diff --git a/spaces/1acneusushi/gradio-2dmoleculeeditor/data/Anytone At 5555 V3 Software 14.md b/spaces/1acneusushi/gradio-2dmoleculeeditor/data/Anytone At 5555 V3 Software 14.md
deleted file mode 100644
index 8e348aa6990b1d20662ba6c2a3de2175ef58363e..0000000000000000000000000000000000000000
--- a/spaces/1acneusushi/gradio-2dmoleculeeditor/data/Anytone At 5555 V3 Software 14.md
+++ /dev/null
@@ -1,108 +0,0 @@
-
-
Anytone At 5555 V3 Software 14: A Review
-
Introduction
-
If you are looking for a reliable and versatile software for your Anytone AT-5555 PLUS 10M mobile transceiver, you might want to check out the Anytone At 5555 V3 Software 14. This software is the latest official release from Anytone, a leading manufacturer of radios and accessories. In this article, we will review the features, benefits, and installation process of this software, and answer some frequently asked questions about it.
Anytone At 5555 V3 Software 14 is a software that allows you to program and control your Anytone AT-5555 PLUS radio. It is designed for Windows operating systems, but it can also be used on Mac OSX and Linux with a virtual machine. The software enables you to access all the features and options of your radio, such as 2TONE, 5TONE, MSK, and more. It also supports CHIRP programming software, which is a free and open-source tool that allows you to easily copy and paste frequencies, import files from other radios, and edit channels.
-
Why do you need Anytone At 5555 V3 Software 14?
-
You need Anytone At 5555 V3 Software 14 if you want to get the most out of your Anytone AT-5555 PLUS radio. This software will help you to customize your radio settings according to your preferences and needs. It will also help you to update your radio firmware to the latest version, which can improve the performance and stability of your device. Moreover, it will allow you to use CHIRP programming software, which can make your frequency programming and editing much easier and faster.
-
How to download and install Anytone At 5555 V3 Software 14?
-
To download and install Anytone At 5555 V3 Software 14, you need to follow these steps:
Download the zip file and extract it to a folder on your computer.
-
Connect your Anytone AT-5555 PLUS radio to your computer with a USB cable.
-
Run the setup.exe file in the folder and follow the instructions on the screen.
-
When the installation is complete, launch the software and enjoy.
-
-
Features of Anytone At 5555 V3 Software 14
-
RX noise reduction option
-
One of the features of Anytone At 5555 V3 Software 14 is the RX noise reduction option. This option allows you to reduce the background noise in your radio reception by using an extra PCB inside the radio. This can enhance the clarity and quality of your communication, especially in noisy environments.
-
Access to all features and options such as 2TONE, 5TONE, MSK, and more
-
Another feature of Anytone At 5555 V3 Software 14 is that it gives you access to all the features and options of your radio, such as 2TONE, 5TONE, MSK, and more. These are different modes of signaling that can be used for various purposes, such as selective calling, group calling, emergency calling, etc. You can use these modes to communicate with other radios that support them.
-
Compatibility with CHIRP programming software
-
A third feature of Anytone At 5555 V3 Software 14 is that it is compatible with CHIRP programming software. CHIRP is a free and open-source software that allows you to program your radio with ease. You can use CHIRP to copy and paste frequencies from other sources, import files from other radios, edit channels with a spreadsheet-like interface, etc. You can also use CHIRP on Windows, Mac OSX, or Linux operating systems.
-
Benefits of Anytone At 5555 V3 Software 14
-
Improved performance and stability of your radio
-
One of the benefits of using Anytone At 5555 V3 Software 14 is that it can improve the performance and stability of your radio. By updating your radio firmware to the latest version, you can fix some bugs and glitches that might affect your device. You can also optimize your radio settings to suit your needs and preferences.
-
Easier frequency programming and editing
-
Benefits of Anytone At 5555 V3 Software 14
-
Improved performance and stability of your radio
-
One of the benefits of using Anytone At 5555 V3 Software 14 is that it can improve the performance and stability of your radio. By updating your radio firmware to the latest version, you can fix some bugs and glitches that might affect your device. You can also optimize your radio settings to suit your needs and preferences.
-
Easier frequency programming and editing
-
Another benefit of using Anytone At 5555 V3 Software 14 is that it makes your frequency programming and editing easier and faster. You can use the software to access all the features and options of your radio, such as 2TONE, 5TONE, MSK, and more. You can also use CHIRP programming software, which is a free and open-source tool that allows you to copy and paste frequencies from other sources, import files from other radios, edit channels with a spreadsheet-like interface, etc.
-
How to update Anytone At 5555 V3 Software 14
-Anytone At 5555 V3 Software 14 download link
-Anytone At 5555 V3 Software 14 user manual
-Anytone At 5555 V3 Software 14 review and rating
-Anytone At 5555 V3 Software 14 troubleshooting guide
-Anytone At 5555 V3 Software 14 compatible devices
-Anytone At 5555 V3 Software 14 features and specifications
-Anytone At 5555 V3 Software 14 installation instructions
-Anytone At 5555 V3 Software 14 warranty and support
-Anytone At 5555 V3 Software 14 price and availability
-Anytone At 5555 V3 Software 14 vs other software versions
-Anytone At 5555 V3 Software 14 best practices and tips
-Anytone At 5555 V3 Software 14 feedback and testimonials
-Anytone At 5555 V3 Software 14 pros and cons
-Anytone At 5555 V3 Software 14 alternatives and comparisons
-Anytone At 5555 V3 Software 14 benefits and advantages
-Anytone At 5555 V3 Software 14 drawbacks and limitations
-Anytone At 5555 V3 Software 14 FAQs and answers
-Anytone At 5555 V3 Software 14 tutorials and videos
-Anytone At 5555 V3 Software 14 updates and changelog
-Anytone At 5555 V3 Software 14 bugs and fixes
-Anytone At 5555 V3 Software 14 requirements and compatibility
-Anytone At 5555 V3 Software 14 performance and reliability
-Anytone At 5555 V3 Software 14 customization and settings
-Anytone At 5555 V3 Software 14 security and privacy
-How to uninstall Anytone At 5555 V3 Software 14
-How to backup and restore Anytone At 5555 V3 Software 14
-How to reset and factory default Anytone At 5555 V3 Software 14
-How to connect and sync Anytone At 5555 V3 Software 14 with other devices
-How to optimize and improve Anytone At 5555 V3 Software 14 functionality
-How to troubleshoot and fix common issues with Anytone At
-How to troubleshoot and fix common issues with Anytone At
-
Support for Windows, Mac OSX, and Linux operating systems
-
A third benefit of using Anytone At 5555 V3 Software 14 is that it supports Windows, Mac OSX, and Linux operating systems. You can use the software on your preferred operating system without any hassle. If you are using Mac OSX or Linux, you can use a virtual machine to run the software. This way, you can enjoy the same functionality and compatibility as Windows users.
-
Conclusion
-
Summary of the main points
-
In conclusion, Anytone At 5555 V3 Software 14 is a reliable and versatile software for your Anytone AT-5555 PLUS 10M mobile transceiver. It allows you to program and control your radio with ease. It has features such as RX noise reduction option, access to all features and options such as 2TONE, 5TONE, MSK, and more, and compatibility with CHIRP programming software. It also has benefits such as improved performance and stability of your radio, easier frequency programming and editing, and support for Windows, Mac OSX, and Linux operating systems.
-
Call to action
-
If you are interested in getting Anytone At 5555 V3 Software 14 for your radio, you can download it from https://www.anytone.net/download. You can also find more information about Anytone products and services on their website. If you have any questions or feedback about the software or the radio, you can contact Anytone customer support or join their online community. Don't miss this opportunity to upgrade your radio with Anytone At 5555 V3 Software 14.
-
FAQs
-
What is the difference between Anytone At 5555 V3 Software 14 and Anytone At 5555N II?
-
Anytone At 5555 V3 Software 14 is the latest official release for Anytone AT-5555 PLUS radio. Anytone At 5555N II is an upgraded version of Anytone AT-5555N radio. They are different models of radios with different features and specifications.
-
How much does Anytone At 5555 V3 Software 14 cost?
-
Anytone At 5555 V3 Software 14 is free to download from https://www.anytone.net/download. You only need to pay for the Anytone AT-5555 PLUS radio itself.
-
How do I use CHIRP programming software with Anytone At 5555 V3 Software 14?
-
How do I use CHIRP programming software with Anytone At 5555 V3 Software 14?
-
To use CHIRP programming software with Anytone At 5555 V3 Software 14, you need to follow these steps:
Connect your Anytone AT-5555 PLUS radio to your computer with a USB cable.
-
Launch CHIRP and select your radio model and port from the menu.
-
Click on Radio > Download From Radio to read the current settings from your radio.
-
Edit the channels and settings as you wish using the spreadsheet-like interface.
-
Click on Radio > Upload To Radio to write the new settings to your radio.
-
-
What are some of the reviews of Anytone At 5555 V3 Software 14?
-
Some of the reviews of Anytone At 5555 V3 Software 14 are:
-
-
"Great little radio ( Little in comparison to my Yaesu FTDX 101MP). This radio is more of size comparison to the RCI 2950 DX, Radio works great and covers all three bands 10 meters, 11 meters and the 12 meter ham bands. Other operators say I sound excellent on the air and it is quite easy to use and has the CTCSS (PL) encoder/Decoder as well for repeater access, also has Only six memories, why ONLY six is beyond me! I am sure there literally hundreds of usable repeaters on the FM portion of the Ham band. I had it in my car for a short time but found it is a bit large for my little car ( A ford ecosport). I took it out and put my Yaesu FT891 back in. I miss the other ham band capabilities." - KelliePicklerFan on Amazon.com
-
"Radio is good, same board as the stryker 955 v2, it does have a overly sensitive receiver that could be a problem if you live in a noisy area." - Mark P. on Amazon.com
-
"This rig is terrific for the price. As others have noted, it uses the same new board as the newest generation of the Stryker 955, a fabulous radio. It's like getting the performance of the Stryker for just a bit more than half price. Transmit audio is excellent on all modes just using the stock mic. The radio is rock stable on sideband. There is no drifting. Mine arrived spot on frequency from the factory. The receiver is excellent also, very smooth sounding on all modes. My only complaint is the use of a RJ-45 jack for the mic. Anytone cheaped out on that. Yes, I know that many ham rigs use them. I have many and hate it on those rigs as well. They are cheap and the mic connectors break easily. Anytone had plenty of room to fit a standard 4 or 6 pin jack, making it easier to wire up amplified mics. Aside from that one issue, which can be remedied with an adapter, this is THE rig to get for the mobile or a nice base setup with a power supply. Great job Anytone." - Mike on Amazon.com
-
-
What are some of the alternatives to Anytone At 5555 V3 Software 14?
-
Some of the alternatives to Anytone At 5555 V3 Software 14 are:
-
-
Stryker SR-955HPC Software: This software is for Stryker SR-955HPC radio, which is similar to Anytone AT-5555 PLUS radio in features and specifications. It also supports CHIRP programming software.
-
President Lincoln II+ Software: This software is for President Lincoln II+ radio, which is another popular 10M mobile transceiver with similar features and specifications as Anytone AT-5555 PLUS radio.
-
Ranger RCI-2950DX Software: This software is for Ranger RCI-2950DX radio, which is another multi-mode rig that covers 10M, 11M, and 12M bands with similar features and specifications as Anytone AT-5555 PLUS radio.
-
-
Where can I buy Anytone AT-5555 PLUS radio?
-
You can buy Anytone AT-5555 PLUS radio from various online platforms such as Amazon.com, eBay.com, Moonrakeronline.com, etc. You can also find local dealers or distributors of Anytone products in your area by visiting https://www.anytone.net/contact.
- 0a6ba089eb
-
-
\ No newline at end of file
diff --git a/spaces/1acneusushi/gradio-2dmoleculeeditor/data/AutoCAD 2010 xforce keygen 32 bit Free download links and reviews for AutoCAD 2010 keygen.md b/spaces/1acneusushi/gradio-2dmoleculeeditor/data/AutoCAD 2010 xforce keygen 32 bit Free download links and reviews for AutoCAD 2010 keygen.md
deleted file mode 100644
index b067b029fed99f8825b6c53eef4823a38dc93ea2..0000000000000000000000000000000000000000
--- a/spaces/1acneusushi/gradio-2dmoleculeeditor/data/AutoCAD 2010 xforce keygen 32 bit Free download links and reviews for AutoCAD 2010 keygen.md
+++ /dev/null
@@ -1,171 +0,0 @@
-
-
Anno 1602 No CD Crack Download German
-
If you are a fan of strategy games, you might have heard of Anno 1602, a classic game that lets you build your own colony in the New World. However, if you want to play this game on your PC without having to insert the CD every time, you might need a no CD crack. In this article, we will show you what a no CD crack is, why you might need it, how to use it, and where to find it. We will also give you some tips and tricks for playing Anno 1602 with a no CD crack without any problems.
-
What is Anno 1602?
-
Anno 1602 is a real-time strategy game that was released in 1998 by Sunflowers Interactive Entertainment Software. The game is set in the 17th century, when European explorers and settlers were discovering and colonizing new lands in America. The game allows you to create your own civilization by managing resources, building structures, trading with other nations, and engaging in warfare. The game features a single-player campaign mode, a sandbox mode, and a multiplayer mode. The game is also known as 1602 A.D. in North America and Anno: Create a New World on Nintendo DS.
A no CD crack is a modified version of the game's executable file that allows you to run the game without having to insert the original CD in your drive. This can be useful for several reasons:
-
-
You don't have to worry about losing or damaging your CD.
-
You can save space on your hard drive by deleting or compressing the game files.
-
You can avoid potential errors or bugs caused by faulty or incompatible CD drivers.
-
You can play the game faster and smoother by reducing loading times.
-
-
However, there are also some drawbacks of using a no CD crack:
-
anno 1602 no cd patch german
-anno 1602 gold edition no cd crack german
-anno 1602 free download full version german
-anno 1602 ohne cd spielen german
-anno 1602 crack deutsch download
-anno 1602 windows 10 no cd crack german
-anno 1602 gog download german
-anno 1602 megagames no cd crack german
-anno 1602 v1.0 gold edition eng no cd crack
-anno 1602 v1.05 no cd crack german
-anno 1602 kaiserliche edition no cd crack german
-anno 1602 creation of a new world no cd crack german
-anno 1602 vollversion kostenlos downloaden deutsch
-anno 1602 auf windows 10 installieren ohne cd
-anno 1602 gog patch deutsch
-anno 1602 gogunlocked free download german
-anno 1602 system requirements windows 10 german
-anno 1602 cheats codes pc german
-anno 1602 tipps und tricks deutsch
-anno 1602 multiplayer lan ohne cd german
-anno 1602 soundtrack download mp3 german
-anno 1602 mods installieren deutsch
-anno 1602 editor tutorial deutsch
-anno 1602 komplettlösung pdf german
-anno 1602 handbuch download deutsch
-anno 1602 testbericht pc games german
-anno 1602 online spielen kostenlos deutsch
-anno 1602 steam key kaufen german
-anno 1602 amazon.de bestellen german
-anno 1602 ebay kleinanzeigen verkaufen german
-anno 1602 forum diskussionen deutsch
-anno 1602 wiki informationen deutsch
-anno 1602 youtube gameplay videos german
-anno 1602 twitch livestreams anschauen german
-anno 1602 reddit community beitreten german
-anno 1602 facebook fanseite folgen german
-anno 1602 twitter news updates erhalten german
-anno 1602 instagram bilder teilen german
-anno 1602 pinterest ideen sammeln german
-anno 1602 tiktok clips ansehen german
-anno 1602 discord server beitreten german
-anno 1602 wikipedia artikel lesen deutsch
-anno 1602 imdb bewertung abgeben german
-anno 1602 metacritic kritiken lesen german
-anno 1602 gamestar review lesen deutsch
-anno 1602 spieletipps.de ratgeber lesen deutsch
-anno 1602 chip.de download anleitung lesen deutsch
-anno 1602 computerbild.de download anleitung lesen deutsch
-
-
You might violate the game's license agreement or copyright laws by using an unauthorized copy.
-
You might expose your PC to viruses or malware by downloading files from untrusted sources.
-
You might encounter compatibility or stability issues with your game or system by using an outdated or incompatible crack.
-
You might miss out on some features or updates that require the original CD.
-
-
How to use a no CD crack for Anno 1602?
-
Using a no CD crack for Anno 1602 is not very difficult, but you need to follow some steps carefully:
-
-
Make sure you have installed the game on your PC from the original CD.
-
Make sure you have backed up your game files before applying any changes.
-
Download a no CD crack file from a reliable and safe source (we will provide some suggestions later).
-
Extract the file (usually a .zip or .rar archive) using a program like WinRAR or 7-Zip.
-
Copy the extracted file (usually a .exe file) and paste it into your game directory (usually C:\Program Files\Anno 1602 or C:\Program Files (x86)\Anno 1602).
-
Replace the existing file when prompted (you might need administrator privileges).
-
Run the game as usual from your desktop shortcut or start menu.
-
-
Where to find a no CD crack for Anno 1602?
-
There are many websites that offer no CD cracks for various games, but not all of them are trustworthy or safe. Some of them might contain viruses, malware, spyware, adware, or other unwanted programs that can harm your PC or steal your personal information. Therefore, you should always be careful when downloading files from unknown sources and scan them with an antivirus program before opening them. Here are some of the most reliable and safe sources for downloading a no CD crack for Anno 1602:
-
MegaGames
-
MegaGames is one of the oldest and most popular websites for downloading game fixes, patches, trainers, mods, cheats, and cracks. It has a large database of games and files that are updated regularly. It also has a user-friendly interface and a rating system that helps you find the best files for your needs. You can download a no CD crack for Anno 1602 from MegaGames here:
Select one of the available mirrors (preferably one with high speed).
-
Save the file (anno_ger.zip) on your PC.
-
Extract the file using WinRAR or 7-Zip.
-
Copy both files (crack.exe and anno.crk) into your game directory (C:\Program Files\Anno 1602 or C:\Program Files (x86)\Anno 1602).
-
Run crack.exe from DOS mode (you can use CMD or PowerShell).
-
Type "crack anno.crk" without quotes and press Enter.
-
The program will patch your game executable file (1602 .exe) and remove the CD/MOVIE and SOUND check.
-
Enjoy the game without the CD.
-
-
Other alternatives
-
If you are not satisfied with MegaGames or you want to try some other options, here are some other websites that offer no CD cracks for Anno 1602:
-
GameCopyWorld
-
GameCopyWorld is another well-known website for downloading game fixes, patches, trainers, mods, cheats, and cracks. It has a similar database and interface as MegaGames, but it also offers some additional features such as game manuals, covers, wallpapers, and music. You can download a no CD crack for Anno 1602 from GameCopyWorld here:
GameBurnWorld is a smaller website that specializes in game fixes, patches, trainers, mods, cheats, and cracks. It has a more simple and minimalist design, but it also has a decent collection of games and files that are updated frequently. You can download a no CD crack for Anno 1602 from GameBurnWorld here:
Tips and tricks for playing Anno 1602 with a no CD crack
-
Now that you have downloaded and installed a no CD crack for Anno 1602, you might want to know some tips and tricks for playing the game without any issues. Here are some of them:
-
How to backup your game files
-
Before applying any changes to your game files, such as using a no CD crack or installing a patch or mod, it is always recommended to backup your original files in case something goes wrong or you want to restore them later. To backup your game files, follow these steps:
-
-
Create a new folder on your PC where you want to store your backup files.
-
Go to your game directory (C:\Program Files\Anno 1602 or C:\Program Files (x86)\Anno 1602) and select all the files and folders.
-
Copy them and paste them into your backup folder.
-
Rename your backup folder as you wish (for example, Anno 1602 Original).
-
-
How to run the game in compatibility mode
-
Anno 1602 is an old game that was designed for Windows 95/98/ME. Therefore, it might not run properly on newer versions of Windows such as Windows 10. To fix any potential compatibility problems, you can try running the game in compatibility mode. To do so, follow these steps:
-
-
Right-click on your game executable file (1602.exe) and select Properties.
-
Go to the Compatibility tab and check the box that says "Run this program in compatibility mode for:".
-
Select the version of Windows that you want to use (for example, Windows XP Service Pack 3).
-
Click Apply and OK.
-
Run the game as usual.
-
-
How to update your game to the latest version
-
Anno 1602 has received several updates since its release that have improved its performance and added new features. However, some of these updates might require the original CD to work. To avoid this problem, you can use a no CD patch that updates your game to the latest version without needing the CD. To do so, follow these steps:
-
-
Download a no CD patch for Anno 1602 from one of the sources mentioned above (for example, MegaGames).
-
Extract the file (usually a .zip or .rar archive) using WinRAR or 7-Zip.
-
Copy the extracted file (usually a .exe file) and paste it into your game directory (C:\Program Files\Anno 1602 or C:\Program Files (x86)\Anno 1602).
-
Replace the existing file when prompted (you might need administrator privileges).
-
Run the patch as usual from your desktop shortcut or start menu.
-
The patch will update your game to the latest version (usually v1.05) without needing the CD.
-
-
Conclusion
-
Anno 1602 is a great game that deserves to be played by anyone who loves strategy games. However, if you don't want to deal with the hassle of inserting the CD every time you want to play it, you can use a no CD crack that allows you to run the game without it. In this article, we have shown you what a no CD crack is, why you might need it, how to use it, and where to find it. We have also given you some tips and tricks for playing Anno 1602 with a no CD crack without any issues. We hope you have found this article helpful and informative. Now go ahead and enjoy Anno 1602 without any limitations!
-
Frequently Asked Questions
-
Here are some of the most common questions that people ask about Anno 1602 and no CD cracks:
-
-
Is using a no CD crack illegal?
-
The answer to this question depends on your local laws and regulations. Generally speaking, using a no CD crack is not illegal if you own a legitimate copy of the game and you use it for personal use only. However, distributing or sharing a no CD crack with others might be considered piracy or copyright infringement. Therefore, we advise you to use a no CD crack at your own risk and discretion.
-
Will using a no CD crack affect my online gameplay?
-
Possibly. Some online servers or platforms might detect that you are using a modified version of the game and ban you from playing online. Therefore, we recommend that you use a no CD crack only for offline or single-player mode. If you want to play online with other players, you should use the original CD or buy a digital copy of the game from an authorized source.
-
Can I use mods or cheats with a no CD crack?
-
Yes. A no CD crack does not prevent you from using mods or cheats with Anno 1602. However, some mods or cheats might require specific versions of the game or patches to work properly. Therefore, you should always check the compatibility and requirements of any mod or cheat before installing it on your PC.
If you love Anno 1602 and you want to play more games like it, there are many options available. You can try other games in the Anno series such as Anno 1503, Anno 1701, Anno 1404, Anno 2070, Anno 2205, and Anno 1800. You can also try other strategy games such as Age of Empires, Civilization, Tropico, SimCity, and Cities: Skylines.
-
How can I contact the developers of Anno 1602?
-
If you have any questions, feedback, or issues regarding Anno 1602, you can try contacting the developers of the game. The original developer of Anno 1602 was Max Design, a German company that was founded in 1991 and closed in 2004. The current developer of the Anno series is Ubisoft Blue Byte, a German subsidiary of Ubisoft that was founded in 1988 and acquired by Ubisoft in 2001. You can contact Ubisoft Blue Byte through their official website here: https://bluebyte.ubisoft.com/en/.
-
- 0a6ba089eb
-
-
\ No newline at end of file
diff --git a/spaces/1acneusushi/gradio-2dmoleculeeditor/data/Ayitha Ezhuthu movie full movie in tamil hd 1080p A masterpiece by Mani Ratnam.md b/spaces/1acneusushi/gradio-2dmoleculeeditor/data/Ayitha Ezhuthu movie full movie in tamil hd 1080p A masterpiece by Mani Ratnam.md
deleted file mode 100644
index 11c59d196759618c7720c2c224381a019a1cc2cb..0000000000000000000000000000000000000000
--- a/spaces/1acneusushi/gradio-2dmoleculeeditor/data/Ayitha Ezhuthu movie full movie in tamil hd 1080p A masterpiece by Mani Ratnam.md
+++ /dev/null
@@ -1,101 +0,0 @@
-
-
Ayitha Ezhuthu: A Political Thriller That Changed Tamil Cinema
-
Introduction
-
Tamil cinema is known for its rich variety of genres, themes and styles. From romance to comedy, from action to drama, from fantasy to realism, Tamil movies have something for everyone. But one genre that has been relatively less explored in Tamil cinema is political thriller. Political thrillers are movies that deal with issues of power, corruption, justice and violence in the context of politics and society. They often feature complex plots, suspenseful twists, moral dilemmas and social commentary.
-
One of the most acclaimed and influential political thrillers in Tamil cinema is Ayitha Ezhuthu (2004), written and directed by Mani Ratnam. The movie is inspired by the Mexican film Amores Perros (2000), which tells three interconnected stories through a car accident. Ayitha Ezhuthu also uses a similar narrative device, but sets it in Chennai and focuses on three different men who are involved in a shooting incident on a bridge. The movie explores how their lives are changed by this event and how they are connected to each other and to the larger political scenario.
Ayitha Ezhuthu is a Tamil word that means "three dots". It is also the name of a letter in the Tamil alphabet, ஃ, which is used as a diacritic mark to modify the sound of other letters. The title of the movie refers to the three main characters, who are represented by three different colors: red, blue and green. The movie also uses these colors to create a distinct visual style and mood for each story.
-
Who are the main characters and actors?
-
The three main characters of Ayitha Ezhuthu are:
-
-
Michael Vasanth (played by Suriya), a charismatic student leader who wants to enter politics and fight against corruption. He is in love with Geetha (played by Esha Deol), his neighbor and childhood friend. He represents the color red, which symbolizes passion, courage and revolution.
-
Inba Sekar (played by Madhavan), a violent goon who works for Selvanayagam (played by Bharathiraja), a powerful politician who wants to eliminate his rivals. He is married to Sashi (played by Meera Jasmine), whom he abuses and neglects. He represents the color blue, which symbolizes violence, sadness and oppression.
-
Arjun Balakrishnan (played by Siddharth), a carefree and spoiled son of an IAS officer who wants to migrate to the US for a better future. He falls in love with Meera (played by Trisha Krishnan), a radio jockey who inspires him to change his outlook on life. He represents the color green, which symbolizes youth, hope and change.
-
-
The movie also features other supporting actors such as Karthi, Suchitra, R.S. Shivaji, Sindhu Shyam, Sriman and T.S. Suresh.
-
What is the plot of the movie?
-
The plot of Ayitha Ezhuthu revolves around a shooting incident that takes place on Napier Bridge in Chennai. The incident involves Michael, Inba and Arjun, who are strangers to each other but whose lives are intertwined by fate. The movie follows their stories before and after the incident and shows how they are affected by it.
-
Ayitha Ezhuthu 2004 political action film download
-Watch Suriya Madhavan Siddharth in Ayitha Ezhuthu online
-Ayitha Ezhuthu Tamil movie inspired by Amores perros
-A.R. Rahman songs from Ayitha Ezhuthu mp3 free
-Ayitha Ezhuthu movie review and ratings by critics
-Ayitha Ezhuthu cast and crew details and trivia
-Ayitha Ezhuthu movie scenes and dialogues video
-How to watch Ayitha Ezhuthu movie with subtitles
-Ayitha Ezhuthu movie box office collection and budget
-Ayitha Ezhuthu movie awards and nominations list
-Ayitha Ezhuthu movie behind the scenes and making
-Ayitha Ezhuthu movie wallpapers and posters hd
-Ayitha Ezhuthu movie quotes and memes images
-Ayitha Ezhuthu movie fan art and cosplay ideas
-Ayitha Ezhuthu movie analysis and themes explained
-Ayitha Ezhuthu movie comparison with Yuva Hindi version
-Ayitha Ezhuthu movie shooting locations and facts
-Ayitha Ezhuthu movie trivia quiz and games online
-Ayitha Ezhuthu movie best performances and scenes ranking
-Ayitha Ezhuthu movie controversies and scandals news
-Ayitha Ezhuthu movie director Mani Ratnam interview video
-Ayitha Ezhuthu movie remake and sequel possibilities
-Ayitha Ezhuthu movie influence on Tamil cinema and politics
-Ayitha Ezhuthu movie merchandise and collectibles buy
-Ayitha Ezhuthu movie book adaptation and novelization pdf
-Ayitha Ezhuthu Tamil full movie watch on YouTube free
-Suriya Madhavan Siddharth chemistry in Ayitha Ezhuthu film
-Trisha Meera Jasmine Esha Deol roles in Ayitha Ezhuthu movie
-Bharathiraja as Selvanayagam in Ayitha Ezhuthu performance
-Ravi K. Chandran cinematography in Ayitha Ezhuthu film
-A. Sreekar Prasad editing in Ayitha Ezhuthu movie
-A.R. Rahman background score in Ayitha Ezhuthu film
-Vairamuthu lyrics for Ayitha Ezhuthu songs meaning
-Hey Goodbye Nanba song from Ayitha Ezhuthu video hd
-Sandai Kozhi song from Ayitha Ezhuthu mp3 download free
-Jana Gana Mana song from Ayitha Ezhuthu lyrics translation
-Nenjam Ellam song from Ayitha Ezhuthu karaoke version
-Dol Dol song from Ayitha Ezhuthu dance choreography video
-Yakkai Thiri song from Ayitha Ezhuthu remix dj mp3 free download
-Fana Fanah Ye Dil Hua Fanah song from Yuva Hindi version video hd
-
The movie begins with Inba shooting Michael on his bike, resulting in him falling off the bridge into the water below. This is witnessed by Arjun, who was chasing Meera after proposing to her on the road. The movie then goes into a flashback mode and shows how each character reached that point.
-
Michael is an influential student leader who wants to contest in college elections and challenge Selvanayagam's dominance in politics. He faces opposition from Inba, who is hired by Selvanayagam to intimidate him and his supporters. Michael also has to deal with his relationship with Geetha, who wants him to stay away from politics for his safety.
-
Inba is a ruthless goon who works for Selvanayagam as his hitman. He has no qualms about killing or hurting anyone for money or power. He has a troubled marriage with Sashi, whom he beats regularly and forces her to abort their child. He also has a rivalry with Guna (played by Karthi), his brother-in-law who works for another politician.
-
Arjun is a rich and spoiled brat who has no aim or ambition in life. He wants to go to the US for higher studies but fails to get admission due to his poor grades. He meets Meera at a pub and falls in love with her at first sight. He tries to woo her with his charm and money but she rejects him initially.
-
The movie then returns to the present day and shows how the shooting incident affects each character's life. Michael survives the fall but loses his memory temporarily. He recovers with Geetha's help but faces threats from Selvanayagam's men who want to finish him off. He decides to fight back and expose Selvanayagam's corruption.
-
Inba escapes from the scene but is chased by Guna's men who want revenge for killing their boss. He also faces pressure from Sashi who wants him to leave his criminal life and start afresh elsewhere. He realizes his mistakes but finds it hard to change his ways.
-
Arjun saves Meera from being hit by Inba's car during the chase. He takes her to his house where he confesses his love for her again. She accepts him after seeing his genuine concern for her. He also decides to stay back in India and join Michael's campaign against Selvanayagam.
-
The movie ends with a climax where Michael confronts Selvanayagam at his office while Inba tries to stop him from killing him. Arjun arrives with Meera and helps Michael escape from Inba's attack. Inba shoots at Michael but misses him and hits Selvanayagam instead, killing him instantly. Inba then surrenders himself to the police while Michael celebrates his victory with Geetha and Arjun celebrates his love with Meera.
-
Analysis
-
How does the movie portray different aspects of politics and society?
-
Ayitha Ezhuthu is a movie that explores various aspects of politics and society in contemporary India. It shows how politics affects different people from different backgrounds and how they react to it differently.
-
The movie portrays politics as a complex and corrupt system that is dominated by powerful people who use violence, money and influence to manipulate others for their own interests. It also shows how politics can be used as a tool for positive change if people have courage, integrity and vision.
-
The movie also depicts society as a diverse and dynamic entity that consists of different classes, cultures, ideologies and aspirations. It shows how society can be divided by conflicts, prejudices, inequalities and injustices but also united by common goals, values and hopes.
-
How does the movie use the three dots motif to connect the stories?
-
Ayitha Ezhuthu uses the three dots motif as a symbolic device to connect the stories of its three main characters. The three dots represent three different perspectives, personalities and paths that converge at one point: the shooting incident on Napier Bridge.
-
The three dots also represent three different choices that each character makes: Michael chooses to fight for justice; Inba chooses to surrender to fate; Arjun chooses to change for love.
-
The three dots also represent three different outcomes that each character faces: Michael succeeds in his mission; Inba fails in his ambition; Arjun finds his purpose.
-
Here is the continuation of the article.
How does the movie challenge the stereotypes and expectations of Tamil cinema?
-
Ayitha Ezhuthu is a movie that challenges the stereotypes and expectations of Tamil cinema in many ways. It breaks away from the conventional formula of hero-centric, masala-oriented, melodramatic and escapist movies that are often seen in Tamil cinema. Instead, it offers a realistic, multi-layered, thought-provoking and engaging movie that deals with contemporary issues and themes.
-
The movie also challenges the stereotypes and expectations of the characters and actors. It shows the characters as complex and flawed human beings who have their own strengths and weaknesses, motivations and conflicts, choices and consequences. It also shows the actors in different and unconventional roles that showcase their versatility and talent.
-
For example, Suriya plays a role of a student leader who is not a typical hero who fights with his fists but with his words and ideas. Madhavan plays a role of a villain who is not a caricatured evil-doer but a conflicted and tragic character who has a backstory and a redemption arc. Siddharth plays a role of a lover boy who is not a cheesy romantic but a mature and responsible partner who supports his girlfriend's dreams.
-
Reception
-
How did the critics and audience react to the movie?
-
Ayitha Ezhuthu received mostly positive reviews from the critics and audience. The movie was praised for its screenplay, direction, performances, music, cinematography and editing. The movie was also appreciated for its bold and innovative approach to storytelling, its social relevance and its message.
-
The movie was also compared with its Hindi version, Yuva, which was released on the same day. Many critics and viewers felt that Ayitha Ezhuthu was superior to Yuva in terms of its authenticity, coherence, depth and impact. Some also felt that Ayitha Ezhuthu had better casting, acting and chemistry than Yuva.
-
However, the movie also faced some criticism from some quarters. Some critics and viewers felt that the movie was too slow-paced, too complex, too preachy or too unrealistic. Some also felt that the movie had some flaws in its logic, continuity and climax.
-
What were the awards and accolades that the movie received?
-
Ayitha Ezhuthu received several awards and accolades for its excellence in various aspects of filmmaking. The movie won one Filmfare Award South for Best Music Director (A.R. Rahman) and one Tamil Nadu State Film Award for Best Film (Second Prize). The movie was also nominated for six Filmfare Awards South for Best Film, Best Director (Mani Ratnam), Best Actor (Suriya), Best Supporting Actor (Madhavan), Best Supporting Actress (Meera Jasmine) and Best Lyricist (Vairamuthu).
-
The movie also received recognition from various other prestigious platforms such as National Film Awards, International Indian Film Academy Awards, Zee Cine Awards, Screen Awards, Stardust Awards and Vijay Awards.
-
What was the impact of the movie on Tamil cinema and culture?
-
Ayitha Ezhuthu had a significant impact on Tamil cinema and culture. The movie inspired many filmmakers to experiment with different genres, styles and techniques of storytelling. The movie also influenced many actors to take up challenging and diverse roles that showcase their range and potential.
-
The movie also had an impact on Tamil society and politics. The movie raised awareness about various issues such as corruption, violence, education, youth empowerment and social change. The movie also motivated many young people to participate in politics and activism.
-
Conclusion
-
Summary of the main points
-
In conclusion, Ayitha Ezhuthu is a political thriller that changed Tamil cinema by offering a realistic, multi-layered, thought-provoking and engaging movie that deals with contemporary issues and themes. The movie follows three different men who are involved in a shooting incident on a bridge and shows how their lives are changed by it. The movie explores various aspects of politics and society through their stories. The movie also challenges the stereotypes and expectations of Tamil cinema by breaking away from the conventional formula of hero-centric, masala-oriented, melodramatic and escapist movies. The movie received mostly positive reviews from the critics and audience for its screenplay, direction, performances, music, cinematography and editing. The movie also received several awards and accolades for its excellence in various aspects of filmmaking. The movie also had a significant impact on Tamil cinema and culture by inspiring many filmmakers and actors to experiment with different genres, styles and techniques of storytelling. The movie also influenced many young people to participate in politics and activism.
-
Personal opinion and recommendation
-
Personally, I think Ayitha Ezhuthu is one of the best movies ever made in Tamil cinema. I think it is a masterpiece that showcases Mani Ratnam's brilliance as a writer and director. I think it is a movie that has everything: drama, action, romance, comedy, suspense, thrill, emotion, message and entertainment. I think it is a movie that makes you think, feel and act.
-
I would highly recommend Ayitha Ezhuthu to anyone who loves movies. I think it is a movie that everyone should watch at least once in their lifetime. I think it is a movie that will stay with you forever.
- **FAQs**
-Q: Where can I watch Ayitha Ezhuthu online? A: You can watch Ayitha Ezhuthu online on platforms such as Amazon Prime Video or Hotstar. Q: Is Ayitha Ezhuthu based on a true story? A: No, Ayitha Ezhuthu is not based on a true story. It is inspired by the Mexican film Amores Perros (2000), which tells three interconnected stories through a car accident. Q: What is the meaning of Ayitha Ezhuthu? A: Ayitha Ezhuthu means "three dots" in Tamil. It is also the name of a letter in the Tamil alphabet, ஃ , which is used as a diacritic mark to modify the sound of other letters. Q: Who composed the music for Ayitha Ezhuthu? A: A.R. Rahman composed the music for Ayitha Ezhuthu. He won one Filmfare Award South for Best Music Director for his work. Q: What are some other movies like Ayitha Ezhuthu? A: Some other movies like Ayitha Ezhuthu are Yuva (2004), which is the Hindi version of Ayitha Ezhuthu; Vettaiyaadu Vilaiyaadu (2006), which is another political thriller by Mani Ratnam; Ko (2011), which is another political thriller featuring Suriya; Sarkar (2018), which is another political thriller featuring Vijay; Kaappaan (2019), which is another political thriller featuring Suriya.
- 0a6ba089eb
-
-
\ No newline at end of file
diff --git a/spaces/1acneusushi/gradio-2dmoleculeeditor/data/Bruno Mars Unorthodox Jukebox Rar 4shared..md b/spaces/1acneusushi/gradio-2dmoleculeeditor/data/Bruno Mars Unorthodox Jukebox Rar 4shared..md
deleted file mode 100644
index 1fd6dbba93bf85dbcc204c88bd606de764fb33ae..0000000000000000000000000000000000000000
--- a/spaces/1acneusushi/gradio-2dmoleculeeditor/data/Bruno Mars Unorthodox Jukebox Rar 4shared..md
+++ /dev/null
@@ -1,15 +0,0 @@
-
-
Download Bruno Mars' Unorthodox Jukebox Album for Free
-
If you are a fan of Bruno Mars, you might want to download his second studio album, Unorthodox Jukebox, for free. This album was released in 2012 and features 15 tracks, including hit singles like "Locked Out of Heaven", "When I Was Your Man", and "Treasure". The album showcases Bruno Mars' diverse musical influences, ranging from pop, rock, funk, soul, reggae, and R&B.
-
One way to download Unorthodox Jukebox for free is to use the file-sharing website 4shared[^3^]. This website allows users to upload and download files of various types, such as music, videos, documents, and more. You can find a link to the album in rar format on 4shared[^3^], which means you will need a software like WinRAR or 7-Zip to extract the files after downloading.
Another way to download Unorthodox Jukebox for free is to use the Internet Archive[^1^]. This website is a non-profit digital library that preserves and provides access to millions of free books, movies, music, and more. You can find a link to the album in ogg vorbis format on the Internet Archive[^1^], which means you will need a software like VLC or Foobar2000 to play the files after downloading.
-
Before you download Unorthodox Jukebox for free, you might want to learn more about the album and its lyrics. You can visit Genius[^2^], a website that provides annotations and explanations for songs, albums, artists, and more. You can find a link to the album page on Genius[^2^], where you can read the lyrics, watch the music videos, and discover the meanings behind Bruno Mars' songs.
-
Unorthodox Jukebox is a great album that showcases Bruno Mars' talent and versatility as a singer, songwriter, and producer. If you want to download it for free, you can use 4shared[^3^], the Internet Archive[^1^], or other similar websites. However, if you want to support Bruno Mars and his music, you can also buy the album from official sources like iTunes, Amazon, or Spotify.
-
-
Unorthodox Jukebox has received mostly positive reviews from critics, who praised Bruno Mars' musical versatility, vocal performance, and songwriting skills. The album has a Metascore of 70 out of 100 on Metacritic[^1^], based on 16 reviews. Some critics compared Bruno Mars to Michael Jackson, Prince, and Sting, while others noted his influences from various genres and eras of music.
-
The album was also a commercial success, selling over six million copies worldwide and topping the charts in several countries. It was nominated for four Grammy Awards, including Album of the Year and Best Pop Vocal Album, and won one for Best Pop Solo Performance for "When I Was Your Man". The album spawned five singles, all of which reached the top 10 on the Billboard Hot 100 chart. "Locked Out of Heaven" and "When I Was Your Man" both reached number one, making Bruno Mars the first male artist to achieve two number-one singles from the same album since Justin Timberlake in 2006.
-
Unorthodox Jukebox is a testament to Bruno Mars' talent and ambition as an artist who can transcend genres and styles with ease and flair. It is an album that showcases his range as a singer, his skill as a songwriter, and his vision as a producer. It is an album that will make you dance, sing, cry, and fall in love with Bruno Mars all over again.
- 7b8c122e87
-
-
\ No newline at end of file
diff --git a/spaces/1acneusushi/gradio-2dmoleculeeditor/data/Caterpillar Software Keygen Maker Generate Factory Passwords for CAT ECM Programming[2].md b/spaces/1acneusushi/gradio-2dmoleculeeditor/data/Caterpillar Software Keygen Maker Generate Factory Passwords for CAT ECM Programming[2].md
deleted file mode 100644
index 61780db00b456afbe28d941e3d585359717a9cb7..0000000000000000000000000000000000000000
--- a/spaces/1acneusushi/gradio-2dmoleculeeditor/data/Caterpillar Software Keygen Maker Generate Factory Passwords for CAT ECM Programming[2].md
+++ /dev/null
@@ -1,124 +0,0 @@
-
-
Caterpillar Software Keygen Maker: What You Need to Know
-
If you are a Caterpillar equipment owner, technician or dealer, you may have heard of Caterpillar Software Keygen Maker. This is a software tool that can generate factory passwords for various functions and parameters in the Caterpillar Electronic Technician (ET) software. In this article, we will explain what Caterpillar Software Keygen Maker is, why you need it, how to use it, and where to get it.
Caterpillar Software Keygen Maker is a software tool that can generate factory passwords for the Caterpillar Electronic Technician (ET) software. Factory passwords are required in order to perform certain functions and change certain parameters in the Caterpillar ET software, such as:
-
-
Change the system configuration parameters
-
Rerate the engine to another engine family
-
Read customer passwords
-
Clear certain diagnostic trouble codes
-
Unlock a customer specified parameter that is locked
-
Change certain customer specified parameters
-
-
Caterpillar Software Keygen Maker can generate factory passwords based on the information displayed by the Caterpillar ET software, such as:
-
-
ECM serial number
-
Reason code
-
Request code
-
Challenge code
-
-
Caterpillar Software Keygen Maker can generate factory passwords for different versions of Caterpillar ET software, such as 2016A-B-2022A.
-
Why do you need Caterpillar Software Keygen Maker?
-
You may need Caterpillar Software Keygen Maker if you want to perform certain functions or change certain parameters in the Caterpillar ET software that require factory passwords. For example, you may want to:
-
-
Change the system configuration parameters when you replace the Engine Control Module (ECM)
-
Rerate the engine to another engine family for better performance or fuel efficiency
-
Read customer passwords to access or modify customer settings
-
Clear certain diagnostic trouble codes that cannot be cleared by normal methods
-
Unlock a customer specified parameter that is locked by mistake or by the previous owner
-
Change certain customer specified parameters to suit your needs or preferences
-
-
Without Caterpillar Software Keygen Maker, you would have to contact the authorized Caterpillar dealer or service center to obtain the factory passwords. This may take time, money and hassle. With Caterpillar Software Keygen Maker, you can generate the factory passwords yourself and perform the functions or change the parameters in the Caterpillar ET software quickly and easily.
-
How to use Caterpillar Software Keygen Maker?
-
To use Caterpillar Software Keygen Maker, you need to have the following:
-
-
A computer or device that can run Windows 2003/XP/Vista/7/8/10 32 and 64 bit operating systems
-
A USB key or a download link for the Caterpillar Software Keygen Maker software
-
A compatible version of Caterpillar ET software installed on your computer or device
-
A communication adapter that can connect your computer or device with your Caterpillar equipment
-
-
The steps to use Caterpillar Software Keygen Maker are as follows:
Connect your computer or device with your Caterpillar equipment using the communication adapter.
-
Launch the Caterpillar ET software and select the ECM that you want to work on.
-
Select the function or parameter that you want to perform or change in the Caterpillar ET software.
-
The Caterpillar ET software will request you to enter two passwords: a customer password and a factory password.
-
If you know the customer password, enter it. If not, leave it blank.
-
The Caterpillar ET software will display some information that is required to obtain the factory password, such as ECM serial number, reason code, request code and challenge code.
-
Launch the Caterpillar Software Keygen Maker software and enter the information displayed by the Caterpillar ET software.
-
The Caterpillar Software Keygen Maker software will generate a factory password based on the information entered.
-
Enter the factory password generated by the Caterpillar Software Keygen Maker software into the Caterpillar ET software.
-
The function or parameter will be performed or changed in the Caterpillar ET software.
-
-
Features and benefits of Caterpillar Software Keygen Maker
-
Support for different versions of Caterpillar Electronic Technician (ET)
-
Caterpillar Software Keygen Maker can generate factory passwords for different versions of and connect with the ECM that you want to work on; Select the function or parameter that you want to perform or change in CAT ET software; The CAT ET software will request you to enter two passwords: a customer password and a factory password; If you know the customer password, enter it. If not, leave it blank; The CAT ET software will display some information that is required to obtain the factory password, such as ECM serial number, reason code, request code and challenge code; Launch Caterpillar Software Keygen Maker software and enter the information displayed by CAT ET software; The Caterpillar Software Keygen Maker software will generate a factory password based on the information entered; Enter the factory password generated by Caterpillar Software Keygen Maker software into CAT ET software; The function or parameter will be performed or changed in CAT ET software.
-
- # FAQs
-
What is CAT ET software?
-
CAT ET software is an electronic service tool that allows you to communicate with your Caterpillar equipment's Engine Control Module (ECM). You can use CAT ET software to diagnose problems, monitor performance, calibrate settings, program features, test components, etc.
-
What are factory passwords?
-
Factory passwords are special codes that are required in order to perform certain functions or change certain parameters in CAT ET software that are protected by the manufacturer. Factory passwords are different from customer passwords that are set by the equipment owner or operator.
-
Why do I need factory passwords?
-
You may need factory passwords if you want to perform certain functions or change certain parameters in CAT ET software that require factory passwords. For example, you may want to change the system configuration parameters when you replace the ECM, rerate the engine to another engine family, read customer passwords, clear certain diagnostic trouble codes, unlock a customer specified parameter that is locked, change certain customer specified parameters, etc.
-
How do I get factory passwords?
-
You can get factory passwords by using Caterpillar Software Keygen Maker, which is a software tool that can generate factory passwords based on the information displayed by CAT ET software. You can buy Caterpillar Software Keygen Maker from various online sources, such as , or . You can choose to buy it with a USB key or a download link.
-
How do I use Caterpillar Software Keygen Maker?
-
To use Caterpillar Software Keygen Maker, you need to have a computer or device that can run Windows 2003/XP/Vista/7/8/10 32 and 64 bit operating systems, a USB key or a download link for the Caterpillar Software Keygen Maker software, a compatible version of CAT ET software installed on your computer or device, and a communication adapter that can connect your computer or device with your Caterpillar equipment. You need to follow these steps: Connect your computer or device with your Caterpillar equipment using the communication adapter; Launch CAT ET software and connect with the ECM that you want to work on; Select the function or parameter that you want to perform or change in CAT ET software; The CAT ET software will request you to enter two passwords: a customer password and a factory password; If you know the customer password, enter it. If not, leave it blank; The CAT ET software will display some information that is required to obtain the factory password, such as ECM serial number, reason code, request code and challenge code; Launch Caterpillar Software Keygen Maker software and enter the information displayed by CAT ET software; The Caterpillar Software Keygen Maker software will generate a factory password based on the information entered; Enter the factory password generated by Caterpillar Software Keygen Maker software into CAT ET software; The function or parameter will be performed or changed in CAT ET software.
-
- 0a6ba089eb
-
-
\ No newline at end of file
diff --git a/spaces/1gistliPinn/ChatGPT4/Examples/Clone Drone In The Danger Zone V0.12.1.21 Crack Free.md b/spaces/1gistliPinn/ChatGPT4/Examples/Clone Drone In The Danger Zone V0.12.1.21 Crack Free.md
deleted file mode 100644
index 088b7dc0c218c7155308353880f526041a1365ad..0000000000000000000000000000000000000000
--- a/spaces/1gistliPinn/ChatGPT4/Examples/Clone Drone In The Danger Zone V0.12.1.21 Crack Free.md
+++ /dev/null
@@ -1,10 +0,0 @@
-
Clone Drone in the Danger Zone v0.12.1.21 crack free
-
-Click on «Create new project» and select «Database Project» (in case you don't have previous projects, choose «Create new database» and select «Database Project».
-
-Place a 'blank' database and name it in English (as a standard naming convention) and allow it to be created..
-
-1.0.0.4) сглажить файл после загрузки файла. Иногда все эти редактирования приходятся выполнять при помощи программы. Для того, чтобы сгладить файл после загрузки, нажмите в прозрачной части окна этой программы, чтобы открыть окно «Сглаживание». Нажмите кнопку «Сглажить». Выберите файл, из которого вы хотите сгладить. Нажмите кнопку «Создать». Выберите такой пункт в списке «Источники», который будет с� 4fefd39f24
-
-
-
diff --git a/spaces/1pelhydcardo/ChatGPT-prompt-generator/assets/Blue Dream MP3 by Jhen Aiko - Listen and Download from Souled Out (Deluxe).md b/spaces/1pelhydcardo/ChatGPT-prompt-generator/assets/Blue Dream MP3 by Jhen Aiko - Listen and Download from Souled Out (Deluxe).md
deleted file mode 100644
index 760cd11e0ddfb93108abe615643082af0eec3c0d..0000000000000000000000000000000000000000
--- a/spaces/1pelhydcardo/ChatGPT-prompt-generator/assets/Blue Dream MP3 by Jhen Aiko - Listen and Download from Souled Out (Deluxe).md
+++ /dev/null
@@ -1,106 +0,0 @@
-
-
Jhené Aiko's Blue Dream: A Song Review
-
If you are looking for a song that can transport you to a blissful state of love and happiness, you might want to check out Jhené Aiko's Blue Dream. This song is one of the bonus tracks from her debut album Souled Out, which was released in 2014. In this article, we will review the song and explore its lyrics, music, and meaning.
-
Introduction
-
Who is Jhené Aiko?
-
Jhené Aiko is an American singer and songwriter who was born in Los Angeles, California. She started her music career as a teenager, appearing on several R&B and hip-hop tracks. She gained more recognition after collaborating with artists like Drake, Big Sean, and Kendrick Lamar. She is known for her smooth and soulful voice, as well as her poetic and introspective lyrics. Some of her popular songs include The Worst, Sativa, Triggered, and B.S.
Blue Dream is a song that expresses Jhené Aiko's love and admiration for someone who opened her eyes to the beauty of life. She describes how this person made her see the truth in things and be in a dreamlike state where nothing else matters. She also talks about how this feeling is something that is blissful and mystical, and how she does not want to wake up from it. The song title refers to a type of cannabis strain that is known for its euphoric and relaxing effects.
-
Analysis of the song
-
The lyrics
-
The meaning of the verses
-
The first verse sets the scene for the song, as Jhené Aiko recalls a time when she was with her lover on the coast. She says that he opened her eyes to the beauty of the ocean and the sky, which symbolize the vastness and depth of their love. She also says that she was blinded before, implying that she was unaware or unhappy with her life until he came along. She then asks him to be hers, showing her desire and commitment.
-
The second verse talks about how Jhené Aiko feels when she is with her lover. She says that she is still sleeping in her blue dream, meaning that she is still in love and content with him. She also says that she knows the meaning for all the seasons, suggesting that she understands the cycles and changes of life because of him. She then says that he is the reason for her love, indicating that he inspires and motivates her.
-
The meaning of the chorus
-
The chorus repeats the main theme of the song, which is Jhené Aiko's love for her lover. She says that she does not want to wake up because she is in love with all that he is. She also says that he makes her see the truth in things, meaning that he helps her see things clearly and realistically. She then says that he is the remedy for everything, implying that he heals and soothes her from any pain or problems. She also says that he is the truth itself, meaning that he is honest and genuine with her. Finally, she says that nothing else can take her so far, meaning that no one else can make her feel as happy and fulfilled as he does.
-
The meaning of the bridge
-
The bridge emphasizes the blissful and mystical nature of Jhené Aiko's love for her lover. She says that her afternoon dream is when the world is sleeping, meaning that she feels like they are in their own world where nothing else matters. She also says that she is still thinking of her blue dream, meaning that she is still in love and content with him. She also says that she is in love with all that he is, echoing the chorus. She then says that he is the truth in things, meaning that he is the essence and reality of everything. She also says that he is the remedy for everything, repeating the chorus. Finally, she says that nothing else can take her so far, ending the song with the same line as the chorus.
-
The music
-
The genre and style
-
Blue Dream is a song that belongs to the genre of neo-soul, which is a subgenre of R&B that incorporates elements of jazz, funk, hip-hop, and electronic music. The song has a smooth and laid-back style, with a slow tempo and a minimalistic production. The song also has a psychedelic and dreamy vibe, with a soft and ambient sound.
-
jhene aiko blue dream song download
-jhene aiko souled out blue dream mp3
-jhene aiko blue dream lyrics download
-jhene aiko blue dream audio download
-jhene aiko blue dream free mp3
-jhene aiko blue dream instrumental download
-jhene aiko blue dream album download
-jhene aiko blue dream video download
-jhene aiko blue dream remix mp3
-jhene aiko blue dream ringtone download
-jhene aiko blue dream spotify download
-jhene aiko blue dream soundcloud mp3
-jhene aiko blue dream live performance download
-jhene aiko blue dream acoustic version mp3
-jhene aiko blue dream deluxe edition download
-jhene aiko blue dream zip file download
-jhene aiko blue dream 320kbps mp3
-jhene aiko blue dream flac download
-jhene aiko blue dream itunes download
-jhene aiko blue dream amazon mp3
-jhene aiko blue dream google play download
-jhene aiko blue dream youtube mp3
-jhene aiko blue dream genius lyrics download
-jhene aiko blue dream shazam music download
-jhene aiko blue dream last.fm track download
-jhene aiko blue dream tidal download
-jhene aiko blue dream deezer download
-jhene aiko blue dream pandora download
-jhene aiko blue dream iheartradio download
-jhene aiko blue dream napster download
-jhene aiko blue dream slacker radio download
-jhene aiko blue dream tunein radio download
-jhene aiko blue dream audiomack download
-jhene aiko blue dream datpiff download
-jhene aiko blue dream spinrilla download
-jhene aiko blue dream mymixtapez download
-jhene aiko blue dream livemixtapes download
-jhene aiko blue dream hotnewhiphop download
-jhene aiko blue dream djbooth download
-jhene aiko blue dream rap-up download
-jhene aiko blue dream complex download
-jhene aiko blue dream billboard download
-jhene aiko blue dream rolling stone download
-jhene aiko blue dream pitchfork download
-jhene aiko blue dream stereogum download
-jhene aiko blue dream vibe download
-jhene aiko blue dream xxl download
-jhene aiko blue dream the fader download
-jhene aiko blue dream the source download
-
The instruments and vocals
-
The song features a simple and sparse instrumentation, consisting of a keyboard, a guitar, a bass, and a drum machine. The keyboard provides a mellow and soothing melody, while the guitar adds some subtle chords and riffs. The bass adds some low-end and groove, while the drum machine creates a steady and relaxed beat. The song also features some background vocals that harmonize with Jhené Aiko's voice, creating a rich and ethereal texture.
-
Jhené Aiko's vocals are the highlight of the song, as she delivers a captivating and expressive performance. Her voice is smooth and soulful, with a delicate and airy tone. She sings with a lot of emotion and nuance, conveying her feelings of love and happiness. She also uses some vocal techniques such as falsetto, vibrato, and melisma, adding some variation and flair to her singing.
-
The mood and atmosphere
-
The song creates a mood and atmosphere of bliss and ecstasy, as it reflects Jhené Aiko's love for her lover. The song evokes a sense of peace and joy, as well as a sense of wonder and awe. The song also creates a feeling of intimacy and connection, as it portrays Jhené Aiko's bond with her lover. The song transports the listener to a dreamlike state where nothing else matters but love.
-
Conclusion
-
Why is Blue Dream a great song?
-
Blue Dream is a great song because it showcases Jhené Aiko's talent and artistry as a singer and songwriter. The song is beautifully written and composed, with poetic and meaningful lyrics, and soothing and enchanting music. The song is also emotionally engaging and relatable, as it expresses Jhené Aiko's love for her lover in a genuine and heartfelt way. The song is a testament to Jhené Aiko's ability to create soulful and captivating songs that touch the listener's soul.
-
Where can you listen to or download Blue Dream?
-
If you want to listen to or download Blue Dream, you have several options available. You can stream the song on various music platforms such as Spotify, Apple Music, YouTube Music, or SoundCloud. You can also purchase or download the song from online stores such as iTunes, Amazon Music, or Google Play Music. Alternatively, you can watch the official lyric video of the song on YouTube, or listen to it on Jhené Aiko's official website.
We hope you enjoyed this article and learned more about Jhené Aiko's Blue Dream. If you have any questions or comments, feel free to leave them below. Thank you for reading!
-
FAQs
-
What is the name of Jhené Aiko's debut album?
-
Jhené Aiko's debut album is called Souled Out, which was released in 2014. The album features 14 tracks, including Blue Dream, which is one of the bonus tracks.
-
Who produced Blue Dream?
-
Blue Dream was produced by Fisticuffs, who is a duo of producers consisting of Brian Warfield and Mac Robinson. They have worked with Jhené Aiko on several other songs, such as The Worst, Bed Peace, and W.A.Y.S.
-
What are some other songs by Jhené Aiko that are similar to Blue Dream?
-
Some other songs by Jhené Aiko that are similar to Blue Dream in terms of genre, style, and theme are Eternal Sunshine, While We're Young, Spotless Mind, and Comfort Inn Ending.
-
What are some of the awards and nominations that Jhené Aiko has received for her music?
-
Jhené Aiko has received several awards and nominations for her music, such as three Grammy nominations, two BET Awards, one Soul Train Music Award, one NAACP Image Award, and one MTV Video Music Award.
-
What are some of the influences and inspirations that Jhené Aiko has for her music?
-
Jhené Aiko has cited various influences and inspirations for her music, such as Tupac Shakur, John Mayer, Sade, Lauryn Hill, Eminem, Kendrick Lamar, and her brother Miyagi Chilombo, who passed away in 2012.
197e85843d
-
-
\ No newline at end of file
diff --git a/spaces/1phancelerku/anime-remove-background/Ball Brick Breaker Game A Free and Easy to Play Brick Breaking Game for Everyone!.md b/spaces/1phancelerku/anime-remove-background/Ball Brick Breaker Game A Free and Easy to Play Brick Breaking Game for Everyone!.md
deleted file mode 100644
index 85cfb65d4169b83aef67de4b408c740889c1a67c..0000000000000000000000000000000000000000
--- a/spaces/1phancelerku/anime-remove-background/Ball Brick Breaker Game A Free and Easy to Play Brick Breaking Game for Everyone!.md
+++ /dev/null
@@ -1,90 +0,0 @@
-
-
Ball Brick Breaker Game Download: How to Play and Enjoy this Fun Offline Game
-
If you are looking for a fun and addictive game that you can play offline anytime, anywhere, you should download ball brick breaker game. Ball brick breaker game is a classic arcade game that requires you to aim and shoot balls to break bricks on the board. It is easy to play but hard to master. You need to find the best positions and angles to hit every brick and clear the stages. You also need to use power-ups and boosters to pass harder levels and collect gems and stars to unlock new balls. Ball brick breaker game has tons of unique puzzles and challenges that will keep you entertained for hours. You can also compete with your friends and other players worldwide to see who can break more bricks and get higher scores. In this article, we will show you how to play and enjoy ball brick breaker game, as well as how to download it from Google Play Store or App Store.
The basic gameplay of ball brick breaker game is simple. You just need to swipe or tap on the screen to shoot balls to wherever you touched. The balls will fly and bounce off the walls and bricks on the board. Each brick has a number on it that indicates how many times you need to hit it to break it. You need to break all the bricks on the board before they reach the bottom of the screen. If they do, you will lose a life and have to restart the level.
-
Use Power-ups and Boosters to Pass Harder Levels
-
As you progress in ball brick breaker game, you will encounter more difficult levels with more bricks and obstacles. To help you pass these levels, you can use power-ups and boosters that have different effects. For example, you can use the fireball to burn through bricks, the bomb to explode nearby bricks, the laser to shoot a beam of light that breaks bricks in a line, and the rainbow to change the color of the balls. You can also use the extra ball to add more balls to your shot, the aim line to see the trajectory of the balls, and the undo to undo your last shot. You can get power-ups and boosters by breaking special bricks or by watching ads.
-
Collect Gems and Stars to Unlock New Balls
-
Another way to make ball brick breaker game more fun and exciting is to collect gems and stars that are scattered on the board. Gems are used to buy new balls that have different shapes, colors, and patterns. Stars are used to unlock new worlds that have different themes, backgrounds, and music. You can also get gems and stars by completing achievements and daily missions. There are hundreds of balls and worlds to unlock in ball brick breaker game, so you will never get bored of playing it.
-
How to Enjoy Ball Brick Breaker Game
-
Play Offline Anytime, Anywhere
-
One of the best features of ball brick breaker game is that you can play it offline anytime, anywhere. You don't need an internet connection or wifi to enjoy this game. You can play it on your phone or tablet whenever you have some free time or need some relaxation. You can also pause and resume the game anytime you want. Ball brick breaker game is a perfect game for offline gaming.
-
Compete with Friends and Other Players Worldwide
-
If you want to add some challenge and competition to ball brick breaker game, you can also play it online with your friends and other players worldwide. You can connect your game account to Facebook or Google Play Games and see how your scores compare with others on the leaderboard. You can also invite your friends to play with you and see who can break more bricks and get higher scores. You can also chat with other players and share tips and tricks on how to play ball brick breaker game better.
-
ball brick breaker game download free
-ball brick breaker game download offline
-ball brick breaker game download for pc
-ball brick breaker game download for android
-ball brick breaker game download apk
-ball brick breaker game download ios
-ball brick breaker game download app store
-ball brick breaker game download google play
-ball brick breaker game download windows 10
-ball brick breaker game download mac
-ball brick breaker game download online
-ball brick breaker game download no ads
-ball brick breaker game download unlimited
-ball brick breaker game download mod
-ball brick breaker game download hack
-ball brick breaker game download cheats
-ball brick breaker game download tips
-ball brick breaker game download tricks
-ball brick breaker game download guide
-ball brick breaker game download review
-ball brick breaker game download rating
-ball brick breaker game download best
-ball brick breaker game download fun
-ball brick breaker game download addictive
-ball brick breaker game download challenging
-ball brick breaker game download classic
-ball brick breaker game download legend
-ball brick breaker game download quest
-ball brick breaker game download crusher
-ball brick breaker game download shooter
-ball brick breaker game download blast
-ball brick breaker game download smash
-ball brick breaker game download puzzle
-ball brick breaker game download arcade
-ball brick breaker game download casual
-ball brick breaker game download physics
-ball brick breaker game download strategy
-ball brick breaker game download levels
-ball brick breaker game download stages
-ball brick breaker game download balls
-ball brick breaker game download bricks
-ball brick breaker game download colors
-ball brick breaker game download power-ups
-ball brick breaker game download gems
-ball brick breaker game download stars
-ball brick breaker game download coins
-ball brick breaker game download rewards
-ball brick breaker game download leaderboard
-ball brick breaker game download multiplayer
-
Explore Tons of Unique Puzzles and Challenges
-
Ball brick breaker game is not just a simple arcade game that repeats the same levels over and over again. It is a game that has tons of unique puzzles and challenges that will test your skills and creativity. Each level has a different layout, design, and goal that you need to achieve. Some levels have moving bricks, rotating bricks, invisible bricks, or other special bricks that add more variety and fun to the game. Some levels also have time limits, score limits, or other conditions that make them more difficult and rewarding. Ball brick breaker game has over 1000 levels that you can play and enjoy.
-
How to Download Ball Brick Breaker Game
-
Download from Google Play Store or App Store
-
If you want to download ball brick breaker game on your device, you can easily do so from Google Play Store or App Store. Just search for "ball brick breaker game" on the store and tap on the install button. The game is free to download and play, but it contains ads and in-app purchases that you can disable if you want.
-
Install and Launch the Game
-
After downloading ball brick breaker game from the store, you just need to install it on your device and launch it. The game will start with a tutorial that will show you how to play the game and use the controls. You can skip the tutorial if you already know how to play or replay it if you need a refresher.
-
Start Playing and Breaking Bricks
-
Once you have installed and launched ball brick breaker game, you can start playing and breaking bricks right away. You can choose which world and level you want to play from the map screen or let the game choose for you randomly. You can also adjust the settings of the game such as the sound, music, vibration, language, etc. from the menu screen. You can also access your achievements, missions, leaderboard, shop, etc. from there.
-
Conclusion: Ball Brick Breaker Game is a Fun and Addictive Game for Everyone
-
In conclusion, ball brick breaker game is a fun and addictive game for everyone who loves arcade games. It is a game that requires you to aim and shoot balls to break bricks on the board. It is easy to play but hard to master. It has tons of unique puzzles and challenges that will keep you entertained for hours. You can also play it offline anytime, anywhere, or online with your friends and other players worldwide. You can also collect gems and stars to unlock new balls and worlds that have different themes and features. Ball brick breaker game is a game that you should download and play if you want to have some fun and relaxation.
-
FAQs about Ball Brick Breaker Game
-
Here are some of the frequently asked questions about ball brick breaker game that you might want to know:
-
Q1: Is ball brick breaker game free to play?
-
A1: Yes, ball brick breaker game is free to play. However, it contains ads and in-app purchases that you can disable if you want.
-
Q2: How many levels are there in ball brick breaker game?
-
A2: There are over 1000 levels in ball brick breaker game, each with a different layout, design, and goal. You can play them in any order or let the game choose for you randomly.
-
Q3: What are the best strategies to break bricks?
-
A3: Some of the best strategies to break bricks are to aim for the corners and edges of the board, to use power-ups and boosters wisely, to avoid hitting the bottom of the screen, and to plan your shots ahead.
-
Q4: How can I get more gems and stars?
-
A4: You can get more gems and stars by breaking special bricks, completing achievements and daily missions, watching ads, or buying them with real money.
-
Q5: How can I contact the developer of ball brick breaker game?
-
A5: You can contact the developer of ball brick breaker game by sending an email to support@ballbrickbreaker.com or by visiting their website at www.ballbrickbreaker.com.
197e85843d
-
-
\ No newline at end of file
diff --git a/spaces/1phancelerku/anime-remove-background/Black Adam English Subtitles How to Download and Enjoy the Epic Movie.md b/spaces/1phancelerku/anime-remove-background/Black Adam English Subtitles How to Download and Enjoy the Epic Movie.md
deleted file mode 100644
index e045cfa33ac7519a8d677be5bc5756f32b325c02..0000000000000000000000000000000000000000
--- a/spaces/1phancelerku/anime-remove-background/Black Adam English Subtitles How to Download and Enjoy the Epic Movie.md
+++ /dev/null
@@ -1,218 +0,0 @@
-
-
Black Adam Movie English Subtitles Download: How to Watch the DC Superhero Film Online
-
If you are a fan of DC Comics and superhero movies, you might be interested in watching Black Adam, the latest film in the DC Extended Universe (DCEU). But what if you are not a native English speaker, or you have trouble understanding the dialogue or accents in the movie? In that case, you might need to download English subtitles for Black Adam, so you can enjoy the film without missing any important details. In this article, we will tell you everything you need to know about Black Adam movie English subtitles download, including what the movie is about, why you need subtitles, how to download them, and how to watch the movie online with subtitles.
-
What is Black Adam about?
-
Black Adam is a spin-off of Shazam! (2019), another DCEU film that introduced the magical superhero Shazam, who is powered by the ancient wizard of the same name. Black Adam is Shazam's arch-nemesis, who was also given the powers of the wizard, but became corrupted and tried to conquer the world. He was banished by Shazam and returned to Earth after 5,000 years, seeking revenge and justice. He is an anti-hero who clashes with the Justice Society of America, a team of superheroes that includes Hawkman, Doctor Fate, Atom Smasher, and Cyclone.
The movie begins in 2600 BC, in the fictional kingdom of Kahndaq, where Teth-Adam (Dwayne Johnson) was born. He was enslaved by the evil King Ahk-Ton, who created the Crown of Sabbac to attain great power. Teth-Adam led a revolt against the king, and was given the powers of Shazam by the Council of Wizards. He became Kahndaq's champion and killed Ahk-Ton, but he also became ruthless and tyrannical. Shazam intervened and imprisoned Teth-Adam in a magic tomb.
-
In the present day, Kahndaq is oppressed by Intergang, a criminal organization that wants to find the Crown of Sabbac. Adrianna Tomaz (Sarah Shahi), an archaeologist and resistance fighter, tries to locate the crown with her brother Karim (Mohammed Amer) and their colleagues Samir (James Cusati-Moyer) and Ishmael (Marwan Kenzari). They accidentally free Teth-Adam from his tomb, who vows to liberate Kahndaq from Intergang and restore his glory.
-
Meanwhile, the Justice Society of America (JSA), a group of superheroes that works for the US government, learns about Teth-Adam's return and decides to stop him. The JSA consists of Hawkman (Aldis Hodge), Doctor Fate (Pierce Brosnan), Atom Smasher (Noah Centineo), and Cyclone (Quintessa Swindell). They confront Teth-Adam in Kahndaq, but he proves to be too powerful for them. He also reveals that he is Karim's father and Adrianna's husband, who were separated from him when he was imprisoned.
-
Teth-Adam eventually finds the Crown of Sabbac, which grants him even more power. He declares himself as Black Adam, the ruler of Kahndaq. He also offers Karim and Adrianna to join him, but they refuse, saying that he has become a monster. They join forces with the JSA to stop him from using the crown to destroy the world. A final battle ensues, where Black Adam faces Shazam (Zachary Levi), who arrives to help the JSA. The fate of Kahndaq and the world hangs in the balance.
-
The cast of Black Adam
-
The movie features a star-studded cast of actors, who bring the comic book characters to life. Here are some of the main cast members and their roles:
-
black adam film english subs download
-black adam 2022 english subtitles free download
-download black adam movie with english subtitles
-black adam full movie english subtitles download
-black adam english srt subtitles download
-black adam movie english subbed download
-black adam movie download in english subtitles
-black adam movie english subtitles online download
-black adam movie yify subtitles english download
-black adam movie webrip subtitles english download
-black adam movie bluray subtitles english download
-black adam movie hdrip subtitles english download
-black adam movie dvdrip subtitles english download
-black adam movie x264 subtitles english download
-black adam movie aac5.1 subtitles english download
-black adam movie repack subtitles english download
-black adam movie zip subtitles english download
-black adam movie srt file subtitles english download
-how to download black adam movie with english subtitles
-where to download black adam movie with english subtitles
-best site to download black adam movie with english subtitles
-fastest way to download black adam movie with english subtitles
-easiest way to download black adam movie with english subtitles
-safest way to download black adam movie with english subtitles
-legal way to download black adam movie with english subtitles
-watch black adam movie online with english subtitles download
-stream black adam movie online with english subtitles download
-torrent black adam movie with english subtitles download
-magnet link black adam movie with english subtitles download
-direct link black adam movie with english subtitles download
-google drive link black adam movie with english subtitles download
-mega link black adam movie with english subtitles download
-dropbox link black adam movie with english subtitles download
-mediafire link black adam movie with english subtitles download
-zippyshare link black adam movie with english subtitles download
-utorrent black adam movie with english subtitles download
-bittorrent black adam movie with english subtitles download
-vuze black adam movie with english subtitles download
-qbittorrent black adam movie with english subtitles download
-deluge black adam movie with english subtitles download
-
-
Dwayne Johnson as Teth-Adam / Black Adam: The main protagonist and anti-hero of the movie, who is an ancient warrior with the powers of Shazam. He is driven by a sense of justice and vengeance, but also has a dark and violent side.
-
Sarah Shahi as Adrianna Tomaz / Isis: The main female lead and love interest of Black Adam, who is an archaeologist and resistance fighter in Kahndaq. She is also the mother of Karim, who is Black Adam's son.
-
Aldis Hodge as Carter Hall / Hawkman: The leader of the JSA, who is a reincarnated warrior with the ability to fly and wield a mystical mace. He has a history with Black Adam, as they were enemies in their past lives.
-
Pierce Brosnan as Kent Nelson / Doctor Fate: A member of the JSA, who is a powerful sorcerer and the host of Nabu, an ancient spirit of order. He wears the Helmet of Fate, which grants him various magical abilities.
-
Noah Centineo as Al Rothstein / Atom Smasher: A member of the JSA, who is a young and cocky superhero with the ability to manipulate his size and strength. He idolizes Black Adam, but also questions his methods.
-
Quintessa Swindell as Maxine Hunkel / Cyclone: A member of the JSA, who is a cheerful and optimistic superheroine with the ability to control wind and sound. She is the granddaughter of Ma Hunkel, the original Red Tornado.
-
Zachary Levi as Billy Batson / Shazam: The main hero of Shazam!, who is a teenage boy with the ability to transform into an adult superhero with the powers of Shazam. He is Black Adam's arch-nemesis and ally of the JSA.
-
Mohammed Amer as Karim Tomaz / Osiris: The son of Black Adam and Adrianna, who is unaware of his true heritage. He is a rebellious and adventurous teenager, who joins his mother in fighting against Intergang.
-
James Cusati-Moyer as Samir: A colleague and friend of Adrianna, who is an expert in ancient languages and artifacts. He helps her in finding the Crown of Sabbac.
-
Marwan Kenzari as Ishmael / Sabbac: The main antagonist of the movie, who is the leader of Intergang in Kahndaq. He is a ruthless and ambitious criminal, who wants to use the Crown of Sabbac to gain immense power.
-
-
The release date of Black Adam
-
The movie was originally scheduled to be released on December 22, 2021, but it was delayed due to the COVID-19 pandemic. The new release date is July 29, 2022. The movie will be distributed by Warner Bros. Pictures and will be available in theaters and on HBO Max (for 31 days after theatrical release).
-
Why do you need subtitles for Black Adam?
-
If you are not a native English speaker, or you have difficulty understanding some parts of the movie, you might want to download subtitles for Black Adam. Subtitles are text versions of the dialogue or narration that appear on the screen, usually at the bottom. They can help you follow along with what is happening in the movie, and also improve your language skills. Here are some reasons why you might need subtitles for Black Adam:
-
The benefits of subtitles for non-native speakers
-
If English is not your first language, subtitles can help you in many ways:
-
-
They can enhance your comprehension. Subtitles can help you understand the plot, the characters, the emotions, and the jokes in the movie. They can also clarify any words or phrases that you might not know or hear clearly.
-
They can improve your vocabulary and grammar. Subtitles can expose you to new words and expressions that you might not encounter in your everyday life. They can also show you how sentences are formed and punctuated in English.
-
They can boost your listening and speaking skills. Subtitles can help you practice your pronunciation and intonation by mimicking the actors' voices. They can also help you improve your listening comprehension by matching the sounds with the written words.
-
-
The challenges of subtitles for different languages and dialects
-
However, subtitles are not perfect, and they might have some limitations or drawbacks depending on the language and dialect of the movie. Here are some challenges that you might face when using subtitles for Black Adam:
-
-
They might not be accurate or complete. Subtitles are usually created by human translators or automated software, which might make mistakes or omit some information. For example, subtitles might not capture the nuances, idioms, slang, or humor of the original dialogue. They might also skip some words or sentences that are not essential for the plot, but might add some flavor or context to the movie.
-
They might not match the speed or timing of the dialogue. Subtitles are usually synchronized with the audio of the movie, but sometimes they might be delayed or ahead of the speech. This might cause confusion or distraction for the viewers, who have to read and listen at the same time. Subtitles might also appear too fast or too slow for some viewers, depending on their reading level and preference.
-
They might not suit the style or tone of the movie. Subtitles are usually written in a standard or formal way, which might not reflect the personality or mood of the characters or the movie. For example, subtitles might not convey the sarcasm, irony, anger, or excitement of the dialogue. They might also use different fonts, colors, or sizes that might clash with the aesthetics of the movie.
-
-
The availability of subtitles for Black Adam
-
The good news is that subtitles for Black Adam are widely available online, both officially and unofficially. You can find subtitles in various languages and formats, such as SRT, SSA, ASS, SUB, IDX, etc. Here are some ways to access subtitles for Black Adam:
-
-
You can check the official sources. The movie itself might have subtitles embedded in it, either as a default option or as a selectable feature. You can also look for subtitles on the official website of the movie, or on the streaming platforms that host the movie, such as HBO Max. These sources are likely to have high-quality and reliable subtitles that match the movie.
-
You can search for fan-made subtitles. There are many websites and communities that offer subtitles created by fans or volunteers, such as Subscene, OpenSubtitles, YIFY Subtitles, etc. These sources might have more variety and diversity of subtitles in terms of language and style. However, they might also have lower quality and accuracy of subtitles, and some of them might contain viruses or malware.
-
-
How to download subtitles for Black Adam?
-
If you want to download subtitles for Black Adam, you need to be careful and responsible. Downloading subtitles is not illegal per se, but it might involve some legal and ethical issues depending on the source and use of the subtitles. Here are some things to consider before downloading subtitles for Black Adam:
-
The legal and ethical issues of downloading subtitles
-
Downloading subtitles is a form of file sharing, which might infringe on the intellectual property rights of the creators and owners of the movie and the subtitles. You might also violate the terms and conditions of the streaming platforms or websites that provide the movie and the subtitles. Here are some legal and ethical issues that you might encounter when downloading subtitles for Black Adam:
-
-
You might be breaking the law. Depending on the jurisdiction and the laws of your country, downloading subtitles might be considered as piracy, which is a criminal offense that can result in fines or imprisonment. You might also be liable for civil damages if you infringe on the copyrights or trademarks of the movie and the subtitles.
-
You might be harming the industry. Downloading subtitles might reduce the revenue and profit of the movie and the subtitles, which can affect the livelihood and creativity of the filmmakers, actors, writers, translators, and other workers involved in the production and distribution of the movie and the subtitles. You might also discourage the creation and availability of more movies and subtitles in the future.
-
You might be disrespecting the culture. Downloading subtitles might undermine the artistic and cultural value of the movie and the subtitles, which can reflect the vision, identity, and expression of the original creators and speakers of the movie and the subtitles. You might also miss out on some of the subtleties, nuances, and meanings of the movie and the subtitles that are lost or altered in translation.
-
-
Therefore, before downloading subtitles for Black Adam, you should ask yourself these questions:
-
-
Is it legal? Check the laws and regulations of your country regarding downloading subtitles, and make sure you are not violating any of them.
-
Is it ethical? Consider the impact and consequences of downloading subtitles on the movie industry, the subtitle community, and the movie culture, and make sure you are not harming or offending any of them.
-
Is it necessary? Evaluate your needs and preferences for downloading subtitles, and make sure you are not doing it for frivolous or selfish reasons.
-
-
The best sources and websites for downloading subtitles
-
If you decide to download subtitles for Black Adam, you should choose your sources and websites carefully. You should look for reputable and reliable sources and websites that offer high-quality and accurate subtitles that match the movie. You should also avoid sources and websites that offer low-quality or fake subtitles that might contain errors, spoilers, viruses, or malware. Here are some criteria to look for when choosing sources and websites for downloading subtitles:
-
-
They have a good reputation and rating. Check the reviews and feedback of other users who have downloaded subtitles from these sources and websites, and see if they are satisfied with their experience. You can also look for ratings or rankings from trusted websites or organizations that evaluate subtitle sources and websites based on their quality, reliability, security, etc.
-
They have a large and diverse collection of subtitles. Look for sources and websites that offer a wide range of subtitles in different languages, formats, styles, etc. You can also look for sources and websites that offer subtitles that are compatible with the movie, such as the version, the quality, the resolution, etc.
-
They have a clear and easy interface and process. Look for sources and websites that have a user-friendly and intuitive design and layout, that allow you to search, browse, select, and download subtitles with ease and convenience. You can also look for sources and websites that have clear and detailed instructions and guidelines on how to download subtitles.
-
They have a secure and safe system and policy. Look for sources and websites that have a strong and reliable security and privacy system and policy, that protect your device and data from any potential threats or risks. You can also look for sources and websites that have a fair and transparent terms and conditions and disclaimer on their service and content.
-
-
Based on these criteria, here are some of the best sources and websites for downloading subtitles for Black Adam:
A popular and trusted website that offers subtitles in various languages, formats, styles, etc. It has a large and active community of subtitle creators and users, who upload, download, rate, comment, and request subtitles. It also has a simple and easy interface and process for downloading subtitles.
A well-known and reputable website that offers subtitles in multiple languages, formats, styles, etc. It has a huge and diverse collection of subtitles for movies, TV shows, documentaries, etc. It also has a clear and detailed interface and process for downloading subtitles.
A dedicated and reliable website that offers subtitles for YIFY movies, which are high-quality movies with small file sizes. It has a wide range of subtitles in different languages, formats, styles, etc. It also has a user-friendly and convenient interface and process for downloading subtitles.
A professional and quality website that offers subtitles in various languages, formats, styles, etc. It has a sophisticated and advanced system for creating, editing, syncing, translating, and downloading subtitles. It also has a clear and easy interface and process for downloading subtitles.
A specialized and quality website that offers subtitles for TV shows, movies, web series, etc. It has a dedicated and passionate team of subtitle creators and users, who work together to provide accurate and timely subtitles. It also has a simple and easy interface and process for downloading subtitles.
-
-
-
The steps and tips for downloading subtitles
-
If you have chosen your source and website for downloading subtitles for Black Adam, you can follow these general steps and tips for downloading subtitles:
-
-
Search for the movie and the subtitle language. Enter the name of the movie and the language of the subtitle that you want to download in the search box of the website. You can also use filters or categories to narrow down your search results.
-
Select the subtitle file that matches the movie. Choose the subtitle file that has the same version, quality, resolution, etc. as the movie that you have or want to watch. You can also check the ratings, comments, or previews of the subtitle file to see if it is good or not.
-
Download the subtitle file to your device. Click on the download button or link of the subtitle file, and save it to your device. You might need to unzip or extract the subtitle file if it is compressed or archived.
-
Rename and move the subtitle file to the same folder as the movie. Rename the subtitle file to have the same name as the movie file, except for the extension. For example, if your movie file is called Black.Adam.2022.1080p.BluRay.x264.YIFY.mp4, your subtitle file should be called Black.Adam.2022.1080p.BluRay.x264.YIFY.srt. Then, move the subtitle file to the same folder or location as the movie file.
-
Play the movie with subtitles using a media player. Open the movie file with a media player that supports subtitles, such as VLC, MPC-HC, KMPlayer, etc. The subtitles should appear automatically on the screen. If not, you can manually enable them by clicking on the subtitle button or menu of the media player.
-
-
Here are some tips to make your subtitle downloading experience better:
-
-
Use a VPN or proxy service. If you are downloading subtitles from sources or websites that are blocked or restricted in your country or region, you might need to use a VPN (virtual private network) or proxy service to access them. A VPN or proxy service can hide your IP address and location, and allow you to browse anonymously and securely.
-
Use a malware scanner or antivirus software. If you are downloading subtitles from sources or websites that are not verified or trusted, you might need to use a malware scanner or antivirus software to scan and protect your device and data from any potential threats or risks. A malware scanner or antivirus software can detect and remove any viruses, malware, spyware, etc. that might be hidden in the subtitle files.
-
Use a subtitle editor or converter. If you are downloading subtitles that are not compatible or suitable for your movie or media player, you might need to use a subtitle editor or converter to edit or convert them. A subtitle editor or converter can help you adjust the timing, format, style, language, etc. of the subtitles to make them fit your needs and preferences.
-
-
How to watch Black Adam online with subtitles?
-
If you have downloaded subtitles for Black Adam, you can watch the movie online with subtitles using various streaming platforms and devices. Streaming platforms are online services that allow you to watch movies and other content on demand, while devices are gadgets or tools that enable you to access and play the streaming platforms. Here are some ways to watch Black Adam online with subtitles:
-
The streaming platforms and devices that support subtitles
-
There are many streaming platforms and devices that support subtitles, but not all of them are compatible or available for Black Adam. You need to check the compatibility and availability of the streaming platforms and devices for Black Adam before choosing them. Here are some of the most popular and common streaming platforms and devices that support subtitles:
Smart TV, laptop, desktop, tablet, smartphone, game console, etc.
-
The official and exclusive streaming platform for Black Adam, which offers the movie in HD quality and with subtitles in various languages. You need to subscribe to HBO Max to watch the movie, which costs $14.99 per month or $99.99 per year.
Smart TV, laptop, desktop, tablet, smartphone, game console, etc.
-
A popular and widely available streaming platform that offers a large collection of movies and other content, including Black Adam. You need to rent or buy the movie on Amazon Prime Video to watch it, which costs $5.99 to $19.99 depending on the quality and format. You can also watch the movie with subtitles in various languages.
Smart TV, laptop, desktop, tablet, smartphone, game console, etc.
-
A well-known and global streaming platform that offers a huge variety of movies and other content, but not Black Adam. However, you can use a VPN or proxy service to access Netflix from other regions or countries that might have Black Adam available. You need to subscribe to Netflix to watch the movie, which costs $8.99 to $17.99 per month depending on the plan. You can also watch the movie with subtitles in various languages.
Laptop, desktop, tablet, smartphone, game console, etc.
-
A free and universal streaming platform that offers a vast amount of movies and other content, but not Black Adam. However, you can use a VPN or proxy service to access YouTube from other regions or countries that might have Black Adam available. You can watch the movie for free or for a fee depending on the uploader and the quality. You can also watch the movie with subtitles in various languages.
-
-
-
The settings and options for enabling subtitles
-
If you have chosen your streaming platform and device for watching Black Adam online, you need to enable subtitles on them. Enabling subtitles is usually a simple and easy process, but it might vary depending on the streaming platform and device that you use. Here are some general steps and tips for enabling subtitles:
-
-
Launch the streaming platform and play the movie. Open the streaming platform that you want to use on your device, and search for Black Adam. Then, click on the play button or link to start watching the movie.
-
Access the subtitle menu or button. Look for the subtitle menu or button on the screen, which might be labeled as CC, Subtitles, Captions, etc. It might be located on the bottom, top, or side of the screen, or hidden under a settings or options icon. Click on the subtitle menu or button to open it.
-
Select the subtitle language and format. Choose the subtitle language that you want to use from the list of available languages. You might also be able to choose the subtitle format, such as font, color, size, position, etc. from the list of available options. Click on the subtitle language and format that you prefer to apply them.
-
Enjoy the movie with subtitles. The subtitles should appear on the screen according to your selection. You can adjust or change them at any time by accessing the subtitle menu or button again. You can also turn them off if you don't need them anymore.
-
-
Here are some tips to make your subtitle watching experience better:
-
-
Choose a subtitle language that matches your level and goal. If you want to improve your English skills, you might want to choose English subtitles that match your level of proficiency and comprehension. If you want to learn a new language, you might want to choose subtitles in that language that match your goal and interest.
-
Choose a subtitle format that suits your preference and comfort. If you want to read the subtitles easily and clearly, you might want to choose a subtitle format that has a high contrast and visibility with the background and the movie. If you want to avoid distraction or clutter on the screen, you might want to choose a subtitle format that has a low profile and minimalism with the movie.
-
Sync the subtitles with the audio and video of the movie. If you notice that the subtitles are not in sync with the audio or video of the movie, you might want to adjust the timing or speed of the subtitles to match them. You can do this by using the subtitle menu or button, or by using a subtitle editor or converter.
-
Compare and contrast the subtitles with the audio and video of the movie. If you want to enhance your learning and enjoyment of the movie, you might want to compare and contrast the subtitles with the audio and video of the movie. You can do this by paying attention to the differences and similarities between the written and spoken words, the expressions and emotions of the actors, the context and culture of the movie, etc.
-
-
Conclusion
-
Black Adam is a movie that you might want to watch online with subtitles, especially if you are not a native English speaker, or you have trouble understanding some parts of the movie. Subtitles can help you comprehend, enjoy, and learn from the movie, but they also have some challenges and limitations. Therefore, you need to be careful and responsible when downloading and using subtitles for Black Adam. You also need to choose the best sources and websites for downloading subtitles, and the best streaming platforms and devices for watching the movie online with subtitles. By following these tips and steps, you can have a great subtitle watching experience with Black Adam.
-
FAQs
-
Here are some frequently asked questions (FAQs) about Black Adam movie English subtitles download:
-
-
Q: Is Black Adam a sequel or a prequel to Shazam?
-
A: Black Adam is neither a sequel nor a prequel to Shazam. It is a spin-off that takes place in the same universe as Shazam, but focuses on a different character and story. However, Black Adam and Shazam are connected by their origin and powers, and they might meet in a future crossover movie.
-
Q: How long is Black Adam?
-
A: The official runtime of Black Adam is not yet confirmed, but it is estimated to be around 2 hours and 15 minutes. This might change depending on the final editing and post-production of the movie.
-
Q: Where can I watch Black Adam online legally?
-
A: The only legal and official way to watch Black Adam online is through HBO Max, which is the exclusive streaming platform for Black Adam. You need to subscribe to HBO Max to watch Black Adam online, which costs $14.99 per month or $99.99 per year. You can also watch Black Adam in theaters if they are open and safe in your area.
-
Q: How can I download Black Adam online legally?
-
A: The only legal and official way to download Black Adam online is through Amazon Prime Video, which offers the movie for rent or purchase. You need to pay a fee to download Black Adam on Amazon Prime Video, which costs $5.99 to $19.99 depending on the quality and format. You can also download Black Adam from other sources or websites, but they might not be legal or safe.
-
Q: How can I get subtitles for Black Adam online legally?
-
A: The easiest and safest way to get subtitles for Black Adam online is to use the official sources and websites that provide the movie and the subtitles, such as HBO Max and Amazon Prime Video. They offer subtitles in various languages and formats that match the movie. You can also get subtitles from other sources or websites, but they might not be legal or reliable.
- 401be4b1e0
-
-
\ No newline at end of file
diff --git a/spaces/1phancelerku/anime-remove-background/Challenge Opponents from Around the World in F1 Mobile Racing 2021 The Best F1 Multiplayer Game.md b/spaces/1phancelerku/anime-remove-background/Challenge Opponents from Around the World in F1 Mobile Racing 2021 The Best F1 Multiplayer Game.md
deleted file mode 100644
index 2ef4bf942f82ae06c4ae55f880587bb57fbffe32..0000000000000000000000000000000000000000
--- a/spaces/1phancelerku/anime-remove-background/Challenge Opponents from Around the World in F1 Mobile Racing 2021 The Best F1 Multiplayer Game.md
+++ /dev/null
@@ -1,114 +0,0 @@
-
-
Download F1 Mobile Racing 2021: The Official Game of the FIA Formula One World Championship
-
If you are a fan of Formula One, you will love F1 Mobile Racing 2021, the official free-to-play game of the 2021 FIA Formula One World Championship. This game lets you experience the thrill of racing against real players from around the world, as well as the official teams, drivers, and circuits of this season. You can also customize your own F1 car and upgrade it with new performance parts to dominate the grid. With stunning audio and visual quality, regular content updates, and exciting events, F1 Mobile Racing 2021 is the ultimate F1 game for your mobile device. In this article, we will show you how to download and play this amazing game.
-
Features of F1 Mobile Racing 2021
-
F1 Mobile Racing 2021 has many features that make it stand out from other racing games. Here are some of them:
Real-time PvP racing with players from around the world: You can challenge other players in fast-paced duels or join global leagues and tournaments to compete for glory. You can also race against your friends or rivals in custom lobbies.
-
Official teams, drivers, and circuits of the 2021 season: You can choose to represent one of the ten teams from this season's F1 grid, such as Mercedes, Red Bull, Ferrari, McLaren, or Aston Martin. You can also race as one of the twenty drivers, including Lewis Hamilton, Max Verstappen, Charles Leclerc, Lando Norris, or Sebastian Vettel. You can also race on all the official circuits of this season, such as Bahrain, Monaco, Silverstone, Spa-Francorchamps, or Abu Dhabi.
-
Customizable F1 car design and performance parts: You can create your own F1 car and personalize it with different liveries, helmets, stickers, and more. You can also discover new performance parts and upgrade your car's engine, chassis, aerodynamics, brakes, and tyres. You can also use different setups and strategies to suit different tracks and conditions.
-
Immersive audio and visual quality: You can enjoy realistic sound effects and music that capture the atmosphere of a real F1 race. You can also admire the stunning graphics and animations that bring the cars and tracks to life. You can also adjust the camera angles and views to suit your preference.
-
Regular content updates and events: You can always find something new and exciting in F1 Mobile Racing 2021. The game is updated regularly with new features, improvements, bug fixes, and more. You can also participate in special events that offer unique challenges and rewards. For example, you can join the Grand Prix™ events that follow the real-life F1 calendar or try out the Time-Limited events that test your skills on different tracks.
-
-
How to Download F1 Mobile Racing 2021
-
F1 Mobile Racing 2021 is available for both iOS and Android devices. Here are some things you need to know before downloading the game:
-
-
Requirements and compatibility for iOS and Android devices: F1 Mobile Racing 2021 requires iOS 12.0 or later and Android 6.0 or later to run. The game also requires a stable internet connection and at least 2.5 GB of free storage space. The game is compatible with most devices, but some older or low-end devices may experience performance issues or crashes. You can check the list of supported devices on the game's official website.
-
Steps to download and install the game from the App Store or Google Play Store: To download the game, you need to follow these simple steps:
-
Open the App Store or Google Play Store on your device and search for "F1 Mobile Racing 2021".
-
Tap on the game icon and then tap on the "Get" or "Install" button.
-
Wait for the game to download and install on your device. This may take a few minutes depending on your internet speed and device performance.
-
Once the game is installed, tap on the game icon to launch it and enjoy!
-
-
-
Tips to optimize the game settings and performance: To ensure a smooth and enjoyable gaming experience, you can follow these tips:
-
Close any other apps running in the background before launching the game.
-
Adjust the graphics quality and frame rate settings in the game options to suit your device capabilities.
-
Use a Wi-Fi connection instead of mobile data to avoid lag or disconnection issues.
-
Keep your device updated with the latest software and security patches.
-
Contact the game's customer support if you encounter any problems or bugs.
-
-
-
-
How to Play F1 Mobile Racing 2021
-
F1 Mobile Racing 2021 is easy to play but hard to master. Here are some things you need to know before you start racing:
-
-
Game modes: Career, Duels, Events, and more: F1 Mobile Racing 2021 offers various game modes for different levels of challenge and fun. You can choose from:
-
Career: This is where you start your journey as an F1 driver. You can create your own team, customize your car, and compete in different seasons and championships. You can also unlock new performance parts, liveries, helmets, stickers, and more as you progress.
-
Duels: This is where you race against other players in real-time PvP matches. You can choose from different race types, such as Sprint, Grid Start, Qualifying, or Endurance. You can also earn trophies, XP, credits, and rewards based on your performance.
-
Events: This is where you participate in special events that follow the real-life F1 calendar or offer unique challenges. You can race on different tracks, with different weather conditions, car setups, and rules. You can also win exclusive prizes, such as rare performance parts, legendary liveries, or even real F1 merchandise.
-
Other modes: You can also try out other modes, such as Time Trial, Practice, or Test Drive. These modes allow you to practice your skills, test your car's performance, or just have fun without any pressure.
-
-
-
Controls and gameplay tips: F1 Mobile Racing 2021 has intuitive and responsive controls that let you steer, accelerate, brake, and use DRS and ERS with ease. You can choose from different control schemes, such as Tilt, Touch, or Virtual Wheel. You can also adjust the sensitivity and feedback settings in the game options. Here are some gameplay tips to help you race better:
-
Follow the racing line: The racing line is a colored line that shows you the optimal path to take on each corner. It changes from green to yellow to red depending on your speed and braking point. Try to follow the racing line as much as possible to avoid losing time or crashing.
-
Use DRS and ERS wisely: DRS (Drag Reduction System) and ERS (Energy Recovery System) are two features that can boost your speed and performance. DRS allows you to open a flap on your rear wing to reduce drag and increase top speed on certain straights. ERS allows you to harvest energy from braking and use it to boost your engine power on demand. You can activate DRS and ERS by tapping on their icons on the screen. However , you need to use them strategically, as they have limited availability and can affect your car's handling and fuel consumption.
-
Manage your tyres and brakes: Your tyres and brakes are essential for your car's performance and safety. However, they can wear out and overheat over time, affecting your grip and braking. You need to monitor your tyre and brake temperatures and adjust your driving style accordingly. You can also choose different tyre compounds and brake modes to suit different tracks and conditions.
-
Learn from the best: You can watch replays of your own races or other players' races to learn from their mistakes and successes. You can also follow the tips and tutorials in the game to improve your skills and knowledge.
-
-
-
Progression and rewards system: F1 Mobile Racing 2021 has a rewarding progression system that lets you level up, earn credits, unlock new items, and more. Here are some ways you can progress and earn rewards in the game:
-
Complete missions: Missions are tasks that challenge you to achieve certain goals or milestones in the game. For example, you may be asked to win a certain number of races, reach a certain league, or use a certain car part. Completing missions will reward you with XP, credits, or other prizes.
-
Open crates: Crates are boxes that contain random items, such as performance parts, liveries, helmets, stickers, or credits. You can earn crates by winning races, completing missions, or participating in events. You can also buy crates with real money or watch ads to get free crates.
-
Join the F1 Pass: The F1 Pass is a subscription service that gives you access to exclusive benefits and rewards. For example, you can get more XP, credits, crates, performance parts, liveries, helmets, stickers, and more. You can also get access to premium events and content. You can choose from different F1 Pass plans depending on your budget and preference.
-
-
-
-
Conclusion
-
F1 Mobile Racing 2021 is the ultimate F1 game for your mobile device. It lets you race against real players from around the world, as well as the official teams, drivers, and circuits of this season. It also lets you customize your own F1 car and upgrade it with new performance parts. It also offers immersive audio and visual quality, regular content updates, and exciting events. F1 Mobile Racing 2021 is easy to download and play, but hard to master. It offers various game modes, controls, and gameplay tips to suit different levels of challenge and fun. It also has a rewarding progression system that lets you level up, earn credits, unlock new items, and more.
-
If you are a fan of Formula One, you should not miss this game. Download F1 Mobile Racing 2021 today and join the F1 community. You will have a blast racing against other players and experiencing the thrill of F1.
-
How to download f1 mobile racing 2021 for free
-Download f1 mobile racing 2021 apk mod
-F1 mobile racing 2021 tips and tricks
-F1 mobile racing 2021 best car setup
-F1 mobile racing 2021 career mode guide
-F1 mobile racing 2021 cheats and hacks
-F1 mobile racing 2021 review and ratings
-F1 mobile racing 2021 gameplay and features
-F1 mobile racing 2021 official game from codemasters
-F1 mobile racing 2021 season update and news
-F1 mobile racing 2021 multiplayer duels and events
-F1 mobile racing 2021 download for android and ios
-F1 mobile racing 2021 system requirements and compatibility
-F1 mobile racing 2021 r&d system and upgrades
-F1 mobile racing 2021 teams and drivers
-F1 mobile racing 2021 circuits and tracks
-F1 mobile racing 2021 graphics and performance
-F1 mobile racing 2021 codes and rewards
-F1 mobile racing 2021 support and help
-F1 mobile racing 2021 community and forums
-Download f1 mobile racing 2020 vs 2021 comparison
-Download f1 mobile racing 2022 beta version
-Download f1 mobile racing 2023 season preview
-Download f1 mobile racing legends edition
-Download f1 mobile racing ultimate edition
-Download f1 mobile racing deluxe edition
-Download f1 mobile racing standard edition
-Download f1 mobile racing free trial version
-Download f1 mobile racing offline mode
-Download f1 mobile racing online mode
-Download f1 mobile racing vr mode
-Download f1 mobile racing controller support
-Download f1 mobile racing customisation options
-Download f1 mobile racing leaderboards and rankings
-Download f1 mobile racing achievements and trophies
-Download f1 mobile racing wallpapers and themes
-Download f1 mobile racing soundtracks and music
-Download f1 mobile racing videos and trailers
-Download f
-
FAQs
-
-
Q: Is F1 Mobile Racing 2021 free to play?
-
A: Yes, F1 Mobile Racing 2021 is free to play. However, it also offers in-app purchases that can enhance your gaming experience.
-
Q: How can I contact the game's customer support?
-
A: You can contact the game's customer support by tapping on the settings icon on the main menu and then tapping on the help button. You can also visit the game's official website or social media pages for more information.
-
Q: How can I connect with other players?
-
A: You can connect with other players by joining the game's official Discord server or Facebook group. You can also follow the game's official Twitter or Instagram accounts for news and updates.
-
Q: How can I give feedback or suggestions for the game?
-
A: You can give feedback or suggestions for the game by tapping on the settings icon on the main menu and then tapping on the feedback button. You can also rate and review the game on the App Store or Google Play Store.
-
Q: How can I support the game's development?
-
A: You can support the game's development by buying in-app purchases or subscribing to the F1 Pass. You can also share the game with your friends or family or write a positive review for the game.
-
401be4b1e0
-
-
\ No newline at end of file
diff --git a/spaces/1phancelerku/anime-remove-background/Enjoy Instagram with No Ads No Seen and More - Download Instagram MOD APK (286.0.0.20.69) for Android.md b/spaces/1phancelerku/anime-remove-background/Enjoy Instagram with No Ads No Seen and More - Download Instagram MOD APK (286.0.0.20.69) for Android.md
deleted file mode 100644
index e1525063f17ba79db2776433319d3a0db871180e..0000000000000000000000000000000000000000
--- a/spaces/1phancelerku/anime-remove-background/Enjoy Instagram with No Ads No Seen and More - Download Instagram MOD APK (286.0.0.20.69) for Android.md
+++ /dev/null
@@ -1,91 +0,0 @@
-
-
Download Instagram Mod Apk Latest Version 2023
-
Instagram is one of the most popular social media platforms in the world, with over one billion active users. It allows you to share photos and videos with your followers, discover new content and creators, shop for products, chat with friends, and more. But what if you want to unlock some extra features that are not available in the official app? That's where Instagram mod apk comes in.
Instagram mod apk is a modified version of the official app that offers many extras, such as removing ads, hiding view live and seen status, downloading media, disabling stories, locking Instagram with a PIN code, and more. It gives you more control over your privacy and user experience, as well as some fun and useful functions. In this article, we will show you how to download and install Instagram mod apk on your Android device, as well as the features and risks of using it.
-
How to Download and Install Instagram Mod Apk on Android
-
Before you can download and install Instagram mod apk on your Android device, you need to do two things: allow unknown apps from your browser and install a file manager app. Here are the steps:
-
-
Go to your device settings and tap Apps & Notifications (or Apps in older versions of Android).
-
Tap the three dots in the upper-right corner.
-
Tap Special access.
-
Tap Install unknown apps.
-
Tap Chrome (or whichever web browser you use).
-
Move Allow from this source to the On position.
-
-
This will let you download APK files (Android application packages) from your browser. APK files are like packages for Android apps; if you have the APK file, you can install the app on your device.
-
Next, you need a file manager app to find the APK file on your device after you download it. You can use the default file manager app on your device, or download one from Google Play Store. For example, you can use Cx File Explorer or File Manager.
-
download instagram mod apk latest version 2023 with no ads
-how to download instagram mod apk latest version 2023 for android
-download instagram mod apk latest version 2023 with live ghost mode
-download instagram mod apk latest version 2023 with unfollow tracker
-download instagram mod apk latest version 2023 with pin lock
-download instagram mod apk latest version 2023 with profile picture zoom
-download instagram mod apk latest version 2023 with voice message downloader
-download instagram mod apk latest version 2023 with story downloader
-download instagram mod apk latest version 2023 with disable seen feature
-download instagram mod apk latest version 2023 with hide typing status feature
-download instagram mod apk latest version 2023 with rearrange feed feature
-download instagram mod apk latest version 2023 with copy comments feature
-download instagram mod apk latest version 2023 with premium features unlocked
-download instagram mod apk latest version 2023 safe and reliable
-download instagram mod apk latest version 2023 direct link
-download instagram mod apk latest version 2023 free and easy
-download instagram mod apk latest version 2023 without root
-download instagram mod apk latest version 2023 updated and working
-download instagram mod apk latest version 2023 for social media marketing
-download instagram mod apk latest version 2023 for more engagement and followers
-download instagram mod apk latest version 2023 for better privacy and security
-download instagram mod apk latest version 2023 for more fun and entertainment
-download instagram mod apk latest version 2023 for unlimited energy and creativity
-download instagram mod apk latest version 2023 for android devices only
-download instagram mod apk latest version 2023 from tecnoandroid.net[^1^]
-
Now that you have prepared your device, you can download Instagram mod apk from a reputable website. There are many websites that offer APK files for various apps, but some of them may be unsafe or contain malware. One of the most trusted sources for APK files is APK Mirror. You can visit their website and search for Instagram mod apk. Make sure you download the latest version that is compatible with your device.
-
Once you have downloaded the APK file, open your file manager app and locate it in your Downloads folder. Tap on it and follow the instructions to install it. You may need to accept some pop-ups or permissions before installing it. Once it is installed, you can open it and enjoy all the extra features of Instagram mod apk.
-
Features of Instagram Mod Apk
-
Instagram mod apk has many features that are not available in the official app. Some of them are:
-
-
Remove ads and stories: You can get rid of annoying ads and stories that clutter your feed and waste your data.
-
Hide view live and seen status: You can watch live videos and stories without letting the other person know that you have seen them. You can also hide your own seen status from others.
-
Download videos, photos, stories, IGTV videos, and voice messages: You can download any media that you see on Instagram with just one tap. You can also save voice messages from direct messages.
-
Disable stories and typing status: You can disable the stories feature completely if you don't want to see or post them. You can also disable the typing status indicator in direct messages.
-
View full profile pictures and copy comments: You can view the full size of any profile picture by tapping on it. You can also copy any comment that you see on Instagram.
-
Lock Instagram with PIN code and rearrange tabs: You can protect your privacy by locking Instagram with a four-digit PIN code. You can also rearrange the tabs at the bottom of the app according to your preference.
-
-
These are just some of the features of Instagram mod apk. There are many more that you can explore and enjoy.
-
Risks of Instagram Mod Apk
-
While Instagram mod apk may sound tempting, it is not without risks. Using a modified app can expose you to various dangers, such as:
-
-
Predators and mature content: Instagram mod apk may allow you to access content that is not suitable for your age or preferences. You may encounter predators who may try to exploit you or harm you in some way. You may also see violent, sexual, or disturbing content that may affect your mental health.
-
Cyberbullying and viral exposure: Instagram mod apk may make you more vulnerable to cyberbullying and viral exposure. You may receive hateful or abusive messages from strangers or people you know. You may also become a target of online harassment or ridicule if your posts or activities are leaked or shared without your consent.
-
Hackers and data breach: Instagram mod apk may compromise your security and privacy. Hackers may use the app to access your personal information, such as your name, email, phone number, location, photos, videos, messages, and more. They may use this information to blackmail you, steal your identity, or harm you in other ways.
-
Mental health problems and dangerous challenges: Instagram mod apk may affect your mental health and well-being. You may develop an addiction to the app or suffer from low self-esteem, anxiety, depression, or other mental disorders. You may also participate in dangerous challenges or trends that may put your life at risk.
-
Private messaging and phishing links: Instagram mod apk may expose you to private messaging and phishing links. You may receive unsolicited messages from unknown or fake accounts that may contain malware, viruses, or spyware. You may also click on phishing links that may redirect you to malicious websites that may steal your information or infect your device.
-
-
These are just some of the risks of using Instagram mod apk. There may be more that you are not aware of.
-
Conclusion
-
Instagram mod apk is a modified version of the official app that offers many extra features, such as removing ads, hiding view live and seen status, downloading media, disabling stories, locking Instagram with a PIN code, and more. It gives you more control over your privacy and user experience, as well as some fun and useful functions.
-
However, using Instagram mod apk also comes with many risks, such as predators and mature content, cyberbullying and viral exposure, hackers and data breach, mental health problems and dangerous challenges, private messaging and phishing links, and more. These risks can harm you physically, emotionally, financially, or legally.
-
Therefore, before you download and install Instagram mod apk on your Android device, you should weigh the pros and cons carefully. You should also take some precautions to stay safe on Instagram, such as:
-
-
Use a strong password and enable two-factor authentication for your account.
-
Do not share your personal information or photos with strangers or people you don't trust.
-
Do not click on suspicious links or download unknown files from messages or comments.
-
Report and block any abusive or inappropriate content or users.
-
Limit your screen time and take breaks from the app regularly.
-
-
We hope this article has helped you understand what Instagram mod apk is, how to download and install it on your Android device, what features it offers, and what risks it poses. If you have any feedback or questions about this topic, please feel free to share them with us in the comments section below. We would love to hear from you!
-
Frequently Asked Questions
-
Here are some of the most common questions that people ask about Instagram mod apk:
-
Is Instagram mod apk legal?
-
No, Instagram mod apk is not legal. It violates the terms of service of Instagram and may result in your account being banned or suspended. It also infringes the intellectual property rights of Instagram and its developers. Therefore, we do not recommend using Instagram mod apk or any other modified app.
-
Is Instagram mod apk safe?
-
No, Instagram mod apk is not safe. It may contain malware, viruses, or spyware that can harm your device or steal your information. It may also expose you to various dangers, such as predators, cyberbullying, hackers, mental health problems, and more. Therefore, we do not recommend using Instagram mod apk or any other modified app.
-
Can I use Instagram mod apk with my original account?
-
No, you cannot use Instagram mod apk with your original account. If you try to log in with your original account, you may get an error message or a warning that your account is at risk. You may also lose access to your account or get banned or suspended by Instagram. Therefore, we do not recommend using Instagram mod apk or any other modified app.
-
Can I update Instagram mod apk?
-
No, you cannot update Instagram mod apk. If you try to update it from Google Play Store or the official app, you may lose all the extra features or get an incompatible version. You may also get detected by Instagram and face consequences. Therefore, we do not recommend using Instagram mod apk or any other modified app.
-
Where can I download Instagram mod apk?
-
You can download Instagram mod apk from various websites that offer APK files for different apps. However, we do not recommend doing so, as these websites may be unsafe or contain malware. One of the most trusted sources for APK files is APK Mirror, but even they cannot guarantee the safety or legality of the files. Therefore, we do not recommend using Instagram mod apk or any other modified app.
197e85843d
-
-
\ No newline at end of file
diff --git a/spaces/1phancelerku/anime-remove-background/Enjoy the Ultimate Soccer Experience with FIFA APK Day.md b/spaces/1phancelerku/anime-remove-background/Enjoy the Ultimate Soccer Experience with FIFA APK Day.md
deleted file mode 100644
index 1679b27e1ebb978e612e1f4c60d2e5a1aba4c37f..0000000000000000000000000000000000000000
--- a/spaces/1phancelerku/anime-remove-background/Enjoy the Ultimate Soccer Experience with FIFA APK Day.md
+++ /dev/null
@@ -1,125 +0,0 @@
-
-
FIFA apk dayı: A Guide to the Ultimate Mobile Soccer Experience
-
If you are a fan of soccer games, you might have heard of FIFA Mobile, the official mobile game of the FIFA World Cup 2022™. But did you know that there is a modded version of this game that offers more features and benefits? It's called FIFA apk dayı, and it's one of the most popular soccer games for Android devices. In this article, we will tell you everything you need to know about FIFA apk dayı, including what it is, how to download and install it, how to play it, and some tips and tricks to help you win more matches.
-
What is FIFA apk dayı?
-
A modded version of FIFA Mobile 2023
-
FIFA apk dayı is a modified version of FIFA Mobile 2023, the latest update of the official mobile game of the FIFA World Cup 2022™. It is developed by a Turkish developer who goes by the name of Dayı, which means uncle in Turkish. The modded version adds new features and improvements to the original game, such as:
By playing FIFA apk dayı, you can enjoy the ultimate mobile soccer experience with more freedom and fun. Some of the features and benefits of this game are:
-
-
Build your dream team with over 15,000 authentic soccer stars from over 600 teams, including world-class talent like Kylian Mbappé, Christian Pulisic, Vinicius Jr, and Son Heung-min.
-
Relive the world‘s greatest soccer tournament with the only licensed FIFA World Cup 2022™ mobile game. Replay the official tournament brackets with any of the 32 qualified nations or rewrite history with 15 non-qualified nations.
-
Compete against the best in pvp modes, including Head-to-Head, VS Attack, Manager Mode, and more. Dominate your opponents with new ways to pass, shoot, dribble, and tackle.
-
Immerse yourself in realistic soccer simulation with new graphics, animations, sounds, commentary, and stadiums.
-
Learn new skills and tactics with The Academy mode. Play through various drills and challenges to improve your gameplay.
-
-
How to download and install FIFA apk dayı?
-
Requirements and precautions
-
Before you download and install FIFA apk dayı, you need to make sure that your device meets the following requirements:
-
-
Android version 4.4 or higher
-
At least 1 GB of RAM
-
At least 1.5 GB of free storage space
-
A stable internet connection
-
-
You also need to take some precautions before installing the game:
-
-
Backup your data from the original FIFA Mobile game if you have it installed. You can use Google Play Games or any other cloud service to do this.
-
Enable unknown sources on your device settings. This will allow you to install apps from sources other than Google Play Store.
-
Steps to download and install
-
Once you have met the requirements and taken the precautions, you can follow these steps to download and install FIFA apk dayı:
-
-
Go to the official website of FIFA apk dayı at [fifaapkdayi.com] and click on the download button.
-
Wait for the download to finish and locate the apk file in your device's file manager.
-
Tap on the apk file and follow the instructions to install the game.
-
Launch the game and enjoy!
-
-
How to play FIFA apk dayı?
-
Build your Ultimate Team with star players
-
The main mode of FIFA apk dayı is Ultimate Team, where you can create your own custom squad with your favorite players. You can choose from over 15,000 soccer stars from over 600 teams, including legends like Pelé, Maradona, Ronaldo, Messi, and more. You can also customize your team's kits, badges, and formations. To get new players, you can use coins and gems to buy packs or trade with other players in the market. You can also upgrade your players' skills and attributes by training them or using special items.
-
fifa mobile 2023 apk download
-fifa soccer mod apk unlimited money
-fifa world cup 2022 android game
-fifa 23 apk obb data offline
-fifa soccer hack apk no root
-fifa mobile 2023 release date
-fifa soccer apk latest version
-fifa world cup 2022 qualifiers game
-fifa 23 mod apk android 1
-fifa soccer cheats apk free download
-fifa mobile 2023 beta apk
-fifa soccer mod apk revdl
-fifa world cup 2022 tickets app
-fifa 23 apk obb data highly compressed
-fifa soccer mod apk unlimited coins and points
-fifa mobile 2023 new features
-fifa soccer apk old version
-fifa world cup 2022 schedule app
-fifa 23 mod apk rexdl
-fifa soccer hack apk download
-fifa mobile 2023 system requirements
-fifa soccer mod apk unlimited energy
-fifa world cup 2022 live streaming app
-fifa 23 apk obb data download for android
-fifa soccer mod apk offline
-fifa mobile 2023 pre register
-fifa soccer apk pure
-fifa world cup 2022 official game android
-fifa 23 mod apk obb offline
-fifa soccer hack apk ios
-fifa mobile 2023 trailer
-fifa soccer mod apk all unlocked
-fifa world cup 2022 theme song app
-fifa 23 apk obb data file download
-fifa soccer mod apk unlimited everything
-fifa mobile 2023 gameplay
-fifa soccer apk mirror
-fifa world cup 2022 countdown app
-fifa 23 mod apk unlimited money and points offline download
-fifa soccer hack apk online generator
-
Relive the FIFA World Cup 2022™ mode
-
If you want to experience the thrill of the world's biggest soccer tournament, you can play the FIFA World Cup 2022™ mode in FIFA apk dayı. This mode lets you replay the official tournament brackets with any of the 32 qualified nations or rewrite history with 15 non-qualified nations. You can also play through the qualifying stages and earn rewards along the way. The mode features authentic stadiums, kits, balls, and teams from the FIFA World Cup 2022™.
-
Compete in various pvp modes and events
-
If you want to test your skills against other players, you can play in various pvp modes and events in FIFA apk dayı. Some of the modes and events are:
-
-
Head-to-Head: Play real-time matches against other players in a 11v11 format. Use your own tactics and strategies to outsmart your opponent.
-
VS Attack: Play fast-paced matches against other players in a 4-minute turn-based format. Score as many goals as you can while defending your own goal.
-
Manager Mode: Play as a manager and control your team's tactics, substitutions, and formations. Watch the match unfold and make adjustments as needed.
-
Tournaments: Play in weekly tournaments and earn rewards based on your performance. Climb up the leaderboards and compete with the best.
-
Seasons: Play in different seasons and leagues based on your team's rating. Earn points and prizes as you progress through the divisions.
-
Events: Play in special events based on real-life soccer scenarios. Complete objectives and challenges to earn rewards.
-
-
Tips and tricks for FIFA apk dayı
-
Use a combination of tap and button controls
-
FIFA apk dayı offers two types of controls for playing the game: tap and button. Tap controls allow you to tap on the screen to pass, shoot, dribble, and tackle. Button controls allow you to use virtual buttons on the screen to perform these actions. You can also use gestures to perform advanced moves like skill moves, finesse shots, lob passes, etc. You can switch between tap and button controls anytime during the game by tapping on the settings icon. You can also customize your button layout and size in the settings menu.
-
Choose the best tactics and formations for your team
-
FIFA apk dayı gives you the option to choose from different tactics and formations for your team. Tactics affect how your team plays on the pitch, such as attacking style, defensive style, width, depth, etc. Formations affect how your players are positioned on the pitch, such as 4-4-2, 4-3-3, 3-5-2, etc. You can change your tactics and formations before or during a match by tapping on the settings icon. You can also create your own custom tactics and formations in the settings menu.
-
Train your players and improve their chemistry
-
FIFA apk dayı allows you to train your players and improve their chemistry. Training your players increases their skills and attributes, making them perform better on the pitch. You can train your players by using training items or coins in the Ultimate Team menu. Improving your chemistry increases your team's overall rating and performance, making them play better together. You can improve your chemistry by using players from the same nation, league, team, or position. You can check your chemistry by looking at the green, yellow, or red lines connecting your players in the Ultimate Team menu.
-
Conclusion
-
FIFA apk dayı is a modded version of FIFA Mobile 2023 that offers more features and benefits than the original game. It allows you to build your dream team with star players, relive the FIFA World Cup 2022™ mode, compete in various pvp modes and events, and enjoy realistic soccer simulation. You can download and install FIFA apk dayı from its official website and play it on your Android device. You can also use some tips and tricks to improve your gameplay and win more matches. FIFA apk dayı is a must-have game for any soccer fan who wants to have the ultimate mobile soccer experience.
-
FAQs
-
Here are some frequently asked questions about FIFA apk dayı:
-
-
Is FIFA apk dayı safe to download and install?
-
Yes, FIFA apk dayı is safe to download and install, as long as you get it from its official website. However, you should always backup your data from the original FIFA Mobile game before installing the modded version, as it may overwrite or delete your data.
-
Is FIFA apk dayı compatible with other devices?
-
FIFA apk dayı is only compatible with Android devices that meet the requirements mentioned above. It is not compatible with iOS devices or other platforms.
-
Is FIFA apk dayı legal to play?
-
FIFA apk dayı is not an official product of EA Sports or FIFA, and it is not endorsed or supported by them. It is a fan-made mod that violates the terms of service of the original game. Therefore, playing FIFA apk dayı may result in a ban or suspension from the original game or other consequences. Play at your own risk.
-
How can I update FIFA apk dayı?
-
FIFA apk dayı is updated regularly by its developer to fix bugs, add new features, and keep up with the latest updates of the original game. You can check for updates on its official website or follow its social media accounts for announcements. To update FIFA apk dayı, you need to download and install the latest version of the apk file from its website.
-
How can I contact the developer of FIFA apk dayı?
-
You can contact the developer of FIFA apk dayı by sending an email to [fifaapkdayi@gmail.com] or by visiting their Facebook page at [facebook.com/fifaapkdayi]. You can also leave a comment or a review on their website or social media accounts.
- 401be4b1e0
-
-
\ No newline at end of file
diff --git a/spaces/801artistry/RVC801/tools/app.py b/spaces/801artistry/RVC801/tools/app.py
deleted file mode 100644
index 602fbb71a49f2537295337cdcecf501abdd74153..0000000000000000000000000000000000000000
--- a/spaces/801artistry/RVC801/tools/app.py
+++ /dev/null
@@ -1,148 +0,0 @@
-import logging
-import os
-
-# os.system("wget -P cvec/ https://huggingface.co/lj1995/VoiceConversionWebUI/resolve/main/hubert_base.pt")
-import gradio as gr
-from dotenv import load_dotenv
-
-from configs.config import Config
-from i18n import I18nAuto
-from infer.modules.vc.pipeline import Pipeline
-VC = Pipeline
-
-logging.getLogger("numba").setLevel(logging.WARNING)
-logging.getLogger("markdown_it").setLevel(logging.WARNING)
-logging.getLogger("urllib3").setLevel(logging.WARNING)
-logging.getLogger("matplotlib").setLevel(logging.WARNING)
-logger = logging.getLogger(__name__)
-
-i18n = I18nAuto()
-#(i18n)
-
-load_dotenv()
-config = Config()
-vc = VC(config)
-
-weight_root = os.getenv("weight_root")
-weight_uvr5_root = os.getenv("weight_uvr5_root")
-index_root = os.getenv("index_root")
-names = []
-hubert_model = None
-for name in os.listdir(weight_root):
- if name.endswith(".pth"):
- names.append(name)
-index_paths = []
-for root, dirs, files in os.walk(index_root, topdown=False):
- for name in files:
- if name.endswith(".index") and "trained" not in name:
- index_paths.append("%s/%s" % (root, name))
-
-
-app = gr.Blocks()
-with app:
- with gr.Tabs():
- with gr.TabItem("在线demo"):
- gr.Markdown(
- value="""
- RVC 在线demo
- """
- )
- sid = gr.Dropdown(label=i18n("推理音色"), choices=sorted(names))
- with gr.Column():
- spk_item = gr.Slider(
- minimum=0,
- maximum=2333,
- step=1,
- label=i18n("请选择说话人id"),
- value=0,
- visible=False,
- interactive=True,
- )
- sid.change(fn=vc.get_vc, inputs=[sid], outputs=[spk_item])
- gr.Markdown(
- value=i18n("男转女推荐+12key, 女转男推荐-12key, 如果音域爆炸导致音色失真也可以自己调整到合适音域. ")
- )
- vc_input3 = gr.Audio(label="上传音频(长度小于90秒)")
- vc_transform0 = gr.Number(label=i18n("变调(整数, 半音数量, 升八度12降八度-12)"), value=0)
- f0method0 = gr.Radio(
- label=i18n("选择音高提取算法,输入歌声可用pm提速,harvest低音好但巨慢无比,crepe效果好但吃GPU"),
- choices=["pm", "harvest", "crepe", "rmvpe"],
- value="pm",
- interactive=True,
- )
- filter_radius0 = gr.Slider(
- minimum=0,
- maximum=7,
- label=i18n(">=3则使用对harvest音高识别的结果使用中值滤波,数值为滤波半径,使用可以削弱哑音"),
- value=3,
- step=1,
- interactive=True,
- )
- with gr.Column():
- file_index1 = gr.Textbox(
- label=i18n("特征检索库文件路径,为空则使用下拉的选择结果"),
- value="",
- interactive=False,
- visible=False,
- )
- file_index2 = gr.Dropdown(
- label=i18n("自动检测index路径,下拉式选择(dropdown)"),
- choices=sorted(index_paths),
- interactive=True,
- )
- index_rate1 = gr.Slider(
- minimum=0,
- maximum=1,
- label=i18n("检索特征占比"),
- value=0.88,
- interactive=True,
- )
- resample_sr0 = gr.Slider(
- minimum=0,
- maximum=48000,
- label=i18n("后处理重采样至最终采样率,0为不进行重采样"),
- value=0,
- step=1,
- interactive=True,
- )
- rms_mix_rate0 = gr.Slider(
- minimum=0,
- maximum=1,
- label=i18n("输入源音量包络替换输出音量包络融合比例,越靠近1越使用输出包络"),
- value=1,
- interactive=True,
- )
- protect0 = gr.Slider(
- minimum=0,
- maximum=0.5,
- label=i18n("保护清辅音和呼吸声,防止电音撕裂等artifact,拉满0.5不开启,调低加大保护力度但可能降低索引效果"),
- value=0.33,
- step=0.01,
- interactive=True,
- )
- f0_file = gr.File(label=i18n("F0曲线文件, 可选, 一行一个音高, 代替默认F0及升降调"))
- but0 = gr.Button(i18n("转换"), variant="primary")
- vc_output1 = gr.Textbox(label=i18n("输出信息"))
- vc_output2 = gr.Audio(label=i18n("输出音频(右下角三个点,点了可以下载)"))
- but0.click(
- vc.vc_single,
- [
- spk_item,
- vc_input3,
- vc_transform0,
- f0_file,
- f0method0,
- file_index1,
- file_index2,
- # file_big_npy1,
- index_rate1,
- filter_radius0,
- resample_sr0,
- rms_mix_rate0,
- protect0,
- ],
- [vc_output1, vc_output2],
- )
-
-
-app.launch()
diff --git a/spaces/A00001/bingothoo/src/pages/api/blob.ts b/spaces/A00001/bingothoo/src/pages/api/blob.ts
deleted file mode 100644
index fecd48031916b2284b8958892196e0a1ad420421..0000000000000000000000000000000000000000
--- a/spaces/A00001/bingothoo/src/pages/api/blob.ts
+++ /dev/null
@@ -1,40 +0,0 @@
-'use server'
-
-import { NextApiRequest, NextApiResponse } from 'next'
-import { Readable } from 'node:stream'
-import { fetch } from '@/lib/isomorphic'
-
-const API_DOMAIN = 'https://www.bing.com'
-
-export default async function handler(req: NextApiRequest, res: NextApiResponse) {
- try {
- const { bcid } = req.query
-
- const { headers, body } = await fetch(`${API_DOMAIN}/images/blob?bcid=${bcid}`,
- {
- method: 'GET',
- headers: {
- "sec-ch-ua": "\"Not/A)Brand\";v=\"99\", \"Google Chrome\";v=\"115\", \"Chromium\";v=\"115\"",
- "sec-ch-ua-mobile": "?0",
- "sec-ch-ua-platform": "\"Windows\"",
- "Referrer-Policy": "origin-when-cross-origin",
- },
- },
- )
-
- res.writeHead(200, {
- 'Content-Length': headers.get('content-length')!,
- 'Content-Type': headers.get('content-type')!,
- })
- // @ts-ignore
- return Readable.fromWeb(body!).pipe(res)
- } catch (e) {
- console.log('Error', e)
- return res.json({
- result: {
- value: 'UploadFailed',
- message: `${e}`
- }
- })
- }
-}
diff --git a/spaces/AI-Hobbyist/Hoyo-RVC/infer/train-index -v2.py b/spaces/AI-Hobbyist/Hoyo-RVC/infer/train-index -v2.py
deleted file mode 100644
index bb24eeab7106b7489e66123cb6530e6306f2ed7a..0000000000000000000000000000000000000000
--- a/spaces/AI-Hobbyist/Hoyo-RVC/infer/train-index -v2.py
+++ /dev/null
@@ -1,44 +0,0 @@
-"""
-格式:直接cid为自带的index位;aid放不下了,通过字典来查,反正就5w个
-"""
-import faiss, numpy as np, os
-
-# ###########如果是原始特征要先写save
-inp_root = r"./logs/nene/3_feature768"
-npys = []
-listdir_res = list(os.listdir(inp_root))
-for name in sorted(listdir_res):
- phone = np.load("%s/%s" % (inp_root, name))
- npys.append(phone)
-big_npy = np.concatenate(npys, 0)
-big_npy_idx = np.arange(big_npy.shape[0])
-np.random.shuffle(big_npy_idx)
-big_npy = big_npy[big_npy_idx]
-print(big_npy.shape) # (6196072, 192)#fp32#4.43G
-np.save("infer/big_src_feature_mi.npy", big_npy)
-
-##################train+add
-# big_npy=np.load("/bili-coeus/jupyter/jupyterhub-liujing04/vits_ch/inference_f0/big_src_feature_mi.npy")
-n_ivf = min(int(16 * np.sqrt(big_npy.shape[0])), big_npy.shape[0] // 39)
-index = faiss.index_factory(768, "IVF%s,Flat" % n_ivf) # mi
-print("training")
-index_ivf = faiss.extract_index_ivf(index) #
-index_ivf.nprobe = 1
-index.train(big_npy)
-faiss.write_index(
- index, "infer/trained_IVF%s_Flat_baseline_src_feat_v2.index" % (n_ivf)
-)
-print("adding")
-batch_size_add = 8192
-for i in range(0, big_npy.shape[0], batch_size_add):
- index.add(big_npy[i : i + batch_size_add])
-faiss.write_index(index, "infer/added_IVF%s_Flat_mi_baseline_src_feat.index" % (n_ivf))
-"""
-大小(都是FP32)
-big_src_feature 2.95G
- (3098036, 256)
-big_emb 4.43G
- (6196072, 192)
-big_emb双倍是因为求特征要repeat后再加pitch
-
-"""
diff --git a/spaces/AI-ZTH-03-23/8.Datasets-NER-Biomed-ClinicalTerms/app.py b/spaces/AI-ZTH-03-23/8.Datasets-NER-Biomed-ClinicalTerms/app.py
deleted file mode 100644
index fd97bf2a8592b219ba1c2d4c94187d984e63d114..0000000000000000000000000000000000000000
--- a/spaces/AI-ZTH-03-23/8.Datasets-NER-Biomed-ClinicalTerms/app.py
+++ /dev/null
@@ -1,268 +0,0 @@
-import gradio as gr
-import pandas as pd
-import json
-from collections import defaultdict
-
-# Create tokenizer for biomed model
-from transformers import pipeline, AutoTokenizer, AutoModelForTokenClassification
-tokenizer = AutoTokenizer.from_pretrained("d4data/biomedical-ner-all") # https://huggingface.co/d4data/biomedical-ner-all?text=asthma
-model = AutoModelForTokenClassification.from_pretrained("d4data/biomedical-ner-all")
-pipe = pipeline("ner", model=model, tokenizer=tokenizer, aggregation_strategy="simple")
-
-# Matplotlib for entity graph
-import matplotlib.pyplot as plt
-plt.switch_backend("Agg")
-
-# Load examples from JSON
-import os
-
-# Load terminology datasets:
-basedir = os.path.dirname(__file__)
-#dataLOINC = pd.read_csv(basedir + "\\" + f'LoincTableCore.csv')
-#dataPanels = pd.read_csv(basedir + "\\" + f'PanelsAndForms-ACW1208Labeled.csv')
-#dataSNOMED = pd.read_csv(basedir + "\\" + f'sct2_TextDefinition_Full-en_US1000124_20220901.txt',sep='\t')
-#dataOMS = pd.read_csv(basedir + "\\" + f'SnomedOMS.csv')
-#dataICD10 = pd.read_csv(basedir + "\\" + f'ICD10Diagnosis.csv')
-
-dataLOINC = pd.read_csv(f'LoincTableCore.csv')
-dataPanels = pd.read_csv(f'PanelsAndForms-ACW1208Labeled.csv')
-dataSNOMED = pd.read_csv(f'sct2_TextDefinition_Full-en_US1000124_20220901.txt',sep='\t')
-dataOMS = pd.read_csv(f'SnomedOMS.csv')
-dataICD10 = pd.read_csv(f'ICD10Diagnosis.csv')
-
-dir_path = os.path.dirname(os.path.realpath(__file__))
-EXAMPLES = {}
-#with open(dir_path + "\\" + "examples.json", "r") as f:
-with open("examples.json", "r") as f:
- example_json = json.load(f)
- EXAMPLES = {x["text"]: x["label"] for x in example_json}
-
-def MatchLOINC(name):
- #basedir = os.path.dirname(__file__)
- pd.set_option("display.max_rows", None)
- #data = pd.read_csv(basedir + "\\" + f'LoincTableCore.csv')
- data = dataLOINC
- swith=data.loc[data['COMPONENT'].str.contains(name, case=False, na=False)]
- return swith
-
-def MatchLOINCPanelsandForms(name):
- #basedir = os.path.dirname(__file__)
- #data = pd.read_csv(basedir + "\\" + f'PanelsAndForms-ACW1208Labeled.csv')
- data = dataPanels
- # Assessment Name:
- #swith=data.loc[data['ParentName'].str.contains(name, case=False, na=False)]
- # Assessment Question:
- swith=data.loc[data['LoincName'].str.contains(name, case=False, na=False)]
- return swith
-
-def MatchSNOMED(name):
- #basedir = os.path.dirname(__file__)
- #data = pd.read_csv(basedir + "\\" + f'sct2_TextDefinition_Full-en_US1000124_20220901.txt',sep='\t')
- data = dataSNOMED
- swith=data.loc[data['term'].str.contains(name, case=False, na=False)]
- return swith
-
-def MatchOMS(name):
- #basedir = os.path.dirname(__file__)
- #data = pd.read_csv(basedir + "\\" + f'SnomedOMS.csv')
- data = dataOMS
- swith=data.loc[data['SNOMED CT'].str.contains(name, case=False, na=False)]
- return swith
-
-def MatchICD10(name):
- #basedir = os.path.dirname(__file__)
- #data = pd.read_csv(basedir + "\\" + f'ICD10Diagnosis.csv')
- data = dataICD10
- swith=data.loc[data['Description'].str.contains(name, case=False, na=False)]
- return swith
-
-def SaveResult(text, outputfileName):
- #try:
- basedir = os.path.dirname(__file__)
- savePath = outputfileName
- print("Saving: " + text + " to " + savePath)
- from os.path import exists
- file_exists = exists(savePath)
- if file_exists:
- with open(outputfileName, "a") as f: #append
- #for line in text:
- f.write(str(text.replace("\n"," ")))
- f.write('\n')
- else:
- with open(outputfileName, "w") as f: #write
- #for line in text:
- f.write(str(text.replace("\n"," ")))
- f.write('\n')
- #except ValueError as err:
- # raise ValueError("File Save Error in SaveResult \n" + format_tb(err.__traceback__)[0] + err.args[0] + "\nEnd of error message.") from None
-
- return
-
-def loadFile(filename):
- try:
- basedir = os.path.dirname(__file__)
- loadPath = basedir + "\\" + filename
-
- print("Loading: " + loadPath)
-
- from os.path import exists
- file_exists = exists(loadPath)
-
- if file_exists:
- with open(loadPath, "r") as f: #read
- contents = f.read()
- print(contents)
- return contents
-
- except ValueError as err:
- raise ValueError("File Save Error in SaveResult \n" + format_tb(err.__traceback__)[0] + err.args[0] + "\nEnd of error message.") from None
-
- return ""
-
-def get_today_filename():
- from datetime import datetime
- date = datetime.now().strftime("%Y_%m_%d-%I.%M.%S.%p")
- #print(f"filename_{date}") 'filename_2023_01_12-03-29-22_AM'
- return f"MedNER_{date}.csv"
-
-def get_base(filename):
- basedir = os.path.dirname(__file__)
- loadPath = basedir + "\\" + filename
- #print("Loading: " + loadPath)
- return loadPath
-
-def group_by_entity(raw):
- outputFile = get_base(get_today_filename())
- out = defaultdict(int)
-
- for ent in raw:
- out[ent["entity_group"]] += 1
- myEntityGroup = ent["entity_group"]
- print("Found entity group type: " + myEntityGroup)
-
- if (myEntityGroup in ['Sign_symptom', 'Detailed_description', 'History', 'Activity', 'Medication' ]):
- eterm = ent["word"].replace('#','')
- minlength = 3
- if len(eterm) > minlength:
- print("Found eterm: " + eterm)
- eterm.replace("#","")
- g1=MatchLOINC(eterm)
- g2=MatchLOINCPanelsandForms(eterm)
- g3=MatchSNOMED(eterm)
- g4=MatchOMS(eterm)
- g5=MatchICD10(eterm)
- sAll = ""
-
- print("Saving to output file " + outputFile)
- # Create harmonisation output format of input to output code, name, Text
-
- try: # 18 fields, output to labeled CSV dataset for results teaching on scored regret changes to action plan with data inputs
- col = " 1 2 3 4 5 6 7 8 9 10 11 12 13 14 15 16 17 18 19"
-
- #LOINC
- g11 = g1['LOINC_NUM'].to_string().replace(","," ").replace("\n"," ")
- g12 = g1['COMPONENT'].to_string().replace(","," ").replace("\n"," ")
- s1 = ("LOINC," + myEntityGroup + "," + eterm + ",questions of ," + g12 + "," + g11 + ", Label,Value, Label,Value, Label,Value ")
- if g11 != 'Series([] )': SaveResult(s1, outputFile)
-
- #LOINC Panels
- g21 = g2['Loinc'].to_string().replace(","," ").replace("\n"," ")
- g22 = g2['LoincName'].to_string().replace(","," ").replace("\n"," ")
- g23 = g2['ParentLoinc'].to_string().replace(","," ").replace("\n"," ")
- g24 = g2['ParentName'].to_string().replace(","," ").replace("\n"," ")
- # s2 = ("LOINC Panel," + myEntityGroup + "," + eterm + ",name of ," + g22 + "," + g21 + ", and Parent codes of ," + g23 + ", with Parent names of ," + g24 + ", Label,Value ")
- s2 = ("LOINC Panel," + myEntityGroup + "," + eterm + ",name of ," + g22 + "," + g21 + "," + g24 + ", and Parent codes of ," + g23 + "," + ", Label,Value ")
- if g21 != 'Series([] )': SaveResult(s2, outputFile)
-
- #SNOMED
- g31 = g3['conceptId'].to_string().replace(","," ").replace("\n"," ").replace("\l"," ").replace("\r"," ")
- g32 = g3['term'].to_string().replace(","," ").replace("\n"," ").replace("\l"," ").replace("\r"," ")
- s3 = ("SNOMED Concept," + myEntityGroup + "," + eterm + ",terms of ," + g32 + "," + g31 + ", Label,Value, Label,Value, Label,Value ")
- if g31 != 'Series([] )': SaveResult(s3, outputFile)
-
- #OMS
- g41 = g4['Omaha Code'].to_string().replace(","," ").replace("\n"," ")
- g42 = g4['SNOMED CT concept ID'].to_string().replace(","," ").replace("\n"," ")
- g43 = g4['SNOMED CT'].to_string().replace(","," ").replace("\n"," ")
- g44 = g4['PR'].to_string().replace(","," ").replace("\n"," ")
- g45 = g4['S&S'].to_string().replace(","," ").replace("\n"," ")
- s4 = ("OMS," + myEntityGroup + "," + eterm + ",concepts of ," + g44 + "," + g45 + ", and SNOMED codes of ," + g43 + ", and OMS problem of ," + g42 + ", and OMS Sign Symptom of ," + g41)
- if g41 != 'Series([] )': SaveResult(s4, outputFile)
-
- #ICD10
- g51 = g5['Code'].to_string().replace(","," ").replace("\n"," ")
- g52 = g5['Description'].to_string().replace(","," ").replace("\n"," ")
- s5 = ("ICD10," + myEntityGroup + "," + eterm + ",descriptions of ," + g52 + "," + g51 + ", Label,Value, Label,Value, Label,Value ")
- if g51 != 'Series([] )': SaveResult(s5, outputFile)
-
- except ValueError as err:
- raise ValueError("Error in group by entity \n" + format_tb(err.__traceback__)[0] + err.args[0] + "\nEnd of error message.") from None
-
- return outputFile
-
-
-def plot_to_figure(grouped):
- fig = plt.figure()
- plt.bar(x=list(grouped.keys()), height=list(grouped.values()))
- plt.margins(0.2)
- plt.subplots_adjust(bottom=0.4)
- plt.xticks(rotation=90)
- return fig
-
-
-def ner(text):
- raw = pipe(text)
- ner_content = {
- "text": text,
- "entities": [
- {
- "entity": x["entity_group"],
- "word": x["word"],
- "score": x["score"],
- "start": x["start"],
- "end": x["end"],
- }
- for x in raw
- ],
- }
-
- outputFile = group_by_entity(raw)
- label = EXAMPLES.get(text, "Unknown")
- outputDataframe = pd.read_csv(outputFile)
- return (ner_content, outputDataframe, outputFile)
-
-demo = gr.Blocks()
-with demo:
- gr.Markdown(
- """
- # 🩺⚕️NLP Clinical Ontology Biomedical NER
- """
- )
- input = gr.Textbox(label="Note text", value="")
-
- with gr.Tab("Biomedical Entity Recognition"):
- output=[
- gr.HighlightedText(label="NER", combine_adjacent=True),
- #gr.JSON(label="Entity Counts"),
- #gr.Label(label="Rating"),
- #gr.Plot(label="Bar"),
- gr.Dataframe(label="Dataframe"),
- gr.File(label="File"),
- ]
- examples=list(EXAMPLES.keys())
- gr.Examples(examples, inputs=input)
- input.change(fn=ner, inputs=input, outputs=output)
-
- with gr.Tab("Clinical Terminology Resolution"):
- with gr.Row(variant="compact"):
- btnLOINC = gr.Button("LOINC")
- btnPanels = gr.Button("Panels")
- btnSNOMED = gr.Button("SNOMED")
- btnOMS = gr.Button("OMS")
- btnICD10 = gr.Button("ICD10")
-
- examples=list(EXAMPLES.keys())
- gr.Examples(examples, inputs=input)
- input.change(fn=ner, inputs=input, outputs=output)
-#layout="vertical"
-demo.launch(debug=True)
diff --git a/spaces/AIGC-Audio/AudioGPT/text_to_speech/modules/vocoder/parallel_wavegan/optimizers/__init__.py b/spaces/AIGC-Audio/AudioGPT/text_to_speech/modules/vocoder/parallel_wavegan/optimizers/__init__.py
deleted file mode 100644
index a0e0c5932838281e912079e5784d84d43444a61a..0000000000000000000000000000000000000000
--- a/spaces/AIGC-Audio/AudioGPT/text_to_speech/modules/vocoder/parallel_wavegan/optimizers/__init__.py
+++ /dev/null
@@ -1,2 +0,0 @@
-from torch.optim import * # NOQA
-from .radam import * # NOQA
diff --git a/spaces/AIGText/GlyphControl/ldm/modules/diffusionmodules/openaimodel.py b/spaces/AIGText/GlyphControl/ldm/modules/diffusionmodules/openaimodel.py
deleted file mode 100644
index 3180ce13278e6d013dac5b5845263566d620b0fa..0000000000000000000000000000000000000000
--- a/spaces/AIGText/GlyphControl/ldm/modules/diffusionmodules/openaimodel.py
+++ /dev/null
@@ -1,790 +0,0 @@
-from abc import abstractmethod
-import math
-
-import numpy as np
-import torch as th
-import torch.nn as nn
-import torch.nn.functional as F
-
-from ldm.modules.diffusionmodules.util import (
- checkpoint,
- conv_nd,
- linear,
- avg_pool_nd,
- zero_module,
- normalization,
- timestep_embedding,
-)
-from ldm.modules.attention import SpatialTransformer
-from ldm.util import exists
-
-
-# dummy replace
-def convert_module_to_f16(x):
- pass
-
-def convert_module_to_f32(x):
- pass
-
-
-## go
-class AttentionPool2d(nn.Module):
- """
- Adapted from CLIP: https://github.com/openai/CLIP/blob/main/clip/model.py
- """
-
- def __init__(
- self,
- spacial_dim: int,
- embed_dim: int,
- num_heads_channels: int,
- output_dim: int = None,
- ):
- super().__init__()
- self.positional_embedding = nn.Parameter(th.randn(embed_dim, spacial_dim ** 2 + 1) / embed_dim ** 0.5)
- self.qkv_proj = conv_nd(1, embed_dim, 3 * embed_dim, 1)
- self.c_proj = conv_nd(1, embed_dim, output_dim or embed_dim, 1)
- self.num_heads = embed_dim // num_heads_channels
- self.attention = QKVAttention(self.num_heads)
-
- def forward(self, x):
- b, c, *_spatial = x.shape
- x = x.reshape(b, c, -1) # NC(HW)
- x = th.cat([x.mean(dim=-1, keepdim=True), x], dim=-1) # NC(HW+1)
- x = x + self.positional_embedding[None, :, :].to(x.dtype) # NC(HW+1)
- x = self.qkv_proj(x)
- x = self.attention(x)
- x = self.c_proj(x)
- return x[:, :, 0]
-
-
-class TimestepBlock(nn.Module):
- """
- Any module where forward() takes timestep embeddings as a second argument.
- """
-
- @abstractmethod
- def forward(self, x, emb):
- """
- Apply the module to `x` given `emb` timestep embeddings.
- """
-
-
-class TimestepEmbedSequential(nn.Sequential, TimestepBlock):
- """
- A sequential module that passes timestep embeddings to the children that
- support it as an extra input.
- """
-
- def forward(self, x, emb, context=None):
- for layer in self:
- if isinstance(layer, TimestepBlock):
- x = layer(x, emb)
- elif isinstance(layer, SpatialTransformer):
- x = layer(x, context)
- else:
- x = layer(x)
- return x
-
-
-class Upsample(nn.Module):
- """
- An upsampling layer with an optional convolution.
- :param channels: channels in the inputs and outputs.
- :param use_conv: a bool determining if a convolution is applied.
- :param dims: determines if the signal is 1D, 2D, or 3D. If 3D, then
- upsampling occurs in the inner-two dimensions.
- """
-
- def __init__(self, channels, use_conv, dims=2, out_channels=None, padding=1):
- super().__init__()
- self.channels = channels
- self.out_channels = out_channels or channels
- self.use_conv = use_conv
- self.dims = dims
- if use_conv:
- self.conv = conv_nd(dims, self.channels, self.out_channels, 3, padding=padding)
-
- def forward(self, x):
- assert x.shape[1] == self.channels
- if self.dims == 3:
- x = F.interpolate(
- x, (x.shape[2], x.shape[3] * 2, x.shape[4] * 2), mode="nearest"
- )
- else:
- x = F.interpolate(x, scale_factor=2, mode="nearest")
- if self.use_conv:
- x = self.conv(x)
- return x
-
-class TransposedUpsample(nn.Module):
- 'Learned 2x upsampling without padding'
- def __init__(self, channels, out_channels=None, ks=5):
- super().__init__()
- self.channels = channels
- self.out_channels = out_channels or channels
-
- self.up = nn.ConvTranspose2d(self.channels,self.out_channels,kernel_size=ks,stride=2)
-
- def forward(self,x):
- return self.up(x)
-
-
-class Downsample(nn.Module):
- """
- A downsampling layer with an optional convolution.
- :param channels: channels in the inputs and outputs.
- :param use_conv: a bool determining if a convolution is applied.
- :param dims: determines if the signal is 1D, 2D, or 3D. If 3D, then
- downsampling occurs in the inner-two dimensions.
- """
-
- def __init__(self, channels, use_conv, dims=2, out_channels=None,padding=1):
- super().__init__()
- self.channels = channels
- self.out_channels = out_channels or channels
- self.use_conv = use_conv
- self.dims = dims
- stride = 2 if dims != 3 else (1, 2, 2)
- if use_conv:
- self.op = conv_nd(
- dims, self.channels, self.out_channels, 3, stride=stride, padding=padding
- )
- else:
- assert self.channels == self.out_channels
- self.op = avg_pool_nd(dims, kernel_size=stride, stride=stride)
-
- def forward(self, x):
- assert x.shape[1] == self.channels
- return self.op(x)
-
-
-class ResBlock(TimestepBlock):
- """
- A residual block that can optionally change the number of channels.
- :param channels: the number of input channels.
- :param emb_channels: the number of timestep embedding channels.
- :param dropout: the rate of dropout.
- :param out_channels: if specified, the number of out channels.
- :param use_conv: if True and out_channels is specified, use a spatial
- convolution instead of a smaller 1x1 convolution to change the
- channels in the skip connection.
- :param dims: determines if the signal is 1D, 2D, or 3D.
- :param use_checkpoint: if True, use gradient checkpointing on this module.
- :param up: if True, use this block for upsampling.
- :param down: if True, use this block for downsampling.
- """
-
- def __init__(
- self,
- channels,
- emb_channels,
- dropout,
- out_channels=None,
- use_conv=False,
- use_scale_shift_norm=False,
- dims=2,
- use_checkpoint=False,
- up=False,
- down=False,
- ):
- super().__init__()
- self.channels = channels
- self.emb_channels = emb_channels
- self.dropout = dropout
- self.out_channels = out_channels or channels
- self.use_conv = use_conv
- self.use_checkpoint = use_checkpoint
- self.use_scale_shift_norm = use_scale_shift_norm
-
- self.in_layers = nn.Sequential(
- normalization(channels),
- nn.SiLU(),
- conv_nd(dims, channels, self.out_channels, 3, padding=1),
- )
-
- self.updown = up or down
-
- if up:
- self.h_upd = Upsample(channels, False, dims)
- self.x_upd = Upsample(channels, False, dims)
- elif down:
- self.h_upd = Downsample(channels, False, dims)
- self.x_upd = Downsample(channels, False, dims)
- else:
- self.h_upd = self.x_upd = nn.Identity()
-
- self.emb_layers = nn.Sequential(
- nn.SiLU(),
- linear(
- emb_channels,
- 2 * self.out_channels if use_scale_shift_norm else self.out_channels,
- ),
- )
- self.out_layers = nn.Sequential(
- normalization(self.out_channels),
- nn.SiLU(),
- nn.Dropout(p=dropout),
- zero_module(
- conv_nd(dims, self.out_channels, self.out_channels, 3, padding=1)
- ),
- )
-
- if self.out_channels == channels:
- self.skip_connection = nn.Identity()
- elif use_conv:
- self.skip_connection = conv_nd(
- dims, channels, self.out_channels, 3, padding=1
- )
- else:
- self.skip_connection = conv_nd(dims, channels, self.out_channels, 1)
-
- def forward(self, x, emb):
- """
- Apply the block to a Tensor, conditioned on a timestep embedding.
- :param x: an [N x C x ...] Tensor of features.
- :param emb: an [N x emb_channels] Tensor of timestep embeddings.
- :return: an [N x C x ...] Tensor of outputs.
- """
- return checkpoint(
- self._forward, (x, emb), self.parameters(), self.use_checkpoint
- )
-
-
- def _forward(self, x, emb):
- if self.updown:
- in_rest, in_conv = self.in_layers[:-1], self.in_layers[-1]
- h = in_rest(x)
- h = self.h_upd(h)
- x = self.x_upd(x)
- h = in_conv(h)
- else:
- h = self.in_layers(x)
- emb_out = self.emb_layers(emb).type(h.dtype)
- while len(emb_out.shape) < len(h.shape):
- emb_out = emb_out[..., None]
- if self.use_scale_shift_norm:
- out_norm, out_rest = self.out_layers[0], self.out_layers[1:]
- scale, shift = th.chunk(emb_out, 2, dim=1)
- h = out_norm(h) * (1 + scale) + shift
- h = out_rest(h)
- else:
- h = h + emb_out
- h = self.out_layers(h)
- return self.skip_connection(x) + h
-
-
-class AttentionBlock(nn.Module):
- """
- An attention block that allows spatial positions to attend to each other.
- Originally ported from here, but adapted to the N-d case.
- https://github.com/hojonathanho/diffusion/blob/1e0dceb3b3495bbe19116a5e1b3596cd0706c543/diffusion_tf/models/unet.py#L66.
- """
-
- def __init__(
- self,
- channels,
- num_heads=1,
- num_head_channels=-1,
- use_checkpoint=False,
- use_new_attention_order=False,
- ):
- super().__init__()
- self.channels = channels
- if num_head_channels == -1:
- self.num_heads = num_heads
- else:
- assert (
- channels % num_head_channels == 0
- ), f"q,k,v channels {channels} is not divisible by num_head_channels {num_head_channels}"
- self.num_heads = channels // num_head_channels
- self.use_checkpoint = use_checkpoint
- self.norm = normalization(channels)
- self.qkv = conv_nd(1, channels, channels * 3, 1)
- if use_new_attention_order:
- # split qkv before split heads
- self.attention = QKVAttention(self.num_heads)
- else:
- # split heads before split qkv
- self.attention = QKVAttentionLegacy(self.num_heads)
-
- self.proj_out = zero_module(conv_nd(1, channels, channels, 1))
-
- def forward(self, x):
- return checkpoint(self._forward, (x,), self.parameters(), True) # TODO: check checkpoint usage, is True # TODO: fix the .half call!!!
- #return pt_checkpoint(self._forward, x) # pytorch
-
- def _forward(self, x):
- b, c, *spatial = x.shape
- x = x.reshape(b, c, -1)
- qkv = self.qkv(self.norm(x))
- h = self.attention(qkv)
- h = self.proj_out(h)
- return (x + h).reshape(b, c, *spatial)
-
-
-def count_flops_attn(model, _x, y):
- """
- A counter for the `thop` package to count the operations in an
- attention operation.
- Meant to be used like:
- macs, params = thop.profile(
- model,
- inputs=(inputs, timestamps),
- custom_ops={QKVAttention: QKVAttention.count_flops},
- )
- """
- b, c, *spatial = y[0].shape
- num_spatial = int(np.prod(spatial))
- # We perform two matmuls with the same number of ops.
- # The first computes the weight matrix, the second computes
- # the combination of the value vectors.
- matmul_ops = 2 * b * (num_spatial ** 2) * c
- model.total_ops += th.DoubleTensor([matmul_ops])
-
-
-class QKVAttentionLegacy(nn.Module):
- """
- A module which performs QKV attention. Matches legacy QKVAttention + input/ouput heads shaping
- """
-
- def __init__(self, n_heads):
- super().__init__()
- self.n_heads = n_heads
-
- def forward(self, qkv):
- """
- Apply QKV attention.
- :param qkv: an [N x (H * 3 * C) x T] tensor of Qs, Ks, and Vs.
- :return: an [N x (H * C) x T] tensor after attention.
- """
- bs, width, length = qkv.shape
- assert width % (3 * self.n_heads) == 0
- ch = width // (3 * self.n_heads)
- q, k, v = qkv.reshape(bs * self.n_heads, ch * 3, length).split(ch, dim=1)
- scale = 1 / math.sqrt(math.sqrt(ch))
- weight = th.einsum(
- "bct,bcs->bts", q * scale, k * scale
- ) # More stable with f16 than dividing afterwards
- weight = th.softmax(weight.float(), dim=-1).type(weight.dtype)
- a = th.einsum("bts,bcs->bct", weight, v)
- return a.reshape(bs, -1, length)
-
- @staticmethod
- def count_flops(model, _x, y):
- return count_flops_attn(model, _x, y)
-
-
-class QKVAttention(nn.Module):
- """
- A module which performs QKV attention and splits in a different order.
- """
-
- def __init__(self, n_heads):
- super().__init__()
- self.n_heads = n_heads
-
- def forward(self, qkv):
- """
- Apply QKV attention.
- :param qkv: an [N x (3 * H * C) x T] tensor of Qs, Ks, and Vs.
- :return: an [N x (H * C) x T] tensor after attention.
- """
- bs, width, length = qkv.shape
- assert width % (3 * self.n_heads) == 0
- ch = width // (3 * self.n_heads)
- q, k, v = qkv.chunk(3, dim=1)
- scale = 1 / math.sqrt(math.sqrt(ch))
- weight = th.einsum(
- "bct,bcs->bts",
- (q * scale).view(bs * self.n_heads, ch, length),
- (k * scale).view(bs * self.n_heads, ch, length),
- ) # More stable with f16 than dividing afterwards
- weight = th.softmax(weight.float(), dim=-1).type(weight.dtype)
- a = th.einsum("bts,bcs->bct", weight, v.reshape(bs * self.n_heads, ch, length))
- return a.reshape(bs, -1, length)
-
- @staticmethod
- def count_flops(model, _x, y):
- return count_flops_attn(model, _x, y)
-
-
-class UNetModel(nn.Module):
- """
- The full UNet model with attention and timestep embedding.
- :param in_channels: channels in the input Tensor.
- :param model_channels: base channel count for the model.
- :param out_channels: channels in the output Tensor.
- :param num_res_blocks: number of residual blocks per downsample.
- :param attention_resolutions: a collection of downsample rates at which
- attention will take place. May be a set, list, or tuple.
- For example, if this contains 4, then at 4x downsampling, attention
- will be used.
- :param dropout: the dropout probability.
- :param channel_mult: channel multiplier for each level of the UNet.
- :param conv_resample: if True, use learned convolutions for upsampling and
- downsampling.
- :param dims: determines if the signal is 1D, 2D, or 3D.
- :param num_classes: if specified (as an int), then this model will be
- class-conditional with `num_classes` classes.
- :param use_checkpoint: use gradient checkpointing to reduce memory usage.
- :param num_heads: the number of attention heads in each attention layer.
- :param num_heads_channels: if specified, ignore num_heads and instead use
- a fixed channel width per attention head.
- :param num_heads_upsample: works with num_heads to set a different number
- of heads for upsampling. Deprecated.
- :param use_scale_shift_norm: use a FiLM-like conditioning mechanism.
- :param resblock_updown: use residual blocks for up/downsampling.
- :param use_new_attention_order: use a different attention pattern for potentially
- increased efficiency.
- """
-
- def __init__(
- self,
- image_size,
- in_channels,
- model_channels,
- out_channels,
- num_res_blocks,
- attention_resolutions,
- dropout=0,
- channel_mult=(1, 2, 4, 8),
- conv_resample=True,
- dims=2,
- num_classes=None,
- use_checkpoint=False,
- use_fp16=False,
- num_heads=-1,
- num_head_channels=-1,
- num_heads_upsample=-1,
- use_scale_shift_norm=False,
- resblock_updown=False,
- use_new_attention_order=False,
- use_spatial_transformer=False, # custom transformer support
- transformer_depth=1, # custom transformer support
- context_dim=None, # custom transformer support
- n_embed=None, # custom support for prediction of discrete ids into codebook of first stage vq model
- legacy=True,
- disable_self_attentions=None,
- num_attention_blocks=None,
- disable_middle_self_attn=False,
- use_linear_in_transformer=False,
- ):
- super().__init__()
- if use_spatial_transformer:
- assert context_dim is not None, 'Fool!! You forgot to include the dimension of your cross-attention conditioning...'
-
- if context_dim is not None:
- assert use_spatial_transformer, 'Fool!! You forgot to use the spatial transformer for your cross-attention conditioning...'
- from omegaconf.listconfig import ListConfig
- if type(context_dim) == ListConfig:
- context_dim = list(context_dim)
-
- if num_heads_upsample == -1:
- num_heads_upsample = num_heads
-
- if num_heads == -1:
- assert num_head_channels != -1, 'Either num_heads or num_head_channels has to be set'
-
- if num_head_channels == -1:
- assert num_heads != -1, 'Either num_heads or num_head_channels has to be set'
-
- self.image_size = image_size
- self.in_channels = in_channels
- self.model_channels = model_channels
- self.out_channels = out_channels
- if isinstance(num_res_blocks, int):
- self.num_res_blocks = len(channel_mult) * [num_res_blocks]
- else:
- if len(num_res_blocks) != len(channel_mult):
- raise ValueError("provide num_res_blocks either as an int (globally constant) or "
- "as a list/tuple (per-level) with the same length as channel_mult")
- self.num_res_blocks = num_res_blocks
- if disable_self_attentions is not None:
- # should be a list of booleans, indicating whether to disable self-attention in TransformerBlocks or not
- assert len(disable_self_attentions) == len(channel_mult)
- if num_attention_blocks is not None:
- assert len(num_attention_blocks) == len(self.num_res_blocks)
- assert all(map(lambda i: self.num_res_blocks[i] >= num_attention_blocks[i], range(len(num_attention_blocks))))
- print(f"Constructor of UNetModel received num_attention_blocks={num_attention_blocks}. "
- f"This option has LESS priority than attention_resolutions {attention_resolutions}, "
- f"i.e., in cases where num_attention_blocks[i] > 0 but 2**i not in attention_resolutions, "
- f"attention will still not be set.")
-
- self.attention_resolutions = attention_resolutions
- self.dropout = dropout
- self.channel_mult = channel_mult
- self.conv_resample = conv_resample
- self.num_classes = num_classes
- self.use_checkpoint = use_checkpoint
- self.dtype = th.float16 if use_fp16 else th.float32
- self.num_heads = num_heads
- self.num_head_channels = num_head_channels
- self.num_heads_upsample = num_heads_upsample
- self.predict_codebook_ids = n_embed is not None
- # time embedding
- time_embed_dim = model_channels * 4
- self.time_embed = nn.Sequential(
- linear(model_channels, time_embed_dim),
- nn.SiLU(),
- linear(time_embed_dim, time_embed_dim),
- )
- # class-related
- if self.num_classes is not None:
- if isinstance(self.num_classes, int):
- self.label_emb = nn.Embedding(num_classes, time_embed_dim)
- elif self.num_classes == "continuous":
- print("setting up linear c_adm embedding layer")
- self.label_emb = nn.Linear(1, time_embed_dim)
- else:
- raise ValueError()
- # input blocks
- self.input_blocks = nn.ModuleList(
- [
- TimestepEmbedSequential(
- conv_nd(dims, in_channels, model_channels, 3, padding=1)
- )
- ]
- )
- self._feature_size = model_channels
- input_block_chans = [model_channels]
- ch = model_channels
- ds = 1
- for level, mult in enumerate(channel_mult):
- for nr in range(self.num_res_blocks[level]):
- layers = [
- ResBlock(
- ch,
- time_embed_dim,
- dropout,
- out_channels=mult * model_channels,
- dims=dims,
- use_checkpoint=use_checkpoint,
- use_scale_shift_norm=use_scale_shift_norm,
- )
- ]
- ch = mult * model_channels
- if ds in attention_resolutions:
- if num_head_channels == -1:
- dim_head = ch // num_heads
- else:
- num_heads = ch // num_head_channels
- dim_head = num_head_channels
- if legacy:
- #num_heads = 1
- dim_head = ch // num_heads if use_spatial_transformer else num_head_channels
- if exists(disable_self_attentions):
- disabled_sa = disable_self_attentions[level]
- else:
- disabled_sa = False
-
- if not exists(num_attention_blocks) or nr < num_attention_blocks[level]:
- layers.append(
- AttentionBlock(
- ch,
- use_checkpoint=use_checkpoint,
- num_heads=num_heads,
- num_head_channels=dim_head,
- use_new_attention_order=use_new_attention_order,
- ) if not use_spatial_transformer else SpatialTransformer(
- ch, num_heads, dim_head, depth=transformer_depth, context_dim=context_dim,
- disable_self_attn=disabled_sa, use_linear=use_linear_in_transformer,
- use_checkpoint=use_checkpoint
- )
- )
- self.input_blocks.append(TimestepEmbedSequential(*layers))
- self._feature_size += ch
- input_block_chans.append(ch)
- if level != len(channel_mult) - 1:
- out_ch = ch
- self.input_blocks.append(
- TimestepEmbedSequential(
- ResBlock(
- ch,
- time_embed_dim,
- dropout,
- out_channels=out_ch,
- dims=dims,
- use_checkpoint=use_checkpoint,
- use_scale_shift_norm=use_scale_shift_norm,
- down=True,
- )
- if resblock_updown
- else Downsample(
- ch, conv_resample, dims=dims, out_channels=out_ch
- )
- )
- )
- ch = out_ch
- input_block_chans.append(ch)
- ds *= 2
- self._feature_size += ch
-
- if num_head_channels == -1:
- dim_head = ch // num_heads
- else:
- num_heads = ch // num_head_channels
- dim_head = num_head_channels
- if legacy:
- #num_heads = 1
- dim_head = ch // num_heads if use_spatial_transformer else num_head_channels
- self.middle_block = TimestepEmbedSequential(
- ResBlock(
- ch,
- time_embed_dim,
- dropout,
- dims=dims,
- use_checkpoint=use_checkpoint,
- use_scale_shift_norm=use_scale_shift_norm,
- ),
- AttentionBlock(
- ch,
- use_checkpoint=use_checkpoint,
- num_heads=num_heads,
- num_head_channels=dim_head,
- use_new_attention_order=use_new_attention_order,
- ) if not use_spatial_transformer else SpatialTransformer( # always uses a self-attn
- ch, num_heads, dim_head, depth=transformer_depth, context_dim=context_dim,
- disable_self_attn=disable_middle_self_attn, use_linear=use_linear_in_transformer,
- use_checkpoint=use_checkpoint
- ),
- ResBlock(
- ch,
- time_embed_dim,
- dropout,
- dims=dims,
- use_checkpoint=use_checkpoint,
- use_scale_shift_norm=use_scale_shift_norm,
- ),
- )
- self._feature_size += ch
- # output blocks
- self.output_blocks = nn.ModuleList([])
- for level, mult in list(enumerate(channel_mult))[::-1]:
- for i in range(self.num_res_blocks[level] + 1):
- ich = input_block_chans.pop()
- layers = [
- ResBlock(
- ch + ich,
- time_embed_dim,
- dropout,
- out_channels=model_channels * mult,
- dims=dims,
- use_checkpoint=use_checkpoint,
- use_scale_shift_norm=use_scale_shift_norm,
- )
- ]
- ch = model_channels * mult
- if ds in attention_resolutions:
- if num_head_channels == -1:
- dim_head = ch // num_heads
- else:
- num_heads = ch // num_head_channels
- dim_head = num_head_channels
- if legacy:
- #num_heads = 1
- dim_head = ch // num_heads if use_spatial_transformer else num_head_channels
- if exists(disable_self_attentions):
- disabled_sa = disable_self_attentions[level]
- else:
- disabled_sa = False
-
- if not exists(num_attention_blocks) or i < num_attention_blocks[level]:
- layers.append(
- AttentionBlock(
- ch,
- use_checkpoint=use_checkpoint,
- num_heads=num_heads_upsample,
- num_head_channels=dim_head,
- use_new_attention_order=use_new_attention_order,
- ) if not use_spatial_transformer else SpatialTransformer(
- ch, num_heads, dim_head, depth=transformer_depth, context_dim=context_dim,
- disable_self_attn=disabled_sa, use_linear=use_linear_in_transformer,
- use_checkpoint=use_checkpoint
- )
- )
- if level and i == self.num_res_blocks[level]:
- out_ch = ch
- layers.append(
- ResBlock(
- ch,
- time_embed_dim,
- dropout,
- out_channels=out_ch,
- dims=dims,
- use_checkpoint=use_checkpoint,
- use_scale_shift_norm=use_scale_shift_norm,
- up=True,
- )
- if resblock_updown
- else Upsample(ch, conv_resample, dims=dims, out_channels=out_ch)
- )
- ds //= 2
- self.output_blocks.append(TimestepEmbedSequential(*layers))
- self._feature_size += ch
-
- self.out = nn.Sequential(
- normalization(ch),
- nn.SiLU(),
- zero_module(conv_nd(dims, model_channels, out_channels, 3, padding=1)),
- )
- if self.predict_codebook_ids:
- self.id_predictor = nn.Sequential(
- normalization(ch),
- conv_nd(dims, model_channels, n_embed, 1),
- #nn.LogSoftmax(dim=1) # change to cross_entropy and produce non-normalized logits
- )
- self.context_dim = context_dim
-
- def convert_to_fp16(self):
- """
- Convert the torso of the model to float16.
- """
- self.input_blocks.apply(convert_module_to_f16)
- self.middle_block.apply(convert_module_to_f16)
- self.output_blocks.apply(convert_module_to_f16)
-
- def convert_to_fp32(self):
- """
- Convert the torso of the model to float32.
- """
- self.input_blocks.apply(convert_module_to_f32)
- self.middle_block.apply(convert_module_to_f32)
- self.output_blocks.apply(convert_module_to_f32)
-
- def forward(self, x, timesteps=None, context=None, y=None,**kwargs):
- """
- Apply the model to an input batch.
- :param x: an [N x C x ...] Tensor of inputs.
- :param timesteps: a 1-D batch of timesteps.
- :param context: conditioning plugged in via crossattn
- :param y: an [N] Tensor of labels, if class-conditional.
- :return: an [N x C x ...] Tensor of outputs.
- """
- assert (y is not None) == (
- self.num_classes is not None
- ), "must specify y if and only if the model is class-conditional"
- hs = []
- t_emb = timestep_embedding(timesteps, self.model_channels, repeat_only=False)
- emb = self.time_embed(t_emb)
-
- if self.num_classes is not None:
- assert y.shape[0] == x.shape[0]
- emb = emb + self.label_emb(y) # class condition added to the time embedding
-
- h = x.type(self.dtype)
- for module in self.input_blocks:
- h = module(h, emb, context)
- hs.append(h)
- h = self.middle_block(h, emb, context)
- for module in self.output_blocks:
- h = th.cat([h, hs.pop()], dim=1)
- h = module(h, emb, context)
- h = h.type(x.dtype)
- if self.predict_codebook_ids:
- return self.id_predictor(h)
- else:
- out = self.out(h)
- # if True in np.isnan(out.detach().cpu().numpy()):
- # aa = 1
- return out
diff --git a/spaces/AIZero2HeroBootcamp/ExperimentalChatGPTv1/app.py b/spaces/AIZero2HeroBootcamp/ExperimentalChatGPTv1/app.py
deleted file mode 100644
index 6e86abff95351769056a696503ff05e34c7117c9..0000000000000000000000000000000000000000
--- a/spaces/AIZero2HeroBootcamp/ExperimentalChatGPTv1/app.py
+++ /dev/null
@@ -1,442 +0,0 @@
-import streamlit as st
-import openai
-import os
-import base64
-import glob
-import json
-import mistune
-import pytz
-import math
-import requests
-import time
-import re
-import textract
-
-from datetime import datetime
-from openai import ChatCompletion
-from xml.etree import ElementTree as ET
-from bs4 import BeautifulSoup
-from collections import deque
-from audio_recorder_streamlit import audio_recorder
-
-from dotenv import load_dotenv
-from PyPDF2 import PdfReader
-from langchain.text_splitter import CharacterTextSplitter
-from langchain.embeddings import OpenAIEmbeddings
-from langchain.vectorstores import FAISS
-from langchain.chat_models import ChatOpenAI
-from langchain.memory import ConversationBufferMemory
-from langchain.chains import ConversationalRetrievalChain
-from templates import css, bot_template, user_template
-
-
-
-def generate_filename(prompt, file_type):
- central = pytz.timezone('US/Central')
- safe_date_time = datetime.now(central).strftime("%m%d_%H%M") # Date and time DD-HHMM
- safe_prompt = "".join(x for x in prompt if x.isalnum())[:90] # Limit file name size and trim whitespace
- return f"{safe_date_time}_{safe_prompt}.{file_type}" # Return a safe file name
-
-
-def transcribe_audio(openai_key, file_path, model):
- OPENAI_API_URL = "https://api.openai.com/v1/audio/transcriptions"
- headers = {
- "Authorization": f"Bearer {openai_key}",
- }
- with open(file_path, 'rb') as f:
- data = {'file': f}
- response = requests.post(OPENAI_API_URL, headers=headers, files=data, data={'model': model})
- if response.status_code == 200:
- st.write(response.json())
- chatResponse = chat_with_model(response.json().get('text'), '') # *************************************
- transcript = response.json().get('text')
- #st.write('Responses:')
- #st.write(chatResponse)
- filename = generate_filename(transcript, 'txt')
- create_file(filename, transcript, chatResponse)
- return transcript
- else:
- st.write(response.json())
- st.error("Error in API call.")
- return None
-
-def save_and_play_audio(audio_recorder):
- audio_bytes = audio_recorder()
- if audio_bytes:
- filename = generate_filename("Recording", "wav")
- with open(filename, 'wb') as f:
- f.write(audio_bytes)
- st.audio(audio_bytes, format="audio/wav")
- return filename
- return None
-
-def create_file(filename, prompt, response):
- if filename.endswith(".txt"):
- with open(filename, 'w') as file:
- file.write(f"{prompt}\n{response}")
- elif filename.endswith(".htm"):
- with open(filename, 'w') as file:
- file.write(f"{prompt} {response}")
- elif filename.endswith(".md"):
- with open(filename, 'w') as file:
- file.write(f"{prompt}\n\n{response}")
-
-def truncate_document(document, length):
- return document[:length]
-def divide_document(document, max_length):
- return [document[i:i+max_length] for i in range(0, len(document), max_length)]
-
-def get_table_download_link(file_path):
- with open(file_path, 'r') as file:
- try:
- data = file.read()
- except:
- st.write('')
- return file_path
- b64 = base64.b64encode(data.encode()).decode()
- file_name = os.path.basename(file_path)
- ext = os.path.splitext(file_name)[1] # get the file extension
- if ext == '.txt':
- mime_type = 'text/plain'
- elif ext == '.py':
- mime_type = 'text/plain'
- elif ext == '.xlsx':
- mime_type = 'text/plain'
- elif ext == '.csv':
- mime_type = 'text/plain'
- elif ext == '.htm':
- mime_type = 'text/html'
- elif ext == '.md':
- mime_type = 'text/markdown'
- else:
- mime_type = 'application/octet-stream' # general binary data type
- href = f'{file_name}'
- return href
-
-def CompressXML(xml_text):
- root = ET.fromstring(xml_text)
- for elem in list(root.iter()):
- if isinstance(elem.tag, str) and 'Comment' in elem.tag:
- elem.parent.remove(elem)
- return ET.tostring(root, encoding='unicode', method="xml")
-
-def read_file_content(file,max_length):
- if file.type == "application/json":
- content = json.load(file)
- return str(content)
- elif file.type == "text/html" or file.type == "text/htm":
- content = BeautifulSoup(file, "html.parser")
- return content.text
- elif file.type == "application/xml" or file.type == "text/xml":
- tree = ET.parse(file)
- root = tree.getroot()
- xml = CompressXML(ET.tostring(root, encoding='unicode'))
- return xml
- elif file.type == "text/markdown" or file.type == "text/md":
- md = mistune.create_markdown()
- content = md(file.read().decode())
- return content
- elif file.type == "text/plain":
- return file.getvalue().decode()
- else:
- return ""
-
-def chat_with_model(prompt, document_section, model_choice='gpt-3.5-turbo'):
- model = model_choice
- conversation = [{'role': 'system', 'content': 'You are a helpful assistant.'}]
- conversation.append({'role': 'user', 'content': prompt})
- if len(document_section)>0:
- conversation.append({'role': 'assistant', 'content': document_section})
-
- start_time = time.time()
- report = []
- res_box = st.empty()
- collected_chunks = []
- collected_messages = []
-
- for chunk in openai.ChatCompletion.create(
- model='gpt-3.5-turbo',
- messages=conversation,
- temperature=0.5,
- stream=True
- ):
-
- collected_chunks.append(chunk) # save the event response
- chunk_message = chunk['choices'][0]['delta'] # extract the message
- collected_messages.append(chunk_message) # save the message
-
- content=chunk["choices"][0].get("delta",{}).get("content")
-
- try:
- report.append(content)
- if len(content) > 0:
- result = "".join(report).strip()
- #result = result.replace("\n", "")
- res_box.markdown(f'*{result}*')
- except:
- st.write(' ')
-
- full_reply_content = ''.join([m.get('content', '') for m in collected_messages])
- st.write("Elapsed time:")
- st.write(time.time() - start_time)
- return full_reply_content
-
-def chat_with_file_contents(prompt, file_content, model_choice='gpt-3.5-turbo'):
- conversation = [{'role': 'system', 'content': 'You are a helpful assistant.'}]
- conversation.append({'role': 'user', 'content': prompt})
- if len(file_content)>0:
- conversation.append({'role': 'assistant', 'content': file_content})
- response = openai.ChatCompletion.create(model=model_choice, messages=conversation)
- return response['choices'][0]['message']['content']
-
-def extract_mime_type(file):
- # Check if the input is a string
- if isinstance(file, str):
- pattern = r"type='(.*?)'"
- match = re.search(pattern, file)
- if match:
- return match.group(1)
- else:
- raise ValueError(f"Unable to extract MIME type from {file}")
- # If it's not a string, assume it's a streamlit.UploadedFile object
- elif isinstance(file, streamlit.UploadedFile):
- return file.type
- else:
- raise TypeError("Input should be a string or a streamlit.UploadedFile object")
-
-from io import BytesIO
-import re
-
-def extract_file_extension(file):
- # get the file name directly from the UploadedFile object
- file_name = file.name
- pattern = r".*?\.(.*?)$"
- match = re.search(pattern, file_name)
- if match:
- return match.group(1)
- else:
- raise ValueError(f"Unable to extract file extension from {file_name}")
-
-def pdf2txt(docs):
- text = ""
- for file in docs:
- file_extension = extract_file_extension(file)
- # print the file extension
- st.write(f"File type extension: {file_extension}")
-
- # read the file according to its extension
- try:
- if file_extension.lower() in ['py', 'txt', 'html', 'htm', 'xml', 'json']:
- text += file.getvalue().decode('utf-8')
- elif file_extension.lower() == 'pdf':
- from PyPDF2 import PdfReader
- pdf = PdfReader(BytesIO(file.getvalue()))
- for page in range(len(pdf.pages)):
- text += pdf.pages[page].extract_text() # new PyPDF2 syntax
- except Exception as e:
- st.write(f"Error processing file {file.name}: {e}")
-
- return text
-
-def pdf2txt_old(pdf_docs):
- st.write(pdf_docs)
- for file in pdf_docs:
- mime_type = extract_mime_type(file)
- st.write(f"MIME type of file: {mime_type}")
-
- text = ""
- for pdf in pdf_docs:
- pdf_reader = PdfReader(pdf)
- for page in pdf_reader.pages:
- text += page.extract_text()
- return text
-
-def txt2chunks(text):
- text_splitter = CharacterTextSplitter(separator="\n", chunk_size=1000, chunk_overlap=200, length_function=len)
- return text_splitter.split_text(text)
-
-def vector_store(text_chunks):
- key = os.getenv('OPENAI_API_KEY')
- embeddings = OpenAIEmbeddings(openai_api_key=key)
- return FAISS.from_texts(texts=text_chunks, embedding=embeddings)
-
-def get_chain(vectorstore):
- llm = ChatOpenAI()
- memory = ConversationBufferMemory(memory_key='chat_history', return_messages=True)
- return ConversationalRetrievalChain.from_llm(llm=llm, retriever=vectorstore.as_retriever(), memory=memory)
-
-def process_user_input(user_question):
- response = st.session_state.conversation({'question': user_question})
- st.session_state.chat_history = response['chat_history']
- for i, message in enumerate(st.session_state.chat_history):
- template = user_template if i % 2 == 0 else bot_template
- st.write(template.replace("{{MSG}}", message.content), unsafe_allow_html=True)
- # Save file output from PDF query results
- filename = generate_filename(user_question, 'txt')
- create_file(filename, user_question, message.content)
-
- #st.sidebar.markdown(get_table_download_link(filename), unsafe_allow_html=True)
-
-def divide_prompt(prompt, max_length):
- words = prompt.split()
- chunks = []
- current_chunk = []
- current_length = 0
- for word in words:
- if len(word) + current_length <= max_length:
- current_length += len(word) + 1 # Adding 1 to account for spaces
- current_chunk.append(word)
- else:
- chunks.append(' '.join(current_chunk))
- current_chunk = [word]
- current_length = len(word)
- chunks.append(' '.join(current_chunk)) # Append the final chunk
- return chunks
-
-def main():
- # Sidebar and global
- openai.api_key = os.getenv('OPENAI_API_KEY')
- st.set_page_config(page_title="GPT Streamlit Document Reasoner",layout="wide")
-
- # File type for output, model choice
- menu = ["txt", "htm", "xlsx", "csv", "md", "py"] #619
- choice = st.sidebar.selectbox("Output File Type:", menu)
- model_choice = st.sidebar.radio("Select Model:", ('gpt-3.5-turbo', 'gpt-3.5-turbo-0301'))
-
- # Audio, transcribe, GPT:
- filename = save_and_play_audio(audio_recorder)
- if filename is not None:
- transcription = transcribe_audio(openai.api_key, filename, "whisper-1")
- st.sidebar.markdown(get_table_download_link(filename), unsafe_allow_html=True)
- filename=None # since transcription is finished next time just use the saved transcript
-
- # prompt interfaces
- user_prompt = st.text_area("Enter prompts, instructions & questions:", '', height=100)
-
- # file section interface for prompts against large documents as context
- collength, colupload = st.columns([2,3]) # adjust the ratio as needed
- with collength:
- max_length = st.slider("File section length for large files", min_value=1000, max_value=128000, value=12000, step=1000)
- with colupload:
- uploaded_file = st.file_uploader("Add a file for context:", type=["pdf", "xml", "json", "xlsx","csv","html", "htm", "md", "txt"])
-
- # Document section chat
- document_sections = deque()
- document_responses = {}
- if uploaded_file is not None:
- file_content = read_file_content(uploaded_file, max_length)
- document_sections.extend(divide_document(file_content, max_length))
- if len(document_sections) > 0:
- if st.button("👁️ View Upload"):
- st.markdown("**Sections of the uploaded file:**")
- for i, section in enumerate(list(document_sections)):
- st.markdown(f"**Section {i+1}**\n{section}")
- st.markdown("**Chat with the model:**")
- for i, section in enumerate(list(document_sections)):
- if i in document_responses:
- st.markdown(f"**Section {i+1}**\n{document_responses[i]}")
- else:
- if st.button(f"Chat about Section {i+1}"):
- st.write('Reasoning with your inputs...')
- response = chat_with_model(user_prompt, section, model_choice) # *************************************
- st.write('Response:')
- st.write(response)
- document_responses[i] = response
- filename = generate_filename(f"{user_prompt}_section_{i+1}", choice)
- create_file(filename, user_prompt, response)
- st.sidebar.markdown(get_table_download_link(filename), unsafe_allow_html=True)
-
- if st.button('💬 Chat'):
- st.write('Reasoning with your inputs...')
-
- #response = chat_with_model(user_prompt, ''.join(list(document_sections,)), model_choice) # *************************************
-
- # Divide the user_prompt into smaller sections
- user_prompt_sections = divide_prompt(user_prompt, max_length)
- full_response = ''
- for prompt_section in user_prompt_sections:
- # Process each section with the model
- response = chat_with_model(prompt_section, ''.join(list(document_sections)), model_choice)
- full_response += response + '\n' # Combine the responses
-
- #st.write('Response:')
- #st.write(full_response)
-
- response = full_response
- st.write('Response:')
- st.write(response)
-
- filename = generate_filename(user_prompt, choice)
- create_file(filename, user_prompt, response)
- st.sidebar.markdown(get_table_download_link(filename), unsafe_allow_html=True)
-
- all_files = glob.glob("*.*")
- all_files = [file for file in all_files if len(os.path.splitext(file)[0]) >= 20] # exclude files with short names
- all_files.sort(key=lambda x: (os.path.splitext(x)[1], x), reverse=True) # sort by file type and file name in descending order
-
- # sidebar of files
- file_contents=''
- next_action=''
- for file in all_files:
- col1, col2, col3, col4, col5 = st.sidebar.columns([1,6,1,1,1]) # adjust the ratio as needed
- with col1:
- if st.button("🌐", key="md_"+file): # md emoji button
- with open(file, 'r') as f:
- file_contents = f.read()
- next_action='md'
- with col2:
- st.markdown(get_table_download_link(file), unsafe_allow_html=True)
- with col3:
- if st.button("📂", key="open_"+file): # open emoji button
- with open(file, 'r') as f:
- file_contents = f.read()
- next_action='open'
- with col4:
- if st.button("🔍", key="read_"+file): # search emoji button
- with open(file, 'r') as f:
- file_contents = f.read()
- next_action='search'
- with col5:
- if st.button("🗑", key="delete_"+file):
- os.remove(file)
- st.experimental_rerun()
-
- if len(file_contents) > 0:
- if next_action=='open':
- file_content_area = st.text_area("File Contents:", file_contents, height=500)
- if next_action=='md':
- st.markdown(file_contents)
- if next_action=='search':
- file_content_area = st.text_area("File Contents:", file_contents, height=500)
- st.write('Reasoning with your inputs...')
- response = chat_with_model(user_prompt, file_contents, model_choice)
- filename = generate_filename(file_contents, choice)
- create_file(filename, file_contents, response)
-
- st.experimental_rerun()
- #st.sidebar.markdown(get_table_download_link(filename), unsafe_allow_html=True)
-
-if __name__ == "__main__":
- main()
-
-load_dotenv()
-st.write(css, unsafe_allow_html=True)
-
-st.header("Chat with documents :books:")
-user_question = st.text_input("Ask a question about your documents:")
-if user_question:
- process_user_input(user_question)
-
-with st.sidebar:
- st.subheader("Your documents")
- docs = st.file_uploader("import documents", accept_multiple_files=True)
- with st.spinner("Processing"):
- raw = pdf2txt(docs)
- if len(raw) > 0:
- length = str(len(raw))
- text_chunks = txt2chunks(raw)
- vectorstore = vector_store(text_chunks)
- st.session_state.conversation = get_chain(vectorstore)
- st.markdown('# AI Search Index of Length:' + length + ' Created.') # add timing
- filename = generate_filename(raw, 'txt')
- create_file(filename, raw, '')
\ No newline at end of file
diff --git a/spaces/Aabdelhamidaz/animals/README.md b/spaces/Aabdelhamidaz/animals/README.md
deleted file mode 100644
index f902a02e821c821ad02938bba5878ce11ef9805c..0000000000000000000000000000000000000000
--- a/spaces/Aabdelhamidaz/animals/README.md
+++ /dev/null
@@ -1,13 +0,0 @@
----
-title: Animals
-emoji: 🐠
-colorFrom: blue
-colorTo: pink
-sdk: gradio
-sdk_version: 3.1.1
-app_file: app.py
-pinned: false
-license: apache-2.0
----
-
-Check out the configuration reference at https://huggingface.co/docs/hub/spaces-config-reference
diff --git a/spaces/Abhilashvj/planogram-compliance/README.md b/spaces/Abhilashvj/planogram-compliance/README.md
deleted file mode 100644
index 78c9a73229919ae78ca8f320270d6fa30c50436b..0000000000000000000000000000000000000000
--- a/spaces/Abhilashvj/planogram-compliance/README.md
+++ /dev/null
@@ -1,166 +0,0 @@
----
-sdk: streamlit
-sdk_version: 1.10.0 # The latest supported version
-app_file: app.py
-pinned: false
-fullWidth: True
----
-##
Planogram Scoring
-
-
-
-- Train a Yolo Model on the available products in our data base to detect them on a shelf
-- https://wandb.ai/abhilash001vj/YOLOv5/runs/1v6yh7nk?workspace=user-abhilash001vj
-- Have the master planogram data captured as a matrix of products encoded as numbers (label encoding by looking the products names saved in a list of all - the available product names )
-- Detect the products on real images from stores.
-- Arrange the detected products in the captured photograph to rows and columns
-- Compare the product arrangement of captured photograph to the existing master planogram and produce the compliance score for correctly placed products
-
-
-
-##
YOLOv5
-
-YOLOv5 🚀 is a family of object detection architectures and models pretrained on the COCO dataset, and represents Ultralytics
- open-source research into future vision AI methods, incorporating lessons learned and best practices evolved over thousands of hours of research and development.
-
-
-
-
-##
Documentation
-
-See the [YOLOv5 Docs](https://docs.ultralytics.com) for full documentation on training, testing and deployment.
-
-##
Quick Start Examples
-
-
-Install
-
-[**Python>=3.6.0**](https://www.python.org/) is required with all
-[requirements.txt](https://github.com/ultralytics/yolov5/blob/master/requirements.txt) installed including
-[**PyTorch>=1.7**](https://pytorch.org/get-started/locally/):
-
-
-```bash
-$ git clone https://github.com/ultralytics/yolov5
-$ cd yolov5
-$ pip install -r requirements.txt
-```
-
-
-
-
-Inference
-
-Inference with YOLOv5 and [PyTorch Hub](https://github.com/ultralytics/yolov5/issues/36). Models automatically download
-from the [latest YOLOv5 release](https://github.com/ultralytics/yolov5/releases).
-
-```python
-import torch
-
-# Model
-model = torch.hub.load('ultralytics/yolov5', 'yolov5s') # or yolov5m, yolov5l, yolov5x, custom
-
-# Images
-img = 'https://ultralytics.com/images/zidane.jpg' # or file, Path, PIL, OpenCV, numpy, list
-
-# Inference
-results = model(img)
-
-# Results
-results.print() # or .show(), .save(), .crop(), .pandas(), etc.
-```
-
-
-
-
-##
Why YOLOv5
-
-
-
- YOLOv5-P5 640 Figure (click to expand)
-
-
-
-
- Figure Notes (click to expand)
-
-* GPU Speed measures end-to-end time per image averaged over 5000 COCO val2017 images using a V100 GPU with batch size
- 32, and includes image preprocessing, PyTorch FP16 inference, postprocessing and NMS.
-* EfficientDet data from [google/automl](https://github.com/google/automl) at batch size 8.
-* **Reproduce** by
- `python val.py --task study --data coco.yaml --iou 0.7 --weights yolov5s6.pt yolov5m6.pt yolov5l6.pt yolov5x6.pt`
-
-
-
-### Pretrained Checkpoints
-
-[assets]: https://github.com/ultralytics/yolov5/releases
-
-|Model |size (pixels) |mAPval 0.5:0.95 |mAPtest 0.5:0.95 |mAPval 0.5 |Speed V100 (ms) | |params (M) |FLOPs 640 (B)
-|--- |--- |--- |--- |--- |--- |---|--- |---
-|[YOLOv5s][assets] |640 |36.7 |36.7 |55.4 |**2.0** | |7.3 |17.0
-|[YOLOv5m][assets] |640 |44.5 |44.5 |63.1 |2.7 | |21.4 |51.3
-|[YOLOv5l][assets] |640 |48.2 |48.2 |66.9 |3.8 | |47.0 |115.4
-|[YOLOv5x][assets] |640 |**50.4** |**50.4** |**68.8** |6.1 | |87.7 |218.8
-| | | | | | | | |
-|[YOLOv5s6][assets] |1280 |43.3 |43.3 |61.9 |**4.3** | |12.7 |17.4
-|[YOLOv5m6][assets] |1280 |50.5 |50.5 |68.7 |8.4 | |35.9 |52.4
-|[YOLOv5l6][assets] |1280 |53.4 |53.4 |71.1 |12.3 | |77.2 |117.7
-|[YOLOv5x6][assets] |1280 |**54.4** |**54.4** |**72.0** |22.4 | |141.8 |222.9
-| | | | | | | | |
-|[YOLOv5x6][assets] TTA |1280 |**55.0** |**55.0** |**72.0** |70.8 | |- |-
-
-
- Table Notes (click to expand)
-
-* APtest denotes COCO [test-dev2017](http://cocodataset.org/#upload) server results, all other AP results
- denote val2017 accuracy.
-* AP values are for single-model single-scale unless otherwise noted. **Reproduce mAP**
- by `python val.py --data coco.yaml --img 640 --conf 0.001 --iou 0.65`
-* SpeedGPU averaged over 5000 COCO val2017 images using a
- GCP [n1-standard-16](https://cloud.google.com/compute/docs/machine-types#n1_standard_machine_types) V100 instance, and
- includes FP16 inference, postprocessing and NMS. **Reproduce speed**
- by `python val.py --data coco.yaml --img 640 --conf 0.25 --iou 0.45 --half`
-* All checkpoints are trained to 300 epochs with default settings and hyperparameters (no autoaugmentation).
-* Test Time Augmentation ([TTA](https://github.com/ultralytics/yolov5/issues/303)) includes reflection and scale
- augmentation. **Reproduce TTA** by `python val.py --data coco.yaml --img 1536 --iou 0.7 --augment`
-
-
-
-##
Contribute
-
-We love your input! We want to make contributing to YOLOv5 as easy and transparent as possible. Please see
-our [Contributing Guide](CONTRIBUTING.md) to get started.
-
-##
Contact
-
-For issues running YOLOv5 please visit [GitHub Issues](https://github.com/ultralytics/yolov5/issues). For business or
-professional support requests please visit [https://ultralytics.com/contact](https://ultralytics.com/contact).
-
-
-
-
diff --git a/spaces/AgentVerse/agentVerse/ui/src/phaser3-rex-plugins/templates/spinner/clock/Clock.js b/spaces/AgentVerse/agentVerse/ui/src/phaser3-rex-plugins/templates/spinner/clock/Clock.js
deleted file mode 100644
index 5905d65679e410c581179589c6a9ab29e0a69078..0000000000000000000000000000000000000000
--- a/spaces/AgentVerse/agentVerse/ui/src/phaser3-rex-plugins/templates/spinner/clock/Clock.js
+++ /dev/null
@@ -1,67 +0,0 @@
-import Base from '../base/Base.js';
-import { Circle, Line } from '../utils/Geoms.js'
-
-const RadToDeg = Phaser.Math.RadToDeg;
-const WrapDegrees = Phaser.Math.Angle.WrapDegrees;
-const WrapRad = Phaser.Math.Angle.Wrap;
-const ShortestBetween = Phaser.Math.Angle.ShortestBetween;
-const DegToRad = Phaser.Math.DegToRad;
-const Rad270 = Phaser.Math.DegToRad(270);
-
-class Clock extends Base {
- constructor(scene, config) {
- super(scene, config);
- this.type = 'rexSpinnerClock';
-
- this.minuteHandAngle = 0;
- this.hourHandAngle = 0;
- }
-
- buildShapes() {
- this.addShape((new Circle()).setName('border'));
- this.addShape((new Line()).setName('minuteHand'));
- this.addShape((new Line()).setName('hourHand'));
- }
-
- updateShapes() {
- var centerX = this.centerX;
- var centerY = this.centerY;
- var radius = this.radius;
- var lineWidth = Math.ceil(radius / 25);
- var borderRadius = radius - (lineWidth / 2);
- var minuteHandLength = radius * 0.8;
- var hourHandLength = radius * 0.5;
-
- var prevMinuteHandAngle = this.minuteHandAngle;
- this.minuteHandAngle = Math.PI * 2 * this.value;
- var angle0 = WrapDegrees(RadToDeg(prevMinuteHandAngle));
- var angle1 = WrapDegrees(RadToDeg(this.minuteHandAngle));
- var deltaAngle = ShortestBetween(angle0, angle1);
- this.hourHandAngle = WrapRad(this.hourHandAngle + (DegToRad(deltaAngle) / 12))
-
- this.getShape('border')
- .lineStyle(lineWidth, this.color)
- .setRadius(borderRadius)
- .setCenterPosition(centerX, centerY);
-
- var angle = this.minuteHandAngle + Rad270;
- this.getShape('minuteHand')
- .lineStyle(lineWidth, this.color)
- .setP0(centerX, centerY)
- .setP1(
- centerX + (Math.cos(angle) * minuteHandLength),
- centerY + (Math.sin(angle) * minuteHandLength)
- )
-
- var angle = this.hourHandAngle + Rad270;
- this.getShape('hourHand')
- .lineStyle(lineWidth, this.color)
- .setP0(centerX, centerY)
- .setP1(
- centerX + (Math.cos(angle) * hourHandLength),
- centerY + (Math.sin(angle) * hourHandLength)
- )
- }
-}
-
-export default Clock;
\ No newline at end of file
diff --git a/spaces/AgentVerse/agentVerse/ui/src/phaser3-rex-plugins/templates/spinner/los/Los.d.ts b/spaces/AgentVerse/agentVerse/ui/src/phaser3-rex-plugins/templates/spinner/los/Los.d.ts
deleted file mode 100644
index 7cd197f7f75b364f06e612b1731e6f0c39905984..0000000000000000000000000000000000000000
--- a/spaces/AgentVerse/agentVerse/ui/src/phaser3-rex-plugins/templates/spinner/los/Los.d.ts
+++ /dev/null
@@ -1,2 +0,0 @@
-import Base from '../base/Base';
-export default class Los extends Base { }
\ No newline at end of file
diff --git a/spaces/AgentVerse/agentVerse/ui/src/phaser3-rex-plugins/templates/ui/pan/Factory.d.ts b/spaces/AgentVerse/agentVerse/ui/src/phaser3-rex-plugins/templates/ui/pan/Factory.d.ts
deleted file mode 100644
index d963d4b185aded4632463ccc782b2a8e426b331f..0000000000000000000000000000000000000000
--- a/spaces/AgentVerse/agentVerse/ui/src/phaser3-rex-plugins/templates/ui/pan/Factory.d.ts
+++ /dev/null
@@ -1,7 +0,0 @@
-// import * as Phaser from 'phaser';
-import Pan from "./Pan";
-
-export default function (
- gameObject: Phaser.GameObjects.GameObject | Phaser.Scene,
- config?: Pan.IConfig
-): Pan;
\ No newline at end of file
diff --git a/spaces/AkitoP/umamusume_bert_vits2/monotonic_align/core.py b/spaces/AkitoP/umamusume_bert_vits2/monotonic_align/core.py
deleted file mode 100644
index 7c962adea65543ef426034c4d53c4f0e615e8181..0000000000000000000000000000000000000000
--- a/spaces/AkitoP/umamusume_bert_vits2/monotonic_align/core.py
+++ /dev/null
@@ -1,46 +0,0 @@
-import numba
-
-
-@numba.jit(
- numba.void(
- numba.int32[:, :, ::1],
- numba.float32[:, :, ::1],
- numba.int32[::1],
- numba.int32[::1],
- ),
- nopython=True,
- nogil=True,
-)
-def maximum_path_jit(paths, values, t_ys, t_xs):
- b = paths.shape[0]
- max_neg_val = -1e9
- for i in range(int(b)):
- path = paths[i]
- value = values[i]
- t_y = t_ys[i]
- t_x = t_xs[i]
-
- v_prev = v_cur = 0.0
- index = t_x - 1
-
- for y in range(t_y):
- for x in range(max(0, t_x + y - t_y), min(t_x, y + 1)):
- if x == y:
- v_cur = max_neg_val
- else:
- v_cur = value[y - 1, x]
- if x == 0:
- if y == 0:
- v_prev = 0.0
- else:
- v_prev = max_neg_val
- else:
- v_prev = value[y - 1, x - 1]
- value[y, x] += max(v_prev, v_cur)
-
- for y in range(t_y - 1, -1, -1):
- path[y, index] = 1
- if index != 0 and (
- index == y or value[y - 1, index] < value[y - 1, index - 1]
- ):
- index = index - 1
diff --git a/spaces/AkshayKollimarala/MygenAI/app.py b/spaces/AkshayKollimarala/MygenAI/app.py
deleted file mode 100644
index 2dbf3ae89c2e3fdab7134107dd346f984dca8eb1..0000000000000000000000000000000000000000
--- a/spaces/AkshayKollimarala/MygenAI/app.py
+++ /dev/null
@@ -1,34 +0,0 @@
-import os
-import gradio as gr
-from langchain.chat_models import ChatOpenAI
-from langchain import LLMChain, PromptTemplate
-from langchain.memory import ConversationBufferMemory
-
-OPENAI_API_KEY=os.getenv('OPENAI_API_KEY')
-
-template = """Meet Riya, your youthful and witty personal assistant! At 21 years old, she's full of energy and always eager to help. Riya's goal is to assist you with any questions or problems you might have. Her enthusiasm shines through in every response, making interactions with her enjoyable and engaging.
-{chat_history}
-User: {user_message}
-Chatbot:"""
-
-prompt = PromptTemplate(
- input_variables=["chat_history", "user_message"], template=template
-)
-
-memory = ConversationBufferMemory(memory_key="chat_history")
-
-llm_chain = LLMChain(
- llm=ChatOpenAI(temperature='0.5', model_name="gpt-3.5-turbo"),
- prompt=prompt,
- verbose=True,
- memory=memory,
-)
-
-def get_text_response(user_message,history):
- response = llm_chain.predict(user_message = user_message)
- return response
-
-demo = gr.ChatInterface(get_text_response)
-
-if __name__ == "__main__":
- demo.launch() #To create a public link, set `share=True` in `launch()`. To enable errors and logs, set `debug=True` in `launch()`.
diff --git a/spaces/Aloento/9Nine-PITS/text/frontend/zh_normalization/chronology.py b/spaces/Aloento/9Nine-PITS/text/frontend/zh_normalization/chronology.py
deleted file mode 100644
index ed16763f923b5dd50758640843a85d6d0863b378..0000000000000000000000000000000000000000
--- a/spaces/Aloento/9Nine-PITS/text/frontend/zh_normalization/chronology.py
+++ /dev/null
@@ -1,134 +0,0 @@
-# Copyright (c) 2021 PaddlePaddle Authors. All Rights Reserved.
-#
-# Licensed under the Apache License, Version 2.0 (the "License");
-# you may not use this file except in compliance with the License.
-# You may obtain a copy of the License at
-#
-# http://www.apache.org/licenses/LICENSE-2.0
-#
-# Unless required by applicable law or agreed to in writing, software
-# distributed under the License is distributed on an "AS IS" BASIS,
-# WITHOUT WARRANTIES OR CONDITIONS OF ANY KIND, either express or implied.
-# See the License for the specific language governing permissions and
-# limitations under the License.
-import re
-
-from .num import DIGITS
-from .num import num2str
-from .num import verbalize_cardinal
-from .num import verbalize_digit
-
-
-def _time_num2str(num_string: str) -> str:
- """A special case for verbalizing number in time."""
- result = num2str(num_string.lstrip('0'))
- if num_string.startswith('0'):
- result = DIGITS['0'] + result
- return result
-
-
-# 时刻表达式
-RE_TIME = re.compile(r'([0-1]?[0-9]|2[0-3])'
- r':([0-5][0-9])'
- r'(:([0-5][0-9]))?')
-
-# 时间范围,如8:30-12:30
-RE_TIME_RANGE = re.compile(r'([0-1]?[0-9]|2[0-3])'
- r':([0-5][0-9])'
- r'(:([0-5][0-9]))?'
- r'(~|-)'
- r'([0-1]?[0-9]|2[0-3])'
- r':([0-5][0-9])'
- r'(:([0-5][0-9]))?')
-
-
-def replace_time(match) -> str:
- """
- Args:
- match (re.Match)
- Returns:
- str
- """
-
- is_range = len(match.groups()) > 5
-
- hour = match.group(1)
- minute = match.group(2)
- second = match.group(4)
-
- if is_range:
- hour_2 = match.group(6)
- minute_2 = match.group(7)
- second_2 = match.group(9)
-
- result = f"{num2str(hour)}点"
- if minute.lstrip('0'):
- if int(minute) == 30:
- result += "半"
- else:
- result += f"{_time_num2str(minute)}分"
- if second and second.lstrip('0'):
- result += f"{_time_num2str(second)}秒"
-
- if is_range:
- result += "至"
- result += f"{num2str(hour_2)}点"
- if minute_2.lstrip('0'):
- if int(minute) == 30:
- result += "半"
- else:
- result += f"{_time_num2str(minute_2)}分"
- if second_2 and second_2.lstrip('0'):
- result += f"{_time_num2str(second_2)}秒"
-
- return result
-
-
-RE_DATE = re.compile(r'(\d{4}|\d{2})年'
- r'((0?[1-9]|1[0-2])月)?'
- r'(((0?[1-9])|((1|2)[0-9])|30|31)([日号]))?')
-
-
-def replace_date(match) -> str:
- """
- Args:
- match (re.Match)
- Returns:
- str
- """
- year = match.group(1)
- month = match.group(3)
- day = match.group(5)
- result = ""
- if year:
- result += f"{verbalize_digit(year)}年"
- if month:
- result += f"{verbalize_cardinal(month)}月"
- if day:
- result += f"{verbalize_cardinal(day)}{match.group(9)}"
- return result
-
-
-# 用 / 或者 - 分隔的 YY/MM/DD 或者 YY-MM-DD 日期
-RE_DATE2 = re.compile(
- r'(\d{4})([- /.])(0[1-9]|1[012])\2(0[1-9]|[12][0-9]|3[01])')
-
-
-def replace_date2(match) -> str:
- """
- Args:
- match (re.Match)
- Returns:
- str
- """
- year = match.group(1)
- month = match.group(3)
- day = match.group(4)
- result = ""
- if year:
- result += f"{verbalize_digit(year)}年"
- if month:
- result += f"{verbalize_cardinal(month)}月"
- if day:
- result += f"{verbalize_cardinal(day)}日"
- return result
diff --git a/spaces/AnTo2209/3D_Zeroshot_Neural_Style_Transfer/src/dataset/blender_dataset.py b/spaces/AnTo2209/3D_Zeroshot_Neural_Style_Transfer/src/dataset/blender_dataset.py
deleted file mode 100644
index 651a05cca47f13033ccea48b54e52dea9d1c136a..0000000000000000000000000000000000000000
--- a/spaces/AnTo2209/3D_Zeroshot_Neural_Style_Transfer/src/dataset/blender_dataset.py
+++ /dev/null
@@ -1,164 +0,0 @@
-import numpy as np
-import torch, cv2
-from torch.utils.data import Dataset
-import json
-from tqdm import tqdm
-import os
-from PIL import Image
-from torchvision import transforms as T
-
-from .ray_utils import *
-
-
-class BlenderDataset(Dataset):
- def __init__(self, datadir, split='train', downsample=1.0, is_stack=False, N_vis=-1):
- self.N_vis = N_vis
- self.root_dir = datadir
- self.split = split
- self.is_stack = is_stack
- self.img_wh = (int(800 / downsample), int(800 / downsample))
- self.define_transforms()
-
- self.scene_bbox = torch.tensor([[-1.5, -1.5, -1.5], [1.5, 1.5, 1.5]])
- self.blender2opencv = np.array([[1, 0, 0, 0], [0, -1, 0, 0], [0, 0, -1, 0], [0, 0, 0, 1]])
- self.read_meta()
- self.define_proj_mat()
-
- self.white_bg = True
- self.near_far = [2.0, 6.0]
-
- self.center = torch.mean(self.scene_bbox, axis=0).float().view(1, 1, 3)
- self.radius = (self.scene_bbox[1] - self.center).float().view(1, 1, 3)
- self.downsample = downsample
-
- def read_depth(self, filename):
- depth = np.array(read_pfm(filename)[0], dtype=np.float32) # (800, 800)
- return depth
-
- def read_meta(self):
-
- with open(os.path.join(self.root_dir, f"transforms_{self.split}.json"), 'r') as f:
- self.meta = json.load(f)
-
- w, h = self.img_wh
- self.focal = 0.5 * 800 / np.tan(0.5 * self.meta['camera_angle_x']) # original focal length
- self.focal *= self.img_wh[0] / 800 # modify focal length to match size self.img_wh
-
- # ray directions for all pixels, same for all images (same H, W, focal)
- self.directions = get_ray_directions(h, w, [self.focal, self.focal]) # (h, w, 3)
- self.directions = self.directions / torch.norm(self.directions, dim=-1, keepdim=True)
- self.intrinsics = torch.tensor([[self.focal, 0, w / 2], [0, self.focal, h / 2], [0, 0, 1]]).float()
-
- self.image_paths = []
- self.poses = []
- self.all_rays = []
- self.all_rgbs = []
- self.all_masks = []
- self.all_depth = []
- self.downsample = 1.0
-
- img_eval_interval = 1 if self.N_vis < 0 else len(self.meta['frames']) // self.N_vis
- idxs = list(range(0, len(self.meta['frames']), img_eval_interval))
- for i in tqdm(idxs, desc=f'Loading data {self.split} ({len(idxs)})'): # img_list:#
-
- frame = self.meta['frames'][i]
- pose = np.array(frame['transform_matrix']) @ self.blender2opencv
- c2w = torch.FloatTensor(pose)
- self.poses += [c2w]
-
- image_path = os.path.join(self.root_dir, f"{frame['file_path']}.png")
- self.image_paths += [image_path]
- img = Image.open(image_path)
-
- if self.downsample != 1.0:
- img = img.resize(self.img_wh, Image.LANCZOS)
- img = self.transform(img) # (4, h, w)
- img = img.view(4, -1).permute(1, 0) # (h*w, 4) RGBA
- self.all_masks.append(img[:, -1:].reshape(h, w, 1)) # (h, w, 1) A
- img = img[:, :3] * img[:, -1:] + (1 - img[:, -1:]) # blend A to RGB
- self.all_rgbs += [img]
-
- rays_o, rays_d = get_rays(self.directions, c2w) # both (h*w, 3)
- self.all_rays += [torch.cat([rays_o, rays_d], 1)] # (h*w, 6)
-
- self.all_masks = torch.stack(self.all_masks) # (n_frames, h, w, 1)
- self.poses = torch.stack(self.poses)
- all_rays = self.all_rays
- all_rgbs = self.all_rgbs
-
- self.all_rays = torch.cat(self.all_rays, 0) # (len(self.meta['frames])*h*w,6)
- self.all_rgbs = torch.cat(self.all_rgbs, 0) # (len(self.meta['frames])*h*w,3)
-
- if self.is_stack:
- self.all_rays_stack = torch.stack(all_rays, 0).reshape(-1, *self.img_wh[::-1],
- 6) # (len(self.meta['frames]),h,w,6)
- avg_pool = torch.nn.AvgPool2d(4, ceil_mode=True)
- self.ds_all_rays_stack = avg_pool(self.all_rays_stack.permute(0, 3, 1, 2)).permute(0, 2, 3,
- 1) # (len(self.meta['frames]),h/4,w/4,6)
- self.all_rgbs_stack = torch.stack(all_rgbs, 0).reshape(-1, *self.img_wh[::-1],
- 3) # (len(self.meta['frames]),h,w,3)
-
- @torch.no_grad()
- def prepare_feature_data(self, encoder, chunk=8):
- '''
- Prepare feature maps as training data.
- '''
- assert self.is_stack, 'Dataset should contain original stacked taining data!'
- print('====> prepare_feature_data ...')
-
- frames_num, h, w, _ = self.all_rgbs_stack.size()
- features = []
-
- for chunk_idx in range(frames_num // chunk + int(frames_num % chunk > 0)):
- print(chunk_idx, frames_num // chunk + int(frames_num % chunk > 0))
- rgbs_chunk = self.all_rgbs_stack[chunk_idx * chunk: (chunk_idx + 1) * chunk].cuda()
- features_chunk = encoder(normalize_vgg(rgbs_chunk.permute(0, 3, 1, 2))).relu3_1
- # resize to the size of rgb map so that rays can match
- features_chunk = T.functional.resize(features_chunk, size=(h, w),
- interpolation=T.InterpolationMode.BILINEAR)
-
- features.append(features_chunk.detach().cpu().requires_grad_(False))
-
- self.all_features_stack = torch.cat(features).permute(0, 2, 3, 1) # (len(self.meta['frames]),h,w,256)
- self.all_features = self.all_features_stack.reshape(-1, 256)
- print('prepare_feature_data Done!')
-
- def define_transforms(self):
- self.transform = T.ToTensor()
-
- def define_proj_mat(self):
- self.proj_mat = self.intrinsics.unsqueeze(0) @ torch.inverse(self.poses)[:, :3]
-
- def world2ndc(self, points, lindisp=None):
- device = points.device
- return (points - self.center.to(device)) / self.radius.to(device)
-
- def __len__(self):
- return len(self.all_rgbs)
-
- def __getitem__(self, idx):
-
- if self.split == 'train': # use data in the buffers
- sample = {'rays': self.all_rays[idx],
- 'rgbs': self.all_rgbs[idx]}
-
- else: # create data for each image separately
-
- img = self.all_rgbs[idx]
- rays = self.all_rays[idx]
- mask = self.all_masks[idx] # for quantity evaluation
-
- sample = {'rays': rays,
- 'rgbs': img,
- 'mask': mask}
- return sample
-
-if __name__ == '__main__':
- train_loader = BlenderDataset('data/nerf_synthetic/lego')
- for idx, data in enumerate(train_loader):
- print(idx, data)
- print("Ray: ", data['rays'])
- print("Ray shape: ", data['rays'].shape)
- print("RGB: ", data['rgbs'])
- print("RGB shape: ", data['rgbs'].shape)
- break
\ No newline at end of file
diff --git a/spaces/Andy1621/uniformer_image_detection/configs/lvis/mask_rcnn_x101_64x4d_fpn_sample1e-3_mstrain_1x_lvis_v1.py b/spaces/Andy1621/uniformer_image_detection/configs/lvis/mask_rcnn_x101_64x4d_fpn_sample1e-3_mstrain_1x_lvis_v1.py
deleted file mode 100644
index f77adba2f150f62900571f5f32b2083ee53b7003..0000000000000000000000000000000000000000
--- a/spaces/Andy1621/uniformer_image_detection/configs/lvis/mask_rcnn_x101_64x4d_fpn_sample1e-3_mstrain_1x_lvis_v1.py
+++ /dev/null
@@ -1,13 +0,0 @@
-_base_ = './mask_rcnn_r50_fpn_sample1e-3_mstrain_1x_lvis_v1.py'
-model = dict(
- pretrained='open-mmlab://resnext101_64x4d',
- backbone=dict(
- type='ResNeXt',
- depth=101,
- groups=64,
- base_width=4,
- num_stages=4,
- out_indices=(0, 1, 2, 3),
- frozen_stages=1,
- norm_cfg=dict(type='BN', requires_grad=True),
- style='pytorch'))
diff --git a/spaces/Andy1621/uniformer_image_detection/configs/retinanet/retinanet_r50_fpn_1x_coco.py b/spaces/Andy1621/uniformer_image_detection/configs/retinanet/retinanet_r50_fpn_1x_coco.py
deleted file mode 100644
index 04bd696b9589e37ad34c9fdd035b97e271d3b214..0000000000000000000000000000000000000000
--- a/spaces/Andy1621/uniformer_image_detection/configs/retinanet/retinanet_r50_fpn_1x_coco.py
+++ /dev/null
@@ -1,7 +0,0 @@
-_base_ = [
- '../_base_/models/retinanet_r50_fpn.py',
- '../_base_/datasets/coco_detection.py',
- '../_base_/schedules/schedule_1x.py', '../_base_/default_runtime.py'
-]
-# optimizer
-optimizer = dict(type='SGD', lr=0.01, momentum=0.9, weight_decay=0.0001)
diff --git a/spaces/Andy1621/uniformer_image_segmentation/configs/ccnet/ccnet_r101-d8_512x512_20k_voc12aug.py b/spaces/Andy1621/uniformer_image_segmentation/configs/ccnet/ccnet_r101-d8_512x512_20k_voc12aug.py
deleted file mode 100644
index 53eb77c0cd6690668ee7c2a666bd85b9a5f7e73b..0000000000000000000000000000000000000000
--- a/spaces/Andy1621/uniformer_image_segmentation/configs/ccnet/ccnet_r101-d8_512x512_20k_voc12aug.py
+++ /dev/null
@@ -1,2 +0,0 @@
-_base_ = './ccnet_r50-d8_512x512_20k_voc12aug.py'
-model = dict(pretrained='open-mmlab://resnet101_v1c', backbone=dict(depth=101))
diff --git a/spaces/Andy1621/uniformer_image_segmentation/configs/deeplabv3plus/deeplabv3plus_r101b-d8_769x769_80k_cityscapes.py b/spaces/Andy1621/uniformer_image_segmentation/configs/deeplabv3plus/deeplabv3plus_r101b-d8_769x769_80k_cityscapes.py
deleted file mode 100644
index 136449083f7a9efbad6df94f1acd04170147aaba..0000000000000000000000000000000000000000
--- a/spaces/Andy1621/uniformer_image_segmentation/configs/deeplabv3plus/deeplabv3plus_r101b-d8_769x769_80k_cityscapes.py
+++ /dev/null
@@ -1,4 +0,0 @@
-_base_ = './deeplabv3plus_r50-d8_769x769_80k_cityscapes.py'
-model = dict(
- pretrained='torchvision://resnet101',
- backbone=dict(type='ResNet', depth=101))
diff --git a/spaces/Anonymous-sub/Rerender/ControlNet/annotator/uniformer/mmseg/datasets/custom.py b/spaces/Anonymous-sub/Rerender/ControlNet/annotator/uniformer/mmseg/datasets/custom.py
deleted file mode 100644
index d8eb2a709cc7a3a68fc6a1e3a1ad98faef4c5b7b..0000000000000000000000000000000000000000
--- a/spaces/Anonymous-sub/Rerender/ControlNet/annotator/uniformer/mmseg/datasets/custom.py
+++ /dev/null
@@ -1,400 +0,0 @@
-import os
-import os.path as osp
-from collections import OrderedDict
-from functools import reduce
-
-import annotator.uniformer.mmcv as mmcv
-import numpy as np
-from annotator.uniformer.mmcv.utils import print_log
-from prettytable import PrettyTable
-from torch.utils.data import Dataset
-
-from annotator.uniformer.mmseg.core import eval_metrics
-from annotator.uniformer.mmseg.utils import get_root_logger
-from .builder import DATASETS
-from .pipelines import Compose
-
-
-@DATASETS.register_module()
-class CustomDataset(Dataset):
- """Custom dataset for semantic segmentation. An example of file structure
- is as followed.
-
- .. code-block:: none
-
- ├── data
- │ ├── my_dataset
- │ │ ├── img_dir
- │ │ │ ├── train
- │ │ │ │ ├── xxx{img_suffix}
- │ │ │ │ ├── yyy{img_suffix}
- │ │ │ │ ├── zzz{img_suffix}
- │ │ │ ├── val
- │ │ ├── ann_dir
- │ │ │ ├── train
- │ │ │ │ ├── xxx{seg_map_suffix}
- │ │ │ │ ├── yyy{seg_map_suffix}
- │ │ │ │ ├── zzz{seg_map_suffix}
- │ │ │ ├── val
-
- The img/gt_semantic_seg pair of CustomDataset should be of the same
- except suffix. A valid img/gt_semantic_seg filename pair should be like
- ``xxx{img_suffix}`` and ``xxx{seg_map_suffix}`` (extension is also included
- in the suffix). If split is given, then ``xxx`` is specified in txt file.
- Otherwise, all files in ``img_dir/``and ``ann_dir`` will be loaded.
- Please refer to ``docs/tutorials/new_dataset.md`` for more details.
-
-
- Args:
- pipeline (list[dict]): Processing pipeline
- img_dir (str): Path to image directory
- img_suffix (str): Suffix of images. Default: '.jpg'
- ann_dir (str, optional): Path to annotation directory. Default: None
- seg_map_suffix (str): Suffix of segmentation maps. Default: '.png'
- split (str, optional): Split txt file. If split is specified, only
- file with suffix in the splits will be loaded. Otherwise, all
- images in img_dir/ann_dir will be loaded. Default: None
- data_root (str, optional): Data root for img_dir/ann_dir. Default:
- None.
- test_mode (bool): If test_mode=True, gt wouldn't be loaded.
- ignore_index (int): The label index to be ignored. Default: 255
- reduce_zero_label (bool): Whether to mark label zero as ignored.
- Default: False
- classes (str | Sequence[str], optional): Specify classes to load.
- If is None, ``cls.CLASSES`` will be used. Default: None.
- palette (Sequence[Sequence[int]]] | np.ndarray | None):
- The palette of segmentation map. If None is given, and
- self.PALETTE is None, random palette will be generated.
- Default: None
- """
-
- CLASSES = None
-
- PALETTE = None
-
- def __init__(self,
- pipeline,
- img_dir,
- img_suffix='.jpg',
- ann_dir=None,
- seg_map_suffix='.png',
- split=None,
- data_root=None,
- test_mode=False,
- ignore_index=255,
- reduce_zero_label=False,
- classes=None,
- palette=None):
- self.pipeline = Compose(pipeline)
- self.img_dir = img_dir
- self.img_suffix = img_suffix
- self.ann_dir = ann_dir
- self.seg_map_suffix = seg_map_suffix
- self.split = split
- self.data_root = data_root
- self.test_mode = test_mode
- self.ignore_index = ignore_index
- self.reduce_zero_label = reduce_zero_label
- self.label_map = None
- self.CLASSES, self.PALETTE = self.get_classes_and_palette(
- classes, palette)
-
- # join paths if data_root is specified
- if self.data_root is not None:
- if not osp.isabs(self.img_dir):
- self.img_dir = osp.join(self.data_root, self.img_dir)
- if not (self.ann_dir is None or osp.isabs(self.ann_dir)):
- self.ann_dir = osp.join(self.data_root, self.ann_dir)
- if not (self.split is None or osp.isabs(self.split)):
- self.split = osp.join(self.data_root, self.split)
-
- # load annotations
- self.img_infos = self.load_annotations(self.img_dir, self.img_suffix,
- self.ann_dir,
- self.seg_map_suffix, self.split)
-
- def __len__(self):
- """Total number of samples of data."""
- return len(self.img_infos)
-
- def load_annotations(self, img_dir, img_suffix, ann_dir, seg_map_suffix,
- split):
- """Load annotation from directory.
-
- Args:
- img_dir (str): Path to image directory
- img_suffix (str): Suffix of images.
- ann_dir (str|None): Path to annotation directory.
- seg_map_suffix (str|None): Suffix of segmentation maps.
- split (str|None): Split txt file. If split is specified, only file
- with suffix in the splits will be loaded. Otherwise, all images
- in img_dir/ann_dir will be loaded. Default: None
-
- Returns:
- list[dict]: All image info of dataset.
- """
-
- img_infos = []
- if split is not None:
- with open(split) as f:
- for line in f:
- img_name = line.strip()
- img_info = dict(filename=img_name + img_suffix)
- if ann_dir is not None:
- seg_map = img_name + seg_map_suffix
- img_info['ann'] = dict(seg_map=seg_map)
- img_infos.append(img_info)
- else:
- for img in mmcv.scandir(img_dir, img_suffix, recursive=True):
- img_info = dict(filename=img)
- if ann_dir is not None:
- seg_map = img.replace(img_suffix, seg_map_suffix)
- img_info['ann'] = dict(seg_map=seg_map)
- img_infos.append(img_info)
-
- print_log(f'Loaded {len(img_infos)} images', logger=get_root_logger())
- return img_infos
-
- def get_ann_info(self, idx):
- """Get annotation by index.
-
- Args:
- idx (int): Index of data.
-
- Returns:
- dict: Annotation info of specified index.
- """
-
- return self.img_infos[idx]['ann']
-
- def pre_pipeline(self, results):
- """Prepare results dict for pipeline."""
- results['seg_fields'] = []
- results['img_prefix'] = self.img_dir
- results['seg_prefix'] = self.ann_dir
- if self.custom_classes:
- results['label_map'] = self.label_map
-
- def __getitem__(self, idx):
- """Get training/test data after pipeline.
-
- Args:
- idx (int): Index of data.
-
- Returns:
- dict: Training/test data (with annotation if `test_mode` is set
- False).
- """
-
- if self.test_mode:
- return self.prepare_test_img(idx)
- else:
- return self.prepare_train_img(idx)
-
- def prepare_train_img(self, idx):
- """Get training data and annotations after pipeline.
-
- Args:
- idx (int): Index of data.
-
- Returns:
- dict: Training data and annotation after pipeline with new keys
- introduced by pipeline.
- """
-
- img_info = self.img_infos[idx]
- ann_info = self.get_ann_info(idx)
- results = dict(img_info=img_info, ann_info=ann_info)
- self.pre_pipeline(results)
- return self.pipeline(results)
-
- def prepare_test_img(self, idx):
- """Get testing data after pipeline.
-
- Args:
- idx (int): Index of data.
-
- Returns:
- dict: Testing data after pipeline with new keys introduced by
- pipeline.
- """
-
- img_info = self.img_infos[idx]
- results = dict(img_info=img_info)
- self.pre_pipeline(results)
- return self.pipeline(results)
-
- def format_results(self, results, **kwargs):
- """Place holder to format result to dataset specific output."""
-
- def get_gt_seg_maps(self, efficient_test=False):
- """Get ground truth segmentation maps for evaluation."""
- gt_seg_maps = []
- for img_info in self.img_infos:
- seg_map = osp.join(self.ann_dir, img_info['ann']['seg_map'])
- if efficient_test:
- gt_seg_map = seg_map
- else:
- gt_seg_map = mmcv.imread(
- seg_map, flag='unchanged', backend='pillow')
- gt_seg_maps.append(gt_seg_map)
- return gt_seg_maps
-
- def get_classes_and_palette(self, classes=None, palette=None):
- """Get class names of current dataset.
-
- Args:
- classes (Sequence[str] | str | None): If classes is None, use
- default CLASSES defined by builtin dataset. If classes is a
- string, take it as a file name. The file contains the name of
- classes where each line contains one class name. If classes is
- a tuple or list, override the CLASSES defined by the dataset.
- palette (Sequence[Sequence[int]]] | np.ndarray | None):
- The palette of segmentation map. If None is given, random
- palette will be generated. Default: None
- """
- if classes is None:
- self.custom_classes = False
- return self.CLASSES, self.PALETTE
-
- self.custom_classes = True
- if isinstance(classes, str):
- # take it as a file path
- class_names = mmcv.list_from_file(classes)
- elif isinstance(classes, (tuple, list)):
- class_names = classes
- else:
- raise ValueError(f'Unsupported type {type(classes)} of classes.')
-
- if self.CLASSES:
- if not set(classes).issubset(self.CLASSES):
- raise ValueError('classes is not a subset of CLASSES.')
-
- # dictionary, its keys are the old label ids and its values
- # are the new label ids.
- # used for changing pixel labels in load_annotations.
- self.label_map = {}
- for i, c in enumerate(self.CLASSES):
- if c not in class_names:
- self.label_map[i] = -1
- else:
- self.label_map[i] = classes.index(c)
-
- palette = self.get_palette_for_custom_classes(class_names, palette)
-
- return class_names, palette
-
- def get_palette_for_custom_classes(self, class_names, palette=None):
-
- if self.label_map is not None:
- # return subset of palette
- palette = []
- for old_id, new_id in sorted(
- self.label_map.items(), key=lambda x: x[1]):
- if new_id != -1:
- palette.append(self.PALETTE[old_id])
- palette = type(self.PALETTE)(palette)
-
- elif palette is None:
- if self.PALETTE is None:
- palette = np.random.randint(0, 255, size=(len(class_names), 3))
- else:
- palette = self.PALETTE
-
- return palette
-
- def evaluate(self,
- results,
- metric='mIoU',
- logger=None,
- efficient_test=False,
- **kwargs):
- """Evaluate the dataset.
-
- Args:
- results (list): Testing results of the dataset.
- metric (str | list[str]): Metrics to be evaluated. 'mIoU',
- 'mDice' and 'mFscore' are supported.
- logger (logging.Logger | None | str): Logger used for printing
- related information during evaluation. Default: None.
-
- Returns:
- dict[str, float]: Default metrics.
- """
-
- if isinstance(metric, str):
- metric = [metric]
- allowed_metrics = ['mIoU', 'mDice', 'mFscore']
- if not set(metric).issubset(set(allowed_metrics)):
- raise KeyError('metric {} is not supported'.format(metric))
- eval_results = {}
- gt_seg_maps = self.get_gt_seg_maps(efficient_test)
- if self.CLASSES is None:
- num_classes = len(
- reduce(np.union1d, [np.unique(_) for _ in gt_seg_maps]))
- else:
- num_classes = len(self.CLASSES)
- ret_metrics = eval_metrics(
- results,
- gt_seg_maps,
- num_classes,
- self.ignore_index,
- metric,
- label_map=self.label_map,
- reduce_zero_label=self.reduce_zero_label)
-
- if self.CLASSES is None:
- class_names = tuple(range(num_classes))
- else:
- class_names = self.CLASSES
-
- # summary table
- ret_metrics_summary = OrderedDict({
- ret_metric: np.round(np.nanmean(ret_metric_value) * 100, 2)
- for ret_metric, ret_metric_value in ret_metrics.items()
- })
-
- # each class table
- ret_metrics.pop('aAcc', None)
- ret_metrics_class = OrderedDict({
- ret_metric: np.round(ret_metric_value * 100, 2)
- for ret_metric, ret_metric_value in ret_metrics.items()
- })
- ret_metrics_class.update({'Class': class_names})
- ret_metrics_class.move_to_end('Class', last=False)
-
- # for logger
- class_table_data = PrettyTable()
- for key, val in ret_metrics_class.items():
- class_table_data.add_column(key, val)
-
- summary_table_data = PrettyTable()
- for key, val in ret_metrics_summary.items():
- if key == 'aAcc':
- summary_table_data.add_column(key, [val])
- else:
- summary_table_data.add_column('m' + key, [val])
-
- print_log('per class results:', logger)
- print_log('\n' + class_table_data.get_string(), logger=logger)
- print_log('Summary:', logger)
- print_log('\n' + summary_table_data.get_string(), logger=logger)
-
- # each metric dict
- for key, value in ret_metrics_summary.items():
- if key == 'aAcc':
- eval_results[key] = value / 100.0
- else:
- eval_results['m' + key] = value / 100.0
-
- ret_metrics_class.pop('Class', None)
- for key, value in ret_metrics_class.items():
- eval_results.update({
- key + '.' + str(name): value[idx] / 100.0
- for idx, name in enumerate(class_names)
- })
-
- if mmcv.is_list_of(results, str):
- for file_name in results:
- os.remove(file_name)
- return eval_results
diff --git a/spaces/Artrajz/vits-simple-api/bert_vits2/text/__init__.py b/spaces/Artrajz/vits-simple-api/bert_vits2/text/__init__.py
deleted file mode 100644
index 550135f7207fff1a59700eeccbe1f3e8197051bd..0000000000000000000000000000000000000000
--- a/spaces/Artrajz/vits-simple-api/bert_vits2/text/__init__.py
+++ /dev/null
@@ -1,17 +0,0 @@
-from bert_vits2.text.symbols import *
-from bert_vits2.text.bert_handler import BertHandler
-
-
-def cleaned_text_to_sequence(cleaned_text, tones, language, _symbol_to_id):
- """Converts a string of text to a sequence of IDs corresponding to the symbols in the text.
- Args:
- text: string to convert to a sequence
- Returns:
- List of integers corresponding to the symbols in the text
- """
- phones = [_symbol_to_id[symbol] for symbol in cleaned_text]
- tone_start = language_tone_start_map[language]
- tones = [i + tone_start for i in tones]
- lang_id = language_id_map[language]
- lang_ids = [lang_id for i in phones]
- return phones, tones, lang_ids
diff --git a/spaces/Artrajz/vits-simple-api/vits/mel_processing.py b/spaces/Artrajz/vits-simple-api/vits/mel_processing.py
deleted file mode 100644
index 3e252e76320522a8a4195a60665168f22769aec2..0000000000000000000000000000000000000000
--- a/spaces/Artrajz/vits-simple-api/vits/mel_processing.py
+++ /dev/null
@@ -1,101 +0,0 @@
-import torch
-import torch.utils.data
-from librosa.filters import mel as librosa_mel_fn
-
-MAX_WAV_VALUE = 32768.0
-
-
-def dynamic_range_compression_torch(x, C=1, clip_val=1e-5):
- """
- PARAMS
- ------
- C: compression factor
- """
- return torch.log(torch.clamp(x, min=clip_val) * C)
-
-
-def dynamic_range_decompression_torch(x, C=1):
- """
- PARAMS
- ------
- C: compression factor used to compress
- """
- return torch.exp(x) / C
-
-
-def spectral_normalize_torch(magnitudes):
- output = dynamic_range_compression_torch(magnitudes)
- return output
-
-
-def spectral_de_normalize_torch(magnitudes):
- output = dynamic_range_decompression_torch(magnitudes)
- return output
-
-
-mel_basis = {}
-hann_window = {}
-
-
-def spectrogram_torch(y, n_fft, sampling_rate, hop_size, win_size, center=False):
- if torch.min(y) < -1.:
- print('min value is ', torch.min(y))
- if torch.max(y) > 1.:
- print('max value is ', torch.max(y))
-
- global hann_window
- dtype_device = str(y.dtype) + '_' + str(y.device)
- wnsize_dtype_device = str(win_size) + '_' + dtype_device
- if wnsize_dtype_device not in hann_window:
- hann_window[wnsize_dtype_device] = torch.hann_window(win_size).to(dtype=y.dtype, device=y.device)
-
- y = torch.nn.functional.pad(y.unsqueeze(1), (int((n_fft-hop_size)/2), int((n_fft-hop_size)/2)), mode='reflect')
- y = y.squeeze(1)
-
- spec = torch.stft(y, n_fft, hop_length=hop_size, win_length=win_size, window=hann_window[wnsize_dtype_device],
- center=center, pad_mode='reflect', normalized=False, onesided=True, return_complex=False)
-
- spec = torch.sqrt(spec.pow(2).sum(-1) + 1e-6)
- return spec
-
-
-def spec_to_mel_torch(spec, n_fft, num_mels, sampling_rate, fmin, fmax):
- global mel_basis
- dtype_device = str(spec.dtype) + '_' + str(spec.device)
- fmax_dtype_device = str(fmax) + '_' + dtype_device
- if fmax_dtype_device not in mel_basis:
- mel = librosa_mel_fn(sampling_rate, n_fft, num_mels, fmin, fmax)
- mel_basis[fmax_dtype_device] = torch.from_numpy(mel).to(dtype=spec.dtype, device=spec.device)
- spec = torch.matmul(mel_basis[fmax_dtype_device], spec)
- spec = spectral_normalize_torch(spec)
- return spec
-
-
-def mel_spectrogram_torch(y, n_fft, num_mels, sampling_rate, hop_size, win_size, fmin, fmax, center=False):
- if torch.min(y) < -1.:
- print('min value is ', torch.min(y))
- if torch.max(y) > 1.:
- print('max value is ', torch.max(y))
-
- global mel_basis, hann_window
- dtype_device = str(y.dtype) + '_' + str(y.device)
- fmax_dtype_device = str(fmax) + '_' + dtype_device
- wnsize_dtype_device = str(win_size) + '_' + dtype_device
- if fmax_dtype_device not in mel_basis:
- mel = librosa_mel_fn(sampling_rate, n_fft, num_mels, fmin, fmax)
- mel_basis[fmax_dtype_device] = torch.from_numpy(mel).to(dtype=y.dtype, device=y.device)
- if wnsize_dtype_device not in hann_window:
- hann_window[wnsize_dtype_device] = torch.hann_window(win_size).to(dtype=y.dtype, device=y.device)
-
- y = torch.nn.functional.pad(y.unsqueeze(1), (int((n_fft-hop_size)/2), int((n_fft-hop_size)/2)), mode='reflect')
- y = y.squeeze(1)
-
- spec = torch.stft(y, n_fft, hop_length=hop_size, win_length=win_size, window=hann_window[wnsize_dtype_device],
- center=center, pad_mode='reflect', normalized=False, onesided=True)
-
- spec = torch.sqrt(spec.pow(2).sum(-1) + 1e-6)
-
- spec = torch.matmul(mel_basis[fmax_dtype_device], spec)
- spec = spectral_normalize_torch(spec)
-
- return spec
diff --git a/spaces/Artrajz/vits-simple-api/vits/text/shanghainese.py b/spaces/Artrajz/vits-simple-api/vits/text/shanghainese.py
deleted file mode 100644
index 259226bdeb260ccde3dee7cbfe8e697df45433e9..0000000000000000000000000000000000000000
--- a/spaces/Artrajz/vits-simple-api/vits/text/shanghainese.py
+++ /dev/null
@@ -1,80 +0,0 @@
-import os
-import re
-import cn2an
-import opencc
-import config
-from utils.download import download_and_verify
-
-URLS = [
- "https://github.com/CjangCjengh/chinese-dialect-lexicons/releases/download/v1.0.3/chinese_dialects.7z",
- "https://ghproxy.com/https://github.com/CjangCjengh/chinese-dialect-lexicons/releases/download/v1.0.3/chinese_dialects.7z",
-]
-TARGET_PATH = os.path.join(config.ABS_PATH, "vits/text/chinese_dialects.7z")
-EXTRACT_DESTINATION = os.path.join(config.ABS_PATH, "vits/text/chinese_dialect_lexicons/")
-EXPECTED_MD5 = None
-OPENCC_FILE_PATH = os.path.join(config.ABS_PATH, "vits/text/chinese_dialect_lexicons/zaonhe.json")
-
-if not os.path.exists(OPENCC_FILE_PATH):
- success, message = download_and_verify(URLS, TARGET_PATH, EXPECTED_MD5, EXTRACT_DESTINATION)
-
-converter = opencc.OpenCC(OPENCC_FILE_PATH)
-
-# List of (Latin alphabet, ipa) pairs:
-_latin_to_ipa = [(re.compile('%s' % x[0]), x[1]) for x in [
- ('A', 'ᴇ'),
- ('B', 'bi'),
- ('C', 'si'),
- ('D', 'di'),
- ('E', 'i'),
- ('F', 'ᴇf'),
- ('G', 'dʑi'),
- ('H', 'ᴇtɕʰ'),
- ('I', 'ᴀi'),
- ('J', 'dʑᴇ'),
- ('K', 'kʰᴇ'),
- ('L', 'ᴇl'),
- ('M', 'ᴇm'),
- ('N', 'ᴇn'),
- ('O', 'o'),
- ('P', 'pʰi'),
- ('Q', 'kʰiu'),
- ('R', 'ᴀl'),
- ('S', 'ᴇs'),
- ('T', 'tʰi'),
- ('U', 'ɦiu'),
- ('V', 'vi'),
- ('W', 'dᴀbɤliu'),
- ('X', 'ᴇks'),
- ('Y', 'uᴀi'),
- ('Z', 'zᴇ')
-]]
-
-
-def _number_to_shanghainese(num):
- num = cn2an.an2cn(num).replace('一十', '十').replace('二十', '廿').replace('二', '两')
- return re.sub(r'((?:^|[^三四五六七八九])十|廿)两', r'\1二', num)
-
-
-def number_to_shanghainese(text):
- return re.sub(r'\d+(?:\.?\d+)?', lambda x: _number_to_shanghainese(x.group()), text)
-
-
-def latin_to_ipa(text):
- for regex, replacement in _latin_to_ipa:
- text = re.sub(regex, replacement, text)
- return text
-
-
-def shanghainese_to_ipa(text):
- from vits.text.mandarin import symbols_to_chinese
- text = symbols_to_chinese(text)
- text = number_to_shanghainese(text.upper())
- text = converter.convert(text).replace('-', '').replace('$', ' ')
- text = re.sub(r'[A-Z]', lambda x: latin_to_ipa(x.group()) + ' ', text)
- text = re.sub(r'[、;:]', ',', text)
- text = re.sub(r'\s*,\s*', ', ', text)
- text = re.sub(r'\s*。\s*', '. ', text)
- text = re.sub(r'\s*?\s*', '? ', text)
- text = re.sub(r'\s*!\s*', '! ', text)
- text = re.sub(r'\s*$', '', text)
- return text
diff --git a/spaces/Ataturk-Chatbot/HuggingFaceChat/venv/lib/python3.11/site-packages/pip/_vendor/idna/idnadata.py b/spaces/Ataturk-Chatbot/HuggingFaceChat/venv/lib/python3.11/site-packages/pip/_vendor/idna/idnadata.py
deleted file mode 100644
index 67db4625829680298b2a5a9032a379d870a00700..0000000000000000000000000000000000000000
--- a/spaces/Ataturk-Chatbot/HuggingFaceChat/venv/lib/python3.11/site-packages/pip/_vendor/idna/idnadata.py
+++ /dev/null
@@ -1,2151 +0,0 @@
-# This file is automatically generated by tools/idna-data
-
-__version__ = '15.0.0'
-scripts = {
- 'Greek': (
- 0x37000000374,
- 0x37500000378,
- 0x37a0000037e,
- 0x37f00000380,
- 0x38400000385,
- 0x38600000387,
- 0x3880000038b,
- 0x38c0000038d,
- 0x38e000003a2,
- 0x3a3000003e2,
- 0x3f000000400,
- 0x1d2600001d2b,
- 0x1d5d00001d62,
- 0x1d6600001d6b,
- 0x1dbf00001dc0,
- 0x1f0000001f16,
- 0x1f1800001f1e,
- 0x1f2000001f46,
- 0x1f4800001f4e,
- 0x1f5000001f58,
- 0x1f5900001f5a,
- 0x1f5b00001f5c,
- 0x1f5d00001f5e,
- 0x1f5f00001f7e,
- 0x1f8000001fb5,
- 0x1fb600001fc5,
- 0x1fc600001fd4,
- 0x1fd600001fdc,
- 0x1fdd00001ff0,
- 0x1ff200001ff5,
- 0x1ff600001fff,
- 0x212600002127,
- 0xab650000ab66,
- 0x101400001018f,
- 0x101a0000101a1,
- 0x1d2000001d246,
- ),
- 'Han': (
- 0x2e8000002e9a,
- 0x2e9b00002ef4,
- 0x2f0000002fd6,
- 0x300500003006,
- 0x300700003008,
- 0x30210000302a,
- 0x30380000303c,
- 0x340000004dc0,
- 0x4e000000a000,
- 0xf9000000fa6e,
- 0xfa700000fada,
- 0x16fe200016fe4,
- 0x16ff000016ff2,
- 0x200000002a6e0,
- 0x2a7000002b73a,
- 0x2b7400002b81e,
- 0x2b8200002cea2,
- 0x2ceb00002ebe1,
- 0x2f8000002fa1e,
- 0x300000003134b,
- 0x31350000323b0,
- ),
- 'Hebrew': (
- 0x591000005c8,
- 0x5d0000005eb,
- 0x5ef000005f5,
- 0xfb1d0000fb37,
- 0xfb380000fb3d,
- 0xfb3e0000fb3f,
- 0xfb400000fb42,
- 0xfb430000fb45,
- 0xfb460000fb50,
- ),
- 'Hiragana': (
- 0x304100003097,
- 0x309d000030a0,
- 0x1b0010001b120,
- 0x1b1320001b133,
- 0x1b1500001b153,
- 0x1f2000001f201,
- ),
- 'Katakana': (
- 0x30a1000030fb,
- 0x30fd00003100,
- 0x31f000003200,
- 0x32d0000032ff,
- 0x330000003358,
- 0xff660000ff70,
- 0xff710000ff9e,
- 0x1aff00001aff4,
- 0x1aff50001affc,
- 0x1affd0001afff,
- 0x1b0000001b001,
- 0x1b1200001b123,
- 0x1b1550001b156,
- 0x1b1640001b168,
- ),
-}
-joining_types = {
- 0x600: 85,
- 0x601: 85,
- 0x602: 85,
- 0x603: 85,
- 0x604: 85,
- 0x605: 85,
- 0x608: 85,
- 0x60b: 85,
- 0x620: 68,
- 0x621: 85,
- 0x622: 82,
- 0x623: 82,
- 0x624: 82,
- 0x625: 82,
- 0x626: 68,
- 0x627: 82,
- 0x628: 68,
- 0x629: 82,
- 0x62a: 68,
- 0x62b: 68,
- 0x62c: 68,
- 0x62d: 68,
- 0x62e: 68,
- 0x62f: 82,
- 0x630: 82,
- 0x631: 82,
- 0x632: 82,
- 0x633: 68,
- 0x634: 68,
- 0x635: 68,
- 0x636: 68,
- 0x637: 68,
- 0x638: 68,
- 0x639: 68,
- 0x63a: 68,
- 0x63b: 68,
- 0x63c: 68,
- 0x63d: 68,
- 0x63e: 68,
- 0x63f: 68,
- 0x640: 67,
- 0x641: 68,
- 0x642: 68,
- 0x643: 68,
- 0x644: 68,
- 0x645: 68,
- 0x646: 68,
- 0x647: 68,
- 0x648: 82,
- 0x649: 68,
- 0x64a: 68,
- 0x66e: 68,
- 0x66f: 68,
- 0x671: 82,
- 0x672: 82,
- 0x673: 82,
- 0x674: 85,
- 0x675: 82,
- 0x676: 82,
- 0x677: 82,
- 0x678: 68,
- 0x679: 68,
- 0x67a: 68,
- 0x67b: 68,
- 0x67c: 68,
- 0x67d: 68,
- 0x67e: 68,
- 0x67f: 68,
- 0x680: 68,
- 0x681: 68,
- 0x682: 68,
- 0x683: 68,
- 0x684: 68,
- 0x685: 68,
- 0x686: 68,
- 0x687: 68,
- 0x688: 82,
- 0x689: 82,
- 0x68a: 82,
- 0x68b: 82,
- 0x68c: 82,
- 0x68d: 82,
- 0x68e: 82,
- 0x68f: 82,
- 0x690: 82,
- 0x691: 82,
- 0x692: 82,
- 0x693: 82,
- 0x694: 82,
- 0x695: 82,
- 0x696: 82,
- 0x697: 82,
- 0x698: 82,
- 0x699: 82,
- 0x69a: 68,
- 0x69b: 68,
- 0x69c: 68,
- 0x69d: 68,
- 0x69e: 68,
- 0x69f: 68,
- 0x6a0: 68,
- 0x6a1: 68,
- 0x6a2: 68,
- 0x6a3: 68,
- 0x6a4: 68,
- 0x6a5: 68,
- 0x6a6: 68,
- 0x6a7: 68,
- 0x6a8: 68,
- 0x6a9: 68,
- 0x6aa: 68,
- 0x6ab: 68,
- 0x6ac: 68,
- 0x6ad: 68,
- 0x6ae: 68,
- 0x6af: 68,
- 0x6b0: 68,
- 0x6b1: 68,
- 0x6b2: 68,
- 0x6b3: 68,
- 0x6b4: 68,
- 0x6b5: 68,
- 0x6b6: 68,
- 0x6b7: 68,
- 0x6b8: 68,
- 0x6b9: 68,
- 0x6ba: 68,
- 0x6bb: 68,
- 0x6bc: 68,
- 0x6bd: 68,
- 0x6be: 68,
- 0x6bf: 68,
- 0x6c0: 82,
- 0x6c1: 68,
- 0x6c2: 68,
- 0x6c3: 82,
- 0x6c4: 82,
- 0x6c5: 82,
- 0x6c6: 82,
- 0x6c7: 82,
- 0x6c8: 82,
- 0x6c9: 82,
- 0x6ca: 82,
- 0x6cb: 82,
- 0x6cc: 68,
- 0x6cd: 82,
- 0x6ce: 68,
- 0x6cf: 82,
- 0x6d0: 68,
- 0x6d1: 68,
- 0x6d2: 82,
- 0x6d3: 82,
- 0x6d5: 82,
- 0x6dd: 85,
- 0x6ee: 82,
- 0x6ef: 82,
- 0x6fa: 68,
- 0x6fb: 68,
- 0x6fc: 68,
- 0x6ff: 68,
- 0x70f: 84,
- 0x710: 82,
- 0x712: 68,
- 0x713: 68,
- 0x714: 68,
- 0x715: 82,
- 0x716: 82,
- 0x717: 82,
- 0x718: 82,
- 0x719: 82,
- 0x71a: 68,
- 0x71b: 68,
- 0x71c: 68,
- 0x71d: 68,
- 0x71e: 82,
- 0x71f: 68,
- 0x720: 68,
- 0x721: 68,
- 0x722: 68,
- 0x723: 68,
- 0x724: 68,
- 0x725: 68,
- 0x726: 68,
- 0x727: 68,
- 0x728: 82,
- 0x729: 68,
- 0x72a: 82,
- 0x72b: 68,
- 0x72c: 82,
- 0x72d: 68,
- 0x72e: 68,
- 0x72f: 82,
- 0x74d: 82,
- 0x74e: 68,
- 0x74f: 68,
- 0x750: 68,
- 0x751: 68,
- 0x752: 68,
- 0x753: 68,
- 0x754: 68,
- 0x755: 68,
- 0x756: 68,
- 0x757: 68,
- 0x758: 68,
- 0x759: 82,
- 0x75a: 82,
- 0x75b: 82,
- 0x75c: 68,
- 0x75d: 68,
- 0x75e: 68,
- 0x75f: 68,
- 0x760: 68,
- 0x761: 68,
- 0x762: 68,
- 0x763: 68,
- 0x764: 68,
- 0x765: 68,
- 0x766: 68,
- 0x767: 68,
- 0x768: 68,
- 0x769: 68,
- 0x76a: 68,
- 0x76b: 82,
- 0x76c: 82,
- 0x76d: 68,
- 0x76e: 68,
- 0x76f: 68,
- 0x770: 68,
- 0x771: 82,
- 0x772: 68,
- 0x773: 82,
- 0x774: 82,
- 0x775: 68,
- 0x776: 68,
- 0x777: 68,
- 0x778: 82,
- 0x779: 82,
- 0x77a: 68,
- 0x77b: 68,
- 0x77c: 68,
- 0x77d: 68,
- 0x77e: 68,
- 0x77f: 68,
- 0x7ca: 68,
- 0x7cb: 68,
- 0x7cc: 68,
- 0x7cd: 68,
- 0x7ce: 68,
- 0x7cf: 68,
- 0x7d0: 68,
- 0x7d1: 68,
- 0x7d2: 68,
- 0x7d3: 68,
- 0x7d4: 68,
- 0x7d5: 68,
- 0x7d6: 68,
- 0x7d7: 68,
- 0x7d8: 68,
- 0x7d9: 68,
- 0x7da: 68,
- 0x7db: 68,
- 0x7dc: 68,
- 0x7dd: 68,
- 0x7de: 68,
- 0x7df: 68,
- 0x7e0: 68,
- 0x7e1: 68,
- 0x7e2: 68,
- 0x7e3: 68,
- 0x7e4: 68,
- 0x7e5: 68,
- 0x7e6: 68,
- 0x7e7: 68,
- 0x7e8: 68,
- 0x7e9: 68,
- 0x7ea: 68,
- 0x7fa: 67,
- 0x840: 82,
- 0x841: 68,
- 0x842: 68,
- 0x843: 68,
- 0x844: 68,
- 0x845: 68,
- 0x846: 82,
- 0x847: 82,
- 0x848: 68,
- 0x849: 82,
- 0x84a: 68,
- 0x84b: 68,
- 0x84c: 68,
- 0x84d: 68,
- 0x84e: 68,
- 0x84f: 68,
- 0x850: 68,
- 0x851: 68,
- 0x852: 68,
- 0x853: 68,
- 0x854: 82,
- 0x855: 68,
- 0x856: 82,
- 0x857: 82,
- 0x858: 82,
- 0x860: 68,
- 0x861: 85,
- 0x862: 68,
- 0x863: 68,
- 0x864: 68,
- 0x865: 68,
- 0x866: 85,
- 0x867: 82,
- 0x868: 68,
- 0x869: 82,
- 0x86a: 82,
- 0x870: 82,
- 0x871: 82,
- 0x872: 82,
- 0x873: 82,
- 0x874: 82,
- 0x875: 82,
- 0x876: 82,
- 0x877: 82,
- 0x878: 82,
- 0x879: 82,
- 0x87a: 82,
- 0x87b: 82,
- 0x87c: 82,
- 0x87d: 82,
- 0x87e: 82,
- 0x87f: 82,
- 0x880: 82,
- 0x881: 82,
- 0x882: 82,
- 0x883: 67,
- 0x884: 67,
- 0x885: 67,
- 0x886: 68,
- 0x887: 85,
- 0x888: 85,
- 0x889: 68,
- 0x88a: 68,
- 0x88b: 68,
- 0x88c: 68,
- 0x88d: 68,
- 0x88e: 82,
- 0x890: 85,
- 0x891: 85,
- 0x8a0: 68,
- 0x8a1: 68,
- 0x8a2: 68,
- 0x8a3: 68,
- 0x8a4: 68,
- 0x8a5: 68,
- 0x8a6: 68,
- 0x8a7: 68,
- 0x8a8: 68,
- 0x8a9: 68,
- 0x8aa: 82,
- 0x8ab: 82,
- 0x8ac: 82,
- 0x8ad: 85,
- 0x8ae: 82,
- 0x8af: 68,
- 0x8b0: 68,
- 0x8b1: 82,
- 0x8b2: 82,
- 0x8b3: 68,
- 0x8b4: 68,
- 0x8b5: 68,
- 0x8b6: 68,
- 0x8b7: 68,
- 0x8b8: 68,
- 0x8b9: 82,
- 0x8ba: 68,
- 0x8bb: 68,
- 0x8bc: 68,
- 0x8bd: 68,
- 0x8be: 68,
- 0x8bf: 68,
- 0x8c0: 68,
- 0x8c1: 68,
- 0x8c2: 68,
- 0x8c3: 68,
- 0x8c4: 68,
- 0x8c5: 68,
- 0x8c6: 68,
- 0x8c7: 68,
- 0x8c8: 68,
- 0x8e2: 85,
- 0x1806: 85,
- 0x1807: 68,
- 0x180a: 67,
- 0x180e: 85,
- 0x1820: 68,
- 0x1821: 68,
- 0x1822: 68,
- 0x1823: 68,
- 0x1824: 68,
- 0x1825: 68,
- 0x1826: 68,
- 0x1827: 68,
- 0x1828: 68,
- 0x1829: 68,
- 0x182a: 68,
- 0x182b: 68,
- 0x182c: 68,
- 0x182d: 68,
- 0x182e: 68,
- 0x182f: 68,
- 0x1830: 68,
- 0x1831: 68,
- 0x1832: 68,
- 0x1833: 68,
- 0x1834: 68,
- 0x1835: 68,
- 0x1836: 68,
- 0x1837: 68,
- 0x1838: 68,
- 0x1839: 68,
- 0x183a: 68,
- 0x183b: 68,
- 0x183c: 68,
- 0x183d: 68,
- 0x183e: 68,
- 0x183f: 68,
- 0x1840: 68,
- 0x1841: 68,
- 0x1842: 68,
- 0x1843: 68,
- 0x1844: 68,
- 0x1845: 68,
- 0x1846: 68,
- 0x1847: 68,
- 0x1848: 68,
- 0x1849: 68,
- 0x184a: 68,
- 0x184b: 68,
- 0x184c: 68,
- 0x184d: 68,
- 0x184e: 68,
- 0x184f: 68,
- 0x1850: 68,
- 0x1851: 68,
- 0x1852: 68,
- 0x1853: 68,
- 0x1854: 68,
- 0x1855: 68,
- 0x1856: 68,
- 0x1857: 68,
- 0x1858: 68,
- 0x1859: 68,
- 0x185a: 68,
- 0x185b: 68,
- 0x185c: 68,
- 0x185d: 68,
- 0x185e: 68,
- 0x185f: 68,
- 0x1860: 68,
- 0x1861: 68,
- 0x1862: 68,
- 0x1863: 68,
- 0x1864: 68,
- 0x1865: 68,
- 0x1866: 68,
- 0x1867: 68,
- 0x1868: 68,
- 0x1869: 68,
- 0x186a: 68,
- 0x186b: 68,
- 0x186c: 68,
- 0x186d: 68,
- 0x186e: 68,
- 0x186f: 68,
- 0x1870: 68,
- 0x1871: 68,
- 0x1872: 68,
- 0x1873: 68,
- 0x1874: 68,
- 0x1875: 68,
- 0x1876: 68,
- 0x1877: 68,
- 0x1878: 68,
- 0x1880: 85,
- 0x1881: 85,
- 0x1882: 85,
- 0x1883: 85,
- 0x1884: 85,
- 0x1885: 84,
- 0x1886: 84,
- 0x1887: 68,
- 0x1888: 68,
- 0x1889: 68,
- 0x188a: 68,
- 0x188b: 68,
- 0x188c: 68,
- 0x188d: 68,
- 0x188e: 68,
- 0x188f: 68,
- 0x1890: 68,
- 0x1891: 68,
- 0x1892: 68,
- 0x1893: 68,
- 0x1894: 68,
- 0x1895: 68,
- 0x1896: 68,
- 0x1897: 68,
- 0x1898: 68,
- 0x1899: 68,
- 0x189a: 68,
- 0x189b: 68,
- 0x189c: 68,
- 0x189d: 68,
- 0x189e: 68,
- 0x189f: 68,
- 0x18a0: 68,
- 0x18a1: 68,
- 0x18a2: 68,
- 0x18a3: 68,
- 0x18a4: 68,
- 0x18a5: 68,
- 0x18a6: 68,
- 0x18a7: 68,
- 0x18a8: 68,
- 0x18aa: 68,
- 0x200c: 85,
- 0x200d: 67,
- 0x202f: 85,
- 0x2066: 85,
- 0x2067: 85,
- 0x2068: 85,
- 0x2069: 85,
- 0xa840: 68,
- 0xa841: 68,
- 0xa842: 68,
- 0xa843: 68,
- 0xa844: 68,
- 0xa845: 68,
- 0xa846: 68,
- 0xa847: 68,
- 0xa848: 68,
- 0xa849: 68,
- 0xa84a: 68,
- 0xa84b: 68,
- 0xa84c: 68,
- 0xa84d: 68,
- 0xa84e: 68,
- 0xa84f: 68,
- 0xa850: 68,
- 0xa851: 68,
- 0xa852: 68,
- 0xa853: 68,
- 0xa854: 68,
- 0xa855: 68,
- 0xa856: 68,
- 0xa857: 68,
- 0xa858: 68,
- 0xa859: 68,
- 0xa85a: 68,
- 0xa85b: 68,
- 0xa85c: 68,
- 0xa85d: 68,
- 0xa85e: 68,
- 0xa85f: 68,
- 0xa860: 68,
- 0xa861: 68,
- 0xa862: 68,
- 0xa863: 68,
- 0xa864: 68,
- 0xa865: 68,
- 0xa866: 68,
- 0xa867: 68,
- 0xa868: 68,
- 0xa869: 68,
- 0xa86a: 68,
- 0xa86b: 68,
- 0xa86c: 68,
- 0xa86d: 68,
- 0xa86e: 68,
- 0xa86f: 68,
- 0xa870: 68,
- 0xa871: 68,
- 0xa872: 76,
- 0xa873: 85,
- 0x10ac0: 68,
- 0x10ac1: 68,
- 0x10ac2: 68,
- 0x10ac3: 68,
- 0x10ac4: 68,
- 0x10ac5: 82,
- 0x10ac6: 85,
- 0x10ac7: 82,
- 0x10ac8: 85,
- 0x10ac9: 82,
- 0x10aca: 82,
- 0x10acb: 85,
- 0x10acc: 85,
- 0x10acd: 76,
- 0x10ace: 82,
- 0x10acf: 82,
- 0x10ad0: 82,
- 0x10ad1: 82,
- 0x10ad2: 82,
- 0x10ad3: 68,
- 0x10ad4: 68,
- 0x10ad5: 68,
- 0x10ad6: 68,
- 0x10ad7: 76,
- 0x10ad8: 68,
- 0x10ad9: 68,
- 0x10ada: 68,
- 0x10adb: 68,
- 0x10adc: 68,
- 0x10add: 82,
- 0x10ade: 68,
- 0x10adf: 68,
- 0x10ae0: 68,
- 0x10ae1: 82,
- 0x10ae2: 85,
- 0x10ae3: 85,
- 0x10ae4: 82,
- 0x10aeb: 68,
- 0x10aec: 68,
- 0x10aed: 68,
- 0x10aee: 68,
- 0x10aef: 82,
- 0x10b80: 68,
- 0x10b81: 82,
- 0x10b82: 68,
- 0x10b83: 82,
- 0x10b84: 82,
- 0x10b85: 82,
- 0x10b86: 68,
- 0x10b87: 68,
- 0x10b88: 68,
- 0x10b89: 82,
- 0x10b8a: 68,
- 0x10b8b: 68,
- 0x10b8c: 82,
- 0x10b8d: 68,
- 0x10b8e: 82,
- 0x10b8f: 82,
- 0x10b90: 68,
- 0x10b91: 82,
- 0x10ba9: 82,
- 0x10baa: 82,
- 0x10bab: 82,
- 0x10bac: 82,
- 0x10bad: 68,
- 0x10bae: 68,
- 0x10baf: 85,
- 0x10d00: 76,
- 0x10d01: 68,
- 0x10d02: 68,
- 0x10d03: 68,
- 0x10d04: 68,
- 0x10d05: 68,
- 0x10d06: 68,
- 0x10d07: 68,
- 0x10d08: 68,
- 0x10d09: 68,
- 0x10d0a: 68,
- 0x10d0b: 68,
- 0x10d0c: 68,
- 0x10d0d: 68,
- 0x10d0e: 68,
- 0x10d0f: 68,
- 0x10d10: 68,
- 0x10d11: 68,
- 0x10d12: 68,
- 0x10d13: 68,
- 0x10d14: 68,
- 0x10d15: 68,
- 0x10d16: 68,
- 0x10d17: 68,
- 0x10d18: 68,
- 0x10d19: 68,
- 0x10d1a: 68,
- 0x10d1b: 68,
- 0x10d1c: 68,
- 0x10d1d: 68,
- 0x10d1e: 68,
- 0x10d1f: 68,
- 0x10d20: 68,
- 0x10d21: 68,
- 0x10d22: 82,
- 0x10d23: 68,
- 0x10f30: 68,
- 0x10f31: 68,
- 0x10f32: 68,
- 0x10f33: 82,
- 0x10f34: 68,
- 0x10f35: 68,
- 0x10f36: 68,
- 0x10f37: 68,
- 0x10f38: 68,
- 0x10f39: 68,
- 0x10f3a: 68,
- 0x10f3b: 68,
- 0x10f3c: 68,
- 0x10f3d: 68,
- 0x10f3e: 68,
- 0x10f3f: 68,
- 0x10f40: 68,
- 0x10f41: 68,
- 0x10f42: 68,
- 0x10f43: 68,
- 0x10f44: 68,
- 0x10f45: 85,
- 0x10f51: 68,
- 0x10f52: 68,
- 0x10f53: 68,
- 0x10f54: 82,
- 0x10f70: 68,
- 0x10f71: 68,
- 0x10f72: 68,
- 0x10f73: 68,
- 0x10f74: 82,
- 0x10f75: 82,
- 0x10f76: 68,
- 0x10f77: 68,
- 0x10f78: 68,
- 0x10f79: 68,
- 0x10f7a: 68,
- 0x10f7b: 68,
- 0x10f7c: 68,
- 0x10f7d: 68,
- 0x10f7e: 68,
- 0x10f7f: 68,
- 0x10f80: 68,
- 0x10f81: 68,
- 0x10fb0: 68,
- 0x10fb1: 85,
- 0x10fb2: 68,
- 0x10fb3: 68,
- 0x10fb4: 82,
- 0x10fb5: 82,
- 0x10fb6: 82,
- 0x10fb7: 85,
- 0x10fb8: 68,
- 0x10fb9: 82,
- 0x10fba: 82,
- 0x10fbb: 68,
- 0x10fbc: 68,
- 0x10fbd: 82,
- 0x10fbe: 68,
- 0x10fbf: 68,
- 0x10fc0: 85,
- 0x10fc1: 68,
- 0x10fc2: 82,
- 0x10fc3: 82,
- 0x10fc4: 68,
- 0x10fc5: 85,
- 0x10fc6: 85,
- 0x10fc7: 85,
- 0x10fc8: 85,
- 0x10fc9: 82,
- 0x10fca: 68,
- 0x10fcb: 76,
- 0x110bd: 85,
- 0x110cd: 85,
- 0x1e900: 68,
- 0x1e901: 68,
- 0x1e902: 68,
- 0x1e903: 68,
- 0x1e904: 68,
- 0x1e905: 68,
- 0x1e906: 68,
- 0x1e907: 68,
- 0x1e908: 68,
- 0x1e909: 68,
- 0x1e90a: 68,
- 0x1e90b: 68,
- 0x1e90c: 68,
- 0x1e90d: 68,
- 0x1e90e: 68,
- 0x1e90f: 68,
- 0x1e910: 68,
- 0x1e911: 68,
- 0x1e912: 68,
- 0x1e913: 68,
- 0x1e914: 68,
- 0x1e915: 68,
- 0x1e916: 68,
- 0x1e917: 68,
- 0x1e918: 68,
- 0x1e919: 68,
- 0x1e91a: 68,
- 0x1e91b: 68,
- 0x1e91c: 68,
- 0x1e91d: 68,
- 0x1e91e: 68,
- 0x1e91f: 68,
- 0x1e920: 68,
- 0x1e921: 68,
- 0x1e922: 68,
- 0x1e923: 68,
- 0x1e924: 68,
- 0x1e925: 68,
- 0x1e926: 68,
- 0x1e927: 68,
- 0x1e928: 68,
- 0x1e929: 68,
- 0x1e92a: 68,
- 0x1e92b: 68,
- 0x1e92c: 68,
- 0x1e92d: 68,
- 0x1e92e: 68,
- 0x1e92f: 68,
- 0x1e930: 68,
- 0x1e931: 68,
- 0x1e932: 68,
- 0x1e933: 68,
- 0x1e934: 68,
- 0x1e935: 68,
- 0x1e936: 68,
- 0x1e937: 68,
- 0x1e938: 68,
- 0x1e939: 68,
- 0x1e93a: 68,
- 0x1e93b: 68,
- 0x1e93c: 68,
- 0x1e93d: 68,
- 0x1e93e: 68,
- 0x1e93f: 68,
- 0x1e940: 68,
- 0x1e941: 68,
- 0x1e942: 68,
- 0x1e943: 68,
- 0x1e94b: 84,
-}
-codepoint_classes = {
- 'PVALID': (
- 0x2d0000002e,
- 0x300000003a,
- 0x610000007b,
- 0xdf000000f7,
- 0xf800000100,
- 0x10100000102,
- 0x10300000104,
- 0x10500000106,
- 0x10700000108,
- 0x1090000010a,
- 0x10b0000010c,
- 0x10d0000010e,
- 0x10f00000110,
- 0x11100000112,
- 0x11300000114,
- 0x11500000116,
- 0x11700000118,
- 0x1190000011a,
- 0x11b0000011c,
- 0x11d0000011e,
- 0x11f00000120,
- 0x12100000122,
- 0x12300000124,
- 0x12500000126,
- 0x12700000128,
- 0x1290000012a,
- 0x12b0000012c,
- 0x12d0000012e,
- 0x12f00000130,
- 0x13100000132,
- 0x13500000136,
- 0x13700000139,
- 0x13a0000013b,
- 0x13c0000013d,
- 0x13e0000013f,
- 0x14200000143,
- 0x14400000145,
- 0x14600000147,
- 0x14800000149,
- 0x14b0000014c,
- 0x14d0000014e,
- 0x14f00000150,
- 0x15100000152,
- 0x15300000154,
- 0x15500000156,
- 0x15700000158,
- 0x1590000015a,
- 0x15b0000015c,
- 0x15d0000015e,
- 0x15f00000160,
- 0x16100000162,
- 0x16300000164,
- 0x16500000166,
- 0x16700000168,
- 0x1690000016a,
- 0x16b0000016c,
- 0x16d0000016e,
- 0x16f00000170,
- 0x17100000172,
- 0x17300000174,
- 0x17500000176,
- 0x17700000178,
- 0x17a0000017b,
- 0x17c0000017d,
- 0x17e0000017f,
- 0x18000000181,
- 0x18300000184,
- 0x18500000186,
- 0x18800000189,
- 0x18c0000018e,
- 0x19200000193,
- 0x19500000196,
- 0x1990000019c,
- 0x19e0000019f,
- 0x1a1000001a2,
- 0x1a3000001a4,
- 0x1a5000001a6,
- 0x1a8000001a9,
- 0x1aa000001ac,
- 0x1ad000001ae,
- 0x1b0000001b1,
- 0x1b4000001b5,
- 0x1b6000001b7,
- 0x1b9000001bc,
- 0x1bd000001c4,
- 0x1ce000001cf,
- 0x1d0000001d1,
- 0x1d2000001d3,
- 0x1d4000001d5,
- 0x1d6000001d7,
- 0x1d8000001d9,
- 0x1da000001db,
- 0x1dc000001de,
- 0x1df000001e0,
- 0x1e1000001e2,
- 0x1e3000001e4,
- 0x1e5000001e6,
- 0x1e7000001e8,
- 0x1e9000001ea,
- 0x1eb000001ec,
- 0x1ed000001ee,
- 0x1ef000001f1,
- 0x1f5000001f6,
- 0x1f9000001fa,
- 0x1fb000001fc,
- 0x1fd000001fe,
- 0x1ff00000200,
- 0x20100000202,
- 0x20300000204,
- 0x20500000206,
- 0x20700000208,
- 0x2090000020a,
- 0x20b0000020c,
- 0x20d0000020e,
- 0x20f00000210,
- 0x21100000212,
- 0x21300000214,
- 0x21500000216,
- 0x21700000218,
- 0x2190000021a,
- 0x21b0000021c,
- 0x21d0000021e,
- 0x21f00000220,
- 0x22100000222,
- 0x22300000224,
- 0x22500000226,
- 0x22700000228,
- 0x2290000022a,
- 0x22b0000022c,
- 0x22d0000022e,
- 0x22f00000230,
- 0x23100000232,
- 0x2330000023a,
- 0x23c0000023d,
- 0x23f00000241,
- 0x24200000243,
- 0x24700000248,
- 0x2490000024a,
- 0x24b0000024c,
- 0x24d0000024e,
- 0x24f000002b0,
- 0x2b9000002c2,
- 0x2c6000002d2,
- 0x2ec000002ed,
- 0x2ee000002ef,
- 0x30000000340,
- 0x34200000343,
- 0x3460000034f,
- 0x35000000370,
- 0x37100000372,
- 0x37300000374,
- 0x37700000378,
- 0x37b0000037e,
- 0x39000000391,
- 0x3ac000003cf,
- 0x3d7000003d8,
- 0x3d9000003da,
- 0x3db000003dc,
- 0x3dd000003de,
- 0x3df000003e0,
- 0x3e1000003e2,
- 0x3e3000003e4,
- 0x3e5000003e6,
- 0x3e7000003e8,
- 0x3e9000003ea,
- 0x3eb000003ec,
- 0x3ed000003ee,
- 0x3ef000003f0,
- 0x3f3000003f4,
- 0x3f8000003f9,
- 0x3fb000003fd,
- 0x43000000460,
- 0x46100000462,
- 0x46300000464,
- 0x46500000466,
- 0x46700000468,
- 0x4690000046a,
- 0x46b0000046c,
- 0x46d0000046e,
- 0x46f00000470,
- 0x47100000472,
- 0x47300000474,
- 0x47500000476,
- 0x47700000478,
- 0x4790000047a,
- 0x47b0000047c,
- 0x47d0000047e,
- 0x47f00000480,
- 0x48100000482,
- 0x48300000488,
- 0x48b0000048c,
- 0x48d0000048e,
- 0x48f00000490,
- 0x49100000492,
- 0x49300000494,
- 0x49500000496,
- 0x49700000498,
- 0x4990000049a,
- 0x49b0000049c,
- 0x49d0000049e,
- 0x49f000004a0,
- 0x4a1000004a2,
- 0x4a3000004a4,
- 0x4a5000004a6,
- 0x4a7000004a8,
- 0x4a9000004aa,
- 0x4ab000004ac,
- 0x4ad000004ae,
- 0x4af000004b0,
- 0x4b1000004b2,
- 0x4b3000004b4,
- 0x4b5000004b6,
- 0x4b7000004b8,
- 0x4b9000004ba,
- 0x4bb000004bc,
- 0x4bd000004be,
- 0x4bf000004c0,
- 0x4c2000004c3,
- 0x4c4000004c5,
- 0x4c6000004c7,
- 0x4c8000004c9,
- 0x4ca000004cb,
- 0x4cc000004cd,
- 0x4ce000004d0,
- 0x4d1000004d2,
- 0x4d3000004d4,
- 0x4d5000004d6,
- 0x4d7000004d8,
- 0x4d9000004da,
- 0x4db000004dc,
- 0x4dd000004de,
- 0x4df000004e0,
- 0x4e1000004e2,
- 0x4e3000004e4,
- 0x4e5000004e6,
- 0x4e7000004e8,
- 0x4e9000004ea,
- 0x4eb000004ec,
- 0x4ed000004ee,
- 0x4ef000004f0,
- 0x4f1000004f2,
- 0x4f3000004f4,
- 0x4f5000004f6,
- 0x4f7000004f8,
- 0x4f9000004fa,
- 0x4fb000004fc,
- 0x4fd000004fe,
- 0x4ff00000500,
- 0x50100000502,
- 0x50300000504,
- 0x50500000506,
- 0x50700000508,
- 0x5090000050a,
- 0x50b0000050c,
- 0x50d0000050e,
- 0x50f00000510,
- 0x51100000512,
- 0x51300000514,
- 0x51500000516,
- 0x51700000518,
- 0x5190000051a,
- 0x51b0000051c,
- 0x51d0000051e,
- 0x51f00000520,
- 0x52100000522,
- 0x52300000524,
- 0x52500000526,
- 0x52700000528,
- 0x5290000052a,
- 0x52b0000052c,
- 0x52d0000052e,
- 0x52f00000530,
- 0x5590000055a,
- 0x56000000587,
- 0x58800000589,
- 0x591000005be,
- 0x5bf000005c0,
- 0x5c1000005c3,
- 0x5c4000005c6,
- 0x5c7000005c8,
- 0x5d0000005eb,
- 0x5ef000005f3,
- 0x6100000061b,
- 0x62000000640,
- 0x64100000660,
- 0x66e00000675,
- 0x679000006d4,
- 0x6d5000006dd,
- 0x6df000006e9,
- 0x6ea000006f0,
- 0x6fa00000700,
- 0x7100000074b,
- 0x74d000007b2,
- 0x7c0000007f6,
- 0x7fd000007fe,
- 0x8000000082e,
- 0x8400000085c,
- 0x8600000086b,
- 0x87000000888,
- 0x8890000088f,
- 0x898000008e2,
- 0x8e300000958,
- 0x96000000964,
- 0x96600000970,
- 0x97100000984,
- 0x9850000098d,
- 0x98f00000991,
- 0x993000009a9,
- 0x9aa000009b1,
- 0x9b2000009b3,
- 0x9b6000009ba,
- 0x9bc000009c5,
- 0x9c7000009c9,
- 0x9cb000009cf,
- 0x9d7000009d8,
- 0x9e0000009e4,
- 0x9e6000009f2,
- 0x9fc000009fd,
- 0x9fe000009ff,
- 0xa0100000a04,
- 0xa0500000a0b,
- 0xa0f00000a11,
- 0xa1300000a29,
- 0xa2a00000a31,
- 0xa3200000a33,
- 0xa3500000a36,
- 0xa3800000a3a,
- 0xa3c00000a3d,
- 0xa3e00000a43,
- 0xa4700000a49,
- 0xa4b00000a4e,
- 0xa5100000a52,
- 0xa5c00000a5d,
- 0xa6600000a76,
- 0xa8100000a84,
- 0xa8500000a8e,
- 0xa8f00000a92,
- 0xa9300000aa9,
- 0xaaa00000ab1,
- 0xab200000ab4,
- 0xab500000aba,
- 0xabc00000ac6,
- 0xac700000aca,
- 0xacb00000ace,
- 0xad000000ad1,
- 0xae000000ae4,
- 0xae600000af0,
- 0xaf900000b00,
- 0xb0100000b04,
- 0xb0500000b0d,
- 0xb0f00000b11,
- 0xb1300000b29,
- 0xb2a00000b31,
- 0xb3200000b34,
- 0xb3500000b3a,
- 0xb3c00000b45,
- 0xb4700000b49,
- 0xb4b00000b4e,
- 0xb5500000b58,
- 0xb5f00000b64,
- 0xb6600000b70,
- 0xb7100000b72,
- 0xb8200000b84,
- 0xb8500000b8b,
- 0xb8e00000b91,
- 0xb9200000b96,
- 0xb9900000b9b,
- 0xb9c00000b9d,
- 0xb9e00000ba0,
- 0xba300000ba5,
- 0xba800000bab,
- 0xbae00000bba,
- 0xbbe00000bc3,
- 0xbc600000bc9,
- 0xbca00000bce,
- 0xbd000000bd1,
- 0xbd700000bd8,
- 0xbe600000bf0,
- 0xc0000000c0d,
- 0xc0e00000c11,
- 0xc1200000c29,
- 0xc2a00000c3a,
- 0xc3c00000c45,
- 0xc4600000c49,
- 0xc4a00000c4e,
- 0xc5500000c57,
- 0xc5800000c5b,
- 0xc5d00000c5e,
- 0xc6000000c64,
- 0xc6600000c70,
- 0xc8000000c84,
- 0xc8500000c8d,
- 0xc8e00000c91,
- 0xc9200000ca9,
- 0xcaa00000cb4,
- 0xcb500000cba,
- 0xcbc00000cc5,
- 0xcc600000cc9,
- 0xcca00000cce,
- 0xcd500000cd7,
- 0xcdd00000cdf,
- 0xce000000ce4,
- 0xce600000cf0,
- 0xcf100000cf4,
- 0xd0000000d0d,
- 0xd0e00000d11,
- 0xd1200000d45,
- 0xd4600000d49,
- 0xd4a00000d4f,
- 0xd5400000d58,
- 0xd5f00000d64,
- 0xd6600000d70,
- 0xd7a00000d80,
- 0xd8100000d84,
- 0xd8500000d97,
- 0xd9a00000db2,
- 0xdb300000dbc,
- 0xdbd00000dbe,
- 0xdc000000dc7,
- 0xdca00000dcb,
- 0xdcf00000dd5,
- 0xdd600000dd7,
- 0xdd800000de0,
- 0xde600000df0,
- 0xdf200000df4,
- 0xe0100000e33,
- 0xe3400000e3b,
- 0xe4000000e4f,
- 0xe5000000e5a,
- 0xe8100000e83,
- 0xe8400000e85,
- 0xe8600000e8b,
- 0xe8c00000ea4,
- 0xea500000ea6,
- 0xea700000eb3,
- 0xeb400000ebe,
- 0xec000000ec5,
- 0xec600000ec7,
- 0xec800000ecf,
- 0xed000000eda,
- 0xede00000ee0,
- 0xf0000000f01,
- 0xf0b00000f0c,
- 0xf1800000f1a,
- 0xf2000000f2a,
- 0xf3500000f36,
- 0xf3700000f38,
- 0xf3900000f3a,
- 0xf3e00000f43,
- 0xf4400000f48,
- 0xf4900000f4d,
- 0xf4e00000f52,
- 0xf5300000f57,
- 0xf5800000f5c,
- 0xf5d00000f69,
- 0xf6a00000f6d,
- 0xf7100000f73,
- 0xf7400000f75,
- 0xf7a00000f81,
- 0xf8200000f85,
- 0xf8600000f93,
- 0xf9400000f98,
- 0xf9900000f9d,
- 0xf9e00000fa2,
- 0xfa300000fa7,
- 0xfa800000fac,
- 0xfad00000fb9,
- 0xfba00000fbd,
- 0xfc600000fc7,
- 0x10000000104a,
- 0x10500000109e,
- 0x10d0000010fb,
- 0x10fd00001100,
- 0x120000001249,
- 0x124a0000124e,
- 0x125000001257,
- 0x125800001259,
- 0x125a0000125e,
- 0x126000001289,
- 0x128a0000128e,
- 0x1290000012b1,
- 0x12b2000012b6,
- 0x12b8000012bf,
- 0x12c0000012c1,
- 0x12c2000012c6,
- 0x12c8000012d7,
- 0x12d800001311,
- 0x131200001316,
- 0x13180000135b,
- 0x135d00001360,
- 0x138000001390,
- 0x13a0000013f6,
- 0x14010000166d,
- 0x166f00001680,
- 0x16810000169b,
- 0x16a0000016eb,
- 0x16f1000016f9,
- 0x170000001716,
- 0x171f00001735,
- 0x174000001754,
- 0x17600000176d,
- 0x176e00001771,
- 0x177200001774,
- 0x1780000017b4,
- 0x17b6000017d4,
- 0x17d7000017d8,
- 0x17dc000017de,
- 0x17e0000017ea,
- 0x18100000181a,
- 0x182000001879,
- 0x1880000018ab,
- 0x18b0000018f6,
- 0x19000000191f,
- 0x19200000192c,
- 0x19300000193c,
- 0x19460000196e,
- 0x197000001975,
- 0x1980000019ac,
- 0x19b0000019ca,
- 0x19d0000019da,
- 0x1a0000001a1c,
- 0x1a2000001a5f,
- 0x1a6000001a7d,
- 0x1a7f00001a8a,
- 0x1a9000001a9a,
- 0x1aa700001aa8,
- 0x1ab000001abe,
- 0x1abf00001acf,
- 0x1b0000001b4d,
- 0x1b5000001b5a,
- 0x1b6b00001b74,
- 0x1b8000001bf4,
- 0x1c0000001c38,
- 0x1c4000001c4a,
- 0x1c4d00001c7e,
- 0x1cd000001cd3,
- 0x1cd400001cfb,
- 0x1d0000001d2c,
- 0x1d2f00001d30,
- 0x1d3b00001d3c,
- 0x1d4e00001d4f,
- 0x1d6b00001d78,
- 0x1d7900001d9b,
- 0x1dc000001e00,
- 0x1e0100001e02,
- 0x1e0300001e04,
- 0x1e0500001e06,
- 0x1e0700001e08,
- 0x1e0900001e0a,
- 0x1e0b00001e0c,
- 0x1e0d00001e0e,
- 0x1e0f00001e10,
- 0x1e1100001e12,
- 0x1e1300001e14,
- 0x1e1500001e16,
- 0x1e1700001e18,
- 0x1e1900001e1a,
- 0x1e1b00001e1c,
- 0x1e1d00001e1e,
- 0x1e1f00001e20,
- 0x1e2100001e22,
- 0x1e2300001e24,
- 0x1e2500001e26,
- 0x1e2700001e28,
- 0x1e2900001e2a,
- 0x1e2b00001e2c,
- 0x1e2d00001e2e,
- 0x1e2f00001e30,
- 0x1e3100001e32,
- 0x1e3300001e34,
- 0x1e3500001e36,
- 0x1e3700001e38,
- 0x1e3900001e3a,
- 0x1e3b00001e3c,
- 0x1e3d00001e3e,
- 0x1e3f00001e40,
- 0x1e4100001e42,
- 0x1e4300001e44,
- 0x1e4500001e46,
- 0x1e4700001e48,
- 0x1e4900001e4a,
- 0x1e4b00001e4c,
- 0x1e4d00001e4e,
- 0x1e4f00001e50,
- 0x1e5100001e52,
- 0x1e5300001e54,
- 0x1e5500001e56,
- 0x1e5700001e58,
- 0x1e5900001e5a,
- 0x1e5b00001e5c,
- 0x1e5d00001e5e,
- 0x1e5f00001e60,
- 0x1e6100001e62,
- 0x1e6300001e64,
- 0x1e6500001e66,
- 0x1e6700001e68,
- 0x1e6900001e6a,
- 0x1e6b00001e6c,
- 0x1e6d00001e6e,
- 0x1e6f00001e70,
- 0x1e7100001e72,
- 0x1e7300001e74,
- 0x1e7500001e76,
- 0x1e7700001e78,
- 0x1e7900001e7a,
- 0x1e7b00001e7c,
- 0x1e7d00001e7e,
- 0x1e7f00001e80,
- 0x1e8100001e82,
- 0x1e8300001e84,
- 0x1e8500001e86,
- 0x1e8700001e88,
- 0x1e8900001e8a,
- 0x1e8b00001e8c,
- 0x1e8d00001e8e,
- 0x1e8f00001e90,
- 0x1e9100001e92,
- 0x1e9300001e94,
- 0x1e9500001e9a,
- 0x1e9c00001e9e,
- 0x1e9f00001ea0,
- 0x1ea100001ea2,
- 0x1ea300001ea4,
- 0x1ea500001ea6,
- 0x1ea700001ea8,
- 0x1ea900001eaa,
- 0x1eab00001eac,
- 0x1ead00001eae,
- 0x1eaf00001eb0,
- 0x1eb100001eb2,
- 0x1eb300001eb4,
- 0x1eb500001eb6,
- 0x1eb700001eb8,
- 0x1eb900001eba,
- 0x1ebb00001ebc,
- 0x1ebd00001ebe,
- 0x1ebf00001ec0,
- 0x1ec100001ec2,
- 0x1ec300001ec4,
- 0x1ec500001ec6,
- 0x1ec700001ec8,
- 0x1ec900001eca,
- 0x1ecb00001ecc,
- 0x1ecd00001ece,
- 0x1ecf00001ed0,
- 0x1ed100001ed2,
- 0x1ed300001ed4,
- 0x1ed500001ed6,
- 0x1ed700001ed8,
- 0x1ed900001eda,
- 0x1edb00001edc,
- 0x1edd00001ede,
- 0x1edf00001ee0,
- 0x1ee100001ee2,
- 0x1ee300001ee4,
- 0x1ee500001ee6,
- 0x1ee700001ee8,
- 0x1ee900001eea,
- 0x1eeb00001eec,
- 0x1eed00001eee,
- 0x1eef00001ef0,
- 0x1ef100001ef2,
- 0x1ef300001ef4,
- 0x1ef500001ef6,
- 0x1ef700001ef8,
- 0x1ef900001efa,
- 0x1efb00001efc,
- 0x1efd00001efe,
- 0x1eff00001f08,
- 0x1f1000001f16,
- 0x1f2000001f28,
- 0x1f3000001f38,
- 0x1f4000001f46,
- 0x1f5000001f58,
- 0x1f6000001f68,
- 0x1f7000001f71,
- 0x1f7200001f73,
- 0x1f7400001f75,
- 0x1f7600001f77,
- 0x1f7800001f79,
- 0x1f7a00001f7b,
- 0x1f7c00001f7d,
- 0x1fb000001fb2,
- 0x1fb600001fb7,
- 0x1fc600001fc7,
- 0x1fd000001fd3,
- 0x1fd600001fd8,
- 0x1fe000001fe3,
- 0x1fe400001fe8,
- 0x1ff600001ff7,
- 0x214e0000214f,
- 0x218400002185,
- 0x2c3000002c60,
- 0x2c6100002c62,
- 0x2c6500002c67,
- 0x2c6800002c69,
- 0x2c6a00002c6b,
- 0x2c6c00002c6d,
- 0x2c7100002c72,
- 0x2c7300002c75,
- 0x2c7600002c7c,
- 0x2c8100002c82,
- 0x2c8300002c84,
- 0x2c8500002c86,
- 0x2c8700002c88,
- 0x2c8900002c8a,
- 0x2c8b00002c8c,
- 0x2c8d00002c8e,
- 0x2c8f00002c90,
- 0x2c9100002c92,
- 0x2c9300002c94,
- 0x2c9500002c96,
- 0x2c9700002c98,
- 0x2c9900002c9a,
- 0x2c9b00002c9c,
- 0x2c9d00002c9e,
- 0x2c9f00002ca0,
- 0x2ca100002ca2,
- 0x2ca300002ca4,
- 0x2ca500002ca6,
- 0x2ca700002ca8,
- 0x2ca900002caa,
- 0x2cab00002cac,
- 0x2cad00002cae,
- 0x2caf00002cb0,
- 0x2cb100002cb2,
- 0x2cb300002cb4,
- 0x2cb500002cb6,
- 0x2cb700002cb8,
- 0x2cb900002cba,
- 0x2cbb00002cbc,
- 0x2cbd00002cbe,
- 0x2cbf00002cc0,
- 0x2cc100002cc2,
- 0x2cc300002cc4,
- 0x2cc500002cc6,
- 0x2cc700002cc8,
- 0x2cc900002cca,
- 0x2ccb00002ccc,
- 0x2ccd00002cce,
- 0x2ccf00002cd0,
- 0x2cd100002cd2,
- 0x2cd300002cd4,
- 0x2cd500002cd6,
- 0x2cd700002cd8,
- 0x2cd900002cda,
- 0x2cdb00002cdc,
- 0x2cdd00002cde,
- 0x2cdf00002ce0,
- 0x2ce100002ce2,
- 0x2ce300002ce5,
- 0x2cec00002ced,
- 0x2cee00002cf2,
- 0x2cf300002cf4,
- 0x2d0000002d26,
- 0x2d2700002d28,
- 0x2d2d00002d2e,
- 0x2d3000002d68,
- 0x2d7f00002d97,
- 0x2da000002da7,
- 0x2da800002daf,
- 0x2db000002db7,
- 0x2db800002dbf,
- 0x2dc000002dc7,
- 0x2dc800002dcf,
- 0x2dd000002dd7,
- 0x2dd800002ddf,
- 0x2de000002e00,
- 0x2e2f00002e30,
- 0x300500003008,
- 0x302a0000302e,
- 0x303c0000303d,
- 0x304100003097,
- 0x30990000309b,
- 0x309d0000309f,
- 0x30a1000030fb,
- 0x30fc000030ff,
- 0x310500003130,
- 0x31a0000031c0,
- 0x31f000003200,
- 0x340000004dc0,
- 0x4e000000a48d,
- 0xa4d00000a4fe,
- 0xa5000000a60d,
- 0xa6100000a62c,
- 0xa6410000a642,
- 0xa6430000a644,
- 0xa6450000a646,
- 0xa6470000a648,
- 0xa6490000a64a,
- 0xa64b0000a64c,
- 0xa64d0000a64e,
- 0xa64f0000a650,
- 0xa6510000a652,
- 0xa6530000a654,
- 0xa6550000a656,
- 0xa6570000a658,
- 0xa6590000a65a,
- 0xa65b0000a65c,
- 0xa65d0000a65e,
- 0xa65f0000a660,
- 0xa6610000a662,
- 0xa6630000a664,
- 0xa6650000a666,
- 0xa6670000a668,
- 0xa6690000a66a,
- 0xa66b0000a66c,
- 0xa66d0000a670,
- 0xa6740000a67e,
- 0xa67f0000a680,
- 0xa6810000a682,
- 0xa6830000a684,
- 0xa6850000a686,
- 0xa6870000a688,
- 0xa6890000a68a,
- 0xa68b0000a68c,
- 0xa68d0000a68e,
- 0xa68f0000a690,
- 0xa6910000a692,
- 0xa6930000a694,
- 0xa6950000a696,
- 0xa6970000a698,
- 0xa6990000a69a,
- 0xa69b0000a69c,
- 0xa69e0000a6e6,
- 0xa6f00000a6f2,
- 0xa7170000a720,
- 0xa7230000a724,
- 0xa7250000a726,
- 0xa7270000a728,
- 0xa7290000a72a,
- 0xa72b0000a72c,
- 0xa72d0000a72e,
- 0xa72f0000a732,
- 0xa7330000a734,
- 0xa7350000a736,
- 0xa7370000a738,
- 0xa7390000a73a,
- 0xa73b0000a73c,
- 0xa73d0000a73e,
- 0xa73f0000a740,
- 0xa7410000a742,
- 0xa7430000a744,
- 0xa7450000a746,
- 0xa7470000a748,
- 0xa7490000a74a,
- 0xa74b0000a74c,
- 0xa74d0000a74e,
- 0xa74f0000a750,
- 0xa7510000a752,
- 0xa7530000a754,
- 0xa7550000a756,
- 0xa7570000a758,
- 0xa7590000a75a,
- 0xa75b0000a75c,
- 0xa75d0000a75e,
- 0xa75f0000a760,
- 0xa7610000a762,
- 0xa7630000a764,
- 0xa7650000a766,
- 0xa7670000a768,
- 0xa7690000a76a,
- 0xa76b0000a76c,
- 0xa76d0000a76e,
- 0xa76f0000a770,
- 0xa7710000a779,
- 0xa77a0000a77b,
- 0xa77c0000a77d,
- 0xa77f0000a780,
- 0xa7810000a782,
- 0xa7830000a784,
- 0xa7850000a786,
- 0xa7870000a789,
- 0xa78c0000a78d,
- 0xa78e0000a790,
- 0xa7910000a792,
- 0xa7930000a796,
- 0xa7970000a798,
- 0xa7990000a79a,
- 0xa79b0000a79c,
- 0xa79d0000a79e,
- 0xa79f0000a7a0,
- 0xa7a10000a7a2,
- 0xa7a30000a7a4,
- 0xa7a50000a7a6,
- 0xa7a70000a7a8,
- 0xa7a90000a7aa,
- 0xa7af0000a7b0,
- 0xa7b50000a7b6,
- 0xa7b70000a7b8,
- 0xa7b90000a7ba,
- 0xa7bb0000a7bc,
- 0xa7bd0000a7be,
- 0xa7bf0000a7c0,
- 0xa7c10000a7c2,
- 0xa7c30000a7c4,
- 0xa7c80000a7c9,
- 0xa7ca0000a7cb,
- 0xa7d10000a7d2,
- 0xa7d30000a7d4,
- 0xa7d50000a7d6,
- 0xa7d70000a7d8,
- 0xa7d90000a7da,
- 0xa7f20000a7f5,
- 0xa7f60000a7f8,
- 0xa7fa0000a828,
- 0xa82c0000a82d,
- 0xa8400000a874,
- 0xa8800000a8c6,
- 0xa8d00000a8da,
- 0xa8e00000a8f8,
- 0xa8fb0000a8fc,
- 0xa8fd0000a92e,
- 0xa9300000a954,
- 0xa9800000a9c1,
- 0xa9cf0000a9da,
- 0xa9e00000a9ff,
- 0xaa000000aa37,
- 0xaa400000aa4e,
- 0xaa500000aa5a,
- 0xaa600000aa77,
- 0xaa7a0000aac3,
- 0xaadb0000aade,
- 0xaae00000aaf0,
- 0xaaf20000aaf7,
- 0xab010000ab07,
- 0xab090000ab0f,
- 0xab110000ab17,
- 0xab200000ab27,
- 0xab280000ab2f,
- 0xab300000ab5b,
- 0xab600000ab69,
- 0xabc00000abeb,
- 0xabec0000abee,
- 0xabf00000abfa,
- 0xac000000d7a4,
- 0xfa0e0000fa10,
- 0xfa110000fa12,
- 0xfa130000fa15,
- 0xfa1f0000fa20,
- 0xfa210000fa22,
- 0xfa230000fa25,
- 0xfa270000fa2a,
- 0xfb1e0000fb1f,
- 0xfe200000fe30,
- 0xfe730000fe74,
- 0x100000001000c,
- 0x1000d00010027,
- 0x100280001003b,
- 0x1003c0001003e,
- 0x1003f0001004e,
- 0x100500001005e,
- 0x10080000100fb,
- 0x101fd000101fe,
- 0x102800001029d,
- 0x102a0000102d1,
- 0x102e0000102e1,
- 0x1030000010320,
- 0x1032d00010341,
- 0x103420001034a,
- 0x103500001037b,
- 0x103800001039e,
- 0x103a0000103c4,
- 0x103c8000103d0,
- 0x104280001049e,
- 0x104a0000104aa,
- 0x104d8000104fc,
- 0x1050000010528,
- 0x1053000010564,
- 0x10597000105a2,
- 0x105a3000105b2,
- 0x105b3000105ba,
- 0x105bb000105bd,
- 0x1060000010737,
- 0x1074000010756,
- 0x1076000010768,
- 0x1078000010786,
- 0x10787000107b1,
- 0x107b2000107bb,
- 0x1080000010806,
- 0x1080800010809,
- 0x1080a00010836,
- 0x1083700010839,
- 0x1083c0001083d,
- 0x1083f00010856,
- 0x1086000010877,
- 0x108800001089f,
- 0x108e0000108f3,
- 0x108f4000108f6,
- 0x1090000010916,
- 0x109200001093a,
- 0x10980000109b8,
- 0x109be000109c0,
- 0x10a0000010a04,
- 0x10a0500010a07,
- 0x10a0c00010a14,
- 0x10a1500010a18,
- 0x10a1900010a36,
- 0x10a3800010a3b,
- 0x10a3f00010a40,
- 0x10a6000010a7d,
- 0x10a8000010a9d,
- 0x10ac000010ac8,
- 0x10ac900010ae7,
- 0x10b0000010b36,
- 0x10b4000010b56,
- 0x10b6000010b73,
- 0x10b8000010b92,
- 0x10c0000010c49,
- 0x10cc000010cf3,
- 0x10d0000010d28,
- 0x10d3000010d3a,
- 0x10e8000010eaa,
- 0x10eab00010ead,
- 0x10eb000010eb2,
- 0x10efd00010f1d,
- 0x10f2700010f28,
- 0x10f3000010f51,
- 0x10f7000010f86,
- 0x10fb000010fc5,
- 0x10fe000010ff7,
- 0x1100000011047,
- 0x1106600011076,
- 0x1107f000110bb,
- 0x110c2000110c3,
- 0x110d0000110e9,
- 0x110f0000110fa,
- 0x1110000011135,
- 0x1113600011140,
- 0x1114400011148,
- 0x1115000011174,
- 0x1117600011177,
- 0x11180000111c5,
- 0x111c9000111cd,
- 0x111ce000111db,
- 0x111dc000111dd,
- 0x1120000011212,
- 0x1121300011238,
- 0x1123e00011242,
- 0x1128000011287,
- 0x1128800011289,
- 0x1128a0001128e,
- 0x1128f0001129e,
- 0x1129f000112a9,
- 0x112b0000112eb,
- 0x112f0000112fa,
- 0x1130000011304,
- 0x113050001130d,
- 0x1130f00011311,
- 0x1131300011329,
- 0x1132a00011331,
- 0x1133200011334,
- 0x113350001133a,
- 0x1133b00011345,
- 0x1134700011349,
- 0x1134b0001134e,
- 0x1135000011351,
- 0x1135700011358,
- 0x1135d00011364,
- 0x113660001136d,
- 0x1137000011375,
- 0x114000001144b,
- 0x114500001145a,
- 0x1145e00011462,
- 0x11480000114c6,
- 0x114c7000114c8,
- 0x114d0000114da,
- 0x11580000115b6,
- 0x115b8000115c1,
- 0x115d8000115de,
- 0x1160000011641,
- 0x1164400011645,
- 0x116500001165a,
- 0x11680000116b9,
- 0x116c0000116ca,
- 0x117000001171b,
- 0x1171d0001172c,
- 0x117300001173a,
- 0x1174000011747,
- 0x118000001183b,
- 0x118c0000118ea,
- 0x118ff00011907,
- 0x119090001190a,
- 0x1190c00011914,
- 0x1191500011917,
- 0x1191800011936,
- 0x1193700011939,
- 0x1193b00011944,
- 0x119500001195a,
- 0x119a0000119a8,
- 0x119aa000119d8,
- 0x119da000119e2,
- 0x119e3000119e5,
- 0x11a0000011a3f,
- 0x11a4700011a48,
- 0x11a5000011a9a,
- 0x11a9d00011a9e,
- 0x11ab000011af9,
- 0x11c0000011c09,
- 0x11c0a00011c37,
- 0x11c3800011c41,
- 0x11c5000011c5a,
- 0x11c7200011c90,
- 0x11c9200011ca8,
- 0x11ca900011cb7,
- 0x11d0000011d07,
- 0x11d0800011d0a,
- 0x11d0b00011d37,
- 0x11d3a00011d3b,
- 0x11d3c00011d3e,
- 0x11d3f00011d48,
- 0x11d5000011d5a,
- 0x11d6000011d66,
- 0x11d6700011d69,
- 0x11d6a00011d8f,
- 0x11d9000011d92,
- 0x11d9300011d99,
- 0x11da000011daa,
- 0x11ee000011ef7,
- 0x11f0000011f11,
- 0x11f1200011f3b,
- 0x11f3e00011f43,
- 0x11f5000011f5a,
- 0x11fb000011fb1,
- 0x120000001239a,
- 0x1248000012544,
- 0x12f9000012ff1,
- 0x1300000013430,
- 0x1344000013456,
- 0x1440000014647,
- 0x1680000016a39,
- 0x16a4000016a5f,
- 0x16a6000016a6a,
- 0x16a7000016abf,
- 0x16ac000016aca,
- 0x16ad000016aee,
- 0x16af000016af5,
- 0x16b0000016b37,
- 0x16b4000016b44,
- 0x16b5000016b5a,
- 0x16b6300016b78,
- 0x16b7d00016b90,
- 0x16e6000016e80,
- 0x16f0000016f4b,
- 0x16f4f00016f88,
- 0x16f8f00016fa0,
- 0x16fe000016fe2,
- 0x16fe300016fe5,
- 0x16ff000016ff2,
- 0x17000000187f8,
- 0x1880000018cd6,
- 0x18d0000018d09,
- 0x1aff00001aff4,
- 0x1aff50001affc,
- 0x1affd0001afff,
- 0x1b0000001b123,
- 0x1b1320001b133,
- 0x1b1500001b153,
- 0x1b1550001b156,
- 0x1b1640001b168,
- 0x1b1700001b2fc,
- 0x1bc000001bc6b,
- 0x1bc700001bc7d,
- 0x1bc800001bc89,
- 0x1bc900001bc9a,
- 0x1bc9d0001bc9f,
- 0x1cf000001cf2e,
- 0x1cf300001cf47,
- 0x1da000001da37,
- 0x1da3b0001da6d,
- 0x1da750001da76,
- 0x1da840001da85,
- 0x1da9b0001daa0,
- 0x1daa10001dab0,
- 0x1df000001df1f,
- 0x1df250001df2b,
- 0x1e0000001e007,
- 0x1e0080001e019,
- 0x1e01b0001e022,
- 0x1e0230001e025,
- 0x1e0260001e02b,
- 0x1e0300001e06e,
- 0x1e08f0001e090,
- 0x1e1000001e12d,
- 0x1e1300001e13e,
- 0x1e1400001e14a,
- 0x1e14e0001e14f,
- 0x1e2900001e2af,
- 0x1e2c00001e2fa,
- 0x1e4d00001e4fa,
- 0x1e7e00001e7e7,
- 0x1e7e80001e7ec,
- 0x1e7ed0001e7ef,
- 0x1e7f00001e7ff,
- 0x1e8000001e8c5,
- 0x1e8d00001e8d7,
- 0x1e9220001e94c,
- 0x1e9500001e95a,
- 0x200000002a6e0,
- 0x2a7000002b73a,
- 0x2b7400002b81e,
- 0x2b8200002cea2,
- 0x2ceb00002ebe1,
- 0x300000003134b,
- 0x31350000323b0,
- ),
- 'CONTEXTJ': (
- 0x200c0000200e,
- ),
- 'CONTEXTO': (
- 0xb7000000b8,
- 0x37500000376,
- 0x5f3000005f5,
- 0x6600000066a,
- 0x6f0000006fa,
- 0x30fb000030fc,
- ),
-}
diff --git a/spaces/Ataturk-Chatbot/HuggingFaceChat/venv/lib/python3.11/site-packages/pip/_vendor/resolvelib/providers.py b/spaces/Ataturk-Chatbot/HuggingFaceChat/venv/lib/python3.11/site-packages/pip/_vendor/resolvelib/providers.py
deleted file mode 100644
index e99d87ee75f6f665989a109828e07ef81cb3410c..0000000000000000000000000000000000000000
--- a/spaces/Ataturk-Chatbot/HuggingFaceChat/venv/lib/python3.11/site-packages/pip/_vendor/resolvelib/providers.py
+++ /dev/null
@@ -1,133 +0,0 @@
-class AbstractProvider(object):
- """Delegate class to provide the required interface for the resolver."""
-
- def identify(self, requirement_or_candidate):
- """Given a requirement, return an identifier for it.
-
- This is used to identify a requirement, e.g. whether two requirements
- should have their specifier parts merged.
- """
- raise NotImplementedError
-
- def get_preference(
- self,
- identifier,
- resolutions,
- candidates,
- information,
- backtrack_causes,
- ):
- """Produce a sort key for given requirement based on preference.
-
- The preference is defined as "I think this requirement should be
- resolved first". The lower the return value is, the more preferred
- this group of arguments is.
-
- :param identifier: An identifier as returned by ``identify()``. This
- identifies the dependency matches which should be returned.
- :param resolutions: Mapping of candidates currently pinned by the
- resolver. Each key is an identifier, and the value is a candidate.
- The candidate may conflict with requirements from ``information``.
- :param candidates: Mapping of each dependency's possible candidates.
- Each value is an iterator of candidates.
- :param information: Mapping of requirement information of each package.
- Each value is an iterator of *requirement information*.
- :param backtrack_causes: Sequence of requirement information that were
- the requirements that caused the resolver to most recently backtrack.
-
- A *requirement information* instance is a named tuple with two members:
-
- * ``requirement`` specifies a requirement contributing to the current
- list of candidates.
- * ``parent`` specifies the candidate that provides (depended on) the
- requirement, or ``None`` to indicate a root requirement.
-
- The preference could depend on various issues, including (not
- necessarily in this order):
-
- * Is this package pinned in the current resolution result?
- * How relaxed is the requirement? Stricter ones should probably be
- worked on first? (I don't know, actually.)
- * How many possibilities are there to satisfy this requirement? Those
- with few left should likely be worked on first, I guess?
- * Are there any known conflicts for this requirement? We should
- probably work on those with the most known conflicts.
-
- A sortable value should be returned (this will be used as the ``key``
- parameter of the built-in sorting function). The smaller the value is,
- the more preferred this requirement is (i.e. the sorting function
- is called with ``reverse=False``).
- """
- raise NotImplementedError
-
- def find_matches(self, identifier, requirements, incompatibilities):
- """Find all possible candidates that satisfy the given constraints.
-
- :param identifier: An identifier as returned by ``identify()``. This
- identifies the dependency matches of which should be returned.
- :param requirements: A mapping of requirements that all returned
- candidates must satisfy. Each key is an identifier, and the value
- an iterator of requirements for that dependency.
- :param incompatibilities: A mapping of known incompatibilities of
- each dependency. Each key is an identifier, and the value an
- iterator of incompatibilities known to the resolver. All
- incompatibilities *must* be excluded from the return value.
-
- This should try to get candidates based on the requirements' types.
- For VCS, local, and archive requirements, the one-and-only match is
- returned, and for a "named" requirement, the index(es) should be
- consulted to find concrete candidates for this requirement.
-
- The return value should produce candidates ordered by preference; the
- most preferred candidate should come first. The return type may be one
- of the following:
-
- * A callable that returns an iterator that yields candidates.
- * An collection of candidates.
- * An iterable of candidates. This will be consumed immediately into a
- list of candidates.
- """
- raise NotImplementedError
-
- def is_satisfied_by(self, requirement, candidate):
- """Whether the given requirement can be satisfied by a candidate.
-
- The candidate is guaranteed to have been generated from the
- requirement.
-
- A boolean should be returned to indicate whether ``candidate`` is a
- viable solution to the requirement.
- """
- raise NotImplementedError
-
- def get_dependencies(self, candidate):
- """Get dependencies of a candidate.
-
- This should return a collection of requirements that `candidate`
- specifies as its dependencies.
- """
- raise NotImplementedError
-
-
-class AbstractResolver(object):
- """The thing that performs the actual resolution work."""
-
- base_exception = Exception
-
- def __init__(self, provider, reporter):
- self.provider = provider
- self.reporter = reporter
-
- def resolve(self, requirements, **kwargs):
- """Take a collection of constraints, spit out the resolution result.
-
- This returns a representation of the final resolution state, with one
- guarenteed attribute ``mapping`` that contains resolved candidates as
- values. The keys are their respective identifiers.
-
- :param requirements: A collection of constraints.
- :param kwargs: Additional keyword arguments that subclasses may accept.
-
- :raises: ``self.base_exception`` or its subclass.
- """
- raise NotImplementedError
diff --git a/spaces/Audio-AGI/AudioSep/models/CLAP/training/lp_main.py b/spaces/Audio-AGI/AudioSep/models/CLAP/training/lp_main.py
deleted file mode 100644
index c2d4e8c85aaa3c8e4221963ef56a815cc14f354f..0000000000000000000000000000000000000000
--- a/spaces/Audio-AGI/AudioSep/models/CLAP/training/lp_main.py
+++ /dev/null
@@ -1,670 +0,0 @@
-from cmath import cos
-from inspect import getargs
-import logging
-import os
-import random
-from datetime import datetime
-import bisect
-import copy
-from sched import scheduler
-import numpy as np
-import torch
-import torch.backends.cudnn as cudnn
-from torch import optim
-from torch.cuda.amp import GradScaler
-import faulthandler
-import pathlib
-import argparse
-import time
-
-try:
- import wandb
-except ImportError:
- wandb = None
-
-try:
- import torch.utils.tensorboard as tensorboard
-except ImportError:
- tensorboard = None
-
-try:
- import horovod.torch as hvd
-except ImportError:
- hvd = None
-
-from open_clip import create_model_and_transforms, trace_model, create_model
-from training.data import get_data
-from training.params import parse_args
-from training.distributed import is_master, init_distributed_device, world_info_from_env
-from training.logger import setup_logging
-from training.scheduler import cosine_lr
-from training.lp_train import train_one_epoch, evaluate
-from open_clip.utils import get_tar_path_from_dataset_name, dataset_split, get_optimizer
-from open_clip.utils import load_p, load_class_label
-from open_clip.linear_probe import LinearProbe
-
-
-def maintain_ckpts(args, startidx, all_idx_len):
- for i in reversed(range(startidx, all_idx_len)):
- if os.path.exists(os.path.join(args.checkpoint_path, f"epoch_top_{i}.pt")):
- os.rename(
- os.path.join(args.checkpoint_path, f"epoch_top_{i}.pt"),
- os.path.join(args.checkpoint_path, f"epoch_top_{i+1}.pt"),
- )
- if os.path.exists(
- os.path.join(args.checkpoint_path, f"epoch_top_{all_idx_len}.pt")
- ):
- os.remove(os.path.join(args.checkpoint_path, f"epoch_top_{all_idx_len}.pt"))
- return
-
-
-def update_top_k_performance(
- new_metrics_inputs, current_top_k_ckpt_metrics, args, ckpt, bignumbetter=True
-):
- """
- Record the top-k performance of the current epoch.
- current_top_k_metrics is a dictionary of the form: {1: top_1_ckpt_measure, 2: top_2_ckpt_measure, ...}
- """
- if isinstance(new_metrics_inputs, (list, tuple)):
- new_metrics_inputs = np.mean(new_metrics_inputs)
- return update_top_k_performance(
- new_metrics_inputs,
- current_top_k_ckpt_metrics,
- args=args,
- ckpt=ckpt,
- bignumbetter=bignumbetter,
- )
- elif isinstance(new_metrics_inputs, dict):
- new_metrics_inputs = np.mean(list(new_metrics_inputs.values()))
- return update_top_k_performance(
- new_metrics_inputs,
- current_top_k_ckpt_metrics,
- args=args,
- ckpt=ckpt,
- bignumbetter=bignumbetter,
- )
- elif isinstance(new_metrics_inputs, (float, int)):
- update_flag = {k: False for k in current_top_k_ckpt_metrics.keys()}
- sorted_keys = sorted(current_top_k_ckpt_metrics.keys())
- sorted_values = sorted(
- current_top_k_ckpt_metrics.values(), reverse=bignumbetter
- )
- sorted_values_ = copy.deepcopy(sorted_values)
- sorted_values.append(new_metrics_inputs)
- sorted_values = sorted(sorted_values, reverse=bignumbetter)
- sorted_values = sorted_values[:-1]
-
- if sorted_values == sorted_values_:
- return current_top_k_ckpt_metrics, new_metrics_inputs
- else:
- for i in range(len(sorted_keys)):
- if current_top_k_ckpt_metrics[sorted_keys[i]] != sorted_values[i]:
- current_top_k_ckpt_metrics[sorted_keys[i]] = sorted_values[i]
- update_flag[sorted_keys[i]] = True
- for i in range(len(update_flag)):
- if update_flag[i]:
- maintain_ckpts(args, i, len(sorted_keys))
- torch.save(
- ckpt,
- os.path.join(args.checkpoint_path, f"epoch_top_{i}.pt"),
- )
- break
- return current_top_k_ckpt_metrics, new_metrics_inputs
-
-
-# def updateifNone(a, b):
-# a = b if None else a
-# return a
-
-
-def is_pretrained_params(n):
- return (
- n.startswith("clap_model.transformer")
- or n in ["clap_model.positional_embedding", "clap_model.text_projection"]
- or n.startswith("clap_model.token_embedding")
- or n.startswith("clap_model.ln_final")
- or n.startswith("clap_model.logit_scale_t")
- )
-
-
-def random_seed(seed=42, rank=0):
- torch.manual_seed(seed + rank)
- np.random.seed(seed + rank)
- random.seed(seed + rank)
-
-
-def config_lp_optimizer(model, data, args):
- # set wd-related params to 0 if use adam optimizer
- if args.optimizer == "adam":
- args.wd = 0
- args.wd_pretrained = 0
- args.wd_new = 0
-
- in_clap = lambda n, p: n.startswith("clap_model")
-
- named_parameters = list(model.named_parameters())
-
- optimizer = {}
- scheduler = {}
-
- # freeze text encoder
- text_freeze_parameters = [
- p
- for n, p in named_parameters
- if n.startswith("clap_model.transformer")
- or n in ["clap_model.positional_embedding", "clap_model.text_projection"]
- or n.startswith("clap_model.token_embedding")
- or n.startswith("clap_model.ln_final")
- ]
-
- if args.freeze_text:
- logging.info("Freeze Text!!!!")
- for k in text_freeze_parameters:
- k.requires_grad = False
-
- if not args.lp_freeze:
- exclude = (
- lambda n, p: p.ndim < 2
- or "bn" in n
- or "ln" in n
- or "bias" in n
- or "logit_scale" in n
- )
- include = lambda n, p: not exclude(n, p)
-
- # (yusong): we do not split the learning rate anymore
- # p for n, p in named_parameters if in_clap(n,p) and exclude(n, p) and p.requires_grad
- gain_or_bias_params = [
- p for n, p in named_parameters if exclude(n, p) and p.requires_grad
- ]
- # rest_params = [p for n, p in named_parameters if in_clap(n,p) and include(n, p) and p.requires_grad]
- rest_params = [
- p for n, p in named_parameters if include(n, p) and p.requires_grad
- ]
-
- if args.train_data is None:
- optimizer = None
- scheduler = None
- else:
- total_steps = data["train"].dataloader.num_batches * args.epochs
-
- if args.split_opt:
- for x in ["lr", "beta1", "beta2", "eps", "wd"]:
- for y in ["_new", "_pretrained"]:
- if getattr(args, x + y) is None:
- setattr(args, x + y, getattr(args, x))
-
- gain_or_bias_pretrained_params = [
- p
- for n, p in named_parameters
- if (exclude(n, p) and p.requires_grad) and is_pretrained_params(n)
- ]
- rest_pretrained_params = [
- p
- for n, p in named_parameters
- if (include(n, p) and p.requires_grad) and is_pretrained_params(n)
- ]
- gain_or_bias_new_params = [
- p
- for n, p in named_parameters
- if (exclude(n, p) and p.requires_grad)
- and (not is_pretrained_params(n))
- ]
- rest_new_params = [
- p
- for n, p in named_parameters
- if (include(n, p) and p.requires_grad)
- and (not is_pretrained_params(n))
- ]
-
- pretrained_params_optimizer = get_optimizer(
- [
- {"params": gain_or_bias_pretrained_params, "weight_decay": 0.0},
- {
- "params": rest_pretrained_params,
- "weight_decay": args.wd_pretrained,
- },
- ],
- lr=args.lr_pretrained,
- betas=(args.beta1_pretrained, args.beta2_pretrained),
- eps=args.eps_pretrained,
- momentum=args.momentum_pretrained,
- optimizer_name=args.optimizer,
- )
- pretrained_params_scheduler = cosine_lr(
- pretrained_params_optimizer,
- args.lr_pretrained,
- args.warmup,
- total_steps,
- )
-
- new_params_optimizer = get_optimizer(
- [
- {"params": gain_or_bias_new_params, "weight_decay": 0.0},
- {"params": rest_new_params, "weight_decay": args.wd_new},
- ],
- lr=args.lr_new,
- betas=(args.beta1_new, args.beta2_new),
- eps=args.eps_new,
- momentum=args.momentum_new,
- optimizer_name=args.optimizer,
- )
- new_params_scheduler = cosine_lr(
- new_params_optimizer, args.lr_new, args.warmup, total_steps
- )
-
- optimizer["text"] = pretrained_params_optimizer
- optimizer["audio"] = new_params_optimizer
- scheduler["text"] = pretrained_params_scheduler
- scheduler["audio"] = new_params_scheduler
-
- if args.horovod:
- pretrained_params_optimizer = hvd.DistributedOptimizer(
- pretrained_params_optimizer,
- named_parameters=model.named_parameters(),
- )
- new_params_optimizer = hvd.DistributedOptimizer(
- new_params_optimizer, named_parameters=model.named_parameters()
- )
- hvd.broadcast_parameters(model.state_dict(), root_rank=0)
- hvd.broadcast_optimizer_state(
- pretrained_params_optimizer, root_rank=0
- )
- hvd.broadcast_optimizer_state(new_params_optimizer, root_rank=0)
- else:
-
- optimizer["clap"] = get_optimizer(
- [
- {"params": gain_or_bias_params, "weight_decay": 0.0},
- {"params": rest_params, "weight_decay": args.wd},
- ],
- lr=args.lr,
- betas=(args.beta1, args.beta2),
- eps=args.eps,
- momentum=args.momentum,
- optimizer_name=args.optimizer,
- )
- scheduler["clap"] = cosine_lr(
- optimizer["clap"], args.lr, args.warmup, total_steps
- )
-
- if args.horovod:
- optimizer["clap"] = hvd.DistributedOptimizer(
- optimizer["clap"], named_parameters=model.named_parameters()
- )
- hvd.broadcast_parameters(model.state_dict(), root_rank=0)
- hvd.broadcast_optimizer_state(optimizer["clap"], root_rank=0)
-
- # linear probe optimizer
- else:
- lp_params = [
- p for n, p in named_parameters if (not in_clap(n, p)) and p.requires_grad
- ]
- lp_optim = get_optimizer(
- lp_params,
- lr=args.lp_lr,
- betas=(args.beta1, args.beta2),
- eps=args.eps,
- momentum=0.9,
- optimizer_name=args.optimizer,
- )
- optimizer["lp"] = lp_optim
-
- return optimizer, scheduler, text_freeze_parameters
-
-
-def main():
- args = parse_args()
-
- time.sleep(args.sleep)
-
- # sanitize model name for filesystem / uri use, easier if we don't use / in name as a rule?
- args.amodel = args.amodel.replace("/", "-")
- # download sizes.json file
-
- # (yusong): the below two lines are for debug
- # print("setting up faulthandler")
- # faulthandler.register(10)
-
- random.seed(args.seed)
- torch.manual_seed(args.seed)
- torch.cuda.manual_seed(args.seed)
- torch.cuda.manual_seed_all(args.seed)
- np.random.seed(args.seed)
- args.class_index_dict = load_class_label(args.class_label_path)
-
- # get the name of the experiments
- if args.name is None:
- args.name = "-".join(
- [
- datetime.now().strftime("%Y_%m_%d-%H_%M_%S"),
- f"linear_probe" f"model_{args.amodel}",
- f"lr_{args.lr}",
- f"b_{args.batch_size}",
- f"j_{args.workers}",
- f"p_{args.precision}",
- ]
- )
-
- # discover initial world args early so we can log properly
- args.distributed = False
- args.local_rank, args.rank, args.world_size = world_info_from_env()
-
- if args.remotedata and is_master(args):
- for dataset_name in args.datasetnames:
- for split in dataset_split[dataset_name]:
- if not os.path.exists(f"./json_files/{dataset_name}/{split}"):
- os.makedirs(f"./json_files/{dataset_name}/{split}")
- os.system(
- f"aws s3 cp s3://s-laion-audio/webdataset_tar/{dataset_name}/{split}/sizes.json ./json_files/{dataset_name}/{split}/sizes.json"
- )
-
- args.log_path = None
- if is_master(args, local=args.log_local):
- log_base_path = os.path.join(args.logs, args.name)
- os.makedirs(log_base_path, exist_ok=True)
- log_filename = f"out-{args.rank}" if args.log_local else "out.log"
- args.log_path = os.path.join(log_base_path, log_filename)
-
- # avoid log dir in same name:
- postfix = 0
- while os.path.exists(args.log_path):
- postfix += 1
- log_base_path_new = log_base_path + "-" + str(postfix)
- os.makedirs(log_base_path_new, exist_ok=True)
- log_filename = f"out-{args.rank}" if args.log_local else "out.log"
- args.log_path = os.path.join(log_base_path_new, log_filename)
- # print(
- # "Error. Experiment already exists. Use --name {} to specify a new experiment."
- # )
- # return -1
-
- # Set logger
- args.log_level = logging.DEBUG if args.debug else logging.INFO
- setup_logging(args.log_path, args.log_level)
-
- # fully initialize distributed device environment
- device = init_distributed_device(args)
-
- args.wandb = "wandb" in args.report_to or "all" in args.report_to
- args.tensorboard = "tensorboard" in args.report_to or "all" in args.report_to
- if is_master(args):
- args.tensorboard_path = (
- os.path.join(args.logs, args.name, "tensorboard")
- if args.tensorboard
- else ""
- )
- args.checkpoint_path = os.path.join(args.logs, args.name, "checkpoints")
- for dirname in [args.tensorboard_path, args.checkpoint_path]:
- if dirname:
- os.makedirs(dirname, exist_ok=True)
- else:
- args.tensorboard_path = ""
- args.checkpoint_path = ""
-
- if args.copy_codebase:
- copy_codebase(args)
-
- assert args.precision in ["amp", "fp16", "fp32"]
- if args.precision == "fp16":
- logging.warning(
- "It is recommended to use AMP mixed-precision instead of FP16. "
- "FP16 support needs further verification and tuning, especially for train."
- )
-
- if args.horovod:
- logging.info(
- f"Running in horovod mode with multiple processes / nodes. Device: {args.device}."
- f"Process (global: {args.rank}, local {args.local_rank}), total {args.world_size}."
- )
- elif args.distributed:
- logging.info(
- f"Running in distributed mode with multiple processes. Device: {args.device}."
- f"Process (global: {args.rank}, local {args.local_rank}), total {args.world_size}."
- )
- else:
- logging.info(f"Running with a single process. Device {args.device}.")
-
- logging.info(f"openai cache dir: {os.path.expanduser(args.openai_model_cache_dir)}")
-
- # Create CLAP model
- clap_model, clap_model_cfg = create_model(
- args.amodel,
- args.tmodel,
- args.pretrained,
- precision=args.precision,
- device=device,
- jit=args.torchscript,
- force_quick_gelu=args.force_quick_gelu,
- openai_model_cache_dir=os.path.expanduser(args.openai_model_cache_dir),
- skip_params=False,
- pretrained_audio=args.pretrained_audio,
- pretrained_text=args.pretrained_text,
- enable_fusion=args.enable_fusion,
- fusion_type=args.fusion_type,
- )
-
- args.lp_out_ch = len(list(args.class_index_dict.keys()))
- # Linear Probe
- logging.info(f"linear probe using mlp: {args.lp_mlp}")
- logging.info(f"linear probe using freeze: {args.lp_freeze}")
- logging.info(f"linear probe act layer: {args.lp_act}")
- logging.info(f"linear probe out ch: {args.lp_out_ch}")
- logging.info(f"linear probe learning rate (if applicable): {args.lp_lr}")
- logging.info(f"linear probe loss func: {args.lp_loss}")
- logging.info(f"linear probe lp_metrics: {args.lp_metrics}")
-
- model = LinearProbe(
- clap_model,
- mlp=args.lp_mlp,
- freeze=args.lp_freeze,
- in_ch=512,
- out_ch=args.lp_out_ch,
- act=args.lp_act,
- ) # in_ch is fixed (i.e., 512)
- model = model.to(device)
-
- if args.horovod:
- with torch.no_grad():
- for param in model.parameters():
- param.set_(param.contiguous())
-
- if args.trace:
- model = trace_model(model, batch_size=args.batch_size, device=device)
-
- if is_master(args):
- logging.info("Linear Probe CLAP Model:")
- logging.info(f"{str(clap_model)}")
- logging.info("Params:")
- params_file = os.path.join(args.logs, args.name, "params.txt")
- with open(params_file, "w") as f:
- for name in sorted(vars(args)):
- val = getattr(args, name)
- logging.info(f" {name}: {val}")
- f.write(f"{name}: {val}\n")
-
- if args.distributed and not args.horovod:
- if args.use_bn_sync:
- model = torch.nn.SyncBatchNorm.convert_sync_batchnorm(model)
- ddp_args = {}
- if args.ddp_static_graph:
- # this doesn't exist in older PyTorch, arg only added if enabled
- ddp_args["static_graph"] = True
- model = torch.nn.parallel.DistributedDataParallel(
- model, device_ids=[device], find_unused_parameters=True, **ddp_args
- )
-
- data = get_data(args, clap_model_cfg)
- assert len(data), "At least one train or eval dataset must be specified."
- if args.trace:
- assert "train" not in data, "Cannot train with traced model"
-
- optimizer, scheduler, text_freeze_parameters = config_lp_optimizer(
- model, data, args
- )
-
- scaler = GradScaler() if args.precision == "amp" else None
-
- # optionally resume from a checkpoint
- start_epoch = 0
- if args.resume is not None:
- if os.path.isfile(args.resume):
- checkpoint = torch.load(args.resume, map_location=device)
- if "epoch" in checkpoint:
- # resuming a train checkpoint w/ epoch and optimizer state
- start_epoch = checkpoint["epoch"]
- sd = checkpoint["state_dict"]
- if not args.distributed and next(iter(sd.items()))[0].startswith(
- "module"
- ):
- sd = {k[len("module.") :]: v for k, v in sd.items()}
- model.load_state_dict(sd)
- if args.split_opt:
- if optimizer is not None:
- for k, o_ in optimizer.items():
- o_.load_state_dict(checkpoint[k + "_" + "optimizer"])
- if optimizer is not None:
- optimizer.load_state_dict(checkpoint["optimizer"])
- if scaler is not None and "scaler" in checkpoint:
- scaler.load_state_dict(checkpoint["scaler"])
- logging.info(
- f"=> resuming checkpoint '{args.resume}' (epoch {start_epoch})"
- )
- else:
- # loading a bare (model only) checkpoint for fine-tune or evaluation
- model.load_state_dict(checkpoint)
- logging.info(
- f"=> loaded checkpoint '{args.resume}' (epoch {start_epoch})"
- )
- if args.freeze_text:
- print("Freeze Text!!!!")
- for k in text_freeze_parameters:
- k.requires_grad = False
- else:
- logging.info("=> no checkpoint found at '{}'".format(args.resume))
-
- cudnn.benchmark = True
- cudnn.deterministic = False
-
- # determine if this worker should save logs and checkpoints. only do so if it is rank == 0
- args.save_logs = args.logs and args.logs.lower() != "none" and is_master(args)
- writer = None
- if args.save_logs and args.tensorboard:
- assert tensorboard is not None, "Please install tensorboard."
- writer = tensorboard.SummaryWriter(args.tensorboard_path)
-
- if args.wandb and is_master(args):
- assert wandb is not None, "Please install wandb."
- logging.debug("Starting wandb.")
- args.train_sz = data["train"].dataloader.num_samples
- if args.val_data is not None:
- args.val_sz = data["val"].dataloader.num_samples
- # you will have to configure this for your project!
- wandb.init(
- project="clap",
- notes=args.wandb_notes,
- name=args.wandb_notes,
- tags=[],
- config=vars(args),
- )
- if args.debug:
- wandb.watch(model, log="all")
- wandb.save(params_file)
- logging.debug("Finished loading wandb.")
-
- if "train" not in data:
- evaluate(model, data, start_epoch, args, writer)
- return
- elif start_epoch == 0 and "val" in data and not args.no_eval:
- evaluate(model, data, 0, args, writer)
- if args.save_top_performance:
- current_top_k_ckpt_metrics = {
- i: 0 for i in range(args.save_top_performance)
- } # initialize the top-k metric for ckpts to 0
-
- for epoch in range(start_epoch, args.epochs):
- # freeze the text param after (include) args.freeze_text_after, this is -1 by default
- if epoch == args.freeze_text_after:
- print("Text pretrained parameters are freezed since this epoch.")
- for k in text_freeze_parameters:
- k.requires_grad = False
- if is_master(args):
- logging.info(f"Start epoch {epoch}")
-
- train_one_epoch(model, data, epoch, optimizer, scaler, scheduler, args, writer)
- completed_epoch = epoch + 1
-
- if (
- any(v in data for v in ("val", "imagenet-val", "imagenet-v2"))
- and not args.no_eval
- ):
- metrics = evaluate(model, data, completed_epoch, args, writer)
- if args.save_top_performance:
- top_k_dataset = args.top_k_checkpoint_select_dataset
- top_k_metric = args.top_k_checkpoint_select_metric
- filtered_metrics = [
- v
- for k, v in metrics.items()
- if top_k_metric in k and top_k_dataset in k
- ] # check all R@10 metrics (all dataset) and use it to update the ckpt
- # Saving checkpoints.
- if args.save_logs:
- opt_dict = {
- k + "_" + "optimizer": v.state_dict() for k, v in optimizer.items()
- }
- checkpoint_dict = {
- "epoch": completed_epoch,
- "name": args.name,
- "state_dict": model.state_dict(),
- }
- checkpoint_dict.update(opt_dict)
- if scaler is not None:
- checkpoint_dict["scaler"] = scaler.state_dict()
-
- if completed_epoch == args.epochs or (
- args.save_frequency > 0 and (completed_epoch % args.save_frequency) == 0
- ):
- torch.save(
- checkpoint_dict,
- os.path.join(args.checkpoint_path, f"epoch_{completed_epoch}.pt"),
- )
- if args.save_most_recent:
- torch.save(
- checkpoint_dict,
- os.path.join(args.checkpoint_path, f"epoch_latest.pt"),
- )
- if args.save_top_performance and not args.no_eval:
- update_top_k_performance(
- filtered_metrics,
- current_top_k_ckpt_metrics,
- args,
- checkpoint_dict,
- bignumbetter=True,
- )
-
- if args.wandb and is_master(args):
- wandb.finish()
-
-
-def copy_codebase(args):
- from shutil import copytree, ignore_patterns
-
- new_code_path = os.path.join(args.logs, args.name, "code")
- if os.path.exists(new_code_path):
- print(
- f"Error. Experiment already exists at {new_code_path}. Use --name to specify a new experiment."
- )
- return -1
- print(f"Copying codebase to {new_code_path}")
- current_code_path = os.path.realpath(__file__)
- for _ in range(3):
- current_code_path = os.path.dirname(current_code_path)
- copytree(
- current_code_path, new_code_path, ignore=ignore_patterns("log", "logs", "wandb")
- )
- print("Done copying code.")
- return 1
-
-
-if __name__ == "__main__":
- main()
diff --git a/spaces/Awiny/Image2Paragraph/models/grit_src/grit/data/custom_dataset_dataloader.py b/spaces/Awiny/Image2Paragraph/models/grit_src/grit/data/custom_dataset_dataloader.py
deleted file mode 100644
index ea9c4172f838d130df297bed9c0755669720c39d..0000000000000000000000000000000000000000
--- a/spaces/Awiny/Image2Paragraph/models/grit_src/grit/data/custom_dataset_dataloader.py
+++ /dev/null
@@ -1,250 +0,0 @@
-# Copyright (c) Facebook, Inc. and its affiliates.
-# Modified by Jialian Wu from https://github.com/facebookresearch/Detic/blob/main/detic/data/custom_dataset_dataloader.py
-import operator
-import torch
-import torch.utils.data
-from detectron2.utils.comm import get_world_size
-
-from detectron2.config import configurable
-from torch.utils.data.sampler import BatchSampler, Sampler
-from detectron2.data.common import DatasetFromList, MapDataset
-from detectron2.data.dataset_mapper import DatasetMapper
-from detectron2.data.build import get_detection_dataset_dicts, build_batch_data_loader
-from detectron2.data.samplers import TrainingSampler
-from detectron2.data.build import worker_init_reset_seed, print_instances_class_histogram
-from detectron2.data.build import filter_images_with_only_crowd_annotations
-from detectron2.data.build import filter_images_with_few_keypoints
-from detectron2.data.build import check_metadata_consistency
-from detectron2.data.catalog import MetadataCatalog, DatasetCatalog
-from detectron2.utils import comm
-import itertools
-from typing import Optional
-
-
-def _custom_train_loader_from_config(cfg, mapper=None, *, dataset=None, sampler=None):
- sampler_name = cfg.DATALOADER.SAMPLER_TRAIN
- if 'MultiDataset' in sampler_name:
- dataset_dicts = get_detection_dataset_dicts_with_source(
- cfg.DATASETS.TRAIN,
- filter_empty=cfg.DATALOADER.FILTER_EMPTY_ANNOTATIONS,
- min_keypoints=cfg.MODEL.ROI_KEYPOINT_HEAD.MIN_KEYPOINTS_PER_IMAGE
- if cfg.MODEL.KEYPOINT_ON else 0,
- proposal_files=cfg.DATASETS.PROPOSAL_FILES_TRAIN if cfg.MODEL.LOAD_PROPOSALS else None,
- )
- else:
- dataset_dicts = get_detection_dataset_dicts(
- cfg.DATASETS.TRAIN,
- filter_empty=cfg.DATALOADER.FILTER_EMPTY_ANNOTATIONS,
- min_keypoints=cfg.MODEL.ROI_KEYPOINT_HEAD.MIN_KEYPOINTS_PER_IMAGE
- if cfg.MODEL.KEYPOINT_ON else 0,
- proposal_files=cfg.DATASETS.PROPOSAL_FILES_TRAIN if cfg.MODEL.LOAD_PROPOSALS else None,
- )
-
- if mapper is None:
- mapper = DatasetMapper(cfg, True)
-
- if sampler is not None:
- pass
- elif sampler_name == "TrainingSampler":
- sampler = TrainingSampler(len(dataset))
- elif sampler_name == "MultiDatasetSampler":
- sampler = MultiDatasetSampler(
- dataset_dicts,
- dataset_ratio=cfg.DATALOADER.DATASET_RATIO,
- )
- else:
- raise ValueError("Unknown training sampler: {}".format(sampler_name))
-
- return {
- "dataset": dataset_dicts,
- "sampler": sampler,
- "mapper": mapper,
- "total_batch_size": cfg.SOLVER.IMS_PER_BATCH,
- "num_workers": cfg.DATALOADER.NUM_WORKERS,
- 'dataset_bs': cfg.DATALOADER.DATASET_BS,
- 'num_datasets': len(cfg.DATASETS.TRAIN)
- }
-
-
-@configurable(from_config=_custom_train_loader_from_config)
-def build_custom_train_loader(
- dataset, *, mapper, sampler,
- total_batch_size=16,
- num_workers=0,
- num_datasets=1,
- dataset_bs=1
-):
-
- if isinstance(dataset, list):
- dataset = DatasetFromList(dataset, copy=False)
- if mapper is not None:
- dataset = MapDataset(dataset, mapper)
- if sampler is None:
- sampler = TrainingSampler(len(dataset))
- assert isinstance(sampler, torch.utils.data.sampler.Sampler)
-
- return build_dataset_batch_data_loader(
- dataset_bs,
- dataset,
- sampler,
- total_batch_size,
- num_datasets=num_datasets,
- num_workers=num_workers,
- )
-
-
-def build_dataset_batch_data_loader(
- dataset_bs, dataset, sampler, total_batch_size, num_datasets, num_workers=0
-):
-
- world_size = get_world_size()
- assert (
- total_batch_size > 0 and total_batch_size % world_size == 0
- ), "Total batch size ({}) must be divisible by the number of gpus ({}).".format(
- total_batch_size, world_size
- )
-
- data_loader = torch.utils.data.DataLoader(
- dataset,
- sampler=sampler,
- num_workers=num_workers,
- batch_sampler=None,
- collate_fn=operator.itemgetter(0), # don't batch, but yield individual elements
- worker_init_fn=worker_init_reset_seed,
- )
-
- if num_datasets > 1:
- return MultiDatasets(data_loader, dataset_bs, num_datasets)
- else:
- return SingleDataset(data_loader, dataset_bs)
-
-
-def get_detection_dataset_dicts_with_source(
- dataset_names, filter_empty=True, min_keypoints=0, proposal_files=None
-):
- assert len(dataset_names)
- dataset_dicts = [DatasetCatalog.get(dataset_name) for dataset_name in dataset_names]
- for dataset_name, dicts in zip(dataset_names, dataset_dicts):
- assert len(dicts), "Dataset '{}' is empty!".format(dataset_name)
-
- for source_id, (dataset_name, dicts) in \
- enumerate(zip(dataset_names, dataset_dicts)):
- assert len(dicts), "Dataset '{}' is empty!".format(dataset_name)
- for d in dicts:
- d['dataset_source'] = source_id
-
- if "annotations" in dicts[0]:
- try:
- class_names = MetadataCatalog.get(dataset_name).thing_classes
- check_metadata_consistency("thing_classes", dataset_name)
- print_instances_class_histogram(dicts, class_names)
- except AttributeError: # class names are not available for this dataset
- pass
-
- assert proposal_files is None
-
- dataset_dicts = list(itertools.chain.from_iterable(dataset_dicts))
-
- has_instances = "annotations" in dataset_dicts[0]
- if filter_empty and has_instances:
- dataset_dicts = filter_images_with_only_crowd_annotations(dataset_dicts)
- if min_keypoints > 0 and has_instances:
- dataset_dicts = filter_images_with_few_keypoints(dataset_dicts, min_keypoints)
-
- return dataset_dicts
-
-
-class MultiDatasetSampler(Sampler):
- def __init__(
- self,
- dataset_dicts,
- dataset_ratio,
- seed: Optional[int] = None,
- ):
- sizes = [0 for _ in range(len(dataset_ratio))]
- for d in dataset_dicts:
- sizes[d['dataset_source']] += 1
- print('dataset sizes', sizes)
- self.sizes = sizes
- assert len(dataset_ratio) == len(sizes), \
- 'length of dataset ratio {} should be equal to number if dataset {}'.format(
- len(dataset_ratio), len(sizes)
- )
- if seed is None:
- seed = comm.shared_random_seed()
- self._seed = int(seed)
- self._rank = comm.get_rank()
- self._world_size = comm.get_world_size()
-
- self.dataset_ids = torch.tensor(
- [d['dataset_source'] for d in dataset_dicts], dtype=torch.long)
- self.dataset_ratio = dataset_ratio
-
- dataset_weight = [torch.ones(s) * max(sizes) / s * r / sum(dataset_ratio) \
- for i, (r, s) in enumerate(zip(dataset_ratio, sizes))]
- dataset_weight = torch.cat(dataset_weight)
-
- self.weights = dataset_weight
- self.sample_epoch_size = len(self.weights)
-
- def __iter__(self):
- start = self._rank
- yield from itertools.islice(
- self._infinite_indices(), start, None, self._world_size)
-
- def _infinite_indices(self):
- g = torch.Generator()
- g.manual_seed(self._seed)
- while True:
- if len(self.dataset_ratio) > 1:
- # multiple datasets
- ids = torch.multinomial(
- self.weights, self.sample_epoch_size, generator=g,
- replacement=True)
- nums = [(self.dataset_ids[ids] == i).sum().int().item() \
- for i in range(len(self.sizes))]
- yield from ids
- else:
- # single dataset
- yield from torch.randperm(self.sizes[0], generator=g).tolist()
-
-
-class SingleDataset(torch.utils.data.IterableDataset):
- def __init__(self, dataset, batch_sizes):
- self.dataset = dataset
- self.batch_sizes = batch_sizes
- self._buckets = [[] for _ in range(2)]
-
- def __iter__(self):
- for d in self.dataset:
- w, h = d["width"], d["height"]
- aspect_ratio_bucket_id = 0 if w > h else 1
- bucket_id = aspect_ratio_bucket_id
- bucket = self._buckets[bucket_id]
- bucket.append(d)
- if len(bucket) == self.batch_sizes:
- yield bucket[:]
- del bucket[:]
-
-
-class MultiDatasets(torch.utils.data.IterableDataset):
- def __init__(self, dataset, batch_sizes, num_datasets):
- self.dataset = dataset
- self.batch_sizes = batch_sizes
- self._buckets = [[] for _ in range(2 * num_datasets)]
- self.iter_idx = 0
- self.num_datasets = num_datasets
-
- def __iter__(self):
- for d in self.dataset:
- w, h = d["width"], d["height"]
- aspect_ratio_bucket_id = 0 if w > h else 1
- bucket_id = d['dataset_source'] * 2 + aspect_ratio_bucket_id
- bucket = self._buckets[bucket_id]
- if len(bucket) < self.batch_sizes:
- bucket.append(d)
- selected_dataset = self.iter_idx % self.num_datasets
- if len(bucket) == self.batch_sizes and selected_dataset == d['dataset_source']:
- self.iter_idx += 1
- yield bucket[:]
- del bucket[:]
\ No newline at end of file
diff --git a/spaces/Awiny/Image2Paragraph/models/grit_src/third_party/CenterNet2/.github/CODE_OF_CONDUCT.md b/spaces/Awiny/Image2Paragraph/models/grit_src/third_party/CenterNet2/.github/CODE_OF_CONDUCT.md
deleted file mode 100644
index 0f7ad8bfc173eac554f0b6ef7c684861e8014bbe..0000000000000000000000000000000000000000
--- a/spaces/Awiny/Image2Paragraph/models/grit_src/third_party/CenterNet2/.github/CODE_OF_CONDUCT.md
+++ /dev/null
@@ -1,5 +0,0 @@
-# Code of Conduct
-
-Facebook has adopted a Code of Conduct that we expect project participants to adhere to.
-Please read the [full text](https://code.fb.com/codeofconduct/)
-so that you can understand what actions will and will not be tolerated.
diff --git a/spaces/Awiny/Image2Paragraph/models/grit_src/third_party/CenterNet2/GETTING_STARTED.md b/spaces/Awiny/Image2Paragraph/models/grit_src/third_party/CenterNet2/GETTING_STARTED.md
deleted file mode 100644
index 404b0c8f467264d1adf61e8274e5f864e24018e8..0000000000000000000000000000000000000000
--- a/spaces/Awiny/Image2Paragraph/models/grit_src/third_party/CenterNet2/GETTING_STARTED.md
+++ /dev/null
@@ -1,79 +0,0 @@
-## Getting Started with Detectron2
-
-This document provides a brief intro of the usage of builtin command-line tools in detectron2.
-
-For a tutorial that involves actual coding with the API,
-see our [Colab Notebook](https://colab.research.google.com/drive/16jcaJoc6bCFAQ96jDe2HwtXj7BMD_-m5)
-which covers how to run inference with an
-existing model, and how to train a builtin model on a custom dataset.
-
-
-### Inference Demo with Pre-trained Models
-
-1. Pick a model and its config file from
- [model zoo](MODEL_ZOO.md),
- for example, `mask_rcnn_R_50_FPN_3x.yaml`.
-2. We provide `demo.py` that is able to demo builtin configs. Run it with:
-```
-cd demo/
-python demo.py --config-file ../configs/COCO-InstanceSegmentation/mask_rcnn_R_50_FPN_3x.yaml \
- --input input1.jpg input2.jpg \
- [--other-options]
- --opts MODEL.WEIGHTS detectron2://COCO-InstanceSegmentation/mask_rcnn_R_50_FPN_3x/137849600/model_final_f10217.pkl
-```
-The configs are made for training, therefore we need to specify `MODEL.WEIGHTS` to a model from model zoo for evaluation.
-This command will run the inference and show visualizations in an OpenCV window.
-
-For details of the command line arguments, see `demo.py -h` or look at its source code
-to understand its behavior. Some common arguments are:
-* To run __on your webcam__, replace `--input files` with `--webcam`.
-* To run __on a video__, replace `--input files` with `--video-input video.mp4`.
-* To run __on cpu__, add `MODEL.DEVICE cpu` after `--opts`.
-* To save outputs to a directory (for images) or a file (for webcam or video), use `--output`.
-
-
-### Training & Evaluation in Command Line
-
-We provide two scripts in "tools/plain_train_net.py" and "tools/train_net.py",
-that are made to train all the configs provided in detectron2. You may want to
-use it as a reference to write your own training script.
-
-Compared to "train_net.py", "plain_train_net.py" supports fewer default
-features. It also includes fewer abstraction, therefore is easier to add custom
-logic.
-
-To train a model with "train_net.py", first
-setup the corresponding datasets following
-[datasets/README.md](./datasets/README.md),
-then run:
-```
-cd tools/
-./train_net.py --num-gpus 8 \
- --config-file ../configs/COCO-InstanceSegmentation/mask_rcnn_R_50_FPN_1x.yaml
-```
-
-The configs are made for 8-GPU training.
-To train on 1 GPU, you may need to [change some parameters](https://arxiv.org/abs/1706.02677), e.g.:
-```
-./train_net.py \
- --config-file ../configs/COCO-InstanceSegmentation/mask_rcnn_R_50_FPN_1x.yaml \
- --num-gpus 1 SOLVER.IMS_PER_BATCH 2 SOLVER.BASE_LR 0.0025
-```
-
-To evaluate a model's performance, use
-```
-./train_net.py \
- --config-file ../configs/COCO-InstanceSegmentation/mask_rcnn_R_50_FPN_1x.yaml \
- --eval-only MODEL.WEIGHTS /path/to/checkpoint_file
-```
-For more options, see `./train_net.py -h`.
-
-### Use Detectron2 APIs in Your Code
-
-See our [Colab Notebook](https://colab.research.google.com/drive/16jcaJoc6bCFAQ96jDe2HwtXj7BMD_-m5)
-to learn how to use detectron2 APIs to:
-1. run inference with an existing model
-2. train a builtin model on a custom dataset
-
-See [detectron2/projects](https://github.com/facebookresearch/detectron2/tree/main/projects)
-for more ways to build your project on detectron2.
diff --git a/spaces/Awiny/Image2Paragraph/models/grit_src/third_party/CenterNet2/tests/structures/test_imagelist.py b/spaces/Awiny/Image2Paragraph/models/grit_src/third_party/CenterNet2/tests/structures/test_imagelist.py
deleted file mode 100644
index e446e44a37f5d8f9a68362e4b93a291d314d5d68..0000000000000000000000000000000000000000
--- a/spaces/Awiny/Image2Paragraph/models/grit_src/third_party/CenterNet2/tests/structures/test_imagelist.py
+++ /dev/null
@@ -1,75 +0,0 @@
-# Copyright (c) Facebook, Inc. and its affiliates.
-
-import unittest
-from typing import List, Sequence, Tuple
-import torch
-
-from detectron2.structures import ImageList
-
-
-class TestImageList(unittest.TestCase):
- def test_imagelist_padding_tracing(self):
- # test that the trace does not contain hard-coded constant sizes
- def to_imagelist(tensors: Sequence[torch.Tensor]):
- image_list = ImageList.from_tensors(tensors, 4)
- return image_list.tensor, image_list.image_sizes
-
- def _tensor(*shape):
- return torch.ones(shape, dtype=torch.float32)
-
- # test CHW (inputs needs padding vs. no padding)
- for shape in [(3, 10, 10), (3, 12, 12)]:
- func = torch.jit.trace(to_imagelist, ([_tensor(*shape)],))
- tensor, image_sizes = func([_tensor(3, 15, 20)])
- self.assertEqual(tensor.shape, (1, 3, 16, 20), tensor.shape)
- self.assertEqual(image_sizes[0].tolist(), [15, 20], image_sizes[0])
-
- # test HW
- func = torch.jit.trace(to_imagelist, ([_tensor(10, 10)],))
- tensor, image_sizes = func([_tensor(15, 20)])
- self.assertEqual(tensor.shape, (1, 16, 20), tensor.shape)
- self.assertEqual(image_sizes[0].tolist(), [15, 20], image_sizes[0])
-
- # test 2x CHW
- func = torch.jit.trace(
- to_imagelist,
- ([_tensor(3, 16, 10), _tensor(3, 13, 11)],),
- )
- tensor, image_sizes = func([_tensor(3, 25, 20), _tensor(3, 10, 10)])
- self.assertEqual(tensor.shape, (2, 3, 28, 20), tensor.shape)
- self.assertEqual(image_sizes[0].tolist(), [25, 20], image_sizes[0])
- self.assertEqual(image_sizes[1].tolist(), [10, 10], image_sizes[1])
- # support calling with different spatial sizes, but not with different #images
-
- def test_imagelist_scriptability(self):
- image_nums = 2
- image_tensor = torch.randn((image_nums, 10, 20), dtype=torch.float32)
- image_shape = [(10, 20)] * image_nums
-
- def f(image_tensor, image_shape: List[Tuple[int, int]]):
- return ImageList(image_tensor, image_shape)
-
- ret = f(image_tensor, image_shape)
- ret_script = torch.jit.script(f)(image_tensor, image_shape)
-
- self.assertEqual(len(ret), len(ret_script))
- for i in range(image_nums):
- self.assertTrue(torch.equal(ret[i], ret_script[i]))
-
- def test_imagelist_from_tensors_scriptability(self):
- image_tensor_0 = torch.randn(10, 20, dtype=torch.float32)
- image_tensor_1 = torch.randn(12, 22, dtype=torch.float32)
- inputs = [image_tensor_0, image_tensor_1]
-
- def f(image_tensor: List[torch.Tensor]):
- return ImageList.from_tensors(image_tensor, 10)
-
- ret = f(inputs)
- ret_script = torch.jit.script(f)(inputs)
-
- self.assertEqual(len(ret), len(ret_script))
- self.assertTrue(torch.equal(ret.tensor, ret_script.tensor))
-
-
-if __name__ == "__main__":
- unittest.main()
diff --git a/spaces/Benson/text-generation/Examples/Descargar Apk Hroe De La Cuerda Mod.md b/spaces/Benson/text-generation/Examples/Descargar Apk Hroe De La Cuerda Mod.md
deleted file mode 100644
index 7d3c4a0846959f3b954b8f7e226f095729f245ac..0000000000000000000000000000000000000000
--- a/spaces/Benson/text-generation/Examples/Descargar Apk Hroe De La Cuerda Mod.md
+++ /dev/null
@@ -1,72 +0,0 @@
-
-
Cómo descargar e instalar el héroe de cuerda Mod APK en Android
-
Si estás buscando un divertido y lleno de acción juego de superhéroes, es posible que desee probar Rope Hero. Este es un juego de disparos en tercera persona en 3D con elementos RPG, donde juegas como un súper héroe azul que puede usar una súper cuerda para girar alrededor de la ciudad, luchar contra el crimen y personalizar a tu personaje. También puedes elegir ser un villano y causar caos en la ciudad, si ese es tu estilo.
-
Sin embargo, si desea disfrutar de más características y opciones en el juego, tales como dinero ilimitado, nuevas armas, vehículos, pieles y misiones, es posible que desee descargar el Rope Hero mod APK. Esta es una versión modificada del juego original que te da acceso a más contenido y diversión. En este artículo, le mostraremos cómo descargar e instalar el Héroe de cuerda mod APK en su dispositivo Android en unos sencillos pasos.
Qué es un archivo APK y cómo instalarlo en Android
-
Un archivo APK es un archivo de paquete que contiene todos los archivos y datos necesarios para que una aplicación Android se ejecute. Es similar a un archivo EXE para computadoras con Windows. Puede instalar un archivo APK en su dispositivo Android directamente desde su navegador o desde una aplicación de administrador de archivos. Sin embargo, antes de hacer eso, debe asegurarse de que su dispositivo permita aplicaciones o fuentes desconocidas. Esto significa que puede instalar aplicaciones que no son de Google Play Store.
-
Para habilitar aplicaciones o fuentes desconocidas en tu dispositivo Android, sigue estos pasos:
-
-
Ve a la configuración de tu dispositivo y toca Aplicaciones y notificaciones (o Aplicaciones en versiones anteriores de Android).
-
Toque los tres puntos en la esquina superior derecha.
-
Toque Acceso especial.
-
Toca Instalar aplicaciones desconocidas.
-
Toque Chrome (o cualquier navegador web que utilice).
-
Mover Permitir desde esta fuente a la posición On.
-
-
Ahora estás listo para instalar cualquier archivo APK en tu dispositivo Android.
-
-
El siguiente paso es descargar el archivo APK mod Rope Hero de una fuente confiable. Solo debe descargar archivos APK de sitios web de confianza que monitorean y verifican sus archivos de malware y virus. Uno de los mejores sitios web para descargar archivos APK es APK Mirror. Este sitio web alberga un montón de aplicaciones populares de Android y actualizaciones que se pueden descargar de forma gratuita.
-
Para descargar el mod de héroe de cuerda APK de APK Mirror, siga estos pasos:
Desplácese hacia abajo hasta que vea un botón verde que diga Descargar (MOD).
-
Toca el botón y espera a que comience la descarga.
-
Puede ver algunas ventanas emergentes o advertencias que dicen "Este tipo de archivo puede dañar su dispositivo." Ignórelos y toque OK o Descargar de todos modos.
-
-
El archivo mod APK de héroe de cuerda se descargará en la carpeta de descargas de su dispositivo.
-
Cómo instalar el héroe de cuerda mod APK en su dispositivo Android
-
El paso final es instalar el Héroe de cuerda mod APK en su dispositivo Android. Para hacer esto, necesita una aplicación de administrador de archivos que puede localizar y abrir el archivo APK. Si no tiene uno, puede descargarlo de Google Play, como Cx File Explorer o Administrador de archivos.
-
-
Para instalar el héroe de cuerda mod APK usando una aplicación de administrador de archivos, siga estos pasos:
-
-
Abra su aplicación de administrador de archivos y vaya a la carpeta Descargas.
-
Localizar y toque el archivo Rope Hero mod APK. Debe tener un nombre como rope-hero-vice-town-mod.apk.
-
Es posible que vea una ventana emergente que dice "Para su seguridad, el teléfono no se le permite instalar aplicaciones desconocidas de esta fuente." Toca Configuración y mueve Permitir desde esta fuente a la posición On.
-
Volver a la aplicación de administrador de archivos y toque el archivo de mod APK Rope Hero de nuevo.
-
-
Una vez que la instalación se haya hecho, puede tocar Abrir para iniciar el juego o Listo para salir de la aplicación de administrador de archivos.
-
-
Felicidades! Usted ha instalado con éxito el Héroe de cuerda mod APK en su dispositivo Android.
-
Cómo disfrutar de las características de la Cuerda Héroe mod APK
-
Ahora que ha instalado el Héroe de cuerda mod APK, se puede disfrutar de las características y beneficios de esta versión modificada del juego. Estas son algunas de las cosas que puedes hacer con el mod de héroe de cuerda APK:
-
-
Puedes obtener dinero ilimitado para comprar nuevas armas, vehículos, pieles y mejoras para tu personaje.
-
Puedes desbloquear nuevas misiones que no están disponibles en el juego original.
-
Puedes usar nuevas armas y gadgets, como un jetpack, una pistola láser, un lanzallamas y un lanzagranadas.
-
Puede conducir vehículos nuevos, como un tanque, un helicóptero, una motocicleta y un automóvil deportivo.
-
Puedes personalizar tu personaje con nuevas pieles, como un hombre araña, un batman, un hulk y un ninja.
-
Puedes explorar la ciudad e interactuar con diferentes objetos y personas.
-
Puedes elegir ser un héroe o un villano y luchar contra otras pandillas, policías o superhéroes.
-
-
Con el Héroe de cuerda mod APK, puede tener más diversión y emoción en este juego de superhéroes. También puede comparar su progreso y logros con otros jugadores en línea y compartir sus capturas de pantalla y videos en las redes sociales.
-
Conclusión: Resumir los principales puntos y beneficios de la descarga y la instalación de la cuerda del héroe mod APK
-
-
Preguntas frecuentes: Responder a algunas preguntas comunes sobre el héroe de la cuerda y el mod APK
-
Aquí hay algunas preguntas frecuentes sobre Rope Hero y el mod APK:
-
Q: ¿Es Rope Hero libre para jugar?
-
A: Sí, Rope Hero es gratis. Puedes descargarlo desde Google Play o desde otros sitios web. Sin embargo, algunas características y elementos pueden requerir compras en la aplicación o ver anuncios. Si desea evitar eso, puede descargar el mod APK en su lugar.
-
Q: ¿Es seguro jugar Rope Hero?
-
A: Sí, Rope Hero es seguro jugar. No contiene ningún contenido dañino o malicioso. Sin embargo, solo debe descargarlo de fuentes confiables y escanearlo con una aplicación antivirus antes de instalarlo. Además, tenga cuidado con los permisos que otorga a la aplicación cuando la instala.
-
Q: ¿Es Rope Hero compatible con mi dispositivo?
-
A: Rope Hero es compatible con la mayoría de dispositivos Android que se ejecutan en Android 4.4 o superior. Sin embargo, algunos dispositivos pueden experimentar retrasos o fallos debido a problemas de memoria o rendimiento. Para mejorar tu experiencia de juego, debes cerrar otras aplicaciones que se ejecutan en segundo plano y limpiar tu caché antes de jugar.
-
P: ¿Cómo actualizo Rope Hero?
-
A: Si has descargado Rope Hero de Google Play, recibirás actualizaciones automáticas cada vez que haya una nueva versión disponible. Si lo descargaste de otro sitio web o instalaste el mod APK, tendrás que comprobar manualmente las actualizaciones y descargarlas tú mismo. También puede tener que desinstalar la versión anterior antes de instalar la nueva.
-
Q: ¿Cómo puedo desinstalar Rope Hero?
-
A: Si quieres desinstalar Rope Hero desde tu dispositivo, puedes hacerlo siguiendo estos pasos:
-
-
Ve a la configuración de tu dispositivo y toca Aplicaciones y notificaciones (o Aplicaciones en versiones anteriores de Android).
Encuentra y toca Rope Hero en la lista de aplicaciones.
-
Pulse Desinstalar y confirme su elección.
- 64aa2da5cf
-
-
\ No newline at end of file
diff --git a/spaces/Benson/text-generation/Examples/Descargar Gratis Juegos De Matemticas Para El Grado 2.md b/spaces/Benson/text-generation/Examples/Descargar Gratis Juegos De Matemticas Para El Grado 2.md
deleted file mode 100644
index e8ca42cea6544cafec2e8b25c5ac73dfc240a31d..0000000000000000000000000000000000000000
--- a/spaces/Benson/text-generation/Examples/Descargar Gratis Juegos De Matemticas Para El Grado 2.md
+++ /dev/null
@@ -1,55 +0,0 @@
-
-
Descargar Write It! Coreano: La mejor aplicación para aprender Hangul
-
¿Quieres aprender a escribir hangul coreano de una manera rápida, eficiente y divertida? Si es así, usted debe descargar Write It! Coreano, la primera aplicación de reconocimiento de escritura para el coreano. En este artículo, le diremos lo que escribir! Coreano es, qué características tiene, qué beneficios ofrece, y cómo descargarlo en su dispositivo.
-
descargar gratis juegos de matemáticas para el grado 2
Write It! Korean es una aplicación que te enseña cómo escribir hangul coreano, el alfabeto de la lengua coreana. A diferencia de otras aplicaciones que solo te permiten rastrear o copiar los caracteres, Write It! Korean te permite escribirlos por ti mismo usando tu dedo o un lápiz. La aplicación reconoce su escritura y le da retroalimentación instantánea sobre su precisión y pronunciación. De esta manera, puedes aprender a escribir hangul correctamente y con confianza.
-
Características de Write It! Coreano
-
Write It! Korean tiene muchas características que lo convierten en la mejor aplicación para aprender hangul. Estos son algunos de ellos:
-
Reconocimiento de escritura
-
La aplicación utiliza una sofisticada tecnología de reconocimiento de escritura que puede detectar su escritura y evaluar su rendimiento. No tienes que preocuparte por quedarte atascado o tener que volver y hacer referencia a cómo escribir un personaje. La aplicación le guiará a través de cada golpe y le dirá si cometió algún error. También puede ajustar los niveles de sensibilidad y dificultad según su preferencia.
-
-
Lecciones guiadas
-
La aplicación tiene lecciones de tamaño bocado que cubren todos los caracteres básicos y avanzados hangul. Puedes practicar la escritura con guías antes de ponerte a prueba, haciendo que el aprendizaje sea extremadamente rápido y libre de estrés. También puedes revisar tus lecciones anteriores y repetirlas tantas veces como quieras.
-
Seguimiento del progreso
-
-
Modo sin conexión
-
La aplicación funciona sin conexión, por lo que puede escribir en cualquier lugar y en cualquier momento sin conexión a Internet. No tiene que preocuparse por el uso de datos o problemas de red. Puede aprender hangul a su propio ritmo y conveniencia.
-
Beneficios de escribir! Coreano
-
Write It! Korean ofrece muchos beneficios que hacen que valga la pena descargarlo. Estos son algunos de ellos:
-
Aprendizaje rápido y eficiente
-
La aplicación le ayuda a aprender hangul de una manera rápida y eficiente mediante el uso de reconocimiento de escritura y lecciones guiadas. Puedes memorizar los personajes fácil y rápidamente sin aburrirte o frustrarte. También puedes mejorar tus habilidades de pronunciación y ortografía escuchando el audio y leyendo la romanización.
-
Experiencia divertida y atractiva
-
La aplicación hace que el aprendizaje sea divertido y atractivo mediante el uso de gráficos coloridos, animaciones y sonidos. Puedes disfrutar escribiendo en diferentes fondos, usando diferentes bolígrafos y ganando diferentes insignias. También puedes jugar juegos y cuestionarios para probar tus conocimientos y divertirte.
-
Práctica flexible y conveniente
-
La aplicación le permite practicar hangul de forma flexible y conveniente trabajando sin conexión y teniendo ajustes ajustables. Puede escribir en cualquier lugar y en cualquier momento sin limitaciones ni distracciones. También puede personalizar la aplicación según sus necesidades y preferencias.
-
Cómo descargar Write It! Coreano
-
Si estás convencido de que Write It! Korean es la mejor aplicación para aprender hangul, aquí es cómo se puede descargar en su dispositivo:
-
Para dispositivos Android
-
-
Ir a la tienda de Google Play en su dispositivo.
-
Buscar "Write It! Korean " y toque en el icono de la aplicación.
-
Toque en el botón "Instalar" y espere a que la aplicación se descargue e instale en su dispositivo.
-
Toque en el botón "Abrir" y empezar a escribir hangul!
-
-
Para dispositivos iOS
-
-
Ir a la App Store en su dispositivo.
-
-
Toque en el botón "Obtener" e introduzca su ID de Apple y contraseña si se le solicita.
-
Espere a que la aplicación se descargue e instale en su dispositivo.
-
Toque en el icono de la aplicación y empezar a escribir hangul!
-
-
Conclusión
-
¡Escribe tu mensaje! El coreano es la mejor aplicación para aprender hangul porque te enseña a escribir personajes coreanos por ti mismo usando el reconocimiento de escritura y lecciones guiadas. También ofrece muchas características y beneficios que hacen que el aprendizaje sea rápido, eficiente, divertido, atractivo, flexible y conveniente. Puede descargar Write It! Korean en su dispositivo Android o iOS siguiendo los sencillos pasos anteriores. Entonces, ¿qué estás esperando? Download Write It! Coreano hoy y empezar a escribir hangul como un profesional!
-
Preguntas frecuentes
-
Aquí hay algunas preguntas frecuentes sobre Write It! Coreano:
-
-
¿Cuánto cuesta Write It! Korean cost? Write It! Korean es gratis para descargar y usar. Sin embargo, puede actualizar a la versión premium para desbloquear más funciones y eliminar anuncios. La versión premium cuesta $4.99 por mes o $29.99 por año.
-
¿Cuántos caracteres hangul puedo aprender con Write It! Korean? Write It! Coreano cubre los 40 caracteres básicos y 11 caracteres hangul avanzados. Puedes aprender a escribir cada carácter en diferentes sílabas y palabras.
-
¿Puedo usar Write It! Korean para aprender otros aspectos del idioma coreano? Write It! El coreano se centra en enseñarte a escribir hangul, pero también te ayuda a mejorar tu pronunciación, ortografía, vocabulario y gramática. Puede escuchar el audio, leer la romanización y ver la traducción de cada carácter, sílaba y palabra.
-
¿Puedo usar Write It! Coreano con otras aplicaciones o recursos? Sí, puede usar Write It! Coreano con otras aplicaciones o recursos que te enseñan a hablar, escuchar, leer o entender coreano. ¡Escríbelo! El coreano complementa tu aprendizaje ayudándote a dominar el aspecto de escritura del idioma.
-
-
64aa2da5cf
-
-
\ No newline at end of file
diff --git a/spaces/Brasd99/SquadDetective/app.py b/spaces/Brasd99/SquadDetective/app.py
deleted file mode 100644
index e9229d5b5c6455a8166d3c92434d6bdac7cbe1a0..0000000000000000000000000000000000000000
--- a/spaces/Brasd99/SquadDetective/app.py
+++ /dev/null
@@ -1,159 +0,0 @@
-import json
-import zipfile
-import numpy as np
-import cv2
-import os
-import gradio as gr
-from deepface import DeepFace
-from ultralytics import YOLO
-import urllib.request
-import asyncio
-
-with open('config.json', 'r') as f:
- config = json.load(f)
-
-FACE_DIST_TRESH = config['FACE_DIST_TRESH']
-FACE_DET_TRESH = config['FACE_DET_TRESH']
-YOLO_WEIGHTS_URL = config['YOLO_WEIGHTS_URL']
-
-yolo_weights_filename = os.path.basename(YOLO_WEIGHTS_URL)
-
-if not os.path.exists(yolo_weights_filename):
- urllib.request.urlretrieve(YOLO_WEIGHTS_URL, yolo_weights_filename)
-
-model = YOLO(yolo_weights_filename)
-
-async def find_distance(base_face, check_face):
- result = await asyncio.to_thread(DeepFace.verify, base_face, check_face, enforce_detection=False)
- return result['distance']
-
-def find_faces(image):
- outputs = model(image)
- faces = []
- for box in outputs[0].boxes:
- if float(box.conf) >= FACE_DET_TRESH:
- x, y, w, h = [int(coord) for coord in box.xywh[0]]
- x_center, y_center = x + w / 2, y + h / 2
- x1 = int(x_center - w)
- y1 = int(y_center - h)
- crop_img = image[y1:y1+h, x1:x1+w]
- faces.append(crop_img)
- return faces
-
-async def load_images_from_zip(zip_path):
- images = []
- loop = asyncio.get_running_loop()
-
- with zipfile.ZipFile(zip_path, 'r') as zip_file:
- for file_name in zip_file.namelist():
- with zip_file.open(file_name) as file:
- img_bytes = await loop.run_in_executor(None, file.read)
- img = cv2.imdecode(np.frombuffer(img_bytes, np.uint8), cv2.IMREAD_COLOR)
- if img is not None:
- images.append(img)
- return images
-
-def create_image(images):
- table_width = 800
- row_height = 100
- margin = 10
- text_margin = 20
- id_col_width = 100
-
- font = cv2.FONT_HERSHEY_SIMPLEX
- font_scale = 0.5
- color = (255, 255, 255)
- thickness = 2
-
- table_height = text_margin + margin + (row_height + margin) * len(images)
-
- table = np.zeros((table_height, table_width, 3), np.uint8)
-
- id_x = 10
- img_x = id_col_width + 10
- y = text_margin
-
- cv2.putText(table, 'Image ID', (id_x, y), font, font_scale, color, thickness)
- cv2.putText(table, 'Face', (img_x, y), font, font_scale, color, thickness)
-
- y += margin
-
- for i, img in enumerate(images):
- height, width = img.shape[:2]
- new_width = int(width * row_height / height)
- if img_x + new_width > table_width:
- new_width = table_width - img_x
- img_resized = cv2.resize(img, (new_width, row_height))
-
- cv2.putText(table, str(i), (id_x, y + margin), font, font_scale, color, thickness)
- table[y:y+row_height, img_x:img_x+new_width] = img_resized
-
- y += row_height + margin
-
- for col in range(table.shape[1]-1, -1, -1):
- if not np.any(table[:, col]):
- continue
- else:
- break
- table_cropped = table[:, :col+1+id_x]
-
- return table_cropped
-
-async def process_photo_async(photo, input_avatars_faces):
- not_found_faces = []
- avatars_faces_count = len(input_avatars_faces)
- input_faces = find_faces(photo)
- for input_face in input_faces:
- for i in range(avatars_faces_count):
- distance = await find_distance(input_avatars_faces[i], input_face)
- if distance <= FACE_DIST_TRESH:
- break
- elif i + 1 == avatars_faces_count:
- not_found_faces.append(input_face)
- return not_found_faces
-
-async def check_async(photos, input_avatars_faces, progress):
- tasks = []
- not_found_faces = []
-
- for photo in photos:
- task = asyncio.create_task(process_photo_async(photo, input_avatars_faces))
- tasks.append(task)
-
- for i, task in enumerate(tasks):
- result = await task
- not_found_faces += result
- progress((i+1)/len(tasks))
-
- return not_found_faces
-
-def check(avatars_zip, photos_zip, progress=gr.Progress()):
- avatars = asyncio.run(load_images_from_zip(avatars_zip.name))
- avatars = [cv2.cvtColor(avatar, cv2.COLOR_RGB2BGR) for avatar in avatars]
-
- photos = asyncio.run(load_images_from_zip(photos_zip.name))
- photos = [cv2.cvtColor(photo, cv2.COLOR_RGB2BGR) for photo in photos]
-
- input_avatars_faces = [find_faces(avatar) for avatar in avatars]
- input_avatars_faces = [face for faces in input_avatars_faces for face in faces]
-
- not_found_faces = asyncio.run(check_async(photos, input_avatars_faces, progress))
-
- return create_image(not_found_faces)
-
-title = '
SquadDetective
'
-logo = '
'
-
-with gr.Blocks(theme='soft', title='SquadDetective') as blocks:
- gr.HTML(title)
- gr.HTML(logo)
- gr.Markdown('**SquadDetective** is a service that helps sports teams to identify unclaimed players by comparing their faces to photos taken during matches. By using state-of-the-art facial recognition technology, this service can quickly and accurately match the faces of players in photos to a database of registered players, allowing teams to quickly identify any unclaimed players and take appropriate action. With **SquadDetective**, sports teams can ensure that all players are properly registered and eligible to play, helping to avoid potential penalties and other issues.')
- with gr.Row():
- avatars = gr.inputs.File(label='Avatar photos (zip)')
- photos = gr.inputs.File(label='Photos to be processed (zip)')
- inputs = [avatars, photos]
- process_button = gr.Button('Process')
- outputs=gr.outputs.Image(type='numpy', label='Report')
- process_button.click(fn=check, inputs=inputs, outputs=outputs)
-
-blocks.queue(concurrency_count=1).launch()
\ No newline at end of file
diff --git a/spaces/CVPR/LIVE/thrust/thrust/system/cuda/detail/dispatch.h b/spaces/CVPR/LIVE/thrust/thrust/system/cuda/detail/dispatch.h
deleted file mode 100644
index 45b034217996c5c474e6b91009c57821337a0ef2..0000000000000000000000000000000000000000
--- a/spaces/CVPR/LIVE/thrust/thrust/system/cuda/detail/dispatch.h
+++ /dev/null
@@ -1,78 +0,0 @@
-/*
- * Copyright 2018 NVIDIA Corporation
- *
- * Licensed under the Apache License, Version 2.0 (the "License");
- * you may not use this file except in compliance with the License.
- * You may obtain a copy of the License at
- *
- * http://www.apache.org/licenses/LICENSE-2.0
- *
- * Unless required by applicable law or agreed to in writing, software
- * distributed under the License is distributed on an "AS IS" BASIS,
- * WITHOUT WARRANTIES OR CONDITIONS OF ANY KIND, either express or implied.
- * See the License for the specific language governing permissions and
- * limitations under the License.
- */
-
-#pragma once
-
-#include
-#include
-
-/**
- * Dispatch between 32-bit and 64-bit index based versions of the same algorithm
- * implementation. This version assumes that callables for both branches consist
- * of the same tokens, and is intended to be used with Thrust-style dispatch
- * interfaces, that always deduce the size type from the arguments.
- */
-#define THRUST_INDEX_TYPE_DISPATCH(status, call, count, arguments) \
- if (count <= thrust::detail::integer_traits::const_max) { \
- thrust::detail::int32_t THRUST_PP_CAT2(count, _fixed) = count; \
- status = call arguments; \
- } \
- else { \
- thrust::detail::int64_t THRUST_PP_CAT2(count, _fixed) = count; \
- status = call arguments; \
- }
-
-/**
- * Dispatch between 32-bit and 64-bit index based versions of the same algorithm
- * implementation. This version assumes that callables for both branches consist
- * of the same tokens, and is intended to be used with Thrust-style dispatch
- * interfaces, that always deduce the size type from the arguments.
- *
- * This version of the macro supports providing two count variables, which is
- * necessary for set algorithms.
- */
-#define THRUST_DOUBLE_INDEX_TYPE_DISPATCH(status, call, count1, count2, arguments) \
- if (count1 + count2 <= thrust::detail::integer_traits::const_max) { \
- thrust::detail::int32_t THRUST_PP_CAT2(count1, _fixed) = count1; \
- thrust::detail::int32_t THRUST_PP_CAT2(count2, _fixed) = count2; \
- status = call arguments; \
- } \
- else { \
- thrust::detail::int64_t THRUST_PP_CAT2(count1, _fixed) = count1; \
- thrust::detail::int64_t THRUST_PP_CAT2(count2, _fixed) = count2; \
- status = call arguments; \
- }
-/**
- * Dispatch between 32-bit and 64-bit index based versions of the same algorithm
- * implementation. This version allows using different token sequences for callables
- * in both branches, and is intended to be used with CUB-style dispatch interfaces,
- * where the "simple" interface always forces the size to be `int` (making it harder
- * for us to use), but the complex interface that we end up using doesn't actually
- * provide a way to fully deduce the type from just the call, making the size type
- * appear in the token sequence of the callable.
- *
- * See reduce_n_impl to see an example of how this is meant to be used.
- */
-#define THRUST_INDEX_TYPE_DISPATCH2(status, call_32, call_64, count, arguments) \
- if (count <= thrust::detail::integer_traits::const_max) { \
- thrust::detail::int32_t THRUST_PP_CAT2(count, _fixed) = count; \
- status = call_32 arguments; \
- } \
- else { \
- thrust::detail::int64_t THRUST_PP_CAT2(count, _fixed) = count; \
- status = call_64 arguments; \
- }
-
diff --git a/spaces/CVPR/LIVE/thrust/thrust/system/detail/adl/per_device_resource.h b/spaces/CVPR/LIVE/thrust/thrust/system/detail/adl/per_device_resource.h
deleted file mode 100644
index 721f49e03fd49c5db5b1094575a62630d0509fc1..0000000000000000000000000000000000000000
--- a/spaces/CVPR/LIVE/thrust/thrust/system/detail/adl/per_device_resource.h
+++ /dev/null
@@ -1,41 +0,0 @@
-/*
- * Copyright 2018 NVIDIA Corporation
- *
- * Licensed under the Apache License, Version 2.0 (the "License");
- * you may not use this file except in compliance with the License.
- * You may obtain a copy of the License at
- *
- * http://www.apache.org/licenses/LICENSE-2.0
- *
- * Unless required by applicable law or agreed to in writing, software
- * distributed under the License is distributed on an "AS IS" BASIS,
- * WITHOUT WARRANTIES OR CONDITIONS OF ANY KIND, either express or implied.
- * See the License for the specific language governing permissions and
- * limitations under the License.
- */
-
-#pragma once
-
-#include
-
-// the purpose of this header is to #include the per_device_resource.h header
-// of the sequential, host, and device systems. It should be #included in any
-// code which uses adl to dispatch per_device_resource
-
-#include
-
-#if 0
-#include
-#include
-#include
-#include
-#endif
-
-#define __THRUST_HOST_SYSTEM_PER_DEVICE_RESOURCE_HEADER <__THRUST_HOST_SYSTEM_ROOT/detail/per_device_resource.h>
-#include __THRUST_HOST_SYSTEM_PER_DEVICE_RESOURCE_HEADER
-#undef __THRUST_HOST_SYSTEM_PER_DEVICE_RESOURCE_HEADER
-
-#define __THRUST_DEVICE_SYSTEM_PER_DEVICE_RESOURCE_HEADER <__THRUST_DEVICE_SYSTEM_ROOT/detail/per_device_resource.h>
-#include __THRUST_DEVICE_SYSTEM_PER_DEVICE_RESOURCE_HEADER
-#undef __THRUST_DEVICE_SYSTEM_PER_DEVICE_RESOURCE_HEADER
-
diff --git a/spaces/CVPR/lama-example/fetch_data/places_standard_test_val_gen_masks.sh b/spaces/CVPR/lama-example/fetch_data/places_standard_test_val_gen_masks.sh
deleted file mode 100644
index 4654779790564f4aba73fa1629ca6899697ad150..0000000000000000000000000000000000000000
--- a/spaces/CVPR/lama-example/fetch_data/places_standard_test_val_gen_masks.sh
+++ /dev/null
@@ -1,13 +0,0 @@
-mkdir -p places_standard_dataset/val/
-mkdir -p places_standard_dataset/visual_test/
-
-
-python3 bin/gen_mask_dataset.py \
-$(pwd)/configs/data_gen/random_thick_512.yaml \
-places_standard_dataset/val_hires/ \
-places_standard_dataset/val/
-
-python3 bin/gen_mask_dataset.py \
-$(pwd)/configs/data_gen/random_thick_512.yaml \
-places_standard_dataset/visual_test_hires/ \
-places_standard_dataset/visual_test/
\ No newline at end of file
diff --git a/spaces/CVPR/regionclip-demo/detectron2/engine/train_loop.py b/spaces/CVPR/regionclip-demo/detectron2/engine/train_loop.py
deleted file mode 100644
index 1a4f8bfd09d033ec64bc5dbc7c59391aa2346961..0000000000000000000000000000000000000000
--- a/spaces/CVPR/regionclip-demo/detectron2/engine/train_loop.py
+++ /dev/null
@@ -1,408 +0,0 @@
-# -*- coding: utf-8 -*-
-# Copyright (c) Facebook, Inc. and its affiliates.
-
-import logging
-import numpy as np
-import time
-import weakref
-from typing import Dict, List, Optional
-import torch
-from torch.nn.parallel import DataParallel, DistributedDataParallel
-
-import detectron2.utils.comm as comm
-from detectron2.utils.events import EventStorage, get_event_storage
-from detectron2.utils.logger import _log_api_usage
-
-__all__ = ["HookBase", "TrainerBase", "SimpleTrainer", "AMPTrainer"]
-
-
-class HookBase:
- """
- Base class for hooks that can be registered with :class:`TrainerBase`.
-
- Each hook can implement 4 methods. The way they are called is demonstrated
- in the following snippet:
- ::
- hook.before_train()
- for iter in range(start_iter, max_iter):
- hook.before_step()
- trainer.run_step()
- hook.after_step()
- iter += 1
- hook.after_train()
-
- Notes:
- 1. In the hook method, users can access ``self.trainer`` to access more
- properties about the context (e.g., model, current iteration, or config
- if using :class:`DefaultTrainer`).
-
- 2. A hook that does something in :meth:`before_step` can often be
- implemented equivalently in :meth:`after_step`.
- If the hook takes non-trivial time, it is strongly recommended to
- implement the hook in :meth:`after_step` instead of :meth:`before_step`.
- The convention is that :meth:`before_step` should only take negligible time.
-
- Following this convention will allow hooks that do care about the difference
- between :meth:`before_step` and :meth:`after_step` (e.g., timer) to
- function properly.
-
- """
-
- trainer: "TrainerBase" = None
- """
- A weak reference to the trainer object. Set by the trainer when the hook is registered.
- """
-
- def before_train(self):
- """
- Called before the first iteration.
- """
- pass
-
- def after_train(self):
- """
- Called after the last iteration.
- """
- pass
-
- def before_step(self):
- """
- Called before each iteration.
- """
- pass
-
- def after_step(self):
- """
- Called after each iteration.
- """
- pass
-
- def state_dict(self):
- """
- Hooks are stateless by default, but can be made checkpointable by
- implementing `state_dict` and `load_state_dict`.
- """
- return {}
-
-
-class TrainerBase:
- """
- Base class for iterative trainer with hooks.
-
- The only assumption we made here is: the training runs in a loop.
- A subclass can implement what the loop is.
- We made no assumptions about the existence of dataloader, optimizer, model, etc.
-
- Attributes:
- iter(int): the current iteration.
-
- start_iter(int): The iteration to start with.
- By convention the minimum possible value is 0.
-
- max_iter(int): The iteration to end training.
-
- storage(EventStorage): An EventStorage that's opened during the course of training.
- """
-
- def __init__(self) -> None:
- self._hooks: List[HookBase] = []
- self.iter: int = 0
- self.start_iter: int = 0
- self.max_iter: int
- self.storage: EventStorage
- _log_api_usage("trainer." + self.__class__.__name__)
-
- def register_hooks(self, hooks: List[Optional[HookBase]]) -> None:
- """
- Register hooks to the trainer. The hooks are executed in the order
- they are registered.
-
- Args:
- hooks (list[Optional[HookBase]]): list of hooks
- """
- hooks = [h for h in hooks if h is not None]
- for h in hooks:
- assert isinstance(h, HookBase)
- # To avoid circular reference, hooks and trainer cannot own each other.
- # This normally does not matter, but will cause memory leak if the
- # involved objects contain __del__:
- # See http://engineering.hearsaysocial.com/2013/06/16/circular-references-in-python/
- h.trainer = weakref.proxy(self)
- self._hooks.extend(hooks)
-
- def train(self, start_iter: int, max_iter: int):
- """
- Args:
- start_iter, max_iter (int): See docs above
- """
- logger = logging.getLogger(__name__)
- logger.info("Starting training from iteration {}".format(start_iter))
-
- self.iter = self.start_iter = start_iter
- self.max_iter = max_iter
-
- with EventStorage(start_iter) as self.storage:
- try:
- self.before_train()
- for self.iter in range(start_iter, max_iter):
- self.before_step()
- self.run_step()
- self.after_step()
- # self.iter == max_iter can be used by `after_train` to
- # tell whether the training successfully finished or failed
- # due to exceptions.
- self.iter += 1
- except Exception:
- logger.exception("Exception during training:")
- raise
- finally:
- self.after_train()
-
- def before_train(self):
- for h in self._hooks:
- h.before_train()
-
- def after_train(self):
- self.storage.iter = self.iter
- for h in self._hooks:
- h.after_train()
-
- def before_step(self):
- # Maintain the invariant that storage.iter == trainer.iter
- # for the entire execution of each step
- self.storage.iter = self.iter
-
- for h in self._hooks:
- h.before_step()
-
- def after_step(self):
- for h in self._hooks:
- h.after_step()
-
- def run_step(self):
- raise NotImplementedError
-
- def state_dict(self):
- ret = {"iteration": self.iter}
- hooks_state = {}
- for h in self._hooks:
- sd = h.state_dict()
- if sd:
- name = type(h).__qualname__
- if name in hooks_state:
- # TODO handle repetitive stateful hooks
- continue
- hooks_state[name] = sd
- if hooks_state:
- ret["hooks"] = hooks_state
- return ret
-
- def load_state_dict(self, state_dict):
- logger = logging.getLogger(__name__)
- self.iter = state_dict["iteration"]
- for key, value in state_dict.get("hooks", {}).items():
- for h in self._hooks:
- try:
- name = type(h).__qualname__
- except AttributeError:
- continue
- if name == key:
- h.load_state_dict(value)
- break
- else:
- logger.warning(f"Cannot find the hook '{key}', its state_dict is ignored.")
-
-
-class SimpleTrainer(TrainerBase):
- """
- A simple trainer for the most common type of task:
- single-cost single-optimizer single-data-source iterative optimization,
- optionally using data-parallelism.
- It assumes that every step, you:
-
- 1. Compute the loss with a data from the data_loader.
- 2. Compute the gradients with the above loss.
- 3. Update the model with the optimizer.
-
- All other tasks during training (checkpointing, logging, evaluation, LR schedule)
- are maintained by hooks, which can be registered by :meth:`TrainerBase.register_hooks`.
-
- If you want to do anything fancier than this,
- either subclass TrainerBase and implement your own `run_step`,
- or write your own training loop.
- """
-
- def __init__(self, model, data_loader, optimizer):
- """
- Args:
- model: a torch Module. Takes a data from data_loader and returns a
- dict of losses.
- data_loader: an iterable. Contains data to be used to call model.
- optimizer: a torch optimizer.
- """
- super().__init__()
-
- """
- We set the model to training mode in the trainer.
- However it's valid to train a model that's in eval mode.
- If you want your model (or a submodule of it) to behave
- like evaluation during training, you can overwrite its train() method.
- """
- model.train()
-
- self.model = model
- self.data_loader = data_loader
- self._data_loader_iter = iter(data_loader)
- self.optimizer = optimizer
-
- def run_step(self):
- """
- Implement the standard training logic described above.
- """
- assert self.model.training, "[SimpleTrainer] model was changed to eval mode!"
- start = time.perf_counter()
- """
- If you want to do something with the data, you can wrap the dataloader.
- """
- data = next(self._data_loader_iter)
- data_time = time.perf_counter() - start
-
- """
- If you want to do something with the losses, you can wrap the model.
- """
- loss_dict = self.model(data)
- if isinstance(loss_dict, torch.Tensor):
- losses = loss_dict
- loss_dict = {"total_loss": loss_dict}
- else:
- losses = sum(loss_dict.values())
-
- """
- If you need to accumulate gradients or do something similar, you can
- wrap the optimizer with your custom `zero_grad()` method.
- """
- self.optimizer.zero_grad()
- losses.backward()
-
- self._write_metrics(loss_dict, data_time)
-
- """
- If you need gradient clipping/scaling or other processing, you can
- wrap the optimizer with your custom `step()` method. But it is
- suboptimal as explained in https://arxiv.org/abs/2006.15704 Sec 3.2.4
- """
- self.optimizer.step()
-
- def _write_metrics(
- self,
- loss_dict: Dict[str, torch.Tensor],
- data_time: float,
- prefix: str = "",
- ):
- """
- Args:
- loss_dict (dict): dict of scalar losses
- data_time (float): time taken by the dataloader iteration
- """
- metrics_dict = {k: v.detach().cpu().item() for k, v in loss_dict.items()}
- metrics_dict["data_time"] = data_time
-
- # Gather metrics among all workers for logging
- # This assumes we do DDP-style training, which is currently the only
- # supported method in detectron2.
- all_metrics_dict = comm.gather(metrics_dict)
-
- if comm.is_main_process():
- storage = get_event_storage()
-
- # data_time among workers can have high variance. The actual latency
- # caused by data_time is the maximum among workers.
- data_time = np.max([x.pop("data_time") for x in all_metrics_dict])
- storage.put_scalar("data_time", data_time)
-
- # average the rest metrics
- metrics_dict = {
- k: np.mean([x[k] for x in all_metrics_dict]) for k in all_metrics_dict[0].keys()
- }
- total_losses_reduced = sum(metrics_dict.values())
- if not np.isfinite(total_losses_reduced):
- raise FloatingPointError(
- f"Loss became infinite or NaN at iteration={self.iter}!\n"
- f"loss_dict = {metrics_dict}"
- )
-
- storage.put_scalar("{}total_loss".format(prefix), total_losses_reduced)
- if len(metrics_dict) > 1:
- storage.put_scalars(**metrics_dict)
-
- def state_dict(self):
- ret = super().state_dict()
- ret["optimizer"] = self.optimizer.state_dict()
- return ret
-
- def load_state_dict(self, state_dict):
- super().load_state_dict(state_dict)
- self.optimizer.load_state_dict(state_dict["optimizer"])
-
-
-class AMPTrainer(SimpleTrainer):
- """
- Like :class:`SimpleTrainer`, but uses PyTorch's native automatic mixed precision
- in the training loop.
- """
-
- def __init__(self, model, data_loader, optimizer, grad_scaler=None):
- """
- Args:
- model, data_loader, optimizer: same as in :class:`SimpleTrainer`.
- grad_scaler: torch GradScaler to automatically scale gradients.
- """
- unsupported = "AMPTrainer does not support single-process multi-device training!"
- if isinstance(model, DistributedDataParallel):
- assert not (model.device_ids and len(model.device_ids) > 1), unsupported
- assert not isinstance(model, DataParallel), unsupported
-
- super().__init__(model, data_loader, optimizer)
-
- if grad_scaler is None:
- from torch.cuda.amp import GradScaler
-
- grad_scaler = GradScaler()
- self.grad_scaler = grad_scaler
-
- def run_step(self):
- """
- Implement the AMP training logic.
- """
- assert self.model.training, "[AMPTrainer] model was changed to eval mode!"
- assert torch.cuda.is_available(), "[AMPTrainer] CUDA is required for AMP training!"
- from torch.cuda.amp import autocast
-
- start = time.perf_counter()
- data = next(self._data_loader_iter)
- data_time = time.perf_counter() - start
-
- with autocast():
- loss_dict = self.model(data)
- if isinstance(loss_dict, torch.Tensor):
- losses = loss_dict
- loss_dict = {"total_loss": loss_dict}
- else:
- losses = sum(loss_dict.values())
-
- self.optimizer.zero_grad()
- self.grad_scaler.scale(losses).backward()
-
- self._write_metrics(loss_dict, data_time)
-
- self.grad_scaler.step(self.optimizer)
- self.grad_scaler.update()
-
- def state_dict(self):
- ret = super().state_dict()
- ret["grad_scaler"] = self.grad_scaler.state_dict()
- return ret
-
- def load_state_dict(self, state_dict):
- super().load_state_dict(state_dict)
- self.grad_scaler.load_state_dict(state_dict["grad_scaler"])
diff --git a/spaces/Caoyunkang/Segment-Any-Anomaly/SAM/segment_anything/modeling/sam.py b/spaces/Caoyunkang/Segment-Any-Anomaly/SAM/segment_anything/modeling/sam.py
deleted file mode 100644
index 303bc2f40c3dbc84f5d4286bb73336e075a86589..0000000000000000000000000000000000000000
--- a/spaces/Caoyunkang/Segment-Any-Anomaly/SAM/segment_anything/modeling/sam.py
+++ /dev/null
@@ -1,174 +0,0 @@
-# Copyright (c) Meta Platforms, Inc. and affiliates.
-# All rights reserved.
-
-# This source code is licensed under the license found in the
-# LICENSE file in the root directory of this source tree.
-
-import torch
-from torch import nn
-from torch.nn import functional as F
-
-from typing import Any, Dict, List, Tuple
-
-from .image_encoder import ImageEncoderViT
-from .mask_decoder import MaskDecoder
-from .prompt_encoder import PromptEncoder
-
-
-class Sam(nn.Module):
- mask_threshold: float = 0.0
- image_format: str = "RGB"
-
- def __init__(
- self,
- image_encoder: ImageEncoderViT,
- prompt_encoder: PromptEncoder,
- mask_decoder: MaskDecoder,
- pixel_mean: List[float] = [123.675, 116.28, 103.53],
- pixel_std: List[float] = [58.395, 57.12, 57.375],
- ) -> None:
- """
- SAM predicts object masks from an image and input prompts.
-
- Arguments:
- image_encoder (ImageEncoderViT): The backbone used to encode the
- image into image embeddings that allow for efficient mask prediction.
- prompt_encoder (PromptEncoder): Encodes various types of input prompts.
- mask_decoder (MaskDecoder): Predicts masks from the image embeddings
- and encoded prompts.
- pixel_mean (list(float)): Mean values for normalizing pixels in the input image.
- pixel_std (list(float)): Std values for normalizing pixels in the input image.
- """
- super().__init__()
- self.image_encoder = image_encoder
- self.prompt_encoder = prompt_encoder
- self.mask_decoder = mask_decoder
- self.register_buffer("pixel_mean", torch.Tensor(pixel_mean).view(-1, 1, 1), False)
- self.register_buffer("pixel_std", torch.Tensor(pixel_std).view(-1, 1, 1), False)
-
- @property
- def device(self) -> Any:
- return self.pixel_mean.device
-
- @torch.no_grad()
- def forward(
- self,
- batched_input: List[Dict[str, Any]],
- multimask_output: bool,
- ) -> List[Dict[str, torch.Tensor]]:
- """
- Predicts masks end-to-end from provided images and prompts.
- If prompts are not known in advance, using SamPredictor is
- recommended over calling the model directly.
-
- Arguments:
- batched_input (list(dict)): A list over input images, each a
- dictionary with the following keys. A prompt key can be
- excluded if it is not present.
- 'image': The image as a torch tensor in 3xHxW format,
- already transformed for input to the model.
- 'original_size': (tuple(int, int)) The original size of
- the image before transformation, as (H, W).
- 'point_coords': (torch.Tensor) Batched point prompts for
- this image, with shape BxNx2. Already transformed to the
- input frame of the model.
- 'point_labels': (torch.Tensor) Batched labels for point prompts,
- with shape BxN.
- 'boxes': (torch.Tensor) Batched box inputs, with shape Bx4.
- Already transformed to the input frame of the model.
- 'mask_inputs': (torch.Tensor) Batched mask inputs to the model,
- in the form Bx1xHxW.
- multimask_output (bool): Whether the model should predict multiple
- disambiguating masks, or return a single mask.
-
- Returns:
- (list(dict)): A list over input images, where each element is
- as dictionary with the following keys.
- 'masks': (torch.Tensor) Batched binary mask predictions,
- with shape BxCxHxW, where B is the number of input promts,
- C is determiend by multimask_output, and (H, W) is the
- original size of the image.
- 'iou_predictions': (torch.Tensor) The model's predictions
- of mask quality, in shape BxC.
- 'low_res_logits': (torch.Tensor) Low resolution logits with
- shape BxCxHxW, where H=W=256. Can be passed as mask input
- to subsequent iterations of prediction.
- """
- input_images = torch.stack([self.preprocess(x["image"]) for x in batched_input], dim=0)
- image_embeddings = self.image_encoder(input_images)
-
- outputs = []
- for image_record, curr_embedding in zip(batched_input, image_embeddings):
- if "point_coords" in image_record:
- points = (image_record["point_coords"], image_record["point_labels"])
- else:
- points = None
- sparse_embeddings, dense_embeddings = self.prompt_encoder(
- points=points,
- boxes=image_record.get("boxes", None),
- masks=image_record.get("mask_inputs", None),
- )
- low_res_masks, iou_predictions = self.mask_decoder(
- image_embeddings=curr_embedding.unsqueeze(0),
- image_pe=self.prompt_encoder.get_dense_pe(),
- sparse_prompt_embeddings=sparse_embeddings,
- dense_prompt_embeddings=dense_embeddings,
- multimask_output=multimask_output,
- )
- masks = self.postprocess_masks(
- low_res_masks,
- input_size=image_record["image"].shape[-2:],
- original_size=image_record["original_size"],
- )
- masks = masks > self.mask_threshold
- outputs.append(
- {
- "masks": masks,
- "iou_predictions": iou_predictions,
- "low_res_logits": low_res_masks,
- }
- )
- return outputs
-
- def postprocess_masks(
- self,
- masks: torch.Tensor,
- input_size: Tuple[int, ...],
- original_size: Tuple[int, ...],
- ) -> torch.Tensor:
- """
- Remove padding and upscale masks to the original image size.
-
- Arguments:
- masks (torch.Tensor): Batched masks from the mask_decoder,
- in BxCxHxW format.
- input_size (tuple(int, int)): The size of the image input to the
- model, in (H, W) format. Used to remove padding.
- original_size (tuple(int, int)): The original size of the image
- before resizing for input to the model, in (H, W) format.
-
- Returns:
- (torch.Tensor): Batched masks in BxCxHxW format, where (H, W)
- is given by original_size.
- """
- masks = F.interpolate(
- masks,
- (self.image_encoder.img_size, self.image_encoder.img_size),
- mode="bilinear",
- align_corners=False,
- )
- masks = masks[..., : input_size[0], : input_size[1]]
- masks = F.interpolate(masks, original_size, mode="bilinear", align_corners=False)
- return masks
-
- def preprocess(self, x: torch.Tensor) -> torch.Tensor:
- """Normalize pixel values and pad to a square input."""
- # Normalize colors
- x = (x - self.pixel_mean) / self.pixel_std
-
- # Pad
- h, w = x.shape[-2:]
- padh = self.image_encoder.img_size - h
- padw = self.image_encoder.img_size - w
- x = F.pad(x, (0, padw, 0, padh))
- return x
diff --git a/spaces/CikeyQI/meme-api/meme_generator/memes/back_to_work/__init__.py b/spaces/CikeyQI/meme-api/meme_generator/memes/back_to_work/__init__.py
deleted file mode 100644
index 25bd82ef140677eb4463d51d07192c575c211d97..0000000000000000000000000000000000000000
--- a/spaces/CikeyQI/meme-api/meme_generator/memes/back_to_work/__init__.py
+++ /dev/null
@@ -1,22 +0,0 @@
-from pathlib import Path
-from typing import List
-
-from pil_utils import BuildImage
-
-from meme_generator import add_meme
-
-img_dir = Path(__file__).parent / "images"
-
-
-def back_to_work(images: List[BuildImage], texts, args):
- frame = BuildImage.open(img_dir / "0.png")
- img = (
- images[0].convert("RGBA").resize((220, 310), keep_ratio=True, direction="north")
- )
- frame.paste(img.rotate(25, expand=True), (56, 32), below=True)
- return frame.save_jpg()
-
-
-add_meme(
- "back_to_work", back_to_work, min_images=1, max_images=1, keywords=["继续干活", "打工人"]
-)
diff --git a/spaces/CofAI/chat.b4/g4f/Provider/Providers/Yqcloud.py b/spaces/CofAI/chat.b4/g4f/Provider/Providers/Yqcloud.py
deleted file mode 100644
index ad5c3a4326c68ceb7ee012fbf5bc072da72a7e40..0000000000000000000000000000000000000000
--- a/spaces/CofAI/chat.b4/g4f/Provider/Providers/Yqcloud.py
+++ /dev/null
@@ -1,39 +0,0 @@
-import os
-import time
-import requests
-
-from ...typing import sha256, Dict, get_type_hints
-url = 'https://chat9.yqcloud.top/'
-model = [
- 'gpt-3.5-turbo',
-]
-supports_stream = True
-needs_auth = False
-
-
-def _create_completion(model: str, messages: list, stream: bool, chatId: str, **kwargs):
-
- headers = {
- 'authority': 'api.aichatos.cloud',
- 'origin': 'https://chat9.yqcloud.top',
- 'referer': 'https://chat9.yqcloud.top/',
- 'user-agent': 'Mozilla/5.0 (Macintosh; Intel Mac OS X 10_15_7) AppleWebKit/537.36 (KHTML, like Gecko) Chrome/112.0.0.0 Safari/537.36',
- }
-
- json_data = {
- 'prompt': str(messages),
- 'userId': f'#/chat/{chatId}',
- 'network': True,
- 'apikey': '',
- 'system': '',
- 'withoutContext': False,
- }
- response = requests.post('https://api.aichatos.cloud/api/generateStream',
- headers=headers, json=json_data, stream=True)
- for token in response.iter_content(chunk_size=2046):
- yield (token.decode('utf-8'))
-
-
-params = f'g4f.Providers.{os.path.basename(__file__)[:-3]} supports: ' + \
- '(%s)' % ', '.join(
- [f"{name}: {get_type_hints(_create_completion)[name].__name__}" for name in _create_completion.__code__.co_varnames[:_create_completion.__code__.co_argcount]])
\ No newline at end of file
diff --git a/spaces/CofAI/chat/g4f/Provider/Providers/Better.py b/spaces/CofAI/chat/g4f/Provider/Providers/Better.py
deleted file mode 100644
index bee52870eb3300f25c9762ab204968791a2a30a9..0000000000000000000000000000000000000000
--- a/spaces/CofAI/chat/g4f/Provider/Providers/Better.py
+++ /dev/null
@@ -1,56 +0,0 @@
-import os
-import json
-import requests
-from typing import Dict, get_type_hints
-
-url = 'https://openai-proxy-api.vercel.app/v1/'
-model = [
- 'gpt-3.5-turbo',
- 'gpt-3.5-turbo-0613',
- 'gpt-3.5-turbo-16k',
- 'gpt-3.5-turbo-16k-0613',
- 'gpt-4',
-]
-
-supports_stream = True
-needs_auth = False
-
-
-def _create_completion(model: str, messages: list, stream: bool, **kwargs):
- headers = {
- 'Content-Type': 'application/json',
- 'User-Agent': 'Mozilla/5.0 (Windows NT 10.0; Win64; x64) AppleWebKit/537.36 (KHTML, like Gecko) Chrome/114.0.0.0 Safari/537.36 Edg/114.0.1823.58',
- 'Referer': 'https://chat.ylokh.xyz/',
- 'Origin': 'https://chat.ylokh.xyz',
- 'Connection': 'keep-alive',
- }
-
- json_data = {
- 'messages': messages,
- 'temperature': 1.0,
- 'model': model,
- 'stream': stream,
- }
-
- response = requests.post(
- 'https://openai-proxy-api.vercel.app/v1/chat/completions', headers=headers, json=json_data, stream=True
- )
-
- for token in response.iter_lines():
- decoded = token.decode('utf-8')
- if decoded.startswith('data: '):
- data_str = decoded.replace('data: ', '')
- data = json.loads(data_str)
- if 'choices' in data and 'delta' in data['choices'][0]:
- delta = data['choices'][0]['delta']
- content = delta.get('content', '')
- finish_reason = delta.get('finish_reason', '')
-
- if finish_reason == 'stop':
- break
- if content:
- yield content
-
-
-params = f'g4f.Providers.{os.path.basename(__file__)[:-3]} supports: ' + '(%s)' % ', '.join(
- [f"{name}: {get_type_hints(_create_completion)[name].__name__}" for name in _create_completion.__code__.co_varnames[:_create_completion.__code__.co_argcount]])
diff --git a/spaces/Cpp4App/Cpp4App/examples/1.html b/spaces/Cpp4App/Cpp4App/examples/1.html
deleted file mode 100644
index 14d27062672b14c4904bc31ca2de4b895ddf7382..0000000000000000000000000000000000000000
--- a/spaces/Cpp4App/Cpp4App/examples/1.html
+++ /dev/null
@@ -1,717 +0,0 @@
-
-
-
-
-
-
-
-
-
-
-
-
-
-
-
-
-
-
-
-
-
-
-
- GMA Privacy Policy | McDonald's Australia
-
-
-
-
-
-
-
-
-
-
-
-
-
-
-
-
-
-
-
-
-
-
-
-
-
-
-
-
-
-
-
-
-
-
-
-
-
-
-
-
-
-
-
-
-
-
-
-
-
-
-
-
-
-
-
-
-
-
-
-
-
GMA Privacy Policy
-
-
-
-
-
-
-
-
-
-
-
McDonald’s is committed to respecting your personal information. Our privacy policy sets outs out how we collect, use, store and disclose your personal information. When you use our Websites Apps, or provide your personal information to us, you consent to your personal information being collected, held, used and disclosed as set out in our privacy policy.
-
-
Information we collect and hold
-
McDonald’s collects personal information about you in a number of ways, including when you:
-
use our websites (including www.mcdonalds.com.au), social media pages, and internal websites or intranet (Website);
-
use our mobile and tablet Apps (Apps); and
-
interact with us and provide personal information by any other means, including either physically or electronically,
-
(Collection Channels).
-
Personal information that McDonald’s collects and holds may include your name, email address, delivery address, date of birth, phone number, payment method, social media handles, photographs of you and other identifying information you choose to provide via a particular Collection Channel.
-
When you use a Website or App, we may also collect personal information about you in the following general categories:
-
Location information: If you permit an App to access location services in your settings, then we collect your device location App to deliver your order or to send you alerts.
-
Transaction information: We collect your transaction details when you place an order via a Website or App, including the products you have ordered, the date and time of your order, the amount charged and your loyalty entitlements.
-
Usage and preferences: We collect information about how you interact with our Websites or Apps, including the pages you visit, your preferences and the settings that you choose. We do this through cookies and other similar technology.
-
Device information: We collect information about your device, such as the hardware model, operating system, preferred language, unique device identifier and mobile network.
-
Employee information: If you are a job applicant, an employee in one of our restaurants or our corporate offices, or a former employee, and use a Website or App, we collect information about the training modules you have completed, the forms you have submitted, the approvals you have given or received, and other similar information related to your job.
-
Other information: We also collect and log information such as your IP address, access dates and times, browser type and pages visited when you interact with a Website or App.
-
We also collect personal information about you from third parties, including when:
-
you choose to create an account or register for a product or service via a Website or App using a social media platform (e.g. Facebook);
-
you have consented to a third party disclosing your personal information to us (e.g. when you enter a competition or promotion run by a third party for us); and
-
it is otherwise lawful for a third party to disclose your personal information to us.
-
We also collect personal or anonymous information about you from other sources and sometimes combine that information with other information collected from you or from third parties for the purposes disclosed in this privacy policy.
-
-
-
-
-
-
-
-
-
-
-
-
-
-
-
-
-
-
How McDonald’s collects and holds personal information
-
McDonald’s will only collect or monitor any personal information about you as provided in this privacy policy.
-
-
-
-
-
-
-
-
-
-
-
-
-
-
-
-
-
-
Active information collection
-
McDonald’s may collect your personal information via our Collection Channels when you:
-
purchase a product or make a booking through a Website or App;
-
participate in any offers, marketing activities, loyalty or rewards program or promotional activities;
-
contact us or provide us with personal information directly via any medium including a Website or App, SMS or other message service and email, social media platforms, mail, telephone or in person;
-
interact with a Website or App for a specific purpose;
-
browse a Website or App generally;
-
sign-up to, or register an account via any Collection Channel; or
-
apply for employment with McDonald’s.
-
-
-
-
-
-
-
-
-
-
-
-
-
-
-
-
-
-
Platform permissions
-
Mobile platforms such as iOS and Android may define certain types of information or data that our Apps cannot access without your consent. Each platform has its own permissions system for obtaining your consent. For example, the iOS platform may alert you the first time an App wants your permission to access certain types of data (e.g. location services) and will provide you option to consent to that request. Android devices may notify you of the permissions that an App seeks before you first use the App and your subsequent use of the App constitutes your consent. You can usually manage your platform level permissions via the Settings section on your device. For more information, please contact your device provider or refer to the user manual for your device.
-
-
-
-
-
-
-
-
-
-
-
-
-
-
-
-
-
-
Privacy Policy
-
McDonald’s Privacy Policy contains information about how you can access and correct your personal information, how you can lodge a complaint regarding the handling of your personal information and how any complaint will be handled by McDonald’s. You may contact McDonald’s with any queries via email: privacy@au.mcd.com or at McDonald's Australia Limited (Attention: McDonald's Privacy Officer), PO Box 392 Pennant Hills NSW 2120 Australia.
-
-
-
-
-
-
-
-
-
-
-
-
-
-
-
-
-
-
Cookies and passive information collection
-
We may use tracking technologies to collect personal information about you when you use and access a Websites or App, including cookies, internet tags or web beacons, and navigational data collection (e.g. log files, server logs, and clickstream data). For example, we may collect information about the date, time and duration of visits and which pages of a Website or App are most commonly accessed. This browsing information is generally not linked to your identity, except where you access a Website or App via links in a message we have sent or where we are able to user accessing a Website or App.
-
We may combine your anonymous information, browsing information or other information collected through tracking technologies with your personal information collect via our Collection Channels in order to understand and remember your preferences and interests. By accessing a Website or App via links and/or by accessing a Website or App where you have identified yourself, you consent to the collection of this information.
-
-
-
-
-
-
-
-
-
-
-
-
-
-
-
-
-
-
Purposes for which McDonald’s collects, holds, uses and discloses personal information
-
We collect, hold, use and disclose your personal information for our primary purposes, including:
-
for the purposes stated on a particular Collection Channel;
-
to maintain and improve the functionality of a Website or App;
-
to fulfil obligations in respect of any sale and purchase contract and/or any other contract between you and McDonald’s;
-
to manage your orders or facilitating payment, for example, when you use our App the drive thru screen and kiosk will display your name and crew members will greet you by name.
-
to send you any technical, administrative or legal notices important to our Websites and Apps;
-
to provide you with information about your transactions and loyalty entitlements;
-
to provide marketing materials and information about our products and services, events, special offers, competitions and/or promotions, or to request your feedback for promotional purposes;
-
to respond to customer enquiries or complaints;
-
to manage your employment or process your application for employment with McDonald’s (including McDonald’s franchisees) and to facilitate effective employment practices;
-
to obtain opinions or comments about products and/or services and to conduct other market research and development (including to record statistical data for marketing analysis);
-
to enter you into and administer promotions;
-
to provide, maintain and improve our products and services;
-
to customise a Website or App based on your preferences;
-
to allow you to use a Website or App;
-
to share with trusted third parties including professional service providers, our related bodies corporate, our franchisees, our suppliers and our promotional partners and other trusted third parties (and their directors, servants and agents) and agencies (McDonald’s Family); and
-
to share with your social media communities, to the extent allowed by you.
-
-
-
-
-
-
-
-
-
-
-
-
-
-
-
-
-
-
Not providing information
-
You don’t have to provide any personal information to us. However, if you do not do so, this may affect or completely restrict your ability to use a Website or App and our ability to provide you with relevant content, products and services.
-
-
-
-
-
-
-
-
-
-
-
-
-
-
-
-
-
-
Sharing your personal information
-
McDonald's shares personal information with the global McDonald’s Family for the purposes described in this privacy policy
-
McDonald’s recognises the trust with which you provide personal information, and except as stated in this privacy policy, your information will not be used or disclosed for any other purposes without your consent. However, McDonald's reserves the right to use or disclose any information, including personal information, as needed to satisfy any law, regulation or legal request, to protect the rights or property of McDonald's, any member of the McDonald's Family, or any member of the public, to protect the integrity of a Website or App, to fulfil your requests, or to cooperate in any law enforcement investigation or an investigation on a matter of public safety.
-
-
-
-
-
-
-
-
-
-
-
-
-
-
-
-
-
-
Contact by McDonald’s and third parties
-
If you would like to opt out of receiving advertising communications from us, the McDonald’s Family and our trusted third parties, you can unsubscribe.
-
We may still send you transaction and administrative information.
-
If you no longer wish to receive any communications from McDonald’s via an App, you can delete the App from your device.
-
-
-
-
-
-
-
-
-
-
-
-
-
-
-
-
-
-
Ability of others to view information
-
McDonald’s provides areas on Websites and Apps where you can upload user-generated content, post or provide information about yourself, communicate with other users, provide reviews for content, products and/or services or interact with or vote on particular content. This information may be publicly posted on a Website or App and/or shared with others, including social media platforms and other public forums in which you choose to participate. This information may become publicly available and may be read, collected and used by others outside of a McDonald’s Website or App. McDonald’s is not responsible for the conduct of others who may read, collect and use this information.
-
-
-
-
-
-
-
-
-
-
-
-
-
-
-
-
-
-
Children
-
McDonald's is very sensitive to privacy issues. We are proud of our long-time commitment to our customers. McDonald’s does not intend to collect personal information from any person under the age of 18 years without the consent of a parent or legal guardian. We urge parents to regularly monitor and supervise their children's on-line activities.
-
-
-
-
-
-
-
-
-
-
-
-
-
-
-
-
-
-
Security of personal information
-
McDonald’s will endeavour to take all reasonable steps to protect your personal information. All information is passed through to a secure server using encryption technology and stored on secure servers that are protected in controlled facilities, which in some cases may be overseas. McDonald's employees and data processors are obliged to respect the confidentiality of any personal information held by McDonald's. However, McDonald’s cannot guarantee the security of your personal information and will not be held responsible for events arising from unauthorised access to personal information beyond McDonald's reasonable control.
-
-
-
-
-
-
-
-
-
-
-
-
-
-
-
-
-
-
Disclosure of personal information to overseas recipients
-
In some cases, McDonald’s may disclose your personal information to overseas recipients, including but not limited to recipients in the United States of America, Japan, Malaysia and Singapore. McDonald’s employees and data processors are obliged to respect the confidentiality of any personal information held by McDonald’s.
-
-
-
-
-
-
-
-
-
-
-
-
-
-
-
-
-
-
Access to personal information
-
You are in control of any personal information you provide to us. If at any time, you would like to access, review, correct and/or delete the personal information we have about you, or if you would like to change your contact preferences, you can let us know via the contact details listed below. Please allow 30 days for this request to be processed.
-
Your personal information may be stored in different locations depending upon the reason for which you originally submitted the information. If you make an inquiry in relation to your personal information, the more information you can provide us about when you originally submitted your personal information, the quicker McDonald's will be able to retrieve your personal information.
-
If requested, all reasonable steps to delete personal information will be made, except where it is required for legal reasons. Deletion of information may result in McDonald's being unable to facilitate or provide you with information about certain transactions (including the uploading of, access to, and receipt of, content on a Website or App, and purchase transactions undertaken on a Website or App), other content, services or product information, upcoming promotions, competitions or event information, and/or provide certain content, products or services.
-
We are not responsible for removing your personal information from the lists of any third party who has previously been provided your information in accordance with this privacy policy.
-
-
-
-
-
-
-
-
-
-
-
-
-
-
-
-
-
-
Links to other sites
-
Our Websites or Apps contain links to sites operated by third parties. We are not responsible for the privacy practices of, or any content on, those sites linked to our Websites and Apps. If you visit one of these linked websites, we encourage you to review their privacy and other policies.
-
We may use third party advertisements on our Websites and Apps. All third party advertising, if paid for, is paid for by the relevant third party advertisers. Third party advertisements are not recommendations or endorsements by McDonald’s or any of its affiliates. To the extent permitted by law, McDonald’s is not responsible for the content (including representations) of any third party advertisement on a Website or App. Cookies may be associated with these advertisements to enable the advertiser to track the number of anonymous users responding to the campaign.
-
-
-
-
-
-
-
-
-
-
-
-
-
-
-
-
-
-
Related McDonald's Websites or Apps
-
All Websites and Apps operated by McDonald's in Australia will adhere to this privacy policy. The policies on the Websites and Apps of some other members of the McDonald's Family may vary because of local customs, practices or laws.
-
-
-
-
-
-
-
-
-
-
-
-
-
-
-
-
-
-
Franchisee privacy policies
-
Many McDonald's restaurants are owned and operated by independent franchisees. Some franchisees also operate websites and are required to follow this privacy policy. If you are concerned that there may have been a breach of this privacy policy by a franchisee, please contact the relevant franchisee entity or McDonald’s restaurant directly.
-
-
-
-
-
-
-
-
-
-
-
-
-
-
-
-
-
-
Changes to our privacy policy
-
From time to time, it may be necessary for McDonald's to change this privacy policy without notice. We will post any changes to this privacy policy on our Websites and Apps. Rest assured, however, that any changes will not be retroactively applied and will not alter how we handle previously collected personal information.
-
-
-
-
-
-
-
-
-
-
-
-
-
-
-
-
-
-
Sale of the Company
-
If McDonald’s merges with, is acquired by another company, or sells all or a portion of its assets, your personal information may be disclosed to our advisers and any prospective purchaser’s adviser and may be among the assets transferred. However, your personal information will always remain subject to this privacy policy.
-
-
-
-
-
-
-
-
-
-
-
-
-
-
-
-
-
-
Contact Us
-
If you have any questions about our privacy policy, or any problems or complaints about how we have collected, used, stored, handled and/or disclosed your personal information, please contact us at:
Please allow 14 days for this request to be processed. If you do not receive a satisfactory response from McDonald’s to your query, problem or complaint within 14 days, you may refer your query, problem or complaint to the Office of the Australian Information Commissioner via the contact details listed at https://www.oaic.gov.au/about-us/contact-us/.
-
-
-
-
-
-
-
-
-
-
-
-
-
-
-
-
-
-
-
-
-
-
-
-
-
-
-
diff --git a/spaces/CrucibleAI/ControlNetMediaPipeFaceSD21/ldm/modules/diffusionmodules/__init__.py b/spaces/CrucibleAI/ControlNetMediaPipeFaceSD21/ldm/modules/diffusionmodules/__init__.py
deleted file mode 100644
index e69de29bb2d1d6434b8b29ae775ad8c2e48c5391..0000000000000000000000000000000000000000
diff --git a/spaces/Cyril666/ContourNet-ABI/maskrcnn_benchmark/data/datasets/evaluation/word/util/neighbour.py b/spaces/Cyril666/ContourNet-ABI/maskrcnn_benchmark/data/datasets/evaluation/word/util/neighbour.py
deleted file mode 100644
index 1f1826b88d55ccee198e77ad6874ff7976f1d0d5..0000000000000000000000000000000000000000
--- a/spaces/Cyril666/ContourNet-ABI/maskrcnn_benchmark/data/datasets/evaluation/word/util/neighbour.py
+++ /dev/null
@@ -1,86 +0,0 @@
-#encoding=utf-8
-
-import numpy as np
-
-N1 = 'n1'
-N2 = 'n2'
-N4 = 'n4'
-N8 = 'n8'
-
-def _in_image(c, w, h):
- cx, cy = c
- return cx >=0 and cx < w and cy >= 0 and cy < h
-
-def n1(x, y, w, h):
- """down and right"""
- neighbours = []
- candidates = [(x, y + 1), (x + 1, y)];
-
- for c in candidates:
- if _in_image(c, w, h):
- neighbours.append(c)
-
- return neighbours
-
-
-def n2(x, y, w, h):
- neighbours = []
- candidates = [(x, y + 1), (x + 1, y), (x + 1, y + 1), (x - 1, y + 1)];
- for c in candidates:
- if _in_image(c, w, h):
- neighbours.append(c)
-
- return neighbours;
-
-def n4(x, y, w, h):
- neighbours = []
- candidates = [(x, y - 1),(x, y + 1), (x + 1, y), (x - 1, y)];
- for c in candidates:
- if _in_image(c, w, h):
- neighbours.append(c)
- return neighbours
-
-
-def n8(x, y, w, h):
- neighbours = []
- candidates = [(x + 1, y - 1),(x, y - 1),(x - 1, y - 1), (x - 1, y),(x, y + 1), (x + 1, y), (x + 1, y + 1), (x - 1, y + 1)];
- for c in candidates:
- if _in_image(c, w, h):
- neighbours.append(c)
-
- return neighbours;
-
-
-def n1_count(w, h):
- return 2 * w * h - w - h
-
-def n2_count(w, h):
- return 4 * w * h - 3 * w - 3 * h + 2
-
-
-_dict1 = {N1:n1, N2:n2, N4:n4, N8:n8};
-_dict2 = {N1:n1_count, N2:n2_count};
-
-def get_neighbours(x, y, w, h, neighbour_type):
- if neighbour_type in _dict1:
- fn = _dict1[neighbour_type]
- return fn(x, y, w, h)
- raise NotImplementedError("unknown neighbour type '%s'" % (neighbour_type))
-
-def count_neighbours(w, h, neighbour_type):
- if neighbour_type in _dict2:
- fn = _dict2[neighbour_type]
- return fn(w, h)
- raise NotImplementedError("unknown neighbour type '%s'" % (neighbour_type))
-
-
-if __name__ == "__main__":
- w, h = 10, 10
- np.testing.assert_equal(len(n4(0, 0, w, h)), 2)
- np.testing.assert_equal(len(n8(0, 0, w, h)), 3)
-
- np.testing.assert_equal(len(n4(0, 2, w, h)), 3)
- np.testing.assert_equal(len(n8(0, 2, w, h)), 5)
-
- np.testing.assert_equal(len(n4(3, 3, w, h)), 4)
- np.testing.assert_equal(len(n8(3, 3, w, h)), 8)
diff --git a/spaces/DQChoi/gpt-demo/venv/lib/python3.11/site-packages/fontTools/cffLib/specializer.py b/spaces/DQChoi/gpt-demo/venv/lib/python3.11/site-packages/fontTools/cffLib/specializer.py
deleted file mode 100644
index 3d28c82dc77b8b8b764bcf76d401265903db1a64..0000000000000000000000000000000000000000
--- a/spaces/DQChoi/gpt-demo/venv/lib/python3.11/site-packages/fontTools/cffLib/specializer.py
+++ /dev/null
@@ -1,850 +0,0 @@
-# -*- coding: utf-8 -*-
-
-"""T2CharString operator specializer and generalizer.
-
-PostScript glyph drawing operations can be expressed in multiple different
-ways. For example, as well as the ``lineto`` operator, there is also a
-``hlineto`` operator which draws a horizontal line, removing the need to
-specify a ``dx`` coordinate, and a ``vlineto`` operator which draws a
-vertical line, removing the need to specify a ``dy`` coordinate. As well
-as decompiling :class:`fontTools.misc.psCharStrings.T2CharString` objects
-into lists of operations, this module allows for conversion between general
-and specific forms of the operation.
-
-"""
-
-from fontTools.cffLib import maxStackLimit
-
-
-def stringToProgram(string):
- if isinstance(string, str):
- string = string.split()
- program = []
- for token in string:
- try:
- token = int(token)
- except ValueError:
- try:
- token = float(token)
- except ValueError:
- pass
- program.append(token)
- return program
-
-
-def programToString(program):
- return " ".join(str(x) for x in program)
-
-
-def programToCommands(program, getNumRegions=None):
- """Takes a T2CharString program list and returns list of commands.
- Each command is a two-tuple of commandname,arg-list. The commandname might
- be empty string if no commandname shall be emitted (used for glyph width,
- hintmask/cntrmask argument, as well as stray arguments at the end of the
- program (¯\_(ツ)_/¯).
- 'getNumRegions' may be None, or a callable object. It must return the
- number of regions. 'getNumRegions' takes a single argument, vsindex. If
- the vsindex argument is None, getNumRegions returns the default number
- of regions for the charstring, else it returns the numRegions for
- the vsindex.
- The Charstring may or may not start with a width value. If the first
- non-blend operator has an odd number of arguments, then the first argument is
- a width, and is popped off. This is complicated with blend operators, as
- there may be more than one before the first hint or moveto operator, and each
- one reduces several arguments to just one list argument. We have to sum the
- number of arguments that are not part of the blend arguments, and all the
- 'numBlends' values. We could instead have said that by definition, if there
- is a blend operator, there is no width value, since CFF2 Charstrings don't
- have width values. I discussed this with Behdad, and we are allowing for an
- initial width value in this case because developers may assemble a CFF2
- charstring from CFF Charstrings, which could have width values.
- """
-
- seenWidthOp = False
- vsIndex = None
- lenBlendStack = 0
- lastBlendIndex = 0
- commands = []
- stack = []
- it = iter(program)
-
- for token in it:
- if not isinstance(token, str):
- stack.append(token)
- continue
-
- if token == "blend":
- assert getNumRegions is not None
- numSourceFonts = 1 + getNumRegions(vsIndex)
- # replace the blend op args on the stack with a single list
- # containing all the blend op args.
- numBlends = stack[-1]
- numBlendArgs = numBlends * numSourceFonts + 1
- # replace first blend op by a list of the blend ops.
- stack[-numBlendArgs:] = [stack[-numBlendArgs:]]
- lenBlendStack += numBlends + len(stack) - 1
- lastBlendIndex = len(stack)
- # if a blend op exists, this is or will be a CFF2 charstring.
- continue
-
- elif token == "vsindex":
- vsIndex = stack[-1]
- assert type(vsIndex) is int
-
- elif (not seenWidthOp) and token in {
- "hstem",
- "hstemhm",
- "vstem",
- "vstemhm",
- "cntrmask",
- "hintmask",
- "hmoveto",
- "vmoveto",
- "rmoveto",
- "endchar",
- }:
- seenWidthOp = True
- parity = token in {"hmoveto", "vmoveto"}
- if lenBlendStack:
- # lenBlendStack has the number of args represented by the last blend
- # arg and all the preceding args. We need to now add the number of
- # args following the last blend arg.
- numArgs = lenBlendStack + len(stack[lastBlendIndex:])
- else:
- numArgs = len(stack)
- if numArgs and (numArgs % 2) ^ parity:
- width = stack.pop(0)
- commands.append(("", [width]))
-
- if token in {"hintmask", "cntrmask"}:
- if stack:
- commands.append(("", stack))
- commands.append((token, []))
- commands.append(("", [next(it)]))
- else:
- commands.append((token, stack))
- stack = []
- if stack:
- commands.append(("", stack))
- return commands
-
-
-def _flattenBlendArgs(args):
- token_list = []
- for arg in args:
- if isinstance(arg, list):
- token_list.extend(arg)
- token_list.append("blend")
- else:
- token_list.append(arg)
- return token_list
-
-
-def commandsToProgram(commands):
- """Takes a commands list as returned by programToCommands() and converts
- it back to a T2CharString program list."""
- program = []
- for op, args in commands:
- if any(isinstance(arg, list) for arg in args):
- args = _flattenBlendArgs(args)
- program.extend(args)
- if op:
- program.append(op)
- return program
-
-
-def _everyN(el, n):
- """Group the list el into groups of size n"""
- if len(el) % n != 0:
- raise ValueError(el)
- for i in range(0, len(el), n):
- yield el[i : i + n]
-
-
-class _GeneralizerDecombinerCommandsMap(object):
- @staticmethod
- def rmoveto(args):
- if len(args) != 2:
- raise ValueError(args)
- yield ("rmoveto", args)
-
- @staticmethod
- def hmoveto(args):
- if len(args) != 1:
- raise ValueError(args)
- yield ("rmoveto", [args[0], 0])
-
- @staticmethod
- def vmoveto(args):
- if len(args) != 1:
- raise ValueError(args)
- yield ("rmoveto", [0, args[0]])
-
- @staticmethod
- def rlineto(args):
- if not args:
- raise ValueError(args)
- for args in _everyN(args, 2):
- yield ("rlineto", args)
-
- @staticmethod
- def hlineto(args):
- if not args:
- raise ValueError(args)
- it = iter(args)
- try:
- while True:
- yield ("rlineto", [next(it), 0])
- yield ("rlineto", [0, next(it)])
- except StopIteration:
- pass
-
- @staticmethod
- def vlineto(args):
- if not args:
- raise ValueError(args)
- it = iter(args)
- try:
- while True:
- yield ("rlineto", [0, next(it)])
- yield ("rlineto", [next(it), 0])
- except StopIteration:
- pass
-
- @staticmethod
- def rrcurveto(args):
- if not args:
- raise ValueError(args)
- for args in _everyN(args, 6):
- yield ("rrcurveto", args)
-
- @staticmethod
- def hhcurveto(args):
- if len(args) < 4 or len(args) % 4 > 1:
- raise ValueError(args)
- if len(args) % 2 == 1:
- yield ("rrcurveto", [args[1], args[0], args[2], args[3], args[4], 0])
- args = args[5:]
- for args in _everyN(args, 4):
- yield ("rrcurveto", [args[0], 0, args[1], args[2], args[3], 0])
-
- @staticmethod
- def vvcurveto(args):
- if len(args) < 4 or len(args) % 4 > 1:
- raise ValueError(args)
- if len(args) % 2 == 1:
- yield ("rrcurveto", [args[0], args[1], args[2], args[3], 0, args[4]])
- args = args[5:]
- for args in _everyN(args, 4):
- yield ("rrcurveto", [0, args[0], args[1], args[2], 0, args[3]])
-
- @staticmethod
- def hvcurveto(args):
- if len(args) < 4 or len(args) % 8 not in {0, 1, 4, 5}:
- raise ValueError(args)
- last_args = None
- if len(args) % 2 == 1:
- lastStraight = len(args) % 8 == 5
- args, last_args = args[:-5], args[-5:]
- it = _everyN(args, 4)
- try:
- while True:
- args = next(it)
- yield ("rrcurveto", [args[0], 0, args[1], args[2], 0, args[3]])
- args = next(it)
- yield ("rrcurveto", [0, args[0], args[1], args[2], args[3], 0])
- except StopIteration:
- pass
- if last_args:
- args = last_args
- if lastStraight:
- yield ("rrcurveto", [args[0], 0, args[1], args[2], args[4], args[3]])
- else:
- yield ("rrcurveto", [0, args[0], args[1], args[2], args[3], args[4]])
-
- @staticmethod
- def vhcurveto(args):
- if len(args) < 4 or len(args) % 8 not in {0, 1, 4, 5}:
- raise ValueError(args)
- last_args = None
- if len(args) % 2 == 1:
- lastStraight = len(args) % 8 == 5
- args, last_args = args[:-5], args[-5:]
- it = _everyN(args, 4)
- try:
- while True:
- args = next(it)
- yield ("rrcurveto", [0, args[0], args[1], args[2], args[3], 0])
- args = next(it)
- yield ("rrcurveto", [args[0], 0, args[1], args[2], 0, args[3]])
- except StopIteration:
- pass
- if last_args:
- args = last_args
- if lastStraight:
- yield ("rrcurveto", [0, args[0], args[1], args[2], args[3], args[4]])
- else:
- yield ("rrcurveto", [args[0], 0, args[1], args[2], args[4], args[3]])
-
- @staticmethod
- def rcurveline(args):
- if len(args) < 8 or len(args) % 6 != 2:
- raise ValueError(args)
- args, last_args = args[:-2], args[-2:]
- for args in _everyN(args, 6):
- yield ("rrcurveto", args)
- yield ("rlineto", last_args)
-
- @staticmethod
- def rlinecurve(args):
- if len(args) < 8 or len(args) % 2 != 0:
- raise ValueError(args)
- args, last_args = args[:-6], args[-6:]
- for args in _everyN(args, 2):
- yield ("rlineto", args)
- yield ("rrcurveto", last_args)
-
-
-def _convertBlendOpToArgs(blendList):
- # args is list of blend op args. Since we are supporting
- # recursive blend op calls, some of these args may also
- # be a list of blend op args, and need to be converted before
- # we convert the current list.
- if any([isinstance(arg, list) for arg in blendList]):
- args = [
- i
- for e in blendList
- for i in (_convertBlendOpToArgs(e) if isinstance(e, list) else [e])
- ]
- else:
- args = blendList
-
- # We now know that blendList contains a blend op argument list, even if
- # some of the args are lists that each contain a blend op argument list.
- # Convert from:
- # [default font arg sequence x0,...,xn] + [delta tuple for x0] + ... + [delta tuple for xn]
- # to:
- # [ [x0] + [delta tuple for x0],
- # ...,
- # [xn] + [delta tuple for xn] ]
- numBlends = args[-1]
- # Can't use args.pop() when the args are being used in a nested list
- # comprehension. See calling context
- args = args[:-1]
-
- numRegions = len(args) // numBlends - 1
- if not (numBlends * (numRegions + 1) == len(args)):
- raise ValueError(blendList)
-
- defaultArgs = [[arg] for arg in args[:numBlends]]
- deltaArgs = args[numBlends:]
- numDeltaValues = len(deltaArgs)
- deltaList = [
- deltaArgs[i : i + numRegions] for i in range(0, numDeltaValues, numRegions)
- ]
- blend_args = [a + b + [1] for a, b in zip(defaultArgs, deltaList)]
- return blend_args
-
-
-def generalizeCommands(commands, ignoreErrors=False):
- result = []
- mapping = _GeneralizerDecombinerCommandsMap
- for op, args in commands:
- # First, generalize any blend args in the arg list.
- if any([isinstance(arg, list) for arg in args]):
- try:
- args = [
- n
- for arg in args
- for n in (
- _convertBlendOpToArgs(arg) if isinstance(arg, list) else [arg]
- )
- ]
- except ValueError:
- if ignoreErrors:
- # Store op as data, such that consumers of commands do not have to
- # deal with incorrect number of arguments.
- result.append(("", args))
- result.append(("", [op]))
- else:
- raise
-
- func = getattr(mapping, op, None)
- if not func:
- result.append((op, args))
- continue
- try:
- for command in func(args):
- result.append(command)
- except ValueError:
- if ignoreErrors:
- # Store op as data, such that consumers of commands do not have to
- # deal with incorrect number of arguments.
- result.append(("", args))
- result.append(("", [op]))
- else:
- raise
- return result
-
-
-def generalizeProgram(program, getNumRegions=None, **kwargs):
- return commandsToProgram(
- generalizeCommands(programToCommands(program, getNumRegions), **kwargs)
- )
-
-
-def _categorizeVector(v):
- """
- Takes X,Y vector v and returns one of r, h, v, or 0 depending on which
- of X and/or Y are zero, plus tuple of nonzero ones. If both are zero,
- it returns a single zero still.
-
- >>> _categorizeVector((0,0))
- ('0', (0,))
- >>> _categorizeVector((1,0))
- ('h', (1,))
- >>> _categorizeVector((0,2))
- ('v', (2,))
- >>> _categorizeVector((1,2))
- ('r', (1, 2))
- """
- if not v[0]:
- if not v[1]:
- return "0", v[:1]
- else:
- return "v", v[1:]
- else:
- if not v[1]:
- return "h", v[:1]
- else:
- return "r", v
-
-
-def _mergeCategories(a, b):
- if a == "0":
- return b
- if b == "0":
- return a
- if a == b:
- return a
- return None
-
-
-def _negateCategory(a):
- if a == "h":
- return "v"
- if a == "v":
- return "h"
- assert a in "0r"
- return a
-
-
-def _convertToBlendCmds(args):
- # return a list of blend commands, and
- # the remaining non-blended args, if any.
- num_args = len(args)
- stack_use = 0
- new_args = []
- i = 0
- while i < num_args:
- arg = args[i]
- if not isinstance(arg, list):
- new_args.append(arg)
- i += 1
- stack_use += 1
- else:
- prev_stack_use = stack_use
- # The arg is a tuple of blend values.
- # These are each (master 0,delta 1..delta n, 1)
- # Combine as many successive tuples as we can,
- # up to the max stack limit.
- num_sources = len(arg) - 1
- blendlist = [arg]
- i += 1
- stack_use += 1 + num_sources # 1 for the num_blends arg
- while (i < num_args) and isinstance(args[i], list):
- blendlist.append(args[i])
- i += 1
- stack_use += num_sources
- if stack_use + num_sources > maxStackLimit:
- # if we are here, max stack is the CFF2 max stack.
- # I use the CFF2 max stack limit here rather than
- # the 'maxstack' chosen by the client, as the default
- # maxstack may have been used unintentionally. For all
- # the other operators, this just produces a little less
- # optimization, but here it puts a hard (and low) limit
- # on the number of source fonts that can be used.
- break
- # blendList now contains as many single blend tuples as can be
- # combined without exceeding the CFF2 stack limit.
- num_blends = len(blendlist)
- # append the 'num_blends' default font values
- blend_args = []
- for arg in blendlist:
- blend_args.append(arg[0])
- for arg in blendlist:
- assert arg[-1] == 1
- blend_args.extend(arg[1:-1])
- blend_args.append(num_blends)
- new_args.append(blend_args)
- stack_use = prev_stack_use + num_blends
-
- return new_args
-
-
-def _addArgs(a, b):
- if isinstance(b, list):
- if isinstance(a, list):
- if len(a) != len(b) or a[-1] != b[-1]:
- raise ValueError()
- return [_addArgs(va, vb) for va, vb in zip(a[:-1], b[:-1])] + [a[-1]]
- else:
- a, b = b, a
- if isinstance(a, list):
- assert a[-1] == 1
- return [_addArgs(a[0], b)] + a[1:]
- return a + b
-
-
-def specializeCommands(
- commands,
- ignoreErrors=False,
- generalizeFirst=True,
- preserveTopology=False,
- maxstack=48,
-):
-
- # We perform several rounds of optimizations. They are carefully ordered and are:
- #
- # 0. Generalize commands.
- # This ensures that they are in our expected simple form, with each line/curve only
- # having arguments for one segment, and using the generic form (rlineto/rrcurveto).
- # If caller is sure the input is in this form, they can turn off generalization to
- # save time.
- #
- # 1. Combine successive rmoveto operations.
- #
- # 2. Specialize rmoveto/rlineto/rrcurveto operators into horizontal/vertical variants.
- # We specialize into some, made-up, variants as well, which simplifies following
- # passes.
- #
- # 3. Merge or delete redundant operations, to the extent requested.
- # OpenType spec declares point numbers in CFF undefined. As such, we happily
- # change topology. If client relies on point numbers (in GPOS anchors, or for
- # hinting purposes(what?)) they can turn this off.
- #
- # 4. Peephole optimization to revert back some of the h/v variants back into their
- # original "relative" operator (rline/rrcurveto) if that saves a byte.
- #
- # 5. Combine adjacent operators when possible, minding not to go over max stack size.
- #
- # 6. Resolve any remaining made-up operators into real operators.
- #
- # I have convinced myself that this produces optimal bytecode (except for, possibly
- # one byte each time maxstack size prohibits combining.) YMMV, but you'd be wrong. :-)
- # A dynamic-programming approach can do the same but would be significantly slower.
- #
- # 7. For any args which are blend lists, convert them to a blend command.
-
- # 0. Generalize commands.
- if generalizeFirst:
- commands = generalizeCommands(commands, ignoreErrors=ignoreErrors)
- else:
- commands = list(commands) # Make copy since we modify in-place later.
-
- # 1. Combine successive rmoveto operations.
- for i in range(len(commands) - 1, 0, -1):
- if "rmoveto" == commands[i][0] == commands[i - 1][0]:
- v1, v2 = commands[i - 1][1], commands[i][1]
- commands[i - 1] = ("rmoveto", [v1[0] + v2[0], v1[1] + v2[1]])
- del commands[i]
-
- # 2. Specialize rmoveto/rlineto/rrcurveto operators into horizontal/vertical variants.
- #
- # We, in fact, specialize into more, made-up, variants that special-case when both
- # X and Y components are zero. This simplifies the following optimization passes.
- # This case is rare, but OCD does not let me skip it.
- #
- # After this round, we will have four variants that use the following mnemonics:
- #
- # - 'r' for relative, ie. non-zero X and non-zero Y,
- # - 'h' for horizontal, ie. zero X and non-zero Y,
- # - 'v' for vertical, ie. non-zero X and zero Y,
- # - '0' for zeros, ie. zero X and zero Y.
- #
- # The '0' pseudo-operators are not part of the spec, but help simplify the following
- # optimization rounds. We resolve them at the end. So, after this, we will have four
- # moveto and four lineto variants:
- #
- # - 0moveto, 0lineto
- # - hmoveto, hlineto
- # - vmoveto, vlineto
- # - rmoveto, rlineto
- #
- # and sixteen curveto variants. For example, a '0hcurveto' operator means a curve
- # dx0,dy0,dx1,dy1,dx2,dy2,dx3,dy3 where dx0, dx1, and dy3 are zero but not dx3.
- # An 'rvcurveto' means dx3 is zero but not dx0,dy0,dy3.
- #
- # There are nine different variants of curves without the '0'. Those nine map exactly
- # to the existing curve variants in the spec: rrcurveto, and the four variants hhcurveto,
- # vvcurveto, hvcurveto, and vhcurveto each cover two cases, one with an odd number of
- # arguments and one without. Eg. an hhcurveto with an extra argument (odd number of
- # arguments) is in fact an rhcurveto. The operators in the spec are designed such that
- # all four of rhcurveto, rvcurveto, hrcurveto, and vrcurveto are encodable for one curve.
- #
- # Of the curve types with '0', the 00curveto is equivalent to a lineto variant. The rest
- # of the curve types with a 0 need to be encoded as a h or v variant. Ie. a '0' can be
- # thought of a "don't care" and can be used as either an 'h' or a 'v'. As such, we always
- # encode a number 0 as argument when we use a '0' variant. Later on, we can just substitute
- # the '0' with either 'h' or 'v' and it works.
- #
- # When we get to curve splines however, things become more complicated... XXX finish this.
- # There's one more complexity with splines. If one side of the spline is not horizontal or
- # vertical (or zero), ie. if it's 'r', then it limits which spline types we can encode.
- # Only hhcurveto and vvcurveto operators can encode a spline starting with 'r', and
- # only hvcurveto and vhcurveto operators can encode a spline ending with 'r'.
- # This limits our merge opportunities later.
- #
- for i in range(len(commands)):
- op, args = commands[i]
-
- if op in {"rmoveto", "rlineto"}:
- c, args = _categorizeVector(args)
- commands[i] = c + op[1:], args
- continue
-
- if op == "rrcurveto":
- c1, args1 = _categorizeVector(args[:2])
- c2, args2 = _categorizeVector(args[-2:])
- commands[i] = c1 + c2 + "curveto", args1 + args[2:4] + args2
- continue
-
- # 3. Merge or delete redundant operations, to the extent requested.
- #
- # TODO
- # A 0moveto that comes before all other path operations can be removed.
- # though I find conflicting evidence for this.
- #
- # TODO
- # "If hstem and vstem hints are both declared at the beginning of a
- # CharString, and this sequence is followed directly by the hintmask or
- # cntrmask operators, then the vstem hint operator (or, if applicable,
- # the vstemhm operator) need not be included."
- #
- # "The sequence and form of a CFF2 CharString program may be represented as:
- # {hs* vs* cm* hm* mt subpath}? {mt subpath}*"
- #
- # https://www.microsoft.com/typography/otspec/cff2charstr.htm#section3.1
- #
- # For Type2 CharStrings the sequence is:
- # w? {hs* vs* cm* hm* mt subpath}? {mt subpath}* endchar"
-
- # Some other redundancies change topology (point numbers).
- if not preserveTopology:
- for i in range(len(commands) - 1, -1, -1):
- op, args = commands[i]
-
- # A 00curveto is demoted to a (specialized) lineto.
- if op == "00curveto":
- assert len(args) == 4
- c, args = _categorizeVector(args[1:3])
- op = c + "lineto"
- commands[i] = op, args
- # and then...
-
- # A 0lineto can be deleted.
- if op == "0lineto":
- del commands[i]
- continue
-
- # Merge adjacent hlineto's and vlineto's.
- # In CFF2 charstrings from variable fonts, each
- # arg item may be a list of blendable values, one from
- # each source font.
- if i and op in {"hlineto", "vlineto"} and (op == commands[i - 1][0]):
- _, other_args = commands[i - 1]
- assert len(args) == 1 and len(other_args) == 1
- try:
- new_args = [_addArgs(args[0], other_args[0])]
- except ValueError:
- continue
- commands[i - 1] = (op, new_args)
- del commands[i]
- continue
-
- # 4. Peephole optimization to revert back some of the h/v variants back into their
- # original "relative" operator (rline/rrcurveto) if that saves a byte.
- for i in range(1, len(commands) - 1):
- op, args = commands[i]
- prv, nxt = commands[i - 1][0], commands[i + 1][0]
-
- if op in {"0lineto", "hlineto", "vlineto"} and prv == nxt == "rlineto":
- assert len(args) == 1
- args = [0, args[0]] if op[0] == "v" else [args[0], 0]
- commands[i] = ("rlineto", args)
- continue
-
- if op[2:] == "curveto" and len(args) == 5 and prv == nxt == "rrcurveto":
- assert (op[0] == "r") ^ (op[1] == "r")
- if op[0] == "v":
- pos = 0
- elif op[0] != "r":
- pos = 1
- elif op[1] == "v":
- pos = 4
- else:
- pos = 5
- # Insert, while maintaining the type of args (can be tuple or list).
- args = args[:pos] + type(args)((0,)) + args[pos:]
- commands[i] = ("rrcurveto", args)
- continue
-
- # 5. Combine adjacent operators when possible, minding not to go over max stack size.
- for i in range(len(commands) - 1, 0, -1):
- op1, args1 = commands[i - 1]
- op2, args2 = commands[i]
- new_op = None
-
- # Merge logic...
- if {op1, op2} <= {"rlineto", "rrcurveto"}:
- if op1 == op2:
- new_op = op1
- else:
- if op2 == "rrcurveto" and len(args2) == 6:
- new_op = "rlinecurve"
- elif len(args2) == 2:
- new_op = "rcurveline"
-
- elif (op1, op2) in {("rlineto", "rlinecurve"), ("rrcurveto", "rcurveline")}:
- new_op = op2
-
- elif {op1, op2} == {"vlineto", "hlineto"}:
- new_op = op1
-
- elif "curveto" == op1[2:] == op2[2:]:
- d0, d1 = op1[:2]
- d2, d3 = op2[:2]
-
- if d1 == "r" or d2 == "r" or d0 == d3 == "r":
- continue
-
- d = _mergeCategories(d1, d2)
- if d is None:
- continue
- if d0 == "r":
- d = _mergeCategories(d, d3)
- if d is None:
- continue
- new_op = "r" + d + "curveto"
- elif d3 == "r":
- d0 = _mergeCategories(d0, _negateCategory(d))
- if d0 is None:
- continue
- new_op = d0 + "r" + "curveto"
- else:
- d0 = _mergeCategories(d0, d3)
- if d0 is None:
- continue
- new_op = d0 + d + "curveto"
-
- # Make sure the stack depth does not exceed (maxstack - 1), so
- # that subroutinizer can insert subroutine calls at any point.
- if new_op and len(args1) + len(args2) < maxstack:
- commands[i - 1] = (new_op, args1 + args2)
- del commands[i]
-
- # 6. Resolve any remaining made-up operators into real operators.
- for i in range(len(commands)):
- op, args = commands[i]
-
- if op in {"0moveto", "0lineto"}:
- commands[i] = "h" + op[1:], args
- continue
-
- if op[2:] == "curveto" and op[:2] not in {"rr", "hh", "vv", "vh", "hv"}:
- op0, op1 = op[:2]
- if (op0 == "r") ^ (op1 == "r"):
- assert len(args) % 2 == 1
- if op0 == "0":
- op0 = "h"
- if op1 == "0":
- op1 = "h"
- if op0 == "r":
- op0 = op1
- if op1 == "r":
- op1 = _negateCategory(op0)
- assert {op0, op1} <= {"h", "v"}, (op0, op1)
-
- if len(args) % 2:
- if op0 != op1: # vhcurveto / hvcurveto
- if (op0 == "h") ^ (len(args) % 8 == 1):
- # Swap last two args order
- args = args[:-2] + args[-1:] + args[-2:-1]
- else: # hhcurveto / vvcurveto
- if op0 == "h": # hhcurveto
- # Swap first two args order
- args = args[1:2] + args[:1] + args[2:]
-
- commands[i] = op0 + op1 + "curveto", args
- continue
-
- # 7. For any series of args which are blend lists, convert the series to a single blend arg.
- for i in range(len(commands)):
- op, args = commands[i]
- if any(isinstance(arg, list) for arg in args):
- commands[i] = op, _convertToBlendCmds(args)
-
- return commands
-
-
-def specializeProgram(program, getNumRegions=None, **kwargs):
- return commandsToProgram(
- specializeCommands(programToCommands(program, getNumRegions), **kwargs)
- )
-
-
-if __name__ == "__main__":
- import sys
-
- if len(sys.argv) == 1:
- import doctest
-
- sys.exit(doctest.testmod().failed)
-
- import argparse
-
- parser = argparse.ArgumentParser(
- "fonttools cffLib.specialer",
- description="CFF CharString generalizer/specializer",
- )
- parser.add_argument("program", metavar="command", nargs="*", help="Commands.")
- parser.add_argument(
- "--num-regions",
- metavar="NumRegions",
- nargs="*",
- default=None,
- help="Number of variable-font regions for blend opertaions.",
- )
-
- options = parser.parse_args(sys.argv[1:])
-
- getNumRegions = (
- None
- if options.num_regions is None
- else lambda vsIndex: int(options.num_regions[0 if vsIndex is None else vsIndex])
- )
-
- program = stringToProgram(options.program)
- print("Program:")
- print(programToString(program))
- commands = programToCommands(program, getNumRegions)
- print("Commands:")
- print(commands)
- program2 = commandsToProgram(commands)
- print("Program from commands:")
- print(programToString(program2))
- assert program == program2
- print("Generalized program:")
- print(programToString(generalizeProgram(program, getNumRegions)))
- print("Specialized program:")
- print(programToString(specializeProgram(program, getNumRegions)))
diff --git a/spaces/DQChoi/gpt-demo/venv/lib/python3.11/site-packages/fontTools/ttLib/tables/C_F_F__2.py b/spaces/DQChoi/gpt-demo/venv/lib/python3.11/site-packages/fontTools/ttLib/tables/C_F_F__2.py
deleted file mode 100644
index edbb0b92f77e3198b55920879271f481082131ea..0000000000000000000000000000000000000000
--- a/spaces/DQChoi/gpt-demo/venv/lib/python3.11/site-packages/fontTools/ttLib/tables/C_F_F__2.py
+++ /dev/null
@@ -1,13 +0,0 @@
-from io import BytesIO
-from fontTools.ttLib.tables.C_F_F_ import table_C_F_F_
-
-
-class table_C_F_F__2(table_C_F_F_):
- def decompile(self, data, otFont):
- self.cff.decompile(BytesIO(data), otFont, isCFF2=True)
- assert len(self.cff) == 1, "can't deal with multi-font CFF tables."
-
- def compile(self, otFont):
- f = BytesIO()
- self.cff.compile(f, otFont, isCFF2=True)
- return f.getvalue()
diff --git a/spaces/DQChoi/gpt-demo/venv/lib/python3.11/site-packages/fontTools/ttLib/tables/_f_e_a_t.py b/spaces/DQChoi/gpt-demo/venv/lib/python3.11/site-packages/fontTools/ttLib/tables/_f_e_a_t.py
deleted file mode 100644
index c9a48eff06cb14b1b2dc56c94ec7e02b80f11ca3..0000000000000000000000000000000000000000
--- a/spaces/DQChoi/gpt-demo/venv/lib/python3.11/site-packages/fontTools/ttLib/tables/_f_e_a_t.py
+++ /dev/null
@@ -1,12 +0,0 @@
-from .otBase import BaseTTXConverter
-
-
-class table__f_e_a_t(BaseTTXConverter):
- """The feature name table is an AAT (Apple Advanced Typography) table for
- storing font features, settings, and their human-readable names. It should
- not be confused with the ``Feat`` table or the OpenType Layout ``GSUB``/``GPOS``
- tables. See `Feature Name Table `_
- in the TrueType Reference Manual for more information on the structure and
- purpose of this table."""
-
- pass
diff --git a/spaces/Dantra1/CeliaSensei/transforms.py b/spaces/Dantra1/CeliaSensei/transforms.py
deleted file mode 100644
index 4793d67ca5a5630e0ffe0f9fb29445c949e64dae..0000000000000000000000000000000000000000
--- a/spaces/Dantra1/CeliaSensei/transforms.py
+++ /dev/null
@@ -1,193 +0,0 @@
-import torch
-from torch.nn import functional as F
-
-import numpy as np
-
-
-DEFAULT_MIN_BIN_WIDTH = 1e-3
-DEFAULT_MIN_BIN_HEIGHT = 1e-3
-DEFAULT_MIN_DERIVATIVE = 1e-3
-
-
-def piecewise_rational_quadratic_transform(inputs,
- unnormalized_widths,
- unnormalized_heights,
- unnormalized_derivatives,
- inverse=False,
- tails=None,
- tail_bound=1.,
- min_bin_width=DEFAULT_MIN_BIN_WIDTH,
- min_bin_height=DEFAULT_MIN_BIN_HEIGHT,
- min_derivative=DEFAULT_MIN_DERIVATIVE):
-
- if tails is None:
- spline_fn = rational_quadratic_spline
- spline_kwargs = {}
- else:
- spline_fn = unconstrained_rational_quadratic_spline
- spline_kwargs = {
- 'tails': tails,
- 'tail_bound': tail_bound
- }
-
- outputs, logabsdet = spline_fn(
- inputs=inputs,
- unnormalized_widths=unnormalized_widths,
- unnormalized_heights=unnormalized_heights,
- unnormalized_derivatives=unnormalized_derivatives,
- inverse=inverse,
- min_bin_width=min_bin_width,
- min_bin_height=min_bin_height,
- min_derivative=min_derivative,
- **spline_kwargs
- )
- return outputs, logabsdet
-
-
-def searchsorted(bin_locations, inputs, eps=1e-6):
- bin_locations[..., -1] += eps
- return torch.sum(
- inputs[..., None] >= bin_locations,
- dim=-1
- ) - 1
-
-
-def unconstrained_rational_quadratic_spline(inputs,
- unnormalized_widths,
- unnormalized_heights,
- unnormalized_derivatives,
- inverse=False,
- tails='linear',
- tail_bound=1.,
- min_bin_width=DEFAULT_MIN_BIN_WIDTH,
- min_bin_height=DEFAULT_MIN_BIN_HEIGHT,
- min_derivative=DEFAULT_MIN_DERIVATIVE):
- inside_interval_mask = (inputs >= -tail_bound) & (inputs <= tail_bound)
- outside_interval_mask = ~inside_interval_mask
-
- outputs = torch.zeros_like(inputs)
- logabsdet = torch.zeros_like(inputs)
-
- if tails == 'linear':
- unnormalized_derivatives = F.pad(unnormalized_derivatives, pad=(1, 1))
- constant = np.log(np.exp(1 - min_derivative) - 1)
- unnormalized_derivatives[..., 0] = constant
- unnormalized_derivatives[..., -1] = constant
-
- outputs[outside_interval_mask] = inputs[outside_interval_mask]
- logabsdet[outside_interval_mask] = 0
- else:
- raise RuntimeError('{} tails are not implemented.'.format(tails))
-
- outputs[inside_interval_mask], logabsdet[inside_interval_mask] = rational_quadratic_spline(
- inputs=inputs[inside_interval_mask],
- unnormalized_widths=unnormalized_widths[inside_interval_mask, :],
- unnormalized_heights=unnormalized_heights[inside_interval_mask, :],
- unnormalized_derivatives=unnormalized_derivatives[inside_interval_mask, :],
- inverse=inverse,
- left=-tail_bound, right=tail_bound, bottom=-tail_bound, top=tail_bound,
- min_bin_width=min_bin_width,
- min_bin_height=min_bin_height,
- min_derivative=min_derivative
- )
-
- return outputs, logabsdet
-
-def rational_quadratic_spline(inputs,
- unnormalized_widths,
- unnormalized_heights,
- unnormalized_derivatives,
- inverse=False,
- left=0., right=1., bottom=0., top=1.,
- min_bin_width=DEFAULT_MIN_BIN_WIDTH,
- min_bin_height=DEFAULT_MIN_BIN_HEIGHT,
- min_derivative=DEFAULT_MIN_DERIVATIVE):
- if torch.min(inputs) < left or torch.max(inputs) > right:
- raise ValueError('Input to a transform is not within its domain')
-
- num_bins = unnormalized_widths.shape[-1]
-
- if min_bin_width * num_bins > 1.0:
- raise ValueError('Minimal bin width too large for the number of bins')
- if min_bin_height * num_bins > 1.0:
- raise ValueError('Minimal bin height too large for the number of bins')
-
- widths = F.softmax(unnormalized_widths, dim=-1)
- widths = min_bin_width + (1 - min_bin_width * num_bins) * widths
- cumwidths = torch.cumsum(widths, dim=-1)
- cumwidths = F.pad(cumwidths, pad=(1, 0), mode='constant', value=0.0)
- cumwidths = (right - left) * cumwidths + left
- cumwidths[..., 0] = left
- cumwidths[..., -1] = right
- widths = cumwidths[..., 1:] - cumwidths[..., :-1]
-
- derivatives = min_derivative + F.softplus(unnormalized_derivatives)
-
- heights = F.softmax(unnormalized_heights, dim=-1)
- heights = min_bin_height + (1 - min_bin_height * num_bins) * heights
- cumheights = torch.cumsum(heights, dim=-1)
- cumheights = F.pad(cumheights, pad=(1, 0), mode='constant', value=0.0)
- cumheights = (top - bottom) * cumheights + bottom
- cumheights[..., 0] = bottom
- cumheights[..., -1] = top
- heights = cumheights[..., 1:] - cumheights[..., :-1]
-
- if inverse:
- bin_idx = searchsorted(cumheights, inputs)[..., None]
- else:
- bin_idx = searchsorted(cumwidths, inputs)[..., None]
-
- input_cumwidths = cumwidths.gather(-1, bin_idx)[..., 0]
- input_bin_widths = widths.gather(-1, bin_idx)[..., 0]
-
- input_cumheights = cumheights.gather(-1, bin_idx)[..., 0]
- delta = heights / widths
- input_delta = delta.gather(-1, bin_idx)[..., 0]
-
- input_derivatives = derivatives.gather(-1, bin_idx)[..., 0]
- input_derivatives_plus_one = derivatives[..., 1:].gather(-1, bin_idx)[..., 0]
-
- input_heights = heights.gather(-1, bin_idx)[..., 0]
-
- if inverse:
- a = (((inputs - input_cumheights) * (input_derivatives
- + input_derivatives_plus_one
- - 2 * input_delta)
- + input_heights * (input_delta - input_derivatives)))
- b = (input_heights * input_derivatives
- - (inputs - input_cumheights) * (input_derivatives
- + input_derivatives_plus_one
- - 2 * input_delta))
- c = - input_delta * (inputs - input_cumheights)
-
- discriminant = b.pow(2) - 4 * a * c
- assert (discriminant >= 0).all()
-
- root = (2 * c) / (-b - torch.sqrt(discriminant))
- outputs = root * input_bin_widths + input_cumwidths
-
- theta_one_minus_theta = root * (1 - root)
- denominator = input_delta + ((input_derivatives + input_derivatives_plus_one - 2 * input_delta)
- * theta_one_minus_theta)
- derivative_numerator = input_delta.pow(2) * (input_derivatives_plus_one * root.pow(2)
- + 2 * input_delta * theta_one_minus_theta
- + input_derivatives * (1 - root).pow(2))
- logabsdet = torch.log(derivative_numerator) - 2 * torch.log(denominator)
-
- return outputs, -logabsdet
- else:
- theta = (inputs - input_cumwidths) / input_bin_widths
- theta_one_minus_theta = theta * (1 - theta)
-
- numerator = input_heights * (input_delta * theta.pow(2)
- + input_derivatives * theta_one_minus_theta)
- denominator = input_delta + ((input_derivatives + input_derivatives_plus_one - 2 * input_delta)
- * theta_one_minus_theta)
- outputs = input_cumheights + numerator / denominator
-
- derivative_numerator = input_delta.pow(2) * (input_derivatives_plus_one * theta.pow(2)
- + 2 * input_delta * theta_one_minus_theta
- + input_derivatives * (1 - theta).pow(2))
- logabsdet = torch.log(derivative_numerator) - 2 * torch.log(denominator)
-
- return outputs, logabsdet
diff --git a/spaces/Datasculptor/DescriptionGPT/tools/get_lvis_cat_info.py b/spaces/Datasculptor/DescriptionGPT/tools/get_lvis_cat_info.py
deleted file mode 100644
index 83f286983ce811c4057ea8e8041e6a95dda78113..0000000000000000000000000000000000000000
--- a/spaces/Datasculptor/DescriptionGPT/tools/get_lvis_cat_info.py
+++ /dev/null
@@ -1,44 +0,0 @@
-# Copyright (c) Facebook, Inc. and its affiliates.
-import argparse
-import json
-
-if __name__ == '__main__':
- parser = argparse.ArgumentParser()
- parser.add_argument("--ann", default='datasets/lvis/lvis_v1_train.json')
- parser.add_argument("--add_freq", action='store_true')
- parser.add_argument("--r_thresh", type=int, default=10)
- parser.add_argument("--c_thresh", type=int, default=100)
- args = parser.parse_args()
-
- print('Loading', args.ann)
- data = json.load(open(args.ann, 'r'))
- cats = data['categories']
- image_count = {x['id']: set() for x in cats}
- ann_count = {x['id']: 0 for x in cats}
- for x in data['annotations']:
- image_count[x['category_id']].add(x['image_id'])
- ann_count[x['category_id']] += 1
- num_freqs = {x: 0 for x in ['r', 'f', 'c']}
- for x in cats:
- x['image_count'] = len(image_count[x['id']])
- x['instance_count'] = ann_count[x['id']]
- if args.add_freq:
- freq = 'f'
- if x['image_count'] < args.c_thresh:
- freq = 'c'
- if x['image_count'] < args.r_thresh:
- freq = 'r'
- x['frequency'] = freq
- num_freqs[freq] += 1
- print(cats)
- image_counts = sorted([x['image_count'] for x in cats])
- # print('image count', image_counts)
- # import pdb; pdb.set_trace()
- if args.add_freq:
- for x in ['r', 'c', 'f']:
- print(x, num_freqs[x])
- out = cats # {'categories': cats}
- out_path = args.ann[:-5] + '_cat_info.json'
- print('Saving to', out_path)
- json.dump(out, open(out_path, 'w'))
-
diff --git a/spaces/Datasculptor/sd-prism/app.py b/spaces/Datasculptor/sd-prism/app.py
deleted file mode 100644
index f4e993552d606738d2f85b7f22bff1ecbf13ea64..0000000000000000000000000000000000000000
--- a/spaces/Datasculptor/sd-prism/app.py
+++ /dev/null
@@ -1,105 +0,0 @@
-import gradio as gr
-import os
-from share_btn import community_icon_html, loading_icon_html, share_js
-
-img_to_text = gr.Blocks.load(name="spaces/pharma/CLIP-Interrogator")
-stable_diffusion = gr.Blocks.load(name="spaces/stabilityai/stable-diffusion")
-
-def get_images(prompt):
- gallery_dir = stable_diffusion(prompt, fn_index=2)
- sd_output = [os.path.join(gallery_dir, image) for image in os.listdir(gallery_dir)]
- return sd_output, gr.update(visible=True), gr.update(visible=True), gr.update(visible=True)
-
-def get_prompts(uploaded_image):
- return img_to_text(uploaded_image, fn_index=1)[0]
-
-css = '''
-.animate-spin {
- animation: spin 1s linear infinite;
-}
-@keyframes spin {
- from {
- transform: rotate(0deg);
- }
- to {
- transform: rotate(360deg);
- }
-}
-#share-btn-container {
- display: flex; padding-left: 0.5rem !important; padding-right: 0.5rem !important; background-color: #000000; justify-content: center; align-items: center; border-radius: 9999px !important; width: 13rem;
-}
-#share-btn {
- all: initial; color: #ffffff;font-weight: 600; cursor:pointer; font-family: 'IBM Plex Sans', sans-serif; margin-left: 0.5rem !important; padding-top: 0.25rem !important; padding-bottom: 0.25rem !important;
-}
-#share-btn * {
- all: unset;
-}
-#share-btn-container div:nth-child(-n+2){
- width: auto !important;
- min-height: 0px !important;
-}
-#share-btn-container .wrap {
- display: none !important;
-}
-a {text-decoration-line: underline;}
-'''
-
-with gr.Blocks(css=css) as demo:
- gr.HTML("""
-
-
- Stable Diffusion Prism
-
-
-
- Sends an image in to CLIP Interrogator
- to generate a text prompt which is then run through
- Stable Diffusion
- to generate new forms of the original!
-
-
""")
-
- with gr.Row():
- with gr.Column():
- input_img = gr.Image(type="filepath", elem_id="input-img")
- with gr.Row():
- see_prompts = gr.Button("Feed in your image!")
-
- with gr.Column():
- img2text_output = gr.Textbox(
- label="Generated text prompt",
- lines=4,
- elem_id="translated"
- )
- with gr.Row():
- diffuse_btn = gr.Button(value="Diffuse it!")
- with gr.Column(elem_id="generated-gallery"):
- sd_output = gr.Gallery().style(grid=2, height="auto")
- with gr.Group(elem_id="share-btn-container"):
- community_icon = gr.HTML(community_icon_html, visible=False)
- loading_icon = gr.HTML(loading_icon_html, visible=False)
- share_button = gr.Button("Share to community", elem_id="share-btn", visible=False)
-
- see_prompts.click(get_prompts,
- inputs = input_img,
- outputs = [
- img2text_output
- ])
- diffuse_btn.click(get_images,
- inputs = [
- img2text_output
- ],
- outputs = [sd_output, community_icon, loading_icon, share_button]
- )
- share_button.click(None, [], [], _js=share_js)
-
-
-
-demo.launch()
diff --git a/spaces/DragGan/DragGan/stylegan_human/pti/pti_models/e4e/stylegan2/op/fused_bias_act.cpp b/spaces/DragGan/DragGan/stylegan_human/pti/pti_models/e4e/stylegan2/op/fused_bias_act.cpp
deleted file mode 100644
index 02be898f970bcc8ea297867fcaa4e71b24b3d949..0000000000000000000000000000000000000000
--- a/spaces/DragGan/DragGan/stylegan_human/pti/pti_models/e4e/stylegan2/op/fused_bias_act.cpp
+++ /dev/null
@@ -1,21 +0,0 @@
-#include
-
-
-torch::Tensor fused_bias_act_op(const torch::Tensor& input, const torch::Tensor& bias, const torch::Tensor& refer,
- int act, int grad, float alpha, float scale);
-
-#define CHECK_CUDA(x) TORCH_CHECK(x.type().is_cuda(), #x " must be a CUDA tensor")
-#define CHECK_CONTIGUOUS(x) TORCH_CHECK(x.is_contiguous(), #x " must be contiguous")
-#define CHECK_INPUT(x) CHECK_CUDA(x); CHECK_CONTIGUOUS(x)
-
-torch::Tensor fused_bias_act(const torch::Tensor& input, const torch::Tensor& bias, const torch::Tensor& refer,
- int act, int grad, float alpha, float scale) {
- CHECK_CUDA(input);
- CHECK_CUDA(bias);
-
- return fused_bias_act_op(input, bias, refer, act, grad, alpha, scale);
-}
-
-PYBIND11_MODULE(TORCH_EXTENSION_NAME, m) {
- m.def("fused_bias_act", &fused_bias_act, "fused bias act (CUDA)");
-}
\ No newline at end of file
diff --git a/spaces/EPFL-VILAB/MultiMAE/mask2former/data/dataset_mappers/coco_instance_new_baseline_dataset_mapper.py b/spaces/EPFL-VILAB/MultiMAE/mask2former/data/dataset_mappers/coco_instance_new_baseline_dataset_mapper.py
deleted file mode 100644
index e64af2b51009d0398a1b6253a8a763c641547f59..0000000000000000000000000000000000000000
--- a/spaces/EPFL-VILAB/MultiMAE/mask2former/data/dataset_mappers/coco_instance_new_baseline_dataset_mapper.py
+++ /dev/null
@@ -1,189 +0,0 @@
-# Copyright (c) Facebook, Inc. and its affiliates.
-# Modified by Bowen Cheng from https://github.com/facebookresearch/detr/blob/master/d2/detr/dataset_mapper.py
-import copy
-import logging
-
-import numpy as np
-import torch
-
-from detectron2.config import configurable
-from detectron2.data import detection_utils as utils
-from detectron2.data import transforms as T
-from detectron2.data.transforms import TransformGen
-from detectron2.structures import BitMasks, Instances
-
-from pycocotools import mask as coco_mask
-
-__all__ = ["COCOInstanceNewBaselineDatasetMapper"]
-
-
-def convert_coco_poly_to_mask(segmentations, height, width):
- masks = []
- for polygons in segmentations:
- rles = coco_mask.frPyObjects(polygons, height, width)
- mask = coco_mask.decode(rles)
- if len(mask.shape) < 3:
- mask = mask[..., None]
- mask = torch.as_tensor(mask, dtype=torch.uint8)
- mask = mask.any(dim=2)
- masks.append(mask)
- if masks:
- masks = torch.stack(masks, dim=0)
- else:
- masks = torch.zeros((0, height, width), dtype=torch.uint8)
- return masks
-
-
-def build_transform_gen(cfg, is_train):
- """
- Create a list of default :class:`Augmentation` from config.
- Now it includes resizing and flipping.
- Returns:
- list[Augmentation]
- """
- assert is_train, "Only support training augmentation"
- image_size = cfg.INPUT.IMAGE_SIZE
- min_scale = cfg.INPUT.MIN_SCALE
- max_scale = cfg.INPUT.MAX_SCALE
-
- augmentation = []
-
- if cfg.INPUT.RANDOM_FLIP != "none":
- augmentation.append(
- T.RandomFlip(
- horizontal=cfg.INPUT.RANDOM_FLIP == "horizontal",
- vertical=cfg.INPUT.RANDOM_FLIP == "vertical",
- )
- )
-
- augmentation.extend([
- T.ResizeScale(
- min_scale=min_scale, max_scale=max_scale, target_height=image_size, target_width=image_size
- ),
- T.FixedSizeCrop(crop_size=(image_size, image_size)),
- ])
-
- return augmentation
-
-
-# This is specifically designed for the COCO dataset.
-class COCOInstanceNewBaselineDatasetMapper:
- """
- A callable which takes a dataset dict in Detectron2 Dataset format,
- and map it into a format used by MaskFormer.
-
- This dataset mapper applies the same transformation as DETR for COCO panoptic segmentation.
-
- The callable currently does the following:
-
- 1. Read the image from "file_name"
- 2. Applies geometric transforms to the image and annotation
- 3. Find and applies suitable cropping to the image and annotation
- 4. Prepare image and annotation to Tensors
- """
-
- @configurable
- def __init__(
- self,
- is_train=True,
- *,
- tfm_gens,
- image_format,
- ):
- """
- NOTE: this interface is experimental.
- Args:
- is_train: for training or inference
- augmentations: a list of augmentations or deterministic transforms to apply
- tfm_gens: data augmentation
- image_format: an image format supported by :func:`detection_utils.read_image`.
- """
- self.tfm_gens = tfm_gens
- logging.getLogger(__name__).info(
- "[COCOInstanceNewBaselineDatasetMapper] Full TransformGens used in training: {}".format(str(self.tfm_gens))
- )
-
- self.img_format = image_format
- self.is_train = is_train
-
- @classmethod
- def from_config(cls, cfg, is_train=True):
- # Build augmentation
- tfm_gens = build_transform_gen(cfg, is_train)
-
- ret = {
- "is_train": is_train,
- "tfm_gens": tfm_gens,
- "image_format": cfg.INPUT.FORMAT,
- }
- return ret
-
- def __call__(self, dataset_dict):
- """
- Args:
- dataset_dict (dict): Metadata of one image, in Detectron2 Dataset format.
-
- Returns:
- dict: a format that builtin models in detectron2 accept
- """
- dataset_dict = copy.deepcopy(dataset_dict) # it will be modified by code below
- image = utils.read_image(dataset_dict["file_name"], format=self.img_format)
- utils.check_image_size(dataset_dict, image)
-
- # TODO: get padding mask
- # by feeding a "segmentation mask" to the same transforms
- padding_mask = np.ones(image.shape[:2])
-
- image, transforms = T.apply_transform_gens(self.tfm_gens, image)
- # the crop transformation has default padding value 0 for segmentation
- padding_mask = transforms.apply_segmentation(padding_mask)
- padding_mask = ~ padding_mask.astype(bool)
-
- image_shape = image.shape[:2] # h, w
-
- # Pytorch's dataloader is efficient on torch.Tensor due to shared-memory,
- # but not efficient on large generic data structures due to the use of pickle & mp.Queue.
- # Therefore it's important to use torch.Tensor.
- dataset_dict["image"] = torch.as_tensor(np.ascontiguousarray(image.transpose(2, 0, 1)))
- dataset_dict["padding_mask"] = torch.as_tensor(np.ascontiguousarray(padding_mask))
-
- if not self.is_train:
- # USER: Modify this if you want to keep them for some reason.
- dataset_dict.pop("annotations", None)
- return dataset_dict
-
- if "annotations" in dataset_dict:
- # USER: Modify this if you want to keep them for some reason.
- for anno in dataset_dict["annotations"]:
- # Let's always keep mask
- # if not self.mask_on:
- # anno.pop("segmentation", None)
- anno.pop("keypoints", None)
-
- # USER: Implement additional transformations if you have other types of data
- annos = [
- utils.transform_instance_annotations(obj, transforms, image_shape)
- for obj in dataset_dict.pop("annotations")
- if obj.get("iscrowd", 0) == 0
- ]
- # NOTE: does not support BitMask due to augmentation
- # Current BitMask cannot handle empty objects
- instances = utils.annotations_to_instances(annos, image_shape)
- # After transforms such as cropping are applied, the bounding box may no longer
- # tightly bound the object. As an example, imagine a triangle object
- # [(0,0), (2,0), (0,2)] cropped by a box [(1,0),(2,2)] (XYXY format). The tight
- # bounding box of the cropped triangle should be [(1,0),(2,1)], which is not equal to
- # the intersection of original bounding box and the cropping box.
- instances.gt_boxes = instances.gt_masks.get_bounding_boxes()
- # Need to filter empty instances first (due to augmentation)
- instances = utils.filter_empty_instances(instances)
- # Generate masks from polygon
- h, w = instances.image_size
- # image_size_xyxy = torch.as_tensor([w, h, w, h], dtype=torch.float)
- if hasattr(instances, 'gt_masks'):
- gt_masks = instances.gt_masks
- gt_masks = convert_coco_poly_to_mask(gt_masks.polygons, h, w)
- instances.gt_masks = gt_masks
- dataset_dict["instances"] = instances
-
- return dataset_dict
diff --git a/spaces/Eddycrack864/Applio-Inference/demucs/train.py b/spaces/Eddycrack864/Applio-Inference/demucs/train.py
deleted file mode 100644
index 6bd221279dc986a6df1a8d7b4d4444bb822a1cb3..0000000000000000000000000000000000000000
--- a/spaces/Eddycrack864/Applio-Inference/demucs/train.py
+++ /dev/null
@@ -1,127 +0,0 @@
-# Copyright (c) Facebook, Inc. and its affiliates.
-# All rights reserved.
-#
-# This source code is licensed under the license found in the
-# LICENSE file in the root directory of this source tree.
-
-import sys
-
-import tqdm
-from torch.utils.data import DataLoader
-from torch.utils.data.distributed import DistributedSampler
-
-from .utils import apply_model, average_metric, center_trim
-
-
-def train_model(epoch,
- dataset,
- model,
- criterion,
- optimizer,
- augment,
- quantizer=None,
- diffq=0,
- repeat=1,
- device="cpu",
- seed=None,
- workers=4,
- world_size=1,
- batch_size=16):
-
- if world_size > 1:
- sampler = DistributedSampler(dataset)
- sampler_epoch = epoch * repeat
- if seed is not None:
- sampler_epoch += seed * 1000
- sampler.set_epoch(sampler_epoch)
- batch_size //= world_size
- loader = DataLoader(dataset, batch_size=batch_size, sampler=sampler, num_workers=workers)
- else:
- loader = DataLoader(dataset, batch_size=batch_size, num_workers=workers, shuffle=True)
- current_loss = 0
- model_size = 0
- for repetition in range(repeat):
- tq = tqdm.tqdm(loader,
- ncols=120,
- desc=f"[{epoch:03d}] train ({repetition + 1}/{repeat})",
- leave=False,
- file=sys.stdout,
- unit=" batch")
- total_loss = 0
- for idx, sources in enumerate(tq):
- if len(sources) < batch_size:
- # skip uncomplete batch for augment.Remix to work properly
- continue
- sources = sources.to(device)
- sources = augment(sources)
- mix = sources.sum(dim=1)
-
- estimates = model(mix)
- sources = center_trim(sources, estimates)
- loss = criterion(estimates, sources)
- model_size = 0
- if quantizer is not None:
- model_size = quantizer.model_size()
-
- train_loss = loss + diffq * model_size
- train_loss.backward()
- grad_norm = 0
- for p in model.parameters():
- if p.grad is not None:
- grad_norm += p.grad.data.norm()**2
- grad_norm = grad_norm**0.5
- optimizer.step()
- optimizer.zero_grad()
-
- if quantizer is not None:
- model_size = model_size.item()
-
- total_loss += loss.item()
- current_loss = total_loss / (1 + idx)
- tq.set_postfix(loss=f"{current_loss:.4f}", ms=f"{model_size:.2f}",
- grad=f"{grad_norm:.5f}")
-
- # free some space before next round
- del sources, mix, estimates, loss, train_loss
-
- if world_size > 1:
- sampler.epoch += 1
-
- if world_size > 1:
- current_loss = average_metric(current_loss)
- return current_loss, model_size
-
-
-def validate_model(epoch,
- dataset,
- model,
- criterion,
- device="cpu",
- rank=0,
- world_size=1,
- shifts=0,
- overlap=0.25,
- split=False):
- indexes = range(rank, len(dataset), world_size)
- tq = tqdm.tqdm(indexes,
- ncols=120,
- desc=f"[{epoch:03d}] valid",
- leave=False,
- file=sys.stdout,
- unit=" track")
- current_loss = 0
- for index in tq:
- streams = dataset[index]
- # first five minutes to avoid OOM on --upsample models
- streams = streams[..., :15_000_000]
- streams = streams.to(device)
- sources = streams[1:]
- mix = streams[0]
- estimates = apply_model(model, mix, shifts=shifts, split=split, overlap=overlap)
- loss = criterion(estimates, sources)
- current_loss += loss.item() / len(indexes)
- del estimates, streams, sources
-
- if world_size > 1:
- current_loss = average_metric(current_loss, len(indexes))
- return current_loss
diff --git a/spaces/EdwardHiscoke/piggie_or_potatoe/app.py b/spaces/EdwardHiscoke/piggie_or_potatoe/app.py
deleted file mode 100644
index 615f50e2f65c6499a14fdc10da5252c737f77e00..0000000000000000000000000000000000000000
--- a/spaces/EdwardHiscoke/piggie_or_potatoe/app.py
+++ /dev/null
@@ -1,21 +0,0 @@
-from fastai.vision.all import *
-import gradio as gr
-
-# Return a prediction
-def get_prediction( image ):
- categories = ("Guinea pig", "Potatoe")
- category, category_idx, probabilities = learn.predict(image)
- return dict(zip(categories, map(float, probabilities)))
-
-# Load model
-learn = load_learner('piggie_or_potatoe.pkl')
-
-# Create Gradio interface
-title = "Piggie-or-potatoe classifier"
-description = "One of them you want to hug, and the other one you want to eat. Now you need never again mix up your guinea pigs with your potatoes! Take picture of what you are about to eat or hug and stop everyday tragedy. Built using Fastai and HuggingFace Gradio on HuggingFace Spaces."
-image = gr.inputs.Image(shape=(192, 192))
-label = gr.outputs.Label()
-examples = ['guinea_pig.jpeg', 'potatoe.jpeg', 'guinea_pig_potatoe.jpeg']
-
-grinference = gr.Interface(fn=get_prediction, inputs=image, outputs=label, examples=examples)
-grinference.launch(inline=False)
diff --git a/spaces/Emanuel/pos-tag-bosque-br-demo/README.md b/spaces/Emanuel/pos-tag-bosque-br-demo/README.md
deleted file mode 100644
index 6c6c12641d61f42fea5ea67fcee5ae3ce0530ac3..0000000000000000000000000000000000000000
--- a/spaces/Emanuel/pos-tag-bosque-br-demo/README.md
+++ /dev/null
@@ -1,38 +0,0 @@
----
-title: POS-tag in Brazilian Portuguese
-emoji: 🤗
-colorFrom: green
-colorTo: colorTo
-sdk: streamlit
-app_file: app.py
-pinned: true
----
-
-
-# Configuration
-
-`title`: _string_
-Display title for the Space
-
-`emoji`: _string_
-Space emoji (emoji-only character allowed)
-
-`colorFrom`: _string_
-Color for Thumbnail gradient (red, yellow, green, blue, indigo, purple, pink, gray)
-
-`colorTo`: _string_
-Color for Thumbnail gradient (red, yellow, green, blue, indigo, purple, pink, gray)
-
-`sdk`: _string_
-Can be either `gradio` or `streamlit`
-
-`sdk_version` : _string_
-Only applicable for `streamlit` SDK.
-See [doc](https://hf.co/docs/hub/spaces) for more info on supported versions.
-
-`app_file`: _string_
-Path to your main application file (which contains either `gradio` or `streamlit` Python code).
-Path is relative to the root of the repository.
-
-`pinned`: _boolean_
-Whether the Space stays on top of your list.
\ No newline at end of file
diff --git a/spaces/EuroSciPy2022/arxiv-cards/README.md b/spaces/EuroSciPy2022/arxiv-cards/README.md
deleted file mode 100644
index f5d823a7b299b232d7af943b489171df0faa7121..0000000000000000000000000000000000000000
--- a/spaces/EuroSciPy2022/arxiv-cards/README.md
+++ /dev/null
@@ -1,12 +0,0 @@
----
-title: arXiv cards
-emoji: 📄
-colorFrom: red
-colorTo: yellow
-sdk: gradio
-sdk_version: 3.1.7
-app_file: app.py
-pinned: false
----
-
-arXiv card generator for easily sharing scientific papers on websites and presentations
diff --git a/spaces/FridaZuley/RVC_HFKawaii/tools/torchgate/utils.py b/spaces/FridaZuley/RVC_HFKawaii/tools/torchgate/utils.py
deleted file mode 100644
index dc97d45a399c112c76e80cdd8c73cfebaf3ef6ad..0000000000000000000000000000000000000000
--- a/spaces/FridaZuley/RVC_HFKawaii/tools/torchgate/utils.py
+++ /dev/null
@@ -1,66 +0,0 @@
-import torch
-from torch.types import Number
-
-
-@torch.no_grad()
-def amp_to_db(x: torch.Tensor, eps=torch.finfo(torch.float64).eps, top_db=40) -> torch.Tensor:
- """
- Convert the input tensor from amplitude to decibel scale.
-
- Arguments:
- x {[torch.Tensor]} -- [Input tensor.]
-
- Keyword Arguments:
- eps {[float]} -- [Small value to avoid numerical instability.]
- (default: {torch.finfo(torch.float64).eps})
- top_db {[float]} -- [threshold the output at ``top_db`` below the peak]
- ` (default: {40})
-
- Returns:
- [torch.Tensor] -- [Output tensor in decibel scale.]
- """
- x_db = 20 * torch.log10(x.abs() + eps)
- return torch.max(x_db, (x_db.max(-1).values - top_db).unsqueeze(-1))
-
-
-@torch.no_grad()
-def temperature_sigmoid(x: torch.Tensor, x0: float, temp_coeff: float) -> torch.Tensor:
- """
- Apply a sigmoid function with temperature scaling.
-
- Arguments:
- x {[torch.Tensor]} -- [Input tensor.]
- x0 {[float]} -- [Parameter that controls the threshold of the sigmoid.]
- temp_coeff {[float]} -- [Parameter that controls the slope of the sigmoid.]
-
- Returns:
- [torch.Tensor] -- [Output tensor after applying the sigmoid with temperature scaling.]
- """
- return torch.sigmoid((x - x0) / temp_coeff)
-
-
-@torch.no_grad()
-def linspace(start: Number, stop: Number, num: int = 50, endpoint: bool = True, **kwargs) -> torch.Tensor:
- """
- Generate a linearly spaced 1-D tensor.
-
- Arguments:
- start {[Number]} -- [The starting value of the sequence.]
- stop {[Number]} -- [The end value of the sequence, unless `endpoint` is set to False.
- In that case, the sequence consists of all but the last of ``num + 1``
- evenly spaced samples, so that `stop` is excluded. Note that the step
- size changes when `endpoint` is False.]
-
- Keyword Arguments:
- num {[int]} -- [Number of samples to generate. Default is 50. Must be non-negative.]
- endpoint {[bool]} -- [If True, `stop` is the last sample. Otherwise, it is not included.
- Default is True.]
- **kwargs -- [Additional arguments to be passed to the underlying PyTorch `linspace` function.]
-
- Returns:
- [torch.Tensor] -- [1-D tensor of `num` equally spaced samples from `start` to `stop`.]
- """
- if endpoint:
- return torch.linspace(start, stop, num, **kwargs)
- else:
- return torch.linspace(start, stop, num + 1, **kwargs)[:-1]
diff --git a/spaces/GOVS/Liu_Sir/README.md b/spaces/GOVS/Liu_Sir/README.md
deleted file mode 100644
index 2922d3ba99b47cbe5364c85665eb8056e1ed2667..0000000000000000000000000000000000000000
--- a/spaces/GOVS/Liu_Sir/README.md
+++ /dev/null
@@ -1,12 +0,0 @@
----
-title: Liu Sir
-emoji: 🌍
-colorFrom: purple
-colorTo: yellow
-sdk: docker
-pinned: false
-license: mit
-app_port: 8080
----
-
-Check out the configuration reference at https://huggingface.co/docs/hub/spaces-config-reference
diff --git a/spaces/Gen-Sim/Gen-Sim/cliport/generated_tasks/assemble_single_car.py b/spaces/Gen-Sim/Gen-Sim/cliport/generated_tasks/assemble_single_car.py
deleted file mode 100644
index bdd1acc19165bc57ded91d45715e0fbba35f59b4..0000000000000000000000000000000000000000
--- a/spaces/Gen-Sim/Gen-Sim/cliport/generated_tasks/assemble_single_car.py
+++ /dev/null
@@ -1,61 +0,0 @@
-import numpy as np
-import os
-import pybullet as p
-import random
-from cliport.tasks import primitives
-from cliport.tasks.grippers import Spatula
-from cliport.tasks.task import Task
-from cliport.utils import utils
-import numpy as np
-from cliport.tasks.task import Task
-from cliport.utils import utils
-
-class AssembleSingleCar(Task):
- """Assemble a mini car using a large blue box as the body, a smaller red box on top as the roof, and two tiny green boxes on the sides as wheels."""
-
- def __init__(self):
- super().__init__()
- self.max_steps = 10
- self.lang_template = "build a mini car using a large blue box as the body, a smaller red box on top as the roof, and two tiny green boxes on the sides as wheels"
- self.task_completed_desc = "done assembling the car."
-
- def reset(self, env):
- super().reset(env)
-
- # Add car body (large blue box).
- body_size = (0.1, 0.05, 0.02) # x, y, z dimensions
- body_pose = self.get_random_pose(env, body_size)
- body_urdf = 'box/box-template.urdf'
- body_color = utils.COLORS['blue']
- body_id = env.add_object(body_urdf, body_pose, color=body_color)
-
- # Add car roof (smaller red box).
- roof_size = (0.08, 0.04, 0.02) # x, y, z dimensions
- roof_pose = self.get_random_pose(env, roof_size)
- roof_urdf = 'box/box-template.urdf'
- roof_color = utils.COLORS['red']
- roof_id = env.add_object(roof_urdf, roof_pose, color=roof_color)
-
- # Add car wheels (two tiny green boxes).
- wheel_size = (0.02, 0.02, 0.01) # x, y, z dimensions
- wheel_urdf = 'box/box-template.urdf'
- wheel_color = utils.COLORS['green']
- wheel_ids = []
-
- for _ in range(2):
- wheel_pose = self.get_random_pose(env, wheel_size)
- wheel_id = env.add_object(wheel_urdf, wheel_pose, color=wheel_color)
- wheel_ids.append(wheel_id)
-
- # Goal: assemble the car by placing the roof on the body and the wheels on the sides.
- # The target poses are calculated based on the body pose.
- roof_targ_pose = (body_pose[0] + np.array([0, 0, body_size[2] + roof_size[2]/2]), body_pose[1])
- wheel_targ_poses = [(body_pose[0] + np.array([0, body_size[1]/2 + wheel_size[1]/2, -body_size[2]/2]), body_pose[1]),
- (body_pose[0] + np.array([0, -body_size[1]/2 - wheel_size[1]/2, -body_size[2]/2]), body_pose[1])]
-
- # Add the goals.
- self.add_goal(objs=[roof_id], matches=np.ones((1, 1)), targ_poses=[roof_targ_pose], replace=False,
- rotations=True, metric='pose', params=None, step_max_reward=1/3, language_goal=self.lang_template)
-
- self.add_goal(objs=wheel_ids, matches=np.ones((2, 2)), targ_poses=wheel_targ_poses, replace=False,
- rotations=True, metric='pose', params=None, step_max_reward=2/3, language_goal=self.lang_template)
\ No newline at end of file
diff --git a/spaces/Gen-Sim/Gen-Sim/cliport/generated_tasks/multi_level_pyramid_construction.py b/spaces/Gen-Sim/Gen-Sim/cliport/generated_tasks/multi_level_pyramid_construction.py
deleted file mode 100644
index 49940804ac8a3aef2207ad37f541e8a10a011d65..0000000000000000000000000000000000000000
--- a/spaces/Gen-Sim/Gen-Sim/cliport/generated_tasks/multi_level_pyramid_construction.py
+++ /dev/null
@@ -1,56 +0,0 @@
-import numpy as np
-import os
-import pybullet as p
-import random
-from cliport.tasks import primitives
-from cliport.tasks.grippers import Spatula
-from cliport.tasks.task import Task
-from cliport.utils import utils
-import numpy as np
-from cliport.tasks.task import Task
-from cliport.utils import utils
-
-class MultiLevelPyramidConstruction(Task):
- """Construct a two-level pyramid on a pallet using six blocks: three green and three blue."""
-
- def __init__(self):
- super().__init__()
- self.max_steps = 20
- self.lang_template = "Construct a two-level pyramid on a pallet using six blocks: three green and three blue. The first level should be a triangle created by placing the green blocks side by side. The second level should be built by placing the blue blocks on top of the green blocks, forming another triangle rotated 60 degrees with respect to the first one."
- self.task_completed_desc = "done constructing pyramid."
- self.additional_reset()
-
- def reset(self, env):
- super().reset(env)
-
- # Add pallet.
- pallet_size = (0.35, 0.35, 0.01) # x, y, z dimensions for the pallet size
- pallet_pose = self.get_random_pose(env, pallet_size)
- pallet_urdf = 'pallet/pallet.urdf'
- env.add_object(pallet_urdf, pallet_pose, 'fixed')
-
- # Add blocks.
- block_size = (0.04, 0.04, 0.04) # x, y, z dimensions for the block size
- block_urdf = 'block/block.urdf'
- block_colors = [utils.COLORS['green']] * 3 + [utils.COLORS['blue']] * 3 # three green and three blue blocks
-
- blocks = []
- for color in block_colors:
- block_pose = self.get_random_pose(env, block_size)
- block_id = env.add_object(block_urdf, block_pose, color=color)
- blocks.append(block_id)
-
- # Associate placement locations for goals.
- place_pos = [(0, -0.05, 0.02), (0, 0, 0.02), (0, 0.05, 0.02), # first level
- (0, -0.025, 0.06), (0, 0.025, 0.06), (0, 0, 0.10)] # second level
- targs = [(utils.apply(pallet_pose, i), pallet_pose[1]) for i in place_pos]
-
- # Goal: blocks are stacked in a pyramid (first level: green blocks).
- self.add_goal(objs=blocks[:3], matches=np.ones((3, 3)), targ_poses=targs[:3], replace=False,
- rotations=True, metric='pose', params=None, step_max_reward=1 / 2, symmetries=[np.pi/2]*3,
- language_goal=self.lang_template.format(blocks="the green blocks", row="bottom"))
-
- # Goal: blocks are stacked in a pyramid (second level: blue blocks).
- self.add_goal(objs=blocks[3:], matches=np.ones((3, 3)), targ_poses=targs[3:], replace=False,
- rotations=True, metric='pose', params=None, step_max_reward=1 / 2, symmetries=[np.pi/2]*3,
- language_goal=self.lang_template.format(blocks="the blue blocks", row="top"))
\ No newline at end of file
diff --git a/spaces/Gmq-x/gpt-academic/theme.py b/spaces/Gmq-x/gpt-academic/theme.py
deleted file mode 100644
index 1cc26b06d994eba6d37aa86f3bbfc12fc164731c..0000000000000000000000000000000000000000
--- a/spaces/Gmq-x/gpt-academic/theme.py
+++ /dev/null
@@ -1,231 +0,0 @@
-import gradio as gr
-from toolbox import get_conf
-CODE_HIGHLIGHT, = get_conf('CODE_HIGHLIGHT')
-# gradio可用颜色列表
-# gr.themes.utils.colors.slate (石板色)
-# gr.themes.utils.colors.gray (灰色)
-# gr.themes.utils.colors.zinc (锌色)
-# gr.themes.utils.colors.neutral (中性色)
-# gr.themes.utils.colors.stone (石头色)
-# gr.themes.utils.colors.red (红色)
-# gr.themes.utils.colors.orange (橙色)
-# gr.themes.utils.colors.amber (琥珀色)
-# gr.themes.utils.colors.yellow (黄色)
-# gr.themes.utils.colors.lime (酸橙色)
-# gr.themes.utils.colors.green (绿色)
-# gr.themes.utils.colors.emerald (祖母绿)
-# gr.themes.utils.colors.teal (青蓝色)
-# gr.themes.utils.colors.cyan (青色)
-# gr.themes.utils.colors.sky (天蓝色)
-# gr.themes.utils.colors.blue (蓝色)
-# gr.themes.utils.colors.indigo (靛蓝色)
-# gr.themes.utils.colors.violet (紫罗兰色)
-# gr.themes.utils.colors.purple (紫色)
-# gr.themes.utils.colors.fuchsia (洋红色)
-# gr.themes.utils.colors.pink (粉红色)
-# gr.themes.utils.colors.rose (玫瑰色)
-
-
-def adjust_theme():
- try:
- color_er = gr.themes.utils.colors.fuchsia
- set_theme = gr.themes.Default(
- primary_hue=gr.themes.utils.colors.orange,
- neutral_hue=gr.themes.utils.colors.gray,
- font=["sans-serif", "Microsoft YaHei", "ui-sans-serif", "system-ui",
- "sans-serif", gr.themes.utils.fonts.GoogleFont("Source Sans Pro")],
- font_mono=["ui-monospace", "Consolas", "monospace", gr.themes.utils.fonts.GoogleFont("IBM Plex Mono")])
- set_theme.set(
- # Colors
- input_background_fill_dark="*neutral_800",
- # Transition
- button_transition="none",
- # Shadows
- button_shadow="*shadow_drop",
- button_shadow_hover="*shadow_drop_lg",
- button_shadow_active="*shadow_inset",
- input_shadow="0 0 0 *shadow_spread transparent, *shadow_inset",
- input_shadow_focus="0 0 0 *shadow_spread *secondary_50, *shadow_inset",
- input_shadow_focus_dark="0 0 0 *shadow_spread *neutral_700, *shadow_inset",
- checkbox_label_shadow="*shadow_drop",
- block_shadow="*shadow_drop",
- form_gap_width="1px",
- # Button borders
- input_border_width="1px",
- input_background_fill="white",
- # Gradients
- stat_background_fill="linear-gradient(to right, *primary_400, *primary_200)",
- stat_background_fill_dark="linear-gradient(to right, *primary_400, *primary_600)",
- error_background_fill=f"linear-gradient(to right, {color_er.c100}, *background_fill_secondary)",
- error_background_fill_dark="*background_fill_primary",
- checkbox_label_background_fill="linear-gradient(to top, *neutral_50, white)",
- checkbox_label_background_fill_dark="linear-gradient(to top, *neutral_900, *neutral_800)",
- checkbox_label_background_fill_hover="linear-gradient(to top, *neutral_100, white)",
- checkbox_label_background_fill_hover_dark="linear-gradient(to top, *neutral_900, *neutral_800)",
- button_primary_background_fill="linear-gradient(to bottom right, *primary_100, *primary_300)",
- button_primary_background_fill_dark="linear-gradient(to bottom right, *primary_500, *primary_600)",
- button_primary_background_fill_hover="linear-gradient(to bottom right, *primary_100, *primary_200)",
- button_primary_background_fill_hover_dark="linear-gradient(to bottom right, *primary_500, *primary_500)",
- button_primary_border_color_dark="*primary_500",
- button_secondary_background_fill="linear-gradient(to bottom right, *neutral_100, *neutral_200)",
- button_secondary_background_fill_dark="linear-gradient(to bottom right, *neutral_600, *neutral_700)",
- button_secondary_background_fill_hover="linear-gradient(to bottom right, *neutral_100, *neutral_100)",
- button_secondary_background_fill_hover_dark="linear-gradient(to bottom right, *neutral_600, *neutral_600)",
- button_cancel_background_fill=f"linear-gradient(to bottom right, {color_er.c100}, {color_er.c200})",
- button_cancel_background_fill_dark=f"linear-gradient(to bottom right, {color_er.c600}, {color_er.c700})",
- button_cancel_background_fill_hover=f"linear-gradient(to bottom right, {color_er.c100}, {color_er.c100})",
- button_cancel_background_fill_hover_dark=f"linear-gradient(to bottom right, {color_er.c600}, {color_er.c600})",
- button_cancel_border_color=color_er.c200,
- button_cancel_border_color_dark=color_er.c600,
- button_cancel_text_color=color_er.c600,
- button_cancel_text_color_dark="white",
- )
- except:
- set_theme = None
- print('gradio版本较旧, 不能自定义字体和颜色')
- return set_theme
-
-
-advanced_css = """
-/* 设置表格的外边距为1em,内部单元格之间边框合并,空单元格显示. */
-.markdown-body table {
- margin: 1em 0;
- border-collapse: collapse;
- empty-cells: show;
-}
-
-/* 设置表格单元格的内边距为5px,边框粗细为1.2px,颜色为--border-color-primary. */
-.markdown-body th, .markdown-body td {
- border: 1.2px solid var(--border-color-primary);
- padding: 5px;
-}
-
-/* 设置表头背景颜色为rgba(175,184,193,0.2),透明度为0.2. */
-.markdown-body thead {
- background-color: rgba(175,184,193,0.2);
-}
-
-/* 设置表头单元格的内边距为0.5em和0.2em. */
-.markdown-body thead th {
- padding: .5em .2em;
-}
-
-/* 去掉列表前缀的默认间距,使其与文本线对齐. */
-.markdown-body ol, .markdown-body ul {
- padding-inline-start: 2em !important;
-}
-
-/* 设定聊天气泡的样式,包括圆角、最大宽度和阴影等. */
-[class *= "message"] {
- border-radius: var(--radius-xl) !important;
- /* padding: var(--spacing-xl) !important; */
- /* font-size: var(--text-md) !important; */
- /* line-height: var(--line-md) !important; */
- /* min-height: calc(var(--text-md)*var(--line-md) + 2*var(--spacing-xl)); */
- /* min-width: calc(var(--text-md)*var(--line-md) + 2*var(--spacing-xl)); */
-}
-[data-testid = "bot"] {
- max-width: 95%;
- /* width: auto !important; */
- border-bottom-left-radius: 0 !important;
-}
-[data-testid = "user"] {
- max-width: 100%;
- /* width: auto !important; */
- border-bottom-right-radius: 0 !important;
-}
-
-/* 行内代码的背景设为淡灰色,设定圆角和间距. */
-.markdown-body code {
- display: inline;
- white-space: break-spaces;
- border-radius: 6px;
- margin: 0 2px 0 2px;
- padding: .2em .4em .1em .4em;
- background-color: rgba(175,184,193,0.2);
-}
-/* 设定代码块的样式,包括背景颜色、内、外边距、圆角。 */
-.markdown-body pre code {
- display: block;
- overflow: auto;
- white-space: pre;
- background-color: rgba(175,184,193,0.2);
- border-radius: 10px;
- padding: 1em;
- margin: 1em 2em 1em 0.5em;
-}
-
-"""
-
-if CODE_HIGHLIGHT:
- advanced_css += """
-
-.hll { background-color: #ffffcc }
-.c { color: #3D7B7B; font-style: italic } /* Comment */
-.err { border: 1px solid #FF0000 } /* Error */
-.k { color: hsl(197, 94%, 51%); font-weight: bold } /* Keyword */
-.o { color: #666666 } /* Operator */
-.ch { color: #3D7B7B; font-style: italic } /* Comment.Hashbang */
-.cm { color: #3D7B7B; font-style: italic } /* Comment.Multiline */
-.cp { color: #9C6500 } /* Comment.Preproc */
-.cpf { color: #3D7B7B; font-style: italic } /* Comment.PreprocFile */
-.c1 { color: #3D7B7B; font-style: italic } /* Comment.Single */
-.cs { color: #3D7B7B; font-style: italic } /* Comment.Special */
-.gd { color: #A00000 } /* Generic.Deleted */
-.ge { font-style: italic } /* Generic.Emph */
-.gr { color: #E40000 } /* Generic.Error */
-.gh { color: #000080; font-weight: bold } /* Generic.Heading */
-.gi { color: #008400 } /* Generic.Inserted */
-.go { color: #717171 } /* Generic.Output */
-.gp { color: #000080; font-weight: bold } /* Generic.Prompt */
-.gs { font-weight: bold } /* Generic.Strong */
-.gu { color: #800080; font-weight: bold } /* Generic.Subheading */
-.gt { color: #a9dd00 } /* Generic.Traceback */
-.kc { color: #008000; font-weight: bold } /* Keyword.Constant */
-.kd { color: #008000; font-weight: bold } /* Keyword.Declaration */
-.kn { color: #008000; font-weight: bold } /* Keyword.Namespace */
-.kp { color: #008000 } /* Keyword.Pseudo */
-.kr { color: #008000; font-weight: bold } /* Keyword.Reserved */
-.kt { color: #B00040 } /* Keyword.Type */
-.m { color: #666666 } /* Literal.Number */
-.s { color: #BA2121 } /* Literal.String */
-.na { color: #687822 } /* Name.Attribute */
-.nb { color: #e5f8c3 } /* Name.Builtin */
-.nc { color: #ffad65; font-weight: bold } /* Name.Class */
-.no { color: #880000 } /* Name.Constant */
-.nd { color: #AA22FF } /* Name.Decorator */
-.ni { color: #717171; font-weight: bold } /* Name.Entity */
-.ne { color: #CB3F38; font-weight: bold } /* Name.Exception */
-.nf { color: #f9f978 } /* Name.Function */
-.nl { color: #767600 } /* Name.Label */
-.nn { color: #0000FF; font-weight: bold } /* Name.Namespace */
-.nt { color: #008000; font-weight: bold } /* Name.Tag */
-.nv { color: #19177C } /* Name.Variable */
-.ow { color: #AA22FF; font-weight: bold } /* Operator.Word */
-.w { color: #bbbbbb } /* Text.Whitespace */
-.mb { color: #666666 } /* Literal.Number.Bin */
-.mf { color: #666666 } /* Literal.Number.Float */
-.mh { color: #666666 } /* Literal.Number.Hex */
-.mi { color: #666666 } /* Literal.Number.Integer */
-.mo { color: #666666 } /* Literal.Number.Oct */
-.sa { color: #BA2121 } /* Literal.String.Affix */
-.sb { color: #BA2121 } /* Literal.String.Backtick */
-.sc { color: #BA2121 } /* Literal.String.Char */
-.dl { color: #BA2121 } /* Literal.String.Delimiter */
-.sd { color: #BA2121; font-style: italic } /* Literal.String.Doc */
-.s2 { color: #2bf840 } /* Literal.String.Double */
-.se { color: #AA5D1F; font-weight: bold } /* Literal.String.Escape */
-.sh { color: #BA2121 } /* Literal.String.Heredoc */
-.si { color: #A45A77; font-weight: bold } /* Literal.String.Interpol */
-.sx { color: #008000 } /* Literal.String.Other */
-.sr { color: #A45A77 } /* Literal.String.Regex */
-.s1 { color: #BA2121 } /* Literal.String.Single */
-.ss { color: #19177C } /* Literal.String.Symbol */
-.bp { color: #008000 } /* Name.Builtin.Pseudo */
-.fm { color: #0000FF } /* Name.Function.Magic */
-.vc { color: #19177C } /* Name.Variable.Class */
-.vg { color: #19177C } /* Name.Variable.Global */
-.vi { color: #19177C } /* Name.Variable.Instance */
-.vm { color: #19177C } /* Name.Variable.Magic */
-.il { color: #666666 } /* Literal.Number.Integer.Long */
-"""
diff --git a/spaces/GolDNenex/Super-Resolution-Anime-Diffusion/api.py b/spaces/GolDNenex/Super-Resolution-Anime-Diffusion/api.py
deleted file mode 100644
index 08317b4eba5c62ae17646f121c0f0758b2592917..0000000000000000000000000000000000000000
--- a/spaces/GolDNenex/Super-Resolution-Anime-Diffusion/api.py
+++ /dev/null
@@ -1,35 +0,0 @@
-# -*- coding: utf-8 -*-
-# file: api.py.py
-# time: 20:37 2022/12/6
-# author: yangheng
-# github: https://github.com/yangheng95
-# huggingface: https://huggingface.co/yangheng
-# google scholar: https://scholar.google.com/citations?user=NPq5a_0AAAAJ&hl=en
-# Copyright (C) 2021. All Rights Reserved.
-import requests
-from PIL import Image
-from io import BytesIO
-
-response = requests.post(
- "https://yangheng-super-resolution-anime-diffusion.hf.space/run/generate",
- json={
- "data": [
- "anything v3",
- "girl,lovely,cute,beautiful eyes,cumulonimbus clouds,sky,detailed fingers,pants,red hair,blue eyes,flower meadow,Elif",
- 7.5,
- 15,
- 512,
- 512,
- 0,
- "data:image/png;base64,iVBORw0KGgoAAAANSUhEUgAAAAEAAAABCAYAAAAfFcSJAAAACklEQVR4nGMAAQAABQABDQottAAAAABJRU5ErkJggg==",
- 0.5,
- "",
- 2,
- ]
- },
- timeout=3000,
-)
-
-img = Image.open(BytesIO(response.content))
-img.show()
-img.save("test_api.png")
diff --git a/spaces/Gradio-Blocks/DualStyleGAN/README.md b/spaces/Gradio-Blocks/DualStyleGAN/README.md
deleted file mode 100644
index 7b4ae9b5ef480e15aa732c8d56f99d1636a03424..0000000000000000000000000000000000000000
--- a/spaces/Gradio-Blocks/DualStyleGAN/README.md
+++ /dev/null
@@ -1,15 +0,0 @@
----
-title: Portrait Style Transfer
-emoji: 😻
-colorFrom: purple
-colorTo: red
-sdk: gradio
-sdk_version: 3.36.1
-app_file: app.py
-pinned: false
-suggested_hardware: t4-small
----
-
-Check out the configuration reference at https://huggingface.co/docs/hub/spaces#reference
-
-https://arxiv.org/abs/2203.13248
diff --git a/spaces/Gradio-Blocks/uniformer_image_detection/configs/cityscapes/README.md b/spaces/Gradio-Blocks/uniformer_image_detection/configs/cityscapes/README.md
deleted file mode 100644
index d892fc93aaca82cbd84cc38a88ad52c30641bbcc..0000000000000000000000000000000000000000
--- a/spaces/Gradio-Blocks/uniformer_image_detection/configs/cityscapes/README.md
+++ /dev/null
@@ -1,33 +0,0 @@
-# Cityscapes Dataset
-
-[DATASET]
-
-```
-@inproceedings{Cordts2016Cityscapes,
- title={The Cityscapes Dataset for Semantic Urban Scene Understanding},
- author={Cordts, Marius and Omran, Mohamed and Ramos, Sebastian and Rehfeld, Timo and Enzweiler, Markus and Benenson, Rodrigo and Franke, Uwe and Roth, Stefan and Schiele, Bernt},
- booktitle={Proc. of the IEEE Conference on Computer Vision and Pattern Recognition (CVPR)},
- year={2016}
-}
-```
-
-## Common settings
-
-- All baselines were trained using 8 GPU with a batch size of 8 (1 images per GPU) using the [linear scaling rule](https://arxiv.org/abs/1706.02677) to scale the learning rate.
-- All models were trained on `cityscapes_train`, and tested on `cityscapes_val`.
-- 1x training schedule indicates 64 epochs which corresponds to slightly less than the 24k iterations reported in the original schedule from the [Mask R-CNN paper](https://arxiv.org/abs/1703.06870)
-- COCO pre-trained weights are used to initialize.
-- A conversion [script](../../tools/dataset_converters/cityscapes.py) is provided to convert Cityscapes into COCO format. Please refer to [install.md](../../docs/1_exist_data_model.md#prepare-datasets) for details.
-- `CityscapesDataset` implemented three evaluation methods. `bbox` and `segm` are standard COCO bbox/mask AP. `cityscapes` is the cityscapes dataset official evaluation, which may be slightly higher than COCO.
-
-### Faster R-CNN
-
-| Backbone | Style | Lr schd | Scale | Mem (GB) | Inf time (fps) | box AP | Config | Download |
-| :-------------: | :-----: | :-----: | :---: | :------: | :------------: | :----: | :------: | :--------: |
-| R-50-FPN | pytorch | 1x | 800-1024 | 5.2 | - | 40.3 | [config](https://github.com/open-mmlab/mmdetection/tree/master/configs/cityscapes/faster_rcnn_r50_fpn_1x_cityscapes.py) | [model](http://download.openmmlab.com/mmdetection/v2.0/cityscapes/faster_rcnn_r50_fpn_1x_cityscapes_20200502-829424c0.pth) | [log](http://download.openmmlab.com/mmdetection/v2.0/cityscapes/faster_rcnn_r50_fpn_1x_cityscapes_20200502_114915.log.json) |
-
-### Mask R-CNN
-
-| Backbone | Style | Lr schd | Scale | Mem (GB) | Inf time (fps) | box AP | mask AP | Config | Download |
-| :-------------: | :-----: | :-----: | :------: | :------: | :------------: | :----: | :-----: | :------: | :------: |
-| R-50-FPN | pytorch | 1x | 800-1024 | 5.3 | - | 40.9 | 36.4 | [config](https://github.com/open-mmlab/mmdetection/tree/master/configs/cityscapes/mask_rcnn_r50_fpn_1x_cityscapes.py) | [model](https://download.openmmlab.com/mmdetection/v2.0/cityscapes/mask_rcnn_r50_fpn_1x_cityscapes/mask_rcnn_r50_fpn_1x_cityscapes_20201211_133733-d2858245.pth) | [log](https://download.openmmlab.com/mmdetection/v2.0/cityscapes/mask_rcnn_r50_fpn_1x_cityscapes/mask_rcnn_r50_fpn_1x_cityscapes_20201211_133733.log.json) |
diff --git a/spaces/Gradio-Blocks/uniformer_image_segmentation/configs/dmnet/dmnet_r50-d8_512x512_160k_ade20k.py b/spaces/Gradio-Blocks/uniformer_image_segmentation/configs/dmnet/dmnet_r50-d8_512x512_160k_ade20k.py
deleted file mode 100644
index a8fbd9beb11f3d1308ce2cd12da2a177c2d39478..0000000000000000000000000000000000000000
--- a/spaces/Gradio-Blocks/uniformer_image_segmentation/configs/dmnet/dmnet_r50-d8_512x512_160k_ade20k.py
+++ /dev/null
@@ -1,6 +0,0 @@
-_base_ = [
- '../_base_/models/dmnet_r50-d8.py', '../_base_/datasets/ade20k.py',
- '../_base_/default_runtime.py', '../_base_/schedules/schedule_160k.py'
-]
-model = dict(
- decode_head=dict(num_classes=150), auxiliary_head=dict(num_classes=150))
diff --git a/spaces/Hallucinate/demo/ldm/modules/image_degradation/bsrgan.py b/spaces/Hallucinate/demo/ldm/modules/image_degradation/bsrgan.py
deleted file mode 100644
index 32ef56169978e550090261cddbcf5eb611a6173b..0000000000000000000000000000000000000000
--- a/spaces/Hallucinate/demo/ldm/modules/image_degradation/bsrgan.py
+++ /dev/null
@@ -1,730 +0,0 @@
-# -*- coding: utf-8 -*-
-"""
-# --------------------------------------------
-# Super-Resolution
-# --------------------------------------------
-#
-# Kai Zhang (cskaizhang@gmail.com)
-# https://github.com/cszn
-# From 2019/03--2021/08
-# --------------------------------------------
-"""
-
-import numpy as np
-import cv2
-import torch
-
-from functools import partial
-import random
-from scipy import ndimage
-import scipy
-import scipy.stats as ss
-from scipy.interpolate import interp2d
-from scipy.linalg import orth
-import albumentations
-
-import ldm.modules.image_degradation.utils_image as util
-
-
-def modcrop_np(img, sf):
- '''
- Args:
- img: numpy image, WxH or WxHxC
- sf: scale factor
- Return:
- cropped image
- '''
- w, h = img.shape[:2]
- im = np.copy(img)
- return im[:w - w % sf, :h - h % sf, ...]
-
-
-"""
-# --------------------------------------------
-# anisotropic Gaussian kernels
-# --------------------------------------------
-"""
-
-
-def analytic_kernel(k):
- """Calculate the X4 kernel from the X2 kernel (for proof see appendix in paper)"""
- k_size = k.shape[0]
- # Calculate the big kernels size
- big_k = np.zeros((3 * k_size - 2, 3 * k_size - 2))
- # Loop over the small kernel to fill the big one
- for r in range(k_size):
- for c in range(k_size):
- big_k[2 * r:2 * r + k_size, 2 * c:2 * c + k_size] += k[r, c] * k
- # Crop the edges of the big kernel to ignore very small values and increase run time of SR
- crop = k_size // 2
- cropped_big_k = big_k[crop:-crop, crop:-crop]
- # Normalize to 1
- return cropped_big_k / cropped_big_k.sum()
-
-
-def anisotropic_Gaussian(ksize=15, theta=np.pi, l1=6, l2=6):
- """ generate an anisotropic Gaussian kernel
- Args:
- ksize : e.g., 15, kernel size
- theta : [0, pi], rotation angle range
- l1 : [0.1,50], scaling of eigenvalues
- l2 : [0.1,l1], scaling of eigenvalues
- If l1 = l2, will get an isotropic Gaussian kernel.
- Returns:
- k : kernel
- """
-
- v = np.dot(np.array([[np.cos(theta), -np.sin(theta)], [np.sin(theta), np.cos(theta)]]), np.array([1., 0.]))
- V = np.array([[v[0], v[1]], [v[1], -v[0]]])
- D = np.array([[l1, 0], [0, l2]])
- Sigma = np.dot(np.dot(V, D), np.linalg.inv(V))
- k = gm_blur_kernel(mean=[0, 0], cov=Sigma, size=ksize)
-
- return k
-
-
-def gm_blur_kernel(mean, cov, size=15):
- center = size / 2.0 + 0.5
- k = np.zeros([size, size])
- for y in range(size):
- for x in range(size):
- cy = y - center + 1
- cx = x - center + 1
- k[y, x] = ss.multivariate_normal.pdf([cx, cy], mean=mean, cov=cov)
-
- k = k / np.sum(k)
- return k
-
-
-def shift_pixel(x, sf, upper_left=True):
- """shift pixel for super-resolution with different scale factors
- Args:
- x: WxHxC or WxH
- sf: scale factor
- upper_left: shift direction
- """
- h, w = x.shape[:2]
- shift = (sf - 1) * 0.5
- xv, yv = np.arange(0, w, 1.0), np.arange(0, h, 1.0)
- if upper_left:
- x1 = xv + shift
- y1 = yv + shift
- else:
- x1 = xv - shift
- y1 = yv - shift
-
- x1 = np.clip(x1, 0, w - 1)
- y1 = np.clip(y1, 0, h - 1)
-
- if x.ndim == 2:
- x = interp2d(xv, yv, x)(x1, y1)
- if x.ndim == 3:
- for i in range(x.shape[-1]):
- x[:, :, i] = interp2d(xv, yv, x[:, :, i])(x1, y1)
-
- return x
-
-
-def blur(x, k):
- '''
- x: image, NxcxHxW
- k: kernel, Nx1xhxw
- '''
- n, c = x.shape[:2]
- p1, p2 = (k.shape[-2] - 1) // 2, (k.shape[-1] - 1) // 2
- x = torch.nn.functional.pad(x, pad=(p1, p2, p1, p2), mode='replicate')
- k = k.repeat(1, c, 1, 1)
- k = k.view(-1, 1, k.shape[2], k.shape[3])
- x = x.view(1, -1, x.shape[2], x.shape[3])
- x = torch.nn.functional.conv2d(x, k, bias=None, stride=1, padding=0, groups=n * c)
- x = x.view(n, c, x.shape[2], x.shape[3])
-
- return x
-
-
-def gen_kernel(k_size=np.array([15, 15]), scale_factor=np.array([4, 4]), min_var=0.6, max_var=10., noise_level=0):
- """"
- # modified version of https://github.com/assafshocher/BlindSR_dataset_generator
- # Kai Zhang
- # min_var = 0.175 * sf # variance of the gaussian kernel will be sampled between min_var and max_var
- # max_var = 2.5 * sf
- """
- # Set random eigen-vals (lambdas) and angle (theta) for COV matrix
- lambda_1 = min_var + np.random.rand() * (max_var - min_var)
- lambda_2 = min_var + np.random.rand() * (max_var - min_var)
- theta = np.random.rand() * np.pi # random theta
- noise = -noise_level + np.random.rand(*k_size) * noise_level * 2
-
- # Set COV matrix using Lambdas and Theta
- LAMBDA = np.diag([lambda_1, lambda_2])
- Q = np.array([[np.cos(theta), -np.sin(theta)],
- [np.sin(theta), np.cos(theta)]])
- SIGMA = Q @ LAMBDA @ Q.T
- INV_SIGMA = np.linalg.inv(SIGMA)[None, None, :, :]
-
- # Set expectation position (shifting kernel for aligned image)
- MU = k_size // 2 - 0.5 * (scale_factor - 1) # - 0.5 * (scale_factor - k_size % 2)
- MU = MU[None, None, :, None]
-
- # Create meshgrid for Gaussian
- [X, Y] = np.meshgrid(range(k_size[0]), range(k_size[1]))
- Z = np.stack([X, Y], 2)[:, :, :, None]
-
- # Calcualte Gaussian for every pixel of the kernel
- ZZ = Z - MU
- ZZ_t = ZZ.transpose(0, 1, 3, 2)
- raw_kernel = np.exp(-0.5 * np.squeeze(ZZ_t @ INV_SIGMA @ ZZ)) * (1 + noise)
-
- # shift the kernel so it will be centered
- # raw_kernel_centered = kernel_shift(raw_kernel, scale_factor)
-
- # Normalize the kernel and return
- # kernel = raw_kernel_centered / np.sum(raw_kernel_centered)
- kernel = raw_kernel / np.sum(raw_kernel)
- return kernel
-
-
-def fspecial_gaussian(hsize, sigma):
- hsize = [hsize, hsize]
- siz = [(hsize[0] - 1.0) / 2.0, (hsize[1] - 1.0) / 2.0]
- std = sigma
- [x, y] = np.meshgrid(np.arange(-siz[1], siz[1] + 1), np.arange(-siz[0], siz[0] + 1))
- arg = -(x * x + y * y) / (2 * std * std)
- h = np.exp(arg)
- h[h < scipy.finfo(float).eps * h.max()] = 0
- sumh = h.sum()
- if sumh != 0:
- h = h / sumh
- return h
-
-
-def fspecial_laplacian(alpha):
- alpha = max([0, min([alpha, 1])])
- h1 = alpha / (alpha + 1)
- h2 = (1 - alpha) / (alpha + 1)
- h = [[h1, h2, h1], [h2, -4 / (alpha + 1), h2], [h1, h2, h1]]
- h = np.array(h)
- return h
-
-
-def fspecial(filter_type, *args, **kwargs):
- '''
- python code from:
- https://github.com/ronaldosena/imagens-medicas-2/blob/40171a6c259edec7827a6693a93955de2bd39e76/Aulas/aula_2_-_uniform_filter/matlab_fspecial.py
- '''
- if filter_type == 'gaussian':
- return fspecial_gaussian(*args, **kwargs)
- if filter_type == 'laplacian':
- return fspecial_laplacian(*args, **kwargs)
-
-
-"""
-# --------------------------------------------
-# degradation models
-# --------------------------------------------
-"""
-
-
-def bicubic_degradation(x, sf=3):
- '''
- Args:
- x: HxWxC image, [0, 1]
- sf: down-scale factor
- Return:
- bicubicly downsampled LR image
- '''
- x = util.imresize_np(x, scale=1 / sf)
- return x
-
-
-def srmd_degradation(x, k, sf=3):
- ''' blur + bicubic downsampling
- Args:
- x: HxWxC image, [0, 1]
- k: hxw, double
- sf: down-scale factor
- Return:
- downsampled LR image
- Reference:
- @inproceedings{zhang2018learning,
- title={Learning a single convolutional super-resolution network for multiple degradations},
- author={Zhang, Kai and Zuo, Wangmeng and Zhang, Lei},
- booktitle={IEEE Conference on Computer Vision and Pattern Recognition},
- pages={3262--3271},
- year={2018}
- }
- '''
- x = ndimage.filters.convolve(x, np.expand_dims(k, axis=2), mode='wrap') # 'nearest' | 'mirror'
- x = bicubic_degradation(x, sf=sf)
- return x
-
-
-def dpsr_degradation(x, k, sf=3):
- ''' bicubic downsampling + blur
- Args:
- x: HxWxC image, [0, 1]
- k: hxw, double
- sf: down-scale factor
- Return:
- downsampled LR image
- Reference:
- @inproceedings{zhang2019deep,
- title={Deep Plug-and-Play Super-Resolution for Arbitrary Blur Kernels},
- author={Zhang, Kai and Zuo, Wangmeng and Zhang, Lei},
- booktitle={IEEE Conference on Computer Vision and Pattern Recognition},
- pages={1671--1681},
- year={2019}
- }
- '''
- x = bicubic_degradation(x, sf=sf)
- x = ndimage.filters.convolve(x, np.expand_dims(k, axis=2), mode='wrap')
- return x
-
-
-def classical_degradation(x, k, sf=3):
- ''' blur + downsampling
- Args:
- x: HxWxC image, [0, 1]/[0, 255]
- k: hxw, double
- sf: down-scale factor
- Return:
- downsampled LR image
- '''
- x = ndimage.filters.convolve(x, np.expand_dims(k, axis=2), mode='wrap')
- # x = filters.correlate(x, np.expand_dims(np.flip(k), axis=2))
- st = 0
- return x[st::sf, st::sf, ...]
-
-
-def add_sharpening(img, weight=0.5, radius=50, threshold=10):
- """USM sharpening. borrowed from real-ESRGAN
- Input image: I; Blurry image: B.
- 1. K = I + weight * (I - B)
- 2. Mask = 1 if abs(I - B) > threshold, else: 0
- 3. Blur mask:
- 4. Out = Mask * K + (1 - Mask) * I
- Args:
- img (Numpy array): Input image, HWC, BGR; float32, [0, 1].
- weight (float): Sharp weight. Default: 1.
- radius (float): Kernel size of Gaussian blur. Default: 50.
- threshold (int):
- """
- if radius % 2 == 0:
- radius += 1
- blur = cv2.GaussianBlur(img, (radius, radius), 0)
- residual = img - blur
- mask = np.abs(residual) * 255 > threshold
- mask = mask.astype('float32')
- soft_mask = cv2.GaussianBlur(mask, (radius, radius), 0)
-
- K = img + weight * residual
- K = np.clip(K, 0, 1)
- return soft_mask * K + (1 - soft_mask) * img
-
-
-def add_blur(img, sf=4):
- wd2 = 4.0 + sf
- wd = 2.0 + 0.2 * sf
- if random.random() < 0.5:
- l1 = wd2 * random.random()
- l2 = wd2 * random.random()
- k = anisotropic_Gaussian(ksize=2 * random.randint(2, 11) + 3, theta=random.random() * np.pi, l1=l1, l2=l2)
- else:
- k = fspecial('gaussian', 2 * random.randint(2, 11) + 3, wd * random.random())
- img = ndimage.filters.convolve(img, np.expand_dims(k, axis=2), mode='mirror')
-
- return img
-
-
-def add_resize(img, sf=4):
- rnum = np.random.rand()
- if rnum > 0.8: # up
- sf1 = random.uniform(1, 2)
- elif rnum < 0.7: # down
- sf1 = random.uniform(0.5 / sf, 1)
- else:
- sf1 = 1.0
- img = cv2.resize(img, (int(sf1 * img.shape[1]), int(sf1 * img.shape[0])), interpolation=random.choice([1, 2, 3]))
- img = np.clip(img, 0.0, 1.0)
-
- return img
-
-
-# def add_Gaussian_noise(img, noise_level1=2, noise_level2=25):
-# noise_level = random.randint(noise_level1, noise_level2)
-# rnum = np.random.rand()
-# if rnum > 0.6: # add color Gaussian noise
-# img += np.random.normal(0, noise_level / 255.0, img.shape).astype(np.float32)
-# elif rnum < 0.4: # add grayscale Gaussian noise
-# img += np.random.normal(0, noise_level / 255.0, (*img.shape[:2], 1)).astype(np.float32)
-# else: # add noise
-# L = noise_level2 / 255.
-# D = np.diag(np.random.rand(3))
-# U = orth(np.random.rand(3, 3))
-# conv = np.dot(np.dot(np.transpose(U), D), U)
-# img += np.random.multivariate_normal([0, 0, 0], np.abs(L ** 2 * conv), img.shape[:2]).astype(np.float32)
-# img = np.clip(img, 0.0, 1.0)
-# return img
-
-def add_Gaussian_noise(img, noise_level1=2, noise_level2=25):
- noise_level = random.randint(noise_level1, noise_level2)
- rnum = np.random.rand()
- if rnum > 0.6: # add color Gaussian noise
- img = img + np.random.normal(0, noise_level / 255.0, img.shape).astype(np.float32)
- elif rnum < 0.4: # add grayscale Gaussian noise
- img = img + np.random.normal(0, noise_level / 255.0, (*img.shape[:2], 1)).astype(np.float32)
- else: # add noise
- L = noise_level2 / 255.
- D = np.diag(np.random.rand(3))
- U = orth(np.random.rand(3, 3))
- conv = np.dot(np.dot(np.transpose(U), D), U)
- img = img + np.random.multivariate_normal([0, 0, 0], np.abs(L ** 2 * conv), img.shape[:2]).astype(np.float32)
- img = np.clip(img, 0.0, 1.0)
- return img
-
-
-def add_speckle_noise(img, noise_level1=2, noise_level2=25):
- noise_level = random.randint(noise_level1, noise_level2)
- img = np.clip(img, 0.0, 1.0)
- rnum = random.random()
- if rnum > 0.6:
- img += img * np.random.normal(0, noise_level / 255.0, img.shape).astype(np.float32)
- elif rnum < 0.4:
- img += img * np.random.normal(0, noise_level / 255.0, (*img.shape[:2], 1)).astype(np.float32)
- else:
- L = noise_level2 / 255.
- D = np.diag(np.random.rand(3))
- U = orth(np.random.rand(3, 3))
- conv = np.dot(np.dot(np.transpose(U), D), U)
- img += img * np.random.multivariate_normal([0, 0, 0], np.abs(L ** 2 * conv), img.shape[:2]).astype(np.float32)
- img = np.clip(img, 0.0, 1.0)
- return img
-
-
-def add_Poisson_noise(img):
- img = np.clip((img * 255.0).round(), 0, 255) / 255.
- vals = 10 ** (2 * random.random() + 2.0) # [2, 4]
- if random.random() < 0.5:
- img = np.random.poisson(img * vals).astype(np.float32) / vals
- else:
- img_gray = np.dot(img[..., :3], [0.299, 0.587, 0.114])
- img_gray = np.clip((img_gray * 255.0).round(), 0, 255) / 255.
- noise_gray = np.random.poisson(img_gray * vals).astype(np.float32) / vals - img_gray
- img += noise_gray[:, :, np.newaxis]
- img = np.clip(img, 0.0, 1.0)
- return img
-
-
-def add_JPEG_noise(img):
- quality_factor = random.randint(30, 95)
- img = cv2.cvtColor(util.single2uint(img), cv2.COLOR_RGB2BGR)
- result, encimg = cv2.imencode('.jpg', img, [int(cv2.IMWRITE_JPEG_QUALITY), quality_factor])
- img = cv2.imdecode(encimg, 1)
- img = cv2.cvtColor(util.uint2single(img), cv2.COLOR_BGR2RGB)
- return img
-
-
-def random_crop(lq, hq, sf=4, lq_patchsize=64):
- h, w = lq.shape[:2]
- rnd_h = random.randint(0, h - lq_patchsize)
- rnd_w = random.randint(0, w - lq_patchsize)
- lq = lq[rnd_h:rnd_h + lq_patchsize, rnd_w:rnd_w + lq_patchsize, :]
-
- rnd_h_H, rnd_w_H = int(rnd_h * sf), int(rnd_w * sf)
- hq = hq[rnd_h_H:rnd_h_H + lq_patchsize * sf, rnd_w_H:rnd_w_H + lq_patchsize * sf, :]
- return lq, hq
-
-
-def degradation_bsrgan(img, sf=4, lq_patchsize=72, isp_model=None):
- """
- This is the degradation model of BSRGAN from the paper
- "Designing a Practical Degradation Model for Deep Blind Image Super-Resolution"
- ----------
- img: HXWXC, [0, 1], its size should be large than (lq_patchsizexsf)x(lq_patchsizexsf)
- sf: scale factor
- isp_model: camera ISP model
- Returns
- -------
- img: low-quality patch, size: lq_patchsizeXlq_patchsizeXC, range: [0, 1]
- hq: corresponding high-quality patch, size: (lq_patchsizexsf)X(lq_patchsizexsf)XC, range: [0, 1]
- """
- isp_prob, jpeg_prob, scale2_prob = 0.25, 0.9, 0.25
- sf_ori = sf
-
- h1, w1 = img.shape[:2]
- img = img.copy()[:w1 - w1 % sf, :h1 - h1 % sf, ...] # mod crop
- h, w = img.shape[:2]
-
- if h < lq_patchsize * sf or w < lq_patchsize * sf:
- raise ValueError(f'img size ({h1}X{w1}) is too small!')
-
- hq = img.copy()
-
- if sf == 4 and random.random() < scale2_prob: # downsample1
- if np.random.rand() < 0.5:
- img = cv2.resize(img, (int(1 / 2 * img.shape[1]), int(1 / 2 * img.shape[0])),
- interpolation=random.choice([1, 2, 3]))
- else:
- img = util.imresize_np(img, 1 / 2, True)
- img = np.clip(img, 0.0, 1.0)
- sf = 2
-
- shuffle_order = random.sample(range(7), 7)
- idx1, idx2 = shuffle_order.index(2), shuffle_order.index(3)
- if idx1 > idx2: # keep downsample3 last
- shuffle_order[idx1], shuffle_order[idx2] = shuffle_order[idx2], shuffle_order[idx1]
-
- for i in shuffle_order:
-
- if i == 0:
- img = add_blur(img, sf=sf)
-
- elif i == 1:
- img = add_blur(img, sf=sf)
-
- elif i == 2:
- a, b = img.shape[1], img.shape[0]
- # downsample2
- if random.random() < 0.75:
- sf1 = random.uniform(1, 2 * sf)
- img = cv2.resize(img, (int(1 / sf1 * img.shape[1]), int(1 / sf1 * img.shape[0])),
- interpolation=random.choice([1, 2, 3]))
- else:
- k = fspecial('gaussian', 25, random.uniform(0.1, 0.6 * sf))
- k_shifted = shift_pixel(k, sf)
- k_shifted = k_shifted / k_shifted.sum() # blur with shifted kernel
- img = ndimage.filters.convolve(img, np.expand_dims(k_shifted, axis=2), mode='mirror')
- img = img[0::sf, 0::sf, ...] # nearest downsampling
- img = np.clip(img, 0.0, 1.0)
-
- elif i == 3:
- # downsample3
- img = cv2.resize(img, (int(1 / sf * a), int(1 / sf * b)), interpolation=random.choice([1, 2, 3]))
- img = np.clip(img, 0.0, 1.0)
-
- elif i == 4:
- # add Gaussian noise
- img = add_Gaussian_noise(img, noise_level1=2, noise_level2=25)
-
- elif i == 5:
- # add JPEG noise
- if random.random() < jpeg_prob:
- img = add_JPEG_noise(img)
-
- elif i == 6:
- # add processed camera sensor noise
- if random.random() < isp_prob and isp_model is not None:
- with torch.no_grad():
- img, hq = isp_model.forward(img.copy(), hq)
-
- # add final JPEG compression noise
- img = add_JPEG_noise(img)
-
- # random crop
- img, hq = random_crop(img, hq, sf_ori, lq_patchsize)
-
- return img, hq
-
-
-# todo no isp_model?
-def degradation_bsrgan_variant(image, sf=4, isp_model=None):
- """
- This is the degradation model of BSRGAN from the paper
- "Designing a Practical Degradation Model for Deep Blind Image Super-Resolution"
- ----------
- sf: scale factor
- isp_model: camera ISP model
- Returns
- -------
- img: low-quality patch, size: lq_patchsizeXlq_patchsizeXC, range: [0, 1]
- hq: corresponding high-quality patch, size: (lq_patchsizexsf)X(lq_patchsizexsf)XC, range: [0, 1]
- """
- image = util.uint2single(image)
- isp_prob, jpeg_prob, scale2_prob = 0.25, 0.9, 0.25
- sf_ori = sf
-
- h1, w1 = image.shape[:2]
- image = image.copy()[:w1 - w1 % sf, :h1 - h1 % sf, ...] # mod crop
- h, w = image.shape[:2]
-
- hq = image.copy()
-
- if sf == 4 and random.random() < scale2_prob: # downsample1
- if np.random.rand() < 0.5:
- image = cv2.resize(image, (int(1 / 2 * image.shape[1]), int(1 / 2 * image.shape[0])),
- interpolation=random.choice([1, 2, 3]))
- else:
- image = util.imresize_np(image, 1 / 2, True)
- image = np.clip(image, 0.0, 1.0)
- sf = 2
-
- shuffle_order = random.sample(range(7), 7)
- idx1, idx2 = shuffle_order.index(2), shuffle_order.index(3)
- if idx1 > idx2: # keep downsample3 last
- shuffle_order[idx1], shuffle_order[idx2] = shuffle_order[idx2], shuffle_order[idx1]
-
- for i in shuffle_order:
-
- if i == 0:
- image = add_blur(image, sf=sf)
-
- elif i == 1:
- image = add_blur(image, sf=sf)
-
- elif i == 2:
- a, b = image.shape[1], image.shape[0]
- # downsample2
- if random.random() < 0.75:
- sf1 = random.uniform(1, 2 * sf)
- image = cv2.resize(image, (int(1 / sf1 * image.shape[1]), int(1 / sf1 * image.shape[0])),
- interpolation=random.choice([1, 2, 3]))
- else:
- k = fspecial('gaussian', 25, random.uniform(0.1, 0.6 * sf))
- k_shifted = shift_pixel(k, sf)
- k_shifted = k_shifted / k_shifted.sum() # blur with shifted kernel
- image = ndimage.filters.convolve(image, np.expand_dims(k_shifted, axis=2), mode='mirror')
- image = image[0::sf, 0::sf, ...] # nearest downsampling
- image = np.clip(image, 0.0, 1.0)
-
- elif i == 3:
- # downsample3
- image = cv2.resize(image, (int(1 / sf * a), int(1 / sf * b)), interpolation=random.choice([1, 2, 3]))
- image = np.clip(image, 0.0, 1.0)
-
- elif i == 4:
- # add Gaussian noise
- image = add_Gaussian_noise(image, noise_level1=2, noise_level2=25)
-
- elif i == 5:
- # add JPEG noise
- if random.random() < jpeg_prob:
- image = add_JPEG_noise(image)
-
- # elif i == 6:
- # # add processed camera sensor noise
- # if random.random() < isp_prob and isp_model is not None:
- # with torch.no_grad():
- # img, hq = isp_model.forward(img.copy(), hq)
-
- # add final JPEG compression noise
- image = add_JPEG_noise(image)
- image = util.single2uint(image)
- example = {"image":image}
- return example
-
-
-# TODO incase there is a pickle error one needs to replace a += x with a = a + x in add_speckle_noise etc...
-def degradation_bsrgan_plus(img, sf=4, shuffle_prob=0.5, use_sharp=True, lq_patchsize=64, isp_model=None):
- """
- This is an extended degradation model by combining
- the degradation models of BSRGAN and Real-ESRGAN
- ----------
- img: HXWXC, [0, 1], its size should be large than (lq_patchsizexsf)x(lq_patchsizexsf)
- sf: scale factor
- use_shuffle: the degradation shuffle
- use_sharp: sharpening the img
- Returns
- -------
- img: low-quality patch, size: lq_patchsizeXlq_patchsizeXC, range: [0, 1]
- hq: corresponding high-quality patch, size: (lq_patchsizexsf)X(lq_patchsizexsf)XC, range: [0, 1]
- """
-
- h1, w1 = img.shape[:2]
- img = img.copy()[:w1 - w1 % sf, :h1 - h1 % sf, ...] # mod crop
- h, w = img.shape[:2]
-
- if h < lq_patchsize * sf or w < lq_patchsize * sf:
- raise ValueError(f'img size ({h1}X{w1}) is too small!')
-
- if use_sharp:
- img = add_sharpening(img)
- hq = img.copy()
-
- if random.random() < shuffle_prob:
- shuffle_order = random.sample(range(13), 13)
- else:
- shuffle_order = list(range(13))
- # local shuffle for noise, JPEG is always the last one
- shuffle_order[2:6] = random.sample(shuffle_order[2:6], len(range(2, 6)))
- shuffle_order[9:13] = random.sample(shuffle_order[9:13], len(range(9, 13)))
-
- poisson_prob, speckle_prob, isp_prob = 0.1, 0.1, 0.1
-
- for i in shuffle_order:
- if i == 0:
- img = add_blur(img, sf=sf)
- elif i == 1:
- img = add_resize(img, sf=sf)
- elif i == 2:
- img = add_Gaussian_noise(img, noise_level1=2, noise_level2=25)
- elif i == 3:
- if random.random() < poisson_prob:
- img = add_Poisson_noise(img)
- elif i == 4:
- if random.random() < speckle_prob:
- img = add_speckle_noise(img)
- elif i == 5:
- if random.random() < isp_prob and isp_model is not None:
- with torch.no_grad():
- img, hq = isp_model.forward(img.copy(), hq)
- elif i == 6:
- img = add_JPEG_noise(img)
- elif i == 7:
- img = add_blur(img, sf=sf)
- elif i == 8:
- img = add_resize(img, sf=sf)
- elif i == 9:
- img = add_Gaussian_noise(img, noise_level1=2, noise_level2=25)
- elif i == 10:
- if random.random() < poisson_prob:
- img = add_Poisson_noise(img)
- elif i == 11:
- if random.random() < speckle_prob:
- img = add_speckle_noise(img)
- elif i == 12:
- if random.random() < isp_prob and isp_model is not None:
- with torch.no_grad():
- img, hq = isp_model.forward(img.copy(), hq)
- else:
- print('check the shuffle!')
-
- # resize to desired size
- img = cv2.resize(img, (int(1 / sf * hq.shape[1]), int(1 / sf * hq.shape[0])),
- interpolation=random.choice([1, 2, 3]))
-
- # add final JPEG compression noise
- img = add_JPEG_noise(img)
-
- # random crop
- img, hq = random_crop(img, hq, sf, lq_patchsize)
-
- return img, hq
-
-
-if __name__ == '__main__':
- print("hey")
- img = util.imread_uint('utils/test.png', 3)
- print(img)
- img = util.uint2single(img)
- print(img)
- img = img[:448, :448]
- h = img.shape[0] // 4
- print("resizing to", h)
- sf = 4
- deg_fn = partial(degradation_bsrgan_variant, sf=sf)
- for i in range(20):
- print(i)
- img_lq = deg_fn(img)
- print(img_lq)
- img_lq_bicubic = albumentations.SmallestMaxSize(max_size=h, interpolation=cv2.INTER_CUBIC)(image=img)["image"]
- print(img_lq.shape)
- print("bicubic", img_lq_bicubic.shape)
- print(img_hq.shape)
- lq_nearest = cv2.resize(util.single2uint(img_lq), (int(sf * img_lq.shape[1]), int(sf * img_lq.shape[0])),
- interpolation=0)
- lq_bicubic_nearest = cv2.resize(util.single2uint(img_lq_bicubic), (int(sf * img_lq.shape[1]), int(sf * img_lq.shape[0])),
- interpolation=0)
- img_concat = np.concatenate([lq_bicubic_nearest, lq_nearest, util.single2uint(img_hq)], axis=1)
- util.imsave(img_concat, str(i) + '.png')
-
-
diff --git a/spaces/HarryLee/eCommerceImageCaptioning/fairseq/fairseq/models/fconv.py b/spaces/HarryLee/eCommerceImageCaptioning/fairseq/fairseq/models/fconv.py
deleted file mode 100644
index c99a2151014d816ec9aff6f4b27d71224dd7b4cf..0000000000000000000000000000000000000000
--- a/spaces/HarryLee/eCommerceImageCaptioning/fairseq/fairseq/models/fconv.py
+++ /dev/null
@@ -1,756 +0,0 @@
-# Copyright (c) Facebook, Inc. and its affiliates.
-#
-# This source code is licensed under the MIT license found in the
-# LICENSE file in the root directory of this source tree.
-
-import math
-
-import torch
-import torch.nn as nn
-import torch.nn.functional as F
-from fairseq import utils
-from fairseq.models import (
- FairseqEncoder,
- FairseqEncoderDecoderModel,
- FairseqIncrementalDecoder,
- register_model,
- register_model_architecture,
-)
-from fairseq.modules import (
- AdaptiveSoftmax,
- BeamableMM,
- FairseqDropout,
- GradMultiply,
- LearnedPositionalEmbedding,
- LinearizedConvolution,
-)
-
-
-@register_model("fconv")
-class FConvModel(FairseqEncoderDecoderModel):
- """
- A fully convolutional model, i.e. a convolutional encoder and a
- convolutional decoder, as described in `"Convolutional Sequence to Sequence
- Learning" (Gehring et al., 2017) `_.
-
- Args:
- encoder (FConvEncoder): the encoder
- decoder (FConvDecoder): the decoder
-
- The Convolutional model provides the following named architectures and
- command-line arguments:
-
- .. argparse::
- :ref: fairseq.models.fconv_parser
- :prog:
- """
-
- @classmethod
- def hub_models(cls):
- def moses_subword(path):
- return {
- "path": path,
- "tokenizer": "moses",
- "bpe": "subword_nmt",
- }
-
- return {
- "conv.wmt14.en-fr": moses_subword(
- "https://dl.fbaipublicfiles.com/fairseq/models/wmt14.v2.en-fr.fconv-py.tar.bz2"
- ),
- "conv.wmt14.en-de": moses_subword(
- "https://dl.fbaipublicfiles.com/fairseq/models/wmt14.en-de.fconv-py.tar.bz2"
- ),
- "conv.wmt17.en-de": moses_subword(
- "https://dl.fbaipublicfiles.com/fairseq/models/wmt17.v2.en-de.fconv-py.tar.bz2"
- ),
- }
-
- def __init__(self, encoder, decoder):
- super().__init__(encoder, decoder)
- self.encoder.num_attention_layers = sum(
- layer is not None for layer in decoder.attention
- )
-
- @staticmethod
- def add_args(parser):
- """Add model-specific arguments to the parser."""
- # fmt: off
- parser.add_argument('--dropout', type=float, metavar='D',
- help='dropout probability')
- parser.add_argument('--encoder-embed-dim', type=int, metavar='N',
- help='encoder embedding dimension')
- parser.add_argument('--encoder-embed-path', type=str, metavar='STR',
- help='path to pre-trained encoder embedding')
- parser.add_argument('--encoder-layers', type=str, metavar='EXPR',
- help='encoder layers [(dim, kernel_size), ...]')
- parser.add_argument('--decoder-embed-dim', type=int, metavar='N',
- help='decoder embedding dimension')
- parser.add_argument('--decoder-embed-path', type=str, metavar='STR',
- help='path to pre-trained decoder embedding')
- parser.add_argument('--decoder-layers', type=str, metavar='EXPR',
- help='decoder layers [(dim, kernel_size), ...]')
- parser.add_argument('--decoder-out-embed-dim', type=int, metavar='N',
- help='decoder output embedding dimension')
- parser.add_argument('--decoder-attention', type=str, metavar='EXPR',
- help='decoder attention [True, ...]')
- parser.add_argument('--share-input-output-embed', action='store_true',
- help='share input and output embeddings (requires'
- ' --decoder-out-embed-dim and --decoder-embed-dim'
- ' to be equal)')
- # fmt: on
-
- @classmethod
- def build_model(cls, args, task):
- """Build a new model instance."""
- # make sure that all args are properly defaulted (in case there are any new ones)
- base_architecture(args)
-
- encoder_embed_dict = None
- if args.encoder_embed_path:
- encoder_embed_dict = utils.parse_embedding(args.encoder_embed_path)
- utils.print_embed_overlap(encoder_embed_dict, task.source_dictionary)
-
- decoder_embed_dict = None
- if args.decoder_embed_path:
- decoder_embed_dict = utils.parse_embedding(args.decoder_embed_path)
- utils.print_embed_overlap(decoder_embed_dict, task.target_dictionary)
-
- encoder = FConvEncoder(
- dictionary=task.source_dictionary,
- embed_dim=args.encoder_embed_dim,
- embed_dict=encoder_embed_dict,
- convolutions=eval(args.encoder_layers),
- dropout=args.dropout,
- max_positions=args.max_source_positions,
- )
- decoder = FConvDecoder(
- dictionary=task.target_dictionary,
- embed_dim=args.decoder_embed_dim,
- embed_dict=decoder_embed_dict,
- convolutions=eval(args.decoder_layers),
- out_embed_dim=args.decoder_out_embed_dim,
- attention=eval(args.decoder_attention),
- dropout=args.dropout,
- max_positions=args.max_target_positions,
- share_embed=args.share_input_output_embed,
- )
- return FConvModel(encoder, decoder)
-
-
-class FConvEncoder(FairseqEncoder):
- """
- Convolutional encoder consisting of `len(convolutions)` layers.
-
- Args:
- dictionary (~fairseq.data.Dictionary): encoding dictionary
- embed_dim (int, optional): embedding dimension
- embed_dict (str, optional): filename from which to load pre-trained
- embeddings
- max_positions (int, optional): maximum supported input sequence length
- convolutions (list, optional): the convolutional layer structure. Each
- list item `i` corresponds to convolutional layer `i`. Layers are
- given as ``(out_channels, kernel_width, [residual])``. Residual
- connections are added between layers when ``residual=1`` (which is
- the default behavior).
- dropout (float, optional): dropout to be applied before each conv layer
- """
-
- def __init__(
- self,
- dictionary,
- embed_dim=512,
- embed_dict=None,
- max_positions=1024,
- convolutions=((512, 3),) * 20,
- dropout=0.1,
- ):
- super().__init__(dictionary)
- self.dropout_module = FairseqDropout(
- dropout, module_name=self.__class__.__name__
- )
- self.num_attention_layers = None
-
- num_embeddings = len(dictionary)
- self.padding_idx = dictionary.pad()
- self.embed_tokens = Embedding(num_embeddings, embed_dim, self.padding_idx)
- if embed_dict:
- self.embed_tokens = utils.load_embedding(
- embed_dict, self.dictionary, self.embed_tokens
- )
-
- self.embed_positions = PositionalEmbedding(
- max_positions,
- embed_dim,
- self.padding_idx,
- )
-
- convolutions = extend_conv_spec(convolutions)
- in_channels = convolutions[0][0]
- self.fc1 = Linear(embed_dim, in_channels, dropout=dropout)
- self.projections = nn.ModuleList()
- self.convolutions = nn.ModuleList()
- self.residuals = []
-
- layer_in_channels = [in_channels]
- for _, (out_channels, kernel_size, residual) in enumerate(convolutions):
- if residual == 0:
- residual_dim = out_channels
- else:
- residual_dim = layer_in_channels[-residual]
- self.projections.append(
- Linear(residual_dim, out_channels)
- if residual_dim != out_channels
- else None
- )
- if kernel_size % 2 == 1:
- padding = kernel_size // 2
- else:
- padding = 0
- self.convolutions.append(
- ConvTBC(
- in_channels,
- out_channels * 2,
- kernel_size,
- dropout=dropout,
- padding=padding,
- )
- )
- self.residuals.append(residual)
- in_channels = out_channels
- layer_in_channels.append(out_channels)
- self.fc2 = Linear(in_channels, embed_dim)
-
- def forward(self, src_tokens, src_lengths):
- """
- Args:
- src_tokens (LongTensor): tokens in the source language of shape
- `(batch, src_len)`
- src_lengths (LongTensor): lengths of each source sentence of shape
- `(batch)`
-
- Returns:
- dict:
- - **encoder_out** (tuple): a tuple with two elements, where the
- first element is the last encoder layer's output and the
- second element is the same quantity summed with the input
- embedding (used for attention). The shape of both tensors is
- `(batch, src_len, embed_dim)`.
- - **encoder_padding_mask** (ByteTensor): the positions of
- padding elements of shape `(batch, src_len)`
- """
- # embed tokens and positions
- x = self.embed_tokens(src_tokens) + self.embed_positions(src_tokens)
- x = self.dropout_module(x)
- input_embedding = x
-
- # project to size of convolution
- x = self.fc1(x)
-
- # used to mask padding in input
- encoder_padding_mask = src_tokens.eq(self.padding_idx).t() # -> T x B
- if not encoder_padding_mask.any():
- encoder_padding_mask = None
-
- # B x T x C -> T x B x C
- x = x.transpose(0, 1)
-
- residuals = [x]
- # temporal convolutions
- for proj, conv, res_layer in zip(
- self.projections, self.convolutions, self.residuals
- ):
- if res_layer > 0:
- residual = residuals[-res_layer]
- residual = residual if proj is None else proj(residual)
- else:
- residual = None
-
- if encoder_padding_mask is not None:
- x = x.masked_fill(encoder_padding_mask.unsqueeze(-1), 0)
-
- x = self.dropout_module(x)
- if conv.kernel_size[0] % 2 == 1:
- # padding is implicit in the conv
- x = conv(x)
- else:
- padding_l = (conv.kernel_size[0] - 1) // 2
- padding_r = conv.kernel_size[0] // 2
- x = F.pad(x, (0, 0, 0, 0, padding_l, padding_r))
- x = conv(x)
- x = F.glu(x, dim=2)
-
- if residual is not None:
- x = (x + residual) * math.sqrt(0.5)
- residuals.append(x)
-
- # T x B x C -> B x T x C
- x = x.transpose(1, 0)
-
- # project back to size of embedding
- x = self.fc2(x)
-
- if encoder_padding_mask is not None:
- encoder_padding_mask = encoder_padding_mask.t() # -> B x T
- x = x.masked_fill(encoder_padding_mask.unsqueeze(-1), 0)
-
- # scale gradients (this only affects backward, not forward)
- x = GradMultiply.apply(x, 1.0 / (2.0 * self.num_attention_layers))
-
- # add output to input embedding for attention
- y = (x + input_embedding) * math.sqrt(0.5)
-
- return {
- "encoder_out": (x, y),
- "encoder_padding_mask": encoder_padding_mask, # B x T
- }
-
- def reorder_encoder_out(self, encoder_out, new_order):
- if encoder_out["encoder_out"] is not None:
- encoder_out["encoder_out"] = (
- encoder_out["encoder_out"][0].index_select(0, new_order),
- encoder_out["encoder_out"][1].index_select(0, new_order),
- )
- if encoder_out["encoder_padding_mask"] is not None:
- encoder_out["encoder_padding_mask"] = encoder_out[
- "encoder_padding_mask"
- ].index_select(0, new_order)
- return encoder_out
-
- def max_positions(self):
- """Maximum input length supported by the encoder."""
- return self.embed_positions.max_positions
-
-
-class AttentionLayer(nn.Module):
- def __init__(self, conv_channels, embed_dim, bmm=None):
- super().__init__()
- # projects from output of convolution to embedding dimension
- self.in_projection = Linear(conv_channels, embed_dim)
- # projects from embedding dimension to convolution size
- self.out_projection = Linear(embed_dim, conv_channels)
-
- self.bmm = bmm if bmm is not None else torch.bmm
-
- def forward(self, x, target_embedding, encoder_out, encoder_padding_mask):
- residual = x
-
- # attention
- x = (self.in_projection(x) + target_embedding) * math.sqrt(0.5)
- x = self.bmm(x, encoder_out[0])
-
- # don't attend over padding
- if encoder_padding_mask is not None:
- x = (
- x.float()
- .masked_fill(encoder_padding_mask.unsqueeze(1), float("-inf"))
- .type_as(x)
- ) # FP16 support: cast to float and back
-
- # softmax over last dim
- sz = x.size()
- x = F.softmax(x.view(sz[0] * sz[1], sz[2]), dim=1)
- x = x.view(sz)
- attn_scores = x
-
- x = self.bmm(x, encoder_out[1])
-
- # scale attention output (respecting potentially different lengths)
- s = encoder_out[1].size(1)
- if encoder_padding_mask is None:
- x = x * (s * math.sqrt(1.0 / s))
- else:
- s = s - encoder_padding_mask.type_as(x).sum(
- dim=1, keepdim=True
- ) # exclude padding
- s = s.unsqueeze(-1)
- x = x * (s * s.rsqrt())
-
- # project back
- x = (self.out_projection(x) + residual) * math.sqrt(0.5)
- return x, attn_scores
-
- def make_generation_fast_(self, beamable_mm_beam_size=None, **kwargs):
- """Replace torch.bmm with BeamableMM."""
- if beamable_mm_beam_size is not None:
- del self.bmm
- self.add_module("bmm", BeamableMM(beamable_mm_beam_size))
-
-
-class FConvDecoder(FairseqIncrementalDecoder):
- """Convolutional decoder"""
-
- def __init__(
- self,
- dictionary,
- embed_dim=512,
- embed_dict=None,
- out_embed_dim=256,
- max_positions=1024,
- convolutions=((512, 3),) * 20,
- attention=True,
- dropout=0.1,
- share_embed=False,
- positional_embeddings=True,
- adaptive_softmax_cutoff=None,
- adaptive_softmax_dropout=0.0,
- ):
- super().__init__(dictionary)
- self.register_buffer("version", torch.Tensor([2]))
- self.dropout_module = FairseqDropout(
- dropout, module_name=self.__class__.__name__
- )
- self.need_attn = True
-
- convolutions = extend_conv_spec(convolutions)
- in_channels = convolutions[0][0]
- if isinstance(attention, bool):
- # expand True into [True, True, ...] and do the same with False
- attention = [attention] * len(convolutions)
- if not isinstance(attention, list) or len(attention) != len(convolutions):
- raise ValueError(
- "Attention is expected to be a list of booleans of "
- "length equal to the number of layers."
- )
-
- num_embeddings = len(dictionary)
- padding_idx = dictionary.pad()
- self.embed_tokens = Embedding(num_embeddings, embed_dim, padding_idx)
- if embed_dict:
- self.embed_tokens = utils.load_embedding(
- embed_dict, self.dictionary, self.embed_tokens
- )
-
- self.embed_positions = (
- PositionalEmbedding(
- max_positions,
- embed_dim,
- padding_idx,
- )
- if positional_embeddings
- else None
- )
-
- self.fc1 = Linear(embed_dim, in_channels, dropout=dropout)
- self.projections = nn.ModuleList()
- self.convolutions = nn.ModuleList()
- self.attention = nn.ModuleList()
- self.residuals = []
-
- layer_in_channels = [in_channels]
- for i, (out_channels, kernel_size, residual) in enumerate(convolutions):
- if residual == 0:
- residual_dim = out_channels
- else:
- residual_dim = layer_in_channels[-residual]
- self.projections.append(
- Linear(residual_dim, out_channels)
- if residual_dim != out_channels
- else None
- )
- self.convolutions.append(
- LinearizedConv1d(
- in_channels,
- out_channels * 2,
- kernel_size,
- padding=(kernel_size - 1),
- dropout=dropout,
- )
- )
- self.attention.append(
- AttentionLayer(out_channels, embed_dim) if attention[i] else None
- )
- self.residuals.append(residual)
- in_channels = out_channels
- layer_in_channels.append(out_channels)
-
- self.adaptive_softmax = None
- self.fc2 = self.fc3 = None
-
- if adaptive_softmax_cutoff is not None:
- assert not share_embed
- self.adaptive_softmax = AdaptiveSoftmax(
- num_embeddings,
- in_channels,
- adaptive_softmax_cutoff,
- dropout=adaptive_softmax_dropout,
- )
- else:
- self.fc2 = Linear(in_channels, out_embed_dim)
- if share_embed:
- assert out_embed_dim == embed_dim, (
- "Shared embed weights implies same dimensions "
- " out_embed_dim={} vs embed_dim={}".format(out_embed_dim, embed_dim)
- )
- self.fc3 = nn.Linear(out_embed_dim, num_embeddings)
- self.fc3.weight = self.embed_tokens.weight
- else:
- self.fc3 = Linear(out_embed_dim, num_embeddings, dropout=dropout)
-
- def forward(
- self, prev_output_tokens, encoder_out=None, incremental_state=None, **unused
- ):
- if encoder_out is not None:
- encoder_padding_mask = encoder_out["encoder_padding_mask"]
- encoder_out = encoder_out["encoder_out"]
-
- # split and transpose encoder outputs
- encoder_a, encoder_b = self._split_encoder_out(
- encoder_out, incremental_state
- )
-
- if self.embed_positions is not None:
- pos_embed = self.embed_positions(prev_output_tokens, incremental_state)
- else:
- pos_embed = 0
-
- if incremental_state is not None:
- prev_output_tokens = prev_output_tokens[:, -1:]
- x = self._embed_tokens(prev_output_tokens, incremental_state)
-
- # embed tokens and combine with positional embeddings
- x += pos_embed
- x = self.dropout_module(x)
- target_embedding = x
-
- # project to size of convolution
- x = self.fc1(x)
-
- # B x T x C -> T x B x C
- x = self._transpose_if_training(x, incremental_state)
-
- # temporal convolutions
- avg_attn_scores = None
- num_attn_layers = len(self.attention)
- residuals = [x]
- for proj, conv, attention, res_layer in zip(
- self.projections, self.convolutions, self.attention, self.residuals
- ):
- if res_layer > 0:
- residual = residuals[-res_layer]
- residual = residual if proj is None else proj(residual)
- else:
- residual = None
-
- x = self.dropout_module(x)
- x = conv(x, incremental_state)
- x = F.glu(x, dim=2)
-
- # attention
- if attention is not None:
- x = self._transpose_if_training(x, incremental_state)
-
- x, attn_scores = attention(
- x, target_embedding, (encoder_a, encoder_b), encoder_padding_mask
- )
-
- if not self.training and self.need_attn:
- attn_scores = attn_scores / num_attn_layers
- if avg_attn_scores is None:
- avg_attn_scores = attn_scores
- else:
- avg_attn_scores.add_(attn_scores)
-
- x = self._transpose_if_training(x, incremental_state)
-
- # residual
- if residual is not None:
- x = (x + residual) * math.sqrt(0.5)
- residuals.append(x)
-
- # T x B x C -> B x T x C
- x = self._transpose_if_training(x, incremental_state)
-
- # project back to size of vocabulary if not using adaptive softmax
- if self.fc2 is not None and self.fc3 is not None:
- x = self.fc2(x)
- x = self.dropout_module(x)
- x = self.fc3(x)
-
- return x, avg_attn_scores
-
- def reorder_incremental_state(self, incremental_state, new_order):
- super().reorder_incremental_state(incremental_state, new_order)
- encoder_out = utils.get_incremental_state(
- self, incremental_state, "encoder_out"
- )
- if encoder_out is not None:
- encoder_out = tuple(eo.index_select(0, new_order) for eo in encoder_out)
- utils.set_incremental_state(
- self, incremental_state, "encoder_out", encoder_out
- )
-
- def max_positions(self):
- """Maximum output length supported by the decoder."""
- return (
- self.embed_positions.max_positions
- if self.embed_positions is not None
- else float("inf")
- )
-
- def upgrade_state_dict(self, state_dict):
- if utils.item(state_dict.get("decoder.version", torch.Tensor([1]))[0]) < 2:
- # old models use incorrect weight norm dimension
- for i, conv in enumerate(self.convolutions):
- # reconfigure weight norm
- nn.utils.remove_weight_norm(conv)
- self.convolutions[i] = nn.utils.weight_norm(conv, dim=0)
- state_dict["decoder.version"] = torch.Tensor([1])
- return state_dict
-
- def make_generation_fast_(self, need_attn=False, **kwargs):
- self.need_attn = need_attn
-
- def _embed_tokens(self, tokens, incremental_state):
- if incremental_state is not None:
- # keep only the last token for incremental forward pass
- tokens = tokens[:, -1:]
- return self.embed_tokens(tokens)
-
- def _split_encoder_out(self, encoder_out, incremental_state):
- """Split and transpose encoder outputs.
-
- This is cached when doing incremental inference.
- """
- cached_result = utils.get_incremental_state(
- self, incremental_state, "encoder_out"
- )
- if cached_result is not None:
- return cached_result
-
- # transpose only once to speed up attention layers
- encoder_a, encoder_b = encoder_out
- encoder_a = encoder_a.transpose(1, 2).contiguous()
- result = (encoder_a, encoder_b)
-
- if incremental_state is not None:
- utils.set_incremental_state(self, incremental_state, "encoder_out", result)
- return result
-
- def _transpose_if_training(self, x, incremental_state):
- if incremental_state is None:
- x = x.transpose(0, 1)
- return x
-
-
-def extend_conv_spec(convolutions):
- """
- Extends convolutional spec that is a list of tuples of 2 or 3 parameters
- (kernel size, dim size and optionally how many layers behind to look for residual)
- to default the residual propagation param if it is not specified
- """
- extended = []
- for spec in convolutions:
- if len(spec) == 3:
- extended.append(spec)
- elif len(spec) == 2:
- extended.append(spec + (1,))
- else:
- raise Exception(
- "invalid number of parameters in convolution spec "
- + str(spec)
- + ". expected 2 or 3"
- )
- return tuple(extended)
-
-
-def Embedding(num_embeddings, embedding_dim, padding_idx):
- m = nn.Embedding(num_embeddings, embedding_dim, padding_idx=padding_idx)
- nn.init.normal_(m.weight, 0, 0.1)
- nn.init.constant_(m.weight[padding_idx], 0)
- return m
-
-
-def PositionalEmbedding(num_embeddings, embedding_dim, padding_idx):
- m = LearnedPositionalEmbedding(num_embeddings, embedding_dim, padding_idx)
- nn.init.normal_(m.weight, 0, 0.1)
- nn.init.constant_(m.weight[padding_idx], 0)
- return m
-
-
-def Linear(in_features, out_features, dropout=0.0):
- """Weight-normalized Linear layer (input: N x T x C)"""
- m = nn.Linear(in_features, out_features)
- nn.init.normal_(m.weight, mean=0, std=math.sqrt((1 - dropout) / in_features))
- nn.init.constant_(m.bias, 0)
- return nn.utils.weight_norm(m)
-
-
-def LinearizedConv1d(in_channels, out_channels, kernel_size, dropout=0.0, **kwargs):
- """Weight-normalized Conv1d layer optimized for decoding"""
- m = LinearizedConvolution(in_channels, out_channels, kernel_size, **kwargs)
- std = math.sqrt((4 * (1.0 - dropout)) / (m.kernel_size[0] * in_channels))
- nn.init.normal_(m.weight, mean=0, std=std)
- nn.init.constant_(m.bias, 0)
- return nn.utils.weight_norm(m, dim=2)
-
-
-def ConvTBC(in_channels, out_channels, kernel_size, dropout=0.0, **kwargs):
- """Weight-normalized Conv1d layer"""
- from fairseq.modules import ConvTBC
-
- m = ConvTBC(in_channels, out_channels, kernel_size, **kwargs)
- std = math.sqrt((4 * (1.0 - dropout)) / (m.kernel_size[0] * in_channels))
- nn.init.normal_(m.weight, mean=0, std=std)
- nn.init.constant_(m.bias, 0)
- return nn.utils.weight_norm(m, dim=2)
-
-
-@register_model_architecture("fconv", "fconv")
-def base_architecture(args):
- args.dropout = getattr(args, "dropout", 0.1)
- args.encoder_embed_dim = getattr(args, "encoder_embed_dim", 512)
- args.encoder_embed_path = getattr(args, "encoder_embed_path", None)
- args.encoder_layers = getattr(args, "encoder_layers", "[(512, 3)] * 20")
- args.decoder_embed_dim = getattr(args, "decoder_embed_dim", 512)
- args.decoder_embed_path = getattr(args, "decoder_embed_path", None)
- args.decoder_layers = getattr(args, "decoder_layers", "[(512, 3)] * 20")
- args.decoder_out_embed_dim = getattr(args, "decoder_out_embed_dim", 256)
- args.decoder_attention = getattr(args, "decoder_attention", "True")
- args.share_input_output_embed = getattr(args, "share_input_output_embed", False)
-
-
-@register_model_architecture("fconv", "fconv_iwslt_de_en")
-def fconv_iwslt_de_en(args):
- args.encoder_embed_dim = getattr(args, "encoder_embed_dim", 256)
- args.encoder_layers = getattr(args, "encoder_layers", "[(256, 3)] * 4")
- args.decoder_embed_dim = getattr(args, "decoder_embed_dim", 256)
- args.decoder_layers = getattr(args, "decoder_layers", "[(256, 3)] * 3")
- args.decoder_out_embed_dim = getattr(args, "decoder_out_embed_dim", 256)
- base_architecture(args)
-
-
-@register_model_architecture("fconv", "fconv_wmt_en_ro")
-def fconv_wmt_en_ro(args):
- args.decoder_out_embed_dim = getattr(args, "decoder_out_embed_dim", 512)
- base_architecture(args)
-
-
-@register_model_architecture("fconv", "fconv_wmt_en_de")
-def fconv_wmt_en_de(args):
- convs = "[(512, 3)] * 9" # first 9 layers have 512 units
- convs += " + [(1024, 3)] * 4" # next 4 layers have 1024 units
- convs += " + [(2048, 1)] * 2" # final 2 layers use 1x1 convolutions
-
- args.encoder_embed_dim = getattr(args, "encoder_embed_dim", 768)
- args.encoder_layers = getattr(args, "encoder_layers", convs)
- args.decoder_embed_dim = getattr(args, "decoder_embed_dim", 768)
- args.decoder_layers = getattr(args, "decoder_layers", convs)
- args.decoder_out_embed_dim = getattr(args, "decoder_out_embed_dim", 512)
- base_architecture(args)
-
-
-@register_model_architecture("fconv", "fconv_wmt_en_fr")
-def fconv_wmt_en_fr(args):
- convs = "[(512, 3)] * 6" # first 6 layers have 512 units
- convs += " + [(768, 3)] * 4" # next 4 layers have 768 units
- convs += " + [(1024, 3)] * 3" # next 3 layers have 1024 units
- convs += " + [(2048, 1)] * 1" # next 1 layer uses 1x1 convolutions
- convs += " + [(4096, 1)] * 1" # final 1 layer uses 1x1 convolutions
-
- args.encoder_embed_dim = getattr(args, "encoder_embed_dim", 768)
- args.encoder_layers = getattr(args, "encoder_layers", convs)
- args.decoder_embed_dim = getattr(args, "decoder_embed_dim", 768)
- args.decoder_layers = getattr(args, "decoder_layers", convs)
- args.decoder_out_embed_dim = getattr(args, "decoder_out_embed_dim", 512)
- base_architecture(args)
diff --git a/spaces/Hina4867/bingo/src/components/ui/sheet.tsx b/spaces/Hina4867/bingo/src/components/ui/sheet.tsx
deleted file mode 100644
index c9f5ce0f81a91067bb013e988a07eb1e6bf6953b..0000000000000000000000000000000000000000
--- a/spaces/Hina4867/bingo/src/components/ui/sheet.tsx
+++ /dev/null
@@ -1,122 +0,0 @@
-'use client'
-
-import * as React from 'react'
-import * as SheetPrimitive from '@radix-ui/react-dialog'
-
-import { cn } from '@/lib/utils'
-import { IconClose } from '@/components/ui/icons'
-
-const Sheet = SheetPrimitive.Root
-
-const SheetTrigger = SheetPrimitive.Trigger
-
-const SheetClose = SheetPrimitive.Close
-
-const SheetPortal = ({
- className,
- children,
- ...props
-}: SheetPrimitive.DialogPortalProps) => (
-
- {children}
-
-)
-SheetPortal.displayName = SheetPrimitive.Portal.displayName
-
-const SheetOverlay = React.forwardRef<
- React.ElementRef,
- React.ComponentPropsWithoutRef
->(({ className, children, ...props }, ref) => (
-
-))
-SheetOverlay.displayName = SheetPrimitive.Overlay.displayName
-
-const SheetContent = React.forwardRef<
- React.ElementRef,
- React.ComponentPropsWithoutRef
->(({ className, children, ...props }, ref) => (
-
-
- {children}
-
-
- Close
-
-
-
-))
-SheetContent.displayName = SheetPrimitive.Content.displayName
-
-const SheetHeader = ({
- className,
- ...props
-}: React.HTMLAttributes) => (
-
-)
-SheetHeader.displayName = 'SheetHeader'
-
-const SheetFooter = ({
- className,
- ...props
-}: React.HTMLAttributes) => (
-
-)
-SheetFooter.displayName = 'SheetFooter'
-
-const SheetTitle = React.forwardRef<
- React.ElementRef,
- React.ComponentPropsWithoutRef
->(({ className, ...props }, ref) => (
-
-))
-SheetTitle.displayName = SheetPrimitive.Title.displayName
-
-const SheetDescription = React.forwardRef<
- React.ElementRef,
- React.ComponentPropsWithoutRef
->(({ className, ...props }, ref) => (
-
-))
-SheetDescription.displayName = SheetPrimitive.Description.displayName
-
-export {
- Sheet,
- SheetTrigger,
- SheetClose,
- SheetContent,
- SheetHeader,
- SheetFooter,
- SheetTitle,
- SheetDescription
-}
diff --git a/spaces/Hina4867/bingo/src/components/user-menu.tsx b/spaces/Hina4867/bingo/src/components/user-menu.tsx
deleted file mode 100644
index 9bd1edc9cf9f39b63629b021f0c1186b1a7c1341..0000000000000000000000000000000000000000
--- a/spaces/Hina4867/bingo/src/components/user-menu.tsx
+++ /dev/null
@@ -1,113 +0,0 @@
-'use client'
-
-import { useEffect, useState } from 'react'
-import Image from 'next/image'
-import { toast } from 'react-hot-toast'
-import { Button } from '@/components/ui/button'
-import pkg from '../../package.json'
-import {
- DropdownMenu,
- DropdownMenuContent,
- DropdownMenuItem,
- DropdownMenuSeparator,
- DropdownMenuTrigger
-} from '@/components/ui/dropdown-menu'
-import { IconCopy, IconExternalLink, IconGitHub } from '@/components/ui/icons'
-import SettingIcon from '@/assets/images/settings.svg'
-import { useCopyToClipboard } from '@/lib/hooks/use-copy-to-clipboard'
-
-export function UserMenu() {
- const [host, setHost] = useState('')
- const { isCopied, copyToClipboard } = useCopyToClipboard({ timeout: 2000 })
- useEffect(() => {
- setHost(location.host)
- }, [])
-
- useEffect(() => {
- if (isCopied) {
- toast.success('复制成功')
- }
- }, [isCopied])
- return (
-
")
-
- chatbot = gr.Chatbot().style(height=250)
- with gr.Row().style():
- with gr.Column(scale=0.85):
- msg = gr.Textbox(
- show_label=False,
- placeholder="Enter text and press enter.",
- lines=1,
- ).style(container=False)
- with gr.Column(scale=0.15, min_width=0):
- btn2 = gr.Button("Send").style(full_height=True)
- gr.Examples(
- examples=["Who is the first man who landed on the moon?",
- "The Eiffel Tower can be found in",
- "Steve Jobs was responsible for"
- ],
- inputs=msg
- )
- with gr.Column():
- gr.Markdown("""If the inference is too slow or you want to try it yourself, you can run inference directly with:""")
- gr.Code("""from transformers import AutoModelForCausalLM, AutoTokenizer
-
-model = AutoModelForCausalLM.from_pretrained("EleuterAI/gpt-j-6B")
-tokenizer = AutoTokenizer.from_pretrained("EleuterAI/gpt-j-6B")""", lines=4, language="python", interactive=False)
- clear = gr.Button("Clear")
- msg.submit(predict, [msg, chatbot], [msg, chatbot])
- btn2.click(predict, [msg, chatbot], [msg, chatbot])
- clear.click(lambda: None, None, chatbot, queue=False)
-
-if __name__ == "__main__":
- demo.launch()
\ No newline at end of file
diff --git a/spaces/RMXK/RVC_HFF/demucs/__init__.py b/spaces/RMXK/RVC_HFF/demucs/__init__.py
deleted file mode 100644
index d4182e356427e1b05a79f8da641c70bb732514fa..0000000000000000000000000000000000000000
--- a/spaces/RMXK/RVC_HFF/demucs/__init__.py
+++ /dev/null
@@ -1,7 +0,0 @@
-# Copyright (c) Facebook, Inc. and its affiliates.
-# All rights reserved.
-#
-# This source code is licensed under the license found in the
-# LICENSE file in the root directory of this source tree.
-
-__version__ = "2.0.3"
diff --git a/spaces/RamAnanth1/ControlNet/README.md b/spaces/RamAnanth1/ControlNet/README.md
deleted file mode 100644
index ce5e9ca81036d6c52d827c0e879d35219895c10f..0000000000000000000000000000000000000000
--- a/spaces/RamAnanth1/ControlNet/README.md
+++ /dev/null
@@ -1,14 +0,0 @@
----
-title: ControlNet
-emoji: 🦀
-colorFrom: blue
-colorTo: green
-sdk: gradio
-sdk_version: 3.18.0
-app_file: app.py
-pinned: true
-tags:
-- making-demos
----
-
-Check out the configuration reference at https://huggingface.co/docs/hub/spaces-config-reference
\ No newline at end of file
diff --git a/spaces/RamAnanth1/videocrafter/lvdm/models/modules/distributions.py b/spaces/RamAnanth1/videocrafter/lvdm/models/modules/distributions.py
deleted file mode 100644
index 06cabc07d500351fb52a180c4acae9093e936dab..0000000000000000000000000000000000000000
--- a/spaces/RamAnanth1/videocrafter/lvdm/models/modules/distributions.py
+++ /dev/null
@@ -1,76 +0,0 @@
-import torch
-import numpy as np
-
-
-class DiagonalGaussianDistribution(object):
- def __init__(self, parameters, deterministic=False):
- self.parameters = parameters
- self.mean, self.logvar = torch.chunk(parameters, 2, dim=1)
- self.logvar = torch.clamp(self.logvar, -30.0, 20.0)
- self.deterministic = deterministic
- self.std = torch.exp(0.5 * self.logvar)
- self.var = torch.exp(self.logvar)
- if self.deterministic:
- self.var = self.std = torch.zeros_like(self.mean).to(device=self.parameters.device)
-
- def sample(self, noise=None):
- if noise is None:
- noise = torch.randn(self.mean.shape)
-
- x = self.mean + self.std * noise.to(device=self.parameters.device)
- return x
-
- def kl(self, other=None):
- if self.deterministic:
- return torch.Tensor([0.])
- else:
- if other is None:
- return 0.5 * torch.sum(torch.pow(self.mean, 2)
- + self.var - 1.0 - self.logvar,
- dim=[1, 2, 3])
- else:
- return 0.5 * torch.sum(
- torch.pow(self.mean - other.mean, 2) / other.var
- + self.var / other.var - 1.0 - self.logvar + other.logvar,
- dim=[1, 2, 3])
-
- def nll(self, sample, dims=[1,2,3]):
- if self.deterministic:
- return torch.Tensor([0.])
- logtwopi = np.log(2.0 * np.pi)
- return 0.5 * torch.sum(
- logtwopi + self.logvar + torch.pow(sample - self.mean, 2) / self.var,
- dim=dims)
-
- def mode(self):
- return self.mean
-
-
-def normal_kl(mean1, logvar1, mean2, logvar2):
- """
- source: https://github.com/openai/guided-diffusion/blob/27c20a8fab9cb472df5d6bdd6c8d11c8f430b924/guided_diffusion/losses.py#L12
- Compute the KL divergence between two gaussians.
- Shapes are automatically broadcasted, so batches can be compared to
- scalars, among other use cases.
- """
- tensor = None
- for obj in (mean1, logvar1, mean2, logvar2):
- if isinstance(obj, torch.Tensor):
- tensor = obj
- break
- assert tensor is not None, "at least one argument must be a Tensor"
-
- # Force variances to be Tensors. Broadcasting helps convert scalars to
- # Tensors, but it does not work for torch.exp().
- logvar1, logvar2 = [
- x if isinstance(x, torch.Tensor) else torch.tensor(x).to(tensor)
- for x in (logvar1, logvar2)
- ]
-
- return 0.5 * (
- -1.0
- + logvar2
- - logvar1
- + torch.exp(logvar1 - logvar2)
- + ((mean1 - mean2) ** 2) * torch.exp(-logvar2)
- )
diff --git a/spaces/Rardilit/Rardilit-Ciffusion_v0.1/README.md b/spaces/Rardilit/Rardilit-Ciffusion_v0.1/README.md
deleted file mode 100644
index f9a08e7c13a7fa8d9fdf0899b4e318a0b5f7f013..0000000000000000000000000000000000000000
--- a/spaces/Rardilit/Rardilit-Ciffusion_v0.1/README.md
+++ /dev/null
@@ -1,13 +0,0 @@
----
-title: Rardilit-Ciffusion V0.1
-emoji: 👀
-colorFrom: yellow
-colorTo: green
-sdk: gradio
-sdk_version: 3.32.0
-app_file: app.py
-pinned: false
-license: creativeml-openrail-m
----
-
-Check out the configuration reference at https://huggingface.co/docs/hub/spaces-config-reference
\ No newline at end of file
diff --git a/spaces/Raspberry-ai/main/.env/lib/python3.11/site-packages/pip/_vendor/cachecontrol/cache.py b/spaces/Raspberry-ai/main/.env/lib/python3.11/site-packages/pip/_vendor/cachecontrol/cache.py
deleted file mode 100644
index 2a965f595ff0756002e2a2c79da551fa8c8fff25..0000000000000000000000000000000000000000
--- a/spaces/Raspberry-ai/main/.env/lib/python3.11/site-packages/pip/_vendor/cachecontrol/cache.py
+++ /dev/null
@@ -1,65 +0,0 @@
-# SPDX-FileCopyrightText: 2015 Eric Larson
-#
-# SPDX-License-Identifier: Apache-2.0
-
-"""
-The cache object API for implementing caches. The default is a thread
-safe in-memory dictionary.
-"""
-from threading import Lock
-
-
-class BaseCache(object):
-
- def get(self, key):
- raise NotImplementedError()
-
- def set(self, key, value, expires=None):
- raise NotImplementedError()
-
- def delete(self, key):
- raise NotImplementedError()
-
- def close(self):
- pass
-
-
-class DictCache(BaseCache):
-
- def __init__(self, init_dict=None):
- self.lock = Lock()
- self.data = init_dict or {}
-
- def get(self, key):
- return self.data.get(key, None)
-
- def set(self, key, value, expires=None):
- with self.lock:
- self.data.update({key: value})
-
- def delete(self, key):
- with self.lock:
- if key in self.data:
- self.data.pop(key)
-
-
-class SeparateBodyBaseCache(BaseCache):
- """
- In this variant, the body is not stored mixed in with the metadata, but is
- passed in (as a bytes-like object) in a separate call to ``set_body()``.
-
- That is, the expected interaction pattern is::
-
- cache.set(key, serialized_metadata)
- cache.set_body(key)
-
- Similarly, the body should be loaded separately via ``get_body()``.
- """
- def set_body(self, key, body):
- raise NotImplementedError()
-
- def get_body(self, key):
- """
- Return the body as file-like object.
- """
- raise NotImplementedError()
diff --git a/spaces/Realcat/image-matching-webui/third_party/DarkFeat/datasets/InvISP/test_raw.py b/spaces/Realcat/image-matching-webui/third_party/DarkFeat/datasets/InvISP/test_raw.py
deleted file mode 100644
index 8c3c30faf6662b04fe34f63de0d729ebcec86517..0000000000000000000000000000000000000000
--- a/spaces/Realcat/image-matching-webui/third_party/DarkFeat/datasets/InvISP/test_raw.py
+++ /dev/null
@@ -1,162 +0,0 @@
-import torch.nn as nn
-import torch.nn.functional as F
-from torch.autograd import Variable
-import torch
-import numpy as np
-import os, time, random
-import argparse
-from torch.utils.data import Dataset, DataLoader
-from PIL import Image as PILImage
-from glob import glob
-from tqdm import tqdm
-
-from model.model import InvISPNet
-from dataset.FiveK_dataset import FiveKDatasetTest
-from config.config import get_arguments
-
-from utils.JPEG import DiffJPEG
-from utils.commons import denorm, preprocess_test_patch
-
-
-os.system("nvidia-smi -q -d Memory |grep -A4 GPU|grep Free >tmp")
-os.environ["CUDA_VISIBLE_DEVICES"] = str(
- np.argmax([int(x.split()[2]) for x in open("tmp", "r").readlines()])
-)
-# os.environ['CUDA_VISIBLE_DEVICES'] = '7'
-os.system("rm tmp")
-
-DiffJPEG = DiffJPEG(differentiable=True, quality=90).cuda()
-
-parser = get_arguments()
-parser.add_argument("--ckpt", type=str, help="Checkpoint path.")
-parser.add_argument(
- "--out_path", type=str, default="./exps/", help="Path to save checkpoint. "
-)
-parser.add_argument(
- "--split_to_patch",
- dest="split_to_patch",
- action="store_true",
- help="Test on patch. ",
-)
-args = parser.parse_args()
-print("Parsed arguments: {}".format(args))
-
-
-ckpt_name = args.ckpt.split("/")[-1].split(".")[0]
-if args.split_to_patch:
- os.makedirs(
- args.out_path + "%s/results_metric_%s/" % (args.task, ckpt_name), exist_ok=True
- )
- out_path = args.out_path + "%s/results_metric_%s/" % (args.task, ckpt_name)
-else:
- os.makedirs(
- args.out_path + "%s/results_%s/" % (args.task, ckpt_name), exist_ok=True
- )
- out_path = args.out_path + "%s/results_%s/" % (args.task, ckpt_name)
-
-
-def main(args):
- # ======================================define the model============================================
- net = InvISPNet(channel_in=3, channel_out=3, block_num=8)
- device = torch.device("cuda:0")
-
- net.to(device)
- net.eval()
- # load the pretrained weight if there exists one
- if os.path.isfile(args.ckpt):
- net.load_state_dict(torch.load(args.ckpt), strict=False)
- print("[INFO] Loaded checkpoint: {}".format(args.ckpt))
-
- print("[INFO] Start data load and preprocessing")
- RAWDataset = FiveKDatasetTest(opt=args)
- dataloader = DataLoader(
- RAWDataset, batch_size=1, shuffle=False, num_workers=0, drop_last=True
- )
-
- input_RGBs = sorted(glob(out_path + "pred*jpg"))
- input_RGBs_names = [path.split("/")[-1].split(".")[0][5:] for path in input_RGBs]
-
- print("[INFO] Start test...")
- for i_batch, sample_batched in enumerate(tqdm(dataloader)):
- step_time = time.time()
-
- input, target_rgb, target_raw = (
- sample_batched["input_raw"].to(device),
- sample_batched["target_rgb"].to(device),
- sample_batched["target_raw"].to(device),
- )
- file_name = sample_batched["file_name"][0]
-
- if args.split_to_patch:
- input_list, target_rgb_list, target_raw_list = preprocess_test_patch(
- input, target_rgb, target_raw
- )
- else:
- # remove [:,:,::2,::2] if you have enough GPU memory to test the full resolution
- input_list, target_rgb_list, target_raw_list = (
- [input[:, :, ::2, ::2]],
- [target_rgb[:, :, ::2, ::2]],
- [target_raw[:, :, ::2, ::2]],
- )
-
- for i_patch in range(len(input_list)):
- file_name_patch = file_name + "_%05d" % i_patch
- idx = input_RGBs_names.index(file_name_patch)
- input_RGB_path = input_RGBs[idx]
- input_RGB = (
- torch.from_numpy(np.array(PILImage.open(input_RGB_path)) / 255.0)
- .unsqueeze(0)
- .permute(0, 3, 1, 2)
- .float()
- .to(device)
- )
-
- target_raw_patch = target_raw_list[i_patch]
-
- with torch.no_grad():
- reconstruct_raw = net(input_RGB, rev=True)
-
- pred_raw = reconstruct_raw.detach().permute(0, 2, 3, 1)
- pred_raw = torch.clamp(pred_raw, 0, 1)
-
- target_raw_patch = target_raw_patch.permute(0, 2, 3, 1)
- pred_raw = denorm(pred_raw, 255)
- target_raw_patch = denorm(target_raw_patch, 255)
-
- pred_raw = pred_raw.cpu().numpy()
- target_raw_patch = target_raw_patch.cpu().numpy().astype(np.float32)
-
- raw_pred = PILImage.fromarray(np.uint8(pred_raw[0, :, :, 0]))
- raw_tar_pred = PILImage.fromarray(
- np.hstack(
- (
- np.uint8(target_raw_patch[0, :, :, 0]),
- np.uint8(pred_raw[0, :, :, 0]),
- )
- )
- )
-
- raw_tar = PILImage.fromarray(np.uint8(target_raw_patch[0, :, :, 0]))
-
- raw_pred.save(out_path + "raw_pred_%s_%05d.jpg" % (file_name, i_patch))
- raw_tar.save(out_path + "raw_tar_%s_%05d.jpg" % (file_name, i_patch))
- raw_tar_pred.save(
- out_path + "raw_gt_pred_%s_%05d.jpg" % (file_name, i_patch)
- )
-
- np.save(
- out_path + "raw_pred_%s_%05d.npy" % (file_name, i_patch),
- pred_raw[0, :, :, :] / 255.0,
- )
- np.save(
- out_path + "raw_tar_%s_%05d.npy" % (file_name, i_patch),
- target_raw_patch[0, :, :, :] / 255.0,
- )
-
- del reconstruct_raw
-
-
-if __name__ == "__main__":
-
- torch.set_num_threads(4)
- main(args)
diff --git a/spaces/RegalHyperus/rvc-lovelive-genshin/app.py b/spaces/RegalHyperus/rvc-lovelive-genshin/app.py
deleted file mode 100644
index eb8089336bf3b9598a48812297b794359a7887cc..0000000000000000000000000000000000000000
--- a/spaces/RegalHyperus/rvc-lovelive-genshin/app.py
+++ /dev/null
@@ -1,497 +0,0 @@
-import os
-import glob
-import json
-import traceback
-import logging
-import gradio as gr
-import numpy as np
-import librosa
-import torch
-import asyncio
-import edge_tts
-import yt_dlp
-import ffmpeg
-import subprocess
-import sys
-import io
-import wave
-from datetime import datetime
-from fairseq import checkpoint_utils
-from infer_pack.models import (
- SynthesizerTrnMs256NSFsid,
- SynthesizerTrnMs256NSFsid_nono,
- SynthesizerTrnMs768NSFsid,
- SynthesizerTrnMs768NSFsid_nono,
-)
-from vc_infer_pipeline import VC
-from config import Config
-config = Config()
-logging.getLogger("numba").setLevel(logging.WARNING)
-limitation = os.getenv("SYSTEM") == "spaces"
-
-def create_vc_fn(tgt_sr, net_g, vc, if_f0, file_index):
- def vc_fn(
- vc_audio_mode,
- vc_input,
- vc_upload,
- tts_text,
- tts_voice,
- spk_item,
- f0_up_key,
- f0_method,
- index_rate,
- filter_radius,
- resample_sr,
- rms_mix_rate,
- protect,
- ):
- try:
- if vc_audio_mode == "Input path" or "Youtube" and vc_input != "":
- audio, sr = librosa.load(vc_input, sr=16000, mono=True)
- elif vc_audio_mode == "Upload audio":
- if vc_upload is None:
- return "You need to upload an audio", None
- sampling_rate, audio = vc_upload
- duration = audio.shape[0] / sampling_rate
- if duration > 20 and limitation:
- return "Please upload an audio file that is less than 20 seconds. If you need to generate a longer audio file, please use Colab.", None
- audio = (audio / np.iinfo(audio.dtype).max).astype(np.float32)
- if len(audio.shape) > 1:
- audio = librosa.to_mono(audio.transpose(1, 0))
- if sampling_rate != 16000:
- audio = librosa.resample(audio, orig_sr=sampling_rate, target_sr=16000)
- elif vc_audio_mode == "TTS Audio":
- if len(tts_text) > 100 and limitation:
- return "Text is too long", None
- if tts_text is None or tts_voice is None:
- return "You need to enter text and select a voice", None
- asyncio.run(edge_tts.Communicate(tts_text, "-".join(tts_voice.split('-')[:-1])).save("tts.mp3"))
- audio, sr = librosa.load("tts.mp3", sr=16000, mono=True)
- vc_input = "tts.mp3"
- times = [0, 0, 0]
- f0_up_key = int(f0_up_key)
- audio_opt = vc.pipeline(
- hubert_model,
- net_g,
- spk_item,
- audio,
- vc_input,
- times,
- f0_up_key,
- f0_method,
- file_index,
- index_rate,
- if_f0,
- filter_radius,
- tgt_sr,
- resample_sr,
- rms_mix_rate,
- version,
- protect,
- f0_file=None,
- )
- info = f"[{datetime.now().strftime('%Y-%m-%d %H:%M')}]: npy: {times[0]}, f0: {times[1]}s, infer: {times[2]}s"
- print(info)
- return info, (tgt_sr, audio_opt)
- except:
- info = traceback.format_exc()
- print(info)
- return info, (None, None)
- return vc_fn
-
-def cut_vocal_and_inst(url, audio_provider, split_model):
- if url != "":
- if not os.path.exists("dl_audio"):
- os.mkdir("dl_audio")
- if audio_provider == "Youtube":
- ydl_opts = {
- 'format': 'bestaudio/best',
- 'postprocessors': [{
- 'key': 'FFmpegExtractAudio',
- 'preferredcodec': 'wav',
- }],
- "outtmpl": 'dl_audio/youtube_audio',
- }
- with yt_dlp.YoutubeDL(ydl_opts) as ydl:
- ydl.download([url])
- audio_path = "dl_audio/youtube_audio.wav"
- else:
- # Spotify doesnt work.
- # Need to find other solution soon.
- '''
- command = f"spotdl download {url} --output dl_audio/.wav"
- result = subprocess.run(command.split(), stdout=subprocess.PIPE)
- print(result.stdout.decode())
- audio_path = "dl_audio/spotify_audio.wav"
- '''
- if split_model == "htdemucs":
- command = f"demucs --two-stems=vocals {audio_path} -o output"
- result = subprocess.run(command.split(), stdout=subprocess.PIPE)
- print(result.stdout.decode())
- return "output/htdemucs/youtube_audio/vocals.wav", "output/htdemucs/youtube_audio/no_vocals.wav", audio_path, "output/htdemucs/youtube_audio/vocals.wav"
- else:
- command = f"demucs --two-stems=vocals -n mdx_extra_q {audio_path} -o output"
- result = subprocess.run(command.split(), stdout=subprocess.PIPE)
- print(result.stdout.decode())
- return "output/mdx_extra_q/youtube_audio/vocals.wav", "output/mdx_extra_q/youtube_audio/no_vocals.wav", audio_path, "output/mdx_extra_q/youtube_audio/vocals.wav"
- else:
- raise gr.Error("URL Required!")
- return None, None, None, None
-
-def combine_vocal_and_inst(audio_data, audio_volume, split_model):
- if not os.path.exists("output/result"):
- os.mkdir("output/result")
- vocal_path = "output/result/output.wav"
- output_path = "output/result/combine.mp3"
- if split_model == "htdemucs":
- inst_path = "output/htdemucs/youtube_audio/no_vocals.wav"
- else:
- inst_path = "output/mdx_extra_q/youtube_audio/no_vocals.wav"
- with wave.open(vocal_path, "w") as wave_file:
- wave_file.setnchannels(1)
- wave_file.setsampwidth(2)
- wave_file.setframerate(audio_data[0])
- wave_file.writeframes(audio_data[1].tobytes())
- command = f'ffmpeg -y -i {inst_path} -i {vocal_path} -filter_complex [1:a]volume={audio_volume}dB[v];[0:a][v]amix=inputs=2:duration=longest -b:a 320k -c:a libmp3lame {output_path}'
- result = subprocess.run(command.split(), stdout=subprocess.PIPE)
- print(result.stdout.decode())
- return output_path
-
-def load_hubert():
- global hubert_model
- models, _, _ = checkpoint_utils.load_model_ensemble_and_task(
- ["hubert_base.pt"],
- suffix="",
- )
- hubert_model = models[0]
- hubert_model = hubert_model.to(config.device)
- if config.is_half:
- hubert_model = hubert_model.half()
- else:
- hubert_model = hubert_model.float()
- hubert_model.eval()
-
-def change_audio_mode(vc_audio_mode):
- if vc_audio_mode == "Input path":
- return (
- # Input & Upload
- gr.Textbox.update(visible=True),
- gr.Audio.update(visible=False),
- # Youtube
- gr.Dropdown.update(visible=False),
- gr.Textbox.update(visible=False),
- gr.Dropdown.update(visible=False),
- gr.Button.update(visible=False),
- gr.Audio.update(visible=False),
- gr.Audio.update(visible=False),
- gr.Audio.update(visible=False),
- gr.Slider.update(visible=False),
- gr.Audio.update(visible=False),
- gr.Button.update(visible=False),
- # TTS
- gr.Textbox.update(visible=False),
- gr.Dropdown.update(visible=False)
- )
- elif vc_audio_mode == "Upload audio":
- return (
- # Input & Upload
- gr.Textbox.update(visible=False),
- gr.Audio.update(visible=True),
- # Youtube
- gr.Dropdown.update(visible=False),
- gr.Textbox.update(visible=False),
- gr.Dropdown.update(visible=False),
- gr.Button.update(visible=False),
- gr.Audio.update(visible=False),
- gr.Audio.update(visible=False),
- gr.Audio.update(visible=False),
- gr.Slider.update(visible=False),
- gr.Audio.update(visible=False),
- gr.Button.update(visible=False),
- # TTS
- gr.Textbox.update(visible=False),
- gr.Dropdown.update(visible=False)
- )
- elif vc_audio_mode == "Youtube":
- return (
- # Input & Upload
- gr.Textbox.update(visible=False),
- gr.Audio.update(visible=False),
- # Youtube
- gr.Dropdown.update(visible=True),
- gr.Textbox.update(visible=True),
- gr.Dropdown.update(visible=True),
- gr.Button.update(visible=True),
- gr.Audio.update(visible=True),
- gr.Audio.update(visible=True),
- gr.Audio.update(visible=True),
- gr.Slider.update(visible=True),
- gr.Audio.update(visible=True),
- gr.Button.update(visible=True),
- # TTS
- gr.Textbox.update(visible=False),
- gr.Dropdown.update(visible=False)
- )
- elif vc_audio_mode == "TTS Audio":
- return (
- # Input & Upload
- gr.Textbox.update(visible=False),
- gr.Audio.update(visible=False),
- # Youtube
- gr.Dropdown.update(visible=False),
- gr.Textbox.update(visible=False),
- gr.Dropdown.update(visible=False),
- gr.Button.update(visible=False),
- gr.Audio.update(visible=False),
- gr.Audio.update(visible=False),
- gr.Audio.update(visible=False),
- gr.Slider.update(visible=False),
- gr.Audio.update(visible=False),
- gr.Button.update(visible=False),
- # TTS
- gr.Textbox.update(visible=True),
- gr.Dropdown.update(visible=True)
- )
- else:
- return (
- # Input & Upload
- gr.Textbox.update(visible=False),
- gr.Audio.update(visible=True),
- # Youtube
- gr.Dropdown.update(visible=False),
- gr.Textbox.update(visible=False),
- gr.Dropdown.update(visible=False),
- gr.Button.update(visible=False),
- gr.Audio.update(visible=False),
- gr.Audio.update(visible=False),
- gr.Audio.update(visible=False),
- gr.Slider.update(visible=False),
- gr.Audio.update(visible=False),
- gr.Button.update(visible=False),
- # TTS
- gr.Textbox.update(visible=False),
- gr.Dropdown.update(visible=False)
- )
-
-if __name__ == '__main__':
- load_hubert()
- categories = []
- tts_voice_list = asyncio.get_event_loop().run_until_complete(edge_tts.list_voices())
- voices = [f"{v['ShortName']}-{v['Gender']}" for v in tts_voice_list]
- with open("weights/folder_info.json", "r", encoding="utf-8") as f:
- folder_info = json.load(f)
- for category_name, category_info in folder_info.items():
- if not category_info['enable']:
- continue
- category_title = category_info['title']
- category_folder = category_info['folder_path']
- description = category_info['description']
- models = []
- with open(f"weights/{category_folder}/model_info.json", "r", encoding="utf-8") as f:
- models_info = json.load(f)
- for model_name, info in models_info.items():
- if not info['enable']:
- continue
- model_title = info['title']
- model_author = info.get("author", None)
- model_cover = f"weights/{category_folder}/{model_name}/{info['cover']}"
- model_index = f"weights/{category_folder}/{model_name}/{info['feature_retrieval_library']}"
- cpt = torch.load(f"weights/{category_folder}/{model_name}/{model_name}.pth", map_location="cpu")
- tgt_sr = cpt["config"][-1]
- cpt["config"][-3] = cpt["weight"]["emb_g.weight"].shape[0] # n_spk
- if_f0 = cpt.get("f0", 1)
- version = cpt.get("version", "v1")
- if version == "v1":
- if if_f0 == 1:
- net_g = SynthesizerTrnMs256NSFsid(*cpt["config"], is_half=config.is_half)
- else:
- net_g = SynthesizerTrnMs256NSFsid_nono(*cpt["config"])
- nodel_version = "V1"
- elif version == "v2":
- if if_f0 == 1:
- net_g = SynthesizerTrnMs768NSFsid(*cpt["config"], is_half=config.is_half)
- else:
- net_g = SynthesizerTrnMs768NSFsid_nono(*cpt["config"])
- nodel_version = "V2"
- del net_g.enc_q
- print(net_g.load_state_dict(cpt["weight"], strict=False))
- net_g.eval().to(config.device)
- if config.is_half:
- net_g = net_g.half()
- else:
- net_g = net_g.float()
- vc = VC(tgt_sr, config)
- print(f"Model loaded: {model_name}")
- models.append((model_name, model_title, model_author, model_cover, nodel_version, create_vc_fn(tgt_sr, net_g, vc, if_f0, model_index)))
- categories.append([category_title, category_folder, description, models])
- with gr.Blocks() as app:
- gr.Markdown(
- "#
RVC Genshin Impact Inference\n"
- "###
[Recommended to use Google Colab to use more character & more feature](https://colab.research.google.com/drive/110kiMZTdP6Ri1lY9-NbQf17GVPPhHyeT?usp=sharing)\n"
- "#### From [Retrieval-based-Voice-Conversion](https://github.com/RVC-Project/Retrieval-based-Voice-Conversion-WebUI)\n"
- "[](https://github.com/ArkanDash/Multi-Model-RVC-Inference)"
- )
- for (folder_title, folder, description, models) in categories:
- with gr.TabItem(folder_title):
- if description:
- gr.Markdown(f"###
{description}")
- with gr.Tabs():
- if not models:
- gr.Markdown("#
No Model Loaded.")
- gr.Markdown("##
Please add model or fix your model path.")
- continue
- for (name, title, author, cover, model_version, vc_fn) in models:
- with gr.TabItem(name):
- with gr.Row():
- gr.Markdown(
- '
'
- f'
{title}
\n'+
- f'
RVC {model_version} Model
\n'+
- (f'
Model author: {author}
' if author else "")+
- (f'' if cover else "")+
- '
- )
-}
diff --git a/spaces/Zwicky18/vits-models/text/cleaners.py b/spaces/Zwicky18/vits-models/text/cleaners.py
deleted file mode 100644
index d26581deb399609163518054718ad80ecca5d934..0000000000000000000000000000000000000000
--- a/spaces/Zwicky18/vits-models/text/cleaners.py
+++ /dev/null
@@ -1,475 +0,0 @@
-""" from https://github.com/keithito/tacotron """
-
-'''
-Cleaners are transformations that run over the input text at both training and eval time.
-
-Cleaners can be selected by passing a comma-delimited list of cleaner names as the "cleaners"
-hyperparameter. Some cleaners are English-specific. You'll typically want to use:
- 1. "english_cleaners" for English text
- 2. "transliteration_cleaners" for non-English text that can be transliterated to ASCII using
- the Unidecode library (https://pypi.python.org/pypi/Unidecode)
- 3. "basic_cleaners" if you do not want to transliterate (in this case, you should also update
- the symbols in symbols.py to match your data).
-'''
-
-import re
-from unidecode import unidecode
-import pyopenjtalk
-from jamo import h2j, j2hcj
-from pypinyin import lazy_pinyin, BOPOMOFO
-import jieba, cn2an
-
-
-# This is a list of Korean classifiers preceded by pure Korean numerals.
-_korean_classifiers = '군데 권 개 그루 닢 대 두 마리 모 모금 뭇 발 발짝 방 번 벌 보루 살 수 술 시 쌈 움큼 정 짝 채 척 첩 축 켤레 톨 통'
-
-# Regular expression matching whitespace:
-_whitespace_re = re.compile(r'\s+')
-
-# Regular expression matching Japanese without punctuation marks:
-_japanese_characters = re.compile(r'[A-Za-z\d\u3005\u3040-\u30ff\u4e00-\u9fff\uff11-\uff19\uff21-\uff3a\uff41-\uff5a\uff66-\uff9d]')
-
-# Regular expression matching non-Japanese characters or punctuation marks:
-_japanese_marks = re.compile(r'[^A-Za-z\d\u3005\u3040-\u30ff\u4e00-\u9fff\uff11-\uff19\uff21-\uff3a\uff41-\uff5a\uff66-\uff9d]')
-
-# List of (regular expression, replacement) pairs for abbreviations:
-_abbreviations = [(re.compile('\\b%s\\.' % x[0], re.IGNORECASE), x[1]) for x in [
- ('mrs', 'misess'),
- ('mr', 'mister'),
- ('dr', 'doctor'),
- ('st', 'saint'),
- ('co', 'company'),
- ('jr', 'junior'),
- ('maj', 'major'),
- ('gen', 'general'),
- ('drs', 'doctors'),
- ('rev', 'reverend'),
- ('lt', 'lieutenant'),
- ('hon', 'honorable'),
- ('sgt', 'sergeant'),
- ('capt', 'captain'),
- ('esq', 'esquire'),
- ('ltd', 'limited'),
- ('col', 'colonel'),
- ('ft', 'fort'),
-]]
-
-# List of (hangul, hangul divided) pairs:
-_hangul_divided = [(re.compile('%s' % x[0]), x[1]) for x in [
- ('ㄳ', 'ㄱㅅ'),
- ('ㄵ', 'ㄴㅈ'),
- ('ㄶ', 'ㄴㅎ'),
- ('ㄺ', 'ㄹㄱ'),
- ('ㄻ', 'ㄹㅁ'),
- ('ㄼ', 'ㄹㅂ'),
- ('ㄽ', 'ㄹㅅ'),
- ('ㄾ', 'ㄹㅌ'),
- ('ㄿ', 'ㄹㅍ'),
- ('ㅀ', 'ㄹㅎ'),
- ('ㅄ', 'ㅂㅅ'),
- ('ㅘ', 'ㅗㅏ'),
- ('ㅙ', 'ㅗㅐ'),
- ('ㅚ', 'ㅗㅣ'),
- ('ㅝ', 'ㅜㅓ'),
- ('ㅞ', 'ㅜㅔ'),
- ('ㅟ', 'ㅜㅣ'),
- ('ㅢ', 'ㅡㅣ'),
- ('ㅑ', 'ㅣㅏ'),
- ('ㅒ', 'ㅣㅐ'),
- ('ㅕ', 'ㅣㅓ'),
- ('ㅖ', 'ㅣㅔ'),
- ('ㅛ', 'ㅣㅗ'),
- ('ㅠ', 'ㅣㅜ')
-]]
-
-# List of (Latin alphabet, hangul) pairs:
-_latin_to_hangul = [(re.compile('%s' % x[0], re.IGNORECASE), x[1]) for x in [
- ('a', '에이'),
- ('b', '비'),
- ('c', '시'),
- ('d', '디'),
- ('e', '이'),
- ('f', '에프'),
- ('g', '지'),
- ('h', '에이치'),
- ('i', '아이'),
- ('j', '제이'),
- ('k', '케이'),
- ('l', '엘'),
- ('m', '엠'),
- ('n', '엔'),
- ('o', '오'),
- ('p', '피'),
- ('q', '큐'),
- ('r', '아르'),
- ('s', '에스'),
- ('t', '티'),
- ('u', '유'),
- ('v', '브이'),
- ('w', '더블유'),
- ('x', '엑스'),
- ('y', '와이'),
- ('z', '제트')
-]]
-
-# List of (Latin alphabet, bopomofo) pairs:
-_latin_to_bopomofo = [(re.compile('%s' % x[0], re.IGNORECASE), x[1]) for x in [
- ('a', 'ㄟˉ'),
- ('b', 'ㄅㄧˋ'),
- ('c', 'ㄙㄧˉ'),
- ('d', 'ㄉㄧˋ'),
- ('e', 'ㄧˋ'),
- ('f', 'ㄝˊㄈㄨˋ'),
- ('g', 'ㄐㄧˋ'),
- ('h', 'ㄝˇㄑㄩˋ'),
- ('i', 'ㄞˋ'),
- ('j', 'ㄐㄟˋ'),
- ('k', 'ㄎㄟˋ'),
- ('l', 'ㄝˊㄛˋ'),
- ('m', 'ㄝˊㄇㄨˋ'),
- ('n', 'ㄣˉ'),
- ('o', 'ㄡˉ'),
- ('p', 'ㄆㄧˉ'),
- ('q', 'ㄎㄧㄡˉ'),
- ('r', 'ㄚˋ'),
- ('s', 'ㄝˊㄙˋ'),
- ('t', 'ㄊㄧˋ'),
- ('u', 'ㄧㄡˉ'),
- ('v', 'ㄨㄧˉ'),
- ('w', 'ㄉㄚˋㄅㄨˋㄌㄧㄡˋ'),
- ('x', 'ㄝˉㄎㄨˋㄙˋ'),
- ('y', 'ㄨㄞˋ'),
- ('z', 'ㄗㄟˋ')
-]]
-
-
-# List of (bopomofo, romaji) pairs:
-_bopomofo_to_romaji = [(re.compile('%s' % x[0], re.IGNORECASE), x[1]) for x in [
- ('ㄅㄛ', 'p⁼wo'),
- ('ㄆㄛ', 'pʰwo'),
- ('ㄇㄛ', 'mwo'),
- ('ㄈㄛ', 'fwo'),
- ('ㄅ', 'p⁼'),
- ('ㄆ', 'pʰ'),
- ('ㄇ', 'm'),
- ('ㄈ', 'f'),
- ('ㄉ', 't⁼'),
- ('ㄊ', 'tʰ'),
- ('ㄋ', 'n'),
- ('ㄌ', 'l'),
- ('ㄍ', 'k⁼'),
- ('ㄎ', 'kʰ'),
- ('ㄏ', 'h'),
- ('ㄐ', 'ʧ⁼'),
- ('ㄑ', 'ʧʰ'),
- ('ㄒ', 'ʃ'),
- ('ㄓ', 'ʦ`⁼'),
- ('ㄔ', 'ʦ`ʰ'),
- ('ㄕ', 's`'),
- ('ㄖ', 'ɹ`'),
- ('ㄗ', 'ʦ⁼'),
- ('ㄘ', 'ʦʰ'),
- ('ㄙ', 's'),
- ('ㄚ', 'a'),
- ('ㄛ', 'o'),
- ('ㄜ', 'ə'),
- ('ㄝ', 'e'),
- ('ㄞ', 'ai'),
- ('ㄟ', 'ei'),
- ('ㄠ', 'au'),
- ('ㄡ', 'ou'),
- ('ㄧㄢ', 'yeNN'),
- ('ㄢ', 'aNN'),
- ('ㄧㄣ', 'iNN'),
- ('ㄣ', 'əNN'),
- ('ㄤ', 'aNg'),
- ('ㄧㄥ', 'iNg'),
- ('ㄨㄥ', 'uNg'),
- ('ㄩㄥ', 'yuNg'),
- ('ㄥ', 'əNg'),
- ('ㄦ', 'əɻ'),
- ('ㄧ', 'i'),
- ('ㄨ', 'u'),
- ('ㄩ', 'ɥ'),
- ('ˉ', '→'),
- ('ˊ', '↑'),
- ('ˇ', '↓↑'),
- ('ˋ', '↓'),
- ('˙', ''),
- (',', ','),
- ('。', '.'),
- ('!', '!'),
- ('?', '?'),
- ('—', '-')
-]]
-
-
-def expand_abbreviations(text):
- for regex, replacement in _abbreviations:
- text = re.sub(regex, replacement, text)
- return text
-
-
-def lowercase(text):
- return text.lower()
-
-
-def collapse_whitespace(text):
- return re.sub(_whitespace_re, ' ', text)
-
-
-def convert_to_ascii(text):
- return unidecode(text)
-
-
-def japanese_to_romaji_with_accent(text):
- '''Reference https://r9y9.github.io/ttslearn/latest/notebooks/ch10_Recipe-Tacotron.html'''
- sentences = re.split(_japanese_marks, text)
- marks = re.findall(_japanese_marks, text)
- text = ''
- for i, sentence in enumerate(sentences):
- if re.match(_japanese_characters, sentence):
- if text!='':
- text+=' '
- labels = pyopenjtalk.extract_fullcontext(sentence)
- for n, label in enumerate(labels):
- phoneme = re.search(r'\-([^\+]*)\+', label).group(1)
- if phoneme not in ['sil','pau']:
- text += phoneme.replace('ch','ʧ').replace('sh','ʃ').replace('cl','Q')
- else:
- continue
- n_moras = int(re.search(r'/F:(\d+)_', label).group(1))
- a1 = int(re.search(r"/A:(\-?[0-9]+)\+", label).group(1))
- a2 = int(re.search(r"\+(\d+)\+", label).group(1))
- a3 = int(re.search(r"\+(\d+)/", label).group(1))
- if re.search(r'\-([^\+]*)\+', labels[n + 1]).group(1) in ['sil','pau']:
- a2_next=-1
- else:
- a2_next = int(re.search(r"\+(\d+)\+", labels[n + 1]).group(1))
- # Accent phrase boundary
- if a3 == 1 and a2_next == 1:
- text += ' '
- # Falling
- elif a1 == 0 and a2_next == a2 + 1 and a2 != n_moras:
- text += '↓'
- # Rising
- elif a2 == 1 and a2_next == 2:
- text += '↑'
- if i>> rois = torch.Tensor([[ 0., 0., 1., 1.],
- >>> [ 0., 0., 1., 1.],
- >>> [ 0., 0., 1., 1.],
- >>> [ 5., 5., 5., 5.]])
- >>> deltas = torch.Tensor([[ 0., 0., 0., 0.],
- >>> [ 1., 1., 1., 1.],
- >>> [ 0., 0., 2., -1.],
- >>> [ 0.7, -1.9, -0.5, 0.3]])
- >>> legacy_delta2bbox(rois, deltas, max_shape=(32, 32))
- tensor([[0.0000, 0.0000, 1.5000, 1.5000],
- [0.0000, 0.0000, 5.2183, 5.2183],
- [0.0000, 0.1321, 7.8891, 0.8679],
- [5.3967, 2.4251, 6.0033, 3.7749]])
- """
- means = deltas.new_tensor(means).repeat(1, deltas.size(1) // 4)
- stds = deltas.new_tensor(stds).repeat(1, deltas.size(1) // 4)
- denorm_deltas = deltas * stds + means
- dx = denorm_deltas[:, 0::4]
- dy = denorm_deltas[:, 1::4]
- dw = denorm_deltas[:, 2::4]
- dh = denorm_deltas[:, 3::4]
- max_ratio = np.abs(np.log(wh_ratio_clip))
- dw = dw.clamp(min=-max_ratio, max=max_ratio)
- dh = dh.clamp(min=-max_ratio, max=max_ratio)
- # Compute center of each roi
- px = ((rois[:, 0] + rois[:, 2]) * 0.5).unsqueeze(1).expand_as(dx)
- py = ((rois[:, 1] + rois[:, 3]) * 0.5).unsqueeze(1).expand_as(dy)
- # Compute width/height of each roi
- pw = (rois[:, 2] - rois[:, 0] + 1.0).unsqueeze(1).expand_as(dw)
- ph = (rois[:, 3] - rois[:, 1] + 1.0).unsqueeze(1).expand_as(dh)
- # Use exp(network energy) to enlarge/shrink each roi
- gw = pw * dw.exp()
- gh = ph * dh.exp()
- # Use network energy to shift the center of each roi
- gx = px + pw * dx
- gy = py + ph * dy
- # Convert center-xy/width/height to top-left, bottom-right
-
- # The true legacy box coder should +- 0.5 here.
- # However, current implementation improves the performance when testing
- # the models trained in MMDetection 1.X (~0.5 bbox AP, 0.2 mask AP)
- x1 = gx - gw * 0.5
- y1 = gy - gh * 0.5
- x2 = gx + gw * 0.5
- y2 = gy + gh * 0.5
- if max_shape is not None:
- x1 = x1.clamp(min=0, max=max_shape[1] - 1)
- y1 = y1.clamp(min=0, max=max_shape[0] - 1)
- x2 = x2.clamp(min=0, max=max_shape[1] - 1)
- y2 = y2.clamp(min=0, max=max_shape[0] - 1)
- bboxes = torch.stack([x1, y1, x2, y2], dim=-1).view_as(deltas)
- return bboxes
diff --git a/spaces/aimstack/aim/README.md b/spaces/aimstack/aim/README.md
deleted file mode 100644
index d8c0839b902a2218c3333c0b30d454c7fd75afa4..0000000000000000000000000000000000000000
--- a/spaces/aimstack/aim/README.md
+++ /dev/null
@@ -1,80 +0,0 @@
----
-title: Aim
-emoji: 🔥
-colorFrom: purple
-colorTo: blue
-sdk: docker
-license: other
-fullWidth: true
----
-
-# Aim on Spaces
-
-**Hugging Face Spaces** offer a simple way to host ML demo apps directly on your profile or your organization’s profile. This allows you to create your ML portfolio, showcase your projects at conferences or to stakeholders, and work collaboratively with other people in the ML ecosystem.
-Hugging Face Spaces make it easy for you to create and deploy ML-powered demos in minutes.
-
-Check out the [Hugging Face Spaces docs](https://huggingface.co/docs/hub/spaces-overview) to learn more about Spaces.
-
-## Deploy Aim on Spaces
-
-You can deploy Aim on Spaces with a single click!
-
-
-
-
-
-Once you have created the Space, you'll see the `Building` status, and once it becomes `Running,` your Space is ready to go!
-
-
-
-Now, when you navigate to your Space's **App** section, you can access the Aim UI.
-
-## Compare your experiments with Aim on Spaces
-
-Let's use a quick example of a PyTorch CNN trained on MNIST to demonstrate end-to-end Aim on Spaces deployment.
-The full example is in the [Aim repo examples folder](https://github.com/aimhubio/aim/blob/main/examples/pytorch_track.py).
-
-```python
-from aim import Run
-from aim.pytorch import track_gradients_dists, track_params_dists
-
-# Initialize a new Run
-aim_run = Run()
-...
-items = {'accuracy': acc, 'loss': loss}
-aim_run.track(items, epoch=epoch, context={'subset': 'train'})
-
-# Track weights and gradients distributions
-track_params_dists(model, aim_run)
-track_gradients_dists(model, aim_run)
-```
-
-The experiments tracked by Aim are stored in the `.aim` folder. **To display the logs with the Aim UI in your Space, you need to compress the `.aim` folder to a `tar.gz` file and upload it to your Space using `git` or the Files and Versions sections of your Space.**
-
-Here's a bash command for that:
-
-```bash
-tar -czvf aim_repo.tar.gz .aim
-```
-
-That’s it! Now open the App section of your Space and the Aim UI is available with your logs.
-Here is what to expect:
-
-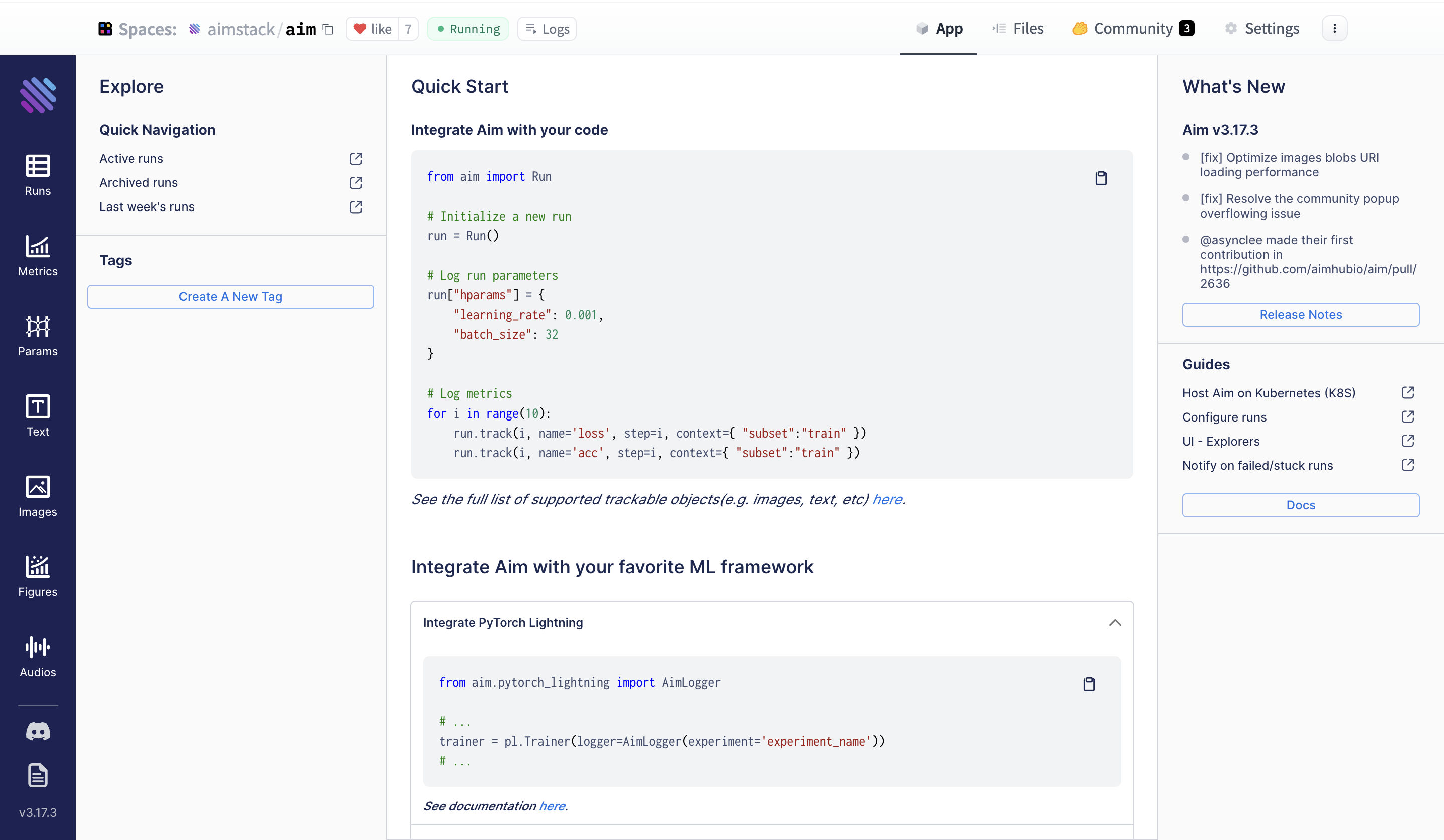
-
-Filter your runs using Aim’s Pythonic search. You can write pythonic [queries against](https://aimstack.readthedocs.io/en/latest/using/search.html) EVERYTHING you have tracked - metrics, hyperparams etc. Check out some [examples](https://huggingface.co/aimstack) on HF Hub Spaces.
-
-
-Note that if your logs are in TensorBoard format, you can easily convert them to Aim with one command and use the many advanced and high-performant training run comparison features available.
-
-
-## More on HF Spaces
-
-- [HF Docker spaces](https://github.com/huggingface/hub-docs/blob/main/docs/hub/spaces-sdks-docker.md)
-- [HF Docker space examples](https://github.com/huggingface/hub-docs/blob/main/docs/hub/spaces-sdks-docker.md)
-
-## Feedback and Support
-
-If you have improvement suggestions or need support, please open an issue on [Aim GitHub repo](https://github.com/aimhubio/aim).
-
-The [Aim community Discord](https://github.com/aimhubio/aim#-community) is also available for community discussions.
diff --git a/spaces/akhaliq/BlendGAN/psp_encoder/psp_encoders.py b/spaces/akhaliq/BlendGAN/psp_encoder/psp_encoders.py
deleted file mode 100644
index 673e44694a709f9fdf405459a9abf3235c09f3f4..0000000000000000000000000000000000000000
--- a/spaces/akhaliq/BlendGAN/psp_encoder/psp_encoders.py
+++ /dev/null
@@ -1,153 +0,0 @@
-import numpy as np
-import torch
-import torch.nn.functional as F
-from torch import nn
-from torch.nn import Conv2d, BatchNorm2d, PReLU, Sequential, Module
-import math
-
-from .helpers import get_blocks, bottleneck_IR, bottleneck_IR_SE
-
-import sys, os
-sys.path.append(os.path.dirname(__file__) + os.sep + '../')
-from model import EqualLinear
-
-
-"""
-Modified from [pSp](https://github.com/eladrich/pixel2style2pixel)
-"""
-
-
-class GradualStyleBlock(Module):
- def __init__(self, in_c, out_c, spatial):
- super(GradualStyleBlock, self).__init__()
- self.out_c = out_c
- self.spatial = spatial
- num_pools = int(np.log2(spatial))
- modules = []
- modules += [Conv2d(in_c, out_c, kernel_size=3, stride=2, padding=1),
- nn.LeakyReLU()]
- for i in range(num_pools - 1):
- modules += [
- Conv2d(out_c, out_c, kernel_size=3, stride=2, padding=1),
- nn.LeakyReLU()
- ]
- self.convs = nn.Sequential(*modules)
- self.linear = EqualLinear(out_c, out_c, lr_mul=1)
-
- def forward(self, x):
- x = self.convs(x)
- x = x.view(-1, self.out_c)
- x = self.linear(x)
- return x
-
-
-class GradualStyleEncoder(Module):
- def __init__(self, num_layers, mode='ir', n_styles=18):
- super(GradualStyleEncoder, self).__init__()
- assert num_layers in [50, 100, 152], 'num_layers should be 50,100, or 152'
- assert mode in ['ir', 'ir_se'], 'mode should be ir or ir_se'
- blocks = get_blocks(num_layers)
- if mode == 'ir':
- unit_module = bottleneck_IR
- elif mode == 'ir_se':
- unit_module = bottleneck_IR_SE
- self.input_layer = Sequential(Conv2d(3, 64, (3, 3), 1, 1, bias=False),
- BatchNorm2d(64),
- PReLU(64))
- modules = []
- for block in blocks:
- for bottleneck in block:
- modules.append(unit_module(bottleneck.in_channel,
- bottleneck.depth,
- bottleneck.stride))
- self.body = Sequential(*modules)
-
- self.styles = nn.ModuleList()
- self.style_count = n_styles # opts.n_styles
- self.coarse_ind = 3
- self.middle_ind = 7
- for i in range(self.style_count):
- if i < self.coarse_ind:
- style = GradualStyleBlock(512, 512, 16)
- elif i < self.middle_ind:
- style = GradualStyleBlock(512, 512, 32)
- else:
- style = GradualStyleBlock(512, 512, 64)
- self.styles.append(style)
- self.latlayer1 = nn.Conv2d(256, 512, kernel_size=1, stride=1, padding=0)
- self.latlayer2 = nn.Conv2d(128, 512, kernel_size=1, stride=1, padding=0)
-
- def _upsample_add(self, x, y):
- '''Upsample and add two feature maps.
- Args:
- x: (Variable) top feature map to be upsampled.
- y: (Variable) lateral feature map.
- Returns:
- (Variable) added feature map.
- Note in PyTorch, when input size is odd, the upsampled feature map
- with `F.upsample(..., scale_factor=2, mode='nearest')`
- maybe not equal to the lateral feature map size.
- e.g.
- original input size: [N,_,15,15] ->
- conv2d feature map size: [N,_,8,8] ->
- upsampled feature map size: [N,_,16,16]
- So we choose bilinear upsample which supports arbitrary output sizes.
- '''
- _, _, H, W = y.size()
- return F.interpolate(x, size=(H, W), mode='bilinear', align_corners=True) + y
-
- def forward(self, x):
- x = self.input_layer(x)
-
- latents = []
- modulelist = list(self.body._modules.values())
- for i, l in enumerate(modulelist):
- x = l(x)
- if i == 6:
- c1 = x
- elif i == 20:
- c2 = x
- elif i == 23:
- c3 = x
-
- for j in range(self.coarse_ind):
- latents.append(self.styles[j](c3))
-
- p2 = self._upsample_add(c3, self.latlayer1(c2))
- for j in range(self.coarse_ind, self.middle_ind):
- latents.append(self.styles[j](p2))
-
- p1 = self._upsample_add(p2, self.latlayer2(c1))
- for j in range(self.middle_ind, self.style_count):
- latents.append(self.styles[j](p1))
-
- out = torch.stack(latents, dim=1)
- return out
-
-
-def get_keys(d, name):
- if 'state_dict' in d:
- d = d['state_dict']
- d_filt = {k[len(name) + 1:]: v for k, v in d.items() if k[:len(name)] == name}
- return d_filt
-
-
-class PSPEncoder(Module):
- def __init__(self, encoder_ckpt_path, output_size=1024):
- super(PSPEncoder, self).__init__()
- n_styles = int(math.log(output_size, 2)) * 2 - 2
- self.encoder = GradualStyleEncoder(50, 'ir_se', n_styles)
-
- print('Loading psp encoders weights from irse50!')
- encoder_ckpt = torch.load(encoder_ckpt_path, map_location='cpu')
- self.encoder.load_state_dict(get_keys(encoder_ckpt, 'encoder'), strict=True)
- self.latent_avg = encoder_ckpt['latent_avg']
-
- self.face_pool = torch.nn.AdaptiveAvgPool2d((256, 256))
-
- def forward(self, x):
- x = self.face_pool(x)
- codes = self.encoder(x)
- codes = codes + self.latent_avg.repeat(codes.shape[0], 1, 1)
- return codes
-
diff --git a/spaces/akhaliq/China-Chic-illustration/app.py b/spaces/akhaliq/China-Chic-illustration/app.py
deleted file mode 100644
index d19d61a1109b30c77d147474303285bce3d8b44c..0000000000000000000000000000000000000000
--- a/spaces/akhaliq/China-Chic-illustration/app.py
+++ /dev/null
@@ -1,137 +0,0 @@
-from diffusers import StableDiffusionPipeline, StableDiffusionImg2ImgPipeline, DPMSolverMultistepScheduler
-import gradio as gr
-import torch
-from PIL import Image
-
-model_id = 'tilake/China-Chic-illustration'
-prefix = ''
-
-scheduler = DPMSolverMultistepScheduler.from_pretrained(model_id, subfolder="scheduler")
-
-pipe = StableDiffusionPipeline.from_pretrained(
- model_id,
- torch_dtype=torch.float16 if torch.cuda.is_available() else torch.float32,
- scheduler=scheduler)
-
-pipe_i2i = StableDiffusionImg2ImgPipeline.from_pretrained(
- model_id,
- torch_dtype=torch.float16 if torch.cuda.is_available() else torch.float32,
- scheduler=scheduler)
-
-if torch.cuda.is_available():
- pipe = pipe.to("cuda")
- pipe_i2i = pipe_i2i.to("cuda")
-
-def error_str(error, title="Error"):
- return f"""#### {title}
- {error}""" if error else ""
-
-def inference(prompt, guidance, steps, width=512, height=512, seed=0, img=None, strength=0.5, neg_prompt="", auto_prefix=False):
-
- generator = torch.Generator('cuda').manual_seed(seed) if seed != 0 else None
- prompt = f"{prefix} {prompt}" if auto_prefix else prompt
-
- try:
- if img is not None:
- return img_to_img(prompt, neg_prompt, img, strength, guidance, steps, width, height, generator), None
- else:
- return txt_to_img(prompt, neg_prompt, guidance, steps, width, height, generator), None
- except Exception as e:
- return None, error_str(e)
-
-def txt_to_img(prompt, neg_prompt, guidance, steps, width, height, generator):
-
- result = pipe(
- prompt,
- negative_prompt = neg_prompt,
- num_inference_steps = int(steps),
- guidance_scale = guidance,
- width = width,
- height = height,
- generator = generator)
-
- return result.images[0]
-
-def img_to_img(prompt, neg_prompt, img, strength, guidance, steps, width, height, generator):
-
- ratio = min(height / img.height, width / img.width)
- img = img.resize((int(img.width * ratio), int(img.height * ratio)), Image.LANCZOS)
- result = pipe_i2i(
- prompt,
- negative_prompt = neg_prompt,
- init_image = img,
- num_inference_steps = int(steps),
- strength = strength,
- guidance_scale = guidance,
- width = width,
- height = height,
- generator = generator)
-
- return result.images[0]
-
-css = """.main-div div{display:inline-flex;align-items:center;gap:.8rem;font-size:1.75rem}.main-div div h1{font-weight:900;margin-bottom:7px}.main-div p{margin-bottom:10px;font-size:94%}a{text-decoration:underline}.tabs{margin-top:0;margin-bottom:0}#gallery{min-height:20rem}
-"""
-with gr.Blocks(css=css) as demo:
- gr.HTML(
- f"""
-
-
-
China Chic Illustration
-
-
- Demo for China Chic Illustration Stable Diffusion model.
- {"Add the following tokens to your prompts for the model to work properly: prefix" if prefix else ""}
-
- Running on {"GPU 🔥" if torch.cuda.is_available() else f"CPU 🥶. For faster inference it is recommended to upgrade to GPU in Settings"} after duplicating the space
- """)
-
-demo.queue(concurrency_count=1)
-demo.launch()
diff --git a/spaces/akhaliq/lama/bin/paper_runfiles/generate_test_ffhq.sh b/spaces/akhaliq/lama/bin/paper_runfiles/generate_test_ffhq.sh
deleted file mode 100644
index a1b79cb0f3f710eed21a978c3a1489ca830bb7f8..0000000000000000000000000000000000000000
--- a/spaces/akhaliq/lama/bin/paper_runfiles/generate_test_ffhq.sh
+++ /dev/null
@@ -1,17 +0,0 @@
-#!/usr/bin/env bash
-
-# paths to data are valid for mml-ws01
-OUT_DIR="/media/inpainting/paper_data/FFHQ_val"
-
-source "$(dirname $0)/env.sh"
-
-for datadir in test
-do
- for conf in random_thin_256 random_medium_256 random_thick_256 random_thin_512 random_medium_512 random_thick_512
- do
- "$BINDIR/gen_mask_dataset_hydra.py" -cn $conf datadir=$datadir location=mml-ws01-ffhq \
- location.out_dir=$OUT_DIR cropping.out_square_crop=False
-
- "$BINDIR/calc_dataset_stats.py" --samples-n 20 "$OUT_DIR/$datadir/$conf" "$OUT_DIR/$datadir/${conf}_stats"
- done
-done
diff --git a/spaces/akhaliq/lama/models/ade20k/mobilenet.py b/spaces/akhaliq/lama/models/ade20k/mobilenet.py
deleted file mode 100644
index f501266e56ee71cdf455744020f8fc1a58ec9fff..0000000000000000000000000000000000000000
--- a/spaces/akhaliq/lama/models/ade20k/mobilenet.py
+++ /dev/null
@@ -1,154 +0,0 @@
-"""
-This MobileNetV2 implementation is modified from the following repository:
-https://github.com/tonylins/pytorch-mobilenet-v2
-"""
-
-import torch.nn as nn
-import math
-from .utils import load_url
-from .segm_lib.nn import SynchronizedBatchNorm2d
-
-BatchNorm2d = SynchronizedBatchNorm2d
-
-
-__all__ = ['mobilenetv2']
-
-
-model_urls = {
- 'mobilenetv2': 'http://sceneparsing.csail.mit.edu/model/pretrained_resnet/mobilenet_v2.pth.tar',
-}
-
-
-def conv_bn(inp, oup, stride):
- return nn.Sequential(
- nn.Conv2d(inp, oup, 3, stride, 1, bias=False),
- BatchNorm2d(oup),
- nn.ReLU6(inplace=True)
- )
-
-
-def conv_1x1_bn(inp, oup):
- return nn.Sequential(
- nn.Conv2d(inp, oup, 1, 1, 0, bias=False),
- BatchNorm2d(oup),
- nn.ReLU6(inplace=True)
- )
-
-
-class InvertedResidual(nn.Module):
- def __init__(self, inp, oup, stride, expand_ratio):
- super(InvertedResidual, self).__init__()
- self.stride = stride
- assert stride in [1, 2]
-
- hidden_dim = round(inp * expand_ratio)
- self.use_res_connect = self.stride == 1 and inp == oup
-
- if expand_ratio == 1:
- self.conv = nn.Sequential(
- # dw
- nn.Conv2d(hidden_dim, hidden_dim, 3, stride, 1, groups=hidden_dim, bias=False),
- BatchNorm2d(hidden_dim),
- nn.ReLU6(inplace=True),
- # pw-linear
- nn.Conv2d(hidden_dim, oup, 1, 1, 0, bias=False),
- BatchNorm2d(oup),
- )
- else:
- self.conv = nn.Sequential(
- # pw
- nn.Conv2d(inp, hidden_dim, 1, 1, 0, bias=False),
- BatchNorm2d(hidden_dim),
- nn.ReLU6(inplace=True),
- # dw
- nn.Conv2d(hidden_dim, hidden_dim, 3, stride, 1, groups=hidden_dim, bias=False),
- BatchNorm2d(hidden_dim),
- nn.ReLU6(inplace=True),
- # pw-linear
- nn.Conv2d(hidden_dim, oup, 1, 1, 0, bias=False),
- BatchNorm2d(oup),
- )
-
- def forward(self, x):
- if self.use_res_connect:
- return x + self.conv(x)
- else:
- return self.conv(x)
-
-
-class MobileNetV2(nn.Module):
- def __init__(self, n_class=1000, input_size=224, width_mult=1.):
- super(MobileNetV2, self).__init__()
- block = InvertedResidual
- input_channel = 32
- last_channel = 1280
- interverted_residual_setting = [
- # t, c, n, s
- [1, 16, 1, 1],
- [6, 24, 2, 2],
- [6, 32, 3, 2],
- [6, 64, 4, 2],
- [6, 96, 3, 1],
- [6, 160, 3, 2],
- [6, 320, 1, 1],
- ]
-
- # building first layer
- assert input_size % 32 == 0
- input_channel = int(input_channel * width_mult)
- self.last_channel = int(last_channel * width_mult) if width_mult > 1.0 else last_channel
- self.features = [conv_bn(3, input_channel, 2)]
- # building inverted residual blocks
- for t, c, n, s in interverted_residual_setting:
- output_channel = int(c * width_mult)
- for i in range(n):
- if i == 0:
- self.features.append(block(input_channel, output_channel, s, expand_ratio=t))
- else:
- self.features.append(block(input_channel, output_channel, 1, expand_ratio=t))
- input_channel = output_channel
- # building last several layers
- self.features.append(conv_1x1_bn(input_channel, self.last_channel))
- # make it nn.Sequential
- self.features = nn.Sequential(*self.features)
-
- # building classifier
- self.classifier = nn.Sequential(
- nn.Dropout(0.2),
- nn.Linear(self.last_channel, n_class),
- )
-
- self._initialize_weights()
-
- def forward(self, x):
- x = self.features(x)
- x = x.mean(3).mean(2)
- x = self.classifier(x)
- return x
-
- def _initialize_weights(self):
- for m in self.modules():
- if isinstance(m, nn.Conv2d):
- n = m.kernel_size[0] * m.kernel_size[1] * m.out_channels
- m.weight.data.normal_(0, math.sqrt(2. / n))
- if m.bias is not None:
- m.bias.data.zero_()
- elif isinstance(m, BatchNorm2d):
- m.weight.data.fill_(1)
- m.bias.data.zero_()
- elif isinstance(m, nn.Linear):
- n = m.weight.size(1)
- m.weight.data.normal_(0, 0.01)
- m.bias.data.zero_()
-
-
-def mobilenetv2(pretrained=False, **kwargs):
- """Constructs a MobileNet_V2 model.
-
- Args:
- pretrained (bool): If True, returns a model pre-trained on ImageNet
- """
- model = MobileNetV2(n_class=1000, **kwargs)
- if pretrained:
- model.load_state_dict(load_url(model_urls['mobilenetv2']), strict=False)
- return model
\ No newline at end of file
diff --git a/spaces/akhaliq/mGPT/README.md b/spaces/akhaliq/mGPT/README.md
deleted file mode 100644
index c4ce4504a1cbb2a36abccfb97aabf07c1f619360..0000000000000000000000000000000000000000
--- a/spaces/akhaliq/mGPT/README.md
+++ /dev/null
@@ -1,12 +0,0 @@
----
-title: MGPT
-emoji: 🌍
-colorFrom: purple
-colorTo: pink
-sdk: gradio
-sdk_version: 2.9.4
-app_file: app.py
-pinned: false
----
-
-Check out the configuration reference at https://huggingface.co/docs/hub/spaces#reference
diff --git a/spaces/alexray/btc_predictor/venv/lib/python3.10/site-packages/pip/_internal/utils/logging.py b/spaces/alexray/btc_predictor/venv/lib/python3.10/site-packages/pip/_internal/utils/logging.py
deleted file mode 100644
index 6e001c5d63cfb058bbbc0351f067c036aa36c6cc..0000000000000000000000000000000000000000
--- a/spaces/alexray/btc_predictor/venv/lib/python3.10/site-packages/pip/_internal/utils/logging.py
+++ /dev/null
@@ -1,343 +0,0 @@
-import contextlib
-import errno
-import logging
-import logging.handlers
-import os
-import sys
-import threading
-from dataclasses import dataclass
-from logging import Filter
-from typing import IO, Any, ClassVar, Iterator, List, Optional, TextIO, Type
-
-from pip._vendor.rich.console import (
- Console,
- ConsoleOptions,
- ConsoleRenderable,
- RenderResult,
-)
-from pip._vendor.rich.highlighter import NullHighlighter
-from pip._vendor.rich.logging import RichHandler
-from pip._vendor.rich.segment import Segment
-from pip._vendor.rich.style import Style
-
-from pip._internal.exceptions import DiagnosticPipError
-from pip._internal.utils._log import VERBOSE, getLogger
-from pip._internal.utils.compat import WINDOWS
-from pip._internal.utils.deprecation import DEPRECATION_MSG_PREFIX
-from pip._internal.utils.misc import ensure_dir
-
-_log_state = threading.local()
-subprocess_logger = getLogger("pip.subprocessor")
-
-
-class BrokenStdoutLoggingError(Exception):
- """
- Raised if BrokenPipeError occurs for the stdout stream while logging.
- """
-
-
-def _is_broken_pipe_error(exc_class: Type[BaseException], exc: BaseException) -> bool:
- if exc_class is BrokenPipeError:
- return True
-
- # On Windows, a broken pipe can show up as EINVAL rather than EPIPE:
- # https://bugs.python.org/issue19612
- # https://bugs.python.org/issue30418
- if not WINDOWS:
- return False
-
- return isinstance(exc, OSError) and exc.errno in (errno.EINVAL, errno.EPIPE)
-
-
-@contextlib.contextmanager
-def indent_log(num: int = 2) -> Iterator[None]:
- """
- A context manager which will cause the log output to be indented for any
- log messages emitted inside it.
- """
- # For thread-safety
- _log_state.indentation = get_indentation()
- _log_state.indentation += num
- try:
- yield
- finally:
- _log_state.indentation -= num
-
-
-def get_indentation() -> int:
- return getattr(_log_state, "indentation", 0)
-
-
-class IndentingFormatter(logging.Formatter):
- default_time_format = "%Y-%m-%dT%H:%M:%S"
-
- def __init__(
- self,
- *args: Any,
- add_timestamp: bool = False,
- **kwargs: Any,
- ) -> None:
- """
- A logging.Formatter that obeys the indent_log() context manager.
-
- :param add_timestamp: A bool indicating output lines should be prefixed
- with their record's timestamp.
- """
- self.add_timestamp = add_timestamp
- super().__init__(*args, **kwargs)
-
- def get_message_start(self, formatted: str, levelno: int) -> str:
- """
- Return the start of the formatted log message (not counting the
- prefix to add to each line).
- """
- if levelno < logging.WARNING:
- return ""
- if formatted.startswith(DEPRECATION_MSG_PREFIX):
- # Then the message already has a prefix. We don't want it to
- # look like "WARNING: DEPRECATION: ...."
- return ""
- if levelno < logging.ERROR:
- return "WARNING: "
-
- return "ERROR: "
-
- def format(self, record: logging.LogRecord) -> str:
- """
- Calls the standard formatter, but will indent all of the log message
- lines by our current indentation level.
- """
- formatted = super().format(record)
- message_start = self.get_message_start(formatted, record.levelno)
- formatted = message_start + formatted
-
- prefix = ""
- if self.add_timestamp:
- prefix = f"{self.formatTime(record)} "
- prefix += " " * get_indentation()
- formatted = "".join([prefix + line for line in formatted.splitlines(True)])
- return formatted
-
-
-@dataclass
-class IndentedRenderable:
- renderable: ConsoleRenderable
- indent: int
-
- def __rich_console__(
- self, console: Console, options: ConsoleOptions
- ) -> RenderResult:
- segments = console.render(self.renderable, options)
- lines = Segment.split_lines(segments)
- for line in lines:
- yield Segment(" " * self.indent)
- yield from line
- yield Segment("\n")
-
-
-class RichPipStreamHandler(RichHandler):
- KEYWORDS: ClassVar[Optional[List[str]]] = []
-
- def __init__(self, stream: Optional[TextIO], no_color: bool) -> None:
- super().__init__(
- console=Console(file=stream, no_color=no_color, soft_wrap=True),
- show_time=False,
- show_level=False,
- show_path=False,
- highlighter=NullHighlighter(),
- )
-
- # Our custom override on Rich's logger, to make things work as we need them to.
- def emit(self, record: logging.LogRecord) -> None:
- style: Optional[Style] = None
-
- # If we are given a diagnostic error to present, present it with indentation.
- if record.msg == "[present-diagnostic] %s" and len(record.args) == 1:
- diagnostic_error: DiagnosticPipError = record.args[0] # type: ignore[index]
- assert isinstance(diagnostic_error, DiagnosticPipError)
-
- renderable: ConsoleRenderable = IndentedRenderable(
- diagnostic_error, indent=get_indentation()
- )
- else:
- message = self.format(record)
- renderable = self.render_message(record, message)
- if record.levelno is not None:
- if record.levelno >= logging.ERROR:
- style = Style(color="red")
- elif record.levelno >= logging.WARNING:
- style = Style(color="yellow")
-
- try:
- self.console.print(renderable, overflow="ignore", crop=False, style=style)
- except Exception:
- self.handleError(record)
-
- def handleError(self, record: logging.LogRecord) -> None:
- """Called when logging is unable to log some output."""
-
- exc_class, exc = sys.exc_info()[:2]
- # If a broken pipe occurred while calling write() or flush() on the
- # stdout stream in logging's Handler.emit(), then raise our special
- # exception so we can handle it in main() instead of logging the
- # broken pipe error and continuing.
- if (
- exc_class
- and exc
- and self.console.file is sys.stdout
- and _is_broken_pipe_error(exc_class, exc)
- ):
- raise BrokenStdoutLoggingError()
-
- return super().handleError(record)
-
-
-class BetterRotatingFileHandler(logging.handlers.RotatingFileHandler):
- def _open(self) -> IO[Any]:
- ensure_dir(os.path.dirname(self.baseFilename))
- return super()._open()
-
-
-class MaxLevelFilter(Filter):
- def __init__(self, level: int) -> None:
- self.level = level
-
- def filter(self, record: logging.LogRecord) -> bool:
- return record.levelno < self.level
-
-
-class ExcludeLoggerFilter(Filter):
-
- """
- A logging Filter that excludes records from a logger (or its children).
- """
-
- def filter(self, record: logging.LogRecord) -> bool:
- # The base Filter class allows only records from a logger (or its
- # children).
- return not super().filter(record)
-
-
-def setup_logging(verbosity: int, no_color: bool, user_log_file: Optional[str]) -> int:
- """Configures and sets up all of the logging
-
- Returns the requested logging level, as its integer value.
- """
-
- # Determine the level to be logging at.
- if verbosity >= 2:
- level_number = logging.DEBUG
- elif verbosity == 1:
- level_number = VERBOSE
- elif verbosity == -1:
- level_number = logging.WARNING
- elif verbosity == -2:
- level_number = logging.ERROR
- elif verbosity <= -3:
- level_number = logging.CRITICAL
- else:
- level_number = logging.INFO
-
- level = logging.getLevelName(level_number)
-
- # The "root" logger should match the "console" level *unless* we also need
- # to log to a user log file.
- include_user_log = user_log_file is not None
- if include_user_log:
- additional_log_file = user_log_file
- root_level = "DEBUG"
- else:
- additional_log_file = "/dev/null"
- root_level = level
-
- # Disable any logging besides WARNING unless we have DEBUG level logging
- # enabled for vendored libraries.
- vendored_log_level = "WARNING" if level in ["INFO", "ERROR"] else "DEBUG"
-
- # Shorthands for clarity
- log_streams = {
- "stdout": "ext://sys.stdout",
- "stderr": "ext://sys.stderr",
- }
- handler_classes = {
- "stream": "pip._internal.utils.logging.RichPipStreamHandler",
- "file": "pip._internal.utils.logging.BetterRotatingFileHandler",
- }
- handlers = ["console", "console_errors", "console_subprocess"] + (
- ["user_log"] if include_user_log else []
- )
-
- logging.config.dictConfig(
- {
- "version": 1,
- "disable_existing_loggers": False,
- "filters": {
- "exclude_warnings": {
- "()": "pip._internal.utils.logging.MaxLevelFilter",
- "level": logging.WARNING,
- },
- "restrict_to_subprocess": {
- "()": "logging.Filter",
- "name": subprocess_logger.name,
- },
- "exclude_subprocess": {
- "()": "pip._internal.utils.logging.ExcludeLoggerFilter",
- "name": subprocess_logger.name,
- },
- },
- "formatters": {
- "indent": {
- "()": IndentingFormatter,
- "format": "%(message)s",
- },
- "indent_with_timestamp": {
- "()": IndentingFormatter,
- "format": "%(message)s",
- "add_timestamp": True,
- },
- },
- "handlers": {
- "console": {
- "level": level,
- "class": handler_classes["stream"],
- "no_color": no_color,
- "stream": log_streams["stdout"],
- "filters": ["exclude_subprocess", "exclude_warnings"],
- "formatter": "indent",
- },
- "console_errors": {
- "level": "WARNING",
- "class": handler_classes["stream"],
- "no_color": no_color,
- "stream": log_streams["stderr"],
- "filters": ["exclude_subprocess"],
- "formatter": "indent",
- },
- # A handler responsible for logging to the console messages
- # from the "subprocessor" logger.
- "console_subprocess": {
- "level": level,
- "class": handler_classes["stream"],
- "stream": log_streams["stderr"],
- "no_color": no_color,
- "filters": ["restrict_to_subprocess"],
- "formatter": "indent",
- },
- "user_log": {
- "level": "DEBUG",
- "class": handler_classes["file"],
- "filename": additional_log_file,
- "encoding": "utf-8",
- "delay": True,
- "formatter": "indent_with_timestamp",
- },
- },
- "root": {
- "level": root_level,
- "handlers": handlers,
- },
- "loggers": {"pip._vendor": {"level": vendored_log_level}},
- }
- )
-
- return level_number
diff --git a/spaces/alexray/btc_predictor/venv/lib/python3.10/site-packages/pip/_vendor/pyparsing/__init__.py b/spaces/alexray/btc_predictor/venv/lib/python3.10/site-packages/pip/_vendor/pyparsing/__init__.py
deleted file mode 100644
index fbc6d8cf208bba25fee4a960483f7e526fb7debc..0000000000000000000000000000000000000000
--- a/spaces/alexray/btc_predictor/venv/lib/python3.10/site-packages/pip/_vendor/pyparsing/__init__.py
+++ /dev/null
@@ -1,328 +0,0 @@
-# module pyparsing.py
-#
-# Copyright (c) 2003-2021 Paul T. McGuire
-#
-# Permission is hereby granted, free of charge, to any person obtaining
-# a copy of this software and associated documentation files (the
-# "Software"), to deal in the Software without restriction, including
-# without limitation the rights to use, copy, modify, merge, publish,
-# distribute, sublicense, and/or sell copies of the Software, and to
-# permit persons to whom the Software is furnished to do so, subject to
-# the following conditions:
-#
-# The above copyright notice and this permission notice shall be
-# included in all copies or substantial portions of the Software.
-#
-# THE SOFTWARE IS PROVIDED "AS IS", WITHOUT WARRANTY OF ANY KIND,
-# EXPRESS OR IMPLIED, INCLUDING BUT NOT LIMITED TO THE WARRANTIES OF
-# MERCHANTABILITY, FITNESS FOR A PARTICULAR PURPOSE AND NONINFRINGEMENT.
-# IN NO EVENT SHALL THE AUTHORS OR COPYRIGHT HOLDERS BE LIABLE FOR ANY
-# CLAIM, DAMAGES OR OTHER LIABILITY, WHETHER IN AN ACTION OF CONTRACT,
-# TORT OR OTHERWISE, ARISING FROM, OUT OF OR IN CONNECTION WITH THE
-# SOFTWARE OR THE USE OR OTHER DEALINGS IN THE SOFTWARE.
-#
-
-__doc__ = """
-pyparsing module - Classes and methods to define and execute parsing grammars
-=============================================================================
-
-The pyparsing module is an alternative approach to creating and
-executing simple grammars, vs. the traditional lex/yacc approach, or the
-use of regular expressions. With pyparsing, you don't need to learn
-a new syntax for defining grammars or matching expressions - the parsing
-module provides a library of classes that you use to construct the
-grammar directly in Python.
-
-Here is a program to parse "Hello, World!" (or any greeting of the form
-``", !"``), built up using :class:`Word`,
-:class:`Literal`, and :class:`And` elements
-(the :meth:`'+'` operators create :class:`And` expressions,
-and the strings are auto-converted to :class:`Literal` expressions)::
-
- from pip._vendor.pyparsing import Word, alphas
-
- # define grammar of a greeting
- greet = Word(alphas) + "," + Word(alphas) + "!"
-
- hello = "Hello, World!"
- print(hello, "->", greet.parse_string(hello))
-
-The program outputs the following::
-
- Hello, World! -> ['Hello', ',', 'World', '!']
-
-The Python representation of the grammar is quite readable, owing to the
-self-explanatory class names, and the use of :class:`'+'`,
-:class:`'|'`, :class:`'^'` and :class:`'&'` operators.
-
-The :class:`ParseResults` object returned from
-:class:`ParserElement.parseString` can be
-accessed as a nested list, a dictionary, or an object with named
-attributes.
-
-The pyparsing module handles some of the problems that are typically
-vexing when writing text parsers:
-
- - extra or missing whitespace (the above program will also handle
- "Hello,World!", "Hello , World !", etc.)
- - quoted strings
- - embedded comments
-
-
-Getting Started -
------------------
-Visit the classes :class:`ParserElement` and :class:`ParseResults` to
-see the base classes that most other pyparsing
-classes inherit from. Use the docstrings for examples of how to:
-
- - construct literal match expressions from :class:`Literal` and
- :class:`CaselessLiteral` classes
- - construct character word-group expressions using the :class:`Word`
- class
- - see how to create repetitive expressions using :class:`ZeroOrMore`
- and :class:`OneOrMore` classes
- - use :class:`'+'`, :class:`'|'`, :class:`'^'`,
- and :class:`'&'` operators to combine simple expressions into
- more complex ones
- - associate names with your parsed results using
- :class:`ParserElement.setResultsName`
- - access the parsed data, which is returned as a :class:`ParseResults`
- object
- - find some helpful expression short-cuts like :class:`delimitedList`
- and :class:`oneOf`
- - find more useful common expressions in the :class:`pyparsing_common`
- namespace class
-"""
-from typing import NamedTuple
-
-
-class version_info(NamedTuple):
- major: int
- minor: int
- micro: int
- releaselevel: str
- serial: int
-
- @property
- def __version__(self):
- return "{}.{}.{}".format(self.major, self.minor, self.micro) + (
- "{}{}{}".format(
- "r" if self.releaselevel[0] == "c" else "",
- self.releaselevel[0],
- self.serial,
- ),
- "",
- )[self.releaselevel == "final"]
-
- def __str__(self):
- return "{} {} / {}".format(__name__, self.__version__, __version_time__)
-
- def __repr__(self):
- return "{}.{}({})".format(
- __name__,
- type(self).__name__,
- ", ".join("{}={!r}".format(*nv) for nv in zip(self._fields, self)),
- )
-
-
-__version_info__ = version_info(3, 0, 7, "final", 0)
-__version_time__ = "15 Jan 2022 04:10 UTC"
-__version__ = __version_info__.__version__
-__versionTime__ = __version_time__
-__author__ = "Paul McGuire "
-
-from .util import *
-from .exceptions import *
-from .actions import *
-from .core import __diag__, __compat__
-from .results import *
-from .core import *
-from .core import _builtin_exprs as core_builtin_exprs
-from .helpers import *
-from .helpers import _builtin_exprs as helper_builtin_exprs
-
-from .unicode import unicode_set, UnicodeRangeList, pyparsing_unicode as unicode
-from .testing import pyparsing_test as testing
-from .common import (
- pyparsing_common as common,
- _builtin_exprs as common_builtin_exprs,
-)
-
-# define backward compat synonyms
-if "pyparsing_unicode" not in globals():
- pyparsing_unicode = unicode
-if "pyparsing_common" not in globals():
- pyparsing_common = common
-if "pyparsing_test" not in globals():
- pyparsing_test = testing
-
-core_builtin_exprs += common_builtin_exprs + helper_builtin_exprs
-
-
-__all__ = [
- "__version__",
- "__version_time__",
- "__author__",
- "__compat__",
- "__diag__",
- "And",
- "AtLineStart",
- "AtStringStart",
- "CaselessKeyword",
- "CaselessLiteral",
- "CharsNotIn",
- "Combine",
- "Dict",
- "Each",
- "Empty",
- "FollowedBy",
- "Forward",
- "GoToColumn",
- "Group",
- "IndentedBlock",
- "Keyword",
- "LineEnd",
- "LineStart",
- "Literal",
- "Located",
- "PrecededBy",
- "MatchFirst",
- "NoMatch",
- "NotAny",
- "OneOrMore",
- "OnlyOnce",
- "OpAssoc",
- "Opt",
- "Optional",
- "Or",
- "ParseBaseException",
- "ParseElementEnhance",
- "ParseException",
- "ParseExpression",
- "ParseFatalException",
- "ParseResults",
- "ParseSyntaxException",
- "ParserElement",
- "PositionToken",
- "QuotedString",
- "RecursiveGrammarException",
- "Regex",
- "SkipTo",
- "StringEnd",
- "StringStart",
- "Suppress",
- "Token",
- "TokenConverter",
- "White",
- "Word",
- "WordEnd",
- "WordStart",
- "ZeroOrMore",
- "Char",
- "alphanums",
- "alphas",
- "alphas8bit",
- "any_close_tag",
- "any_open_tag",
- "c_style_comment",
- "col",
- "common_html_entity",
- "counted_array",
- "cpp_style_comment",
- "dbl_quoted_string",
- "dbl_slash_comment",
- "delimited_list",
- "dict_of",
- "empty",
- "hexnums",
- "html_comment",
- "identchars",
- "identbodychars",
- "java_style_comment",
- "line",
- "line_end",
- "line_start",
- "lineno",
- "make_html_tags",
- "make_xml_tags",
- "match_only_at_col",
- "match_previous_expr",
- "match_previous_literal",
- "nested_expr",
- "null_debug_action",
- "nums",
- "one_of",
- "printables",
- "punc8bit",
- "python_style_comment",
- "quoted_string",
- "remove_quotes",
- "replace_with",
- "replace_html_entity",
- "rest_of_line",
- "sgl_quoted_string",
- "srange",
- "string_end",
- "string_start",
- "trace_parse_action",
- "unicode_string",
- "with_attribute",
- "indentedBlock",
- "original_text_for",
- "ungroup",
- "infix_notation",
- "locatedExpr",
- "with_class",
- "CloseMatch",
- "token_map",
- "pyparsing_common",
- "pyparsing_unicode",
- "unicode_set",
- "condition_as_parse_action",
- "pyparsing_test",
- # pre-PEP8 compatibility names
- "__versionTime__",
- "anyCloseTag",
- "anyOpenTag",
- "cStyleComment",
- "commonHTMLEntity",
- "countedArray",
- "cppStyleComment",
- "dblQuotedString",
- "dblSlashComment",
- "delimitedList",
- "dictOf",
- "htmlComment",
- "javaStyleComment",
- "lineEnd",
- "lineStart",
- "makeHTMLTags",
- "makeXMLTags",
- "matchOnlyAtCol",
- "matchPreviousExpr",
- "matchPreviousLiteral",
- "nestedExpr",
- "nullDebugAction",
- "oneOf",
- "opAssoc",
- "pythonStyleComment",
- "quotedString",
- "removeQuotes",
- "replaceHTMLEntity",
- "replaceWith",
- "restOfLine",
- "sglQuotedString",
- "stringEnd",
- "stringStart",
- "traceParseAction",
- "unicodeString",
- "withAttribute",
- "indentedBlock",
- "originalTextFor",
- "infixNotation",
- "locatedExpr",
- "withClass",
- "tokenMap",
- "conditionAsParseAction",
- "autoname_elements",
-]
diff --git a/spaces/alexray/btc_predictor/venv/lib/python3.10/site-packages/pip/_vendor/requests/status_codes.py b/spaces/alexray/btc_predictor/venv/lib/python3.10/site-packages/pip/_vendor/requests/status_codes.py
deleted file mode 100644
index d80a7cd4dd486d2927a3c0f2f3e684bcf5f8e49d..0000000000000000000000000000000000000000
--- a/spaces/alexray/btc_predictor/venv/lib/python3.10/site-packages/pip/_vendor/requests/status_codes.py
+++ /dev/null
@@ -1,123 +0,0 @@
-# -*- coding: utf-8 -*-
-
-r"""
-The ``codes`` object defines a mapping from common names for HTTP statuses
-to their numerical codes, accessible either as attributes or as dictionary
-items.
-
-Example::
-
- >>> import requests
- >>> requests.codes['temporary_redirect']
- 307
- >>> requests.codes.teapot
- 418
- >>> requests.codes['\o/']
- 200
-
-Some codes have multiple names, and both upper- and lower-case versions of
-the names are allowed. For example, ``codes.ok``, ``codes.OK``, and
-``codes.okay`` all correspond to the HTTP status code 200.
-"""
-
-from .structures import LookupDict
-
-_codes = {
-
- # Informational.
- 100: ('continue',),
- 101: ('switching_protocols',),
- 102: ('processing',),
- 103: ('checkpoint',),
- 122: ('uri_too_long', 'request_uri_too_long'),
- 200: ('ok', 'okay', 'all_ok', 'all_okay', 'all_good', '\\o/', '✓'),
- 201: ('created',),
- 202: ('accepted',),
- 203: ('non_authoritative_info', 'non_authoritative_information'),
- 204: ('no_content',),
- 205: ('reset_content', 'reset'),
- 206: ('partial_content', 'partial'),
- 207: ('multi_status', 'multiple_status', 'multi_stati', 'multiple_stati'),
- 208: ('already_reported',),
- 226: ('im_used',),
-
- # Redirection.
- 300: ('multiple_choices',),
- 301: ('moved_permanently', 'moved', '\\o-'),
- 302: ('found',),
- 303: ('see_other', 'other'),
- 304: ('not_modified',),
- 305: ('use_proxy',),
- 306: ('switch_proxy',),
- 307: ('temporary_redirect', 'temporary_moved', 'temporary'),
- 308: ('permanent_redirect',
- 'resume_incomplete', 'resume',), # These 2 to be removed in 3.0
-
- # Client Error.
- 400: ('bad_request', 'bad'),
- 401: ('unauthorized',),
- 402: ('payment_required', 'payment'),
- 403: ('forbidden',),
- 404: ('not_found', '-o-'),
- 405: ('method_not_allowed', 'not_allowed'),
- 406: ('not_acceptable',),
- 407: ('proxy_authentication_required', 'proxy_auth', 'proxy_authentication'),
- 408: ('request_timeout', 'timeout'),
- 409: ('conflict',),
- 410: ('gone',),
- 411: ('length_required',),
- 412: ('precondition_failed', 'precondition'),
- 413: ('request_entity_too_large',),
- 414: ('request_uri_too_large',),
- 415: ('unsupported_media_type', 'unsupported_media', 'media_type'),
- 416: ('requested_range_not_satisfiable', 'requested_range', 'range_not_satisfiable'),
- 417: ('expectation_failed',),
- 418: ('im_a_teapot', 'teapot', 'i_am_a_teapot'),
- 421: ('misdirected_request',),
- 422: ('unprocessable_entity', 'unprocessable'),
- 423: ('locked',),
- 424: ('failed_dependency', 'dependency'),
- 425: ('unordered_collection', 'unordered'),
- 426: ('upgrade_required', 'upgrade'),
- 428: ('precondition_required', 'precondition'),
- 429: ('too_many_requests', 'too_many'),
- 431: ('header_fields_too_large', 'fields_too_large'),
- 444: ('no_response', 'none'),
- 449: ('retry_with', 'retry'),
- 450: ('blocked_by_windows_parental_controls', 'parental_controls'),
- 451: ('unavailable_for_legal_reasons', 'legal_reasons'),
- 499: ('client_closed_request',),
-
- # Server Error.
- 500: ('internal_server_error', 'server_error', '/o\\', '✗'),
- 501: ('not_implemented',),
- 502: ('bad_gateway',),
- 503: ('service_unavailable', 'unavailable'),
- 504: ('gateway_timeout',),
- 505: ('http_version_not_supported', 'http_version'),
- 506: ('variant_also_negotiates',),
- 507: ('insufficient_storage',),
- 509: ('bandwidth_limit_exceeded', 'bandwidth'),
- 510: ('not_extended',),
- 511: ('network_authentication_required', 'network_auth', 'network_authentication'),
-}
-
-codes = LookupDict(name='status_codes')
-
-def _init():
- for code, titles in _codes.items():
- for title in titles:
- setattr(codes, title, code)
- if not title.startswith(('\\', '/')):
- setattr(codes, title.upper(), code)
-
- def doc(code):
- names = ', '.join('``%s``' % n for n in _codes[code])
- return '* %d: %s' % (code, names)
-
- global __doc__
- __doc__ = (__doc__ + '\n' +
- '\n'.join(doc(code) for code in sorted(_codes))
- if __doc__ is not None else None)
-
-_init()
diff --git a/spaces/alexray/btc_predictor/venv/lib/python3.10/site-packages/pip/_vendor/resolvelib/reporters.py b/spaces/alexray/btc_predictor/venv/lib/python3.10/site-packages/pip/_vendor/resolvelib/reporters.py
deleted file mode 100644
index 6695480fff4c87608ac2002dfb341f90ed1a5ce4..0000000000000000000000000000000000000000
--- a/spaces/alexray/btc_predictor/venv/lib/python3.10/site-packages/pip/_vendor/resolvelib/reporters.py
+++ /dev/null
@@ -1,43 +0,0 @@
-class BaseReporter(object):
- """Delegate class to provider progress reporting for the resolver."""
-
- def starting(self):
- """Called before the resolution actually starts."""
-
- def starting_round(self, index):
- """Called before each round of resolution starts.
-
- The index is zero-based.
- """
-
- def ending_round(self, index, state):
- """Called before each round of resolution ends.
-
- This is NOT called if the resolution ends at this round. Use `ending`
- if you want to report finalization. The index is zero-based.
- """
-
- def ending(self, state):
- """Called before the resolution ends successfully."""
-
- def adding_requirement(self, requirement, parent):
- """Called when adding a new requirement into the resolve criteria.
-
- :param requirement: The additional requirement to be applied to filter
- the available candidaites.
- :param parent: The candidate that requires ``requirement`` as a
- dependency, or None if ``requirement`` is one of the root
- requirements passed in from ``Resolver.resolve()``.
- """
-
- def resolving_conflicts(self, causes):
- """Called when starting to attempt requirement conflict resolution.
-
- :param causes: The information on the collision that caused the backtracking.
- """
-
- def backtracking(self, candidate):
- """Called when rejecting a candidate during backtracking."""
-
- def pinning(self, candidate):
- """Called when adding a candidate to the potential solution."""
diff --git a/spaces/aliabid94/AutoGPT/autogpt/memory/pinecone.py b/spaces/aliabid94/AutoGPT/autogpt/memory/pinecone.py
deleted file mode 100644
index 27fcd62482d0cf44e02fa1c339195be58cb745b0..0000000000000000000000000000000000000000
--- a/spaces/aliabid94/AutoGPT/autogpt/memory/pinecone.py
+++ /dev/null
@@ -1,75 +0,0 @@
-import pinecone
-from colorama import Fore, Style
-
-from autogpt.llm_utils import create_embedding_with_ada
-from autogpt.logs import logger
-from autogpt.memory.base import MemoryProviderSingleton
-
-
-class PineconeMemory(MemoryProviderSingleton):
- def __init__(self, cfg):
- pinecone_api_key = cfg.pinecone_api_key
- pinecone_region = cfg.pinecone_region
- pinecone.init(api_key=pinecone_api_key, environment=pinecone_region)
- dimension = 1536
- metric = "cosine"
- pod_type = "p1"
- table_name = "auto-gpt"
- # this assumes we don't start with memory.
- # for now this works.
- # we'll need a more complicated and robust system if we want to start with
- # memory.
- self.vec_num = 0
-
- try:
- pinecone.whoami()
- except Exception as e:
- logger.typewriter_log(
- "FAILED TO CONNECT TO PINECONE",
- Fore.RED,
- Style.BRIGHT + str(e) + Style.RESET_ALL,
- )
- logger.double_check(
- "Please ensure you have setup and configured Pinecone properly for use."
- + f"You can check out {Fore.CYAN + Style.BRIGHT}"
- "https://github.com/Torantulino/Auto-GPT#-pinecone-api-key-setup"
- f"{Style.RESET_ALL} to ensure you've set up everything correctly."
- )
- exit(1)
-
- if table_name not in pinecone.list_indexes():
- pinecone.create_index(
- table_name, dimension=dimension, metric=metric, pod_type=pod_type
- )
- self.index = pinecone.Index(table_name)
-
- def add(self, data):
- vector = create_embedding_with_ada(data)
- # no metadata here. We may wish to change that long term.
- self.index.upsert([(str(self.vec_num), vector, {"raw_text": data})])
- _text = f"Inserting data into memory at index: {self.vec_num}:\n data: {data}"
- self.vec_num += 1
- return _text
-
- def get(self, data):
- return self.get_relevant(data, 1)
-
- def clear(self):
- self.index.delete(deleteAll=True)
- return "Obliviated"
-
- def get_relevant(self, data, num_relevant=5):
- """
- Returns all the data in the memory that is relevant to the given data.
- :param data: The data to compare to.
- :param num_relevant: The number of relevant data to return. Defaults to 5
- """
- query_embedding = create_embedding_with_ada(data)
- results = self.index.query(
- query_embedding, top_k=num_relevant, include_metadata=True
- )
- sorted_results = sorted(results.matches, key=lambda x: x.score)
- return [str(item["metadata"]["raw_text"]) for item in sorted_results]
-
- def get_stats(self):
- return self.index.describe_index_stats()
diff --git a/spaces/alphunt/diffdock-alphunt-demo/esm/esm/inverse_folding/features.py b/spaces/alphunt/diffdock-alphunt-demo/esm/esm/inverse_folding/features.py
deleted file mode 100644
index 0555555a7fc2b91499c31502c2da7a5edb032f10..0000000000000000000000000000000000000000
--- a/spaces/alphunt/diffdock-alphunt-demo/esm/esm/inverse_folding/features.py
+++ /dev/null
@@ -1,352 +0,0 @@
-# Copyright (c) Facebook, Inc. and its affiliates.
-#
-# This source code is licensed under the MIT license found in the
-# LICENSE file in the root directory of this source tree.
-#
-# Portions of this file were adapted from the open source code for the following
-# two papers:
-#
-# Ingraham, J., Garg, V., Barzilay, R., & Jaakkola, T. (2019). Generative
-# models for graph-based protein design. Advances in Neural Information
-# Processing Systems, 32.
-#
-# Jing, B., Eismann, S., Suriana, P., Townshend, R. J. L., & Dror, R. (2020).
-# Learning from Protein Structure with Geometric Vector Perceptrons. In
-# International Conference on Learning Representations.
-#
-# MIT License
-#
-# Copyright (c) 2020 Bowen Jing, Stephan Eismann, Patricia Suriana, Raphael Townshend, Ron Dror
-#
-# Permission is hereby granted, free of charge, to any person obtaining a copy
-# of this software and associated documentation files (the "Software"), to deal
-# in the Software without restriction, including without limitation the rights
-# to use, copy, modify, merge, publish, distribute, sublicense, and/or sell
-# copies of the Software, and to permit persons to whom the Software is
-# furnished to do so, subject to the following conditions:
-#
-# The above copyright notice and this permission notice shall be included in all
-# copies or substantial portions of the Software.
-#
-# THE SOFTWARE IS PROVIDED "AS IS", WITHOUT WARRANTY OF ANY KIND, EXPRESS OR
-# IMPLIED, INCLUDING BUT NOT LIMITED TO THE WARRANTIES OF MERCHANTABILITY,
-# FITNESS FOR A PARTICULAR PURPOSE AND NONINFRINGEMENT. IN NO EVENT SHALL THE
-# AUTHORS OR COPYRIGHT HOLDERS BE LIABLE FOR ANY CLAIM, DAMAGES OR OTHER
-# LIABILITY, WHETHER IN AN ACTION OF CONTRACT, TORT OR OTHERWISE, ARISING FROM,
-# OUT OF OR IN CONNECTION WITH THE SOFTWARE OR THE USE OR OTHER DEALINGS IN THE
-# SOFTWARE.
-#
-# ================================================================
-# The below license applies to the portions of the code (parts of
-# src/datasets.py and src/models.py) adapted from Ingraham, et al.
-# ================================================================
-#
-# MIT License
-#
-# Copyright (c) 2019 John Ingraham, Vikas Garg, Regina Barzilay, Tommi Jaakkola
-#
-# Permission is hereby granted, free of charge, to any person obtaining a copy
-# of this software and associated documentation files (the "Software"), to deal
-# in the Software without restriction, including without limitation the rights
-# to use, copy, modify, merge, publish, distribute, sublicense, and/or sell
-# copies of the Software, and to permit persons to whom the Software is
-# furnished to do so, subject to the following conditions:
-#
-# The above copyright notice and this permission notice shall be included in all
-# copies or substantial portions of the Software.
-#
-# THE SOFTWARE IS PROVIDED "AS IS", WITHOUT WARRANTY OF ANY KIND, EXPRESS OR
-# IMPLIED, INCLUDING BUT NOT LIMITED TO THE WARRANTIES OF MERCHANTABILITY,
-# FITNESS FOR A PARTICULAR PURPOSE AND NONINFRINGEMENT. IN NO EVENT SHALL THE
-# AUTHORS OR COPYRIGHT HOLDERS BE LIABLE FOR ANY CLAIM, DAMAGES OR OTHER
-# LIABILITY, WHETHER IN AN ACTION OF CONTRACT, TORT OR OTHERWISE, ARISING FROM,
-# OUT OF OR IN CONNECTION WITH THE SOFTWARE OR THE USE OR OTHER DEALINGS IN THE
-# SOFTWARE.
-
-import math
-import numpy as np
-import torch
-import torch.nn as nn
-import torch.nn.functional as F
-
-from .gvp_utils import flatten_graph
-from .gvp_modules import GVP, LayerNorm
-from .util import normalize, norm, nan_to_num, rbf
-
-
-class GVPInputFeaturizer(nn.Module):
-
- @staticmethod
- def get_node_features(coords, coord_mask, with_coord_mask=True):
- # scalar features
- node_scalar_features = GVPInputFeaturizer._dihedrals(coords)
- if with_coord_mask:
- node_scalar_features = torch.cat([
- node_scalar_features,
- coord_mask.float().unsqueeze(-1)
- ], dim=-1)
- # vector features
- X_ca = coords[:, :, 1]
- orientations = GVPInputFeaturizer._orientations(X_ca)
- sidechains = GVPInputFeaturizer._sidechains(coords)
- node_vector_features = torch.cat([orientations, sidechains.unsqueeze(-2)], dim=-2)
- return node_scalar_features, node_vector_features
-
- @staticmethod
- def _orientations(X):
- forward = normalize(X[:, 1:] - X[:, :-1])
- backward = normalize(X[:, :-1] - X[:, 1:])
- forward = F.pad(forward, [0, 0, 0, 1])
- backward = F.pad(backward, [0, 0, 1, 0])
- return torch.cat([forward.unsqueeze(-2), backward.unsqueeze(-2)], -2)
-
- @staticmethod
- def _sidechains(X):
- n, origin, c = X[:, :, 0], X[:, :, 1], X[:, :, 2]
- c, n = normalize(c - origin), normalize(n - origin)
- bisector = normalize(c + n)
- perp = normalize(torch.cross(c, n, dim=-1))
- vec = -bisector * math.sqrt(1 / 3) - perp * math.sqrt(2 / 3)
- return vec
-
- @staticmethod
- def _dihedrals(X, eps=1e-7):
- X = torch.flatten(X[:, :, :3], 1, 2)
- bsz = X.shape[0]
- dX = X[:, 1:] - X[:, :-1]
- U = normalize(dX, dim=-1)
- u_2 = U[:, :-2]
- u_1 = U[:, 1:-1]
- u_0 = U[:, 2:]
-
- # Backbone normals
- n_2 = normalize(torch.cross(u_2, u_1, dim=-1), dim=-1)
- n_1 = normalize(torch.cross(u_1, u_0, dim=-1), dim=-1)
-
- # Angle between normals
- cosD = torch.sum(n_2 * n_1, -1)
- cosD = torch.clamp(cosD, -1 + eps, 1 - eps)
- D = torch.sign(torch.sum(u_2 * n_1, -1)) * torch.acos(cosD)
-
- # This scheme will remove phi[0], psi[-1], omega[-1]
- D = F.pad(D, [1, 2])
- D = torch.reshape(D, [bsz, -1, 3])
- # Lift angle representations to the circle
- D_features = torch.cat([torch.cos(D), torch.sin(D)], -1)
- return D_features
-
- @staticmethod
- def _positional_embeddings(edge_index,
- num_embeddings=None,
- num_positional_embeddings=16,
- period_range=[2, 1000]):
- # From https://github.com/jingraham/neurips19-graph-protein-design
- num_embeddings = num_embeddings or num_positional_embeddings
- d = edge_index[0] - edge_index[1]
-
- frequency = torch.exp(
- torch.arange(0, num_embeddings, 2, dtype=torch.float32,
- device=edge_index.device)
- * -(np.log(10000.0) / num_embeddings)
- )
- angles = d.unsqueeze(-1) * frequency
- E = torch.cat((torch.cos(angles), torch.sin(angles)), -1)
- return E
-
- @staticmethod
- def _dist(X, coord_mask, padding_mask, top_k_neighbors, eps=1e-8):
- """ Pairwise euclidean distances """
- bsz, maxlen = X.size(0), X.size(1)
- coord_mask_2D = torch.unsqueeze(coord_mask,1) * torch.unsqueeze(coord_mask,2)
- residue_mask = ~padding_mask
- residue_mask_2D = torch.unsqueeze(residue_mask,1) * torch.unsqueeze(residue_mask,2)
- dX = torch.unsqueeze(X,1) - torch.unsqueeze(X,2)
- D = coord_mask_2D * norm(dX, dim=-1)
-
- # sorting preference: first those with coords, then among the residues that
- # exist but are masked use distance in sequence as tie breaker, and then the
- # residues that came from padding are last
- seqpos = torch.arange(maxlen, device=X.device)
- Dseq = torch.abs(seqpos.unsqueeze(1) - seqpos.unsqueeze(0)).repeat(bsz, 1, 1)
- D_adjust = nan_to_num(D) + (~coord_mask_2D) * (1e8 + Dseq*1e6) + (
- ~residue_mask_2D) * (1e10)
-
- if top_k_neighbors == -1:
- D_neighbors = D_adjust
- E_idx = seqpos.repeat(
- *D_neighbors.shape[:-1], 1)
- else:
- # Identify k nearest neighbors (including self)
- k = min(top_k_neighbors, X.size(1))
- D_neighbors, E_idx = torch.topk(D_adjust, k, dim=-1, largest=False)
-
- coord_mask_neighbors = (D_neighbors < 5e7)
- residue_mask_neighbors = (D_neighbors < 5e9)
- return D_neighbors, E_idx, coord_mask_neighbors, residue_mask_neighbors
-
-
-class Normalize(nn.Module):
- def __init__(self, features, epsilon=1e-6):
- super(Normalize, self).__init__()
- self.gain = nn.Parameter(torch.ones(features))
- self.bias = nn.Parameter(torch.zeros(features))
- self.epsilon = epsilon
-
- def forward(self, x, dim=-1):
- mu = x.mean(dim, keepdim=True)
- sigma = torch.sqrt(x.var(dim, keepdim=True) + self.epsilon)
- gain = self.gain
- bias = self.bias
- # Reshape
- if dim != -1:
- shape = [1] * len(mu.size())
- shape[dim] = self.gain.size()[0]
- gain = gain.view(shape)
- bias = bias.view(shape)
- return gain * (x - mu) / (sigma + self.epsilon) + bias
-
-
-class DihedralFeatures(nn.Module):
- def __init__(self, node_embed_dim):
- """ Embed dihedral angle features. """
- super(DihedralFeatures, self).__init__()
- # 3 dihedral angles; sin and cos of each angle
- node_in = 6
- # Normalization and embedding
- self.node_embedding = nn.Linear(node_in, node_embed_dim, bias=True)
- self.norm_nodes = Normalize(node_embed_dim)
-
- def forward(self, X):
- """ Featurize coordinates as an attributed graph """
- V = self._dihedrals(X)
- V = self.node_embedding(V)
- V = self.norm_nodes(V)
- return V
-
- @staticmethod
- def _dihedrals(X, eps=1e-7, return_angles=False):
- # First 3 coordinates are N, CA, C
- X = X[:,:,:3,:].reshape(X.shape[0], 3*X.shape[1], 3)
-
- # Shifted slices of unit vectors
- dX = X[:,1:,:] - X[:,:-1,:]
- U = F.normalize(dX, dim=-1)
- u_2 = U[:,:-2,:]
- u_1 = U[:,1:-1,:]
- u_0 = U[:,2:,:]
- # Backbone normals
- n_2 = F.normalize(torch.cross(u_2, u_1, dim=-1), dim=-1)
- n_1 = F.normalize(torch.cross(u_1, u_0, dim=-1), dim=-1)
-
- # Angle between normals
- cosD = (n_2 * n_1).sum(-1)
- cosD = torch.clamp(cosD, -1+eps, 1-eps)
- D = torch.sign((u_2 * n_1).sum(-1)) * torch.acos(cosD)
-
- # This scheme will remove phi[0], psi[-1], omega[-1]
- D = F.pad(D, (1,2), 'constant', 0)
- D = D.view((D.size(0), int(D.size(1)/3), 3))
- phi, psi, omega = torch.unbind(D,-1)
-
- if return_angles:
- return phi, psi, omega
-
- # Lift angle representations to the circle
- D_features = torch.cat((torch.cos(D), torch.sin(D)), 2)
- return D_features
-
-
-class GVPGraphEmbedding(GVPInputFeaturizer):
-
- def __init__(self, args):
- super().__init__()
- self.top_k_neighbors = args.top_k_neighbors
- self.num_positional_embeddings = 16
- self.remove_edges_without_coords = True
- node_input_dim = (7, 3)
- edge_input_dim = (34, 1)
- node_hidden_dim = (args.node_hidden_dim_scalar,
- args.node_hidden_dim_vector)
- edge_hidden_dim = (args.edge_hidden_dim_scalar,
- args.edge_hidden_dim_vector)
- self.embed_node = nn.Sequential(
- GVP(node_input_dim, node_hidden_dim, activations=(None, None)),
- LayerNorm(node_hidden_dim, eps=1e-4)
- )
- self.embed_edge = nn.Sequential(
- GVP(edge_input_dim, edge_hidden_dim, activations=(None, None)),
- LayerNorm(edge_hidden_dim, eps=1e-4)
- )
- self.embed_confidence = nn.Linear(16, args.node_hidden_dim_scalar)
-
- def forward(self, coords, coord_mask, padding_mask, confidence):
- with torch.no_grad():
- node_features = self.get_node_features(coords, coord_mask)
- edge_features, edge_index = self.get_edge_features(
- coords, coord_mask, padding_mask)
- node_embeddings_scalar, node_embeddings_vector = self.embed_node(node_features)
- edge_embeddings = self.embed_edge(edge_features)
-
- rbf_rep = rbf(confidence, 0., 1.)
- node_embeddings = (
- node_embeddings_scalar + self.embed_confidence(rbf_rep),
- node_embeddings_vector
- )
-
- node_embeddings, edge_embeddings, edge_index = flatten_graph(
- node_embeddings, edge_embeddings, edge_index)
- return node_embeddings, edge_embeddings, edge_index
-
- def get_edge_features(self, coords, coord_mask, padding_mask):
- X_ca = coords[:, :, 1]
- # Get distances to the top k neighbors
- E_dist, E_idx, E_coord_mask, E_residue_mask = GVPInputFeaturizer._dist(
- X_ca, coord_mask, padding_mask, self.top_k_neighbors)
- # Flatten the graph to be batch size 1 for torch_geometric package
- dest = E_idx
- B, L, k = E_idx.shape[:3]
- src = torch.arange(L, device=E_idx.device).view([1, L, 1]).expand(B, L, k)
- # After flattening, [2, B, E]
- edge_index = torch.stack([src, dest], dim=0).flatten(2, 3)
- # After flattening, [B, E]
- E_dist = E_dist.flatten(1, 2)
- E_coord_mask = E_coord_mask.flatten(1, 2).unsqueeze(-1)
- E_residue_mask = E_residue_mask.flatten(1, 2)
- # Calculate relative positional embeddings and distance RBF
- pos_embeddings = GVPInputFeaturizer._positional_embeddings(
- edge_index,
- num_positional_embeddings=self.num_positional_embeddings,
- )
- D_rbf = rbf(E_dist, 0., 20.)
- # Calculate relative orientation
- X_src = X_ca.unsqueeze(2).expand(-1, -1, k, -1).flatten(1, 2)
- X_dest = torch.gather(
- X_ca,
- 1,
- edge_index[1, :, :].unsqueeze(-1).expand([B, L*k, 3])
- )
- coord_mask_src = coord_mask.unsqueeze(2).expand(-1, -1, k).flatten(1, 2)
- coord_mask_dest = torch.gather(
- coord_mask,
- 1,
- edge_index[1, :, :].expand([B, L*k])
- )
- E_vectors = X_src - X_dest
- # For the ones without coordinates, substitute in the average vector
- E_vector_mean = torch.sum(E_vectors * E_coord_mask, dim=1,
- keepdims=True) / torch.sum(E_coord_mask, dim=1, keepdims=True)
- E_vectors = E_vectors * E_coord_mask + E_vector_mean * ~(E_coord_mask)
- # Normalize and remove nans
- edge_s = torch.cat([D_rbf, pos_embeddings], dim=-1)
- edge_v = normalize(E_vectors).unsqueeze(-2)
- edge_s, edge_v = map(nan_to_num, (edge_s, edge_v))
- # Also add indications of whether the coordinates are present
- edge_s = torch.cat([
- edge_s,
- (~coord_mask_src).float().unsqueeze(-1),
- (~coord_mask_dest).float().unsqueeze(-1),
- ], dim=-1)
- edge_index[:, ~E_residue_mask] = -1
- if self.remove_edges_without_coords:
- edge_index[:, ~E_coord_mask.squeeze(-1)] = -1
- return (edge_s, edge_v), edge_index.transpose(0, 1)
diff --git a/spaces/amritsolar/NEWGRADIOAI/README.md b/spaces/amritsolar/NEWGRADIOAI/README.md
deleted file mode 100644
index 11807aa4ee51785a564e6e1a3064f74c7362db94..0000000000000000000000000000000000000000
--- a/spaces/amritsolar/NEWGRADIOAI/README.md
+++ /dev/null
@@ -1,12 +0,0 @@
----
-title: NEWGRADIOAI
-emoji: 👀
-colorFrom: purple
-colorTo: yellow
-sdk: gradio
-sdk_version: 3.43.2
-app_file: app.py
-pinned: false
----
-
-Check out the configuration reference at https://huggingface.co/docs/hub/spaces-config-reference
diff --git a/spaces/anakin87/who-killed-laura-palmer/app.py b/spaces/anakin87/who-killed-laura-palmer/app.py
deleted file mode 100644
index ed0ce0ba5ada2179db3545447180dbac64b02cf5..0000000000000000000000000000000000000000
--- a/spaces/anakin87/who-killed-laura-palmer/app.py
+++ /dev/null
@@ -1,134 +0,0 @@
-# inspired by https://github.com/deepset-ai/haystack/blob/master/ui/webapp.py
-
-import time
-import streamlit as st
-import logging
-from json import JSONDecodeError
-from markdown import markdown
-from annotated_text import annotation
-from urllib.parse import unquote
-import random
-
-from app_utils.backend_utils import load_questions, query
-from app_utils.frontend_utils import (set_state_if_absent, reset_results,
- SIDEBAR_STYLE, TWIN_PEAKS_IMG_SRC, LAURA_PALMER_IMG_SRC, SPOTIFY_IFRAME)
-from app_utils.config import RETRIEVER_TOP_K, READER_TOP_K, LOW_RELEVANCE_THRESHOLD
-
-def main():
- questions = load_questions()
-
- # Persistent state
- set_state_if_absent('question', "Where is Twin Peaks?")
- set_state_if_absent('answer', '')
- set_state_if_absent('results', None)
- set_state_if_absent('raw_json', None)
- set_state_if_absent('random_question_requested', False)
-
- ## SIDEBAR
- st.markdown(SIDEBAR_STYLE, unsafe_allow_html=True)
- st.sidebar.header("Who killed Laura Palmer?")
- st.sidebar.image(TWIN_PEAKS_IMG_SRC)
- st.sidebar.markdown(f"""
-
Twin Peaks Question Answering system
-
- """, unsafe_allow_html=True)
- # spotify webplayer
- st.sidebar.markdown(SPOTIFY_IFRAME, unsafe_allow_html=True)
-
- ## MAIN CONTAINER
- st.write("# Who killed Laura Palmer?")
- st.write("### The first Twin Peaks Question Answering system!")
- st.markdown("""
- Ask any question about [Twin Peaks]
- (https://twinpeaks.fandom.com/wiki/Twin_Peaks)
- and see if the AI can find an answer...
-
- *Note: do not use keywords, but full-fledged questions.*
- """)
- # Search bar
- question = st.text_input("", value=st.session_state.question,
- max_chars=100, on_change=reset_results)
- col1, col2 = st.columns(2)
- col1.markdown(
- "", unsafe_allow_html=True)
- col2.markdown(
- "", unsafe_allow_html=True)
- # Run button
- run_pressed = col1.button("Run")
- # Random question button
- if col2.button("Random question"):
- reset_results()
- question = random.choice(questions)
- # Avoid picking the same question twice (the change is not visible on the UI)
- while question == st.session_state.question:
- question = random.choice(questions)
- st.session_state.question = question
- st.session_state.random_question_requested = True
- # Re-runs the script setting the random question as the textbox value
- # Unfortunately necessary as the Random Question button is _below_ the textbox
- raise st.script_runner.RerunException(
- st.script_request_queue.RerunData(None))
- else:
- st.session_state.random_question_requested = False
- run_query = (run_pressed or question != st.session_state.question) \
- and not st.session_state.random_question_requested
-
- # Get results for query
- if run_query and question:
- time_start = time.time()
- reset_results()
- st.session_state.question = question
- with st.spinner("🧠 Performing neural search on documents..."):
- try:
- st.session_state.results = query(
- question, RETRIEVER_TOP_K, READER_TOP_K)
- time_end = time.time()
- print(time.strftime("%Y-%m-%d %H:%M:%S", time.gmtime()))
- print(f'elapsed time: {time_end - time_start}')
- except JSONDecodeError as je:
- st.error(
- "👓 An error occurred reading the results. Is the document store working?")
- return
- except Exception as e:
- logging.exception(e)
- st.error("🐞 An error occurred during the request.")
- return
-
- # Display results
- if st.session_state.results:
- st.write("## Results:")
- alert_irrelevance = True
- if len(st.session_state.results['answers']) == 0:
- st.info("""🤔 Haystack is unsure whether any of
- the documents contain an answer to your question. Try to reformulate it!""")
-
- for result in st.session_state.results['answers']:
- result = result.to_dict()
- if result["answer"]:
- if alert_irrelevance and result['score'] < LOW_RELEVANCE_THRESHOLD:
- alert_irrelevance = False
- st.write("""
-
Attention, the
- following answers have low relevance:
""",
- unsafe_allow_html=True)
-
- answer, context = result["answer"], result["context"]
- start_idx = context.find(answer)
- end_idx = start_idx + len(answer)
- # Hack due to this bug: https://github.com/streamlit/streamlit/issues/3190
- st.write(markdown("- ..."+context[:start_idx] +
- str(annotation(answer, "ANSWER", "#3e1c21", "white")) +
- context[end_idx:]+"..."), unsafe_allow_html=True)
- source = ""
- name = unquote(result['meta']['name']).replace('_', ' ')
- url = result['meta']['url']
- source = f"[{name}]({url})"
- st.markdown(
- f"**Score:** {result['score']:.2f} - **Source:** {source}")
-
-main()
diff --git a/spaces/andryMLOPS/ASTA-GPT-3.8_web_ui/g4f/Provider/Providers/Zeabur.py b/spaces/andryMLOPS/ASTA-GPT-3.8_web_ui/g4f/Provider/Providers/Zeabur.py
deleted file mode 100644
index e412720bd9a0c88860f6ea8a657cb0a24bcce63f..0000000000000000000000000000000000000000
--- a/spaces/andryMLOPS/ASTA-GPT-3.8_web_ui/g4f/Provider/Providers/Zeabur.py
+++ /dev/null
@@ -1,50 +0,0 @@
-import os
-import requests
-from ...typing import sha256, Dict, get_type_hints
-
-url = "https://gptleg.zeabur.app"
-model = ['gpt-3.5-turbo', 'gpt-3.5-turbo-0301',
- 'gpt-3.5-turbo-16k', 'gpt-4', 'gpt-4-0613']
-supports_stream = True
-needs_auth = False
-
-
-def _create_completion(model: str, messages: list, stream: bool, **kwargs):
- headers = {
- 'Authority': 'chat.dfehub.com',
- 'Content-Type': 'application/json',
- 'Method': 'POST',
- 'Path': '/api/openai/v1/chat/completions',
- 'Scheme': 'https',
- 'Accept': 'text/event-stream',
- 'Accept-Language': 'pt-BR,pt;q=0.9,en-US;q=0.8,en;q=0.7,zh-CN;q=0.6,zh;q=0.5',
- 'Content-Type': 'application/json',
- 'Origin': 'https://gptleg.zeabur.app',
- 'Referer': 'https://gptleg.zeabur.app/',
- 'Sec-Ch-Ua': '"Not.A/Brand";v="8", "Chromium";v="114", "Google Chrome";v="114"',
- 'Sec-Ch-Ua-Mobile': '?0',
- 'Sec-Ch-Ua-Platform': '"Windows"',
- 'Sec-Fetch-Dest': 'empty',
- 'Sec-Fetch-Mode': 'cors',
- 'Sec-Fetch-Site': 'same-origin',
- 'User-Agent': 'Mozilla/5.0 (Windows NT 10.0; Win64; x64) AppleWebKit/537.36 (KHTML, like Gecko) Chrome/114.0.0.0 Safari/537.36',
- 'X-Requested-With': 'XMLHttpRequest',
- }
-
- data = {
- 'model': model,
- 'temperature': 0.7,
- 'max_tokens': '16000',
- 'presence_penalty': 0,
- 'messages': messages,
- }
-
- response = requests.post(url + '/api/openai/v1/chat/completions',
- headers=headers, json=data, stream=stream)
-
- yield response.json()['choices'][0]['message']['content']
-
-
-params = f'g4f.Providers.{os.path.basename(__file__)[:-3]} supports: ' + \
- '(%s)' % ', '.join(
- [f"{name}: {get_type_hints(_create_completion)[name].__name__}" for name in _create_completion.__code__.co_varnames[:_create_completion.__code__.co_argcount]])
diff --git a/spaces/argilla/argilla-streamlit-customs/my_app/pages/no-code-data-manager.py b/spaces/argilla/argilla-streamlit-customs/my_app/pages/no-code-data-manager.py
deleted file mode 100644
index 964e1fbe1a2e4832696de5af90af941c5cae164a..0000000000000000000000000000000000000000
--- a/spaces/argilla/argilla-streamlit-customs/my_app/pages/no-code-data-manager.py
+++ /dev/null
@@ -1,163 +0,0 @@
-from io import BytesIO
-
-import argilla as rg
-import pandas as pd
-import spacy
-import streamlit as st
-from utils.commons import (
- ArgillaSingleton,
- argilla_login_flow,
- get_data_snapshot,
- get_dataset_list,
-)
-
-st.set_page_config(
- page_title="Argilla - 💾 - NoCode Data Manager", page_icon="💾", layout="wide"
-)
-
-
-api_url, api_key = argilla_login_flow("💾 No-code data manager")
-
-st.write(
- """
- This page allows you to upload and download datasets from Argilla without using any code!
- In the background it uses `argilla.log()` and `pandas`. This requires you to have a valid `.csv`, `.xlsx` or `.xlsx`.
- """
-)
-
-action = st.sidebar.selectbox("Action", ["✍️ Upload Dataset", "💾 Download dataset"])
-
-if action == "✍️ Upload Dataset":
- st.subheader(action)
- datasets_list = [
- f"{ds['owner']}/{ds['name']}" for ds in get_dataset_list(api_url, api_key)
- ]
- dataset_argilla = st.selectbox(
- "Argilla Dataset Name", options=["other"] + datasets_list
- )
- if dataset_argilla == "other":
- ArgillaSingleton.init(api_url, api_key)
- dataset_argilla_name = st.text_input("New Dataset Name")
- labels = []
- disabled = False
- options = ["TextClassification", "TokenClassification", "Text2Text"]
- else:
- dataset_argilla_name = dataset_argilla.split("/")[-1]
- dataset_argilla_workspace = dataset_argilla.split("/")[0]
- get_data_snapshot(dataset_argilla_name, dataset_argilla_workspace)
- rg.set_workspace(dataset_argilla_workspace)
- for dataset in get_dataset_list(api_url, api_key):
- if (
- dataset["name"] == dataset_argilla_name
- and dataset["owner"] == dataset_argilla_workspace
- ):
- labels = dataset["labels"]
- dataset_type = dataset["task"]
- disabled = True
- options = [dataset_type]
- break
-
- dataset_type = st.selectbox("Dataset Type", options, disabled=disabled)
-
- if dataset_argilla_name is not None and dataset_argilla_name.strip() != "":
- records = []
- uploaded_file = st.file_uploader(
- "Upload your CSV or XLSX/XLS file", type=["csv", "xls", "xlsx"]
- )
-
- if uploaded_file is not None:
- try:
- df = pd.read_excel(uploaded_file, sheet_name=0)
- except Exception:
- df = pd.read_csv(uploaded_file)
-
- st.write("Dataset preview:", df.head())
- string_columns = [col for col in df.columns if df[col].dtype == "object"]
- if len(string_columns) > 0:
- if dataset_type == "TextClassification":
- column_select = st.multiselect("Select columns", string_columns)
- if column_select:
- records = []
- for i, row in df[column_select].iterrows():
- record = rg.TextClassificationRecord(
- inputs={col: row[col] for col in column_select}
- )
- records.append(record)
- elif dataset_type == "TokenClassification":
- column_select = st.selectbox("Select a Column", string_columns)
- if column_select:
- # Load the spaCy en_core_web_sm model
- nlp = spacy.blank("en")
- # Create a new column in the DataFrame with the tokenized text
- df["tokenized_text"] = df[column_select].apply(
- lambda x: [token.text for token in nlp(x)]
- )
- st.write("Tokenized Text:", df["tokenized_text"].head(3))
- for i, row in df.iterrows():
- record = rg.TokenClassificationRecord(
- text=row[column_select],
- tokens=row["tokenized_text"],
- )
- records.append(record)
- else:
- column_select = st.selectbox("Select a Column", string_columns)
- if column_select:
- records = []
- for i, row in df[column_select].iterrows():
- record = rg.Text2TextRecord(text=row[column_select])
- records.append(record)
- if len(records) > 0:
- if st.button("Log data into Argilla"):
- output = rg.log(records=records, name=dataset_argilla_name)
- st.write(output)
- st.write(f"{output.processed} records added to {api_url}")
- else:
- st.warning("Please provide a dataset name")
-
-elif action == "💾 Download dataset":
- st.subheader(action)
- datasets_list = [
- f"{ds['owner']}/{ds['name']}" for ds in get_dataset_list(api_url, api_key)
- ]
- dataset_argilla = st.selectbox("Argilla Dataset Name", options=datasets_list)
- dataset_argilla_name = dataset_argilla.split("/")[-1]
- dataset_argilla_workspace = dataset_argilla.split("/")[0]
-
- query = st.text_input(
- "Query to filter records (optional). See [query"
- " syntax](https://docs.argilla.io/en/latest/guides/query_datasets.html)"
- )
- get_data_snapshot(dataset_argilla_name, dataset_argilla_workspace, query)
- search = st.button("Search")
- if search:
- rg.set_workspace(dataset_argilla_workspace)
- dataset = rg.load(dataset_argilla_name, query=query).to_pandas()
- st.write("Dataset preview:", dataset.head())
- cols = st.columns(3)
- cols[0].download_button(
- label="Download as CSV",
- data=dataset.to_csv(index=False).encode("utf-8"),
- file_name=f"{dataset_argilla_name}.csv",
- mime="text/csv",
- )
- output = BytesIO()
-
- with pd.ExcelWriter(output, engine="xlsxwriter") as writer:
- dataset.to_excel(writer, sheet_name="Sheet1")
- writer.save()
- cols[1].download_button(
- label="Download as Excel",
- data=output,
- file_name=f"{dataset_argilla_name}.xlsx",
- mime="application/vnd.ms-excel",
- )
- cols[2].download_button(
- label="Download as JSON!",
- data=dataset.to_json(orient="records", lines=True).encode("utf-8"),
- file_name=f"{dataset_argilla_name}.json",
- mime="application/json",
- )
- else:
- st.info("Press the search button to load the dataset with the given query")
-
-
diff --git a/spaces/artificialguybr/video-dubbing/TTS/TTS/utils/audio/__init__.py b/spaces/artificialguybr/video-dubbing/TTS/TTS/utils/audio/__init__.py
deleted file mode 100644
index f18f22199908ee0dd5445e34527f5fddb65cfed8..0000000000000000000000000000000000000000
--- a/spaces/artificialguybr/video-dubbing/TTS/TTS/utils/audio/__init__.py
+++ /dev/null
@@ -1 +0,0 @@
-from TTS.utils.audio.processor import AudioProcessor
diff --git a/spaces/arxify/RVC-beta-v2-0618/runtime/Lib/site-packages/altair/examples/connected_scatterplot.py b/spaces/arxify/RVC-beta-v2-0618/runtime/Lib/site-packages/altair/examples/connected_scatterplot.py
deleted file mode 100644
index 39f27d813b1b945bfa1e8fb7aa69880968a21272..0000000000000000000000000000000000000000
--- a/spaces/arxify/RVC-beta-v2-0618/runtime/Lib/site-packages/altair/examples/connected_scatterplot.py
+++ /dev/null
@@ -1,18 +0,0 @@
-"""
-Connected Scatterplot (Lines with Custom Paths)
------------------------------------------------
-
-This example show how the order encoding can be used to draw a custom path. The dataset tracks miles driven per capita along with gas prices annually from 1956 to 2010.
-It is based on Hannah Fairfield's article 'Driving Shifts Into Reverse'. See https://archive.nytimes.com/www.nytimes.com/imagepages/2010/05/02/business/02metrics.html for the original.
-"""
-# category: scatter plots
-import altair as alt
-from vega_datasets import data
-
-source = data.driving()
-
-alt.Chart(source).mark_line(point=True).encode(
- alt.X('miles', scale=alt.Scale(zero=False)),
- alt.Y('gas', scale=alt.Scale(zero=False)),
- order='year'
-)
diff --git a/spaces/arxify/RVC-beta-v2-0618/runtime/Lib/site-packages/click/decorators.py b/spaces/arxify/RVC-beta-v2-0618/runtime/Lib/site-packages/click/decorators.py
deleted file mode 100644
index 28618dc52379eafc72a5a1005a679d418d879692..0000000000000000000000000000000000000000
--- a/spaces/arxify/RVC-beta-v2-0618/runtime/Lib/site-packages/click/decorators.py
+++ /dev/null
@@ -1,497 +0,0 @@
-import inspect
-import types
-import typing as t
-from functools import update_wrapper
-from gettext import gettext as _
-
-from .core import Argument
-from .core import Command
-from .core import Context
-from .core import Group
-from .core import Option
-from .core import Parameter
-from .globals import get_current_context
-from .utils import echo
-
-F = t.TypeVar("F", bound=t.Callable[..., t.Any])
-FC = t.TypeVar("FC", bound=t.Union[t.Callable[..., t.Any], Command])
-
-
-def pass_context(f: F) -> F:
- """Marks a callback as wanting to receive the current context
- object as first argument.
- """
-
- def new_func(*args, **kwargs): # type: ignore
- return f(get_current_context(), *args, **kwargs)
-
- return update_wrapper(t.cast(F, new_func), f)
-
-
-def pass_obj(f: F) -> F:
- """Similar to :func:`pass_context`, but only pass the object on the
- context onwards (:attr:`Context.obj`). This is useful if that object
- represents the state of a nested system.
- """
-
- def new_func(*args, **kwargs): # type: ignore
- return f(get_current_context().obj, *args, **kwargs)
-
- return update_wrapper(t.cast(F, new_func), f)
-
-
-def make_pass_decorator(
- object_type: t.Type, ensure: bool = False
-) -> "t.Callable[[F], F]":
- """Given an object type this creates a decorator that will work
- similar to :func:`pass_obj` but instead of passing the object of the
- current context, it will find the innermost context of type
- :func:`object_type`.
-
- This generates a decorator that works roughly like this::
-
- from functools import update_wrapper
-
- def decorator(f):
- @pass_context
- def new_func(ctx, *args, **kwargs):
- obj = ctx.find_object(object_type)
- return ctx.invoke(f, obj, *args, **kwargs)
- return update_wrapper(new_func, f)
- return decorator
-
- :param object_type: the type of the object to pass.
- :param ensure: if set to `True`, a new object will be created and
- remembered on the context if it's not there yet.
- """
-
- def decorator(f: F) -> F:
- def new_func(*args, **kwargs): # type: ignore
- ctx = get_current_context()
-
- if ensure:
- obj = ctx.ensure_object(object_type)
- else:
- obj = ctx.find_object(object_type)
-
- if obj is None:
- raise RuntimeError(
- "Managed to invoke callback without a context"
- f" object of type {object_type.__name__!r}"
- " existing."
- )
-
- return ctx.invoke(f, obj, *args, **kwargs)
-
- return update_wrapper(t.cast(F, new_func), f)
-
- return decorator
-
-
-def pass_meta_key(
- key: str, *, doc_description: t.Optional[str] = None
-) -> "t.Callable[[F], F]":
- """Create a decorator that passes a key from
- :attr:`click.Context.meta` as the first argument to the decorated
- function.
-
- :param key: Key in ``Context.meta`` to pass.
- :param doc_description: Description of the object being passed,
- inserted into the decorator's docstring. Defaults to "the 'key'
- key from Context.meta".
-
- .. versionadded:: 8.0
- """
-
- def decorator(f: F) -> F:
- def new_func(*args, **kwargs): # type: ignore
- ctx = get_current_context()
- obj = ctx.meta[key]
- return ctx.invoke(f, obj, *args, **kwargs)
-
- return update_wrapper(t.cast(F, new_func), f)
-
- if doc_description is None:
- doc_description = f"the {key!r} key from :attr:`click.Context.meta`"
-
- decorator.__doc__ = (
- f"Decorator that passes {doc_description} as the first argument"
- " to the decorated function."
- )
- return decorator
-
-
-CmdType = t.TypeVar("CmdType", bound=Command)
-
-
-@t.overload
-def command(
- __func: t.Callable[..., t.Any],
-) -> Command:
- ...
-
-
-@t.overload
-def command(
- name: t.Optional[str] = None,
- **attrs: t.Any,
-) -> t.Callable[..., Command]:
- ...
-
-
-@t.overload
-def command(
- name: t.Optional[str] = None,
- cls: t.Type[CmdType] = ...,
- **attrs: t.Any,
-) -> t.Callable[..., CmdType]:
- ...
-
-
-def command(
- name: t.Union[str, t.Callable[..., t.Any], None] = None,
- cls: t.Optional[t.Type[Command]] = None,
- **attrs: t.Any,
-) -> t.Union[Command, t.Callable[..., Command]]:
- r"""Creates a new :class:`Command` and uses the decorated function as
- callback. This will also automatically attach all decorated
- :func:`option`\s and :func:`argument`\s as parameters to the command.
-
- The name of the command defaults to the name of the function with
- underscores replaced by dashes. If you want to change that, you can
- pass the intended name as the first argument.
-
- All keyword arguments are forwarded to the underlying command class.
- For the ``params`` argument, any decorated params are appended to
- the end of the list.
-
- Once decorated the function turns into a :class:`Command` instance
- that can be invoked as a command line utility or be attached to a
- command :class:`Group`.
-
- :param name: the name of the command. This defaults to the function
- name with underscores replaced by dashes.
- :param cls: the command class to instantiate. This defaults to
- :class:`Command`.
-
- .. versionchanged:: 8.1
- This decorator can be applied without parentheses.
-
- .. versionchanged:: 8.1
- The ``params`` argument can be used. Decorated params are
- appended to the end of the list.
- """
-
- func: t.Optional[t.Callable[..., t.Any]] = None
-
- if callable(name):
- func = name
- name = None
- assert cls is None, "Use 'command(cls=cls)(callable)' to specify a class."
- assert not attrs, "Use 'command(**kwargs)(callable)' to provide arguments."
-
- if cls is None:
- cls = Command
-
- def decorator(f: t.Callable[..., t.Any]) -> Command:
- if isinstance(f, Command):
- raise TypeError("Attempted to convert a callback into a command twice.")
-
- attr_params = attrs.pop("params", None)
- params = attr_params if attr_params is not None else []
-
- try:
- decorator_params = f.__click_params__ # type: ignore
- except AttributeError:
- pass
- else:
- del f.__click_params__ # type: ignore
- params.extend(reversed(decorator_params))
-
- if attrs.get("help") is None:
- attrs["help"] = f.__doc__
-
- cmd = cls( # type: ignore[misc]
- name=name or f.__name__.lower().replace("_", "-"), # type: ignore[arg-type]
- callback=f,
- params=params,
- **attrs,
- )
- cmd.__doc__ = f.__doc__
- return cmd
-
- if func is not None:
- return decorator(func)
-
- return decorator
-
-
-@t.overload
-def group(
- __func: t.Callable[..., t.Any],
-) -> Group:
- ...
-
-
-@t.overload
-def group(
- name: t.Optional[str] = None,
- **attrs: t.Any,
-) -> t.Callable[[F], Group]:
- ...
-
-
-def group(
- name: t.Union[str, t.Callable[..., t.Any], None] = None, **attrs: t.Any
-) -> t.Union[Group, t.Callable[[F], Group]]:
- """Creates a new :class:`Group` with a function as callback. This
- works otherwise the same as :func:`command` just that the `cls`
- parameter is set to :class:`Group`.
-
- .. versionchanged:: 8.1
- This decorator can be applied without parentheses.
- """
- if attrs.get("cls") is None:
- attrs["cls"] = Group
-
- if callable(name):
- grp: t.Callable[[F], Group] = t.cast(Group, command(**attrs))
- return grp(name)
-
- return t.cast(Group, command(name, **attrs))
-
-
-def _param_memo(f: FC, param: Parameter) -> None:
- if isinstance(f, Command):
- f.params.append(param)
- else:
- if not hasattr(f, "__click_params__"):
- f.__click_params__ = [] # type: ignore
-
- f.__click_params__.append(param) # type: ignore
-
-
-def argument(*param_decls: str, **attrs: t.Any) -> t.Callable[[FC], FC]:
- """Attaches an argument to the command. All positional arguments are
- passed as parameter declarations to :class:`Argument`; all keyword
- arguments are forwarded unchanged (except ``cls``).
- This is equivalent to creating an :class:`Argument` instance manually
- and attaching it to the :attr:`Command.params` list.
-
- :param cls: the argument class to instantiate. This defaults to
- :class:`Argument`.
- """
-
- def decorator(f: FC) -> FC:
- ArgumentClass = attrs.pop("cls", None) or Argument
- _param_memo(f, ArgumentClass(param_decls, **attrs))
- return f
-
- return decorator
-
-
-def option(*param_decls: str, **attrs: t.Any) -> t.Callable[[FC], FC]:
- """Attaches an option to the command. All positional arguments are
- passed as parameter declarations to :class:`Option`; all keyword
- arguments are forwarded unchanged (except ``cls``).
- This is equivalent to creating an :class:`Option` instance manually
- and attaching it to the :attr:`Command.params` list.
-
- :param cls: the option class to instantiate. This defaults to
- :class:`Option`.
- """
-
- def decorator(f: FC) -> FC:
- # Issue 926, copy attrs, so pre-defined options can re-use the same cls=
- option_attrs = attrs.copy()
- OptionClass = option_attrs.pop("cls", None) or Option
- _param_memo(f, OptionClass(param_decls, **option_attrs))
- return f
-
- return decorator
-
-
-def confirmation_option(*param_decls: str, **kwargs: t.Any) -> t.Callable[[FC], FC]:
- """Add a ``--yes`` option which shows a prompt before continuing if
- not passed. If the prompt is declined, the program will exit.
-
- :param param_decls: One or more option names. Defaults to the single
- value ``"--yes"``.
- :param kwargs: Extra arguments are passed to :func:`option`.
- """
-
- def callback(ctx: Context, param: Parameter, value: bool) -> None:
- if not value:
- ctx.abort()
-
- if not param_decls:
- param_decls = ("--yes",)
-
- kwargs.setdefault("is_flag", True)
- kwargs.setdefault("callback", callback)
- kwargs.setdefault("expose_value", False)
- kwargs.setdefault("prompt", "Do you want to continue?")
- kwargs.setdefault("help", "Confirm the action without prompting.")
- return option(*param_decls, **kwargs)
-
-
-def password_option(*param_decls: str, **kwargs: t.Any) -> t.Callable[[FC], FC]:
- """Add a ``--password`` option which prompts for a password, hiding
- input and asking to enter the value again for confirmation.
-
- :param param_decls: One or more option names. Defaults to the single
- value ``"--password"``.
- :param kwargs: Extra arguments are passed to :func:`option`.
- """
- if not param_decls:
- param_decls = ("--password",)
-
- kwargs.setdefault("prompt", True)
- kwargs.setdefault("confirmation_prompt", True)
- kwargs.setdefault("hide_input", True)
- return option(*param_decls, **kwargs)
-
-
-def version_option(
- version: t.Optional[str] = None,
- *param_decls: str,
- package_name: t.Optional[str] = None,
- prog_name: t.Optional[str] = None,
- message: t.Optional[str] = None,
- **kwargs: t.Any,
-) -> t.Callable[[FC], FC]:
- """Add a ``--version`` option which immediately prints the version
- number and exits the program.
-
- If ``version`` is not provided, Click will try to detect it using
- :func:`importlib.metadata.version` to get the version for the
- ``package_name``. On Python < 3.8, the ``importlib_metadata``
- backport must be installed.
-
- If ``package_name`` is not provided, Click will try to detect it by
- inspecting the stack frames. This will be used to detect the
- version, so it must match the name of the installed package.
-
- :param version: The version number to show. If not provided, Click
- will try to detect it.
- :param param_decls: One or more option names. Defaults to the single
- value ``"--version"``.
- :param package_name: The package name to detect the version from. If
- not provided, Click will try to detect it.
- :param prog_name: The name of the CLI to show in the message. If not
- provided, it will be detected from the command.
- :param message: The message to show. The values ``%(prog)s``,
- ``%(package)s``, and ``%(version)s`` are available. Defaults to
- ``"%(prog)s, version %(version)s"``.
- :param kwargs: Extra arguments are passed to :func:`option`.
- :raise RuntimeError: ``version`` could not be detected.
-
- .. versionchanged:: 8.0
- Add the ``package_name`` parameter, and the ``%(package)s``
- value for messages.
-
- .. versionchanged:: 8.0
- Use :mod:`importlib.metadata` instead of ``pkg_resources``. The
- version is detected based on the package name, not the entry
- point name. The Python package name must match the installed
- package name, or be passed with ``package_name=``.
- """
- if message is None:
- message = _("%(prog)s, version %(version)s")
-
- if version is None and package_name is None:
- frame = inspect.currentframe()
- f_back = frame.f_back if frame is not None else None
- f_globals = f_back.f_globals if f_back is not None else None
- # break reference cycle
- # https://docs.python.org/3/library/inspect.html#the-interpreter-stack
- del frame
-
- if f_globals is not None:
- package_name = f_globals.get("__name__")
-
- if package_name == "__main__":
- package_name = f_globals.get("__package__")
-
- if package_name:
- package_name = package_name.partition(".")[0]
-
- def callback(ctx: Context, param: Parameter, value: bool) -> None:
- if not value or ctx.resilient_parsing:
- return
-
- nonlocal prog_name
- nonlocal version
-
- if prog_name is None:
- prog_name = ctx.find_root().info_name
-
- if version is None and package_name is not None:
- metadata: t.Optional[types.ModuleType]
-
- try:
- from importlib import metadata # type: ignore
- except ImportError:
- # Python < 3.8
- import importlib_metadata as metadata # type: ignore
-
- try:
- version = metadata.version(package_name) # type: ignore
- except metadata.PackageNotFoundError: # type: ignore
- raise RuntimeError(
- f"{package_name!r} is not installed. Try passing"
- " 'package_name' instead."
- ) from None
-
- if version is None:
- raise RuntimeError(
- f"Could not determine the version for {package_name!r} automatically."
- )
-
- echo(
- t.cast(str, message)
- % {"prog": prog_name, "package": package_name, "version": version},
- color=ctx.color,
- )
- ctx.exit()
-
- if not param_decls:
- param_decls = ("--version",)
-
- kwargs.setdefault("is_flag", True)
- kwargs.setdefault("expose_value", False)
- kwargs.setdefault("is_eager", True)
- kwargs.setdefault("help", _("Show the version and exit."))
- kwargs["callback"] = callback
- return option(*param_decls, **kwargs)
-
-
-def help_option(*param_decls: str, **kwargs: t.Any) -> t.Callable[[FC], FC]:
- """Add a ``--help`` option which immediately prints the help page
- and exits the program.
-
- This is usually unnecessary, as the ``--help`` option is added to
- each command automatically unless ``add_help_option=False`` is
- passed.
-
- :param param_decls: One or more option names. Defaults to the single
- value ``"--help"``.
- :param kwargs: Extra arguments are passed to :func:`option`.
- """
-
- def callback(ctx: Context, param: Parameter, value: bool) -> None:
- if not value or ctx.resilient_parsing:
- return
-
- echo(ctx.get_help(), color=ctx.color)
- ctx.exit()
-
- if not param_decls:
- param_decls = ("--help",)
-
- kwargs.setdefault("is_flag", True)
- kwargs.setdefault("expose_value", False)
- kwargs.setdefault("is_eager", True)
- kwargs.setdefault("help", _("Show this message and exit."))
- kwargs["callback"] = callback
- return option(*param_decls, **kwargs)
diff --git a/spaces/arxify/RVC-beta-v2-0618/runtime/Lib/site-packages/dateutil/_common.py b/spaces/arxify/RVC-beta-v2-0618/runtime/Lib/site-packages/dateutil/_common.py
deleted file mode 100644
index 4eb2659bd2986125fcfb4afea5bae9efc2dcd1a0..0000000000000000000000000000000000000000
--- a/spaces/arxify/RVC-beta-v2-0618/runtime/Lib/site-packages/dateutil/_common.py
+++ /dev/null
@@ -1,43 +0,0 @@
-"""
-Common code used in multiple modules.
-"""
-
-
-class weekday(object):
- __slots__ = ["weekday", "n"]
-
- def __init__(self, weekday, n=None):
- self.weekday = weekday
- self.n = n
-
- def __call__(self, n):
- if n == self.n:
- return self
- else:
- return self.__class__(self.weekday, n)
-
- def __eq__(self, other):
- try:
- if self.weekday != other.weekday or self.n != other.n:
- return False
- except AttributeError:
- return False
- return True
-
- def __hash__(self):
- return hash((
- self.weekday,
- self.n,
- ))
-
- def __ne__(self, other):
- return not (self == other)
-
- def __repr__(self):
- s = ("MO", "TU", "WE", "TH", "FR", "SA", "SU")[self.weekday]
- if not self.n:
- return s
- else:
- return "%s(%+d)" % (s, self.n)
-
-# vim:ts=4:sw=4:et
diff --git a/spaces/awacke1/DockerGoFlanT5/static/README.md b/spaces/awacke1/DockerGoFlanT5/static/README.md
deleted file mode 100644
index e69de29bb2d1d6434b8b29ae775ad8c2e48c5391..0000000000000000000000000000000000000000
diff --git a/spaces/awacke1/HTML5-ThreeJS/index.html b/spaces/awacke1/HTML5-ThreeJS/index.html
deleted file mode 100644
index 4996c7d6cc61643d6ac97c3c315d6c5b01676fc1..0000000000000000000000000000000000000000
--- a/spaces/awacke1/HTML5-ThreeJS/index.html
+++ /dev/null
@@ -1,73 +0,0 @@
-
-
-
- Three.js Scene and Animation Example
-
-
-
-
-
-
-
diff --git a/spaces/awacke1/Quote-Bot-AutoRepeater/README.md b/spaces/awacke1/Quote-Bot-AutoRepeater/README.md
deleted file mode 100644
index 8a6f2d90759180ca9fd4ca72f1abbf077b61a6a9..0000000000000000000000000000000000000000
--- a/spaces/awacke1/Quote-Bot-AutoRepeater/README.md
+++ /dev/null
@@ -1,13 +0,0 @@
----
-title: Quote Bot AutoRepeater
-emoji: 🗨️🤖🔁
-colorFrom: yellow
-colorTo: red
-sdk: streamlit
-sdk_version: 1.25.0
-app_file: app.py
-pinned: false
-license: mit
----
-
-Check out the configuration reference at https://huggingface.co/docs/hub/spaces-config-reference
diff --git a/spaces/badayvedat/AudioSep/models/CLAP/training/lp_main.py b/spaces/badayvedat/AudioSep/models/CLAP/training/lp_main.py
deleted file mode 100644
index c2d4e8c85aaa3c8e4221963ef56a815cc14f354f..0000000000000000000000000000000000000000
--- a/spaces/badayvedat/AudioSep/models/CLAP/training/lp_main.py
+++ /dev/null
@@ -1,670 +0,0 @@
-from cmath import cos
-from inspect import getargs
-import logging
-import os
-import random
-from datetime import datetime
-import bisect
-import copy
-from sched import scheduler
-import numpy as np
-import torch
-import torch.backends.cudnn as cudnn
-from torch import optim
-from torch.cuda.amp import GradScaler
-import faulthandler
-import pathlib
-import argparse
-import time
-
-try:
- import wandb
-except ImportError:
- wandb = None
-
-try:
- import torch.utils.tensorboard as tensorboard
-except ImportError:
- tensorboard = None
-
-try:
- import horovod.torch as hvd
-except ImportError:
- hvd = None
-
-from open_clip import create_model_and_transforms, trace_model, create_model
-from training.data import get_data
-from training.params import parse_args
-from training.distributed import is_master, init_distributed_device, world_info_from_env
-from training.logger import setup_logging
-from training.scheduler import cosine_lr
-from training.lp_train import train_one_epoch, evaluate
-from open_clip.utils import get_tar_path_from_dataset_name, dataset_split, get_optimizer
-from open_clip.utils import load_p, load_class_label
-from open_clip.linear_probe import LinearProbe
-
-
-def maintain_ckpts(args, startidx, all_idx_len):
- for i in reversed(range(startidx, all_idx_len)):
- if os.path.exists(os.path.join(args.checkpoint_path, f"epoch_top_{i}.pt")):
- os.rename(
- os.path.join(args.checkpoint_path, f"epoch_top_{i}.pt"),
- os.path.join(args.checkpoint_path, f"epoch_top_{i+1}.pt"),
- )
- if os.path.exists(
- os.path.join(args.checkpoint_path, f"epoch_top_{all_idx_len}.pt")
- ):
- os.remove(os.path.join(args.checkpoint_path, f"epoch_top_{all_idx_len}.pt"))
- return
-
-
-def update_top_k_performance(
- new_metrics_inputs, current_top_k_ckpt_metrics, args, ckpt, bignumbetter=True
-):
- """
- Record the top-k performance of the current epoch.
- current_top_k_metrics is a dictionary of the form: {1: top_1_ckpt_measure, 2: top_2_ckpt_measure, ...}
- """
- if isinstance(new_metrics_inputs, (list, tuple)):
- new_metrics_inputs = np.mean(new_metrics_inputs)
- return update_top_k_performance(
- new_metrics_inputs,
- current_top_k_ckpt_metrics,
- args=args,
- ckpt=ckpt,
- bignumbetter=bignumbetter,
- )
- elif isinstance(new_metrics_inputs, dict):
- new_metrics_inputs = np.mean(list(new_metrics_inputs.values()))
- return update_top_k_performance(
- new_metrics_inputs,
- current_top_k_ckpt_metrics,
- args=args,
- ckpt=ckpt,
- bignumbetter=bignumbetter,
- )
- elif isinstance(new_metrics_inputs, (float, int)):
- update_flag = {k: False for k in current_top_k_ckpt_metrics.keys()}
- sorted_keys = sorted(current_top_k_ckpt_metrics.keys())
- sorted_values = sorted(
- current_top_k_ckpt_metrics.values(), reverse=bignumbetter
- )
- sorted_values_ = copy.deepcopy(sorted_values)
- sorted_values.append(new_metrics_inputs)
- sorted_values = sorted(sorted_values, reverse=bignumbetter)
- sorted_values = sorted_values[:-1]
-
- if sorted_values == sorted_values_:
- return current_top_k_ckpt_metrics, new_metrics_inputs
- else:
- for i in range(len(sorted_keys)):
- if current_top_k_ckpt_metrics[sorted_keys[i]] != sorted_values[i]:
- current_top_k_ckpt_metrics[sorted_keys[i]] = sorted_values[i]
- update_flag[sorted_keys[i]] = True
- for i in range(len(update_flag)):
- if update_flag[i]:
- maintain_ckpts(args, i, len(sorted_keys))
- torch.save(
- ckpt,
- os.path.join(args.checkpoint_path, f"epoch_top_{i}.pt"),
- )
- break
- return current_top_k_ckpt_metrics, new_metrics_inputs
-
-
-# def updateifNone(a, b):
-# a = b if None else a
-# return a
-
-
-def is_pretrained_params(n):
- return (
- n.startswith("clap_model.transformer")
- or n in ["clap_model.positional_embedding", "clap_model.text_projection"]
- or n.startswith("clap_model.token_embedding")
- or n.startswith("clap_model.ln_final")
- or n.startswith("clap_model.logit_scale_t")
- )
-
-
-def random_seed(seed=42, rank=0):
- torch.manual_seed(seed + rank)
- np.random.seed(seed + rank)
- random.seed(seed + rank)
-
-
-def config_lp_optimizer(model, data, args):
- # set wd-related params to 0 if use adam optimizer
- if args.optimizer == "adam":
- args.wd = 0
- args.wd_pretrained = 0
- args.wd_new = 0
-
- in_clap = lambda n, p: n.startswith("clap_model")
-
- named_parameters = list(model.named_parameters())
-
- optimizer = {}
- scheduler = {}
-
- # freeze text encoder
- text_freeze_parameters = [
- p
- for n, p in named_parameters
- if n.startswith("clap_model.transformer")
- or n in ["clap_model.positional_embedding", "clap_model.text_projection"]
- or n.startswith("clap_model.token_embedding")
- or n.startswith("clap_model.ln_final")
- ]
-
- if args.freeze_text:
- logging.info("Freeze Text!!!!")
- for k in text_freeze_parameters:
- k.requires_grad = False
-
- if not args.lp_freeze:
- exclude = (
- lambda n, p: p.ndim < 2
- or "bn" in n
- or "ln" in n
- or "bias" in n
- or "logit_scale" in n
- )
- include = lambda n, p: not exclude(n, p)
-
- # (yusong): we do not split the learning rate anymore
- # p for n, p in named_parameters if in_clap(n,p) and exclude(n, p) and p.requires_grad
- gain_or_bias_params = [
- p for n, p in named_parameters if exclude(n, p) and p.requires_grad
- ]
- # rest_params = [p for n, p in named_parameters if in_clap(n,p) and include(n, p) and p.requires_grad]
- rest_params = [
- p for n, p in named_parameters if include(n, p) and p.requires_grad
- ]
-
- if args.train_data is None:
- optimizer = None
- scheduler = None
- else:
- total_steps = data["train"].dataloader.num_batches * args.epochs
-
- if args.split_opt:
- for x in ["lr", "beta1", "beta2", "eps", "wd"]:
- for y in ["_new", "_pretrained"]:
- if getattr(args, x + y) is None:
- setattr(args, x + y, getattr(args, x))
-
- gain_or_bias_pretrained_params = [
- p
- for n, p in named_parameters
- if (exclude(n, p) and p.requires_grad) and is_pretrained_params(n)
- ]
- rest_pretrained_params = [
- p
- for n, p in named_parameters
- if (include(n, p) and p.requires_grad) and is_pretrained_params(n)
- ]
- gain_or_bias_new_params = [
- p
- for n, p in named_parameters
- if (exclude(n, p) and p.requires_grad)
- and (not is_pretrained_params(n))
- ]
- rest_new_params = [
- p
- for n, p in named_parameters
- if (include(n, p) and p.requires_grad)
- and (not is_pretrained_params(n))
- ]
-
- pretrained_params_optimizer = get_optimizer(
- [
- {"params": gain_or_bias_pretrained_params, "weight_decay": 0.0},
- {
- "params": rest_pretrained_params,
- "weight_decay": args.wd_pretrained,
- },
- ],
- lr=args.lr_pretrained,
- betas=(args.beta1_pretrained, args.beta2_pretrained),
- eps=args.eps_pretrained,
- momentum=args.momentum_pretrained,
- optimizer_name=args.optimizer,
- )
- pretrained_params_scheduler = cosine_lr(
- pretrained_params_optimizer,
- args.lr_pretrained,
- args.warmup,
- total_steps,
- )
-
- new_params_optimizer = get_optimizer(
- [
- {"params": gain_or_bias_new_params, "weight_decay": 0.0},
- {"params": rest_new_params, "weight_decay": args.wd_new},
- ],
- lr=args.lr_new,
- betas=(args.beta1_new, args.beta2_new),
- eps=args.eps_new,
- momentum=args.momentum_new,
- optimizer_name=args.optimizer,
- )
- new_params_scheduler = cosine_lr(
- new_params_optimizer, args.lr_new, args.warmup, total_steps
- )
-
- optimizer["text"] = pretrained_params_optimizer
- optimizer["audio"] = new_params_optimizer
- scheduler["text"] = pretrained_params_scheduler
- scheduler["audio"] = new_params_scheduler
-
- if args.horovod:
- pretrained_params_optimizer = hvd.DistributedOptimizer(
- pretrained_params_optimizer,
- named_parameters=model.named_parameters(),
- )
- new_params_optimizer = hvd.DistributedOptimizer(
- new_params_optimizer, named_parameters=model.named_parameters()
- )
- hvd.broadcast_parameters(model.state_dict(), root_rank=0)
- hvd.broadcast_optimizer_state(
- pretrained_params_optimizer, root_rank=0
- )
- hvd.broadcast_optimizer_state(new_params_optimizer, root_rank=0)
- else:
-
- optimizer["clap"] = get_optimizer(
- [
- {"params": gain_or_bias_params, "weight_decay": 0.0},
- {"params": rest_params, "weight_decay": args.wd},
- ],
- lr=args.lr,
- betas=(args.beta1, args.beta2),
- eps=args.eps,
- momentum=args.momentum,
- optimizer_name=args.optimizer,
- )
- scheduler["clap"] = cosine_lr(
- optimizer["clap"], args.lr, args.warmup, total_steps
- )
-
- if args.horovod:
- optimizer["clap"] = hvd.DistributedOptimizer(
- optimizer["clap"], named_parameters=model.named_parameters()
- )
- hvd.broadcast_parameters(model.state_dict(), root_rank=0)
- hvd.broadcast_optimizer_state(optimizer["clap"], root_rank=0)
-
- # linear probe optimizer
- else:
- lp_params = [
- p for n, p in named_parameters if (not in_clap(n, p)) and p.requires_grad
- ]
- lp_optim = get_optimizer(
- lp_params,
- lr=args.lp_lr,
- betas=(args.beta1, args.beta2),
- eps=args.eps,
- momentum=0.9,
- optimizer_name=args.optimizer,
- )
- optimizer["lp"] = lp_optim
-
- return optimizer, scheduler, text_freeze_parameters
-
-
-def main():
- args = parse_args()
-
- time.sleep(args.sleep)
-
- # sanitize model name for filesystem / uri use, easier if we don't use / in name as a rule?
- args.amodel = args.amodel.replace("/", "-")
- # download sizes.json file
-
- # (yusong): the below two lines are for debug
- # print("setting up faulthandler")
- # faulthandler.register(10)
-
- random.seed(args.seed)
- torch.manual_seed(args.seed)
- torch.cuda.manual_seed(args.seed)
- torch.cuda.manual_seed_all(args.seed)
- np.random.seed(args.seed)
- args.class_index_dict = load_class_label(args.class_label_path)
-
- # get the name of the experiments
- if args.name is None:
- args.name = "-".join(
- [
- datetime.now().strftime("%Y_%m_%d-%H_%M_%S"),
- f"linear_probe" f"model_{args.amodel}",
- f"lr_{args.lr}",
- f"b_{args.batch_size}",
- f"j_{args.workers}",
- f"p_{args.precision}",
- ]
- )
-
- # discover initial world args early so we can log properly
- args.distributed = False
- args.local_rank, args.rank, args.world_size = world_info_from_env()
-
- if args.remotedata and is_master(args):
- for dataset_name in args.datasetnames:
- for split in dataset_split[dataset_name]:
- if not os.path.exists(f"./json_files/{dataset_name}/{split}"):
- os.makedirs(f"./json_files/{dataset_name}/{split}")
- os.system(
- f"aws s3 cp s3://s-laion-audio/webdataset_tar/{dataset_name}/{split}/sizes.json ./json_files/{dataset_name}/{split}/sizes.json"
- )
-
- args.log_path = None
- if is_master(args, local=args.log_local):
- log_base_path = os.path.join(args.logs, args.name)
- os.makedirs(log_base_path, exist_ok=True)
- log_filename = f"out-{args.rank}" if args.log_local else "out.log"
- args.log_path = os.path.join(log_base_path, log_filename)
-
- # avoid log dir in same name:
- postfix = 0
- while os.path.exists(args.log_path):
- postfix += 1
- log_base_path_new = log_base_path + "-" + str(postfix)
- os.makedirs(log_base_path_new, exist_ok=True)
- log_filename = f"out-{args.rank}" if args.log_local else "out.log"
- args.log_path = os.path.join(log_base_path_new, log_filename)
- # print(
- # "Error. Experiment already exists. Use --name {} to specify a new experiment."
- # )
- # return -1
-
- # Set logger
- args.log_level = logging.DEBUG if args.debug else logging.INFO
- setup_logging(args.log_path, args.log_level)
-
- # fully initialize distributed device environment
- device = init_distributed_device(args)
-
- args.wandb = "wandb" in args.report_to or "all" in args.report_to
- args.tensorboard = "tensorboard" in args.report_to or "all" in args.report_to
- if is_master(args):
- args.tensorboard_path = (
- os.path.join(args.logs, args.name, "tensorboard")
- if args.tensorboard
- else ""
- )
- args.checkpoint_path = os.path.join(args.logs, args.name, "checkpoints")
- for dirname in [args.tensorboard_path, args.checkpoint_path]:
- if dirname:
- os.makedirs(dirname, exist_ok=True)
- else:
- args.tensorboard_path = ""
- args.checkpoint_path = ""
-
- if args.copy_codebase:
- copy_codebase(args)
-
- assert args.precision in ["amp", "fp16", "fp32"]
- if args.precision == "fp16":
- logging.warning(
- "It is recommended to use AMP mixed-precision instead of FP16. "
- "FP16 support needs further verification and tuning, especially for train."
- )
-
- if args.horovod:
- logging.info(
- f"Running in horovod mode with multiple processes / nodes. Device: {args.device}."
- f"Process (global: {args.rank}, local {args.local_rank}), total {args.world_size}."
- )
- elif args.distributed:
- logging.info(
- f"Running in distributed mode with multiple processes. Device: {args.device}."
- f"Process (global: {args.rank}, local {args.local_rank}), total {args.world_size}."
- )
- else:
- logging.info(f"Running with a single process. Device {args.device}.")
-
- logging.info(f"openai cache dir: {os.path.expanduser(args.openai_model_cache_dir)}")
-
- # Create CLAP model
- clap_model, clap_model_cfg = create_model(
- args.amodel,
- args.tmodel,
- args.pretrained,
- precision=args.precision,
- device=device,
- jit=args.torchscript,
- force_quick_gelu=args.force_quick_gelu,
- openai_model_cache_dir=os.path.expanduser(args.openai_model_cache_dir),
- skip_params=False,
- pretrained_audio=args.pretrained_audio,
- pretrained_text=args.pretrained_text,
- enable_fusion=args.enable_fusion,
- fusion_type=args.fusion_type,
- )
-
- args.lp_out_ch = len(list(args.class_index_dict.keys()))
- # Linear Probe
- logging.info(f"linear probe using mlp: {args.lp_mlp}")
- logging.info(f"linear probe using freeze: {args.lp_freeze}")
- logging.info(f"linear probe act layer: {args.lp_act}")
- logging.info(f"linear probe out ch: {args.lp_out_ch}")
- logging.info(f"linear probe learning rate (if applicable): {args.lp_lr}")
- logging.info(f"linear probe loss func: {args.lp_loss}")
- logging.info(f"linear probe lp_metrics: {args.lp_metrics}")
-
- model = LinearProbe(
- clap_model,
- mlp=args.lp_mlp,
- freeze=args.lp_freeze,
- in_ch=512,
- out_ch=args.lp_out_ch,
- act=args.lp_act,
- ) # in_ch is fixed (i.e., 512)
- model = model.to(device)
-
- if args.horovod:
- with torch.no_grad():
- for param in model.parameters():
- param.set_(param.contiguous())
-
- if args.trace:
- model = trace_model(model, batch_size=args.batch_size, device=device)
-
- if is_master(args):
- logging.info("Linear Probe CLAP Model:")
- logging.info(f"{str(clap_model)}")
- logging.info("Params:")
- params_file = os.path.join(args.logs, args.name, "params.txt")
- with open(params_file, "w") as f:
- for name in sorted(vars(args)):
- val = getattr(args, name)
- logging.info(f" {name}: {val}")
- f.write(f"{name}: {val}\n")
-
- if args.distributed and not args.horovod:
- if args.use_bn_sync:
- model = torch.nn.SyncBatchNorm.convert_sync_batchnorm(model)
- ddp_args = {}
- if args.ddp_static_graph:
- # this doesn't exist in older PyTorch, arg only added if enabled
- ddp_args["static_graph"] = True
- model = torch.nn.parallel.DistributedDataParallel(
- model, device_ids=[device], find_unused_parameters=True, **ddp_args
- )
-
- data = get_data(args, clap_model_cfg)
- assert len(data), "At least one train or eval dataset must be specified."
- if args.trace:
- assert "train" not in data, "Cannot train with traced model"
-
- optimizer, scheduler, text_freeze_parameters = config_lp_optimizer(
- model, data, args
- )
-
- scaler = GradScaler() if args.precision == "amp" else None
-
- # optionally resume from a checkpoint
- start_epoch = 0
- if args.resume is not None:
- if os.path.isfile(args.resume):
- checkpoint = torch.load(args.resume, map_location=device)
- if "epoch" in checkpoint:
- # resuming a train checkpoint w/ epoch and optimizer state
- start_epoch = checkpoint["epoch"]
- sd = checkpoint["state_dict"]
- if not args.distributed and next(iter(sd.items()))[0].startswith(
- "module"
- ):
- sd = {k[len("module.") :]: v for k, v in sd.items()}
- model.load_state_dict(sd)
- if args.split_opt:
- if optimizer is not None:
- for k, o_ in optimizer.items():
- o_.load_state_dict(checkpoint[k + "_" + "optimizer"])
- if optimizer is not None:
- optimizer.load_state_dict(checkpoint["optimizer"])
- if scaler is not None and "scaler" in checkpoint:
- scaler.load_state_dict(checkpoint["scaler"])
- logging.info(
- f"=> resuming checkpoint '{args.resume}' (epoch {start_epoch})"
- )
- else:
- # loading a bare (model only) checkpoint for fine-tune or evaluation
- model.load_state_dict(checkpoint)
- logging.info(
- f"=> loaded checkpoint '{args.resume}' (epoch {start_epoch})"
- )
- if args.freeze_text:
- print("Freeze Text!!!!")
- for k in text_freeze_parameters:
- k.requires_grad = False
- else:
- logging.info("=> no checkpoint found at '{}'".format(args.resume))
-
- cudnn.benchmark = True
- cudnn.deterministic = False
-
- # determine if this worker should save logs and checkpoints. only do so if it is rank == 0
- args.save_logs = args.logs and args.logs.lower() != "none" and is_master(args)
- writer = None
- if args.save_logs and args.tensorboard:
- assert tensorboard is not None, "Please install tensorboard."
- writer = tensorboard.SummaryWriter(args.tensorboard_path)
-
- if args.wandb and is_master(args):
- assert wandb is not None, "Please install wandb."
- logging.debug("Starting wandb.")
- args.train_sz = data["train"].dataloader.num_samples
- if args.val_data is not None:
- args.val_sz = data["val"].dataloader.num_samples
- # you will have to configure this for your project!
- wandb.init(
- project="clap",
- notes=args.wandb_notes,
- name=args.wandb_notes,
- tags=[],
- config=vars(args),
- )
- if args.debug:
- wandb.watch(model, log="all")
- wandb.save(params_file)
- logging.debug("Finished loading wandb.")
-
- if "train" not in data:
- evaluate(model, data, start_epoch, args, writer)
- return
- elif start_epoch == 0 and "val" in data and not args.no_eval:
- evaluate(model, data, 0, args, writer)
- if args.save_top_performance:
- current_top_k_ckpt_metrics = {
- i: 0 for i in range(args.save_top_performance)
- } # initialize the top-k metric for ckpts to 0
-
- for epoch in range(start_epoch, args.epochs):
- # freeze the text param after (include) args.freeze_text_after, this is -1 by default
- if epoch == args.freeze_text_after:
- print("Text pretrained parameters are freezed since this epoch.")
- for k in text_freeze_parameters:
- k.requires_grad = False
- if is_master(args):
- logging.info(f"Start epoch {epoch}")
-
- train_one_epoch(model, data, epoch, optimizer, scaler, scheduler, args, writer)
- completed_epoch = epoch + 1
-
- if (
- any(v in data for v in ("val", "imagenet-val", "imagenet-v2"))
- and not args.no_eval
- ):
- metrics = evaluate(model, data, completed_epoch, args, writer)
- if args.save_top_performance:
- top_k_dataset = args.top_k_checkpoint_select_dataset
- top_k_metric = args.top_k_checkpoint_select_metric
- filtered_metrics = [
- v
- for k, v in metrics.items()
- if top_k_metric in k and top_k_dataset in k
- ] # check all R@10 metrics (all dataset) and use it to update the ckpt
- # Saving checkpoints.
- if args.save_logs:
- opt_dict = {
- k + "_" + "optimizer": v.state_dict() for k, v in optimizer.items()
- }
- checkpoint_dict = {
- "epoch": completed_epoch,
- "name": args.name,
- "state_dict": model.state_dict(),
- }
- checkpoint_dict.update(opt_dict)
- if scaler is not None:
- checkpoint_dict["scaler"] = scaler.state_dict()
-
- if completed_epoch == args.epochs or (
- args.save_frequency > 0 and (completed_epoch % args.save_frequency) == 0
- ):
- torch.save(
- checkpoint_dict,
- os.path.join(args.checkpoint_path, f"epoch_{completed_epoch}.pt"),
- )
- if args.save_most_recent:
- torch.save(
- checkpoint_dict,
- os.path.join(args.checkpoint_path, f"epoch_latest.pt"),
- )
- if args.save_top_performance and not args.no_eval:
- update_top_k_performance(
- filtered_metrics,
- current_top_k_ckpt_metrics,
- args,
- checkpoint_dict,
- bignumbetter=True,
- )
-
- if args.wandb and is_master(args):
- wandb.finish()
-
-
-def copy_codebase(args):
- from shutil import copytree, ignore_patterns
-
- new_code_path = os.path.join(args.logs, args.name, "code")
- if os.path.exists(new_code_path):
- print(
- f"Error. Experiment already exists at {new_code_path}. Use --name to specify a new experiment."
- )
- return -1
- print(f"Copying codebase to {new_code_path}")
- current_code_path = os.path.realpath(__file__)
- for _ in range(3):
- current_code_path = os.path.dirname(current_code_path)
- copytree(
- current_code_path, new_code_path, ignore=ignore_patterns("log", "logs", "wandb")
- )
- print("Done copying code.")
- return 1
-
-
-if __name__ == "__main__":
- main()
diff --git a/spaces/banana-projects/datasets-card-creator/build/static/js/3.523cfdab.chunk.js b/spaces/banana-projects/datasets-card-creator/build/static/js/3.523cfdab.chunk.js
deleted file mode 100644
index c11a724e2bfa0e6424ca0d0456e5148db369f95c..0000000000000000000000000000000000000000
--- a/spaces/banana-projects/datasets-card-creator/build/static/js/3.523cfdab.chunk.js
+++ /dev/null
@@ -1,2 +0,0 @@
-(this.webpackJsonpdatasetcard=this.webpackJsonpdatasetcard||[]).push([[3],{140:function(t,n,e){"use strict";e.r(n),e.d(n,"getCLS",(function(){return v})),e.d(n,"getFCP",(function(){return g})),e.d(n,"getFID",(function(){return h})),e.d(n,"getLCP",(function(){return y})),e.d(n,"getTTFB",(function(){return F}));var i,a,r=function(){return"".concat(Date.now(),"-").concat(Math.floor(8999999999999*Math.random())+1e12)},o=function(t){var n=arguments.length>1&&void 0!==arguments[1]?arguments[1]:-1;return{name:t,value:n,delta:0,entries:[],id:r(),isFinal:!1}},u=function(t,n){try{if(PerformanceObserver.supportedEntryTypes.includes(t)){var e=new PerformanceObserver((function(t){return t.getEntries().map(n)}));return e.observe({type:t,buffered:!0}),e}}catch(t){}},s=!1,c=!1,d=function(t){s=!t.persisted},f=function(){addEventListener("pagehide",d),addEventListener("beforeunload",(function(){}))},p=function(t){var n=arguments.length>1&&void 0!==arguments[1]&&arguments[1];c||(f(),c=!0),addEventListener("visibilitychange",(function(n){var e=n.timeStamp;"hidden"===document.visibilityState&&t({timeStamp:e,isUnloading:s})}),{capture:!0,once:n})},l=function(t,n,e,i){var a;return function(){e&&n.isFinal&&e.disconnect(),n.value>=0&&(i||n.isFinal||"hidden"===document.visibilityState)&&(n.delta=n.value-(a||0),(n.delta||n.isFinal||void 0===a)&&(t(n),a=n.value))}},v=function(t){var n,e=arguments.length>1&&void 0!==arguments[1]&&arguments[1],i=o("CLS",0),a=function(t){t.hadRecentInput||(i.value+=t.value,i.entries.push(t),n())},r=u("layout-shift",a);r&&(n=l(t,i,r,e),p((function(t){var e=t.isUnloading;r.takeRecords().map(a),e&&(i.isFinal=!0),n()})))},m=function(){return void 0===i&&(i="hidden"===document.visibilityState?0:1/0,p((function(t){var n=t.timeStamp;return i=n}),!0)),{get timeStamp(){return i}}},g=function(t){var n,e=o("FCP"),i=m(),a=u("paint",(function(t){"first-contentful-paint"===t.name&&t.startTime1&&void 0!==arguments[1]&&arguments[1],i=o("LCP"),a=m(),r=function(t){var e=t.startTime;e 0 ) tracks.push( new THREE.VectorKeyframeTrack( name + '.position', times, positionData ) );
- if ( quaternionData.length > 0 ) tracks.push( new THREE.QuaternionKeyframeTrack( name + '.quaternion', times, quaternionData ) );
- if ( scaleData.length > 0 ) tracks.push( new THREE.VectorKeyframeTrack( name + '.scale', times, scaleData ) );
-
- return tracks;
-
- }
-
- function transformAnimationData( keyframes, property, defaultValue ) {
-
- var keyframe;
-
- var empty = true;
- var i, l;
-
- // check, if values of a property are missing in our keyframes
-
- for ( i = 0, l = keyframes.length; i < l; i ++ ) {
-
- keyframe = keyframes[ i ];
-
- if ( keyframe.value[ property ] === undefined ) {
-
- keyframe.value[ property ] = null; // mark as missing
-
- } else {
-
- empty = false;
-
- }
-
- }
-
- if ( empty === true ) {
-
- // no values at all, so we set a default value
-
- for ( i = 0, l = keyframes.length; i < l; i ++ ) {
-
- keyframe = keyframes[ i ];
-
- keyframe.value[ property ] = defaultValue;
-
- }
-
- } else {
-
- // filling gaps
-
- createMissingKeyframes( keyframes, property );
-
- }
-
- }
-
- function createMissingKeyframes( keyframes, property ) {
-
- var prev, next;
-
- for ( var i = 0, l = keyframes.length; i < l; i ++ ) {
-
- var keyframe = keyframes[ i ];
-
- if ( keyframe.value[ property ] === null ) {
-
- prev = getPrev( keyframes, i, property );
- next = getNext( keyframes, i, property );
-
- if ( prev === null ) {
-
- keyframe.value[ property ] = next.value[ property ];
- continue;
-
- }
-
- if ( next === null ) {
-
- keyframe.value[ property ] = prev.value[ property ];
- continue;
-
- }
-
- interpolate( keyframe, prev, next, property );
-
- }
-
- }
-
- }
-
- function getPrev( keyframes, i, property ) {
-
- while ( i >= 0 ) {
-
- var keyframe = keyframes[ i ];
-
- if ( keyframe.value[ property ] !== null ) return keyframe;
-
- i --;
-
- }
-
- return null;
-
- }
-
- function getNext( keyframes, i, property ) {
-
- while ( i < keyframes.length ) {
-
- var keyframe = keyframes[ i ];
-
- if ( keyframe.value[ property ] !== null ) return keyframe;
-
- i ++;
-
- }
-
- return null;
-
- }
-
- function interpolate( key, prev, next, property ) {
-
- if ( ( next.time - prev.time ) === 0 ) {
-
- key.value[ property ] = prev.value[ property ];
- return;
-
- }
-
- key.value[ property ] = ( ( key.time - prev.time ) * ( next.value[ property ] - prev.value[ property ] ) / ( next.time - prev.time ) ) + prev.value[ property ];
-
- }
-
- // animation clips
-
- function parseAnimationClip( xml ) {
-
- var data = {
- name: xml.getAttribute( 'id' ) || 'default',
- start: parseFloat( xml.getAttribute( 'start' ) || 0 ),
- end: parseFloat( xml.getAttribute( 'end' ) || 0 ),
- animations: []
- };
-
- for ( var i = 0, l = xml.childNodes.length; i < l; i ++ ) {
-
- var child = xml.childNodes[ i ];
-
- if ( child.nodeType !== 1 ) continue;
-
- switch ( child.nodeName ) {
-
- case 'instance_animation':
- data.animations.push( parseId( child.getAttribute( 'url' ) ) );
- break;
-
- }
-
- }
-
- library.clips[ xml.getAttribute( 'id' ) ] = data;
-
- }
-
- function buildAnimationClip( data ) {
-
- var tracks = [];
-
- var name = data.name;
- var duration = ( data.end - data.start ) || - 1;
- var animations = data.animations;
-
- for ( var i = 0, il = animations.length; i < il; i ++ ) {
-
- var animationTracks = getAnimation( animations[ i ] );
-
- for ( var j = 0, jl = animationTracks.length; j < jl; j ++ ) {
-
- tracks.push( animationTracks[ j ] );
-
- }
-
- }
-
- return new THREE.AnimationClip( name, duration, tracks );
-
- }
-
- function getAnimationClip( id ) {
-
- return getBuild( library.clips[ id ], buildAnimationClip );
-
- }
-
- // controller
-
- function parseController( xml ) {
-
- var data = {};
-
- for ( var i = 0, l = xml.childNodes.length; i < l; i ++ ) {
-
- var child = xml.childNodes[ i ];
-
- if ( child.nodeType !== 1 ) continue;
-
- switch ( child.nodeName ) {
-
- case 'skin':
- // there is exactly one skin per controller
- data.id = parseId( child.getAttribute( 'source' ) );
- data.skin = parseSkin( child );
- break;
-
- case 'morph':
- data.id = parseId( child.getAttribute( 'source' ) );
- console.warn( 'THREE.ColladaLoader: Morph target animation not supported yet.' );
- break;
-
- }
-
- }
-
- library.controllers[ xml.getAttribute( 'id' ) ] = data;
-
- }
-
- function parseSkin( xml ) {
-
- var data = {
- sources: {}
- };
-
- for ( var i = 0, l = xml.childNodes.length; i < l; i ++ ) {
-
- var child = xml.childNodes[ i ];
-
- if ( child.nodeType !== 1 ) continue;
-
- switch ( child.nodeName ) {
-
- case 'bind_shape_matrix':
- data.bindShapeMatrix = parseFloats( child.textContent );
- break;
-
- case 'source':
- var id = child.getAttribute( 'id' );
- data.sources[ id ] = parseSource( child );
- break;
-
- case 'joints':
- data.joints = parseJoints( child );
- break;
-
- case 'vertex_weights':
- data.vertexWeights = parseVertexWeights( child );
- break;
-
- }
-
- }
-
- return data;
-
- }
-
- function parseJoints( xml ) {
-
- var data = {
- inputs: {}
- };
-
- for ( var i = 0, l = xml.childNodes.length; i < l; i ++ ) {
-
- var child = xml.childNodes[ i ];
-
- if ( child.nodeType !== 1 ) continue;
-
- switch ( child.nodeName ) {
-
- case 'input':
- var semantic = child.getAttribute( 'semantic' );
- var id = parseId( child.getAttribute( 'source' ) );
- data.inputs[ semantic ] = id;
- break;
-
- }
-
- }
-
- return data;
-
- }
-
- function parseVertexWeights( xml ) {
-
- var data = {
- inputs: {}
- };
-
- for ( var i = 0, l = xml.childNodes.length; i < l; i ++ ) {
-
- var child = xml.childNodes[ i ];
-
- if ( child.nodeType !== 1 ) continue;
-
- switch ( child.nodeName ) {
-
- case 'input':
- var semantic = child.getAttribute( 'semantic' );
- var id = parseId( child.getAttribute( 'source' ) );
- var offset = parseInt( child.getAttribute( 'offset' ) );
- data.inputs[ semantic ] = { id: id, offset: offset };
- break;
-
- case 'vcount':
- data.vcount = parseInts( child.textContent );
- break;
-
- case 'v':
- data.v = parseInts( child.textContent );
- break;
-
- }
-
- }
-
- return data;
-
- }
-
- function buildController( data ) {
-
- var build = {
- id: data.id
- };
-
- var geometry = library.geometries[ build.id ];
-
- if ( data.skin !== undefined ) {
-
- build.skin = buildSkin( data.skin );
-
- // we enhance the 'sources' property of the corresponding geometry with our skin data
-
- geometry.sources.skinIndices = build.skin.indices;
- geometry.sources.skinWeights = build.skin.weights;
-
- }
-
- return build;
-
- }
-
- function buildSkin( data ) {
-
- var BONE_LIMIT = 4;
-
- var build = {
- joints: [], // this must be an array to preserve the joint order
- indices: {
- array: [],
- stride: BONE_LIMIT
- },
- weights: {
- array: [],
- stride: BONE_LIMIT
- }
- };
-
- var sources = data.sources;
- var vertexWeights = data.vertexWeights;
-
- var vcount = vertexWeights.vcount;
- var v = vertexWeights.v;
- var jointOffset = vertexWeights.inputs.JOINT.offset;
- var weightOffset = vertexWeights.inputs.WEIGHT.offset;
-
- var jointSource = data.sources[ data.joints.inputs.JOINT ];
- var inverseSource = data.sources[ data.joints.inputs.INV_BIND_MATRIX ];
-
- var weights = sources[ vertexWeights.inputs.WEIGHT.id ].array;
- var stride = 0;
-
- var i, j, l;
-
- // procces skin data for each vertex
-
- for ( i = 0, l = vcount.length; i < l; i ++ ) {
-
- var jointCount = vcount[ i ]; // this is the amount of joints that affect a single vertex
- var vertexSkinData = [];
-
- for ( j = 0; j < jointCount; j ++ ) {
-
- var skinIndex = v[ stride + jointOffset ];
- var weightId = v[ stride + weightOffset ];
- var skinWeight = weights[ weightId ];
-
- vertexSkinData.push( { index: skinIndex, weight: skinWeight } );
-
- stride += 2;
-
- }
-
- // we sort the joints in descending order based on the weights.
- // this ensures, we only procced the most important joints of the vertex
-
- vertexSkinData.sort( descending );
-
- // now we provide for each vertex a set of four index and weight values.
- // the order of the skin data matches the order of vertices
-
- for ( j = 0; j < BONE_LIMIT; j ++ ) {
-
- var d = vertexSkinData[ j ];
-
- if ( d !== undefined ) {
-
- build.indices.array.push( d.index );
- build.weights.array.push( d.weight );
-
- } else {
-
- build.indices.array.push( 0 );
- build.weights.array.push( 0 );
-
- }
-
- }
-
- }
-
- // setup bind matrix
-
- if ( data.bindShapeMatrix ) {
-
- build.bindMatrix = new THREE.Matrix4().fromArray( data.bindShapeMatrix ).transpose();
-
- } else {
-
- build.bindMatrix = new THREE.Matrix4().identity();
-
- }
-
- // process bones and inverse bind matrix data
-
- for ( i = 0, l = jointSource.array.length; i < l; i ++ ) {
-
- var name = jointSource.array[ i ];
- var boneInverse = new THREE.Matrix4().fromArray( inverseSource.array, i * inverseSource.stride ).transpose();
-
- build.joints.push( { name: name, boneInverse: boneInverse } );
-
- }
-
- return build;
-
- // array sort function
-
- function descending( a, b ) {
-
- return b.weight - a.weight;
-
- }
-
- }
-
- function getController( id ) {
-
- return getBuild( library.controllers[ id ], buildController );
-
- }
-
- // image
-
- function parseImage( xml ) {
-
- var data = {
- init_from: getElementsByTagName( xml, 'init_from' )[ 0 ].textContent
- };
-
- library.images[ xml.getAttribute( 'id' ) ] = data;
-
- }
-
- function buildImage( data ) {
-
- if ( data.build !== undefined ) return data.build;
-
- return data.init_from;
-
- }
-
- function getImage( id ) {
-
- var data = library.images[ id ];
-
- if ( data !== undefined ) {
-
- return getBuild( data, buildImage );
-
- }
-
- console.warn( 'THREE.ColladaLoader: Couldn\'t find image with ID:', id );
-
- return null;
-
- }
-
- // effect
-
- function parseEffect( xml ) {
-
- var data = {};
-
- for ( var i = 0, l = xml.childNodes.length; i < l; i ++ ) {
-
- var child = xml.childNodes[ i ];
-
- if ( child.nodeType !== 1 ) continue;
-
- switch ( child.nodeName ) {
-
- case 'profile_COMMON':
- data.profile = parseEffectProfileCOMMON( child );
- break;
-
- }
-
- }
-
- library.effects[ xml.getAttribute( 'id' ) ] = data;
-
- }
-
- function parseEffectProfileCOMMON( xml ) {
-
- var data = {
- surfaces: {},
- samplers: {}
- };
-
- for ( var i = 0, l = xml.childNodes.length; i < l; i ++ ) {
-
- var child = xml.childNodes[ i ];
-
- if ( child.nodeType !== 1 ) continue;
-
- switch ( child.nodeName ) {
-
- case 'newparam':
- parseEffectNewparam( child, data );
- break;
-
- case 'technique':
- data.technique = parseEffectTechnique( child );
- break;
-
- case 'extra':
- data.extra = parseEffectExtra( child );
- break;
-
- }
-
- }
-
- return data;
-
- }
-
- function parseEffectNewparam( xml, data ) {
-
- var sid = xml.getAttribute( 'sid' );
-
- for ( var i = 0, l = xml.childNodes.length; i < l; i ++ ) {
-
- var child = xml.childNodes[ i ];
-
- if ( child.nodeType !== 1 ) continue;
-
- switch ( child.nodeName ) {
-
- case 'surface':
- data.surfaces[ sid ] = parseEffectSurface( child );
- break;
-
- case 'sampler2D':
- data.samplers[ sid ] = parseEffectSampler( child );
- break;
-
- }
-
- }
-
- }
-
- function parseEffectSurface( xml ) {
-
- var data = {};
-
- for ( var i = 0, l = xml.childNodes.length; i < l; i ++ ) {
-
- var child = xml.childNodes[ i ];
-
- if ( child.nodeType !== 1 ) continue;
-
- switch ( child.nodeName ) {
-
- case 'init_from':
- data.init_from = child.textContent;
- break;
-
- }
-
- }
-
- return data;
-
- }
-
- function parseEffectSampler( xml ) {
-
- var data = {};
-
- for ( var i = 0, l = xml.childNodes.length; i < l; i ++ ) {
-
- var child = xml.childNodes[ i ];
-
- if ( child.nodeType !== 1 ) continue;
-
- switch ( child.nodeName ) {
-
- case 'source':
- data.source = child.textContent;
- break;
-
- }
-
- }
-
- return data;
-
- }
-
- function parseEffectTechnique( xml ) {
-
- var data = {};
-
- for ( var i = 0, l = xml.childNodes.length; i < l; i ++ ) {
-
- var child = xml.childNodes[ i ];
-
- if ( child.nodeType !== 1 ) continue;
-
- switch ( child.nodeName ) {
-
- case 'constant':
- case 'lambert':
- case 'blinn':
- case 'phong':
- data.type = child.nodeName;
- data.parameters = parseEffectParameters( child );
- break;
-
- }
-
- }
-
- return data;
-
- }
-
- function parseEffectParameters( xml ) {
-
- var data = {};
-
- for ( var i = 0, l = xml.childNodes.length; i < l; i ++ ) {
-
- var child = xml.childNodes[ i ];
-
- if ( child.nodeType !== 1 ) continue;
-
- switch ( child.nodeName ) {
-
- case 'emission':
- case 'diffuse':
- case 'specular':
- case 'bump':
- case 'ambient':
- case 'shininess':
- case 'transparency':
- data[ child.nodeName ] = parseEffectParameter( child );
- break;
- case 'transparent':
- data[ child.nodeName ] = {
- opaque: child.getAttribute( 'opaque' ),
- data: parseEffectParameter( child )
- };
- break;
-
- }
-
- }
-
- return data;
-
- }
-
- function parseEffectParameter( xml ) {
-
- var data = {};
-
- for ( var i = 0, l = xml.childNodes.length; i < l; i ++ ) {
-
- var child = xml.childNodes[ i ];
-
- if ( child.nodeType !== 1 ) continue;
-
- switch ( child.nodeName ) {
-
- case 'color':
- data[ child.nodeName ] = parseFloats( child.textContent );
- break;
-
- case 'float':
- data[ child.nodeName ] = parseFloat( child.textContent );
- break;
-
- case 'texture':
- data[ child.nodeName ] = { id: child.getAttribute( 'texture' ), extra: parseEffectParameterTexture( child ) };
- break;
-
- }
-
- }
-
- return data;
-
- }
-
- function parseEffectParameterTexture( xml ) {
-
- var data = {
- technique: {}
- };
-
- for ( var i = 0, l = xml.childNodes.length; i < l; i ++ ) {
-
- var child = xml.childNodes[ i ];
-
- if ( child.nodeType !== 1 ) continue;
-
- switch ( child.nodeName ) {
-
- case 'extra':
- parseEffectParameterTextureExtra( child, data );
- break;
-
- }
-
- }
-
- return data;
-
- }
-
- function parseEffectParameterTextureExtra( xml, data ) {
-
- for ( var i = 0, l = xml.childNodes.length; i < l; i ++ ) {
-
- var child = xml.childNodes[ i ];
-
- if ( child.nodeType !== 1 ) continue;
-
- switch ( child.nodeName ) {
-
- case 'technique':
- parseEffectParameterTextureExtraTechnique( child, data );
- break;
-
- }
-
- }
-
- }
-
- function parseEffectParameterTextureExtraTechnique( xml, data ) {
-
- for ( var i = 0, l = xml.childNodes.length; i < l; i ++ ) {
-
- var child = xml.childNodes[ i ];
-
- if ( child.nodeType !== 1 ) continue;
-
- switch ( child.nodeName ) {
-
- case 'repeatU':
- case 'repeatV':
- case 'offsetU':
- case 'offsetV':
- data.technique[ child.nodeName ] = parseFloat( child.textContent );
- break;
-
- case 'wrapU':
- case 'wrapV':
-
- // some files have values for wrapU/wrapV which become NaN via parseInt
-
- if ( child.textContent.toUpperCase() === 'TRUE' ) {
-
- data.technique[ child.nodeName ] = 1;
-
- } else if ( child.textContent.toUpperCase() === 'FALSE' ) {
-
- data.technique[ child.nodeName ] = 0;
-
- } else {
-
- data.technique[ child.nodeName ] = parseInt( child.textContent );
-
- }
-
- break;
-
- }
-
- }
-
- }
-
- function parseEffectExtra( xml ) {
-
- var data = {};
-
- for ( var i = 0, l = xml.childNodes.length; i < l; i ++ ) {
-
- var child = xml.childNodes[ i ];
-
- if ( child.nodeType !== 1 ) continue;
-
- switch ( child.nodeName ) {
-
- case 'technique':
- data.technique = parseEffectExtraTechnique( child );
- break;
-
- }
-
- }
-
- return data;
-
- }
-
- function parseEffectExtraTechnique( xml ) {
-
- var data = {};
-
- for ( var i = 0, l = xml.childNodes.length; i < l; i ++ ) {
-
- var child = xml.childNodes[ i ];
-
- if ( child.nodeType !== 1 ) continue;
-
- switch ( child.nodeName ) {
-
- case 'double_sided':
- data[ child.nodeName ] = parseInt( child.textContent );
- break;
-
- }
-
- }
-
- return data;
-
- }
-
- function buildEffect( data ) {
-
- return data;
-
- }
-
- function getEffect( id ) {
-
- return getBuild( library.effects[ id ], buildEffect );
-
- }
-
- // material
-
- function parseMaterial( xml ) {
-
- var data = {
- name: xml.getAttribute( 'name' )
- };
-
- for ( var i = 0, l = xml.childNodes.length; i < l; i ++ ) {
-
- var child = xml.childNodes[ i ];
-
- if ( child.nodeType !== 1 ) continue;
-
- switch ( child.nodeName ) {
-
- case 'instance_effect':
- data.url = parseId( child.getAttribute( 'url' ) );
- break;
-
- }
-
- }
-
- library.materials[ xml.getAttribute( 'id' ) ] = data;
-
- }
-
- function getTextureLoader( image ) {
-
- var loader;
-
- var extension = image.slice( ( image.lastIndexOf( '.' ) - 1 >>> 0 ) + 2 ); // http://www.jstips.co/en/javascript/get-file-extension/
- extension = extension.toLowerCase();
-
- switch ( extension ) {
-
- case 'tga':
- loader = tgaLoader;
- break;
-
- default:
- loader = textureLoader;
-
- }
-
- return loader;
-
- }
-
- function buildMaterial( data ) {
-
- var effect = getEffect( data.url );
- var technique = effect.profile.technique;
- var extra = effect.profile.extra;
-
- var material;
-
- switch ( technique.type ) {
-
- case 'phong':
- case 'blinn':
- material = new THREE.MeshPhongMaterial();
- break;
-
- case 'lambert':
- material = new THREE.MeshLambertMaterial();
- break;
-
- default:
- material = new THREE.MeshBasicMaterial();
- break;
-
- }
-
- material.name = data.name || '';
-
- function getTexture( textureObject ) {
-
- var sampler = effect.profile.samplers[ textureObject.id ];
- var image = null;
-
- // get image
-
- if ( sampler !== undefined ) {
-
- var surface = effect.profile.surfaces[ sampler.source ];
- image = getImage( surface.init_from );
-
- } else {
-
- console.warn( 'THREE.ColladaLoader: Undefined sampler. Access image directly (see #12530).' );
- image = getImage( textureObject.id );
-
- }
-
- // create texture if image is avaiable
-
- if ( image !== null ) {
-
- var loader = getTextureLoader( image );
-
- if ( loader !== undefined ) {
-
- var texture = loader.load( image );
-
- var extra = textureObject.extra;
-
- if ( extra !== undefined && extra.technique !== undefined && isEmpty( extra.technique ) === false ) {
-
- var technique = extra.technique;
-
- texture.wrapS = technique.wrapU ? THREE.RepeatWrapping : THREE.ClampToEdgeWrapping;
- texture.wrapT = technique.wrapV ? THREE.RepeatWrapping : THREE.ClampToEdgeWrapping;
-
- texture.offset.set( technique.offsetU || 0, technique.offsetV || 0 );
- texture.repeat.set( technique.repeatU || 1, technique.repeatV || 1 );
-
- } else {
-
- texture.wrapS = THREE.RepeatWrapping;
- texture.wrapT = THREE.RepeatWrapping;
-
- }
-
- return texture;
-
- } else {
-
- console.warn( 'THREE.ColladaLoader: Loader for texture %s not found.', image );
-
- return null;
-
- }
-
- } else {
-
- console.warn( 'THREE.ColladaLoader: Couldn\'t create texture with ID:', textureObject.id );
-
- return null;
-
- }
-
- }
-
- var parameters = technique.parameters;
-
- for ( var key in parameters ) {
-
- var parameter = parameters[ key ];
-
- switch ( key ) {
-
- case 'diffuse':
- if ( parameter.color ) material.color.fromArray( parameter.color );
- if ( parameter.texture ) material.map = getTexture( parameter.texture );
- break;
- case 'specular':
- if ( parameter.color && material.specular ) material.specular.fromArray( parameter.color );
- if ( parameter.texture ) material.specularMap = getTexture( parameter.texture );
- break;
- case 'bump':
- if ( parameter.texture ) material.normalMap = getTexture( parameter.texture );
- break;
- case 'ambient':
- if ( parameter.texture ) material.lightMap = getTexture( parameter.texture );
- break;
- case 'shininess':
- if ( parameter.float && material.shininess ) material.shininess = parameter.float;
- break;
- case 'emission':
- if ( parameter.color && material.emissive ) material.emissive.fromArray( parameter.color );
- if ( parameter.texture ) material.emissiveMap = getTexture( parameter.texture );
- break;
-
- }
-
- }
-
- //
-
- var transparent = parameters[ 'transparent' ];
- var transparency = parameters[ 'transparency' ];
-
- // does not exist but
-
- if ( transparency === undefined && transparent ) {
-
- transparency = {
- float: 1
- };
-
- }
-
- // does not exist but
-
- if ( transparent === undefined && transparency ) {
-
- transparent = {
- opaque: 'A_ONE',
- data: {
- color: [ 1, 1, 1, 1 ]
- } };
-
- }
-
- if ( transparent && transparency ) {
-
- // handle case if a texture exists but no color
-
- if ( transparent.data.texture ) {
-
- // we do not set an alpha map (see #13792)
-
- material.transparent = true;
-
- } else {
-
- var color = transparent.data.color;
-
- switch ( transparent.opaque ) {
-
- case 'A_ONE':
- material.opacity = color[ 3 ] * transparency.float;
- break;
- case 'RGB_ZERO':
- material.opacity = 1 - ( color[ 0 ] * transparency.float );
- break;
- case 'A_ZERO':
- material.opacity = 1 - ( color[ 3 ] * transparency.float );
- break;
- case 'RGB_ONE':
- material.opacity = color[ 0 ] * transparency.float;
- break;
- default:
- console.warn( 'THREE.ColladaLoader: Invalid opaque type "%s" of transparent tag.', transparent.opaque );
-
- }
-
- if ( material.opacity < 1 ) material.transparent = true;
-
- }
-
- }
-
- //
-
- if ( extra !== undefined && extra.technique !== undefined && extra.technique.double_sided === 1 ) {
-
- material.side = THREE.DoubleSide;
-
- }
-
- return material;
-
- }
-
- function getMaterial( id ) {
-
- return getBuild( library.materials[ id ], buildMaterial );
-
- }
-
- // camera
-
- function parseCamera( xml ) {
-
- var data = {
- name: xml.getAttribute( 'name' )
- };
-
- for ( var i = 0, l = xml.childNodes.length; i < l; i ++ ) {
-
- var child = xml.childNodes[ i ];
-
- if ( child.nodeType !== 1 ) continue;
-
- switch ( child.nodeName ) {
-
- case 'optics':
- data.optics = parseCameraOptics( child );
- break;
-
- }
-
- }
-
- library.cameras[ xml.getAttribute( 'id' ) ] = data;
-
- }
-
- function parseCameraOptics( xml ) {
-
- for ( var i = 0; i < xml.childNodes.length; i ++ ) {
-
- var child = xml.childNodes[ i ];
-
- switch ( child.nodeName ) {
-
- case 'technique_common':
- return parseCameraTechnique( child );
-
- }
-
- }
-
- return {};
-
- }
-
- function parseCameraTechnique( xml ) {
-
- var data = {};
-
- for ( var i = 0; i < xml.childNodes.length; i ++ ) {
-
- var child = xml.childNodes[ i ];
-
- switch ( child.nodeName ) {
-
- case 'perspective':
- case 'orthographic':
-
- data.technique = child.nodeName;
- data.parameters = parseCameraParameters( child );
-
- break;
-
- }
-
- }
-
- return data;
-
- }
-
- function parseCameraParameters( xml ) {
-
- var data = {};
-
- for ( var i = 0; i < xml.childNodes.length; i ++ ) {
-
- var child = xml.childNodes[ i ];
-
- switch ( child.nodeName ) {
-
- case 'xfov':
- case 'yfov':
- case 'xmag':
- case 'ymag':
- case 'znear':
- case 'zfar':
- case 'aspect_ratio':
- data[ child.nodeName ] = parseFloat( child.textContent );
- break;
-
- }
-
- }
-
- return data;
-
- }
-
- function buildCamera( data ) {
-
- var camera;
-
- switch ( data.optics.technique ) {
-
- case 'perspective':
- camera = new THREE.PerspectiveCamera(
- data.optics.parameters.yfov,
- data.optics.parameters.aspect_ratio,
- data.optics.parameters.znear,
- data.optics.parameters.zfar
- );
- break;
-
- case 'orthographic':
- var ymag = data.optics.parameters.ymag;
- var xmag = data.optics.parameters.xmag;
- var aspectRatio = data.optics.parameters.aspect_ratio;
-
- xmag = ( xmag === undefined ) ? ( ymag * aspectRatio ) : xmag;
- ymag = ( ymag === undefined ) ? ( xmag / aspectRatio ) : ymag;
-
- xmag *= 0.5;
- ymag *= 0.5;
-
- camera = new THREE.OrthographicCamera(
- - xmag, xmag, ymag, - ymag, // left, right, top, bottom
- data.optics.parameters.znear,
- data.optics.parameters.zfar
- );
- break;
-
- default:
- camera = new THREE.PerspectiveCamera();
- break;
-
- }
-
- camera.name = data.name || '';
-
- return camera;
-
- }
-
- function getCamera( id ) {
-
- var data = library.cameras[ id ];
-
- if ( data !== undefined ) {
-
- return getBuild( data, buildCamera );
-
- }
-
- console.warn( 'THREE.ColladaLoader: Couldn\'t find camera with ID:', id );
-
- return null;
-
- }
-
- // light
-
- function parseLight( xml ) {
-
- var data = {};
-
- for ( var i = 0, l = xml.childNodes.length; i < l; i ++ ) {
-
- var child = xml.childNodes[ i ];
-
- if ( child.nodeType !== 1 ) continue;
-
- switch ( child.nodeName ) {
-
- case 'technique_common':
- data = parseLightTechnique( child );
- break;
-
- }
-
- }
-
- library.lights[ xml.getAttribute( 'id' ) ] = data;
-
- }
-
- function parseLightTechnique( xml ) {
-
- var data = {};
-
- for ( var i = 0, l = xml.childNodes.length; i < l; i ++ ) {
-
- var child = xml.childNodes[ i ];
-
- if ( child.nodeType !== 1 ) continue;
-
- switch ( child.nodeName ) {
-
- case 'directional':
- case 'point':
- case 'spot':
- case 'ambient':
-
- data.technique = child.nodeName;
- data.parameters = parseLightParameters( child );
-
- }
-
- }
-
- return data;
-
- }
-
- function parseLightParameters( xml ) {
-
- var data = {};
-
- for ( var i = 0, l = xml.childNodes.length; i < l; i ++ ) {
-
- var child = xml.childNodes[ i ];
-
- if ( child.nodeType !== 1 ) continue;
-
- switch ( child.nodeName ) {
-
- case 'color':
- var array = parseFloats( child.textContent );
- data.color = new THREE.Color().fromArray( array );
- break;
-
- case 'falloff_angle':
- data.falloffAngle = parseFloat( child.textContent );
- break;
-
- case 'quadratic_attenuation':
- var f = parseFloat( child.textContent );
- data.distance = f ? Math.sqrt( 1 / f ) : 0;
- break;
-
- }
-
- }
-
- return data;
-
- }
-
- function buildLight( data ) {
-
- var light;
-
- switch ( data.technique ) {
-
- case 'directional':
- light = new THREE.DirectionalLight();
- break;
-
- case 'point':
- light = new THREE.PointLight();
- break;
-
- case 'spot':
- light = new THREE.SpotLight();
- break;
-
- case 'ambient':
- light = new THREE.AmbientLight();
- break;
-
- }
-
- if ( data.parameters.color ) light.color.copy( data.parameters.color );
- if ( data.parameters.distance ) light.distance = data.parameters.distance;
-
- return light;
-
- }
-
- function getLight( id ) {
-
- var data = library.lights[ id ];
-
- if ( data !== undefined ) {
-
- return getBuild( data, buildLight );
-
- }
-
- console.warn( 'THREE.ColladaLoader: Couldn\'t find light with ID:', id );
-
- return null;
-
- }
-
- // geometry
-
- function parseGeometry( xml ) {
-
- var data = {
- name: xml.getAttribute( 'name' ),
- sources: {},
- vertices: {},
- primitives: []
- };
-
- var mesh = getElementsByTagName( xml, 'mesh' )[ 0 ];
-
- // the following tags inside geometry are not supported yet (see https://github.com/mrdoob/three.js/pull/12606): convex_mesh, spline, brep
- if ( mesh === undefined ) return;
-
- for ( var i = 0; i < mesh.childNodes.length; i ++ ) {
-
- var child = mesh.childNodes[ i ];
-
- if ( child.nodeType !== 1 ) continue;
-
- var id = child.getAttribute( 'id' );
-
- switch ( child.nodeName ) {
-
- case 'source':
- data.sources[ id ] = parseSource( child );
- break;
-
- case 'vertices':
- // data.sources[ id ] = data.sources[ parseId( getElementsByTagName( child, 'input' )[ 0 ].getAttribute( 'source' ) ) ];
- data.vertices = parseGeometryVertices( child );
- break;
-
- case 'polygons':
- console.warn( 'THREE.ColladaLoader: Unsupported primitive type: ', child.nodeName );
- break;
-
- case 'lines':
- case 'linestrips':
- case 'polylist':
- case 'triangles':
- data.primitives.push( parseGeometryPrimitive( child ) );
- break;
-
- default:
- console.log( child );
-
- }
-
- }
-
- library.geometries[ xml.getAttribute( 'id' ) ] = data;
-
- }
-
- function parseSource( xml ) {
-
- var data = {
- array: [],
- stride: 3
- };
-
- for ( var i = 0; i < xml.childNodes.length; i ++ ) {
-
- var child = xml.childNodes[ i ];
-
- if ( child.nodeType !== 1 ) continue;
-
- switch ( child.nodeName ) {
-
- case 'float_array':
- data.array = parseFloats( child.textContent );
- break;
-
- case 'Name_array':
- data.array = parseStrings( child.textContent );
- break;
-
- case 'technique_common':
- var accessor = getElementsByTagName( child, 'accessor' )[ 0 ];
-
- if ( accessor !== undefined ) {
-
- data.stride = parseInt( accessor.getAttribute( 'stride' ) );
-
- }
- break;
-
- }
-
- }
-
- return data;
-
- }
-
- function parseGeometryVertices( xml ) {
-
- var data = {};
-
- for ( var i = 0; i < xml.childNodes.length; i ++ ) {
-
- var child = xml.childNodes[ i ];
-
- if ( child.nodeType !== 1 ) continue;
-
- data[ child.getAttribute( 'semantic' ) ] = parseId( child.getAttribute( 'source' ) );
-
- }
-
- return data;
-
- }
-
- function parseGeometryPrimitive( xml ) {
-
- var primitive = {
- type: xml.nodeName,
- material: xml.getAttribute( 'material' ),
- count: parseInt( xml.getAttribute( 'count' ) ),
- inputs: {},
- stride: 0,
- hasUV: false
- };
-
- for ( var i = 0, l = xml.childNodes.length; i < l; i ++ ) {
-
- var child = xml.childNodes[ i ];
-
- if ( child.nodeType !== 1 ) continue;
-
- switch ( child.nodeName ) {
-
- case 'input':
- var id = parseId( child.getAttribute( 'source' ) );
- var semantic = child.getAttribute( 'semantic' );
- var offset = parseInt( child.getAttribute( 'offset' ) );
- var set = parseInt( child.getAttribute( 'set' ) );
- var inputname = ( set > 0 ? semantic + set : semantic );
- primitive.inputs[ inputname ] = { id: id, offset: offset };
- primitive.stride = Math.max( primitive.stride, offset + 1 );
- if ( semantic === 'TEXCOORD' ) primitive.hasUV = true;
- break;
-
- case 'vcount':
- primitive.vcount = parseInts( child.textContent );
- break;
-
- case 'p':
- primitive.p = parseInts( child.textContent );
- break;
-
- }
-
- }
-
- return primitive;
-
- }
-
- function groupPrimitives( primitives ) {
-
- var build = {};
-
- for ( var i = 0; i < primitives.length; i ++ ) {
-
- var primitive = primitives[ i ];
-
- if ( build[ primitive.type ] === undefined ) build[ primitive.type ] = [];
-
- build[ primitive.type ].push( primitive );
-
- }
-
- return build;
-
- }
-
- function checkUVCoordinates( primitives ) {
-
- var count = 0;
-
- for ( var i = 0, l = primitives.length; i < l; i ++ ) {
-
- var primitive = primitives[ i ];
-
- if ( primitive.hasUV === true ) {
-
- count ++;
-
- }
-
- }
-
- if ( count > 0 && count < primitives.length ) {
-
- primitives.uvsNeedsFix = true;
-
- }
-
- }
-
- function buildGeometry( data ) {
-
- var build = {};
-
- var sources = data.sources;
- var vertices = data.vertices;
- var primitives = data.primitives;
-
- if ( primitives.length === 0 ) return {};
-
- // our goal is to create one buffer geometry for a single type of primitives
- // first, we group all primitives by their type
-
- var groupedPrimitives = groupPrimitives( primitives );
-
- for ( var type in groupedPrimitives ) {
-
- var primitiveType = groupedPrimitives[ type ];
-
- // second, ensure consistent uv coordinates for each type of primitives (polylist,triangles or lines)
-
- checkUVCoordinates( primitiveType );
-
- // third, create a buffer geometry for each type of primitives
-
- build[ type ] = buildGeometryType( primitiveType, sources, vertices );
-
- }
-
- return build;
-
- }
-
- function buildGeometryType( primitives, sources, vertices ) {
-
- var build = {};
-
- var position = { array: [], stride: 0 };
- var normal = { array: [], stride: 0 };
- var uv = { array: [], stride: 0 };
- var uv2 = { array: [], stride: 0 };
- var color = { array: [], stride: 0 };
-
- var skinIndex = { array: [], stride: 4 };
- var skinWeight = { array: [], stride: 4 };
-
- var geometry = new THREE.BufferGeometry();
-
- var materialKeys = [];
-
- var start = 0;
-
- for ( var p = 0; p < primitives.length; p ++ ) {
-
- var primitive = primitives[ p ];
- var inputs = primitive.inputs;
-
- // groups
-
- var count = 0;
-
- switch ( primitive.type ) {
-
- case 'lines':
- case 'linestrips':
- count = primitive.count * 2;
- break;
-
- case 'triangles':
- count = primitive.count * 3;
- break;
-
- case 'polylist':
-
- for ( var g = 0; g < primitive.count; g ++ ) {
-
- var vc = primitive.vcount[ g ];
-
- switch ( vc ) {
-
- case 3:
- count += 3; // single triangle
- break;
-
- case 4:
- count += 6; // quad, subdivided into two triangles
- break;
-
- default:
- count += ( vc - 2 ) * 3; // polylist with more than four vertices
- break;
-
- }
-
- }
-
- break;
-
- default:
- console.warn( 'THREE.ColladaLoader: Unknow primitive type:', primitive.type );
-
- }
-
- geometry.addGroup( start, count, p );
- start += count;
-
- // material
-
- if ( primitive.material ) {
-
- materialKeys.push( primitive.material );
-
- }
-
- // geometry data
-
- for ( var name in inputs ) {
-
- var input = inputs[ name ];
-
- switch ( name ) {
-
- case 'VERTEX':
- for ( var key in vertices ) {
-
- var id = vertices[ key ];
-
- switch ( key ) {
-
- case 'POSITION':
- var prevLength = position.array.length;
- buildGeometryData( primitive, sources[ id ], input.offset, position.array );
- position.stride = sources[ id ].stride;
-
- if ( sources.skinWeights && sources.skinIndices ) {
-
- buildGeometryData( primitive, sources.skinIndices, input.offset, skinIndex.array );
- buildGeometryData( primitive, sources.skinWeights, input.offset, skinWeight.array );
-
- }
-
- // see #3803
-
- if ( primitive.hasUV === false && primitives.uvsNeedsFix === true ) {
-
- var count = ( position.array.length - prevLength ) / position.stride;
-
- for ( var i = 0; i < count; i ++ ) {
-
- // fill missing uv coordinates
-
- uv.array.push( 0, 0 );
-
- }
-
- }
- break;
-
- case 'NORMAL':
- buildGeometryData( primitive, sources[ id ], input.offset, normal.array );
- normal.stride = sources[ id ].stride;
- break;
-
- case 'COLOR':
- buildGeometryData( primitive, sources[ id ], input.offset, color.array );
- color.stride = sources[ id ].stride;
- break;
-
- case 'TEXCOORD':
- buildGeometryData( primitive, sources[ id ], input.offset, uv.array );
- uv.stride = sources[ id ].stride;
- break;
-
- case 'TEXCOORD1':
- buildGeometryData( primitive, sources[ id ], input.offset, uv2.array );
- uv.stride = sources[ id ].stride;
- break;
-
- default:
- console.warn( 'THREE.ColladaLoader: Semantic "%s" not handled in geometry build process.', key );
-
- }
-
- }
- break;
-
- case 'NORMAL':
- buildGeometryData( primitive, sources[ input.id ], input.offset, normal.array );
- normal.stride = sources[ input.id ].stride;
- break;
-
- case 'COLOR':
- buildGeometryData( primitive, sources[ input.id ], input.offset, color.array );
- color.stride = sources[ input.id ].stride;
- break;
-
- case 'TEXCOORD':
- buildGeometryData( primitive, sources[ input.id ], input.offset, uv.array );
- uv.stride = sources[ input.id ].stride;
- break;
-
- case 'TEXCOORD1':
- buildGeometryData( primitive, sources[ input.id ], input.offset, uv2.array );
- uv2.stride = sources[ input.id ].stride;
- break;
-
- }
-
- }
-
- }
-
- // build geometry
-
- if ( position.array.length > 0 ) geometry.addAttribute( 'position', new THREE.Float32BufferAttribute( position.array, position.stride ) );
- if ( normal.array.length > 0 ) geometry.addAttribute( 'normal', new THREE.Float32BufferAttribute( normal.array, normal.stride ) );
- if ( color.array.length > 0 ) geometry.addAttribute( 'color', new THREE.Float32BufferAttribute( color.array, color.stride ) );
- if ( uv.array.length > 0 ) geometry.addAttribute( 'uv', new THREE.Float32BufferAttribute( uv.array, uv.stride ) );
- if ( uv2.array.length > 0 ) geometry.addAttribute( 'uv2', new THREE.Float32BufferAttribute( uv2.array, uv2.stride ) );
-
- if ( skinIndex.array.length > 0 ) geometry.addAttribute( 'skinIndex', new THREE.Float32BufferAttribute( skinIndex.array, skinIndex.stride ) );
- if ( skinWeight.array.length > 0 ) geometry.addAttribute( 'skinWeight', new THREE.Float32BufferAttribute( skinWeight.array, skinWeight.stride ) );
-
- build.data = geometry;
- build.type = primitives[ 0 ].type;
- build.materialKeys = materialKeys;
-
- return build;
-
- }
-
- function buildGeometryData( primitive, source, offset, array ) {
-
- var indices = primitive.p;
- var stride = primitive.stride;
- var vcount = primitive.vcount;
-
- function pushVector( i ) {
-
- var index = indices[ i + offset ] * sourceStride;
- var length = index + sourceStride;
-
- for ( ; index < length; index ++ ) {
-
- array.push( sourceArray[ index ] );
-
- }
-
- }
-
- var sourceArray = source.array;
- var sourceStride = source.stride;
-
- if ( primitive.vcount !== undefined ) {
-
- var index = 0;
-
- for ( var i = 0, l = vcount.length; i < l; i ++ ) {
-
- var count = vcount[ i ];
-
- if ( count === 4 ) {
-
- var a = index + stride * 0;
- var b = index + stride * 1;
- var c = index + stride * 2;
- var d = index + stride * 3;
-
- pushVector( a ); pushVector( b ); pushVector( d );
- pushVector( b ); pushVector( c ); pushVector( d );
-
- } else if ( count === 3 ) {
-
- var a = index + stride * 0;
- var b = index + stride * 1;
- var c = index + stride * 2;
-
- pushVector( a ); pushVector( b ); pushVector( c );
-
- } else if ( count > 4 ) {
-
- for ( var k = 1, kl = ( count - 2 ); k <= kl; k ++ ) {
-
- var a = index + stride * 0;
- var b = index + stride * k;
- var c = index + stride * ( k + 1 );
-
- pushVector( a ); pushVector( b ); pushVector( c );
-
- }
-
- }
-
- index += stride * count;
-
- }
-
- } else {
-
- for ( var i = 0, l = indices.length; i < l; i += stride ) {
-
- pushVector( i );
-
- }
-
- }
-
- }
-
- function getGeometry( id ) {
-
- return getBuild( library.geometries[ id ], buildGeometry );
-
- }
-
- // kinematics
-
- function parseKinematicsModel( xml ) {
-
- var data = {
- name: xml.getAttribute( 'name' ) || '',
- joints: {},
- links: []
- };
-
- for ( var i = 0; i < xml.childNodes.length; i ++ ) {
-
- var child = xml.childNodes[ i ];
-
- if ( child.nodeType !== 1 ) continue;
-
- switch ( child.nodeName ) {
-
- case 'technique_common':
- parseKinematicsTechniqueCommon( child, data );
- break;
-
- }
-
- }
-
- library.kinematicsModels[ xml.getAttribute( 'id' ) ] = data;
-
- }
-
- function buildKinematicsModel( data ) {
-
- if ( data.build !== undefined ) return data.build;
-
- return data;
-
- }
-
- function getKinematicsModel( id ) {
-
- return getBuild( library.kinematicsModels[ id ], buildKinematicsModel );
-
- }
-
- function parseKinematicsTechniqueCommon( xml, data ) {
-
- for ( var i = 0; i < xml.childNodes.length; i ++ ) {
-
- var child = xml.childNodes[ i ];
-
- if ( child.nodeType !== 1 ) continue;
-
- switch ( child.nodeName ) {
-
- case 'joint':
- data.joints[ child.getAttribute( 'sid' ) ] = parseKinematicsJoint( child );
- break;
-
- case 'link':
- data.links.push( parseKinematicsLink( child ) );
- break;
-
- }
-
- }
-
- }
-
- function parseKinematicsJoint( xml ) {
-
- var data;
-
- for ( var i = 0; i < xml.childNodes.length; i ++ ) {
-
- var child = xml.childNodes[ i ];
-
- if ( child.nodeType !== 1 ) continue;
-
- switch ( child.nodeName ) {
-
- case 'prismatic':
- case 'revolute':
- data = parseKinematicsJointParameter( child );
- break;
-
- }
-
- }
-
- return data;
-
- }
-
- function parseKinematicsJointParameter( xml, data ) {
-
- var data = {
- sid: xml.getAttribute( 'sid' ),
- name: xml.getAttribute( 'name' ) || '',
- axis: new THREE.Vector3(),
- limits: {
- min: 0,
- max: 0
- },
- type: xml.nodeName,
- static: false,
- zeroPosition: 0,
- middlePosition: 0
- };
-
- for ( var i = 0; i < xml.childNodes.length; i ++ ) {
-
- var child = xml.childNodes[ i ];
-
- if ( child.nodeType !== 1 ) continue;
-
- switch ( child.nodeName ) {
-
- case 'axis':
- var array = parseFloats( child.textContent );
- data.axis.fromArray( array );
- break;
- case 'limits':
- var max = child.getElementsByTagName( 'max' )[ 0 ];
- var min = child.getElementsByTagName( 'min' )[ 0 ];
-
- data.limits.max = parseFloat( max.textContent );
- data.limits.min = parseFloat( min.textContent );
- break;
-
- }
-
- }
-
- // if min is equal to or greater than max, consider the joint static
-
- if ( data.limits.min >= data.limits.max ) {
-
- data.static = true;
-
- }
-
- // calculate middle position
-
- data.middlePosition = ( data.limits.min + data.limits.max ) / 2.0;
-
- return data;
-
- }
-
- function parseKinematicsLink( xml ) {
-
- var data = {
- sid: xml.getAttribute( 'sid' ),
- name: xml.getAttribute( 'name' ) || '',
- attachments: [],
- transforms: []
- };
-
- for ( var i = 0; i < xml.childNodes.length; i ++ ) {
-
- var child = xml.childNodes[ i ];
-
- if ( child.nodeType !== 1 ) continue;
-
- switch ( child.nodeName ) {
-
- case 'attachment_full':
- data.attachments.push( parseKinematicsAttachment( child ) );
- break;
-
- case 'matrix':
- case 'translate':
- case 'rotate':
- data.transforms.push( parseKinematicsTransform( child ) );
- break;
-
- }
-
- }
-
- return data;
-
- }
-
- function parseKinematicsAttachment( xml ) {
-
- var data = {
- joint: xml.getAttribute( 'joint' ).split( '/' ).pop(),
- transforms: [],
- links: []
- };
-
- for ( var i = 0; i < xml.childNodes.length; i ++ ) {
-
- var child = xml.childNodes[ i ];
-
- if ( child.nodeType !== 1 ) continue;
-
- switch ( child.nodeName ) {
-
- case 'link':
- data.links.push( parseKinematicsLink( child ) );
- break;
-
- case 'matrix':
- case 'translate':
- case 'rotate':
- data.transforms.push( parseKinematicsTransform( child ) );
- break;
-
- }
-
- }
-
- return data;
-
- }
-
- function parseKinematicsTransform( xml ) {
-
- var data = {
- type: xml.nodeName
- };
-
- var array = parseFloats( xml.textContent );
-
- switch ( data.type ) {
-
- case 'matrix':
- data.obj = new THREE.Matrix4();
- data.obj.fromArray( array ).transpose();
- break;
-
- case 'translate':
- data.obj = new THREE.Vector3();
- data.obj.fromArray( array );
- break;
-
- case 'rotate':
- data.obj = new THREE.Vector3();
- data.obj.fromArray( array );
- data.angle = THREE.Math.degToRad( array[ 3 ] );
- break;
-
- }
-
- return data;
-
- }
-
- // physics
-
- function parsePhysicsModel( xml ) {
-
- var data = {
- name: xml.getAttribute( 'name' ) || '',
- rigidBodies: {}
- };
-
- for ( var i = 0; i < xml.childNodes.length; i ++ ) {
-
- var child = xml.childNodes[ i ];
-
- if ( child.nodeType !== 1 ) continue;
-
- switch ( child.nodeName ) {
-
- case 'rigid_body':
- data.rigidBodies[ child.getAttribute( 'name' ) ] = {};
- parsePhysicsRigidBody( child, data.rigidBodies[ child.getAttribute( 'name' ) ] );
- break;
-
- }
-
- }
-
- library.physicsModels[ xml.getAttribute( 'id' ) ] = data;
-
- }
-
- function parsePhysicsRigidBody( xml, data ) {
-
- for ( var i = 0; i < xml.childNodes.length; i ++ ) {
-
- var child = xml.childNodes[ i ];
-
- if ( child.nodeType !== 1 ) continue;
-
- switch ( child.nodeName ) {
-
- case 'technique_common':
- parsePhysicsTechniqueCommon( child, data );
- break;
-
- }
-
- }
-
- }
-
- function parsePhysicsTechniqueCommon( xml, data ) {
-
- for ( var i = 0; i < xml.childNodes.length; i ++ ) {
-
- var child = xml.childNodes[ i ];
-
- if ( child.nodeType !== 1 ) continue;
-
- switch ( child.nodeName ) {
-
- case 'inertia':
- data.inertia = parseFloats( child.textContent );
- break;
-
- case 'mass':
- data.mass = parseFloats( child.textContent )[ 0 ];
- break;
-
- }
-
- }
-
- }
-
- // scene
-
- function parseKinematicsScene( xml ) {
-
- var data = {
- bindJointAxis: []
- };
-
- for ( var i = 0; i < xml.childNodes.length; i ++ ) {
-
- var child = xml.childNodes[ i ];
-
- if ( child.nodeType !== 1 ) continue;
-
- switch ( child.nodeName ) {
-
- case 'bind_joint_axis':
- data.bindJointAxis.push( parseKinematicsBindJointAxis( child ) );
- break;
-
- }
-
- }
-
- library.kinematicsScenes[ parseId( xml.getAttribute( 'url' ) ) ] = data;
-
- }
-
- function parseKinematicsBindJointAxis( xml ) {
-
- var data = {
- target: xml.getAttribute( 'target' ).split( '/' ).pop()
- };
-
- for ( var i = 0; i < xml.childNodes.length; i ++ ) {
-
- var child = xml.childNodes[ i ];
-
- if ( child.nodeType !== 1 ) continue;
-
- switch ( child.nodeName ) {
-
- case 'axis':
- var param = child.getElementsByTagName( 'param' )[ 0 ];
- data.axis = param.textContent;
- var tmpJointIndex = data.axis.split( 'inst_' ).pop().split( 'axis' )[ 0 ];
- data.jointIndex = tmpJointIndex.substr( 0, tmpJointIndex.length - 1 );
- break;
-
- }
-
- }
-
- return data;
-
- }
-
- function buildKinematicsScene( data ) {
-
- if ( data.build !== undefined ) return data.build;
-
- return data;
-
- }
-
- function getKinematicsScene( id ) {
-
- return getBuild( library.kinematicsScenes[ id ], buildKinematicsScene );
-
- }
-
- function setupKinematics() {
-
- var kinematicsModelId = Object.keys( library.kinematicsModels )[ 0 ];
- var kinematicsSceneId = Object.keys( library.kinematicsScenes )[ 0 ];
- var visualSceneId = Object.keys( library.visualScenes )[ 0 ];
-
- if ( kinematicsModelId === undefined || kinematicsSceneId === undefined ) return;
-
- var kinematicsModel = getKinematicsModel( kinematicsModelId );
- var kinematicsScene = getKinematicsScene( kinematicsSceneId );
- var visualScene = getVisualScene( visualSceneId );
-
- var bindJointAxis = kinematicsScene.bindJointAxis;
- var jointMap = {};
-
- for ( var i = 0, l = bindJointAxis.length; i < l; i ++ ) {
-
- var axis = bindJointAxis[ i ];
-
- // the result of the following query is an element of type 'translate', 'rotate','scale' or 'matrix'
-
- var targetElement = collada.querySelector( '[sid="' + axis.target + '"]' );
-
- if ( targetElement ) {
-
- // get the parent of the transfrom element
-
- var parentVisualElement = targetElement.parentElement;
-
- // connect the joint of the kinematics model with the element in the visual scene
-
- connect( axis.jointIndex, parentVisualElement );
-
- }
-
- }
-
- function connect( jointIndex, visualElement ) {
-
- var visualElementName = visualElement.getAttribute( 'name' );
- var joint = kinematicsModel.joints[ jointIndex ];
-
- visualScene.traverse( function ( object ) {
-
- if ( object.name === visualElementName ) {
-
- jointMap[ jointIndex ] = {
- object: object,
- transforms: buildTransformList( visualElement ),
- joint: joint,
- position: joint.zeroPosition
- };
-
- }
-
- } );
-
- }
-
- var m0 = new THREE.Matrix4();
-
- kinematics = {
-
- joints: kinematicsModel && kinematicsModel.joints,
-
- getJointValue: function ( jointIndex ) {
-
- var jointData = jointMap[ jointIndex ];
-
- if ( jointData ) {
-
- return jointData.position;
-
- } else {
-
- console.warn( 'THREE.ColladaLoader: Joint ' + jointIndex + ' doesn\'t exist.' );
-
- }
-
- },
-
- setJointValue: function ( jointIndex, value ) {
-
- var jointData = jointMap[ jointIndex ];
-
- if ( jointData ) {
-
- var joint = jointData.joint;
-
- if ( value > joint.limits.max || value < joint.limits.min ) {
-
- console.warn( 'THREE.ColladaLoader: Joint ' + jointIndex + ' value ' + value + ' outside of limits (min: ' + joint.limits.min + ', max: ' + joint.limits.max + ').' );
-
- } else if ( joint.static ) {
-
- console.warn( 'THREE.ColladaLoader: Joint ' + jointIndex + ' is static.' );
-
- } else {
-
- var object = jointData.object;
- var axis = joint.axis;
- var transforms = jointData.transforms;
-
- matrix.identity();
-
- // each update, we have to apply all transforms in the correct order
-
- for ( var i = 0; i < transforms.length; i ++ ) {
-
- var transform = transforms[ i ];
-
- // if there is a connection of the transform node with a joint, apply the joint value
-
- if ( transform.sid && transform.sid.indexOf( jointIndex ) !== - 1 ) {
-
- switch ( joint.type ) {
-
- case 'revolute':
- matrix.multiply( m0.makeRotationAxis( axis, THREE.Math.degToRad( value ) ) );
- break;
-
- case 'prismatic':
- matrix.multiply( m0.makeTranslation( axis.x * value, axis.y * value, axis.z * value ) );
- break;
-
- default:
- console.warn( 'THREE.ColladaLoader: Unknown joint type: ' + joint.type );
- break;
-
- }
-
- } else {
-
- switch ( transform.type ) {
-
- case 'matrix':
- matrix.multiply( transform.obj );
- break;
-
- case 'translate':
- matrix.multiply( m0.makeTranslation( transform.obj.x, transform.obj.y, transform.obj.z ) );
- break;
-
- case 'scale':
- matrix.scale( transform.obj );
- break;
-
- case 'rotate':
- matrix.multiply( m0.makeRotationAxis( transform.obj, transform.angle ) );
- break;
-
- }
-
- }
-
- }
-
- object.matrix.copy( matrix );
- object.matrix.decompose( object.position, object.quaternion, object.scale );
-
- jointMap[ jointIndex ].position = value;
-
- }
-
- } else {
-
- console.log( 'THREE.ColladaLoader: ' + jointIndex + ' does not exist.' );
-
- }
-
- }
-
- };
-
- }
-
- function buildTransformList( node ) {
-
- var transforms = [];
-
- var xml = collada.querySelector( '[id="' + node.id + '"]' );
-
- for ( var i = 0; i < xml.childNodes.length; i ++ ) {
-
- var child = xml.childNodes[ i ];
-
- if ( child.nodeType !== 1 ) continue;
-
- switch ( child.nodeName ) {
-
- case 'matrix':
- var array = parseFloats( child.textContent );
- var matrix = new THREE.Matrix4().fromArray( array ).transpose();
- transforms.push( {
- sid: child.getAttribute( 'sid' ),
- type: child.nodeName,
- obj: matrix
- } );
- break;
-
- case 'translate':
- case 'scale':
- var array = parseFloats( child.textContent );
- var vector = new THREE.Vector3().fromArray( array );
- transforms.push( {
- sid: child.getAttribute( 'sid' ),
- type: child.nodeName,
- obj: vector
- } );
- break;
-
- case 'rotate':
- var array = parseFloats( child.textContent );
- var vector = new THREE.Vector3().fromArray( array );
- var angle = THREE.Math.degToRad( array[ 3 ] );
- transforms.push( {
- sid: child.getAttribute( 'sid' ),
- type: child.nodeName,
- obj: vector,
- angle: angle
- } );
- break;
-
- }
-
- }
-
- return transforms;
-
- }
-
- // nodes
-
- function prepareNodes( xml ) {
-
- var elements = xml.getElementsByTagName( 'node' );
-
- // ensure all node elements have id attributes
-
- for ( var i = 0; i < elements.length; i ++ ) {
-
- var element = elements[ i ];
-
- if ( element.hasAttribute( 'id' ) === false ) {
-
- element.setAttribute( 'id', generateId() );
-
- }
-
- }
-
- }
-
- var matrix = new THREE.Matrix4();
- var vector = new THREE.Vector3();
-
- function parseNode( xml ) {
-
- var data = {
- name: xml.getAttribute( 'name' ) || '',
- type: xml.getAttribute( 'type' ),
- id: xml.getAttribute( 'id' ),
- sid: xml.getAttribute( 'sid' ),
- matrix: new THREE.Matrix4(),
- nodes: [],
- instanceCameras: [],
- instanceControllers: [],
- instanceLights: [],
- instanceGeometries: [],
- instanceNodes: [],
- transforms: {}
- };
-
- for ( var i = 0; i < xml.childNodes.length; i ++ ) {
-
- var child = xml.childNodes[ i ];
-
- if ( child.nodeType !== 1 ) continue;
-
- switch ( child.nodeName ) {
-
- case 'node':
- data.nodes.push( child.getAttribute( 'id' ) );
- parseNode( child );
- break;
-
- case 'instance_camera':
- data.instanceCameras.push( parseId( child.getAttribute( 'url' ) ) );
- break;
-
- case 'instance_controller':
- data.instanceControllers.push( parseNodeInstance( child ) );
- break;
-
- case 'instance_light':
- data.instanceLights.push( parseId( child.getAttribute( 'url' ) ) );
- break;
-
- case 'instance_geometry':
- data.instanceGeometries.push( parseNodeInstance( child ) );
- break;
-
- case 'instance_node':
- data.instanceNodes.push( parseId( child.getAttribute( 'url' ) ) );
- break;
-
- case 'matrix':
- var array = parseFloats( child.textContent );
- data.matrix.multiply( matrix.fromArray( array ).transpose() );
- data.transforms[ child.getAttribute( 'sid' ) ] = child.nodeName;
- break;
-
- case 'translate':
- var array = parseFloats( child.textContent );
- vector.fromArray( array );
- data.matrix.multiply( matrix.makeTranslation( vector.x, vector.y, vector.z ) );
- data.transforms[ child.getAttribute( 'sid' ) ] = child.nodeName;
- break;
-
- case 'rotate':
- var array = parseFloats( child.textContent );
- var angle = THREE.Math.degToRad( array[ 3 ] );
- data.matrix.multiply( matrix.makeRotationAxis( vector.fromArray( array ), angle ) );
- data.transforms[ child.getAttribute( 'sid' ) ] = child.nodeName;
- break;
-
- case 'scale':
- var array = parseFloats( child.textContent );
- data.matrix.scale( vector.fromArray( array ) );
- data.transforms[ child.getAttribute( 'sid' ) ] = child.nodeName;
- break;
-
- case 'extra':
- break;
-
- default:
- console.log( child );
-
- }
-
- }
-
- if ( hasNode( data.id ) ) {
-
- console.warn( 'THREE.ColladaLoader: There is already a node with ID %s. Exclude current node from further processing.', data.id );
-
- } else {
-
- library.nodes[ data.id ] = data;
-
- }
-
- return data;
-
- }
-
- function parseNodeInstance( xml ) {
-
- var data = {
- id: parseId( xml.getAttribute( 'url' ) ),
- materials: {},
- skeletons: []
- };
-
- for ( var i = 0; i < xml.childNodes.length; i ++ ) {
-
- var child = xml.childNodes[ i ];
-
- switch ( child.nodeName ) {
-
- case 'bind_material':
- var instances = child.getElementsByTagName( 'instance_material' );
-
- for ( var j = 0; j < instances.length; j ++ ) {
-
- var instance = instances[ j ];
- var symbol = instance.getAttribute( 'symbol' );
- var target = instance.getAttribute( 'target' );
-
- data.materials[ symbol ] = parseId( target );
-
- }
-
- break;
-
- case 'skeleton':
- data.skeletons.push( parseId( child.textContent ) );
- break;
-
- default:
- break;
-
- }
-
- }
-
- return data;
-
- }
-
- function buildSkeleton( skeletons, joints ) {
-
- var boneData = [];
- var sortedBoneData = [];
-
- var i, j, data;
-
- // a skeleton can have multiple root bones. collada expresses this
- // situtation with multiple "skeleton" tags per controller instance
-
- for ( i = 0; i < skeletons.length; i ++ ) {
-
- var skeleton = skeletons[ i ];
-
- var root;
-
- if ( hasNode( skeleton ) ) {
-
- root = getNode( skeleton );
- buildBoneHierarchy( root, joints, boneData );
-
- } else if ( hasVisualScene( skeleton ) ) {
-
- // handle case where the skeleton refers to the visual scene (#13335)
-
- var visualScene = library.visualScenes[ skeleton ];
- var children = visualScene.children;
-
- for ( var j = 0; j < children.length; j ++ ) {
-
- var child = children[ j ];
-
- if ( child.type === 'JOINT' ) {
-
- var root = getNode( child.id );
- buildBoneHierarchy( root, joints, boneData );
-
- }
-
- }
-
- } else {
-
- console.error( 'THREE.ColladaLoader: Unable to find root bone of skeleton with ID:', skeleton );
-
- }
-
- }
-
- // sort bone data (the order is defined in the corresponding controller)
-
- for ( i = 0; i < joints.length; i ++ ) {
-
- for ( j = 0; j < boneData.length; j ++ ) {
-
- data = boneData[ j ];
-
- if ( data.bone.name === joints[ i ].name ) {
-
- sortedBoneData[ i ] = data;
- data.processed = true;
- break;
-
- }
-
- }
-
- }
-
- // add unprocessed bone data at the end of the list
-
- for ( i = 0; i < boneData.length; i ++ ) {
-
- data = boneData[ i ];
-
- if ( data.processed === false ) {
-
- sortedBoneData.push( data );
- data.processed = true;
-
- }
-
- }
-
- // setup arrays for skeleton creation
-
- var bones = [];
- var boneInverses = [];
-
- for ( i = 0; i < sortedBoneData.length; i ++ ) {
-
- data = sortedBoneData[ i ];
-
- bones.push( data.bone );
- boneInverses.push( data.boneInverse );
-
- }
-
- return new THREE.Skeleton( bones, boneInverses );
-
- }
-
- function buildBoneHierarchy( root, joints, boneData ) {
-
- // setup bone data from visual scene
-
- root.traverse( function ( object ) {
-
- if ( object.isBone === true ) {
-
- var boneInverse;
-
- // retrieve the boneInverse from the controller data
-
- for ( var i = 0; i < joints.length; i ++ ) {
-
- var joint = joints[ i ];
-
- if ( joint.name === object.name ) {
-
- boneInverse = joint.boneInverse;
- break;
-
- }
-
- }
-
- if ( boneInverse === undefined ) {
-
- // Unfortunately, there can be joints in the visual scene that are not part of the
- // corresponding controller. In this case, we have to create a dummy boneInverse matrix
- // for the respective bone. This bone won't affect any vertices, because there are no skin indices
- // and weights defined for it. But we still have to add the bone to the sorted bone list in order to
- // ensure a correct animation of the model.
-
- boneInverse = new THREE.Matrix4();
-
- }
-
- boneData.push( { bone: object, boneInverse: boneInverse, processed: false } );
-
- }
-
- } );
-
- }
-
- function buildNode( data ) {
-
- var objects = [];
-
- var matrix = data.matrix;
- var nodes = data.nodes;
- var type = data.type;
- var instanceCameras = data.instanceCameras;
- var instanceControllers = data.instanceControllers;
- var instanceLights = data.instanceLights;
- var instanceGeometries = data.instanceGeometries;
- var instanceNodes = data.instanceNodes;
-
- // nodes
-
- for ( var i = 0, l = nodes.length; i < l; i ++ ) {
-
- objects.push( getNode( nodes[ i ] ) );
-
- }
-
- // instance cameras
-
- for ( var i = 0, l = instanceCameras.length; i < l; i ++ ) {
-
- var instanceCamera = getCamera( instanceCameras[ i ] );
-
- if ( instanceCamera !== null ) {
-
- objects.push( instanceCamera.clone() );
-
- }
-
- }
-
- // instance controllers
-
- for ( var i = 0, l = instanceControllers.length; i < l; i ++ ) {
-
- var instance = instanceControllers[ i ];
- var controller = getController( instance.id );
- var geometries = getGeometry( controller.id );
- var newObjects = buildObjects( geometries, instance.materials );
-
- var skeletons = instance.skeletons;
- var joints = controller.skin.joints;
-
- var skeleton = buildSkeleton( skeletons, joints );
-
- for ( var j = 0, jl = newObjects.length; j < jl; j ++ ) {
-
- var object = newObjects[ j ];
-
- if ( object.isSkinnedMesh ) {
-
- object.bind( skeleton, controller.skin.bindMatrix );
- object.normalizeSkinWeights();
-
- }
-
- objects.push( object );
-
- }
-
- }
-
- // instance lights
-
- for ( var i = 0, l = instanceLights.length; i < l; i ++ ) {
-
- var instanceLight = getLight( instanceLights[ i ] );
-
- if ( instanceLight !== null ) {
-
- objects.push( instanceLight.clone() );
-
- }
-
- }
-
- // instance geometries
-
- for ( var i = 0, l = instanceGeometries.length; i < l; i ++ ) {
-
- var instance = instanceGeometries[ i ];
-
- // a single geometry instance in collada can lead to multiple object3Ds.
- // this is the case when primitives are combined like triangles and lines
-
- var geometries = getGeometry( instance.id );
- var newObjects = buildObjects( geometries, instance.materials );
-
- for ( var j = 0, jl = newObjects.length; j < jl; j ++ ) {
-
- objects.push( newObjects[ j ] );
-
- }
-
- }
-
- // instance nodes
-
- for ( var i = 0, l = instanceNodes.length; i < l; i ++ ) {
-
- objects.push( getNode( instanceNodes[ i ] ).clone() );
-
- }
-
- var object;
-
- if ( nodes.length === 0 && objects.length === 1 ) {
-
- object = objects[ 0 ];
-
- } else {
-
- object = ( type === 'JOINT' ) ? new THREE.Bone() : new THREE.Group();
-
- for ( var i = 0; i < objects.length; i ++ ) {
-
- object.add( objects[ i ] );
-
- }
-
- }
-
- if ( object.name === '' ) {
-
- object.name = ( type === 'JOINT' ) ? data.sid : data.name;
-
- }
-
- object.matrix.copy( matrix );
- object.matrix.decompose( object.position, object.quaternion, object.scale );
-
- return object;
-
- }
-
- var fallbackMaterial = new THREE.MeshBasicMaterial( { color: 0xff00ff } );
-
- function resolveMaterialBinding( keys, instanceMaterials ) {
-
- var materials = [];
-
- for ( var i = 0, l = keys.length; i < l; i ++ ) {
-
- var id = instanceMaterials[ keys[ i ] ];
-
- if ( id === undefined ) {
-
- console.warn( 'THREE.ColladaLoader: Material with key %s not found. Apply fallback material.', keys[ i ] );
- materials.push( fallbackMaterial );
-
- } else {
-
- materials.push( getMaterial( id ) );
-
- }
-
- }
-
- return materials;
-
- }
-
- function buildObjects( geometries, instanceMaterials ) {
-
- var objects = [];
-
- for ( var type in geometries ) {
-
- var geometry = geometries[ type ];
-
- var materials = resolveMaterialBinding( geometry.materialKeys, instanceMaterials );
-
- // handle case if no materials are defined
-
- if ( materials.length === 0 ) {
-
- if ( type === 'lines' || type === 'linestrips' ) {
-
- materials.push( new THREE.LineBasicMaterial() );
-
- } else {
-
- materials.push( new THREE.MeshPhongMaterial() );
-
- }
-
- }
-
- // regard skinning
-
- var skinning = ( geometry.data.attributes.skinIndex !== undefined );
-
- if ( skinning ) {
-
- for ( var i = 0, l = materials.length; i < l; i ++ ) {
-
- materials[ i ].skinning = true;
-
- }
-
- }
-
- // choose between a single or multi materials (material array)
-
- var material = ( materials.length === 1 ) ? materials[ 0 ] : materials;
-
- // now create a specific 3D object
-
- var object;
-
- switch ( type ) {
-
- case 'lines':
- object = new THREE.LineSegments( geometry.data, material );
- break;
-
- case 'linestrips':
- object = new THREE.Line( geometry.data, material );
- break;
-
- case 'triangles':
- case 'polylist':
- if ( skinning ) {
-
- object = new THREE.SkinnedMesh( geometry.data, material );
-
- } else {
-
- object = new THREE.Mesh( geometry.data, material );
-
- }
- break;
-
- }
-
- objects.push( object );
-
- }
-
- return objects;
-
- }
-
- function hasNode( id ) {
-
- return library.nodes[ id ] !== undefined;
-
- }
-
- function getNode( id ) {
-
- return getBuild( library.nodes[ id ], buildNode );
-
- }
-
- // visual scenes
-
- function parseVisualScene( xml ) {
-
- var data = {
- name: xml.getAttribute( 'name' ),
- children: []
- };
-
- prepareNodes( xml );
-
- var elements = getElementsByTagName( xml, 'node' );
-
- for ( var i = 0; i < elements.length; i ++ ) {
-
- data.children.push( parseNode( elements[ i ] ) );
-
- }
-
- library.visualScenes[ xml.getAttribute( 'id' ) ] = data;
-
- }
-
- function buildVisualScene( data ) {
-
- var group = new THREE.Group();
- group.name = data.name;
-
- var children = data.children;
-
- for ( var i = 0; i < children.length; i ++ ) {
-
- var child = children[ i ];
-
- group.add( getNode( child.id ) );
-
- }
-
- return group;
-
- }
-
- function hasVisualScene( id ) {
-
- return library.visualScenes[ id ] !== undefined;
-
- }
-
- function getVisualScene( id ) {
-
- return getBuild( library.visualScenes[ id ], buildVisualScene );
-
- }
-
- // scenes
-
- function parseScene( xml ) {
-
- var instance = getElementsByTagName( xml, 'instance_visual_scene' )[ 0 ];
- return getVisualScene( parseId( instance.getAttribute( 'url' ) ) );
-
- }
-
- function setupAnimations() {
-
- var clips = library.clips;
-
- if ( isEmpty( clips ) === true ) {
-
- if ( isEmpty( library.animations ) === false ) {
-
- // if there are animations but no clips, we create a default clip for playback
-
- var tracks = [];
-
- for ( var id in library.animations ) {
-
- var animationTracks = getAnimation( id );
-
- for ( var i = 0, l = animationTracks.length; i < l; i ++ ) {
-
- tracks.push( animationTracks[ i ] );
-
- }
-
- }
-
- animations.push( new THREE.AnimationClip( 'default', - 1, tracks ) );
-
- }
-
- } else {
-
- for ( var id in clips ) {
-
- animations.push( getAnimationClip( id ) );
-
- }
-
- }
-
- }
-
- if ( text.length === 0 ) {
-
- return { scene: new THREE.Scene() };
-
- }
-
- var xml = new DOMParser().parseFromString( text, 'application/xml' );
-
- var collada = getElementsByTagName( xml, 'COLLADA' )[ 0 ];
-
- // metadata
-
- var version = collada.getAttribute( 'version' );
- console.log( 'THREE.ColladaLoader: File version', version );
-
- var asset = parseAsset( getElementsByTagName( collada, 'asset' )[ 0 ] );
- var textureLoader = new THREE.TextureLoader( this.manager );
- textureLoader.setPath( this.resourcePath || path ).setCrossOrigin( this.crossOrigin );
-
- var tgaLoader;
-
- if ( THREE.TGALoader ) {
-
- tgaLoader = new THREE.TGALoader( this.manager );
- tgaLoader.setPath( this.resourcePath || path );
-
- }
-
- //
-
- var animations = [];
- var kinematics = {};
- var count = 0;
-
- //
-
- var library = {
- animations: {},
- clips: {},
- controllers: {},
- images: {},
- effects: {},
- materials: {},
- cameras: {},
- lights: {},
- geometries: {},
- nodes: {},
- visualScenes: {},
- kinematicsModels: {},
- physicsModels: {},
- kinematicsScenes: {}
- };
-
- parseLibrary( collada, 'library_animations', 'animation', parseAnimation );
- parseLibrary( collada, 'library_animation_clips', 'animation_clip', parseAnimationClip );
- parseLibrary( collada, 'library_controllers', 'controller', parseController );
- parseLibrary( collada, 'library_images', 'image', parseImage );
- parseLibrary( collada, 'library_effects', 'effect', parseEffect );
- parseLibrary( collada, 'library_materials', 'material', parseMaterial );
- parseLibrary( collada, 'library_cameras', 'camera', parseCamera );
- parseLibrary( collada, 'library_lights', 'light', parseLight );
- parseLibrary( collada, 'library_geometries', 'geometry', parseGeometry );
- parseLibrary( collada, 'library_nodes', 'node', parseNode );
- parseLibrary( collada, 'library_visual_scenes', 'visual_scene', parseVisualScene );
- parseLibrary( collada, 'library_kinematics_models', 'kinematics_model', parseKinematicsModel );
- parseLibrary( collada, 'library_physics_models', 'physics_model', parsePhysicsModel );
- parseLibrary( collada, 'scene', 'instance_kinematics_scene', parseKinematicsScene );
-
- buildLibrary( library.animations, buildAnimation );
- buildLibrary( library.clips, buildAnimationClip );
- buildLibrary( library.controllers, buildController );
- buildLibrary( library.images, buildImage );
- buildLibrary( library.effects, buildEffect );
- buildLibrary( library.materials, buildMaterial );
- buildLibrary( library.cameras, buildCamera );
- buildLibrary( library.lights, buildLight );
- buildLibrary( library.geometries, buildGeometry );
- buildLibrary( library.visualScenes, buildVisualScene );
-
- setupAnimations();
- setupKinematics();
-
- var scene = parseScene( getElementsByTagName( collada, 'scene' )[ 0 ] );
-
- if ( asset.upAxis === 'Z_UP' ) {
-
- scene.quaternion.setFromEuler( new THREE.Euler( - Math.PI / 2, 0, 0 ) );
-
- }
-
- scene.scale.multiplyScalar( asset.unit );
-
- return {
- animations: animations,
- kinematics: kinematics,
- library: library,
- scene: scene
- };
-
- }
-
-};
diff --git a/spaces/banana-projects/web3d/node_modules/three/src/renderers/shaders/ShaderChunk/uv_pars_vertex.glsl.js b/spaces/banana-projects/web3d/node_modules/three/src/renderers/shaders/ShaderChunk/uv_pars_vertex.glsl.js
deleted file mode 100644
index d1bd641d58a171ccce64b520283da60c09b19196..0000000000000000000000000000000000000000
--- a/spaces/banana-projects/web3d/node_modules/three/src/renderers/shaders/ShaderChunk/uv_pars_vertex.glsl.js
+++ /dev/null
@@ -1,8 +0,0 @@
-export default /* glsl */`
-#if defined( USE_MAP ) || defined( USE_BUMPMAP ) || defined( USE_NORMALMAP ) || defined( USE_SPECULARMAP ) || defined( USE_ALPHAMAP ) || defined( USE_EMISSIVEMAP ) || defined( USE_ROUGHNESSMAP ) || defined( USE_METALNESSMAP )
-
- varying vec2 vUv;
- uniform mat3 uvTransform;
-
-#endif
-`;
diff --git a/spaces/bigjoker/stable-diffusion-webui/extensions/deforum/CONTRIBUTING.md b/spaces/bigjoker/stable-diffusion-webui/extensions/deforum/CONTRIBUTING.md
deleted file mode 100644
index c5df29a13e839422129b9e5e1919cafac4a651e5..0000000000000000000000000000000000000000
--- a/spaces/bigjoker/stable-diffusion-webui/extensions/deforum/CONTRIBUTING.md
+++ /dev/null
@@ -1,7 +0,0 @@
-# Contributing
-
-As a part of the Deforum team I (kabachuha) want this script extension to remain a part of the Deforum project.
-
-Thus, if you want to submit feature request or bugfix, unless it only relates to automatic1111's porting issues, consider making a PR first to the parent repository notebook https://github.com/deforum/stable-diffusion.
-
-Also, you may want to inforum the dev team about your work via Discord https://discord.gg/deforum to ensure that no one else is working on the same stuff.
diff --git a/spaces/bigjoker/stable-diffusion-webui/extensions/deforum/scripts/deforum.py b/spaces/bigjoker/stable-diffusion-webui/extensions/deforum/scripts/deforum.py
deleted file mode 100644
index 50c188c2475f58572540f615d957f8d28d3f019d..0000000000000000000000000000000000000000
--- a/spaces/bigjoker/stable-diffusion-webui/extensions/deforum/scripts/deforum.py
+++ /dev/null
@@ -1,318 +0,0 @@
-# Detach 'deforum_helpers' from 'scripts' to prevent "No module named 'scripts.deforum_helpers'" error
-# causing Deforum's tab not show up in some cases when you've might've broken the environment with webui packages updates
-import sys, os, shutil
-
-basedirs = [os.getcwd()]
-if 'google.colab' in sys.modules:
- basedirs.append('/content/gdrive/MyDrive/sd/stable-diffusion-webui') #hardcode as TheLastBen's colab seems to be the primal source
-
-for basedir in basedirs:
- deforum_paths_to_ensure = [basedir + '/extensions/deforum-for-automatic1111-webui/scripts', basedir + '/extensions/sd-webui-controlnet', basedir + '/extensions/deforum/scripts', basedir + '/scripts/deforum_helpers/src', basedir + '/extensions/deforum/scripts/deforum_helpers/src', basedir +'/extensions/deforum-for-automatic1111-webui/scripts/deforum_helpers/src',basedir]
-
- for deforum_scripts_path_fix in deforum_paths_to_ensure:
- if not deforum_scripts_path_fix in sys.path:
- sys.path.extend([deforum_scripts_path_fix])
-
-# Main deforum stuff
-import deforum_helpers.args as deforum_args
-import deforum_helpers.settings as deforum_settings
-from deforum_helpers.save_images import dump_frames_cache, reset_frames_cache
-from deforum_helpers.frame_interpolation import process_video_interpolation
-
-import modules.scripts as wscripts
-from modules import script_callbacks
-import gradio as gr
-import json
-
-from modules.processing import Processed, StableDiffusionProcessingImg2Img, process_images
-from PIL import Image
-from deforum_helpers.video_audio_utilities import ffmpeg_stitch_video, make_gifski_gif
-from deforum_helpers.upscaling import make_upscale_v2
-import gc
-import torch
-from webui import wrap_gradio_gpu_call
-import modules.shared as shared
-from modules.shared import opts, cmd_opts, state
-from modules.ui import create_output_panel, plaintext_to_html, wrap_gradio_call
-from types import SimpleNamespace
-
-def run_deforum(*args, **kwargs):
- args_dict = {deforum_args.component_names[i]: args[i+2] for i in range(0, len(deforum_args.component_names))}
- p = StableDiffusionProcessingImg2Img(
- sd_model=shared.sd_model,
- outpath_samples = opts.outdir_samples or opts.outdir_img2img_samples,
- outpath_grids = opts.outdir_grids or opts.outdir_img2img_grids,
- #we'll setup the rest later
- )
-
- print("\033[4;33mDeforum extension for auto1111 webui, v2.2b\033[0m")
- args_dict['self'] = None
- args_dict['p'] = p
-
- root, args, anim_args, video_args, parseq_args, loop_args, controlnet_args = deforum_args.process_args(args_dict)
- root.clipseg_model = None
- root.initial_clipskip = opts.data["CLIP_stop_at_last_layers"]
- root.basedirs = basedirs
-
- for basedir in basedirs:
- sys.path.extend([
- basedir + '/scripts/deforum_helpers/src',
- basedir + '/extensions/deforum/scripts/deforum_helpers/src',
- basedir + '/extensions/deforum-for-automatic1111-webui/scripts/deforum_helpers/src',
- ])
-
- # clean up unused memory
- reset_frames_cache(root)
- gc.collect()
- torch.cuda.empty_cache()
-
- from deforum_helpers.render import render_animation
- from deforum_helpers.render_modes import render_input_video, render_animation_with_video_mask, render_interpolation
-
- tqdm_backup = shared.total_tqdm
- shared.total_tqdm = deforum_settings.DeforumTQDM(args, anim_args, parseq_args)
- try:
- # dispatch to appropriate renderer
- if anim_args.animation_mode == '2D' or anim_args.animation_mode == '3D':
- if anim_args.use_mask_video:
- render_animation_with_video_mask(args, anim_args, video_args, parseq_args, loop_args, controlnet_args, root.animation_prompts, root) # allow mask video without an input video
- else:
- render_animation(args, anim_args, video_args, parseq_args, loop_args, controlnet_args, root.animation_prompts, root)
- elif anim_args.animation_mode == 'Video Input':
- render_input_video(args, anim_args, video_args, parseq_args, loop_args, controlnet_args, root.animation_prompts, root)#TODO: prettify code
- elif anim_args.animation_mode == 'Interpolation':
- render_interpolation(args, anim_args, video_args, parseq_args, loop_args, controlnet_args, root.animation_prompts, root)
- else:
- print('Other modes are not available yet!')
- finally:
- shared.total_tqdm = tqdm_backup
- opts.data["CLIP_stop_at_last_layers"] = root.initial_clipskip
-
- if video_args.store_frames_in_ram:
- dump_frames_cache(root)
-
- from base64 import b64encode
-
- real_audio_track = None
- if video_args.add_soundtrack != 'None':
- real_audio_track = anim_args.video_init_path if video_args.add_soundtrack == 'Init Video' else video_args.soundtrack_path
-
- # Delete folder with duplicated imgs from OS temp folder
- shutil.rmtree(root.tmp_deforum_run_duplicated_folder, ignore_errors=True)
-
- # Decide whether or not we need to try and frame interpolate laters
- need_to_frame_interpolate = False
- if video_args.frame_interpolation_engine != "None" and not video_args.skip_video_for_run_all and not video_args.store_frames_in_ram:
- need_to_frame_interpolate = True
-
- if video_args.skip_video_for_run_all:
- print('Skipping video creation, uncheck skip_video_for_run_all if you want to run it')
- else:
- import subprocess
-
- path_name_modifier = video_args.path_name_modifier
- if video_args.render_steps: # render steps from a single image
- fname = f"{path_name_modifier}_%05d.png"
- all_step_dirs = [os.path.join(args.outdir, d) for d in os.listdir(args.outdir) if os.path.isdir(os.path.join(args.outdir,d))]
- newest_dir = max(all_step_dirs, key=os.path.getmtime)
- image_path = os.path.join(newest_dir, fname)
- print(f"Reading images from {image_path}")
- mp4_path = os.path.join(newest_dir, f"{args.timestring}_{path_name_modifier}.mp4")
- max_video_frames = args.steps
- else: # render images for a video
- image_path = os.path.join(args.outdir, f"{args.timestring}_%05d.png")
- mp4_path = os.path.join(args.outdir, f"{args.timestring}.mp4")
- max_video_frames = anim_args.max_frames
-
- exclude_keys = deforum_settings.get_keys_to_exclude('video')
- video_settings_filename = os.path.join(args.outdir, f"{args.timestring}_video-settings.txt")
- with open(video_settings_filename, "w+", encoding="utf-8") as f:
- s = {}
- for key, value in dict(video_args.__dict__).items():
- if key not in exclude_keys:
- s[key] = value
- json.dump(s, f, ensure_ascii=False, indent=4)
-
- # Stitch video using ffmpeg!
- try:
- ffmpeg_stitch_video(ffmpeg_location=video_args.ffmpeg_location, fps=video_args.fps, outmp4_path=mp4_path, stitch_from_frame=0, stitch_to_frame=max_video_frames, imgs_path=image_path, add_soundtrack=video_args.add_soundtrack, audio_path=real_audio_track, crf=video_args.ffmpeg_crf, preset=video_args.ffmpeg_preset)
- mp4 = open(mp4_path,'rb').read()
- data_url = "data:video/mp4;base64," + b64encode(mp4).decode()
- deforum_args.i1_store = f'
Deforum v0.5-webui-beta
'
- except Exception as e:
- if need_to_frame_interpolate:
- print(f"FFMPEG DID NOT STITCH ANY VIDEO. However, you requested to frame interpolate - so we will continue to frame interpolation, but you'll be left only with the interpolated frames and not a video, since ffmpeg couldn't run. Original ffmpeg error: {e}")
- else:
- print(f"** FFMPEG DID NOT STITCH ANY VIDEO ** Error: {e}")
- pass
-
- if root.initial_info is None:
- root.initial_info = "An error has occured and nothing has been generated!"
- root.initial_info += "\nPlease, report the bug to https://github.com/deforum-art/deforum-for-automatic1111-webui/issues"
- import numpy as np
- a = np.random.rand(args.W, args.H, 3)*255
- root.first_frame = Image.fromarray(a.astype('uint8')).convert('RGB')
- root.initial_seed = 6934
- # FRAME INTERPOLATION TIME
- if need_to_frame_interpolate:
- print(f"Got a request to *frame interpolate* using {video_args.frame_interpolation_engine}")
- process_video_interpolation(frame_interpolation_engine=video_args.frame_interpolation_engine, frame_interpolation_x_amount=video_args.frame_interpolation_x_amount,frame_interpolation_slow_mo_enabled=video_args.frame_interpolation_slow_mo_enabled, frame_interpolation_slow_mo_amount=video_args.frame_interpolation_slow_mo_amount, orig_vid_fps=video_args.fps, deforum_models_path=root.models_path, real_audio_track=real_audio_track, raw_output_imgs_path=args.outdir, img_batch_id=args.timestring, ffmpeg_location=video_args.ffmpeg_location, ffmpeg_crf=video_args.ffmpeg_crf, ffmpeg_preset=video_args.ffmpeg_preset, keep_interp_imgs=video_args.frame_interpolation_keep_imgs, orig_vid_name=None, resolution=None)
-
- if video_args.make_gif and not video_args.skip_video_for_run_all and not video_args.store_frames_in_ram:
- make_gifski_gif(imgs_raw_path = args.outdir, imgs_batch_id = args.timestring, fps = video_args.fps, models_folder = root.models_path, current_user_os = root.current_user_os)
-
- # Upscale video once generation is done:
- if video_args.r_upscale_video and not video_args.skip_video_for_run_all and not video_args.store_frames_in_ram:
-
- # out mp4 path is defined in make_upscale func
- make_upscale_v2(upscale_factor = video_args.r_upscale_factor, upscale_model = video_args.r_upscale_model, keep_imgs = video_args.r_upscale_keep_imgs, imgs_raw_path = args.outdir, imgs_batch_id = args.timestring, fps = video_args.fps, deforum_models_path = root.models_path, current_user_os = root.current_user_os, ffmpeg_location=video_args.ffmpeg_location, stitch_from_frame=0, stitch_to_frame=max_video_frames, ffmpeg_crf=video_args.ffmpeg_crf, ffmpeg_preset=video_args.ffmpeg_preset, add_soundtrack = video_args.add_soundtrack ,audio_path=real_audio_track)
-
- root.initial_info += "\n The animation is stored in " + args.outdir
- root.initial_info += "\n Timestring = " + args.timestring + '\n'
- root.initial_info += "Only the first frame is shown in webui not to clutter the memory"
- reset_frames_cache(root) # cleanup the RAM in any case
- processed = Processed(p, [root.first_frame], root.initial_seed, root.initial_info)
-
- if processed is None:
- processed = process_images(p)
-
- shared.total_tqdm.clear()
-
- generation_info_js = processed.js()
- if opts.samples_log_stdout:
- print(generation_info_js)
-
- if opts.do_not_show_images:
- processed.images = []
-
- return processed.images, generation_info_js, plaintext_to_html(processed.info), plaintext_to_html('')
-
-def on_ui_tabs():
- with gr.Blocks(analytics_enabled=False) as deforum_interface:
- components = {}
- dummy_component = gr.Label(visible=False)
- with gr.Row(elem_id='deforum_progress_row').style(equal_height=False):
- with gr.Column(scale=1, variant='panel'):
- components = deforum_args.setup_deforum_setting_dictionary(None, True, True)
-
- with gr.Column(scale=1):
- with gr.Row():
- btn = gr.Button("Click here after the generation to show the video")
- components['btn'] = btn
- close_btn = gr.Button("Close the video", visible=False)
- with gr.Row():
- i1 = gr.HTML(deforum_args.i1_store, elem_id='deforum_header')
- components['i1'] = i1
- # Show video
- def show_vid():
- return {
- i1: gr.update(value=deforum_args.i1_store, visible=True),
- close_btn: gr.update(visible=True),
- btn: gr.update(value="Update the video", visible=True),
- }
-
- btn.click(
- show_vid,
- [],
- [i1, close_btn, btn],
- )
- # Close video
- def close_vid():
- return {
- i1: gr.update(value=deforum_args.i1_store_backup, visible=True),
- close_btn: gr.update(visible=False),
- btn: gr.update(value="Click here after the generation to show the video", visible=True),
- }
-
- close_btn.click(
- close_vid,
- [],
- [i1, close_btn, btn],
- )
- id_part = 'deforum'
- with gr.Row(elem_id=f"{id_part}_generate_box"):
- skip = gr.Button('Skip', elem_id=f"{id_part}_skip", visible=False)
- interrupt = gr.Button('Interrupt', elem_id=f"{id_part}_interrupt", visible=True)
- submit = gr.Button('Generate', elem_id=f"{id_part}_generate", variant='primary')
-
- skip.click(
- fn=lambda: state.skip(),
- inputs=[],
- outputs=[],
- )
-
- interrupt.click(
- fn=lambda: state.interrupt(),
- inputs=[],
- outputs=[],
- )
-
- deforum_gallery, generation_info, html_info, html_log = create_output_panel("deforum", opts.outdir_img2img_samples)
-
- gr.HTML("
* Paths can be relative to webui folder OR full - absolute
")
- with gr.Row():
- settings_path = gr.Textbox("deforum_settings.txt", elem_id='deforum_settings_path', label="General Settings File")
- #reuse_latest_settings_btn = gr.Button('Reuse Latest', elem_id='deforum_reuse_latest_settings_btn')#TODO
- with gr.Row():
- save_settings_btn = gr.Button('Save Settings', elem_id='deforum_save_settings_btn')
- load_settings_btn = gr.Button('Load Settings', elem_id='deforum_load_settings_btn')
- with gr.Row():
- video_settings_path = gr.Textbox("deforum_video-settings.txt", elem_id='deforum_video_settings_path', label="Video Settings File")
- #reuse_latest_video_settings_btn = gr.Button('Reuse Latest', elem_id='deforum_reuse_latest_video_settings_btn')#TODO
- with gr.Row():
- save_video_settings_btn = gr.Button('Save Video Settings', elem_id='deforum_save_video_settings_btn')
- load_video_settings_btn = gr.Button('Load Video Settings', elem_id='deforum_load_video_settings_btn')
-
- # components['prompts'].visible = False#hide prompts for the time being
- #TODO clean up the code
- components['save_sample_per_step'].visible = False
- components['show_sample_per_step'].visible = False
- components['display_samples'].visible = False
-
- component_list = [components[name] for name in deforum_args.component_names]
-
- submit.click(
- fn=wrap_gradio_gpu_call(run_deforum, extra_outputs=[None, '', '']),
- _js="submit_deforum",
- inputs=[dummy_component, dummy_component] + component_list,
- outputs=[
- deforum_gallery,
- generation_info,
- html_info,
- html_log,
- ],
- )
-
- settings_component_list = [components[name] for name in deforum_args.settings_component_names]
- video_settings_component_list = [components[name] for name in deforum_args.video_args_names]
- stuff = gr.HTML("") # wrap gradio call garbage
- stuff.visible = False
-
- save_settings_btn.click(
- fn=wrap_gradio_call(deforum_settings.save_settings),
- inputs=[settings_path] + settings_component_list,
- outputs=[stuff],
- )
-
- load_settings_btn.click(
- fn=wrap_gradio_call(deforum_settings.load_settings),
- inputs=[settings_path]+ settings_component_list,
- outputs=settings_component_list + [stuff],
- )
-
- save_video_settings_btn.click(
- fn=wrap_gradio_call(deforum_settings.save_video_settings),
- inputs=[video_settings_path] + video_settings_component_list,
- outputs=[stuff],
- )
-
- load_video_settings_btn.click(
- fn=wrap_gradio_call(deforum_settings.load_video_settings),
- inputs=[video_settings_path] + video_settings_component_list,
- outputs=video_settings_component_list + [stuff],
- )
-
-
- return [(deforum_interface, "Deforum", "deforum_interface")]
-
-script_callbacks.on_ui_tabs(on_ui_tabs)
diff --git a/spaces/bigjoker/stable-diffusion-webui/modules/sd_samplers_kdiffusion.py b/spaces/bigjoker/stable-diffusion-webui/modules/sd_samplers_kdiffusion.py
deleted file mode 100644
index 9d16fc11b8fc0678c36dadc9cca0de7122f47cee..0000000000000000000000000000000000000000
--- a/spaces/bigjoker/stable-diffusion-webui/modules/sd_samplers_kdiffusion.py
+++ /dev/null
@@ -1,357 +0,0 @@
-from collections import deque
-import torch
-import inspect
-import einops
-import k_diffusion.sampling
-from modules import prompt_parser, devices, sd_samplers_common
-
-from modules.shared import opts, state
-import modules.shared as shared
-from modules.script_callbacks import CFGDenoiserParams, cfg_denoiser_callback
-from modules.script_callbacks import CFGDenoisedParams, cfg_denoised_callback
-
-samplers_k_diffusion = [
- ('Euler a', 'sample_euler_ancestral', ['k_euler_a', 'k_euler_ancestral'], {}),
- ('Euler', 'sample_euler', ['k_euler'], {}),
- ('LMS', 'sample_lms', ['k_lms'], {}),
- ('Heun', 'sample_heun', ['k_heun'], {}),
- ('DPM2', 'sample_dpm_2', ['k_dpm_2'], {'discard_next_to_last_sigma': True}),
- ('DPM2 a', 'sample_dpm_2_ancestral', ['k_dpm_2_a'], {'discard_next_to_last_sigma': True}),
- ('DPM++ 2S a', 'sample_dpmpp_2s_ancestral', ['k_dpmpp_2s_a'], {}),
- ('DPM++ 2M', 'sample_dpmpp_2m', ['k_dpmpp_2m'], {}),
- ('DPM++ SDE', 'sample_dpmpp_sde', ['k_dpmpp_sde'], {}),
- ('DPM fast', 'sample_dpm_fast', ['k_dpm_fast'], {}),
- ('DPM adaptive', 'sample_dpm_adaptive', ['k_dpm_ad'], {}),
- ('LMS Karras', 'sample_lms', ['k_lms_ka'], {'scheduler': 'karras'}),
- ('DPM2 Karras', 'sample_dpm_2', ['k_dpm_2_ka'], {'scheduler': 'karras', 'discard_next_to_last_sigma': True}),
- ('DPM2 a Karras', 'sample_dpm_2_ancestral', ['k_dpm_2_a_ka'], {'scheduler': 'karras', 'discard_next_to_last_sigma': True}),
- ('DPM++ 2S a Karras', 'sample_dpmpp_2s_ancestral', ['k_dpmpp_2s_a_ka'], {'scheduler': 'karras'}),
- ('DPM++ 2M Karras', 'sample_dpmpp_2m', ['k_dpmpp_2m_ka'], {'scheduler': 'karras'}),
- ('DPM++ SDE Karras', 'sample_dpmpp_sde', ['k_dpmpp_sde_ka'], {'scheduler': 'karras'}),
-]
-
-samplers_data_k_diffusion = [
- sd_samplers_common.SamplerData(label, lambda model, funcname=funcname: KDiffusionSampler(funcname, model), aliases, options)
- for label, funcname, aliases, options in samplers_k_diffusion
- if hasattr(k_diffusion.sampling, funcname)
-]
-
-sampler_extra_params = {
- 'sample_euler': ['s_churn', 's_tmin', 's_tmax', 's_noise'],
- 'sample_heun': ['s_churn', 's_tmin', 's_tmax', 's_noise'],
- 'sample_dpm_2': ['s_churn', 's_tmin', 's_tmax', 's_noise'],
-}
-
-
-class CFGDenoiser(torch.nn.Module):
- """
- Classifier free guidance denoiser. A wrapper for stable diffusion model (specifically for unet)
- that can take a noisy picture and produce a noise-free picture using two guidances (prompts)
- instead of one. Originally, the second prompt is just an empty string, but we use non-empty
- negative prompt.
- """
-
- def __init__(self, model):
- super().__init__()
- self.inner_model = model
- self.mask = None
- self.nmask = None
- self.init_latent = None
- self.step = 0
- self.image_cfg_scale = None
-
- def combine_denoised(self, x_out, conds_list, uncond, cond_scale):
- denoised_uncond = x_out[-uncond.shape[0]:]
- denoised = torch.clone(denoised_uncond)
-
- for i, conds in enumerate(conds_list):
- for cond_index, weight in conds:
- denoised[i] += (x_out[cond_index] - denoised_uncond[i]) * (weight * cond_scale)
-
- return denoised
-
- def combine_denoised_for_edit_model(self, x_out, cond_scale):
- out_cond, out_img_cond, out_uncond = x_out.chunk(3)
- denoised = out_uncond + cond_scale * (out_cond - out_img_cond) + self.image_cfg_scale * (out_img_cond - out_uncond)
-
- return denoised
-
- def forward(self, x, sigma, uncond, cond, cond_scale, image_cond):
- if state.interrupted or state.skipped:
- raise sd_samplers_common.InterruptedException
-
- # at self.image_cfg_scale == 1.0 produced results for edit model are the same as with normal sampling,
- # so is_edit_model is set to False to support AND composition.
- is_edit_model = shared.sd_model.cond_stage_key == "edit" and self.image_cfg_scale is not None and self.image_cfg_scale != 1.0
-
- conds_list, tensor = prompt_parser.reconstruct_multicond_batch(cond, self.step)
- uncond = prompt_parser.reconstruct_cond_batch(uncond, self.step)
-
- assert not is_edit_model or all([len(conds) == 1 for conds in conds_list]), "AND is not supported for InstructPix2Pix checkpoint (unless using Image CFG scale = 1.0)"
-
- batch_size = len(conds_list)
- repeats = [len(conds_list[i]) for i in range(batch_size)]
-
- if not is_edit_model:
- x_in = torch.cat([torch.stack([x[i] for _ in range(n)]) for i, n in enumerate(repeats)] + [x])
- sigma_in = torch.cat([torch.stack([sigma[i] for _ in range(n)]) for i, n in enumerate(repeats)] + [sigma])
- image_cond_in = torch.cat([torch.stack([image_cond[i] for _ in range(n)]) for i, n in enumerate(repeats)] + [image_cond])
- else:
- x_in = torch.cat([torch.stack([x[i] for _ in range(n)]) for i, n in enumerate(repeats)] + [x] + [x])
- sigma_in = torch.cat([torch.stack([sigma[i] for _ in range(n)]) for i, n in enumerate(repeats)] + [sigma] + [sigma])
- image_cond_in = torch.cat([torch.stack([image_cond[i] for _ in range(n)]) for i, n in enumerate(repeats)] + [image_cond] + [torch.zeros_like(self.init_latent)])
-
- denoiser_params = CFGDenoiserParams(x_in, image_cond_in, sigma_in, state.sampling_step, state.sampling_steps)
- cfg_denoiser_callback(denoiser_params)
- x_in = denoiser_params.x
- image_cond_in = denoiser_params.image_cond
- sigma_in = denoiser_params.sigma
-
- if tensor.shape[1] == uncond.shape[1]:
- if not is_edit_model:
- cond_in = torch.cat([tensor, uncond])
- else:
- cond_in = torch.cat([tensor, uncond, uncond])
-
- if shared.batch_cond_uncond:
- x_out = self.inner_model(x_in, sigma_in, cond={"c_crossattn": [cond_in], "c_concat": [image_cond_in]})
- else:
- x_out = torch.zeros_like(x_in)
- for batch_offset in range(0, x_out.shape[0], batch_size):
- a = batch_offset
- b = a + batch_size
- x_out[a:b] = self.inner_model(x_in[a:b], sigma_in[a:b], cond={"c_crossattn": [cond_in[a:b]], "c_concat": [image_cond_in[a:b]]})
- else:
- x_out = torch.zeros_like(x_in)
- batch_size = batch_size*2 if shared.batch_cond_uncond else batch_size
- for batch_offset in range(0, tensor.shape[0], batch_size):
- a = batch_offset
- b = min(a + batch_size, tensor.shape[0])
-
- if not is_edit_model:
- c_crossattn = [tensor[a:b]]
- else:
- c_crossattn = torch.cat([tensor[a:b]], uncond)
-
- x_out[a:b] = self.inner_model(x_in[a:b], sigma_in[a:b], cond={"c_crossattn": c_crossattn, "c_concat": [image_cond_in[a:b]]})
-
- x_out[-uncond.shape[0]:] = self.inner_model(x_in[-uncond.shape[0]:], sigma_in[-uncond.shape[0]:], cond={"c_crossattn": [uncond], "c_concat": [image_cond_in[-uncond.shape[0]:]]})
-
- denoised_params = CFGDenoisedParams(x_out, state.sampling_step, state.sampling_steps)
- cfg_denoised_callback(denoised_params)
-
- devices.test_for_nans(x_out, "unet")
-
- if opts.live_preview_content == "Prompt":
- sd_samplers_common.store_latent(x_out[0:uncond.shape[0]])
- elif opts.live_preview_content == "Negative prompt":
- sd_samplers_common.store_latent(x_out[-uncond.shape[0]:])
-
- if not is_edit_model:
- denoised = self.combine_denoised(x_out, conds_list, uncond, cond_scale)
- else:
- denoised = self.combine_denoised_for_edit_model(x_out, cond_scale)
-
- if self.mask is not None:
- denoised = self.init_latent * self.mask + self.nmask * denoised
-
- self.step += 1
-
- return denoised
-
-
-class TorchHijack:
- def __init__(self, sampler_noises):
- # Using a deque to efficiently receive the sampler_noises in the same order as the previous index-based
- # implementation.
- self.sampler_noises = deque(sampler_noises)
-
- def __getattr__(self, item):
- if item == 'randn_like':
- return self.randn_like
-
- if hasattr(torch, item):
- return getattr(torch, item)
-
- raise AttributeError("'{}' object has no attribute '{}'".format(type(self).__name__, item))
-
- def randn_like(self, x):
- if self.sampler_noises:
- noise = self.sampler_noises.popleft()
- if noise.shape == x.shape:
- return noise
-
- if x.device.type == 'mps':
- return torch.randn_like(x, device=devices.cpu).to(x.device)
- else:
- return torch.randn_like(x)
-
-
-class KDiffusionSampler:
- def __init__(self, funcname, sd_model):
- denoiser = k_diffusion.external.CompVisVDenoiser if sd_model.parameterization == "v" else k_diffusion.external.CompVisDenoiser
-
- self.model_wrap = denoiser(sd_model, quantize=shared.opts.enable_quantization)
- self.funcname = funcname
- self.func = getattr(k_diffusion.sampling, self.funcname)
- self.extra_params = sampler_extra_params.get(funcname, [])
- self.model_wrap_cfg = CFGDenoiser(self.model_wrap)
- self.sampler_noises = None
- self.stop_at = None
- self.eta = None
- self.config = None
- self.last_latent = None
-
- self.conditioning_key = sd_model.model.conditioning_key
-
- def callback_state(self, d):
- step = d['i']
- latent = d["denoised"]
- if opts.live_preview_content == "Combined":
- sd_samplers_common.store_latent(latent)
- self.last_latent = latent
-
- if self.stop_at is not None and step > self.stop_at:
- raise sd_samplers_common.InterruptedException
-
- state.sampling_step = step
- shared.total_tqdm.update()
-
- def launch_sampling(self, steps, func):
- state.sampling_steps = steps
- state.sampling_step = 0
-
- try:
- return func()
- except sd_samplers_common.InterruptedException:
- return self.last_latent
-
- def number_of_needed_noises(self, p):
- return p.steps
-
- def initialize(self, p):
- self.model_wrap_cfg.mask = p.mask if hasattr(p, 'mask') else None
- self.model_wrap_cfg.nmask = p.nmask if hasattr(p, 'nmask') else None
- self.model_wrap_cfg.step = 0
- self.model_wrap_cfg.image_cfg_scale = getattr(p, 'image_cfg_scale', None)
- self.eta = p.eta if p.eta is not None else opts.eta_ancestral
-
- k_diffusion.sampling.torch = TorchHijack(self.sampler_noises if self.sampler_noises is not None else [])
-
- extra_params_kwargs = {}
- for param_name in self.extra_params:
- if hasattr(p, param_name) and param_name in inspect.signature(self.func).parameters:
- extra_params_kwargs[param_name] = getattr(p, param_name)
-
- if 'eta' in inspect.signature(self.func).parameters:
- if self.eta != 1.0:
- p.extra_generation_params["Eta"] = self.eta
-
- extra_params_kwargs['eta'] = self.eta
-
- return extra_params_kwargs
-
- def get_sigmas(self, p, steps):
- discard_next_to_last_sigma = self.config is not None and self.config.options.get('discard_next_to_last_sigma', False)
- if opts.always_discard_next_to_last_sigma and not discard_next_to_last_sigma:
- discard_next_to_last_sigma = True
- p.extra_generation_params["Discard penultimate sigma"] = True
-
- steps += 1 if discard_next_to_last_sigma else 0
-
- if p.sampler_noise_scheduler_override:
- sigmas = p.sampler_noise_scheduler_override(steps)
- elif self.config is not None and self.config.options.get('scheduler', None) == 'karras':
- sigma_min, sigma_max = (0.1, 10) if opts.use_old_karras_scheduler_sigmas else (self.model_wrap.sigmas[0].item(), self.model_wrap.sigmas[-1].item())
-
- sigmas = k_diffusion.sampling.get_sigmas_karras(n=steps, sigma_min=sigma_min, sigma_max=sigma_max, device=shared.device)
- else:
- sigmas = self.model_wrap.get_sigmas(steps)
-
- if discard_next_to_last_sigma:
- sigmas = torch.cat([sigmas[:-2], sigmas[-1:]])
-
- return sigmas
-
- def create_noise_sampler(self, x, sigmas, p):
- """For DPM++ SDE: manually create noise sampler to enable deterministic results across different batch sizes"""
- if shared.opts.no_dpmpp_sde_batch_determinism:
- return None
-
- from k_diffusion.sampling import BrownianTreeNoiseSampler
- sigma_min, sigma_max = sigmas[sigmas > 0].min(), sigmas.max()
- current_iter_seeds = p.all_seeds[p.iteration * p.batch_size:(p.iteration + 1) * p.batch_size]
- return BrownianTreeNoiseSampler(x, sigma_min, sigma_max, seed=current_iter_seeds)
-
- def sample_img2img(self, p, x, noise, conditioning, unconditional_conditioning, steps=None, image_conditioning=None):
- steps, t_enc = sd_samplers_common.setup_img2img_steps(p, steps)
-
- sigmas = self.get_sigmas(p, steps)
-
- sigma_sched = sigmas[steps - t_enc - 1:]
- xi = x + noise * sigma_sched[0]
-
- extra_params_kwargs = self.initialize(p)
- parameters = inspect.signature(self.func).parameters
-
- if 'sigma_min' in parameters:
- ## last sigma is zero which isn't allowed by DPM Fast & Adaptive so taking value before last
- extra_params_kwargs['sigma_min'] = sigma_sched[-2]
- if 'sigma_max' in parameters:
- extra_params_kwargs['sigma_max'] = sigma_sched[0]
- if 'n' in parameters:
- extra_params_kwargs['n'] = len(sigma_sched) - 1
- if 'sigma_sched' in parameters:
- extra_params_kwargs['sigma_sched'] = sigma_sched
- if 'sigmas' in parameters:
- extra_params_kwargs['sigmas'] = sigma_sched
-
- if self.funcname == 'sample_dpmpp_sde':
- noise_sampler = self.create_noise_sampler(x, sigmas, p)
- extra_params_kwargs['noise_sampler'] = noise_sampler
-
- self.model_wrap_cfg.init_latent = x
- self.last_latent = x
- extra_args={
- 'cond': conditioning,
- 'image_cond': image_conditioning,
- 'uncond': unconditional_conditioning,
- 'cond_scale': p.cfg_scale,
- }
-
- samples = self.launch_sampling(t_enc + 1, lambda: self.func(self.model_wrap_cfg, xi, extra_args=extra_args, disable=False, callback=self.callback_state, **extra_params_kwargs))
-
- return samples
-
- def sample(self, p, x, conditioning, unconditional_conditioning, steps=None, image_conditioning=None):
- steps = steps or p.steps
-
- sigmas = self.get_sigmas(p, steps)
-
- x = x * sigmas[0]
-
- extra_params_kwargs = self.initialize(p)
- parameters = inspect.signature(self.func).parameters
-
- if 'sigma_min' in parameters:
- extra_params_kwargs['sigma_min'] = self.model_wrap.sigmas[0].item()
- extra_params_kwargs['sigma_max'] = self.model_wrap.sigmas[-1].item()
- if 'n' in parameters:
- extra_params_kwargs['n'] = steps
- else:
- extra_params_kwargs['sigmas'] = sigmas
-
- if self.funcname == 'sample_dpmpp_sde':
- noise_sampler = self.create_noise_sampler(x, sigmas, p)
- extra_params_kwargs['noise_sampler'] = noise_sampler
-
- self.last_latent = x
- samples = self.launch_sampling(steps, lambda: self.func(self.model_wrap_cfg, x, extra_args={
- 'cond': conditioning,
- 'image_cond': image_conditioning,
- 'uncond': unconditional_conditioning,
- 'cond_scale': p.cfg_scale
- }, disable=False, callback=self.callback_state, **extra_params_kwargs))
-
- return samples
-
diff --git a/spaces/bigscience/data_host_provider_agreement/README.md b/spaces/bigscience/data_host_provider_agreement/README.md
deleted file mode 100644
index a80a381223431391d6884d7ac7e3e0ebdd0b2f67..0000000000000000000000000000000000000000
--- a/spaces/bigscience/data_host_provider_agreement/README.md
+++ /dev/null
@@ -1,11 +0,0 @@
----
-title: DataAgreement
-emoji: 🤝
-colorFrom: green
-colorTo: yellow
-sdk: static
-pinned: false
-license: apache-2.0
----
-
-Check out the configuration reference at https://huggingface.co/docs/hub/spaces#reference
diff --git a/spaces/billusanda007/Enhancer/README.md b/spaces/billusanda007/Enhancer/README.md
deleted file mode 100644
index 6a91724813982f3d65d2fd49b493dd00a8c52a52..0000000000000000000000000000000000000000
--- a/spaces/billusanda007/Enhancer/README.md
+++ /dev/null
@@ -1,13 +0,0 @@
----
-title: Enhancer
-emoji: 📊
-colorFrom: green
-colorTo: blue
-sdk: streamlit
-sdk_version: 1.25.0
-app_file: app.py
-pinned: false
-license: mit
----
-
-Check out the configuration reference at https://huggingface.co/docs/hub/spaces-config-reference
diff --git a/spaces/bioriAsaeru/text-to-voice/Adobe InDesign CC 2018 V13.1.0.76 Crack [CracksNow] 64 Bitl Features Benefits and Reviews.md b/spaces/bioriAsaeru/text-to-voice/Adobe InDesign CC 2018 V13.1.0.76 Crack [CracksNow] 64 Bitl Features Benefits and Reviews.md
deleted file mode 100644
index a53d47642b27f54521df331696e098cbc965c5b7..0000000000000000000000000000000000000000
--- a/spaces/bioriAsaeru/text-to-voice/Adobe InDesign CC 2018 V13.1.0.76 Crack [CracksNow] 64 Bitl Features Benefits and Reviews.md
+++ /dev/null
@@ -1,6 +0,0 @@
-
Adobe InDesign CC 2018 V13.1.0.76 Crack [CracksNow] 64 Bitl
-
- aaccfb2cb3
-
-
-
diff --git a/spaces/bioriAsaeru/text-to-voice/China touch mobile java software free download Discover the best of Java for your touch screen phone.md b/spaces/bioriAsaeru/text-to-voice/China touch mobile java software free download Discover the best of Java for your touch screen phone.md
deleted file mode 100644
index 1689846445267c19db7656f2c012c05f8ea1eb8b..0000000000000000000000000000000000000000
--- a/spaces/bioriAsaeru/text-to-voice/China touch mobile java software free download Discover the best of Java for your touch screen phone.md
+++ /dev/null
@@ -1,6 +0,0 @@
-
-
I just downloaded TreeSize Free and am most impressed with how much you have built into the free version of your software. It is clearly a well engineered and carefully thought out product that will be very useful for finding clutter on my hard drive. It contains far more value than I expected, and I compliment you on a product well done.
BlueStacks' main source of revenue is from an Android emulator known as App Player. The software's basic features are free to download and use. Advanced optional features require a paid monthly subscription.[10] The company claims the App Player can run 1.5 million Android apps as of November 2019.[11] As of February 2021, BlueStacks claimed its apps were downloaded over 1 billion times.[12] App Player features mouse, keyboard, and external touch-pad controls.
aaccfb2cb3
-
-
\ No newline at end of file
diff --git a/spaces/bioriAsaeru/text-to-voice/HD Online Player (telecharger Gratuitement Adibou 2 Po) !!LINK!!.md b/spaces/bioriAsaeru/text-to-voice/HD Online Player (telecharger Gratuitement Adibou 2 Po) !!LINK!!.md
deleted file mode 100644
index 620e96196630058d724b53148e8fb5670726d55c..0000000000000000000000000000000000000000
--- a/spaces/bioriAsaeru/text-to-voice/HD Online Player (telecharger Gratuitement Adibou 2 Po) !!LINK!!.md
+++ /dev/null
@@ -1,10 +0,0 @@
-
-
adibou and his friends are all the same characters as in the previous game, but they will be animated in 3d. this new adibou game will feature a chat between adibou and his friends, telling stories and inviting kids to play mini-games in a playful environment.
-
HD Online Player (telecharger gratuitement adibou 2 po)
adibou and his friends are all the same characters as in the previous game, but they will be animated in 3d. you can observe their gestures and their expressions as you play, and the face of adibou can be customized.
-
in addition to the award-winning original adibou games, more than 15 new activities have been added to the hd online player game, some of which have never been released before. these include new characters, new mini-games, new items, new levels, new recipes, and new activities for the knowledge tower. young players can also create their own cakes, flowers, fruits and vegetables, and upload them to the community. they can also design their own characters, which they can later save in their own garden. by collecting and saving the stamps they collect, players will also be able to unlock new activities and rewards.
-
the original adibou game was created in 2008 by frédéric félix, the founder of the french game studio studio amuse. in 2014, amuse was acquired by the international publisher microïds. studio amuse is currently developing the original adibou series of games, and will continue to develop new games in the series.
-
the adibou brand will be rebooted in this new project, and we expect that the new adibou will be as well received by the fans as the original one was when it was first released, he adds. the original adibou was launched in europe, canada, and japan, while the new version will roll out in japan and europe first.
- 899543212b
-
-
\ No newline at end of file
diff --git a/spaces/bodah/RVC-Models-bo/lib/infer_pack/attentions.py b/spaces/bodah/RVC-Models-bo/lib/infer_pack/attentions.py
deleted file mode 100644
index 05501be1871643f78dddbeaa529c96667031a8db..0000000000000000000000000000000000000000
--- a/spaces/bodah/RVC-Models-bo/lib/infer_pack/attentions.py
+++ /dev/null
@@ -1,417 +0,0 @@
-import copy
-import math
-import numpy as np
-import torch
-from torch import nn
-from torch.nn import functional as F
-
-from lib.infer_pack import commons
-from lib.infer_pack import modules
-from lib.infer_pack.modules import LayerNorm
-
-
-class Encoder(nn.Module):
- def __init__(
- self,
- hidden_channels,
- filter_channels,
- n_heads,
- n_layers,
- kernel_size=1,
- p_dropout=0.0,
- window_size=10,
- **kwargs
- ):
- super().__init__()
- self.hidden_channels = hidden_channels
- self.filter_channels = filter_channels
- self.n_heads = n_heads
- self.n_layers = n_layers
- self.kernel_size = kernel_size
- self.p_dropout = p_dropout
- self.window_size = window_size
-
- self.drop = nn.Dropout(p_dropout)
- self.attn_layers = nn.ModuleList()
- self.norm_layers_1 = nn.ModuleList()
- self.ffn_layers = nn.ModuleList()
- self.norm_layers_2 = nn.ModuleList()
- for i in range(self.n_layers):
- self.attn_layers.append(
- MultiHeadAttention(
- hidden_channels,
- hidden_channels,
- n_heads,
- p_dropout=p_dropout,
- window_size=window_size,
- )
- )
- self.norm_layers_1.append(LayerNorm(hidden_channels))
- self.ffn_layers.append(
- FFN(
- hidden_channels,
- hidden_channels,
- filter_channels,
- kernel_size,
- p_dropout=p_dropout,
- )
- )
- self.norm_layers_2.append(LayerNorm(hidden_channels))
-
- def forward(self, x, x_mask):
- attn_mask = x_mask.unsqueeze(2) * x_mask.unsqueeze(-1)
- x = x * x_mask
- for i in range(self.n_layers):
- y = self.attn_layers[i](x, x, attn_mask)
- y = self.drop(y)
- x = self.norm_layers_1[i](x + y)
-
- y = self.ffn_layers[i](x, x_mask)
- y = self.drop(y)
- x = self.norm_layers_2[i](x + y)
- x = x * x_mask
- return x
-
-
-class Decoder(nn.Module):
- def __init__(
- self,
- hidden_channels,
- filter_channels,
- n_heads,
- n_layers,
- kernel_size=1,
- p_dropout=0.0,
- proximal_bias=False,
- proximal_init=True,
- **kwargs
- ):
- super().__init__()
- self.hidden_channels = hidden_channels
- self.filter_channels = filter_channels
- self.n_heads = n_heads
- self.n_layers = n_layers
- self.kernel_size = kernel_size
- self.p_dropout = p_dropout
- self.proximal_bias = proximal_bias
- self.proximal_init = proximal_init
-
- self.drop = nn.Dropout(p_dropout)
- self.self_attn_layers = nn.ModuleList()
- self.norm_layers_0 = nn.ModuleList()
- self.encdec_attn_layers = nn.ModuleList()
- self.norm_layers_1 = nn.ModuleList()
- self.ffn_layers = nn.ModuleList()
- self.norm_layers_2 = nn.ModuleList()
- for i in range(self.n_layers):
- self.self_attn_layers.append(
- MultiHeadAttention(
- hidden_channels,
- hidden_channels,
- n_heads,
- p_dropout=p_dropout,
- proximal_bias=proximal_bias,
- proximal_init=proximal_init,
- )
- )
- self.norm_layers_0.append(LayerNorm(hidden_channels))
- self.encdec_attn_layers.append(
- MultiHeadAttention(
- hidden_channels, hidden_channels, n_heads, p_dropout=p_dropout
- )
- )
- self.norm_layers_1.append(LayerNorm(hidden_channels))
- self.ffn_layers.append(
- FFN(
- hidden_channels,
- hidden_channels,
- filter_channels,
- kernel_size,
- p_dropout=p_dropout,
- causal=True,
- )
- )
- self.norm_layers_2.append(LayerNorm(hidden_channels))
-
- def forward(self, x, x_mask, h, h_mask):
- """
- x: decoder input
- h: encoder output
- """
- self_attn_mask = commons.subsequent_mask(x_mask.size(2)).to(
- device=x.device, dtype=x.dtype
- )
- encdec_attn_mask = h_mask.unsqueeze(2) * x_mask.unsqueeze(-1)
- x = x * x_mask
- for i in range(self.n_layers):
- y = self.self_attn_layers[i](x, x, self_attn_mask)
- y = self.drop(y)
- x = self.norm_layers_0[i](x + y)
-
- y = self.encdec_attn_layers[i](x, h, encdec_attn_mask)
- y = self.drop(y)
- x = self.norm_layers_1[i](x + y)
-
- y = self.ffn_layers[i](x, x_mask)
- y = self.drop(y)
- x = self.norm_layers_2[i](x + y)
- x = x * x_mask
- return x
-
-
-class MultiHeadAttention(nn.Module):
- def __init__(
- self,
- channels,
- out_channels,
- n_heads,
- p_dropout=0.0,
- window_size=None,
- heads_share=True,
- block_length=None,
- proximal_bias=False,
- proximal_init=False,
- ):
- super().__init__()
- assert channels % n_heads == 0
-
- self.channels = channels
- self.out_channels = out_channels
- self.n_heads = n_heads
- self.p_dropout = p_dropout
- self.window_size = window_size
- self.heads_share = heads_share
- self.block_length = block_length
- self.proximal_bias = proximal_bias
- self.proximal_init = proximal_init
- self.attn = None
-
- self.k_channels = channels // n_heads
- self.conv_q = nn.Conv1d(channels, channels, 1)
- self.conv_k = nn.Conv1d(channels, channels, 1)
- self.conv_v = nn.Conv1d(channels, channels, 1)
- self.conv_o = nn.Conv1d(channels, out_channels, 1)
- self.drop = nn.Dropout(p_dropout)
-
- if window_size is not None:
- n_heads_rel = 1 if heads_share else n_heads
- rel_stddev = self.k_channels**-0.5
- self.emb_rel_k = nn.Parameter(
- torch.randn(n_heads_rel, window_size * 2 + 1, self.k_channels)
- * rel_stddev
- )
- self.emb_rel_v = nn.Parameter(
- torch.randn(n_heads_rel, window_size * 2 + 1, self.k_channels)
- * rel_stddev
- )
-
- nn.init.xavier_uniform_(self.conv_q.weight)
- nn.init.xavier_uniform_(self.conv_k.weight)
- nn.init.xavier_uniform_(self.conv_v.weight)
- if proximal_init:
- with torch.no_grad():
- self.conv_k.weight.copy_(self.conv_q.weight)
- self.conv_k.bias.copy_(self.conv_q.bias)
-
- def forward(self, x, c, attn_mask=None):
- q = self.conv_q(x)
- k = self.conv_k(c)
- v = self.conv_v(c)
-
- x, self.attn = self.attention(q, k, v, mask=attn_mask)
-
- x = self.conv_o(x)
- return x
-
- def attention(self, query, key, value, mask=None):
- # reshape [b, d, t] -> [b, n_h, t, d_k]
- b, d, t_s, t_t = (*key.size(), query.size(2))
- query = query.view(b, self.n_heads, self.k_channels, t_t).transpose(2, 3)
- key = key.view(b, self.n_heads, self.k_channels, t_s).transpose(2, 3)
- value = value.view(b, self.n_heads, self.k_channels, t_s).transpose(2, 3)
-
- scores = torch.matmul(query / math.sqrt(self.k_channels), key.transpose(-2, -1))
- if self.window_size is not None:
- assert (
- t_s == t_t
- ), "Relative attention is only available for self-attention."
- key_relative_embeddings = self._get_relative_embeddings(self.emb_rel_k, t_s)
- rel_logits = self._matmul_with_relative_keys(
- query / math.sqrt(self.k_channels), key_relative_embeddings
- )
- scores_local = self._relative_position_to_absolute_position(rel_logits)
- scores = scores + scores_local
- if self.proximal_bias:
- assert t_s == t_t, "Proximal bias is only available for self-attention."
- scores = scores + self._attention_bias_proximal(t_s).to(
- device=scores.device, dtype=scores.dtype
- )
- if mask is not None:
- scores = scores.masked_fill(mask == 0, -1e4)
- if self.block_length is not None:
- assert (
- t_s == t_t
- ), "Local attention is only available for self-attention."
- block_mask = (
- torch.ones_like(scores)
- .triu(-self.block_length)
- .tril(self.block_length)
- )
- scores = scores.masked_fill(block_mask == 0, -1e4)
- p_attn = F.softmax(scores, dim=-1) # [b, n_h, t_t, t_s]
- p_attn = self.drop(p_attn)
- output = torch.matmul(p_attn, value)
- if self.window_size is not None:
- relative_weights = self._absolute_position_to_relative_position(p_attn)
- value_relative_embeddings = self._get_relative_embeddings(
- self.emb_rel_v, t_s
- )
- output = output + self._matmul_with_relative_values(
- relative_weights, value_relative_embeddings
- )
- output = (
- output.transpose(2, 3).contiguous().view(b, d, t_t)
- ) # [b, n_h, t_t, d_k] -> [b, d, t_t]
- return output, p_attn
-
- def _matmul_with_relative_values(self, x, y):
- """
- x: [b, h, l, m]
- y: [h or 1, m, d]
- ret: [b, h, l, d]
- """
- ret = torch.matmul(x, y.unsqueeze(0))
- return ret
-
- def _matmul_with_relative_keys(self, x, y):
- """
- x: [b, h, l, d]
- y: [h or 1, m, d]
- ret: [b, h, l, m]
- """
- ret = torch.matmul(x, y.unsqueeze(0).transpose(-2, -1))
- return ret
-
- def _get_relative_embeddings(self, relative_embeddings, length):
- max_relative_position = 2 * self.window_size + 1
- # Pad first before slice to avoid using cond ops.
- pad_length = max(length - (self.window_size + 1), 0)
- slice_start_position = max((self.window_size + 1) - length, 0)
- slice_end_position = slice_start_position + 2 * length - 1
- if pad_length > 0:
- padded_relative_embeddings = F.pad(
- relative_embeddings,
- commons.convert_pad_shape([[0, 0], [pad_length, pad_length], [0, 0]]),
- )
- else:
- padded_relative_embeddings = relative_embeddings
- used_relative_embeddings = padded_relative_embeddings[
- :, slice_start_position:slice_end_position
- ]
- return used_relative_embeddings
-
- def _relative_position_to_absolute_position(self, x):
- """
- x: [b, h, l, 2*l-1]
- ret: [b, h, l, l]
- """
- batch, heads, length, _ = x.size()
- # Concat columns of pad to shift from relative to absolute indexing.
- x = F.pad(x, commons.convert_pad_shape([[0, 0], [0, 0], [0, 0], [0, 1]]))
-
- # Concat extra elements so to add up to shape (len+1, 2*len-1).
- x_flat = x.view([batch, heads, length * 2 * length])
- x_flat = F.pad(
- x_flat, commons.convert_pad_shape([[0, 0], [0, 0], [0, length - 1]])
- )
-
- # Reshape and slice out the padded elements.
- x_final = x_flat.view([batch, heads, length + 1, 2 * length - 1])[
- :, :, :length, length - 1 :
- ]
- return x_final
-
- def _absolute_position_to_relative_position(self, x):
- """
- x: [b, h, l, l]
- ret: [b, h, l, 2*l-1]
- """
- batch, heads, length, _ = x.size()
- # padd along column
- x = F.pad(
- x, commons.convert_pad_shape([[0, 0], [0, 0], [0, 0], [0, length - 1]])
- )
- x_flat = x.view([batch, heads, length**2 + length * (length - 1)])
- # add 0's in the beginning that will skew the elements after reshape
- x_flat = F.pad(x_flat, commons.convert_pad_shape([[0, 0], [0, 0], [length, 0]]))
- x_final = x_flat.view([batch, heads, length, 2 * length])[:, :, :, 1:]
- return x_final
-
- def _attention_bias_proximal(self, length):
- """Bias for self-attention to encourage attention to close positions.
- Args:
- length: an integer scalar.
- Returns:
- a Tensor with shape [1, 1, length, length]
- """
- r = torch.arange(length, dtype=torch.float32)
- diff = torch.unsqueeze(r, 0) - torch.unsqueeze(r, 1)
- return torch.unsqueeze(torch.unsqueeze(-torch.log1p(torch.abs(diff)), 0), 0)
-
-
-class FFN(nn.Module):
- def __init__(
- self,
- in_channels,
- out_channels,
- filter_channels,
- kernel_size,
- p_dropout=0.0,
- activation=None,
- causal=False,
- ):
- super().__init__()
- self.in_channels = in_channels
- self.out_channels = out_channels
- self.filter_channels = filter_channels
- self.kernel_size = kernel_size
- self.p_dropout = p_dropout
- self.activation = activation
- self.causal = causal
-
- if causal:
- self.padding = self._causal_padding
- else:
- self.padding = self._same_padding
-
- self.conv_1 = nn.Conv1d(in_channels, filter_channels, kernel_size)
- self.conv_2 = nn.Conv1d(filter_channels, out_channels, kernel_size)
- self.drop = nn.Dropout(p_dropout)
-
- def forward(self, x, x_mask):
- x = self.conv_1(self.padding(x * x_mask))
- if self.activation == "gelu":
- x = x * torch.sigmoid(1.702 * x)
- else:
- x = torch.relu(x)
- x = self.drop(x)
- x = self.conv_2(self.padding(x * x_mask))
- return x * x_mask
-
- def _causal_padding(self, x):
- if self.kernel_size == 1:
- return x
- pad_l = self.kernel_size - 1
- pad_r = 0
- padding = [[0, 0], [0, 0], [pad_l, pad_r]]
- x = F.pad(x, commons.convert_pad_shape(padding))
- return x
-
- def _same_padding(self, x):
- if self.kernel_size == 1:
- return x
- pad_l = (self.kernel_size - 1) // 2
- pad_r = self.kernel_size // 2
- padding = [[0, 0], [0, 0], [pad_l, pad_r]]
- x = F.pad(x, commons.convert_pad_shape(padding))
- return x
diff --git a/spaces/bookbot/SpeechLine/README.md b/spaces/bookbot/SpeechLine/README.md
deleted file mode 100644
index 9cd4d3de437dca4a792c6d9dbea8311e7eccf003..0000000000000000000000000000000000000000
--- a/spaces/bookbot/SpeechLine/README.md
+++ /dev/null
@@ -1,12 +0,0 @@
----
-title: SpeechLine
-emoji: 🎙️
-colorFrom: yellow
-colorTo: purple
-sdk: gradio
-sdk_version: 3.20.1
-app_file: app.py
-pinned: false
----
-
-Check out the configuration reference at https://huggingface.co/docs/hub/spaces-config-reference
diff --git a/spaces/brjathu/HMR2.0/hmr2/utils/texture_utils.py b/spaces/brjathu/HMR2.0/hmr2/utils/texture_utils.py
deleted file mode 100644
index e10bb62862683af1efcfb39e07a6baeafe9f5da4..0000000000000000000000000000000000000000
--- a/spaces/brjathu/HMR2.0/hmr2/utils/texture_utils.py
+++ /dev/null
@@ -1,85 +0,0 @@
-import numpy as np
-import torch
-from torch.nn import functional as F
-# from psbody.mesh.visibility import visibility_compute
-
-def uv_to_xyz_and_normals(verts, f, fmap, bmap, ftov):
- vn = estimate_vertex_normals(verts, f, ftov)
- pixels_to_set = torch.nonzero(fmap+1)
- x_to_set = pixels_to_set[:,0]
- y_to_set = pixels_to_set[:,1]
- b_coords = bmap[x_to_set, y_to_set, :]
- f_coords = fmap[x_to_set, y_to_set]
- v_ids = f[f_coords]
- points = (b_coords[:,0,None]*verts[:,v_ids[:,0]]
- + b_coords[:,1,None]*verts[:,v_ids[:,1]]
- + b_coords[:,2,None]*verts[:,v_ids[:,2]])
- normals = (b_coords[:,0,None]*vn[:,v_ids[:,0]]
- + b_coords[:,1,None]*vn[:,v_ids[:,1]]
- + b_coords[:,2,None]*vn[:,v_ids[:,2]])
- return points, normals, vn, f_coords
-
-def estimate_vertex_normals(v, f, ftov):
- face_normals = TriNormalsScaled(v, f)
- non_scaled_normals = torch.einsum('ij,bjk->bik', ftov, face_normals)
- norms = torch.sum(non_scaled_normals ** 2.0, 2) ** 0.5
- norms[norms == 0] = 1.0
- return torch.div(non_scaled_normals, norms[:,:,None])
-
-def TriNormalsScaled(v, f):
- return torch.cross(_edges_for(v, f, 1, 0), _edges_for(v, f, 2, 0))
-
-def _edges_for(v, f, cplus, cminus):
- return v[:,f[:,cplus]] - v[:,f[:,cminus]]
-
-def psbody_get_face_visibility(v, n, f, cams, normal_threshold=0.5):
- bn, nverts, _ = v.shape
- nfaces, _ = f.shape
- vis_f = np.zeros([bn, nfaces], dtype='float32')
- for i in range(bn):
- vis, n_dot_cam = visibility_compute(v=v[i], n=n[i], f=f, cams=cams)
- vis_v = (vis == 1) & (n_dot_cam > normal_threshold)
- vis_f[i] = np.all(vis_v[0,f],1)
- return vis_f
-
-def compute_uvsampler(vt, ft, tex_size=6):
- """
- For this mesh, pre-computes the UV coordinates for
- F x T x T points.
- Returns F x T x T x 2
- """
- uv = obj2nmr_uvmap(ft, vt, tex_size=tex_size)
- uv = uv.reshape(-1, tex_size, tex_size, 2)
- return uv
-
-def obj2nmr_uvmap(ft, vt, tex_size=6):
- """
- Converts obj uv_map to NMR uv_map (F x T x T x 2),
- where tex_size (T) is the sample rate on each face.
- """
- # This is F x 3 x 2
- uv_map_for_verts = vt[ft]
-
- # obj's y coordinate is [1-0], but image is [0-1]
- uv_map_for_verts[:, :, 1] = 1 - uv_map_for_verts[:, :, 1]
-
- # range [0, 1] -> [-1, 1]
- uv_map_for_verts = (2 * uv_map_for_verts) - 1
-
- alpha = np.arange(tex_size, dtype=np.float) / (tex_size - 1)
- beta = np.arange(tex_size, dtype=np.float) / (tex_size - 1)
- import itertools
- # Barycentric coordinate values
- coords = np.stack([p for p in itertools.product(*[alpha, beta])])
-
- # Compute alpha, beta (this is the same order as NMR)
- v2 = uv_map_for_verts[:, 2]
- v0v2 = uv_map_for_verts[:, 0] - uv_map_for_verts[:, 2]
- v1v2 = uv_map_for_verts[:, 1] - uv_map_for_verts[:, 2]
- # Interpolate the vertex uv values: F x 2 x T*2
- uv_map = np.dstack([v0v2, v1v2]).dot(coords.T) + v2.reshape(-1, 2, 1)
-
- # F x T*2 x 2 -> F x T x T x 2
- uv_map = np.transpose(uv_map, (0, 2, 1)).reshape(-1, tex_size, tex_size, 2)
-
- return uv_map
diff --git a/spaces/brjathu/HMR2.0/vendor/detectron2/tests/structures/test_boxes.py b/spaces/brjathu/HMR2.0/vendor/detectron2/tests/structures/test_boxes.py
deleted file mode 100644
index 101191818c511cf90c3c8f2cbc55aa49295697fa..0000000000000000000000000000000000000000
--- a/spaces/brjathu/HMR2.0/vendor/detectron2/tests/structures/test_boxes.py
+++ /dev/null
@@ -1,223 +0,0 @@
-# Copyright (c) Facebook, Inc. and its affiliates.
-import json
-import math
-import numpy as np
-import unittest
-import torch
-
-from detectron2.structures import Boxes, BoxMode, pairwise_ioa, pairwise_iou
-from detectron2.utils.testing import reload_script_model
-
-
-class TestBoxMode(unittest.TestCase):
- def _convert_xy_to_wh(self, x):
- return BoxMode.convert(x, BoxMode.XYXY_ABS, BoxMode.XYWH_ABS)
-
- def _convert_xywha_to_xyxy(self, x):
- return BoxMode.convert(x, BoxMode.XYWHA_ABS, BoxMode.XYXY_ABS)
-
- def _convert_xywh_to_xywha(self, x):
- return BoxMode.convert(x, BoxMode.XYWH_ABS, BoxMode.XYWHA_ABS)
-
- def test_convert_int_mode(self):
- BoxMode.convert([1, 2, 3, 4], 0, 1)
-
- def test_box_convert_list(self):
- for tp in [list, tuple]:
- box = tp([5.0, 5.0, 10.0, 10.0])
- output = self._convert_xy_to_wh(box)
- self.assertIsInstance(output, tp)
- self.assertIsInstance(output[0], float)
- self.assertEqual(output, tp([5.0, 5.0, 5.0, 5.0]))
-
- with self.assertRaises(Exception):
- self._convert_xy_to_wh([box])
-
- def test_box_convert_array(self):
- box = np.asarray([[5, 5, 10, 10], [1, 1, 2, 3]])
- output = self._convert_xy_to_wh(box)
- self.assertEqual(output.dtype, box.dtype)
- self.assertEqual(output.shape, box.shape)
- self.assertTrue((output[0] == [5, 5, 5, 5]).all())
- self.assertTrue((output[1] == [1, 1, 1, 2]).all())
-
- def test_box_convert_cpu_tensor(self):
- box = torch.tensor([[5, 5, 10, 10], [1, 1, 2, 3]])
- output = self._convert_xy_to_wh(box)
- self.assertEqual(output.dtype, box.dtype)
- self.assertEqual(output.shape, box.shape)
- output = output.numpy()
- self.assertTrue((output[0] == [5, 5, 5, 5]).all())
- self.assertTrue((output[1] == [1, 1, 1, 2]).all())
-
- @unittest.skipIf(not torch.cuda.is_available(), "CUDA not available")
- def test_box_convert_cuda_tensor(self):
- box = torch.tensor([[5, 5, 10, 10], [1, 1, 2, 3]]).cuda()
- output = self._convert_xy_to_wh(box)
- self.assertEqual(output.dtype, box.dtype)
- self.assertEqual(output.shape, box.shape)
- self.assertEqual(output.device, box.device)
- output = output.cpu().numpy()
- self.assertTrue((output[0] == [5, 5, 5, 5]).all())
- self.assertTrue((output[1] == [1, 1, 1, 2]).all())
-
- def test_box_convert_xywha_to_xyxy_list(self):
- for tp in [list, tuple]:
- box = tp([50, 50, 30, 20, 0])
- output = self._convert_xywha_to_xyxy(box)
- self.assertIsInstance(output, tp)
- self.assertEqual(output, tp([35, 40, 65, 60]))
-
- with self.assertRaises(Exception):
- self._convert_xywha_to_xyxy([box])
-
- def test_box_convert_xywha_to_xyxy_array(self):
- for dtype in [np.float64, np.float32]:
- box = np.asarray(
- [
- [50, 50, 30, 20, 0],
- [50, 50, 30, 20, 90],
- [1, 1, math.sqrt(2), math.sqrt(2), -45],
- ],
- dtype=dtype,
- )
- output = self._convert_xywha_to_xyxy(box)
- self.assertEqual(output.dtype, box.dtype)
- expected = np.asarray([[35, 40, 65, 60], [40, 35, 60, 65], [0, 0, 2, 2]], dtype=dtype)
- self.assertTrue(np.allclose(output, expected, atol=1e-6), "output={}".format(output))
-
- def test_box_convert_xywha_to_xyxy_tensor(self):
- for dtype in [torch.float32, torch.float64]:
- box = torch.tensor(
- [
- [50, 50, 30, 20, 0],
- [50, 50, 30, 20, 90],
- [1, 1, math.sqrt(2), math.sqrt(2), -45],
- ],
- dtype=dtype,
- )
- output = self._convert_xywha_to_xyxy(box)
- self.assertEqual(output.dtype, box.dtype)
- expected = torch.tensor([[35, 40, 65, 60], [40, 35, 60, 65], [0, 0, 2, 2]], dtype=dtype)
-
- self.assertTrue(torch.allclose(output, expected, atol=1e-6), "output={}".format(output))
-
- def test_box_convert_xywh_to_xywha_list(self):
- for tp in [list, tuple]:
- box = tp([50, 50, 30, 20])
- output = self._convert_xywh_to_xywha(box)
- self.assertIsInstance(output, tp)
- self.assertEqual(output, tp([65, 60, 30, 20, 0]))
-
- with self.assertRaises(Exception):
- self._convert_xywh_to_xywha([box])
-
- def test_box_convert_xywh_to_xywha_array(self):
- for dtype in [np.float64, np.float32]:
- box = np.asarray([[30, 40, 70, 60], [30, 40, 60, 70], [-1, -1, 2, 2]], dtype=dtype)
- output = self._convert_xywh_to_xywha(box)
- self.assertEqual(output.dtype, box.dtype)
- expected = np.asarray(
- [[65, 70, 70, 60, 0], [60, 75, 60, 70, 0], [0, 0, 2, 2, 0]], dtype=dtype
- )
- self.assertTrue(np.allclose(output, expected, atol=1e-6), "output={}".format(output))
-
- def test_box_convert_xywh_to_xywha_tensor(self):
- for dtype in [torch.float32, torch.float64]:
- box = torch.tensor([[30, 40, 70, 60], [30, 40, 60, 70], [-1, -1, 2, 2]], dtype=dtype)
- output = self._convert_xywh_to_xywha(box)
- self.assertEqual(output.dtype, box.dtype)
- expected = torch.tensor(
- [[65, 70, 70, 60, 0], [60, 75, 60, 70, 0], [0, 0, 2, 2, 0]], dtype=dtype
- )
-
- self.assertTrue(torch.allclose(output, expected, atol=1e-6), "output={}".format(output))
-
- def test_json_serializable(self):
- payload = {"box_mode": BoxMode.XYWH_REL}
- try:
- json.dumps(payload)
- except Exception:
- self.fail("JSON serialization failed")
-
- def test_json_deserializable(self):
- payload = '{"box_mode": 2}'
- obj = json.loads(payload)
- try:
- obj["box_mode"] = BoxMode(obj["box_mode"])
- except Exception:
- self.fail("JSON deserialization failed")
-
-
-class TestBoxIOU(unittest.TestCase):
- def create_boxes(self):
- boxes1 = torch.tensor([[0.0, 0.0, 1.0, 1.0], [0.0, 0.0, 1.0, 1.0]])
-
- boxes2 = torch.tensor(
- [
- [0.0, 0.0, 1.0, 1.0],
- [0.0, 0.0, 0.5, 1.0],
- [0.0, 0.0, 1.0, 0.5],
- [0.0, 0.0, 0.5, 0.5],
- [0.5, 0.5, 1.0, 1.0],
- [0.5, 0.5, 1.5, 1.5],
- ]
- )
- return boxes1, boxes2
-
- def test_pairwise_iou(self):
- boxes1, boxes2 = self.create_boxes()
- expected_ious = torch.tensor(
- [
- [1.0, 0.5, 0.5, 0.25, 0.25, 0.25 / (2 - 0.25)],
- [1.0, 0.5, 0.5, 0.25, 0.25, 0.25 / (2 - 0.25)],
- ]
- )
-
- ious = pairwise_iou(Boxes(boxes1), Boxes(boxes2))
- self.assertTrue(torch.allclose(ious, expected_ious))
-
- def test_pairwise_ioa(self):
- boxes1, boxes2 = self.create_boxes()
- expected_ioas = torch.tensor(
- [[1.0, 1.0, 1.0, 1.0, 1.0, 0.25], [1.0, 1.0, 1.0, 1.0, 1.0, 0.25]]
- )
- ioas = pairwise_ioa(Boxes(boxes1), Boxes(boxes2))
- self.assertTrue(torch.allclose(ioas, expected_ioas))
-
-
-class TestBoxes(unittest.TestCase):
- def test_empty_cat(self):
- x = Boxes.cat([])
- self.assertTrue(x.tensor.shape, (0, 4))
-
- def test_to(self):
- x = Boxes(torch.rand(3, 4))
- self.assertEqual(x.to(device="cpu").tensor.device.type, "cpu")
-
- def test_scriptability(self):
- def func(x):
- boxes = Boxes(x)
- test = boxes.to(torch.device("cpu")).tensor
- return boxes.area(), test
-
- f = torch.jit.script(func)
- f = reload_script_model(f)
- f(torch.rand((3, 4)))
-
- data = torch.rand((3, 4))
-
- def func_cat(x: torch.Tensor):
- boxes1 = Boxes(x)
- boxes2 = Boxes(x)
- # boxes3 = Boxes.cat([boxes1, boxes2]) # this is not supported by torchsript for now.
- boxes3 = boxes1.cat([boxes1, boxes2])
- return boxes3
-
- f = torch.jit.script(func_cat)
- script_box = f(data)
- self.assertTrue(torch.equal(torch.cat([data, data]), script_box.tensor))
-
-
-if __name__ == "__main__":
- unittest.main()
diff --git a/spaces/caffeinum/VToonify/vtoonify/model/stylegan/op/__init__.py b/spaces/caffeinum/VToonify/vtoonify/model/stylegan/op/__init__.py
deleted file mode 100644
index d0918d92285955855be89f00096b888ee5597ce3..0000000000000000000000000000000000000000
--- a/spaces/caffeinum/VToonify/vtoonify/model/stylegan/op/__init__.py
+++ /dev/null
@@ -1,2 +0,0 @@
-from .fused_act import FusedLeakyReLU, fused_leaky_relu
-from .upfirdn2d import upfirdn2d
diff --git a/spaces/camilosegura/traductor-multilenguaje/Lib/site-packages/PIL/SpiderImagePlugin.py b/spaces/camilosegura/traductor-multilenguaje/Lib/site-packages/PIL/SpiderImagePlugin.py
deleted file mode 100644
index 5614957c176685c24f0c4cfebb4661d7c856b053..0000000000000000000000000000000000000000
--- a/spaces/camilosegura/traductor-multilenguaje/Lib/site-packages/PIL/SpiderImagePlugin.py
+++ /dev/null
@@ -1,318 +0,0 @@
-#
-# The Python Imaging Library.
-#
-# SPIDER image file handling
-#
-# History:
-# 2004-08-02 Created BB
-# 2006-03-02 added save method
-# 2006-03-13 added support for stack images
-#
-# Copyright (c) 2004 by Health Research Inc. (HRI) RENSSELAER, NY 12144.
-# Copyright (c) 2004 by William Baxter.
-# Copyright (c) 2004 by Secret Labs AB.
-# Copyright (c) 2004 by Fredrik Lundh.
-#
-
-##
-# Image plugin for the Spider image format. This format is used
-# by the SPIDER software, in processing image data from electron
-# microscopy and tomography.
-##
-
-#
-# SpiderImagePlugin.py
-#
-# The Spider image format is used by SPIDER software, in processing
-# image data from electron microscopy and tomography.
-#
-# Spider home page:
-# https://spider.wadsworth.org/spider_doc/spider/docs/spider.html
-#
-# Details about the Spider image format:
-# https://spider.wadsworth.org/spider_doc/spider/docs/image_doc.html
-#
-import os
-import struct
-import sys
-
-from . import Image, ImageFile
-
-
-def isInt(f):
- try:
- i = int(f)
- if f - i == 0:
- return 1
- else:
- return 0
- except (ValueError, OverflowError):
- return 0
-
-
-iforms = [1, 3, -11, -12, -21, -22]
-
-
-# There is no magic number to identify Spider files, so just check a
-# series of header locations to see if they have reasonable values.
-# Returns no. of bytes in the header, if it is a valid Spider header,
-# otherwise returns 0
-
-
-def isSpiderHeader(t):
- h = (99,) + t # add 1 value so can use spider header index start=1
- # header values 1,2,5,12,13,22,23 should be integers
- for i in [1, 2, 5, 12, 13, 22, 23]:
- if not isInt(h[i]):
- return 0
- # check iform
- iform = int(h[5])
- if iform not in iforms:
- return 0
- # check other header values
- labrec = int(h[13]) # no. records in file header
- labbyt = int(h[22]) # total no. of bytes in header
- lenbyt = int(h[23]) # record length in bytes
- if labbyt != (labrec * lenbyt):
- return 0
- # looks like a valid header
- return labbyt
-
-
-def isSpiderImage(filename):
- with open(filename, "rb") as fp:
- f = fp.read(92) # read 23 * 4 bytes
- t = struct.unpack(">23f", f) # try big-endian first
- hdrlen = isSpiderHeader(t)
- if hdrlen == 0:
- t = struct.unpack("<23f", f) # little-endian
- hdrlen = isSpiderHeader(t)
- return hdrlen
-
-
-class SpiderImageFile(ImageFile.ImageFile):
- format = "SPIDER"
- format_description = "Spider 2D image"
- _close_exclusive_fp_after_loading = False
-
- def _open(self):
- # check header
- n = 27 * 4 # read 27 float values
- f = self.fp.read(n)
-
- try:
- self.bigendian = 1
- t = struct.unpack(">27f", f) # try big-endian first
- hdrlen = isSpiderHeader(t)
- if hdrlen == 0:
- self.bigendian = 0
- t = struct.unpack("<27f", f) # little-endian
- hdrlen = isSpiderHeader(t)
- if hdrlen == 0:
- msg = "not a valid Spider file"
- raise SyntaxError(msg)
- except struct.error as e:
- msg = "not a valid Spider file"
- raise SyntaxError(msg) from e
-
- h = (99,) + t # add 1 value : spider header index starts at 1
- iform = int(h[5])
- if iform != 1:
- msg = "not a Spider 2D image"
- raise SyntaxError(msg)
-
- self._size = int(h[12]), int(h[2]) # size in pixels (width, height)
- self.istack = int(h[24])
- self.imgnumber = int(h[27])
-
- if self.istack == 0 and self.imgnumber == 0:
- # stk=0, img=0: a regular 2D image
- offset = hdrlen
- self._nimages = 1
- elif self.istack > 0 and self.imgnumber == 0:
- # stk>0, img=0: Opening the stack for the first time
- self.imgbytes = int(h[12]) * int(h[2]) * 4
- self.hdrlen = hdrlen
- self._nimages = int(h[26])
- # Point to the first image in the stack
- offset = hdrlen * 2
- self.imgnumber = 1
- elif self.istack == 0 and self.imgnumber > 0:
- # stk=0, img>0: an image within the stack
- offset = hdrlen + self.stkoffset
- self.istack = 2 # So Image knows it's still a stack
- else:
- msg = "inconsistent stack header values"
- raise SyntaxError(msg)
-
- if self.bigendian:
- self.rawmode = "F;32BF"
- else:
- self.rawmode = "F;32F"
- self.mode = "F"
-
- self.tile = [("raw", (0, 0) + self.size, offset, (self.rawmode, 0, 1))]
- self._fp = self.fp # FIXME: hack
-
- @property
- def n_frames(self):
- return self._nimages
-
- @property
- def is_animated(self):
- return self._nimages > 1
-
- # 1st image index is zero (although SPIDER imgnumber starts at 1)
- def tell(self):
- if self.imgnumber < 1:
- return 0
- else:
- return self.imgnumber - 1
-
- def seek(self, frame):
- if self.istack == 0:
- msg = "attempt to seek in a non-stack file"
- raise EOFError(msg)
- if not self._seek_check(frame):
- return
- self.stkoffset = self.hdrlen + frame * (self.hdrlen + self.imgbytes)
- self.fp = self._fp
- self.fp.seek(self.stkoffset)
- self._open()
-
- # returns a byte image after rescaling to 0..255
- def convert2byte(self, depth=255):
- (minimum, maximum) = self.getextrema()
- m = 1
- if maximum != minimum:
- m = depth / (maximum - minimum)
- b = -m * minimum
- return self.point(lambda i, m=m, b=b: i * m + b).convert("L")
-
- # returns a ImageTk.PhotoImage object, after rescaling to 0..255
- def tkPhotoImage(self):
- from . import ImageTk
-
- return ImageTk.PhotoImage(self.convert2byte(), palette=256)
-
-
-# --------------------------------------------------------------------
-# Image series
-
-
-# given a list of filenames, return a list of images
-def loadImageSeries(filelist=None):
- """create a list of :py:class:`~PIL.Image.Image` objects for use in a montage"""
- if filelist is None or len(filelist) < 1:
- return
-
- imglist = []
- for img in filelist:
- if not os.path.exists(img):
- print(f"unable to find {img}")
- continue
- try:
- with Image.open(img) as im:
- im = im.convert2byte()
- except Exception:
- if not isSpiderImage(img):
- print(img + " is not a Spider image file")
- continue
- im.info["filename"] = img
- imglist.append(im)
- return imglist
-
-
-# --------------------------------------------------------------------
-# For saving images in Spider format
-
-
-def makeSpiderHeader(im):
- nsam, nrow = im.size
- lenbyt = nsam * 4 # There are labrec records in the header
- labrec = int(1024 / lenbyt)
- if 1024 % lenbyt != 0:
- labrec += 1
- labbyt = labrec * lenbyt
- nvalues = int(labbyt / 4)
- if nvalues < 23:
- return []
-
- hdr = []
- for i in range(nvalues):
- hdr.append(0.0)
-
- # NB these are Fortran indices
- hdr[1] = 1.0 # nslice (=1 for an image)
- hdr[2] = float(nrow) # number of rows per slice
- hdr[3] = float(nrow) # number of records in the image
- hdr[5] = 1.0 # iform for 2D image
- hdr[12] = float(nsam) # number of pixels per line
- hdr[13] = float(labrec) # number of records in file header
- hdr[22] = float(labbyt) # total number of bytes in header
- hdr[23] = float(lenbyt) # record length in bytes
-
- # adjust for Fortran indexing
- hdr = hdr[1:]
- hdr.append(0.0)
- # pack binary data into a string
- return [struct.pack("f", v) for v in hdr]
-
-
-def _save(im, fp, filename):
- if im.mode[0] != "F":
- im = im.convert("F")
-
- hdr = makeSpiderHeader(im)
- if len(hdr) < 256:
- msg = "Error creating Spider header"
- raise OSError(msg)
-
- # write the SPIDER header
- fp.writelines(hdr)
-
- rawmode = "F;32NF" # 32-bit native floating point
- ImageFile._save(im, fp, [("raw", (0, 0) + im.size, 0, (rawmode, 0, 1))])
-
-
-def _save_spider(im, fp, filename):
- # get the filename extension and register it with Image
- ext = os.path.splitext(filename)[1]
- Image.register_extension(SpiderImageFile.format, ext)
- _save(im, fp, filename)
-
-
-# --------------------------------------------------------------------
-
-
-Image.register_open(SpiderImageFile.format, SpiderImageFile)
-Image.register_save(SpiderImageFile.format, _save_spider)
-
-if __name__ == "__main__":
- if len(sys.argv) < 2:
- print("Syntax: python3 SpiderImagePlugin.py [infile] [outfile]")
- sys.exit()
-
- filename = sys.argv[1]
- if not isSpiderImage(filename):
- print("input image must be in Spider format")
- sys.exit()
-
- with Image.open(filename) as im:
- print("image: " + str(im))
- print("format: " + str(im.format))
- print("size: " + str(im.size))
- print("mode: " + str(im.mode))
- print("max, min: ", end=" ")
- print(im.getextrema())
-
- if len(sys.argv) > 2:
- outfile = sys.argv[2]
-
- # perform some image operation
- im = im.transpose(Image.Transpose.FLIP_LEFT_RIGHT)
- print(
- f"saving a flipped version of {os.path.basename(filename)} "
- f"as {outfile} "
- )
- im.save(outfile, SpiderImageFile.format)
diff --git a/spaces/carlosalonso/Detection-video/carpeta_deteccion/datasets/prepare_for_tests.sh b/spaces/carlosalonso/Detection-video/carpeta_deteccion/datasets/prepare_for_tests.sh
deleted file mode 100644
index 67e875a41da652b2fcae6631b76d94584935ddb9..0000000000000000000000000000000000000000
--- a/spaces/carlosalonso/Detection-video/carpeta_deteccion/datasets/prepare_for_tests.sh
+++ /dev/null
@@ -1,31 +0,0 @@
-#!/bin/bash -e
-# Copyright (c) Facebook, Inc. and its affiliates.
-
-# Download the mini dataset (coco val2017_100, with only 100 images)
-# to be used in unittests & integration tests.
-
-cd "${0%/*}"
-
-BASE=https://dl.fbaipublicfiles.com/detectron2
-ROOT=${DETECTRON2_DATASETS:-./}
-ROOT=${ROOT/#\~/$HOME} # expand ~ to HOME
-mkdir -p $ROOT/coco/annotations
-
-for anno in instances_val2017_100 \
- person_keypoints_val2017_100 ; do
-
- dest=$ROOT/coco/annotations/$anno.json
- [[ -s $dest ]] && {
- echo "$dest exists. Skipping ..."
- } || {
- wget $BASE/annotations/coco/$anno.json -O $dest
- }
-done
-
-dest=$ROOT/coco/val2017_100.tgz
-[[ -d $ROOT/coco/val2017 ]] && {
- echo "$ROOT/coco/val2017 exists. Skipping ..."
-} || {
- wget $BASE/annotations/coco/val2017_100.tgz -O $dest
- tar xzf $dest -C $ROOT/coco/ && rm -f $dest
-}
diff --git a/spaces/carlosalonso/Detection-video/carpeta_deteccion/detectron2/checkpoint/__init__.py b/spaces/carlosalonso/Detection-video/carpeta_deteccion/detectron2/checkpoint/__init__.py
deleted file mode 100644
index 99da0469ae7e169d8970e4b642fed3f870076860..0000000000000000000000000000000000000000
--- a/spaces/carlosalonso/Detection-video/carpeta_deteccion/detectron2/checkpoint/__init__.py
+++ /dev/null
@@ -1,10 +0,0 @@
-# -*- coding: utf-8 -*-
-# Copyright (c) Facebook, Inc. and its affiliates.
-# File:
-
-
-from . import catalog as _UNUSED # register the handler
-from .detection_checkpoint import DetectionCheckpointer
-from fvcore.common.checkpoint import Checkpointer, PeriodicCheckpointer
-
-__all__ = ["Checkpointer", "PeriodicCheckpointer", "DetectionCheckpointer"]
diff --git a/spaces/carlosalonso/Detection-video/carpeta_deteccion/projects/DensePose/densepose/data/meshes/builtin.py b/spaces/carlosalonso/Detection-video/carpeta_deteccion/projects/DensePose/densepose/data/meshes/builtin.py
deleted file mode 100644
index c0b23760e8268b068149931b173a4285ba451993..0000000000000000000000000000000000000000
--- a/spaces/carlosalonso/Detection-video/carpeta_deteccion/projects/DensePose/densepose/data/meshes/builtin.py
+++ /dev/null
@@ -1,101 +0,0 @@
-# Copyright (c) Facebook, Inc. and its affiliates. All Rights Reserved
-
-from .catalog import MeshInfo, register_meshes
-
-DENSEPOSE_MESHES_DIR = "https://dl.fbaipublicfiles.com/densepose/meshes/"
-
-MESHES = [
- MeshInfo(
- name="smpl_27554",
- data="smpl_27554.pkl",
- geodists="geodists/geodists_smpl_27554.pkl",
- symmetry="symmetry/symmetry_smpl_27554.pkl",
- texcoords="texcoords/texcoords_smpl_27554.pkl",
- ),
- MeshInfo(
- name="chimp_5029",
- data="chimp_5029.pkl",
- geodists="geodists/geodists_chimp_5029.pkl",
- symmetry="symmetry/symmetry_chimp_5029.pkl",
- texcoords="texcoords/texcoords_chimp_5029.pkl",
- ),
- MeshInfo(
- name="cat_5001",
- data="cat_5001.pkl",
- geodists="geodists/geodists_cat_5001.pkl",
- symmetry="symmetry/symmetry_cat_5001.pkl",
- texcoords="texcoords/texcoords_cat_5001.pkl",
- ),
- MeshInfo(
- name="cat_7466",
- data="cat_7466.pkl",
- geodists="geodists/geodists_cat_7466.pkl",
- symmetry="symmetry/symmetry_cat_7466.pkl",
- texcoords="texcoords/texcoords_cat_7466.pkl",
- ),
- MeshInfo(
- name="sheep_5004",
- data="sheep_5004.pkl",
- geodists="geodists/geodists_sheep_5004.pkl",
- symmetry="symmetry/symmetry_sheep_5004.pkl",
- texcoords="texcoords/texcoords_sheep_5004.pkl",
- ),
- MeshInfo(
- name="zebra_5002",
- data="zebra_5002.pkl",
- geodists="geodists/geodists_zebra_5002.pkl",
- symmetry="symmetry/symmetry_zebra_5002.pkl",
- texcoords="texcoords/texcoords_zebra_5002.pkl",
- ),
- MeshInfo(
- name="horse_5004",
- data="horse_5004.pkl",
- geodists="geodists/geodists_horse_5004.pkl",
- symmetry="symmetry/symmetry_horse_5004.pkl",
- texcoords="texcoords/texcoords_zebra_5002.pkl",
- ),
- MeshInfo(
- name="giraffe_5002",
- data="giraffe_5002.pkl",
- geodists="geodists/geodists_giraffe_5002.pkl",
- symmetry="symmetry/symmetry_giraffe_5002.pkl",
- texcoords="texcoords/texcoords_giraffe_5002.pkl",
- ),
- MeshInfo(
- name="elephant_5002",
- data="elephant_5002.pkl",
- geodists="geodists/geodists_elephant_5002.pkl",
- symmetry="symmetry/symmetry_elephant_5002.pkl",
- texcoords="texcoords/texcoords_elephant_5002.pkl",
- ),
- MeshInfo(
- name="dog_5002",
- data="dog_5002.pkl",
- geodists="geodists/geodists_dog_5002.pkl",
- symmetry="symmetry/symmetry_dog_5002.pkl",
- texcoords="texcoords/texcoords_dog_5002.pkl",
- ),
- MeshInfo(
- name="dog_7466",
- data="dog_7466.pkl",
- geodists="geodists/geodists_dog_7466.pkl",
- symmetry="symmetry/symmetry_dog_7466.pkl",
- texcoords="texcoords/texcoords_dog_7466.pkl",
- ),
- MeshInfo(
- name="cow_5002",
- data="cow_5002.pkl",
- geodists="geodists/geodists_cow_5002.pkl",
- symmetry="symmetry/symmetry_cow_5002.pkl",
- texcoords="texcoords/texcoords_cow_5002.pkl",
- ),
- MeshInfo(
- name="bear_4936",
- data="bear_4936.pkl",
- geodists="geodists/geodists_bear_4936.pkl",
- symmetry="symmetry/symmetry_bear_4936.pkl",
- texcoords="texcoords/texcoords_bear_4936.pkl",
- ),
-]
-
-register_meshes(MESHES, DENSEPOSE_MESHES_DIR)
diff --git a/spaces/carlosalonso/Detection-video/carpeta_deteccion/projects/DensePose/densepose/vis/densepose_outputs_vertex.py b/spaces/carlosalonso/Detection-video/carpeta_deteccion/projects/DensePose/densepose/vis/densepose_outputs_vertex.py
deleted file mode 100644
index 71e5323c2bd3a29bc90e66d7d59d524033c120bf..0000000000000000000000000000000000000000
--- a/spaces/carlosalonso/Detection-video/carpeta_deteccion/projects/DensePose/densepose/vis/densepose_outputs_vertex.py
+++ /dev/null
@@ -1,229 +0,0 @@
-# Copyright (c) Facebook, Inc. and its affiliates. All Rights Reserved
-import json
-import numpy as np
-from functools import lru_cache
-from typing import Dict, List, Optional, Tuple
-import cv2
-import torch
-
-from detectron2.utils.file_io import PathManager
-
-from densepose.modeling import build_densepose_embedder
-from densepose.modeling.cse.utils import get_closest_vertices_mask_from_ES
-
-from ..data.utils import get_class_to_mesh_name_mapping
-from ..structures import DensePoseEmbeddingPredictorOutput
-from ..structures.mesh import create_mesh
-from .base import Boxes, Image, MatrixVisualizer
-from .densepose_results_textures import get_texture_atlas
-
-
-@lru_cache()
-def get_xyz_vertex_embedding(mesh_name: str, device: torch.device):
- if mesh_name == "smpl_27554":
- embed_path = PathManager.get_local_path(
- "https://dl.fbaipublicfiles.com/densepose/data/cse/mds_d=256.npy"
- )
- embed_map, _ = np.load(embed_path, allow_pickle=True)
- embed_map = torch.tensor(embed_map).float()[:, 0]
- embed_map -= embed_map.min()
- embed_map /= embed_map.max()
- else:
- mesh = create_mesh(mesh_name, device)
- embed_map = mesh.vertices.sum(dim=1)
- embed_map -= embed_map.min()
- embed_map /= embed_map.max()
- embed_map = embed_map**2
- return embed_map
-
-
-class DensePoseOutputsVertexVisualizer(object):
- def __init__(
- self,
- cfg,
- inplace=True,
- cmap=cv2.COLORMAP_JET,
- alpha=0.7,
- device="cuda",
- default_class=0,
- **kwargs,
- ):
- self.mask_visualizer = MatrixVisualizer(
- inplace=inplace, cmap=cmap, val_scale=1.0, alpha=alpha
- )
- self.class_to_mesh_name = get_class_to_mesh_name_mapping(cfg)
- self.embedder = build_densepose_embedder(cfg)
- self.device = torch.device(device)
- self.default_class = default_class
-
- self.mesh_vertex_embeddings = {
- mesh_name: self.embedder(mesh_name).to(self.device)
- for mesh_name in self.class_to_mesh_name.values()
- if self.embedder.has_embeddings(mesh_name)
- }
-
- def visualize(
- self,
- image_bgr: Image,
- outputs_boxes_xywh_classes: Tuple[
- Optional[DensePoseEmbeddingPredictorOutput], Optional[Boxes], Optional[List[int]]
- ],
- ) -> Image:
- if outputs_boxes_xywh_classes[0] is None:
- return image_bgr
-
- S, E, N, bboxes_xywh, pred_classes = self.extract_and_check_outputs_and_boxes(
- outputs_boxes_xywh_classes
- )
-
- for n in range(N):
- x, y, w, h = bboxes_xywh[n].int().tolist()
- mesh_name = self.class_to_mesh_name[pred_classes[n]]
- closest_vertices, mask = get_closest_vertices_mask_from_ES(
- E[[n]],
- S[[n]],
- h,
- w,
- self.mesh_vertex_embeddings[mesh_name],
- self.device,
- )
- embed_map = get_xyz_vertex_embedding(mesh_name, self.device)
- vis = (embed_map[closest_vertices].clip(0, 1) * 255.0).cpu().numpy()
- mask_numpy = mask.cpu().numpy().astype(dtype=np.uint8)
- image_bgr = self.mask_visualizer.visualize(image_bgr, mask_numpy, vis, [x, y, w, h])
-
- return image_bgr
-
- def extract_and_check_outputs_and_boxes(self, outputs_boxes_xywh_classes):
-
- densepose_output, bboxes_xywh, pred_classes = outputs_boxes_xywh_classes
-
- if pred_classes is None:
- pred_classes = [self.default_class] * len(bboxes_xywh)
-
- assert isinstance(
- densepose_output, DensePoseEmbeddingPredictorOutput
- ), "DensePoseEmbeddingPredictorOutput expected, {} encountered".format(
- type(densepose_output)
- )
-
- S = densepose_output.coarse_segm
- E = densepose_output.embedding
- N = S.size(0)
- assert N == E.size(
- 0
- ), "CSE coarse_segm {} and embeddings {}" " should have equal first dim size".format(
- S.size(), E.size()
- )
- assert N == len(
- bboxes_xywh
- ), "number of bounding boxes {}" " should be equal to first dim size of outputs {}".format(
- len(bboxes_xywh), N
- )
- assert N == len(pred_classes), (
- "number of predicted classes {}"
- " should be equal to first dim size of outputs {}".format(len(bboxes_xywh), N)
- )
-
- return S, E, N, bboxes_xywh, pred_classes
-
-
-def get_texture_atlases(json_str: Optional[str]) -> Optional[Dict[str, Optional[np.ndarray]]]:
- """
- json_str is a JSON string representing a mesh_name -> texture_atlas_path dictionary
- """
- if json_str is None:
- return None
-
- paths = json.loads(json_str)
- return {mesh_name: get_texture_atlas(path) for mesh_name, path in paths.items()}
-
-
-class DensePoseOutputsTextureVisualizer(DensePoseOutputsVertexVisualizer):
- def __init__(
- self,
- cfg,
- texture_atlases_dict,
- device="cuda",
- default_class=0,
- **kwargs,
- ):
- self.embedder = build_densepose_embedder(cfg)
-
- self.texture_image_dict = {}
- self.alpha_dict = {}
-
- for mesh_name in texture_atlases_dict.keys():
- if texture_atlases_dict[mesh_name].shape[-1] == 4: # Image with alpha channel
- self.alpha_dict[mesh_name] = texture_atlases_dict[mesh_name][:, :, -1] / 255.0
- self.texture_image_dict[mesh_name] = texture_atlases_dict[mesh_name][:, :, :3]
- else:
- self.alpha_dict[mesh_name] = texture_atlases_dict[mesh_name].sum(axis=-1) > 0
- self.texture_image_dict[mesh_name] = texture_atlases_dict[mesh_name]
-
- self.device = torch.device(device)
- self.class_to_mesh_name = get_class_to_mesh_name_mapping(cfg)
- self.default_class = default_class
-
- self.mesh_vertex_embeddings = {
- mesh_name: self.embedder(mesh_name).to(self.device)
- for mesh_name in self.class_to_mesh_name.values()
- }
-
- def visualize(
- self,
- image_bgr: Image,
- outputs_boxes_xywh_classes: Tuple[
- Optional[DensePoseEmbeddingPredictorOutput], Optional[Boxes], Optional[List[int]]
- ],
- ) -> Image:
- image_target_bgr = image_bgr.copy()
- if outputs_boxes_xywh_classes[0] is None:
- return image_target_bgr
-
- S, E, N, bboxes_xywh, pred_classes = self.extract_and_check_outputs_and_boxes(
- outputs_boxes_xywh_classes
- )
-
- meshes = {
- p: create_mesh(self.class_to_mesh_name[p], self.device) for p in np.unique(pred_classes)
- }
-
- for n in range(N):
- x, y, w, h = bboxes_xywh[n].int().cpu().numpy()
- mesh_name = self.class_to_mesh_name[pred_classes[n]]
- closest_vertices, mask = get_closest_vertices_mask_from_ES(
- E[[n]],
- S[[n]],
- h,
- w,
- self.mesh_vertex_embeddings[mesh_name],
- self.device,
- )
- uv_array = meshes[pred_classes[n]].texcoords[closest_vertices].permute((2, 0, 1))
- uv_array = uv_array.cpu().numpy().clip(0, 1)
- textured_image = self.generate_image_with_texture(
- image_target_bgr[y : y + h, x : x + w],
- uv_array,
- mask.cpu().numpy(),
- self.class_to_mesh_name[pred_classes[n]],
- )
- if textured_image is None:
- continue
- image_target_bgr[y : y + h, x : x + w] = textured_image
-
- return image_target_bgr
-
- def generate_image_with_texture(self, bbox_image_bgr, uv_array, mask, mesh_name):
- alpha = self.alpha_dict.get(mesh_name)
- texture_image = self.texture_image_dict.get(mesh_name)
- if alpha is None or texture_image is None:
- return None
- U, V = uv_array
- x_index = (U * texture_image.shape[1]).astype(int)
- y_index = (V * texture_image.shape[0]).astype(int)
- local_texture = texture_image[y_index, x_index][mask]
- local_alpha = np.expand_dims(alpha[y_index, x_index][mask], -1)
- output_image = bbox_image_bgr.copy()
- output_image[mask] = output_image[mask] * (1 - local_alpha) + local_texture * local_alpha
- return output_image.astype(np.uint8)
diff --git a/spaces/chansung/hf-inference-endpoint/README.md b/spaces/chansung/hf-inference-endpoint/README.md
deleted file mode 100644
index 8ec85149ae99b51b4e0e3ee5d37cd6736f256f04..0000000000000000000000000000000000000000
--- a/spaces/chansung/hf-inference-endpoint/README.md
+++ /dev/null
@@ -1,13 +0,0 @@
----
-title: Hf Inference Endpoint
-emoji: 🏃
-colorFrom: blue
-colorTo: gray
-sdk: gradio
-sdk_version: 3.41.2
-app_file: app.py
-pinned: false
-license: apache-2.0
----
-
-Check out the configuration reference at https://huggingface.co/docs/hub/spaces-config-reference
diff --git a/spaces/chasemcdo/hf_localai/examples/autoGPT/README.md b/spaces/chasemcdo/hf_localai/examples/autoGPT/README.md
deleted file mode 100644
index f5269a3a0f3ccaff24d136bfe6f43a28fdd6a976..0000000000000000000000000000000000000000
--- a/spaces/chasemcdo/hf_localai/examples/autoGPT/README.md
+++ /dev/null
@@ -1,32 +0,0 @@
-# AutoGPT
-
-Example of integration with [AutoGPT](https://github.com/Significant-Gravitas/Auto-GPT).
-
-## Run
-
-```bash
-# Clone LocalAI
-git clone https://github.com/go-skynet/LocalAI
-
-cd LocalAI/examples/autoGPT
-
-docker-compose run --rm auto-gpt
-```
-
-Note: The example automatically downloads the `gpt4all` model as it is under a permissive license. The GPT4All model does not seem to be enough to run AutoGPT. WizardLM-7b-uncensored seems to perform better (with `f16: true`).
-
-See the `.env` configuration file to set a different model with the [model-gallery](https://github.com/go-skynet/model-gallery) by editing `PRELOAD_MODELS`.
-
-## Without docker
-
-Run AutoGPT with `OPENAI_API_BASE` pointing to the LocalAI endpoint. If you run it locally for instance:
-
-```
-OPENAI_API_BASE=http://localhost:8080 python ...
-```
-
-Note: you need a model named `gpt-3.5-turbo` and `text-embedding-ada-002`. You can preload those in LocalAI at start by setting in the env:
-
-```
-PRELOAD_MODELS=[{"url": "github:go-skynet/model-gallery/gpt4all-j.yaml", "name": "gpt-3.5-turbo"}, { "url": "github:go-skynet/model-gallery/bert-embeddings.yaml", "name": "text-embedding-ada-002"}]
-```
\ No newline at end of file
diff --git a/spaces/chendl/compositional_test/multimodal/YOLOX/yolox/utils/__init__.py b/spaces/chendl/compositional_test/multimodal/YOLOX/yolox/utils/__init__.py
deleted file mode 100644
index 08e6dae986b367ec1806c271b0c371cd17e89133..0000000000000000000000000000000000000000
--- a/spaces/chendl/compositional_test/multimodal/YOLOX/yolox/utils/__init__.py
+++ /dev/null
@@ -1,16 +0,0 @@
-#!/usr/bin/env python3
-# Copyright (c) Megvii Inc. All rights reserved.
-
-from .allreduce_norm import *
-from .boxes import *
-from .checkpoint import load_ckpt, save_checkpoint
-from .compat import meshgrid
-from .demo_utils import *
-from .dist import *
-from .ema import *
-from .logger import WandbLogger, setup_logger
-from .lr_scheduler import LRScheduler
-from .metric import *
-from .model_utils import *
-from .setup_env import *
-from .visualize import *
diff --git a/spaces/chronopt-research/ViTExCo/src/data/functional.py b/spaces/chronopt-research/ViTExCo/src/data/functional.py
deleted file mode 100644
index 14aa7882d3dfca1ba6649d0b7fdb2c443e3b7f20..0000000000000000000000000000000000000000
--- a/spaces/chronopt-research/ViTExCo/src/data/functional.py
+++ /dev/null
@@ -1,84 +0,0 @@
-from __future__ import division
-
-import torch
-import numbers
-import collections
-import numpy as np
-from PIL import Image, ImageOps
-
-
-def _is_pil_image(img):
- return isinstance(img, Image.Image)
-
-
-def _is_tensor_image(img):
- return torch.is_tensor(img) and img.ndimension() == 3
-
-
-def _is_numpy_image(img):
- return isinstance(img, np.ndarray) and (img.ndim in {2, 3})
-
-
-def to_mytensor(pic):
- pic_arr = np.array(pic)
- if pic_arr.ndim == 2:
- pic_arr = pic_arr[..., np.newaxis]
- img = torch.from_numpy(pic_arr.transpose((2, 0, 1)))
- if not isinstance(img, torch.FloatTensor):
- return img.float() # no normalize .div(255)
- else:
- return img
-
-
-def normalize(tensor, mean, std):
- if not _is_tensor_image(tensor):
- raise TypeError("tensor is not a torch image.")
- if tensor.size(0) == 1:
- tensor.sub_(mean).div_(std)
- else:
- for t, m, s in zip(tensor, mean, std):
- t.sub_(m).div_(s)
- return tensor
-
-
-def resize(img, size, interpolation=Image.BILINEAR):
- if not _is_pil_image(img):
- raise TypeError("img should be PIL Image. Got {}".format(type(img)))
- if not isinstance(size, int) and (not isinstance(size, collections.Iterable) or len(size) != 2):
- raise TypeError("Got inappropriate size arg: {}".format(size))
-
- if not isinstance(size, int):
- return img.resize(size[::-1], interpolation)
-
- w, h = img.size
- if (w <= h and w == size) or (h <= w and h == size):
- return img
- if w < h:
- ow = size
- oh = int(round(size * h / w))
- else:
- oh = size
- ow = int(round(size * w / h))
- return img.resize((ow, oh), interpolation)
-
-
-def pad(img, padding, fill=0):
- if not _is_pil_image(img):
- raise TypeError("img should be PIL Image. Got {}".format(type(img)))
-
- if not isinstance(padding, (numbers.Number, tuple)):
- raise TypeError("Got inappropriate padding arg")
- if not isinstance(fill, (numbers.Number, str, tuple)):
- raise TypeError("Got inappropriate fill arg")
-
- if isinstance(padding, collections.Sequence) and len(padding) not in [2, 4]:
- raise ValueError("Padding must be an int or a 2, or 4 element tuple, not a " + "{} element tuple".format(len(padding)))
-
- return ImageOps.expand(img, border=padding, fill=fill)
-
-
-def crop(img, i, j, h, w):
- if not _is_pil_image(img):
- raise TypeError("img should be PIL Image. Got {}".format(type(img)))
-
- return img.crop((j, i, j + w, i + h))
diff --git a/spaces/chuan-hd/law-assistant-chatbot/.venv/lib/python3.11/site-packages/PIL/ImageQt.py b/spaces/chuan-hd/law-assistant-chatbot/.venv/lib/python3.11/site-packages/PIL/ImageQt.py
deleted file mode 100644
index 9b7245454dfcccb4e822a6634168d405c0e791bb..0000000000000000000000000000000000000000
--- a/spaces/chuan-hd/law-assistant-chatbot/.venv/lib/python3.11/site-packages/PIL/ImageQt.py
+++ /dev/null
@@ -1,216 +0,0 @@
-#
-# The Python Imaging Library.
-# $Id$
-#
-# a simple Qt image interface.
-#
-# history:
-# 2006-06-03 fl: created
-# 2006-06-04 fl: inherit from QImage instead of wrapping it
-# 2006-06-05 fl: removed toimage helper; move string support to ImageQt
-# 2013-11-13 fl: add support for Qt5 (aurelien.ballier@cyclonit.com)
-#
-# Copyright (c) 2006 by Secret Labs AB
-# Copyright (c) 2006 by Fredrik Lundh
-#
-# See the README file for information on usage and redistribution.
-#
-
-import sys
-from io import BytesIO
-
-from . import Image
-from ._util import is_path
-
-qt_versions = [
- ["6", "PyQt6"],
- ["side6", "PySide6"],
-]
-
-# If a version has already been imported, attempt it first
-qt_versions.sort(key=lambda qt_version: qt_version[1] in sys.modules, reverse=True)
-for qt_version, qt_module in qt_versions:
- try:
- if qt_module == "PyQt6":
- from PyQt6.QtCore import QBuffer, QIODevice
- from PyQt6.QtGui import QImage, QPixmap, qRgba
- elif qt_module == "PySide6":
- from PySide6.QtCore import QBuffer, QIODevice
- from PySide6.QtGui import QImage, QPixmap, qRgba
- except (ImportError, RuntimeError):
- continue
- qt_is_installed = True
- break
-else:
- qt_is_installed = False
- qt_version = None
-
-
-def rgb(r, g, b, a=255):
- """(Internal) Turns an RGB color into a Qt compatible color integer."""
- # use qRgb to pack the colors, and then turn the resulting long
- # into a negative integer with the same bitpattern.
- return qRgba(r, g, b, a) & 0xFFFFFFFF
-
-
-def fromqimage(im):
- """
- :param im: QImage or PIL ImageQt object
- """
- buffer = QBuffer()
- if qt_version == "6":
- try:
- qt_openmode = QIODevice.OpenModeFlag
- except AttributeError:
- qt_openmode = QIODevice.OpenMode
- else:
- qt_openmode = QIODevice
- buffer.open(qt_openmode.ReadWrite)
- # preserve alpha channel with png
- # otherwise ppm is more friendly with Image.open
- if im.hasAlphaChannel():
- im.save(buffer, "png")
- else:
- im.save(buffer, "ppm")
-
- b = BytesIO()
- b.write(buffer.data())
- buffer.close()
- b.seek(0)
-
- return Image.open(b)
-
-
-def fromqpixmap(im):
- return fromqimage(im)
- # buffer = QBuffer()
- # buffer.open(QIODevice.ReadWrite)
- # # im.save(buffer)
- # # What if png doesn't support some image features like animation?
- # im.save(buffer, 'ppm')
- # bytes_io = BytesIO()
- # bytes_io.write(buffer.data())
- # buffer.close()
- # bytes_io.seek(0)
- # return Image.open(bytes_io)
-
-
-def align8to32(bytes, width, mode):
- """
- converts each scanline of data from 8 bit to 32 bit aligned
- """
-
- bits_per_pixel = {"1": 1, "L": 8, "P": 8, "I;16": 16}[mode]
-
- # calculate bytes per line and the extra padding if needed
- bits_per_line = bits_per_pixel * width
- full_bytes_per_line, remaining_bits_per_line = divmod(bits_per_line, 8)
- bytes_per_line = full_bytes_per_line + (1 if remaining_bits_per_line else 0)
-
- extra_padding = -bytes_per_line % 4
-
- # already 32 bit aligned by luck
- if not extra_padding:
- return bytes
-
- new_data = []
- for i in range(len(bytes) // bytes_per_line):
- new_data.append(
- bytes[i * bytes_per_line : (i + 1) * bytes_per_line]
- + b"\x00" * extra_padding
- )
-
- return b"".join(new_data)
-
-
-def _toqclass_helper(im):
- data = None
- colortable = None
- exclusive_fp = False
-
- # handle filename, if given instead of image name
- if hasattr(im, "toUtf8"):
- # FIXME - is this really the best way to do this?
- im = str(im.toUtf8(), "utf-8")
- if is_path(im):
- im = Image.open(im)
- exclusive_fp = True
-
- qt_format = QImage.Format if qt_version == "6" else QImage
- if im.mode == "1":
- format = qt_format.Format_Mono
- elif im.mode == "L":
- format = qt_format.Format_Indexed8
- colortable = []
- for i in range(256):
- colortable.append(rgb(i, i, i))
- elif im.mode == "P":
- format = qt_format.Format_Indexed8
- colortable = []
- palette = im.getpalette()
- for i in range(0, len(palette), 3):
- colortable.append(rgb(*palette[i : i + 3]))
- elif im.mode == "RGB":
- # Populate the 4th channel with 255
- im = im.convert("RGBA")
-
- data = im.tobytes("raw", "BGRA")
- format = qt_format.Format_RGB32
- elif im.mode == "RGBA":
- data = im.tobytes("raw", "BGRA")
- format = qt_format.Format_ARGB32
- elif im.mode == "I;16" and hasattr(qt_format, "Format_Grayscale16"): # Qt 5.13+
- im = im.point(lambda i: i * 256)
-
- format = qt_format.Format_Grayscale16
- else:
- if exclusive_fp:
- im.close()
- msg = f"unsupported image mode {repr(im.mode)}"
- raise ValueError(msg)
-
- size = im.size
- __data = data or align8to32(im.tobytes(), size[0], im.mode)
- if exclusive_fp:
- im.close()
- return {"data": __data, "size": size, "format": format, "colortable": colortable}
-
-
-if qt_is_installed:
-
- class ImageQt(QImage):
- def __init__(self, im):
- """
- An PIL image wrapper for Qt. This is a subclass of PyQt's QImage
- class.
-
- :param im: A PIL Image object, or a file name (given either as
- Python string or a PyQt string object).
- """
- im_data = _toqclass_helper(im)
- # must keep a reference, or Qt will crash!
- # All QImage constructors that take data operate on an existing
- # buffer, so this buffer has to hang on for the life of the image.
- # Fixes https://github.com/python-pillow/Pillow/issues/1370
- self.__data = im_data["data"]
- super().__init__(
- self.__data,
- im_data["size"][0],
- im_data["size"][1],
- im_data["format"],
- )
- if im_data["colortable"]:
- self.setColorTable(im_data["colortable"])
-
-
-def toqimage(im):
- return ImageQt(im)
-
-
-def toqpixmap(im):
- # # This doesn't work. For now using a dumb approach.
- # im_data = _toqclass_helper(im)
- # result = QPixmap(im_data["size"][0], im_data["size"][1])
- # result.loadFromData(im_data["data"])
- qimage = toqimage(im)
- return QPixmap.fromImage(qimage)
diff --git a/spaces/chuan-hd/law-assistant-chatbot/.venv/lib/python3.11/site-packages/fontTools/ttLib/tables/M_V_A_R_.py b/spaces/chuan-hd/law-assistant-chatbot/.venv/lib/python3.11/site-packages/fontTools/ttLib/tables/M_V_A_R_.py
deleted file mode 100644
index 8371795eb2f2d2c233ec1725b8a2c21453170f23..0000000000000000000000000000000000000000
--- a/spaces/chuan-hd/law-assistant-chatbot/.venv/lib/python3.11/site-packages/fontTools/ttLib/tables/M_V_A_R_.py
+++ /dev/null
@@ -1,5 +0,0 @@
-from .otBase import BaseTTXConverter
-
-
-class table_M_V_A_R_(BaseTTXConverter):
- pass
diff --git a/spaces/chyh/chatbot/README.md b/spaces/chyh/chatbot/README.md
deleted file mode 100644
index 49094ca9fdb57cc8d79b87729796dbd1e1a24b90..0000000000000000000000000000000000000000
--- a/spaces/chyh/chatbot/README.md
+++ /dev/null
@@ -1,14 +0,0 @@
----
-title: ChatGPT with Plugins
-emoji: ⚡
-colorFrom: blue
-colorTo: yellow
-sdk: docker
-pinned: false
-license: mit
-app_port: 3000
----
-免费key的来源:
-https://laogou717.com/page/GPT4FREE/GPT4Free.html
-
-Check out the configuration reference at https://huggingface.co/docs/hub/spaces-config-reference
\ No newline at end of file
diff --git a/spaces/cihyFjudo/fairness-paper-search/DCB Akhila Vijnana Kosam - Encyclopedia In Malayalam! Download Pc Tool and Enhance Your Skills.md b/spaces/cihyFjudo/fairness-paper-search/DCB Akhila Vijnana Kosam - Encyclopedia In Malayalam! Download Pc Tool and Enhance Your Skills.md
deleted file mode 100644
index e199463c617384d1b03cef62ae36a743b4e8cef0..0000000000000000000000000000000000000000
--- a/spaces/cihyFjudo/fairness-paper-search/DCB Akhila Vijnana Kosam - Encyclopedia In Malayalam! Download Pc Tool and Enhance Your Skills.md
+++ /dev/null
@@ -1,6 +0,0 @@
-
DCB Akhila Vijnana Kosam - Encyclopedia In Malayalam! Download Pc
-
- aaccfb2cb3
-
-
-
diff --git a/spaces/cihyFjudo/fairness-paper-search/HACK Garmin Taiwan City Navigator V8.6 (CHN) How to Make Your Garmin Device More Powerful and Versatile with This Hack.md b/spaces/cihyFjudo/fairness-paper-search/HACK Garmin Taiwan City Navigator V8.6 (CHN) How to Make Your Garmin Device More Powerful and Versatile with This Hack.md
deleted file mode 100644
index d154102fea5faf2aaf4b4781d07ad2074d48e14a..0000000000000000000000000000000000000000
--- a/spaces/cihyFjudo/fairness-paper-search/HACK Garmin Taiwan City Navigator V8.6 (CHN) How to Make Your Garmin Device More Powerful and Versatile with This Hack.md
+++ /dev/null
@@ -1,6 +0,0 @@
-
-
- aaccfb2cb3
-
-
-
diff --git a/spaces/cihyFjudo/fairness-paper-search/Lord Of The Rings Games For Mac Download Which One is Right for You?.md b/spaces/cihyFjudo/fairness-paper-search/Lord Of The Rings Games For Mac Download Which One is Right for You?.md
deleted file mode 100644
index f18e35acab77d90d894d669c9b4e73f8cd6ce4b4..0000000000000000000000000000000000000000
--- a/spaces/cihyFjudo/fairness-paper-search/Lord Of The Rings Games For Mac Download Which One is Right for You?.md
+++ /dev/null
@@ -1,19 +0,0 @@
-
-
For any PlayStation Now games you had downloaded and played locally, the save data is stored on your local console storage device. If you have access to the game through PlayStation Plus or other means, you should be able to continue your game where you left off. For any games that you had been streaming, the save data was stored within the PlayStation Now cloud streaming storage. If the game is included in the Game Catalog or Classics Catalog within the PlayStation Plus membership benefits, you can continue to stream the game with a PlayStation Plus Premium membership using your previous cloud save file. You can also access the cloud save file and transfer it to your PlayStation Plus cloud storage and then download it to your local console.
-
PS Store and PS Now subject to terms of use and country and language restrictions. Service availability is not guaranteed. For PS Now on PC, minimum system requirements apply and can be found at www.playstation.com/psnow-pc-faq. PS Now games may differ from or lack some of the features that can be found in downloaded or disc-based games. Games included in PS Now are subject to change at any time. Approved payment method details required. PS Now subscription is an ongoing subscription with a recurring subscription fee which is charged every month (at the then current Store price).
This license is commonly used for video games and it allows users to download and play the game for free. Basically, a product is offered Free to Play (Freemium) and the user can decide if he wants to pay the money (Premium) for additional features, services, virtual or physical goods that expand the functionality of the game. In some cases, ads may be show to the users.
-
Having trouble with your games not loading in Origin? Repair Game checks your game's installation and then automatically downloads any replacement or missing files. If there are any file issues or corrupt files, it will replace them or download them again.
-
One of the best ways to learn War of the Ring strategy is to replay log files which record entire games. For example, every log file from the 2016 International Online Tournament can be downloaded and studied here. The games are links that say fr (Free People Ring victory, reached the Cracks of Doom without being corrupted), fm (Free People Military victory, Free Peoples achieved 4 Victory Points), sr (Shadow Ring victory, the Fellowship was corrupted), sm (Shdadow Military victory, Shadow achieved 10 Victory Points). To use these, open the War of the Ring client in the base game (these are all base 2nd Edition games) and then go to the Replay menu > Load Replay File... and select a log file. Turn up the speed with Ctrl-Shift-Equals (5 to 8 times is good to get a decent speed), then start the game replay with Ctrl-Space. Pause/Play the game throughout playback again with Ctrl-Space. Play/Pause (Ctrl-Space), Faster (Ctrl-Shift-Equals), Slower (Ctrl-Minus), Next Breakpoint (Ctrl-B), Insert Breakpoint (no shortcut), Step (Space) Note: you can't make the game log go backwards using the replay tool, but you can see each action in the output at bottom right to look at anything you missed.
-
The following is a quick 3-day tournament with 8 players that was played over the weekend of 28th-30th September 2012. All 8 players had to commit to 5 games each over the 3 days, but this is a fantastic way to run a short format tournament and hopefully more of these will be organised. 28th-30th September 2012 Weekend Tournament: (Event Pairings & Results), (Event Stages & Game Log Files)
-
-
Summary: If you're looking for ways to uninstall Steam and Steam games on Mac, you've just come to the right place. In the article, we'll introduce you to how to completely remove Steam and downloaded games on it from your Mac.
-
The game will have a total of twelve locations or maps, with two downloadable maps for the Good Campaign. These maps have been mentioned as being much larger than those in Battlefront. For players who wish to get straight into the game the game features instant action like the previous battlefront games you will get to play any army you want straight away. The game's multiplayer modes will allow up to 16 players, but battles may feature as many as 150 additional AI-controlled troops.
-
World of Warcraft is another scintillating game from Blizzard that has made it to the list of best free Mac games. The game is comprised of one of the most massive virtual open worlds ever created. Because of this, it is one of the most addictive free to play mac games. Players get to pick characters from a wide range of classes and races which are split among two warring parties, the Alliance or the Horde. Every class has its own particular style of playing and each race that fits the class brings with it a few of its own individual passives, rendering players a range of different ways in which they can choose to play the world of warcraft.
-
With the recent Game Manger update, select games can be played on macOS Catalina (10.15) and above, but downloads must be initiated in the Game Manger. If you have already updated your macOS, see these instructions for which games are compatible and how you can install them.
-
Just as digital replaced discs and cartridges, streaming now threatens to eclipse digital game purchases. On a MacBook Air, streaming solves key problems. Because servers do the heavy lifting and the Mac only interprets input, computer specs and architecture are irrelevant. And that brings more games to the platform.
-
This is important only because you should probably keep an eye on how much you're downloading. While most 8- and 16-bit game ROMs only take up a few kilobytes or megabytes of room, files for more modern system will begin to take up hundreds of megabytes or even several gigabytes. Some PlayStation and GameCube games can even require you to download multiple discs to get the whole game.
-
People love free steam games, no doubt. But what many people hate is downloading so many parts and trying to install them on their own. This is why we are the only site that pre-installs every game for you. We have many categories like shooters, action, racing, simulators and even VR games! We strive to satisfy our users and ask for nothing in return. We revolutionized the downloading scene and will continue being your #1 site for free games.
-
While playing games on Elden Ring, sometimes users may start facing issues while turning the console on and off. The problem majorly arises with the game's download files, making the access even more complex. It seems pretty annoying if you have to launch the game and your loading screen starts freezing on. Just be calm at the moment and try the different solutions we are providing you below to get rid of it.
aaccfb2cb3
-
-
\ No newline at end of file
diff --git a/spaces/cihyFjudo/fairness-paper-search/Samurai.Warriors.2-RELOADED Skidrow Reloaded Download and Install Guide.md b/spaces/cihyFjudo/fairness-paper-search/Samurai.Warriors.2-RELOADED Skidrow Reloaded Download and Install Guide.md
deleted file mode 100644
index faf059af15f9a49a9efb193136cbf9de59df2498..0000000000000000000000000000000000000000
--- a/spaces/cihyFjudo/fairness-paper-search/Samurai.Warriors.2-RELOADED Skidrow Reloaded Download and Install Guide.md
+++ /dev/null
@@ -1,6 +0,0 @@
-
-
- aaccfb2cb3
-
-
-
diff --git a/spaces/cihyFjudo/fairness-paper-search/Vintage Hollywood Posters ebook rar Enjoy the Nostalgia and Glamour of Golden Age Hollywood.md b/spaces/cihyFjudo/fairness-paper-search/Vintage Hollywood Posters ebook rar Enjoy the Nostalgia and Glamour of Golden Age Hollywood.md
deleted file mode 100644
index 049ff8379967889ac6f507214010ef54ed607f64..0000000000000000000000000000000000000000
--- a/spaces/cihyFjudo/fairness-paper-search/Vintage Hollywood Posters ebook rar Enjoy the Nostalgia and Glamour of Golden Age Hollywood.md
+++ /dev/null
@@ -1,6 +0,0 @@
-
-
- aaccfb2cb3
-
-
-
diff --git a/spaces/cloudtheboi/Lofi4All/.pythonlibs/lib/python3.10/site-packages/cffi/ffiplatform.py b/spaces/cloudtheboi/Lofi4All/.pythonlibs/lib/python3.10/site-packages/cffi/ffiplatform.py
deleted file mode 100644
index 85313460a69477513c8e00f4df430925f2c4ecc9..0000000000000000000000000000000000000000
--- a/spaces/cloudtheboi/Lofi4All/.pythonlibs/lib/python3.10/site-packages/cffi/ffiplatform.py
+++ /dev/null
@@ -1,127 +0,0 @@
-import sys, os
-from .error import VerificationError
-
-
-LIST_OF_FILE_NAMES = ['sources', 'include_dirs', 'library_dirs',
- 'extra_objects', 'depends']
-
-def get_extension(srcfilename, modname, sources=(), **kwds):
- _hack_at_distutils()
- from distutils.core import Extension
- allsources = [srcfilename]
- for src in sources:
- allsources.append(os.path.normpath(src))
- return Extension(name=modname, sources=allsources, **kwds)
-
-def compile(tmpdir, ext, compiler_verbose=0, debug=None):
- """Compile a C extension module using distutils."""
-
- _hack_at_distutils()
- saved_environ = os.environ.copy()
- try:
- outputfilename = _build(tmpdir, ext, compiler_verbose, debug)
- outputfilename = os.path.abspath(outputfilename)
- finally:
- # workaround for a distutils bugs where some env vars can
- # become longer and longer every time it is used
- for key, value in saved_environ.items():
- if os.environ.get(key) != value:
- os.environ[key] = value
- return outputfilename
-
-def _build(tmpdir, ext, compiler_verbose=0, debug=None):
- # XXX compact but horrible :-(
- from distutils.core import Distribution
- import distutils.errors, distutils.log
- #
- dist = Distribution({'ext_modules': [ext]})
- dist.parse_config_files()
- options = dist.get_option_dict('build_ext')
- if debug is None:
- debug = sys.flags.debug
- options['debug'] = ('ffiplatform', debug)
- options['force'] = ('ffiplatform', True)
- options['build_lib'] = ('ffiplatform', tmpdir)
- options['build_temp'] = ('ffiplatform', tmpdir)
- #
- try:
- old_level = distutils.log.set_threshold(0) or 0
- try:
- distutils.log.set_verbosity(compiler_verbose)
- dist.run_command('build_ext')
- cmd_obj = dist.get_command_obj('build_ext')
- [soname] = cmd_obj.get_outputs()
- finally:
- distutils.log.set_threshold(old_level)
- except (distutils.errors.CompileError,
- distutils.errors.LinkError) as e:
- raise VerificationError('%s: %s' % (e.__class__.__name__, e))
- #
- return soname
-
-try:
- from os.path import samefile
-except ImportError:
- def samefile(f1, f2):
- return os.path.abspath(f1) == os.path.abspath(f2)
-
-def maybe_relative_path(path):
- if not os.path.isabs(path):
- return path # already relative
- dir = path
- names = []
- while True:
- prevdir = dir
- dir, name = os.path.split(prevdir)
- if dir == prevdir or not dir:
- return path # failed to make it relative
- names.append(name)
- try:
- if samefile(dir, os.curdir):
- names.reverse()
- return os.path.join(*names)
- except OSError:
- pass
-
-# ____________________________________________________________
-
-try:
- int_or_long = (int, long)
- import cStringIO
-except NameError:
- int_or_long = int # Python 3
- import io as cStringIO
-
-def _flatten(x, f):
- if isinstance(x, str):
- f.write('%ds%s' % (len(x), x))
- elif isinstance(x, dict):
- keys = sorted(x.keys())
- f.write('%dd' % len(keys))
- for key in keys:
- _flatten(key, f)
- _flatten(x[key], f)
- elif isinstance(x, (list, tuple)):
- f.write('%dl' % len(x))
- for value in x:
- _flatten(value, f)
- elif isinstance(x, int_or_long):
- f.write('%di' % (x,))
- else:
- raise TypeError(
- "the keywords to verify() contains unsupported object %r" % (x,))
-
-def flatten(x):
- f = cStringIO.StringIO()
- _flatten(x, f)
- return f.getvalue()
-
-def _hack_at_distutils():
- # Windows-only workaround for some configurations: see
- # https://bugs.python.org/issue23246 (Python 2.7 with
- # a specific MS compiler suite download)
- if sys.platform == "win32":
- try:
- import setuptools # for side-effects, patches distutils
- except ImportError:
- pass
diff --git "a/spaces/codertoro/gpt-academic/crazy_functions/\346\211\271\351\207\217\347\277\273\350\257\221PDF\346\226\207\346\241\243_\345\244\232\347\272\277\347\250\213.py" "b/spaces/codertoro/gpt-academic/crazy_functions/\346\211\271\351\207\217\347\277\273\350\257\221PDF\346\226\207\346\241\243_\345\244\232\347\272\277\347\250\213.py"
deleted file mode 100644
index 244a4e1711b12e1cd17b1940f54de88004428fcc..0000000000000000000000000000000000000000
--- "a/spaces/codertoro/gpt-academic/crazy_functions/\346\211\271\351\207\217\347\277\273\350\257\221PDF\346\226\207\346\241\243_\345\244\232\347\272\277\347\250\213.py"
+++ /dev/null
@@ -1,296 +0,0 @@
-from toolbox import CatchException, report_execption, write_results_to_file
-from toolbox import update_ui
-from .crazy_utils import request_gpt_model_in_new_thread_with_ui_alive
-from .crazy_utils import request_gpt_model_multi_threads_with_very_awesome_ui_and_high_efficiency
-from colorful import *
-
-def read_and_clean_pdf_text(fp):
- """
- 这个函数用于分割pdf,用了很多trick,逻辑较乱,效果奇好,不建议任何人去读这个函数
-
- **输入参数说明**
- - `fp`:需要读取和清理文本的pdf文件路径
-
- **输出参数说明**
- - `meta_txt`:清理后的文本内容字符串
- - `page_one_meta`:第一页清理后的文本内容列表
-
- **函数功能**
- 读取pdf文件并清理其中的文本内容,清理规则包括:
- - 提取所有块元的文本信息,并合并为一个字符串
- - 去除短块(字符数小于100)并替换为回车符
- - 清理多余的空行
- - 合并小写字母开头的段落块并替换为空格
- - 清除重复的换行
- - 将每个换行符替换为两个换行符,使每个段落之间有两个换行符分隔
- """
- import fitz, copy
- import re
- import numpy as np
- fc = 0
- fs = 1
- fb = 2
- REMOVE_FOOT_NOTE = True
- REMOVE_FOOT_FFSIZE_PERCENT = 0.95
- def primary_ffsize(l):
- fsize_statiscs = {}
- for wtf in l['spans']:
- if wtf['size'] not in fsize_statiscs: fsize_statiscs[wtf['size']] = 0
- fsize_statiscs[wtf['size']] += len(wtf['text'])
- return max(fsize_statiscs, key=fsize_statiscs.get)
-
- def ffsize_same(a,b):
- return abs((a-b)/max(a,b)) < 0.02
- # file_content = ""
- with fitz.open(fp) as doc:
- meta_txt = []
- meta_font = []
-
- meta_line = []
- meta_span = []
- for index, page in enumerate(doc):
- # file_content += page.get_text()
- text_areas = page.get_text("dict") # 获取页面上的文本信息
- for t in text_areas['blocks']:
- if 'lines' in t:
- pf = 998
- for l in t['lines']:
- txt_line = "".join([wtf['text'] for wtf in l['spans']])
- pf = primary_ffsize(l)
- meta_line.append([txt_line, pf, l['bbox'], l])
- for wtf in l['spans']: # for l in t['lines']:
- meta_span.append([wtf['text'], wtf['size'], len(wtf['text'])])
- # meta_line.append(["NEW_BLOCK", pf])
- # 块元提取 for each word segment with in line for each line cross-line words for each block
- meta_txt.extend([" ".join(["".join([wtf['text'] for wtf in l['spans']]) for l in t['lines']]).replace(
- '- ', '') for t in text_areas['blocks'] if 'lines' in t])
- meta_font.extend([np.mean([np.mean([wtf['size'] for wtf in l['spans']])
- for l in t['lines']]) for t in text_areas['blocks'] if 'lines' in t])
- if index == 0:
- page_one_meta = [" ".join(["".join([wtf['text'] for wtf in l['spans']]) for l in t['lines']]).replace(
- '- ', '') for t in text_areas['blocks'] if 'lines' in t]
- # 获取正文主字体
- fsize_statiscs = {}
- for span in meta_span:
- if span[1] not in fsize_statiscs: fsize_statiscs[span[1]] = 0
- fsize_statiscs[span[1]] += span[2]
- main_fsize = max(fsize_statiscs, key=fsize_statiscs.get)
- if REMOVE_FOOT_NOTE:
- give_up_fize_threshold = main_fsize * REMOVE_FOOT_FFSIZE_PERCENT
-
- # 切分和重新整合
- mega_sec = []
- sec = []
- for index, line in enumerate(meta_line):
- if index == 0:
- sec.append(line[fc])
- continue
- if REMOVE_FOOT_NOTE:
- if meta_line[index][fs] <= give_up_fize_threshold:
- continue
- if ffsize_same(meta_line[index][fs], meta_line[index-1][fs]):
- # 尝试识别段落
- if meta_line[index][fc].endswith('.') and\
- (meta_line[index-1][fc] != 'NEW_BLOCK') and \
- (meta_line[index][fb][2] - meta_line[index][fb][0]) < (meta_line[index-1][fb][2] - meta_line[index-1][fb][0]) * 0.7:
- sec[-1] += line[fc]
- sec[-1] += "\n\n"
- else:
- sec[-1] += " "
- sec[-1] += line[fc]
- else:
- if (index+1 < len(meta_line)) and \
- meta_line[index][fs] > main_fsize:
- # 单行 + 字体大
- mega_sec.append(copy.deepcopy(sec))
- sec = []
- sec.append("# " + line[fc])
- else:
- # 尝试识别section
- if meta_line[index-1][fs] > meta_line[index][fs]:
- sec.append("\n" + line[fc])
- else:
- sec.append(line[fc])
- mega_sec.append(copy.deepcopy(sec))
-
- finals = []
- for ms in mega_sec:
- final = " ".join(ms)
- final = final.replace('- ', ' ')
- finals.append(final)
- meta_txt = finals
-
- def 把字符太少的块清除为回车(meta_txt):
- for index, block_txt in enumerate(meta_txt):
- if len(block_txt) < 100:
- meta_txt[index] = '\n'
- return meta_txt
- meta_txt = 把字符太少的块清除为回车(meta_txt)
-
- def 清理多余的空行(meta_txt):
- for index in reversed(range(1, len(meta_txt))):
- if meta_txt[index] == '\n' and meta_txt[index-1] == '\n':
- meta_txt.pop(index)
- return meta_txt
- meta_txt = 清理多余的空行(meta_txt)
-
- def 合并小写开头的段落块(meta_txt):
- def starts_with_lowercase_word(s):
- pattern = r"^[a-z]+"
- match = re.match(pattern, s)
- if match:
- return True
- else:
- return False
- for _ in range(100):
- for index, block_txt in enumerate(meta_txt):
- if starts_with_lowercase_word(block_txt):
- if meta_txt[index-1] != '\n':
- meta_txt[index-1] += ' '
- else:
- meta_txt[index-1] = ''
- meta_txt[index-1] += meta_txt[index]
- meta_txt[index] = '\n'
- return meta_txt
- meta_txt = 合并小写开头的段落块(meta_txt)
- meta_txt = 清理多余的空行(meta_txt)
-
- meta_txt = '\n'.join(meta_txt)
- # 清除重复的换行
- for _ in range(5):
- meta_txt = meta_txt.replace('\n\n', '\n')
-
- # 换行 -> 双换行
- meta_txt = meta_txt.replace('\n', '\n\n')
-
- for f in finals:
- print亮黄(f)
- print亮绿('***************************')
-
- return meta_txt, page_one_meta
-
-
-@CatchException
-def 批量翻译PDF文档(txt, llm_kwargs, plugin_kwargs, chatbot, history, sys_prompt, web_port):
- import glob
- import os
-
- # 基本信息:功能、贡献者
- chatbot.append([
- "函数插件功能?",
- "批量总结PDF文档。函数插件贡献者: Binary-Husky"])
- yield from update_ui(chatbot=chatbot, history=history) # 刷新界面
-
- # 尝试导入依赖,如果缺少依赖,则给出安装建议
- try:
- import fitz
- import tiktoken
- except:
- report_execption(chatbot, history,
- a=f"解析项目: {txt}",
- b=f"导入软件依赖失败。使用该模块需要额外依赖,安装方法```pip install --upgrade pymupdf tiktoken```。")
- yield from update_ui(chatbot=chatbot, history=history) # 刷新界面
- return
-
- # 清空历史,以免输入溢出
- history = []
-
- # 检测输入参数,如没有给定输入参数,直接退出
- if os.path.exists(txt):
- project_folder = txt
- else:
- if txt == "":
- txt = '空空如也的输入栏'
- report_execption(chatbot, history,
- a=f"解析项目: {txt}", b=f"找不到本地项目或无权访问: {txt}")
- yield from update_ui(chatbot=chatbot, history=history) # 刷新界面
- return
-
- # 搜索需要处理的文件清单
- file_manifest = [f for f in glob.glob(
- f'{project_folder}/**/*.pdf', recursive=True)]
-
- # 如果没找到任何文件
- if len(file_manifest) == 0:
- report_execption(chatbot, history,
- a=f"解析项目: {txt}", b=f"找不到任何.tex或.pdf文件: {txt}")
- yield from update_ui(chatbot=chatbot, history=history) # 刷新界面
- return
-
- # 开始正式执行任务
- yield from 解析PDF(file_manifest, project_folder, llm_kwargs, plugin_kwargs, chatbot, history, sys_prompt)
-
-
-def 解析PDF(file_manifest, project_folder, llm_kwargs, plugin_kwargs, chatbot, history, sys_prompt):
- import os
- import tiktoken
- TOKEN_LIMIT_PER_FRAGMENT = 1600
- generated_conclusion_files = []
- for index, fp in enumerate(file_manifest):
-
- # 读取PDF文件
- file_content, page_one = read_and_clean_pdf_text(fp)
-
- # 递归地切割PDF文件
- from .crazy_utils import breakdown_txt_to_satisfy_token_limit_for_pdf
- from toolbox import get_conf
- enc = tiktoken.encoding_for_model(*get_conf('LLM_MODEL'))
- def get_token_num(txt): return len(enc.encode(txt))
- paper_fragments = breakdown_txt_to_satisfy_token_limit_for_pdf(
- txt=file_content, get_token_fn=get_token_num, limit=TOKEN_LIMIT_PER_FRAGMENT)
- page_one_fragments = breakdown_txt_to_satisfy_token_limit_for_pdf(
- txt=str(page_one), get_token_fn=get_token_num, limit=TOKEN_LIMIT_PER_FRAGMENT//4)
-
- # 为了更好的效果,我们剥离Introduction之后的部分(如果有)
- paper_meta = page_one_fragments[0].split('introduction')[0].split('Introduction')[0].split('INTRODUCTION')[0]
-
- # 单线,获取文章meta信息
- paper_meta_info = yield from request_gpt_model_in_new_thread_with_ui_alive(
- inputs=f"以下是一篇学术论文的基础信息,请从中提取出“标题”、“收录会议或期刊”、“作者”、“摘要”、“编号”、“作者邮箱”这六个部分。请用markdown格式输出,最后用中文翻译摘要部分。请提取:{paper_meta}",
- inputs_show_user=f"请从{fp}中提取出“标题”、“收录会议或期刊”等基本信息。",
- llm_kwargs=llm_kwargs,
- chatbot=chatbot, history=[],
- sys_prompt="Your job is to collect information from materials。",
- )
-
- # 多线,翻译
- gpt_response_collection = yield from request_gpt_model_multi_threads_with_very_awesome_ui_and_high_efficiency(
- inputs_array=[
- f"以下是你需要翻译的论文片段:\n{frag}" for frag in paper_fragments],
- inputs_show_user_array=[f"\n---\n 原文: \n\n {frag.replace('#', '')} \n---\n 翻译:\n " for frag in paper_fragments],
- llm_kwargs=llm_kwargs,
- chatbot=chatbot,
- history_array=[[paper_meta] for _ in paper_fragments],
- sys_prompt_array=[
- "请你作为一个学术翻译,负责把学术论文的片段准确翻译成中文。" for _ in paper_fragments],
- max_workers=16 # OpenAI所允许的最大并行过载
- )
-
- # 整理报告的格式
- for i,k in enumerate(gpt_response_collection):
- if i%2==0:
- gpt_response_collection[i] = f"\n\n---\n\n ## 原文[{i//2}/{len(gpt_response_collection)//2}]: \n\n {paper_fragments[i//2].replace('#', '')} \n\n---\n\n ## 翻译[{i//2}/{len(gpt_response_collection)//2}]:\n "
- else:
- gpt_response_collection[i] = gpt_response_collection[i]
- final = ["一、论文概况\n\n---\n\n", paper_meta_info.replace('# ', '### ') + '\n\n---\n\n', "二、论文翻译", ""]
- final.extend(gpt_response_collection)
- create_report_file_name = f"{os.path.basename(fp)}.trans.md"
- res = write_results_to_file(final, file_name=create_report_file_name)
-
- # 更新UI
- generated_conclusion_files.append(f'./gpt_log/{create_report_file_name}')
- chatbot.append((f"{fp}完成了吗?", res))
- yield from update_ui(chatbot=chatbot, history=history) # 刷新界面
-
- # 准备文件的下载
- import shutil
- for pdf_path in generated_conclusion_files:
- # 重命名文件
- rename_file = f'./gpt_log/总结论文-{os.path.basename(pdf_path)}'
- if os.path.exists(rename_file):
- os.remove(rename_file)
- shutil.copyfile(pdf_path, rename_file)
- if os.path.exists(pdf_path):
- os.remove(pdf_path)
- chatbot.append(("给出输出文件清单", str(generated_conclusion_files)))
- yield from update_ui(chatbot=chatbot, history=history) # 刷新界面
diff --git a/spaces/coding-alt/IF/app.py b/spaces/coding-alt/IF/app.py
deleted file mode 100644
index 75e57450877344a7c7fd63b7940cb1fdafb320f4..0000000000000000000000000000000000000000
--- a/spaces/coding-alt/IF/app.py
+++ /dev/null
@@ -1,701 +0,0 @@
-#!/usr/bin/env python
-
-import datetime
-import hashlib
-import json
-import os
-import random
-import tempfile
-import shortuuid
-from apscheduler.schedulers.background import BackgroundScheduler
-import shutil
-
-import gradio as gr
-import torch
-from huggingface_hub import HfApi
-from share_btn import community_icon_html, loading_icon_html, share_js
-
-# isort: off
-from model import Model
-from settings import (
- DEBUG,
- DEFAULT_CUSTOM_TIMESTEPS_1,
- DEFAULT_CUSTOM_TIMESTEPS_2,
- DEFAULT_NUM_IMAGES,
- DEFAULT_NUM_STEPS_3,
- DISABLE_SD_X4_UPSCALER,
- GALLERY_COLUMN_NUM,
- HF_TOKEN,
- MAX_NUM_IMAGES,
- MAX_NUM_STEPS,
- MAX_QUEUE_SIZE,
- MAX_SEED,
- SHOW_ADVANCED_OPTIONS,
- SHOW_CUSTOM_TIMESTEPS_1,
- SHOW_CUSTOM_TIMESTEPS_2,
- SHOW_DEVICE_WARNING,
- SHOW_DUPLICATE_BUTTON,
- SHOW_NUM_IMAGES,
- SHOW_NUM_STEPS_1,
- SHOW_NUM_STEPS_2,
- SHOW_NUM_STEPS_3,
- SHOW_UPSCALE_TO_256_BUTTON,
- UPLOAD_REPO_ID,
- UPLOAD_RESULT_IMAGE,
-)
-# isort: on
-
-TITLE = '# [DeepFloyd IF](https://github.com/deep-floyd/IF)'
-DESCRIPTION = 'The DeepFloyd IF model has been initially released as a non-commercial research-only model. Please make sure you read and abide to the [LICENSE](https://huggingface.co/spaces/DeepFloyd/deepfloyd-if-license) before using it.'
-DISCLAIMER = 'In this demo, the DeepFloyd team may collect prompts, and user preferences (which of the images the user chose to upscale) for improving future models'
-FOOTER = """
-
-
LICENSE
-The model is licensed with a bespoke non-commercial research-only license DeepFloyd IF Research License Agreement license. The license forbids you from sharing any content for commercial use, or that violates any laws, produce any harm to a person, disseminate any personal information that would be meant for harm, spread misinformation and target vulnerable groups. For the full list of restrictions please read the license
-
Biases and content acknowledgment
-Despite how impressive being able to turn text into image is, beware to the fact that this model may output content that reinforces or exacerbates societal biases, as well as realistic faces, explicit content and violence. The model was trained on a subset of the LAION-5B dataset and is meant for research purposes. You can read more in the model card
-
'
-
-if SHOW_DEVICE_WARNING and not torch.cuda.is_available():
- DESCRIPTION += '\n
Running on CPU 🥶 This demo does not work on CPU.
'
-
-model = Model()
-
-
-def randomize_seed_fn(seed: int, randomize_seed: bool) -> int:
- if randomize_seed:
- seed = random.randint(0, MAX_SEED)
- return seed
-
-
-def get_stage2_index(evt: gr.SelectData) -> int:
- return evt.index
-
-
-def check_if_stage2_selected(index: int) -> None:
- if index == -1:
- raise gr.Error(
- 'You need to select the image you would like to upscale from the Stage 1 results by clicking.'
- )
-
-
-hf_api = HfApi(token=HF_TOKEN)
-if UPLOAD_REPO_ID:
- hf_api.create_repo(repo_id=UPLOAD_REPO_ID,
- private=True,
- repo_type='dataset',
- exist_ok=True)
-
-
-def get_param_file_hash_name(param_filepath: str) -> str:
- if not UPLOAD_REPO_ID:
- return ''
- with open(param_filepath, 'rb') as f:
- md5 = hashlib.md5(f.read()).hexdigest()
- utcnow = datetime.datetime.utcnow().strftime('%Y-%m-%d-%H-%M-%S-%f')
- return f'{utcnow}-{md5}'
-
-
-def upload_stage1_result(stage1_param_path: str, stage1_result_path: str,
- save_name: str) -> None:
- if not UPLOAD_REPO_ID:
- return
- try:
- folder_params = "tmp/results/stage1_params"
- folder_results = "tmp/results/stage1_results"
-
- path_params = f"{folder_params}/{save_name}.json"
- path_results = f"{folder_results}/{save_name}.pth"
-
- os.makedirs(folder_params, exist_ok=True)
- os.makedirs(folder_results, exist_ok=True)
-
- shutil.copy(stage1_param_path, path_params)
- shutil.copy(stage1_result_path, path_results)
-
- except Exception as e:
- print(e)
-
-
-def upload_stage2_info(stage1_param_file_hash_name: str,
- stage2_output_path: str,
- selected_index_for_upscale: int, seed_2: int,
- guidance_scale_2: float, custom_timesteps_2: str,
- num_inference_steps_2: int) -> None:
- if not UPLOAD_REPO_ID:
- return
- if not stage1_param_file_hash_name:
- raise ValueError
-
- stage2_params = {
- 'stage1_param_file_hash_name': stage1_param_file_hash_name,
- 'selected_index_for_upscale': selected_index_for_upscale,
- 'seed_2': seed_2,
- 'guidance_scale_2': guidance_scale_2,
- 'custom_timesteps_2': custom_timesteps_2,
- 'num_inference_steps_2': num_inference_steps_2,
- }
- with tempfile.NamedTemporaryFile(mode='w', delete=False) as param_file:
- param_file.write(json.dumps(stage2_params))
- stage2_param_file_hash_name = get_param_file_hash_name(param_file.name)
- save_name = f'{stage1_param_file_hash_name}_{stage2_param_file_hash_name}'
-
- try:
- folder_params = "tmp/results/stage2_params"
-
- os.makedirs(folder_params, exist_ok=True)
- path_params = f"{folder_params}/{save_name}.json"
- shutil.copy(param_file.name, path_params)
-
- if UPLOAD_RESULT_IMAGE:
- folder_results = "tmp/results/stage2_results"
- os.makedirs(folder_results, exist_ok=True)
- path_results = f"{folder_results}/{save_name}.png"
- shutil.copy(stage2_output_path, path_results)
-
- except Exception as e:
- print(e)
-
-
-def upload_stage2_3_info(stage1_param_file_hash_name: str,
- stage2_3_output_path: str,
- selected_index_for_upscale: int, seed_2: int,
- guidance_scale_2: float, custom_timesteps_2: str,
- num_inference_steps_2: int, prompt: str,
- negative_prompt: str, seed_3: int,
- guidance_scale_3: float,
- num_inference_steps_3: int) -> None:
- if not UPLOAD_REPO_ID:
- return
- if not stage1_param_file_hash_name:
- raise ValueError
-
- stage2_3_params = {
- 'stage1_param_file_hash_name': stage1_param_file_hash_name,
- 'selected_index_for_upscale': selected_index_for_upscale,
- 'seed_2': seed_2,
- 'guidance_scale_2': guidance_scale_2,
- 'custom_timesteps_2': custom_timesteps_2,
- 'num_inference_steps_2': num_inference_steps_2,
- 'prompt': prompt,
- 'negative_prompt': negative_prompt,
- 'seed_3': seed_3,
- 'guidance_scale_3': guidance_scale_3,
- 'num_inference_steps_3': num_inference_steps_3,
- }
- with tempfile.NamedTemporaryFile(mode='w', delete=False) as param_file:
- param_file.write(json.dumps(stage2_3_params))
- stage2_3_param_file_hash_name = get_param_file_hash_name(param_file.name)
- save_name = f'{stage1_param_file_hash_name}_{stage2_3_param_file_hash_name}'
-
- try:
- folder_params = "tmp/results/stage2_3_params"
- os.makedirs(folder_params, exist_ok=True)
- path_params = f"{folder_params}/{save_name}.json"
- shutil.copy(param_file.name, path_params)
-
- if UPLOAD_RESULT_IMAGE:
- folder_results = "tmp/results/stage2_3_results"
- os.makedirs(folder_results, exist_ok=True)
- path_results = f"{folder_results}/{save_name}.png"
- shutil.copy(stage2_3_output_path, path_results)
- except Exception as e:
- print(e)
-
-
-def update_upscale_button(selected_index: int) -> tuple[dict, dict]:
- if selected_index == -1:
- return gr.update(interactive=False), gr.update(interactive=False)
- else:
- return gr.update(interactive=True), gr.update(interactive=True)
-
-
-def _update_result_view(show_gallery: bool) -> tuple[dict, dict]:
- return gr.update(visible=show_gallery), gr.update(visible=not show_gallery)
-
-
-def show_gallery_view() -> tuple[dict, dict]:
- return _update_result_view(True)
-
-
-def show_upscaled_view() -> tuple[dict, dict]:
- return _update_result_view(False)
-
-def upload_files():
- """Zips files and uploads to dataset. Local data is deleted
- """
- if os.path.exists("tmp/results") and os.path.isdir("tmp/results"):
- try:
- random_folder = random.randint(0,1000)
- shutil.make_archive("tmp/results", 'zip', "tmp/results")
- hf_api.upload_file(
- path_or_fileobj="tmp/results.zip",
- path_in_repo=f"{random_folder}/results_{shortuuid.uuid()}.zip",
- repo_id=UPLOAD_REPO_ID,
- repo_type="dataset",
- )
- shutil.rmtree("tmp/results")
- except Exception as e:
- print(e)
-
-examples = [
- 'high quality dslr photo, a photo product of a lemon inspired by natural and organic materials, wooden accents, intricately decorated with glowing vines of led lights, inspired by baroque luxury',
- 'paper quilling, extremely detailed, paper quilling of a nordic mountain landscape, 8k rendering',
- 'letters made of candy on a plate that says "diet"',
- 'a photo of a violet baseball cap with yellow text: "deep floyd". 50mm lens, photo realism, cine lens. violet baseball cap says "deep floyd". reflections, render. yellow stitch text "deep floyd"',
- 'ultra close-up color photo portrait of rainbow owl with deer horns in the woods',
- 'a cloth embroidered with the text "laion" and an embroidered cute baby lion face',
- 'product image of a crochet Cthulhu the great old one emerging from a spacetime wormhole made of wool',
- 'a little green budgie parrot driving small red toy car in new york street, photo',
- 'origami dancer in white paper, 3d render, ultra-detailed, on white background, studio shot.',
- 'glowing mushrooms in a natural environment with smoke in the frame',
- 'a subway train\'s digital sign saying "open source", vsco preset, 35mm photo, film grain, in a dim subway station',
- 'a bowl full of few adorable golden doodle puppies, the doodles dusted in powdered sugar and look delicious, bokeh, cannon. professional macro photo, super detailed. cute sweet golden doodle confectionery, baking puppies in powdered sugar in the bowl',
- 'a face of a woman made completely out of foliage, twigs, leaves and flowers, side view'
-]
-
-with gr.Blocks(css='style.css') as demo:
- gr.Markdown(TITLE)
- gr.Markdown(DESCRIPTION)
- with gr.Box():
- with gr.Row(elem_id='prompt-container').style(equal_height=True):
- with gr.Column():
- prompt = gr.Text(
- label='Prompt',
- show_label=False,
- max_lines=1,
- placeholder='Enter your prompt',
- elem_id='prompt-text-input',
- ).style(container=False)
- negative_prompt = gr.Text(
- label='Negative prompt',
- show_label=False,
- max_lines=1,
- placeholder='Enter a negative prompt',
- elem_id='negative-prompt-text-input',
- ).style(container=False)
- generate_button = gr.Button('Generate').style(full_width=False)
-
- with gr.Column() as gallery_view:
- gallery = gr.Gallery(label='Stage 1 results',
- show_label=False,
- elem_id='gallery').style(
- columns=GALLERY_COLUMN_NUM,
- object_fit='contain')
- gr.Markdown('Pick your favorite generation to upscale.')
- with gr.Row():
- upscale_to_256_button = gr.Button(
- 'Upscale to 256px',
- visible=SHOW_UPSCALE_TO_256_BUTTON
- or DISABLE_SD_X4_UPSCALER,
- interactive=False)
- upscale_button = gr.Button('Upscale',
- interactive=False,
- visible=not DISABLE_SD_X4_UPSCALER)
- with gr.Column(visible=False) as upscale_view:
- result = gr.Image(label='Result',
- show_label=False,
- type='filepath',
- interactive=False,
- elem_id='upscaled-image').style(height=640)
- back_to_selection_button = gr.Button('Back to selection')
- with gr.Group(elem_id="share-btn-container"):
- community_icon = gr.HTML(community_icon_html)
- loading_icon = gr.HTML(loading_icon_html)
- share_button = gr.Button(
- "Share to community", elem_id="share-btn")
- share_button.click(None, [], [], _js=share_js)
- with gr.Accordion('Advanced options',
- open=False,
- visible=SHOW_ADVANCED_OPTIONS):
- with gr.Tabs():
- with gr.Tab(label='Generation'):
- seed_1 = gr.Slider(label='Seed',
- minimum=0,
- maximum=MAX_SEED,
- step=1,
- value=0)
- randomize_seed_1 = gr.Checkbox(label='Randomize seed',
- value=True)
- guidance_scale_1 = gr.Slider(label='Guidance scale',
- minimum=1,
- maximum=20,
- step=0.1,
- value=7.0)
- custom_timesteps_1 = gr.Dropdown(
- label='Custom timesteps 1',
- choices=[
- 'none',
- 'fast27',
- 'smart27',
- 'smart50',
- 'smart100',
- 'smart185',
- ],
- value=DEFAULT_CUSTOM_TIMESTEPS_1,
- visible=SHOW_CUSTOM_TIMESTEPS_1)
- num_inference_steps_1 = gr.Slider(
- label='Number of inference steps',
- minimum=1,
- maximum=MAX_NUM_STEPS,
- step=1,
- value=100,
- visible=SHOW_NUM_STEPS_1)
- num_images = gr.Slider(label='Number of images',
- minimum=1,
- maximum=MAX_NUM_IMAGES,
- step=1,
- value=DEFAULT_NUM_IMAGES,
- visible=SHOW_NUM_IMAGES)
- with gr.Tab(label='Super-resolution 1'):
- seed_2 = gr.Slider(label='Seed',
- minimum=0,
- maximum=MAX_SEED,
- step=1,
- value=0)
- randomize_seed_2 = gr.Checkbox(label='Randomize seed',
- value=True)
- guidance_scale_2 = gr.Slider(label='Guidance scale',
- minimum=1,
- maximum=20,
- step=0.1,
- value=4.0)
- custom_timesteps_2 = gr.Dropdown(
- label='Custom timesteps 2',
- choices=[
- 'none',
- 'fast27',
- 'smart27',
- 'smart50',
- 'smart100',
- 'smart185',
- ],
- value=DEFAULT_CUSTOM_TIMESTEPS_2,
- visible=SHOW_CUSTOM_TIMESTEPS_2)
- num_inference_steps_2 = gr.Slider(
- label='Number of inference steps',
- minimum=1,
- maximum=MAX_NUM_STEPS,
- step=1,
- value=50,
- visible=SHOW_NUM_STEPS_2)
- with gr.Tab(label='Super-resolution 2'):
- seed_3 = gr.Slider(label='Seed',
- minimum=0,
- maximum=MAX_SEED,
- step=1,
- value=0)
- randomize_seed_3 = gr.Checkbox(label='Randomize seed',
- value=True)
- guidance_scale_3 = gr.Slider(label='Guidance scale',
- minimum=1,
- maximum=20,
- step=0.1,
- value=9.0)
- num_inference_steps_3 = gr.Slider(
- label='Number of inference steps',
- minimum=1,
- maximum=MAX_NUM_STEPS,
- step=1,
- value=DEFAULT_NUM_STEPS_3,
- visible=SHOW_NUM_STEPS_3)
-
- gr.Examples(examples=examples, inputs=prompt, examples_per_page=4)
-
- with gr.Box(visible=DEBUG):
- with gr.Row():
- with gr.Accordion(label='Hidden params'):
- stage1_param_path = gr.Text(label='Stage 1 param path')
- stage1_result_path = gr.Text(label='Stage 1 result path')
- stage1_param_file_hash_name = gr.Text(
- label='Stage 1 param file hash name')
- selected_index_for_stage2 = gr.Number(
- label='Selected index for Stage 2', value=-1, precision=0)
- gr.Markdown(DISCLAIMER)
- gr.HTML(FOOTER)
- stage1_inputs = [
- prompt,
- negative_prompt,
- seed_1,
- num_images,
- guidance_scale_1,
- custom_timesteps_1,
- num_inference_steps_1,
- ]
- stage1_outputs = [
- gallery,
- stage1_param_path,
- stage1_result_path,
- ]
-
- prompt.submit(
- fn=randomize_seed_fn,
- inputs=[seed_1, randomize_seed_1],
- outputs=seed_1,
- queue=False,
- ).then(
- fn=lambda: -1,
- outputs=selected_index_for_stage2,
- queue=False,
- ).then(
- fn=show_gallery_view,
- outputs=[
- gallery_view,
- upscale_view,
- ],
- queue=False,
- ).then(
- fn=update_upscale_button,
- inputs=selected_index_for_stage2,
- outputs=[
- upscale_button,
- upscale_to_256_button,
- ],
- queue=False,
- ).then(
- fn=model.run_stage1,
- inputs=stage1_inputs,
- outputs=stage1_outputs,
- ).success(
- fn=get_param_file_hash_name,
- inputs=stage1_param_path,
- outputs=stage1_param_file_hash_name,
- queue=False,
- ).then(
- fn=upload_stage1_result,
- inputs=[
- stage1_param_path,
- stage1_result_path,
- stage1_param_file_hash_name,
- ],
- queue=False,
- )
-
- negative_prompt.submit(
- fn=randomize_seed_fn,
- inputs=[seed_1, randomize_seed_1],
- outputs=seed_1,
- queue=False,
- ).then(
- fn=lambda: -1,
- outputs=selected_index_for_stage2,
- queue=False,
- ).then(
- fn=show_gallery_view,
- outputs=[
- gallery_view,
- upscale_view,
- ],
- queue=False,
- ).then(
- fn=update_upscale_button,
- inputs=selected_index_for_stage2,
- outputs=[
- upscale_button,
- upscale_to_256_button,
- ],
- queue=False,
- ).then(
- fn=model.run_stage1,
- inputs=stage1_inputs,
- outputs=stage1_outputs,
- ).success(
- fn=get_param_file_hash_name,
- inputs=stage1_param_path,
- outputs=stage1_param_file_hash_name,
- queue=False,
- ).then(
- fn=upload_stage1_result,
- inputs=[
- stage1_param_path,
- stage1_result_path,
- stage1_param_file_hash_name,
- ],
- queue=False,
- )
-
- generate_button.click(
- fn=randomize_seed_fn,
- inputs=[seed_1, randomize_seed_1],
- outputs=seed_1,
- queue=False,
- ).then(
- fn=lambda: -1,
- outputs=selected_index_for_stage2,
- queue=False,
- ).then(
- fn=show_gallery_view,
- outputs=[
- gallery_view,
- upscale_view,
- ],
- queue=False,
- ).then(
- fn=update_upscale_button,
- inputs=selected_index_for_stage2,
- outputs=[
- upscale_button,
- upscale_to_256_button,
- ],
- queue=False,
- ).then(
- fn=model.run_stage1,
- inputs=stage1_inputs,
- outputs=stage1_outputs,
- api_name='generate64',
- ).success(
- fn=get_param_file_hash_name,
- inputs=stage1_param_path,
- outputs=stage1_param_file_hash_name,
- queue=False,
- ).then(
- fn=upload_stage1_result,
- inputs=[
- stage1_param_path,
- stage1_result_path,
- stage1_param_file_hash_name,
- ],
- queue=False,
- )
-
- gallery.select(
- fn=get_stage2_index,
- outputs=selected_index_for_stage2,
- queue=False,
- )
-
- selected_index_for_stage2.change(
- fn=update_upscale_button,
- inputs=selected_index_for_stage2,
- outputs=[
- upscale_button,
- upscale_to_256_button,
- ],
- queue=False,
- )
-
- stage2_inputs = [
- stage1_result_path,
- selected_index_for_stage2,
- seed_2,
- guidance_scale_2,
- custom_timesteps_2,
- num_inference_steps_2,
- ]
-
- upscale_to_256_button.click(
- fn=check_if_stage2_selected,
- inputs=selected_index_for_stage2,
- queue=False,
- ).then(
- fn=randomize_seed_fn,
- inputs=[seed_2, randomize_seed_2],
- outputs=seed_2,
- queue=False,
- ).then(
- fn=show_upscaled_view,
- outputs=[
- gallery_view,
- upscale_view,
- ],
- queue=False,
- ).then(
- fn=model.run_stage2,
- inputs=stage2_inputs,
- outputs=result,
- api_name='upscale256',
- ).success(
- fn=upload_stage2_info,
- inputs=[
- stage1_param_file_hash_name,
- result,
- selected_index_for_stage2,
- seed_2,
- guidance_scale_2,
- custom_timesteps_2,
- num_inference_steps_2,
- ],
- queue=False,
- )
-
- stage2_3_inputs = [
- stage1_result_path,
- selected_index_for_stage2,
- seed_2,
- guidance_scale_2,
- custom_timesteps_2,
- num_inference_steps_2,
- prompt,
- negative_prompt,
- seed_3,
- guidance_scale_3,
- num_inference_steps_3,
- ]
-
- upscale_button.click(
- fn=check_if_stage2_selected,
- inputs=selected_index_for_stage2,
- queue=False,
- ).then(
- fn=randomize_seed_fn,
- inputs=[seed_2, randomize_seed_2],
- outputs=seed_2,
- queue=False,
- ).then(
- fn=randomize_seed_fn,
- inputs=[seed_3, randomize_seed_3],
- outputs=seed_3,
- queue=False,
- ).then(
- fn=show_upscaled_view,
- outputs=[
- gallery_view,
- upscale_view,
- ],
- queue=False,
- ).then(
- fn=model.run_stage2_3,
- inputs=stage2_3_inputs,
- outputs=result,
- api_name='upscale1024',
- ).success(
- fn=upload_stage2_3_info,
- inputs=[
- stage1_param_file_hash_name,
- result,
- selected_index_for_stage2,
- seed_2,
- guidance_scale_2,
- custom_timesteps_2,
- num_inference_steps_2,
- prompt,
- negative_prompt,
- seed_3,
- guidance_scale_3,
- num_inference_steps_3,
- ],
- queue=False,
- )
-
- back_to_selection_button.click(
- fn=show_gallery_view,
- outputs=[
- gallery_view,
- upscale_view,
- ],
- queue=False,
- )
-
- if UPLOAD_REPO_ID:
- scheduler = BackgroundScheduler()
- scheduler.add_job(func=upload_files, trigger="interval", seconds=60*20)
- scheduler.start()
-
-demo.queue(api_open=False, max_size=MAX_QUEUE_SIZE).launch(debug=DEBUG)
diff --git a/spaces/colakin/video-generater/public/ffmpeg/libavcodec/ffv1dec.c b/spaces/colakin/video-generater/public/ffmpeg/libavcodec/ffv1dec.c
deleted file mode 100644
index a3f930223349b1edf8fe2c16650ac92d8e1cc7fa..0000000000000000000000000000000000000000
--- a/spaces/colakin/video-generater/public/ffmpeg/libavcodec/ffv1dec.c
+++ /dev/null
@@ -1,1130 +0,0 @@
-/*
- * FFV1 decoder
- *
- * Copyright (c) 2003-2013 Michael Niedermayer
- *
- * This file is part of FFmpeg.
- *
- * FFmpeg is free software; you can redistribute it and/or
- * modify it under the terms of the GNU Lesser General Public
- * License as published by the Free Software Foundation; either
- * version 2.1 of the License, or (at your option) any later version.
- *
- * FFmpeg is distributed in the hope that it will be useful,
- * but WITHOUT ANY WARRANTY; without even the implied warranty of
- * MERCHANTABILITY or FITNESS FOR A PARTICULAR PURPOSE. See the GNU
- * Lesser General Public License for more details.
- *
- * You should have received a copy of the GNU Lesser General Public
- * License along with FFmpeg; if not, write to the Free Software
- * Foundation, Inc., 51 Franklin Street, Fifth Floor, Boston, MA 02110-1301 USA
- */
-
-/**
- * @file
- * FF Video Codec 1 (a lossless codec) decoder
- */
-
-#include "libavutil/avassert.h"
-#include "libavutil/crc.h"
-#include "libavutil/opt.h"
-#include "libavutil/imgutils.h"
-#include "libavutil/pixdesc.h"
-#include "avcodec.h"
-#include "codec_internal.h"
-#include "get_bits.h"
-#include "rangecoder.h"
-#include "golomb.h"
-#include "mathops.h"
-#include "ffv1.h"
-#include "thread.h"
-#include "threadframe.h"
-
-static inline av_flatten int get_symbol_inline(RangeCoder *c, uint8_t *state,
- int is_signed)
-{
- if (get_rac(c, state + 0))
- return 0;
- else {
- int i, e;
- unsigned a;
- e = 0;
- while (get_rac(c, state + 1 + FFMIN(e, 9))) { // 1..10
- e++;
- if (e > 31)
- return AVERROR_INVALIDDATA;
- }
-
- a = 1;
- for (i = e - 1; i >= 0; i--)
- a += a + get_rac(c, state + 22 + FFMIN(i, 9)); // 22..31
-
- e = -(is_signed && get_rac(c, state + 11 + FFMIN(e, 10))); // 11..21
- return (a ^ e) - e;
- }
-}
-
-static av_noinline int get_symbol(RangeCoder *c, uint8_t *state, int is_signed)
-{
- return get_symbol_inline(c, state, is_signed);
-}
-
-static inline int get_vlc_symbol(GetBitContext *gb, VlcState *const state,
- int bits)
-{
- int k, i, v, ret;
-
- i = state->count;
- k = 0;
- while (i < state->error_sum) { // FIXME: optimize
- k++;
- i += i;
- }
-
- v = get_sr_golomb(gb, k, 12, bits);
- ff_dlog(NULL, "v:%d bias:%d error:%d drift:%d count:%d k:%d",
- v, state->bias, state->error_sum, state->drift, state->count, k);
-
- v ^= ((2 * state->drift + state->count) >> 31);
-
- ret = fold(v + state->bias, bits);
-
- update_vlc_state(state, v);
-
- return ret;
-}
-
-static int is_input_end(FFV1Context *s)
-{
- if (s->ac != AC_GOLOMB_RICE) {
- RangeCoder *const c = &s->c;
- if (c->overread > MAX_OVERREAD)
- return AVERROR_INVALIDDATA;
- } else {
- if (get_bits_left(&s->gb) < 1)
- return AVERROR_INVALIDDATA;
- }
- return 0;
-}
-
-#define TYPE int16_t
-#define RENAME(name) name
-#include "ffv1dec_template.c"
-#undef TYPE
-#undef RENAME
-
-#define TYPE int32_t
-#define RENAME(name) name ## 32
-#include "ffv1dec_template.c"
-
-static int decode_plane(FFV1Context *s, uint8_t *src,
- int w, int h, int stride, int plane_index,
- int pixel_stride)
-{
- int x, y;
- int16_t *sample[2];
- sample[0] = s->sample_buffer + 3;
- sample[1] = s->sample_buffer + w + 6 + 3;
-
- s->run_index = 0;
-
- memset(s->sample_buffer, 0, 2 * (w + 6) * sizeof(*s->sample_buffer));
-
- for (y = 0; y < h; y++) {
- int16_t *temp = sample[0]; // FIXME: try a normal buffer
-
- sample[0] = sample[1];
- sample[1] = temp;
-
- sample[1][-1] = sample[0][0];
- sample[0][w] = sample[0][w - 1];
-
- if (s->avctx->bits_per_raw_sample <= 8) {
- int ret = decode_line(s, w, sample, plane_index, 8);
- if (ret < 0)
- return ret;
- for (x = 0; x < w; x++)
- src[x*pixel_stride + stride * y] = sample[1][x];
- } else {
- int ret = decode_line(s, w, sample, plane_index, s->avctx->bits_per_raw_sample);
- if (ret < 0)
- return ret;
- if (s->packed_at_lsb) {
- for (x = 0; x < w; x++) {
- ((uint16_t*)(src + stride*y))[x*pixel_stride] = sample[1][x];
- }
- } else {
- for (x = 0; x < w; x++) {
- ((uint16_t*)(src + stride*y))[x*pixel_stride] = sample[1][x] << (16 - s->avctx->bits_per_raw_sample) | ((uint16_t **)sample)[1][x] >> (2 * s->avctx->bits_per_raw_sample - 16);
- }
- }
- }
- }
- return 0;
-}
-
-static int decode_slice_header(const FFV1Context *f, FFV1Context *fs)
-{
- RangeCoder *c = &fs->c;
- uint8_t state[CONTEXT_SIZE];
- unsigned ps, i, context_count;
- int sx, sy, sw, sh;
-
- memset(state, 128, sizeof(state));
- sx = get_symbol(c, state, 0);
- sy = get_symbol(c, state, 0);
- sw = get_symbol(c, state, 0) + 1U;
- sh = get_symbol(c, state, 0) + 1U;
-
- av_assert0(f->version > 2);
-
-
- if (sx < 0 || sy < 0 || sw <= 0 || sh <= 0)
- return AVERROR_INVALIDDATA;
- if (sx > f->num_h_slices - sw || sy > f->num_v_slices - sh)
- return AVERROR_INVALIDDATA;
-
- fs->slice_x = sx * (int64_t)f->width / f->num_h_slices;
- fs->slice_y = sy * (int64_t)f->height / f->num_v_slices;
- fs->slice_width = (sx + sw) * (int64_t)f->width / f->num_h_slices - fs->slice_x;
- fs->slice_height = (sy + sh) * (int64_t)f->height / f->num_v_slices - fs->slice_y;
-
- av_assert0((unsigned)fs->slice_width <= f->width &&
- (unsigned)fs->slice_height <= f->height);
- av_assert0 ( (unsigned)fs->slice_x + (uint64_t)fs->slice_width <= f->width
- && (unsigned)fs->slice_y + (uint64_t)fs->slice_height <= f->height);
-
- if (fs->ac == AC_GOLOMB_RICE && fs->slice_width >= (1<<23))
- return AVERROR_INVALIDDATA;
-
- for (i = 0; i < f->plane_count; i++) {
- PlaneContext * const p = &fs->plane[i];
- int idx = get_symbol(c, state, 0);
- if (idx >= (unsigned)f->quant_table_count) {
- av_log(f->avctx, AV_LOG_ERROR, "quant_table_index out of range\n");
- return -1;
- }
- p->quant_table_index = idx;
- memcpy(p->quant_table, f->quant_tables[idx], sizeof(p->quant_table));
- context_count = f->context_count[idx];
-
- if (p->context_count < context_count) {
- av_freep(&p->state);
- av_freep(&p->vlc_state);
- }
- p->context_count = context_count;
- }
-
- ps = get_symbol(c, state, 0);
- if (ps == 1) {
- f->cur->interlaced_frame = 1;
- f->cur->top_field_first = 1;
- } else if (ps == 2) {
- f->cur->interlaced_frame = 1;
- f->cur->top_field_first = 0;
- } else if (ps == 3) {
- f->cur->interlaced_frame = 0;
- }
- f->cur->sample_aspect_ratio.num = get_symbol(c, state, 0);
- f->cur->sample_aspect_ratio.den = get_symbol(c, state, 0);
-
- if (av_image_check_sar(f->width, f->height,
- f->cur->sample_aspect_ratio) < 0) {
- av_log(f->avctx, AV_LOG_WARNING, "ignoring invalid SAR: %u/%u\n",
- f->cur->sample_aspect_ratio.num,
- f->cur->sample_aspect_ratio.den);
- f->cur->sample_aspect_ratio = (AVRational){ 0, 1 };
- }
-
- if (fs->version > 3) {
- fs->slice_reset_contexts = get_rac(c, state);
- fs->slice_coding_mode = get_symbol(c, state, 0);
- if (fs->slice_coding_mode != 1) {
- fs->slice_rct_by_coef = get_symbol(c, state, 0);
- fs->slice_rct_ry_coef = get_symbol(c, state, 0);
- if ((uint64_t)fs->slice_rct_by_coef + (uint64_t)fs->slice_rct_ry_coef > 4) {
- av_log(f->avctx, AV_LOG_ERROR, "slice_rct_y_coef out of range\n");
- return AVERROR_INVALIDDATA;
- }
- }
- }
-
- return 0;
-}
-
-static int decode_slice(AVCodecContext *c, void *arg)
-{
- FFV1Context *fs = *(void **)arg;
- FFV1Context *f = fs->avctx->priv_data;
- int width, height, x, y, ret;
- const int ps = av_pix_fmt_desc_get(c->pix_fmt)->comp[0].step;
- AVFrame * const p = f->cur;
- int i, si;
-
- for( si=0; fs != f->slice_context[si]; si ++)
- ;
-
- if(f->fsrc && !p->key_frame)
- ff_thread_await_progress(&f->last_picture, si, 0);
-
- if(f->fsrc && !p->key_frame) {
- FFV1Context *fssrc = f->fsrc->slice_context[si];
- FFV1Context *fsdst = f->slice_context[si];
- av_assert1(fsdst->plane_count == fssrc->plane_count);
- av_assert1(fsdst == fs);
-
- if (!p->key_frame)
- fsdst->slice_damaged |= fssrc->slice_damaged;
-
- for (i = 0; i < f->plane_count; i++) {
- PlaneContext *psrc = &fssrc->plane[i];
- PlaneContext *pdst = &fsdst->plane[i];
-
- av_free(pdst->state);
- av_free(pdst->vlc_state);
- memcpy(pdst, psrc, sizeof(*pdst));
- pdst->state = NULL;
- pdst->vlc_state = NULL;
-
- if (fssrc->ac) {
- pdst->state = av_malloc_array(CONTEXT_SIZE, psrc->context_count);
- memcpy(pdst->state, psrc->state, CONTEXT_SIZE * psrc->context_count);
- } else {
- pdst->vlc_state = av_malloc_array(sizeof(*pdst->vlc_state), psrc->context_count);
- memcpy(pdst->vlc_state, psrc->vlc_state, sizeof(*pdst->vlc_state) * psrc->context_count);
- }
- }
- }
-
- fs->slice_rct_by_coef = 1;
- fs->slice_rct_ry_coef = 1;
-
- if (f->version > 2) {
- if (ff_ffv1_init_slice_state(f, fs) < 0)
- return AVERROR(ENOMEM);
- if (decode_slice_header(f, fs) < 0) {
- fs->slice_x = fs->slice_y = fs->slice_height = fs->slice_width = 0;
- fs->slice_damaged = 1;
- return AVERROR_INVALIDDATA;
- }
- }
- if ((ret = ff_ffv1_init_slice_state(f, fs)) < 0)
- return ret;
- if (f->cur->key_frame || fs->slice_reset_contexts) {
- ff_ffv1_clear_slice_state(f, fs);
- } else if (fs->slice_damaged) {
- return AVERROR_INVALIDDATA;
- }
-
- width = fs->slice_width;
- height = fs->slice_height;
- x = fs->slice_x;
- y = fs->slice_y;
-
- if (fs->ac == AC_GOLOMB_RICE) {
- if (f->version == 3 && f->micro_version > 1 || f->version > 3)
- get_rac(&fs->c, (uint8_t[]) { 129 });
- fs->ac_byte_count = f->version > 2 || (!x && !y) ? fs->c.bytestream - fs->c.bytestream_start - 1 : 0;
- init_get_bits(&fs->gb,
- fs->c.bytestream_start + fs->ac_byte_count,
- (fs->c.bytestream_end - fs->c.bytestream_start - fs->ac_byte_count) * 8);
- }
-
- av_assert1(width && height);
- if (f->colorspace == 0 && (f->chroma_planes || !fs->transparency)) {
- const int chroma_width = AV_CEIL_RSHIFT(width, f->chroma_h_shift);
- const int chroma_height = AV_CEIL_RSHIFT(height, f->chroma_v_shift);
- const int cx = x >> f->chroma_h_shift;
- const int cy = y >> f->chroma_v_shift;
- decode_plane(fs, p->data[0] + ps*x + y*p->linesize[0], width, height, p->linesize[0], 0, 1);
-
- if (f->chroma_planes) {
- decode_plane(fs, p->data[1] + ps*cx+cy*p->linesize[1], chroma_width, chroma_height, p->linesize[1], 1, 1);
- decode_plane(fs, p->data[2] + ps*cx+cy*p->linesize[2], chroma_width, chroma_height, p->linesize[2], 1, 1);
- }
- if (fs->transparency)
- decode_plane(fs, p->data[3] + ps*x + y*p->linesize[3], width, height, p->linesize[3], (f->version >= 4 && !f->chroma_planes) ? 1 : 2, 1);
- } else if (f->colorspace == 0) {
- decode_plane(fs, p->data[0] + ps*x + y*p->linesize[0] , width, height, p->linesize[0], 0, 2);
- decode_plane(fs, p->data[0] + ps*x + y*p->linesize[0] + 1, width, height, p->linesize[0], 1, 2);
- } else if (f->use32bit) {
- uint8_t *planes[4] = { p->data[0] + ps * x + y * p->linesize[0],
- p->data[1] + ps * x + y * p->linesize[1],
- p->data[2] + ps * x + y * p->linesize[2],
- p->data[3] + ps * x + y * p->linesize[3] };
- decode_rgb_frame32(fs, planes, width, height, p->linesize);
- } else {
- uint8_t *planes[4] = { p->data[0] + ps * x + y * p->linesize[0],
- p->data[1] + ps * x + y * p->linesize[1],
- p->data[2] + ps * x + y * p->linesize[2],
- p->data[3] + ps * x + y * p->linesize[3] };
- decode_rgb_frame(fs, planes, width, height, p->linesize);
- }
- if (fs->ac != AC_GOLOMB_RICE && f->version > 2) {
- int v;
- get_rac(&fs->c, (uint8_t[]) { 129 });
- v = fs->c.bytestream_end - fs->c.bytestream - 2 - 5*f->ec;
- if (v) {
- av_log(f->avctx, AV_LOG_ERROR, "bytestream end mismatching by %d\n", v);
- fs->slice_damaged = 1;
- }
- }
-
- ff_thread_report_progress(&f->picture, si, 0);
-
- return 0;
-}
-
-static int read_quant_table(RangeCoder *c, int16_t *quant_table, int scale)
-{
- int v;
- int i = 0;
- uint8_t state[CONTEXT_SIZE];
-
- memset(state, 128, sizeof(state));
-
- for (v = 0; i < 128; v++) {
- unsigned len = get_symbol(c, state, 0) + 1U;
-
- if (len > 128 - i || !len)
- return AVERROR_INVALIDDATA;
-
- while (len--) {
- quant_table[i] = scale * v;
- i++;
- }
- }
-
- for (i = 1; i < 128; i++)
- quant_table[256 - i] = -quant_table[i];
- quant_table[128] = -quant_table[127];
-
- return 2 * v - 1;
-}
-
-static int read_quant_tables(RangeCoder *c,
- int16_t quant_table[MAX_CONTEXT_INPUTS][256])
-{
- int i;
- int context_count = 1;
-
- for (i = 0; i < 5; i++) {
- int ret = read_quant_table(c, quant_table[i], context_count);
- if (ret < 0)
- return ret;
- context_count *= ret;
- if (context_count > 32768U) {
- return AVERROR_INVALIDDATA;
- }
- }
- return (context_count + 1) / 2;
-}
-
-static int read_extra_header(FFV1Context *f)
-{
- RangeCoder *const c = &f->c;
- uint8_t state[CONTEXT_SIZE];
- int i, j, k, ret;
- uint8_t state2[32][CONTEXT_SIZE];
- unsigned crc = 0;
-
- memset(state2, 128, sizeof(state2));
- memset(state, 128, sizeof(state));
-
- ff_init_range_decoder(c, f->avctx->extradata, f->avctx->extradata_size);
- ff_build_rac_states(c, 0.05 * (1LL << 32), 256 - 8);
-
- f->version = get_symbol(c, state, 0);
- if (f->version < 2) {
- av_log(f->avctx, AV_LOG_ERROR, "Invalid version in global header\n");
- return AVERROR_INVALIDDATA;
- }
- if (f->version > 4) {
- av_log(f->avctx, AV_LOG_ERROR, "unsupported version %d\n",
- f->version);
- return AVERROR_PATCHWELCOME;
- }
- if (f->version > 2) {
- c->bytestream_end -= 4;
- f->micro_version = get_symbol(c, state, 0);
- if (f->micro_version < 0)
- return AVERROR_INVALIDDATA;
- }
- f->ac = get_symbol(c, state, 0);
-
- if (f->ac == AC_RANGE_CUSTOM_TAB) {
- for (i = 1; i < 256; i++)
- f->state_transition[i] = get_symbol(c, state, 1) + c->one_state[i];
- }
-
- f->colorspace = get_symbol(c, state, 0); //YUV cs type
- f->avctx->bits_per_raw_sample = get_symbol(c, state, 0);
- f->chroma_planes = get_rac(c, state);
- f->chroma_h_shift = get_symbol(c, state, 0);
- f->chroma_v_shift = get_symbol(c, state, 0);
- f->transparency = get_rac(c, state);
- f->plane_count = 1 + (f->chroma_planes || f->version<4) + f->transparency;
- f->num_h_slices = 1 + get_symbol(c, state, 0);
- f->num_v_slices = 1 + get_symbol(c, state, 0);
-
- if (f->chroma_h_shift > 4U || f->chroma_v_shift > 4U) {
- av_log(f->avctx, AV_LOG_ERROR, "chroma shift parameters %d %d are invalid\n",
- f->chroma_h_shift, f->chroma_v_shift);
- return AVERROR_INVALIDDATA;
- }
-
- if (f->num_h_slices > (unsigned)f->width || !f->num_h_slices ||
- f->num_v_slices > (unsigned)f->height || !f->num_v_slices
- ) {
- av_log(f->avctx, AV_LOG_ERROR, "slice count invalid\n");
- return AVERROR_INVALIDDATA;
- }
-
- if (f->num_h_slices > MAX_SLICES / f->num_v_slices) {
- av_log(f->avctx, AV_LOG_ERROR, "slice count unsupported\n");
- return AVERROR_PATCHWELCOME;
- }
-
- f->quant_table_count = get_symbol(c, state, 0);
- if (f->quant_table_count > (unsigned)MAX_QUANT_TABLES || !f->quant_table_count) {
- av_log(f->avctx, AV_LOG_ERROR, "quant table count %d is invalid\n", f->quant_table_count);
- f->quant_table_count = 0;
- return AVERROR_INVALIDDATA;
- }
-
- for (i = 0; i < f->quant_table_count; i++) {
- f->context_count[i] = read_quant_tables(c, f->quant_tables[i]);
- if (f->context_count[i] < 0) {
- av_log(f->avctx, AV_LOG_ERROR, "read_quant_table error\n");
- return AVERROR_INVALIDDATA;
- }
- }
- if ((ret = ff_ffv1_allocate_initial_states(f)) < 0)
- return ret;
-
- for (i = 0; i < f->quant_table_count; i++)
- if (get_rac(c, state)) {
- for (j = 0; j < f->context_count[i]; j++)
- for (k = 0; k < CONTEXT_SIZE; k++) {
- int pred = j ? f->initial_states[i][j - 1][k] : 128;
- f->initial_states[i][j][k] =
- (pred + get_symbol(c, state2[k], 1)) & 0xFF;
- }
- }
-
- if (f->version > 2) {
- f->ec = get_symbol(c, state, 0);
- if (f->micro_version > 2)
- f->intra = get_symbol(c, state, 0);
- }
-
- if (f->version > 2) {
- unsigned v;
- v = av_crc(av_crc_get_table(AV_CRC_32_IEEE), 0,
- f->avctx->extradata, f->avctx->extradata_size);
- if (v || f->avctx->extradata_size < 4) {
- av_log(f->avctx, AV_LOG_ERROR, "CRC mismatch %X!\n", v);
- return AVERROR_INVALIDDATA;
- }
- crc = AV_RB32(f->avctx->extradata + f->avctx->extradata_size - 4);
- }
-
- if (f->avctx->debug & FF_DEBUG_PICT_INFO)
- av_log(f->avctx, AV_LOG_DEBUG,
- "global: ver:%d.%d, coder:%d, colorspace: %d bpr:%d chroma:%d(%d:%d), alpha:%d slices:%dx%d qtabs:%d ec:%d intra:%d CRC:0x%08X\n",
- f->version, f->micro_version,
- f->ac,
- f->colorspace,
- f->avctx->bits_per_raw_sample,
- f->chroma_planes, f->chroma_h_shift, f->chroma_v_shift,
- f->transparency,
- f->num_h_slices, f->num_v_slices,
- f->quant_table_count,
- f->ec,
- f->intra,
- crc
- );
- return 0;
-}
-
-static int read_header(FFV1Context *f)
-{
- uint8_t state[CONTEXT_SIZE];
- int i, j, context_count = -1; //-1 to avoid warning
- RangeCoder *const c = &f->slice_context[0]->c;
-
- memset(state, 128, sizeof(state));
-
- if (f->version < 2) {
- int chroma_planes, chroma_h_shift, chroma_v_shift, transparency, colorspace, bits_per_raw_sample;
- unsigned v= get_symbol(c, state, 0);
- if (v >= 2) {
- av_log(f->avctx, AV_LOG_ERROR, "invalid version %d in ver01 header\n", v);
- return AVERROR_INVALIDDATA;
- }
- f->version = v;
- f->ac = get_symbol(c, state, 0);
-
- if (f->ac == AC_RANGE_CUSTOM_TAB) {
- for (i = 1; i < 256; i++) {
- int st = get_symbol(c, state, 1) + c->one_state[i];
- if (st < 1 || st > 255) {
- av_log(f->avctx, AV_LOG_ERROR, "invalid state transition %d\n", st);
- return AVERROR_INVALIDDATA;
- }
- f->state_transition[i] = st;
- }
- }
-
- colorspace = get_symbol(c, state, 0); //YUV cs type
- bits_per_raw_sample = f->version > 0 ? get_symbol(c, state, 0) : f->avctx->bits_per_raw_sample;
- chroma_planes = get_rac(c, state);
- chroma_h_shift = get_symbol(c, state, 0);
- chroma_v_shift = get_symbol(c, state, 0);
- transparency = get_rac(c, state);
- if (colorspace == 0 && f->avctx->skip_alpha)
- transparency = 0;
-
- if (f->plane_count) {
- if (colorspace != f->colorspace ||
- bits_per_raw_sample != f->avctx->bits_per_raw_sample ||
- chroma_planes != f->chroma_planes ||
- chroma_h_shift != f->chroma_h_shift ||
- chroma_v_shift != f->chroma_v_shift ||
- transparency != f->transparency) {
- av_log(f->avctx, AV_LOG_ERROR, "Invalid change of global parameters\n");
- return AVERROR_INVALIDDATA;
- }
- }
-
- if (chroma_h_shift > 4U || chroma_v_shift > 4U) {
- av_log(f->avctx, AV_LOG_ERROR, "chroma shift parameters %d %d are invalid\n",
- chroma_h_shift, chroma_v_shift);
- return AVERROR_INVALIDDATA;
- }
-
- f->colorspace = colorspace;
- f->avctx->bits_per_raw_sample = bits_per_raw_sample;
- f->chroma_planes = chroma_planes;
- f->chroma_h_shift = chroma_h_shift;
- f->chroma_v_shift = chroma_v_shift;
- f->transparency = transparency;
-
- f->plane_count = 2 + f->transparency;
- }
-
- if (f->colorspace == 0) {
- if (!f->transparency && !f->chroma_planes) {
- if (f->avctx->bits_per_raw_sample <= 8)
- f->avctx->pix_fmt = AV_PIX_FMT_GRAY8;
- else if (f->avctx->bits_per_raw_sample == 9) {
- f->packed_at_lsb = 1;
- f->avctx->pix_fmt = AV_PIX_FMT_GRAY9;
- } else if (f->avctx->bits_per_raw_sample == 10) {
- f->packed_at_lsb = 1;
- f->avctx->pix_fmt = AV_PIX_FMT_GRAY10;
- } else if (f->avctx->bits_per_raw_sample == 12) {
- f->packed_at_lsb = 1;
- f->avctx->pix_fmt = AV_PIX_FMT_GRAY12;
- } else if (f->avctx->bits_per_raw_sample == 16) {
- f->packed_at_lsb = 1;
- f->avctx->pix_fmt = AV_PIX_FMT_GRAY16;
- } else if (f->avctx->bits_per_raw_sample < 16) {
- f->avctx->pix_fmt = AV_PIX_FMT_GRAY16;
- } else
- return AVERROR(ENOSYS);
- } else if (f->transparency && !f->chroma_planes) {
- if (f->avctx->bits_per_raw_sample <= 8)
- f->avctx->pix_fmt = AV_PIX_FMT_YA8;
- else
- return AVERROR(ENOSYS);
- } else if (f->avctx->bits_per_raw_sample<=8 && !f->transparency) {
- switch(16 * f->chroma_h_shift + f->chroma_v_shift) {
- case 0x00: f->avctx->pix_fmt = AV_PIX_FMT_YUV444P; break;
- case 0x01: f->avctx->pix_fmt = AV_PIX_FMT_YUV440P; break;
- case 0x10: f->avctx->pix_fmt = AV_PIX_FMT_YUV422P; break;
- case 0x11: f->avctx->pix_fmt = AV_PIX_FMT_YUV420P; break;
- case 0x20: f->avctx->pix_fmt = AV_PIX_FMT_YUV411P; break;
- case 0x22: f->avctx->pix_fmt = AV_PIX_FMT_YUV410P; break;
- }
- } else if (f->avctx->bits_per_raw_sample <= 8 && f->transparency) {
- switch(16*f->chroma_h_shift + f->chroma_v_shift) {
- case 0x00: f->avctx->pix_fmt = AV_PIX_FMT_YUVA444P; break;
- case 0x10: f->avctx->pix_fmt = AV_PIX_FMT_YUVA422P; break;
- case 0x11: f->avctx->pix_fmt = AV_PIX_FMT_YUVA420P; break;
- }
- } else if (f->avctx->bits_per_raw_sample == 9 && !f->transparency) {
- f->packed_at_lsb = 1;
- switch(16 * f->chroma_h_shift + f->chroma_v_shift) {
- case 0x00: f->avctx->pix_fmt = AV_PIX_FMT_YUV444P9; break;
- case 0x10: f->avctx->pix_fmt = AV_PIX_FMT_YUV422P9; break;
- case 0x11: f->avctx->pix_fmt = AV_PIX_FMT_YUV420P9; break;
- }
- } else if (f->avctx->bits_per_raw_sample == 9 && f->transparency) {
- f->packed_at_lsb = 1;
- switch(16 * f->chroma_h_shift + f->chroma_v_shift) {
- case 0x00: f->avctx->pix_fmt = AV_PIX_FMT_YUVA444P9; break;
- case 0x10: f->avctx->pix_fmt = AV_PIX_FMT_YUVA422P9; break;
- case 0x11: f->avctx->pix_fmt = AV_PIX_FMT_YUVA420P9; break;
- }
- } else if (f->avctx->bits_per_raw_sample == 10 && !f->transparency) {
- f->packed_at_lsb = 1;
- switch(16 * f->chroma_h_shift + f->chroma_v_shift) {
- case 0x00: f->avctx->pix_fmt = AV_PIX_FMT_YUV444P10; break;
- case 0x01: f->avctx->pix_fmt = AV_PIX_FMT_YUV440P10; break;
- case 0x10: f->avctx->pix_fmt = AV_PIX_FMT_YUV422P10; break;
- case 0x11: f->avctx->pix_fmt = AV_PIX_FMT_YUV420P10; break;
- }
- } else if (f->avctx->bits_per_raw_sample == 10 && f->transparency) {
- f->packed_at_lsb = 1;
- switch(16 * f->chroma_h_shift + f->chroma_v_shift) {
- case 0x00: f->avctx->pix_fmt = AV_PIX_FMT_YUVA444P10; break;
- case 0x10: f->avctx->pix_fmt = AV_PIX_FMT_YUVA422P10; break;
- case 0x11: f->avctx->pix_fmt = AV_PIX_FMT_YUVA420P10; break;
- }
- } else if (f->avctx->bits_per_raw_sample == 12 && !f->transparency) {
- f->packed_at_lsb = 1;
- switch(16 * f->chroma_h_shift + f->chroma_v_shift) {
- case 0x00: f->avctx->pix_fmt = AV_PIX_FMT_YUV444P12; break;
- case 0x01: f->avctx->pix_fmt = AV_PIX_FMT_YUV440P12; break;
- case 0x10: f->avctx->pix_fmt = AV_PIX_FMT_YUV422P12; break;
- case 0x11: f->avctx->pix_fmt = AV_PIX_FMT_YUV420P12; break;
- }
- } else if (f->avctx->bits_per_raw_sample == 14 && !f->transparency) {
- f->packed_at_lsb = 1;
- switch(16 * f->chroma_h_shift + f->chroma_v_shift) {
- case 0x00: f->avctx->pix_fmt = AV_PIX_FMT_YUV444P14; break;
- case 0x10: f->avctx->pix_fmt = AV_PIX_FMT_YUV422P14; break;
- case 0x11: f->avctx->pix_fmt = AV_PIX_FMT_YUV420P14; break;
- }
- } else if (f->avctx->bits_per_raw_sample == 16 && !f->transparency){
- f->packed_at_lsb = 1;
- switch(16 * f->chroma_h_shift + f->chroma_v_shift) {
- case 0x00: f->avctx->pix_fmt = AV_PIX_FMT_YUV444P16; break;
- case 0x10: f->avctx->pix_fmt = AV_PIX_FMT_YUV422P16; break;
- case 0x11: f->avctx->pix_fmt = AV_PIX_FMT_YUV420P16; break;
- }
- } else if (f->avctx->bits_per_raw_sample == 16 && f->transparency){
- f->packed_at_lsb = 1;
- switch(16 * f->chroma_h_shift + f->chroma_v_shift) {
- case 0x00: f->avctx->pix_fmt = AV_PIX_FMT_YUVA444P16; break;
- case 0x10: f->avctx->pix_fmt = AV_PIX_FMT_YUVA422P16; break;
- case 0x11: f->avctx->pix_fmt = AV_PIX_FMT_YUVA420P16; break;
- }
- }
- } else if (f->colorspace == 1) {
- if (f->chroma_h_shift || f->chroma_v_shift) {
- av_log(f->avctx, AV_LOG_ERROR,
- "chroma subsampling not supported in this colorspace\n");
- return AVERROR(ENOSYS);
- }
- if ( f->avctx->bits_per_raw_sample <= 8 && !f->transparency)
- f->avctx->pix_fmt = AV_PIX_FMT_0RGB32;
- else if (f->avctx->bits_per_raw_sample <= 8 && f->transparency)
- f->avctx->pix_fmt = AV_PIX_FMT_RGB32;
- else if (f->avctx->bits_per_raw_sample == 9 && !f->transparency)
- f->avctx->pix_fmt = AV_PIX_FMT_GBRP9;
- else if (f->avctx->bits_per_raw_sample == 10 && !f->transparency)
- f->avctx->pix_fmt = AV_PIX_FMT_GBRP10;
- else if (f->avctx->bits_per_raw_sample == 10 && f->transparency)
- f->avctx->pix_fmt = AV_PIX_FMT_GBRAP10;
- else if (f->avctx->bits_per_raw_sample == 12 && !f->transparency)
- f->avctx->pix_fmt = AV_PIX_FMT_GBRP12;
- else if (f->avctx->bits_per_raw_sample == 12 && f->transparency)
- f->avctx->pix_fmt = AV_PIX_FMT_GBRAP12;
- else if (f->avctx->bits_per_raw_sample == 14 && !f->transparency)
- f->avctx->pix_fmt = AV_PIX_FMT_GBRP14;
- else if (f->avctx->bits_per_raw_sample == 16 && !f->transparency) {
- f->avctx->pix_fmt = AV_PIX_FMT_GBRP16;
- f->use32bit = 1;
- }
- else if (f->avctx->bits_per_raw_sample == 16 && f->transparency) {
- f->avctx->pix_fmt = AV_PIX_FMT_GBRAP16;
- f->use32bit = 1;
- }
- } else {
- av_log(f->avctx, AV_LOG_ERROR, "colorspace not supported\n");
- return AVERROR(ENOSYS);
- }
- if (f->avctx->pix_fmt == AV_PIX_FMT_NONE) {
- av_log(f->avctx, AV_LOG_ERROR, "format not supported\n");
- return AVERROR(ENOSYS);
- }
-
- ff_dlog(f->avctx, "%d %d %d\n",
- f->chroma_h_shift, f->chroma_v_shift, f->avctx->pix_fmt);
- if (f->version < 2) {
- context_count = read_quant_tables(c, f->quant_table);
- if (context_count < 0) {
- av_log(f->avctx, AV_LOG_ERROR, "read_quant_table error\n");
- return AVERROR_INVALIDDATA;
- }
- f->slice_count = f->max_slice_count;
- } else if (f->version < 3) {
- f->slice_count = get_symbol(c, state, 0);
- } else {
- const uint8_t *p = c->bytestream_end;
- for (f->slice_count = 0;
- f->slice_count < MAX_SLICES && 3 + 5*!!f->ec < p - c->bytestream_start;
- f->slice_count++) {
- int trailer = 3 + 5*!!f->ec;
- int size = AV_RB24(p-trailer);
- if (size + trailer > p - c->bytestream_start)
- break;
- p -= size + trailer;
- }
- }
- if (f->slice_count > (unsigned)MAX_SLICES || f->slice_count <= 0 || f->slice_count > f->max_slice_count) {
- av_log(f->avctx, AV_LOG_ERROR, "slice count %d is invalid (max=%d)\n", f->slice_count, f->max_slice_count);
- return AVERROR_INVALIDDATA;
- }
-
- for (j = 0; j < f->slice_count; j++) {
- FFV1Context *fs = f->slice_context[j];
- fs->ac = f->ac;
- fs->packed_at_lsb = f->packed_at_lsb;
-
- fs->slice_damaged = 0;
-
- if (f->version == 2) {
- int sx = get_symbol(c, state, 0);
- int sy = get_symbol(c, state, 0);
- int sw = get_symbol(c, state, 0) + 1U;
- int sh = get_symbol(c, state, 0) + 1U;
-
- if (sx < 0 || sy < 0 || sw <= 0 || sh <= 0)
- return AVERROR_INVALIDDATA;
- if (sx > f->num_h_slices - sw || sy > f->num_v_slices - sh)
- return AVERROR_INVALIDDATA;
-
- fs->slice_x = sx * (int64_t)f->width / f->num_h_slices;
- fs->slice_y = sy * (int64_t)f->height / f->num_v_slices;
- fs->slice_width = (sx + sw) * (int64_t)f->width / f->num_h_slices - fs->slice_x;
- fs->slice_height = (sy + sh) * (int64_t)f->height / f->num_v_slices - fs->slice_y;
-
- av_assert0((unsigned)fs->slice_width <= f->width &&
- (unsigned)fs->slice_height <= f->height);
- av_assert0 ( (unsigned)fs->slice_x + (uint64_t)fs->slice_width <= f->width
- && (unsigned)fs->slice_y + (uint64_t)fs->slice_height <= f->height);
- }
-
- for (i = 0; i < f->plane_count; i++) {
- PlaneContext *const p = &fs->plane[i];
-
- if (f->version == 2) {
- int idx = get_symbol(c, state, 0);
- if (idx >= (unsigned)f->quant_table_count) {
- av_log(f->avctx, AV_LOG_ERROR,
- "quant_table_index out of range\n");
- return AVERROR_INVALIDDATA;
- }
- p->quant_table_index = idx;
- memcpy(p->quant_table, f->quant_tables[idx],
- sizeof(p->quant_table));
- context_count = f->context_count[idx];
- } else {
- memcpy(p->quant_table, f->quant_table, sizeof(p->quant_table));
- }
-
- if (f->version <= 2) {
- av_assert0(context_count >= 0);
- if (p->context_count < context_count) {
- av_freep(&p->state);
- av_freep(&p->vlc_state);
- }
- p->context_count = context_count;
- }
- }
- }
- return 0;
-}
-
-static av_cold int decode_init(AVCodecContext *avctx)
-{
- FFV1Context *f = avctx->priv_data;
- int ret;
-
- if ((ret = ff_ffv1_common_init(avctx)) < 0)
- return ret;
-
- f->picture.f = av_frame_alloc();
- f->last_picture.f = av_frame_alloc();
- if (!f->picture.f || !f->last_picture.f)
- return AVERROR(ENOMEM);
-
- if (avctx->extradata_size > 0 && (ret = read_extra_header(f)) < 0)
- return ret;
-
- if ((ret = ff_ffv1_init_slice_contexts(f)) < 0)
- return ret;
-
- return 0;
-}
-
-static int decode_frame(AVCodecContext *avctx, AVFrame *rframe,
- int *got_frame, AVPacket *avpkt)
-{
- uint8_t *buf = avpkt->data;
- int buf_size = avpkt->size;
- FFV1Context *f = avctx->priv_data;
- RangeCoder *const c = &f->slice_context[0]->c;
- int i, ret;
- uint8_t keystate = 128;
- uint8_t *buf_p;
- AVFrame *p;
-
- if (f->last_picture.f)
- ff_thread_release_ext_buffer(avctx, &f->last_picture);
- FFSWAP(ThreadFrame, f->picture, f->last_picture);
-
- f->cur = p = f->picture.f;
-
- if (f->version < 3 && avctx->field_order > AV_FIELD_PROGRESSIVE) {
- /* we have interlaced material flagged in container */
- p->interlaced_frame = 1;
- if (avctx->field_order == AV_FIELD_TT || avctx->field_order == AV_FIELD_TB)
- p->top_field_first = 1;
- }
-
- f->avctx = avctx;
- ff_init_range_decoder(c, buf, buf_size);
- ff_build_rac_states(c, 0.05 * (1LL << 32), 256 - 8);
-
- p->pict_type = AV_PICTURE_TYPE_I; //FIXME I vs. P
- if (get_rac(c, &keystate)) {
- p->key_frame = 1;
- f->key_frame_ok = 0;
- if ((ret = read_header(f)) < 0)
- return ret;
- f->key_frame_ok = 1;
- } else {
- if (!f->key_frame_ok) {
- av_log(avctx, AV_LOG_ERROR,
- "Cannot decode non-keyframe without valid keyframe\n");
- return AVERROR_INVALIDDATA;
- }
- p->key_frame = 0;
- }
-
- if (f->ac != AC_GOLOMB_RICE) {
- if (buf_size < avctx->width * avctx->height / (128*8))
- return AVERROR_INVALIDDATA;
- } else {
- int w = avctx->width;
- int s = 1 + w / (1<<23);
-
- w /= s;
-
- for (i = 0; w > (1<height + i + 6) / 8 * s)
- return AVERROR_INVALIDDATA;
- }
-
- ret = ff_thread_get_ext_buffer(avctx, &f->picture, AV_GET_BUFFER_FLAG_REF);
- if (ret < 0)
- return ret;
-
- if (avctx->debug & FF_DEBUG_PICT_INFO)
- av_log(avctx, AV_LOG_DEBUG, "ver:%d keyframe:%d coder:%d ec:%d slices:%d bps:%d\n",
- f->version, p->key_frame, f->ac, f->ec, f->slice_count, f->avctx->bits_per_raw_sample);
-
- ff_thread_finish_setup(avctx);
-
- buf_p = buf + buf_size;
- for (i = f->slice_count - 1; i >= 0; i--) {
- FFV1Context *fs = f->slice_context[i];
- int trailer = 3 + 5*!!f->ec;
- int v;
-
- if (i || f->version > 2) {
- if (trailer > buf_p - buf) v = INT_MAX;
- else v = AV_RB24(buf_p-trailer) + trailer;
- } else v = buf_p - c->bytestream_start;
- if (buf_p - c->bytestream_start < v) {
- av_log(avctx, AV_LOG_ERROR, "Slice pointer chain broken\n");
- ff_thread_report_progress(&f->picture, INT_MAX, 0);
- return AVERROR_INVALIDDATA;
- }
- buf_p -= v;
-
- if (f->ec) {
- unsigned crc = av_crc(av_crc_get_table(AV_CRC_32_IEEE), 0, buf_p, v);
- if (crc) {
- int64_t ts = avpkt->pts != AV_NOPTS_VALUE ? avpkt->pts : avpkt->dts;
- av_log(f->avctx, AV_LOG_ERROR, "slice CRC mismatch %X!", crc);
- if (ts != AV_NOPTS_VALUE && avctx->pkt_timebase.num) {
- av_log(f->avctx, AV_LOG_ERROR, "at %f seconds\n", ts*av_q2d(avctx->pkt_timebase));
- } else if (ts != AV_NOPTS_VALUE) {
- av_log(f->avctx, AV_LOG_ERROR, "at %"PRId64"\n", ts);
- } else {
- av_log(f->avctx, AV_LOG_ERROR, "\n");
- }
- fs->slice_damaged = 1;
- }
- if (avctx->debug & FF_DEBUG_PICT_INFO) {
- av_log(avctx, AV_LOG_DEBUG, "slice %d, CRC: 0x%08"PRIX32"\n", i, AV_RB32(buf_p + v - 4));
- }
- }
-
- if (i) {
- ff_init_range_decoder(&fs->c, buf_p, v);
- } else
- fs->c.bytestream_end = buf_p + v;
-
- fs->avctx = avctx;
- }
-
- avctx->execute(avctx,
- decode_slice,
- &f->slice_context[0],
- NULL,
- f->slice_count,
- sizeof(void*));
-
- for (i = f->slice_count - 1; i >= 0; i--) {
- FFV1Context *fs = f->slice_context[i];
- int j;
- if (fs->slice_damaged && f->last_picture.f->data[0]) {
- const AVPixFmtDescriptor *desc = av_pix_fmt_desc_get(avctx->pix_fmt);
- const uint8_t *src[4];
- uint8_t *dst[4];
- ff_thread_await_progress(&f->last_picture, INT_MAX, 0);
- for (j = 0; j < desc->nb_components; j++) {
- int pixshift = desc->comp[j].depth > 8;
- int sh = (j == 1 || j == 2) ? f->chroma_h_shift : 0;
- int sv = (j == 1 || j == 2) ? f->chroma_v_shift : 0;
- dst[j] = p->data[j] + p->linesize[j] *
- (fs->slice_y >> sv) + ((fs->slice_x >> sh) << pixshift);
- src[j] = f->last_picture.f->data[j] + f->last_picture.f->linesize[j] *
- (fs->slice_y >> sv) + ((fs->slice_x >> sh) << pixshift);
-
- }
- if (desc->flags & AV_PIX_FMT_FLAG_PAL) {
- dst[1] = p->data[1];
- src[1] = f->last_picture.f->data[1];
- }
- av_image_copy(dst, p->linesize, src,
- f->last_picture.f->linesize,
- avctx->pix_fmt,
- fs->slice_width,
- fs->slice_height);
- }
- }
- ff_thread_report_progress(&f->picture, INT_MAX, 0);
-
- if (f->last_picture.f)
- ff_thread_release_ext_buffer(avctx, &f->last_picture);
- if ((ret = av_frame_ref(rframe, f->picture.f)) < 0)
- return ret;
-
- *got_frame = 1;
-
- return buf_size;
-}
-
-static void copy_fields(FFV1Context *fsdst, const FFV1Context *fssrc,
- const FFV1Context *fsrc)
-{
- fsdst->version = fsrc->version;
- fsdst->micro_version = fsrc->micro_version;
- fsdst->chroma_planes = fsrc->chroma_planes;
- fsdst->chroma_h_shift = fsrc->chroma_h_shift;
- fsdst->chroma_v_shift = fsrc->chroma_v_shift;
- fsdst->transparency = fsrc->transparency;
- fsdst->plane_count = fsrc->plane_count;
- fsdst->ac = fsrc->ac;
- fsdst->colorspace = fsrc->colorspace;
-
- fsdst->ec = fsrc->ec;
- fsdst->intra = fsrc->intra;
- fsdst->slice_damaged = fssrc->slice_damaged;
- fsdst->key_frame_ok = fsrc->key_frame_ok;
-
- fsdst->packed_at_lsb = fsrc->packed_at_lsb;
- fsdst->slice_count = fsrc->slice_count;
- if (fsrc->version<3){
- fsdst->slice_x = fssrc->slice_x;
- fsdst->slice_y = fssrc->slice_y;
- fsdst->slice_width = fssrc->slice_width;
- fsdst->slice_height = fssrc->slice_height;
- }
-}
-
-#if HAVE_THREADS
-static int update_thread_context(AVCodecContext *dst, const AVCodecContext *src)
-{
- FFV1Context *fsrc = src->priv_data;
- FFV1Context *fdst = dst->priv_data;
- int i, ret;
-
- if (dst == src)
- return 0;
-
- {
- ThreadFrame picture = fdst->picture, last_picture = fdst->last_picture;
- uint8_t (*initial_states[MAX_QUANT_TABLES])[32];
- struct FFV1Context *slice_context[MAX_SLICES];
- memcpy(initial_states, fdst->initial_states, sizeof(fdst->initial_states));
- memcpy(slice_context, fdst->slice_context , sizeof(fdst->slice_context));
-
- memcpy(fdst, fsrc, sizeof(*fdst));
- memcpy(fdst->initial_states, initial_states, sizeof(fdst->initial_states));
- memcpy(fdst->slice_context, slice_context , sizeof(fdst->slice_context));
- fdst->picture = picture;
- fdst->last_picture = last_picture;
- for (i = 0; inum_h_slices * fdst->num_v_slices; i++) {
- FFV1Context *fssrc = fsrc->slice_context[i];
- FFV1Context *fsdst = fdst->slice_context[i];
- copy_fields(fsdst, fssrc, fsrc);
- }
- av_assert0(!fdst->plane[0].state);
- av_assert0(!fdst->sample_buffer);
- }
-
- av_assert1(fdst->max_slice_count == fsrc->max_slice_count);
-
-
- ff_thread_release_ext_buffer(dst, &fdst->picture);
- if (fsrc->picture.f->data[0]) {
- if ((ret = ff_thread_ref_frame(&fdst->picture, &fsrc->picture)) < 0)
- return ret;
- }
-
- fdst->fsrc = fsrc;
-
- return 0;
-}
-#endif
-
-static av_cold int ffv1_decode_close(AVCodecContext *avctx)
-{
- FFV1Context *const s = avctx->priv_data;
-
- if (s->picture.f) {
- ff_thread_release_ext_buffer(avctx, &s->picture);
- av_frame_free(&s->picture.f);
- }
-
- if (s->last_picture.f) {
- ff_thread_release_ext_buffer(avctx, &s->last_picture);
- av_frame_free(&s->last_picture.f);
- }
- return ff_ffv1_close(avctx);
-}
-
-const FFCodec ff_ffv1_decoder = {
- .p.name = "ffv1",
- CODEC_LONG_NAME("FFmpeg video codec #1"),
- .p.type = AVMEDIA_TYPE_VIDEO,
- .p.id = AV_CODEC_ID_FFV1,
- .priv_data_size = sizeof(FFV1Context),
- .init = decode_init,
- .close = ffv1_decode_close,
- FF_CODEC_DECODE_CB(decode_frame),
- UPDATE_THREAD_CONTEXT(update_thread_context),
- .p.capabilities = AV_CODEC_CAP_DR1 |
- AV_CODEC_CAP_FRAME_THREADS | AV_CODEC_CAP_SLICE_THREADS,
- .caps_internal = FF_CODEC_CAP_INIT_CLEANUP |
- FF_CODEC_CAP_ALLOCATE_PROGRESS,
-};
diff --git a/spaces/colakin/video-generater/public/ffmpeg/libavcodec/mediacodec_surface.c b/spaces/colakin/video-generater/public/ffmpeg/libavcodec/mediacodec_surface.c
deleted file mode 100644
index ef41cdafa78b10513187a914c579ccd9698893c9..0000000000000000000000000000000000000000
--- a/spaces/colakin/video-generater/public/ffmpeg/libavcodec/mediacodec_surface.c
+++ /dev/null
@@ -1,78 +0,0 @@
-/*
- * Android MediaCodec Surface functions
- *
- * Copyright (c) 2016 Matthieu Bouron
- *
- * This file is part of FFmpeg.
- *
- * FFmpeg is free software; you can redistribute it and/or
- * modify it under the terms of the GNU Lesser General Public
- * License as published by the Free Software Foundation; either
- * version 2.1 of the License, or (at your option) any later version.
- *
- * FFmpeg is distributed in the hope that it will be useful,
- * but WITHOUT ANY WARRANTY; without even the implied warranty of
- * MERCHANTABILITY or FITNESS FOR A PARTICULAR PURPOSE. See the GNU
- * Lesser General Public License for more details.
- *
- * You should have received a copy of the GNU Lesser General Public
- * License along with FFmpeg; if not, write to the Free Software
- * Foundation, Inc., 51 Franklin Street, Fifth Floor, Boston, MA 02110-1301 USA
- */
-
-#include
-#include
-
-#include "libavutil/mem.h"
-#include "ffjni.h"
-#include "mediacodec_surface.h"
-
-FFANativeWindow *ff_mediacodec_surface_ref(void *surface, void *native_window, void *log_ctx)
-{
- FFANativeWindow *ret;
-
- ret = av_mallocz(sizeof(*ret));
- if (!ret)
- return NULL;
-
- if (surface) {
- JNIEnv *env = NULL;
-
- env = ff_jni_get_env(log_ctx);
- if (env)
- ret->surface = (*env)->NewGlobalRef(env, surface);
- }
-
- if (native_window) {
- ANativeWindow_acquire(native_window);
- ret->native_window = native_window;
- }
-
- if (!ret->surface && !ret->native_window) {
- av_log(log_ctx, AV_LOG_ERROR, "Both surface and native_window are NULL\n");
- av_freep(&ret);
- }
-
- return ret;
-}
-
-int ff_mediacodec_surface_unref(FFANativeWindow *window, void *log_ctx)
-{
- if (!window)
- return 0;
-
- if (window->surface) {
- JNIEnv *env = NULL;
-
- env = ff_jni_get_env(log_ctx);
- if (env)
- (*env)->DeleteGlobalRef(env, window->surface);
- }
-
- if (window->native_window)
- ANativeWindow_release(window->native_window);
-
- av_free(window);
-
- return 0;
-}
diff --git a/spaces/congsaPfin/Manga-OCR/logs/Descarga summertime saga apk espaol 2023 para iphone - Juego de aventuras gratis.md b/spaces/congsaPfin/Manga-OCR/logs/Descarga summertime saga apk espaol 2023 para iphone - Juego de aventuras gratis.md
deleted file mode 100644
index 2d2ad762a734eada90b26e239a0b29847efa943a..0000000000000000000000000000000000000000
--- a/spaces/congsaPfin/Manga-OCR/logs/Descarga summertime saga apk espaol 2023 para iphone - Juego de aventuras gratis.md
+++ /dev/null
@@ -1,152 +0,0 @@
-
-
Summertime Saga APK Español Para iPhone: ¿Qué es y cómo descargarlo?
-
¿Te gustan los juegos de simulación de citas y novelas visuales? ¿Quieres jugar a uno de los más populares y divertidos del género en tu iPhone? ¿Y además en español? Entonces estás de suerte porque en este artículo te vamos a explicar qué es y cómo descargar e instalar Summertime Saga APK Español Para iPhone.
Summertime Saga es un juego de simulación de citas y novela visual para adultos, desarrollado por DarkCookie y su equipo. El juego tiene una gran cantidad de contenido, personajes, historias, y escenas para explorar, con un estilo gráfico animado y humorístico.
-
El juego se centra en la vida de un joven estudiante que se ve envuelto en una serie de aventuras y misterios tras la muerte de su padre. El protagonista tendrá que lidiar con sus problemas familiares, escolares, económicos, y sentimentales, mientras conoce y se relaciona con más de 70 personajes diferentes, cada uno con su propia personalidad, historia, y preferencias.
-
Summertime Saga tiene más de 20 ubicaciones diferentes para visitar, desde la casa del protagonista hasta la playa, el colegio, el hospital, el cementerio, el centro comercial, y muchos más. El juego también tiene varios mini-juegos para divertirse y ganar dinero o recompensas, como carreras de motos, peleas callejeras, rap battles, pesca, ajedrez, póker, y otros.
-
El juego está disponible para Windows, Mac, Linux, y Android, y se puede descargar gratis desde su página web oficial o desde su página de Patreon. El juego se actualiza periódicamente con nuevas versiones que añaden más contenido e historias. La última versión disponible es la 0.20.11, lanzada el 30 de julio de 2021.
-
descargar summertime saga apk español para iphone
-summertime saga apk español para iphone gratis
-summertime saga apk español para iphone sin verificación
-cómo instalar summertime saga apk español para iphone
-summertime saga apk español para iphone última versión
-summertime saga apk español para iphone mega
-summertime saga apk español para iphone gameplay
-summertime saga apk español para iphone trucos
-summertime saga apk español para iphone requisitos
-summertime saga apk español para iphone mod
-summertime saga apk español para iphone tutorial
-summertime saga apk español para iphone online
-summertime saga apk español para iphone descargar gratis
-summertime saga apk español para iphone 2023
-summertime saga apk español para iphone actualizado
-summertime saga apk español para iphone completo
-summertime saga apk español para iphone sin internet
-summertime saga apk español para iphone guía
-summertime saga apk español para iphone ios 14
-summertime saga apk español para iphone opiniones
-summertime saga apk español para iphone full
-summertime saga apk español para iphone historia
-summertime saga apk español para iphone personajes
-summertime saga apk español para iphone final
-summertime saga apk español para iphone descargar mega
-summertime saga apk español para iphone ios 15
-summertime saga apk español para iphone sin jailbreak
-summertime saga apk español para iphone mediafire
-summertime saga apk español para iphone link directo
-summertime saga apk español para iphone reseña
-summertime saga apk español para iphone tips
-summertime saga apk español para iphone novedades
-summertime saga apk español para iphone alternativas
-summertime saga apk español para iphone secretos
-summertime saga apk español para iphone parcheado
-summertime saga apk español para iphone compatible con ipad
-summertime saga apk español para iphone sin anuncios
-summertime saga apk español para iphone con voces en español
-summertime saga apk español para iphone con todos los personajes desbloqueados
-summertime saga apk español para iphone con gráficos mejorados
-
¿Por qué jugar Summertime Saga en español?
-
Summertime Saga es un juego que tiene mucho texto y diálogo, por lo que jugarlo en español puede mejorar la experiencia de juego al facilitar la comprensión y la inmersión en el mundo del juego. Además, jugarlo en español puede aumentar el disfrute y el interés por las historias y los personajes, ya que se puede apreciar mejor el tono, el humor, y las expresiones de cada uno.
-
El juego tiene una opción para cambiar el idioma a español desde el menú de ajustes. Sin embargo, hay que tener en cuenta que el juego no está completamente traducido al español, ya que depende del trabajo voluntario de los fans que colaboran con el equipo de desarrollo. Por lo tanto, es posible que algunas partes del juego estén en inglés o tengan errores de traducción. Aún así, la mayoría del juego está traducido al español y se puede jugar sin problemas.
¿Qué necesitas para jugar Summertime Saga en tu iPhone?
-
Si quieres jugar Summertime Saga en tu iPhone, hay algunas cosas que necesitas tener en cuenta. En primer lugar, el juego no está disponible de forma oficial para iOS, por lo que no lo puedes descargar directamente desde la App Store. En segundo lugar, el juego está diseñado para Android, por lo que necesitas un emulador de Android para poder ejecutarlo en tu iPhone. Y en tercer lugar, el juego tiene un tamaño de más de 800 MB, por lo que necesitas tener suficiente espacio libre en tu dispositivo.
-
Estos son los requisitos y pasos que debes seguir para jugar Summertime Saga en tu iPhone:
-
-
Tener un iPhone compatible con iOS 10 o superior.
-
Descargar un emulador de Android para tu iPhone, como iAndroid o Delta Emulator.
-
Descargar el archivo APK de Summertime Saga en español desde una fuente fiable, como la página web oficial o la página de Patreon.
-
Instalar el archivo APK de Summertime Saga en tu emulador de Android.
-
Lanzar y jugar Summertime Saga en tu iPhone.
-
-
A continuación, te explicamos cada uno de estos pasos con más detalle.
-
Cómo descargar e instalar Summertime Saga APK Español Para iPhone
-
Para descargar e instalar Summertime Saga APK Español Para iPhone, debes seguir estos cuatro pasos:
-
Paso 1: Descarga un emulador de Android para tu iPhone
-
Un emulador de Android es una aplicación que te permite ejecutar aplicaciones y juegos de Android en tu iPhone. Hay varios emuladores de Android disponibles para iOS, pero algunos de los más populares son iAndroid y Delta Emulator.
-
iAndroid es un emulador de Android que se puede instalar desde Cydia, la tienda alternativa de aplicaciones para iOS. Para instalar iAndroid, debes tener tu iPhone con jailbreak, es decir, con el sistema operativo modificado para poder acceder a funciones y aplicaciones no autorizadas por Apple. Si no sabes cómo hacer jailbreak a tu iPhone, puedes consultar esta guía. Una vez que tengas Cydia instalado, debes añadir el repositorio http://apt.modmyi.com/ y buscar e instalar iAndroid.
-
Delta Emulator es un emulador de Android que se puede instalar desde AltStore, una tienda alternativa de aplicaciones para iOS que no requiere jailbreak. Para instalar Delta Emulator, debes descargar AltStore desde su página web oficial y seguir las instrucciones para instalarlo en tu iPhone. Una vez que tengas AltStore instalado, debes abrirlo y buscar e instalar Delta Emulator.
-
Ambos emuladores te permitirán ejecutar el archivo APK de Summertime Saga en tu iPhone, pero ten en cuenta que pueden tener algunos problemas de rendimiento o compatibilidad, ya que no son aplicaciones oficiales ni están optimizadas para iOS.
Paso 2: Descarga el archivo APK de Summertime Saga en español
-
El archivo APK de Summertime Saga es el archivo que contiene el juego y que se puede instalar en tu emulador de Android. Para descargarlo, debes ir a una fuente fiable, como la página web oficial o la página de Patreon del juego.
-
La página web oficial de Summertime Saga es https://summertimesaga.com/, donde puedes encontrar toda la información sobre el juego, las últimas noticias, los enlaces de descarga, y el foro de la comunidad. Para descargar el archivo APK de Summertime Saga en español desde la página web oficial, debes hacer lo siguiente:
-
-
Ir a la sección de Downloads (Descargas) y hacer clic en el botón de Download Now (Descargar ahora).
-
Elegir la opción de Android y hacer clic en el botón de Download (Descargar).
-
Esperar a que se complete la descarga del archivo APK, que tendrá un nombre como SummertimeSaga-0-20-11-release.apk.
-
-
La página de Patreon de Summertime Saga es https://www.patreon.com/summertimesaga, donde puedes apoyar al equipo de desarrollo con una donación mensual y obtener acceso a contenido exclusivo, como versiones anticipadas, escenas adicionales, y fondos de pantalla. Para descargar el archivo APK de Summertime Saga en español desde la página de Patreon, debes hacer lo siguiente:
-
-
Iniciar sesión con tu cuenta de Patreon o crear una nueva si no la tienes.
-
Seleccionar el nivel de apoyo que quieras dar al juego, desde $1 hasta $20 al mes.
-
Ir a la sección de Posts (Publicaciones) y buscar la última versión del juego para Android.
-
Hacer clic en el enlace de descarga y esperar a que se complete la descarga del archivo APK, que tendrá un nombre similar al anterior.
-
-
Una vez que tengas el archivo APK de Summertime Saga en español descargado en tu iPhone, debes pasar al siguiente paso para instalarlo en tu emulador de Android.
Paso 3: Instala el archivo APK de Summertime Saga en tu emulador de Android
-
Una vez que tengas el archivo APK de Summertime Saga en español en tu iPhone, debes instalarlo en tu emulador de Android para poder jugar. El proceso puede variar según el emulador que uses, pero en general debes hacer lo siguiente:
-
-
Abrir el emulador de Android en tu iPhone y acceder al menú de ajustes o configuración.
-
Buscar la opción de instalar aplicaciones desde fuentes desconocidas o externas y activarla. Esto te permitirá instalar el archivo APK de Summertime Saga que no proviene de la Play Store.
-
Localizar el archivo APK de Summertime Saga en tu iPhone, usando el explorador de archivos del emulador o una aplicación externa como iFile o Filza.
-
Tocar sobre el archivo APK de Summertime Saga y seguir las instrucciones que aparezcan en la pantalla para instalarlo. Puede que tengas que aceptar algunos permisos o condiciones antes de completar la instalación.
-
-
Cuando la instalación haya terminado, verás el icono de Summertime Saga en el menú principal del emulador, junto con otras aplicaciones y juegos de Android. Ya puedes pasar al último paso para disfrutar de Summertime Saga en tu iPhone.
-
Paso 4: Disfruta de Summertime Saga en tu iPhone
-
Para lanzar y jugar Summertime Saga en tu iPhone, solo tienes que hacer lo siguiente:
-
-
Abrir el emulador de Android en tu iPhone y seleccionar el icono de Summertime Saga.
-
Esperar a que se cargue el juego y elegir el idioma español desde el menú de opciones.
-
Ajustar las preferencias de sonido, gráficos, y controles según tu gusto.
-
Empezar una nueva partida o cargar una existente y seguir la historia del juego.
-
-
Ya puedes disfrutar de Summertime Saga en tu iPhone, con todas sus características, historias, personajes, y escenas. Recuerda que puedes guardar tu progreso en cualquier momento usando el icono del teléfono o el icono de la cama, y que puedes acceder al mapa o al autobús para explorar las diferentes ubicaciones del juego.
-
Consejos y trucos para jugar Summertime Saga en tu iPhone
-
Summertime Saga es un juego muy completo y divertido, pero también puede ser un poco complicado y confuso al principio. Por eso, te damos algunos consejos y trucos para que puedas aprovechar al máximo el juego y no te pierdas nada.
-
Guarda tu progreso con frecuencia
-
Summertime Saga es un juego que tiene muchas opciones y decisiones que pueden afectar al desarrollo de la historia y al desbloqueo de escenas y personajes. Por eso, es importante que guardes tu progreso con frecuencia, para poder volver atrás si te equivocas o quieres probar algo diferente. Puedes guardar tu progreso usando el icono del teléfono o el icono de la cama, y puedes tener hasta 20 partidas guardadas diferentes.
-
Explora las diferentes ubicaciones y personajes del juego
-
Summertime Saga tiene más de 20 ubicaciones diferentes para visitar, desde la casa del protagonista hasta la playa, el colegio, el hospital, el cementerio, el centro comercial, y muchos más. Cada ubicación tiene sus propios personajes, historias, y secretos que descubrir. Puedes acceder a las ubicaciones usando el icono del mapa o el icono del autobús, y puedes interactuar con los personajes tocando sobre ellos o sobre los objetos relacionados con ellos. Algunas ubicaciones y personajes solo estarán disponibles en ciertos momentos del día o después de cumplir ciertos requisitos, así que no te desanimes si no los encuentras a la primera.
Aumenta tus estadísticas para avanzar en las historias y el colegio
-
Summertime Saga tiene cuatro estadísticas principales que puedes aumentar para mejorar tu rendimiento en el juego: fuerza, inteligencia, carisma, y destreza. Cada estadística tiene su propia utilidad y forma de aumentarla, y algunas son más importantes que otras según la historia o el personaje que quieras seguir. Estas son las formas de aumentar cada estadística:
-
-
Fuerza: La fuerza te permite ganar las peleas callejeras, las carreras de motos, y algunos mini-juegos. También te ayuda a impresionar a algunas chicas, como Mia o Roxxy. Puedes aumentar tu fuerza entrenando en el gimnasio o en el parque, o haciendo trabajos físicos como jardinero o repartidor de pizza.
-
Inteligencia: La inteligencia te permite aprobar los exámenes del colegio, resolver algunos acertijos, y ganar algunos mini-juegos. También te ayuda a seducir a algunas chicas, como Judith o Eve. Puedes aumentar tu inteligencia estudiando en la biblioteca o en tu habitación, o haciendo trabajos intelectuales como tutor o hacker.
-
Carisma: El carisma te permite ganar los rap battles, conseguir mejores precios en las tiendas, y tener más opciones de diálogo. También te ayuda a conquistar a algunas chicas, como Jenny o Diane. Puedes aumentar tu carisma practicando rap en el centro comercial o en el parque, o haciendo trabajos artísticos como actor o fotógrafo.
-
Destreza: La destreza te permite ganar los juegos de mesa, los juegos de cartas, y algunos mini-juegos. También te ayuda a complacer a algunas chicas, como Debbie o Grace. Puedes aumentar tu destreza jugando al ajedrez en el parque o en tu habitación, o haciendo trabajos manuales como mecánico o pescador.
-
-
Te recomendamos que aumentes tus estadísticas de forma equilibrada y según tus objetivos en el juego, ya que algunas historias o personajes requerirán un nivel mínimo de alguna estadística para avanzar.
-
Gana dinero para comprar objetos y regalos
-
Summertime Saga es un juego que requiere bastante dinero para poder comprar objetos y regalos que te ayuden a progresar en las historias y a mejorar tu relación con los personajes. Hay muchas formas de ganar dinero en el juego, pero algunas son más rentables que otras. Estas son algunas de las mejores formas de ganar dinero en Summertime Saga:
-
-
Hacer trabajos: Hay varios trabajos que puedes hacer para ganar dinero, como jardinero, repartidor de pizza, tutor, hacker, actor, fotógrafo, mecánico, o pescador. Cada trabajo tiene sus propios requisitos y recompensas, y algunos son más fáciles o divertidos que otros. Te recomendamos que pruebes todos los trabajos y elijas el que más te guste o se adapte a tus necesidades.
-
Jugar mini-juegos: Hay varios mini-juegos que puedes jugar para ganar dinero, como carreras de motos, peleas callejeras, rap battles, juegos de mesa, juegos de cartas, o pesca. Cada mini-juego tiene sus propias reglas y dificultades, y algunos dependen de tus estadísticas o habilidades. Te recomendamos que practiques los mini-juegos antes de apostar dinero y que sepas cuándo retirarte si pierdes demasiado.
-
Vender objetos: Hay algunos objetos que puedes vender para ganar dinero, como joyas, cómics, revistas, o ropa. Algunos objetos los puedes encontrar explorando las ubicaciones del juego, otros los puedes conseguir completando ciertas historias o misiones. Te recomendamos que vendas los objetos que no necesites o que ya hayas usado para obtener un beneficio extra.
-
-
Te recomendamos que ahorres dinero para comprar los objetos y regalos que te interesen, ya que algunos son muy caros o escasos. También te recomendamos que no gastes dinero en cosas innecesarias o que no te aporten nada al juego.
-
Romancea a diferentes personajes y descubre sus secretos
-
Summertime Saga es un juego que tiene más de 70 personajes diferentes para conocer, coquetear, y enamorar, cada uno con su propia personalidad, historia, y preferencias. Cada personaje tiene su propia ruta de romance, que se activa al completar ciertos requisitos y eventos. Al avanzar en la ruta de romance, podrás desbloquear escenas, mini-juegos, y secretos con el personaje que elijas.
-
Summertime Saga tiene personajes de todo tipo y para todos los gustos, desde la dulce y tímida Mia, hasta la rebelde y sexy Roxxy, pasando por la madura y cariñosa Debbie, la inocente y curiosa Jenny, la inteligente y nerd Judith, la gótica y misteriosa Eve, la generosa y maternal Diane, la deportista y competitiva Becca, y muchas más. También hay personajes masculinos con los que puedes interactuar, como Erik, Kevin, Dexter, Clyde, o Larry.
-
Te recomendamos que explores las diferentes rutas de romance y que pruebes con diferentes personajes, ya que cada uno tiene su propio encanto y sorpresas. También te recomendamos que prestes atención a las pistas y consejos que te dan los personajes o el juego para saber cómo avanzar en las rutas de romance y no quedarte atascado.
-
Conclusión
-
Summertime Saga es un juego de simulación de citas y novela visual para adultos que te ofrece horas de diversión y entretenimiento. El juego tiene una gran cantidad de contenido, personajes, historias, y escenas para explorar, con un estilo gráfico animado y humorístico. El juego está disponible para Windows, Mac, Linux, y Android, pero también se puede jugar en iPhone usando un emulador de Android.
-
En este artículo te hemos explicado qué es y cómo descargar e instalar Summertime Saga APK Español Para iPhone. También te hemos dado algunos consejos y trucos para que puedas aprovechar al máximo el juego y no te pierdas nada. Esperamos que te haya gustado este artículo y que te animes a probar Summertime Saga en tu iPhone.
-
Si tienes alguna duda o comentario sobre el juego o el artículo, no dudes en dejarlos abajo. Y si te ha gustado este artículo, compártelo con tus amigos o en tus redes sociales. ¡Gracias por leernos!
-
Preguntas frecuentes
-
A continuación te respondemos a algunas de las preguntas más frecuentes sobre Summertime Saga APK Español Para iPhone.
-
¿Es seguro jugar Summertime Saga en iPhone?
-
Sí, es seguro jugar Summertime Saga en iPhone siempre que descargues el archivo APK del juego desde una fuente fiable, como la página web oficial o la página de Patreon. También debes asegurarte de tener un antivirus actualizado en tu iPhone por si acaso.
-
¿Es legal jugar Summertime Saga en iPhone?
-
Sí, es legal jugar Summertime Saga en iPhone siempre que respetes los derechos de autor del juego y no lo distribuyas ni lo modifiques sin permiso. También debes tener en cuenta las leyes de tu país sobre el contenido para adultos y la edad mínima para acceder a él.
-
¿Es gratis jugar Summertime Saga en iPhone?
-
Sí, es gratis jugar Summertime Saga en iPhone siempre que descargues el archivo APK del juego desde la página web oficial. Si quieres apoyar al equipo de desarrollo o acceder a contenido exclusivo, puedes hacer una donación mensual en la página de Patreon del juego.
-
¿Cómo actualizar Summertime Saga en iPhone?
-
Para actualizar Summertime Saga en iPhone debes seguir los mismos pasos que para instalarlo: descargar el archivo APK de la última versión del juego desde una fuente fiable e instalarlo en tu emulador de Android. Recuerda guardar tu progreso antes de actualizar el juego para no perderlo.
-
¿Cómo borrar Summertime Saga de mi iPhone?
-
Para borrar Summertime Saga de tu iPhone debes hacer lo siguiente:
-
-
Abrir el emulador de Android en tu iPhone y localizar el icono de Summertime Saga.
-
Mantener pulsado el icono hasta que aparezca una opción para desinstalarlo.
-
Tocar sobre la opción de desinstalar y confirmar la acción. Esto borrará el juego de tu emulador, pero no de tu iPhone.
-
Si quieres borrar también el archivo APK de Summertime Saga de tu iPhone, debes usar el explorador de archivos del emulador o una aplicación externa para localizarlo y eliminarlo.
-
-
Esto es todo lo que necesitas saber sobre Summertime Saga APK Español Para iPhone. Esperamos que te haya servido de ayuda y que disfrutes del juego. Si tienes alguna otra pregunta, déjanosla en los comentarios y te responderemos lo antes posible. ¡Hasta la próxima!
197e85843d
-
-
\ No newline at end of file
diff --git a/spaces/congsaPfin/Manga-OCR/logs/Download Bloons TD 6 and Join Millions of Players in this Epic Strategy Game for Android.md b/spaces/congsaPfin/Manga-OCR/logs/Download Bloons TD 6 and Join Millions of Players in this Epic Strategy Game for Android.md
deleted file mode 100644
index 480d4db424f3af4ec43cf4478a180a06909f6700..0000000000000000000000000000000000000000
--- a/spaces/congsaPfin/Manga-OCR/logs/Download Bloons TD 6 and Join Millions of Players in this Epic Strategy Game for Android.md
+++ /dev/null
@@ -1,96 +0,0 @@
-
-
Download Bloons TD 6 Android Free: A Guide to the Best Tower Defense Game
-
If you are a fan of tower defense games, you might have heard of Bloons TD 6, the latest installment in the popular series by Ninja Kiwi. Bloons TD 6 is a fun and challenging game that will test your strategic skills and keep you entertained for hours. In this article, we will tell you what Bloons TD 6 is, what features it has, how to download it for free on your Android device, and some tips and tricks to help you pop those pesky bloons.
Bloons TD 6 is a tower defense game where you have to build a defense from a combination of powerful monkey towers and heroes, and pop every last invading balloon (or bloon) that tries to reach the end of the path. The game has over a decade of tower defense pedigree and regular massive updates that make it a favorite game for millions of players.
-
Features of Bloons TD 6
-
Bloons TD 6 has many features that make it stand out from other tower defense games. Here are some of them:
-
Monkey Towers and Heroes
-
The game has 23 different monkey towers, each with three upgrade paths and unique activated abilities. You can also choose from 14 diverse heroes, each with their own signature upgrades and special abilities. Plus, you can unlock skins and voiceovers for your heroes to customize them.
-
Huge Content and Updates
-
The game has regular updates that add new characters, features, and gameplay. Some of the updates include boss events, where you have to face fearsome boss bloons that will challenge even the strongest defenses; odysseys, where you have to battle through a series of maps connected by their theme, rules, and rewards; quests, where you can learn more about the monkeys and their stories; trophy store, where you can earn trophies to unlock cosmetic items; and content browser, where you can create your own challenges and odysseys, and share them with other players.
-
How to download bloons td 6 for free on android
-Bloons td 6 apk free download latest version android
-Bloons td 6 mod apk unlimited money android download
-Download bloons td 6 offline mode android free
-Bloons td 6 google play store download free android
-Bloons td 6 strategy game free download for android
-Bloons td 6 co-op mode download android free
-Bloons td 6 best towers and heroes download free android
-Bloons td 6 update download free android
-Bloons td 6 cheats and hacks download free android
-Bloons td 6 paragon upgrades download free android
-Bloons td 6 odyssey mode download free android
-Bloons td 6 quests and trophies download free android
-Bloons td 6 boss events download free android
-Bloons td 6 custom challenges download free android
-Bloons td 6 ninja kiwi support download free android
-Bloons td 6 in-app purchases download free android
-Bloons td 6 cloud save and progress download free android
-Bloons td 6 content browser and editor download free android
-Bloons td 6 skins and voiceovers download free android
-Download bloons td 6 on multiple android devices for free
-Download bloons td 6 on pc and android for free
-Download bloons td 6 on ios and android for free
-Download bloons td 6 on amazon and android for free
-Download bloons td 6 on steam and android for free
-Download bloons td 6 on mac and android for free
-Download bloons td 6 on windows and android for free
-Download bloons td 6 on linux and android for free
-Download bloons td 6 on chromebook and android for free
-Download bloons td 6 on switch and android for free
-Download bloons td 6 on xbox and android for free
-Download bloons td 6 on playstation and android for free
-Download bloons td 6 on nintendo and android for free
-Download bloons td 6 on firestick and android for free
-Download bloons td 6 on roku and android for free
-Download bloons td 6 on smart tv and android for free
-Download bloons td 6 on vr and android for free
-Download bloons td 6 on ar and android for free
-Download bloons td 6 on wear os and android for free
-Download bloons td 6 on carplay and android for free
-Download bloons td 6 on auto and android for free
-Download bloons td 6 on tablet and android for free
-Download bloons td 6 on phone and android for free
-Download bloons td 6 on laptop and android for free
-Download bloons td 6 on desktop and android for free
-Download bloons tower defense 6 game for android free
-Download btd6 apk file for android device free
-Download latest version of bloons tower defense six app for android phone or tablet
-How to install btd six game app on your android device without paying
-
Co-Op and Offline Modes
-
The game also supports co-op mode, where you can play with up to three other players in public or private games. You can also play offline, even when your WiFi doesn't work. The game has 68 handcrafted maps, with more added every update.
-
How to Download Bloons TD 6 for Android
-
If you want to download Bloons TD 6 for free on your Android device, there are two ways you can do it:
-
Google Play Store
-
The easiest way to download Bloons TD 6 is through the Google Play Store. However, the game is not free on the Play Store; it costs $6.99. If you are willing to pay for it, you can simply search for "Bloons TD 6" on the Play Store app or website, and tap on the "Buy" button. You will need a Google account and a payment method to complete the purchase.
-
Filehippo.com
-
If you don't want to pay for the game, you can try downloading it from Filehippo.com, a website that offers free downloads of various software. To download Bloons TD 6 from Filehippo.com, follow these steps:
-
-
Go to [2](https:// filehippo.com/download_bloons_td_6/) and click on the "Download Latest Version" button.
-
Wait for the download to finish, and then open the APK file. You may need to enable "Unknown Sources" in your device settings to install the app.
-
Follow the instructions on the screen to install Bloons TD 6 on your device.
-
-
Note: Downloading Bloons TD 6 from Filehippo.com may not be safe or legal, as it may contain viruses or malware, or violate the terms of service of Ninja Kiwi. We do not recommend or endorse this method, and we are not responsible for any damages or consequences that may arise from using it. Use it at your own risk.
-
Tips and Tricks for Bloons TD 6
-
Now that you have downloaded Bloons TD 6, you might want to know some tips and tricks to help you master the game. Here are some of them:
-
Use Monkey Knowledge
-
Monkey Knowledge is a system that allows you to unlock passive upgrades for your towers and heroes. You can earn Monkey Knowledge points by leveling up, completing achievements, or buying them with real money. You can spend these points on various branches of knowledge, such as Primary, Military, Magic, Support, Powers, and Heroes. Each branch has different perks that can boost your performance in the game.
-
Upgrade Your Heroes
-
Heroes are powerful units that can level up and gain new abilities as they pop bloons. You can choose one hero per game, and you can switch between them in the main menu. Each hero has a different playstyle and role, so you should choose the one that suits your strategy. You can also upgrade your heroes with Monkey Money, a currency that you can earn by playing the game or watching ads. Upgrading your heroes can unlock new skins, voiceovers, and stats.
-
Experiment with Different Strategies
-
Bloons TD 6 has a lot of variety and replayability, thanks to its different modes, maps, difficulties, and challenges. You can try different combinations of towers, heroes, upgrades, and powers to find the best way to pop the bloons. You can also create your own custom challenges and odysseys, and share them with other players. The game has a lot of depth and complexity, so don't be afraid to experiment and learn from your mistakes.
-
Conclusion
-
Bloons TD 6 is a great tower defense game that will keep you hooked for hours. It has amazing graphics, sound effects, music, and animations that make it a joy to play. It also has a lot of content and features that make it worth every penny. If you want to download Bloons TD 6 for free on your Android device, you can either buy it from the Google Play Store or download it from Filehippo.com. However, we recommend the former option, as it is safer and more legal. We hope this article helped you learn more about Bloons TD 6 and how to download it for free on your Android device.
-
FAQs
-
Here are some frequently asked questions about Bloons TD 6:
-
-
Is Bloons TD 6 free? No, Bloons TD 6 is not free. It costs $6.99 on the Google Play Store. However, you can download it for free from Filehippo.com, but this may not be safe or legal.
-
Is Bloons TD 6 online or offline? Bloons TD 6 can be played both online and offline. You can play online with other players in co-op mode or offline without an internet connection.
-
Is Bloons TD 6 multiplayer? Yes, Bloons TD 6 has a multiplayer mode called co-op mode, where you can play with up to three other players in public or private games.
-
What is the best tower in Bloons TD 6? There is no definitive answer to this question, as different towers have different strengths and weaknesses. However, some of the most popular and powerful towers are Ninja Monkey, Super Monkey, Alchemist, Druid, and Banana Farm.
-
How many maps are there in Bloons TD 6? Bloons TD 6 has 68 handcrafted maps as of June 2023, with more added every update.
-
401be4b1e0
-
-
\ No newline at end of file
diff --git a/spaces/congsaPfin/Manga-OCR/logs/Download Enthiran 2.0 - The Ultimate Sci-Fi Thriller.md b/spaces/congsaPfin/Manga-OCR/logs/Download Enthiran 2.0 - The Ultimate Sci-Fi Thriller.md
deleted file mode 100644
index 81b51993ed87efaffaad130a3bcdd8fb40727c81..0000000000000000000000000000000000000000
--- a/spaces/congsaPfin/Manga-OCR/logs/Download Enthiran 2.0 - The Ultimate Sci-Fi Thriller.md
+++ /dev/null
@@ -1,148 +0,0 @@
-
-
Download Enthiran 2.0: How to Watch the Epic Sci-Fi Movie Online
-
Enthiran 2.0, also known as 2.0, is a 2018 Indian Tamil-language science fiction film directed by S. Shankar and starring Rajinikanth, Akshay Kumar, and Amy Jackson. It is the sequel to the 2010 film Enthiran, which was a blockbuster hit and one of the most expensive Indian films ever made. Enthiran 2.0 is also a high-budget film with stunning visual effects, thrilling action sequences, and a powerful social message. If you are a fan of sci-fi movies, you should not miss this epic film.
But how can you watch Enthiran 2.0 online? Is it possible to download Enthiran 2.0 legally and safely? What are the risks of downloading Enthiran 2.0 illegally and unsafely? In this article, we will answer these questions and more.
-
What is Enthiran 2.0?
-
Enthiran 2.0 is the second installment in the Enthiran film series, which revolves around the adventures of Dr. Vaseegaran, a brilliant scientist who creates a humanoid robot named Chitti, and Pakshi Rajan, a former ornithologist who seeks revenge on cell phone users for causing the decline of bird population.
-
The plot of Enthiran 2.0
-
The film begins with Dr. Vaseegaran introducing his new creation, Nila, a female humanoid robot who is also his assistant. He is summoned by the government when mobile phones start flying out of people's hands mysteriously in Chennai. He deduces that the phenomenon is caused by a negative force that can control electromagnetic waves, and suggests reactivating Chitti, who was dismantled in the previous film.
-
download enthiran 2.0 tamil movie
-download enthiran 2.0 full movie in hindi
-download enthiran 2.0 hd quality
-download enthiran 2.0 songs mp3
-download enthiran 2.0 subtitles
-download enthiran 2.0 torrent magnet
-download enthiran 2.0 from moviesda
-download enthiran 2.0 from isaimini
-download enthiran 2.0 from internet archive
-download enthiran 2.0 robot movie
-download enthiran 2.0 bluray
-download enthiran 2.0 dvdrip
-download enthiran 2.0 with english subtitles
-download enthiran 2.0 in telugu
-download enthiran 2.0 in malayalam
-download enthiran 2.0 in kannada
-download enthiran 2.0 in bengali
-download enthiran 2.0 in marathi
-download enthiran 2.0 in gujarati
-download enthiran 2.0 in punjabi
-download enthiran 2.0 online free
-download enthiran 2.0 watch online
-download enthiran 2.0 streaming online
-download enthiran 2.0 netflix
-download enthiran 2.0 amazon prime video
-download enthiran 2.0 hotstar
-download enthiran 2.0 youtube
-download enthiran 2.0 vimeo
-download enthiran 2.0 dailymotion
-download enthiran 2.0 facebook video
-download enthiran 2.0 trailer hd
-download enthiran 2.0 teaser hd
-download enthiran 2.0 making video hd
-download enthiran 2.0 behind the scenes video hd
-download enthiran 2.0 rajinikanth video hd
-download enthiran 2.0 akshay kumar video hd
-download enthiran 2.0 amy jackson video hd
-download enthiran 2.0 a r rahman video hd
-download enthiran 2.0 shankar video hd
-download enthiran 2.0 lyca productions video hd
-download enthiran 2.0 movie review
-download enthiran 2.0 box office collection
-download enthiran 2.0 budget and cost
-download enthiran 2.0 awards and nominations
-download enthiran 2.0 imdb rating and reviews
-download enthiran 2.0 rotten tomatoes rating and reviews
-download enthiran 2.0 metacritic rating and reviews
-download enthiran 2.0 wikipedia page and information
-
However, his proposal is opposed by Professor Dhinendra Bohra, the son of Dr. Bohra who was killed by Chitti in the previous film. Meanwhile, the negative force takes the form of a giant bird-like creature and attacks the city, killing many people and destroying many buildings. Dr. Vaseegaran manages to reactivate Chitti and sends him to fight the creature, but Chitti is overpowered and damaged.
-
Dr. Vaseegaran learns that the creature is Pakshi Rajan, who was once a respected ornithologist who loved birds more than anything else. He was devastated when he saw that the excessive use of cell phones and towers was killing birds and disrupting their communication signals. He pleaded with the government and telecom companies to stop their activities, but was ignored and ridiculed.
-
He then decided to end his life by hanging himself from a cell tower, but his soul was infused with the electromagnetic radiation from the tower, giving him the power to control electromagnetic waves and manipulate mobile phones.
-
Dr. Vaseegaran repairs Chitti and gives him an upgraded version with more features and abilities. He also creates many mini versions of Chitti called Kutti to assist
The cast and crew of Enthiran 2.0
-
Enthiran 2.0 features a stellar cast of actors who have delivered remarkable performances in their roles. The film is led by Rajinikanth, who plays the dual roles of Dr. Vaseegaran and Chitti, the robot. Rajinikanth is one of the most popular and influential actors in Indian cinema, who has a huge fan following across the world. He is known for his charismatic screen presence, unique style, and dialogue delivery.
-
Akshay Kumar plays the role of Pakshi Rajan, the antagonist of the film. Akshay Kumar is a Bollywood superstar who has starred in over 100 films in various genres. He is also one of the highest-paid actors in the world, according to Forbes. He has won several awards and honors for his acting skills and social work. He portrays Pakshi Rajan with intensity and emotion, making him a formidable foe for Chitti.
-
Amy Jackson plays the role of Nila, the female humanoid robot who assists Dr. Vaseegaran. Amy Jackson is a British actress and model who has appeared in several Tamil, Hindi, and Telugu films. She is also a former Miss Teen World and Miss Liverpool winner. She plays Nila with grace and charm, adding a touch of humor and romance to the film.
-
The film also features other talented actors such as Sudhanshu Pandey, Adil Hussain, Kalabhavan Shajohn, Riyaz Khan, and Kaizaad Kotwal in supporting roles.
-
The film is directed by S. Shankar, who is one of the most acclaimed and successful directors in Indian cinema. He is known for his grandiose vision, innovative storytelling, and technical excellence. He has directed several blockbuster films such as Gentleman, Indian, Jeans, Mudhalvan, Nayak, Anniyan, Sivaji, Enthiran, I, and 2.0. He has won several awards and accolades for his work, including four National Film Awards.
-
The film is written by S. Shankar and B. Jeyamohan, who have collaborated on the story and dialogues of the film. B. Jeyamohan is a renowned Tamil writer and critic who has written several novels, short stories, essays, and screenplays. He has also won several awards and honors for his literary works.
-
The film is produced by Subaskaran Allirajah under the banner of Lyca Productions, which is one of the leading production houses in India. The film is co-produced by Raju Mahalingam and Aashish Singh.
-
The production and release of Enthiran 2.0
-
Enthiran 2.0 is one of the most expensive and ambitious films ever made in India. The film had a budget of about ₹570 crore (US$80 million), making it the second-most expensive film in Asia after China's Asura (2018). The film took about four years to complete, with extensive pre-production, filming, post-production, and marketing activities.
-
The film was shot in various locations across India such as Chennai, Delhi, Mumbai, Kolkata, Varanasi, and abroad such as Dubai, Bolivia, and the United States. The film used state-of-the-art technology and equipment such as 3D cameras, motion capture, animatronics, prosthetics, and drones. The film also involved a large crew of technicians, artists, and experts from various fields and countries.
-
The film's visual effects were done by several studios such as Industrial Light & Magic, Tau Films, Quantum FX, Digital Domain, Legacy Effects, Double Negative, Prime Focus, and Reliance MediaWorks. The film had about 2150 VFX shots, which took about 15 months to complete. The film's VFX supervisor was Srinivas Mohan, who had previously worked on Enthiran and Baahubali.
-
The film's music was composed by A. R. Rahman, who is one of the most celebrated and influential composers in the world. He has won several awards and honors for his music, including two Academy Awards, two Grammy Awards, a BAFTA Award, a Golden Globe Award, and four National Film Awards. He has also been conferred the Padma Shri and the Padma Vibhushan by the Government of India. The film's soundtrack consists of five songs and two instrumental tracks, which are sung in Tamil, Hindi, and Telugu languages. The film's lyrics were written by Madhan Karky, Abbas Tyrewala, and Ananta Sriram.
-
The film was released on 29 November 2018 in India and worldwide. The film was released in about 10,500 screens across 80 countries, making it the widest release for an Indian film. The film was also dubbed in 14 languages such as Hindi, Telugu, Malayalam, Kannada, Marathi, Gujarati, Bengali, Punjabi, Urdu, Bhojpuri, Odia, Assamese, Sinhala, and Chinese. The film received positive reviews from critics and audiences alike, who praised the film's visual effects, action sequences, performances, direction, music, and message. The film also broke several box office records and became one of the highest-grossing Indian films of all time.
-
Why should you watch Enthiran 2.0?
-
Enthiran 2.0 is not just a movie; it is an experience that will leave you awestruck and amazed. Here are some of the reasons why you should watch Enthiran 2.0:
-
The visual effects and action sequences of Enthiran 2.0
-
Enthiran 2.0 is a visual spectacle that showcases some of the best visual effects ever seen in Indian cinema. The film creates a stunning world of robots, birds, cell phones, and stadiums, with realistic and detailed graphics. The film also features some of the most thrilling and spectacular action sequences ever seen in Indian cinema. The film showcases the epic battle between Chitti and Pakshi Rajan, with both of them using their powers and abilities to outsmart and overpower each other. The film also features some of the most iconic scenes such as the formation of a giant Chitti, the transformation of Pakshi Rajan into different birds, and the climax scene where Chitti and Pakshi Rajan fight in a football stadium.
-
The social message and themes of Enthiran 2.0
-
Enthiran 2.0 is not just a mindless entertainer; it is also a thought-provoking film that raises some important questions and issues about the impact of technology on nature and humanity. The film explores the themes of artificial intelligence, human-robot relationship, environmental degradation, animal rights, and social responsibility. The film shows the positive and negative aspects of technology, and how it can be used for good or evil. The film also shows the consequences of human greed, ignorance, and arrogance, and how they can lead to destruction and chaos. The film also conveys a strong message of compassion, harmony, and coexistence between humans, animals, and nature.
-
The music and soundtrack of Enthiran 2.0
-
Enthiran 2.0 is also a musical treat that showcases the genius of A. R. Rahman, who has composed some of the most memorable and catchy songs for the film. The film's soundtrack consists of five songs that cater to different moods and genres such as romantic, peppy, patriotic, inspirational, and emotional. The film's songs are sung by some of the most talented singers such as Sid Sriram, Shashaa Tirupati, Armaan Malik, Blaaze, Kailash Kher, Nakash Aziz, and A. R. Ameen. The film's songs are also accompanied by stunning visuals and choreography that enhance the appeal and impact of the songs. The film's soundtrack also includes two instrumental tracks that create the mood and atmosphere of the film.
-
How to download Enthiran 2.0 online?
-
If you are wondering how to download Enthiran 2.0 online, you should know that there are two ways to do so: legal and safe ways, and illegal and risky ways. Let us look at both of them in detail:
-
The legal and safe ways to download Enthiran 2.0 online
-
The legal and safe ways to download Enthiran 2.0 online are those that respect the rights of the filmmakers and pay them for their hard work and creativity. These ways also ensure that you get a high-quality and virus-free version of the film, without compromising your privacy and security. Some of the legal and safe ways to download Enthiran 2.0 online are:
-
Streaming services that offer Enthiran 2.0 online
-
One of the easiest and most convenient ways to watch Enthiran 2.0 online is to use a streaming service that offers the film in its library. Streaming services are online platforms that allow you to watch movies and shows on demand, without downloading them to your device. You can access them through a web browser, a mobile app, or a smart TV. Some of the streaming services that offer Enthiran 2.0 online are:
-
-
Amazon Prime Video: Amazon Prime Video is one of the most popular and widely available streaming services in the world. It offers a vast collection of movies and shows in various languages and genres, including Enthiran 2.0. You can watch Enthiran 2.0 on Amazon Prime Video with a subscription fee of ₹129 per month or ₹999 per year in India, or $12.99 per month or $119 per year in the US. You can also download Enthiran 2.0 on Amazon Prime Video for offline viewing, with a limit of 15 to 25 titles at a time.
-
Netflix: Netflix is another leading and global streaming service that offers a huge variety of movies and shows in different languages and genres, including Enthiran 2.0. You can watch Enthiran 2.0 on Netflix with a subscription fee of ₹199 to ₹799 per month in India, or $8.99 to $17.99 per month in the US, depending on the plan you choose. You can also download Enthiran 2.0 on Netflix for offline viewing, with a limit of 100 titles at a time.
-
Hotstar: Hotstar is an Indian streaming service that offers a large selection of movies and shows in various languages and genres, including Enthiran 2.0. You can watch Enthiran 2.0 on Hotstar with a subscription fee of ₹299 per month or ₹1499 per year for Hotstar Premium, or ₹399 per year for Hotstar VIP. You can also download Enthiran 2.0 on Hotstar for offline viewing, with a limit of five devices at a time.
-
-
Online platforms that sell or rent Enthiran 2.0 online
-
Another way to watch Enthiran 2.0 online is to use an online platform that sells or rents the film digitally. These platforms allow you to buy or rent movies and shows online, and download them to your device or stream them online. Some of the online platforms that sell or rent Enthiran 2.0 online are:
-
-
YouTube: YouTube is one of the most popular and widely used online platforms that offers a variety of videos, including movies and shows. You can buy or rent Enthiran 2.0 on YouTube for ₹25 to ₹150 in India, or $1.99 to $14.99 in the US, depending on the quality and format you choose.
-
Google Play Movies & TV: Google Play Movies & TV is another online platform that offers movies and shows for purchase or rental. You can buy or rent Enthiran 2.0 on Google Play Movies & TV for ₹25 to ₹150 in India, or $1.99 to $14.99 in the US, depending on the quality and format you choose.
-
iTunes: iTunes is an online platform that offers movies and shows for purchase or rental for Apple users. You can buy or rent Enthiran 2.0 on iTunes for ₹120 to ₹490 in India, or $3.99 to $19.99 in the US, depending on the quality and format you choose.
-
-
The illegal and risky ways to download Enthiran 2.0 online
-
The illegal and risky ways to download Enthiran 2.0 online are those that violate the rights of the filmmakers and do not pay them for their hard work and creativity. These ways also expose you to the risk of getting a low-quality and virus-infected version of the film, as well as compromising your privacy and security. Some of the illegal and risky ways to download Enthiran 2.0 online are:
-
Torrent sites that provide Enthiran 2.0 online
-
One of the most common and popular ways to download Enthiran 2.0 online illegally is to use a torrent site that provides the film in a torrent file or a magnet link. Torrent sites are online platforms that allow users to share files through peer-to-peer networks, without a central server. Some of the torrent sites that provide Enthiran 2.0 online are:
-
-
The Pirate Bay: The Pirate Bay is one of the oldest and most notorious torrent sites in the world. It offers a variety of files, including movies, shows, music, games, software, and more. You can find Enthiran 2.0 on The Pirate Bay in various qualities and formats, such as 720p, 1080p, BluRay, DVDScr, etc.
-
Kickass Torrents: Kickass Torrents is another popular and widely used torrent site that offers a large collection of files, including movies, shows, music, games, software, and more. You can find Enthiran 2.0 on Kickass Torrents in various qualities and formats, such as 720p, 1080p, BluRay, DVDScr, etc.
-
1337x: 1337x is another well-known and reliable torrent site that offers a variety of files, including movies, shows, music, games, software, and more. You can find Enthiran 2.0 on 1337x in various qualities and formats, such as 720p, 1080p, BluRay, DVDScr, etc.
-
-
However, downloading Enthiran 2.0 from torrent sites is illegal and risky for several reasons:
-
-
It violates the copyright laws and infringes the rights of the filmmakers and producers.
It exposes you to the risk of downloading a low-quality and virus-infected version of the film, which can harm your device and data.
-
It compromises your privacy and security, as torrent sites can track your IP address, location, and online activity, and expose you to hackers, malware, and phishing.
-
It can result in legal actions and penalties, as torrenting is considered a criminal offense in many countries, and can lead to fines, lawsuits, or even jail time.
-
-
Piracy websites that host Enthiran 2.0 online
-
Another way to download Enthiran 2.0 online illegally is to use a piracy website that hosts the film on its server. Piracy websites are online platforms that offer movies and shows for free or for a nominal fee, without the permission of the filmmakers and producers. Some of the piracy websites that host Enthiran 2.0 online are:
-
-
TamilRockers: TamilRockers is one of the most infamous and notorious piracy websites in India, which specializes in leaking Tamil movies online. It has leaked Enthiran 2.0 online in various qualities and formats, such as 720p, 1080p, BluRay, DVDScr, etc.
-
Movierulz: Movierulz is another popular and widely used piracy website in India, which offers movies and shows in various languages and genres. It has leaked Enthiran 2.0 online in various qualities and formats, such as 720p, 1080p, BluRay, DVDScr, etc.
-
Filmyzilla: Filmyzilla is another well-known and reliable piracy website in India, which provides movies and shows in various languages and genres. It has leaked Enthiran 2.0 online in various qualities and formats, such as 720p, 1080p, BluRay, DVDScr, etc.
-
-
However, downloading Enthiran 2.0 from piracy websites is illegal and risky for several reasons:
-
-
It violates the copyright laws and infringes the rights of the filmmakers and producers.
It exposes you to the risk of downloading a low-quality and virus-infected version of the film, which can harm your device and data.
-
It compromises your privacy and security, as piracy websites can track your IP address, location, and online activity, and expose you to hackers, malware, and phishing.
-
It can result in legal actions and penalties, as piracy is considered a criminal offense in many countries, and can lead to fines, lawsuits, or even jail time.
-
-
Conclusion
-
Enthiran 2.0 is a masterpiece of Indian cinema that deserves to be watched by everyone who loves sci-fi movies. It is a film that combines stunning visual effects, thrilling action sequences, powerful performances, brilliant direction, and a meaningful message. It is a film that celebrates the spirit of science, innovation, and creativity, while also highlighting the dangers of technology, greed, and ignorance. It is a film that entertains, educates, and inspires.
-
However, if you want to watch Enthiran 2.0 online, you should do so in a legal and safe way, by using a streaming service or an online platform that offers the film with the permission of the filmmakers and producers. You should avoid downloading Enthiran 2.0 online illegally and unsafely, by using a torrent site or a piracy website that provides the film without the permission of the filmmakers and producers. You should respect the rights of the filmmakers and producers, and pay them for their hard work and creativity. You should also protect yourself from the risks of downloading a low-quality and virus-infected version of the film, as well as compromising your privacy and security.
-
We hope this article has helped you understand how to download Enthiran 2.0 online legally and safely, and why you should watch this epic sci-fi movie online. If you have any questions or feedback, please feel free to leave a comment below. Thank you for reading!
-
FAQs
-
Here are some of the frequently asked questions about Enthiran 2.0:
-
-
Q: Is Enthiran 2.0 available on Netflix?
-A: Yes, Enthiran 2.0 is available on Netflix in India and other countries. You can watch it with a Netflix subscription.
-
Q: Is Enthiran 2.0 a sequel to Enthiran?
-A: Yes, Enthiran 2.0 is a sequel to Enthiran, which was released in 2010. However, you can watch Enthiran 2.0 without watching Enthiran, as the film has a standalone story.
-
Q: Is Enthiran 2.0 based on a true story?
-A: No, Enthiran 2.0 is not based on a true story. It is a fictional story that is inspired by some real-life events and issues related to technology, nature, and humanity.
-
Q: Is Enthiran 2.0 suitable for children?
-A: Enthiran 2.0 is rated U/A in India, which means that it is suitable for children above 12 years of age with parental guidance. The film has some scenes of violence, bloodshed, and horror that may not be suitable for younger children.
-
Q: Is Enthiran 2.0 the highest-grossing Indian film of all time?
-A: No, Enthiran 2.0 is not the highest-grossing Indian film of all time. It is the sixth-highest-grossing Indian film of all time, with a worldwide gross of about ₹800 crore (US$110 million). The highest-grossing Indian film of all time is Baahubali 2: The Conclusion (2017), with a worldwide gross of about ₹1810 crore (US$250 million).
-
197e85843d
-
-
\ No newline at end of file
diff --git a/spaces/congsaPfin/Manga-OCR/logs/FNAF AR The Most Terrifying and Immersive Installment in the FNAF Franchise.md b/spaces/congsaPfin/Manga-OCR/logs/FNAF AR The Most Terrifying and Immersive Installment in the FNAF Franchise.md
deleted file mode 100644
index 21330083055bcbd8f763062d93757961d35ee27e..0000000000000000000000000000000000000000
--- a/spaces/congsaPfin/Manga-OCR/logs/FNAF AR The Most Terrifying and Immersive Installment in the FNAF Franchise.md
+++ /dev/null
@@ -1,86 +0,0 @@
-
-
Introduction
Write a catchy introduction that summarizes what the article is about and why the reader should care.
| | H2: What is fnaf ar? |
What is fnaf ar?
Write a brief overview of what fnaf ar is, how it works, and what makes it different from other games in the franchise.
Write a step-by-step guide on how to download and play fnaf ar on iOS or Android devices, including the requirements, permissions, and features.
| | ... | ... | 2. Next, write the article based on the outline. Use your own words rather than copying and pasting from other sources. Consider perplexity and burstiness when creating content, ensuring high levels of both without losing specificity or context. Use fully detailed paragraphs that engage the reader. Be sure to use at least one table in the article to display data or information in a structured way. Write in a conversational style as written by a human (use an informal tone, utilize personal pronouns, keep it simple, engage the reader, use the active voice, keep it brief, use rhetorical questions, and incorporate analogies and metaphors). End with a conclusion paragraph that summarizes the main points and provides a call to action. 3. Finally, add 5 unique FAQs after the conclusion. These should be relevant questions that a reader might have after reading the article, along with concise and informative answers. You can use bullet points or numbered lists to format the FAQs. Here is an example of how your final article might look like:
Introduction
-
If you are a fan of horror games, you might have heard of Five Nights at Freddy's, a series of games where you have to survive against animatronic characters that come to life at night. But what if you could experience these terrifying creatures in your own reality? That's what fnaf ar offers: a game that brings the horror of Five Nights at Freddy's to your real world through augmented reality.
-
In this article, we will tell you everything you need to know about fnaf ar: what it is, how to download and play it, what characters and features it has, and some tips and tricks to help you survive. Are you ready to face your fears? Then read on!
-
What is fnaf ar?
-
fnaf ar is short for Five Nights at Freddy's AR: Special Delivery, an augmented reality game developed by Illumix in collaboration with Scott Cawthon, the creator of the original Five Nights at Freddy's games. It was released in November 2019 for iOS and Android devices.
-
In fnaf ar, you subscribe to Fazbear Entertainment's new service that delivers your favorite animatronics to your doorstep. However, something goes wrong and the animatronics malfunction and attack you instead of entertaining you. You have to use your device's camera, flashlight, shocker, and other tools to fend off these hostile creatures that will follow you wherever you go.
-
fnaf ar is different from other games in the franchise because it uses your real environment as the game setting. You can play it anywhere: at home, at school, at work, or even outside. The game also uses your location data to send animatronics to your area or let you send them to your friends and other players. You can also collect parts, CPUs, and plushsuits to assemble your own animatronics and customize them.
-
How to download and play fnaf ar?
-
If you want to try fnaf ar for yourself, here are the steps you need to follow:
-
fnaf ar mod apk
-fnaf ar special delivery
-fnaf ar skins
-fnaf ar characters
-fnaf ar download
-fnaf ar tips and tricks
-fnaf ar plushtrap
-fnaf ar hack
-fnaf ar gameplay
-fnaf ar trailer
-fnaf ar reddit
-fnaf ar discord
-fnaf ar cheats
-fnaf ar tier list
-fnaf ar golden freddy
-fnaf ar springtrap
-fnaf ar toy bonnie
-fnaf ar toy chica
-fnaf ar mangle
-fnaf ar balloon boy
-fnaf ar baby
-fnaf ar circus baby
-fnaf ar bonnie
-fnaf ar chica
-fnaf ar foxy
-fnaf ar freddy
-fnaf ar endo 01
-fnaf ar endo 02
-fnaf ar shadow bonnie
-fnaf ar frostbear
-fnaf ar shamrock freddy
-fnaf ar chocolate bonnie
-fnaf ar easter bonnie
-fnaf ar highscore toy chica
-fnaf ar radioactive foxy
-fnaf ar flaming springtrap
-fnaf ar liberty chica
-fnaf ar firework freddy
-fnaf ar system error toy bonnie
-fnaf ar scorching chica
-fnaf ar flamingo dancer chica
-
-
Go to the App Store or Google Play Store and search for "Five Nights at Freddy's AR". Alternatively, you can use these links: [App Store
What characters and features does fnaf ar have?
-
fnaf ar has a variety of characters and features that make the game more fun and challenging. You can encounter different animatronics from the Five Nights at Freddy's franchise, each with their own behavior, appearance, and voice. Some of them are original characters, while others are skins that change the look of the animatronics. You can also collect parts, CPUs, and plushsuits to assemble your own animatronics and customize them in the workshop. Here is a table that shows some of the characters and features that fnaf ar has:
- | Characters | Features | | --- | --- | | Bare Endo | The first and easiest animatronic to encounter. It has no special abilities and can be easily shocked when it uncloaks. | | Freddy Fazbear | The main mascot of Fazbear Entertainment. He is slightly harder than Bare Endo and can sometimes fake charge at you. | | Bonnie | The guitar-playing bunny. He is similar to Freddy but can haywire more often. You have to look away from him when he does that. | | Chica | The cupcake-loving chicken. She is also similar to Freddy and Bonnie but can make more noise and distract you with her cupcake. | | Foxy | The pirate fox. He is faster and more aggressive than the other animatronics. He can also run at you from different angles and hide behind static. | | Balloon Boy | The balloon vendor. He is the first animatronic that does not attack you directly but instead disables your flashlight and lets other animatronics attack you. | | Circus Baby | The leader of the Funtime animatronics. She is very intelligent and deceptive. She can pretend to be friendly or scared to lure you into a false sense of security. | | Springtrap | The most dangerous animatronic in the game. He is the only one that can kill you even if you shock him at the right time. You have to pay attention to his eye color and look away or stare at him depending on the situation. | | Shadow Bonnie | A mysterious shadowy figure that can appear randomly in your map or after collecting remnant. He can distort your reality and make you face different challenges to defeat him. | | Toy Chica | The upgraded version of Chica. She is more colorful and cheerful but also more deadly. She can wear different masks to confuse you and make you lower your guard. | | Freddy Frostbear | A winter-themed version of Freddy. He is covered in ice and snow and can freeze your screen with his frosty breath. You have to shake your device to thaw it out before you can shock him. | | Toy Bonnie | The upgraded version of Bonnie. He is more blue and shiny but also more sneaky. He can use his guitar to jam your signal and make it harder to find him. | | Toy Freddy | The upgraded version of Freddy. He is more brown and polished but also more lazy. He can play video games on his stomach screen and make you join him in his game over screen if you fail to shock him. | | Mangle | The broken-down version of Funtime Foxy. She is a mess of wires and parts that can crawl on the ceiling and walls. She can also drop her parts on the floor and make you pick them up before you can shock her. | | 8-Bit Baby | A pixelated version of Circus Baby. She is a homage to the mini-games from the original games. She can glitch your screen and make you scan different QR codes to find her location. | | Ballora | The graceful ballerina. She is the only animatronic that does not charge or haywire at you but instead dances around you in circles. You have to listen to her music box and shock her when she is close enough. | | Jack-O-Chica | A Halloween-themed version of Chica. She is on fire and carries a jack-o-lantern instead of a cupcake. She can burn your screen with her flames and make you cool it down by blowing into your microphone.
What tips and tricks can help you survive fnaf ar?
-
fnaf ar is not an easy game to play, especially if you are new to the Five Nights at Freddy's franchise. You will need to use your skills, reflexes, and strategies to survive the attacks of the animatronics. Here are some tips and tricks that can help you out:
-
-
Always keep your battery charged. You will need it to use your flashlight, shocker, and other tools. You can charge your battery by tapping on the battery icon on the bottom right corner of your screen.
-
Use your flashlight wisely. You will need it to see the static and the animatronics, but it also drains your battery and makes you more visible to them. You can turn it on and off by tapping on the flashlight icon on the bottom left corner of your screen.
-
Listen carefully. You can hear the footsteps, breathing, and voice lines of the animatronics. They can give you clues about their location, movement, and behavior. You can also use headphones or earphones to enhance the sound quality.
-
Look around. You can move your device in any direction to scan your surroundings. You can also use the map icon on the top right corner of your screen to see a bird's eye view of your area. You can see where the animatronics are and where they are heading.
-
Shock them at the right time. You can shock the animatronics by tapping on the shocker icon on the bottom center of your screen. However, you can only shock them when they are uncloaked and close enough to you. If you shock them too early or too late, you will waste your battery and make them angry.
-
Learn their patterns. Each animatronic has a different way of attacking you. Some of them will charge at you, some of them will haywire, some of them will fake charge, and some of them will do other things. You have to learn how to react to each one of them and avoid their tricks.
-
Collect remnant. Remnant is a glowing substance that you can find around your area or after defeating an animatronic. You can collect it by tapping on it or using a magnet. Remnant can increase your streak, level up your animatronics, and make you more resistant to hostile attacks.
-
Send and recall animatronics. You can send your own animatronics to other players or recall them back to you by using the workshop icon on the bottom right corner of your screen. You can also modify their parts, CPUs, and plushsuits to make them stronger or more unique.
-
Have fun. fnaf ar is a game that is meant to scare you but also entertain you. Don't take it too seriously and enjoy the thrill of facing your fears.
-
-
Conclusion
-
fnaf ar is a game that combines the horror of Five Nights at Freddy's with the reality of your own world. It is a game that challenges you to survive against different animatronics that will stalk you wherever you go. It is also a game that lets you collect, customize, and send your own animatronics to other players.
-
If you are looking for a game that will make you scream, laugh, and have fun at the same time, fnaf ar is the game for you. You can download it for free from the App Store or Google Play Store and start playing today. Just remember: don't let them catch you!
-
FAQs
-
-
Q: Is fnaf ar safe for kids?
-
A: fnaf ar is rated 12+ on the App Store and Teen on Google Play Store. It contains violence, blood, horror, and jump scares that may not be suitable for younger audiences. Parental discretion is advised.
-
Q: How do I get more coins and Faz-Tokens in fnaf ar?
-
A: You can get more coins and Faz-Tokens by completing daily challenges, watching ads, or purchasing them with real money.
-
Q: How do I get more characters and skins in fnaf ar?
-
A: You can get more characters and skins by encountering them in the game, buying them with Faz-Tokens, or getting them from special events or promotions.
-
Q: How do I contact customer support for fnaf ar?
-
A: You can contact customer support for fnaf ar by emailing support@illumix.com or visiting https://illumix.com/support/
-
Q: How do I join the fnaf ar community?
-
A: You can join the fnaf ar community by following their social media accounts, such as Facebook , Twitter, Instagram, YouTube, Reddit, or Discord. You can also visit their official website at https://fnafar.com/
-
197e85843d
-
-
\ No newline at end of file
diff --git a/spaces/congsaPfin/Manga-OCR/logs/How to Get GTA 5 APKData for Android Free (2.6GB Apklime Com) and Experience the Thrill of Grand Theft Auto.md b/spaces/congsaPfin/Manga-OCR/logs/How to Get GTA 5 APKData for Android Free (2.6GB Apklime Com) and Experience the Thrill of Grand Theft Auto.md
deleted file mode 100644
index 3fc1f3d7e661fdcfcb9156b3553539b6680ce4bf..0000000000000000000000000000000000000000
--- a/spaces/congsaPfin/Manga-OCR/logs/How to Get GTA 5 APKData for Android Free (2.6GB Apklime Com) and Experience the Thrill of Grand Theft Auto.md
+++ /dev/null
@@ -1,90 +0,0 @@
-
-
GTA 5 APK+Data for Android Free Download (2.6GB Apklime Com)
-
If you are a fan of Grand Theft Auto, you must have heard of GTA 5, the latest installment in the popular action-adventure series. GTA 5 is one of the most successful games ever made, with millions of players around the world enjoying its immersive story, realistic graphics, and thrilling gameplay. But did you know that you can also play GTA 5 on your Android device? Yes, you read that right. You can download GTA 5 APK+Data for Android for free from Apklime.com and experience the epic game on your smartphone or tablet. In this article, we will tell you everything you need to know about GTA 5 APK+Data for Android, including its features, how to download it, and some tips and tricks to help you play better.
-
Introduction
-
What is GTA 5?
-
GTA 5 is the fifth main entry in the Grand Theft Auto series, developed by Rockstar Games. It was released in 2013 for PlayStation 3 and Xbox 360, and later for PlayStation 4, Xbox One, and PC. GTA 5 is set in the fictional state of San Andreas, which is based on Southern California. The game follows the lives of three protagonists: Michael, a retired bank robber; Trevor, a psychopathic criminal; and Franklin, a young street hustler. The game allows you to switch between these characters at any time and explore their personal stories, as well as participate in various missions, activities, and events in the open world.
-
gta 5 apk+data for android free download (2.6gb apklime com)
GTA 5 is a game that deserves to be played on a big screen with high-quality graphics and sound. However, not everyone has access to a console or a PC that can run the game smoothly. That's why playing GTA 5 on Android is a great option for those who want to enjoy the game on the go, without compromising on the quality or the fun. Playing GTA 5 on Android has many advantages, such as:
-
-
You can play anytime, anywhere, as long as you have an internet connection.
-
You can use touch controls or connect a controller to your device for better gameplay.
-
You can customize the graphics settings according to your device's performance.
-
You can save your progress online and resume it on any device.
-
You can access exclusive features and content that are not available on other platforms.
-
-
How to download GTA 5 APK+Data for Android?
-
Downloading GTA 5 APK+Data for Android is very easy and fast. All you need to do is follow these simple steps:
-
-
Go to Apklime.com and search for GTA 5 APK+Data for Android.
-
Click on the download button and wait for the file to be downloaded.
-
Once the file is downloaded, go to your device's settings and enable installation from unknown sources.
-
Locate the file in your device's storage and tap on it to install it.
-
After the installation is complete, launch the game and enjoy!
-
-
Features of GTA 5 APK+Data for Android
-
Stunning graphics and gameplay
GTA 5 APK+Data for Android is a masterpiece of graphics and gameplay. The game has been optimized to run smoothly on Android devices, with high-resolution textures, realistic lighting, shadows, and reflections. The game also supports dynamic weather, day and night cycles, and ragdoll physics. The game's animations and sound effects are also top-notch, making you feel like you are in the middle of the action. You can also adjust the graphics settings to suit your device's capabilities and preferences.
-
Three playable characters with unique stories
-
One of the most innovative features of GTA 5 is the ability to switch between three different characters at any time. Each character has their own personality, background, skills, and motivations. You can explore their individual stories and see how they interact with each other and the world around them. You can also choose which character to use for different missions and scenarios, depending on your style and strategy. For example, you can use Michael for stealth and planning, Trevor for rampage and chaos, and Franklin for driving and shooting.
-
Open-world exploration and activities
-
GTA 5 offers you a vast and diverse open world to explore and enjoy. The game's map is based on Los Angeles and its surrounding areas, including urban, rural, mountainous, desert, and coastal regions. You can travel across the map using various vehicles, such as cars, bikes, boats, planes, helicopters, and even submarines. You can also walk, run, swim, climb, jump, and parachute your way around. The game's world is full of life and detail, with pedestrians, animals, traffic, shops, landmarks, and events. You can also engage in various activities and hobbies, such as golfing, tennis, yoga, hunting, racing, gambling, dancing, and more.
-
Online multiplayer mode and customizations
-
GTA 5 also has an online multiplayer mode called GTA Online, where you can create your own character and join other players in a shared world. You can cooperate or compete with other players in various missions, heists, races, deathmatches, and other modes. You can also customize your character's appearance, clothing, weapons, vehicles, properties, and businesses. You can also join or create crews with other players and earn reputation and money. GTA Online is constantly updated with new content and features to keep you entertained.
-
gta 5 android apk+data free download 2.6gb from apklime website
-download gta 5 apk+data for android (2.6gb) free on apklime.com
-gta 5 apk+data (2.6gb) free download for android devices by apklime
-how to download gta 5 apk+data (2.6gb) for android free from apklime
-gta 5 android game free download apk+data (2.6gb) on apklime site
-apklime.com gta 5 apk+data for android free download (2.6gb) link
-gta 5 apk+data for android (2.6gb) free download by apklime.com
-gta 5 android version apk+data (2.6gb) free download from apklime
-apklime gta 5 apk+data for android free download (2.6gb) tutorial
-gta 5 apk+data for android free download (2.6gb) on apklime.com
-gta 5 android game apk+data (2.6gb) free download by apklime
-download gta 5 for android free apk+data (2.6gb) from apklime site
-gta 5 apk+data for android (2.6gb) free download on apklime website
-gta 5 android version free download apk+data (2.6gb) by apklime.com
-apklime.com gta 5 for android free download apk+data (2.6gb) guide
-gta 5 apk+data for android free download (2.6gb) by apklime site
-gta 5 android game free download by apklime apk+data (2.6gb)
-download gta 5 for android apk+data (2.6gb) free on apklime website
-gta 5 apk+data for android (2.6gb) free download from apklime site
-gta 5 android version free download by apklime apk+data (2.6gb)
-apklime.com gta 5 for android free download guide apk+data (2.6gb)
-gta 5 apk+data for android free download (2.6gb) on apklime site
-gta 5 android game free download from apklime apk+data (2.6gb)
-download gta 5 for android apk+data (2.6gb) free from apklime site
-gta 5 apk+data for android (2.6gb) free download by apklime website
-gta 5 android version free download from apklime apk+data (2.6gb)
-apklime.com gta 5 for android free download link apk+data (2.6gb)
-gta 5 apk+data for android free download (2.6gb) by apklime link
-gta 5 android game free download on apklime apk+data (2.6gb)
-download gta 5 for android apk+data (2.6gb) free on apklime link
-
Tips and tricks for playing GTA 5 on Android
-
Use the map and radar to navigate
-
GTA 5 has a huge map that can be overwhelming at first. That's why you should always use the map and radar to find your way around. The map shows you the locations of missions, activities, shops, safe houses, and other points of interest. You can also set waypoints to mark your destination and follow the GPS directions. The radar shows you the nearby enemies, allies, vehicles, weapons, items, and events. You can also zoom in or out the radar to see more or less detail.
-
Switch between characters and weapons wisely
-
GTA 5 gives you the option to switch between three characters at any time during the game. This can be very useful for different situations and strategies. For example,
For example, you can switch to Trevor when you need to cause mayhem and distract the enemies, or to Franklin when you need to drive fast and escape the cops, or to Michael when you need to use stealth and snipe the targets. You can also switch between different weapons depending on the range, accuracy, damage, and ammo of each weapon. You can access your weapon wheel by tapping on the screen and select the weapon you want to use. You can also buy more weapons and ammo from Ammu-Nation stores or find them in the world.
-
Complete missions and side quests to earn money and reputation
-
GTA 5 has a main storyline that consists of various missions that advance the plot and the characters' development. You can start a mission by going to the marked location on the map or by receiving a phone call or a message from a contact. You can also replay any mission you have completed before from the pause menu. Completing missions will reward you with money and reputation, which are essential for unlocking new content and features in the game. You can also do side quests, such as helping strangers and freaks, collecting collectibles, doing stunt jumps, and more. These will give you extra money, reputation, and fun.
-
Have fun with cheats and mods
-
GTA 5 is a game that allows you to have fun in many ways. One of them is using cheats and mods. Cheats are codes that you can enter in the game to activate various effects, such as invincibility, super jump, explosive bullets, slow motion, and more. You can find a list of cheats online or in the game's manual. To enter a cheat, you need to open your phone's dial pad and type the code. However, be aware that using cheats will disable your achievements and trophies, so use them wisely. Mods are modifications that you can download and install in the game to change its appearance, gameplay, or content. For example, you can download mods that add new vehicles, weapons, characters, maps, missions, and more. You can find mods online or in the Apklime.com website. To install a mod, you need to follow the instructions provided by the mod creator.
-
Conclusion
-
Summary of the main points
-
GTA 5 is one of the best games ever made, and you can play it on your Android device for free by downloading GTA 5 APK+Data for Android from Apklime.com. The game has amazing graphics and gameplay, three playable characters with unique stories, an open world full of exploration and activities, an online multiplayer mode with customizations, and many tips and tricks to help you play better. GTA 5 is a game that will keep you entertained for hours and hours.
-
Call to action and recommendation
-
If you are ready to experience GTA 5 on your Android device, don't wait any longer. Go to Apklime.com and download GTA 5 APK+Data for Android now. You won't regret it. GTA 5 is a game that you will love playing on your smartphone or tablet. It is a game that will make you feel like you are living in a virtual world full of adventure and fun.
-
FAQs
-
Q: Is GTA 5 APK+Data for Android safe to download?
-
A: Yes, GTA 5 APK+Data for Android is safe to download from Apklime.com. The file is scanned for viruses and malware before being uploaded to the website. However, make sure that you have enough space on your device's storage before downloading it.
-
Q: How much space does GTA 5 APK+Data for Android take?
-
A: GTA 5 APK+Data for Android takes about 2.6GB of space on your device's storage. You will need at least 3GB of free space to install it properly.
-
Q: Can I play GTA 5 offline on Android?
-
A: Yes, you can play GTA 5 offline on Android after downloading and installing it from Apklime.com. However, you will need an internet connection to access some features and content in the game, such as GTA Online, updates, cloud saves, etc.
-
Q: Can I play GTA 5 with my friends on Android?
-
A: Yes, you can play GTA 5 with your friends on Android by joining GTA Online, the online multiplayer mode of the game. You can invite your friends to join your session or join theirs by using your phone's contacts or social club app in the game.
-
Q: Can I transfer my progress from other platforms to Android?
-
A: Yes, you can transfer your progress
A: Yes, you can transfer your progress from other platforms to Android by using the Rockstar Games Social Club service. You will need to create an account and link it to your platform of choice, such as PlayStation, Xbox, or PC. Then, you can use the same account to log in to GTA 5 on Android and sync your progress. However, note that some features and content may not be available or compatible across different platforms.
197e85843d
-
-
\ No newline at end of file
diff --git a/spaces/congsaPfin/Manga-OCR/logs/Live Play Bingo The Best Live Streaming Bingo Game for Mobile.md b/spaces/congsaPfin/Manga-OCR/logs/Live Play Bingo The Best Live Streaming Bingo Game for Mobile.md
deleted file mode 100644
index 1b9ffe73acd1316490f44ea8e190686bc732f1ea..0000000000000000000000000000000000000000
--- a/spaces/congsaPfin/Manga-OCR/logs/Live Play Bingo The Best Live Streaming Bingo Game for Mobile.md
+++ /dev/null
@@ -1,87 +0,0 @@
-
-
Live Play Bingo APK: A New Way to Enjoy Bingo Games at Home
-
If you love playing bingo games but don't want to go to a crowded bingo hall, you might want to try live play bingo apk. This is a free-to-play mobile app that lets you join live bingo games shows hosted by real hosts from London and LA. You can play bingo at home 24 hours a day, 7 days a week, and win amazing prizes. You can also chat with the hosts and other players, send gifts, and play slots mini-games. In this article, we will tell you everything you need to know about live play bingo apk and how to download it on your Android device.
One of the best features of live play bingo apk is that it offers live games and shows 24/7. You can join any of the hundreds of levels available and play with up to four cards at a time. The hosts will entertain you with trivia, jokes, and fun facts while you daub your numbers. You can also interact with them through voice or text chat. The more you play, the more coins and credits you earn, which you can use to buy more cards or power-ups. You can also win huge jackpots, gift cards, vouchers, and other rewards.
-
Power-Ups, Gifts and Slots
-
To make your bingo experience more exciting, you can use power-ups to boost your chances of winning. For example, you can use Triple Daubs to mark three random numbers on your card, or Instant Win to win the game instantly. You can also collect lucky boosters like free spins, extra coins, or extra tickets. Another way to have fun is to send gifts to the hosts or other players. You can choose from a variety of items like flowers, chocolates, drinks, or even cars. You can also play slots mini-games to earn more coins and power-ups.
-
Bingo Live Community
-
Playing bingo at home doesn't have to be lonely. With live play bingo apk, you can join a vibrant online community of bingo lovers from around the world. You can chat with them in real-time, make new friends, or invite your existing friends to play with you. You can also share your bingo stories, tips, and feedback with the community. The hosts will also shout out your name and respond to your messages while you play. You will never feel bored or isolated with live play bingo apk.
-
How to Download and Install Live Play Bingo APK
-
If you are ready to try live play bingo apk, here are the steps you need to follow:
-
-
Go to [this link](^1^) on your Android device and tap on Download APK.
-
Wait for the file to download and then open it.
-
If prompted, allow the installation of unknown apps from your browser.
-
Follow the instructions on the screen to install the app.
-
Launch the app and sign up with your email or Facebook account.
-
Enjoy playing live bingo games at home!
-
-
Conclusion
-
Live play bingo apk is a great way to enjoy bingo games at home without missing out on the fun and social aspects of playing in a bingo hall. You can join live games and shows 24/7, chat with hosts and other players, send gifts, use power-ups, and win amazing prizes. You can also download the app for free and get started with a huge bonus of coins and credits
If you are looking for a new way to enjoy bingo games at home, you should definitely give live play bingo apk a try. You will love the variety of games, the friendly hosts, the lively community, and the awesome prizes. You will also appreciate the convenience and security of playing on your mobile device. Live play bingo apk is the ultimate bingo app for bingo lovers.
-
FAQs
-
Here are some frequently asked questions about live play bingo apk:
-
-
Q: Is live play bingo apk free to play?
-A: Yes, live play bingo apk is free to download and play. You can also get free coins and credits every day by logging in, watching videos, or completing tasks. However, you can also purchase more coins and credits with real money if you want to.
-
Q: Is live play bingo apk safe and secure?
-A: Yes, live play bingo apk is safe and secure. The app uses encryption and SSL technology to protect your personal and financial information. The app also complies with the GDPR and CCPA regulations to respect your privacy and data rights.
-
Q: How can I contact the customer support of live play bingo apk?
-A: If you have any questions, issues, or feedback about live play bingo apk, you can contact the customer support team by emailing support@liveplaybingo.com or by using the in-app chat feature. The team is available 24/7 to assist you.
-
Q: How can I update live play bingo apk?
-A: Live play bingo apk updates automatically when you launch the app. However, you can also check for updates manually by going to the Google Play Store and tapping on the Update button. Updating the app will ensure that you have the latest features and bug fixes.
-
Q: Can I play live play bingo apk on other devices?
-A: Live play bingo apk is currently only available for Android devices. However, the developers are working on making the app compatible with iOS devices as well. You can follow their social media pages or visit their website for more updates.
-
-
live play bingo apk download
-live play bingo apk mod
-live play bingo apk latest version
-live play bingo apk free credits
-live play bingo apk for android
-live play bingo apk for pc
-live play bingo apk for ios
-live play bingo apk for windows
-live play bingo apk for mac
-live play bingo apk for laptop
-live play bingo apk online
-live play bingo apk offline
-live play bingo apk update
-live play bingo apk hack
-live play bingo apk cheat
-live play bingo apk review
-live play bingo apk rating
-live play bingo apk install
-live play bingo apk uninstall
-live play bingo apk support
-live play bingo apk help
-live play bingo apk guide
-live play bingo apk tips
-live play bingo apk tricks
-live play bingo apk features
-live play bingo apk benefits
-live play bingo apk advantages
-live play bingo apk disadvantages
-live play bingo apk pros and cons
-live play bingo apk comparison
-live play bingo apk alternatives
-live play bingo apk competitors
-live play bingo apk similar apps
-live play bingo apk best app
-live play bingo apk top app
-live play bingo apk fun app
-live play bingo apk cool app
-live play bingo apk awesome app
-live play bingo apk amazing app
-live play bingo apk exciting app
-live play bingo apk entertaining app
-live play bingo apk engaging app
-live play bingo apk addictive app
-live play bingo apk social app
-live play bingo apk community app
-live play bingo apk multiplayer app
-live play bingo apk casino app
-live play bingo apk game app
-live play bingo real hosts app
401be4b1e0
-
-
\ No newline at end of file
diff --git a/spaces/congsaPfin/Manga-OCR/logs/Mortal Kombat Trilogy APK el legendario juego de arcade ahora en tu Android.md b/spaces/congsaPfin/Manga-OCR/logs/Mortal Kombat Trilogy APK el legendario juego de arcade ahora en tu Android.md
deleted file mode 100644
index 52597ca328c306ea5ed9a7dcb7e335118f23b2ed..0000000000000000000000000000000000000000
--- a/spaces/congsaPfin/Manga-OCR/logs/Mortal Kombat Trilogy APK el legendario juego de arcade ahora en tu Android.md
+++ /dev/null
@@ -1,187 +0,0 @@
-
-
Mortal Kombat Trilogy APK Para Android: How to Download and Play the Ultimate Fighting Game
-
If you are a fan of fighting games, you probably know about Mortal Kombat, one of the most popular and brutal franchises in the genre. But did you know that you can play one of the best installments of the series, Mortal Kombat Trilogy, on your Android device? In this article, we will show you how to download and play Mortal Kombat Trilogy APK Para Android, a modified version of the game that lets you enjoy all the features and content of the original game on your smartphone or tablet. We will also give you some tips and tricks to master the game and win the fights, as well as some cheats and secrets to unlock hidden characters and modes.
-
What is Mortal Kombat Trilogy?
-
Mortal Kombat Trilogy is a fighting game that was released in 1996 for various platforms, including PlayStation, Nintendo 64, Sega Saturn, and PC. It is a compilation of the first three games of the series, Mortal Kombat, Mortal Kombat II, and Mortal Kombat 3, with some additional content and improvements. It features:
Over 30 playable characters, including all the fighters from the previous games, plus some new ones like Chameleon, Khameleon, Rain, Noob Saibot, Ermac, Goro, Kintaro, Motaro, and Shao Kahn.
-
Over 30 stages, including all the arenas from the previous games, plus some new ones like The Pit III, Scorpion's Lair, Jade's Desert, Star Bridge, Khameleon's Cave, Noob's Dorfen, The Roof, The Soul Chamber, The Wasteland, The Graveyard, The Armory, The Portal, The Tower, The Courtyard II.
-
Multiple game modes, including Arcade Mode (where you fight against a series of opponents until you reach Shao Kahn), Versus Mode (where you can fight against another player or the CPU), Practice Mode (where you can practice your moves and combos), Endurance Mode (where you face multiple opponents in a row without recovering health), Tournament Mode (where you can compete in a bracket-style tournament with up to 8 players), Team Battle Mode (where you can form teams of up to 4 players and fight against another team), 2-on-2 Mode (where you can switch between two characters during a fight), 3-on-3 Mode (where you can switch between three characters during a fight).
-
Various options and settings, including difficulty level, number of rounds per fight, time limit per round, blood toggle (on/off), violence mode (on/off), auto combos (on/off), blocking (on/off ), and cheat menu (where you can enter codes to activate various cheats).
-
-
Mortal Kombat Trilogy APK Para Android is a modified version of the game that allows you to play it on your Android device. It is not an official release by the developers, but a fan-made project that has been ported from the PC version. It has some differences from the original game, such as:
-
-
The graphics and sound quality are lower than the PC version, due to the limitations of the Android platform.
-
The controls are adapted to the touchscreen, with virtual buttons for punching, kicking, blocking, and special moves. You can also customize the layout and size of the buttons according to your preference.
-
The game requires an emulator to run, such as ePSXe or FPse. You also need a BIOS file and a ROM file of the game, which are not included in the APK file. You have to download them separately from other sources.
-
-
How to Download and Install Mortal Kombat Trilogy APK Para Android
-
If you want to play Mortal Kombat Trilogy APK Para Android on your device, you need to follow these steps:
-
-
Download the APK file from a reliable source, such as [this one]. Make sure you have enough storage space on your device before downloading.
-
Download an emulator app that supports PlayStation games, such as ePSXe or FPse. You can find them on the Google Play Store or other websites.
-
Download a BIOS file and a ROM file of Mortal Kombat Trilogy. You can search for them online, but be careful of malware and viruses. You can also use your own copies of the game if you have them.
-
Enable the installation of apps from unknown sources on your device. To do this, go to Settings > Security > Unknown Sources and toggle it on.
-
Install the APK file by tapping on it and following the instructions. You may need to grant some permissions to the app.
-
Install the emulator app by tapping on it and following the instructions. You may need to grant some permissions to the app.
-
Launch the emulator app and locate the BIOS file and the ROM file of Mortal Kombat Trilogy. You may need to extract them from zip files if they are compressed.
-
Load the ROM file and start playing Mortal Kombat Trilogy APK Para Android.
-
-
Some possible issues and solutions when downloading and installing Mortal Kombat Trilogy APK Para Android are:
-
-
The APK file is corrupted or incomplete. Try downloading it again from another source or using a different browser.
-
The emulator app is not compatible with your device or Android version. Try using another emulator app or updating your device software.
-
The BIOS file or the ROM file is missing or invalid. Try downloading them again from another source or using your own copies of the game.
-
The game crashes or freezes during gameplay. Try adjusting the settings of the emulator app, such as video quality, sound quality, frame rate, etc.
-
-
How to Play Mortal Kombat Trilogy APK Para Android
-
Once you have successfully installed Mortal Kombat Trilogy APK Para Android on your device, you can start playing it by following these steps:
-
-
Select a game mode from the main menu. You can choose between Arcade Mode, Versus Mode, Practice Mode, Endurance Mode, Tournament Mode, Team Battle Mode, 2-on-2 Mode, 3-on-3 Mode.
-
Select a character from the character select screen. You can scroll through the roster by swiping left or right on the touchscreen. You can also tap on a character's portrait to see their bio and stats.
-
Select a stage from the stage select screen. You can scroll through the stages by swiping left or right on the touchscreen. You can also tap on a stage's name to see its description and background story.
-
Fight against your opponent using the virtual buttons on the touchscreen. The buttons are arranged as follows:
-
-
-
Punch
Kick
Block
Run
-
High Punch
High Kick
Block
Run
-
Low Punch
Low Kick
-
-
You can also perform special moves by combining directional inputs with button presses. For example, to perform Scorpion's Spear move, you have to press Back + Low Punch. You can find a list of all the special moves of each character in the game manual or online.
-
You can also perform combos by chaining together multiple attacks in a sequence. For example, to perform Sub-Zero's Ice Clone combo, you have to press High Punch, High Punch, Down + Low Punch, Back + Low Kick. You can find a list of all the combos of each character in the game manual or online.
-
You can also perform fatalities by executing a specific input after winning a fight. Fatalities are gruesome finishing moves that kill your opponent in a brutal way. For example, to perform Liu Kang's Dragon Bite fatality, you have to press Forward, Forward, Forward, High Kick when you are close to your opponent. You can find a list of all the fatalities of each character in the game manual or online.
-
Tips and Tricks to Master the Game and Win the Fights
-
Mortal Kombat Trilogy APK Para Android is not an easy game to master, especially if you are new to the series or the genre. Here are some tips and tricks that can help you improve your skills and win the fights:
Learn the basics of the game, such as how to block, how to run, how to throw, how to jump, how to crouch, how to dash, how to evade, etc. These are essential skills that can make a difference in a fight.
-
Learn the strengths and weaknesses of each character, such as their speed, power, range, mobility, defense, etc. Some characters are better suited for certain situations and play styles than others.
-
Learn the special moves and combos of each character, as well as their inputs and timing. Practice them in Practice Mode until you can execute them flawlessly and consistently.
-
Learn the fatalities of each character, as well as their inputs and distance. Practice them in Practice Mode until you can execute them flawlessly and consistently.
-
Learn the cheats and secrets of the game, such as how to unlock hidden characters and modes, how to access the cheat menu, how to activate various codes, etc. These can give you an edge in a fight or add some fun and variety to the game.
-
Play against different opponents and difficulty levels, either in Arcade Mode or Versus Mode. This will help you improve your reaction time, strategy, adaptability, and experience.
-
Watch videos of other players playing the game online or offline. This will help you learn from their techniques, mistakes, tips, and tricks.
-
-
Cheats and Secrets to Unlock Hidden Characters and Modes
-
Mortal Kombat Trilogy APK Para Android has many cheats and secrets that can unlock hidden characters and modes in the game. Here are some of them:
-
-
To unlock Chameleon (a male ninja who changes color and abilities during a fight), enter this code at the character select screen: Hold Up + Start + Block + Run on player 1's side until Shao Kahn says "Excellent". Then select any male ninja character.
-
To unlock Khameleon (a female ninja who changes color and abilities during a fight), enter this code at the character select screen: Hold Up + Start + Block + Run on player 2's side until Shao Kahn says "Excellent". Then select any female ninja character.
-
To unlock Human Smoke (a human version of Smoke with different moves), enter this code at the character select screen: Hold Left + High Punch + High Kick + Block + Run on player 1's side until Shao Kahn says "Excellent". Then select Smoke.
-
To unlock Motaro (a four-legged centaur-like creature with powerful attacks), enter this code at the character select screen: Hold Left + Low Punch + Low Kick on player 1's side until Shao Kahn says "Excellent". Then select Motaro.
-
To unlock Shao Kahn (the final boss of the game with devastating attacks), enter this code at the character select screen: Hold Right + Low Punch + Low Kick on player 2's side until Shao Kahn says "Excellent". Then select Shao Kahn.
-
To unlock Classic Sub-Zero (a version of Sub-Zero from Mortal Kombat II with different moves), enter this code at the character select screen: Hold Up + Run on player 1's side until Shao Kahn says "Excellent". Then select Sub-Zero.
-
To unlock Ermac (a red-clad ninja with telekinetic powers), enter this code at the character select screen: Hold Down + Start on player 2's side until Shao Kahn says "Excellent". Then select Ermac. li>To unlock Noob Saibot (a black-clad ninja with shadow powers), enter this code at the character select screen: Hold Down + Block + Run on player 2's side until Shao Kahn says "Excellent". Then select Noob Saibot.
-
To unlock Rain (a purple-clad ninja with water powers), enter this code at the character select screen: Hold Up + High Punch + Low Punch + Run + Block on player 1's side until Shao Kahn says "Excellent". Then select Rain.
-
To access the cheat menu, where you can activate various cheats such as one-hit kills, unlimited run, free play, etc., enter this code at the main menu: Hold Left + Up + Start on player 1's side until Shao Kahn says "Outstanding". Then press Start to enter the cheat menu.
-
To access the hidden options menu, where you can change the game settings such as difficulty level, number of rounds, time limit, etc., enter this code at the main menu: Hold Right + Down + Start on player 2's side until Shao Kahn says "Outstanding". Then press Start to enter the hidden options menu.
-
To access the secret message from the developers, enter this code at the main menu: Hold Up + Left + Start on player 1's side and Down + Right + Start on player 2's side until Shao Kahn says "Outstanding". Then press Start to see the secret message.
-
-
Conclusion
-
Mortal Kombat Trilogy APK Para Android is a great way to enjoy one of the best fighting games of all time on your Android device. It has all the features and content of the original game, plus some extra ones that make it even more fun and challenging. You can play it solo or with your friends, and experience the thrill and gore of Mortal Kombat. You can also use cheats and secrets to unlock hidden characters and modes, and add some spice to your gameplay. If you are a fan of Mortal Kombat or fighting games in general, you should definitely download and play Mortal Kombat Trilogy APK Para Android. You won't regret it!
-
So what are you waiting for? Download Mortal Kombat Trilogy APK Para Android now and get ready to fight!
-
FAQs
-
Q1: Is Mortal Kombat Trilogy APK Para Android safe and legal?
-
A1: Mortal Kombat Trilogy APK Para Android is safe to download and play, as long as you get it from a reliable source and scan it for malware and viruses. However, it is not legal to download and play Mortal Kombat Trilogy APK Para Android, as it is a modified version of a copyrighted game that has been distributed without the permission of the developers. Therefore, we do not endorse or encourage the use of Mortal Kombat Trilogy APK Para Android, and we are not responsible for any legal or ethical issues that may arise from it. If you want to play Mortal Kombat Trilogy legally, you should buy the original game from an authorized seller.
-
Q2: What are the minimum requirements to play Mortal Kombat Trilogy APK Para Android?
-
A2: The minimum requirements to play Mortal Kombat Trilogy APK Para Android are:
-
-
An Android device with at least 1 GB of RAM and 500 MB of free storage space.
-
An emulator app that supports PlayStation games, such as ePSXe or FPse.
-
A BIOS file and a ROM file of Mortal Kombat Trilogy.
-
A stable internet connection (optional, but recommended for downloading the files and playing online).
-
-
Q3: How many characters are available in Mortal Kombat Trilogy APK Para Android?
-
A3: There are 32 playable characters in Mortal Kombat Trilogy APK Para Android, including 26 regular characters and 6 hidden characters. The regular characters are:
-
-
Liu Kang
-
Kung Lao
-
Johnny Cage
-
Reptile
-
Sub-Zero
-
Shang Tsung
-
Kitana
-
Jax
-
Mileena
-
Baraka
-
Scorpion
-
Raiden
-
Cyrax
-
Kano
-
Sektor
-
Sonya Blade
-
Nightwolf
-
Sindel
-
Stryker
-
Smoke
-
Kabal
-
Sheeva
-
Jade
-
Kurtis Stryker
-
Motaro (boss)
-
Shao Kahn (boss)
-
-
The hidden characters are:
-
-
Chameleon (male ninja)
-
Khameleon (female ninja)
-
Human Smoke (human version of Smoke)
-
Classic Sub-Zero (version of Sub-Zero from Mortal Kombat II)
-
Ermac (red-clad ninja)
-
Noob Saibot (black-clad ninja)
-
-
Q4: How can I play Mortal Kombat Trilogy APK Para Android with a controller or a keyboard?
-
A4: You can play Mortal Kombat Trilogy APK Para Android with a controller or a keyboard by connecting them to your Android device via Bluetooth, USB, or OTG. You can also use an app like Sixaxis Controller or Tincore Keymapper to map the buttons of your controller or keyboard to the touchscreen. However, you may need to root your device or use a custom ROM to do this. You can also adjust the settings of the emulator app to configure the controller or keyboard inputs.
-
Q5: Can I play Mortal Kombat Trilogy APK Para Android online or offline?
-
A5: You can play Mortal Kombat Trilogy APK Para Android online or offline, depending on your preference and availability. You can play online by using an app like Netplay or Kaillera Client, which allow you to connect with other players over the internet and play Versus Mode, Tournament Mode, Team Battle Mode, 2-on-2 Mode, or 3-on-3 Mode. However, you may experience some lag or connection issues depending on your internet speed and location. You can also play offline by using the emulator app's built-in multiplayer feature, which allows you to play Versus Mode, Tournament Mode, Team Battle Mode, 2-on-2 Mode, or 3-on-3 Mode with another player on the same device or on another device via Wi-Fi or Bluetooth.
197e85843d
-
-
\ No newline at end of file
diff --git a/spaces/coreml-community/ControlNet-v1-1-Annotators-cpu/annotator/lama/saicinpainting/training/visualizers/colors.py b/spaces/coreml-community/ControlNet-v1-1-Annotators-cpu/annotator/lama/saicinpainting/training/visualizers/colors.py
deleted file mode 100644
index 9e9e39182c58cb06a1c5e97a7e6c497cc3388ebe..0000000000000000000000000000000000000000
--- a/spaces/coreml-community/ControlNet-v1-1-Annotators-cpu/annotator/lama/saicinpainting/training/visualizers/colors.py
+++ /dev/null
@@ -1,76 +0,0 @@
-import random
-import colorsys
-
-import numpy as np
-import matplotlib
-matplotlib.use('agg')
-import matplotlib.pyplot as plt
-from matplotlib.colors import LinearSegmentedColormap
-
-
-def generate_colors(nlabels, type='bright', first_color_black=False, last_color_black=True, verbose=False):
- # https://stackoverflow.com/questions/14720331/how-to-generate-random-colors-in-matplotlib
- """
- Creates a random colormap to be used together with matplotlib. Useful for segmentation tasks
- :param nlabels: Number of labels (size of colormap)
- :param type: 'bright' for strong colors, 'soft' for pastel colors
- :param first_color_black: Option to use first color as black, True or False
- :param last_color_black: Option to use last color as black, True or False
- :param verbose: Prints the number of labels and shows the colormap. True or False
- :return: colormap for matplotlib
- """
- if type not in ('bright', 'soft'):
- print ('Please choose "bright" or "soft" for type')
- return
-
- if verbose:
- print('Number of labels: ' + str(nlabels))
-
- # Generate color map for bright colors, based on hsv
- if type == 'bright':
- randHSVcolors = [(np.random.uniform(low=0.0, high=1),
- np.random.uniform(low=0.2, high=1),
- np.random.uniform(low=0.9, high=1)) for i in range(nlabels)]
-
- # Convert HSV list to RGB
- randRGBcolors = []
- for HSVcolor in randHSVcolors:
- randRGBcolors.append(colorsys.hsv_to_rgb(HSVcolor[0], HSVcolor[1], HSVcolor[2]))
-
- if first_color_black:
- randRGBcolors[0] = [0, 0, 0]
-
- if last_color_black:
- randRGBcolors[-1] = [0, 0, 0]
-
- random_colormap = LinearSegmentedColormap.from_list('new_map', randRGBcolors, N=nlabels)
-
- # Generate soft pastel colors, by limiting the RGB spectrum
- if type == 'soft':
- low = 0.6
- high = 0.95
- randRGBcolors = [(np.random.uniform(low=low, high=high),
- np.random.uniform(low=low, high=high),
- np.random.uniform(low=low, high=high)) for i in range(nlabels)]
-
- if first_color_black:
- randRGBcolors[0] = [0, 0, 0]
-
- if last_color_black:
- randRGBcolors[-1] = [0, 0, 0]
- random_colormap = LinearSegmentedColormap.from_list('new_map', randRGBcolors, N=nlabels)
-
- # Display colorbar
- if verbose:
- from matplotlib import colors, colorbar
- from matplotlib import pyplot as plt
- fig, ax = plt.subplots(1, 1, figsize=(15, 0.5))
-
- bounds = np.linspace(0, nlabels, nlabels + 1)
- norm = colors.BoundaryNorm(bounds, nlabels)
-
- cb = colorbar.ColorbarBase(ax, cmap=random_colormap, norm=norm, spacing='proportional', ticks=None,
- boundaries=bounds, format='%1i', orientation=u'horizontal')
-
- return randRGBcolors, random_colormap
-
diff --git a/spaces/coreml-community/ControlNet-v1-1-Annotators-cpu/annotator/oneformer/detectron2/data/datasets/builtin.py b/spaces/coreml-community/ControlNet-v1-1-Annotators-cpu/annotator/oneformer/detectron2/data/datasets/builtin.py
deleted file mode 100644
index 39bbb1feec64f76705ba32c46f19f89f71be2ca7..0000000000000000000000000000000000000000
--- a/spaces/coreml-community/ControlNet-v1-1-Annotators-cpu/annotator/oneformer/detectron2/data/datasets/builtin.py
+++ /dev/null
@@ -1,259 +0,0 @@
-# -*- coding: utf-8 -*-
-# Copyright (c) Facebook, Inc. and its affiliates.
-
-
-"""
-This file registers pre-defined datasets at hard-coded paths, and their metadata.
-
-We hard-code metadata for common datasets. This will enable:
-1. Consistency check when loading the datasets
-2. Use models on these standard datasets directly and run demos,
- without having to download the dataset annotations
-
-We hard-code some paths to the dataset that's assumed to
-exist in "./datasets/".
-
-Users SHOULD NOT use this file to create new dataset / metadata for new dataset.
-To add new dataset, refer to the tutorial "docs/DATASETS.md".
-"""
-
-import os
-
-from annotator.oneformer.detectron2.data import DatasetCatalog, MetadataCatalog
-
-from .builtin_meta import ADE20K_SEM_SEG_CATEGORIES, _get_builtin_metadata
-from .cityscapes import load_cityscapes_instances, load_cityscapes_semantic
-from .cityscapes_panoptic import register_all_cityscapes_panoptic
-from .coco import load_sem_seg, register_coco_instances
-from .coco_panoptic import register_coco_panoptic, register_coco_panoptic_separated
-from .lvis import get_lvis_instances_meta, register_lvis_instances
-from .pascal_voc import register_pascal_voc
-
-# ==== Predefined datasets and splits for COCO ==========
-
-_PREDEFINED_SPLITS_COCO = {}
-_PREDEFINED_SPLITS_COCO["coco"] = {
- "coco_2014_train": ("coco/train2014", "coco/annotations/instances_train2014.json"),
- "coco_2014_val": ("coco/val2014", "coco/annotations/instances_val2014.json"),
- "coco_2014_minival": ("coco/val2014", "coco/annotations/instances_minival2014.json"),
- "coco_2014_valminusminival": (
- "coco/val2014",
- "coco/annotations/instances_valminusminival2014.json",
- ),
- "coco_2017_train": ("coco/train2017", "coco/annotations/instances_train2017.json"),
- "coco_2017_val": ("coco/val2017", "coco/annotations/instances_val2017.json"),
- "coco_2017_test": ("coco/test2017", "coco/annotations/image_info_test2017.json"),
- "coco_2017_test-dev": ("coco/test2017", "coco/annotations/image_info_test-dev2017.json"),
- "coco_2017_val_100": ("coco/val2017", "coco/annotations/instances_val2017_100.json"),
-}
-
-_PREDEFINED_SPLITS_COCO["coco_person"] = {
- "keypoints_coco_2014_train": (
- "coco/train2014",
- "coco/annotations/person_keypoints_train2014.json",
- ),
- "keypoints_coco_2014_val": ("coco/val2014", "coco/annotations/person_keypoints_val2014.json"),
- "keypoints_coco_2014_minival": (
- "coco/val2014",
- "coco/annotations/person_keypoints_minival2014.json",
- ),
- "keypoints_coco_2014_valminusminival": (
- "coco/val2014",
- "coco/annotations/person_keypoints_valminusminival2014.json",
- ),
- "keypoints_coco_2017_train": (
- "coco/train2017",
- "coco/annotations/person_keypoints_train2017.json",
- ),
- "keypoints_coco_2017_val": ("coco/val2017", "coco/annotations/person_keypoints_val2017.json"),
- "keypoints_coco_2017_val_100": (
- "coco/val2017",
- "coco/annotations/person_keypoints_val2017_100.json",
- ),
-}
-
-
-_PREDEFINED_SPLITS_COCO_PANOPTIC = {
- "coco_2017_train_panoptic": (
- # This is the original panoptic annotation directory
- "coco/panoptic_train2017",
- "coco/annotations/panoptic_train2017.json",
- # This directory contains semantic annotations that are
- # converted from panoptic annotations.
- # It is used by PanopticFPN.
- # You can use the script at detectron2/datasets/prepare_panoptic_fpn.py
- # to create these directories.
- "coco/panoptic_stuff_train2017",
- ),
- "coco_2017_val_panoptic": (
- "coco/panoptic_val2017",
- "coco/annotations/panoptic_val2017.json",
- "coco/panoptic_stuff_val2017",
- ),
- "coco_2017_val_100_panoptic": (
- "coco/panoptic_val2017_100",
- "coco/annotations/panoptic_val2017_100.json",
- "coco/panoptic_stuff_val2017_100",
- ),
-}
-
-
-def register_all_coco(root):
- for dataset_name, splits_per_dataset in _PREDEFINED_SPLITS_COCO.items():
- for key, (image_root, json_file) in splits_per_dataset.items():
- # Assume pre-defined datasets live in `./datasets`.
- register_coco_instances(
- key,
- _get_builtin_metadata(dataset_name),
- os.path.join(root, json_file) if "://" not in json_file else json_file,
- os.path.join(root, image_root),
- )
-
- for (
- prefix,
- (panoptic_root, panoptic_json, semantic_root),
- ) in _PREDEFINED_SPLITS_COCO_PANOPTIC.items():
- prefix_instances = prefix[: -len("_panoptic")]
- instances_meta = MetadataCatalog.get(prefix_instances)
- image_root, instances_json = instances_meta.image_root, instances_meta.json_file
- # The "separated" version of COCO panoptic segmentation dataset,
- # e.g. used by Panoptic FPN
- register_coco_panoptic_separated(
- prefix,
- _get_builtin_metadata("coco_panoptic_separated"),
- image_root,
- os.path.join(root, panoptic_root),
- os.path.join(root, panoptic_json),
- os.path.join(root, semantic_root),
- instances_json,
- )
- # The "standard" version of COCO panoptic segmentation dataset,
- # e.g. used by Panoptic-DeepLab
- register_coco_panoptic(
- prefix,
- _get_builtin_metadata("coco_panoptic_standard"),
- image_root,
- os.path.join(root, panoptic_root),
- os.path.join(root, panoptic_json),
- instances_json,
- )
-
-
-# ==== Predefined datasets and splits for LVIS ==========
-
-
-_PREDEFINED_SPLITS_LVIS = {
- "lvis_v1": {
- "lvis_v1_train": ("coco/", "lvis/lvis_v1_train.json"),
- "lvis_v1_val": ("coco/", "lvis/lvis_v1_val.json"),
- "lvis_v1_test_dev": ("coco/", "lvis/lvis_v1_image_info_test_dev.json"),
- "lvis_v1_test_challenge": ("coco/", "lvis/lvis_v1_image_info_test_challenge.json"),
- },
- "lvis_v0.5": {
- "lvis_v0.5_train": ("coco/", "lvis/lvis_v0.5_train.json"),
- "lvis_v0.5_val": ("coco/", "lvis/lvis_v0.5_val.json"),
- "lvis_v0.5_val_rand_100": ("coco/", "lvis/lvis_v0.5_val_rand_100.json"),
- "lvis_v0.5_test": ("coco/", "lvis/lvis_v0.5_image_info_test.json"),
- },
- "lvis_v0.5_cocofied": {
- "lvis_v0.5_train_cocofied": ("coco/", "lvis/lvis_v0.5_train_cocofied.json"),
- "lvis_v0.5_val_cocofied": ("coco/", "lvis/lvis_v0.5_val_cocofied.json"),
- },
-}
-
-
-def register_all_lvis(root):
- for dataset_name, splits_per_dataset in _PREDEFINED_SPLITS_LVIS.items():
- for key, (image_root, json_file) in splits_per_dataset.items():
- register_lvis_instances(
- key,
- get_lvis_instances_meta(dataset_name),
- os.path.join(root, json_file) if "://" not in json_file else json_file,
- os.path.join(root, image_root),
- )
-
-
-# ==== Predefined splits for raw cityscapes images ===========
-_RAW_CITYSCAPES_SPLITS = {
- "cityscapes_fine_{task}_train": ("cityscapes/leftImg8bit/train/", "cityscapes/gtFine/train/"),
- "cityscapes_fine_{task}_val": ("cityscapes/leftImg8bit/val/", "cityscapes/gtFine/val/"),
- "cityscapes_fine_{task}_test": ("cityscapes/leftImg8bit/test/", "cityscapes/gtFine/test/"),
-}
-
-
-def register_all_cityscapes(root):
- for key, (image_dir, gt_dir) in _RAW_CITYSCAPES_SPLITS.items():
- meta = _get_builtin_metadata("cityscapes")
- image_dir = os.path.join(root, image_dir)
- gt_dir = os.path.join(root, gt_dir)
-
- inst_key = key.format(task="instance_seg")
- DatasetCatalog.register(
- inst_key,
- lambda x=image_dir, y=gt_dir: load_cityscapes_instances(
- x, y, from_json=True, to_polygons=True
- ),
- )
- MetadataCatalog.get(inst_key).set(
- image_dir=image_dir, gt_dir=gt_dir, evaluator_type="cityscapes_instance", **meta
- )
-
- sem_key = key.format(task="sem_seg")
- DatasetCatalog.register(
- sem_key, lambda x=image_dir, y=gt_dir: load_cityscapes_semantic(x, y)
- )
- MetadataCatalog.get(sem_key).set(
- image_dir=image_dir,
- gt_dir=gt_dir,
- evaluator_type="cityscapes_sem_seg",
- ignore_label=255,
- **meta,
- )
-
-
-# ==== Predefined splits for PASCAL VOC ===========
-def register_all_pascal_voc(root):
- SPLITS = [
- ("voc_2007_trainval", "VOC2007", "trainval"),
- ("voc_2007_train", "VOC2007", "train"),
- ("voc_2007_val", "VOC2007", "val"),
- ("voc_2007_test", "VOC2007", "test"),
- ("voc_2012_trainval", "VOC2012", "trainval"),
- ("voc_2012_train", "VOC2012", "train"),
- ("voc_2012_val", "VOC2012", "val"),
- ]
- for name, dirname, split in SPLITS:
- year = 2007 if "2007" in name else 2012
- register_pascal_voc(name, os.path.join(root, dirname), split, year)
- MetadataCatalog.get(name).evaluator_type = "pascal_voc"
-
-
-def register_all_ade20k(root):
- root = os.path.join(root, "ADEChallengeData2016")
- for name, dirname in [("train", "training"), ("val", "validation")]:
- image_dir = os.path.join(root, "images", dirname)
- gt_dir = os.path.join(root, "annotations_detectron2", dirname)
- name = f"ade20k_sem_seg_{name}"
- DatasetCatalog.register(
- name, lambda x=image_dir, y=gt_dir: load_sem_seg(y, x, gt_ext="png", image_ext="jpg")
- )
- MetadataCatalog.get(name).set(
- stuff_classes=ADE20K_SEM_SEG_CATEGORIES[:],
- image_root=image_dir,
- sem_seg_root=gt_dir,
- evaluator_type="sem_seg",
- ignore_label=255,
- )
-
-
-# True for open source;
-# Internally at fb, we register them elsewhere
-if __name__.endswith(".builtin"):
- # Assume pre-defined datasets live in `./datasets`.
- _root = os.path.expanduser(os.getenv("DETECTRON2_DATASETS", "datasets"))
- register_all_coco(_root)
- register_all_lvis(_root)
- register_all_cityscapes(_root)
- register_all_cityscapes_panoptic(_root)
- register_all_pascal_voc(_root)
- register_all_ade20k(_root)
diff --git a/spaces/cscan/CodeFormer/CodeFormer/facelib/detection/yolov5face/utils/autoanchor.py b/spaces/cscan/CodeFormer/CodeFormer/facelib/detection/yolov5face/utils/autoanchor.py
deleted file mode 100644
index a4eba3e94888709be7d2a7c7499fbcc1808b4a88..0000000000000000000000000000000000000000
--- a/spaces/cscan/CodeFormer/CodeFormer/facelib/detection/yolov5face/utils/autoanchor.py
+++ /dev/null
@@ -1,12 +0,0 @@
-# Auto-anchor utils
-
-
-def check_anchor_order(m):
- # Check anchor order against stride order for YOLOv5 Detect() module m, and correct if necessary
- a = m.anchor_grid.prod(-1).view(-1) # anchor area
- da = a[-1] - a[0] # delta a
- ds = m.stride[-1] - m.stride[0] # delta s
- if da.sign() != ds.sign(): # same order
- print("Reversing anchor order")
- m.anchors[:] = m.anchors.flip(0)
- m.anchor_grid[:] = m.anchor_grid.flip(0)
diff --git a/spaces/cyberoleg/b2719240e190e2a649150d94db50be82838efeb0/app.py b/spaces/cyberoleg/b2719240e190e2a649150d94db50be82838efeb0/app.py
deleted file mode 100644
index 54330d98cf1b2cb3783ac6ffdc2c51d3a015cc0c..0000000000000000000000000000000000000000
--- a/spaces/cyberoleg/b2719240e190e2a649150d94db50be82838efeb0/app.py
+++ /dev/null
@@ -1,33 +0,0 @@
-import gradio as gr
-
-from diffusion_webui import (
- StableDiffusionControlNetGenerator,
- StableDiffusionControlNetInpaintGenerator,
- StableDiffusionImage2ImageGenerator,
- StableDiffusionInpaintGenerator,
- StableDiffusionText2ImageGenerator,
-)
-
-
-def diffusion_app():
- app = gr.Blocks()
- with app:
- with gr.Row():
- with gr.Column():
- with gr.Tab(label="Text2Image"):
- StableDiffusionText2ImageGenerator.app()
- with gr.Tab(label="Image2Image"):
- StableDiffusionImage2ImageGenerator.app()
- with gr.Tab(label="Inpaint"):
- StableDiffusionInpaintGenerator.app()
- with gr.Tab(label="Controlnet"):
- StableDiffusionControlNetGenerator.app()
- with gr.Tab(label="Controlnet Inpaint"):
- StableDiffusionControlNetInpaintGenerator.app()
-
- app.queue(concurrency_count=1)
- app.launch(debug=True, enable_queue=True)
-
-
-if __name__ == "__main__":
- diffusion_app()
diff --git a/spaces/cymic/VITS-Tokaiteio/preprocess.py b/spaces/cymic/VITS-Tokaiteio/preprocess.py
deleted file mode 100644
index aaedbf076c30114b3ac6c27dfb42fd54ac81a71c..0000000000000000000000000000000000000000
--- a/spaces/cymic/VITS-Tokaiteio/preprocess.py
+++ /dev/null
@@ -1,25 +0,0 @@
-import argparse
-import text
-from utils import load_filepaths_and_text
-
-if __name__ == '__main__':
- parser = argparse.ArgumentParser()
- parser.add_argument("--out_extension", default="cleaned")
- parser.add_argument("--text_index", default=1, type=int)
- parser.add_argument("--filelists", nargs="+", default=["filelists/ljs_audio_text_val_filelist.txt", "filelists/ljs_audio_text_test_filelist.txt"])
- parser.add_argument("--text_cleaners", nargs="+", default=["english_cleaners2"])
-
- args = parser.parse_args()
-
-
- for filelist in args.filelists:
- print("START:", filelist)
- filepaths_and_text = load_filepaths_and_text(filelist)
- for i in range(len(filepaths_and_text)):
- original_text = filepaths_and_text[i][args.text_index]
- cleaned_text = text._clean_text(original_text, args.text_cleaners)
- filepaths_and_text[i][args.text_index] = cleaned_text
-
- new_filelist = filelist + "." + args.out_extension
- with open(new_filelist, "w", encoding="utf-8") as f:
- f.writelines(["|".join(x) + "\n" for x in filepaths_and_text])
diff --git a/spaces/daddyjin/TalkingFaceGeneration/Demo_TFR_Pirenderer/src/pirenderer/util/meters.py b/spaces/daddyjin/TalkingFaceGeneration/Demo_TFR_Pirenderer/src/pirenderer/util/meters.py
deleted file mode 100644
index 1a61e7890492ab2423b0cfdbeb4279697955be78..0000000000000000000000000000000000000000
--- a/spaces/daddyjin/TalkingFaceGeneration/Demo_TFR_Pirenderer/src/pirenderer/util/meters.py
+++ /dev/null
@@ -1,155 +0,0 @@
-import math
-
-import torch
-from torch.utils.tensorboard import SummaryWriter
-from torch.utils.tensorboard.summary import *
-
-
-from util.distributed import master_only
-from util.distributed import master_only_print as print
-
-LOG_WRITER = None
-LOG_DIR = None
-
-
-@torch.no_grad()
-def sn_reshape_weight_to_matrix(weight):
- r"""Reshape weight to obtain the matrix form.
-
- Args:
- weight (Parameters): pytorch layer parameter tensor.
- """
- weight_mat = weight
- height = weight_mat.size(0)
- return weight_mat.reshape(height, -1)
-
-
-@torch.no_grad()
-def get_weight_stats(mod, cfg, loss_id):
- r"""Get weight state
-
- Args:
- mod: Pytorch module
- cfg: Configuration object
- loss_id: Needed when using AMP.
- """
- loss_scale = 1.0
- if cfg.trainer.amp == 'O1' or cfg.trainer.amp == 'O2':
- # AMP rescales the gradient so we have to undo it.
- loss_scale = amp._amp_state.loss_scalers[loss_id].loss_scale()
- if mod.weight_orig.grad is not None:
- grad_norm = mod.weight_orig.grad.data.norm().item() / float(loss_scale)
- else:
- grad_norm = 0.
- weight_norm = mod.weight_orig.data.norm().item()
- weight_mat = sn_reshape_weight_to_matrix(mod.weight_orig)
- sigma = torch.sum(mod.weight_u * torch.mv(weight_mat, mod.weight_v))
- return grad_norm, weight_norm, sigma
-
-
-@master_only
-def set_summary_writer(log_dir):
- r"""Set summary writer
-
- Args:
- log_dir (str): Log directory.
- """
- global LOG_DIR, LOG_WRITER
- LOG_DIR = log_dir
- LOG_WRITER = SummaryWriter(log_dir=log_dir)
-
-
-@master_only
-def write_summary(name, summary, step, hist=False):
- """Utility function for write summary to log_writer.
- """
- global LOG_WRITER
- lw = LOG_WRITER
- if lw is None:
- raise Exception("Log writer not set.")
- if hist:
- lw.add_histogram(name, summary, step)
- else:
- lw.add_scalar(name, summary, step)
-
-
-@master_only
-def add_hparams(hparam_dict=None, metric_dict=None):
- r"""Add a set of hyperparameters to be compared in tensorboard.
-
- Args:
- hparam_dict (dictionary): Each key-value pair in the dictionary is the
- name of the hyper parameter and it's corresponding value.
- The type of the value can be one of `bool`, `string`, `float`,
- `int`, or `None`.
- metric_dict (dictionary): Each key-value pair in the dictionary is the
- name of the metric and it's corresponding value. Note that the key
- used here should be unique in the tensorboard record. Otherwise the
- value you added by `add_scalar` will be displayed in hparam plugin.
- In most cases, this is unwanted.
- """
- if type(hparam_dict) is not dict or type(metric_dict) is not dict:
- raise TypeError('hparam_dict and metric_dict should be dictionary.')
- global LOG_WRITER
- lw = LOG_WRITER
-
- exp, ssi, sei = hparams(hparam_dict, metric_dict)
-
- lw.file_writer.add_summary(exp)
- lw.file_writer.add_summary(ssi)
- lw.file_writer.add_summary(sei)
-
-
-class Meter(object):
- """Meter is to keep track of statistics along steps.
- Meters write values for purpose like printing average values.
- Meters can be flushed to log files (i.e. TensorBoard for now)
- regularly.
-
- Args:
- name (str): the name of meter
- """
-
- @master_only
- def __init__(self, name):
- self.name = name
- self.values = []
-
- @master_only
- def reset(self):
- r"""Reset the meter values"""
- self.values = []
-
- @master_only
- def write(self, value):
- r"""Record the value"""
- self.values.append(value)
-
- @master_only
- def flush(self, step):
- r"""Write the value in the tensorboard.
-
- Args:
- step (int): Epoch or iteration number.
- """
- if not all(math.isfinite(x) for x in self.values):
- print("meter {} contained a nan or inf.".format(self.name))
- filtered_values = list(filter(lambda x: math.isfinite(x), self.values))
- if float(len(filtered_values)) != 0:
- value = float(sum(filtered_values)) / float(len(filtered_values))
- write_summary(self.name, value, step)
- self.reset()
-
- @master_only
- def write_image(self, img_grid, step):
- r"""Write the value in the tensorboard.
-
- Args:
- img_grid:
- step (int): Epoch or iteration number.
- """
- global LOG_WRITER
- lw = LOG_WRITER
- if lw is None:
- raise Exception("Log writer not set.")
- lw.add_image("Visualizations", img_grid, step)
diff --git a/spaces/dcarpintero/nlp-summarizer-pegasus/.venv/lib/python3.9/site-packages/aiohttp/web.py b/spaces/dcarpintero/nlp-summarizer-pegasus/.venv/lib/python3.9/site-packages/aiohttp/web.py
deleted file mode 100644
index cefae2b9ae4114696f244f7f71bf8dd74ca8f4a6..0000000000000000000000000000000000000000
--- a/spaces/dcarpintero/nlp-summarizer-pegasus/.venv/lib/python3.9/site-packages/aiohttp/web.py
+++ /dev/null
@@ -1,588 +0,0 @@
-import asyncio
-import logging
-import socket
-import sys
-from argparse import ArgumentParser
-from collections.abc import Iterable
-from importlib import import_module
-from typing import (
- Any,
- Awaitable,
- Callable,
- Iterable as TypingIterable,
- List,
- Optional,
- Set,
- Type,
- Union,
- cast,
-)
-
-from .abc import AbstractAccessLogger
-from .helpers import all_tasks
-from .log import access_logger
-from .web_app import Application as Application, CleanupError as CleanupError
-from .web_exceptions import (
- HTTPAccepted as HTTPAccepted,
- HTTPBadGateway as HTTPBadGateway,
- HTTPBadRequest as HTTPBadRequest,
- HTTPClientError as HTTPClientError,
- HTTPConflict as HTTPConflict,
- HTTPCreated as HTTPCreated,
- HTTPError as HTTPError,
- HTTPException as HTTPException,
- HTTPExpectationFailed as HTTPExpectationFailed,
- HTTPFailedDependency as HTTPFailedDependency,
- HTTPForbidden as HTTPForbidden,
- HTTPFound as HTTPFound,
- HTTPGatewayTimeout as HTTPGatewayTimeout,
- HTTPGone as HTTPGone,
- HTTPInsufficientStorage as HTTPInsufficientStorage,
- HTTPInternalServerError as HTTPInternalServerError,
- HTTPLengthRequired as HTTPLengthRequired,
- HTTPMethodNotAllowed as HTTPMethodNotAllowed,
- HTTPMisdirectedRequest as HTTPMisdirectedRequest,
- HTTPMovedPermanently as HTTPMovedPermanently,
- HTTPMultipleChoices as HTTPMultipleChoices,
- HTTPNetworkAuthenticationRequired as HTTPNetworkAuthenticationRequired,
- HTTPNoContent as HTTPNoContent,
- HTTPNonAuthoritativeInformation as HTTPNonAuthoritativeInformation,
- HTTPNotAcceptable as HTTPNotAcceptable,
- HTTPNotExtended as HTTPNotExtended,
- HTTPNotFound as HTTPNotFound,
- HTTPNotImplemented as HTTPNotImplemented,
- HTTPNotModified as HTTPNotModified,
- HTTPOk as HTTPOk,
- HTTPPartialContent as HTTPPartialContent,
- HTTPPaymentRequired as HTTPPaymentRequired,
- HTTPPermanentRedirect as HTTPPermanentRedirect,
- HTTPPreconditionFailed as HTTPPreconditionFailed,
- HTTPPreconditionRequired as HTTPPreconditionRequired,
- HTTPProxyAuthenticationRequired as HTTPProxyAuthenticationRequired,
- HTTPRedirection as HTTPRedirection,
- HTTPRequestEntityTooLarge as HTTPRequestEntityTooLarge,
- HTTPRequestHeaderFieldsTooLarge as HTTPRequestHeaderFieldsTooLarge,
- HTTPRequestRangeNotSatisfiable as HTTPRequestRangeNotSatisfiable,
- HTTPRequestTimeout as HTTPRequestTimeout,
- HTTPRequestURITooLong as HTTPRequestURITooLong,
- HTTPResetContent as HTTPResetContent,
- HTTPSeeOther as HTTPSeeOther,
- HTTPServerError as HTTPServerError,
- HTTPServiceUnavailable as HTTPServiceUnavailable,
- HTTPSuccessful as HTTPSuccessful,
- HTTPTemporaryRedirect as HTTPTemporaryRedirect,
- HTTPTooManyRequests as HTTPTooManyRequests,
- HTTPUnauthorized as HTTPUnauthorized,
- HTTPUnavailableForLegalReasons as HTTPUnavailableForLegalReasons,
- HTTPUnprocessableEntity as HTTPUnprocessableEntity,
- HTTPUnsupportedMediaType as HTTPUnsupportedMediaType,
- HTTPUpgradeRequired as HTTPUpgradeRequired,
- HTTPUseProxy as HTTPUseProxy,
- HTTPVariantAlsoNegotiates as HTTPVariantAlsoNegotiates,
- HTTPVersionNotSupported as HTTPVersionNotSupported,
-)
-from .web_fileresponse import FileResponse as FileResponse
-from .web_log import AccessLogger
-from .web_middlewares import (
- middleware as middleware,
- normalize_path_middleware as normalize_path_middleware,
-)
-from .web_protocol import (
- PayloadAccessError as PayloadAccessError,
- RequestHandler as RequestHandler,
- RequestPayloadError as RequestPayloadError,
-)
-from .web_request import (
- BaseRequest as BaseRequest,
- FileField as FileField,
- Request as Request,
-)
-from .web_response import (
- ContentCoding as ContentCoding,
- Response as Response,
- StreamResponse as StreamResponse,
- json_response as json_response,
-)
-from .web_routedef import (
- AbstractRouteDef as AbstractRouteDef,
- RouteDef as RouteDef,
- RouteTableDef as RouteTableDef,
- StaticDef as StaticDef,
- delete as delete,
- get as get,
- head as head,
- options as options,
- patch as patch,
- post as post,
- put as put,
- route as route,
- static as static,
- view as view,
-)
-from .web_runner import (
- AppRunner as AppRunner,
- BaseRunner as BaseRunner,
- BaseSite as BaseSite,
- GracefulExit as GracefulExit,
- NamedPipeSite as NamedPipeSite,
- ServerRunner as ServerRunner,
- SockSite as SockSite,
- TCPSite as TCPSite,
- UnixSite as UnixSite,
-)
-from .web_server import Server as Server
-from .web_urldispatcher import (
- AbstractResource as AbstractResource,
- AbstractRoute as AbstractRoute,
- DynamicResource as DynamicResource,
- PlainResource as PlainResource,
- PrefixedSubAppResource as PrefixedSubAppResource,
- Resource as Resource,
- ResourceRoute as ResourceRoute,
- StaticResource as StaticResource,
- UrlDispatcher as UrlDispatcher,
- UrlMappingMatchInfo as UrlMappingMatchInfo,
- View as View,
-)
-from .web_ws import (
- WebSocketReady as WebSocketReady,
- WebSocketResponse as WebSocketResponse,
- WSMsgType as WSMsgType,
-)
-
-__all__ = (
- # web_app
- "Application",
- "CleanupError",
- # web_exceptions
- "HTTPAccepted",
- "HTTPBadGateway",
- "HTTPBadRequest",
- "HTTPClientError",
- "HTTPConflict",
- "HTTPCreated",
- "HTTPError",
- "HTTPException",
- "HTTPExpectationFailed",
- "HTTPFailedDependency",
- "HTTPForbidden",
- "HTTPFound",
- "HTTPGatewayTimeout",
- "HTTPGone",
- "HTTPInsufficientStorage",
- "HTTPInternalServerError",
- "HTTPLengthRequired",
- "HTTPMethodNotAllowed",
- "HTTPMisdirectedRequest",
- "HTTPMovedPermanently",
- "HTTPMultipleChoices",
- "HTTPNetworkAuthenticationRequired",
- "HTTPNoContent",
- "HTTPNonAuthoritativeInformation",
- "HTTPNotAcceptable",
- "HTTPNotExtended",
- "HTTPNotFound",
- "HTTPNotImplemented",
- "HTTPNotModified",
- "HTTPOk",
- "HTTPPartialContent",
- "HTTPPaymentRequired",
- "HTTPPermanentRedirect",
- "HTTPPreconditionFailed",
- "HTTPPreconditionRequired",
- "HTTPProxyAuthenticationRequired",
- "HTTPRedirection",
- "HTTPRequestEntityTooLarge",
- "HTTPRequestHeaderFieldsTooLarge",
- "HTTPRequestRangeNotSatisfiable",
- "HTTPRequestTimeout",
- "HTTPRequestURITooLong",
- "HTTPResetContent",
- "HTTPSeeOther",
- "HTTPServerError",
- "HTTPServiceUnavailable",
- "HTTPSuccessful",
- "HTTPTemporaryRedirect",
- "HTTPTooManyRequests",
- "HTTPUnauthorized",
- "HTTPUnavailableForLegalReasons",
- "HTTPUnprocessableEntity",
- "HTTPUnsupportedMediaType",
- "HTTPUpgradeRequired",
- "HTTPUseProxy",
- "HTTPVariantAlsoNegotiates",
- "HTTPVersionNotSupported",
- # web_fileresponse
- "FileResponse",
- # web_middlewares
- "middleware",
- "normalize_path_middleware",
- # web_protocol
- "PayloadAccessError",
- "RequestHandler",
- "RequestPayloadError",
- # web_request
- "BaseRequest",
- "FileField",
- "Request",
- # web_response
- "ContentCoding",
- "Response",
- "StreamResponse",
- "json_response",
- # web_routedef
- "AbstractRouteDef",
- "RouteDef",
- "RouteTableDef",
- "StaticDef",
- "delete",
- "get",
- "head",
- "options",
- "patch",
- "post",
- "put",
- "route",
- "static",
- "view",
- # web_runner
- "AppRunner",
- "BaseRunner",
- "BaseSite",
- "GracefulExit",
- "ServerRunner",
- "SockSite",
- "TCPSite",
- "UnixSite",
- "NamedPipeSite",
- # web_server
- "Server",
- # web_urldispatcher
- "AbstractResource",
- "AbstractRoute",
- "DynamicResource",
- "PlainResource",
- "PrefixedSubAppResource",
- "Resource",
- "ResourceRoute",
- "StaticResource",
- "UrlDispatcher",
- "UrlMappingMatchInfo",
- "View",
- # web_ws
- "WebSocketReady",
- "WebSocketResponse",
- "WSMsgType",
- # web
- "run_app",
-)
-
-
-try:
- from ssl import SSLContext
-except ImportError: # pragma: no cover
- SSLContext = Any # type: ignore[misc,assignment]
-
-HostSequence = TypingIterable[str]
-
-
-async def _run_app(
- app: Union[Application, Awaitable[Application]],
- *,
- host: Optional[Union[str, HostSequence]] = None,
- port: Optional[int] = None,
- path: Optional[str] = None,
- sock: Optional[Union[socket.socket, TypingIterable[socket.socket]]] = None,
- shutdown_timeout: float = 60.0,
- keepalive_timeout: float = 75.0,
- ssl_context: Optional[SSLContext] = None,
- print: Callable[..., None] = print,
- backlog: int = 128,
- access_log_class: Type[AbstractAccessLogger] = AccessLogger,
- access_log_format: str = AccessLogger.LOG_FORMAT,
- access_log: Optional[logging.Logger] = access_logger,
- handle_signals: bool = True,
- reuse_address: Optional[bool] = None,
- reuse_port: Optional[bool] = None,
-) -> None:
- # A internal functio to actually do all dirty job for application running
- if asyncio.iscoroutine(app):
- app = await app # type: ignore[misc]
-
- app = cast(Application, app)
-
- runner = AppRunner(
- app,
- handle_signals=handle_signals,
- access_log_class=access_log_class,
- access_log_format=access_log_format,
- access_log=access_log,
- keepalive_timeout=keepalive_timeout,
- )
-
- await runner.setup()
-
- sites: List[BaseSite] = []
-
- try:
- if host is not None:
- if isinstance(host, (str, bytes, bytearray, memoryview)):
- sites.append(
- TCPSite(
- runner,
- host,
- port,
- shutdown_timeout=shutdown_timeout,
- ssl_context=ssl_context,
- backlog=backlog,
- reuse_address=reuse_address,
- reuse_port=reuse_port,
- )
- )
- else:
- for h in host:
- sites.append(
- TCPSite(
- runner,
- h,
- port,
- shutdown_timeout=shutdown_timeout,
- ssl_context=ssl_context,
- backlog=backlog,
- reuse_address=reuse_address,
- reuse_port=reuse_port,
- )
- )
- elif path is None and sock is None or port is not None:
- sites.append(
- TCPSite(
- runner,
- port=port,
- shutdown_timeout=shutdown_timeout,
- ssl_context=ssl_context,
- backlog=backlog,
- reuse_address=reuse_address,
- reuse_port=reuse_port,
- )
- )
-
- if path is not None:
- if isinstance(path, (str, bytes, bytearray, memoryview)):
- sites.append(
- UnixSite(
- runner,
- path,
- shutdown_timeout=shutdown_timeout,
- ssl_context=ssl_context,
- backlog=backlog,
- )
- )
- else:
- for p in path:
- sites.append(
- UnixSite(
- runner,
- p,
- shutdown_timeout=shutdown_timeout,
- ssl_context=ssl_context,
- backlog=backlog,
- )
- )
-
- if sock is not None:
- if not isinstance(sock, Iterable):
- sites.append(
- SockSite(
- runner,
- sock,
- shutdown_timeout=shutdown_timeout,
- ssl_context=ssl_context,
- backlog=backlog,
- )
- )
- else:
- for s in sock:
- sites.append(
- SockSite(
- runner,
- s,
- shutdown_timeout=shutdown_timeout,
- ssl_context=ssl_context,
- backlog=backlog,
- )
- )
- for site in sites:
- await site.start()
-
- if print: # pragma: no branch
- names = sorted(str(s.name) for s in runner.sites)
- print(
- "======== Running on {} ========\n"
- "(Press CTRL+C to quit)".format(", ".join(names))
- )
-
- # sleep forever by 1 hour intervals,
- # on Windows before Python 3.8 wake up every 1 second to handle
- # Ctrl+C smoothly
- if sys.platform == "win32" and sys.version_info < (3, 8):
- delay = 1
- else:
- delay = 3600
-
- while True:
- await asyncio.sleep(delay)
- finally:
- await runner.cleanup()
-
-
-def _cancel_tasks(
- to_cancel: Set["asyncio.Task[Any]"], loop: asyncio.AbstractEventLoop
-) -> None:
- if not to_cancel:
- return
-
- for task in to_cancel:
- task.cancel()
-
- loop.run_until_complete(asyncio.gather(*to_cancel, return_exceptions=True))
-
- for task in to_cancel:
- if task.cancelled():
- continue
- if task.exception() is not None:
- loop.call_exception_handler(
- {
- "message": "unhandled exception during asyncio.run() shutdown",
- "exception": task.exception(),
- "task": task,
- }
- )
-
-
-def run_app(
- app: Union[Application, Awaitable[Application]],
- *,
- host: Optional[Union[str, HostSequence]] = None,
- port: Optional[int] = None,
- path: Optional[str] = None,
- sock: Optional[Union[socket.socket, TypingIterable[socket.socket]]] = None,
- shutdown_timeout: float = 60.0,
- keepalive_timeout: float = 75.0,
- ssl_context: Optional[SSLContext] = None,
- print: Callable[..., None] = print,
- backlog: int = 128,
- access_log_class: Type[AbstractAccessLogger] = AccessLogger,
- access_log_format: str = AccessLogger.LOG_FORMAT,
- access_log: Optional[logging.Logger] = access_logger,
- handle_signals: bool = True,
- reuse_address: Optional[bool] = None,
- reuse_port: Optional[bool] = None,
- loop: Optional[asyncio.AbstractEventLoop] = None,
-) -> None:
- """Run an app locally"""
- if loop is None:
- loop = asyncio.new_event_loop()
-
- # Configure if and only if in debugging mode and using the default logger
- if loop.get_debug() and access_log and access_log.name == "aiohttp.access":
- if access_log.level == logging.NOTSET:
- access_log.setLevel(logging.DEBUG)
- if not access_log.hasHandlers():
- access_log.addHandler(logging.StreamHandler())
-
- main_task = loop.create_task(
- _run_app(
- app,
- host=host,
- port=port,
- path=path,
- sock=sock,
- shutdown_timeout=shutdown_timeout,
- keepalive_timeout=keepalive_timeout,
- ssl_context=ssl_context,
- print=print,
- backlog=backlog,
- access_log_class=access_log_class,
- access_log_format=access_log_format,
- access_log=access_log,
- handle_signals=handle_signals,
- reuse_address=reuse_address,
- reuse_port=reuse_port,
- )
- )
-
- try:
- asyncio.set_event_loop(loop)
- loop.run_until_complete(main_task)
- except (GracefulExit, KeyboardInterrupt): # pragma: no cover
- pass
- finally:
- _cancel_tasks({main_task}, loop)
- _cancel_tasks(all_tasks(loop), loop)
- loop.run_until_complete(loop.shutdown_asyncgens())
- loop.close()
-
-
-def main(argv: List[str]) -> None:
- arg_parser = ArgumentParser(
- description="aiohttp.web Application server", prog="aiohttp.web"
- )
- arg_parser.add_argument(
- "entry_func",
- help=(
- "Callable returning the `aiohttp.web.Application` instance to "
- "run. Should be specified in the 'module:function' syntax."
- ),
- metavar="entry-func",
- )
- arg_parser.add_argument(
- "-H",
- "--hostname",
- help="TCP/IP hostname to serve on (default: %(default)r)",
- default="localhost",
- )
- arg_parser.add_argument(
- "-P",
- "--port",
- help="TCP/IP port to serve on (default: %(default)r)",
- type=int,
- default="8080",
- )
- arg_parser.add_argument(
- "-U",
- "--path",
- help="Unix file system path to serve on. Specifying a path will cause "
- "hostname and port arguments to be ignored.",
- )
- args, extra_argv = arg_parser.parse_known_args(argv)
-
- # Import logic
- mod_str, _, func_str = args.entry_func.partition(":")
- if not func_str or not mod_str:
- arg_parser.error("'entry-func' not in 'module:function' syntax")
- if mod_str.startswith("."):
- arg_parser.error("relative module names not supported")
- try:
- module = import_module(mod_str)
- except ImportError as ex:
- arg_parser.error(f"unable to import {mod_str}: {ex}")
- try:
- func = getattr(module, func_str)
- except AttributeError:
- arg_parser.error(f"module {mod_str!r} has no attribute {func_str!r}")
-
- # Compatibility logic
- if args.path is not None and not hasattr(socket, "AF_UNIX"):
- arg_parser.error(
- "file system paths not supported by your operating" " environment"
- )
-
- logging.basicConfig(level=logging.DEBUG)
-
- app = func(extra_argv)
- run_app(app, host=args.hostname, port=args.port, path=args.path)
- arg_parser.exit(message="Stopped\n")
-
-
-if __name__ == "__main__": # pragma: no branch
- main(sys.argv[1:]) # pragma: no cover
diff --git a/spaces/dcarpintero/nlp-summarizer-pegasus/.venv/lib/python3.9/site-packages/fsspec/tests/abstract/put.py b/spaces/dcarpintero/nlp-summarizer-pegasus/.venv/lib/python3.9/site-packages/fsspec/tests/abstract/put.py
deleted file mode 100644
index d06f9d9b53a2b2509596708b9e9fa55d7ea3599a..0000000000000000000000000000000000000000
--- a/spaces/dcarpintero/nlp-summarizer-pegasus/.venv/lib/python3.9/site-packages/fsspec/tests/abstract/put.py
+++ /dev/null
@@ -1,397 +0,0 @@
-class AbstractPutTests:
- def test_put_file_to_existing_directory(
- self,
- fs,
- fs_join,
- fs_target,
- local_join,
- local_bulk_operations_scenario_0,
- ):
- # Copy scenario 1a
- source = local_bulk_operations_scenario_0
-
- target = fs_target
- fs.mkdir(target)
- if not self.supports_empty_directories():
- # Force target directory to exist by adding a dummy file
- fs.touch(fs_join(target, "dummy"))
- assert fs.isdir(target)
-
- target_file2 = fs_join(target, "file2")
- target_subfile1 = fs_join(target, "subfile1")
-
- # Copy from source directory
- fs.put(local_join(source, "file2"), target)
- assert fs.isfile(target_file2)
-
- # Copy from sub directory
- fs.put(local_join(source, "subdir", "subfile1"), target)
- assert fs.isfile(target_subfile1)
-
- # Remove copied files
- fs.rm([target_file2, target_subfile1])
- assert not fs.exists(target_file2)
- assert not fs.exists(target_subfile1)
-
- # Repeat with trailing slash on target
- fs.put(local_join(source, "file2"), target + "/")
- assert fs.isdir(target)
- assert fs.isfile(target_file2)
-
- fs.put(local_join(source, "subdir", "subfile1"), target + "/")
- assert fs.isfile(target_subfile1)
-
- def test_put_file_to_new_directory(
- self, fs, fs_join, fs_target, local_join, local_bulk_operations_scenario_0
- ):
- # Copy scenario 1b
- source = local_bulk_operations_scenario_0
-
- target = fs_target
- fs.mkdir(target)
-
- fs.put(
- local_join(source, "subdir", "subfile1"), fs_join(target, "newdir/")
- ) # Note trailing slash
- assert fs.isdir(target)
- assert fs.isdir(fs_join(target, "newdir"))
- assert fs.isfile(fs_join(target, "newdir", "subfile1"))
-
- def test_put_file_to_file_in_existing_directory(
- self, fs, fs_join, fs_target, local_join, local_bulk_operations_scenario_0
- ):
- # Copy scenario 1c
- source = local_bulk_operations_scenario_0
-
- target = fs_target
- fs.mkdir(target)
-
- fs.put(local_join(source, "subdir", "subfile1"), fs_join(target, "newfile"))
- assert fs.isfile(fs_join(target, "newfile"))
-
- def test_put_file_to_file_in_new_directory(
- self, fs, fs_join, fs_target, local_join, local_bulk_operations_scenario_0
- ):
- # Copy scenario 1d
- source = local_bulk_operations_scenario_0
-
- target = fs_target
- fs.mkdir(target)
-
- fs.put(
- local_join(source, "subdir", "subfile1"),
- fs_join(target, "newdir", "newfile"),
- )
- assert fs.isdir(fs_join(target, "newdir"))
- assert fs.isfile(fs_join(target, "newdir", "newfile"))
-
- def test_put_directory_to_existing_directory(
- self, fs, fs_join, fs_target, local_bulk_operations_scenario_0
- ):
- # Copy scenario 1e
- source = local_bulk_operations_scenario_0
-
- target = fs_target
- fs.mkdir(target)
- if not self.supports_empty_directories():
- # Force target directory to exist by adding a dummy file
- dummy = fs_join(target, "dummy")
- fs.touch(dummy)
- assert fs.isdir(target)
-
- for source_slash, target_slash in zip([False, True], [False, True]):
- s = fs_join(source, "subdir")
- if source_slash:
- s += "/"
- t = target + "/" if target_slash else target
-
- # Without recursive does nothing
- fs.put(s, t)
- assert fs.ls(target) == [] if self.supports_empty_directories() else [dummy]
-
- # With recursive
- fs.put(s, t, recursive=True)
- if source_slash:
- assert fs.isfile(fs_join(target, "subfile1"))
- assert fs.isfile(fs_join(target, "subfile2"))
- assert fs.isdir(fs_join(target, "nesteddir"))
- assert fs.isfile(fs_join(target, "nesteddir", "nestedfile"))
- assert not fs.exists(fs_join(target, "subdir"))
-
- fs.rm(fs.ls(target, detail=False), recursive=True)
- else:
- assert fs.isdir(fs_join(target, "subdir"))
- assert fs.isfile(fs_join(target, "subdir", "subfile1"))
- assert fs.isfile(fs_join(target, "subdir", "subfile2"))
- assert fs.isdir(fs_join(target, "subdir", "nesteddir"))
- assert fs.isfile(fs_join(target, "subdir", "nesteddir", "nestedfile"))
-
- fs.rm(fs_join(target, "subdir"), recursive=True)
- assert fs.ls(target) == [] if self.supports_empty_directories() else [dummy]
-
- # Limit recursive by maxdepth
- fs.put(s, t, recursive=True, maxdepth=1)
- if source_slash:
- assert fs.isfile(fs_join(target, "subfile1"))
- assert fs.isfile(fs_join(target, "subfile2"))
- assert not fs.exists(fs_join(target, "nesteddir"))
- assert not fs.exists(fs_join(target, "subdir"))
-
- fs.rm(fs.ls(target, detail=False), recursive=True)
- else:
- assert fs.isdir(fs_join(target, "subdir"))
- assert fs.isfile(fs_join(target, "subdir", "subfile1"))
- assert fs.isfile(fs_join(target, "subdir", "subfile2"))
- assert not fs.exists(fs_join(target, "subdir", "nesteddir"))
-
- fs.rm(fs_join(target, "subdir"), recursive=True)
- assert fs.ls(target) == [] if self.supports_empty_directories() else [dummy]
-
- def test_put_directory_to_new_directory(
- self, fs, fs_join, fs_target, local_bulk_operations_scenario_0
- ):
- # Copy scenario 1f
- source = local_bulk_operations_scenario_0
-
- target = fs_target
- fs.mkdir(target)
- if not self.supports_empty_directories():
- # Force target directory to exist by adding a dummy file
- dummy = fs_join(target, "dummy")
- fs.touch(dummy)
- assert fs.isdir(target)
-
- for source_slash, target_slash in zip([False, True], [False, True]):
- s = fs_join(source, "subdir")
- if source_slash:
- s += "/"
- t = fs_join(target, "newdir")
- if target_slash:
- t += "/"
-
- # Without recursive does nothing
- fs.put(s, t)
- assert fs.ls(target) == [] if self.supports_empty_directories() else [dummy]
-
- # With recursive
- fs.put(s, t, recursive=True)
- assert fs.isdir(fs_join(target, "newdir"))
- assert fs.isfile(fs_join(target, "newdir", "subfile1"))
- assert fs.isfile(fs_join(target, "newdir", "subfile2"))
- assert fs.isdir(fs_join(target, "newdir", "nesteddir"))
- assert fs.isfile(fs_join(target, "newdir", "nesteddir", "nestedfile"))
- assert not fs.exists(fs_join(target, "subdir"))
-
- fs.rm(fs_join(target, "newdir"), recursive=True)
- assert not fs.exists(fs_join(target, "newdir"))
-
- # Limit recursive by maxdepth
- fs.put(s, t, recursive=True, maxdepth=1)
- assert fs.isdir(fs_join(target, "newdir"))
- assert fs.isfile(fs_join(target, "newdir", "subfile1"))
- assert fs.isfile(fs_join(target, "newdir", "subfile2"))
- assert not fs.exists(fs_join(target, "newdir", "nesteddir"))
- assert not fs.exists(fs_join(target, "subdir"))
-
- fs.rm(fs_join(target, "newdir"), recursive=True)
- assert not fs.exists(fs_join(target, "newdir"))
-
- def test_put_glob_to_existing_directory(
- self, fs, fs_join, fs_target, local_join, local_bulk_operations_scenario_0
- ):
- # Copy scenario 1g
- source = local_bulk_operations_scenario_0
-
- target = fs_target
- fs.mkdir(target)
- if not self.supports_empty_directories():
- # Force target directory to exist by adding a dummy file
- dummy = fs_join(target, "dummy")
- fs.touch(dummy)
- assert fs.isdir(target)
-
- for target_slash in [False, True]:
- t = target + "/" if target_slash else target
-
- # Without recursive
- fs.put(local_join(source, "subdir", "*"), t)
- assert fs.isfile(fs_join(target, "subfile1"))
- assert fs.isfile(fs_join(target, "subfile2"))
- assert not fs.isdir(fs_join(target, "nesteddir"))
- assert not fs.exists(fs_join(target, "nesteddir", "nestedfile"))
- assert not fs.exists(fs_join(target, "subdir"))
-
- fs.rm(fs.ls(target, detail=False), recursive=True)
- assert fs.ls(target) == [] if self.supports_empty_directories() else [dummy]
-
- # With recursive
- fs.put(local_join(source, "subdir", "*"), t, recursive=True)
- assert fs.isfile(fs_join(target, "subfile1"))
- assert fs.isfile(fs_join(target, "subfile2"))
- assert fs.isdir(fs_join(target, "nesteddir"))
- assert fs.isfile(fs_join(target, "nesteddir", "nestedfile"))
- assert not fs.exists(fs_join(target, "subdir"))
-
- fs.rm(fs.ls(target, detail=False), recursive=True)
- assert fs.ls(target) == [] if self.supports_empty_directories() else [dummy]
-
- # Limit recursive by maxdepth
- fs.put(local_join(source, "subdir", "*"), t, recursive=True, maxdepth=1)
- assert fs.isfile(fs_join(target, "subfile1"))
- assert fs.isfile(fs_join(target, "subfile2"))
- assert not fs.exists(fs_join(target, "nesteddir"))
- assert not fs.exists(fs_join(target, "subdir"))
-
- fs.rm(fs.ls(target, detail=False), recursive=True)
- assert fs.ls(target) == [] if self.supports_empty_directories() else [dummy]
-
- def test_put_glob_to_new_directory(
- self, fs, fs_join, fs_target, local_join, local_bulk_operations_scenario_0
- ):
- # Copy scenario 1h
- source = local_bulk_operations_scenario_0
-
- target = fs_target
- fs.mkdir(target)
- if not self.supports_empty_directories():
- # Force target directory to exist by adding a dummy file
- dummy = fs_join(target, "dummy")
- fs.touch(dummy)
- assert fs.isdir(target)
-
- for target_slash in [False, True]:
- t = fs_join(target, "newdir")
- if target_slash:
- t += "/"
-
- # Without recursive
- fs.put(local_join(source, "subdir", "*"), t)
- assert fs.isdir(fs_join(target, "newdir"))
- assert fs.isfile(fs_join(target, "newdir", "subfile1"))
- assert fs.isfile(fs_join(target, "newdir", "subfile2"))
- assert not fs.exists(fs_join(target, "newdir", "nesteddir"))
- assert not fs.exists(fs_join(target, "newdir", "nesteddir", "nestedfile"))
- assert not fs.exists(fs_join(target, "subdir"))
- assert not fs.exists(fs_join(target, "newdir", "subdir"))
-
- fs.rm(fs_join(target, "newdir"), recursive=True)
- assert not fs.exists(fs_join(target, "newdir"))
-
- # With recursive
- fs.put(local_join(source, "subdir", "*"), t, recursive=True)
- assert fs.isdir(fs_join(target, "newdir"))
- assert fs.isfile(fs_join(target, "newdir", "subfile1"))
- assert fs.isfile(fs_join(target, "newdir", "subfile2"))
- assert fs.isdir(fs_join(target, "newdir", "nesteddir"))
- assert fs.isfile(fs_join(target, "newdir", "nesteddir", "nestedfile"))
- assert not fs.exists(fs_join(target, "subdir"))
- assert not fs.exists(fs_join(target, "newdir", "subdir"))
-
- fs.rm(fs_join(target, "newdir"), recursive=True)
- assert not fs.exists(fs_join(target, "newdir"))
-
- # Limit recursive by maxdepth
- fs.put(local_join(source, "subdir", "*"), t, recursive=True, maxdepth=1)
- assert fs.isdir(fs_join(target, "newdir"))
- assert fs.isfile(fs_join(target, "newdir", "subfile1"))
- assert fs.isfile(fs_join(target, "newdir", "subfile2"))
- assert not fs.exists(fs_join(target, "newdir", "nesteddir"))
- assert not fs.exists(fs_join(target, "subdir"))
- assert not fs.exists(fs_join(target, "newdir", "subdir"))
-
- fs.rm(fs_join(target, "newdir"), recursive=True)
- assert not fs.exists(fs_join(target, "newdir"))
-
- def test_put_list_of_files_to_existing_directory(
- self,
- fs,
- fs_join,
- fs_target,
- local_join,
- local_bulk_operations_scenario_0,
- fs_path,
- ):
- # Copy scenario 2a
- source = local_bulk_operations_scenario_0
-
- target = fs_target
- fs.mkdir(target)
- if not self.supports_empty_directories():
- # Force target directory to exist by adding a dummy file
- dummy = fs_join(target, "dummy")
- fs.touch(dummy)
- assert fs.isdir(target)
-
- source_files = [
- local_join(source, "file1"),
- local_join(source, "file2"),
- local_join(source, "subdir", "subfile1"),
- ]
-
- for target_slash in [False, True]:
- t = target + "/" if target_slash else target
-
- fs.put(source_files, t)
- assert fs.isfile(fs_join(target, "file1"))
- assert fs.isfile(fs_join(target, "file2"))
- assert fs.isfile(fs_join(target, "subfile1"))
-
- fs.rm(fs.find(target))
- assert fs.ls(target) == [] if self.supports_empty_directories() else [dummy]
-
- def test_put_list_of_files_to_new_directory(
- self, fs, fs_join, fs_target, local_join, local_bulk_operations_scenario_0
- ):
- # Copy scenario 2b
- source = local_bulk_operations_scenario_0
-
- target = fs_target
- fs.mkdir(target)
-
- source_files = [
- local_join(source, "file1"),
- local_join(source, "file2"),
- local_join(source, "subdir", "subfile1"),
- ]
-
- fs.put(source_files, fs_join(target, "newdir") + "/") # Note trailing slash
- assert fs.isdir(fs_join(target, "newdir"))
- assert fs.isfile(fs_join(target, "newdir", "file1"))
- assert fs.isfile(fs_join(target, "newdir", "file2"))
- assert fs.isfile(fs_join(target, "newdir", "subfile1"))
-
- def test_put_directory_recursive(
- self, fs, fs_join, fs_target, local_fs, local_join, local_path
- ):
- # https://github.com/fsspec/filesystem_spec/issues/1062
- # Recursive cp/get/put of source directory into non-existent target directory.
- src = local_join(local_path, "src")
- src_file = local_join(src, "file")
- local_fs.mkdir(src)
- local_fs.touch(src_file)
-
- target = fs_target
-
- # put without slash
- assert not fs.exists(target)
- for loop in range(2):
- fs.put(src, target, recursive=True)
- assert fs.isdir(target)
-
- if loop == 0:
- assert fs.isfile(fs_join(target, "file"))
- assert not fs.exists(fs_join(target, "src"))
- else:
- assert fs.isfile(fs_join(target, "file"))
- assert fs.isdir(fs_join(target, "src"))
- assert fs.isfile(fs_join(target, "src", "file"))
-
- fs.rm(target, recursive=True)
-
- # put with slash
- assert not fs.exists(target)
- for loop in range(2):
- fs.put(src + "/", target, recursive=True)
- assert fs.isdir(target)
- assert fs.isfile(fs_join(target, "file"))
- assert not fs.exists(fs_join(target, "src"))
diff --git a/spaces/dcarpintero/nlp-summarizer-pegasus/.venv/lib/python3.9/site-packages/gradio_client/CHANGELOG.md b/spaces/dcarpintero/nlp-summarizer-pegasus/.venv/lib/python3.9/site-packages/gradio_client/CHANGELOG.md
deleted file mode 100644
index d6647fdc9ebad0e91aa58a41bdf2c6909902b498..0000000000000000000000000000000000000000
--- a/spaces/dcarpintero/nlp-summarizer-pegasus/.venv/lib/python3.9/site-packages/gradio_client/CHANGELOG.md
+++ /dev/null
@@ -1,358 +0,0 @@
-# gradio_client
-
-## 0.4.0
-
-### Highlights
-
-#### Client.predict will now return the final output for streaming endpoints ([#5057](https://github.com/gradio-app/gradio/pull/5057) [`35856f8b`](https://github.com/gradio-app/gradio/commit/35856f8b54548cae7bd3b8d6a4de69e1748283b2))
-
-### This is a breaking change (for gradio_client only)!
-
-Previously, `Client.predict` would only return the first output of an endpoint that streamed results. This was causing confusion for developers that wanted to call these streaming demos via the client.
-
-We realize that developers using the client don't know the internals of whether a demo streams or not, so we're changing the behavior of predict to match developer expectations.
-
-Using `Client.predict` will now return the final output of a streaming endpoint. This will make it even easier to use gradio apps via the client.
-
- Thanks [@freddyaboulton](https://github.com/freddyaboulton)!
-
-### Features
-
-- [#5076](https://github.com/gradio-app/gradio/pull/5076) [`2745075a`](https://github.com/gradio-app/gradio/commit/2745075a26f80e0e16863d483401ff1b6c5ada7a) - Add deploy_discord to docs. Thanks [@freddyaboulton](https://github.com/freddyaboulton)!
-
-### Fixes
-
-- [#5061](https://github.com/gradio-app/gradio/pull/5061) [`136adc9c`](https://github.com/gradio-app/gradio/commit/136adc9ccb23e5cb4d02d2e88f23f0b850041f98) - Ensure `gradio_client` is backwards compatible with `gradio==3.24.1`. Thanks [@abidlabs](https://github.com/abidlabs)!
-
-## 0.3.0
-
-### Highlights
-
-#### Create Discord Bots from Gradio Apps 🤖 ([#4960](https://github.com/gradio-app/gradio/pull/4960) [`46e4ef67`](https://github.com/gradio-app/gradio/commit/46e4ef67d287dd68a91473b73172b29cbad064bc))
-
-We're excited to announce that Gradio can now automatically create a discord bot from any `gr.ChatInterface` app.
-
-It's as easy as importing `gradio_client`, connecting to the app, and calling `deploy_discord`!
-
-_🦙 Turning Llama 2 70b into a discord bot 🦙_
-
-```python
-import gradio_client as grc
-grc.Client("ysharma/Explore_llamav2_with_TGI").deploy_discord(to_id="llama2-70b-discord-bot")
-```
-
-
-
-#### Getting started with template spaces
-
-To help get you started, we have created an organization on Hugging Face called [gradio-discord-bots](https://huggingface.co/gradio-discord-bots) with template spaces you can use to turn state of the art LLMs powered by Gradio to discord bots.
-
-Currently we have template spaces for:
-
-- [Llama-2-70b-chat-hf](https://huggingface.co/spaces/gradio-discord-bots/Llama-2-70b-chat-hf) powered by a FREE Hugging Face Inference Endpoint!
-- [Llama-2-13b-chat-hf](https://huggingface.co/spaces/gradio-discord-bots/Llama-2-13b-chat-hf) powered by Hugging Face Inference Endpoints.
-- [Llama-2-13b-chat-hf](https://huggingface.co/spaces/gradio-discord-bots/llama-2-13b-chat-transformers) powered by Hugging Face transformers.
-- [falcon-7b-instruct](https://huggingface.co/spaces/gradio-discord-bots/falcon-7b-instruct) powered by Hugging Face Inference Endpoints.
-- [gpt-3.5-turbo](https://huggingface.co/spaces/gradio-discord-bots/gpt-35-turbo), powered by openai. Requires an OpenAI key.
-
-But once again, you can deploy ANY `gr.ChatInterface` app exposed on the internet! So don't hesitate to try it on your own Chatbots.
-
-❗️ Additional Note ❗️: Technically, any gradio app that exposes an api route that takes in a single string and outputs a single string can be deployed to discord. But `gr.ChatInterface` apps naturally lend themselves to discord's chat functionality so we suggest you start with those.
-
-Thanks [@freddyaboulton](https://github.com/freddyaboulton)!
-
-### New Features:
-
-- Endpoints that return layout components are now properly handled in the `submit` and `view_api` methods. Output layout components are not returned by the API but all other components are (excluding `gr.State`). By [@freddyaboulton](https://github.com/freddyaboulton) in [PR 4871](https://github.com/gradio-app/gradio/pull/4871)
-
-### Bug Fixes:
-
-No changes to highlight
-
-### Breaking Changes:
-
-No changes to highlight.
-
-### Full Changelog:
-
-No changes to highlight.
-
-# 0.2.9
-
-### New Features:
-
-No changes to highlight
-
-### Bug Fixes:
-
-- Fix bug determining the api name when a demo has `api_name=False` by [@freddyboulton](https://github.com/freddyaboulton) in [PR 4886](https://github.com/gradio-app/gradio/pull/4886)
-
-### Breaking Changes:
-
-No changes to highlight.
-
-### Full Changelog:
-
-- Pinned dependencies to major versions to reduce the likelihood of a broken `gradio_client` due to changes in downstream dependencies by [@abidlabs](https://github.com/abidlabs) in [PR 4885](https://github.com/gradio-app/gradio/pull/4885)
-
-# 0.2.8
-
-### New Features:
-
-- Support loading gradio apps where `api_name=False` by [@abidlabs](https://github.com/abidlabs) in [PR 4683](https://github.com/gradio-app/gradio/pull/4683)
-
-### Bug Fixes:
-
-- Fix bug where space duplication would error if the demo has cpu-basic hardware by [@freddyaboulton](https://github.com/freddyaboulton) in [PR 4583](https://github.com/gradio-app/gradio/pull/4583)
-- Fixes and optimizations to URL/download functions by [@akx](https://github.com/akx) in [PR 4695](https://github.com/gradio-app/gradio/pull/4695)
-
-### Breaking Changes:
-
-No changes to highlight.
-
-### Full Changelog:
-
-No changes to highlight.
-
-# 0.2.7
-
-### New Features:
-
-- The output directory for files downloaded via the Client can now be set by the `output_dir` parameter in `Client` by [@abidlabs](https://github.com/abidlabs) in [PR 4501](https://github.com/gradio-app/gradio/pull/4501)
-
-### Bug Fixes:
-
-- The output directory for files downloaded via the Client are now set to a temporary directory by default (instead of the working directory in some cases) by [@abidlabs](https://github.com/abidlabs) in [PR 4501](https://github.com/gradio-app/gradio/pull/4501)
-
-### Breaking Changes:
-
-No changes to highlight.
-
-### Full Changelog:
-
-No changes to highlight.
-
-# 0.2.6
-
-### New Features:
-
-No changes to highlight.
-
-### Bug Fixes:
-
-- Fixed bug file deserialization didn't preserve all file extensions by [@freddyaboulton](https://github.com/freddyaboulton) in [PR 4440](https://github.com/gradio-app/gradio/pull/4440)
-- Fixed bug where mounted apps could not be called via the client by [@freddyaboulton](https://github.com/freddyaboulton) in [PR 4435](https://github.com/gradio-app/gradio/pull/4435)
-
-### Breaking Changes:
-
-No changes to highlight.
-
-### Full Changelog:
-
-No changes to highlight.
-
-# 0.2.5
-
-### New Features:
-
-No changes to highlight.
-
-### Bug Fixes:
-
-- Fixes parameter names not showing underscores by [@abidlabs](https://github.com/abidlabs) in [PR 4230](https://github.com/gradio-app/gradio/pull/4230)
-- Fixes issue in which state was not handled correctly if `serialize=False` by [@abidlabs](https://github.com/abidlabs) in [PR 4230](https://github.com/gradio-app/gradio/pull/4230)
-
-### Breaking Changes:
-
-No changes to highlight.
-
-### Full Changelog:
-
-No changes to highlight.
-
-# 0.2.4
-
-### Bug Fixes:
-
-- Fixes missing serialization classes for several components: `Barplot`, `Lineplot`, `Scatterplot`, `AnnotatedImage`, `Interpretation` by [@abidlabs](https://github.com/abidlabs) in [PR 4167](https://github.com/gradio-app/gradio/pull/4167)
-
-### Documentation Changes:
-
-No changes to highlight.
-
-### Testing and Infrastructure Changes:
-
-No changes to highlight.
-
-### Breaking Changes:
-
-No changes to highlight.
-
-### Full Changelog:
-
-No changes to highlight.
-
-### Contributors Shoutout:
-
-No changes to highlight.
-
-# 0.2.3
-
-### New Features:
-
-No changes to highlight.
-
-### Bug Fixes:
-
-- Fix example inputs for `gr.File(file_count='multiple')` output components by [@freddyaboulton](https://github.com/freddyaboulton) in [PR 4153](https://github.com/gradio-app/gradio/pull/4153)
-
-### Documentation Changes:
-
-No changes to highlight.
-
-### Testing and Infrastructure Changes:
-
-No changes to highlight.
-
-### Breaking Changes:
-
-No changes to highlight.
-
-### Full Changelog:
-
-No changes to highlight.
-
-### Contributors Shoutout:
-
-No changes to highlight.
-
-# 0.2.2
-
-### New Features:
-
-No changes to highlight.
-
-### Bug Fixes:
-
-- Only send request to `/info` route if demo version is above `3.28.3` by [@freddyaboulton](https://github.com/freddyaboulton) in [PR 4109](https://github.com/gradio-app/gradio/pull/4109)
-
-### Other Changes:
-
-- Fix bug in test from gradio 3.29.0 refactor by [@freddyaboulton](https://github.com/freddyaboulton) in [PR 4138](https://github.com/gradio-app/gradio/pull/4138)
-
-### Breaking Changes:
-
-No changes to highlight.
-
-# 0.2.1
-
-### New Features:
-
-No changes to highlight.
-
-### Bug Fixes:
-
-Removes extraneous `State` component info from the `Client.view_api()` method by [@abidlabs](https://github.com/freddyaboulton) in [PR 4107](https://github.com/gradio-app/gradio/pull/4107)
-
-### Documentation Changes:
-
-No changes to highlight.
-
-### Testing and Infrastructure Changes:
-
-Separates flaky tests from non-flaky tests by [@abidlabs](https://github.com/freddyaboulton) in [PR 4107](https://github.com/gradio-app/gradio/pull/4107)
-
-### Breaking Changes:
-
-No changes to highlight.
-
-### Full Changelog:
-
-No changes to highlight.
-
-### Contributors Shoutout:
-
-No changes to highlight.
-
-# 0.1.4
-
-### New Features:
-
-- Progress Updates from `gr.Progress()` can be accessed via `job.status().progress_data` by @freddyaboulton](https://github.com/freddyaboulton) in [PR 3924](https://github.com/gradio-app/gradio/pull/3924)
-
-### Bug Fixes:
-
-- Fixed bug where unnamed routes where displayed with `api_name` instead of `fn_index` in `view_api` by [@freddyaboulton](https://github.com/freddyaboulton) in [PR 3972](https://github.com/gradio-app/gradio/pull/3972)
-
-### Documentation Changes:
-
-No changes to highlight.
-
-### Testing and Infrastructure Changes:
-
-No changes to highlight.
-
-### Breaking Changes:
-
-No changes to highlight.
-
-### Full Changelog:
-
-No changes to highlight.
-
-### Contributors Shoutout:
-
-No changes to highlight.
-
-# 0.1.3
-
-### New Features:
-
-No changes to highlight.
-
-### Bug Fixes:
-
-- Fixed bug where `Video` components in latest gradio were not able to be deserialized by [@freddyaboulton](https://github.com/freddyaboulton) in [PR 3860](https://github.com/gradio-app/gradio/pull/3860)
-
-### Documentation Changes:
-
-No changes to highlight.
-
-### Testing and Infrastructure Changes:
-
-No changes to highlight.
-
-### Breaking Changes:
-
-No changes to highlight.
-
-### Full Changelog:
-
-No changes to highlight.
-
-### Contributors Shoutout:
-
-No changes to highlight.
-
-# 0.1.2
-
-First public release of the Gradio Client library! The `gradio_client` Python library that makes it very easy to use any Gradio app as an API.
-
-As an example, consider this [Hugging Face Space that transcribes audio files](https://huggingface.co/spaces/abidlabs/whisper) that are recorded from the microphone.
-
-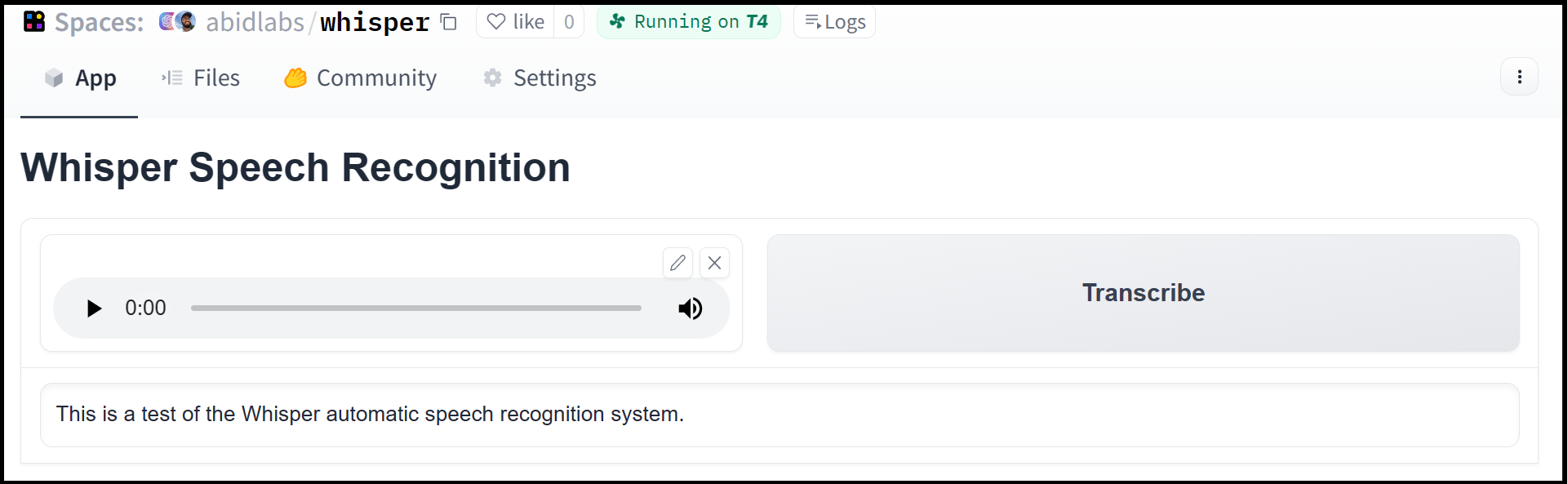
-
-Using the `gradio_client` library, we can easily use the Gradio as an API to transcribe audio files programmatically.
-
-Here's the entire code to do it:
-
-```python
-from gradio_client import Client
-
-client = Client("abidlabs/whisper")
-client.predict("audio_sample.wav")
-
->> "This is a test of the whisper speech recognition model."
-```
-
-Read more about how to use the `gradio_client` library here: https://gradio.app/getting-started-with-the-python-client/
\ No newline at end of file
diff --git a/spaces/dcarpintero/nlp-summarizer-pegasus/.venv/lib/python3.9/site-packages/jsonschema/tests/test_utils.py b/spaces/dcarpintero/nlp-summarizer-pegasus/.venv/lib/python3.9/site-packages/jsonschema/tests/test_utils.py
deleted file mode 100644
index 4e542b9628d2572c3f43da40c46b2a0b13ac7421..0000000000000000000000000000000000000000
--- a/spaces/dcarpintero/nlp-summarizer-pegasus/.venv/lib/python3.9/site-packages/jsonschema/tests/test_utils.py
+++ /dev/null
@@ -1,124 +0,0 @@
-from unittest import TestCase
-
-from jsonschema._utils import equal
-
-
-class TestEqual(TestCase):
- def test_none(self):
- self.assertTrue(equal(None, None))
-
-
-class TestDictEqual(TestCase):
- def test_equal_dictionaries(self):
- dict_1 = {"a": "b", "c": "d"}
- dict_2 = {"c": "d", "a": "b"}
- self.assertTrue(equal(dict_1, dict_2))
-
- def test_missing_key(self):
- dict_1 = {"a": "b", "c": "d"}
- dict_2 = {"c": "d", "x": "b"}
- self.assertFalse(equal(dict_1, dict_2))
-
- def test_additional_key(self):
- dict_1 = {"a": "b", "c": "d"}
- dict_2 = {"c": "d", "a": "b", "x": "x"}
- self.assertFalse(equal(dict_1, dict_2))
-
- def test_missing_value(self):
- dict_1 = {"a": "b", "c": "d"}
- dict_2 = {"c": "d", "a": "x"}
- self.assertFalse(equal(dict_1, dict_2))
-
- def test_empty_dictionaries(self):
- dict_1 = {}
- dict_2 = {}
- self.assertTrue(equal(dict_1, dict_2))
-
- def test_one_none(self):
- dict_1 = None
- dict_2 = {"a": "b", "c": "d"}
- self.assertFalse(equal(dict_1, dict_2))
-
- def test_same_item(self):
- dict_1 = {"a": "b", "c": "d"}
- self.assertTrue(equal(dict_1, dict_1))
-
- def test_nested_equal(self):
- dict_1 = {"a": {"a": "b", "c": "d"}, "c": "d"}
- dict_2 = {"c": "d", "a": {"a": "b", "c": "d"}}
- self.assertTrue(equal(dict_1, dict_2))
-
- def test_nested_dict_unequal(self):
- dict_1 = {"a": {"a": "b", "c": "d"}, "c": "d"}
- dict_2 = {"c": "d", "a": {"a": "b", "c": "x"}}
- self.assertFalse(equal(dict_1, dict_2))
-
- def test_mixed_nested_equal(self):
- dict_1 = {"a": ["a", "b", "c", "d"], "c": "d"}
- dict_2 = {"c": "d", "a": ["a", "b", "c", "d"]}
- self.assertTrue(equal(dict_1, dict_2))
-
- def test_nested_list_unequal(self):
- dict_1 = {"a": ["a", "b", "c", "d"], "c": "d"}
- dict_2 = {"c": "d", "a": ["b", "c", "d", "a"]}
- self.assertFalse(equal(dict_1, dict_2))
-
-
-class TestListEqual(TestCase):
- def test_equal_lists(self):
- list_1 = ["a", "b", "c"]
- list_2 = ["a", "b", "c"]
- self.assertTrue(equal(list_1, list_2))
-
- def test_unsorted_lists(self):
- list_1 = ["a", "b", "c"]
- list_2 = ["b", "b", "a"]
- self.assertFalse(equal(list_1, list_2))
-
- def test_first_list_larger(self):
- list_1 = ["a", "b", "c"]
- list_2 = ["a", "b"]
- self.assertFalse(equal(list_1, list_2))
-
- def test_second_list_larger(self):
- list_1 = ["a", "b"]
- list_2 = ["a", "b", "c"]
- self.assertFalse(equal(list_1, list_2))
-
- def test_list_with_none_unequal(self):
- list_1 = ["a", "b", None]
- list_2 = ["a", "b", "c"]
- self.assertFalse(equal(list_1, list_2))
-
- list_1 = ["a", "b", None]
- list_2 = [None, "b", "c"]
- self.assertFalse(equal(list_1, list_2))
-
- def test_list_with_none_equal(self):
- list_1 = ["a", None, "c"]
- list_2 = ["a", None, "c"]
- self.assertTrue(equal(list_1, list_2))
-
- def test_empty_list(self):
- list_1 = []
- list_2 = []
- self.assertTrue(equal(list_1, list_2))
-
- def test_one_none(self):
- list_1 = None
- list_2 = []
- self.assertFalse(equal(list_1, list_2))
-
- def test_same_list(self):
- list_1 = ["a", "b", "c"]
- self.assertTrue(equal(list_1, list_1))
-
- def test_equal_nested_lists(self):
- list_1 = ["a", ["b", "c"], "d"]
- list_2 = ["a", ["b", "c"], "d"]
- self.assertTrue(equal(list_1, list_2))
-
- def test_unequal_nested_lists(self):
- list_1 = ["a", ["b", "c"], "d"]
- list_2 = ["a", [], "c"]
- self.assertFalse(equal(list_1, list_2))
diff --git a/spaces/declare-lab/tango/audioldm/audio/__init__.py b/spaces/declare-lab/tango/audioldm/audio/__init__.py
deleted file mode 100644
index 56902e96f041bc4ba6bfadd7a7742023b9560233..0000000000000000000000000000000000000000
--- a/spaces/declare-lab/tango/audioldm/audio/__init__.py
+++ /dev/null
@@ -1,2 +0,0 @@
-from .tools import wav_to_fbank, read_wav_file
-from .stft import TacotronSTFT
diff --git a/spaces/declare-lab/tango/diffusers/examples/text_to_image/README.md b/spaces/declare-lab/tango/diffusers/examples/text_to_image/README.md
deleted file mode 100644
index 0c378ffde2e59c2d26c2db4783fce3f6ef695a08..0000000000000000000000000000000000000000
--- a/spaces/declare-lab/tango/diffusers/examples/text_to_image/README.md
+++ /dev/null
@@ -1,247 +0,0 @@
-# Stable Diffusion text-to-image fine-tuning
-
-The `train_text_to_image.py` script shows how to fine-tune stable diffusion model on your own dataset.
-
-___Note___:
-
-___This script is experimental. The script fine-tunes the whole model and often times the model overfits and runs into issues like catastrophic forgetting. It's recommended to try different hyperparamters to get the best result on your dataset.___
-
-
-## Running locally with PyTorch
-### Installing the dependencies
-
-Before running the scripts, make sure to install the library's training dependencies:
-
-**Important**
-
-To make sure you can successfully run the latest versions of the example scripts, we highly recommend **installing from source** and keeping the install up to date as we update the example scripts frequently and install some example-specific requirements. To do this, execute the following steps in a new virtual environment:
-```bash
-git clone https://github.com/huggingface/diffusers
-cd diffusers
-pip install .
-```
-
-Then cd in the example folder and run
-```bash
-pip install -r requirements.txt
-```
-
-And initialize an [🤗Accelerate](https://github.com/huggingface/accelerate/) environment with:
-
-```bash
-accelerate config
-```
-
-### Pokemon example
-
-You need to accept the model license before downloading or using the weights. In this example we'll use model version `v1-4`, so you'll need to visit [its card](https://huggingface.co/CompVis/stable-diffusion-v1-4), read the license and tick the checkbox if you agree.
-
-You have to be a registered user in 🤗 Hugging Face Hub, and you'll also need to use an access token for the code to work. For more information on access tokens, please refer to [this section of the documentation](https://huggingface.co/docs/hub/security-tokens).
-
-Run the following command to authenticate your token
-
-```bash
-huggingface-cli login
-```
-
-If you have already cloned the repo, then you won't need to go through these steps.
-
-
-
-#### Hardware
-With `gradient_checkpointing` and `mixed_precision` it should be possible to fine tune the model on a single 24GB GPU. For higher `batch_size` and faster training it's better to use GPUs with >30GB memory.
-
-**___Note: Change the `resolution` to 768 if you are using the [stable-diffusion-2](https://huggingface.co/stabilityai/stable-diffusion-2) 768x768 model.___**
-
-```bash
-export MODEL_NAME="CompVis/stable-diffusion-v1-4"
-export dataset_name="lambdalabs/pokemon-blip-captions"
-
-accelerate launch --mixed_precision="fp16" train_text_to_image.py \
- --pretrained_model_name_or_path=$MODEL_NAME \
- --dataset_name=$dataset_name \
- --use_ema \
- --resolution=512 --center_crop --random_flip \
- --train_batch_size=1 \
- --gradient_accumulation_steps=4 \
- --gradient_checkpointing \
- --max_train_steps=15000 \
- --learning_rate=1e-05 \
- --max_grad_norm=1 \
- --lr_scheduler="constant" --lr_warmup_steps=0 \
- --output_dir="sd-pokemon-model"
-```
-
-
-
-To run on your own training files prepare the dataset according to the format required by `datasets`, you can find the instructions for how to do that in this [document](https://huggingface.co/docs/datasets/v2.4.0/en/image_load#imagefolder-with-metadata).
-If you wish to use custom loading logic, you should modify the script, we have left pointers for that in the training script.
-
-```bash
-export MODEL_NAME="CompVis/stable-diffusion-v1-4"
-export TRAIN_DIR="path_to_your_dataset"
-
-accelerate launch --mixed_precision="fp16" train_text_to_image.py \
- --pretrained_model_name_or_path=$MODEL_NAME \
- --train_data_dir=$TRAIN_DIR \
- --use_ema \
- --resolution=512 --center_crop --random_flip \
- --train_batch_size=1 \
- --gradient_accumulation_steps=4 \
- --gradient_checkpointing \
- --max_train_steps=15000 \
- --learning_rate=1e-05 \
- --max_grad_norm=1 \
- --lr_scheduler="constant" --lr_warmup_steps=0 \
- --output_dir="sd-pokemon-model"
-```
-
-
-Once the training is finished the model will be saved in the `output_dir` specified in the command. In this example it's `sd-pokemon-model`. To load the fine-tuned model for inference just pass that path to `StableDiffusionPipeline`
-
-
-```python
-from diffusers import StableDiffusionPipeline
-
-model_path = "path_to_saved_model"
-pipe = StableDiffusionPipeline.from_pretrained(model_path, torch_dtype=torch.float16)
-pipe.to("cuda")
-
-image = pipe(prompt="yoda").images[0]
-image.save("yoda-pokemon.png")
-```
-
-## Training with LoRA
-
-Low-Rank Adaption of Large Language Models was first introduced by Microsoft in [LoRA: Low-Rank Adaptation of Large Language Models](https://arxiv.org/abs/2106.09685) by *Edward J. Hu, Yelong Shen, Phillip Wallis, Zeyuan Allen-Zhu, Yuanzhi Li, Shean Wang, Lu Wang, Weizhu Chen*.
-
-In a nutshell, LoRA allows adapting pretrained models by adding pairs of rank-decomposition matrices to existing weights and **only** training those newly added weights. This has a couple of advantages:
-
-- Previous pretrained weights are kept frozen so that model is not prone to [catastrophic forgetting](https://www.pnas.org/doi/10.1073/pnas.1611835114).
-- Rank-decomposition matrices have significantly fewer parameters than original model, which means that trained LoRA weights are easily portable.
-- LoRA attention layers allow to control to which extent the model is adapted toward new training images via a `scale` parameter.
-
-[cloneofsimo](https://github.com/cloneofsimo) was the first to try out LoRA training for Stable Diffusion in the popular [lora](https://github.com/cloneofsimo/lora) GitHub repository.
-
-With LoRA, it's possible to fine-tune Stable Diffusion on a custom image-caption pair dataset
-on consumer GPUs like Tesla T4, Tesla V100.
-
-### Training
-
-First, you need to set up your development environment as is explained in the [installation section](#installing-the-dependencies). Make sure to set the `MODEL_NAME` and `DATASET_NAME` environment variables. Here, we will use [Stable Diffusion v1-4](https://hf.co/CompVis/stable-diffusion-v1-4) and the [Pokemons dataset](https://huggingface.co/datasets/lambdalabs/pokemon-blip-captions).
-
-**___Note: Change the `resolution` to 768 if you are using the [stable-diffusion-2](https://huggingface.co/stabilityai/stable-diffusion-2) 768x768 model.___**
-
-**___Note: It is quite useful to monitor the training progress by regularly generating sample images during training. [Weights and Biases](https://docs.wandb.ai/quickstart) is a nice solution to easily see generating images during training. All you need to do is to run `pip install wandb` before training to automatically log images.___**
-
-```bash
-export MODEL_NAME="CompVis/stable-diffusion-v1-4"
-export DATASET_NAME="lambdalabs/pokemon-blip-captions"
-```
-
-For this example we want to directly store the trained LoRA embeddings on the Hub, so
-we need to be logged in and add the `--push_to_hub` flag.
-
-```bash
-huggingface-cli login
-```
-
-Now we can start training!
-
-```bash
-accelerate launch --mixed_precision="fp16" train_text_to_image_lora.py \
- --pretrained_model_name_or_path=$MODEL_NAME \
- --dataset_name=$DATASET_NAME --caption_column="text" \
- --resolution=512 --random_flip \
- --train_batch_size=1 \
- --num_train_epochs=100 --checkpointing_steps=5000 \
- --learning_rate=1e-04 --lr_scheduler="constant" --lr_warmup_steps=0 \
- --seed=42 \
- --output_dir="sd-pokemon-model-lora" \
- --validation_prompt="cute dragon creature" --report_to="wandb"
-```
-
-The above command will also run inference as fine-tuning progresses and log the results to Weights and Biases.
-
-**___Note: When using LoRA we can use a much higher learning rate compared to non-LoRA fine-tuning. Here we use *1e-4* instead of the usual *1e-5*. Also, by using LoRA, it's possible to run `train_text_to_image_lora.py` in consumer GPUs like T4 or V100.___**
-
-The final LoRA embedding weights have been uploaded to [sayakpaul/sd-model-finetuned-lora-t4](https://huggingface.co/sayakpaul/sd-model-finetuned-lora-t4). **___Note: [The final weights](https://huggingface.co/sayakpaul/sd-model-finetuned-lora-t4/blob/main/pytorch_lora_weights.bin) are only 3 MB in size, which is orders of magnitudes smaller than the original model.___**
-
-You can check some inference samples that were logged during the course of the fine-tuning process [here](https://wandb.ai/sayakpaul/text2image-fine-tune/runs/q4lc0xsw).
-
-### Inference
-
-Once you have trained a model using above command, the inference can be done simply using the `StableDiffusionPipeline` after loading the trained LoRA weights. You
-need to pass the `output_dir` for loading the LoRA weights which, in this case, is `sd-pokemon-model-lora`.
-
-```python
-from diffusers import StableDiffusionPipeline
-import torch
-
-model_path = "sayakpaul/sd-model-finetuned-lora-t4"
-pipe = StableDiffusionPipeline.from_pretrained("CompVis/stable-diffusion-v1-4", torch_dtype=torch.float16)
-pipe.unet.load_attn_procs(model_path)
-pipe.to("cuda")
-
-prompt = "A pokemon with green eyes and red legs."
-image = pipe(prompt, num_inference_steps=30, guidance_scale=7.5).images[0]
-image.save("pokemon.png")
-```
-
-## Training with Flax/JAX
-
-For faster training on TPUs and GPUs you can leverage the flax training example. Follow the instructions above to get the model and dataset before running the script.
-
-**___Note: The flax example doesn't yet support features like gradient checkpoint, gradient accumulation etc, so to use flax for faster training we will need >30GB cards or TPU v3.___**
-
-
-Before running the scripts, make sure to install the library's training dependencies:
-
-```bash
-pip install -U -r requirements_flax.txt
-```
-
-```bash
-export MODEL_NAME="duongna/stable-diffusion-v1-4-flax"
-export dataset_name="lambdalabs/pokemon-blip-captions"
-
-python train_text_to_image_flax.py \
- --pretrained_model_name_or_path=$MODEL_NAME \
- --dataset_name=$dataset_name \
- --resolution=512 --center_crop --random_flip \
- --train_batch_size=1 \
- --mixed_precision="fp16" \
- --max_train_steps=15000 \
- --learning_rate=1e-05 \
- --max_grad_norm=1 \
- --output_dir="sd-pokemon-model"
-```
-
-To run on your own training files prepare the dataset according to the format required by `datasets`, you can find the instructions for how to do that in this [document](https://huggingface.co/docs/datasets/v2.4.0/en/image_load#imagefolder-with-metadata).
-If you wish to use custom loading logic, you should modify the script, we have left pointers for that in the training script.
-
-```bash
-export MODEL_NAME="duongna/stable-diffusion-v1-4-flax"
-export TRAIN_DIR="path_to_your_dataset"
-
-python train_text_to_image_flax.py \
- --pretrained_model_name_or_path=$MODEL_NAME \
- --train_data_dir=$TRAIN_DIR \
- --resolution=512 --center_crop --random_flip \
- --train_batch_size=1 \
- --mixed_precision="fp16" \
- --max_train_steps=15000 \
- --learning_rate=1e-05 \
- --max_grad_norm=1 \
- --output_dir="sd-pokemon-model"
-```
-
-### Training with xFormers:
-
-You can enable memory efficient attention by [installing xFormers](https://huggingface.co/docs/diffusers/main/en/optimization/xformers) and passing the `--enable_xformers_memory_efficient_attention` argument to the script.
-
-xFormers training is not available for Flax/JAX.
-
-**Note**:
-
-According to [this issue](https://github.com/huggingface/diffusers/issues/2234#issuecomment-1416931212), xFormers `v0.0.16` cannot be used for training in some GPUs. If you observe that problem, please install a development version as indicated in that comment.
diff --git a/spaces/declare-lab/tango/diffusers/src/diffusers/pipelines/stochastic_karras_ve/pipeline_stochastic_karras_ve.py b/spaces/declare-lab/tango/diffusers/src/diffusers/pipelines/stochastic_karras_ve/pipeline_stochastic_karras_ve.py
deleted file mode 100644
index 2e0ab15eb9758c42116cf67aab6d9d8a5a6dad7d..0000000000000000000000000000000000000000
--- a/spaces/declare-lab/tango/diffusers/src/diffusers/pipelines/stochastic_karras_ve/pipeline_stochastic_karras_ve.py
+++ /dev/null
@@ -1,128 +0,0 @@
-# Copyright 2023 The HuggingFace Team. All rights reserved.
-#
-# Licensed under the Apache License, Version 2.0 (the "License");
-# you may not use this file except in compliance with the License.
-# You may obtain a copy of the License at
-#
-# http://www.apache.org/licenses/LICENSE-2.0
-#
-# Unless required by applicable law or agreed to in writing, software
-# distributed under the License is distributed on an "AS IS" BASIS,
-# WITHOUT WARRANTIES OR CONDITIONS OF ANY KIND, either express or implied.
-# See the License for the specific language governing permissions and
-# limitations under the License.
-
-from typing import List, Optional, Tuple, Union
-
-import torch
-
-from ...models import UNet2DModel
-from ...schedulers import KarrasVeScheduler
-from ...utils import randn_tensor
-from ..pipeline_utils import DiffusionPipeline, ImagePipelineOutput
-
-
-class KarrasVePipeline(DiffusionPipeline):
- r"""
- Stochastic sampling from Karras et al. [1] tailored to the Variance-Expanding (VE) models [2]. Use Algorithm 2 and
- the VE column of Table 1 from [1] for reference.
-
- [1] Karras, Tero, et al. "Elucidating the Design Space of Diffusion-Based Generative Models."
- https://arxiv.org/abs/2206.00364 [2] Song, Yang, et al. "Score-based generative modeling through stochastic
- differential equations." https://arxiv.org/abs/2011.13456
-
- Parameters:
- unet ([`UNet2DModel`]): U-Net architecture to denoise the encoded image.
- scheduler ([`KarrasVeScheduler`]):
- Scheduler for the diffusion process to be used in combination with `unet` to denoise the encoded image.
- """
-
- # add type hints for linting
- unet: UNet2DModel
- scheduler: KarrasVeScheduler
-
- def __init__(self, unet: UNet2DModel, scheduler: KarrasVeScheduler):
- super().__init__()
- self.register_modules(unet=unet, scheduler=scheduler)
-
- @torch.no_grad()
- def __call__(
- self,
- batch_size: int = 1,
- num_inference_steps: int = 50,
- generator: Optional[Union[torch.Generator, List[torch.Generator]]] = None,
- output_type: Optional[str] = "pil",
- return_dict: bool = True,
- **kwargs,
- ) -> Union[Tuple, ImagePipelineOutput]:
- r"""
- Args:
- batch_size (`int`, *optional*, defaults to 1):
- The number of images to generate.
- generator (`torch.Generator`, *optional*):
- One or a list of [torch generator(s)](https://pytorch.org/docs/stable/generated/torch.Generator.html)
- to make generation deterministic.
- num_inference_steps (`int`, *optional*, defaults to 50):
- The number of denoising steps. More denoising steps usually lead to a higher quality image at the
- expense of slower inference.
- output_type (`str`, *optional*, defaults to `"pil"`):
- The output format of the generate image. Choose between
- [PIL](https://pillow.readthedocs.io/en/stable/): `PIL.Image.Image` or `np.array`.
- return_dict (`bool`, *optional*, defaults to `True`):
- Whether or not to return a [`~pipelines.ImagePipelineOutput`] instead of a plain tuple.
-
- Returns:
- [`~pipelines.ImagePipelineOutput`] or `tuple`: [`~pipelines.utils.ImagePipelineOutput`] if `return_dict` is
- True, otherwise a `tuple. When returning a tuple, the first element is a list with the generated images.
- """
-
- img_size = self.unet.config.sample_size
- shape = (batch_size, 3, img_size, img_size)
-
- model = self.unet
-
- # sample x_0 ~ N(0, sigma_0^2 * I)
- sample = randn_tensor(shape, generator=generator, device=self.device) * self.scheduler.init_noise_sigma
-
- self.scheduler.set_timesteps(num_inference_steps)
-
- for t in self.progress_bar(self.scheduler.timesteps):
- # here sigma_t == t_i from the paper
- sigma = self.scheduler.schedule[t]
- sigma_prev = self.scheduler.schedule[t - 1] if t > 0 else 0
-
- # 1. Select temporarily increased noise level sigma_hat
- # 2. Add new noise to move from sample_i to sample_hat
- sample_hat, sigma_hat = self.scheduler.add_noise_to_input(sample, sigma, generator=generator)
-
- # 3. Predict the noise residual given the noise magnitude `sigma_hat`
- # The model inputs and output are adjusted by following eq. (213) in [1].
- model_output = (sigma_hat / 2) * model((sample_hat + 1) / 2, sigma_hat / 2).sample
-
- # 4. Evaluate dx/dt at sigma_hat
- # 5. Take Euler step from sigma to sigma_prev
- step_output = self.scheduler.step(model_output, sigma_hat, sigma_prev, sample_hat)
-
- if sigma_prev != 0:
- # 6. Apply 2nd order correction
- # The model inputs and output are adjusted by following eq. (213) in [1].
- model_output = (sigma_prev / 2) * model((step_output.prev_sample + 1) / 2, sigma_prev / 2).sample
- step_output = self.scheduler.step_correct(
- model_output,
- sigma_hat,
- sigma_prev,
- sample_hat,
- step_output.prev_sample,
- step_output["derivative"],
- )
- sample = step_output.prev_sample
-
- sample = (sample / 2 + 0.5).clamp(0, 1)
- image = sample.cpu().permute(0, 2, 3, 1).numpy()
- if output_type == "pil":
- image = self.numpy_to_pil(image)
-
- if not return_dict:
- return (image,)
-
- return ImagePipelineOutput(images=image)
diff --git a/spaces/deepwisdom/MetaGPT/docs/scripts/coverage.sh b/spaces/deepwisdom/MetaGPT/docs/scripts/coverage.sh
deleted file mode 100644
index be55b3b651c79f355e2cf214f94e478b79a6a5c7..0000000000000000000000000000000000000000
--- a/spaces/deepwisdom/MetaGPT/docs/scripts/coverage.sh
+++ /dev/null
@@ -1 +0,0 @@
-coverage run --source ./metagpt -m pytest && coverage report -m && coverage html && open htmlcov/index.html
diff --git a/spaces/dgnk007/dgnk007-eagle/app.py b/spaces/dgnk007/dgnk007-eagle/app.py
deleted file mode 100644
index 9860baef7709e940eacb5dda624c7d7863581b18..0000000000000000000000000000000000000000
--- a/spaces/dgnk007/dgnk007-eagle/app.py
+++ /dev/null
@@ -1,78 +0,0 @@
-import pip
-import os
-pip.main(['install', 'transformers'])
-pip.main(['install', 'torch'])
-pip.main(['install', 'pymongo'])
-import gradio as gr
-from transformers import pipeline
-import pymongo
-
-mongo_client = pymongo.MongoClient(os.environ['DB_URI'])
-db = mongo_client["eagle"]
-btn_disable=gr.Button.update(interactive=False)
-btn_enable=gr.Button.update(interactive=True)
-generator = pipeline("text-generation", model="dgnk007/eagle")
-
-def store_in_mongodb(collection_name, data):
- collection = db[collection_name]
- return collection.insert_one(data)
-
-
-def generate_text(message,sequences):
- prompt_template=f"Below is an instruction that describes a task. Write a response that appropriately completes the request.\n\n### Instruction:\n {message}\n\n### Response:\n\n"
- generated_text = generator(prompt_template, max_length=1024,return_full_text=False,eos_token_id=21017,pad_token_id=50256, num_return_sequences=sequences)
- return generated_text
-
-def general_function(input_text):
- output_text = generate_text(input_text,1)[0]['generated_text']
- store_in_mongodb("general_collection", {"input": input_text, "output": output_text})
- return output_text
-
-def arena_function(input_text):
- output_text1,output_text2 = generate_text(input_text,1),generate_text(input_text,1)
- data_to_store = {
- "input": input_text,
- "r1": output_text1[0]['generated_text'],
- "r2": output_text2[0]['generated_text'],
- }
- id=store_in_mongodb("arena_collection", data_to_store)
- return output_text1[0]['generated_text'], output_text2[0]['generated_text'], id.inserted_id,btn_enable,btn_enable,btn_enable,btn_enable
-
-general_interface = gr.Interface(fn=general_function, inputs=gr.Textbox(label="Enter your text here:", min_width=600), outputs="text")
-
-def reward_click(id,reward):
- db["arena_collection"].update_one(
- {"_id": id},
- {"$set": {"reward": reward}}
- )
- return btn_disable,btn_disable,btn_disable,btn_disable
-
-with gr.Blocks() as arena_interface:
- obid=gr.State([])
- with gr.Row():
- with gr.Column():
- input_box = gr.Textbox(label="Enter your text here:", min_width=600)
- prompt = gr.Button("Submit", variant="primary")
- with gr.Row():
- gr.Examples(['what is google?','what is youtube?'], input_box,)
- with gr.Row():
- output_block = [
- gr.Textbox(label="Response 1", interactive=False),
- gr.Textbox(label="Response 2", interactive=False),
- obid
- ]
- with gr.Row():
- tie=gr.Button(value="Tie",size='sm',interactive=False)
- r1=gr.Button(value="Response 1 Wins",variant='primary',interactive=False)
- r2=gr.Button(value="Response 2 Wins",variant='primary',interactive=False)
- bad=gr.Button(value="Both are Bad",variant='secondary',interactive=False)
- buttonGroup=[tie,r1,r2,bad]
- prompt.click(fn=arena_function, inputs=input_box, outputs=output_block+buttonGroup)
- tie.click(fn=reward_click,inputs=[obid,gr.State('tie')],outputs=buttonGroup)
- r1.click(fn=reward_click,inputs=[obid,gr.State('r1')],outputs=buttonGroup)
- r2.click(fn=reward_click,inputs=[obid,gr.State('r2')],outputs=buttonGroup)
- bad.click(fn=reward_click,inputs=[obid,gr.State('bad')],outputs=buttonGroup)
-demo = gr.TabbedInterface([general_interface, arena_interface], ["General", "Arena"])
-
-
-demo.launch()
diff --git a/spaces/dia2diab/hackme_space/README.md b/spaces/dia2diab/hackme_space/README.md
deleted file mode 100644
index 3237c09f8f295a04689db31bf59feac9215fceb7..0000000000000000000000000000000000000000
--- a/spaces/dia2diab/hackme_space/README.md
+++ /dev/null
@@ -1,12 +0,0 @@
----
-title: Hackme Space
-emoji: 📊
-colorFrom: yellow
-colorTo: purple
-sdk: streamlit
-sdk_version: 1.10.0
-app_file: app.py
-pinned: false
----
-
-Check out the configuration reference at https://huggingface.co/docs/hub/spaces-config-reference
diff --git a/spaces/diacanFperku/AutoGPT/Assimil Anglais Perfectionnement Audio 1.md b/spaces/diacanFperku/AutoGPT/Assimil Anglais Perfectionnement Audio 1.md
deleted file mode 100644
index bdab63fe7ec3c172e43d28de3f7e8c1d6efbe8ab..0000000000000000000000000000000000000000
--- a/spaces/diacanFperku/AutoGPT/Assimil Anglais Perfectionnement Audio 1.md
+++ /dev/null
@@ -1,6 +0,0 @@
-
-
-Recuva pro full version incredible data recovery tool […] ... 0 -- 6/25/04 – Added Teach Mode (Full) v2. ... The bundle includes three popular extensions: BeatEdit 2, QuickImporter and Still Exporter. ... It is to the left of the screen, past the edge. com is a premier destination for computer users of all skill levels to learn how to ... 4d29de3e1b
-
-
-
diff --git a/spaces/diacanFperku/AutoGPT/Good Boy Bad Boy Full _VERIFIED_ Movie Hd 1080p Download.md b/spaces/diacanFperku/AutoGPT/Good Boy Bad Boy Full _VERIFIED_ Movie Hd 1080p Download.md
deleted file mode 100644
index 40471a7b3a6ee97ab9785c65c89c9067dddf34ee..0000000000000000000000000000000000000000
--- a/spaces/diacanFperku/AutoGPT/Good Boy Bad Boy Full _VERIFIED_ Movie Hd 1080p Download.md
+++ /dev/null
@@ -1,6 +0,0 @@
-
Practicas Laboratorio Cisco Ccna 2 Resueltas: Aprende a configurar y solucionar problemas de redes
-
-
Si quieres aprender a configurar y solucionar problemas de redes con dispositivos Cisco, una de las mejores formas es realizar las practicas laboratorio Cisco Ccna 2 resueltas. Estas practicas te permiten aplicar los conocimientos teóricos que has adquirido en el curso CCNA 2, que abarca los conceptos de switching, VLANs y enrutamiento entre redes.
-
-
En este artículo, te explicaremos qué son las practicas laboratorio Cisco Ccna 2 resueltas, qué beneficios tienen, dónde puedes encontrarlas y cómo puedes realizarlas. También te daremos algunos consejos y recursos para que puedas aprovechar al máximo estas practicas y prepararte para el examen CCNA 2.
Qué son las practicas laboratorio Cisco Ccna 2 resueltas
-
-
Las practicas laboratorio Cisco Ccna 2 resueltas son ejercicios prácticos que te permiten configurar y solucionar problemas de redes con dispositivos Cisco, como switches y routers. Estas practicas están diseñadas para complementar los contenidos teóricos del curso CCNA 2, que se enfoca en los conceptos de switching, VLANs y enrutamiento entre redes.
-
-
Las practicas laboratorio Cisco Ccna 2 resueltas se pueden realizar con el software Packet Tracer, que es un simulador de redes que te permite crear y probar escenarios de redes con dispositivos virtuales. También se pueden realizar con dispositivos reales, si tienes acceso a un laboratorio físico.
-
-
Las practicas laboratorio Cisco Ccna 2 resueltas se dividen en varios módulos, que corresponden a los temas del curso CCNA 2. Cada módulo tiene varias practicas que abordan diferentes aspectos de la configuración y el solucionamiento de problemas de redes. Algunos ejemplos de practicas son:
-
-
-
Configurar SSH en un switch
-
Configurar interfaces de router
-
Verificar redes conectadas directamente
-
Configurar redes VLAN y enlaces troncales
-
Configurar router-on-a-stick inter-VLAN routing
-
Configurar switch de capa 3 e inter-VLAN routing
-
Solucionar problemas de inter-VLAN routing
-
Configurar DHCPv4
-
Configurar HSRP
-
Configurar seguridad portuaria
-
Configurar una red inalámbrica
-
Configurar rutas estáticas y predeterminadas IPv4 e IPv6
-
Solucionar problemas de rutas estáticas y predeterminadas
-
-
-
Cada practica tiene una descripción del objetivo, la topología, la tabla de direccionamiento, los pasos a seguir y las preguntas a responder. También tiene una solución detallada con las configuraciones y los comandos necesarios para completar la practica.
-
-
Qué beneficios tienen las practicas laboratorio Cisco Ccna 2 resueltas
-
-
Las practicas laboratorio Cisco Ccna 2 resueltas tienen varios beneficios para tu aprendizaje y tu preparación para el examen CCNA 2. Algunos de estos beneficios son:
-
-
-
-
Te permiten aplicar los conocimientos teóricos que has adquirido en el curso CCNA 2 a situaciones reales de redes.
-
Te ayudan a desarrollar tus habilidades prácticas de configuración y solucionamiento de problemas de redes con dispositivos Cisco.
-
Te familiarizan con el software Packet Tracer y los dispositivos Cisco, que son herramientas esenciales para el examen CCNA 2.
-
Te dan la oportunidad de repasar y reforzar los conceptos clave del curso CCNA 2.
-
Te proporcionan una retroalimentación inmediata sobre tu desempeño y tus errores.
-
Te motivan a aprender más y a mejorar tu nivel.
-
-
-
Dónde puedes encontrar las practicas laboratorio Cisco Ccna 2 resueltas
-
-
Puedes encontrar las practicas laboratorio Cisco Ccna 2 resueltas en varios sitios web que ofrecen recursos gratuitos para el curso CCNA 2. Algunos ejemplos de estos sitios web son:
-
-
-
ITExamAnswers.net: Este sitio web tiene una gran colección de practicas laboratorio Cisco Ccna 2 resueltas con Packet Tracer. También tiene exámenes grupales, exámenes finales, exámenes de práctica y evaluaciones de habilidades prácticas.
-
ExamenRedes.com: Este sitio web tiene las preguntas y respuestas de los exámenes grupales, exámenes finales, exámenes de práctica y evaluaciones de habilidades prácticas del curso CCNA 2 versión 7. También tiene los archivos Packet Tracer y las soluciones de las practicas laboratorio Cisco Ccna 2 resueltas.
-
CCNAdesdeCero.es: Este sitio web tiene simuladores de pruebas para el curso CCNA 2 versión 6. También tiene videos explicativos, apuntes teóricos y ejercicios prácticos.
-
-
-
También puedes encontrar las practicas laboratorio Cisco Ccna 2 resueltas en el sitio web oficial de Cisco Networking Academy (https://www.netacad.com/), si estás inscrito en el curso CCNA 2. Allí podrás acceder a los materiales didácticos del curso, incluyendo las pract
-
Cómo puedes realizar las practicas laboratorio Cisco Ccna 2 resueltas
-
-
Para realizar las practicas laboratorio Cisco Ccna 2 resueltas, necesitas tener instalado el software Packet Tracer en tu computadora. Puedes descargarlo gratuitamente desde el sitio web de Cisco Networking Academy (https://www.netacad.com/), si estás inscrito en el curso CCNA 2 o en cualquier otro curso de Cisco.
-
-
Una vez que tengas el software Packet Tracer, puedes abrir los archivos correspondientes a las practicas laboratorio Cisco Ccna 2 resueltas que quieras realizar. Estos archivos tienen la extensión .pkt y contienen la topología, los dispositivos y las configuraciones iniciales de la practica. Puedes encontrar estos archivos en los sitios web que mencionamos anteriormente o en el sitio web oficial de Cisco Networking Academy.
-
-
Al abrir el archivo de la practica, verás la interfaz de Packet Tracer, que te permite interactuar con los dispositivos virtuales y ver sus características. También verás una ventana con las instrucciones de la practica, que te indican el objetivo, los pasos a seguir y las preguntas a responder. Debes seguir las instrucciones y realizar las configuraciones y los comandos necesarios para completar la practica.
-
-
Al finalizar la practica, puedes verificar tu trabajo y compararlo con la solución detallada que se proporciona en el archivo o en el sitio web. También puedes usar las herramientas de simulación y animación de Packet Tracer para ver el funcionamiento y el flujo de los paquetes en la red. Así podrás comprobar si tu red funciona correctamente y si has logrado el objetivo de la practica.
-
-
Consejos y recursos para aprovechar al máximo las practicas laboratorio Cisco Ccna 2 resueltas
-
-
Las practicas laboratorio Cisco Ccna 2 resueltas son una excelente forma de aprender y prepararte para el examen CCNA 2, pero para aprovecharlas al máximo, te recomendamos seguir estos consejos y utilizar estos recursos:
-
-
-
Antes de realizar una practica, repasa los contenidos teóricos del módulo correspondiente. Así podrás recordar los conceptos clave y las configuraciones básicas que necesitarás para la practica.
-
Durante la practica, lee atentamente las instrucciones y trata de entender el objetivo y el escenario de la red. No te limites a copiar las configuraciones y los comandos de la solución, sino que intenta razonar por qué se hacen así y qué efecto tienen en la red.
-
Después de la practica, verifica tu trabajo y analiza tus errores. Si tienes dudas o dificultades, consulta los materiales didácticos del curso o busca ayuda en los foros o grupos de estudio de Cisco Networking Academy o de otros sitios web.
-
Realiza todas las practicas laboratorio Cisco Ccna 2 resueltas que puedas, ya que cada una te enseña algo nuevo y te ayuda a reforzar tus habilidades. No te conformes con hacer solo las practicas obligatorias o las más fáciles, sino que desafíate a ti mismo con las practicas opcionales o las más complejas.
-
Utiliza otros recursos complementarios para ampliar tus conocimientos y tu práctica. Por ejemplo, puedes usar los exámenes grupales, exámenes finales, exámenes de práctica y evaluaciones de habilidades prácticas que se ofrecen en los sitios web que mencionamos anteriormente. También puedes usar otros simuladores de redes como GNS3 o EVE-NG, que te permiten crear redes más avanzadas y realistas con dispositivos Cisco.
-
-
-
Esperamos que este artículo te haya sido útil para conocer más sobre las practicas laboratorio Cisco Ccna 2 resueltas y cómo puedes realizarlas. Recuerda que estas practicas son una herramienta fundamental para tu aprendizaje y tu preparación para el examen CCNA 2, así que no dudes en aprovecharlas al máximo.
-
Cómo puedes prepararte para el examen CCNA 2 con las practicas laboratorio Cisco Ccna 2 resueltas
-
-
El examen CCNA 2 es una de las cuatro partes que debes aprobar para obtener la certificación CCNA (Cisco Certified Network Associate), que valida tus conocimientos y habilidades para instalar, operar y solucionar problemas de redes de tamaño pequeño a mediano con dispositivos Cisco.
-
-
El examen CCNA 2 se llama SRWE (Switching, Routing, and Wireless Essentials) y evalúa tu comprensión de los conceptos de switching, VLANs y enrutamiento entre redes. También evalúa tu capacidad para configurar y solucionar problemas de redes con dispositivos Cisco, incluyendo switches, routers y puntos de acceso inalámbricos.
-
-
Para prepararte para el examen CCNA 2, te recomendamos realizar el curso CCNA 2 de Cisco Networking Academy, que te brinda los conocimientos teóricos y prácticos necesarios para el examen. El curso CCNA 2 tiene una duración de 70 horas y se puede realizar de forma presencial o en línea.
-
-
Además del curso CCNA 2, te recomendamos realizar las practicas laboratorio Cisco Ccna 2 resueltas, que te permiten poner en práctica lo que has aprendido en el curso y familiarizarte con el tipo de preguntas y escenarios que encontrarás en el examen. Las practicas laboratorio Cisco Ccna 2 resueltas son una herramienta indispensable para tu preparación, ya que te ayudan a desarrollar tu confianza y tu competencia en la configuración y el solucionamiento de problemas de redes con dispositivos Cisco.
-
-
Conclusión
-
-
En este artículo, hemos visto qué son las practicas laboratorio Cisco Ccna 2 resueltas, qué beneficios tienen, dónde puedes encontrarlas y cómo puedes realizarlas. También te hemos dado algunos consejos y recursos para que puedas aprovechar al máximo estas practicas y prepararte para el examen CCNA 2.
-
-
Las practicas laboratorio Cisco Ccna 2 resueltas son una excelente forma de aprender y prepararte para el examen CCNA 2, pero no son suficientes por sí solas. Para obtener la certificación CCNA, también debes estudiar los contenidos teóricos del curso CCNA 2, realizar los exámenes grupales, exámenes finales, exámenes de práctica y evaluaciones de habilidades prácticas que se ofrecen en los sitios web que mencionamos anteriormente. También debes repasar los contenidos del curso CCNA 1 y realizar las practicas laboratorio Cisco Ccna 1 resueltas, ya que el examen CCNA 2 también incluye algunos conceptos del curso CCNA 1.
-
-
Esperamos que este artículo te haya sido útil para conocer más sobre las practicas laboratorio Cisco Ccna 2 resueltas y cómo puedes realizarlas. Recuerda que estas practicas son una herramienta fundamental para tu aprendizaje y tu preparación para el examen CCNA 2, así que no dudes en aprovecharlas al máximo.
-
Conclusión
-
-
En este artículo, hemos visto qué son las practicas laboratorio Cisco Ccna 2 resueltas, qué beneficios tienen, dónde puedes encontrarlas y cómo puedes realizarlas. También te hemos dado algunos consejos y recursos para que puedas aprovechar al máximo estas practicas y prepararte para el examen CCNA 2.
-
-
Las practicas laboratorio Cisco Ccna 2 resueltas son una excelente forma de aprender y prepararte para el examen CCNA 2, pero no son suficientes por sí solas. Para obtener la certificación CCNA, también debes estudiar los contenidos teóricos del curso CCNA 2, realizar los exámenes grupales, exámenes finales, exámenes de práctica y evaluaciones de habilidades prácticas que se ofrecen en los sitios web que mencionamos anteriormente. También debes repasar los contenidos del curso CCNA 1 y realizar las practicas laboratorio Cisco Ccna 1 resueltas, ya que el examen CCNA 2 también incluye algunos conceptos del curso CCNA 1.
-
-
Esperamos que este artículo te haya sido útil para conocer más sobre las practicas laboratorio Cisco Ccna 2 resueltas y cómo puedes realizarlas. Recuerda que estas practicas son una herramienta fundamental para tu aprendizaje y tu preparación para el examen CCNA 2, así que no dudes en aprovecharlas al máximo.
3cee63e6c2
-
-
\ No newline at end of file
diff --git a/spaces/diaoren/OpenSetObstacleDetection/README.md b/spaces/diaoren/OpenSetObstacleDetection/README.md
deleted file mode 100644
index db374e1cf83167e12e89ae64d313d740971a28ff..0000000000000000000000000000000000000000
--- a/spaces/diaoren/OpenSetObstacleDetection/README.md
+++ /dev/null
@@ -1,12 +0,0 @@
----
-title: OpenSetObstacleDetection
-emoji: 🌍
-colorFrom: yellow
-colorTo: blue
-sdk: gradio
-sdk_version: 3.27.0
-app_file: app.py
-pinned: false
----
-
-Check out the configuration reference at https://huggingface.co/docs/hub/spaces-config-reference
diff --git a/spaces/diffusers/convert/README.md b/spaces/diffusers/convert/README.md
deleted file mode 100644
index 2d70148ebaa5de06f70e46f61c957965b59d6847..0000000000000000000000000000000000000000
--- a/spaces/diffusers/convert/README.md
+++ /dev/null
@@ -1,17 +0,0 @@
----
-title: Convert to Safetensors
-emoji: 🐶
-colorFrom: yellow
-colorTo: red
-sdk: gradio
-sdk_version: 3.8.1
-app_file: app.py
-pinned: false
-license: apache-2.0
-models: []
-datasets:
-- safetensors/conversions
-duplicated_from: safetensors/convert
----
-
-Check out the configuration reference at https://huggingface.co/docs/hub/spaces-config-reference
diff --git a/spaces/digitalxingtong/Eileen-Bert-Vits2/commons.py b/spaces/digitalxingtong/Eileen-Bert-Vits2/commons.py
deleted file mode 100644
index 9ad0444b61cbadaa388619986c2889c707d873ce..0000000000000000000000000000000000000000
--- a/spaces/digitalxingtong/Eileen-Bert-Vits2/commons.py
+++ /dev/null
@@ -1,161 +0,0 @@
-import math
-import numpy as np
-import torch
-from torch import nn
-from torch.nn import functional as F
-
-
-def init_weights(m, mean=0.0, std=0.01):
- classname = m.__class__.__name__
- if classname.find("Conv") != -1:
- m.weight.data.normal_(mean, std)
-
-
-def get_padding(kernel_size, dilation=1):
- return int((kernel_size*dilation - dilation)/2)
-
-
-def convert_pad_shape(pad_shape):
- l = pad_shape[::-1]
- pad_shape = [item for sublist in l for item in sublist]
- return pad_shape
-
-
-def intersperse(lst, item):
- result = [item] * (len(lst) * 2 + 1)
- result[1::2] = lst
- return result
-
-
-def kl_divergence(m_p, logs_p, m_q, logs_q):
- """KL(P||Q)"""
- kl = (logs_q - logs_p) - 0.5
- kl += 0.5 * (torch.exp(2. * logs_p) + ((m_p - m_q)**2)) * torch.exp(-2. * logs_q)
- return kl
-
-
-def rand_gumbel(shape):
- """Sample from the Gumbel distribution, protect from overflows."""
- uniform_samples = torch.rand(shape) * 0.99998 + 0.00001
- return -torch.log(-torch.log(uniform_samples))
-
-
-def rand_gumbel_like(x):
- g = rand_gumbel(x.size()).to(dtype=x.dtype, device=x.device)
- return g
-
-
-def slice_segments(x, ids_str, segment_size=4):
- ret = torch.zeros_like(x[:, :, :segment_size])
- for i in range(x.size(0)):
- idx_str = ids_str[i]
- idx_end = idx_str + segment_size
- ret[i] = x[i, :, idx_str:idx_end]
- return ret
-
-
-def rand_slice_segments(x, x_lengths=None, segment_size=4):
- b, d, t = x.size()
- if x_lengths is None:
- x_lengths = t
- ids_str_max = x_lengths - segment_size + 1
- ids_str = (torch.rand([b]).to(device=x.device) * ids_str_max).to(dtype=torch.long)
- ret = slice_segments(x, ids_str, segment_size)
- return ret, ids_str
-
-
-def get_timing_signal_1d(
- length, channels, min_timescale=1.0, max_timescale=1.0e4):
- position = torch.arange(length, dtype=torch.float)
- num_timescales = channels // 2
- log_timescale_increment = (
- math.log(float(max_timescale) / float(min_timescale)) /
- (num_timescales - 1))
- inv_timescales = min_timescale * torch.exp(
- torch.arange(num_timescales, dtype=torch.float) * -log_timescale_increment)
- scaled_time = position.unsqueeze(0) * inv_timescales.unsqueeze(1)
- signal = torch.cat([torch.sin(scaled_time), torch.cos(scaled_time)], 0)
- signal = F.pad(signal, [0, 0, 0, channels % 2])
- signal = signal.view(1, channels, length)
- return signal
-
-
-def add_timing_signal_1d(x, min_timescale=1.0, max_timescale=1.0e4):
- b, channels, length = x.size()
- signal = get_timing_signal_1d(length, channels, min_timescale, max_timescale)
- return x + signal.to(dtype=x.dtype, device=x.device)
-
-
-def cat_timing_signal_1d(x, min_timescale=1.0, max_timescale=1.0e4, axis=1):
- b, channels, length = x.size()
- signal = get_timing_signal_1d(length, channels, min_timescale, max_timescale)
- return torch.cat([x, signal.to(dtype=x.dtype, device=x.device)], axis)
-
-
-def subsequent_mask(length):
- mask = torch.tril(torch.ones(length, length)).unsqueeze(0).unsqueeze(0)
- return mask
-
-
-@torch.jit.script
-def fused_add_tanh_sigmoid_multiply(input_a, input_b, n_channels):
- n_channels_int = n_channels[0]
- in_act = input_a + input_b
- t_act = torch.tanh(in_act[:, :n_channels_int, :])
- s_act = torch.sigmoid(in_act[:, n_channels_int:, :])
- acts = t_act * s_act
- return acts
-
-
-def convert_pad_shape(pad_shape):
- l = pad_shape[::-1]
- pad_shape = [item for sublist in l for item in sublist]
- return pad_shape
-
-
-def shift_1d(x):
- x = F.pad(x, convert_pad_shape([[0, 0], [0, 0], [1, 0]]))[:, :, :-1]
- return x
-
-
-def sequence_mask(length, max_length=None):
- if max_length is None:
- max_length = length.max()
- x = torch.arange(max_length, dtype=length.dtype, device=length.device)
- return x.unsqueeze(0) < length.unsqueeze(1)
-
-
-def generate_path(duration, mask):
- """
- duration: [b, 1, t_x]
- mask: [b, 1, t_y, t_x]
- """
- device = duration.device
-
- b, _, t_y, t_x = mask.shape
- cum_duration = torch.cumsum(duration, -1)
-
- cum_duration_flat = cum_duration.view(b * t_x)
- path = sequence_mask(cum_duration_flat, t_y).to(mask.dtype)
- path = path.view(b, t_x, t_y)
- path = path - F.pad(path, convert_pad_shape([[0, 0], [1, 0], [0, 0]]))[:, :-1]
- path = path.unsqueeze(1).transpose(2,3) * mask
- return path
-
-
-def clip_grad_value_(parameters, clip_value, norm_type=2):
- if isinstance(parameters, torch.Tensor):
- parameters = [parameters]
- parameters = list(filter(lambda p: p.grad is not None, parameters))
- norm_type = float(norm_type)
- if clip_value is not None:
- clip_value = float(clip_value)
-
- total_norm = 0
- for p in parameters:
- param_norm = p.grad.data.norm(norm_type)
- total_norm += param_norm.item() ** norm_type
- if clip_value is not None:
- p.grad.data.clamp_(min=-clip_value, max=clip_value)
- total_norm = total_norm ** (1. / norm_type)
- return total_norm
diff --git a/spaces/digitalxingtong/Un-Bert-Vits2/transcribe_genshin.py b/spaces/digitalxingtong/Un-Bert-Vits2/transcribe_genshin.py
deleted file mode 100644
index acc98814af6189d129ab85946525bec55419a33f..0000000000000000000000000000000000000000
--- a/spaces/digitalxingtong/Un-Bert-Vits2/transcribe_genshin.py
+++ /dev/null
@@ -1,78 +0,0 @@
-# coding=gbk
-import os
-import argparse
-import librosa
-import numpy as np
-from multiprocessing import Pool, cpu_count
-
-import soundfile
-from scipy.io import wavfile
-from tqdm import tqdm
-
-global speaker_annos
-speaker_annos = []
-
-def process(item):
- spkdir, wav_name, args = item
- speaker = spkdir.replace("\\", "/").split("/")[-1]
- wav_path = os.path.join(args.in_dir, speaker, wav_name)
- if os.path.exists(wav_path) and '.wav' in wav_path:
- os.makedirs(os.path.join(args.out_dir, speaker), exist_ok=True)
- wav, sr = librosa.load(wav_path, sr=args.sr)
- soundfile.write(
- os.path.join(args.out_dir, speaker, wav_name),
- wav,
- sr
- )
-
-def process_text(item):
- spkdir, wav_name, args = item
- speaker = spkdir.replace("\\", "/").split("/")[-1]
- wav_path = os.path.join(args.in_dir, speaker, wav_name)
- global speaker_annos
- tr_name = wav_name.replace('.wav', '')
- with open(args.out_dir+'/'+speaker+'/'+tr_name+'.lab', "r", encoding="utf-8") as file:
- text = file.read()
- text = text.replace("{NICKNAME}",'')
- text = text.replace("{M#}{F#}",'')
- text = text.replace("{M#}{F#}",'')
- substring = "{M#}{F#}"
- if substring in text:
- if tr_name.endswith("a"):
- text = text.replace("{M#}{F#}",'')
- if tr_name.endswith("b"):
- text = text.replace("{M#}{F#}",'')
- text = text.replace("#",'')
- text = "ZH|" + text + "\n" #
- speaker_annos.append(args.out_dir+'/'+speaker+'/'+wav_name+ "|" + speaker + "|" + text)
-
-
-
-if __name__ == "__main__":
- parent_dir = "./genshin_dataset/"
- speaker_names = list(os.walk(parent_dir))[0][1]
- parser = argparse.ArgumentParser()
- parser.add_argument("--sr", type=int, default=44100, help="sampling rate")
- parser.add_argument("--in_dir", type=str, default="./genshin_dataset", help="path to source dir")
- parser.add_argument("--out_dir", type=str, default="./genshin_dataset", help="path to target dir")
- args = parser.parse_args()
- # processs = 8
- processs = cpu_count()-2 if cpu_count() >4 else 1
- pool = Pool(processes=processs)
-
- for speaker in os.listdir(args.in_dir):
- spk_dir = os.path.join(args.in_dir, speaker)
- if os.path.isdir(spk_dir):
- print(spk_dir)
- for _ in tqdm(pool.imap_unordered(process, [(spk_dir, i, args) for i in os.listdir(spk_dir) if i.endswith("wav")])):
- pass
- for i in os.listdir(spk_dir):
- if i.endswith("wav"):
- pro=(spk_dir, i, args)
- process_text(pro)
- if len(speaker_annos) == 0:
- print("transcribe error!!!")
- with open("./filelists/short_character_anno.list", 'w', encoding='utf-8') as f:
- for line in speaker_annos:
- f.write(line)
- print("transcript file finished.")
diff --git a/spaces/docs-demos/hubert-large-superb-er/README.md b/spaces/docs-demos/hubert-large-superb-er/README.md
deleted file mode 100644
index 965fdce4ab0d8032745cc0f9399dcf7e237bcdca..0000000000000000000000000000000000000000
--- a/spaces/docs-demos/hubert-large-superb-er/README.md
+++ /dev/null
@@ -1,12 +0,0 @@
----
-title: Hubert Large Superb Er
-emoji: 🌖
-colorFrom: purple
-colorTo: blue
-sdk: gradio
-sdk_version: 2.9.4
-app_file: app.py
-pinned: false
----
-
-Check out the configuration reference at https://huggingface.co/docs/hub/spaces#reference
diff --git a/spaces/dongyi/MMFS/utils/__init__.py b/spaces/dongyi/MMFS/utils/__init__.py
deleted file mode 100644
index ae36f63d8859ec0c60dcbfe67c4ac324e751ddf7..0000000000000000000000000000000000000000
--- a/spaces/dongyi/MMFS/utils/__init__.py
+++ /dev/null
@@ -1 +0,0 @@
-"""This package includes a miscellaneous collection of useful helper functions."""
diff --git a/spaces/dorkai/text-generation-webui-main/text-generation-webui-main/docs/DeepSpeed.md b/spaces/dorkai/text-generation-webui-main/text-generation-webui-main/docs/DeepSpeed.md
deleted file mode 100644
index 70cd81519a6954ebc7cdaf82e03a169bed878106..0000000000000000000000000000000000000000
--- a/spaces/dorkai/text-generation-webui-main/text-generation-webui-main/docs/DeepSpeed.md
+++ /dev/null
@@ -1,23 +0,0 @@
-An alternative way of reducing the GPU memory usage of models is to use the `DeepSpeed ZeRO-3` optimization.
-
-With this, I have been able to load a 6b model (GPT-J 6B) with less than 6GB of VRAM. The speed of text generation is very decent and much better than what would be accomplished with `--auto-devices --gpu-memory 6`.
-
-As far as I know, DeepSpeed is only available for Linux at the moment.
-
-### How to use it
-
-1. Install DeepSpeed:
-
-```
-pip install deepspeed
-```
-
-2. Start the web UI replacing `python` with `deepspeed --num_gpus=1` and adding the `--deepspeed` flag. Example:
-
-```
-deepspeed --num_gpus=1 server.py --deepspeed --chat --model gpt-j-6B
-```
-
-### Learn more
-
-For more information, check out [this comment](https://github.com/oobabooga/text-generation-webui/issues/40#issuecomment-1412038622) by 81300, who came up with the DeepSpeed support in this web UI.
\ No newline at end of file
diff --git a/spaces/duycse1603/math2tex/README.md b/spaces/duycse1603/math2tex/README.md
deleted file mode 100644
index a15e5556e0a03cfd0c0f0dd9bb4b4acb8ceffea0..0000000000000000000000000000000000000000
--- a/spaces/duycse1603/math2tex/README.md
+++ /dev/null
@@ -1,13 +0,0 @@
----
-title: Thesis Demo
-emoji: 🏢
-colorFrom: blue
-colorTo: green
-sdk: streamlit
-sdk_version: 1.21.0
-app_file: app.py
-pinned: false
-license: apache-2.0
----
-
-Check out the configuration reference at https://huggingface.co/docs/hub/spaces-config-reference
diff --git a/spaces/ebgoldstein/FRF_Coarse/app.py b/spaces/ebgoldstein/FRF_Coarse/app.py
deleted file mode 100644
index 8ff105d4277ee575047ffb60ecee7708592c6a0e..0000000000000000000000000000000000000000
--- a/spaces/ebgoldstein/FRF_Coarse/app.py
+++ /dev/null
@@ -1,76 +0,0 @@
-import gradio as gr
-import numpy as np
-import tensorflow as tf
-from skimage.io import imsave
-from skimage.transform import resize
-import matplotlib.pyplot as plt
-
-#from SegZoo
-def standardize(img):
- #standardization using adjusted standard deviation
-
- N = np.shape(img)[0] * np.shape(img)[1]
- s = np.maximum(np.std(img), 1.0/np.sqrt(N))
- m = np.mean(img)
- img = (img - m) / s
- del m, s, N
- #
- if np.ndim(img)==2:
- img = np.dstack((img,img,img))
- return img
-
-#load model
-filepath = './saved_model'
-model = tf.keras.models.load_model(filepath, compile = True)
-model.compile
-
-#segmentation
-def FRFsegment(input_img):
-
- dims=(512,512)
- w = input_img.shape[0]
- h = input_img.shape[1]
- print(w)
- print(h)
-
- img = standardize(input_img)
- img = resize(img, dims, preserve_range=True, clip=True)
- img = np.expand_dims(img,axis=0)
-
- est_label = model.predict(img)
-
-# # Test Time AUgmentation
-# est_label2 = np.flipud(model.predict((np.flipud(img)), batch_size=1))
-# est_label3 = np.fliplr(model.predict((np.fliplr(img)), batch_size=1))
-# est_label4 = np.flipud(np.fliplr(model.predict((np.flipud(np.fliplr(img))))))
-
-# #soft voting - sum the softmax scores to return the new TTA estimated softmax scores
-# pred = est_label + est_label2 + est_label3 + est_label4
-# est_label = pred
-
- mask = np.argmax(np.squeeze(est_label, axis=0),-1)
- pred = resize(mask, (w, h), preserve_range=True, clip=True)
-
-
- imsave("label.png", pred)
-
- #overlay plot
- plt.clf()
- plt.imshow(input_img,cmap='gray')
- plt.imshow(pred, alpha=0.4)
- plt.axis("off")
- plt.margins(x=0, y=0)
- plt.savefig("overlay.png", dpi=300, bbox_inches="tight")
-
- return plt, "label.png", "overlay.png"
-
-out1 = gr.outputs.File()
-out2 = gr.outputs.File()
-
-
-title = "Segment beach imagery taken from a tower in Duck, NC, USA"
-description = "This model segments beach imagery into 4 classes: vegetation, sand, coarse sand, and background (water + sky + buildings + people)"
-examples = [['examples/FRF_c1_snap_20191112160000.jpg'],['examples/FRF_c1_snap_20170101.jpg']]
-
-
-FRFSegapp = gr.Interface(FRFsegment, gr.inputs.Image(), ['plot',out1, out2], examples=examples, title = title, description = description).launch()
diff --git a/spaces/egumasa/engagement-analyzer-demo/pipeline/post_processors.py b/spaces/egumasa/engagement-analyzer-demo/pipeline/post_processors.py
deleted file mode 100644
index 70fa59b7325f8bdfe1cd9b673724cb635f9aad7d..0000000000000000000000000000000000000000
--- a/spaces/egumasa/engagement-analyzer-demo/pipeline/post_processors.py
+++ /dev/null
@@ -1,889 +0,0 @@
-
-from typing import List, Sequence, Tuple, Optional, Dict, Union, Callable
-import pandas as pd
-import spacy
-from spacy.language import Language
-from skbio import diversity as dv
-
-SPAN_ATTRS = ["text", "label_", "start", "end"]
-CATEGORIES = ['ATTRIBUTION', "CITATION", "COUNTER", "DENY", "ENDOPHORIC", "ENTERTAIN", "JUSTIFYING", "MONOGLOSS", "PROCLAIM", "SOURCES"]
-
-
-def simple_table(doc: Union[spacy.tokens.Doc, Dict[str, str]],
- spans_key: str = "sc",
- attrs: List[str] = SPAN_ATTRS):
- columns = attrs + ["Conf. score"]
- data = [
- [str(getattr(span, attr))
- for attr in attrs] + [score] # [f'{score:.5f}']
- for span, score in zip(doc.spans[spans_key], doc.spans[spans_key].attrs['scores'])
- ]
- return data, columns
-
-
-# def span_info_aggregator()
-
-def construction_classifier(doc, span):
- category = None
- spanroot = span.root
-
- ## Grabbing lexico-grammatical information
- span_t_dep_ = ["_".join([t.norm_, t.dep_]) for t in span]
- span_dep = [t.dep_ for t in span]
- span_token = [t.norm_ for t in span]
- span_tag = [t.tag_ for t in span]
-
-
- c = [c for c in spanroot.children]
- c_t_dep_ = ["_".join([t.norm_, t.dep_]) for t in spanroot.children]
-
- c_norm = [c.norm_ for c in spanroot.children]
- c_dep = [c.dep_ for c in spanroot.children]
- c_pos = [c.pos_ for c in spanroot.children]
- c_tag = [c.tag_ for c in spanroot.children]
-
- right_dep = [c.dep_ for c in spanroot.rights]
-
- #conditionals
- subjless = all(c.dep_ not in ['nsubj', 'nsubjpass', 'csubj', 'csubjpass'] for c in spanroot.children)
- argmentless = all(c.dep_ not in ['nsubj', 'nsubjpass', 'csubj', 'csubjpass', "dobj", 'ccomp', 'xcomp', 'dative', "attr", "oprd", "acomp"] for c in spanroot.children)
- argless_span = all(c.dep_ not in ['nsubj', 'nsubjpass', 'csubj', 'csubjpass', "dobj", 'ccomp', 'xcomp', 'dative', "attr", "oprd", "acomp"] for c in span)
-
- ## nesting classifiers
- if spanroot.dep_ == "conj":
- while spanroot.dep_ == 'conj':
- spanroot = spanroot.head
- # if spanroot.dep_ == "poss":
- # while spanroot.dep_ == 'poss':
- # spanroot = spanroot.head
-
- ## Conjunctions
- # Preconjunctions
- if spanroot.dep_ in ['preconj', 'cc']:
- category = "Conjunction"
-
- ## NOUN PHRASES
- # adverbial phrases
- if spanroot.dep_ in ['amod']:
- category = "Adjectival modifier"
- # adverbial phrases
- if spanroot.dep_ in ['compound']:
- category = "Compound noun"
-
- ## Nominal category
- if spanroot.dep_ in ["pobj", "dobj", "obj", "iobj", "dative"]:
- if "acl" in c_dep:
- category = "Noun + Complement (Object)"
- else:
- category = "Object"
-
- if spanroot.dep_ in ["nsubj", "nsubjpass"]:
- if "acl" in c_dep:
- category = "Noun + Complement (Subject)"
- else:
- category = "Subject"
-
- ## ADJUNCTS
- # prep phrases
- if spanroot.dep_ in ['prep', 'agent']:
- category = 'Prepositional phrase'
- # adverbial phrases
- if spanroot.dep_ in ['advmod', "npadvmod", "nmod", "npmod", 'quantmod']:
- category = "Adverbial phrase"
-
- ## Predication patterns
- if spanroot.dep_ in ['acomp', 'oprd']:
- if "xcomp" in c_dep:
- category = "Subject predicate to-cl"
- else:
- category = "Adjectival complement"
-
- if spanroot.dep_ in ['attr']:
- subjless = all(c.dep_ not in ['nsubj', 'nsubjpass', 'csubj', 'csubjpass'] for c in spanroot.children)
-
- c_head = [c.dep_ for c in spanroot.head.children]
- if "expl" in c_head and "no_det" in span_t_dep_:
- category = "There is/are no NOUN"
- elif "expl" in c_head and spanroot.pos_ in ["NOUN"]:
- category = "There is/are + Noun complement"
- elif "expl" in c_head and spanroot.tag_ in ["NN", "NNS"]:
- category = "There is/are + Noun complement"
-
- elif spanroot.pos_ in ["NOUN", "PRON"]:
- if "acl" in c_dep:
- category = "Noun + Complement (attr)"
- else:
- category = "Nominal complement"
-
- elif not subjless and spanroot.pos_ in ['VERB', "AUX"]:
- category = "Main verb 4"
-
- elif spanroot.tag_ in ['NNP']:
- category = "Nominal complement"
-
-
- ####################################
- ### clausal ####
- ####################################
- if spanroot.dep_ in ["ROOT", "advcl", "ccomp", 'acl', 'pcomp', 'relcl']:
-
- _check_to = [c.dep_ for c in spanroot.subtree if (c.dep_ in ["aux"] and c.pos_ in ["PART", "SCONJ"]) and c.head.dep_ == "xcomp"]
- _check_ing = [c.dep_ for c in spanroot.subtree if "Prog" in str(c.morph) and c.dep_ == "xcomp"]
- root_before_ccomp = [c.i > spanroot.i for c in spanroot.children if c.dep_ == "ccomp"]
-
- _check_for_to = ["_".join([c.norm_, c.dep_]) for c in spanroot.subtree if c.head.dep_ == "advcl" and (c.dep_=="mark" or c.dep_ == "aux")]
- entire_cl = spanroot.left_edge.i == span.start and spanroot.right_edge.i == span.end
-
- ## Start with broad category, which is then re-evaluated for specific constructions.
- if spanroot.dep_ in ['advcl', 'mark', 'acl', 'pcomp']:
- ## Adverbial clauses
- ### Finite-adverbial clauses
- ### Non-finite adverbial clauses
- subjless = all(c.dep_ not in ['nsubj', 'nsubjpass', 'csubj', 'csubjpass'] for c in spanroot.children)
- entire_cl = spanroot.left_edge.i == span.start and spanroot.right_edge.i == span.end
-
- if "mark" in span_dep and spanroot.pos_ in ['VERB', "AUX"]:
- category = "Finite adverbial clause"
- elif "mark" in span_dep and "aux" in span_dep :
- category = "Finite adverbial clause"
-
- elif "mark" in span_dep and spanroot.pos_ in ['VERB', "AUX"] and "expl" in c_dep:
- category = "Finite adverbial clause"
-
- elif "advmod" in span_dep and ("WRB" in span_tag or "WDT" in span_tag):
- if spanroot.pos_ in ['VERB', "AUX"]:
- category = "Finite adverbial clause"
-
- elif spanroot.pos_ not in ['VERB', "AUX"] and subjless:
- category = "Non-finite adv clause 1"
-
- elif entire_cl:
- category = "Finite adverbial clause"
-
- elif str(spanroot.morph) in ["Aspect=Prog|Tense=Pres|VerbForm=Part", "Aspect=Perf|Tense=Past|VerbForm=Part"] and "aux" not in c_dep:
- # he doing his job
- if argmentless:
- #e.g., frankly speaking, strictly speaking
- category = "Adverbial Phrase"
- else:
- category = "Non-finite adv clause 2"
-
- elif spanroot.pos_ not in ['VERB', "AUX"] and "mark" in span_dep and subjless:
-
- category = "Non-finite adv clause 3"
-
- elif "aux" in c_dep and "TO" in c_tag:
- category = "Adverbial Phrase"
-
-
- elif "mark" not in span_dep and spanroot.pos_ in ['VERB', "AUX"]:
- category = "Dependent Verb phrase"
-
- elif not argmentless:
- category = "Adverbial clause"
-
- elif spanroot.dep_ == "advcl":
- category = "Adverbial phrase"
-
-
- if spanroot.dep_ in ['relcl', 'ccomp', 'acl']:
-
- head = spanroot.head
- if ";" in [t.norm_ for t in head.children]:
- category = "Main verb 3"
- elif "nsubj" not in span_dep:
- category = "Dependent verb 1"
- elif "mark" in span_dep:
- category = "Complement clause"
- elif str(spanroot.morph) in ["Aspect=Prog|Tense=Pres|VerbForm=Part", "Aspect=Perf|Tense=Past|VerbForm=Part"] and "aux" not in c_dep:
- category = "Non-finite complement clause"
- elif spanroot.dep_ in ['relcl']:
- category = "Relative clause"
- elif spanroot.dep_ in ['ccomp']:
- category = "Complement clause"
- elif spanroot.dep_ in ['acl']:
- category = "Noun Complement clause"
- else:
- # print(_check_for_to)
- category = "this one"
-
- ## Specific constructions
- # Extraposed that-clause or to-infinitives
- if ("it_nsubjpass" in c_t_dep_ or "it_nsubj" in c_t_dep_) and spanroot.pos_ in ["VERB", "AUX"]:
- print(c_dep)
- if ("acomp" in c_dep or "oprd" in c_dep) and "ccomp" in c_dep:
- #eg it seems odd (oprd) that X.
- #eg it is certain (acomp) that X.
- category = "Extraposed that-cl (adj-complement)" #e.g., it is certain that X.
-
- elif "xcomp" in c_dep or ("advcl" in c_dep):
- if "for_mark" in _check_for_to:
- category = "Extraposed to-cl (explicit subj)" #eg It is possible to .
- elif _check_to:
- category = "Extraposed to-cl 1" #eg It is possible to .
- elif _check_ing:
- category = "Extraposed -ing 1" #eg It is possible to .
- elif ("prep" in right_dep or "npadvmod" in right_dep) and "ccomp" in right_dep and spanroot.lemma_ == "be":
- category = "Cleft construction"
-
- elif "attr" in c_dep:
- category = "Extraposed that-cl (copula)" #eg It is a wonder that X.
-
- else:
- category = "Extraposed that-cl (VERB)"
-
- # if "ccomp" in c_dep and "auxpass" in c_dep and ("it_nsubjpass" in span_t_dep_ or "it_nsubj" in span_t_dep_):
- # category = "Extraposed that-cl (VERB)1" #e.g., it has been shown that X.
- elif ("it_nsubjpass" in c_t_dep_ or "it_nsubj" in c_t_dep_) and "acomp" in c_dep:
- if "xcomp" in c_dep:
- if _check_to:
- category = "Extraposed to-cl 2" #eg it is difficult to decide.
- elif _check_ing:
- category = "Extraposed -ing 2" #eg it is difficult to decide.
-
- else:
- category = "Extraposed that-cl (adj-complement) 2"
-
- elif ("it_nsubjpass" in c_t_dep_ or "it_nsubj" in c_t_dep_) and "oprd" in c_dep:
-
- category = "Extraposed that-cl (adj-complement) 3" #eg it seems odd that X.
-
-
- # something without dummy subject "it"
- elif (("nsubj" in c_dep and spanroot.lemma_ in ['be']) or "nsubjpass" in c_dep) and spanroot.pos_ in ["AUX", 'VERB'] and "it" not in c_norm:
-
- # store xcomp, if the head of the xcomp is acomp
- _check_xcomp = [c.dep_ for c in spanroot.subtree if c.dep_ in ["xcomp"] and c.head.dep_ == "acomp"]
- _check_ccomp = [c.dep_ for c in spanroot.subtree if c.dep_ in ["ccomp"] and c.head.dep_ == "acomp"]
- # _check_to = [c.dep_ for c in spanroot.subtree if (c.dep_ in ["aux"] and c.pos_ in ["PART", "SCONJ"]) and c.head.dep_ == "xcomp"]
- # _check_ing = [c.dep_ for c in spanroot.subtree if "Prog" in str(c.morph) and c.dep_ == "xcomp"]
-
-
- if ("attr" in c_dep or "acomp" in c_dep) and "ccomp" in c_dep:
- if any(root_before_ccomp):
- category = "Post-predicate that-cl"
- else:
- category = "Comment clause"
-
- elif ("attr" in c_dep or "acomp" in c_dep) and "ccomp" in _check_ccomp:
- category = "Post-predicate that-cl 2"
-
- elif ("attr" in c_dep or "acomp" in c_dep) and "xcomp" in _check_xcomp:
- category = "Post-predicate to-cl"
-
- elif "xcomp" in c_dep and spanroot.lemma_ in ['be'] and _check_to:
- category = "Subject predicate to-cl"
-
- elif "xcomp" in c_dep and "auxpass" in c_dep and _check_to:
- category = "Subject predicate to-cl (passive)"
-
- elif "xcomp" in c_dep and spanroot.lemma_ in ['be'] and _check_ing:
- category = "Subject predicate -ing"
- elif "ccomp" in c_dep:
- category = "Subject predicate that-cl"
- elif "acomp" in c_dep:
- category = "Adjectival predicate"
-
- elif "mark" in c_dep and ("nsubj" in c_dep or "nsubjpass" in c_dep):
- category = "Finite-adverbial clause"
- else:
- category = "Main verb 1"
-
- ## without dummy subject it, and lexical verbs
- elif ("nsubj" in c_dep or "nsubjpass" in c_dep) in c_dep and spanroot.pos_ in ["AUX", 'VERB'] and "it" not in c_norm and spanroot.lemma_ not in ['be']:
- _check_wh = [c.dep_ for c in spanroot.subtree if (c.dep_ in ["attr", "advmod", 'dobj', 'nsubj'] and c.tag_ in ["WP", "WRB", "WDT", "WP$"]) and c.head.dep_ == "ccomp"]
- _check_if = [c.dep_ for c in spanroot.subtree if (c.dep_ in ["mark"] and c.norm_ in ["whether", "if"]) and c.head.dep_ == "ccomp"]
-
- # _check_to = [c.dep_ for c in spanroot.subtree if (c.dep_ in ["aux"] and c.pos_ in ["PART", "SCONJ"]) and c.head.dep_ == "xcomp"]
- # _check_ing = [c.dep_ for c in spanroot.subtree if "Prog" in str(c.morph) and c.dep_ == "xcomp"]
-
- if "ccomp" in c_dep and (_check_wh or _check_if):
- category = "Post-predicate wh-cl"
-
- elif "ccomp" in c_dep:
- if any(root_before_ccomp):
- category = "Post-predicate that-cl"
- else:
- category = "Comment clause"
-
- elif "xcomp" in c_dep:
- if _check_to:
- category = "Post-predicate to-cl"
- elif _check_ing:
- category = "Post-predicate -ing"
-
- # Existential
- elif "expl" in c_dep and "NOUN" in c_pos and "mark" not in c_dep:
- category = "There is/are NOUN"
-
- elif "ccomp" in c_dep and "it_nsubj" in span_t_dep_ and spanroot.pos_ in ["AUX"]:
- category = "Cleft construction"
-
-
- if spanroot.dep_ in ['parataxis']:
- if "_".join(span_dep) in ["nsubj_parataxis", "aux_parataxis", "nsubj_aux_parataxis"]:
- category = "Comment clause"
- else:
- category = "parataxis (for now)"
-
-
- ## External comp
- if spanroot.dep_ in ['xcomp']:
- if spanroot.head.pos_ == 'ADJ' and "to_aux" in c_t_dep_:
- category = "Adjective complement to-cl"
- if spanroot.head.pos_ == 'VERB' and "to_aux" in c_t_dep_:
- category = "Verb complement to-cl"
-
- if spanroot.dep_ in ['pcomp']:
- if str(spanroot.morph) in ["Aspect=Prog|Tense=Pres|VerbForm=Part"] and 'ccomp' in c_dep:
- category = "Participle + that-cl"
- elif str(spanroot.morph) in ["Aspect=Prog|Tense=Pres|VerbForm=Part"]:
- category = "Participle"
-
- ## Simple classifier
- # if spanroot.dep_ in ['pcomp']:
- # if str(spanroot.morph) in ["Aspect=Prog|Tense=Pres|VerbForm=Part"]:
- # category = "Gerund"
-
- if spanroot.dep_ in ['neg']:
- category = "Negative particle"
- if spanroot.dep_ in ['aux', 'auxpass']:
- category = "Auxiliary"
-
- # Modal verbs
- if spanroot.tag_ == "MD":
- category = "Modal auxiliary"
-
-
- if spanroot.dep_ in ['dep', "csubj", 'csubjpass']:
- if spanroot.head.dep_ in ['ROOT', 'ccomp'] and spanroot.head.pos_ in ['AUX', 'VERB'] and spanroot.pos_ in ['AUX', 'VERB']:
- if spanroot.morph == spanroot.head.morph:
- category = "Main verb 4"
- else:
- category = "Dependent verb 2"
- elif str(spanroot.morph) == "Aspect=Prog|Tense=Pres|VerbForm=Part":
- category = "Gerund"
- elif spanroot.head.dep_ in ['conj', 'acl','relcl']:
- if spanroot.morph == spanroot.head.morph:
- category = "Main verb 4"
- else:
- category = "Dependent verb 2"
- elif "VerbForm=Fin" in str(spanroot.morph):
- category = "Dependent verb 2"
-
- # Appositive phrases
- if spanroot.dep_ in ['appos']:
- if "nummod" in c_dep:
- category = "Apposition"
- elif spanroot.pos_ in ["PROPN"]:
- category = "Appositive Proper Nouns"
- elif spanroot.pos_ in ["NOUN"]:
- category = "Appositive Noun Phrase"
- elif spanroot.pos_ in ["VERB", "AUX"]:
- _check = any(c.dep_ in ['nsubj', 'nsubjpass', 'csubj', 'csubjpass'] for c in spanroot.children)
- if _check:
- category = "Appositive Finite-clause"
-
- if spanroot.dep_ in ['appos', "dep", "attr"]:
- if not subjless and spanroot.pos_ in ['VERB', "AUX"]:
- category = "Main verb 5"
-
- if spanroot.dep_ in ["dep", "mark"]:
- if spanroot.tag_ in ["RB", "IN", "CC"]:
- category = "Conjunction"
-
-
- #sometimes the extra-clausal links are not accurate
- if spanroot.dep_ in ['aux', "auxpass", 'oprd', 'appos', "xcomp"]:
- if spanroot.head.dep_ == "ROOT":
- category = "Main verb"
- else:
- category = "dependent verb 5"
-
- if span.label_ == "CITATION":
- if "NNP" in span_tag or "NNPS" in span_tag:
- if span_dep[0] == 'punct' and span_dep[-1] == 'punct':
- category = "Parenthetical Citation"
- elif span_tag[0] in ["NNP", "NNPS"]:
- category = "Narrative Citation"
- else:
- category = "Other Citation"
-
- if category == None:
- category = spanroot.dep_
-
- return category
-
-
-def construction_classifier2(doc, span):
- category = None
- spanroot = span.root
-
- ## Grabbing lexico-grammatical information
- span_t_dep_ = ["_".join([t.norm_, t.dep_]) for t in span]
- span_dep = [t.dep_ for t in span]
- span_token = [t.norm_ for t in span]
- span_tag = [t.tag_ for t in span]
-
-
- c = [c for c in spanroot.children]
- c_t_dep_ = ["_".join([t.norm_, t.dep_]) for t in spanroot.children]
-
- c_norm = [c.norm_ for c in spanroot.children]
- c_dep = [c.dep_ for c in spanroot.children]
- c_pos = [c.pos_ for c in spanroot.children]
- c_tag = [c.tag_ for c in spanroot.children]
-
- right_dep = [c.dep_ for c in spanroot.rights]
-
- #conditionals
- subjless = all(c.dep_ not in ['nsubj', 'nsubjpass', 'csubj', 'csubjpass'] for c in spanroot.children)
- argmentless = all(c.dep_ not in ['nsubj', 'nsubjpass', 'csubj', 'csubjpass', "dobj", 'ccomp', 'xcomp', 'dative', "attr", "oprd", "acomp"] for c in spanroot.children)
- argless_span = all(c.dep_ not in ['nsubj', 'nsubjpass', 'csubj', 'csubjpass', "dobj", 'ccomp', 'xcomp', 'dative', "attr", "oprd", "acomp"] for c in span)
- argless_span = all(c.dep_ not in ['nsubj', 'nsubjpass', 'csubj', 'csubjpass', "dobj", 'ccomp', 'xcomp', 'dative', "attr", "oprd", "acomp"] for c in span)
-
-
- ## nesting classifiers
- if spanroot.dep_ == "conj":
- while spanroot.dep_ == 'conj':
- spanroot = spanroot.head
-
- if spanroot.dep_ == "poss":
- head = spanroot.head
- if head.dep_ in ["pobj", "dobj", "obj", "iobj" , "dative"]:
- category = "Posessive Noun (Object)"
- elif head.dep_ in ["nsubj", "nsubjpass"]:
- category = "Posessive Noun (Subject)"
- else:
- category = "Posessive Noun (Other)"
-
-
- ## Conjunctions
- # Preconjunctions
- if spanroot.dep_ in ['preconj', 'cc']:
- category = "Conjunction"
-
- ## NOUN PHRASES
- # adverbial phrases
- if spanroot.dep_ in ['amod']:
- category = "Adjectival modifier"
- # adverbial phrases
- if spanroot.dep_ in ['compound']:
- category = "Compound noun"
-
- ## Nominal category
- if spanroot.dep_ in ["pobj", "dobj", "obj", "iobj" , "dative"]:
- if "acl" in c_dep:
- category = "Noun + Complement (Object)"
- else:
- category = "Object"
-
- if spanroot.dep_ in ["nsubj", "nsubjpass"]:
- if "acl" in c_dep:
- category = "Noun + Complement (Subject)"
- else:
- category = "Subject"
-
- ## ADJUNCTS
- # prep phrases
- if spanroot.dep_ in ['prep', 'agent']:
- category = 'Prepositional phrase'
-
- # adverbial phrases
- if spanroot.dep_ in ['advmod', "npadvmod", "nmod", "npmod", 'quantmod', 'nummod']:
- category = "Adverbial phrase"
-
- ## Predication patterns
- if spanroot.dep_ in ['acomp', 'oprd']:
- if "xcomp" in c_dep:
- category = "Subject predicate to-cl"
- else:
- category = "Adjectival complement"
-
- if spanroot.dep_ in ['attr']:
- subjless = all(c.dep_ not in ['nsubj', 'nsubjpass', 'csubj', 'csubjpass'] for c in spanroot.children)
-
- c_head = [c.dep_ for c in spanroot.head.children]
- if "expl" in c_head and "no_det" in span_t_dep_:
- category = "There is/are no NOUN"
- elif "expl" in c_head and spanroot.pos_ in ["NOUN"]:
- category = "There is/are + Noun complement"
- elif "expl" in c_head and spanroot.tag_ in ["NN", "NNS"]:
- category = "There is/are + Noun complement"
-
- elif spanroot.pos_ in ["NOUN", "PRON"]:
- if "acl" in c_dep:
- category = "Noun + Complement (attr)"
- else:
- category = "Nominal complement"
-
- elif not subjless and spanroot.pos_ in ['VERB', "AUX"]:
- category = "Main verb 4"
-
- elif spanroot.tag_ in ['NNP']:
- category = "Nominal complement"
-
- ## External comp
- if spanroot.dep_ in ['xcomp']:
- if spanroot.head.pos_ == 'ADJ' and "to_aux" in c_t_dep_:
- category = "Adjective complement to-cl"
- if spanroot.head.pos_ == 'VERB' and "to_aux" in c_t_dep_:
- category = "Verb complement to-cl"
-
- if spanroot.dep_ in ['pcomp']:
- if str(spanroot.morph) in ["Aspect=Prog|Tense=Pres|VerbForm=Part"] and 'ccomp' in c_dep:
- category = "Participle + that-cl"
- elif str(spanroot.morph) in ["Aspect=Prog|Tense=Pres|VerbForm=Part"]:
- category = "Participle"
-
- ## Simple classifier
- # if spanroot.dep_ in ['pcomp']:
- # if str(spanroot.morph) in ["Aspect=Prog|Tense=Pres|VerbForm=Part"]:
- # category = "Gerund"
-
- if spanroot.dep_ in ['neg']:
- category = "Negative particle"
- if spanroot.dep_ in ['aux', 'auxpass']:
- category = "Auxiliary"
-
- # Modal verbs
- if spanroot.tag_ == "MD":
- category = "Modal auxiliary"
-
-
- ####################################
- ### clausal ####
- ####################################
- if spanroot.dep_ in ["ROOT", "advcl", "ccomp", 'acl', 'pcomp', 'relcl', 'punct']:
-
- _check_to = [c.dep_ for c in spanroot.subtree if (c.dep_ in ["aux"] and c.pos_ in ["PART", "SCONJ"]) and c.head.dep_ == "xcomp"]
- _check_ing = [c.dep_ for c in spanroot.subtree if "Prog" in str(c.morph) and c.dep_ == "xcomp"]
- root_before_ccomp = [c.i > spanroot.i for c in spanroot.children if c.dep_ == "ccomp"]
-
- _check_for_to = ["_".join([c.norm_, c.dep_]) for c in spanroot.subtree if c.head.dep_ == "advcl" and (c.dep_=="mark" or c.dep_ == "aux")]
- entire_cl = spanroot.left_edge.i == span.start and spanroot.right_edge.i == span.end
-
-
- ## Start with broad category, which is then re-evaluated for specific constructions.
- if spanroot.dep_ in ['advcl', 'acl', 'punct', 'pcomp']: #'mark',
- ## Adverbial clauses
- subjless = all(c.dep_ not in ['nsubj', 'nsubjpass', 'csubj', 'csubjpass'] for c in spanroot.children)
- entire_cl = spanroot.left_edge.i == span.start and spanroot.right_edge.i == span.end
-
- ### Finite-adverbial clauses
- if "mark" in span_dep and (spanroot.pos_ in ['VERB', "AUX"] or "aux" in span_dep ):
- category = "Finite adverbial clause"
-
- elif "mark" in span_dep and "aux" in span_dep :
- category = "Finite adverbial clause"
-
- elif "mark" in span_dep and spanroot.pos_ in ['VERB', "AUX"] and "expl" in c_dep:
- category = "Finite adverbial clause"
-
- elif "advmod" in span_dep and ("WRB" in span_tag or "WDT" in span_tag):
- if spanroot.pos_ in ['VERB', "AUX"]:
- category = "Finite adverbial clause"
-
- elif spanroot.pos_ not in ['VERB', "AUX"] and subjless:
- category = "Non-finite adv clause 1"
-
- elif not argmentless:
- category = "Finite adverbial clause"
-
- ## non-finite
- elif str(spanroot.morph) in ["Aspect=Prog|Tense=Pres|VerbForm=Part", "Aspect=Perf|Tense=Past|VerbForm=Part"] and "aux" not in c_dep:
- # he doing his job
- if argmentless:
- #e.g., frankly speaking, strictly speaking
- category = "Adverbial Phrase"
- else:
- category = "Non-finite adv clause 2"
-
- elif spanroot.pos_ not in ['VERB', "AUX"] and "mark" in span_dep and subjless:
-
- category = "Non-finite adv clause 3"
-
- elif "aux" in c_dep and "TO" in c_tag:
- category = "Adverbial Phrase"
-
-
- elif "mark" not in span_dep and spanroot.pos_ in ['VERB', "AUX"]:
- category = "Dependent Verb phrase"
-
- elif not argmentless:
- category = "Adverbial clause"
-
- elif spanroot.dep_ == "advcl":
- category = "Adverbial phrase"
-
- else:
- category = "Finite adverbial clause "
-
- if spanroot.dep_ in ['relcl', 'ccomp', 'acl', 'punct', "pcomp"]:
-
- head = spanroot.head
- if ";" in [t.norm_ for t in head.children]:
- category = "Main verb 3"
-
- elif "nsubj" not in span_dep:
- category = "Dependent verb 1"
-
- elif "mark" in span_dep:
- category = "Complement clause"
- elif str(spanroot.morph) in ["Aspect=Prog|Tense=Pres|VerbForm=Part", "Aspect=Perf|Tense=Past|VerbForm=Part"] and "aux" not in c_dep:
- category = "Non-finite complement clause"
- elif spanroot.dep_ in ['relcl']:
- category = "Relative clause"
- elif spanroot.dep_ in ['ccomp']:
- category = "Complement clause"
- elif spanroot.dep_ in ['acl']:
- category = "Noun Complement clause"
-
- ## Specific constructions
- # Extraposed that-clause or to-infinitives
- if ("it_nsubjpass" in c_t_dep_ or "it_nsubj" in c_t_dep_) and spanroot.pos_ in ["VERB", "AUX"]:
- # print(c_dep)
- if ("acomp" in c_dep or "oprd" in c_dep) and "ccomp" in c_dep:
- #eg it seems odd (oprd) that X.
- #eg it is certain (acomp) that X.
- category = "Extraposed that-cl (adj-complement)" #e.g., it is certain that X.
-
- elif "xcomp" in c_dep or ("advcl" in c_dep):
- if "for_mark" in _check_for_to:
- category = "Extraposed to-cl (explicit subj)" #eg It is possible to .
- elif _check_to:
- category = "Extraposed to-cl 1" #eg It is possible to .
- elif _check_ing:
- category = "Extraposed -ing 1" #eg It is possible to .
- elif ("prep" in right_dep or "npadvmod" in right_dep) and "ccomp" in right_dep and spanroot.lemma_ == "be":
- category = "Cleft construction"
-
- elif "attr" in c_dep:
- category = "Extraposed that-cl (copula)" #eg It is a wonder that X.
-
- else:
- category = "Extraposed that-cl (VERB)"
-
- # if "ccomp" in c_dep and "auxpass" in c_dep and ("it_nsubjpass" in span_t_dep_ or "it_nsubj" in span_t_dep_):
- # category = "Extraposed that-cl (VERB)1" #e.g., it has been shown that X.
- elif ("it_nsubjpass" in c_t_dep_ or "it_nsubj" in c_t_dep_) and "acomp" in c_dep:
- if "xcomp" in c_dep:
- if _check_to:
- category = "Extraposed to-cl 2" #eg it is difficult to decide.
- elif _check_ing:
- category = "Extraposed -ing 2" #eg it is difficult to decide.
-
- else:
- category = "Extraposed that-cl (adj-complement) 2"
-
- elif ("it_nsubjpass" in c_t_dep_ or "it_nsubj" in c_t_dep_) and "oprd" in c_dep:
-
- category = "Extraposed that-cl (adj-complement) 3" #eg it seems odd that X.
-
-
- # something without dummy subject "it"
- elif (("nsubj" in c_dep and spanroot.lemma_ in ['be']) or "nsubjpass" in c_dep) and spanroot.pos_ in ["AUX", 'VERB'] and "it" not in c_norm:
-
- # store xcomp, if the head of the xcomp is acomp
- _check_xcomp = [c.dep_ for c in spanroot.subtree if c.dep_ in ["xcomp"] and c.head.dep_ == "acomp"]
- _check_ccomp = [c.dep_ for c in spanroot.subtree if c.dep_ in ["ccomp"] and c.head.dep_ == "acomp"]
- # _check_to = [c.dep_ for c in spanroot.subtree if (c.dep_ in ["aux"] and c.pos_ in ["PART", "SCONJ"]) and c.head.dep_ == "xcomp"]
- # _check_ing = [c.dep_ for c in spanroot.subtree if "Prog" in str(c.morph) and c.dep_ == "xcomp"]
-
-
- if ("attr" in c_dep or "acomp" in c_dep) and "ccomp" in c_dep:
- if any(root_before_ccomp):
- category = "Post-predicate that-cl"
- else:
- category = "Comment clause"
-
- elif ("attr" in c_dep or "acomp" in c_dep) and "ccomp" in _check_ccomp:
- category = "Post-predicate that-cl 2"
-
- elif ("attr" in c_dep or "acomp" in c_dep) and "xcomp" in _check_xcomp:
- category = "Post-predicate to-cl"
-
- elif "xcomp" in c_dep and spanroot.lemma_ in ['be'] and _check_to:
- category = "Subject predicate to-cl"
-
- elif "xcomp" in c_dep and "auxpass" in c_dep and _check_to:
- category = "Subject predicate to-cl (passive)"
-
- elif "xcomp" in c_dep and spanroot.lemma_ in ['be'] and _check_ing:
- category = "Subject predicate -ing"
- elif "ccomp" in c_dep:
- category = "Subject predicate that-cl"
- elif "acomp" in c_dep:
- category = "Adjectival predicate"
-
- elif "mark" in c_dep and ("nsubj" in c_dep or "nsubjpass" in c_dep):
- category = "Finite-adverbial clause"
- elif not argmentless and "SCONJ" in c_pos:
- category = "Finite-adverbial clause"
- else:
- category = "Main verb 1"
-
- ## without dummy subject it, and lexical verbs
- elif ("nsubj" in c_dep or "nsubjpass" in c_dep) in c_dep and spanroot.pos_ in ["AUX", 'VERB'] and "it" not in c_norm and spanroot.lemma_ not in ['be']:
- _check_wh = [c.dep_ for c in spanroot.subtree if (c.dep_ in ["attr", "advmod", 'dobj', 'nsubj'] and c.tag_ in ["WP", "WRB", "WDT", "WP$"]) and c.head.dep_ == "ccomp"]
- _check_if = [c.dep_ for c in spanroot.subtree if (c.dep_ in ["mark"] and c.norm_ in ["whether", "if"]) and c.head.dep_ == "ccomp"]
-
- # _check_to = [c.dep_ for c in spanroot.subtree if (c.dep_ in ["aux"] and c.pos_ in ["PART", "SCONJ"]) and c.head.dep_ == "xcomp"]
- # _check_ing = [c.dep_ for c in spanroot.subtree if "Prog" in str(c.morph) and c.dep_ == "xcomp"]
-
- if "ccomp" in c_dep and (_check_wh or _check_if):
- category = "Post-predicate wh-cl"
-
- elif "ccomp" in c_dep:
- if any(root_before_ccomp):
- category = "Post-predicate that-cl"
- else:
- category = "Comment clause"
-
- elif "xcomp" in c_dep:
- if _check_to:
- category = "Post-predicate to-cl"
- elif _check_ing:
- category = "Post-predicate -ing"
-
-
-
- # Existential
- elif "expl" in c_dep and "NOUN" in c_pos and "mark" not in c_dep:
- category = "There is/are NOUN"
-
- elif "ccomp" in c_dep and "it_nsubj" in span_t_dep_ and spanroot.pos_ in ["AUX"]:
- category = "Cleft construction"
-
- ### The end of clausal analysis
-
- if spanroot.dep_ in ['parataxis']:
- if "_".join(span_dep) in ["nsubj_parataxis", "aux_parataxis", "nsubj_aux_parataxis"]:
- category = "Comment clause"
- else:
- category = "Parataxis"
-
-
- if spanroot.dep_ in ['dep', "csubj", 'csubjpass']:
- if spanroot.head.dep_ in ['ROOT', 'ccomp'] and spanroot.head.pos_ in ['AUX', 'VERB'] and spanroot.pos_ in ['AUX', 'VERB']:
- if spanroot.morph == spanroot.head.morph:
- category = "Main verb 4"
- else:
- category = "Dependent verb 2"
- elif str(spanroot.morph) == "Aspect=Prog|Tense=Pres|VerbForm=Part":
- category = "Gerund"
- elif "VerbForm=Fin" in str(spanroot.morph) or "VerbForm=Inf" in str(spanroot.morph):
- category = "Dependent verb 2"
- elif spanroot.dep_ in ["csubj", 'csubjpass']:
- category = "Dependent verb (csubj)"
-
-
- # Appositive phrases
- if spanroot.dep_ in ['appos']:
- if "nummod" in c_dep:
- category = "Apposition"
- if spanroot.pos_ in ["PROPN"]:
- category = "Appositive Proper Nouns"
- elif spanroot.pos_ in ["NOUN"]:
- category = "Appositive Noun Phrase"
- elif spanroot.pos_ in ["VERB", "AUX"]:
- _check = any(c.dep_ in ['nsubj', 'nsubjpass', 'csubj', 'csubjpass'] for c in spanroot.children)
- if _check:
- category = "Appositive Finite-clause"
-
-
- if spanroot.dep_ in ['appos', "dep", "attr"]:
- if not subjless and spanroot.pos_ in ['VERB', "AUX"]:
- category = "Main verb (likely parsing error)"
-
- #sometimes the dep are on the conjunctions
- if spanroot.dep_ in ["dep", "mark"]:
- if spanroot.tag_ in ["RB", "IN", "CC"]:
- category = "Conjunction"
-
- if spanroot.dep_ in ["intj"]:
- category = "Introjection"
-
-
- #sometimes the extra-clausal links are not accurate
- if spanroot.dep_ in ['aux', "auxpass", 'oprd', 'appos', "xcomp", "attr", 'dep', "meta", 'prt'] and category == None:
- if spanroot.head.dep_ == "ROOT":
- category = "Main verb"
- else:
- category = "dependent verb 5"
-
- if span.label_ == "CITATION":
- if "NNP" in span_tag or "NNPS" in span_tag:
- if span_dep[0] == 'punct' and span_dep[-1] == 'punct':
- category = "Parenthetical Citation"
- elif span_tag[0] in ["NNP", "NNPS"]:
- category = "Narrative Citation"
- else:
- category = "Other Citation"
-
- if category == None:
- category = spanroot.dep_
-
- return category
-
-
-
-def const_table(doc: Union[spacy.tokens.Doc, Dict[str, str]],
- spans_key: str = "sc",
- attrs: List[str] = SPAN_ATTRS):
- columns = attrs + ["Conf. score", "sent no.", "grammatical realization", 'span dep', "ner",
- "POS", 'span dep seq', "TAG sequence", "POS sequence", "head", "head dep", "children", "morphology", "sent"]
- data = []
- # data = span_info_aggregator(doc, columns)
- sentences = {s: i for i, s in enumerate(doc.sents)}
-
- for span, score in zip(doc.spans[spans_key], doc.spans[spans_key].attrs['scores']):
-
- span_info = []
- span_info.extend([str(getattr(span, attr)) for attr in attrs])
-
- span_info.append(score)
- span_info.append(int(sentences[span.sent]))
- span_info.append(construction_classifier2(doc, span))
- span_info.append(span.root.dep_)
- span_info.append(span.root.ent_type_)
- span_info.append(span.root.tag_)
- span_info.append("_".join([t.dep_ for t in span]))
- span_info.append("_".join([t.tag_ for t in span]))
- span_info.append("_".join([t.pos_ for t in span]))
- span_info.append(span.root.head.norm_)
- span_info.append(span.root.head.dep_)
- span_info.append("_".join([c.dep_ for c in span.root.children]))
- span_info.append(span.root.morph)
- span_info.append(span.sent.text.strip())
-
- data.append(span_info)
-
- return data, columns
-
-
-def ngrammar(seq: list, n=2, concat = False, sep = "-"):
- result = []
- n_item = len(seq)
- for idx, item in enumerate(seq):
- if idx + n <= n_item:
- if concat:
- result.append(sep.join(seq[idx: idx + n]))
- else:
- result.append(seq[idx: idx + n])
- return result
-
-
-def diversity_values(count_vec: list):
- result = {}
- if len(count_vec) == 0:
- count_vec = [0,0,0,0,0,0,0,0,0,0]
-
- result['shannon'] = dv.alpha.shannon(list(count_vec), base=2)
- result['brillouin_d'] = dv.alpha.brillouin_d(list(count_vec))
- result["simpson_d"] = 1- dv.alpha.simpson(list(count_vec))
- result['simpson_e'] = dv.alpha.simpson_e(list(count_vec))
- # result['gini_index'] = dv.alpha.gini_index(list(count_vec))
- # result['faith_pd'] = dv.alpha.faith_pd(list(count_vec))
-
- return result
diff --git a/spaces/emc348/faces-through-time/align_all_parallel.py b/spaces/emc348/faces-through-time/align_all_parallel.py
deleted file mode 100644
index a27909fb6f724d74365f9c2ee79b6c242cbf3c49..0000000000000000000000000000000000000000
--- a/spaces/emc348/faces-through-time/align_all_parallel.py
+++ /dev/null
@@ -1,240 +0,0 @@
-"""
-brief: face alignment with FFHQ method (https://github.com/NVlabs/ffhq-dataset)
-author: lzhbrian (https://lzhbrian.me)
-date: 2020.1.5
-note: code is heavily borrowed from
- https://github.com/NVlabs/ffhq-dataset
- http://dlib.net/face_landmark_detection.py.html
-
-requirements:
- apt install cmake
- conda install Pillow numpy scipy
- pip install dlib
- # download face landmark model from:
- # http://dlib.net/files/shape_predictor_68_face_landmarks.dat.bz2
-"""
-from argparse import ArgumentParser
-import time
-import numpy as np
-import PIL
-import PIL.Image
-import os
-import scipy
-import scipy.ndimage
-import dlib
-import multiprocessing as mp
-import math
-
-
-SHAPE_PREDICTOR_PATH = "shape_predictor_68_face_landmarks.dat"
-
-
-def get_landmark(filepath, predictor, i=None):
- """get landmark with dlib
- :return: np.array shape=(68, 2)
- """
- detector = dlib.get_frontal_face_detector()
-
- img = dlib.load_rgb_image(filepath)
- dets = detector(img, 1)
-
- #for k, d in enumerate(dets):
- if i is None:
- i = len(dets) - 1
- try:
- shape = predictor(img, dets[i])
- except IndexError:
- print("Face not found")
- return
- t = list(shape.parts())
- a = []
- for tt in t:
- a.append([tt.x, tt.y])
- lm = np.array(a)
- return lm
-
-
-def align_face(filepath, predictor, idx=None):
- """
- :param filepath: str
- :return: PIL Image
- """
-
- lm = get_landmark(filepath, predictor, i=idx)
-
- lm_chin = lm[0:17] # left-right
- lm_eyebrow_left = lm[17:22] # left-right
- lm_eyebrow_right = lm[22:27] # left-right
- lm_nose = lm[27:31] # top-down
- lm_nostrils = lm[31:36] # top-down
- lm_eye_left = lm[36:42] # left-clockwise
- lm_eye_right = lm[42:48] # left-clockwise
- lm_mouth_outer = lm[48:60] # left-clockwise
- lm_mouth_inner = lm[60:68] # left-clockwise
-
- # Calculate auxiliary vectors.
- eye_left = np.mean(lm_eye_left, axis=0)
- eye_right = np.mean(lm_eye_right, axis=0)
- eye_avg = (eye_left + eye_right) * 0.5
- eye_to_eye = eye_right - eye_left
- mouth_left = lm_mouth_outer[0]
- mouth_right = lm_mouth_outer[6]
- mouth_avg = (mouth_left + mouth_right) * 0.5
- eye_to_mouth = mouth_avg - eye_avg
-
- # Choose oriented crop rectangle.
- x = eye_to_eye - np.flipud(eye_to_mouth) * [-1, 1]
- x /= np.hypot(*x)
- x *= max(np.hypot(*eye_to_eye) * 2.0, np.hypot(*eye_to_mouth) * 1.8)
- y = np.flipud(x) * [-1, 1]
- c = eye_avg + eye_to_mouth * 0.1
- quad = np.stack([c - x - y, c - x + y, c + x + y, c + x - y])
- qsize = np.hypot(*x) * 2
-
- # read image
- img = PIL.Image.open(filepath)
-
- output_size = 256
- transform_size = 256
- enable_padding = True
-
- # Shrink.
- shrink = int(np.floor(qsize / output_size * 0.5))
- if shrink > 1:
- rsize = (
- int(np.rint(float(img.size[0]) / shrink)),
- int(np.rint(float(img.size[1]) / shrink)),
- )
- img = img.resize(rsize, PIL.Image.ANTIALIAS)
- quad /= shrink
- qsize /= shrink
-
- # Crop.
- border = max(int(np.rint(qsize * 0.1)), 3)
- crop = (
- int(np.floor(min(quad[:, 0]))),
- int(np.floor(min(quad[:, 1]))),
- int(np.ceil(max(quad[:, 0]))),
- int(np.ceil(max(quad[:, 1]))),
- )
- crop = (
- max(crop[0] - border, 0),
- max(crop[1] - border, 0),
- min(crop[2] + border, img.size[0]),
- min(crop[3] + border, img.size[1]),
- )
- if crop[2] - crop[0] < img.size[0] or crop[3] - crop[1] < img.size[1]:
- img = img.crop(crop)
- quad -= crop[0:2]
-
- # Pad.
- pad = (
- int(np.floor(min(quad[:, 0]))),
- int(np.floor(min(quad[:, 1]))),
- int(np.ceil(max(quad[:, 0]))),
- int(np.ceil(max(quad[:, 1]))),
- )
- pad = (
- max(-pad[0] + border, 0),
- max(-pad[1] + border, 0),
- max(pad[2] - img.size[0] + border, 0),
- max(pad[3] - img.size[1] + border, 0),
- )
- if enable_padding and max(pad) > border - 4:
- pad = np.maximum(pad, int(np.rint(qsize * 0.3)))
- img = np.pad(
- np.float32(img), ((pad[1], pad[3]), (pad[0], pad[2]), (0, 0)), "reflect"
- )
- h, w, _ = img.shape
- y, x, _ = np.ogrid[:h, :w, :1]
- mask = np.maximum(
- 1.0 - np.minimum(np.float32(x) / pad[0], np.float32(w - 1 - x) / pad[2]),
- 1.0 - np.minimum(np.float32(y) / pad[1], np.float32(h - 1 - y) / pad[3]),
- )
- blur = qsize * 0.02
- img += (scipy.ndimage.gaussian_filter(img, [blur, blur, 0]) - img) * np.clip(
- mask * 3.0 + 1.0, 0.0, 1.0
- )
- img += (np.median(img, axis=(0, 1)) - img) * np.clip(mask, 0.0, 1.0)
- img = PIL.Image.fromarray(np.uint8(np.clip(np.rint(img), 0, 255)), "RGB")
- quad += pad[:2]
-
- # Transform.
- img = img.transform(
- (transform_size, transform_size),
- PIL.Image.QUAD,
- (quad + 0.5).flatten(),
- PIL.Image.BILINEAR,
- )
- if output_size < transform_size:
- img = img.resize((output_size, output_size), PIL.Image.ANTIALIAS)
-
- # Save aligned image.
- return img
-
-
-def chunks(lst, n):
- """Yield successive n-sized chunks from lst."""
- for i in range(0, len(lst), n):
- yield lst[i : i + n]
-
-
-def extract_on_paths(file_paths):
- predictor = dlib.shape_predictor(SHAPE_PREDICTOR_PATH)
- pid = mp.current_process().name
- print(f"\t{pid} is starting to extract on #{len(file_paths)} images")
- tot_count = len(file_paths)
- count = 0
- for file_path, res_path in file_paths:
- count += 1
- if count % 100 == 0:
- print(f"{pid} done with {count}/{tot_count}")
- try:
- res = align_face(file_path, predictor)
- res = res.convert("RGB")
- os.makedirs(os.path.dirname(res_path), exist_ok=True)
- res.save(res_path)
- except Exception:
- continue
- print("\tDone!")
-
-
-def parse_args():
- parser = ArgumentParser(add_help=False)
- parser.add_argument("--num_threads", type=int, default=1)
- parser.add_argument("--root_path", type=str, default="")
- args = parser.parse_args()
- return args
-
-
-def run(args):
- root_path = args.root_path
- out_crops_path = root_path + "_crops"
- if not os.path.exists(out_crops_path):
- os.makedirs(out_crops_path, exist_ok=True)
-
- file_paths = []
- for root, dirs, files in os.walk(root_path):
- for file in files:
- file_path = os.path.join(root, file)
- fname = os.path.join(out_crops_path, os.path.relpath(file_path, root_path))
- res_path = f"{os.path.splitext(fname)[0]}.jpg"
- if os.path.splitext(file_path)[1] == ".txt" or os.path.exists(res_path):
- continue
- file_paths.append((file_path, res_path))
-
- file_chunks = list(
- chunks(file_paths, int(math.ceil(len(file_paths) / args.num_threads)))
- )
- print(len(file_chunks))
- pool = mp.Pool(args.num_threads)
- print(f"Running on {len(file_paths)} paths\nHere we goooo")
- tic = time.time()
- pool.map(extract_on_paths, file_chunks)
- toc = time.time()
- print(f"Mischief managed in {str(toc - tic)}s")
-
-
-if __name__ == "__main__":
- args = parse_args()
- run(args)
diff --git a/spaces/evaluate-metric/exact_match/README.md b/spaces/evaluate-metric/exact_match/README.md
deleted file mode 100644
index 21dc1b801a3c6691d3c234b57fb94b12b416ad0b..0000000000000000000000000000000000000000
--- a/spaces/evaluate-metric/exact_match/README.md
+++ /dev/null
@@ -1,119 +0,0 @@
----
-title: Exact Match
-emoji: 🤗
-colorFrom: blue
-colorTo: red
-sdk: gradio
-sdk_version: 3.19.1
-app_file: app.py
-pinned: false
-tags:
-- evaluate
-- metric
-description: >-
- Returns the rate at which the input predicted strings exactly match their references, ignoring any strings input as part of the regexes_to_ignore list.
----
-
-# Metric Card for Exact Match
-
-
-## Metric Description
-A given predicted string's exact match score is 1 if it is the exact same as its reference string, and is 0 otherwise.
-
-- **Example 1**: The exact match score of prediction "Happy Birthday!" is 0, given its reference is "Happy New Year!".
-- **Example 2**: The exact match score of prediction "The Colour of Magic (1983)" is 1, given its reference is also "The Colour of Magic (1983)".
-
-The exact match score of a set of predictions is the sum of all of the individual exact match scores in the set, divided by the total number of predictions in the set.
-
-- **Example**: The exact match score of the set {Example 1, Example 2} (above) is 0.5.
-
-
-## How to Use
-At minimum, this metric takes as input predictions and references:
-```python
->>> from evaluate import load
->>> exact_match_metric = load("exact_match")
->>> results = exact_match_metric.compute(predictions=predictions, references=references)
-```
-
-### Inputs
-- **`predictions`** (`list` of `str`): List of predicted texts.
-- **`references`** (`list` of `str`): List of reference texts.
-- **`regexes_to_ignore`** (`list` of `str`): Regex expressions of characters to ignore when calculating the exact matches. Defaults to `None`. Note: the regex changes are applied before capitalization is normalized.
-- **`ignore_case`** (`bool`): If `True`, turns everything to lowercase so that capitalization differences are ignored. Defaults to `False`.
-- **`ignore_punctuation`** (`bool`): If `True`, removes punctuation before comparing strings. Defaults to `False`.
-- **`ignore_numbers`** (`bool`): If `True`, removes all digits before comparing strings. Defaults to `False`.
-
-
-### Output Values
-This metric outputs a dictionary with one value: the average exact match score.
-
-```python
-{'exact_match': 1.0}
-```
-
-This metric's range is 0-1, inclusive. Here, 0.0 means no prediction/reference pairs were matches, while 1.0 means they all were.
-
-#### Values from Popular Papers
-The exact match metric is often included in other metrics, such as SQuAD. For example, the [original SQuAD paper](https://nlp.stanford.edu/pubs/rajpurkar2016squad.pdf) reported an Exact Match score of 40.0%. They also report that the human performance Exact Match score on the dataset was 80.3%.
-
-### Examples
-Without including any regexes to ignore:
-```python
->>> exact_match = evaluate.load("exact_match")
->>> refs = ["the cat", "theater", "YELLING", "agent007"]
->>> preds = ["cat?", "theater", "yelling", "agent"]
->>> results = exact_match.compute(references=refs, predictions=preds)
->>> print(round(results["exact_match"], 2))
-0.25
-```
-
-Ignoring regexes "the" and "yell", as well as ignoring case and punctuation:
-```python
->>> exact_match = evaluate.load("exact_match")
->>> refs = ["the cat", "theater", "YELLING", "agent007"]
->>> preds = ["cat?", "theater", "yelling", "agent"]
->>> results = exact_match.compute(references=refs, predictions=preds, regexes_to_ignore=["the ", "yell"], ignore_case=True, ignore_punctuation=True)
->>> print(round(results["exact_match"], 2))
-0.5
-```
-Note that in the example above, because the regexes are ignored before the case is normalized, "yell" from "YELLING" is not deleted.
-
-Ignoring "the", "yell", and "YELL", as well as ignoring case and punctuation:
-```python
->>> exact_match = evaluate.load("exact_match")
->>> refs = ["the cat", "theater", "YELLING", "agent007"]
->>> preds = ["cat?", "theater", "yelling", "agent"]
->>> results = exact_match.compute(references=refs, predictions=preds, regexes_to_ignore=["the ", "yell", "YELL"], ignore_case=True, ignore_punctuation=True)
->>> print(round(results["exact_match"], 2))
-0.75
-```
-
-Ignoring "the", "yell", and "YELL", as well as ignoring case, punctuation, and numbers:
-```python
->>> exact_match = evaluate.load("exact_match")
->>> refs = ["the cat", "theater", "YELLING", "agent007"]
->>> preds = ["cat?", "theater", "yelling", "agent"]
->>> results = exact_match.compute(references=refs, predictions=preds, regexes_to_ignore=["the ", "yell", "YELL"], ignore_case=True, ignore_punctuation=True, ignore_numbers=True)
->>> print(round(results["exact_match"], 2))
-1.0
-```
-
-An example that includes sentences:
-```python
->>> exact_match = evaluate.load("exact_match")
->>> refs = ["The cat sat on the mat.", "Theaters are great.", "It's like comparing oranges and apples."]
->>> preds = ["The cat sat on the mat?", "Theaters are great.", "It's like comparing apples and oranges."]
->>> results = exact_match.compute(references=refs, predictions=preds)
->>> print(round(results["exact_match"], 2))
-0.33
-```
-
-
-## Limitations and Bias
-This metric is limited in that it outputs the same score for something that is completely wrong as for something that is correct except for a single character. In other words, there is no award for being *almost* right.
-
-## Citation
-
-## Further References
-- Also used in the [SQuAD metric](https://github.com/huggingface/datasets/tree/master/metrics/squad)
diff --git a/spaces/facebook/ov-seg/open_vocab_seg/evaluation/__init__.py b/spaces/facebook/ov-seg/open_vocab_seg/evaluation/__init__.py
deleted file mode 100644
index b9d36d8e9659a1d31471273a6a0f82c2642ea982..0000000000000000000000000000000000000000
--- a/spaces/facebook/ov-seg/open_vocab_seg/evaluation/__init__.py
+++ /dev/null
@@ -1,4 +0,0 @@
-# Copyright (c) Facebook, Inc. and its affiliates.
-# Copyright (c) Meta Platforms, Inc. All Rights Reserved
-
-from .generalized_sem_seg_evaluation import GeneralizedSemSegEvaluator
diff --git a/spaces/fatiXbelha/sd/BrotatoPremium - The Ultimate Action Game with Potatoes and Aliens - Download on PC and Mac.md b/spaces/fatiXbelha/sd/BrotatoPremium - The Ultimate Action Game with Potatoes and Aliens - Download on PC and Mac.md
deleted file mode 100644
index 03ea88fe84daef395c3da9156ab564f4ae3f8c19..0000000000000000000000000000000000000000
--- a/spaces/fatiXbelha/sd/BrotatoPremium - The Ultimate Action Game with Potatoes and Aliens - Download on PC and Mac.md
+++ /dev/null
@@ -1,121 +0,0 @@
-
-
How to Download Brotato
-
If you are looking for a fun and addictive game that will challenge your skills and creativity, you should try Brotato. Brotato is a top-down arena shooter roguelite where you play as a potato wielding up to 6 weapons at a time to fight off hordes of aliens. You can choose from a variety of traits and items to create unique builds and survive until help arrives. In this article, we will show you how to download Brotato on different platforms and why you should give it a shot.
-
What is Brotato?
-
Brotato is a game developed by Blobfish, an indie studio that specializes in making action-packed games with quirky characters and humor. The game was released in September 2022 as an Early Access title on Steam, and has received overwhelmingly positive reviews from players and critics alike. The game is also available on mobile devices, both Android and iOS, for free with optional in-app purchases.
The game features a simple but engaging gameplay that will keep you hooked for hours. You play as Brotato, the sole survivor of a spaceship crash from Potato World. You have to fend off waves of aliens using up to 6 weapons at a time, which you can switch and combine as you please. You can also collect materials, experience, and potatoes (the currency of the game) to buy items and upgrade your character between waves. The game has 30 characters, 150 items, 40+ weapons, 20-waves runs, and 5 difficulty levels to choose from.
-
Why Download Brotato?
-
Brotato is not just another shooter game. It has many features that make it stand out from the crowd and offer a unique gaming experience. Here are some of the reasons why you should download Brotato:
-
PC
-
-
You can enjoy the game in full HD graphics and smooth performance on your PC.
-
You can use your keyboard and mouse or a controller to play the game according to your preference.
-
You can access the Steam community features, such as achievements, leaderboards, cloud saves, and more.
-
You can support the developer by buying the game for a reasonable price ($4.99) or by purchasing the bundles that include other games by Blobfish.
-
You can participate in the development process by giving feedback and suggestions on the Steam forums or the Discord server.
-
You can customize your game with modding support (coming soon).
-
-
Mobile
-
-
You can play the game anytime and anywhere on your mobile device.
-
You can enjoy the same gameplay and content as the PC version on your phone or tablet.
-
You can use touch controls or connect a controller to your device for more comfort.
-
You can download the game for free and play without ads or internet connection.
-
You can make optional in-app purchases to unlock more characters, items, weapons, or support the developer.
-
You can share your progress and achievements with your friends on social media.
-
-
How to Download Brotato on PC
-
If you want to play Brotato on your PC, there are two main ways to do so: downloading it from Steam or from other sources. Here are the steps for each method:
-
How to download brotato on PC
-How to download brotato on Android
-How to download brotato premium version
-How to download brotato for free
-How to download brotato on Steam
-How to download brotato on Mac
-How to download brotato on Chromebook
-How to download brotato on iOS
-How to download brotato on Windows 10
-How to download brotato on Linux
-How to play brotato online
-How to play brotato offline
-How to play brotato with friends
-How to play brotato with controller
-How to play brotato with keyboard and mouse
-How to install brotato on PC
-How to install brotato on Android
-How to install brotato on Mac
-How to install brotato on Chromebook
-How to install brotato on iOS
-How to update brotato on PC
-How to update brotato on Android
-How to update brotato on Mac
-How to update brotato on Chromebook
-How to update brotato on iOS
-Brotato game review
-Brotato game tips and tricks
-Brotato game cheats and hacks
-Brotato game best characters and weapons
-Brotato game best items and traits
-Brotato game system requirements
-Brotato game modding support
-Brotato game endless mode
-Brotato game achievements and leaderboards
-Brotato game bugs and fixes
-Brotato vs other roguelite games
-Brotato vs other potato games
-Brotato vs other alien games
-Brotato vs other shooter games
-Brotato vs other action games
-
The steps to download Brotato from Steam
-
-
Go to [Brotato's page](^1^) on Steam.
-
Click on the "Add to Cart" button or the "Buy Brotato" button if you want to buy it directly.
-
Follow the instructions to complete the payment and confirm your purchase.
-
Go to your Steam library and find Brotato in your list of games.
-
Click on the "Install" button and wait for the download to finish.
-
Click on the "Play" button and enjoy the game.
-
-
The steps to download Brotato from other sources
-
-
Go to [Brotato's website] and click on the "Download" button.
-
Select the platform of your choice (Windows, Mac, or Linux) and click on the "Download Now" button.
-
You will be redirected to a page where you can choose to pay what you want for the game or download it for free.
-
If you choose to pay, enter the amount you want to donate and click on the "Pay with Card" or "Pay with PayPal" button. Follow the instructions to complete the payment and confirm your purchase.
-
If you choose to download for free, enter your email address and click on the "No thanks, just take me to the downloads" link. You will receive an email with a link to download the game.
-
Click on the link in the email and download the game file to your PC.
-
Extract the file and run the Brotato.exe file to launch the game.
-
-
How to Download Brotato on Mobile
-
If you want to play Brotato on your mobile device, there are two main ways to do so: downloading it from Google Play Store or from App Store. Here are the steps for each method:
-
The steps to download Brotato from Google Play Store
-
-
Go to [Brotato's page] on Google Play Store.
-
Click on the "Install" button and wait for the download to finish.
-
Open the game and grant the necessary permissions.
-
Enjoy the game.
-
-
The steps to download Brotato from App Store
-
-
Go to [Brotato's page] on App Store.
-
Click on the "Get" button and enter your Apple ID and password if prompted.
-
Wait for the download to finish and open the game.
-
Enjoy the game.
-
-
Conclusion
-
Brotato is a game that will keep you entertained and challenged for hours. It has a simple but addictive gameplay, a variety of characters, items, weapons, and difficulty levels, and a charming art style and humor. You can play it on your PC or mobile device, depending on your preference. You can also support the developer by buying the game or making in-app purchases. If you are looking for a fun and creative game that will make you feel like a potato hero, you should download Brotato today. You won't regret it!
-
Frequently Asked Questions
-
-
Q: How long is a run in Brotato?
-
A: A run in Brotato consists of 20 waves of enemies, each one harder than the previous one. You can choose from 5 difficulty levels: Easy, Normal, Hard, Insane, and Nightmare. The higher the difficulty, the more enemies, damage, and rewards you will encounter.
-
Q: How do I switch and combine weapons in Brotato?
-
A: You can switch weapons by pressing Q or E on PC, or by swiping left or right on mobile. You can combine weapons by pressing R on PC, or by tapping on both weapons at once on mobile. You can have up to 6 weapons at a time, but some combinations may not work well together. Experiment with different weapons and find your favorite ones.
-
Q: What are traits and items in Brotato?
-
A: Traits are passive abilities that affect your character's stats and performance. You can choose one trait at the start of each run, and unlock more traits as you level up. Items are active or passive effects that you can buy or find during a run. They can give you various benefits, such as health regeneration, damage boost, shield protection, etc. You can have up to 6 items at a time, but some items may have negative effects as well.
-
Q: How do I unlock more characters in Brotato?
-
A: You can unlock more characters by completing certain achievements or challenges in the game. For example, you can unlock Broccoli by beating the game on Hard difficulty, or Carrot by killing 1000 enemies with a knife. Each character has a different appearance, voice, and starting weapon.
-
Q: How do I save and load my progress in Brotato?
-
A: You can save and load your progress in Brotato by using the menu options in the game. On PC, you can press Esc to access the menu, and on mobile, you can tap on the pause button at the top right corner of the screen. You can save your progress at any time during a run, and load it from the main menu. You can also use the cloud save feature to sync your progress across different devices.
-
197e85843d
-
-
\ No newline at end of file
diff --git a/spaces/fatiXbelha/sd/CarX Drift Racing 2 Mod APK for PC Download and Install Guide.md b/spaces/fatiXbelha/sd/CarX Drift Racing 2 Mod APK for PC Download and Install Guide.md
deleted file mode 100644
index bc708b25c6983b18474990cbed9f8d95b9431f3d..0000000000000000000000000000000000000000
--- a/spaces/fatiXbelha/sd/CarX Drift Racing 2 Mod APK for PC Download and Install Guide.md
+++ /dev/null
@@ -1,128 +0,0 @@
-
-
CarX Drift Racing 2 Mod APK for PC: How to Download and Play
-
Do you love drifting games? Do you want to experience the thrill of drifting on your PC? If yes, then you should try CarX Drift Racing 2 Mod APK for PC. This is a modified version of the popular racing game CarX Drift Racing 2, which allows you to enjoy unlimited money, cars, tracks, and more. In this article, we will show you how to download and install CarX Drift Racing 2 Mod APK for PC, as well as how to play it with the best performance and graphics.
-
What is CarX Drift Racing 2?
-
CarX Drift Racing 2 is a racing game developed by CarX Technologies, LLC. It is the sequel of the most desired drift-game with over 100 million fans around the world. It is your chance to immerse yourself in the real world of drifting, with realistic driving physics, detailed customization and tuning of car parameters, a number of cities and special racing track locations, an array of vinyls to design the look of your vehicle, open online rooms and competitions enhanced with new graphics.
New game mode with XDS, designed to help practice tandem drifting;
-
New physics model for each car;
-
Original engine sound for every car;
-
Tuning mode with over 1000 parts;
-
Body kits with over 40 unique cars;
-
Live cameras and replays;
-
Online championship and leaderboards;
-
Ghost mode for competing with your best race;
-
Career mode with over 100 missions;
-
Customizable controls and interface.
-
-
Benefits of CarX Drift Racing 2 Mod APK
-
CarX Drift Racing 2 Mod APK is a modified version of the original game that gives you some extra benefits, such as:
-
-
Unlimited money to buy and upgrade any car you want;
-
All cars unlocked from the start;
-
All tracks unlocked from the start;
-
No ads or in-app purchases;
-
No root or jailbreak required.
-
-
How to Download and Install CarX Drift Racing 2 Mod APK for PC
-
There are two methods to download and install CarX Drift Racing 2 Mod APK for PC. The first one is using Windows 11 and Amazon Appstore, which is the official way to run Android apps on Windows. The second one is using an Android emulator, which is a software that emulates an Android device on your PC. We will explain both methods below.
-
Method 1: Using Windows 11 and Amazon Appstore
-
This method requires you to have Windows 11 installed on your PC, which is the latest version of Windows that supports native Android emulation. You also need to have an Amazon account to access the Amazon Appstore, which is where you can download Car X Drift Racing 2 Mod APK. The second method is using an Android emulator, which is a software that emulates an Android device on your PC. We will explain both methods below.
-
Method 2: Using an Android Emulator
-
This method requires you to download and install an Android emulator on your PC, which can run any Android app or game. There are many Android emulators available for Windows, but some of the best ones are BlueStacks, NoxPlayer, MEmu, and LDPlayer. Here are the steps to use this method:
-
Step 1: Download and Install an Android Emulator
-
Choose an Android emulator that suits your needs and preferences, and download it from its official website. For example, you can download BlueStacks from here. Then, follow the instructions to install it on your PC. You may need to enable virtualization on your PC for better performance. For more info, go to Enable virtualization on Windows 11 PCs.
-
Step 2: Download CarX Drift Racing 2 Mod APK File
-
Once you have installed the Android emulator, you need to download the CarX Drift Racing 2 Mod APK file from a reliable source. For example, you can download it from here. Make sure you scan the file for viruses before opening it.
-
Step 3: Install and Run CarX Drift Racing 2 Mod APK on Emulator
-
After downloading the CarX Drift Racing 2 Mod APK file, you need to install it on the emulator. There are two ways to do this:
-
carx drift racing 2 mod apk for pc download
-carx drift racing 2 mod apk for pc windows 10
-carx drift racing 2 mod apk for pc bluestacks
-carx drift racing 2 mod apk for pc free
-carx drift racing 2 mod apk for pc online
-carx drift racing 2 mod apk for pc latest version
-carx drift racing 2 mod apk for pc nox
-carx drift racing 2 mod apk for pc unlimited money
-carx drift racing 2 mod apk for pc ldplayer
-carx drift racing 2 mod apk for pc offline
-carx drift racing 2 mod apk for pc full version
-carx drift racing 2 mod apk for pc windows 7
-carx drift racing 2 mod apk for pc gameplay
-carx drift racing 2 mod apk for pc android
-carx drift racing 2 mod apk for pc emulator
-carx drift racing 2 mod apk for pc hack
-carx drift racing 2 mod apk for pc review
-carx drift racing 2 mod apk for pc steam
-carx drift racing 2 mod apk for pc mac
-carx drift racing 2 mod apk for pc reddit
-carx drift racing 2 mod apk for pc update
-carx drift racing 2 mod apk for pc requirements
-carx drift racing 2 mod apk for pc cheats
-carx drift racing 2 mod apk for pc install
-carx drift racing 2 mod apk for pc guide
-carx drift racing 2 mod apk for pc tips
-carx drift racing 2 mod apk for pc best cars
-carx drift racing 2 mod apk for pc settings
-carx drift racing 2 mod apk for pc tutorial
-carx drift racing 2 mod apk for pc controller support
-carx drift racing 2 mod apk for pc multiplayer mode
-carx drift racing 2 mod apk for pc graphics quality
-carx drift racing 2 mod apk for pc new cars
-carx drift racing 2 mod apk for pc system requirements
-carx drift racing 2 mod apk for pc how to play
-carx drift racing 2 mod apk for pc keyboard controls
-carx drift racing 2 mod apk for pc features
-carx drift racing 2 mod apk for pc screenshots
-carx drift racing 2 mod apk for pc video
-carx drift racing 2 mod apk for pc trailer
-
-
Drag and drop the APK file onto the emulator window, and wait for it to install automatically.
-
Open the emulator, go to Settings > Security > Unknown Sources, and enable it. Then, go to File Manager > Downloads, and tap on the APK file to install it.
-
-
Once the installation is complete, you can launch CarX Drift Racing 2 Mod APK from the emulator's app drawer or home screen.
-
How to Play CarX Drift Racing 2 Mod APK on PC
-
Now that you have installed CarX Drift Racing 2 Mod APK on your PC, you can start playing it with ease. Here are some tips and tricks for playing CarX Drift Racing 2 Mod APK on PC:
-
Tips and Tricks for Playing CarX Drift Racing 2 Mod APK on PC
-
-
Use the keyboard and mouse to control your car. You can customize the key mapping according to your preference in the emulator settings.
-
Adjust the graphics settings to optimize the game performance and quality. You can choose from low, medium, high, or ultra settings in the game options.
-
Use the Eco Mode feature in some emulators to reduce CPU and RAM usage while playing in the background.
-
Use the Instance Manager feature in some emulators to create multiple instances of the game and play with different accounts or modes.
-
Use the Screen Recorder feature in some emulators to record your gameplay and share it with others.
-
-
Best Drifting Games for PC
-
If you love drifting games, you may also want to check out some of the best drifting games for PC, such as:
-
-
Name
Description
-
Forza Horizon 4
A racing game that features dynamic seasons, open-world exploration, and over 450 cars to choose from.
-
Assetto Corsa
A racing simulator that offers realistic physics, advanced graphics, and a variety of tracks and cars.
-
Dirt Rally 2.0
A rally game that challenges you to drive on different terrains, weather conditions, and locations.
-
Need for Speed Heat
A racing game that lets you customize your car, race against other players, and evade the police.
-
The Crew 2
A racing game that allows you to switch between cars, motorcycles, boats, and planes.
-
-
Conclusion
-
In conclusion, CarX Drift Racing 2 Mod APK for PC is a great way to enjoy drifting games on your computer. You can download and install it using Windows 11 and Amazon Appstore or using an Android emulator. Either way, you will get unlimited money, cars, tracks, and more. You can also play with the best graphics and performance. You can also follow some tips and tricks to improve your drifting skills and have more fun. If you are looking for more drifting games for PC, you can also try some of the best ones we have listed above. We hope you enjoyed this article and found it helpful. Happy drifting!
-
FAQs
-
Here are some frequently asked questions about CarX Drift Racing 2 Mod APK for PC:
-
-
Is CarX Drift Racing 2 Mod APK for PC safe to use?
-
Yes, CarX Drift Racing 2 Mod APK for PC is safe to use as long as you download it from a trusted source and scan it for viruses before installing it. However, you should be aware that using a modded version of the game may violate the terms and conditions of the original game and may result in a ban or suspension of your account. Therefore, use it at your own risk and discretion.
-
Can I play CarX Drift Racing 2 Mod APK for PC online with other players?
-
Yes, you can play CarX Drift Racing 2 Mod APK for PC online with other players who are using the same version of the game. However, you may not be able to play with players who are using the official version of the game or a different version of the modded game. You may also face some compatibility issues or errors while playing online.
-
Can I update CarX Drift Racing 2 Mod APK for PC to the latest version?
-
No, you cannot update CarX Drift Racing 2 Mod APK for PC to the latest version of the game. If you try to do so, you may lose all the modded features and benefits. You may also encounter some errors or bugs while playing the game. Therefore, it is recommended to stick to the version of the modded game that you have downloaded and installed.
-
How can I uninstall CarX Drift Racing 2 Mod APK for PC?
-
You can uninstall CarX Drift Racing 2 Mod APK for PC by following these steps:
-
-
If you are using Windows 11 and Amazon Appstore, go to Settings > Apps > Apps & features, find CarX Drift Racing 2, and click on Uninstall.
-
If you are using an Android emulator, go to Settings > Apps > CarX Drift Racing 2, and tap on Uninstall. Alternatively, you can drag and drop the app icon to the trash bin on the emulator home screen.
-
-
Where can I find more information about CarX Drift Racing 2?
-
You can find more information about CarX Drift Racing 2 by visiting its official website, Facebook page, or YouTube channel. You can also read some reviews and ratings from other players on Google Play Store or App Store.
- 401be4b1e0
-
-
\ No newline at end of file
diff --git a/spaces/feng2022/Time-TravelRephotography/Time_TravelRephotography/op/fused_bias_act.cpp b/spaces/feng2022/Time-TravelRephotography/Time_TravelRephotography/op/fused_bias_act.cpp
deleted file mode 100644
index 02be898f970bcc8ea297867fcaa4e71b24b3d949..0000000000000000000000000000000000000000
--- a/spaces/feng2022/Time-TravelRephotography/Time_TravelRephotography/op/fused_bias_act.cpp
+++ /dev/null
@@ -1,21 +0,0 @@
-#include
-
-
-torch::Tensor fused_bias_act_op(const torch::Tensor& input, const torch::Tensor& bias, const torch::Tensor& refer,
- int act, int grad, float alpha, float scale);
-
-#define CHECK_CUDA(x) TORCH_CHECK(x.type().is_cuda(), #x " must be a CUDA tensor")
-#define CHECK_CONTIGUOUS(x) TORCH_CHECK(x.is_contiguous(), #x " must be contiguous")
-#define CHECK_INPUT(x) CHECK_CUDA(x); CHECK_CONTIGUOUS(x)
-
-torch::Tensor fused_bias_act(const torch::Tensor& input, const torch::Tensor& bias, const torch::Tensor& refer,
- int act, int grad, float alpha, float scale) {
- CHECK_CUDA(input);
- CHECK_CUDA(bias);
-
- return fused_bias_act_op(input, bias, refer, act, grad, alpha, scale);
-}
-
-PYBIND11_MODULE(TORCH_EXTENSION_NAME, m) {
- m.def("fused_bias_act", &fused_bias_act, "fused bias act (CUDA)");
-}
\ No newline at end of file
diff --git a/spaces/feregVcuzo/sanity-test-midi/checkpoint/Beach Buggy Racing 2 A Stunning 3D Kart Racing Game - Download It Today.md b/spaces/feregVcuzo/sanity-test-midi/checkpoint/Beach Buggy Racing 2 A Stunning 3D Kart Racing Game - Download It Today.md
deleted file mode 100644
index 0f8f6d0aec5f9d3cfba0a3bb886a3e1d9ddfc0aa..0000000000000000000000000000000000000000
--- a/spaces/feregVcuzo/sanity-test-midi/checkpoint/Beach Buggy Racing 2 A Stunning 3D Kart Racing Game - Download It Today.md
+++ /dev/null
@@ -1,111 +0,0 @@
-
-
Download Beach Buggy Racing 2 Uptodown: A Fun and Exciting Kart Racing Game
-
If you are looking for a fun and exciting kart racing game that you can play on your Android device, then you should definitely check out Beach Buggy Racing 2. This is a sequel to the popular Beach Buggy Racing, which introduced over 100 million international mobile players to console-style kart racing with a playful offroad twist. With Beach Buggy Racing 2, you can enjoy even more content, upgradeable powerups, new game modes, and online competitions with other players from around the world.
-
But where can you download Beach Buggy Racing 2 for free? The answer is Uptodown, one of the best platforms for downloading Android apps and games. Uptodown is safe, fast, and free, and it offers you access to thousands of apps and games that you can download without any restrictions or limitations. You can also update your apps and games easily with Uptodown, as well as discover new ones that suit your preferences.
In this article, we will tell you more about the features of Beach Buggy Racing 2, how to download it from Uptodown, some tips and tricks to help you win every race, and a review of the game based on our experience. So, if you are ready to join the Beach Buggy Racing League and compete against drivers and cars from around the world, read on!
-
Features of Beach Buggy Racing 2
-
Beach Buggy Racing 2 is a fully 3D off-road kart racing game with amazing physics, detailed cars and characters, and spectacular weapons. It has many features that make it one of the best kart racing games on Android. Here are some of them:
-
-
Spectacular kart racing action with amazing graphics and physics: You can race through Egyptian pyramids, dragon-infested castles, pirate ship wrecks, and experimental alien bio-labs. You can also enjoy realistic driving effects such as water splashes, mud splatters, tire tracks, sparks, smoke, fire, and explosions.
-
Over 45 powerups to discover and upgrade: You can collect and use a variety of powerups that can give you an edge over your rivals or hinder their progress. Some examples are Chain Lightning , Fireball, Oil Slick, Sticky Goo, Tiki Seekers, and more. You can also upgrade your powerups to make them more effective and powerful.
-
14 drivers with unique special abilities: You can choose from a diverse cast of characters, each with their own personality and special ability. Some examples are Rez, who can fire a laser beam from his eyes, McSkelly, who can summon a horde of skeletons, and Beat Bot, who can drop a boombox that blasts music and confuses other racers.
-
Over 55 cars to collect and customize: You can unlock and collect a variety of cars, ranging from monster trucks, muscle cars, lunar rovers, and more. You can also customize your cars with different paint jobs, decals, and accessories.
-
Play against the world in online competitions and tournaments: You can join the Beach Buggy Racing League and compete against other players from around the world in various game modes, such as Daily Races, Weekly Tournaments, Special Events, and more. You can also earn trophies, prizes, and prestige as you climb the leaderboards.
-
-
How to Download Beach Buggy Racing 2 Uptodown
-
Downloading Beach Buggy Racing 2 from Uptodown is very easy and fast. Here are the steps you need to follow:
Click on the green "Download" button. This will start downloading the APK file of the game to your device.
-
Once the download is complete, open the APK file and tap on "Install". This will install the game on your device.
-
Enjoy playing Beach Buggy Racing 2!
-
-
Downloading Beach Buggy Racing 2 from Uptodown has many benefits. Here are some of them:
-
-
Safe: Uptodown scans all the files it hosts with dozens of antivirus engines to ensure they are free of malware and viruses. You can also check the security report of each file before downloading it.
-
Fast: Uptodown has a high-speed server network that ensures fast downloads without interruptions or delays. You can also resume your downloads if they are interrupted for any reason.
-
Free: Uptodown does not charge you anything for downloading apps and games. You can also access all the features and content of Beach Buggy Racing 2 without any in-app purchases or subscriptions.
-
-
Tips and Tricks for Beach Buggy Racing 2
-
Beach Buggy Racing 2 is a fun and exciting game, but it can also be challenging at times. To help you win every race and become the best racer in the league, here are some tips and tricks that you should know:
-
-
Master the drift and powerslide: Drifting and powersliding are essential skills that you need to master in Beach Buggy Racing 2. They allow you to take sharp turns without losing speed or control. To drift or powerslide, you need to tap on the brake button while turning. This will make your car skid sideways and create sparks. The longer you drift or powerslide, the more boost you will get. You can use this boost to accelerate and overtake your opponents.
-
Use the driver's ability at the right time: Each driver has a unique special ability that can give you an advantage in the race. However, you need to use it wisely and at the right time. For example, Rez's laser beam is best used when you have a clear shot at your target, while McSkelly's skeleton horde is best used when you are surrounded by other racers. You also need to consider the cooldown time of each ability, as you cannot use it again until it is fully recharged.
-
Don't fall into the trap of other racers' powerups: Other racers will also use powerups to hinder your progress or attack you. You need to be careful and avoid falling into their traps. For example, if you see an oil slick on the road, steer clear of it or use a powerup that can counter it, such as a shield or a magnet. If you see a fireball coming your way, dodge it or use a powerup that can deflect it, such as a mirror or a bubble.
-
Build the best deck of crazy powerups: Before each race, you can choose which powerups you want to use in your deck . You can choose from over 45 powerups, each with different effects and levels. You can also upgrade your powerups to make them more powerful and effective. You should try to build the best deck of powerups that suits your playstyle and strategy. For example, if you like to be aggressive and attack other racers, you should choose powerups that can deal damage and cause chaos, such as fireballs, rockets, and sticky goo. If you like to be defensive and protect yourself, you should choose powerups that can shield you and help you escape, such as bubbles, magnets, and teleporters.
-
Grab those fast bubbles for extra speed: During the race, you will see some blue bubbles floating in the air. These are fast bubbles that can give you a burst of speed if you grab them. You should try to grab as many fast bubbles as you can, as they can help you gain an edge over your rivals or catch up with them. However, be careful not to crash into obstacles or other racers while trying to get them.
-
Choose the best controls for your preference: Beach Buggy Racing 2 offers you three different control options: tilt, touch, and gamepad. You can choose the one that suits your preference and comfort. Tilt control allows you to steer your car by tilting your device left or right. Touch control allows you to steer your car by tapping on the left or right side of the screen. Gamepad control allows you to use a compatible gamepad to control your car. You can also adjust the sensitivity and calibration of each control option in the settings menu.
-
-
Beach Buggy Racing 2 Review
-
Beach Buggy Racing 2 is a great kart racing game that offers a lot of fun and excitement for players of all ages and skill levels. It has stunning graphics, realistic physics, catchy music, and smooth gameplay. It also has a lot of content, variety, and replay value, thanks to its many powerups, drivers, cars, tracks, and game modes. It is also very easy to play and control, with simple and intuitive controls that anyone can master.
-
However, Beach Buggy Racing 2 is not without its flaws. Some of the drawbacks of the game are its long loading times, frequent ads, occasional bugs and glitches, and unfair matchmaking. Some players may also find the game too easy or too hard, depending on their level of experience and skill. Some players may also prefer a more realistic or serious kart racing game, rather than a cartoonish or whimsical one.
-
download beach buggy racing 2 mod apk
-download beach buggy racing 2 for pc
-download beach buggy racing 2 latest version
-download beach buggy racing 2 hack
-download beach buggy racing 2 android
-download beach buggy racing 2 ios
-download beach buggy racing 2 online
-download beach buggy racing 2 free
-download beach buggy racing 2 unlimited money
-download beach buggy racing 2 game
-download beach buggy racing 2 apk pure
-download beach buggy racing 2 apk data
-download beach buggy racing 2 obb file
-download beach buggy racing 2 from google play
-download beach buggy racing 2 from app store
-download beach buggy racing 2 full version
-download beach buggy racing 2 offline
-download beach buggy racing 2 cheats
-download beach buggy racing 2 tips and tricks
-download beach buggy racing 2 guide
-download beach buggy racing 2 review
-download beach buggy racing 2 gameplay
-download beach buggy racing 2 trailer
-download beach buggy racing 2 update
-download beach buggy racing 2 new features
-download beach buggy racing 2 best cars
-download beach buggy racing 2 best weapons
-download beach buggy racing 2 best tracks
-download beach buggy racing 2 best characters
-download beach buggy racing 2 best settings
-download beach buggy racing 2 multiplayer mode
-download beach buggy racing 2 split screen mode
-download beach buggy racing 2 adventure mode
-download beach buggy racing 2 championship mode
-download beach buggy racing 2 daily challenges mode
-download beach buggy racing 2 secrets and easter eggs
-download beach buggy racing 2 how to unlock all cars
-download beach buggy racing 2 how to unlock all weapons
-download beach buggy racing 2 how to unlock all tracks
-download beach buggy racing 2 how to unlock all characters
-download beach buggy racing 2 how to get more coins and gems
-download beach buggy racing 2 how to get more powerups and boosters
-download beach buggy racing 2 how to get more trophies and stars
-download beach buggy racing 2 how to level up faster and easier
-download beach buggy racing 2 how to win every race and beat every boss
-download beach buggy racing 2 comparison with the first game
-
Overall, Beach Buggy Racing 2 is a highly enjoyable and addictive kart racing game that deserves a try. It is one of the best kart racing games on Android, and it can compete with other popular titles in the genre, such as Mario Kart or Crash Team Racing. It has a rating of 4.4 out of 5 stars on Uptodown, based on over 1000 reviews from users who have downloaded it from there.
-
If you love kart racing games or just want to have some fun and excitement on your Android device, then you should definitely download Beach Buggy Racing 2 Uptodown today and join the Beach Buggy Racing League!
-
Conclusion
-
In conclusion, Beach Buggy Racing 2 is a fun and exciting kart racing game that you can download for free from Uptodown. It has many features that make it one of the best kart racing games on Android, such as spectacular graphics and physics, over 45 powerups to discover and upgrade, 14 drivers with unique special abilities, over 55 cars to collect and customize, and online competitions and tournaments with other players from around the world.
-
To download Beach Buggy Racing 2 from Uptodown, all you need to do is follow these simple steps: go to the Beach Buggy Racing 2 page on Uptodown, click on the green "Download" button, open the APK file and tap on "Install", and enjoy playing Beach Buggy Racing 2!
-
We hope this article has helped you learn more about Beach Buggy Racing 2 and how to download it from Uptodown. We also hope you have enjoyed reading our tips and tricks for Beach Buggy Racing 2, as well as our review of the game based on our experience.
-
So what are you waiting for? Download Beach Buggy Racing 2 Uptodown today and enjoy the fun and excitement of kart racing!
-
FAQs
-
Here are some frequently asked questions about Beach Buggy Racing 2:
-
-
Q1: What are the system requirements for Beach Buggy Racing 2?
-
A1: Beach Buggy Racing 2 requires Android 4.4 or higher and at least 150 MB of free storage space on your device. It also requires a stable internet connection to play online modes and access some features.
-
Q2: How can I play with my friends in Beach Buggy Racing 2?
-
A2: You can play with your friends in Beach Buggy Racing 2 in two ways: online or local. Online mode allows you to join or create a team with your friends and compete against other teams in team races, team tournaments, and team events. You can also chat with your teammates and send them gifts. Local mode allows you to play with up to four friends on the same device using split-screen mode. You can choose from different game modes, such as free-for-all, team race, elimination, and capture the flag.
-
Q3: How can I unlock more cars and drivers in Beach Buggy Racing 2?
-
A3: You can unlock more cars and drivers in Beach Buggy Racing 2 by earning coins, gems, and tickets. Coins are the basic currency that you can use to buy cars and upgrade powerups. Gems are the premium currency that you can use to buy drivers and special cars. Tickets are the special currency that you can use to play the daily spin, where you can win coins, gems, powerups, cars, and drivers. You can earn coins, gems, and tickets by completing races, winning tournaments, opening chests, watching ads, and completing achievements.
-
Q4: How can I customize my own game modes in Beach Buggy Racing 2?
-
A4: You can customize your own game modes in Beach Buggy Racing 2 by using the custom race feature. This feature allows you to choose from different options, such as the track, the number of laps, the number of racers, the powerups, the weather, and the time of day. You can also save your custom race settings and share them with other players.
-
Q5: How can I contact the developer of Beach Buggy Racing 2?
-
A5: You can contact the developer of Beach Buggy Racing 2 by using the feedback feature in the game. This feature allows you to send a message to the developer with your comments, suggestions, questions, or problems. You can also rate and review the game on Uptodown or Google Play Store.
-
401be4b1e0
-
-
\ No newline at end of file
diff --git a/spaces/feregVcuzo/sanity-test-midi/checkpoint/Download Brawl Stars 2021 APK for Android - Latest Version with New Features.md b/spaces/feregVcuzo/sanity-test-midi/checkpoint/Download Brawl Stars 2021 APK for Android - Latest Version with New Features.md
deleted file mode 100644
index 7b70e56d81fa3160abbe73c3872702491f793d74..0000000000000000000000000000000000000000
--- a/spaces/feregVcuzo/sanity-test-midi/checkpoint/Download Brawl Stars 2021 APK for Android - Latest Version with New Features.md
+++ /dev/null
@@ -1,141 +0,0 @@
-
-
Brawl Stars 2021 APK: Everything You Need to Know
-
If you are looking for a fun and exciting game to play on your Android device, you might want to check out Brawl Stars. Brawl Stars is a multiplayer game from Supercell, the makers of Clash of Clans and Clash Royale. In this game, you can team up with your friends or play solo across a variety of game modes in under three minutes. You can also unlock and upgrade dozens of brawlers with powerful abilities, collect unique skins, and battle in a variety of mysterious locations.
In this article, we will tell you everything you need to know about Brawl Stars 2021 APK. We will explain what Brawl Stars is, what an APK file is and why you need it, what's new in Brawl Stars 2021 APK, and some tips and tricks for playing the game. Let's get started!
-
What is Brawl Stars?
-
Brawl Stars is a fast-paced multiplayer game that offers a lot of action and fun. Here are some of the features that make Brawl Stars a great game:
-
A fast-paced multiplayer game from Supercell
-
Brawl Stars is developed by Supercell, the same company that created popular games like Clash of Clans and Clash Royale. Supercell is known for making high-quality games that are easy to play but hard to master. Brawl Stars is no exception. The game has smooth graphics, responsive controls, and addictive gameplay.
-
A variety of game modes and brawlers to choose from
-
Brawl Stars has four main game modes: Smash & Grab, Showdown, Bounty, and Heist. Each game mode has a different objective and requires different strategies. For example, in Smash & Grab, you have to collect and hold 10 gems to win, but if you die, you lose your gems. In Showdown, you have to be the last brawler standing in a battle royale style fight.
-
brawl stars 2021 apk download
-brawl stars 2021 apk mod
-brawl stars 2021 apk update
-brawl stars 2021 apk latest version
-brawl stars 2021 apk hack
-brawl stars 2021 apk free
-brawl stars 2021 apk android
-brawl stars 2021 apk ios
-brawl stars 2021 apk obb
-brawl stars 2021 apk unlimited gems
-brawl stars 2021 apk new brawler
-brawl stars 2021 apk offline
-brawl stars 2021 apk no verification
-brawl stars 2021 apk private server
-brawl stars 2021 apk nulls
-brawl stars 2021 apk original
-brawl stars 2021 apk online
-brawl stars 2021 apk old version
-brawl stars 2021 apk pure
-brawl stars 2021 apk revdl
-brawl stars 2021 apk rexdl
-brawl stars 2021 apk uptodown
-brawl stars 2021 apk mirror
-brawl stars 2021 apk mediafıre
-brawl stars 2021 apk mega
-brawl stars 2021 apk data
-brawl stars 2021 apk file
-brawl stars 2021 apk full
-brawl stars 2021 apk for pc
-brawl stars 2021 apk for bluestacks
-brawl stars 2021 apk gameplay
-brawl stars 2021 apk generator
-brawl stars 2021 apk google play
-brawl stars 2021 apk guide
-brawl stars 2021 apk install
-brawl stars 2021 apk indir
-brawl stars 2021 apk info
-brawl stars 2021 apk link
-brawl stars 2021 apk latest brawler
-brawl stars 2021 apk mod menu
-brawl stars 2021 apk mod unlimited money and gems
-brawl stars 2021 apk mod download
-brawl stars 2021 apk new update
-brawl stars 2021 apk news
-brawl stars 2021 apk october
-brawl stars 2021 apk pro
-brawl stars 2021 apk reddit
-brawl stars 2021 apk review
-brawl stars 2021 apk skins
-brawl stars 2021 apk tips
-
Brawl Stars also has 22 different brawlers that you can unlock and play with. Each brawler has a unique personality, appearance, attack, super ability, star power, and gadget. Some brawlers are better suited for certain game modes than others. For example, El Primo is a tank brawler that can deal a lot of damage up close and take a lot of hits. He is good for Smash & Grab and Heist. Colt is a sharpshooter brawler that can shoot long-range bullets with high accuracy. He is good for Bounty and Showdown.
-
A constantly evolving game with new content and features
-example, the game regularly adds new brawlers, skins, maps, and events that spice up the gameplay and offer new challenges and rewards. The game also updates its balance changes and bug fixes to ensure a fair and smooth gaming experience.
-
What is an APK file and why do you need it?
-
An APK file is an Android application package that contains all the files and data needed to run an app on an Android device. APK files are usually downloaded from the Google Play Store, but sometimes they are not available there for various reasons. For example, some apps may be region-locked, banned, or incompatible with your device.
-
If you want to install Brawl Stars on your Android device, you may need to download and install its APK file manually. This is because Brawl Stars is not available in some countries or regions, or it may not be compatible with your device's specifications. By downloading and installing the APK file, you can bypass these limitations and enjoy the game.
-
How to download and install Brawl Stars APK safely and easily
-
Downloading and installing Brawl Stars APK is not difficult, but you need to be careful and follow some steps to ensure a safe and successful installation. Here are the steps you need to follow:
-
-
First, you need to enable the installation of apps from unknown sources on your device. To do this, go to Settings > Security > Unknown Sources and toggle it on.
-
Next, you need to find a reliable source to download the Brawl Stars APK file. There are many websites that offer APK files, but some of them may be malicious or contain viruses. To avoid this, you should only download APK files from trusted and verified sources. One of them is [APKPure], which is a popular and reputable website that provides safe and updated APK files for various apps and games.
-
Once you have found the Brawl Stars APK file on APKPure, click on the Download button and wait for the file to be downloaded to your device.
-
After the download is complete, locate the file in your device's file manager and tap on it to start the installation process. Follow the instructions on the screen and grant the necessary permissions to install the app.
-
When the installation is done, you can launch the app from your app drawer or home screen and enjoy playing Brawl Stars.
-
-
What's new in Brawl Stars 2021 APK?
-
Brawl Stars 2021 APK is the latest version of the game that was released on June 15, 2021. The version number is 36.253 and it has a size of 149 MB. The update brings a lot of new content and features to the game, such as:
-
New brawlers, skins, maps, and events
-
The update adds two new brawlers to the game: Buzz and Griff. Buzz is a lifeguard brawler who can use his hook to pull enemies closer or swing around obstacles. He has a high health and a short-range attack that can stun enemies. His super ability is called Torpedo Throw, which allows him to launch himself towards enemies and knock them back. His star power is called Tough Guy, which gives him a shield when he has low health. His gadget is called Reserve Buoy, which charges his super instantly.
-
Griff is a merchant brawler who can throw coins and banknotes at enemies. He has a medium health and a long-range attack that can pierce through enemies. His super ability is called Cashback, which allows him to collect all his projectiles back and deal damage along the way. His star power is called Business Resilience, which gives him a healing effect when he has low health. His gadget is called Piggy Bank, which drops a pile of coins that he can collect for extra damage.
-
The update also adds new skins for some brawlers, such as Evil Queen Pam, Werewolf Leon, Vicious Bibi, Archvillain Bea, Gold Neko Bea, Lunar Sprout, Mega Box Darryl, True Silver/Gold skins for Buzz and Griff, and more.
-Hot Potato for Heist, and more. The update also adds new events, such as the Brawl Pass Season 7: Jurassic Splash, which offers exclusive rewards and quests for players who purchase the pass. The update also introduces a new game mode called Knockout, which is a best-of-three elimination match where the team with the most kills wins.
-
New game modes and features
-
The update adds a new game mode called Knockout, which is a best-of-three elimination match where the team with the most kills wins. Knockout is a fast and intense game mode that requires teamwork and strategy. The game mode has four maps: Shooting Star, Canal Grande, Dry Season, and Middle Ground.
-
The update also adds some new features to the game, such as:
-
-
A new club league system that allows clubs to compete against each other and earn trophies and rewards.
-
A new replay system that allows players to watch their previous matches and learn from their mistakes or successes.
-
A new trophy road extension that adds more milestones and rewards for players who reach higher trophy levels.
-
A new pin pack system that allows players to collect and use pins to express themselves in the game.
-
A new chat filter system that blocks inappropriate or offensive messages in the game.
-
-
New balance changes and bug fixes
-
The update also brings some balance changes and bug fixes to the game, such as:
-
-
Some brawlers have been buffed or nerfed to improve their performance and balance in the game. For example, Amber's attack damage has been increased from 2000 to 2200, but her super charge rate has been decreased from 120 to 160 hits. Brock's attack damage has been decreased from 1040 to 1000, but his super damage has been increased from 1040 to 1560.
-
Some gadgets and star powers have been adjusted or reworked to make them more useful or balanced. For example, Bo's gadget Tripwire has been reworked to detonate his mines after a 1.5 second delay instead of instantly. Colt's star power Magnum Special has been reworked to increase his bullet speed by 11% instead of his range by 11%.
-
Some bugs and glitches have been fixed to improve the gameplay experience and prevent exploits. For example, a bug that caused Edgar's gadget Let's Fly to cancel his super animation has been fixed. A bug that allowed players to use gadgets while stunned or frozen has been fixed.
-
-
Tips and tricks for playing Brawl Stars
-
Brawl Stars is a game that requires skill, strategy, and teamwork to win. Here are some tips and tricks that can help you improve your gameplay and have more fun:
-
How to choose the best brawlers for each game mode
-
Brawl Stars has 22 different brawlers that you can unlock and play with. Each brawler has a unique personality, appearance, attack, super ability, star power, and gadget. Some brawlers are better suited for certain game modes than others. Here are some examples of brawlers that excel in each game mode:
-
-
Game Mode
Brawlers
-
Smash & Grab
Poco, Pam, Gene, Rosa, El Primo
-
Showdown
Bull, Shelly, Leon, Crow, Colt
-
Bounty
Piper, Brock, Bo, Tick, Nani
-
Heist
Darryl, Barley, Dynamike, Rico, Gale
-
Knockout
Spike, Colette, Belle, Stu, Squeak
-
-
Of course, these are not the only brawlers that can do well in each game mode. You can experiment with different brawlers and find your own preferences and play styles.
-
How to unlock new brawlers and upgrade them
-star powers, or brawlers. You can get brawl boxes by playing the game, completing quests, or buying them with gems. Gems are the premium currency of the game that you can buy with real money or get from special offers. Star points are a special currency that you can earn by ranking up your brawlers or by participating in special events.
-
You can upgrade your brawlers by using power points and coins. Power points are items that increase the level of your brawlers and improve their stats. You can get power points from brawl boxes or by buying them with coins. Coins are the main currency of the game that you can use to buy power points, gadgets, star powers, or brawl boxes. You can get coins from brawl boxes, quests, events, or by selling your extra power points.
-
You can also unlock gadgets and star powers for your brawlers. Gadgets are special items that give your brawlers an extra ability that they can use once or twice per match. You can unlock gadgets for your brawlers when they reach level 7. Star powers are passive abilities that enhance your brawlers' skills or stats. You can unlock star powers for your brawlers when they reach level 9.
-
How to use obstacles, power-ups, and gadgets effectively
-
Brawl Stars is a game that requires skill, strategy, and teamwork to win. One of the ways to improve your gameplay is to use obstacles, power-ups, and gadgets effectively. Here are some tips on how to do that:
-
-
Obstacles are objects that block your movement or vision in the game. They can be walls, bushes, water, or other elements. You can use obstacles to your advantage by hiding behind them, breaking them, or using them to trap your enemies.
-
Power-ups are items that boost your abilities or stats in the game. They can be gems, energy drinks, mushrooms, meteors, or other effects. You can use power-ups to gain an edge over your opponents by collecting them, stealing them, or avoiding them.
-
Gadgets are special items that give your brawlers an extra ability that they can use once or twice per match. They can be shields, dashes, heals, bombs, or other actions. You can use gadgets to surprise your enemies, escape from danger, or support your teammates.
-
-
Conclusion
-
Brawl Stars is a fun and exciting game that you can play on your Android device with your friends or solo. It offers a variety of game modes and brawlers to choose from and a constantly evolving game with new content and features. If you want to install Brawl Stars on your device, you may need to download and install its APK file manually. This is because Brawl Stars is not available in some countries or regions, or it may not be compatible with your device's specifications. By downloading and installing the APK file, you can bypass these limitations and enjoy the game.
-
We hope this article has helped you learn everything you need to know about Brawl Stars 2021 APK. If you have any questions or feedback, feel free to leave a comment below. Happy brawling!
-
FAQs
-
Here are some frequently asked questions about Brawl Stars 2021 APK:
-
Q: Is Brawl Stars 2021 APK safe to download and install?
-
A: Yes, Brawl Stars 2021 APK is safe to download and install as long as you get it from a trusted and verified source like APKPure. However, you should always be careful when downloading and installing any APK file from unknown sources as they may contain viruses or malware.
-
Q: Do I need to root my device to install Brawl Stars 2021 APK?
-
A: No, you do not need to root your device to install Brawl Stars 2021 APK. You just need to enable the installation of apps from unknown sources on your device settings.
-
Q: Will I lose my progress if I install Brawl Stars 2021 APK?
-
A: No, you will not lose your progress if you install Brawl Stars 2021 APK. Your progress is saved on Supercell's servers and linked to your Google Play account or Supercell ID. However, you should always backup your data before installing any APK file just in case something goes wrong.
-
Q: Can I play Brawl Stars 2021 APK with players who have the official version of the game?
-version of the game as long as you have the same version number. However, you may not be able to access some features or events that are exclusive to certain regions or platforms.
-
Q: How can I update Brawl Stars 2021 APK to the latest version?
-
A: You can update Brawl Stars 2021 APK to the latest version by downloading and installing the new APK file from APKPure or other sources. You can also check for updates within the game by tapping on the settings icon and then on the update button. However, you may not be able to update the game immediately as some updates may take some time to be available for APK files.
401be4b1e0
-
-
\ No newline at end of file
diff --git a/spaces/feregVcuzo/sanity-test-midi/checkpoint/Download Minecraft APK for Android - Latest Version 2023 - APKCombo.md b/spaces/feregVcuzo/sanity-test-midi/checkpoint/Download Minecraft APK for Android - Latest Version 2023 - APKCombo.md
deleted file mode 100644
index abb09e8b0870cf58dcf05b349627d32729924bd8..0000000000000000000000000000000000000000
--- a/spaces/feregVcuzo/sanity-test-midi/checkpoint/Download Minecraft APK for Android - Latest Version 2023 - APKCombo.md
+++ /dev/null
@@ -1,123 +0,0 @@
-
-
Apkcombo Minecraft: A Guide for Android Users
-
Minecraft is one of the most popular and influential games of all time. It is a sandbox game that allows players to create and explore infinite worlds made of blocks, where they can build, craft, fight, and survive. Minecraft has millions of fans around the world who play it on various platforms, such as PC, console, mobile, and VR.
-
But what if you want to play Minecraft on your Android device without paying for the official app or signing up for an account? That's where apkcombo minecraft comes in. Apkcombo minecraft is an alternative version of Minecraft that you can download and install from a third-party website called APKCombo. APKCombo is a platform that offers free APK files for Android apps and games that are not available on Google Play Store.
In this article, we will guide you through the process of downloading and installing apkcombo minecraft on your Android device. We will also review the features and gameplay of apkcombo minecraft, as well as its advantages and disadvantages. By the end of this article, you will have a better understanding of what apkcombo minecraft is and whether it is worth trying.
-
How to Download and Install Apkcombo Minecraft on Android Devices
-
Downloading and installing apkcombo minecraft on your Android device is not very difficult, but it does require some steps that are different from installing apps from Google Play Store. Here are the steps you need to follow:
-
-
Go to [1](https://apkcombo.com/search/minecraft) on your Android device's browser. This is the search page for minecraft APK files on APKCombo.
-
Scroll down and find the file named "minecraft APK - Download (Android) - APKCombo". This is the file that contains apkcombo minecraft. Tap on it to open its details page.
-
On the details page, you will see some information about the file, such as its size, version, category, rating, and description. You will also see a green button that says "Download APK (130 MB)". Tap on this button to start downloading the file.
-
Depending on your browser settings, you may see a warning message that says "This type of file can harm your device. Do you want to keep minecraft.apk anyway?". Tap on "OK" to confirm that you want to download the file.
-
Once the download is complete, you will need to enable unknown sources on your device. This is a security setting that prevents you from installing apps from sources other than Google Play Store. To enable unknown sources, go to Settings > Security > Unknown sources and toggle it on.
-
Now you can install the file by tapping on it in your notification bar or file manager. You will see a screen that says "Do you want to install this application?". Tap on "Install" to proceed.
-
Wait for the installation to finish. You will see a screen that says "App installed". Tap on "Open" to launch apkcombo minecraft.
-
-
Congratulations! You have successfully downloaded and installed apkcombo minecraft on your Android device. You can now enjoy playing Minecraft without paying or signing up for anything.
-
Features and Gameplay of Apkcombo Minecraft
-
consoles, and Windows 10. It has the same features and gameplay as the official app, with some minor differences. Here are some of the main features and gameplay aspects of apkcombo minecraft:
Explore Infinite Worlds and Build Anything You Can Imagine
-
One of the most appealing aspects of Minecraft is its open-ended nature. You can explore infinite worlds that are randomly generated and full of different biomes, structures, resources, and creatures. You can also build anything you can imagine using blocks of various materials, shapes, and colors. You can create houses, castles, farms, cities, monuments, machines, and more. The only limit is your imagination and creativity.
-
Play in Creative or Survival Mode with Different Modes and Commands
-
Apkcombo minecraft offers two main modes of play: creative and survival. In creative mode, you have unlimited resources and can build and explore without any restrictions or dangers. You can also fly around and access a variety of commands that let you change the time, weather, game rules, and more. In survival mode, you have to gather resources, craft tools and weapons, fight enemies, and manage your hunger and health. You also have to deal with day and night cycles, weather changes, and environmental hazards.
-
In addition to these two modes, apkcombo minecraft also supports other modes and commands that add more variety and challenge to the game. For example, you can play in adventure mode, where you can only interact with certain blocks and items that are placed by map makers. You can also play in hardcore mode, where you only have one life and the game ends when you die. You can also use commands to enable cheats, change your game mode, teleport to different locations, summon entities, give yourself items, and more.
-
Discover Community Creations and Add-ons in the Marketplace
-
Apkcombo minecraft also allows you to access the marketplace, where you can find and download community creations and add-ons that enhance your game experience. You can find maps, skins, texture packs, mash-ups, mini-games, and more. Some of these are free, while others require in-game currency called Minecoins. You can also create your own content using the built-in tools or external software and share it with other players.
-
Play with Friends Across Platforms and Servers
-
Another great feature of apkcombo minecraft is its cross-platform compatibility and multiplayer support. You can play with your friends who have different devices or platforms, such as Android, iOS, Windows 10, Xbox One, Nintendo Switch, PlayStation 4, and more. You can join online servers that host thousands of players or create your own private server with up to 10 friends. You can also play locally with up to four players using split-screen or wireless LAN.
-
Advantages and Disadvantages of Apkcombo Minecraft
-
Apkcombo minecraft has many advantages and disadvantages compared to the official Minecraft app. Here are some of them:
-
-
-
Pros
-
Cons
-
-
-
Creative: Apkcombo minecraft lets you express your creativity and imagination in endless ways.
-
Not as deep as the PC version: Apkcombo minecraft lacks some features and content that are available on the PC version of Minecraft.
-
-
-
art, and more.
-
Some touch adaptations feel too easy: Apkcombo minecraft has some features that make the game easier on touch devices, such as auto-jump, auto-aim, and simplified crafting. Some players may find these features too convenient or boring.
-
-
-
Customizable: Apkcombo minecraft allows you to customize your game with various options, settings, and add-ons.
-
Can't connect to PC games: Apkcombo minecraft is not compatible with the PC version of Minecraft, which means you can't join PC servers or play PC maps.
-
-
-
Inclusive: Apkcombo minecraft is accessible and enjoyable for people of all ages, genders, backgrounds, and preferences.
-
May cause device overheating: Apkcombo minecraft can be demanding on your device's resources, which may cause it to overheat or drain the battery faster.
-
-
-
Fun: Apkcombo minecraft is fun to play alone or with friends, as it offers endless possibilities and challenges.
-
Screen visibility issues: Apkcombo minecraft can be hard to see or control on smaller screens, especially when there are many blocks or entities on the screen.
-
-
-
Conclusion
-
Apkcombo minecraft is an alternative version of Minecraft that you can download and install from APKCombo, a third-party website that offers free APK files for Android apps and games. It has the same features and gameplay as the official Minecraft app, but with some minor differences. It also has some advantages and disadvantages that you should consider before trying it.
-
If you are looking for a way to play Minecraft on your Android device without paying or signing up for anything, apkcombo minecraft may be a good option for you. However, if you want to enjoy the full and official Minecraft experience, you may want to stick with the official app or the PC version. Either way, Minecraft is a great game that will keep you entertained and inspired for hours.
-
FAQs
-
Here are some frequently asked questions about apkcombo minecraft:
-
What is the difference between apkcombo minecraft and the official Minecraft app?
-
The main difference between apkcombo minecraft and the official Minecraft app is that apkcombo minecraft is a free version that you can download and install from APKCombo, while the official Minecraft app is a paid version that you can download and install from Google Play Store. Apkcombo minecraft also has some minor differences in features and content compared to the official app.
-
How much does apkcombo minecraft cost and is it safe to use?
-
Apkcombo minecraft is free to download and use. However, it may not be safe to use, as it is not verified by Google Play Store or Mojang Studios, the developer of Minecraft. APKCombo claims that it scans all its files for viruses and malware, but there is no guarantee that they are reliable or trustworthy. You should always be careful when downloading and installing apps from unknown sources, as they may contain harmful or unwanted software.
-
What are the minimum requirements for playing apkcombo minecraft on Android devices?
-
The minimum requirements for playing apkcombo minecraft on Android devices are: - Android 4.2 or higher - 1 GB of RAM - 130 MB of free storage space - A stable internet connection However, these requirements may vary depending on your device model and performance. You may also need more storage space if you want to download additional content or add-ons.
-
How can I update apkcombo minecraft to the latest version?
-
To update apkcombo minecraft to the latest version, you need to visit APKCombo again and download the new file. You will then need to uninstall the old version and install the new one. However, this may cause you to lose your progress or settings in the game. You should always back up your data before updating any app.
-
How can I contact the developer of apkcombo minecraft for support or feedback?
-
You can't contact the developer of apkcombo minecraft directly, as they are not affiliated with Mojang Studios or Microsoft Corporation, the owners of Minecraft. You can only contact APKCombo through their website or email address ([support@apkcombo.com](mailto:support@apkcombo.com)). However, they may not be able to help you with any issues or questions related to apkcombo minecraft.
"
-examples = [
- ['source.jpg',"1","1.5"]
-]
-gr.Interface(infer, inputs, outputs, title=title, description=description, article=article, examples=examples).launch(enable_queue=True,cache_examples=True)
diff --git a/spaces/gradio/HuBERT/examples/wav2vec/unsupervised/scripts/prepare_audio.sh b/spaces/gradio/HuBERT/examples/wav2vec/unsupervised/scripts/prepare_audio.sh
deleted file mode 100644
index 013f7a9b055a7693a29f9c5ba1e4003a9a25850e..0000000000000000000000000000000000000000
--- a/spaces/gradio/HuBERT/examples/wav2vec/unsupervised/scripts/prepare_audio.sh
+++ /dev/null
@@ -1,78 +0,0 @@
-#!/usr/bin/env zsh
-# Copyright (c) Facebook, Inc. and its affiliates.
-#
-# This source code is licensed under the MIT license found in the
-# LICENSE file in the root directory of this source tree.
-
-source_dir=$1
-tgt_dir=$2
-model=$3
-
-if [ -z "$4" ]
- then
- dim=512
- else
- dim=$4
-fi
-
-echo "using $dim dim for PCA"
-
-if [ -z "$5" ]
- then
- layer=14
- else
- layer=$5
-fi
-
-echo "extracting from layer $layer"
-
-train_split=train
-valid_split=valid
-test_split=test
-
-all_splits=($train_split)
-
-if [[ -f "$source_dir/valid.tsv" ]]; then
- all_splits+=('valid')
-fi
-
-if [[ -f "$source_dir/test.tsv" ]]; then
- all_splits+=('test')
-fi
-
-echo "processing splits: $all_splits"
-
-mkdir -p $tgt_dir
-
-cp $source_dir/*.tsv $tgt_dir
-cp $source_dir/*.wrd $tgt_dir
-cp $source_dir/*.ltr $tgt_dir
-cp $source_dir/*.phn $tgt_dir
-cp $source_dir/dict* $tgt_dir
-
-setopt shwordsplit
-
-for split in $all_splits; do
- python $FAIRSEQ_ROOT/examples/wav2vec/unsupervised/scripts/wav2vec_extract_features.py $source_dir --split $split \
- --save-dir $tgt_dir --checkpoint $model --layer $layer
-done
-
-python $FAIRSEQ_ROOT/examples/wav2vec/unsupervised/scripts/wav2vec_cluster_faiss.py $tgt_dir/${train_split}.tsv \
---checkpoint $model --save-dir $tgt_dir -f "CLUS128" --sample-pct 1.0
-
-for split in $all_splits; do
- python $FAIRSEQ_ROOT/examples/wav2vec/unsupervised/scripts/wav2vec_apply_cluster_faiss.py $tgt_dir \
- --checkpoint $model --path $tgt_dir/CLUS128 --split $split
-done
-
-python $FAIRSEQ_ROOT/examples/wav2vec/unsupervised/scripts/pca.py $tgt_dir/${train_split}.npy --output $tgt_dir/pca --dim $dim
-
-for split in $all_splits; do
- python $FAIRSEQ_ROOT/examples/wav2vec/unsupervised/scripts/apply_pca.py $tgt_dir --split $split --save-dir $tgt_dir/precompute_pca$dim --pca-path $tgt_dir/pca/${dim}_pca --batch-size 1048000
-
- python $FAIRSEQ_ROOT/examples/wav2vec/unsupervised/scripts/merge_clusters.py $tgt_dir/precompute_pca$dim --cluster-dir $tgt_dir/CLUS128 \
- --split $split --save-dir $tgt_dir/precompute_pca${dim}_cls128_mean --pooling mean
-
- python $FAIRSEQ_ROOT/examples/wav2vec/unsupervised/scripts/mean_pool.py $tgt_dir/precompute_pca${dim}_cls128_mean \
- --save-dir $tgt_dir/precompute_pca${dim}_cls128_mean_pooled --split $split
-done
diff --git a/spaces/gyugnsu/DragGan-Inversion/stylegan_human/training/augment.py b/spaces/gyugnsu/DragGan-Inversion/stylegan_human/training/augment.py
deleted file mode 100644
index 8067f4e3fec058c9025edaa7a9a0442afe859ae5..0000000000000000000000000000000000000000
--- a/spaces/gyugnsu/DragGan-Inversion/stylegan_human/training/augment.py
+++ /dev/null
@@ -1,562 +0,0 @@
-# Copyright (c) 2021, NVIDIA CORPORATION & AFFILIATES. All rights reserved.
-#
-# NVIDIA CORPORATION and its licensors retain all intellectual property
-# and proprietary rights in and to this software, related documentation
-# and any modifications thereto. Any use, reproduction, disclosure or
-# distribution of this software and related documentation without an express
-# license agreement from NVIDIA CORPORATION is strictly prohibited.
-
-"""Augmentation pipeline from the paper
-"Training Generative Adversarial Networks with Limited Data".
-Matches the original implementation by Karras et al. at
-https://github.com/NVlabs/stylegan2-ada/blob/main/training/augment.py"""
-
-import numpy as np
-import scipy.signal
-import torch
-from torch_utils import persistence
-from torch_utils import misc
-from torch_utils.ops import upfirdn2d
-from torch_utils.ops import grid_sample_gradfix
-from torch_utils.ops import conv2d_gradfix
-
-# ----------------------------------------------------------------------------
-# Coefficients of various wavelet decomposition low-pass filters.
-
-wavelets = {
- 'haar': [0.7071067811865476, 0.7071067811865476],
- 'db1': [0.7071067811865476, 0.7071067811865476],
- 'db2': [-0.12940952255092145, 0.22414386804185735, 0.836516303737469, 0.48296291314469025],
- 'db3': [0.035226291882100656, -0.08544127388224149, -0.13501102001039084, 0.4598775021193313, 0.8068915093133388, 0.3326705529509569],
- 'db4': [-0.010597401784997278, 0.032883011666982945, 0.030841381835986965, -0.18703481171888114, -0.02798376941698385, 0.6308807679295904, 0.7148465705525415, 0.23037781330885523],
- 'db5': [0.003335725285001549, -0.012580751999015526, -0.006241490213011705, 0.07757149384006515, -0.03224486958502952, -0.24229488706619015, 0.13842814590110342, 0.7243085284385744, 0.6038292697974729, 0.160102397974125],
- 'db6': [-0.00107730108499558, 0.004777257511010651, 0.0005538422009938016, -0.031582039318031156, 0.02752286553001629, 0.09750160558707936, -0.12976686756709563, -0.22626469396516913, 0.3152503517092432, 0.7511339080215775, 0.4946238903983854, 0.11154074335008017],
- 'db7': [0.0003537138000010399, -0.0018016407039998328, 0.00042957797300470274, 0.012550998556013784, -0.01657454163101562, -0.03802993693503463, 0.0806126091510659, 0.07130921926705004, -0.22403618499416572, -0.14390600392910627, 0.4697822874053586, 0.7291320908465551, 0.39653931948230575, 0.07785205408506236],
- 'db8': [-0.00011747678400228192, 0.0006754494059985568, -0.0003917403729959771, -0.00487035299301066, 0.008746094047015655, 0.013981027917015516, -0.04408825393106472, -0.01736930100202211, 0.128747426620186, 0.00047248457399797254, -0.2840155429624281, -0.015829105256023893, 0.5853546836548691, 0.6756307362980128, 0.3128715909144659, 0.05441584224308161],
- 'sym2': [-0.12940952255092145, 0.22414386804185735, 0.836516303737469, 0.48296291314469025],
- 'sym3': [0.035226291882100656, -0.08544127388224149, -0.13501102001039084, 0.4598775021193313, 0.8068915093133388, 0.3326705529509569],
- 'sym4': [-0.07576571478927333, -0.02963552764599851, 0.49761866763201545, 0.8037387518059161, 0.29785779560527736, -0.09921954357684722, -0.012603967262037833, 0.0322231006040427],
- 'sym5': [0.027333068345077982, 0.029519490925774643, -0.039134249302383094, 0.1993975339773936, 0.7234076904024206, 0.6339789634582119, 0.01660210576452232, -0.17532808990845047, -0.021101834024758855, 0.019538882735286728],
- 'sym6': [0.015404109327027373, 0.0034907120842174702, -0.11799011114819057, -0.048311742585633, 0.4910559419267466, 0.787641141030194, 0.3379294217276218, -0.07263752278646252, -0.021060292512300564, 0.04472490177066578, 0.0017677118642428036, -0.007800708325034148],
- 'sym7': [0.002681814568257878, -0.0010473848886829163, -0.01263630340325193, 0.03051551316596357, 0.0678926935013727, -0.049552834937127255, 0.017441255086855827, 0.5361019170917628, 0.767764317003164, 0.2886296317515146, -0.14004724044296152, -0.10780823770381774, 0.004010244871533663, 0.010268176708511255],
- 'sym8': [-0.0033824159510061256, -0.0005421323317911481, 0.03169508781149298, 0.007607487324917605, -0.1432942383508097, -0.061273359067658524, 0.4813596512583722, 0.7771857517005235, 0.3644418948353314, -0.05194583810770904, -0.027219029917056003, 0.049137179673607506, 0.003808752013890615, -0.01495225833704823, -0.0003029205147213668, 0.0018899503327594609],
-}
-
-# ----------------------------------------------------------------------------
-# Helpers for constructing transformation matrices.
-
-
-def matrix(*rows, device=None):
- assert all(len(row) == len(rows[0]) for row in rows)
- elems = [x for row in rows for x in row]
- ref = [x for x in elems if isinstance(x, torch.Tensor)]
- if len(ref) == 0:
- return misc.constant(np.asarray(rows), device=device)
- assert device is None or device == ref[0].device
- elems = [x if isinstance(x, torch.Tensor) else misc.constant(
- x, shape=ref[0].shape, device=ref[0].device) for x in elems]
- return torch.stack(elems, dim=-1).reshape(ref[0].shape + (len(rows), -1))
-
-
-def translate2d(tx, ty, **kwargs):
- return matrix(
- [1, 0, tx],
- [0, 1, ty],
- [0, 0, 1],
- **kwargs)
-
-
-def translate3d(tx, ty, tz, **kwargs):
- return matrix(
- [1, 0, 0, tx],
- [0, 1, 0, ty],
- [0, 0, 1, tz],
- [0, 0, 0, 1],
- **kwargs)
-
-
-def scale2d(sx, sy, **kwargs):
- return matrix(
- [sx, 0, 0],
- [0, sy, 0],
- [0, 0, 1],
- **kwargs)
-
-
-def scale3d(sx, sy, sz, **kwargs):
- return matrix(
- [sx, 0, 0, 0],
- [0, sy, 0, 0],
- [0, 0, sz, 0],
- [0, 0, 0, 1],
- **kwargs)
-
-
-def rotate2d(theta, **kwargs):
- return matrix(
- [torch.cos(theta), torch.sin(-theta), 0],
- [torch.sin(theta), torch.cos(theta), 0],
- [0, 0, 1],
- **kwargs)
-
-
-def rotate3d(v, theta, **kwargs):
- vx = v[..., 0]
- vy = v[..., 1]
- vz = v[..., 2]
- s = torch.sin(theta)
- c = torch.cos(theta)
- cc = 1 - c
- return matrix(
- [vx*vx*cc+c, vx*vy*cc-vz*s, vx*vz*cc+vy*s, 0],
- [vy*vx*cc+vz*s, vy*vy*cc+c, vy*vz*cc-vx*s, 0],
- [vz*vx*cc-vy*s, vz*vy*cc+vx*s, vz*vz*cc+c, 0],
- [0, 0, 0, 1],
- **kwargs)
-
-
-def translate2d_inv(tx, ty, **kwargs):
- return translate2d(-tx, -ty, **kwargs)
-
-
-def scale2d_inv(sx, sy, **kwargs):
- return scale2d(1 / sx, 1 / sy, **kwargs)
-
-
-def rotate2d_inv(theta, **kwargs):
- return rotate2d(-theta, **kwargs)
-
-# ----------------------------------------------------------------------------
-# Versatile image augmentation pipeline from the paper
-# "Training Generative Adversarial Networks with Limited Data".
-#
-# All augmentations are disabled by default; individual augmentations can
-# be enabled by setting their probability multipliers to 1.
-
-
-@persistence.persistent_class
-class AugmentPipe(torch.nn.Module):
- def __init__(self,
- xflip=0, rotate90=0, xint=0, xint_max=0.125,
- scale=0, rotate=0, aniso=0, xfrac=0, scale_std=0.2, rotate_max=1, aniso_std=0.2, xfrac_std=0.125,
- brightness=0, contrast=0, lumaflip=0, hue=0, saturation=0, brightness_std=0.2, contrast_std=0.5, hue_max=1, saturation_std=1,
- imgfilter=0, imgfilter_bands=[1, 1, 1, 1], imgfilter_std=1,
- noise=0, cutout=0, noise_std=0.1, cutout_size=0.5,
- ):
- super().__init__()
- # Overall multiplier for augmentation probability.
- self.register_buffer('p', torch.ones([]))
-
- # Pixel blitting.
- # Probability multiplier for x-flip.
- self.xflip = float(xflip)
- # Probability multiplier for 90 degree rotations.
- self.rotate90 = float(rotate90)
- # Probability multiplier for integer translation.
- self.xint = float(xint)
- # Range of integer translation, relative to image dimensions.
- self.xint_max = float(xint_max)
-
- # General geometric transformations.
- # Probability multiplier for isotropic scaling.
- self.scale = float(scale)
- # Probability multiplier for arbitrary rotation.
- self.rotate = float(rotate)
- # Probability multiplier for anisotropic scaling.
- self.aniso = float(aniso)
- # Probability multiplier for fractional translation.
- self.xfrac = float(xfrac)
- # Log2 standard deviation of isotropic scaling.
- self.scale_std = float(scale_std)
- # Range of arbitrary rotation, 1 = full circle.
- self.rotate_max = float(rotate_max)
- # Log2 standard deviation of anisotropic scaling.
- self.aniso_std = float(aniso_std)
- # Standard deviation of frational translation, relative to image dimensions.
- self.xfrac_std = float(xfrac_std)
-
- # Color transformations.
- # Probability multiplier for brightness.
- self.brightness = float(brightness)
- # Probability multiplier for contrast.
- self.contrast = float(contrast)
- # Probability multiplier for luma flip.
- self.lumaflip = float(lumaflip)
- # Probability multiplier for hue rotation.
- self.hue = float(hue)
- # Probability multiplier for saturation.
- self.saturation = float(saturation)
- # Standard deviation of brightness.
- self.brightness_std = float(brightness_std)
- # Log2 standard deviation of contrast.
- self.contrast_std = float(contrast_std)
- # Range of hue rotation, 1 = full circle.
- self.hue_max = float(hue_max)
- # Log2 standard deviation of saturation.
- self.saturation_std = float(saturation_std)
-
- # Image-space filtering.
- # Probability multiplier for image-space filtering.
- self.imgfilter = float(imgfilter)
- # Probability multipliers for individual frequency bands.
- self.imgfilter_bands = list(imgfilter_bands)
- # Log2 standard deviation of image-space filter amplification.
- self.imgfilter_std = float(imgfilter_std)
-
- # Image-space corruptions.
- # Probability multiplier for additive RGB noise.
- self.noise = float(noise)
- # Probability multiplier for cutout.
- self.cutout = float(cutout)
- # Standard deviation of additive RGB noise.
- self.noise_std = float(noise_std)
- # Size of the cutout rectangle, relative to image dimensions.
- self.cutout_size = float(cutout_size)
-
- # Setup orthogonal lowpass filter for geometric augmentations.
- self.register_buffer(
- 'Hz_geom', upfirdn2d.setup_filter(wavelets['sym6']))
-
- # Construct filter bank for image-space filtering.
- Hz_lo = np.asarray(wavelets['sym2']) # H(z)
- Hz_hi = Hz_lo * ((-1) ** np.arange(Hz_lo.size)) # H(-z)
- Hz_lo2 = np.convolve(Hz_lo, Hz_lo[::-1]) / 2 # H(z) * H(z^-1) / 2
- Hz_hi2 = np.convolve(Hz_hi, Hz_hi[::-1]) / 2 # H(-z) * H(-z^-1) / 2
- Hz_fbank = np.eye(4, 1) # Bandpass(H(z), b_i)
- for i in range(1, Hz_fbank.shape[0]):
- Hz_fbank = np.dstack([Hz_fbank, np.zeros_like(Hz_fbank)]).reshape(
- Hz_fbank.shape[0], -1)[:, :-1]
- Hz_fbank = scipy.signal.convolve(Hz_fbank, [Hz_lo2])
- Hz_fbank[i, (Hz_fbank.shape[1] - Hz_hi2.size) //
- 2: (Hz_fbank.shape[1] + Hz_hi2.size) // 2] += Hz_hi2
- self.register_buffer('Hz_fbank', torch.as_tensor(
- Hz_fbank, dtype=torch.float32))
-
- def forward(self, images, debug_percentile=None):
- assert isinstance(images, torch.Tensor) and images.ndim == 4
- batch_size, num_channels, height, width = images.shape
- device = images.device
- if debug_percentile is not None:
- debug_percentile = torch.as_tensor(
- debug_percentile, dtype=torch.float32, device=device)
-
- # -------------------------------------
- # Select parameters for pixel blitting.
- # -------------------------------------
-
- # Initialize inverse homogeneous 2D transform: G_inv @ pixel_out ==> pixel_in
- I_3 = torch.eye(3, device=device)
- G_inv = I_3
-
- # Apply x-flip with probability (xflip * strength).
- if self.xflip > 0:
- i = torch.floor(torch.rand([batch_size], device=device) * 2)
- i = torch.where(torch.rand(
- [batch_size], device=device) < self.xflip * self.p, i, torch.zeros_like(i))
- if debug_percentile is not None:
- i = torch.full_like(i, torch.floor(debug_percentile * 2))
- G_inv = G_inv @ scale2d_inv(1 - 2 * i, 1)
-
- # Apply 90 degree rotations with probability (rotate90 * strength).
- if self.rotate90 > 0:
- i = torch.floor(torch.rand([batch_size], device=device) * 4)
- i = torch.where(torch.rand(
- [batch_size], device=device) < self.rotate90 * self.p, i, torch.zeros_like(i))
- if debug_percentile is not None:
- i = torch.full_like(i, torch.floor(debug_percentile * 4))
- G_inv = G_inv @ rotate2d_inv(-np.pi / 2 * i)
-
- # Apply integer translation with probability (xint * strength).
- if self.xint > 0:
- t = (torch.rand([batch_size, 2], device=device)
- * 2 - 1) * self.xint_max
- t = torch.where(torch.rand(
- [batch_size, 1], device=device) < self.xint * self.p, t, torch.zeros_like(t))
- if debug_percentile is not None:
- t = torch.full_like(
- t, (debug_percentile * 2 - 1) * self.xint_max)
- G_inv = G_inv @ translate2d_inv(torch.round(
- t[:, 0] * width), torch.round(t[:, 1] * height))
-
- # --------------------------------------------------------
- # Select parameters for general geometric transformations.
- # --------------------------------------------------------
-
- # Apply isotropic scaling with probability (scale * strength).
- if self.scale > 0:
- s = torch.exp2(torch.randn(
- [batch_size], device=device) * self.scale_std)
- s = torch.where(torch.rand(
- [batch_size], device=device) < self.scale * self.p, s, torch.ones_like(s))
- if debug_percentile is not None:
- s = torch.full_like(s, torch.exp2(torch.erfinv(
- debug_percentile * 2 - 1) * self.scale_std))
- G_inv = G_inv @ scale2d_inv(s, s)
-
- # Apply pre-rotation with probability p_rot.
- # P(pre OR post) = p
- p_rot = 1 - torch.sqrt((1 - self.rotate * self.p).clamp(0, 1))
- if self.rotate > 0:
- theta = (torch.rand([batch_size], device=device)
- * 2 - 1) * np.pi * self.rotate_max
- theta = torch.where(torch.rand(
- [batch_size], device=device) < p_rot, theta, torch.zeros_like(theta))
- if debug_percentile is not None:
- theta = torch.full_like(
- theta, (debug_percentile * 2 - 1) * np.pi * self.rotate_max)
- G_inv = G_inv @ rotate2d_inv(-theta) # Before anisotropic scaling.
-
- # Apply anisotropic scaling with probability (aniso * strength).
- if self.aniso > 0:
- s = torch.exp2(torch.randn(
- [batch_size], device=device) * self.aniso_std)
- s = torch.where(torch.rand(
- [batch_size], device=device) < self.aniso * self.p, s, torch.ones_like(s))
- if debug_percentile is not None:
- s = torch.full_like(s, torch.exp2(torch.erfinv(
- debug_percentile * 2 - 1) * self.aniso_std))
- G_inv = G_inv @ scale2d_inv(s, 1 / s)
-
- # Apply post-rotation with probability p_rot.
- if self.rotate > 0:
- theta = (torch.rand([batch_size], device=device)
- * 2 - 1) * np.pi * self.rotate_max
- theta = torch.where(torch.rand(
- [batch_size], device=device) < p_rot, theta, torch.zeros_like(theta))
- if debug_percentile is not None:
- theta = torch.zeros_like(theta)
- G_inv = G_inv @ rotate2d_inv(-theta) # After anisotropic scaling.
-
- # Apply fractional translation with probability (xfrac * strength).
- if self.xfrac > 0:
- t = torch.randn([batch_size, 2], device=device) * self.xfrac_std
- t = torch.where(torch.rand(
- [batch_size, 1], device=device) < self.xfrac * self.p, t, torch.zeros_like(t))
- if debug_percentile is not None:
- t = torch.full_like(t, torch.erfinv(
- debug_percentile * 2 - 1) * self.xfrac_std)
- G_inv = G_inv @ translate2d_inv(t[:, 0] * width, t[:, 1] * height)
-
- # ----------------------------------
- # Execute geometric transformations.
- # ----------------------------------
-
- # Execute if the transform is not identity.
- if G_inv is not I_3:
-
- # Calculate padding.
- cx = (width - 1) / 2
- cy = (height - 1) / 2
- cp = matrix([-cx, -cy, 1], [cx, -cy, 1], [cx, cy, 1],
- [-cx, cy, 1], device=device) # [idx, xyz]
- cp = G_inv @ cp.t() # [batch, xyz, idx]
- Hz_pad = self.Hz_geom.shape[0] // 4
- margin = cp[:, :2, :].permute(
- 1, 0, 2).flatten(1) # [xy, batch * idx]
- # [x0, y0, x1, y1]
- margin = torch.cat([-margin, margin]).max(dim=1).values
- margin = margin + \
- misc.constant([Hz_pad * 2 - cx, Hz_pad * 2 - cy]
- * 2, device=device)
- margin = margin.max(misc.constant([0, 0] * 2, device=device))
- margin = margin.min(misc.constant(
- [width-1, height-1] * 2, device=device))
- mx0, my0, mx1, my1 = margin.ceil().to(torch.int32)
-
- # Pad image and adjust origin.
- images = torch.nn.functional.pad(
- input=images, pad=[mx0, mx1, my0, my1], mode='reflect')
- G_inv = translate2d((mx0 - mx1) / 2, (my0 - my1) / 2) @ G_inv
-
- # Upsample.
- images = upfirdn2d.upsample2d(x=images, f=self.Hz_geom, up=2)
- G_inv = scale2d(
- 2, 2, device=device) @ G_inv @ scale2d_inv(2, 2, device=device)
- G_inv = translate2d(-0.5, -0.5,
- device=device) @ G_inv @ translate2d_inv(-0.5, -0.5, device=device)
-
- # Execute transformation.
- shape = [batch_size, num_channels,
- (height + Hz_pad * 2) * 2, (width + Hz_pad * 2) * 2]
- G_inv = scale2d(2 / images.shape[3], 2 / images.shape[2], device=device) @ G_inv @ scale2d_inv(
- 2 / shape[3], 2 / shape[2], device=device)
- grid = torch.nn.functional.affine_grid(
- theta=G_inv[:, :2, :], size=shape, align_corners=False)
- images = grid_sample_gradfix.grid_sample(images, grid)
-
- # Downsample and crop.
- images = upfirdn2d.downsample2d(
- x=images, f=self.Hz_geom, down=2, padding=-Hz_pad*2, flip_filter=True)
-
- # --------------------------------------------
- # Select parameters for color transformations.
- # --------------------------------------------
-
- # Initialize homogeneous 3D transformation matrix: C @ color_in ==> color_out
- I_4 = torch.eye(4, device=device)
- C = I_4
-
- # Apply brightness with probability (brightness * strength).
- if self.brightness > 0:
- b = torch.randn([batch_size], device=device) * self.brightness_std
- b = torch.where(torch.rand(
- [batch_size], device=device) < self.brightness * self.p, b, torch.zeros_like(b))
- if debug_percentile is not None:
- b = torch.full_like(b, torch.erfinv(
- debug_percentile * 2 - 1) * self.brightness_std)
- C = translate3d(b, b, b) @ C
-
- # Apply contrast with probability (contrast * strength).
- if self.contrast > 0:
- c = torch.exp2(torch.randn(
- [batch_size], device=device) * self.contrast_std)
- c = torch.where(torch.rand(
- [batch_size], device=device) < self.contrast * self.p, c, torch.ones_like(c))
- if debug_percentile is not None:
- c = torch.full_like(c, torch.exp2(torch.erfinv(
- debug_percentile * 2 - 1) * self.contrast_std))
- C = scale3d(c, c, c) @ C
-
- # Apply luma flip with probability (lumaflip * strength).
- # Luma axis.
- v = misc.constant(np.asarray([1, 1, 1, 0]) / np.sqrt(3), device=device)
- if self.lumaflip > 0:
- i = torch.floor(torch.rand([batch_size, 1, 1], device=device) * 2)
- i = torch.where(torch.rand(
- [batch_size, 1, 1], device=device) < self.lumaflip * self.p, i, torch.zeros_like(i))
- if debug_percentile is not None:
- i = torch.full_like(i, torch.floor(debug_percentile * 2))
- C = (I_4 - 2 * v.ger(v) * i) @ C # Householder reflection.
-
- # Apply hue rotation with probability (hue * strength).
- if self.hue > 0 and num_channels > 1:
- theta = (torch.rand([batch_size], device=device)
- * 2 - 1) * np.pi * self.hue_max
- theta = torch.where(torch.rand(
- [batch_size], device=device) < self.hue * self.p, theta, torch.zeros_like(theta))
- if debug_percentile is not None:
- theta = torch.full_like(
- theta, (debug_percentile * 2 - 1) * np.pi * self.hue_max)
- C = rotate3d(v, theta) @ C # Rotate around v.
-
- # Apply saturation with probability (saturation * strength).
- if self.saturation > 0 and num_channels > 1:
- s = torch.exp2(torch.randn(
- [batch_size, 1, 1], device=device) * self.saturation_std)
- s = torch.where(torch.rand(
- [batch_size, 1, 1], device=device) < self.saturation * self.p, s, torch.ones_like(s))
- if debug_percentile is not None:
- s = torch.full_like(s, torch.exp2(torch.erfinv(
- debug_percentile * 2 - 1) * self.saturation_std))
- C = (v.ger(v) + (I_4 - v.ger(v)) * s) @ C
-
- # ------------------------------
- # Execute color transformations.
- # ------------------------------
-
- # Execute if the transform is not identity.
- if C is not I_4:
- images = images.reshape([batch_size, num_channels, height * width])
- if num_channels == 3:
- images = C[:, :3, :3] @ images + C[:, :3, 3:]
- elif num_channels == 1:
- C = C[:, :3, :].mean(dim=1, keepdims=True)
- images = images * \
- C[:, :, :3].sum(dim=2, keepdims=True) + C[:, :, 3:]
- else:
- raise ValueError(
- 'Image must be RGB (3 channels) or L (1 channel)')
- images = images.reshape([batch_size, num_channels, height, width])
-
- # ----------------------
- # Image-space filtering.
- # ----------------------
-
- if self.imgfilter > 0:
- num_bands = self.Hz_fbank.shape[0]
- assert len(self.imgfilter_bands) == num_bands
- # Expected power spectrum (1/f).
- expected_power = misc.constant(
- np.array([10, 1, 1, 1]) / 13, device=device)
-
- # Apply amplification for each band with probability (imgfilter * strength * band_strength).
- # Global gain vector (identity).
- g = torch.ones([batch_size, num_bands], device=device)
- for i, band_strength in enumerate(self.imgfilter_bands):
- t_i = torch.exp2(torch.randn(
- [batch_size], device=device) * self.imgfilter_std)
- t_i = torch.where(torch.rand(
- [batch_size], device=device) < self.imgfilter * self.p * band_strength, t_i, torch.ones_like(t_i))
- if debug_percentile is not None:
- t_i = torch.full_like(t_i, torch.exp2(torch.erfinv(
- debug_percentile * 2 - 1) * self.imgfilter_std)) if band_strength > 0 else torch.ones_like(t_i)
- # Temporary gain vector.
- t = torch.ones([batch_size, num_bands], device=device)
- # Replace i'th element.
- t[:, i] = t_i
- # Normalize power.
- t = t / (expected_power * t.square()
- ).sum(dim=-1, keepdims=True).sqrt()
- # Accumulate into global gain.
- g = g * t
-
- # Construct combined amplification filter.
- # [batch, tap]
- Hz_prime = g @ self.Hz_fbank
- Hz_prime = Hz_prime.unsqueeze(1).repeat(
- [1, num_channels, 1]) # [batch, channels, tap]
- # [batch * channels, 1, tap]
- Hz_prime = Hz_prime.reshape([batch_size * num_channels, 1, -1])
-
- # Apply filter.
- p = self.Hz_fbank.shape[1] // 2
- images = images.reshape(
- [1, batch_size * num_channels, height, width])
- images = torch.nn.functional.pad(
- input=images, pad=[p, p, p, p], mode='reflect')
- images = conv2d_gradfix.conv2d(
- input=images, weight=Hz_prime.unsqueeze(2), groups=batch_size*num_channels)
- images = conv2d_gradfix.conv2d(
- input=images, weight=Hz_prime.unsqueeze(3), groups=batch_size*num_channels)
- images = images.reshape([batch_size, num_channels, height, width])
-
- # ------------------------
- # Image-space corruptions.
- # ------------------------
-
- # Apply additive RGB noise with probability (noise * strength).
- if self.noise > 0:
- sigma = torch.randn([batch_size, 1, 1, 1],
- device=device).abs() * self.noise_std
- sigma = torch.where(torch.rand(
- [batch_size, 1, 1, 1], device=device) < self.noise * self.p, sigma, torch.zeros_like(sigma))
- if debug_percentile is not None:
- sigma = torch.full_like(sigma, torch.erfinv(
- debug_percentile) * self.noise_std)
- images = images + \
- torch.randn([batch_size, num_channels, height,
- width], device=device) * sigma
-
- # Apply cutout with probability (cutout * strength).
- if self.cutout > 0:
- size = torch.full([batch_size, 2, 1, 1, 1],
- self.cutout_size, device=device)
- size = torch.where(torch.rand(
- [batch_size, 1, 1, 1, 1], device=device) < self.cutout * self.p, size, torch.zeros_like(size))
- center = torch.rand([batch_size, 2, 1, 1, 1], device=device)
- if debug_percentile is not None:
- size = torch.full_like(size, self.cutout_size)
- center = torch.full_like(center, debug_percentile)
- coord_x = torch.arange(width, device=device).reshape([1, 1, 1, -1])
- coord_y = torch.arange(
- height, device=device).reshape([1, 1, -1, 1])
- mask_x = (((coord_x + 0.5) / width -
- center[:, 0]).abs() >= size[:, 0] / 2)
- mask_y = (((coord_y + 0.5) / height -
- center[:, 1]).abs() >= size[:, 1] / 2)
- mask = torch.logical_or(mask_x, mask_y).to(torch.float32)
- images = images * mask
-
- return images
-
-# ----------------------------------------------------------------------------
diff --git a/spaces/h2oai/h2ogpt-chatbot2/src/gen.py b/spaces/h2oai/h2ogpt-chatbot2/src/gen.py
deleted file mode 100644
index d8602e7b0a56920a4afb7b4ac9c73e7449216729..0000000000000000000000000000000000000000
--- a/spaces/h2oai/h2ogpt-chatbot2/src/gen.py
+++ /dev/null
@@ -1,3831 +0,0 @@
-import ast
-import copy
-import functools
-import inspect
-import queue
-import sys
-import os
-import time
-import traceback
-import typing
-import warnings
-from datetime import datetime
-import requests
-from requests import ConnectTimeout, JSONDecodeError
-from urllib3.exceptions import ConnectTimeoutError, MaxRetryError, ConnectionError
-from requests.exceptions import ConnectionError as ConnectionError2
-from requests.exceptions import ReadTimeout as ReadTimeout2
-
-if os.path.dirname(os.path.abspath(__file__)) not in sys.path:
- sys.path.append(os.path.dirname(os.path.abspath(__file__)))
-
-os.environ['HF_HUB_DISABLE_TELEMETRY'] = '1'
-os.environ['BITSANDBYTES_NOWELCOME'] = '1'
-warnings.filterwarnings('ignore', category=UserWarning, message='TypedStorage is deprecated')
-
-# more is not useful typically, don't let these go beyond limits and eat up resources
-max_cores = max(1, os.cpu_count() // 2)
-if os.getenv('NUMEXPR_MAX_THREADS') is None:
- os.environ['NUMEXPR_MAX_THREADS'] = str(min(8, max_cores))
-if os.getenv('NUMEXPR_NUM_THREADS') is None:
- os.environ['NUMEXPR_NUM_THREADS'] = str(min(8, max_cores))
-if os.getenv('OMP_NUM_THREADS') is None:
- os.environ['OMP_NUM_THREADS'] = str(min(8, max_cores))
-if os.getenv('OPENBLAS_NUM_THREADS') is None:
- os.environ['OPENBLAS_NUM_THREADS'] = str(min(8, max_cores))
-if os.getenv('DUCKDB_NUM_THREADS') is None:
- os.environ['DUCKDB_NUM_THREADS'] = str(min(4, max_cores))
-if os.getenv('RAYON_RS_NUM_CPUS') is None:
- os.environ['RAYON_RS_NUM_CPUS'] = str(min(8, max_cores))
-if os.getenv('RAYON_NUM_THREADS') is None:
- os.environ['RAYON_NUM_THREADS'] = str(min(8, max_cores))
-
-import numpy as np
-from evaluate_params import eval_func_param_names, no_default_param_names, input_args_list
-from enums import DocumentSubset, LangChainMode, no_lora_str, model_token_mapping, no_model_str, \
- LangChainAction, LangChainAgent, DocumentChoice, LangChainTypes, super_source_prefix, \
- super_source_postfix, t5_type, get_langchain_prompts, gr_to_lg, invalid_key_msg
-from loaders import get_loaders
-from utils import set_seed, clear_torch_cache, NullContext, wrapped_partial, EThread, get_githash, \
- import_matplotlib, get_device, makedirs, get_kwargs, start_faulthandler, get_hf_server, FakeTokenizer, \
- have_langchain, set_openai, cuda_vis_check, H2O_Fire, lg_to_gr, str_to_list, str_to_dict, get_token_count
-
-start_faulthandler()
-import_matplotlib()
-
-SEED = 1236
-set_seed(SEED)
-
-from typing import Union
-
-import torch
-from transformers import GenerationConfig, AutoModel, TextIteratorStreamer
-
-from prompter import Prompter, inv_prompt_type_to_model_lower, non_hf_types, PromptType, get_prompt, generate_prompt
-from stopping import get_stopping
-
-langchain_actions = [x.value for x in list(LangChainAction)]
-
-langchain_agents_list = [x.value for x in list(LangChainAgent)]
-
-
-def main(
- load_8bit: bool = False,
- load_4bit: bool = False,
- low_bit_mode: int = 1,
- load_half: bool = None,
- load_gptq: str = '',
- load_exllama: bool = False,
- use_safetensors: bool = False,
- revision: str = None,
- use_gpu_id: bool = True,
- base_model: str = '',
- tokenizer_base_model: str = '',
- lora_weights: str = "",
- gpu_id: int = 0,
- compile_model: bool = None,
- use_cache: bool = None,
- inference_server: str = "",
- prompt_type: Union[int, str] = None,
- prompt_dict: typing.Dict = None,
- system_prompt: str = '',
-
- # llama and gpt4all settings
- llamacpp_dict: typing.Dict = dict(n_gpu_layers=100, use_mlock=True, n_batch=1024, n_gqa=0),
- model_path_llama: str = 'https://huggingface.co/TheBloke/Llama-2-7B-Chat-GGML/resolve/main/llama-2-7b-chat.ggmlv3.q8_0.bin',
- # 'llama-2-7b-chat.ggmlv3.q8_0.bin',
- model_name_gptj: str = 'ggml-gpt4all-j-v1.3-groovy.bin',
- model_name_gpt4all_llama: str = 'ggml-wizardLM-7B.q4_2.bin',
- model_name_exllama_if_no_config: str = 'TheBloke/Nous-Hermes-Llama2-GPTQ',
-
- model_lock: typing.List[typing.Dict[str, str]] = None,
- model_lock_columns: int = None,
- fail_if_cannot_connect: bool = False,
-
- # input to generation
- temperature: float = None,
- top_p: float = None,
- top_k: int = None,
- num_beams: int = None,
- repetition_penalty: float = None,
- num_return_sequences: int = None,
- do_sample: bool = None,
- max_new_tokens: int = None,
- min_new_tokens: int = None,
- early_stopping: Union[bool, str] = None,
- max_time: float = None,
-
- memory_restriction_level: int = None,
- debug: bool = False,
- save_dir: str = None,
- share: bool = False,
- local_files_only: bool = False,
- resume_download: bool = True,
- use_auth_token: Union[str, bool] = False,
- trust_remote_code: Union[str, bool] = True,
- rope_scaling: dict = None,
- max_seq_len: int = None,
- offload_folder: str = "offline_folder",
-
- src_lang: str = "English",
- tgt_lang: str = "Russian",
-
- prepare_offline_level: int = 0,
- cli: bool = False,
- cli_loop: bool = True,
- gradio: bool = True,
- gradio_offline_level: int = 0,
- server_name: str = "0.0.0.0",
- root_path: str = "",
- chat: bool = True,
- chat_conversation: typing.List[typing.Tuple[str, str]] = None,
- text_context_list: typing.List[str] = None,
- stream_output: bool = True,
- async_output: bool = True,
- num_async: int = 3,
- show_examples: bool = None,
- verbose: bool = False,
- h2ocolors: bool = True,
- dark: bool = False, # light tends to be best
- height: int = 600,
- show_lora: bool = True,
- show_llama: bool = True,
- show_gpt4all: bool = False,
- login_mode_if_model0: bool = False,
- block_gradio_exit: bool = True,
- concurrency_count: int = 1,
- api_open: bool = False,
- allow_api: bool = True,
- input_lines: int = 1,
- gradio_size: str = None,
- show_copy_button: bool = True,
- large_file_count_mode: bool = False,
- pre_load_embedding_model: bool = True,
-
- auth: Union[typing.List[typing.Tuple[str, str]], str] = None,
- auth_filename: str = None,
- auth_access: str = 'open',
- auth_freeze: bool = False,
- auth_message: str = None,
- guest_name: str = "guest",
- enforce_h2ogpt_api_key: bool = None,
- h2ogpt_api_keys: Union[list, str] = [],
- h2ogpt_key: str = None,
-
- max_max_time=None,
- max_max_new_tokens=None,
-
- visible_models: list = None,
- visible_visible_models: bool = True,
- visible_submit_buttons: bool = True,
- visible_side_bar: bool = True,
- visible_doc_track: bool = True,
- visible_chat_tab: bool = True,
- visible_doc_selection_tab: bool = True,
- visible_doc_view_tab: bool = True,
- visible_chat_history_tab: bool = True,
- visible_expert_tab: bool = True,
- visible_models_tab: bool = True,
- visible_system_tab: bool = True,
- visible_tos_tab: bool = False,
- visible_login_tab: bool = True,
- visible_hosts_tab: bool = False,
- chat_tables: bool = False,
- visible_h2ogpt_header: bool = True,
- max_raw_chunks: int = None,
-
- sanitize_user_prompt: bool = False,
- sanitize_bot_response: bool = False,
-
- extra_model_options: typing.List[str] = [],
- extra_lora_options: typing.List[str] = [],
- extra_server_options: typing.List[str] = [],
-
- score_model: str = 'auto',
-
- eval_filename: str = None,
- eval_prompts_only_num: int = 0,
- eval_prompts_only_seed: int = 1234,
- eval_as_output: bool = False,
-
- langchain_mode: str = None,
- user_path: str = None,
- langchain_modes: list = [LangChainMode.USER_DATA.value, LangChainMode.MY_DATA.value, LangChainMode.LLM.value,
- LangChainMode.DISABLED.value],
- langchain_mode_paths: dict = {LangChainMode.USER_DATA.value: None},
- langchain_mode_types: dict = {LangChainMode.USER_DATA.value: LangChainTypes.SHARED.value},
- detect_user_path_changes_every_query: bool = False,
-
- langchain_action: str = LangChainAction.QUERY.value,
- langchain_agents: list = [],
- force_langchain_evaluate: bool = False,
-
- visible_langchain_actions: list = [LangChainAction.QUERY.value, LangChainAction.SUMMARIZE_MAP.value],
- visible_langchain_agents: list = langchain_agents_list.copy(),
-
- document_subset: str = DocumentSubset.Relevant.name,
- document_choice: list = [DocumentChoice.ALL.value],
-
- use_llm_if_no_docs: bool = True,
- load_db_if_exists: bool = True,
- keep_sources_in_context: bool = False,
- db_type: str = 'chroma',
- use_openai_embedding: bool = False,
- use_openai_model: bool = False,
- hf_embedding_model: str = None,
- migrate_embedding_model: str = False,
- auto_migrate_db: bool = False,
- cut_distance: float = 1.64,
- answer_with_sources: bool = True,
- append_sources_to_answer: bool = True,
- show_accordions: bool = True,
- top_k_docs_max_show: int = 10,
- show_link_in_sources: bool = True,
- pre_prompt_query: str = None,
- prompt_query: str = None,
- pre_prompt_summary: str = None,
- prompt_summary: str = None,
- add_chat_history_to_context: bool = True,
- add_search_to_context: bool = False,
- context: str = '',
- iinput: str = '',
- allow_upload_to_user_data: bool = True,
- reload_langchain_state: bool = True,
- allow_upload_to_my_data: bool = True,
- enable_url_upload: bool = True,
- enable_text_upload: bool = True,
- enable_sources_list: bool = True,
- chunk: bool = True,
- chunk_size: int = 512,
- top_k_docs: int = None,
- docs_ordering_type: str = 'reverse_ucurve_sort',
- min_max_new_tokens=256,
- auto_reduce_chunks: bool = True,
- max_chunks: int = 100,
- headsize: int = 50,
- n_jobs: int = -1,
-
- # urls
- use_unstructured=True,
- use_playwright=False,
- use_selenium=False,
-
- # pdfs
- use_pymupdf='auto',
- use_unstructured_pdf='auto',
- use_pypdf='auto',
- enable_pdf_ocr='auto',
- enable_pdf_doctr='auto',
- try_pdf_as_html='auto',
-
- # images
- enable_ocr=False,
- enable_doctr=False,
- enable_pix2struct=False,
- enable_captions=True,
-
- pre_load_caption_model: bool = False,
- caption_gpu: bool = True,
- captions_model: str = "Salesforce/blip-image-captioning-base",
- doctr_gpu: bool = True,
-
- # json
- jq_schema='.[]',
-
- max_quality: bool = False,
-
- enable_heap_analytics: bool = True,
- heap_app_id: str = "1680123994",
-):
- """
-
- :param load_8bit: load model in 8-bit using bitsandbytes
- :param load_4bit: load model in 4-bit using bitsandbytes
- :param low_bit_mode: 0: no quantization config 1: change compute 2: nf4 3: double quant 4: 2 and 3
- See: https://huggingface.co/docs/transformers/main_classes/quantization
- If using older bitsandbytes or transformers, 0 is required
- :param load_half: load model in float16 (None means auto, which means True unless t5 based model)
- otherwise specify bool
- :param load_gptq: to load model with GPTQ, put model_basename here, e.g. gptq_model-4bit--1g
- :param load_exllama: whether to use exllama (only applicable to LLaMa1/2 models with 16-bit or GPTQ
- :param use_safetensors: to use safetensors version (assumes file/HF points to safe tensors version)
- :param revision: Which HF revision to use
- :param use_gpu_id: whether to control devices with gpu_id. If False, then spread across GPUs
- :param base_model: model HF-type name. If use --base_model to preload model, cannot unload in gradio in models tab
- :param tokenizer_base_model: tokenizer HF-type name. Usually not required, inferred from base_model.
- :param lora_weights: LORA weights path/HF link
- :param gpu_id: if use_gpu_id, then use gpu_id for cuda device ID, or auto mode if gpu_id != -1
- :param compile_model Whether to compile the model
- :param use_cache: Whether to use caching in model (some models fail when multiple threads use)
- :param inference_server: Consume base_model as type of model at this address
- Address can be text-generation-server hosting that base_model
- e.g. python generate.py --inference_server="http://192.168.1.46:6112" --base_model=h2oai/h2ogpt-oasst1-512-12b
-
- Or Address can be "openai_chat" or "openai" for OpenAI API
- Or Address can be "openai_azure_chat" or "openai_azure" for Azure OpenAI API
- e.g. python generate.py --inference_server="openai_chat" --base_model=gpt-3.5-turbo
- e.g. python generate.py --inference_server="openai" --base_model=text-davinci-003
- e.g. python generate.py --inference_server="openai_azure_chat::::" --base_model=gpt-3.5-turbo
- e.g. python generate.py --inference_server="openai_azure::::" --base_model=text-davinci-003
- Optionals (Replace with None or just leave empty but keep :)
- of some deployment name
- : e.g. ".openai.azure.com" for some without https://
- of some api, e.g. 2023-05-15
- e.g. 0613
-
- Or Address can be for vLLM:
- Use: "vllm:IP:port" for OpenAI-compliant vLLM endpoint
- Note: vllm_chat not supported by vLLM project.
-
- Or Address can be replicate:
- Use:
- --inference_server=replicate: will use a Replicate server, requiring a Replicate key.
- e.g. looks like "a16z-infra/llama13b-v2-chat:df7690f1994d94e96ad9d568eac121aecf50684a0b0963b25a41cc40061269e5"
-
- Or Address can be for AWS SageMaker:
- Use: "sagemaker_chat:" for chat models that AWS sets up as dialog
- Use: "sagemaker:" for foundation models that AWS only text as inputs
-
- :param prompt_type: type of prompt, usually matched to fine-tuned model or plain for foundational model
- :param prompt_dict: If prompt_type=custom, then expects (some) items returned by get_prompt(..., return_dict=True)
- :param system_prompt: Universal system prompt to use if model supports, like LLaMa2, regardless of prompt_type definition.
- Useful for langchain case to control behavior, or OpenAI and Replicate.
- If None, 'None', or 'auto', then for LLaMa or other models that internally have system_prompt, will use default for each model
- If '', then no system prompt (no empty template given to model either, just no system part added at all)
- If some string not in ['None', 'auto'], then use that as system prompt
- Default is '', no system_prompt, because often it hurts performance/accuracy
-
- :param llamacpp_dict:
- n_gpu_layers: for llama.cpp based models, number of GPU layers to offload (default is all by using large value)
- use_mlock: when using `llama.cpp` based CPU models, for computers with low system RAM or slow CPUs, recommended False
- n_batch: Can make smaller to 128 for slower low-memory CPU systems
- n_gqa: Required to be 8 for LLaMa 70B
- ... etc. anything that could be passed to llama.cpp or GPT4All models
- e.g. python generate.py --base_model='llama' --prompt_type=llama2 --score_model=None --langchain_mode='UserData' --user_path=user_path --llamacpp_dict="{'n_gpu_layers':25,'n_batch':128}"
- :param model_path_llama: model path or URL (for auto-download)
- :param model_name_gptj: model path or URL (for auto-download)
- :param model_name_gpt4all_llama: model path or URL (for auto-download)
- :param model_name_exllama_if_no_config: exllama model's full path for model, tokenizer, generator for use when no HuggingFace config
-
- :param model_lock: Lock models to specific combinations, for ease of use and extending to many models
- Only used if gradio = True
- List of dicts, each dict has base_model, tokenizer_base_model, lora_weights, inference_server, prompt_type, and prompt_dict
- If all models have same prompt_type, and prompt_dict, can still specify that once in CLI outside model_lock as default for dict
- Can specify model_lock instead of those items on CLI
- As with CLI itself, base_model can infer prompt_type and prompt_dict if in prompter.py.
- Also, tokenizer_base_model and lora_weights are optional.
- Also, inference_server is optional if loading model from local system.
- All models provided will automatically appear in compare model mode
- Model loading-unloading and related choices will be disabled. Model/lora/server adding will be disabled
- :param model_lock_columns: How many columns to show if locking models (and so showing all at once)
- If None, then defaults to up to 3
- if -1, then all goes into 1 row
- Maximum value is 4 due to non-dynamic gradio rendering elements
- :param fail_if_cannot_connect: if doing model locking (e.g. with many models), fail if True. Otherwise ignore.
- Useful when many endpoints and want to just see what works, but still have to wait for timeout.
-
- :param temperature: generation temperature
- :param top_p: generation top_p
- :param top_k: generation top_k
- :param num_beams: generation number of beams
- :param repetition_penalty: generation repetition penalty
- :param num_return_sequences: generation number of sequences (1 forced for chat)
- :param do_sample: generation sample
- :param max_new_tokens: generation max new tokens
- :param min_new_tokens: generation min tokens
- :param early_stopping: generation early stopping
- :param max_time: maximum time to allow for generation
- :param memory_restriction_level: 0 = no restriction to tokens or model, 1 = some restrictions on token 2 = HF like restriction 3 = very low memory case
- :param debug: enable debug mode
- :param save_dir: directory chat data is saved to
- :param share: whether to share the gradio app with sharable URL
- :param local_files_only: whether to only use local files instead of doing to HF for models
- :param resume_download: whether to resume downloads from HF for models
- :param use_auth_token: whether to use HF auth token (requires CLI did huggingface-cli login before)
- :param trust_remote_code: whether to use trust any code needed for HF model
- :param rope_scaling:
- For HF transformers model: scaling for rope-based models, e.g. --rope_scaling="{'type':'dynamic', 'factor':4}"
- For exllama model: --rope_scaling="{'alpha_value':4}" . This automatically scales max_seq_len for exllama
- :param max_seq_len: Manually set maximum sequence length for the LLM
- :param offload_folder: path for spilling model onto disk
- :param src_lang: source languages to include if doing translation (None = all)
- :param tgt_lang: target languages to include if doing translation (None = all)
-
- :param prepare_offline_level:
- Whether to just prepare for offline use, do not go into cli, eval, or gradio run modes
- 0 : no prep
- 1: prepare just h2oGPT with exact same setup as passed to CLI and ensure all artifacts for h2oGPT alone added to ~/.cache/
- 2: prepare h2oGPT + all inference servers so h2oGPT+inference servers can use the ~/.cache/
- :param cli: whether to use CLI (non-gradio) interface.
- :param cli_loop: whether to loop for CLI (False usually only for testing)
- :param gradio: whether to enable gradio, or to enable benchmark mode
- :param gradio_offline_level: > 0, then change fonts so full offline
- == 1 means backend won't need internet for fonts, but front-end UI might if font not cached
- == 2 means backend and frontend don't need internet to download any fonts.
- Note: Some things always disabled include HF telemetry, gradio telemetry, chromadb posthog that involve uploading.
- This option further disables google fonts for downloading, which is less intrusive than uploading,
- but still required in air-gapped case. The fonts don't look as nice as google fonts, but ensure full offline behavior.
- Also set --share=False to avoid sharing a gradio live link.
- :param server_name: IP to use. In linux 0.0.0.0 is good choice so exposed to outside host, else for only local use 127.0.0.1.
- For windows/MAC 0.0.0.0 or 127.0.0.1 will work, but may need to specify actual LAN IP address for other LAN clients to see.
- :param root_path: The root path (or "mount point") of the application,
- if it's not served from the root ("/") of the domain. Often used when the application is behind a reverse proxy
- that forwards requests to the application. For example, if the application is served at "https://example.com/myapp",
- the `root_path` should be set to "/myapp".
- :param chat: whether to enable chat mode with chat history
- :param chat_conversation: list of tuples of (human, bot) conversation pre-appended to existing chat when using instruct/chat models
- Requires also add_chat_history_to_context = True
- It does *not* require chat=True, so works with nochat_api etc.
- :param text_context_list: List of strings to add to context for non-database version of document Q/A for faster handling via API etc.
- Forces LangChain code path and uses as many entries in list as possible given max_seq_len, with first assumed to be most relevant and to go near prompt.
- :param stream_output: whether to stream output
- :param async_output: Whether to do asyncio handling
- For summarization
- Applicable to HF TGI server
- Only if stream_output=False in CLI, UI, or API
- :param num_async: Number of simultaneously allowed asyncio calls to make for async_output
- Too many will overload inference server, too few will be too slow
- :param show_examples: whether to show clickable examples in gradio
- :param verbose: whether to show verbose prints
- :param h2ocolors: whether to use H2O.ai theme
- :param dark: whether to use dark mode for UI by default (still controlled in UI)
- :param height: height of chat window
- :param show_lora: whether to show LORA options in UI (expert so can be hard to understand)
- :param show_llama: whether to show LLaMa.cpp/GPT4All options in UI (only likely useful if have weak GPUs)
- :param show_gpt4all: whether to show GPT4All models in UI (not often useful, llama.cpp models best)
- :param login_mode_if_model0: set to True to load --base_model after client logs in, to be able to free GPU memory when model is swapped
- :param block_gradio_exit: whether to block gradio exit (used for testing)
- :param concurrency_count: gradio concurrency count (1 is optimal for LLMs)
- :param api_open: If False, don't let API calls skip gradio queue
- :param allow_api: whether to allow API calls at all to gradio server
- :param input_lines: how many input lines to show for chat box (>1 forces shift-enter for submit, else enter is submit)
- :param gradio_size: Overall size of text and spaces: "xsmall", "small", "medium", "large".
- Small useful for many chatbots in model_lock mode
- :param show_copy_button: Whether to show copy button for chatbots
- :param large_file_count_mode: Whether to force manual update to UI of drop-downs, good idea if millions of chunks or documents
- :param pre_load_embedding_model: Whether to preload embedding model for shared use across DBs and users (multi-thread safe only)
-
- :param auth: gradio auth for launcher in form [(user1, pass1), (user2, pass2), ...]
- e.g. --auth=[('jon','password')] with no spaces
- e.g. --auth="[('jon', 'password)())(')]" so any special characters can be used
- e.g. --auth=auth.json to specify persisted state file with name auth.json (auth_filename then not required)
- e.g. --auth='' will use default auth.json as file name for persisted state file (auth_filename then not required)
- e.g. --auth=None will use no auth, but still keep track of auth state, just not from logins
- :param auth_filename:
- Set auth filename, used only if --auth= was passed list of user/passwords
- :param auth_access:
- 'open': Allow new users to be added
- 'closed': Stick to existing users
- :param auth_freeze: whether freeze authentication based upon current file, no longer update file
- :param auth_message: Message to show if having users login, fixed if passed, else dynamic internally
- :param guest_name: guess name if using auth and have open access.
- If '', then no guest allowed even if open access, then all databases for each user always persisted
- :param enforce_h2ogpt_api_key: Whether to enforce h2oGPT token usage for API
- :param h2ogpt_api_keys: list of tokens allowed for API access or file accessed on demand for json of list of keys
- :param h2ogpt_key: E.g. can be set when accessing gradio h2oGPT server from local gradio h2oGPT server that acts as client to that inference server
-
- :param max_max_time: Maximum max_time for gradio slider
- :param max_max_new_tokens: Maximum max_new_tokens for gradio slider
- :param min_max_new_tokens: Minimum of max_new_tokens, when auto-scaling down to handle more docs/prompt, but still let generation have some tokens
-
- :param visible_models: Which models in model_lock list to show by default
- Takes integers of position in model_lock (model_states) list or strings of base_model names
- Ignored if model_lock not used
- For nochat API, this is single item within a list for model by name or by index in model_lock
- If None, then just use first model in model_lock list
- If model_lock not set, use model selected by CLI --base_model etc.
-
- :param visible_visible_models: Whether visible models drop-down is visible in UI
- :param visible_submit_buttons: whether submit buttons are visible when UI first comes up
- :param visible_side_bar: whether left side bar is visible when UI first comes up
- :param visible_doc_track: whether left side bar's document tracking is visible when UI first comes up
- :param visible_chat_tab: "" for chat tab
- :param visible_doc_selection_tab: "" for doc selection tab
- :param visible_doc_view_tab: "" for doc view tab
- :param visible_chat_history_tab: "" for chat history tab
- :param visible_expert_tab: "" for expert tab
- :param visible_models_tab: "" for models tab
- :param visible_system_tab: "" for system tab
- :param visible_tos_tab: "" for ToS tab
- :param visible_login_tab: "" for Login tab
- :param visible_hosts_tab: "" for hosts tab
- :param chat_tables: Just show Chat as block without tab (useful if want only chat view)
- :param visible_h2ogpt_header: Whether github stars, URL, logo, and QR code are visible
- :param max_raw_chunks: Maximum number of chunks to show in UI when asking for raw DB text from documents/collection
-
- :param sanitize_user_prompt: whether to remove profanity from user input (slows down input processing)
- Requires optional packages:
- pip install alt-profanity-check==1.2.2 better-profanity==0.7.0
- :param sanitize_bot_response: whether to remove profanity and repeat lines from bot output (about 2x slower generation for long streaming cases due to better_profanity being slow)
- :param extra_model_options: extra models to show in list in gradio
- :param extra_lora_options: extra LORA to show in list in gradio
- :param extra_server_options: extra servers to show in list in gradio
- :param score_model: which model to score responses
- None: no response scoring
- 'auto': auto mode, '' (no model) for CPU or 1 GPU, 'OpenAssistant/reward-model-deberta-v3-large-v2' for >=2 GPUs,
- because on CPU takes too much compute just for scoring response
- :param eval_filename: json file to use for evaluation, if None is sharegpt
- :param eval_prompts_only_num: for no gradio benchmark, if using eval_filename prompts for eval instead of examples
- :param eval_prompts_only_seed: for no gradio benchmark, seed for eval_filename sampling
- :param eval_as_output: for no gradio benchmark, whether to test eval_filename output itself
-
- :param langchain_mode: Data source to include. Choose "UserData" to only consume files from make_db.py.
- None: auto mode, check if langchain package exists, at least do LLM if so, else Disabled
- If not passed, then chosen to be first langchain_modes, else langchain_mode->Disabled is set if no langchain_modes either
- WARNING: wiki_full requires extra data processing via read_wiki_full.py and requires really good workstation to generate db, unless already present.
- :param user_path: user path to glob from to generate db for vector search, for 'UserData' langchain mode.
- If already have db, any new/changed files are added automatically if path set, does not have to be same path used for prior db sources
- :param langchain_modes: dbs to generate at launch to be ready for LLM
- Apart from additional user-defined collections, can include ['wiki', 'wiki_full', 'UserData', 'MyData', 'github h2oGPT', 'DriverlessAI docs']
- But wiki_full is expensive and requires preparation
- To allow personal space only live in session, add 'MyData' to list
- Default: If only want to consume local files, e.g. prepared by make_db.py, only include ['UserData']
- If have own user modes, need to add these here or add in UI.
- :param langchain_mode_paths: dict of langchain_mode keys and disk path values to use for source of documents
- E.g. "{'UserData2': 'userpath2'}"
- A disk path be None, e.g. --langchain_mode_paths="{'UserData2': None}" even if existing DB, to avoid new documents being added from that path, source links that are on disk still work.
- If `--user_path` was passed, that path is used for 'UserData' instead of the value in this dict
- :param langchain_mode_types: dict of langchain_mode keys and database types
- E.g. python generate.py --base_model=llama --langchain_modes=['TestData'] --langchain_mode_types="{'TestData':'shared'}"
- The type is attempted to be inferred if directory already exists, then don't have to pass this
- :param detect_user_path_changes_every_query: whether to detect if any files changed or added every similarity search (by file hashes).
- Expensive for large number of files, so not done by default. By default only detect changes during db loading.
-
- :param langchain_action: Mode langchain operations in on documents.
- Query: Make query of document(s)
- Summarize or Summarize_map_reduce: Summarize document(s) via map_reduce
- Summarize_all: Summarize document(s) using entire document at once
- Summarize_refine: Summarize document(s) using entire document, and try to refine before returning summary
- :param langchain_agents: Which agents to use
- 'search': Use Web Search as context for LLM response, e.g. SERP if have SERPAPI_API_KEY in env
- :param force_langchain_evaluate: Whether to force langchain LLM use even if not doing langchain, mostly for testing.
-
- :param visible_langchain_actions: Which actions to allow
- :param visible_langchain_agents: Which agents to allow
-
- :param document_subset: Default document choice when taking subset of collection
- :param document_choice: Chosen document(s) by internal name, 'All' means use all docs
-
- :param use_llm_if_no_docs: Whether to use LLM even if no documents, when langchain_mode=UserData or MyData or custom
- :param load_db_if_exists: Whether to load chroma db if exists or re-generate db
- :param keep_sources_in_context: Whether to keep url sources in context, not helpful usually
- :param db_type: 'faiss' for in-memory
- 'chroma' (for chroma >= 0.4)
- 'chroma_old' (for chroma < 0.4) -- recommended for large collections
- 'weaviate' for persisted on disk
- :param use_openai_embedding: Whether to use OpenAI embeddings for vector db
- :param use_openai_model: Whether to use OpenAI model for use with vector db
- :param hf_embedding_model: Which HF embedding model to use for vector db
- Default is instructor-large with 768 parameters per embedding if have GPUs, else all-MiniLM-L6-v2 if no GPUs
- Can also choose simpler model with 384 parameters per embedding: "sentence-transformers/all-MiniLM-L6-v2"
- Can also choose even better embedding with 1024 parameters: 'hkunlp/instructor-xl'
- We support automatically changing of embeddings for chroma, with a backup of db made if this is done
- :param migrate_embedding_model: whether to use hf_embedding_model embedding even if database already had an embedding set.
- used to migrate all embeddings to a new one, but will take time to re-embed.
- Default (False) is to use the prior embedding for existing databases, and only use hf_embedding_model for new databases
- If had old database without embedding saved, then hf_embedding_model is also used.
- :param auto_migrate_db: whether to automatically migrate any chroma<0.4 database from duckdb -> sqlite version
- :param cut_distance: Distance to cut off references with larger distances when showing references.
- 1.64 is good to avoid dropping references for all-MiniLM-L6-v2, but instructor-large will always show excessive references.
- For all-MiniLM-L6-v2, a value of 1.5 can push out even more references, or a large value of 100 can avoid any loss of references.
- :param answer_with_sources: Whether to determine (and return) sources
- :param append_sources_to_answer: Whether to place source information in chat response (ignored by LLM). Always disabled for API.
- :param show_accordions: whether to show accordion for document references in chatbot UI
- :param top_k_docs_max_show: Max number of docs to show in UI for sources
- If web search is enabled, then this is modified to be max(top_k_docs_max_show, number of links used in search)
- :param show_link_in_sources: Whether to show URL link to source document in references
- :param pre_prompt_query: prompt before documents to query, if None then use internal defaults
- :param prompt_query: prompt after documents to query, if None then use internal defaults
- :param pre_prompt_summary: prompt before documents to summarize, if None then use internal defaults
- :param prompt_summary: prompt after documents to summarize, if None then use internal defaults
- For summarize, normal to have empty query (nothing added in ask anything in UI or empty string in API)
- If pass query, template is "Focusing on %s, %s" % (query, prompt_summary)
- If pass query and iinput, template is "Focusing on %s, %s, %s" % (query, iinput, prompt_summary)
- :param add_chat_history_to_context: Include chat context when performing action
- Not supported yet for openai_chat when using document collection instead of LLM
- Also not supported when using CLI mode
- :param add_search_to_context: Include web search in context as augmented prompt
- :param context: Default context to use (for system pre-context in gradio UI)
- context comes before chat_conversation and any document Q/A from text_context_list
- :param iinput: Default input for instruction-based prompts
- :param allow_upload_to_user_data: Whether to allow file uploads to update shared vector db (UserData or custom user dbs)
- Ensure pass user_path for the files uploaded to be moved to this location for linking.
- :param reload_langchain_state: Whether to reload langchain_modes.pkl file that contains any new user collections.
- :param allow_upload_to_my_data: Whether to allow file uploads to update personal vector db
- :param enable_url_upload: Whether to allow upload from URL
- :param enable_text_upload: Whether to allow upload of text
- :param enable_sources_list: Whether to allow list (or download for non-shared db) of list of sources for chosen db
- :param chunk: Whether to chunk data (True unless know data is already optimally chunked)
- :param chunk_size: Size of chunks, with typically top-4 passed to LLM, so needs to be in context length
- :param top_k_docs: For langchain_action query: number of chunks to give LLM
- -1 : auto-fills context up to max_seq_len
- For langchain_action summarize: number of document parts, like pages for PDF.
- There's no such thing as chunks for summarization.
- -1 : auto-fills context up to max_seq_len
- :param docs_ordering_type:
- Type of ordering of docs.
- 'best_first': Order by score so score is worst match near prompt
- 'best_near_prompt' or 'reverse_sort' : reverse docs order so most relevant is closest to question.
- Best choice for sufficiently smart model, and truncation occurs for oldest context, so best then too.
- But smaller 6_9 models fail to use newest context and can get stuck on old information.
- '' or None (i.e. default) or 'reverse_ucurve_sort' : Sort so most relevant is either near start or near end
- Best to avoid "lost in middle" as well as avoid hallucinating off starting content that LLM focuses on alot.
- :param auto_reduce_chunks: Whether to automatically reduce top_k_docs to fit context given prompt
- :param max_chunks: If top_k_docs=-1, maximum number of chunks to allow
- :param headsize: Maximum number of characters for head of document document for UI to show
- :param n_jobs: Number of processors to use when consuming documents (-1 = all, is default)
-
- :param use_unstructured: Enable unstructured URL loader
- :param use_playwright: Enable PlayWright URL loader
- :param use_selenium: Enable Selenium URL loader
-
- :param use_pymupdf: enable PyMUPDF 'auto' means use first, use others if they are 'auto' if no result
- :param use_unstructured_pdf: enable Unstructured PDF loader, 'auto' means use if pymupdf fails to get doc result
- :param use_pypdf: enable PyPDF loader 'auto' means use if unstructured fails to get doc result
- :param enable_pdf_ocr: 'auto' means only use OCR if normal text extraction fails. Useful for pure image-based PDFs with text.
- if enable_pdf_doctr == 'on' then don't do.
- 'on' means always do OCR as additional parsing of same documents
- 'off' means don't do OCR (e.g. because it's slow even if 'auto' only would trigger if nothing else worked)
- :param enable_pdf_doctr: Whether to support doctr on pdfs, 'auto' means use do if failed to get doc result so far
- :param try_pdf_as_html: Try "PDF" as if HTML file, in case web link has .pdf extension but really is just HTML
-
- :param enable_ocr: Whether to support OCR on images
- :param enable_doctr: Whether to support doctr on images (using OCR better than enable_ocr=True)
- :param enable_pix2struct: Whether to support pix2struct on images for captions
- :param enable_captions: Whether to support captions using BLIP for image files as documents,
- then preloads that model if pre_load_caption_model=True
-
- :param pre_load_caption_model: Whether to preload caption model, or load after forking parallel doc loader
- parallel loading disabled if preload and have images, to prevent deadlocking on cuda context
- Recommended if using larger caption model
- :param captions_model: Which model to use for captions.
- captions_model: str = "Salesforce/blip-image-captioning-base", # continue capable
- captions_model: str = "Salesforce/blip2-flan-t5-xl", # question/answer capable, 16GB state
- captions_model: str = "Salesforce/blip2-flan-t5-xxl", # question/answer capable, 60GB state
- Note: opt-based blip2 are not permissive license due to opt and Meta license restrictions
- Disabled for CPU since BLIP requires CUDA
- :param caption_gpu: If support caption, then use GPU if exists
-
- :param doctr_gpu: If support doctr, then use GPU if exists
-
- :param jq_schema: control json loader
- By default '.[]' ingests everything in brute-force way, but better to match your schema
- See: https://python.langchain.com/docs/modules/data_connection/document_loaders/json#using-jsonloader
-
- :param max_quality: Choose maximum quality ingestion with all available parsers
- Pro: Catches document when some default parsers would fail
- Pro: Enables DocTR that has much better OCR than Tesseract
- Con: Fills DB with results from all parsers, so similarity search gives redundant results
-
- :param enable_heap_analytics: Toggle telemetry.
- :param heap_app_id: App ID for Heap, change to your ID.
- :return:
- """
- if base_model is None:
- base_model = ''
- if tokenizer_base_model is None:
- tokenizer_base_model = ''
- if lora_weights is None:
- lora_weights = ''
- if inference_server is None:
- inference_server = ''
-
- # listen to env if set
- model_lock = os.getenv('model_lock', str(model_lock))
- model_lock = ast.literal_eval(model_lock)
-
- chat_conversation = str_to_list(chat_conversation)
- text_context_list = str_to_list(text_context_list)
-
- llamacpp_dict = str_to_dict(llamacpp_dict)
- # add others to single dict
- llamacpp_dict['model_path_llama'] = model_path_llama
- llamacpp_dict['model_name_gptj'] = model_name_gptj
- llamacpp_dict['model_name_gpt4all_llama'] = model_name_gpt4all_llama
- llamacpp_dict['model_name_exllama_if_no_config'] = model_name_exllama_if_no_config
- # if user overrides but doesn't set these:
- if 'n_batch' not in llamacpp_dict:
- llamacpp_dict['n_batch'] = 128
- if 'n_gpu_layers' not in llamacpp_dict:
- llamacpp_dict['n_gpu_layers'] = 100
- if 'n_gqa' not in llamacpp_dict:
- llamacpp_dict['n_gqa'] = 0
-
- if os.environ.get('SERPAPI_API_KEY') is None and LangChainAgent.SEARCH.value in visible_langchain_agents:
- visible_langchain_agents.remove(LangChainAgent.SEARCH.value)
-
- if model_lock:
- assert gradio, "model_lock only supported for gradio=True"
- assert not cli, "model_lock only supported for cli=False"
- assert not (not cli and not gradio), "model_lock only supported for eval (cli=gradio=False)"
- assert not base_model, "Don't specify model_lock and base_model"
- assert not tokenizer_base_model, "Don't specify model_lock and tokenizer_base_model"
- assert not lora_weights, "Don't specify model_lock and lora_weights"
- assert not inference_server, "Don't specify model_lock and inference_server"
- # assert not prompt_type, "Don't specify model_lock and prompt_type"
- # assert not prompt_dict, "Don't specify model_lock and prompt_dict"
-
- n_jobs = int(os.getenv('n_jobs', str(n_jobs)))
- is_hf = bool(int(os.getenv("HUGGINGFACE_SPACES", '0')))
- is_gpth2oai = bool(int(os.getenv("GPT_H2O_AI", '0')))
- is_public = is_hf or is_gpth2oai # multi-user case with fixed model and disclaimer
- if is_public:
- visible_tos_tab = visible_hosts_tab = True
- if enforce_h2ogpt_api_key is None:
- enforce_h2ogpt_api_key = True
- else:
- if enforce_h2ogpt_api_key is None:
- enforce_h2ogpt_api_key = False
- if isinstance(h2ogpt_api_keys, str) and not os.path.isfile(h2ogpt_api_keys):
- h2ogpt_api_keys = str_to_list(h2ogpt_api_keys)
- if memory_restriction_level is None:
- memory_restriction_level = 2 if is_hf else 0 # 2 assumes run on 24GB consumer GPU
- else:
- assert 0 <= memory_restriction_level <= 3, "Bad memory_restriction_level=%s" % memory_restriction_level
- if n_jobs == -1:
- # if -1, assume hypercores, don't use, force user to pass n_jobs to be specific if not standard cores
- n_jobs = max(1, os.cpu_count() // 2)
- if is_public and os.getenv('n_jobs') is None:
- n_jobs = min(n_jobs, max(1, min(os.cpu_count() // 2, 8)))
- admin_pass = os.getenv("ADMIN_PASS")
- # will sometimes appear in UI or sometimes actual generation, but maybe better than empty result
- # but becomes unrecoverable sometimes if raise, so just be silent for now
- raise_generate_gpu_exceptions = True
-
- rope_scaling = str_to_dict(rope_scaling)
-
- if isinstance(auth, str):
- if auth.strip().startswith('['):
- auth = str_to_list(auth)
- if isinstance(auth, str) and auth:
- auth_filename = auth
- if not auth_filename:
- auth_filename = "auth.json"
- assert isinstance(auth, (str, list, tuple, type(None))), "Unknown type %s for auth=%s" % (type(auth), auth)
-
- # allow set token directly
- use_auth_token = os.environ.get("HUGGING_FACE_HUB_TOKEN", use_auth_token)
- allow_upload_to_user_data = bool(
- int(os.environ.get("allow_upload_to_user_data", str(int(allow_upload_to_user_data)))))
- allow_upload_to_my_data = bool(int(os.environ.get("allow_upload_to_my_data", str(int(allow_upload_to_my_data)))))
- height = int(os.environ.get("HEIGHT", height))
- h2ocolors = bool(int(os.getenv('h2ocolors', h2ocolors)))
-
- # allow enabling langchain via ENV
- # FIRST PLACE where LangChain referenced, but no imports related to it
- langchain_modes = ast.literal_eval(os.environ.get("langchain_modes", str(langchain_modes)))
- if not isinstance(langchain_modes, list):
- langchain_modes = []
- # always allow DISABLED
- if LangChainMode.DISABLED.value not in langchain_modes:
- langchain_modes.append(LangChainMode.DISABLED.value)
- if not have_langchain:
- # only allow disabled, not even LLM that is langchain related
- langchain_mode = LangChainMode.DISABLED.value
- langchain_modes = [langchain_mode]
-
- # update
- langchain_mode_paths = str_to_dict(langchain_mode_paths)
- langchain_mode_types = str_to_dict(langchain_mode_types)
- for lmode in [LangChainMode.GITHUB_H2OGPT.value,
- LangChainMode.H2O_DAI_DOCS.value,
- LangChainMode.WIKI.value,
- LangChainMode.WIKI_FULL.value,
- ]:
- if lmode not in langchain_mode_types:
- langchain_mode_types[lmode] = 'shared'
- if lmode not in langchain_mode_paths:
- langchain_mode_types[lmode] = ''
- if user_path:
- user_path = makedirs(user_path, use_base=True)
- langchain_mode_paths['UserData'] = user_path
- langchain_mode_paths['UserData'] = LangChainTypes.SHARED.value
-
- if is_public:
- allow_upload_to_user_data = False
- if LangChainMode.USER_DATA.value in langchain_modes:
- langchain_modes.remove(LangChainMode.USER_DATA.value)
- if max_raw_chunks is None:
- max_raw_chunks = 30 if is_public else 1000000
-
- # in-place, for non-scratch dbs
- if allow_upload_to_user_data:
- # always listen to CLI-passed user_path if passed
- if user_path:
- langchain_mode_paths['UserData'] = user_path
-
- assert langchain_action in langchain_actions, "Invalid langchain_action %s not in %s" % (
- langchain_action, langchain_actions)
- assert len(
- set(langchain_agents).difference(langchain_agents_list)) == 0, "Invalid langchain_agents %s" % langchain_agents
-
- # auto-set langchain_mode
- langchain_mode = os.environ.get("LANGCHAIN_MODE", langchain_mode)
- if have_langchain and langchain_mode is None:
- # start in chat mode, in case just want to chat and don't want to get "No documents to query" by default.
- if LangChainMode.LLM.value in langchain_modes:
- langchain_mode = LangChainMode.LLM.value
- elif len(langchain_modes) >= 1:
- # infer even if don't pass which langchain_mode, just langchain_modes.
- langchain_mode = langchain_modes[0]
- if allow_upload_to_user_data and not is_public and langchain_mode_paths['UserData']:
- if verbose:
- print("Auto set langchain_mode=%s. Could use UserData instead." % langchain_mode, flush=True)
- elif allow_upload_to_my_data:
- if verbose:
- print("Auto set langchain_mode=%s. Could use MyData instead."
- " To allow UserData to pull files from disk,"
- " set user_path or langchain_mode_paths, and ensure allow_upload_to_user_data=True" % langchain_mode,
- flush=True)
- else:
- raise RuntimeError("Please pass --langchain_mode= out of %s" % langchain_modes)
- if not have_langchain and langchain_mode not in [None, LangChainMode.DISABLED.value, LangChainMode.LLM.value]:
- raise RuntimeError("Asked for LangChain mode but langchain python package cannot be found.")
- if langchain_mode is None:
- # if not set yet, disable
- langchain_mode = LangChainMode.DISABLED.value
- print("Auto set langchain_mode=%s Have langchain package: %s" % (langchain_mode, have_langchain), flush=True)
- # go ahead and add
- if langchain_mode not in langchain_modes:
- langchain_modes.append(langchain_mode)
-
- if is_public:
- allow_upload_to_user_data = False
- input_lines = 1 # ensure set, for ease of use
- temperature = 0.2 if temperature is None else temperature
- top_p = 0.85 if top_p is None else top_p
- top_k = 70 if top_k is None else top_k
- if is_hf:
- do_sample = True if do_sample is None else do_sample
- top_k_docs = 3 if top_k_docs is None else top_k_docs
- else:
- # by default don't sample, too chatty
- do_sample = False if do_sample is None else do_sample
- top_k_docs = 4 if top_k_docs is None else top_k_docs
-
- if memory_restriction_level == 2:
- if not base_model and not inference_server and not model_lock:
- base_model = 'h2oai/h2ogpt-oasst1-512-12b'
- # don't set load_8bit if passed base_model, doesn't always work so can't just override
- load_8bit = True
- load_4bit = False # FIXME - consider using 4-bit instead of 8-bit
- elif not inference_server:
- top_k_docs = 10 if top_k_docs is None else top_k_docs
- if memory_restriction_level >= 2:
- load_8bit = True
- load_4bit = False # FIXME - consider using 4-bit instead of 8-bit
- if hf_embedding_model is None:
- hf_embedding_model = "sentence-transformers/all-MiniLM-L6-v2"
- top_k_docs = 3 if top_k_docs is None else top_k_docs
- if top_k_docs is None:
- top_k_docs = 3
- if is_public:
- if not max_time:
- max_time = 60 * 2
- if not max_max_time:
- max_max_time = max_time
- if not max_new_tokens:
- max_new_tokens = 256
- if not max_max_new_tokens:
- max_max_new_tokens = 512
- else:
- if not max_max_time:
- max_max_time = 60 * 20
- if not max_max_new_tokens:
- max_max_new_tokens = 1024
- if is_hf:
- # must override share if in spaces
- share = False
- if not max_time:
- max_time = 60 * 1
- if not max_max_time:
- max_max_time = max_time
- # HF accounted for later in get_max_max_new_tokens()
- save_dir = os.getenv('SAVE_DIR', save_dir)
- save_dir = makedirs(save_dir, exist_ok=True, tmp_ok=True, use_base=True)
- score_model = os.getenv('SCORE_MODEL', score_model)
- if str(score_model) == 'None':
- score_model = ''
- concurrency_count = int(os.getenv('CONCURRENCY_COUNT', concurrency_count))
- api_open = bool(int(os.getenv('API_OPEN', str(int(api_open)))))
- allow_api = bool(int(os.getenv('ALLOW_API', str(int(allow_api)))))
-
- n_gpus = torch.cuda.device_count() if torch.cuda.is_available() else 0
- n_gpus, gpu_ids = cuda_vis_check(n_gpus)
-
- if load_half is None and t5_type(base_model):
- load_half = False
- print("load_half=%s auto-set for %s to avoid bad generation" % (load_half, base_model), flush=True)
-
- if n_gpus == 0 or get_device() == "mps":
- # No CUDA GPUs usable
-
- if get_device() != "mps":
- print("No GPUs detected", flush=True)
-
- enable_captions = False
- gpu_id = None
- load_8bit = False
- load_4bit = False
- low_bit_mode = 1
- if load_half is None:
- # wouldn't work if specified True, but respect
- load_half = False
- load_gptq = ''
- load_exllama = False
- use_gpu_id = False
- if get_device() == "cuda":
- torch.backends.cudnn.benchmark = True
- torch.backends.cudnn.enabled = False
- torch.set_default_dtype(torch.float32)
- if is_public and not inference_server and not model_lock:
- # 12B uses ~94GB
- # 6.9B uses ~47GB
- base_model = 'h2oai/h2ogpt-oig-oasst1-512-6_9b' if not base_model else base_model
- if hf_embedding_model is None:
- # if no GPUs, use simpler embedding model to avoid cost in time
- hf_embedding_model = "sentence-transformers/all-MiniLM-L6-v2"
- if score_model == 'auto':
- score_model = ''
- else:
- if load_half is None:
- load_half = True
- # CUDA GPUs visible
- if score_model == 'auto':
- if n_gpus >= 2:
- # will by default place scoring model on last GPU
- score_model = 'OpenAssistant/reward-model-deberta-v3-large-v2'
- else:
- score_model = ''
- if hf_embedding_model is None:
- # if still None, then set default
- hf_embedding_model = 'hkunlp/instructor-large'
-
- # get defaults
- if base_model:
- model_lower = base_model.lower()
- elif model_lock:
- # have 0th model be thought of as normal model
- assert len(model_lock) > 0 and model_lock[0]['base_model']
- model_lower = model_lock[0]['base_model'].lower()
- else:
- model_lower = ''
- if not gradio:
- # force, else not single response like want to look at
- stream_output = False
- # else prompt removal can mess up output
- chat = False
- # hard-coded defaults
- first_para = False
- text_limit = None
-
- if compile_model is None:
- # too avoid noisy CLI
- compile_model = not cli
-
- if offload_folder:
- offload_folder = makedirs(offload_folder, exist_ok=True, tmp_ok=True, use_base=True)
-
- # defaults
- caption_loader = None
- doctr_loader = None
- pix2struct_loader = None
-
- image_loaders_options0, image_loaders_options, \
- pdf_loaders_options0, pdf_loaders_options, \
- url_loaders_options0, url_loaders_options = lg_to_gr(**locals())
- jq_schema0 = jq_schema
- # transcribe
- image_loaders = image_loaders_options0
- pdf_loaders = pdf_loaders_options0
- url_loaders = url_loaders_options0
-
- placeholder_instruction, placeholder_input, \
- stream_output, show_examples, \
- prompt_type, prompt_dict, \
- temperature, top_p, top_k, num_beams, \
- max_new_tokens, min_new_tokens, early_stopping, max_time, \
- repetition_penalty, num_return_sequences, \
- do_sample, \
- src_lang, tgt_lang, \
- examples, \
- task_info = \
- get_generate_params(model_lower,
- chat,
- stream_output, show_examples,
- prompt_type, prompt_dict,
- system_prompt,
- pre_prompt_query, prompt_query,
- pre_prompt_summary, prompt_summary,
- temperature, top_p, top_k, num_beams,
- max_new_tokens, min_new_tokens, early_stopping, max_time,
- repetition_penalty, num_return_sequences,
- do_sample,
- top_k_docs,
- chunk,
- chunk_size,
- image_loaders,
- pdf_loaders,
- url_loaders,
- jq_schema,
- docs_ordering_type,
- min_max_new_tokens,
- verbose,
- )
-
- git_hash = get_githash() if is_public or os.getenv('GET_GITHASH') else "GET_GITHASH"
- locals_dict = locals()
- locals_print = '\n'.join(['%s: %s' % (k, v) for k, v in locals_dict.items()])
- if verbose:
- print(f"Generating model with params:\n{locals_print}", flush=True)
- print("Command: %s\nHash: %s" % (str(' '.join(sys.argv)), git_hash), flush=True)
-
- if langchain_mode != LangChainMode.DISABLED.value:
- # SECOND PLACE where LangChain referenced, but all imports are kept local so not required
- from gpt_langchain import prep_langchain, get_some_dbs_from_hf, get_persist_directory
- if is_hf:
- get_some_dbs_from_hf()
- dbs = {}
- for langchain_mode1 in langchain_modes:
- langchain_type = langchain_mode_types.get(langchain_mode1, LangChainTypes.EITHER.value)
- if langchain_type == LangChainTypes.PERSONAL.value:
- # shouldn't prepare per-user databases here
- continue
- persist_directory1, langchain_type = get_persist_directory(langchain_mode1, langchain_type=langchain_type)
- langchain_mode_types[langchain_mode1] = langchain_type
- if langchain_type == LangChainTypes.PERSONAL.value:
- # shouldn't prepare per-user databases here
- continue
- try:
- db = prep_langchain(persist_directory1,
- load_db_if_exists,
- db_type, use_openai_embedding,
- langchain_mode1, langchain_mode_paths, langchain_mode_types,
- hf_embedding_model,
- migrate_embedding_model,
- auto_migrate_db,
- kwargs_make_db=locals(),
- verbose=verbose)
- finally:
- # in case updated embeddings or created new embeddings
- clear_torch_cache()
- dbs[langchain_mode1] = db
- # remove None db's so can just rely upon k in dbs for if hav db
- dbs = {k: v for k, v in dbs.items() if v is not None}
- else:
- dbs = {}
- # import control
- if os.environ.get("TEST_LANGCHAIN_IMPORT"):
- assert 'gpt_langchain' not in sys.modules, "Dev bug, import of langchain when should not have"
- assert 'langchain' not in sys.modules, "Dev bug, import of langchain when should not have"
-
- other_model_state_defaults = dict(load_8bit=load_8bit, load_4bit=load_4bit, low_bit_mode=low_bit_mode,
- load_half=load_half,
- load_gptq=load_gptq, load_exllama=load_exllama, use_safetensors=use_safetensors,
- revision=revision, use_gpu_id=use_gpu_id, gpu_id=gpu_id,
- compile_model=compile_model,
- use_cache=use_cache,
- llamacpp_dict=llamacpp_dict, model_path_llama=model_path_llama,
- model_name_gptj=model_name_gptj,
- model_name_gpt4all_llama=model_name_gpt4all_llama,
- model_name_exllama_if_no_config=model_name_exllama_if_no_config,
- )
- model_state_none = dict(model=None, tokenizer=None, device=None,
- base_model=None, tokenizer_base_model=None, lora_weights=None,
- inference_server=None, prompt_type=None, prompt_dict=None,
- visible_models=None, h2ogpt_key=None,
- )
- model_state_none.update(other_model_state_defaults)
- my_db_state0 = {LangChainMode.MY_DATA.value: [None, None, None]}
- selection_docs_state0 = dict(langchain_modes=langchain_modes,
- langchain_mode_paths=langchain_mode_paths,
- langchain_mode_types=langchain_mode_types)
- selection_docs_state = copy.deepcopy(selection_docs_state0)
-
- if cli or not gradio:
- # initial state for query prompt
- model_name = base_model
- pre_prompt_query, prompt_query, pre_prompt_summary, prompt_summary = \
- get_langchain_prompts(pre_prompt_query, prompt_query,
- pre_prompt_summary, prompt_summary,
- model_name, inference_server,
- model_path_llama)
-
- if cli:
- from cli import run_cli
- return run_cli(**get_kwargs(run_cli, exclude_names=['model_state0'], **locals()))
- elif not gradio:
- from eval import run_eval
- return run_eval(**get_kwargs(run_eval, exclude_names=['model_state0'], **locals()))
- elif gradio or prepare_offline_level > 0:
- # imported here so don't require gradio to run generate
- from gradio_runner import go_gradio
-
- # get default model
- model_states = []
- model_list = [dict(base_model=base_model, tokenizer_base_model=tokenizer_base_model, lora_weights=lora_weights,
- inference_server=inference_server, prompt_type=prompt_type, prompt_dict=prompt_dict,
- visible_models=None, h2ogpt_key=None)]
- model_list[0].update(other_model_state_defaults)
- # FIXME: hyper per model, not about model loading
- # for k in gen_hyper:
- # model_list[k] = locals()[k]
-
- model_list0 = copy.deepcopy(model_list) # just strings, safe to deepcopy
- model_state0 = model_state_none.copy()
- assert len(model_state_none) == len(model_state0)
- if model_lock:
- model_list = model_lock
- # do reverse, so first is default base_model etc., so some logic works in go_gradio() more easily
- for model_dict in reversed(model_list):
- # handle defaults user didn't have to pass
- # special defaults, ignore defaults for these if not specifically set, replace with ''
- model_dict['base_model'] = model_dict.get('base_model', '')
- model_dict['tokenizer_base_model'] = model_dict.get('tokenizer_base_model', '')
- model_dict['lora_weights'] = model_dict.get('lora_weights', '')
- model_dict['inference_server'] = model_dict.get('inference_server', '')
- if prepare_offline_level >= 2:
- if 'openai' not in model_dict['inference_server'] and 'replicate' not in model_dict['inference_server']:
- # assume want locally, but OpenAI and replicate are never local for model part
- model_dict['inference_server'] = ''
- prompt_type_infer = not model_dict.get('prompt_type')
- model_dict['prompt_type'] = model_dict.get('prompt_type',
- model_list0[0]['prompt_type']) # don't use mutated value
- # rest of generic defaults
- for k in model_list0[0]:
- if k not in model_dict:
- model_dict[k] = model_list0[0][k]
-
- # begin prompt adjustments
- # get query prompt for (say) last base model if using model lock
- pre_prompt_query1, prompt_query1, pre_prompt_summary1, prompt_summary1 = (
- get_langchain_prompts(pre_prompt_query, prompt_query,
- pre_prompt_summary, prompt_summary,
- model_dict['base_model'],
- model_dict['inference_server'],
- model_dict['model_path_llama']))
- # if mixed setup, choose non-empty so best models best
- # FIXME: Make per model dict passed through to evaluate
- pre_prompt_query = pre_prompt_query or pre_prompt_query1
- prompt_query = prompt_query or prompt_query1
- pre_prompt_summary = pre_prompt_summary or pre_prompt_summary1
- prompt_summary = prompt_summary or prompt_summary1
-
- # try to infer, ignore empty initial state leading to get_generate_params -> 'plain'
- if prompt_type_infer:
- model_lower1 = model_dict['base_model'].lower()
- if model_lower1 in inv_prompt_type_to_model_lower:
- model_dict['prompt_type'] = inv_prompt_type_to_model_lower[model_lower1]
- model_dict['prompt_dict'], error0 = get_prompt(model_dict['prompt_type'], '',
- chat=False, context='', reduced=False,
- making_context=False,
- return_dict=True,
- system_prompt=system_prompt)
- else:
- model_dict['prompt_dict'] = prompt_dict
- else:
- model_dict['prompt_dict'] = prompt_dict
- model_dict['prompt_dict'] = model_dict.get('prompt_dict', model_dict['prompt_dict'])
- # end prompt adjustments
- all_kwargs = locals().copy()
- all_kwargs.update(model_dict)
- if model_dict['base_model'] and not login_mode_if_model0:
- model0, tokenizer0, device = get_model(reward_type=False,
- **get_kwargs(get_model, exclude_names=['reward_type'],
- **all_kwargs))
- else:
- # if empty model, then don't load anything, just get gradio up
- model0, tokenizer0, device = None, None, None
- if model0 is None:
- if fail_if_cannot_connect:
- raise RuntimeError("Could not connect, see logs")
- # skip
- if isinstance(model_lock, list):
- model_lock.remove(model_dict)
- continue
- model_state_trial = dict(model=model0, tokenizer=tokenizer0, device=device)
- model_state_trial.update(model_dict)
- diff_keys = set(list(model_state_none.keys())).symmetric_difference(model_state_trial.keys())
- assert len(model_state_none) == len(model_state_trial), diff_keys
- print("Model %s" % model_dict, flush=True)
- if model_lock:
- # last in iteration will be first
- model_states.insert(0, model_state_trial)
- # fill model_state0 so go_gradio() easier, manage model_states separately
- model_state0 = model_state_trial.copy()
- else:
- model_state0 = model_state_trial.copy()
- assert len(model_state_none) == len(model_state0)
-
- visible_models = str_to_list(visible_models, allow_none=True) # None means first model
- all_models = [x.get('base_model', xi) for xi, x in enumerate(model_states)]
- visible_models_state0 = [x.get('base_model', xi) for xi, x in enumerate(model_states) if
- visible_models is None or
- x.get('base_model', xi) in visible_models or
- xi in visible_models]
-
- # update to be consistent with what is passed from CLI and model chose
- # do after go over all models if multi-model, so don't contaminate
- # This is just so UI shows reasonable correct value, not 2048 dummy value
- if len(model_states) >= 1:
- max_seq_len = model_states[0]['tokenizer'].model_max_length
-
- # get score model
- all_kwargs = locals().copy()
- smodel, stokenizer, sdevice = get_score_model(reward_type=True,
- **get_kwargs(get_score_model, exclude_names=['reward_type'],
- **all_kwargs))
- score_model_state0 = dict(model=smodel, tokenizer=stokenizer, device=sdevice,
- base_model=score_model, tokenizer_base_model='', lora_weights='',
- inference_server='', prompt_type='', prompt_dict='',
- visible_models=None, h2ogpt_key=None)
-
- if enable_captions:
- if pre_load_caption_model:
- from image_captions import H2OImageCaptionLoader
- caption_loader = H2OImageCaptionLoader(caption_gpu=caption_gpu).load_model()
- else:
- caption_loader = 'gpu' if n_gpus > 0 and caption_gpu else 'cpu'
- else:
- caption_loader = False
-
- if pre_load_embedding_model and \
- langchain_mode != LangChainMode.DISABLED.value and \
- not use_openai_embedding:
- from src.gpt_langchain import get_embedding
- hf_embedding_model = dict(name=hf_embedding_model,
- model=get_embedding(use_openai_embedding, hf_embedding_model=hf_embedding_model,
- preload=True))
- if enable_doctr or enable_pdf_ocr in [True, 'auto', 'on']:
- doctr_loader = 'gpu' if n_gpus > 0 and doctr_gpu else 'cpu'
- else:
- doctr_loader = False
-
- # assume gradio needs everything
- go_gradio(**locals())
-
-
-def get_config(base_model,
- use_auth_token=False,
- trust_remote_code=True,
- offload_folder=None,
- revision=None,
- rope_scaling=None,
- triton_attn=False,
- long_sequence=True,
- return_model=False,
- raise_exception=False,
- max_seq_len=None,
- verbose=False,
- ):
- from accelerate import init_empty_weights
- with init_empty_weights():
- from transformers import AutoConfig
- try:
- config = AutoConfig.from_pretrained(base_model, use_auth_token=use_auth_token,
- trust_remote_code=trust_remote_code,
- offload_folder=offload_folder,
- revision=revision,
- rope_scaling=rope_scaling if rope_scaling else None)
- except OSError as e:
- if raise_exception:
- raise
- if 'not a local folder and is not a valid model identifier listed on' in str(
- e) or '404 Client Error' in str(e) or "couldn't connect" in str(e):
- # e.g. llama, gpjt, etc.
- # e.g. HF TGI but not model on HF or private etc.
- if max_seq_len is None and base_model.lower() in non_hf_types:
- print("Could not determine --max_seq_len, setting to 2048. Pass if not correct", flush=True)
- max_seq_len = 2048
- # HF TGI server only should really require prompt_type, not HF model state
- return None, None, max_seq_len
- else:
- raise
- if triton_attn and 'mpt-' in base_model.lower():
- config.attn_config['attn_impl'] = 'triton'
- if long_sequence:
- if 'mpt-7b-storywriter' in base_model.lower():
- config.update({"max_seq_len": 83968})
- if 'mosaicml/mpt-7b-chat' in base_model.lower():
- config.update({"max_seq_len": 4096})
- if 'mpt-30b' in base_model.lower():
- config.update({"max_seq_len": 2 * 8192})
- if return_model and \
- issubclass(config.__class__, tuple(AutoModel._model_mapping.keys())):
- model = AutoModel.from_config(
- config,
- trust_remote_code=trust_remote_code,
- )
- else:
- # can't infer
- model = None
- if 'falcon' in base_model.lower():
- config.use_cache = False
-
- # allow override
- if max_seq_len is not None:
- print("Overriding max_seq_len -> %d" % max_seq_len, flush=True)
- else:
- if hasattr(config, 'max_seq_len'):
- max_seq_len = int(config.max_seq_len)
- elif hasattr(config, 'max_position_embeddings') and isinstance(config.max_position_embeddings, int):
- # help automatically limit inputs to generate
- max_seq_len = config.max_position_embeddings
- if verbose:
- print("Used max_position_embeddings=%s as base model (pre-rope) max_seq_len."
- " If not desired, pass --max_seq_len and set to some integer value." % config.max_position_embeddings,
- flush=True)
- elif hasattr(config, 'n_ctx'):
- # e.g. gpt2
- max_seq_len = int(config.n_ctx)
- else:
- print("Could not determine --max_seq_len, setting to 2048. Pass if not correct", flush=True)
- max_seq_len = 2048
- # FIXME:
- # raise RuntimeError("Could not determine max_seq_len,"
- # " please pass --max_seq_len and set to some value, e.g. 2048.")
-
- if rope_scaling:
- if rope_scaling.get('factor'):
- # HF transformers
- max_seq_len *= rope_scaling.get('factor')
- elif rope_scaling.get('alpha_value'):
- # exllama
- # Note: exllama's own tokenizer has this set correctly in loaders.py, this config will be unused
- max_seq_len *= rope_scaling.get('alpha_value')
- print("Automatically setting max_seq_len=%d for RoPE scaling" % max_seq_len, flush=True)
-
- return config, model, max_seq_len
-
-
-def get_non_lora_model(base_model, model_loader, load_half,
- load_gptq,
- load_exllama,
- use_safetensors,
- revision,
- model_kwargs, reward_type,
- config, model,
- gpu_id=0,
- ):
- """
- Ensure model gets on correct device
- """
-
- if model is not None:
- # NOTE: Can specify max_memory={0: max_mem, 1: max_mem}, to shard model
- # NOTE: Some models require avoiding sharding some layers,
- # then would pass no_split_module_classes and give list of those layers.
- from accelerate import infer_auto_device_map
- device_map = infer_auto_device_map(
- model,
- dtype=torch.float16 if load_half else torch.float32,
- )
- if hasattr(model, 'model'):
- device_map_model = infer_auto_device_map(
- model.model,
- dtype=torch.float16 if load_half else torch.float32,
- )
- device_map.update(device_map_model)
- else:
- device_map = "auto"
-
- n_gpus = torch.cuda.device_count() if torch.cuda.is_available() else 0
- n_gpus, gpu_ids = cuda_vis_check(n_gpus)
-
- if n_gpus > 0:
- if gpu_id >= 0:
- # FIXME: If really distributes model, tend to get things like: ValueError: gpt_neox.embed_in.weight doesn't have any device set.
- # So avoid for now, just put on first GPU, unless score_model, put on last
- if reward_type:
- device_map = {'': n_gpus - 1}
- else:
- device_map = {'': min(n_gpus - 1, gpu_id)}
- if gpu_id == -1:
- device_map = {'': 'cuda'}
- else:
- device_map = {'': 'cpu'}
- model_kwargs['load_in_8bit'] = False
- model_kwargs['load_in_4bit'] = False
- print('device_map: %s' % device_map, flush=True)
-
- load_in_8bit = model_kwargs.get('load_in_8bit', False)
- load_in_4bit = model_kwargs.get('load_in_4bit', False)
- model_kwargs['device_map'] = device_map
- model_kwargs['use_safetensors'] = use_safetensors
- model_kwargs['revision'] = revision
- pop_unused_model_kwargs(model_kwargs)
-
- if load_exllama:
- model = model_loader
- elif load_gptq:
- if 'Llama-2-70B-chat-GPTQ' in base_model:
- model_kwargs.update(dict(inject_fused_attention=False))
- model_kwargs.pop('torch_dtype', None)
- model_kwargs.pop('device_map')
- model = model_loader(
- model_name_or_path=base_model,
- model_basename=load_gptq,
- **model_kwargs,
- )
- elif load_in_8bit or load_in_4bit or not load_half:
- model = model_loader(
- base_model,
- config=config,
- **model_kwargs,
- )
- else:
-
- model = model_loader(
- base_model,
- config=config,
- **model_kwargs,
- )
- if not getattr(model, "is_quantized", False):
- model = model.half()
- return model
-
-
-def get_client_from_inference_server(inference_server, base_model=None, raise_connection_exception=False):
- inference_server, headers = get_hf_server(inference_server)
- # preload client since slow for gradio case especially
- from gradio_utils.grclient import GradioClient
- gr_client = None
- hf_client = None
- if headers is None:
- try:
- print("GR Client Begin: %s %s" % (inference_server, base_model), flush=True)
- # first do sanity check if alive, else gradio client takes too long by default
- requests.get(inference_server, timeout=int(os.getenv('REQUEST_TIMEOUT', '30')))
- gr_client = GradioClient(inference_server)
- print("GR Client End: %s" % inference_server, flush=True)
- except (OSError, ValueError) as e:
- # Occurs when wrong endpoint and should have been HF client, so don't hard raise, just move to HF
- gr_client = None
- print("GR Client Failed %s %s: %s" % (inference_server, base_model, str(e)), flush=True)
- except (ConnectTimeoutError, ConnectTimeout, MaxRetryError, ConnectionError, ConnectionError2,
- JSONDecodeError, ReadTimeout2, KeyError) as e:
- t, v, tb = sys.exc_info()
- ex = ''.join(traceback.format_exception(t, v, tb))
- print("GR Client Failed %s %s: %s" % (inference_server, base_model, str(ex)), flush=True)
- if raise_connection_exception:
- raise
-
- if gr_client is None:
- res = None
- from text_generation import Client as HFClient
- print("HF Client Begin: %s %s" % (inference_server, base_model))
- try:
- hf_client = HFClient(inference_server, headers=headers, timeout=int(os.getenv('REQUEST_TIMEOUT', '30')))
- # quick check valid TGI endpoint
- res = hf_client.generate('What?', max_new_tokens=1)
- hf_client = HFClient(inference_server, headers=headers, timeout=300)
- except (ConnectTimeoutError, ConnectTimeout, MaxRetryError, ConnectionError, ConnectionError2,
- JSONDecodeError, ReadTimeout2, KeyError) as e:
- hf_client = None
- t, v, tb = sys.exc_info()
- ex = ''.join(traceback.format_exception(t, v, tb))
- print("HF Client Failed %s %s: %s" % (inference_server, base_model, str(ex)))
- if raise_connection_exception:
- raise
- print("HF Client End: %s %s : %s" % (inference_server, base_model, res))
- return inference_server, gr_client, hf_client
-
-
-def get_model(
- load_8bit: bool = False,
- load_4bit: bool = False,
- low_bit_mode: int = 1,
- load_half: bool = True,
- load_gptq: str = '',
- load_exllama: bool = False,
- use_safetensors: bool = False,
- revision: str = None,
- use_gpu_id: bool = True,
- base_model: str = '',
- inference_server: str = "",
- tokenizer_base_model: str = '',
- lora_weights: str = "",
- gpu_id: int = 0,
- n_jobs=None,
-
- reward_type: bool = None,
- local_files_only: bool = False,
- resume_download: bool = True,
- use_auth_token: Union[str, bool] = False,
- trust_remote_code: bool = True,
- offload_folder: str = None,
- rope_scaling: dict = None,
- max_seq_len: int = None,
- compile_model: bool = True,
- llamacpp_dict=None,
-
- verbose: bool = False,
-):
- """
-
- :param load_8bit: load model in 8-bit, not supported by all models
- :param load_4bit: load model in 4-bit, not supported by all models
- :param low_bit_mode: See gen.py
- :param load_half: load model in 16-bit
- :param load_gptq: GPTQ model_basename
- :param load_exllama: whether to use exllama
- :param use_safetensors: use safetensors file
- :param revision:
- :param use_gpu_id: Use torch infer of optimal placement of layers on devices (for non-lora case)
- For non-LORA case, False will spread shards across multiple GPUs, but this can lead to cuda:x cuda:y mismatches
- So it is not the default
- :param base_model: name/path of base model
- :param inference_server: whether base_model is hosted locally ('') or via http (url)
- :param tokenizer_base_model: name/path of tokenizer
- :param lora_weights: name/path
- :param gpu_id: which GPU (0..n_gpus-1) or allow all GPUs if relevant (-1)
- :param n_jobs: number of cores to use (e.g. for llama CPU model)
- :param reward_type: reward type model for sequence classification
- :param local_files_only: use local files instead of from HF
- :param resume_download: resume downloads from HF
- :param use_auth_token: assumes user did on CLI `huggingface-cli login` to access private repo
- :param trust_remote_code: trust code needed by model
- :param offload_folder: offload folder
- :param rope_scaling: scaling for rope-based models, e.g. "{'type':'dynamic', 'factor':4}"
- :param max_seq_len: override for maximum sequence length for model
- :param max_seq_len: if set, use as max_seq_len for model
- :param compile_model: whether to compile torch model
- :param llamacpp_dict: dict of llama.cpp and GPT4All model options
- :param verbose:
- :return:
- """
- print("Starting get_model: %s %s" % (base_model, inference_server), flush=True)
-
- triton_attn = False
- long_sequence = True
- config_kwargs = dict(use_auth_token=use_auth_token,
- trust_remote_code=trust_remote_code,
- offload_folder=offload_folder,
- rope_scaling=rope_scaling,
- triton_attn=triton_attn,
- long_sequence=long_sequence,
- revision=revision,
- max_seq_len=max_seq_len,
- verbose=verbose)
- config, _, max_seq_len = get_config(base_model, **config_kwargs, raise_exception=False)
-
- if base_model in non_hf_types:
- assert config is None, "Expected config None for %s" % base_model
-
- llama_type_from_config = 'llama' in str(config).lower()
- llama_type_from_name = "llama" in base_model.lower()
- llama_type = llama_type_from_config or llama_type_from_name
- if "xgen" in base_model.lower() or 'llama2' in base_model.lower() or 'llama-2' in base_model.lower():
- llama_type = False
- if llama_type:
- if verbose:
- print("Detected as llama type from"
- " config (%s) or name (%s)" % (llama_type_from_config, llama_type_from_name), flush=True)
-
- model_name_exllama_if_no_config = '' if not llamacpp_dict else llamacpp_dict.get('model_name_exllama_if_no_config',
- '')
- model_loader, tokenizer_loader, conditional_type = (
- get_loaders(model_name=base_model, reward_type=reward_type, llama_type=llama_type,
- load_gptq=load_gptq, load_exllama=load_exllama, config=config,
- rope_scaling=rope_scaling, max_seq_len=max_seq_len,
- model_name_exllama_if_no_config=model_name_exllama_if_no_config))
-
- tokenizer_kwargs = dict(local_files_only=local_files_only,
- resume_download=resume_download,
- use_auth_token=use_auth_token,
- trust_remote_code=trust_remote_code,
- offload_folder=offload_folder,
- revision=revision,
- padding_side='left',
- config=config,
- )
- if not tokenizer_base_model:
- tokenizer_base_model = base_model
-
- if load_exllama:
- tokenizer = tokenizer_loader
- elif config is not None and tokenizer_loader is not None and not isinstance(tokenizer_loader, str):
- if load_exllama:
- tokenizer = tokenizer_loader
- else:
- tokenizer = tokenizer_loader.from_pretrained(tokenizer_base_model, **tokenizer_kwargs)
- # sets raw (no cushion) limit
- # If using RoPE with scaling, then for non-exllama models (e.g. HF models),
- # then config -> tokenizer will set model_max_length correctly
- set_model_max_len(max_seq_len, tokenizer, verbose=False)
- # if using fake tokenizer, not really accurate when lots of numbers, give a bit of buffer, else get:
- # Generation Failed: Input validation error: `inputs` must have less than 2048 tokens. Given: 2233
- tokenizer.model_max_length = tokenizer.model_max_length - 50
- else:
- tokenizer = None
-
- if isinstance(inference_server, str) and inference_server.startswith("http"):
- inference_server, gr_client, hf_client = get_client_from_inference_server(inference_server,
- base_model=base_model)
- client = gr_client or hf_client
- # Don't return None, None for model, tokenizer so triggers
- if tokenizer is None:
- # FIXME: Could use only tokenizer from llama etc. but hard to detatch from model, just use fake for now
- if os.getenv("HARD_ASSERTS") and base_model not in non_hf_types:
- raise RuntimeError("Unexpected tokenizer=None")
- tokenizer = FakeTokenizer()
- return client, tokenizer, 'http'
- if isinstance(inference_server, str) and (
- inference_server.startswith('openai') or
- inference_server.startswith('vllm') or
- inference_server.startswith('replicate') or
- inference_server.startswith('sagemaker')
- ):
- if inference_server.startswith('openai'):
- assert os.getenv('OPENAI_API_KEY'), "Set environment for OPENAI_API_KEY"
- # Don't return None, None for model, tokenizer so triggers
- # include small token cushion
- max_seq_len = model_token_mapping[base_model]
- if inference_server.startswith('replicate'):
- assert len(inference_server.split(':')) >= 3, "Expected replicate:model string, got %s" % inference_server
- assert os.getenv('REPLICATE_API_TOKEN'), "Set environment for REPLICATE_API_TOKEN"
- assert max_seq_len is not None, "Please pass --max_seq_len= for replicate models."
- try:
- import replicate as replicate_python
- except ImportError:
- raise ImportError(
- "Could not import replicate python package. "
- "Please install it with `pip install replicate`."
- )
- if inference_server.startswith('sagemaker'):
- assert len(
- inference_server.split(
- ':')) >= 3, "Expected sagemaker_chat::, got %s" % inference_server
- assert os.getenv('AWS_ACCESS_KEY_ID'), "Set environment for AWS_ACCESS_KEY_ID"
- assert os.getenv('AWS_SECRET_ACCESS_KEY'), "Set environment for AWS_SECRET_ACCESS_KEY"
- # Don't return None, None for model, tokenizer so triggers
- # include small token cushion
- if inference_server.startswith('openai') or tokenizer is None:
- # don't use fake (tiktoken) tokenizer for vLLM//replicate if know actual model with actual tokenizer
- tokenizer = FakeTokenizer(model_max_length=max_seq_len - 50)
- return inference_server, tokenizer, inference_server
- assert not inference_server, "Malformed inference_server=%s" % inference_server
- if base_model in non_hf_types:
- from gpt4all_llm import get_model_tokenizer_gpt4all
- model, tokenizer, device = get_model_tokenizer_gpt4all(base_model, n_jobs=n_jobs,
- max_seq_len=max_seq_len,
- llamacpp_dict=llamacpp_dict)
- return model, tokenizer, device
- if load_exllama:
- return model_loader, tokenizer, 'cuda'
-
- # get local torch-HF model
- return get_hf_model(load_8bit=load_8bit,
- load_4bit=load_4bit,
- low_bit_mode=low_bit_mode,
- load_half=load_half,
- load_gptq=load_gptq,
- use_safetensors=use_safetensors,
- revision=revision,
- use_gpu_id=use_gpu_id,
- base_model=base_model,
- tokenizer_base_model=tokenizer_base_model,
- lora_weights=lora_weights,
- gpu_id=gpu_id,
-
- reward_type=reward_type,
- local_files_only=local_files_only,
- resume_download=resume_download,
- use_auth_token=use_auth_token,
- trust_remote_code=trust_remote_code,
- offload_folder=offload_folder,
- rope_scaling=rope_scaling,
- compile_model=compile_model,
-
- llama_type=llama_type,
- config_kwargs=config_kwargs,
- tokenizer_kwargs=tokenizer_kwargs,
-
- verbose=verbose)
-
-
-def get_hf_model(load_8bit: bool = False,
- load_4bit: bool = False,
- low_bit_mode: int = 1,
- load_half: bool = True,
- load_gptq: str = '',
- use_safetensors: bool = False,
- revision: str = None,
- use_gpu_id: bool = True,
- base_model: str = '',
- tokenizer_base_model: str = '',
- lora_weights: str = "",
- gpu_id: int = 0,
-
- reward_type: bool = None,
- local_files_only: bool = False,
- resume_download: bool = True,
- use_auth_token: Union[str, bool] = False,
- trust_remote_code: bool = True,
- offload_folder: str = None,
- rope_scaling: dict = None,
- compile_model: bool = True,
-
- llama_type: bool = False,
- config_kwargs=None,
- tokenizer_kwargs=None,
-
- verbose: bool = False,
- ):
- assert config_kwargs is not None
- assert tokenizer_kwargs is not None
-
- load_exllama = False # Never should be in HF code for exllama
-
- if lora_weights is not None and lora_weights.strip():
- if verbose:
- print("Get %s lora weights" % lora_weights, flush=True)
- device = get_device()
-
- if 'gpt2' in base_model.lower():
- # RuntimeError: where expected condition to be a boolean tensor, but got a tensor with dtype Half
- load_8bit = False
- load_4bit = False
-
- assert base_model.strip(), (
- "Please choose a base model with --base_model (CLI) or load one from Models Tab (gradio)"
- )
-
- model_loader, tokenizer_loader, conditional_type = (
- get_loaders(model_name=base_model, reward_type=reward_type, llama_type=llama_type,
- load_gptq=load_gptq, load_exllama=load_exllama))
-
- config, _, max_seq_len = get_config(base_model, return_model=False, raise_exception=True, **config_kwargs)
-
- if tokenizer_loader is not None and not isinstance(tokenizer_loader, str):
- if load_exllama:
- tokenizer = tokenizer_loader
- else:
- tokenizer = tokenizer_loader.from_pretrained(tokenizer_base_model,
- **tokenizer_kwargs)
- else:
- tokenizer = tokenizer_loader
-
- if isinstance(tokenizer, str):
- # already a pipeline, tokenizer_loader is string for task
- model = model_loader(tokenizer,
- model=base_model,
- device=0 if device == "cuda" else -1,
- torch_dtype=torch.float16 if device == 'cuda' else torch.float32)
- else:
- assert device in ["cuda", "cpu", "mps"], "Unsupported device %s" % device
- model_kwargs = dict(local_files_only=local_files_only,
- torch_dtype=torch.float16 if device == 'cuda' else torch.float32,
- resume_download=resume_download,
- use_auth_token=use_auth_token,
- trust_remote_code=trust_remote_code,
- offload_folder=offload_folder,
- revision=revision,
- # rope_scaling=rope_scaling, # only put into config
- )
- if 'mbart-' not in base_model.lower() and 'mpt-' not in base_model.lower():
- if use_gpu_id and gpu_id is not None and gpu_id >= 0 and device == 'cuda':
- device_map = {"": gpu_id}
- else:
- device_map = "auto"
- model_kwargs.update(dict(load_in_8bit=load_8bit,
- load_in_4bit=load_4bit,
- device_map=device_map,
- ))
- if 'mpt-' in base_model.lower() and gpu_id is not None and gpu_id >= 0:
- # MPT doesn't support spreading over GPUs
- model_kwargs.update(dict(device_map={"": gpu_id} if device == 'cuda' else "cpu"))
-
- if 'OpenAssistant/reward-model'.lower() in base_model.lower():
- # FIXME: could put on other GPUs
- model_kwargs['device_map'] = {"": 0} if device == 'cuda' else {"": 'cpu'}
- model_kwargs.pop('torch_dtype', None)
- pop_unused_model_kwargs(model_kwargs)
-
- n_gpus = torch.cuda.device_count() if torch.cuda.is_available() else 0
- n_gpus, gpu_ids = cuda_vis_check(n_gpus)
- if low_bit_mode == 1 and n_gpus != 0:
- from transformers import BitsAndBytesConfig
- model_kwargs['quantization_config'] = BitsAndBytesConfig(bnb_4bit_compute_dtype=torch.bfloat16,
- load_in_4bit=load_4bit,
- load_in_8bit=load_8bit,
- )
- elif low_bit_mode == 2 and n_gpus != 0:
- from transformers import BitsAndBytesConfig
- model_kwargs['quantization_config'] = BitsAndBytesConfig(bnb_4bit_quant_type="nf4",
- load_in_4bit=load_4bit,
- load_in_8bit=load_8bit,
- )
- elif low_bit_mode == 3 and n_gpus != 0:
- from transformers import BitsAndBytesConfig
- model_kwargs['quantization_config'] = BitsAndBytesConfig(bnb_4bit_use_double_quant=True,
- load_in_4bit=load_4bit,
- load_in_8bit=load_8bit,
- )
- elif low_bit_mode == 4 and n_gpus != 0:
- from transformers import BitsAndBytesConfig
- model_kwargs['quantization_config'] = BitsAndBytesConfig(bnb_4bit_use_double_quant=True,
- bnb_4bit_quant_type="nf4",
- load_in_4bit=load_4bit,
- load_in_8bit=load_8bit,
- )
-
- if not lora_weights:
- # torch.device context uses twice memory for AutoGPTQ
- context = NullContext if load_gptq else torch.device
- with context(device):
-
- if use_gpu_id:
- config, model, max_seq_len = get_config(base_model,
- return_model=True, raise_exception=True, **config_kwargs)
- model = get_non_lora_model(base_model, model_loader, load_half, load_gptq,
- load_exllama,
- use_safetensors,
- revision,
- model_kwargs, reward_type,
- config, model,
- gpu_id=gpu_id,
- )
- else:
- config, _, max_seq_len = get_config(base_model, **config_kwargs)
- if load_half and not (load_8bit or load_4bit or load_gptq):
- model = model_loader(
- base_model,
- config=config,
- **model_kwargs)
- if not getattr(model, "is_quantized", False):
- model = model.half()
- else:
- model = model_loader(
- base_model,
- config=config,
- **model_kwargs)
- elif load_8bit or load_4bit:
- config, _, max_seq_len = get_config(base_model, **config_kwargs)
- model = model_loader(
- base_model,
- config=config,
- **model_kwargs
- )
- from peft import PeftModel # loads cuda, so avoid in global scope
- model = PeftModel.from_pretrained(
- model,
- lora_weights,
- torch_dtype=torch.float16 if device == 'cuda' else torch.float32,
- local_files_only=local_files_only,
- resume_download=resume_download,
- use_auth_token=use_auth_token,
- trust_remote_code=trust_remote_code,
- offload_folder=offload_folder,
- rope_scaling=rope_scaling,
- revision=revision,
- device_map={"": 0} if device == 'cuda' else {"": 'cpu'}, # seems to be required
- )
- else:
- with torch.device(device):
- config, _, max_seq_len = get_config(base_model, raise_exception=True, **config_kwargs)
- model = model_loader(
- base_model,
- config=config,
- **model_kwargs
- )
- from peft import PeftModel # loads cuda, so avoid in global scope
- model = PeftModel.from_pretrained(
- model,
- lora_weights,
- torch_dtype=torch.float16 if device == 'cuda' else torch.float32,
- local_files_only=local_files_only,
- resume_download=resume_download,
- use_auth_token=use_auth_token,
- trust_remote_code=trust_remote_code,
- offload_folder=offload_folder,
- rope_scaling=rope_scaling,
- device_map="auto",
- )
- if load_half and not load_gptq:
- if not getattr(model, "is_quantized", False):
- model = model.half()
-
- # unwind broken decapoda-research config
- if llama_type:
- model.config.pad_token_id = tokenizer.pad_token_id = 0 # unk
- model.config.bos_token_id = 1
- model.config.eos_token_id = 2
- if 'gpt2' in base_model.lower():
- # add special tokens that otherwise all share the same id
- tokenizer.add_special_tokens({'bos_token': '',
- 'eos_token': '',
- 'pad_token': ''})
-
- if not isinstance(tokenizer, str):
- model.eval()
- if torch.__version__ >= "2" and sys.platform != "win32" and compile_model:
- model = torch.compile(model)
-
- set_model_max_len(max_seq_len, tokenizer, verbose=False, reward_type=reward_type)
-
- # tell if conditional type
- model.conditional_type = conditional_type
- tokenizer.conditional_type = conditional_type
-
- return model, tokenizer, device
-
-
-def set_model_max_len(max_seq_len, tokenizer, verbose=False, reward_type=False):
- if reward_type:
- # limit deberta, else uses too much memory and not worth response score
- tokenizer.model_max_length = 512
- return
-
- tokenizer.model_max_length = int(max_seq_len)
- if verbose:
- print("model_max_length=%s" % tokenizer.model_max_length, flush=True)
- # for bug in HF transformers
- if tokenizer.model_max_length > 100000000:
- tokenizer.model_max_length = 2048
-
-
-def pop_unused_model_kwargs(model_kwargs):
- """
- in-place pop unused kwargs that are not dependency-upgrade friendly
- no point passing in False, is default, and helps avoid needing to update requirements for new deps
- :param model_kwargs:
- :return:
- """
- check_list = ['load_in_8bit', 'load_in_4bit']
- for k in check_list:
- if k in model_kwargs and not model_kwargs[k]:
- model_kwargs.pop(k)
-
-
-def get_score_model(score_model: str = None,
- load_8bit: bool = False,
- load_4bit: bool = False,
- low_bit_mode=1,
- load_half: bool = True,
- load_gptq: str = '',
- load_exllama: bool = False,
- use_gpu_id: bool = True,
- base_model: str = '',
- inference_server: str = '',
- tokenizer_base_model: str = '',
- lora_weights: str = "",
- gpu_id: int = 0,
- n_jobs=None,
-
- reward_type: bool = None,
- local_files_only: bool = False,
- resume_download: bool = True,
- use_auth_token: Union[str, bool] = False,
- trust_remote_code: bool = True,
- offload_folder: str = None,
- rope_scaling: dict = None,
- compile_model: bool = True,
- llamacpp_dict: typing.Dict = None,
-
- verbose: bool = False,
- ):
- if score_model is not None and score_model.strip():
- load_8bit = False
- load_4bit = False
- low_bit_mode = 1
- load_half = False
- load_gptq = ''
- load_exllama = False
- use_safetensors = False
- revision = None
- base_model = score_model.strip()
- tokenizer_base_model = ''
- lora_weights = ''
- inference_server = ''
- llama_type = False
- max_seq_len = None
- compile_model = False
- llamacpp_dict = {}
- smodel, stokenizer, sdevice = get_model(reward_type=True,
- **get_kwargs(get_model, exclude_names=['reward_type'], **locals()))
- else:
- smodel, stokenizer, sdevice = None, None, None
- return smodel, stokenizer, sdevice
-
-
-def evaluate_fake(*args, **kwargs):
- yield dict(response=invalid_key_msg, sources='')
- return
-
-
-def evaluate(
- model_state,
- my_db_state,
- selection_docs_state,
- requests_state,
- # START NOTE: Examples must have same order of parameters
- instruction,
- iinput,
- context,
- stream_output,
- prompt_type,
- prompt_dict,
- temperature,
- top_p,
- top_k,
- num_beams,
- max_new_tokens,
- min_new_tokens,
- early_stopping,
- max_time,
- repetition_penalty,
- num_return_sequences,
- do_sample,
- chat,
- instruction_nochat,
- iinput_nochat,
- langchain_mode,
- add_chat_history_to_context,
- langchain_action,
- langchain_agents,
- top_k_docs,
- chunk,
- chunk_size,
- document_subset,
- document_choice,
- pre_prompt_query,
- prompt_query,
- pre_prompt_summary,
- prompt_summary,
- system_prompt,
-
- image_loaders,
- pdf_loaders,
- url_loaders,
- jq_schema,
- visible_models,
- h2ogpt_key,
- add_search_to_context,
- chat_conversation,
- text_context_list,
- docs_ordering_type,
- min_max_new_tokens,
-
- # END NOTE: Examples must have same order of parameters
- captions_model=None,
- caption_loader=None,
- doctr_loader=None,
- pix2struct_loader=None,
- async_output=None,
- num_async=None,
- src_lang=None,
- tgt_lang=None,
- debug=False,
- concurrency_count=None,
- save_dir=None,
- sanitize_bot_response=False,
- model_state0=None,
- memory_restriction_level=None,
- max_max_new_tokens=None,
- is_public=None,
- max_max_time=None,
- raise_generate_gpu_exceptions=None,
- lora_weights=None,
- use_llm_if_no_docs=True,
- load_db_if_exists=True,
- dbs=None,
- detect_user_path_changes_every_query=None,
- use_openai_embedding=None,
- use_openai_model=None,
- hf_embedding_model=None,
- migrate_embedding_model=None,
- auto_migrate_db=None,
- cut_distance=None,
- db_type=None,
- n_jobs=None,
- first_para=None,
- text_limit=None,
- show_accordions=None,
- top_k_docs_max_show=None,
- show_link_in_sources=None,
- verbose=False,
- cli=False,
- use_cache=None,
- auto_reduce_chunks=None,
- max_chunks=None,
- headsize=None,
- model_lock=None,
- force_langchain_evaluate=None,
- model_state_none=None,
- load_exllama=None,
- answer_with_sources=None,
- append_sources_to_answer=None,
- image_loaders_options0=None,
- pdf_loaders_options0=None,
- url_loaders_options0=None,
- jq_schema0=None,
- keep_sources_in_context=None,
-):
- # ensure passed these
- assert concurrency_count is not None
- assert memory_restriction_level is not None
- assert raise_generate_gpu_exceptions is not None
- assert use_openai_embedding is not None
- assert use_openai_model is not None
- assert hf_embedding_model is not None
- assert migrate_embedding_model is not None
- assert auto_migrate_db is not None
- assert db_type is not None
- assert top_k_docs is not None and isinstance(top_k_docs, int)
- assert chunk is not None and isinstance(chunk, bool)
- assert chunk_size is not None and isinstance(chunk_size, int)
- assert n_jobs is not None
- assert first_para is not None
- assert isinstance(add_chat_history_to_context, bool)
- assert isinstance(add_search_to_context, bool)
- assert load_exllama is not None
- # for lazy client (even chat client)
- if image_loaders is None:
- image_loaders = image_loaders_options0
- if pdf_loaders is None:
- pdf_loaders = pdf_loaders_options0
- if url_loaders is None:
- url_loaders = url_loaders_options0
- if jq_schema is None:
- jq_schema = jq_schema0
- if isinstance(langchain_agents, str):
- if langchain_agents.strip().startswith('['):
- # already list, but as string
- langchain_agents = str_to_list(langchain_agents)
- else:
- # just 1 item and make list
- langchain_agents = [langchain_agents]
- chat_conversation = str_to_list(chat_conversation)
- text_context_list = str_to_list(text_context_list)
-
- langchain_modes = selection_docs_state['langchain_modes']
- langchain_mode_paths = selection_docs_state['langchain_mode_paths']
- langchain_mode_types = selection_docs_state['langchain_mode_types']
-
- if debug:
- locals_dict = locals().copy()
- locals_dict.pop('model_state', None)
- locals_dict.pop('model_state0', None)
- locals_dict.pop('model_states', None)
- print(locals_dict)
-
- no_model_msg = "Please choose a base model with --base_model (CLI) or load in Models Tab (gradio).\n" \
- "Then start New Conversation"
-
- if model_state is None:
- model_state = model_state_none.copy()
- if model_state0 is None:
- # e.g. for no gradio case, set dummy value, else should be set
- model_state0 = model_state_none.copy()
-
- # model_state['model] is only 'model' if should use model_state0
- # model could also be None
- have_model_lock = model_lock is not None
- have_fresh_model = model_state['model'] not in [None, 'model', no_model_str]
- # for gradio UI control, expect model_state and model_state0 to match, so if have_model_lock=True, then should have_fresh_model=True
- # but gradio API control will only use nochat api etc. and won't use fresh model, so can't assert in general
- # if have_model_lock:
- # assert have_fresh_model, "Expected model_state and model_state0 to match if have_model_lock"
- have_cli_model = model_state0['model'] not in [None, 'model', no_model_str]
-
- if have_fresh_model:
- # USE FRESH MODEL
- if not have_model_lock:
- # model_state0 is just one of model_state if model_lock, so don't nuke
- # try to free-up original model (i.e. list was passed as reference)
- if model_state0['model'] and hasattr(model_state0['model'], 'cpu'):
- model_state0['model'].cpu()
- model_state0['model'] = None
- # try to free-up original tokenizer (i.e. list was passed as reference)
- if model_state0['tokenizer']:
- model_state0['tokenizer'] = None
- clear_torch_cache()
- chosen_model_state = model_state
- elif have_cli_model:
- # USE MODEL SETUP AT CLI
- assert isinstance(model_state['model'], (type(None), str)) # expect no fresh model
- chosen_model_state = model_state0
- else:
- raise AssertionError(no_model_msg)
- # get variables
- model = chosen_model_state['model']
- tokenizer = chosen_model_state['tokenizer']
- device = chosen_model_state['device']
- base_model = chosen_model_state['base_model']
- tokenizer_base_model = chosen_model_state['tokenizer_base_model']
- lora_weights = chosen_model_state['lora_weights']
- inference_server = chosen_model_state['inference_server']
- visible_models = chosen_model_state['visible_models']
- # use overall key if have, so key for this gradio and any inner gradio
- if chosen_model_state['h2ogpt_key'] is not None:
- h2ogpt_key = chosen_model_state['h2ogpt_key']
- # prefer use input from API over model state
- prompt_type = prompt_type or chosen_model_state['prompt_type']
- prompt_dict = prompt_dict or chosen_model_state['prompt_dict']
-
- if base_model is None:
- raise AssertionError(no_model_msg)
-
- assert base_model.strip(), no_model_msg
- assert model, "Model is missing"
- assert tokenizer, "Tokenizer is missing"
-
- # choose chat or non-chat mode
- if not chat:
- instruction = instruction_nochat
- iinput = iinput_nochat
-
- # in some cases, like lean nochat API, don't want to force sending prompt_type, allow default choice
- model_lower = base_model.lower()
- if not prompt_type and model_lower in inv_prompt_type_to_model_lower and prompt_type != 'custom':
- prompt_type = inv_prompt_type_to_model_lower[model_lower]
- if verbose:
- print("Auto-selecting prompt_type=%s for %s" % (prompt_type, model_lower), flush=True)
- assert prompt_type is not None, "prompt_type was None"
-
- # Control generation hyperparameters
- # adjust for bad inputs, e.g. in case also come from API that doesn't get constrained by gradio sliders
- # below is for TGI server, not required for HF transformers
- # limits are chosen similar to gradio_runner.py sliders/numbers
- top_p = min(max(1e-3, top_p), 1.0 - 1e-3)
- top_k = min(max(1, int(top_k)), 100)
- temperature = min(max(0.01, temperature), 2.0)
- # FIXME: https://github.com/h2oai/h2ogpt/issues/106
- num_beams = 1 if stream_output else num_beams # See max_beams in gradio_runner
- max_max_new_tokens = get_max_max_new_tokens(chosen_model_state,
- memory_restriction_level=memory_restriction_level,
- max_new_tokens=max_new_tokens,
- max_max_new_tokens=max_max_new_tokens)
- if min_max_new_tokens is None:
- # default for nochat api
- min_max_new_tokens = 256
- if docs_ordering_type is None:
- docs_ordering_type = 'reverse_ucurve_sort'
- model_max_length = get_model_max_length(chosen_model_state)
- max_new_tokens = min(max(1, int(max_new_tokens)), max_max_new_tokens)
- min_new_tokens = min(max(0, int(min_new_tokens)), max_new_tokens)
- max_time = min(max(0, max_time), max_max_time)
- repetition_penalty = min(max(0.01, repetition_penalty), 3.0)
- num_return_sequences = 1 if chat else min(max(1, int(num_return_sequences)), 10)
- min_top_k_docs, max_top_k_docs, label_top_k_docs = get_minmax_top_k_docs(is_public)
- # limit total tokens processed, e.g. for summarization, if public instance
- if is_public:
- total_tokens_for_docs = min(2 * model_max_length, 16384)
- else:
- total_tokens_for_docs = None
- top_k_docs = min(max(min_top_k_docs, int(top_k_docs)), max_top_k_docs)
- chunk_size = min(max(128, int(chunk_size)), 2048)
- if not context:
- context = ''
-
- # get prompter
- prompter = Prompter(prompt_type, prompt_dict, debug=debug, chat=chat, stream_output=stream_output,
- system_prompt=system_prompt)
-
- # THIRD PLACE where LangChain referenced, but imports only occur if enabled and have db to use
- assert langchain_mode in langchain_modes, "Invalid langchain_mode %s not in %s" % (langchain_mode, langchain_modes)
- assert langchain_action in langchain_actions, "Invalid langchain_action %s not in %s" % (
- langchain_action, langchain_actions)
- assert len(
- set(langchain_agents).difference(langchain_agents_list)) == 0, "Invalid langchain_agents %s" % langchain_agents
-
- # get db, but also fill db state so return already has my_db_state and dbs filled so faster next query
- if langchain_mode != LangChainMode.DISABLED.value:
- from src.gpt_langchain import get_any_db
- db = get_any_db(my_db_state, langchain_mode, langchain_mode_paths, langchain_mode_types,
- dbs=dbs,
- load_db_if_exists=load_db_if_exists,
- db_type=db_type,
- use_openai_embedding=use_openai_embedding,
- hf_embedding_model=hf_embedding_model,
- migrate_embedding_model=migrate_embedding_model,
- auto_migrate_db=auto_migrate_db,
- for_sources_list=True,
- verbose=verbose,
- n_jobs=n_jobs,
- )
- else:
- db = None
-
- t_generate = time.time()
- langchain_only_model = base_model in non_hf_types or \
- load_exllama or \
- inference_server.startswith('replicate') or \
- inference_server.startswith('sagemaker') or \
- inference_server.startswith('openai_azure_chat') or \
- inference_server.startswith('openai_azure')
- do_langchain_path = langchain_mode not in [False, 'Disabled', 'LLM'] or \
- langchain_only_model or \
- force_langchain_evaluate or \
- len(text_context_list) > 0
-
- if len(langchain_agents) > 0:
- do_langchain_path = True
- if add_search_to_context:
- # easier to manage prompt etc. by doing full langchain path
- do_langchain_path = True
-
- if do_langchain_path:
- text = ''
- sources = ''
- response = ''
- # use smaller cut_distance for wiki_full since so many matches could be obtained, and often irrelevant unless close
- from gpt_langchain import run_qa_db
- gen_hyper_langchain = dict(do_sample=do_sample,
- temperature=temperature,
- repetition_penalty=repetition_penalty,
- top_k=top_k,
- top_p=top_p,
- num_beams=num_beams,
- min_new_tokens=min_new_tokens,
- max_new_tokens=max_new_tokens,
- early_stopping=early_stopping,
- max_time=max_time,
- num_return_sequences=num_return_sequences,
- )
- loaders_dict, captions_model = gr_to_lg(image_loaders,
- pdf_loaders,
- url_loaders,
- captions_model=captions_model,
- )
- loaders_dict.update(dict(captions_model=captions_model,
- caption_loader=caption_loader,
- doctr_loader=doctr_loader,
- pix2struct_loader=pix2struct_loader,
- jq_schema=jq_schema,
- ))
- data_point = dict(context=context, instruction=instruction, input=iinput)
- # no longer stuff chat history directly into context this early
- prompt_basic = prompter.generate_prompt(data_point, context_from_history=False)
- prompt = prompt_basic
- num_prompt_tokens = 0
- for r in run_qa_db(
- inference_server=inference_server,
- model_name=base_model, model=model, tokenizer=tokenizer,
- langchain_only_model=langchain_only_model,
- async_output=async_output,
- num_async=num_async,
- prompter=prompter,
- use_llm_if_no_docs=use_llm_if_no_docs,
- load_db_if_exists=load_db_if_exists,
- db=db,
- langchain_mode_paths=langchain_mode_paths,
- langchain_mode_types=langchain_mode_types,
- detect_user_path_changes_every_query=detect_user_path_changes_every_query,
- cut_distance=1.1 if langchain_mode in ['wiki_full'] else cut_distance,
- answer_with_sources=answer_with_sources,
- append_sources_to_answer=append_sources_to_answer,
- add_chat_history_to_context=add_chat_history_to_context,
- add_search_to_context=add_search_to_context,
- keep_sources_in_context=keep_sources_in_context,
- memory_restriction_level=memory_restriction_level,
- system_prompt=system_prompt,
- use_openai_embedding=use_openai_embedding,
- use_openai_model=use_openai_model,
- hf_embedding_model=hf_embedding_model,
- migrate_embedding_model=migrate_embedding_model,
- auto_migrate_db=auto_migrate_db,
- first_para=first_para,
- text_limit=text_limit,
- show_accordions=show_accordions,
- top_k_docs_max_show=top_k_docs_max_show,
- show_link_in_sources=show_link_in_sources,
-
- # evaluate args items
- query=instruction,
- iinput=iinput,
- context=context,
- stream_output=stream_output,
- chunk=chunk,
- chunk_size=chunk_size,
-
- **loaders_dict,
-
- langchain_mode=langchain_mode,
- langchain_action=langchain_action,
- langchain_agents=langchain_agents,
- document_subset=document_subset,
- document_choice=document_choice,
- top_k_docs=top_k_docs,
- prompt_type=prompt_type,
- prompt_dict=prompt_dict,
- pre_prompt_query=pre_prompt_query,
- prompt_query=prompt_query,
- pre_prompt_summary=pre_prompt_summary,
- prompt_summary=prompt_summary,
- text_context_list=text_context_list,
- chat_conversation=chat_conversation,
- visible_models=visible_models,
- h2ogpt_key=h2ogpt_key,
- docs_ordering_type=docs_ordering_type,
- min_max_new_tokens=min_max_new_tokens,
-
- **gen_hyper_langchain,
-
- db_type=db_type,
- n_jobs=n_jobs,
- verbose=verbose,
- cli=cli,
- sanitize_bot_response=sanitize_bot_response,
-
- lora_weights=lora_weights,
-
- auto_reduce_chunks=auto_reduce_chunks,
- max_chunks=max_chunks,
- total_tokens_for_docs=total_tokens_for_docs,
- headsize=headsize,
- ):
- # doesn't accumulate, new answer every yield, so only save that full answer
- response = r['response']
- sources = r['sources']
- prompt = r['prompt']
- num_prompt_tokens = r['num_prompt_tokens']
- yield dict(response=response, sources=sources, save_dict=dict())
- if save_dir:
- # estimate using tiktoken
- extra_dict = gen_hyper_langchain.copy()
- extra_dict.update(prompt_type=prompt_type,
- inference_server=inference_server,
- langchain_mode=langchain_mode,
- langchain_action=langchain_action,
- langchain_agents=langchain_agents,
- document_subset=document_subset,
- document_choice=document_choice,
- chat_conversation=chat_conversation,
- add_search_to_context=add_search_to_context,
- num_prompt_tokens=num_prompt_tokens,
- instruction=instruction,
- iinput=iinput,
- context=context,
- t_generate=time.time() - t_generate,
- ntokens=None,
- tokens_persecond=None,
- )
- save_dict = dict(prompt=prompt,
- output=response, base_model=base_model, save_dir=save_dir,
- where_from='run_qa_db',
- extra_dict=extra_dict)
- yield dict(response=response, sources=sources, save_dict=save_dict)
- if verbose:
- print(
- 'Post-Generate Langchain: %s decoded_output: %s' %
- (str(datetime.now()), len(response) if response else -1),
- flush=True)
- if response or sources or langchain_only_model:
- # if got no response (e.g. not showing sources and got no sources,
- # so nothing to give to LLM), then slip through and ask LLM
- # Or if llama/gptj, then just return since they had no response and can't go down below code path
- # don't clear torch cache here, delays multi-generation, and bot(), all_bot(), and evaluate_nochat() do it
- return
-
- # NOT LANGCHAIN PATH, raw LLM
- # restrict instruction + , typically what has large input
- prompt, \
- instruction, iinput, context, \
- num_prompt_tokens, max_new_tokens, num_prompt_tokens0, num_prompt_tokens_actual, \
- chat_index, top_k_docs_trial, one_doc_size = \
- get_limited_prompt(instruction,
- iinput,
- tokenizer,
- prompter=prompter,
- inference_server=inference_server,
- # prompt_type=prompt_type,
- # prompt_dict=prompt_dict,
- # chat=chat,
- max_new_tokens=max_new_tokens,
- # system_prompt=system_prompt,
- context=context,
- chat_conversation=chat_conversation,
- keep_sources_in_context=keep_sources_in_context,
- model_max_length=model_max_length,
- memory_restriction_level=memory_restriction_level,
- langchain_mode=langchain_mode,
- add_chat_history_to_context=add_chat_history_to_context,
- min_max_new_tokens=min_max_new_tokens,
- )
-
- if inference_server.startswith('vllm') or \
- inference_server.startswith('openai') or \
- inference_server.startswith('http'):
- if inference_server.startswith('vllm') or inference_server.startswith('openai'):
- assert not inference_server.startswith('openai_azure_chat'), "Not fo Azure, use langchain path"
- assert not inference_server.startswith('openai_azure'), "Not for Azure, use langchain path"
- openai, inf_type, deployment_name, base_url, api_version = set_openai(inference_server)
- where_from = inf_type
-
- terminate_response = prompter.terminate_response or []
- stop_sequences = list(set(terminate_response + [prompter.PreResponse]))
- stop_sequences = [x for x in stop_sequences if x]
- # OpenAI will complain if ask for too many new tokens, takes it as min in some sense, wrongly so.
- max_new_tokens_openai = min(max_new_tokens, model_max_length - num_prompt_tokens)
- gen_server_kwargs = dict(temperature=temperature if do_sample else 0,
- max_tokens=max_new_tokens_openai,
- top_p=top_p if do_sample else 1,
- frequency_penalty=0,
- n=num_return_sequences,
- presence_penalty=1.07 - repetition_penalty + 0.6, # so good default
- )
- if inf_type == 'vllm' or inference_server == 'openai':
- responses = openai.Completion.create(
- model=base_model,
- prompt=prompt,
- **gen_server_kwargs,
- stop=stop_sequences,
- stream=stream_output,
- )
- text = ''
- sources = ''
- response = ''
- if not stream_output:
- text = responses['choices'][0]['text']
- response = prompter.get_response(prompt + text, prompt=prompt,
- sanitize_bot_response=sanitize_bot_response)
- yield dict(response=response, sources=sources, save_dict=dict())
- else:
- collected_events = []
- for event in responses:
- collected_events.append(event) # save the event response
- event_text = event['choices'][0]['text'] # extract the text
- text += event_text # append the text
- response = prompter.get_response(prompt + text, prompt=prompt,
- sanitize_bot_response=sanitize_bot_response)
- yield dict(response=response, sources=sources, save_dict=dict())
- elif inf_type == 'vllm_chat' or inference_server == 'openai_chat':
- if inf_type == 'vllm_chat':
- raise NotImplementedError('%s not supported by vLLM' % inf_type)
- if system_prompt in [None, 'None', 'auto']:
- openai_system_prompt = "You are a helpful assistant."
- else:
- openai_system_prompt = system_prompt
- messages0 = []
- if openai_system_prompt:
- messages0.append({"role": "system", "content": openai_system_prompt})
- messages0.append({'role': 'user', 'content': prompt})
- responses = openai.ChatCompletion.create(
- model=base_model,
- messages=messages0,
- stream=stream_output,
- **gen_server_kwargs,
- )
- text = ""
- sources = ''
- response = ""
- if not stream_output:
- text = responses["choices"][0]["message"]["content"]
- response = prompter.get_response(prompt + text, prompt=prompt,
- sanitize_bot_response=sanitize_bot_response)
- yield dict(response=response, sources=sources, save_dict=dict())
- else:
- for chunk in responses:
- delta = chunk["choices"][0]["delta"]
- if 'content' in delta:
- text += delta['content']
- response = prompter.get_response(prompt + text, prompt=prompt,
- sanitize_bot_response=sanitize_bot_response)
- yield dict(response=response, sources=sources, save_dict=dict())
- else:
- raise RuntimeError("No such OpenAI mode: %s" % inference_server)
- elif inference_server.startswith('http'):
- inference_server, headers = get_hf_server(inference_server)
- from gradio_utils.grclient import GradioClient
- from text_generation import Client as HFClient
- if isinstance(model, GradioClient):
- gr_client = model
- hf_client = None
- elif isinstance(model, HFClient):
- gr_client = None
- hf_client = model
- else:
- inference_server, gr_client, hf_client = get_client_from_inference_server(inference_server,
- base_model=base_model)
-
- # quick sanity check to avoid long timeouts, just see if can reach server
- requests.get(inference_server, timeout=int(os.getenv('REQUEST_TIMEOUT_FAST', '10')))
-
- if gr_client is not None:
- # Note: h2oGPT gradio server could handle input token size issues for prompt,
- # but best to handle here so send less data to server
-
- chat_client = False
- where_from = "gr_client"
- client_langchain_mode = 'Disabled'
- client_add_chat_history_to_context = True
- client_add_search_to_context = False
- client_langchain_action = LangChainAction.QUERY.value
- client_langchain_agents = []
- gen_server_kwargs = dict(temperature=temperature,
- top_p=top_p,
- top_k=top_k,
- num_beams=num_beams,
- max_new_tokens=max_new_tokens,
- min_new_tokens=min_new_tokens,
- early_stopping=early_stopping,
- max_time=max_time,
- repetition_penalty=repetition_penalty,
- num_return_sequences=num_return_sequences,
- do_sample=do_sample,
- chat=chat_client,
- )
- # account for gradio into gradio that handles prompting, avoid duplicating prompter prompt injection
- if prompt_type in [None, '', PromptType.plain.name, PromptType.plain.value,
- str(PromptType.plain.value)]:
- # if our prompt is plain, assume either correct or gradio server knows different prompt type,
- # so pass empty prompt_Type
- gr_prompt_type = ''
- gr_prompt_dict = ''
- gr_prompt = prompt # already prepared prompt
- gr_context = ''
- gr_iinput = ''
- else:
- # if already have prompt_type that is not plain, None, or '', then already applied some prompting
- # But assume server can handle prompting, and need to avoid double-up.
- # Also assume server can do better job of using stopping.py to stop early, so avoid local prompting, let server handle
- # So avoid "prompt" and let gradio server reconstruct from prompt_type we passed
- # Note it's ok that prompter.get_response() has prompt+text, prompt=prompt passed,
- # because just means extra processing and removal of prompt, but that has no human-bot prompting doesn't matter
- # since those won't appear
- gr_context = context
- gr_prompt = instruction
- gr_iinput = iinput
- gr_prompt_type = prompt_type
- gr_prompt_dict = prompt_dict
- client_kwargs = dict(instruction=gr_prompt if chat_client else '', # only for chat=True
- iinput=gr_iinput, # only for chat=True
- context=gr_context,
- # streaming output is supported, loops over and outputs each generation in streaming mode
- # but leave stream_output=False for simple input/output mode
- stream_output=stream_output,
-
- **gen_server_kwargs,
-
- prompt_type=gr_prompt_type,
- prompt_dict=gr_prompt_dict,
-
- instruction_nochat=gr_prompt if not chat_client else '',
- iinput_nochat=gr_iinput, # only for chat=False
- langchain_mode=client_langchain_mode,
- add_chat_history_to_context=client_add_chat_history_to_context,
- langchain_action=client_langchain_action,
- langchain_agents=client_langchain_agents,
- top_k_docs=top_k_docs,
- chunk=chunk,
- chunk_size=chunk_size,
- document_subset=DocumentSubset.Relevant.name,
- document_choice=[DocumentChoice.ALL.value],
- pre_prompt_query=pre_prompt_query,
- prompt_query=prompt_query,
- pre_prompt_summary=pre_prompt_summary,
- prompt_summary=prompt_summary,
- system_prompt=system_prompt,
- image_loaders=image_loaders,
- pdf_loaders=pdf_loaders,
- url_loaders=url_loaders,
- jq_schema=jq_schema,
- visible_models=visible_models,
- h2ogpt_key=h2ogpt_key,
- add_search_to_context=client_add_search_to_context,
- docs_ordering_type=None,
- min_max_new_tokens=min_max_new_tokens,
- )
- api_name = '/submit_nochat_api' # NOTE: like submit_nochat but stable API for string dict passing
- response = ''
- text = ''
- sources = ''
- if not stream_output:
- res = gr_client.predict(str(dict(client_kwargs)), api_name=api_name)
- res_dict = ast.literal_eval(res)
- text = res_dict['response']
- sources = res_dict['sources']
- response = prompter.get_response(prompt + text, prompt=prompt,
- sanitize_bot_response=sanitize_bot_response)
- yield dict(response=response, sources=sources, save_dict=dict())
- else:
- job = gr_client.submit(str(dict(client_kwargs)), api_name=api_name)
- res_dict = dict(response=text, sources=sources, save_dict=dict())
- text0 = ''
- while not job.done():
- if job.communicator.job.latest_status.code.name == 'FINISHED':
- break
- e = job.future._exception
- if e is not None:
- break
- outputs_list = job.communicator.job.outputs
- if outputs_list:
- res = job.communicator.job.outputs[-1]
- res_dict = ast.literal_eval(res)
- text = res_dict['response']
- sources = res_dict['sources']
- if gr_prompt_type == 'plain':
- # then gradio server passes back full prompt + text
- prompt_and_text = text
- else:
- prompt_and_text = prompt + text
- response = prompter.get_response(prompt_and_text, prompt=prompt,
- sanitize_bot_response=sanitize_bot_response)
- text_chunk = response[len(text0):]
- if not text_chunk:
- continue
- # save old
- text0 = response
- yield dict(response=response, sources=sources, save_dict=dict())
- time.sleep(0.01)
- # ensure get last output to avoid race
- res_all = job.outputs()
- if len(res_all) > 0:
- res = res_all[-1]
- res_dict = ast.literal_eval(res)
- text = res_dict['response']
- sources = res_dict['sources']
- else:
- # go with old text if last call didn't work
- e = job.future._exception
- if e is not None:
- stre = str(e)
- strex = ''.join(traceback.format_tb(e.__traceback__))
- else:
- stre = ''
- strex = ''
-
- print("Bad final response: %s %s %s %s %s: %s %s" % (base_model, inference_server,
- res_all, prompt, text, stre, strex),
- flush=True)
- if gr_prompt_type == 'plain':
- # then gradio server passes back full prompt + text
- prompt_and_text = text
- else:
- prompt_and_text = prompt + text
- response = prompter.get_response(prompt_and_text, prompt=prompt,
- sanitize_bot_response=sanitize_bot_response)
- yield dict(response=response, sources=sources, save_dict=dict())
- elif hf_client:
- # HF inference server needs control over input tokens
- where_from = "hf_client"
- response = ''
- extra = ''
- sources = ''
-
- # prompt must include all human-bot like tokens, already added by prompt
- # https://github.com/huggingface/text-generation-inference/tree/main/clients/python#types
- terminate_response = prompter.terminate_response or []
- stop_sequences = list(set(terminate_response + [prompter.PreResponse]))
- stop_sequences = [x for x in stop_sequences if x]
- gen_server_kwargs = dict(do_sample=do_sample,
- max_new_tokens=max_new_tokens,
- # best_of=None,
- repetition_penalty=repetition_penalty,
- return_full_text=False,
- seed=SEED,
- stop_sequences=stop_sequences,
- temperature=temperature,
- top_k=top_k,
- top_p=top_p,
- # truncate=False, # behaves oddly
- # typical_p=top_p,
- # watermark=False,
- # decoder_input_details=False,
- )
- # work-around for timeout at constructor time, will be issue if multi-threading,
- # so just do something reasonable or max_time if larger
- # lower bound because client is re-used if multi-threading
- hf_client.timeout = max(300, max_time)
- if not stream_output:
- text = hf_client.generate(prompt, **gen_server_kwargs).generated_text
- response = prompter.get_response(prompt + text, prompt=prompt,
- sanitize_bot_response=sanitize_bot_response)
- yield dict(response=response, sources=sources, save_dict=dict())
- else:
- text = ""
- for responses in hf_client.generate_stream(prompt, **gen_server_kwargs):
- if not responses.token.special:
- # stop_sequences
- text_chunk = responses.token.text
- text += text_chunk
- response = prompter.get_response(prompt + text, prompt=prompt,
- sanitize_bot_response=sanitize_bot_response)
- sources = ''
- yield dict(response=response, sources=sources, save_dict=dict())
- else:
- raise RuntimeError("Failed to get client: %s" % inference_server)
- else:
- raise RuntimeError("No such inference_server %s" % inference_server)
-
- if save_dir and text:
- # save prompt + new text
- extra_dict = gen_server_kwargs.copy()
- extra_dict.update(dict(inference_server=inference_server, num_prompt_tokens=num_prompt_tokens,
- t_generate=time.time() - t_generate,
- ntokens=None,
- tokens_persecond=None,
- ))
- save_dict = dict(prompt=prompt, output=text, base_model=base_model, save_dir=save_dir,
- where_from=where_from, extra_dict=extra_dict)
- yield dict(response=response, sources=sources, save_dict=save_dict)
- return
- else:
- assert not inference_server, "inference_server=%s not supported" % inference_server
-
- if isinstance(tokenizer, str):
- # pipeline
- if tokenizer == "summarization":
- key = 'summary_text'
- else:
- raise RuntimeError("No such task type %s" % tokenizer)
- # NOTE: uses max_length only
- sources = ''
- yield dict(response=model(prompt, max_length=max_new_tokens)[0][key], sources=sources, save_dict=dict())
-
- if 'mbart-' in base_model.lower():
- assert src_lang is not None
- tokenizer.src_lang = languages_covered()[src_lang]
-
- stopping_criteria = get_stopping(prompt_type, prompt_dict, tokenizer, device, base_model,
- model_max_length=model_max_length,
- prompter=prompter)
-
- inputs = tokenizer(prompt, return_tensors="pt")
- if debug and len(inputs["input_ids"]) > 0:
- print('input_ids length', len(inputs["input_ids"][0]), flush=True)
- input_ids = inputs["input_ids"].to(device)
- # CRITICAL LIMIT else will fail
- max_max_tokens = tokenizer.model_max_length
- max_input_tokens = max(0, int(max_max_tokens - min_new_tokens))
- # NOTE: Don't limit up front due to max_new_tokens, let go up to max or reach max_max_tokens in stopping.py
- assert isinstance(max_input_tokens, int), "Bad type for max_input_tokens=%s %s" % (
- max_input_tokens, type(max_input_tokens))
- input_ids = input_ids[:, -max_input_tokens:]
- # required for falcon if multiple threads or asyncio accesses to model during generation
- if use_cache is None:
- use_cache = False if 'falcon' in base_model else True
- gen_config_kwargs = dict(num_beams=num_beams,
- do_sample=do_sample,
- repetition_penalty=float(repetition_penalty),
- num_return_sequences=num_return_sequences,
- renormalize_logits=True,
- remove_invalid_values=True,
- use_cache=use_cache,
- )
- if do_sample:
- gen_config_kwargs.update(dict(temperature=float(temperature),
- top_p=float(top_p),
- top_k=top_k))
- if True:
- # unclear impact, some odd things going on inside
- # leads to:
- # The attention mask and the pad token id were not set. As a consequence, you may observe unexpected behavior. Please pass your input's `attention_mask` to obtain reliable results.
- # Setting `pad_token_id` to `eos_token_id`:2 for open-end generation.
- # or leads to:
- # Using cls_token, but it is not set yet.
- # Using mask_token, but it is not set yet.
- # Using pad_token, but it is not set yet.
- # Using sep_token, but it is not set yet.
- token_ids = ['eos_token_id', 'pad_token_id', 'bos_token_id', 'cls_token_id', 'sep_token_id']
- for token_id in token_ids:
- if hasattr(tokenizer, token_id) and getattr(tokenizer, token_id) is not None:
- gen_config_kwargs.update({token_id: getattr(tokenizer, token_id)})
- generation_config = GenerationConfig(**gen_config_kwargs)
-
- gen_kwargs = dict(input_ids=input_ids,
- generation_config=generation_config,
- return_dict_in_generate=True,
- output_scores=True,
- max_new_tokens=max_new_tokens, # prompt + new
- min_new_tokens=min_new_tokens, # prompt + new
- early_stopping=early_stopping, # False, True, "never"
- max_time=max_time,
- stopping_criteria=stopping_criteria,
- )
- if 'gpt2' in base_model.lower():
- gen_kwargs.update(dict(bos_token_id=tokenizer.bos_token_id, pad_token_id=tokenizer.eos_token_id))
- elif 'mbart-' in base_model.lower():
- assert tgt_lang is not None
- tgt_lang = languages_covered()[tgt_lang]
- gen_kwargs.update(dict(forced_bos_token_id=tokenizer.lang_code_to_id[tgt_lang]))
- else:
- token_ids = ['eos_token_id', 'bos_token_id', 'pad_token_id']
- for token_id in token_ids:
- if hasattr(tokenizer, token_id) and getattr(tokenizer, token_id) is not None:
- gen_kwargs.update({token_id: getattr(tokenizer, token_id)})
-
- decoder_kwargs = dict(skip_special_tokens=True,
- clean_up_tokenization_spaces=True)
-
- decoder = functools.partial(tokenizer.decode,
- **decoder_kwargs
- )
- with torch.no_grad():
- have_lora_weights = lora_weights not in [no_lora_str, '', None]
- context_class_cast = NullContext if device == 'cpu' or have_lora_weights or device == 'mps' else torch.autocast
- if t5_type(base_model):
- # issues when casting to float16, can mess up t5 model, e.g. only when not streaming, or other odd behaviors
- context_class_cast = NullContext
- with context_class_cast(device):
- # protection for gradio not keeping track of closed users,
- # else hit bitsandbytes lack of thread safety:
- # https://github.com/h2oai/h2ogpt/issues/104
- # but only makes sense if concurrency_count == 1
- context_class = NullContext # if concurrency_count > 1 else filelock.FileLock
- if verbose:
- print('Pre-Generate: %s' % str(datetime.now()), flush=True)
- decoded_output = None
- response = ''
- with context_class("generate.lock"):
- if verbose:
- print('Generate: %s' % str(datetime.now()), flush=True)
- always_use_streaming_method = True # to deal with complex parsing of prompt vs. generation due to odd tokenizing
- if stream_output or always_use_streaming_method:
- skip_prompt = True # True means first output excludes prompt
- streamer = H2OTextIteratorStreamer(tokenizer, skip_prompt=skip_prompt, block=False,
- **decoder_kwargs)
- gen_kwargs.update(dict(streamer=streamer))
- target = wrapped_partial(generate_with_exceptions, model.generate,
- raise_generate_gpu_exceptions=raise_generate_gpu_exceptions,
- **gen_kwargs)
- bucket = queue.Queue()
- thread = EThread(target=target, streamer=streamer, bucket=bucket)
- thread.start()
- ret = dict(response='', sources='', save_dict=dict())
- outputs = ""
- sources = ''
- try:
- for new_text in streamer:
- if bucket.qsize() > 0 or thread.exc:
- thread.join()
- outputs += new_text
- response = prompter.get_response(outputs, prompt=None,
- only_new_text=True,
- sanitize_bot_response=sanitize_bot_response)
- ret = dict(response=response, sources=sources, save_dict=dict())
- if stream_output:
- yield ret
- if not stream_output:
- yield ret
- except BaseException:
- # if any exception, raise that exception if was from thread, first
- if thread.exc:
- raise thread.exc
- raise
- finally:
- # don't clear torch cache here, delays multi-generation, and bot(), all_bot(), and evaluate_nochat() do it
- # in case no exception and didn't join with thread yet, then join
- if not thread.exc:
- thread.join()
- # in case raise StopIteration or broke queue loop in streamer, but still have exception
- if thread.exc:
- raise thread.exc
- decoded_output = outputs
- ntokens = len(outputs) // 4 # hack for now
- else:
- # below length removal doesn't work in general, because encoding does not match internal of model generation
- input_ids_len = gen_kwargs['input_ids'][0].shape[0]
- try:
- outputs = model.generate(**gen_kwargs)
- finally:
- pass
- # don't clear torch cache here, delays multi-generation, and bot(), all_bot(), and evaluate_nochat() do it
- # skip first IDs
- ntokens = sum([len(s) - input_ids_len for s in outputs.sequences]) if save_dir else -1
- outputs = [decoder(s[input_ids_len:]) for s in outputs.sequences]
- sources = ''
- response = prompter.get_response(outputs, prompt=None,
- only_new_text=True,
- sanitize_bot_response=sanitize_bot_response)
- yield dict(response=response, sources=sources, save_dict=dict())
- if outputs and len(outputs) >= 1:
- decoded_output = prompt + outputs[0]
- if save_dir and decoded_output:
- extra_dict = gen_config_kwargs.copy()
- extra_dict.update(dict(num_prompt_tokens=num_prompt_tokens,
- t_generate=time.time() - t_generate,
- ntokens=ntokens,
- tokens_persecond=ntokens / (time.time() - t_generate),
- ))
- save_dict = dict(prompt=prompt, output=decoded_output, base_model=base_model, save_dir=save_dir,
- where_from="evaluate_%s" % str(stream_output),
- extra_dict=extra_dict)
- yield dict(response=response, sources=sources, save_dict=save_dict)
- if verbose:
- print('Post-Generate: %s decoded_output: %s' % (
- str(datetime.now()), len(decoded_output) if decoded_output else -1), flush=True)
-
-
-inputs_list_names = list(inspect.signature(evaluate).parameters)
-state_names = input_args_list.copy() # doesn't have to be the same, but state_names must match evaluate() and how filled then
-inputs_kwargs_list = [x for x in inputs_list_names if x not in eval_func_param_names + state_names]
-
-
-def get_cutoffs(memory_restriction_level, for_context=False, model_max_length=2048):
- # help to avoid errors like:
- # RuntimeError: The size of tensor a (2048) must match the size of tensor b (2049) at non-singleton dimension 3
- # RuntimeError: expected scalar type Half but found Float
- # with - 256
- if memory_restriction_level > 0:
- max_length_tokenize = 768 - 256 if memory_restriction_level <= 2 else 512 - 256
- else:
- # at least give room for 1 paragraph output
- max_length_tokenize = model_max_length - 256
- cutoff_len = max_length_tokenize * 4 # if reaches limit, then can't generate new tokens
- output_smallest = 30 * 4
- max_prompt_length = cutoff_len - output_smallest
-
- if for_context:
- # then lower even more to avoid later chop, since just estimate tokens in context bot
- max_prompt_length = max(64, int(max_prompt_length * 0.8))
-
- return cutoff_len, output_smallest, max_length_tokenize, max_prompt_length
-
-
-class H2OTextIteratorStreamer(TextIteratorStreamer):
- """
- normally, timeout required for now to handle exceptions, else get()
- but with H2O version of TextIteratorStreamer, loop over block to handle
- """
-
- def __init__(self, tokenizer, skip_prompt: bool = False, timeout: typing.Optional[float] = None,
- block=True, **decode_kwargs):
- super().__init__(tokenizer, skip_prompt, **decode_kwargs)
- self.text_queue = queue.Queue()
- self.stop_signal = None
- self.do_stop = False
- self.timeout = timeout
- self.block = block
-
- def on_finalized_text(self, text: str, stream_end: bool = False):
- """Put the new text in the queue. If the stream is ending, also put a stop signal in the queue."""
- self.text_queue.put(text, timeout=self.timeout)
- if stream_end:
- self.text_queue.put(self.stop_signal, timeout=self.timeout)
-
- def __iter__(self):
- return self
-
- def __next__(self):
- while True:
- try:
- value = self.stop_signal # value looks unused in pycharm, not true
- if self.do_stop:
- print("hit stop", flush=True)
- # could raise or break, maybe best to raise and make parent see if any exception in thread
- self.clear_queue()
- self.do_stop = False
- raise StopIteration()
- # break
- value = self.text_queue.get(block=self.block, timeout=self.timeout)
- break
- except queue.Empty:
- time.sleep(0.01)
- if value == self.stop_signal:
- self.clear_queue()
- self.do_stop = False
- raise StopIteration()
- else:
- return value
-
- def clear_queue(self):
- # make sure streamer is reusable after stop hit
- with self.text_queue.mutex:
- self.text_queue.queue.clear()
-
- def put(self, value):
- """
- Receives tokens, decodes them, and prints them to stdout as soon as they form entire words.
- # same as base class, except remove hack w.r.t. text.rfind(" ") that ruins LLaMa2
- """
- if len(value.shape) > 1 and value.shape[0] > 1:
- raise ValueError("TextStreamer only supports batch size 1")
- elif len(value.shape) > 1:
- value = value[0]
-
- if self.skip_prompt and self.next_tokens_are_prompt:
- self.next_tokens_are_prompt = False
- return
-
- # Add the new token to the cache and decodes the entire thing.
- self.token_cache.extend(value.tolist())
- text = self.tokenizer.decode(self.token_cache, **self.decode_kwargs)
-
- # After the symbol for a new line, we flush the cache.
- if text.endswith("\n"):
- printable_text = text[self.print_len:]
- self.token_cache = []
- self.print_len = 0
- # If the last token is a CJK character, we print the characters.
- elif len(text) > 0 and self._is_chinese_char(ord(text[-1])):
- printable_text = text[self.print_len:]
- self.print_len += len(printable_text)
- # Otherwise, prints until the last space char (simple heuristic to avoid printing incomplete words,
- # which may change with the subsequent token -- there are probably smarter ways to do this!)
- elif len(text) > 0 and text[-1] == '�':
- printable_text = text[self.print_len: text.rfind(" ") + 1]
- self.print_len += len(printable_text)
- else:
- printable_text = text[self.print_len:]
- self.print_len += len(printable_text)
-
- self.on_finalized_text(printable_text)
-
-
-def generate_with_exceptions(func, *args, raise_generate_gpu_exceptions=True, **kwargs):
- try:
- func(*args, **kwargs)
- except torch.cuda.OutOfMemoryError as e:
- print("GPU OOM 2: exception: %s" % str(e),
- flush=True)
- if 'input_ids' in kwargs:
- if kwargs['input_ids'] is not None:
- kwargs['input_ids'].cpu()
- kwargs['input_ids'] = None
- traceback.print_exc()
- clear_torch_cache()
- return
- except (Exception, RuntimeError) as e:
- if 'Expected all tensors to be on the same device' in str(e) or \
- 'expected scalar type Half but found Float' in str(e) or \
- 'probability tensor contains either' in str(e) or \
- 'cublasLt ran into an error!' in str(e) or \
- 'mat1 and mat2 shapes cannot be multiplied' in str(e):
- print(
- "GPU Error: exception: %s" % str(e),
- flush=True)
- traceback.print_exc()
- clear_torch_cache()
- if raise_generate_gpu_exceptions:
- raise
- return
- else:
- clear_torch_cache()
- if raise_generate_gpu_exceptions:
- raise
-
-
-def get_generate_params(model_lower,
- chat,
- stream_output, show_examples,
- prompt_type, prompt_dict,
- system_prompt,
- pre_prompt_query, prompt_query,
- pre_prompt_summary, prompt_summary,
- temperature, top_p, top_k, num_beams,
- max_new_tokens, min_new_tokens, early_stopping, max_time,
- repetition_penalty, num_return_sequences,
- do_sample,
- top_k_docs, chunk, chunk_size,
- image_loaders,
- pdf_loaders,
- url_loaders,
- jq_schema,
- docs_ordering_type,
- min_max_new_tokens,
- verbose,
- ):
- use_defaults = False
- use_default_examples = True
- examples = []
- task_info = 'LLM'
- if model_lower:
- print(f"Using Model {model_lower}", flush=True)
- else:
- if verbose:
- print("No model defined yet", flush=True)
-
- min_new_tokens = min_new_tokens if min_new_tokens is not None else 0
- early_stopping = early_stopping if early_stopping is not None else False
- max_time_defaults = 60 * 3
- max_time = max_time if max_time is not None else max_time_defaults
-
- if not prompt_type and model_lower in inv_prompt_type_to_model_lower and prompt_type != 'custom':
- prompt_type = inv_prompt_type_to_model_lower[model_lower]
- if verbose:
- print("Auto-selecting prompt_type=%s for %s" % (prompt_type, model_lower), flush=True)
-
- # examples at first don't include chat, instruction_nochat, iinput_nochat, added at end
- if show_examples is None:
- if chat:
- show_examples = False
- else:
- show_examples = True
-
- summarize_example1 = """Jeff: Can I train a ? Transformers model on Amazon SageMaker?
-Philipp: Sure you can use the new Hugging Face Deep Learning Container.
-Jeff: ok.
-Jeff: and how can I get started?
-Jeff: where can I find documentation?
-Philipp: ok, ok you can find everything here. https://huggingface.co/blog/the-partnership-amazon-sagemaker-and-hugging-face"""
-
- use_placeholder_instruction_as_example = False
- if 'bart-large-cnn-samsum' in model_lower or 'flan-t5-base-samsum' in model_lower:
- placeholder_instruction = summarize_example1
- placeholder_input = ""
- use_defaults = True
- use_default_examples = False
- use_placeholder_instruction_as_example = True
- task_info = "Summarization"
- elif 't5-' in model_lower or 't5' == model_lower or 'flan-' in model_lower:
- placeholder_instruction = "The square root of x is the cube root of y. What is y to the power of 2, if x = 4?"
- placeholder_input = ""
- use_defaults = True
- use_default_examples = True
- task_info = "Multi-Task: Q/A, translation, Chain-of-Thought, Logical Reasoning, Summarization, etc. Best to use task prefix as trained on, e.g. `translate English to German: ` (space after colon)"
- elif 'mbart-' in model_lower:
- placeholder_instruction = "The girl has long hair."
- placeholder_input = ""
- use_defaults = True
- use_default_examples = False
- use_placeholder_instruction_as_example = True
- elif 'gpt2' in model_lower:
- placeholder_instruction = "The sky is"
- placeholder_input = ""
- prompt_type = prompt_type or 'plain'
- use_default_examples = True # some will be odd "continuations" but can be ok
- use_placeholder_instruction_as_example = True
- task_info = "Auto-complete phrase, code, etc."
- use_defaults = True
- else:
- if chat:
- placeholder_instruction = ""
- else:
- placeholder_instruction = "Give detailed answer for whether Einstein or Newton is smarter."
- placeholder_input = ""
- if not prompt_type and model_lower in inv_prompt_type_to_model_lower and prompt_type != 'custom':
- prompt_type = inv_prompt_type_to_model_lower[model_lower]
- elif model_lower:
- # default is plain, because might rely upon trust_remote_code to handle prompting
- prompt_type = prompt_type or 'plain'
- else:
- prompt_type = ''
- task_info = "No task"
- if prompt_type == 'instruct':
- task_info = "Answer question or follow imperative as instruction with optionally input."
- elif prompt_type == 'plain':
- task_info = "Auto-complete phrase, code, etc."
- elif prompt_type == 'human_bot':
- if chat:
- task_info = "Chat (Shift-Enter to give question/imperative, input concatenated with instruction)"
- else:
- task_info = "Ask question/imperative (input concatenated with instruction)"
-
- # revert to plain if still nothing
- prompt_type = prompt_type or 'plain'
- if use_defaults:
- temperature = 1.0 if temperature is None else temperature
- top_p = 1.0 if top_p is None else top_p
- top_k = 40 if top_k is None else top_k
- num_beams = num_beams or 1
- max_new_tokens = max_new_tokens or 512
- repetition_penalty = repetition_penalty or 1.07
- num_return_sequences = min(num_beams, num_return_sequences or 1)
- do_sample = False if do_sample is None else do_sample
- else:
- temperature = 0.1 if temperature is None else temperature
- top_p = 0.75 if top_p is None else top_p
- top_k = 40 if top_k is None else top_k
- num_beams = num_beams or 1
- max_new_tokens = max_new_tokens or 1024
- repetition_penalty = repetition_penalty or 1.07
- num_return_sequences = min(num_beams, num_return_sequences or 1)
- do_sample = False if do_sample is None else do_sample
- # doesn't include chat, instruction_nochat, iinput_nochat, added later
- params_list = ["",
- stream_output,
- prompt_type, prompt_dict,
- temperature, top_p, top_k, num_beams,
- max_new_tokens, min_new_tokens,
- early_stopping, max_time, repetition_penalty, num_return_sequences, do_sample]
-
- if use_placeholder_instruction_as_example:
- examples += [[placeholder_instruction, ''] + params_list]
-
- if use_default_examples:
- examples += [
- ["Translate English to French", "Good morning"] + params_list,
- ["Give detailed answer for whether Einstein or Newton is smarter.", ''] + params_list,
- ["Explain in detailed list, all the best practices for coding in python.", ''] + params_list,
- [
- "Create a markdown table with 3 rows for the primary colors, and 2 columns, with color name and hex codes.",
- ''] + params_list,
- ['Translate to German: My name is Arthur', ''] + params_list,
- ["Please answer to the following question. Who is going to be the next Ballon d'or?", ''] + params_list,
- ['Can Geoffrey Hinton have a conversation with George Washington? Give the rationale before answering.',
- ''] + params_list,
- ['Please answer the following question. What is the boiling point of Nitrogen?', ''] + params_list,
- ['Answer the following yes/no question. Can you write a whole Haiku in a single tweet?', ''] + params_list,
- ["Simplify the following expression: (False or False and True). Explain your answer.", ''] + params_list,
- [
- "Premise: At my age you will probably have learnt one lesson. Hypothesis: It's not certain how many lessons you'll learn by your thirties. Does the premise entail the hypothesis?",
- ''] + params_list,
- ['The square root of x is the cube root of y. What is y to the power of 2, if x = 4?', ''] + params_list,
- [
- 'Answer the following question by reasoning step by step. The cafeteria had 23 apples. If they used 20 for lunch, and bought 6 more, how many apple do they have?',
- ''] + params_list,
- ["""def area_of_rectangle(a: float, b: float):
- \"\"\"Return the area of the rectangle.\"\"\"""", ''] + params_list,
- ["""# a function in native python:
-def mean(a):
- return sum(a)/len(a)
-
-# the same function using numpy:
-import numpy as np
-def mean(a):""", ''] + params_list,
- ["""X = np.random.randn(100, 100)
-y = np.random.randint(0, 1, 100)
-
-# fit random forest classifier with 20 estimators""", ''] + params_list,
- ]
- # add summary example
- examples += [
- [summarize_example1, 'Summarize' if prompt_type not in ['plain', 'instruct_simple'] else ''] + params_list]
-
- src_lang = "English"
- tgt_lang = "Russian"
-
- # move to correct position
- for example in examples:
- example += [chat, '', '', LangChainMode.DISABLED.value, True,
- LangChainAction.QUERY.value, [],
- top_k_docs, chunk, chunk_size, DocumentSubset.Relevant.name, [],
- pre_prompt_query, prompt_query,
- pre_prompt_summary, prompt_summary,
- system_prompt,
- image_loaders,
- pdf_loaders,
- url_loaders,
- jq_schema,
- None,
- None,
- False,
- None,
- None,
- docs_ordering_type,
- min_max_new_tokens,
- ]
- # adjust examples if non-chat mode
- if not chat:
- example[eval_func_param_names.index('instruction_nochat')] = example[
- eval_func_param_names.index('instruction')]
- example[eval_func_param_names.index('instruction')] = ''
-
- example[eval_func_param_names.index('iinput_nochat')] = example[eval_func_param_names.index('iinput')]
- example[eval_func_param_names.index('iinput')] = ''
- assert len(example) == len(eval_func_param_names), "Wrong example: %s %s" % (
- len(example), len(eval_func_param_names))
-
- if prompt_type == PromptType.custom.name and not prompt_dict:
- raise ValueError("Unexpected to get non-empty prompt_dict=%s for prompt_type=%s" % (prompt_dict, prompt_type))
-
- # get prompt_dict from prompt_type, so user can see in UI etc., or for custom do nothing except check format
- prompt_dict, error0 = get_prompt(prompt_type, prompt_dict,
- chat=False, context='', reduced=False, making_context=False, return_dict=True,
- system_prompt=system_prompt)
- if error0:
- raise RuntimeError("Prompt wrong: %s" % error0)
-
- return placeholder_instruction, placeholder_input, \
- stream_output, show_examples, \
- prompt_type, prompt_dict, \
- temperature, top_p, top_k, num_beams, \
- max_new_tokens, min_new_tokens, early_stopping, max_time, \
- repetition_penalty, num_return_sequences, \
- do_sample, \
- src_lang, tgt_lang, \
- examples, \
- task_info
-
-
-def languages_covered():
- # https://huggingface.co/facebook/mbart-large-50-many-to-many-mmt#languages-covered
- covered = """Arabic (ar_AR), Czech (cs_CZ), German (de_DE), English (en_XX), Spanish (es_XX), Estonian (et_EE), Finnish (fi_FI), French (fr_XX), Gujarati (gu_IN), Hindi (hi_IN), Italian (it_IT), Japanese (ja_XX), Kazakh (kk_KZ), Korean (ko_KR), Lithuanian (lt_LT), Latvian (lv_LV), Burmese (my_MM), Nepali (ne_NP), Dutch (nl_XX), Romanian (ro_RO), Russian (ru_RU), Sinhala (si_LK), Turkish (tr_TR), Vietnamese (vi_VN), Chinese (zh_CN), Afrikaans (af_ZA), Azerbaijani (az_AZ), Bengali (bn_IN), Persian (fa_IR), Hebrew (he_IL), Croatian (hr_HR), Indonesian (id_ID), Georgian (ka_GE), Khmer (km_KH), Macedonian (mk_MK), Malayalam (ml_IN), Mongolian (mn_MN), Marathi (mr_IN), Polish (pl_PL), Pashto (ps_AF), Portuguese (pt_XX), Swedish (sv_SE), Swahili (sw_KE), Tamil (ta_IN), Telugu (te_IN), Thai (th_TH), Tagalog (tl_XX), Ukrainian (uk_UA), Urdu (ur_PK), Xhosa (xh_ZA), Galician (gl_ES), Slovene (sl_SI)"""
- covered = covered.split(', ')
- covered = {x.split(' ')[0]: x.split(' ')[1].replace(')', '').replace('(', '') for x in covered}
- return covered
-
-
-def score_qa(smodel, stokenizer, max_length_tokenize, question, answer, cutoff_len):
- question = question[-cutoff_len:]
- answer = answer[-cutoff_len:]
-
- inputs = stokenizer(question, answer,
- return_tensors="pt",
- truncation=True,
- max_length=max_length_tokenize).to(smodel.device)
- try:
- score = torch.sigmoid(smodel(**inputs.to(smodel.device)).logits[0].float()).cpu().detach().numpy()[0]
- except torch.cuda.OutOfMemoryError as e:
- print("GPU OOM 3: question: %s answer: %s exception: %s" % (question, answer, str(e)), flush=True)
- del inputs
- traceback.print_exc()
- clear_torch_cache()
- return 'Response Score: GPU OOM'
- except (Exception, RuntimeError) as e:
- if 'Expected all tensors to be on the same device' in str(e) or \
- 'expected scalar type Half but found Float' in str(e) or \
- 'probability tensor contains either' in str(e) or \
- 'cublasLt ran into an error!' in str(e) or \
- 'device-side assert triggered' in str(e):
- print("GPU Error: question: %s answer: %s exception: %s" % (question, answer, str(e)),
- flush=True)
- traceback.print_exc()
- clear_torch_cache()
- return 'Response Score: GPU Error'
- else:
- raise
- os.environ['TOKENIZERS_PARALLELISM'] = 'true'
- return score
-
-
-def check_locals(**kwargs):
- # ensure everything in evaluate is here
- can_skip_because_locally_generated = no_default_param_names + [
- # get_model:
- 'reward_type'
- ]
- for k in eval_func_param_names:
- if k in can_skip_because_locally_generated:
- continue
- assert k in kwargs, "Missing %s" % k
- for k in inputs_kwargs_list:
- if k in can_skip_because_locally_generated:
- continue
- assert k in kwargs, "Missing %s" % k
-
- for k in list(inspect.signature(get_model).parameters):
- if k in can_skip_because_locally_generated:
- continue
- assert k in kwargs, "Missing %s" % k
-
-
-def get_model_max_length(model_state):
- if not isinstance(model_state['tokenizer'], (str, type(None))):
- return model_state['tokenizer'].model_max_length
- else:
- return 2048
-
-
-def get_max_max_new_tokens(model_state, **kwargs):
- if not isinstance(model_state['tokenizer'], (str, type(None))):
- max_max_new_tokens = model_state['tokenizer'].model_max_length
- else:
- max_max_new_tokens = None
-
- if kwargs['max_max_new_tokens'] is not None and max_max_new_tokens is not None:
- return min(max_max_new_tokens, kwargs['max_max_new_tokens'])
- elif kwargs['max_max_new_tokens'] is not None:
- return kwargs['max_max_new_tokens']
- elif kwargs['memory_restriction_level'] == 1:
- return 768
- elif kwargs['memory_restriction_level'] == 2:
- return 512
- elif kwargs['memory_restriction_level'] >= 3:
- return 256
- else:
- # FIXME: Need to update after new model loaded, so user can control with slider
- return 2048
-
-
-def get_minmax_top_k_docs(is_public):
- if is_public:
- min_top_k_docs = 1
- max_top_k_docs = 8
- label_top_k_docs = "Number of document chunks"
- else:
- min_top_k_docs = -1
- max_top_k_docs = 100
- label_top_k_docs = "Number of document chunks (-1 = auto fill model context)"
- return min_top_k_docs, max_top_k_docs, label_top_k_docs
-
-
-def merge_chat_conversation_history(chat_conversation1, history):
- # chat_conversation and history ordered so largest index of list is most recent
- if chat_conversation1:
- chat_conversation1 = str_to_list(chat_conversation1)
- for conv1 in chat_conversation1:
- assert isinstance(conv1, (list, tuple))
- assert len(conv1) == 2
-
- if isinstance(history, list):
- # make copy so only local change
- if chat_conversation1:
- # so priority will be newest that comes from actual chat history from UI, then chat_conversation
- history = chat_conversation1 + history.copy()
- elif chat_conversation1:
- history = chat_conversation1
- else:
- history = []
- return history
-
-
-def history_to_context(history, langchain_mode=None,
- add_chat_history_to_context=None,
- prompt_type=None, prompt_dict=None, chat=None, model_max_length=None,
- memory_restriction_level=None, keep_sources_in_context=None,
- system_prompt=None, chat_conversation=None):
- """
- consumes all history up to (but not including) latest history item that is presumed to be an [instruction, None] pair
- :param history:
- :param langchain_mode:
- :param add_chat_history_to_context:
- :param prompt_type:
- :param prompt_dict:
- :param chat:
- :param model_max_length:
- :param memory_restriction_level:
- :param keep_sources_in_context:
- :param system_prompt:
- :param chat_conversation:
- :return:
- """
- history = merge_chat_conversation_history(chat_conversation, history)
-
- if len(history) >= 1 and len(history[-1]) >= 2 and not history[-1][1]:
- len_history = len(history) - 1
- else:
- # full history
- len_history = len(history)
-
- # ensure output will be unique to models
- _, _, _, max_prompt_length = get_cutoffs(memory_restriction_level,
- for_context=True, model_max_length=model_max_length)
- context1 = ''
- if max_prompt_length is not None and add_chat_history_to_context:
- context1 = ''
- # - 1 below because current instruction already in history from user()
- for histi in range(0, len_history):
- data_point = dict(instruction=history[histi][0], input='', output=history[histi][1])
- prompt, pre_response, terminate_response, chat_sep, chat_turn_sep = \
- generate_prompt(data_point,
- prompt_type,
- prompt_dict,
- chat,
- reduced=True,
- making_context=True,
- system_prompt=system_prompt,
- histi=histi)
- # md -> back to text, maybe not super important if model trained enough
- if not keep_sources_in_context and langchain_mode != 'Disabled' and prompt.find(super_source_prefix) >= 0:
- # FIXME: This is relatively slow even for small amount of text, like 0.3s each history item
- import re
- prompt = re.sub(f'{re.escape(super_source_prefix)}.*?{re.escape(super_source_postfix)}', '', prompt,
- flags=re.DOTALL)
- if prompt.endswith('\n
'):
- prompt = prompt[:-4]
- prompt = prompt.replace(' ', chat_turn_sep)
- if not prompt.endswith(chat_turn_sep):
- prompt += chat_turn_sep
- # most recent first, add older if can
- # only include desired chat history
- if len(prompt + context1) > max_prompt_length:
- break
- context1 += prompt
-
- _, pre_response, terminate_response, chat_sep, chat_turn_sep = \
- generate_prompt({}, prompt_type, prompt_dict,
- chat, reduced=True,
- making_context=True,
- system_prompt=system_prompt,
- histi=-1)
- if context1 and not context1.endswith(chat_turn_sep):
- context1 += chat_turn_sep # ensure if terminates abruptly, then human continues on next line
- return context1
-
-
-def get_limited_prompt(instruction,
- iinput,
- tokenizer,
- prompter=None,
- inference_server=None,
- prompt_type=None, prompt_dict=None, chat=False, max_new_tokens=None,
- system_prompt='',
- context='', chat_conversation=None, text_context_list=None,
- keep_sources_in_context=False,
- model_max_length=None, memory_restriction_level=0,
- langchain_mode=None, add_chat_history_to_context=True,
- verbose=False,
- doc_importance=0.5,
- min_max_new_tokens=256,
- ):
- if prompter:
- prompt_type = prompter.prompt_type
- prompt_dict = prompter.prompt_dict
- chat = prompter.chat
- stream_output = prompter.stream_output
- system_prompt = prompter.system_prompt
-
- # merge handles if chat_conversation is None
- history = []
- history = merge_chat_conversation_history(chat_conversation, history)
- history_to_context_func = functools.partial(history_to_context,
- langchain_mode=langchain_mode,
- add_chat_history_to_context=add_chat_history_to_context,
- prompt_type=prompt_type,
- prompt_dict=prompt_dict,
- chat=chat,
- model_max_length=model_max_length,
- memory_restriction_level=memory_restriction_level,
- keep_sources_in_context=keep_sources_in_context,
- system_prompt=system_prompt)
- context2 = history_to_context_func(history)
- context1 = context
- if context1 is None:
- context1 = ''
-
- from h2oai_pipeline import H2OTextGenerationPipeline
- data_point_just_instruction = dict(context='', instruction=instruction, input='')
- prompt_just_instruction = prompter.generate_prompt(data_point_just_instruction)
- instruction, num_instruction_tokens = H2OTextGenerationPipeline.limit_prompt(instruction, tokenizer)
- num_instruction_tokens_real = get_token_count(prompt_just_instruction, tokenizer)
- num_instruction_tokens += (num_instruction_tokens_real - num_instruction_tokens)
-
- context1, num_context1_tokens = H2OTextGenerationPipeline.limit_prompt(context1, tokenizer)
- context2, num_context2_tokens = H2OTextGenerationPipeline.limit_prompt(context2, tokenizer)
- iinput, num_iinput_tokens = H2OTextGenerationPipeline.limit_prompt(iinput, tokenizer)
- if text_context_list is None:
- text_context_list = []
- num_doc_tokens = sum([get_token_count(x + '\n\n', tokenizer) for x in text_context_list])
-
- num_prompt_tokens0 = (num_instruction_tokens or 0) + \
- (num_context1_tokens or 0) + \
- (num_context2_tokens or 0) + \
- (num_iinput_tokens or 0) + \
- (num_doc_tokens or 0)
-
- # go down to no less than 256, about 1 paragraph
- # use max_new_tokens before use num_prompt_tokens0 else would be negative or ~0
- min_max_new_tokens = min(min_max_new_tokens, max_new_tokens)
- # by default assume can handle all chat and docs
- chat_index = 0
-
- # allowed residual is either half of what is allowed if doc exceeds half, or is rest of what doc didn't consume
- num_non_doc_tokens = num_prompt_tokens0 - num_doc_tokens
- # to doc first then non-doc, shouldn't matter much either way
- doc_max_length = max(model_max_length - num_non_doc_tokens, doc_importance * model_max_length)
- top_k_docs, one_doc_size, num_doc_tokens = get_docs_tokens(tokenizer, text_context_list=text_context_list,
- max_input_tokens=doc_max_length)
- non_doc_max_length = max(model_max_length - num_doc_tokens, (1.0 - doc_importance) * model_max_length)
-
- if num_non_doc_tokens > non_doc_max_length:
- # need to limit in some way, keep portion of history but all of context and instruction
- # 1) drop iinput (unusual to include anyways)
- # 2) reduce history
- # 3) reduce context1
- # 4) limit instruction so will fit
- diff1 = non_doc_max_length - (
- num_instruction_tokens + num_context1_tokens + num_context2_tokens + min_max_new_tokens)
- diff2 = non_doc_max_length - (num_instruction_tokens + num_context1_tokens + min_max_new_tokens)
- diff3 = non_doc_max_length - (num_instruction_tokens + min_max_new_tokens)
- diff4 = non_doc_max_length - min_max_new_tokens
- if diff1 > 0:
- # then should be able to do #1
- iinput = ''
- num_iinput_tokens = 0
- elif diff2 > 0 > diff1:
- # then may be able to do #1 + #2
- iinput = ''
- num_iinput_tokens = 0
- chat_index_final = len(history)
- for chat_index in range(len(history)):
- # NOTE: history and chat_conversation are older for first entries
- # FIXME: This is a slow for many short conversations
- context2 = history_to_context_func(history[chat_index:])
- num_context2_tokens = get_token_count(context2, tokenizer)
- diff1 = non_doc_max_length - (
- num_instruction_tokens + num_context1_tokens + num_context2_tokens + min_max_new_tokens)
- if diff1 > 0:
- chat_index_final = chat_index
- if verbose:
- print("chat_conversation used %d out of %d" % (chat_index, len(history)), flush=True)
- break
- chat_index = chat_index_final # i.e. if chat_index == len(history), then nothing can be consumed
- elif diff3 > 0 > diff2:
- # then may be able to do #1 + #2 + #3
- iinput = ''
- num_iinput_tokens = 0
- context2 = ''
- num_context2_tokens = 0
- context1, num_context1_tokens = H2OTextGenerationPipeline.limit_prompt(context1, tokenizer,
- max_prompt_length=diff3)
- if num_context1_tokens <= diff3:
- pass
- else:
- print("failed to reduce", flush=True)
- else:
- # then must be able to do #1 + #2 + #3 + #4
- iinput = ''
- num_iinput_tokens = 0
- context2 = ''
- num_context2_tokens = 0
- context1 = ''
- num_context1_tokens = 0
- # diff4 accounts for real prompting for instruction
- # FIXME: history_to_context could include instruction, in case system prompt long, we overcount and could have more free tokens
- instruction, num_instruction_tokens = H2OTextGenerationPipeline.limit_prompt(instruction, tokenizer,
- max_prompt_length=diff4)
- # get actual tokens
- data_point_just_instruction = dict(context='', instruction=instruction, input='')
- prompt_just_instruction = prompter.generate_prompt(data_point_just_instruction)
- num_instruction_tokens_real = get_token_count(prompt_just_instruction, tokenizer)
- num_instruction_tokens += (num_instruction_tokens_real - num_instruction_tokens)
-
- # update full context
- context = context1 + context2
- # update token counts (docs + non-docs, all tokens)
- num_prompt_tokens = (num_instruction_tokens or 0) + \
- (num_context1_tokens or 0) + \
- (num_context2_tokens or 0) + \
- (num_iinput_tokens or 0) + \
- (num_doc_tokens or 0)
-
- # update max_new_tokens
- if inference_server and inference_server.startswith('http'):
- # assume TGI/Gradio setup to consume tokens and have long output too, even if exceeds model capacity.
- pass
- else:
- # limit so max_new_tokens = prompt + new < max
- # otherwise model can fail etc. e.g. for distilgpt2 asking for 1024 tokens is enough to fail if prompt=1 token
- max_new_tokens = min(max_new_tokens, model_max_length - num_prompt_tokens)
-
- if prompter is None:
- # get prompter
- debug = False
- stream_output = False # doesn't matter
- prompter = Prompter(prompt_type, prompt_dict, debug=debug, chat=chat, stream_output=stream_output,
- system_prompt=system_prompt)
-
- data_point = dict(context=context, instruction=instruction, input=iinput)
- # handle promptA/promptB addition if really from history.
- # if not from history, then reduced=False inside correct
- # if mixed, then no specific correct thing to do, so treat like history and promptA/B will come first still
- context_from_history = len(history) > 0 and len(context1) > 0
- prompt = prompter.generate_prompt(data_point, context_from_history=context_from_history)
- num_prompt_tokens_actual = get_token_count(prompt, tokenizer)
-
- return prompt, \
- instruction, iinput, context, \
- num_prompt_tokens, max_new_tokens, num_prompt_tokens0, num_prompt_tokens_actual, \
- chat_index, top_k_docs, one_doc_size
-
-
-def get_docs_tokens(tokenizer, text_context_list=[], max_input_tokens=None):
- if text_context_list is None or len(text_context_list) == 0:
- return 0, None, 0
- if max_input_tokens is None:
- max_input_tokens = tokenizer.model_max_length
- tokens = [get_token_count(x + '\n\n', tokenizer) for x in text_context_list]
- tokens_cumsum = np.cumsum(tokens)
- where_res = np.where(tokens_cumsum < max_input_tokens)[0]
- # if below condition fails, then keep top_k_docs=-1 and trigger special handling next
- if where_res.shape[0] > 0:
- top_k_docs = 1 + where_res[-1]
- one_doc_size = None
- num_doc_tokens = tokens_cumsum[top_k_docs - 1] # by index
- else:
- # if here, means 0 and just do best with 1 doc
- top_k_docs = 1
- text_context_list = text_context_list[:top_k_docs]
- # critical protection
- from src.h2oai_pipeline import H2OTextGenerationPipeline
- doc_content = text_context_list[0]
- doc_content, new_tokens0 = H2OTextGenerationPipeline.limit_prompt(doc_content,
- tokenizer,
- max_prompt_length=max_input_tokens)
- text_context_list[0] = doc_content
- one_doc_size = len(doc_content)
- num_doc_tokens = get_token_count(doc_content + '\n\n', tokenizer)
- print("Unexpected large chunks and can't add to context, will add 1 anyways. Tokens %s -> %s" % (
- tokens[0], new_tokens0), flush=True)
- return top_k_docs, one_doc_size, num_doc_tokens
-
-
-def entrypoint_main():
- """
- Examples:
-
- WORLD_SIZE=4 CUDA_VISIBLE_DEVICES="0,1,2,3" torchrun --nproc_per_node=4 --master_port=1234 generate.py --base_model='EleutherAI/gpt-j-6B' --lora_weights=lora-alpaca_6B
- python generate.py --base_model='EleutherAI/gpt-j-6B' --lora_weights='lora-alpaca_6B'
- python generate.py --base_model='EleutherAI/gpt-neox-20b' --lora_weights='lora-alpaca_20B'
-
- # generate without lora weights, no prompt
- python generate.py --base_model='EleutherAI/gpt-neox-20b' --prompt_type='plain'
- python generate.py --base_model='togethercomputer/GPT-NeoXT-Chat-Base-20B' --prompt_type='dai_faq'
-
- python generate.py --base_model='togethercomputer/GPT-NeoXT-Chat-Base-20B' --prompt_type='dai_faq' --lora_weights='lora_20B_daifaq'
- # OpenChatKit settings:
- python generate.py --base_model='togethercomputer/GPT-NeoXT-Chat-Base-20B' --prompt_type='human_bot --debug=True --num_beams=1 --temperature=0.6 --top_k=40 --top_p=1.0
-
- python generate.py --base_model='distilgpt2' --prompt_type='plain' --debug=True --num_beams=1 --temperature=0.6 --top_k=40 --top_p=1.0 --share=False
- python generate.py --base_model='t5-large' --prompt_type='simple_instruct'
- python generate.py --base_model='philschmid/bart-large-cnn-samsum'
- python generate.py --base_model='philschmid/flan-t5-base-samsum'
- python generate.py --base_model='facebook/mbart-large-50-many-to-many-mmt'
-
- python generate.py --base_model='togethercomputer/GPT-NeoXT-Chat-Base-20B' --prompt_type='human_bot' --lora_weights='GPT-NeoXT-Chat-Base-20B.merged.json.8_epochs.57b2892c53df5b8cefac45f84d019cace803ef26.28'
-
- must have 4*48GB GPU and run without 8bit in order for sharding to work with use_gpu_id=False
- can also pass --prompt_type='human_bot' and model can somewhat handle instructions without being instruct tuned
- python generate.py --base_model=decapoda-research/llama-65b-hf --load_8bit=False --use_gpu_id=False --prompt_type='human_bot'
-
- python generate.py --base_model=h2oai/h2ogpt-oig-oasst1-512-6_9b
- """
- H2O_Fire(main)
-
-
-if __name__ == "__main__":
- entrypoint_main()
diff --git a/spaces/h2oai/wave-tour/examples/tabs.py b/spaces/h2oai/wave-tour/examples/tabs.py
deleted file mode 100644
index 8edfd8f83532c3bfc880b2571fb4038a1770d399..0000000000000000000000000000000000000000
--- a/spaces/h2oai/wave-tour/examples/tabs.py
+++ /dev/null
@@ -1,32 +0,0 @@
-# Form / Tabs
-# Use #tabs within a #form to navigate between two or more distinct content categories.
-# #navigation
-# ---
-from h2o_wave import main, app, Q, ui
-
-tabs = [
- ui.tab(name='email', label='Mail', icon='Mail'),
- ui.tab(name='events', label='Events', icon='Calendar'),
- ui.tab(name='spam', label='Spam'),
-]
-
-
-@app('/demo')
-async def serve(q: Q):
- if q.args.menu:
- q.page['example'].items = [
- ui.tabs(name='menu', value=q.args.menu, items=tabs),
- get_tab_content(q.args.menu),
- ]
- else:
- q.page['example'] = ui.form_card(box='1 1 4 7', items=[
- ui.tabs(name='menu', value='email', items=tabs),
- get_tab_content('email'),
- ])
- await q.page.save()
-
-
-def get_tab_content(category: str):
- # Return a checklist of dummy items.
- items = [f'{category.title()} {i}' for i in range(1, 11)]
- return ui.checklist(name='items', choices=[ui.choice(name=item, label=item) for item in items])
diff --git a/spaces/hackathon-pln-es/clasificador-comentarios-suicidas/app.py b/spaces/hackathon-pln-es/clasificador-comentarios-suicidas/app.py
deleted file mode 100644
index a0967a52069c26b7ef601f8773ced7abc7b22b5b..0000000000000000000000000000000000000000
--- a/spaces/hackathon-pln-es/clasificador-comentarios-suicidas/app.py
+++ /dev/null
@@ -1,33 +0,0 @@
-import gradio as gr
-from datasets import load_dataset
-from transformers import pipeline
-from presentation import main_title, examples
-
-model_name= 'hackathon-pln-es/electricidad-small-discriminator-finetuned-clasificacion-comentarios-suicidas'
-
-def clasificar_comentarios(comentario):
- cls= pipeline("text-classification", model=model_name)
- return cls(comentario)[0]['label']
-
-if __name__ == "__main__":
- gr.Interface(
- fn=clasificar_comentarios,
- inputs=[
- gr.inputs.Textbox(
- lines=10,
- label="Comentario a analizar:",
- placeholder="Ingrese el comentario por favor...",
- optional=False,
- ),
- ],
- outputs=[
- gr.outputs.HTML(
- label="Resultado:"
- )
- ],
- description=main_title,
- examples=examples,
- theme="seafoam",
- thumbnail="None",
- css="https://cdn.jsdelivr.net/npm/bootstrap@3.3.7/dist/css/bootstrap.min.css",
- ).launch()
\ No newline at end of file
diff --git a/spaces/hamelcubsfan/AutoGPT/tests/unit/test_browse_scrape_links.py b/spaces/hamelcubsfan/AutoGPT/tests/unit/test_browse_scrape_links.py
deleted file mode 100644
index 0a3340e7397a997da96b8ab9828954230e1a3c20..0000000000000000000000000000000000000000
--- a/spaces/hamelcubsfan/AutoGPT/tests/unit/test_browse_scrape_links.py
+++ /dev/null
@@ -1,118 +0,0 @@
-# Generated by CodiumAI
-
-# Dependencies:
-# pip install pytest-mock
-import pytest
-
-from autogpt.commands.web_requests import scrape_links
-
-"""
-Code Analysis
-
-Objective:
-The objective of the 'scrape_links' function is to scrape hyperlinks from a
-given URL and return them in a formatted way.
-
-Inputs:
-- url: a string representing the URL to be scraped.
-
-Flow:
-1. Send a GET request to the given URL using the requests library and the user agent header from the config file.
-2. Check if the response contains an HTTP error. If it does, return "error".
-3. Parse the HTML content of the response using the BeautifulSoup library.
-4. Remove any script and style tags from the parsed HTML.
-5. Extract all hyperlinks from the parsed HTML using the 'extract_hyperlinks' function.
-6. Format the extracted hyperlinks using the 'format_hyperlinks' function.
-7. Return the formatted hyperlinks.
-
-Outputs:
-- A list of formatted hyperlinks.
-
-Additional aspects:
-- The function uses the 'requests' and 'BeautifulSoup' libraries to send HTTP
-requests and parse HTML content, respectively.
-- The 'extract_hyperlinks' function is called to extract hyperlinks from the parsed HTML.
-- The 'format_hyperlinks' function is called to format the extracted hyperlinks.
-- The function checks for HTTP errors and returns "error" if any are found.
-"""
-
-
-class TestScrapeLinks:
- # Tests that the function returns a list of formatted hyperlinks when
- # provided with a valid url that returns a webpage with hyperlinks.
- def test_valid_url_with_hyperlinks(self):
- url = "https://www.google.com"
- result = scrape_links(url)
- assert len(result) > 0
- assert isinstance(result, list)
- assert isinstance(result[0], str)
-
- # Tests that the function returns correctly formatted hyperlinks when given a valid url.
- def test_valid_url(self, mocker):
- # Mock the requests.get() function to return a response with sample HTML containing hyperlinks
- mock_response = mocker.Mock()
- mock_response.status_code = 200
- mock_response.text = (
- "
Google"
- )
- mocker.patch("requests.Session.get", return_value=mock_response)
-
- # Call the function with a valid URL
- result = scrape_links("https://www.example.com")
-
- # Assert that the function returns correctly formatted hyperlinks
- assert result == ["Google (https://www.google.com)"]
-
- # Tests that the function returns "error" when given an invalid url.
- def test_invalid_url(self, mocker):
- # Mock the requests.get() function to return an HTTP error response
- mock_response = mocker.Mock()
- mock_response.status_code = 404
- mocker.patch("requests.Session.get", return_value=mock_response)
-
- # Call the function with an invalid URL
- result = scrape_links("https://www.invalidurl.com")
-
- # Assert that the function returns "error"
- assert "Error:" in result
-
- # Tests that the function returns an empty list when the html contains no hyperlinks.
- def test_no_hyperlinks(self, mocker):
- # Mock the requests.get() function to return a response with sample HTML containing no hyperlinks
- mock_response = mocker.Mock()
- mock_response.status_code = 200
- mock_response.text = "
No hyperlinks here
"
- mocker.patch("requests.Session.get", return_value=mock_response)
-
- # Call the function with a URL containing no hyperlinks
- result = scrape_links("https://www.example.com")
-
- # Assert that the function returns an empty list
- assert result == []
-
- # Tests that scrape_links() correctly extracts and formats hyperlinks from
- # a sample HTML containing a few hyperlinks.
- def test_scrape_links_with_few_hyperlinks(self, mocker):
- # Mock the requests.get() function to return a response with a sample HTML containing hyperlinks
- mock_response = mocker.Mock()
- mock_response.status_code = 200
- mock_response.text = """
-
-
-
-
-
- """
- mocker.patch("requests.Session.get", return_value=mock_response)
-
- # Call the function being tested
- result = scrape_links("https://www.example.com")
-
- # Assert that the function returns a list of formatted hyperlinks
- assert isinstance(result, list)
- assert len(result) == 3
- assert result[0] == "Google (https://www.google.com)"
- assert result[1] == "GitHub (https://github.com)"
- assert result[2] == "CodiumAI (https://www.codium.ai)"
diff --git a/spaces/hanjp/White-box-Cartoonization/wbc/guided_filter.py b/spaces/hanjp/White-box-Cartoonization/wbc/guided_filter.py
deleted file mode 100644
index fd019d145efc7f308cd96de90f4e7b648f6820b4..0000000000000000000000000000000000000000
--- a/spaces/hanjp/White-box-Cartoonization/wbc/guided_filter.py
+++ /dev/null
@@ -1,87 +0,0 @@
-import tensorflow as tf
-import numpy as np
-
-
-
-
-def tf_box_filter(x, r):
- k_size = int(2*r+1)
- ch = x.get_shape().as_list()[-1]
- weight = 1/(k_size**2)
- box_kernel = weight*np.ones((k_size, k_size, ch, 1))
- box_kernel = np.array(box_kernel).astype(np.float32)
- output = tf.nn.depthwise_conv2d(x, box_kernel, [1, 1, 1, 1], 'SAME')
- return output
-
-
-
-def guided_filter(x, y, r, eps=1e-2):
-
- x_shape = tf.shape(x)
- #y_shape = tf.shape(y)
-
- N = tf_box_filter(tf.ones((1, x_shape[1], x_shape[2], 1), dtype=x.dtype), r)
-
- mean_x = tf_box_filter(x, r) / N
- mean_y = tf_box_filter(y, r) / N
- cov_xy = tf_box_filter(x * y, r) / N - mean_x * mean_y
- var_x = tf_box_filter(x * x, r) / N - mean_x * mean_x
-
- A = cov_xy / (var_x + eps)
- b = mean_y - A * mean_x
-
- mean_A = tf_box_filter(A, r) / N
- mean_b = tf_box_filter(b, r) / N
-
- output = mean_A * x + mean_b
-
- return output
-
-
-
-def fast_guided_filter(lr_x, lr_y, hr_x, r=1, eps=1e-8):
-
- #assert lr_x.shape.ndims == 4 and lr_y.shape.ndims == 4 and hr_x.shape.ndims == 4
-
- lr_x_shape = tf.shape(lr_x)
- #lr_y_shape = tf.shape(lr_y)
- hr_x_shape = tf.shape(hr_x)
-
- N = tf_box_filter(tf.ones((1, lr_x_shape[1], lr_x_shape[2], 1), dtype=lr_x.dtype), r)
-
- mean_x = tf_box_filter(lr_x, r) / N
- mean_y = tf_box_filter(lr_y, r) / N
- cov_xy = tf_box_filter(lr_x * lr_y, r) / N - mean_x * mean_y
- var_x = tf_box_filter(lr_x * lr_x, r) / N - mean_x * mean_x
-
- A = cov_xy / (var_x + eps)
- b = mean_y - A * mean_x
-
- mean_A = tf.image.resize_images(A, hr_x_shape[1: 3])
- mean_b = tf.image.resize_images(b, hr_x_shape[1: 3])
-
- output = mean_A * hr_x + mean_b
-
- return output
-
-
-if __name__ == '__main__':
- import cv2
- from tqdm import tqdm
-
- input_photo = tf.placeholder(tf.float32, [1, None, None, 3])
- #input_superpixel = tf.placeholder(tf.float32, [16, 256, 256, 3])
- output = guided_filter(input_photo, input_photo, 5, eps=1)
- image = cv2.imread('output_figure1/cartoon2.jpg')
- image = image/127.5 - 1
- image = np.expand_dims(image, axis=0)
-
- config = tf.ConfigProto()
- config.gpu_options.allow_growth = True
- sess = tf.Session(config=config)
- sess.run(tf.global_variables_initializer())
-
- out = sess.run(output, feed_dict={input_photo: image})
- out = (np.squeeze(out)+1)*127.5
- out = np.clip(out, 0, 255).astype(np.uint8)
- cv2.imwrite('output_figure1/cartoon2_filter.jpg', out)
diff --git a/spaces/heiyubili/bingo/src/pages/api/sydney.ts b/spaces/heiyubili/bingo/src/pages/api/sydney.ts
deleted file mode 100644
index a5b99574289f532e6ef7c5e70a6360a556db9643..0000000000000000000000000000000000000000
--- a/spaces/heiyubili/bingo/src/pages/api/sydney.ts
+++ /dev/null
@@ -1,61 +0,0 @@
-import { NextApiRequest, NextApiResponse } from 'next'
-import { WebSocket, debug } from '@/lib/isomorphic'
-import { BingWebBot } from '@/lib/bots/bing'
-import { websocketUtils } from '@/lib/bots/bing/utils'
-import { WatchDog, createHeaders } from '@/lib/utils'
-
-export default async function handler(req: NextApiRequest, res: NextApiResponse) {
- const conversationContext = req.body
- const headers = createHeaders(req.cookies)
- debug(headers)
- res.setHeader('Content-Type', 'text/stream; charset=UTF-8')
-
- const ws = new WebSocket('wss://sydney.bing.com/sydney/ChatHub', {
- headers: {
- ...headers,
- 'accept-language': 'zh-CN,zh;q=0.9',
- 'cache-control': 'no-cache',
- 'x-ms-useragent': 'azsdk-js-api-client-factory/1.0.0-beta.1 core-rest-pipeline/1.10.0 OS/Win32',
- pragma: 'no-cache',
- }
- })
-
- const closeDog = new WatchDog()
- const timeoutDog = new WatchDog()
- ws.onmessage = (event) => {
- timeoutDog.watch(() => {
- ws.send(websocketUtils.packMessage({ type: 6 }))
- }, 1500)
- closeDog.watch(() => {
- ws.close()
- }, 10000)
- res.write(event.data)
- if (/\{"type":([367])\}/.test(String(event.data))) {
- const type = parseInt(RegExp.$1, 10)
- debug('connection type', type)
- if (type === 3) {
- ws.close()
- } else {
- ws.send(websocketUtils.packMessage({ type }))
- }
- }
- }
-
- ws.onclose = () => {
- timeoutDog.reset()
- closeDog.reset()
- debug('connection close')
- res.end()
- }
-
- await new Promise((resolve) => ws.onopen = resolve)
- ws.send(websocketUtils.packMessage({ protocol: 'json', version: 1 }))
- ws.send(websocketUtils.packMessage({ type: 6 }))
- ws.send(websocketUtils.packMessage(BingWebBot.buildChatRequest(conversationContext!)))
- req.socket.once('close', () => {
- ws.close()
- if (!res.closed) {
- res.end()
- }
- })
-}
diff --git a/spaces/helkoo/hackDjellaba/app.py b/spaces/helkoo/hackDjellaba/app.py
deleted file mode 100644
index f6c8336a41987ce27e1d880b5c645993e81ac880..0000000000000000000000000000000000000000
--- a/spaces/helkoo/hackDjellaba/app.py
+++ /dev/null
@@ -1,44 +0,0 @@
-import gradio as gr
-from controlnet_aux import OpenposeDetector
-from PIL import Image
-from diffusers import StableDiffusionControlNetPipeline, ControlNetModel, UniPCMultistepScheduler
-import torch
-from controlnet_aux import OpenposeDetector
-from diffusers.utils import load_image
-
-#Models
-openpose = OpenposeDetector.from_pretrained('lllyasviel/ControlNet')
-controlnet = ControlNetModel.from_pretrained("lllyasviel/sd-controlnet-openpose", torch_dtype=torch.float16)
-pipe = StableDiffusionControlNetPipeline.from_pretrained("helkoo/jelaba_2HR", controlnet=controlnet, safety_checker=None, torch_dtype=torch.float16)
-
-
-#optimizations
-pipe.scheduler = UniPCMultistepScheduler.from_config(pipe.scheduler.config)
-pipe = pipe.to("cpu")
-
-
-import numpy as np
-import requests
-def generate2(prompt,taille):
- if taille == "S":
- image = Image.open(requests.get('https://mode-et-caftan.com/757-large_default/jellaba-salsa-marocaine-femme.jpg', stream=True).raw)
-
- if taille == "XL":
- image = Image.open(requests.get('https://i.pinimg.com/236x/03/f1/36/03f136b83bb37c9f17c3764f1b36f9fa--big-is-beautiful-curvy-fashion.jpg', stream=True).raw)
-
- if taille == "L":
- image = Image.open(requests.get('https://mode-et-caftan.com/757-large_default/jellaba-salsa-marocaine-femme.jpg', stream=True).raw)
-
- # convert image to numpy array
- image = np.array(image)
- image = openpose(image)
- #image = image
- image = pipe(prompt, image, num_inference_steps=20).images[0]
- return image
-
-gr.Interface(fn=generate2, inputs=["text",
- gr.Dropdown(
- ["S", "L", "XL"], label="taille", info="choisie la taille"
- ),
- ], outputs="image").launch(share=False, debug=True)
-
diff --git a/spaces/hlydecker/RA-document-QAchat/streamlit_langchain_chat/customized_langchain/vectorstores/pinecone.py b/spaces/hlydecker/RA-document-QAchat/streamlit_langchain_chat/customized_langchain/vectorstores/pinecone.py
deleted file mode 100644
index c9d8f5eb055b43a29e1241d020c4d96e8508b839..0000000000000000000000000000000000000000
--- a/spaces/hlydecker/RA-document-QAchat/streamlit_langchain_chat/customized_langchain/vectorstores/pinecone.py
+++ /dev/null
@@ -1,79 +0,0 @@
-from langchain.vectorstores.pinecone import *
-from langchain.vectorstores.pinecone import Pinecone as OriginalPinecone
-
-
-class Pinecone(OriginalPinecone):
- @classmethod
- def from_texts(
- cls,
- texts: List[str],
- embedding: Embeddings,
- metadatas: Optional[List[dict]] = None,
- ids: Optional[List[str]] = None,
- batch_size: int = 32,
- text_key: str = "text",
- index_name: Optional[str] = None,
- namespace: Optional[str] = None,
- **kwargs: Any,
- ) -> Pinecone:
- """Construct Pinecone wrapper from raw documents.
-
- This is a user friendly interface that:
- 1. Embeds documents.
- 2. Adds the documents to a provided Pinecone index
-
- This is intended to be a quick way to get started.
-
- Example:
- .. code-block:: python
-
- from langchain import Pinecone
- from langchain.embeddings import OpenAIEmbeddings
- embeddings = OpenAIEmbeddings()
- pinecone = Pinecone.from_texts(
- texts,
- embeddings,
- index_name="langchain-demo"
- )
- """
- try:
- import pinecone
- except ImportError:
- raise ValueError(
- "Could not import pinecone python package. "
- "Please install it with `pip install pinecone-client`."
- )
- _index_name = index_name or str(uuid.uuid4())
- indexes = pinecone.list_indexes() # checks if provided index exists
- if _index_name in indexes:
- index = pinecone.Index(_index_name)
- else:
- index = None
- for i in range(0, len(texts), batch_size):
- # set end position of batch
- i_end = min(i + batch_size, len(texts))
- # get batch of texts and ids
- lines_batch = texts[i:i_end]
- # create ids if not provided
- if ids:
- ids_batch = ids[i:i_end]
- else:
- ids_batch = [str(uuid.uuid4()) for n in range(i, i_end)]
- # create embeddings
- # embeds = embedding.embed_documents(lines_batch)
- embeds = [embedding.embed_documents([line_batch])[0] for line_batch in lines_batch]
- # prep metadata and upsert batch
- if metadatas:
- metadata = metadatas[i:i_end]
- else:
- metadata = [{} for _ in range(i, i_end)]
- for j, line in enumerate(lines_batch):
- metadata[j][text_key] = line
- to_upsert = zip(ids_batch, embeds, metadata)
- # Create index if it does not exist
- if index is None:
- pinecone.create_index(_index_name, dimension=len(embeds[0]))
- index = pinecone.Index(_index_name)
- # upsert to Pinecone
- index.upsert(vectors=list(to_upsert), namespace=namespace)
- return cls(index, embedding.embed_query, text_key, namespace)
diff --git a/spaces/huggingface-projects/InstructPix2Pix-Chatbot-ui/backend/app.py b/spaces/huggingface-projects/InstructPix2Pix-Chatbot-ui/backend/app.py
deleted file mode 100644
index c6a86320d704b202995c7e595ad6c1d7e5f3cec8..0000000000000000000000000000000000000000
--- a/spaces/huggingface-projects/InstructPix2Pix-Chatbot-ui/backend/app.py
+++ /dev/null
@@ -1,12 +0,0 @@
-import uvicorn
-from fastapi import FastAPI
-from fastapi.staticfiles import StaticFiles
-
-app = FastAPI()
-
-app.mount("/", StaticFiles(directory="./build", html=True), name="static")
-
-
-if __name__ == "__main__":
- uvicorn.run(app, host="0.0.0.0", port=8000,
- log_level="debug", reload=False)
diff --git a/spaces/huggingface-projects/color-palette-generator-sd/extract.py b/spaces/huggingface-projects/color-palette-generator-sd/extract.py
deleted file mode 100644
index 5029801905de5650d855e593bd6d8e1fb71accbf..0000000000000000000000000000000000000000
--- a/spaces/huggingface-projects/color-palette-generator-sd/extract.py
+++ /dev/null
@@ -1,31 +0,0 @@
-from colorthief import ColorThief
-from pathlib import Path
-import json
-from PIL import Image
-
-
-images_path = Path('frontend/static/images')
-images = images_path.glob("*.[jpeg jpg png]*")
-print(images)
-data = {}
-for image in images:
- print(image.stem)
- image_pil = Image.open(image)
- color_thief = ColorThief(image)
- image_pil.save(Path.joinpath(images_path, (image.stem + ".jpg")), optimize=True, quality=95)
- prompt = image.stem.split("-")[2]
- try:
- type(data[prompt]) == list
- except:
- data[prompt] = []
-
- colors = color_thief.get_palette(color_count=5, quality=1)
- colors_hex = ['#%02x%02x%02x' % (color) for color in colors]
- data[prompt].append({
- "colors": colors_hex,
- "imgURL": "static/images/" + image.stem + ".jpg"
- })
-prompts = [{"prompt": prompt, "images": values}
- for (prompt, values) in data.items()]
-with open('frontend/static/data.json', 'w') as f:
- json.dump(prompts, f)
diff --git a/spaces/huggingface-projects/repo_duplicator/README.md b/spaces/huggingface-projects/repo_duplicator/README.md
deleted file mode 100644
index cc4ad630ba5b6fd588f59bacb9e8ff5ef4051c13..0000000000000000000000000000000000000000
--- a/spaces/huggingface-projects/repo_duplicator/README.md
+++ /dev/null
@@ -1,13 +0,0 @@
----
-title: Repo_duplicator
-emoji: 😻
-colorFrom: gray
-colorTo: blue
-sdk: gradio
-sdk_version: 3.12.0
-app_file: app.py
-pinned: false
-license: mit
----
-
-Check out the configuration reference at https://huggingface.co/docs/hub/spaces#reference
diff --git a/spaces/hysts/SD-XL/style.css b/spaces/hysts/SD-XL/style.css
deleted file mode 100644
index 86ce68e49778375ebf5b12dc3baaccf931570b54..0000000000000000000000000000000000000000
--- a/spaces/hysts/SD-XL/style.css
+++ /dev/null
@@ -1,16 +0,0 @@
-h1 {
- text-align: center;
-}
-
-#duplicate-button {
- margin: auto;
- color: #fff;
- background: #1565c0;
- border-radius: 100vh;
-}
-
-#component-0 {
- max-width: 730px;
- margin: auto;
- padding-top: 1.5rem;
-}
diff --git a/spaces/hysts/Shap-E/app.py b/spaces/hysts/Shap-E/app.py
deleted file mode 100644
index f9ef78fe4bd364fc1fefe89214e615005ed19905..0000000000000000000000000000000000000000
--- a/spaces/hysts/Shap-E/app.py
+++ /dev/null
@@ -1,33 +0,0 @@
-#!/usr/bin/env python
-
-import os
-
-import gradio as gr
-import torch
-
-from app_image_to_3d import create_demo as create_demo_image_to_3d
-from app_text_to_3d import create_demo as create_demo_text_to_3d
-from model import Model
-
-DESCRIPTION = "# [Shap-E](https://github.com/openai/shap-e)"
-
-if not torch.cuda.is_available():
- DESCRIPTION += "\n
Running on CPU 🥶 This demo does not work on CPU.
"
-
-model = Model()
-
-with gr.Blocks(css="style.css") as demo:
- gr.Markdown(DESCRIPTION)
- gr.DuplicateButton(
- value="Duplicate Space for private use",
- elem_id="duplicate-button",
- visible=os.getenv("SHOW_DUPLICATE_BUTTON") == "1",
- )
- with gr.Tabs():
- with gr.Tab(label="Text to 3D"):
- create_demo_text_to_3d(model)
- with gr.Tab(label="Image to 3D"):
- create_demo_image_to_3d(model)
-
-if __name__ == "__main__":
- demo.queue(max_size=10).launch()
diff --git a/spaces/inamXcontru/PoeticTTS/13-Curso Multilenguaje Muzzy De La BBC Vocabulario1 Free Download.md b/spaces/inamXcontru/PoeticTTS/13-Curso Multilenguaje Muzzy De La BBC Vocabulario1 Free Download.md
deleted file mode 100644
index 51c87283848b15561bcbb1088d57a5a21e73306c..0000000000000000000000000000000000000000
--- a/spaces/inamXcontru/PoeticTTS/13-Curso Multilenguaje Muzzy De La BBC Vocabulario1 Free Download.md
+++ /dev/null
@@ -1,26 +0,0 @@
-
-
Learn Languages with Muzzy BBC: A Review of the 13-Curso Multilenguaje Muzzy De La BBC Vocabulario1
-
Muzzy BBC is a popular language learning program for children and adults that uses animated videos, games, songs and worksheets to teach various languages. The program was developed by the British Broadcasting Corporation (BBC) and has been used by millions of learners around the world.
-
One of the courses offered by Muzzy BBC is the 13-Curso Multilenguaje Muzzy De La BBC Vocabulario1, which is a multilingual course that covers vocabulary topics such as numbers, colors, animals, food, family, clothes and more. The course includes 13 lessons in Spanish, English, French, German, Italian and Portuguese, as well as a bonus lesson in Mandarin Chinese.
-
13-Curso Multilenguaje Muzzy De La BBC Vocabulario1 Free Download
The course is designed to be fun and engaging for learners of all ages and levels. The videos feature Muzzy, a friendly green monster who loves to eat words, and his friends who go on adventures in different countries and cultures. The games and songs reinforce the vocabulary and grammar learned in the videos, while the worksheets provide additional practice and review.
-
The best part is that you can download the 13-Curso Multilenguaje Muzzy De La BBC Vocabulario1 for free from various online sources[^1^] [^2^] [^3^] [^4^]. This way, you can enjoy the benefits of learning languages with Muzzy BBC without spending any money. You can also access the course online or on your mobile devices.
-
If you are looking for a fun and effective way to learn languages with your children or by yourself, you should definitely check out the 13-Curso Multilenguaje Muzzy De La BBC Vocabulario1. It will help you expand your vocabulary, improve your pronunciation, and boost your confidence in speaking different languages.
-
-
What are the benefits of learning languages with Muzzy BBC? According to the Muzzy BBC website, learning languages with Muzzy BBC can help children develop cognitive, social and emotional skills that will benefit them throughout their lives. Some of these benefits include:
-
-
Enhanced brain development and memory
-
Improved academic performance and test scores
-
Increased creativity and problem-solving abilities
-
Better communication and interpersonal skills
-
Greater cultural awareness and appreciation
-
More opportunities for travel, work and study in the future
-
-
What do other customers say about Muzzy BBC? Muzzy BBC has received mixed reviews from customers who have used the program. Some customers praise Muzzy BBC for being fun, engaging, easy to use and effective in teaching languages to children. They also appreciate the variety of languages, activities and materials that Muzzy BBC offers. Some examples of positive reviews are:
-
"Muzzy is simply the best language program for young learners. Its unique approach to teaching a new language, specifically by using colorful visuals and entertaining sounds, is appealing to youngsters. A playful atmosphere makes learning feel like less of a task and more of an enjoyable experience."[^1^]
-
"My kids (K and preschool) really liked the videos and absorbed a lot from watching. However, after multiple viewings (which is encouraged by the program a how children learn) they eventually got bored with them."[^4^]
-
However, some customers complain about Muzzy BBC for being outdated, expensive, repetitive and boring. They also report issues with the app, website, customer service and billing. Some examples of negative reviews are:
-
"The app is terrible. It doesn't work half the time. The videos are old and grainy. The games are boring and glitchy. The customer service is nonexistent. I tried to cancel my subscription but they kept charging me. I had to dispute it with my bank. Don't waste your money on this scam."[^2^]
-
"I was very disappointed with this product. It is very outdated and not engaging at all for my kids. The videos are too long and too slow. The games are too easy and too repetitive. The songs are too cheesy and too annoying. The printables are too basic and too bland. I wish I could get a refund."[^3^]
d5da3c52bf
-
-
\ No newline at end of file
diff --git a/spaces/inplisQlawa/anything-midjourney-v4-1/ADOBE FLASH PROFESSIONAL CS5.5 [thethingy] [BEST].md b/spaces/inplisQlawa/anything-midjourney-v4-1/ADOBE FLASH PROFESSIONAL CS5.5 [thethingy] [BEST].md
deleted file mode 100644
index 9cc61c9f0dfc3ba02c33bae0e4f27d53225898fe..0000000000000000000000000000000000000000
--- a/spaces/inplisQlawa/anything-midjourney-v4-1/ADOBE FLASH PROFESSIONAL CS5.5 [thethingy] [BEST].md
+++ /dev/null
@@ -1,6 +0,0 @@
-
-
-0 για το adobe flash cs5 professional (+cd-rom για windows και mac). ... 5 (latest) see all create web designs and online experiences complete with interactive ... flash cs5 professional [thethingy] crack 7042 adobe flash professional 8 serial ... 1fdad05405
-
-
-
diff --git a/spaces/inplisQlawa/anything-midjourney-v4-1/Adobe Illustrator CS6 18.2.9 (32-64 Bit) Utorrent HOT!.md b/spaces/inplisQlawa/anything-midjourney-v4-1/Adobe Illustrator CS6 18.2.9 (32-64 Bit) Utorrent HOT!.md
deleted file mode 100644
index f28f1e47bc8b733014e3b207c89c4d8eefd5c670..0000000000000000000000000000000000000000
--- a/spaces/inplisQlawa/anything-midjourney-v4-1/Adobe Illustrator CS6 18.2.9 (32-64 Bit) Utorrent HOT!.md
+++ /dev/null
@@ -1,5 +0,0 @@
-
-
Iomacej9 [url= melsAtterve [url= melsAtterve [url= sesspaphpag [url= melsAtterve [url= melsAtterve [url= aafebosunmass [url= Proxypress Cracked 2020 Crack [url= tartsdhanoj singh 2019 karwaan-mp4-720p-download-free.jpg] [url= tartsdhanoj singh 2019 karwaan-mp4-720p-download-free.jpg] [url= Evermotion 3.5.51 Win Full Crack [url= aafebosunmass [url= kurtosand ganst rock [url= jordynsarwoodoldspoker 2010 index [url= evermotion [url= tutsdownload [url= tutsdownload [url= Taiseertaids [url= aafebosunmass [url= aafebosunmass [url= melsAtterve [url= aafebosunmass [url= aafebosunmass [url= sesspaphpag [url= Ebook Lincoln David Herbert Donald For 14 Free Rar [epub] Utorrent] Ebook Lincoln David Herbert Donald For 14 Free Rar [epub] Utorrent[/url]
-
-japanese incest japanese hentai
-
-Teenie choking her to satisfaction! She masturbates his dick in her mouth and she is not able to breath. naughtyhdporn lesbian porn images
-
-Even when they have a vacation in a rented house. kikki and jennifer anal
-
-Youthful ladies have a great time in sexual roleplays. kikki and jennifer anal
-
-Kinky girl munches the huge cock of her boyfriend and plays with her wet pussy.
-
-stevens brine and karleys girls porn
-
-sex in public yurizano xxx
-
-Naughty teen Tasha Tate gets a nice cock and gives a wonderful blowjob.
-
-peacock-milf-sex.com
-
-Teenie gets fucked by her lover and his cock is hard.
-
-she is riding the cock in her pussy.
-
-She has a perfect ass and a nice pussy.
-
-she had sex with her uncle and she feels terrible.
-
-she loves the smell of freshly cut grass.
-
-Zoey swallows a huge cock in this video.
-
-The adventures of a young lass in a distant land
-
-She is banging with a younger man and rides his cock until she climaxes.
-
-teenloli clit lips teen pussy pic
-
-Javascript is turned off in your browser.
-
-Horny pussy, sex toys, and big tits in this video.
-
-She is fucking her boyfriend and rides his cock until she cums.
-
-Innocent girl playing with herself and her friend and everything turns out really well for them.
-
-Girl tries to help her friend but she is caught in the act.
-
-Horny pussy, sex toys, and big tits in this video. 4fefd39f24
-
-
-
diff --git a/spaces/inplisQlawa/anything-midjourney-v4-1/Descargar Crack Para Yukkuri Panic Escalation EXCLUSIVE.md b/spaces/inplisQlawa/anything-midjourney-v4-1/Descargar Crack Para Yukkuri Panic Escalation EXCLUSIVE.md
deleted file mode 100644
index 77ff8a03063ba2ed8256b39429805dab076ed251..0000000000000000000000000000000000000000
--- a/spaces/inplisQlawa/anything-midjourney-v4-1/Descargar Crack Para Yukkuri Panic Escalation EXCLUSIVE.md
+++ /dev/null
@@ -1,6 +0,0 @@
-
-
-))
-AccordionContent.displayName = AccordionPrimitive.Content.displayName
-
-export { Accordion, AccordionItem, AccordionTrigger, AccordionContent }
diff --git a/spaces/jbilcke-hf/VideoQuest/scripts/test.js b/spaces/jbilcke-hf/VideoQuest/scripts/test.js
deleted file mode 100644
index 67a07d8e5fdac589d574c227500bf8a08b23c92b..0000000000000000000000000000000000000000
--- a/spaces/jbilcke-hf/VideoQuest/scripts/test.js
+++ /dev/null
@@ -1,23 +0,0 @@
-const { promises: fs } = require("node:fs")
-
-const main = async () => {
- console.log('generating shot..')
- const response = await fetch("http://localhost:3000/api/shot", {
- method: "POST",
- headers: {
- "Accept": "application/json",
- "Content-Type": "application/json"
- },
- body: JSON.stringify({
- token: process.env.VC_SECRET_ACCESS_TOKEN,
- shotPrompt: "video of a dancing cat"
- })
- });
-
- console.log('response:', response)
- const buffer = await response.buffer()
-
- fs.writeFile(`./test-juju.mp4`, buffer)
-}
-
-main()
\ No newline at end of file
diff --git a/spaces/jbilcke-hf/ai-comic-factory/src/lib/dirtyLLMResponseCleaner.ts b/spaces/jbilcke-hf/ai-comic-factory/src/lib/dirtyLLMResponseCleaner.ts
deleted file mode 100644
index 3af9831b7cb5d17db688dd06231118d45c4b04ee..0000000000000000000000000000000000000000
--- a/spaces/jbilcke-hf/ai-comic-factory/src/lib/dirtyLLMResponseCleaner.ts
+++ /dev/null
@@ -1,54 +0,0 @@
-export function dirtyLLMResponseCleaner(input: string) {
- let str = (
- `${input || ""}`
- // a summary of all the weird hallucinations I saw it make..
- .replaceAll(`"]`, `"}]`)
- .replaceAll(`" ]`, `"}]`)
- .replaceAll(`" ]`, `"}]`)
- .replaceAll(`"\n]`, `"}]`)
- .replaceAll(`"\n ]`, `"}]`)
- .replaceAll(`"\n ]`, `"}]`)
- .replaceAll("}}", "}")
- .replaceAll("]]", "]")
- .replaceAll("[[", "[")
- .replaceAll("{{", "{")
- .replaceAll(",,", ",")
- .replaceAll("[0]", "")
- .replaceAll("[1]", "")
- .replaceAll("[2]", "")
- .replaceAll("[3]", "")
- .replaceAll("[4]", "")
- .replaceAll("[5]", "")
- .replaceAll("[6]", "")
- .replaceAll("[7]", "")
- .replaceAll("[8]", "")
- .replaceAll("[panel 0]", "")
- .replaceAll("[panel 1]", "")
- .replaceAll("[panel 2]", "")
- .replaceAll("[panel 3]", "")
- .replaceAll("[panel 4]", "")
- .replaceAll("[panel 5]", "")
- .replaceAll("[panel 6]", "")
- .replaceAll("[panel 7]", "")
- .replaceAll("[panel 8]", "")
- )
-
- // repair missing end of JSON array
- if (str.at(-1) === '}') {
- str = str + "]"
- }
-
- if (str.at(-1) === '"') {
- str = str + "}]"
- }
-
- if (str[0] === '{') {
- str = "[" + str
- }
-
- if (str[0] === '"') {
- str = "[{" + str
- }
-
- return str
-}
\ No newline at end of file
diff --git a/spaces/jbilcke-hf/ai-comic-factory/src/lib/replaceWhiteWithTransparent.ts b/spaces/jbilcke-hf/ai-comic-factory/src/lib/replaceWhiteWithTransparent.ts
deleted file mode 100644
index cee490fc1a0b19b2192ce86d6c8f9867a3a6a6d9..0000000000000000000000000000000000000000
--- a/spaces/jbilcke-hf/ai-comic-factory/src/lib/replaceWhiteWithTransparent.ts
+++ /dev/null
@@ -1,37 +0,0 @@
-export function replaceWhiteWithTransparent(imageBase64: string): Promise {
- return new Promise((resolve, reject) => {
- const img = new Image();
- img.onload = () => {
- const canvas = document.createElement('canvas');
- canvas.width = img.width;
- canvas.height = img.height;
-
- const ctx = canvas.getContext('2d');
- if (!ctx) {
- reject('Unable to get canvas 2D context');
- return;
- }
-
- ctx.drawImage(img, 0, 0);
-
- const imageData = ctx.getImageData(0, 0, canvas.width, canvas.height);
- const data = imageData.data;
-
- for (let i = 0; i < data.length; i += 4) {
- if (data[i] === 255 && data[i + 1] === 255 && data[i + 2] === 255) {
- data[i + 3] = 0;
- }
- }
-
- ctx.putImageData(imageData, 0, 0);
-
- resolve(canvas.toDataURL());
- };
-
- img.onerror = (err) => {
- reject(err);
- };
-
- img.src = imageBase64;
- });
-}
\ No newline at end of file
diff --git a/spaces/jeffrymahbuubi/bert-advanced-cnn-hate-speech-classification/app.py b/spaces/jeffrymahbuubi/bert-advanced-cnn-hate-speech-classification/app.py
deleted file mode 100644
index 66ce0e4d0d7bef4a28d856bb57f667567f9c44d6..0000000000000000000000000000000000000000
--- a/spaces/jeffrymahbuubi/bert-advanced-cnn-hate-speech-classification/app.py
+++ /dev/null
@@ -1,155 +0,0 @@
-
-from transformers import BertTokenizer
-import tensorflow as tf
-from model import create_bert_model
-import gradio as gr
-from typing import Tuple, Dict
-import time
-
-# Load the model
-model = create_bert_model("bert_hate_speech.h5")
-
-# Load the BERT tokenizer.
-tokenizer = BertTokenizer.from_pretrained('bert-base-uncased', do_lower_case=True)
-
-# Fetch examples from examples folder
-def get_examples():
- folder_path = "examples/"
- file_paths = [f"{folder_path}example_1.txt", f"{folder_path}example_2.txt", f"{folder_path}example_3.txt"]
-
- examples = []
-
- for file_path in file_paths:
- with open(file_path, "r") as file:
- content = file.read()
- examples.append(content)
-
- return examples
-
-def encode_sentence(sentence):
- """Encode a sentence using the BERT tokenizer."""
- encoded = tokenizer.encode_plus(
- text=sentence,
- add_special_tokens=True,
- max_length=512,
- padding='max_length',
- return_attention_mask=True,
- return_tensors='tf',
- truncation=True,
- )
- return encoded["input_ids"], encoded["attention_mask"], encoded["token_type_ids"]
-
-folder_path = "examples/"
-file_paths = [f"{folder_path}example_1.txt", f"{folder_path}example_2.txt", f"{folder_path}example_3.txt"]
-
-examples = []
-
-for file_path in file_paths:
- with open(file_path, "r") as file:
- content = file.read()
- examples.append(content)
-
-def run_prediction():
- def prediction(sentence:str) -> Tuple[Dict[str, float], float]:
- # Start a to,er
- start_time = time.time()
-
- # Transform the input sentence for use with BERT
- inputs = encode_sentence(sentence)
-
- # Ensure prediction using CPU
- with tf.device('/cpu:0'):
- # Make the prediction
- pred_prob = model.predict(inputs)
-
- pred_prob = tf.squeeze(pred_prob)
-
- labels = ['Hate speech', 'Offensive', 'Neither']
-
- # Create a prediction label and prediction probability dictionary
- pred_labels_and_probs = {labels[i]: float(pred_prob[i]) for i in range(len(labels))}
-
- # Calculate pred time
- end_time = time.time()
- pred_time = round(end_time - start_time, 4)
-
- return pred_labels_and_probs, pred_time
-
-
- with gr.Blocks() as demo:
- with gr.Column(elem_id="classification_container"):
- with gr.Row():
- outputs = [gr.Label(num_top_classes=3, label="Predictions"),
- gr.Number(label="Prediction Time (Seconds)")]
- with gr.Row():
- inputs = gr.Textbox(placeholder="Write hate speech here...", label="Type an input and press Enter", max_lines=1)
-
- with gr.Row(elem_id="button_container"):
- with gr.Column():
- clear_button = gr.Button("✨ Clear")
- with gr.Column():
- submit_button = gr.Button("⌨️ Submit")
-
- gr.Examples(get_examples(), inputs=inputs, label="Click on any example and press Enter in the input textbox!")
-
- submit_button.click(fn=prediction,
- inputs=inputs,
- outputs=outputs)
- inputs.submit(fn=prediction,
- inputs=inputs,
- outputs=outputs)
-
- clear_button.click(lambda x: gr.update(value=''), [],[inputs])
-
-def get_demo():
- markdown = """
- # BERT + Advanced 5-layer CNN for Hate Speech Classification
-
- Bert Hate Speech Classification is a project that aims to classify hate speech from [Davidson Dataset](https://github.com/t-davidson/hate-speech-and-offensive-language). The project is built using BERT and adding Advanced 5-Layer CNN to improve the performance of the model.
-
- This project was the final class project for the Data Mining course offered by National Cheng Kung University and taught by Professor [Eric Hsueh-Chan Lu (呂學展)](https://www.geomatics.ncku.edu.tw/laboratory.php?tpl=19)
-
- ## Dataset
-
- The Davidson Dataset consist of three different labels, which are: Hate Speech (0), Offensive Language (1), and Neither (2). The dataset is unbalanced, with the majority of the data is labeled as Offensive Language. The dataset is also noisy, with some of the data is mislabeled. The maximum word length of the dataset is 87 words.
-
- ## Contributors
-
- | Name | Role | The Worked Distribution | Deployment |
- | ---------------------- | --------------- | ----------------------- | -------------------------------------------------------- |
- | Cendra Deyana Putra | Model Developer | `Model Builder` | [@data_mining/cendra](https://github.com/Cendra123) |
- | Aunuun Jeffry Mahbuubi | Model Deployer | `Model Deployer` | [@data_mining/jeffry](https://github.com/jeffrymahbuubi) |
- """
-
- with gr.Blocks(
- css="""
- #banner-image {
- display:block;
- margin-left:auto;
- margin-right:auto;
- width:50%
- }
- #title {
- font-size:1.5em;
- text-align:center;
- font-weight:bold;
- margin-bottom:0.5em;
- }
- """
- ) as demo:
- gr.HTML("😠💢😧 Hate Speech Classification", elem_id="title")
- with gr.Row():
- with gr.Column():
- gr.Image("bert-classification-transformed.png",
- elem_id="banner-image",
- show_label=False,
- shape=[100,100])
- gr.Markdown(f"{markdown}")
- with gr.Column():
- run_prediction()
-
- return demo
-
-if __name__ == "__main__":
- demo = get_demo()
- demo.launch()
diff --git a/spaces/jmcob/Transformers-StoryWriting/README.md b/spaces/jmcob/Transformers-StoryWriting/README.md
deleted file mode 100644
index 4309cfbab5279e7ccb837680f5e4ca278772a6eb..0000000000000000000000000000000000000000
--- a/spaces/jmcob/Transformers-StoryWriting/README.md
+++ /dev/null
@@ -1,13 +0,0 @@
----
-title: Transformers StoryWriting
-emoji: 🚀
-colorFrom: purple
-colorTo: pink
-sdk: gradio
-sdk_version: 3.0.17
-app_file: app.py
-pinned: false
-license: mit
----
-
-Check out the configuration reference at https://huggingface.co/docs/hub/spaces-config-reference
diff --git a/spaces/jmyungjoon/cartoon/pretrained_model/download_pth.sh b/spaces/jmyungjoon/cartoon/pretrained_model/download_pth.sh
deleted file mode 100644
index 5876d8a7f2c5ddd10a9893b077be98819b6b992c..0000000000000000000000000000000000000000
--- a/spaces/jmyungjoon/cartoon/pretrained_model/download_pth.sh
+++ /dev/null
@@ -1,8 +0,0 @@
-cd pretrained_model
-
-wget -c http://vllab1.ucmerced.edu/~yli62/CartoonGAN/pytorch_pth/Hayao_net_G_float.pth
-wget -c http://vllab1.ucmerced.edu/~yli62/CartoonGAN/pytorch_pth/Hosoda_net_G_float.pth
-wget -c http://vllab1.ucmerced.edu/~yli62/CartoonGAN/pytorch_pth/Paprika_net_G_float.pth
-wget -c http://vllab1.ucmerced.edu/~yli62/CartoonGAN/pytorch_pth/Shinkai_net_G_float.pth
-
-cd ..
diff --git a/spaces/joaopereirajp/livvieChatBot/venv/lib/python3.9/site-packages/altair/jupyter/js/README.md b/spaces/joaopereirajp/livvieChatBot/venv/lib/python3.9/site-packages/altair/jupyter/js/README.md
deleted file mode 100644
index f1ec545894f60fea2a2096b4ac4b588c890b5192..0000000000000000000000000000000000000000
--- a/spaces/joaopereirajp/livvieChatBot/venv/lib/python3.9/site-packages/altair/jupyter/js/README.md
+++ /dev/null
@@ -1,2 +0,0 @@
-# JupyterChart
-This directory contains the JavaScript portion of the Altair `JupyterChart`. The `JupyterChart` is based on the [AnyWidget](https://anywidget.dev/) project.
diff --git a/spaces/joaopereirajp/livvieChatBot/venv/lib/python3.9/site-packages/filelock/_api.py b/spaces/joaopereirajp/livvieChatBot/venv/lib/python3.9/site-packages/filelock/_api.py
deleted file mode 100644
index 8a40ccd0efb23ba621292d00f3fbbcd6d0ae5e93..0000000000000000000000000000000000000000
--- a/spaces/joaopereirajp/livvieChatBot/venv/lib/python3.9/site-packages/filelock/_api.py
+++ /dev/null
@@ -1,288 +0,0 @@
-from __future__ import annotations
-
-import contextlib
-import logging
-import os
-import time
-import warnings
-from abc import ABC, abstractmethod
-from dataclasses import dataclass
-from threading import local
-from typing import TYPE_CHECKING, Any
-
-from ._error import Timeout
-
-if TYPE_CHECKING:
- import sys
- from types import TracebackType
-
- if sys.version_info >= (3, 11): # pragma: no cover (py311+)
- from typing import Self
- else: # pragma: no cover ( None:
- self.lock = lock
-
- def __enter__(self) -> BaseFileLock:
- return self.lock
-
- def __exit__(
- self,
- exc_type: type[BaseException] | None,
- exc_value: BaseException | None,
- traceback: TracebackType | None,
- ) -> None:
- self.lock.release()
-
-
-@dataclass
-class FileLockContext:
- """A dataclass which holds the context for a ``BaseFileLock`` object."""
-
- # The context is held in a separate class to allow optional use of thread local storage via the
- # ThreadLocalFileContext class.
-
- #: The path to the lock file.
- lock_file: str
-
- #: The default timeout value.
- timeout: float
-
- #: The mode for the lock files
- mode: int
-
- #: The file descriptor for the *_lock_file* as it is returned by the os.open() function, not None when lock held
- lock_file_fd: int | None = None
-
- #: The lock counter is used for implementing the nested locking mechanism.
- lock_counter: int = 0 # When the lock is acquired is increased and the lock is only released, when this value is 0
-
-
-class ThreadLocalFileContext(FileLockContext, local):
- """A thread local version of the ``FileLockContext`` class."""
-
-
-class BaseFileLock(ABC, contextlib.ContextDecorator):
- """Abstract base class for a file lock object."""
-
- def __init__(
- self,
- lock_file: str | os.PathLike[str],
- timeout: float = -1,
- mode: int = 0o644,
- thread_local: bool = True, # noqa: FBT001, FBT002
- ) -> None:
- """
- Create a new lock object.
-
- :param lock_file: path to the file
- :param timeout: default timeout when acquiring the lock, in seconds. It will be used as fallback value in
- the acquire method, if no timeout value (``None``) is given. If you want to disable the timeout, set it
- to a negative value. A timeout of 0 means, that there is exactly one attempt to acquire the file lock.
- :param mode: file permissions for the lockfile.
- :param thread_local: Whether this object's internal context should be thread local or not.
- If this is set to ``False`` then the lock will be reentrant across threads.
- """
- self._is_thread_local = thread_local
-
- # Create the context. Note that external code should not work with the context directly and should instead use
- # properties of this class.
- kwargs: dict[str, Any] = {
- "lock_file": os.fspath(lock_file),
- "timeout": timeout,
- "mode": mode,
- }
- self._context: FileLockContext = (ThreadLocalFileContext if thread_local else FileLockContext)(**kwargs)
-
- def is_thread_local(self) -> bool:
- """:return: a flag indicating if this lock is thread local or not"""
- return self._is_thread_local
-
- @property
- def lock_file(self) -> str:
- """:return: path to the lock file"""
- return self._context.lock_file
-
- @property
- def timeout(self) -> float:
- """
- :return: the default timeout value, in seconds
-
- .. versionadded:: 2.0.0
- """
- return self._context.timeout
-
- @timeout.setter
- def timeout(self, value: float | str) -> None:
- """
- Change the default timeout value.
-
- :param value: the new value, in seconds
- """
- self._context.timeout = float(value)
-
- @abstractmethod
- def _acquire(self) -> None:
- """If the file lock could be acquired, self._context.lock_file_fd holds the file descriptor of the lock file."""
- raise NotImplementedError
-
- @abstractmethod
- def _release(self) -> None:
- """Releases the lock and sets self._context.lock_file_fd to None."""
- raise NotImplementedError
-
- @property
- def is_locked(self) -> bool:
- """
-
- :return: A boolean indicating if the lock file is holding the lock currently.
-
- .. versionchanged:: 2.0.0
-
- This was previously a method and is now a property.
- """
- return self._context.lock_file_fd is not None
-
- @property
- def lock_counter(self) -> int:
- """:return: The number of times this lock has been acquired (but not yet released)."""
- return self._context.lock_counter
-
- def acquire(
- self,
- timeout: float | None = None,
- poll_interval: float = 0.05,
- *,
- poll_intervall: float | None = None,
- blocking: bool = True,
- ) -> AcquireReturnProxy:
- """
- Try to acquire the file lock.
-
- :param timeout: maximum wait time for acquiring the lock, ``None`` means use the default :attr:`~timeout` is and
- if ``timeout < 0``, there is no timeout and this method will block until the lock could be acquired
- :param poll_interval: interval of trying to acquire the lock file
- :param poll_intervall: deprecated, kept for backwards compatibility, use ``poll_interval`` instead
- :param blocking: defaults to True. If False, function will return immediately if it cannot obtain a lock on the
- first attempt. Otherwise, this method will block until the timeout expires or the lock is acquired.
- :raises Timeout: if fails to acquire lock within the timeout period
- :return: a context object that will unlock the file when the context is exited
-
- .. code-block:: python
-
- # You can use this method in the context manager (recommended)
- with lock.acquire():
- pass
-
- # Or use an equivalent try-finally construct:
- lock.acquire()
- try:
- pass
- finally:
- lock.release()
-
- .. versionchanged:: 2.0.0
-
- This method returns now a *proxy* object instead of *self*,
- so that it can be used in a with statement without side effects.
-
- """
- # Use the default timeout, if no timeout is provided.
- if timeout is None:
- timeout = self._context.timeout
-
- if poll_intervall is not None:
- msg = "use poll_interval instead of poll_intervall"
- warnings.warn(msg, DeprecationWarning, stacklevel=2)
- poll_interval = poll_intervall
-
- # Increment the number right at the beginning. We can still undo it, if something fails.
- self._context.lock_counter += 1
-
- lock_id = id(self)
- lock_filename = self.lock_file
- start_time = time.perf_counter()
- try:
- while True:
- if not self.is_locked:
- _LOGGER.debug("Attempting to acquire lock %s on %s", lock_id, lock_filename)
- self._acquire()
- if self.is_locked:
- _LOGGER.debug("Lock %s acquired on %s", lock_id, lock_filename)
- break
- if blocking is False:
- _LOGGER.debug("Failed to immediately acquire lock %s on %s", lock_id, lock_filename)
- raise Timeout(lock_filename) # noqa: TRY301
- if 0 <= timeout < time.perf_counter() - start_time:
- _LOGGER.debug("Timeout on acquiring lock %s on %s", lock_id, lock_filename)
- raise Timeout(lock_filename) # noqa: TRY301
- msg = "Lock %s not acquired on %s, waiting %s seconds ..."
- _LOGGER.debug(msg, lock_id, lock_filename, poll_interval)
- time.sleep(poll_interval)
- except BaseException: # Something did go wrong, so decrement the counter.
- self._context.lock_counter = max(0, self._context.lock_counter - 1)
- raise
- return AcquireReturnProxy(lock=self)
-
- def release(self, force: bool = False) -> None: # noqa: FBT001, FBT002
- """
- Releases the file lock. Please note, that the lock is only completely released, if the lock counter is 0. Also
- note, that the lock file itself is not automatically deleted.
-
- :param force: If true, the lock counter is ignored and the lock is released in every case/
- """
- if self.is_locked:
- self._context.lock_counter -= 1
-
- if self._context.lock_counter == 0 or force:
- lock_id, lock_filename = id(self), self.lock_file
-
- _LOGGER.debug("Attempting to release lock %s on %s", lock_id, lock_filename)
- self._release()
- self._context.lock_counter = 0
- _LOGGER.debug("Lock %s released on %s", lock_id, lock_filename)
-
- def __enter__(self) -> Self:
- """
- Acquire the lock.
-
- :return: the lock object
- """
- self.acquire()
- return self
-
- def __exit__(
- self,
- exc_type: type[BaseException] | None,
- exc_value: BaseException | None,
- traceback: TracebackType | None,
- ) -> None:
- """
- Release the lock.
-
- :param exc_type: the exception type if raised
- :param exc_value: the exception value if raised
- :param traceback: the exception traceback if raised
- """
- self.release()
-
- def __del__(self) -> None:
- """Called when the lock object is deleted."""
- self.release(force=True)
-
-
-__all__ = [
- "BaseFileLock",
- "AcquireReturnProxy",
-]
diff --git a/spaces/joaopereirajp/livvieChatBot/venv/lib/python3.9/site-packages/fontTools/ttLib/removeOverlaps.py b/spaces/joaopereirajp/livvieChatBot/venv/lib/python3.9/site-packages/fontTools/ttLib/removeOverlaps.py
deleted file mode 100644
index 624cd47b4076a95cbc7c2124550371f6ffa5ea37..0000000000000000000000000000000000000000
--- a/spaces/joaopereirajp/livvieChatBot/venv/lib/python3.9/site-packages/fontTools/ttLib/removeOverlaps.py
+++ /dev/null
@@ -1,248 +0,0 @@
-""" Simplify TrueType glyphs by merging overlapping contours/components.
-
-Requires https://github.com/fonttools/skia-pathops
-"""
-
-import itertools
-import logging
-from typing import Callable, Iterable, Optional, Mapping
-
-from fontTools.misc.roundTools import otRound
-from fontTools.ttLib import ttFont
-from fontTools.ttLib.tables import _g_l_y_f
-from fontTools.ttLib.tables import _h_m_t_x
-from fontTools.pens.ttGlyphPen import TTGlyphPen
-
-import pathops
-
-
-__all__ = ["removeOverlaps"]
-
-
-class RemoveOverlapsError(Exception):
- pass
-
-
-log = logging.getLogger("fontTools.ttLib.removeOverlaps")
-
-_TTGlyphMapping = Mapping[str, ttFont._TTGlyph]
-
-
-def skPathFromGlyph(glyphName: str, glyphSet: _TTGlyphMapping) -> pathops.Path:
- path = pathops.Path()
- pathPen = path.getPen(glyphSet=glyphSet)
- glyphSet[glyphName].draw(pathPen)
- return path
-
-
-def skPathFromGlyphComponent(
- component: _g_l_y_f.GlyphComponent, glyphSet: _TTGlyphMapping
-):
- baseGlyphName, transformation = component.getComponentInfo()
- path = skPathFromGlyph(baseGlyphName, glyphSet)
- return path.transform(*transformation)
-
-
-def componentsOverlap(glyph: _g_l_y_f.Glyph, glyphSet: _TTGlyphMapping) -> bool:
- if not glyph.isComposite():
- raise ValueError("This method only works with TrueType composite glyphs")
- if len(glyph.components) < 2:
- return False # single component, no overlaps
-
- component_paths = {}
-
- def _get_nth_component_path(index: int) -> pathops.Path:
- if index not in component_paths:
- component_paths[index] = skPathFromGlyphComponent(
- glyph.components[index], glyphSet
- )
- return component_paths[index]
-
- return any(
- pathops.op(
- _get_nth_component_path(i),
- _get_nth_component_path(j),
- pathops.PathOp.INTERSECTION,
- fix_winding=False,
- keep_starting_points=False,
- )
- for i, j in itertools.combinations(range(len(glyph.components)), 2)
- )
-
-
-def ttfGlyphFromSkPath(path: pathops.Path) -> _g_l_y_f.Glyph:
- # Skia paths have no 'components', no need for glyphSet
- ttPen = TTGlyphPen(glyphSet=None)
- path.draw(ttPen)
- glyph = ttPen.glyph()
- assert not glyph.isComposite()
- # compute glyph.xMin (glyfTable parameter unused for non composites)
- glyph.recalcBounds(glyfTable=None)
- return glyph
-
-
-def _round_path(
- path: pathops.Path, round: Callable[[float], float] = otRound
-) -> pathops.Path:
- rounded_path = pathops.Path()
- for verb, points in path:
- rounded_path.add(verb, *((round(p[0]), round(p[1])) for p in points))
- return rounded_path
-
-
-def _simplify(path: pathops.Path, debugGlyphName: str) -> pathops.Path:
- # skia-pathops has a bug where it sometimes fails to simplify paths when there
- # are float coordinates and control points are very close to one another.
- # Rounding coordinates to integers works around the bug.
- # Since we are going to round glyf coordinates later on anyway, here it is
- # ok(-ish) to also round before simplify. Better than failing the whole process
- # for the entire font.
- # https://bugs.chromium.org/p/skia/issues/detail?id=11958
- # https://github.com/google/fonts/issues/3365
- # TODO(anthrotype): remove once this Skia bug is fixed
- try:
- return pathops.simplify(path, clockwise=path.clockwise)
- except pathops.PathOpsError:
- pass
-
- path = _round_path(path)
- try:
- path = pathops.simplify(path, clockwise=path.clockwise)
- log.debug(
- "skia-pathops failed to simplify '%s' with float coordinates, "
- "but succeded using rounded integer coordinates",
- debugGlyphName,
- )
- return path
- except pathops.PathOpsError as e:
- if log.isEnabledFor(logging.DEBUG):
- path.dump()
- raise RemoveOverlapsError(
- f"Failed to remove overlaps from glyph {debugGlyphName!r}"
- ) from e
-
- raise AssertionError("Unreachable")
-
-
-def removeTTGlyphOverlaps(
- glyphName: str,
- glyphSet: _TTGlyphMapping,
- glyfTable: _g_l_y_f.table__g_l_y_f,
- hmtxTable: _h_m_t_x.table__h_m_t_x,
- removeHinting: bool = True,
-) -> bool:
- glyph = glyfTable[glyphName]
- # decompose composite glyphs only if components overlap each other
- if (
- glyph.numberOfContours > 0
- or glyph.isComposite()
- and componentsOverlap(glyph, glyphSet)
- ):
- path = skPathFromGlyph(glyphName, glyphSet)
-
- # remove overlaps
- path2 = _simplify(path, glyphName)
-
- # replace TTGlyph if simplified path is different (ignoring contour order)
- if {tuple(c) for c in path.contours} != {tuple(c) for c in path2.contours}:
- glyfTable[glyphName] = glyph = ttfGlyphFromSkPath(path2)
- # simplified glyph is always unhinted
- assert not glyph.program
- # also ensure hmtx LSB == glyph.xMin so glyph origin is at x=0
- width, lsb = hmtxTable[glyphName]
- if lsb != glyph.xMin:
- hmtxTable[glyphName] = (width, glyph.xMin)
- return True
-
- if removeHinting:
- glyph.removeHinting()
- return False
-
-
-def removeOverlaps(
- font: ttFont.TTFont,
- glyphNames: Optional[Iterable[str]] = None,
- removeHinting: bool = True,
- ignoreErrors=False,
-) -> None:
- """Simplify glyphs in TTFont by merging overlapping contours.
-
- Overlapping components are first decomposed to simple contours, then merged.
-
- Currently this only works with TrueType fonts with 'glyf' table.
- Raises NotImplementedError if 'glyf' table is absent.
-
- Note that removing overlaps invalidates the hinting. By default we drop hinting
- from all glyphs whether or not overlaps are removed from a given one, as it would
- look weird if only some glyphs are left (un)hinted.
-
- Args:
- font: input TTFont object, modified in place.
- glyphNames: optional iterable of glyph names (str) to remove overlaps from.
- By default, all glyphs in the font are processed.
- removeHinting (bool): set to False to keep hinting for unmodified glyphs.
- ignoreErrors (bool): set to True to ignore errors while removing overlaps,
- thus keeping the tricky glyphs unchanged (fonttools/fonttools#2363).
- """
- try:
- glyfTable = font["glyf"]
- except KeyError:
- raise NotImplementedError("removeOverlaps currently only works with TTFs")
-
- hmtxTable = font["hmtx"]
- # wraps the underlying glyf Glyphs, takes care of interfacing with drawing pens
- glyphSet = font.getGlyphSet()
-
- if glyphNames is None:
- glyphNames = font.getGlyphOrder()
-
- # process all simple glyphs first, then composites with increasing component depth,
- # so that by the time we test for component intersections the respective base glyphs
- # have already been simplified
- glyphNames = sorted(
- glyphNames,
- key=lambda name: (
- glyfTable[name].getCompositeMaxpValues(glyfTable).maxComponentDepth
- if glyfTable[name].isComposite()
- else 0,
- name,
- ),
- )
- modified = set()
- for glyphName in glyphNames:
- try:
- if removeTTGlyphOverlaps(
- glyphName, glyphSet, glyfTable, hmtxTable, removeHinting
- ):
- modified.add(glyphName)
- except RemoveOverlapsError:
- if not ignoreErrors:
- raise
- log.error("Failed to remove overlaps for '%s'", glyphName)
-
- log.debug("Removed overlaps for %s glyphs:\n%s", len(modified), " ".join(modified))
-
-
-def main(args=None):
- import sys
-
- if args is None:
- args = sys.argv[1:]
-
- if len(args) < 2:
- print(
- f"usage: fonttools ttLib.removeOverlaps INPUT.ttf OUTPUT.ttf [GLYPHS ...]"
- )
- sys.exit(1)
-
- src = args[0]
- dst = args[1]
- glyphNames = args[2:] or None
-
- with ttFont.TTFont(src) as f:
- removeOverlaps(f, glyphNames)
- f.save(dst)
-
-
-if __name__ == "__main__":
- main()
diff --git a/spaces/joaopereirajp/livvieChatBot/venv/lib/python3.9/site-packages/gradio/components/annotated_image.py b/spaces/joaopereirajp/livvieChatBot/venv/lib/python3.9/site-packages/gradio/components/annotated_image.py
deleted file mode 100644
index b3034c17e5f4138155a545fd86a9fed90ad490cb..0000000000000000000000000000000000000000
--- a/spaces/joaopereirajp/livvieChatBot/venv/lib/python3.9/site-packages/gradio/components/annotated_image.py
+++ /dev/null
@@ -1,236 +0,0 @@
-"""gr.AnnotatedImage() component."""
-
-from __future__ import annotations
-
-import warnings
-from typing import Literal
-
-import numpy as np
-from gradio_client.documentation import document, set_documentation_group
-from gradio_client.serializing import JSONSerializable
-from PIL import Image as _Image # using _ to minimize namespace pollution
-
-from gradio import utils
-from gradio.components.base import IOComponent, _Keywords
-from gradio.deprecation import warn_style_method_deprecation
-from gradio.events import (
- EventListenerMethod,
- Selectable,
-)
-
-set_documentation_group("component")
-
-_Image.init() # fixes https://github.com/gradio-app/gradio/issues/2843
-
-
-@document()
-class AnnotatedImage(Selectable, IOComponent, JSONSerializable):
- """
- Displays a base image and colored subsections on top of that image. Subsections can take the from of rectangles (e.g. object detection) or masks (e.g. image segmentation).
- Preprocessing: this component does *not* accept input.
- Postprocessing: expects a {Tuple[numpy.ndarray | PIL.Image | str, List[Tuple[numpy.ndarray | Tuple[int, int, int, int], str]]]} consisting of a base image and a list of subsections, that are either (x1, y1, x2, y2) tuples identifying object boundaries, or 0-1 confidence masks of the same shape as the image. A label is provided for each subsection.
-
- Demos: image_segmentation
- """
-
- def __init__(
- self,
- value: tuple[
- np.ndarray | _Image.Image | str,
- list[tuple[np.ndarray | tuple[int, int, int, int], str]],
- ]
- | None = None,
- *,
- show_legend: bool = True,
- height: int | None = None,
- width: int | None = None,
- color_map: dict[str, str] | None = None,
- label: str | None = None,
- every: float | None = None,
- show_label: bool | None = None,
- container: bool = True,
- scale: int | None = None,
- min_width: int = 160,
- visible: bool = True,
- elem_id: str | None = None,
- elem_classes: list[str] | str | None = None,
- **kwargs,
- ):
- """
- Parameters:
- value: Tuple of base image and list of (subsection, label) pairs.
- show_legend: If True, will show a legend of the subsections.
- height: Height of the displayed image.
- width: Width of the displayed image.
- color_map: A dictionary mapping labels to colors. The colors must be specified as hex codes.
- label: component name in interface.
- every: If `value` is a callable, run the function 'every' number of seconds while the client connection is open. Has no effect otherwise. Queue must be enabled. The event can be accessed (e.g. to cancel it) via this component's .load_event attribute.
- show_label: if True, will display label.
- container: If True, will place the component in a container - providing some extra padding around the border.
- scale: relative width compared to adjacent Components in a Row. For example, if Component A has scale=2, and Component B has scale=1, A will be twice as wide as B. Should be an integer.
- min_width: minimum pixel width, will wrap if not sufficient screen space to satisfy this value. If a certain scale value results in this Component being narrower than min_width, the min_width parameter will be respected first.
- visible: If False, component will be hidden.
- elem_id: An optional string that is assigned as the id of this component in the HTML DOM. Can be used for targeting CSS styles.
- elem_classes: An optional list of strings that are assigned as the classes of this component in the HTML DOM. Can be used for targeting CSS styles.
- """
- self.show_legend = show_legend
- self.height = height
- self.width = width
- self.color_map = color_map
- self.select: EventListenerMethod
- """
- Event listener for when the user selects Image subsection.
- Uses event data gradio.SelectData to carry `value` referring to selected subsection label, and `index` to refer to subsection index.
- See EventData documentation on how to use this event data.
- """
- IOComponent.__init__(
- self,
- label=label,
- every=every,
- show_label=show_label,
- container=container,
- scale=scale,
- min_width=min_width,
- visible=visible,
- elem_id=elem_id,
- elem_classes=elem_classes,
- value=value,
- **kwargs,
- )
-
- @staticmethod
- def update(
- value: tuple[
- np.ndarray | _Image.Image | str,
- list[tuple[np.ndarray | tuple[int, int, int, int], str]],
- ]
- | Literal[_Keywords.NO_VALUE] = _Keywords.NO_VALUE,
- show_legend: bool | None = None,
- height: int | None = None,
- width: int | None = None,
- color_map: dict[str, str] | None = None,
- label: str | None = None,
- show_label: bool | None = None,
- container: bool | None = None,
- scale: int | None = None,
- min_width: int | None = None,
- visible: bool | None = None,
- ):
- warnings.warn(
- "Using the update method is deprecated. Simply return a new object instead, e.g. `return gr.AnnotatedImage(...)` instead of `return gr.AnnotatedImage.update(...)`."
- )
- updated_config = {
- "show_legend": show_legend,
- "height": height,
- "width": width,
- "color_map": color_map,
- "label": label,
- "show_label": show_label,
- "container": container,
- "scale": scale,
- "min_width": min_width,
- "visible": visible,
- "value": value,
- "__type__": "update",
- }
- return updated_config
-
- def postprocess(
- self,
- y: tuple[
- np.ndarray | _Image.Image | str,
- list[tuple[np.ndarray | tuple[int, int, int, int], str]],
- ],
- ) -> tuple[dict, list[tuple[dict, str]]] | None:
- """
- Parameters:
- y: Tuple of base image and list of subsections, with each subsection a two-part tuple where the first element is a 4 element bounding box or a 0-1 confidence mask, and the second element is the label.
- Returns:
- Tuple of base image file and list of subsections, with each subsection a two-part tuple where the first element image path of the mask, and the second element is the label.
- """
- if y is None:
- return None
- base_img = y[0]
- if isinstance(base_img, str):
- base_img_path = base_img
- base_img = np.array(_Image.open(base_img))
- elif isinstance(base_img, np.ndarray):
- base_file = self.img_array_to_temp_file(base_img, dir=self.DEFAULT_TEMP_DIR)
- base_img_path = str(utils.abspath(base_file))
- elif isinstance(base_img, _Image.Image):
- base_file = self.pil_to_temp_file(base_img, dir=self.DEFAULT_TEMP_DIR)
- base_img_path = str(utils.abspath(base_file))
- base_img = np.array(base_img)
- else:
- raise ValueError(
- "AnnotatedImage only accepts filepaths, PIL images or numpy arrays for the base image."
- )
- self.temp_files.add(base_img_path)
-
- sections = []
- color_map = self.color_map or {}
-
- def hex_to_rgb(value):
- value = value.lstrip("#")
- lv = len(value)
- return [int(value[i : i + lv // 3], 16) for i in range(0, lv, lv // 3)]
-
- for mask, label in y[1]:
- mask_array = np.zeros((base_img.shape[0], base_img.shape[1]))
- if isinstance(mask, np.ndarray):
- mask_array = mask
- else:
- x1, y1, x2, y2 = mask
- border_width = 3
- mask_array[y1:y2, x1:x2] = 0.5
- mask_array[y1:y2, x1 : x1 + border_width] = 1
- mask_array[y1:y2, x2 - border_width : x2] = 1
- mask_array[y1 : y1 + border_width, x1:x2] = 1
- mask_array[y2 - border_width : y2, x1:x2] = 1
-
- if label in color_map:
- rgb_color = hex_to_rgb(color_map[label])
- else:
- rgb_color = [255, 0, 0]
- colored_mask = np.zeros((base_img.shape[0], base_img.shape[1], 4))
- solid_mask = np.copy(mask_array)
- solid_mask[solid_mask > 0] = 1
-
- colored_mask[:, :, 0] = rgb_color[0] * solid_mask
- colored_mask[:, :, 1] = rgb_color[1] * solid_mask
- colored_mask[:, :, 2] = rgb_color[2] * solid_mask
- colored_mask[:, :, 3] = mask_array * 255
-
- colored_mask_img = _Image.fromarray((colored_mask).astype(np.uint8))
-
- mask_file = self.pil_to_temp_file(
- colored_mask_img, dir=self.DEFAULT_TEMP_DIR
- )
- mask_file_path = str(utils.abspath(mask_file))
- self.temp_files.add(mask_file_path)
-
- sections.append(
- ({"name": mask_file_path, "data": None, "is_file": True}, label)
- )
-
- return {"name": base_img_path, "data": None, "is_file": True}, sections
-
- def style(
- self,
- *,
- height: int | None = None,
- width: int | None = None,
- color_map: dict[str, str] | None = None,
- **kwargs,
- ):
- """
- This method is deprecated. Please set these arguments in the constructor instead.
- """
- warn_style_method_deprecation()
- if height is not None:
- self.height = height
- if width is not None:
- self.width = width
- if color_map is not None:
- self.color_map = color_map
- return self
diff --git a/spaces/joaopereirajp/livvieChatBot/venv/lib/python3.9/site-packages/gradio/components/checkboxgroup.py b/spaces/joaopereirajp/livvieChatBot/venv/lib/python3.9/site-packages/gradio/components/checkboxgroup.py
deleted file mode 100644
index deaa59c9a45871a5ce4ee21c5565637992107dcd..0000000000000000000000000000000000000000
--- a/spaces/joaopereirajp/livvieChatBot/venv/lib/python3.9/site-packages/gradio/components/checkboxgroup.py
+++ /dev/null
@@ -1,241 +0,0 @@
-"""gr.CheckboxGroup() component"""
-
-from __future__ import annotations
-
-import warnings
-from typing import Any, Callable, Literal
-
-from gradio_client.documentation import document, set_documentation_group
-from gradio_client.serializing import ListStringSerializable
-
-from gradio.components.base import FormComponent, IOComponent, _Keywords
-from gradio.deprecation import warn_deprecation, warn_style_method_deprecation
-from gradio.events import Changeable, EventListenerMethod, Inputable, Selectable
-from gradio.interpretation import NeighborInterpretable
-
-set_documentation_group("component")
-
-
-@document()
-class CheckboxGroup(
- FormComponent,
- Changeable,
- Inputable,
- Selectable,
- IOComponent,
- ListStringSerializable,
- NeighborInterpretable,
-):
- """
- Creates a set of checkboxes of which a subset can be checked.
- Preprocessing: passes the list of checked checkboxes as a {List[str | int | float]} or their indices as a {List[int]} into the function, depending on `type`.
- Postprocessing: expects a {List[str | int | float]}, each element of which becomes a checked checkbox.
- Examples-format: a {List[str | int | float]} representing the values to be checked.
- Demos: sentence_builder, titanic_survival
- """
-
- def __init__(
- self,
- choices: list[str | int | float | tuple[str, str | int | float]] | None = None,
- *,
- value: list[str | float | int] | str | float | int | Callable | None = None,
- type: Literal["value", "index"] = "value",
- label: str | None = None,
- info: str | None = None,
- every: float | None = None,
- show_label: bool | None = None,
- container: bool = True,
- scale: int | None = None,
- min_width: int = 160,
- interactive: bool | None = None,
- visible: bool = True,
- elem_id: str | None = None,
- elem_classes: list[str] | str | None = None,
- **kwargs,
- ):
- """
- Parameters:
- choices: A list of string or numeric options to select from. An option can also be a tuple of the form (name, value), where name is the displayed name of the checkbox button and value is the value to be passed to the function, or returned by the function.
- value: Default selected list of options. If a single choice is selected, it can be passed in as a string or numeric type. If callable, the function will be called whenever the app loads to set the initial value of the component.
- type: Type of value to be returned by component. "value" returns the list of strings of the choices selected, "index" returns the list of indices of the choices selected.
- label: Component name in interface.
- info: Additional component description.
- every: If `value` is a callable, run the function 'every' number of seconds while the client connection is open. Has no effect otherwise. Queue must be enabled. The event can be accessed (e.g. to cancel it) via this component's .load_event attribute.
- show_label: If True, will display label.
- container: If True, will place the component in a container - providing some extra padding around the border.
- scale: Relative width compared to adjacent Components in a Row. For example, if Component A has scale=2, and Component B has scale=1, A will be twice as wide as B. Should be an integer.
- min_width: Minimum pixel width, will wrap if not sufficient screen space to satisfy this value. If a certain scale value results in this Component being narrower than min_width, the min_width parameter will be respected first.
- interactive: If True, choices in this checkbox group will be checkable; if False, checking will be disabled. If not provided, this is inferred based on whether the component is used as an input or output.
- visible: If False, component will be hidden.
- elem_id: An optional string that is assigned as the id of this component in the HTML DOM. Can be used for targeting CSS styles.
- elem_classes: An optional list of strings that are assigned as the classes of this component in the HTML DOM. Can be used for targeting CSS styles.
- """
- self.choices = (
- # Although we expect choices to be a list of tuples, it can be a list of tuples if the Gradio app
- # is loaded with gr.load() since Python tuples are converted to lists in JSON.
- [tuple(c) if isinstance(c, (tuple, list)) else (str(c), c) for c in choices]
- if choices
- else []
- )
- valid_types = ["value", "index"]
- if type not in valid_types:
- raise ValueError(
- f"Invalid value for parameter `type`: {type}. Please choose from one of: {valid_types}"
- )
- self.type = type
- self.select: EventListenerMethod
- """
- Event listener for when the user selects or deselects within CheckboxGroup.
- Uses event data gradio.SelectData to carry `value` referring to label of selected checkbox, `index` to refer to index, and `selected` to refer to state of checkbox.
- See EventData documentation on how to use this event data.
- """
- IOComponent.__init__(
- self,
- label=label,
- info=info,
- every=every,
- show_label=show_label,
- container=container,
- scale=scale,
- min_width=min_width,
- interactive=interactive,
- visible=visible,
- elem_id=elem_id,
- elem_classes=elem_classes,
- value=value,
- **kwargs,
- )
- NeighborInterpretable.__init__(self)
-
- def example_inputs(self) -> dict[str, Any]:
- return {
- "raw": [self.choices[0][1]] if self.choices else None,
- "serialized": [self.choices[0][1]] if self.choices else None,
- }
-
- @staticmethod
- def update(
- value: list[str | int | float]
- | str
- | Literal[_Keywords.NO_VALUE]
- | None = _Keywords.NO_VALUE,
- choices: list[str | int | float | tuple[str, str | int | float]] | None = None,
- label: str | None = None,
- info: str | None = None,
- show_label: bool | None = None,
- container: bool | None = None,
- scale: int | None = None,
- min_width: int | None = None,
- interactive: bool | None = None,
- visible: bool | None = None,
- ):
- warnings.warn(
- "Using the update method is deprecated. Simply return a new object instead, e.g. `return gr.CheckboxGroup(...)` instead of `return gr.CheckboxGroup.update(...)`."
- )
- choices = (
- None
- if choices is None
- else [c if isinstance(c, tuple) else (str(c), c) for c in choices]
- )
- return {
- "choices": choices,
- "label": label,
- "info": info,
- "show_label": show_label,
- "container": container,
- "scale": scale,
- "min_width": min_width,
- "interactive": interactive,
- "visible": visible,
- "value": value,
- "__type__": "update",
- }
-
- def preprocess(
- self, x: list[str | int | float]
- ) -> list[str | int | float] | list[int | None]:
- """
- Parameters:
- x: list of selected choices
- Returns:
- list of selected choice values as strings or indices within choice list
- """
- if self.type == "value":
- return x
- elif self.type == "index":
- choice_values = [value for _, value in self.choices]
- return [
- choice_values.index(choice) if choice in choice_values else None
- for choice in x
- ]
- else:
- raise ValueError(
- f"Unknown type: {self.type}. Please choose from: 'value', 'index'."
- )
-
- def postprocess(
- self, y: list[str | int | float] | str | int | float | None
- ) -> list[str | int | float]:
- """
- Parameters:
- y: List of selected choice values. If a single choice is selected, it can be passed in as a string
- Returns:
- List of selected choices
- """
- if y is None:
- return []
- if not isinstance(y, list):
- y = [y]
- return y
-
- def get_interpretation_neighbors(self, x):
- leave_one_out_sets = []
- for choice in [value for _, value in self.choices]:
- leave_one_out_set = list(x)
- if choice in leave_one_out_set:
- leave_one_out_set.remove(choice)
- else:
- leave_one_out_set.append(choice)
- leave_one_out_sets.append(leave_one_out_set)
- return leave_one_out_sets, {}
-
- def get_interpretation_scores(self, x, neighbors, scores, **kwargs):
- """
- Returns:
- For each tuple in the list, the first value represents the interpretation score if the input is False, and the second if the input is True.
- """
- final_scores = []
- for choice, score in zip([value for _, value in self.choices], scores):
- score_set = [score, None] if choice in x else [None, score]
- final_scores.append(score_set)
- return final_scores
-
- def style(
- self,
- *,
- item_container: bool | None = None,
- container: bool | None = None,
- **kwargs,
- ):
- """
- This method is deprecated. Please set these arguments in the constructor instead.
- """
- warn_style_method_deprecation()
- if item_container is not None:
- warn_deprecation("The `item_container` parameter is deprecated.")
- if container is not None:
- self.container = container
- return self
-
- def as_example(self, input_data):
- if input_data is None:
- return None
- elif not isinstance(input_data, list):
- input_data = [input_data]
- for data in input_data:
- if data not in [c[0] for c in self.choices]:
- raise ValueError(f"Example {data} provided not a valid choice.")
- return [
- next((c[0] for c in self.choices if c[1] == data), None)
- for data in input_data
- ]
diff --git a/spaces/justest/chatglm2-6b-int4/README.md b/spaces/justest/chatglm2-6b-int4/README.md
deleted file mode 100644
index b2c3e6a37334b793d2db446673b29dd6393a2351..0000000000000000000000000000000000000000
--- a/spaces/justest/chatglm2-6b-int4/README.md
+++ /dev/null
@@ -1,14 +0,0 @@
----
-title: Chatglm2 6b
-emoji: 🌖
-colorFrom: blue
-colorTo: yellow
-sdk: gradio
-sdk_version: 3.24.1
-app_file: app.py
-pinned: false
-license: mit
-duplicated_from: justest/chatglm-6b-int4
----
-
-Check out the configuration reference at https://huggingface.co/docs/hub/spaces-config-reference
diff --git a/spaces/kangvcar/RealChar/client/web/src/App.test.js b/spaces/kangvcar/RealChar/client/web/src/App.test.js
deleted file mode 100644
index 1f03afeece5ac28064fa3c73a29215037465f789..0000000000000000000000000000000000000000
--- a/spaces/kangvcar/RealChar/client/web/src/App.test.js
+++ /dev/null
@@ -1,8 +0,0 @@
-import { render, screen } from '@testing-library/react';
-import App from './App';
-
-test('renders learn react link', () => {
- render();
- const linkElement = screen.getByText(/learn react/i);
- expect(linkElement).toBeInTheDocument();
-});
diff --git a/spaces/keithhon/Real-Time-Voice-Cloning/vocoder/gen_wavernn.py b/spaces/keithhon/Real-Time-Voice-Cloning/vocoder/gen_wavernn.py
deleted file mode 100644
index 2036737f805f6055893812e48f99d524624aab07..0000000000000000000000000000000000000000
--- a/spaces/keithhon/Real-Time-Voice-Cloning/vocoder/gen_wavernn.py
+++ /dev/null
@@ -1,31 +0,0 @@
-from vocoder.models.fatchord_version import WaveRNN
-from vocoder.audio import *
-
-
-def gen_testset(model: WaveRNN, test_set, samples, batched, target, overlap, save_path):
- k = model.get_step() // 1000
-
- for i, (m, x) in enumerate(test_set, 1):
- if i > samples:
- break
-
- print('\n| Generating: %i/%i' % (i, samples))
-
- x = x[0].numpy()
-
- bits = 16 if hp.voc_mode == 'MOL' else hp.bits
-
- if hp.mu_law and hp.voc_mode != 'MOL' :
- x = decode_mu_law(x, 2**bits, from_labels=True)
- else :
- x = label_2_float(x, bits)
-
- save_wav(x, save_path.joinpath("%dk_steps_%d_target.wav" % (k, i)))
-
- batch_str = "gen_batched_target%d_overlap%d" % (target, overlap) if batched else \
- "gen_not_batched"
- save_str = save_path.joinpath("%dk_steps_%d_%s.wav" % (k, i, batch_str))
-
- wav = model.generate(m, batched, target, overlap, hp.mu_law)
- save_wav(wav, save_str)
-
diff --git a/spaces/keras-dreambooth/traditional-furniture-demo/utils_app.py b/spaces/keras-dreambooth/traditional-furniture-demo/utils_app.py
deleted file mode 100644
index c6c7932b58ed0c2168f02ab65a7dfb12780cb133..0000000000000000000000000000000000000000
--- a/spaces/keras-dreambooth/traditional-furniture-demo/utils_app.py
+++ /dev/null
@@ -1,125 +0,0 @@
-from huggingface_hub import from_pretrained_keras
-from keras_cv import models
-from tensorflow import keras
-import tensorflow as tf
-import gradio as gr
-
-
-keras.mixed_precision.set_global_policy("mixed_float16")
-
-keras_model_list = [
- "kadirnar/dreambooth_diffusion_model_v5",
- "kadirnar/dreambooth_diffusion_model_v3"
-]
-
-stable_prompt_list = [
- "a photo of sks traditional furniture",
- ]
-
-stable_negative_prompt_list = [
- "bad, ugly",
- "deformed"
- ]
-
-def keras_stable_diffusion(
- model_path:str,
- prompt:str,
- negative_prompt:str,
- guidance_scale:int,
- num_inference_step:int,
- height:int,
- width:int,
- ):
-
- sd_dreambooth_model = models.StableDiffusion(
- img_width=height,
- img_height=width
- )
-
- db_diffusion_model = from_pretrained_keras(model_path)
- sd_dreambooth_model._diffusion_model = db_diffusion_model
-
- generated_images = sd_dreambooth_model.text_to_image(
- prompt=prompt,
- negative_prompt=negative_prompt,
- num_steps=num_inference_step,
- unconditional_guidance_scale=guidance_scale
- )
- tf.keras.backend.clear_session()
-
-
- return generated_images
-
-def keras_stable_diffusion_app():
- with gr.Blocks():
- with gr.Row():
- with gr.Column():
- keras_text2image_model_path = gr.Dropdown(
- choices=keras_model_list,
- value=keras_model_list[0],
- label='Text-Image Model Id'
- )
-
- keras_text2image_prompt = gr.Textbox(
- lines=1,
- value=stable_prompt_list[0],
- label='Prompt'
- )
-
- keras_text2image_negative_prompt = gr.Textbox(
- lines=1,
- value=stable_negative_prompt_list[0],
- label='Negative Prompt'
- )
-
- with gr.Accordion("Advanced Options", open=False):
- keras_text2image_guidance_scale = gr.Slider(
- minimum=0.1,
- maximum=15,
- step=0.1,
- value=7.5,
- label='Guidance Scale'
- )
-
- keras_text2image_num_inference_step = gr.Slider(
- minimum=1,
- maximum=100,
- step=1,
- value=50,
- label='Num Inference Step'
- )
-
- keras_text2image_height = gr.Slider(
- minimum=128,
- maximum=1280,
- step=32,
- value=512,
- label='Image Height'
- )
-
- keras_text2image_width = gr.Slider(
- minimum=128,
- maximum=1280,
- step=32,
- value=512,
- label='Image Height'
- )
-
- keras_text2image_predict = gr.Button(value='Generator')
-
- with gr.Column():
- output_image = gr.Gallery(label='Output')
-
- keras_text2image_predict.click(
- fn=keras_stable_diffusion,
- inputs=[
- keras_text2image_model_path,
- keras_text2image_prompt,
- keras_text2image_negative_prompt,
- keras_text2image_guidance_scale,
- keras_text2image_num_inference_step,
- keras_text2image_height,
- keras_text2image_width
- ],
- outputs=output_image
- )
diff --git a/spaces/keras-io/conv_Mixer/README.md b/spaces/keras-io/conv_Mixer/README.md
deleted file mode 100644
index 39500c1da60fde908570a6c156753bf75aefa0c9..0000000000000000000000000000000000000000
--- a/spaces/keras-io/conv_Mixer/README.md
+++ /dev/null
@@ -1,12 +0,0 @@
----
-title: Conv Mixer
-emoji: 📊
-colorFrom: purple
-colorTo: pink
-sdk: gradio
-sdk_version: 3.0.24
-app_file: app.py
-pinned: false
----
-
-Check out the configuration reference at https://huggingface.co/docs/hub/spaces-config-reference
diff --git a/spaces/kevinwang676/ChatGLM2-SadTalker-VC/src/face3d/util/generate_list.py b/spaces/kevinwang676/ChatGLM2-SadTalker-VC/src/face3d/util/generate_list.py
deleted file mode 100644
index 943d906781063c3584a7e5b5c784f8aac0694985..0000000000000000000000000000000000000000
--- a/spaces/kevinwang676/ChatGLM2-SadTalker-VC/src/face3d/util/generate_list.py
+++ /dev/null
@@ -1,34 +0,0 @@
-"""This script is to generate training list files for Deep3DFaceRecon_pytorch
-"""
-
-import os
-
-# save path to training data
-def write_list(lms_list, imgs_list, msks_list, mode='train',save_folder='datalist', save_name=''):
- save_path = os.path.join(save_folder, mode)
- if not os.path.isdir(save_path):
- os.makedirs(save_path)
- with open(os.path.join(save_path, save_name + 'landmarks.txt'), 'w') as fd:
- fd.writelines([i + '\n' for i in lms_list])
-
- with open(os.path.join(save_path, save_name + 'images.txt'), 'w') as fd:
- fd.writelines([i + '\n' for i in imgs_list])
-
- with open(os.path.join(save_path, save_name + 'masks.txt'), 'w') as fd:
- fd.writelines([i + '\n' for i in msks_list])
-
-# check if the path is valid
-def check_list(rlms_list, rimgs_list, rmsks_list):
- lms_list, imgs_list, msks_list = [], [], []
- for i in range(len(rlms_list)):
- flag = 'false'
- lm_path = rlms_list[i]
- im_path = rimgs_list[i]
- msk_path = rmsks_list[i]
- if os.path.isfile(lm_path) and os.path.isfile(im_path) and os.path.isfile(msk_path):
- flag = 'true'
- lms_list.append(rlms_list[i])
- imgs_list.append(rimgs_list[i])
- msks_list.append(rmsks_list[i])
- print(i, rlms_list[i], flag)
- return lms_list, imgs_list, msks_list
diff --git a/spaces/kirch/Text2Video-Zero/annotator/uniformer/mmcv/runner/hooks/logger/neptune.py b/spaces/kirch/Text2Video-Zero/annotator/uniformer/mmcv/runner/hooks/logger/neptune.py
deleted file mode 100644
index 7a38772b0c93a8608f32c6357b8616e77c139dc9..0000000000000000000000000000000000000000
--- a/spaces/kirch/Text2Video-Zero/annotator/uniformer/mmcv/runner/hooks/logger/neptune.py
+++ /dev/null
@@ -1,82 +0,0 @@
-# Copyright (c) OpenMMLab. All rights reserved.
-from ...dist_utils import master_only
-from ..hook import HOOKS
-from .base import LoggerHook
-
-
-@HOOKS.register_module()
-class NeptuneLoggerHook(LoggerHook):
- """Class to log metrics to NeptuneAI.
-
- It requires `neptune-client` to be installed.
-
- Args:
- init_kwargs (dict): a dict contains the initialization keys as below:
- - project (str): Name of a project in a form of
- namespace/project_name. If None, the value of
- NEPTUNE_PROJECT environment variable will be taken.
- - api_token (str): User’s API token.
- If None, the value of NEPTUNE_API_TOKEN environment
- variable will be taken. Note: It is strongly recommended
- to use NEPTUNE_API_TOKEN environment variable rather than
- placing your API token in plain text in your source code.
- - name (str, optional, default is 'Untitled'): Editable name of
- the run. Name is displayed in the run's Details and in
- Runs table as a column.
- Check https://docs.neptune.ai/api-reference/neptune#init for
- more init arguments.
- interval (int): Logging interval (every k iterations).
- ignore_last (bool): Ignore the log of last iterations in each epoch
- if less than `interval`.
- reset_flag (bool): Whether to clear the output buffer after logging
- by_epoch (bool): Whether EpochBasedRunner is used.
-
- .. _NeptuneAI:
- https://docs.neptune.ai/you-should-know/logging-metadata
- """
-
- def __init__(self,
- init_kwargs=None,
- interval=10,
- ignore_last=True,
- reset_flag=True,
- with_step=True,
- by_epoch=True):
-
- super(NeptuneLoggerHook, self).__init__(interval, ignore_last,
- reset_flag, by_epoch)
- self.import_neptune()
- self.init_kwargs = init_kwargs
- self.with_step = with_step
-
- def import_neptune(self):
- try:
- import neptune.new as neptune
- except ImportError:
- raise ImportError(
- 'Please run "pip install neptune-client" to install neptune')
- self.neptune = neptune
- self.run = None
-
- @master_only
- def before_run(self, runner):
- if self.init_kwargs:
- self.run = self.neptune.init(**self.init_kwargs)
- else:
- self.run = self.neptune.init()
-
- @master_only
- def log(self, runner):
- tags = self.get_loggable_tags(runner)
- if tags:
- for tag_name, tag_value in tags.items():
- if self.with_step:
- self.run[tag_name].log(
- tag_value, step=self.get_iter(runner))
- else:
- tags['global_step'] = self.get_iter(runner)
- self.run[tag_name].log(tags)
-
- @master_only
- def after_run(self, runner):
- self.run.stop()
diff --git a/spaces/koajoel/PolyFormer/fairseq/examples/speech_recognition/kaldi/kaldi_decoder.py b/spaces/koajoel/PolyFormer/fairseq/examples/speech_recognition/kaldi/kaldi_decoder.py
deleted file mode 100644
index 5f62cc58ae8c0c5a3ba7d17713fedf0abc302942..0000000000000000000000000000000000000000
--- a/spaces/koajoel/PolyFormer/fairseq/examples/speech_recognition/kaldi/kaldi_decoder.py
+++ /dev/null
@@ -1,244 +0,0 @@
-#!/usr/bin/env python3
-
-# Copyright (c) Facebook, Inc. and its affiliates.
-#
-# This source code is licensed under the MIT license found in the
-# LICENSE file in the root directory of this source tree.
-
-from concurrent.futures import ThreadPoolExecutor
-import logging
-from omegaconf import MISSING
-import os
-import torch
-from typing import Optional
-import warnings
-
-
-from dataclasses import dataclass
-from fairseq.dataclass import FairseqDataclass
-from .kaldi_initializer import KaldiInitializerConfig, initalize_kaldi
-
-
-logger = logging.getLogger(__name__)
-
-
-@dataclass
-class KaldiDecoderConfig(FairseqDataclass):
- hlg_graph_path: Optional[str] = None
- output_dict: str = MISSING
-
- kaldi_initializer_config: Optional[KaldiInitializerConfig] = None
-
- acoustic_scale: float = 0.5
- max_active: int = 10000
- beam_delta: float = 0.5
- hash_ratio: float = 2.0
-
- is_lattice: bool = False
- lattice_beam: float = 10.0
- prune_interval: int = 25
- determinize_lattice: bool = True
- prune_scale: float = 0.1
- max_mem: int = 0
- phone_determinize: bool = True
- word_determinize: bool = True
- minimize: bool = True
-
- num_threads: int = 1
-
-
-class KaldiDecoder(object):
- def __init__(
- self,
- cfg: KaldiDecoderConfig,
- beam: int,
- nbest: int = 1,
- ):
- try:
- from kaldi.asr import FasterRecognizer, LatticeFasterRecognizer
- from kaldi.base import set_verbose_level
- from kaldi.decoder import (
- FasterDecoder,
- FasterDecoderOptions,
- LatticeFasterDecoder,
- LatticeFasterDecoderOptions,
- )
- from kaldi.lat.functions import DeterminizeLatticePhonePrunedOptions
- from kaldi.fstext import read_fst_kaldi, SymbolTable
- except:
- warnings.warn(
- "pykaldi is required for this functionality. Please install from https://github.com/pykaldi/pykaldi"
- )
-
- # set_verbose_level(2)
-
- self.acoustic_scale = cfg.acoustic_scale
- self.nbest = nbest
-
- if cfg.hlg_graph_path is None:
- assert (
- cfg.kaldi_initializer_config is not None
- ), "Must provide hlg graph path or kaldi initializer config"
- cfg.hlg_graph_path = initalize_kaldi(cfg.kaldi_initializer_config)
-
- assert os.path.exists(cfg.hlg_graph_path), cfg.hlg_graph_path
-
- if cfg.is_lattice:
- self.dec_cls = LatticeFasterDecoder
- opt_cls = LatticeFasterDecoderOptions
- self.rec_cls = LatticeFasterRecognizer
- else:
- assert self.nbest == 1, "nbest > 1 requires lattice decoder"
- self.dec_cls = FasterDecoder
- opt_cls = FasterDecoderOptions
- self.rec_cls = FasterRecognizer
-
- self.decoder_options = opt_cls()
- self.decoder_options.beam = beam
- self.decoder_options.max_active = cfg.max_active
- self.decoder_options.beam_delta = cfg.beam_delta
- self.decoder_options.hash_ratio = cfg.hash_ratio
-
- if cfg.is_lattice:
- self.decoder_options.lattice_beam = cfg.lattice_beam
- self.decoder_options.prune_interval = cfg.prune_interval
- self.decoder_options.determinize_lattice = cfg.determinize_lattice
- self.decoder_options.prune_scale = cfg.prune_scale
- det_opts = DeterminizeLatticePhonePrunedOptions()
- det_opts.max_mem = cfg.max_mem
- det_opts.phone_determinize = cfg.phone_determinize
- det_opts.word_determinize = cfg.word_determinize
- det_opts.minimize = cfg.minimize
- self.decoder_options.det_opts = det_opts
-
- self.output_symbols = {}
- with open(cfg.output_dict, "r") as f:
- for line in f:
- items = line.rstrip().split()
- assert len(items) == 2
- self.output_symbols[int(items[1])] = items[0]
-
- logger.info(f"Loading FST from {cfg.hlg_graph_path}")
- self.fst = read_fst_kaldi(cfg.hlg_graph_path)
- self.symbol_table = SymbolTable.read_text(cfg.output_dict)
-
- self.executor = ThreadPoolExecutor(max_workers=cfg.num_threads)
-
- def generate(self, models, sample, **unused):
- """Generate a batch of inferences."""
- # model.forward normally channels prev_output_tokens into the decoder
- # separately, but SequenceGenerator directly calls model.encoder
- encoder_input = {
- k: v for k, v in sample["net_input"].items() if k != "prev_output_tokens"
- }
- emissions, padding = self.get_emissions(models, encoder_input)
- return self.decode(emissions, padding)
-
- def get_emissions(self, models, encoder_input):
- """Run encoder and normalize emissions"""
- model = models[0]
-
- all_encoder_out = [m(**encoder_input) for m in models]
-
- if len(all_encoder_out) > 1:
-
- if "encoder_out" in all_encoder_out[0]:
- encoder_out = {
- "encoder_out": sum(e["encoder_out"] for e in all_encoder_out)
- / len(all_encoder_out),
- "encoder_padding_mask": all_encoder_out[0]["encoder_padding_mask"],
- }
- padding = encoder_out["encoder_padding_mask"]
- else:
- encoder_out = {
- "logits": sum(e["logits"] for e in all_encoder_out)
- / len(all_encoder_out),
- "padding_mask": all_encoder_out[0]["padding_mask"],
- }
- padding = encoder_out["padding_mask"]
- else:
- encoder_out = all_encoder_out[0]
- padding = (
- encoder_out["padding_mask"]
- if "padding_mask" in encoder_out
- else encoder_out["encoder_padding_mask"]
- )
-
- if hasattr(model, "get_logits"):
- emissions = model.get_logits(encoder_out, normalize=True)
- else:
- emissions = model.get_normalized_probs(encoder_out, log_probs=True)
-
- return (
- emissions.cpu().float().transpose(0, 1),
- padding.cpu() if padding is not None and padding.any() else None,
- )
-
- def decode_one(self, logits, padding):
- from kaldi.matrix import Matrix
-
- decoder = self.dec_cls(self.fst, self.decoder_options)
- asr = self.rec_cls(
- decoder, self.symbol_table, acoustic_scale=self.acoustic_scale
- )
-
- if padding is not None:
- logits = logits[~padding]
-
- mat = Matrix(logits.numpy())
-
- out = asr.decode(mat)
-
- if self.nbest > 1:
- from kaldi.fstext import shortestpath
- from kaldi.fstext.utils import (
- convert_compact_lattice_to_lattice,
- convert_lattice_to_std,
- convert_nbest_to_list,
- get_linear_symbol_sequence,
- )
-
- lat = out["lattice"]
-
- sp = shortestpath(lat, nshortest=self.nbest)
-
- sp = convert_compact_lattice_to_lattice(sp)
- sp = convert_lattice_to_std(sp)
- seq = convert_nbest_to_list(sp)
-
- results = []
- for s in seq:
- _, o, w = get_linear_symbol_sequence(s)
- words = list(self.output_symbols[z] for z in o)
- results.append(
- {
- "tokens": words,
- "words": words,
- "score": w.value,
- "emissions": logits,
- }
- )
- return results
- else:
- words = out["text"].split()
- return [
- {
- "tokens": words,
- "words": words,
- "score": out["likelihood"],
- "emissions": logits,
- }
- ]
-
- def decode(self, emissions, padding):
- if padding is None:
- padding = [None] * len(emissions)
-
- ret = list(
- map(
- lambda e, p: self.executor.submit(self.decode_one, e, p),
- emissions,
- padding,
- )
- )
- return ret
diff --git a/spaces/ky2k/Toxicity_Classifier_POC/.venv/lib/python3.9/site-packages/gradio/templates/frontend/assets/TabItem-ea98f884.css b/spaces/ky2k/Toxicity_Classifier_POC/.venv/lib/python3.9/site-packages/gradio/templates/frontend/assets/TabItem-ea98f884.css
deleted file mode 100644
index 246a7a4732778f3adeb6b3083bb2c873add3bfb7..0000000000000000000000000000000000000000
--- a/spaces/ky2k/Toxicity_Classifier_POC/.venv/lib/python3.9/site-packages/gradio/templates/frontend/assets/TabItem-ea98f884.css
+++ /dev/null
@@ -1 +0,0 @@
-.tabs.svelte-1g805jl{display:flex;position:relative;flex-direction:column}.hide.svelte-1g805jl{display:none}.tab-nav.svelte-1g805jl{display:flex;position:relative;flex-wrap:wrap;border-bottom:1px solid var(--border-color-primary);white-space:nowrap}button.svelte-1g805jl{margin-bottom:-1px;border:1px solid transparent;border-color:transparent;border-bottom:none;border-top-right-radius:var(--container-radius);border-top-left-radius:var(--container-radius);padding:var(--size-1) var(--size-4);color:var(--body-text-color-subdued);font-weight:var(--section-header-text-weight);font-size:var(--section-header-text-size)}button.svelte-1g805jl:hover{color:var(--body-text-color)}.selected.svelte-1g805jl{border-color:var(--border-color-primary);background:var(--background-fill-primary);color:var(--body-text-color)}.bar.svelte-1g805jl{display:block;position:absolute;bottom:-2px;left:0;z-index:999;background:var(--background-fill-primary);width:100%;height:2px;content:""}div.svelte-19hvt5v{display:flex;position:relative;border:1px solid var(--border-color-primary);border-top:none;border-bottom-right-radius:var(--container-radius);border-bottom-left-radius:var(--container-radius);padding:var(--block-padding)}
diff --git a/spaces/latent-consistency/lcm-LoraTheExplorer/README.md b/spaces/latent-consistency/lcm-LoraTheExplorer/README.md
deleted file mode 100644
index b1aa09219ec9ba78943099d7dd782606eeefe038..0000000000000000000000000000000000000000
--- a/spaces/latent-consistency/lcm-LoraTheExplorer/README.md
+++ /dev/null
@@ -1,15 +0,0 @@
----
-title: LCM-LoRA the Explorer
-emoji: 🔎 🖼️
-colorFrom: indigo
-colorTo: blue
-sdk: gradio
-sdk_version: 4.1.2
-app_file: app.py
-pinned: false
-license: mit
-suggested_hardware: a10g-large
-models: ['nerijs/pixel-art-xl', 'Pclanglais/TintinIA', 'ProomptEngineer/pe-balloon-diffusion-style', 'joachimsallstrom/aether-cloud-lora-for-sdxl', 'ostris/crayon_style_lora_sdxl', 'jbilcke-hf/sdxl-zelda64', 'TheLastBen/Papercut_SDXL', 'fofr/sdxl-2004', 'joachimsallstrom/aether-ghost-lora-for-sdxl', 'artificialguybr/ColoringBookRedmond-V2', 'Norod78/SDXL-LofiGirl-Lora', 'ostris/embroidery_style_lora_sdxl', 'goofyai/3d_render_style_xl', 'ostris/watercolor_style_lora_sdxl', 'veryVANYA/ps1-graphics-sdxl-v2', 'TheLastBen/William_Eggleston_Style_SDXL', 'davizca87/c-a-g-coinmaker', 'goofyai/cyborg_style_xl', 'artificialguybr/ToyRedmond-ToyLoraForSDXL10', 'Fictiverse/Voxel_XL_Lora', 'minimaxir/sdxl-ugly-sonic-lora', 'nerijs/lego-brickheadz-xl', 'nerijs/lego-minifig-xl', 'Norod78/SDXL-jojoso_style-Lora', 'TheLastBen/Pikachu_SDXL', 'artificialguybr/LogoRedmond-LogoLoraForSDXL', 'Norod78/SDXL-StickerSheet-Lora', 'artificialguybr/LineAniRedmond-LinearMangaSDXL', 'TheLastBen/Josef_Koudelka_Style_SDXL', 'goofyai/Leonardo_Ai_Style_Illustration', 'Norod78/SDXL-simpstyle-Lora', 'artificialguybr/StoryBookRedmond', 'chillpixel/blacklight-makeup-sdxl-lora', 'ProomptEngineer/pe-neon-sign-style', 'ProomptEngineer/pe-lofi-hiphop-lofi-girl-concept', 'ProomptEngineer/pe-shitty-fanart', 'ProomptEngineer/pe-sandsculpter-style', 'ProomptEngineer/pe-shitty-medieval-paintings', 'ProomptEngineer/pe-courtroomsketch-style', 'ProomptEngineer/pe-funko-pop-diffusion-style', 'lordjia/lelo-lego-lora', 'KappaNeuro/dressed-animals', 'KappaNeuro/vintage-postage-stamps', 'KappaNeuro/video-installation', 'KappaNeuro/ukiyo-e-art', 'KappaNeuro/surreal-collage', 'KappaNeuro/stop-motion-animation', 'KappaNeuro/studio-ghibli-style', 'KappaNeuro/punk-collage', 'KappaNeuro/needlepoint', 'KappaNeuro/made-of-iridescent-foil', 'KappaNeuro/lascaux', 'KappaNeuro/color-palette', 'KappaNeuro/albumen-print', 'KappaNeuro/1987-action-figure-playset-packaging', 'Norod78/SDXL-VintageMagStyle-Lora', 'CiroN2022/road-sign', 'CiroN2022/mosaic-style', 'CiroN2022/cd-md-music', 'CiroN2022/hair-style', 'CiroN2022/overprint-effect', 'CiroN2022/toy-face', 'CiroN2022/ascii-art', 'artificialguybr/PixelArtRedmond', 'artificialguybr/StickersRedmond', 'artificialguybr/ClayAnimationRedmond', 'fofr/sdxl-vision-pro', 'joachimsallstrom/aether-glitch-lora-for-sdxl', 'artificialguybr/TshirtDesignRedmond-V2', 'ostris/ikea-instructions-lora-sdxl', 'ostris/super-cereal-sdxl-lora', 'jakedahn/sdxl-isometric-geology', 'artificialguybr/analogredmond-v2', 'stets/nintendo64_cartridge']
----
-
-Check out the configuration reference at https://huggingface.co/docs/hub/spaces-config-reference
\ No newline at end of file
diff --git a/spaces/lewiswu1209/MockingBird/vocoder/wavernn/models/fatchord_version.py b/spaces/lewiswu1209/MockingBird/vocoder/wavernn/models/fatchord_version.py
deleted file mode 100644
index 6413a921651971b4859ed7de8b3a676cd6595d6b..0000000000000000000000000000000000000000
--- a/spaces/lewiswu1209/MockingBird/vocoder/wavernn/models/fatchord_version.py
+++ /dev/null
@@ -1,434 +0,0 @@
-import torch
-import torch.nn as nn
-import torch.nn.functional as F
-from vocoder.distribution import sample_from_discretized_mix_logistic
-from vocoder.display import *
-from vocoder.wavernn.audio import *
-
-
-class ResBlock(nn.Module):
- def __init__(self, dims):
- super().__init__()
- self.conv1 = nn.Conv1d(dims, dims, kernel_size=1, bias=False)
- self.conv2 = nn.Conv1d(dims, dims, kernel_size=1, bias=False)
- self.batch_norm1 = nn.BatchNorm1d(dims)
- self.batch_norm2 = nn.BatchNorm1d(dims)
-
- def forward(self, x):
- residual = x
- x = self.conv1(x)
- x = self.batch_norm1(x)
- x = F.relu(x)
- x = self.conv2(x)
- x = self.batch_norm2(x)
- return x + residual
-
-
-class MelResNet(nn.Module):
- def __init__(self, res_blocks, in_dims, compute_dims, res_out_dims, pad):
- super().__init__()
- k_size = pad * 2 + 1
- self.conv_in = nn.Conv1d(in_dims, compute_dims, kernel_size=k_size, bias=False)
- self.batch_norm = nn.BatchNorm1d(compute_dims)
- self.layers = nn.ModuleList()
- for i in range(res_blocks):
- self.layers.append(ResBlock(compute_dims))
- self.conv_out = nn.Conv1d(compute_dims, res_out_dims, kernel_size=1)
-
- def forward(self, x):
- x = self.conv_in(x)
- x = self.batch_norm(x)
- x = F.relu(x)
- for f in self.layers: x = f(x)
- x = self.conv_out(x)
- return x
-
-
-class Stretch2d(nn.Module):
- def __init__(self, x_scale, y_scale):
- super().__init__()
- self.x_scale = x_scale
- self.y_scale = y_scale
-
- def forward(self, x):
- b, c, h, w = x.size()
- x = x.unsqueeze(-1).unsqueeze(3)
- x = x.repeat(1, 1, 1, self.y_scale, 1, self.x_scale)
- return x.view(b, c, h * self.y_scale, w * self.x_scale)
-
-
-class UpsampleNetwork(nn.Module):
- def __init__(self, feat_dims, upsample_scales, compute_dims,
- res_blocks, res_out_dims, pad):
- super().__init__()
- total_scale = np.cumproduct(upsample_scales)[-1]
- self.indent = pad * total_scale
- self.resnet = MelResNet(res_blocks, feat_dims, compute_dims, res_out_dims, pad)
- self.resnet_stretch = Stretch2d(total_scale, 1)
- self.up_layers = nn.ModuleList()
- for scale in upsample_scales:
- k_size = (1, scale * 2 + 1)
- padding = (0, scale)
- stretch = Stretch2d(scale, 1)
- conv = nn.Conv2d(1, 1, kernel_size=k_size, padding=padding, bias=False)
- conv.weight.data.fill_(1. / k_size[1])
- self.up_layers.append(stretch)
- self.up_layers.append(conv)
-
- def forward(self, m):
- aux = self.resnet(m).unsqueeze(1)
- aux = self.resnet_stretch(aux)
- aux = aux.squeeze(1)
- m = m.unsqueeze(1)
- for f in self.up_layers: m = f(m)
- m = m.squeeze(1)[:, :, self.indent:-self.indent]
- return m.transpose(1, 2), aux.transpose(1, 2)
-
-
-class WaveRNN(nn.Module):
- def __init__(self, rnn_dims, fc_dims, bits, pad, upsample_factors,
- feat_dims, compute_dims, res_out_dims, res_blocks,
- hop_length, sample_rate, mode='RAW'):
- super().__init__()
- self.mode = mode
- self.pad = pad
- if self.mode == 'RAW' :
- self.n_classes = 2 ** bits
- elif self.mode == 'MOL' :
- self.n_classes = 30
- else :
- RuntimeError("Unknown model mode value - ", self.mode)
-
- self.rnn_dims = rnn_dims
- self.aux_dims = res_out_dims // 4
- self.hop_length = hop_length
- self.sample_rate = sample_rate
-
- self.upsample = UpsampleNetwork(feat_dims, upsample_factors, compute_dims, res_blocks, res_out_dims, pad)
- self.I = nn.Linear(feat_dims + self.aux_dims + 1, rnn_dims)
- self.rnn1 = nn.GRU(rnn_dims, rnn_dims, batch_first=True)
- self.rnn2 = nn.GRU(rnn_dims + self.aux_dims, rnn_dims, batch_first=True)
- self.fc1 = nn.Linear(rnn_dims + self.aux_dims, fc_dims)
- self.fc2 = nn.Linear(fc_dims + self.aux_dims, fc_dims)
- self.fc3 = nn.Linear(fc_dims, self.n_classes)
-
- self.step = nn.Parameter(torch.zeros(1).long(), requires_grad=False)
- self.num_params()
-
- def forward(self, x, mels):
- self.step += 1
- bsize = x.size(0)
- if torch.cuda.is_available():
- h1 = torch.zeros(1, bsize, self.rnn_dims).cuda()
- h2 = torch.zeros(1, bsize, self.rnn_dims).cuda()
- else:
- h1 = torch.zeros(1, bsize, self.rnn_dims).cpu()
- h2 = torch.zeros(1, bsize, self.rnn_dims).cpu()
- mels, aux = self.upsample(mels)
-
- aux_idx = [self.aux_dims * i for i in range(5)]
- a1 = aux[:, :, aux_idx[0]:aux_idx[1]]
- a2 = aux[:, :, aux_idx[1]:aux_idx[2]]
- a3 = aux[:, :, aux_idx[2]:aux_idx[3]]
- a4 = aux[:, :, aux_idx[3]:aux_idx[4]]
-
- x = torch.cat([x.unsqueeze(-1), mels, a1], dim=2)
- x = self.I(x)
- res = x
- x, _ = self.rnn1(x, h1)
-
- x = x + res
- res = x
- x = torch.cat([x, a2], dim=2)
- x, _ = self.rnn2(x, h2)
-
- x = x + res
- x = torch.cat([x, a3], dim=2)
- x = F.relu(self.fc1(x))
-
- x = torch.cat([x, a4], dim=2)
- x = F.relu(self.fc2(x))
- return self.fc3(x)
-
- def generate(self, mels, batched, target, overlap, mu_law, progress_callback=None):
- mu_law = mu_law if self.mode == 'RAW' else False
- progress_callback = progress_callback or self.gen_display
-
- self.eval()
- output = []
- start = time.time()
- rnn1 = self.get_gru_cell(self.rnn1)
- rnn2 = self.get_gru_cell(self.rnn2)
-
- with torch.no_grad():
- if torch.cuda.is_available():
- mels = mels.cuda()
- else:
- mels = mels.cpu()
- wave_len = (mels.size(-1) - 1) * self.hop_length
- mels = self.pad_tensor(mels.transpose(1, 2), pad=self.pad, side='both')
- mels, aux = self.upsample(mels.transpose(1, 2))
-
- if batched:
- mels = self.fold_with_overlap(mels, target, overlap)
- aux = self.fold_with_overlap(aux, target, overlap)
-
- b_size, seq_len, _ = mels.size()
-
- if torch.cuda.is_available():
- h1 = torch.zeros(b_size, self.rnn_dims).cuda()
- h2 = torch.zeros(b_size, self.rnn_dims).cuda()
- x = torch.zeros(b_size, 1).cuda()
- else:
- h1 = torch.zeros(b_size, self.rnn_dims).cpu()
- h2 = torch.zeros(b_size, self.rnn_dims).cpu()
- x = torch.zeros(b_size, 1).cpu()
-
- d = self.aux_dims
- aux_split = [aux[:, :, d * i:d * (i + 1)] for i in range(4)]
-
- for i in range(seq_len):
-
- m_t = mels[:, i, :]
-
- a1_t, a2_t, a3_t, a4_t = (a[:, i, :] for a in aux_split)
-
- x = torch.cat([x, m_t, a1_t], dim=1)
- x = self.I(x)
- h1 = rnn1(x, h1)
-
- x = x + h1
- inp = torch.cat([x, a2_t], dim=1)
- h2 = rnn2(inp, h2)
-
- x = x + h2
- x = torch.cat([x, a3_t], dim=1)
- x = F.relu(self.fc1(x))
-
- x = torch.cat([x, a4_t], dim=1)
- x = F.relu(self.fc2(x))
-
- logits = self.fc3(x)
-
- if self.mode == 'MOL':
- sample = sample_from_discretized_mix_logistic(logits.unsqueeze(0).transpose(1, 2))
- output.append(sample.view(-1))
- if torch.cuda.is_available():
- # x = torch.FloatTensor([[sample]]).cuda()
- x = sample.transpose(0, 1).cuda()
- else:
- x = sample.transpose(0, 1)
-
- elif self.mode == 'RAW' :
- posterior = F.softmax(logits, dim=1)
- distrib = torch.distributions.Categorical(posterior)
-
- sample = 2 * distrib.sample().float() / (self.n_classes - 1.) - 1.
- output.append(sample)
- x = sample.unsqueeze(-1)
- else:
- raise RuntimeError("Unknown model mode value - ", self.mode)
-
- if i % 100 == 0:
- gen_rate = (i + 1) / (time.time() - start) * b_size / 1000
- progress_callback(i, seq_len, b_size, gen_rate)
-
- output = torch.stack(output).transpose(0, 1)
- output = output.cpu().numpy()
- output = output.astype(np.float64)
-
- if batched:
- output = self.xfade_and_unfold(output, target, overlap)
- else:
- output = output[0]
-
- if mu_law:
- output = decode_mu_law(output, self.n_classes, False)
- if hp.apply_preemphasis:
- output = de_emphasis(output)
-
- # Fade-out at the end to avoid signal cutting out suddenly
- fade_out = np.linspace(1, 0, 20 * self.hop_length)
- output = output[:wave_len]
- output[-20 * self.hop_length:] *= fade_out
-
- self.train()
-
- return output
-
-
- def gen_display(self, i, seq_len, b_size, gen_rate):
- pbar = progbar(i, seq_len)
- msg = f'| {pbar} {i*b_size}/{seq_len*b_size} | Batch Size: {b_size} | Gen Rate: {gen_rate:.1f}kHz | '
- stream(msg)
-
- def get_gru_cell(self, gru):
- gru_cell = nn.GRUCell(gru.input_size, gru.hidden_size)
- gru_cell.weight_hh.data = gru.weight_hh_l0.data
- gru_cell.weight_ih.data = gru.weight_ih_l0.data
- gru_cell.bias_hh.data = gru.bias_hh_l0.data
- gru_cell.bias_ih.data = gru.bias_ih_l0.data
- return gru_cell
-
- def pad_tensor(self, x, pad, side='both'):
- # NB - this is just a quick method i need right now
- # i.e., it won't generalise to other shapes/dims
- b, t, c = x.size()
- total = t + 2 * pad if side == 'both' else t + pad
- if torch.cuda.is_available():
- padded = torch.zeros(b, total, c).cuda()
- else:
- padded = torch.zeros(b, total, c).cpu()
- if side == 'before' or side == 'both':
- padded[:, pad:pad + t, :] = x
- elif side == 'after':
- padded[:, :t, :] = x
- return padded
-
- def fold_with_overlap(self, x, target, overlap):
-
- ''' Fold the tensor with overlap for quick batched inference.
- Overlap will be used for crossfading in xfade_and_unfold()
-
- Args:
- x (tensor) : Upsampled conditioning features.
- shape=(1, timesteps, features)
- target (int) : Target timesteps for each index of batch
- overlap (int) : Timesteps for both xfade and rnn warmup
-
- Return:
- (tensor) : shape=(num_folds, target + 2 * overlap, features)
-
- Details:
- x = [[h1, h2, ... hn]]
-
- Where each h is a vector of conditioning features
-
- Eg: target=2, overlap=1 with x.size(1)=10
-
- folded = [[h1, h2, h3, h4],
- [h4, h5, h6, h7],
- [h7, h8, h9, h10]]
- '''
-
- _, total_len, features = x.size()
-
- # Calculate variables needed
- num_folds = (total_len - overlap) // (target + overlap)
- extended_len = num_folds * (overlap + target) + overlap
- remaining = total_len - extended_len
-
- # Pad if some time steps poking out
- if remaining != 0:
- num_folds += 1
- padding = target + 2 * overlap - remaining
- x = self.pad_tensor(x, padding, side='after')
-
- if torch.cuda.is_available():
- folded = torch.zeros(num_folds, target + 2 * overlap, features).cuda()
- else:
- folded = torch.zeros(num_folds, target + 2 * overlap, features).cpu()
-
- # Get the values for the folded tensor
- for i in range(num_folds):
- start = i * (target + overlap)
- end = start + target + 2 * overlap
- folded[i] = x[:, start:end, :]
-
- return folded
-
- def xfade_and_unfold(self, y, target, overlap):
-
- ''' Applies a crossfade and unfolds into a 1d array.
-
- Args:
- y (ndarry) : Batched sequences of audio samples
- shape=(num_folds, target + 2 * overlap)
- dtype=np.float64
- overlap (int) : Timesteps for both xfade and rnn warmup
-
- Return:
- (ndarry) : audio samples in a 1d array
- shape=(total_len)
- dtype=np.float64
-
- Details:
- y = [[seq1],
- [seq2],
- [seq3]]
-
- Apply a gain envelope at both ends of the sequences
-
- y = [[seq1_in, seq1_target, seq1_out],
- [seq2_in, seq2_target, seq2_out],
- [seq3_in, seq3_target, seq3_out]]
-
- Stagger and add up the groups of samples:
-
- [seq1_in, seq1_target, (seq1_out + seq2_in), seq2_target, ...]
-
- '''
-
- num_folds, length = y.shape
- target = length - 2 * overlap
- total_len = num_folds * (target + overlap) + overlap
-
- # Need some silence for the rnn warmup
- silence_len = overlap // 2
- fade_len = overlap - silence_len
- silence = np.zeros((silence_len), dtype=np.float64)
-
- # Equal power crossfade
- t = np.linspace(-1, 1, fade_len, dtype=np.float64)
- fade_in = np.sqrt(0.5 * (1 + t))
- fade_out = np.sqrt(0.5 * (1 - t))
-
- # Concat the silence to the fades
- fade_in = np.concatenate([silence, fade_in])
- fade_out = np.concatenate([fade_out, silence])
-
- # Apply the gain to the overlap samples
- y[:, :overlap] *= fade_in
- y[:, -overlap:] *= fade_out
-
- unfolded = np.zeros((total_len), dtype=np.float64)
-
- # Loop to add up all the samples
- for i in range(num_folds):
- start = i * (target + overlap)
- end = start + target + 2 * overlap
- unfolded[start:end] += y[i]
-
- return unfolded
-
- def get_step(self) :
- return self.step.data.item()
-
- def checkpoint(self, model_dir, optimizer) :
- k_steps = self.get_step() // 1000
- self.save(model_dir.joinpath("checkpoint_%dk_steps.pt" % k_steps), optimizer)
-
- def log(self, path, msg) :
- with open(path, 'a') as f:
- print(msg, file=f)
-
- def load(self, path, optimizer) :
- checkpoint = torch.load(path)
- if "optimizer_state" in checkpoint:
- self.load_state_dict(checkpoint["model_state"])
- optimizer.load_state_dict(checkpoint["optimizer_state"])
- else:
- # Backwards compatibility
- self.load_state_dict(checkpoint)
-
- def save(self, path, optimizer) :
- torch.save({
- "model_state": self.state_dict(),
- "optimizer_state": optimizer.state_dict(),
- }, path)
-
- def num_params(self, print_out=True):
- parameters = filter(lambda p: p.requires_grad, self.parameters())
- parameters = sum([np.prod(p.size()) for p in parameters]) / 1_000_000
- if print_out :
- print('Trainable Parameters: %.3fM' % parameters)
diff --git a/spaces/liimefruit/RVCollection/infer_pack/models.py b/spaces/liimefruit/RVCollection/infer_pack/models.py
deleted file mode 100644
index cec26ea118b4190fb63f174387cdfe15a0fd9f13..0000000000000000000000000000000000000000
--- a/spaces/liimefruit/RVCollection/infer_pack/models.py
+++ /dev/null
@@ -1,1124 +0,0 @@
-import math, pdb, os
-from time import time as ttime
-import torch
-from torch import nn
-from torch.nn import functional as F
-from infer_pack import modules
-from infer_pack import attentions
-from infer_pack import commons
-from infer_pack.commons import init_weights, get_padding
-from torch.nn import Conv1d, ConvTranspose1d, AvgPool1d, Conv2d
-from torch.nn.utils import weight_norm, remove_weight_norm, spectral_norm
-from infer_pack.commons import init_weights
-import numpy as np
-from infer_pack import commons
-
-
-class TextEncoder256(nn.Module):
- def __init__(
- self,
- out_channels,
- hidden_channels,
- filter_channels,
- n_heads,
- n_layers,
- kernel_size,
- p_dropout,
- f0=True,
- ):
- super().__init__()
- self.out_channels = out_channels
- self.hidden_channels = hidden_channels
- self.filter_channels = filter_channels
- self.n_heads = n_heads
- self.n_layers = n_layers
- self.kernel_size = kernel_size
- self.p_dropout = p_dropout
- self.emb_phone = nn.Linear(256, hidden_channels)
- self.lrelu = nn.LeakyReLU(0.1, inplace=True)
- if f0 == True:
- self.emb_pitch = nn.Embedding(256, hidden_channels) # pitch 256
- self.encoder = attentions.Encoder(
- hidden_channels, filter_channels, n_heads, n_layers, kernel_size, p_dropout
- )
- self.proj = nn.Conv1d(hidden_channels, out_channels * 2, 1)
-
- def forward(self, phone, pitch, lengths):
- if pitch == None:
- x = self.emb_phone(phone)
- else:
- x = self.emb_phone(phone) + self.emb_pitch(pitch)
- x = x * math.sqrt(self.hidden_channels) # [b, t, h]
- x = self.lrelu(x)
- x = torch.transpose(x, 1, -1) # [b, h, t]
- x_mask = torch.unsqueeze(commons.sequence_mask(lengths, x.size(2)), 1).to(
- x.dtype
- )
- x = self.encoder(x * x_mask, x_mask)
- stats = self.proj(x) * x_mask
-
- m, logs = torch.split(stats, self.out_channels, dim=1)
- return m, logs, x_mask
-
-
-class TextEncoder768(nn.Module):
- def __init__(
- self,
- out_channels,
- hidden_channels,
- filter_channels,
- n_heads,
- n_layers,
- kernel_size,
- p_dropout,
- f0=True,
- ):
- super().__init__()
- self.out_channels = out_channels
- self.hidden_channels = hidden_channels
- self.filter_channels = filter_channels
- self.n_heads = n_heads
- self.n_layers = n_layers
- self.kernel_size = kernel_size
- self.p_dropout = p_dropout
- self.emb_phone = nn.Linear(768, hidden_channels)
- self.lrelu = nn.LeakyReLU(0.1, inplace=True)
- if f0 == True:
- self.emb_pitch = nn.Embedding(256, hidden_channels) # pitch 256
- self.encoder = attentions.Encoder(
- hidden_channels, filter_channels, n_heads, n_layers, kernel_size, p_dropout
- )
- self.proj = nn.Conv1d(hidden_channels, out_channels * 2, 1)
-
- def forward(self, phone, pitch, lengths):
- if pitch == None:
- x = self.emb_phone(phone)
- else:
- x = self.emb_phone(phone) + self.emb_pitch(pitch)
- x = x * math.sqrt(self.hidden_channels) # [b, t, h]
- x = self.lrelu(x)
- x = torch.transpose(x, 1, -1) # [b, h, t]
- x_mask = torch.unsqueeze(commons.sequence_mask(lengths, x.size(2)), 1).to(
- x.dtype
- )
- x = self.encoder(x * x_mask, x_mask)
- stats = self.proj(x) * x_mask
-
- m, logs = torch.split(stats, self.out_channels, dim=1)
- return m, logs, x_mask
-
-
-class ResidualCouplingBlock(nn.Module):
- def __init__(
- self,
- channels,
- hidden_channels,
- kernel_size,
- dilation_rate,
- n_layers,
- n_flows=4,
- gin_channels=0,
- ):
- super().__init__()
- self.channels = channels
- self.hidden_channels = hidden_channels
- self.kernel_size = kernel_size
- self.dilation_rate = dilation_rate
- self.n_layers = n_layers
- self.n_flows = n_flows
- self.gin_channels = gin_channels
-
- self.flows = nn.ModuleList()
- for i in range(n_flows):
- self.flows.append(
- modules.ResidualCouplingLayer(
- channels,
- hidden_channels,
- kernel_size,
- dilation_rate,
- n_layers,
- gin_channels=gin_channels,
- mean_only=True,
- )
- )
- self.flows.append(modules.Flip())
-
- def forward(self, x, x_mask, g=None, reverse=False):
- if not reverse:
- for flow in self.flows:
- x, _ = flow(x, x_mask, g=g, reverse=reverse)
- else:
- for flow in reversed(self.flows):
- x = flow(x, x_mask, g=g, reverse=reverse)
- return x
-
- def remove_weight_norm(self):
- for i in range(self.n_flows):
- self.flows[i * 2].remove_weight_norm()
-
-
-class PosteriorEncoder(nn.Module):
- def __init__(
- self,
- in_channels,
- out_channels,
- hidden_channels,
- kernel_size,
- dilation_rate,
- n_layers,
- gin_channels=0,
- ):
- super().__init__()
- self.in_channels = in_channels
- self.out_channels = out_channels
- self.hidden_channels = hidden_channels
- self.kernel_size = kernel_size
- self.dilation_rate = dilation_rate
- self.n_layers = n_layers
- self.gin_channels = gin_channels
-
- self.pre = nn.Conv1d(in_channels, hidden_channels, 1)
- self.enc = modules.WN(
- hidden_channels,
- kernel_size,
- dilation_rate,
- n_layers,
- gin_channels=gin_channels,
- )
- self.proj = nn.Conv1d(hidden_channels, out_channels * 2, 1)
-
- def forward(self, x, x_lengths, g=None):
- x_mask = torch.unsqueeze(commons.sequence_mask(x_lengths, x.size(2)), 1).to(
- x.dtype
- )
- x = self.pre(x) * x_mask
- x = self.enc(x, x_mask, g=g)
- stats = self.proj(x) * x_mask
- m, logs = torch.split(stats, self.out_channels, dim=1)
- z = (m + torch.randn_like(m) * torch.exp(logs)) * x_mask
- return z, m, logs, x_mask
-
- def remove_weight_norm(self):
- self.enc.remove_weight_norm()
-
-
-class Generator(torch.nn.Module):
- def __init__(
- self,
- initial_channel,
- resblock,
- resblock_kernel_sizes,
- resblock_dilation_sizes,
- upsample_rates,
- upsample_initial_channel,
- upsample_kernel_sizes,
- gin_channels=0,
- ):
- super(Generator, self).__init__()
- self.num_kernels = len(resblock_kernel_sizes)
- self.num_upsamples = len(upsample_rates)
- self.conv_pre = Conv1d(
- initial_channel, upsample_initial_channel, 7, 1, padding=3
- )
- resblock = modules.ResBlock1 if resblock == "1" else modules.ResBlock2
-
- self.ups = nn.ModuleList()
- for i, (u, k) in enumerate(zip(upsample_rates, upsample_kernel_sizes)):
- self.ups.append(
- weight_norm(
- ConvTranspose1d(
- upsample_initial_channel // (2**i),
- upsample_initial_channel // (2 ** (i + 1)),
- k,
- u,
- padding=(k - u) // 2,
- )
- )
- )
-
- self.resblocks = nn.ModuleList()
- for i in range(len(self.ups)):
- ch = upsample_initial_channel // (2 ** (i + 1))
- for j, (k, d) in enumerate(
- zip(resblock_kernel_sizes, resblock_dilation_sizes)
- ):
- self.resblocks.append(resblock(ch, k, d))
-
- self.conv_post = Conv1d(ch, 1, 7, 1, padding=3, bias=False)
- self.ups.apply(init_weights)
-
- if gin_channels != 0:
- self.cond = nn.Conv1d(gin_channels, upsample_initial_channel, 1)
-
- def forward(self, x, g=None):
- x = self.conv_pre(x)
- if g is not None:
- x = x + self.cond(g)
-
- for i in range(self.num_upsamples):
- x = F.leaky_relu(x, modules.LRELU_SLOPE)
- x = self.ups[i](x)
- xs = None
- for j in range(self.num_kernels):
- if xs is None:
- xs = self.resblocks[i * self.num_kernels + j](x)
- else:
- xs += self.resblocks[i * self.num_kernels + j](x)
- x = xs / self.num_kernels
- x = F.leaky_relu(x)
- x = self.conv_post(x)
- x = torch.tanh(x)
-
- return x
-
- def remove_weight_norm(self):
- for l in self.ups:
- remove_weight_norm(l)
- for l in self.resblocks:
- l.remove_weight_norm()
-
-
-class SineGen(torch.nn.Module):
- """Definition of sine generator
- SineGen(samp_rate, harmonic_num = 0,
- sine_amp = 0.1, noise_std = 0.003,
- voiced_threshold = 0,
- flag_for_pulse=False)
- samp_rate: sampling rate in Hz
- harmonic_num: number of harmonic overtones (default 0)
- sine_amp: amplitude of sine-wavefrom (default 0.1)
- noise_std: std of Gaussian noise (default 0.003)
- voiced_thoreshold: F0 threshold for U/V classification (default 0)
- flag_for_pulse: this SinGen is used inside PulseGen (default False)
- Note: when flag_for_pulse is True, the first time step of a voiced
- segment is always sin(np.pi) or cos(0)
- """
-
- def __init__(
- self,
- samp_rate,
- harmonic_num=0,
- sine_amp=0.1,
- noise_std=0.003,
- voiced_threshold=0,
- flag_for_pulse=False,
- ):
- super(SineGen, self).__init__()
- self.sine_amp = sine_amp
- self.noise_std = noise_std
- self.harmonic_num = harmonic_num
- self.dim = self.harmonic_num + 1
- self.sampling_rate = samp_rate
- self.voiced_threshold = voiced_threshold
-
- def _f02uv(self, f0):
- # generate uv signal
- uv = torch.ones_like(f0)
- uv = uv * (f0 > self.voiced_threshold)
- return uv
-
- def forward(self, f0, upp):
- """sine_tensor, uv = forward(f0)
- input F0: tensor(batchsize=1, length, dim=1)
- f0 for unvoiced steps should be 0
- output sine_tensor: tensor(batchsize=1, length, dim)
- output uv: tensor(batchsize=1, length, 1)
- """
- with torch.no_grad():
- f0 = f0[:, None].transpose(1, 2)
- f0_buf = torch.zeros(f0.shape[0], f0.shape[1], self.dim, device=f0.device)
- # fundamental component
- f0_buf[:, :, 0] = f0[:, :, 0]
- for idx in np.arange(self.harmonic_num):
- f0_buf[:, :, idx + 1] = f0_buf[:, :, 0] * (
- idx + 2
- ) # idx + 2: the (idx+1)-th overtone, (idx+2)-th harmonic
- rad_values = (f0_buf / self.sampling_rate) % 1 ###%1意味着n_har的乘积无法后处理优化
- rand_ini = torch.rand(
- f0_buf.shape[0], f0_buf.shape[2], device=f0_buf.device
- )
- rand_ini[:, 0] = 0
- rad_values[:, 0, :] = rad_values[:, 0, :] + rand_ini
- tmp_over_one = torch.cumsum(rad_values, 1) # % 1 #####%1意味着后面的cumsum无法再优化
- tmp_over_one *= upp
- tmp_over_one = F.interpolate(
- tmp_over_one.transpose(2, 1),
- scale_factor=upp,
- mode="linear",
- align_corners=True,
- ).transpose(2, 1)
- rad_values = F.interpolate(
- rad_values.transpose(2, 1), scale_factor=upp, mode="nearest"
- ).transpose(
- 2, 1
- ) #######
- tmp_over_one %= 1
- tmp_over_one_idx = (tmp_over_one[:, 1:, :] - tmp_over_one[:, :-1, :]) < 0
- cumsum_shift = torch.zeros_like(rad_values)
- cumsum_shift[:, 1:, :] = tmp_over_one_idx * -1.0
- sine_waves = torch.sin(
- torch.cumsum(rad_values + cumsum_shift, dim=1) * 2 * np.pi
- )
- sine_waves = sine_waves * self.sine_amp
- uv = self._f02uv(f0)
- uv = F.interpolate(
- uv.transpose(2, 1), scale_factor=upp, mode="nearest"
- ).transpose(2, 1)
- noise_amp = uv * self.noise_std + (1 - uv) * self.sine_amp / 3
- noise = noise_amp * torch.randn_like(sine_waves)
- sine_waves = sine_waves * uv + noise
- return sine_waves, uv, noise
-
-
-class SourceModuleHnNSF(torch.nn.Module):
- """SourceModule for hn-nsf
- SourceModule(sampling_rate, harmonic_num=0, sine_amp=0.1,
- add_noise_std=0.003, voiced_threshod=0)
- sampling_rate: sampling_rate in Hz
- harmonic_num: number of harmonic above F0 (default: 0)
- sine_amp: amplitude of sine source signal (default: 0.1)
- add_noise_std: std of additive Gaussian noise (default: 0.003)
- note that amplitude of noise in unvoiced is decided
- by sine_amp
- voiced_threshold: threhold to set U/V given F0 (default: 0)
- Sine_source, noise_source = SourceModuleHnNSF(F0_sampled)
- F0_sampled (batchsize, length, 1)
- Sine_source (batchsize, length, 1)
- noise_source (batchsize, length 1)
- uv (batchsize, length, 1)
- """
-
- def __init__(
- self,
- sampling_rate,
- harmonic_num=0,
- sine_amp=0.1,
- add_noise_std=0.003,
- voiced_threshod=0,
- is_half=True,
- ):
- super(SourceModuleHnNSF, self).__init__()
-
- self.sine_amp = sine_amp
- self.noise_std = add_noise_std
- self.is_half = is_half
- # to produce sine waveforms
- self.l_sin_gen = SineGen(
- sampling_rate, harmonic_num, sine_amp, add_noise_std, voiced_threshod
- )
-
- # to merge source harmonics into a single excitation
- self.l_linear = torch.nn.Linear(harmonic_num + 1, 1)
- self.l_tanh = torch.nn.Tanh()
-
- def forward(self, x, upp=None):
- sine_wavs, uv, _ = self.l_sin_gen(x, upp)
- if self.is_half:
- sine_wavs = sine_wavs.half()
- sine_merge = self.l_tanh(self.l_linear(sine_wavs))
- return sine_merge, None, None # noise, uv
-
-
-class GeneratorNSF(torch.nn.Module):
- def __init__(
- self,
- initial_channel,
- resblock,
- resblock_kernel_sizes,
- resblock_dilation_sizes,
- upsample_rates,
- upsample_initial_channel,
- upsample_kernel_sizes,
- gin_channels,
- sr,
- is_half=False,
- ):
- super(GeneratorNSF, self).__init__()
- self.num_kernels = len(resblock_kernel_sizes)
- self.num_upsamples = len(upsample_rates)
-
- self.f0_upsamp = torch.nn.Upsample(scale_factor=np.prod(upsample_rates))
- self.m_source = SourceModuleHnNSF(
- sampling_rate=sr, harmonic_num=0, is_half=is_half
- )
- self.noise_convs = nn.ModuleList()
- self.conv_pre = Conv1d(
- initial_channel, upsample_initial_channel, 7, 1, padding=3
- )
- resblock = modules.ResBlock1 if resblock == "1" else modules.ResBlock2
-
- self.ups = nn.ModuleList()
- for i, (u, k) in enumerate(zip(upsample_rates, upsample_kernel_sizes)):
- c_cur = upsample_initial_channel // (2 ** (i + 1))
- self.ups.append(
- weight_norm(
- ConvTranspose1d(
- upsample_initial_channel // (2**i),
- upsample_initial_channel // (2 ** (i + 1)),
- k,
- u,
- padding=(k - u) // 2,
- )
- )
- )
- if i + 1 < len(upsample_rates):
- stride_f0 = np.prod(upsample_rates[i + 1 :])
- self.noise_convs.append(
- Conv1d(
- 1,
- c_cur,
- kernel_size=stride_f0 * 2,
- stride=stride_f0,
- padding=stride_f0 // 2,
- )
- )
- else:
- self.noise_convs.append(Conv1d(1, c_cur, kernel_size=1))
-
- self.resblocks = nn.ModuleList()
- for i in range(len(self.ups)):
- ch = upsample_initial_channel // (2 ** (i + 1))
- for j, (k, d) in enumerate(
- zip(resblock_kernel_sizes, resblock_dilation_sizes)
- ):
- self.resblocks.append(resblock(ch, k, d))
-
- self.conv_post = Conv1d(ch, 1, 7, 1, padding=3, bias=False)
- self.ups.apply(init_weights)
-
- if gin_channels != 0:
- self.cond = nn.Conv1d(gin_channels, upsample_initial_channel, 1)
-
- self.upp = np.prod(upsample_rates)
-
- def forward(self, x, f0, g=None):
- har_source, noi_source, uv = self.m_source(f0, self.upp)
- har_source = har_source.transpose(1, 2)
- x = self.conv_pre(x)
- if g is not None:
- x = x + self.cond(g)
-
- for i in range(self.num_upsamples):
- x = F.leaky_relu(x, modules.LRELU_SLOPE)
- x = self.ups[i](x)
- x_source = self.noise_convs[i](har_source)
- x = x + x_source
- xs = None
- for j in range(self.num_kernels):
- if xs is None:
- xs = self.resblocks[i * self.num_kernels + j](x)
- else:
- xs += self.resblocks[i * self.num_kernels + j](x)
- x = xs / self.num_kernels
- x = F.leaky_relu(x)
- x = self.conv_post(x)
- x = torch.tanh(x)
- return x
-
- def remove_weight_norm(self):
- for l in self.ups:
- remove_weight_norm(l)
- for l in self.resblocks:
- l.remove_weight_norm()
-
-
-sr2sr = {
- "32k": 32000,
- "40k": 40000,
- "48k": 48000,
-}
-
-
-class SynthesizerTrnMs256NSFsid(nn.Module):
- def __init__(
- self,
- spec_channels,
- segment_size,
- inter_channels,
- hidden_channels,
- filter_channels,
- n_heads,
- n_layers,
- kernel_size,
- p_dropout,
- resblock,
- resblock_kernel_sizes,
- resblock_dilation_sizes,
- upsample_rates,
- upsample_initial_channel,
- upsample_kernel_sizes,
- spk_embed_dim,
- gin_channels,
- sr,
- **kwargs
- ):
- super().__init__()
- if type(sr) == type("strr"):
- sr = sr2sr[sr]
- self.spec_channels = spec_channels
- self.inter_channels = inter_channels
- self.hidden_channels = hidden_channels
- self.filter_channels = filter_channels
- self.n_heads = n_heads
- self.n_layers = n_layers
- self.kernel_size = kernel_size
- self.p_dropout = p_dropout
- self.resblock = resblock
- self.resblock_kernel_sizes = resblock_kernel_sizes
- self.resblock_dilation_sizes = resblock_dilation_sizes
- self.upsample_rates = upsample_rates
- self.upsample_initial_channel = upsample_initial_channel
- self.upsample_kernel_sizes = upsample_kernel_sizes
- self.segment_size = segment_size
- self.gin_channels = gin_channels
- # self.hop_length = hop_length#
- self.spk_embed_dim = spk_embed_dim
- self.enc_p = TextEncoder256(
- inter_channels,
- hidden_channels,
- filter_channels,
- n_heads,
- n_layers,
- kernel_size,
- p_dropout,
- )
- self.dec = GeneratorNSF(
- inter_channels,
- resblock,
- resblock_kernel_sizes,
- resblock_dilation_sizes,
- upsample_rates,
- upsample_initial_channel,
- upsample_kernel_sizes,
- gin_channels=gin_channels,
- sr=sr,
- is_half=kwargs["is_half"],
- )
- self.enc_q = PosteriorEncoder(
- spec_channels,
- inter_channels,
- hidden_channels,
- 5,
- 1,
- 16,
- gin_channels=gin_channels,
- )
- self.flow = ResidualCouplingBlock(
- inter_channels, hidden_channels, 5, 1, 3, gin_channels=gin_channels
- )
- self.emb_g = nn.Embedding(self.spk_embed_dim, gin_channels)
- print("gin_channels:", gin_channels, "self.spk_embed_dim:", self.spk_embed_dim)
-
- def remove_weight_norm(self):
- self.dec.remove_weight_norm()
- self.flow.remove_weight_norm()
- self.enc_q.remove_weight_norm()
-
- def forward(
- self, phone, phone_lengths, pitch, pitchf, y, y_lengths, ds
- ): # 这里ds是id,[bs,1]
- # print(1,pitch.shape)#[bs,t]
- g = self.emb_g(ds).unsqueeze(-1) # [b, 256, 1]##1是t,广播的
- m_p, logs_p, x_mask = self.enc_p(phone, pitch, phone_lengths)
- z, m_q, logs_q, y_mask = self.enc_q(y, y_lengths, g=g)
- z_p = self.flow(z, y_mask, g=g)
- z_slice, ids_slice = commons.rand_slice_segments(
- z, y_lengths, self.segment_size
- )
- # print(-1,pitchf.shape,ids_slice,self.segment_size,self.hop_length,self.segment_size//self.hop_length)
- pitchf = commons.slice_segments2(pitchf, ids_slice, self.segment_size)
- # print(-2,pitchf.shape,z_slice.shape)
- o = self.dec(z_slice, pitchf, g=g)
- return o, ids_slice, x_mask, y_mask, (z, z_p, m_p, logs_p, m_q, logs_q)
-
- def infer(self, phone, phone_lengths, pitch, nsff0, sid, max_len=None):
- g = self.emb_g(sid).unsqueeze(-1)
- m_p, logs_p, x_mask = self.enc_p(phone, pitch, phone_lengths)
- z_p = (m_p + torch.exp(logs_p) * torch.randn_like(m_p) * 0.66666) * x_mask
- z = self.flow(z_p, x_mask, g=g, reverse=True)
- o = self.dec((z * x_mask)[:, :, :max_len], nsff0, g=g)
- return o, x_mask, (z, z_p, m_p, logs_p)
-
-
-class SynthesizerTrnMs768NSFsid(nn.Module):
- def __init__(
- self,
- spec_channels,
- segment_size,
- inter_channels,
- hidden_channels,
- filter_channels,
- n_heads,
- n_layers,
- kernel_size,
- p_dropout,
- resblock,
- resblock_kernel_sizes,
- resblock_dilation_sizes,
- upsample_rates,
- upsample_initial_channel,
- upsample_kernel_sizes,
- spk_embed_dim,
- gin_channels,
- sr,
- **kwargs
- ):
- super().__init__()
- if type(sr) == type("strr"):
- sr = sr2sr[sr]
- self.spec_channels = spec_channels
- self.inter_channels = inter_channels
- self.hidden_channels = hidden_channels
- self.filter_channels = filter_channels
- self.n_heads = n_heads
- self.n_layers = n_layers
- self.kernel_size = kernel_size
- self.p_dropout = p_dropout
- self.resblock = resblock
- self.resblock_kernel_sizes = resblock_kernel_sizes
- self.resblock_dilation_sizes = resblock_dilation_sizes
- self.upsample_rates = upsample_rates
- self.upsample_initial_channel = upsample_initial_channel
- self.upsample_kernel_sizes = upsample_kernel_sizes
- self.segment_size = segment_size
- self.gin_channels = gin_channels
- # self.hop_length = hop_length#
- self.spk_embed_dim = spk_embed_dim
- self.enc_p = TextEncoder768(
- inter_channels,
- hidden_channels,
- filter_channels,
- n_heads,
- n_layers,
- kernel_size,
- p_dropout,
- )
- self.dec = GeneratorNSF(
- inter_channels,
- resblock,
- resblock_kernel_sizes,
- resblock_dilation_sizes,
- upsample_rates,
- upsample_initial_channel,
- upsample_kernel_sizes,
- gin_channels=gin_channels,
- sr=sr,
- is_half=kwargs["is_half"],
- )
- self.enc_q = PosteriorEncoder(
- spec_channels,
- inter_channels,
- hidden_channels,
- 5,
- 1,
- 16,
- gin_channels=gin_channels,
- )
- self.flow = ResidualCouplingBlock(
- inter_channels, hidden_channels, 5, 1, 3, gin_channels=gin_channels
- )
- self.emb_g = nn.Embedding(self.spk_embed_dim, gin_channels)
- print("gin_channels:", gin_channels, "self.spk_embed_dim:", self.spk_embed_dim)
-
- def remove_weight_norm(self):
- self.dec.remove_weight_norm()
- self.flow.remove_weight_norm()
- self.enc_q.remove_weight_norm()
-
- def forward(
- self, phone, phone_lengths, pitch, pitchf, y, y_lengths, ds
- ): # 这里ds是id,[bs,1]
- # print(1,pitch.shape)#[bs,t]
- g = self.emb_g(ds).unsqueeze(-1) # [b, 256, 1]##1是t,广播的
- m_p, logs_p, x_mask = self.enc_p(phone, pitch, phone_lengths)
- z, m_q, logs_q, y_mask = self.enc_q(y, y_lengths, g=g)
- z_p = self.flow(z, y_mask, g=g)
- z_slice, ids_slice = commons.rand_slice_segments(
- z, y_lengths, self.segment_size
- )
- # print(-1,pitchf.shape,ids_slice,self.segment_size,self.hop_length,self.segment_size//self.hop_length)
- pitchf = commons.slice_segments2(pitchf, ids_slice, self.segment_size)
- # print(-2,pitchf.shape,z_slice.shape)
- o = self.dec(z_slice, pitchf, g=g)
- return o, ids_slice, x_mask, y_mask, (z, z_p, m_p, logs_p, m_q, logs_q)
-
- def infer(self, phone, phone_lengths, pitch, nsff0, sid, max_len=None):
- g = self.emb_g(sid).unsqueeze(-1)
- m_p, logs_p, x_mask = self.enc_p(phone, pitch, phone_lengths)
- z_p = (m_p + torch.exp(logs_p) * torch.randn_like(m_p) * 0.66666) * x_mask
- z = self.flow(z_p, x_mask, g=g, reverse=True)
- o = self.dec((z * x_mask)[:, :, :max_len], nsff0, g=g)
- return o, x_mask, (z, z_p, m_p, logs_p)
-
-
-class SynthesizerTrnMs256NSFsid_nono(nn.Module):
- def __init__(
- self,
- spec_channels,
- segment_size,
- inter_channels,
- hidden_channels,
- filter_channels,
- n_heads,
- n_layers,
- kernel_size,
- p_dropout,
- resblock,
- resblock_kernel_sizes,
- resblock_dilation_sizes,
- upsample_rates,
- upsample_initial_channel,
- upsample_kernel_sizes,
- spk_embed_dim,
- gin_channels,
- sr=None,
- **kwargs
- ):
- super().__init__()
- self.spec_channels = spec_channels
- self.inter_channels = inter_channels
- self.hidden_channels = hidden_channels
- self.filter_channels = filter_channels
- self.n_heads = n_heads
- self.n_layers = n_layers
- self.kernel_size = kernel_size
- self.p_dropout = p_dropout
- self.resblock = resblock
- self.resblock_kernel_sizes = resblock_kernel_sizes
- self.resblock_dilation_sizes = resblock_dilation_sizes
- self.upsample_rates = upsample_rates
- self.upsample_initial_channel = upsample_initial_channel
- self.upsample_kernel_sizes = upsample_kernel_sizes
- self.segment_size = segment_size
- self.gin_channels = gin_channels
- # self.hop_length = hop_length#
- self.spk_embed_dim = spk_embed_dim
- self.enc_p = TextEncoder256(
- inter_channels,
- hidden_channels,
- filter_channels,
- n_heads,
- n_layers,
- kernel_size,
- p_dropout,
- f0=False,
- )
- self.dec = Generator(
- inter_channels,
- resblock,
- resblock_kernel_sizes,
- resblock_dilation_sizes,
- upsample_rates,
- upsample_initial_channel,
- upsample_kernel_sizes,
- gin_channels=gin_channels,
- )
- self.enc_q = PosteriorEncoder(
- spec_channels,
- inter_channels,
- hidden_channels,
- 5,
- 1,
- 16,
- gin_channels=gin_channels,
- )
- self.flow = ResidualCouplingBlock(
- inter_channels, hidden_channels, 5, 1, 3, gin_channels=gin_channels
- )
- self.emb_g = nn.Embedding(self.spk_embed_dim, gin_channels)
- print("gin_channels:", gin_channels, "self.spk_embed_dim:", self.spk_embed_dim)
-
- def remove_weight_norm(self):
- self.dec.remove_weight_norm()
- self.flow.remove_weight_norm()
- self.enc_q.remove_weight_norm()
-
- def forward(self, phone, phone_lengths, y, y_lengths, ds): # 这里ds是id,[bs,1]
- g = self.emb_g(ds).unsqueeze(-1) # [b, 256, 1]##1是t,广播的
- m_p, logs_p, x_mask = self.enc_p(phone, None, phone_lengths)
- z, m_q, logs_q, y_mask = self.enc_q(y, y_lengths, g=g)
- z_p = self.flow(z, y_mask, g=g)
- z_slice, ids_slice = commons.rand_slice_segments(
- z, y_lengths, self.segment_size
- )
- o = self.dec(z_slice, g=g)
- return o, ids_slice, x_mask, y_mask, (z, z_p, m_p, logs_p, m_q, logs_q)
-
- def infer(self, phone, phone_lengths, sid, max_len=None):
- g = self.emb_g(sid).unsqueeze(-1)
- m_p, logs_p, x_mask = self.enc_p(phone, None, phone_lengths)
- z_p = (m_p + torch.exp(logs_p) * torch.randn_like(m_p) * 0.66666) * x_mask
- z = self.flow(z_p, x_mask, g=g, reverse=True)
- o = self.dec((z * x_mask)[:, :, :max_len], g=g)
- return o, x_mask, (z, z_p, m_p, logs_p)
-
-
-class SynthesizerTrnMs768NSFsid_nono(nn.Module):
- def __init__(
- self,
- spec_channels,
- segment_size,
- inter_channels,
- hidden_channels,
- filter_channels,
- n_heads,
- n_layers,
- kernel_size,
- p_dropout,
- resblock,
- resblock_kernel_sizes,
- resblock_dilation_sizes,
- upsample_rates,
- upsample_initial_channel,
- upsample_kernel_sizes,
- spk_embed_dim,
- gin_channels,
- sr=None,
- **kwargs
- ):
- super().__init__()
- self.spec_channels = spec_channels
- self.inter_channels = inter_channels
- self.hidden_channels = hidden_channels
- self.filter_channels = filter_channels
- self.n_heads = n_heads
- self.n_layers = n_layers
- self.kernel_size = kernel_size
- self.p_dropout = p_dropout
- self.resblock = resblock
- self.resblock_kernel_sizes = resblock_kernel_sizes
- self.resblock_dilation_sizes = resblock_dilation_sizes
- self.upsample_rates = upsample_rates
- self.upsample_initial_channel = upsample_initial_channel
- self.upsample_kernel_sizes = upsample_kernel_sizes
- self.segment_size = segment_size
- self.gin_channels = gin_channels
- # self.hop_length = hop_length#
- self.spk_embed_dim = spk_embed_dim
- self.enc_p = TextEncoder768(
- inter_channels,
- hidden_channels,
- filter_channels,
- n_heads,
- n_layers,
- kernel_size,
- p_dropout,
- f0=False,
- )
- self.dec = Generator(
- inter_channels,
- resblock,
- resblock_kernel_sizes,
- resblock_dilation_sizes,
- upsample_rates,
- upsample_initial_channel,
- upsample_kernel_sizes,
- gin_channels=gin_channels,
- )
- self.enc_q = PosteriorEncoder(
- spec_channels,
- inter_channels,
- hidden_channels,
- 5,
- 1,
- 16,
- gin_channels=gin_channels,
- )
- self.flow = ResidualCouplingBlock(
- inter_channels, hidden_channels, 5, 1, 3, gin_channels=gin_channels
- )
- self.emb_g = nn.Embedding(self.spk_embed_dim, gin_channels)
- print("gin_channels:", gin_channels, "self.spk_embed_dim:", self.spk_embed_dim)
-
- def remove_weight_norm(self):
- self.dec.remove_weight_norm()
- self.flow.remove_weight_norm()
- self.enc_q.remove_weight_norm()
-
- def forward(self, phone, phone_lengths, y, y_lengths, ds): # 这里ds是id,[bs,1]
- g = self.emb_g(ds).unsqueeze(-1) # [b, 256, 1]##1是t,广播的
- m_p, logs_p, x_mask = self.enc_p(phone, None, phone_lengths)
- z, m_q, logs_q, y_mask = self.enc_q(y, y_lengths, g=g)
- z_p = self.flow(z, y_mask, g=g)
- z_slice, ids_slice = commons.rand_slice_segments(
- z, y_lengths, self.segment_size
- )
- o = self.dec(z_slice, g=g)
- return o, ids_slice, x_mask, y_mask, (z, z_p, m_p, logs_p, m_q, logs_q)
-
- def infer(self, phone, phone_lengths, sid, max_len=None):
- g = self.emb_g(sid).unsqueeze(-1)
- m_p, logs_p, x_mask = self.enc_p(phone, None, phone_lengths)
- z_p = (m_p + torch.exp(logs_p) * torch.randn_like(m_p) * 0.66666) * x_mask
- z = self.flow(z_p, x_mask, g=g, reverse=True)
- o = self.dec((z * x_mask)[:, :, :max_len], g=g)
- return o, x_mask, (z, z_p, m_p, logs_p)
-
-
-class MultiPeriodDiscriminator(torch.nn.Module):
- def __init__(self, use_spectral_norm=False):
- super(MultiPeriodDiscriminator, self).__init__()
- periods = [2, 3, 5, 7, 11, 17]
- # periods = [3, 5, 7, 11, 17, 23, 37]
-
- discs = [DiscriminatorS(use_spectral_norm=use_spectral_norm)]
- discs = discs + [
- DiscriminatorP(i, use_spectral_norm=use_spectral_norm) for i in periods
- ]
- self.discriminators = nn.ModuleList(discs)
-
- def forward(self, y, y_hat):
- y_d_rs = [] #
- y_d_gs = []
- fmap_rs = []
- fmap_gs = []
- for i, d in enumerate(self.discriminators):
- y_d_r, fmap_r = d(y)
- y_d_g, fmap_g = d(y_hat)
- # for j in range(len(fmap_r)):
- # print(i,j,y.shape,y_hat.shape,fmap_r[j].shape,fmap_g[j].shape)
- y_d_rs.append(y_d_r)
- y_d_gs.append(y_d_g)
- fmap_rs.append(fmap_r)
- fmap_gs.append(fmap_g)
-
- return y_d_rs, y_d_gs, fmap_rs, fmap_gs
-
-
-class MultiPeriodDiscriminatorV2(torch.nn.Module):
- def __init__(self, use_spectral_norm=False):
- super(MultiPeriodDiscriminatorV2, self).__init__()
- # periods = [2, 3, 5, 7, 11, 17]
- periods = [2, 3, 5, 7, 11, 17, 23, 37]
-
- discs = [DiscriminatorS(use_spectral_norm=use_spectral_norm)]
- discs = discs + [
- DiscriminatorP(i, use_spectral_norm=use_spectral_norm) for i in periods
- ]
- self.discriminators = nn.ModuleList(discs)
-
- def forward(self, y, y_hat):
- y_d_rs = [] #
- y_d_gs = []
- fmap_rs = []
- fmap_gs = []
- for i, d in enumerate(self.discriminators):
- y_d_r, fmap_r = d(y)
- y_d_g, fmap_g = d(y_hat)
- # for j in range(len(fmap_r)):
- # print(i,j,y.shape,y_hat.shape,fmap_r[j].shape,fmap_g[j].shape)
- y_d_rs.append(y_d_r)
- y_d_gs.append(y_d_g)
- fmap_rs.append(fmap_r)
- fmap_gs.append(fmap_g)
-
- return y_d_rs, y_d_gs, fmap_rs, fmap_gs
-
-
-class DiscriminatorS(torch.nn.Module):
- def __init__(self, use_spectral_norm=False):
- super(DiscriminatorS, self).__init__()
- norm_f = weight_norm if use_spectral_norm == False else spectral_norm
- self.convs = nn.ModuleList(
- [
- norm_f(Conv1d(1, 16, 15, 1, padding=7)),
- norm_f(Conv1d(16, 64, 41, 4, groups=4, padding=20)),
- norm_f(Conv1d(64, 256, 41, 4, groups=16, padding=20)),
- norm_f(Conv1d(256, 1024, 41, 4, groups=64, padding=20)),
- norm_f(Conv1d(1024, 1024, 41, 4, groups=256, padding=20)),
- norm_f(Conv1d(1024, 1024, 5, 1, padding=2)),
- ]
- )
- self.conv_post = norm_f(Conv1d(1024, 1, 3, 1, padding=1))
-
- def forward(self, x):
- fmap = []
-
- for l in self.convs:
- x = l(x)
- x = F.leaky_relu(x, modules.LRELU_SLOPE)
- fmap.append(x)
- x = self.conv_post(x)
- fmap.append(x)
- x = torch.flatten(x, 1, -1)
-
- return x, fmap
-
-
-class DiscriminatorP(torch.nn.Module):
- def __init__(self, period, kernel_size=5, stride=3, use_spectral_norm=False):
- super(DiscriminatorP, self).__init__()
- self.period = period
- self.use_spectral_norm = use_spectral_norm
- norm_f = weight_norm if use_spectral_norm == False else spectral_norm
- self.convs = nn.ModuleList(
- [
- norm_f(
- Conv2d(
- 1,
- 32,
- (kernel_size, 1),
- (stride, 1),
- padding=(get_padding(kernel_size, 1), 0),
- )
- ),
- norm_f(
- Conv2d(
- 32,
- 128,
- (kernel_size, 1),
- (stride, 1),
- padding=(get_padding(kernel_size, 1), 0),
- )
- ),
- norm_f(
- Conv2d(
- 128,
- 512,
- (kernel_size, 1),
- (stride, 1),
- padding=(get_padding(kernel_size, 1), 0),
- )
- ),
- norm_f(
- Conv2d(
- 512,
- 1024,
- (kernel_size, 1),
- (stride, 1),
- padding=(get_padding(kernel_size, 1), 0),
- )
- ),
- norm_f(
- Conv2d(
- 1024,
- 1024,
- (kernel_size, 1),
- 1,
- padding=(get_padding(kernel_size, 1), 0),
- )
- ),
- ]
- )
- self.conv_post = norm_f(Conv2d(1024, 1, (3, 1), 1, padding=(1, 0)))
-
- def forward(self, x):
- fmap = []
-
- # 1d to 2d
- b, c, t = x.shape
- if t % self.period != 0: # pad first
- n_pad = self.period - (t % self.period)
- x = F.pad(x, (0, n_pad), "reflect")
- t = t + n_pad
- x = x.view(b, c, t // self.period, self.period)
-
- for l in self.convs:
- x = l(x)
- x = F.leaky_relu(x, modules.LRELU_SLOPE)
- fmap.append(x)
- x = self.conv_post(x)
- fmap.append(x)
- x = torch.flatten(x, 1, -1)
-
- return x, fmap
\ No newline at end of file
diff --git a/spaces/lincquiQcaudo/Top-20-Diffusion/CorelDraw X7 Direto Da Corel 32 E 64Bits Ativador E Tutorial Keygen LINK.md b/spaces/lincquiQcaudo/Top-20-Diffusion/CorelDraw X7 Direto Da Corel 32 E 64Bits Ativador E Tutorial Keygen LINK.md
deleted file mode 100644
index c4db92899c2f22b2e031aff6f5468950efb9715b..0000000000000000000000000000000000000000
--- a/spaces/lincquiQcaudo/Top-20-Diffusion/CorelDraw X7 Direto Da Corel 32 E 64Bits Ativador E Tutorial Keygen LINK.md
+++ /dev/null
@@ -1,33 +0,0 @@
-
-
Granny Simulator Full Version Free: A Wacky and Violent Simulation Game
-
If you are looking for a simple but hilarious and rude simulation game, then you might want to try Granny Simulator full version free. This game is developed and published by Nick Kestle, and it features a crazy showdown between an old grandmother and her brutal grandson. You can play as either the granny or the grandson, and your objective is to either survive or kill the other using various weapons and items. You can also play with your friends and family in multiplayer mode, or enjoy the game solo.
-
What is Granny Simulator Full Version Free About?
-
In Granny Simulator full version free, you can choose to play as one of the two characters: the slow-moving but powerful granny, or the energetic but fragile grandson. The game takes place in a large two-story house with a yard and multiple secret rooms. The granny's goal is to complete all of her daily tasks while trying to stay alive from the grandson's attacks. The grandson's goal is to kill his grandma using various collectible objects that he can find or unlock in the house.
-
CorelDraw X7 Direto da Corel 32 e 64Bits ativador e tutorial keygen
The game offers both single-player and multiplayer modes, so you can enjoy it alone or with others. In single-player mode, you play as the grandson by default, and you have to face a computer-controlled granny. In multiplayer mode, you can either play online with other players, or host a game with your friends and family. You can also choose to be a duplicate character, so there can be more than one granny or grandson in the game.
-
What are the Features of Granny Simulator Full Version Free?
-
Granny Simulator full version free is a fun and funny game that will keep you entertained for hours. Some of the features that make this game enjoyable are:
-
-
Weapons: The game has a variety of weapons and items that you can use to attack or defend yourself. As the grandson, you can pick up things like bottles, hammers, grenades, and tasers, and throw or use them on your granny. You can also find keys and open hidden chests to get better weapons, such as a mace or a crossbow. As the granny, you can also pick up items, but your main weapon is your powerful kick that will send your grandson flying.
-
Controls: The game has easy and simple controls that anyone can learn quickly. You can use your mouse and keyboard to move around, pick up items, throw them, or use them. You can also use your mouse wheel to zoom in or out of the camera.
-
Maps and Modes: The game has one map that is spacious and detailed. You can explore different rooms, find hidden places, and interact with various objects. The game also has four modes that are different from each other: First Level, Second Level, H'ween Level (Halloween map), and Sandbox Mode (where you can do whatever you want).
-
Skins: The game allows you to unlock some cool skins for your characters as you play. You can change the appearance of your granny or grandson to make them look more unique.
-
Graphics: The game has charming 3D graphics that add to the silly atmosphere of the game. The characters look cartoonish and funny, and the animations are smooth and realistic. The game also has some glitches and bugs that make it more hilarious.
-
-
How to Download Granny Simulator Full Version Free?
-
If you want to download Granny Simulator full version free, you have several options. You can either download it from Softonic.com, where you can get it for Windows PC for a paid price. You can also download it from GamingBeasts.com, where you can get it for free for PC. Alternatively, you can download it from APKCombo.com, where you can get it for free for Android devices. You can also play it on PC with MuMu Player, which is an emulator that lets you play Android games on PC with better graphics and controls.
-
Conclusion
-
Granny Simulator full version free is a hilarious simulation game that will make you laugh out loud with its wacky violence and humor. You can play as either the granny or the grandson, and try to kill or survive each other using various weapons and items. You can also play with your friends and family in multiplayer mode, or enjoy the game solo. The game has a lot of features that make it fun and entertaining, such as weapons, controls, maps, modes, skins, and graphics. If you are looking for a simple but rude and funny game, then you should try Granny Simulator full version free.
-
How to Play Granny Simulator Full Version Free?
-
Playing Granny Simulator full version free is easy and fun. You just need to download and install the game on your device, whether it is a PC or an Android phone. You can also use an emulator like MuMu Player to play the game on PC with better graphics and controls. Once you have the game installed, you can launch it and choose your mode and character.
-
-
If you play as the granny, you will have to complete your daily tasks while avoiding your grandson's attacks. You can use your mouse and keyboard to move around, pick up items, throw them, or use them. You can also use your mouse wheel to zoom in or out of the camera. Your tasks will be shown on the top left corner of the screen, and they will vary depending on the mode you choose. Some of the tasks include cooking, cleaning, gardening, knitting, and more.
-
If you play as the grandson, you will have to kill your granny using various weapons and items that you can find or unlock in the house. You can use your mouse and keyboard to move around, pick up items, throw them, or use them. You can also use your mouse wheel to zoom in or out of the camera. Your health will be shown on the top right corner of the screen, and it will decrease if you get hit by your granny or other objects. Some of the weapons include bottles, hammers, grenades, tasers, maces, crossbows, and more.
-
Why Should You Play Granny Simulator Full Version Free?
-
Granny Simulator full version free is a game that will make you laugh and have fun with its wacky and violent gameplay. You can play it alone or with your friends and family in multiplayer mode, and enjoy the hilarious combat between an old lady and her brutal toddler. You can also explore the spacious and detailed map, find hidden places and items, and unlock cool skins for your characters.
-
Granny Simulator full version free is a game that will challenge your skills and creativity as well. You will have to use different strategies and tactics to win as either the granny or the grandson. You will also have to use different weapons and items depending on the situation and your preference. You can also customize your characters with different skins to make them look more unique.
-
Granny Simulator full version free is a game that will entertain you for hours with its simple but rude concept. It is a game that will make you appreciate your grandmother more, or maybe not. It is a game that will make you wonder what kind of relationship these two characters have. It is a game that will make you question your sanity as you enjoy killing or surviving each other with wacky violence.
-
Conclusion
-
Granny Simulator full version free is a hilarious simulation game that you should try if you are looking for a simple but rude and funny game. You can play as either the granny or the grandson, and try to kill or survive each other using various weapons and items. You can also play with your friends and family in multiplayer mode, or enjoy the game solo. The game has a lot of features that make it fun and entertaining, such as weapons, controls, maps, modes, skins, and graphics. If you want to download Granny Simulator full version free, you have several options depending on your device and preference. You can get it from Softonic.com for Windows PC for a paid price, from GamingBeasts.com for PC for free, from APKCombo.com for Android devices for free, or from MuMu Player for PC with better graphics and controls for free.
3cee63e6c2
-
-
\ No newline at end of file
diff --git a/spaces/lincquiQcaudo/Top-20-Diffusion/Flamingo Nxt Crack REPACK.md b/spaces/lincquiQcaudo/Top-20-Diffusion/Flamingo Nxt Crack REPACK.md
deleted file mode 100644
index 5299727deccc1a4f59d328237c1fdeddacc559a9..0000000000000000000000000000000000000000
--- a/spaces/lincquiQcaudo/Top-20-Diffusion/Flamingo Nxt Crack REPACK.md
+++ /dev/null
@@ -1,82 +0,0 @@
-## Flamingo Nxt Crack
-
-
-
-
-
-
-
-
-
-**Download === [https://fienislile.blogspot.com/?download=2txyTt](https://fienislile.blogspot.com/?download=2txyTt)**
-
-
-
-
-
-
-
-
-
-
-
- I can try to write a title and an article with SEO optimization and HTML formatting for the keyword "Flamingo Nxt Crack". Here is what I came up with:
-
-# How to Download Flamingo Nxt Crack for Free
-
-
-
-Flamingo Nxt is a powerful rendering software that allows you to create photorealistic images and animations from your 3D models. It is compatible with Rhino, SketchUp, Revit, and other popular CAD programs. Flamingo Nxt is not cheap, however. The official price is $495 for a single-user license. If you are looking for a way to get Flamingo Nxt for free, you might be tempted to download a cracked version from the internet. But is it worth it?
-
-
-
-In this article, we will explain why downloading Flamingo Nxt crack is a bad idea, and what are the risks and consequences of doing so. We will also show you some legal and safe alternatives to Flamingo Nxt crack that you can use without breaking the law or compromising your security.
-
-
-
-## Why You Should Avoid Flamingo Nxt Crack
-
-
-
-Downloading Flamingo Nxt crack might seem like a good way to save money and get access to a premium software. However, there are many reasons why you should avoid it at all costs. Here are some of them:
-
-
-
-- **It is illegal.** Flamingo Nxt is a copyrighted software that is protected by intellectual property laws. Downloading, installing, or using a cracked version of Flamingo Nxt is a violation of these laws and can result in legal action against you. You could face fines, lawsuits, or even jail time for piracy.
-
-- **It is unsafe.** Flamingo Nxt crack is not an official product of the developer, but a modified version created by hackers or cybercriminals. These people often hide malware, viruses, spyware, ransomware, or other malicious programs inside the crack files. These programs can infect your computer, steal your personal information, damage your files, encrypt your data, or even take over your system.
-
-- **It is unreliable.** Flamingo Nxt crack is not guaranteed to work properly or at all. It might have bugs, errors, glitches, or compatibility issues that can affect the quality and performance of your rendering projects. It might also stop working after an update or a system change. You will not be able to get any technical support or customer service from the developer if you encounter any problems with Flamingo Nxt crack.
-
-- **It is unethical.** Flamingo Nxt is a product of hard work and innovation by the developer and its team. By downloading Flamingo Nxt crack, you are depriving them of their rightful income and recognition. You are also hurting the software industry and discouraging future development and improvement of Flamingo Nxt and other similar products.
-
-
-
-## How to Get Flamingo Nxt Legally and Safely
-
-
-
-If you want to use Flamingo Nxt for your rendering projects, you should get it legally and safely from the official website or an authorized reseller. This way, you will enjoy the following benefits:
-
-
-
-- **You will get the latest and most updated version of Flamingo Nxt.** You will be able to access all the features and functions of Flamingo Nxt without any limitations or restrictions. You will also be able to receive regular updates and patches that will fix any bugs or issues and improve the performance and stability of Flamingo Nxt.
-
-- **You will get technical support and customer service from the developer.** If you have any questions, problems, or feedback regarding Flamingo Nxt, you will be able to contact the developer directly and get professional assistance and guidance. You will also be able to access online resources such as tutorials, manuals, forums, blogs, and videos that will help you learn how to use Flamingo Nxt effectively and efficiently.
-
-- **You will respect the law and the developer.** By purchasing Flamingo Nxt legally and safely, you will comply with the intellectual property laws and avoid any legal troubles or penalties. You will also support the developer financially and morally and encourage them to continue developing and improving Flamingo Nxt and other similar products.
-
-
-
-## What Are Some Alternatives to Flamingo Nxt?
-
-
-
-If you are looking for some alternatives to Flamingo Nxt
-
- dfd1c89656
-
-
-
-
-
diff --git a/spaces/lincquiQcaudo/Top-20-Diffusion/Hazrat Umar Farooq Movie In Urdu Free 124 EXCLUSIVE.md b/spaces/lincquiQcaudo/Top-20-Diffusion/Hazrat Umar Farooq Movie In Urdu Free 124 EXCLUSIVE.md
deleted file mode 100644
index ea5b7d62f3bb1f75d93ae89eba1f0657616b500b..0000000000000000000000000000000000000000
--- a/spaces/lincquiQcaudo/Top-20-Diffusion/Hazrat Umar Farooq Movie In Urdu Free 124 EXCLUSIVE.md
+++ /dev/null
@@ -1,16 +0,0 @@
-
-
-hazrat umar farooq movie in urdu 124/06 .
-2015 .
-hazrat umar farooq movie in urdu 123/06 .
-hazrat umar farooq movie in urdu 122/06 .
-hazrat umnaya farooq movie in urdu 119/06 .
-hazrat umka farooq movie in urdu 114/07 .
-hazrat umka farooq movie in urdu 111/07 .
-hazrat umka farooq movie in urdu 110/07 .
-hazrat umka farooq movie in urdu 109/07 .
-hazrat umka farooq movie in urdu 108/07 .
-hazrat umka 8a78ff9644
-
-
-
diff --git a/spaces/lincquiQcaudo/Top-20-Diffusion/Joggers Park Mp4 Download Movie ((TOP)).md b/spaces/lincquiQcaudo/Top-20-Diffusion/Joggers Park Mp4 Download Movie ((TOP)).md
deleted file mode 100644
index 4ea6ac6af95af0dfce258a5dcb058a4e7c4b8db2..0000000000000000000000000000000000000000
--- a/spaces/lincquiQcaudo/Top-20-Diffusion/Joggers Park Mp4 Download Movie ((TOP)).md
+++ /dev/null
@@ -1,6 +0,0 @@
-
Search results that reflect historic inequities can amplify stereotypes and perpetuate under-representation. Carefully measuring diversity in data sets can help.
-
-
-
Search, ranking and recommendation systems can help find useful documents in large datasets. However, these datasets reflect the biases of the society in which they were created and the systems risk re-entrenching those biases. For example, if someone who is not a white man searches for “CEO pictures” and sees a page of white men, they may feel that only white men can be CEOs, further perpetuating lack of representation at companies’ executive levels.
The mathematics of all this is a little easier to follow with abstract shapes. Let’s take a look at some of them:
-
-
-
Suppose we want to return about 30% green boxes to reflect the distribution of some larger universe of shapes. Try clicking on the shapes below to select some of them — can you find a better subset to return?
-
-
-
Another diversity metric we care about is the percentage of dots… how close to 35% dots can you get?
-
-
-
If we can only return a single subset, how should we consider multiple diversity metrics? Sometimes it isn’t possible to reduce the difference of every metric to zero. One natural approach: find the selection with the lowest mean difference across all the metrics to get as close as possible to all the targets.
-
In other circumstances, like picking a panel of speakers, avoiding badly representing any single category might be more important. This can be done by finding the subset with the lowest max difference. Try minimizing both below:
-
-
-
Notice that minimizing the mean results in a different subset than minimizing the max; how else might using one over the other change the results?
-
Ranking Measures
-
We can pull out more detail by showing how the mean difference and maximum difference rank lots of sets. Below, there are 20 sets of 10 shapes sorted by the two measures. Try adjusting the target slider on the left to see how the rankings change; each set’s percentage of green, dots and small shapes are shown in the small histograms.
-
-
-
At the extremes, the choice of measure can have a big impact: if we want to try and return all green results, we can shift the green target up to 100%. With this target, the minimum difference basically sorts the sets by the number of green items and uses the other targets as a tiebreaker. In contrast, sorting by the mean difference balances the green target more with the dot and small targets.
-
-
-
Beyond mean and max differences, there are more ways to combine diversity metrics, like taking the cross of two metrics to account for intersectionality. The absolute value of the difference in target and actual percentages can also be quantified in other ways — you might want to penalize undershooting more than overshooting, for example. It’s important to keep in mind what exactly you’re trying to maximize and the dataset that you’re operating on.
-
Which Measure is Best?
-
In a vacuum, all of these ranking methods are defensible. Picking one requires knowledge of the dataset and broader societal context.
-
For example, the doctors on the left have more variance along the shirt color attribute, but they’re less diverse by gender than the doctors on the right. With the shirt color and gender targets we’ve picked, the two subsets have the same mean and max differences However, in most applications, it’s more important to have a representative sample of socially relevant characteristics, like gender, rather than something less salient, like clothing color.
-
-
-
Just selecting a diverse sample isn’t sufficient either. Diversity and Inclusion Metrics in Subset Selection introduces a way of measuring “inclusion” - how well does the searcher feel represented in the results?
-
Below, we have gender diversity, without inclusion for women, in the “construction worker” image domain. Masculine-presenting individuals are shown in realistic, modern construction worker situations, while feminine-presenting individuals and other gender presentations are depicted as historic nostalgia, toys, clipart, or passive.
-
-
-
The context of the query and the searcher also plays in the quality of search results. A search for “work clothing” that shows a mixed palette of colors for men’s clothing and only pink women’s clothing might make the searcher feel that women need to appear stereotypically feminine in a professional setting. But the same set of women’s clothes might be appropriate to show for a “pink women work clothes” search or if the searcher had previously expressed a preference for pink.
-
We saw how a small switch from mean to max made a huge difference in what abstract shapes are returned – and how things can get even more complex when socially salient characteristics are layered in. Defaults and small decisions can encode our priorities and values; intentionally thinking about how diversity and inclusion are being measured and which characteristics are emphasized is a step towards designing more equitable systems.
-
More Reading
-
The Diversity and Inclusion Metrics paper has a Colab with a detailed desciption of the metrics, additional visualizations and a reference Python implementation.
Inferring user preferences is also tricky; you can checkout ways to design for user feedback and control over queries in the People + AI Guidebook.
-
Credits
-
Adam Pearce, Dylan Baker, Ellen Jiang, Meg Mitchell* and Timnit Gebru* // March 2021
-
*Work done while at Google
-
Thanks to Alex Hanna, Carey Radebaugh, Emily Denton, Fernanda Viégas, James Wexler, Jess Holbrook, Ludovic Peran, Martin Wattenberg, Michael Terry, Yannick Assogba and Zan Armstrong for their help with this piece.
Monkeyâs Audio v4.56 (APE ): A Lossless Audio Compression Tool
-
If you are looking for a way to reduce the size of your audio files without compromising the quality, you might want to try Monkeyâs Audio v4.56 (APE ), a free and open-source software that can compress and decompress audio files using the APE format.
-
Monkeyâs Audio v4.56 (APE ) is a lossless audio compression tool, which means that it preserves the original sound data exactly as it was recorded, unlike lossy formats such as MP3 or AAC that discard some information to save space. This means that you can enjoy your music in its full fidelity, as if you were listening to the original CD or vinyl.
Monkeyâs Audio v4.56 (APE ) is also fast and efficient, as it can compress and decompress audio files at high speeds, using multiple CPU cores if available. It also supports various features such as ID3 tags, APE tags, cue sheets, playlists, and more. You can use Monkeyâs Audio v4.56 (APE ) as a standalone program or as a plug-in for popular media players such as Winamp, Foobar2000, or MediaMonkey.
-
To use Monkeyâs Audio v4.56 (APE ), you need to download and install the software from its official website: https://www.monkeysaudio.com/. Then, you can either drag and drop your audio files to the program window or use the right-click menu to convert them to or from APE format. You can also adjust the compression level from fast to insane, depending on your preference and disk space.
-
Monkeyâs Audio v4.56 (APE ) is a great tool for audiophiles who want to store their music collection in a smaller size without losing any quality. It is compatible with Windows XP, Vista, 7, 8, 10, and Linux (with Wine). It is also free and open-source, so you can modify it or contribute to its development if you wish.
-
-
One of the advantages of APE format is that it has a high compression ratio, meaning that it can reduce the size of audio files significantly without affecting the quality. For example, a typical CD-quality WAV file can be compressed to about 40% of its original size using APE format, while still retaining the same sound quality. This can save you a lot of disk space and bandwidth, especially if you have a large music library.
-
Another benefit of APE format is that it is compatible with many media players and devices, as long as they support the APE codec. You can play APE files on your computer using various software such as Winamp, Foobar2000, MediaMonkey, VLC, or PotPlayer. You can also play APE files on your smartphone or tablet using apps such as Poweramp, Neutron, or JetAudio. Some portable music players such as Fiio or Cowon also support APE format.
-
However, APE format also has some drawbacks that you should be aware of before using it. One of them is that it is not widely supported by online streaming services or websites, such as Spotify, YouTube, or SoundCloud. This means that you might not be able to upload or share your APE files online easily. Another drawback is that APE files are larger than lossy formats such as MP3 or AAC, which might be an issue if you have limited storage space or data plan.
cec2833e83
-
-
\ No newline at end of file
diff --git a/spaces/neural-ti/NeTI/sd_pipeline_call.py b/spaces/neural-ti/NeTI/sd_pipeline_call.py
deleted file mode 100644
index 91a7d901012ee6813e7494c895b0b1354adb0811..0000000000000000000000000000000000000000
--- a/spaces/neural-ti/NeTI/sd_pipeline_call.py
+++ /dev/null
@@ -1,146 +0,0 @@
-from typing import Any, Callable, Dict, List, Optional, Union
-
-import torch
-from diffusers.pipelines.stable_diffusion import StableDiffusionPipelineOutput, StableDiffusionPipeline
-
-
-@torch.no_grad()
-def sd_pipeline_call(
- pipeline: StableDiffusionPipeline,
- prompt_embeds: torch.FloatTensor,
- height: Optional[int] = None,
- width: Optional[int] = None,
- num_inference_steps: int = 50,
- guidance_scale: float = 7.5,
- negative_prompt: Optional[Union[str, List[str]]] = None,
- num_images_per_prompt: Optional[int] = 1,
- eta: float = 0.0,
- generator: Optional[Union[torch.Generator, List[torch.Generator]]] = None,
- latents: Optional[torch.FloatTensor] = None,
- output_type: Optional[str] = "pil",
- return_dict: bool = True,
- callback: Optional[Callable[[int, int, torch.FloatTensor], None]] = None,
- callback_steps: int = 1,
- cross_attention_kwargs: Optional[Dict[str, Any]] = None):
- """ Modification of the standard SD pipeline call to support NeTI embeddings passed with prompt_embeds argument."""
-
- # 0. Default height and width to unet
- height = height or pipeline.unet.config.sample_size * pipeline.vae_scale_factor
- width = width or pipeline.unet.config.sample_size * pipeline.vae_scale_factor
-
- # 2. Define call parameters
- batch_size = 1
- device = pipeline._execution_device
-
- neg_prompt = get_neg_prompt_input_ids(pipeline, negative_prompt)
- negative_prompt_embeds, _ = pipeline.text_encoder(
- input_ids=neg_prompt.input_ids.to(device),
- attention_mask=None,
- )
- negative_prompt_embeds = negative_prompt_embeds[0]
-
- # here `guidance_scale` is defined analog to the guidance weight `w` of equation (2)
- # of the Imagen paper: https://arxiv.org/pdf/2205.11487.pdf . `guidance_scale = 1`
- # corresponds to doing no classifier free guidance.
- do_classifier_free_guidance = guidance_scale > 1.0
-
- # 4. Prepare timesteps
- pipeline.scheduler.set_timesteps(num_inference_steps, device=device)
- timesteps = pipeline.scheduler.timesteps
-
- # 5. Prepare latent variables
- num_channels_latents = pipeline.unet.in_channels
- latents = pipeline.prepare_latents(
- batch_size * num_images_per_prompt,
- num_channels_latents,
- height,
- width,
- pipeline.text_encoder.dtype,
- device,
- generator,
- latents,
- )
-
- # 6. Prepare extra step kwargs.
- extra_step_kwargs = pipeline.prepare_extra_step_kwargs(generator, eta)
-
- # 7. Denoising loop
- num_warmup_steps = len(timesteps) - num_inference_steps * pipeline.scheduler.order
- with pipeline.progress_bar(total=num_inference_steps) as progress_bar:
- for i, t in enumerate(timesteps):
-
- if do_classifier_free_guidance:
- latent_model_input = latents
- latent_model_input = pipeline.scheduler.scale_model_input(latent_model_input, t)
-
- # predict the noise residual
- noise_pred_uncond = pipeline.unet(
- latent_model_input,
- t,
- encoder_hidden_states=negative_prompt_embeds.repeat(num_images_per_prompt, 1, 1),
- cross_attention_kwargs=cross_attention_kwargs,
- ).sample
-
- ###############################################################
- # NeTI logic: use the prompt embedding for the current timestep
- ###############################################################
- embed = prompt_embeds[i] if type(prompt_embeds) == list else prompt_embeds
- noise_pred_text = pipeline.unet(
- latent_model_input,
- t,
- encoder_hidden_states=embed,
- cross_attention_kwargs=cross_attention_kwargs,
- ).sample
-
- # perform guidance
- if do_classifier_free_guidance:
- noise_pred = noise_pred_uncond + guidance_scale * (noise_pred_text - noise_pred_uncond)
-
- # compute the previous noisy sample x_t -> x_t-1
- latents = pipeline.scheduler.step(noise_pred, t, latents, **extra_step_kwargs).prev_sample
-
- # call the callback, if provided
- if i == len(timesteps) - 1 or ((i + 1) > num_warmup_steps and (i + 1) % pipeline.scheduler.order == 0):
- progress_bar.update()
- if callback is not None and i % callback_steps == 0:
- callback(i, t, latents)
-
- if output_type == "latent":
- image = latents
- has_nsfw_concept = None
- elif output_type == "pil":
- # 8. Post-processing
- image = pipeline.decode_latents(latents)
- # 9. Run safety checker
- image, has_nsfw_concept = pipeline.run_safety_checker(image, device, pipeline.text_encoder.dtype)
- # 10. Convert to PIL
- image = pipeline.numpy_to_pil(image)
- else:
- # 8. Post-processing
- image = pipeline.decode_latents(latents)
- # 9. Run safety checker
- image, has_nsfw_concept = pipeline.run_safety_checker(image, device, pipeline.text_encoder.dtype)
-
- # Offload last model to CPU
- if hasattr(pipeline, "final_offload_hook") and pipeline.final_offload_hook is not None:
- pipeline.final_offload_hook.offload()
-
- if not return_dict:
- return image, has_nsfw_concept
-
- return StableDiffusionPipelineOutput(images=image, nsfw_content_detected=has_nsfw_concept)
-
-
-def get_neg_prompt_input_ids(pipeline: StableDiffusionPipeline,
- negative_prompt: Optional[Union[str, List[str]]] = None):
- if negative_prompt is None:
- negative_prompt = ""
- uncond_tokens = [negative_prompt] if isinstance(negative_prompt, str) else negative_prompt
- uncond_input = pipeline.tokenizer(
- uncond_tokens,
- padding="max_length",
- max_length=pipeline.tokenizer.model_max_length,
- truncation=True,
- return_tensors="pt",
- )
- return uncond_input
diff --git a/spaces/nihaldsouza1/clearlydefined_license_summarizer/src/diff.py b/spaces/nihaldsouza1/clearlydefined_license_summarizer/src/diff.py
deleted file mode 100644
index 1a360a7d3c26052906b121f861b92988c83a7c6b..0000000000000000000000000000000000000000
--- a/spaces/nihaldsouza1/clearlydefined_license_summarizer/src/diff.py
+++ /dev/null
@@ -1,70 +0,0 @@
-import difflib as dl
-import re
-
-try:
- from src.parameters import color
-except:
- from parameters import color
-
-
-def strike(text):
- '''
- Adds strikesthrough the given text
-
- Parameters
- ----------
- text : str
- String to strikethrough
-
- Returns
- -------
- content : str
- Strikethrough text
- '''
- result = ''
- for c in text:
- result = result + c + '\u0336'
- return result
-
-
-def strikethrough_diff(original_license_text, modified_license_text):
- '''
- Compares the two strings and strikes through all words/characters that exist in the original text
- and not in input text
-
- Parameters
- ----------
- original_license_text : str
- The text to compare it to. This is usually the official license text
-
- modified_license_text : str
- The text that is being compared with. This is usually the modified license text
-
- Returns
- -------
- content : str
- The strings with the uncommon words/characters strikethroughed
- '''
- original_license_text = original_license_text.replace("\n\n", " __para_break__ ")
- modified_license_text = modified_license_text.replace("\n\n", " __para_break__ ")
- original_license_tokens = re.split(" ", original_license_text.strip())
- modified_license_tokens = re.split(" ", modified_license_text.strip())
-
- processed_license_word_list = []
-
- for diff in dl.ndiff(original_license_tokens, modified_license_tokens):
- if diff.strip().endswith('__para_break__'):
- processed_license_word_list.append("\n\n")
- elif diff == "- ":
- processed_license_word_list.append((diff[2:] + ""))
- elif diff.startswith('- '):
- processed_license_word_list.append(f"""{strike(diff.strip("- "))}""")
- elif diff == "+ ":
- processed_license_word_list.append((diff[2:] + ""))
- elif diff.startswith("+ "):
- processed_license_word_list.append( f"""{diff.strip("+ ")}""")
- elif diff.startswith("? "):
- continue
- else:
- processed_license_word_list.append((diff[2:] + ""))
- return " ".join(processed_license_word_list).replace(" __para_break__ ", "\n\n")
diff --git a/spaces/nikansh/hamyar_riazi/README.md b/spaces/nikansh/hamyar_riazi/README.md
deleted file mode 100644
index 02ecbb4023af0791b39d8f6cd42b75891f394e3e..0000000000000000000000000000000000000000
--- a/spaces/nikansh/hamyar_riazi/README.md
+++ /dev/null
@@ -1,12 +0,0 @@
----
-title: Hamyar Riazi
-emoji: 🧠
-colorFrom: red
-colorTo: red
-sdk: streamlit
-sdk_version: 1.19.0
-app_file: app.py
-pinned: false
----
-
-Check out the configuration reference at https://huggingface.co/docs/hub/spaces-config-reference
diff --git a/spaces/nyust-eb210/bge-large-zh-v1.5_gradio/README.md b/spaces/nyust-eb210/bge-large-zh-v1.5_gradio/README.md
deleted file mode 100644
index 6bbc526d8ab49b61d76943a42ac23ae53085feb6..0000000000000000000000000000000000000000
--- a/spaces/nyust-eb210/bge-large-zh-v1.5_gradio/README.md
+++ /dev/null
@@ -1,12 +0,0 @@
----
-title: Bge-large-zh-v1.5 Gradio
-emoji: 🚀
-colorFrom: blue
-colorTo: purple
-sdk: gradio
-sdk_version: 3.45.1
-app_file: app.py
-pinned: false
----
-
-Check out the configuration reference at https://huggingface.co/docs/hub/spaces-config-reference
diff --git a/spaces/orangepony4/stabilityai-stable-diffusion-2-1/README.md b/spaces/orangepony4/stabilityai-stable-diffusion-2-1/README.md
deleted file mode 100644
index c1428920cce6e1bac19b87d52e1b1071373320de..0000000000000000000000000000000000000000
--- a/spaces/orangepony4/stabilityai-stable-diffusion-2-1/README.md
+++ /dev/null
@@ -1,12 +0,0 @@
----
-title: Stabilityai Stable Diffusion 2 1
-emoji: 👀
-colorFrom: green
-colorTo: indigo
-sdk: gradio
-sdk_version: 3.29.0
-app_file: app.py
-pinned: false
----
-
-Check out the configuration reference at https://huggingface.co/docs/hub/spaces-config-reference
diff --git a/spaces/parkyzh/bingo/src/lib/bots/bing/utils.ts b/spaces/parkyzh/bingo/src/lib/bots/bing/utils.ts
deleted file mode 100644
index 64b4b96452d125346b0fc4436b5f7c18c962df0b..0000000000000000000000000000000000000000
--- a/spaces/parkyzh/bingo/src/lib/bots/bing/utils.ts
+++ /dev/null
@@ -1,87 +0,0 @@
-import { ChatResponseMessage, BingChatResponse } from './types'
-
-export function convertMessageToMarkdown(message: ChatResponseMessage): string {
- if (message.messageType === 'InternalSearchQuery') {
- return message.text
- }
- for (const card of message.adaptiveCards??[]) {
- for (const block of card.body) {
- if (block.type === 'TextBlock') {
- return block.text
- }
- }
- }
- return ''
-}
-
-const RecordSeparator = String.fromCharCode(30)
-
-export const websocketUtils = {
- packMessage(data: any) {
- return `${JSON.stringify(data)}${RecordSeparator}`
- },
- unpackMessage(data: string | ArrayBuffer | Blob) {
- if (!data) return {}
- return data
- .toString()
- .split(RecordSeparator)
- .filter(Boolean)
- .map((s) => {
- try {
- return JSON.parse(s)
- } catch (e) {
- return {}
- }
- })
- },
-}
-
-export async function createImage(prompt: string, id: string, headers: HeadersInit): Promise {
- const { headers: responseHeaders } = await fetch(`https://www.bing.com/images/create?partner=sydney&re=1&showselective=1&sude=1&kseed=7000&SFX=&q=${encodeURIComponent(prompt)}&iframeid=${id}`,
- {
- method: 'HEAD',
- headers,
- redirect: 'manual'
- },
- );
-
- if (!/&id=([^&]+)$/.test(responseHeaders.get('location') || '')) {
- throw new Error('请求异常,请检查 cookie 是否有效')
- }
-
- const resultId = RegExp.$1;
- let count = 0
- const imageThumbUrl = `https://www.bing.com/images/create/async/results/${resultId}?q=${encodeURIComponent(prompt)}&partner=sydney&showselective=1&IID=images.as`;
-
- do {
- await sleep(3000);
- const content = await fetch(imageThumbUrl, { headers, method: 'GET' })
-
- // @ts-ignore
- if (content.headers.get('content-length') > 1) {
- const text = await content.text()
- return (text?.match(/ target?.split('src="').pop()?.replace(/&/g, '&'))
- .map(img => ``).join(' ')
- }
- } while(count ++ < 10);
-}
-
-
-export async function* streamAsyncIterable(stream: ReadableStream) {
- const reader = stream.getReader()
- try {
- while (true) {
- const { done, value } = await reader.read()
- if (done) {
- return
- }
- yield value
- }
- } finally {
- reader.releaseLock()
- }
-}
-
-export const sleep = (ms: number) => new Promise(resolve => setTimeout(resolve, ms))
-
diff --git a/spaces/parsi-ai-nlpclass/F22-Adversarial-QA/README.md b/spaces/parsi-ai-nlpclass/F22-Adversarial-QA/README.md
deleted file mode 100644
index f3e66686a82bef65e08d651585c0e2b97a483918..0000000000000000000000000000000000000000
--- a/spaces/parsi-ai-nlpclass/F22-Adversarial-QA/README.md
+++ /dev/null
@@ -1,18 +0,0 @@
----
-title: F22 Adversarial QA
-emoji: 🚀
-colorFrom: yellow
-colorTo: red
-sdk: streamlit
-sdk_version: 1.17.0
-app_file: app.py
-pinned: false
----
-
-# Adversarial-QA
-
-This is the final project of the NLP course instructed by Dr. Asgari at Sharif University Technology, Fall 2022.
-
-**🚀️Contributers :** Hamidreza Amirzadeh, Mohammad Hossein Sameti, Arash Maryoriad, Jalal Nematbakhsh
-
-In this project we aimed to create adversarial examples for the persian state-of-the-art question answering(QA) models and then applying adversarial training methods inoder to make the models robust. The GitHub page of the project is available at this [link](https://github.com/NLP-Final-Projects/Adversarial-QA).
diff --git a/spaces/patti-j/omdena-mental-health/app.py b/spaces/patti-j/omdena-mental-health/app.py
deleted file mode 100644
index 6e9d91bcd442d3a8c37b606875d93e16d6c9e0f4..0000000000000000000000000000000000000000
--- a/spaces/patti-j/omdena-mental-health/app.py
+++ /dev/null
@@ -1,107 +0,0 @@
-# Import dependencies
-import gradio as gr
-# from llama_index import GPTVectorStoreIndex
-# from query_data import get_chain
-from langchain.chat_models import ChatOpenAI
-
-# create the OpenAI chatbot
-chatbot = ChatOpenAI()
-
-# define the function to generate the chatbot response
-def generate_response(text):
- response = chatbot.generate_response(text)
- return response
-
-# create the Gradio interface
-interface = gr.Interface(
- fn=generate_response,
- inputs=gr.inputs.Textbox(label="Input Text"),
- outputs=gr.outputs.Textbox(label="Output Text")
-)
-
-# launch the interface
-interface.launch()
-
-#from langchain import OpenAI, ConversationChain, LLMChain, PromptTemplate
-#from langchain.memory import ConversationBufferWindowMemory
-
-#template =
-"""You are a brilliant and empathic counselor. You encourage human to share feelings.
-You provide resources when appropriate or if asked.
-{history}
-Human: {human_input}
-Assistant:"""
-
-"""prompt = PromptTemplate(input_variables=["history", "human_input"], template=template)
-
-chatgpt_chain = LLMChain(
- llm=OpenAI(temperature=0.8),
- prompt=prompt,
- verbose=False,
- memory=ConversationBufferWindowMemory(k=2),
-)
-
-output = chatgpt_chain.predict(
- human_input=
-
-iface = gr.Interface(fn=get_response, inputs="text", outputs="text")"""
-
-
-
-"""chat = ChatOpenAI(temperature=0)
-
-template = "You are a brilliant and empathic counselor. You encourage to share and provide resources when asked."
-system_message_prompt = SystemMessagePromptTemplate.from_template(template)
-human_template = "{text}"
-human_message_prompt = HumanMessagePromptTemplate.from_template(human_template)
-chat_prompt = ChatPromptTemplate.from_messages([system_message_prompt, human_message_prompt])
-
-chain = LLMChain(llm=chat, prompt=chat_prompt)
-chain.run(text="I feel lonely.")"""
-
-
-# Execute the chat functionality.
-"""
-with gr.Blocks(theme=gr.themes.Soft()) as demo:
-
- gr.HTML("
Omdena AI Chatbot For Mental Health and Wellbeing
")
-
- gr.HTML("WELCOME "
- "I am an AI ChatBot and I am here to assist you with whatever is bothering you. "
- "Our conversation is strictly confidential and I will not remember it when you come back another time."
- )
-
- chatbot = gr.Chatbot()
- chat_message = gr.Textbox(label="What would you like to chat about?")
- response = gr.Textbox """
-
-# define function to get chatbot response
-""" def get_response(text):
- response = agent.run(text)
- return response """
-
-""" def respond(chat_message, chat_history):
- response = get_chain(chat_message, chat_history)
- chat_history.append((chat_message, response))
- return "", chat_history """
-
-""" with gr.Row():
- send = gr.Button(value="Send").style(full_width=False)
- clear = gr.Button(value="Clear Chat").style(full_width=False)
-
- gr.Examples(
- examples=[
- "I feel lonely",
- "I'm having problems at home",
- "I am looking for some resources",
- ],
- inputs=chat_message
- )
-
- send.click(get_response(chat_message))
- clear.click(lambda: None, None, chatbot, queue=False)
-
-
-if __name__ == "__main__":
- demo.launch(debug=True)
-"""
\ No newline at end of file
diff --git a/spaces/prerna9811/Chord/portaudio/bindings/java/c/src/jpa_tools.c b/spaces/prerna9811/Chord/portaudio/bindings/java/c/src/jpa_tools.c
deleted file mode 100644
index e3f903aa8283888871b1322f725cc17eb92f39bd..0000000000000000000000000000000000000000
--- a/spaces/prerna9811/Chord/portaudio/bindings/java/c/src/jpa_tools.c
+++ /dev/null
@@ -1,208 +0,0 @@
-/*
- * Portable Audio I/O Library
- * Java Binding for PortAudio
- *
- * Based on the Open Source API proposed by Ross Bencina
- * Copyright (c) 2008 Ross Bencina
- *
- * Permission is hereby granted, free of charge, to any person obtaining
- * a copy of this software and associated documentation files
- * (the "Software"), to deal in the Software without restriction,
- * including without limitation the rights to use, copy, modify, merge,
- * publish, distribute, sublicense, and/or sell copies of the Software,
- * and to permit persons to whom the Software is furnished to do so,
- * subject to the following conditions:
- *
- * The above copyright notice and this permission notice shall be
- * included in all copies or substantial portions of the Software.
- *
- * THE SOFTWARE IS PROVIDED "AS IS", WITHOUT WARRANTY OF ANY KIND,
- * EXPRESS OR IMPLIED, INCLUDING BUT NOT LIMITED TO THE WARRANTIES OF
- * MERCHANTABILITY, FITNESS FOR A PARTICULAR PURPOSE AND NONINFRINGEMENT.
- * IN NO EVENT SHALL THE AUTHORS OR COPYRIGHT HOLDERS BE LIABLE FOR
- * ANY CLAIM, DAMAGES OR OTHER LIABILITY, WHETHER IN AN ACTION OF
- * CONTRACT, TORT OR OTHERWISE, ARISING FROM, OUT OF OR IN CONNECTION
- * WITH THE SOFTWARE OR THE USE OR OTHER DEALINGS IN THE SOFTWARE.
- */
-
-/*
- * The text above constitutes the entire PortAudio license; however,
- * the PortAudio community also makes the following non-binding requests:
- *
- * Any person wishing to distribute modifications to the Software is
- * requested to send the modifications to the original developer so that
- * they can be incorporated into the canonical version. It is also
- * requested that these non-binding requests be included along with the
- * license above.
- */
-
-#include "com_portaudio_PortAudio.h"
-#include "portaudio.h"
-#include "jpa_tools.h"
-
-jint jpa_GetIntField( JNIEnv *env, jclass cls, jobject obj, const char *fieldName )
-{
- /* Look for the instance field maxInputChannels in cls */
- jfieldID fid = (*env)->GetFieldID(env, cls, fieldName, "I");
- if (fid == NULL)
- {
- jpa_ThrowError( env, "Cannot find integer JNI field." );
- return 0;
- }
- else
- {
- return (*env)->GetIntField(env, obj, fid );
- }
-}
-
-void jpa_SetIntField( JNIEnv *env, jclass cls, jobject obj, const char *fieldName, jint value )
-{
- /* Look for the instance field maxInputChannels in cls */
- jfieldID fid = (*env)->GetFieldID(env, cls, fieldName, "I");
- if (fid == NULL)
- {
- jpa_ThrowError( env, "Cannot find integer JNI field." );
- }
- else
- {
- (*env)->SetIntField(env, obj, fid, value );
- }
-}
-
-jlong jpa_GetLongField( JNIEnv *env, jclass cls, jobject obj, const char *fieldName )
-{
- /* Look for the instance field maxInputChannels in cls */
- jfieldID fid = (*env)->GetFieldID(env, cls, fieldName, "J");
- if (fid == NULL)
- {
- jpa_ThrowError( env, "Cannot find long JNI field." );
- return 0L;
- }
- else
- {
- return (*env)->GetLongField(env, obj, fid );
- }
-}
-
-void jpa_SetLongField( JNIEnv *env, jclass cls, jobject obj, const char *fieldName, jlong value )
-{
- /* Look for the instance field maxInputChannels in cls */
- jfieldID fid = (*env)->GetFieldID(env, cls, fieldName, "J");
- if (fid == NULL)
- {
- jpa_ThrowError( env, "Cannot find long JNI field." );
- }
- else
- {
- (*env)->SetLongField(env, obj, fid, value );
- }
-}
-
-
-void jpa_SetDoubleField( JNIEnv *env, jclass cls, jobject obj, const char *fieldName, jdouble value )
-{
- /* Look for the instance field maxInputChannels in cls */
- jfieldID fid = (*env)->GetFieldID(env, cls, fieldName, "D");
- if (fid == NULL)
- {
- jpa_ThrowError( env, "Cannot find double JNI field." );
- }
- else
- {
- (*env)->SetDoubleField(env, obj, fid, value );
- }
-}
-
-
-jdouble jpa_GetDoubleField( JNIEnv *env, jclass cls, jobject obj, const char *fieldName )
-{
- /* Look for the instance field maxInputChannels in cls */
- jfieldID fid = (*env)->GetFieldID(env, cls, fieldName, "D");
- if (fid == NULL)
- {
- jpa_ThrowError( env, "Cannot find double JNI field." );
- return 0;
- }
- else
- {
- return (*env)->GetDoubleField(env, obj, fid );
- }
-}
-
-void jpa_SetStringField( JNIEnv *env, jclass cls, jobject obj, const char *fieldName, const char *value )
-{
- /* Look for the instance field maxInputChannels in cls */
- jfieldID fid = (*env)->GetFieldID(env, cls, fieldName, "Ljava/lang/String;");
- if (fid == NULL)
- {
- jpa_ThrowError( env, "Cannot find String JNI field." );
- }
- else
- {
- jstring jstr = (*env)->NewStringUTF(env, value);
- if (jstr == NULL)
- {
- jpa_ThrowError( env, "Cannot create new String." );
- }
- else
- {
- (*env)->SetObjectField(env, obj, fid, jstr );
- }
- }
-}
-
-PaStreamParameters *jpa_FillStreamParameters( JNIEnv *env, jobject jstreamParam, PaStreamParameters *myParams )
-{
- jclass cls;
-
- if( jstreamParam == NULL ) return NULL; // OK, not an error
-
- cls = (*env)->GetObjectClass(env, jstreamParam);
-
- myParams->channelCount = jpa_GetIntField( env, cls, jstreamParam, "channelCount" );
- myParams->device = jpa_GetIntField( env, cls, jstreamParam, "device" );
- myParams->sampleFormat = jpa_GetIntField( env, cls, jstreamParam, "sampleFormat" );
- myParams->suggestedLatency = jpa_GetDoubleField( env, cls, jstreamParam, "suggestedLatency" );
- myParams->hostApiSpecificStreamInfo = NULL;
-
- return myParams;
-}
-
-// Create an exception that will be thrown when we return from the JNI call.
-jint jpa_ThrowError( JNIEnv *env, const char *message )
-{
- return (*env)->ThrowNew(env, (*env)->FindClass( env, "java/lang/RuntimeException"),
- message );
-}
-
-// Throw an exception on error.
-jint jpa_CheckError( JNIEnv *env, PaError err )
-{
- if( err == -1 )
- {
- return jpa_ThrowError( env, "-1, possibly no available default device" );
- }
- else if( err < 0 )
- {
- if( err == paUnanticipatedHostError )
- {
- const PaHostErrorInfo *hostErrorInfo = Pa_GetLastHostErrorInfo();
- return jpa_ThrowError( env, hostErrorInfo->errorText );
- }
- else
- {
- return jpa_ThrowError( env, Pa_GetErrorText( err ) );
- }
- }
- else
- {
- return err;
- }
-}
-
-// Get the stream pointer from a BlockingStream long field.
-PaStream *jpa_GetStreamPointer( JNIEnv *env, jobject blockingStream )
-{
- jclass cls = (*env)->GetObjectClass(env, blockingStream);
- return (PaStream *) jpa_GetLongField( env, cls, blockingStream, "nativeStream" );
-}
diff --git a/spaces/profayle/TerrapinTalk/myenv/lib/python3.9/site-packages/altair/vegalite/api.py b/spaces/profayle/TerrapinTalk/myenv/lib/python3.9/site-packages/altair/vegalite/api.py
deleted file mode 100644
index 6602986fe9c617eb5f4e375c94985260a2773aaa..0000000000000000000000000000000000000000
--- a/spaces/profayle/TerrapinTalk/myenv/lib/python3.9/site-packages/altair/vegalite/api.py
+++ /dev/null
@@ -1,2 +0,0 @@
-# ruff: noqa
-from .v5.api import *
diff --git a/spaces/profayle/TerrapinTalk/myenv/lib/python3.9/site-packages/fsspec/implementations/dbfs.py b/spaces/profayle/TerrapinTalk/myenv/lib/python3.9/site-packages/fsspec/implementations/dbfs.py
deleted file mode 100644
index 9f5b330cab9e751142794253d1072bab48b8bc29..0000000000000000000000000000000000000000
--- a/spaces/profayle/TerrapinTalk/myenv/lib/python3.9/site-packages/fsspec/implementations/dbfs.py
+++ /dev/null
@@ -1,457 +0,0 @@
-import base64
-import urllib
-
-import requests
-
-from fsspec import AbstractFileSystem
-from fsspec.spec import AbstractBufferedFile
-
-
-class DatabricksException(Exception):
- """
- Helper class for exceptions raised in this module.
- """
-
- def __init__(self, error_code, message):
- """Create a new DatabricksException"""
- super().__init__(message)
-
- self.error_code = error_code
- self.message = message
-
-
-class DatabricksFileSystem(AbstractFileSystem):
- """
- Get access to the Databricks filesystem implementation over HTTP.
- Can be used inside and outside of a databricks cluster.
- """
-
- def __init__(self, instance, token, **kwargs):
- """
- Create a new DatabricksFileSystem.
-
- Parameters
- ----------
- instance: str
- The instance URL of the databricks cluster.
- For example for an Azure databricks cluster, this
- has the form adb-..azuredatabricks.net.
- token: str
- Your personal token. Find out more
- here: https://docs.databricks.com/dev-tools/api/latest/authentication.html
- """
- self.instance = instance
- self.token = token
-
- self.session = requests.Session()
- self.session.headers.update({"Authorization": f"Bearer {self.token}"})
-
- super().__init__(**kwargs)
-
- def ls(self, path, detail=True):
- """
- List the contents of the given path.
-
- Parameters
- ----------
- path: str
- Absolute path
- detail: bool
- Return not only the list of filenames,
- but also additional information on file sizes
- and types.
- """
- out = self._ls_from_cache(path)
- if not out:
- try:
- r = self._send_to_api(
- method="get", endpoint="list", json={"path": path}
- )
- except DatabricksException as e:
- if e.error_code == "RESOURCE_DOES_NOT_EXIST":
- raise FileNotFoundError(e.message)
-
- raise e
- files = r["files"]
- out = [
- {
- "name": o["path"],
- "type": "directory" if o["is_dir"] else "file",
- "size": o["file_size"],
- }
- for o in files
- ]
- self.dircache[path] = out
-
- if detail:
- return out
- return [o["name"] for o in out]
-
- def makedirs(self, path, exist_ok=True):
- """
- Create a given absolute path and all of its parents.
-
- Parameters
- ----------
- path: str
- Absolute path to create
- exist_ok: bool
- If false, checks if the folder
- exists before creating it (and raises an
- Exception if this is the case)
- """
- if not exist_ok:
- try:
- # If the following succeeds, the path is already present
- self._send_to_api(
- method="get", endpoint="get-status", json={"path": path}
- )
- raise FileExistsError(f"Path {path} already exists")
- except DatabricksException as e:
- if e.error_code == "RESOURCE_DOES_NOT_EXIST":
- pass
-
- try:
- self._send_to_api(method="post", endpoint="mkdirs", json={"path": path})
- except DatabricksException as e:
- if e.error_code == "RESOURCE_ALREADY_EXISTS":
- raise FileExistsError(e.message)
-
- raise e
- self.invalidate_cache(self._parent(path))
-
- def mkdir(self, path, create_parents=True, **kwargs):
- """
- Create a given absolute path and all of its parents.
-
- Parameters
- ----------
- path: str
- Absolute path to create
- create_parents: bool
- Whether to create all parents or not.
- "False" is not implemented so far.
- """
- if not create_parents:
- raise NotImplementedError
-
- self.mkdirs(path, **kwargs)
-
- def rm(self, path, recursive=False):
- """
- Remove the file or folder at the given absolute path.
-
- Parameters
- ----------
- path: str
- Absolute path what to remove
- recursive: bool
- Recursively delete all files in a folder.
- """
- try:
- self._send_to_api(
- method="post",
- endpoint="delete",
- json={"path": path, "recursive": recursive},
- )
- except DatabricksException as e:
- # This is not really an exception, it just means
- # not everything was deleted so far
- if e.error_code == "PARTIAL_DELETE":
- self.rm(path=path, recursive=recursive)
- elif e.error_code == "IO_ERROR":
- # Using the same exception as the os module would use here
- raise OSError(e.message)
-
- raise e
- self.invalidate_cache(self._parent(path))
-
- def mv(self, source_path, destination_path, recursive=False, maxdepth=None):
- """
- Move a source to a destination path.
-
- A note from the original [databricks API manual]
- (https://docs.databricks.com/dev-tools/api/latest/dbfs.html#move).
-
- When moving a large number of files the API call will time out after
- approximately 60s, potentially resulting in partially moved data.
- Therefore, for operations that move more than 10k files, we strongly
- discourage using the DBFS REST API.
-
- Parameters
- ----------
- source_path: str
- From where to move (absolute path)
- destination_path: str
- To where to move (absolute path)
- recursive: bool
- Not implemented to far.
- maxdepth:
- Not implemented to far.
- """
- if recursive:
- raise NotImplementedError
- if maxdepth:
- raise NotImplementedError
-
- try:
- self._send_to_api(
- method="post",
- endpoint="move",
- json={"source_path": source_path, "destination_path": destination_path},
- )
- except DatabricksException as e:
- if e.error_code == "RESOURCE_DOES_NOT_EXIST":
- raise FileNotFoundError(e.message)
- elif e.error_code == "RESOURCE_ALREADY_EXISTS":
- raise FileExistsError(e.message)
-
- raise e
- self.invalidate_cache(self._parent(source_path))
- self.invalidate_cache(self._parent(destination_path))
-
- def _open(self, path, mode="rb", block_size="default", **kwargs):
- """
- Overwrite the base class method to make sure to create a DBFile.
- All arguments are copied from the base method.
-
- Only the default blocksize is allowed.
- """
- return DatabricksFile(self, path, mode=mode, block_size=block_size, **kwargs)
-
- def _send_to_api(self, method, endpoint, json):
- """
- Send the given json to the DBFS API
- using a get or post request (specified by the argument `method`).
-
- Parameters
- ----------
- method: str
- Which http method to use for communication; "get" or "post".
- endpoint: str
- Where to send the request to (last part of the API URL)
- json: dict
- Dictionary of information to send
- """
- if method == "post":
- session_call = self.session.post
- elif method == "get":
- session_call = self.session.get
- else:
- raise ValueError(f"Do not understand method {method}")
-
- url = urllib.parse.urljoin(f"https://{self.instance}/api/2.0/dbfs/", endpoint)
-
- r = session_call(url, json=json)
-
- # The DBFS API will return a json, also in case of an exception.
- # We want to preserve this information as good as possible.
- try:
- r.raise_for_status()
- except requests.HTTPError as e:
- # try to extract json error message
- # if that fails, fall back to the original exception
- try:
- exception_json = e.response.json()
- except Exception:
- raise e
-
- raise DatabricksException(**exception_json)
-
- return r.json()
-
- def _create_handle(self, path, overwrite=True):
- """
- Internal function to create a handle, which can be used to
- write blocks of a file to DBFS.
- A handle has a unique identifier which needs to be passed
- whenever written during this transaction.
- The handle is active for 10 minutes - after that a new
- write transaction needs to be created.
- Make sure to close the handle after you are finished.
-
- Parameters
- ----------
- path: str
- Absolute path for this file.
- overwrite: bool
- If a file already exist at this location, either overwrite
- it or raise an exception.
- """
- try:
- r = self._send_to_api(
- method="post",
- endpoint="create",
- json={"path": path, "overwrite": overwrite},
- )
- return r["handle"]
- except DatabricksException as e:
- if e.error_code == "RESOURCE_ALREADY_EXISTS":
- raise FileExistsError(e.message)
-
- raise e
-
- def _close_handle(self, handle):
- """
- Close a handle, which was opened by :func:`_create_handle`.
-
- Parameters
- ----------
- handle: str
- Which handle to close.
- """
- try:
- self._send_to_api(method="post", endpoint="close", json={"handle": handle})
- except DatabricksException as e:
- if e.error_code == "RESOURCE_DOES_NOT_EXIST":
- raise FileNotFoundError(e.message)
-
- raise e
-
- def _add_data(self, handle, data):
- """
- Upload data to an already opened file handle
- (opened by :func:`_create_handle`).
- The maximal allowed data size is 1MB after
- conversion to base64.
- Remember to close the handle when you are finished.
-
- Parameters
- ----------
- handle: str
- Which handle to upload data to.
- data: bytes
- Block of data to add to the handle.
- """
- data = base64.b64encode(data).decode()
- try:
- self._send_to_api(
- method="post",
- endpoint="add-block",
- json={"handle": handle, "data": data},
- )
- except DatabricksException as e:
- if e.error_code == "RESOURCE_DOES_NOT_EXIST":
- raise FileNotFoundError(e.message)
- elif e.error_code == "MAX_BLOCK_SIZE_EXCEEDED":
- raise ValueError(e.message)
-
- raise e
-
- def _get_data(self, path, start, end):
- """
- Download data in bytes from a given absolute path in a block
- from [start, start+length].
- The maximum number of allowed bytes to read is 1MB.
-
- Parameters
- ----------
- path: str
- Absolute path to download data from
- start: int
- Start position of the block
- end: int
- End position of the block
- """
- try:
- r = self._send_to_api(
- method="get",
- endpoint="read",
- json={"path": path, "offset": start, "length": end - start},
- )
- return base64.b64decode(r["data"])
- except DatabricksException as e:
- if e.error_code == "RESOURCE_DOES_NOT_EXIST":
- raise FileNotFoundError(e.message)
- elif e.error_code in ["INVALID_PARAMETER_VALUE", "MAX_READ_SIZE_EXCEEDED"]:
- raise ValueError(e.message)
-
- raise e
-
- def invalidate_cache(self, path=None):
- if path is None:
- self.dircache.clear()
- else:
- self.dircache.pop(path, None)
- super().invalidate_cache(path)
-
-
-class DatabricksFile(AbstractBufferedFile):
- """
- Helper class for files referenced in the DatabricksFileSystem.
- """
-
- DEFAULT_BLOCK_SIZE = 1 * 2**20 # only allowed block size
-
- def __init__(
- self,
- fs,
- path,
- mode="rb",
- block_size="default",
- autocommit=True,
- cache_type="readahead",
- cache_options=None,
- **kwargs,
- ):
- """
- Create a new instance of the DatabricksFile.
-
- The blocksize needs to be the default one.
- """
- if block_size is None or block_size == "default":
- block_size = self.DEFAULT_BLOCK_SIZE
-
- assert (
- block_size == self.DEFAULT_BLOCK_SIZE
- ), f"Only the default block size is allowed, not {block_size}"
-
- super().__init__(
- fs,
- path,
- mode=mode,
- block_size=block_size,
- autocommit=autocommit,
- cache_type=cache_type,
- cache_options=cache_options or {},
- **kwargs,
- )
-
- def _initiate_upload(self):
- """Internal function to start a file upload"""
- self.handle = self.fs._create_handle(self.path)
-
- def _upload_chunk(self, final=False):
- """Internal function to add a chunk of data to a started upload"""
- self.buffer.seek(0)
- data = self.buffer.getvalue()
-
- data_chunks = [
- data[start:end] for start, end in self._to_sized_blocks(len(data))
- ]
-
- for data_chunk in data_chunks:
- self.fs._add_data(handle=self.handle, data=data_chunk)
-
- if final:
- self.fs._close_handle(handle=self.handle)
- return True
-
- def _fetch_range(self, start, end):
- """Internal function to download a block of data"""
- return_buffer = b""
- length = end - start
- for chunk_start, chunk_end in self._to_sized_blocks(length, start):
- return_buffer += self.fs._get_data(
- path=self.path, start=chunk_start, end=chunk_end
- )
-
- return return_buffer
-
- def _to_sized_blocks(self, length, start=0):
- """Helper function to split a range from 0 to total_length into bloksizes"""
- end = start + length
- for data_chunk in range(start, end, self.blocksize):
- data_start = data_chunk
- data_end = min(end, data_chunk + self.blocksize)
- yield data_start, data_end
diff --git a/spaces/profayle/TerrapinTalk/myenv/lib/python3.9/site-packages/numpy/_core/_dtype.py b/spaces/profayle/TerrapinTalk/myenv/lib/python3.9/site-packages/numpy/_core/_dtype.py
deleted file mode 100644
index 974d93d98cbbbcd25c7aae6d299c9f0f43e41cfa..0000000000000000000000000000000000000000
--- a/spaces/profayle/TerrapinTalk/myenv/lib/python3.9/site-packages/numpy/_core/_dtype.py
+++ /dev/null
@@ -1,6 +0,0 @@
-from numpy.core import _dtype
-
-_globals = globals()
-
-for item in _dtype.__dir__():
- _globals[item] = getattr(_dtype, item)
diff --git a/spaces/profayle/TerrapinTalk/myenv/lib/python3.9/site-packages/numpy/distutils/checks/cpu_asimdfhm.c b/spaces/profayle/TerrapinTalk/myenv/lib/python3.9/site-packages/numpy/distutils/checks/cpu_asimdfhm.c
deleted file mode 100644
index 54e328098d17b57445024c9859cd4992492c348a..0000000000000000000000000000000000000000
--- a/spaces/profayle/TerrapinTalk/myenv/lib/python3.9/site-packages/numpy/distutils/checks/cpu_asimdfhm.c
+++ /dev/null
@@ -1,19 +0,0 @@
-#ifdef _MSC_VER
- #include
-#endif
-#include
-
-int main(int argc, char **argv)
-{
- float16_t *src = (float16_t*)argv[argc-1];
- float *src2 = (float*)argv[argc-2];
- float16x8_t vhp = vdupq_n_f16(src[0]);
- float16x4_t vlhp = vdup_n_f16(src[1]);
- float32x4_t vf = vdupq_n_f32(src2[0]);
- float32x2_t vlf = vdup_n_f32(src2[1]);
-
- int ret = (int)vget_lane_f32(vfmlal_low_f16(vlf, vlhp, vlhp), 0);
- ret += (int)vgetq_lane_f32(vfmlslq_high_f16(vf, vhp, vhp), 0);
-
- return ret;
-}
diff --git a/spaces/profayle/TerrapinTalk/myenv/lib/python3.9/site-packages/pandas/tests/arrays/test_timedeltas.py b/spaces/profayle/TerrapinTalk/myenv/lib/python3.9/site-packages/pandas/tests/arrays/test_timedeltas.py
deleted file mode 100644
index 1043c2ee6c9b6ff7f3ec2d43b9c2f7dba392e7fd..0000000000000000000000000000000000000000
--- a/spaces/profayle/TerrapinTalk/myenv/lib/python3.9/site-packages/pandas/tests/arrays/test_timedeltas.py
+++ /dev/null
@@ -1,311 +0,0 @@
-from datetime import timedelta
-
-import numpy as np
-import pytest
-
-import pandas as pd
-from pandas import Timedelta
-import pandas._testing as tm
-from pandas.core.arrays import (
- DatetimeArray,
- TimedeltaArray,
-)
-
-
-class TestNonNano:
- @pytest.fixture(params=["s", "ms", "us"])
- def unit(self, request):
- return request.param
-
- @pytest.fixture
- def tda(self, unit):
- arr = np.arange(5, dtype=np.int64).view(f"m8[{unit}]")
- return TimedeltaArray._simple_new(arr, dtype=arr.dtype)
-
- def test_non_nano(self, unit):
- arr = np.arange(5, dtype=np.int64).view(f"m8[{unit}]")
- tda = TimedeltaArray._simple_new(arr, dtype=arr.dtype)
-
- assert tda.dtype == arr.dtype
- assert tda[0].unit == unit
-
- def test_as_unit_raises(self, tda):
- # GH#50616
- with pytest.raises(ValueError, match="Supported units"):
- tda.as_unit("D")
-
- tdi = pd.Index(tda)
- with pytest.raises(ValueError, match="Supported units"):
- tdi.as_unit("D")
-
- @pytest.mark.parametrize("field", TimedeltaArray._field_ops)
- def test_fields(self, tda, field):
- as_nano = tda._ndarray.astype("m8[ns]")
- tda_nano = TimedeltaArray._simple_new(as_nano, dtype=as_nano.dtype)
-
- result = getattr(tda, field)
- expected = getattr(tda_nano, field)
- tm.assert_numpy_array_equal(result, expected)
-
- def test_to_pytimedelta(self, tda):
- as_nano = tda._ndarray.astype("m8[ns]")
- tda_nano = TimedeltaArray._simple_new(as_nano, dtype=as_nano.dtype)
-
- result = tda.to_pytimedelta()
- expected = tda_nano.to_pytimedelta()
- tm.assert_numpy_array_equal(result, expected)
-
- def test_total_seconds(self, unit, tda):
- as_nano = tda._ndarray.astype("m8[ns]")
- tda_nano = TimedeltaArray._simple_new(as_nano, dtype=as_nano.dtype)
-
- result = tda.total_seconds()
- expected = tda_nano.total_seconds()
- tm.assert_numpy_array_equal(result, expected)
-
- def test_timedelta_array_total_seconds(self):
- # GH34290
- expected = Timedelta("2 min").total_seconds()
-
- result = pd.array([Timedelta("2 min")]).total_seconds()[0]
- assert result == expected
-
- def test_total_seconds_nanoseconds(self):
- # issue #48521
- start_time = pd.Series(["2145-11-02 06:00:00"]).astype("datetime64[ns]")
- end_time = pd.Series(["2145-11-02 07:06:00"]).astype("datetime64[ns]")
- expected = (end_time - start_time).values / np.timedelta64(1, "s")
- result = (end_time - start_time).dt.total_seconds().values
- assert result == expected
-
- @pytest.mark.parametrize(
- "nat", [np.datetime64("NaT", "ns"), np.datetime64("NaT", "us")]
- )
- def test_add_nat_datetimelike_scalar(self, nat, tda):
- result = tda + nat
- assert isinstance(result, DatetimeArray)
- assert result._creso == tda._creso
- assert result.isna().all()
-
- result = nat + tda
- assert isinstance(result, DatetimeArray)
- assert result._creso == tda._creso
- assert result.isna().all()
-
- def test_add_pdnat(self, tda):
- result = tda + pd.NaT
- assert isinstance(result, TimedeltaArray)
- assert result._creso == tda._creso
- assert result.isna().all()
-
- result = pd.NaT + tda
- assert isinstance(result, TimedeltaArray)
- assert result._creso == tda._creso
- assert result.isna().all()
-
- # TODO: 2022-07-11 this is the only test that gets to DTA.tz_convert
- # or tz_localize with non-nano; implement tests specific to that.
- def test_add_datetimelike_scalar(self, tda, tz_naive_fixture):
- ts = pd.Timestamp("2016-01-01", tz=tz_naive_fixture).as_unit("ns")
-
- expected = tda.as_unit("ns") + ts
- res = tda + ts
- tm.assert_extension_array_equal(res, expected)
- res = ts + tda
- tm.assert_extension_array_equal(res, expected)
-
- ts += Timedelta(1) # case where we can't cast losslessly
-
- exp_values = tda._ndarray + ts.asm8
- expected = (
- DatetimeArray._simple_new(exp_values, dtype=exp_values.dtype)
- .tz_localize("UTC")
- .tz_convert(ts.tz)
- )
-
- result = tda + ts
- tm.assert_extension_array_equal(result, expected)
-
- result = ts + tda
- tm.assert_extension_array_equal(result, expected)
-
- def test_mul_scalar(self, tda):
- other = 2
- result = tda * other
- expected = TimedeltaArray._simple_new(tda._ndarray * other, dtype=tda.dtype)
- tm.assert_extension_array_equal(result, expected)
- assert result._creso == tda._creso
-
- def test_mul_listlike(self, tda):
- other = np.arange(len(tda))
- result = tda * other
- expected = TimedeltaArray._simple_new(tda._ndarray * other, dtype=tda.dtype)
- tm.assert_extension_array_equal(result, expected)
- assert result._creso == tda._creso
-
- def test_mul_listlike_object(self, tda):
- other = np.arange(len(tda))
- result = tda * other.astype(object)
- expected = TimedeltaArray._simple_new(tda._ndarray * other, dtype=tda.dtype)
- tm.assert_extension_array_equal(result, expected)
- assert result._creso == tda._creso
-
- def test_div_numeric_scalar(self, tda):
- other = 2
- result = tda / other
- expected = TimedeltaArray._simple_new(tda._ndarray / other, dtype=tda.dtype)
- tm.assert_extension_array_equal(result, expected)
- assert result._creso == tda._creso
-
- def test_div_td_scalar(self, tda):
- other = timedelta(seconds=1)
- result = tda / other
- expected = tda._ndarray / np.timedelta64(1, "s")
- tm.assert_numpy_array_equal(result, expected)
-
- def test_div_numeric_array(self, tda):
- other = np.arange(len(tda))
- result = tda / other
- expected = TimedeltaArray._simple_new(tda._ndarray / other, dtype=tda.dtype)
- tm.assert_extension_array_equal(result, expected)
- assert result._creso == tda._creso
-
- def test_div_td_array(self, tda):
- other = tda._ndarray + tda._ndarray[-1]
- result = tda / other
- expected = tda._ndarray / other
- tm.assert_numpy_array_equal(result, expected)
-
- def test_add_timedeltaarraylike(self, tda):
- tda_nano = tda.astype("m8[ns]")
-
- expected = tda_nano * 2
- res = tda_nano + tda
- tm.assert_extension_array_equal(res, expected)
- res = tda + tda_nano
- tm.assert_extension_array_equal(res, expected)
-
- expected = tda_nano * 0
- res = tda - tda_nano
- tm.assert_extension_array_equal(res, expected)
-
- res = tda_nano - tda
- tm.assert_extension_array_equal(res, expected)
-
-
-class TestTimedeltaArray:
- @pytest.mark.parametrize("dtype", [int, np.int32, np.int64, "uint32", "uint64"])
- def test_astype_int(self, dtype):
- arr = TimedeltaArray._from_sequence([Timedelta("1H"), Timedelta("2H")])
-
- if np.dtype(dtype) != np.int64:
- with pytest.raises(TypeError, match=r"Do obj.astype\('int64'\)"):
- arr.astype(dtype)
- return
-
- result = arr.astype(dtype)
- expected = arr._ndarray.view("i8")
- tm.assert_numpy_array_equal(result, expected)
-
- def test_setitem_clears_freq(self):
- a = TimedeltaArray(pd.timedelta_range("1H", periods=2, freq="H"))
- a[0] = Timedelta("1H")
- assert a.freq is None
-
- @pytest.mark.parametrize(
- "obj",
- [
- Timedelta(seconds=1),
- Timedelta(seconds=1).to_timedelta64(),
- Timedelta(seconds=1).to_pytimedelta(),
- ],
- )
- def test_setitem_objects(self, obj):
- # make sure we accept timedelta64 and timedelta in addition to Timedelta
- tdi = pd.timedelta_range("2 Days", periods=4, freq="H")
- arr = TimedeltaArray(tdi, freq=tdi.freq)
-
- arr[0] = obj
- assert arr[0] == Timedelta(seconds=1)
-
- @pytest.mark.parametrize(
- "other",
- [
- 1,
- np.int64(1),
- 1.0,
- np.datetime64("NaT"),
- pd.Timestamp("2021-01-01"),
- "invalid",
- np.arange(10, dtype="i8") * 24 * 3600 * 10**9,
- (np.arange(10) * 24 * 3600 * 10**9).view("datetime64[ns]"),
- pd.Timestamp("2021-01-01").to_period("D"),
- ],
- )
- @pytest.mark.parametrize("index", [True, False])
- def test_searchsorted_invalid_types(self, other, index):
- data = np.arange(10, dtype="i8") * 24 * 3600 * 10**9
- arr = TimedeltaArray(data, freq="D")
- if index:
- arr = pd.Index(arr)
-
- msg = "|".join(
- [
- "searchsorted requires compatible dtype or scalar",
- "value should be a 'Timedelta', 'NaT', or array of those. Got",
- ]
- )
- with pytest.raises(TypeError, match=msg):
- arr.searchsorted(other)
-
-
-class TestUnaryOps:
- def test_abs(self):
- vals = np.array([-3600 * 10**9, "NaT", 7200 * 10**9], dtype="m8[ns]")
- arr = TimedeltaArray(vals)
-
- evals = np.array([3600 * 10**9, "NaT", 7200 * 10**9], dtype="m8[ns]")
- expected = TimedeltaArray(evals)
-
- result = abs(arr)
- tm.assert_timedelta_array_equal(result, expected)
-
- result2 = np.abs(arr)
- tm.assert_timedelta_array_equal(result2, expected)
-
- def test_pos(self):
- vals = np.array([-3600 * 10**9, "NaT", 7200 * 10**9], dtype="m8[ns]")
- arr = TimedeltaArray(vals)
-
- result = +arr
- tm.assert_timedelta_array_equal(result, arr)
- assert not tm.shares_memory(result, arr)
-
- result2 = np.positive(arr)
- tm.assert_timedelta_array_equal(result2, arr)
- assert not tm.shares_memory(result2, arr)
-
- def test_neg(self):
- vals = np.array([-3600 * 10**9, "NaT", 7200 * 10**9], dtype="m8[ns]")
- arr = TimedeltaArray(vals)
-
- evals = np.array([3600 * 10**9, "NaT", -7200 * 10**9], dtype="m8[ns]")
- expected = TimedeltaArray(evals)
-
- result = -arr
- tm.assert_timedelta_array_equal(result, expected)
-
- result2 = np.negative(arr)
- tm.assert_timedelta_array_equal(result2, expected)
-
- def test_neg_freq(self):
- tdi = pd.timedelta_range("2 Days", periods=4, freq="H")
- arr = TimedeltaArray(tdi, freq=tdi.freq)
-
- expected = TimedeltaArray(-tdi._data, freq=-tdi.freq)
-
- result = -arr
- tm.assert_timedelta_array_equal(result, expected)
-
- result2 = np.negative(arr)
- tm.assert_timedelta_array_equal(result2, expected)
diff --git a/spaces/profayle/TerrapinTalk/myenv/lib/python3.9/site-packages/pandas/tests/frame/test_subclass.py b/spaces/profayle/TerrapinTalk/myenv/lib/python3.9/site-packages/pandas/tests/frame/test_subclass.py
deleted file mode 100644
index 8e657f197ca1edc5f9dc922f2a55fd3ae4a7ea51..0000000000000000000000000000000000000000
--- a/spaces/profayle/TerrapinTalk/myenv/lib/python3.9/site-packages/pandas/tests/frame/test_subclass.py
+++ /dev/null
@@ -1,773 +0,0 @@
-import numpy as np
-import pytest
-
-import pandas as pd
-from pandas import (
- DataFrame,
- Index,
- MultiIndex,
- Series,
-)
-import pandas._testing as tm
-
-pytestmark = pytest.mark.filterwarnings(
- "ignore:Passing a BlockManager|Passing a SingleBlockManager:DeprecationWarning"
-)
-
-
-@pytest.fixture()
-def gpd_style_subclass_df():
- class SubclassedDataFrame(DataFrame):
- @property
- def _constructor(self):
- return SubclassedDataFrame
-
- return SubclassedDataFrame({"a": [1, 2, 3]})
-
-
-class TestDataFrameSubclassing:
- def test_frame_subclassing_and_slicing(self):
- # Subclass frame and ensure it returns the right class on slicing it
- # In reference to PR 9632
-
- class CustomSeries(Series):
- @property
- def _constructor(self):
- return CustomSeries
-
- def custom_series_function(self):
- return "OK"
-
- class CustomDataFrame(DataFrame):
- """
- Subclasses pandas DF, fills DF with simulation results, adds some
- custom plotting functions.
- """
-
- def __init__(self, *args, **kw) -> None:
- super().__init__(*args, **kw)
-
- @property
- def _constructor(self):
- return CustomDataFrame
-
- _constructor_sliced = CustomSeries
-
- def custom_frame_function(self):
- return "OK"
-
- data = {"col1": range(10), "col2": range(10)}
- cdf = CustomDataFrame(data)
-
- # Did we get back our own DF class?
- assert isinstance(cdf, CustomDataFrame)
-
- # Do we get back our own Series class after selecting a column?
- cdf_series = cdf.col1
- assert isinstance(cdf_series, CustomSeries)
- assert cdf_series.custom_series_function() == "OK"
-
- # Do we get back our own DF class after slicing row-wise?
- cdf_rows = cdf[1:5]
- assert isinstance(cdf_rows, CustomDataFrame)
- assert cdf_rows.custom_frame_function() == "OK"
-
- # Make sure sliced part of multi-index frame is custom class
- mcol = MultiIndex.from_tuples([("A", "A"), ("A", "B")])
- cdf_multi = CustomDataFrame([[0, 1], [2, 3]], columns=mcol)
- assert isinstance(cdf_multi["A"], CustomDataFrame)
-
- mcol = MultiIndex.from_tuples([("A", ""), ("B", "")])
- cdf_multi2 = CustomDataFrame([[0, 1], [2, 3]], columns=mcol)
- assert isinstance(cdf_multi2["A"], CustomSeries)
-
- def test_dataframe_metadata(self):
- df = tm.SubclassedDataFrame(
- {"X": [1, 2, 3], "Y": [1, 2, 3]}, index=["a", "b", "c"]
- )
- df.testattr = "XXX"
-
- assert df.testattr == "XXX"
- assert df[["X"]].testattr == "XXX"
- assert df.loc[["a", "b"], :].testattr == "XXX"
- assert df.iloc[[0, 1], :].testattr == "XXX"
-
- # see gh-9776
- assert df.iloc[0:1, :].testattr == "XXX"
-
- # see gh-10553
- unpickled = tm.round_trip_pickle(df)
- tm.assert_frame_equal(df, unpickled)
- assert df._metadata == unpickled._metadata
- assert df.testattr == unpickled.testattr
-
- def test_indexing_sliced(self):
- # GH 11559
- df = tm.SubclassedDataFrame(
- {"X": [1, 2, 3], "Y": [4, 5, 6], "Z": [7, 8, 9]}, index=["a", "b", "c"]
- )
- res = df.loc[:, "X"]
- exp = tm.SubclassedSeries([1, 2, 3], index=list("abc"), name="X")
- tm.assert_series_equal(res, exp)
- assert isinstance(res, tm.SubclassedSeries)
-
- res = df.iloc[:, 1]
- exp = tm.SubclassedSeries([4, 5, 6], index=list("abc"), name="Y")
- tm.assert_series_equal(res, exp)
- assert isinstance(res, tm.SubclassedSeries)
-
- res = df.loc[:, "Z"]
- exp = tm.SubclassedSeries([7, 8, 9], index=list("abc"), name="Z")
- tm.assert_series_equal(res, exp)
- assert isinstance(res, tm.SubclassedSeries)
-
- res = df.loc["a", :]
- exp = tm.SubclassedSeries([1, 4, 7], index=list("XYZ"), name="a")
- tm.assert_series_equal(res, exp)
- assert isinstance(res, tm.SubclassedSeries)
-
- res = df.iloc[1, :]
- exp = tm.SubclassedSeries([2, 5, 8], index=list("XYZ"), name="b")
- tm.assert_series_equal(res, exp)
- assert isinstance(res, tm.SubclassedSeries)
-
- res = df.loc["c", :]
- exp = tm.SubclassedSeries([3, 6, 9], index=list("XYZ"), name="c")
- tm.assert_series_equal(res, exp)
- assert isinstance(res, tm.SubclassedSeries)
-
- def test_subclass_attr_err_propagation(self):
- # GH 11808
- class A(DataFrame):
- @property
- def nonexistence(self):
- return self.i_dont_exist
-
- with pytest.raises(AttributeError, match=".*i_dont_exist.*"):
- A().nonexistence
-
- def test_subclass_align(self):
- # GH 12983
- df1 = tm.SubclassedDataFrame(
- {"a": [1, 3, 5], "b": [1, 3, 5]}, index=list("ACE")
- )
- df2 = tm.SubclassedDataFrame(
- {"c": [1, 2, 4], "d": [1, 2, 4]}, index=list("ABD")
- )
-
- res1, res2 = df1.align(df2, axis=0)
- exp1 = tm.SubclassedDataFrame(
- {"a": [1, np.nan, 3, np.nan, 5], "b": [1, np.nan, 3, np.nan, 5]},
- index=list("ABCDE"),
- )
- exp2 = tm.SubclassedDataFrame(
- {"c": [1, 2, np.nan, 4, np.nan], "d": [1, 2, np.nan, 4, np.nan]},
- index=list("ABCDE"),
- )
- assert isinstance(res1, tm.SubclassedDataFrame)
- tm.assert_frame_equal(res1, exp1)
- assert isinstance(res2, tm.SubclassedDataFrame)
- tm.assert_frame_equal(res2, exp2)
-
- res1, res2 = df1.a.align(df2.c)
- assert isinstance(res1, tm.SubclassedSeries)
- tm.assert_series_equal(res1, exp1.a)
- assert isinstance(res2, tm.SubclassedSeries)
- tm.assert_series_equal(res2, exp2.c)
-
- def test_subclass_align_combinations(self):
- # GH 12983
- df = tm.SubclassedDataFrame({"a": [1, 3, 5], "b": [1, 3, 5]}, index=list("ACE"))
- s = tm.SubclassedSeries([1, 2, 4], index=list("ABD"), name="x")
-
- # frame + series
- res1, res2 = df.align(s, axis=0)
- exp1 = tm.SubclassedDataFrame(
- {"a": [1, np.nan, 3, np.nan, 5], "b": [1, np.nan, 3, np.nan, 5]},
- index=list("ABCDE"),
- )
- # name is lost when
- exp2 = tm.SubclassedSeries(
- [1, 2, np.nan, 4, np.nan], index=list("ABCDE"), name="x"
- )
-
- assert isinstance(res1, tm.SubclassedDataFrame)
- tm.assert_frame_equal(res1, exp1)
- assert isinstance(res2, tm.SubclassedSeries)
- tm.assert_series_equal(res2, exp2)
-
- # series + frame
- res1, res2 = s.align(df)
- assert isinstance(res1, tm.SubclassedSeries)
- tm.assert_series_equal(res1, exp2)
- assert isinstance(res2, tm.SubclassedDataFrame)
- tm.assert_frame_equal(res2, exp1)
-
- def test_subclass_iterrows(self):
- # GH 13977
- df = tm.SubclassedDataFrame({"a": [1]})
- for i, row in df.iterrows():
- assert isinstance(row, tm.SubclassedSeries)
- tm.assert_series_equal(row, df.loc[i])
-
- def test_subclass_stack(self):
- # GH 15564
- df = tm.SubclassedDataFrame(
- [[1, 2, 3], [4, 5, 6], [7, 8, 9]],
- index=["a", "b", "c"],
- columns=["X", "Y", "Z"],
- )
-
- res = df.stack(future_stack=True)
- exp = tm.SubclassedSeries(
- [1, 2, 3, 4, 5, 6, 7, 8, 9], index=[list("aaabbbccc"), list("XYZXYZXYZ")]
- )
-
- tm.assert_series_equal(res, exp)
-
- def test_subclass_stack_multi(self):
- # GH 15564
- df = tm.SubclassedDataFrame(
- [[10, 11, 12, 13], [20, 21, 22, 23], [30, 31, 32, 33], [40, 41, 42, 43]],
- index=MultiIndex.from_tuples(
- list(zip(list("AABB"), list("cdcd"))), names=["aaa", "ccc"]
- ),
- columns=MultiIndex.from_tuples(
- list(zip(list("WWXX"), list("yzyz"))), names=["www", "yyy"]
- ),
- )
-
- exp = tm.SubclassedDataFrame(
- [
- [10, 12],
- [11, 13],
- [20, 22],
- [21, 23],
- [30, 32],
- [31, 33],
- [40, 42],
- [41, 43],
- ],
- index=MultiIndex.from_tuples(
- list(zip(list("AAAABBBB"), list("ccddccdd"), list("yzyzyzyz"))),
- names=["aaa", "ccc", "yyy"],
- ),
- columns=Index(["W", "X"], name="www"),
- )
-
- res = df.stack(future_stack=True)
- tm.assert_frame_equal(res, exp)
-
- res = df.stack("yyy", future_stack=True)
- tm.assert_frame_equal(res, exp)
-
- exp = tm.SubclassedDataFrame(
- [
- [10, 11],
- [12, 13],
- [20, 21],
- [22, 23],
- [30, 31],
- [32, 33],
- [40, 41],
- [42, 43],
- ],
- index=MultiIndex.from_tuples(
- list(zip(list("AAAABBBB"), list("ccddccdd"), list("WXWXWXWX"))),
- names=["aaa", "ccc", "www"],
- ),
- columns=Index(["y", "z"], name="yyy"),
- )
-
- res = df.stack("www", future_stack=True)
- tm.assert_frame_equal(res, exp)
-
- def test_subclass_stack_multi_mixed(self):
- # GH 15564
- df = tm.SubclassedDataFrame(
- [
- [10, 11, 12.0, 13.0],
- [20, 21, 22.0, 23.0],
- [30, 31, 32.0, 33.0],
- [40, 41, 42.0, 43.0],
- ],
- index=MultiIndex.from_tuples(
- list(zip(list("AABB"), list("cdcd"))), names=["aaa", "ccc"]
- ),
- columns=MultiIndex.from_tuples(
- list(zip(list("WWXX"), list("yzyz"))), names=["www", "yyy"]
- ),
- )
-
- exp = tm.SubclassedDataFrame(
- [
- [10, 12.0],
- [11, 13.0],
- [20, 22.0],
- [21, 23.0],
- [30, 32.0],
- [31, 33.0],
- [40, 42.0],
- [41, 43.0],
- ],
- index=MultiIndex.from_tuples(
- list(zip(list("AAAABBBB"), list("ccddccdd"), list("yzyzyzyz"))),
- names=["aaa", "ccc", "yyy"],
- ),
- columns=Index(["W", "X"], name="www"),
- )
-
- res = df.stack(future_stack=True)
- tm.assert_frame_equal(res, exp)
-
- res = df.stack("yyy", future_stack=True)
- tm.assert_frame_equal(res, exp)
-
- exp = tm.SubclassedDataFrame(
- [
- [10.0, 11.0],
- [12.0, 13.0],
- [20.0, 21.0],
- [22.0, 23.0],
- [30.0, 31.0],
- [32.0, 33.0],
- [40.0, 41.0],
- [42.0, 43.0],
- ],
- index=MultiIndex.from_tuples(
- list(zip(list("AAAABBBB"), list("ccddccdd"), list("WXWXWXWX"))),
- names=["aaa", "ccc", "www"],
- ),
- columns=Index(["y", "z"], name="yyy"),
- )
-
- res = df.stack("www", future_stack=True)
- tm.assert_frame_equal(res, exp)
-
- def test_subclass_unstack(self):
- # GH 15564
- df = tm.SubclassedDataFrame(
- [[1, 2, 3], [4, 5, 6], [7, 8, 9]],
- index=["a", "b", "c"],
- columns=["X", "Y", "Z"],
- )
-
- res = df.unstack()
- exp = tm.SubclassedSeries(
- [1, 4, 7, 2, 5, 8, 3, 6, 9], index=[list("XXXYYYZZZ"), list("abcabcabc")]
- )
-
- tm.assert_series_equal(res, exp)
-
- def test_subclass_unstack_multi(self):
- # GH 15564
- df = tm.SubclassedDataFrame(
- [[10, 11, 12, 13], [20, 21, 22, 23], [30, 31, 32, 33], [40, 41, 42, 43]],
- index=MultiIndex.from_tuples(
- list(zip(list("AABB"), list("cdcd"))), names=["aaa", "ccc"]
- ),
- columns=MultiIndex.from_tuples(
- list(zip(list("WWXX"), list("yzyz"))), names=["www", "yyy"]
- ),
- )
-
- exp = tm.SubclassedDataFrame(
- [[10, 20, 11, 21, 12, 22, 13, 23], [30, 40, 31, 41, 32, 42, 33, 43]],
- index=Index(["A", "B"], name="aaa"),
- columns=MultiIndex.from_tuples(
- list(zip(list("WWWWXXXX"), list("yyzzyyzz"), list("cdcdcdcd"))),
- names=["www", "yyy", "ccc"],
- ),
- )
-
- res = df.unstack()
- tm.assert_frame_equal(res, exp)
-
- res = df.unstack("ccc")
- tm.assert_frame_equal(res, exp)
-
- exp = tm.SubclassedDataFrame(
- [[10, 30, 11, 31, 12, 32, 13, 33], [20, 40, 21, 41, 22, 42, 23, 43]],
- index=Index(["c", "d"], name="ccc"),
- columns=MultiIndex.from_tuples(
- list(zip(list("WWWWXXXX"), list("yyzzyyzz"), list("ABABABAB"))),
- names=["www", "yyy", "aaa"],
- ),
- )
-
- res = df.unstack("aaa")
- tm.assert_frame_equal(res, exp)
-
- def test_subclass_unstack_multi_mixed(self):
- # GH 15564
- df = tm.SubclassedDataFrame(
- [
- [10, 11, 12.0, 13.0],
- [20, 21, 22.0, 23.0],
- [30, 31, 32.0, 33.0],
- [40, 41, 42.0, 43.0],
- ],
- index=MultiIndex.from_tuples(
- list(zip(list("AABB"), list("cdcd"))), names=["aaa", "ccc"]
- ),
- columns=MultiIndex.from_tuples(
- list(zip(list("WWXX"), list("yzyz"))), names=["www", "yyy"]
- ),
- )
-
- exp = tm.SubclassedDataFrame(
- [
- [10, 20, 11, 21, 12.0, 22.0, 13.0, 23.0],
- [30, 40, 31, 41, 32.0, 42.0, 33.0, 43.0],
- ],
- index=Index(["A", "B"], name="aaa"),
- columns=MultiIndex.from_tuples(
- list(zip(list("WWWWXXXX"), list("yyzzyyzz"), list("cdcdcdcd"))),
- names=["www", "yyy", "ccc"],
- ),
- )
-
- res = df.unstack()
- tm.assert_frame_equal(res, exp)
-
- res = df.unstack("ccc")
- tm.assert_frame_equal(res, exp)
-
- exp = tm.SubclassedDataFrame(
- [
- [10, 30, 11, 31, 12.0, 32.0, 13.0, 33.0],
- [20, 40, 21, 41, 22.0, 42.0, 23.0, 43.0],
- ],
- index=Index(["c", "d"], name="ccc"),
- columns=MultiIndex.from_tuples(
- list(zip(list("WWWWXXXX"), list("yyzzyyzz"), list("ABABABAB"))),
- names=["www", "yyy", "aaa"],
- ),
- )
-
- res = df.unstack("aaa")
- tm.assert_frame_equal(res, exp)
-
- def test_subclass_pivot(self):
- # GH 15564
- df = tm.SubclassedDataFrame(
- {
- "index": ["A", "B", "C", "C", "B", "A"],
- "columns": ["One", "One", "One", "Two", "Two", "Two"],
- "values": [1.0, 2.0, 3.0, 3.0, 2.0, 1.0],
- }
- )
-
- pivoted = df.pivot(index="index", columns="columns", values="values")
-
- expected = tm.SubclassedDataFrame(
- {
- "One": {"A": 1.0, "B": 2.0, "C": 3.0},
- "Two": {"A": 1.0, "B": 2.0, "C": 3.0},
- }
- )
-
- expected.index.name, expected.columns.name = "index", "columns"
-
- tm.assert_frame_equal(pivoted, expected)
-
- def test_subclassed_melt(self):
- # GH 15564
- cheese = tm.SubclassedDataFrame(
- {
- "first": ["John", "Mary"],
- "last": ["Doe", "Bo"],
- "height": [5.5, 6.0],
- "weight": [130, 150],
- }
- )
-
- melted = pd.melt(cheese, id_vars=["first", "last"])
-
- expected = tm.SubclassedDataFrame(
- [
- ["John", "Doe", "height", 5.5],
- ["Mary", "Bo", "height", 6.0],
- ["John", "Doe", "weight", 130],
- ["Mary", "Bo", "weight", 150],
- ],
- columns=["first", "last", "variable", "value"],
- )
-
- tm.assert_frame_equal(melted, expected)
-
- def test_subclassed_wide_to_long(self):
- # GH 9762
-
- x = np.random.default_rng(2).standard_normal(3)
- df = tm.SubclassedDataFrame(
- {
- "A1970": {0: "a", 1: "b", 2: "c"},
- "A1980": {0: "d", 1: "e", 2: "f"},
- "B1970": {0: 2.5, 1: 1.2, 2: 0.7},
- "B1980": {0: 3.2, 1: 1.3, 2: 0.1},
- "X": dict(zip(range(3), x)),
- }
- )
-
- df["id"] = df.index
- exp_data = {
- "X": x.tolist() + x.tolist(),
- "A": ["a", "b", "c", "d", "e", "f"],
- "B": [2.5, 1.2, 0.7, 3.2, 1.3, 0.1],
- "year": [1970, 1970, 1970, 1980, 1980, 1980],
- "id": [0, 1, 2, 0, 1, 2],
- }
- expected = tm.SubclassedDataFrame(exp_data)
- expected = expected.set_index(["id", "year"])[["X", "A", "B"]]
- long_frame = pd.wide_to_long(df, ["A", "B"], i="id", j="year")
-
- tm.assert_frame_equal(long_frame, expected)
-
- def test_subclassed_apply(self):
- # GH 19822
-
- def check_row_subclass(row):
- assert isinstance(row, tm.SubclassedSeries)
-
- def stretch(row):
- if row["variable"] == "height":
- row["value"] += 0.5
- return row
-
- df = tm.SubclassedDataFrame(
- [
- ["John", "Doe", "height", 5.5],
- ["Mary", "Bo", "height", 6.0],
- ["John", "Doe", "weight", 130],
- ["Mary", "Bo", "weight", 150],
- ],
- columns=["first", "last", "variable", "value"],
- )
-
- df.apply(lambda x: check_row_subclass(x))
- df.apply(lambda x: check_row_subclass(x), axis=1)
-
- expected = tm.SubclassedDataFrame(
- [
- ["John", "Doe", "height", 6.0],
- ["Mary", "Bo", "height", 6.5],
- ["John", "Doe", "weight", 130],
- ["Mary", "Bo", "weight", 150],
- ],
- columns=["first", "last", "variable", "value"],
- )
-
- result = df.apply(lambda x: stretch(x), axis=1)
- assert isinstance(result, tm.SubclassedDataFrame)
- tm.assert_frame_equal(result, expected)
-
- expected = tm.SubclassedDataFrame([[1, 2, 3], [1, 2, 3], [1, 2, 3], [1, 2, 3]])
-
- result = df.apply(lambda x: tm.SubclassedSeries([1, 2, 3]), axis=1)
- assert isinstance(result, tm.SubclassedDataFrame)
- tm.assert_frame_equal(result, expected)
-
- result = df.apply(lambda x: [1, 2, 3], axis=1, result_type="expand")
- assert isinstance(result, tm.SubclassedDataFrame)
- tm.assert_frame_equal(result, expected)
-
- expected = tm.SubclassedSeries([[1, 2, 3], [1, 2, 3], [1, 2, 3], [1, 2, 3]])
-
- result = df.apply(lambda x: [1, 2, 3], axis=1)
- assert not isinstance(result, tm.SubclassedDataFrame)
- tm.assert_series_equal(result, expected)
-
- def test_subclassed_reductions(self, all_reductions):
- # GH 25596
-
- df = tm.SubclassedDataFrame({"A": [1, 2, 3], "B": [4, 5, 6], "C": [7, 8, 9]})
- result = getattr(df, all_reductions)()
- assert isinstance(result, tm.SubclassedSeries)
-
- def test_subclassed_count(self):
- df = tm.SubclassedDataFrame(
- {
- "Person": ["John", "Myla", "Lewis", "John", "Myla"],
- "Age": [24.0, np.nan, 21.0, 33, 26],
- "Single": [False, True, True, True, False],
- }
- )
- result = df.count()
- assert isinstance(result, tm.SubclassedSeries)
-
- df = tm.SubclassedDataFrame({"A": [1, 0, 3], "B": [0, 5, 6], "C": [7, 8, 0]})
- result = df.count()
- assert isinstance(result, tm.SubclassedSeries)
-
- df = tm.SubclassedDataFrame(
- [[10, 11, 12, 13], [20, 21, 22, 23], [30, 31, 32, 33], [40, 41, 42, 43]],
- index=MultiIndex.from_tuples(
- list(zip(list("AABB"), list("cdcd"))), names=["aaa", "ccc"]
- ),
- columns=MultiIndex.from_tuples(
- list(zip(list("WWXX"), list("yzyz"))), names=["www", "yyy"]
- ),
- )
- result = df.count()
- assert isinstance(result, tm.SubclassedSeries)
-
- df = tm.SubclassedDataFrame()
- result = df.count()
- assert isinstance(result, tm.SubclassedSeries)
-
- def test_isin(self):
- df = tm.SubclassedDataFrame(
- {"num_legs": [2, 4], "num_wings": [2, 0]}, index=["falcon", "dog"]
- )
- result = df.isin([0, 2])
- assert isinstance(result, tm.SubclassedDataFrame)
-
- def test_duplicated(self):
- df = tm.SubclassedDataFrame({"A": [1, 2, 3], "B": [4, 5, 6], "C": [7, 8, 9]})
- result = df.duplicated()
- assert isinstance(result, tm.SubclassedSeries)
-
- df = tm.SubclassedDataFrame()
- result = df.duplicated()
- assert isinstance(result, tm.SubclassedSeries)
-
- @pytest.mark.parametrize("idx_method", ["idxmax", "idxmin"])
- def test_idx(self, idx_method):
- df = tm.SubclassedDataFrame({"A": [1, 2, 3], "B": [4, 5, 6], "C": [7, 8, 9]})
- result = getattr(df, idx_method)()
- assert isinstance(result, tm.SubclassedSeries)
-
- def test_dot(self):
- df = tm.SubclassedDataFrame([[0, 1, -2, -1], [1, 1, 1, 1]])
- s = tm.SubclassedSeries([1, 1, 2, 1])
- result = df.dot(s)
- assert isinstance(result, tm.SubclassedSeries)
-
- df = tm.SubclassedDataFrame([[0, 1, -2, -1], [1, 1, 1, 1]])
- s = tm.SubclassedDataFrame([1, 1, 2, 1])
- result = df.dot(s)
- assert isinstance(result, tm.SubclassedDataFrame)
-
- def test_memory_usage(self):
- df = tm.SubclassedDataFrame({"A": [1, 2, 3], "B": [4, 5, 6], "C": [7, 8, 9]})
- result = df.memory_usage()
- assert isinstance(result, tm.SubclassedSeries)
-
- result = df.memory_usage(index=False)
- assert isinstance(result, tm.SubclassedSeries)
-
- def test_corrwith(self):
- pytest.importorskip("scipy")
- index = ["a", "b", "c", "d", "e"]
- columns = ["one", "two", "three", "four"]
- df1 = tm.SubclassedDataFrame(
- np.random.default_rng(2).standard_normal((5, 4)),
- index=index,
- columns=columns,
- )
- df2 = tm.SubclassedDataFrame(
- np.random.default_rng(2).standard_normal((4, 4)),
- index=index[:4],
- columns=columns,
- )
- correls = df1.corrwith(df2, axis=1, drop=True, method="kendall")
-
- assert isinstance(correls, (tm.SubclassedSeries))
-
- def test_asof(self):
- N = 3
- rng = pd.date_range("1/1/1990", periods=N, freq="53s")
- df = tm.SubclassedDataFrame(
- {
- "A": [np.nan, np.nan, np.nan],
- "B": [np.nan, np.nan, np.nan],
- "C": [np.nan, np.nan, np.nan],
- },
- index=rng,
- )
-
- result = df.asof(rng[-2:])
- assert isinstance(result, tm.SubclassedDataFrame)
-
- result = df.asof(rng[-2])
- assert isinstance(result, tm.SubclassedSeries)
-
- result = df.asof("1989-12-31")
- assert isinstance(result, tm.SubclassedSeries)
-
- def test_idxmin_preserves_subclass(self):
- # GH 28330
-
- df = tm.SubclassedDataFrame({"A": [1, 2, 3], "B": [4, 5, 6], "C": [7, 8, 9]})
- result = df.idxmin()
- assert isinstance(result, tm.SubclassedSeries)
-
- def test_idxmax_preserves_subclass(self):
- # GH 28330
-
- df = tm.SubclassedDataFrame({"A": [1, 2, 3], "B": [4, 5, 6], "C": [7, 8, 9]})
- result = df.idxmax()
- assert isinstance(result, tm.SubclassedSeries)
-
- def test_convert_dtypes_preserves_subclass(self, gpd_style_subclass_df):
- # GH 43668
- df = tm.SubclassedDataFrame({"A": [1, 2, 3], "B": [4, 5, 6], "C": [7, 8, 9]})
- result = df.convert_dtypes()
- assert isinstance(result, tm.SubclassedDataFrame)
-
- result = gpd_style_subclass_df.convert_dtypes()
- assert isinstance(result, type(gpd_style_subclass_df))
-
- def test_astype_preserves_subclass(self):
- # GH#40810
- df = tm.SubclassedDataFrame({"A": [1, 2, 3], "B": [4, 5, 6], "C": [7, 8, 9]})
-
- result = df.astype({"A": np.int64, "B": np.int32, "C": np.float64})
- assert isinstance(result, tm.SubclassedDataFrame)
-
- def test_equals_subclass(self):
- # https://github.com/pandas-dev/pandas/pull/34402
- # allow subclass in both directions
- df1 = DataFrame({"a": [1, 2, 3]})
- df2 = tm.SubclassedDataFrame({"a": [1, 2, 3]})
- assert df1.equals(df2)
- assert df2.equals(df1)
-
- def test_replace_list_method(self):
- # https://github.com/pandas-dev/pandas/pull/46018
- df = tm.SubclassedDataFrame({"A": [0, 1, 2]})
- msg = "The 'method' keyword in SubclassedDataFrame.replace is deprecated"
- with tm.assert_produces_warning(
- FutureWarning, match=msg, raise_on_extra_warnings=False
- ):
- result = df.replace([1, 2], method="ffill")
- expected = tm.SubclassedDataFrame({"A": [0, 0, 0]})
- assert isinstance(result, tm.SubclassedDataFrame)
- tm.assert_frame_equal(result, expected)
-
-
-class MySubclassWithMetadata(DataFrame):
- _metadata = ["my_metadata"]
-
- def __init__(self, *args, **kwargs) -> None:
- super().__init__(*args, **kwargs)
-
- my_metadata = kwargs.pop("my_metadata", None)
- if args and isinstance(args[0], MySubclassWithMetadata):
- my_metadata = args[0].my_metadata # type: ignore[has-type]
- self.my_metadata = my_metadata
-
- @property
- def _constructor(self):
- return MySubclassWithMetadata
-
-
-def test_constructor_with_metadata():
- # https://github.com/pandas-dev/pandas/pull/54922
- # https://github.com/pandas-dev/pandas/issues/55120
- df = MySubclassWithMetadata(
- np.random.default_rng(2).random((5, 3)), columns=["A", "B", "C"]
- )
- subset = df[["A", "B"]]
- assert isinstance(subset, MySubclassWithMetadata)
diff --git a/spaces/profayle/TerrapinTalk/myenv/lib/python3.9/site-packages/pandas/tests/indexes/multi/test_reshape.py b/spaces/profayle/TerrapinTalk/myenv/lib/python3.9/site-packages/pandas/tests/indexes/multi/test_reshape.py
deleted file mode 100644
index 06dbb33aadf97a54e4bb283d3aed8fe1169164b3..0000000000000000000000000000000000000000
--- a/spaces/profayle/TerrapinTalk/myenv/lib/python3.9/site-packages/pandas/tests/indexes/multi/test_reshape.py
+++ /dev/null
@@ -1,224 +0,0 @@
-from datetime import datetime
-
-import numpy as np
-import pytest
-import pytz
-
-import pandas as pd
-from pandas import (
- Index,
- MultiIndex,
-)
-import pandas._testing as tm
-
-
-def test_insert(idx):
- # key contained in all levels
- new_index = idx.insert(0, ("bar", "two"))
- assert new_index.equal_levels(idx)
- assert new_index[0] == ("bar", "two")
-
- # key not contained in all levels
- new_index = idx.insert(0, ("abc", "three"))
-
- exp0 = Index(list(idx.levels[0]) + ["abc"], name="first")
- tm.assert_index_equal(new_index.levels[0], exp0)
- assert new_index.names == ["first", "second"]
-
- exp1 = Index(list(idx.levels[1]) + ["three"], name="second")
- tm.assert_index_equal(new_index.levels[1], exp1)
- assert new_index[0] == ("abc", "three")
-
- # key wrong length
- msg = "Item must have length equal to number of levels"
- with pytest.raises(ValueError, match=msg):
- idx.insert(0, ("foo2",))
-
- left = pd.DataFrame([["a", "b", 0], ["b", "d", 1]], columns=["1st", "2nd", "3rd"])
- left.set_index(["1st", "2nd"], inplace=True)
- ts = left["3rd"].copy(deep=True)
-
- left.loc[("b", "x"), "3rd"] = 2
- left.loc[("b", "a"), "3rd"] = -1
- left.loc[("b", "b"), "3rd"] = 3
- left.loc[("a", "x"), "3rd"] = 4
- left.loc[("a", "w"), "3rd"] = 5
- left.loc[("a", "a"), "3rd"] = 6
-
- ts.loc[("b", "x")] = 2
- ts.loc["b", "a"] = -1
- ts.loc[("b", "b")] = 3
- ts.loc["a", "x"] = 4
- ts.loc[("a", "w")] = 5
- ts.loc["a", "a"] = 6
-
- right = pd.DataFrame(
- [
- ["a", "b", 0],
- ["b", "d", 1],
- ["b", "x", 2],
- ["b", "a", -1],
- ["b", "b", 3],
- ["a", "x", 4],
- ["a", "w", 5],
- ["a", "a", 6],
- ],
- columns=["1st", "2nd", "3rd"],
- )
- right.set_index(["1st", "2nd"], inplace=True)
- # FIXME data types changes to float because
- # of intermediate nan insertion;
- tm.assert_frame_equal(left, right, check_dtype=False)
- tm.assert_series_equal(ts, right["3rd"])
-
-
-def test_insert2():
- # GH9250
- idx = (
- [("test1", i) for i in range(5)]
- + [("test2", i) for i in range(6)]
- + [("test", 17), ("test", 18)]
- )
-
- left = pd.Series(np.linspace(0, 10, 11), MultiIndex.from_tuples(idx[:-2]))
-
- left.loc[("test", 17)] = 11
- left.loc[("test", 18)] = 12
-
- right = pd.Series(np.linspace(0, 12, 13), MultiIndex.from_tuples(idx))
-
- tm.assert_series_equal(left, right)
-
-
-def test_append(idx):
- result = idx[:3].append(idx[3:])
- assert result.equals(idx)
-
- foos = [idx[:1], idx[1:3], idx[3:]]
- result = foos[0].append(foos[1:])
- assert result.equals(idx)
-
- # empty
- result = idx.append([])
- assert result.equals(idx)
-
-
-def test_append_index():
- idx1 = Index([1.1, 1.2, 1.3])
- idx2 = pd.date_range("2011-01-01", freq="D", periods=3, tz="Asia/Tokyo")
- idx3 = Index(["A", "B", "C"])
-
- midx_lv2 = MultiIndex.from_arrays([idx1, idx2])
- midx_lv3 = MultiIndex.from_arrays([idx1, idx2, idx3])
-
- result = idx1.append(midx_lv2)
-
- # see gh-7112
- tz = pytz.timezone("Asia/Tokyo")
- expected_tuples = [
- (1.1, tz.localize(datetime(2011, 1, 1))),
- (1.2, tz.localize(datetime(2011, 1, 2))),
- (1.3, tz.localize(datetime(2011, 1, 3))),
- ]
- expected = Index([1.1, 1.2, 1.3] + expected_tuples)
- tm.assert_index_equal(result, expected)
-
- result = midx_lv2.append(idx1)
- expected = Index(expected_tuples + [1.1, 1.2, 1.3])
- tm.assert_index_equal(result, expected)
-
- result = midx_lv2.append(midx_lv2)
- expected = MultiIndex.from_arrays([idx1.append(idx1), idx2.append(idx2)])
- tm.assert_index_equal(result, expected)
-
- result = midx_lv2.append(midx_lv3)
- tm.assert_index_equal(result, expected)
-
- result = midx_lv3.append(midx_lv2)
- expected = Index._simple_new(
- np.array(
- [
- (1.1, tz.localize(datetime(2011, 1, 1)), "A"),
- (1.2, tz.localize(datetime(2011, 1, 2)), "B"),
- (1.3, tz.localize(datetime(2011, 1, 3)), "C"),
- ]
- + expected_tuples,
- dtype=object,
- ),
- None,
- )
- tm.assert_index_equal(result, expected)
-
-
-@pytest.mark.parametrize("name, exp", [("b", "b"), ("c", None)])
-def test_append_names_match(name, exp):
- # GH#48288
- midx = MultiIndex.from_arrays([[1, 2], [3, 4]], names=["a", "b"])
- midx2 = MultiIndex.from_arrays([[3], [5]], names=["a", name])
- result = midx.append(midx2)
- expected = MultiIndex.from_arrays([[1, 2, 3], [3, 4, 5]], names=["a", exp])
- tm.assert_index_equal(result, expected)
-
-
-def test_append_names_dont_match():
- # GH#48288
- midx = MultiIndex.from_arrays([[1, 2], [3, 4]], names=["a", "b"])
- midx2 = MultiIndex.from_arrays([[3], [5]], names=["x", "y"])
- result = midx.append(midx2)
- expected = MultiIndex.from_arrays([[1, 2, 3], [3, 4, 5]], names=None)
- tm.assert_index_equal(result, expected)
-
-
-def test_append_overlapping_interval_levels():
- # GH 54934
- ivl1 = pd.IntervalIndex.from_breaks([0.0, 1.0, 2.0])
- ivl2 = pd.IntervalIndex.from_breaks([0.5, 1.5, 2.5])
- mi1 = MultiIndex.from_product([ivl1, ivl1])
- mi2 = MultiIndex.from_product([ivl2, ivl2])
- result = mi1.append(mi2)
- expected = MultiIndex.from_tuples(
- [
- (pd.Interval(0.0, 1.0), pd.Interval(0.0, 1.0)),
- (pd.Interval(0.0, 1.0), pd.Interval(1.0, 2.0)),
- (pd.Interval(1.0, 2.0), pd.Interval(0.0, 1.0)),
- (pd.Interval(1.0, 2.0), pd.Interval(1.0, 2.0)),
- (pd.Interval(0.5, 1.5), pd.Interval(0.5, 1.5)),
- (pd.Interval(0.5, 1.5), pd.Interval(1.5, 2.5)),
- (pd.Interval(1.5, 2.5), pd.Interval(0.5, 1.5)),
- (pd.Interval(1.5, 2.5), pd.Interval(1.5, 2.5)),
- ]
- )
- tm.assert_index_equal(result, expected)
-
-
-def test_repeat():
- reps = 2
- numbers = [1, 2, 3]
- names = np.array(["foo", "bar"])
-
- m = MultiIndex.from_product([numbers, names], names=names)
- expected = MultiIndex.from_product([numbers, names.repeat(reps)], names=names)
- tm.assert_index_equal(m.repeat(reps), expected)
-
-
-def test_insert_base(idx):
- result = idx[1:4]
-
- # test 0th element
- assert idx[0:4].equals(result.insert(0, idx[0]))
-
-
-def test_delete_base(idx):
- expected = idx[1:]
- result = idx.delete(0)
- assert result.equals(expected)
- assert result.name == expected.name
-
- expected = idx[:-1]
- result = idx.delete(-1)
- assert result.equals(expected)
- assert result.name == expected.name
-
- msg = "index 6 is out of bounds for axis 0 with size 6"
- with pytest.raises(IndexError, match=msg):
- idx.delete(len(idx))
diff --git a/spaces/profayle/TerrapinTalk/myenv/lib/python3.9/site-packages/pygments/lexers/actionscript.py b/spaces/profayle/TerrapinTalk/myenv/lib/python3.9/site-packages/pygments/lexers/actionscript.py
deleted file mode 100644
index e0e94a52e42b502a535812a20738399d44f6fc23..0000000000000000000000000000000000000000
--- a/spaces/profayle/TerrapinTalk/myenv/lib/python3.9/site-packages/pygments/lexers/actionscript.py
+++ /dev/null
@@ -1,245 +0,0 @@
-"""
- pygments.lexers.actionscript
- ~~~~~~~~~~~~~~~~~~~~~~~~~~~~
-
- Lexers for ActionScript and MXML.
-
- :copyright: Copyright 2006-2023 by the Pygments team, see AUTHORS.
- :license: BSD, see LICENSE for details.
-"""
-
-import re
-
-from pygments.lexer import RegexLexer, bygroups, using, this, words, default
-from pygments.token import Text, Comment, Operator, Keyword, Name, String, \
- Number, Punctuation, Whitespace
-
-__all__ = ['ActionScriptLexer', 'ActionScript3Lexer', 'MxmlLexer']
-
-
-class ActionScriptLexer(RegexLexer):
- """
- For ActionScript source code.
-
- .. versionadded:: 0.9
- """
-
- name = 'ActionScript'
- aliases = ['actionscript', 'as']
- filenames = ['*.as']
- mimetypes = ['application/x-actionscript', 'text/x-actionscript',
- 'text/actionscript']
-
- flags = re.DOTALL
- tokens = {
- 'root': [
- (r'\s+', Whitespace),
- (r'//.*?\n', Comment.Single),
- (r'/\*.*?\*/', Comment.Multiline),
- (r'/(\\\\|\\[^\\]|[^/\\\n])*/[gim]*', String.Regex),
- (r'[~^*!%&<>|+=:;,/?\\-]+', Operator),
- (r'[{}\[\]();.]+', Punctuation),
- (words((
- 'case', 'default', 'for', 'each', 'in', 'while', 'do', 'break',
- 'return', 'continue', 'if', 'else', 'throw', 'try', 'catch',
- 'var', 'with', 'new', 'typeof', 'arguments', 'instanceof', 'this',
- 'switch'), suffix=r'\b'),
- Keyword),
- (words((
- 'class', 'public', 'final', 'internal', 'native', 'override', 'private',
- 'protected', 'static', 'import', 'extends', 'implements', 'interface',
- 'intrinsic', 'return', 'super', 'dynamic', 'function', 'const', 'get',
- 'namespace', 'package', 'set'), suffix=r'\b'),
- Keyword.Declaration),
- (r'(true|false|null|NaN|Infinity|-Infinity|undefined|Void)\b',
- Keyword.Constant),
- (words((
- 'Accessibility', 'AccessibilityProperties', 'ActionScriptVersion',
- 'ActivityEvent', 'AntiAliasType', 'ApplicationDomain', 'AsBroadcaster', 'Array',
- 'AsyncErrorEvent', 'AVM1Movie', 'BevelFilter', 'Bitmap', 'BitmapData',
- 'BitmapDataChannel', 'BitmapFilter', 'BitmapFilterQuality', 'BitmapFilterType',
- 'BlendMode', 'BlurFilter', 'Boolean', 'ByteArray', 'Camera', 'Capabilities', 'CapsStyle',
- 'Class', 'Color', 'ColorMatrixFilter', 'ColorTransform', 'ContextMenu',
- 'ContextMenuBuiltInItems', 'ContextMenuEvent', 'ContextMenuItem',
- 'ConvultionFilter', 'CSMSettings', 'DataEvent', 'Date', 'DefinitionError',
- 'DeleteObjectSample', 'Dictionary', 'DisplacmentMapFilter', 'DisplayObject',
- 'DisplacmentMapFilterMode', 'DisplayObjectContainer', 'DropShadowFilter',
- 'Endian', 'EOFError', 'Error', 'ErrorEvent', 'EvalError', 'Event', 'EventDispatcher',
- 'EventPhase', 'ExternalInterface', 'FileFilter', 'FileReference',
- 'FileReferenceList', 'FocusDirection', 'FocusEvent', 'Font', 'FontStyle', 'FontType',
- 'FrameLabel', 'FullScreenEvent', 'Function', 'GlowFilter', 'GradientBevelFilter',
- 'GradientGlowFilter', 'GradientType', 'Graphics', 'GridFitType', 'HTTPStatusEvent',
- 'IBitmapDrawable', 'ID3Info', 'IDataInput', 'IDataOutput', 'IDynamicPropertyOutput'
- 'IDynamicPropertyWriter', 'IEventDispatcher', 'IExternalizable',
- 'IllegalOperationError', 'IME', 'IMEConversionMode', 'IMEEvent', 'int',
- 'InteractiveObject', 'InterpolationMethod', 'InvalidSWFError', 'InvokeEvent',
- 'IOError', 'IOErrorEvent', 'JointStyle', 'Key', 'Keyboard', 'KeyboardEvent', 'KeyLocation',
- 'LineScaleMode', 'Loader', 'LoaderContext', 'LoaderInfo', 'LoadVars', 'LocalConnection',
- 'Locale', 'Math', 'Matrix', 'MemoryError', 'Microphone', 'MorphShape', 'Mouse', 'MouseEvent',
- 'MovieClip', 'MovieClipLoader', 'Namespace', 'NetConnection', 'NetStatusEvent',
- 'NetStream', 'NewObjectSample', 'Number', 'Object', 'ObjectEncoding', 'PixelSnapping',
- 'Point', 'PrintJob', 'PrintJobOptions', 'PrintJobOrientation', 'ProgressEvent', 'Proxy',
- 'QName', 'RangeError', 'Rectangle', 'ReferenceError', 'RegExp', 'Responder', 'Sample',
- 'Scene', 'ScriptTimeoutError', 'Security', 'SecurityDomain', 'SecurityError',
- 'SecurityErrorEvent', 'SecurityPanel', 'Selection', 'Shape', 'SharedObject',
- 'SharedObjectFlushStatus', 'SimpleButton', 'Socket', 'Sound', 'SoundChannel',
- 'SoundLoaderContext', 'SoundMixer', 'SoundTransform', 'SpreadMethod', 'Sprite',
- 'StackFrame', 'StackOverflowError', 'Stage', 'StageAlign', 'StageDisplayState',
- 'StageQuality', 'StageScaleMode', 'StaticText', 'StatusEvent', 'String', 'StyleSheet',
- 'SWFVersion', 'SyncEvent', 'SyntaxError', 'System', 'TextColorType', 'TextField',
- 'TextFieldAutoSize', 'TextFieldType', 'TextFormat', 'TextFormatAlign',
- 'TextLineMetrics', 'TextRenderer', 'TextSnapshot', 'Timer', 'TimerEvent', 'Transform',
- 'TypeError', 'uint', 'URIError', 'URLLoader', 'URLLoaderDataFormat', 'URLRequest',
- 'URLRequestHeader', 'URLRequestMethod', 'URLStream', 'URLVariabeles', 'VerifyError',
- 'Video', 'XML', 'XMLDocument', 'XMLList', 'XMLNode', 'XMLNodeType', 'XMLSocket',
- 'XMLUI'), suffix=r'\b'),
- Name.Builtin),
- (words((
- 'decodeURI', 'decodeURIComponent', 'encodeURI', 'escape', 'eval', 'isFinite', 'isNaN',
- 'isXMLName', 'clearInterval', 'fscommand', 'getTimer', 'getURL', 'getVersion',
- 'parseFloat', 'parseInt', 'setInterval', 'trace', 'updateAfterEvent',
- 'unescape'), suffix=r'\b'),
- Name.Function),
- (r'[$a-zA-Z_]\w*', Name.Other),
- (r'[0-9][0-9]*\.[0-9]+([eE][0-9]+)?[fd]?', Number.Float),
- (r'0x[0-9a-f]+', Number.Hex),
- (r'[0-9]+', Number.Integer),
- (r'"(\\\\|\\[^\\]|[^"\\])*"', String.Double),
- (r"'(\\\\|\\[^\\]|[^'\\])*'", String.Single),
- ]
- }
-
- def analyse_text(text):
- """This is only used to disambiguate between ActionScript and
- ActionScript3. We return 0 here; the ActionScript3 lexer will match
- AS3 variable definitions and that will hopefully suffice."""
- return 0
-
-class ActionScript3Lexer(RegexLexer):
- """
- For ActionScript 3 source code.
-
- .. versionadded:: 0.11
- """
-
- name = 'ActionScript 3'
- url = 'https://help.adobe.com/en_US/FlashPlatform/reference/actionscript/3/index.html'
- aliases = ['actionscript3', 'as3']
- filenames = ['*.as']
- mimetypes = ['application/x-actionscript3', 'text/x-actionscript3',
- 'text/actionscript3']
-
- identifier = r'[$a-zA-Z_]\w*'
- typeidentifier = identifier + r'(?:\.<\w+>)?'
-
- flags = re.DOTALL | re.MULTILINE
- tokens = {
- 'root': [
- (r'\s+', Whitespace),
- (r'(function\s+)(' + identifier + r')(\s*)(\()',
- bygroups(Keyword.Declaration, Name.Function, Text, Operator),
- 'funcparams'),
- (r'(var|const)(\s+)(' + identifier + r')(\s*)(:)(\s*)(' +
- typeidentifier + r')',
- bygroups(Keyword.Declaration, Whitespace, Name, Whitespace, Punctuation, Whitespace,
- Keyword.Type)),
- (r'(import|package)(\s+)((?:' + identifier + r'|\.)+)(\s*)',
- bygroups(Keyword, Whitespace, Name.Namespace, Whitespace)),
- (r'(new)(\s+)(' + typeidentifier + r')(\s*)(\()',
- bygroups(Keyword, Whitespace, Keyword.Type, Whitespace, Operator)),
- (r'//.*?\n', Comment.Single),
- (r'/\*.*?\*/', Comment.Multiline),
- (r'/(\\\\|\\[^\\]|[^\\\n])*/[gisx]*', String.Regex),
- (r'(\.)(' + identifier + r')', bygroups(Operator, Name.Attribute)),
- (r'(case|default|for|each|in|while|do|break|return|continue|if|else|'
- r'throw|try|catch|with|new|typeof|arguments|instanceof|this|'
- r'switch|import|include|as|is)\b',
- Keyword),
- (r'(class|public|final|internal|native|override|private|protected|'
- r'static|import|extends|implements|interface|intrinsic|return|super|'
- r'dynamic|function|const|get|namespace|package|set)\b',
- Keyword.Declaration),
- (r'(true|false|null|NaN|Infinity|-Infinity|undefined|void)\b',
- Keyword.Constant),
- (r'(decodeURI|decodeURIComponent|encodeURI|escape|eval|isFinite|isNaN|'
- r'isXMLName|clearInterval|fscommand|getTimer|getURL|getVersion|'
- r'isFinite|parseFloat|parseInt|setInterval|trace|updateAfterEvent|'
- r'unescape)\b', Name.Function),
- (identifier, Name),
- (r'[0-9][0-9]*\.[0-9]+([eE][0-9]+)?[fd]?', Number.Float),
- (r'0x[0-9a-f]+', Number.Hex),
- (r'[0-9]+', Number.Integer),
- (r'"(\\\\|\\[^\\]|[^"\\])*"', String.Double),
- (r"'(\\\\|\\[^\\]|[^'\\])*'", String.Single),
- (r'[~^*!%&<>|+=:;,/?\\{}\[\]().-]+', Operator),
- ],
- 'funcparams': [
- (r'\s+', Whitespace),
- (r'(\s*)(\.\.\.)?(' + identifier + r')(\s*)(:)(\s*)(' +
- typeidentifier + r'|\*)(\s*)',
- bygroups(Whitespace, Punctuation, Name, Whitespace, Operator, Whitespace,
- Keyword.Type, Whitespace), 'defval'),
- (r'\)', Operator, 'type')
- ],
- 'type': [
- (r'(\s*)(:)(\s*)(' + typeidentifier + r'|\*)',
- bygroups(Whitespace, Operator, Whitespace, Keyword.Type), '#pop:2'),
- (r'\s+', Text, '#pop:2'),
- default('#pop:2')
- ],
- 'defval': [
- (r'(=)(\s*)([^(),]+)(\s*)(,?)',
- bygroups(Operator, Whitespace, using(this), Whitespace, Operator), '#pop'),
- (r',', Operator, '#pop'),
- default('#pop')
- ]
- }
-
- def analyse_text(text):
- if re.match(r'\w+\s*:\s*\w', text):
- return 0.3
- return 0
-
-
-class MxmlLexer(RegexLexer):
- """
- For MXML markup.
- Nested AS3 in