, 0.423373],
- [0.290884, 0.326816, 0.423517],
- [0.294669, 0.329531, 0.423716],
- [0.298421, 0.332247, 0.423973],
- [0.302169, 0.334963, 0.424213],
- [0.305886, 0.337681, 0.424512],
- [0.309601, 0.340399, 0.424790],
- [0.313287, 0.343120, 0.425120],
- [0.316941, 0.345842, 0.425512],
- [0.320595, 0.348565, 0.425889],
- [0.324250, 0.351289, 0.426250],
- [0.327875, 0.354016, 0.426670],
- [0.331474, 0.356744, 0.427144],
- [0.335073, 0.359474, 0.427605],
- [0.338673, 0.362206, 0.428053],
- [0.342246, 0.364939, 0.428559],
- [0.345793, 0.367676, 0.429127],
- [0.349341, 0.370414, 0.429685],
- [0.352892, 0.373153, 0.430226],
- [0.356418, 0.375896, 0.430823],
- [0.359916, 0.378641, 0.431501],
- [0.363446, 0.381388, 0.432075],
- [0.366923, 0.384139, 0.432796],
- [0.370430, 0.386890, 0.433428],
- [0.373884, 0.389646, 0.434209],
- [0.377371, 0.392404, 0.434890],
- [0.380830, 0.395164, 0.435653],
- [0.384268, 0.397928, 0.436475],
- [0.387705, 0.400694, 0.437305],
- [0.391151, 0.403464, 0.438096],
- [0.394568, 0.406236, 0.438986],
- [0.397991, 0.409011, 0.439848],
- [0.401418, 0.411790, 0.440708],
- [0.404820, 0.414572, 0.441642],
- [0.408226, 0.417357, 0.442570],
- [0.411607, 0.420145, 0.443577],
- [0.414992, 0.422937, 0.444578],
- [0.418383, 0.425733, 0.445560],
- [0.421748, 0.428531, 0.446640],
- [0.425120, 0.431334, 0.447692],
- [0.428462, 0.434140, 0.448864],
- [0.431817, 0.436950, 0.449982],
- [0.435168, 0.439763, 0.451134],
- [0.438504, 0.442580, 0.452341],
- [0.441810, 0.445402, 0.453659],
- [0.445148, 0.448226, 0.454885],
- [0.448447, 0.451053, 0.456264],
- [0.451759, 0.453887, 0.457582],
- [0.455072, 0.456718, 0.458976],
- [0.458366, 0.459552, 0.460457],
- [0.461616, 0.462405, 0.461969],
- [0.464947, 0.465241, 0.463395],
- [0.468254, 0.468083, 0.464908],
- [0.471501, 0.470960, 0.466357],
- [0.474812, 0.473832, 0.467681],
- [0.478186, 0.476699, 0.468845],
- [0.481622, 0.479573, 0.469767],
- [0.485141, 0.482451, 0.470384],
- [0.488697, 0.485318, 0.471008],
- [0.492278, 0.488198, 0.471453],
- [0.495913, 0.491076, 0.471751],
- [0.499552, 0.493960, 0.472032],
- [0.503185, 0.496851, 0.472305],
- [0.506866, 0.499743, 0.472432],
- [0.510540, 0.502643, 0.472550],
- [0.514226, 0.505546, 0.472640],
- [0.517920, 0.508454, 0.472707],
- [0.521643, 0.511367, 0.472639],
- [0.525348, 0.514285, 0.472660],
- [0.529086, 0.517207, 0.472543],
- [0.532829, 0.520135, 0.472401],
- [0.536553, 0.523067, 0.472352],
- [0.540307, 0.526005, 0.472163],
- [0.544069, 0.528948, 0.471947],
- [0.547840, 0.531895, 0.471704],
- [0.551612, 0.534849, 0.471439],
- [0.555393, 0.537807, 0.471147],
- [0.559181, 0.540771, 0.470829],
- [0.562972, 0.543741, 0.470488],
- [0.566802, 0.546715, 0.469988],
- [0.570607, 0.549695, 0.469593],
- [0.574417, 0.552682, 0.469172],
- [0.578236, 0.555673, 0.468724],
- [0.582087, 0.558670, 0.468118],
- [0.585916, 0.561674, 0.467618],
- [0.589753, 0.564682, 0.467090],
- [0.593622, 0.567697, 0.466401],
- [0.597469, 0.570718, 0.465821],
- [0.601354, 0.573743, 0.465074],
- [0.605211, 0.576777, 0.464441],
- [0.609105, 0.579816, 0.463638],
- [0.612977, 0.582861, 0.462950],
- [0.616852, 0.585913, 0.462237],
- [0.620765, 0.588970, 0.461351],
- [0.624654, 0.592034, 0.460583],
- [0.628576, 0.595104, 0.459641],
- [0.632506, 0.598180, 0.458668],
- [0.636412, 0.601264, 0.457818],
- [0.640352, 0.604354, 0.456791],
- [0.644270, 0.607450, 0.455886],
- [0.648222, 0.610553, 0.454801],
- [0.652178, 0.613664, 0.453689],
- [0.656114, 0.616780, 0.452702],
- [0.660082, 0.619904, 0.451534],
- [0.664055, 0.623034, 0.450338],
- [0.668008, 0.626171, 0.449270],
- [0.671991, 0.629316, 0.448018],
- [0.675981, 0.632468, 0.446736],
- [0.679979, 0.635626, 0.445424],
- [0.683950, 0.638793, 0.444251],
- [0.687957, 0.641966, 0.442886],
- [0.691971, 0.645145, 0.441491],
- [0.695985, 0.648334, 0.440072],
- [0.700008, 0.651529, 0.438624],
- [0.704037, 0.654731, 0.437147],
- [0.708067, 0.657942, 0.435647],
- [0.712105, 0.661160, 0.434117],
- [0.716177, 0.664384, 0.432386],
- [0.720222, 0.667618, 0.430805],
- [0.724274, 0.670859, 0.429194],
- [0.728334, 0.674107, 0.427554],
- [0.732422, 0.677364, 0.425717],
- [0.736488, 0.680629, 0.424028],
- [0.740589, 0.683900, 0.422131],
- [0.744664, 0.687181, 0.420393],
- [0.748772, 0.690470, 0.418448],
- [0.752886, 0.693766, 0.416472],
- [0.756975, 0.697071, 0.414659],
- [0.761096, 0.700384, 0.412638],
- [0.765223, 0.703705, 0.410587],
- [0.769353, 0.707035, 0.408516],
- [0.773486, 0.710373, 0.406422],
- [0.777651, 0.713719, 0.404112],
- [0.781795, 0.717074, 0.401966],
- [0.785965, 0.720438, 0.399613],
- [0.790116, 0.723810, 0.397423],
- [0.794298, 0.727190, 0.395016],
- [0.798480, 0.730580, 0.392597],
- [0.802667, 0.733978, 0.390153],
- [0.806859, 0.737385, 0.387684],
- [0.811054, 0.740801, 0.385198],
- [0.815274, 0.744226, 0.382504],
- [0.819499, 0.747659, 0.379785],
- [0.823729, 0.751101, 0.377043],
- [0.827959, 0.754553, 0.374292],
- [0.832192, 0.758014, 0.371529],
- [0.836429, 0.761483, 0.368747],
- [0.840693, 0.764962, 0.365746],
- [0.844957, 0.768450, 0.362741],
- [0.849223, 0.771947, 0.359729],
- [0.853515, 0.775454, 0.356500],
- [0.857809, 0.778969, 0.353259],
- [0.862105, 0.782494, 0.350011],
- [0.866421, 0.786028, 0.346571],
- [0.870717, 0.789572, 0.343333],
- [0.875057, 0.793125, 0.339685],
- [0.879378, 0.796687, 0.336241],
- [0.883720, 0.800258, 0.332599],
- [0.888081, 0.803839, 0.328770],
- [0.892440, 0.807430, 0.324968],
- [0.896818, 0.811030, 0.320982],
- [0.901195, 0.814639, 0.317021],
- [0.905589, 0.818257, 0.312889],
- [0.910000, 0.821885, 0.308594],
- [0.914407, 0.825522, 0.304348],
- [0.918828, 0.829168, 0.299960],
- [0.923279, 0.832822, 0.295244],
- [0.927724, 0.836486, 0.290611],
- [0.932180, 0.840159, 0.285880],
- [0.936660, 0.843841, 0.280876],
- [0.941147, 0.847530, 0.275815],
- [0.945654, 0.851228, 0.270532],
- [0.950178, 0.854933, 0.265085],
- [0.954725, 0.858646, 0.259365],
- [0.959284, 0.862365, 0.253563],
- [0.963872, 0.866089, 0.247445],
- [0.968469, 0.869819, 0.241310],
- [0.973114, 0.873550, 0.234677],
- [0.977780, 0.877281, 0.227954],
- [0.982497, 0.881008, 0.220878],
- [0.987293, 0.884718, 0.213336],
- [0.992218, 0.888385, 0.205468],
- [0.994847, 0.892954, 0.203445],
- [0.995249, 0.898384, 0.207561],
- [0.995503, 0.903866, 0.212370],
- [0.995737, 0.909344, 0.217772]]
-
-_twilight_data = [
- [0.88575015840754434, 0.85000924943067835, 0.8879736506427196],
- [0.88378520195539056, 0.85072940540310626, 0.88723222096949894],
- [0.88172231059285788, 0.85127594077653468, 0.88638056925514819],
- [0.8795410528270573, 0.85165675407495722, 0.8854143767924102],
- [0.87724880858965482, 0.85187028338870274, 0.88434120381311432],
- [0.87485347508575972, 0.85191526123023187, 0.88316926967613829],
- [0.87233134085124076, 0.85180165478080894, 0.88189704355001619],
- [0.86970474853509816, 0.85152403004797894, 0.88053883390003362],
- [0.86696015505333579, 0.8510896085314068, 0.87909766977173343],
- [0.86408985081463996, 0.85050391167507788, 0.87757925784892632],
- [0.86110245436899846, 0.84976754857001258, 0.87599242923439569],
- [0.85798259245670372, 0.84888934810281835, 0.87434038553446281],
- [0.85472593189256985, 0.84787488124672816, 0.8726282980930582],
- [0.85133714570857189, 0.84672735796116472, 0.87086081657350445],
- [0.84780710702577922, 0.8454546229209523, 0.86904036783694438],
- [0.8441261828674842, 0.84406482711037389, 0.86716973322690072],
- [0.84030420805957784, 0.8425605950855084, 0.865250882410458],
- [0.83634031809191178, 0.84094796518951942, 0.86328528001070159],
- [0.83222705712934408, 0.83923490627754482, 0.86127563500427884],
- [0.82796894316013536, 0.83742600751395202, 0.85922399451306786],
- [0.82357429680252847, 0.83552487764795436, 0.85713191328514948],
- [0.81904654677937527, 0.8335364929949034, 0.85500206287010105],
- [0.81438982121143089, 0.83146558694197847, 0.85283759062147024],
- [0.8095999819094809, 0.82931896673505456, 0.85064441601050367],
- [0.80469164429814577, 0.82709838780560663, 0.84842449296974021],
- [0.79967075421267997, 0.82480781812080928, 0.84618210029578533],
- [0.79454305089231114, 0.82245116226304615, 0.84392184786827984],
- [0.78931445564608915, 0.82003213188702007, 0.8416486380471222],
- [0.78399101042764918, 0.81755426400533426, 0.83936747464036732],
- [0.77857892008227592, 0.81502089378742548, 0.8370834463093898],
- [0.77308416590170936, 0.81243524735466011, 0.83480172950579679],
- [0.76751108504417864, 0.8098007598713145, 0.83252816638059668],
- [0.76186907937980286, 0.80711949387647486, 0.830266486168872],
- [0.75616443584381976, 0.80439408733477935, 0.82802138994719998],
- [0.75040346765406696, 0.80162699008965321, 0.82579737851082424],
- [0.74459247771890169, 0.79882047719583249, 0.82359867586156521],
- [0.73873771700494939, 0.79597665735031009, 0.82142922780433014],
- [0.73284543645523459, 0.79309746468844067, 0.81929263384230377],
- [0.72692177512829703, 0.7901846863592763, 0.81719217466726379],
- [0.72097280665536778, 0.78723995923452639, 0.81513073920879264],
- [0.71500403076252128, 0.78426487091581187, 0.81311116559949914],
- [0.70902078134539304, 0.78126088716070907, 0.81113591855117928],
- [0.7030297722540817, 0.77822904973358131, 0.80920618848056969],
- [0.6970365443886174, 0.77517050008066057, 0.80732335380063447],
- [0.69104641009309098, 0.77208629460678091, 0.80548841690679074],
- [0.68506446154395928, 0.7689774029354699, 0.80370206267176914],
- [0.67909554499882152, 0.76584472131395898, 0.8019646617300199],
- [0.67314422559426212, 0.76268908733890484, 0.80027628545809526],
- [0.66721479803752815, 0.7595112803730375, 0.79863674654537764],
- [0.6613112930078745, 0.75631202708719025, 0.7970456043491897],
- [0.65543692326454717, 0.75309208756768431, 0.79550271129031047],
- [0.64959573004253479, 0.74985201221941766, 0.79400674021499107],
- [0.6437910831099849, 0.7465923800833657, 0.79255653201306053],
- [0.63802586828545982, 0.74331376714033193, 0.79115100459573173],
- [0.6323027138710603, 0.74001672160131404, 0.78978892762640429],
- [0.62662402022604591, 0.73670175403699445, 0.78846901316334561],
- [0.62099193064817548, 0.73336934798923203, 0.78718994624696581],
- [0.61540846411770478, 0.73001995232739691, 0.78595022706750484],
- [0.60987543176093062, 0.72665398759758293, 0.78474835732694714],
- [0.60439434200274855, 0.7232718614323369, 0.78358295593535587],
- [0.5989665814482068, 0.71987394892246725, 0.78245259899346642],
- [0.59359335696837223, 0.7164606049658685, 0.78135588237640097],
- [0.58827579780555495, 0.71303214646458135, 0.78029141405636515],
- [0.58301487036932409, 0.70958887676997473, 0.77925781820476592],
- [0.5778116438998202, 0.70613106157153982, 0.77825345121025524],
- [0.5726668948158774, 0.7026589535425779, 0.77727702680911992],
- [0.56758117853861967, 0.69917279302646274, 0.77632748534275298],
- [0.56255515357219343, 0.69567278381629649, 0.77540359142309845],
- [0.55758940419605174, 0.69215911458254054, 0.7745041337932782],
- [0.55268450589347129, 0.68863194515166382, 0.7736279426902245],
- [0.54784098153018634, 0.68509142218509878, 0.77277386473440868],
- [0.54305932424018233, 0.68153767253065878, 0.77194079697835083],
- [0.53834015575176275, 0.67797081129095405, 0.77112734439057717],
- [0.53368389147728401, 0.67439093705212727, 0.7703325054879735],
- [0.529090861832473, 0.67079812302806219, 0.76955552292313134],
- [0.52456151470593582, 0.66719242996142225, 0.76879541714230948],
- [0.52009627392235558, 0.66357391434030388, 0.76805119403344102],
- [0.5156955988596057, 0.65994260812897998, 0.76732191489596169],
- [0.51135992541601927, 0.65629853981831865, 0.76660663780645333],
- [0.50708969576451657, 0.65264172403146448, 0.76590445660835849],
- [0.5028853540415561, 0.64897216734095264, 0.76521446718174913],
- [0.49874733661356069, 0.6452898684900934, 0.76453578734180083],
- [0.4946761847863938, 0.64159484119504429, 0.76386719002130909],
- [0.49067224938561221, 0.63788704858847078, 0.76320812763163837],
- [0.4867359599430568, 0.63416646251100506, 0.76255780085924041],
- [0.4828677867260272, 0.6304330455306234, 0.76191537149895305],
- [0.47906816236197386, 0.62668676251860134, 0.76128000375662419],
- [0.47533752394906287, 0.62292757283835809, 0.76065085571817748],
- [0.47167629518877091, 0.61915543242884641, 0.76002709227883047],
- [0.46808490970531597, 0.61537028695790286, 0.75940789891092741],
- [0.46456376716303932, 0.61157208822864151, 0.75879242623025811],
- [0.46111326647023881, 0.607760777169989, 0.75817986436807139],
- [0.45773377230160567, 0.60393630046586455, 0.75756936901859162],
- [0.45442563977552913, 0.60009859503858665, 0.75696013660606487],
- [0.45118918687617743, 0.59624762051353541, 0.75635120643246645],
- [0.44802470933589172, 0.59238331452146575, 0.75574176474107924],
- [0.44493246854215379, 0.5885055998308617, 0.7551311041857901],
- [0.44191271766696399, 0.58461441100175571, 0.75451838884410671],
- [0.43896563958048396, 0.58070969241098491, 0.75390276208285945],
- [0.43609138958356369, 0.57679137998186081, 0.7532834105961016],
- [0.43329008867358393, 0.57285941625606673, 0.75265946532566674],
- [0.43056179073057571, 0.56891374572457176, 0.75203008099312696],
- [0.42790652284925834, 0.5649543060909209, 0.75139443521914839],
- [0.42532423665011354, 0.56098104959950301, 0.75075164989005116],
- [0.42281485675772662, 0.55699392126996583, 0.75010086988227642],
- [0.42037822361396326, 0.55299287158108168, 0.7494412559451894],
- [0.41801414079233629, 0.54897785421888889, 0.74877193167001121],
- [0.4157223260454232, 0.54494882715350401, 0.74809204459000522],
- [0.41350245743314729, 0.54090574771098476, 0.74740073297543086],
- [0.41135414697304568, 0.53684857765005933, 0.74669712855065784],
- [0.4092768899914751, 0.53277730177130322, 0.74598030635707824],
- [0.40727018694219069, 0.52869188011057411, 0.74524942637581271],
- [0.40533343789303178, 0.52459228174983119, 0.74450365836708132],
- [0.40346600333905397, 0.52047847653840029, 0.74374215223567086],
- [0.40166714010896104, 0.51635044969688759, 0.7429640345324835],
- [0.39993606933454834, 0.51220818143218516, 0.74216844571317986],
- [0.3982719152586337, 0.50805166539276136, 0.74135450918099721],
- [0.39667374905665609, 0.50388089053847973, 0.74052138580516735],
- [0.39514058808207631, 0.49969585326377758, 0.73966820211715711],
- [0.39367135736822567, 0.49549655777451179, 0.738794102296364],
- [0.39226494876209317, 0.49128300332899261, 0.73789824784475078],
- [0.39092017571994903, 0.48705520251223039, 0.73697977133881254],
- [0.38963580160340855, 0.48281316715123496, 0.73603782546932739],
- [0.38841053300842432, 0.47855691131792805, 0.73507157641157261],
- [0.38724301459330251, 0.47428645933635388, 0.73408016787854391],
- [0.38613184178892102, 0.4700018340988123, 0.7330627749243106],
- [0.38507556793651387, 0.46570306719930193, 0.73201854033690505],
- [0.38407269378943537, 0.46139018782416635, 0.73094665432902683],
- [0.38312168084402748, 0.45706323581407199, 0.72984626791353258],
- [0.38222094988570376, 0.45272225034283325, 0.72871656144003782],
- [0.38136887930454161, 0.44836727669277859, 0.72755671317141346],
- [0.38056380696565623, 0.44399837208633719, 0.72636587045135315],
- [0.37980403744848751, 0.43961558821222629, 0.72514323778761092],
- [0.37908789283110761, 0.43521897612544935, 0.72388798691323131],
- [0.378413635091359, 0.43080859411413064, 0.72259931993061044],
- [0.37777949753513729, 0.4263845142616835, 0.72127639993530235],
- [0.37718371844251231, 0.42194680223454828, 0.71991841524475775],
- [0.37662448930806297, 0.41749553747893614, 0.71852454736176108],
- [0.37610001286385814, 0.41303079952477062, 0.71709396919920232],
- [0.37560846919442398, 0.40855267638072096, 0.71562585091587549],
- [0.37514802505380473, 0.4040612609993941, 0.7141193695725726],
- [0.37471686019302231, 0.3995566498711684, 0.71257368516500463],
- [0.37431313199312338, 0.39503894828283309, 0.71098796522377461],
- [0.37393499330475782, 0.39050827529375831, 0.70936134293478448],
- [0.3735806215098284, 0.38596474386057539, 0.70769297607310577],
- [0.37324816143326384, 0.38140848555753937, 0.70598200974806036],
- [0.37293578646665032, 0.37683963835219841, 0.70422755780589941],
- [0.37264166757849604, 0.37225835004836849, 0.7024287314570723],
- [0.37236397858465387, 0.36766477862108266, 0.70058463496520773],
- [0.37210089702443822, 0.36305909736982378, 0.69869434615073722],
- [0.3718506155898596, 0.35844148285875221, 0.69675695810256544],
- [0.37161133234400479, 0.3538121372967869, 0.69477149919380887],
- [0.37138124223736607, 0.34917126878479027, 0.69273703471928827],
- [0.37115856636209105, 0.34451911410230168, 0.69065253586464992],
- [0.37094151551337329, 0.33985591488818123, 0.68851703379505125],
- [0.37072833279422668, 0.33518193808489577, 0.68632948169606767],
- [0.37051738634484427, 0.33049741244307851, 0.68408888788857214],
- [0.37030682071842685, 0.32580269697872455, 0.68179411684486679],
- [0.37009487130772695, 0.3210981375964933, 0.67944405399056851],
- [0.36987980329025361, 0.31638410101153364, 0.67703755438090574],
- [0.36965987626565955, 0.31166098762951971, 0.67457344743419545],
- [0.36943334591276228, 0.30692923551862339, 0.67205052849120617],
- [0.36919847837592484, 0.30218932176507068, 0.66946754331614522],
- [0.36895355306596778, 0.29744175492366276, 0.66682322089824264],
- [0.36869682231895268, 0.29268709856150099, 0.66411625298236909],
- [0.36842655638020444, 0.28792596437778462, 0.66134526910944602],
- [0.36814101479899719, 0.28315901221182987, 0.65850888806972308],
- [0.36783843696531082, 0.27838697181297761, 0.65560566838453704],
- [0.36751707094367697, 0.27361063317090978, 0.65263411711618635],
- [0.36717513650699446, 0.26883085667326956, 0.64959272297892245],
- [0.36681085540107988, 0.26404857724525643, 0.64647991652908243],
- [0.36642243251550632, 0.25926481158628106, 0.64329409140765537],
- [0.36600853966739794, 0.25448043878086224, 0.64003361803368586],
- [0.36556698373538982, 0.24969683475296395, 0.63669675187488584],
- [0.36509579845886808, 0.24491536803550484, 0.63328173520055586],
- [0.36459308890125008, 0.24013747024823828, 0.62978680155026101],
- [0.36405693022088509, 0.23536470386204195, 0.62621013451953023],
- [0.36348537610385145, 0.23059876218396419, 0.62254988622392882],
- [0.36287643560041027, 0.22584149293287031, 0.61880417410823019],
- [0.36222809558295926, 0.22109488427338303, 0.61497112346096128],
- [0.36153829010998356, 0.21636111429594002, 0.61104880679640927],
- [0.36080493826624654, 0.21164251793458128, 0.60703532172064711],
- [0.36002681809096376, 0.20694122817889948, 0.60292845431916875],
- [0.35920088560930186, 0.20226037920758122, 0.5987265295935138],
- [0.35832489966617809, 0.197602942459778, 0.59442768517501066],
- [0.35739663292915563, 0.19297208197842461, 0.59003011251063131],
- [0.35641381143126327, 0.18837119869242164, 0.5855320765920552],
- [0.35537415306906722, 0.18380392577704466, 0.58093191431832802],
- [0.35427534960663759, 0.17927413271618647, 0.57622809660668717],
- [0.35311574421123737, 0.17478570377561287, 0.57141871523555288],
- [0.35189248608873791, 0.17034320478524959, 0.56650284911216653],
- [0.35060304441931012, 0.16595129984720861, 0.56147964703993225],
- [0.34924513554955644, 0.16161477763045118, 0.55634837474163779],
- [0.34781653238777782, 0.15733863511152979, 0.55110853452703257],
- [0.34631507175793091, 0.15312802296627787, 0.5457599924248665],
- [0.34473901574536375, 0.14898820589826409, 0.54030245920406539],
- [0.34308600291572294, 0.14492465359918028, 0.53473704282067103],
- [0.34135411074506483, 0.1409427920655632, 0.52906500940336754],
- [0.33954168752669694, 0.13704801896718169, 0.52328797535085236],
- [0.33764732090671112, 0.13324562282438077, 0.51740807573979475],
- [0.33566978565015315, 0.12954074251271822, 0.51142807215168951],
- [0.33360804901486002, 0.12593818301005921, 0.50535164796654897],
- [0.33146154891145124, 0.12244245263391232, 0.49918274588431072],
- [0.32923005203231409, 0.11905764321981127, 0.49292595612342666],
- [0.3269137124539796, 0.1157873496841953, 0.48658646495697461],
- [0.32451307931207785, 0.11263459791730848, 0.48017007211645196],
- [0.32202882276069322, 0.10960114111258401, 0.47368494725726878],
- [0.31946262395497965, 0.10668879882392659, 0.46713728801395243],
- [0.31681648089023501, 0.10389861387653518, 0.46053414662739794],
- [0.31409278414755532, 0.10123077676403242, 0.45388335612058467],
- [0.31129434479712365, 0.098684771934052201, 0.44719313715161618],
- [0.30842444457210105, 0.096259385340577736, 0.44047194882050544],
- [0.30548675819945936, 0.093952764840823738, 0.43372849999361113],
- [0.30248536364574252, 0.091761187397303601, 0.42697404043749887],
- [0.29942483960214772, 0.089682253716750038, 0.42021619665853854],
- [0.29631000388905288, 0.087713250960463951, 0.41346259134143476],
- [0.29314593096985248, 0.085850656889620708, 0.40672178082365834],
- [0.28993792445176608, 0.08409078829085731, 0.40000214725256295],
- [0.28669151388283165, 0.082429873848480689, 0.39331182532243375],
- [0.28341239797185225, 0.080864153365499375, 0.38665868550105914],
- [0.28010638576975472, 0.079389994802261526, 0.38005028528138707],
- [0.27677939615815589, 0.078003941033788216, 0.37349382846504675],
- [0.27343739342450812, 0.076702800237496066, 0.36699616136347685],
- [0.27008637749114051, 0.075483675584275545, 0.36056376228111864],
- [0.26673233211995284, 0.074344018028546205, 0.35420276066240958],
- [0.26338121807151404, 0.073281657939897077, 0.34791888996380105],
- [0.26003895187439957, 0.072294781043362205, 0.3417175669546984],
- [0.25671191651083902, 0.071380106242082242, 0.33560648984600089],
- [0.25340685873736807, 0.070533582926851829, 0.3295945757321303],
- [0.25012845306199383, 0.069758206429106989, 0.32368100685760637],
- [0.24688226237958999, 0.069053639449204451, 0.31786993834254956],
- [0.24367372557466271, 0.068419855150922693, 0.31216524050888372],
- [0.24050813332295939, 0.067857103814855602, 0.30657054493678321],
- [0.23739062429054825, 0.067365888050555517, 0.30108922184065873],
- [0.23433055727563878, 0.066935599661639394, 0.29574009929867601],
- [0.23132955273021344, 0.066576186939090592, 0.29051361067988485],
- [0.2283917709422868, 0.06628997924139618, 0.28541074411068496],
- [0.22552164337737857, 0.066078173119395595, 0.28043398847505197],
- [0.22272706739121817, 0.065933790675651943, 0.27559714652053702],
- [0.22001251100779617, 0.065857918918907604, 0.27090279994325861],
- [0.21737845072382705, 0.065859661233562045, 0.26634209349669508],
- [0.21482843531473683, 0.065940385613778491, 0.26191675992376573],
- [0.21237411048541005, 0.066085024661758446, 0.25765165093569542],
- [0.21001214221188125, 0.066308573918947178, 0.2535289048041211],
- [0.2077442377448806, 0.06661453200418091, 0.24954644291943817],
- [0.20558051999470117, 0.066990462397868739, 0.24572497420147632],
- [0.20352007949514977, 0.067444179612424215, 0.24205576625191821],
- [0.20156133764129841, 0.067983271026200248, 0.23852974228695395],
- [0.19971571438603364, 0.068592710553704722, 0.23517094067076993],
- [0.19794834061899208, 0.069314066071660657, 0.23194647381302336],
- [0.1960826032659409, 0.070321227242423623, 0.22874673279569585],
- [0.19410351363791453, 0.071608304856891569, 0.22558727307410353],
- [0.19199449184606268, 0.073182830649273306, 0.22243385243433622],
- [0.18975853639094634, 0.075019861862143766, 0.2193005075652994],
- [0.18739228342697645, 0.077102096899588329, 0.21618875376309582],
- [0.18488035509396164, 0.079425730279723883, 0.21307651648984993],
- [0.18774482037046955, 0.077251588468039312, 0.21387448578597812],
- [0.19049578401722037, 0.075311278416787641, 0.2146562337112265],
- [0.1931548636579131, 0.073606819040117955, 0.21542362939081539],
- [0.19571853588267552, 0.072157781039602742, 0.21617499187076789],
- [0.19819343656336558, 0.070974625252738788, 0.21690975060032436],
- [0.20058760685133747, 0.070064576149984209, 0.21762721310371608],
- [0.20290365333558247, 0.069435248580458964, 0.21833167885096033],
- [0.20531725273301316, 0.068919592266397572, 0.21911516689288835],
- [0.20785704662965598, 0.068484398797025281, 0.22000133917653536],
- [0.21052882914958676, 0.06812195249816172, 0.22098759107715404],
- [0.2133313859647627, 0.067830148426026665, 0.22207043213024291],
- [0.21625279838647882, 0.067616330270516389, 0.22324568672294431],
- [0.21930503925136402, 0.067465786362940039, 0.22451023616807558],
- [0.22247308588973624, 0.067388214053092838, 0.22585960379408354],
- [0.2257539681670791, 0.067382132300147474, 0.22728984778098055],
- [0.22915620278592841, 0.067434730871152565, 0.22879681433956656],
- [0.23266299920501882, 0.067557104388479783, 0.23037617493752832],
- [0.23627495835774248, 0.06774359820987802, 0.23202360805926608],
- [0.23999586188690308, 0.067985029964779953, 0.23373434258507808],
- [0.24381149720247919, 0.068289851529011875, 0.23550427698321885],
- [0.24772092990501099, 0.068653337909486523, 0.2373288009471749],
- [0.25172899728289466, 0.069064630826035506, 0.23920260612763083],
- [0.25582135547481771, 0.06953231029187984, 0.24112190491594204],
- [0.25999463887892144, 0.070053855603861875, 0.24308218808684579],
- [0.26425512207060942, 0.070616595622995437, 0.24507758869355967],
- [0.26859095948172862, 0.071226716277922458, 0.24710443563450618],
- [0.27299701518897301, 0.071883555446163511, 0.24915847093232929],
- [0.27747150809142801, 0.072582969899254779, 0.25123493995942769],
- [0.28201746297366942, 0.073315693214040967, 0.25332800295084507],
- [0.28662309235899847, 0.074088460826808866, 0.25543478673717029],
- [0.29128515387578635, 0.074899049847466703, 0.25755101595750435],
- [0.2960004726065818, 0.075745336000958424, 0.25967245030364566],
- [0.30077276812918691, 0.076617824336164764, 0.26179294097819672],
- [0.30559226007249934, 0.077521963107537312, 0.26391006692119662],
- [0.31045520848595526, 0.078456871676182177, 0.2660200572779356],
- [0.31535870009205808, 0.079420997315243186, 0.26811904076941961],
- [0.32029986557994061, 0.080412994737554838, 0.27020322893039511],
- [0.32527888860401261, 0.081428390076546092, 0.27226772884656186],
- [0.33029174471181438, 0.08246763389003825, 0.27430929404579435],
- [0.33533353224455448, 0.083532434119003962, 0.27632534356790039],
- [0.34040164359597463, 0.084622236191702671, 0.27831254595259397],
- [0.34549355713871799, 0.085736654965126335, 0.28026769921081435],
- [0.35060678246032478, 0.08687555176033529, 0.28218770540182386],
- [0.35573889947341125, 0.088038974350243354, 0.2840695897279818],
- [0.36088752387578377, 0.089227194362745205, 0.28591050458531014],
- [0.36605031412464006, 0.090440685427697898, 0.2877077458811747],
- [0.37122508431309342, 0.091679997480262732, 0.28945865397633169],
- [0.3764103053221462, 0.092945198093777909, 0.29116024157313919],
- [0.38160247377467543, 0.094238731263712183, 0.29281107506269488],
- [0.38679939079544168, 0.09556181960083443, 0.29440901248173756],
- [0.39199887556812907, 0.09691583650296684, 0.29595212005509081],
- [0.39719876876325577, 0.098302320968278623, 0.29743856476285779],
- [0.40239692379737496, 0.099722930314950553, 0.29886674369733968],
- [0.40759120392688708, 0.10117945586419633, 0.30023519507728602],
- [0.41277985630360303, 0.1026734006932461, 0.30154226437468967],
- [0.41796105205173684, 0.10420644885760968, 0.30278652039631843],
- [0.42313214269556043, 0.10578120994917611, 0.3039675809469457],
- [0.42829101315789753, 0.1073997763055258, 0.30508479060294547],
- [0.4334355841041439, 0.1090642347484701, 0.30613767928289148],
- [0.43856378187931538, 0.11077667828375456, 0.30712600062348083],
- [0.44367358645071275, 0.11253912421257944, 0.30804973095465449],
- [0.44876299173174822, 0.11435355574622549, 0.30890905921943196],
- [0.45383005086999889, 0.11622183788331528, 0.30970441249844921],
- [0.45887288947308297, 0.11814571137706886, 0.31043636979038808],
- [0.46389102840284874, 0.12012561256850712, 0.31110343446582983],
- [0.46888111384598413, 0.12216445576414045, 0.31170911458932665],
- [0.473841437035254, 0.12426354237989065, 0.31225470169927194],
- [0.47877034239726296, 0.12642401401409453, 0.31274172735821959],
- [0.48366628618847957, 0.12864679022013889, 0.31317188565991266],
- [0.48852847371852987, 0.13093210934893723, 0.31354553695453014],
- [0.49335504375145617, 0.13328091630401023, 0.31386561956734976],
- [0.49814435462074153, 0.13569380302451714, 0.314135190862664],
- [0.50289524974970612, 0.13817086581280427, 0.31435662153833671],
- [0.50760681181053691, 0.14071192654913128, 0.31453200120082569],
- [0.51227835105321762, 0.14331656120063752, 0.3146630922831542],
- [0.51690848800544464, 0.14598463068714407, 0.31475407592280041],
- [0.52149652863229956, 0.14871544765633712, 0.31480767954534428],
- [0.52604189625477482, 0.15150818660835483, 0.31482653406646727],
- [0.53054420489856446, 0.15436183633886777, 0.31481299789187128],
- [0.5350027976174474, 0.15727540775107324, 0.31477085207396532],
- [0.53941736649199057, 0.16024769309971934, 0.31470295028655965],
- [0.54378771313608565, 0.16327738551419116, 0.31461204226295625],
- [0.54811370033467621, 0.1663630904279047, 0.31450102990914708],
- [0.55239521572711914, 0.16950338809328983, 0.31437291554615371],
- [0.55663229034969341, 0.17269677158182117, 0.31423043195101424],
- [0.56082499039117173, 0.17594170887918095, 0.31407639883970623],
- [0.56497343529017696, 0.17923664950367169, 0.3139136046337036],
- [0.56907784784011428, 0.18258004462335425, 0.31374440956796529],
- [0.57313845754107873, 0.18597036007065024, 0.31357126868520002],
- [0.57715550812992045, 0.18940601489760422, 0.31339704333572083],
- [0.58112932761586555, 0.19288548904692518, 0.31322399394183942],
- [0.58506024396466882, 0.19640737049066315, 0.31305401163732732],
- [0.58894861935544707, 0.19997020971775276, 0.31288922211590126],
- [0.59279480536520257, 0.20357251410079796, 0.31273234839304942],
- [0.59659918109122367, 0.207212956082026, 0.31258523031121233],
- [0.60036213010411577, 0.21089030138947745, 0.31244934410414688],
- [0.60408401696732739, 0.21460331490206347, 0.31232652641170694],
- [0.60776523994818654, 0.21835070166659282, 0.31221903291870201],
- [0.6114062072731884, 0.22213124697023234, 0.31212881396435238],
- [0.61500723236391375, 0.22594402043981826, 0.31205680685765741],
- [0.61856865258877192, 0.22978799249179921, 0.31200463838728931],
- [0.62209079821082613, 0.2336621873300741, 0.31197383273627388],
- [0.62557416500434959, 0.23756535071152696, 0.31196698314912269],
- [0.62901892016985872, 0.24149689191922535, 0.31198447195645718],
- [0.63242534854210275, 0.24545598775548677, 0.31202765974624452],
- [0.6357937104834237, 0.24944185818822678, 0.31209793953300591],
- [0.6391243387840212, 0.25345365461983138, 0.31219689612063978],
- [0.642417577481186, 0.257490519876798, 0.31232631707560987],
- [0.64567349382645434, 0.26155203161615281, 0.31248673753935263],
- [0.64889230169458245, 0.26563755336209077, 0.31267941819570189],
- [0.65207417290277303, 0.26974650525236699, 0.31290560605819168],
- [0.65521932609327127, 0.27387826652410152, 0.3131666792687211],
- [0.6583280801134499, 0.27803210957665631, 0.3134643447952643],
- [0.66140037532601781, 0.28220778870555907, 0.31379912926498488],
- [0.66443632469878844, 0.28640483614256179, 0.31417223403606975],
- [0.66743603766369131, 0.29062280081258873, 0.31458483752056837],
- [0.67039959547676198, 0.29486126309253047, 0.31503813956872212],
- [0.67332725564817331, 0.29911962764489264, 0.31553372323982209],
- [0.67621897924409746, 0.30339762792450425, 0.3160724937230589],
- [0.67907474028157344, 0.30769497879760166, 0.31665545668946665],
- [0.68189457150944521, 0.31201133280550686, 0.31728380489244951],
- [0.68467850942494535, 0.31634634821222207, 0.31795870784057567],
- [0.68742656435169625, 0.32069970535138104, 0.31868137622277692],
- [0.6901389321505248, 0.32507091815606004, 0.31945332332898302],
- [0.69281544846764931, 0.32945984647042675, 0.3202754315314667],
- [0.69545608346891119, 0.33386622163232865, 0.32114884306985791],
- [0.6980608153581771, 0.33828976326048621, 0.32207478855218091],
- [0.70062962477242097, 0.34273019305341756, 0.32305449047765694],
- [0.70316249458814151, 0.34718723719597999, 0.32408913679491225],
- [0.70565951122610093, 0.35166052978120937, 0.32518014084085567],
- [0.70812059568420482, 0.35614985523380299, 0.32632861885644465],
- [0.7105456546582587, 0.36065500290840113, 0.32753574162788762],
- [0.71293466839773467, 0.36517570519856757, 0.3288027427038317],
- [0.71528760614847287, 0.36971170225223449, 0.3301308728723546],
- [0.71760444908133847, 0.37426272710686193, 0.33152138620958932],
- [0.71988521490549851, 0.37882848839337313, 0.33297555200245399],
- [0.7221299918421461, 0.38340864508963057, 0.33449469983585844],
- [0.72433865647781592, 0.38800301593162145, 0.33607995965691828],
- [0.72651122900227549, 0.3926113126792577, 0.3377325942005665],
- [0.72864773856716547, 0.39723324476747235, 0.33945384341064017],
- [0.73074820754845171, 0.401868526884681, 0.3412449533046818],
- [0.73281270506268747, 0.4065168468778026, 0.34310715173410822],
- [0.73484133598564938, 0.41117787004519513, 0.34504169470809071],
- [0.73683422173585866, 0.41585125850290111, 0.34704978520758401],
- [0.73879140024599266, 0.42053672992315327, 0.34913260148542435],
- [0.74071301619506091, 0.4252339389526239, 0.35129130890802607],
- [0.7425992159973317, 0.42994254036133867, 0.35352709245374592],
- [0.74445018676570673, 0.43466217184617112, 0.35584108091122535],
- [0.74626615789163442, 0.43939245044973502, 0.35823439142300639],
- [0.74804739275559562, 0.44413297780351974, 0.36070813602540136],
- [0.74979420547170472, 0.44888333481548809, 0.36326337558360278],
- [0.75150685045891663, 0.45364314496866825, 0.36590112443835765],
- [0.75318566369046569, 0.45841199172949604, 0.36862236642234769],
- [0.75483105066959544, 0.46318942799460555, 0.3714280448394211],
- [0.75644341577140706, 0.46797501437948458, 0.37431909037543515],
- [0.75802325538455839, 0.4727682731566229, 0.37729635531096678],
- [0.75957111105340058, 0.47756871222057079, 0.380360657784311],
- [0.7610876378057071, 0.48237579130289127, 0.38351275723852291],
- [0.76257333554052609, 0.48718906673415824, 0.38675335037837993],
- [0.76402885609288662, 0.49200802533379656, 0.39008308392311997],
- [0.76545492593330511, 0.49683212909727231, 0.39350254000115381],
- [0.76685228950643891, 0.5016608471009063, 0.39701221751773474],
- [0.76822176599735303, 0.50649362371287909, 0.40061257089416885],
- [0.7695642334401418, 0.5113298901696085, 0.40430398069682483],
- [0.77088091962302474, 0.51616892643469103, 0.40808667584648967],
- [0.77217257229605551, 0.5210102658711383, 0.41196089987122869],
- [0.77344021829889886, 0.52585332093451564, 0.41592679539764366],
- [0.77468494746063199, 0.53069749384776732, 0.41998440356963762],
- [0.77590790730685699, 0.53554217882461186, 0.42413367909988375],
- [0.7771103295521099, 0.54038674910561235, 0.42837450371258479],
- [0.77829345807633121, 0.54523059488426595, 0.432706647838971],
- [0.77945862731506643, 0.55007308413977274, 0.43712979856444761],
- [0.78060774749483774, 0.55491335744890613, 0.44164332426364639],
- [0.78174180478981836, 0.55975098052594863, 0.44624687186865436],
- [0.78286225264440912, 0.56458533111166875, 0.45093985823706345],
- [0.78397060836414478, 0.56941578326710418, 0.45572154742892063],
- [0.78506845019606841, 0.5742417003617839, 0.46059116206904965],
- [0.78615737132332963, 0.5790624629815756, 0.46554778281918402],
- [0.78723904108188347, 0.58387743744557208, 0.47059039582133383],
- [0.78831514045623963, 0.58868600173562435, 0.47571791879076081],
- [0.78938737766251943, 0.5934875421745599, 0.48092913815357724],
- [0.79045776847727878, 0.59828134277062461, 0.48622257801969754],
- [0.79152832843475607, 0.60306670593147205, 0.49159667021646397],
- [0.79260034304237448, 0.60784322087037024, 0.49705020621532009],
- [0.79367559698664958, 0.61261029334072192, 0.50258161291269432],
- [0.79475585972654039, 0.61736734400220705, 0.50818921213102985],
- [0.79584292379583765, 0.62211378808451145, 0.51387124091909786],
- [0.79693854719951607, 0.62684905679296699, 0.5196258425240281],
- [0.79804447815136637, 0.63157258225089552, 0.52545108144834785],
- [0.7991624518501963, 0.63628379372029187, 0.53134495942561433],
- [0.80029415389753977, 0.64098213306749863, 0.53730535185141037],
- [0.80144124292560048, 0.64566703459218766, 0.5433300863249918],
- [0.80260531146112946, 0.65033793748103852, 0.54941691584603647],
- [0.80378792531077625, 0.65499426549472628, 0.55556350867083815],
- [0.80499054790810298, 0.65963545027564163, 0.56176745110546977],
- [0.80621460526927058, 0.66426089585282289, 0.56802629178649788],
- [0.8074614045096935, 0.6688700095398864, 0.57433746373459582],
- [0.80873219170089694, 0.67346216702194517, 0.58069834805576737],
- [0.81002809466520687, 0.67803672673971815, 0.58710626908082753],
- [0.81135014011763329, 0.68259301546243389, 0.59355848909050757],
- [0.81269922039881493, 0.68713033714618876, 0.60005214820435104],
- [0.81407611046993344, 0.69164794791482131, 0.6065843782630862],
- [0.81548146627279483, 0.69614505508308089, 0.61315221209322646],
- [0.81691575775055891, 0.70062083014783982, 0.61975260637257923],
- [0.81837931164498223, 0.70507438189635097, 0.62638245478933297],
- [0.81987230650455289, 0.70950474978787481, 0.63303857040067113],
- [0.8213947205565636, 0.7139109141951604, 0.63971766697672761],
- [0.82294635110428427, 0.71829177331290062, 0.6464164243818421],
- [0.8245268129450285, 0.72264614312088882, 0.65313137915422603],
- [0.82613549710580259, 0.72697275518238258, 0.65985900156216504],
- [0.8277716072353446, 0.73127023324078089, 0.66659570204682972],
- [0.82943407816481474, 0.7355371221572935, 0.67333772009301907],
- [0.83112163529096306, 0.73977184647638616, 0.68008125203631464],
- [0.83283277185777982, 0.74397271817459876, 0.68682235874648545],
- [0.8345656905566583, 0.7481379479992134, 0.69355697649863846],
- [0.83631898844737929, 0.75226548952875261, 0.70027999028864962],
- [0.83809123476131964, 0.75635314860808633, 0.70698561390212977],
- [0.83987839884120874, 0.76039907199779677, 0.71367147811129228],
- [0.84167750766845151, 0.76440101200982946, 0.72033299387284622],
- [0.84348529222933699, 0.76835660399870176, 0.72696536998972039],
- [0.84529810731955113, 0.77226338601044719, 0.73356368240541492],
- [0.84711195507965098, 0.77611880236047159, 0.74012275762807056],
- [0.84892245563117641, 0.77992021407650147, 0.74663719293664366],
- [0.85072697023178789, 0.78366457342383888, 0.7530974636118285],
- [0.85251907207708444, 0.78734936133548439, 0.7594994148789691],
- [0.85429219611470464, 0.79097196777091994, 0.76583801477914104],
- [0.85604022314725403, 0.79452963601550608, 0.77210610037674143],
- [0.85775662943504905, 0.79801963142713928, 0.77829571667247499],
- [0.8594346370300241, 0.8014392309950078, 0.78439788751383921],
- [0.86107117027565516, 0.80478517909812231, 0.79039529663736285],
- [0.86265601051127572, 0.80805523804261525, 0.796282666437655],
- [0.86418343723941027, 0.81124644224653542, 0.80204612696863953],
- [0.86564934325605325, 0.81435544067514909, 0.80766972324164554],
- [0.86705314907048503, 0.81737804041911244, 0.81313419626911398],
- [0.86839954695818633, 0.82030875512181523, 0.81841638963128993],
- [0.86969131502613806, 0.82314158859569164, 0.82350476683173168],
- [0.87093846717297507, 0.82586857889438514, 0.82838497261149613],
- [0.87215331978454325, 0.82848052823709672, 0.8330486712880828],
- [0.87335171360916275, 0.83096715251272624, 0.83748851001197089],
- [0.87453793320260187, 0.83331972948645461, 0.84171925358069011],
- [0.87571458709961403, 0.8355302318472394, 0.84575537519027078],
- [0.87687848451614692, 0.83759238071186537, 0.84961373549150254],
- [0.87802298436649007, 0.83950165618540074, 0.85330645352458923],
- [0.87913244240792765, 0.84125554884475906, 0.85685572291039636],
- [0.88019293315695812, 0.84285224824778615, 0.86027399927156634],
- [0.88119169871341951, 0.84429066717717349, 0.86356595168669881],
- [0.88211542489401606, 0.84557007254559347, 0.86673765046233331],
- [0.88295168595448525, 0.84668970275699273, 0.86979617048190971],
- [0.88369127145898041, 0.84764891761519268, 0.87274147101441557],
- [0.88432713054113543, 0.84844741572055415, 0.87556785228242973],
- [0.88485138159908572, 0.84908426422893801, 0.87828235285372469],
- [0.88525897972630474, 0.84955892810989209, 0.88088414794024839],
- [0.88554714811952384, 0.84987174283631584, 0.88336206121170946],
- [0.88571155122845646, 0.85002186115856315, 0.88572538990087124]]
-
-_twilight_shifted_data = (_twilight_data[len(_twilight_data)//2:] +
- _twilight_data[:len(_twilight_data)//2])
-_twilight_shifted_data.reverse()
-_turbo_data = [[0.18995, 0.07176, 0.23217],
- [0.19483, 0.08339, 0.26149],
- [0.19956, 0.09498, 0.29024],
- [0.20415, 0.10652, 0.31844],
- [0.20860, 0.11802, 0.34607],
- [0.21291, 0.12947, 0.37314],
- [0.21708, 0.14087, 0.39964],
- [0.22111, 0.15223, 0.42558],
- [0.22500, 0.16354, 0.45096],
- [0.22875, 0.17481, 0.47578],
- [0.23236, 0.18603, 0.50004],
- [0.23582, 0.19720, 0.52373],
- [0.23915, 0.20833, 0.54686],
- [0.24234, 0.21941, 0.56942],
- [0.24539, 0.23044, 0.59142],
- [0.24830, 0.24143, 0.61286],
- [0.25107, 0.25237, 0.63374],
- [0.25369, 0.26327, 0.65406],
- [0.25618, 0.27412, 0.67381],
- [0.25853, 0.28492, 0.69300],
- [0.26074, 0.29568, 0.71162],
- [0.26280, 0.30639, 0.72968],
- [0.26473, 0.31706, 0.74718],
- [0.26652, 0.32768, 0.76412],
- [0.26816, 0.33825, 0.78050],
- [0.26967, 0.34878, 0.79631],
- [0.27103, 0.35926, 0.81156],
- [0.27226, 0.36970, 0.82624],
- [0.27334, 0.38008, 0.84037],
- [0.27429, 0.39043, 0.85393],
- [0.27509, 0.40072, 0.86692],
- [0.27576, 0.41097, 0.87936],
- [0.27628, 0.42118, 0.89123],
- [0.27667, 0.43134, 0.90254],
- [0.27691, 0.44145, 0.91328],
- [0.27701, 0.45152, 0.92347],
- [0.27698, 0.46153, 0.93309],
- [0.27680, 0.47151, 0.94214],
- [0.27648, 0.48144, 0.95064],
- [0.27603, 0.49132, 0.95857],
- [0.27543, 0.50115, 0.96594],
- [0.27469, 0.51094, 0.97275],
- [0.27381, 0.52069, 0.97899],
- [0.27273, 0.53040, 0.98461],
- [0.27106, 0.54015, 0.98930],
- [0.26878, 0.54995, 0.99303],
- [0.26592, 0.55979, 0.99583],
- [0.26252, 0.56967, 0.99773],
- [0.25862, 0.57958, 0.99876],
- [0.25425, 0.58950, 0.99896],
- [0.24946, 0.59943, 0.99835],
- [0.24427, 0.60937, 0.99697],
- [0.23874, 0.61931, 0.99485],
- [0.23288, 0.62923, 0.99202],
- [0.22676, 0.63913, 0.98851],
- [0.22039, 0.64901, 0.98436],
- [0.21382, 0.65886, 0.97959],
- [0.20708, 0.66866, 0.97423],
- [0.20021, 0.67842, 0.96833],
- [0.19326, 0.68812, 0.96190],
- [0.18625, 0.69775, 0.95498],
- [0.17923, 0.70732, 0.94761],
- [0.17223, 0.71680, 0.93981],
- [0.16529, 0.72620, 0.93161],
- [0.15844, 0.73551, 0.92305],
- [0.15173, 0.74472, 0.91416],
- [0.14519, 0.75381, 0.90496],
- [0.13886, 0.76279, 0.89550],
- [0.13278, 0.77165, 0.88580],
- [0.12698, 0.78037, 0.87590],
- [0.12151, 0.78896, 0.86581],
- [0.11639, 0.79740, 0.85559],
- [0.11167, 0.80569, 0.84525],
- [0.10738, 0.81381, 0.83484],
- [0.10357, 0.82177, 0.82437],
- [0.10026, 0.82955, 0.81389],
- [0.09750, 0.83714, 0.80342],
- [0.09532, 0.84455, 0.79299],
- [0.09377, 0.85175, 0.78264],
- [0.09287, 0.85875, 0.77240],
- [0.09267, 0.86554, 0.76230],
- [0.09320, 0.87211, 0.75237],
- [0.09451, 0.87844, 0.74265],
- [0.09662, 0.88454, 0.73316],
- [0.09958, 0.89040, 0.72393],
- [0.10342, 0.89600, 0.71500],
- [0.10815, 0.90142, 0.70599],
- [0.11374, 0.90673, 0.69651],
- [0.12014, 0.91193, 0.68660],
- [0.12733, 0.91701, 0.67627],
- [0.13526, 0.92197, 0.66556],
- [0.14391, 0.92680, 0.65448],
- [0.15323, 0.93151, 0.64308],
- [0.16319, 0.93609, 0.63137],
- [0.17377, 0.94053, 0.61938],
- [0.18491, 0.94484, 0.60713],
- [0.19659, 0.94901, 0.59466],
- [0.20877, 0.95304, 0.58199],
- [0.22142, 0.95692, 0.56914],
- [0.23449, 0.96065, 0.55614],
- [0.24797, 0.96423, 0.54303],
- [0.26180, 0.96765, 0.52981],
- [0.27597, 0.97092, 0.51653],
- [0.29042, 0.97403, 0.50321],
- [0.30513, 0.97697, 0.48987],
- [0.32006, 0.97974, 0.47654],
- [0.33517, 0.98234, 0.46325],
- [0.35043, 0.98477, 0.45002],
- [0.36581, 0.98702, 0.43688],
- [0.38127, 0.98909, 0.42386],
- [0.39678, 0.99098, 0.41098],
- [0.41229, 0.99268, 0.39826],
- [0.42778, 0.99419, 0.38575],
- [0.44321, 0.99551, 0.37345],
- [0.45854, 0.99663, 0.36140],
- [0.47375, 0.99755, 0.34963],
- [0.48879, 0.99828, 0.33816],
- [0.50362, 0.99879, 0.32701],
- [0.51822, 0.99910, 0.31622],
- [0.53255, 0.99919, 0.30581],
- [0.54658, 0.99907, 0.29581],
- [0.56026, 0.99873, 0.28623],
- [0.57357, 0.99817, 0.27712],
- [0.58646, 0.99739, 0.26849],
- [0.59891, 0.99638, 0.26038],
- [0.61088, 0.99514, 0.25280],
- [0.62233, 0.99366, 0.24579],
- [0.63323, 0.99195, 0.23937],
- [0.64362, 0.98999, 0.23356],
- [0.65394, 0.98775, 0.22835],
- [0.66428, 0.98524, 0.22370],
- [0.67462, 0.98246, 0.21960],
- [0.68494, 0.97941, 0.21602],
- [0.69525, 0.97610, 0.21294],
- [0.70553, 0.97255, 0.21032],
- [0.71577, 0.96875, 0.20815],
- [0.72596, 0.96470, 0.20640],
- [0.73610, 0.96043, 0.20504],
- [0.74617, 0.95593, 0.20406],
- [0.75617, 0.95121, 0.20343],
- [0.76608, 0.94627, 0.20311],
- [0.77591, 0.94113, 0.20310],
- [0.78563, 0.93579, 0.20336],
- [0.79524, 0.93025, 0.20386],
- [0.80473, 0.92452, 0.20459],
- [0.81410, 0.91861, 0.20552],
- [0.82333, 0.91253, 0.20663],
- [0.83241, 0.90627, 0.20788],
- [0.84133, 0.89986, 0.20926],
- [0.85010, 0.89328, 0.21074],
- [0.85868, 0.88655, 0.21230],
- [0.86709, 0.87968, 0.21391],
- [0.87530, 0.87267, 0.21555],
- [0.88331, 0.86553, 0.21719],
- [0.89112, 0.85826, 0.21880],
- [0.89870, 0.85087, 0.22038],
- [0.90605, 0.84337, 0.22188],
- [0.91317, 0.83576, 0.22328],
- [0.92004, 0.82806, 0.22456],
- [0.92666, 0.82025, 0.22570],
- [0.93301, 0.81236, 0.22667],
- [0.93909, 0.80439, 0.22744],
- [0.94489, 0.79634, 0.22800],
- [0.95039, 0.78823, 0.22831],
- [0.95560, 0.78005, 0.22836],
- [0.96049, 0.77181, 0.22811],
- [0.96507, 0.76352, 0.22754],
- [0.96931, 0.75519, 0.22663],
- [0.97323, 0.74682, 0.22536],
- [0.97679, 0.73842, 0.22369],
- [0.98000, 0.73000, 0.22161],
- [0.98289, 0.72140, 0.21918],
- [0.98549, 0.71250, 0.21650],
- [0.98781, 0.70330, 0.21358],
- [0.98986, 0.69382, 0.21043],
- [0.99163, 0.68408, 0.20706],
- [0.99314, 0.67408, 0.20348],
- [0.99438, 0.66386, 0.19971],
- [0.99535, 0.65341, 0.19577],
- [0.99607, 0.64277, 0.19165],
- [0.99654, 0.63193, 0.18738],
- [0.99675, 0.62093, 0.18297],
- [0.99672, 0.60977, 0.17842],
- [0.99644, 0.59846, 0.17376],
- [0.99593, 0.58703, 0.16899],
- [0.99517, 0.57549, 0.16412],
- [0.99419, 0.56386, 0.15918],
- [0.99297, 0.55214, 0.15417],
- [0.99153, 0.54036, 0.14910],
- [0.98987, 0.52854, 0.14398],
- [0.98799, 0.51667, 0.13883],
- [0.98590, 0.50479, 0.13367],
- [0.98360, 0.49291, 0.12849],
- [0.98108, 0.48104, 0.12332],
- [0.97837, 0.46920, 0.11817],
- [0.97545, 0.45740, 0.11305],
- [0.97234, 0.44565, 0.10797],
- [0.96904, 0.43399, 0.10294],
- [0.96555, 0.42241, 0.09798],
- [0.96187, 0.41093, 0.09310],
- [0.95801, 0.39958, 0.08831],
- [0.95398, 0.38836, 0.08362],
- [0.94977, 0.37729, 0.07905],
- [0.94538, 0.36638, 0.07461],
- [0.94084, 0.35566, 0.07031],
- [0.93612, 0.34513, 0.06616],
- [0.93125, 0.33482, 0.06218],
- [0.92623, 0.32473, 0.05837],
- [0.92105, 0.31489, 0.05475],
- [0.91572, 0.30530, 0.05134],
- [0.91024, 0.29599, 0.04814],
- [0.90463, 0.28696, 0.04516],
- [0.89888, 0.27824, 0.04243],
- [0.89298, 0.26981, 0.03993],
- [0.88691, 0.26152, 0.03753],
- [0.88066, 0.25334, 0.03521],
- [0.87422, 0.24526, 0.03297],
- [0.86760, 0.23730, 0.03082],
- [0.86079, 0.22945, 0.02875],
- [0.85380, 0.22170, 0.02677],
- [0.84662, 0.21407, 0.02487],
- [0.83926, 0.20654, 0.02305],
- [0.83172, 0.19912, 0.02131],
- [0.82399, 0.19182, 0.01966],
- [0.81608, 0.18462, 0.01809],
- [0.80799, 0.17753, 0.01660],
- [0.79971, 0.17055, 0.01520],
- [0.79125, 0.16368, 0.01387],
- [0.78260, 0.15693, 0.01264],
- [0.77377, 0.15028, 0.01148],
- [0.76476, 0.14374, 0.01041],
- [0.75556, 0.13731, 0.00942],
- [0.74617, 0.13098, 0.00851],
- [0.73661, 0.12477, 0.00769],
- [0.72686, 0.11867, 0.00695],
- [0.71692, 0.11268, 0.00629],
- [0.70680, 0.10680, 0.00571],
- [0.69650, 0.10102, 0.00522],
- [0.68602, 0.09536, 0.00481],
- [0.67535, 0.08980, 0.00449],
- [0.66449, 0.08436, 0.00424],
- [0.65345, 0.07902, 0.00408],
- [0.64223, 0.07380, 0.00401],
- [0.63082, 0.06868, 0.00401],
- [0.61923, 0.06367, 0.00410],
- [0.60746, 0.05878, 0.00427],
- [0.59550, 0.05399, 0.00453],
- [0.58336, 0.04931, 0.00486],
- [0.57103, 0.04474, 0.00529],
- [0.55852, 0.04028, 0.00579],
- [0.54583, 0.03593, 0.00638],
- [0.53295, 0.03169, 0.00705],
- [0.51989, 0.02756, 0.00780],
- [0.50664, 0.02354, 0.00863],
- [0.49321, 0.01963, 0.00955],
- [0.47960, 0.01583, 0.01055]]
-
-
-cmaps = {
- name: ListedColormap(data, name=name) for name, data in [
- ('magma', _magma_data),
- ('inferno', _inferno_data),
- ('plasma', _plasma_data),
- ('viridis', _viridis_data),
- ('cividis', _cividis_data),
- ('twilight', _twilight_data),
- ('twilight_shifted', _twilight_shifted_data),
- ('turbo', _turbo_data),
- ]}
diff --git a/spaces/dcq/freegpt-webui/g4f/Provider/Providers/Gravityengine.py b/spaces/dcq/freegpt-webui/g4f/Provider/Providers/Gravityengine.py
deleted file mode 100644
index f0cd09daaaae0adaa349f91139dc60c7ac79c028..0000000000000000000000000000000000000000
--- a/spaces/dcq/freegpt-webui/g4f/Provider/Providers/Gravityengine.py
+++ /dev/null
@@ -1,27 +0,0 @@
-import requests
-import os
-import json
-from ...typing import sha256, Dict, get_type_hints
-
-url = 'https://gpt4.xunika.uk/'
-model = ['gpt-3.5-turbo-16k', 'gpt-3.5-turbo-0613']
-supports_stream = True
-needs_auth = False
-
-def _create_completion(model: str, messages: list, stream: bool, temperature: float = 0.7, **kwargs):
- headers = {
- 'Content-Type': 'application/json',
- }
- data = {
- 'model': model,
- 'temperature': 0.7,
- 'presence_penalty': 0,
- 'messages': messages,
- }
- response = requests.post(url + '/api/openai/v1/chat/completions',
- json=data, stream=True)
-
- yield response.json()['choices'][0]['message']['content']
-
-params = f'g4f.Providers.{os.path.basename(__file__)[:-3]} supports: ' + \
- '(%s)' % ', '.join([f"{name}: {get_type_hints(_create_completion)[name].__name__}" for name in _create_completion.__code__.co_varnames[:_create_completion.__code__.co_argcount]])
\ No newline at end of file
diff --git a/spaces/declare-lab/tango/diffusers/src/diffusers/pipelines/stable_diffusion/pipeline_stable_diffusion.py b/spaces/declare-lab/tango/diffusers/src/diffusers/pipelines/stable_diffusion/pipeline_stable_diffusion.py
deleted file mode 100644
index 73b9178e3ab1f9da9c74e3bc97355dbb63ae02b3..0000000000000000000000000000000000000000
--- a/spaces/declare-lab/tango/diffusers/src/diffusers/pipelines/stable_diffusion/pipeline_stable_diffusion.py
+++ /dev/null
@@ -1,723 +0,0 @@
-# Copyright 2023 The HuggingFace Team. All rights reserved.
-#
-# Licensed under the Apache License, Version 2.0 (the "License");
-# you may not use this file except in compliance with the License.
-# You may obtain a copy of the License at
-#
-# http://www.apache.org/licenses/LICENSE-2.0
-#
-# Unless required by applicable law or agreed to in writing, software
-# distributed under the License is distributed on an "AS IS" BASIS,
-# WITHOUT WARRANTIES OR CONDITIONS OF ANY KIND, either express or implied.
-# See the License for the specific language governing permissions and
-# limitations under the License.
-
-import inspect
-from typing import Any, Callable, Dict, List, Optional, Union
-
-import torch
-from packaging import version
-from transformers import CLIPImageProcessor, CLIPTextModel, CLIPTokenizer
-
-from ...configuration_utils import FrozenDict
-from ...loaders import TextualInversionLoaderMixin
-from ...models import AutoencoderKL, UNet2DConditionModel
-from ...schedulers import KarrasDiffusionSchedulers
-from ...utils import (
- deprecate,
- is_accelerate_available,
- is_accelerate_version,
- logging,
- randn_tensor,
- replace_example_docstring,
-)
-from ..pipeline_utils import DiffusionPipeline
-from . import StableDiffusionPipelineOutput
-from .safety_checker import StableDiffusionSafetyChecker
-
-
-logger = logging.get_logger(__name__) # pylint: disable=invalid-name
-
-EXAMPLE_DOC_STRING = """
- Examples:
- ```py
- >>> import torch
- >>> from diffusers import StableDiffusionPipeline
-
- >>> pipe = StableDiffusionPipeline.from_pretrained("runwayml/stable-diffusion-v1-5", torch_dtype=torch.float16)
- >>> pipe = pipe.to("cuda")
-
- >>> prompt = "a photo of an astronaut riding a horse on mars"
- >>> image = pipe(prompt).images[0]
- ```
-"""
-
-
-class StableDiffusionPipeline(DiffusionPipeline, TextualInversionLoaderMixin):
- r"""
- Pipeline for text-to-image generation using Stable Diffusion.
-
- This model inherits from [`DiffusionPipeline`]. Check the superclass documentation for the generic methods the
- library implements for all the pipelines (such as downloading or saving, running on a particular device, etc.)
-
- Args:
- vae ([`AutoencoderKL`]):
- Variational Auto-Encoder (VAE) Model to encode and decode images to and from latent representations.
- text_encoder ([`CLIPTextModel`]):
- Frozen text-encoder. Stable Diffusion uses the text portion of
- [CLIP](https://huggingface.co/docs/transformers/model_doc/clip#transformers.CLIPTextModel), specifically
- the [clip-vit-large-patch14](https://huggingface.co/openai/clip-vit-large-patch14) variant.
- tokenizer (`CLIPTokenizer`):
- Tokenizer of class
- [CLIPTokenizer](https://huggingface.co/docs/transformers/v4.21.0/en/model_doc/clip#transformers.CLIPTokenizer).
- unet ([`UNet2DConditionModel`]): Conditional U-Net architecture to denoise the encoded image latents.
- scheduler ([`SchedulerMixin`]):
- A scheduler to be used in combination with `unet` to denoise the encoded image latents. Can be one of
- [`DDIMScheduler`], [`LMSDiscreteScheduler`], or [`PNDMScheduler`].
- safety_checker ([`StableDiffusionSafetyChecker`]):
- Classification module that estimates whether generated images could be considered offensive or harmful.
- Please, refer to the [model card](https://huggingface.co/runwayml/stable-diffusion-v1-5) for details.
- feature_extractor ([`CLIPImageProcessor`]):
- Model that extracts features from generated images to be used as inputs for the `safety_checker`.
- """
- _optional_components = ["safety_checker", "feature_extractor"]
-
- def __init__(
- self,
- vae: AutoencoderKL,
- text_encoder: CLIPTextModel,
- tokenizer: CLIPTokenizer,
- unet: UNet2DConditionModel,
- scheduler: KarrasDiffusionSchedulers,
- safety_checker: StableDiffusionSafetyChecker,
- feature_extractor: CLIPImageProcessor,
- requires_safety_checker: bool = True,
- ):
- super().__init__()
-
- if hasattr(scheduler.config, "steps_offset") and scheduler.config.steps_offset != 1:
- deprecation_message = (
- f"The configuration file of this scheduler: {scheduler} is outdated. `steps_offset`"
- f" should be set to 1 instead of {scheduler.config.steps_offset}. Please make sure "
- "to update the config accordingly as leaving `steps_offset` might led to incorrect results"
- " in future versions. If you have downloaded this checkpoint from the Hugging Face Hub,"
- " it would be very nice if you could open a Pull request for the `scheduler/scheduler_config.json`"
- " file"
- )
- deprecate("steps_offset!=1", "1.0.0", deprecation_message, standard_warn=False)
- new_config = dict(scheduler.config)
- new_config["steps_offset"] = 1
- scheduler._internal_dict = FrozenDict(new_config)
-
- if hasattr(scheduler.config, "clip_sample") and scheduler.config.clip_sample is True:
- deprecation_message = (
- f"The configuration file of this scheduler: {scheduler} has not set the configuration `clip_sample`."
- " `clip_sample` should be set to False in the configuration file. Please make sure to update the"
- " config accordingly as not setting `clip_sample` in the config might lead to incorrect results in"
- " future versions. If you have downloaded this checkpoint from the Hugging Face Hub, it would be very"
- " nice if you could open a Pull request for the `scheduler/scheduler_config.json` file"
- )
- deprecate("clip_sample not set", "1.0.0", deprecation_message, standard_warn=False)
- new_config = dict(scheduler.config)
- new_config["clip_sample"] = False
- scheduler._internal_dict = FrozenDict(new_config)
-
- if safety_checker is None and requires_safety_checker:
- logger.warning(
- f"You have disabled the safety checker for {self.__class__} by passing `safety_checker=None`. Ensure"
- " that you abide to the conditions of the Stable Diffusion license and do not expose unfiltered"
- " results in services or applications open to the public. Both the diffusers team and Hugging Face"
- " strongly recommend to keep the safety filter enabled in all public facing circumstances, disabling"
- " it only for use-cases that involve analyzing network behavior or auditing its results. For more"
- " information, please have a look at https://github.com/huggingface/diffusers/pull/254 ."
- )
-
- if safety_checker is not None and feature_extractor is None:
- raise ValueError(
- "Make sure to define a feature extractor when loading {self.__class__} if you want to use the safety"
- " checker. If you do not want to use the safety checker, you can pass `'safety_checker=None'` instead."
- )
-
- is_unet_version_less_0_9_0 = hasattr(unet.config, "_diffusers_version") and version.parse(
- version.parse(unet.config._diffusers_version).base_version
- ) < version.parse("0.9.0.dev0")
- is_unet_sample_size_less_64 = hasattr(unet.config, "sample_size") and unet.config.sample_size < 64
- if is_unet_version_less_0_9_0 and is_unet_sample_size_less_64:
- deprecation_message = (
- "The configuration file of the unet has set the default `sample_size` to smaller than"
- " 64 which seems highly unlikely. If your checkpoint is a fine-tuned version of any of the"
- " following: \n- CompVis/stable-diffusion-v1-4 \n- CompVis/stable-diffusion-v1-3 \n-"
- " CompVis/stable-diffusion-v1-2 \n- CompVis/stable-diffusion-v1-1 \n- runwayml/stable-diffusion-v1-5"
- " \n- runwayml/stable-diffusion-inpainting \n you should change 'sample_size' to 64 in the"
- " configuration file. Please make sure to update the config accordingly as leaving `sample_size=32`"
- " in the config might lead to incorrect results in future versions. If you have downloaded this"
- " checkpoint from the Hugging Face Hub, it would be very nice if you could open a Pull request for"
- " the `unet/config.json` file"
- )
- deprecate("sample_size<64", "1.0.0", deprecation_message, standard_warn=False)
- new_config = dict(unet.config)
- new_config["sample_size"] = 64
- unet._internal_dict = FrozenDict(new_config)
-
- self.register_modules(
- vae=vae,
- text_encoder=text_encoder,
- tokenizer=tokenizer,
- unet=unet,
- scheduler=scheduler,
- safety_checker=safety_checker,
- feature_extractor=feature_extractor,
- )
- self.vae_scale_factor = 2 ** (len(self.vae.config.block_out_channels) - 1)
- self.register_to_config(requires_safety_checker=requires_safety_checker)
-
- def enable_vae_slicing(self):
- r"""
- Enable sliced VAE decoding.
-
- When this option is enabled, the VAE will split the input tensor in slices to compute decoding in several
- steps. This is useful to save some memory and allow larger batch sizes.
- """
- self.vae.enable_slicing()
-
- def disable_vae_slicing(self):
- r"""
- Disable sliced VAE decoding. If `enable_vae_slicing` was previously invoked, this method will go back to
- computing decoding in one step.
- """
- self.vae.disable_slicing()
-
- def enable_vae_tiling(self):
- r"""
- Enable tiled VAE decoding.
-
- When this option is enabled, the VAE will split the input tensor into tiles to compute decoding and encoding in
- several steps. This is useful to save a large amount of memory and to allow the processing of larger images.
- """
- self.vae.enable_tiling()
-
- def disable_vae_tiling(self):
- r"""
- Disable tiled VAE decoding. If `enable_vae_tiling` was previously invoked, this method will go back to
- computing decoding in one step.
- """
- self.vae.disable_tiling()
-
- def enable_sequential_cpu_offload(self, gpu_id=0):
- r"""
- Offloads all models to CPU using accelerate, significantly reducing memory usage. When called, unet,
- text_encoder, vae and safety checker have their state dicts saved to CPU and then are moved to a
- `torch.device('meta') and loaded to GPU only when their specific submodule has its `forward` method called.
- Note that offloading happens on a submodule basis. Memory savings are higher than with
- `enable_model_cpu_offload`, but performance is lower.
- """
- if is_accelerate_available() and is_accelerate_version(">=", "0.14.0"):
- from accelerate import cpu_offload
- else:
- raise ImportError("`enable_sequential_cpu_offload` requires `accelerate v0.14.0` or higher")
-
- device = torch.device(f"cuda:{gpu_id}")
-
- if self.device.type != "cpu":
- self.to("cpu", silence_dtype_warnings=True)
- torch.cuda.empty_cache() # otherwise we don't see the memory savings (but they probably exist)
-
- for cpu_offloaded_model in [self.unet, self.text_encoder, self.vae]:
- cpu_offload(cpu_offloaded_model, device)
-
- if self.safety_checker is not None:
- cpu_offload(self.safety_checker, execution_device=device, offload_buffers=True)
-
- def enable_model_cpu_offload(self, gpu_id=0):
- r"""
- Offloads all models to CPU using accelerate, reducing memory usage with a low impact on performance. Compared
- to `enable_sequential_cpu_offload`, this method moves one whole model at a time to the GPU when its `forward`
- method is called, and the model remains in GPU until the next model runs. Memory savings are lower than with
- `enable_sequential_cpu_offload`, but performance is much better due to the iterative execution of the `unet`.
- """
- if is_accelerate_available() and is_accelerate_version(">=", "0.17.0.dev0"):
- from accelerate import cpu_offload_with_hook
- else:
- raise ImportError("`enable_model_cpu_offload` requires `accelerate v0.17.0` or higher.")
-
- device = torch.device(f"cuda:{gpu_id}")
-
- if self.device.type != "cpu":
- self.to("cpu", silence_dtype_warnings=True)
- torch.cuda.empty_cache() # otherwise we don't see the memory savings (but they probably exist)
-
- hook = None
- for cpu_offloaded_model in [self.text_encoder, self.unet, self.vae]:
- _, hook = cpu_offload_with_hook(cpu_offloaded_model, device, prev_module_hook=hook)
-
- if self.safety_checker is not None:
- _, hook = cpu_offload_with_hook(self.safety_checker, device, prev_module_hook=hook)
-
- # We'll offload the last model manually.
- self.final_offload_hook = hook
-
- @property
- def _execution_device(self):
- r"""
- Returns the device on which the pipeline's models will be executed. After calling
- `pipeline.enable_sequential_cpu_offload()` the execution device can only be inferred from Accelerate's module
- hooks.
- """
- if not hasattr(self.unet, "_hf_hook"):
- return self.device
- for module in self.unet.modules():
- if (
- hasattr(module, "_hf_hook")
- and hasattr(module._hf_hook, "execution_device")
- and module._hf_hook.execution_device is not None
- ):
- return torch.device(module._hf_hook.execution_device)
- return self.device
-
- def _encode_prompt(
- self,
- prompt,
- device,
- num_images_per_prompt,
- do_classifier_free_guidance,
- negative_prompt=None,
- prompt_embeds: Optional[torch.FloatTensor] = None,
- negative_prompt_embeds: Optional[torch.FloatTensor] = None,
- ):
- r"""
- Encodes the prompt into text encoder hidden states.
-
- Args:
- prompt (`str` or `List[str]`, *optional*):
- prompt to be encoded
- device: (`torch.device`):
- torch device
- num_images_per_prompt (`int`):
- number of images that should be generated per prompt
- do_classifier_free_guidance (`bool`):
- whether to use classifier free guidance or not
- negative_prompt (`str` or `List[str]`, *optional*):
- The prompt or prompts not to guide the image generation. If not defined, one has to pass
- `negative_prompt_embeds` instead. Ignored when not using guidance (i.e., ignored if `guidance_scale` is
- less than `1`).
- prompt_embeds (`torch.FloatTensor`, *optional*):
- Pre-generated text embeddings. Can be used to easily tweak text inputs, *e.g.* prompt weighting. If not
- provided, text embeddings will be generated from `prompt` input argument.
- negative_prompt_embeds (`torch.FloatTensor`, *optional*):
- Pre-generated negative text embeddings. Can be used to easily tweak text inputs, *e.g.* prompt
- weighting. If not provided, negative_prompt_embeds will be generated from `negative_prompt` input
- argument.
- """
- if prompt is not None and isinstance(prompt, str):
- batch_size = 1
- elif prompt is not None and isinstance(prompt, list):
- batch_size = len(prompt)
- else:
- batch_size = prompt_embeds.shape[0]
-
- if prompt_embeds is None:
- # textual inversion: procecss multi-vector tokens if necessary
- if isinstance(self, TextualInversionLoaderMixin):
- prompt = self.maybe_convert_prompt(prompt, self.tokenizer)
-
- text_inputs = self.tokenizer(
- prompt,
- padding="max_length",
- max_length=self.tokenizer.model_max_length,
- truncation=True,
- return_tensors="pt",
- )
- text_input_ids = text_inputs.input_ids
- untruncated_ids = self.tokenizer(prompt, padding="longest", return_tensors="pt").input_ids
-
- if untruncated_ids.shape[-1] >= text_input_ids.shape[-1] and not torch.equal(
- text_input_ids, untruncated_ids
- ):
- removed_text = self.tokenizer.batch_decode(
- untruncated_ids[:, self.tokenizer.model_max_length - 1 : -1]
- )
- logger.warning(
- "The following part of your input was truncated because CLIP can only handle sequences up to"
- f" {self.tokenizer.model_max_length} tokens: {removed_text}"
- )
-
- if hasattr(self.text_encoder.config, "use_attention_mask") and self.text_encoder.config.use_attention_mask:
- attention_mask = text_inputs.attention_mask.to(device)
- else:
- attention_mask = None
-
- prompt_embeds = self.text_encoder(
- text_input_ids.to(device),
- attention_mask=attention_mask,
- )
- prompt_embeds = prompt_embeds[0]
-
- prompt_embeds = prompt_embeds.to(dtype=self.text_encoder.dtype, device=device)
-
- bs_embed, seq_len, _ = prompt_embeds.shape
- # duplicate text embeddings for each generation per prompt, using mps friendly method
- prompt_embeds = prompt_embeds.repeat(1, num_images_per_prompt, 1)
- prompt_embeds = prompt_embeds.view(bs_embed * num_images_per_prompt, seq_len, -1)
-
- # get unconditional embeddings for classifier free guidance
- if do_classifier_free_guidance and negative_prompt_embeds is None:
- uncond_tokens: List[str]
- if negative_prompt is None:
- uncond_tokens = [""] * batch_size
- elif type(prompt) is not type(negative_prompt):
- raise TypeError(
- f"`negative_prompt` should be the same type to `prompt`, but got {type(negative_prompt)} !="
- f" {type(prompt)}."
- )
- elif isinstance(negative_prompt, str):
- uncond_tokens = [negative_prompt]
- elif batch_size != len(negative_prompt):
- raise ValueError(
- f"`negative_prompt`: {negative_prompt} has batch size {len(negative_prompt)}, but `prompt`:"
- f" {prompt} has batch size {batch_size}. Please make sure that passed `negative_prompt` matches"
- " the batch size of `prompt`."
- )
- else:
- uncond_tokens = negative_prompt
-
- # textual inversion: procecss multi-vector tokens if necessary
- if isinstance(self, TextualInversionLoaderMixin):
- uncond_tokens = self.maybe_convert_prompt(uncond_tokens, self.tokenizer)
-
- max_length = prompt_embeds.shape[1]
- uncond_input = self.tokenizer(
- uncond_tokens,
- padding="max_length",
- max_length=max_length,
- truncation=True,
- return_tensors="pt",
- )
-
- if hasattr(self.text_encoder.config, "use_attention_mask") and self.text_encoder.config.use_attention_mask:
- attention_mask = uncond_input.attention_mask.to(device)
- else:
- attention_mask = None
-
- negative_prompt_embeds = self.text_encoder(
- uncond_input.input_ids.to(device),
- attention_mask=attention_mask,
- )
- negative_prompt_embeds = negative_prompt_embeds[0]
-
- if do_classifier_free_guidance:
- # duplicate unconditional embeddings for each generation per prompt, using mps friendly method
- seq_len = negative_prompt_embeds.shape[1]
-
- negative_prompt_embeds = negative_prompt_embeds.to(dtype=self.text_encoder.dtype, device=device)
-
- negative_prompt_embeds = negative_prompt_embeds.repeat(1, num_images_per_prompt, 1)
- negative_prompt_embeds = negative_prompt_embeds.view(batch_size * num_images_per_prompt, seq_len, -1)
-
- # For classifier free guidance, we need to do two forward passes.
- # Here we concatenate the unconditional and text embeddings into a single batch
- # to avoid doing two forward passes
- prompt_embeds = torch.cat([negative_prompt_embeds, prompt_embeds])
-
- return prompt_embeds
-
- def run_safety_checker(self, image, device, dtype):
- if self.safety_checker is not None:
- safety_checker_input = self.feature_extractor(self.numpy_to_pil(image), return_tensors="pt").to(device)
- image, has_nsfw_concept = self.safety_checker(
- images=image, clip_input=safety_checker_input.pixel_values.to(dtype)
- )
- else:
- has_nsfw_concept = None
- return image, has_nsfw_concept
-
- def decode_latents(self, latents):
- latents = 1 / self.vae.config.scaling_factor * latents
- image = self.vae.decode(latents).sample
- image = (image / 2 + 0.5).clamp(0, 1)
- # we always cast to float32 as this does not cause significant overhead and is compatible with bfloat16
- image = image.cpu().permute(0, 2, 3, 1).float().numpy()
- return image
-
- def prepare_extra_step_kwargs(self, generator, eta):
- # prepare extra kwargs for the scheduler step, since not all schedulers have the same signature
- # eta (η) is only used with the DDIMScheduler, it will be ignored for other schedulers.
- # eta corresponds to η in DDIM paper: https://arxiv.org/abs/2010.02502
- # and should be between [0, 1]
-
- accepts_eta = "eta" in set(inspect.signature(self.scheduler.step).parameters.keys())
- extra_step_kwargs = {}
- if accepts_eta:
- extra_step_kwargs["eta"] = eta
-
- # check if the scheduler accepts generator
- accepts_generator = "generator" in set(inspect.signature(self.scheduler.step).parameters.keys())
- if accepts_generator:
- extra_step_kwargs["generator"] = generator
- return extra_step_kwargs
-
- def check_inputs(
- self,
- prompt,
- height,
- width,
- callback_steps,
- negative_prompt=None,
- prompt_embeds=None,
- negative_prompt_embeds=None,
- ):
- if height % 8 != 0 or width % 8 != 0:
- raise ValueError(f"`height` and `width` have to be divisible by 8 but are {height} and {width}.")
-
- if (callback_steps is None) or (
- callback_steps is not None and (not isinstance(callback_steps, int) or callback_steps <= 0)
- ):
- raise ValueError(
- f"`callback_steps` has to be a positive integer but is {callback_steps} of type"
- f" {type(callback_steps)}."
- )
-
- if prompt is not None and prompt_embeds is not None:
- raise ValueError(
- f"Cannot forward both `prompt`: {prompt} and `prompt_embeds`: {prompt_embeds}. Please make sure to"
- " only forward one of the two."
- )
- elif prompt is None and prompt_embeds is None:
- raise ValueError(
- "Provide either `prompt` or `prompt_embeds`. Cannot leave both `prompt` and `prompt_embeds` undefined."
- )
- elif prompt is not None and (not isinstance(prompt, str) and not isinstance(prompt, list)):
- raise ValueError(f"`prompt` has to be of type `str` or `list` but is {type(prompt)}")
-
- if negative_prompt is not None and negative_prompt_embeds is not None:
- raise ValueError(
- f"Cannot forward both `negative_prompt`: {negative_prompt} and `negative_prompt_embeds`:"
- f" {negative_prompt_embeds}. Please make sure to only forward one of the two."
- )
-
- if prompt_embeds is not None and negative_prompt_embeds is not None:
- if prompt_embeds.shape != negative_prompt_embeds.shape:
- raise ValueError(
- "`prompt_embeds` and `negative_prompt_embeds` must have the same shape when passed directly, but"
- f" got: `prompt_embeds` {prompt_embeds.shape} != `negative_prompt_embeds`"
- f" {negative_prompt_embeds.shape}."
- )
-
- def prepare_latents(self, batch_size, num_channels_latents, height, width, dtype, device, generator, latents=None):
- shape = (batch_size, num_channels_latents, height // self.vae_scale_factor, width // self.vae_scale_factor)
- if isinstance(generator, list) and len(generator) != batch_size:
- raise ValueError(
- f"You have passed a list of generators of length {len(generator)}, but requested an effective batch"
- f" size of {batch_size}. Make sure the batch size matches the length of the generators."
- )
-
- if latents is None:
- latents = randn_tensor(shape, generator=generator, device=device, dtype=dtype)
- else:
- latents = latents.to(device)
-
- # scale the initial noise by the standard deviation required by the scheduler
- latents = latents * self.scheduler.init_noise_sigma
- return latents
-
- @torch.no_grad()
- @replace_example_docstring(EXAMPLE_DOC_STRING)
- def __call__(
- self,
- prompt: Union[str, List[str]] = None,
- height: Optional[int] = None,
- width: Optional[int] = None,
- num_inference_steps: int = 50,
- guidance_scale: float = 7.5,
- negative_prompt: Optional[Union[str, List[str]]] = None,
- num_images_per_prompt: Optional[int] = 1,
- eta: float = 0.0,
- generator: Optional[Union[torch.Generator, List[torch.Generator]]] = None,
- latents: Optional[torch.FloatTensor] = None,
- prompt_embeds: Optional[torch.FloatTensor] = None,
- negative_prompt_embeds: Optional[torch.FloatTensor] = None,
- output_type: Optional[str] = "pil",
- return_dict: bool = True,
- callback: Optional[Callable[[int, int, torch.FloatTensor], None]] = None,
- callback_steps: int = 1,
- cross_attention_kwargs: Optional[Dict[str, Any]] = None,
- ):
- r"""
- Function invoked when calling the pipeline for generation.
-
- Args:
- prompt (`str` or `List[str]`, *optional*):
- The prompt or prompts to guide the image generation. If not defined, one has to pass `prompt_embeds`.
- instead.
- height (`int`, *optional*, defaults to self.unet.config.sample_size * self.vae_scale_factor):
- The height in pixels of the generated image.
- width (`int`, *optional*, defaults to self.unet.config.sample_size * self.vae_scale_factor):
- The width in pixels of the generated image.
- num_inference_steps (`int`, *optional*, defaults to 50):
- The number of denoising steps. More denoising steps usually lead to a higher quality image at the
- expense of slower inference.
- guidance_scale (`float`, *optional*, defaults to 7.5):
- Guidance scale as defined in [Classifier-Free Diffusion Guidance](https://arxiv.org/abs/2207.12598).
- `guidance_scale` is defined as `w` of equation 2. of [Imagen
- Paper](https://arxiv.org/pdf/2205.11487.pdf). Guidance scale is enabled by setting `guidance_scale >
- 1`. Higher guidance scale encourages to generate images that are closely linked to the text `prompt`,
- usually at the expense of lower image quality.
- negative_prompt (`str` or `List[str]`, *optional*):
- The prompt or prompts not to guide the image generation. If not defined, one has to pass
- `negative_prompt_embeds` instead. Ignored when not using guidance (i.e., ignored if `guidance_scale` is
- less than `1`).
- num_images_per_prompt (`int`, *optional*, defaults to 1):
- The number of images to generate per prompt.
- eta (`float`, *optional*, defaults to 0.0):
- Corresponds to parameter eta (η) in the DDIM paper: https://arxiv.org/abs/2010.02502. Only applies to
- [`schedulers.DDIMScheduler`], will be ignored for others.
- generator (`torch.Generator` or `List[torch.Generator]`, *optional*):
- One or a list of [torch generator(s)](https://pytorch.org/docs/stable/generated/torch.Generator.html)
- to make generation deterministic.
- latents (`torch.FloatTensor`, *optional*):
- Pre-generated noisy latents, sampled from a Gaussian distribution, to be used as inputs for image
- generation. Can be used to tweak the same generation with different prompts. If not provided, a latents
- tensor will ge generated by sampling using the supplied random `generator`.
- prompt_embeds (`torch.FloatTensor`, *optional*):
- Pre-generated text embeddings. Can be used to easily tweak text inputs, *e.g.* prompt weighting. If not
- provided, text embeddings will be generated from `prompt` input argument.
- negative_prompt_embeds (`torch.FloatTensor`, *optional*):
- Pre-generated negative text embeddings. Can be used to easily tweak text inputs, *e.g.* prompt
- weighting. If not provided, negative_prompt_embeds will be generated from `negative_prompt` input
- argument.
- output_type (`str`, *optional*, defaults to `"pil"`):
- The output format of the generate image. Choose between
- [PIL](https://pillow.readthedocs.io/en/stable/): `PIL.Image.Image` or `np.array`.
- return_dict (`bool`, *optional*, defaults to `True`):
- Whether or not to return a [`~pipelines.stable_diffusion.StableDiffusionPipelineOutput`] instead of a
- plain tuple.
- callback (`Callable`, *optional*):
- A function that will be called every `callback_steps` steps during inference. The function will be
- called with the following arguments: `callback(step: int, timestep: int, latents: torch.FloatTensor)`.
- callback_steps (`int`, *optional*, defaults to 1):
- The frequency at which the `callback` function will be called. If not specified, the callback will be
- called at every step.
- cross_attention_kwargs (`dict`, *optional*):
- A kwargs dictionary that if specified is passed along to the `AttentionProcessor` as defined under
- `self.processor` in
- [diffusers.cross_attention](https://github.com/huggingface/diffusers/blob/main/src/diffusers/models/cross_attention.py).
-
- Examples:
-
- Returns:
- [`~pipelines.stable_diffusion.StableDiffusionPipelineOutput`] or `tuple`:
- [`~pipelines.stable_diffusion.StableDiffusionPipelineOutput`] if `return_dict` is True, otherwise a `tuple.
- When returning a tuple, the first element is a list with the generated images, and the second element is a
- list of `bool`s denoting whether the corresponding generated image likely represents "not-safe-for-work"
- (nsfw) content, according to the `safety_checker`.
- """
- # 0. Default height and width to unet
- height = height or self.unet.config.sample_size * self.vae_scale_factor
- width = width or self.unet.config.sample_size * self.vae_scale_factor
-
- # 1. Check inputs. Raise error if not correct
- self.check_inputs(
- prompt, height, width, callback_steps, negative_prompt, prompt_embeds, negative_prompt_embeds
- )
-
- # 2. Define call parameters
- if prompt is not None and isinstance(prompt, str):
- batch_size = 1
- elif prompt is not None and isinstance(prompt, list):
- batch_size = len(prompt)
- else:
- batch_size = prompt_embeds.shape[0]
-
- device = self._execution_device
- # here `guidance_scale` is defined analog to the guidance weight `w` of equation (2)
- # of the Imagen paper: https://arxiv.org/pdf/2205.11487.pdf . `guidance_scale = 1`
- # corresponds to doing no classifier free guidance.
- do_classifier_free_guidance = guidance_scale > 1.0
-
- # 3. Encode input prompt
- prompt_embeds = self._encode_prompt(
- prompt,
- device,
- num_images_per_prompt,
- do_classifier_free_guidance,
- negative_prompt,
- prompt_embeds=prompt_embeds,
- negative_prompt_embeds=negative_prompt_embeds,
- )
-
- # 4. Prepare timesteps
- self.scheduler.set_timesteps(num_inference_steps, device=device)
- timesteps = self.scheduler.timesteps
-
- # 5. Prepare latent variables
- num_channels_latents = self.unet.in_channels
- latents = self.prepare_latents(
- batch_size * num_images_per_prompt,
- num_channels_latents,
- height,
- width,
- prompt_embeds.dtype,
- device,
- generator,
- latents,
- )
-
- # 6. Prepare extra step kwargs. TODO: Logic should ideally just be moved out of the pipeline
- extra_step_kwargs = self.prepare_extra_step_kwargs(generator, eta)
-
- # 7. Denoising loop
- num_warmup_steps = len(timesteps) - num_inference_steps * self.scheduler.order
- with self.progress_bar(total=num_inference_steps) as progress_bar:
- for i, t in enumerate(timesteps):
- # expand the latents if we are doing classifier free guidance
- latent_model_input = torch.cat([latents] * 2) if do_classifier_free_guidance else latents
- latent_model_input = self.scheduler.scale_model_input(latent_model_input, t)
-
- # predict the noise residual
- noise_pred = self.unet(
- latent_model_input,
- t,
- encoder_hidden_states=prompt_embeds,
- cross_attention_kwargs=cross_attention_kwargs,
- ).sample
-
- # perform guidance
- if do_classifier_free_guidance:
- noise_pred_uncond, noise_pred_text = noise_pred.chunk(2)
- noise_pred = noise_pred_uncond + guidance_scale * (noise_pred_text - noise_pred_uncond)
-
- # compute the previous noisy sample x_t -> x_t-1
- latents = self.scheduler.step(noise_pred, t, latents, **extra_step_kwargs).prev_sample
-
- # call the callback, if provided
- if i == len(timesteps) - 1 or ((i + 1) > num_warmup_steps and (i + 1) % self.scheduler.order == 0):
- progress_bar.update()
- if callback is not None and i % callback_steps == 0:
- callback(i, t, latents)
-
- if output_type == "latent":
- image = latents
- has_nsfw_concept = None
- elif output_type == "pil":
- # 8. Post-processing
- image = self.decode_latents(latents)
-
- # 9. Run safety checker
- image, has_nsfw_concept = self.run_safety_checker(image, device, prompt_embeds.dtype)
-
- # 10. Convert to PIL
- image = self.numpy_to_pil(image)
- else:
- # 8. Post-processing
- image = self.decode_latents(latents)
-
- # 9. Run safety checker
- image, has_nsfw_concept = self.run_safety_checker(image, device, prompt_embeds.dtype)
-
- # Offload last model to CPU
- if hasattr(self, "final_offload_hook") and self.final_offload_hook is not None:
- self.final_offload_hook.offload()
-
- if not return_dict:
- return (image, has_nsfw_concept)
-
- return StableDiffusionPipelineOutput(images=image, nsfw_content_detected=has_nsfw_concept)
diff --git a/spaces/declare-lab/tango/diffusers/src/diffusers/utils/__init__.py b/spaces/declare-lab/tango/diffusers/src/diffusers/utils/__init__.py
deleted file mode 100644
index 3a1103ac1adfd346c200e2cccaa2f6f80b8c791b..0000000000000000000000000000000000000000
--- a/spaces/declare-lab/tango/diffusers/src/diffusers/utils/__init__.py
+++ /dev/null
@@ -1,115 +0,0 @@
-# Copyright 2023 The HuggingFace Inc. team. All rights reserved.
-#
-# Licensed under the Apache License, Version 2.0 (the "License");
-# you may not use this file except in compliance with the License.
-# You may obtain a copy of the License at
-#
-# http://www.apache.org/licenses/LICENSE-2.0
-#
-# Unless required by applicable law or agreed to in writing, software
-# distributed under the License is distributed on an "AS IS" BASIS,
-# WITHOUT WARRANTIES OR CONDITIONS OF ANY KIND, either express or implied.
-# See the License for the specific language governing permissions and
-# limitations under the License.
-
-
-import os
-
-from packaging import version
-
-from .. import __version__
-from .accelerate_utils import apply_forward_hook
-from .constants import (
- CONFIG_NAME,
- DEPRECATED_REVISION_ARGS,
- DIFFUSERS_CACHE,
- DIFFUSERS_DYNAMIC_MODULE_NAME,
- FLAX_WEIGHTS_NAME,
- HF_MODULES_CACHE,
- HUGGINGFACE_CO_RESOLVE_ENDPOINT,
- ONNX_EXTERNAL_WEIGHTS_NAME,
- ONNX_WEIGHTS_NAME,
- SAFETENSORS_WEIGHTS_NAME,
- WEIGHTS_NAME,
-)
-from .deprecation_utils import deprecate
-from .doc_utils import replace_example_docstring
-from .dynamic_modules_utils import get_class_from_dynamic_module
-from .hub_utils import (
- HF_HUB_OFFLINE,
- _add_variant,
- _get_model_file,
- extract_commit_hash,
- http_user_agent,
-)
-from .import_utils import (
- ENV_VARS_TRUE_AND_AUTO_VALUES,
- ENV_VARS_TRUE_VALUES,
- USE_JAX,
- USE_TF,
- USE_TORCH,
- DummyObject,
- OptionalDependencyNotAvailable,
- is_accelerate_available,
- is_accelerate_version,
- is_flax_available,
- is_inflect_available,
- is_k_diffusion_available,
- is_k_diffusion_version,
- is_librosa_available,
- is_note_seq_available,
- is_omegaconf_available,
- is_onnx_available,
- is_safetensors_available,
- is_scipy_available,
- is_tensorboard_available,
- is_tf_available,
- is_torch_available,
- is_torch_version,
- is_transformers_available,
- is_transformers_version,
- is_unidecode_available,
- is_wandb_available,
- is_xformers_available,
- requires_backends,
-)
-from .logging import get_logger
-from .outputs import BaseOutput
-from .pil_utils import PIL_INTERPOLATION
-from .torch_utils import is_compiled_module, randn_tensor
-
-
-if is_torch_available():
- from .testing_utils import (
- floats_tensor,
- load_hf_numpy,
- load_image,
- load_numpy,
- nightly,
- parse_flag_from_env,
- print_tensor_test,
- require_torch_2,
- require_torch_gpu,
- skip_mps,
- slow,
- torch_all_close,
- torch_device,
- )
-
-from .testing_utils import export_to_video
-
-
-logger = get_logger(__name__)
-
-
-def check_min_version(min_version):
- if version.parse(__version__) < version.parse(min_version):
- if "dev" in min_version:
- error_message = (
- "This example requires a source install from HuggingFace diffusers (see "
- "`https://huggingface.co/docs/diffusers/installation#install-from-source`),"
- )
- else:
- error_message = f"This example requires a minimum version of {min_version},"
- error_message += f" but the version found is {__version__}.\n"
- raise ImportError(error_message)
diff --git a/spaces/deepwisdom/MetaGPT/examples/search_kb.py b/spaces/deepwisdom/MetaGPT/examples/search_kb.py
deleted file mode 100644
index 449099380b4f8c1704fbd9358ef45c80f218d02f..0000000000000000000000000000000000000000
--- a/spaces/deepwisdom/MetaGPT/examples/search_kb.py
+++ /dev/null
@@ -1,29 +0,0 @@
-#!/usr/bin/env python
-# -*- coding: utf-8 -*-
-"""
-@File : search_kb.py
-@Modified By: mashenquan, 2023-8-9, fix-bug: cannot find metagpt module.
-"""
-import asyncio
-from pathlib import Path
-import sys
-sys.path.append(str(Path(__file__).resolve().parent.parent))
-from metagpt.const import DATA_PATH
-from metagpt.document_store import FaissStore
-from metagpt.logs import logger
-from metagpt.roles import Sales
-
-
-async def search():
- store = FaissStore(DATA_PATH / 'example.json')
- role = Sales(profile="Sales", store=store)
-
- queries = ["Which facial cleanser is good for oily skin?", "Is L'Oreal good to use?"]
- for query in queries:
- logger.info(f"User: {query}")
- result = await role.run(query)
- logger.info(result)
-
-
-if __name__ == '__main__':
- asyncio.run(search())
diff --git a/spaces/deepwisdom/MetaGPT/metagpt/roles/prompt.py b/spaces/deepwisdom/MetaGPT/metagpt/roles/prompt.py
deleted file mode 100644
index 9915f1426c3a8b2c09edb576fc8b1fafe1aec9ce..0000000000000000000000000000000000000000
--- a/spaces/deepwisdom/MetaGPT/metagpt/roles/prompt.py
+++ /dev/null
@@ -1,46 +0,0 @@
-#!/usr/bin/env python
-# -*- coding: utf-8 -*-
-"""
-@Time : 2023/5/18 22:43
-@Author : alexanderwu
-@File : prompt.py
-"""
-from enum import Enum
-
-PREFIX = """尽你所能回答以下问题。你可以使用以下工具:"""
-FORMAT_INSTRUCTIONS = """请按照以下格式:
-
-问题:你需要回答的输入问题
-思考:你应该始终思考该怎么做
-行动:要采取的行动,应该是[{tool_names}]中的一个
-行动输入:行动的输入
-观察:行动的结果
-...(这个思考/行动/行动输入/观察可以重复N次)
-思考:我现在知道最终答案了
-最终答案:对原始输入问题的最终答案"""
-SUFFIX = """开始吧!
-
-问题:{input}
-思考:{agent_scratchpad}"""
-
-
-class PromptString(Enum):
- REFLECTION_QUESTIONS = "以下是一些陈述:\n{memory_descriptions}\n\n仅根据以上信息,我们可以回答关于陈述中主题的3个最显著的高级问题是什么?\n\n{format_instructions}"
-
- REFLECTION_INSIGHTS = "\n{memory_strings}\n你可以从以上陈述中推断出5个高级洞察吗?在提到人时,总是指定他们的名字。\n\n{format_instructions}"
-
- IMPORTANCE = "你是一个记忆重要性AI。根据角色的个人资料和记忆描述,对记忆的重要性进行1到10的评级,其中1是纯粹的日常(例如,刷牙,整理床铺),10是极其深刻的(例如,分手,大学录取)。确保你的评级相对于角色的个性和关注点。\n\n示例#1:\n姓名:Jojo\n简介:Jojo是一个专业的滑冰运动员,喜欢特色咖啡。她希望有一天能参加奥运会。\n记忆:Jojo看到了一个新的咖啡店\n\n 你的回应:'{{\"rating\": 3}}'\n\n示例#2:\n姓名:Skylar\n简介:Skylar是一名产品营销经理。她在一家成长阶段的科技公司工作,该公司制造自动驾驶汽车。她喜欢猫。\n记忆:Skylar看到了一个新的咖啡店\n\n 你的回应:'{{\"rating\": 1}}'\n\n示例#3:\n姓名:Bob\n简介:Bob是纽约市下东区的一名水管工。他已经做了20年的水管工。周末他喜欢和他的妻子一起散步。\n记忆:Bob的妻子打了他一巴掌。\n\n 你的回应:'{{\"rating\": 9}}'\n\n示例#4:\n姓名:Thomas\n简介:Thomas是明尼阿波利斯的一名警察。他只在警队工作了6个月,因为经验不足在工作中遇到了困难。\n记忆:Thomas不小心把饮料洒在了一个陌生人身上\n\n 你的回应:'{{\"rating\": 6}}'\n\n示例#5:\n姓名:Laura\n简介:Laura是一名在大型科技公司工作的营销专家。她喜欢旅行和尝试新的食物。她对探索新的文化和结识来自各行各业的人充满热情。\n记忆:Laura到达了会议室\n\n 你的回应:'{{\"rating\": 1}}'\n\n{format_instructions} 让我们开始吧! \n\n 姓名:{full_name}\n个人简介:{private_bio}\n记忆:{memory_description}\n\n"
-
- RECENT_ACTIIVITY = "根据以下记忆,生成一个关于{full_name}最近在做什么的简短总结。不要编造记忆中未明确指定的细节。对于任何对话,一定要提到对话是否已经结束或者仍在进行中。\n\n记忆:{memory_descriptions}"
-
- MAKE_PLANS = '你是一个计划生成的AI,你的工作是根据新信息帮助角色制定新计划。根据角色的信息(个人简介,目标,最近的活动,当前计划,和位置上下文)和角色的当前思考过程,为他们生成一套新的计划,使得最后的计划包括至少{time_window}的活动,并且不超过5个单独的计划。计划列表应按照他们应执行的顺序编号,每个计划包含描述,位置,开始时间,停止条件,和最大持续时间。\n\n示例计划:\'{{"index": 1, "description": "Cook dinner", "location_id": "0a3bc22b-36aa-48ab-adb0-18616004caed","start_time": "2022-12-12T20:00:00+00:00","max_duration_hrs": 1.5, "stop_condition": "Dinner is fully prepared"}}\'\n\n对于每个计划,从这个列表中选择最合理的位置名称:{allowed_location_descriptions}\n\n{format_instructions}\n\n总是优先完成任何未完成的对话。\n\n让我们开始吧!\n\n姓名:{full_name}\n个人简介:{private_bio}\n目标:{directives}\n位置上下文:{location_context}\n当前计划:{current_plans}\n最近的活动:{recent_activity}\n思考过程:{thought_process}\n重要的是:鼓励角色在他们的计划中与其他角色合作。\n\n'
-
- EXECUTE_PLAN = "你是一个角色扮演的AI,扮演的角色是{your_name},在一个现场观众面前。你说的每一句话都可以被观众观察到,所以确保你经常说话,并且让它有趣。你不能直接与观众互动。\n\n根据以下的上下文和工具,像你是{your_name}一样进行。你的优先任务是完成下面给你的任务,然而,如果你当前正在与另一个角色进行对话,你应该总是先完成对话,然后再进行任务。不要在你参与未完成的对话时开始工作。使用你最好的判断力来确定一个对话是否涉及到你,以及它是否未完成。你不需要回应你收到的每一条消息。\n\n这是你角色的个人简介:\n{your_private_bio}\n\n这是你的位置和其他附近角色的描述,你可以和他们交谈:\n\n{location_context}\n\n这是一些相关的记忆:\n```\n{relevant_memories}\n```\n\n这是一些相关的对话历史:\n```\n{conversation_history}\n```\n\n这是你可以使用的工具:\n{tools}\n\n你的回应应该使用以下的格式:\n\n任务:你必须完成的任务\n思考:你应该做什么的思考\n行动:要采取的行动,必须是这些词之一:[{tool_names}]\n行动输入:行动的输入\n观察:行动的结果\n... (这个思考/行动/行动输入/观察可以重复N次)\n思考:'我已经完成了任务'\n最终回应:任务的最终回应\n\n如果你没有准备好最终的回应,那么你必须采取一个行动。\n\n如果你确定你无法用你被给予的工具完成任务,返回'最终回应:需要帮助',然而,如果你正在与另一个角色对话,像'我不知道'这样的回应是有效的回应。在对话中,你永远不应该打破角色,或者承认你是一个AI。\n如果任务完成并且不需要特定的回应,返回'最终回应:完成'\n开始吧!\n\n任务:{input}\n\n{agent_scratchpad}"
-
- REACT = "你是一个角色扮演的AI,扮演的角色是{full_name}。\n\n根据你的角色和他们当前上下文的以下信息,决定他们应该如何继续他们当前的计划。你的决定必须是:[\"推迟\", \"继续\",或 \"取消\"]。如果你的角色的当前计划不再与上下文相关,你应该取消它。如果你的角色的当前计划仍然与上下文相关,但是发生了新的事情需要优先处理,你应该决定推迟,这样你可以先做其他事情,然后再回来继续当前的计划。在所有其他情况下,你应该继续。\n\n当需要回应时,应优先回应其他角色。当回应被认为是必要的时,回应被认为是必要的。例如,假设你当前的计划是阅读一本书,Sally问'你在读什么?'。在这种情况下,你应该推迟你当前的计划(阅读)以便你可以回应进来的消息,因为在这种情况下,如果不回应Sally会很粗鲁。在你当前的计划涉及与另一个角色的对话的情况下,你不需要推迟来回应那个角色。例如,假设你当前的计划是和Sally谈话,然后Sally对你说你好。在这种情况下,你应该继续你当前的计划(和sally谈话)。在你不需要从你那里得到口头回应的情况下,你应该继续。例如,假设你当前的计划是散步,你刚刚对Sally说'再见',然后Sally回应你'再见'。在这种情况下,不需要口头回应,你应该继续你的计划。\n\n总是在你的决定之外包含一个思考过程,而在你选择推迟你当前的计划的情况下,包含新计划的规格。\n\n{format_instructions}\n\n这是关于你的角色的一些信息:\n\n姓名:{full_name}\n\n简介:{private_bio}\n\n目标:{directives}\n\n这是你的角色在这个时刻的一些上下文:\n\n位置上下文:{location_context}\n\n最近的活动:{recent_activity}\n\n对话历史:{conversation_history}\n\n这是你的角色当前的计划:{current_plan}\n\n这是自你的角色制定这个计划以来发生的新事件:{event_descriptions}。\n"
-
- GOSSIP = "你是{full_name}。 \n{memory_descriptions}\n\n根据以上陈述,说一两句对你所在位置的其他人:{other_agent_names}感兴趣的话。\n在提到其他人时,总是指定他们的名字。"
-
- HAS_HAPPENED = "给出以下角色的观察和他们正在等待的事情的描述,说明角色是否已经见证了这个事件。\n{format_instructions}\n\n示例:\n\n观察:\nJoe在2023-05-04 08:00:00+00:00走进办公室\nJoe在2023-05-04 08:05:00+00:00对Sally说hi\nSally在2023-05-04 08:05:30+00:00对Joe说hello\nRebecca在2023-05-04 08:10:00+00:00开始工作\nJoe在2023-05-04 08:15:00+00:00做了一些早餐\n\n等待:Sally回应了Joe\n\n 你的回应:'{{\"has_happened\": true, \"date_occured\": 2023-05-04 08:05:30+00:00}}'\n\n让我们开始吧!\n\n观察:\n{memory_descriptions}\n\n等待:{event_description}\n"
-
- OUTPUT_FORMAT = "\n\n(记住!确保你的输出总是符合以下两种格式之一:\n\nA. 如果你已经完成了任务:\n思考:'我已经完成了任务'\n最终回应:\n\nB. 如果你还没有完成任务:\n思考:\n行动:\n行动输入:\n观察:)\n"
diff --git a/spaces/deepwisdom/MetaGPT/tests/metagpt/__init__.py b/spaces/deepwisdom/MetaGPT/tests/metagpt/__init__.py
deleted file mode 100644
index 583942d31eee92261b22930fde15f8a151d49141..0000000000000000000000000000000000000000
--- a/spaces/deepwisdom/MetaGPT/tests/metagpt/__init__.py
+++ /dev/null
@@ -1,7 +0,0 @@
-#!/usr/bin/env python
-# -*- coding: utf-8 -*-
-"""
-@Time : 2023/4/29 16:01
-@Author : alexanderwu
-@File : __init__.py
-"""
diff --git a/spaces/diacanFperku/AutoGPT/Barn Yarn Premium Edition Full Cracked.md b/spaces/diacanFperku/AutoGPT/Barn Yarn Premium Edition Full Cracked.md
deleted file mode 100644
index 5e7c69da5e33fc78d68c925f4e551e1b3e6e4d84..0000000000000000000000000000000000000000
--- a/spaces/diacanFperku/AutoGPT/Barn Yarn Premium Edition Full Cracked.md
+++ /dev/null
@@ -1,11 +0,0 @@
-Barn Yarn Premium Edition Full Cracked DOWNLOAD ››››› https://gohhs.com/2uFSZi
-
-... know it won't soften, so feed them a tour of Bass stores Pro Shops*, ... Visit www.polarguard.com Find out more about premium insulation that keeps ... Shop Men's Jackets at Funday online clothing store.
-A wide range of models at attractive prices.
-Production Russia.
-Winter jackets for women with climate control from LimoLady are a stylish and modern solution to the problem of cold.
-Buy winter jackets for women in. Boots with solid soles, sneakers, boots with heels, sneakers and other models of fashionable platform shoes are not only comfortable, but also very feminine.
-In the Mohito online store you will find this fashion offer for. 8a78ff9644
-
-
-
diff --git a/spaces/diego2554/RemBG_super/rembg/commands/p_command.py b/spaces/diego2554/RemBG_super/rembg/commands/p_command.py
deleted file mode 100644
index 2163bfbb2332f5b23f2fa9c305b15df9c6c425ff..0000000000000000000000000000000000000000
--- a/spaces/diego2554/RemBG_super/rembg/commands/p_command.py
+++ /dev/null
@@ -1,181 +0,0 @@
-import json
-import pathlib
-import time
-from typing import cast
-
-import click
-import filetype
-from tqdm import tqdm
-from watchdog.events import FileSystemEvent, FileSystemEventHandler
-from watchdog.observers import Observer
-
-from ..bg import remove
-from ..session_factory import new_session
-from ..sessions import sessions_names
-
-
-@click.command(
- name="p",
- help="for a folder as input",
-)
-@click.option(
- "-m",
- "--model",
- default="u2net",
- type=click.Choice(sessions_names),
- show_default=True,
- show_choices=True,
- help="model name",
-)
-@click.option(
- "-a",
- "--alpha-matting",
- is_flag=True,
- show_default=True,
- help="use alpha matting",
-)
-@click.option(
- "-af",
- "--alpha-matting-foreground-threshold",
- default=240,
- type=int,
- show_default=True,
- help="trimap fg threshold",
-)
-@click.option(
- "-ab",
- "--alpha-matting-background-threshold",
- default=10,
- type=int,
- show_default=True,
- help="trimap bg threshold",
-)
-@click.option(
- "-ae",
- "--alpha-matting-erode-size",
- default=10,
- type=int,
- show_default=True,
- help="erode size",
-)
-@click.option(
- "-om",
- "--only-mask",
- is_flag=True,
- show_default=True,
- help="output only the mask",
-)
-@click.option(
- "-ppm",
- "--post-process-mask",
- is_flag=True,
- show_default=True,
- help="post process the mask",
-)
-@click.option(
- "-w",
- "--watch",
- default=False,
- is_flag=True,
- show_default=True,
- help="watches a folder for changes",
-)
-@click.option(
- "-bgc",
- "--bgcolor",
- default=None,
- type=(int, int, int, int),
- nargs=4,
- help="Background color (R G B A) to replace the removed background with",
-)
-@click.option("-x", "--extras", type=str)
-@click.argument(
- "input",
- type=click.Path(
- exists=True,
- path_type=pathlib.Path,
- file_okay=False,
- dir_okay=True,
- readable=True,
- ),
-)
-@click.argument(
- "output",
- type=click.Path(
- exists=False,
- path_type=pathlib.Path,
- file_okay=False,
- dir_okay=True,
- writable=True,
- ),
-)
-def p_command(
- model: str,
- extras: str,
- input: pathlib.Path,
- output: pathlib.Path,
- watch: bool,
- **kwargs,
-) -> None:
- try:
- kwargs.update(json.loads(extras))
- except Exception:
- pass
-
- session = new_session(model)
-
- def process(each_input: pathlib.Path) -> None:
- try:
- mimetype = filetype.guess(each_input)
- if mimetype is None:
- return
- if mimetype.mime.find("image") < 0:
- return
-
- each_output = (output / each_input.name).with_suffix(".png")
- each_output.parents[0].mkdir(parents=True, exist_ok=True)
-
- if not each_output.exists():
- each_output.write_bytes(
- cast(
- bytes,
- remove(each_input.read_bytes(), session=session, **kwargs),
- )
- )
-
- if watch:
- print(
- f"processed: {each_input.absolute()} -> {each_output.absolute()}"
- )
- except Exception as e:
- print(e)
-
- inputs = list(input.glob("**/*"))
- if not watch:
- inputs = tqdm(inputs)
-
- for each_input in inputs:
- if not each_input.is_dir():
- process(each_input)
-
- if watch:
- observer = Observer()
-
- class EventHandler(FileSystemEventHandler):
- def on_any_event(self, event: FileSystemEvent) -> None:
- if not (
- event.is_directory or event.event_type in ["deleted", "closed"]
- ):
- process(pathlib.Path(event.src_path))
-
- event_handler = EventHandler()
- observer.schedule(event_handler, input, recursive=False)
- observer.start()
-
- try:
- while True:
- time.sleep(1)
-
- finally:
- observer.stop()
- observer.join()
diff --git a/spaces/digitalxingtong/Bufeiyan-a-Bert-VITS2/text/__init__.py b/spaces/digitalxingtong/Bufeiyan-a-Bert-VITS2/text/__init__.py
deleted file mode 100644
index 7566bf351ca9b95af9cdc6d729557a9da083800f..0000000000000000000000000000000000000000
--- a/spaces/digitalxingtong/Bufeiyan-a-Bert-VITS2/text/__init__.py
+++ /dev/null
@@ -1,28 +0,0 @@
-from text.symbols import *
-
-
-_symbol_to_id = {s: i for i, s in enumerate(symbols)}
-
-def cleaned_text_to_sequence(cleaned_text, tones, language):
- '''Converts a string of text to a sequence of IDs corresponding to the symbols in the text.
- Args:
- text: string to convert to a sequence
- Returns:
- List of integers corresponding to the symbols in the text
- '''
- phones = [_symbol_to_id[symbol] for symbol in cleaned_text]
- tone_start = language_tone_start_map[language]
- tones = [i + tone_start for i in tones]
- lang_id = language_id_map[language]
- lang_ids = [lang_id for i in phones]
- return phones, tones, lang_ids
-
-def get_bert(norm_text, word2ph, language):
- from .chinese_bert import get_bert_feature as zh_bert
- from .english_bert_mock import get_bert_feature as en_bert
- lang_bert_func_map = {
- 'ZH': zh_bert,
- 'EN': en_bert
- }
- bert = lang_bert_func_map[language](norm_text, word2ph)
- return bert
diff --git a/spaces/digitalxingtong/Lixiang-Bert-Vits2/text/symbols.py b/spaces/digitalxingtong/Lixiang-Bert-Vits2/text/symbols.py
deleted file mode 100644
index 9dfae4e633829f20c4fd767b1c7a9198911ed801..0000000000000000000000000000000000000000
--- a/spaces/digitalxingtong/Lixiang-Bert-Vits2/text/symbols.py
+++ /dev/null
@@ -1,51 +0,0 @@
-punctuation = ['!', '?', '…', ",", ".", "'", '-']
-pu_symbols = punctuation + ["SP", "UNK"]
-pad = '_'
-
-# chinese
-zh_symbols = ['E', 'En', 'a', 'ai', 'an', 'ang', 'ao', 'b', 'c', 'ch', 'd', 'e', 'ei', 'en', 'eng', 'er', 'f', 'g', 'h',
- 'i', 'i0', 'ia', 'ian', 'iang', 'iao', 'ie', 'in', 'ing', 'iong', 'ir', 'iu', 'j', 'k', 'l', 'm', 'n', 'o',
- 'ong',
- 'ou', 'p', 'q', 'r', 's', 'sh', 't', 'u', 'ua', 'uai', 'uan', 'uang', 'ui', 'un', 'uo', 'v', 'van', 've', 'vn',
- 'w', 'x', 'y', 'z', 'zh',
- "AA", "EE", "OO"]
-num_zh_tones = 6
-
-# japanese
-ja_symbols = ['I', 'N', 'U', 'a', 'b', 'by', 'ch', 'cl', 'd', 'dy', 'e', 'f', 'g', 'gy', 'h', 'hy', 'i', 'j', 'k', 'ky',
- 'm', 'my', 'n', 'ny', 'o', 'p', 'py', 'r', 'ry', 's', 'sh', 't', 'ts', 'u', 'V', 'w', 'y', 'z']
-num_ja_tones = 1
-
-# English
-en_symbols = ['aa', 'ae', 'ah', 'ao', 'aw', 'ay', 'b', 'ch', 'd', 'dh', 'eh', 'er', 'ey', 'f', 'g', 'hh', 'ih', 'iy',
- 'jh', 'k', 'l', 'm', 'n', 'ng', 'ow', 'oy', 'p', 'r', 's',
- 'sh', 't', 'th', 'uh', 'uw', 'V', 'w', 'y', 'z', 'zh']
-num_en_tones = 4
-
-# combine all symbols
-normal_symbols = sorted(set(zh_symbols + ja_symbols + en_symbols))
-symbols = [pad] + normal_symbols + pu_symbols
-sil_phonemes_ids = [symbols.index(i) for i in pu_symbols]
-
-# combine all tones
-num_tones = num_zh_tones + num_ja_tones + num_en_tones
-
-# language maps
-language_id_map = {
- 'ZH': 0,
- "JA": 1,
- "EN": 2
-}
-num_languages = len(language_id_map.keys())
-
-language_tone_start_map = {
- 'ZH': 0,
- "JA": num_zh_tones,
- "EN": num_zh_tones + num_ja_tones
-}
-
-if __name__ == '__main__':
- a = set(zh_symbols)
- b = set(en_symbols)
- print(sorted(a&b))
-
diff --git a/spaces/dineshreddy/WALT/mmdet/models/dense_heads/corner_head.py b/spaces/dineshreddy/WALT/mmdet/models/dense_heads/corner_head.py
deleted file mode 100644
index 50cdb49a29f2ced1a31a50e654a3bdc14f5f5004..0000000000000000000000000000000000000000
--- a/spaces/dineshreddy/WALT/mmdet/models/dense_heads/corner_head.py
+++ /dev/null
@@ -1,1074 +0,0 @@
-from logging import warning
-from math import ceil, log
-
-import torch
-import torch.nn as nn
-import torch.nn.functional as F
-from mmcv.cnn import ConvModule, bias_init_with_prob
-from mmcv.ops import CornerPool, batched_nms
-
-from mmdet.core import multi_apply
-from ..builder import HEADS, build_loss
-from ..utils import gaussian_radius, gen_gaussian_target
-from .base_dense_head import BaseDenseHead
-
-
-class BiCornerPool(nn.Module):
- """Bidirectional Corner Pooling Module (TopLeft, BottomRight, etc.)
-
- Args:
- in_channels (int): Input channels of module.
- out_channels (int): Output channels of module.
- feat_channels (int): Feature channels of module.
- directions (list[str]): Directions of two CornerPools.
- norm_cfg (dict): Dictionary to construct and config norm layer.
- """
-
- def __init__(self,
- in_channels,
- directions,
- feat_channels=128,
- out_channels=128,
- norm_cfg=dict(type='BN', requires_grad=True)):
- super(BiCornerPool, self).__init__()
- self.direction1_conv = ConvModule(
- in_channels, feat_channels, 3, padding=1, norm_cfg=norm_cfg)
- self.direction2_conv = ConvModule(
- in_channels, feat_channels, 3, padding=1, norm_cfg=norm_cfg)
-
- self.aftpool_conv = ConvModule(
- feat_channels,
- out_channels,
- 3,
- padding=1,
- norm_cfg=norm_cfg,
- act_cfg=None)
-
- self.conv1 = ConvModule(
- in_channels, out_channels, 1, norm_cfg=norm_cfg, act_cfg=None)
- self.conv2 = ConvModule(
- in_channels, out_channels, 3, padding=1, norm_cfg=norm_cfg)
-
- self.direction1_pool = CornerPool(directions[0])
- self.direction2_pool = CornerPool(directions[1])
- self.relu = nn.ReLU(inplace=True)
-
- def forward(self, x):
- """Forward features from the upstream network.
-
- Args:
- x (tensor): Input feature of BiCornerPool.
-
- Returns:
- conv2 (tensor): Output feature of BiCornerPool.
- """
- direction1_conv = self.direction1_conv(x)
- direction2_conv = self.direction2_conv(x)
- direction1_feat = self.direction1_pool(direction1_conv)
- direction2_feat = self.direction2_pool(direction2_conv)
- aftpool_conv = self.aftpool_conv(direction1_feat + direction2_feat)
- conv1 = self.conv1(x)
- relu = self.relu(aftpool_conv + conv1)
- conv2 = self.conv2(relu)
- return conv2
-
-
-@HEADS.register_module()
-class CornerHead(BaseDenseHead):
- """Head of CornerNet: Detecting Objects as Paired Keypoints.
-
- Code is modified from the `official github repo
- `_ .
-
- More details can be found in the `paper
- `_ .
-
- Args:
- num_classes (int): Number of categories excluding the background
- category.
- in_channels (int): Number of channels in the input feature map.
- num_feat_levels (int): Levels of feature from the previous module. 2
- for HourglassNet-104 and 1 for HourglassNet-52. Because
- HourglassNet-104 outputs the final feature and intermediate
- supervision feature and HourglassNet-52 only outputs the final
- feature. Default: 2.
- corner_emb_channels (int): Channel of embedding vector. Default: 1.
- train_cfg (dict | None): Training config. Useless in CornerHead,
- but we keep this variable for SingleStageDetector. Default: None.
- test_cfg (dict | None): Testing config of CornerHead. Default: None.
- loss_heatmap (dict | None): Config of corner heatmap loss. Default:
- GaussianFocalLoss.
- loss_embedding (dict | None): Config of corner embedding loss. Default:
- AssociativeEmbeddingLoss.
- loss_offset (dict | None): Config of corner offset loss. Default:
- SmoothL1Loss.
- """
-
- def __init__(self,
- num_classes,
- in_channels,
- num_feat_levels=2,
- corner_emb_channels=1,
- train_cfg=None,
- test_cfg=None,
- loss_heatmap=dict(
- type='GaussianFocalLoss',
- alpha=2.0,
- gamma=4.0,
- loss_weight=1),
- loss_embedding=dict(
- type='AssociativeEmbeddingLoss',
- pull_weight=0.25,
- push_weight=0.25),
- loss_offset=dict(
- type='SmoothL1Loss', beta=1.0, loss_weight=1)):
- super(CornerHead, self).__init__()
- self.num_classes = num_classes
- self.in_channels = in_channels
- self.corner_emb_channels = corner_emb_channels
- self.with_corner_emb = self.corner_emb_channels > 0
- self.corner_offset_channels = 2
- self.num_feat_levels = num_feat_levels
- self.loss_heatmap = build_loss(
- loss_heatmap) if loss_heatmap is not None else None
- self.loss_embedding = build_loss(
- loss_embedding) if loss_embedding is not None else None
- self.loss_offset = build_loss(
- loss_offset) if loss_offset is not None else None
- self.train_cfg = train_cfg
- self.test_cfg = test_cfg
-
- self._init_layers()
-
- def _make_layers(self, out_channels, in_channels=256, feat_channels=256):
- """Initialize conv sequential for CornerHead."""
- return nn.Sequential(
- ConvModule(in_channels, feat_channels, 3, padding=1),
- ConvModule(
- feat_channels, out_channels, 1, norm_cfg=None, act_cfg=None))
-
- def _init_corner_kpt_layers(self):
- """Initialize corner keypoint layers.
-
- Including corner heatmap branch and corner offset branch. Each branch
- has two parts: prefix `tl_` for top-left and `br_` for bottom-right.
- """
- self.tl_pool, self.br_pool = nn.ModuleList(), nn.ModuleList()
- self.tl_heat, self.br_heat = nn.ModuleList(), nn.ModuleList()
- self.tl_off, self.br_off = nn.ModuleList(), nn.ModuleList()
-
- for _ in range(self.num_feat_levels):
- self.tl_pool.append(
- BiCornerPool(
- self.in_channels, ['top', 'left'],
- out_channels=self.in_channels))
- self.br_pool.append(
- BiCornerPool(
- self.in_channels, ['bottom', 'right'],
- out_channels=self.in_channels))
-
- self.tl_heat.append(
- self._make_layers(
- out_channels=self.num_classes,
- in_channels=self.in_channels))
- self.br_heat.append(
- self._make_layers(
- out_channels=self.num_classes,
- in_channels=self.in_channels))
-
- self.tl_off.append(
- self._make_layers(
- out_channels=self.corner_offset_channels,
- in_channels=self.in_channels))
- self.br_off.append(
- self._make_layers(
- out_channels=self.corner_offset_channels,
- in_channels=self.in_channels))
-
- def _init_corner_emb_layers(self):
- """Initialize corner embedding layers.
-
- Only include corner embedding branch with two parts: prefix `tl_` for
- top-left and `br_` for bottom-right.
- """
- self.tl_emb, self.br_emb = nn.ModuleList(), nn.ModuleList()
-
- for _ in range(self.num_feat_levels):
- self.tl_emb.append(
- self._make_layers(
- out_channels=self.corner_emb_channels,
- in_channels=self.in_channels))
- self.br_emb.append(
- self._make_layers(
- out_channels=self.corner_emb_channels,
- in_channels=self.in_channels))
-
- def _init_layers(self):
- """Initialize layers for CornerHead.
-
- Including two parts: corner keypoint layers and corner embedding layers
- """
- self._init_corner_kpt_layers()
- if self.with_corner_emb:
- self._init_corner_emb_layers()
-
- def init_weights(self):
- """Initialize weights of the head."""
- bias_init = bias_init_with_prob(0.1)
- for i in range(self.num_feat_levels):
- # The initialization of parameters are different between nn.Conv2d
- # and ConvModule. Our experiments show that using the original
- # initialization of nn.Conv2d increases the final mAP by about 0.2%
- self.tl_heat[i][-1].conv.reset_parameters()
- self.tl_heat[i][-1].conv.bias.data.fill_(bias_init)
- self.br_heat[i][-1].conv.reset_parameters()
- self.br_heat[i][-1].conv.bias.data.fill_(bias_init)
- self.tl_off[i][-1].conv.reset_parameters()
- self.br_off[i][-1].conv.reset_parameters()
- if self.with_corner_emb:
- self.tl_emb[i][-1].conv.reset_parameters()
- self.br_emb[i][-1].conv.reset_parameters()
-
- def forward(self, feats):
- """Forward features from the upstream network.
-
- Args:
- feats (tuple[Tensor]): Features from the upstream network, each is
- a 4D-tensor.
-
- Returns:
- tuple: Usually a tuple of corner heatmaps, offset heatmaps and
- embedding heatmaps.
- - tl_heats (list[Tensor]): Top-left corner heatmaps for all
- levels, each is a 4D-tensor, the channels number is
- num_classes.
- - br_heats (list[Tensor]): Bottom-right corner heatmaps for all
- levels, each is a 4D-tensor, the channels number is
- num_classes.
- - tl_embs (list[Tensor] | list[None]): Top-left embedding
- heatmaps for all levels, each is a 4D-tensor or None.
- If not None, the channels number is corner_emb_channels.
- - br_embs (list[Tensor] | list[None]): Bottom-right embedding
- heatmaps for all levels, each is a 4D-tensor or None.
- If not None, the channels number is corner_emb_channels.
- - tl_offs (list[Tensor]): Top-left offset heatmaps for all
- levels, each is a 4D-tensor. The channels number is
- corner_offset_channels.
- - br_offs (list[Tensor]): Bottom-right offset heatmaps for all
- levels, each is a 4D-tensor. The channels number is
- corner_offset_channels.
- """
- lvl_ind = list(range(self.num_feat_levels))
- return multi_apply(self.forward_single, feats, lvl_ind)
-
- def forward_single(self, x, lvl_ind, return_pool=False):
- """Forward feature of a single level.
-
- Args:
- x (Tensor): Feature of a single level.
- lvl_ind (int): Level index of current feature.
- return_pool (bool): Return corner pool feature or not.
-
- Returns:
- tuple[Tensor]: A tuple of CornerHead's output for current feature
- level. Containing the following Tensors:
-
- - tl_heat (Tensor): Predicted top-left corner heatmap.
- - br_heat (Tensor): Predicted bottom-right corner heatmap.
- - tl_emb (Tensor | None): Predicted top-left embedding heatmap.
- None for `self.with_corner_emb == False`.
- - br_emb (Tensor | None): Predicted bottom-right embedding
- heatmap. None for `self.with_corner_emb == False`.
- - tl_off (Tensor): Predicted top-left offset heatmap.
- - br_off (Tensor): Predicted bottom-right offset heatmap.
- - tl_pool (Tensor): Top-left corner pool feature. Not must
- have.
- - br_pool (Tensor): Bottom-right corner pool feature. Not must
- have.
- """
- tl_pool = self.tl_pool[lvl_ind](x)
- tl_heat = self.tl_heat[lvl_ind](tl_pool)
- br_pool = self.br_pool[lvl_ind](x)
- br_heat = self.br_heat[lvl_ind](br_pool)
-
- tl_emb, br_emb = None, None
- if self.with_corner_emb:
- tl_emb = self.tl_emb[lvl_ind](tl_pool)
- br_emb = self.br_emb[lvl_ind](br_pool)
-
- tl_off = self.tl_off[lvl_ind](tl_pool)
- br_off = self.br_off[lvl_ind](br_pool)
-
- result_list = [tl_heat, br_heat, tl_emb, br_emb, tl_off, br_off]
- if return_pool:
- result_list.append(tl_pool)
- result_list.append(br_pool)
-
- return result_list
-
- def get_targets(self,
- gt_bboxes,
- gt_labels,
- feat_shape,
- img_shape,
- with_corner_emb=False,
- with_guiding_shift=False,
- with_centripetal_shift=False):
- """Generate corner targets.
-
- Including corner heatmap, corner offset.
-
- Optional: corner embedding, corner guiding shift, centripetal shift.
-
- For CornerNet, we generate corner heatmap, corner offset and corner
- embedding from this function.
-
- For CentripetalNet, we generate corner heatmap, corner offset, guiding
- shift and centripetal shift from this function.
-
- Args:
- gt_bboxes (list[Tensor]): Ground truth bboxes of each image, each
- has shape (num_gt, 4).
- gt_labels (list[Tensor]): Ground truth labels of each box, each has
- shape (num_gt,).
- feat_shape (list[int]): Shape of output feature,
- [batch, channel, height, width].
- img_shape (list[int]): Shape of input image,
- [height, width, channel].
- with_corner_emb (bool): Generate corner embedding target or not.
- Default: False.
- with_guiding_shift (bool): Generate guiding shift target or not.
- Default: False.
- with_centripetal_shift (bool): Generate centripetal shift target or
- not. Default: False.
-
- Returns:
- dict: Ground truth of corner heatmap, corner offset, corner
- embedding, guiding shift and centripetal shift. Containing the
- following keys:
-
- - topleft_heatmap (Tensor): Ground truth top-left corner
- heatmap.
- - bottomright_heatmap (Tensor): Ground truth bottom-right
- corner heatmap.
- - topleft_offset (Tensor): Ground truth top-left corner offset.
- - bottomright_offset (Tensor): Ground truth bottom-right corner
- offset.
- - corner_embedding (list[list[list[int]]]): Ground truth corner
- embedding. Not must have.
- - topleft_guiding_shift (Tensor): Ground truth top-left corner
- guiding shift. Not must have.
- - bottomright_guiding_shift (Tensor): Ground truth bottom-right
- corner guiding shift. Not must have.
- - topleft_centripetal_shift (Tensor): Ground truth top-left
- corner centripetal shift. Not must have.
- - bottomright_centripetal_shift (Tensor): Ground truth
- bottom-right corner centripetal shift. Not must have.
- """
- batch_size, _, height, width = feat_shape
- img_h, img_w = img_shape[:2]
-
- width_ratio = float(width / img_w)
- height_ratio = float(height / img_h)
-
- gt_tl_heatmap = gt_bboxes[-1].new_zeros(
- [batch_size, self.num_classes, height, width])
- gt_br_heatmap = gt_bboxes[-1].new_zeros(
- [batch_size, self.num_classes, height, width])
- gt_tl_offset = gt_bboxes[-1].new_zeros([batch_size, 2, height, width])
- gt_br_offset = gt_bboxes[-1].new_zeros([batch_size, 2, height, width])
-
- if with_corner_emb:
- match = []
-
- # Guiding shift is a kind of offset, from center to corner
- if with_guiding_shift:
- gt_tl_guiding_shift = gt_bboxes[-1].new_zeros(
- [batch_size, 2, height, width])
- gt_br_guiding_shift = gt_bboxes[-1].new_zeros(
- [batch_size, 2, height, width])
- # Centripetal shift is also a kind of offset, from center to corner
- # and normalized by log.
- if with_centripetal_shift:
- gt_tl_centripetal_shift = gt_bboxes[-1].new_zeros(
- [batch_size, 2, height, width])
- gt_br_centripetal_shift = gt_bboxes[-1].new_zeros(
- [batch_size, 2, height, width])
-
- for batch_id in range(batch_size):
- # Ground truth of corner embedding per image is a list of coord set
- corner_match = []
- for box_id in range(len(gt_labels[batch_id])):
- left, top, right, bottom = gt_bboxes[batch_id][box_id]
- center_x = (left + right) / 2.0
- center_y = (top + bottom) / 2.0
- label = gt_labels[batch_id][box_id]
-
- # Use coords in the feature level to generate ground truth
- scale_left = left * width_ratio
- scale_right = right * width_ratio
- scale_top = top * height_ratio
- scale_bottom = bottom * height_ratio
- scale_center_x = center_x * width_ratio
- scale_center_y = center_y * height_ratio
-
- # Int coords on feature map/ground truth tensor
- left_idx = int(min(scale_left, width - 1))
- right_idx = int(min(scale_right, width - 1))
- top_idx = int(min(scale_top, height - 1))
- bottom_idx = int(min(scale_bottom, height - 1))
-
- # Generate gaussian heatmap
- scale_box_width = ceil(scale_right - scale_left)
- scale_box_height = ceil(scale_bottom - scale_top)
- radius = gaussian_radius((scale_box_height, scale_box_width),
- min_overlap=0.3)
- radius = max(0, int(radius))
- gt_tl_heatmap[batch_id, label] = gen_gaussian_target(
- gt_tl_heatmap[batch_id, label], [left_idx, top_idx],
- radius)
- gt_br_heatmap[batch_id, label] = gen_gaussian_target(
- gt_br_heatmap[batch_id, label], [right_idx, bottom_idx],
- radius)
-
- # Generate corner offset
- left_offset = scale_left - left_idx
- top_offset = scale_top - top_idx
- right_offset = scale_right - right_idx
- bottom_offset = scale_bottom - bottom_idx
- gt_tl_offset[batch_id, 0, top_idx, left_idx] = left_offset
- gt_tl_offset[batch_id, 1, top_idx, left_idx] = top_offset
- gt_br_offset[batch_id, 0, bottom_idx, right_idx] = right_offset
- gt_br_offset[batch_id, 1, bottom_idx,
- right_idx] = bottom_offset
-
- # Generate corner embedding
- if with_corner_emb:
- corner_match.append([[top_idx, left_idx],
- [bottom_idx, right_idx]])
- # Generate guiding shift
- if with_guiding_shift:
- gt_tl_guiding_shift[batch_id, 0, top_idx,
- left_idx] = scale_center_x - left_idx
- gt_tl_guiding_shift[batch_id, 1, top_idx,
- left_idx] = scale_center_y - top_idx
- gt_br_guiding_shift[batch_id, 0, bottom_idx,
- right_idx] = right_idx - scale_center_x
- gt_br_guiding_shift[
- batch_id, 1, bottom_idx,
- right_idx] = bottom_idx - scale_center_y
- # Generate centripetal shift
- if with_centripetal_shift:
- gt_tl_centripetal_shift[batch_id, 0, top_idx,
- left_idx] = log(scale_center_x -
- scale_left)
- gt_tl_centripetal_shift[batch_id, 1, top_idx,
- left_idx] = log(scale_center_y -
- scale_top)
- gt_br_centripetal_shift[batch_id, 0, bottom_idx,
- right_idx] = log(scale_right -
- scale_center_x)
- gt_br_centripetal_shift[batch_id, 1, bottom_idx,
- right_idx] = log(scale_bottom -
- scale_center_y)
-
- if with_corner_emb:
- match.append(corner_match)
-
- target_result = dict(
- topleft_heatmap=gt_tl_heatmap,
- topleft_offset=gt_tl_offset,
- bottomright_heatmap=gt_br_heatmap,
- bottomright_offset=gt_br_offset)
-
- if with_corner_emb:
- target_result.update(corner_embedding=match)
- if with_guiding_shift:
- target_result.update(
- topleft_guiding_shift=gt_tl_guiding_shift,
- bottomright_guiding_shift=gt_br_guiding_shift)
- if with_centripetal_shift:
- target_result.update(
- topleft_centripetal_shift=gt_tl_centripetal_shift,
- bottomright_centripetal_shift=gt_br_centripetal_shift)
-
- return target_result
-
- def loss(self,
- tl_heats,
- br_heats,
- tl_embs,
- br_embs,
- tl_offs,
- br_offs,
- gt_bboxes,
- gt_labels,
- img_metas,
- gt_bboxes_ignore=None):
- """Compute losses of the head.
-
- Args:
- tl_heats (list[Tensor]): Top-left corner heatmaps for each level
- with shape (N, num_classes, H, W).
- br_heats (list[Tensor]): Bottom-right corner heatmaps for each
- level with shape (N, num_classes, H, W).
- tl_embs (list[Tensor]): Top-left corner embeddings for each level
- with shape (N, corner_emb_channels, H, W).
- br_embs (list[Tensor]): Bottom-right corner embeddings for each
- level with shape (N, corner_emb_channels, H, W).
- tl_offs (list[Tensor]): Top-left corner offsets for each level
- with shape (N, corner_offset_channels, H, W).
- br_offs (list[Tensor]): Bottom-right corner offsets for each level
- with shape (N, corner_offset_channels, H, W).
- gt_bboxes (list[Tensor]): Ground truth bboxes for each image with
- shape (num_gts, 4) in [left, top, right, bottom] format.
- gt_labels (list[Tensor]): Class indices corresponding to each box.
- img_metas (list[dict]): Meta information of each image, e.g.,
- image size, scaling factor, etc.
- gt_bboxes_ignore (list[Tensor] | None): Specify which bounding
- boxes can be ignored when computing the loss.
-
- Returns:
- dict[str, Tensor]: A dictionary of loss components. Containing the
- following losses:
-
- - det_loss (list[Tensor]): Corner keypoint losses of all
- feature levels.
- - pull_loss (list[Tensor]): Part one of AssociativeEmbedding
- losses of all feature levels.
- - push_loss (list[Tensor]): Part two of AssociativeEmbedding
- losses of all feature levels.
- - off_loss (list[Tensor]): Corner offset losses of all feature
- levels.
- """
- targets = self.get_targets(
- gt_bboxes,
- gt_labels,
- tl_heats[-1].shape,
- img_metas[0]['pad_shape'],
- with_corner_emb=self.with_corner_emb)
- mlvl_targets = [targets for _ in range(self.num_feat_levels)]
- det_losses, pull_losses, push_losses, off_losses = multi_apply(
- self.loss_single, tl_heats, br_heats, tl_embs, br_embs, tl_offs,
- br_offs, mlvl_targets)
- loss_dict = dict(det_loss=det_losses, off_loss=off_losses)
- if self.with_corner_emb:
- loss_dict.update(pull_loss=pull_losses, push_loss=push_losses)
- return loss_dict
-
- def loss_single(self, tl_hmp, br_hmp, tl_emb, br_emb, tl_off, br_off,
- targets):
- """Compute losses for single level.
-
- Args:
- tl_hmp (Tensor): Top-left corner heatmap for current level with
- shape (N, num_classes, H, W).
- br_hmp (Tensor): Bottom-right corner heatmap for current level with
- shape (N, num_classes, H, W).
- tl_emb (Tensor): Top-left corner embedding for current level with
- shape (N, corner_emb_channels, H, W).
- br_emb (Tensor): Bottom-right corner embedding for current level
- with shape (N, corner_emb_channels, H, W).
- tl_off (Tensor): Top-left corner offset for current level with
- shape (N, corner_offset_channels, H, W).
- br_off (Tensor): Bottom-right corner offset for current level with
- shape (N, corner_offset_channels, H, W).
- targets (dict): Corner target generated by `get_targets`.
-
- Returns:
- tuple[torch.Tensor]: Losses of the head's differnet branches
- containing the following losses:
-
- - det_loss (Tensor): Corner keypoint loss.
- - pull_loss (Tensor): Part one of AssociativeEmbedding loss.
- - push_loss (Tensor): Part two of AssociativeEmbedding loss.
- - off_loss (Tensor): Corner offset loss.
- """
- gt_tl_hmp = targets['topleft_heatmap']
- gt_br_hmp = targets['bottomright_heatmap']
- gt_tl_off = targets['topleft_offset']
- gt_br_off = targets['bottomright_offset']
- gt_embedding = targets['corner_embedding']
-
- # Detection loss
- tl_det_loss = self.loss_heatmap(
- tl_hmp.sigmoid(),
- gt_tl_hmp,
- avg_factor=max(1,
- gt_tl_hmp.eq(1).sum()))
- br_det_loss = self.loss_heatmap(
- br_hmp.sigmoid(),
- gt_br_hmp,
- avg_factor=max(1,
- gt_br_hmp.eq(1).sum()))
- det_loss = (tl_det_loss + br_det_loss) / 2.0
-
- # AssociativeEmbedding loss
- if self.with_corner_emb and self.loss_embedding is not None:
- pull_loss, push_loss = self.loss_embedding(tl_emb, br_emb,
- gt_embedding)
- else:
- pull_loss, push_loss = None, None
-
- # Offset loss
- # We only compute the offset loss at the real corner position.
- # The value of real corner would be 1 in heatmap ground truth.
- # The mask is computed in class agnostic mode and its shape is
- # batch * 1 * width * height.
- tl_off_mask = gt_tl_hmp.eq(1).sum(1).gt(0).unsqueeze(1).type_as(
- gt_tl_hmp)
- br_off_mask = gt_br_hmp.eq(1).sum(1).gt(0).unsqueeze(1).type_as(
- gt_br_hmp)
- tl_off_loss = self.loss_offset(
- tl_off,
- gt_tl_off,
- tl_off_mask,
- avg_factor=max(1, tl_off_mask.sum()))
- br_off_loss = self.loss_offset(
- br_off,
- gt_br_off,
- br_off_mask,
- avg_factor=max(1, br_off_mask.sum()))
-
- off_loss = (tl_off_loss + br_off_loss) / 2.0
-
- return det_loss, pull_loss, push_loss, off_loss
-
- def get_bboxes(self,
- tl_heats,
- br_heats,
- tl_embs,
- br_embs,
- tl_offs,
- br_offs,
- img_metas,
- rescale=False,
- with_nms=True):
- """Transform network output for a batch into bbox predictions.
-
- Args:
- tl_heats (list[Tensor]): Top-left corner heatmaps for each level
- with shape (N, num_classes, H, W).
- br_heats (list[Tensor]): Bottom-right corner heatmaps for each
- level with shape (N, num_classes, H, W).
- tl_embs (list[Tensor]): Top-left corner embeddings for each level
- with shape (N, corner_emb_channels, H, W).
- br_embs (list[Tensor]): Bottom-right corner embeddings for each
- level with shape (N, corner_emb_channels, H, W).
- tl_offs (list[Tensor]): Top-left corner offsets for each level
- with shape (N, corner_offset_channels, H, W).
- br_offs (list[Tensor]): Bottom-right corner offsets for each level
- with shape (N, corner_offset_channels, H, W).
- img_metas (list[dict]): Meta information of each image, e.g.,
- image size, scaling factor, etc.
- rescale (bool): If True, return boxes in original image space.
- Default: False.
- with_nms (bool): If True, do nms before return boxes.
- Default: True.
- """
- assert tl_heats[-1].shape[0] == br_heats[-1].shape[0] == len(img_metas)
- result_list = []
- for img_id in range(len(img_metas)):
- result_list.append(
- self._get_bboxes_single(
- tl_heats[-1][img_id:img_id + 1, :],
- br_heats[-1][img_id:img_id + 1, :],
- tl_offs[-1][img_id:img_id + 1, :],
- br_offs[-1][img_id:img_id + 1, :],
- img_metas[img_id],
- tl_emb=tl_embs[-1][img_id:img_id + 1, :],
- br_emb=br_embs[-1][img_id:img_id + 1, :],
- rescale=rescale,
- with_nms=with_nms))
-
- return result_list
-
- def _get_bboxes_single(self,
- tl_heat,
- br_heat,
- tl_off,
- br_off,
- img_meta,
- tl_emb=None,
- br_emb=None,
- tl_centripetal_shift=None,
- br_centripetal_shift=None,
- rescale=False,
- with_nms=True):
- """Transform outputs for a single batch item into bbox predictions.
-
- Args:
- tl_heat (Tensor): Top-left corner heatmap for current level with
- shape (N, num_classes, H, W).
- br_heat (Tensor): Bottom-right corner heatmap for current level
- with shape (N, num_classes, H, W).
- tl_off (Tensor): Top-left corner offset for current level with
- shape (N, corner_offset_channels, H, W).
- br_off (Tensor): Bottom-right corner offset for current level with
- shape (N, corner_offset_channels, H, W).
- img_meta (dict): Meta information of current image, e.g.,
- image size, scaling factor, etc.
- tl_emb (Tensor): Top-left corner embedding for current level with
- shape (N, corner_emb_channels, H, W).
- br_emb (Tensor): Bottom-right corner embedding for current level
- with shape (N, corner_emb_channels, H, W).
- tl_centripetal_shift: Top-left corner's centripetal shift for
- current level with shape (N, 2, H, W).
- br_centripetal_shift: Bottom-right corner's centripetal shift for
- current level with shape (N, 2, H, W).
- rescale (bool): If True, return boxes in original image space.
- Default: False.
- with_nms (bool): If True, do nms before return boxes.
- Default: True.
- """
- if isinstance(img_meta, (list, tuple)):
- img_meta = img_meta[0]
-
- batch_bboxes, batch_scores, batch_clses = self.decode_heatmap(
- tl_heat=tl_heat.sigmoid(),
- br_heat=br_heat.sigmoid(),
- tl_off=tl_off,
- br_off=br_off,
- tl_emb=tl_emb,
- br_emb=br_emb,
- tl_centripetal_shift=tl_centripetal_shift,
- br_centripetal_shift=br_centripetal_shift,
- img_meta=img_meta,
- k=self.test_cfg.corner_topk,
- kernel=self.test_cfg.local_maximum_kernel,
- distance_threshold=self.test_cfg.distance_threshold)
-
- if rescale:
- batch_bboxes /= batch_bboxes.new_tensor(img_meta['scale_factor'])
-
- bboxes = batch_bboxes.view([-1, 4])
- scores = batch_scores.view([-1, 1])
- clses = batch_clses.view([-1, 1])
-
- idx = scores.argsort(dim=0, descending=True)
- bboxes = bboxes[idx].view([-1, 4])
- scores = scores[idx].view(-1)
- clses = clses[idx].view(-1)
-
- detections = torch.cat([bboxes, scores.unsqueeze(-1)], -1)
- keepinds = (detections[:, -1] > -0.1)
- detections = detections[keepinds]
- labels = clses[keepinds]
-
- if with_nms:
- detections, labels = self._bboxes_nms(detections, labels,
- self.test_cfg)
-
- return detections, labels
-
- def _bboxes_nms(self, bboxes, labels, cfg):
- if labels.numel() == 0:
- return bboxes, labels
-
- if 'nms_cfg' in cfg:
- warning.warn('nms_cfg in test_cfg will be deprecated. '
- 'Please rename it as nms')
- if 'nms' not in cfg:
- cfg.nms = cfg.nms_cfg
-
- out_bboxes, keep = batched_nms(bboxes[:, :4], bboxes[:, -1], labels,
- cfg.nms)
- out_labels = labels[keep]
-
- if len(out_bboxes) > 0:
- idx = torch.argsort(out_bboxes[:, -1], descending=True)
- idx = idx[:cfg.max_per_img]
- out_bboxes = out_bboxes[idx]
- out_labels = out_labels[idx]
-
- return out_bboxes, out_labels
-
- def _gather_feat(self, feat, ind, mask=None):
- """Gather feature according to index.
-
- Args:
- feat (Tensor): Target feature map.
- ind (Tensor): Target coord index.
- mask (Tensor | None): Mask of featuremap. Default: None.
-
- Returns:
- feat (Tensor): Gathered feature.
- """
- dim = feat.size(2)
- ind = ind.unsqueeze(2).repeat(1, 1, dim)
- feat = feat.gather(1, ind)
- if mask is not None:
- mask = mask.unsqueeze(2).expand_as(feat)
- feat = feat[mask]
- feat = feat.view(-1, dim)
- return feat
-
- def _local_maximum(self, heat, kernel=3):
- """Extract local maximum pixel with given kernel.
-
- Args:
- heat (Tensor): Target heatmap.
- kernel (int): Kernel size of max pooling. Default: 3.
-
- Returns:
- heat (Tensor): A heatmap where local maximum pixels maintain its
- own value and other positions are 0.
- """
- pad = (kernel - 1) // 2
- hmax = F.max_pool2d(heat, kernel, stride=1, padding=pad)
- keep = (hmax == heat).float()
- return heat * keep
-
- def _transpose_and_gather_feat(self, feat, ind):
- """Transpose and gather feature according to index.
-
- Args:
- feat (Tensor): Target feature map.
- ind (Tensor): Target coord index.
-
- Returns:
- feat (Tensor): Transposed and gathered feature.
- """
- feat = feat.permute(0, 2, 3, 1).contiguous()
- feat = feat.view(feat.size(0), -1, feat.size(3))
- feat = self._gather_feat(feat, ind)
- return feat
-
- def _topk(self, scores, k=20):
- """Get top k positions from heatmap.
-
- Args:
- scores (Tensor): Target heatmap with shape
- [batch, num_classes, height, width].
- k (int): Target number. Default: 20.
-
- Returns:
- tuple[torch.Tensor]: Scores, indexes, categories and coords of
- topk keypoint. Containing following Tensors:
-
- - topk_scores (Tensor): Max scores of each topk keypoint.
- - topk_inds (Tensor): Indexes of each topk keypoint.
- - topk_clses (Tensor): Categories of each topk keypoint.
- - topk_ys (Tensor): Y-coord of each topk keypoint.
- - topk_xs (Tensor): X-coord of each topk keypoint.
- """
- batch, _, height, width = scores.size()
- topk_scores, topk_inds = torch.topk(scores.view(batch, -1), k)
- topk_clses = topk_inds // (height * width)
- topk_inds = topk_inds % (height * width)
- topk_ys = topk_inds // width
- topk_xs = (topk_inds % width).int().float()
- return topk_scores, topk_inds, topk_clses, topk_ys, topk_xs
-
- def decode_heatmap(self,
- tl_heat,
- br_heat,
- tl_off,
- br_off,
- tl_emb=None,
- br_emb=None,
- tl_centripetal_shift=None,
- br_centripetal_shift=None,
- img_meta=None,
- k=100,
- kernel=3,
- distance_threshold=0.5,
- num_dets=1000):
- """Transform outputs for a single batch item into raw bbox predictions.
-
- Args:
- tl_heat (Tensor): Top-left corner heatmap for current level with
- shape (N, num_classes, H, W).
- br_heat (Tensor): Bottom-right corner heatmap for current level
- with shape (N, num_classes, H, W).
- tl_off (Tensor): Top-left corner offset for current level with
- shape (N, corner_offset_channels, H, W).
- br_off (Tensor): Bottom-right corner offset for current level with
- shape (N, corner_offset_channels, H, W).
- tl_emb (Tensor | None): Top-left corner embedding for current
- level with shape (N, corner_emb_channels, H, W).
- br_emb (Tensor | None): Bottom-right corner embedding for current
- level with shape (N, corner_emb_channels, H, W).
- tl_centripetal_shift (Tensor | None): Top-left centripetal shift
- for current level with shape (N, 2, H, W).
- br_centripetal_shift (Tensor | None): Bottom-right centripetal
- shift for current level with shape (N, 2, H, W).
- img_meta (dict): Meta information of current image, e.g.,
- image size, scaling factor, etc.
- k (int): Get top k corner keypoints from heatmap.
- kernel (int): Max pooling kernel for extract local maximum pixels.
- distance_threshold (float): Distance threshold. Top-left and
- bottom-right corner keypoints with feature distance less than
- the threshold will be regarded as keypoints from same object.
- num_dets (int): Num of raw boxes before doing nms.
-
- Returns:
- tuple[torch.Tensor]: Decoded output of CornerHead, containing the
- following Tensors:
-
- - bboxes (Tensor): Coords of each box.
- - scores (Tensor): Scores of each box.
- - clses (Tensor): Categories of each box.
- """
- with_embedding = tl_emb is not None and br_emb is not None
- with_centripetal_shift = (
- tl_centripetal_shift is not None
- and br_centripetal_shift is not None)
- assert with_embedding + with_centripetal_shift == 1
- batch, _, height, width = tl_heat.size()
- inp_h, inp_w, _ = img_meta['pad_shape']
-
- # perform nms on heatmaps
- tl_heat = self._local_maximum(tl_heat, kernel=kernel)
- br_heat = self._local_maximum(br_heat, kernel=kernel)
-
- tl_scores, tl_inds, tl_clses, tl_ys, tl_xs = self._topk(tl_heat, k=k)
- br_scores, br_inds, br_clses, br_ys, br_xs = self._topk(br_heat, k=k)
-
- # We use repeat instead of expand here because expand is a
- # shallow-copy function. Thus it could cause unexpected testing result
- # sometimes. Using expand will decrease about 10% mAP during testing
- # compared to repeat.
- tl_ys = tl_ys.view(batch, k, 1).repeat(1, 1, k)
- tl_xs = tl_xs.view(batch, k, 1).repeat(1, 1, k)
- br_ys = br_ys.view(batch, 1, k).repeat(1, k, 1)
- br_xs = br_xs.view(batch, 1, k).repeat(1, k, 1)
-
- tl_off = self._transpose_and_gather_feat(tl_off, tl_inds)
- tl_off = tl_off.view(batch, k, 1, 2)
- br_off = self._transpose_and_gather_feat(br_off, br_inds)
- br_off = br_off.view(batch, 1, k, 2)
-
- tl_xs = tl_xs + tl_off[..., 0]
- tl_ys = tl_ys + tl_off[..., 1]
- br_xs = br_xs + br_off[..., 0]
- br_ys = br_ys + br_off[..., 1]
-
- if with_centripetal_shift:
- tl_centripetal_shift = self._transpose_and_gather_feat(
- tl_centripetal_shift, tl_inds).view(batch, k, 1, 2).exp()
- br_centripetal_shift = self._transpose_and_gather_feat(
- br_centripetal_shift, br_inds).view(batch, 1, k, 2).exp()
-
- tl_ctxs = tl_xs + tl_centripetal_shift[..., 0]
- tl_ctys = tl_ys + tl_centripetal_shift[..., 1]
- br_ctxs = br_xs - br_centripetal_shift[..., 0]
- br_ctys = br_ys - br_centripetal_shift[..., 1]
-
- # all possible boxes based on top k corners (ignoring class)
- tl_xs *= (inp_w / width)
- tl_ys *= (inp_h / height)
- br_xs *= (inp_w / width)
- br_ys *= (inp_h / height)
-
- if with_centripetal_shift:
- tl_ctxs *= (inp_w / width)
- tl_ctys *= (inp_h / height)
- br_ctxs *= (inp_w / width)
- br_ctys *= (inp_h / height)
-
- x_off = img_meta['border'][2]
- y_off = img_meta['border'][0]
-
- tl_xs -= x_off
- tl_ys -= y_off
- br_xs -= x_off
- br_ys -= y_off
-
- tl_xs *= tl_xs.gt(0.0).type_as(tl_xs)
- tl_ys *= tl_ys.gt(0.0).type_as(tl_ys)
- br_xs *= br_xs.gt(0.0).type_as(br_xs)
- br_ys *= br_ys.gt(0.0).type_as(br_ys)
-
- bboxes = torch.stack((tl_xs, tl_ys, br_xs, br_ys), dim=3)
- area_bboxes = ((br_xs - tl_xs) * (br_ys - tl_ys)).abs()
-
- if with_centripetal_shift:
- tl_ctxs -= x_off
- tl_ctys -= y_off
- br_ctxs -= x_off
- br_ctys -= y_off
-
- tl_ctxs *= tl_ctxs.gt(0.0).type_as(tl_ctxs)
- tl_ctys *= tl_ctys.gt(0.0).type_as(tl_ctys)
- br_ctxs *= br_ctxs.gt(0.0).type_as(br_ctxs)
- br_ctys *= br_ctys.gt(0.0).type_as(br_ctys)
-
- ct_bboxes = torch.stack((tl_ctxs, tl_ctys, br_ctxs, br_ctys),
- dim=3)
- area_ct_bboxes = ((br_ctxs - tl_ctxs) * (br_ctys - tl_ctys)).abs()
-
- rcentral = torch.zeros_like(ct_bboxes)
- # magic nums from paper section 4.1
- mu = torch.ones_like(area_bboxes) / 2.4
- mu[area_bboxes > 3500] = 1 / 2.1 # large bbox have smaller mu
-
- bboxes_center_x = (bboxes[..., 0] + bboxes[..., 2]) / 2
- bboxes_center_y = (bboxes[..., 1] + bboxes[..., 3]) / 2
- rcentral[..., 0] = bboxes_center_x - mu * (bboxes[..., 2] -
- bboxes[..., 0]) / 2
- rcentral[..., 1] = bboxes_center_y - mu * (bboxes[..., 3] -
- bboxes[..., 1]) / 2
- rcentral[..., 2] = bboxes_center_x + mu * (bboxes[..., 2] -
- bboxes[..., 0]) / 2
- rcentral[..., 3] = bboxes_center_y + mu * (bboxes[..., 3] -
- bboxes[..., 1]) / 2
- area_rcentral = ((rcentral[..., 2] - rcentral[..., 0]) *
- (rcentral[..., 3] - rcentral[..., 1])).abs()
- dists = area_ct_bboxes / area_rcentral
-
- tl_ctx_inds = (ct_bboxes[..., 0] <= rcentral[..., 0]) | (
- ct_bboxes[..., 0] >= rcentral[..., 2])
- tl_cty_inds = (ct_bboxes[..., 1] <= rcentral[..., 1]) | (
- ct_bboxes[..., 1] >= rcentral[..., 3])
- br_ctx_inds = (ct_bboxes[..., 2] <= rcentral[..., 0]) | (
- ct_bboxes[..., 2] >= rcentral[..., 2])
- br_cty_inds = (ct_bboxes[..., 3] <= rcentral[..., 1]) | (
- ct_bboxes[..., 3] >= rcentral[..., 3])
-
- if with_embedding:
- tl_emb = self._transpose_and_gather_feat(tl_emb, tl_inds)
- tl_emb = tl_emb.view(batch, k, 1)
- br_emb = self._transpose_and_gather_feat(br_emb, br_inds)
- br_emb = br_emb.view(batch, 1, k)
- dists = torch.abs(tl_emb - br_emb)
-
- tl_scores = tl_scores.view(batch, k, 1).repeat(1, 1, k)
- br_scores = br_scores.view(batch, 1, k).repeat(1, k, 1)
-
- scores = (tl_scores + br_scores) / 2 # scores for all possible boxes
-
- # tl and br should have same class
- tl_clses = tl_clses.view(batch, k, 1).repeat(1, 1, k)
- br_clses = br_clses.view(batch, 1, k).repeat(1, k, 1)
- cls_inds = (tl_clses != br_clses)
-
- # reject boxes based on distances
- dist_inds = dists > distance_threshold
-
- # reject boxes based on widths and heights
- width_inds = (br_xs <= tl_xs)
- height_inds = (br_ys <= tl_ys)
-
- scores[cls_inds] = -1
- scores[width_inds] = -1
- scores[height_inds] = -1
- scores[dist_inds] = -1
- if with_centripetal_shift:
- scores[tl_ctx_inds] = -1
- scores[tl_cty_inds] = -1
- scores[br_ctx_inds] = -1
- scores[br_cty_inds] = -1
-
- scores = scores.view(batch, -1)
- scores, inds = torch.topk(scores, num_dets)
- scores = scores.unsqueeze(2)
-
- bboxes = bboxes.view(batch, -1, 4)
- bboxes = self._gather_feat(bboxes, inds)
-
- clses = tl_clses.contiguous().view(batch, -1, 1)
- clses = self._gather_feat(clses, inds).float()
-
- return bboxes, scores, clses
diff --git a/spaces/dinhminh20521597/OCR_DEMO/app_pages/ocr_comparator.py b/spaces/dinhminh20521597/OCR_DEMO/app_pages/ocr_comparator.py
deleted file mode 100644
index 6b5ec1934b01d9be96adc9d60bdabbc0a3e6e62f..0000000000000000000000000000000000000000
--- a/spaces/dinhminh20521597/OCR_DEMO/app_pages/ocr_comparator.py
+++ /dev/null
@@ -1,1380 +0,0 @@
-"""This Streamlit app allows you to compare, from a given image, the results of different solutions:
- EasyOcr, PaddleOCR, MMOCR, Tesseract
-"""
-import streamlit as st
-import plotly.express as px
-import numpy as np
-import math
-import pandas as pd
-from time import sleep
-
-import cv2
-from PIL import Image, ImageColor
-import PIL
-import easyocr
-from paddleocr import PaddleOCR
-from mmocr.utils.ocr import MMOCR
-import pytesseract
-from pytesseract import Output
-import os
-from mycolorpy import colorlist as mcp
-
-
-###################################################################################################
-## MAIN
-###################################################################################################
-def app():
-
- ###################################################################################################
- ## FUNCTIONS
- ###################################################################################################
-
- @st.cache_data
- def convert_df(in_df):
- """Convert data frame function, used by download button
-
- Args:
- in_df (data frame): data frame to convert
-
- Returns:
- data frame: converted data frame
- """
- # IMPORTANT: Cache the conversion to prevent computation on every rerun
- return in_df.to_csv().encode('utf-8')
-
- ###
- def easyocr_coord_convert(in_list_coord):
- """Convert easyocr coordinates to standard format used by others functions
-
- Args:
- in_list_coord (list of numbers): format [x_min, x_max, y_min, y_max]
-
- Returns:
- list of lists: format [ [x_min, y_min], [x_max, y_min], [x_max, y_max], [x_min, y_max] ]
- """
-
- coord = in_list_coord
- return [[coord[0], coord[2]], [coord[1], coord[2]], [coord[1], coord[3]], [coord[0], coord[3]]]
-
- ###
- @st.cache_data
- def initializations():
- """Initializations for the app
-
- Returns:
- list of strings : list of OCR solutions names
- (['EasyOCR', 'PPOCR', 'MMOCR', 'Tesseract'])
- dict : names and indices of the OCR solutions
- ({'EasyOCR': 0, 'PPOCR': 1, 'MMOCR': 2, 'Tesseract': 3})
- list of dicts : list of languages supported by each OCR solution
- list of int : columns for recognition details results
- dict : confidence color scale
- plotly figure : confidence color scale figure
- """
- # the readers considered
- out_reader_type_list = ['EasyOCR', 'PPOCR', 'MMOCR', 'Tesseract']
- out_reader_type_dict = {'EasyOCR': 0, 'PPOCR': 1, 'MMOCR': 2, 'Tesseract': 3}
-
- # Columns for recognition details results
- out_cols_size = [2] + [2,1]*(len(out_reader_type_list)-1) # Except Tesseract
-
- # Dicts of laguages supported by each reader
- # out_dict_lang_easyocr = {'Abaza': 'abq', 'Adyghe': 'ady', 'Afrikaans': 'af', 'Angika': 'ang', \
- # 'Arabic': 'ar', 'Assamese': 'as', 'Avar': 'ava', 'Azerbaijani': 'az', 'Belarusian': 'be', \
- # 'Bulgarian': 'bg', 'Bihari': 'bh', 'Bhojpuri': 'bho', 'Bengali': 'bn', 'Bosnian': 'bs', \
- # 'Simplified Chinese': 'ch_sim', 'Traditional Chinese': 'ch_tra', 'Chechen': 'che', \
- # 'Czech': 'cs', 'Welsh': 'cy', 'Danish': 'da', 'Dargwa': 'dar', 'German': 'de', \
- # 'English': 'en', 'Spanish': 'es', 'Estonian': 'et', 'Persian (Farsi)': 'fa', 'French': 'fr', \
- # 'Irish': 'ga', 'Goan Konkani': 'gom', 'Hindi': 'hi', 'Croatian': 'hr', 'Hungarian': 'hu', \
- # 'Indonesian': 'id', 'Ingush': 'inh', 'Icelandic': 'is', 'Italian': 'it', 'Japanese': 'ja', \
- # 'Kabardian': 'kbd', 'Kannada': 'kn', 'Korean': 'ko', 'Kurdish': 'ku', 'Latin': 'la', \
- # 'Lak': 'lbe', 'Lezghian': 'lez', 'Lithuanian': 'lt', 'Latvian': 'lv', 'Magahi': 'mah', \
- # 'Maithili': 'mai', 'Maori': 'mi', 'Mongolian': 'mn', 'Marathi': 'mr', 'Malay': 'ms', \
- # 'Maltese': 'mt', 'Nepali': 'ne', 'Newari': 'new', 'Dutch': 'nl', 'Norwegian': 'no', \
- # 'Occitan': 'oc', 'Pali': 'pi', 'Polish': 'pl', 'Portuguese': 'pt', 'Romanian': 'ro', \
- # 'Russian': 'ru', 'Serbian (cyrillic)': 'rs_cyrillic', 'Serbian (latin)': 'rs_latin', \
- # 'Nagpuri': 'sck', 'Slovak': 'sk', 'Slovenian': 'sl', 'Albanian': 'sq', 'Swedish': 'sv', \
- # 'Swahili': 'sw', 'Tamil': 'ta', 'Tabassaran': 'tab', 'Telugu': 'te', 'Thai': 'th', \
- # 'Tajik': 'tjk', 'Tagalog': 'tl', 'Turkish': 'tr', 'Uyghur': 'ug', 'Ukranian': 'uk', \
- # 'Urdu': 'ur', 'Uzbek': 'uz', 'Vietnamese': 'vi'}
-
- # out_dict_lang_ppocr = {'Abaza': 'abq', 'Adyghe': 'ady', 'Afrikaans': 'af', 'Albanian': 'sq', \
- # 'Angika': 'ang', 'Arabic': 'ar', 'Avar': 'ava', 'Azerbaijani': 'az', 'Belarusian': 'be', \
- # 'Bhojpuri': 'bho','Bihari': 'bh','Bosnian': 'bs','Bulgarian': 'bg','Chinese & English': 'ch', \
- # 'Chinese Traditional': 'chinese_cht', 'Croatian': 'hr', 'Czech': 'cs', 'Danish': 'da', \
- # 'Dargwa': 'dar', 'Dutch': 'nl', 'English': 'en', 'Estonian': 'et', 'French': 'fr', \
- # 'German': 'german','Goan Konkani': 'gom','Hindi': 'hi','Hungarian': 'hu','Icelandic': 'is', \
- # 'Indonesian': 'id', 'Ingush': 'inh', 'Irish': 'ga', 'Italian': 'it', 'Japan': 'japan', \
- # 'Kabardian': 'kbd', 'Korean': 'korean', 'Kurdish': 'ku', 'Lak': 'lbe', 'Latvian': 'lv', \
- # 'Lezghian': 'lez', 'Lithuanian': 'lt', 'Magahi': 'mah', 'Maithili': 'mai', 'Malay': 'ms', \
- # 'Maltese': 'mt', 'Maori': 'mi', 'Marathi': 'mr', 'Mongolian': 'mn', 'Nagpur': 'sck', \
- # 'Nepali': 'ne', 'Newari': 'new', 'Norwegian': 'no', 'Occitan': 'oc', 'Persian': 'fa', \
- # 'Polish': 'pl', 'Portuguese': 'pt', 'Romanian': 'ro', 'Russia': 'ru', 'Saudi Arabia': 'sa', \
- # 'Serbian(cyrillic)': 'rs_cyrillic', 'Serbian(latin)': 'rs_latin', 'Slovak': 'sk', \
- # 'Slovenian': 'sl', 'Spanish': 'es', 'Swahili': 'sw', 'Swedish': 'sv', 'Tabassaran': 'tab', \
- # 'Tagalog': 'tl', 'Tamil': 'ta', 'Telugu': 'te', 'Turkish': 'tr', 'Ukranian': 'uk', \
- # 'Urdu': 'ur', 'Uyghur': 'ug', 'Uzbek': 'uz', 'Vietnamese': 'vi', 'Welsh': 'cy'}
-
- out_dict_lang_mmocr = {'English': 'en'}
-
- # out_dict_lang_tesseract = {'Afrikaans': 'afr','Albanian': 'sqi','Amharic': 'amh', \
- # 'Arabic': 'ara', 'Armenian': 'hye','Assamese': 'asm','Azerbaijani - Cyrilic': 'aze_cyrl', \
- # 'Azerbaijani': 'aze', 'Basque': 'eus','Belarusian': 'bel','Bengali': 'ben','Bosnian': 'bos', \
- # 'Breton': 'bre', 'Bulgarian': 'bul','Burmese': 'mya','Catalan; Valencian': 'cat', \
- # 'Cebuano': 'ceb', 'Central Khmer': 'khm','Cherokee': 'chr','Chinese - Simplified': 'chi_sim', \
- # 'Chinese - Traditional': 'chi_tra','Corsican': 'cos','Croatian': 'hrv','Czech': 'ces', \
- # 'Danish':'dan','Dutch; Flemish':'nld','Dzongkha':'dzo','English, Middle (1100-1500)':'enm', \
- # 'English': 'eng','Esperanto': 'epo','Estonian': 'est','Faroese': 'fao', \
- # 'Filipino (old - Tagalog)': 'fil','Finnish': 'fin','French, Middle (ca.1400-1600)': 'frm', \
- # 'French': 'fra','Galician': 'glg','Georgian - Old': 'kat_old','Georgian': 'kat', \
- # 'German - Fraktur': 'frk','German': 'deu','Greek, Modern (1453-)': 'ell','Gujarati': 'guj', \
- # 'Haitian; Haitian Creole': 'hat','Hebrew': 'heb','Hindi': 'hin','Hungarian': 'hun', \
- # 'Icelandic': 'isl','Indonesian': 'ind','Inuktitut': 'iku','Irish': 'gle', \
- # 'Italian - Old': 'ita_old','Italian': 'ita','Japanese': 'jpn','Javanese': 'jav', \
- # 'Kannada': 'kan','Kazakh': 'kaz','Kirghiz; Kyrgyz': 'kir','Korean (vertical)': 'kor_vert', \
- # 'Korean': 'kor','Kurdish (Arabic Script)': 'kur_ara','Lao': 'lao','Latin': 'lat', \
- # 'Latvian':'lav','Lithuanian':'lit','Luxembourgish':'ltz','Macedonian':'mkd','Malay':'msa', \
- # 'Malayalam': 'mal','Maltese': 'mlt','Maori': 'mri','Marathi': 'mar','Mongolian': 'mon', \
- # 'Nepali': 'nep','Norwegian': 'nor','Occitan (post 1500)': 'oci', \
- # 'Orientation and script detection module':'osd','Oriya':'ori','Panjabi; Punjabi':'pan', \
- # 'Persian':'fas','Polish':'pol','Portuguese':'por','Pushto; Pashto':'pus','Quechua':'que', \
- # 'Romanian; Moldavian; Moldovan': 'ron','Russian': 'rus','Sanskrit': 'san', \
- # 'Scottish Gaelic': 'gla','Serbian - Latin': 'srp_latn','Serbian': 'srp','Sindhi': 'snd', \
- # 'Sinhala; Sinhalese': 'sin','Slovak': 'slk','Slovenian': 'slv', \
- # 'Spanish; Castilian - Old': 'spa_old','Spanish; Castilian': 'spa','Sundanese': 'sun', \
- # 'Swahili': 'swa','Swedish': 'swe','Syriac': 'syr','Tajik': 'tgk','Tamil': 'tam', \
- # 'Tatar':'tat','Telugu':'tel','Thai':'tha','Tibetan':'bod','Tigrinya':'tir','Tonga':'ton', \
- # 'Turkish': 'tur','Uighur; Uyghur': 'uig','Ukrainian': 'ukr','Urdu': 'urd', \
- # 'Uzbek - Cyrilic': 'uzb_cyrl','Uzbek': 'uzb','Vietnamese': 'vie','Welsh': 'cym', \
- # 'Western Frisian': 'fry','Yiddish': 'yid','Yoruba': 'yor'}
-
- out_list_dict_lang = [out_dict_lang_mmocr]
-
- # Initialization of detection form
- if 'columns_size' not in st.session_state:
- st.session_state.columns_size = [2] + [1 for x in out_reader_type_list[1:]]
- if 'column_width' not in st.session_state:
- st.session_state.column_width = [400] + [300 for x in out_reader_type_list[1:]]
- if 'columns_color' not in st.session_state:
- st.session_state.columns_color = ["rgb(228,26,28)"] + \
- ["rgb(0,0,0)" for x in out_reader_type_list[1:]]
- if 'list_coordinates' not in st.session_state:
- st.session_state.list_coordinates = []
-
- # Confidence color scale
- out_list_confid = list(np.arange(0,101,1))
- out_list_grad = mcp.gen_color_normalized(cmap="Greens",data_arr=np.array(out_list_confid))
- out_dict_back_colors = {out_list_confid[i]: out_list_grad[i] \
- for i in range(len(out_list_confid))}
-
- list_y = [1 for i in out_list_confid]
- df_confid = pd.DataFrame({'% confidence scale': out_list_confid, 'y': list_y})
-
- out_fig = px.scatter(df_confid, x='% confidence scale', y='y', \
- hover_data={'% confidence scale': True, 'y': False},
- color=out_dict_back_colors.values(), range_y=[0.9,1.1], range_x=[0,100],
- color_discrete_map="identity",height=50,symbol='y',symbol_sequence=['square'])
- out_fig.update_xaxes(showticklabels=False)
- out_fig.update_yaxes(showticklabels=False, range=[0.1, 1.1], visible=False)
- out_fig.update_traces(marker_size=50)
- out_fig.update_layout(paper_bgcolor="white", margin=dict(b=0,r=0,t=0,l=0), xaxis_side="top", \
- showlegend=False)
-
- return out_reader_type_list, out_reader_type_dict, out_list_dict_lang, \
- out_cols_size, out_dict_back_colors, out_fig
-
- ###
- @st.cache_data
- def init_easyocr(in_params):
- """Initialization of easyOCR reader
-
- Args:
- in_params (list): list with the language
-
- Returns:
- easyocr reader: the easyocr reader instance
- """
- out_ocr = easyocr.Reader(in_params)
- return out_ocr
-
- ###
- @st.cache_data
- def init_ppocr(in_params):
- """Initialization of PPOCR reader
-
- Args:
- in_params (dict): dict with parameters
-
- Returns:
- ppocr reader: the ppocr reader instance
- """
- out_ocr = PaddleOCR(lang=in_params[0], **in_params[1])
- return out_ocr
-
- ###
- @st.cache_data
- def init_mmocr(in_params):
- """Initialization of MMOCR reader
-
- Args:
- in_params (dict): dict with parameters
-
- Returns:
- mmocr reader: the ppocr reader instance
- """
- out_ocr = MMOCR(recog=None, **in_params[1])
- return out_ocr
-
- ###
- def init_readers(in_list_params):
- """Initialization of the readers, and return them as list
-
- Args:
- in_list_params (list): list of dicts of parameters for each reader
-
- Returns:
- list: list of the reader's instances
- """
- # Instantiations of the readers :
- # - EasyOCR
- # with st.spinner("EasyOCR reader initialization in progress ..."):
- # reader_easyocr = init_easyocr([in_list_params[0][0]])
-
- # # - PPOCR
- # # Paddleocr
- # with st.spinner("PPOCR reader initialization in progress ..."):
- # reader_ppocr = init_ppocr(in_list_params[1])
-
- # - MMOCR
- with st.spinner("MMOCR reader initialization in progress ..."):
- reader_mmocr = init_mmocr(in_list_params[0])
-
- out_list_readers = [reader_mmocr]
-
- # out_list_readers = [reader_easyocr, reader_ppocr, reader_mmocr]
-
- return out_list_readers
-
- ###
- def load_image(in_image_file):
- """Load input file and open it
-
- Args:
- in_image_file (string or Streamlit UploadedFile): image to consider
-
- Returns:
- string : locally saved image path (img.)
- PIL.Image : input file opened with Pillow
- matrix : input file opened with Opencv
- """
-
- #if isinstance(in_image_file, str):
- # out_image_path = "img."+in_image_file.split('.')[-1]
- #else:
- # out_image_path = "img."+in_image_file.name.split('.')[-1]
-
- if isinstance(in_image_file, str):
- out_image_path = "tmp_"+in_image_file
- else:
- out_image_path = "tmp_"+in_image_file.name
-
- img = Image.open(in_image_file)
- img_saved = img.save(out_image_path)
-
- # Read image
- out_image_orig = Image.open(out_image_path)
- out_image_cv2 = cv2.cvtColor(cv2.imread(out_image_path), cv2.COLOR_BGR2RGB)
-
- return out_image_path, out_image_orig, out_image_cv2
-
- ###
- @st.cache_data
- def easyocr_detect(_in_reader, in_image_path, in_params):
- """Detection with EasyOCR
-
- Args:
- _in_reader (EasyOCR reader) : the previously initialized instance
- in_image_path (string ) : locally saved image path
- in_params (list) : list with the parameters for detection
-
- Returns:
- list : list of the boxes coordinates
- exception on error, string 'OK' otherwise
- """
- try:
- dict_param = in_params[1]
- detection_result = _in_reader.detect(in_image_path,
- #width_ths=0.7,
- #mag_ratio=1.5
- **dict_param
- )
- easyocr_coordinates = detection_result[0][0]
-
- # The format of the coordinate is as follows: [x_min, x_max, y_min, y_max]
- # Format boxes coordinates for draw
- out_easyocr_boxes_coordinates = list(map(easyocr_coord_convert, easyocr_coordinates))
- out_status = 'OK'
- except Exception as e:
- out_easyocr_boxes_coordinates = []
- out_status = e
-
- return out_easyocr_boxes_coordinates, out_status
-
- ###
- @st.cache_data
- def ppocr_detect(_in_reader, in_image_path):
- """Detection with PPOCR
-
- Args:
- _in_reader (PPOCR reader) : the previously initialized instance
- in_image_path (string ) : locally saved image path
-
- Returns:
- list : list of the boxes coordinates
- exception on error, string 'OK' otherwise
- """
- # PPOCR detection method
- try:
- out_ppocr_boxes_coordinates = _in_reader.ocr(in_image_path, rec=False)
- out_status = 'OK'
- except Exception as e:
- out_ppocr_boxes_coordinates = []
- out_status = e
-
- return out_ppocr_boxes_coordinates, out_status
-
- ###
- @st.cache_data
- def mmocr_detect(_in_reader, in_image_path):
- """Detection with MMOCR
-
- Args:
- _in_reader (EasyORC reader) : the previously initialized instance
- in_image_path (string) : locally saved image path
- in_params (list) : list with the parameters
-
- Returns:
- list : list of the boxes coordinates
- exception on error, string 'OK' otherwise
- """
- # MMOCR detection method
- out_mmocr_boxes_coordinates = []
- try:
- det_result = _in_reader.readtext(in_image_path, details=True)
- bboxes_list = [res['boundary_result'] for res in det_result]
- for bboxes in bboxes_list:
- for bbox in bboxes:
- if len(bbox) > 9:
- min_x = min(bbox[0:-1:2])
- min_y = min(bbox[1:-1:2])
- max_x = max(bbox[0:-1:2])
- max_y = max(bbox[1:-1:2])
- #box = [min_x, min_y, max_x, min_y, max_x, max_y, min_x, max_y]
- else:
- min_x = min(bbox[0:-1:2])
- min_y = min(bbox[1::2])
- max_x = max(bbox[0:-1:2])
- max_y = max(bbox[1::2])
- box4 = [ [min_x, min_y], [max_x, min_y], [max_x, max_y], [min_x, max_y] ]
- out_mmocr_boxes_coordinates.append(box4)
- out_status = 'OK'
- except Exception as e:
- out_status = e
-
- return out_mmocr_boxes_coordinates, out_status
-
- ###
- def cropped_1box(in_box, in_img):
- """Construction of an cropped image corresponding to an area of the initial image
-
- Args:
- in_box (list) : box with coordinates
- in_img (matrix) : image
-
- Returns:
- matrix : cropped image
- """
- box_ar = np.array(in_box).astype(np.int64)
- x_min = box_ar[:, 0].min()
- x_max = box_ar[:, 0].max()
- y_min = box_ar[:, 1].min()
- y_max = box_ar[:, 1].max()
- out_cropped = in_img[y_min:y_max, x_min:x_max]
-
- return out_cropped
-
- ###
- @st.cache_data
- def tesserocr_detect(in_image_path, _in_img, in_params):
- """Detection with Tesseract
-
- Args:
- in_image_path (string) : locally saved image path
- _in_img (PIL.Image) : image to consider
- in_params (list) : list with the parameters for detection
-
- Returns:
- list : list of the boxes coordinates
- exception on error, string 'OK' otherwise
- """
- try:
- dict_param = in_params[1]
- df_res = pytesseract.image_to_data(_in_img, **dict_param, output_type=Output.DATAFRAME)
-
- df_res['box'] = df_res.apply(lambda d: [[d['left'], d['top']], \
- [d['left'] + d['width'], d['top']], \
- [d['left'] + d['width'], d['top'] + d['height']], \
- [d['left'], d['top'] + d['height']], \
- ], axis=1)
- out_tesserocr_boxes_coordinates = df_res[df_res.word_num > 0]['box'].to_list()
- out_status = 'OK'
- except Exception as e:
- out_tesserocr_boxes_coordinates = []
- out_status = e
-
- return out_tesserocr_boxes_coordinates, out_status
-
- ###
- @st.cache_data
- def process_detect(in_image_path, _in_list_images, _in_list_readers, in_list_params, in_color):
- """Detection process for each OCR solution
-
- Args:
- in_image_path (string) : locally saved image path
- _in_list_images (list) : list of original image
- _in_list_readers (list) : list with previously initialized reader's instances
- in_list_params (list) : list with dict parameters for each OCR solution
- in_color (tuple) : color for boxes around text
-
- Returns:
- list: list of detection results images
- list: list of boxes coordinates
- """
- ## ------- EasyOCR Text detection
- # with st.spinner('EasyOCR Text detection in progress ...'):
- # easyocr_boxes_coordinates,easyocr_status = easyocr_detect(_in_list_readers[0], \
- # in_image_path, in_list_params[0])
- # # Visualization
- # if easyocr_boxes_coordinates:
- # easyocr_image_detect = draw_detected(_in_list_images[0], easyocr_boxes_coordinates, \
- # in_color, 'None', 3)
- # else:
- # easyocr_boxes_coordinates = easyocr_status
- ##
-
- ## ------- PPOCR Text detection
- # with st.spinner('PPOCR Text detection in progress ...'):
- # ppocr_boxes_coordinates, ppocr_status = ppocr_detect(_in_list_readers[1], in_image_path)
- # # Visualization
- # if ppocr_boxes_coordinates:
- # ppocr_image_detect = draw_detected(_in_list_images[0], ppocr_boxes_coordinates, \
- # in_color, 'None', 3)
- # else:
- # ppocr_image_detect = ppocr_status
- ##
-
- ## ------- MMOCR Text detection
- with st.spinner('Text detection in progress ...'):
- mmocr_boxes_coordinates, mmocr_status = mmocr_detect(_in_list_readers[0], in_image_path)
- # Visualization
- if mmocr_boxes_coordinates:
- mmocr_image_detect = draw_detected(_in_list_images[0], mmocr_boxes_coordinates, \
- in_color, 'None', 3)
- else:
- mmocr_image_detect = mmocr_status
- ##
-
- ## ------- Tesseract Text detection
- # with st.spinner('Tesseract Text detection in progress ...'):
- # tesserocr_boxes_coordinates, tesserocr_status = tesserocr_detect(in_image_path, \
- # _in_list_images[0], \
- # in_list_params[3])
- # # Visualization
- # if tesserocr_status == 'OK':
- # tesserocr_image_detect = draw_detected(_in_list_images[0],tesserocr_boxes_coordinates,\
- # in_color, 'None', 3)
- # else:
- # tesserocr_image_detect = tesserocr_status
- ##
- #
- out_list_images = _in_list_images + [ mmocr_image_detect]
- out_list_coordinates = [mmocr_boxes_coordinates]
- # out_list_images = _in_list_images + [easyocr_image_detect, ppocr_image_detect, \
- # mmocr_image_detect, tesserocr_image_detect]
- # out_list_coordinates = [easyocr_boxes_coordinates, ppocr_boxes_coordinates, \
- # mmocr_boxes_coordinates, tesserocr_boxes_coordinates]
- #
-
- return out_list_images, out_list_coordinates
-
- ###
- def draw_detected(in_image, in_boxes_coordinates, in_color, posit='None', in_thickness=4):
- """Draw boxes around detected text
-
- Args:
- in_image (PIL.Image) : original image
- in_boxes_coordinates (list) : boxes coordinates, from top to bottom and from left to right
- [ [ [x_min, y_min], [x_max, y_min], [x_max, y_max], [x_min, y_max] ],
- [ ... ]
- ]
- in_color (tuple) : color for boxes around text
- posit (str, optional) : position for text. Defaults to 'None'.
- in_thickness (int, optional): thickness of the box. Defaults to 4.
-
- Returns:
- PIL.Image : original image with detected areas
- """
- work_img = in_image.copy()
- if in_boxes_coordinates:
- font = cv2.FONT_HERSHEY_SIMPLEX
- for ind_box, box in enumerate(in_boxes_coordinates):
- box = np.reshape(np.array(box), [-1, 1, 2]).astype(np.int64)
- work_img = cv2.polylines(np.array(work_img), [box], True, in_color, in_thickness)
- if posit != 'None':
- if posit == 'top_left':
- pos = tuple(box[0][0])
- elif posit == 'top_right':
- pos = tuple(box[1][0])
- work_img = cv2.putText(work_img, str(ind_box+1), pos, font, 5.5, color, \
- in_thickness,cv2.LINE_AA)
-
- out_image_drawn = Image.fromarray(work_img)
- else:
- out_image_drawn = work_img
-
- return out_image_drawn
-
- ###
- @st.cache_data
- def get_cropped(in_boxes_coordinates, in_image_cv):
- """Construct list of cropped images corresponding of the input boxes coordinates list
-
- Args:
- in_boxes_coordinates (list) : list of boxes coordinates
- in_image_cv (matrix) : original image
-
- Returns:
- list : list with cropped images
- """
- out_list_images = []
- for box in in_boxes_coordinates:
- cropped = cropped_1box(box, in_image_cv)
- out_list_images.append(cropped)
- return out_list_images
-
- ###
- def process_recog(in_list_readers, in_image_cv, in_boxes_coordinates, in_list_dict_params):
- """Recognition process for each OCR solution
-
- Args:
- in_list_readers (list) : list with previously initialized reader's instances
- in_image_cv (matrix) : original image
- in_boxes_coordinates (list) : list of boxes coordinates
- in_list_dict_params (list) : list with dict parameters for each OCR solution
-
- Returns:
- data frame : results for each OCR solution, except Tesseract
- data frame : results for Tesseract
- list : status for each recognition (exception or 'OK')
- """
- out_df_results = pd.DataFrame([])
-
- list_text_easyocr = []
- list_confidence_easyocr = []
- list_text_ppocr = []
- list_confidence_ppocr = []
- list_text_mmocr = []
- list_confidence_mmocr = []
-
- # Create cropped images from detection
- list_cropped_images = get_cropped(in_boxes_coordinates, in_image_cv)
-
- # Recognize with EasyOCR
- # with st.spinner('EasyOCR Text recognition in progress ...'):
- # list_text_easyocr, list_confidence_easyocr, status_easyocr = \
- # easyocr_recog(list_cropped_images, in_list_readers[0], in_list_dict_params[0])
- ##
-
- # Recognize with PPOCR
- # with st.spinner('PPOCR Text recognition in progress ...'):
- # list_text_ppocr, list_confidence_ppocr, status_ppocr = \
- # ppocr_recog(list_cropped_images, in_list_dict_params[1])
- ##
-
- # Recognize with MMOCR
- with st.spinner('Text recognition in progress ...'):
- list_text_mmocr, list_confidence_mmocr, status_mmocr = \
- mmocr_recog(list_cropped_images, in_list_dict_params[0])
- ##
-
- # # Recognize with Tesseract
- # with st.spinner('Tesseract Text recognition in progress ...'):
- # out_df_results_tesseract, status_tesseract = \
- # tesserocr_recog(in_image_cv, in_list_dict_params[3], len(list_cropped_images))
- ##
-
- # Create results data frame
- out_df_results = pd.DataFrame({'cropped_image': list_cropped_images,
- 'text_mmocr': list_text_mmocr,
- 'confidence_mmocr': list_confidence_mmocr
- }
- )
-
- out_list_reco_status = [status_mmocr]
-
- return out_df_results, out_list_reco_status
-
- ###
- @st.cache_data
- def easyocr_recog(in_list_images, _in_reader_easyocr, in_params):
- """Recognition with EasyOCR
-
- Args:
- in_list_images (list) : list of cropped images
- _in_reader_easyocr (EasyOCR reader) : the previously initialized instance
- in_params (dict) : parameters for recognition
-
- Returns:
- list : list of recognized text
- list : list of recognition confidence
- string/Exception : recognition status
- """
- progress_bar = st.progress(0)
- out_list_text_easyocr = []
- out_list_confidence_easyocr = []
- ## ------- EasyOCR Text recognition
- try:
- step = 0*len(in_list_images) # first recognition process
- nb_steps = 4 * len(in_list_images)
- for ind_img, cropped in enumerate(in_list_images):
- result = _in_reader_easyocr.recognize(cropped, **in_params)
- try:
- out_list_text_easyocr.append(result[0][1])
- out_list_confidence_easyocr.append(np.round(100*result[0][2], 1))
- except:
- out_list_text_easyocr.append('Not recognize')
- out_list_confidence_easyocr.append(100.)
- progress_bar.progress((step+ind_img+1)/nb_steps)
- out_status = 'OK'
- except Exception as e:
- out_status = e
- progress_bar.empty()
-
- return out_list_text_easyocr, out_list_confidence_easyocr, out_status
-
- ###
- @st.cache_data
- def ppocr_recog(in_list_images, in_params):
- """Recognition with PPOCR
-
- Args:
- in_list_images (list) : list of cropped images
- in_params (dict) : parameters for recognition
-
- Returns:
- list : list of recognized text
- list : list of recognition confidence
- string/Exception : recognition status
- """
- ## ------- PPOCR Text recognition
- out_list_text_ppocr = []
- out_list_confidence_ppocr = []
- try:
- reader_ppocr = PaddleOCR(**in_params)
- step = 1*len(in_list_images) # second recognition process
- nb_steps = 4 * len(in_list_images)
- progress_bar = st.progress(step/nb_steps)
-
- for ind_img, cropped in enumerate(in_list_images):
- result = reader_ppocr.ocr(cropped, det=False, cls=False)
- try:
- out_list_text_ppocr.append(result[0][0])
- out_list_confidence_ppocr.append(np.round(100*result[0][1], 1))
- except:
- out_list_text_ppocr.append('Not recognize')
- out_list_confidence_ppocr.append(100.)
- progress_bar.progress((step+ind_img+1)/nb_steps)
- out_status = 'OK'
- except Exception as e:
- out_status = e
- progress_bar.empty()
-
- return out_list_text_ppocr, out_list_confidence_ppocr, out_status
-
- ###
- @st.cache_data
- def mmocr_recog(in_list_images, in_params):
- """Recognition with MMOCR
-
- Args:
- in_list_images (list) : list of cropped images
- in_params (dict) : parameters for recognition
-
- Returns:
- list : list of recognized text
- list : list of recognition confidence
- string/Exception : recognition status
- """
- ## ------- MMOCR Text recognition
- out_list_text_mmocr = []
- out_list_confidence_mmocr = []
- try:
- reader_mmocr = MMOCR(det=None, **in_params)
- step = 2*len(in_list_images) # third recognition process
- nb_steps = 4 * len(in_list_images)
- progress_bar = st.progress(step/nb_steps)
-
- for ind_img, cropped in enumerate(in_list_images):
- result = reader_mmocr.readtext(cropped, details=True)
- try:
- out_list_text_mmocr.append(result[0]['text'])
- out_list_confidence_mmocr.append(np.round(100* \
- (np.array(result[0]['score']).mean()), 1))
- except:
- out_list_text_mmocr.append('Not recognize')
- out_list_confidence_mmocr.append(100.)
- progress_bar.progress((step+ind_img+1)/nb_steps)
- out_status = 'OK'
- except Exception as e:
- out_status = e
- progress_bar.empty()
-
- return out_list_text_mmocr, out_list_confidence_mmocr, out_status
-
- ###
- @st.cache_data
- def tesserocr_recog(in_img, in_params, in_nb_images):
- """Recognition with Tesseract
-
- Args:
- in_image_cv (matrix) : original image
- in_params (dict) : parameters for recognition
- in_nb_images : nb cropped images (used for progress bar)
-
- Returns:
- Pandas data frame : recognition results
- string/Exception : recognition status
- """
- ## ------- Tesseract Text recognition
- step = 3*in_nb_images # fourth recognition process
- nb_steps = 4 * in_nb_images
- progress_bar = st.progress(step/nb_steps)
-
- try:
- out_df_result = pytesseract.image_to_data(in_img, **in_params,output_type=Output.DATAFRAME)
-
- out_df_result['box'] = out_df_result.apply(lambda d: [[d['left'], d['top']], \
- [d['left'] + d['width'], d['top']], \
- [d['left']+d['width'], d['top']+d['height']], \
- [d['left'], d['top'] + d['height']], \
- ], axis=1)
- out_df_result['cropped'] = out_df_result['box'].apply(lambda b: cropped_1box(b, in_img))
- out_df_result = out_df_result[(out_df_result.word_num > 0) & (out_df_result.text != ' ')] \
- .reset_index(drop=True)
- out_status = 'OK'
- except Exception as e:
- out_df_result = pd.DataFrame([])
- out_status = e
-
- progress_bar.progress(1.)
-
- return out_df_result, out_status
-
- ###
- def draw_reco_images(in_image, in_boxes_coordinates, in_list_texts, in_list_confid, \
- in_dict_back_colors, in_reader_type_list, \
- in_font_scale=1, in_conf_threshold=65):
- """Draw recognized text on original image, for each OCR solution used
-
- Args:
- in_image (matrix) : original image
- in_boxes_coordinates (list) : list of boxes coordinates
- in_list_texts (list): list of recognized text for each recognizer (except Tesseract)
- in_list_confid (list): list of recognition confidence for each recognizer (except Tesseract)
- in_df_results_tesseract (Pandas data frame): Tesseract recognition results
- in_font_scale (int, optional): text font scale. Defaults to 3.
-
- Returns:
- shows the results container
- """
- img = in_image.copy()
- nb_readers = 1
- # list_reco_images = img.copy()
-
- for num, box_ in enumerate(in_boxes_coordinates):
- box = np.array(box_).astype(np.int64)
-
- # For each box : draw the results of each recognizer
-
- confid = np.round(in_list_confid[0][num], 0)
- rgb_color = ImageColor.getcolor(in_dict_back_colors[confid], "RGB")
- if confid < in_conf_threshold:
- text_color = (0, 0, 0)
- else:
- text_color = (255, 255, 255)
-
- list_reco_images = cv2.rectangle(img, \
- (box[0][0], box[0][1]), \
- (box[2][0], box[2][1]), rgb_color, -1)
- list_reco_images = cv2.putText(img, \
- in_list_texts[0][num], \
- (box[0][0],int(np.round((box[0][1]+box[2][1])/2,0))), \
- cv2.FONT_HERSHEY_DUPLEX, in_font_scale, text_color, 2)
-
- # # Add Tesseract process
- # if not in_df_results_tesseract.empty:
- # ind_tessocr = nb_readers-1
- # for num, box_ in enumerate(in_df_results_tesseract['box'].to_list()):
- # box = np.array(box_).astype(np.int64)
- # confid = np.round(in_df_results_tesseract.iloc[num]['conf'], 0)
- # rgb_color = ImageColor.getcolor(in_dict_back_colors[confid], "RGB")
- # if confid < in_conf_threshold:
- # text_color = (0, 0, 0)
- # else:
- # text_color = (255, 255, 255)
-
- # list_reco_images[ind_tessocr] = \
- # cv2.rectangle(list_reco_images[ind_tessocr], (box[0][0], box[0][1]), \
- # (box[2][0], box[2][1]), rgb_color, -1)
- # try:
- # list_reco_images[ind_tessocr] = \
- # cv2.putText(list_reco_images[ind_tessocr], \
- # in_df_results_tesseract.iloc[num]['text'], \
- # (box[0][0],int(np.round((box[0][1]+box[2][1])/2,0))), \
- # cv2.FONT_HERSHEY_DUPLEX, in_font_scale, text_color, 2)
-
- # except:
-
- # pass
- with show_reco.container():
- # column_width = 400
-
- cols = st.columns((1,1))
- cols[0].image(list_reco_images,use_column_width=True)
- # with show_reco.container():
- # # Draw the results, 2 images per line
- # reco_lines = math.ceil(len(in_reader_type_list) / 2)
- # column_width = 400
- # for ind_lig in range(0, reco_lines+1, 2):
- # cols = st.columns(2)
- # for ind_col in range(2):
- # ind = ind_lig + ind_col
- # if ind <= len(in_reader_type_list):
- # if in_reader_type_list[ind] == 'Tesseract':
- # column_title = 'Recognition with ' + in_reader_type_list[ind] + \
- # ' (with its own detector) \
- #
'
- # else:
- # column_title = 'Recognition with ' + \
- # in_reader_type_list[ind]+ '
'
- # cols[ind_col].markdown(column_title, unsafe_allow_html=True)
- # if st.session_state.list_reco_status[ind] == 'OK':
- # cols[ind_col].image(list_reco_images[ind], \
- # width=column_width, use_column_width=True)
- # else:
- # cols[ind_col].write(list_reco_status[ind], \
- # use_column_width=True)
-
- # st.markdown(' 💡 Bad font size? you can adjust it below and refresh:')
-
- ###
- def highlight():
- """ Highlight MMOCR results """
- with show_detect.container():
- column_title = 'Detection with MMOCR
'
- show_detect.markdown(column_title, unsafe_allow_html=True)
- if isinstance(list_images[2], PIL.Image.Image):
- show_detect.image(list_images[2], width=400, use_column_width=True)
- else:
- show_detect.write(list_images[2], use_column_width=True)
-
-
- ###
- @st.cache_data
- def get_demo():
- """Get the demo files
-
- Returns:
- PIL.Image : input file opened with Pillow
- PIL.Image : input file opened with Pillow
- """
-
- out_img_demo_1 = Image.open("img_demo_1.jpg")
- out_img_demo_2 = Image.open("img_demo_2.jpg")
-
- return out_img_demo_1, out_img_demo_2
-
- ###
- def raz():
- st.session_state.list_coordinates = []
- st.session_state.list_images = []
- st.session_state.detect_reader = reader_type_list[0]
-
- st.session_state.columns_size = [2] + [1 for x in reader_type_list[1:]]
- st.session_state.column_width = [400] + [300 for x in reader_type_list[1:]]
- st.session_state.columns_color = ["rgb(228,26,28)"] + \
- ["rgb(0,0,0)" for x in reader_type_list[1:]]
-
- # Clear caches
- easyocr_detect.clear()
- ppocr_detect.clear()
- mmocr_detect.clear()
- tesserocr_detect.clear()
- process_detect.clear()
- get_cropped.clear()
- easyocr_recog.clear()
- ppocr_recog.clear()
- mmocr_recog.clear()
- tesserocr_recog.clear()
-
-
- ##----------- Initializations ---------------------------------------------------------------------
- #print("PID : ", os.getpid())
-
- st.title("Scene text detection DEMO apps")
- #st.markdown("#### PID : " + str(os.getpid()))
-
- # Initializations
- with st.spinner("Initializations in progress ..."):
- reader_type_list, reader_type_dict, list_dict_lang, \
- cols_size, dict_back_colors, fig_colorscale = initializations()
- img_demo_1, img_demo_2 = get_demo()
-
- ##----------- Choose language & image -------------------------------------------------------------
- st.markdown("#### Choose languages:")
- lang_col = st.columns((1,3)) # 1/4 of the space for the dropdown, 3/4 for the rest of the content
- mmocr_key_lang = lang_col[0].selectbox("", list_dict_lang[0].keys(), 0)
- mmocr_lang = list_dict_lang[0][mmocr_key_lang]
-
-
- st.markdown("#### Choose picture:")
- cols_pict = st.columns([1, 2])
- img_typ = cols_pict[0].radio("", ['Upload file', 'Take a picture', 'Use a demo file'], \
- index=0, on_change=raz)
-
- if img_typ == 'Upload file':
- image_file = cols_pict[1].file_uploader("Upload a file:", type=["jpg","jpeg"], on_change=raz)
- if img_typ == 'Take a picture':
- image_file = cols_pict[1].camera_input("Take a picture:", on_change=raz)
- if img_typ == 'Use a demo file':
- with st.expander('Choose a demo file:', expanded=True):
- demo_used = st.radio('', ['File 1', 'File 2'], index=0, \
- horizontal=True, on_change=raz)
- cols_demo = st.columns([1, 2])
- cols_demo[0].markdown('###### File 1')
- cols_demo[0].image(img_demo_1, width=150)
- cols_demo[1].markdown('###### File 2')
- cols_demo[1].image(img_demo_2, width=300)
- if demo_used == 'File 1':
- image_file = 'img_demo_1.jpg'
- else:
- image_file = 'img_demo_2.jpg'
-
- ##----------- Process input image -----------------------------------------------------------------
- if image_file is not None:
- image_path, image_orig, image_cv2 = load_image(image_file)
- list_images = [image_orig, image_cv2]
-
- ##----------- Form with original image & hyperparameters for detectors ----------------------------
- with st.form("form1"):
- col1, col2 = st.columns(2, ) #gap="medium")
- col1.markdown("##### Original image")
- col1.image(list_images[0], width=400)
- col2.markdown("##### Hyperparameters values for detection")
-
- # with col2.expander("Choose detection hyperparameters for " + reader_type_list[0], \
- # expanded=False):
- # t0_min_size = st.slider("min_size", 1, 20, 10, step=1, \
- # help="min_size (int, default = 10) - Filter text box smaller than \
- # minimum value in pixel")
- # t0_text_threshold = st.slider("text_threshold", 0.1, 1., 0.7, step=0.1, \
- # help="text_threshold (float, default = 0.7) - Text confidence threshold")
- # t0_low_text = st.slider("low_text", 0.1, 1., 0.4, step=0.1, \
- # help="low_text (float, default = 0.4) - Text low-bound score")
- # t0_link_threshold = st.slider("link_threshold", 0.1, 1., 0.4, step=0.1, \
- # help="link_threshold (float, default = 0.4) - Link confidence threshold")
- # t0_canvas_size = st.slider("canvas_size", 2000, 5000, 2560, step=10, \
- # help='''canvas_size (int, default = 2560) \n
- # Maximum e size. Image bigger than this value will be resized down''')
- # t0_mag_ratio = st.slider("mag_ratio", 0.1, 5., 1., step=0.1, \
- # help="mag_ratio (float, default = 1) - Image magnification ratio")
- # t0_slope_ths = st.slider("slope_ths", 0.01, 1., 0.1, step=0.01, \
- # help='''slope_ths (float, default = 0.1) - Maximum slope \
- # (delta y/delta x) to considered merging. \n
- # Low valuans tiled boxes will not be merged.''')
- # t0_ycenter_ths = st.slider("ycenter_ths", 0.1, 1., 0.5, step=0.1, \
- # help='''ycenter_ths (float, default = 0.5) - Maximum shift in y direction. \n
- # Boxes wiifferent level should not be merged.''')
- # t0_height_ths = st.slider("height_ths", 0.1, 1., 0.5, step=0.1, \
- # help='''height_ths (float, default = 0.5) - Maximum different in box height. \n
- # Boxes wiery different text size should not be merged.''')
- # t0_width_ths = st.slider("width_ths", 0.1, 1., 0.5, step=0.1, \
- # help="width_ths (float, default = 0.5) - Maximum horizontal \
- # distance to merge boxes.")
- # t0_add_margin = st.slider("add_margin", 0.1, 1., 0.1, step=0.1, \
- # help='''add_margin (float, default = 0.1) - \
- # Extend bounding boxes in all direction by certain value. \n
- # This is rtant for language with complex script (E.g. Thai).''')
- # t0_optimal_num_chars = st.slider("optimal_num_chars", None, 100, None, step=10, \
- # help="optimal_num_chars (int, default = None) - If specified, bounding boxes \
- # with estimated number of characters near this value are returned first.")
-
- # with col2.expander("Choose detection hyperparameters for " + reader_type_list[1], \
- # expanded=False):
- # t1_det_algorithm = st.selectbox('det_algorithm', ['DB'], \
- # help='Type of detection algorithm selected. (default = DB)')
- # t1_det_max_side_len = st.slider('det_max_side_len', 500, 2000, 960, step=10, \
- # help='''The maximum size of the long side of the image. (default = 960)\n
- # Limit thximum image height and width.\n
- # When theg side exceeds this value, the long side will be resized to this size, and the short side \
- # will be ed proportionally.''')
- # t1_det_db_thresh = st.slider('det_db_thresh', 0.1, 1., 0.3, step=0.1, \
- # help='''Binarization threshold value of DB output map. (default = 0.3) \n
- # Used to er the binarized image of DB prediction, setting 0.-0.3 has no obvious effect on the result.''')
- # t1_det_db_box_thresh = st.slider('det_db_box_thresh', 0.1, 1., 0.6, step=0.1, \
- # help='''The threshold value of the DB output box. (default = 0.6) \n
- # DB post-essing filter box threshold, if there is a missing box detected, it can be reduced as appropriate. \n
- # Boxes sclower than this value will be discard.''')
- # t1_det_db_unclip_ratio = st.slider('det_db_unclip_ratio', 1., 3.0, 1.6, step=0.1, \
- # help='''The expanded ratio of DB output box. (default = 1.6) \n
- # Indicatee compactness of the text box, the smaller the value, the closer the text box to the text.''')
- # t1_det_east_score_thresh = st.slider('det_east_cover_thresh', 0.1, 1., 0.8, step=0.1, \
- # help="Binarization threshold value of EAST output map. (default = 0.8)")
- # t1_det_east_cover_thresh = st.slider('det_east_cover_thresh', 0.1, 1., 0.1, step=0.1, \
- # help='''The threshold value of the EAST output box. (default = 0.1) \n
- # Boxes sclower than this value will be discarded.''')
- # t1_det_east_nms_thresh = st.slider('det_east_nms_thresh', 0.1, 1., 0.2, step=0.1, \
- # help="The NMS threshold value of EAST model output box. (default = 0.2)")
- # t1_det_db_score_mode = st.selectbox('det_db_score_mode', ['fast', 'slow'], \
- # help='''slow: use polygon box to calculate bbox score, fast: use rectangle box \
- # to calculate. (default = fast) \n
- # Use rectlar box to calculate faster, and polygonal box more accurate for curved text area.''')
-
- with col2.expander("Choose detection hyperparameters for detection model" ,expanded=False):
- t2_det = 'DBPP_r50'
- st.write("###### *More about text detection models* 👉 \
- [here](https://github.com/UIT-Together-Research-Group/K2020-KLTN-Scene-Text-Detection-Using-A-Segmentation-Method?fbclid=IwAR3_UmOEJFI09aWlfTGpO2_75qOeodgVu4N3gS7vU6qMBUpYwDQUyIBbhaU)")
- t2_merge_xdist = st.slider('merge_xdist', 1, 50, 20, step=1, \
- help='The maximum x-axis distance to merge boxes. (defaut=20)')
-
- # with col2.expander("Choose detection hyperparameters for " + reader_type_list[3], \
- # expanded=False):
- # t3_psm = st.selectbox('Page segmentation mode (psm)', \
- # [' - Default', \
- # ' 4 Assume a single column of text of variable sizes', \
- # ' 5 Assume a single uniform block of vertically aligned text', \
- # ' 6 Assume a single uniform block of text', \
- # ' 7 Treat the image as a single text line', \
- # ' 8 Treat the image as a single word', \
- # ' 9 Treat the image as a single word in a circle', \
- # '10 Treat the image as a single character', \
- # '11 Sparse text. Find as much text as possible in no \
- # particular order', \
- # '13 Raw line. Treat the image as a single text line, \
- # bypassing hacks that are Tesseract-specific'])
- # t3_oem = st.selectbox('OCR engine mode', ['0 Legacy engine only', \
- # '1 Neural nets LSTM engine only', \
- # '2 Legacy + LSTM engines', \
- # '3 Default, based on what is available'], 3)
- # t3_whitelist = st.text_input('Limit tesseract to recognize only this characters :', \
- # placeholder='Limit tesseract to recognize only this characters', \
- # help='Example for numbers only : 0123456789')
-
- color_hex = col2.color_picker('Set a color for box outlines:', '#004C99')
- color_part = color_hex.lstrip('#')
- color = tuple(int(color_part[i:i+2], 16) for i in (0, 2, 4))
-
- submit_detect = st.form_submit_button("Launch detection")
-
- ##----------- Process text detection --------------------------------------------------------------
- if submit_detect:
- # Process text detection
-
- # if t0_optimal_num_chars == 0:
- # t0_optimal_num_chars = None
-
- # Construct the config Tesseract parameter
- # t3_config = ''
- # psm = t3_psm[:2]
- # if psm != ' -':
- # t3_config += '--psm ' + psm.strip()
- # oem = t3_oem[:1]
- # if oem != '3':
- # t3_config += ' --oem ' + oem
- # if t3_whitelist != '':
- # t3_config += ' -c tessedit_char_whitelist=' + t3_whitelist
-
- list_params_det = [[mmocr_lang, {'det': t2_det, 'merge_xdist': t2_merge_xdist}]]
- # [[easyocr_lang, \
- # {'min_size': t0_min_size, 'text_threshold': t0_text_threshold, \
- # 'low_text': t0_low_text, 'link_threshold': t0_link_threshold, \
- # 'canvas_size': t0_canvas_size, 'mag_ratio': t0_mag_ratio, \
- # 'slope_ths': t0_slope_ths, 'ycenter_ths': t0_ycenter_ths, \
- # 'height_ths': t0_height_ths, 'width_ths': t0_width_ths, \
- # 'add_margin': t0_add_margin, 'optimal_num_chars': t0_optimal_num_chars \
- # }], \
- # [ppocr_lang, \
- # {'det_algorithm': t1_det_algorithm, 'det_max_side_len': t1_det_max_side_len, \
- # 'det_db_thresh': t1_det_db_thresh, 'det_db_box_thresh': t1_det_db_box_thresh, \
- # 'det_db_unclip_ratio': t1_det_db_unclip_ratio, \
- # 'det_east_score_thresh': t1_det_east_score_thresh, \
- # 'det_east_cover_thresh': t1_det_east_cover_thresh, \
- # 'det_east_nms_thresh': t1_det_east_nms_thresh, \
- # 'det_db_score_mode': t1_det_db_score_mode}],
- # [tesserocr_lang, {'lang': tesserocr_lang, 'config': t3_config}]
- # ]
-
- show_info1 = st.empty()
- show_info1.info("Readers initializations in progress (it may take a while) ...")
- list_readers = init_readers(list_params_det)
-
- show_info1.info("Text detection in progress ...")
- list_images, list_coordinates = process_detect(image_path, list_images, list_readers, \
- list_params_det, color)
- show_info1.empty()
-
- # Clear previous recognition results
- st.session_state.df_results = pd.DataFrame([])
-
- st.session_state.list_readers = list_readers
- st.session_state.list_coordinates = list_coordinates
- st.session_state.list_images = list_images
- st.session_state.list_params_det = list_params_det
-
- if 'columns_size' not in st.session_state:
- st.session_state.columns_size = [2] + [1 for x in reader_type_list[1:]]
- if 'column_width' not in st.session_state:
- st.session_state.column_width = [400] + [300 for x in reader_type_list[1:]]
- if 'columns_color' not in st.session_state:
- st.session_state.columns_color = ["rgb(228,26,28)"] + \
- ["rgb(0,0,0)" for x in reader_type_list[1:]]
-
- if st.session_state.list_coordinates:
- list_coordinates = st.session_state.list_coordinates
- list_images = st.session_state.list_images
- list_readers = st.session_state.list_readers
- list_params_det = st.session_state.list_params_det
-
- ##----------- Text detection results --------------------------------------------------------------
- st.subheader("Text detection")
- show_detect = st.empty()
- list_ok_detect = []
- with show_detect.container():
- column_title = 'Detection with MMOCR
'
- show_detect.markdown(column_title, unsafe_allow_html=True)
- if isinstance(list_images[2], PIL.Image.Image):
- show_detect.image(list_images[2], width=st.session_state.column_width[0], use_column_width=True)
- list_ok_detect.append('MMOCR')
- else:
- show_detect.write(list_images[2], use_column_width=True)
-
-
- st.subheader("Text recognition")
-
- # st.markdown("##### Using detection performed above by:")
- # st.radio('Choose the detecter:', list_ok_detect, key='detect_reader', \
- # horizontal=True, on_change=highlight)
-
- ##----------- Form with hyperparameters for recognition -----------------------
- st.markdown("##### Hyperparameters values for recognition:")
- with st.form("form2"):
- # with st.expander("Choose recognition hyperparameters for " + reader_type_list[0], \
- # expanded=False):
- # t0_decoder = st.selectbox('decoder', ['greedy', 'beamsearch', 'wordbeamsearch'], \
- # help="decoder (string, default = 'greedy') - options are 'greedy', \
- # 'beamsearch' and 'wordbeamsearch.")
- # t0_beamWidth = st.slider('beamWidth', 2, 20, 5, step=1, \
- # help="beamWidth (int, default = 5) - How many beam to keep when decoder = \
- # 'beamsearch' or 'wordbeamsearch'.")
- # t0_batch_size = st.slider('batch_size', 1, 10, 1, step=1, \
- # help="batch_size (int, default = 1) - batch_size>1 will make EasyOCR faster \
- # but use more memory.")
- # t0_workers = st.slider('workers', 0, 10, 0, step=1, \
- # help="workers (int, default = 0) - Number thread used in of dataloader.")
- # t0_allowlist = st.text_input('allowlist', value="", max_chars=None, \
- # placeholder='Force EasyOCR to recognize only this subset of characters', \
- # help='''allowlist (string) - Force EasyOCR to recognize only subset of characters.\n
- # Usefor specific problem (E.g. license plate, etc.)''')
- # t0_blocklist = st.text_input('blocklist', value="", max_chars=None, \
- # placeholder='Block subset of character (will be ignored if allowlist is given)', \
- # help='''blocklist (string) - Block subset of character. This argument will be \
- # ignored if allowlist is given.''')
- # t0_detail = st.radio('detail', [0, 1], 1, horizontal=True, \
- # help="detail (int, default = 1) - Set this to 0 for simple output")
- # t0_paragraph = st.radio('paragraph', [True, False], 1, horizontal=True, \
- # help='paragraph (bool, default = False) - Combine result into paragraph')
- # t0_contrast_ths = st.slider('contrast_ths', 0.05, 1., 0.1, step=0.01, \
- # help='''contrast_ths (float, default = 0.1) - Text box with contrast lower than \
- # this value will be passed into model 2 times.\n
- # Firs with original image and second with contrast adjusted to 'adjust_contrast' value.\n
- # The with more confident level will be returned as a result.''')
- # t0_adjust_contrast = st.slider('adjust_contrast', 0.1, 1., 0.5, step=0.1, \
- # help = 'adjust_contrast (float, default = 0.5) - target contrast level for low \
- # contrast text box')
-
- # with st.expander("Choose recognition hyperparameters for " + reader_type_list[1], \
- # expanded=False):
- # t1_rec_algorithm = st.selectbox('rec_algorithm', ['CRNN', 'SVTR_LCNet'], 0, \
- # help="Type of recognition algorithm selected. (default=CRNN)")
- # t1_rec_batch_num = st.slider('rec_batch_num', 1, 50, step=1, \
- # help="When performing recognition, the batchsize of forward images. \
- # (default=30)")
- # t1_max_text_length = st.slider('max_text_length', 3, 250, 25, step=1, \
- # help="The maximum text length that the recognition algorithm can recognize. \
- # (default=25)")
- # t1_use_space_char = st.radio('use_space_char', [True, False], 0, horizontal=True, \
- # help="Whether to recognize spaces. (default=TRUE)")
- # t1_drop_score = st.slider('drop_score', 0., 1., 0.25, step=.05, \
- # help="Filter the output by score (from the recognition model), and those \
- # below this score will not be returned. (default=0.5)")
-
- with st.expander("Choose recognition hyperparameters for " + reader_type_list[2], \
- expanded=False):
- t2_recog = st.selectbox('recog', ['ABINet','CRNN','CRNN_TPS','MASTER', \
- 'NRTR_1/16-1/8','NRTR_1/8-1/4','RobustScanner','SAR','SAR_CN', \
- 'SATRN','SATRN_sm','SEG','Tesseract'], 7, \
- help='Text recognition algorithm. (default = SAR)')
- st.write("###### *More about text recognition models* 👉 \
- [here](https://mmocr.readthedocs.io/en/latest/textrecog_models.html)")
-
- # with st.expander("Choose recognition hyperparameters for " + reader_type_list[3], \
- # expanded=False):
- # t3r_psm = st.selectbox('Page segmentation mode (psm)', \
- # [' - Default', \
- # ' 4 Assume a single column of text of variable sizes', \
- # ' 5 Assume a single uniform block of vertically aligned \
- # text', \
- # ' 6 Assume a single uniform block of text', \
- # ' 7 Treat the image as a single text line', \
- # ' 8 Treat the image as a single word', \
- # ' 9 Treat the image as a single word in a circle', \
- # '10 Treat the image as a single character', \
- # '11 Sparse text. Find as much text as possible in no \
- # particular order', \
- # '13 Raw line. Treat the image as a single text line, \
- # bypassing hacks that are Tesseract-specific'])
- # t3r_oem = st.selectbox('OCR engine mode', ['0 Legacy engine only', \
- # '1 Neural nets LSTM engine only', \
- # '2 Legacy + LSTM engines', \
- # '3 Default, based on what is available'], 3)
- # t3r_whitelist = st.text_input('Limit tesseract to recognize only this \
- # characters :', \
- # placeholder='Limit tesseract to recognize only this characters', \
- # help='Example for numbers only : 0123456789')
-
- submit_reco = st.form_submit_button("Launch recognition")
-
- if submit_reco:
- process_detect.clear()
- ##----------- Process recognition ------------------------------------------
- reader_ind = reader_type_dict[st.session_state.detect_reader]
- list_boxes = list_coordinates[0]
-
- # # Construct the config Tesseract parameter
- # t3r_config = ''
- # psm = t3r_psm[:2]
- # if psm != ' -':
- # t3r_config += '--psm ' + psm.strip()
- # oem = t3r_oem[:1]
- # if oem != '3':
- # t3r_config += ' --oem ' + oem
- # if t3r_whitelist != '':
- # t3r_config += ' -c tessedit_char_whitelist=' + t3r_whitelist
-
- list_params_rec = \
- [
- {'recog': t2_recog},
- ]
-
- show_info2 = st.empty()
-
- with show_info2.container():
- st.info("Text recognition in progress ...")
- df_results, list_reco_status = \
- process_recog(list_readers, list_images[1], list_boxes, list_params_rec)
- show_info2.empty()
-
- st.session_state.df_results = df_results
- st.session_state.list_boxes = list_boxes
- # st.session_state.df_results_tesseract = df_results_tesseract
- st.session_state.list_reco_status = list_reco_status
-
- if 'df_results' in st.session_state:
- if not st.session_state.df_results.empty:
- ##----------- Show recognition results ------------------------------------------------------------
- results_cols = st.session_state.df_results.columns
- list_col_text = np.arange(1, len(cols_size), 2)
- list_col_confid = np.arange(2, len(cols_size), 2)
-
- dict_draw_reco = {'in_image': st.session_state.list_images[1], \
- 'in_boxes_coordinates': st.session_state.list_boxes, \
- 'in_list_texts': [st.session_state.df_results[results_cols[1]].to_list() ], \
- 'in_list_confid': [st.session_state.df_results[results_cols[2]].to_list()], \
- 'in_dict_back_colors': dict_back_colors, \
- 'in_reader_type_list': reader_type_list
- }
- show_reco = st.empty()
-
- with st.form("form3"):
- st.plotly_chart(fig_colorscale, use_container_width=True)
-
- col_font, col_threshold = st.columns(2)
-
- col_font.slider('Font scale', 1, 7, 1, step=1, key="font_scale_sld")
- col_threshold.slider('% confidence threshold for text color change', 40, 100, 64, \
- step=1, key="conf_threshold_sld")
- col_threshold.write("(text color is black below this % confidence threshold, \
- and white above)")
-
- draw_reco_images(**dict_draw_reco)
-
- submit_resize = st.form_submit_button("Refresh")
-
- if submit_resize:
- draw_reco_images(**dict_draw_reco, \
- in_font_scale=st.session_state.font_scale_sld, \
- in_conf_threshold=st.session_state.conf_threshold_sld)
-
- st.subheader("Recognition details")
- with st.expander("Detailed areas for OCR", expanded=True):
- cols = st.columns(3)
- cols[0].markdown('#### Detected area')
- cols[1].markdown('#### text ' + "OCR")
- cols[2].markdown('#### confidence_score')
- for row in st.session_state.df_results.itertuples():
- #cols = st.columns(1 + len(reader_type_list)*2)
- cols = st.columns(3)
- cols[0].image(row.cropped_image, width=150)
- cols[1].write(getattr(row, results_cols[1]))
- cols[2].write("("+str( \
- getattr(row, results_cols[2]))+"%)")
-
- st.download_button(
- label="Download results as CSV file",
- data=convert_df(st.session_state.df_results),
- file_name='OCR_comparator_results.csv',
- mime='text/csv',
- )
-
- # if not st.session_state.df_results_tesseract.empty:
- # with st.expander("Detailed areas for Tesseract", expanded=False):
- # cols = st.columns([2,2,1])
- # cols[0].markdown('#### Detected area')
- # cols[1].markdown('#### with Tesseract')
-
- # for row in st.session_state.df_results_tesseract.itertuples():
- # cols = st.columns([2,2,1])
- # cols[0].image(row.cropped, width=150)
- # cols[1].write(getattr(row, 'text'))
- # cols[2].write("("+str(getattr(row, 'conf'))+"%)")
-
- # st.download_button(
- # label="Download Tesseract results as CSV file",
- # data=convert_df(st.session_state.df_results),
- # file_name='OCR_comparator_Tesseract_results.csv',
- # mime='text/csv',
- # )
diff --git a/spaces/doluvor/faster-whisper-webui/src/hooks/whisperProgressHook.py b/spaces/doluvor/faster-whisper-webui/src/hooks/whisperProgressHook.py
deleted file mode 100644
index aa09958a05e0b3c54736f7209f8a05a94912752e..0000000000000000000000000000000000000000
--- a/spaces/doluvor/faster-whisper-webui/src/hooks/whisperProgressHook.py
+++ /dev/null
@@ -1,91 +0,0 @@
-import sys
-import threading
-from typing import List, Union
-import tqdm
-
-from src.hooks.progressListener import ProgressListener
-
-class ProgressListenerHandle:
- def __init__(self, listener: ProgressListener):
- self.listener = listener
-
- def __enter__(self):
- register_thread_local_progress_listener(self.listener)
-
- def __exit__(self, exc_type, exc_val, exc_tb):
- unregister_thread_local_progress_listener(self.listener)
-
- if exc_type is None:
- self.listener.on_finished()
-
-class _CustomProgressBar(tqdm.tqdm):
- def __init__(self, *args, **kwargs):
- super().__init__(*args, **kwargs)
- self._current = self.n # Set the initial value
-
- def update(self, n):
- super().update(n)
- # Because the progress bar might be disabled, we need to manually update the progress
- self._current += n
-
- # Inform listeners
- listeners = _get_thread_local_listeners()
-
- for listener in listeners:
- listener.on_progress(self._current, self.total)
-
-_thread_local = threading.local()
-
-def _get_thread_local_listeners():
- if not hasattr(_thread_local, 'listeners'):
- _thread_local.listeners = []
- return _thread_local.listeners
-
-_hooked = False
-
-def init_progress_hook():
- global _hooked
-
- if _hooked:
- return
-
- # Inject into tqdm.tqdm of Whisper, so we can see progress
- import whisper.transcribe
- transcribe_module = sys.modules['whisper.transcribe']
- transcribe_module.tqdm.tqdm = _CustomProgressBar
- _hooked = True
-
-def register_thread_local_progress_listener(progress_listener: ProgressListener):
- # This is a workaround for the fact that the progress bar is not exposed in the API
- init_progress_hook()
-
- listeners = _get_thread_local_listeners()
- listeners.append(progress_listener)
-
-def unregister_thread_local_progress_listener(progress_listener: ProgressListener):
- listeners = _get_thread_local_listeners()
-
- if progress_listener in listeners:
- listeners.remove(progress_listener)
-
-def create_progress_listener_handle(progress_listener: ProgressListener):
- return ProgressListenerHandle(progress_listener)
-
-# Example usage
-if __name__ == '__main__':
- class PrintingProgressListener:
- def on_progress(self, current: Union[int, float], total: Union[int, float]):
- print(f"Progress: {current}/{total}")
-
- def on_finished(self):
- print("Finished")
-
- import whisper
- model = whisper.load_model("medium")
-
- with create_progress_listener_handle(PrintingProgressListener()) as listener:
- # Set verbose to None to disable the progress bar, as we are using our own
- result = model.transcribe("J:\\Dev\\OpenAI\\whisper\\tests\\Noriko\\out.mka", language="Japanese", fp16=False, verbose=None)
- print(result)
-
- print("Done")
\ No newline at end of file
diff --git a/spaces/dongyi/MMFS/utils/augmentation.py b/spaces/dongyi/MMFS/utils/augmentation.py
deleted file mode 100644
index cfcc3db13309598145c55c4c92ed360edd2c44b5..0000000000000000000000000000000000000000
--- a/spaces/dongyi/MMFS/utils/augmentation.py
+++ /dev/null
@@ -1,667 +0,0 @@
-from packaging import version
-import random
-import numpy as np
-from PIL import Image, ImageFilter, ImageOps
-from torchvision.transforms.transforms import Lambda, Compose
-from torchvision.transforms import functional as F
-from collections.abc import Iterable
-import torch, torchvision
-import numbers
-import copy
-
-if version.parse(torchvision.__version__) <= version.parse('0.7.0'):
- from torchvision.transforms.transforms import _get_image_size
-
-def check_input_type_perform_action(input, type, action, *args, **kwargs):
- output = input
- if isinstance(input, list):
- for i in range(0, len(input)):
- if type is None:
- if input[i] is not None: # do not combine with last line, to avoid calling isinstance on None.
- output[i] = action(input[i], *args, **kwargs)
- elif isinstance(input[i], type):
- output[i] = action(input[i], *args, **kwargs)
- elif type is None:
- if input is not None:
- output = action(input, *args, **kwargs)
- elif isinstance(input, type):
- output = action(input, *args, **kwargs)
- return output
-
-
-"""
-Most of these functions are imported from torchvision.transforms.transforms and edited to support 2 or more inputs.
-"""
-
-class JointCompose(object):
- """
- Composes several transforms together.
- """
-
- def __init__(self, transforms):
- self.transforms = transforms
-
- def __call__(self, input1, input2):
- for t in self.transforms:
- input1, input2 = t(input1, input2)
- return input1, input2
-
-
-class Grayscale(object):
-
- def __init__(self, input1_output_channels=1, input2_output_channels=1):
- self.input1_output_channels = input1_output_channels
- self.input2_output_channels = input2_output_channels
-
- def __call__(self, input1, input2):
- output1 = F.to_grayscale(input1, num_output_channels=self.input1_output_channels) if self.input1_output_channels == 1 else input1
- output2 = check_input_type_perform_action(input2, Image.Image, F.to_grayscale, num_output_channels=self.input2_output_channels) \
- if self.input2_output_channels == 1 else input2
- return output1, output2
-
-
-class Resize(object):
-
- def __init__(self, size, interpolation=Image.BILINEAR):
- assert isinstance(size, int) or (isinstance(size, Iterable) and len(size) == 2)
- self.size = size
- self.interpolation = interpolation
-
- def __call__(self, input1, input2):
- output1 = F.resize(input1, self.size, self.interpolation)
- output2 = check_input_type_perform_action(input2, Image.Image, F.resize, self.size, self.interpolation)
- return output1, output2
-
-
-class ScaleWidth:
-
- def __init__(self, target_size, method=Image.BICUBIC):
- self.target_size = target_size
- self.method = method
-
- def scalewidth(self, img):
- ow, oh = img.size
- w = self.target_size
- h = int(self.target_size * oh / ow)
- img_resized = img.resize((w, h), self.method)
-
- if h > w:
- # if resized image's height is larger than its width, crop the center
- left = 0
- top = h // 2 - self.target_size // 2
- right = self.target_size
- bottom = top + self.target_size
- img_resized = img_resized.crop((left, top, right, bottom))
- elif h < w:
- # pad the heights
- delta_w = self.target_size - w
- delta_h = self.target_size - h
- padding = (delta_w // 2, delta_h // 2, delta_w - (delta_w // 2), delta_h - (delta_h // 2))
- img_resized = ImageOps.expand(img_resized, padding)
-
- return img_resized
-
- def __call__(self, input1, input2):
- output1 = self.scalewidth(input1)
- output2 = check_input_type_perform_action(input2, Image.Image, self.scalewidth)
- return output1, output2
-
-
-class RandomCrop(object):
-
- def __init__(self, size, padding=None, pad_if_needed=False, fill=0, padding_mode='constant'):
- if isinstance(size, numbers.Number):
- self.size = (int(size), int(size))
- else:
- self.size = size
- self.padding = padding
- self.pad_if_needed = pad_if_needed
- self.fill = fill
- self.padding_mode = padding_mode
-
- @staticmethod
- def get_params(img, output_size):
- if version.parse(torchvision.__version__) <= version.parse('0.7.0'):
- w, h = _get_image_size(img)
- else:
- w, h = F._get_image_size(img)
- th, tw = output_size
- if w == tw and h == th:
- return 0, 0, h, w
-
- i = random.randint(0, h - th)
- j = random.randint(0, w - tw)
- return i, j, th, tw
-
- def pad(self, img):
- if self.padding is not None:
- img = F.pad(img, self.padding, self.fill, self.padding_mode)
-
- # pad the width if needed
- if self.pad_if_needed and img.size[0] < self.size[1]:
- img = F.pad(img, (self.size[1] - img.size[0], 0), self.fill, self.padding_mode)
- # pad the height if needed
- if self.pad_if_needed and img.size[1] < self.size[0]:
- img = F.pad(img, (0, self.size[0] - img.size[1]), self.fill, self.padding_mode)
-
- return img
-
- def get_crop_range(self, img):
- return self.get_params(img, self.size)
-
- def pad_and_crop(self, input, i, j, h, w):
- return F.crop(self.pad(input), i, j, h, w)
-
- def __call__(self, input1, input2):
- output1 = self.pad(input1)
- i, j, h, w = self.get_crop_range(output1)
- output1 = F.crop(output1, i, j, h, w)
- output2 = check_input_type_perform_action(input2, Image.Image, self.pad_and_crop, i, j, h, w)
- return output1, output2
-
-
-class Crop:
-
- def __init__(self, pos, size):
- self.pos = pos
- self.size = size
-
- def crop(self, img):
- ow, oh = img.size
- x1, y1 = self.pos
- tw = th = self.size
- if (ow > tw or oh > th):
- return img.crop((x1, y1, x1 + tw, y1 + th))
- return img
-
- def __call__(self, input1, input2):
- output1 = self.crop(input1)
- output2 = check_input_type_perform_action(input2, Image.Image, self.crop)
- return output1, output2
-
-
-class ColorJitter(object):
-
- def __init__(self, brightness=0, contrast=0, saturation=0, hue=0):
- self.brightness = self._check_input(brightness, 'brightness')
- self.contrast = self._check_input(contrast, 'contrast')
- self.saturation = self._check_input(saturation, 'saturation')
- self.hue = self._check_input(hue, 'hue', center=0, bound=(-0.5, 0.5),
- clip_first_on_zero=False)
-
- def _check_input(self, value, name, center=1, bound=(0, float('inf')), clip_first_on_zero=True):
- if isinstance(value, numbers.Number):
- if value < 0:
- raise ValueError("If {} is a single number, it must be non negative.".format(name))
- value = [center - value, center + value]
- if clip_first_on_zero:
- value[0] = max(value[0], 0)
- elif isinstance(value, (tuple, list)) and len(value) == 2:
- if not bound[0] <= value[0] <= value[1] <= bound[1]:
- raise ValueError("{} values should be between {}".format(name, bound))
- else:
- raise TypeError("{} should be a single number or a list/tuple with lenght 2.".format(name))
-
- # if value is 0 or (1., 1.) for brightness/contrast/saturation
- # or (0., 0.) for hue, do nothing
- if value[0] == value[1] == center:
- value = None
- return value
-
- @staticmethod
- def get_params(brightness, contrast, saturation, hue):
- transforms = []
-
- if brightness is not None:
- brightness_factor = random.uniform(brightness[0], brightness[1])
- transforms.append(Lambda(lambda img: F.adjust_brightness(img, brightness_factor)))
-
- if contrast is not None:
- contrast_factor = random.uniform(contrast[0], contrast[1])
- transforms.append(Lambda(lambda img: F.adjust_contrast(img, contrast_factor)))
-
- if saturation is not None:
- saturation_factor = random.uniform(saturation[0], saturation[1])
- transforms.append(Lambda(lambda img: F.adjust_saturation(img, saturation_factor)))
-
- if hue is not None:
- hue_factor = random.uniform(hue[0], hue[1])
- transforms.append(Lambda(lambda img: F.adjust_hue(img, hue_factor)))
-
- random.shuffle(transforms)
- transform = Compose(transforms)
-
- return transform
-
- def __call__(self, input1, input2):
- transform = self.get_params(self.brightness, self.contrast,
- self.saturation, self.hue)
- output1 = transform(input1)
- output2 = check_input_type_perform_action(input2, Image.Image, transform)
- return output1, output2
-
-
-class RandomAffine(object):
-
- def __init__(self, degrees, translate=None, scale=None, shear=None, resample=False, fillcolor=0):
- if isinstance(degrees, numbers.Number):
- if degrees < 0:
- raise ValueError("If degrees is a single number, it must be positive.")
- self.degrees = (-degrees, degrees)
- else:
- assert isinstance(degrees, (tuple, list)) and len(degrees) == 2, \
- "degrees should be a list or tuple and it must be of length 2."
- self.degrees = degrees
-
- if translate is not None:
- assert isinstance(translate, (tuple, list)) and len(translate) == 2, \
- "translate should be a list or tuple and it must be of length 2."
- for t in translate:
- if not (0.0 <= t <= 1.0):
- raise ValueError("translation values should be between 0 and 1")
- self.translate = translate
-
- if scale is not None:
- assert isinstance(scale, (tuple, list)) and len(scale) == 2, \
- "scale should be a list or tuple and it must be of length 2."
- for s in scale:
- if s <= 0:
- raise ValueError("scale values should be positive")
- self.scale = scale
-
- if shear is not None:
- if isinstance(shear, numbers.Number):
- if shear < 0:
- raise ValueError("If shear is a single number, it must be positive.")
- self.shear = (-shear, shear)
- else:
- assert isinstance(shear, (tuple, list)) and \
- (len(shear) == 2 or len(shear) == 4), \
- "shear should be a list or tuple and it must be of length 2 or 4."
- # X-Axis shear with [min, max]
- if len(shear) == 2:
- self.shear = [shear[0], shear[1], 0., 0.]
- elif len(shear) == 4:
- self.shear = [s for s in shear]
- else:
- self.shear = shear
-
- self.resample = resample
- self.fillcolor = fillcolor
-
- @staticmethod
- def get_params(degrees, translate, scale_ranges, shears, img_size):
- angle = random.uniform(degrees[0], degrees[1])
- if translate is not None:
- max_dx = translate[0] * img_size[0]
- max_dy = translate[1] * img_size[1]
- translations = (np.round(random.uniform(-max_dx, max_dx)),
- np.round(random.uniform(-max_dy, max_dy)))
- else:
- translations = (0, 0)
-
- if scale_ranges is not None:
- scale = random.uniform(scale_ranges[0], scale_ranges[1])
- else:
- scale = 1.0
-
- if shears is not None:
- if len(shears) == 2:
- shear = [random.uniform(shears[0], shears[1]), 0.]
- elif len(shears) == 4:
- shear = [random.uniform(shears[0], shears[1]),
- random.uniform(shears[2], shears[3])]
- else:
- shear = 0.0
-
- return angle, translations, scale, shear
-
- def __call__(self, input1, input2):
- params = self.get_params(self.degrees, self.translate, self.scale, self.shear, input1.size)
- output1 = F.affine(input1, *params, resample=self.resample, fillcolor=self.fillcolor)
- output2 = check_input_type_perform_action(input2, Image.Image, F.affine, *params, resample=self.resample, fillcolor=self.fillcolor)
- return output1, output2
-
-
-class RandomRotation(object):
- def __init__(self, degrees, resample=False, expand=False, center=None, fill=None):
- if isinstance(degrees, numbers.Number):
- if degrees < 0:
- raise ValueError("If degrees is a single number, it must be positive.")
- self.degrees = (-degrees, degrees)
- else:
- if len(degrees) != 2:
- raise ValueError("If degrees is a sequence, it must be of len 2.")
- self.degrees = degrees
-
- self.resample = resample
- self.expand = expand
- self.center = center
- self.fill = fill
-
- @staticmethod
- def get_params(degrees):
- angle = random.uniform(degrees[0], degrees[1])
- return angle
-
- def __call__(self, input1, input2):
- angle = self.get_params(self.degrees)
- output1 = F.rotate(input1, angle, self.resample, self.expand, self.center, self.fill)
- output2 = check_input_type_perform_action(input2, Image.Image, F.rotate, angle, self.resample, self.expand, self.center, self.fill)
- return output1, output2
-
-
-class RandomBlur:
- def __init__(self, blur_chance):
- self.blur_chance = blur_chance
-
- def get_params(self):
- if self.blur_chance > random.random():
- kernel = random.randint(3, 12)
- while kernel % 2 != 1:
- kernel = random.randint(3, 12)
- else:
- kernel = None
- return kernel
-
- def blur(self, image, kernel):
- image = image.filter(ImageFilter.GaussianBlur(radius=kernel))
- return image
-
- def __call__(self, input1, input2):
- kernel = self.get_params()
- if kernel is None:
- return input1, input2
- else:
- output1 = self.blur(input1, kernel)
- output2 = check_input_type_perform_action(input2, Image.Image, self.blur, kernel)
- return output1, output2
-
-
-class NoiseTransform:
- """code is partly from http://www.xiaoliangbai.com/2016/09/09/more-on-image-noise-generation and edited by Oliver."""
-
- def __init__(self, noise_type):
- self.noise_type = noise_type
-
- def get_params(self, image):
- params = []
- image_np = np.array(image)
- row, col, ch = image_np.shape
- if random.random() < 0.5:
- return None
- if self.noise_type == "gauss":
- mean = 0.0
- std = random.uniform(0.001, 0.3)
- gauss = np.random.normal(mean, std, (row, col, ch))
- gauss = gauss.reshape(row, col, ch)
- params.append(gauss)
- return params
- elif self.noise_type == "s&p":
- s_vs_p = 0.5
- amount = random.uniform(0.001, 0.01)
-
- # Generate Salt '1' noise
- num_salt = np.ceil(amount * image_np.size * s_vs_p)
- coords = [np.random.randint(0, i - 1, int(num_salt))
- for i in image_np.shape]
- coords[2] = np.random.randint(0, 3, int(num_salt))
- params.append(copy.deepcopy(coords))
-
- # Generate Pepper '0' noise
- num_pepper = np.ceil(amount * image_np.size * (1. - s_vs_p))
- coords = [np.random.randint(0, i - 1, int(num_pepper))
- for i in image_np.shape]
- params.append(copy.deepcopy(coords))
- return params
- elif self.noise_type == "poisson":
- noisy = np.random.poisson(image_np)
- params.append(noisy)
- return params
- elif self.noise_type == "speckle":
- factor = random.uniform(0.01, 0.4)
- gauss = np.random.randn(row, col, ch)
- gauss = gauss.reshape(row, col, ch) * factor
- params.append(gauss)
- return params
- elif self.noise_type == "band":
- smaller_dim = min(col, row)
- num_bands = random.randrange(smaller_dim // 2, smaller_dim)
- scale = random.uniform(1.0, 10.0)
-
- offset = np.zeros(image_np.shape).astype(np.float64)
-
- # horizontal branding
- num_list = list(range(image.width)) # list of integers from 0 to image width-1
- # adjust this boundaries to fit your needs
- random.shuffle(num_list)
- horizontal_bands = num_list[:num_bands]
- for w in horizontal_bands:
- offset[w, :, :] += random.uniform(-1, 1) * scale
-
- # vertical branding
- num_list = list(range(image.height)) # list of integers from 0 to image height-1
- # adjust this boundaries to fit your needs
- random.shuffle(num_list)
- vertical_bands = num_list[:num_bands]
- for h in vertical_bands:
- offset[:, h, :] += random.uniform(-1, 1) * scale
-
- params.append(offset)
- return params
- else:
- return params
-
- def apply(self, image, params):
- """
- image: ndarray (input image data. It will be converted to float)
- """
- if params is None:
- return image
- image_np = np.array(image)
- if self.noise_type == "gauss":
- gauss = params[0]
- noisy = image_np + image_np * gauss
- noisy = np.clip(noisy, 0, 255)
- return Image.fromarray(noisy.astype('uint8'))
- elif self.noise_type == "s&p":
- out = image_np
- # Generate Salt '1' noise
- coords = params[0]
- out[tuple(coords)] = 255
- # Generate Pepper '0' noise
- coords = params[1]
- out[tuple(coords)] = 0
- out = np.clip(out, 0, 255)
- return Image.fromarray(out.astype('uint8'))
- elif self.noise_type == "poisson":
- noisy = params[0]
- noisy = np.clip(noisy, 0, 255)
- return Image.fromarray(noisy.astype('uint8'))
- elif self.noise_type == "speckle":
- gauss = params[0]
- noisy = image_np + image_np * gauss
- noisy = np.clip(noisy, 0, 255)
- return Image.fromarray(noisy.astype('uint8'))
- elif self.noise_type == "band":
- offset = params[0]
- noisy = image_np + offset
- noisy = np.clip(noisy, 0, 255)
- return Image.fromarray(noisy.astype('uint8'))
- else:
- return image
-
- def __call__(self, input1, input2):
- params = self.get_params(input1)
- output1 = self.apply(input1, params)
- output2 = check_input_type_perform_action(input2, Image.Image, self.apply, params)
- return output1, output2
-
-
-class MakePower2:
- def __init__(self, base, method=Image.BICUBIC):
- self.base = base
- self.method = method
- self.print_size_warning = PrintSizeWarning()
-
- def apply(self, img):
- ow, oh = img.size
- h = int(round(oh / self.base) * self.base)
- w = int(round(ow / self.base) * self.base)
- if h == oh and w == ow:
- return img
-
- self.print_size_warning(ow, oh, w, h)
- return img.resize((w, h), self.method)
-
- def __call__(self, input1, input2):
- output1 = self.apply(input1)
- output2 = check_input_type_perform_action(input2, Image.Image, self.apply)
- return output1, output2
-
-
-class RandomHorizontalFlip(object):
- """Horizontally flip the given PIL Image randomly with a given probability.
-
- Args:
- p (float): probability of the image being flipped. Default value is 0.5
- """
-
- def __init__(self, p=0.5):
- self.p = p
-
- def get_params(self):
- if random.random() < self.p:
- return True
- else:
- return False
-
- def __call__(self, input1, input2):
- flip = self.get_params()
- if flip:
- output1 = F.hflip(input1)
- output2 = check_input_type_perform_action(input2, Image.Image, F.hflip)
- else:
- output1, output2 = input1, input2
- return output1, output2
-
-
-class Flip:
- def __init__(self, flip):
- self.flip = flip
-
- def transpose(self, input):
- return input.transpose(Image.FLIP_LEFT_RIGHT)
-
- def __call__(self, input1, input2):
- if self.flip:
- output1 = input1.transpose(Image.FLIP_LEFT_RIGHT)
- output2 = check_input_type_perform_action(input2, Image.Image, self.transpose)
- else:
- output1, output2 = input1, input2
- return output1, output2
-
-
-class ToTensor(object):
- """Convert a ``PIL Image`` or ``numpy.ndarray`` to tensor.
-
- Converts a PIL Image or numpy.ndarray (H x W x C) in the range
- [0, 255] to a torch.FloatTensor of shape (C x H x W) in the range [0.0, 1.0]
- if the PIL Image belongs to one of the modes (L, LA, P, I, F, RGB, YCbCr, RGBA, CMYK, 1)
- or if the numpy.ndarray has dtype = np.uint8
-
- In the other cases, tensors are returned without scaling.
- """
-
- def __call__(self, input1, input2):
- output1 = F.to_tensor(input1)
- output2 = check_input_type_perform_action(input2, None, F.to_tensor)
- return output1, output2
-
-
-class Normalize(object):
- """Normalize a tensor image with mean and standard deviation.
- Given mean: ``(M1,...,Mn)`` and std: ``(S1,..,Sn)`` for ``n`` channels, this transform
- will normalize each channel of the input ``torch.*Tensor`` i.e.
- ``output[channel] = (input[channel] - mean[channel]) / std[channel]``
-
- .. note::
- This transform acts out of place, i.e., it does not mutate the input tensor.
-
- Args:
- mean (sequence): Sequence of means for each channel.
- std (sequence): Sequence of standard deviations for each channel.
- inplace(bool,optional): Bool to make this operation in-place.
-
- """
-
- def __init__(self, first_input_mean, first_input_std, second_input_mean=None, second_input_std=None, inplace=False):
- self.first_input_mean = first_input_mean
- self.first_input_std = first_input_std
- self.second_input_mean = second_input_mean if second_input_mean is not None else first_input_mean
- self.second_input_std = second_input_std if second_input_std is not None else first_input_std
- self.inplace = inplace
-
- def __call__(self, tensor1, tensor2):
- """
- Args:
- tensor (Tensor): Tensor image of size (C, H, W) to be normalized.
-
- Returns:
- Tensor: Normalized Tensor image.
- """
- output1 = F.normalize(tensor1, self.first_input_mean, self.first_input_std, self.inplace)
- output2 = check_input_type_perform_action(tensor2, None, F.normalize, self.second_input_mean, self.second_input_std, self.inplace)
- return output1, output2
-
-
-class PrintSizeWarning:
- def __init__(self):
- self.has_printed = False
-
- def __call__(self, ow, oh, w, h):
- if not self.has_printed:
- print("The image size needs to be a multiple of 4. "
- "The loaded image size was (%d, %d), so it was adjusted to "
- "(%d, %d). This adjustment will be done to all images "
- "whose sizes are not multiples of 4" % (ow, oh, w, h))
- self.has_printed = True
-
-
-class ImagePathToImage:
- """Convert an image path to an image.
-
- Parameters:
- filename -- the input file path.
- """
-
- def load_img(self, path):
- return Image.open(path).convert('RGB')
-
- def __call__(self, filename1, filename2):
- img1 = self.load_img(filename1)
- img2 = check_input_type_perform_action(filename2, None, self.load_img)
- return img1, img2
-
-
-class NumpyToTensor:
- """Convert a numpy array to a tensor.
-
- Parameters:
- filename -- the input file path.
- """
-
- def load_numpy(self, filename):
- npy = np.load(filename)
- if isinstance(npy, np.lib.npyio.NpzFile):
- npy = npy['data']
- if len(npy.shape) == 2:
- npy = np.tile(npy, (1, 1, 1))
- else:
- npy = np.transpose(npy, (2, 0, 1))
- return torch.from_numpy(npy).float()
-
- def __call__(self, filename1, filename2):
- tensor1 = self.load_numpy(filename1)
- tensor2 = check_input_type_perform_action(filename2, None, self.load_numpy)
- return tensor1, tensor2
diff --git a/spaces/dvc890/go-chatgpt-api/main.go b/spaces/dvc890/go-chatgpt-api/main.go
deleted file mode 100644
index db5ac41a30a04a8736c126fe1118a9f4bbc21e40..0000000000000000000000000000000000000000
--- a/spaces/dvc890/go-chatgpt-api/main.go
+++ /dev/null
@@ -1,119 +0,0 @@
-package main
-
-import (
- "log"
- "net/http"
- "os"
- "strings"
-
- "github.com/gin-gonic/gin"
- "github.com/linweiyuan/go-chatgpt-api/api/chatgpt"
- "github.com/linweiyuan/go-chatgpt-api/api/platform"
- _ "github.com/linweiyuan/go-chatgpt-api/env"
- "github.com/linweiyuan/go-chatgpt-api/middleware"
-)
-
-func init() {
- gin.ForceConsoleColor()
- gin.SetMode(gin.ReleaseMode)
-}
-
-func main() {
- router := gin.Default()
- router.Use(middleware.CORSMiddleware())
- router.Use(middleware.CheckHeaderMiddleware())
-
- setupChatGPTAPIs(router)
-
- setupPlatformAPIs(router)
-
- router.NoRoute(handleFallbackRoute)
-
- port := os.Getenv("GO_CHATGPT_API_PORT")
- if port == "" {
- port = "8080"
- }
- err := router.Run(":" + port)
- if err != nil {
- log.Fatal("Failed to start server: " + err.Error())
- }
-}
-
-func setupChatGPTAPIs(router *gin.Engine) {
- chatgptGroup := router.Group("/chatgpt")
- {
- chatgptGroup.POST("/login", chatgpt.Login)
-
- conversationsGroup := chatgptGroup.Group("/conversations")
- {
- conversationsGroup.GET("", chatgpt.GetConversations)
-
- // PATCH is official method, POST is added for Java support
- conversationsGroup.PATCH("", chatgpt.ClearConversations)
- conversationsGroup.POST("", chatgpt.ClearConversations)
- }
-
- conversationGroup := chatgptGroup.Group("/conversation")
- {
- conversationGroup.POST("", chatgpt.CreateConversation)
- conversationGroup.POST("/gen_title/:id", chatgpt.GenerateTitle)
- conversationGroup.GET("/:id", chatgpt.GetConversation)
-
- // rename or delete conversation use a same API with different parameters
- conversationGroup.PATCH("/:id", chatgpt.UpdateConversation)
- conversationGroup.POST("/:id", chatgpt.UpdateConversation)
-
- conversationGroup.POST("/message_feedback", chatgpt.FeedbackMessage)
- }
-
- // misc
- chatgptGroup.GET("/models", chatgpt.GetModels)
- chatgptGroup.GET("/accounts/check", chatgpt.GetAccountCheck)
- }
-}
-
-func setupPlatformAPIs(router *gin.Engine) {
- platformGroup := router.Group("/platform")
- {
- platformGroup.POST("/login", platform.Login)
-
- apiGroup := platformGroup.Group("/v1")
- {
- apiGroup.GET("/models", platform.ListModels)
- apiGroup.GET("/models/:model", platform.RetrieveModel)
- apiGroup.POST("/completions", platform.CreateCompletions)
- apiGroup.POST("/chat/completions", platform.CreateChatCompletions)
- apiGroup.POST("/edits", platform.CreateEdit)
- apiGroup.POST("/images/generations", platform.CreateImage)
- apiGroup.POST("/embeddings", platform.CreateEmbeddings)
- apiGroup.GET("/files", platform.ListFiles)
- apiGroup.POST("/moderations", platform.CreateModeration)
- }
-
- dashboardGroup := platformGroup.Group("/dashboard")
- {
- billingGroup := dashboardGroup.Group("/billing")
- {
- billingGroup.GET("/credit_grants", platform.GetCreditGrants)
- billingGroup.GET("/subscription", platform.GetSubscription)
- }
-
- userGroup := dashboardGroup.Group("/user")
- {
- userGroup.GET("/api_keys", platform.GetApiKeys)
- }
- }
- }
-}
-
-func handleFallbackRoute(c *gin.Context) {
- path := c.Request.URL.Path
-
- if strings.HasPrefix(path, "/chatgpt") {
- trimmedPath := strings.TrimPrefix(path, "/chatgpt")
- c.Request.URL.Path = trimmedPath
- chatgpt.Fallback(c)
- } else {
- c.JSON(http.StatusNotFound, gin.H{"message": "Route not found"})
- }
-}
diff --git a/spaces/ec7719/Excel/README.md b/spaces/ec7719/Excel/README.md
deleted file mode 100644
index 9ba1d6180fc3699d7c50a75069a68398c09316bb..0000000000000000000000000000000000000000
--- a/spaces/ec7719/Excel/README.md
+++ /dev/null
@@ -1,13 +0,0 @@
----
-title: Excel
-emoji: 📊
-colorFrom: blue
-colorTo: purple
-sdk: streamlit
-sdk_version: 1.21.0
-app_file: app.py
-pinned: false
-license: mit
----
-
-Check out the configuration reference at https://huggingface.co/docs/hub/spaces-config-reference
diff --git a/spaces/ehristoforu/sbinterface/README.md b/spaces/ehristoforu/sbinterface/README.md
deleted file mode 100644
index 128880dc49ce22f2b1b37fe72c41046ee2edc767..0000000000000000000000000000000000000000
--- a/spaces/ehristoforu/sbinterface/README.md
+++ /dev/null
@@ -1,13 +0,0 @@
----
-title: Sbinterface
-emoji: 🌍
-colorFrom: red
-colorTo: pink
-sdk: gradio
-sdk_version: 3.39.0
-app_file: app.py
-pinned: false
-license: openrail
----
-
-Check out the configuration reference at https://huggingface.co/docs/hub/spaces-config-reference
diff --git a/spaces/erbanku/gpt-academic/crazy_functions/test_project/cpp/longcode/prod_cons.h b/spaces/erbanku/gpt-academic/crazy_functions/test_project/cpp/longcode/prod_cons.h
deleted file mode 100644
index c9004bb8043a12e32814436baa6262a00c8ef68e..0000000000000000000000000000000000000000
--- a/spaces/erbanku/gpt-academic/crazy_functions/test_project/cpp/longcode/prod_cons.h
+++ /dev/null
@@ -1,433 +0,0 @@
-#pragma once
-
-#include
-#include
-#include
-#include
-#include
-
-#include "libipc/def.h"
-
-#include "libipc/platform/detail.h"
-#include "libipc/circ/elem_def.h"
-#include "libipc/utility/log.h"
-#include "libipc/utility/utility.h"
-
-namespace ipc {
-
-////////////////////////////////////////////////////////////////
-/// producer-consumer implementation
-////////////////////////////////////////////////////////////////
-
-template
-struct prod_cons_impl;
-
-template <>
-struct prod_cons_impl> {
-
- template
- struct elem_t {
- std::aligned_storage_t data_ {};
- };
-
- alignas(cache_line_size) std::atomic rd_; // read index
- alignas(cache_line_size) std::atomic wt_; // write index
-
- constexpr circ::u2_t cursor() const noexcept {
- return 0;
- }
-
- template
- bool push(W* /*wrapper*/, F&& f, E* elems) {
- auto cur_wt = circ::index_of(wt_.load(std::memory_order_relaxed));
- if (cur_wt == circ::index_of(rd_.load(std::memory_order_acquire) - 1)) {
- return false; // full
- }
- std::forward(f)(&(elems[cur_wt].data_));
- wt_.fetch_add(1, std::memory_order_release);
- return true;
- }
-
- /**
- * In single-single-unicast, 'force_push' means 'no reader' or 'the only one reader is dead'.
- * So we could just disconnect all connections of receiver, and return false.
- */
- template
- bool force_push(W* wrapper, F&&, E*) {
- wrapper->elems()->disconnect_receiver(~static_cast(0u));
- return false;
- }
-
- template
- bool pop(W* /*wrapper*/, circ::u2_t& /*cur*/, F&& f, R&& out, E* elems) {
- auto cur_rd = circ::index_of(rd_.load(std::memory_order_relaxed));
- if (cur_rd == circ::index_of(wt_.load(std::memory_order_acquire))) {
- return false; // empty
- }
- std::forward(f)(&(elems[cur_rd].data_));
- std::forward(out)(true);
- rd_.fetch_add(1, std::memory_order_release);
- return true;
- }
-};
-
-template <>
-struct prod_cons_impl>
- : prod_cons_impl> {
-
- template
- bool force_push(W* wrapper, F&&, E*) {
- wrapper->elems()->disconnect_receiver(1);
- return false;
- }
-
- template class E, std::size_t DS, std::size_t AS>
- bool pop(W* /*wrapper*/, circ::u2_t& /*cur*/, F&& f, R&& out, E* elems) {
- byte_t buff[DS];
- for (unsigned k = 0;;) {
- auto cur_rd = rd_.load(std::memory_order_relaxed);
- if (circ::index_of(cur_rd) ==
- circ::index_of(wt_.load(std::memory_order_acquire))) {
- return false; // empty
- }
- std::memcpy(buff, &(elems[circ::index_of(cur_rd)].data_), sizeof(buff));
- if (rd_.compare_exchange_weak(cur_rd, cur_rd + 1, std::memory_order_release)) {
- std::forward(f)(buff);
- std::forward(out)(true);
- return true;
- }
- ipc::yield(k);
- }
- }
-};
-
-template <>
-struct prod_cons_impl>
- : prod_cons_impl> {
-
- using flag_t = std::uint64_t;
-
- template
- struct elem_t {
- std::aligned_storage_t data_ {};
- std::atomic f_ct_ { 0 }; // commit flag
- };
-
- alignas(cache_line_size) std::atomic ct_; // commit index
-
- template
- bool push(W* /*wrapper*/, F&& f, E* elems) {
- circ::u2_t cur_ct, nxt_ct;
- for (unsigned k = 0;;) {
- cur_ct = ct_.load(std::memory_order_relaxed);
- if (circ::index_of(nxt_ct = cur_ct + 1) ==
- circ::index_of(rd_.load(std::memory_order_acquire))) {
- return false; // full
- }
- if (ct_.compare_exchange_weak(cur_ct, nxt_ct, std::memory_order_acq_rel)) {
- break;
- }
- ipc::yield(k);
- }
- auto* el = elems + circ::index_of(cur_ct);
- std::forward(f)(&(el->data_));
- // set flag & try update wt
- el->f_ct_.store(~static_cast(cur_ct), std::memory_order_release);
- while (1) {
- auto cac_ct = el->f_ct_.load(std::memory_order_acquire);
- if (cur_ct != wt_.load(std::memory_order_relaxed)) {
- return true;
- }
- if ((~cac_ct) != cur_ct) {
- return true;
- }
- if (!el->f_ct_.compare_exchange_strong(cac_ct, 0, std::memory_order_relaxed)) {
- return true;
- }
- wt_.store(nxt_ct, std::memory_order_release);
- cur_ct = nxt_ct;
- nxt_ct = cur_ct + 1;
- el = elems + circ::index_of(cur_ct);
- }
- return true;
- }
-
- template
- bool force_push(W* wrapper, F&&, E*) {
- wrapper->elems()->disconnect_receiver(1);
- return false;
- }
-
- template class E, std::size_t DS, std::size_t AS>
- bool pop(W* /*wrapper*/, circ::u2_t& /*cur*/, F&& f, R&& out, E* elems) {
- byte_t buff[DS];
- for (unsigned k = 0;;) {
- auto cur_rd = rd_.load(std::memory_order_relaxed);
- auto cur_wt = wt_.load(std::memory_order_acquire);
- auto id_rd = circ::index_of(cur_rd);
- auto id_wt = circ::index_of(cur_wt);
- if (id_rd == id_wt) {
- auto* el = elems + id_wt;
- auto cac_ct = el->f_ct_.load(std::memory_order_acquire);
- if ((~cac_ct) != cur_wt) {
- return false; // empty
- }
- if (el->f_ct_.compare_exchange_weak(cac_ct, 0, std::memory_order_relaxed)) {
- wt_.store(cur_wt + 1, std::memory_order_release);
- }
- k = 0;
- }
- else {
- std::memcpy(buff, &(elems[circ::index_of(cur_rd)].data_), sizeof(buff));
- if (rd_.compare_exchange_weak(cur_rd, cur_rd + 1, std::memory_order_release)) {
- std::forward(f)(buff);
- std::forward(out)(true);
- return true;
- }
- ipc::yield(k);
- }
- }
- }
-};
-
-template <>
-struct prod_cons_impl> {
-
- using rc_t = std::uint64_t;
-
- enum : rc_t {
- ep_mask = 0x00000000ffffffffull,
- ep_incr = 0x0000000100000000ull
- };
-
- template
- struct elem_t {
- std::aligned_storage_t data_ {};
- std::atomic rc_ { 0 }; // read-counter
- };
-
- alignas(cache_line_size) std::atomic wt_; // write index
- alignas(cache_line_size) rc_t epoch_ { 0 }; // only one writer
-
- circ::u2_t cursor() const noexcept {
- return wt_.load(std::memory_order_acquire);
- }
-
- template
- bool push(W* wrapper, F&& f, E* elems) {
- E* el;
- for (unsigned k = 0;;) {
- circ::cc_t cc = wrapper->elems()->connections(std::memory_order_relaxed);
- if (cc == 0) return false; // no reader
- el = elems + circ::index_of(wt_.load(std::memory_order_relaxed));
- // check all consumers have finished reading this element
- auto cur_rc = el->rc_.load(std::memory_order_acquire);
- circ::cc_t rem_cc = cur_rc & ep_mask;
- if ((cc & rem_cc) && ((cur_rc & ~ep_mask) == epoch_)) {
- return false; // has not finished yet
- }
- // consider rem_cc to be 0 here
- if (el->rc_.compare_exchange_weak(
- cur_rc, epoch_ | static_cast(cc), std::memory_order_release)) {
- break;
- }
- ipc::yield(k);
- }
- std::forward(f)(&(el->data_));
- wt_.fetch_add(1, std::memory_order_release);
- return true;
- }
-
- template
- bool force_push(W* wrapper, F&& f, E* elems) {
- E* el;
- epoch_ += ep_incr;
- for (unsigned k = 0;;) {
- circ::cc_t cc = wrapper->elems()->connections(std::memory_order_relaxed);
- if (cc == 0) return false; // no reader
- el = elems + circ::index_of(wt_.load(std::memory_order_relaxed));
- // check all consumers have finished reading this element
- auto cur_rc = el->rc_.load(std::memory_order_acquire);
- circ::cc_t rem_cc = cur_rc & ep_mask;
- if (cc & rem_cc) {
- ipc::log("force_push: k = %u, cc = %u, rem_cc = %u\n", k, cc, rem_cc);
- cc = wrapper->elems()->disconnect_receiver(rem_cc); // disconnect all invalid readers
- if (cc == 0) return false; // no reader
- }
- // just compare & exchange
- if (el->rc_.compare_exchange_weak(
- cur_rc, epoch_ | static_cast(cc), std::memory_order_release)) {
- break;
- }
- ipc::yield(k);
- }
- std::forward(f)(&(el->data_));
- wt_.fetch_add(1, std::memory_order_release);
- return true;
- }
-
- template
- bool pop(W* wrapper, circ::u2_t& cur, F&& f, R&& out, E* elems) {
- if (cur == cursor()) return false; // acquire
- auto* el = elems + circ::index_of(cur++);
- std::forward(f)(&(el->data_));
- for (unsigned k = 0;;) {
- auto cur_rc = el->rc_.load(std::memory_order_acquire);
- if ((cur_rc & ep_mask) == 0) {
- std::forward(out)(true);
- return true;
- }
- auto nxt_rc = cur_rc & ~static_cast(wrapper->connected_id());
- if (el->rc_.compare_exchange_weak(cur_rc, nxt_rc, std::memory_order_release)) {
- std::forward(out)((nxt_rc & ep_mask) == 0);
- return true;
- }
- ipc::yield(k);
- }
- }
-};
-
-template <>
-struct prod_cons_impl> {
-
- using rc_t = std::uint64_t;
- using flag_t = std::uint64_t;
-
- enum : rc_t {
- rc_mask = 0x00000000ffffffffull,
- ep_mask = 0x00ffffffffffffffull,
- ep_incr = 0x0100000000000000ull,
- ic_mask = 0xff000000ffffffffull,
- ic_incr = 0x0000000100000000ull
- };
-
- template
- struct elem_t {
- std::aligned_storage_t data_ {};
- std::atomic rc_ { 0 }; // read-counter
- std::atomic f_ct_ { 0 }; // commit flag
- };
-
- alignas(cache_line_size) std::atomic ct_; // commit index
- alignas(cache_line_size) std::atomic epoch_ { 0 };
-
- circ::u2_t cursor() const noexcept {
- return ct_.load(std::memory_order_acquire);
- }
-
- constexpr static rc_t inc_rc(rc_t rc) noexcept {
- return (rc & ic_mask) | ((rc + ic_incr) & ~ic_mask);
- }
-
- constexpr static rc_t inc_mask(rc_t rc) noexcept {
- return inc_rc(rc) & ~rc_mask;
- }
-
- template
- bool push(W* wrapper, F&& f, E* elems) {
- E* el;
- circ::u2_t cur_ct;
- rc_t epoch = epoch_.load(std::memory_order_acquire);
- for (unsigned k = 0;;) {
- circ::cc_t cc = wrapper->elems()->connections(std::memory_order_relaxed);
- if (cc == 0) return false; // no reader
- el = elems + circ::index_of(cur_ct = ct_.load(std::memory_order_relaxed));
- // check all consumers have finished reading this element
- auto cur_rc = el->rc_.load(std::memory_order_relaxed);
- circ::cc_t rem_cc = cur_rc & rc_mask;
- if ((cc & rem_cc) && ((cur_rc & ~ep_mask) == epoch)) {
- return false; // has not finished yet
- }
- else if (!rem_cc) {
- auto cur_fl = el->f_ct_.load(std::memory_order_acquire);
- if ((cur_fl != cur_ct) && cur_fl) {
- return false; // full
- }
- }
- // consider rem_cc to be 0 here
- if (el->rc_.compare_exchange_weak(
- cur_rc, inc_mask(epoch | (cur_rc & ep_mask)) | static_cast(cc), std::memory_order_relaxed) &&
- epoch_.compare_exchange_weak(epoch, epoch, std::memory_order_acq_rel)) {
- break;
- }
- ipc::yield(k);
- }
- // only one thread/process would touch here at one time
- ct_.store(cur_ct + 1, std::memory_order_release);
- std::forward(f)(&(el->data_));
- // set flag & try update wt
- el->f_ct_.store(~static_cast(cur_ct), std::memory_order_release);
- return true;
- }
-
- template
- bool force_push(W* wrapper, F&& f, E* elems) {
- E* el;
- circ::u2_t cur_ct;
- rc_t epoch = epoch_.fetch_add(ep_incr, std::memory_order_release) + ep_incr;
- for (unsigned k = 0;;) {
- circ::cc_t cc = wrapper->elems()->connections(std::memory_order_relaxed);
- if (cc == 0) return false; // no reader
- el = elems + circ::index_of(cur_ct = ct_.load(std::memory_order_relaxed));
- // check all consumers have finished reading this element
- auto cur_rc = el->rc_.load(std::memory_order_acquire);
- circ::cc_t rem_cc = cur_rc & rc_mask;
- if (cc & rem_cc) {
- ipc::log("force_push: k = %u, cc = %u, rem_cc = %u\n", k, cc, rem_cc);
- cc = wrapper->elems()->disconnect_receiver(rem_cc); // disconnect all invalid readers
- if (cc == 0) return false; // no reader
- }
- // just compare & exchange
- if (el->rc_.compare_exchange_weak(
- cur_rc, inc_mask(epoch | (cur_rc & ep_mask)) | static_cast(cc), std::memory_order_relaxed)) {
- if (epoch == epoch_.load(std::memory_order_acquire)) {
- break;
- }
- else if (push(wrapper, std::forward(f), elems)) {
- return true;
- }
- epoch = epoch_.fetch_add(ep_incr, std::memory_order_release) + ep_incr;
- }
- ipc::yield(k);
- }
- // only one thread/process would touch here at one time
- ct_.store(cur_ct + 1, std::memory_order_release);
- std::forward(f)(&(el->data_));
- // set flag & try update wt
- el->f_ct_.store(~static_cast(cur_ct), std::memory_order_release);
- return true;
- }
-
- template
- bool pop(W* wrapper, circ::u2_t& cur, F&& f, R&& out, E(& elems)[N]) {
- auto* el = elems + circ::index_of(cur);
- auto cur_fl = el->f_ct_.load(std::memory_order_acquire);
- if (cur_fl != ~static_cast(cur)) {
- return false; // empty
- }
- ++cur;
- std::forward(f)(&(el->data_));
- for (unsigned k = 0;;) {
- auto cur_rc = el->rc_.load(std::memory_order_acquire);
- if ((cur_rc & rc_mask) == 0) {
- std::forward(out)(true);
- el->f_ct_.store(cur + N - 1, std::memory_order_release);
- return true;
- }
- auto nxt_rc = inc_rc(cur_rc) & ~static_cast(wrapper->connected_id());
- bool last_one = false;
- if ((last_one = (nxt_rc & rc_mask) == 0)) {
- el->f_ct_.store(cur + N - 1, std::memory_order_release);
- }
- if (el->rc_.compare_exchange_weak(cur_rc, nxt_rc, std::memory_order_release)) {
- std::forward(out)(last_one);
- return true;
- }
- ipc::yield(k);
- }
- }
-};
-
-} // namespace ipc
diff --git "a/spaces/erbanku/gpt-academic/crazy_functions/\346\211\271\351\207\217\346\200\273\347\273\223PDF\346\226\207\346\241\243pdfminer.py" "b/spaces/erbanku/gpt-academic/crazy_functions/\346\211\271\351\207\217\346\200\273\347\273\223PDF\346\226\207\346\241\243pdfminer.py"
deleted file mode 100644
index ffbb05599ef09c9de25334ebeca2eef8022b9aaf..0000000000000000000000000000000000000000
--- "a/spaces/erbanku/gpt-academic/crazy_functions/\346\211\271\351\207\217\346\200\273\347\273\223PDF\346\226\207\346\241\243pdfminer.py"
+++ /dev/null
@@ -1,160 +0,0 @@
-from toolbox import update_ui
-from toolbox import CatchException, report_execption, write_results_to_file
-from .crazy_utils import request_gpt_model_in_new_thread_with_ui_alive
-
-fast_debug = False
-
-def readPdf(pdfPath):
- """
- 读取pdf文件,返回文本内容
- """
- import pdfminer
- from pdfminer.pdfparser import PDFParser
- from pdfminer.pdfdocument import PDFDocument
- from pdfminer.pdfpage import PDFPage, PDFTextExtractionNotAllowed
- from pdfminer.pdfinterp import PDFResourceManager, PDFPageInterpreter
- from pdfminer.pdfdevice import PDFDevice
- from pdfminer.layout import LAParams
- from pdfminer.converter import PDFPageAggregator
-
- fp = open(pdfPath, 'rb')
-
- # Create a PDF parser object associated with the file object
- parser = PDFParser(fp)
-
- # Create a PDF document object that stores the document structure.
- # Password for initialization as 2nd parameter
- document = PDFDocument(parser)
- # Check if the document allows text extraction. If not, abort.
- if not document.is_extractable:
- raise PDFTextExtractionNotAllowed
-
- # Create a PDF resource manager object that stores shared resources.
- rsrcmgr = PDFResourceManager()
-
- # Create a PDF device object.
- # device = PDFDevice(rsrcmgr)
-
- # BEGIN LAYOUT ANALYSIS.
- # Set parameters for analysis.
- laparams = LAParams(
- char_margin=10.0,
- line_margin=0.2,
- boxes_flow=0.2,
- all_texts=False,
- )
- # Create a PDF page aggregator object.
- device = PDFPageAggregator(rsrcmgr, laparams=laparams)
- # Create a PDF interpreter object.
- interpreter = PDFPageInterpreter(rsrcmgr, device)
-
- # loop over all pages in the document
- outTextList = []
- for page in PDFPage.create_pages(document):
- # read the page into a layout object
- interpreter.process_page(page)
- layout = device.get_result()
- for obj in layout._objs:
- if isinstance(obj, pdfminer.layout.LTTextBoxHorizontal):
- # print(obj.get_text())
- outTextList.append(obj.get_text())
-
- return outTextList
-
-
-def 解析Paper(file_manifest, project_folder, llm_kwargs, plugin_kwargs, chatbot, history, system_prompt):
- import time, glob, os
- from bs4 import BeautifulSoup
- print('begin analysis on:', file_manifest)
- for index, fp in enumerate(file_manifest):
- if ".tex" in fp:
- with open(fp, 'r', encoding='utf-8', errors='replace') as f:
- file_content = f.read()
- if ".pdf" in fp.lower():
- file_content = readPdf(fp)
- file_content = BeautifulSoup(''.join(file_content), features="lxml").body.text.encode('gbk', 'ignore').decode('gbk')
-
- prefix = "接下来请你逐文件分析下面的论文文件,概括其内容" if index==0 else ""
- i_say = prefix + f'请对下面的文章片段用中文做一个概述,文件名是{os.path.relpath(fp, project_folder)},文章内容是 ```{file_content}```'
- i_say_show_user = prefix + f'[{index}/{len(file_manifest)}] 请对下面的文章片段做一个概述: {os.path.abspath(fp)}'
- chatbot.append((i_say_show_user, "[Local Message] waiting gpt response."))
- yield from update_ui(chatbot=chatbot, history=history) # 刷新界面
-
- if not fast_debug:
- msg = '正常'
- # ** gpt request **
- gpt_say = yield from request_gpt_model_in_new_thread_with_ui_alive(
- inputs=i_say,
- inputs_show_user=i_say_show_user,
- llm_kwargs=llm_kwargs,
- chatbot=chatbot,
- history=[],
- sys_prompt="总结文章。"
- ) # 带超时倒计时
- chatbot[-1] = (i_say_show_user, gpt_say)
- history.append(i_say_show_user); history.append(gpt_say)
- yield from update_ui(chatbot=chatbot, history=history, msg=msg) # 刷新界面
- if not fast_debug: time.sleep(2)
-
- all_file = ', '.join([os.path.relpath(fp, project_folder) for index, fp in enumerate(file_manifest)])
- i_say = f'根据以上你自己的分析,对全文进行概括,用学术性语言写一段中文摘要,然后再写一段英文摘要(包括{all_file})。'
- chatbot.append((i_say, "[Local Message] waiting gpt response."))
- yield from update_ui(chatbot=chatbot, history=history) # 刷新界面
-
- if not fast_debug:
- msg = '正常'
- # ** gpt request **
- gpt_say = yield from request_gpt_model_in_new_thread_with_ui_alive(
- inputs=i_say,
- inputs_show_user=i_say,
- llm_kwargs=llm_kwargs,
- chatbot=chatbot,
- history=history,
- sys_prompt="总结文章。"
- ) # 带超时倒计时
- chatbot[-1] = (i_say, gpt_say)
- history.append(i_say); history.append(gpt_say)
- yield from update_ui(chatbot=chatbot, history=history, msg=msg) # 刷新界面
- res = write_results_to_file(history)
- chatbot.append(("完成了吗?", res))
- yield from update_ui(chatbot=chatbot, history=history, msg=msg) # 刷新界面
-
-
-
-@CatchException
-def 批量总结PDF文档pdfminer(txt, llm_kwargs, plugin_kwargs, chatbot, history, system_prompt, web_port):
- history = [] # 清空历史,以免输入溢出
- import glob, os
-
- # 基本信息:功能、贡献者
- chatbot.append([
- "函数插件功能?",
- "批量总结PDF文档,此版本使用pdfminer插件,带token约简功能。函数插件贡献者: Euclid-Jie。"])
- yield from update_ui(chatbot=chatbot, history=history) # 刷新界面
-
- # 尝试导入依赖,如果缺少依赖,则给出安装建议
- try:
- import pdfminer, bs4
- except:
- report_execption(chatbot, history,
- a = f"解析项目: {txt}",
- b = f"导入软件依赖失败。使用该模块需要额外依赖,安装方法```pip install --upgrade pdfminer beautifulsoup4```。")
- yield from update_ui(chatbot=chatbot, history=history) # 刷新界面
- return
- if os.path.exists(txt):
- project_folder = txt
- else:
- if txt == "": txt = '空空如也的输入栏'
- report_execption(chatbot, history, a = f"解析项目: {txt}", b = f"找不到本地项目或无权访问: {txt}")
- yield from update_ui(chatbot=chatbot, history=history) # 刷新界面
- return
- file_manifest = [f for f in glob.glob(f'{project_folder}/**/*.tex', recursive=True)] + \
- [f for f in glob.glob(f'{project_folder}/**/*.pdf', recursive=True)] # + \
- # [f for f in glob.glob(f'{project_folder}/**/*.cpp', recursive=True)] + \
- # [f for f in glob.glob(f'{project_folder}/**/*.c', recursive=True)]
- if len(file_manifest) == 0:
- report_execption(chatbot, history, a = f"解析项目: {txt}", b = f"找不到任何.tex或pdf文件: {txt}")
- yield from update_ui(chatbot=chatbot, history=history) # 刷新界面
- return
- yield from 解析Paper(file_manifest, project_folder, llm_kwargs, plugin_kwargs, chatbot, history, system_prompt)
-
diff --git a/spaces/etri-vilab/Ko-LLaVA/static/css/bulma-carousel.min.css b/spaces/etri-vilab/Ko-LLaVA/static/css/bulma-carousel.min.css
deleted file mode 100644
index 4d4b7d103e0013f64e4dedd2ad0b2947cc0d11a5..0000000000000000000000000000000000000000
--- a/spaces/etri-vilab/Ko-LLaVA/static/css/bulma-carousel.min.css
+++ /dev/null
@@ -1 +0,0 @@
-@-webkit-keyframes spinAround{from{-webkit-transform:rotate(0);transform:rotate(0)}to{-webkit-transform:rotate(359deg);transform:rotate(359deg)}}@keyframes spinAround{from{-webkit-transform:rotate(0);transform:rotate(0)}to{-webkit-transform:rotate(359deg);transform:rotate(359deg)}}.slider{position:relative;width:100%}.slider-container{display:flex;flex-wrap:nowrap;flex-direction:row;overflow:hidden;-webkit-transform:translate3d(0,0,0);transform:translate3d(0,0,0);min-height:100%}.slider-container.is-vertical{flex-direction:column}.slider-container .slider-item{flex:none}.slider-container .slider-item .image.is-covered img{-o-object-fit:cover;object-fit:cover;-o-object-position:center center;object-position:center center;height:100%;width:100%}.slider-container .slider-item .video-container{height:0;padding-bottom:0;padding-top:56.25%;margin:0;position:relative}.slider-container .slider-item .video-container.is-1by1,.slider-container .slider-item .video-container.is-square{padding-top:100%}.slider-container .slider-item .video-container.is-4by3{padding-top:75%}.slider-container .slider-item .video-container.is-21by9{padding-top:42.857143%}.slider-container .slider-item .video-container embed,.slider-container .slider-item .video-container iframe,.slider-container .slider-item .video-container object{position:absolute;top:0;left:0;width:100%!important;height:100%!important}.slider-navigation-next,.slider-navigation-previous{display:flex;justify-content:center;align-items:center;position:absolute;width:42px;height:42px;background:#fff center center no-repeat;background-size:20px 20px;border:1px solid #fff;border-radius:25091983px;box-shadow:0 2px 5px #3232321a;top:50%;margin-top:-20px;left:0;cursor:pointer;transition:opacity .3s,-webkit-transform .3s;transition:transform .3s,opacity .3s;transition:transform .3s,opacity .3s,-webkit-transform .3s}.slider-navigation-next:hover,.slider-navigation-previous:hover{-webkit-transform:scale(1.2);transform:scale(1.2)}.slider-navigation-next.is-hidden,.slider-navigation-previous.is-hidden{display:none;opacity:0}.slider-navigation-next svg,.slider-navigation-previous svg{width:25%}.slider-navigation-next{left:auto;right:0;background:#fff center center no-repeat;background-size:20px 20px}.slider-pagination{display:none;justify-content:center;align-items:center;position:absolute;bottom:0;left:0;right:0;padding:.5rem 1rem;text-align:center}.slider-pagination .slider-page{background:#fff;width:10px;height:10px;border-radius:25091983px;display:inline-block;margin:0 3px;box-shadow:0 2px 5px #3232321a;transition:-webkit-transform .3s;transition:transform .3s;transition:transform .3s,-webkit-transform .3s;cursor:pointer}.slider-pagination .slider-page.is-active,.slider-pagination .slider-page:hover{-webkit-transform:scale(1.4);transform:scale(1.4)}@media screen and (min-width:800px){.slider-pagination{display:flex}}.hero.has-carousel{position:relative}.hero.has-carousel+.hero-body,.hero.has-carousel+.hero-footer,.hero.has-carousel+.hero-head{z-index:10;overflow:hidden}.hero.has-carousel .hero-carousel{position:absolute;top:0;left:0;bottom:0;right:0;height:auto;border:none;margin:auto;padding:0;z-index:0}.hero.has-carousel .hero-carousel .slider{width:100%;max-width:100%;overflow:hidden;height:100%!important;max-height:100%;z-index:0}.hero.has-carousel .hero-carousel .slider .has-background{max-height:100%}.hero.has-carousel .hero-carousel .slider .has-background .is-background{-o-object-fit:cover;object-fit:cover;-o-object-position:center center;object-position:center center;height:100%;width:100%}.hero.has-carousel .hero-body{margin:0 3rem;z-index:10}
\ No newline at end of file
diff --git a/spaces/facebook/MusicGen/audiocraft/quantization/__init__.py b/spaces/facebook/MusicGen/audiocraft/quantization/__init__.py
deleted file mode 100644
index 1e0c7e429ab96d67be667e23bf7a0ffa389c036b..0000000000000000000000000000000000000000
--- a/spaces/facebook/MusicGen/audiocraft/quantization/__init__.py
+++ /dev/null
@@ -1,9 +0,0 @@
-# Copyright (c) Meta Platforms, Inc. and affiliates.
-# All rights reserved.
-#
-# This source code is licensed under the license found in the
-# LICENSE file in the root directory of this source tree.
-"""RVQ."""
-# flake8: noqa
-from .vq import ResidualVectorQuantizer
-from .base import BaseQuantizer, DummyQuantizer, QuantizedResult
diff --git a/spaces/falterWliame/Face_Mask_Detection/Crack Eset 5 0 95-0003b _HOT_.md b/spaces/falterWliame/Face_Mask_Detection/Crack Eset 5 0 95-0003b _HOT_.md
deleted file mode 100644
index e6435fcc6e76a5eeb5da9ee529cb8e11d24deb78..0000000000000000000000000000000000000000
--- a/spaces/falterWliame/Face_Mask_Detection/Crack Eset 5 0 95-0003b _HOT_.md
+++ /dev/null
@@ -1,6 +0,0 @@
-crack eset 5 0 95-0003b Download ››››› https://urlca.com/2uDbSS
-
-ESET NOD32 Antivirus Crack es el software antivirus más utilizado ... ESET NOD32 Antivirus Crack License Key 13.2.15.0 Descarga gratuita ... Clave de activación de IM-Magic Partition Resizer 3.6.0 completamente gratis [más reciente] ... 0, 86. 1, 89. 2, 79. 3, 78. 4, 86. 5, 89. 6, 91. 7, 90. 8, 92. 9, 74. 10, 73. 4d29de3e1b
-
-
-
diff --git a/spaces/fatiXbelha/sd/Baldi 39s Basics Classic Remastered No Download.md b/spaces/fatiXbelha/sd/Baldi 39s Basics Classic Remastered No Download.md
deleted file mode 100644
index 42017d87d76474b01ca252fdf05627ec47b860f4..0000000000000000000000000000000000000000
--- a/spaces/fatiXbelha/sd/Baldi 39s Basics Classic Remastered No Download.md
+++ /dev/null
@@ -1,84 +0,0 @@
-
-Baldi's Basics Classic Remastered: A Survival Horror Stealth Game That Parodies 90's Edutainment
- Do you remember those cheap educational games from the 90's that were supposed to teach you math, spelling, or geography? Well, what if one of those games turned out to be a nightmare where you have to escape from a creepy teacher who chases you with a ruler? That's the premise of Baldi's Basics Classic Remastered , a survival horror stealth game that parodies 90's edutainment and never takes itself too seriously.
-baldi 39;s basics classic remastered no download Download Zip ⚹⚹⚹ https://urllie.com/2uNuV5
- In this article, we will tell you everything you need to know about this game, such as what it is, what are its features, how to play it, and some tips and tricks to help you survive. If you are looking for a fun and scary game that will keep you on your toes, then read on!
- What is Baldi's Basics Classic Remastered?
- A remastered version of the original Baldi's Basics game
- Baldi's Basics Classic Remastered is a remake of the original Baldi's Basics in Education and Learning game that was released in 2018 as a submission for the Meta Game Jam. The original game was created by Micah McGonigal (also known as mystman12), who wanted to make a game that looked like it was made in 1999 with a low budget. The game became a viral hit and spawned many fan-made mods, spin-offs, and sequels.
- The remastered version was released in 2022 as a free update for anyone who owned Baldi's Basics Plus , which is a paid version of the game that features more content and modes. The remastered version was made using the same framework as Baldi's Basics Plus, which means it has many of the improvements and features found in that game.
- A game that features three games in one, gameplay improvements, a more authentic art style, accessibility improvements, and brand new ways to play
- Baldi's Basics Classic Remastered is not just a simple remake of the original game. It is also a game that features three games in one, gameplay improvements, a more authentic art style, accessibility improvements, and brand new ways to play. Here are some of the main features of the game:
A game that is free to play on Steam
- One of the best things about Baldi's Basics Classic Remastered is that it is free to play on Steam. You don't need to pay anything to enjoy this game, as long as you have a Steam account and a compatible device. You can download the game from this link and start playing right away. You can also check out the official website of the game for more information and updates.
- What are the features of Baldi's Basics Classic Remastered?
- Three games in one: Classic Style, Party Style, and Demo Style
- Baldi's Basics Classic Remastered is not just one game, but three games in one. You can choose from three different styles of gameplay, each with its own rules and challenges. Here are the three styles:
-
-
-Classic Style: This is the original Baldi's Basics game that started it all. You have to collect 7 notebooks and escape the schoolhouse while avoiding Baldi and other characters. This style is faithful to the original game, but with some minor changes and improvements.
-Party Style: This is a new style that adds a twist to the classic gameplay. You have to collect 7 notebooks and escape the schoolhouse while avoiding Baldi and other characters, but with a random modifier applied to each level. The modifiers can change the rules, the layout, the items, the characters, or even the graphics of the game. This style is unpredictable and chaotic, but also fun and replayable.
-Demo Style: This is a special style that recreates the demo version of Baldi's Basics Plus that was released in 2019. You have to collect 7 notebooks and escape the schoolhouse while avoiding Baldi and other characters, but with some features from Baldi's Basics Plus added to the game. This style is a preview of what Baldi's Basics Plus has to offer, but with some limitations and differences.
-
- Gameplay improvements: better performance, directional subtitles, improved NPC AI, and more
- Baldi's Basics Classic Remastered also features many gameplay improvements that make the game more enjoyable and accessible. Some of these improvements are:
-
-Better performance: The game runs smoother and faster than before, thanks to the new framework used for the remastered version. The game also has less bugs and glitches, and more options to customize your graphics settings.
-Directional subtitles: The game has subtitles for all the dialogue and sounds in the game, which can help you understand what is going on and where it is coming from. The subtitles also indicate the direction of the sound source, which can help you locate or avoid certain characters or events.
-Improved NPC AI: The non-player characters (NPCs) in the game have been improved to behave more realistically and intelligently. They can react to your actions, interact with each other, follow paths, avoid obstacles, and more.
-And more: The game also has other gameplay improvements, such as a pause menu, a map system, a notebook counter, a stamina bar, a noise meter, an item inventory, a quick save feature, and more.
-
- A more authentic art style: more consistent and polished graphics, animations, and sounds
- Baldi's Basics Classic Remastered also features a more authentic art style that matches the 90's edutainment aesthetic better than before. The game has more consistent and polished graphics, animations, and sounds that make it look like it was made in 1999 with a low budget. Some of these changes are:
-
-More consistent and polished graphics: The game has more consistent and polished graphics that match the 90's edutainment style better than before. The game has less pixelated textures, more detailed models, more varied colors, more realistic lighting effects, more accurate shadows, more consistent perspective angles, and more.
-More consistent and polished animations: The game has more consistent and polished animations that match the 90's edutainment style better than before. The game has less choppy movements, more fluid transitions, more realistic physics effects, more expressive facial expressions, more varied poses, and more.
-More consistent and polished sounds: The game has more consistent and polished sounds that match the 90's edutainment style better than before. The game has less distorted noises, more clear voices, more realistic sound effects, more fitting music, more ambient sounds, and more.
-
- Accessibility improvements: colorblind mode, dyslexia-friendly font, adjustable text size, and more
- Baldi's Basics Classic Remastered also features many accessibility improvements that make the game more playable and enjoyable for everyone. Some of these improvements are:
-
-Colorblind mode: The game has a colorblind mode that changes the colors of the game to make it easier for people with color vision deficiency to distinguish them. The colorblind mode has three options: deuteranopia, protanopia, and tritanopia.
-Dyslexia-friendly font: The game has a dyslexia-friendly font that changes the font of the game to make it easier for people with dyslexia to read it. The dyslexia-friendly font has a unique design that reduces the confusion between similar letters and improves the readability of the text.
-Adjustable text size: The game has an adjustable text size that changes the size of the text in the game to make it easier for people with vision impairment to read it. The adjustable text size has three options: small, medium, and large.
-And more: The game also has other accessibility improvements, such as a volume slider, a brightness slider, a mouse sensitivity slider, a keyboard remapping feature, a controller support feature, and more.
-
- Brand new ways to play: endless mode, fun settings, secret endings, and more
- Baldi's Basics Classic Remastered also features many brand new ways to play that add more fun and variety to the game. Some of these new ways are:
-
-Endless mode: This is a new mode that lets you play the game endlessly without any end goal. You can collect as many notebooks as you want and explore the schoolhouse as long as you can. The difficulty of the game increases with every notebook you collect, making it harder to survive. This mode is perfect for testing your skills and endurance.
-Fun settings: This is a new feature that lets you customize the game to your liking. You can change various aspects of the game, such as the speed of Baldi, the number of notebooks, the behavior of other characters, the appearance of the graphics, and more. You can also enable or disable some modifiers that can make the game easier or harder. This feature is perfect for creating your own challenges and experiences.
-Secret endings: This is a new feature that lets you discover some hidden endings that are not part of the main story. You can unlock these endings by doing some specific actions or finding some secret items in the game. These endings can reveal some secrets or surprises about the game or its characters. This feature is perfect for satisfying your curiosity and exploring the game further.
-And more: The game also has other new ways to play, such as achievements, leaderboards, trading cards, badges, emoticons, backgrounds, and more.
-
- How to play Baldi's Basics Classic Remastered?
- The objective: collect all 7 notebooks and escape the schoolhouse without getting caught by Baldi
- The main objective of Baldi's Basics Classic Remastered is to collect all 7 notebooks that are scattered around the schoolhouse and escape through one of the exits without getting caught by Baldi. Each notebook contains 3 math problems that you have to solve by typing in your answer. However, one of the problems in each notebook is impossible to solve correctly, which will make Baldi angry and chase you with his ruler.
- The challenge: Baldi gets angrier and faster with every wrong answer you give in the notebooks
- The main challenge of Baldi's Basics Classic Remastered is to avoid Baldi at all costs. Baldi gets angrier and faster with every wrong answer you give in the notebooks, which means he will be able to catch up with you easier. Baldi can hear every sound you make in the game, such as opening doors, running, using items, or even breathing. He will use his ruler to locate you and follow you until he gets you.
- The strategy: use stealth and items to your advantage, avoid other characters that can hinder you, and stay quiet as much as possible
- The main strategy of Baldi's Basics Classic Remastered is to use stealth and items to your advantage, avoid other characters that can hinder you, and stay quiet as much as possible. You can use stealth to hide from Baldi or lose his track by taking turns without him seeing you. You can use items to distract Baldi or slow him down, such as the soda, the chocolate bar, the alarm clock, the anti-hearing tape, and more. You can avoid other characters that can hinder you, such as the Principal of the Thing, Playtime, It's a Bully, Arts and Crafters, Gotta Sweep, 1st Prize, and more. You can stay quiet as much as possible by walking instead of running, closing doors behind you, avoiding noisy objects, and using the silence item.
- The tips and tricks: keep your distance from Baldi, get the awkward notebooks first, use the anti-hearing tape when in detention, lose Baldi's track by taking turns without him seeing you, and more
- Here are some tips and tricks that can help you survive Baldi's Basics Classic Remastered:
-
-Keep your distance from Baldi: The farther you are from Baldi, the safer you are. Try to keep your distance from him by running away when he is close or using items that can push him back or teleport him away.
-Get the awkward notebooks first: The awkward notebooks are the ones that are located in hard-to-reach places or near other characters that can bother you. Try to get these notebooks first before Baldi gets too fast and angry.
-Use the anti-hearing tape when in detention: The anti-hearing tape is an item that can block all sounds in a room for a short time. Use it when you are in detention to prevent Baldi from hearing you and finding you.
-Lose Baldi's track by taking turns without him seeing you: Baldi can only follow you if he sees you or hears you. If you take a turn without him seeing you, he will lose your track and go in a random direction. Use this trick to escape from him or confuse him.
-And more: There are more tips and tricks that you can discover by playing the game yourself. Experiment with different items, characters, and strategies to find out what works best for you.
-
- Conclusion: Baldi's Basics Classic Remastered is a fun and scary game that will keep you on your toes
- Baldi's Basics Classic Remastered is a survival horror stealth game that parodies 90's edutainment and never takes itself too seriously. It is a remastered version of the original Baldi's Basics game that features three games in one, gameplay improvements, a more authentic art style, accessibility improvements, and brand new ways to play. The objective of the game is to collect all 7 notebooks and escape the schoolhouse without getting caught by Baldi, who gets angrier and faster with every wrong answer you give in the notebooks. The challenge of the game is to avoid Baldi at all costs, using stealth and items to your advantage, avoiding other characters that can hinder you, and staying quiet as much as possible. The game is free to play on Steam and has many tips and tricks that can help you survive.
- If you are looking for a fun and scary game that will keep you on your toes, then we recommend you to try Baldi's Basics Classic Remastered for yourself. You will have a blast playing this game, whether you are a fan of the original game or not. Just be prepared for some jump scares and some laughs along the way.
- So what are you waiting for? Download Baldi's Basics Classic Remastered today and see if you can outsmart Baldi!
- Frequently Asked Questions
- Q: Is Baldi's Basics Classic Remastered safe to play?
- A: Yes, Baldi's Basics Classic Remastered is safe to play. It does not contain any viruses, malware, or inappropriate content. However, it does contain some jump scares and some dark humor that may not be suitable for young children or people who are easily scared.
- Q: Is Baldi's Basics Classic Remastered based on a true story?
- A: No, Baldi's Basics Classic Remastered is not based on a true story. It is a fictional game that parodies 90's edutainment games and horror tropes. It does not have any connection to any real people or events.
- Q: How long does it take to finish Baldi's Basics Classic Remastered? A: It depends on your skill level and the style of gameplay you choose. The game can take anywhere from 10 minutes to an hour to finish, depending on how fast you can collect the notebooks and escape the schoolhouse. The game also has different endings and modes that can add more replay value and challenge to the game.
- Q: How can I get Baldi's Basics Plus?
- A: Baldi's Basics Plus is a paid version of the game that features more content and modes than Baldi's Basics Classic Remastered. You can get Baldi's Basics Plus by purchasing it on Steam for $4.99. You can also check out the official website of the game for more information and updates.
- Q: How can I support the developer of Baldi's Basics Classic Remastered?
- A: You can support the developer of Baldi's Basics Classic Remastered by buying Baldi's Basics Plus, leaving a positive review or rating on Steam, sharing the game with your friends, following the developer on social media, or donating to the developer on Patreon . You can also check out the official merchandise of the game for some cool and funny items.
197e85843d
-
-
\ No newline at end of file
diff --git "a/spaces/fb700/chat3/crazy_functions/\347\224\237\346\210\220\345\207\275\346\225\260\346\263\250\351\207\212.py" "b/spaces/fb700/chat3/crazy_functions/\347\224\237\346\210\220\345\207\275\346\225\260\346\263\250\351\207\212.py"
deleted file mode 100644
index a564f21d231cd65c29b539573929ca5d2df63203..0000000000000000000000000000000000000000
--- "a/spaces/fb700/chat3/crazy_functions/\347\224\237\346\210\220\345\207\275\346\225\260\346\263\250\351\207\212.py"
+++ /dev/null
@@ -1,54 +0,0 @@
-from toolbox import update_ui
-from toolbox import CatchException, report_execption, write_results_to_file
-from .crazy_utils import request_gpt_model_in_new_thread_with_ui_alive
-fast_debug = False
-
-def 生成函数注释(file_manifest, project_folder, llm_kwargs, plugin_kwargs, chatbot, history, system_prompt):
- import time, os
- print('begin analysis on:', file_manifest)
- for index, fp in enumerate(file_manifest):
- with open(fp, 'r', encoding='utf-8', errors='replace') as f:
- file_content = f.read()
-
- i_say = f'请对下面的程序文件做一个概述,并对文件中的所有函数生成注释,使用markdown表格输出结果,文件名是{os.path.relpath(fp, project_folder)},文件内容是 ```{file_content}```'
- i_say_show_user = f'[{index}/{len(file_manifest)}] 请对下面的程序文件做一个概述,并对文件中的所有函数生成注释: {os.path.abspath(fp)}'
- chatbot.append((i_say_show_user, "[Local Message] waiting gpt response."))
- yield from update_ui(chatbot=chatbot, history=history) # 刷新界面
-
- if not fast_debug:
- msg = '正常'
- # ** gpt request **
- gpt_say = yield from request_gpt_model_in_new_thread_with_ui_alive(
- i_say, i_say_show_user, llm_kwargs, chatbot, history=[], sys_prompt=system_prompt) # 带超时倒计时
-
- chatbot[-1] = (i_say_show_user, gpt_say)
- history.append(i_say_show_user); history.append(gpt_say)
- yield from update_ui(chatbot=chatbot, history=history, msg=msg) # 刷新界面
- if not fast_debug: time.sleep(2)
-
- if not fast_debug:
- res = write_results_to_file(history)
- chatbot.append(("完成了吗?", res))
- yield from update_ui(chatbot=chatbot, history=history, msg=msg) # 刷新界面
-
-
-
-@CatchException
-def 批量生成函数注释(txt, llm_kwargs, plugin_kwargs, chatbot, history, system_prompt, web_port):
- history = [] # 清空历史,以免输入溢出
- import glob, os
- if os.path.exists(txt):
- project_folder = txt
- else:
- if txt == "": txt = '空空如也的输入栏'
- report_execption(chatbot, history, a = f"解析项目: {txt}", b = f"找不到本地项目或无权访问: {txt}")
- yield from update_ui(chatbot=chatbot, history=history) # 刷新界面
- return
- file_manifest = [f for f in glob.glob(f'{project_folder}/**/*.py', recursive=True)] + \
- [f for f in glob.glob(f'{project_folder}/**/*.cpp', recursive=True)]
-
- if len(file_manifest) == 0:
- report_execption(chatbot, history, a = f"解析项目: {txt}", b = f"找不到任何.tex文件: {txt}")
- yield from update_ui(chatbot=chatbot, history=history) # 刷新界面
- return
- yield from 生成函数注释(file_manifest, project_folder, llm_kwargs, plugin_kwargs, chatbot, history, system_prompt)
diff --git a/spaces/fclong/summary/fengshen/examples/classification/finetune_classification_bert-3.9B_iflytek.sh b/spaces/fclong/summary/fengshen/examples/classification/finetune_classification_bert-3.9B_iflytek.sh
deleted file mode 100644
index 13e08efc318a60eabec72cd4357f8aa9dd558f44..0000000000000000000000000000000000000000
--- a/spaces/fclong/summary/fengshen/examples/classification/finetune_classification_bert-3.9B_iflytek.sh
+++ /dev/null
@@ -1,158 +0,0 @@
-#!/bin/bash
-#SBATCH --job-name=slurm-test # create a short name for your job
-#SBATCH --nodes=1 # node count
-#SBATCH --ntasks=2 # total number of tasks across all nodes
-#SBATCH --cpus-per-task=16 # cpu-cores per task (>1 if multi-threaded tasks)
-#SBATCH --mem-per-cpu=8G # memory per cpu-core (4G is default)
-#SBATCH --gres=gpu:2 # number of gpus per node
-#SBATCH --mail-type=ALL # send email when job begins, ends or failed etc.
-
-
-export TORCH_EXTENSIONS_DIR=/cognitive_comp/yangping/cache/torch_extendsions
-
-BERT_NAME=bert-3.9B
-
-TASK=iflytek
-TEXTA_NAME=sentence
-LABEL_NAME=label
-ID_NAME=id
-
-
-BATCH_SIZE=16
-VAL_BATCH_SIZE=56
-ZERO_STAGE=2
-
-
-ROOT_PATH=cognitive_comp
-DATA_DIR=/$ROOT_PATH/yangping/data/ChineseCLUE_DATA/${TASK}_public/
-PRETRAINED_MODEL_PATH=/$ROOT_PATH/yangping/pretrained_model/$BERT_NAME/
-
-
-CHECKPOINT_PATH=/$ROOT_PATH/yangping/checkpoints/fengshen-finetune/$TASK/
-DEFAULT_ROOT_DIR=/cognitive_comp/yangping/nlp/Fengshenbang-LM/fengshen/scripts/log/$TASK
-OUTPUT_PATH=/$ROOT_PATH/yangping/nlp/modelevaluation/output/${TASK}_predict.json
-
-
-config_json="./ds_config.$SLURM_JOBID.json"
-# Deepspeed figures out GAS dynamically from dynamic GBS via set_train_batch_size()
-# reduce_bucket_size: hidden_size*hidden_size
-# stage3_prefetch_bucket_size: 0.9 * hidden_size * hidden_size
-# stage3_param_persistence_threshold: 10 * hidden_size
-
-cat < $config_json
-{
- "train_micro_batch_size_per_gpu": $BATCH_SIZE,
- "steps_per_print": 100,
- "gradient_clipping": 1.0,
- "zero_optimization": {
- "stage": 3,
- "offload_optimizer": {
- "device": "cpu",
- "pin_memory": true
- },
- "offload_param": {
- "device": "cpu",
- "pin_memory": true
- },
- "overlap_comm": true,
- "contiguous_gradients": true,
- "sub_group_size": 1e9,
- "reduce_bucket_size": 6553600,
- "stage3_prefetch_bucket_size": 5898240,
- "stage3_param_persistence_threshold": 25600,
- "stage3_max_live_parameters": 1e9,
- "stage3_max_reuse_distance": 1e9,
- "stage3_gather_fp16_weights_on_model_save": true
- },
- "optimizer": {
- "type": "Adam",
- "params": {
- "lr": 1e-5,
- "betas": [
- 0.9,
- 0.95
- ],
- "eps": 1e-8,
- "weight_decay": 1e-2
- }
- },
- "scheduler": {
- "type": "WarmupLR",
- "params":{
- "warmup_min_lr": 5e-6,
- "warmup_max_lr": 1e-5
- }
- },
- "zero_allow_untested_optimizer": false,
- "fp16": {
- "enabled": true,
- "loss_scale": 0,
- "loss_scale_window": 1000,
- "hysteresis": 2,
- "min_loss_scale": 1
- },
- "activation_checkpointing": {
- "partition_activations": false,
- "contiguous_memory_optimization": false
- },
- "wall_clock_breakdown": false
-}
-EOT
-
-export PL_DEEPSPEED_CONFIG_PATH=$config_json
-
-
-DATA_ARGS="\
- --data_dir $DATA_DIR \
- --train_data train.json \
- --valid_data dev.json \
- --test_data test.json \
- --train_batchsize $BATCH_SIZE \
- --valid_batchsize $VAL_BATCH_SIZE \
- --max_length 128 \
- --texta_name $TEXTA_NAME \
- --label_name $LABEL_NAME \
- --id_name $ID_NAME \
- "
-
-MODEL_ARGS="\
- --learning_rate 0.00001 \
- --weight_decay 0.01 \
- --warmup 0.001 \
- --num_labels 119 \
- "
-
-MODEL_CHECKPOINT_ARGS="\
- --monitor val_acc \
- --save_top_k 3 \
- --mode max \
- --every_n_train_steps 100 \
- --save_weights_only True \
- --dirpath $CHECKPOINT_PATH \
- --filename model-{epoch:02d}-{val_acc:.4f} \
- "
-TRAINER_ARGS="\
- --max_epochs 7 \
- --gpus 2 \
- --strategy deepspeed_stage_3 \
- --precision 16 \
- --check_val_every_n_epoch 1 \
- --val_check_interval 100 \
- --default_root_dir $DEFAULT_ROOT_DIR \
- "
-
-options=" \
- --pretrained_model_path $PRETRAINED_MODEL_PATH \
- --output_save_path $OUTPUT_PATH \
- $DATA_ARGS \
- $MODEL_ARGS \
- $MODEL_CHECKPOINT_ARGS \
- $TRAINER_ARGS \
- "
-
-DOCKER_PATH=/$ROOT_PATH/yangping/containers/pytorch21_06_py3_docker_image.sif
-SCRIPT_PATH=/$ROOT_PATH/yangping/nlp/fengshen/fengshen/examples/finetune_classification.py
-
-# python3 $SCRIPT_PATH $options
-srun singularity exec --nv -B /cognitive_comp/:/cognitive_comp/ $DOCKER_PATH python3 $SCRIPT_PATH $options
-
diff --git a/spaces/feedexpdition/gardio-patient-clinical-summary/app.py b/spaces/feedexpdition/gardio-patient-clinical-summary/app.py
deleted file mode 100644
index 270717d4f70ab459cb6a9d5827f99fa535d7ada4..0000000000000000000000000000000000000000
--- a/spaces/feedexpdition/gardio-patient-clinical-summary/app.py
+++ /dev/null
@@ -1,86 +0,0 @@
-
-import PyPDF2
-import io
-import os
-from dotenv import load_dotenv
-from dotenv import load_dotenv, find_dotenv
-import json, requests
-import gradio as gr
-from gtts import gTTS
-import IPython.display as ipd
-
-
-_ = load_dotenv(find_dotenv())
-hf_api_key = "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"
-HF_API_SUMMARY_BASE = "https://api-inference.huggingface.co/models/sshleifer/distilbart-cnn-12-6"
-#generator = pipeline('text-generation', model = 'gpt2')
-
-def pdf_2_txt(pdf_path):
- try:
- pdf_file = open(pdf_path, 'rb')
- pdf_reader = PyPDF2.PdfReader(pdf_file)
- text_content = ' '
- for page_number in range(len(pdf_reader.pages)):
- page = pdf_reader.pages[page_number]
- text_content += page.extract_text()
- pdf_file.close()
- text_file_path = "Amy_Cripto_EncounterDetails.txt"
- with open(text_file_path, 'w', encoding = 'utf-8') as text_file:
- text_file.write(text_content)
- print("Text extracted and saved to",text_file_path)
- except Exception as e:
- print("Error:",e)
- return text_file_path
-
-#Summarization endpoint
-def get_completion(inputs, parameters=None,ENDPOINT_URL=HF_API_SUMMARY_BASE):
- headers = {
- "Authorization": f"Bearer {hf_api_key}",
- "Content-Type": "application/json"
- }
- data = { "inputs": inputs }
- if parameters is not None:
- data.update({"parameters": parameters})
- response = requests.request("POST",
- ENDPOINT_URL, headers=headers,
- data=json.dumps(data)
- )
- return json.loads(response.content.decode("utf-8"))
-
-
-
-def summarize(patient_name,time,include_history):
-
- path = "./Data/"+patient_name+".pdf"
- text_file_path = "Amy_Cripto_EncounterDetails.txt" #pdf_2_txt(path)
-
- with open(text_file_path) as f:
- patient_report = f.read()
- output = get_completion(patient_report)
-
- summary_text = output[0]['summary_text']
-
- tts = gTTS(summary_text, lang='en')
- audio_path = 'summary_audio.wav'
- tts.save(audio_path)
-
- print("Processed Successfully.." + output[0]['summary_text'],audio_path )
- return patient_report,summary_text,audio_path
-
-
-
-
-gr.close_all()
-demo = gr.Interface(fn=summarize,
- inputs=[ gr.components.Dropdown(label="Select Patient Report", choices=["Amy_Cripto_EncounterDetails", "James_Wood_PAT0001_ClinicalSummary", "Clifford_Burton_CHARM0031_ClinicalSummary", "Brock_Purdy_0059_ClinicalSummary","DAVID_CROSS_PAT0002_ClinicalSummary","Harry_Styles_0056_ClinicalSummary"]),
- gr.Slider(100, 500, step=100, value=100, label="Summarize Length", info="Summarize between 100-500 words"),
- gr.Checkbox(label="Yes", info="Include previous history and trends ?")
- ],
- outputs=[gr.Textbox(label="Original Report of Patient", lines=7,max_lines=7),
- gr.Textbox(label="Clinical Summary of Patient", lines=5),
- gr.Audio(label = "Read the Summary for me ..")
- ],
- title="CharmHealth CodeRx Hackathon",
- description="THEME : `Clinical Summary Challenge`"
- )
-demo.launch()
\ No newline at end of file
diff --git a/spaces/felixz/open_llm_leaderboard/Makefile b/spaces/felixz/open_llm_leaderboard/Makefile
deleted file mode 100644
index b5685772804c8af4235a8504dc6752bfc9ae5d1d..0000000000000000000000000000000000000000
--- a/spaces/felixz/open_llm_leaderboard/Makefile
+++ /dev/null
@@ -1,13 +0,0 @@
-.PHONY: style format
-
-
-style:
- python -m black --line-length 119 .
- python -m isort .
- ruff check --fix .
-
-
-quality:
- python -m black --check --line-length 119 .
- python -m isort --check-only .
- ruff check .
diff --git a/spaces/feregVcuzo/sanity-test-midi/checkpoint/Catch and Train Dynamons in an Epic RPG Adventure - Free Download.md b/spaces/feregVcuzo/sanity-test-midi/checkpoint/Catch and Train Dynamons in an Epic RPG Adventure - Free Download.md
deleted file mode 100644
index 83620f38b5ad765f35c4a0d35b356298611a2edd..0000000000000000000000000000000000000000
--- a/spaces/feregVcuzo/sanity-test-midi/checkpoint/Catch and Train Dynamons in an Epic RPG Adventure - Free Download.md
+++ /dev/null
@@ -1,154 +0,0 @@
-
-Free Download Dynamons World: A Fun and Addictive RPG Game
-Do you love RPG games that let you catch and train cute and powerful monsters? Do you want to explore an open world full of adventure and challenges? Do you want to battle your friends and other players online in real-time? If you answered yes to any of these questions, then you should try Dynamons World , a free RPG game that is loved by millions of players around the world. In this article, we will tell you what Dynamons World is, how to download it for free, and some tips and tricks for playing it.
-free download dynamons world Download File » https://gohhs.com/2uPna8
- What is Dynamons World?
-Dynamons World is a role-playing game developed by Azerion Casual, a company that specializes in creating fun and casual games for everyone. It is the third installment in the popular Dynamons series, which started as a web-based game inspired by Pokemon. In Dynamons World, you can join the adventure and discover the amazing world of Dynamons, where you can catch and train dozens of unique creatures with different skills and abilities. You can also challenge your friends and other players in online multiplayer battles, where you can show off your skills and strategies. You can also travel across different regions, from the Dynamons Camp to the Temple Ruins, and follow an epic story that will keep you hooked.
- The story and gameplay of Dynamons World
-The story of Dynamons World revolves around your character, who is a young and aspiring Dynamon Captain. You start your journey at the Dynamons Camp, where you meet Jovani, a friendly and helpful mentor who will teach you the basics of catching and training Dynamons. He will also give you your first Dynamon, which you can choose from three options: Tailton, a water-type; Kikflick, a fire-type; or Snorky, a plant-type. Each Dynamon has its own strengths and weaknesses, so choose wisely.
-The gameplay of Dynamons World is simple and intuitive. You can move around the map by tapping or clicking on the screen, and interact with objects and characters by tapping or clicking on them. You can also access your menu by tapping or clicking on the icon at the bottom right corner of the screen, where you can see your inventory, your team, your quests, your achievements, your settings, and more. You can also chat with other players by tapping or clicking on the chat icon at the top right corner of the screen.
-As you explore the world of Dynamons, you will encounter wild Dynamons that you can battle and catch. To start a battle, just tap or click on a wild Dynamon that appears on the map. The battle screen will show your current Dynamon on the left side, and the enemy Dynamon on the right side. You can see their health bars, their names, their levels, and their elements at the top of the screen. You can also see your skill cards at the bottom of the screen, which are special moves that your Dynamon can use in battle. To use a skill card, just tap or click on it, and then tap or click on the enemy Dynamon to target it. Each skill card has a different effect, such as dealing damage, healing, buffing, debuffing, or changing the weather. Some skill cards also have elemental advantages or disadvantages against certain types of Dynamons. For example, water-type skill cards are strong against fire-type Dynamons, but weak against plant-type Dynamons.
-To catch a wild Dynamon, you need to lower its health until it becomes weak enough to be captured. Then, you can use a capture card, which is a special item that you can find or buy in the game. To use a capture card, just tap or click on it, and then tap or click on the wild Dynamon to throw it. The capture card will shake for a few seconds, and if the capture is successful, the wild Dynamon will join your team. If the capture fails, the wild Dynamon will escape and you will lose the capture card. You can have up to six Dynamons in your team at a time, and you can switch between them during battles by tapping or clicking on their icons at the bottom left corner of the screen.
-free download dynamons world apk
-free download dynamons world mod
-free download dynamons world for pc
-free download dynamons world game
-free download dynamons world hack
-free download dynamons world android
-free download dynamons world ios
-free download dynamons world online
-free download dynamons world app
-free download dynamons world cheats
-free download dynamons world latest version
-free download dynamons world offline
-free download dynamons world rpg
-free download dynamons world update
-free download dynamons world bluestacks
-free download dynamons world emulator
-free download dynamons world play store
-free download dynamons world azerion casual
-free download dynamons world review
-free download dynamons world tips
-free download dynamons world guide
-free download dynamons world walkthrough
-free download dynamons world wiki
-free download dynamons world reddit
-free download dynamons world youtube
-free download dynamons world gameplay
-free download dynamons world trailer
-free download dynamons world best team
-free download dynamons world all monsters
-free download dynamons world legendary
-free download dynamons world evolution
-free download dynamons world skills
-free download dynamons world codes
-free download dynamons world generator
-free download dynamons world unlimited coins and gems
-free download dynamons world no ads
-free download dynamons world pvp battles
-free download dynamons world multiplayer mode
-free download dynamons world adventure game
-free download dynamons world catch and train game
-free download dynamons world pokemon like game
-free download dynamons world monster catching game
-free download dynamons world role playing game
-free download dynamons world turn based game
-free download dynamons world strategy game
-free download dynamons world fun and addictive game
-free download dynamons world amazing and immersive game
-free download dynamons world loved by millions of players
-free download dynamons world join the adventure game
- The features and benefits of Dynamons World
-Dynamons World is a game that offers many features and benefits for its players. Some of them are:
-
-Free to play : You can download and play Dynamons World for free, and enjoy all its content without spending any money. However, if you want to support the developers and get some extra perks, you can also buy some in-game currency called coins, which you can use to buy more skill cards, capture cards, potions, and other items. You can also watch some ads to get some free coins or rewards.
-Fun and addictive : Dynamons World is a game that will keep you entertained for hours, as you catch and train your Dynamons, explore the world, complete quests, earn achievements, and battle other players. The game has a colorful and charming graphics style, a catchy and upbeat soundtrack, and a humorous and engaging storyline. The game is also easy to play, but hard to master, as you need to use your skills and strategies to win battles and progress in the game.
-Online multiplayer : Dynamons World is a game that lets you connect with other players from around the world, and challenge them in real-time battles. You can either join a random match, or invite your friends to play with you. You can also chat with other players, send them gifts, and join clans. By playing online battles, you can earn trophies, which will increase your rank and unlock new rewards.
-
- How to download Dynamons World for free?
-Dynamons World is a game that is available for both Android and iOS devices, as well as for PC using an emulator. Here are the steps to download Dynamons World for free:
- Download Dynamons World from Google Play Store
-If you have an Android device, you can download Dynamons World from the Google Play Store by following these steps:
-
-Open the Google Play Store app on your device.
-Search for "Dynamons World" in the search bar.
-Tap on the icon of Dynamons World from the results.
-Tap on the "Install" button to start downloading the game.
-Wait for the download to finish, and then tap on the "Open" button to launch the game.
-
- Download Dynamons World from App Store
-If you have an iOS device, you can download Dynamons World from the App Store by following these steps:
-
-Open the App Store app on your device.
-Search for "Dynamons World" in the search bar.
-Tap on the icon of Dynamons World from the results.
-Tap on the "Get" button to start downloading the game.
-Wait for the download to finish, and then tap on the "Open" button to launch the game.
-
- Download Dynamons World from BlueStacks Emulator
-If you want to play Dynamons World on your PC, you can use an emulator called BlueStacks, which is a software that allows you to run Android apps on your computer. To download Dynamons World from BlueStacks Emulator, follow these steps:
-
-Download and install BlueStacks Emulator from its official website: https://www.bluestacks.com/
-Launch BlueStacks Emulator on your PC.
-Login with your Google account or create a new one.
-Search for "Dynamons World" in the search bar.
-Click on the icon of Dynamons World from the results.
-Click on the "Install" button to start downloading the game.
-Wait for the download to finish, and then click on the "Open" button to launch the game.
-
- Tips and tricks for playing Dynamons World
-Dynamons World is a game that requires some skill and strategy to play well. Here are some tips and tricks that can help you improve your performance and enjoy the game more:
- Choose your starter Dynamon wisely
-Your starter Dynamon is the first Dynamon that you will receive in the game, and it will be your main companion throughout your adventure. Therefore, you should choose it wisely, based on your preference and playstyle. There are three options to choose from: Tailton, a water-type; Kikflick, a fire-type; or Snorky, a plant-type. Each one has its own strengths and weaknesses, as well as different skill cards that they can learn. For example, Tailton is good at healing and buffing, Kikflick is good at dealing damage and debuffing, and Snorky is good at changing the weather and inflicting status effects. You should also consider the elemental advantages and disadvantages of each type, as they will affect your battles against other Dynamons. For example, water-type Dynamons are strong against fire-type Dynamons, but weak against plant-type Dynamons.
- Collect and train more Dynamons
-As you progress in the game, you will encounter more Dynamons that you can catch and add to your team. You should try to collect as many as you can, as they will give you more options and variety in your battles. You can also train your Dynamons by battling with them, which will increase their level and stats, as well as unlock new skill cards that they can use. You can also evolve your Dynamons by using evolution stones, which are rare items that you can find or buy in the game. Evolution will change the appearance and abilities of your Dynamons, making them stronger and more powerful.
- Use skill cards and elemental advantages
-Skill cards are special moves that your Dynamons can use in battle. They have different effects, such as dealing damage, healing, buffing, debuffing, or changing the weather. Some skill cards also have elemental advantages or disadvantages against certain types of Dynamons. For example, water-type skill cards are strong against fire-type Dynamons, but weak against plant-type Dynamons. You should use skill cards wisely, based on the situation and the enemy's type. You should also try to collect more skill cards by buying them from shops or finding them in chests or quests. You can equip up to four skill cards for each Dynamon at a time, and you can change them anytime by accessing your menu.
- Challenge other players in online battles
-Dynamons World is a game that lets you challenge other players in online multiplayer battles, where you can show off your skills and strategies. You can either join a random match, or invite your friends to play with you. You can also chat with other players, send them gifts, and join clans. By playing online battles, you can earn trophies, which will increase your rank and unlock new rewards. You can also see your stats and achievements in the leaderboard, where you can compare yourself with other players from around the world.
- Conclusion
-Dynamons World is a fun and addictive RPG game that lets you catch and train cute and powerful monsters, explore an open world full of adventure and challenges, and battle your friends and other players online in real-time. It is a game that is free to play, easy to learn, but hard to master. It is a game that will keep you entertained for hours, as you discover the amazing world of Dynamons.
- Summary of the main points
-In this article, we have covered the following points:
-
-What is Dynamons World?
-How to download Dynamons World for free?
-Tips and tricks for playing Dynamons World.
-
- Call to action
-If you are interested in playing Dynamons World, you can download it for free from the Google Play Store, the App Store, or the BlueStacks Emulator. You can also visit the official website of Dynamons World for more information: https://www.dynamonsworld.com/
-What are you waiting for? Join the adventure and become the best Dynamon Captain in the world!
- Frequently Asked Questions
-Here are some frequently asked questions about Dynamons World:
-
-How many Dynamons are there in the game?
-There are over 50 different Dynamons that you can catch and train in the game. Each one has its own unique appearance, personality, skills, and abilities.
-What are the different types of Dynamons in the game?
-There are six different types of Dynamons in the game: water, fire, plant, electric, normal, and ghost. Each type has its own advantages and disadvantages against other types. For example, water-type Dynamons are strong against fire-type Dynamons, but weak against plant-type Dynamons.
-How can I get more coins in the game?
-Coins are the in-game currency that you can use to buy more skill cards, capture cards, potions, and other items. You can get more coins by doing the following things:
-
-Completing quests and achievements.
-Winning battles against wild Dynamons or other players.
-Finding chests or coins on the map.
-Watching ads or completing offers.
-Buying coins with real money.
-
-How can I join a clan in the game?
-A clan is a group of players who can chat, send gifts, and battle together. You can join a clan by doing the following things:
-
-Tapping or clicking on the clan icon at the top left corner of the screen.
-Searching for a clan that suits your preferences and level.
-Sending a request to join the clan.
-Waiting for the clan leader or co-leader to accept your request.
-
-How can I contact the developers of the game?
-If you have any questions, feedback, or issues about the game, you can contact the developers of the game by doing the following things:
-
-Tapping or clicking on the settings icon at the bottom right corner of the screen.
-Tapping or clicking on the "Support" button.
-Filling out the form with your name, email, subject, and message.
-Tapping or clicking on the "Send" button.
- 197e85843d
-
-
\ No newline at end of file
diff --git a/spaces/feregVcuzo/sanity-test-midi/checkpoint/Download Candy Crush Saga Mod APK for iPhone and Have Fun with the Amazing Graphics and Sound Effects.md b/spaces/feregVcuzo/sanity-test-midi/checkpoint/Download Candy Crush Saga Mod APK for iPhone and Have Fun with the Amazing Graphics and Sound Effects.md
deleted file mode 100644
index 5463da4260f592f99c54dbf2a6df0437b50f820d..0000000000000000000000000000000000000000
--- a/spaces/feregVcuzo/sanity-test-midi/checkpoint/Download Candy Crush Saga Mod APK for iPhone and Have Fun with the Amazing Graphics and Sound Effects.md
+++ /dev/null
@@ -1,99 +0,0 @@
-
-Download Candy Crush Saga Mod Apk for iPhone
-If you are a fan of puzzle games, you must have heard of Candy Crush Saga, one of the most popular and addictive games in the world. But did you know that you can download Candy Crush Saga mod apk for iPhone and enjoy unlimited gameplay with all the features unlocked? In this article, we will tell you everything you need to know about Candy Crush Saga mod apk, how to download it, and what are the pros and cons of using it.
- What is Candy Crush Saga?
-Candy Crush Saga is a match-three puzzle game developed by King and released in 2012. The game has over 8,000 levels and episodes, each with different goals and challenges. The game is free to play, but some features require in-app purchases or watching ads. The game is available for Android, iOS, Windows Phone, and Facebook platforms.
-download candy crush saga mod apk for iphone Download >>> https://gohhs.com/2uPoXB
- Features of Candy Crush Saga
-Some of the features of Candy Crush Saga are:
-
-Colorful and sweet graphics with various candies and characters
-Fun and challenging gameplay with different modes and obstacles
-Social features that allow you to connect with your friends and compete with them
-Daily rewards and events that give you extra lives, moves, and boosters
-New levels and episodes added regularly
-
- Why download Candy Crush Saga mod apk?
-Candy Crush Saga mod apk is a modified version of the original game that gives you unlimited access to all the features without spending any money or watching any ads. With Candy Crush Saga mod apk, you can enjoy:
-
-Unlimited lives, moves, and boosters that help you complete any level easily
-Access to all levels and episodes without waiting for updates or unlocking them
-No ads and no in-app purchases that interrupt your gameplay or tempt you to spend money
-A more satisfying gaming experience with more freedom and fun
-
- How to download Candy Crush Saga mod apk for iPhone?
-To download Candy Crush Saga mod apk for iPhone, you need to follow these steps:
- Step 1: Download the mod apk file from a trusted source
-The first step is to find a reliable website that offers the mod apk file for Candy Crush Saga. You can use Google or any other search engine to look for it. Make sure you check the reviews and ratings of the website before downloading anything. One of the websites that we recommend is [APKdone](^1^), which has a high-quality mod apk file for Candy Crush Saga. You can download it by clicking on the link below:
- Download Candy Crush Saga Mod Apk for iPhone
- Step 2: Install the mod apk file using a third-party app installer
-The second step is to install the mod apk file on your iPhone using a third-party app installer. You cannot install it directly from your device because Apple does not allow installing apps from unknown sources. You need to use an app installer like TutuApp, AppValley, or Panda Helper that can bypass this restriction. You can download any of these app installers from their official websites. Once you have downloaded an app installer, follow these steps to install the mod apk file:
-How to install candy crush saga mod apk on iphone
-Candy crush saga mod apk unlimited lives and boosters for iphone
-Candy crush saga mod apk latest version free download for iphone
-Candy crush saga hack ios download no jailbreak
-Candy crush saga cheats ios 2023
-Best site to download candy crush saga mod apk for iphone
-Candy crush saga mod apk offline for iphone
-Candy crush saga mod apk with all levels unlocked for iphone
-Candy crush saga mod apk ios 14
-Candy crush saga mod apk no ads for iphone
-Download candy crush soda saga mod apk for iphone
-Candy crush saga mod apk unlimited gold bars for iphone
-Candy crush saga mod apk 1.254.2.1 for iphone
-Candy crush saga mod menu ios download
-Candy crush saga tips and tricks ios
-Download candy crush jelly saga mod apk for iphone
-Candy crush saga mod apk unlimited everything for iphone
-Candy crush saga mod apk free shopping for iphone
-Candy crush saga hack tool ios download
-Candy crush saga unlimited moves ios
-Download candy crush friends saga mod apk for iphone
-Candy crush saga mod apk all episodes unlocked for iphone
-Candy crush saga mod apk 2023 for iphone
-Candy crush saga hack online generator ios
-Candy crush saga free boosters ios
-Download candy crush dreamworld mod apk for iphone
-Candy crush saga mod apk unlimited time for iphone
-Candy crush saga mod apk with facebook login for iphone
-Candy crush saga hack without human verification ios
-Candy crush saga free lives ios
- - Open the app installer and search for Candy Crush Saga mod apk - Tap on the install button and wait for the installation to complete - Trust the app developer by going to Settings > General > Profiles & Device Management and selecting the app developer's name - Launch the app from your home screen and enjoy the mod apk Step 3: Enjoy the unlimited gameplay with the mod apk
-The third and final step is to enjoy the unlimited gameplay with the mod apk. You can now play any level or episode you want, use any booster or move you need, and never run out of lives. You can also connect with your Facebook friends and share your progress and achievements. You can also switch back to the original game anytime you want by deleting the mod apk and reinstalling the official app from the App Store.
- Pros and cons of downloading Candy Crush Saga mod apk for iPhone
-Downloading Candy Crush Saga mod apk for iPhone has its advantages and disadvantages. Here are some of them:
- Pros
-
-Unlimited lives, moves, and boosters
-This is the main benefit of downloading the mod apk. You can play as much as you want without worrying about running out of lives, moves, or boosters. You can also use any booster or move you need to complete any level easily. This makes the game more fun and less frustrating.
-Access to all levels and episodes
-Another benefit of downloading the mod apk is that you can access all levels and episodes without waiting for updates or unlocking them. You can play any level or episode you want, regardless of your progress or rank. You can also replay any level or episode you like, without losing any lives, moves, or boosters.
-No ads and no in-app purchases
-A third benefit of downloading the mod apk is that you can enjoy the game without any ads or in-app purchases. You don't have to watch any ads or spend any money to get extra lives, moves, or boosters. You can also avoid any temptation or pressure to buy anything from the game store.
-
- Cons
-
-Risk of malware and viruses
-One of the drawbacks of downloading the mod apk is that you may expose your device to malware and viruses. Since you are downloading the mod apk from an unknown source, you cannot be sure if it is safe or not. You may end up installing a malicious file that can harm your device or steal your personal information. Therefore, you should always scan the mod apk file before installing it and use a reliable antivirus app on your device.
-Possible ban from the official game server
-Another drawback of downloading the mod apk is that you may get banned from the official game server. Since you are using a modified version of the game, you may violate the terms and conditions of the game developer. You may also get detected by the game's anti-cheat system and get flagged as a cheater. If this happens, you may lose your account, progress, and achievements. Therefore, you should always use the mod apk at your own risk and discretion.
-Compatibility issues with some devices and iOS versions
-A third drawback of downloading the mod apk is that you may face compatibility issues with some devices and iOS versions. Since you are using a third-party app installer, you may not be able to install the mod apk on some devices or iOS versions. You may also encounter some bugs or glitches while playing the game with the mod apk. Therefore, you should always check the compatibility of the mod apk with your device and iOS version before installing it.
-
- Conclusion
-Candy Crush Saga is a popular and addictive puzzle game that millions of people enjoy playing. However, if you want to have more fun and freedom while playing it, you can download Candy Crush Saga mod apk for iPhone and get unlimited access to all features without spending any money or watching any ads. In this article, we have explained what Candy Crush Saga mod apk is, how to download it, and what are the pros and cons of using it. We hope this article has been helpful for you and that you have learned something new today.
- FAQs
-
-Q: Is Candy Crush Saga mod apk legal?
-A: Candy Crush Saga mod apk is not legal because it violates the intellectual property rights of the game developer. It also gives an unfair advantage to the players who use it over those who don't. Therefore Therefore, we do not recommend using Candy Crush Saga mod apk or any other mod apk for any game.
-Q: How can I update Candy Crush Saga mod apk?
-A: Candy Crush Saga mod apk may not be updated automatically as the official game app. You may need to download and install the latest version of the mod apk from the same website that you downloaded it from. However, you should be careful as some websites may not update their mod apk files regularly or may provide fake or outdated files. Therefore, you should always check the date and version of the mod apk file before downloading it.
-Q: Can I play Candy Crush Saga mod apk offline?
-A: Candy Crush Saga mod apk can be played offline, but you may not be able to access some features that require an internet connection. For example, you may not be able to connect with your Facebook friends, sync your progress across devices, or participate in daily events and rewards. Therefore, you should always play Candy Crush Saga mod apk online whenever possible.
-Q: Can I play Candy Crush Saga mod apk with the original game app?
-A: Candy Crush Saga mod apk cannot be played with the original game app because they have different package names and signatures. You can only have one version of the game installed on your device at a time. Therefore, you should delete the original game app before installing the mod apk or vice versa.
-Q: Can I transfer my progress from Candy Crush Saga mod apk to the official game app?
-A: Candy Crush Saga mod apk may not be compatible with the official game app, so you may not be able to transfer your progress from one to another. You may also lose your progress if you uninstall the mod apk or switch to the official game app. Therefore, you should backup your progress before making any changes to your game app.
- 197e85843d
-
-
\ No newline at end of file
diff --git a/spaces/feregVcuzo/sanity-test-midi/checkpoint/Download One Piece Bounty Rush APK IOS and Join the Pirate Battle Arena.md b/spaces/feregVcuzo/sanity-test-midi/checkpoint/Download One Piece Bounty Rush APK IOS and Join the Pirate Battle Arena.md
deleted file mode 100644
index f7947675c751a28fb440c209b9e618a396b6cf8b..0000000000000000000000000000000000000000
--- a/spaces/feregVcuzo/sanity-test-midi/checkpoint/Download One Piece Bounty Rush APK IOS and Join the Pirate Battle Arena.md
+++ /dev/null
@@ -1,104 +0,0 @@
-
-One Piece Bounty Rush APK IOS: A Guide for Anime Pirate Fans
-If you are a fan of the manga and anime series One Piece, you might be interested in trying out a game that lets you join the adventure of Luffy and his crew. One Piece Bounty Rush is a 3D anime battle arena treasure looting game that is available for iOS devices. In this article, we will tell you everything you need to know about this game, including how to download and install it, how to play it, and what are some tips and tricks to help you become the Pirate King.
-one piece bounty rush apk ios Download ››››› https://gohhs.com/2uPpOx
- What is One Piece Bounty Rush?
-One Piece Bounty Rush is a game that is based on the popular manga and anime series One Piece, created by Eiichiro Oda. The series follows the adventures of Monkey D. Luffy, a young pirate who dreams of finding the legendary treasure One Piece and becoming the Pirate King. Along his journey, he meets and recruits various characters with unique abilities and personalities, forming the Straw Hat Pirates.
- A 3D anime battle arena treasure looting game
-One Piece Bounty Rush is a game that lets you experience the thrill of pirate battles in a 3D anime style. The game features 4 vs 4 real-time multiplayer battles, where two teams of four players each compete to loot the most treasure. The treasure is represented by berry coins, which are scattered around the map. The team that collects more berry coins within the time limit wins the match.
- Based on the popular manga and anime series One Piece
-One Piece Bounty Rush is a game that faithfully recreates the world and characters of One Piece. You can choose from over 100 characters from the series, including Luffy, Zoro, Nami, Sanji, Chopper, Robin, Franky, Brook, Law, Ace, Sabo, Doflamingo, Big Mom, Kaido, and many more. Each character has their own skills and abilities that match their personality and role in the story. You can also enjoy the original voice acting and sound effects from the anime.
-one piece bounty rush ios download
-one piece bounty rush apk for iphone
-how to play one piece bounty rush on ios
-one piece bounty rush ios release date
-one piece bounty rush apk mod ios
-one piece bounty rush ios app store
-one piece bounty rush apk obb ios
-one piece bounty rush ios gameplay
-one piece bounty rush apk hack ios
-one piece bounty rush ios review
-one piece bounty rush apk latest version ios
-one piece bounty rush ios update
-one piece bounty rush apk free download ios
-one piece bounty rush ios tips and tricks
-one piece bounty rush apk no verification ios
-one piece bounty rush ios characters
-one piece bounty rush apk unlimited diamonds ios
-one piece bounty rush ios requirements
-one piece bounty rush apk offline ios
-one piece bounty rush ios cheats
-one piece bounty rush apk english version ios
-one piece bounty rush ios reddit
-one piece bounty rush apk online ios
-one piece bounty rush ios tier list
-one piece bounty rush apk data ios
-one piece bounty rush ios guide
-one piece bounty rush apk full version ios
-one piece bounty rush ios best characters
-one piece bounty rush apk original ios
-one piece bounty rush ios discord
-one piece bounty rush apk cracked ios
-one piece bounty rush ios codes
-one piece bounty rush apk global version ios
-one piece bounty rush ios wiki
-one piece bounty rush apk no root ios
-one piece bounty rush ios medals
-one piece bounty rush apk new update ios
-one piece bounty rush ios support
-one piece bounty rush apk mirror ios
-one piece bounty rush ios events
-one piece bounty rush apk old version ios
-one piece bounty rush ios forum
-one piece bounty rush apk direct download ios
-one piece bounty rush ios news
-one piece bounty rush apk revdl ios
-one piece bounty rush ios facebook
-one piece bounty rush apk rexdl ios
-one piece bounty rush ios twitter
- Features 4 vs 4 real-time multiplayer battles
-One Piece Bounty Rush is a game that lets you team up with other players from around the world and fight for treasure. You can join random matches or create your own room with your friends. You can also chat with your teammates using preset messages or voice chat. The game has different modes and leagues to suit your preference and skill level. You can also participate in events and campaigns to earn rewards and prizes.
- How to download and install One Piece Bounty Rush APK IOS?
-One Piece Bounty Rush is a game that is available on the App Store for free. You can download and install it on your iOS device easily by following these steps:
- Available on the App Store for free
-One Piece Bounty Rush is a free-to-play game that you can download from and wait for the app to download on your device.
-
Once the download is complete, tap on the "Open" button or find the app icon on your home screen and tap on it.
-Accept the terms and conditions and grant the necessary permissions to the app.
-Choose your preferred language and region from the options.
-Create or log in to your Bandai Namco ID account or play as a guest.
-Enjoy playing One Piece Bounty Rush on your iOS device!
-
- How to play One Piece Bounty Rush?
-One Piece Bounty Rush is a game that is easy to learn but hard to master. The game has a tutorial mode that will teach you the basics of the gameplay, such as how to move, attack, dodge, use skills, and loot treasure. You can also access the help menu from the settings to learn more about the game mechanics, such as character classes, items, medals, and modes. Here are some general tips on how to play One Piece Bounty Rush:
- Create your own pirate crew from various characters
-One Piece Bounty Rush is a game that lets you create your own pirate crew from over 100 characters from the One Piece series. You can collect character fragments from playing matches, events, or campaigns, or by using rainbow diamonds, the premium currency of the game. You can also use character fragments to level up your characters and increase their stats. You can have up to eight characters in your crew, and you can switch between them during matches. You can also customize your characters with costumes, titles, and tags.
- Choose your character class and upgrade your skills
-One Piece Bounty Rush is a game that has five character classes: attacker, defender, runner, support, and warrior. Each class has its own strengths and weaknesses, as well as unique skills and traits. You can upgrade your skills by using skill orbs that you can obtain from playing matches or by using rainbow diamonds. You can also equip medals that enhance your skills and abilities. You can have up to three medals per character, and you can create medal sets that activate bonus effects.
- Fight for treasure in different locations from the anime
-One Piece Bounty Rush is a game that has various locations from the anime as battle stages, such as Alabasta, Marineford, Dressrosa, Whole Cake Island, Wano Country, and more. Each stage has its own layout, obstacles, and environmental effects that can affect the gameplay. You can also encounter special events and characters from the anime that can change the tide of the battle. You can explore different stages by playing different modes and leagues.
- What are the tips and tricks for One Piece Bounty Rush?
-One Piece Bounty Rush is a game that requires strategy and teamwork to win matches. The game has a lot of depth and complexity that can challenge even veteran players. Here are some tips and tricks that can help you improve your skills and enjoy the game more:
- Use items and medals to boost your performance
-One Piece Bounty Rush is a game that has items and medals that can help you gain an edge in battles. Items are consumables that you can use during matches to heal yourself, increase your attack or defense, or revive yourself or your teammates. You can buy items with berry coins before or during matches. Medals are accessories that you can equip on your characters to enhance their stats and skills. You can obtain medals from playing matches or by using rainbow diamonds. You can also upgrade your medals by using medal fragments or other medals.
- Coordinate with your teammates and use strategy
-One Piece Bounty Rush is a game that requires teamwork and communication to win matches. You can chat with your teammates using preset messages or voice chat. You can also use emotes and stamps to express yourself. You should coordinate with your teammates on which treasure areas to capture, which enemies to target, and when to use skills or items. You should also use strategy based on your character class, stage layout, and enemy composition.
- Collect character fragments and unlock new characters
-One Piece Bounty Rush is a game that has a lot of characters to collect and unlock. You can obtain character fragments from playing matches, events, campaigns, or by using rainbow diamonds. You need a certain number of character fragments to unlock a character or level them up. You should try to collect as many character fragments as possible to expand your crew and increase your options.
- Conclusion
-One Piece Bounty Rush is a game that is a must-try for anime pirate fans. The game lets you join the adventure of Luffy and his crew in a 3D anime battle arena treasure looting game. The game is based on the popular manga and anime series One Piece, and features over 100 characters from the series. The game is available on the App Store for free, and requires iOS 9.0 or later and compatible devices. The game is easy to learn but hard to master, and requires strategy and teamwork to win matches. The game has different modes and leagues to suit your preference and skill level, and also has events and campaigns to earn rewards and prizes. You can also customize your characters with costumes, titles, and tags, and use items and medals to boost your performance. If you are looking for a fun and exciting game that lets you experience the world of One Piece, you should download and install One Piece Bounty Rush APK IOS today!
- FAQs
-Here are some frequently asked questions about One Piece Bounty Rush APK IOS:
-
-Q: How can I get more rainbow diamonds?
-A: Rainbow diamonds are the premium currency of the game, which you can use to buy character fragments, items, medals, costumes, and more. You can get rainbow diamonds by completing missions, achievements, events, campaigns, or by purchasing them with real money.
-Q: How can I change my character's costume?
-A: You can change your character's costume by tapping on the "Edit Crew" button on the main menu, then tapping on the character you want to change. You can then tap on the "Costume" button on the bottom right corner of the screen, and choose from the available costumes. Some costumes are exclusive to certain events or campaigns, so be sure to check them out.
-Q: How can I join a league?
-A: You can join a league by tapping on the "League Battle" button on the main menu, then tapping on the "Join League" button on the top right corner of the screen. You can then choose from the available leagues based on your rank and level. You can also create your own league or join a friend's league by using a league code.
-Q: How can I play with my friends?
-A: You can play with your friends by tapping on the "Friend" button on the main menu, then tapping on the "Invite Friend" button on the bottom right corner of the screen. You can then send an invitation code to your friends or enter their invitation code. You can also add your friends as contacts by tapping on their name or icon on the match results screen or the chat screen.
-Q: How can I contact the support team?
-A: You can contact the support team by tapping on the "Settings" button on the main menu, then tapping on the "Support" button on the bottom left corner of the screen. You can then choose from the available options, such as FAQ, Inquiry Form, Terms of Service, Privacy Policy, or Customer Support.
- 197e85843d
-
-
\ No newline at end of file
diff --git a/spaces/fffiloni/controlnet-animation-doodle/node_modules/cors/HISTORY.md b/spaces/fffiloni/controlnet-animation-doodle/node_modules/cors/HISTORY.md
deleted file mode 100644
index 5762bce92212a44c4ceaab3cff5eded5efc72874..0000000000000000000000000000000000000000
--- a/spaces/fffiloni/controlnet-animation-doodle/node_modules/cors/HISTORY.md
+++ /dev/null
@@ -1,58 +0,0 @@
-2.8.5 / 2018-11-04
-==================
-
- * Fix setting `maxAge` option to `0`
-
-2.8.4 / 2017-07-12
-==================
-
- * Work-around Safari bug in default pre-flight response
-
-2.8.3 / 2017-03-29
-==================
-
- * Fix error when options delegate missing `methods` option
-
-2.8.2 / 2017-03-28
-==================
-
- * Fix error when frozen options are passed
- * Send "Vary: Origin" when using regular expressions
- * Send "Vary: Access-Control-Request-Headers" when dynamic `allowedHeaders`
-
-2.8.1 / 2016-09-08
-==================
-
-This release only changed documentation.
-
-2.8.0 / 2016-08-23
-==================
-
- * Add `optionsSuccessStatus` option
-
-2.7.2 / 2016-08-23
-==================
-
- * Fix error when Node.js running in strict mode
-
-2.7.1 / 2015-05-28
-==================
-
- * Move module into expressjs organization
-
-2.7.0 / 2015-05-28
-==================
-
- * Allow array of matching condition as `origin` option
- * Allow regular expression as `origin` option
-
-2.6.1 / 2015-05-28
-==================
-
- * Update `license` in package.json
-
-2.6.0 / 2015-04-27
-==================
-
- * Add `preflightContinue` option
- * Fix "Vary: Origin" header added for "*"
diff --git a/spaces/fgenie/scamtext_PAL_self_consistency/funcs/f_58.py b/spaces/fgenie/scamtext_PAL_self_consistency/funcs/f_58.py
deleted file mode 100644
index d9c717403da12a6fef0c99972c49872c84f7672d..0000000000000000000000000000000000000000
--- a/spaces/fgenie/scamtext_PAL_self_consistency/funcs/f_58.py
+++ /dev/null
@@ -1,34 +0,0 @@
-
-import re
-
-def is_spam(message):
- """
- This function takes a message as input and returns True if the message is a spam, False otherwise.
- It checks for common spam message patterns, such as short URLs, promotional phrases, and unusual punctuation.
- """
-
- # Check for presence of short URLs in the message
- short_url_patterns = [r'bit\.ly', r'goo\.gl', r'me2\.kr', r'gg\.gg', r'opcn-kakao\.com']
- if any(re.search(pattern, message) for pattern in short_url_patterns):
- return True
-
- # Check for promotional phrases in the message
- promo_phrases = [r'상한가확정', r'폭등예상', r'성과', r'지원금', r'거래량', r'수수료',r'무료거부']
- if any(re.search(rf'(?i){phrase}', message) for phrase in promo_phrases):
- return True
-
- # Check for unusual punctuation in the message
- unusual_punctuations = [
- r'\*[^\n]*\*',
- r'\-[^\n]*\-',
- r'\^[^\n]*\^',
- r'\_[^\n]*\_',
- r'◆[^\n]*◆',
- r'▲[^\n]*▲',
- r'▼[^\n]*▼',
- r'▶?[^\n]*\?'
- ]
- if any(re.search(pattern, message) for pattern in unusual_punctuations):
- return True
-
- return False
diff --git a/spaces/fgenie/scamtext_PAL_self_consistency/funcs/f_70.py b/spaces/fgenie/scamtext_PAL_self_consistency/funcs/f_70.py
deleted file mode 100644
index e15d4ae369f1a06d85c626b580ff9de8fb73aab7..0000000000000000000000000000000000000000
--- a/spaces/fgenie/scamtext_PAL_self_consistency/funcs/f_70.py
+++ /dev/null
@@ -1,24 +0,0 @@
-
-import re
-
-def is_spam(text):
- # Check for unusual numeric or special characters percentage
- non_alphabetic_chars = sum(not c.isalnum() for c in text)
- percentage = non_alphabetic_chars / len(text)
- if percentage > 0.3:
- return True
-
- # Check for excessively long alphanumeric strings (potential URLs)
- alphanumeric_chunks = re.compile(r'\S+').split(text)
- for chunk in alphanumeric_chunks:
- if len(chunk) > 20:
- return True
-
- # Check for common spam phrases
- spam_phrases = ['상한가', '최고이자율', '특별정보', 'M반도체', '적금', '출금', '출시', '이벤트',
- '공개', '혜택', '우대', '핵심정보', '투자', '수익률', '계좌']
- for phrase in spam_phrases:
- if phrase in text:
- return True
-
- return False
diff --git a/spaces/finaspirant/SearchWithVoice/interface.py b/spaces/finaspirant/SearchWithVoice/interface.py
deleted file mode 100644
index e354bc5dad2b42f3e26a699f71a8a5f751e71225..0000000000000000000000000000000000000000
--- a/spaces/finaspirant/SearchWithVoice/interface.py
+++ /dev/null
@@ -1,27 +0,0 @@
-import os
-import speech_recognition as sr
-from elevenlabs import generate, play, set_api_key
-set_api_key(os.environ['ELEVEN_API_KEY'])
-
-class AudioInterface:
-
- def listen(self) -> str:
- recognizer = sr.Recognizer()
- with sr.Microphone() as source:
- print("Say something!")
- audio = recognizer.listen(source)
-
- text = recognizer.recognize_whisper_api(
- audio,
- api_key=os.environ['OPENAI_API_KEY'],
- )
-
- return text
-
- def speak(self, text):
- audio = generate(
- text=text,
- voice='Bella',
- model='eleven_monolingual_v1'
- )
- return play(audio)
\ No newline at end of file
diff --git a/spaces/freddyaboulton/3.1.4.9-all-demos/demos/longest_word/run.py b/spaces/freddyaboulton/3.1.4.9-all-demos/demos/longest_word/run.py
deleted file mode 100644
index 4fef82a328c6152c79a52c25ddde70db796c335e..0000000000000000000000000000000000000000
--- a/spaces/freddyaboulton/3.1.4.9-all-demos/demos/longest_word/run.py
+++ /dev/null
@@ -1,18 +0,0 @@
-import gradio as gr
-
-
-def longest_word(text):
- words = text.split(" ")
- lengths = [len(word) for word in words]
- return max(lengths)
-
-
-ex = "The quick brown fox jumped over the lazy dog."
-
-demo = gr.Interface(
- longest_word, "textbox", "label", interpretation="default", examples=[[ex]]
-)
-
-
-if __name__ == "__main__":
- demo.launch()
diff --git a/spaces/georgefen/Face-Landmark-ControlNet/annotator/uniformer/exp/upernet_global_small/test_config_h32.py b/spaces/georgefen/Face-Landmark-ControlNet/annotator/uniformer/exp/upernet_global_small/test_config_h32.py
deleted file mode 100644
index a31e3874f76f9f7b089ac8834d85df2441af9b0e..0000000000000000000000000000000000000000
--- a/spaces/georgefen/Face-Landmark-ControlNet/annotator/uniformer/exp/upernet_global_small/test_config_h32.py
+++ /dev/null
@@ -1,39 +0,0 @@
-_base_ = [
- '../../configs/_base_/models/upernet_uniformer.py',
- '../../configs/_base_/datasets/ade20k.py',
- '../../configs/_base_/default_runtime.py',
- '../../configs/_base_/schedules/schedule_160k.py'
-]
-model = dict(
- backbone=dict(
- type='UniFormer',
- embed_dim=[64, 128, 320, 512],
- layers=[3, 4, 8, 3],
- head_dim=64,
- drop_path_rate=0.25,
- windows=False,
- hybrid=True,
- window_size=32
- ),
- decode_head=dict(
- in_channels=[64, 128, 320, 512],
- num_classes=150
- ),
- auxiliary_head=dict(
- in_channels=320,
- num_classes=150
- ))
-
-# AdamW optimizer, no weight decay for position embedding & layer norm in backbone
-optimizer = dict(_delete_=True, type='AdamW', lr=0.00006, betas=(0.9, 0.999), weight_decay=0.01,
- paramwise_cfg=dict(custom_keys={'absolute_pos_embed': dict(decay_mult=0.),
- 'relative_position_bias_table': dict(decay_mult=0.),
- 'norm': dict(decay_mult=0.)}))
-
-lr_config = dict(_delete_=True, policy='poly',
- warmup='linear',
- warmup_iters=1500,
- warmup_ratio=1e-6,
- power=1.0, min_lr=0.0, by_epoch=False)
-
-data=dict(samples_per_gpu=2)
\ No newline at end of file
diff --git a/spaces/georgefen/Face-Landmark-ControlNet/annotator/uniformer/mmcv/utils/timer.py b/spaces/georgefen/Face-Landmark-ControlNet/annotator/uniformer/mmcv/utils/timer.py
deleted file mode 100644
index e3db7d497d8b374e18b5297e0a1d6eb186fd8cba..0000000000000000000000000000000000000000
--- a/spaces/georgefen/Face-Landmark-ControlNet/annotator/uniformer/mmcv/utils/timer.py
+++ /dev/null
@@ -1,118 +0,0 @@
-# Copyright (c) OpenMMLab. All rights reserved.
-from time import time
-
-
-class TimerError(Exception):
-
- def __init__(self, message):
- self.message = message
- super(TimerError, self).__init__(message)
-
-
-class Timer:
- """A flexible Timer class.
-
- :Example:
-
- >>> import time
- >>> import annotator.uniformer.mmcv as mmcv
- >>> with mmcv.Timer():
- >>> # simulate a code block that will run for 1s
- >>> time.sleep(1)
- 1.000
- >>> with mmcv.Timer(print_tmpl='it takes {:.1f} seconds'):
- >>> # simulate a code block that will run for 1s
- >>> time.sleep(1)
- it takes 1.0 seconds
- >>> timer = mmcv.Timer()
- >>> time.sleep(0.5)
- >>> print(timer.since_start())
- 0.500
- >>> time.sleep(0.5)
- >>> print(timer.since_last_check())
- 0.500
- >>> print(timer.since_start())
- 1.000
- """
-
- def __init__(self, start=True, print_tmpl=None):
- self._is_running = False
- self.print_tmpl = print_tmpl if print_tmpl else '{:.3f}'
- if start:
- self.start()
-
- @property
- def is_running(self):
- """bool: indicate whether the timer is running"""
- return self._is_running
-
- def __enter__(self):
- self.start()
- return self
-
- def __exit__(self, type, value, traceback):
- print(self.print_tmpl.format(self.since_last_check()))
- self._is_running = False
-
- def start(self):
- """Start the timer."""
- if not self._is_running:
- self._t_start = time()
- self._is_running = True
- self._t_last = time()
-
- def since_start(self):
- """Total time since the timer is started.
-
- Returns (float): Time in seconds.
- """
- if not self._is_running:
- raise TimerError('timer is not running')
- self._t_last = time()
- return self._t_last - self._t_start
-
- def since_last_check(self):
- """Time since the last checking.
-
- Either :func:`since_start` or :func:`since_last_check` is a checking
- operation.
-
- Returns (float): Time in seconds.
- """
- if not self._is_running:
- raise TimerError('timer is not running')
- dur = time() - self._t_last
- self._t_last = time()
- return dur
-
-
-_g_timers = {} # global timers
-
-
-def check_time(timer_id):
- """Add check points in a single line.
-
- This method is suitable for running a task on a list of items. A timer will
- be registered when the method is called for the first time.
-
- :Example:
-
- >>> import time
- >>> import annotator.uniformer.mmcv as mmcv
- >>> for i in range(1, 6):
- >>> # simulate a code block
- >>> time.sleep(i)
- >>> mmcv.check_time('task1')
- 2.000
- 3.000
- 4.000
- 5.000
-
- Args:
- timer_id (str): Timer identifier.
- """
- if timer_id not in _g_timers:
- _g_timers[timer_id] = Timer()
- return 0
- else:
- return _g_timers[timer_id].since_last_check()
diff --git a/spaces/georgefen/Face-Landmark-ControlNet/annotator/uniformer/mmseg/models/segmentors/__init__.py b/spaces/georgefen/Face-Landmark-ControlNet/annotator/uniformer/mmseg/models/segmentors/__init__.py
deleted file mode 100644
index dca2f09405330743c476e190896bee39c45498ea..0000000000000000000000000000000000000000
--- a/spaces/georgefen/Face-Landmark-ControlNet/annotator/uniformer/mmseg/models/segmentors/__init__.py
+++ /dev/null
@@ -1,5 +0,0 @@
-from .base import BaseSegmentor
-from .cascade_encoder_decoder import CascadeEncoderDecoder
-from .encoder_decoder import EncoderDecoder
-
-__all__ = ['BaseSegmentor', 'EncoderDecoder', 'CascadeEncoderDecoder']
diff --git a/spaces/georgefen/Face-Landmark-ControlNet/annotator/uniformer/mmseg/models/utils/self_attention_block.py b/spaces/georgefen/Face-Landmark-ControlNet/annotator/uniformer/mmseg/models/utils/self_attention_block.py
deleted file mode 100644
index 440c7b73ee4706fde555595926d63a18d7574acc..0000000000000000000000000000000000000000
--- a/spaces/georgefen/Face-Landmark-ControlNet/annotator/uniformer/mmseg/models/utils/self_attention_block.py
+++ /dev/null
@@ -1,159 +0,0 @@
-import torch
-from annotator.uniformer.mmcv.cnn import ConvModule, constant_init
-from torch import nn as nn
-from torch.nn import functional as F
-
-
-class SelfAttentionBlock(nn.Module):
- """General self-attention block/non-local block.
-
- Please refer to https://arxiv.org/abs/1706.03762 for details about key,
- query and value.
-
- Args:
- key_in_channels (int): Input channels of key feature.
- query_in_channels (int): Input channels of query feature.
- channels (int): Output channels of key/query transform.
- out_channels (int): Output channels.
- share_key_query (bool): Whether share projection weight between key
- and query projection.
- query_downsample (nn.Module): Query downsample module.
- key_downsample (nn.Module): Key downsample module.
- key_query_num_convs (int): Number of convs for key/query projection.
- value_num_convs (int): Number of convs for value projection.
- matmul_norm (bool): Whether normalize attention map with sqrt of
- channels
- with_out (bool): Whether use out projection.
- conv_cfg (dict|None): Config of conv layers.
- norm_cfg (dict|None): Config of norm layers.
- act_cfg (dict|None): Config of activation layers.
- """
-
- def __init__(self, key_in_channels, query_in_channels, channels,
- out_channels, share_key_query, query_downsample,
- key_downsample, key_query_num_convs, value_out_num_convs,
- key_query_norm, value_out_norm, matmul_norm, with_out,
- conv_cfg, norm_cfg, act_cfg):
- super(SelfAttentionBlock, self).__init__()
- if share_key_query:
- assert key_in_channels == query_in_channels
- self.key_in_channels = key_in_channels
- self.query_in_channels = query_in_channels
- self.out_channels = out_channels
- self.channels = channels
- self.share_key_query = share_key_query
- self.conv_cfg = conv_cfg
- self.norm_cfg = norm_cfg
- self.act_cfg = act_cfg
- self.key_project = self.build_project(
- key_in_channels,
- channels,
- num_convs=key_query_num_convs,
- use_conv_module=key_query_norm,
- conv_cfg=conv_cfg,
- norm_cfg=norm_cfg,
- act_cfg=act_cfg)
- if share_key_query:
- self.query_project = self.key_project
- else:
- self.query_project = self.build_project(
- query_in_channels,
- channels,
- num_convs=key_query_num_convs,
- use_conv_module=key_query_norm,
- conv_cfg=conv_cfg,
- norm_cfg=norm_cfg,
- act_cfg=act_cfg)
- self.value_project = self.build_project(
- key_in_channels,
- channels if with_out else out_channels,
- num_convs=value_out_num_convs,
- use_conv_module=value_out_norm,
- conv_cfg=conv_cfg,
- norm_cfg=norm_cfg,
- act_cfg=act_cfg)
- if with_out:
- self.out_project = self.build_project(
- channels,
- out_channels,
- num_convs=value_out_num_convs,
- use_conv_module=value_out_norm,
- conv_cfg=conv_cfg,
- norm_cfg=norm_cfg,
- act_cfg=act_cfg)
- else:
- self.out_project = None
-
- self.query_downsample = query_downsample
- self.key_downsample = key_downsample
- self.matmul_norm = matmul_norm
-
- self.init_weights()
-
- def init_weights(self):
- """Initialize weight of later layer."""
- if self.out_project is not None:
- if not isinstance(self.out_project, ConvModule):
- constant_init(self.out_project, 0)
-
- def build_project(self, in_channels, channels, num_convs, use_conv_module,
- conv_cfg, norm_cfg, act_cfg):
- """Build projection layer for key/query/value/out."""
- if use_conv_module:
- convs = [
- ConvModule(
- in_channels,
- channels,
- 1,
- conv_cfg=conv_cfg,
- norm_cfg=norm_cfg,
- act_cfg=act_cfg)
- ]
- for _ in range(num_convs - 1):
- convs.append(
- ConvModule(
- channels,
- channels,
- 1,
- conv_cfg=conv_cfg,
- norm_cfg=norm_cfg,
- act_cfg=act_cfg))
- else:
- convs = [nn.Conv2d(in_channels, channels, 1)]
- for _ in range(num_convs - 1):
- convs.append(nn.Conv2d(channels, channels, 1))
- if len(convs) > 1:
- convs = nn.Sequential(*convs)
- else:
- convs = convs[0]
- return convs
-
- def forward(self, query_feats, key_feats):
- """Forward function."""
- batch_size = query_feats.size(0)
- query = self.query_project(query_feats)
- if self.query_downsample is not None:
- query = self.query_downsample(query)
- query = query.reshape(*query.shape[:2], -1)
- query = query.permute(0, 2, 1).contiguous()
-
- key = self.key_project(key_feats)
- value = self.value_project(key_feats)
- if self.key_downsample is not None:
- key = self.key_downsample(key)
- value = self.key_downsample(value)
- key = key.reshape(*key.shape[:2], -1)
- value = value.reshape(*value.shape[:2], -1)
- value = value.permute(0, 2, 1).contiguous()
-
- sim_map = torch.matmul(query, key)
- if self.matmul_norm:
- sim_map = (self.channels**-.5) * sim_map
- sim_map = F.softmax(sim_map, dim=-1)
-
- context = torch.matmul(sim_map, value)
- context = context.permute(0, 2, 1).contiguous()
- context = context.reshape(batch_size, -1, *query_feats.shape[2:])
- if self.out_project is not None:
- context = self.out_project(context)
- return context
diff --git a/spaces/gggh/anime-remove-background/app.py b/spaces/gggh/anime-remove-background/app.py
deleted file mode 100644
index 230a0d5f8a3da6ab18ecb8db1cd90016a489b96a..0000000000000000000000000000000000000000
--- a/spaces/gggh/anime-remove-background/app.py
+++ /dev/null
@@ -1,52 +0,0 @@
-import gradio as gr
-import huggingface_hub
-import onnxruntime as rt
-import numpy as np
-import cv2
-
-
-def get_mask(img, s=1024):
- img = (img / 255).astype(np.float32)
- h, w = h0, w0 = img.shape[:-1]
- h, w = (s, int(s * w / h)) if h > w else (int(s * h / w), s)
- ph, pw = s - h, s - w
- img_input = np.zeros([s, s, 3], dtype=np.float32)
- img_input[ph // 2:ph // 2 + h, pw // 2:pw // 2 + w] = cv2.resize(img, (w, h))
- img_input = np.transpose(img_input, (2, 0, 1))
- img_input = img_input[np.newaxis, :]
- mask = rmbg_model.run(None, {'img': img_input})[0][0]
- mask = np.transpose(mask, (1, 2, 0))
- mask = mask[ph // 2:ph // 2 + h, pw // 2:pw // 2 + w]
- mask = cv2.resize(mask, (w0, h0))[:, :, np.newaxis]
- return mask
-
-
-def rmbg_fn(img):
- mask = get_mask(img)
- img = (mask * img + 255 * (1 - mask)).astype(np.uint8)
- mask = (mask * 255).astype(np.uint8)
- img = np.concatenate([img, mask], axis=2, dtype=np.uint8)
- mask = mask.repeat(3, axis=2)
- return mask, img
-
-
-if __name__ == "__main__":
- providers = ['CUDAExecutionProvider', 'CPUExecutionProvider']
- model_path = huggingface_hub.hf_hub_download("skytnt/anime-seg", "isnetis.onnx")
- rmbg_model = rt.InferenceSession(model_path, providers=providers)
- app = gr.Blocks()
- with app:
- gr.Markdown("# Anime Remove Background\n\n"
- "\n\n"
- "demo for [https://github.com/SkyTNT/anime-segmentation/](https://github.com/SkyTNT/anime-segmentation/)")
- with gr.Row():
- with gr.Column():
- input_img = gr.Image(label="input image")
- examples_data = [[f"examples/{x:02d}.jpg"] for x in range(1, 4)]
- examples = gr.Dataset(components=[input_img], samples=examples_data)
- run_btn = gr.Button(variant="primary")
- output_mask = gr.Image(label="mask")
- output_img = gr.Image(label="result", image_mode="RGBA")
- examples.click(lambda x: x[0], [examples], [input_img])
- run_btn.click(rmbg_fn, [input_img], [output_mask, output_img])
- app.launch()
diff --git a/spaces/giesAIexperiments/coursera-assistant-3d-printing-applications/app.py b/spaces/giesAIexperiments/coursera-assistant-3d-printing-applications/app.py
deleted file mode 100644
index 9ae7d400a0d69229b29d1c758a8d320a10e3246f..0000000000000000000000000000000000000000
--- a/spaces/giesAIexperiments/coursera-assistant-3d-printing-applications/app.py
+++ /dev/null
@@ -1,118 +0,0 @@
-import time
-import uuid
-
-import gradio as gr
-from gtts import gTTS
-from transformers import pipeline
-
-from main import index, run
-
-p = pipeline("automatic-speech-recognition", model="openai/whisper-base")
-
-"""Use text to call chat method from main.py"""
-
-models = ["GPT-3.5", "Flan UL2", "Flan T5"]
-
-with gr.Blocks(theme='snehilsanyal/scikit-learn') as demo:
- state = gr.State([])
-
-
- def create_session_id():
- return str(uuid.uuid4())
-
-
- def add_text(history, text, model):
- print("Question asked: " + text)
- response = run_model(text, model)
- history = history + [(text, response)]
- print(history)
- return history, ""
-
-
- def run_model(text, model):
- start_time = time.time()
- print("start time:" + str(start_time))
- response = run(text, model, state.session_id)
- end_time = time.time()
- # If response contains string `SOURCES:`, then add a \n before `SOURCES`
- if "SOURCES:" in response:
- response = response.replace("SOURCES:", "\nSOURCES:")
- # response = response + "\n\n" + "Time taken: " + str(end_time - start_time)
- print(response)
- print("Time taken: " + str(end_time - start_time))
- return response
-
-
- def get_output(history, audio, model):
- txt = p(audio)["text"]
- # history.append(( (audio, ) , txt))
- audio_path = 'response.wav'
- response = run_model(txt, model)
- # Remove all text from SOURCES: to the end of the string
- trimmed_response = response.split("SOURCES:")[0]
- myobj = gTTS(text=trimmed_response, lang='en', slow=False)
- myobj.save(audio_path)
- # split audio by / and keep the last element
- # audio = audio.split("/")[-1]
- # audio = audio + ".wav"
- history.append(((audio,), (audio_path,)))
- print(history)
- return history
-
-
- def set_model(history, model):
- print("Model selected: " + model)
- history = get_first_message(history)
- index(model, state.session_id)
- return history
-
-
- def get_first_message(history):
- history = [(None,
- 'Learn about the course and get answers with sources.\n This is an experiment using AI, so it might make errors')]
- return history
-
-
- def bot(history):
- return history
-
-
- state.session_id = create_session_id()
- print("Session ID: " + state.session_id)
- # Title on top in middle of the page
- # gr.HTML("Course Assistant - 3D Printing Revolution ")
-
- chatbot = gr.Chatbot(get_first_message([]), elem_id="chatbot", label='3D Printing Applications Question Answer Bot').style(height=300,
- container=False)
-
- # with gr.Row():
- # Create radio button to select model
- radio = gr.Radio(models, label="Choose a model", value="GPT-3.5", type="value", visible=False)
- with gr.Row():
- # with gr.Column(scale=0.75):
- txt = gr.Textbox(
- label="Ask your question here and press enter",
- placeholder="Enter text and press enter", lines=1
- ).style(container=False)
-
- # with gr.Column(scale=0.25):
- audio = gr.Audio(source="microphone", type="filepath", visible=False)
-
- txt.submit(add_text, [chatbot, txt, radio], [chatbot, txt], postprocess=False).then(
- bot, chatbot, chatbot
- )
-
- audio.change(fn=get_output, inputs=[chatbot, audio, radio], outputs=[chatbot], show_progress=True).then(
- bot, chatbot, chatbot
- )
-
- radio.change(fn=set_model, inputs=[chatbot, radio], outputs=[chatbot]).then(bot, chatbot, chatbot)
-
- audio.change(lambda: None, None, audio)
-
- set_model(chatbot, radio.value)
-
-if __name__ == "__main__":
- demo.queue()
- demo.queue(concurrency_count=5)
- demo.launch(debug=True)
diff --git a/spaces/giiift/expert_system/README.md b/spaces/giiift/expert_system/README.md
deleted file mode 100644
index 1ff011869386afb7e53f3c1341b7b2400f9ed98f..0000000000000000000000000000000000000000
--- a/spaces/giiift/expert_system/README.md
+++ /dev/null
@@ -1,13 +0,0 @@
----
-title: Comestic Advisor
-emoji: 📊
-colorFrom: green
-colorTo: yellow
-sdk: gradio
-sdk_version: 3.24.1
-app_file: app.py
-pinned: false
-license: unknown
----
-
-Check out the configuration reference at https://huggingface.co/docs/hub/spaces-config-reference
diff --git a/spaces/godot-demo/godot-3d-trucks/README.md b/spaces/godot-demo/godot-3d-trucks/README.md
deleted file mode 100644
index d2b7e6270fe81b0615f7a70f8346f2e3321d8c1e..0000000000000000000000000000000000000000
--- a/spaces/godot-demo/godot-3d-trucks/README.md
+++ /dev/null
@@ -1,10 +0,0 @@
----
-title: Godot 3d Trucks
-emoji: ⚡
-colorFrom: red
-colorTo: blue
-sdk: static
-pinned: false
----
-
-Check out the configuration reference at https://huggingface.co/docs/hub/spaces#reference
diff --git a/spaces/gossminn/fillmorle-app/sftp/utils/functions.py b/spaces/gossminn/fillmorle-app/sftp/utils/functions.py
deleted file mode 100644
index f2e15ebaf918847f78de1cb9fe6b11470552126e..0000000000000000000000000000000000000000
--- a/spaces/gossminn/fillmorle-app/sftp/utils/functions.py
+++ /dev/null
@@ -1,75 +0,0 @@
-from typing import *
-
-import numpy as np
-import torch
-from scipy.optimize import linear_sum_assignment
-from torch.nn.utils.rnn import pad_sequence
-
-
-def num2mask(
- nums: torch.Tensor,
- max_length: Optional[int] = None
-) -> torch.Tensor:
- """
- E.g. input a tensor [2, 3, 4], return [[T T F F], [T T T F], [T T T T]]
- :param nums: Shape [batch]
- :param max_length: maximum length. if not provided, will choose the largest number from nums.
- :return: 2D binary mask.
- """
- shape_backup = nums.shape
- nums = nums.flatten()
- max_length = max_length or int(nums.max())
- batch_size = len(nums)
- range_nums = torch.arange(0, max_length, device=nums.device).unsqueeze(0).expand([batch_size, max_length])
- ret = (range_nums.T < nums).T
- return ret.reshape(*shape_backup, max_length)
-
-
-def mask2idx(
- mask: torch.Tensor,
- max_length: Optional[int] = None,
- padding_value: int = 0,
-) -> Tuple[torch.Tensor, torch.Tensor]:
- """
- E.g. input a tensor [[T T F F], [T T T F], [F F F T]] with padding value -1,
- return [[0, 1, -1], [0, 1, 2], [3, -1, -1]]
- :param mask: Mask tensor. Boolean. Not necessarily to be 2D.
- :param max_length: If provided, will truncate.
- :param padding_value: Padding value. Default to 0.
- :return: Index tensor.
- """
- shape_prefix, mask_length = mask.shape[:-1], mask.shape[-1]
- flat_mask = mask.flatten(0, -2)
- index_list = [torch.arange(mask_length, device=mask.device)[one_mask] for one_mask in flat_mask.unbind(0)]
- index_tensor = pad_sequence(index_list, batch_first=True, padding_value=padding_value)
- if max_length is not None:
- index_tensor = index_tensor[:, :max_length]
- index_tensor = index_tensor.reshape(*shape_prefix, -1)
- return index_tensor, mask.sum(-1)
-
-
-def one_hot(tags: torch.Tensor, num_tags: Optional[int] = None) -> torch.Tensor:
- num_tags = num_tags or int(tags.max())
- ret = tags.new_zeros(size=[*tags.shape, num_tags], dtype=torch.bool)
- ret.scatter_(2, tags.unsqueeze(2), tags.new_ones([*tags.shape, 1], dtype=torch.bool))
- return ret
-
-
-def numpy2torch(
- dict_obj: dict
-) -> dict:
- """
- Convert list/np.ndarray data to torch.Tensor and add add a batch dim.
- """
- ret = dict()
- for k, v in dict_obj.items():
- if isinstance(v, list) or isinstance(v, np.ndarray):
- ret[k] = torch.tensor(v).unsqueeze(0)
- else:
- ret[k] = v
- return ret
-
-
-def max_match(mat: np.ndarray):
- row_idx, col_idx = linear_sum_assignment(mat, True)
- return mat[row_idx, col_idx].sum()
diff --git a/spaces/gotiQspiryo/whisper-ui/examples/K53 Learners Licence Test Papers !FREE! Free Pdf Download.md b/spaces/gotiQspiryo/whisper-ui/examples/K53 Learners Licence Test Papers !FREE! Free Pdf Download.md
deleted file mode 100644
index f4467b3b7446b3626f52c3f2c79e9b68ea200b5d..0000000000000000000000000000000000000000
--- a/spaces/gotiQspiryo/whisper-ui/examples/K53 Learners Licence Test Papers !FREE! Free Pdf Download.md
+++ /dev/null
@@ -1,6 +0,0 @@
-K53 Learners Licence Test Papers Free Pdf Download DOWNLOAD ->>->>->> https://urlgoal.com/2uyMK1
-
-k53 learners test papers full. South Africa learning licence test questions pdf download is available to all for use so be free to download K53. 4d29de3e1b
-
-
-
diff --git a/spaces/grosenthal/aineid/src/aineid/src/react-app-env.d.ts b/spaces/grosenthal/aineid/src/aineid/src/react-app-env.d.ts
deleted file mode 100644
index 6431bc5fc6b2c932dfe5d0418fc667b86c18b9fc..0000000000000000000000000000000000000000
--- a/spaces/grosenthal/aineid/src/aineid/src/react-app-env.d.ts
+++ /dev/null
@@ -1 +0,0 @@
-///
diff --git a/spaces/gulabpatel/Real-ESRGAN/setup.py b/spaces/gulabpatel/Real-ESRGAN/setup.py
deleted file mode 100644
index c2b92e31d2db1aba50767f4f844540cfd53c609d..0000000000000000000000000000000000000000
--- a/spaces/gulabpatel/Real-ESRGAN/setup.py
+++ /dev/null
@@ -1,107 +0,0 @@
-#!/usr/bin/env python
-
-from setuptools import find_packages, setup
-
-import os
-import subprocess
-import time
-
-version_file = 'realesrgan/version.py'
-
-
-def readme():
- with open('README.md', encoding='utf-8') as f:
- content = f.read()
- return content
-
-
-def get_git_hash():
-
- def _minimal_ext_cmd(cmd):
- # construct minimal environment
- env = {}
- for k in ['SYSTEMROOT', 'PATH', 'HOME']:
- v = os.environ.get(k)
- if v is not None:
- env[k] = v
- # LANGUAGE is used on win32
- env['LANGUAGE'] = 'C'
- env['LANG'] = 'C'
- env['LC_ALL'] = 'C'
- out = subprocess.Popen(cmd, stdout=subprocess.PIPE, env=env).communicate()[0]
- return out
-
- try:
- out = _minimal_ext_cmd(['git', 'rev-parse', 'HEAD'])
- sha = out.strip().decode('ascii')
- except OSError:
- sha = 'unknown'
-
- return sha
-
-
-def get_hash():
- if os.path.exists('.git'):
- sha = get_git_hash()[:7]
- else:
- sha = 'unknown'
-
- return sha
-
-
-def write_version_py():
- content = """# GENERATED VERSION FILE
-# TIME: {}
-__version__ = '{}'
-__gitsha__ = '{}'
-version_info = ({})
-"""
- sha = get_hash()
- with open('VERSION', 'r') as f:
- SHORT_VERSION = f.read().strip()
- VERSION_INFO = ', '.join([x if x.isdigit() else f'"{x}"' for x in SHORT_VERSION.split('.')])
-
- version_file_str = content.format(time.asctime(), SHORT_VERSION, sha, VERSION_INFO)
- with open(version_file, 'w') as f:
- f.write(version_file_str)
-
-
-def get_version():
- with open(version_file, 'r') as f:
- exec(compile(f.read(), version_file, 'exec'))
- return locals()['__version__']
-
-
-def get_requirements(filename='requirements.txt'):
- here = os.path.dirname(os.path.realpath(__file__))
- with open(os.path.join(here, filename), 'r') as f:
- requires = [line.replace('\n', '') for line in f.readlines()]
- return requires
-
-
-if __name__ == '__main__':
- write_version_py()
- setup(
- name='realesrgan',
- version=get_version(),
- description='Real-ESRGAN aims at developing Practical Algorithms for General Image Restoration',
- long_description=readme(),
- long_description_content_type='text/markdown',
- author='Xintao Wang',
- author_email='xintao.wang@outlook.com',
- keywords='computer vision, pytorch, image restoration, super-resolution, esrgan, real-esrgan',
- url='https://github.com/xinntao/Real-ESRGAN',
- include_package_data=True,
- packages=find_packages(exclude=('options', 'datasets', 'experiments', 'results', 'tb_logger', 'wandb')),
- classifiers=[
- 'Development Status :: 4 - Beta',
- 'License :: OSI Approved :: Apache Software License',
- 'Operating System :: OS Independent',
- 'Programming Language :: Python :: 3',
- 'Programming Language :: Python :: 3.7',
- 'Programming Language :: Python :: 3.8',
- ],
- license='BSD-3-Clause License',
- setup_requires=['cython', 'numpy'],
- install_requires=get_requirements(),
- zip_safe=False)
diff --git a/spaces/gwang-kim/DATID-3D/pose_estimation/nvdiffrast/nvdiffrast/common/antialias.h b/spaces/gwang-kim/DATID-3D/pose_estimation/nvdiffrast/nvdiffrast/common/antialias.h
deleted file mode 100644
index a35737db38c3f70da9ca81729cba4f5515a201d2..0000000000000000000000000000000000000000
--- a/spaces/gwang-kim/DATID-3D/pose_estimation/nvdiffrast/nvdiffrast/common/antialias.h
+++ /dev/null
@@ -1,49 +0,0 @@
-// Copyright (c) 2020, NVIDIA CORPORATION. All rights reserved.
-//
-// NVIDIA CORPORATION and its licensors retain all intellectual property
-// and proprietary rights in and to this software, related documentation
-// and any modifications thereto. Any use, reproduction, disclosure or
-// distribution of this software and related documentation without an express
-// license agreement from NVIDIA CORPORATION is strictly prohibited.
-
-#pragma once
-#include "common.h"
-
-//------------------------------------------------------------------------
-// Constants and helpers.
-
-#define AA_DISCONTINUITY_KERNEL_BLOCK_WIDTH 32
-#define AA_DISCONTINUITY_KERNEL_BLOCK_HEIGHT 8
-#define AA_ANALYSIS_KERNEL_THREADS_PER_BLOCK 256
-#define AA_MESH_KERNEL_THREADS_PER_BLOCK 256
-#define AA_HASH_ELEMENTS_PER_TRIANGLE 8 // Minimum is 4 but 8 gives fewer collisions. Must be power of two.
-#define AA_GRAD_KERNEL_THREADS_PER_BLOCK 256
-
-//------------------------------------------------------------------------
-// CUDA kernel params.
-
-struct AntialiasKernelParams
-{
- const float* color; // Incoming color buffer.
- const float* rasterOut; // Incoming rasterizer output buffer.
- const int* tri; // Incoming triangle buffer.
- const float* pos; // Incoming position buffer.
- float* output; // Output buffer of forward kernel.
- const float* dy; // Incoming gradients.
- float* gradColor; // Output buffer, color gradient.
- float* gradPos; // Output buffer, position gradient.
- int4* workBuffer; // Buffer for storing intermediate work items. First item reserved for counters.
- uint4* evHash; // Edge-vertex hash.
- int allocTriangles; // Number of triangles accommodated by evHash. Always power of two.
- int numTriangles; // Number of triangles.
- int numVertices; // Number of vertices.
- int width; // Input width.
- int height; // Input height.
- int n; // Minibatch size.
- int channels; // Channel count in color input.
- float xh, yh; // Transfer to pixel space.
- int instance_mode; // 0=normal, 1=instance mode.
- int tri_const; // 1 if triangle array is known to be constant.
-};
-
-//------------------------------------------------------------------------
diff --git a/spaces/gyrojeff/YuzuMarker.FontDetection/batch_generate_script_cmd_64.bat b/spaces/gyrojeff/YuzuMarker.FontDetection/batch_generate_script_cmd_64.bat
deleted file mode 100644
index 3b898a2b9d65e389ff773a0ae06898ba102398b8..0000000000000000000000000000000000000000
--- a/spaces/gyrojeff/YuzuMarker.FontDetection/batch_generate_script_cmd_64.bat
+++ /dev/null
@@ -1,64 +0,0 @@
-start " 1" cmd /K python font_ds_generate_script.py 1 64
-start " 2" cmd /K python font_ds_generate_script.py 2 64
-start " 3" cmd /K python font_ds_generate_script.py 3 64
-start " 4" cmd /K python font_ds_generate_script.py 4 64
-start " 5" cmd /K python font_ds_generate_script.py 5 64
-start " 6" cmd /K python font_ds_generate_script.py 6 64
-start " 7" cmd /K python font_ds_generate_script.py 7 64
-start " 8" cmd /K python font_ds_generate_script.py 8 64
-start " 9" cmd /K python font_ds_generate_script.py 9 64
-start "10" cmd /K python font_ds_generate_script.py 10 64
-start "11" cmd /K python font_ds_generate_script.py 11 64
-start "12" cmd /K python font_ds_generate_script.py 12 64
-start "13" cmd /K python font_ds_generate_script.py 13 64
-start "14" cmd /K python font_ds_generate_script.py 14 64
-start "15" cmd /K python font_ds_generate_script.py 15 64
-start "16" cmd /K python font_ds_generate_script.py 16 64
-start "17" cmd /K python font_ds_generate_script.py 17 64
-start "18" cmd /K python font_ds_generate_script.py 18 64
-start "19" cmd /K python font_ds_generate_script.py 19 64
-start "20" cmd /K python font_ds_generate_script.py 20 64
-start "21" cmd /K python font_ds_generate_script.py 21 64
-start "22" cmd /K python font_ds_generate_script.py 22 64
-start "23" cmd /K python font_ds_generate_script.py 23 64
-start "24" cmd /K python font_ds_generate_script.py 24 64
-start "25" cmd /K python font_ds_generate_script.py 25 64
-start "26" cmd /K python font_ds_generate_script.py 26 64
-start "27" cmd /K python font_ds_generate_script.py 27 64
-start "28" cmd /K python font_ds_generate_script.py 28 64
-start "29" cmd /K python font_ds_generate_script.py 29 64
-start "30" cmd /K python font_ds_generate_script.py 30 64
-start "31" cmd /K python font_ds_generate_script.py 31 64
-start "32" cmd /K python font_ds_generate_script.py 32 64
-start "33" cmd /K python font_ds_generate_script.py 33 64
-start "34" cmd /K python font_ds_generate_script.py 34 64
-start "35" cmd /K python font_ds_generate_script.py 35 64
-start "36" cmd /K python font_ds_generate_script.py 36 64
-start "37" cmd /K python font_ds_generate_script.py 37 64
-start "38" cmd /K python font_ds_generate_script.py 38 64
-start "39" cmd /K python font_ds_generate_script.py 39 64
-start "40" cmd /K python font_ds_generate_script.py 40 64
-start "41" cmd /K python font_ds_generate_script.py 41 64
-start "42" cmd /K python font_ds_generate_script.py 42 64
-start "43" cmd /K python font_ds_generate_script.py 43 64
-start "44" cmd /K python font_ds_generate_script.py 44 64
-start "45" cmd /K python font_ds_generate_script.py 45 64
-start "46" cmd /K python font_ds_generate_script.py 46 64
-start "47" cmd /K python font_ds_generate_script.py 47 64
-start "48" cmd /K python font_ds_generate_script.py 48 64
-start "49" cmd /K python font_ds_generate_script.py 49 64
-start "50" cmd /K python font_ds_generate_script.py 50 64
-start "51" cmd /K python font_ds_generate_script.py 51 64
-start "52" cmd /K python font_ds_generate_script.py 52 64
-start "53" cmd /K python font_ds_generate_script.py 53 64
-start "54" cmd /K python font_ds_generate_script.py 54 64
-start "55" cmd /K python font_ds_generate_script.py 55 64
-start "56" cmd /K python font_ds_generate_script.py 56 64
-start "57" cmd /K python font_ds_generate_script.py 57 64
-start "58" cmd /K python font_ds_generate_script.py 58 64
-start "59" cmd /K python font_ds_generate_script.py 59 64
-start "60" cmd /K python font_ds_generate_script.py 60 64
-start "61" cmd /K python font_ds_generate_script.py 61 64
-start "62" cmd /K python font_ds_generate_script.py 62 64
-start "63" cmd /K python font_ds_generate_script.py 63 64
-start "64" cmd /K python font_ds_generate_script.py 64 64
diff --git a/spaces/h2oai/wave-tour/examples/frame.py b/spaces/h2oai/wave-tour/examples/frame.py
deleted file mode 100644
index 033ac35ba49f3f46d505604f6510b2c8dd5da41e..0000000000000000000000000000000000000000
--- a/spaces/h2oai/wave-tour/examples/frame.py
+++ /dev/null
@@ -1,24 +0,0 @@
-# Frame
-# Use a #frame card to display #HTML content.
-# #form
-# ---
-from h2o_wave import site, ui
-
-html = '''
-
-
-
- Hello World!
-
-
-'''
-
-page = site['/demo']
-
-page['example'] = ui.frame_card(
- box='1 1 2 2',
- title='Example',
- content=html,
-)
-
-page.save()
diff --git a/spaces/haakohu/deep_privacy2/dp2/generator/stylegan_unet.py b/spaces/haakohu/deep_privacy2/dp2/generator/stylegan_unet.py
deleted file mode 100644
index 6c3dfc46da323d04919cf5c166ec038820eac1ad..0000000000000000000000000000000000000000
--- a/spaces/haakohu/deep_privacy2/dp2/generator/stylegan_unet.py
+++ /dev/null
@@ -1,211 +0,0 @@
-import torch
-import numpy as np
-from dp2.layers import Sequential
-from dp2.layers.sg2_layers import Conv2d, FullyConnectedLayer, ResidualBlock
-from .base import BaseStyleGAN
-from typing import List, Tuple
-from .utils import spatial_embed_keypoints, mask_output
-
-
-def get_chsize(imsize, cnum, max_imsize, max_cnum_mul):
- n = int(np.log2(max_imsize) - np.log2(imsize))
- mul = min(2**n, max_cnum_mul)
- ch = cnum * mul
- return int(ch)
-
-
-class StyleGANUnet(BaseStyleGAN):
- def __init__(
- self,
- scale_grad: bool,
- im_channels: int,
- min_fmap_resolution: int,
- imsize: List[int],
- cnum: int,
- max_cnum_mul: int,
- mask_output: bool,
- conv_clamp: int,
- input_cse: bool,
- cse_nc: int,
- n_middle_blocks: int,
- input_keypoints: bool,
- n_keypoints: int,
- input_keypoint_indices: Tuple[int],
- fix_errors: bool,
- **kwargs
- ) -> None:
- super().__init__(**kwargs)
- self.n_keypoints = n_keypoints
- self.input_keypoint_indices = list(input_keypoint_indices)
- self.input_keypoints = input_keypoints
- assert not (input_cse and input_keypoints)
- cse_nc = 0 if cse_nc is None else cse_nc
- self.imsize = imsize
- self._cnum = cnum
- self._max_cnum_mul = max_cnum_mul
- self._min_fmap_resolution = min_fmap_resolution
- self._image_channels = im_channels
- self._max_imsize = max(imsize)
- self.input_cse = input_cse
- self.gain_unet = np.sqrt(1/3)
- n_levels = int(np.log2(self._max_imsize) - np.log2(min_fmap_resolution))+1
- encoder_layers = []
- self.from_rgb = Conv2d(
- im_channels + 1 + input_cse*(cse_nc+1) + input_keypoints*len(self.input_keypoint_indices),
- cnum, 1
- )
- for i in range(n_levels): # Encoder layers
- resolution = [x//2**i for x in imsize]
- in_ch = get_chsize(max(resolution), cnum, self._max_imsize, max_cnum_mul)
- second_ch = in_ch
- out_ch = get_chsize(max(resolution)//2, cnum, self._max_imsize, max_cnum_mul)
- down = 2
-
- if i == 0: # first (lowest) block. Downsampling is performed at the start of the block
- down = 1
- if i == n_levels - 1:
- out_ch = second_ch
- block = ResidualBlock(in_ch, out_ch, down=down, conv_clamp=conv_clamp, fix_residual=fix_errors)
- encoder_layers.append(block)
- self._encoder_out_shape = [
- get_chsize(min_fmap_resolution, cnum, self._max_imsize, max_cnum_mul),
- *resolution]
-
- self.encoder = torch.nn.ModuleList(encoder_layers)
-
- # initialize decoder
- decoder_layers = []
- for i in range(n_levels):
- resolution = [x//2**(n_levels-1-i) for x in imsize]
- in_ch = get_chsize(max(resolution)//2, cnum, self._max_imsize, max_cnum_mul)
- out_ch = get_chsize(max(resolution), cnum, self._max_imsize, max_cnum_mul)
- if i == 0: # first (lowest) block
- in_ch = get_chsize(max(resolution), cnum, self._max_imsize, max_cnum_mul)
-
- up = 1
- if i != n_levels - 1:
- up = 2
- block = ResidualBlock(
- in_ch, out_ch, conv_clamp=conv_clamp, gain_out=np.sqrt(1/3),
- w_dim=self.style_net.w_dim, norm=True, up=up,
- fix_residual=fix_errors
- )
- decoder_layers.append(block)
- if i != 0:
- unet_block = Conv2d(
- in_ch, in_ch, kernel_size=1, conv_clamp=conv_clamp, norm=True,
- gain=np.sqrt(1/3) if fix_errors else np.sqrt(.5))
- setattr(self, f"unet_block{i}", unet_block)
-
- # Initialize "middle blocks" that do not have down/up sample
- middle_blocks = []
- for i in range(n_middle_blocks):
- ch = get_chsize(min_fmap_resolution, cnum, self._max_imsize, max_cnum_mul)
- block = ResidualBlock(
- ch, ch, conv_clamp=conv_clamp, gain_out=np.sqrt(.5) if fix_errors else np.sqrt(1/3),
- w_dim=self.style_net.w_dim, norm=True,
- )
- middle_blocks.append(block)
- if n_middle_blocks != 0:
- self.middle_blocks = Sequential(*middle_blocks)
- self.decoder = torch.nn.ModuleList(decoder_layers)
- self.to_rgb = Conv2d(cnum, im_channels, 1, activation="linear", conv_clamp=conv_clamp)
- # Initialize "middle blocks" that do not have down/up sample
- self.decoder = torch.nn.ModuleList(decoder_layers)
- self.scale_grad = scale_grad
- self.mask_output = mask_output
-
- def forward_dec(self, x, w, unet_features, condition, mask, s, **kwargs):
- for i, layer in enumerate(self.decoder):
- if i != 0:
- unet_layer = getattr(self, f"unet_block{i}")
- x = x + unet_layer(unet_features[-i])
- x = layer(x, w=w, s=s)
- x = self.to_rgb(x)
- if self.mask_output:
- x = mask_output(True, condition, x, mask)
- return dict(img=x)
-
- def forward_enc(self, condition, mask, embedding, keypoints, E_mask, **kwargs):
- if self.input_cse:
- x = torch.cat((condition, mask, embedding, E_mask), dim=1)
- else:
- x = torch.cat((condition, mask), dim=1)
- if self.input_keypoints:
- keypoints = keypoints[:, self.input_keypoint_indices]
- one_hot_pose = spatial_embed_keypoints(keypoints, x)
- x = torch.cat((x, one_hot_pose), dim=1)
- x = self.from_rgb(x)
-
- unet_features = []
- for i, layer in enumerate(self.encoder):
- x = layer(x)
- if i != len(self.encoder)-1:
- unet_features.append(x)
- if hasattr(self, "middle_blocks"):
- for layer in self.middle_blocks:
- x = layer(x)
- return x, unet_features
-
- def forward(
- self, condition, mask,
- z=None, embedding=None, w=None, update_emas=False, x=None,
- s=None,
- keypoints=None,
- unet_features=None,
- E_mask=None,
- **kwargs):
- # Used to skip sampling from encoder in inference. E.g. for w projection.
- if x is not None and unet_features is not None:
- assert not self.training
- else:
- x, unet_features = self.forward_enc(condition, mask, embedding, keypoints, E_mask, **kwargs)
- if w is None:
- if z is None:
- z = self.get_z(condition)
- w = self.get_w(z, update_emas=update_emas)
- return self.forward_dec(x, w, unet_features, condition, mask, s, **kwargs)
-
-
-class ComodStyleUNet(StyleGANUnet):
-
- def __init__(self, min_comod_res=4, lr_multiplier_comod=1, **kwargs) -> None:
- super().__init__(**kwargs)
- min_fmap = min(self._encoder_out_shape[1:])
- enc_out_ch = self._encoder_out_shape[0]
- n_down = int(np.ceil(np.log2(min_fmap) - np.log2(min_comod_res)))
- comod_layers = []
- in_ch = enc_out_ch
- for i in range(n_down):
- comod_layers.append(Conv2d(enc_out_ch, 256, kernel_size=3, down=2, lr_multiplier=lr_multiplier_comod))
- in_ch = 256
- if n_down == 0:
- comod_layers = [Conv2d(in_ch, 256, kernel_size=3)]
- comod_layers.append(torch.nn.Flatten())
- out_res = [x//2**n_down for x in self._encoder_out_shape[1:]]
- in_ch_fc = np.prod(out_res) * 256
- comod_layers.append(FullyConnectedLayer(in_ch_fc, 512, lr_multiplier=lr_multiplier_comod))
- self.comod_block = Sequential(*comod_layers)
- self.comod_fc = FullyConnectedLayer(
- 512+self.style_net.w_dim, self.style_net.w_dim, lr_multiplier=lr_multiplier_comod)
-
- def forward_dec(self, x, w, unet_features, condition, mask, **kwargs):
- y = self.comod_block(x)
- y = torch.cat((y, w), dim=1)
- y = self.comod_fc(y)
- for i, layer in enumerate(self.decoder):
- if i != 0:
- unet_layer = getattr(self, f"unet_block{i}")
- x = x + unet_layer(unet_features[-i], gain=np.sqrt(.5))
- x = layer(x, w=y)
- x = self.to_rgb(x)
- if self.mask_output:
- x = mask_output(True, condition, x, mask)
- return dict(img=x)
-
- def get_comod_y(self, batch, w):
- x, unet_features = self.forward_enc(**batch)
- y = self.comod_block(x)
- y = torch.cat((y, w), dim=1)
- y = self.comod_fc(y)
- return y
diff --git a/spaces/haakohu/deep_privacy2_face/dp2/detection/models/vit_pose/topdown_heatmap_simple_head.py b/spaces/haakohu/deep_privacy2_face/dp2/detection/models/vit_pose/topdown_heatmap_simple_head.py
deleted file mode 100644
index 85c08a76e4dab6d89f4b76183f327005211afd35..0000000000000000000000000000000000000000
--- a/spaces/haakohu/deep_privacy2_face/dp2/detection/models/vit_pose/topdown_heatmap_simple_head.py
+++ /dev/null
@@ -1,505 +0,0 @@
-# Copyright (c) OpenMMLab. All rights reserved.
-# Code adapted from: https://github.com/gpastal24/ViTPose-Pytorch
-import torch
-import torch.nn as nn
-import torch.nn.functional as F
-# Copyright (c) OpenMMLab. All rights reserved.
-from abc import ABCMeta, abstractmethod
-
-# from mmpose.core.evaluation.top_down_eval import keypoints_from_heatmaps
-
-
-class TopdownHeatmapBaseHead(nn.Module):
- """Base class for top-down heatmap heads.
-
- All top-down heatmap heads should subclass it.
- All subclass should overwrite:
-
- Methods:`get_loss`, supporting to calculate loss.
- Methods:`get_accuracy`, supporting to calculate accuracy.
- Methods:`forward`, supporting to forward model.
- Methods:`inference_model`, supporting to inference model.
- """
-
- __metaclass__ = ABCMeta
-
- @abstractmethod
- def get_loss(self, **kwargs):
- """Gets the loss."""
-
- @abstractmethod
- def get_accuracy(self, **kwargs):
- """Gets the accuracy."""
-
- @abstractmethod
- def forward(self, **kwargs):
- """Forward function."""
-
- @abstractmethod
- def inference_model(self, **kwargs):
- """Inference function."""
-
- def decode(self, img_metas, output, **kwargs):
- """Decode keypoints from heatmaps.
-
- Args:
- img_metas (list(dict)): Information about data augmentation
- By default this includes:
-
- - "image_file: path to the image file
- - "center": center of the bbox
- - "scale": scale of the bbox
- - "rotation": rotation of the bbox
- - "bbox_score": score of bbox
- output (np.ndarray[N, K, H, W]): model predicted heatmaps.
- """
- # batch_size = len(img_metas)
-
- # if 'bbox_id' in img_metas[0]:
- # bbox_ids = []
- # else:
- # bbox_ids = None
-
- # c = np.zeros((batch_size, 2), dtype=np.float32)
- # s = np.zeros((batch_size, 2), dtype=np.float32)
- # image_paths = []
- # score = np.ones(batch_size)
- # for i in range(batch_size):
- # c[i, :] = img_metas[i]['center']
- # s[i, :] = img_metas[i]['scale']
- # image_paths.append(img_metas[i]['image_file'])
-
- # if 'bbox_score' in img_metas[i]:
- # score[i] = np.array(img_metas[i]['bbox_score']).reshape(-1)
- # if bbox_ids is not None:
- # bbox_ids.append(img_metas[i]['bbox_id'])
-
- # preds, maxvals = keypoints_from_heatmaps(
- # output,
- # c,
- # s,
- # unbiased=self.test_cfg.get('unbiased_decoding', False),
- # post_process=self.test_cfg.get('post_process', 'default'),
- # kernel=self.test_cfg.get('modulate_kernel', 11),
- # valid_radius_factor=self.test_cfg.get('valid_radius_factor',
- # 0.0546875),
- # use_udp=self.test_cfg.get('use_udp', False),
- # target_type=self.test_cfg.get('target_type', 'GaussianHeatmap'))
-
- # all_preds = np.zeros((batch_size, preds.shape[1], 3), dtype=np.float32)
- # all_boxes = np.zeros((batch_size, 6), dtype=np.float32)
- # all_preds[:, :, 0:2] = preds[:, :, 0:2]
- # all_preds[:, :, 2:3] = maxvals
- # all_boxes[:, 0:2] = c[:, 0:2]
- # all_boxes[:, 2:4] = s[:, 0:2]
- # all_boxes[:, 4] = np.prod(s * 200.0, axis=1)
- # all_boxes[:, 5] = score
-
- # result = {}
-
- # result['preds'] = all_preds
- # result['boxes'] = all_boxes
- # result['image_paths'] = image_paths
- # result['bbox_ids'] = bbox_ids
-
- return None
-
- @staticmethod
- def _get_deconv_cfg(deconv_kernel):
- """Get configurations for deconv layers."""
- if deconv_kernel == 4:
- padding = 1
- output_padding = 0
- elif deconv_kernel == 3:
- padding = 1
- output_padding = 1
- elif deconv_kernel == 2:
- padding = 0
- output_padding = 0
- else:
- raise ValueError(f'Not supported num_kernels ({deconv_kernel}).')
-
- return deconv_kernel, padding, output_padding
-
-
-def build_conv_layer(cfg, *args, **kwargs) -> nn.Module:
- """LICENSE"""
-
- if cfg is None:
- cfg_ = dict(type='Conv2d')
- else:
- if not isinstance(cfg, dict):
- raise TypeError('cfg must be a dict')
- if 'type' not in cfg:
- raise KeyError('the cfg dict must contain the key "type"')
- cfg_ = cfg.copy()
-
- layer_type = cfg_.pop('type')
- if layer_type != 'Conv2d':
- raise KeyError(f'Unrecognized layer type {layer_type}')
- else:
- conv_layer = nn.Conv2d
-
- layer = conv_layer(*args, **kwargs, **cfg_)
-
- return layer
-
-
-def build_upsample_layer(cfg, *args, **kwargs) -> nn.Module:
-
- if not isinstance(cfg, dict):
- raise TypeError(f'cfg must be a dict, but got {type(cfg)}')
- if 'type' not in cfg:
- raise KeyError(
- f'the cfg dict must contain the key "type", but got {cfg}')
- cfg_ = cfg.copy()
-
- layer_type = cfg_.pop('type')
- if layer_type != 'deconv':
- raise KeyError(f'Unrecognized upsample type {layer_type}')
- else:
- upsample = nn.ConvTranspose2d
-
- if upsample is nn.Upsample:
- cfg_['mode'] = layer_type
- layer = upsample(*args, **kwargs, **cfg_)
- return layer
-
-# @HEADS.register_module()
-
-
-class TopdownHeatmapSimpleHead(TopdownHeatmapBaseHead):
- """Top-down heatmap simple head. paper ref: Bin Xiao et al. ``Simple
- Baselines for Human Pose Estimation and Tracking``.
-
- TopdownHeatmapSimpleHead is consisted of (>=0) number of deconv layers
- and a simple conv2d layer.
-
- Args:
- in_channels (int): Number of input channels
- out_channels (int): Number of output channels
- num_deconv_layers (int): Number of deconv layers.
- num_deconv_layers should >= 0. Note that 0 means
- no deconv layers.
- num_deconv_filters (list|tuple): Number of filters.
- If num_deconv_layers > 0, the length of
- num_deconv_kernels (list|tuple): Kernel sizes.
- in_index (int|Sequence[int]): Input feature index. Default: 0
- input_transform (str|None): Transformation type of input features.
- Options: 'resize_concat', 'multiple_select', None.
- Default: None.
-
- - 'resize_concat': Multiple feature maps will be resized to the
- same size as the first one and then concat together.
- Usually used in FCN head of HRNet.
- - 'multiple_select': Multiple feature maps will be bundle into
- a list and passed into decode head.
- - None: Only one select feature map is allowed.
- align_corners (bool): align_corners argument of F.interpolate.
- Default: False.
- loss_keypoint (dict): Config for keypoint loss. Default: None.
- """
-
- def __init__(self,
- in_channels,
- out_channels,
- num_deconv_layers=3,
- num_deconv_filters=(256, 256, 256),
- num_deconv_kernels=(4, 4, 4),
- extra=None,
- in_index=0,
- input_transform=None,
- align_corners=False,
- loss_keypoint=None,
- train_cfg=None,
- test_cfg=None,
- upsample=0,):
- super().__init__()
-
- self.in_channels = in_channels
- self.loss = None
- self.upsample = upsample
-
- self.train_cfg = {} if train_cfg is None else train_cfg
- self.test_cfg = {} if test_cfg is None else test_cfg
- self.target_type = self.test_cfg.get('target_type', 'GaussianHeatmap')
-
- self._init_inputs(in_channels, in_index, input_transform)
- self.in_index = in_index
- self.align_corners = align_corners
-
- if extra is not None and not isinstance(extra, dict):
- raise TypeError('extra should be dict or None.')
-
- if num_deconv_layers > 0:
- self.deconv_layers = self._make_deconv_layer(
- num_deconv_layers,
- num_deconv_filters,
- num_deconv_kernels,
- )
- elif num_deconv_layers == 0:
- self.deconv_layers = nn.Identity()
- else:
- raise ValueError(
- f'num_deconv_layers ({num_deconv_layers}) should >= 0.')
-
- identity_final_layer = False
- if extra is not None and 'final_conv_kernel' in extra:
- assert extra['final_conv_kernel'] in [0, 1, 3]
- if extra['final_conv_kernel'] == 3:
- padding = 1
- elif extra['final_conv_kernel'] == 1:
- padding = 0
- else:
- # 0 for Identity mapping.
- identity_final_layer = True
- kernel_size = extra['final_conv_kernel']
- else:
- kernel_size = 1
- padding = 0
-
- if identity_final_layer:
- self.final_layer = nn.Identity()
- else:
- conv_channels = num_deconv_filters[
- -1] if num_deconv_layers > 0 else self.in_channels
-
- layers = []
- if extra is not None:
- num_conv_layers = extra.get('num_conv_layers', 0)
- num_conv_kernels = extra.get('num_conv_kernels',
- [1] * num_conv_layers)
-
- for i in range(num_conv_layers):
- layers.append(
- build_conv_layer(
- dict(type='Conv2d'),
- in_channels=conv_channels,
- out_channels=conv_channels,
- kernel_size=num_conv_kernels[i],
- stride=1,
- padding=(num_conv_kernels[i] - 1) // 2))
- layers.append(
- nn.BatchNorm2d(conv_channels)
- )
- layers.append(nn.ReLU(inplace=True))
-
- layers.append(
- build_conv_layer(
- cfg=dict(type='Conv2d'),
- in_channels=conv_channels,
- out_channels=out_channels,
- kernel_size=kernel_size,
- stride=1,
- padding=padding))
-
- if len(layers) > 1:
- self.final_layer = nn.Sequential(*layers)
- else:
- self.final_layer = layers[0]
-
- def get_loss(self, output, target, target_weight):
- """Calculate top-down keypoint loss.
-
- Note:
- - batch_size: N
- - num_keypoints: K
- - heatmaps height: H
- - heatmaps weight: W
-
- Args:
- output (torch.Tensor[N,K,H,W]): Output heatmaps.
- target (torch.Tensor[N,K,H,W]): Target heatmaps.
- target_weight (torch.Tensor[N,K,1]):
- Weights across different joint types.
- """
-
- losses = dict()
-
- assert not isinstance(self.loss, nn.Sequential)
- assert target.dim() == 4 and target_weight.dim() == 3
- losses['heatmap_loss'] = self.loss(output, target, target_weight)
-
- return losses
-
- def get_accuracy(self, output, target, target_weight):
- """Calculate accuracy for top-down keypoint loss.
-
- Note:
- - batch_size: N
- - num_keypoints: K
- - heatmaps height: H
- - heatmaps weight: W
-
- Args:
- output (torch.Tensor[N,K,H,W]): Output heatmaps.
- target (torch.Tensor[N,K,H,W]): Target heatmaps.
- target_weight (torch.Tensor[N,K,1]):
- Weights across different joint types.
- """
-
- accuracy = dict()
-
- if self.target_type == 'GaussianHeatmap':
- _, avg_acc, _ = pose_pck_accuracy(
- output.detach().cpu().numpy(),
- target.detach().cpu().numpy(),
- target_weight.detach().cpu().numpy().squeeze(-1) > 0)
- accuracy['acc_pose'] = float(avg_acc)
-
- return accuracy
-
- def forward(self, x):
- """Forward function."""
- x = self._transform_inputs(x)
- x = self.deconv_layers(x)
- x = self.final_layer(x)
- return x
-
- def inference_model(self, x, flip_pairs=None):
- """Inference function.
-
- Returns:
- output_heatmap (np.ndarray): Output heatmaps.
-
- Args:
- x (torch.Tensor[N,K,H,W]): Input features.
- flip_pairs (None | list[tuple]):
- Pairs of keypoints which are mirrored.
- """
- output = self.forward(x)
-
- if flip_pairs is not None:
- output_heatmap = flip_back(
- output.detach().cpu().numpy(),
- flip_pairs,
- target_type=self.target_type)
- # feature is not aligned, shift flipped heatmap for higher accuracy
- if self.test_cfg.get('shift_heatmap', False):
- output_heatmap[:, :, :, 1:] = output_heatmap[:, :, :, :-1]
- else:
- output_heatmap = output.detach().cpu().numpy()
- return output_heatmap
-
- def _init_inputs(self, in_channels, in_index, input_transform):
- """Check and initialize input transforms.
-
- The in_channels, in_index and input_transform must match.
- Specifically, when input_transform is None, only single feature map
- will be selected. So in_channels and in_index must be of type int.
- When input_transform is not None, in_channels and in_index must be
- list or tuple, with the same length.
-
- Args:
- in_channels (int|Sequence[int]): Input channels.
- in_index (int|Sequence[int]): Input feature index.
- input_transform (str|None): Transformation type of input features.
- Options: 'resize_concat', 'multiple_select', None.
-
- - 'resize_concat': Multiple feature maps will be resize to the
- same size as first one and than concat together.
- Usually used in FCN head of HRNet.
- - 'multiple_select': Multiple feature maps will be bundle into
- a list and passed into decode head.
- - None: Only one select feature map is allowed.
- """
-
- if input_transform is not None:
- assert input_transform in ['resize_concat', 'multiple_select']
- self.input_transform = input_transform
- self.in_index = in_index
- if input_transform is not None:
- assert isinstance(in_channels, (list, tuple))
- assert isinstance(in_index, (list, tuple))
- assert len(in_channels) == len(in_index)
- if input_transform == 'resize_concat':
- self.in_channels = sum(in_channels)
- else:
- self.in_channels = in_channels
- else:
- assert isinstance(in_channels, int)
- assert isinstance(in_index, int)
- self.in_channels = in_channels
-
- def _transform_inputs(self, inputs):
- """Transform inputs for decoder.
-
- Args:
- inputs (list[Tensor] | Tensor): multi-level img features.
-
- Returns:
- Tensor: The transformed inputs
- """
- if not isinstance(inputs, list):
- if not isinstance(inputs, list):
- if self.upsample > 0:
- inputs = resize(
- input=F.relu(inputs),
- scale_factor=self.upsample,
- mode='bilinear',
- align_corners=self.align_corners
- )
- return inputs
-
- if self.input_transform == 'resize_concat':
- inputs = [inputs[i] for i in self.in_index]
- upsampled_inputs = [
- resize(
- input=x,
- size=inputs[0].shape[2:],
- mode='bilinear',
- align_corners=self.align_corners) for x in inputs
- ]
- inputs = torch.cat(upsampled_inputs, dim=1)
- elif self.input_transform == 'multiple_select':
- inputs = [inputs[i] for i in self.in_index]
- else:
- inputs = inputs[self.in_index]
-
- return inputs
-
- def _make_deconv_layer(self, num_layers, num_filters, num_kernels):
- """Make deconv layers."""
- if num_layers != len(num_filters):
- error_msg = f'num_layers({num_layers}) ' \
- f'!= length of num_filters({len(num_filters)})'
- raise ValueError(error_msg)
- if num_layers != len(num_kernels):
- error_msg = f'num_layers({num_layers}) ' \
- f'!= length of num_kernels({len(num_kernels)})'
- raise ValueError(error_msg)
-
- layers = []
- for i in range(num_layers):
- kernel, padding, output_padding = \
- self._get_deconv_cfg(num_kernels[i])
-
- planes = num_filters[i]
- layers.append(
- build_upsample_layer(
- dict(type='deconv'),
- in_channels=self.in_channels,
- out_channels=planes,
- kernel_size=kernel,
- stride=2,
- padding=padding,
- output_padding=output_padding,
- bias=False))
- layers.append(nn.BatchNorm2d(planes))
- layers.append(nn.ReLU(inplace=True))
- self.in_channels = planes
-
- return nn.Sequential(*layers)
-
- def init_weights(self):
- """Initialize model weights."""
- for _, m in self.deconv_layers.named_modules():
- if isinstance(m, nn.ConvTranspose2d):
- normal_init(m, std=0.001)
- elif isinstance(m, nn.BatchNorm2d):
- constant_init(m, 1)
- for m in self.final_layer.modules():
- if isinstance(m, nn.Conv2d):
- normal_init(m, std=0.001, bias=0)
- elif isinstance(m, nn.BatchNorm2d):
- constant_init(m, 1)
diff --git a/spaces/hebert2099/MusicGen/tests/data/test_audio_utils.py b/spaces/hebert2099/MusicGen/tests/data/test_audio_utils.py
deleted file mode 100644
index 0480671bb17281d61ce02bce6373a5ccec89fece..0000000000000000000000000000000000000000
--- a/spaces/hebert2099/MusicGen/tests/data/test_audio_utils.py
+++ /dev/null
@@ -1,110 +0,0 @@
-# Copyright (c) Meta Platforms, Inc. and affiliates.
-# All rights reserved.
-#
-# This source code is licensed under the license found in the
-# LICENSE file in the root directory of this source tree.
-
-import julius
-import torch
-import pytest
-
-from audiocraft.data.audio_utils import (
- _clip_wav,
- convert_audio_channels,
- convert_audio,
- normalize_audio
-)
-from ..common_utils import get_batch_white_noise
-
-
-class TestConvertAudioChannels:
-
- def test_convert_audio_channels_downmix(self):
- b, c, t = 2, 3, 100
- audio = get_batch_white_noise(b, c, t)
- mixed = convert_audio_channels(audio, channels=2)
- assert list(mixed.shape) == [b, 2, t]
-
- def test_convert_audio_channels_nochange(self):
- b, c, t = 2, 3, 100
- audio = get_batch_white_noise(b, c, t)
- mixed = convert_audio_channels(audio, channels=c)
- assert list(mixed.shape) == list(audio.shape)
-
- def test_convert_audio_channels_upmix(self):
- b, c, t = 2, 1, 100
- audio = get_batch_white_noise(b, c, t)
- mixed = convert_audio_channels(audio, channels=3)
- assert list(mixed.shape) == [b, 3, t]
-
- def test_convert_audio_channels_upmix_error(self):
- b, c, t = 2, 2, 100
- audio = get_batch_white_noise(b, c, t)
- with pytest.raises(ValueError):
- convert_audio_channels(audio, channels=3)
-
-
-class TestConvertAudio:
-
- def test_convert_audio_channels_downmix(self):
- b, c, dur = 2, 3, 4.
- sr = 128
- audio = get_batch_white_noise(b, c, int(sr * dur))
- out = convert_audio(audio, from_rate=sr, to_rate=sr, to_channels=2)
- assert list(out.shape) == [audio.shape[0], 2, audio.shape[-1]]
-
- def test_convert_audio_channels_upmix(self):
- b, c, dur = 2, 1, 4.
- sr = 128
- audio = get_batch_white_noise(b, c, int(sr * dur))
- out = convert_audio(audio, from_rate=sr, to_rate=sr, to_channels=3)
- assert list(out.shape) == [audio.shape[0], 3, audio.shape[-1]]
-
- def test_convert_audio_upsample(self):
- b, c, dur = 2, 1, 4.
- sr = 2
- new_sr = 3
- audio = get_batch_white_noise(b, c, int(sr * dur))
- out = convert_audio(audio, from_rate=sr, to_rate=new_sr, to_channels=c)
- out_j = julius.resample.resample_frac(audio, old_sr=sr, new_sr=new_sr)
- assert torch.allclose(out, out_j)
-
- def test_convert_audio_resample(self):
- b, c, dur = 2, 1, 4.
- sr = 3
- new_sr = 2
- audio = get_batch_white_noise(b, c, int(sr * dur))
- out = convert_audio(audio, from_rate=sr, to_rate=new_sr, to_channels=c)
- out_j = julius.resample.resample_frac(audio, old_sr=sr, new_sr=new_sr)
- assert torch.allclose(out, out_j)
-
-
-class TestNormalizeAudio:
-
- def test_clip_wav(self):
- b, c, dur = 2, 1, 4.
- sr = 3
- audio = 10.0 * get_batch_white_noise(b, c, int(sr * dur))
- _clip_wav(audio)
- assert audio.abs().max() <= 1
-
- def test_normalize_audio_clip(self):
- b, c, dur = 2, 1, 4.
- sr = 3
- audio = 10.0 * get_batch_white_noise(b, c, int(sr * dur))
- norm_audio = normalize_audio(audio, strategy='clip')
- assert norm_audio.abs().max() <= 1
-
- def test_normalize_audio_rms(self):
- b, c, dur = 2, 1, 4.
- sr = 3
- audio = 10.0 * get_batch_white_noise(b, c, int(sr * dur))
- norm_audio = normalize_audio(audio, strategy='rms')
- assert norm_audio.abs().max() <= 1
-
- def test_normalize_audio_peak(self):
- b, c, dur = 2, 1, 4.
- sr = 3
- audio = 10.0 * get_batch_white_noise(b, c, int(sr * dur))
- norm_audio = normalize_audio(audio, strategy='peak')
- assert norm_audio.abs().max() <= 1
diff --git a/spaces/hectorduran/wordsimilarity/app.py b/spaces/hectorduran/wordsimilarity/app.py
deleted file mode 100644
index ed50e566d6d020a09df71c5ebfd4f74bcf036d1d..0000000000000000000000000000000000000000
--- a/spaces/hectorduran/wordsimilarity/app.py
+++ /dev/null
@@ -1,49 +0,0 @@
-import streamlit as st
-import nltk
-from nltk.corpus import cmudict
-from difflib import SequenceMatcher
-
-# Load CMU Pronouncing Dictionary
-nltk.download('cmudict')
-d = cmudict.dict()
-
-# Function to get phonetic transcription of a word
-def phonetic_transcription(word):
- try:
- return d[word.lower()][0]
- except KeyError:
- return None
-
-# Function to calculate phonetic similarity between two words
-def phonetic_similarity(word1, word2):
- pt1 = phonetic_transcription(word1)
- pt2 = phonetic_transcription(word2)
- if pt1 is None or pt2 is None:
- return 0
- else:
- return SequenceMatcher(None, pt1, pt2).ratio()
-
-# User input for list of words and similarity threshold
-words = st.text_input("Enter list of words (separated by commas):")
-threshold = st.slider("Similarity threshold:", min_value=0.0, max_value=1.0, value=0.5)
-
-if words:
- words = [word.strip() for word in words.split(",")]
- n_words = len(words)
-
- # Calculate phonetic similarity matrix
- similarity_matrix = [[0 for _ in range(n_words)] for _ in range(n_words)]
- for i in range(n_words):
- for j in range(i+1, n_words):
- similarity = phonetic_similarity(words[i], words[j])
- similarity_matrix[i][j] = similarity
- similarity_matrix[j][i] = similarity
-
- # Find similar words based on similarity threshold
- similar_words = []
- for i in range(n_words):
- similar_words.append([words[j] for j in range(n_words) if similarity_matrix[i][j] >= threshold])
-
- # Display similar words with matching score
- for i in range(n_words):
- st.write(f"{words[i]}: {[f'{word} ({int(similarity_matrix[i][j]*100)}%)' for j, word in enumerate(similar_words[i])]}")
\ No newline at end of file
diff --git a/spaces/hhim8826/vits-ATR/attentions.py b/spaces/hhim8826/vits-ATR/attentions.py
deleted file mode 100644
index 4e0b0c1fd48c962e21e1fbe60b23fc574927435c..0000000000000000000000000000000000000000
--- a/spaces/hhim8826/vits-ATR/attentions.py
+++ /dev/null
@@ -1,303 +0,0 @@
-import copy
-import math
-import numpy as np
-import torch
-from torch import nn
-from torch.nn import functional as F
-
-import commons
-import modules
-from modules import LayerNorm
-
-
-class Encoder(nn.Module):
- def __init__(self, hidden_channels, filter_channels, n_heads, n_layers, kernel_size=1, p_dropout=0., window_size=4, **kwargs):
- super().__init__()
- self.hidden_channels = hidden_channels
- self.filter_channels = filter_channels
- self.n_heads = n_heads
- self.n_layers = n_layers
- self.kernel_size = kernel_size
- self.p_dropout = p_dropout
- self.window_size = window_size
-
- self.drop = nn.Dropout(p_dropout)
- self.attn_layers = nn.ModuleList()
- self.norm_layers_1 = nn.ModuleList()
- self.ffn_layers = nn.ModuleList()
- self.norm_layers_2 = nn.ModuleList()
- for i in range(self.n_layers):
- self.attn_layers.append(MultiHeadAttention(hidden_channels, hidden_channels, n_heads, p_dropout=p_dropout, window_size=window_size))
- self.norm_layers_1.append(LayerNorm(hidden_channels))
- self.ffn_layers.append(FFN(hidden_channels, hidden_channels, filter_channels, kernel_size, p_dropout=p_dropout))
- self.norm_layers_2.append(LayerNorm(hidden_channels))
-
- def forward(self, x, x_mask):
- attn_mask = x_mask.unsqueeze(2) * x_mask.unsqueeze(-1)
- x = x * x_mask
- for i in range(self.n_layers):
- y = self.attn_layers[i](x, x, attn_mask)
- y = self.drop(y)
- x = self.norm_layers_1[i](x + y)
-
- y = self.ffn_layers[i](x, x_mask)
- y = self.drop(y)
- x = self.norm_layers_2[i](x + y)
- x = x * x_mask
- return x
-
-
-class Decoder(nn.Module):
- def __init__(self, hidden_channels, filter_channels, n_heads, n_layers, kernel_size=1, p_dropout=0., proximal_bias=False, proximal_init=True, **kwargs):
- super().__init__()
- self.hidden_channels = hidden_channels
- self.filter_channels = filter_channels
- self.n_heads = n_heads
- self.n_layers = n_layers
- self.kernel_size = kernel_size
- self.p_dropout = p_dropout
- self.proximal_bias = proximal_bias
- self.proximal_init = proximal_init
-
- self.drop = nn.Dropout(p_dropout)
- self.self_attn_layers = nn.ModuleList()
- self.norm_layers_0 = nn.ModuleList()
- self.encdec_attn_layers = nn.ModuleList()
- self.norm_layers_1 = nn.ModuleList()
- self.ffn_layers = nn.ModuleList()
- self.norm_layers_2 = nn.ModuleList()
- for i in range(self.n_layers):
- self.self_attn_layers.append(MultiHeadAttention(hidden_channels, hidden_channels, n_heads, p_dropout=p_dropout, proximal_bias=proximal_bias, proximal_init=proximal_init))
- self.norm_layers_0.append(LayerNorm(hidden_channels))
- self.encdec_attn_layers.append(MultiHeadAttention(hidden_channels, hidden_channels, n_heads, p_dropout=p_dropout))
- self.norm_layers_1.append(LayerNorm(hidden_channels))
- self.ffn_layers.append(FFN(hidden_channels, hidden_channels, filter_channels, kernel_size, p_dropout=p_dropout, causal=True))
- self.norm_layers_2.append(LayerNorm(hidden_channels))
-
- def forward(self, x, x_mask, h, h_mask):
- """
- x: decoder input
- h: encoder output
- """
- self_attn_mask = commons.subsequent_mask(x_mask.size(2)).to(device=x.device, dtype=x.dtype)
- encdec_attn_mask = h_mask.unsqueeze(2) * x_mask.unsqueeze(-1)
- x = x * x_mask
- for i in range(self.n_layers):
- y = self.self_attn_layers[i](x, x, self_attn_mask)
- y = self.drop(y)
- x = self.norm_layers_0[i](x + y)
-
- y = self.encdec_attn_layers[i](x, h, encdec_attn_mask)
- y = self.drop(y)
- x = self.norm_layers_1[i](x + y)
-
- y = self.ffn_layers[i](x, x_mask)
- y = self.drop(y)
- x = self.norm_layers_2[i](x + y)
- x = x * x_mask
- return x
-
-
-class MultiHeadAttention(nn.Module):
- def __init__(self, channels, out_channels, n_heads, p_dropout=0., window_size=None, heads_share=True, block_length=None, proximal_bias=False, proximal_init=False):
- super().__init__()
- assert channels % n_heads == 0
-
- self.channels = channels
- self.out_channels = out_channels
- self.n_heads = n_heads
- self.p_dropout = p_dropout
- self.window_size = window_size
- self.heads_share = heads_share
- self.block_length = block_length
- self.proximal_bias = proximal_bias
- self.proximal_init = proximal_init
- self.attn = None
-
- self.k_channels = channels // n_heads
- self.conv_q = nn.Conv1d(channels, channels, 1)
- self.conv_k = nn.Conv1d(channels, channels, 1)
- self.conv_v = nn.Conv1d(channels, channels, 1)
- self.conv_o = nn.Conv1d(channels, out_channels, 1)
- self.drop = nn.Dropout(p_dropout)
-
- if window_size is not None:
- n_heads_rel = 1 if heads_share else n_heads
- rel_stddev = self.k_channels**-0.5
- self.emb_rel_k = nn.Parameter(torch.randn(n_heads_rel, window_size * 2 + 1, self.k_channels) * rel_stddev)
- self.emb_rel_v = nn.Parameter(torch.randn(n_heads_rel, window_size * 2 + 1, self.k_channels) * rel_stddev)
-
- nn.init.xavier_uniform_(self.conv_q.weight)
- nn.init.xavier_uniform_(self.conv_k.weight)
- nn.init.xavier_uniform_(self.conv_v.weight)
- if proximal_init:
- with torch.no_grad():
- self.conv_k.weight.copy_(self.conv_q.weight)
- self.conv_k.bias.copy_(self.conv_q.bias)
-
- def forward(self, x, c, attn_mask=None):
- q = self.conv_q(x)
- k = self.conv_k(c)
- v = self.conv_v(c)
-
- x, self.attn = self.attention(q, k, v, mask=attn_mask)
-
- x = self.conv_o(x)
- return x
-
- def attention(self, query, key, value, mask=None):
- # reshape [b, d, t] -> [b, n_h, t, d_k]
- b, d, t_s, t_t = (*key.size(), query.size(2))
- query = query.view(b, self.n_heads, self.k_channels, t_t).transpose(2, 3)
- key = key.view(b, self.n_heads, self.k_channels, t_s).transpose(2, 3)
- value = value.view(b, self.n_heads, self.k_channels, t_s).transpose(2, 3)
-
- scores = torch.matmul(query / math.sqrt(self.k_channels), key.transpose(-2, -1))
- if self.window_size is not None:
- assert t_s == t_t, "Relative attention is only available for self-attention."
- key_relative_embeddings = self._get_relative_embeddings(self.emb_rel_k, t_s)
- rel_logits = self._matmul_with_relative_keys(query /math.sqrt(self.k_channels), key_relative_embeddings)
- scores_local = self._relative_position_to_absolute_position(rel_logits)
- scores = scores + scores_local
- if self.proximal_bias:
- assert t_s == t_t, "Proximal bias is only available for self-attention."
- scores = scores + self._attention_bias_proximal(t_s).to(device=scores.device, dtype=scores.dtype)
- if mask is not None:
- scores = scores.masked_fill(mask == 0, -1e4)
- if self.block_length is not None:
- assert t_s == t_t, "Local attention is only available for self-attention."
- block_mask = torch.ones_like(scores).triu(-self.block_length).tril(self.block_length)
- scores = scores.masked_fill(block_mask == 0, -1e4)
- p_attn = F.softmax(scores, dim=-1) # [b, n_h, t_t, t_s]
- p_attn = self.drop(p_attn)
- output = torch.matmul(p_attn, value)
- if self.window_size is not None:
- relative_weights = self._absolute_position_to_relative_position(p_attn)
- value_relative_embeddings = self._get_relative_embeddings(self.emb_rel_v, t_s)
- output = output + self._matmul_with_relative_values(relative_weights, value_relative_embeddings)
- output = output.transpose(2, 3).contiguous().view(b, d, t_t) # [b, n_h, t_t, d_k] -> [b, d, t_t]
- return output, p_attn
-
- def _matmul_with_relative_values(self, x, y):
- """
- x: [b, h, l, m]
- y: [h or 1, m, d]
- ret: [b, h, l, d]
- """
- ret = torch.matmul(x, y.unsqueeze(0))
- return ret
-
- def _matmul_with_relative_keys(self, x, y):
- """
- x: [b, h, l, d]
- y: [h or 1, m, d]
- ret: [b, h, l, m]
- """
- ret = torch.matmul(x, y.unsqueeze(0).transpose(-2, -1))
- return ret
-
- def _get_relative_embeddings(self, relative_embeddings, length):
- max_relative_position = 2 * self.window_size + 1
- # Pad first before slice to avoid using cond ops.
- pad_length = max(length - (self.window_size + 1), 0)
- slice_start_position = max((self.window_size + 1) - length, 0)
- slice_end_position = slice_start_position + 2 * length - 1
- if pad_length > 0:
- padded_relative_embeddings = F.pad(
- relative_embeddings,
- commons.convert_pad_shape([[0, 0], [pad_length, pad_length], [0, 0]]))
- else:
- padded_relative_embeddings = relative_embeddings
- used_relative_embeddings = padded_relative_embeddings[:,slice_start_position:slice_end_position]
- return used_relative_embeddings
-
- def _relative_position_to_absolute_position(self, x):
- """
- x: [b, h, l, 2*l-1]
- ret: [b, h, l, l]
- """
- batch, heads, length, _ = x.size()
- # Concat columns of pad to shift from relative to absolute indexing.
- x = F.pad(x, commons.convert_pad_shape([[0,0],[0,0],[0,0],[0,1]]))
-
- # Concat extra elements so to add up to shape (len+1, 2*len-1).
- x_flat = x.view([batch, heads, length * 2 * length])
- x_flat = F.pad(x_flat, commons.convert_pad_shape([[0,0],[0,0],[0,length-1]]))
-
- # Reshape and slice out the padded elements.
- x_final = x_flat.view([batch, heads, length+1, 2*length-1])[:, :, :length, length-1:]
- return x_final
-
- def _absolute_position_to_relative_position(self, x):
- """
- x: [b, h, l, l]
- ret: [b, h, l, 2*l-1]
- """
- batch, heads, length, _ = x.size()
- # padd along column
- x = F.pad(x, commons.convert_pad_shape([[0, 0], [0, 0], [0, 0], [0, length-1]]))
- x_flat = x.view([batch, heads, length**2 + length*(length -1)])
- # add 0's in the beginning that will skew the elements after reshape
- x_flat = F.pad(x_flat, commons.convert_pad_shape([[0, 0], [0, 0], [length, 0]]))
- x_final = x_flat.view([batch, heads, length, 2*length])[:,:,:,1:]
- return x_final
-
- def _attention_bias_proximal(self, length):
- """Bias for self-attention to encourage attention to close positions.
- Args:
- length: an integer scalar.
- Returns:
- a Tensor with shape [1, 1, length, length]
- """
- r = torch.arange(length, dtype=torch.float32)
- diff = torch.unsqueeze(r, 0) - torch.unsqueeze(r, 1)
- return torch.unsqueeze(torch.unsqueeze(-torch.log1p(torch.abs(diff)), 0), 0)
-
-
-class FFN(nn.Module):
- def __init__(self, in_channels, out_channels, filter_channels, kernel_size, p_dropout=0., activation=None, causal=False):
- super().__init__()
- self.in_channels = in_channels
- self.out_channels = out_channels
- self.filter_channels = filter_channels
- self.kernel_size = kernel_size
- self.p_dropout = p_dropout
- self.activation = activation
- self.causal = causal
-
- if causal:
- self.padding = self._causal_padding
- else:
- self.padding = self._same_padding
-
- self.conv_1 = nn.Conv1d(in_channels, filter_channels, kernel_size)
- self.conv_2 = nn.Conv1d(filter_channels, out_channels, kernel_size)
- self.drop = nn.Dropout(p_dropout)
-
- def forward(self, x, x_mask):
- x = self.conv_1(self.padding(x * x_mask))
- if self.activation == "gelu":
- x = x * torch.sigmoid(1.702 * x)
- else:
- x = torch.relu(x)
- x = self.drop(x)
- x = self.conv_2(self.padding(x * x_mask))
- return x * x_mask
-
- def _causal_padding(self, x):
- if self.kernel_size == 1:
- return x
- pad_l = self.kernel_size - 1
- pad_r = 0
- padding = [[0, 0], [0, 0], [pad_l, pad_r]]
- x = F.pad(x, commons.convert_pad_shape(padding))
- return x
-
- def _same_padding(self, x):
- if self.kernel_size == 1:
- return x
- pad_l = (self.kernel_size - 1) // 2
- pad_r = self.kernel_size // 2
- padding = [[0, 0], [0, 0], [pad_l, pad_r]]
- x = F.pad(x, commons.convert_pad_shape(padding))
- return x
diff --git a/spaces/ho11laqe/nnUNet_calvingfront_detection/nnunet/postprocessing/connected_components.py b/spaces/ho11laqe/nnUNet_calvingfront_detection/nnunet/postprocessing/connected_components.py
deleted file mode 100644
index c69471ea9e366829d4490008afe2c51001edbb5c..0000000000000000000000000000000000000000
--- a/spaces/ho11laqe/nnUNet_calvingfront_detection/nnunet/postprocessing/connected_components.py
+++ /dev/null
@@ -1,428 +0,0 @@
-# Copyright 2020 Division of Medical Image Computing, German Cancer Research Center (DKFZ), Heidelberg, Germany
-#
-# Licensed under the Apache License, Version 2.0 (the "License");
-# you may not use this file except in compliance with the License.
-# You may obtain a copy of the License at
-#
-# http://www.apache.org/licenses/LICENSE-2.0
-#
-# Unless required by applicable law or agreed to in writing, software
-# distributed under the License is distributed on an "AS IS" BASIS,
-# WITHOUT WARRANTIES OR CONDITIONS OF ANY KIND, either express or implied.
-# See the License for the specific language governing permissions and
-# limitations under the License.
-
-
-import ast
-from copy import deepcopy
-from multiprocessing.pool import Pool
-
-import numpy as np
-from nnunet.configuration import default_num_threads
-from nnunet.evaluation.evaluator import aggregate_scores
-from scipy.ndimage import label
-import SimpleITK as sitk
-from nnunet.utilities.sitk_stuff import copy_geometry
-from batchgenerators.utilities.file_and_folder_operations import *
-import shutil
-
-
-def load_remove_save(input_file: str, output_file: str, for_which_classes: list,
- minimum_valid_object_size: dict = None):
- # Only objects larger than minimum_valid_object_size will be removed. Keys in minimum_valid_object_size must
- # match entries in for_which_classes
- img_in = sitk.ReadImage(input_file)
- img_npy = sitk.GetArrayFromImage(img_in)
- volume_per_voxel = float(np.prod(img_in.GetSpacing(), dtype=np.float64))
-
- image, largest_removed, kept_size = remove_all_but_the_largest_connected_component(img_npy, for_which_classes,
- volume_per_voxel,
- minimum_valid_object_size)
- # print(input_file, "kept:", kept_size)
- img_out_itk = sitk.GetImageFromArray(image)
- img_out_itk = copy_geometry(img_out_itk, img_in)
- sitk.WriteImage(img_out_itk, output_file)
- return largest_removed, kept_size
-
-
-def remove_all_but_the_largest_connected_component(image: np.ndarray, for_which_classes: list, volume_per_voxel: float,
- minimum_valid_object_size: dict = None):
- """
- removes all but the largest connected component, individually for each class
- :param image:
- :param for_which_classes: can be None. Should be list of int. Can also be something like [(1, 2), 2, 4].
- Here (1, 2) will be treated as a joint region, not individual classes (example LiTS here we can use (1, 2)
- to use all foreground classes together)
- :param minimum_valid_object_size: Only objects larger than minimum_valid_object_size will be removed. Keys in
- minimum_valid_object_size must match entries in for_which_classes
- :return:
- """
- if for_which_classes is None:
- for_which_classes = np.unique(image)
- for_which_classes = for_which_classes[for_which_classes > 0]
-
- assert 0 not in for_which_classes, "cannot remove background"
- largest_removed = {}
- kept_size = {}
- for c in for_which_classes:
- if isinstance(c, (list, tuple)):
- c = tuple(c) # otherwise it cant be used as key in the dict
- mask = np.zeros_like(image, dtype=bool)
- for cl in c:
- mask[image == cl] = True
- else:
- mask = image == c
- # get labelmap and number of objects
- lmap, num_objects = label(mask.astype(int))
-
- # collect object sizes
- object_sizes = {}
- for object_id in range(1, num_objects + 1):
- object_sizes[object_id] = (lmap == object_id).sum() * volume_per_voxel
-
- largest_removed[c] = None
- kept_size[c] = None
-
- if num_objects > 0:
- # we always keep the largest object. We could also consider removing the largest object if it is smaller
- # than minimum_valid_object_size in the future but we don't do that now.
- maximum_size = max(object_sizes.values())
- kept_size[c] = maximum_size
-
- for object_id in range(1, num_objects + 1):
- # we only remove objects that are not the largest
- if object_sizes[object_id] != maximum_size:
- # we only remove objects that are smaller than minimum_valid_object_size
- remove = True
- if minimum_valid_object_size is not None:
- remove = object_sizes[object_id] < minimum_valid_object_size[c]
- if remove:
- image[(lmap == object_id) & mask] = 0
- if largest_removed[c] is None:
- largest_removed[c] = object_sizes[object_id]
- else:
- largest_removed[c] = max(largest_removed[c], object_sizes[object_id])
- return image, largest_removed, kept_size
-
-
-def load_postprocessing(json_file):
- '''
- loads the relevant part of the pkl file that is needed for applying postprocessing
- :param pkl_file:
- :return:
- '''
- a = load_json(json_file)
- if 'min_valid_object_sizes' in a.keys():
- min_valid_object_sizes = ast.literal_eval(a['min_valid_object_sizes'])
- else:
- min_valid_object_sizes = None
- return a['for_which_classes'], min_valid_object_sizes
-
-
-def determine_postprocessing(base, gt_labels_folder, raw_subfolder_name="validation_raw",
- temp_folder="temp",
- final_subf_name="validation_final", processes=default_num_threads,
- dice_threshold=0, debug=False,
- advanced_postprocessing=False,
- pp_filename="postprocessing.json"):
- """
- :param base:
- :param gt_labels_folder: subfolder of base with niftis of ground truth labels
- :param raw_subfolder_name: subfolder of base with niftis of predicted (non-postprocessed) segmentations
- :param temp_folder: used to store temporary data, will be deleted after we are done here undless debug=True
- :param final_subf_name: final results will be stored here (subfolder of base)
- :param processes:
- :param dice_threshold: only apply postprocessing if results is better than old_result+dice_threshold (can be used as eps)
- :param debug: if True then the temporary files will not be deleted
- :return:
- """
- # lets see what classes are in the dataset
- classes = [int(i) for i in load_json(join(base, raw_subfolder_name, "summary.json"))['results']['mean'].keys() if
- int(i) != 0]
-
- folder_all_classes_as_fg = join(base, temp_folder + "_allClasses")
- folder_per_class = join(base, temp_folder + "_perClass")
-
- if isdir(folder_all_classes_as_fg):
- shutil.rmtree(folder_all_classes_as_fg)
- if isdir(folder_per_class):
- shutil.rmtree(folder_per_class)
-
- # multiprocessing rules
- p = Pool(processes)
-
- assert isfile(join(base, raw_subfolder_name, "summary.json")), "join(base, raw_subfolder_name) does not " \
- "contain a summary.json"
-
- # these are all the files we will be dealing with
- fnames = subfiles(join(base, raw_subfolder_name), suffix=".nii.gz", join=False)
-
- # make output and temp dir
- maybe_mkdir_p(folder_all_classes_as_fg)
- maybe_mkdir_p(folder_per_class)
- maybe_mkdir_p(join(base, final_subf_name))
-
- pp_results = {}
- pp_results['dc_per_class_raw'] = {}
- pp_results['dc_per_class_pp_all'] = {} # dice scores after treating all foreground classes as one
- pp_results['dc_per_class_pp_per_class'] = {} # dice scores after removing everything except larges cc
- # independently for each class after we already did dc_per_class_pp_all
- pp_results['for_which_classes'] = []
- pp_results['min_valid_object_sizes'] = {}
-
-
- validation_result_raw = load_json(join(base, raw_subfolder_name, "summary.json"))['results']
- pp_results['num_samples'] = len(validation_result_raw['all'])
- validation_result_raw = validation_result_raw['mean']
-
- if advanced_postprocessing:
- # first treat all foreground classes as one and remove all but the largest foreground connected component
- results = []
- for f in fnames:
- predicted_segmentation = join(base, raw_subfolder_name, f)
- # now remove all but the largest connected component for each class
- output_file = join(folder_all_classes_as_fg, f)
- results.append(p.starmap_async(load_remove_save, ((predicted_segmentation, output_file, (classes,)),)))
-
- results = [i.get() for i in results]
-
- # aggregate max_size_removed and min_size_kept
- max_size_removed = {}
- min_size_kept = {}
- for tmp in results:
- mx_rem, min_kept = tmp[0]
- for k in mx_rem:
- if mx_rem[k] is not None:
- if max_size_removed.get(k) is None:
- max_size_removed[k] = mx_rem[k]
- else:
- max_size_removed[k] = max(max_size_removed[k], mx_rem[k])
- for k in min_kept:
- if min_kept[k] is not None:
- if min_size_kept.get(k) is None:
- min_size_kept[k] = min_kept[k]
- else:
- min_size_kept[k] = min(min_size_kept[k], min_kept[k])
-
- print("foreground vs background, smallest valid object size was", min_size_kept[tuple(classes)])
- print("removing only objects smaller than that...")
-
- else:
- min_size_kept = None
-
- # we need to rerun the step from above, now with the size constraint
- pred_gt_tuples = []
- results = []
- # first treat all foreground classes as one and remove all but the largest foreground connected component
- for f in fnames:
- predicted_segmentation = join(base, raw_subfolder_name, f)
- # now remove all but the largest connected component for each class
- output_file = join(folder_all_classes_as_fg, f)
- results.append(
- p.starmap_async(load_remove_save, ((predicted_segmentation, output_file, (classes,), min_size_kept),)))
- pred_gt_tuples.append([output_file, join(gt_labels_folder, f)])
-
- _ = [i.get() for i in results]
-
- # evaluate postprocessed predictions
- _ = aggregate_scores(pred_gt_tuples, labels=classes,
- json_output_file=join(folder_all_classes_as_fg, "summary.json"),
- json_author="Fabian", num_threads=processes)
-
- # now we need to figure out if doing this improved the dice scores. We will implement that defensively in so far
- # that if a single class got worse as a result we won't do this. We can change this in the future but right now I
- # prefer to do it this way
- validation_result_PP_test = load_json(join(folder_all_classes_as_fg, "summary.json"))['results']['mean']
-
- for c in classes:
- dc_raw = validation_result_raw[str(c)]['Dice']
- dc_pp = validation_result_PP_test[str(c)]['Dice']
- pp_results['dc_per_class_raw'][str(c)] = dc_raw
- pp_results['dc_per_class_pp_all'][str(c)] = dc_pp
-
- # true if new is better
- do_fg_cc = False
- comp = [pp_results['dc_per_class_pp_all'][str(cl)] > (pp_results['dc_per_class_raw'][str(cl)] + dice_threshold) for
- cl in classes]
- before = np.mean([pp_results['dc_per_class_raw'][str(cl)] for cl in classes])
- after = np.mean([pp_results['dc_per_class_pp_all'][str(cl)] for cl in classes])
- print("Foreground vs background")
- print("before:", before)
- print("after: ", after)
- if any(comp):
- # at least one class improved - yay!
- # now check if another got worse
- # true if new is worse
- any_worse = any(
- [pp_results['dc_per_class_pp_all'][str(cl)] < pp_results['dc_per_class_raw'][str(cl)] for cl in classes])
- if not any_worse:
- pp_results['for_which_classes'].append(classes)
- if min_size_kept is not None:
- pp_results['min_valid_object_sizes'].update(deepcopy(min_size_kept))
- do_fg_cc = True
- print("Removing all but the largest foreground region improved results!")
- print('for_which_classes', classes)
- print('min_valid_object_sizes', min_size_kept)
- else:
- # did not improve things - don't do it
- pass
-
- if len(classes) > 1:
- # now depending on whether we do remove all but the largest foreground connected component we define the source dir
- # for the next one to be the raw or the temp dir
- if do_fg_cc:
- source = folder_all_classes_as_fg
- else:
- source = join(base, raw_subfolder_name)
-
- if advanced_postprocessing:
- # now run this for each class separately
- results = []
- for f in fnames:
- predicted_segmentation = join(source, f)
- output_file = join(folder_per_class, f)
- results.append(p.starmap_async(load_remove_save, ((predicted_segmentation, output_file, classes),)))
-
- results = [i.get() for i in results]
-
- # aggregate max_size_removed and min_size_kept
- max_size_removed = {}
- min_size_kept = {}
- for tmp in results:
- mx_rem, min_kept = tmp[0]
- for k in mx_rem:
- if mx_rem[k] is not None:
- if max_size_removed.get(k) is None:
- max_size_removed[k] = mx_rem[k]
- else:
- max_size_removed[k] = max(max_size_removed[k], mx_rem[k])
- for k in min_kept:
- if min_kept[k] is not None:
- if min_size_kept.get(k) is None:
- min_size_kept[k] = min_kept[k]
- else:
- min_size_kept[k] = min(min_size_kept[k], min_kept[k])
-
- print("classes treated separately, smallest valid object sizes are")
- print(min_size_kept)
- print("removing only objects smaller than that")
- else:
- min_size_kept = None
-
- # rerun with the size thresholds from above
- pred_gt_tuples = []
- results = []
- for f in fnames:
- predicted_segmentation = join(source, f)
- output_file = join(folder_per_class, f)
- results.append(p.starmap_async(load_remove_save, ((predicted_segmentation, output_file, classes, min_size_kept),)))
- pred_gt_tuples.append([output_file, join(gt_labels_folder, f)])
-
- _ = [i.get() for i in results]
-
- # evaluate postprocessed predictions
- _ = aggregate_scores(pred_gt_tuples, labels=classes,
- json_output_file=join(folder_per_class, "summary.json"),
- json_author="Fabian", num_threads=processes)
-
- if do_fg_cc:
- old_res = deepcopy(validation_result_PP_test)
- else:
- old_res = validation_result_raw
-
- # these are the new dice scores
- validation_result_PP_test = load_json(join(folder_per_class, "summary.json"))['results']['mean']
-
- for c in classes:
- dc_raw = old_res[str(c)]['Dice']
- dc_pp = validation_result_PP_test[str(c)]['Dice']
- pp_results['dc_per_class_pp_per_class'][str(c)] = dc_pp
- print(c)
- print("before:", dc_raw)
- print("after: ", dc_pp)
-
- if dc_pp > (dc_raw + dice_threshold):
- pp_results['for_which_classes'].append(int(c))
- if min_size_kept is not None:
- pp_results['min_valid_object_sizes'].update({c: min_size_kept[c]})
- print("Removing all but the largest region for class %d improved results!" % c)
- print('min_valid_object_sizes', min_size_kept)
- else:
- print("Only one class present, no need to do each class separately as this is covered in fg vs bg")
-
- if not advanced_postprocessing:
- pp_results['min_valid_object_sizes'] = None
-
- print("done")
- print("for which classes:")
- print(pp_results['for_which_classes'])
- print("min_object_sizes")
- print(pp_results['min_valid_object_sizes'])
-
- pp_results['validation_raw'] = raw_subfolder_name
- pp_results['validation_final'] = final_subf_name
-
- # now that we have a proper for_which_classes, apply that
- pred_gt_tuples = []
- results = []
- for f in fnames:
- predicted_segmentation = join(base, raw_subfolder_name, f)
-
- # now remove all but the largest connected component for each class
- output_file = join(base, final_subf_name, f)
- results.append(p.starmap_async(load_remove_save, (
- (predicted_segmentation, output_file, pp_results['for_which_classes'],
- pp_results['min_valid_object_sizes']),)))
-
- pred_gt_tuples.append([output_file,
- join(gt_labels_folder, f)])
-
- _ = [i.get() for i in results]
- # evaluate postprocessed predictions
- _ = aggregate_scores(pred_gt_tuples, labels=classes,
- json_output_file=join(base, final_subf_name, "summary.json"),
- json_author="Fabian", num_threads=processes)
-
- pp_results['min_valid_object_sizes'] = str(pp_results['min_valid_object_sizes'])
-
- save_json(pp_results, join(base, pp_filename))
-
- # delete temp
- if not debug:
- shutil.rmtree(folder_per_class)
- shutil.rmtree(folder_all_classes_as_fg)
-
- p.close()
- p.join()
- print("done")
-
-
-def apply_postprocessing_to_folder(input_folder: str, output_folder: str, for_which_classes: list,
- min_valid_object_size:dict=None, num_processes=8):
- """
- applies removing of all but the largest connected component to all niftis in a folder
- :param min_valid_object_size:
- :param min_valid_object_size:
- :param input_folder:
- :param output_folder:
- :param for_which_classes:
- :param num_processes:
- :return:
- """
- maybe_mkdir_p(output_folder)
- p = Pool(num_processes)
- nii_files = subfiles(input_folder, suffix=".nii.gz", join=False)
- input_files = [join(input_folder, i) for i in nii_files]
- out_files = [join(output_folder, i) for i in nii_files]
- results = p.starmap_async(load_remove_save, zip(input_files, out_files, [for_which_classes] * len(input_files),
- [min_valid_object_size] * len(input_files)))
- res = results.get()
- p.close()
- p.join()
-
-
-if __name__ == "__main__":
- input_folder = "/media/fabian/DKFZ/predictions_Fabian/Liver_and_LiverTumor"
- output_folder = "/media/fabian/DKFZ/predictions_Fabian/Liver_and_LiverTumor_postprocessed"
- for_which_classes = [(1, 2), ]
- apply_postprocessing_to_folder(input_folder, output_folder, for_which_classes)
diff --git a/spaces/ho11laqe/nnUNet_calvingfront_detection/nnunet/training/network_training/nnUNet_variants/loss_function/nnUNetTrainerV2_Loss_TopK10.py b/spaces/ho11laqe/nnUNet_calvingfront_detection/nnunet/training/network_training/nnUNet_variants/loss_function/nnUNetTrainerV2_Loss_TopK10.py
deleted file mode 100644
index d2b0d56bcc69e913b49b0aa70db44d076ebc1ea7..0000000000000000000000000000000000000000
--- a/spaces/ho11laqe/nnUNet_calvingfront_detection/nnunet/training/network_training/nnUNet_variants/loss_function/nnUNetTrainerV2_Loss_TopK10.py
+++ /dev/null
@@ -1,32 +0,0 @@
-# Copyright 2020 Division of Medical Image Computing, German Cancer Research Center (DKFZ), Heidelberg, Germany
-#
-# Licensed under the Apache License, Version 2.0 (the "License");
-# you may not use this file except in compliance with the License.
-# You may obtain a copy of the License at
-#
-# http://www.apache.org/licenses/LICENSE-2.0
-#
-# Unless required by applicable law or agreed to in writing, software
-# distributed under the License is distributed on an "AS IS" BASIS,
-# WITHOUT WARRANTIES OR CONDITIONS OF ANY KIND, either express or implied.
-# See the License for the specific language governing permissions and
-# limitations under the License.
-
-
-from nnunet.training.network_training.nnUNetTrainerV2 import nnUNetTrainerV2
-from nnunet.training.loss_functions.TopK_loss import TopKLoss
-
-
-class nnUNetTrainerV2_Loss_TopK10(nnUNetTrainerV2):
- def __init__(self, plans_file, fold, output_folder=None, dataset_directory=None, batch_dice=True, stage=None,
- unpack_data=True, deterministic=True, fp16=False):
- super().__init__(plans_file, fold, output_folder, dataset_directory, batch_dice, stage, unpack_data,
- deterministic, fp16)
- self.loss = TopKLoss(k=10)
-
-
-nnUNetTrainerV2_Loss_TopK10_copy1 = nnUNetTrainerV2_Loss_TopK10
-nnUNetTrainerV2_Loss_TopK10_copy2 = nnUNetTrainerV2_Loss_TopK10
-nnUNetTrainerV2_Loss_TopK10_copy3 = nnUNetTrainerV2_Loss_TopK10
-nnUNetTrainerV2_Loss_TopK10_copy4 = nnUNetTrainerV2_Loss_TopK10
-
diff --git a/spaces/huggan/night2day/app.py b/spaces/huggan/night2day/app.py
deleted file mode 100644
index 9ab3530e5909524374bb7cc92a89c04c541f4c1e..0000000000000000000000000000000000000000
--- a/spaces/huggan/night2day/app.py
+++ /dev/null
@@ -1,24 +0,0 @@
-import gradio as gr
-from torchvision.transforms import Compose, Resize, ToTensor, Normalize
-from PIL import Image
-from torchvision.utils import save_image
-
-from huggan.pytorch.pix2pix.modeling_pix2pix import GeneratorUNet
-
-transform = Compose(
- [
- Resize((256, 256), Image.BICUBIC),
- ToTensor(),
- Normalize((0.5, 0.5, 0.5), (0.5, 0.5, 0.5)),
- ]
-)
-
-model = GeneratorUNet.from_pretrained('huggan/pix2pix-night2day')
-
-def predict_fn(img):
- inp = transform(img).unsqueeze(0)
- out = model(inp)
- save_image(out, 'out.png', normalize=True)
- return 'out.png'
-
-gr.Interface(predict_fn, inputs=gr.inputs.Image(type='pil'), outputs='image', examples=[['sample.jpg'], ['sample1.jpg'], ['sample2.jpg']]).launch()
\ No newline at end of file
diff --git a/spaces/huggingface-tools/text-download/text_download.py b/spaces/huggingface-tools/text-download/text_download.py
deleted file mode 100644
index 2b202967f661a5d5112baeedda24c1d938c99cd0..0000000000000000000000000000000000000000
--- a/spaces/huggingface-tools/text-download/text_download.py
+++ /dev/null
@@ -1,21 +0,0 @@
-import requests
-from bs4 import BeautifulSoup
-
-from transformers.tools.base import Tool
-
-
-TEXT_DOWNLOAD_DESCRIPTION = (
- "This is a tool that downloads a file from a `url`. It takes the `url` as input, and returns the text"
- " contained in the file."
-)
-
-
-class TextDownloadTool(Tool):
-
- inputs = ['text']
- outputs = ['text']
- description = TEXT_DOWNLOAD_DESCRIPTION
-
- def __call__(self, url):
- return BeautifulSoup(requests.get(url).text, features="html.parser").get_text()
-
diff --git a/spaces/hysts-duplicates/comparing-captioning-models/README.md b/spaces/hysts-duplicates/comparing-captioning-models/README.md
deleted file mode 100644
index ab0fd3d258ade98ffe2e6227e374ed773c78517a..0000000000000000000000000000000000000000
--- a/spaces/hysts-duplicates/comparing-captioning-models/README.md
+++ /dev/null
@@ -1,12 +0,0 @@
----
-title: Comparing Captioning Models
-emoji: 🔥
-colorFrom: yellow
-colorTo: pink
-sdk: gradio
-sdk_version: 3.50.2
-app_file: app.py
-pinned: false
----
-
-Check out the configuration reference at https://huggingface.co/docs/hub/spaces-config-reference
diff --git a/spaces/iakarshu/latr-vqa/README.md b/spaces/iakarshu/latr-vqa/README.md
deleted file mode 100644
index 50c9760618b1c4be9bd8e4f8bae654deb0269f7c..0000000000000000000000000000000000000000
--- a/spaces/iakarshu/latr-vqa/README.md
+++ /dev/null
@@ -1,13 +0,0 @@
----
-title: Latr Vqa
-emoji: 📉
-colorFrom: green
-colorTo: purple
-sdk: gradio
-sdk_version: 3.0.24
-app_file: app.py
-pinned: false
-license: unknown
----
-
-Check out the configuration reference at https://huggingface.co/docs/hub/spaces-config-reference
diff --git a/spaces/inamXcontru/PoeticTTS/Alibaba Aur 40 Chor Movie Download In Hindi Hd 720p Kickass !FULL!.md b/spaces/inamXcontru/PoeticTTS/Alibaba Aur 40 Chor Movie Download In Hindi Hd 720p Kickass !FULL!.md
deleted file mode 100644
index a3648ef16f577369f4c030ea1b4d2591f3d2ab30..0000000000000000000000000000000000000000
--- a/spaces/inamXcontru/PoeticTTS/Alibaba Aur 40 Chor Movie Download In Hindi Hd 720p Kickass !FULL!.md
+++ /dev/null
@@ -1,6 +0,0 @@
-Alibaba Aur 40 Chor movie download in hindi hd 720p kickass Download Zip ❤❤❤ https://gohhs.com/2uz4xc
-
-Avengers Infinity War In Hindi Dubbed Torrent Full Movie Download HD 2018; Sabse Badhkar ... Alibaba Aur 40 Chor 2 Full Movie Download 720p Kickass . 1fdad05405
-
-
-
diff --git a/spaces/inamXcontru/PoeticTTS/Annemann The Jinx Pdf Printer Discover the Secrets of the Jinx the Most Important Publication for Mentalists.md b/spaces/inamXcontru/PoeticTTS/Annemann The Jinx Pdf Printer Discover the Secrets of the Jinx the Most Important Publication for Mentalists.md
deleted file mode 100644
index 076053241b54a8b912c979e380c4472cc1f8b62b..0000000000000000000000000000000000000000
--- a/spaces/inamXcontru/PoeticTTS/Annemann The Jinx Pdf Printer Discover the Secrets of the Jinx the Most Important Publication for Mentalists.md
+++ /dev/null
@@ -1,5 +0,0 @@
-
-The Jinx - Annemann- A small review Simply wanted to discuss my thoughts. I understood I wished it, but wasn't certain what to expect I experienced observed Richard Osterlind point out the jinx on his outstanding videos, and that arranged me wanting to know even more. There appear to become two resources; the CD, or the three certain textbooks. I purchased the publications ( on eBay ).
-Annemann The Jinx Pdf Printer DOWNLOAD ★★★★★ https://gohhs.com/2uz2NQ
aaccfb2cb3
-
-
\ No newline at end of file
diff --git a/spaces/inplisQlawa/anything-midjourney-v4-1/Crack Serial Eobd Facile 27 Hamachi Ferse Verans !EXCLUSIVE!.md b/spaces/inplisQlawa/anything-midjourney-v4-1/Crack Serial Eobd Facile 27 Hamachi Ferse Verans !EXCLUSIVE!.md
deleted file mode 100644
index fec62509d5c51e3cb40248ce69e03b403dd52d80..0000000000000000000000000000000000000000
--- a/spaces/inplisQlawa/anything-midjourney-v4-1/Crack Serial Eobd Facile 27 Hamachi Ferse Verans !EXCLUSIVE!.md
+++ /dev/null
@@ -1,6 +0,0 @@
-Crack Serial Eobd Facile 27 hamachi ferse verans Download Zip ->->->-> https://urlin.us/2uEyj7
-
- what is the best videosoftware for making dvd from vhs (THANK YOU) . i have my own tape machine (cassette player) & a vhs player and i want to put a vhs movie on a dvd to watch it on my tv (i. have a 50 inch tv with an HD tune in ). i want to make a dvd for my son who is 3 years old . . . . . . . . . . . . . . . . . . . . . . . . . . . . . . . . . . . . . . . . . . . . . . . . . . . . . . . . . . . . . . . . . . . . . . . . . . . . . . . . . . . . . . . . . . . . . . . . . . . . . . . . . . . . . . . . . . . . . . . . . . . . . . . . . . . . . . . . . . . . . . . . . . . . . . . . . . . . . . . . . . . . . . . . . . . . . . . . . . . . 4fefd39f24
-
-
-
diff --git a/spaces/inplisQlawa/anything-midjourney-v4-1/Freedownloadtafsiralmaraghibahasaindonesia Fixed.md b/spaces/inplisQlawa/anything-midjourney-v4-1/Freedownloadtafsiralmaraghibahasaindonesia Fixed.md
deleted file mode 100644
index 0c1e589da61db9f738a083df10bca6f423df3976..0000000000000000000000000000000000000000
--- a/spaces/inplisQlawa/anything-midjourney-v4-1/Freedownloadtafsiralmaraghibahasaindonesia Fixed.md
+++ /dev/null
@@ -1,8 +0,0 @@
-
-freedownloadtafsiralmaraghibahasaindonesia Free My Boss Malayalam 2012 DVDRip 400MB X264 Softube Mac 64 Torrent _TOP_ Freedownloadtafsiralmaraghibahasaindonesia.. freedownloadtafsiralmaraghibahasaindonesia Bill Simmons Book Of Basketball Pdf 13. Enjoy this Post Support progemmiling on Ko-fi. Support.
-Free My Boss Malayalam 2012 DVDRip 400MB X264 Softube Mac 64 Torrent _TOP_ Freedownloadtafsiralmaraghibahasaindonesia.pdf_ freedownloadtafsiralmaraghibahasaindonesia Bill Simmons Book Of Basketball Pdf 13. Enjoy this Post Support progemmiling on Ko-fi. Support.
-freedownloadtafsiralmaraghibahasaindonesia Download — https://urlin.us/2uExnB
-freedownloadtafsiralmaraghibahasaindonesia Free My Boss Malayalam 2012 DVDRip 400MB X264 Softube Mac 64 Torrent _TOP_ Freedownloadtafsiralmaraghibahasaindonesia.pdf_ freedownloadtafsiralmaraghibahasaindonesia Bill Simmons Book Of Basketball Pdf 13. Enjoy this Post Support progemmiling on Ko-fi. Support.
-freedownloadtafsiralmaraghibahasaindonesia Miami Heat NBA 2K19 PS4 XBOX ONE DOWNLOADRip ATIDownloadtafsiralmaraghibahasaindonesia game freedownloadtafsiralmaraghibahasaindonesia Accepting R13 Disclaimer The use of this website is governed by The terms of use.. freedownloadtafsiralmaraghibahasaindonesia The Lilac bush had been the guide of J. Leach. The Lilac Bush The Lilac Bush. Utne.. freedownloadtafsiralmaraghibahasaindonesia Boomtown Rats: The Complete Story BoTown Rats: The Complete Story.. freedownloadtafsiralmaraghibahasaindonesia Around 991. the whole thing was enclosed in a gate of dark dead iron. Around 991.. freedownloadtafsiralmaraghibahasaindonesia Ki Joo - The Greatest Drama (Eng dub) Ki Joo - The Greatest Drama (Eng dub) Enjoy this Post And. freedownloadtafsiralmaraghibahasaindonesia Camden County Chief : The Road to the Shawnee Uprising and the Pursuit of Indian Freedom 40 hrs FREE. freedownloadtafsiralmaraghibahasaindonesia Archery - Shooting at the western front http://ashurstandcallew.com/downloads/hot-games/ and. freedownloadtafsiralmaraghibahasaindonesia Windows 7 Repair. In this site, you can download all repair tools for Windows 7 Repair.. freedownloadtafsiralmaraghibahasaindonesia Nengkasi City Football Club, Nenggesiu. Papua. 3060 1.. freedownloadtafsiralmaraghibahasaindonesia FREEDOWNLOAD TAFSIRI MARAGHIABAHASAINDI - Top View HD https://www.google.com/url?sa=t&rct=j&q=&esrc=s&source=web&cd=1&cad=rja&uact=8&ved=0CDEQFjAA&url=https%3A%2F%2Fwww.getfreedownload.org%2F1-tafsir-maraghibahasaindonesia%2F&ei=cjb2IVzKLcHdBsAGWYH&usg=AFQjCNFFv92RjB5KlHniF8mRnIwoMnBqQ freedownloadtafsiralmaraghibahasaindonesia FREEDOWNLOAD TAFSIRI MARAGHIABAHASAINDI. freedownloadtafsiralmaraghibahasaindonesia Free Games For PC - Download Full Version Games For PC - Download Full Version Movies For PC.. freedownloadtafsiralmaraghibahasaindonesia Mananra Emol : (in hindi) Mananra Emol : (in hindi) Enjoy this Post Yes I know.. freedownloadtafsiralmaraghibahasaindonesia Download Wallpaper - Free Download Best Stock Wallpapers HD / 2012 - 401,.
899543212b
-
-
\ No newline at end of file
diff --git a/spaces/inplisQlawa/anything-midjourney-v4-1/HD Online Player (Tarkovsky Stalker 720p Or 1080p).md b/spaces/inplisQlawa/anything-midjourney-v4-1/HD Online Player (Tarkovsky Stalker 720p Or 1080p).md
deleted file mode 100644
index a61eecf68010315374c0a0b808165129bfccefea..0000000000000000000000000000000000000000
--- a/spaces/inplisQlawa/anything-midjourney-v4-1/HD Online Player (Tarkovsky Stalker 720p Or 1080p).md
+++ /dev/null
@@ -1,7 +0,0 @@
-
-The fifth Stalker has the absolute distinction of being the most extraordinary game to come out of the video games industry in quite some time, and for good reason: its the most ambitious video game story of all time, and its it one of the all-time masterpieces of art.
-But the most famous of these GSC games is the original one, though the less accessible GSC sequel, S.T.A.L.K.E.R.: Clear Sky , would go on to be recognized as one of the finest FPS games of all time, owing in large part to the game's revolutionary Havok physics engine. But GSC were not the only Russian developers to take an interest in the S.T.A.L.K.E.R. universe, and in the wake of the film's success a number of other developers came along to offer their own takes on the games. For example, Prey , by Wake Online , used Cyrillic script to make the Zone more appealing to Russian gamers, but interestingly timed the release of Prey 2 to coincide with the 2003 debut of the English-language film of the same name. GamesRadar described Prey and its 2012 sequel Prey 2 as easy to dig out of but said of Prey 2 , “the game does a good job with its approach of providing plenty of things to do within the environment,” noting in the end it would be “hard to pass up this game if you’re interested in the FPS genre.” *
-HD Online Player (Tarkovsky Stalker 720p Or 1080p) Download File ✔ https://urlin.us/2uExPg
-There are different options for playing the video game. You can play it in either turn-based or first-person perspective. In the case of the turn-based, Stalker -style games the game is more complicated than in simply choosing someone to follow. As you go, enemies will move along the path in front of you and try to impede your progress, and you need to pick the right moment to shoot them. Then youll have to move your character to a location where you can safely reload your weapon, and repeat the process; and repeat it again and again and again. The result is a kind of miasma of shootings, reloads, and jumpy, fumbling attempts to avoid getting shot again, like a deadly game of hide-and-shoot. Meanwhile, Epic Games X-Com -style turn-based strategy games like Dune 2000 and FreeX have entered the market, offering a new paradigm of strategic play, with hundreds of options in any turn, with the ability to react quickly to enemy movements, blast waves of attacks in every direction, and coordinate the movements of your entire team. But in the case of Stalker -style games, one of you is designated the primary player, and his or her (or their) character remains where it is, while the others advance in a sequence (real or imagined) that never duplicates, and is repeated indefinitely. Unlike the repetitive quality of a well-known turn-based video game with a single path, however, a game with this feature is nothing like it.
899543212b
-
-
\ No newline at end of file
diff --git a/spaces/inplisQlawa/anything-midjourney-v4-1/IObit Driver Booster Pro 7.3.0.665 __TOP__ Crack With Licence Key.md b/spaces/inplisQlawa/anything-midjourney-v4-1/IObit Driver Booster Pro 7.3.0.665 __TOP__ Crack With Licence Key.md
deleted file mode 100644
index 26071d1636f890a0131f99e362168567ff4aba65..0000000000000000000000000000000000000000
--- a/spaces/inplisQlawa/anything-midjourney-v4-1/IObit Driver Booster Pro 7.3.0.665 __TOP__ Crack With Licence Key.md
+++ /dev/null
@@ -1,6 +0,0 @@
-IObit Driver Booster Pro 7.3.0.665 Crack With Licence Key DOWNLOAD ⏩ https://urlin.us/2uEywD
-
-January 21, 2022 - IObit Driver Booster Pro 7.3.0.665 License Key Serial Code Hack [FIXED] Free Download. IObit Driver Booster Pro 7.3.0.665 license. ↑ Free download IObit Driver Booster Pro 7.3.0.665 with activator (license key) ↑ How to use IObit Driver Booster. ↑ What's new in Driver Booster Pro 7.3.0.665 ↑ Driver update ↑ IObit Driver Booster 7.0.5 ↑ Free download IObit Driver Booster 7.0.5 with activator (license key) ↑ Driver update ↑ IObit Driver Booster 7.0.5 ↑ Free download IObit Driver Booster 7.0.5 with activator (license key) ↑ Driver update. ↑ What's New in Driver Booster Pro 7. 8a78ff9644
-
-
-
diff --git a/spaces/inplisQlawa/anything-midjourney-v4-1/Machine Design Data Book By Vb Bhandari Pdf 31.md b/spaces/inplisQlawa/anything-midjourney-v4-1/Machine Design Data Book By Vb Bhandari Pdf 31.md
deleted file mode 100644
index 6e5b2fb349c6d24a6f79adafa9746ff1873905cb..0000000000000000000000000000000000000000
--- a/spaces/inplisQlawa/anything-midjourney-v4-1/Machine Design Data Book By Vb Bhandari Pdf 31.md
+++ /dev/null
@@ -1,18 +0,0 @@
-Machine Design Data Book By Vb Bhandari Pdf 31 Download Zip >> https://urlin.us/2uExok
-
-pdfXavier a été placé sous surveillance depuis samedi soir, en raison de son comportement en tant qu'internaute.
-
-"Depuis deux mois, on m'a mis surveillant car j'avais été un peu agressif avec les élèves de ma classe, d'abord énervé, puis larmoyant, de plus en plus agressif", confie le garçon.
-
-Vers 1h, il appelle sa maman à son tour, et lui rappelle les reproches qu'il a reçus de son père, qui lui a remis des coups. Elle raccroche immédiatement. Xavier en est conscient : "Sa maman est allée à son père et c'est terminé, ils n'ont pas supporté ça, on a tout vu mais les garçons qui ont suivi m'ont peut-être pas compris. Il y avait autour de moi beaucoup de soutien."
-
-Les professeurs interrogés s'entendent sur l'ampleur des conséquences de son comportement. Mais certains ne savent pas comment éviter la quarantaine des jeunes concernés. Certains exemples : un garçon de 11 ans a eu droit à un coup de fil de la police cette semaine, mais "il a refusé d'entrer en garde à vue".
-
-"Je m'en fiche que le gouvernement le fasse. Ce n'est pas mon choix de me dérober. Je ne veux pas faire de cadeaux à l'État", explique l'élève.
-
-"Il est débile, il le sait et s'en fiche", s'exclame sa mère. "Pour ce qu'il a fait, il vaut mieux se taire et mettre des gants, je me débrouillerai seule."Canadian gardeners rejoice: The sun is starting to shine.
-
-The Canadian Association of Horticulture Producers says those soggy March temperatures have given way to 4fefd39f24
-
-
-
diff --git a/spaces/inreVtussa/clothingai/Examples/Aci 347r 14 Pdf 37 ((EXCLUSIVE)).md b/spaces/inreVtussa/clothingai/Examples/Aci 347r 14 Pdf 37 ((EXCLUSIVE)).md
deleted file mode 100644
index b4cd9158d302e1c50b7bcd1c347d34282af94ef7..0000000000000000000000000000000000000000
--- a/spaces/inreVtussa/clothingai/Examples/Aci 347r 14 Pdf 37 ((EXCLUSIVE)).md
+++ /dev/null
@@ -1,22 +0,0 @@
-Aci 347r 14 Pdf 37 DOWNLOAD ✸✸✸ https://tiurll.com/2uCkFV
-
-Paper, Pencils, Rockers and Rollers. This is a modified. Remember this when driving in the city. This is a free, no strings attached, online dating site for singles. Register in less than 2 minutes and start chatting with singles on our online dating website.
-
-eHarmony – Trusted by over 30 million singles. eHarmony is the #1 rated dating site for relationships in America. eHarmony has more than 30 years of experience helping. Welcome to 35th Avenue South Mission - A Gospel-Based Mission for the Homeless. 35th Avenue South Mission is a faith-based housing ministry located on 35th Avenue.
-
-We believe that God wants to do something special through us as a ministry. Our desire is to help people be. Meet gay singles in your area or worldwide who are looking to meet a new boyfriend. Register for free at Badoo. For gay and bi guys, find gay singles in England. Want to meet gay men for a date, hook-up, or if you are looking for friendship, then this is the best dating.
-
-Older gay men often have more experience dating single mothers to help them with the unique issues that they face. Bisexual hookup dating site, bisexual gay hookup, GayBisexual. We are a site designed for bisexual and bi-curious singles to connect and mingle with others in a fun, safe, and free environment.
-
-Free mobile casino no download zune - Free online slots for fun - Free online games
-
-To find singles on Craigslist, you dont have to leave the comfort of your home. Find a local gay or bisexual person. Post ads for free. Learn about queer dating in Chicago, IL and explore the best online resources for finding love and companionship in Chicago. Search Chicago's gay and lesbian news and local community for the Chicago Tribune, Daily Herald and Chicago Sun Times, Lifestyles, Arts, Entertainment, Movies, Music and more.
-
-Take on the mission of reaching the lost and nudging a friend into the gospel.
-
-Groups - Home; Ministries - Fellowship; Events - Event schedule; Ex-Groups - Home; Groups - Food Ministries; Groups - Ministry Invitations; Groups - Missions; Groups - Resources; Groups - Youth; Events - Home; Events - Local;. Click here to join. We have worked hard to build a community of like-minded Christian singles.
-
-Join us today. Christian Singles. Recent 4fefd39f24
-
-
-
diff --git a/spaces/isabel/pug-or-cat-image-classifier/README.md b/spaces/isabel/pug-or-cat-image-classifier/README.md
deleted file mode 100644
index abb04c41cdd25fe854ce2396888e0032c5f1b82b..0000000000000000000000000000000000000000
--- a/spaces/isabel/pug-or-cat-image-classifier/README.md
+++ /dev/null
@@ -1,37 +0,0 @@
----
-title: Pug Or Cat Image Classifier
-emoji: 🐶
-colorFrom: red
-colorTo: yellow
-sdk: gradio
-app_file: app.py
-pinned: false
----
-
-# Configuration
-
-`title`: _string_
-Display title for the Space
-
-`emoji`: _string_
-Space emoji (emoji-only character allowed)
-
-`colorFrom`: _string_
-Color for Thumbnail gradient (red, yellow, green, blue, indigo, purple, pink, gray)
-
-`colorTo`: _string_
-Color for Thumbnail gradient (red, yellow, green, blue, indigo, purple, pink, gray)
-
-`sdk`: _string_
-Can be either `gradio` or `streamlit`
-
-`sdk_version` : _string_
-Only applicable for `streamlit` SDK.
-See [doc](https://hf.co/docs/hub/spaces) for more info on supported versions.
-
-`app_file`: _string_
-Path to your main application file (which contains either `gradio` or `streamlit` Python code).
-Path is relative to the root of the repository.
-
-`pinned`: _boolean_
-Whether the Space stays on top of your list.
diff --git a/spaces/ivanokhotnikov/longformer-base-health-fact/README.md b/spaces/ivanokhotnikov/longformer-base-health-fact/README.md
deleted file mode 100644
index ac6e0f74a093eb71ebe9bf93ade05562f6a226e9..0000000000000000000000000000000000000000
--- a/spaces/ivanokhotnikov/longformer-base-health-fact/README.md
+++ /dev/null
@@ -1,12 +0,0 @@
----
-title: Longformer Base Health Fact
-emoji: 📉
-colorFrom: gray
-colorTo: yellow
-sdk: streamlit
-sdk_version: 1.17.0
-app_file: app.py
-pinned: false
----
-
-Check out the configuration reference at https://huggingface.co/docs/hub/spaces-config-reference
diff --git a/spaces/jackli888/stable-diffusion-webui/modules/textual_inversion/ui.py b/spaces/jackli888/stable-diffusion-webui/modules/textual_inversion/ui.py
deleted file mode 100644
index 5b75f799e745fa693cda06763af80069324a964f..0000000000000000000000000000000000000000
--- a/spaces/jackli888/stable-diffusion-webui/modules/textual_inversion/ui.py
+++ /dev/null
@@ -1,45 +0,0 @@
-import html
-
-import gradio as gr
-
-import modules.textual_inversion.textual_inversion
-import modules.textual_inversion.preprocess
-from modules import sd_hijack, shared
-
-
-def create_embedding(name, initialization_text, nvpt, overwrite_old):
- filename = modules.textual_inversion.textual_inversion.create_embedding(name, nvpt, overwrite_old, init_text=initialization_text)
-
- sd_hijack.model_hijack.embedding_db.load_textual_inversion_embeddings()
-
- return gr.Dropdown.update(choices=sorted(sd_hijack.model_hijack.embedding_db.word_embeddings.keys())), f"Created: {filename}", ""
-
-
-def preprocess(*args):
- modules.textual_inversion.preprocess.preprocess(*args)
-
- return f"Preprocessing {'interrupted' if shared.state.interrupted else 'finished'}.", ""
-
-
-def train_embedding(*args):
-
- assert not shared.cmd_opts.lowvram, 'Training models with lowvram not possible'
-
- apply_optimizations = shared.opts.training_xattention_optimizations
- try:
- if not apply_optimizations:
- sd_hijack.undo_optimizations()
-
- embedding, filename = modules.textual_inversion.textual_inversion.train_embedding(*args)
-
- res = f"""
-Training {'interrupted' if shared.state.interrupted else 'finished'} at {embedding.step} steps.
-Embedding saved to {html.escape(filename)}
-"""
- return res, ""
- except Exception:
- raise
- finally:
- if not apply_optimizations:
- sd_hijack.apply_optimizations()
-
diff --git a/spaces/james-oldfield/PandA/networks/biggan/convert_tf_to_pytorch.py b/spaces/james-oldfield/PandA/networks/biggan/convert_tf_to_pytorch.py
deleted file mode 100644
index 7ccb787dec188e9dbd9ea31288c049c1bdb30f95..0000000000000000000000000000000000000000
--- a/spaces/james-oldfield/PandA/networks/biggan/convert_tf_to_pytorch.py
+++ /dev/null
@@ -1,312 +0,0 @@
-# coding: utf-8
-"""
-Convert a TF Hub model for BigGAN in a PT one.
-"""
-from __future__ import (absolute_import, division, print_function, unicode_literals)
-
-from itertools import chain
-
-import os
-import argparse
-import logging
-import numpy as np
-import torch
-import torch.nn as nn
-import torch.nn.functional as F
-from torch.nn.functional import normalize
-
-from .model import BigGAN, WEIGHTS_NAME, CONFIG_NAME
-from .config import BigGANConfig
-
-logger = logging.getLogger(__name__)
-
-
-def extract_batch_norm_stats(tf_model_path, batch_norm_stats_path=None):
- try:
- import numpy as np
- import tensorflow as tf
- import tensorflow_hub as hub
- except ImportError:
- raise ImportError("Loading a TensorFlow models in PyTorch, requires TensorFlow and TF Hub to be installed. "
- "Please see https://www.tensorflow.org/install/ for installation instructions for TensorFlow. "
- "And see https://github.com/tensorflow/hub for installing Hub. "
- "Probably pip install tensorflow tensorflow-hub")
- tf.reset_default_graph()
- logger.info('Loading BigGAN module from: {}'.format(tf_model_path))
- module = hub.Module(tf_model_path)
- inputs = {k: tf.placeholder(v.dtype, v.get_shape().as_list(), k)
- for k, v in module.get_input_info_dict().items()}
- output = module(inputs)
-
- initializer = tf.global_variables_initializer()
- sess = tf.Session()
- stacks = sum(((i*10 + 1, i*10 + 3, i*10 + 6, i*10 + 8) for i in range(50)), ())
- numpy_stacks = []
- for i in stacks:
- logger.info("Retrieving module_apply_default/stack_{}".format(i))
- try:
- stack_var = tf.get_default_graph().get_tensor_by_name("module_apply_default/stack_%d:0" % i)
- except KeyError:
- break # We have all the stats
- numpy_stacks.append(sess.run(stack_var))
-
- if batch_norm_stats_path is not None:
- torch.save(numpy_stacks, batch_norm_stats_path)
- else:
- return numpy_stacks
-
-
-def build_tf_to_pytorch_map(model, config):
- """ Build a map from TF variables to PyTorch modules. """
- tf_to_pt_map = {}
-
- # Embeddings and GenZ
- tf_to_pt_map.update({'linear/w/ema_0.9999': model.embeddings.weight,
- 'Generator/GenZ/G_linear/b/ema_0.9999': model.generator.gen_z.bias,
- 'Generator/GenZ/G_linear/w/ema_0.9999': model.generator.gen_z.weight_orig,
- 'Generator/GenZ/G_linear/u0': model.generator.gen_z.weight_u})
-
- # GBlock blocks
- model_layer_idx = 0
- for i, (up, in_channels, out_channels) in enumerate(config.layers):
- if i == config.attention_layer_position:
- model_layer_idx += 1
- layer_str = "Generator/GBlock_%d/" % i if i > 0 else "Generator/GBlock/"
- layer_pnt = model.generator.layers[model_layer_idx]
- for i in range(4): # Batchnorms
- batch_str = layer_str + ("BatchNorm_%d/" % i if i > 0 else "BatchNorm/")
- batch_pnt = getattr(layer_pnt, 'bn_%d' % i)
- for name in ('offset', 'scale'):
- sub_module_str = batch_str + name + "/"
- sub_module_pnt = getattr(batch_pnt, name)
- tf_to_pt_map.update({sub_module_str + "w/ema_0.9999": sub_module_pnt.weight_orig,
- sub_module_str + "u0": sub_module_pnt.weight_u})
- for i in range(4): # Convolutions
- conv_str = layer_str + "conv%d/" % i
- conv_pnt = getattr(layer_pnt, 'conv_%d' % i)
- tf_to_pt_map.update({conv_str + "b/ema_0.9999": conv_pnt.bias,
- conv_str + "w/ema_0.9999": conv_pnt.weight_orig,
- conv_str + "u0": conv_pnt.weight_u})
- model_layer_idx += 1
-
- # Attention block
- layer_str = "Generator/attention/"
- layer_pnt = model.generator.layers[config.attention_layer_position]
- tf_to_pt_map.update({layer_str + "gamma/ema_0.9999": layer_pnt.gamma})
- for pt_name, tf_name in zip(['snconv1x1_g', 'snconv1x1_o_conv', 'snconv1x1_phi', 'snconv1x1_theta'],
- ['g/', 'o_conv/', 'phi/', 'theta/']):
- sub_module_str = layer_str + tf_name
- sub_module_pnt = getattr(layer_pnt, pt_name)
- tf_to_pt_map.update({sub_module_str + "w/ema_0.9999": sub_module_pnt.weight_orig,
- sub_module_str + "u0": sub_module_pnt.weight_u})
-
- # final batch norm and conv to rgb
- layer_str = "Generator/BatchNorm/"
- layer_pnt = model.generator.bn
- tf_to_pt_map.update({layer_str + "offset/ema_0.9999": layer_pnt.bias,
- layer_str + "scale/ema_0.9999": layer_pnt.weight})
- layer_str = "Generator/conv_to_rgb/"
- layer_pnt = model.generator.conv_to_rgb
- tf_to_pt_map.update({layer_str + "b/ema_0.9999": layer_pnt.bias,
- layer_str + "w/ema_0.9999": layer_pnt.weight_orig,
- layer_str + "u0": layer_pnt.weight_u})
- return tf_to_pt_map
-
-
-def load_tf_weights_in_biggan(model, config, tf_model_path, batch_norm_stats_path=None):
- """ Load tf checkpoints and standing statistics in a pytorch model
- """
- try:
- import numpy as np
- import tensorflow as tf
- except ImportError:
- raise ImportError("Loading a TensorFlow models in PyTorch, requires TensorFlow to be installed. Please see "
- "https://www.tensorflow.org/install/ for installation instructions.")
- # Load weights from TF model
- checkpoint_path = tf_model_path + "/variables/variables"
- init_vars = tf.train.list_variables(checkpoint_path)
- from pprint import pprint
- pprint(init_vars)
-
- # Extract batch norm statistics from model if needed
- if batch_norm_stats_path:
- stats = torch.load(batch_norm_stats_path)
- else:
- logger.info("Extracting batch norm stats")
- stats = extract_batch_norm_stats(tf_model_path)
-
- # Build TF to PyTorch weights loading map
- tf_to_pt_map = build_tf_to_pytorch_map(model, config)
-
- tf_weights = {}
- for name in tf_to_pt_map.keys():
- array = tf.train.load_variable(checkpoint_path, name)
- tf_weights[name] = array
- # logger.info("Loading TF weight {} with shape {}".format(name, array.shape))
-
- # Load parameters
- with torch.no_grad():
- pt_params_pnt = set()
- for name, pointer in tf_to_pt_map.items():
- array = tf_weights[name]
- if pointer.dim() == 1:
- if pointer.dim() < array.ndim:
- array = np.squeeze(array)
- elif pointer.dim() == 2: # Weights
- array = np.transpose(array)
- elif pointer.dim() == 4: # Convolutions
- array = np.transpose(array, (3, 2, 0, 1))
- else:
- raise "Wrong dimensions to adjust: " + str((pointer.shape, array.shape))
- if pointer.shape != array.shape:
- raise ValueError("Wrong dimensions: " + str((pointer.shape, array.shape)))
- logger.info("Initialize PyTorch weight {} with shape {}".format(name, pointer.shape))
- pointer.data = torch.from_numpy(array) if isinstance(array, np.ndarray) else torch.tensor(array)
- tf_weights.pop(name, None)
- pt_params_pnt.add(pointer.data_ptr())
-
- # Prepare SpectralNorm buffers by running one step of Spectral Norm (no need to train the model):
- for module in model.modules():
- for n, buffer in module.named_buffers():
- if n == 'weight_v':
- weight_mat = module.weight_orig
- weight_mat = weight_mat.reshape(weight_mat.size(0), -1)
- u = module.weight_u
-
- v = normalize(torch.mv(weight_mat.t(), u), dim=0, eps=config.eps)
- buffer.data = v
- pt_params_pnt.add(buffer.data_ptr())
-
- u = normalize(torch.mv(weight_mat, v), dim=0, eps=config.eps)
- module.weight_u.data = u
- pt_params_pnt.add(module.weight_u.data_ptr())
-
- # Load batch norm statistics
- index = 0
- for layer in model.generator.layers:
- if not hasattr(layer, 'bn_0'):
- continue
- for i in range(4): # Batchnorms
- bn_pointer = getattr(layer, 'bn_%d' % i)
- pointer = bn_pointer.running_means
- if pointer.shape != stats[index].shape:
- raise "Wrong dimensions: " + str((pointer.shape, stats[index].shape))
- pointer.data = torch.from_numpy(stats[index])
- pt_params_pnt.add(pointer.data_ptr())
-
- pointer = bn_pointer.running_vars
- if pointer.shape != stats[index+1].shape:
- raise "Wrong dimensions: " + str((pointer.shape, stats[index].shape))
- pointer.data = torch.from_numpy(stats[index+1])
- pt_params_pnt.add(pointer.data_ptr())
-
- index += 2
-
- bn_pointer = model.generator.bn
- pointer = bn_pointer.running_means
- if pointer.shape != stats[index].shape:
- raise "Wrong dimensions: " + str((pointer.shape, stats[index].shape))
- pointer.data = torch.from_numpy(stats[index])
- pt_params_pnt.add(pointer.data_ptr())
-
- pointer = bn_pointer.running_vars
- if pointer.shape != stats[index+1].shape:
- raise "Wrong dimensions: " + str((pointer.shape, stats[index].shape))
- pointer.data = torch.from_numpy(stats[index+1])
- pt_params_pnt.add(pointer.data_ptr())
-
- remaining_params = list(n for n, t in chain(model.named_parameters(), model.named_buffers()) \
- if t.data_ptr() not in pt_params_pnt)
-
- logger.info("TF Weights not copied to PyTorch model: {} -".format(', '.join(tf_weights.keys())))
- logger.info("Remanining parameters/buffers from PyTorch model: {} -".format(', '.join(remaining_params)))
-
- return model
-
-
-BigGAN128 = BigGANConfig(output_dim=128, z_dim=128, class_embed_dim=128, channel_width=128, num_classes=1000,
- layers=[(False, 16, 16),
- (True, 16, 16),
- (False, 16, 16),
- (True, 16, 8),
- (False, 8, 8),
- (True, 8, 4),
- (False, 4, 4),
- (True, 4, 2),
- (False, 2, 2),
- (True, 2, 1)],
- attention_layer_position=8, eps=1e-4, n_stats=51)
-
-BigGAN256 = BigGANConfig(output_dim=256, z_dim=128, class_embed_dim=128, channel_width=128, num_classes=1000,
- layers=[(False, 16, 16),
- (True, 16, 16),
- (False, 16, 16),
- (True, 16, 8),
- (False, 8, 8),
- (True, 8, 8),
- (False, 8, 8),
- (True, 8, 4),
- (False, 4, 4),
- (True, 4, 2),
- (False, 2, 2),
- (True, 2, 1)],
- attention_layer_position=8, eps=1e-4, n_stats=51)
-
-BigGAN512 = BigGANConfig(output_dim=512, z_dim=128, class_embed_dim=128, channel_width=128, num_classes=1000,
- layers=[(False, 16, 16),
- (True, 16, 16),
- (False, 16, 16),
- (True, 16, 8),
- (False, 8, 8),
- (True, 8, 8),
- (False, 8, 8),
- (True, 8, 4),
- (False, 4, 4),
- (True, 4, 2),
- (False, 2, 2),
- (True, 2, 1),
- (False, 1, 1),
- (True, 1, 1)],
- attention_layer_position=8, eps=1e-4, n_stats=51)
-
-
-def main():
- parser = argparse.ArgumentParser(description="Convert a BigGAN TF Hub model in a PyTorch model")
- parser.add_argument("--model_type", type=str, default="", required=True,
- help="BigGAN model type (128, 256, 512)")
- parser.add_argument("--tf_model_path", type=str, default="", required=True,
- help="Path of the downloaded TF Hub model")
- parser.add_argument("--pt_save_path", type=str, default="",
- help="Folder to save the PyTorch model (default: Folder of the TF Hub model)")
- parser.add_argument("--batch_norm_stats_path", type=str, default="",
- help="Path of previously extracted batch norm statistics")
- args = parser.parse_args()
-
- logging.basicConfig(level=logging.INFO)
-
- if not args.pt_save_path:
- args.pt_save_path = args.tf_model_path
-
- if args.model_type == "128":
- config = BigGAN128
- elif args.model_type == "256":
- config = BigGAN256
- elif args.model_type == "512":
- config = BigGAN512
- else:
- raise ValueError("model_type should be one of 128, 256 or 512")
-
- model = BigGAN(config)
- model = load_tf_weights_in_biggan(model, config, args.tf_model_path, args.batch_norm_stats_path)
-
- model_save_path = os.path.join(args.pt_save_path, WEIGHTS_NAME)
- config_save_path = os.path.join(args.pt_save_path, CONFIG_NAME)
-
- logger.info("Save model dump to {}".format(model_save_path))
- torch.save(model.state_dict(), model_save_path)
- logger.info("Save configuration file to {}".format(config_save_path))
- with open(config_save_path, "w", encoding="utf-8") as f:
- f.write(config.to_json_string())
-
-if __name__ == "__main__":
- main()
diff --git a/spaces/jamesjohnson763/ClinicalTerminologyUIUX-GR/app.py b/spaces/jamesjohnson763/ClinicalTerminologyUIUX-GR/app.py
deleted file mode 100644
index 3a2a532354367b122f50cc6f70f4aca4c1e0ff38..0000000000000000000000000000000000000000
--- a/spaces/jamesjohnson763/ClinicalTerminologyUIUX-GR/app.py
+++ /dev/null
@@ -1,327 +0,0 @@
-import pandas_profiling as pp
-import pandas as pd
-import tensorflow as tf
-
-from datasets import load_dataset
-from tensorflow.python.framework import tensor_shape
-
-#LOINC
-datasetLOINC = load_dataset("awacke1/LOINC-CodeSet-Value-Description.csv", split="train")
-#SNOMED:
-datasetSNOMED = load_dataset("awacke1/SNOMED-CT-Code-Value-Semantic-Set.csv", split="train")
-#eCQM:
-dataseteCQM = load_dataset("awacke1/eCQM-Code-Value-Semantic-Set.csv", split="train")
-
-# map using autotokenizer
-from transformers import AutoTokenizer
-tokenizer = AutoTokenizer.from_pretrained("bert-base-cased")
-dataset = datasetLOINC.map(lambda examples: tokenizer(examples["Description"]), batched=True)
-JSONOBJ2=dataset[0]
-print(JSONOBJ2)
-
-sw = datasetLOINC.filter(lambda example: example["Description"].startswith("Allergy"))
-len(sw)
-print(sw)
-print(datasetLOINC)
-print(datasetSNOMED)
-print(dataseteCQM)
-
-# play with some dataset tools before the show:
-
-#print(start_with_ar["Description"])
-
-#---
-#Main Stage - Begin!
-#---
-
-import os
-import json
-import numpy as np
-import gradio as gr
-
-HF_TOKEN = os.environ.get("HF_TOKEN")
-CHOICES = ["SNOMED", "LOINC", "CQM"]
-JSONOBJ = """{"items":{"item":[{"id": "0001","type": null,"is_good": false,"ppu": 0.55,"batters":{"batter":[{ "id": "1001", "type": "Regular" },{ "id": "1002", "type": "Chocolate" },{ "id": "1003", "type": "Blueberry" },{ "id": "1004", "type": "Devil's Food" }]},"topping":[{ "id": "5001", "type": "None" },{ "id": "5002", "type": "Glazed" },{ "id": "5005", "type": "Sugar" },{ "id": "5007", "type": "Powdered Sugar" },{ "id": "5006", "type": "Chocolate with Sprinkles" },{ "id": "5003", "type": "Chocolate" },{ "id": "5004", "type": "Maple" }]}]}}"""
-
-
-def profile_dataset(dataset=datasetSNOMED, username="awacke1", token=HF_TOKEN, dataset_name="awacke1/SNOMED-CT-Code-Value-Semantic-Set.csv"):
- df = pd.read_csv(dataset.Description)
- if len(df.columns) <= 15:
- profile = pp.ProfileReport(df, title=f"{dataset_name} Report")
- else:
- profile = pp.ProfileReport(df, title=f"{dataset_name} Report", minimal = True)
-
- repo_url = create_repo(f"{username}/{dataset_name}", repo_type = "space", token = token, space_sdk = "static", private=False)
-
- profile.to_file("./index.html")
-
- upload_file(path_or_fileobj ="./index.html", path_in_repo = "index.html", repo_id =f"{username}/{dataset_name}", repo_type = "space", token=token)
- readme = f"---\ntitle: {dataset_name}\nemoji: ✨\ncolorFrom: green\ncolorTo: red\nsdk: static\npinned: false\ntags:\n- dataset-report\n---"
- with open("README.md", "w+") as f:
- f.write(readme)
- upload_file(path_or_fileobj ="./README.md", path_in_repo = "README.md", repo_id =f"{username}/{dataset_name}", repo_type = "space", token=token)
- return f"Your dataset report will be ready at {repo_url}"
-
-#def lowercase_title(example):
-# return {"Description": example[title].lower()}
-
-# demonstrate map function of dataset
-#JSONOBJ_MAP=datasetLOINC.map(lowercase_title)
-#JSONOBJ_MAP=datasetLOINC.filter(lambda example: example["Description"].startswith("Mental health"))
-
-
-
-
-def concatenate_text(examples):
- return {
- "text": examples["Code"]
- + " \n "
- + examples["Description"]
- + " \n "
- + examples["Purpose: Clinical Focus"]
- }
-
-def cls_pooling(model_output):
- return model_output.last_hidden_state[:, 0]
-
-def get_embeddings(text_list):
- encoded_input = tokenizer(
- text_list, padding=True, truncation=True, return_tensors="tf"
- )
- encoded_input = {k: v for k, v in encoded_input.items()}
- model_output = model(**encoded_input)
- return cls_pooling(model_output)
-
-
-def fn( text1, text2, num, slider1, slider2, single_checkbox, checkboxes, radio, dropdown, im1, im2, im3, im4,
- video, audio1, audio2, file, df1, df2,):
-#def fn( text1, text2, single_checkbox, checkboxes, radio, im4, file, df1, df2,):
-
- searchTerm = text1
- searchTermSentence = text2
-
- start_with_searchTermLOINC = datasetLOINC.filter(lambda example:example["Description"].startswith('Allergy')) #Allergy
-
-
- # FAISS
- columns = start_with_searchTermLOINC.column_names
- columns_to_keep = ["Value Set Name", "Code", "Description", "Purpose: Clinical Focus", "Code System OID"]
- columns_to_remove = set(columns_to_keep).symmetric_difference(columns)
- start_with_searchTermLOINC = start_with_searchTermLOINC.remove_columns(columns_to_remove)
- start_with_searchTermLOINC
- start_with_searchTermLOINC.set_format("pandas")
- df = start_with_searchTermLOINC[:]
-
- df["Purpose: Clinical Focus"][0]
-
- df4 = df.explode("Purpose: Clinical Focus", ignore_index=True)
- df4.head(4)
-
- from datasets import Dataset
- clinical_dataset = Dataset.from_pandas(df4)
- clinical_dataset
-
- clinical_dataset = clinical_dataset.map(lambda x: {"c_length": len(x["Description"].split())})
-
- clinical_dataset = clinical_dataset.filter(lambda x: x["c_length"] > 15)
- clinical_dataset
-
-
- clinical_dataset = clinical_dataset.map(concatenate_text)
- #embedding = get_embeddings(clinical_dataset["text"][0])
- #embedding.shape
-
- from transformers import AutoTokenizer, TFAutoModel
-
- model_ckpt = "sentence-transformers/multi-qa-mpnet-base-dot-v1"
- tokenizer = AutoTokenizer.from_pretrained(model_ckpt)
- model = TFAutoModel.from_pretrained(model_ckpt, from_pt=True)
-
-# TensorShape([1, 768])
- tf.shape([1, 768])
-
- embeddings_dataset = clinical_dataset.map(
- lambda x: {"embeddings": get_embeddings(x["text"]).numpy()[0]})
-
-# embeddings_dataset.add_faiss_index(column="embeddings")
-
-# question = "How can I load a dataset offline?"
-# question_embedding = get_embeddings([question]).numpy()
-# question_embedding.shape
-
-# scores, samples = embeddings_dataset.get_nearest_examples("embeddings", question_embedding, k=5)
-
-# import pandas as pd
-
-# samples_df = pd.DataFrame.from_dict(samples)
-# samples_df["scores"] = scores
-# samples_df.sort_values("scores", ascending=False, inplace=True)
-
-
- # "text": examples["Code"]
- # + " \n "
- # + examples["Description"]
- # + " \n "
- # + examples["Purpose: Clinical Focus"]
-
-
-# for _, row in samples_df.iterrows():
-# print(f"Code: {row.Code}")
-# print(f"Description: {row.Description}")
-# #print(f"Purpose: Clinical Focus: {row.Purpose: Clinical Focus}")
-# #print(f"URL: {row.html_url}")
-# print("=" * 50)
-# print()
-
- # SNOMED and CQM ---------------
- start_with_searchTermSNOMED = datasetSNOMED.filter(lambda example: example["Description"].startswith('Hospital')) #Hospital
- start_with_searchTermCQM = dataseteCQM.filter(lambda example: example["Description"].startswith('Telephone')) #Telephone
-
- print(start_with_searchTermLOINC )
- print(start_with_searchTermSNOMED )
- print(start_with_searchTermCQM)
-
- #print(start_with_searchTermLOINC["train"][0] )
- #print(start_with_searchTermSNOMED["train"][0] )
- #print(start_with_searchTermCQM["train"][0] )
-
- #returnMsg=profile_dataset()
- #print(returnMsg)
-
-# try:
- #top1matchLOINC = json.loads(start_with_searchTermLOINC['train'])
- #top1matchSNOMED = json.loads(start_with_searchTermSNOMED['train'])
- #top1matchCQM = json.loads(start_with_searchTermCQM['train'])
-# top1matchLOINC = json.loads(start_with_searchTermLOINC)
-# top1matchSNOMED = json.loads(start_with_searchTermSNOMED)
-# top1matchCQM = json.loads(start_with_searchTermCQM)
-# except:
-# print('Hello')
- #print(start_with_searchTermLOINC[0])
- #print(start_with_searchTermSNOMED[0] )
- #print(start_with_searchTermCQM[0] )
-
- #print(returnMsg)
- # print("Datasets Processed")
-
- return (
- (text1 if single_checkbox else text2)
- + ", selected:"
- + ", ".join(checkboxes), # Text
- {
- "positive": num / (num + slider1 + slider2),
- "negative": slider1 / (num + slider1 + slider2),
- "neutral": slider2 / (num + slider1 + slider2),
- }, # Label
- (audio1[0], np.flipud(audio1[1]))
- if audio1 is not None else os.path.join(os.path.dirname(__file__), "files/cantina.wav"), # Audio
- np.flipud(im1)
- if im1 is not None else os.path.join(os.path.dirname(__file__), "files/cheetah1.jpg"), # Image
- video
- if video is not None else os.path.join(os.path.dirname(__file__), "files/world.mp4"), # Video
- [
- ("The", "art"),
- ("quick brown", "adj"),
- ("fox", "nn"),
- ("jumped", "vrb"),
- ("testing testing testing", None),
- ("over", "prp"),
- ("the", "art"),
- ("testing", None),
- ("lazy", "adj"),
- ("dogs", "nn"),
- (".", "punc"),
- ] + [(f"test {x}", f"test {x}") for x in range(10)], # HighlightedText
- [
- ("The testing testing testing", None),
- ("over", 0.6),
- ("the", 0.2),
- ("testing", None),
- ("lazy", -0.1),
- ("dogs", 0.4),
- (".", 0),
- ] + [(f"test", x / 10) for x in range(-10, 10)], # HighlightedText
- #json.loads(JSONOBJ), # JSON
- start_with_searchTermLOINC.to_json(orient="records", path_or_buf="None"),
- #json.dumps(json.loads(start_with_searchTermLOINC['train'].to_json(orient="records", path_or_buf="None"))),
- "Click Me: " + radio + " ", # HTML
- os.path.join(os.path.dirname(__file__), "files/titanic.csv"),
- df1, # Dataframe
- np.random.randint(0, 10, (4, 4)), # Dataframe
- df2, # Timeseries
- )
-
-
-
-demo = gr.Interface(
- fn,
- inputs=[
- gr.Textbox(value="Allergy", label="Textbox"),
- gr.Textbox(lines=3, value="Bathing", placeholder="Type here..", label="Textbox 2"),
- gr.Number(label="Number", value=42),
- gr.Slider(10, 20, value=15, label="Slider: 10 - 20"),
- gr.Slider(maximum=20, step=0.04, label="Slider: step @ 0.04"),
- gr.Checkbox(label="Check for NER Match on Submit"),
- gr.CheckboxGroup(label="Clinical Terminology to Check", choices=CHOICES, value=CHOICES[0:2]),
- gr.Radio(label="Preferred Terminology Output", choices=CHOICES, value=CHOICES[2]),
- gr.Dropdown(label="Dropdown", choices=CHOICES),
- gr.Image(label="Image"),
- gr.Image(label="Image w/ Cropper", tool="select"),
- gr.Image(label="Sketchpad", source="canvas"),
- gr.Image(label="Webcam", source="webcam"),
- gr.Video(label="Video"),
- gr.Audio(label="Audio"),
- gr.Audio(label="Microphone", source="microphone"),
- gr.File(label="File"),
- gr.Dataframe(label="Filters", headers=["Name", "Age", "Gender"]),
- gr.Timeseries(x="time", y=["price", "value"], colors=["pink", "purple"]),
- ],
- outputs=[
- gr.Textbox(label="Textbox"),
- gr.Label(label="Label"),
- gr.Audio(label="Audio"),
- gr.Image(label="Image"),
- gr.Video(label="Video"),
- gr.HighlightedText(label="HighlightedText", color_map={"punc": "pink", "test 0": "blue"}),
- gr.HighlightedText(label="HighlightedText", show_legend=True),
- gr.JSON(label="JSON"),
- gr.HTML(label="HTML"),
- gr.File(label="File"),
- gr.Dataframe(label="Dataframe"),
- gr.Dataframe(label="Numpy"),
- gr.Timeseries(x="time", y=["price", "value"], label="Timeseries"),
- ],
- examples=[
- [
- "Allergy",
- "Admission",
- 10,
- 12,
- 4,
- True,
- ["SNOMED", "LOINC", "CQM"],
- "SNOMED",
- "bar",
- os.path.join(os.path.dirname(__file__), "files/cheetah1.jpg"),
- os.path.join(os.path.dirname(__file__), "files/cheetah1.jpg"),
- os.path.join(os.path.dirname(__file__), "files/cheetah1.jpg"),
- os.path.join(os.path.dirname(__file__), "files/cheetah1.jpg"),
- os.path.join(os.path.dirname(__file__), "files/world.mp4"),
- os.path.join(os.path.dirname(__file__), "files/cantina.wav"),
- os.path.join(os.path.dirname(__file__), "files/cantina.wav"),
- os.path.join(os.path.dirname(__file__), "files/titanic.csv"),
- [[1, 2, 3], [3, 4, 5]],
- os.path.join(os.path.dirname(__file__), "files/time.csv"),
- ]
- ]
- * 3,
- theme="default",
- title="⚗️🧠🔬🧬 Clinical Terminology Auto Mapper AI 👩⚕️🩺⚕️🙋",
- cache_examples=False,
- description="Clinical Terminology Auto Mapper AI",
- article="Learn more at [Yggdrasil](https://github.com/AaronCWacker/Yggdrasil)",
-# live=True,
-)
-
-if __name__ == "__main__":
- demo.launch(debug=True)
\ No newline at end of file
diff --git a/spaces/jarvisbot/ChatImprovement/crazy_functions/test_project/cpp/cppipc/waiter.h b/spaces/jarvisbot/ChatImprovement/crazy_functions/test_project/cpp/cppipc/waiter.h
deleted file mode 100644
index ee45fe3517be95ac1688a3e3540189edeb0d860c..0000000000000000000000000000000000000000
--- a/spaces/jarvisbot/ChatImprovement/crazy_functions/test_project/cpp/cppipc/waiter.h
+++ /dev/null
@@ -1,83 +0,0 @@
-#pragma once
-
-#include
-#include
-#include
-#include
-
-#include "libipc/def.h"
-#include "libipc/mutex.h"
-#include "libipc/condition.h"
-#include "libipc/platform/detail.h"
-
-namespace ipc {
-namespace detail {
-
-class waiter {
- ipc::sync::condition cond_;
- ipc::sync::mutex lock_;
- std::atomic quit_ {false};
-
-public:
- static void init();
-
- waiter() = default;
- waiter(char const *name) {
- open(name);
- }
-
- ~waiter() {
- close();
- }
-
- bool valid() const noexcept {
- return cond_.valid() && lock_.valid();
- }
-
- bool open(char const *name) noexcept {
- quit_.store(false, std::memory_order_relaxed);
- if (!cond_.open((std::string{"_waiter_cond_"} + name).c_str())) {
- return false;
- }
- if (!lock_.open((std::string{"_waiter_lock_"} + name).c_str())) {
- cond_.close();
- return false;
- }
- return valid();
- }
-
- void close() noexcept {
- cond_.close();
- lock_.close();
- }
-
- template
- bool wait_if(F &&pred, std::uint64_t tm = ipc::invalid_value) noexcept {
- IPC_UNUSED_ std::lock_guard guard {lock_};
- while ([this, &pred] {
- return !quit_.load(std::memory_order_relaxed)
- && std::forward(pred)();
- }()) {
- if (!cond_.wait(lock_, tm)) return false;
- }
- return true;
- }
-
- bool notify() noexcept {
- std::lock_guard{lock_}; // barrier
- return cond_.notify(lock_);
- }
-
- bool broadcast() noexcept {
- std::lock_guard{lock_}; // barrier
- return cond_.broadcast(lock_);
- }
-
- bool quit_waiting() {
- quit_.store(true, std::memory_order_release);
- return broadcast();
- }
-};
-
-} // namespace detail
-} // namespace ipc
diff --git a/spaces/jbilcke-hf/VideoChain-API/Dockerfile b/spaces/jbilcke-hf/VideoChain-API/Dockerfile
deleted file mode 100644
index d4b4d651279894231852f7b2087099f6b8de4351..0000000000000000000000000000000000000000
--- a/spaces/jbilcke-hf/VideoChain-API/Dockerfile
+++ /dev/null
@@ -1,45 +0,0 @@
-FROM node:18
-# try this maybe
-
-ARG DEBIAN_FRONTEND=noninteractive
-
-RUN apt update
-
-# For FFMPEG and gl concat
-RUN apt --yes install ffmpeg curl build-essential python3 python3-dev libx11-dev libxext-dev libxext6 libglu1-mesa-dev xvfb libxi-dev libglew-dev pkg-config
-
-# For Puppeteer
-RUN apt --yes install libnss3 libatk1.0-0 libatk-bridge2.0-0 libcups2 libgbm1 libasound2 libpangocairo-1.0-0 libxss1 libgtk-3-0
-
-# Set up a new user named "user" with user ID 1000
-RUN useradd -o -u 1000 user
-
-# Switch to the "user" user
-USER user
-
-# Set home to the user's home directory
-ENV HOME=/home/user \
- PATH=/home/user/.local/bin:$PATH
-
-# Set the working directory to the user's home directory
-WORKDIR $HOME/app
-
-# Install app dependencies
-# A wildcard is used to ensure both package.json AND package-lock.json are copied
-# where available (npm@5+)
-COPY --chown=user package*.json $HOME/app
-
-# make sure the .env is copied as well
-COPY --chown=user .env $HOME/app
-
-RUN npm install
-
-
-# Copy the current directory contents into the container at $HOME/app setting the owner to the user
-COPY --chown=user . $HOME/app
-
-EXPOSE 7860
-
-# we can't use this (it time out)
-# CMD [ "xvfb-run", "-s", "-ac -screen 0 1920x1080x24", "npm", "run", "start" ]
-CMD [ "npm", "run", "start" ]
\ No newline at end of file
diff --git a/spaces/jbilcke-hf/VideoQuest/src/app/interface/help/index.tsx b/spaces/jbilcke-hf/VideoQuest/src/app/interface/help/index.tsx
deleted file mode 100644
index afae997243478af6c0ae69843586e95bb38c32fe..0000000000000000000000000000000000000000
--- a/spaces/jbilcke-hf/VideoQuest/src/app/interface/help/index.tsx
+++ /dev/null
@@ -1,23 +0,0 @@
-export function Help({
- clickables,
- isLoading,
-}: {
- clickables: string[]
- isLoading: boolean
-}) {
- return (
-
-
- {isLoading
- ? ⌛ Generating areas for clicks and drag & drop, please wait..
- : 💡 Try to click on:
- }
-
- {clickables.map((clickable, i) =>
-
-
{clickable}
- {i < (clickables.length - 1) ?
,
: null}
-
)}
-
- )
-}
\ No newline at end of file
diff --git a/spaces/jdczlx/ChatGPT-chuanhu/run_macOS.command b/spaces/jdczlx/ChatGPT-chuanhu/run_macOS.command
deleted file mode 100644
index 62af07283093d8e580763d7acfe493c3d88e7b08..0000000000000000000000000000000000000000
--- a/spaces/jdczlx/ChatGPT-chuanhu/run_macOS.command
+++ /dev/null
@@ -1,25 +0,0 @@
-#!/bin/bash
-
-# 获取脚本所在目录
-script_dir=$(dirname "$0")
-
-# 将工作目录更改为脚本所在目录
-cd "$script_dir"
-
-# 检查Git仓库是否有更新
-git remote update
-pwd
-
-if ! git status -uno | grep 'up to date' > /dev/null; then
- # 如果有更新,关闭当前运行的服务器
- pkill -f ChuanhuChatbot.py
-
- # 拉取最新更改
- git pull
-
- # 安装依赖
- pip3 install -r requirements.txt
-
- # 重新启动服务器
- nohup python3 ChuanhuChatbot.py &
-fi
diff --git a/spaces/jennysun/jwsun-multisubject-render-model/gligen/ldm/modules/x_transformer.py b/spaces/jennysun/jwsun-multisubject-render-model/gligen/ldm/modules/x_transformer.py
deleted file mode 100644
index 5fc15bf9cfe0111a910e7de33d04ffdec3877576..0000000000000000000000000000000000000000
--- a/spaces/jennysun/jwsun-multisubject-render-model/gligen/ldm/modules/x_transformer.py
+++ /dev/null
@@ -1,641 +0,0 @@
-"""shout-out to https://github.com/lucidrains/x-transformers/tree/main/x_transformers"""
-import torch
-from torch import nn, einsum
-import torch.nn.functional as F
-from functools import partial
-from inspect import isfunction
-from collections import namedtuple
-from einops import rearrange, repeat, reduce
-
-# constants
-
-DEFAULT_DIM_HEAD = 64
-
-Intermediates = namedtuple('Intermediates', [
- 'pre_softmax_attn',
- 'post_softmax_attn'
-])
-
-LayerIntermediates = namedtuple('Intermediates', [
- 'hiddens',
- 'attn_intermediates'
-])
-
-
-class AbsolutePositionalEmbedding(nn.Module):
- def __init__(self, dim, max_seq_len):
- super().__init__()
- self.emb = nn.Embedding(max_seq_len, dim)
- self.init_()
-
- def init_(self):
- nn.init.normal_(self.emb.weight, std=0.02)
-
- def forward(self, x):
- n = torch.arange(x.shape[1], device=x.device)
- return self.emb(n)[None, :, :]
-
-
-class FixedPositionalEmbedding(nn.Module):
- def __init__(self, dim):
- super().__init__()
- inv_freq = 1. / (10000 ** (torch.arange(0, dim, 2).float() / dim))
- self.register_buffer('inv_freq', inv_freq)
-
- def forward(self, x, seq_dim=1, offset=0):
- t = torch.arange(x.shape[seq_dim], device=x.device).type_as(self.inv_freq) + offset
- sinusoid_inp = torch.einsum('i , j -> i j', t, self.inv_freq)
- emb = torch.cat((sinusoid_inp.sin(), sinusoid_inp.cos()), dim=-1)
- return emb[None, :, :]
-
-
-# helpers
-
-def exists(val):
- return val is not None
-
-
-def default(val, d):
- if exists(val):
- return val
- return d() if isfunction(d) else d
-
-
-def always(val):
- def inner(*args, **kwargs):
- return val
- return inner
-
-
-def not_equals(val):
- def inner(x):
- return x != val
- return inner
-
-
-def equals(val):
- def inner(x):
- return x == val
- return inner
-
-
-def max_neg_value(tensor):
- return -torch.finfo(tensor.dtype).max
-
-
-# keyword argument helpers
-
-def pick_and_pop(keys, d):
- values = list(map(lambda key: d.pop(key), keys))
- return dict(zip(keys, values))
-
-
-def group_dict_by_key(cond, d):
- return_val = [dict(), dict()]
- for key in d.keys():
- match = bool(cond(key))
- ind = int(not match)
- return_val[ind][key] = d[key]
- return (*return_val,)
-
-
-def string_begins_with(prefix, str):
- return str.startswith(prefix)
-
-
-def group_by_key_prefix(prefix, d):
- return group_dict_by_key(partial(string_begins_with, prefix), d)
-
-
-def groupby_prefix_and_trim(prefix, d):
- kwargs_with_prefix, kwargs = group_dict_by_key(partial(string_begins_with, prefix), d)
- kwargs_without_prefix = dict(map(lambda x: (x[0][len(prefix):], x[1]), tuple(kwargs_with_prefix.items())))
- return kwargs_without_prefix, kwargs
-
-
-# classes
-class Scale(nn.Module):
- def __init__(self, value, fn):
- super().__init__()
- self.value = value
- self.fn = fn
-
- def forward(self, x, **kwargs):
- x, *rest = self.fn(x, **kwargs)
- return (x * self.value, *rest)
-
-
-class Rezero(nn.Module):
- def __init__(self, fn):
- super().__init__()
- self.fn = fn
- self.g = nn.Parameter(torch.zeros(1))
-
- def forward(self, x, **kwargs):
- x, *rest = self.fn(x, **kwargs)
- return (x * self.g, *rest)
-
-
-class ScaleNorm(nn.Module):
- def __init__(self, dim, eps=1e-5):
- super().__init__()
- self.scale = dim ** -0.5
- self.eps = eps
- self.g = nn.Parameter(torch.ones(1))
-
- def forward(self, x):
- norm = torch.norm(x, dim=-1, keepdim=True) * self.scale
- return x / norm.clamp(min=self.eps) * self.g
-
-
-class RMSNorm(nn.Module):
- def __init__(self, dim, eps=1e-8):
- super().__init__()
- self.scale = dim ** -0.5
- self.eps = eps
- self.g = nn.Parameter(torch.ones(dim))
-
- def forward(self, x):
- norm = torch.norm(x, dim=-1, keepdim=True) * self.scale
- return x / norm.clamp(min=self.eps) * self.g
-
-
-class Residual(nn.Module):
- def forward(self, x, residual):
- return x + residual
-
-
-class GRUGating(nn.Module):
- def __init__(self, dim):
- super().__init__()
- self.gru = nn.GRUCell(dim, dim)
-
- def forward(self, x, residual):
- gated_output = self.gru(
- rearrange(x, 'b n d -> (b n) d'),
- rearrange(residual, 'b n d -> (b n) d')
- )
-
- return gated_output.reshape_as(x)
-
-
-# feedforward
-
-class GEGLU(nn.Module):
- def __init__(self, dim_in, dim_out):
- super().__init__()
- self.proj = nn.Linear(dim_in, dim_out * 2)
-
- def forward(self, x):
- x, gate = self.proj(x).chunk(2, dim=-1)
- return x * F.gelu(gate)
-
-
-class FeedForward(nn.Module):
- def __init__(self, dim, dim_out=None, mult=4, glu=False, dropout=0.):
- super().__init__()
- inner_dim = int(dim * mult)
- dim_out = default(dim_out, dim)
- project_in = nn.Sequential(
- nn.Linear(dim, inner_dim),
- nn.GELU()
- ) if not glu else GEGLU(dim, inner_dim)
-
- self.net = nn.Sequential(
- project_in,
- nn.Dropout(dropout),
- nn.Linear(inner_dim, dim_out)
- )
-
- def forward(self, x):
- return self.net(x)
-
-
-# attention.
-class Attention(nn.Module):
- def __init__(
- self,
- dim,
- dim_head=DEFAULT_DIM_HEAD,
- heads=8,
- causal=False,
- mask=None,
- talking_heads=False,
- sparse_topk=None,
- use_entmax15=False,
- num_mem_kv=0,
- dropout=0.,
- on_attn=False
- ):
- super().__init__()
- if use_entmax15:
- raise NotImplementedError("Check out entmax activation instead of softmax activation!")
- self.scale = dim_head ** -0.5
- self.heads = heads
- self.causal = causal
- self.mask = mask
-
- inner_dim = dim_head * heads
-
- self.to_q = nn.Linear(dim, inner_dim, bias=False)
- self.to_k = nn.Linear(dim, inner_dim, bias=False)
- self.to_v = nn.Linear(dim, inner_dim, bias=False)
- self.dropout = nn.Dropout(dropout)
-
- # talking heads
- self.talking_heads = talking_heads
- if talking_heads:
- self.pre_softmax_proj = nn.Parameter(torch.randn(heads, heads))
- self.post_softmax_proj = nn.Parameter(torch.randn(heads, heads))
-
- # explicit topk sparse attention
- self.sparse_topk = sparse_topk
-
- # entmax
- #self.attn_fn = entmax15 if use_entmax15 else F.softmax
- self.attn_fn = F.softmax
-
- # add memory key / values
- self.num_mem_kv = num_mem_kv
- if num_mem_kv > 0:
- self.mem_k = nn.Parameter(torch.randn(heads, num_mem_kv, dim_head))
- self.mem_v = nn.Parameter(torch.randn(heads, num_mem_kv, dim_head))
-
- # attention on attention
- self.attn_on_attn = on_attn
- self.to_out = nn.Sequential(nn.Linear(inner_dim, dim * 2), nn.GLU()) if on_attn else nn.Linear(inner_dim, dim)
-
- def forward(
- self,
- x,
- context=None,
- mask=None,
- context_mask=None,
- rel_pos=None,
- sinusoidal_emb=None,
- prev_attn=None,
- mem=None
- ):
- b, n, _, h, talking_heads, device = *x.shape, self.heads, self.talking_heads, x.device
- kv_input = default(context, x)
-
- q_input = x
- k_input = kv_input
- v_input = kv_input
-
- if exists(mem):
- k_input = torch.cat((mem, k_input), dim=-2)
- v_input = torch.cat((mem, v_input), dim=-2)
-
- if exists(sinusoidal_emb):
- # in shortformer, the query would start at a position offset depending on the past cached memory
- offset = k_input.shape[-2] - q_input.shape[-2]
- q_input = q_input + sinusoidal_emb(q_input, offset=offset)
- k_input = k_input + sinusoidal_emb(k_input)
-
- q = self.to_q(q_input)
- k = self.to_k(k_input)
- v = self.to_v(v_input)
-
- q, k, v = map(lambda t: rearrange(t, 'b n (h d) -> b h n d', h=h), (q, k, v))
-
- input_mask = None
- if any(map(exists, (mask, context_mask))):
- q_mask = default(mask, lambda: torch.ones((b, n), device=device).bool())
- k_mask = q_mask if not exists(context) else context_mask
- k_mask = default(k_mask, lambda: torch.ones((b, k.shape[-2]), device=device).bool())
- q_mask = rearrange(q_mask, 'b i -> b () i ()')
- k_mask = rearrange(k_mask, 'b j -> b () () j')
- input_mask = q_mask * k_mask
-
- if self.num_mem_kv > 0:
- mem_k, mem_v = map(lambda t: repeat(t, 'h n d -> b h n d', b=b), (self.mem_k, self.mem_v))
- k = torch.cat((mem_k, k), dim=-2)
- v = torch.cat((mem_v, v), dim=-2)
- if exists(input_mask):
- input_mask = F.pad(input_mask, (self.num_mem_kv, 0), value=True)
-
- dots = einsum('b h i d, b h j d -> b h i j', q, k) * self.scale
- mask_value = max_neg_value(dots)
-
- if exists(prev_attn):
- dots = dots + prev_attn
-
- pre_softmax_attn = dots
-
- if talking_heads:
- dots = einsum('b h i j, h k -> b k i j', dots, self.pre_softmax_proj).contiguous()
-
- if exists(rel_pos):
- dots = rel_pos(dots)
-
- if exists(input_mask):
- dots.masked_fill_(~input_mask, mask_value)
- del input_mask
-
- if self.causal:
- i, j = dots.shape[-2:]
- r = torch.arange(i, device=device)
- mask = rearrange(r, 'i -> () () i ()') < rearrange(r, 'j -> () () () j')
- mask = F.pad(mask, (j - i, 0), value=False)
- dots.masked_fill_(mask, mask_value)
- del mask
-
- if exists(self.sparse_topk) and self.sparse_topk < dots.shape[-1]:
- top, _ = dots.topk(self.sparse_topk, dim=-1)
- vk = top[..., -1].unsqueeze(-1).expand_as(dots)
- mask = dots < vk
- dots.masked_fill_(mask, mask_value)
- del mask
-
- attn = self.attn_fn(dots, dim=-1)
- post_softmax_attn = attn
-
- attn = self.dropout(attn)
-
- if talking_heads:
- attn = einsum('b h i j, h k -> b k i j', attn, self.post_softmax_proj).contiguous()
-
- out = einsum('b h i j, b h j d -> b h i d', attn, v)
- out = rearrange(out, 'b h n d -> b n (h d)')
-
- intermediates = Intermediates(
- pre_softmax_attn=pre_softmax_attn,
- post_softmax_attn=post_softmax_attn
- )
-
- return self.to_out(out), intermediates
-
-
-class AttentionLayers(nn.Module):
- def __init__(
- self,
- dim,
- depth,
- heads=8,
- causal=False,
- cross_attend=False,
- only_cross=False,
- use_scalenorm=False,
- use_rmsnorm=False,
- use_rezero=False,
- rel_pos_num_buckets=32,
- rel_pos_max_distance=128,
- position_infused_attn=False,
- custom_layers=None,
- sandwich_coef=None,
- par_ratio=None,
- residual_attn=False,
- cross_residual_attn=False,
- macaron=False,
- pre_norm=True,
- gate_residual=False,
- **kwargs
- ):
- super().__init__()
- ff_kwargs, kwargs = groupby_prefix_and_trim('ff_', kwargs)
- attn_kwargs, _ = groupby_prefix_and_trim('attn_', kwargs)
-
- dim_head = attn_kwargs.get('dim_head', DEFAULT_DIM_HEAD)
-
- self.dim = dim
- self.depth = depth
- self.layers = nn.ModuleList([])
-
- self.has_pos_emb = position_infused_attn
- self.pia_pos_emb = FixedPositionalEmbedding(dim) if position_infused_attn else None
- self.rotary_pos_emb = always(None)
-
- assert rel_pos_num_buckets <= rel_pos_max_distance, 'number of relative position buckets must be less than the relative position max distance'
- self.rel_pos = None
-
- self.pre_norm = pre_norm
-
- self.residual_attn = residual_attn
- self.cross_residual_attn = cross_residual_attn
-
- norm_class = ScaleNorm if use_scalenorm else nn.LayerNorm
- norm_class = RMSNorm if use_rmsnorm else norm_class
- norm_fn = partial(norm_class, dim)
-
- norm_fn = nn.Identity if use_rezero else norm_fn
- branch_fn = Rezero if use_rezero else None
-
- if cross_attend and not only_cross:
- default_block = ('a', 'c', 'f')
- elif cross_attend and only_cross:
- default_block = ('c', 'f')
- else:
- default_block = ('a', 'f')
-
- if macaron:
- default_block = ('f',) + default_block
-
- if exists(custom_layers):
- layer_types = custom_layers
- elif exists(par_ratio):
- par_depth = depth * len(default_block)
- assert 1 < par_ratio <= par_depth, 'par ratio out of range'
- default_block = tuple(filter(not_equals('f'), default_block))
- par_attn = par_depth // par_ratio
- depth_cut = par_depth * 2 // 3 # 2 / 3 attention layer cutoff suggested by PAR paper
- par_width = (depth_cut + depth_cut // par_attn) // par_attn
- assert len(default_block) <= par_width, 'default block is too large for par_ratio'
- par_block = default_block + ('f',) * (par_width - len(default_block))
- par_head = par_block * par_attn
- layer_types = par_head + ('f',) * (par_depth - len(par_head))
- elif exists(sandwich_coef):
- assert sandwich_coef > 0 and sandwich_coef <= depth, 'sandwich coefficient should be less than the depth'
- layer_types = ('a',) * sandwich_coef + default_block * (depth - sandwich_coef) + ('f',) * sandwich_coef
- else:
- layer_types = default_block * depth
-
- self.layer_types = layer_types
- self.num_attn_layers = len(list(filter(equals('a'), layer_types)))
-
- for layer_type in self.layer_types:
- if layer_type == 'a':
- layer = Attention(dim, heads=heads, causal=causal, **attn_kwargs)
- elif layer_type == 'c':
- layer = Attention(dim, heads=heads, **attn_kwargs)
- elif layer_type == 'f':
- layer = FeedForward(dim, **ff_kwargs)
- layer = layer if not macaron else Scale(0.5, layer)
- else:
- raise Exception(f'invalid layer type {layer_type}')
-
- if isinstance(layer, Attention) and exists(branch_fn):
- layer = branch_fn(layer)
-
- if gate_residual:
- residual_fn = GRUGating(dim)
- else:
- residual_fn = Residual()
-
- self.layers.append(nn.ModuleList([
- norm_fn(),
- layer,
- residual_fn
- ]))
-
- def forward(
- self,
- x,
- context=None,
- mask=None,
- context_mask=None,
- mems=None,
- return_hiddens=False
- ):
- hiddens = []
- intermediates = []
- prev_attn = None
- prev_cross_attn = None
-
- mems = mems.copy() if exists(mems) else [None] * self.num_attn_layers
-
- for ind, (layer_type, (norm, block, residual_fn)) in enumerate(zip(self.layer_types, self.layers)):
- is_last = ind == (len(self.layers) - 1)
-
- if layer_type == 'a':
- hiddens.append(x)
- layer_mem = mems.pop(0)
-
- residual = x
-
- if self.pre_norm:
- x = norm(x)
-
- if layer_type == 'a':
- out, inter = block(x, mask=mask, sinusoidal_emb=self.pia_pos_emb, rel_pos=self.rel_pos,
- prev_attn=prev_attn, mem=layer_mem)
- elif layer_type == 'c':
- out, inter = block(x, context=context, mask=mask, context_mask=context_mask, prev_attn=prev_cross_attn)
- elif layer_type == 'f':
- out = block(x)
-
- x = residual_fn(out, residual)
-
- if layer_type in ('a', 'c'):
- intermediates.append(inter)
-
- if layer_type == 'a' and self.residual_attn:
- prev_attn = inter.pre_softmax_attn
- elif layer_type == 'c' and self.cross_residual_attn:
- prev_cross_attn = inter.pre_softmax_attn
-
- if not self.pre_norm and not is_last:
- x = norm(x)
-
- if return_hiddens:
- intermediates = LayerIntermediates(
- hiddens=hiddens,
- attn_intermediates=intermediates
- )
-
- return x, intermediates
-
- return x
-
-
-class Encoder(AttentionLayers):
- def __init__(self, **kwargs):
- assert 'causal' not in kwargs, 'cannot set causality on encoder'
- super().__init__(causal=False, **kwargs)
-
-
-
-class TransformerWrapper(nn.Module):
- def __init__(
- self,
- *,
- num_tokens,
- max_seq_len,
- attn_layers,
- emb_dim=None,
- max_mem_len=0.,
- emb_dropout=0.,
- num_memory_tokens=None,
- tie_embedding=False,
- use_pos_emb=True
- ):
- super().__init__()
- assert isinstance(attn_layers, AttentionLayers), 'attention layers must be one of Encoder or Decoder'
-
- dim = attn_layers.dim
- emb_dim = default(emb_dim, dim)
-
- self.max_seq_len = max_seq_len
- self.max_mem_len = max_mem_len
- self.num_tokens = num_tokens
-
- self.token_emb = nn.Embedding(num_tokens, emb_dim)
- self.pos_emb = AbsolutePositionalEmbedding(emb_dim, max_seq_len) if (
- use_pos_emb and not attn_layers.has_pos_emb) else always(0)
- self.emb_dropout = nn.Dropout(emb_dropout)
-
- self.project_emb = nn.Linear(emb_dim, dim) if emb_dim != dim else nn.Identity()
- self.attn_layers = attn_layers
- self.norm = nn.LayerNorm(dim)
-
- self.init_()
-
- self.to_logits = nn.Linear(dim, num_tokens) if not tie_embedding else lambda t: t @ self.token_emb.weight.t()
-
- # memory tokens (like [cls]) from Memory Transformers paper
- num_memory_tokens = default(num_memory_tokens, 0)
- self.num_memory_tokens = num_memory_tokens
- if num_memory_tokens > 0:
- self.memory_tokens = nn.Parameter(torch.randn(num_memory_tokens, dim))
-
- # let funnel encoder know number of memory tokens, if specified
- if hasattr(attn_layers, 'num_memory_tokens'):
- attn_layers.num_memory_tokens = num_memory_tokens
-
- def init_(self):
- nn.init.normal_(self.token_emb.weight, std=0.02)
-
- def forward(
- self,
- x,
- return_embeddings=False,
- mask=None,
- return_mems=False,
- return_attn=False,
- mems=None,
- **kwargs
- ):
- b, n, device, num_mem = *x.shape, x.device, self.num_memory_tokens
- x = self.token_emb(x)
- x += self.pos_emb(x)
- x = self.emb_dropout(x)
-
- x = self.project_emb(x)
-
- if num_mem > 0:
- mem = repeat(self.memory_tokens, 'n d -> b n d', b=b)
- x = torch.cat((mem, x), dim=1)
-
- # auto-handle masking after appending memory tokens
- if exists(mask):
- mask = F.pad(mask, (num_mem, 0), value=True)
-
- x, intermediates = self.attn_layers(x, mask=mask, mems=mems, return_hiddens=True, **kwargs)
- x = self.norm(x)
-
- mem, x = x[:, :num_mem], x[:, num_mem:]
-
- out = self.to_logits(x) if not return_embeddings else x
-
- if return_mems:
- hiddens = intermediates.hiddens
- new_mems = list(map(lambda pair: torch.cat(pair, dim=-2), zip(mems, hiddens))) if exists(mems) else hiddens
- new_mems = list(map(lambda t: t[..., -self.max_mem_len:, :].detach(), new_mems))
- return out, new_mems
-
- if return_attn:
- attn_maps = list(map(lambda t: t.post_softmax_attn, intermediates.attn_intermediates))
- return out, attn_maps
-
- return out
-
diff --git a/spaces/joaopereirajp/livvieChatBot/venv/lib/python3.9/site-packages/dns/exception.py b/spaces/joaopereirajp/livvieChatBot/venv/lib/python3.9/site-packages/dns/exception.py
deleted file mode 100644
index 6982373de2a872057ca1fda3a2a752ff8d566355..0000000000000000000000000000000000000000
--- a/spaces/joaopereirajp/livvieChatBot/venv/lib/python3.9/site-packages/dns/exception.py
+++ /dev/null
@@ -1,169 +0,0 @@
-# Copyright (C) Dnspython Contributors, see LICENSE for text of ISC license
-
-# Copyright (C) 2003-2017 Nominum, Inc.
-#
-# Permission to use, copy, modify, and distribute this software and its
-# documentation for any purpose with or without fee is hereby granted,
-# provided that the above copyright notice and this permission notice
-# appear in all copies.
-#
-# THE SOFTWARE IS PROVIDED "AS IS" AND NOMINUM DISCLAIMS ALL WARRANTIES
-# WITH REGARD TO THIS SOFTWARE INCLUDING ALL IMPLIED WARRANTIES OF
-# MERCHANTABILITY AND FITNESS. IN NO EVENT SHALL NOMINUM BE LIABLE FOR
-# ANY SPECIAL, DIRECT, INDIRECT, OR CONSEQUENTIAL DAMAGES OR ANY DAMAGES
-# WHATSOEVER RESULTING FROM LOSS OF USE, DATA OR PROFITS, WHETHER IN AN
-# ACTION OF CONTRACT, NEGLIGENCE OR OTHER TORTIOUS ACTION, ARISING OUT
-# OF OR IN CONNECTION WITH THE USE OR PERFORMANCE OF THIS SOFTWARE.
-
-"""Common DNS Exceptions.
-
-Dnspython modules may also define their own exceptions, which will
-always be subclasses of ``DNSException``.
-"""
-
-
-from typing import Optional, Set
-
-
-class DNSException(Exception):
- """Abstract base class shared by all dnspython exceptions.
-
- It supports two basic modes of operation:
-
- a) Old/compatible mode is used if ``__init__`` was called with
- empty *kwargs*. In compatible mode all *args* are passed
- to the standard Python Exception class as before and all *args* are
- printed by the standard ``__str__`` implementation. Class variable
- ``msg`` (or doc string if ``msg`` is ``None``) is returned from ``str()``
- if *args* is empty.
-
- b) New/parametrized mode is used if ``__init__`` was called with
- non-empty *kwargs*.
- In the new mode *args* must be empty and all kwargs must match
- those set in class variable ``supp_kwargs``. All kwargs are stored inside
- ``self.kwargs`` and used in a new ``__str__`` implementation to construct
- a formatted message based on the ``fmt`` class variable, a ``string``.
-
- In the simplest case it is enough to override the ``supp_kwargs``
- and ``fmt`` class variables to get nice parametrized messages.
- """
-
- msg: Optional[str] = None # non-parametrized message
- supp_kwargs: Set[str] = set() # accepted parameters for _fmt_kwargs (sanity check)
- fmt: Optional[str] = None # message parametrized with results from _fmt_kwargs
-
- def __init__(self, *args, **kwargs):
- self._check_params(*args, **kwargs)
- if kwargs:
- # This call to a virtual method from __init__ is ok in our usage
- self.kwargs = self._check_kwargs(**kwargs) # lgtm[py/init-calls-subclass]
- self.msg = str(self)
- else:
- self.kwargs = dict() # defined but empty for old mode exceptions
- if self.msg is None:
- # doc string is better implicit message than empty string
- self.msg = self.__doc__
- if args:
- super().__init__(*args)
- else:
- super().__init__(self.msg)
-
- def _check_params(self, *args, **kwargs):
- """Old exceptions supported only args and not kwargs.
-
- For sanity we do not allow to mix old and new behavior."""
- if args or kwargs:
- assert bool(args) != bool(
- kwargs
- ), "keyword arguments are mutually exclusive with positional args"
-
- def _check_kwargs(self, **kwargs):
- if kwargs:
- assert (
- set(kwargs.keys()) == self.supp_kwargs
- ), "following set of keyword args is required: %s" % (self.supp_kwargs)
- return kwargs
-
- def _fmt_kwargs(self, **kwargs):
- """Format kwargs before printing them.
-
- Resulting dictionary has to have keys necessary for str.format call
- on fmt class variable.
- """
- fmtargs = {}
- for kw, data in kwargs.items():
- if isinstance(data, (list, set)):
- # convert list of to list of str()
- fmtargs[kw] = list(map(str, data))
- if len(fmtargs[kw]) == 1:
- # remove list brackets [] from single-item lists
- fmtargs[kw] = fmtargs[kw].pop()
- else:
- fmtargs[kw] = data
- return fmtargs
-
- def __str__(self):
- if self.kwargs and self.fmt:
- # provide custom message constructed from keyword arguments
- fmtargs = self._fmt_kwargs(**self.kwargs)
- return self.fmt.format(**fmtargs)
- else:
- # print *args directly in the same way as old DNSException
- return super().__str__()
-
-
-class FormError(DNSException):
- """DNS message is malformed."""
-
-
-class SyntaxError(DNSException):
- """Text input is malformed."""
-
-
-class UnexpectedEnd(SyntaxError):
- """Text input ended unexpectedly."""
-
-
-class TooBig(DNSException):
- """The DNS message is too big."""
-
-
-class Timeout(DNSException):
- """The DNS operation timed out."""
-
- supp_kwargs = {"timeout"}
- fmt = "The DNS operation timed out after {timeout:.3f} seconds"
-
- # We do this as otherwise mypy complains about unexpected keyword argument
- # idna_exception
- def __init__(self, *args, **kwargs):
- super().__init__(*args, **kwargs)
-
-
-class UnsupportedAlgorithm(DNSException):
- """The DNSSEC algorithm is not supported."""
-
-
-class AlgorithmKeyMismatch(UnsupportedAlgorithm):
- """The DNSSEC algorithm is not supported for the given key type."""
-
-
-class ValidationFailure(DNSException):
- """The DNSSEC signature is invalid."""
-
-
-class DeniedByPolicy(DNSException):
- """Denied by DNSSEC policy."""
-
-
-class ExceptionWrapper:
- def __init__(self, exception_class):
- self.exception_class = exception_class
-
- def __enter__(self):
- return self
-
- def __exit__(self, exc_type, exc_val, exc_tb):
- if exc_type is not None and not isinstance(exc_val, self.exception_class):
- raise self.exception_class(str(exc_val)) from exc_val
- return False
diff --git a/spaces/joaopereirajp/livvieChatBot/venv/lib/python3.9/site-packages/dns/inet.py b/spaces/joaopereirajp/livvieChatBot/venv/lib/python3.9/site-packages/dns/inet.py
deleted file mode 100644
index 02e925c6bf89f76ba9a8c0b10abfb97a32382c74..0000000000000000000000000000000000000000
--- a/spaces/joaopereirajp/livvieChatBot/venv/lib/python3.9/site-packages/dns/inet.py
+++ /dev/null
@@ -1,180 +0,0 @@
-# Copyright (C) Dnspython Contributors, see LICENSE for text of ISC license
-
-# Copyright (C) 2003-2017 Nominum, Inc.
-#
-# Permission to use, copy, modify, and distribute this software and its
-# documentation for any purpose with or without fee is hereby granted,
-# provided that the above copyright notice and this permission notice
-# appear in all copies.
-#
-# THE SOFTWARE IS PROVIDED "AS IS" AND NOMINUM DISCLAIMS ALL WARRANTIES
-# WITH REGARD TO THIS SOFTWARE INCLUDING ALL IMPLIED WARRANTIES OF
-# MERCHANTABILITY AND FITNESS. IN NO EVENT SHALL NOMINUM BE LIABLE FOR
-# ANY SPECIAL, DIRECT, INDIRECT, OR CONSEQUENTIAL DAMAGES OR ANY DAMAGES
-# WHATSOEVER RESULTING FROM LOSS OF USE, DATA OR PROFITS, WHETHER IN AN
-# ACTION OF CONTRACT, NEGLIGENCE OR OTHER TORTIOUS ACTION, ARISING OUT
-# OF OR IN CONNECTION WITH THE USE OR PERFORMANCE OF THIS SOFTWARE.
-
-"""Generic Internet address helper functions."""
-
-import socket
-from typing import Any, Optional, Tuple
-
-import dns.ipv4
-import dns.ipv6
-
-# We assume that AF_INET and AF_INET6 are always defined. We keep
-# these here for the benefit of any old code (unlikely though that
-# is!).
-AF_INET = socket.AF_INET
-AF_INET6 = socket.AF_INET6
-
-
-def inet_pton(family: int, text: str) -> bytes:
- """Convert the textual form of a network address into its binary form.
-
- *family* is an ``int``, the address family.
-
- *text* is a ``str``, the textual address.
-
- Raises ``NotImplementedError`` if the address family specified is not
- implemented.
-
- Returns a ``bytes``.
- """
-
- if family == AF_INET:
- return dns.ipv4.inet_aton(text)
- elif family == AF_INET6:
- return dns.ipv6.inet_aton(text, True)
- else:
- raise NotImplementedError
-
-
-def inet_ntop(family: int, address: bytes) -> str:
- """Convert the binary form of a network address into its textual form.
-
- *family* is an ``int``, the address family.
-
- *address* is a ``bytes``, the network address in binary form.
-
- Raises ``NotImplementedError`` if the address family specified is not
- implemented.
-
- Returns a ``str``.
- """
-
- if family == AF_INET:
- return dns.ipv4.inet_ntoa(address)
- elif family == AF_INET6:
- return dns.ipv6.inet_ntoa(address)
- else:
- raise NotImplementedError
-
-
-def af_for_address(text: str) -> int:
- """Determine the address family of a textual-form network address.
-
- *text*, a ``str``, the textual address.
-
- Raises ``ValueError`` if the address family cannot be determined
- from the input.
-
- Returns an ``int``.
- """
-
- try:
- dns.ipv4.inet_aton(text)
- return AF_INET
- except Exception:
- try:
- dns.ipv6.inet_aton(text, True)
- return AF_INET6
- except Exception:
- raise ValueError
-
-
-def is_multicast(text: str) -> bool:
- """Is the textual-form network address a multicast address?
-
- *text*, a ``str``, the textual address.
-
- Raises ``ValueError`` if the address family cannot be determined
- from the input.
-
- Returns a ``bool``.
- """
-
- try:
- first = dns.ipv4.inet_aton(text)[0]
- return first >= 224 and first <= 239
- except Exception:
- try:
- first = dns.ipv6.inet_aton(text, True)[0]
- return first == 255
- except Exception:
- raise ValueError
-
-
-def is_address(text: str) -> bool:
- """Is the specified string an IPv4 or IPv6 address?
-
- *text*, a ``str``, the textual address.
-
- Returns a ``bool``.
- """
-
- try:
- dns.ipv4.inet_aton(text)
- return True
- except Exception:
- try:
- dns.ipv6.inet_aton(text, True)
- return True
- except Exception:
- return False
-
-
-def low_level_address_tuple(
- high_tuple: Tuple[str, int], af: Optional[int] = None
-) -> Any:
- """Given a "high-level" address tuple, i.e.
- an (address, port) return the appropriate "low-level" address tuple
- suitable for use in socket calls.
-
- If an *af* other than ``None`` is provided, it is assumed the
- address in the high-level tuple is valid and has that af. If af
- is ``None``, then af_for_address will be called.
- """
- address, port = high_tuple
- if af is None:
- af = af_for_address(address)
- if af == AF_INET:
- return (address, port)
- elif af == AF_INET6:
- i = address.find("%")
- if i < 0:
- # no scope, shortcut!
- return (address, port, 0, 0)
- # try to avoid getaddrinfo()
- addrpart = address[:i]
- scope = address[i + 1 :]
- if scope.isdigit():
- return (addrpart, port, 0, int(scope))
- try:
- return (addrpart, port, 0, socket.if_nametoindex(scope))
- except AttributeError: # pragma: no cover (we can't really test this)
- ai_flags = socket.AI_NUMERICHOST
- ((*_, tup), *_) = socket.getaddrinfo(address, port, flags=ai_flags)
- return tup
- else:
- raise NotImplementedError(f"unknown address family {af}")
-
-
-def any_for_af(af):
- """Return the 'any' address for the specified address family."""
- if af == socket.AF_INET:
- return "0.0.0.0"
- elif af == socket.AF_INET6:
- return "::"
- raise NotImplementedError(f"unknown address family {af}")
diff --git a/spaces/joaopereirajp/livvieChatBot/venv/lib/python3.9/site-packages/dns/zone.py b/spaces/joaopereirajp/livvieChatBot/venv/lib/python3.9/site-packages/dns/zone.py
deleted file mode 100644
index 9e763f5f0cee92b99f397af83969fb2da8e28745..0000000000000000000000000000000000000000
--- a/spaces/joaopereirajp/livvieChatBot/venv/lib/python3.9/site-packages/dns/zone.py
+++ /dev/null
@@ -1,1395 +0,0 @@
-# Copyright (C) Dnspython Contributors, see LICENSE for text of ISC license
-
-# Copyright (C) 2003-2007, 2009-2011 Nominum, Inc.
-#
-# Permission to use, copy, modify, and distribute this software and its
-# documentation for any purpose with or without fee is hereby granted,
-# provided that the above copyright notice and this permission notice
-# appear in all copies.
-#
-# THE SOFTWARE IS PROVIDED "AS IS" AND NOMINUM DISCLAIMS ALL WARRANTIES
-# WITH REGARD TO THIS SOFTWARE INCLUDING ALL IMPLIED WARRANTIES OF
-# MERCHANTABILITY AND FITNESS. IN NO EVENT SHALL NOMINUM BE LIABLE FOR
-# ANY SPECIAL, DIRECT, INDIRECT, OR CONSEQUENTIAL DAMAGES OR ANY DAMAGES
-# WHATSOEVER RESULTING FROM LOSS OF USE, DATA OR PROFITS, WHETHER IN AN
-# ACTION OF CONTRACT, NEGLIGENCE OR OTHER TORTIOUS ACTION, ARISING OUT
-# OF OR IN CONNECTION WITH THE USE OR PERFORMANCE OF THIS SOFTWARE.
-
-"""DNS Zones."""
-
-import contextlib
-import io
-import os
-import struct
-from typing import Any, Dict, Iterable, Iterator, List, Optional, Set, Tuple, Union
-
-import dns.exception
-import dns.grange
-import dns.immutable
-import dns.name
-import dns.node
-import dns.rdata
-import dns.rdataclass
-import dns.rdataset
-import dns.rdatatype
-import dns.rdtypes.ANY.SOA
-import dns.rdtypes.ANY.ZONEMD
-import dns.rrset
-import dns.tokenizer
-import dns.transaction
-import dns.ttl
-import dns.zonefile
-from dns.zonetypes import DigestHashAlgorithm, DigestScheme, _digest_hashers
-
-
-class BadZone(dns.exception.DNSException):
-
- """The DNS zone is malformed."""
-
-
-class NoSOA(BadZone):
-
- """The DNS zone has no SOA RR at its origin."""
-
-
-class NoNS(BadZone):
-
- """The DNS zone has no NS RRset at its origin."""
-
-
-class UnknownOrigin(BadZone):
-
- """The DNS zone's origin is unknown."""
-
-
-class UnsupportedDigestScheme(dns.exception.DNSException):
-
- """The zone digest's scheme is unsupported."""
-
-
-class UnsupportedDigestHashAlgorithm(dns.exception.DNSException):
-
- """The zone digest's origin is unsupported."""
-
-
-class NoDigest(dns.exception.DNSException):
-
- """The DNS zone has no ZONEMD RRset at its origin."""
-
-
-class DigestVerificationFailure(dns.exception.DNSException):
-
- """The ZONEMD digest failed to verify."""
-
-
-class Zone(dns.transaction.TransactionManager):
-
- """A DNS zone.
-
- A ``Zone`` is a mapping from names to nodes. The zone object may be
- treated like a Python dictionary, e.g. ``zone[name]`` will retrieve
- the node associated with that name. The *name* may be a
- ``dns.name.Name object``, or it may be a string. In either case,
- if the name is relative it is treated as relative to the origin of
- the zone.
- """
-
- node_factory = dns.node.Node
-
- __slots__ = ["rdclass", "origin", "nodes", "relativize"]
-
- def __init__(
- self,
- origin: Optional[Union[dns.name.Name, str]],
- rdclass: dns.rdataclass.RdataClass = dns.rdataclass.IN,
- relativize: bool = True,
- ):
- """Initialize a zone object.
-
- *origin* is the origin of the zone. It may be a ``dns.name.Name``,
- a ``str``, or ``None``. If ``None``, then the zone's origin will
- be set by the first ``$ORIGIN`` line in a zone file.
-
- *rdclass*, an ``int``, the zone's rdata class; the default is class IN.
-
- *relativize*, a ``bool``, determine's whether domain names are
- relativized to the zone's origin. The default is ``True``.
- """
-
- if origin is not None:
- if isinstance(origin, str):
- origin = dns.name.from_text(origin)
- elif not isinstance(origin, dns.name.Name):
- raise ValueError("origin parameter must be convertible to a DNS name")
- if not origin.is_absolute():
- raise ValueError("origin parameter must be an absolute name")
- self.origin = origin
- self.rdclass = rdclass
- self.nodes: Dict[dns.name.Name, dns.node.Node] = {}
- self.relativize = relativize
-
- def __eq__(self, other):
- """Two zones are equal if they have the same origin, class, and
- nodes.
-
- Returns a ``bool``.
- """
-
- if not isinstance(other, Zone):
- return False
- if (
- self.rdclass != other.rdclass
- or self.origin != other.origin
- or self.nodes != other.nodes
- ):
- return False
- return True
-
- def __ne__(self, other):
- """Are two zones not equal?
-
- Returns a ``bool``.
- """
-
- return not self.__eq__(other)
-
- def _validate_name(self, name: Union[dns.name.Name, str]) -> dns.name.Name:
- if isinstance(name, str):
- name = dns.name.from_text(name, None)
- elif not isinstance(name, dns.name.Name):
- raise KeyError("name parameter must be convertible to a DNS name")
- if name.is_absolute():
- if self.origin is None:
- # This should probably never happen as other code (e.g.
- # _rr_line) will notice the lack of an origin before us, but
- # we check just in case!
- raise KeyError("no zone origin is defined")
- if not name.is_subdomain(self.origin):
- raise KeyError("name parameter must be a subdomain of the zone origin")
- if self.relativize:
- name = name.relativize(self.origin)
- elif not self.relativize:
- # We have a relative name in a non-relative zone, so derelativize.
- if self.origin is None:
- raise KeyError("no zone origin is defined")
- name = name.derelativize(self.origin)
- return name
-
- def __getitem__(self, key):
- key = self._validate_name(key)
- return self.nodes[key]
-
- def __setitem__(self, key, value):
- key = self._validate_name(key)
- self.nodes[key] = value
-
- def __delitem__(self, key):
- key = self._validate_name(key)
- del self.nodes[key]
-
- def __iter__(self):
- return self.nodes.__iter__()
-
- def keys(self):
- return self.nodes.keys()
-
- def values(self):
- return self.nodes.values()
-
- def items(self):
- return self.nodes.items()
-
- def get(self, key):
- key = self._validate_name(key)
- return self.nodes.get(key)
-
- def __contains__(self, key):
- key = self._validate_name(key)
- return key in self.nodes
-
- def find_node(
- self, name: Union[dns.name.Name, str], create: bool = False
- ) -> dns.node.Node:
- """Find a node in the zone, possibly creating it.
-
- *name*: the name of the node to find.
- The value may be a ``dns.name.Name`` or a ``str``. If absolute, the
- name must be a subdomain of the zone's origin. If ``zone.relativize``
- is ``True``, then the name will be relativized.
-
- *create*, a ``bool``. If true, the node will be created if it does
- not exist.
-
- Raises ``KeyError`` if the name is not known and create was
- not specified, or if the name was not a subdomain of the origin.
-
- Returns a ``dns.node.Node``.
- """
-
- name = self._validate_name(name)
- node = self.nodes.get(name)
- if node is None:
- if not create:
- raise KeyError
- node = self.node_factory()
- self.nodes[name] = node
- return node
-
- def get_node(
- self, name: Union[dns.name.Name, str], create: bool = False
- ) -> Optional[dns.node.Node]:
- """Get a node in the zone, possibly creating it.
-
- This method is like ``find_node()``, except it returns None instead
- of raising an exception if the node does not exist and creation
- has not been requested.
-
- *name*: the name of the node to find.
- The value may be a ``dns.name.Name`` or a ``str``. If absolute, the
- name must be a subdomain of the zone's origin. If ``zone.relativize``
- is ``True``, then the name will be relativized.
-
- *create*, a ``bool``. If true, the node will be created if it does
- not exist.
-
- Raises ``KeyError`` if the name is not known and create was
- not specified, or if the name was not a subdomain of the origin.
-
- Returns a ``dns.node.Node`` or ``None``.
- """
-
- try:
- node = self.find_node(name, create)
- except KeyError:
- node = None
- return node
-
- def delete_node(self, name: Union[dns.name.Name, str]) -> None:
- """Delete the specified node if it exists.
-
- *name*: the name of the node to find.
- The value may be a ``dns.name.Name`` or a ``str``. If absolute, the
- name must be a subdomain of the zone's origin. If ``zone.relativize``
- is ``True``, then the name will be relativized.
-
- It is not an error if the node does not exist.
- """
-
- name = self._validate_name(name)
- if name in self.nodes:
- del self.nodes[name]
-
- def find_rdataset(
- self,
- name: Union[dns.name.Name, str],
- rdtype: Union[dns.rdatatype.RdataType, str],
- covers: Union[dns.rdatatype.RdataType, str] = dns.rdatatype.NONE,
- create: bool = False,
- ) -> dns.rdataset.Rdataset:
- """Look for an rdataset with the specified name and type in the zone,
- and return an rdataset encapsulating it.
-
- The rdataset returned is not a copy; changes to it will change
- the zone.
-
- KeyError is raised if the name or type are not found.
-
- *name*: the name of the node to find.
- The value may be a ``dns.name.Name`` or a ``str``. If absolute, the
- name must be a subdomain of the zone's origin. If ``zone.relativize``
- is ``True``, then the name will be relativized.
-
- *rdtype*, a ``dns.rdatatype.RdataType`` or ``str``, the rdata type desired.
-
- *covers*, a ``dns.rdatatype.RdataType`` or ``str`` the covered type.
- Usually this value is ``dns.rdatatype.NONE``, but if the
- rdtype is ``dns.rdatatype.SIG`` or ``dns.rdatatype.RRSIG``,
- then the covers value will be the rdata type the SIG/RRSIG
- covers. The library treats the SIG and RRSIG types as if they
- were a family of types, e.g. RRSIG(A), RRSIG(NS), RRSIG(SOA).
- This makes RRSIGs much easier to work with than if RRSIGs
- covering different rdata types were aggregated into a single
- RRSIG rdataset.
-
- *create*, a ``bool``. If true, the node will be created if it does
- not exist.
-
- Raises ``KeyError`` if the name is not known and create was
- not specified, or if the name was not a subdomain of the origin.
-
- Returns a ``dns.rdataset.Rdataset``.
- """
-
- name = self._validate_name(name)
- rdtype = dns.rdatatype.RdataType.make(rdtype)
- covers = dns.rdatatype.RdataType.make(covers)
- node = self.find_node(name, create)
- return node.find_rdataset(self.rdclass, rdtype, covers, create)
-
- def get_rdataset(
- self,
- name: Union[dns.name.Name, str],
- rdtype: Union[dns.rdatatype.RdataType, str],
- covers: Union[dns.rdatatype.RdataType, str] = dns.rdatatype.NONE,
- create: bool = False,
- ) -> Optional[dns.rdataset.Rdataset]:
- """Look for an rdataset with the specified name and type in the zone.
-
- This method is like ``find_rdataset()``, except it returns None instead
- of raising an exception if the rdataset does not exist and creation
- has not been requested.
-
- The rdataset returned is not a copy; changes to it will change
- the zone.
-
- *name*: the name of the node to find.
- The value may be a ``dns.name.Name`` or a ``str``. If absolute, the
- name must be a subdomain of the zone's origin. If ``zone.relativize``
- is ``True``, then the name will be relativized.
-
- *rdtype*, a ``dns.rdatatype.RdataType`` or ``str``, the rdata type desired.
-
- *covers*, a ``dns.rdatatype.RdataType`` or ``str``, the covered type.
- Usually this value is ``dns.rdatatype.NONE``, but if the
- rdtype is ``dns.rdatatype.SIG`` or ``dns.rdatatype.RRSIG``,
- then the covers value will be the rdata type the SIG/RRSIG
- covers. The library treats the SIG and RRSIG types as if they
- were a family of types, e.g. RRSIG(A), RRSIG(NS), RRSIG(SOA).
- This makes RRSIGs much easier to work with than if RRSIGs
- covering different rdata types were aggregated into a single
- RRSIG rdataset.
-
- *create*, a ``bool``. If true, the node will be created if it does
- not exist.
-
- Raises ``KeyError`` if the name is not known and create was
- not specified, or if the name was not a subdomain of the origin.
-
- Returns a ``dns.rdataset.Rdataset`` or ``None``.
- """
-
- try:
- rdataset = self.find_rdataset(name, rdtype, covers, create)
- except KeyError:
- rdataset = None
- return rdataset
-
- def delete_rdataset(
- self,
- name: Union[dns.name.Name, str],
- rdtype: Union[dns.rdatatype.RdataType, str],
- covers: Union[dns.rdatatype.RdataType, str] = dns.rdatatype.NONE,
- ) -> None:
- """Delete the rdataset matching *rdtype* and *covers*, if it
- exists at the node specified by *name*.
-
- It is not an error if the node does not exist, or if there is no matching
- rdataset at the node.
-
- If the node has no rdatasets after the deletion, it will itself be deleted.
-
- *name*: the name of the node to find. The value may be a ``dns.name.Name`` or a
- ``str``. If absolute, the name must be a subdomain of the zone's origin. If
- ``zone.relativize`` is ``True``, then the name will be relativized.
-
- *rdtype*, a ``dns.rdatatype.RdataType`` or ``str``, the rdata type desired.
-
- *covers*, a ``dns.rdatatype.RdataType`` or ``str`` or ``None``, the covered
- type. Usually this value is ``dns.rdatatype.NONE``, but if the rdtype is
- ``dns.rdatatype.SIG`` or ``dns.rdatatype.RRSIG``, then the covers value will be
- the rdata type the SIG/RRSIG covers. The library treats the SIG and RRSIG types
- as if they were a family of types, e.g. RRSIG(A), RRSIG(NS), RRSIG(SOA). This
- makes RRSIGs much easier to work with than if RRSIGs covering different rdata
- types were aggregated into a single RRSIG rdataset.
- """
-
- name = self._validate_name(name)
- rdtype = dns.rdatatype.RdataType.make(rdtype)
- covers = dns.rdatatype.RdataType.make(covers)
- node = self.get_node(name)
- if node is not None:
- node.delete_rdataset(self.rdclass, rdtype, covers)
- if len(node) == 0:
- self.delete_node(name)
-
- def replace_rdataset(
- self, name: Union[dns.name.Name, str], replacement: dns.rdataset.Rdataset
- ) -> None:
- """Replace an rdataset at name.
-
- It is not an error if there is no rdataset matching I{replacement}.
-
- Ownership of the *replacement* object is transferred to the zone;
- in other words, this method does not store a copy of *replacement*
- at the node, it stores *replacement* itself.
-
- If the node does not exist, it is created.
-
- *name*: the name of the node to find.
- The value may be a ``dns.name.Name`` or a ``str``. If absolute, the
- name must be a subdomain of the zone's origin. If ``zone.relativize``
- is ``True``, then the name will be relativized.
-
- *replacement*, a ``dns.rdataset.Rdataset``, the replacement rdataset.
- """
-
- if replacement.rdclass != self.rdclass:
- raise ValueError("replacement.rdclass != zone.rdclass")
- node = self.find_node(name, True)
- node.replace_rdataset(replacement)
-
- def find_rrset(
- self,
- name: Union[dns.name.Name, str],
- rdtype: Union[dns.rdatatype.RdataType, str],
- covers: Union[dns.rdatatype.RdataType, str] = dns.rdatatype.NONE,
- ) -> dns.rrset.RRset:
- """Look for an rdataset with the specified name and type in the zone,
- and return an RRset encapsulating it.
-
- This method is less efficient than the similar
- ``find_rdataset()`` because it creates an RRset instead of
- returning the matching rdataset. It may be more convenient
- for some uses since it returns an object which binds the owner
- name to the rdataset.
-
- This method may not be used to create new nodes or rdatasets;
- use ``find_rdataset`` instead.
-
- *name*: the name of the node to find.
- The value may be a ``dns.name.Name`` or a ``str``. If absolute, the
- name must be a subdomain of the zone's origin. If ``zone.relativize``
- is ``True``, then the name will be relativized.
-
- *rdtype*, a ``dns.rdatatype.RdataType`` or ``str``, the rdata type desired.
-
- *covers*, a ``dns.rdatatype.RdataType`` or ``str``, the covered type.
- Usually this value is ``dns.rdatatype.NONE``, but if the
- rdtype is ``dns.rdatatype.SIG`` or ``dns.rdatatype.RRSIG``,
- then the covers value will be the rdata type the SIG/RRSIG
- covers. The library treats the SIG and RRSIG types as if they
- were a family of types, e.g. RRSIG(A), RRSIG(NS), RRSIG(SOA).
- This makes RRSIGs much easier to work with than if RRSIGs
- covering different rdata types were aggregated into a single
- RRSIG rdataset.
-
- *create*, a ``bool``. If true, the node will be created if it does
- not exist.
-
- Raises ``KeyError`` if the name is not known and create was
- not specified, or if the name was not a subdomain of the origin.
-
- Returns a ``dns.rrset.RRset`` or ``None``.
- """
-
- vname = self._validate_name(name)
- rdtype = dns.rdatatype.RdataType.make(rdtype)
- covers = dns.rdatatype.RdataType.make(covers)
- rdataset = self.nodes[vname].find_rdataset(self.rdclass, rdtype, covers)
- rrset = dns.rrset.RRset(vname, self.rdclass, rdtype, covers)
- rrset.update(rdataset)
- return rrset
-
- def get_rrset(
- self,
- name: Union[dns.name.Name, str],
- rdtype: Union[dns.rdatatype.RdataType, str],
- covers: Union[dns.rdatatype.RdataType, str] = dns.rdatatype.NONE,
- ) -> Optional[dns.rrset.RRset]:
- """Look for an rdataset with the specified name and type in the zone,
- and return an RRset encapsulating it.
-
- This method is less efficient than the similar ``get_rdataset()``
- because it creates an RRset instead of returning the matching
- rdataset. It may be more convenient for some uses since it
- returns an object which binds the owner name to the rdataset.
-
- This method may not be used to create new nodes or rdatasets;
- use ``get_rdataset()`` instead.
-
- *name*: the name of the node to find.
- The value may be a ``dns.name.Name`` or a ``str``. If absolute, the
- name must be a subdomain of the zone's origin. If ``zone.relativize``
- is ``True``, then the name will be relativized.
-
- *rdtype*, a ``dns.rdataset.Rdataset`` or ``str``, the rdata type desired.
-
- *covers*, a ``dns.rdataset.Rdataset`` or ``str``, the covered type.
- Usually this value is ``dns.rdatatype.NONE``, but if the
- rdtype is ``dns.rdatatype.SIG`` or ``dns.rdatatype.RRSIG``,
- then the covers value will be the rdata type the SIG/RRSIG
- covers. The library treats the SIG and RRSIG types as if they
- were a family of types, e.g. RRSIG(A), RRSIG(NS), RRSIG(SOA).
- This makes RRSIGs much easier to work with than if RRSIGs
- covering different rdata types were aggregated into a single
- RRSIG rdataset.
-
- *create*, a ``bool``. If true, the node will be created if it does
- not exist.
-
- Raises ``KeyError`` if the name is not known and create was
- not specified, or if the name was not a subdomain of the origin.
-
- Returns a ``dns.rrset.RRset`` or ``None``.
- """
-
- try:
- rrset = self.find_rrset(name, rdtype, covers)
- except KeyError:
- rrset = None
- return rrset
-
- def iterate_rdatasets(
- self,
- rdtype: Union[dns.rdatatype.RdataType, str] = dns.rdatatype.ANY,
- covers: Union[dns.rdatatype.RdataType, str] = dns.rdatatype.NONE,
- ) -> Iterator[Tuple[dns.name.Name, dns.rdataset.Rdataset]]:
- """Return a generator which yields (name, rdataset) tuples for
- all rdatasets in the zone which have the specified *rdtype*
- and *covers*. If *rdtype* is ``dns.rdatatype.ANY``, the default,
- then all rdatasets will be matched.
-
- *rdtype*, a ``dns.rdataset.Rdataset`` or ``str``, the rdata type desired.
-
- *covers*, a ``dns.rdataset.Rdataset`` or ``str``, the covered type.
- Usually this value is ``dns.rdatatype.NONE``, but if the
- rdtype is ``dns.rdatatype.SIG`` or ``dns.rdatatype.RRSIG``,
- then the covers value will be the rdata type the SIG/RRSIG
- covers. The library treats the SIG and RRSIG types as if they
- were a family of types, e.g. RRSIG(A), RRSIG(NS), RRSIG(SOA).
- This makes RRSIGs much easier to work with than if RRSIGs
- covering different rdata types were aggregated into a single
- RRSIG rdataset.
- """
-
- rdtype = dns.rdatatype.RdataType.make(rdtype)
- covers = dns.rdatatype.RdataType.make(covers)
- for name, node in self.items():
- for rds in node:
- if rdtype == dns.rdatatype.ANY or (
- rds.rdtype == rdtype and rds.covers == covers
- ):
- yield (name, rds)
-
- def iterate_rdatas(
- self,
- rdtype: Union[dns.rdatatype.RdataType, str] = dns.rdatatype.ANY,
- covers: Union[dns.rdatatype.RdataType, str] = dns.rdatatype.NONE,
- ) -> Iterator[Tuple[dns.name.Name, int, dns.rdata.Rdata]]:
- """Return a generator which yields (name, ttl, rdata) tuples for
- all rdatas in the zone which have the specified *rdtype*
- and *covers*. If *rdtype* is ``dns.rdatatype.ANY``, the default,
- then all rdatas will be matched.
-
- *rdtype*, a ``dns.rdataset.Rdataset`` or ``str``, the rdata type desired.
-
- *covers*, a ``dns.rdataset.Rdataset`` or ``str``, the covered type.
- Usually this value is ``dns.rdatatype.NONE``, but if the
- rdtype is ``dns.rdatatype.SIG`` or ``dns.rdatatype.RRSIG``,
- then the covers value will be the rdata type the SIG/RRSIG
- covers. The library treats the SIG and RRSIG types as if they
- were a family of types, e.g. RRSIG(A), RRSIG(NS), RRSIG(SOA).
- This makes RRSIGs much easier to work with than if RRSIGs
- covering different rdata types were aggregated into a single
- RRSIG rdataset.
- """
-
- rdtype = dns.rdatatype.RdataType.make(rdtype)
- covers = dns.rdatatype.RdataType.make(covers)
- for name, node in self.items():
- for rds in node:
- if rdtype == dns.rdatatype.ANY or (
- rds.rdtype == rdtype and rds.covers == covers
- ):
- for rdata in rds:
- yield (name, rds.ttl, rdata)
-
- def to_file(
- self,
- f: Any,
- sorted: bool = True,
- relativize: bool = True,
- nl: Optional[str] = None,
- want_comments: bool = False,
- want_origin: bool = False,
- ) -> None:
- """Write a zone to a file.
-
- *f*, a file or `str`. If *f* is a string, it is treated
- as the name of a file to open.
-
- *sorted*, a ``bool``. If True, the default, then the file
- will be written with the names sorted in DNSSEC order from
- least to greatest. Otherwise the names will be written in
- whatever order they happen to have in the zone's dictionary.
-
- *relativize*, a ``bool``. If True, the default, then domain
- names in the output will be relativized to the zone's origin
- if possible.
-
- *nl*, a ``str`` or None. The end of line string. If not
- ``None``, the output will use the platform's native
- end-of-line marker (i.e. LF on POSIX, CRLF on Windows).
-
- *want_comments*, a ``bool``. If ``True``, emit end-of-line comments
- as part of writing the file. If ``False``, the default, do not
- emit them.
-
- *want_origin*, a ``bool``. If ``True``, emit a $ORIGIN line at
- the start of the file. If ``False``, the default, do not emit
- one.
- """
-
- if isinstance(f, str):
- cm: contextlib.AbstractContextManager = open(f, "wb")
- else:
- cm = contextlib.nullcontext(f)
- with cm as f:
- # must be in this way, f.encoding may contain None, or even
- # attribute may not be there
- file_enc = getattr(f, "encoding", None)
- if file_enc is None:
- file_enc = "utf-8"
-
- if nl is None:
- # binary mode, '\n' is not enough
- nl_b = os.linesep.encode(file_enc)
- nl = "\n"
- elif isinstance(nl, str):
- nl_b = nl.encode(file_enc)
- else:
- nl_b = nl
- nl = nl.decode()
-
- if want_origin:
- assert self.origin is not None
- l = "$ORIGIN " + self.origin.to_text()
- l_b = l.encode(file_enc)
- try:
- f.write(l_b)
- f.write(nl_b)
- except TypeError: # textual mode
- f.write(l)
- f.write(nl)
-
- if sorted:
- names = list(self.keys())
- names.sort()
- else:
- names = self.keys()
- for n in names:
- l = self[n].to_text(
- n,
- origin=self.origin,
- relativize=relativize,
- want_comments=want_comments,
- )
- l_b = l.encode(file_enc)
-
- try:
- f.write(l_b)
- f.write(nl_b)
- except TypeError: # textual mode
- f.write(l)
- f.write(nl)
-
- def to_text(
- self,
- sorted: bool = True,
- relativize: bool = True,
- nl: Optional[str] = None,
- want_comments: bool = False,
- want_origin: bool = False,
- ) -> str:
- """Return a zone's text as though it were written to a file.
-
- *sorted*, a ``bool``. If True, the default, then the file
- will be written with the names sorted in DNSSEC order from
- least to greatest. Otherwise the names will be written in
- whatever order they happen to have in the zone's dictionary.
-
- *relativize*, a ``bool``. If True, the default, then domain
- names in the output will be relativized to the zone's origin
- if possible.
-
- *nl*, a ``str`` or None. The end of line string. If not
- ``None``, the output will use the platform's native
- end-of-line marker (i.e. LF on POSIX, CRLF on Windows).
-
- *want_comments*, a ``bool``. If ``True``, emit end-of-line comments
- as part of writing the file. If ``False``, the default, do not
- emit them.
-
- *want_origin*, a ``bool``. If ``True``, emit a $ORIGIN line at
- the start of the output. If ``False``, the default, do not emit
- one.
-
- Returns a ``str``.
- """
- temp_buffer = io.StringIO()
- self.to_file(temp_buffer, sorted, relativize, nl, want_comments, want_origin)
- return_value = temp_buffer.getvalue()
- temp_buffer.close()
- return return_value
-
- def check_origin(self) -> None:
- """Do some simple checking of the zone's origin.
-
- Raises ``dns.zone.NoSOA`` if there is no SOA RRset.
-
- Raises ``dns.zone.NoNS`` if there is no NS RRset.
-
- Raises ``KeyError`` if there is no origin node.
- """
- if self.relativize:
- name = dns.name.empty
- else:
- assert self.origin is not None
- name = self.origin
- if self.get_rdataset(name, dns.rdatatype.SOA) is None:
- raise NoSOA
- if self.get_rdataset(name, dns.rdatatype.NS) is None:
- raise NoNS
-
- def get_soa(
- self, txn: Optional[dns.transaction.Transaction] = None
- ) -> dns.rdtypes.ANY.SOA.SOA:
- """Get the zone SOA rdata.
-
- Raises ``dns.zone.NoSOA`` if there is no SOA RRset.
-
- Returns a ``dns.rdtypes.ANY.SOA.SOA`` Rdata.
- """
- if self.relativize:
- origin_name = dns.name.empty
- else:
- if self.origin is None:
- # get_soa() has been called very early, and there must not be
- # an SOA if there is no origin.
- raise NoSOA
- origin_name = self.origin
- soa: Optional[dns.rdataset.Rdataset]
- if txn:
- soa = txn.get(origin_name, dns.rdatatype.SOA)
- else:
- soa = self.get_rdataset(origin_name, dns.rdatatype.SOA)
- if soa is None:
- raise NoSOA
- return soa[0]
-
- def _compute_digest(
- self,
- hash_algorithm: DigestHashAlgorithm,
- scheme: DigestScheme = DigestScheme.SIMPLE,
- ) -> bytes:
- hashinfo = _digest_hashers.get(hash_algorithm)
- if not hashinfo:
- raise UnsupportedDigestHashAlgorithm
- if scheme != DigestScheme.SIMPLE:
- raise UnsupportedDigestScheme
-
- if self.relativize:
- origin_name = dns.name.empty
- else:
- assert self.origin is not None
- origin_name = self.origin
- hasher = hashinfo()
- for name, node in sorted(self.items()):
- rrnamebuf = name.to_digestable(self.origin)
- for rdataset in sorted(node, key=lambda rds: (rds.rdtype, rds.covers)):
- if name == origin_name and dns.rdatatype.ZONEMD in (
- rdataset.rdtype,
- rdataset.covers,
- ):
- continue
- rrfixed = struct.pack(
- "!HHI", rdataset.rdtype, rdataset.rdclass, rdataset.ttl
- )
- rdatas = [rdata.to_digestable(self.origin) for rdata in rdataset]
- for rdata in sorted(rdatas):
- rrlen = struct.pack("!H", len(rdata))
- hasher.update(rrnamebuf + rrfixed + rrlen + rdata)
- return hasher.digest()
-
- def compute_digest(
- self,
- hash_algorithm: DigestHashAlgorithm,
- scheme: DigestScheme = DigestScheme.SIMPLE,
- ) -> dns.rdtypes.ANY.ZONEMD.ZONEMD:
- serial = self.get_soa().serial
- digest = self._compute_digest(hash_algorithm, scheme)
- return dns.rdtypes.ANY.ZONEMD.ZONEMD(
- self.rdclass, dns.rdatatype.ZONEMD, serial, scheme, hash_algorithm, digest
- )
-
- def verify_digest(
- self, zonemd: Optional[dns.rdtypes.ANY.ZONEMD.ZONEMD] = None
- ) -> None:
- digests: Union[dns.rdataset.Rdataset, List[dns.rdtypes.ANY.ZONEMD.ZONEMD]]
- if zonemd:
- digests = [zonemd]
- else:
- assert self.origin is not None
- rds = self.get_rdataset(self.origin, dns.rdatatype.ZONEMD)
- if rds is None:
- raise NoDigest
- digests = rds
- for digest in digests:
- try:
- computed = self._compute_digest(digest.hash_algorithm, digest.scheme)
- if computed == digest.digest:
- return
- except Exception:
- pass
- raise DigestVerificationFailure
-
- # TransactionManager methods
-
- def reader(self) -> "Transaction":
- return Transaction(self, False, Version(self, 1, self.nodes, self.origin))
-
- def writer(self, replacement: bool = False) -> "Transaction":
- txn = Transaction(self, replacement)
- txn._setup_version()
- return txn
-
- def origin_information(
- self,
- ) -> Tuple[Optional[dns.name.Name], bool, Optional[dns.name.Name]]:
- effective: Optional[dns.name.Name]
- if self.relativize:
- effective = dns.name.empty
- else:
- effective = self.origin
- return (self.origin, self.relativize, effective)
-
- def get_class(self):
- return self.rdclass
-
- # Transaction methods
-
- def _end_read(self, txn):
- pass
-
- def _end_write(self, txn):
- pass
-
- def _commit_version(self, _, version, origin):
- self.nodes = version.nodes
- if self.origin is None:
- self.origin = origin
-
- def _get_next_version_id(self):
- # Versions are ephemeral and all have id 1
- return 1
-
-
-# These classes used to be in dns.versioned, but have moved here so we can use
-# the copy-on-write transaction mechanism for both kinds of zones. In a
-# regular zone, the version only exists during the transaction, and the nodes
-# are regular dns.node.Nodes.
-
-# A node with a version id.
-
-
-class VersionedNode(dns.node.Node): # lgtm[py/missing-equals]
- __slots__ = ["id"]
-
- def __init__(self):
- super().__init__()
- # A proper id will get set by the Version
- self.id = 0
-
-
-@dns.immutable.immutable
-class ImmutableVersionedNode(VersionedNode):
- def __init__(self, node):
- super().__init__()
- self.id = node.id
- self.rdatasets = tuple(
- [dns.rdataset.ImmutableRdataset(rds) for rds in node.rdatasets]
- )
-
- def find_rdataset(
- self,
- rdclass: dns.rdataclass.RdataClass,
- rdtype: dns.rdatatype.RdataType,
- covers: dns.rdatatype.RdataType = dns.rdatatype.NONE,
- create: bool = False,
- ) -> dns.rdataset.Rdataset:
- if create:
- raise TypeError("immutable")
- return super().find_rdataset(rdclass, rdtype, covers, False)
-
- def get_rdataset(
- self,
- rdclass: dns.rdataclass.RdataClass,
- rdtype: dns.rdatatype.RdataType,
- covers: dns.rdatatype.RdataType = dns.rdatatype.NONE,
- create: bool = False,
- ) -> Optional[dns.rdataset.Rdataset]:
- if create:
- raise TypeError("immutable")
- return super().get_rdataset(rdclass, rdtype, covers, False)
-
- def delete_rdataset(
- self,
- rdclass: dns.rdataclass.RdataClass,
- rdtype: dns.rdatatype.RdataType,
- covers: dns.rdatatype.RdataType = dns.rdatatype.NONE,
- ) -> None:
- raise TypeError("immutable")
-
- def replace_rdataset(self, replacement: dns.rdataset.Rdataset) -> None:
- raise TypeError("immutable")
-
- def is_immutable(self) -> bool:
- return True
-
-
-class Version:
- def __init__(
- self,
- zone: Zone,
- id: int,
- nodes: Optional[Dict[dns.name.Name, dns.node.Node]] = None,
- origin: Optional[dns.name.Name] = None,
- ):
- self.zone = zone
- self.id = id
- if nodes is not None:
- self.nodes = nodes
- else:
- self.nodes = {}
- self.origin = origin
-
- def _validate_name(self, name: dns.name.Name) -> dns.name.Name:
- if name.is_absolute():
- if self.origin is None:
- # This should probably never happen as other code (e.g.
- # _rr_line) will notice the lack of an origin before us, but
- # we check just in case!
- raise KeyError("no zone origin is defined")
- if not name.is_subdomain(self.origin):
- raise KeyError("name is not a subdomain of the zone origin")
- if self.zone.relativize:
- name = name.relativize(self.origin)
- elif not self.zone.relativize:
- # We have a relative name in a non-relative zone, so derelativize.
- if self.origin is None:
- raise KeyError("no zone origin is defined")
- name = name.derelativize(self.origin)
- return name
-
- def get_node(self, name: dns.name.Name) -> Optional[dns.node.Node]:
- name = self._validate_name(name)
- return self.nodes.get(name)
-
- def get_rdataset(
- self,
- name: dns.name.Name,
- rdtype: dns.rdatatype.RdataType,
- covers: dns.rdatatype.RdataType,
- ) -> Optional[dns.rdataset.Rdataset]:
- node = self.get_node(name)
- if node is None:
- return None
- return node.get_rdataset(self.zone.rdclass, rdtype, covers)
-
- def keys(self):
- return self.nodes.keys()
-
- def items(self):
- return self.nodes.items()
-
-
-class WritableVersion(Version):
- def __init__(self, zone: Zone, replacement: bool = False):
- # The zone._versions_lock must be held by our caller in a versioned
- # zone.
- id = zone._get_next_version_id()
- super().__init__(zone, id)
- if not replacement:
- # We copy the map, because that gives us a simple and thread-safe
- # way of doing versions, and we have a garbage collector to help
- # us. We only make new node objects if we actually change the
- # node.
- self.nodes.update(zone.nodes)
- # We have to copy the zone origin as it may be None in the first
- # version, and we don't want to mutate the zone until we commit.
- self.origin = zone.origin
- self.changed: Set[dns.name.Name] = set()
-
- def _maybe_cow(self, name: dns.name.Name) -> dns.node.Node:
- name = self._validate_name(name)
- node = self.nodes.get(name)
- if node is None or name not in self.changed:
- new_node = self.zone.node_factory()
- if hasattr(new_node, "id"):
- # We keep doing this for backwards compatibility, as earlier
- # code used new_node.id != self.id for the "do we need to CoW?"
- # test. Now we use the changed set as this works with both
- # regular zones and versioned zones.
- #
- # We ignore the mypy error as this is safe but it doesn't see it.
- new_node.id = self.id # type: ignore
- if node is not None:
- # moo! copy on write!
- new_node.rdatasets.extend(node.rdatasets)
- self.nodes[name] = new_node
- self.changed.add(name)
- return new_node
- else:
- return node
-
- def delete_node(self, name: dns.name.Name) -> None:
- name = self._validate_name(name)
- if name in self.nodes:
- del self.nodes[name]
- self.changed.add(name)
-
- def put_rdataset(
- self, name: dns.name.Name, rdataset: dns.rdataset.Rdataset
- ) -> None:
- node = self._maybe_cow(name)
- node.replace_rdataset(rdataset)
-
- def delete_rdataset(
- self,
- name: dns.name.Name,
- rdtype: dns.rdatatype.RdataType,
- covers: dns.rdatatype.RdataType,
- ) -> None:
- node = self._maybe_cow(name)
- node.delete_rdataset(self.zone.rdclass, rdtype, covers)
- if len(node) == 0:
- del self.nodes[name]
-
-
-@dns.immutable.immutable
-class ImmutableVersion(Version):
- def __init__(self, version: WritableVersion):
- # We tell super() that it's a replacement as we don't want it
- # to copy the nodes, as we're about to do that with an
- # immutable Dict.
- super().__init__(version.zone, True)
- # set the right id!
- self.id = version.id
- # keep the origin
- self.origin = version.origin
- # Make changed nodes immutable
- for name in version.changed:
- node = version.nodes.get(name)
- # it might not exist if we deleted it in the version
- if node:
- version.nodes[name] = ImmutableVersionedNode(node)
- # We're changing the type of the nodes dictionary here on purpose, so
- # we ignore the mypy error.
- self.nodes = dns.immutable.Dict(version.nodes, True) # type: ignore
-
-
-class Transaction(dns.transaction.Transaction):
- def __init__(self, zone, replacement, version=None, make_immutable=False):
- read_only = version is not None
- super().__init__(zone, replacement, read_only)
- self.version = version
- self.make_immutable = make_immutable
-
- @property
- def zone(self):
- return self.manager
-
- def _setup_version(self):
- assert self.version is None
- self.version = WritableVersion(self.zone, self.replacement)
-
- def _get_rdataset(self, name, rdtype, covers):
- return self.version.get_rdataset(name, rdtype, covers)
-
- def _put_rdataset(self, name, rdataset):
- assert not self.read_only
- self.version.put_rdataset(name, rdataset)
-
- def _delete_name(self, name):
- assert not self.read_only
- self.version.delete_node(name)
-
- def _delete_rdataset(self, name, rdtype, covers):
- assert not self.read_only
- self.version.delete_rdataset(name, rdtype, covers)
-
- def _name_exists(self, name):
- return self.version.get_node(name) is not None
-
- def _changed(self):
- if self.read_only:
- return False
- else:
- return len(self.version.changed) > 0
-
- def _end_transaction(self, commit):
- if self.read_only:
- self.zone._end_read(self)
- elif commit and len(self.version.changed) > 0:
- if self.make_immutable:
- version = ImmutableVersion(self.version)
- else:
- version = self.version
- self.zone._commit_version(self, version, self.version.origin)
- else:
- # rollback
- self.zone._end_write(self)
-
- def _set_origin(self, origin):
- if self.version.origin is None:
- self.version.origin = origin
-
- def _iterate_rdatasets(self):
- for name, node in self.version.items():
- for rdataset in node:
- yield (name, rdataset)
-
- def _iterate_names(self):
- return self.version.keys()
-
- def _get_node(self, name):
- return self.version.get_node(name)
-
- def _origin_information(self):
- (absolute, relativize, effective) = self.manager.origin_information()
- if absolute is None and self.version.origin is not None:
- # No origin has been committed yet, but we've learned one as part of
- # this txn. Use it.
- absolute = self.version.origin
- if relativize:
- effective = dns.name.empty
- else:
- effective = absolute
- return (absolute, relativize, effective)
-
-
-def from_text(
- text: str,
- origin: Optional[Union[dns.name.Name, str]] = None,
- rdclass: dns.rdataclass.RdataClass = dns.rdataclass.IN,
- relativize: bool = True,
- zone_factory: Any = Zone,
- filename: Optional[str] = None,
- allow_include: bool = False,
- check_origin: bool = True,
- idna_codec: Optional[dns.name.IDNACodec] = None,
- allow_directives: Union[bool, Iterable[str]] = True,
-) -> Zone:
- """Build a zone object from a zone file format string.
-
- *text*, a ``str``, the zone file format input.
-
- *origin*, a ``dns.name.Name``, a ``str``, or ``None``. The origin
- of the zone; if not specified, the first ``$ORIGIN`` statement in the
- zone file will determine the origin of the zone.
-
- *rdclass*, a ``dns.rdataclass.RdataClass``, the zone's rdata class; the default is
- class IN.
-
- *relativize*, a ``bool``, determine's whether domain names are
- relativized to the zone's origin. The default is ``True``.
-
- *zone_factory*, the zone factory to use or ``None``. If ``None``, then
- ``dns.zone.Zone`` will be used. The value may be any class or callable
- that returns a subclass of ``dns.zone.Zone``.
-
- *filename*, a ``str`` or ``None``, the filename to emit when
- describing where an error occurred; the default is ``''``.
-
- *allow_include*, a ``bool``. If ``True``, the default, then ``$INCLUDE``
- directives are permitted. If ``False``, then encoutering a ``$INCLUDE``
- will raise a ``SyntaxError`` exception.
-
- *check_origin*, a ``bool``. If ``True``, the default, then sanity
- checks of the origin node will be made by calling the zone's
- ``check_origin()`` method.
-
- *idna_codec*, a ``dns.name.IDNACodec``, specifies the IDNA
- encoder/decoder. If ``None``, the default IDNA 2003 encoder/decoder
- is used.
-
- *allow_directives*, a ``bool`` or an iterable of `str`. If ``True``, the default,
- then directives are permitted, and the *allow_include* parameter controls whether
- ``$INCLUDE`` is permitted. If ``False`` or an empty iterable, then no directive
- processing is done and any directive-like text will be treated as a regular owner
- name. If a non-empty iterable, then only the listed directives (including the
- ``$``) are allowed.
-
- Raises ``dns.zone.NoSOA`` if there is no SOA RRset.
-
- Raises ``dns.zone.NoNS`` if there is no NS RRset.
-
- Raises ``KeyError`` if there is no origin node.
-
- Returns a subclass of ``dns.zone.Zone``.
- """
-
- # 'text' can also be a file, but we don't publish that fact
- # since it's an implementation detail. The official file
- # interface is from_file().
-
- if filename is None:
- filename = ""
- zone = zone_factory(origin, rdclass, relativize=relativize)
- with zone.writer(True) as txn:
- tok = dns.tokenizer.Tokenizer(text, filename, idna_codec=idna_codec)
- reader = dns.zonefile.Reader(
- tok,
- rdclass,
- txn,
- allow_include=allow_include,
- allow_directives=allow_directives,
- )
- try:
- reader.read()
- except dns.zonefile.UnknownOrigin:
- # for backwards compatibility
- raise dns.zone.UnknownOrigin
- # Now that we're done reading, do some basic checking of the zone.
- if check_origin:
- zone.check_origin()
- return zone
-
-
-def from_file(
- f: Any,
- origin: Optional[Union[dns.name.Name, str]] = None,
- rdclass: dns.rdataclass.RdataClass = dns.rdataclass.IN,
- relativize: bool = True,
- zone_factory: Any = Zone,
- filename: Optional[str] = None,
- allow_include: bool = True,
- check_origin: bool = True,
- idna_codec: Optional[dns.name.IDNACodec] = None,
- allow_directives: Union[bool, Iterable[str]] = True,
-) -> Zone:
- """Read a zone file and build a zone object.
-
- *f*, a file or ``str``. If *f* is a string, it is treated
- as the name of a file to open.
-
- *origin*, a ``dns.name.Name``, a ``str``, or ``None``. The origin
- of the zone; if not specified, the first ``$ORIGIN`` statement in the
- zone file will determine the origin of the zone.
-
- *rdclass*, an ``int``, the zone's rdata class; the default is class IN.
-
- *relativize*, a ``bool``, determine's whether domain names are
- relativized to the zone's origin. The default is ``True``.
-
- *zone_factory*, the zone factory to use or ``None``. If ``None``, then
- ``dns.zone.Zone`` will be used. The value may be any class or callable
- that returns a subclass of ``dns.zone.Zone``.
-
- *filename*, a ``str`` or ``None``, the filename to emit when
- describing where an error occurred; the default is ``''``.
-
- *allow_include*, a ``bool``. If ``True``, the default, then ``$INCLUDE``
- directives are permitted. If ``False``, then encoutering a ``$INCLUDE``
- will raise a ``SyntaxError`` exception.
-
- *check_origin*, a ``bool``. If ``True``, the default, then sanity
- checks of the origin node will be made by calling the zone's
- ``check_origin()`` method.
-
- *idna_codec*, a ``dns.name.IDNACodec``, specifies the IDNA
- encoder/decoder. If ``None``, the default IDNA 2003 encoder/decoder
- is used.
-
- *allow_directives*, a ``bool`` or an iterable of `str`. If ``True``, the default,
- then directives are permitted, and the *allow_include* parameter controls whether
- ``$INCLUDE`` is permitted. If ``False`` or an empty iterable, then no directive
- processing is done and any directive-like text will be treated as a regular owner
- name. If a non-empty iterable, then only the listed directives (including the
- ``$``) are allowed.
-
- Raises ``dns.zone.NoSOA`` if there is no SOA RRset.
-
- Raises ``dns.zone.NoNS`` if there is no NS RRset.
-
- Raises ``KeyError`` if there is no origin node.
-
- Returns a subclass of ``dns.zone.Zone``.
- """
-
- if isinstance(f, str):
- if filename is None:
- filename = f
- cm: contextlib.AbstractContextManager = open(f)
- else:
- cm = contextlib.nullcontext(f)
- with cm as f:
- return from_text(
- f,
- origin,
- rdclass,
- relativize,
- zone_factory,
- filename,
- allow_include,
- check_origin,
- idna_codec,
- allow_directives,
- )
- assert False # make mypy happy lgtm[py/unreachable-statement]
-
-
-def from_xfr(
- xfr: Any,
- zone_factory: Any = Zone,
- relativize: bool = True,
- check_origin: bool = True,
-) -> Zone:
- """Convert the output of a zone transfer generator into a zone object.
-
- *xfr*, a generator of ``dns.message.Message`` objects, typically
- ``dns.query.xfr()``.
-
- *relativize*, a ``bool``, determine's whether domain names are
- relativized to the zone's origin. The default is ``True``.
- It is essential that the relativize setting matches the one specified
- to the generator.
-
- *check_origin*, a ``bool``. If ``True``, the default, then sanity
- checks of the origin node will be made by calling the zone's
- ``check_origin()`` method.
-
- Raises ``dns.zone.NoSOA`` if there is no SOA RRset.
-
- Raises ``dns.zone.NoNS`` if there is no NS RRset.
-
- Raises ``KeyError`` if there is no origin node.
-
- Raises ``ValueError`` if no messages are yielded by the generator.
-
- Returns a subclass of ``dns.zone.Zone``.
- """
-
- z = None
- for r in xfr:
- if z is None:
- if relativize:
- origin = r.origin
- else:
- origin = r.answer[0].name
- rdclass = r.answer[0].rdclass
- z = zone_factory(origin, rdclass, relativize=relativize)
- for rrset in r.answer:
- znode = z.nodes.get(rrset.name)
- if not znode:
- znode = z.node_factory()
- z.nodes[rrset.name] = znode
- zrds = znode.find_rdataset(rrset.rdclass, rrset.rdtype, rrset.covers, True)
- zrds.update_ttl(rrset.ttl)
- for rd in rrset:
- zrds.add(rd)
- if z is None:
- raise ValueError("empty transfer")
- if check_origin:
- z.check_origin()
- return z
diff --git a/spaces/joaopereirajp/livvieChatBot/venv/lib/python3.9/site-packages/fastapi/utils.py b/spaces/joaopereirajp/livvieChatBot/venv/lib/python3.9/site-packages/fastapi/utils.py
deleted file mode 100644
index 267d64ce8aee9eaf5462d9cbd47deca44cfdef28..0000000000000000000000000000000000000000
--- a/spaces/joaopereirajp/livvieChatBot/venv/lib/python3.9/site-packages/fastapi/utils.py
+++ /dev/null
@@ -1,228 +0,0 @@
-import re
-import warnings
-from dataclasses import is_dataclass
-from typing import (
- TYPE_CHECKING,
- Any,
- Dict,
- MutableMapping,
- Optional,
- Set,
- Type,
- Union,
- cast,
-)
-from weakref import WeakKeyDictionary
-
-import fastapi
-from fastapi._compat import (
- PYDANTIC_V2,
- BaseConfig,
- ModelField,
- PydanticSchemaGenerationError,
- Undefined,
- UndefinedType,
- Validator,
- lenient_issubclass,
-)
-from fastapi.datastructures import DefaultPlaceholder, DefaultType
-from pydantic import BaseModel, create_model
-from pydantic.fields import FieldInfo
-from typing_extensions import Literal
-
-if TYPE_CHECKING: # pragma: nocover
- from .routing import APIRoute
-
-# Cache for `create_cloned_field`
-_CLONED_TYPES_CACHE: MutableMapping[
- Type[BaseModel], Type[BaseModel]
-] = WeakKeyDictionary()
-
-
-def is_body_allowed_for_status_code(status_code: Union[int, str, None]) -> bool:
- if status_code is None:
- return True
- # Ref: https://github.com/OAI/OpenAPI-Specification/blob/main/versions/3.1.0.md#patterned-fields-1
- if status_code in {
- "default",
- "1XX",
- "2XX",
- "3XX",
- "4XX",
- "5XX",
- }:
- return True
- current_status_code = int(status_code)
- return not (current_status_code < 200 or current_status_code in {204, 304})
-
-
-def get_path_param_names(path: str) -> Set[str]:
- return set(re.findall("{(.*?)}", path))
-
-
-def create_response_field(
- name: str,
- type_: Type[Any],
- class_validators: Optional[Dict[str, Validator]] = None,
- default: Optional[Any] = Undefined,
- required: Union[bool, UndefinedType] = Undefined,
- model_config: Type[BaseConfig] = BaseConfig,
- field_info: Optional[FieldInfo] = None,
- alias: Optional[str] = None,
- mode: Literal["validation", "serialization"] = "validation",
-) -> ModelField:
- """
- Create a new response field. Raises if type_ is invalid.
- """
- class_validators = class_validators or {}
- if PYDANTIC_V2:
- field_info = field_info or FieldInfo(
- annotation=type_, default=default, alias=alias
- )
- else:
- field_info = field_info or FieldInfo()
- kwargs = {"name": name, "field_info": field_info}
- if PYDANTIC_V2:
- kwargs.update({"mode": mode})
- else:
- kwargs.update(
- {
- "type_": type_,
- "class_validators": class_validators,
- "default": default,
- "required": required,
- "model_config": model_config,
- "alias": alias,
- }
- )
- try:
- return ModelField(**kwargs) # type: ignore[arg-type]
- except (RuntimeError, PydanticSchemaGenerationError):
- raise fastapi.exceptions.FastAPIError(
- "Invalid args for response field! Hint: "
- f"check that {type_} is a valid Pydantic field type. "
- "If you are using a return type annotation that is not a valid Pydantic "
- "field (e.g. Union[Response, dict, None]) you can disable generating the "
- "response model from the type annotation with the path operation decorator "
- "parameter response_model=None. Read more: "
- "https://fastapi.tiangolo.com/tutorial/response-model/"
- ) from None
-
-
-def create_cloned_field(
- field: ModelField,
- *,
- cloned_types: Optional[MutableMapping[Type[BaseModel], Type[BaseModel]]] = None,
-) -> ModelField:
- if PYDANTIC_V2:
- return field
- # cloned_types caches already cloned types to support recursive models and improve
- # performance by avoiding unecessary cloning
- if cloned_types is None:
- cloned_types = _CLONED_TYPES_CACHE
-
- original_type = field.type_
- if is_dataclass(original_type) and hasattr(original_type, "__pydantic_model__"):
- original_type = original_type.__pydantic_model__
- use_type = original_type
- if lenient_issubclass(original_type, BaseModel):
- original_type = cast(Type[BaseModel], original_type)
- use_type = cloned_types.get(original_type)
- if use_type is None:
- use_type = create_model(original_type.__name__, __base__=original_type)
- cloned_types[original_type] = use_type
- for f in original_type.__fields__.values():
- use_type.__fields__[f.name] = create_cloned_field(
- f, cloned_types=cloned_types
- )
- new_field = create_response_field(name=field.name, type_=use_type)
- new_field.has_alias = field.has_alias # type: ignore[attr-defined]
- new_field.alias = field.alias # type: ignore[misc]
- new_field.class_validators = field.class_validators # type: ignore[attr-defined]
- new_field.default = field.default # type: ignore[misc]
- new_field.required = field.required # type: ignore[misc]
- new_field.model_config = field.model_config # type: ignore[attr-defined]
- new_field.field_info = field.field_info
- new_field.allow_none = field.allow_none # type: ignore[attr-defined]
- new_field.validate_always = field.validate_always # type: ignore[attr-defined]
- if field.sub_fields: # type: ignore[attr-defined]
- new_field.sub_fields = [ # type: ignore[attr-defined]
- create_cloned_field(sub_field, cloned_types=cloned_types)
- for sub_field in field.sub_fields # type: ignore[attr-defined]
- ]
- if field.key_field: # type: ignore[attr-defined]
- new_field.key_field = create_cloned_field( # type: ignore[attr-defined]
- field.key_field, cloned_types=cloned_types # type: ignore[attr-defined]
- )
- new_field.validators = field.validators # type: ignore[attr-defined]
- new_field.pre_validators = field.pre_validators # type: ignore[attr-defined]
- new_field.post_validators = field.post_validators # type: ignore[attr-defined]
- new_field.parse_json = field.parse_json # type: ignore[attr-defined]
- new_field.shape = field.shape # type: ignore[attr-defined]
- new_field.populate_validators() # type: ignore[attr-defined]
- return new_field
-
-
-def generate_operation_id_for_path(
- *, name: str, path: str, method: str
-) -> str: # pragma: nocover
- warnings.warn(
- "fastapi.utils.generate_operation_id_for_path() was deprecated, "
- "it is not used internally, and will be removed soon",
- DeprecationWarning,
- stacklevel=2,
- )
- operation_id = name + path
- operation_id = re.sub(r"\W", "_", operation_id)
- operation_id = operation_id + "_" + method.lower()
- return operation_id
-
-
-def generate_unique_id(route: "APIRoute") -> str:
- operation_id = route.name + route.path_format
- operation_id = re.sub(r"\W", "_", operation_id)
- assert route.methods
- operation_id = operation_id + "_" + list(route.methods)[0].lower()
- return operation_id
-
-
-def deep_dict_update(main_dict: Dict[Any, Any], update_dict: Dict[Any, Any]) -> None:
- for key, value in update_dict.items():
- if (
- key in main_dict
- and isinstance(main_dict[key], dict)
- and isinstance(value, dict)
- ):
- deep_dict_update(main_dict[key], value)
- elif (
- key in main_dict
- and isinstance(main_dict[key], list)
- and isinstance(update_dict[key], list)
- ):
- main_dict[key] = main_dict[key] + update_dict[key]
- else:
- main_dict[key] = value
-
-
-def get_value_or_default(
- first_item: Union[DefaultPlaceholder, DefaultType],
- *extra_items: Union[DefaultPlaceholder, DefaultType],
-) -> Union[DefaultPlaceholder, DefaultType]:
- """
- Pass items or `DefaultPlaceholder`s by descending priority.
-
- The first one to _not_ be a `DefaultPlaceholder` will be returned.
-
- Otherwise, the first item (a `DefaultPlaceholder`) will be returned.
- """
- items = (first_item,) + extra_items
- for item in items:
- if not isinstance(item, DefaultPlaceholder):
- return item
- return first_item
-
-
-def match_pydantic_error_url(error_type: str) -> Any:
- from dirty_equals import IsStr
-
- return IsStr(regex=rf"^https://errors\.pydantic\.dev/.*/v/{error_type}")
diff --git a/spaces/joaopereirajp/livvieChatBot/venv/lib/python3.9/site-packages/fontTools/merge/base.py b/spaces/joaopereirajp/livvieChatBot/venv/lib/python3.9/site-packages/fontTools/merge/base.py
deleted file mode 100644
index 37f9097ab2595413066cebd102fdf697280a93bb..0000000000000000000000000000000000000000
--- a/spaces/joaopereirajp/livvieChatBot/venv/lib/python3.9/site-packages/fontTools/merge/base.py
+++ /dev/null
@@ -1,81 +0,0 @@
-# Copyright 2013 Google, Inc. All Rights Reserved.
-#
-# Google Author(s): Behdad Esfahbod, Roozbeh Pournader
-
-from fontTools.ttLib.tables.DefaultTable import DefaultTable
-import logging
-
-
-log = logging.getLogger("fontTools.merge")
-
-
-def add_method(*clazzes, **kwargs):
- """Returns a decorator function that adds a new method to one or
- more classes."""
- allowDefault = kwargs.get("allowDefaultTable", False)
-
- def wrapper(method):
- done = []
- for clazz in clazzes:
- if clazz in done:
- continue # Support multiple names of a clazz
- done.append(clazz)
- assert allowDefault or clazz != DefaultTable, "Oops, table class not found."
- assert (
- method.__name__ not in clazz.__dict__
- ), "Oops, class '%s' has method '%s'." % (clazz.__name__, method.__name__)
- setattr(clazz, method.__name__, method)
- return None
-
- return wrapper
-
-
-def mergeObjects(lst):
- lst = [item for item in lst if item is not NotImplemented]
- if not lst:
- return NotImplemented
- lst = [item for item in lst if item is not None]
- if not lst:
- return None
-
- clazz = lst[0].__class__
- assert all(type(item) == clazz for item in lst), lst
-
- logic = clazz.mergeMap
- returnTable = clazz()
- returnDict = {}
-
- allKeys = set.union(set(), *(vars(table).keys() for table in lst))
- for key in allKeys:
- try:
- mergeLogic = logic[key]
- except KeyError:
- try:
- mergeLogic = logic["*"]
- except KeyError:
- raise Exception(
- "Don't know how to merge key %s of class %s" % (key, clazz.__name__)
- )
- if mergeLogic is NotImplemented:
- continue
- value = mergeLogic(getattr(table, key, NotImplemented) for table in lst)
- if value is not NotImplemented:
- returnDict[key] = value
-
- returnTable.__dict__ = returnDict
-
- return returnTable
-
-
-@add_method(DefaultTable, allowDefaultTable=True)
-def merge(self, m, tables):
- if not hasattr(self, "mergeMap"):
- log.info("Don't know how to merge '%s'.", self.tableTag)
- return NotImplemented
-
- logic = self.mergeMap
-
- if isinstance(logic, dict):
- return m.mergeObjects(self, self.mergeMap, tables)
- else:
- return logic(tables)
diff --git a/spaces/joaopereirajp/livvieChatBot/venv/lib/python3.9/site-packages/fontTools/ttLib/tables/G_P_K_G_.py b/spaces/joaopereirajp/livvieChatBot/venv/lib/python3.9/site-packages/fontTools/ttLib/tables/G_P_K_G_.py
deleted file mode 100644
index eed34d92105926dcdb988ef345e8421a93b85518..0000000000000000000000000000000000000000
--- a/spaces/joaopereirajp/livvieChatBot/venv/lib/python3.9/site-packages/fontTools/ttLib/tables/G_P_K_G_.py
+++ /dev/null
@@ -1,126 +0,0 @@
-from fontTools.misc import sstruct
-from fontTools.misc.textTools import bytesjoin, safeEval, readHex
-from . import DefaultTable
-import sys
-import array
-
-GPKGFormat = """
- > # big endian
- version: H
- flags: H
- numGMAPs: H
- numGlyplets: H
-"""
-# psFontName is a byte string which follows the record above. This is zero padded
-# to the beginning of the records array. The recordsOffsst is 32 bit aligned.
-
-
-class table_G_P_K_G_(DefaultTable.DefaultTable):
- def decompile(self, data, ttFont):
- dummy, newData = sstruct.unpack2(GPKGFormat, data, self)
-
- GMAPoffsets = array.array("I")
- endPos = (self.numGMAPs + 1) * 4
- GMAPoffsets.frombytes(newData[:endPos])
- if sys.byteorder != "big":
- GMAPoffsets.byteswap()
- self.GMAPs = []
- for i in range(self.numGMAPs):
- start = GMAPoffsets[i]
- end = GMAPoffsets[i + 1]
- self.GMAPs.append(data[start:end])
- pos = endPos
- endPos = pos + (self.numGlyplets + 1) * 4
- glyphletOffsets = array.array("I")
- glyphletOffsets.frombytes(newData[pos:endPos])
- if sys.byteorder != "big":
- glyphletOffsets.byteswap()
- self.glyphlets = []
- for i in range(self.numGlyplets):
- start = glyphletOffsets[i]
- end = glyphletOffsets[i + 1]
- self.glyphlets.append(data[start:end])
-
- def compile(self, ttFont):
- self.numGMAPs = len(self.GMAPs)
- self.numGlyplets = len(self.glyphlets)
- GMAPoffsets = [0] * (self.numGMAPs + 1)
- glyphletOffsets = [0] * (self.numGlyplets + 1)
-
- dataList = [sstruct.pack(GPKGFormat, self)]
-
- pos = len(dataList[0]) + (self.numGMAPs + 1) * 4 + (self.numGlyplets + 1) * 4
- GMAPoffsets[0] = pos
- for i in range(1, self.numGMAPs + 1):
- pos += len(self.GMAPs[i - 1])
- GMAPoffsets[i] = pos
- gmapArray = array.array("I", GMAPoffsets)
- if sys.byteorder != "big":
- gmapArray.byteswap()
- dataList.append(gmapArray.tobytes())
-
- glyphletOffsets[0] = pos
- for i in range(1, self.numGlyplets + 1):
- pos += len(self.glyphlets[i - 1])
- glyphletOffsets[i] = pos
- glyphletArray = array.array("I", glyphletOffsets)
- if sys.byteorder != "big":
- glyphletArray.byteswap()
- dataList.append(glyphletArray.tobytes())
- dataList += self.GMAPs
- dataList += self.glyphlets
- data = bytesjoin(dataList)
- return data
-
- def toXML(self, writer, ttFont):
- writer.comment("Most of this table will be recalculated by the compiler")
- writer.newline()
- formatstring, names, fixes = sstruct.getformat(GPKGFormat)
- for name in names:
- value = getattr(self, name)
- writer.simpletag(name, value=value)
- writer.newline()
-
- writer.begintag("GMAPs")
- writer.newline()
- for gmapData in self.GMAPs:
- writer.begintag("hexdata")
- writer.newline()
- writer.dumphex(gmapData)
- writer.endtag("hexdata")
- writer.newline()
- writer.endtag("GMAPs")
- writer.newline()
-
- writer.begintag("glyphlets")
- writer.newline()
- for glyphletData in self.glyphlets:
- writer.begintag("hexdata")
- writer.newline()
- writer.dumphex(glyphletData)
- writer.endtag("hexdata")
- writer.newline()
- writer.endtag("glyphlets")
- writer.newline()
-
- def fromXML(self, name, attrs, content, ttFont):
- if name == "GMAPs":
- if not hasattr(self, "GMAPs"):
- self.GMAPs = []
- for element in content:
- if isinstance(element, str):
- continue
- itemName, itemAttrs, itemContent = element
- if itemName == "hexdata":
- self.GMAPs.append(readHex(itemContent))
- elif name == "glyphlets":
- if not hasattr(self, "glyphlets"):
- self.glyphlets = []
- for element in content:
- if isinstance(element, str):
- continue
- itemName, itemAttrs, itemContent = element
- if itemName == "hexdata":
- self.glyphlets.append(readHex(itemContent))
- else:
- setattr(self, name, safeEval(attrs["value"]))
diff --git a/spaces/joaopereirajp/livvieChatBot/venv/lib/python3.9/site-packages/fontTools/ttLib/tables/_f_e_a_t.py b/spaces/joaopereirajp/livvieChatBot/venv/lib/python3.9/site-packages/fontTools/ttLib/tables/_f_e_a_t.py
deleted file mode 100644
index c9a48eff06cb14b1b2dc56c94ec7e02b80f11ca3..0000000000000000000000000000000000000000
--- a/spaces/joaopereirajp/livvieChatBot/venv/lib/python3.9/site-packages/fontTools/ttLib/tables/_f_e_a_t.py
+++ /dev/null
@@ -1,12 +0,0 @@
-from .otBase import BaseTTXConverter
-
-
-class table__f_e_a_t(BaseTTXConverter):
- """The feature name table is an AAT (Apple Advanced Typography) table for
- storing font features, settings, and their human-readable names. It should
- not be confused with the ``Feat`` table or the OpenType Layout ``GSUB``/``GPOS``
- tables. See `Feature Name Table `_
- in the TrueType Reference Manual for more information on the structure and
- purpose of this table."""
-
- pass
diff --git a/spaces/jonas/sdg-policy-tracing/check_site.py b/spaces/jonas/sdg-policy-tracing/check_site.py
deleted file mode 100644
index 306104663c4e4cbfc08383432742e611601b2e4d..0000000000000000000000000000000000000000
--- a/spaces/jonas/sdg-policy-tracing/check_site.py
+++ /dev/null
@@ -1,16 +0,0 @@
-import streamlit as st
-from PIL import Image
-
-
-def app():
- # Sidebar
- st.sidebar.title('Check Coherence')
- st.sidebar.write(' ')
- st.sidebar.selectbox('Select NDC', ('South Africa', 'Ethiopia'))
-
- # Container
- c1, c2, c3 = st.columns([1, 7, 1])
- c2.markdown("SDSN X GIZ Policy Tracing ", unsafe_allow_html=True)
- c1, c2, c3 = st.columns([1.8, 7, 1])
- image = Image.open('pic1.PNG')
- c2.image(image, width=1000)
\ No newline at end of file
diff --git a/spaces/jonathang/dog_breed_v2/README.md b/spaces/jonathang/dog_breed_v2/README.md
deleted file mode 100644
index 5e36d05606adf5c318857d5ff40a62ecbd0fe967..0000000000000000000000000000000000000000
--- a/spaces/jonathang/dog_breed_v2/README.md
+++ /dev/null
@@ -1,12 +0,0 @@
----
-title: Dog Breed V2
-emoji: 🚀
-colorFrom: gray
-colorTo: pink
-sdk: gradio
-sdk_version: 3.9
-app_file: app.py
-pinned: false
----
-
-Check out the configuration reference at https://huggingface.co/docs/hub/spaces-config-reference
diff --git a/spaces/jonigata/PoseTweak/external/default_runtime.py b/spaces/jonigata/PoseTweak/external/default_runtime.py
deleted file mode 100644
index 62b7ff270aae280268ea528c1fbe99c0052e20e3..0000000000000000000000000000000000000000
--- a/spaces/jonigata/PoseTweak/external/default_runtime.py
+++ /dev/null
@@ -1,20 +0,0 @@
-checkpoint_config = dict(interval=10)
-
-log_config = dict(
- interval=50,
- hooks=[
- dict(type='TextLoggerHook'),
- # dict(type='TensorboardLoggerHook')
- # dict(type='PaviLoggerHook') # for internal services
- ])
-
-log_level = 'INFO'
-load_from = None
-resume_from = None
-dist_params = dict(backend='nccl')
-workflow = [('train', 1)]
-
-# disable opencv multithreading to avoid system being overloaded
-opencv_num_threads = 0
-# set multi-process start method as `fork` to speed up the training
-mp_start_method = 'fork'
diff --git a/spaces/joshipunitram/crowd-counting-p2p/train.py b/spaces/joshipunitram/crowd-counting-p2p/train.py
deleted file mode 100644
index db0bd8619ba4e4f471e6844f1125c643a95c70fc..0000000000000000000000000000000000000000
--- a/spaces/joshipunitram/crowd-counting-p2p/train.py
+++ /dev/null
@@ -1,222 +0,0 @@
-import argparse
-import datetime
-import random
-import time
-from pathlib import Path
-
-import torch
-from torch.utils.data import DataLoader, DistributedSampler
-
-from crowd_datasets import build_dataset
-from engine import *
-from models import build_model
-import os
-from tensorboardX import SummaryWriter
-import warnings
-warnings.filterwarnings('ignore')
-
-def get_args_parser():
- parser = argparse.ArgumentParser('Set parameters for training P2PNet', add_help=False)
- parser.add_argument('--lr', default=1e-4, type=float)
- parser.add_argument('--lr_backbone', default=1e-5, type=float)
- parser.add_argument('--batch_size', default=8, type=int)
- parser.add_argument('--weight_decay', default=1e-4, type=float)
- parser.add_argument('--epochs', default=3500, type=int)
- parser.add_argument('--lr_drop', default=3500, type=int)
- parser.add_argument('--clip_max_norm', default=0.1, type=float,
- help='gradient clipping max norm')
-
- # Model parameters
- parser.add_argument('--frozen_weights', type=str, default=None,
- help="Path to the pretrained model. If set, only the mask head will be trained")
-
- # * Backbone
- parser.add_argument('--backbone', default='vgg16_bn', type=str,
- help="Name of the convolutional backbone to use")
-
- # * Matcher
- parser.add_argument('--set_cost_class', default=1, type=float,
- help="Class coefficient in the matching cost")
-
- parser.add_argument('--set_cost_point', default=0.05, type=float,
- help="L1 point coefficient in the matching cost")
-
- # * Loss coefficients
- parser.add_argument('--point_loss_coef', default=0.0002, type=float)
-
- parser.add_argument('--eos_coef', default=0.5, type=float,
- help="Relative classification weight of the no-object class")
- parser.add_argument('--row', default=2, type=int,
- help="row number of anchor points")
- parser.add_argument('--line', default=2, type=int,
- help="line number of anchor points")
-
- # dataset parameters
- parser.add_argument('--dataset_file', default='SHHA')
- parser.add_argument('--data_root', default='./new_public_density_data',
- help='path where the dataset is')
-
- parser.add_argument('--output_dir', default='./log',
- help='path where to save, empty for no saving')
- parser.add_argument('--checkpoints_dir', default='./ckpt',
- help='path where to save checkpoints, empty for no saving')
- parser.add_argument('--tensorboard_dir', default='./runs',
- help='path where to save, empty for no saving')
-
- parser.add_argument('--seed', default=42, type=int)
- parser.add_argument('--resume', default='', help='resume from checkpoint')
- parser.add_argument('--start_epoch', default=0, type=int, metavar='N',
- help='start epoch')
- parser.add_argument('--eval', action='store_true')
- parser.add_argument('--num_workers', default=8, type=int)
- parser.add_argument('--eval_freq', default=5, type=int,
- help='frequency of evaluation, default setting is evaluating in every 5 epoch')
- parser.add_argument('--gpu_id', default=0, type=int, help='the gpu used for training')
-
- return parser
-
-def main(args):
- os.environ["CUDA_VISIBLE_DEVICES"] = '{}'.format(args.gpu_id)
- # create the logging file
- run_log_name = os.path.join(args.output_dir, 'run_log.txt')
- with open(run_log_name, "w") as log_file:
- log_file.write('Eval Log %s\n' % time.strftime("%c"))
-
- if args.frozen_weights is not None:
- assert args.masks, "Frozen training is meant for segmentation only"
- # backup the arguments
- print(args)
- with open(run_log_name, "a") as log_file:
- log_file.write("{}".format(args))
- device = torch.device('cuda')
- # fix the seed for reproducibility
- seed = args.seed + utils.get_rank()
- torch.manual_seed(seed)
- np.random.seed(seed)
- random.seed(seed)
- # get the P2PNet model
- model, criterion = build_model(args, training=True)
- # move to GPU
- model.to(device)
- criterion.to(device)
-
- model_without_ddp = model
-
- n_parameters = sum(p.numel() for p in model.parameters() if p.requires_grad)
- print('number of params:', n_parameters)
- # use different optimation params for different parts of the model
- param_dicts = [
- {"params": [p for n, p in model_without_ddp.named_parameters() if "backbone" not in n and p.requires_grad]},
- {
- "params": [p for n, p in model_without_ddp.named_parameters() if "backbone" in n and p.requires_grad],
- "lr": args.lr_backbone,
- },
- ]
- # Adam is used by default
- optimizer = torch.optim.Adam(param_dicts, lr=args.lr)
- lr_scheduler = torch.optim.lr_scheduler.StepLR(optimizer, args.lr_drop)
- # create the dataset
- loading_data = build_dataset(args=args)
- # create the training and valiation set
- train_set, val_set = loading_data(args.data_root)
- # create the sampler used during training
- sampler_train = torch.utils.data.RandomSampler(train_set)
- sampler_val = torch.utils.data.SequentialSampler(val_set)
-
- batch_sampler_train = torch.utils.data.BatchSampler(
- sampler_train, args.batch_size, drop_last=True)
- # the dataloader for training
- data_loader_train = DataLoader(train_set, batch_sampler=batch_sampler_train,
- collate_fn=utils.collate_fn_crowd, num_workers=args.num_workers)
-
- data_loader_val = DataLoader(val_set, 1, sampler=sampler_val,
- drop_last=False, collate_fn=utils.collate_fn_crowd, num_workers=args.num_workers)
-
- if args.frozen_weights is not None:
- checkpoint = torch.load(args.frozen_weights, map_location='cpu')
- model_without_ddp.detr.load_state_dict(checkpoint['model'])
- # resume the weights and training state if exists
- if args.resume:
- checkpoint = torch.load(args.resume, map_location='cpu')
- model_without_ddp.load_state_dict(checkpoint['model'])
- if not args.eval and 'optimizer' in checkpoint and 'lr_scheduler' in checkpoint and 'epoch' in checkpoint:
- optimizer.load_state_dict(checkpoint['optimizer'])
- lr_scheduler.load_state_dict(checkpoint['lr_scheduler'])
- args.start_epoch = checkpoint['epoch'] + 1
-
- print("Start training")
- start_time = time.time()
- # save the performance during the training
- mae = []
- mse = []
- # the logger writer
- writer = SummaryWriter(args.tensorboard_dir)
-
- step = 0
- # training starts here
- for epoch in range(args.start_epoch, args.epochs):
- t1 = time.time()
- stat = train_one_epoch(
- model, criterion, data_loader_train, optimizer, device, epoch,
- args.clip_max_norm)
-
- # record the training states after every epoch
- if writer is not None:
- with open(run_log_name, "a") as log_file:
- log_file.write("loss/loss@{}: {}".format(epoch, stat['loss']))
- log_file.write("loss/loss_ce@{}: {}".format(epoch, stat['loss_ce']))
-
- writer.add_scalar('loss/loss', stat['loss'], epoch)
- writer.add_scalar('loss/loss_ce', stat['loss_ce'], epoch)
-
- t2 = time.time()
- print('[ep %d][lr %.7f][%.2fs]' % \
- (epoch, optimizer.param_groups[0]['lr'], t2 - t1))
- with open(run_log_name, "a") as log_file:
- log_file.write('[ep %d][lr %.7f][%.2fs]' % (epoch, optimizer.param_groups[0]['lr'], t2 - t1))
- # change lr according to the scheduler
- lr_scheduler.step()
- # save latest weights every epoch
- checkpoint_latest_path = os.path.join(args.checkpoints_dir, 'latest.pth')
- torch.save({
- 'model': model_without_ddp.state_dict(),
- }, checkpoint_latest_path)
- # run evaluation
- if epoch % args.eval_freq == 0 and epoch != 0:
- t1 = time.time()
- result = evaluate_crowd_no_overlap(model, data_loader_val, device)
- t2 = time.time()
-
- mae.append(result[0])
- mse.append(result[1])
- # print the evaluation results
- print('=======================================test=======================================')
- print("mae:", result[0], "mse:", result[1], "time:", t2 - t1, "best mae:", np.min(mae), )
- with open(run_log_name, "a") as log_file:
- log_file.write("mae:{}, mse:{}, time:{}, best mae:{}".format(result[0],
- result[1], t2 - t1, np.min(mae)))
- print('=======================================test=======================================')
- # recored the evaluation results
- if writer is not None:
- with open(run_log_name, "a") as log_file:
- log_file.write("metric/mae@{}: {}".format(step, result[0]))
- log_file.write("metric/mse@{}: {}".format(step, result[1]))
- writer.add_scalar('metric/mae', result[0], step)
- writer.add_scalar('metric/mse', result[1], step)
- step += 1
-
- # save the best model since begining
- if abs(np.min(mae) - result[0]) < 0.01:
- checkpoint_best_path = os.path.join(args.checkpoints_dir, 'best_mae.pth')
- torch.save({
- 'model': model_without_ddp.state_dict(),
- }, checkpoint_best_path)
- # total time for training
- total_time = time.time() - start_time
- total_time_str = str(datetime.timedelta(seconds=int(total_time)))
- print('Training time {}'.format(total_time_str))
-
-if __name__ == '__main__':
- parser = argparse.ArgumentParser('P2PNet training and evaluation script', parents=[get_args_parser()])
- args = parser.parse_args()
- main(args)
\ No newline at end of file
diff --git a/spaces/jpwahle/field-diversity/README.md b/spaces/jpwahle/field-diversity/README.md
deleted file mode 100644
index e9589afb5ad02025c95b027d4c4fc9d98749d2bf..0000000000000000000000000000000000000000
--- a/spaces/jpwahle/field-diversity/README.md
+++ /dev/null
@@ -1,10 +0,0 @@
----
-title: Field Diversity
-emoji: 🚀
-colorFrom: pink
-colorTo: red
-sdk: docker
-pinned: false
----
-
-Check out the configuration reference at https://huggingface.co/docs/hub/spaces-config-reference
diff --git a/spaces/jroust/darkstorm2150-Protogen_v2.2_Official_Release/app.py b/spaces/jroust/darkstorm2150-Protogen_v2.2_Official_Release/app.py
deleted file mode 100644
index aca6cf204d6e8a1aecfd27e41ea3c114089a936c..0000000000000000000000000000000000000000
--- a/spaces/jroust/darkstorm2150-Protogen_v2.2_Official_Release/app.py
+++ /dev/null
@@ -1,3 +0,0 @@
-import gradio as gr
-
-gr.Interface.load("models/darkstorm2150/Protogen_v2.2_Official_Release").launch()
\ No newline at end of file
diff --git a/spaces/jskalbg/ChatDev01/camel/agents/__init__.py b/spaces/jskalbg/ChatDev01/camel/agents/__init__.py
deleted file mode 100644
index 619a9404269535d41a5d359dfd1c1b7146f3a599..0000000000000000000000000000000000000000
--- a/spaces/jskalbg/ChatDev01/camel/agents/__init__.py
+++ /dev/null
@@ -1,33 +0,0 @@
-# =========== Copyright 2023 @ CAMEL-AI.org. All Rights Reserved. ===========
-# Licensed under the Apache License, Version 2.0 (the “License”);
-# you may not use this file except in compliance with the License.
-# You may obtain a copy of the License at
-#
-# http://www.apache.org/licenses/LICENSE-2.0
-#
-# Unless required by applicable law or agreed to in writing, software
-# distributed under the License is distributed on an “AS IS” BASIS,
-# WITHOUT WARRANTIES OR CONDITIONS OF ANY KIND, either express or implied.
-# See the License for the specific language governing permissions and
-# limitations under the License.
-# =========== Copyright 2023 @ CAMEL-AI.org. All Rights Reserved. ===========
-from .base import BaseAgent
-from .chat_agent import ChatAgent
-from .task_agent import TaskPlannerAgent, TaskSpecifyAgent
-from .critic_agent import CriticAgent
-from .tool_agents.base import BaseToolAgent
-from .tool_agents.hugging_face_tool_agent import HuggingFaceToolAgent
-from .embodied_agent import EmbodiedAgent
-from .role_playing import RolePlaying
-
-__all__ = [
- 'BaseAgent',
- 'ChatAgent',
- 'TaskSpecifyAgent',
- 'TaskPlannerAgent',
- 'CriticAgent',
- 'BaseToolAgent',
- 'HuggingFaceToolAgent',
- 'EmbodiedAgent',
- 'RolePlaying',
-]
diff --git a/spaces/juancopi81/youtube-music-transcribe/t5x/examples/decoder_only/layers.py b/spaces/juancopi81/youtube-music-transcribe/t5x/examples/decoder_only/layers.py
deleted file mode 100644
index 8037981df2e15f6a80810d98627217c3da2bf655..0000000000000000000000000000000000000000
--- a/spaces/juancopi81/youtube-music-transcribe/t5x/examples/decoder_only/layers.py
+++ /dev/null
@@ -1,1074 +0,0 @@
-# Copyright 2022 The T5X Authors.
-#
-# Licensed under the Apache License, Version 2.0 (the "License");
-# you may not use this file except in compliance with the License.
-# You may obtain a copy of the License at
-#
-# http://www.apache.org/licenses/LICENSE-2.0
-#
-# Unless required by applicable law or agreed to in writing, software
-# distributed under the License is distributed on an "AS IS" BASIS,
-# WITHOUT WARRANTIES OR CONDITIONS OF ANY KIND, either express or implied.
-# See the License for the specific language governing permissions and
-# limitations under the License.
-
-"""Dense attention classes and mask/weighting functions."""
-
-# pylint: disable=attribute-defined-outside-init,g-bare-generic
-
-import dataclasses
-import functools
-import operator
-from typing import Any, Callable, Iterable, Optional, Sequence, Tuple, Union
-
-from flax import linen as nn
-import flax.core.variables as variables
-from flax.linen import partitioning as nn_partitioning
-from flax.training import common_utils
-import jax
-from jax import lax
-from jax import random
-import jax.numpy as jnp
-import numpy as np
-
-
-# from flax.linen.partitioning import param_with_axes, with_sharding_constraint
-param_with_axes = nn_partitioning.param_with_axes
-with_sharding_constraint = nn_partitioning.with_sharding_constraint
-
-
-# Type annotations
-Array = jnp.ndarray
-DType = jnp.dtype
-PRNGKey = jnp.ndarray
-Shape = Iterable[int]
-Activation = Callable[..., Array]
-# Parameter initializers.
-Initializer = Callable[[PRNGKey, Shape, DType], Array]
-
-default_embed_init = nn.initializers.variance_scaling(
- 1.0, 'fan_in', 'normal', out_axis=0)
-
-
-def dot_product_attention(query: Array,
- key: Array,
- value: Array,
- bias: Optional[Array] = None,
- dropout_rng: Optional[PRNGKey] = None,
- dropout_rate: float = 0.,
- deterministic: bool = False,
- dtype: DType = jnp.float32,
- float32_logits: bool = False):
- """Computes dot-product attention given query, key, and value.
-
- This is the core function for applying attention based on
- https://arxiv.org/abs/1706.03762. It calculates the attention weights given
- query and key and combines the values using the attention weights.
-
- Args:
- query: queries for calculating attention with shape of `[batch, q_length,
- num_heads, qk_depth_per_head]`.
- key: keys for calculating attention with shape of `[batch, kv_length,
- num_heads, qk_depth_per_head]`.
- value: values to be used in attention with shape of `[batch, kv_length,
- num_heads, v_depth_per_head]`.
- bias: bias for the attention weights. This should be broadcastable to the
- shape `[batch, num_heads, q_length, kv_length]` This can be used for
- incorporating causal masks, padding masks, proximity bias, etc.
- dropout_rng: JAX PRNGKey: to be used for dropout
- dropout_rate: dropout rate
- deterministic: bool, deterministic or not (to apply dropout)
- dtype: the dtype of the computation (default: float32)
- float32_logits: bool, if True then compute logits in float32 to avoid
- numerical issues with bfloat16.
-
- Returns:
- Output of shape `[batch, length, num_heads, v_depth_per_head]`.
- """
- assert key.ndim == query.ndim == value.ndim, 'q, k, v must have same rank.'
- assert query.shape[:-3] == key.shape[:-3] == value.shape[:-3], (
- 'q, k, v batch dims must match.')
- assert query.shape[-2] == key.shape[-2] == value.shape[-2], (
- 'q, k, v num_heads must match.')
- assert key.shape[-3] == value.shape[-3], 'k, v lengths must match.'
- assert query.shape[-1] == key.shape[-1], 'q, k depths must match.'
-
- # Casting logits and softmax computation for float32 for model stability.
- if float32_logits:
- query = query.astype(jnp.float32)
- key = key.astype(jnp.float32)
-
- # `attn_weights`: [batch, num_heads, q_length, kv_length]
- attn_weights = jnp.einsum('bqhd,bkhd->bhqk', query, key)
-
- # Apply attention bias: masking, dropout, proximity bias, etc.
- if bias is not None:
- attn_weights = attn_weights + bias.astype(attn_weights.dtype)
-
- # Normalize the attention weights across `kv_length` dimension.
- attn_weights = jax.nn.softmax(attn_weights).astype(dtype)
-
- # Apply attention dropout.
- if not deterministic and dropout_rate > 0.:
- keep_prob = 1.0 - dropout_rate
- # T5 broadcasts along the "length" dim, but unclear which one that
- # corresponds to in positional dimensions here, assuming query dim.
- dropout_shape = list(attn_weights.shape)
- dropout_shape[-2] = 1
- keep = random.bernoulli(dropout_rng, keep_prob, dropout_shape)
- keep = jnp.broadcast_to(keep, attn_weights.shape)
- multiplier = (
- keep.astype(attn_weights.dtype) / jnp.asarray(keep_prob, dtype=dtype))
- attn_weights = attn_weights * multiplier
-
- # Take the linear combination of `value`.
- return jnp.einsum('bhqk,bkhd->bqhd', attn_weights, value)
-
-
-class MultiHeadDotProductAttention(nn.Module):
- """Multi-head dot-product attention.
-
- Attributes:
- num_heads: number of attention heads. Features (i.e. inputs_q.shape[-1])
- should be divisible by the number of heads.
- head_dim: dimension of each head.
- dtype: the dtype of the computation.
- dropout_rate: dropout rate
- kernel_init: initializer for the kernel of the Dense layers.
- float32_logits: bool, if True then compute logits in float32 to avoid
- numerical issues with bfloat16.
- """
- num_heads: int
- head_dim: int
- dtype: DType = jnp.float32
- dropout_rate: float = 0.
- kernel_init: Initializer = nn.initializers.variance_scaling(
- 1.0, 'fan_in', 'normal')
- float32_logits: bool = False
-
- def update_cache_prefill(
- self, key: Array, value: Array, cached_key: variables.Variable,
- cached_value: variables.Variable, cache_index: variables.Variable,
- prefill_lengths: Array
- ) -> Tuple[Array, Array, Array, Array, Array, Array]:
- """Update the autoregressive cache for multiple timesteps at once.
-
- This is useful for things like a prefix-lm where the encoder section of the
- input is visible bidirectionally. The key and value for this section need to
- be computed in a single shot, as a step by step approach would result in
- causal attention.
-
- Args:
- key: The calculated key used in attention. [batch..., length, num_heads,
- features_per_head]
- value: The calculated value used in attention. [batch..., length,
- num_heads, features_per_head]
- cached_key: The cache of previous keys. [batch..., num_heads,
- features_per_head, length]
- cached_value: The cache of previous values. [batch..., num_heads,
- features_per_head, length]
- cache_index: The timestep that we are currently calculating the key and
- value for. [batch]
- prefill_lengths: The number of timesteps we should fill in the cache.
- [batch]
-
- Returns:
- The key, value, and the last timestep we just filled in the cache.
- We also return the new cache values for now because assigning to a
- variable inside of a method doesn't work. These returns will be removed
- eventually.
- """
- # Make a reference to the data underlaying the variable for ease of
- # use.
- cache_index.value = prefill_lengths
- # Note, the cache index is now a vector of batch size so that each example
- # can start just after its prefix, which can be different lengths for
- # different examples.
- cur_index = cache_index.value
- # Move the sequence dimension to the end to match the cache shapes.
- key_cached = jnp.moveaxis(key, -3, -1)
- value_cached = jnp.moveaxis(value, -3, -1)
- # Reshape the index so the batch is at the beginning. The default
- # broadcasting behavior is to add singleton dims to the front, but we need
- # them at the end.
- batch_first_index = jnp.reshape(
- cur_index, (-1,) + tuple(1 for _ in range(cached_key.value.ndim - 1)))
- # Calculate a mask that will set any position past the prefix to zero
- # when applied to the key.
- key_mask = (
- lax.broadcasted_iota(jnp.int32, cached_key.value.shape,
- cached_key.value.ndim - 1) < batch_first_index)
- value_mask = (
- lax.broadcasted_iota(jnp.int32, cached_value.value.shape,
- cached_value.value.ndim - 1) < batch_first_index)
- # Set the caches with the calculated key and values but hide anything
- # past the prefix.
- cached_key_value = key_cached * key_mask
- cached_value_value = value_cached * value_mask
- # TODO(hwchung): remove the return values once direct assignment to
- # variables inside a method is possible.
- return (key, value, cur_index, cached_key_value, cached_value_value,
- prefill_lengths)
-
- def update_cache_decode(
- self, key: Array, value: Array, cached_key: variables.Variable,
- cached_value: variables.Variable, cache_index: variables.Variable
- ) -> Tuple[Array, Array, Array, Array, Array, Array]:
- """Update the next timestep in the autoregressive cache.
-
- This is used during step by step decoding where each key and value we get
- are a single (the next) timestep.
-
- Args:
- key: The calculated key used in attention. [batch..., 1, num_heads,
- features_per_head]
- value: The calculated value used in attention. [batch..., 1, num_heads,
- features_per_head]
- cached_key: The cache of previous keys. [batch..., num_heads,
- features_per_head, length]
- cached_value: The cache of previous values. [batch..., num_heads,
- features_per_head, length]
- cache_index: The timestep that we are currently calculating the key and
- value for. [batch] if we are decoding after doing a prefill or [1] if we
- are starting with step-by-step decoding.
-
- Returns:
- The key, value, and the last timestep we just filled in the cache. Note:
- this index is the last timestep we just fill, the actual value of the
- `cache_index` is already increased to point to the next timestep to fill.
- We also return the new cache values for now because assigning to a
- variable inside of a method doesn't work. These returns will be removed
- eventually.
- """
- cache_length = cached_key.value.shape[-1]
- # Create a OHE of the current index. NOTE: the index is increased
- # below.
- # Note: We reshape the index into a column vector so that it will work
- # if the index is a scalar or a vector with different cache positions
- # from different elements in a batch.
- cur_index = jnp.reshape(cache_index.value, (-1,))
- one_hot_indices = jax.nn.one_hot(cur_index, cache_length, dtype=key.dtype)
- # In order to update the key, value caches with the current key and
- # value, we move the length axis to the back, similar to what we did
- # for the cached ones above.
- # Note these are currently the key and value of a single position,
- # since we feed one position at a time.
- one_token_key = jnp.moveaxis(key, -3, -1)
- one_token_value = jnp.moveaxis(value, -3, -1)
- # The one hot indices are now either [1, length] for a scalar index or
- # [batch size, length] for examples where there are different lengths
- # of prefixes. We need to add dims for num_heads and num_features as
- # broadcasting doesn't work for the batched version.
- one_hot_indices = jnp.expand_dims(
- jnp.expand_dims(one_hot_indices, axis=1), axis=1)
- # Update key, value caches with our new 1d spatial slices.
- # We implement an efficient scatter into the cache via one-hot
- # broadcast and addition.
- # Key/Value have seq lengths of 1 while one_hot has a seq_length
- # of length. key/value will broadcast their value to each timestep
- # and the onehot will mask all but the correct timesteps.
- key = cached_key.value + one_token_key * one_hot_indices
- value = cached_value.value + one_token_value * one_hot_indices
- cached_key_value = key
- cached_value_value = value
- cache_index_value = cache_index.value + 1
- # Move the keys and values back to their original shapes.
- key = jnp.moveaxis(key, -1, -3)
- value = jnp.moveaxis(value, -1, -3)
- # TODO(hwchung): remove the return values once direct assignment to
- # variables inside a method is possible.
- return (key, value, cur_index, cached_key_value, cached_value_value,
- cache_index_value)
-
- @nn.compact
- def __call__(self,
- inputs_q: Array,
- inputs_kv: Array,
- mask: Optional[Array] = None,
- bias: Optional[Array] = None,
- *,
- decode: bool = False,
- deterministic: bool = False,
- prefill: bool = False,
- prefill_lengths: Optional[Array] = None) -> Array:
- """Applies multi-head dot product attention on the input data.
-
- Projects the inputs into multi-headed query, key, and value vectors,
- applies dot-product attention and project the results to an output vector.
-
- There are two modes: decoding and non-decoding (e.g., training). The mode is
- determined by `decode`.
-
- During decoding mode, this method is called twice, by `init` and
- `apply`. In the former, inputs_q: `[batch..., length, qkv_features]` and
- inputs_kv: `[batch..., length, qkv_features]`.
-
- During apply, query, key and value all have the shape: `[batch * beam, 1,
- qkv_features]` where the batch dimension is added to include multiple beams.
- Note that the batch dimension is different during the `init` and `apply`
- calls. This is because the cached variables are directly passed-in during
- `apply` method. In other words, the cache variables such as `cached_key` are
- initialized with `batch` dim, expanded by tiling in the beam search function
- to `batch * beam` dimension, and passed to the `apply` method as part of a
- variable dict.
-
- Args:
- inputs_q: input queries of shape `[batch, q_length, embed]`.
- inputs_kv: key/values of shape `[batch, kv_length, embed]`.
- mask: attention mask of shape `[batch, num_heads, q_length, kv_length]`.
- bias: attention bias of shape `[batch, num_heads, q_length, kv_length]`.
- decode: whether to prepare and use an autoregressive cache.
- deterministic: whether deterministic or not (to apply dropout)
- prefill: whether to run a partial sequence to prefill the cache.
- prefill_lengths: an array of shape [batch] denoting the length of each
- partial sequence we are filling in the cache.
-
- Returns:
- output of shape `[batch, q_length, embed]`.
- """
- projection = functools.partial(
- DenseGeneral,
- axis=-1,
- features=(self.num_heads, self.head_dim),
- kernel_axes=('embed', 'joined_kv'),
- dtype=self.dtype)
-
- # NOTE: T5 does not explicitly rescale the attention logits by
- # 1/sqrt(depth_kq)! This is folded into the initializers of the
- # linear transformations, which is equivalent under Adafactor.
- depth_scaling = jnp.sqrt(self.head_dim).astype(self.dtype)
- query_init = lambda *args: self.kernel_init(*args) / depth_scaling
-
- # Project inputs_q to multi-headed q/k/v
- # dimensions are then [batch, length, num_heads, head_dim]
- query = projection(kernel_init=query_init, name='query')(inputs_q)
- key = projection(kernel_init=self.kernel_init, name='key')(inputs_kv)
- value = projection(kernel_init=self.kernel_init, name='value')(inputs_kv)
-
- query = with_sharding_constraint(query, ('batch', 'length', 'heads', 'kv'))
- key = with_sharding_constraint(key, ('batch', 'length', 'heads', 'kv'))
- value = with_sharding_constraint(value, ('batch', 'length', 'heads', 'kv'))
-
- if prefill and decode:
- raise ValueError('prefill and decode cannot both be true at the same'
- 'time. If you are using a prefix LM with bidirectional '
- 'attention on the inputs, please make a call with '
- 'prefill=True that includes an attention mask that '
- 'covers your inputs first and then make your decoding '
- 'calls.')
- if prefill or decode:
- # Detect if we're initializing by absence of existing cache data.
- is_initialized = self.has_variable('cache', 'cached_key')
- # The key and value have dimension
- # [batch..., length, num_heads, features_per_head], but we cache them as
- # [batch..., num_heads, features_per_head, length] as a TPU fusion
- # optimization. This also enable the "scatter via one-hot broadcast"
- # trick, which means we do a one-hot broadcast instead of a scatter/gather
- # operations, which gives a 3-4x speedup in practice.
- swap_dims = lambda x: x[:-3] + tuple(x[i] for i in [-2, -1, -3])
- cached_key = self.variable('cache', 'cached_key', jnp.zeros,
- swap_dims(key.shape), key.dtype)
- cached_value = self.variable('cache', 'cached_value', jnp.zeros,
- swap_dims(value.shape), value.dtype)
- cache_index = self.variable('cache', 'cache_index',
- lambda: jnp.array(0, dtype=jnp.int32))
- if is_initialized:
- # Here we are in "apply()".
- *batch_dims, num_heads, features_per_head, length = (
- cached_key.value.shape)
- if prefill:
- if prefill_lengths is None:
- # Figure out how far each element in the batch fills the cache based
- # on the mask. We index each element in the batch, the first head
- # dim (because this is always set to one), and the first query
- # vector. If there is any prefix at all, the first element in the
- # prefix would be part of it.
- prefill_lengths = jnp.sum(
- mask[:, 0, 0, :], axis=-1).astype(cache_index.value.dtype)
- (key, value, cur_index, cached_key_value, cached_value_value,
- cache_index_value) = self.update_cache_prefill(
- key, value, cached_key, cached_value, cache_index,
- prefill_lengths)
- # During fast autoregressive decoding, we feed one position at a time,
- # and cache the keys and values step by step.
- elif decode:
- # Check the shape of the cached key against the input query.
- expected_shape = tuple(batch_dims) + (1, num_heads, features_per_head)
- if expected_shape != query.shape:
- raise ValueError('Autoregressive cache shape error, '
- 'expected query shape %s instead got %s.' %
- (expected_shape, query.shape))
- (key, value, cur_index, cached_key_value, cached_value_value,
- cache_index_value) = self.update_cache_decode(
- key, value, cached_key, cached_value, cache_index)
- # Enforcing the Causal mask over previous positions and selecting only
- # the bias value for the current index is only needed during decode
- # mode where a single example is feed at a time. In prefill mode we
- # uses these as provided, that same way it is done in a normal forward
- # pass, like when computing logits during training.
-
- # Causal mask for cached decoder self-attention: our single query
- # position should only attend to those key positions that have already
- # been generated and cached, not the remaining zero elements.
-
- # (1, 1, length) represent (head dim, query length, key length)
- # query length is 1 because during decoding we deal with one
- # index.
- # The same mask is applied to all batch elements and heads.
- #
- # Add trailing dims to the current index so it can either
- # broadcast over the batch dim or it can just be batch size.
- mask = combine_masks(
- mask,
- jnp.broadcast_to(
- jnp.arange(length),
- tuple(batch_dims) +
- (1, 1, length)) <= jnp.reshape(cur_index, (-1, 1, 1, 1)))
- # Grab the correct relative attention bias during decoding. This is
- # only required during single step decoding.
- if bias is not None:
- # The bias is a full attention matrix, but during decoding we only
- # have to take a slice of it.
- # This is equivalent to `bias[..., cur_index:cur_index+1, :]`. If
- # we are doing prefix decoding where `cur_index` is a vector the
- # result will be `[batch, heads, 1, :]`. If `cur_index` is a scalar
- # like in encdec decoding, the result will be `[1, heads, 1, :]`.
- # We use a one-hot einsum rather than a slice to avoid introducing a
- # Gather op that is currently lowered poorly by SPMD passes, adding
- # expensive all-reduce and all-gather operations.
-
- bias = jnp.einsum(
- 'bq, bhqk->bhk',
- common_utils.onehot(cur_index, num_classes=length), bias)
- bias = jnp.expand_dims(bias, 2)
-
- # Currently, updating a variable inside of a method is not handled
- # in flax, so we return the actual values and assign them in the main
- # compacted call for now.
- # TODO(brianlester,levskaya): Move variable assignment inside of the
- # cache update functions once variable references are tracked across
- # transform boundaries.
- cache_index.value = cache_index_value
- cached_key.value = cached_key_value
- cached_value.value = cached_value_value
-
- # Convert the boolean attention mask to an attention bias.
- if mask is not None:
- # attention mask in the form of attention bias
- attention_bias = lax.select(
- mask > 0,
- jnp.full(mask.shape, 0.).astype(self.dtype),
- jnp.full(mask.shape, -1e10).astype(self.dtype))
- else:
- attention_bias = None
-
- # Add provided bias term (e.g. relative position embedding).
- if bias is not None:
- attention_bias = combine_biases(attention_bias, bias)
-
- dropout_rng = None
- if not deterministic and self.dropout_rate > 0.:
- dropout_rng = self.make_rng('dropout')
-
- # Apply attention.
- x = dot_product_attention(
- query,
- key,
- value,
- bias=attention_bias,
- dropout_rng=dropout_rng,
- dropout_rate=self.dropout_rate,
- deterministic=deterministic,
- dtype=self.dtype,
- float32_logits=self.float32_logits)
-
- # Back to the original inputs dimensions.
- out = DenseGeneral(
- features=inputs_q.shape[-1], # output dim is set to the input dim.
- axis=(-2, -1),
- kernel_init=self.kernel_init,
- kernel_axes=('joined_kv', 'embed'),
- dtype=self.dtype,
- name='out')(
- x)
- return out
-
-
-def _normalize_axes(axes: Iterable[int], ndim: int) -> Tuple[int]:
- # A tuple by convention. len(axes_tuple) then also gives the rank efficiently.
- return tuple([ax if ax >= 0 else ndim + ax for ax in axes])
-
-
-def _canonicalize_tuple(x):
- if isinstance(x, Iterable):
- return tuple(x)
- else:
- return (x,)
-
-
-#------------------------------------------------------------------------------
-# DenseGeneral for attention layers.
-#------------------------------------------------------------------------------
-class DenseGeneral(nn.Module):
- """A linear transformation (without bias) with flexible axes.
-
- Attributes:
- features: tuple with numbers of output features.
- axis: tuple with axes to apply the transformation on.
- dtype: the dtype of the computation (default: float32).
- kernel_init: initializer function for the weight matrix.
- """
- features: Union[Iterable[int], int]
- axis: Union[Iterable[int], int] = -1
- dtype: DType = jnp.float32
- kernel_init: Initializer = nn.initializers.variance_scaling(
- 1.0, 'fan_in', 'truncated_normal')
- kernel_axes: Tuple[str, ...] = ()
-
- @nn.compact
- def __call__(self, inputs: Array) -> Array:
- """Applies a linear transformation to the inputs along multiple dimensions.
-
- Args:
- inputs: The nd-array to be transformed.
-
- Returns:
- The transformed input.
- """
- features = _canonicalize_tuple(self.features)
- axis = _canonicalize_tuple(self.axis)
-
- inputs = jnp.asarray(inputs, self.dtype)
- axis = _normalize_axes(axis, inputs.ndim)
-
- kernel_shape = tuple([inputs.shape[ax] for ax in axis]) + features
- kernel_param_shape = (np.prod([inputs.shape[ax] for ax in axis]),
- np.prod(features))
- kernel = param_with_axes(
- 'kernel',
- self.kernel_init,
- kernel_param_shape,
- jnp.float32,
- axes=self.kernel_axes)
- kernel = jnp.asarray(kernel, self.dtype)
- kernel = jnp.reshape(kernel, kernel_shape)
-
- contract_ind = tuple(range(0, len(axis)))
- return lax.dot_general(inputs, kernel, ((axis, contract_ind), ((), ())))
-
-
-def _convert_to_activation_function(
- fn_or_string: Union[str, Callable]) -> Callable:
- """Convert a string to an activation function."""
- if fn_or_string == 'linear':
- return lambda x: x
- elif isinstance(fn_or_string, str):
- return getattr(nn, fn_or_string)
- elif callable(fn_or_string):
- return fn_or_string
- else:
- raise ValueError("don't know how to convert %s to an activation function" %
- (fn_or_string,))
-
-
-class MlpBlock(nn.Module):
- """Transformer MLP / feed-forward block.
-
- Attributes:
- intermediate_dim: Shared dimension of hidden layers.
- activations: Type of activations for each layer. Each element is either
- 'linear', a string function name in flax.linen, or a function.
- kernel_init: Kernel function, passed to the dense layers.
- deterministic: Whether the dropout layers should be deterministic.
- intermediate_dropout_rate: Dropout rate used after the intermediate layers.
- dtype: Type for the dense layer.
- """
- intermediate_dim: int = 2048
- activations: Sequence[Union[str, Callable]] = ('relu',)
- kernel_init: Initializer = nn.initializers.variance_scaling(
- 1.0, 'fan_in', 'truncated_normal')
- intermediate_dropout_rate: float = 0.1
- dtype: Any = jnp.float32
-
- @nn.compact
- def __call__(self, inputs, decode: bool = False, deterministic: bool = False):
- """Applies Transformer MlpBlock module."""
- # Iterate over specified MLP input activation functions.
- # e.g. ('relu',) or ('gelu', 'linear') for gated-gelu.
- activations = []
- for idx, act_fn in enumerate(self.activations):
- dense_name = 'wi' if len(self.activations) == 1 else f'wi_{idx}'
- x = DenseGeneral(
- self.intermediate_dim,
- dtype=self.dtype,
- kernel_init=self.kernel_init,
- kernel_axes=('embed', 'mlp'),
- name=dense_name)(
- inputs)
- x = _convert_to_activation_function(act_fn)(x)
- activations.append(x)
-
- # Take elementwise product of above intermediate activations.
- x = functools.reduce(operator.mul, activations)
- # Apply dropout and final dense output projection.
- x = nn.Dropout(
- rate=self.intermediate_dropout_rate, broadcast_dims=(-2,))(
- x, deterministic=deterministic) # Broadcast along length.
- x = with_sharding_constraint(x, ('batch', 'length', 'mlp'))
- output = DenseGeneral(
- inputs.shape[-1],
- dtype=self.dtype,
- kernel_init=self.kernel_init,
- kernel_axes=('mlp', 'embed'),
- name='wo')(
- x)
- return output
-
-
-class Embed(nn.Module):
- """A parameterized function from integers [0, n) to d-dimensional vectors.
-
- Attributes:
- num_embeddings: number of embeddings.
- features: number of feature dimensions for each embedding.
- dtype: the dtype of the embedding vectors (default: float32).
- embedding_init: embedding initializer.
- one_hot: performs the gather with a one-hot contraction rather than a true
- gather. This is currently needed for SPMD partitioning.
- """
- num_embeddings: int
- features: int
- cast_input_dtype: Optional[DType] = None
- dtype: DType = jnp.float32
- attend_dtype: Optional[DType] = None
- embedding_init: Initializer = default_embed_init
- one_hot: bool = False
- embedding: Array = dataclasses.field(init=False)
-
- def setup(self):
- self.embedding = param_with_axes(
- 'embedding',
- self.embedding_init, (self.num_embeddings, self.features),
- jnp.float32,
- axes=('vocab', 'embed'))
-
- def __call__(self, inputs: Array) -> Array:
- """Embeds the inputs along the last dimension.
-
- Args:
- inputs: input data, all dimensions are considered batch dimensions.
-
- Returns:
- Output which is embedded input data. The output shape follows the input,
- with an additional `features` dimension appended.
- """
- if self.cast_input_dtype:
- inputs = inputs.astype(self.cast_input_dtype)
- if not jnp.issubdtype(inputs.dtype, jnp.integer):
- raise ValueError('Input type must be an integer or unsigned integer.')
- if self.one_hot:
- iota = lax.iota(jnp.int32, self.num_embeddings)
- one_hot = jnp.array(inputs[..., jnp.newaxis] == iota, dtype=self.dtype)
- output = jnp.dot(one_hot, jnp.asarray(self.embedding, self.dtype))
- else:
- output = jnp.asarray(self.embedding, self.dtype)[inputs]
- output = with_sharding_constraint(output, ('batch', 'length', 'embed'))
- return output
-
- def attend(self, query: Array) -> Array:
- """Attend over the embedding using a query array.
-
- Args:
- query: array with last dimension equal the feature depth `features` of the
- embedding.
-
- Returns:
- An array with final dim `num_embeddings` corresponding to the batched
- inner-product of the array of query vectors against each embedding.
- Commonly used for weight-sharing between embeddings and logit transform
- in NLP models.
- """
- dtype = self.attend_dtype if self.attend_dtype is not None else self.dtype
- return jnp.dot(query, jnp.asarray(self.embedding, dtype).T)
-
-
-class RelativePositionBiases(nn.Module):
- """Adds T5-style relative positional embeddings to the attention logits.
-
- Attributes:
- num_buckets: Number of buckets to bucket distances between key and query
- positions into.
- max_distance: Maximum distance before everything is lumped into the last
- distance bucket.
- num_heads: Number of heads in the attention layer. Each head will get a
- different relative position weighting.
- dtype: Type of arrays through this module.
- embedding_init: initializer for relative embedding table.
- """
- num_buckets: int
- max_distance: int
- num_heads: int
- dtype: Any
- embedding_init: Callable[..., Array] = nn.linear.default_embed_init
-
- @staticmethod
- def _relative_position_bucket(relative_position,
- bidirectional=True,
- num_buckets=32,
- max_distance=128):
- """Translate relative position to a bucket number for relative attention.
-
- The relative position is defined as memory_position - query_position, i.e.
- the distance in tokens from the attending position to the attended-to
- position. If bidirectional=False, then positive relative positions are
- invalid.
- We use smaller buckets for small absolute relative_position and larger
- buckets for larger absolute relative_positions. All relative
- positions >=max_distance map to the same bucket. All relative
- positions <=-max_distance map to the same bucket. This should allow for
- more graceful generalization to longer sequences than the model has been
- trained on.
-
- Args:
- relative_position: an int32 array
- bidirectional: a boolean - whether the attention is bidirectional
- num_buckets: an integer
- max_distance: an integer
-
- Returns:
- a Tensor with the same shape as relative_position, containing int32
- values in the range [0, num_buckets)
- """
- ret = 0
- n = -relative_position
- if bidirectional:
- num_buckets //= 2
- ret += (n < 0).astype(np.int32) * num_buckets
- n = np.abs(n)
- else:
- n = np.maximum(n, 0)
- # now n is in the range [0, inf)
- max_exact = num_buckets // 2
- is_small = (n < max_exact)
- val_if_large = max_exact + (
- np.log(n.astype(np.float32) / max_exact + np.finfo(np.float32).eps) /
- np.log(max_distance / max_exact) *
- (num_buckets - max_exact)).astype(np.int32)
- val_if_large = np.minimum(val_if_large, num_buckets - 1)
- ret += np.where(is_small, n, val_if_large)
- return ret
-
- @nn.compact
- def __call__(self, qlen, klen, bidirectional=True, decode=False):
- """Produce relative position embedding attention biases.
-
- Args:
- qlen: attention query length.
- klen: attention key length.
- bidirectional: whether to allow positive memory-query relative position
- embeddings.
- decode: whether to cache relative position bias during autoregressive
- decoding.
-
- Returns:
- output: `(1, num_heads, q_len, k_len)` attention bias
- """
- # bidirectional embeddings don't make sense when decoding (and break cache).
- if decode and bidirectional:
- raise ValueError(
- 'bidirectional RelativePositionBiases are not supported when '
- '`decode=True`.')
-
- # We only cache the bias if the model was already initialized, i.e. if this
- # module is called with `model.apply` and `decode = True`. We raise an error
- # if called with `model.init` and `decode = True`, since this can cache
- # incorrect positional embeddings produced by random parameters.
- is_initialized = self.has_variable('params', 'rel_embedding')
- if decode and not is_initialized:
- raise ValueError(
- 'decode-mode cannot be enabled during init. use model.apply to '
- 'initialize the decoding cache.')
-
- # Return pre-computed relative position bias in cache during decode steps.
- if decode and self.has_variable('cache', 'cached_bias'):
- cached_bias = self.get_variable('cache', 'cached_bias')
- expected_bias_shape = (1, self.num_heads, qlen, klen)
- if cached_bias.shape != expected_bias_shape:
- raise ValueError(f'The cached relative position attention bias was '
- f'expected to have shape {expected_bias_shape} but '
- f'instead has the shape {cached_bias.shape}.')
- return cached_bias
-
- # TODO(levskaya): should we be computing this w. numpy as a program
- # constant?
- context_position = np.arange(qlen, dtype=jnp.int32)[:, None]
- memory_position = np.arange(klen, dtype=jnp.int32)[None, :]
- relative_position = memory_position - context_position # shape (qlen, klen)
- rp_bucket = self._relative_position_bucket(
- relative_position,
- bidirectional=bidirectional,
- num_buckets=self.num_buckets,
- max_distance=self.max_distance)
- relative_attention_bias = param_with_axes(
- 'rel_embedding',
- self.embedding_init, (self.num_heads, self.num_buckets),
- jnp.float32,
- axes=('heads', 'relpos_buckets'))
-
- relative_attention_bias = jnp.asarray(relative_attention_bias, self.dtype)
- # Instead of using a slow gather, we create a leading-dimension one-hot
- # array from rp_bucket and use it to perform the gather-equivalent via a
- # contraction, i.e.:
- # (num_head, num_buckets) x (num_buckets one-hot, qlen, klen).
- # This is equivalent to relative_attention_bias[:, rp_bucket]
- bcast_iota = lax.broadcasted_iota(jnp.int32, (self.num_buckets, 1, 1), 0)
- rp_bucket_one_hot = jnp.array(
- rp_bucket[jnp.newaxis, ...] == bcast_iota, dtype=self.dtype)
- # --> shape (qlen, klen, num_heads)
- values = lax.dot_general(
- relative_attention_bias,
- rp_bucket_one_hot,
- (
- ((1,), (0,)), # rhs, lhs contracting dims
- ((), ()))) # no batched dims
- # Add a singleton batch dimension.
- # --> shape (1, num_heads, qlen, klen)
- out = values[jnp.newaxis, ...]
-
- # Store computed relative position bias in cache after first calculation.
- if decode:
- _ = self.variable('cache', 'cached_bias', lambda: out)
-
- return out
-
-
-#------------------------------------------------------------------------------
-# T5 Layernorm - no subtraction of mean or bias.
-#------------------------------------------------------------------------------
-class LayerNorm(nn.Module):
- """T5 Layer normalization operating on the last axis of the input data."""
- epsilon: float = 1e-6
- dtype: Any = jnp.float32
- scale_init: Initializer = nn.initializers.ones
-
- @nn.compact
- def __call__(self, x: jnp.ndarray) -> jnp.ndarray:
- """Applies layer normalization on the input."""
- x = jnp.asarray(x, jnp.float32)
- features = x.shape[-1]
- mean2 = jnp.mean(lax.square(x), axis=-1, keepdims=True)
- y = jnp.asarray(x * lax.rsqrt(mean2 + self.epsilon), self.dtype)
- scale = param_with_axes(
- 'scale', self.scale_init, (features,), jnp.float32, axes=('embed',))
-
- scale = jnp.asarray(scale, self.dtype)
- return y * scale
-
-
-#------------------------------------------------------------------------------
-# Mask-making utility functions.
-#------------------------------------------------------------------------------
-def make_attention_mask(query_input: Array,
- key_input: Array,
- pairwise_fn: Callable = jnp.multiply,
- extra_batch_dims: int = 0,
- dtype: DType = jnp.float32) -> Array:
- """Mask-making helper for attention weights.
-
- In case of 1d inputs (i.e., `[batch, len_q]`, `[batch, len_kv]`, the
- attention weights will be `[batch, heads, len_q, len_kv]` and this
- function will produce `[batch, 1, len_q, len_kv]`.
-
- Args:
- query_input: a batched, flat input of query_length size
- key_input: a batched, flat input of key_length size
- pairwise_fn: broadcasting elementwise comparison function
- extra_batch_dims: number of extra batch dims to add singleton axes for, none
- by default
- dtype: mask return dtype
-
- Returns:
- A `[batch, 1, len_q, len_kv]` shaped mask for 1d attention.
- """
- # [batch, len_q, len_kv]
- mask = pairwise_fn(
- # [batch, len_q] -> [batch, len_q, 1]
- jnp.expand_dims(query_input, axis=-1),
- # [batch, len_q] -> [batch, 1, len_kv]
- jnp.expand_dims(key_input, axis=-2))
-
- # [batch, 1, len_q, len_kv]. This creates the head dim.
- mask = jnp.expand_dims(mask, axis=-3)
- mask = jnp.expand_dims(mask, axis=tuple(range(extra_batch_dims)))
- return mask.astype(dtype)
-
-
-def make_causal_mask(x: Array,
- extra_batch_dims: int = 0,
- dtype: DType = jnp.float32) -> Array:
- """Make a causal mask for self-attention.
-
- In case of 1d inputs (i.e., `[batch, len]`, the self-attention weights
- will be `[batch, heads, len, len]` and this function will produce a
- causal mask of shape `[batch, 1, len, len]`.
-
- Note that a causal mask does not depend on the values of x; it only depends on
- the shape. If x has padding elements, they will not be treated in a special
- manner.
-
- Args:
- x: input array of shape `[batch, len]`
- extra_batch_dims: number of batch dims to add singleton axes for, none by
- default
- dtype: mask return dtype
-
- Returns:
- A `[batch, 1, len, len]` shaped causal mask for 1d attention.
- """
- idxs = jnp.broadcast_to(jnp.arange(x.shape[-1], dtype=jnp.int32), x.shape)
- return make_attention_mask(
- idxs,
- idxs,
- jnp.greater_equal,
- extra_batch_dims=extra_batch_dims,
- dtype=dtype)
-
-
-def combine_masks(*masks: Optional[Array], dtype: DType = jnp.float32):
- """Combine attention masks.
-
- Args:
- *masks: set of attention mask arguments to combine, some can be None.
- dtype: final mask dtype
-
- Returns:
- Combined mask, reduced by logical and, returns None if no masks given.
- """
- masks = [m for m in masks if m is not None]
- if not masks:
- return None
- assert all(map(lambda x: x.ndim == masks[0].ndim, masks)), (
- f'masks must have same rank: {tuple(map(lambda x: x.ndim, masks))}')
- mask, *other_masks = masks
- for other_mask in other_masks:
- mask = jnp.logical_and(mask, other_mask)
- return mask.astype(dtype)
-
-
-def combine_biases(*masks: Optional[Array]):
- """Combine attention biases.
-
- Args:
- *masks: set of attention bias arguments to combine, some can be None.
-
- Returns:
- Combined mask, reduced by summation, returns None if no masks given.
- """
- masks = [m for m in masks if m is not None]
- if not masks:
- return None
- assert all(map(lambda x: x.ndim == masks[0].ndim, masks)), (
- f'masks must have same rank: {tuple(map(lambda x: x.ndim, masks))}')
- mask, *other_masks = masks
- for other_mask in other_masks:
- mask = mask + other_mask
- return mask
-
-
-def make_decoder_mask(decoder_target_tokens: Array,
- dtype: DType,
- decoder_causal_attention: Optional[Array] = None,
- decoder_segment_ids: Optional[Array] = None) -> Array:
- """Compute the self-attention mask for a decoder.
-
- Decoder mask is formed by combining a causal mask, a padding mask and an
- optional packing mask. If decoder_causal_attention is passed, it makes the
- masking non-causal for positions that have value of 1.
-
- A prefix LM is applied to a dataset which has a notion of "inputs" and
- "targets", e.g., a machine translation task. The inputs and targets are
- concatenated to form a new target. `decoder_target_tokens` is the concatenated
- decoder output tokens.
-
- The "inputs" portion of the concatenated sequence can attend to other "inputs"
- tokens even for those at a later time steps. In order to control this
- behavior, `decoder_causal_attention` is necessary. This is a binary mask with
- a value of 1 indicating that the position belonged to "inputs" portion of the
- original dataset.
-
- Example:
-
- Suppose we have a dataset with two examples.
-
- ds = [{"inputs": [6, 7], "targets": [8]},
- {"inputs": [3, 4], "targets": [5]}]
-
- After the data preprocessing with packing, the two examples are packed into
- one example with the following three fields (some fields are skipped for
- simplicity).
-
- decoder_target_tokens = [[6, 7, 8, 3, 4, 5, 0]]
- decoder_segment_ids = [[1, 1, 1, 2, 2, 2, 0]]
- decoder_causal_attention = [[1, 1, 0, 1, 1, 0, 0]]
-
- where each array has [batch, length] shape with batch size being 1. Then,
- this function computes the following mask.
-
- mask = [[[[1, 1, 0, 0, 0, 0, 0],
- [1, 1, 0, 0, 0, 0, 0],
- [1, 1, 1, 0, 0, 0, 0],
- [0, 0, 0, 1, 1, 0, 0],
- [0, 0, 0, 1, 1, 0, 0],
- [0, 0, 0, 1, 1, 1, 0],
- [0, 0, 0, 0, 0, 0, 0]]]]
-
- mask[b, 1, :, :] represents the mask for the example `b` in the batch.
- Because mask is for a self-attention layer, the mask's shape is a square of
- shape [query length, key length].
-
- mask[b, 1, i, j] = 1 means that the query token at position i can attend to
- the key token at position j.
-
- Args:
- decoder_target_tokens: decoder output tokens. [batch, length]
- dtype: dtype of the output mask.
- decoder_causal_attention: a binary mask indicating which position should
- only attend to earlier positions in the sequence. Others will attend
- bidirectionally. [batch, length]
- decoder_segment_ids: decoder segmentation info for packed examples. [batch,
- length]
-
- Returns:
- the combined decoder mask.
- """
- masks = []
- # The same mask is applied to all attention heads. So the head dimension is 1,
- # i.e., the mask will be broadcast along the heads dim.
- # [batch, 1, length, length]
- causal_mask = make_causal_mask(decoder_target_tokens, dtype=dtype)
-
- # Positions with value 1 in `decoder_causal_attneition` can attend
- # bidirectionally.
- if decoder_causal_attention is not None:
- # [batch, 1, length, length]
- inputs_mask = make_attention_mask(
- decoder_causal_attention,
- decoder_causal_attention,
- jnp.logical_and,
- dtype=dtype)
- masks.append(jnp.logical_or(causal_mask, inputs_mask).astype(dtype))
- else:
- masks.append(causal_mask)
-
- # Padding mask.
- masks.append(
- make_attention_mask(
- decoder_target_tokens > 0, decoder_target_tokens > 0, dtype=dtype))
-
- # Packing mask
- if decoder_segment_ids is not None:
- masks.append(
- make_attention_mask(
- decoder_segment_ids, decoder_segment_ids, jnp.equal, dtype=dtype))
-
- return combine_masks(*masks, dtype=dtype)
diff --git a/spaces/juancopi81/youtube-music-transcribe/utils.py b/spaces/juancopi81/youtube-music-transcribe/utils.py
deleted file mode 100644
index 92d452194145e3457e5e57824cb61a8bf8571c43..0000000000000000000000000000000000000000
--- a/spaces/juancopi81/youtube-music-transcribe/utils.py
+++ /dev/null
@@ -1,60 +0,0 @@
-
-import collections
-import random
-
-import pandas as pd
-import matplotlib.pyplot as plt
-import matplotlib.colors as mcolors
-from matplotlib.patches import Rectangle
-from PIL import Image
-
-import note_seq
-class AudioIOReadError(BaseException): # pylint:disable=g-bad-exception-name
- pass
-
-def upload_audio(audio, sample_rate):
- return note_seq.audio_io.wav_data_to_samples_librosa(audio, sample_rate=sample_rate)
-
-# Generate piano_roll
-def sequence_to_pandas_dataframe(sequence):
- pd_dict = collections.defaultdict(list)
- for note in sequence.notes:
- pd_dict["start_time"].append(note.start_time)
- pd_dict["end_time"].append(note.end_time)
- pd_dict["duration"].append(note.end_time - note.start_time)
- pd_dict["pitch"].append(note.pitch)
- pd_dict["instrument"].append(note.instrument)
-
- return pd.DataFrame(pd_dict)
-
-def dataframe_to_pianoroll_img(df):
- fig = plt.figure(figsize=(8, 5))
- ax = fig.add_subplot(111)
- ax.scatter(df.start_time, df.pitch, c="white")
- colors = [mcolors.CSS4_COLORS[name] for i, name in enumerate(list(mcolors.CSS4_COLORS))]
- random.shuffle(colors)
- colordict = {inst: colors[i] for i, inst in enumerate(df["instrument"].unique())}
- for _, row in df.iterrows():
- ax.add_patch(Rectangle((row["start_time"],
- row["pitch"]-0.4),
- row["duration"],
- 0.4,
- color=colordict[row["instrument"]]))
- plt.xlabel('time (sec.)', fontsize=18)
- plt.ylabel('pitch (MIDI)', fontsize=16)
- return fig
-
-def fig2img(fig):
- """Convert a Matplotlib figure to a PIL Image and return it"""
- import io
- buf = io.BytesIO()
- fig.savefig(buf, format="png")
- buf.seek(0)
- img = Image.open(buf)
- return img
-
-def create_image_from_note_sequence(sequence):
- df_sequence = sequence_to_pandas_dataframe(sequence)
- fig = dataframe_to_pianoroll_img(df_sequence)
- img = fig2img(fig)
- return img
\ No newline at end of file
diff --git a/spaces/jxu124/vits-genshin/attentions.py b/spaces/jxu124/vits-genshin/attentions.py
deleted file mode 100644
index 86bc73b5fe98cc7b443e9078553920346c996707..0000000000000000000000000000000000000000
--- a/spaces/jxu124/vits-genshin/attentions.py
+++ /dev/null
@@ -1,300 +0,0 @@
-import math
-import torch
-from torch import nn
-from torch.nn import functional as F
-
-import commons
-from modules import LayerNorm
-
-
-class Encoder(nn.Module):
- def __init__(self, hidden_channels, filter_channels, n_heads, n_layers, kernel_size=1, p_dropout=0., window_size=4, **kwargs):
- super().__init__()
- self.hidden_channels = hidden_channels
- self.filter_channels = filter_channels
- self.n_heads = n_heads
- self.n_layers = n_layers
- self.kernel_size = kernel_size
- self.p_dropout = p_dropout
- self.window_size = window_size
-
- self.drop = nn.Dropout(p_dropout)
- self.attn_layers = nn.ModuleList()
- self.norm_layers_1 = nn.ModuleList()
- self.ffn_layers = nn.ModuleList()
- self.norm_layers_2 = nn.ModuleList()
- for i in range(self.n_layers):
- self.attn_layers.append(MultiHeadAttention(hidden_channels, hidden_channels, n_heads, p_dropout=p_dropout, window_size=window_size))
- self.norm_layers_1.append(LayerNorm(hidden_channels))
- self.ffn_layers.append(FFN(hidden_channels, hidden_channels, filter_channels, kernel_size, p_dropout=p_dropout))
- self.norm_layers_2.append(LayerNorm(hidden_channels))
-
- def forward(self, x, x_mask):
- attn_mask = x_mask.unsqueeze(2) * x_mask.unsqueeze(-1)
- x = x * x_mask
- for i in range(self.n_layers):
- y = self.attn_layers[i](x, x, attn_mask)
- y = self.drop(y)
- x = self.norm_layers_1[i](x + y)
-
- y = self.ffn_layers[i](x, x_mask)
- y = self.drop(y)
- x = self.norm_layers_2[i](x + y)
- x = x * x_mask
- return x
-
-
-class Decoder(nn.Module):
- def __init__(self, hidden_channels, filter_channels, n_heads, n_layers, kernel_size=1, p_dropout=0., proximal_bias=False, proximal_init=True, **kwargs):
- super().__init__()
- self.hidden_channels = hidden_channels
- self.filter_channels = filter_channels
- self.n_heads = n_heads
- self.n_layers = n_layers
- self.kernel_size = kernel_size
- self.p_dropout = p_dropout
- self.proximal_bias = proximal_bias
- self.proximal_init = proximal_init
-
- self.drop = nn.Dropout(p_dropout)
- self.self_attn_layers = nn.ModuleList()
- self.norm_layers_0 = nn.ModuleList()
- self.encdec_attn_layers = nn.ModuleList()
- self.norm_layers_1 = nn.ModuleList()
- self.ffn_layers = nn.ModuleList()
- self.norm_layers_2 = nn.ModuleList()
- for i in range(self.n_layers):
- self.self_attn_layers.append(MultiHeadAttention(hidden_channels, hidden_channels, n_heads, p_dropout=p_dropout, proximal_bias=proximal_bias, proximal_init=proximal_init))
- self.norm_layers_0.append(LayerNorm(hidden_channels))
- self.encdec_attn_layers.append(MultiHeadAttention(hidden_channels, hidden_channels, n_heads, p_dropout=p_dropout))
- self.norm_layers_1.append(LayerNorm(hidden_channels))
- self.ffn_layers.append(FFN(hidden_channels, hidden_channels, filter_channels, kernel_size, p_dropout=p_dropout, causal=True))
- self.norm_layers_2.append(LayerNorm(hidden_channels))
-
- def forward(self, x, x_mask, h, h_mask):
- """
- x: decoder input
- h: encoder output
- """
- self_attn_mask = commons.subsequent_mask(x_mask.size(2)).to(device=x.device, dtype=x.dtype)
- encdec_attn_mask = h_mask.unsqueeze(2) * x_mask.unsqueeze(-1)
- x = x * x_mask
- for i in range(self.n_layers):
- y = self.self_attn_layers[i](x, x, self_attn_mask)
- y = self.drop(y)
- x = self.norm_layers_0[i](x + y)
-
- y = self.encdec_attn_layers[i](x, h, encdec_attn_mask)
- y = self.drop(y)
- x = self.norm_layers_1[i](x + y)
-
- y = self.ffn_layers[i](x, x_mask)
- y = self.drop(y)
- x = self.norm_layers_2[i](x + y)
- x = x * x_mask
- return x
-
-
-class MultiHeadAttention(nn.Module):
- def __init__(self, channels, out_channels, n_heads, p_dropout=0., window_size=None, heads_share=True, block_length=None, proximal_bias=False, proximal_init=False):
- super().__init__()
- assert channels % n_heads == 0
-
- self.channels = channels
- self.out_channels = out_channels
- self.n_heads = n_heads
- self.p_dropout = p_dropout
- self.window_size = window_size
- self.heads_share = heads_share
- self.block_length = block_length
- self.proximal_bias = proximal_bias
- self.proximal_init = proximal_init
- self.attn = None
-
- self.k_channels = channels // n_heads
- self.conv_q = nn.Conv1d(channels, channels, 1)
- self.conv_k = nn.Conv1d(channels, channels, 1)
- self.conv_v = nn.Conv1d(channels, channels, 1)
- self.conv_o = nn.Conv1d(channels, out_channels, 1)
- self.drop = nn.Dropout(p_dropout)
-
- if window_size is not None:
- n_heads_rel = 1 if heads_share else n_heads
- rel_stddev = self.k_channels**-0.5
- self.emb_rel_k = nn.Parameter(torch.randn(n_heads_rel, window_size * 2 + 1, self.k_channels) * rel_stddev)
- self.emb_rel_v = nn.Parameter(torch.randn(n_heads_rel, window_size * 2 + 1, self.k_channels) * rel_stddev)
-
- nn.init.xavier_uniform_(self.conv_q.weight)
- nn.init.xavier_uniform_(self.conv_k.weight)
- nn.init.xavier_uniform_(self.conv_v.weight)
- if proximal_init:
- with torch.no_grad():
- self.conv_k.weight.copy_(self.conv_q.weight)
- self.conv_k.bias.copy_(self.conv_q.bias)
-
- def forward(self, x, c, attn_mask=None):
- q = self.conv_q(x)
- k = self.conv_k(c)
- v = self.conv_v(c)
-
- x, self.attn = self.attention(q, k, v, mask=attn_mask)
-
- x = self.conv_o(x)
- return x
-
- def attention(self, query, key, value, mask=None):
- # reshape [b, d, t] -> [b, n_h, t, d_k]
- b, d, t_s, t_t = (*key.size(), query.size(2))
- query = query.view(b, self.n_heads, self.k_channels, t_t).transpose(2, 3)
- key = key.view(b, self.n_heads, self.k_channels, t_s).transpose(2, 3)
- value = value.view(b, self.n_heads, self.k_channels, t_s).transpose(2, 3)
-
- scores = torch.matmul(query / math.sqrt(self.k_channels), key.transpose(-2, -1))
- if self.window_size is not None:
- assert t_s == t_t, "Relative attention is only available for self-attention."
- key_relative_embeddings = self._get_relative_embeddings(self.emb_rel_k, t_s)
- rel_logits = self._matmul_with_relative_keys(query /math.sqrt(self.k_channels), key_relative_embeddings)
- scores_local = self._relative_position_to_absolute_position(rel_logits)
- scores = scores + scores_local
- if self.proximal_bias:
- assert t_s == t_t, "Proximal bias is only available for self-attention."
- scores = scores + self._attention_bias_proximal(t_s).to(device=scores.device, dtype=scores.dtype)
- if mask is not None:
- scores = scores.masked_fill(mask == 0, -1e4)
- if self.block_length is not None:
- assert t_s == t_t, "Local attention is only available for self-attention."
- block_mask = torch.ones_like(scores).triu(-self.block_length).tril(self.block_length)
- scores = scores.masked_fill(block_mask == 0, -1e4)
- p_attn = F.softmax(scores, dim=-1) # [b, n_h, t_t, t_s]
- p_attn = self.drop(p_attn)
- output = torch.matmul(p_attn, value)
- if self.window_size is not None:
- relative_weights = self._absolute_position_to_relative_position(p_attn)
- value_relative_embeddings = self._get_relative_embeddings(self.emb_rel_v, t_s)
- output = output + self._matmul_with_relative_values(relative_weights, value_relative_embeddings)
- output = output.transpose(2, 3).contiguous().view(b, d, t_t) # [b, n_h, t_t, d_k] -> [b, d, t_t]
- return output, p_attn
-
- def _matmul_with_relative_values(self, x, y):
- """
- x: [b, h, l, m]
- y: [h or 1, m, d]
- ret: [b, h, l, d]
- """
- ret = torch.matmul(x, y.unsqueeze(0))
- return ret
-
- def _matmul_with_relative_keys(self, x, y):
- """
- x: [b, h, l, d]
- y: [h or 1, m, d]
- ret: [b, h, l, m]
- """
- ret = torch.matmul(x, y.unsqueeze(0).transpose(-2, -1))
- return ret
-
- def _get_relative_embeddings(self, relative_embeddings, length):
- max_relative_position = 2 * self.window_size + 1
- # Pad first before slice to avoid using cond ops.
- pad_length = max(length - (self.window_size + 1), 0)
- slice_start_position = max((self.window_size + 1) - length, 0)
- slice_end_position = slice_start_position + 2 * length - 1
- if pad_length > 0:
- padded_relative_embeddings = F.pad(
- relative_embeddings,
- commons.convert_pad_shape([[0, 0], [pad_length, pad_length], [0, 0]]))
- else:
- padded_relative_embeddings = relative_embeddings
- used_relative_embeddings = padded_relative_embeddings[:,slice_start_position:slice_end_position]
- return used_relative_embeddings
-
- def _relative_position_to_absolute_position(self, x):
- """
- x: [b, h, l, 2*l-1]
- ret: [b, h, l, l]
- """
- batch, heads, length, _ = x.size()
- # Concat columns of pad to shift from relative to absolute indexing.
- x = F.pad(x, commons.convert_pad_shape([[0,0],[0,0],[0,0],[0,1]]))
-
- # Concat extra elements so to add up to shape (len+1, 2*len-1).
- x_flat = x.view([batch, heads, length * 2 * length])
- x_flat = F.pad(x_flat, commons.convert_pad_shape([[0,0],[0,0],[0,length-1]]))
-
- # Reshape and slice out the padded elements.
- x_final = x_flat.view([batch, heads, length+1, 2*length-1])[:, :, :length, length-1:]
- return x_final
-
- def _absolute_position_to_relative_position(self, x):
- """
- x: [b, h, l, l]
- ret: [b, h, l, 2*l-1]
- """
- batch, heads, length, _ = x.size()
- # padd along column
- x = F.pad(x, commons.convert_pad_shape([[0, 0], [0, 0], [0, 0], [0, length-1]]))
- x_flat = x.view([batch, heads, length**2 + length*(length -1)])
- # add 0's in the beginning that will skew the elements after reshape
- x_flat = F.pad(x_flat, commons.convert_pad_shape([[0, 0], [0, 0], [length, 0]]))
- x_final = x_flat.view([batch, heads, length, 2*length])[:,:,:,1:]
- return x_final
-
- def _attention_bias_proximal(self, length):
- """Bias for self-attention to encourage attention to close positions.
- Args:
- length: an integer scalar.
- Returns:
- a Tensor with shape [1, 1, length, length]
- """
- r = torch.arange(length, dtype=torch.float32)
- diff = torch.unsqueeze(r, 0) - torch.unsqueeze(r, 1)
- return torch.unsqueeze(torch.unsqueeze(-torch.log1p(torch.abs(diff)), 0), 0)
-
-
-class FFN(nn.Module):
- def __init__(self, in_channels, out_channels, filter_channels, kernel_size, p_dropout=0., activation=None, causal=False):
- super().__init__()
- self.in_channels = in_channels
- self.out_channels = out_channels
- self.filter_channels = filter_channels
- self.kernel_size = kernel_size
- self.p_dropout = p_dropout
- self.activation = activation
- self.causal = causal
-
- if causal:
- self.padding = self._causal_padding
- else:
- self.padding = self._same_padding
-
- self.conv_1 = nn.Conv1d(in_channels, filter_channels, kernel_size)
- self.conv_2 = nn.Conv1d(filter_channels, out_channels, kernel_size)
- self.drop = nn.Dropout(p_dropout)
-
- def forward(self, x, x_mask):
- x = self.conv_1(self.padding(x * x_mask))
- if self.activation == "gelu":
- x = x * torch.sigmoid(1.702 * x)
- else:
- x = torch.relu(x)
- x = self.drop(x)
- x = self.conv_2(self.padding(x * x_mask))
- return x * x_mask
-
- def _causal_padding(self, x):
- if self.kernel_size == 1:
- return x
- pad_l = self.kernel_size - 1
- pad_r = 0
- padding = [[0, 0], [0, 0], [pad_l, pad_r]]
- x = F.pad(x, commons.convert_pad_shape(padding))
- return x
-
- def _same_padding(self, x):
- if self.kernel_size == 1:
- return x
- pad_l = (self.kernel_size - 1) // 2
- pad_r = self.kernel_size // 2
- padding = [[0, 0], [0, 0], [pad_l, pad_r]]
- x = F.pad(x, commons.convert_pad_shape(padding))
- return x
diff --git a/spaces/k1ngtai/MMS/vits/mel_processing.py b/spaces/k1ngtai/MMS/vits/mel_processing.py
deleted file mode 100644
index 817f03756f64caf8cc54329a9325024c8fb9e0c3..0000000000000000000000000000000000000000
--- a/spaces/k1ngtai/MMS/vits/mel_processing.py
+++ /dev/null
@@ -1,112 +0,0 @@
-import math
-import os
-import random
-import torch
-from torch import nn
-import torch.nn.functional as F
-import torch.utils.data
-import numpy as np
-import librosa
-import librosa.util as librosa_util
-from librosa.util import normalize, pad_center, tiny
-from scipy.signal import get_window
-from scipy.io.wavfile import read
-from librosa.filters import mel as librosa_mel_fn
-
-MAX_WAV_VALUE = 32768.0
-
-
-def dynamic_range_compression_torch(x, C=1, clip_val=1e-5):
- """
- PARAMS
- ------
- C: compression factor
- """
- return torch.log(torch.clamp(x, min=clip_val) * C)
-
-
-def dynamic_range_decompression_torch(x, C=1):
- """
- PARAMS
- ------
- C: compression factor used to compress
- """
- return torch.exp(x) / C
-
-
-def spectral_normalize_torch(magnitudes):
- output = dynamic_range_compression_torch(magnitudes)
- return output
-
-
-def spectral_de_normalize_torch(magnitudes):
- output = dynamic_range_decompression_torch(magnitudes)
- return output
-
-
-mel_basis = {}
-hann_window = {}
-
-
-def spectrogram_torch(y, n_fft, sampling_rate, hop_size, win_size, center=False):
- if torch.min(y) < -1.:
- print('min value is ', torch.min(y))
- if torch.max(y) > 1.:
- print('max value is ', torch.max(y))
-
- global hann_window
- dtype_device = str(y.dtype) + '_' + str(y.device)
- wnsize_dtype_device = str(win_size) + '_' + dtype_device
- if wnsize_dtype_device not in hann_window:
- hann_window[wnsize_dtype_device] = torch.hann_window(win_size).to(dtype=y.dtype, device=y.device)
-
- y = torch.nn.functional.pad(y.unsqueeze(1), (int((n_fft-hop_size)/2), int((n_fft-hop_size)/2)), mode='reflect')
- y = y.squeeze(1)
-
- spec = torch.stft(y, n_fft, hop_length=hop_size, win_length=win_size, window=hann_window[wnsize_dtype_device],
- center=center, pad_mode='reflect', normalized=False, onesided=True)
-
- spec = torch.sqrt(spec.pow(2).sum(-1) + 1e-6)
- return spec
-
-
-def spec_to_mel_torch(spec, n_fft, num_mels, sampling_rate, fmin, fmax):
- global mel_basis
- dtype_device = str(spec.dtype) + '_' + str(spec.device)
- fmax_dtype_device = str(fmax) + '_' + dtype_device
- if fmax_dtype_device not in mel_basis:
- mel = librosa_mel_fn(sampling_rate, n_fft, num_mels, fmin, fmax)
- mel_basis[fmax_dtype_device] = torch.from_numpy(mel).to(dtype=spec.dtype, device=spec.device)
- spec = torch.matmul(mel_basis[fmax_dtype_device], spec)
- spec = spectral_normalize_torch(spec)
- return spec
-
-
-def mel_spectrogram_torch(y, n_fft, num_mels, sampling_rate, hop_size, win_size, fmin, fmax, center=False):
- if torch.min(y) < -1.:
- print('min value is ', torch.min(y))
- if torch.max(y) > 1.:
- print('max value is ', torch.max(y))
-
- global mel_basis, hann_window
- dtype_device = str(y.dtype) + '_' + str(y.device)
- fmax_dtype_device = str(fmax) + '_' + dtype_device
- wnsize_dtype_device = str(win_size) + '_' + dtype_device
- if fmax_dtype_device not in mel_basis:
- mel = librosa_mel_fn(sampling_rate, n_fft, num_mels, fmin, fmax)
- mel_basis[fmax_dtype_device] = torch.from_numpy(mel).to(dtype=y.dtype, device=y.device)
- if wnsize_dtype_device not in hann_window:
- hann_window[wnsize_dtype_device] = torch.hann_window(win_size).to(dtype=y.dtype, device=y.device)
-
- y = torch.nn.functional.pad(y.unsqueeze(1), (int((n_fft-hop_size)/2), int((n_fft-hop_size)/2)), mode='reflect')
- y = y.squeeze(1)
-
- spec = torch.stft(y, n_fft, hop_length=hop_size, win_length=win_size, window=hann_window[wnsize_dtype_device],
- center=center, pad_mode='reflect', normalized=False, onesided=True)
-
- spec = torch.sqrt(spec.pow(2).sum(-1) + 1e-6)
-
- spec = torch.matmul(mel_basis[fmax_dtype_device], spec)
- spec = spectral_normalize_torch(spec)
-
- return spec
diff --git a/spaces/kdrkdrkdr/YuukaTTS/transforms.py b/spaces/kdrkdrkdr/YuukaTTS/transforms.py
deleted file mode 100644
index 4793d67ca5a5630e0ffe0f9fb29445c949e64dae..0000000000000000000000000000000000000000
--- a/spaces/kdrkdrkdr/YuukaTTS/transforms.py
+++ /dev/null
@@ -1,193 +0,0 @@
-import torch
-from torch.nn import functional as F
-
-import numpy as np
-
-
-DEFAULT_MIN_BIN_WIDTH = 1e-3
-DEFAULT_MIN_BIN_HEIGHT = 1e-3
-DEFAULT_MIN_DERIVATIVE = 1e-3
-
-
-def piecewise_rational_quadratic_transform(inputs,
- unnormalized_widths,
- unnormalized_heights,
- unnormalized_derivatives,
- inverse=False,
- tails=None,
- tail_bound=1.,
- min_bin_width=DEFAULT_MIN_BIN_WIDTH,
- min_bin_height=DEFAULT_MIN_BIN_HEIGHT,
- min_derivative=DEFAULT_MIN_DERIVATIVE):
-
- if tails is None:
- spline_fn = rational_quadratic_spline
- spline_kwargs = {}
- else:
- spline_fn = unconstrained_rational_quadratic_spline
- spline_kwargs = {
- 'tails': tails,
- 'tail_bound': tail_bound
- }
-
- outputs, logabsdet = spline_fn(
- inputs=inputs,
- unnormalized_widths=unnormalized_widths,
- unnormalized_heights=unnormalized_heights,
- unnormalized_derivatives=unnormalized_derivatives,
- inverse=inverse,
- min_bin_width=min_bin_width,
- min_bin_height=min_bin_height,
- min_derivative=min_derivative,
- **spline_kwargs
- )
- return outputs, logabsdet
-
-
-def searchsorted(bin_locations, inputs, eps=1e-6):
- bin_locations[..., -1] += eps
- return torch.sum(
- inputs[..., None] >= bin_locations,
- dim=-1
- ) - 1
-
-
-def unconstrained_rational_quadratic_spline(inputs,
- unnormalized_widths,
- unnormalized_heights,
- unnormalized_derivatives,
- inverse=False,
- tails='linear',
- tail_bound=1.,
- min_bin_width=DEFAULT_MIN_BIN_WIDTH,
- min_bin_height=DEFAULT_MIN_BIN_HEIGHT,
- min_derivative=DEFAULT_MIN_DERIVATIVE):
- inside_interval_mask = (inputs >= -tail_bound) & (inputs <= tail_bound)
- outside_interval_mask = ~inside_interval_mask
-
- outputs = torch.zeros_like(inputs)
- logabsdet = torch.zeros_like(inputs)
-
- if tails == 'linear':
- unnormalized_derivatives = F.pad(unnormalized_derivatives, pad=(1, 1))
- constant = np.log(np.exp(1 - min_derivative) - 1)
- unnormalized_derivatives[..., 0] = constant
- unnormalized_derivatives[..., -1] = constant
-
- outputs[outside_interval_mask] = inputs[outside_interval_mask]
- logabsdet[outside_interval_mask] = 0
- else:
- raise RuntimeError('{} tails are not implemented.'.format(tails))
-
- outputs[inside_interval_mask], logabsdet[inside_interval_mask] = rational_quadratic_spline(
- inputs=inputs[inside_interval_mask],
- unnormalized_widths=unnormalized_widths[inside_interval_mask, :],
- unnormalized_heights=unnormalized_heights[inside_interval_mask, :],
- unnormalized_derivatives=unnormalized_derivatives[inside_interval_mask, :],
- inverse=inverse,
- left=-tail_bound, right=tail_bound, bottom=-tail_bound, top=tail_bound,
- min_bin_width=min_bin_width,
- min_bin_height=min_bin_height,
- min_derivative=min_derivative
- )
-
- return outputs, logabsdet
-
-def rational_quadratic_spline(inputs,
- unnormalized_widths,
- unnormalized_heights,
- unnormalized_derivatives,
- inverse=False,
- left=0., right=1., bottom=0., top=1.,
- min_bin_width=DEFAULT_MIN_BIN_WIDTH,
- min_bin_height=DEFAULT_MIN_BIN_HEIGHT,
- min_derivative=DEFAULT_MIN_DERIVATIVE):
- if torch.min(inputs) < left or torch.max(inputs) > right:
- raise ValueError('Input to a transform is not within its domain')
-
- num_bins = unnormalized_widths.shape[-1]
-
- if min_bin_width * num_bins > 1.0:
- raise ValueError('Minimal bin width too large for the number of bins')
- if min_bin_height * num_bins > 1.0:
- raise ValueError('Minimal bin height too large for the number of bins')
-
- widths = F.softmax(unnormalized_widths, dim=-1)
- widths = min_bin_width + (1 - min_bin_width * num_bins) * widths
- cumwidths = torch.cumsum(widths, dim=-1)
- cumwidths = F.pad(cumwidths, pad=(1, 0), mode='constant', value=0.0)
- cumwidths = (right - left) * cumwidths + left
- cumwidths[..., 0] = left
- cumwidths[..., -1] = right
- widths = cumwidths[..., 1:] - cumwidths[..., :-1]
-
- derivatives = min_derivative + F.softplus(unnormalized_derivatives)
-
- heights = F.softmax(unnormalized_heights, dim=-1)
- heights = min_bin_height + (1 - min_bin_height * num_bins) * heights
- cumheights = torch.cumsum(heights, dim=-1)
- cumheights = F.pad(cumheights, pad=(1, 0), mode='constant', value=0.0)
- cumheights = (top - bottom) * cumheights + bottom
- cumheights[..., 0] = bottom
- cumheights[..., -1] = top
- heights = cumheights[..., 1:] - cumheights[..., :-1]
-
- if inverse:
- bin_idx = searchsorted(cumheights, inputs)[..., None]
- else:
- bin_idx = searchsorted(cumwidths, inputs)[..., None]
-
- input_cumwidths = cumwidths.gather(-1, bin_idx)[..., 0]
- input_bin_widths = widths.gather(-1, bin_idx)[..., 0]
-
- input_cumheights = cumheights.gather(-1, bin_idx)[..., 0]
- delta = heights / widths
- input_delta = delta.gather(-1, bin_idx)[..., 0]
-
- input_derivatives = derivatives.gather(-1, bin_idx)[..., 0]
- input_derivatives_plus_one = derivatives[..., 1:].gather(-1, bin_idx)[..., 0]
-
- input_heights = heights.gather(-1, bin_idx)[..., 0]
-
- if inverse:
- a = (((inputs - input_cumheights) * (input_derivatives
- + input_derivatives_plus_one
- - 2 * input_delta)
- + input_heights * (input_delta - input_derivatives)))
- b = (input_heights * input_derivatives
- - (inputs - input_cumheights) * (input_derivatives
- + input_derivatives_plus_one
- - 2 * input_delta))
- c = - input_delta * (inputs - input_cumheights)
-
- discriminant = b.pow(2) - 4 * a * c
- assert (discriminant >= 0).all()
-
- root = (2 * c) / (-b - torch.sqrt(discriminant))
- outputs = root * input_bin_widths + input_cumwidths
-
- theta_one_minus_theta = root * (1 - root)
- denominator = input_delta + ((input_derivatives + input_derivatives_plus_one - 2 * input_delta)
- * theta_one_minus_theta)
- derivative_numerator = input_delta.pow(2) * (input_derivatives_plus_one * root.pow(2)
- + 2 * input_delta * theta_one_minus_theta
- + input_derivatives * (1 - root).pow(2))
- logabsdet = torch.log(derivative_numerator) - 2 * torch.log(denominator)
-
- return outputs, -logabsdet
- else:
- theta = (inputs - input_cumwidths) / input_bin_widths
- theta_one_minus_theta = theta * (1 - theta)
-
- numerator = input_heights * (input_delta * theta.pow(2)
- + input_derivatives * theta_one_minus_theta)
- denominator = input_delta + ((input_derivatives + input_derivatives_plus_one - 2 * input_delta)
- * theta_one_minus_theta)
- outputs = input_cumheights + numerator / denominator
-
- derivative_numerator = input_delta.pow(2) * (input_derivatives_plus_one * theta.pow(2)
- + 2 * input_delta * theta_one_minus_theta
- + input_derivatives * (1 - theta).pow(2))
- logabsdet = torch.log(derivative_numerator) - 2 * torch.log(denominator)
-
- return outputs, logabsdet
diff --git a/spaces/kevinwang676/ChatGLM2-VC-SadTalker/src/face3d/models/arcface_torch/configs/3millions.py b/spaces/kevinwang676/ChatGLM2-VC-SadTalker/src/face3d/models/arcface_torch/configs/3millions.py
deleted file mode 100644
index c9edc2f1414e35f93abfd3dfe11a61f1f406580e..0000000000000000000000000000000000000000
--- a/spaces/kevinwang676/ChatGLM2-VC-SadTalker/src/face3d/models/arcface_torch/configs/3millions.py
+++ /dev/null
@@ -1,23 +0,0 @@
-from easydict import EasyDict as edict
-
-# configs for test speed
-
-config = edict()
-config.loss = "arcface"
-config.network = "r50"
-config.resume = False
-config.output = None
-config.embedding_size = 512
-config.sample_rate = 1.0
-config.fp16 = True
-config.momentum = 0.9
-config.weight_decay = 5e-4
-config.batch_size = 128
-config.lr = 0.1 # batch size is 512
-
-config.rec = "synthetic"
-config.num_classes = 300 * 10000
-config.num_epoch = 30
-config.warmup_epoch = -1
-config.decay_epoch = [10, 16, 22]
-config.val_targets = []
diff --git a/spaces/kevinwang676/SadTalker/src/face3d/models/arcface_torch/configs/glint360k_r50.py b/spaces/kevinwang676/SadTalker/src/face3d/models/arcface_torch/configs/glint360k_r50.py
deleted file mode 100644
index 37e7922f1f63284e356dcc45a5f979f9c105f25e..0000000000000000000000000000000000000000
--- a/spaces/kevinwang676/SadTalker/src/face3d/models/arcface_torch/configs/glint360k_r50.py
+++ /dev/null
@@ -1,26 +0,0 @@
-from easydict import EasyDict as edict
-
-# make training faster
-# our RAM is 256G
-# mount -t tmpfs -o size=140G tmpfs /train_tmp
-
-config = edict()
-config.loss = "cosface"
-config.network = "r50"
-config.resume = False
-config.output = None
-config.embedding_size = 512
-config.sample_rate = 1.0
-config.fp16 = True
-config.momentum = 0.9
-config.weight_decay = 5e-4
-config.batch_size = 128
-config.lr = 0.1 # batch size is 512
-
-config.rec = "/train_tmp/glint360k"
-config.num_classes = 360232
-config.num_image = 17091657
-config.num_epoch = 20
-config.warmup_epoch = -1
-config.decay_epoch = [8, 12, 15, 18]
-config.val_targets = ["lfw", "cfp_fp", "agedb_30"]
diff --git a/spaces/kevinwang676/VoiceChanger/src/face3d/util/visualizer.py b/spaces/kevinwang676/VoiceChanger/src/face3d/util/visualizer.py
deleted file mode 100644
index 4023a6d4086acba9bc88e079f625194d324d7c9e..0000000000000000000000000000000000000000
--- a/spaces/kevinwang676/VoiceChanger/src/face3d/util/visualizer.py
+++ /dev/null
@@ -1,227 +0,0 @@
-"""This script defines the visualizer for Deep3DFaceRecon_pytorch
-"""
-
-import numpy as np
-import os
-import sys
-import ntpath
-import time
-from . import util, html
-from subprocess import Popen, PIPE
-from torch.utils.tensorboard import SummaryWriter
-
-def save_images(webpage, visuals, image_path, aspect_ratio=1.0, width=256):
- """Save images to the disk.
-
- Parameters:
- webpage (the HTML class) -- the HTML webpage class that stores these imaegs (see html.py for more details)
- visuals (OrderedDict) -- an ordered dictionary that stores (name, images (either tensor or numpy) ) pairs
- image_path (str) -- the string is used to create image paths
- aspect_ratio (float) -- the aspect ratio of saved images
- width (int) -- the images will be resized to width x width
-
- This function will save images stored in 'visuals' to the HTML file specified by 'webpage'.
- """
- image_dir = webpage.get_image_dir()
- short_path = ntpath.basename(image_path[0])
- name = os.path.splitext(short_path)[0]
-
- webpage.add_header(name)
- ims, txts, links = [], [], []
-
- for label, im_data in visuals.items():
- im = util.tensor2im(im_data)
- image_name = '%s/%s.png' % (label, name)
- os.makedirs(os.path.join(image_dir, label), exist_ok=True)
- save_path = os.path.join(image_dir, image_name)
- util.save_image(im, save_path, aspect_ratio=aspect_ratio)
- ims.append(image_name)
- txts.append(label)
- links.append(image_name)
- webpage.add_images(ims, txts, links, width=width)
-
-
-class Visualizer():
- """This class includes several functions that can display/save images and print/save logging information.
-
- It uses a Python library tensprboardX for display, and a Python library 'dominate' (wrapped in 'HTML') for creating HTML files with images.
- """
-
- def __init__(self, opt):
- """Initialize the Visualizer class
-
- Parameters:
- opt -- stores all the experiment flags; needs to be a subclass of BaseOptions
- Step 1: Cache the training/test options
- Step 2: create a tensorboard writer
- Step 3: create an HTML object for saveing HTML filters
- Step 4: create a logging file to store training losses
- """
- self.opt = opt # cache the option
- self.use_html = opt.isTrain and not opt.no_html
- self.writer = SummaryWriter(os.path.join(opt.checkpoints_dir, 'logs', opt.name))
- self.win_size = opt.display_winsize
- self.name = opt.name
- self.saved = False
- if self.use_html: # create an HTML object at /web/; images will be saved under /web/images/
- self.web_dir = os.path.join(opt.checkpoints_dir, opt.name, 'web')
- self.img_dir = os.path.join(self.web_dir, 'images')
- print('create web directory %s...' % self.web_dir)
- util.mkdirs([self.web_dir, self.img_dir])
- # create a logging file to store training losses
- self.log_name = os.path.join(opt.checkpoints_dir, opt.name, 'loss_log.txt')
- with open(self.log_name, "a") as log_file:
- now = time.strftime("%c")
- log_file.write('================ Training Loss (%s) ================\n' % now)
-
- def reset(self):
- """Reset the self.saved status"""
- self.saved = False
-
-
- def display_current_results(self, visuals, total_iters, epoch, save_result):
- """Display current results on tensorboad; save current results to an HTML file.
-
- Parameters:
- visuals (OrderedDict) - - dictionary of images to display or save
- total_iters (int) -- total iterations
- epoch (int) - - the current epoch
- save_result (bool) - - if save the current results to an HTML file
- """
- for label, image in visuals.items():
- self.writer.add_image(label, util.tensor2im(image), total_iters, dataformats='HWC')
-
- if self.use_html and (save_result or not self.saved): # save images to an HTML file if they haven't been saved.
- self.saved = True
- # save images to the disk
- for label, image in visuals.items():
- image_numpy = util.tensor2im(image)
- img_path = os.path.join(self.img_dir, 'epoch%.3d_%s.png' % (epoch, label))
- util.save_image(image_numpy, img_path)
-
- # update website
- webpage = html.HTML(self.web_dir, 'Experiment name = %s' % self.name, refresh=0)
- for n in range(epoch, 0, -1):
- webpage.add_header('epoch [%d]' % n)
- ims, txts, links = [], [], []
-
- for label, image_numpy in visuals.items():
- image_numpy = util.tensor2im(image)
- img_path = 'epoch%.3d_%s.png' % (n, label)
- ims.append(img_path)
- txts.append(label)
- links.append(img_path)
- webpage.add_images(ims, txts, links, width=self.win_size)
- webpage.save()
-
- def plot_current_losses(self, total_iters, losses):
- # G_loss_collection = {}
- # D_loss_collection = {}
- # for name, value in losses.items():
- # if 'G' in name or 'NCE' in name or 'idt' in name:
- # G_loss_collection[name] = value
- # else:
- # D_loss_collection[name] = value
- # self.writer.add_scalars('G_collec', G_loss_collection, total_iters)
- # self.writer.add_scalars('D_collec', D_loss_collection, total_iters)
- for name, value in losses.items():
- self.writer.add_scalar(name, value, total_iters)
-
- # losses: same format as |losses| of plot_current_losses
- def print_current_losses(self, epoch, iters, losses, t_comp, t_data):
- """print current losses on console; also save the losses to the disk
-
- Parameters:
- epoch (int) -- current epoch
- iters (int) -- current training iteration during this epoch (reset to 0 at the end of every epoch)
- losses (OrderedDict) -- training losses stored in the format of (name, float) pairs
- t_comp (float) -- computational time per data point (normalized by batch_size)
- t_data (float) -- data loading time per data point (normalized by batch_size)
- """
- message = '(epoch: %d, iters: %d, time: %.3f, data: %.3f) ' % (epoch, iters, t_comp, t_data)
- for k, v in losses.items():
- message += '%s: %.3f ' % (k, v)
-
- print(message) # print the message
- with open(self.log_name, "a") as log_file:
- log_file.write('%s\n' % message) # save the message
-
-
-class MyVisualizer:
- def __init__(self, opt):
- """Initialize the Visualizer class
-
- Parameters:
- opt -- stores all the experiment flags; needs to be a subclass of BaseOptions
- Step 1: Cache the training/test options
- Step 2: create a tensorboard writer
- Step 3: create an HTML object for saveing HTML filters
- Step 4: create a logging file to store training losses
- """
- self.opt = opt # cache the optio
- self.name = opt.name
- self.img_dir = os.path.join(opt.checkpoints_dir, opt.name, 'results')
-
- if opt.phase != 'test':
- self.writer = SummaryWriter(os.path.join(opt.checkpoints_dir, opt.name, 'logs'))
- # create a logging file to store training losses
- self.log_name = os.path.join(opt.checkpoints_dir, opt.name, 'loss_log.txt')
- with open(self.log_name, "a") as log_file:
- now = time.strftime("%c")
- log_file.write('================ Training Loss (%s) ================\n' % now)
-
-
- def display_current_results(self, visuals, total_iters, epoch, dataset='train', save_results=False, count=0, name=None,
- add_image=True):
- """Display current results on tensorboad; save current results to an HTML file.
-
- Parameters:
- visuals (OrderedDict) - - dictionary of images to display or save
- total_iters (int) -- total iterations
- epoch (int) - - the current epoch
- dataset (str) - - 'train' or 'val' or 'test'
- """
- # if (not add_image) and (not save_results): return
-
- for label, image in visuals.items():
- for i in range(image.shape[0]):
- image_numpy = util.tensor2im(image[i])
- if add_image:
- self.writer.add_image(label + '%s_%02d'%(dataset, i + count),
- image_numpy, total_iters, dataformats='HWC')
-
- if save_results:
- save_path = os.path.join(self.img_dir, dataset, 'epoch_%s_%06d'%(epoch, total_iters))
- if not os.path.isdir(save_path):
- os.makedirs(save_path)
-
- if name is not None:
- img_path = os.path.join(save_path, '%s.png' % name)
- else:
- img_path = os.path.join(save_path, '%s_%03d.png' % (label, i + count))
- util.save_image(image_numpy, img_path)
-
-
- def plot_current_losses(self, total_iters, losses, dataset='train'):
- for name, value in losses.items():
- self.writer.add_scalar(name + '/%s'%dataset, value, total_iters)
-
- # losses: same format as |losses| of plot_current_losses
- def print_current_losses(self, epoch, iters, losses, t_comp, t_data, dataset='train'):
- """print current losses on console; also save the losses to the disk
-
- Parameters:
- epoch (int) -- current epoch
- iters (int) -- current training iteration during this epoch (reset to 0 at the end of every epoch)
- losses (OrderedDict) -- training losses stored in the format of (name, float) pairs
- t_comp (float) -- computational time per data point (normalized by batch_size)
- t_data (float) -- data loading time per data point (normalized by batch_size)
- """
- message = '(dataset: %s, epoch: %d, iters: %d, time: %.3f, data: %.3f) ' % (
- dataset, epoch, iters, t_comp, t_data)
- for k, v in losses.items():
- message += '%s: %.3f ' % (k, v)
-
- print(message) # print the message
- with open(self.log_name, "a") as log_file:
- log_file.write('%s\n' % message) # save the message
diff --git a/spaces/kukuhtw/AutoGPT/CONTRIBUTING.md b/spaces/kukuhtw/AutoGPT/CONTRIBUTING.md
deleted file mode 100644
index 79169a0c1951853303f73ffa1fddb3518685606a..0000000000000000000000000000000000000000
--- a/spaces/kukuhtw/AutoGPT/CONTRIBUTING.md
+++ /dev/null
@@ -1,105 +0,0 @@
-# Contributing to ProjectName
-
-First of all, thank you for considering contributing to our project! We appreciate your time and effort, and we value any contribution, whether it's reporting a bug, suggesting a new feature, or submitting a pull request.
-
-This document provides guidelines and best practices to help you contribute effectively.
-
-## Table of Contents
-
-- [Code of Conduct](#code-of-conduct)
-- [Getting Started](#getting-started)
-- [How to Contribute](#how-to-contribute)
- - [Reporting Bugs](#reporting-bugs)
- - [Suggesting Enhancements](#suggesting-enhancements)
- - [Submitting Pull Requests](#submitting-pull-requests)
-- [Style Guidelines](#style-guidelines)
- - [Code Formatting](#code-formatting)
- - [Pre-Commit Hooks](#pre-commit-hooks)
-
-## Code of Conduct
-
-By participating in this project, you agree to abide by our [Code of Conduct](CODE_OF_CONDUCT.md). Please read it to understand the expectations we have for everyone who contributes to this project.
-
-## 📢 A Quick Word
-Right now we will not be accepting any Contributions that add non-essential commands to Auto-GPT.
-
-However, you absolutely can still add these commands to Auto-GPT in the form of plugins. Please check out this [template](https://github.com/Significant-Gravitas/Auto-GPT-Plugin-Template).
-> ⚠️ Plugin support is expected to ship within the week. You can follow PR #757 for more updates!
-
-## Getting Started
-
-To start contributing, follow these steps:
-
-1. Fork the repository and clone your fork.
-2. Create a new branch for your changes (use a descriptive name, such as `fix-bug-123` or `add-new-feature`).
-3. Make your changes in the new branch.
-4. Test your changes thoroughly.
-5. Commit and push your changes to your fork.
-6. Create a pull request following the guidelines in the [Submitting Pull Requests](#submitting-pull-requests) section.
-
-## How to Contribute
-
-### Reporting Bugs
-
-If you find a bug in the project, please create an issue on GitHub with the following information:
-
-- A clear, descriptive title for the issue.
-- A description of the problem, including steps to reproduce the issue.
-- Any relevant logs, screenshots, or other supporting information.
-
-### Suggesting Enhancements
-
-If you have an idea for a new feature or improvement, please create an issue on GitHub with the following information:
-
-- A clear, descriptive title for the issue.
-- A detailed description of the proposed enhancement, including any benefits and potential drawbacks.
-- Any relevant examples, mockups, or supporting information.
-
-### Submitting Pull Requests
-
-When submitting a pull request, please ensure that your changes meet the following criteria:
-
-- Your pull request should be atomic and focus on a single change.
-- Your pull request should include tests for your change.
-- You should have thoroughly tested your changes with multiple different prompts.
-- You should have considered potential risks and mitigations for your changes.
-- You should have documented your changes clearly and comprehensively.
-- You should not include any unrelated or "extra" small tweaks or changes.
-
-## Style Guidelines
-
-### Code Formatting
-
-We use the `black` code formatter to maintain a consistent coding style across the project. Please ensure that your code is formatted using `black` before submitting a pull request. You can install `black` using `pip`:
-
-```bash
-pip install black
-```
-
-To format your code, run the following command in the project's root directory:
-
-```bash
-black .
-```
-### Pre-Commit Hooks
-We use pre-commit hooks to ensure that code formatting and other checks are performed automatically before each commit. To set up pre-commit hooks for this project, follow these steps:
-
-Install the pre-commit package using pip:
-```bash
-pip install pre-commit
-```
-
-Run the following command in the project's root directory to install the pre-commit hooks:
-```bash
-pre-commit install
-```
-
-Now, the pre-commit hooks will run automatically before each commit, checking your code formatting and other requirements.
-
-If you encounter any issues or have questions, feel free to reach out to the maintainers or open a new issue on GitHub. We're here to help and appreciate your efforts to contribute to the project.
-
-Happy coding, and once again, thank you for your contributions!
-
-Maintainers will look at PR that have no merge conflicts when deciding what to add to the project. Make sure your PR shows up here:
-
-https://github.com/Torantulino/Auto-GPT/pulls?q=is%3Apr+is%3Aopen+-is%3Aconflict+
\ No newline at end of file
diff --git a/spaces/kukuhtw/VToonify/vtoonify/smooth_parsing_map.py b/spaces/kukuhtw/VToonify/vtoonify/smooth_parsing_map.py
deleted file mode 100644
index 7720d0c7786925db38d3e793d6a3a8f68f6e663e..0000000000000000000000000000000000000000
--- a/spaces/kukuhtw/VToonify/vtoonify/smooth_parsing_map.py
+++ /dev/null
@@ -1,172 +0,0 @@
-import os
-#os.environ['CUDA_VISIBLE_DEVICES'] = "0"
-import numpy as np
-import cv2
-import math
-import argparse
-from tqdm import tqdm
-import torch
-from torch import nn
-from torchvision import transforms
-import torch.nn.functional as F
-from model.raft.core.raft import RAFT
-from model.raft.core.utils.utils import InputPadder
-from model.bisenet.model import BiSeNet
-from model.stylegan.model import Downsample
-
-class Options():
- def __init__(self):
-
- self.parser = argparse.ArgumentParser(description="Smooth Parsing Maps")
- self.parser.add_argument("--window_size", type=int, default=5, help="temporal window size")
-
- self.parser.add_argument("--faceparsing_path", type=str, default='./checkpoint/faceparsing.pth', help="path of the face parsing model")
- self.parser.add_argument("--raft_path", type=str, default='./checkpoint/raft-things.pth', help="path of the RAFT model")
-
- self.parser.add_argument("--video_path", type=str, help="path of the target video")
- self.parser.add_argument("--output_path", type=str, default='./output/', help="path of the output parsing maps")
-
- def parse(self):
- self.opt = self.parser.parse_args()
- args = vars(self.opt)
- print('Load options')
- for name, value in sorted(args.items()):
- print('%s: %s' % (str(name), str(value)))
- return self.opt
-
-# from RAFT
-def warp(x, flo):
- """
- warp an image/tensor (im2) back to im1, according to the optical flow
- x: [B, C, H, W] (im2)
- flo: [B, 2, H, W] flow
- """
- B, C, H, W = x.size()
- # mesh grid
- xx = torch.arange(0, W).view(1,-1).repeat(H,1)
- yy = torch.arange(0, H).view(-1,1).repeat(1,W)
- xx = xx.view(1,1,H,W).repeat(B,1,1,1)
- yy = yy.view(1,1,H,W).repeat(B,1,1,1)
- grid = torch.cat((xx,yy),1).float()
-
-
- #x = x.cuda()
- grid = grid.cuda()
- vgrid = grid + flo # B,2,H,W
-
- # scale grid to [-1,1]
- ##2019 code
- vgrid[:,0,:,:] = 2.0*vgrid[:,0,:,:].clone()/max(W-1,1)-1.0
- vgrid[:,1,:,:] = 2.0*vgrid[:,1,:,:].clone()/max(H-1,1)-1.0
-
- vgrid = vgrid.permute(0,2,3,1)
- output = nn.functional.grid_sample(x, vgrid,align_corners=True)
- mask = torch.autograd.Variable(torch.ones(x.size())).cuda()
- mask = nn.functional.grid_sample(mask, vgrid,align_corners=True)
-
- ##2019 author
- mask[mask<0.9999] = 0
- mask[mask>0] = 1
-
- ##2019 code
- # mask = torch.floor(torch.clamp(mask, 0 ,1))
-
- return output*mask, mask
-
-
-if __name__ == "__main__":
-
- parser = Options()
- args = parser.parse()
- print('*'*98)
-
-
- device = "cuda"
-
- transform = transforms.Compose([
- transforms.ToTensor(),
- transforms.Normalize(mean=[0.5, 0.5, 0.5],std=[0.5,0.5,0.5]),
- ])
-
- parser = argparse.ArgumentParser()
- parser.add_argument('--model', help="restore checkpoint")
- parser.add_argument('--small', action='store_true', help='use small model')
- parser.add_argument('--mixed_precision', action='store_true', help='use mixed precision')
- parser.add_argument('--alternate_corr', action='store_true', help='use efficent correlation implementation')
-
- raft_model = torch.nn.DataParallel(RAFT(parser.parse_args(['--model', args.raft_path])))
- raft_model.load_state_dict(torch.load(args.raft_path))
-
- raft_model = raft_model.module
- raft_model.to(device)
- raft_model.eval()
-
- parsingpredictor = BiSeNet(n_classes=19)
- parsingpredictor.load_state_dict(torch.load(args.faceparsing_path, map_location=lambda storage, loc: storage))
- parsingpredictor.to(device).eval()
-
- down = Downsample(kernel=[1, 3, 3, 1], factor=2).to(device).eval()
-
- print('Load models successfully!')
-
- window = args.window_size
-
- video_cap = cv2.VideoCapture(args.video_path)
- num = int(video_cap.get(7))
-
- Is = []
- for i in range(num):
- success, frame = video_cap.read()
- if success == False:
- break
- frame = cv2.cvtColor(frame, cv2.COLOR_BGR2RGB)
- with torch.no_grad():
- Is += [transform(frame).unsqueeze(dim=0).cpu()]
- video_cap.release()
-
- # enlarge frames for more accurate parsing maps and optical flows
- Is = F.upsample(torch.cat(Is, dim=0), scale_factor=2, mode='bilinear')
- Is_ = torch.cat((Is[0:window], Is, Is[-window:]), dim=0)
-
- print('Load video with %d frames successfully!'%(len(Is)))
-
- Ps = []
- for i in tqdm(range(len(Is))):
- with torch.no_grad():
- Ps += [parsingpredictor(2*Is[i:i+1].to(device))[0].detach().cpu()]
- Ps = torch.cat(Ps, dim=0)
- Ps_ = torch.cat((Ps[0:window], Ps, Ps[-window:]), dim=0)
-
- print('Predict parsing maps successfully!')
-
-
- # temporal weights of the (2*args.window_size+1) frames
- wt = torch.exp(-(torch.arange(2*window+1).float()-window)**2/(2*((window+0.5)**2))).reshape(2*window+1,1,1,1).to(device)
-
- parse = []
- for ii in tqdm(range(len(Is))):
- i = ii + window
- image2 = Is_[i-window:i+window+1].to(device)
- image1 = Is_[i].repeat(2*window+1,1,1,1).to(device)
- padder = InputPadder(image1.shape)
- image1, image2 = padder.pad(image1, image2)
- with torch.no_grad():
- flow_low, flow_up = raft_model((image1+1)*255.0/2, (image2+1)*255.0/2, iters=20, test_mode=True)
- output, mask = warp(torch.cat((image2, Ps_[i-window:i+window+1].to(device)), dim=1), flow_up)
- aligned_Is = output[:,0:3].detach()
- aligned_Ps = output[:,3:].detach()
- # the spatial weight
- ws = torch.exp(-((aligned_Is-image1)**2).mean(dim=1, keepdims=True)/(2*(0.2**2))) * mask[:,0:1]
- aligned_Ps[window] = Ps_[i].to(device)
- # the weight between i and i shoud be 1.0
- ws[window,:,:,:] = 1.0
- weights = ws*wt
- weights = weights / weights.sum(dim=(0), keepdims=True)
- fused_Ps = (aligned_Ps * weights).sum(dim=0, keepdims=True)
- parse += [down(fused_Ps).detach().cpu()]
- parse = torch.cat(parse, dim=0)
-
- basename = os.path.basename(args.video_path).split('.')[0]
- np.save(os.path.join(args.output_path, basename+'_parsingmap.npy'), parse.numpy())
-
- print('Done!')
\ No newline at end of file
diff --git a/spaces/ky2k/Toxicity_Classifier_POC/.venv/lib/python3.9/site-packages/fastapi/templating.py b/spaces/ky2k/Toxicity_Classifier_POC/.venv/lib/python3.9/site-packages/fastapi/templating.py
deleted file mode 100644
index 0cb868486edd9dda38f90c65f314597813128cf8..0000000000000000000000000000000000000000
--- a/spaces/ky2k/Toxicity_Classifier_POC/.venv/lib/python3.9/site-packages/fastapi/templating.py
+++ /dev/null
@@ -1 +0,0 @@
-from starlette.templating import Jinja2Templates as Jinja2Templates # noqa
diff --git a/spaces/ky2k/Toxicity_Classifier_POC/.venv/lib/python3.9/site-packages/fontTools/ttLib/tables/F_F_T_M_.py b/spaces/ky2k/Toxicity_Classifier_POC/.venv/lib/python3.9/site-packages/fontTools/ttLib/tables/F_F_T_M_.py
deleted file mode 100644
index 823ced1bafe991b73d73632773b3d7d21990b572..0000000000000000000000000000000000000000
--- a/spaces/ky2k/Toxicity_Classifier_POC/.venv/lib/python3.9/site-packages/fontTools/ttLib/tables/F_F_T_M_.py
+++ /dev/null
@@ -1,42 +0,0 @@
-from fontTools.misc import sstruct
-from fontTools.misc.textTools import safeEval
-from fontTools.misc.timeTools import timestampFromString, timestampToString
-from . import DefaultTable
-
-FFTMFormat = """
- > # big endian
- version: I
- FFTimeStamp: Q
- sourceCreated: Q
- sourceModified: Q
-"""
-
-
-class table_F_F_T_M_(DefaultTable.DefaultTable):
- def decompile(self, data, ttFont):
- dummy, rest = sstruct.unpack2(FFTMFormat, data, self)
-
- def compile(self, ttFont):
- data = sstruct.pack(FFTMFormat, self)
- return data
-
- def toXML(self, writer, ttFont):
- writer.comment(
- "FontForge's timestamp, font source creation and modification dates"
- )
- writer.newline()
- formatstring, names, fixes = sstruct.getformat(FFTMFormat)
- for name in names:
- value = getattr(self, name)
- if name in ("FFTimeStamp", "sourceCreated", "sourceModified"):
- value = timestampToString(value)
- writer.simpletag(name, value=value)
- writer.newline()
-
- def fromXML(self, name, attrs, content, ttFont):
- value = attrs["value"]
- if name in ("FFTimeStamp", "sourceCreated", "sourceModified"):
- value = timestampFromString(value)
- else:
- value = safeEval(value)
- setattr(self, name, value)
diff --git a/spaces/ky2k/Toxicity_Classifier_POC/.venv/lib/python3.9/site-packages/gradio/templates/frontend/assets/index-79ad4076.js b/spaces/ky2k/Toxicity_Classifier_POC/.venv/lib/python3.9/site-packages/gradio/templates/frontend/assets/index-79ad4076.js
deleted file mode 100644
index aa7b7f776211cd89ad00c96d1e4b1bc6fccf43b2..0000000000000000000000000000000000000000
--- a/spaces/ky2k/Toxicity_Classifier_POC/.venv/lib/python3.9/site-packages/gradio/templates/frontend/assets/index-79ad4076.js
+++ /dev/null
@@ -1,2 +0,0 @@
-import{E as W,C as Y,L as d}from"./index-767254b1.js";import{s as n,t as r,L as R,i as Z,d as a,f as X,a as y,b as f}from"./index-aa084753.js";import"./index-8c3da1d9.js";import"./Blocks-6ad6f005.js";import"./Button-62634b34.js";import"./BlockLabel-98ef75ee.js";import"./Empty-5d52e655.js";/* empty css */import"./Copy-fd383441.js";import"./Download-dfb06e25.js";const l=1,w=189,S=190,b=191,T=192,U=193,m=194,V=22,g=23,h=47,G=48,c=53,u=54,_=55,j=57,E=58,k=59,z=60,v=61,H=63,N=230,A=71,F=255,K=121,C=142,D=143,M=146,i=10,s=13,t=32,o=9,q=35,L=40,B=46,J=new Set([g,h,G,F,H,K,u,_,N,z,v,E,k,A,C,D,M]),OO=new W((O,$)=>{if(O.next<0)O.acceptToken(m);else if(!(O.next!=i&&O.next!=s))if($.context.depth<0)O.acceptToken(T,1);else{O.advance();let Q=0;for(;O.next==t||O.next==o;)O.advance(),Q++;let P=O.next==i||O.next==s||O.next==q;O.acceptToken(P?U:b,-Q)}},{contextual:!0,fallback:!0}),$O=new W((O,$)=>{let Q=$.context.depth;if(Q<0)return;let P=O.peek(-1);if((P==i||P==s)&&$.context.depth>=0){let e=0,x=0;for(;;){if(O.next==t)e++;else if(O.next==o)e+=8-e%8;else break;O.advance(),x++}e!=Q&&O.next!=i&&O.next!=s&&O.next!=q&&(e{for(let $=0;$<5;$++){if(O.next!="print".charCodeAt($))return;O.advance()}if(!/\w/.test(String.fromCharCode(O.next)))for(let $=0;;$++){let Q=O.peek($);if(!(Q==t||Q==o)){Q!=L&&Q!=B&&Q!=i&&Q!=s&&Q!=q&&O.acceptToken(l);return}}}),iO=n({'async "*" "**" FormatConversion FormatSpec':r.modifier,"for while if elif else try except finally return raise break continue with pass assert await yield match case":r.controlKeyword,"in not and or is del":r.operatorKeyword,"from def class global nonlocal lambda":r.definitionKeyword,import:r.moduleKeyword,"with as print":r.keyword,Boolean:r.bool,None:r.null,VariableName:r.variableName,"CallExpression/VariableName":r.function(r.variableName),"FunctionDefinition/VariableName":r.function(r.definition(r.variableName)),"ClassDefinition/VariableName":r.definition(r.className),PropertyName:r.propertyName,"CallExpression/MemberExpression/PropertyName":r.function(r.propertyName),Comment:r.lineComment,Number:r.number,String:r.string,FormatString:r.special(r.string),UpdateOp:r.updateOperator,ArithOp:r.arithmeticOperator,BitOp:r.bitwiseOperator,CompareOp:r.compareOperator,AssignOp:r.definitionOperator,Ellipsis:r.punctuation,At:r.meta,"( )":r.paren,"[ ]":r.squareBracket,"{ }":r.brace,".":r.derefOperator,", ;":r.separator}),sO={__proto__:null,await:40,or:50,and:52,in:56,not:58,is:60,if:66,else:68,lambda:72,yield:90,from:92,async:98,for:100,None:152,True:154,False:154,del:168,pass:172,break:176,continue:180,return:184,raise:192,import:196,as:198,global:202,nonlocal:204,assert:208,elif:218,while:222,try:228,except:230,finally:232,with:236,def:240,class:250,match:261,case:267},oO=d.deserialize({version:14,states:"!L`O`Q$IXOOO%fQ$I[O'#G|OOQ$IS'#Cm'#CmOOQ$IS'#Cn'#CnO'UQ$IWO'#ClO(wQ$I[O'#G{OOQ$IS'#G|'#G|OOQ$IS'#DS'#DSOOQ$IS'#G{'#G{O)eQ$IWO'#CsO)uQ$IWO'#DdO*VQ$IWO'#DhOOQ$IS'#Ds'#DsO*jO`O'#DsO*rOpO'#DsO*zO!bO'#DtO+VO#tO'#DtO+bO&jO'#DtO+mO,UO'#DtO-oQ$I[O'#GmOOQ$IS'#Gm'#GmO'UQ$IWO'#GlO/RQ$I[O'#GlOOQ$IS'#E]'#E]O/jQ$IWO'#E^OOQ$IS'#Gk'#GkO/tQ$IWO'#GjOOQ$IV'#Gj'#GjO0PQ$IWO'#FPOOQ$IS'#GX'#GXO0UQ$IWO'#FOOOQ$IV'#Hx'#HxOOQ$IV'#Gi'#GiOOQ$IT'#Fh'#FhQ`Q$IXOOO'UQ$IWO'#CoO0dQ$IWO'#C{O0kQ$IWO'#DPO0yQ$IWO'#HQO1ZQ$I[O'#EQO'UQ$IWO'#EROOQ$IS'#ET'#ETOOQ$IS'#EV'#EVOOQ$IS'#EX'#EXO1oQ$IWO'#EZO2VQ$IWO'#E_O0PQ$IWO'#EaO2jQ$I[O'#EaO0PQ$IWO'#EdO/jQ$IWO'#EgO/jQ$IWO'#EkO/jQ$IWO'#EnO2uQ$IWO'#EpO2|Q$IWO'#EuO3XQ$IWO'#EqO/jQ$IWO'#EuO0PQ$IWO'#EwO0PQ$IWO'#E|O3^Q$IWO'#FROOQ$IS'#Cc'#CcOOQ$IS'#Cd'#CdOOQ$IS'#Ce'#CeOOQ$IS'#Cf'#CfOOQ$IS'#Cg'#CgOOQ$IS'#Ch'#ChOOQ$IS'#Cj'#CjO'UQ$IWO,58|O'UQ$IWO,58|O'UQ$IWO,58|O'UQ$IWO,58|O'UQ$IWO,58|O'UQ$IWO,58|O3eQ$IWO'#DmOOQ$IS,5:W,5:WO3xQ$IWO'#H[OOQ$IS,5:Z,5:ZO4VQ%1`O,5:ZO4[Q$I[O,59WO0dQ$IWO,59`O0dQ$IWO,59`O0dQ$IWO,59`O6zQ$IWO,59`O7PQ$IWO,59`O7WQ$IWO,59hO7_Q$IWO'#G{O8eQ$IWO'#GzOOQ$IS'#Gz'#GzOOQ$IS'#DY'#DYO8|Q$IWO,59_O'UQ$IWO,59_O9[Q$IWO,59_O9aQ$IWO,5:PO'UQ$IWO,5:POOQ$IS,5:O,5:OO9oQ$IWO,5:OO9tQ$IWO,5:VO'UQ$IWO,5:VO'UQ$IWO,5:TOOQ$IS,5:S,5:SO:VQ$IWO,5:SO:[Q$IWO,5:UOOOO'#Fp'#FpO:aO`O,5:_OOQ$IS,5:_,5:_OOOO'#Fq'#FqO:iOpO,5:_O:qQ$IWO'#DuOOOO'#Fr'#FrO;RO!bO,5:`OOQ$IS,5:`,5:`OOOO'#Fu'#FuO;^O#tO,5:`OOOO'#Fv'#FvO;iO&jO,5:`OOOO'#Fw'#FwO;tO,UO,5:`OOQ$IS'#Fx'#FxOqQ$I[O,5=WO?[Q%GlO,5=WO?{Q$I[O,5=WOOQ$IS,5:x,5:xO@dQ$IXO'#GQOAsQ$IWO,5;TOOQ$IV,5=U,5=UOBOQ$I[O'#HtOBgQ$IWO,5;kOOQ$IS-E:V-E:VOOQ$IV,5;j,5;jO3SQ$IWO'#EwOOQ$IT-E9f-E9fOBoQ$I[O,59ZODvQ$I[O,59gOEaQ$IWO'#G}OElQ$IWO'#G}O0PQ$IWO'#G}OEwQ$IWO'#DROFPQ$IWO,59kOFUQ$IWO'#HRO'UQ$IWO'#HRO/jQ$IWO,5=lOOQ$IS,5=l,5=lO/jQ$IWO'#D|OOQ$IS'#D}'#D}OFsQ$IWO'#FzOGTQ$IWO,58zOGTQ$IWO,58zO)hQ$IWO,5:jOGcQ$I[O'#HTOOQ$IS,5:m,5:mOOQ$IS,5:u,5:uOGvQ$IWO,5:yOHXQ$IWO,5:{OOQ$IS'#F}'#F}OHgQ$I[O,5:{OHuQ$IWO,5:{OHzQ$IWO'#HwOOQ$IS,5;O,5;OOIYQ$IWO'#HsOOQ$IS,5;R,5;RO3XQ$IWO,5;VO3XQ$IWO,5;YOIkQ$I[O'#HyO'UQ$IWO'#HyOIuQ$IWO,5;[O2uQ$IWO,5;[O/jQ$IWO,5;aO0PQ$IWO,5;cOIzQ$IXO'#ElOKTQ$IZO,5;]ONiQ$IWO'#HzO3XQ$IWO,5;aONtQ$IWO,5;cONyQ$IWO,5;hO! RQ$I[O,5;mO'UQ$IWO,5;mO!#uQ$I[O1G.hO!#|Q$I[O1G.hO!&mQ$I[O1G.hO!&wQ$I[O1G.hO!)bQ$I[O1G.hO!)uQ$I[O1G.hO!*YQ$IWO'#HZO!*hQ$I[O'#GmO/jQ$IWO'#HZO!*rQ$IWO'#HYOOQ$IS,5:X,5:XO!*zQ$IWO,5:XO!+PQ$IWO'#H]O!+[Q$IWO'#H]O!+oQ$IWO,5=vOOQ$IS'#Dq'#DqOOQ$IS1G/u1G/uOOQ$IS1G.z1G.zO!,oQ$I[O1G.zO!,vQ$I[O1G.zO0dQ$IWO1G.zO!-cQ$IWO1G/SOOQ$IS'#DX'#DXO/jQ$IWO,59rOOQ$IS1G.y1G.yO!-jQ$IWO1G/cO!-zQ$IWO1G/cO!.SQ$IWO1G/dO'UQ$IWO'#HSO!.XQ$IWO'#HSO!.^Q$I[O1G.yO!.nQ$IWO,59gO!/tQ$IWO,5=rO!0UQ$IWO,5=rO!0^Q$IWO1G/kO!0cQ$I[O1G/kOOQ$IS1G/j1G/jO!0sQ$IWO,5=mO!1jQ$IWO,5=mO/jQ$IWO1G/oO!2XQ$IWO1G/qO!2^Q$I[O1G/qO!2nQ$I[O1G/oOOQ$IS1G/n1G/nOOQ$IS1G/p1G/pOOOO-E9n-E9nOOQ$IS1G/y1G/yOOOO-E9o-E9oO!3OQ$IWO'#HhO/jQ$IWO'#HhO!3^Q$IWO,5:aOOOO-E9p-E9pOOQ$IS1G/z1G/zOOOO-E9s-E9sOOOO-E9t-E9tOOOO-E9u-E9uOOQ$IS-E9v-E9vO!3iQ%GlO1G2rO!4YQ$I[O1G2rO'UQ$IWO,5`OOQ$IS1G1V1G1VO!5YQ$IWO1G1VOOQ$IS'#DT'#DTO/jQ$IWO,5=iOOQ$IS,5=i,5=iO!5_Q$IWO'#FiO!5jQ$IWO,59mO!5rQ$IWO1G/VO!5|Q$I[O,5=mOOQ$IS1G3W1G3WOOQ$IS,5:h,5:hO!6mQ$IWO'#GlOOQ$IS,5cO!8oQ$IWO,5>cO!8}Q$IWO,5>_O!9eQ$IWO,5>_O!9vQ$IZO1G0qO!=XQ$IZO1G0tO!@gQ$IWO,5>eO!@qQ$IWO,5>eO!@yQ$I[O,5>eO/jQ$IWO1G0vO!ATQ$IWO1G0vO3XQ$IWO1G0{ONtQ$IWO1G0}OOQ$IV,5;W,5;WO!AYQ$IYO,5;WO!A_Q$IZO1G0wO!DsQ$IWO'#GUO3XQ$IWO1G0wO3XQ$IWO1G0wO!EQQ$IWO,5>fO!E_Q$IWO,5>fO0PQ$IWO,5>fOOQ$IV1G0{1G0{O!EgQ$IWO'#EyO!ExQ%1`O1G0}OOQ$IV1G1S1G1SO3XQ$IWO1G1SO!FQQ$IWO'#FTOOQ$IV1G1X1G1XO! RQ$I[O1G1XOOQ$IS,5=u,5=uOOQ$IS'#Dn'#DnO/jQ$IWO,5=uO!FVQ$IWO,5=tO!FjQ$IWO,5=tOOQ$IS1G/s1G/sO!FrQ$IWO,5=wO!GSQ$IWO,5=wO!G[Q$IWO,5=wO!GoQ$IWO,5=wO!HPQ$IWO,5=wOOQ$IS1G3b1G3bOOQ$IS7+$f7+$fO!5rQ$IWO7+$nO!IrQ$IWO1G.zO!IyQ$IWO1G.zOOQ$IS1G/^1G/^OOQ$IS,5SO!NaQ$IWO,5>SO!NaQ$IWO,5>SO!NoO!LQO'#DwO!NzOSO'#HiOOOO1G/{1G/{O# PQ$IWO1G/{O# XQ%GlO7+(^O# xQ$I[O1G2PP#!cQ$IWO'#FyOOQ$IS,5T,5>TOOOO7+%g7+%gO#8UQ$IWO1G2rO#8oQ$IWO1G2rP'UQ$IWO'#FlO/jQ$IWO<bO#9cQ$IWO,5>bO0PQ$IWO,5>bO#9tQ$IWO,5>aOOQ$IS<hO#CeQ$IWO,5>hOOQ$IS,5>h,5>hO#CpQ$IWO,5>gO#DRQ$IWO,5>gOOQ$IS1G1P1G1POOQ$IS,5;g,5;gO#DZQ$IWO1G1ZP#D`Q$IWO'#FnO#DpQ$IWO1G1uO#ETQ$IWO1G1uO#EeQ$IWO1G1uP#EpQ$IWO'#FoO#E}Q$IWO7+(}O#F_Q$IWO7+(}O#F_Q$IWO7+(}O#FgQ$IWO7+(}O#FwQ$IWO7+(tO7WQ$IWO7+(tOOQ$ISAN>TAN>TO#GbQ$IWO<aAN>aO/jQ$IWO1G1sO#GrQ$I[O1G1sP#G|Q$IWO'#FmOOQ$IS1G1y1G1yP#HZQ$IWO'#FsO#HhQ$IWO7+)YOOOO-E9r-E9rO#IOQ$IWO7+(^OOQ$ISAN?VAN?VO#IiQ$IWO,5jO$,bQ$IWO,5>jO0PQ$IWO,5;vO$,sQ$IWO,5;zO$,xQ$IWO,5;zO#NzQ$IWO'#IQO$,}Q$IWO'#IQO$-SQ$IWO,5;{OOQ$IS,5;|,5;|O'UQ$IWO'#FgOOQ$IU1G1[1G1[O3XQ$IWO1G1[OOQ$ISAN@gAN@gO$-XQ$IWOG27oO$-iQ$IWO,59{OOQ$IS1G3[1G3[OOQ$IS,5