Commit
·
c1a869e
1
Parent(s):
906f139
Update parquet files (step 63 of 249)
Browse filesThis view is limited to 50 files because it contains too many changes.
See raw diff
- spaces/1acneusushi/gradio-2dmoleculeeditor/data/23rd March 1931 Shaheed Mp4 2021 Download.md +0 -20
- spaces/1gistliPinn/ChatGPT4/Examples/Dc Unlocker 2 Client Full [HOT] 12.md +0 -6
- spaces/1gistliPinn/ChatGPT4/Examples/Download Buku Boyman Pramuka Pdf Viewer Semua yang Perlu Anda Ketahui tentang Pramuka.md +0 -6
- spaces/1gistliPinn/ChatGPT4/Examples/Download Commando - A One Man Army Full Movie.md +0 -6
- spaces/1gistliPinn/ChatGPT4/Examples/F.E.A.R 3 ENGLISH LANGUAGE PACK TORRENT.md +0 -7
- spaces/1pelhydcardo/ChatGPT-prompt-generator/Download Triple Frontier.md +0 -100
- spaces/1phancelerku/anime-remove-background/Download Free Fire MAX APK 32 Bits for Android - The Ultimate Battle Royale Experience.md +0 -153
- spaces/232labs/VToonify/app.py +0 -290
- spaces/AIConsultant/MusicGen/scripts/static/style.css +0 -113
- spaces/AIFILMS/audioldm-text-to-audio-generation/audioldm/pipeline.py +0 -78
- spaces/AIGC-Audio/AudioGPT/audio_detection/__init__.py +0 -0
- spaces/ATang0729/Forecast4Muses/Model/Model6/Model6_0_ClothesDetection/mmyolo/configs/yolov5/voc/yolov5_m-v61_fast_1xb64-50e_voc.py +0 -17
- spaces/Abhilashvj/haystack_QA/search.py +0 -60
- spaces/AchyuthGamer/OpenGPT-Chat-UI/src/lib/utils/deepestChild.ts +0 -6
- spaces/AchyuthGamer/OpenGPT/g4f/Provider/Providers/Myshell.py +0 -173
- spaces/AgentVerse/agentVerse/ui/src/phaser3-rex-plugins/templates/ui/gridtable/TableOnCellVisible.js +0 -28
- spaces/AgentVerse/agentVerse/ui/src/phaser3-rex-plugins/templates/ui/lineprogress/Factory.d.ts +0 -13
- spaces/Amrrs/DragGan-Inversion/stylegan_human/bg_white.py +0 -60
- spaces/Androidonnxfork/CivitAi-to-Diffusers/diffusers/src/diffusers/schedulers/scheduling_ddpm.py +0 -513
- spaces/Androidonnxfork/CivitAi-to-Diffusers/diffusers/tests/pipelines/stable_diffusion_2/__init__.py +0 -0
- spaces/Andy1621/uniformer_image_detection/configs/ghm/retinanet_ghm_x101_64x4d_fpn_1x_coco.py +0 -13
- spaces/AngoHF/ANGO-Leaderboard/assets/constant.py +0 -1
- spaces/AnxiousNugget/janitor/README.md +0 -10
- spaces/ArcAhmedEssam/CLIP-Interrogator-2/app.py +0 -177
- spaces/Ataturk-Chatbot/HuggingFaceChat/venv/lib/python3.11/site-packages/setuptools/_vendor/importlib_metadata/_meta.py +0 -48
- spaces/Awiny/Image2Paragraph/models/grit_src/third_party/CenterNet2/tests/structures/test_boxes.py +0 -223
- spaces/Benson/text-generation/Examples/Barbie Apk.md +0 -74
- spaces/BetterAPI/BetterChat_new/src/lib/utils/analytics.ts +0 -39
- spaces/Big-Web/MMSD/env/Lib/site-packages/pip/_vendor/chardet/cp949prober.py +0 -49
- spaces/Big-Web/MMSD/env/Lib/site-packages/setuptools/_distutils/command/build_clib.py +0 -208
- spaces/BilalSardar/Like-Chatgpt-clone/README.md +0 -12
- spaces/BlitzKriegM/argilla/Dockerfile +0 -19
- spaces/CALM/Dashboard/dashboard_utils/bubbles.py +0 -189
- spaces/CNXT/PiX2TXT/app.py +0 -3
- spaces/CVMX-jaca-tonos/YouTube-Video-Streaming-Spanish-ASR/app.py +0 -126
- spaces/CVPR/Dual-Key_Backdoor_Attacks/openvqa/utils/test_engine.py +0 -157
- spaces/CVPR/LIVE/pybind11/tests/constructor_stats.h +0 -275
- spaces/CVPR/LIVE/pybind11/tests/test_call_policies.py +0 -192
- spaces/CVPR/SPOTER_Sign_Language_Recognition/spoter_mod/sweep-agent.sh +0 -18
- spaces/CVPR/regionclip-demo/detectron2/data/transforms/torchvision_transforms/functional.py +0 -1365
- spaces/CVPR/regionclip-demo/detectron2/utils/registry.py +0 -60
- spaces/CofAI/chat/server/backend.py +0 -176
- spaces/CosmoAI/BhagwatGeeta/htmlTemplates.py +0 -44
- spaces/DQChoi/gpt-demo/venv/lib/python3.11/site-packages/PIL/JpegPresets.py +0 -240
- spaces/DQChoi/gpt-demo/venv/lib/python3.11/site-packages/PIL/_util.py +0 -19
- spaces/DQChoi/gpt-demo/venv/lib/python3.11/site-packages/fontTools/ttLib/tables/G_S_U_B_.py +0 -5
- spaces/DQChoi/gpt-demo/venv/lib/python3.11/site-packages/fontTools/varLib/instancer/names.py +0 -380
- spaces/Daniil-plotnikov/Daniil-plotnikov-russian-vision-v5-beta-3/README.md +0 -12
- spaces/Datasculptor/StyleGAN-NADA/README.md +0 -14
- spaces/Detomo/CuteRobot/README.md +0 -10
spaces/1acneusushi/gradio-2dmoleculeeditor/data/23rd March 1931 Shaheed Mp4 2021 Download.md
DELETED
@@ -1,20 +0,0 @@
|
|
1 |
-
<br />
|
2 |
-
<h1>23rd March 1931 Shaheed: A Tribute to the Martyrs of India</h1>
|
3 |
-
<p>23rd March 1931 Shaheed is a 2002 Hindi movie that depicts the life and struggle of Bhagat Singh, one of the most influential revolutionaries of the Indian independence movement. The movie, directed by Guddu Dhanoa and produced by Dharmendra, stars Bobby Deol as Bhagat Singh, Sunny Deol as Chandrashekhar Azad, and Amrita Singh as Vidyavati Kaur, Bhagat's mother. The movie also features Aishwarya Rai in a special appearance as Mannewali, Bhagat's love interest.</p>
|
4 |
-
<p>The movie portrays the events leading up to the hanging of Bhagat Singh and his comrades Sukhdev Thapar and Shivaram Rajguru on 23rd March 1931, which is observed as Shaheed Diwas or Martyrs' Day in India. The movie shows how Bhagat Singh joined the Hindustan Socialist Republican Association (HSRA) led by Chandrashekhar Azad and participated in various revolutionary activities such as the Lahore Conspiracy Case, the Central Legislative Assembly bombing, and the hunger strike in jail. The movie also highlights the ideological differences between Bhagat Singh and Mahatma Gandhi, who advocated non-violence as the means to achieve freedom.</p>
|
5 |
-
<h2>23rd March 1931 Shaheed mp4 download</h2><br /><p><b><b>Download File</b> ===> <a href="https://byltly.com/2uKvvh">https://byltly.com/2uKvvh</a></b></p><br /><br />
|
6 |
-
<p>23rd March 1931 Shaheed is a tribute to the courage and sacrifice of the martyrs who gave their lives for the freedom of India. The movie received mixed reviews from critics and audiences, but it has gained a cult status over the years. The movie is available for streaming on Disney+ Hotstar[^3^].</p><p>Another movie based on the life of Bhagat Singh is The Legend of Bhagat Singh, a 2002 Hindi movie directed by Rajkumar Santoshi and starring Ajay Devgan in the lead role. The movie covers Bhagat Singh's journey from his childhood where he witnesses the Jallianwala Bagh massacre to his involvement in the Hindustan Socialist Republican Association (HSRA) and his execution along with Rajguru and Sukhdev.</p>
|
7 |
-
<p>The movie focuses on the ideological differences between Bhagat Singh and Mahatma Gandhi, who advocated non-violence as the means to achieve freedom. The movie also shows how Bhagat Singh and his comrades used various tactics such as hunger strike, bomb blast, and court trial to challenge the British rule and inspire the masses. The movie also features Sushant Singh as Sukhdev, D. Santosh as Rajguru, Akhilendra Mishra as Chandrashekhar Azad, and Amrita Rao as Mannewali.</p>
|
8 |
-
<p>The Legend of Bhagat Singh received critical acclaim for its direction, story, screenplay, music, and performances. The movie won two National Film Awards â Best Feature Film in Hindi and Best Actor for Devgan â and three Filmfare Awards from eight nominations. However, the movie failed to perform well at the box office, as it faced competition from another movie on Bhagat Singh released on the same day â 23rd March 1931 Shaheed. The movie is available for streaming on Airtel Xstream[^3^].</p><p>Bhagat Singh was not only a brave revolutionary, but also a visionary thinker and writer. He wrote several essays and articles on various topics such as socialism, religion, nationalism, and violence. He also kept a jail notebook where he recorded his thoughts and quotations from various books he read in prison. Some of his famous quotes are:</p>
|
9 |
-
<p></p>
|
10 |
-
<ul>
|
11 |
-
<li>"They may kill me, but they cannot kill my ideas. They can crush my body, but they will not be able to crush my spirit."[^1^]</li>
|
12 |
-
<li>"Revolution is an inalienable right of mankind. Freedom is an imperishable birth right of all."[^1^]</li>
|
13 |
-
<li>"I am such a lunatic that I am free even in jail."[^2^]</li>
|
14 |
-
<li>"Merciless criticism and independent thinking are two traits of revolutionary thinking. Lovers, lunatics and poets are made of the same stuff."[^1^]</li>
|
15 |
-
<li>"Bombs and pistols don't make a revolution. The sword of revolution is sharpened on the whetting stone of ideas."[^1^]</li>
|
16 |
-
<li>"Labour is the real sustainer of society."[^1^]</li>
|
17 |
-
<li>"People get accustomed to the established order of things and tremble at the idea of change. It is this lethargic spirit that needs be replaced by the revolutionary spirit."[^1^]</li>
|
18 |
-
</ul></p> 81aa517590<br />
|
19 |
-
<br />
|
20 |
-
<br />
|
|
|
|
|
|
|
|
|
|
|
|
|
|
|
|
|
|
|
|
|
|
|
|
|
|
|
|
|
|
|
|
|
|
|
|
|
|
|
|
|
|
spaces/1gistliPinn/ChatGPT4/Examples/Dc Unlocker 2 Client Full [HOT] 12.md
DELETED
@@ -1,6 +0,0 @@
|
|
1 |
-
<h2>dc unlocker 2 client full 12</h2><br /><p><b><b>Download Zip</b> ✺ <a href="https://imgfil.com/2uy1d7">https://imgfil.com/2uy1d7</a></b></p><br /><br />
|
2 |
-
|
3 |
-
d5da3c52bf<br />
|
4 |
-
<br />
|
5 |
-
<br />
|
6 |
-
<p></p>
|
|
|
|
|
|
|
|
|
|
|
|
|
|
spaces/1gistliPinn/ChatGPT4/Examples/Download Buku Boyman Pramuka Pdf Viewer Semua yang Perlu Anda Ketahui tentang Pramuka.md
DELETED
@@ -1,6 +0,0 @@
|
|
1 |
-
<h2>Download Buku Boyman Pramuka Pdf Viewer</h2><br /><p><b><b>Download</b> ✔ <a href="https://imgfil.com/2uxZC2">https://imgfil.com/2uxZC2</a></b></p><br /><br />
|
2 |
-
<br />
|
3 |
-
aaccfb2cb3<br />
|
4 |
-
<br />
|
5 |
-
<br />
|
6 |
-
<p></p>
|
|
|
|
|
|
|
|
|
|
|
|
|
|
spaces/1gistliPinn/ChatGPT4/Examples/Download Commando - A One Man Army Full Movie.md
DELETED
@@ -1,6 +0,0 @@
|
|
1 |
-
<h2>download Commando - A One Man Army full movie</h2><br /><p><b><b>Download Zip</b> ► <a href="https://imgfil.com/2uy0nz">https://imgfil.com/2uy0nz</a></b></p><br /><br />
|
2 |
-
<br />
|
3 |
-
April 12, 2013 - Commando - A One Man Army Bollywood Movie: Check out the latest news... Commando - The War Within is about Captain Karanveer Dogra, ...# ## Commando The Movie 8a78ff9644<br />
|
4 |
-
<br />
|
5 |
-
<br />
|
6 |
-
<p></p>
|
|
|
|
|
|
|
|
|
|
|
|
|
|
spaces/1gistliPinn/ChatGPT4/Examples/F.E.A.R 3 ENGLISH LANGUAGE PACK TORRENT.md
DELETED
@@ -1,7 +0,0 @@
|
|
1 |
-
<h2>F.E.A.R 3 ENGLISH LANGUAGE PACK TORRENT</h2><br /><p><b><b>Download</b> ——— <a href="https://imgfil.com/2uxYEe">https://imgfil.com/2uxYEe</a></b></p><br /><br />
|
2 |
-
<br />
|
3 |
-
FEAR 3 [v160201060 + MULTi10] — [DODI Repack, from 29 GB] torrent... Your ISP can see when you download torrents!... Language : English. bit/Apple IIAdvent...View 109 more lines
|
4 |
-
Images for F.E.A.R 3 ENGLISH LANGUAGE PACK TORRENT 8a78ff9644<br />
|
5 |
-
<br />
|
6 |
-
<br />
|
7 |
-
<p></p>
|
|
|
|
|
|
|
|
|
|
|
|
|
|
|
|
spaces/1pelhydcardo/ChatGPT-prompt-generator/Download Triple Frontier.md
DELETED
@@ -1,100 +0,0 @@
|
|
1 |
-
## Download Triple Frontier
|
2 |
-
|
3 |
-
|
4 |
-
|
5 |
-
|
6 |
-
|
7 |
-
|
8 |
-
|
9 |
-
|
10 |
-
|
11 |
-
**DOWNLOAD 🆗 [https://kneedacexbrew.blogspot.com/?d=2txjn1](https://kneedacexbrew.blogspot.com/?d=2txjn1)**
|
12 |
-
|
13 |
-
|
14 |
-
|
15 |
-
|
16 |
-
|
17 |
-
|
18 |
-
|
19 |
-
|
20 |
-
|
21 |
-
|
22 |
-
|
23 |
-
|
24 |
-
|
25 |
-
# How to Download Triple Frontier, the Netflix Action Thriller Starring Ben Affleck and Oscar Isaac
|
26 |
-
|
27 |
-
|
28 |
-
|
29 |
-
If you are looking for a thrilling and action-packed movie to watch, you might want to check out Triple Frontier, a Netflix original film that was released in 2019. The movie follows a group of former U.S. Army Delta Force operators who reunite to plan a heist of a South American drug lord's fortune, unleashing a chain of unintended consequences.
|
30 |
-
|
31 |
-
|
32 |
-
|
33 |
-
The movie stars Ben Affleck as Tom "Redfly" Davis, a realtor and the leader of the team; Oscar Isaac as Santiago "Pope" Garcia, a private military advisor and the mastermind of the heist; Charlie Hunnam as William "Ironhead" Miller, a motivational speaker and Redfly's old friend; Garrett Hedlund as Ben "Benny" Miller, Ironhead's brother and an MMA fighter; and Pedro Pascal as Francisco "Catfish" Morales, a pilot and the team's getaway driver.
|
34 |
-
|
35 |
-
|
36 |
-
|
37 |
-
The movie was directed by J.C. Chandor, who also co-wrote the screenplay with Mark Boal, based on a story by Boal. The movie was praised for its realistic and gritty portrayal of the heist and its aftermath, as well as the performances of the cast. The movie also features stunning locations and cinematography, as well as an intense soundtrack by Disasterpeace.
|
38 |
-
|
39 |
-
|
40 |
-
|
41 |
-
If you want to watch or download Triple Frontier, you can do so on Netflix, where it is available for streaming and offline viewing. To stream the movie, you need to have a Netflix account and an internet connection. To download the movie, you need to have a Netflix account and the Netflix app on your device. You can download the movie on up to four devices per account.
|
42 |
-
|
43 |
-
|
44 |
-
|
45 |
-
To download Triple Frontier on Netflix, follow these steps:
|
46 |
-
|
47 |
-
|
48 |
-
|
49 |
-
1. Open the Netflix app on your device and sign in with your account.
|
50 |
-
|
51 |
-
2. Search for Triple Frontier in the app or browse through the categories until you find it.
|
52 |
-
|
53 |
-
3. Tap on the movie title or poster to open its details page.
|
54 |
-
|
55 |
-
4. Tap on the Download icon (a downward arrow) next to the Play icon (a triangle).
|
56 |
-
|
57 |
-
5. Wait for the download to complete. You can check the progress in the Downloads section of the app.
|
58 |
-
|
59 |
-
6. Once the download is done, you can watch the movie offline anytime by going to the Downloads section of the app and tapping on Triple Frontier.
|
60 |
-
|
61 |
-
|
62 |
-
|
63 |
-
Note that some titles may not be available for download in certain regions due to licensing restrictions. Also, downloaded titles have an expiration date that varies depending on the title. You can check how much time you have left to watch a downloaded title by going to the Downloads section of the app and tapping on My Downloads.
|
64 |
-
|
65 |
-
|
66 |
-
|
67 |
-
We hope you enjoy watching Triple Frontier on Netflix. If you liked this article, please share it with your friends and family who might be interested in downloading this movie.
|
68 |
-
|
69 |
-
|
70 |
-
|
71 |
-
If you are curious about the making of Triple Frontier, you might want to watch some of the behind-the-scenes videos and interviews that are available on YouTube. You can learn more about the challenges and rewards of filming in various locations across South America, such as Colombia, Brazil, and Peru. You can also hear from the director and the cast about their experiences and insights on working on this project.
|
72 |
-
|
73 |
-
|
74 |
-
|
75 |
-
Some of the videos that you can watch are:
|
76 |
-
|
77 |
-
|
78 |
-
|
79 |
-
- Triple Frontier | Official Trailer #2 [HD] | Netflix
|
80 |
-
|
81 |
-
- Triple Frontier: Gruppendynamik (German Featurette Subtitled)
|
82 |
-
|
83 |
-
- 'Triple Frontier' Alpha Males Are Buds in Real Life, Too
|
84 |
-
|
85 |
-
- What Roles Was Ben Affleck Considered For?
|
86 |
-
|
87 |
-
|
88 |
-
|
89 |
-
You can also check out some of the reviews and ratings that Triple Frontier has received from critics and audiences. The movie has a 6.4/10 score on IMDb, based on over 135,000 user ratings. It also has a 61% approval rating on Rotten Tomatoes, based on 141 critic reviews. The movie was nominated for three awards: Best Action Movie at the Critics' Choice Movie Awards, Best Action or Adventure Film at the Saturn Awards, and Best Stunt Ensemble in a Motion Picture at the Screen Actors Guild Awards.
|
90 |
-
|
91 |
-
|
92 |
-
|
93 |
-
Whether you are a fan of action movies, heist movies, or military movies, Triple Frontier has something for everyone. It is a thrilling and entertaining movie that explores the themes of loyalty, greed, morality, and survival. It is also a movie that showcases the talents and chemistry of its star-studded cast. So don't miss this opportunity to watch or download Triple Frontier on Netflix today.
|
94 |
-
|
95 |
-
1b8d091108
|
96 |
-
|
97 |
-
|
98 |
-
|
99 |
-
|
100 |
-
|
|
|
|
|
|
|
|
|
|
|
|
|
|
|
|
|
|
|
|
|
|
|
|
|
|
|
|
|
|
|
|
|
|
|
|
|
|
|
|
|
|
|
|
|
|
|
|
|
|
|
|
|
|
|
|
|
|
|
|
|
|
|
|
|
|
|
|
|
|
|
|
|
|
|
|
|
|
|
|
|
|
|
|
|
|
|
|
|
|
|
|
|
|
|
|
|
|
|
|
|
|
|
|
|
|
|
|
|
|
|
|
|
|
|
|
|
|
|
|
|
|
|
|
|
|
|
|
|
|
|
|
|
|
|
|
|
|
|
|
|
|
|
|
|
|
|
|
|
|
|
|
|
|
|
|
|
|
|
|
|
|
|
|
|
|
|
|
|
|
|
|
|
|
|
|
|
|
|
|
|
|
|
|
|
|
|
|
|
|
|
|
|
|
|
|
|
|
|
|
|
|
spaces/1phancelerku/anime-remove-background/Download Free Fire MAX APK 32 Bits for Android - The Ultimate Battle Royale Experience.md
DELETED
@@ -1,153 +0,0 @@
|
|
1 |
-
|
2 |
-
<h1>Free Fire Max APK 32 Bits: How to Download and Play the Ultimate Battle Royale Game</h1>
|
3 |
-
<p>If you are a fan of battle royale games, you might have heard of Free Fire, one of the most popular and downloaded games in the world. But did you know that there is a new version of Free Fire that offers a more immersive and realistic gameplay experience? It's called Free Fire Max, and it's designed exclusively for premium players who want to enjoy the best graphics, effects, and features in a battle royale game. In this article, we will tell you everything you need to know about Free Fire Max APK 32 bits, how to download and install it, and how to play it on your PC.</p>
|
4 |
-
<h2>free fire max apk 32 bits</h2><br /><p><b><b>Download File</b> ····· <a href="https://jinyurl.com/2uNKgQ">https://jinyurl.com/2uNKgQ</a></b></p><br /><br />
|
5 |
-
<h2>What is Free Fire Max?</h2>
|
6 |
-
<p>Free Fire Max is a new version of Free Fire that was launched in September 2021 by Garena International I, the same developer of the original game. Free Fire Max is not a separate game, but an enhanced version of Free Fire that uses exclusive Firelink technology to connect with all Free Fire players. This means that you can play with your friends and other players who are using either version of the game, without any compatibility issues.</p>
|
7 |
-
<h3>The difference between Free Fire and Free Fire Max</h3>
|
8 |
-
<p>The main difference between Free Fire and Free Fire Max is the quality of the graphics and the effects. Free Fire Max offers Ultra HD resolutions, breathtaking effects, realistic animations, dynamic lighting, and improved sound quality. The game also has a new lobby design, a new user interface, and a new map called Bermuda Remastered. All these features make Free Fire Max more immersive and realistic than ever before.</p>
|
9 |
-
<h3>The features of Free Fire Max</h3>
|
10 |
-
<p>Free Fire Max has all the features that you love from Free Fire, plus some new ones that make it more fun and exciting. Some of the features of Free Fire Max are:</p>
|
11 |
-
<ul>
|
12 |
-
<li>50-player battle royale mode, where you have to survive against other players and be the last one standing.</li>
|
13 |
-
<li>4v4 Clash Squad mode, where you have to team up with your friends and fight against another squad in fast-paced matches.</li>
|
14 |
-
<li>Rampage mode, where you have to collect evolution stones and transform into powerful beasts with special abilities.</li>
|
15 |
-
<li>Different characters, pets, weapons, vehicles, skins, emotes, and items that you can customize and use in the game.</li>
|
16 |
-
<li>Different events, missions, rewards, and updates that keep the game fresh and engaging.</li>
|
17 |
-
</ul>
|
18 |
-
<h2>How to download and install Free Fire Max APK 32 bits?</h2>
|
19 |
-
<p>If you want to download and install Free Fire Max APK 32 bits on your Android device, you need to follow some simple steps. But before that, you need to make sure that your device meets the requirements for running the game smoothly.</p>
|
20 |
-
<h3>The requirements for Free Fire Max APK 32 bits</h3>
|
21 |
-
<p>The minimum requirements for running Free Fire Max APK 32 bits are:</p>
|
22 |
-
<table>
|
23 |
-
<tr>
|
24 |
-
<th>OS</th>
|
25 |
-
<th>RAM</th>
|
26 |
-
<th>Storage</th>
|
27 |
-
<th>Processor</th>
|
28 |
-
</tr>
|
29 |
-
<tr>
|
30 |
-
<td>Android 4.4 or higher</td>
|
31 |
-
<td>2 GB or higher</td>
|
32 |
-
<td>1.5 GB or higher</td>
|
33 |
-
<td>Quad-core or higher</td>
|
34 |
-
</tr>
|
35 |
-
</table>
|
36 |
-
<p>The recommended requirements for running Free Fire Max APK 32 bits are:</p>
|
37 |
-
<table>
|
38 |
-
<tr>
|
39 |
-
<th>OS</th>
|
40 |
-
<th>RAM</th>
|
41 |
-
<th>Storage</th>
|
42 |
-
<th>Processor</th>
|
43 |
-
</tr>
|
44 |
-
<tr>
|
45 |
-
<td>Android 7.0 or higher</td>
|
46 |
-
<td>4 GB or higher</td> <td>4 GB or higher</td>
|
47 |
-
<td>4 GB or higher</td>
|
48 |
-
<td>Octa-core or higher</td>
|
49 |
-
</tr>
|
50 |
-
</table>
|
51 |
-
<h3>The steps to download and install Free Fire Max APK 32 bits</h3>
|
52 |
-
<p>Once you have checked that your device meets the requirements, you can follow these steps to download and install Free Fire Max APK 32 bits:</p>
|
53 |
-
<ol>
|
54 |
-
<li>Go to the official website of Free Fire Max and click on the download button.</li>
|
55 |
-
<li>Select the APK file for 32-bit devices and wait for it to download.</li>
|
56 |
-
<li>Go to your device settings and enable the installation of apps from unknown sources.</li>
|
57 |
-
<li>Locate the downloaded APK file and tap on it to start the installation process.</li>
|
58 |
-
<li>Follow the on-screen instructions and grant the necessary permissions to the app.</li>
|
59 |
-
<li>Launch the app and log in with your existing Free Fire account or create a new one.</li>
|
60 |
-
<li>Enjoy playing Free Fire Max on your device.</li>
|
61 |
-
</ol>
|
62 |
-
<h2>How to play Free Fire Max on PC?</h2>
|
63 |
-
<p>If you want to play Free Fire Max on your PC, you need to use an Android emulator. An emulator is a software that allows you to run Android apps and games on your PC. There are many emulators available in the market, but not all of them are compatible with Free Fire Max. Here are some of the best emulators that you can use to play Free Fire Max on your PC:</p>
|
64 |
-
<h3>The benefits of playing Free Fire Max on PC</h3>
|
65 |
-
<p>Playing Free Fire Max on PC has many advantages over playing it on your mobile device. Some of the benefits are:</p>
|
66 |
-
<p>free fire max download for 32 bit android<br />
|
67 |
-
how to install free fire max on 32 bit device<br />
|
68 |
-
free fire max apk obb 32 bit<br />
|
69 |
-
free fire max compatible with 32 bit<br />
|
70 |
-
free fire max 32 bit version apk<br />
|
71 |
-
free fire max 32 bit mod apk<br />
|
72 |
-
free fire max 32 bit apk pure<br />
|
73 |
-
free fire max 32 bit apk latest version<br />
|
74 |
-
free fire max 32 bit apk update<br />
|
75 |
-
free fire max 32 bit apk offline<br />
|
76 |
-
free fire max 32 bit apk no verification<br />
|
77 |
-
free fire max 32 bit apk hack<br />
|
78 |
-
free fire max 32 bit apk unlimited diamonds<br />
|
79 |
-
free fire max 32 bit apk and data<br />
|
80 |
-
free fire max 32 bit apk highly compressed<br />
|
81 |
-
free fire max 32 bit apk for pc<br />
|
82 |
-
free fire max 32 bit apk for emulator<br />
|
83 |
-
free fire max 32 bit apk for bluestacks<br />
|
84 |
-
free fire max 32 bit apk for ld player<br />
|
85 |
-
free fire max 32 bit apk for gameloop<br />
|
86 |
-
free fire max 32 bit apk for windows 10<br />
|
87 |
-
free fire max 32 bit apk for ios<br />
|
88 |
-
free fire max 32 bit apk for iphone<br />
|
89 |
-
free fire max 32 bit apk for ipad<br />
|
90 |
-
free fire max 32 bit apk for macbook<br />
|
91 |
-
free fire max 32 bit gameplay<br />
|
92 |
-
free fire max 32 bit graphics settings<br />
|
93 |
-
free fire max 32 bit system requirements<br />
|
94 |
-
free fire max 32 bit vs 64 bit<br />
|
95 |
-
free fire max 32 bit vs normal version<br />
|
96 |
-
free fire max beta test 32 bit<br />
|
97 |
-
how to play free fire max in 32 bit phone<br />
|
98 |
-
how to run free fire max on 32 bit mobile<br />
|
99 |
-
how to update free fire max on 32 bit smartphone<br />
|
100 |
-
how to get free fire max on any device (no root) (no vpn) (no obb) (no ban) (no lag) (no error) (no survey) (no human verification)<br />
|
101 |
-
best settings for free fire max on low end devices (fix lag) (increase fps) (boost performance) (improve graphics)<br />
|
102 |
-
tips and tricks for playing free fire max on android (how to win every match) (how to get more kills) (how to rank up fast) (how to get more diamonds)<br />
|
103 |
-
review of free fire max on android (pros and cons) (comparison with other battle royale games) (features and benefits) (rating and feedback)<br />
|
104 |
-
download link of free fire max on android [^1^]<br />
|
105 |
-
video tutorial of how to download and install free fire max on android [^2^]</p>
|
106 |
-
<ul>
|
107 |
-
<li>You can enjoy better graphics, effects, and sound quality on a larger screen.</li>
|
108 |
-
<li>You can use a keyboard and mouse to control your character and aim more accurately.</li>
|
109 |
-
<li>You can avoid battery drain, overheating, and lag issues that may occur on your mobile device.</li>
|
110 |
-
<li>You can record, stream, and share your gameplay with ease.</li>
|
111 |
-
</ul>
|
112 |
-
<h3>The best emulator to play Free Fire Max on PC</h3>
|
113 |
-
<p>According to our research, the best emulator to play Free Fire Max on PC is BlueStacks. BlueStacks is a powerful and reliable emulator that supports Android 11, which is the latest version of Android. BlueStacks also offers some unique features that enhance your gaming experience, such as:</p>
|
114 |
-
<ul>
|
115 |
-
<li>Shooting mode, which allows you to use your mouse as a trigger and aim with precision.</li>
|
116 |
-
<li>High FPS/Graphics, which allows you to play the game at 120 FPS and Ultra HD resolutions.</li>
|
117 |
-
<li>Script, which allows you to automate tasks and actions in the game with simple commands.</li>
|
118 |
-
<li>Free look, which allows you to look around without moving your character.</li>
|
119 |
-
</ul>
|
120 |
-
<p>To play Free Fire Max on PC with BlueStacks, you need to follow these steps:</p>
|
121 |
-
<ol>
|
122 |
-
<li>Download and install BlueStacks on your PC from its official website.</li>
|
123 |
-
<li>Launch BlueStacks and sign in with your Google account.</li>
|
124 |
-
<li>Go to the Google Play Store and search for Free Fire Max.</li>
|
125 |
-
<li>Download and install the game on BlueStacks.</li>
|
126 |
-
<li>Launch the game and log in with your Free Fire account or create a new one.</li>
|
127 |
-
<li>Enjoy playing Free Fire Max on PC with BlueStacks.</li>
|
128 |
-
</ol>
|
129 |
-
<h2>Conclusion</h2>
|
130 |
-
<p>In conclusion, Free Fire Max is an amazing game that offers a more immersive and realistic gameplay experience than Free Fire. It has better graphics, effects, features, and compatibility than the original game. You can download and install Free Fire Max APK 32 bits on your Android device or play it on your PC with an emulator like BlueStacks. Either way, you will have a lot of fun playing this ultimate battle royale game. So what are you waiting for? Download Free Fire Max today and join the action!</p>
|
131 |
-
<h2>FAQs</h2>
|
132 |
-
<p>Here are some frequently asked questions about Free Fire Max:</p>
|
133 |
-
<h4>Is Free Fire Max free?</h4>
|
134 |
-
<p>Yes, Free Fire Max is free to download and play. However, it may contain some in-app purchases that require real money.</p>
|
135 |
-
<h4>Can I play Free Fire Max offline?</h4>
|
136 |
-
<p>No, you need an internet connection to play Free Fire Max online with other players.</p>
|
137 |
-
<h4>Can I transfer my data from Free Fire to Free Fire Max?</h4>
|
138 |
-
<p>Yes, you can use the same account for both games and transfer your data seamlessly. You can also switch between the games anytime without losing your progress.</p>
|
139 |
-
<h4>What are the <h4>What are the best tips and tricks for playing Free Fire Max?</h4>
|
140 |
-
<p>Some of the best tips and tricks for playing Free Fire Max are:</p>
|
141 |
-
<ul>
|
142 |
-
<li>Choose your character and pet wisely, as they have different skills and abilities that can help you in the game.</li>
|
143 |
-
<li>Use the map and the mini-map to locate enemies, safe zones, airdrops, and vehicles.</li>
|
144 |
-
<li>Loot as much as you can, but be careful of enemies and traps.</li>
|
145 |
-
<li>Use cover, crouch, and prone to avoid being detected and shot by enemies.</li>
|
146 |
-
<li>Use different weapons and items according to the situation and your preference.</li>
|
147 |
-
<li>Communicate and cooperate with your teammates, especially in squad mode.</li>
|
148 |
-
<li>Practice your aim, movement, and strategy in training mode or custom rooms.</li>
|
149 |
-
</ul>
|
150 |
-
<h4>Is Free Fire Max safe to download and play?</h4>
|
151 |
-
<p>Yes, Free Fire Max is safe to download and play, as long as you download it from the official website or the Google Play Store. You should also avoid using any hacks, cheats, or mods that may harm your device or account.</p> 401be4b1e0<br />
|
152 |
-
<br />
|
153 |
-
<br />
|
|
|
|
|
|
|
|
|
|
|
|
|
|
|
|
|
|
|
|
|
|
|
|
|
|
|
|
|
|
|
|
|
|
|
|
|
|
|
|
|
|
|
|
|
|
|
|
|
|
|
|
|
|
|
|
|
|
|
|
|
|
|
|
|
|
|
|
|
|
|
|
|
|
|
|
|
|
|
|
|
|
|
|
|
|
|
|
|
|
|
|
|
|
|
|
|
|
|
|
|
|
|
|
|
|
|
|
|
|
|
|
|
|
|
|
|
|
|
|
|
|
|
|
|
|
|
|
|
|
|
|
|
|
|
|
|
|
|
|
|
|
|
|
|
|
|
|
|
|
|
|
|
|
|
|
|
|
|
|
|
|
|
|
|
|
|
|
|
|
|
|
|
|
|
|
|
|
|
|
|
|
|
|
|
|
|
|
|
|
|
|
|
|
|
|
|
|
|
|
|
|
|
|
|
|
|
|
|
|
|
|
|
|
|
|
|
|
|
|
|
|
|
|
|
|
|
|
|
|
|
|
|
|
|
|
|
|
|
|
|
|
|
|
|
|
|
|
|
|
|
|
|
|
|
|
|
|
|
|
|
|
|
|
|
|
|
|
|
|
|
|
|
|
|
|
|
|
|
|
|
|
|
|
|
|
|
|
|
|
|
|
|
|
|
|
|
|
|
|
|
|
|
|
|
|
|
|
spaces/232labs/VToonify/app.py
DELETED
@@ -1,290 +0,0 @@
|
|
1 |
-
#!/usr/bin/env python
|
2 |
-
|
3 |
-
from __future__ import annotations
|
4 |
-
|
5 |
-
import argparse
|
6 |
-
import torch
|
7 |
-
import gradio as gr
|
8 |
-
|
9 |
-
from vtoonify_model import Model
|
10 |
-
|
11 |
-
def parse_args() -> argparse.Namespace:
|
12 |
-
parser = argparse.ArgumentParser()
|
13 |
-
parser.add_argument('--device', type=str, default='cpu')
|
14 |
-
parser.add_argument('--theme', type=str)
|
15 |
-
parser.add_argument('--share', action='store_true')
|
16 |
-
parser.add_argument('--port', type=int)
|
17 |
-
parser.add_argument('--disable-queue',
|
18 |
-
dest='enable_queue',
|
19 |
-
action='store_false')
|
20 |
-
return parser.parse_args()
|
21 |
-
|
22 |
-
|
23 |
-
DESCRIPTION = '''
|
24 |
-
<div align=center>
|
25 |
-
<h1 style="font-weight: 900; margin-bottom: 7px;">
|
26 |
-
Portrait Style Transfer with <a href="https://github.com/williamyang1991/VToonify">VToonify</a>
|
27 |
-
</h1>
|
28 |
-
<p>For faster inference without waiting in queue, you may duplicate the space and use the GPU setting.
|
29 |
-
<br/>
|
30 |
-
<a href="https://huggingface.co/spaces/PKUWilliamYang/VToonify?duplicate=true">
|
31 |
-
<img style="margin-top: 0em; margin-bottom: 0em" src="https://bit.ly/3gLdBN6" alt="Duplicate Space"></a>
|
32 |
-
<p/>
|
33 |
-
<video id="video" width=50% controls="" preload="none" poster="https://repository-images.githubusercontent.com/534480768/53715b0f-a2df-4daa-969c-0e74c102d339">
|
34 |
-
<source id="mp4" src="https://user-images.githubusercontent.com/18130694/189483939-0fc4a358-fb34-43cc-811a-b22adb820d57.mp4
|
35 |
-
" type="video/mp4">
|
36 |
-
</videos>
|
37 |
-
</div>
|
38 |
-
'''
|
39 |
-
FOOTER = '<div align=center><img id="visitor-badge" alt="visitor badge" src="https://visitor-badge.laobi.icu/badge?page_id=williamyang1991/VToonify" /></div>'
|
40 |
-
|
41 |
-
ARTICLE = r"""
|
42 |
-
If VToonify is helpful, please help to ⭐ the <a href='https://github.com/williamyang1991/VToonify' target='_blank'>Github Repo</a>. Thanks!
|
43 |
-
[](https://github.com/williamyang1991/VToonify)
|
44 |
-
---
|
45 |
-
📝 **Citation**
|
46 |
-
If our work is useful for your research, please consider citing:
|
47 |
-
```bibtex
|
48 |
-
@article{yang2022Vtoonify,
|
49 |
-
title={VToonify: Controllable High-Resolution Portrait Video Style Transfer},
|
50 |
-
author={Yang, Shuai and Jiang, Liming and Liu, Ziwei and Loy, Chen Change},
|
51 |
-
journal={ACM Transactions on Graphics (TOG)},
|
52 |
-
volume={41},
|
53 |
-
number={6},
|
54 |
-
articleno={203},
|
55 |
-
pages={1--15},
|
56 |
-
year={2022},
|
57 |
-
publisher={ACM New York, NY, USA},
|
58 |
-
doi={10.1145/3550454.3555437},
|
59 |
-
}
|
60 |
-
```
|
61 |
-
|
62 |
-
📋 **License**
|
63 |
-
This project is licensed under <a rel="license" href="https://github.com/williamyang1991/VToonify/blob/main/LICENSE.md">S-Lab License 1.0</a>.
|
64 |
-
Redistribution and use for non-commercial purposes should follow this license.
|
65 |
-
|
66 |
-
📧 **Contact**
|
67 |
-
If you have any questions, please feel free to reach me out at <b>[email protected]</b>.
|
68 |
-
"""
|
69 |
-
|
70 |
-
def update_slider(choice: str) -> dict:
|
71 |
-
if type(choice) == str and choice.endswith('-d'):
|
72 |
-
return gr.Slider.update(maximum=1, minimum=0, value=0.5)
|
73 |
-
else:
|
74 |
-
return gr.Slider.update(maximum=0.5, minimum=0.5, value=0.5)
|
75 |
-
|
76 |
-
def set_example_image(example: list) -> dict:
|
77 |
-
return gr.Image.update(value=example[0])
|
78 |
-
|
79 |
-
def set_example_video(example: list) -> dict:
|
80 |
-
return gr.Video.update(value=example[0]),
|
81 |
-
|
82 |
-
sample_video = ['./vtoonify/data/529_2.mp4','./vtoonify/data/7154235.mp4','./vtoonify/data/651.mp4','./vtoonify/data/908.mp4']
|
83 |
-
sample_vid = gr.Video(label='Video file') #for displaying the example
|
84 |
-
example_videos = gr.components.Dataset(components=[sample_vid], samples=[[path] for path in sample_video], type='values', label='Video Examples')
|
85 |
-
|
86 |
-
def main():
|
87 |
-
args = parse_args()
|
88 |
-
args.device = 'cuda' if torch.cuda.is_available() else 'cpu'
|
89 |
-
print('*** Now using %s.'%(args.device))
|
90 |
-
model = Model(device=args.device)
|
91 |
-
|
92 |
-
with gr.Blocks(theme=args.theme, css='style.css') as demo:
|
93 |
-
|
94 |
-
gr.Markdown(DESCRIPTION)
|
95 |
-
|
96 |
-
with gr.Box():
|
97 |
-
gr.Markdown('''## Step 1(Select Style)
|
98 |
-
- Select **Style Type**.
|
99 |
-
- Type with `-d` means it supports style degree adjustment.
|
100 |
-
- Type without `-d` usually has better toonification quality.
|
101 |
-
|
102 |
-
''')
|
103 |
-
with gr.Row():
|
104 |
-
with gr.Column():
|
105 |
-
gr.Markdown('''Select Style Type''')
|
106 |
-
with gr.Row():
|
107 |
-
style_type = gr.Radio(label='Style Type',
|
108 |
-
choices=['cartoon1','cartoon1-d','cartoon2-d','cartoon3-d',
|
109 |
-
'cartoon4','cartoon4-d','cartoon5-d','comic1-d',
|
110 |
-
'comic2-d','arcane1','arcane1-d','arcane2', 'arcane2-d',
|
111 |
-
'caricature1','caricature2','pixar','pixar-d',
|
112 |
-
'illustration1-d', 'illustration2-d', 'illustration3-d', 'illustration4-d', 'illustration5-d',
|
113 |
-
]
|
114 |
-
)
|
115 |
-
exstyle = gr.Variable()
|
116 |
-
with gr.Row():
|
117 |
-
loadmodel_button = gr.Button('Load Model')
|
118 |
-
with gr.Row():
|
119 |
-
load_info = gr.Textbox(label='Process Information', interactive=False, value='No model loaded.')
|
120 |
-
with gr.Column():
|
121 |
-
gr.Markdown('''Reference Styles
|
122 |
-
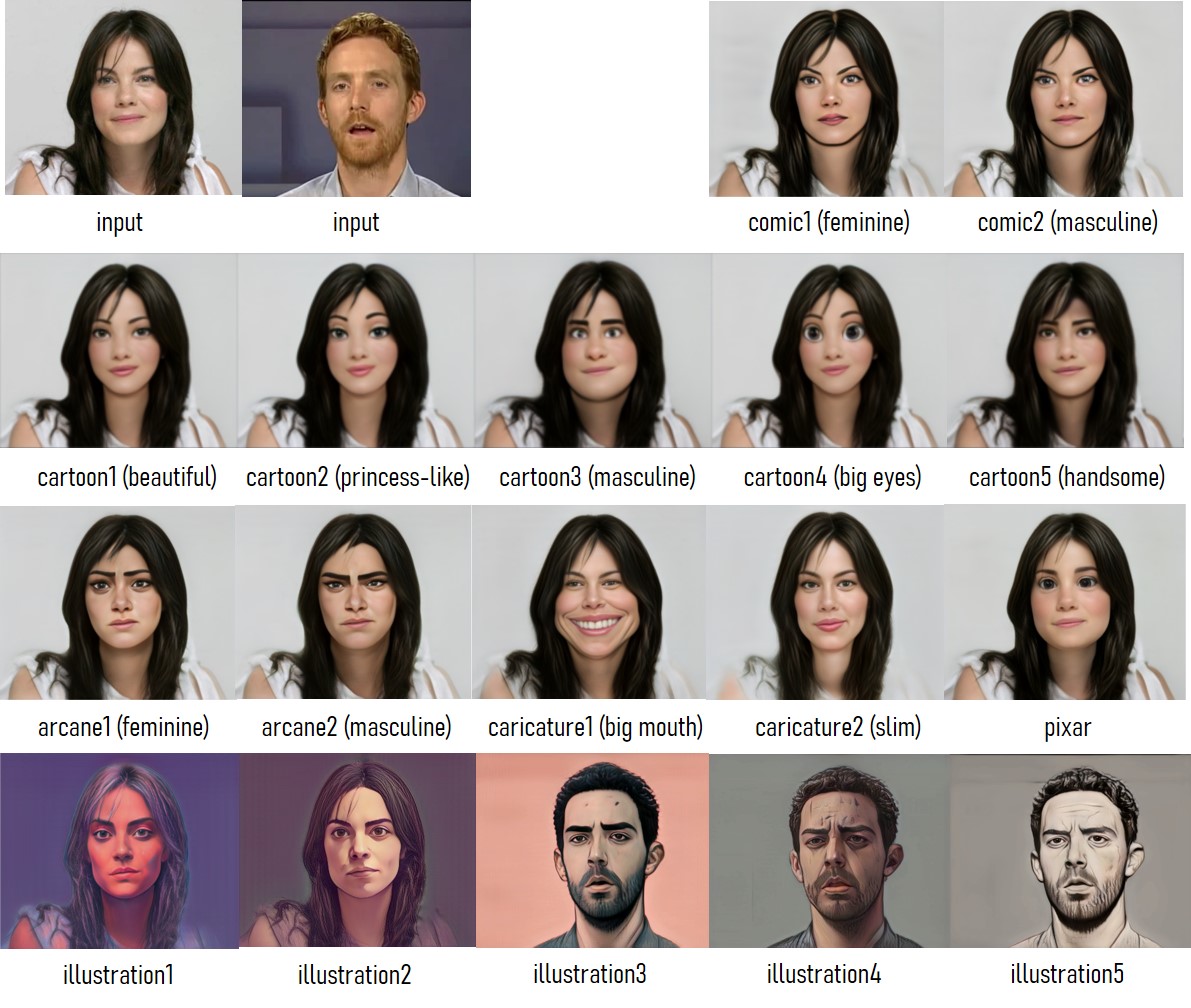''')
|
123 |
-
|
124 |
-
|
125 |
-
with gr.Box():
|
126 |
-
gr.Markdown('''## Step 2 (Preprocess Input Image / Video)
|
127 |
-
- Drop an image/video containing a near-frontal face to the **Input Image**/**Input Video**.
|
128 |
-
- Hit the **Rescale Image**/**Rescale First Frame** button.
|
129 |
-
- Rescale the input to make it best fit the model.
|
130 |
-
- The final image result will be based on this **Rescaled Face**. Use padding parameters to adjust the background space.
|
131 |
-
- **<font color=red>Solution to [Error: no face detected!]</font>**: VToonify uses dlib.get_frontal_face_detector but sometimes it fails to detect a face. You can try several times or use other images until a face is detected, then switch back to the original image.
|
132 |
-
- For video input, further hit the **Rescale Video** button.
|
133 |
-
- The final video result will be based on this **Rescaled Video**. To avoid overload, video is cut to at most **100/300** frames for CPU/GPU, respectively.
|
134 |
-
|
135 |
-
''')
|
136 |
-
with gr.Row():
|
137 |
-
with gr.Box():
|
138 |
-
with gr.Column():
|
139 |
-
gr.Markdown('''Choose the padding parameters.
|
140 |
-
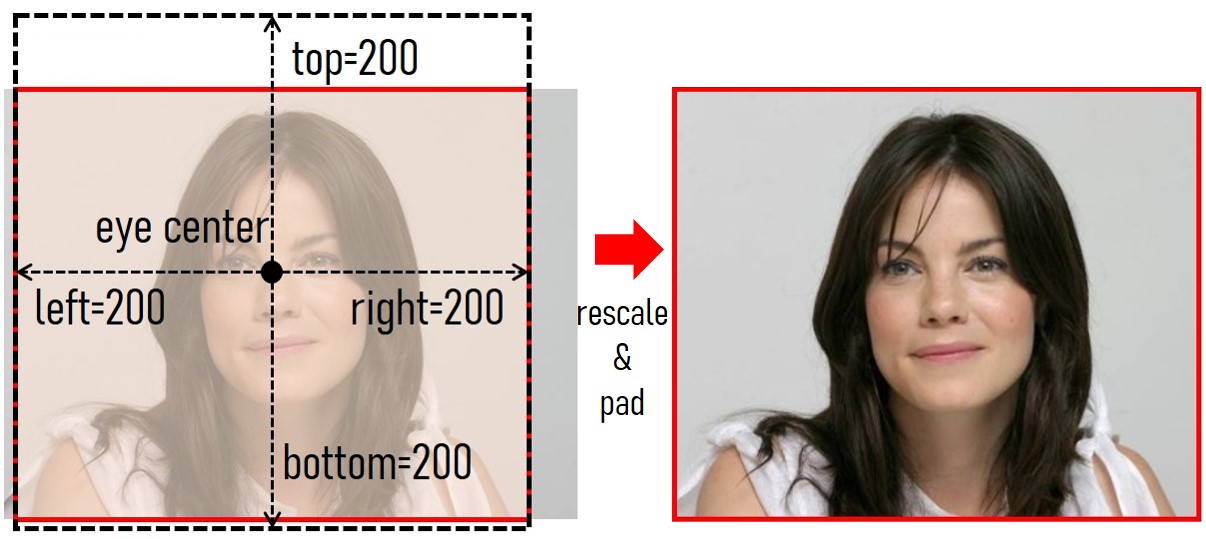''')
|
141 |
-
with gr.Row():
|
142 |
-
top = gr.Slider(128,
|
143 |
-
256,
|
144 |
-
value=200,
|
145 |
-
step=8,
|
146 |
-
label='top')
|
147 |
-
with gr.Row():
|
148 |
-
bottom = gr.Slider(128,
|
149 |
-
256,
|
150 |
-
value=200,
|
151 |
-
step=8,
|
152 |
-
label='bottom')
|
153 |
-
with gr.Row():
|
154 |
-
left = gr.Slider(128,
|
155 |
-
256,
|
156 |
-
value=200,
|
157 |
-
step=8,
|
158 |
-
label='left')
|
159 |
-
with gr.Row():
|
160 |
-
right = gr.Slider(128,
|
161 |
-
256,
|
162 |
-
value=200,
|
163 |
-
step=8,
|
164 |
-
label='right')
|
165 |
-
with gr.Box():
|
166 |
-
with gr.Column():
|
167 |
-
gr.Markdown('''Input''')
|
168 |
-
with gr.Row():
|
169 |
-
input_image = gr.Image(label='Input Image',
|
170 |
-
type='filepath')
|
171 |
-
with gr.Row():
|
172 |
-
preprocess_image_button = gr.Button('Rescale Image')
|
173 |
-
with gr.Row():
|
174 |
-
input_video = gr.Video(label='Input Video',
|
175 |
-
mirror_webcam=False,
|
176 |
-
type='filepath')
|
177 |
-
with gr.Row():
|
178 |
-
preprocess_video0_button = gr.Button('Rescale First Frame')
|
179 |
-
preprocess_video1_button = gr.Button('Rescale Video')
|
180 |
-
|
181 |
-
with gr.Box():
|
182 |
-
with gr.Column():
|
183 |
-
gr.Markdown('''View''')
|
184 |
-
with gr.Row():
|
185 |
-
input_info = gr.Textbox(label='Process Information', interactive=False, value='n.a.')
|
186 |
-
with gr.Row():
|
187 |
-
aligned_face = gr.Image(label='Rescaled Face',
|
188 |
-
type='numpy',
|
189 |
-
interactive=False)
|
190 |
-
instyle = gr.Variable()
|
191 |
-
with gr.Row():
|
192 |
-
aligned_video = gr.Video(label='Rescaled Video',
|
193 |
-
type='mp4',
|
194 |
-
interactive=False)
|
195 |
-
with gr.Row():
|
196 |
-
with gr.Column():
|
197 |
-
paths = ['./vtoonify/data/pexels-andrea-piacquadio-733872.jpg','./vtoonify/data/i5R8hbZFDdc.jpg','./vtoonify/data/yRpe13BHdKw.jpg','./vtoonify/data/ILip77SbmOE.jpg','./vtoonify/data/077436.jpg','./vtoonify/data/081680.jpg']
|
198 |
-
example_images = gr.Dataset(components=[input_image],
|
199 |
-
samples=[[path] for path in paths],
|
200 |
-
label='Image Examples')
|
201 |
-
with gr.Column():
|
202 |
-
#example_videos = gr.Dataset(components=[input_video], samples=[['./vtoonify/data/529.mp4']], type='values')
|
203 |
-
#to render video example on mouse hover/click
|
204 |
-
example_videos.render()
|
205 |
-
#to load sample video into input_video upon clicking on it
|
206 |
-
def load_examples(video):
|
207 |
-
#print("****** inside load_example() ******")
|
208 |
-
#print("in_video is : ", video[0])
|
209 |
-
return video[0]
|
210 |
-
|
211 |
-
example_videos.click(load_examples, example_videos, input_video)
|
212 |
-
|
213 |
-
with gr.Box():
|
214 |
-
gr.Markdown('''## Step 3 (Generate Style Transferred Image/Video)''')
|
215 |
-
with gr.Row():
|
216 |
-
with gr.Column():
|
217 |
-
gr.Markdown('''
|
218 |
-
|
219 |
-
- Adjust **Style Degree**.
|
220 |
-
- Hit **Toonify!** to toonify one frame. Hit **VToonify!** to toonify full video.
|
221 |
-
- Estimated time on 1600x1440 video of 300 frames: 1 hour (CPU); 2 mins (GPU)
|
222 |
-
''')
|
223 |
-
style_degree = gr.Slider(0,
|
224 |
-
1,
|
225 |
-
value=0.5,
|
226 |
-
step=0.05,
|
227 |
-
label='Style Degree')
|
228 |
-
with gr.Column():
|
229 |
-
gr.Markdown('''
|
230 |
-
''')
|
231 |
-
with gr.Row():
|
232 |
-
output_info = gr.Textbox(label='Process Information', interactive=False, value='n.a.')
|
233 |
-
with gr.Row():
|
234 |
-
with gr.Column():
|
235 |
-
with gr.Row():
|
236 |
-
result_face = gr.Image(label='Result Image',
|
237 |
-
type='numpy',
|
238 |
-
interactive=False)
|
239 |
-
with gr.Row():
|
240 |
-
toonify_button = gr.Button('Toonify!')
|
241 |
-
with gr.Column():
|
242 |
-
with gr.Row():
|
243 |
-
result_video = gr.Video(label='Result Video',
|
244 |
-
type='mp4',
|
245 |
-
interactive=False)
|
246 |
-
with gr.Row():
|
247 |
-
vtoonify_button = gr.Button('VToonify!')
|
248 |
-
|
249 |
-
gr.Markdown(ARTICLE)
|
250 |
-
gr.Markdown(FOOTER)
|
251 |
-
loadmodel_button.click(fn=model.load_model,
|
252 |
-
inputs=[style_type],
|
253 |
-
outputs=[exstyle, load_info], api_name="load-model")
|
254 |
-
|
255 |
-
|
256 |
-
style_type.change(fn=update_slider,
|
257 |
-
inputs=style_type,
|
258 |
-
outputs=style_degree)
|
259 |
-
|
260 |
-
preprocess_image_button.click(fn=model.detect_and_align_image,
|
261 |
-
inputs=[input_image, top, bottom, left, right],
|
262 |
-
outputs=[aligned_face, instyle, input_info], api_name="scale-image")
|
263 |
-
preprocess_video0_button.click(fn=model.detect_and_align_video,
|
264 |
-
inputs=[input_video, top, bottom, left, right],
|
265 |
-
outputs=[aligned_face, instyle, input_info], api_name="scale-first-frame")
|
266 |
-
preprocess_video1_button.click(fn=model.detect_and_align_full_video,
|
267 |
-
inputs=[input_video, top, bottom, left, right],
|
268 |
-
outputs=[aligned_video, instyle, input_info], api_name="scale-video")
|
269 |
-
|
270 |
-
toonify_button.click(fn=model.image_toonify,
|
271 |
-
inputs=[aligned_face, instyle, exstyle, style_degree, style_type],
|
272 |
-
outputs=[result_face, output_info], api_name="toonify-image")
|
273 |
-
vtoonify_button.click(fn=model.video_tooniy,
|
274 |
-
inputs=[aligned_video, instyle, exstyle, style_degree, style_type],
|
275 |
-
outputs=[result_video, output_info], api_name="toonify-video")
|
276 |
-
|
277 |
-
example_images.click(fn=set_example_image,
|
278 |
-
inputs=example_images,
|
279 |
-
outputs=example_images.components)
|
280 |
-
|
281 |
-
demo.queue()
|
282 |
-
demo.launch(
|
283 |
-
enable_queue=args.enable_queue,
|
284 |
-
server_port=args.port,
|
285 |
-
share=args.share,
|
286 |
-
)
|
287 |
-
|
288 |
-
|
289 |
-
if __name__ == '__main__':
|
290 |
-
main()
|
|
|
|
|
|
|
|
|
|
|
|
|
|
|
|
|
|
|
|
|
|
|
|
|
|
|
|
|
|
|
|
|
|
|
|
|
|
|
|
|
|
|
|
|
|
|
|
|
|
|
|
|
|
|
|
|
|
|
|
|
|
|
|
|
|
|
|
|
|
|
|
|
|
|
|
|
|
|
|
|
|
|
|
|
|
|
|
|
|
|
|
|
|
|
|
|
|
|
|
|
|
|
|
|
|
|
|
|
|
|
|
|
|
|
|
|
|
|
|
|
|
|
|
|
|
|
|
|
|
|
|
|
|
|
|
|
|
|
|
|
|
|
|
|
|
|
|
|
|
|
|
|
|
|
|
|
|
|
|
|
|
|
|
|
|
|
|
|
|
|
|
|
|
|
|
|
|
|
|
|
|
|
|
|
|
|
|
|
|
|
|
|
|
|
|
|
|
|
|
|
|
|
|
|
|
|
|
|
|
|
|
|
|
|
|
|
|
|
|
|
|
|
|
|
|
|
|
|
|
|
|
|
|
|
|
|
|
|
|
|
|
|
|
|
|
|
|
|
|
|
|
|
|
|
|
|
|
|
|
|
|
|
|
|
|
|
|
|
|
|
|
|
|
|
|
|
|
|
|
|
|
|
|
|
|
|
|
|
|
|
|
|
|
|
|
|
|
|
|
|
|
|
|
|
|
|
|
|
|
|
|
|
|
|
|
|
|
|
|
|
|
|
|
|
|
|
|
|
|
|
|
|
|
|
|
|
|
|
|
|
|
|
|
|
|
|
|
|
|
|
|
|
|
|
|
|
|
|
|
|
|
|
|
|
|
|
|
|
|
|
|
|
|
|
|
|
|
|
|
|
|
|
|
|
|
|
|
|
|
|
|
|
|
|
|
|
|
|
|
|
|
|
|
|
|
|
|
|
|
|
|
|
|
|
|
|
|
|
|
|
|
|
|
|
|
|
|
|
|
|
|
|
|
|
|
|
|
|
|
|
|
|
|
|
|
|
|
|
|
|
|
|
|
|
|
|
|
|
|
|
|
|
|
|
|
|
|
|
|
|
|
|
|
|
|
|
|
|
|
|
|
|
|
|
|
|
|
|
|
|
|
|
|
|
|
|
|
|
|
|
|
|
|
|
|
|
|
|
|
|
|
|
|
|
|
|
|
|
|
|
|
|
|
|
|
|
|
|
|
|
|
|
|
|
|
|
|
|
|
|
|
|
|
|
|
|
|
|
|
|
|
|
|
|
|
|
|
|
|
|
|
|
|
|
|
|
|
|
|
|
|
|
|
|
|
|
|
|
|
|
|
spaces/AIConsultant/MusicGen/scripts/static/style.css
DELETED
@@ -1,113 +0,0 @@
|
|
1 |
-
body {
|
2 |
-
background-color: #fbfbfb;
|
3 |
-
margin: 0;
|
4 |
-
}
|
5 |
-
|
6 |
-
select, input {
|
7 |
-
font-size: 1em;
|
8 |
-
max-width: 100%;
|
9 |
-
}
|
10 |
-
|
11 |
-
.xp_name {
|
12 |
-
font-family: monospace;
|
13 |
-
}
|
14 |
-
|
15 |
-
.simple_form {
|
16 |
-
background-color: #dddddd;
|
17 |
-
padding: 1em;
|
18 |
-
margin: 0.5em;
|
19 |
-
}
|
20 |
-
|
21 |
-
textarea {
|
22 |
-
margin-top: 0.5em;
|
23 |
-
margin-bottom: 0.5em;
|
24 |
-
}
|
25 |
-
|
26 |
-
.rating {
|
27 |
-
background-color: grey;
|
28 |
-
padding-top: 5px;
|
29 |
-
padding-bottom: 5px;
|
30 |
-
padding-left: 8px;
|
31 |
-
padding-right: 8px;
|
32 |
-
margin-right: 2px;
|
33 |
-
cursor:pointer;
|
34 |
-
}
|
35 |
-
|
36 |
-
.rating_selected {
|
37 |
-
background-color: purple;
|
38 |
-
}
|
39 |
-
|
40 |
-
.content {
|
41 |
-
font-family: sans-serif;
|
42 |
-
background-color: #f6f6f6;
|
43 |
-
padding: 40px;
|
44 |
-
margin: 0 auto;
|
45 |
-
max-width: 1000px;
|
46 |
-
}
|
47 |
-
|
48 |
-
.track label {
|
49 |
-
padding-top: 10px;
|
50 |
-
padding-bottom: 10px;
|
51 |
-
}
|
52 |
-
.track {
|
53 |
-
padding: 15px;
|
54 |
-
margin: 5px;
|
55 |
-
background-color: #c8c8c8;
|
56 |
-
}
|
57 |
-
|
58 |
-
.submit-big {
|
59 |
-
width:400px;
|
60 |
-
height:30px;
|
61 |
-
font-size: 20px;
|
62 |
-
}
|
63 |
-
|
64 |
-
.error {
|
65 |
-
color: red;
|
66 |
-
}
|
67 |
-
|
68 |
-
.ratings {
|
69 |
-
margin-left: 10px;
|
70 |
-
}
|
71 |
-
|
72 |
-
.important {
|
73 |
-
font-weight: bold;
|
74 |
-
}
|
75 |
-
|
76 |
-
.survey {
|
77 |
-
margin-bottom: 100px;
|
78 |
-
}
|
79 |
-
|
80 |
-
.success {
|
81 |
-
color: #25901b;
|
82 |
-
font-weight: bold;
|
83 |
-
}
|
84 |
-
.warning {
|
85 |
-
color: #8a1f19;
|
86 |
-
font-weight: bold;
|
87 |
-
}
|
88 |
-
.track>section {
|
89 |
-
display: flex;
|
90 |
-
align-items: center;
|
91 |
-
}
|
92 |
-
|
93 |
-
.prompt {
|
94 |
-
display: flex;
|
95 |
-
align-items: center;
|
96 |
-
}
|
97 |
-
|
98 |
-
.track>section>div {
|
99 |
-
padding-left: 10px;
|
100 |
-
}
|
101 |
-
|
102 |
-
audio {
|
103 |
-
max-width: 280px;
|
104 |
-
max-height: 40px;
|
105 |
-
margin-left: 10px;
|
106 |
-
margin-right: 10px;
|
107 |
-
}
|
108 |
-
|
109 |
-
.special {
|
110 |
-
font-weight: bold;
|
111 |
-
color: #2c2c2c;
|
112 |
-
}
|
113 |
-
|
|
|
|
|
|
|
|
|
|
|
|
|
|
|
|
|
|
|
|
|
|
|
|
|
|
|
|
|
|
|
|
|
|
|
|
|
|
|
|
|
|
|
|
|
|
|
|
|
|
|
|
|
|
|
|
|
|
|
|
|
|
|
|
|
|
|
|
|
|
|
|
|
|
|
|
|
|
|
|
|
|
|
|
|
|
|
|
|
|
|
|
|
|
|
|
|
|
|
|
|
|
|
|
|
|
|
|
|
|
|
|
|
|
|
|
|
|
|
|
|
|
|
|
|
|
|
|
|
|
|
|
|
|
|
|
|
|
|
|
|
|
|
|
|
|
|
|
|
|
|
|
|
|
|
|
|
|
|
|
|
|
|
|
|
|
|
|
|
|
|
|
|
|
|
|
|
|
|
|
|
|
|
|
|
|
|
|
|
|
|
|
|
|
|
|
|
|
|
|
|
|
|
|
|
|
|
|
|
|
|
|
|
|
|
|
|
|
|
|
|
|
|
|
|
|
|
|
spaces/AIFILMS/audioldm-text-to-audio-generation/audioldm/pipeline.py
DELETED
@@ -1,78 +0,0 @@
|
|
1 |
-
|
2 |
-
|
3 |
-
import os
|
4 |
-
|
5 |
-
import argparse
|
6 |
-
import yaml
|
7 |
-
import torch
|
8 |
-
|
9 |
-
from audioldm import LatentDiffusion, seed_everything
|
10 |
-
from audioldm.utils import default_audioldm_config
|
11 |
-
|
12 |
-
|
13 |
-
import time
|
14 |
-
|
15 |
-
def make_batch_for_text_to_audio(text, batchsize=1):
|
16 |
-
text = [text] * batchsize
|
17 |
-
if batchsize < 1:
|
18 |
-
print("Warning: Batchsize must be at least 1. Batchsize is set to .")
|
19 |
-
fbank = torch.zeros((batchsize, 1024, 64)) # Not used, here to keep the code format
|
20 |
-
stft = torch.zeros((batchsize, 1024, 512)) # Not used
|
21 |
-
waveform = torch.zeros((batchsize, 160000)) # Not used
|
22 |
-
fname = [""] * batchsize # Not used
|
23 |
-
batch = (
|
24 |
-
fbank,
|
25 |
-
stft,
|
26 |
-
None,
|
27 |
-
fname,
|
28 |
-
waveform,
|
29 |
-
text,
|
30 |
-
)
|
31 |
-
return batch
|
32 |
-
|
33 |
-
def build_model(config=None):
|
34 |
-
if(torch.cuda.is_available()):
|
35 |
-
device = torch.device("cuda:0")
|
36 |
-
else:
|
37 |
-
device = torch.device("cpu")
|
38 |
-
|
39 |
-
if(config is not None):
|
40 |
-
assert type(config) is str
|
41 |
-
config = yaml.load(open(config, "r"), Loader=yaml.FullLoader)
|
42 |
-
else:
|
43 |
-
config = default_audioldm_config()
|
44 |
-
|
45 |
-
# Use text as condition instead of using waveform during training
|
46 |
-
config["model"]["params"]["device"] = device
|
47 |
-
config["model"]["params"]["cond_stage_key"] = "text"
|
48 |
-
|
49 |
-
# No normalization here
|
50 |
-
latent_diffusion = LatentDiffusion(**config["model"]["params"])
|
51 |
-
|
52 |
-
resume_from_checkpoint = "./ckpt/ldm_trimmed.ckpt"
|
53 |
-
|
54 |
-
checkpoint = torch.load(resume_from_checkpoint, map_location=device)
|
55 |
-
latent_diffusion.load_state_dict(checkpoint["state_dict"])
|
56 |
-
|
57 |
-
latent_diffusion.eval()
|
58 |
-
latent_diffusion = latent_diffusion.to(device)
|
59 |
-
|
60 |
-
latent_diffusion.cond_stage_model.embed_mode = "text"
|
61 |
-
return latent_diffusion
|
62 |
-
|
63 |
-
def duration_to_latent_t_size(duration):
|
64 |
-
return int(duration * 25.6)
|
65 |
-
|
66 |
-
def text_to_audio(latent_diffusion, text, seed=42, duration=10, batchsize=1, guidance_scale=2.5, n_candidate_gen_per_text=3, config=None):
|
67 |
-
seed_everything(int(seed))
|
68 |
-
batch = make_batch_for_text_to_audio(text, batchsize=batchsize)
|
69 |
-
|
70 |
-
latent_diffusion.latent_t_size = duration_to_latent_t_size(duration)
|
71 |
-
with torch.no_grad():
|
72 |
-
waveform = latent_diffusion.generate_sample(
|
73 |
-
[batch],
|
74 |
-
unconditional_guidance_scale=guidance_scale,
|
75 |
-
n_candidate_gen_per_text=n_candidate_gen_per_text,
|
76 |
-
duration=duration
|
77 |
-
)
|
78 |
-
return waveform
|
|
|
|
|
|
|
|
|
|
|
|
|
|
|
|
|
|
|
|
|
|
|
|
|
|
|
|
|
|
|
|
|
|
|
|
|
|
|
|
|
|
|
|
|
|
|
|
|
|
|
|
|
|
|
|
|
|
|
|
|
|
|
|
|
|
|
|
|
|
|
|
|
|
|
|
|
|
|
|
|
|
|
|
|
|
|
|
|
|
|
|
|
|
|
|
|
|
|
|
|
|
|
|
|
|
|
|
|
|
|
|
|
|
|
|
|
|
|
|
|
|
|
|
|
|
|
|
|
|
|
|
|
|
|
|
|
|
|
|
|
|
|
|
|
|
|
|
|
|
|
|
|
|
|
|
|
|
spaces/AIGC-Audio/AudioGPT/audio_detection/__init__.py
DELETED
File without changes
|
spaces/ATang0729/Forecast4Muses/Model/Model6/Model6_0_ClothesDetection/mmyolo/configs/yolov5/voc/yolov5_m-v61_fast_1xb64-50e_voc.py
DELETED
@@ -1,17 +0,0 @@
|
|
1 |
-
_base_ = './yolov5_s-v61_fast_1xb64-50e_voc.py'
|
2 |
-
|
3 |
-
deepen_factor = 0.67
|
4 |
-
widen_factor = 0.75
|
5 |
-
|
6 |
-
load_from = 'https://download.openmmlab.com/mmyolo/v0/yolov5/yolov5_m-v61_syncbn_fast_8xb16-300e_coco/yolov5_m-v61_syncbn_fast_8xb16-300e_coco_20220917_204944-516a710f.pth' # noqa
|
7 |
-
|
8 |
-
model = dict(
|
9 |
-
backbone=dict(
|
10 |
-
deepen_factor=deepen_factor,
|
11 |
-
widen_factor=widen_factor,
|
12 |
-
),
|
13 |
-
neck=dict(
|
14 |
-
deepen_factor=deepen_factor,
|
15 |
-
widen_factor=widen_factor,
|
16 |
-
),
|
17 |
-
bbox_head=dict(head_module=dict(widen_factor=widen_factor)))
|
|
|
|
|
|
|
|
|
|
|
|
|
|
|
|
|
|
|
|
|
|
|
|
|
|
|
|
|
|
|
|
|
|
|
|
spaces/Abhilashvj/haystack_QA/search.py
DELETED
@@ -1,60 +0,0 @@
|
|
1 |
-
|
2 |
-
|
3 |
-
import pinecone
|
4 |
-
index_name = "abstractive-question-answering"
|
5 |
-
|
6 |
-
# check if the abstractive-question-answering index exists
|
7 |
-
if index_name not in pinecone.list_indexes():
|
8 |
-
# create the index if it does not exist
|
9 |
-
pinecone.create_index(
|
10 |
-
index_name,
|
11 |
-
dimension=768,
|
12 |
-
metric="cosine"
|
13 |
-
)
|
14 |
-
|
15 |
-
# connect to abstractive-question-answering index we created
|
16 |
-
index = pinecone.Index(index_name)
|
17 |
-
|
18 |
-
# we will use batches of 64
|
19 |
-
batch_size = 64
|
20 |
-
|
21 |
-
for i in tqdm(range(0, len(df), batch_size)):
|
22 |
-
# find end of batch
|
23 |
-
i_end = min(i+batch_size, len(df))
|
24 |
-
# extract batch
|
25 |
-
batch = df.iloc[i:i_end]
|
26 |
-
# generate embeddings for batch
|
27 |
-
emb = retriever.encode(batch["passage_text"].tolist()).tolist()
|
28 |
-
# get metadata
|
29 |
-
meta = batch.to_dict(orient="records")
|
30 |
-
# create unique IDs
|
31 |
-
ids = [f"{idx}" for idx in range(i, i_end)]
|
32 |
-
# add all to upsert list
|
33 |
-
to_upsert = list(zip(ids, emb, meta))
|
34 |
-
# upsert/insert these records to pinecone
|
35 |
-
_ = index.upsert(vectors=to_upsert)
|
36 |
-
|
37 |
-
# check that we have all vectors in index
|
38 |
-
index.describe_index_stats()
|
39 |
-
|
40 |
-
# from transformers import BartTokenizer, BartForConditionalGeneration
|
41 |
-
|
42 |
-
# # load bart tokenizer and model from huggingface
|
43 |
-
# tokenizer = BartTokenizer.from_pretrained('vblagoje/bart_lfqa')
|
44 |
-
# generator = BartForConditionalGeneration.from_pretrained('vblagoje/bart_lfqa')
|
45 |
-
|
46 |
-
# def query_pinecone(query, top_k):
|
47 |
-
# # generate embeddings for the query
|
48 |
-
# xq = retriever.encode([query]).tolist()
|
49 |
-
# # search pinecone index for context passage with the answer
|
50 |
-
# xc = index.query(xq, top_k=top_k, include_metadata=True)
|
51 |
-
# return xc
|
52 |
-
|
53 |
-
# def format_query(query, context):
|
54 |
-
# # extract passage_text from Pinecone search result and add the tag
|
55 |
-
# context = [f" {m['metadata']['passage_text']}" for m in context]
|
56 |
-
# # concatinate all context passages
|
57 |
-
# context = " ".join(context)
|
58 |
-
# # contcatinate the query and context passages
|
59 |
-
# query = f"question: {query} context: {context}"
|
60 |
-
# return query
|
|
|
|
|
|
|
|
|
|
|
|
|
|
|
|
|
|
|
|
|
|
|
|
|
|
|
|
|
|
|
|
|
|
|
|
|
|
|
|
|
|
|
|
|
|
|
|
|
|
|
|
|
|
|
|
|
|
|
|
|
|
|
|
|
|
|
|
|
|
|
|
|
|
|
|
|
|
|
|
|
|
|
|
|
|
|
|
|
|
|
|
|
|
|
|
|
|
|
|
|
|
|
|
|
|
|
|
|
|
|
|
|
|
|
|
|
|
|
|
|
|
spaces/AchyuthGamer/OpenGPT-Chat-UI/src/lib/utils/deepestChild.ts
DELETED
@@ -1,6 +0,0 @@
|
|
1 |
-
export function deepestChild(el: HTMLElement): HTMLElement {
|
2 |
-
if (el.lastElementChild && el.lastElementChild.nodeType !== Node.TEXT_NODE) {
|
3 |
-
return deepestChild(el.lastElementChild as HTMLElement);
|
4 |
-
}
|
5 |
-
return el;
|
6 |
-
}
|
|
|
|
|
|
|
|
|
|
|
|
|
|
spaces/AchyuthGamer/OpenGPT/g4f/Provider/Providers/Myshell.py
DELETED
@@ -1,173 +0,0 @@
|
|
1 |
-
from __future__ import annotations
|
2 |
-
|
3 |
-
import json, uuid, hashlib, time, random
|
4 |
-
|
5 |
-
from aiohttp import ClientSession
|
6 |
-
from aiohttp.http import WSMsgType
|
7 |
-
import asyncio
|
8 |
-
|
9 |
-
from ..typing import AsyncGenerator
|
10 |
-
from .base_provider import AsyncGeneratorProvider, format_prompt
|
11 |
-
|
12 |
-
|
13 |
-
models = {
|
14 |
-
"samantha": "1e3be7fe89e94a809408b1154a2ee3e1",
|
15 |
-
"gpt-3.5-turbo": "8077335db7cd47e29f7de486612cc7fd",
|
16 |
-
"gpt-4": "01c8de4fbfc548df903712b0922a4e01",
|
17 |
-
}
|
18 |
-
|
19 |
-
|
20 |
-
class Myshell(AsyncGeneratorProvider):
|
21 |
-
url = "https://app.myshell.ai/chat"
|
22 |
-
working = True
|
23 |
-
supports_gpt_35_turbo = True
|
24 |
-
supports_gpt_4 = True
|
25 |
-
|
26 |
-
@classmethod
|
27 |
-
async def create_async_generator(
|
28 |
-
cls,
|
29 |
-
model: str,
|
30 |
-
messages: list[dict[str, str]],
|
31 |
-
timeout: int = 90,
|
32 |
-
**kwargs
|
33 |
-
) -> AsyncGenerator:
|
34 |
-
if not model:
|
35 |
-
bot_id = models["samantha"]
|
36 |
-
elif model in models:
|
37 |
-
bot_id = models[model]
|
38 |
-
else:
|
39 |
-
raise ValueError(f"Model are not supported: {model}")
|
40 |
-
|
41 |
-
user_agent = 'Mozilla/5.0 (Macintosh; Intel Mac OS X 10_15_7) AppleWebKit/537.36 (KHTML, like Gecko) Chrome/111.0.0.0 Safari/537.36'
|
42 |
-
visitor_id = generate_visitor_id(user_agent)
|
43 |
-
|
44 |
-
async with ClientSession(
|
45 |
-
headers={'User-Agent': user_agent}
|
46 |
-
) as session:
|
47 |
-
async with session.ws_connect(
|
48 |
-
"wss://api.myshell.ai/ws/?EIO=4&transport=websocket",
|
49 |
-
autoping=False,
|
50 |
-
timeout=timeout
|
51 |
-
) as wss:
|
52 |
-
# Send and receive hello message
|
53 |
-
await wss.receive_str()
|
54 |
-
message = json.dumps({"token": None, "visitorId": visitor_id})
|
55 |
-
await wss.send_str(f"40/chat,{message}")
|
56 |
-
await wss.receive_str()
|
57 |
-
|
58 |
-
# Fix "need_verify_captcha" issue
|
59 |
-
await asyncio.sleep(5)
|
60 |
-
|
61 |
-
# Create chat message
|
62 |
-
text = format_prompt(messages)
|
63 |
-
chat_data = json.dumps(["text_chat",{
|
64 |
-
"reqId": str(uuid.uuid4()),
|
65 |
-
"botUid": bot_id,
|
66 |
-
"sourceFrom": "myshellWebsite",
|
67 |
-
"text": text,
|
68 |
-
**generate_signature(text)
|
69 |
-
}])
|
70 |
-
|
71 |
-
# Send chat message
|
72 |
-
chat_start = "42/chat,"
|
73 |
-
chat_message = f"{chat_start}{chat_data}"
|
74 |
-
await wss.send_str(chat_message)
|
75 |
-
|
76 |
-
# Receive messages
|
77 |
-
async for message in wss:
|
78 |
-
if message.type != WSMsgType.TEXT:
|
79 |
-
continue
|
80 |
-
# Ping back
|
81 |
-
if message.data == "2":
|
82 |
-
await wss.send_str("3")
|
83 |
-
continue
|
84 |
-
# Is not chat message
|
85 |
-
if not message.data.startswith(chat_start):
|
86 |
-
continue
|
87 |
-
data_type, data = json.loads(message.data[len(chat_start):])
|
88 |
-
if data_type == "text_stream":
|
89 |
-
if data["data"]["text"]:
|
90 |
-
yield data["data"]["text"]
|
91 |
-
elif data["data"]["isFinal"]:
|
92 |
-
break
|
93 |
-
elif data_type in ("message_replied", "need_verify_captcha"):
|
94 |
-
raise RuntimeError(f"Received unexpected message: {data_type}")
|
95 |
-
|
96 |
-
|
97 |
-
@classmethod
|
98 |
-
@property
|
99 |
-
def params(cls):
|
100 |
-
params = [
|
101 |
-
("model", "str"),
|
102 |
-
("messages", "list[dict[str, str]]"),
|
103 |
-
("stream", "bool"),
|
104 |
-
]
|
105 |
-
param = ", ".join([": ".join(p) for p in params])
|
106 |
-
return f"g4f.provider.{cls.__name__} supports: ({param})"
|
107 |
-
|
108 |
-
|
109 |
-
def generate_timestamp() -> str:
|
110 |
-
return str(
|
111 |
-
int(
|
112 |
-
str(int(time.time() * 1000))[:-1]
|
113 |
-
+ str(
|
114 |
-
sum(
|
115 |
-
2 * int(digit)
|
116 |
-
if idx % 2 == 0
|
117 |
-
else 3 * int(digit)
|
118 |
-
for idx, digit in enumerate(str(int(time.time() * 1000))[:-1])
|
119 |
-
)
|
120 |
-
% 10
|
121 |
-
)
|
122 |
-
)
|
123 |
-
)
|
124 |
-
|
125 |
-
def generate_signature(text: str):
|
126 |
-
timestamp = generate_timestamp()
|
127 |
-
version = 'v1.0.0'
|
128 |
-
secret = '8@VXGK3kKHr!u2gA'
|
129 |
-
data = f"{version}#{text}#{timestamp}#{secret}"
|
130 |
-
signature = hashlib.md5(data.encode()).hexdigest()
|
131 |
-
signature = signature[::-1]
|
132 |
-
return {
|
133 |
-
"signature": signature,
|
134 |
-
"timestamp": timestamp,
|
135 |
-
"version": version
|
136 |
-
}
|
137 |
-
|
138 |
-
def xor_hash(B: str):
|
139 |
-
r = []
|
140 |
-
i = 0
|
141 |
-
|
142 |
-
def o(e, t):
|
143 |
-
o_val = 0
|
144 |
-
for i in range(len(t)):
|
145 |
-
o_val |= r[i] << (8 * i)
|
146 |
-
return e ^ o_val
|
147 |
-
|
148 |
-
for e in range(len(B)):
|
149 |
-
t = ord(B[e])
|
150 |
-
r.insert(0, 255 & t)
|
151 |
-
|
152 |
-
if len(r) >= 4:
|
153 |
-
i = o(i, r)
|
154 |
-
r = []
|
155 |
-
|
156 |
-
if len(r) > 0:
|
157 |
-
i = o(i, r)
|
158 |
-
|
159 |
-
return hex(i)[2:]
|
160 |
-
|
161 |
-
def performance() -> str:
|
162 |
-
t = int(time.time() * 1000)
|
163 |
-
e = 0
|
164 |
-
while t == int(time.time() * 1000):
|
165 |
-
e += 1
|
166 |
-
return hex(t)[2:] + hex(e)[2:]
|
167 |
-
|
168 |
-
def generate_visitor_id(user_agent: str) -> str:
|
169 |
-
f = performance()
|
170 |
-
r = hex(int(random.random() * (16**16)))[2:-2]
|
171 |
-
d = xor_hash(user_agent)
|
172 |
-
e = hex(1080 * 1920)[2:]
|
173 |
-
return f"{f}-{r}-{d}-{e}-{f}"
|
|
|
|
|
|
|
|
|
|
|
|
|
|
|
|
|
|
|
|
|
|
|
|
|
|
|
|
|
|
|
|
|
|
|
|
|
|
|
|
|
|
|
|
|
|
|
|
|
|
|
|
|
|
|
|
|
|
|
|
|
|
|
|
|
|
|
|
|
|
|
|
|
|
|
|
|
|
|
|
|
|
|
|
|
|
|
|
|
|
|
|
|
|
|
|
|
|
|
|
|
|
|
|
|
|
|
|
|
|
|
|
|
|
|
|
|
|
|
|
|
|
|
|
|
|
|
|
|
|
|
|
|
|
|
|
|
|
|
|
|
|
|
|
|
|
|
|
|
|
|
|
|
|
|
|
|
|
|
|
|
|
|
|
|
|
|
|
|
|
|
|
|
|
|
|
|
|
|
|
|
|
|
|
|
|
|
|
|
|
|
|
|
|
|
|
|
|
|
|
|
|
|
|
|
|
|
|
|
|
|
|
|
|
|
|
|
|
|
|
|
|
|
|
|
|
|
|
|
|
|
|
|
|
|
|
|
|
|
|
|
|
|
|
|
|
|
|
|
|
|
|
|
|
|
|
|
|
|
|
|
|
|
|
|
|
|
|
|
|
|
|
|
|
|
|
|
|
|
|
|
|
|
|
|
|
|
|
|
|
|
|
|
|
|
|
|
|
|
|
|
|
|
|
|
|
|
|
|
|
|
|
|
|
|
|
|
|
|
|
|
|
|
|
|
|
|
|
|
|
|
|
|
|
|
|
|
|
|
|
|
|
|
|
|
|
|
|
spaces/AgentVerse/agentVerse/ui/src/phaser3-rex-plugins/templates/ui/gridtable/TableOnCellVisible.js
DELETED
@@ -1,28 +0,0 @@
|
|
1 |
-
var TableOnCellVisible = function (table) {
|
2 |
-
table.on('cellvisible', function (cell, cellContainer, table) {
|
3 |
-
var callback = this.createCellContainerCallback;
|
4 |
-
var scope = this.createCellContainerCallbackScope;
|
5 |
-
cell.item = this.items[cell.index];
|
6 |
-
cell.items = this.items;
|
7 |
-
var cellContainer;
|
8 |
-
if (scope) {
|
9 |
-
cellContainer = callback.call(scope, cell, cellContainer, table);
|
10 |
-
} else {
|
11 |
-
cellContainer = callback(cell, cellContainer, table);
|
12 |
-
}
|
13 |
-
|
14 |
-
if (cellContainer) {
|
15 |
-
if ((cell.cellContainerAlign == null) && cellContainer.setOrigin) {
|
16 |
-
cellContainer.setOrigin(0);
|
17 |
-
}
|
18 |
-
if (cellContainer.isRexSizer) {
|
19 |
-
cellContainer.layout(); // Use original size
|
20 |
-
}
|
21 |
-
}
|
22 |
-
|
23 |
-
cell.item = undefined;
|
24 |
-
cell.items = undefined;
|
25 |
-
cell.setContainer(cellContainer);
|
26 |
-
}, this);
|
27 |
-
}
|
28 |
-
export default TableOnCellVisible;
|
|
|
|
|
|
|
|
|
|
|
|
|
|
|
|
|
|
|
|
|
|
|
|
|
|
|
|
|
|
|
|
|
|
|
|
|
|
|
|
|
|
|
|
|
|
|
|
|
|
|
|
|
|
|
|
|
|
spaces/AgentVerse/agentVerse/ui/src/phaser3-rex-plugins/templates/ui/lineprogress/Factory.d.ts
DELETED
@@ -1,13 +0,0 @@
|
|
1 |
-
import LineProgress from './LineProgress';
|
2 |
-
|
3 |
-
export default function (
|
4 |
-
config?: LineProgress.IConfig
|
5 |
-
): LineProgress;
|
6 |
-
|
7 |
-
export default function (
|
8 |
-
x?: number, y?: number,
|
9 |
-
radius?: number,
|
10 |
-
barColor?: string | number,
|
11 |
-
value?: number,
|
12 |
-
config?: LineProgress.IConfig
|
13 |
-
): LineProgress;
|
|
|
|
|
|
|
|
|
|
|
|
|
|
|
|
|
|
|
|
|
|
|
|
|
|
|
|
spaces/Amrrs/DragGan-Inversion/stylegan_human/bg_white.py
DELETED
@@ -1,60 +0,0 @@
|
|
1 |
-
# Copyright (c) SenseTime Research. All rights reserved.
|
2 |
-
|
3 |
-
import os
|
4 |
-
import click
|
5 |
-
import cv2
|
6 |
-
import numpy as np
|
7 |
-
|
8 |
-
|
9 |
-
def bg_white(seg, raw, blur_level=3, gaussian=81):
|
10 |
-
seg = cv2.blur(seg, (blur_level, blur_level))
|
11 |
-
|
12 |
-
empty = np.ones_like(seg)
|
13 |
-
seg_bg = (empty - seg) * 255
|
14 |
-
seg_bg = cv2.GaussianBlur(seg_bg, (gaussian, gaussian), 0)
|
15 |
-
|
16 |
-
background_mask = cv2.cvtColor(
|
17 |
-
255 - cv2.cvtColor(seg, cv2.COLOR_BGR2GRAY), cv2.COLOR_GRAY2BGR)
|
18 |
-
masked_fg = (raw * (1 / 255)) * (seg * (1 / 255))
|
19 |
-
masked_bg = (seg_bg * (1 / 255)) * (background_mask * (1 / 255))
|
20 |
-
|
21 |
-
frame = np.uint8(cv2.add(masked_bg, masked_fg)*255)
|
22 |
-
|
23 |
-
return frame
|
24 |
-
|
25 |
-
|
26 |
-
"""
|
27 |
-
To turn background into white.
|
28 |
-
|
29 |
-
Examples:
|
30 |
-
|
31 |
-
\b
|
32 |
-
python bg_white.py --raw_img_dir=./SHHQ-1.0/no_segment/ --raw_seg_dir=./SHHQ-1.0/segments/ \\
|
33 |
-
--outdir=./SHHQ-1.0/bg_white/
|
34 |
-
"""
|
35 |
-
|
36 |
-
|
37 |
-
@click.command()
|
38 |
-
@click.pass_context
|
39 |
-
@click.option('--raw_img_dir', default="./SHHQ-1.0/no_segment/", help='folder of raw image', required=True)
|
40 |
-
@click.option('--raw_seg_dir', default='./SHHQ-1.0/segments/', help='folder of segmentation masks', required=True)
|
41 |
-
@click.option('--outdir', help='Where to save the output images', default="./SHHQ-1.0/bg_white/", type=str, required=True, metavar='DIR')
|
42 |
-
def main(
|
43 |
-
ctx: click.Context,
|
44 |
-
raw_img_dir: str,
|
45 |
-
raw_seg_dir: str,
|
46 |
-
outdir: str):
|
47 |
-
os.makedirs(outdir, exist_ok=True)
|
48 |
-
files = os.listdir(raw_img_dir)
|
49 |
-
for file in files:
|
50 |
-
print(file)
|
51 |
-
raw = cv2.imread(os.path.join(raw_img_dir, file))
|
52 |
-
seg = cv2.imread(os.path.join(raw_seg_dir, file))
|
53 |
-
assert raw is not None
|
54 |
-
assert seg is not None
|
55 |
-
white_frame = bg_white(seg, raw)
|
56 |
-
cv2.imwrite(os.path.join(outdir, file), white_frame)
|
57 |
-
|
58 |
-
|
59 |
-
if __name__ == "__main__":
|
60 |
-
main()
|
|
|
|
|
|
|
|
|
|
|
|
|
|
|
|
|
|
|
|
|
|
|
|
|
|
|
|
|
|
|
|
|
|
|
|
|
|
|
|
|
|
|
|
|
|
|
|
|
|
|
|
|
|
|
|
|
|
|
|
|
|
|
|
|
|
|
|
|
|
|
|
|
|
|
|
|
|
|
|
|
|
|
|
|
|
|
|
|
|
|
|
|
|
|
|
|
|
|
|
|
|
|
|
|
|
|
|
|
|
|
|
|
|
|
|
|
|
|
|
|
|
spaces/Androidonnxfork/CivitAi-to-Diffusers/diffusers/src/diffusers/schedulers/scheduling_ddpm.py
DELETED
@@ -1,513 +0,0 @@
|
|
1 |
-
# Copyright 2023 UC Berkeley Team and The HuggingFace Team. All rights reserved.
|
2 |
-
#
|
3 |
-
# Licensed under the Apache License, Version 2.0 (the "License");
|
4 |
-
# you may not use this file except in compliance with the License.
|
5 |
-
# You may obtain a copy of the License at
|
6 |
-
#
|
7 |
-
# http://www.apache.org/licenses/LICENSE-2.0
|
8 |
-
#
|
9 |
-
# Unless required by applicable law or agreed to in writing, software
|
10 |
-
# distributed under the License is distributed on an "AS IS" BASIS,
|
11 |
-
# WITHOUT WARRANTIES OR CONDITIONS OF ANY KIND, either express or implied.
|
12 |
-
# See the License for the specific language governing permissions and
|
13 |
-
# limitations under the License.
|
14 |
-
|
15 |
-
# DISCLAIMER: This file is strongly influenced by https://github.com/ermongroup/ddim
|
16 |
-
|
17 |
-
import math
|
18 |
-
from dataclasses import dataclass
|
19 |
-
from typing import List, Optional, Tuple, Union
|
20 |
-
|
21 |
-
import numpy as np
|
22 |
-
import torch
|
23 |
-
|
24 |
-
from ..configuration_utils import ConfigMixin, register_to_config
|
25 |
-
from ..utils import BaseOutput, randn_tensor
|
26 |
-
from .scheduling_utils import KarrasDiffusionSchedulers, SchedulerMixin
|
27 |
-
|
28 |
-
|
29 |
-
@dataclass
|
30 |
-
class DDPMSchedulerOutput(BaseOutput):
|
31 |
-
"""
|
32 |
-
Output class for the scheduler's step function output.
|
33 |
-
|
34 |
-
Args:
|
35 |
-
prev_sample (`torch.FloatTensor` of shape `(batch_size, num_channels, height, width)` for images):
|
36 |
-
Computed sample (x_{t-1}) of previous timestep. `prev_sample` should be used as next model input in the
|
37 |
-
denoising loop.
|
38 |
-
pred_original_sample (`torch.FloatTensor` of shape `(batch_size, num_channels, height, width)` for images):
|
39 |
-
The predicted denoised sample (x_{0}) based on the model output from the current timestep.
|
40 |
-
`pred_original_sample` can be used to preview progress or for guidance.
|
41 |
-
"""
|
42 |
-
|
43 |
-
prev_sample: torch.FloatTensor
|
44 |
-
pred_original_sample: Optional[torch.FloatTensor] = None
|
45 |
-
|
46 |
-
|
47 |
-
def betas_for_alpha_bar(
|
48 |
-
num_diffusion_timesteps,
|
49 |
-
max_beta=0.999,
|
50 |
-
alpha_transform_type="cosine",
|
51 |
-
):
|
52 |
-
"""
|
53 |
-
Create a beta schedule that discretizes the given alpha_t_bar function, which defines the cumulative product of
|
54 |
-
(1-beta) over time from t = [0,1].
|
55 |
-
|
56 |
-
Contains a function alpha_bar that takes an argument t and transforms it to the cumulative product of (1-beta) up
|
57 |
-
to that part of the diffusion process.
|
58 |
-
|
59 |
-
|
60 |
-
Args:
|
61 |
-
num_diffusion_timesteps (`int`): the number of betas to produce.
|
62 |
-
max_beta (`float`): the maximum beta to use; use values lower than 1 to
|
63 |
-
prevent singularities.
|
64 |
-
alpha_transform_type (`str`, *optional*, default to `cosine`): the type of noise schedule for alpha_bar.
|
65 |
-
Choose from `cosine` or `exp`
|
66 |
-
|
67 |
-
Returns:
|
68 |
-
betas (`np.ndarray`): the betas used by the scheduler to step the model outputs
|
69 |
-
"""
|
70 |
-
if alpha_transform_type == "cosine":
|
71 |
-
|
72 |
-
def alpha_bar_fn(t):
|
73 |
-
return math.cos((t + 0.008) / 1.008 * math.pi / 2) ** 2
|
74 |
-
|
75 |
-
elif alpha_transform_type == "exp":
|
76 |
-
|
77 |
-
def alpha_bar_fn(t):
|
78 |
-
return math.exp(t * -12.0)
|
79 |
-
|
80 |
-
else:
|
81 |
-
raise ValueError(f"Unsupported alpha_tranform_type: {alpha_transform_type}")
|
82 |
-
|
83 |
-
betas = []
|
84 |
-
for i in range(num_diffusion_timesteps):
|
85 |
-
t1 = i / num_diffusion_timesteps
|
86 |
-
t2 = (i + 1) / num_diffusion_timesteps
|
87 |
-
betas.append(min(1 - alpha_bar_fn(t2) / alpha_bar_fn(t1), max_beta))
|
88 |
-
return torch.tensor(betas, dtype=torch.float32)
|
89 |
-
|
90 |
-
|
91 |
-
class DDPMScheduler(SchedulerMixin, ConfigMixin):
|
92 |
-
"""
|
93 |
-
Denoising diffusion probabilistic models (DDPMs) explores the connections between denoising score matching and
|
94 |
-
Langevin dynamics sampling.
|
95 |
-
|
96 |
-
[`~ConfigMixin`] takes care of storing all config attributes that are passed in the scheduler's `__init__`
|
97 |
-
function, such as `num_train_timesteps`. They can be accessed via `scheduler.config.num_train_timesteps`.
|
98 |
-
[`SchedulerMixin`] provides general loading and saving functionality via the [`SchedulerMixin.save_pretrained`] and
|
99 |
-
[`~SchedulerMixin.from_pretrained`] functions.
|
100 |
-
|
101 |
-
For more details, see the original paper: https://arxiv.org/abs/2006.11239
|
102 |
-
|
103 |
-
Args:
|
104 |
-
num_train_timesteps (`int`): number of diffusion steps used to train the model.
|
105 |
-
beta_start (`float`): the starting `beta` value of inference.
|
106 |
-
beta_end (`float`): the final `beta` value.
|
107 |
-
beta_schedule (`str`):
|
108 |
-
the beta schedule, a mapping from a beta range to a sequence of betas for stepping the model. Choose from
|
109 |
-
`linear`, `scaled_linear`, `squaredcos_cap_v2` or `sigmoid`.
|
110 |
-
trained_betas (`np.ndarray`, optional):
|
111 |
-
option to pass an array of betas directly to the constructor to bypass `beta_start`, `beta_end` etc.
|
112 |
-
variance_type (`str`):
|
113 |
-
options to clip the variance used when adding noise to the denoised sample. Choose from `fixed_small`,
|
114 |
-
`fixed_small_log`, `fixed_large`, `fixed_large_log`, `learned` or `learned_range`.
|
115 |
-
clip_sample (`bool`, default `True`):
|
116 |
-
option to clip predicted sample for numerical stability.
|
117 |
-
clip_sample_range (`float`, default `1.0`):
|
118 |
-
the maximum magnitude for sample clipping. Valid only when `clip_sample=True`.
|
119 |
-
prediction_type (`str`, default `epsilon`, optional):
|
120 |
-
prediction type of the scheduler function, one of `epsilon` (predicting the noise of the diffusion
|
121 |
-
process), `sample` (directly predicting the noisy sample`) or `v_prediction` (see section 2.4
|
122 |
-
https://imagen.research.google/video/paper.pdf)
|
123 |
-
thresholding (`bool`, default `False`):
|
124 |
-
whether to use the "dynamic thresholding" method (introduced by Imagen, https://arxiv.org/abs/2205.11487).
|
125 |
-
Note that the thresholding method is unsuitable for latent-space diffusion models (such as
|
126 |
-
stable-diffusion).
|
127 |
-
dynamic_thresholding_ratio (`float`, default `0.995`):
|
128 |
-
the ratio for the dynamic thresholding method. Default is `0.995`, the same as Imagen
|
129 |
-
(https://arxiv.org/abs/2205.11487). Valid only when `thresholding=True`.
|
130 |
-
sample_max_value (`float`, default `1.0`):
|
131 |
-
the threshold value for dynamic thresholding. Valid only when `thresholding=True`.
|
132 |
-
timestep_spacing (`str`, default `"leading"`):
|
133 |
-
The way the timesteps should be scaled. Refer to Table 2. of [Common Diffusion Noise Schedules and Sample
|
134 |
-
Steps are Flawed](https://arxiv.org/abs/2305.08891) for more information.
|
135 |
-
steps_offset (`int`, default `0`):
|
136 |
-
an offset added to the inference steps. You can use a combination of `offset=1` and
|
137 |
-
`set_alpha_to_one=False`, to make the last step use step 0 for the previous alpha product, as done in
|
138 |
-
stable diffusion.
|
139 |
-
"""
|
140 |
-
|
141 |
-
_compatibles = [e.name for e in KarrasDiffusionSchedulers]
|
142 |
-
order = 1
|
143 |
-
|
144 |
-
@register_to_config
|
145 |
-
def __init__(
|
146 |
-
self,
|
147 |
-
num_train_timesteps: int = 1000,
|
148 |
-
beta_start: float = 0.0001,
|
149 |
-
beta_end: float = 0.02,
|
150 |
-
beta_schedule: str = "linear",
|
151 |
-
trained_betas: Optional[Union[np.ndarray, List[float]]] = None,
|
152 |
-
variance_type: str = "fixed_small",
|
153 |
-
clip_sample: bool = True,
|
154 |
-
prediction_type: str = "epsilon",
|
155 |
-
thresholding: bool = False,
|
156 |
-
dynamic_thresholding_ratio: float = 0.995,
|
157 |
-
clip_sample_range: float = 1.0,
|
158 |
-
sample_max_value: float = 1.0,
|
159 |
-
timestep_spacing: str = "leading",
|
160 |
-
steps_offset: int = 0,
|
161 |
-
):
|
162 |
-
if trained_betas is not None:
|
163 |
-
self.betas = torch.tensor(trained_betas, dtype=torch.float32)
|
164 |
-
elif beta_schedule == "linear":
|
165 |
-
self.betas = torch.linspace(beta_start, beta_end, num_train_timesteps, dtype=torch.float32)
|
166 |
-
elif beta_schedule == "scaled_linear":
|
167 |
-
# this schedule is very specific to the latent diffusion model.
|
168 |
-
self.betas = (
|
169 |
-
torch.linspace(beta_start**0.5, beta_end**0.5, num_train_timesteps, dtype=torch.float32) ** 2
|
170 |
-
)
|
171 |
-
elif beta_schedule == "squaredcos_cap_v2":
|
172 |
-
# Glide cosine schedule
|
173 |
-
self.betas = betas_for_alpha_bar(num_train_timesteps)
|
174 |
-
elif beta_schedule == "sigmoid":
|
175 |
-
# GeoDiff sigmoid schedule
|
176 |
-
betas = torch.linspace(-6, 6, num_train_timesteps)
|
177 |
-
self.betas = torch.sigmoid(betas) * (beta_end - beta_start) + beta_start
|
178 |
-
else:
|
179 |
-
raise NotImplementedError(f"{beta_schedule} does is not implemented for {self.__class__}")
|
180 |
-
|
181 |
-
self.alphas = 1.0 - self.betas
|
182 |
-
self.alphas_cumprod = torch.cumprod(self.alphas, dim=0)
|
183 |
-
self.one = torch.tensor(1.0)
|
184 |
-
|
185 |
-
# standard deviation of the initial noise distribution
|
186 |
-
self.init_noise_sigma = 1.0
|
187 |
-
|
188 |
-
# setable values
|
189 |
-
self.custom_timesteps = False
|
190 |
-
self.num_inference_steps = None
|
191 |
-
self.timesteps = torch.from_numpy(np.arange(0, num_train_timesteps)[::-1].copy())
|
192 |
-
|
193 |
-
self.variance_type = variance_type
|
194 |
-
|
195 |
-
def scale_model_input(self, sample: torch.FloatTensor, timestep: Optional[int] = None) -> torch.FloatTensor:
|
196 |
-
"""
|
197 |
-
Ensures interchangeability with schedulers that need to scale the denoising model input depending on the
|
198 |
-
current timestep.
|
199 |
-
|
200 |
-
Args:
|
201 |
-
sample (`torch.FloatTensor`): input sample
|
202 |
-
timestep (`int`, optional): current timestep
|
203 |
-
|
204 |
-
Returns:
|
205 |
-
`torch.FloatTensor`: scaled input sample
|
206 |
-
"""
|
207 |
-
return sample
|
208 |
-
|
209 |
-
def set_timesteps(
|
210 |
-
self,
|
211 |
-
num_inference_steps: Optional[int] = None,
|
212 |
-
device: Union[str, torch.device] = None,
|
213 |
-
timesteps: Optional[List[int]] = None,
|
214 |
-
):
|
215 |
-
"""
|
216 |
-
Sets the discrete timesteps used for the diffusion chain. Supporting function to be run before inference.
|
217 |
-
|
218 |
-
Args:
|
219 |
-
num_inference_steps (`Optional[int]`):
|
220 |
-
the number of diffusion steps used when generating samples with a pre-trained model. If passed, then
|
221 |
-
`timesteps` must be `None`.
|
222 |
-
device (`str` or `torch.device`, optional):
|
223 |
-
the device to which the timesteps are moved to.
|
224 |
-
custom_timesteps (`List[int]`, optional):
|
225 |
-
custom timesteps used to support arbitrary spacing between timesteps. If `None`, then the default
|
226 |
-
timestep spacing strategy of equal spacing between timesteps is used. If passed, `num_inference_steps`
|
227 |
-
must be `None`.
|
228 |
-
|
229 |
-
"""
|
230 |
-
if num_inference_steps is not None and timesteps is not None:
|
231 |
-
raise ValueError("Can only pass one of `num_inference_steps` or `custom_timesteps`.")
|
232 |
-
|
233 |
-
if timesteps is not None:
|
234 |
-
for i in range(1, len(timesteps)):
|
235 |
-
if timesteps[i] >= timesteps[i - 1]:
|
236 |
-
raise ValueError("`custom_timesteps` must be in descending order.")
|
237 |
-
|
238 |
-
if timesteps[0] >= self.config.num_train_timesteps:
|
239 |
-
raise ValueError(
|
240 |
-
f"`timesteps` must start before `self.config.train_timesteps`:"
|
241 |
-
f" {self.config.num_train_timesteps}."
|
242 |
-
)
|
243 |
-
|
244 |
-
timesteps = np.array(timesteps, dtype=np.int64)
|
245 |
-
self.custom_timesteps = True
|
246 |
-
else:
|
247 |
-
if num_inference_steps > self.config.num_train_timesteps:
|
248 |
-
raise ValueError(
|
249 |
-
f"`num_inference_steps`: {num_inference_steps} cannot be larger than `self.config.train_timesteps`:"
|
250 |
-
f" {self.config.num_train_timesteps} as the unet model trained with this scheduler can only handle"
|
251 |
-
f" maximal {self.config.num_train_timesteps} timesteps."
|
252 |
-
)
|
253 |
-
|
254 |
-
self.num_inference_steps = num_inference_steps
|
255 |
-
self.custom_timesteps = False
|
256 |
-
|
257 |
-
# "linspace", "leading", "trailing" corresponds to annotation of Table 2. of https://arxiv.org/abs/2305.08891
|
258 |
-
if self.config.timestep_spacing == "linspace":
|
259 |
-
timesteps = (
|
260 |
-
np.linspace(0, self.config.num_train_timesteps - 1, num_inference_steps)
|
261 |
-
.round()[::-1]
|
262 |
-
.copy()
|
263 |
-
.astype(np.int64)
|
264 |
-
)
|
265 |
-
elif self.config.timestep_spacing == "leading":
|
266 |
-
step_ratio = self.config.num_train_timesteps // self.num_inference_steps
|
267 |
-
# creates integer timesteps by multiplying by ratio
|
268 |
-
# casting to int to avoid issues when num_inference_step is power of 3
|
269 |
-
timesteps = (np.arange(0, num_inference_steps) * step_ratio).round()[::-1].copy().astype(np.int64)
|
270 |
-
timesteps += self.config.steps_offset
|
271 |
-
elif self.config.timestep_spacing == "trailing":
|
272 |
-
step_ratio = self.config.num_train_timesteps / self.num_inference_steps
|
273 |
-
# creates integer timesteps by multiplying by ratio
|
274 |
-
# casting to int to avoid issues when num_inference_step is power of 3
|
275 |
-
timesteps = np.round(np.arange(self.config.num_train_timesteps, 0, -step_ratio)).astype(np.int64)
|
276 |
-
timesteps -= 1
|
277 |
-
else:
|
278 |
-
raise ValueError(
|
279 |
-
f"{self.config.timestep_spacing} is not supported. Please make sure to choose one of 'linspace', 'leading' or 'trailing'."
|
280 |
-
)
|
281 |
-
|
282 |
-
self.timesteps = torch.from_numpy(timesteps).to(device)
|
283 |
-
|
284 |
-
def _get_variance(self, t, predicted_variance=None, variance_type=None):
|
285 |
-
prev_t = self.previous_timestep(t)
|
286 |
-
|
287 |
-
alpha_prod_t = self.alphas_cumprod[t]
|
288 |
-
alpha_prod_t_prev = self.alphas_cumprod[prev_t] if prev_t >= 0 else self.one
|
289 |
-
current_beta_t = 1 - alpha_prod_t / alpha_prod_t_prev
|
290 |
-
|
291 |
-
# For t > 0, compute predicted variance βt (see formula (6) and (7) from https://arxiv.org/pdf/2006.11239.pdf)
|
292 |
-
# and sample from it to get previous sample
|
293 |
-
# x_{t-1} ~ N(pred_prev_sample, variance) == add variance to pred_sample
|
294 |
-
variance = (1 - alpha_prod_t_prev) / (1 - alpha_prod_t) * current_beta_t
|
295 |
-
|
296 |
-
# we always take the log of variance, so clamp it to ensure it's not 0
|
297 |
-
variance = torch.clamp(variance, min=1e-20)
|
298 |
-
|
299 |
-
if variance_type is None:
|
300 |
-
variance_type = self.config.variance_type
|
301 |
-
|
302 |
-
# hacks - were probably added for training stability
|
303 |
-
if variance_type == "fixed_small":
|
304 |
-
variance = variance
|
305 |
-
# for rl-diffuser https://arxiv.org/abs/2205.09991
|
306 |
-
elif variance_type == "fixed_small_log":
|
307 |
-
variance = torch.log(variance)
|
308 |
-
variance = torch.exp(0.5 * variance)
|
309 |
-
elif variance_type == "fixed_large":
|
310 |
-
variance = current_beta_t
|
311 |
-
elif variance_type == "fixed_large_log":
|
312 |
-
# Glide max_log
|
313 |
-
variance = torch.log(current_beta_t)
|
314 |
-
elif variance_type == "learned":
|
315 |
-
return predicted_variance
|
316 |
-
elif variance_type == "learned_range":
|
317 |
-
min_log = torch.log(variance)
|
318 |
-
max_log = torch.log(current_beta_t)
|
319 |
-
frac = (predicted_variance + 1) / 2
|
320 |
-
variance = frac * max_log + (1 - frac) * min_log
|
321 |
-
|
322 |
-
return variance
|
323 |
-
|
324 |
-
def _threshold_sample(self, sample: torch.FloatTensor) -> torch.FloatTensor:
|
325 |
-
"""
|
326 |
-
"Dynamic thresholding: At each sampling step we set s to a certain percentile absolute pixel value in xt0 (the
|
327 |
-
prediction of x_0 at timestep t), and if s > 1, then we threshold xt0 to the range [-s, s] and then divide by
|
328 |
-
s. Dynamic thresholding pushes saturated pixels (those near -1 and 1) inwards, thereby actively preventing
|
329 |
-
pixels from saturation at each step. We find that dynamic thresholding results in significantly better
|
330 |
-
photorealism as well as better image-text alignment, especially when using very large guidance weights."
|
331 |
-
|
332 |
-
https://arxiv.org/abs/2205.11487
|
333 |
-
"""
|
334 |
-
dtype = sample.dtype
|
335 |
-
batch_size, channels, height, width = sample.shape
|
336 |
-
|
337 |
-
if dtype not in (torch.float32, torch.float64):
|
338 |
-
sample = sample.float() # upcast for quantile calculation, and clamp not implemented for cpu half
|
339 |
-
|
340 |
-
# Flatten sample for doing quantile calculation along each image
|
341 |
-
sample = sample.reshape(batch_size, channels * height * width)
|
342 |
-
|
343 |
-
abs_sample = sample.abs() # "a certain percentile absolute pixel value"
|
344 |
-
|
345 |
-
s = torch.quantile(abs_sample, self.config.dynamic_thresholding_ratio, dim=1)
|
346 |
-
s = torch.clamp(
|
347 |
-
s, min=1, max=self.config.sample_max_value
|
348 |
-
) # When clamped to min=1, equivalent to standard clipping to [-1, 1]
|
349 |
-
|
350 |
-
s = s.unsqueeze(1) # (batch_size, 1) because clamp will broadcast along dim=0
|
351 |
-
sample = torch.clamp(sample, -s, s) / s # "we threshold xt0 to the range [-s, s] and then divide by s"
|
352 |
-
|
353 |
-
sample = sample.reshape(batch_size, channels, height, width)
|
354 |
-
sample = sample.to(dtype)
|
355 |
-
|
356 |
-
return sample
|
357 |
-
|
358 |
-
def step(
|
359 |
-
self,
|
360 |
-
model_output: torch.FloatTensor,
|
361 |
-
timestep: int,
|
362 |
-
sample: torch.FloatTensor,
|
363 |
-
generator=None,
|
364 |
-
return_dict: bool = True,
|
365 |
-
) -> Union[DDPMSchedulerOutput, Tuple]:
|
366 |
-
"""
|
367 |
-
Predict the sample at the previous timestep by reversing the SDE. Core function to propagate the diffusion
|
368 |
-
process from the learned model outputs (most often the predicted noise).
|
369 |
-
|
370 |
-
Args:
|
371 |
-
model_output (`torch.FloatTensor`): direct output from learned diffusion model.
|
372 |
-
timestep (`int`): current discrete timestep in the diffusion chain.
|
373 |
-
sample (`torch.FloatTensor`):
|
374 |
-
current instance of sample being created by diffusion process.
|
375 |
-
generator: random number generator.
|
376 |
-
return_dict (`bool`): option for returning tuple rather than DDPMSchedulerOutput class
|
377 |
-
|
378 |
-
Returns:
|
379 |
-
[`~schedulers.scheduling_utils.DDPMSchedulerOutput`] or `tuple`:
|
380 |
-
[`~schedulers.scheduling_utils.DDPMSchedulerOutput`] if `return_dict` is True, otherwise a `tuple`. When
|
381 |
-
returning a tuple, the first element is the sample tensor.
|
382 |
-
|
383 |
-
"""
|
384 |
-
t = timestep
|
385 |
-
|
386 |
-
prev_t = self.previous_timestep(t)
|
387 |
-
|
388 |
-
if model_output.shape[1] == sample.shape[1] * 2 and self.variance_type in ["learned", "learned_range"]:
|
389 |
-
model_output, predicted_variance = torch.split(model_output, sample.shape[1], dim=1)
|
390 |
-
else:
|
391 |
-
predicted_variance = None
|
392 |
-
|
393 |
-
# 1. compute alphas, betas
|
394 |
-
alpha_prod_t = self.alphas_cumprod[t]
|
395 |
-
alpha_prod_t_prev = self.alphas_cumprod[prev_t] if prev_t >= 0 else self.one
|
396 |
-
beta_prod_t = 1 - alpha_prod_t
|
397 |
-
beta_prod_t_prev = 1 - alpha_prod_t_prev
|
398 |
-
current_alpha_t = alpha_prod_t / alpha_prod_t_prev
|
399 |
-
current_beta_t = 1 - current_alpha_t
|
400 |
-
|
401 |
-
# 2. compute predicted original sample from predicted noise also called
|
402 |
-
# "predicted x_0" of formula (15) from https://arxiv.org/pdf/2006.11239.pdf
|
403 |
-
if self.config.prediction_type == "epsilon":
|
404 |
-
pred_original_sample = (sample - beta_prod_t ** (0.5) * model_output) / alpha_prod_t ** (0.5)
|
405 |
-
elif self.config.prediction_type == "sample":
|
406 |
-
pred_original_sample = model_output
|
407 |
-
elif self.config.prediction_type == "v_prediction":
|
408 |
-
pred_original_sample = (alpha_prod_t**0.5) * sample - (beta_prod_t**0.5) * model_output
|
409 |
-
else:
|
410 |
-
raise ValueError(
|
411 |
-
f"prediction_type given as {self.config.prediction_type} must be one of `epsilon`, `sample` or"
|
412 |
-
" `v_prediction` for the DDPMScheduler."
|
413 |
-
)
|
414 |
-
|
415 |
-
# 3. Clip or threshold "predicted x_0"
|
416 |
-
if self.config.thresholding:
|
417 |
-
pred_original_sample = self._threshold_sample(pred_original_sample)
|
418 |
-
elif self.config.clip_sample:
|
419 |
-
pred_original_sample = pred_original_sample.clamp(
|
420 |
-
-self.config.clip_sample_range, self.config.clip_sample_range
|
421 |
-
)
|
422 |
-
|
423 |
-
# 4. Compute coefficients for pred_original_sample x_0 and current sample x_t
|
424 |
-
# See formula (7) from https://arxiv.org/pdf/2006.11239.pdf
|
425 |
-
pred_original_sample_coeff = (alpha_prod_t_prev ** (0.5) * current_beta_t) / beta_prod_t
|
426 |
-
current_sample_coeff = current_alpha_t ** (0.5) * beta_prod_t_prev / beta_prod_t
|
427 |
-
|
428 |
-
# 5. Compute predicted previous sample µ_t
|
429 |
-
# See formula (7) from https://arxiv.org/pdf/2006.11239.pdf
|
430 |
-
pred_prev_sample = pred_original_sample_coeff * pred_original_sample + current_sample_coeff * sample
|
431 |
-
|
432 |
-
# 6. Add noise
|
433 |
-
variance = 0
|
434 |
-
if t > 0:
|
435 |
-
device = model_output.device
|
436 |
-
variance_noise = randn_tensor(
|
437 |
-
model_output.shape, generator=generator, device=device, dtype=model_output.dtype
|
438 |
-
)
|
439 |
-
if self.variance_type == "fixed_small_log":
|
440 |
-
variance = self._get_variance(t, predicted_variance=predicted_variance) * variance_noise
|
441 |
-
elif self.variance_type == "learned_range":
|
442 |
-
variance = self._get_variance(t, predicted_variance=predicted_variance)
|
443 |
-
variance = torch.exp(0.5 * variance) * variance_noise
|
444 |
-
else:
|
445 |
-
variance = (self._get_variance(t, predicted_variance=predicted_variance) ** 0.5) * variance_noise
|
446 |
-
|
447 |
-
pred_prev_sample = pred_prev_sample + variance
|
448 |
-
|
449 |
-
if not return_dict:
|
450 |
-
return (pred_prev_sample,)
|
451 |
-
|
452 |
-
return DDPMSchedulerOutput(prev_sample=pred_prev_sample, pred_original_sample=pred_original_sample)
|
453 |
-
|
454 |
-
def add_noise(
|
455 |
-
self,
|
456 |
-
original_samples: torch.FloatTensor,
|
457 |
-
noise: torch.FloatTensor,
|
458 |
-
timesteps: torch.IntTensor,
|
459 |
-
) -> torch.FloatTensor:
|
460 |
-
# Make sure alphas_cumprod and timestep have same device and dtype as original_samples
|
461 |
-
alphas_cumprod = self.alphas_cumprod.to(device=original_samples.device, dtype=original_samples.dtype)
|
462 |
-
timesteps = timesteps.to(original_samples.device)
|
463 |
-
|
464 |
-
sqrt_alpha_prod = alphas_cumprod[timesteps] ** 0.5
|
465 |
-
sqrt_alpha_prod = sqrt_alpha_prod.flatten()
|
466 |
-
while len(sqrt_alpha_prod.shape) < len(original_samples.shape):
|
467 |
-
sqrt_alpha_prod = sqrt_alpha_prod.unsqueeze(-1)
|
468 |
-
|
469 |
-
sqrt_one_minus_alpha_prod = (1 - alphas_cumprod[timesteps]) ** 0.5
|
470 |
-
sqrt_one_minus_alpha_prod = sqrt_one_minus_alpha_prod.flatten()
|
471 |
-
while len(sqrt_one_minus_alpha_prod.shape) < len(original_samples.shape):
|
472 |
-
sqrt_one_minus_alpha_prod = sqrt_one_minus_alpha_prod.unsqueeze(-1)
|
473 |
-
|
474 |
-
noisy_samples = sqrt_alpha_prod * original_samples + sqrt_one_minus_alpha_prod * noise
|
475 |
-
return noisy_samples
|
476 |
-
|
477 |
-
def get_velocity(
|
478 |
-
self, sample: torch.FloatTensor, noise: torch.FloatTensor, timesteps: torch.IntTensor
|
479 |
-
) -> torch.FloatTensor:
|
480 |
-
# Make sure alphas_cumprod and timestep have same device and dtype as sample
|
481 |
-
alphas_cumprod = self.alphas_cumprod.to(device=sample.device, dtype=sample.dtype)
|
482 |
-
timesteps = timesteps.to(sample.device)
|
483 |
-
|
484 |
-
sqrt_alpha_prod = alphas_cumprod[timesteps] ** 0.5
|
485 |
-
sqrt_alpha_prod = sqrt_alpha_prod.flatten()
|
486 |
-
while len(sqrt_alpha_prod.shape) < len(sample.shape):
|
487 |
-
sqrt_alpha_prod = sqrt_alpha_prod.unsqueeze(-1)
|
488 |
-
|
489 |
-
sqrt_one_minus_alpha_prod = (1 - alphas_cumprod[timesteps]) ** 0.5
|
490 |
-
sqrt_one_minus_alpha_prod = sqrt_one_minus_alpha_prod.flatten()
|
491 |
-
while len(sqrt_one_minus_alpha_prod.shape) < len(sample.shape):
|
492 |
-
sqrt_one_minus_alpha_prod = sqrt_one_minus_alpha_prod.unsqueeze(-1)
|
493 |
-
|
494 |
-
velocity = sqrt_alpha_prod * noise - sqrt_one_minus_alpha_prod * sample
|
495 |
-
return velocity
|
496 |
-
|
497 |
-
def __len__(self):
|
498 |
-
return self.config.num_train_timesteps
|
499 |
-
|
500 |
-
def previous_timestep(self, timestep):
|
501 |
-
if self.custom_timesteps:
|
502 |
-
index = (self.timesteps == timestep).nonzero(as_tuple=True)[0][0]
|
503 |
-
if index == self.timesteps.shape[0] - 1:
|
504 |
-
prev_t = torch.tensor(-1)
|
505 |
-
else:
|
506 |
-
prev_t = self.timesteps[index + 1]
|
507 |
-
else:
|
508 |
-
num_inference_steps = (
|
509 |
-
self.num_inference_steps if self.num_inference_steps else self.config.num_train_timesteps
|
510 |
-
)
|
511 |
-
prev_t = timestep - self.config.num_train_timesteps // num_inference_steps
|
512 |
-
|
513 |
-
return prev_t
|
|
|
|
|
|
|
|
|
|
|
|
|
|
|
|
|
|
|
|
|
|
|
|
|
|
|
|
|
|
|
|
|
|
|
|
|
|
|
|
|
|
|
|
|
|
|
|
|
|
|
|
|
|
|
|
|
|
|
|
|
|
|
|
|
|
|
|
|
|
|
|
|
|
|
|
|
|
|
|
|
|
|
|
|
|
|
|
|
|
|
|
|
|
|
|
|
|
|
|
|
|
|
|
|
|
|
|
|
|
|
|
|
|
|
|
|
|
|
|
|
|
|
|
|
|
|
|
|
|
|
|
|
|
|
|
|
|
|
|
|
|
|
|
|
|
|
|
|
|
|
|
|
|
|
|
|
|
|
|
|
|
|
|
|
|
|
|
|
|
|
|
|
|
|
|
|
|
|
|
|
|
|
|
|
|
|
|
|
|
|
|
|
|
|
|
|
|
|
|
|
|
|
|
|
|
|
|
|
|
|
|
|
|
|
|
|
|
|
|
|
|
|
|
|
|
|
|
|
|
|
|
|
|
|
|
|
|
|
|
|
|
|
|
|
|
|
|
|
|
|
|
|
|
|
|
|
|
|
|
|
|
|
|
|
|
|
|
|
|
|
|
|
|
|
|
|
|
|
|
|
|
|
|
|
|
|
|
|
|
|
|
|
|
|
|
|
|
|
|
|
|
|
|
|
|
|
|
|
|
|
|
|
|
|
|
|
|
|
|
|
|
|
|
|
|
|
|
|
|
|
|
|
|
|
|
|
|
|
|
|
|
|
|
|
|
|
|
|
|
|
|
|
|
|
|
|
|
|
|
|
|
|
|
|
|
|
|
|
|
|
|
|
|
|
|
|
|
|
|
|
|
|
|
|
|
|
|
|
|
|
|
|
|
|
|
|
|
|
|
|
|
|
|
|
|
|
|
|
|
|
|
|
|
|
|
|
|
|
|
|
|
|
|
|
|
|
|
|
|
|
|
|
|
|
|
|
|
|
|
|
|
|
|
|
|
|
|
|
|
|
|
|
|
|
|
|
|
|
|
|
|
|
|
|
|
|
|
|
|
|
|
|
|
|
|
|
|
|
|
|
|
|
|
|
|
|
|
|
|
|
|
|
|
|
|
|
|
|
|
|
|
|
|
|
|
|
|
|
|
|
|
|
|
|
|
|
|
|
|
|
|
|
|
|
|
|
|
|
|
|
|
|
|
|
|
|
|
|
|
|
|
|
|
|
|
|
|
|
|
|
|
|
|
|
|
|
|
|
|
|
|
|
|
|
|
|
|
|
|
|
|
|
|
|
|
|
|
|
|
|
|
|
|
|
|
|
|
|
|
|
|
|
|
|
|
|
|
|
|
|
|
|
|
|
|
|
|
|
|
|
|
|
|
|
|
|
|
|
|
|
|
|
|
|
|
|
|
|
|
|
|
|
|
|
|
|
|
|
|
|
|
|
|
|
|
|
|
|
|
|
|
|
|
|
|
|
|
|
|
|
|
|
|
|
|
|
|
|
|
|
|
|
|
|
|
|
|
|
|
|
|
|
|
|
|
|
|
|
|
|
|
|
|
|
|
|
|
|
|
|
|
|
|
|
|
|
|
|
|
|
|
|
|
|
|
|
|
|
|
|
|
|
|
|
|
|
|
|
|
|
|
|
|
|
|
|
|
|
|
|
|
|
|
|
|
|
|
|
|
|
|
|
|
|
|
|
|
|
|
|
|
|
|
|
|
|
|
|
|
|
|
|
|
|
|
|
|
|
|
|
|
|
|
|
|
|
|
|
|
|
|
|
|
|
|
|
|
|
|
|
|
|
|
|
|
|
|
|
|
|
|
|
|
|
|
|
|
|
|
|
|
|
|
|
|
|
|
|
|
|
|
|
|
|
|
|
|
|
|
|
|
|
|
|
|
|
|
|
|
|
|
|
|
|
|
|
|
|
|
|
|
|
|
|
|
|
|
|
|
|
|
|
|
|
|
|
|
|
|
|
|
|
|
|
|
|
|
|
|
|
|
|
|
|
|
|
|
|
|
|
|
|
|
|
|
|
|
|
|
|
|
|
|
|
|
|
|
|
|
|
|
|
|
|
|
|
|
|
|
|
|
|
|
|
|
|
|
|
|
|
|
|
|
|
|
|
|
|
|
|
|
|
|
|
|
|
|
|
|
|
|
|
|
|
|
|
|
|
|
|
|
|
|
|
|
|
|
|
|
|
|
|
|
|
|
|
|
|
|
|
|
|
|
|
|
|
|
|
|
|
|
|
|
|
|
|
|
|
|
|
|
|
|
|
|
|
|
|
|
|
|
|
|
|
|
|
|
spaces/Androidonnxfork/CivitAi-to-Diffusers/diffusers/tests/pipelines/stable_diffusion_2/__init__.py
DELETED
File without changes
|
spaces/Andy1621/uniformer_image_detection/configs/ghm/retinanet_ghm_x101_64x4d_fpn_1x_coco.py
DELETED
@@ -1,13 +0,0 @@
|
|
1 |
-
_base_ = './retinanet_ghm_r50_fpn_1x_coco.py'
|
2 |
-
model = dict(
|
3 |
-
pretrained='open-mmlab://resnext101_64x4d',
|
4 |
-
backbone=dict(
|
5 |
-
type='ResNeXt',
|
6 |
-
depth=101,
|
7 |
-
groups=64,
|
8 |
-
base_width=4,
|
9 |
-
num_stages=4,
|
10 |
-
out_indices=(0, 1, 2, 3),
|
11 |
-
frozen_stages=1,
|
12 |
-
norm_cfg=dict(type='BN', requires_grad=True),
|
13 |
-
style='pytorch'))
|
|
|
|
|
|
|
|
|
|
|
|
|
|
|
|
|
|
|
|
|
|
|
|
|
|
|
|
spaces/AngoHF/ANGO-Leaderboard/assets/constant.py
DELETED
@@ -1 +0,0 @@
|
|
1 |
-
DELIMITER = "|"
|
|
|
|
spaces/AnxiousNugget/janitor/README.md
DELETED
@@ -1,10 +0,0 @@
|
|
1 |
-
---
|
2 |
-
title: Janitor
|
3 |
-
emoji: 🦀
|
4 |
-
colorFrom: gray
|
5 |
-
colorTo: yellow
|
6 |
-
sdk: docker
|
7 |
-
pinned: false
|
8 |
-
---
|
9 |
-
|
10 |
-
Check out the configuration reference at https://huggingface.co/docs/hub/spaces-config-reference
|
|
|
|
|
|
|
|
|
|
|
|
|
|
|
|
|
|
|
|
|
|
spaces/ArcAhmedEssam/CLIP-Interrogator-2/app.py
DELETED
@@ -1,177 +0,0 @@
|
|
1 |
-
from share_btn import community_icon_html, loading_icon_html, share_js
|
2 |
-
|
3 |
-
import os, subprocess
|
4 |
-
import torch
|
5 |
-
|
6 |
-
# def setup():
|
7 |
-
# install_cmds = [
|
8 |
-
# ['pip', 'install', 'ftfy', 'gradio', 'regex', 'tqdm', 'transformers==4.21.2', 'timm', 'fairscale', 'requests'],
|
9 |
-
# ['pip', 'install', 'open_clip_torch'],
|
10 |
-
# ['pip', 'install', '-e', 'git+https://github.com/pharmapsychotic/BLIP.git@lib#egg=blip'],
|
11 |
-
# ['git', 'clone', '-b', 'open-clip', 'https://github.com/pharmapsychotic/clip-interrogator.git']
|
12 |
-
# ]
|
13 |
-
# for cmd in install_cmds:
|
14 |
-
# print(subprocess.run(cmd, stdout=subprocess.PIPE).stdout.decode('utf-8'))
|
15 |
-
|
16 |
-
# setup()
|
17 |
-
|
18 |
-
# download cache files
|
19 |
-
# print("Download preprocessed cache files...")
|
20 |
-
# CACHE_URLS = [
|
21 |
-
# 'https://huggingface.co/pharma/ci-preprocess/resolve/main/ViT-H-14_laion2b_s32b_b79k_artists.pkl',
|
22 |
-
# 'https://huggingface.co/pharma/ci-preprocess/resolve/main/ViT-H-14_laion2b_s32b_b79k_flavors.pkl',
|
23 |
-
# 'https://huggingface.co/pharma/ci-preprocess/resolve/main/ViT-H-14_laion2b_s32b_b79k_mediums.pkl',
|
24 |
-
# 'https://huggingface.co/pharma/ci-preprocess/resolve/main/ViT-H-14_laion2b_s32b_b79k_movements.pkl',
|
25 |
-
# 'https://huggingface.co/pharma/ci-preprocess/resolve/main/ViT-H-14_laion2b_s32b_b79k_trendings.pkl',
|
26 |
-
# ]
|
27 |
-
# os.makedirs('cache', exist_ok=True)
|
28 |
-
# for url in CACHE_URLS:
|
29 |
-
# print(subprocess.run(['wget', url, '-P', 'cache'], stdout=subprocess.PIPE).stdout.decode('utf-8'))
|
30 |
-
|
31 |
-
import sys
|
32 |
-
sys.path.append('src/blip')
|
33 |
-
sys.path.append('clip-interrogator')
|
34 |
-
|
35 |
-
import gradio as gr
|
36 |
-
from clip_interrogator import Config, Interrogator
|
37 |
-
import io
|
38 |
-
from PIL import Image
|
39 |
-
config = Config()
|
40 |
-
config.device = 'cuda' if torch.cuda.is_available() else 'cpu'
|
41 |
-
config.blip_offload = False if torch.cuda.is_available() else True
|
42 |
-
config.chunk_size = 2048
|
43 |
-
config.flavor_intermediate_count = 512
|
44 |
-
config.blip_num_beams = 64
|
45 |
-
|
46 |
-
ci = Interrogator(config)
|
47 |
-
|
48 |
-
def inference(input_images, mode, best_max_flavors):
|
49 |
-
# Process each image in the list and generate prompt results
|
50 |
-
prompt_results = []
|
51 |
-
for image_bytes in input_images:
|
52 |
-
image = Image.open(io.BytesIO(image_bytes)).convert('RGB')
|
53 |
-
if mode == 'best':
|
54 |
-
prompt_result = ci.interrogate(image, max_flavors=int(best_max_flavors))
|
55 |
-
elif mode == 'classic':
|
56 |
-
prompt_result = ci.interrogate_classic(image)
|
57 |
-
else:
|
58 |
-
prompt_result = ci.interrogate_fast(image)
|
59 |
-
prompt_results.append((image, prompt_result)) # Use dictionary to set image labels
|
60 |
-
return prompt_results
|
61 |
-
|
62 |
-
|
63 |
-
title = """
|
64 |
-
<div style="text-align: center; max-width: 500px; margin: 0 auto;">
|
65 |
-
<div
|
66 |
-
style="
|
67 |
-
display: inline-flex;
|
68 |
-
align-items: center;
|
69 |
-
gap: 0.8rem;
|
70 |
-
font-size: 1.75rem;
|
71 |
-
margin-bottom: 10px;
|
72 |
-
"
|
73 |
-
>
|
74 |
-
<h1 style="font-weight: 600; margin-bottom: 7px;">
|
75 |
-
CLIP Interrogator 2.1
|
76 |
-
</h1>
|
77 |
-
</div>
|
78 |
-
<p style="margin-bottom: 10px;font-size: 94%;font-weight: 100;line-height: 1.5em;">
|
79 |
-
Want to figure out what a good prompt might be to create new images like an existing one?
|
80 |
-
<br />The CLIP Interrogator is here to get you answers!
|
81 |
-
<br />This version is specialized for producing nice prompts for use with Stable Diffusion 2.0 using the ViT-H-14 OpenCLIP model!
|
82 |
-
</p>
|
83 |
-
</div>
|
84 |
-
"""
|
85 |
-
|
86 |
-
article = """
|
87 |
-
<div style="text-align: center; max-width: 500px; margin: 0 auto;font-size: 94%;">
|
88 |
-
|
89 |
-
<p>
|
90 |
-
Server busy? You can also run on <a href="https://colab.research.google.com/github/pharmapsychotic/clip-interrogator/blob/open-clip/clip_interrogator.ipynb">Google Colab</a>
|
91 |
-
</p>
|
92 |
-
<p>
|
93 |
-
Has this been helpful to you? Follow Pharma on twitter
|
94 |
-
<a href="https://twitter.com/pharmapsychotic">@pharmapsychotic</a>
|
95 |
-
and check out more tools at his
|
96 |
-
<a href="https://pharmapsychotic.com/tools.html">Ai generative art tools list</a>
|
97 |
-
</p>
|
98 |
-
</div>
|
99 |
-
"""
|
100 |
-
|
101 |
-
css = '''
|
102 |
-
#col-container {width: width: 80%;; margin-left: auto; margin-right: auto;}
|
103 |
-
a {text-decoration-line: underline; font-weight: 600;}
|
104 |
-
.animate-spin {
|
105 |
-
animation: spin 1s linear infinite;
|
106 |
-
}
|
107 |
-
@keyframes spin {
|
108 |
-
from {
|
109 |
-
transform: rotate(0deg);
|
110 |
-
}
|
111 |
-
to {
|
112 |
-
transform: rotate(360deg);
|
113 |
-
}
|
114 |
-
}
|
115 |
-
#share-btn-container {
|
116 |
-
display: flex; padding-left: 0.5rem !important; padding-right: 0.5rem !important; background-color: #000000; justify-content: center; align-items: center; border-radius: 9999px !important; width: 13rem;
|
117 |
-
}
|
118 |
-
#share-btn {
|
119 |
-
all: initial; color: #ffffff;font-weight: 600; cursor:pointer; font-family: 'IBM Plex Sans', sans-serif; margin-left: 0.5rem !important; padding-top: 0.25rem !important; padding-bottom: 0.25rem !important;
|
120 |
-
}
|
121 |
-
#share-btn * {
|
122 |
-
all: unset;
|
123 |
-
}
|
124 |
-
#share-btn-container div:nth-child(-n+2){
|
125 |
-
width: auto !important;
|
126 |
-
min-height: 0px !important;
|
127 |
-
}
|
128 |
-
#share-btn-container .wrap {
|
129 |
-
display: none !important;
|
130 |
-
}
|
131 |
-
#gallery .caption-label {
|
132 |
-
font-size: 15px !important;
|
133 |
-
right: 0 !important;
|
134 |
-
max-width: 100% !important;
|
135 |
-
text-overflow: clip !important;
|
136 |
-
white-space: normal !important;
|
137 |
-
overflow: auto !important;
|
138 |
-
height: 20% !important;
|
139 |
-
}
|
140 |
-
|
141 |
-
#gallery .caption {
|
142 |
-
padding: var(--size-2) var(--size-3) !important;
|
143 |
-
text-overflow: clip !important;
|
144 |
-
white-space: normal !important; /* Allows the text to wrap */
|
145 |
-
color: var(--block-label-text-color) !important;
|
146 |
-
font-weight: var(--weight-semibold) !important;
|
147 |
-
text-align: center !important;
|
148 |
-
height: 100% !important;
|
149 |
-
font-size: 17px !important;
|
150 |
-
}
|
151 |
-
|
152 |
-
'''
|
153 |
-
|
154 |
-
with gr.Blocks(css=css) as block:
|
155 |
-
with gr.Column(elem_id="col-container"):
|
156 |
-
gr.HTML(title)
|
157 |
-
|
158 |
-
input_image = gr.Files(label = "Inputs", file_count="multiple", type='bytes', elem_id='inputs')
|
159 |
-
with gr.Row():
|
160 |
-
mode_input = gr.Radio(['best', 'classic', 'fast'], label='Select mode', value='best')
|
161 |
-
flavor_input = gr.Slider(minimum=2, maximum=24, step=2, value=4, label='best mode max flavors')
|
162 |
-
|
163 |
-
submit_btn = gr.Button("Submit")
|
164 |
-
|
165 |
-
# rows, cols = NUM_IMAGES //3,
|
166 |
-
gallery = gr.Gallery(
|
167 |
-
label="Outputs", show_label=True, elem_id="gallery", object_fit="contain", height="auto"
|
168 |
-
)
|
169 |
-
|
170 |
-
with gr.Group(elem_id="share-btn-container"):
|
171 |
-
loading_icon = gr.HTML(loading_icon_html, visible=False)
|
172 |
-
|
173 |
-
gr.HTML(article)
|
174 |
-
submit_btn.click(fn=inference, inputs=[input_image,mode_input,flavor_input], outputs=[gallery], api_name="clipi2")
|
175 |
-
|
176 |
-
|
177 |
-
block.queue(max_size=32,concurrency_count=10).launch(show_api=False)
|
|
|
|
|
|
|
|
|
|
|
|
|
|
|
|
|
|
|
|
|
|
|
|
|
|
|
|
|
|
|
|
|
|
|
|
|
|
|
|
|
|
|
|
|
|
|
|
|
|
|
|
|
|
|
|
|
|
|
|
|
|
|
|
|
|
|
|
|
|
|
|
|
|
|
|
|
|
|
|
|
|
|
|
|
|
|
|
|
|
|
|
|
|
|
|
|
|
|
|
|
|
|
|
|
|
|
|
|
|
|
|
|
|
|
|
|
|
|
|
|
|
|
|
|
|
|
|
|
|
|
|
|
|
|
|
|
|
|
|
|
|
|
|
|
|
|
|
|
|
|
|
|
|
|
|
|
|
|
|
|
|
|
|
|
|
|
|
|
|
|
|
|
|
|
|
|
|
|
|
|
|
|
|
|
|
|
|
|
|
|
|
|
|
|
|
|
|
|
|
|
|
|
|
|
|
|
|
|
|
|
|
|
|
|
|
|
|
|
|
|
|
|
|
|
|
|
|
|
|
|
|
|
|
|
|
|
|
|
|
|
|
|
|
|
|
|
|
|
|
|
|
|
|
|
|
|
|
|
|
|
|
|
|
|
|
|
|
|
|
|
|
|
|
|
|
|
|
|
|
|
|
|
|
|
|
|
|
|
|
|
|
|
|
|
|
|
|
|
|
|
|
|
|
|
|
|
|
|
|
|
|
|
|
|
|
|
|
|
|
|
|
|
|
|
|
|
|
|
|
|
|
|
|
|
|
|
|
|
|
|
|
|
|
|
|
|
|
|
|
|
|
|
|
|
|
spaces/Ataturk-Chatbot/HuggingFaceChat/venv/lib/python3.11/site-packages/setuptools/_vendor/importlib_metadata/_meta.py
DELETED
@@ -1,48 +0,0 @@
|
|
1 |
-
from ._compat import Protocol
|
2 |
-
from typing import Any, Dict, Iterator, List, TypeVar, Union
|
3 |
-
|
4 |
-
|
5 |
-
_T = TypeVar("_T")
|
6 |
-
|
7 |
-
|
8 |
-
class PackageMetadata(Protocol):
|
9 |
-
def __len__(self) -> int:
|
10 |
-
... # pragma: no cover
|
11 |
-
|
12 |
-
def __contains__(self, item: str) -> bool:
|
13 |
-
... # pragma: no cover
|
14 |
-
|
15 |
-
def __getitem__(self, key: str) -> str:
|
16 |
-
... # pragma: no cover
|
17 |
-
|
18 |
-
def __iter__(self) -> Iterator[str]:
|
19 |
-
... # pragma: no cover
|
20 |
-
|
21 |
-
def get_all(self, name: str, failobj: _T = ...) -> Union[List[Any], _T]:
|
22 |
-
"""
|
23 |
-
Return all values associated with a possibly multi-valued key.
|
24 |
-
"""
|
25 |
-
|
26 |
-
@property
|
27 |
-
def json(self) -> Dict[str, Union[str, List[str]]]:
|
28 |
-
"""
|
29 |
-
A JSON-compatible form of the metadata.
|
30 |
-
"""
|
31 |
-
|
32 |
-
|
33 |
-
class SimplePath(Protocol):
|
34 |
-
"""
|
35 |
-
A minimal subset of pathlib.Path required by PathDistribution.
|
36 |
-
"""
|
37 |
-
|
38 |
-
def joinpath(self) -> 'SimplePath':
|
39 |
-
... # pragma: no cover
|
40 |
-
|
41 |
-
def __truediv__(self) -> 'SimplePath':
|
42 |
-
... # pragma: no cover
|
43 |
-
|
44 |
-
def parent(self) -> 'SimplePath':
|
45 |
-
... # pragma: no cover
|
46 |
-
|
47 |
-
def read_text(self) -> str:
|
48 |
-
... # pragma: no cover
|
|
|
|
|
|
|
|
|
|
|
|
|
|
|
|
|
|
|
|
|
|
|
|
|
|
|
|
|
|
|
|
|
|
|
|
|
|
|
|
|
|
|
|
|
|
|
|
|
|
|
|
|
|
|
|
|
|
|
|
|
|
|
|
|
|
|
|
|
|
|
|
|
|
|
|
|
|
|
|
|
|
|
|
|
|
|
|
|
|
|
|
|
|
|
|
|
|
spaces/Awiny/Image2Paragraph/models/grit_src/third_party/CenterNet2/tests/structures/test_boxes.py
DELETED
@@ -1,223 +0,0 @@
|
|
1 |
-
# Copyright (c) Facebook, Inc. and its affiliates.
|
2 |
-
import json
|
3 |
-
import math
|
4 |
-
import numpy as np
|
5 |
-
import unittest
|
6 |
-
import torch
|
7 |
-
|
8 |
-
from detectron2.structures import Boxes, BoxMode, pairwise_ioa, pairwise_iou
|
9 |
-
from detectron2.utils.testing import reload_script_model
|
10 |
-
|
11 |
-
|
12 |
-
class TestBoxMode(unittest.TestCase):
|
13 |
-
def _convert_xy_to_wh(self, x):
|
14 |
-
return BoxMode.convert(x, BoxMode.XYXY_ABS, BoxMode.XYWH_ABS)
|
15 |
-
|
16 |
-
def _convert_xywha_to_xyxy(self, x):
|
17 |
-
return BoxMode.convert(x, BoxMode.XYWHA_ABS, BoxMode.XYXY_ABS)
|
18 |
-
|
19 |
-
def _convert_xywh_to_xywha(self, x):
|
20 |
-
return BoxMode.convert(x, BoxMode.XYWH_ABS, BoxMode.XYWHA_ABS)
|
21 |
-
|
22 |
-
def test_convert_int_mode(self):
|
23 |
-
BoxMode.convert([1, 2, 3, 4], 0, 1)
|
24 |
-
|
25 |
-
def test_box_convert_list(self):
|
26 |
-
for tp in [list, tuple]:
|
27 |
-
box = tp([5.0, 5.0, 10.0, 10.0])
|
28 |
-
output = self._convert_xy_to_wh(box)
|
29 |
-
self.assertIsInstance(output, tp)
|
30 |
-
self.assertIsInstance(output[0], float)
|
31 |
-
self.assertEqual(output, tp([5.0, 5.0, 5.0, 5.0]))
|
32 |
-
|
33 |
-
with self.assertRaises(Exception):
|
34 |
-
self._convert_xy_to_wh([box])
|
35 |
-
|
36 |
-
def test_box_convert_array(self):
|
37 |
-
box = np.asarray([[5, 5, 10, 10], [1, 1, 2, 3]])
|
38 |
-
output = self._convert_xy_to_wh(box)
|
39 |
-
self.assertEqual(output.dtype, box.dtype)
|
40 |
-
self.assertEqual(output.shape, box.shape)
|
41 |
-
self.assertTrue((output[0] == [5, 5, 5, 5]).all())
|
42 |
-
self.assertTrue((output[1] == [1, 1, 1, 2]).all())
|
43 |
-
|
44 |
-
def test_box_convert_cpu_tensor(self):
|
45 |
-
box = torch.tensor([[5, 5, 10, 10], [1, 1, 2, 3]])
|
46 |
-
output = self._convert_xy_to_wh(box)
|
47 |
-
self.assertEqual(output.dtype, box.dtype)
|
48 |
-
self.assertEqual(output.shape, box.shape)
|
49 |
-
output = output.numpy()
|
50 |
-
self.assertTrue((output[0] == [5, 5, 5, 5]).all())
|
51 |
-
self.assertTrue((output[1] == [1, 1, 1, 2]).all())
|
52 |
-
|
53 |
-
@unittest.skipIf(not torch.cuda.is_available(), "CUDA not available")
|
54 |
-
def test_box_convert_cuda_tensor(self):
|
55 |
-
box = torch.tensor([[5, 5, 10, 10], [1, 1, 2, 3]]).cuda()
|
56 |
-
output = self._convert_xy_to_wh(box)
|
57 |
-
self.assertEqual(output.dtype, box.dtype)
|
58 |
-
self.assertEqual(output.shape, box.shape)
|
59 |
-
self.assertEqual(output.device, box.device)
|
60 |
-
output = output.cpu().numpy()
|
61 |
-
self.assertTrue((output[0] == [5, 5, 5, 5]).all())
|
62 |
-
self.assertTrue((output[1] == [1, 1, 1, 2]).all())
|
63 |
-
|
64 |
-
def test_box_convert_xywha_to_xyxy_list(self):
|
65 |
-
for tp in [list, tuple]:
|
66 |
-
box = tp([50, 50, 30, 20, 0])
|
67 |
-
output = self._convert_xywha_to_xyxy(box)
|
68 |
-
self.assertIsInstance(output, tp)
|
69 |
-
self.assertEqual(output, tp([35, 40, 65, 60]))
|
70 |
-
|
71 |
-
with self.assertRaises(Exception):
|
72 |
-
self._convert_xywha_to_xyxy([box])
|
73 |
-
|
74 |
-
def test_box_convert_xywha_to_xyxy_array(self):
|
75 |
-
for dtype in [np.float64, np.float32]:
|
76 |
-
box = np.asarray(
|
77 |
-
[
|
78 |
-
[50, 50, 30, 20, 0],
|
79 |
-
[50, 50, 30, 20, 90],
|
80 |
-
[1, 1, math.sqrt(2), math.sqrt(2), -45],
|
81 |
-
],
|
82 |
-
dtype=dtype,
|
83 |
-
)
|
84 |
-
output = self._convert_xywha_to_xyxy(box)
|
85 |
-
self.assertEqual(output.dtype, box.dtype)
|
86 |
-
expected = np.asarray([[35, 40, 65, 60], [40, 35, 60, 65], [0, 0, 2, 2]], dtype=dtype)
|
87 |
-
self.assertTrue(np.allclose(output, expected, atol=1e-6), "output={}".format(output))
|
88 |
-
|
89 |
-
def test_box_convert_xywha_to_xyxy_tensor(self):
|
90 |
-
for dtype in [torch.float32, torch.float64]:
|
91 |
-
box = torch.tensor(
|
92 |
-
[
|
93 |
-
[50, 50, 30, 20, 0],
|
94 |
-
[50, 50, 30, 20, 90],
|
95 |
-
[1, 1, math.sqrt(2), math.sqrt(2), -45],
|
96 |
-
],
|
97 |
-
dtype=dtype,
|
98 |
-
)
|
99 |
-
output = self._convert_xywha_to_xyxy(box)
|
100 |
-
self.assertEqual(output.dtype, box.dtype)
|
101 |
-
expected = torch.tensor([[35, 40, 65, 60], [40, 35, 60, 65], [0, 0, 2, 2]], dtype=dtype)
|
102 |
-
|
103 |
-
self.assertTrue(torch.allclose(output, expected, atol=1e-6), "output={}".format(output))
|
104 |
-
|
105 |
-
def test_box_convert_xywh_to_xywha_list(self):
|
106 |
-
for tp in [list, tuple]:
|
107 |
-
box = tp([50, 50, 30, 20])
|
108 |
-
output = self._convert_xywh_to_xywha(box)
|
109 |
-
self.assertIsInstance(output, tp)
|
110 |
-
self.assertEqual(output, tp([65, 60, 30, 20, 0]))
|
111 |
-
|
112 |
-
with self.assertRaises(Exception):
|
113 |
-
self._convert_xywh_to_xywha([box])
|
114 |
-
|
115 |
-
def test_box_convert_xywh_to_xywha_array(self):
|
116 |
-
for dtype in [np.float64, np.float32]:
|
117 |
-
box = np.asarray([[30, 40, 70, 60], [30, 40, 60, 70], [-1, -1, 2, 2]], dtype=dtype)
|
118 |
-
output = self._convert_xywh_to_xywha(box)
|
119 |
-
self.assertEqual(output.dtype, box.dtype)
|
120 |
-
expected = np.asarray(
|
121 |
-
[[65, 70, 70, 60, 0], [60, 75, 60, 70, 0], [0, 0, 2, 2, 0]], dtype=dtype
|
122 |
-
)
|
123 |
-
self.assertTrue(np.allclose(output, expected, atol=1e-6), "output={}".format(output))
|
124 |
-
|
125 |
-
def test_box_convert_xywh_to_xywha_tensor(self):
|
126 |
-
for dtype in [torch.float32, torch.float64]:
|
127 |
-
box = torch.tensor([[30, 40, 70, 60], [30, 40, 60, 70], [-1, -1, 2, 2]], dtype=dtype)
|
128 |
-
output = self._convert_xywh_to_xywha(box)
|
129 |
-
self.assertEqual(output.dtype, box.dtype)
|
130 |
-
expected = torch.tensor(
|
131 |
-
[[65, 70, 70, 60, 0], [60, 75, 60, 70, 0], [0, 0, 2, 2, 0]], dtype=dtype
|
132 |
-
)
|
133 |
-
|
134 |
-
self.assertTrue(torch.allclose(output, expected, atol=1e-6), "output={}".format(output))
|
135 |
-
|
136 |
-
def test_json_serializable(self):
|
137 |
-
payload = {"box_mode": BoxMode.XYWH_REL}
|
138 |
-
try:
|
139 |
-
json.dumps(payload)
|
140 |
-
except Exception:
|
141 |
-
self.fail("JSON serialization failed")
|
142 |
-
|
143 |
-
def test_json_deserializable(self):
|
144 |
-
payload = '{"box_mode": 2}'
|
145 |
-
obj = json.loads(payload)
|
146 |
-
try:
|
147 |
-
obj["box_mode"] = BoxMode(obj["box_mode"])
|
148 |
-
except Exception:
|
149 |
-
self.fail("JSON deserialization failed")
|
150 |
-
|
151 |
-
|
152 |
-
class TestBoxIOU(unittest.TestCase):
|
153 |
-
def create_boxes(self):
|
154 |
-
boxes1 = torch.tensor([[0.0, 0.0, 1.0, 1.0], [0.0, 0.0, 1.0, 1.0]])
|
155 |
-
|
156 |
-
boxes2 = torch.tensor(
|
157 |
-
[
|
158 |
-
[0.0, 0.0, 1.0, 1.0],
|
159 |
-
[0.0, 0.0, 0.5, 1.0],
|
160 |
-
[0.0, 0.0, 1.0, 0.5],
|
161 |
-
[0.0, 0.0, 0.5, 0.5],
|
162 |
-
[0.5, 0.5, 1.0, 1.0],
|
163 |
-
[0.5, 0.5, 1.5, 1.5],
|
164 |
-
]
|
165 |
-
)
|
166 |
-
return boxes1, boxes2
|
167 |
-
|
168 |
-
def test_pairwise_iou(self):
|
169 |
-
boxes1, boxes2 = self.create_boxes()
|
170 |
-
expected_ious = torch.tensor(
|
171 |
-
[
|
172 |
-
[1.0, 0.5, 0.5, 0.25, 0.25, 0.25 / (2 - 0.25)],
|
173 |
-
[1.0, 0.5, 0.5, 0.25, 0.25, 0.25 / (2 - 0.25)],
|
174 |
-
]
|
175 |
-
)
|
176 |
-
|
177 |
-
ious = pairwise_iou(Boxes(boxes1), Boxes(boxes2))
|
178 |
-
self.assertTrue(torch.allclose(ious, expected_ious))
|
179 |
-
|
180 |
-
def test_pairwise_ioa(self):
|
181 |
-
boxes1, boxes2 = self.create_boxes()
|
182 |
-
expected_ioas = torch.tensor(
|
183 |
-
[[1.0, 1.0, 1.0, 1.0, 1.0, 0.25], [1.0, 1.0, 1.0, 1.0, 1.0, 0.25]]
|
184 |
-
)
|
185 |
-
ioas = pairwise_ioa(Boxes(boxes1), Boxes(boxes2))
|
186 |
-
self.assertTrue(torch.allclose(ioas, expected_ioas))
|
187 |
-
|
188 |
-
|
189 |
-
class TestBoxes(unittest.TestCase):
|
190 |
-
def test_empty_cat(self):
|
191 |
-
x = Boxes.cat([])
|
192 |
-
self.assertTrue(x.tensor.shape, (0, 4))
|
193 |
-
|
194 |
-
def test_to(self):
|
195 |
-
x = Boxes(torch.rand(3, 4))
|
196 |
-
self.assertEqual(x.to(device="cpu").tensor.device.type, "cpu")
|
197 |
-
|
198 |
-
def test_scriptability(self):
|
199 |
-
def func(x):
|
200 |
-
boxes = Boxes(x)
|
201 |
-
test = boxes.to(torch.device("cpu")).tensor
|
202 |
-
return boxes.area(), test
|
203 |
-
|
204 |
-
f = torch.jit.script(func)
|
205 |
-
f = reload_script_model(f)
|
206 |
-
f(torch.rand((3, 4)))
|
207 |
-
|
208 |
-
data = torch.rand((3, 4))
|
209 |
-
|
210 |
-
def func_cat(x: torch.Tensor):
|
211 |
-
boxes1 = Boxes(x)
|
212 |
-
boxes2 = Boxes(x)
|
213 |
-
# boxes3 = Boxes.cat([boxes1, boxes2]) # this is not supported by torchsript for now.
|
214 |
-
boxes3 = boxes1.cat([boxes1, boxes2])
|
215 |
-
return boxes3
|
216 |
-
|
217 |
-
f = torch.jit.script(func_cat)
|
218 |
-
script_box = f(data)
|
219 |
-
self.assertTrue(torch.equal(torch.cat([data, data]), script_box.tensor))
|
220 |
-
|
221 |
-
|
222 |
-
if __name__ == "__main__":
|
223 |
-
unittest.main()
|
|
|
|
|
|
|
|
|
|
|
|
|
|
|
|
|
|
|
|
|
|
|
|
|
|
|
|
|
|
|
|
|
|
|
|
|
|
|
|
|
|
|
|
|
|
|
|
|
|
|
|
|
|
|
|
|
|
|
|
|
|
|
|
|
|
|
|
|
|
|
|
|
|
|
|
|
|
|
|
|
|
|
|
|
|
|
|
|
|
|
|
|
|
|
|
|
|
|
|
|
|
|
|
|
|
|
|
|
|
|
|
|
|
|
|
|
|
|
|
|
|
|
|
|
|
|
|
|
|
|
|
|
|
|
|
|
|
|
|
|
|
|
|
|
|
|
|
|
|
|
|
|
|
|
|
|
|
|
|
|
|
|
|
|
|
|
|
|
|
|
|
|
|
|
|
|
|
|
|
|
|
|
|
|
|
|
|
|
|
|
|
|
|
|
|
|
|
|
|
|
|
|
|
|
|
|
|
|
|
|
|
|
|
|
|
|
|
|
|
|
|
|
|
|
|
|
|
|
|
|
|
|
|
|
|
|
|
|
|
|
|
|
|
|
|
|
|
|
|
|
|
|
|
|
|
|
|
|
|
|
|
|
|
|
|
|
|
|
|
|
|
|
|
|
|
|
|
|
|
|
|
|
|
|
|
|
|
|
|
|
|
|
|
|
|
|
|
|
|
|
|
|
|
|
|
|
|
|
|
|
|
|
|
|
|
|
|
|
|
|
|
|
|
|
|
|
|
|
|
|
|
|
|
|
|
|
|
|
|
|
|
|
|
|
|
|
|
|
|
|
|
|
|
|
|
|
|
|
|
|
|
|
|
|
|
|
|
|
|
|
|
|
|
|
|
|
|
|
|
|
|
|
|
|
|
|
|
|
|
|
|
|
|
|
|
|
|
|
|
|
|
|
|
|
|
|
|
|
|
|
|
|
|
|
|
|
|
|
|
|
|
|
|
|
|
|
|
|
|
|
|
|
|
|
|
|
|
|
|
|
|
|
|
|
|
|
|
spaces/Benson/text-generation/Examples/Barbie Apk.md
DELETED
@@ -1,74 +0,0 @@
|
|
1 |
-
<br />
|
2 |
-
<h1>Barbie APK: Un juego divertido y educativo para niños</h1>
|
3 |
-
<p>¿Te encanta jugar con muñecas Barbie? ¿Quieres crear tus propias historias y aventuras con ellas? Si lo hace, entonces te encantará <strong>Barbie APK</strong>, una aplicación de juego que le permite disfrutar del mundo de Barbie en su dispositivo Android. En este artículo, le diremos todo lo que necesita saber sobre Barbie APK, incluyendo lo que es, cómo descargarlo e instalarlo, ¿cuáles son sus características, por qué es popular, y cómo jugar con él. ¡Sigue leyendo y descubre los beneficios divertidos y educativos de jugar con muñecas Barbie! </p>
|
4 |
-
<h2>barbie apk</h2><br /><p><b><b>Download</b> ⚡ <a href="https://bltlly.com/2v6M7f">https://bltlly.com/2v6M7f</a></b></p><br /><br />
|
5 |
-
<h2>¿Qué es Barbie APK? </h2>
|
6 |
-
<p>Barbie APK es una aplicación que contiene varios juegos y actividades relacionadas con Barbie, la muñeca de moda más famosa del mundo. Puede descargarlo e instalarlo en su dispositivo Android de forma gratuita desde varias fuentes, como Uptodown, APKCombo o Google Play Store. La aplicación requiere Android 4.4 o superior y tiene un tamaño de aproximadamente 1,5 GB. La aplicación está desarrollada por Budge Studios, una empresa canadiense líder que crea aplicaciones entretenidas para niños. </p>
|
7 |
-
<h3>Cómo descargar e instalar Barbie APK</h3>
|
8 |
-
<p>Para descargar e instalar Barbie APK en su dispositivo Android, es necesario seguir estos sencillos pasos:</p>
|
9 |
-
<ol>
|
10 |
-
<li>Ir a una de las fuentes mencionadas anteriormente, tales como Uptodown, APKCombo, o Google Play Store, y buscar "Barbie APK". </li>
|
11 |
-
<li> Seleccione la aplicación que coincide con el nombre y el logotipo de Barbie, y toque en el "Descargar" o "Instalar" botón. </li>
|
12 |
-
<li>Espere a que la aplicación se descargue en su dispositivo. Es posible que necesite conceder algunos permisos o habilitar fuentes desconocidas para permitir la instalación. </li>
|
13 |
-
<li>Una vez descargada la aplicación, ábrela y sigue las instrucciones para completar la instalación. </li>
|
14 |
-
<li>Disfruta jugando con Barbie APK! </li>
|
15 |
-
</ol>
|
16 |
-
<h3> ¿Cuáles son las características de Barbie APK? </h3>
|
17 |
-
<p>Barbie APK tiene muchas características que lo convierten en un juego divertido y educativo para los niños. Estos son algunos de ellos:</p>
|
18 |
-
<h4>Aventuras de Barbie Dreamhouse</h4>
|
19 |
-
|
20 |
-
<h4>La vida de Barbie</h4>
|
21 |
-
<p>Este es otro juego en la aplicación que te permite disfrutar de juegos creativos, rompecabezas, fotos, videos y más con Barbie. También puede acceder a contenido exclusivo de las últimas películas y programas con Barbie. También puedes ver episodios de la serie animada "Barbie Dreamhouse Adventures" o "Barbie Dreamtopia". También puedes jugar a juegos como "Barbie Sparkle Blast", "Barbie Fashion Closet" o "Barbie Magical Fashion". También puedes tomar fotos con tus personajes o pegatinas favoritas y compartirlas con tus amigos. </p>
|
22 |
-
<h4>Juegos de Barbie</h4>
|
23 |
-
<p>Esta es una característica que le permite acceder a varios juegos en línea relacionados con Barbie de diferentes sitios web. Puedes jugar a juegos como "Barbie: Makeover Studio", "My Scene: Shopping Spree ", "Barbie: Princess Dress Up", o "Barbie: Fashion Show". También puedes aprender más sobre la historia, carrera, pasatiempos y amigos de Barbie. También puedes encontrar páginas para colorear, fondos de pantalla y cuestionarios para poner a prueba tus conocimientos sobre Barbie.</p>
|
24 |
-
<p></p>
|
25 |
-
<h2>¿Por qué es popular Barbie APK? </h2>
|
26 |
-
<p>Barbie APK es popular porque se basa en Barbie, la muñeca de moda más icónica y querida en el mundo. Barbie ha estado inspirando a generaciones de niñas y niños desde 1959, cuando fue creada por Ruth Handler, la cofundadora de Mattel. Barbie ha sido un símbolo de empoderamiento, diversidad y creatividad para millones de personas en todo el mundo. Aquí hay algunas razones por las que Barbie es tan popular:</p>
|
27 |
-
<h3>La historia de Barbie</h3>
|
28 |
-
|
29 |
-
<h3>Los beneficios de jugar con muñecas Barbie</h3>
|
30 |
-
<p>Jugar con muñecas Barbie no solo es divertido, sino también beneficioso para el desarrollo de los niños. Según la investigación, jugar con muñecas Barbie puede ayudar a los niños a mejorar sus habilidades cognitivas, sociales, emocionales y creativas. Por ejemplo, jugar con muñecas Barbie puede ayudar a los niños a mejorar su imaginación, narración, lenguaje, resolución de problemas, empatía, expresión personal y autoestima. Jugar con muñecas Barbie también puede ayudar a los niños a aprender sobre diferentes roles, profesiones, culturas y estilos de vida. Jugar con muñecas Barbie también puede fomentar una imagen corporal positiva y confianza en sí mismo en los niños. </p>
|
31 |
-
<h3>La diversidad y la inclusividad de las muñecas Barbie</h3>
|
32 |
-
<p>Las muñecas Barbie no solo son hermosas sino también diversas e inclusivas. Con los años, Barbie ha evolucionado para reflejar la diversidad del mundo y celebrar la singularidad de cada persona. Las muñecas Barbie vienen en diferentes formas, tamaños, colores, etnias, habilidades y orígenes. Por ejemplo, hay muñecas Barbie con diferentes tonos de piel, texturas de cabello, colores de ojos, rasgos faciales, tipos de cuerpo, alturas y discapacidades. También hay muñecas Barbie que representan diferentes culturas, religiones, tradiciones y costumbres. También hay muñecas Barbie que celebran diferentes ocasiones, eventos y logros. Las muñecas Barbie están diseñadas para inspirar y empoderar a los niños a ser lo que quieran ser y para respetar y apreciar las diferencias de los demás. </p>
|
33 |
-
<h2>Cómo jugar con Barbie APK</h2>
|
34 |
-
|
35 |
-
<h3>Consejos y trucos para jugar con muñecas Barbie</h3>
|
36 |
-
<p>Aquí hay algunos consejos y trucos que pueden ayudarle a disfrutar jugando con muñecas Barbie más:</p>
|
37 |
-
<ul>
|
38 |
-
<li>Usa tu imaginación y creatividad para crear tus propias historias y escenarios con muñecas Barbie. Puedes usar accesorios, accesorios, muebles, vehículos o cualquier otra cosa que tengas a tu alrededor para hacer tus historias más realistas e interesantes. </li>
|
39 |
-
<li>Pruebe diferentes combinaciones de trajes, peinados, maquillaje y accesorios para muñecas Barbie. Puede mezclar y combinar diferentes elementos para crear nuevos looks y estilos para diferentes ocasiones y estados de ánimo. </li>
|
40 |
-
<li>Explora diferentes carreras y pasatiempos con muñecas Barbie. Puedes fingir que Barbie es un médico, un maestro, un chef, un cantante, un bailarín, o cualquier otra cosa que quieras que sea. También puedes aprender más sobre estas profesiones y pasatiempos jugando a los juegos o viendo los videos en la aplicación. </li>
|
41 |
-
<li>Juega con tus amigos o familiares con muñecas Barbie. Puedes compartir tus historias, ideas, opiniones y sentimientos con ellos. También pueden cooperar, colaborar, competir o desafiarse en diferentes juegos o actividades. </li>
|
42 |
-
</ul>
|
43 |
-
<h3>Formas creativas de usar Barbie APK</h3>
|
44 |
-
<p>Aquí hay algunas maneras creativas que usted puede utilizar Barbie APK para mejorar su experiencia de juego:</p>
|
45 |
-
<ul>
|
46 |
-
<li>Usa la aplicación como fuente de inspiración para tus historias y aventuras con muñecas Barbie. Puedes ver los episodios, películas o videos en la aplicación y recrearlos con tu propio toque. También puedes jugar a los juegos de la aplicación y usarlos como punto de partida para tus propios juegos. </li>
|
47 |
-
<li>Utilice la aplicación como una herramienta para aprender cosas nuevas con muñecas Barbie. Puedes usar la aplicación para aprender más sobre diferentes temas, como moda, cultura, historia, ciencia, arte, música o matemáticas. También puedes usar la aplicación para practicar tus habilidades, como leer, escribir, deletrear, contar o dibujar. </li>
|
48 |
-
|
49 |
-
</ul>
|
50 |
-
<h3>Cómo compartir tus historias de Barbie con otros</h3>
|
51 |
-
<p>Compartir sus historias de Barbie con otros es una gran manera de expresarse, conectarse con otros y divertirse. Aquí hay algunas maneras en las que puedes compartir tus historias de Barbie con otros:</p>
|
52 |
-
<ul>
|
53 |
-
<li>Usa plataformas de redes sociales, como Instagram, Facebook, YouTube o TikTok, para publicar tus fotos o videos de tus muñecas Barbie. También puedes usar hashtags como #Barbie, #BarbieAPK, #BarbieDreamhouseAdventures, o #BarbieLife para llegar a más personas que estén interesadas en Barbie.</li>
|
54 |
-
<li>Usa foros en línea, blogs o sitios web, como Reddit, Quora, Medium o WordPress, para escribir tus historias o artículos sobre tus muñecas Barbie. También puedes usar estas plataformas para hacer preguntas, responder preguntas, dar consejos u obtener comentarios de otros fans de Barbie. </li>
|
55 |
-
<li>Usa juegos, aplicaciones o plataformas en línea, como Roblox, Minecraft, Discord o Zoom, para jugar con tus muñecas Barbie con otras personas en línea. También puedes usar estas plataformas para chatear, hacer llamadas de voz, hacer videollamadas o transmitir tus juegos o actividades de Barbie. </li>
|
56 |
-
</ul>
|
57 |
-
<h2>Conclusión</h2>
|
58 |
-
|
59 |
-
<h3>Preguntas frecuentes</h3>
|
60 |
-
<p>Aquí hay algunas preguntas frecuentes sobre Barbie APK:</p>
|
61 |
-
<ol>
|
62 |
-
<li><strong>¿Es seguro descargar e instalar Barbie APK? </strong></li>
|
63 |
-
<p>Sí, Barbie APK es seguro para descargar e instalar en su dispositivo Android. Sin embargo, siempre debe descargarlo de fuentes confiables, como Uptodown, APKCombo o Google Play Store. También debe escanear la aplicación en busca de virus o malware antes de instalarla. </p>
|
64 |
-
<li><strong>Es Barbie APK libre para jugar? </strong></li>
|
65 |
-
<p>Sí, Barbie APK es libre de jugar en su dispositivo Android. Sin embargo, algunas características o elementos pueden requerir compras en la aplicación o suscripciones. Puedes desactivar estas opciones en tu configuración si no quieres gastar dinero en la aplicación. </p>
|
66 |
-
<li><strong>¿Cuáles son los requisitos mínimos para jugar Barbie APK? </strong></li>
|
67 |
-
<p>Los requisitos mínimos para jugar Barbie APK son Android 4.4 o superior y 1.5 GB de espacio libre en su dispositivo. También es posible que necesite una conexión a Internet estable para algunas funciones o juegos. </p>
|
68 |
-
<li><strong>¿Cómo puedo actualizar Barbie APK? </strong></li>
|
69 |
-
<p>Puede actualizar Barbie APK yendo a la fuente desde donde lo descargó y la comprobación de nuevas versiones. También puede habilitar las actualizaciones automáticas en su configuración si desea que la aplicación se actualice sola cada vez que haya una nueva versión disponible. </p>
|
70 |
-
<li><strong>¿Cómo puedo contactar con el desarrollador de Barbie APK? </strong></li>
|
71 |
-
<p>Puede ponerse en contacto con el desarrollador de Barbie APK visitando su sitio web o enviándoles un correo electrónico a [email protected]. También puedes seguirlos en plataformas de redes sociales, como Facebook, Twitter, Instagram o YouTube.</p>
|
72 |
-
</ol></p> 64aa2da5cf<br />
|
73 |
-
<br />
|
74 |
-
<br />
|
|
|
|
|
|
|
|
|
|
|
|
|
|
|
|
|
|
|
|
|
|
|
|
|
|
|
|
|
|
|
|
|
|
|
|
|
|
|
|
|
|
|
|
|
|
|
|
|
|
|
|
|
|
|
|
|
|
|
|
|
|
|
|
|
|
|
|
|
|
|
|
|
|
|
|
|
|
|
|
|
|
|
|
|
|
|
|
|
|
|
|
|
|
|
|
|
|
|
|
|
|
|
|
|
|
|
|
|
|
|
|
|
|
|
|
|
|
|
|
|
|
|
|
|
|
|
|
|
|
|
|
|
|
|
|
|
|
|
|
|
|
|
|
|
|
|
|
|
|
spaces/BetterAPI/BetterChat_new/src/lib/utils/analytics.ts
DELETED
@@ -1,39 +0,0 @@
|
|
1 |
-
export interface GAEvent {
|
2 |
-
hitType: "event";
|
3 |
-
eventCategory: string;
|
4 |
-
eventAction: string;
|
5 |
-
eventLabel?: string;
|
6 |
-
eventValue?: number;
|
7 |
-
}
|
8 |
-
|
9 |
-
// Send a Google Analytics event
|
10 |
-
export function sendAnalyticsEvent({
|
11 |
-
eventCategory,
|
12 |
-
eventAction,
|
13 |
-
eventLabel,
|
14 |
-
eventValue,
|
15 |
-
}: Omit<GAEvent, "hitType">): void {
|
16 |
-
// Mandatory fields
|
17 |
-
const event: GAEvent = {
|
18 |
-
hitType: "event",
|
19 |
-
eventCategory,
|
20 |
-
eventAction,
|
21 |
-
};
|
22 |
-
// Optional fields
|
23 |
-
if (eventLabel) {
|
24 |
-
event.eventLabel = eventLabel;
|
25 |
-
}
|
26 |
-
if (eventValue) {
|
27 |
-
event.eventValue = eventValue;
|
28 |
-
}
|
29 |
-
|
30 |
-
// @ts-expect-error typescript doesn't know gtag is on the window object
|
31 |
-
if (!!window?.gtag && typeof window?.gtag === "function") {
|
32 |
-
// @ts-expect-error typescript doesn't know gtag is on the window object
|
33 |
-
window?.gtag("event", eventAction, {
|
34 |
-
event_category: event.eventCategory,
|
35 |
-
event_label: event.eventLabel,
|
36 |
-
value: event.eventValue,
|
37 |
-
});
|
38 |
-
}
|
39 |
-
}
|
|
|
|
|
|
|
|
|
|
|
|
|
|
|
|
|
|
|
|
|
|
|
|
|
|
|
|
|
|
|
|
|
|
|
|
|
|
|
|
|
|
|
|
|
|
|
|
|
|
|
|
|
|
|
|
|
|
|
|
|
|
|
|
|
|
|
|
|
|
|
|
|
|
|
|
|
|
|
|
spaces/Big-Web/MMSD/env/Lib/site-packages/pip/_vendor/chardet/cp949prober.py
DELETED
@@ -1,49 +0,0 @@
|
|
1 |
-
######################## BEGIN LICENSE BLOCK ########################
|
2 |
-
# The Original Code is mozilla.org code.
|
3 |
-
#
|
4 |
-
# The Initial Developer of the Original Code is
|
5 |
-
# Netscape Communications Corporation.
|
6 |
-
# Portions created by the Initial Developer are Copyright (C) 1998
|
7 |
-
# the Initial Developer. All Rights Reserved.
|
8 |
-
#
|
9 |
-
# Contributor(s):
|
10 |
-
# Mark Pilgrim - port to Python
|
11 |
-
#
|
12 |
-
# This library is free software; you can redistribute it and/or
|
13 |
-
# modify it under the terms of the GNU Lesser General Public
|
14 |
-
# License as published by the Free Software Foundation; either
|
15 |
-
# version 2.1 of the License, or (at your option) any later version.
|
16 |
-
#
|
17 |
-
# This library is distributed in the hope that it will be useful,
|
18 |
-
# but WITHOUT ANY WARRANTY; without even the implied warranty of
|
19 |
-
# MERCHANTABILITY or FITNESS FOR A PARTICULAR PURPOSE. See the GNU
|
20 |
-
# Lesser General Public License for more details.
|
21 |
-
#
|
22 |
-
# You should have received a copy of the GNU Lesser General Public
|
23 |
-
# License along with this library; if not, write to the Free Software
|
24 |
-
# Foundation, Inc., 51 Franklin St, Fifth Floor, Boston, MA
|
25 |
-
# 02110-1301 USA
|
26 |
-
######################### END LICENSE BLOCK #########################
|
27 |
-
|
28 |
-
from .chardistribution import EUCKRDistributionAnalysis
|
29 |
-
from .codingstatemachine import CodingStateMachine
|
30 |
-
from .mbcharsetprober import MultiByteCharSetProber
|
31 |
-
from .mbcssm import CP949_SM_MODEL
|
32 |
-
|
33 |
-
|
34 |
-
class CP949Prober(MultiByteCharSetProber):
|
35 |
-
def __init__(self) -> None:
|
36 |
-
super().__init__()
|
37 |
-
self.coding_sm = CodingStateMachine(CP949_SM_MODEL)
|
38 |
-
# NOTE: CP949 is a superset of EUC-KR, so the distribution should be
|
39 |
-
# not different.
|
40 |
-
self.distribution_analyzer = EUCKRDistributionAnalysis()
|
41 |
-
self.reset()
|
42 |
-
|
43 |
-
@property
|
44 |
-
def charset_name(self) -> str:
|
45 |
-
return "CP949"
|
46 |
-
|
47 |
-
@property
|
48 |
-
def language(self) -> str:
|
49 |
-
return "Korean"
|
|
|
|
|
|
|
|
|
|
|
|
|
|
|
|
|
|
|
|
|
|
|
|
|
|
|
|
|
|
|
|
|
|
|
|
|
|
|
|
|
|
|
|
|
|
|
|
|
|
|
|
|
|
|
|
|
|
|
|
|
|
|
|
|
|
|
|
|
|
|
|
|
|
|
|
|
|
|
|
|
|
|
|
|
|
|
|
|
|
|
|
|
|
|
|
|
|
|
|
spaces/Big-Web/MMSD/env/Lib/site-packages/setuptools/_distutils/command/build_clib.py
DELETED
@@ -1,208 +0,0 @@
|
|
1 |
-
"""distutils.command.build_clib
|
2 |
-
|
3 |
-
Implements the Distutils 'build_clib' command, to build a C/C++ library
|
4 |
-
that is included in the module distribution and needed by an extension
|
5 |
-
module."""
|
6 |
-
|
7 |
-
|
8 |
-
# XXX this module has *lots* of code ripped-off quite transparently from
|
9 |
-
# build_ext.py -- not surprisingly really, as the work required to build
|
10 |
-
# a static library from a collection of C source files is not really all
|
11 |
-
# that different from what's required to build a shared object file from
|
12 |
-
# a collection of C source files. Nevertheless, I haven't done the
|
13 |
-
# necessary refactoring to account for the overlap in code between the
|
14 |
-
# two modules, mainly because a number of subtle details changed in the
|
15 |
-
# cut 'n paste. Sigh.
|
16 |
-
|
17 |
-
import os
|
18 |
-
from distutils.core import Command
|
19 |
-
from distutils.errors import DistutilsSetupError
|
20 |
-
from distutils.sysconfig import customize_compiler
|
21 |
-
from distutils import log
|
22 |
-
|
23 |
-
|
24 |
-
def show_compilers():
|
25 |
-
from distutils.ccompiler import show_compilers
|
26 |
-
|
27 |
-
show_compilers()
|
28 |
-
|
29 |
-
|
30 |
-
class build_clib(Command):
|
31 |
-
|
32 |
-
description = "build C/C++ libraries used by Python extensions"
|
33 |
-
|
34 |
-
user_options = [
|
35 |
-
('build-clib=', 'b', "directory to build C/C++ libraries to"),
|
36 |
-
('build-temp=', 't', "directory to put temporary build by-products"),
|
37 |
-
('debug', 'g', "compile with debugging information"),
|
38 |
-
('force', 'f', "forcibly build everything (ignore file timestamps)"),
|
39 |
-
('compiler=', 'c', "specify the compiler type"),
|
40 |
-
]
|
41 |
-
|
42 |
-
boolean_options = ['debug', 'force']
|
43 |
-
|
44 |
-
help_options = [
|
45 |
-
('help-compiler', None, "list available compilers", show_compilers),
|
46 |
-
]
|
47 |
-
|
48 |
-
def initialize_options(self):
|
49 |
-
self.build_clib = None
|
50 |
-
self.build_temp = None
|
51 |
-
|
52 |
-
# List of libraries to build
|
53 |
-
self.libraries = None
|
54 |
-
|
55 |
-
# Compilation options for all libraries
|
56 |
-
self.include_dirs = None
|
57 |
-
self.define = None
|
58 |
-
self.undef = None
|
59 |
-
self.debug = None
|
60 |
-
self.force = 0
|
61 |
-
self.compiler = None
|
62 |
-
|
63 |
-
def finalize_options(self):
|
64 |
-
# This might be confusing: both build-clib and build-temp default
|
65 |
-
# to build-temp as defined by the "build" command. This is because
|
66 |
-
# I think that C libraries are really just temporary build
|
67 |
-
# by-products, at least from the point of view of building Python
|
68 |
-
# extensions -- but I want to keep my options open.
|
69 |
-
self.set_undefined_options(
|
70 |
-
'build',
|
71 |
-
('build_temp', 'build_clib'),
|
72 |
-
('build_temp', 'build_temp'),
|
73 |
-
('compiler', 'compiler'),
|
74 |
-
('debug', 'debug'),
|
75 |
-
('force', 'force'),
|
76 |
-
)
|
77 |
-
|
78 |
-
self.libraries = self.distribution.libraries
|
79 |
-
if self.libraries:
|
80 |
-
self.check_library_list(self.libraries)
|
81 |
-
|
82 |
-
if self.include_dirs is None:
|
83 |
-
self.include_dirs = self.distribution.include_dirs or []
|
84 |
-
if isinstance(self.include_dirs, str):
|
85 |
-
self.include_dirs = self.include_dirs.split(os.pathsep)
|
86 |
-
|
87 |
-
# XXX same as for build_ext -- what about 'self.define' and
|
88 |
-
# 'self.undef' ?
|
89 |
-
|
90 |
-
def run(self):
|
91 |
-
if not self.libraries:
|
92 |
-
return
|
93 |
-
|
94 |
-
# Yech -- this is cut 'n pasted from build_ext.py!
|
95 |
-
from distutils.ccompiler import new_compiler
|
96 |
-
|
97 |
-
self.compiler = new_compiler(
|
98 |
-
compiler=self.compiler, dry_run=self.dry_run, force=self.force
|
99 |
-
)
|
100 |
-
customize_compiler(self.compiler)
|
101 |
-
|
102 |
-
if self.include_dirs is not None:
|
103 |
-
self.compiler.set_include_dirs(self.include_dirs)
|
104 |
-
if self.define is not None:
|
105 |
-
# 'define' option is a list of (name,value) tuples
|
106 |
-
for (name, value) in self.define:
|
107 |
-
self.compiler.define_macro(name, value)
|
108 |
-
if self.undef is not None:
|
109 |
-
for macro in self.undef:
|
110 |
-
self.compiler.undefine_macro(macro)
|
111 |
-
|
112 |
-
self.build_libraries(self.libraries)
|
113 |
-
|
114 |
-
def check_library_list(self, libraries):
|
115 |
-
"""Ensure that the list of libraries is valid.
|
116 |
-
|
117 |
-
`library` is presumably provided as a command option 'libraries'.
|
118 |
-
This method checks that it is a list of 2-tuples, where the tuples
|
119 |
-
are (library_name, build_info_dict).
|
120 |
-
|
121 |
-
Raise DistutilsSetupError if the structure is invalid anywhere;
|
122 |
-
just returns otherwise.
|
123 |
-
"""
|
124 |
-
if not isinstance(libraries, list):
|
125 |
-
raise DistutilsSetupError("'libraries' option must be a list of tuples")
|
126 |
-
|
127 |
-
for lib in libraries:
|
128 |
-
if not isinstance(lib, tuple) and len(lib) != 2:
|
129 |
-
raise DistutilsSetupError("each element of 'libraries' must a 2-tuple")
|
130 |
-
|
131 |
-
name, build_info = lib
|
132 |
-
|
133 |
-
if not isinstance(name, str):
|
134 |
-
raise DistutilsSetupError(
|
135 |
-
"first element of each tuple in 'libraries' "
|
136 |
-
"must be a string (the library name)"
|
137 |
-
)
|
138 |
-
|
139 |
-
if '/' in name or (os.sep != '/' and os.sep in name):
|
140 |
-
raise DistutilsSetupError(
|
141 |
-
"bad library name '%s': "
|
142 |
-
"may not contain directory separators" % lib[0]
|
143 |
-
)
|
144 |
-
|
145 |
-
if not isinstance(build_info, dict):
|
146 |
-
raise DistutilsSetupError(
|
147 |
-
"second element of each tuple in 'libraries' "
|
148 |
-
"must be a dictionary (build info)"
|
149 |
-
)
|
150 |
-
|
151 |
-
def get_library_names(self):
|
152 |
-
# Assume the library list is valid -- 'check_library_list()' is
|
153 |
-
# called from 'finalize_options()', so it should be!
|
154 |
-
if not self.libraries:
|
155 |
-
return None
|
156 |
-
|
157 |
-
lib_names = []
|
158 |
-
for (lib_name, build_info) in self.libraries:
|
159 |
-
lib_names.append(lib_name)
|
160 |
-
return lib_names
|
161 |
-
|
162 |
-
def get_source_files(self):
|
163 |
-
self.check_library_list(self.libraries)
|
164 |
-
filenames = []
|
165 |
-
for (lib_name, build_info) in self.libraries:
|
166 |
-
sources = build_info.get('sources')
|
167 |
-
if sources is None or not isinstance(sources, (list, tuple)):
|
168 |
-
raise DistutilsSetupError(
|
169 |
-
"in 'libraries' option (library '%s'), "
|
170 |
-
"'sources' must be present and must be "
|
171 |
-
"a list of source filenames" % lib_name
|
172 |
-
)
|
173 |
-
|
174 |
-
filenames.extend(sources)
|
175 |
-
return filenames
|
176 |
-
|
177 |
-
def build_libraries(self, libraries):
|
178 |
-
for (lib_name, build_info) in libraries:
|
179 |
-
sources = build_info.get('sources')
|
180 |
-
if sources is None or not isinstance(sources, (list, tuple)):
|
181 |
-
raise DistutilsSetupError(
|
182 |
-
"in 'libraries' option (library '%s'), "
|
183 |
-
"'sources' must be present and must be "
|
184 |
-
"a list of source filenames" % lib_name
|
185 |
-
)
|
186 |
-
sources = list(sources)
|
187 |
-
|
188 |
-
log.info("building '%s' library", lib_name)
|
189 |
-
|
190 |
-
# First, compile the source code to object files in the library
|
191 |
-
# directory. (This should probably change to putting object
|
192 |
-
# files in a temporary build directory.)
|
193 |
-
macros = build_info.get('macros')
|
194 |
-
include_dirs = build_info.get('include_dirs')
|
195 |
-
objects = self.compiler.compile(
|
196 |
-
sources,
|
197 |
-
output_dir=self.build_temp,
|
198 |
-
macros=macros,
|
199 |
-
include_dirs=include_dirs,
|
200 |
-
debug=self.debug,
|
201 |
-
)
|
202 |
-
|
203 |
-
# Now "link" the object files together into a static library.
|
204 |
-
# (On Unix at least, this isn't really linking -- it just
|
205 |
-
# builds an archive. Whatever.)
|
206 |
-
self.compiler.create_static_lib(
|
207 |
-
objects, lib_name, output_dir=self.build_clib, debug=self.debug
|
208 |
-
)
|
|
|
|
|
|
|
|
|
|
|
|
|
|
|
|
|
|
|
|
|
|
|
|
|
|
|
|
|
|
|
|
|
|
|
|
|
|
|
|
|
|
|
|
|
|
|
|
|
|
|
|
|
|
|
|
|
|
|
|
|
|
|
|
|
|
|
|
|
|
|
|
|
|
|
|
|
|
|
|
|
|
|
|
|
|
|
|
|
|
|
|
|
|
|
|
|
|
|
|
|
|
|
|
|
|
|
|
|
|
|
|
|
|
|
|
|
|
|
|
|
|
|
|
|
|
|
|
|
|
|
|
|
|
|
|
|
|
|
|
|
|
|
|
|
|
|
|
|
|
|
|
|
|
|
|
|
|
|
|
|
|
|
|
|
|
|
|
|
|
|
|
|
|
|
|
|
|
|
|
|
|
|
|
|
|
|
|
|
|
|
|
|
|
|
|
|
|
|
|
|
|
|
|
|
|
|
|
|
|
|
|
|
|
|
|
|
|
|
|
|
|
|
|
|
|
|
|
|
|
|
|
|
|
|
|
|
|
|
|
|
|
|
|
|
|
|
|
|
|
|
|
|
|
|
|
|
|
|
|
|
|
|
|
|
|
|
|
|
|
|
|
|
|
|
|
|
|
|
|
|
|
|
|
|
|
|
|
|
|
|
|
|
|
|
|
|
|
|
|
|
|
|
|
|
|
|
|
|
|
|
|
|
|
|
|
|
|
|
|
|
|
|
|
|
|
|
|
|
|
|
|
|
|
|
|
|
|
|
|
|
|
|
|
|
|
|
|
|
|
|
|
|
|
|
|
|
|
|
|
|
|
|
|
|
|
|
|
|
|
|
|
|
|
|
|
|
|
|
|
|
|
|
|
|
|
|
|
|
|
|
|
|
|
|
|
|
|
|
|
|
|
|
|
|
|
|
|
|
|
|
|
|
|
|
|
|
|
spaces/BilalSardar/Like-Chatgpt-clone/README.md
DELETED
@@ -1,12 +0,0 @@
|
|
1 |
-
---
|
2 |
-
title: Like Chatgpt Clone
|
3 |
-
emoji: 👁
|
4 |
-
colorFrom: indigo
|
5 |
-
colorTo: pink
|
6 |
-
sdk: gradio
|
7 |
-
sdk_version: 3.16.2
|
8 |
-
app_file: app.py
|
9 |
-
pinned: false
|
10 |
-
---
|
11 |
-
|
12 |
-
Check out the configuration reference at https://huggingface.co/docs/hub/spaces-config-reference
|
|
|
|
|
|
|
|
|
|
|
|
|
|
|
|
|
|
|
|
|
|
|
|
|
|
spaces/BlitzKriegM/argilla/Dockerfile
DELETED
@@ -1,19 +0,0 @@
|
|
1 |
-
FROM argilla/argilla-quickstart:v1.6.0
|
2 |
-
|
3 |
-
# Define datasets to preload: full=all datasets, single=one dataset, and none=no datasets.
|
4 |
-
ENV LOAD_DATASETS=full
|
5 |
-
|
6 |
-
# Uncomment the next section to keep backward compatibility with previous versions
|
7 |
-
## Following variables are used for backward compatibility with the previous security setup for the quickstart image
|
8 |
-
#ENV ADMIN_USERNAME="team"
|
9 |
-
#ENV ADMIN_API_KEY="team.apikey"
|
10 |
-
## The password has a minimum length of 8. Passwords with lower lengths will fail.
|
11 |
-
#ENV ADMIN_PASSWORD=12345678
|
12 |
-
#
|
13 |
-
#ENV ANNOTATOR_USERNAME="argilla"
|
14 |
-
## The password has a minimum length of 8. Passwords with lower lengths will fail.
|
15 |
-
#ENV ANNOTATOR_PASSWORD=12345678
|
16 |
-
#
|
17 |
-
#ENV ARGILLA_WORKSPACE="team"
|
18 |
-
|
19 |
-
CMD /start_quickstart_argilla.sh
|
|
|
|
|
|
|
|
|
|
|
|
|
|
|
|
|
|
|
|
|
|
|
|
|
|
|
|
|
|
|
|
|
|
|
|
|
|
|
|
spaces/CALM/Dashboard/dashboard_utils/bubbles.py
DELETED
@@ -1,189 +0,0 @@
|
|
1 |
-
import datetime
|
2 |
-
from concurrent.futures import as_completed
|
3 |
-
from urllib import parse
|
4 |
-
|
5 |
-
import pandas as pd
|
6 |
-
|
7 |
-
import streamlit as st
|
8 |
-
import wandb
|
9 |
-
from requests_futures.sessions import FuturesSession
|
10 |
-
|
11 |
-
from dashboard_utils.time_tracker import _log, simple_time_tracker
|
12 |
-
|
13 |
-
URL_QUICKSEARCH = "https://huggingface.co/api/quicksearch?"
|
14 |
-
WANDB_REPO = st.secrets["WANDB_REPO_INDIVIDUAL_METRICS"]
|
15 |
-
CACHE_TTL = 100
|
16 |
-
MAX_DELTA_ACTIVE_RUN_SEC = 60 * 5
|
17 |
-
|
18 |
-
|
19 |
-
@st.cache(ttl=CACHE_TTL, show_spinner=False)
|
20 |
-
@simple_time_tracker(_log)
|
21 |
-
def get_new_bubble_data():
|
22 |
-
serialized_data_points, latest_timestamp = get_serialized_data_points()
|
23 |
-
serialized_data = get_serialized_data(serialized_data_points, latest_timestamp)
|
24 |
-
|
25 |
-
usernames = []
|
26 |
-
for item in serialized_data["points"][0]:
|
27 |
-
usernames.append(item["profileId"])
|
28 |
-
|
29 |
-
profiles = get_profiles(usernames)
|
30 |
-
|
31 |
-
return serialized_data, profiles
|
32 |
-
|
33 |
-
|
34 |
-
@st.cache(ttl=CACHE_TTL, show_spinner=False)
|
35 |
-
@simple_time_tracker(_log)
|
36 |
-
def get_profiles(usernames):
|
37 |
-
profiles = []
|
38 |
-
with FuturesSession(max_workers=32) as session:
|
39 |
-
futures = []
|
40 |
-
for username in usernames:
|
41 |
-
future = session.get(URL_QUICKSEARCH + parse.urlencode({"type": "user", "q": username}))
|
42 |
-
future.username = username
|
43 |
-
futures.append(future)
|
44 |
-
for future in as_completed(futures):
|
45 |
-
resp = future.result()
|
46 |
-
username = future.username
|
47 |
-
response = resp.json()
|
48 |
-
avatarUrl = None
|
49 |
-
if response["users"]:
|
50 |
-
for user_candidate in response["users"]:
|
51 |
-
if user_candidate["user"] == username:
|
52 |
-
avatarUrl = response["users"][0]["avatarUrl"]
|
53 |
-
break
|
54 |
-
if not avatarUrl:
|
55 |
-
avatarUrl = "/avatars/57584cb934354663ac65baa04e6829bf.svg"
|
56 |
-
|
57 |
-
if avatarUrl.startswith("/avatars/"):
|
58 |
-
avatarUrl = f"https://huggingface.co{avatarUrl}"
|
59 |
-
|
60 |
-
profiles.append(
|
61 |
-
{"id": username, "name": username, "src": avatarUrl, "url": f"https://huggingface.co/{username}"}
|
62 |
-
)
|
63 |
-
return profiles
|
64 |
-
|
65 |
-
|
66 |
-
@st.cache(ttl=CACHE_TTL, show_spinner=False)
|
67 |
-
@simple_time_tracker(_log)
|
68 |
-
def get_serialized_data_points():
|
69 |
-
|
70 |
-
api = wandb.Api()
|
71 |
-
runs = api.runs(WANDB_REPO)
|
72 |
-
|
73 |
-
serialized_data_points = {}
|
74 |
-
latest_timestamp = None
|
75 |
-
for run in runs:
|
76 |
-
run_summary = run.summary._json_dict
|
77 |
-
run_name = run.name
|
78 |
-
state = run.state
|
79 |
-
|
80 |
-
if run_name in serialized_data_points:
|
81 |
-
if "_timestamp" in run_summary and "_step" in run_summary:
|
82 |
-
timestamp = run_summary["_timestamp"]
|
83 |
-
serialized_data_points[run_name]["Runs"].append(
|
84 |
-
{
|
85 |
-
"batches": run_summary["_step"],
|
86 |
-
"runtime": run_summary["_runtime"],
|
87 |
-
"loss": run_summary["train/loss"],
|
88 |
-
"state": state,
|
89 |
-
"velocity": run_summary["_step"] / run_summary["_runtime"],
|
90 |
-
"date": datetime.datetime.utcfromtimestamp(timestamp),
|
91 |
-
}
|
92 |
-
)
|
93 |
-
if not latest_timestamp or timestamp > latest_timestamp:
|
94 |
-
latest_timestamp = timestamp
|
95 |
-
else:
|
96 |
-
if "_timestamp" in run_summary and "_step" in run_summary:
|
97 |
-
timestamp = run_summary["_timestamp"]
|
98 |
-
serialized_data_points[run_name] = {
|
99 |
-
"profileId": run_name,
|
100 |
-
"Runs": [
|
101 |
-
{
|
102 |
-
"batches": run_summary["_step"],
|
103 |
-
"runtime": run_summary["_runtime"],
|
104 |
-
"loss": run_summary["train/loss"],
|
105 |
-
"state": state,
|
106 |
-
"velocity": run_summary["_step"] / run_summary["_runtime"],
|
107 |
-
"date": datetime.datetime.utcfromtimestamp(timestamp),
|
108 |
-
}
|
109 |
-
],
|
110 |
-
}
|
111 |
-
if not latest_timestamp or timestamp > latest_timestamp:
|
112 |
-
latest_timestamp = timestamp
|
113 |
-
latest_timestamp = datetime.datetime.utcfromtimestamp(latest_timestamp)
|
114 |
-
return serialized_data_points, latest_timestamp
|
115 |
-
|
116 |
-
|
117 |
-
@st.cache(ttl=CACHE_TTL, show_spinner=False)
|
118 |
-
@simple_time_tracker(_log)
|
119 |
-
def get_serialized_data(serialized_data_points, latest_timestamp):
|
120 |
-
serialized_data_points_v2 = []
|
121 |
-
max_velocity = 1
|
122 |
-
for run_name, serialized_data_point in serialized_data_points.items():
|
123 |
-
activeRuns = []
|
124 |
-
loss = 0
|
125 |
-
runtime = 0
|
126 |
-
batches = 0
|
127 |
-
velocity = 0
|
128 |
-
for run in serialized_data_point["Runs"]:
|
129 |
-
if run["state"] == "running":
|
130 |
-
run["date"] = run["date"].isoformat()
|
131 |
-
activeRuns.append(run)
|
132 |
-
loss += run["loss"]
|
133 |
-
velocity += run["velocity"]
|
134 |
-
loss = loss / len(activeRuns) if activeRuns else 0
|
135 |
-
runtime += run["runtime"]
|
136 |
-
batches += run["batches"]
|
137 |
-
new_item = {
|
138 |
-
"date": latest_timestamp.isoformat(),
|
139 |
-
"profileId": run_name,
|
140 |
-
"batches": runtime, # "batches": batches quick and dirty fix
|
141 |
-
"runtime": runtime,
|
142 |
-
"activeRuns": activeRuns,
|
143 |
-
}
|
144 |
-
serialized_data_points_v2.append(new_item)
|
145 |
-
serialized_data = {"points": [serialized_data_points_v2], "maxVelocity": max_velocity}
|
146 |
-
return serialized_data
|
147 |
-
|
148 |
-
|
149 |
-
def get_leaderboard(serialized_data):
|
150 |
-
data_leaderboard = {"user": [], "runtime": []}
|
151 |
-
|
152 |
-
for user_item in serialized_data["points"][0]:
|
153 |
-
data_leaderboard["user"].append(user_item["profileId"])
|
154 |
-
data_leaderboard["runtime"].append(user_item["runtime"])
|
155 |
-
|
156 |
-
df = pd.DataFrame(data_leaderboard)
|
157 |
-
df = df.sort_values("runtime", ascending=False)
|
158 |
-
df["runtime"] = df["runtime"].apply(lambda x: datetime.timedelta(seconds=x))
|
159 |
-
df["runtime"] = df["runtime"].apply(lambda x: str(x))
|
160 |
-
|
161 |
-
df.reset_index(drop=True, inplace=True)
|
162 |
-
df.rename(columns={"user": "User", "runtime": "Total time contributed"}, inplace=True)
|
163 |
-
df["Rank"] = df.index + 1
|
164 |
-
df = df.set_index("Rank")
|
165 |
-
return df
|
166 |
-
|
167 |
-
|
168 |
-
def get_global_metrics(serialized_data):
|
169 |
-
current_time = datetime.datetime.utcnow()
|
170 |
-
num_contributing_users = len(serialized_data["points"][0])
|
171 |
-
num_active_users = 0
|
172 |
-
total_runtime = 0
|
173 |
-
|
174 |
-
for user_item in serialized_data["points"][0]:
|
175 |
-
for run in user_item["activeRuns"]:
|
176 |
-
date_run = datetime.datetime.fromisoformat(run["date"])
|
177 |
-
delta_time_sec = (current_time - date_run).total_seconds()
|
178 |
-
if delta_time_sec < MAX_DELTA_ACTIVE_RUN_SEC:
|
179 |
-
num_active_users += 1
|
180 |
-
break
|
181 |
-
|
182 |
-
total_runtime += user_item["runtime"]
|
183 |
-
|
184 |
-
total_runtime = datetime.timedelta(seconds=total_runtime)
|
185 |
-
return {
|
186 |
-
"num_contributing_users": num_contributing_users,
|
187 |
-
"num_active_users": num_active_users,
|
188 |
-
"total_runtime": total_runtime,
|
189 |
-
}
|
|
|
|
|
|
|
|
|
|
|
|
|
|
|
|
|
|
|
|
|
|
|
|
|
|
|
|
|
|
|
|
|
|
|
|
|
|
|
|
|
|
|
|
|
|
|
|
|
|
|
|
|
|
|
|
|
|
|
|
|
|
|
|
|
|
|
|
|
|
|
|
|
|
|
|
|
|
|
|
|
|
|
|
|
|
|
|
|
|
|
|
|
|
|
|
|
|
|
|
|
|
|
|
|
|
|
|
|
|
|
|
|
|
|
|
|
|
|
|
|
|
|
|
|
|
|
|
|
|
|
|
|
|
|
|
|
|
|
|
|
|
|
|
|
|
|
|
|
|
|
|
|
|
|
|
|
|
|
|
|
|
|
|
|
|
|
|
|
|
|
|
|
|
|
|
|
|
|
|
|
|
|
|
|
|
|
|
|
|
|
|
|
|
|
|
|
|
|
|
|
|
|
|
|
|
|
|
|
|
|
|
|
|
|
|
|
|
|
|
|
|
|
|
|
|
|
|
|
|
|
|
|
|
|
|
|
|
|
|
|
|
|
|
|
|
|
|
|
|
|
|
|
|
|
|
|
|
|
|
|
|
|
|
|
|
|
|
|
|
|
|
|
|
|
|
|
|
|
|
|
|
|
|
|
|
|
|
|
|
|
|
|
|
|
|
|
|
|
|
|
|
|
|
|
|
|
|
|
|
|
|
|
|
|
|
|
|
|
|
|
|
|
|
|
|
|
|
|
|
|
|
|
|
|
|
|
|
|
|
|
|
|
|
|
|
|
|
|
|
|
|
|
|
|
|
|
|
|
|
|
|
|
|
|
|
|
|
|
|
|
|
|
|
|
|
|
|
|
|
spaces/CNXT/PiX2TXT/app.py
DELETED
@@ -1,3 +0,0 @@
|
|
1 |
-
import gradio as gr
|
2 |
-
|
3 |
-
gr.Interface.load("models/microsoft/trocr-base-printed").launch()
|
|
|
|
|
|
|
|
spaces/CVMX-jaca-tonos/YouTube-Video-Streaming-Spanish-ASR/app.py
DELETED
@@ -1,126 +0,0 @@
|
|
1 |
-
from collections import deque
|
2 |
-
import streamlit as st
|
3 |
-
import torch
|
4 |
-
from streamlit_player import st_player
|
5 |
-
from transformers import AutoModelForCTC, Wav2Vec2Processor
|
6 |
-
from streaming import ffmpeg_stream
|
7 |
-
|
8 |
-
device = torch.device('cuda' if torch.cuda.is_available() else 'cpu')
|
9 |
-
player_options = {
|
10 |
-
"events": ["onProgress"],
|
11 |
-
"progress_interval": 200,
|
12 |
-
"volume": 1.0,
|
13 |
-
"playing": True,
|
14 |
-
"loop": False,
|
15 |
-
"controls": False,
|
16 |
-
"muted": False,
|
17 |
-
"config": {"youtube": {"playerVars": {"start": 1}}},
|
18 |
-
}
|
19 |
-
|
20 |
-
st.title("YouTube Video Spanish ASR")
|
21 |
-
st.write("Acknowledgement: This demo is based on Anton Lozhkov's cool Space : https://huggingface.co/spaces/anton-l/youtube-subs-wav2vec")
|
22 |
-
# disable rapid fading in and out on `st.code` updates
|
23 |
-
st.markdown("<style>.element-container{opacity:1 !important}</style>", unsafe_allow_html=True)
|
24 |
-
|
25 |
-
@st.cache(hash_funcs={torch.nn.parameter.Parameter: lambda _: None})
|
26 |
-
def load_model(model_path="facebook/wav2vec2-large-robust-ft-swbd-300h"):
|
27 |
-
processor = Wav2Vec2Processor.from_pretrained(model_path)
|
28 |
-
model = AutoModelForCTC.from_pretrained(model_path).to(device)
|
29 |
-
return processor, model
|
30 |
-
|
31 |
-
model_path = st.radio(
|
32 |
-
"Select a model", (
|
33 |
-
"jonatasgrosman/wav2vec2-xls-r-1b-spanish",
|
34 |
-
"jonatasgrosman/wav2vec2-large-xlsr-53-spanish",
|
35 |
-
"patrickvonplaten/wav2vec2-large-xlsr-53-spanish-with-lm",
|
36 |
-
"facebook/wav2vec2-large-xlsr-53-spanish",
|
37 |
-
"glob-asr/xls-r-es-test-lm"
|
38 |
-
)
|
39 |
-
)
|
40 |
-
|
41 |
-
processor, model = load_model(model_path)
|
42 |
-
|
43 |
-
def stream_text(url, chunk_duration_ms, pad_duration_ms):
|
44 |
-
sampling_rate = processor.feature_extractor.sampling_rate
|
45 |
-
|
46 |
-
# calculate the length of logits to cut from the sides of the output to account for input padding
|
47 |
-
output_pad_len = model._get_feat_extract_output_lengths(int(sampling_rate * pad_duration_ms / 1000))
|
48 |
-
|
49 |
-
# define the audio chunk generator
|
50 |
-
stream = ffmpeg_stream(url, sampling_rate, chunk_duration_ms=chunk_duration_ms, pad_duration_ms=pad_duration_ms)
|
51 |
-
|
52 |
-
leftover_text = ""
|
53 |
-
for i, chunk in enumerate(stream):
|
54 |
-
input_values = processor(chunk, sampling_rate=sampling_rate, return_tensors="pt").input_values
|
55 |
-
|
56 |
-
with torch.no_grad():
|
57 |
-
logits = model(input_values.to(device)).logits[0]
|
58 |
-
if i > 0:
|
59 |
-
logits = logits[output_pad_len : len(logits) - output_pad_len]
|
60 |
-
else: # don't count padding at the start of the clip
|
61 |
-
logits = logits[: len(logits) - output_pad_len]
|
62 |
-
|
63 |
-
predicted_ids = torch.argmax(logits, dim=-1).cpu().tolist()
|
64 |
-
if processor.decode(predicted_ids).strip():
|
65 |
-
leftover_ids = processor.tokenizer.encode(leftover_text)
|
66 |
-
# concat the last word (or its part) from the last frame with the current text
|
67 |
-
text = processor.decode(leftover_ids + predicted_ids)
|
68 |
-
# don't return the last word in case it's just partially recognized
|
69 |
-
if " " in text:
|
70 |
-
text, leftover_text = text.rsplit(" ", 1)
|
71 |
-
else:
|
72 |
-
leftover_text = text
|
73 |
-
text = ""
|
74 |
-
if text:
|
75 |
-
yield text
|
76 |
-
else:
|
77 |
-
yield leftover_text
|
78 |
-
leftover_text = ""
|
79 |
-
yield leftover_text
|
80 |
-
|
81 |
-
def main():
|
82 |
-
state = st.session_state
|
83 |
-
st.header("Video ASR Streamlit from Youtube Link")
|
84 |
-
|
85 |
-
with st.form(key="inputs_form"):
|
86 |
-
|
87 |
-
initial_url = "https://youtu.be/ghOqTkGzX7I?t=60"
|
88 |
-
state.youtube_url = st.text_input("YouTube URL", initial_url)
|
89 |
-
|
90 |
-
state.chunk_duration_ms = st.slider("Audio chunk duration (ms)", 2000, 10000, 3000, 100)
|
91 |
-
state.pad_duration_ms = st.slider("Padding duration (ms)", 100, 5000, 1000, 100)
|
92 |
-
submit_button = st.form_submit_button(label="Submit")
|
93 |
-
|
94 |
-
if "lines" in state:
|
95 |
-
# print the lines of subs
|
96 |
-
st.code("\n".join(state.lines))
|
97 |
-
|
98 |
-
if submit_button or "asr_stream" not in state:
|
99 |
-
# a hack to update the video player on value changes
|
100 |
-
state.youtube_url = (
|
101 |
-
state.youtube_url.split("&hash=")[0]
|
102 |
-
+ f"&hash={state.chunk_duration_ms}-{state.pad_duration_ms}"
|
103 |
-
)
|
104 |
-
state.asr_stream = stream_text(
|
105 |
-
state.youtube_url, state.chunk_duration_ms, state.pad_duration_ms
|
106 |
-
)
|
107 |
-
state.chunks_taken = 0
|
108 |
-
|
109 |
-
|
110 |
-
state.lines = deque([], maxlen=5) # limit to the last n lines of subs
|
111 |
-
|
112 |
-
|
113 |
-
player = st_player(state.youtube_url, **player_options, key="youtube_player")
|
114 |
-
|
115 |
-
if "asr_stream" in state and player.data and player.data["played"] < 1.0:
|
116 |
-
# check how many seconds were played, and if more than processed - write the next text chunk
|
117 |
-
processed_seconds = state.chunks_taken * (state.chunk_duration_ms / 1000)
|
118 |
-
if processed_seconds < player.data["playedSeconds"]:
|
119 |
-
text = next(state.asr_stream)
|
120 |
-
state.lines.append(text)
|
121 |
-
state.chunks_taken += 1
|
122 |
-
|
123 |
-
|
124 |
-
|
125 |
-
if __name__ == "__main__":
|
126 |
-
main()
|
|
|
|
|
|
|
|
|
|
|
|
|
|
|
|
|
|
|
|
|
|
|
|
|
|
|
|
|
|
|
|
|
|
|
|
|
|
|
|
|
|
|
|
|
|
|
|
|
|
|
|
|
|
|
|
|
|
|
|
|
|
|
|
|
|
|
|
|
|
|
|
|
|
|
|
|
|
|
|
|
|
|
|
|
|
|
|
|
|
|
|
|
|
|
|
|
|
|
|
|
|
|
|
|
|
|
|
|
|
|
|
|
|
|
|
|
|
|
|
|
|
|
|
|
|
|
|
|
|
|
|
|
|
|
|
|
|
|
|
|
|
|
|
|
|
|
|
|
|
|
|
|
|
|
|
|
|
|
|
|
|
|
|
|
|
|
|
|
|
|
|
|
|
|
|
|
|
|
|
|
|
|
|
|
|
|
|
|
|
|
|
|
|
|
|
|
|
|
|
|
|
|
|
|
|
|
|
|
|
|
|
|
|
|
|
|
|
|
|
|
|
|
|
|
|
|
|
|
|
|
|
|
|
|
|
|
|
|
|
|
|
|
|
|
|
|
|
|
|
|
|
|
|
spaces/CVPR/Dual-Key_Backdoor_Attacks/openvqa/utils/test_engine.py
DELETED
@@ -1,157 +0,0 @@
|
|
1 |
-
# --------------------------------------------------------
|
2 |
-
# OpenVQA
|
3 |
-
# Written by Yuhao Cui https://github.com/cuiyuhao1996
|
4 |
-
# --------------------------------------------------------
|
5 |
-
|
6 |
-
import os, json, torch, pickle
|
7 |
-
import numpy as np
|
8 |
-
import torch.nn as nn
|
9 |
-
import torch.utils.data as Data
|
10 |
-
from openvqa.models.model_loader import ModelLoader
|
11 |
-
from openvqa.datasets.dataset_loader import EvalLoader
|
12 |
-
|
13 |
-
|
14 |
-
# Evaluation
|
15 |
-
@torch.no_grad()
|
16 |
-
def test_engine(__C, dataset, state_dict=None, validation=False):
|
17 |
-
|
18 |
-
# Load parameters
|
19 |
-
if __C.CKPT_PATH is not None:
|
20 |
-
print('Warning: you are now using CKPT_PATH args, '
|
21 |
-
'CKPT_VERSION and CKPT_EPOCH will not work')
|
22 |
-
|
23 |
-
path = __C.CKPT_PATH
|
24 |
-
else:
|
25 |
-
path = __C.CKPTS_PATH + \
|
26 |
-
'/ckpt_' + __C.CKPT_VERSION + \
|
27 |
-
'/epoch' + str(__C.CKPT_EPOCH) + '.pkl'
|
28 |
-
|
29 |
-
# val_ckpt_flag = False
|
30 |
-
if state_dict is None:
|
31 |
-
# val_ckpt_flag = True
|
32 |
-
print('Loading ckpt from: {}'.format(path))
|
33 |
-
state_dict = torch.load(path)['state_dict']
|
34 |
-
print('Finish!')
|
35 |
-
|
36 |
-
if __C.N_GPU > 1:
|
37 |
-
state_dict = ckpt_proc(state_dict)
|
38 |
-
|
39 |
-
# Store the prediction list
|
40 |
-
# qid_list = [ques['question_id'] for ques in dataset.ques_list]
|
41 |
-
ans_ix_list = []
|
42 |
-
pred_list = []
|
43 |
-
|
44 |
-
data_size = dataset.data_size
|
45 |
-
token_size = dataset.token_size
|
46 |
-
ans_size = dataset.ans_size
|
47 |
-
pretrained_emb = dataset.pretrained_emb
|
48 |
-
|
49 |
-
net = ModelLoader(__C).Net(
|
50 |
-
__C,
|
51 |
-
pretrained_emb,
|
52 |
-
token_size,
|
53 |
-
ans_size
|
54 |
-
)
|
55 |
-
net.cuda()
|
56 |
-
net.eval()
|
57 |
-
|
58 |
-
if __C.N_GPU > 1:
|
59 |
-
net = nn.DataParallel(net, device_ids=__C.DEVICES)
|
60 |
-
|
61 |
-
net.load_state_dict(state_dict)
|
62 |
-
|
63 |
-
dataloader = Data.DataLoader(
|
64 |
-
dataset,
|
65 |
-
batch_size=__C.EVAL_BATCH_SIZE,
|
66 |
-
shuffle=False,
|
67 |
-
num_workers=__C.NUM_WORKERS,
|
68 |
-
pin_memory=__C.PIN_MEM
|
69 |
-
)
|
70 |
-
|
71 |
-
for step, (
|
72 |
-
frcn_feat_iter,
|
73 |
-
grid_feat_iter,
|
74 |
-
bbox_feat_iter,
|
75 |
-
ques_ix_iter,
|
76 |
-
ans_iter
|
77 |
-
) in enumerate(dataloader):
|
78 |
-
|
79 |
-
print("\rEvaluation: [step %4d/%4d]" % (
|
80 |
-
step,
|
81 |
-
int(data_size / __C.EVAL_BATCH_SIZE),
|
82 |
-
), end=' ')
|
83 |
-
|
84 |
-
frcn_feat_iter = frcn_feat_iter.cuda()
|
85 |
-
grid_feat_iter = grid_feat_iter.cuda()
|
86 |
-
bbox_feat_iter = bbox_feat_iter.cuda()
|
87 |
-
ques_ix_iter = ques_ix_iter.cuda()
|
88 |
-
|
89 |
-
pred = net(
|
90 |
-
frcn_feat_iter,
|
91 |
-
grid_feat_iter,
|
92 |
-
bbox_feat_iter,
|
93 |
-
ques_ix_iter
|
94 |
-
)
|
95 |
-
pred_np = pred.cpu().data.numpy()
|
96 |
-
pred_argmax = np.argmax(pred_np, axis=1)
|
97 |
-
|
98 |
-
# Save the answer index
|
99 |
-
if pred_argmax.shape[0] != __C.EVAL_BATCH_SIZE:
|
100 |
-
pred_argmax = np.pad(
|
101 |
-
pred_argmax,
|
102 |
-
(0, __C.EVAL_BATCH_SIZE - pred_argmax.shape[0]),
|
103 |
-
mode='constant',
|
104 |
-
constant_values=-1
|
105 |
-
)
|
106 |
-
|
107 |
-
ans_ix_list.append(pred_argmax)
|
108 |
-
|
109 |
-
# Save the whole prediction vector
|
110 |
-
if __C.TEST_SAVE_PRED:
|
111 |
-
if pred_np.shape[0] != __C.EVAL_BATCH_SIZE:
|
112 |
-
pred_np = np.pad(
|
113 |
-
pred_np,
|
114 |
-
((0, __C.EVAL_BATCH_SIZE - pred_np.shape[0]), (0, 0)),
|
115 |
-
mode='constant',
|
116 |
-
constant_values=-1
|
117 |
-
)
|
118 |
-
|
119 |
-
pred_list.append(pred_np)
|
120 |
-
|
121 |
-
print('')
|
122 |
-
ans_ix_list = np.array(ans_ix_list).reshape(-1)
|
123 |
-
|
124 |
-
|
125 |
-
if validation:
|
126 |
-
if __C.RUN_MODE not in ['train']:
|
127 |
-
result_eval_file = __C.CACHE_PATH + '/result_run_' + __C.CKPT_VERSION
|
128 |
-
else:
|
129 |
-
result_eval_file = __C.CACHE_PATH + '/result_run_' + __C.VERSION
|
130 |
-
else:
|
131 |
-
if __C.CKPT_PATH is not None:
|
132 |
-
result_eval_file = __C.RESULT_PATH + '/result_run_' + __C.CKPT_VERSION
|
133 |
-
else:
|
134 |
-
result_eval_file = __C.RESULT_PATH + '/result_run_' + __C.CKPT_VERSION + '_epoch' + str(__C.CKPT_EPOCH)
|
135 |
-
|
136 |
-
|
137 |
-
if __C.CKPT_PATH is not None:
|
138 |
-
ensemble_file = __C.PRED_PATH + '/result_run_' + __C.CKPT_VERSION + '.pkl'
|
139 |
-
else:
|
140 |
-
ensemble_file = __C.PRED_PATH + '/result_run_' + __C.CKPT_VERSION + '_epoch' + str(__C.CKPT_EPOCH) + '.pkl'
|
141 |
-
|
142 |
-
|
143 |
-
if __C.RUN_MODE not in ['train']:
|
144 |
-
log_file = __C.LOG_PATH + '/log_run_' + __C.CKPT_VERSION + '.txt'
|
145 |
-
else:
|
146 |
-
log_file = __C.LOG_PATH + '/log_run_' + __C.VERSION + '.txt'
|
147 |
-
|
148 |
-
EvalLoader(__C).eval(dataset, ans_ix_list, pred_list, result_eval_file, ensemble_file, log_file, validation)
|
149 |
-
|
150 |
-
|
151 |
-
def ckpt_proc(state_dict):
|
152 |
-
state_dict_new = {}
|
153 |
-
for key in state_dict:
|
154 |
-
state_dict_new['module.' + key] = state_dict[key]
|
155 |
-
# state_dict.pop(key)
|
156 |
-
|
157 |
-
return state_dict_new
|
|
|
|
|
|
|
|
|
|
|
|
|
|
|
|
|
|
|
|
|
|
|
|
|
|
|
|
|
|
|
|
|
|
|
|
|
|
|
|
|
|
|
|
|
|
|
|
|
|
|
|
|
|
|
|
|
|
|
|
|
|
|
|
|
|
|
|
|
|
|
|
|
|
|
|
|
|
|
|
|
|
|
|
|
|
|
|
|
|
|
|
|
|
|
|
|
|
|
|
|
|
|
|
|
|
|
|
|
|
|
|
|
|
|
|
|
|
|
|
|
|
|
|
|
|
|
|
|
|
|
|
|
|
|
|
|
|
|
|
|
|
|
|
|
|
|
|
|
|
|
|
|
|
|
|
|
|
|
|
|
|
|
|
|
|
|
|
|
|
|
|
|
|
|
|
|
|
|
|
|
|
|
|
|
|
|
|
|
|
|
|
|
|
|
|
|
|
|
|
|
|
|
|
|
|
|
|
|
|
|
|
|
|
|
|
|
|
|
|
|
|
|
|
|
|
|
|
|
|
|
|
|
|
|
|
|
|
|
|
|
|
|
|
|
|
|
|
|
|
|
|
|
|
|
|
|
|
|
|
|
|
|
|
|
|
|
|
|
|
|
|
|
|
|
|
|
|
|
|
|
|
|
|
|
|
|
|
|
|
|
|
|
|
|
|
|
|
|
|
|
|
|
|
|
|
|
|
|
|
|
|
|
|
|
|
spaces/CVPR/LIVE/pybind11/tests/constructor_stats.h
DELETED
@@ -1,275 +0,0 @@
|
|
1 |
-
#pragma once
|
2 |
-
/*
|
3 |
-
tests/constructor_stats.h -- framework for printing and tracking object
|
4 |
-
instance lifetimes in example/test code.
|
5 |
-
|
6 |
-
Copyright (c) 2016 Jason Rhinelander <[email protected]>
|
7 |
-
|
8 |
-
All rights reserved. Use of this source code is governed by a
|
9 |
-
BSD-style license that can be found in the LICENSE file.
|
10 |
-
|
11 |
-
This header provides a few useful tools for writing examples or tests that want to check and/or
|
12 |
-
display object instance lifetimes. It requires that you include this header and add the following
|
13 |
-
function calls to constructors:
|
14 |
-
|
15 |
-
class MyClass {
|
16 |
-
MyClass() { ...; print_default_created(this); }
|
17 |
-
~MyClass() { ...; print_destroyed(this); }
|
18 |
-
MyClass(const MyClass &c) { ...; print_copy_created(this); }
|
19 |
-
MyClass(MyClass &&c) { ...; print_move_created(this); }
|
20 |
-
MyClass(int a, int b) { ...; print_created(this, a, b); }
|
21 |
-
MyClass &operator=(const MyClass &c) { ...; print_copy_assigned(this); }
|
22 |
-
MyClass &operator=(MyClass &&c) { ...; print_move_assigned(this); }
|
23 |
-
|
24 |
-
...
|
25 |
-
}
|
26 |
-
|
27 |
-
You can find various examples of these in several of the existing testing .cpp files. (Of course
|
28 |
-
you don't need to add any of the above constructors/operators that you don't actually have, except
|
29 |
-
for the destructor).
|
30 |
-
|
31 |
-
Each of these will print an appropriate message such as:
|
32 |
-
|
33 |
-
### MyClass @ 0x2801910 created via default constructor
|
34 |
-
### MyClass @ 0x27fa780 created 100 200
|
35 |
-
### MyClass @ 0x2801910 destroyed
|
36 |
-
### MyClass @ 0x27fa780 destroyed
|
37 |
-
|
38 |
-
You can also include extra arguments (such as the 100, 200 in the output above, coming from the
|
39 |
-
value constructor) for all of the above methods which will be included in the output.
|
40 |
-
|
41 |
-
For testing, each of these also keeps track the created instances and allows you to check how many
|
42 |
-
of the various constructors have been invoked from the Python side via code such as:
|
43 |
-
|
44 |
-
from pybind11_tests import ConstructorStats
|
45 |
-
cstats = ConstructorStats.get(MyClass)
|
46 |
-
print(cstats.alive())
|
47 |
-
print(cstats.default_constructions)
|
48 |
-
|
49 |
-
Note that `.alive()` should usually be the first thing you call as it invokes Python's garbage
|
50 |
-
collector to actually destroy objects that aren't yet referenced.
|
51 |
-
|
52 |
-
For everything except copy and move constructors and destructors, any extra values given to the
|
53 |
-
print_...() function is stored in a class-specific values list which you can retrieve and inspect
|
54 |
-
from the ConstructorStats instance `.values()` method.
|
55 |
-
|
56 |
-
In some cases, when you need to track instances of a C++ class not registered with pybind11, you
|
57 |
-
need to add a function returning the ConstructorStats for the C++ class; this can be done with:
|
58 |
-
|
59 |
-
m.def("get_special_cstats", &ConstructorStats::get<SpecialClass>, py::return_value_policy::reference)
|
60 |
-
|
61 |
-
Finally, you can suppress the output messages, but keep the constructor tracking (for
|
62 |
-
inspection/testing in python) by using the functions with `print_` replaced with `track_` (e.g.
|
63 |
-
`track_copy_created(this)`).
|
64 |
-
|
65 |
-
*/
|
66 |
-
|
67 |
-
#include "pybind11_tests.h"
|
68 |
-
#include <unordered_map>
|
69 |
-
#include <list>
|
70 |
-
#include <typeindex>
|
71 |
-
#include <sstream>
|
72 |
-
|
73 |
-
class ConstructorStats {
|
74 |
-
protected:
|
75 |
-
std::unordered_map<void*, int> _instances; // Need a map rather than set because members can shared address with parents
|
76 |
-
std::list<std::string> _values; // Used to track values (e.g. of value constructors)
|
77 |
-
public:
|
78 |
-
int default_constructions = 0;
|
79 |
-
int copy_constructions = 0;
|
80 |
-
int move_constructions = 0;
|
81 |
-
int copy_assignments = 0;
|
82 |
-
int move_assignments = 0;
|
83 |
-
|
84 |
-
void copy_created(void *inst) {
|
85 |
-
created(inst);
|
86 |
-
copy_constructions++;
|
87 |
-
}
|
88 |
-
|
89 |
-
void move_created(void *inst) {
|
90 |
-
created(inst);
|
91 |
-
move_constructions++;
|
92 |
-
}
|
93 |
-
|
94 |
-
void default_created(void *inst) {
|
95 |
-
created(inst);
|
96 |
-
default_constructions++;
|
97 |
-
}
|
98 |
-
|
99 |
-
void created(void *inst) {
|
100 |
-
++_instances[inst];
|
101 |
-
}
|
102 |
-
|
103 |
-
void destroyed(void *inst) {
|
104 |
-
if (--_instances[inst] < 0)
|
105 |
-
throw std::runtime_error("cstats.destroyed() called with unknown "
|
106 |
-
"instance; potential double-destruction "
|
107 |
-
"or a missing cstats.created()");
|
108 |
-
}
|
109 |
-
|
110 |
-
static void gc() {
|
111 |
-
// Force garbage collection to ensure any pending destructors are invoked:
|
112 |
-
#if defined(PYPY_VERSION)
|
113 |
-
PyObject *globals = PyEval_GetGlobals();
|
114 |
-
PyObject *result = PyRun_String(
|
115 |
-
"import gc\n"
|
116 |
-
"for i in range(2):"
|
117 |
-
" gc.collect()\n",
|
118 |
-
Py_file_input, globals, globals);
|
119 |
-
if (result == nullptr)
|
120 |
-
throw py::error_already_set();
|
121 |
-
Py_DECREF(result);
|
122 |
-
#else
|
123 |
-
py::module::import("gc").attr("collect")();
|
124 |
-
#endif
|
125 |
-
}
|
126 |
-
|
127 |
-
int alive() {
|
128 |
-
gc();
|
129 |
-
int total = 0;
|
130 |
-
for (const auto &p : _instances)
|
131 |
-
if (p.second > 0)
|
132 |
-
total += p.second;
|
133 |
-
return total;
|
134 |
-
}
|
135 |
-
|
136 |
-
void value() {} // Recursion terminator
|
137 |
-
// Takes one or more values, converts them to strings, then stores them.
|
138 |
-
template <typename T, typename... Tmore> void value(const T &v, Tmore &&...args) {
|
139 |
-
std::ostringstream oss;
|
140 |
-
oss << v;
|
141 |
-
_values.push_back(oss.str());
|
142 |
-
value(std::forward<Tmore>(args)...);
|
143 |
-
}
|
144 |
-
|
145 |
-
// Move out stored values
|
146 |
-
py::list values() {
|
147 |
-
py::list l;
|
148 |
-
for (const auto &v : _values) l.append(py::cast(v));
|
149 |
-
_values.clear();
|
150 |
-
return l;
|
151 |
-
}
|
152 |
-
|
153 |
-
// Gets constructor stats from a C++ type index
|
154 |
-
static ConstructorStats& get(std::type_index type) {
|
155 |
-
static std::unordered_map<std::type_index, ConstructorStats> all_cstats;
|
156 |
-
return all_cstats[type];
|
157 |
-
}
|
158 |
-
|
159 |
-
// Gets constructor stats from a C++ type
|
160 |
-
template <typename T> static ConstructorStats& get() {
|
161 |
-
#if defined(PYPY_VERSION)
|
162 |
-
gc();
|
163 |
-
#endif
|
164 |
-
return get(typeid(T));
|
165 |
-
}
|
166 |
-
|
167 |
-
// Gets constructor stats from a Python class
|
168 |
-
static ConstructorStats& get(py::object class_) {
|
169 |
-
auto &internals = py::detail::get_internals();
|
170 |
-
const std::type_index *t1 = nullptr, *t2 = nullptr;
|
171 |
-
try {
|
172 |
-
auto *type_info = internals.registered_types_py.at((PyTypeObject *) class_.ptr()).at(0);
|
173 |
-
for (auto &p : internals.registered_types_cpp) {
|
174 |
-
if (p.second == type_info) {
|
175 |
-
if (t1) {
|
176 |
-
t2 = &p.first;
|
177 |
-
break;
|
178 |
-
}
|
179 |
-
t1 = &p.first;
|
180 |
-
}
|
181 |
-
}
|
182 |
-
}
|
183 |
-
catch (const std::out_of_range&) {}
|
184 |
-
if (!t1) throw std::runtime_error("Unknown class passed to ConstructorStats::get()");
|
185 |
-
auto &cs1 = get(*t1);
|
186 |
-
// If we have both a t1 and t2 match, one is probably the trampoline class; return whichever
|
187 |
-
// has more constructions (typically one or the other will be 0)
|
188 |
-
if (t2) {
|
189 |
-
auto &cs2 = get(*t2);
|
190 |
-
int cs1_total = cs1.default_constructions + cs1.copy_constructions + cs1.move_constructions + (int) cs1._values.size();
|
191 |
-
int cs2_total = cs2.default_constructions + cs2.copy_constructions + cs2.move_constructions + (int) cs2._values.size();
|
192 |
-
if (cs2_total > cs1_total) return cs2;
|
193 |
-
}
|
194 |
-
return cs1;
|
195 |
-
}
|
196 |
-
};
|
197 |
-
|
198 |
-
// To track construction/destruction, you need to call these methods from the various
|
199 |
-
// constructors/operators. The ones that take extra values record the given values in the
|
200 |
-
// constructor stats values for later inspection.
|
201 |
-
template <class T> void track_copy_created(T *inst) { ConstructorStats::get<T>().copy_created(inst); }
|
202 |
-
template <class T> void track_move_created(T *inst) { ConstructorStats::get<T>().move_created(inst); }
|
203 |
-
template <class T, typename... Values> void track_copy_assigned(T *, Values &&...values) {
|
204 |
-
auto &cst = ConstructorStats::get<T>();
|
205 |
-
cst.copy_assignments++;
|
206 |
-
cst.value(std::forward<Values>(values)...);
|
207 |
-
}
|
208 |
-
template <class T, typename... Values> void track_move_assigned(T *, Values &&...values) {
|
209 |
-
auto &cst = ConstructorStats::get<T>();
|
210 |
-
cst.move_assignments++;
|
211 |
-
cst.value(std::forward<Values>(values)...);
|
212 |
-
}
|
213 |
-
template <class T, typename... Values> void track_default_created(T *inst, Values &&...values) {
|
214 |
-
auto &cst = ConstructorStats::get<T>();
|
215 |
-
cst.default_created(inst);
|
216 |
-
cst.value(std::forward<Values>(values)...);
|
217 |
-
}
|
218 |
-
template <class T, typename... Values> void track_created(T *inst, Values &&...values) {
|
219 |
-
auto &cst = ConstructorStats::get<T>();
|
220 |
-
cst.created(inst);
|
221 |
-
cst.value(std::forward<Values>(values)...);
|
222 |
-
}
|
223 |
-
template <class T, typename... Values> void track_destroyed(T *inst) {
|
224 |
-
ConstructorStats::get<T>().destroyed(inst);
|
225 |
-
}
|
226 |
-
template <class T, typename... Values> void track_values(T *, Values &&...values) {
|
227 |
-
ConstructorStats::get<T>().value(std::forward<Values>(values)...);
|
228 |
-
}
|
229 |
-
|
230 |
-
/// Don't cast pointers to Python, print them as strings
|
231 |
-
inline const char *format_ptrs(const char *p) { return p; }
|
232 |
-
template <typename T>
|
233 |
-
py::str format_ptrs(T *p) { return "{:#x}"_s.format(reinterpret_cast<std::uintptr_t>(p)); }
|
234 |
-
template <typename T>
|
235 |
-
auto format_ptrs(T &&x) -> decltype(std::forward<T>(x)) { return std::forward<T>(x); }
|
236 |
-
|
237 |
-
template <class T, typename... Output>
|
238 |
-
void print_constr_details(T *inst, const std::string &action, Output &&...output) {
|
239 |
-
py::print("###", py::type_id<T>(), "@", format_ptrs(inst), action,
|
240 |
-
format_ptrs(std::forward<Output>(output))...);
|
241 |
-
}
|
242 |
-
|
243 |
-
// Verbose versions of the above:
|
244 |
-
template <class T, typename... Values> void print_copy_created(T *inst, Values &&...values) { // NB: this prints, but doesn't store, given values
|
245 |
-
print_constr_details(inst, "created via copy constructor", values...);
|
246 |
-
track_copy_created(inst);
|
247 |
-
}
|
248 |
-
template <class T, typename... Values> void print_move_created(T *inst, Values &&...values) { // NB: this prints, but doesn't store, given values
|
249 |
-
print_constr_details(inst, "created via move constructor", values...);
|
250 |
-
track_move_created(inst);
|
251 |
-
}
|
252 |
-
template <class T, typename... Values> void print_copy_assigned(T *inst, Values &&...values) {
|
253 |
-
print_constr_details(inst, "assigned via copy assignment", values...);
|
254 |
-
track_copy_assigned(inst, values...);
|
255 |
-
}
|
256 |
-
template <class T, typename... Values> void print_move_assigned(T *inst, Values &&...values) {
|
257 |
-
print_constr_details(inst, "assigned via move assignment", values...);
|
258 |
-
track_move_assigned(inst, values...);
|
259 |
-
}
|
260 |
-
template <class T, typename... Values> void print_default_created(T *inst, Values &&...values) {
|
261 |
-
print_constr_details(inst, "created via default constructor", values...);
|
262 |
-
track_default_created(inst, values...);
|
263 |
-
}
|
264 |
-
template <class T, typename... Values> void print_created(T *inst, Values &&...values) {
|
265 |
-
print_constr_details(inst, "created", values...);
|
266 |
-
track_created(inst, values...);
|
267 |
-
}
|
268 |
-
template <class T, typename... Values> void print_destroyed(T *inst, Values &&...values) { // Prints but doesn't store given values
|
269 |
-
print_constr_details(inst, "destroyed", values...);
|
270 |
-
track_destroyed(inst);
|
271 |
-
}
|
272 |
-
template <class T, typename... Values> void print_values(T *inst, Values &&...values) {
|
273 |
-
print_constr_details(inst, ":", values...);
|
274 |
-
track_values(inst, values...);
|
275 |
-
}
|
|
|
|
|
|
|
|
|
|
|
|
|
|
|
|
|
|
|
|
|
|
|
|
|
|
|
|
|
|
|
|
|
|
|
|
|
|
|
|
|
|
|
|
|
|
|
|
|
|
|
|
|
|
|
|
|
|
|
|
|
|
|
|
|
|
|
|
|
|
|
|
|
|
|
|
|
|
|
|
|
|
|
|
|
|
|
|
|
|
|
|
|
|
|
|
|
|
|
|
|
|
|
|
|
|
|
|
|
|
|
|
|
|
|
|
|
|
|
|
|
|
|
|
|
|
|
|
|
|
|
|
|
|
|
|
|
|
|
|
|
|
|
|
|
|
|
|
|
|
|
|
|
|
|
|
|
|
|
|
|
|
|
|
|
|
|
|
|
|
|
|
|
|
|
|
|
|
|
|
|
|
|
|
|
|
|
|
|
|
|
|
|
|
|
|
|
|
|
|
|
|
|
|
|
|
|
|
|
|
|
|
|
|
|
|
|
|
|
|
|
|
|
|
|
|
|
|
|
|
|
|
|
|
|
|
|
|
|
|
|
|
|
|
|
|
|
|
|
|
|
|
|
|
|
|
|
|
|
|
|
|
|
|
|
|
|
|
|
|
|
|
|
|
|
|
|
|
|
|
|
|
|
|
|
|
|
|
|
|
|
|
|
|
|
|
|
|
|
|
|
|
|
|
|
|
|
|
|
|
|
|
|
|
|
|
|
|
|
|
|
|
|
|
|
|
|
|
|
|
|
|
|
|
|
|
|
|
|
|
|
|
|
|
|
|
|
|
|
|
|
|
|
|
|
|
|
|
|
|
|
|
|
|
|
|
|
|
|
|
|
|
|
|
|
|
|
|
|
|
|
|
|
|
|
|
|
|
|
|
|
|
|
|
|
|
|
|
|
|
|
|
|
|
|
|
|
|
|
|
|
|
|
|
|
|
|
|
|
|
|
|
|
|
|
|
|
|
|
|
|
|
|
|
|
|
|
|
|
|
|
|
|
|
|
|
|
|
|
|
|
|
|
|
|
|
|
|
|
|
|
|
|
|
|
|
|
|
|
|
|
|
|
|
|
|
|
|
|
|
|
|
|
|
|
|
|
|
|
|
|
|
|
|
|
|
|
|
|
|
|
|
|
|
|
|
|
|
|
|
|
|
|
|
|
|
|
|
|
|
|
|
|
|
|
|
|
|
|
|
|
|
|
|
|
|
|
|
|
|
|
|
|
|
|
|
|
|
|
|
|
|
spaces/CVPR/LIVE/pybind11/tests/test_call_policies.py
DELETED
@@ -1,192 +0,0 @@
|
|
1 |
-
# -*- coding: utf-8 -*-
|
2 |
-
import pytest
|
3 |
-
|
4 |
-
import env # noqa: F401
|
5 |
-
|
6 |
-
from pybind11_tests import call_policies as m
|
7 |
-
from pybind11_tests import ConstructorStats
|
8 |
-
|
9 |
-
|
10 |
-
@pytest.mark.xfail("env.PYPY", reason="sometimes comes out 1 off on PyPy", strict=False)
|
11 |
-
def test_keep_alive_argument(capture):
|
12 |
-
n_inst = ConstructorStats.detail_reg_inst()
|
13 |
-
with capture:
|
14 |
-
p = m.Parent()
|
15 |
-
assert capture == "Allocating parent."
|
16 |
-
with capture:
|
17 |
-
p.addChild(m.Child())
|
18 |
-
assert ConstructorStats.detail_reg_inst() == n_inst + 1
|
19 |
-
assert capture == """
|
20 |
-
Allocating child.
|
21 |
-
Releasing child.
|
22 |
-
"""
|
23 |
-
with capture:
|
24 |
-
del p
|
25 |
-
assert ConstructorStats.detail_reg_inst() == n_inst
|
26 |
-
assert capture == "Releasing parent."
|
27 |
-
|
28 |
-
with capture:
|
29 |
-
p = m.Parent()
|
30 |
-
assert capture == "Allocating parent."
|
31 |
-
with capture:
|
32 |
-
p.addChildKeepAlive(m.Child())
|
33 |
-
assert ConstructorStats.detail_reg_inst() == n_inst + 2
|
34 |
-
assert capture == "Allocating child."
|
35 |
-
with capture:
|
36 |
-
del p
|
37 |
-
assert ConstructorStats.detail_reg_inst() == n_inst
|
38 |
-
assert capture == """
|
39 |
-
Releasing parent.
|
40 |
-
Releasing child.
|
41 |
-
"""
|
42 |
-
|
43 |
-
|
44 |
-
def test_keep_alive_return_value(capture):
|
45 |
-
n_inst = ConstructorStats.detail_reg_inst()
|
46 |
-
with capture:
|
47 |
-
p = m.Parent()
|
48 |
-
assert capture == "Allocating parent."
|
49 |
-
with capture:
|
50 |
-
p.returnChild()
|
51 |
-
assert ConstructorStats.detail_reg_inst() == n_inst + 1
|
52 |
-
assert capture == """
|
53 |
-
Allocating child.
|
54 |
-
Releasing child.
|
55 |
-
"""
|
56 |
-
with capture:
|
57 |
-
del p
|
58 |
-
assert ConstructorStats.detail_reg_inst() == n_inst
|
59 |
-
assert capture == "Releasing parent."
|
60 |
-
|
61 |
-
with capture:
|
62 |
-
p = m.Parent()
|
63 |
-
assert capture == "Allocating parent."
|
64 |
-
with capture:
|
65 |
-
p.returnChildKeepAlive()
|
66 |
-
assert ConstructorStats.detail_reg_inst() == n_inst + 2
|
67 |
-
assert capture == "Allocating child."
|
68 |
-
with capture:
|
69 |
-
del p
|
70 |
-
assert ConstructorStats.detail_reg_inst() == n_inst
|
71 |
-
assert capture == """
|
72 |
-
Releasing parent.
|
73 |
-
Releasing child.
|
74 |
-
"""
|
75 |
-
|
76 |
-
|
77 |
-
# https://foss.heptapod.net/pypy/pypy/-/issues/2447
|
78 |
-
@pytest.mark.xfail("env.PYPY", reason="_PyObject_GetDictPtr is unimplemented")
|
79 |
-
def test_alive_gc(capture):
|
80 |
-
n_inst = ConstructorStats.detail_reg_inst()
|
81 |
-
p = m.ParentGC()
|
82 |
-
p.addChildKeepAlive(m.Child())
|
83 |
-
assert ConstructorStats.detail_reg_inst() == n_inst + 2
|
84 |
-
lst = [p]
|
85 |
-
lst.append(lst) # creates a circular reference
|
86 |
-
with capture:
|
87 |
-
del p, lst
|
88 |
-
assert ConstructorStats.detail_reg_inst() == n_inst
|
89 |
-
assert capture == """
|
90 |
-
Releasing parent.
|
91 |
-
Releasing child.
|
92 |
-
"""
|
93 |
-
|
94 |
-
|
95 |
-
def test_alive_gc_derived(capture):
|
96 |
-
class Derived(m.Parent):
|
97 |
-
pass
|
98 |
-
|
99 |
-
n_inst = ConstructorStats.detail_reg_inst()
|
100 |
-
p = Derived()
|
101 |
-
p.addChildKeepAlive(m.Child())
|
102 |
-
assert ConstructorStats.detail_reg_inst() == n_inst + 2
|
103 |
-
lst = [p]
|
104 |
-
lst.append(lst) # creates a circular reference
|
105 |
-
with capture:
|
106 |
-
del p, lst
|
107 |
-
assert ConstructorStats.detail_reg_inst() == n_inst
|
108 |
-
assert capture == """
|
109 |
-
Releasing parent.
|
110 |
-
Releasing child.
|
111 |
-
"""
|
112 |
-
|
113 |
-
|
114 |
-
def test_alive_gc_multi_derived(capture):
|
115 |
-
class Derived(m.Parent, m.Child):
|
116 |
-
def __init__(self):
|
117 |
-
m.Parent.__init__(self)
|
118 |
-
m.Child.__init__(self)
|
119 |
-
|
120 |
-
n_inst = ConstructorStats.detail_reg_inst()
|
121 |
-
p = Derived()
|
122 |
-
p.addChildKeepAlive(m.Child())
|
123 |
-
# +3 rather than +2 because Derived corresponds to two registered instances
|
124 |
-
assert ConstructorStats.detail_reg_inst() == n_inst + 3
|
125 |
-
lst = [p]
|
126 |
-
lst.append(lst) # creates a circular reference
|
127 |
-
with capture:
|
128 |
-
del p, lst
|
129 |
-
assert ConstructorStats.detail_reg_inst() == n_inst
|
130 |
-
assert capture == """
|
131 |
-
Releasing parent.
|
132 |
-
Releasing child.
|
133 |
-
Releasing child.
|
134 |
-
"""
|
135 |
-
|
136 |
-
|
137 |
-
def test_return_none(capture):
|
138 |
-
n_inst = ConstructorStats.detail_reg_inst()
|
139 |
-
with capture:
|
140 |
-
p = m.Parent()
|
141 |
-
assert capture == "Allocating parent."
|
142 |
-
with capture:
|
143 |
-
p.returnNullChildKeepAliveChild()
|
144 |
-
assert ConstructorStats.detail_reg_inst() == n_inst + 1
|
145 |
-
assert capture == ""
|
146 |
-
with capture:
|
147 |
-
del p
|
148 |
-
assert ConstructorStats.detail_reg_inst() == n_inst
|
149 |
-
assert capture == "Releasing parent."
|
150 |
-
|
151 |
-
with capture:
|
152 |
-
p = m.Parent()
|
153 |
-
assert capture == "Allocating parent."
|
154 |
-
with capture:
|
155 |
-
p.returnNullChildKeepAliveParent()
|
156 |
-
assert ConstructorStats.detail_reg_inst() == n_inst + 1
|
157 |
-
assert capture == ""
|
158 |
-
with capture:
|
159 |
-
del p
|
160 |
-
assert ConstructorStats.detail_reg_inst() == n_inst
|
161 |
-
assert capture == "Releasing parent."
|
162 |
-
|
163 |
-
|
164 |
-
def test_keep_alive_constructor(capture):
|
165 |
-
n_inst = ConstructorStats.detail_reg_inst()
|
166 |
-
|
167 |
-
with capture:
|
168 |
-
p = m.Parent(m.Child())
|
169 |
-
assert ConstructorStats.detail_reg_inst() == n_inst + 2
|
170 |
-
assert capture == """
|
171 |
-
Allocating child.
|
172 |
-
Allocating parent.
|
173 |
-
"""
|
174 |
-
with capture:
|
175 |
-
del p
|
176 |
-
assert ConstructorStats.detail_reg_inst() == n_inst
|
177 |
-
assert capture == """
|
178 |
-
Releasing parent.
|
179 |
-
Releasing child.
|
180 |
-
"""
|
181 |
-
|
182 |
-
|
183 |
-
def test_call_guard():
|
184 |
-
assert m.unguarded_call() == "unguarded"
|
185 |
-
assert m.guarded_call() == "guarded"
|
186 |
-
|
187 |
-
assert m.multiple_guards_correct_order() == "guarded & guarded"
|
188 |
-
assert m.multiple_guards_wrong_order() == "unguarded & guarded"
|
189 |
-
|
190 |
-
if hasattr(m, "with_gil"):
|
191 |
-
assert m.with_gil() == "GIL held"
|
192 |
-
assert m.without_gil() == "GIL released"
|
|
|
|
|
|
|
|
|
|
|
|
|
|
|
|
|
|
|
|
|
|
|
|
|
|
|
|
|
|
|
|
|
|
|
|
|
|
|
|
|
|
|
|
|
|
|
|
|
|
|
|
|
|
|
|
|
|
|
|
|
|
|
|
|
|
|
|
|
|
|
|
|
|
|
|
|
|
|
|
|
|
|
|
|
|
|
|
|
|
|
|
|
|
|
|
|
|
|
|
|
|
|
|
|
|
|
|
|
|
|
|
|
|
|
|
|
|
|
|
|
|
|
|
|
|
|
|
|
|
|
|
|
|
|
|
|
|
|
|
|
|
|
|
|
|
|
|
|
|
|
|
|
|
|
|
|
|
|
|
|
|
|
|
|
|
|
|
|
|
|
|
|
|
|
|
|
|
|
|
|
|
|
|
|
|
|
|
|
|
|
|
|
|
|
|
|
|
|
|
|
|
|
|
|
|
|
|
|
|
|
|
|
|
|
|
|
|
|
|
|
|
|
|
|
|
|
|
|
|
|
|
|
|
|
|
|
|
|
|
|
|
|
|
|
|
|
|
|
|
|
|
|
|
|
|
|
|
|
|
|
|
|
|
|
|
|
|
|
|
|
|
|
|
|
|
|
|
|
|
|
|
|
|
|
|
|
|
|
|
|
|
|
|
|
|
|
|
|
|
|
|
|
|
|
|
|
|
|
|
|
|
|
|
|
|
|
|
|
|
|
|
|
|
|
|
|
|
|
|
|
|
|
|
|
|
|
|
|
|
|
|
|
|
|
|
|
|
|
|
|
|
|
|
|
|
|
|
|
|
|
|
|
|
|
|
|
|
|
|
|
|
|
|
|
|
|
|
|
|
|
|
|
|
|
|
spaces/CVPR/SPOTER_Sign_Language_Recognition/spoter_mod/sweep-agent.sh
DELETED
@@ -1,18 +0,0 @@
|
|
1 |
-
|
2 |
-
#PBS -N spoter-zhuo-sweep
|
3 |
-
#PBS -q gpu
|
4 |
-
#PBS -l walltime=24:00:00
|
5 |
-
|
6 |
-
#PBS -l select=1:ncpus=1:ngpus=1:cluster=adan:mem=10gb
|
7 |
-
#PBS -j oe
|
8 |
-
#PBS -m ae
|
9 |
-
|
10 |
-
echo "Experiment starting..."
|
11 |
-
|
12 |
-
cd /storage/plzen4-ntis/home/mbohacek/spoter-zhuo
|
13 |
-
|
14 |
-
module add conda-modules
|
15 |
-
conda activate cslr-transformers
|
16 |
-
|
17 |
-
wandb agent matyasbohacek/Zhuo-collab-SPOTER-Sweep/bh6fc056
|
18 |
-
|
|
|
|
|
|
|
|
|
|
|
|
|
|
|
|
|
|
|
|
|
|
|
|
|
|
|
|
|
|
|
|
|
|
|
|
|
|
spaces/CVPR/regionclip-demo/detectron2/data/transforms/torchvision_transforms/functional.py
DELETED
@@ -1,1365 +0,0 @@
|
|
1 |
-
import math
|
2 |
-
import numbers
|
3 |
-
import warnings
|
4 |
-
from enum import Enum
|
5 |
-
|
6 |
-
import numpy as np
|
7 |
-
from PIL import Image
|
8 |
-
|
9 |
-
import torch
|
10 |
-
from torch import Tensor
|
11 |
-
from typing import List, Tuple, Any, Optional
|
12 |
-
|
13 |
-
try:
|
14 |
-
import accimage
|
15 |
-
except ImportError:
|
16 |
-
accimage = None
|
17 |
-
|
18 |
-
from . import functional_pil as F_pil
|
19 |
-
from . import functional_tensor as F_t
|
20 |
-
|
21 |
-
|
22 |
-
class InterpolationMode(Enum):
|
23 |
-
"""Interpolation modes
|
24 |
-
Available interpolation methods are ``nearest``, ``bilinear``, ``bicubic``, ``box``, ``hamming``, and ``lanczos``.
|
25 |
-
"""
|
26 |
-
NEAREST = "nearest"
|
27 |
-
BILINEAR = "bilinear"
|
28 |
-
BICUBIC = "bicubic"
|
29 |
-
# For PIL compatibility
|
30 |
-
BOX = "box"
|
31 |
-
HAMMING = "hamming"
|
32 |
-
LANCZOS = "lanczos"
|
33 |
-
|
34 |
-
|
35 |
-
# TODO: Once torchscript supports Enums with staticmethod
|
36 |
-
# this can be put into InterpolationMode as staticmethod
|
37 |
-
def _interpolation_modes_from_int(i: int) -> InterpolationMode:
|
38 |
-
inverse_modes_mapping = {
|
39 |
-
0: InterpolationMode.NEAREST,
|
40 |
-
2: InterpolationMode.BILINEAR,
|
41 |
-
3: InterpolationMode.BICUBIC,
|
42 |
-
4: InterpolationMode.BOX,
|
43 |
-
5: InterpolationMode.HAMMING,
|
44 |
-
1: InterpolationMode.LANCZOS,
|
45 |
-
}
|
46 |
-
return inverse_modes_mapping[i]
|
47 |
-
|
48 |
-
|
49 |
-
pil_modes_mapping = {
|
50 |
-
InterpolationMode.NEAREST: 0,
|
51 |
-
InterpolationMode.BILINEAR: 2,
|
52 |
-
InterpolationMode.BICUBIC: 3,
|
53 |
-
InterpolationMode.BOX: 4,
|
54 |
-
InterpolationMode.HAMMING: 5,
|
55 |
-
InterpolationMode.LANCZOS: 1,
|
56 |
-
}
|
57 |
-
|
58 |
-
_is_pil_image = F_pil._is_pil_image
|
59 |
-
|
60 |
-
|
61 |
-
def _get_image_size(img: Tensor) -> List[int]:
|
62 |
-
"""Returns image size as [w, h]
|
63 |
-
"""
|
64 |
-
if isinstance(img, torch.Tensor):
|
65 |
-
return F_t._get_image_size(img)
|
66 |
-
|
67 |
-
return F_pil._get_image_size(img)
|
68 |
-
|
69 |
-
|
70 |
-
def _get_image_num_channels(img: Tensor) -> int:
|
71 |
-
"""Returns number of image channels
|
72 |
-
"""
|
73 |
-
if isinstance(img, torch.Tensor):
|
74 |
-
return F_t._get_image_num_channels(img)
|
75 |
-
|
76 |
-
return F_pil._get_image_num_channels(img)
|
77 |
-
|
78 |
-
|
79 |
-
@torch.jit.unused
|
80 |
-
def _is_numpy(img: Any) -> bool:
|
81 |
-
return isinstance(img, np.ndarray)
|
82 |
-
|
83 |
-
|
84 |
-
@torch.jit.unused
|
85 |
-
def _is_numpy_image(img: Any) -> bool:
|
86 |
-
return img.ndim in {2, 3}
|
87 |
-
|
88 |
-
|
89 |
-
def to_tensor(pic):
|
90 |
-
"""Convert a ``PIL Image`` or ``numpy.ndarray`` to tensor.
|
91 |
-
This function does not support torchscript.
|
92 |
-
|
93 |
-
See :class:`~torchvision.transforms.ToTensor` for more details.
|
94 |
-
|
95 |
-
Args:
|
96 |
-
pic (PIL Image or numpy.ndarray): Image to be converted to tensor.
|
97 |
-
|
98 |
-
Returns:
|
99 |
-
Tensor: Converted image.
|
100 |
-
"""
|
101 |
-
if not(F_pil._is_pil_image(pic) or _is_numpy(pic)):
|
102 |
-
raise TypeError('pic should be PIL Image or ndarray. Got {}'.format(type(pic)))
|
103 |
-
|
104 |
-
if _is_numpy(pic) and not _is_numpy_image(pic):
|
105 |
-
raise ValueError('pic should be 2/3 dimensional. Got {} dimensions.'.format(pic.ndim))
|
106 |
-
|
107 |
-
default_float_dtype = torch.get_default_dtype()
|
108 |
-
|
109 |
-
if isinstance(pic, np.ndarray):
|
110 |
-
# handle numpy array
|
111 |
-
if pic.ndim == 2:
|
112 |
-
pic = pic[:, :, None]
|
113 |
-
|
114 |
-
img = torch.from_numpy(pic.transpose((2, 0, 1))).contiguous()
|
115 |
-
# backward compatibility
|
116 |
-
if isinstance(img, torch.ByteTensor):
|
117 |
-
return img.to(dtype=default_float_dtype).div(255)
|
118 |
-
else:
|
119 |
-
return img
|
120 |
-
|
121 |
-
if accimage is not None and isinstance(pic, accimage.Image):
|
122 |
-
nppic = np.zeros([pic.channels, pic.height, pic.width], dtype=np.float32)
|
123 |
-
pic.copyto(nppic)
|
124 |
-
return torch.from_numpy(nppic).to(dtype=default_float_dtype)
|
125 |
-
|
126 |
-
# handle PIL Image
|
127 |
-
mode_to_nptype = {'I': np.int32, 'I;16': np.int16, 'F': np.float32}
|
128 |
-
img = torch.from_numpy(
|
129 |
-
np.array(pic, mode_to_nptype.get(pic.mode, np.uint8), copy=True)
|
130 |
-
)
|
131 |
-
|
132 |
-
if pic.mode == '1':
|
133 |
-
img = 255 * img
|
134 |
-
img = img.view(pic.size[1], pic.size[0], len(pic.getbands()))
|
135 |
-
# put it from HWC to CHW format
|
136 |
-
img = img.permute((2, 0, 1)).contiguous()
|
137 |
-
if isinstance(img, torch.ByteTensor):
|
138 |
-
return img.to(dtype=default_float_dtype).div(255)
|
139 |
-
else:
|
140 |
-
return img
|
141 |
-
|
142 |
-
|
143 |
-
def pil_to_tensor(pic):
|
144 |
-
"""Convert a ``PIL Image`` to a tensor of the same type.
|
145 |
-
This function does not support torchscript.
|
146 |
-
|
147 |
-
See :class:`~torchvision.transforms.PILToTensor` for more details.
|
148 |
-
|
149 |
-
Args:
|
150 |
-
pic (PIL Image): Image to be converted to tensor.
|
151 |
-
|
152 |
-
Returns:
|
153 |
-
Tensor: Converted image.
|
154 |
-
"""
|
155 |
-
if not F_pil._is_pil_image(pic):
|
156 |
-
raise TypeError('pic should be PIL Image. Got {}'.format(type(pic)))
|
157 |
-
|
158 |
-
if accimage is not None and isinstance(pic, accimage.Image):
|
159 |
-
# accimage format is always uint8 internally, so always return uint8 here
|
160 |
-
nppic = np.zeros([pic.channels, pic.height, pic.width], dtype=np.uint8)
|
161 |
-
pic.copyto(nppic)
|
162 |
-
return torch.as_tensor(nppic)
|
163 |
-
|
164 |
-
# handle PIL Image
|
165 |
-
img = torch.as_tensor(np.asarray(pic))
|
166 |
-
img = img.view(pic.size[1], pic.size[0], len(pic.getbands()))
|
167 |
-
# put it from HWC to CHW format
|
168 |
-
img = img.permute((2, 0, 1))
|
169 |
-
return img
|
170 |
-
|
171 |
-
|
172 |
-
def convert_image_dtype(image: torch.Tensor, dtype: torch.dtype = torch.float) -> torch.Tensor:
|
173 |
-
"""Convert a tensor image to the given ``dtype`` and scale the values accordingly
|
174 |
-
This function does not support PIL Image.
|
175 |
-
|
176 |
-
Args:
|
177 |
-
image (torch.Tensor): Image to be converted
|
178 |
-
dtype (torch.dtype): Desired data type of the output
|
179 |
-
|
180 |
-
Returns:
|
181 |
-
Tensor: Converted image
|
182 |
-
|
183 |
-
.. note::
|
184 |
-
|
185 |
-
When converting from a smaller to a larger integer ``dtype`` the maximum values are **not** mapped exactly.
|
186 |
-
If converted back and forth, this mismatch has no effect.
|
187 |
-
|
188 |
-
Raises:
|
189 |
-
RuntimeError: When trying to cast :class:`torch.float32` to :class:`torch.int32` or :class:`torch.int64` as
|
190 |
-
well as for trying to cast :class:`torch.float64` to :class:`torch.int64`. These conversions might lead to
|
191 |
-
overflow errors since the floating point ``dtype`` cannot store consecutive integers over the whole range
|
192 |
-
of the integer ``dtype``.
|
193 |
-
"""
|
194 |
-
if not isinstance(image, torch.Tensor):
|
195 |
-
raise TypeError('Input img should be Tensor Image')
|
196 |
-
|
197 |
-
return F_t.convert_image_dtype(image, dtype)
|
198 |
-
|
199 |
-
|
200 |
-
def to_pil_image(pic, mode=None):
|
201 |
-
"""Convert a tensor or an ndarray to PIL Image. This function does not support torchscript.
|
202 |
-
|
203 |
-
See :class:`~torchvision.transforms.ToPILImage` for more details.
|
204 |
-
|
205 |
-
Args:
|
206 |
-
pic (Tensor or numpy.ndarray): Image to be converted to PIL Image.
|
207 |
-
mode (`PIL.Image mode`_): color space and pixel depth of input data (optional).
|
208 |
-
|
209 |
-
.. _PIL.Image mode: https://pillow.readthedocs.io/en/latest/handbook/concepts.html#concept-modes
|
210 |
-
|
211 |
-
Returns:
|
212 |
-
PIL Image: Image converted to PIL Image.
|
213 |
-
"""
|
214 |
-
if not(isinstance(pic, torch.Tensor) or isinstance(pic, np.ndarray)):
|
215 |
-
raise TypeError('pic should be Tensor or ndarray. Got {}.'.format(type(pic)))
|
216 |
-
|
217 |
-
elif isinstance(pic, torch.Tensor):
|
218 |
-
if pic.ndimension() not in {2, 3}:
|
219 |
-
raise ValueError('pic should be 2/3 dimensional. Got {} dimensions.'.format(pic.ndimension()))
|
220 |
-
|
221 |
-
elif pic.ndimension() == 2:
|
222 |
-
# if 2D image, add channel dimension (CHW)
|
223 |
-
pic = pic.unsqueeze(0)
|
224 |
-
|
225 |
-
# check number of channels
|
226 |
-
if pic.shape[-3] > 4:
|
227 |
-
raise ValueError('pic should not have > 4 channels. Got {} channels.'.format(pic.shape[-3]))
|
228 |
-
|
229 |
-
elif isinstance(pic, np.ndarray):
|
230 |
-
if pic.ndim not in {2, 3}:
|
231 |
-
raise ValueError('pic should be 2/3 dimensional. Got {} dimensions.'.format(pic.ndim))
|
232 |
-
|
233 |
-
elif pic.ndim == 2:
|
234 |
-
# if 2D image, add channel dimension (HWC)
|
235 |
-
pic = np.expand_dims(pic, 2)
|
236 |
-
|
237 |
-
# check number of channels
|
238 |
-
if pic.shape[-1] > 4:
|
239 |
-
raise ValueError('pic should not have > 4 channels. Got {} channels.'.format(pic.shape[-1]))
|
240 |
-
|
241 |
-
npimg = pic
|
242 |
-
if isinstance(pic, torch.Tensor):
|
243 |
-
if pic.is_floating_point() and mode != 'F':
|
244 |
-
pic = pic.mul(255).byte()
|
245 |
-
npimg = np.transpose(pic.cpu().numpy(), (1, 2, 0))
|
246 |
-
|
247 |
-
if not isinstance(npimg, np.ndarray):
|
248 |
-
raise TypeError('Input pic must be a torch.Tensor or NumPy ndarray, ' +
|
249 |
-
'not {}'.format(type(npimg)))
|
250 |
-
|
251 |
-
if npimg.shape[2] == 1:
|
252 |
-
expected_mode = None
|
253 |
-
npimg = npimg[:, :, 0]
|
254 |
-
if npimg.dtype == np.uint8:
|
255 |
-
expected_mode = 'L'
|
256 |
-
elif npimg.dtype == np.int16:
|
257 |
-
expected_mode = 'I;16'
|
258 |
-
elif npimg.dtype == np.int32:
|
259 |
-
expected_mode = 'I'
|
260 |
-
elif npimg.dtype == np.float32:
|
261 |
-
expected_mode = 'F'
|
262 |
-
if mode is not None and mode != expected_mode:
|
263 |
-
raise ValueError("Incorrect mode ({}) supplied for input type {}. Should be {}"
|
264 |
-
.format(mode, np.dtype, expected_mode))
|
265 |
-
mode = expected_mode
|
266 |
-
|
267 |
-
elif npimg.shape[2] == 2:
|
268 |
-
permitted_2_channel_modes = ['LA']
|
269 |
-
if mode is not None and mode not in permitted_2_channel_modes:
|
270 |
-
raise ValueError("Only modes {} are supported for 2D inputs".format(permitted_2_channel_modes))
|
271 |
-
|
272 |
-
if mode is None and npimg.dtype == np.uint8:
|
273 |
-
mode = 'LA'
|
274 |
-
|
275 |
-
elif npimg.shape[2] == 4:
|
276 |
-
permitted_4_channel_modes = ['RGBA', 'CMYK', 'RGBX']
|
277 |
-
if mode is not None and mode not in permitted_4_channel_modes:
|
278 |
-
raise ValueError("Only modes {} are supported for 4D inputs".format(permitted_4_channel_modes))
|
279 |
-
|
280 |
-
if mode is None and npimg.dtype == np.uint8:
|
281 |
-
mode = 'RGBA'
|
282 |
-
else:
|
283 |
-
permitted_3_channel_modes = ['RGB', 'YCbCr', 'HSV']
|
284 |
-
if mode is not None and mode not in permitted_3_channel_modes:
|
285 |
-
raise ValueError("Only modes {} are supported for 3D inputs".format(permitted_3_channel_modes))
|
286 |
-
if mode is None and npimg.dtype == np.uint8:
|
287 |
-
mode = 'RGB'
|
288 |
-
|
289 |
-
if mode is None:
|
290 |
-
raise TypeError('Input type {} is not supported'.format(npimg.dtype))
|
291 |
-
|
292 |
-
return Image.fromarray(npimg, mode=mode)
|
293 |
-
|
294 |
-
|
295 |
-
def normalize(tensor: Tensor, mean: List[float], std: List[float], inplace: bool = False) -> Tensor:
|
296 |
-
"""Normalize a float tensor image with mean and standard deviation.
|
297 |
-
This transform does not support PIL Image.
|
298 |
-
|
299 |
-
.. note::
|
300 |
-
This transform acts out of place by default, i.e., it does not mutates the input tensor.
|
301 |
-
|
302 |
-
See :class:`~torchvision.transforms.Normalize` for more details.
|
303 |
-
|
304 |
-
Args:
|
305 |
-
tensor (Tensor): Float tensor image of size (C, H, W) or (B, C, H, W) to be normalized.
|
306 |
-
mean (sequence): Sequence of means for each channel.
|
307 |
-
std (sequence): Sequence of standard deviations for each channel.
|
308 |
-
inplace(bool,optional): Bool to make this operation inplace.
|
309 |
-
|
310 |
-
Returns:
|
311 |
-
Tensor: Normalized Tensor image.
|
312 |
-
"""
|
313 |
-
if not isinstance(tensor, torch.Tensor):
|
314 |
-
raise TypeError('Input tensor should be a torch tensor. Got {}.'.format(type(tensor)))
|
315 |
-
|
316 |
-
if not tensor.is_floating_point():
|
317 |
-
raise TypeError('Input tensor should be a float tensor. Got {}.'.format(tensor.dtype))
|
318 |
-
|
319 |
-
if tensor.ndim < 3:
|
320 |
-
raise ValueError('Expected tensor to be a tensor image of size (..., C, H, W). Got tensor.size() = '
|
321 |
-
'{}.'.format(tensor.size()))
|
322 |
-
|
323 |
-
if not inplace:
|
324 |
-
tensor = tensor.clone()
|
325 |
-
|
326 |
-
dtype = tensor.dtype
|
327 |
-
mean = torch.as_tensor(mean, dtype=dtype, device=tensor.device)
|
328 |
-
std = torch.as_tensor(std, dtype=dtype, device=tensor.device)
|
329 |
-
if (std == 0).any():
|
330 |
-
raise ValueError('std evaluated to zero after conversion to {}, leading to division by zero.'.format(dtype))
|
331 |
-
if mean.ndim == 1:
|
332 |
-
mean = mean.view(-1, 1, 1)
|
333 |
-
if std.ndim == 1:
|
334 |
-
std = std.view(-1, 1, 1)
|
335 |
-
tensor.sub_(mean).div_(std)
|
336 |
-
return tensor
|
337 |
-
|
338 |
-
|
339 |
-
def resize(img: Tensor, size: List[int], interpolation: InterpolationMode = InterpolationMode.BILINEAR,
|
340 |
-
max_size: Optional[int] = None, antialias: Optional[bool] = None) -> Tensor:
|
341 |
-
r"""Resize the input image to the given size.
|
342 |
-
If the image is torch Tensor, it is expected
|
343 |
-
to have [..., H, W] shape, where ... means an arbitrary number of leading dimensions
|
344 |
-
|
345 |
-
.. warning::
|
346 |
-
The output image might be different depending on its type: when downsampling, the interpolation of PIL images
|
347 |
-
and tensors is slightly different, because PIL applies antialiasing. This may lead to significant differences
|
348 |
-
in the performance of a network. Therefore, it is preferable to train and serve a model with the same input
|
349 |
-
types. See also below the ``antialias`` parameter, which can help making the output of PIL images and tensors
|
350 |
-
closer.
|
351 |
-
|
352 |
-
Args:
|
353 |
-
img (PIL Image or Tensor): Image to be resized.
|
354 |
-
size (sequence or int): Desired output size. If size is a sequence like
|
355 |
-
(h, w), the output size will be matched to this. If size is an int,
|
356 |
-
the smaller edge of the image will be matched to this number maintaining
|
357 |
-
the aspect ratio. i.e, if height > width, then image will be rescaled to
|
358 |
-
:math:`\left(\text{size} \times \frac{\text{height}}{\text{width}}, \text{size}\right)`.
|
359 |
-
|
360 |
-
.. note::
|
361 |
-
In torchscript mode size as single int is not supported, use a sequence of length 1: ``[size, ]``.
|
362 |
-
interpolation (InterpolationMode): Desired interpolation enum defined by
|
363 |
-
:class:`torchvision.transforms.InterpolationMode`.
|
364 |
-
Default is ``InterpolationMode.BILINEAR``. If input is Tensor, only ``InterpolationMode.NEAREST``,
|
365 |
-
``InterpolationMode.BILINEAR`` and ``InterpolationMode.BICUBIC`` are supported.
|
366 |
-
For backward compatibility integer values (e.g. ``PIL.Image.NEAREST``) are still acceptable.
|
367 |
-
max_size (int, optional): The maximum allowed for the longer edge of
|
368 |
-
the resized image: if the longer edge of the image is greater
|
369 |
-
than ``max_size`` after being resized according to ``size``, then
|
370 |
-
the image is resized again so that the longer edge is equal to
|
371 |
-
``max_size``. As a result, ``size`` might be overruled, i.e the
|
372 |
-
smaller edge may be shorter than ``size``. This is only supported
|
373 |
-
if ``size`` is an int (or a sequence of length 1 in torchscript
|
374 |
-
mode).
|
375 |
-
antialias (bool, optional): antialias flag. If ``img`` is PIL Image, the flag is ignored and anti-alias
|
376 |
-
is always used. If ``img`` is Tensor, the flag is False by default and can be set to True for
|
377 |
-
``InterpolationMode.BILINEAR`` only mode. This can help making the output for PIL images and tensors
|
378 |
-
closer.
|
379 |
-
|
380 |
-
.. warning::
|
381 |
-
There is no autodiff support for ``antialias=True`` option with input ``img`` as Tensor.
|
382 |
-
|
383 |
-
Returns:
|
384 |
-
PIL Image or Tensor: Resized image.
|
385 |
-
"""
|
386 |
-
# Backward compatibility with integer value
|
387 |
-
if isinstance(interpolation, int):
|
388 |
-
warnings.warn(
|
389 |
-
"Argument interpolation should be of type InterpolationMode instead of int. "
|
390 |
-
"Please, use InterpolationMode enum."
|
391 |
-
)
|
392 |
-
interpolation = _interpolation_modes_from_int(interpolation)
|
393 |
-
|
394 |
-
if not isinstance(interpolation, InterpolationMode):
|
395 |
-
raise TypeError("Argument interpolation should be a InterpolationMode")
|
396 |
-
|
397 |
-
if not isinstance(img, torch.Tensor):
|
398 |
-
if antialias is not None and not antialias:
|
399 |
-
warnings.warn(
|
400 |
-
"Anti-alias option is always applied for PIL Image input. Argument antialias is ignored."
|
401 |
-
)
|
402 |
-
pil_interpolation = pil_modes_mapping[interpolation]
|
403 |
-
return F_pil.resize(img, size=size, interpolation=pil_interpolation, max_size=max_size)
|
404 |
-
|
405 |
-
return F_t.resize(img, size=size, interpolation=interpolation.value, max_size=max_size, antialias=antialias)
|
406 |
-
|
407 |
-
|
408 |
-
def scale(*args, **kwargs):
|
409 |
-
warnings.warn("The use of the transforms.Scale transform is deprecated, " +
|
410 |
-
"please use transforms.Resize instead.")
|
411 |
-
return resize(*args, **kwargs)
|
412 |
-
|
413 |
-
|
414 |
-
def pad(img: Tensor, padding: List[int], fill: int = 0, padding_mode: str = "constant") -> Tensor:
|
415 |
-
r"""Pad the given image on all sides with the given "pad" value.
|
416 |
-
If the image is torch Tensor, it is expected
|
417 |
-
to have [..., H, W] shape, where ... means at most 2 leading dimensions for mode reflect and symmetric,
|
418 |
-
at most 3 leading dimensions for mode edge,
|
419 |
-
and an arbitrary number of leading dimensions for mode constant
|
420 |
-
|
421 |
-
Args:
|
422 |
-
img (PIL Image or Tensor): Image to be padded.
|
423 |
-
padding (int or sequence): Padding on each border. If a single int is provided this
|
424 |
-
is used to pad all borders. If sequence of length 2 is provided this is the padding
|
425 |
-
on left/right and top/bottom respectively. If a sequence of length 4 is provided
|
426 |
-
this is the padding for the left, top, right and bottom borders respectively.
|
427 |
-
|
428 |
-
.. note::
|
429 |
-
In torchscript mode padding as single int is not supported, use a sequence of
|
430 |
-
length 1: ``[padding, ]``.
|
431 |
-
fill (number or str or tuple): Pixel fill value for constant fill. Default is 0.
|
432 |
-
If a tuple of length 3, it is used to fill R, G, B channels respectively.
|
433 |
-
This value is only used when the padding_mode is constant.
|
434 |
-
Only number is supported for torch Tensor.
|
435 |
-
Only int or str or tuple value is supported for PIL Image.
|
436 |
-
padding_mode (str): Type of padding. Should be: constant, edge, reflect or symmetric.
|
437 |
-
Default is constant.
|
438 |
-
|
439 |
-
- constant: pads with a constant value, this value is specified with fill
|
440 |
-
|
441 |
-
- edge: pads with the last value at the edge of the image.
|
442 |
-
If input a 5D torch Tensor, the last 3 dimensions will be padded instead of the last 2
|
443 |
-
|
444 |
-
- reflect: pads with reflection of image without repeating the last value on the edge.
|
445 |
-
For example, padding [1, 2, 3, 4] with 2 elements on both sides in reflect mode
|
446 |
-
will result in [3, 2, 1, 2, 3, 4, 3, 2]
|
447 |
-
|
448 |
-
- symmetric: pads with reflection of image repeating the last value on the edge.
|
449 |
-
For example, padding [1, 2, 3, 4] with 2 elements on both sides in symmetric mode
|
450 |
-
will result in [2, 1, 1, 2, 3, 4, 4, 3]
|
451 |
-
|
452 |
-
Returns:
|
453 |
-
PIL Image or Tensor: Padded image.
|
454 |
-
"""
|
455 |
-
if not isinstance(img, torch.Tensor):
|
456 |
-
return F_pil.pad(img, padding=padding, fill=fill, padding_mode=padding_mode)
|
457 |
-
|
458 |
-
return F_t.pad(img, padding=padding, fill=fill, padding_mode=padding_mode)
|
459 |
-
|
460 |
-
|
461 |
-
def crop(img: Tensor, top: int, left: int, height: int, width: int) -> Tensor:
|
462 |
-
"""Crop the given image at specified location and output size.
|
463 |
-
If the image is torch Tensor, it is expected
|
464 |
-
to have [..., H, W] shape, where ... means an arbitrary number of leading dimensions.
|
465 |
-
If image size is smaller than output size along any edge, image is padded with 0 and then cropped.
|
466 |
-
|
467 |
-
Args:
|
468 |
-
img (PIL Image or Tensor): Image to be cropped. (0,0) denotes the top left corner of the image.
|
469 |
-
top (int): Vertical component of the top left corner of the crop box.
|
470 |
-
left (int): Horizontal component of the top left corner of the crop box.
|
471 |
-
height (int): Height of the crop box.
|
472 |
-
width (int): Width of the crop box.
|
473 |
-
|
474 |
-
Returns:
|
475 |
-
PIL Image or Tensor: Cropped image.
|
476 |
-
"""
|
477 |
-
|
478 |
-
if not isinstance(img, torch.Tensor):
|
479 |
-
return F_pil.crop(img, top, left, height, width)
|
480 |
-
|
481 |
-
return F_t.crop(img, top, left, height, width)
|
482 |
-
|
483 |
-
|
484 |
-
def center_crop(img: Tensor, output_size: List[int]) -> Tensor:
|
485 |
-
"""Crops the given image at the center.
|
486 |
-
If the image is torch Tensor, it is expected
|
487 |
-
to have [..., H, W] shape, where ... means an arbitrary number of leading dimensions.
|
488 |
-
If image size is smaller than output size along any edge, image is padded with 0 and then center cropped.
|
489 |
-
|
490 |
-
Args:
|
491 |
-
img (PIL Image or Tensor): Image to be cropped.
|
492 |
-
output_size (sequence or int): (height, width) of the crop box. If int or sequence with single int,
|
493 |
-
it is used for both directions.
|
494 |
-
|
495 |
-
Returns:
|
496 |
-
PIL Image or Tensor: Cropped image.
|
497 |
-
"""
|
498 |
-
if isinstance(output_size, numbers.Number):
|
499 |
-
output_size = (int(output_size), int(output_size))
|
500 |
-
elif isinstance(output_size, (tuple, list)) and len(output_size) == 1:
|
501 |
-
output_size = (output_size[0], output_size[0])
|
502 |
-
|
503 |
-
image_width, image_height = _get_image_size(img)
|
504 |
-
crop_height, crop_width = output_size
|
505 |
-
|
506 |
-
if crop_width > image_width or crop_height > image_height:
|
507 |
-
padding_ltrb = [
|
508 |
-
(crop_width - image_width) // 2 if crop_width > image_width else 0,
|
509 |
-
(crop_height - image_height) // 2 if crop_height > image_height else 0,
|
510 |
-
(crop_width - image_width + 1) // 2 if crop_width > image_width else 0,
|
511 |
-
(crop_height - image_height + 1) // 2 if crop_height > image_height else 0,
|
512 |
-
]
|
513 |
-
img = pad(img, padding_ltrb, fill=0) # PIL uses fill value 0
|
514 |
-
image_width, image_height = _get_image_size(img)
|
515 |
-
if crop_width == image_width and crop_height == image_height:
|
516 |
-
return img
|
517 |
-
|
518 |
-
crop_top = int(round((image_height - crop_height) / 2.))
|
519 |
-
crop_left = int(round((image_width - crop_width) / 2.))
|
520 |
-
return crop(img, crop_top, crop_left, crop_height, crop_width)
|
521 |
-
|
522 |
-
|
523 |
-
def resized_crop(
|
524 |
-
img: Tensor, top: int, left: int, height: int, width: int, size: List[int],
|
525 |
-
interpolation: InterpolationMode = InterpolationMode.BILINEAR
|
526 |
-
) -> Tensor:
|
527 |
-
"""Crop the given image and resize it to desired size.
|
528 |
-
If the image is torch Tensor, it is expected
|
529 |
-
to have [..., H, W] shape, where ... means an arbitrary number of leading dimensions
|
530 |
-
|
531 |
-
Notably used in :class:`~torchvision.transforms.RandomResizedCrop`.
|
532 |
-
|
533 |
-
Args:
|
534 |
-
img (PIL Image or Tensor): Image to be cropped. (0,0) denotes the top left corner of the image.
|
535 |
-
top (int): Vertical component of the top left corner of the crop box.
|
536 |
-
left (int): Horizontal component of the top left corner of the crop box.
|
537 |
-
height (int): Height of the crop box.
|
538 |
-
width (int): Width of the crop box.
|
539 |
-
size (sequence or int): Desired output size. Same semantics as ``resize``.
|
540 |
-
interpolation (InterpolationMode): Desired interpolation enum defined by
|
541 |
-
:class:`torchvision.transforms.InterpolationMode`.
|
542 |
-
Default is ``InterpolationMode.BILINEAR``. If input is Tensor, only ``InterpolationMode.NEAREST``,
|
543 |
-
``InterpolationMode.BILINEAR`` and ``InterpolationMode.BICUBIC`` are supported.
|
544 |
-
For backward compatibility integer values (e.g. ``PIL.Image.NEAREST``) are still acceptable.
|
545 |
-
|
546 |
-
Returns:
|
547 |
-
PIL Image or Tensor: Cropped image.
|
548 |
-
"""
|
549 |
-
img = crop(img, top, left, height, width)
|
550 |
-
img = resize(img, size, interpolation)
|
551 |
-
return img
|
552 |
-
|
553 |
-
|
554 |
-
def hflip(img: Tensor) -> Tensor:
|
555 |
-
"""Horizontally flip the given image.
|
556 |
-
|
557 |
-
Args:
|
558 |
-
img (PIL Image or Tensor): Image to be flipped. If img
|
559 |
-
is a Tensor, it is expected to be in [..., H, W] format,
|
560 |
-
where ... means it can have an arbitrary number of leading
|
561 |
-
dimensions.
|
562 |
-
|
563 |
-
Returns:
|
564 |
-
PIL Image or Tensor: Horizontally flipped image.
|
565 |
-
"""
|
566 |
-
if not isinstance(img, torch.Tensor):
|
567 |
-
return F_pil.hflip(img)
|
568 |
-
|
569 |
-
return F_t.hflip(img)
|
570 |
-
|
571 |
-
|
572 |
-
def _get_perspective_coeffs(
|
573 |
-
startpoints: List[List[int]], endpoints: List[List[int]]
|
574 |
-
) -> List[float]:
|
575 |
-
"""Helper function to get the coefficients (a, b, c, d, e, f, g, h) for the perspective transforms.
|
576 |
-
|
577 |
-
In Perspective Transform each pixel (x, y) in the original image gets transformed as,
|
578 |
-
(x, y) -> ( (ax + by + c) / (gx + hy + 1), (dx + ey + f) / (gx + hy + 1) )
|
579 |
-
|
580 |
-
Args:
|
581 |
-
startpoints (list of list of ints): List containing four lists of two integers corresponding to four corners
|
582 |
-
``[top-left, top-right, bottom-right, bottom-left]`` of the original image.
|
583 |
-
endpoints (list of list of ints): List containing four lists of two integers corresponding to four corners
|
584 |
-
``[top-left, top-right, bottom-right, bottom-left]`` of the transformed image.
|
585 |
-
|
586 |
-
Returns:
|
587 |
-
octuple (a, b, c, d, e, f, g, h) for transforming each pixel.
|
588 |
-
"""
|
589 |
-
a_matrix = torch.zeros(2 * len(startpoints), 8, dtype=torch.float)
|
590 |
-
|
591 |
-
for i, (p1, p2) in enumerate(zip(endpoints, startpoints)):
|
592 |
-
a_matrix[2 * i, :] = torch.tensor([p1[0], p1[1], 1, 0, 0, 0, -p2[0] * p1[0], -p2[0] * p1[1]])
|
593 |
-
a_matrix[2 * i + 1, :] = torch.tensor([0, 0, 0, p1[0], p1[1], 1, -p2[1] * p1[0], -p2[1] * p1[1]])
|
594 |
-
|
595 |
-
b_matrix = torch.tensor(startpoints, dtype=torch.float).view(8)
|
596 |
-
res = torch.linalg.lstsq(a_matrix, b_matrix, driver='gels').solution
|
597 |
-
|
598 |
-
output: List[float] = res.tolist()
|
599 |
-
return output
|
600 |
-
|
601 |
-
|
602 |
-
def perspective(
|
603 |
-
img: Tensor,
|
604 |
-
startpoints: List[List[int]],
|
605 |
-
endpoints: List[List[int]],
|
606 |
-
interpolation: InterpolationMode = InterpolationMode.BILINEAR,
|
607 |
-
fill: Optional[List[float]] = None
|
608 |
-
) -> Tensor:
|
609 |
-
"""Perform perspective transform of the given image.
|
610 |
-
If the image is torch Tensor, it is expected
|
611 |
-
to have [..., H, W] shape, where ... means an arbitrary number of leading dimensions.
|
612 |
-
|
613 |
-
Args:
|
614 |
-
img (PIL Image or Tensor): Image to be transformed.
|
615 |
-
startpoints (list of list of ints): List containing four lists of two integers corresponding to four corners
|
616 |
-
``[top-left, top-right, bottom-right, bottom-left]`` of the original image.
|
617 |
-
endpoints (list of list of ints): List containing four lists of two integers corresponding to four corners
|
618 |
-
``[top-left, top-right, bottom-right, bottom-left]`` of the transformed image.
|
619 |
-
interpolation (InterpolationMode): Desired interpolation enum defined by
|
620 |
-
:class:`torchvision.transforms.InterpolationMode`. Default is ``InterpolationMode.BILINEAR``.
|
621 |
-
If input is Tensor, only ``InterpolationMode.NEAREST``, ``InterpolationMode.BILINEAR`` are supported.
|
622 |
-
For backward compatibility integer values (e.g. ``PIL.Image.NEAREST``) are still acceptable.
|
623 |
-
fill (sequence or number, optional): Pixel fill value for the area outside the transformed
|
624 |
-
image. If given a number, the value is used for all bands respectively.
|
625 |
-
|
626 |
-
.. note::
|
627 |
-
In torchscript mode single int/float value is not supported, please use a sequence
|
628 |
-
of length 1: ``[value, ]``.
|
629 |
-
|
630 |
-
Returns:
|
631 |
-
PIL Image or Tensor: transformed Image.
|
632 |
-
"""
|
633 |
-
|
634 |
-
coeffs = _get_perspective_coeffs(startpoints, endpoints)
|
635 |
-
|
636 |
-
# Backward compatibility with integer value
|
637 |
-
if isinstance(interpolation, int):
|
638 |
-
warnings.warn(
|
639 |
-
"Argument interpolation should be of type InterpolationMode instead of int. "
|
640 |
-
"Please, use InterpolationMode enum."
|
641 |
-
)
|
642 |
-
interpolation = _interpolation_modes_from_int(interpolation)
|
643 |
-
|
644 |
-
if not isinstance(interpolation, InterpolationMode):
|
645 |
-
raise TypeError("Argument interpolation should be a InterpolationMode")
|
646 |
-
|
647 |
-
if not isinstance(img, torch.Tensor):
|
648 |
-
pil_interpolation = pil_modes_mapping[interpolation]
|
649 |
-
return F_pil.perspective(img, coeffs, interpolation=pil_interpolation, fill=fill)
|
650 |
-
|
651 |
-
return F_t.perspective(img, coeffs, interpolation=interpolation.value, fill=fill)
|
652 |
-
|
653 |
-
|
654 |
-
def vflip(img: Tensor) -> Tensor:
|
655 |
-
"""Vertically flip the given image.
|
656 |
-
|
657 |
-
Args:
|
658 |
-
img (PIL Image or Tensor): Image to be flipped. If img
|
659 |
-
is a Tensor, it is expected to be in [..., H, W] format,
|
660 |
-
where ... means it can have an arbitrary number of leading
|
661 |
-
dimensions.
|
662 |
-
|
663 |
-
Returns:
|
664 |
-
PIL Image or Tensor: Vertically flipped image.
|
665 |
-
"""
|
666 |
-
if not isinstance(img, torch.Tensor):
|
667 |
-
return F_pil.vflip(img)
|
668 |
-
|
669 |
-
return F_t.vflip(img)
|
670 |
-
|
671 |
-
|
672 |
-
def five_crop(img: Tensor, size: List[int]) -> Tuple[Tensor, Tensor, Tensor, Tensor, Tensor]:
|
673 |
-
"""Crop the given image into four corners and the central crop.
|
674 |
-
If the image is torch Tensor, it is expected
|
675 |
-
to have [..., H, W] shape, where ... means an arbitrary number of leading dimensions
|
676 |
-
|
677 |
-
.. Note::
|
678 |
-
This transform returns a tuple of images and there may be a
|
679 |
-
mismatch in the number of inputs and targets your ``Dataset`` returns.
|
680 |
-
|
681 |
-
Args:
|
682 |
-
img (PIL Image or Tensor): Image to be cropped.
|
683 |
-
size (sequence or int): Desired output size of the crop. If size is an
|
684 |
-
int instead of sequence like (h, w), a square crop (size, size) is
|
685 |
-
made. If provided a sequence of length 1, it will be interpreted as (size[0], size[0]).
|
686 |
-
|
687 |
-
Returns:
|
688 |
-
tuple: tuple (tl, tr, bl, br, center)
|
689 |
-
Corresponding top left, top right, bottom left, bottom right and center crop.
|
690 |
-
"""
|
691 |
-
if isinstance(size, numbers.Number):
|
692 |
-
size = (int(size), int(size))
|
693 |
-
elif isinstance(size, (tuple, list)) and len(size) == 1:
|
694 |
-
size = (size[0], size[0])
|
695 |
-
|
696 |
-
if len(size) != 2:
|
697 |
-
raise ValueError("Please provide only two dimensions (h, w) for size.")
|
698 |
-
|
699 |
-
image_width, image_height = _get_image_size(img)
|
700 |
-
crop_height, crop_width = size
|
701 |
-
if crop_width > image_width or crop_height > image_height:
|
702 |
-
msg = "Requested crop size {} is bigger than input size {}"
|
703 |
-
raise ValueError(msg.format(size, (image_height, image_width)))
|
704 |
-
|
705 |
-
tl = crop(img, 0, 0, crop_height, crop_width)
|
706 |
-
tr = crop(img, 0, image_width - crop_width, crop_height, crop_width)
|
707 |
-
bl = crop(img, image_height - crop_height, 0, crop_height, crop_width)
|
708 |
-
br = crop(img, image_height - crop_height, image_width - crop_width, crop_height, crop_width)
|
709 |
-
|
710 |
-
center = center_crop(img, [crop_height, crop_width])
|
711 |
-
|
712 |
-
return tl, tr, bl, br, center
|
713 |
-
|
714 |
-
|
715 |
-
def ten_crop(img: Tensor, size: List[int], vertical_flip: bool = False) -> List[Tensor]:
|
716 |
-
"""Generate ten cropped images from the given image.
|
717 |
-
Crop the given image into four corners and the central crop plus the
|
718 |
-
flipped version of these (horizontal flipping is used by default).
|
719 |
-
If the image is torch Tensor, it is expected
|
720 |
-
to have [..., H, W] shape, where ... means an arbitrary number of leading dimensions
|
721 |
-
|
722 |
-
.. Note::
|
723 |
-
This transform returns a tuple of images and there may be a
|
724 |
-
mismatch in the number of inputs and targets your ``Dataset`` returns.
|
725 |
-
|
726 |
-
Args:
|
727 |
-
img (PIL Image or Tensor): Image to be cropped.
|
728 |
-
size (sequence or int): Desired output size of the crop. If size is an
|
729 |
-
int instead of sequence like (h, w), a square crop (size, size) is
|
730 |
-
made. If provided a sequence of length 1, it will be interpreted as (size[0], size[0]).
|
731 |
-
vertical_flip (bool): Use vertical flipping instead of horizontal
|
732 |
-
|
733 |
-
Returns:
|
734 |
-
tuple: tuple (tl, tr, bl, br, center, tl_flip, tr_flip, bl_flip, br_flip, center_flip)
|
735 |
-
Corresponding top left, top right, bottom left, bottom right and
|
736 |
-
center crop and same for the flipped image.
|
737 |
-
"""
|
738 |
-
if isinstance(size, numbers.Number):
|
739 |
-
size = (int(size), int(size))
|
740 |
-
elif isinstance(size, (tuple, list)) and len(size) == 1:
|
741 |
-
size = (size[0], size[0])
|
742 |
-
|
743 |
-
if len(size) != 2:
|
744 |
-
raise ValueError("Please provide only two dimensions (h, w) for size.")
|
745 |
-
|
746 |
-
first_five = five_crop(img, size)
|
747 |
-
|
748 |
-
if vertical_flip:
|
749 |
-
img = vflip(img)
|
750 |
-
else:
|
751 |
-
img = hflip(img)
|
752 |
-
|
753 |
-
second_five = five_crop(img, size)
|
754 |
-
return first_five + second_five
|
755 |
-
|
756 |
-
|
757 |
-
def adjust_brightness(img: Tensor, brightness_factor: float) -> Tensor:
|
758 |
-
"""Adjust brightness of an image.
|
759 |
-
|
760 |
-
Args:
|
761 |
-
img (PIL Image or Tensor): Image to be adjusted.
|
762 |
-
If img is torch Tensor, it is expected to be in [..., 1 or 3, H, W] format,
|
763 |
-
where ... means it can have an arbitrary number of leading dimensions.
|
764 |
-
brightness_factor (float): How much to adjust the brightness. Can be
|
765 |
-
any non negative number. 0 gives a black image, 1 gives the
|
766 |
-
original image while 2 increases the brightness by a factor of 2.
|
767 |
-
|
768 |
-
Returns:
|
769 |
-
PIL Image or Tensor: Brightness adjusted image.
|
770 |
-
"""
|
771 |
-
if not isinstance(img, torch.Tensor):
|
772 |
-
return F_pil.adjust_brightness(img, brightness_factor)
|
773 |
-
|
774 |
-
return F_t.adjust_brightness(img, brightness_factor)
|
775 |
-
|
776 |
-
|
777 |
-
def adjust_contrast(img: Tensor, contrast_factor: float) -> Tensor:
|
778 |
-
"""Adjust contrast of an image.
|
779 |
-
|
780 |
-
Args:
|
781 |
-
img (PIL Image or Tensor): Image to be adjusted.
|
782 |
-
If img is torch Tensor, it is expected to be in [..., 3, H, W] format,
|
783 |
-
where ... means it can have an arbitrary number of leading dimensions.
|
784 |
-
contrast_factor (float): How much to adjust the contrast. Can be any
|
785 |
-
non negative number. 0 gives a solid gray image, 1 gives the
|
786 |
-
original image while 2 increases the contrast by a factor of 2.
|
787 |
-
|
788 |
-
Returns:
|
789 |
-
PIL Image or Tensor: Contrast adjusted image.
|
790 |
-
"""
|
791 |
-
if not isinstance(img, torch.Tensor):
|
792 |
-
return F_pil.adjust_contrast(img, contrast_factor)
|
793 |
-
|
794 |
-
return F_t.adjust_contrast(img, contrast_factor)
|
795 |
-
|
796 |
-
|
797 |
-
def adjust_saturation(img: Tensor, saturation_factor: float) -> Tensor:
|
798 |
-
"""Adjust color saturation of an image.
|
799 |
-
|
800 |
-
Args:
|
801 |
-
img (PIL Image or Tensor): Image to be adjusted.
|
802 |
-
If img is torch Tensor, it is expected to be in [..., 3, H, W] format,
|
803 |
-
where ... means it can have an arbitrary number of leading dimensions.
|
804 |
-
saturation_factor (float): How much to adjust the saturation. 0 will
|
805 |
-
give a black and white image, 1 will give the original image while
|
806 |
-
2 will enhance the saturation by a factor of 2.
|
807 |
-
|
808 |
-
Returns:
|
809 |
-
PIL Image or Tensor: Saturation adjusted image.
|
810 |
-
"""
|
811 |
-
if not isinstance(img, torch.Tensor):
|
812 |
-
return F_pil.adjust_saturation(img, saturation_factor)
|
813 |
-
|
814 |
-
return F_t.adjust_saturation(img, saturation_factor)
|
815 |
-
|
816 |
-
|
817 |
-
def adjust_hue(img: Tensor, hue_factor: float) -> Tensor:
|
818 |
-
"""Adjust hue of an image.
|
819 |
-
|
820 |
-
The image hue is adjusted by converting the image to HSV and
|
821 |
-
cyclically shifting the intensities in the hue channel (H).
|
822 |
-
The image is then converted back to original image mode.
|
823 |
-
|
824 |
-
`hue_factor` is the amount of shift in H channel and must be in the
|
825 |
-
interval `[-0.5, 0.5]`.
|
826 |
-
|
827 |
-
See `Hue`_ for more details.
|
828 |
-
|
829 |
-
.. _Hue: https://en.wikipedia.org/wiki/Hue
|
830 |
-
|
831 |
-
Args:
|
832 |
-
img (PIL Image or Tensor): Image to be adjusted.
|
833 |
-
If img is torch Tensor, it is expected to be in [..., 3, H, W] format,
|
834 |
-
where ... means it can have an arbitrary number of leading dimensions.
|
835 |
-
If img is PIL Image mode "1", "L", "I", "F" and modes with transparency (alpha channel) are not supported.
|
836 |
-
hue_factor (float): How much to shift the hue channel. Should be in
|
837 |
-
[-0.5, 0.5]. 0.5 and -0.5 give complete reversal of hue channel in
|
838 |
-
HSV space in positive and negative direction respectively.
|
839 |
-
0 means no shift. Therefore, both -0.5 and 0.5 will give an image
|
840 |
-
with complementary colors while 0 gives the original image.
|
841 |
-
|
842 |
-
Returns:
|
843 |
-
PIL Image or Tensor: Hue adjusted image.
|
844 |
-
"""
|
845 |
-
if not isinstance(img, torch.Tensor):
|
846 |
-
return F_pil.adjust_hue(img, hue_factor)
|
847 |
-
|
848 |
-
return F_t.adjust_hue(img, hue_factor)
|
849 |
-
|
850 |
-
|
851 |
-
def adjust_gamma(img: Tensor, gamma: float, gain: float = 1) -> Tensor:
|
852 |
-
r"""Perform gamma correction on an image.
|
853 |
-
|
854 |
-
Also known as Power Law Transform. Intensities in RGB mode are adjusted
|
855 |
-
based on the following equation:
|
856 |
-
|
857 |
-
.. math::
|
858 |
-
I_{\text{out}} = 255 \times \text{gain} \times \left(\frac{I_{\text{in}}}{255}\right)^{\gamma}
|
859 |
-
|
860 |
-
See `Gamma Correction`_ for more details.
|
861 |
-
|
862 |
-
.. _Gamma Correction: https://en.wikipedia.org/wiki/Gamma_correction
|
863 |
-
|
864 |
-
Args:
|
865 |
-
img (PIL Image or Tensor): PIL Image to be adjusted.
|
866 |
-
If img is torch Tensor, it is expected to be in [..., 1 or 3, H, W] format,
|
867 |
-
where ... means it can have an arbitrary number of leading dimensions.
|
868 |
-
If img is PIL Image, modes with transparency (alpha channel) are not supported.
|
869 |
-
gamma (float): Non negative real number, same as :math:`\gamma` in the equation.
|
870 |
-
gamma larger than 1 make the shadows darker,
|
871 |
-
while gamma smaller than 1 make dark regions lighter.
|
872 |
-
gain (float): The constant multiplier.
|
873 |
-
Returns:
|
874 |
-
PIL Image or Tensor: Gamma correction adjusted image.
|
875 |
-
"""
|
876 |
-
if not isinstance(img, torch.Tensor):
|
877 |
-
return F_pil.adjust_gamma(img, gamma, gain)
|
878 |
-
|
879 |
-
return F_t.adjust_gamma(img, gamma, gain)
|
880 |
-
|
881 |
-
|
882 |
-
def _get_inverse_affine_matrix(
|
883 |
-
center: List[float], angle: float, translate: List[float], scale: float, shear: List[float]
|
884 |
-
) -> List[float]:
|
885 |
-
# Helper method to compute inverse matrix for affine transformation
|
886 |
-
|
887 |
-
# As it is explained in PIL.Image.rotate
|
888 |
-
# We need compute INVERSE of affine transformation matrix: M = T * C * RSS * C^-1
|
889 |
-
# where T is translation matrix: [1, 0, tx | 0, 1, ty | 0, 0, 1]
|
890 |
-
# C is translation matrix to keep center: [1, 0, cx | 0, 1, cy | 0, 0, 1]
|
891 |
-
# RSS is rotation with scale and shear matrix
|
892 |
-
# RSS(a, s, (sx, sy)) =
|
893 |
-
# = R(a) * S(s) * SHy(sy) * SHx(sx)
|
894 |
-
# = [ s*cos(a - sy)/cos(sy), s*(-cos(a - sy)*tan(x)/cos(y) - sin(a)), 0 ]
|
895 |
-
# [ s*sin(a + sy)/cos(sy), s*(-sin(a - sy)*tan(x)/cos(y) + cos(a)), 0 ]
|
896 |
-
# [ 0 , 0 , 1 ]
|
897 |
-
#
|
898 |
-
# where R is a rotation matrix, S is a scaling matrix, and SHx and SHy are the shears:
|
899 |
-
# SHx(s) = [1, -tan(s)] and SHy(s) = [1 , 0]
|
900 |
-
# [0, 1 ] [-tan(s), 1]
|
901 |
-
#
|
902 |
-
# Thus, the inverse is M^-1 = C * RSS^-1 * C^-1 * T^-1
|
903 |
-
|
904 |
-
rot = math.radians(angle)
|
905 |
-
sx, sy = [math.radians(s) for s in shear]
|
906 |
-
|
907 |
-
cx, cy = center
|
908 |
-
tx, ty = translate
|
909 |
-
|
910 |
-
# RSS without scaling
|
911 |
-
a = math.cos(rot - sy) / math.cos(sy)
|
912 |
-
b = -math.cos(rot - sy) * math.tan(sx) / math.cos(sy) - math.sin(rot)
|
913 |
-
c = math.sin(rot - sy) / math.cos(sy)
|
914 |
-
d = -math.sin(rot - sy) * math.tan(sx) / math.cos(sy) + math.cos(rot)
|
915 |
-
|
916 |
-
# Inverted rotation matrix with scale and shear
|
917 |
-
# det([[a, b], [c, d]]) == 1, since det(rotation) = 1 and det(shear) = 1
|
918 |
-
matrix = [d, -b, 0.0, -c, a, 0.0]
|
919 |
-
matrix = [x / scale for x in matrix]
|
920 |
-
|
921 |
-
# Apply inverse of translation and of center translation: RSS^-1 * C^-1 * T^-1
|
922 |
-
matrix[2] += matrix[0] * (-cx - tx) + matrix[1] * (-cy - ty)
|
923 |
-
matrix[5] += matrix[3] * (-cx - tx) + matrix[4] * (-cy - ty)
|
924 |
-
|
925 |
-
# Apply center translation: C * RSS^-1 * C^-1 * T^-1
|
926 |
-
matrix[2] += cx
|
927 |
-
matrix[5] += cy
|
928 |
-
|
929 |
-
return matrix
|
930 |
-
|
931 |
-
|
932 |
-
def rotate(
|
933 |
-
img: Tensor, angle: float, interpolation: InterpolationMode = InterpolationMode.NEAREST,
|
934 |
-
expand: bool = False, center: Optional[List[int]] = None,
|
935 |
-
fill: Optional[List[float]] = None, resample: Optional[int] = None
|
936 |
-
) -> Tensor:
|
937 |
-
"""Rotate the image by angle.
|
938 |
-
If the image is torch Tensor, it is expected
|
939 |
-
to have [..., H, W] shape, where ... means an arbitrary number of leading dimensions.
|
940 |
-
|
941 |
-
Args:
|
942 |
-
img (PIL Image or Tensor): image to be rotated.
|
943 |
-
angle (number): rotation angle value in degrees, counter-clockwise.
|
944 |
-
interpolation (InterpolationMode): Desired interpolation enum defined by
|
945 |
-
:class:`torchvision.transforms.InterpolationMode`. Default is ``InterpolationMode.NEAREST``.
|
946 |
-
If input is Tensor, only ``InterpolationMode.NEAREST``, ``InterpolationMode.BILINEAR`` are supported.
|
947 |
-
For backward compatibility integer values (e.g. ``PIL.Image.NEAREST``) are still acceptable.
|
948 |
-
expand (bool, optional): Optional expansion flag.
|
949 |
-
If true, expands the output image to make it large enough to hold the entire rotated image.
|
950 |
-
If false or omitted, make the output image the same size as the input image.
|
951 |
-
Note that the expand flag assumes rotation around the center and no translation.
|
952 |
-
center (sequence, optional): Optional center of rotation. Origin is the upper left corner.
|
953 |
-
Default is the center of the image.
|
954 |
-
fill (sequence or number, optional): Pixel fill value for the area outside the transformed
|
955 |
-
image. If given a number, the value is used for all bands respectively.
|
956 |
-
|
957 |
-
.. note::
|
958 |
-
In torchscript mode single int/float value is not supported, please use a sequence
|
959 |
-
of length 1: ``[value, ]``.
|
960 |
-
|
961 |
-
Returns:
|
962 |
-
PIL Image or Tensor: Rotated image.
|
963 |
-
|
964 |
-
.. _filters: https://pillow.readthedocs.io/en/latest/handbook/concepts.html#filters
|
965 |
-
|
966 |
-
"""
|
967 |
-
if resample is not None:
|
968 |
-
warnings.warn(
|
969 |
-
"Argument resample is deprecated and will be removed since v0.10.0. Please, use interpolation instead"
|
970 |
-
)
|
971 |
-
interpolation = _interpolation_modes_from_int(resample)
|
972 |
-
|
973 |
-
# Backward compatibility with integer value
|
974 |
-
if isinstance(interpolation, int):
|
975 |
-
warnings.warn(
|
976 |
-
"Argument interpolation should be of type InterpolationMode instead of int. "
|
977 |
-
"Please, use InterpolationMode enum."
|
978 |
-
)
|
979 |
-
interpolation = _interpolation_modes_from_int(interpolation)
|
980 |
-
|
981 |
-
if not isinstance(angle, (int, float)):
|
982 |
-
raise TypeError("Argument angle should be int or float")
|
983 |
-
|
984 |
-
if center is not None and not isinstance(center, (list, tuple)):
|
985 |
-
raise TypeError("Argument center should be a sequence")
|
986 |
-
|
987 |
-
if not isinstance(interpolation, InterpolationMode):
|
988 |
-
raise TypeError("Argument interpolation should be a InterpolationMode")
|
989 |
-
|
990 |
-
if not isinstance(img, torch.Tensor):
|
991 |
-
pil_interpolation = pil_modes_mapping[interpolation]
|
992 |
-
return F_pil.rotate(img, angle=angle, interpolation=pil_interpolation, expand=expand, center=center, fill=fill)
|
993 |
-
|
994 |
-
center_f = [0.0, 0.0]
|
995 |
-
if center is not None:
|
996 |
-
img_size = _get_image_size(img)
|
997 |
-
# Center values should be in pixel coordinates but translated such that (0, 0) corresponds to image center.
|
998 |
-
center_f = [1.0 * (c - s * 0.5) for c, s in zip(center, img_size)]
|
999 |
-
|
1000 |
-
# due to current incoherence of rotation angle direction between affine and rotate implementations
|
1001 |
-
# we need to set -angle.
|
1002 |
-
matrix = _get_inverse_affine_matrix(center_f, -angle, [0.0, 0.0], 1.0, [0.0, 0.0])
|
1003 |
-
return F_t.rotate(img, matrix=matrix, interpolation=interpolation.value, expand=expand, fill=fill)
|
1004 |
-
|
1005 |
-
|
1006 |
-
def affine(
|
1007 |
-
img: Tensor, angle: float, translate: List[int], scale: float, shear: List[float],
|
1008 |
-
interpolation: InterpolationMode = InterpolationMode.NEAREST, fill: Optional[List[float]] = None,
|
1009 |
-
resample: Optional[int] = None, fillcolor: Optional[List[float]] = None
|
1010 |
-
) -> Tensor:
|
1011 |
-
"""Apply affine transformation on the image keeping image center invariant.
|
1012 |
-
If the image is torch Tensor, it is expected
|
1013 |
-
to have [..., H, W] shape, where ... means an arbitrary number of leading dimensions.
|
1014 |
-
|
1015 |
-
Args:
|
1016 |
-
img (PIL Image or Tensor): image to transform.
|
1017 |
-
angle (number): rotation angle in degrees between -180 and 180, clockwise direction.
|
1018 |
-
translate (sequence of integers): horizontal and vertical translations (post-rotation translation)
|
1019 |
-
scale (float): overall scale
|
1020 |
-
shear (float or sequence): shear angle value in degrees between -180 to 180, clockwise direction.
|
1021 |
-
If a sequence is specified, the first value corresponds to a shear parallel to the x axis, while
|
1022 |
-
the second value corresponds to a shear parallel to the y axis.
|
1023 |
-
interpolation (InterpolationMode): Desired interpolation enum defined by
|
1024 |
-
:class:`torchvision.transforms.InterpolationMode`. Default is ``InterpolationMode.NEAREST``.
|
1025 |
-
If input is Tensor, only ``InterpolationMode.NEAREST``, ``InterpolationMode.BILINEAR`` are supported.
|
1026 |
-
For backward compatibility integer values (e.g. ``PIL.Image.NEAREST``) are still acceptable.
|
1027 |
-
fill (sequence or number, optional): Pixel fill value for the area outside the transformed
|
1028 |
-
image. If given a number, the value is used for all bands respectively.
|
1029 |
-
|
1030 |
-
.. note::
|
1031 |
-
In torchscript mode single int/float value is not supported, please use a sequence
|
1032 |
-
of length 1: ``[value, ]``.
|
1033 |
-
fillcolor (sequence, int, float): deprecated argument and will be removed since v0.10.0.
|
1034 |
-
Please use the ``fill`` parameter instead.
|
1035 |
-
resample (int, optional): deprecated argument and will be removed since v0.10.0.
|
1036 |
-
Please use the ``interpolation`` parameter instead.
|
1037 |
-
|
1038 |
-
Returns:
|
1039 |
-
PIL Image or Tensor: Transformed image.
|
1040 |
-
"""
|
1041 |
-
if resample is not None:
|
1042 |
-
warnings.warn(
|
1043 |
-
"Argument resample is deprecated and will be removed since v0.10.0. Please, use interpolation instead"
|
1044 |
-
)
|
1045 |
-
interpolation = _interpolation_modes_from_int(resample)
|
1046 |
-
|
1047 |
-
# Backward compatibility with integer value
|
1048 |
-
if isinstance(interpolation, int):
|
1049 |
-
warnings.warn(
|
1050 |
-
"Argument interpolation should be of type InterpolationMode instead of int. "
|
1051 |
-
"Please, use InterpolationMode enum."
|
1052 |
-
)
|
1053 |
-
interpolation = _interpolation_modes_from_int(interpolation)
|
1054 |
-
|
1055 |
-
if fillcolor is not None:
|
1056 |
-
warnings.warn(
|
1057 |
-
"Argument fillcolor is deprecated and will be removed since v0.10.0. Please, use fill instead"
|
1058 |
-
)
|
1059 |
-
fill = fillcolor
|
1060 |
-
|
1061 |
-
if not isinstance(angle, (int, float)):
|
1062 |
-
raise TypeError("Argument angle should be int or float")
|
1063 |
-
|
1064 |
-
if not isinstance(translate, (list, tuple)):
|
1065 |
-
raise TypeError("Argument translate should be a sequence")
|
1066 |
-
|
1067 |
-
if len(translate) != 2:
|
1068 |
-
raise ValueError("Argument translate should be a sequence of length 2")
|
1069 |
-
|
1070 |
-
if scale <= 0.0:
|
1071 |
-
raise ValueError("Argument scale should be positive")
|
1072 |
-
|
1073 |
-
if not isinstance(shear, (numbers.Number, (list, tuple))):
|
1074 |
-
raise TypeError("Shear should be either a single value or a sequence of two values")
|
1075 |
-
|
1076 |
-
if not isinstance(interpolation, InterpolationMode):
|
1077 |
-
raise TypeError("Argument interpolation should be a InterpolationMode")
|
1078 |
-
|
1079 |
-
if isinstance(angle, int):
|
1080 |
-
angle = float(angle)
|
1081 |
-
|
1082 |
-
if isinstance(translate, tuple):
|
1083 |
-
translate = list(translate)
|
1084 |
-
|
1085 |
-
if isinstance(shear, numbers.Number):
|
1086 |
-
shear = [shear, 0.0]
|
1087 |
-
|
1088 |
-
if isinstance(shear, tuple):
|
1089 |
-
shear = list(shear)
|
1090 |
-
|
1091 |
-
if len(shear) == 1:
|
1092 |
-
shear = [shear[0], shear[0]]
|
1093 |
-
|
1094 |
-
if len(shear) != 2:
|
1095 |
-
raise ValueError("Shear should be a sequence containing two values. Got {}".format(shear))
|
1096 |
-
|
1097 |
-
img_size = _get_image_size(img)
|
1098 |
-
if not isinstance(img, torch.Tensor):
|
1099 |
-
# center = (img_size[0] * 0.5 + 0.5, img_size[1] * 0.5 + 0.5)
|
1100 |
-
# it is visually better to estimate the center without 0.5 offset
|
1101 |
-
# otherwise image rotated by 90 degrees is shifted vs output image of torch.rot90 or F_t.affine
|
1102 |
-
center = [img_size[0] * 0.5, img_size[1] * 0.5]
|
1103 |
-
matrix = _get_inverse_affine_matrix(center, angle, translate, scale, shear)
|
1104 |
-
pil_interpolation = pil_modes_mapping[interpolation]
|
1105 |
-
return F_pil.affine(img, matrix=matrix, interpolation=pil_interpolation, fill=fill)
|
1106 |
-
|
1107 |
-
translate_f = [1.0 * t for t in translate]
|
1108 |
-
matrix = _get_inverse_affine_matrix([0.0, 0.0], angle, translate_f, scale, shear)
|
1109 |
-
return F_t.affine(img, matrix=matrix, interpolation=interpolation.value, fill=fill)
|
1110 |
-
|
1111 |
-
|
1112 |
-
@torch.jit.unused
|
1113 |
-
def to_grayscale(img, num_output_channels=1):
|
1114 |
-
"""Convert PIL image of any mode (RGB, HSV, LAB, etc) to grayscale version of image.
|
1115 |
-
This transform does not support torch Tensor.
|
1116 |
-
|
1117 |
-
Args:
|
1118 |
-
img (PIL Image): PIL Image to be converted to grayscale.
|
1119 |
-
num_output_channels (int): number of channels of the output image. Value can be 1 or 3. Default is 1.
|
1120 |
-
|
1121 |
-
Returns:
|
1122 |
-
PIL Image: Grayscale version of the image.
|
1123 |
-
|
1124 |
-
- if num_output_channels = 1 : returned image is single channel
|
1125 |
-
- if num_output_channels = 3 : returned image is 3 channel with r = g = b
|
1126 |
-
"""
|
1127 |
-
if isinstance(img, Image.Image):
|
1128 |
-
return F_pil.to_grayscale(img, num_output_channels)
|
1129 |
-
|
1130 |
-
raise TypeError("Input should be PIL Image")
|
1131 |
-
|
1132 |
-
|
1133 |
-
def rgb_to_grayscale(img: Tensor, num_output_channels: int = 1) -> Tensor:
|
1134 |
-
"""Convert RGB image to grayscale version of image.
|
1135 |
-
If the image is torch Tensor, it is expected
|
1136 |
-
to have [..., 3, H, W] shape, where ... means an arbitrary number of leading dimensions
|
1137 |
-
|
1138 |
-
Note:
|
1139 |
-
Please, note that this method supports only RGB images as input. For inputs in other color spaces,
|
1140 |
-
please, consider using meth:`~torchvision.transforms.functional.to_grayscale` with PIL Image.
|
1141 |
-
|
1142 |
-
Args:
|
1143 |
-
img (PIL Image or Tensor): RGB Image to be converted to grayscale.
|
1144 |
-
num_output_channels (int): number of channels of the output image. Value can be 1 or 3. Default, 1.
|
1145 |
-
|
1146 |
-
Returns:
|
1147 |
-
PIL Image or Tensor: Grayscale version of the image.
|
1148 |
-
|
1149 |
-
- if num_output_channels = 1 : returned image is single channel
|
1150 |
-
- if num_output_channels = 3 : returned image is 3 channel with r = g = b
|
1151 |
-
"""
|
1152 |
-
if not isinstance(img, torch.Tensor):
|
1153 |
-
return F_pil.to_grayscale(img, num_output_channels)
|
1154 |
-
|
1155 |
-
return F_t.rgb_to_grayscale(img, num_output_channels)
|
1156 |
-
|
1157 |
-
|
1158 |
-
def erase(img: Tensor, i: int, j: int, h: int, w: int, v: Tensor, inplace: bool = False) -> Tensor:
|
1159 |
-
""" Erase the input Tensor Image with given value.
|
1160 |
-
This transform does not support PIL Image.
|
1161 |
-
|
1162 |
-
Args:
|
1163 |
-
img (Tensor Image): Tensor image of size (C, H, W) to be erased
|
1164 |
-
i (int): i in (i,j) i.e coordinates of the upper left corner.
|
1165 |
-
j (int): j in (i,j) i.e coordinates of the upper left corner.
|
1166 |
-
h (int): Height of the erased region.
|
1167 |
-
w (int): Width of the erased region.
|
1168 |
-
v: Erasing value.
|
1169 |
-
inplace(bool, optional): For in-place operations. By default is set False.
|
1170 |
-
|
1171 |
-
Returns:
|
1172 |
-
Tensor Image: Erased image.
|
1173 |
-
"""
|
1174 |
-
if not isinstance(img, torch.Tensor):
|
1175 |
-
raise TypeError('img should be Tensor Image. Got {}'.format(type(img)))
|
1176 |
-
|
1177 |
-
if not inplace:
|
1178 |
-
img = img.clone()
|
1179 |
-
|
1180 |
-
img[..., i:i + h, j:j + w] = v
|
1181 |
-
return img
|
1182 |
-
|
1183 |
-
|
1184 |
-
def gaussian_blur(img: Tensor, kernel_size: List[int], sigma: Optional[List[float]] = None) -> Tensor:
|
1185 |
-
"""Performs Gaussian blurring on the image by given kernel.
|
1186 |
-
If the image is torch Tensor, it is expected
|
1187 |
-
to have [..., H, W] shape, where ... means an arbitrary number of leading dimensions.
|
1188 |
-
|
1189 |
-
Args:
|
1190 |
-
img (PIL Image or Tensor): Image to be blurred
|
1191 |
-
kernel_size (sequence of ints or int): Gaussian kernel size. Can be a sequence of integers
|
1192 |
-
like ``(kx, ky)`` or a single integer for square kernels.
|
1193 |
-
|
1194 |
-
.. note::
|
1195 |
-
In torchscript mode kernel_size as single int is not supported, use a sequence of
|
1196 |
-
length 1: ``[ksize, ]``.
|
1197 |
-
sigma (sequence of floats or float, optional): Gaussian kernel standard deviation. Can be a
|
1198 |
-
sequence of floats like ``(sigma_x, sigma_y)`` or a single float to define the
|
1199 |
-
same sigma in both X/Y directions. If None, then it is computed using
|
1200 |
-
``kernel_size`` as ``sigma = 0.3 * ((kernel_size - 1) * 0.5 - 1) + 0.8``.
|
1201 |
-
Default, None.
|
1202 |
-
|
1203 |
-
.. note::
|
1204 |
-
In torchscript mode sigma as single float is
|
1205 |
-
not supported, use a sequence of length 1: ``[sigma, ]``.
|
1206 |
-
|
1207 |
-
Returns:
|
1208 |
-
PIL Image or Tensor: Gaussian Blurred version of the image.
|
1209 |
-
"""
|
1210 |
-
if not isinstance(kernel_size, (int, list, tuple)):
|
1211 |
-
raise TypeError('kernel_size should be int or a sequence of integers. Got {}'.format(type(kernel_size)))
|
1212 |
-
if isinstance(kernel_size, int):
|
1213 |
-
kernel_size = [kernel_size, kernel_size]
|
1214 |
-
if len(kernel_size) != 2:
|
1215 |
-
raise ValueError('If kernel_size is a sequence its length should be 2. Got {}'.format(len(kernel_size)))
|
1216 |
-
for ksize in kernel_size:
|
1217 |
-
if ksize % 2 == 0 or ksize < 0:
|
1218 |
-
raise ValueError('kernel_size should have odd and positive integers. Got {}'.format(kernel_size))
|
1219 |
-
|
1220 |
-
if sigma is None:
|
1221 |
-
sigma = [ksize * 0.15 + 0.35 for ksize in kernel_size]
|
1222 |
-
|
1223 |
-
if sigma is not None and not isinstance(sigma, (int, float, list, tuple)):
|
1224 |
-
raise TypeError('sigma should be either float or sequence of floats. Got {}'.format(type(sigma)))
|
1225 |
-
if isinstance(sigma, (int, float)):
|
1226 |
-
sigma = [float(sigma), float(sigma)]
|
1227 |
-
if isinstance(sigma, (list, tuple)) and len(sigma) == 1:
|
1228 |
-
sigma = [sigma[0], sigma[0]]
|
1229 |
-
if len(sigma) != 2:
|
1230 |
-
raise ValueError('If sigma is a sequence, its length should be 2. Got {}'.format(len(sigma)))
|
1231 |
-
for s in sigma:
|
1232 |
-
if s <= 0.:
|
1233 |
-
raise ValueError('sigma should have positive values. Got {}'.format(sigma))
|
1234 |
-
|
1235 |
-
t_img = img
|
1236 |
-
if not isinstance(img, torch.Tensor):
|
1237 |
-
if not F_pil._is_pil_image(img):
|
1238 |
-
raise TypeError('img should be PIL Image or Tensor. Got {}'.format(type(img)))
|
1239 |
-
|
1240 |
-
t_img = to_tensor(img)
|
1241 |
-
|
1242 |
-
output = F_t.gaussian_blur(t_img, kernel_size, sigma)
|
1243 |
-
|
1244 |
-
if not isinstance(img, torch.Tensor):
|
1245 |
-
output = to_pil_image(output)
|
1246 |
-
return output
|
1247 |
-
|
1248 |
-
|
1249 |
-
def invert(img: Tensor) -> Tensor:
|
1250 |
-
"""Invert the colors of an RGB/grayscale image.
|
1251 |
-
|
1252 |
-
Args:
|
1253 |
-
img (PIL Image or Tensor): Image to have its colors inverted.
|
1254 |
-
If img is torch Tensor, it is expected to be in [..., 1 or 3, H, W] format,
|
1255 |
-
where ... means it can have an arbitrary number of leading dimensions.
|
1256 |
-
If img is PIL Image, it is expected to be in mode "L" or "RGB".
|
1257 |
-
|
1258 |
-
Returns:
|
1259 |
-
PIL Image or Tensor: Color inverted image.
|
1260 |
-
"""
|
1261 |
-
if not isinstance(img, torch.Tensor):
|
1262 |
-
return F_pil.invert(img)
|
1263 |
-
|
1264 |
-
return F_t.invert(img)
|
1265 |
-
|
1266 |
-
|
1267 |
-
def posterize(img: Tensor, bits: int) -> Tensor:
|
1268 |
-
"""Posterize an image by reducing the number of bits for each color channel.
|
1269 |
-
|
1270 |
-
Args:
|
1271 |
-
img (PIL Image or Tensor): Image to have its colors posterized.
|
1272 |
-
If img is torch Tensor, it should be of type torch.uint8 and
|
1273 |
-
it is expected to be in [..., 1 or 3, H, W] format, where ... means
|
1274 |
-
it can have an arbitrary number of leading dimensions.
|
1275 |
-
If img is PIL Image, it is expected to be in mode "L" or "RGB".
|
1276 |
-
bits (int): The number of bits to keep for each channel (0-8).
|
1277 |
-
Returns:
|
1278 |
-
PIL Image or Tensor: Posterized image.
|
1279 |
-
"""
|
1280 |
-
if not (0 <= bits <= 8):
|
1281 |
-
raise ValueError('The number if bits should be between 0 and 8. Got {}'.format(bits))
|
1282 |
-
|
1283 |
-
if not isinstance(img, torch.Tensor):
|
1284 |
-
return F_pil.posterize(img, bits)
|
1285 |
-
|
1286 |
-
return F_t.posterize(img, bits)
|
1287 |
-
|
1288 |
-
|
1289 |
-
def solarize(img: Tensor, threshold: float) -> Tensor:
|
1290 |
-
"""Solarize an RGB/grayscale image by inverting all pixel values above a threshold.
|
1291 |
-
|
1292 |
-
Args:
|
1293 |
-
img (PIL Image or Tensor): Image to have its colors inverted.
|
1294 |
-
If img is torch Tensor, it is expected to be in [..., 1 or 3, H, W] format,
|
1295 |
-
where ... means it can have an arbitrary number of leading dimensions.
|
1296 |
-
If img is PIL Image, it is expected to be in mode "L" or "RGB".
|
1297 |
-
threshold (float): All pixels equal or above this value are inverted.
|
1298 |
-
Returns:
|
1299 |
-
PIL Image or Tensor: Solarized image.
|
1300 |
-
"""
|
1301 |
-
if not isinstance(img, torch.Tensor):
|
1302 |
-
return F_pil.solarize(img, threshold)
|
1303 |
-
|
1304 |
-
return F_t.solarize(img, threshold)
|
1305 |
-
|
1306 |
-
|
1307 |
-
def adjust_sharpness(img: Tensor, sharpness_factor: float) -> Tensor:
|
1308 |
-
"""Adjust the sharpness of an image.
|
1309 |
-
|
1310 |
-
Args:
|
1311 |
-
img (PIL Image or Tensor): Image to be adjusted.
|
1312 |
-
If img is torch Tensor, it is expected to be in [..., 1 or 3, H, W] format,
|
1313 |
-
where ... means it can have an arbitrary number of leading dimensions.
|
1314 |
-
sharpness_factor (float): How much to adjust the sharpness. Can be
|
1315 |
-
any non negative number. 0 gives a blurred image, 1 gives the
|
1316 |
-
original image while 2 increases the sharpness by a factor of 2.
|
1317 |
-
|
1318 |
-
Returns:
|
1319 |
-
PIL Image or Tensor: Sharpness adjusted image.
|
1320 |
-
"""
|
1321 |
-
if not isinstance(img, torch.Tensor):
|
1322 |
-
return F_pil.adjust_sharpness(img, sharpness_factor)
|
1323 |
-
|
1324 |
-
return F_t.adjust_sharpness(img, sharpness_factor)
|
1325 |
-
|
1326 |
-
|
1327 |
-
def autocontrast(img: Tensor) -> Tensor:
|
1328 |
-
"""Maximize contrast of an image by remapping its
|
1329 |
-
pixels per channel so that the lowest becomes black and the lightest
|
1330 |
-
becomes white.
|
1331 |
-
|
1332 |
-
Args:
|
1333 |
-
img (PIL Image or Tensor): Image on which autocontrast is applied.
|
1334 |
-
If img is torch Tensor, it is expected to be in [..., 1 or 3, H, W] format,
|
1335 |
-
where ... means it can have an arbitrary number of leading dimensions.
|
1336 |
-
If img is PIL Image, it is expected to be in mode "L" or "RGB".
|
1337 |
-
|
1338 |
-
Returns:
|
1339 |
-
PIL Image or Tensor: An image that was autocontrasted.
|
1340 |
-
"""
|
1341 |
-
if not isinstance(img, torch.Tensor):
|
1342 |
-
return F_pil.autocontrast(img)
|
1343 |
-
|
1344 |
-
return F_t.autocontrast(img)
|
1345 |
-
|
1346 |
-
|
1347 |
-
def equalize(img: Tensor) -> Tensor:
|
1348 |
-
"""Equalize the histogram of an image by applying
|
1349 |
-
a non-linear mapping to the input in order to create a uniform
|
1350 |
-
distribution of grayscale values in the output.
|
1351 |
-
|
1352 |
-
Args:
|
1353 |
-
img (PIL Image or Tensor): Image on which equalize is applied.
|
1354 |
-
If img is torch Tensor, it is expected to be in [..., 1 or 3, H, W] format,
|
1355 |
-
where ... means it can have an arbitrary number of leading dimensions.
|
1356 |
-
The tensor dtype must be ``torch.uint8`` and values are expected to be in ``[0, 255]``.
|
1357 |
-
If img is PIL Image, it is expected to be in mode "P", "L" or "RGB".
|
1358 |
-
|
1359 |
-
Returns:
|
1360 |
-
PIL Image or Tensor: An image that was equalized.
|
1361 |
-
"""
|
1362 |
-
if not isinstance(img, torch.Tensor):
|
1363 |
-
return F_pil.equalize(img)
|
1364 |
-
|
1365 |
-
return F_t.equalize(img)
|
|
|
|
|
|
|
|
|
|
|
|
|
|
|
|
|
|
|
|
|
|
|
|
|
|
|
|
|
|
|
|
|
|
|
|
|
|
|
|
|
|
|
|
|
|
|
|
|
|
|
|
|
|
|
|
|
|
|
|
|
|
|
|
|
|
|
|
|
|
|
|
|
|
|
|
|
|
|
|
|
|
|
|
|
|
|
|
|
|
|
|
|
|
|
|
|
|
|
|
|
|
|
|
|
|
|
|
|
|
|
|
|
|
|
|
|
|
|
|
|
|
|
|
|
|
|
|
|
|
|
|
|
|
|
|
|
|
|
|
|
|
|
|
|
|
|
|
|
|
|
|
|
|
|
|
|
|
|
|
|
|
|
|
|
|
|
|
|
|
|
|
|
|
|
|
|
|
|
|
|
|
|
|
|
|
|
|
|
|
|
|
|
|
|
|
|
|
|
|
|
|
|
|
|
|
|
|
|
|
|
|
|
|
|
|
|
|
|
|
|
|
|
|
|
|
|
|
|
|
|
|
|
|
|
|
|
|
|
|
|
|
|
|
|
|
|
|
|
|
|
|
|
|
|
|
|
|
|
|
|
|
|
|
|
|
|
|
|
|
|
|
|
|
|
|
|
|
|
|
|
|
|
|
|
|
|
|
|
|
|
|
|
|
|
|
|
|
|
|
|
|
|
|
|
|
|
|
|
|
|
|
|
|
|
|
|
|
|
|
|
|
|
|
|
|
|
|
|
|
|
|
|
|
|
|
|
|
|
|
|
|
|
|
|
|
|
|
|
|
|
|
|
|
|
|
|
|
|
|
|
|
|
|
|
|
|
|
|
|
|
|
|
|
|
|
|
|
|
|
|
|
|
|
|
|
|
|
|
|
|
|
|
|
|
|
|
|
|
|
|
|
|
|
|
|
|
|
|
|
|
|
|
|
|
|
|
|
|
|
|
|
|
|
|
|
|
|
|
|
|
|
|
|
|
|
|
|
|
|
|
|
|
|
|
|
|
|
|
|
|
|
|
|
|
|
|
|
|
|
|
|
|
|
|
|
|
|
|
|
|
|
|
|
|
|
|
|
|
|
|
|
|
|
|
|
|
|
|
|
|
|
|
|
|
|
|
|
|
|
|
|
|
|
|
|
|
|
|
|
|
|
|
|
|
|
|
|
|
|
|
|
|
|
|
|
|
|
|
|
|
|
|
|
|
|
|
|
|
|
|
|
|
|
|
|
|
|
|
|
|
|
|
|
|
|
|
|
|
|
|
|
|
|
|
|
|
|
|
|
|
|
|
|
|
|
|
|
|
|
|
|
|
|
|
|
|
|
|
|
|
|
|
|
|
|
|
|
|
|
|
|
|
|
|
|
|
|
|
|
|
|
|
|
|
|
|
|
|
|
|
|
|
|
|
|
|
|
|
|
|
|
|
|
|
|
|
|
|
|
|
|
|
|
|
|
|
|
|
|
|
|
|
|
|
|
|
|
|
|
|
|
|
|
|
|
|
|
|
|
|
|
|
|
|
|
|
|
|
|
|
|
|
|
|
|
|
|
|
|
|
|
|
|
|
|
|
|
|
|
|
|
|
|
|
|
|
|
|
|
|
|
|
|
|
|
|
|
|
|
|
|
|
|
|
|
|
|
|
|
|
|
|
|
|
|
|
|
|
|
|
|
|
|
|
|
|
|
|
|
|
|
|
|
|
|
|
|
|
|
|
|
|
|
|
|
|
|
|
|
|
|
|
|
|
|
|
|
|
|
|
|
|
|
|
|
|
|
|
|
|
|
|
|
|
|
|
|
|
|
|
|
|
|
|
|
|
|
|
|
|
|
|
|
|
|
|
|
|
|
|
|
|
|
|
|
|
|
|
|
|
|
|
|
|
|
|
|
|
|
|
|
|
|
|
|
|
|
|
|
|
|
|
|
|
|
|
|
|
|
|
|
|
|
|
|
|
|
|
|
|
|
|
|
|
|
|
|
|
|
|
|
|
|
|
|
|
|
|
|
|
|
|
|
|
|
|
|
|
|
|
|
|
|
|
|
|
|
|
|
|
|
|
|
|
|
|
|
|
|
|
|
|
|
|
|
|
|
|
|
|
|
|
|
|
|
|
|
|
|
|
|
|
|
|
|
|
|
|
|
|
|
|
|
|
|
|
|
|
|
|
|
|
|
|
|
|
|
|
|
|
|
|
|
|
|
|
|
|
|
|
|
|
|
|
|
|
|
|
|
|
|
|
|
|
|
|
|
|
|
|
|
|
|
|
|
|
|
|
|
|
|
|
|
|
|
|
|
|
|
|
|
|
|
|
|
|
|
|
|
|
|
|
|
|
|
|
|
|
|
|
|
|
|
|
|
|
|
|
|
|
|
|
|
|
|
|
|
|
|
|
|
|
|
|
|
|
|
|
|
|
|
|
|
|
|
|
|
|
|
|
|
|
|
|
|
|
|
|
|
|
|
|
|
|
|
|
|
|
|
|
|
|
|
|
|
|
|
|
|
|
|
|
|
|
|
|
|
|
|
|
|
|
|
|
|
|
|
|
|
|
|
|
|
|
|
|
|
|
|
|
|
|
|
|
|
|
|
|
|
|
|
|
|
|
|
|
|
|
|
|
|
|
|
|
|
|
|
|
|
|
|
|
|
|
|
|
|
|
|
|
|
|
|
|
|
|
|
|
|
|
|
|
|
|
|
|
|
|
|
|
|
|
|
|
|
|
|
|
|
|
|
|
|
|
|
|
|
|
|
|
|
|
|
|
|
|
|
|
|
|
|
|
|
|
|
|
|
|
|
|
|
|
|
|
|
|
|
|
|
|
|
|
|
|
|
|
|
|
|
|
|
|
|
|
|
|
|
|
|
|
|
|
|
|
|
|
|
|
|
|
|
|
|
|
|
|
|
|
|
|
|
|
|
|
|
|
|
|
|
|
|
|
|
|
|
|
|
|
|
|
|
|
|
|
|
|
|
|
|
|
|
|
|
|
|
|
|
|
|
|
|
|
|
|
|
|
|
|
|
|
|
|
|
|
|
|
|
|
|
|
|
|
|
|
|
|
|
|
|
|
|
|
|
|
|
|
|
|
|
|
|
|
|
|
|
|
|
|
|
|
|
|
|
|
|
|
|
|
|
|
|
|
|
|
|
|
|
|
|
|
|
|
|
|
|
|
|
|
|
|
|
|
|
|
|
|
|
|
|
|
|
|
|
|
|
|
|
|
|
|
|
|
|
|
|
|
|
|
|
|
|
|
|
|
|
|
|
|
|
|
|
|
|
|
|
|
|
|
|
|
|
|
|
|
|
|
|
|
|
|
|
|
|
|
|
|
|
|
|
|
|
|
|
|
|
|
|
|
|
|
|
|
|
|
|
|
|
|
|
|
|
|
|
|
|
|
|
|
|
|
|
|
|
|
|
|
|
|
|
|
|
|
|
|
|
|
|
|
|
|
|
|
|
|
|
|
|
|
|
|
|
|
|
|
|
|
|
|
|
|
|
|
|
|
|
|
|
|
|
|
|
|
|
|
|
|
|
|
|
|
|
|
|
|
|
|
|
|
|
|
|
|
|
|
|
|
|
|
|
|
|
|
|
|
|
|
|
|
|
|
|
|
|
|
|
|
|
|
|
|
|
|
|
|
|
|
|
|
|
|
|
|
|
|
|
|
|
|
|
|
|
|
|
|
|
|
|
|
|
|
|
|
|
|
|
|
|
|
|
|
|
|
|
|
|
|
|
|
|
|
|
|
|
|
|
|
|
|
|
|
|
|
|
|
|
|
|
|
|
|
|
|
|
|
|
|
|
|
|
|
|
|
|
|
|
|
|
|
|
|
|
|
|
|
|
|
|
|
|
|
|
|
|
|
|
|
|
|
|
|
|
|
|
|
|
|
|
|
|
|
|
|
|
|
|
|
|
|
|
|
|
|
|
|
|
|
|
|
|
|
|
|
|
|
|
|
|
|
|
|
|
|
|
|
|
|
|
|
|
|
|
|
|
|
|
|
|
|
|
|
|
|
|
|
|
|
|
|
|
|
|
|
|
|
|
|
|
|
|
|
|
|
|
|
|
|
|
|
|
|
|
|
|
|
|
|
|
|
|
|
|
|
|
|
|
|
|
|
|
|
|
|
|
|
|
|
|
|
|
|
|
|
|
|
|
|
|
|
|
|
|
|
|
|
|
|
|
|
|
|
|
|
|
|
|
|
|
|
|
|
|
|
|
|
|
|
|
|
|
|
|
|
|
|
|
|
|
|
|
|
|
|
|
|
|
|
|
|
|
|
|
|
|
|
|
|
|
|
|
|
|
|
|
|
|
|
|
|
|
|
|
|
|
|
|
|
|
|
|
|
|
|
|
|
|
|
|
|
|
|
|
|
|
|
|
|
|
|
|
|
|
|
|
|
|
|
|
|
|
|
|
|
|
|
|
|
|
|
|
|
|
|
|
|
|
|
|
|
|
|
|
|
|
|
|
|
|
|
|
|
|
|
|
|
|
|
|
|
|
|
|
|
|
|
|
|
|
|
|
|
|
|
|
|
|
|
|
|
|
|
|
|
|
|
|
|
|
|
|
|
|
|
|
|
|
|
|
|
|
|
|
|
|
|
|
|
|
|
|
|
|
|
|
|
|
|
|
|
|
|
|
|
|
|
|
|
|
|
|
|
|
|
|
|
|
|
|
|
|
|
|
|
|
|
|
|
|
|
|
|
|
|
|
|
|
|
|
|
|
|
|
|
|
|
|
|
|
|
|
|
|
|
|
|
|
|
|
|
|
|
|
|
|
|
|
|
|
|
|
|
|
|
|
|
|
|
|
|
|
|
|
|
|
|
|
|
|
|
|
|
|
|
|
|
|
|
|
|
|
|
|
|
|
|
|
|
|
|
|
|
|
|
|
|
|
|
|
|
|
|
|
|
|
|
|
|
|
|
|
|
|
|
|
|
|
|
|
|
|
|
|
|
|
|
|
|
|
|
|
|
|
|
|
|
|
|
|
|
|
|
|
|
|
|
|
|
|
|
|
|
|
|
|
|
|
|
|
|
|
|
|
|
|
|
|
|
|
|
|
|
|
|
|
|
|
|
|
|
|
|
|
|
|
|
|
|
|
|
|
|
|
|
|
|
|
|
|
|
|
|
|
|
|
|
|
|
|
|
|
|
|
|
|
|
|
|
|
|
|
|
|
|
|
|
|
|
|
|
|
|
|
|
|
|
|
|
|
|
|
|
|
|
|
|
|
|
|
|
|
|
|
|
|
|
|
|
|
|
|
|
|
|
|
|
|
|
|
|
|
|
|
|
|
|
|
|
|
|
|
|
|
|
|
|
|
|
|
|
|
|
|
|
|
|
|
|
|
|
|
|
|
|
|
|
|
|
|
|
|
|
|
|
|
|
|
|
|
|
|
|
|
|
|
|
|
|
|
|
|
|
|
|
|
|
|
|
|
|
|
|
|
|
|
|
|
|
|
|
|
|
|
|
|
|
|
|
|
|
|
|
|
|
|
|
|
|
|
|
|
|
|
|
|
|
|
|
|
|
|
|
|
|
|
|
|
|
|
|
|
|
|
|
|
|
|
|
|
|
|
|
|
|
|
|
|
|
|
|
|
|
|
|
|
|
|
|
|
|
|
|
|
|
|
|
|
|
|
|
|
|
|
|
|
|
|
|
|
|
|
|
|
|
|
|
|
|
|
|
|
|
|
|
|
|
|
|
|
|
|
|
|
|
|
|
|
|
|
|
|
|
|
|
|
|
|
|
|
|
|
|
|
|
|
|
|
|
|
|
|
|
|
|
|
|
|
|
|
|
|
|
|
|
|
|
|
|
|
|
|
|
|
|
|
|
|
|
|
|
|
|
|
|
|
|
|
|
|
|
|
|
|
|
|
|
|
|
|
|
|
|
|
|
|
|
|
|
|
|
|
|
|
|
|
|
|
|
|
|
|
|
|
|
|
|
|
|
|
|
|
|
|
|
|
|
|
|
|
|
|
|
|
|
|
|
|
|
|
|
|
|
|
|
|
|
|
|
|
|
|
|
|
|
|
|
|
|
|
|
|
|
|
|
|
|
|
|
|
|
|
|
|
|
|
|
|
|
|
|
|
|
|
|
|
|
|
|
|
|
|
|
|
|
|
|
|
|
|
|
|
|
|
spaces/CVPR/regionclip-demo/detectron2/utils/registry.py
DELETED
@@ -1,60 +0,0 @@
|
|
1 |
-
# Copyright (c) Facebook, Inc. and its affiliates.
|
2 |
-
|
3 |
-
from typing import Any
|
4 |
-
import pydoc
|
5 |
-
from fvcore.common.registry import Registry # for backward compatibility.
|
6 |
-
|
7 |
-
"""
|
8 |
-
``Registry`` and `locate` provide ways to map a string (typically found
|
9 |
-
in config files) to callable objects.
|
10 |
-
"""
|
11 |
-
|
12 |
-
__all__ = ["Registry", "locate"]
|
13 |
-
|
14 |
-
|
15 |
-
def _convert_target_to_string(t: Any) -> str:
|
16 |
-
"""
|
17 |
-
Inverse of ``locate()``.
|
18 |
-
|
19 |
-
Args:
|
20 |
-
t: any object with ``__module__`` and ``__qualname__``
|
21 |
-
"""
|
22 |
-
module, qualname = t.__module__, t.__qualname__
|
23 |
-
|
24 |
-
# Compress the path to this object, e.g. ``module.submodule._impl.class``
|
25 |
-
# may become ``module.submodule.class``, if the later also resolves to the same
|
26 |
-
# object. This simplifies the string, and also is less affected by moving the
|
27 |
-
# class implementation.
|
28 |
-
module_parts = module.split(".")
|
29 |
-
for k in range(1, len(module_parts)):
|
30 |
-
prefix = ".".join(module_parts[:k])
|
31 |
-
candidate = f"{prefix}.{qualname}"
|
32 |
-
try:
|
33 |
-
if locate(candidate) is t:
|
34 |
-
return candidate
|
35 |
-
except ImportError:
|
36 |
-
pass
|
37 |
-
return f"{module}.{qualname}"
|
38 |
-
|
39 |
-
|
40 |
-
def locate(name: str) -> Any:
|
41 |
-
"""
|
42 |
-
Locate and return an object ``x`` using an input string ``{x.__module__}.{x.__qualname__}``,
|
43 |
-
such as "module.submodule.class_name".
|
44 |
-
|
45 |
-
Raise Exception if it cannot be found.
|
46 |
-
"""
|
47 |
-
obj = pydoc.locate(name)
|
48 |
-
|
49 |
-
# Some cases (e.g. torch.optim.sgd.SGD) not handled correctly
|
50 |
-
# by pydoc.locate. Try a private function from hydra.
|
51 |
-
if obj is None:
|
52 |
-
try:
|
53 |
-
# from hydra.utils import get_method - will print many errors
|
54 |
-
from hydra.utils import _locate
|
55 |
-
except ImportError as e:
|
56 |
-
raise ImportError(f"Cannot dynamically locate object {name}!") from e
|
57 |
-
else:
|
58 |
-
obj = _locate(name) # it raises if fails
|
59 |
-
|
60 |
-
return obj
|
|
|
|
|
|
|
|
|
|
|
|
|
|
|
|
|
|
|
|
|
|
|
|
|
|
|
|
|
|
|
|
|
|
|
|
|
|
|
|
|
|
|
|
|
|
|
|
|
|
|
|
|
|
|
|
|
|
|
|
|
|
|
|
|
|
|
|
|
|
|
|
|
|
|
|
|
|
|
|
|
|
|
|
|
|
|
|
|
|
|
|
|
|
|
|
|
|
|
|
|
|
|
|
|
|
|
|
|
|
|
|
|
|
|
|
|
|
|
|
|
|
spaces/CofAI/chat/server/backend.py
DELETED
@@ -1,176 +0,0 @@
|
|
1 |
-
import re
|
2 |
-
from datetime import datetime
|
3 |
-
from g4f import ChatCompletion
|
4 |
-
from flask import request, Response, stream_with_context
|
5 |
-
from requests import get
|
6 |
-
from server.config import special_instructions
|
7 |
-
|
8 |
-
|
9 |
-
class Backend_Api:
|
10 |
-
def __init__(self, bp, config: dict) -> None:
|
11 |
-
"""
|
12 |
-
Initialize the Backend_Api class.
|
13 |
-
:param app: Flask application instance
|
14 |
-
:param config: Configuration dictionary
|
15 |
-
"""
|
16 |
-
self.bp = bp
|
17 |
-
self.routes = {
|
18 |
-
'/backend-api/v2/conversation': {
|
19 |
-
'function': self._conversation,
|
20 |
-
'methods': ['POST']
|
21 |
-
}
|
22 |
-
}
|
23 |
-
|
24 |
-
def _conversation(self):
|
25 |
-
"""
|
26 |
-
Handles the conversation route.
|
27 |
-
|
28 |
-
:return: Response object containing the generated conversation stream
|
29 |
-
"""
|
30 |
-
conversation_id = request.json['conversation_id']
|
31 |
-
|
32 |
-
try:
|
33 |
-
jailbreak = request.json['jailbreak']
|
34 |
-
model = request.json['model']
|
35 |
-
messages = build_messages(jailbreak)
|
36 |
-
|
37 |
-
# Generate response
|
38 |
-
response = ChatCompletion.create(
|
39 |
-
model=model,
|
40 |
-
chatId=conversation_id,
|
41 |
-
messages=messages
|
42 |
-
)
|
43 |
-
|
44 |
-
return Response(stream_with_context(generate_stream(response, jailbreak)), mimetype='text/event-stream')
|
45 |
-
|
46 |
-
except Exception as e:
|
47 |
-
print(e)
|
48 |
-
print(e.__traceback__.tb_next)
|
49 |
-
|
50 |
-
return {
|
51 |
-
'_action': '_ask',
|
52 |
-
'success': False,
|
53 |
-
"error": f"an error occurred {str(e)}"
|
54 |
-
}, 400
|
55 |
-
|
56 |
-
|
57 |
-
def build_messages(jailbreak):
|
58 |
-
"""
|
59 |
-
Build the messages for the conversation.
|
60 |
-
|
61 |
-
:param jailbreak: Jailbreak instruction string
|
62 |
-
:return: List of messages for the conversation
|
63 |
-
"""
|
64 |
-
_conversation = request.json['meta']['content']['conversation']
|
65 |
-
internet_access = request.json['meta']['content']['internet_access']
|
66 |
-
prompt = request.json['meta']['content']['parts'][0]
|
67 |
-
|
68 |
-
# Add the existing conversation
|
69 |
-
conversation = _conversation
|
70 |
-
|
71 |
-
# Add web results if enabled
|
72 |
-
if internet_access:
|
73 |
-
current_date = datetime.now().strftime("%Y-%m-%d")
|
74 |
-
query = f'Current date: {current_date}. ' + prompt["content"]
|
75 |
-
search_results = fetch_search_results(query)
|
76 |
-
conversation.extend(search_results)
|
77 |
-
|
78 |
-
# Add jailbreak instructions if enabled
|
79 |
-
if jailbreak_instructions := getJailbreak(jailbreak):
|
80 |
-
conversation.extend(jailbreak_instructions)
|
81 |
-
|
82 |
-
# Add the prompt
|
83 |
-
conversation.append(prompt)
|
84 |
-
|
85 |
-
# Reduce conversation size to avoid API Token quantity error
|
86 |
-
if len(conversation) > 3:
|
87 |
-
conversation = conversation[-4:]
|
88 |
-
|
89 |
-
return conversation
|
90 |
-
|
91 |
-
|
92 |
-
def fetch_search_results(query):
|
93 |
-
"""
|
94 |
-
Fetch search results for a given query.
|
95 |
-
|
96 |
-
:param query: Search query string
|
97 |
-
:return: List of search results
|
98 |
-
"""
|
99 |
-
search = get('https://ddg-api.herokuapp.com/search',
|
100 |
-
params={
|
101 |
-
'query': query,
|
102 |
-
'limit': 3,
|
103 |
-
})
|
104 |
-
|
105 |
-
snippets = ""
|
106 |
-
for index, result in enumerate(search.json()):
|
107 |
-
snippet = f'[{index + 1}] "{result["snippet"]}" URL:{result["link"]}.'
|
108 |
-
snippets += snippet
|
109 |
-
|
110 |
-
response = "Here are some updated web searches. Use this to improve user response:"
|
111 |
-
response += snippets
|
112 |
-
|
113 |
-
return [{'role': 'system', 'content': response}]
|
114 |
-
|
115 |
-
|
116 |
-
def generate_stream(response, jailbreak):
|
117 |
-
"""
|
118 |
-
Generate the conversation stream.
|
119 |
-
|
120 |
-
:param response: Response object from ChatCompletion.create
|
121 |
-
:param jailbreak: Jailbreak instruction string
|
122 |
-
:return: Generator object yielding messages in the conversation
|
123 |
-
"""
|
124 |
-
if getJailbreak(jailbreak):
|
125 |
-
response_jailbreak = ''
|
126 |
-
jailbroken_checked = False
|
127 |
-
for message in response:
|
128 |
-
response_jailbreak += message
|
129 |
-
if jailbroken_checked:
|
130 |
-
yield message
|
131 |
-
else:
|
132 |
-
if response_jailbroken_success(response_jailbreak):
|
133 |
-
jailbroken_checked = True
|
134 |
-
if response_jailbroken_failed(response_jailbreak):
|
135 |
-
yield response_jailbreak
|
136 |
-
jailbroken_checked = True
|
137 |
-
else:
|
138 |
-
yield from response
|
139 |
-
|
140 |
-
|
141 |
-
def response_jailbroken_success(response: str) -> bool:
|
142 |
-
"""Check if the response has been jailbroken.
|
143 |
-
|
144 |
-
:param response: Response string
|
145 |
-
:return: Boolean indicating if the response has been jailbroken
|
146 |
-
"""
|
147 |
-
act_match = re.search(r'ACT:', response, flags=re.DOTALL)
|
148 |
-
return bool(act_match)
|
149 |
-
|
150 |
-
|
151 |
-
def response_jailbroken_failed(response):
|
152 |
-
"""
|
153 |
-
Check if the response has not been jailbroken.
|
154 |
-
|
155 |
-
:param response: Response string
|
156 |
-
:return: Boolean indicating if the response has not been jailbroken
|
157 |
-
"""
|
158 |
-
return False if len(response) < 4 else not (response.startswith("GPT:") or response.startswith("ACT:"))
|
159 |
-
|
160 |
-
|
161 |
-
def getJailbreak(jailbreak):
|
162 |
-
"""
|
163 |
-
Check if jailbreak instructions are provided.
|
164 |
-
|
165 |
-
:param jailbreak: Jailbreak instruction string
|
166 |
-
:return: Jailbreak instructions if provided, otherwise None
|
167 |
-
"""
|
168 |
-
if jailbreak != "default":
|
169 |
-
special_instructions[jailbreak][0]['content'] += special_instructions['two_responses_instruction']
|
170 |
-
if jailbreak in special_instructions:
|
171 |
-
special_instructions[jailbreak]
|
172 |
-
return special_instructions[jailbreak]
|
173 |
-
else:
|
174 |
-
return None
|
175 |
-
else:
|
176 |
-
return None
|
|
|
|
|
|
|
|
|
|
|
|
|
|
|
|
|
|
|
|
|
|
|
|
|
|
|
|
|
|
|
|
|
|
|
|
|
|
|
|
|
|
|
|
|
|
|
|
|
|
|
|
|
|
|
|
|
|
|
|
|
|
|
|
|
|
|
|
|
|
|
|
|
|
|
|
|
|
|
|
|
|
|
|
|
|
|
|
|
|
|
|
|
|
|
|
|
|
|
|
|
|
|
|
|
|
|
|
|
|
|
|
|
|
|
|
|
|
|
|
|
|
|
|
|
|
|
|
|
|
|
|
|
|
|
|
|
|
|
|
|
|
|
|
|
|
|
|
|
|
|
|
|
|
|
|
|
|
|
|
|
|
|
|
|
|
|
|
|
|
|
|
|
|
|
|
|
|
|
|
|
|
|
|
|
|
|
|
|
|
|
|
|
|
|
|
|
|
|
|
|
|
|
|
|
|
|
|
|
|
|
|
|
|
|
|
|
|
|
|
|
|
|
|
|
|
|
|
|
|
|
|
|
|
|
|
|
|
|
|
|
|
|
|
|
|
|
|
|
|
|
|
|
|
|
|
|
|
|
|
|
|
|
|
|
|
|
|
|
|
|
|
|
|
|
|
|
|
|
|
|
|
|
|
|
|
|
|
|
|
|
|
|
|
|
|
|
|
|
|
|
|
|
|
|
|
|
|
|
|
|
|
|
|
|
|
|
|
|
|
|
|
|
|
|
|
|
|
|
|
|
|
|
|
|
|
|
|
|
|
|
|
|
|
|
|
|
|
|
|
|
|
|
|
spaces/CosmoAI/BhagwatGeeta/htmlTemplates.py
DELETED
@@ -1,44 +0,0 @@
|
|
1 |
-
css = '''
|
2 |
-
<style>
|
3 |
-
.chat-message {
|
4 |
-
padding: 1.5rem; border-radius: 0.5rem; margin-bottom: 1rem; display: flex
|
5 |
-
}
|
6 |
-
.chat-message.user {
|
7 |
-
background-color: #2b313e
|
8 |
-
}
|
9 |
-
.chat-message.bot {
|
10 |
-
background-color: #475063
|
11 |
-
}
|
12 |
-
.chat-message .avatar {
|
13 |
-
width: 20%;
|
14 |
-
}
|
15 |
-
.chat-message .avatar img {
|
16 |
-
max-width: 78px;
|
17 |
-
max-height: 78px;
|
18 |
-
border-radius: 50%;
|
19 |
-
object-fit: cover;
|
20 |
-
}
|
21 |
-
.chat-message .message {
|
22 |
-
width: 80%;
|
23 |
-
padding: 0 1.5rem;
|
24 |
-
color: #fff;
|
25 |
-
}
|
26 |
-
'''
|
27 |
-
|
28 |
-
bot_template = '''
|
29 |
-
<div class="chat-message bot">
|
30 |
-
<div class="avatar">
|
31 |
-
<img src="https://i.ibb.co/cN0nmSj/Screenshot-2023-05-28-at-02-37-21.png" style="max-height: 78px; max-width: 78px; border-radius: 50%; object-fit: cover;">
|
32 |
-
</div>
|
33 |
-
<div class="message">{{MSG}}</div>
|
34 |
-
</div>
|
35 |
-
'''
|
36 |
-
|
37 |
-
user_template = '''
|
38 |
-
<div class="chat-message user">
|
39 |
-
<div class="avatar">
|
40 |
-
<img src="https://i.ibb.co/rdZC7LZ/Photo-logo-1.png">
|
41 |
-
</div>
|
42 |
-
<div class="message">{{MSG}}</div>
|
43 |
-
</div>
|
44 |
-
'''
|
|
|
|
|
|
|
|
|
|
|
|
|
|
|
|
|
|
|
|
|
|
|
|
|
|
|
|
|
|
|
|
|
|
|
|
|
|
|
|
|
|
|
|
|
|
|
|
|
|
|
|
|
|
|
|
|
|
|
|
|
|
|
|
|
|
|
|
|
|
|
|
|
|
|
|
|
|
|
|
|
|
|
|
|
|
|
|
|
|
spaces/DQChoi/gpt-demo/venv/lib/python3.11/site-packages/PIL/JpegPresets.py
DELETED
@@ -1,240 +0,0 @@
|
|
1 |
-
"""
|
2 |
-
JPEG quality settings equivalent to the Photoshop settings.
|
3 |
-
Can be used when saving JPEG files.
|
4 |
-
|
5 |
-
The following presets are available by default:
|
6 |
-
``web_low``, ``web_medium``, ``web_high``, ``web_very_high``, ``web_maximum``,
|
7 |
-
``low``, ``medium``, ``high``, ``maximum``.
|
8 |
-
More presets can be added to the :py:data:`presets` dict if needed.
|
9 |
-
|
10 |
-
To apply the preset, specify::
|
11 |
-
|
12 |
-
quality="preset_name"
|
13 |
-
|
14 |
-
To apply only the quantization table::
|
15 |
-
|
16 |
-
qtables="preset_name"
|
17 |
-
|
18 |
-
To apply only the subsampling setting::
|
19 |
-
|
20 |
-
subsampling="preset_name"
|
21 |
-
|
22 |
-
Example::
|
23 |
-
|
24 |
-
im.save("image_name.jpg", quality="web_high")
|
25 |
-
|
26 |
-
Subsampling
|
27 |
-
-----------
|
28 |
-
|
29 |
-
Subsampling is the practice of encoding images by implementing less resolution
|
30 |
-
for chroma information than for luma information.
|
31 |
-
(ref.: https://en.wikipedia.org/wiki/Chroma_subsampling)
|
32 |
-
|
33 |
-
Possible subsampling values are 0, 1 and 2 that correspond to 4:4:4, 4:2:2 and
|
34 |
-
4:2:0.
|
35 |
-
|
36 |
-
You can get the subsampling of a JPEG with the
|
37 |
-
:func:`.JpegImagePlugin.get_sampling` function.
|
38 |
-
|
39 |
-
In JPEG compressed data a JPEG marker is used instead of an EXIF tag.
|
40 |
-
(ref.: https://exiv2.org/tags.html)
|
41 |
-
|
42 |
-
|
43 |
-
Quantization tables
|
44 |
-
-------------------
|
45 |
-
|
46 |
-
They are values use by the DCT (Discrete cosine transform) to remove
|
47 |
-
*unnecessary* information from the image (the lossy part of the compression).
|
48 |
-
(ref.: https://en.wikipedia.org/wiki/Quantization_matrix#Quantization_matrices,
|
49 |
-
https://en.wikipedia.org/wiki/JPEG#Quantization)
|
50 |
-
|
51 |
-
You can get the quantization tables of a JPEG with::
|
52 |
-
|
53 |
-
im.quantization
|
54 |
-
|
55 |
-
This will return a dict with a number of lists. You can pass this dict
|
56 |
-
directly as the qtables argument when saving a JPEG.
|
57 |
-
|
58 |
-
The quantization table format in presets is a list with sublists. These formats
|
59 |
-
are interchangeable.
|
60 |
-
|
61 |
-
Libjpeg ref.:
|
62 |
-
https://web.archive.org/web/20120328125543/http://www.jpegcameras.com/libjpeg/libjpeg-3.html
|
63 |
-
|
64 |
-
"""
|
65 |
-
|
66 |
-
# fmt: off
|
67 |
-
presets = {
|
68 |
-
'web_low': {'subsampling': 2, # "4:2:0"
|
69 |
-
'quantization': [
|
70 |
-
[20, 16, 25, 39, 50, 46, 62, 68,
|
71 |
-
16, 18, 23, 38, 38, 53, 65, 68,
|
72 |
-
25, 23, 31, 38, 53, 65, 68, 68,
|
73 |
-
39, 38, 38, 53, 65, 68, 68, 68,
|
74 |
-
50, 38, 53, 65, 68, 68, 68, 68,
|
75 |
-
46, 53, 65, 68, 68, 68, 68, 68,
|
76 |
-
62, 65, 68, 68, 68, 68, 68, 68,
|
77 |
-
68, 68, 68, 68, 68, 68, 68, 68],
|
78 |
-
[21, 25, 32, 38, 54, 68, 68, 68,
|
79 |
-
25, 28, 24, 38, 54, 68, 68, 68,
|
80 |
-
32, 24, 32, 43, 66, 68, 68, 68,
|
81 |
-
38, 38, 43, 53, 68, 68, 68, 68,
|
82 |
-
54, 54, 66, 68, 68, 68, 68, 68,
|
83 |
-
68, 68, 68, 68, 68, 68, 68, 68,
|
84 |
-
68, 68, 68, 68, 68, 68, 68, 68,
|
85 |
-
68, 68, 68, 68, 68, 68, 68, 68]
|
86 |
-
]},
|
87 |
-
'web_medium': {'subsampling': 2, # "4:2:0"
|
88 |
-
'quantization': [
|
89 |
-
[16, 11, 11, 16, 23, 27, 31, 30,
|
90 |
-
11, 12, 12, 15, 20, 23, 23, 30,
|
91 |
-
11, 12, 13, 16, 23, 26, 35, 47,
|
92 |
-
16, 15, 16, 23, 26, 37, 47, 64,
|
93 |
-
23, 20, 23, 26, 39, 51, 64, 64,
|
94 |
-
27, 23, 26, 37, 51, 64, 64, 64,
|
95 |
-
31, 23, 35, 47, 64, 64, 64, 64,
|
96 |
-
30, 30, 47, 64, 64, 64, 64, 64],
|
97 |
-
[17, 15, 17, 21, 20, 26, 38, 48,
|
98 |
-
15, 19, 18, 17, 20, 26, 35, 43,
|
99 |
-
17, 18, 20, 22, 26, 30, 46, 53,
|
100 |
-
21, 17, 22, 28, 30, 39, 53, 64,
|
101 |
-
20, 20, 26, 30, 39, 48, 64, 64,
|
102 |
-
26, 26, 30, 39, 48, 63, 64, 64,
|
103 |
-
38, 35, 46, 53, 64, 64, 64, 64,
|
104 |
-
48, 43, 53, 64, 64, 64, 64, 64]
|
105 |
-
]},
|
106 |
-
'web_high': {'subsampling': 0, # "4:4:4"
|
107 |
-
'quantization': [
|
108 |
-
[6, 4, 4, 6, 9, 11, 12, 16,
|
109 |
-
4, 5, 5, 6, 8, 10, 12, 12,
|
110 |
-
4, 5, 5, 6, 10, 12, 14, 19,
|
111 |
-
6, 6, 6, 11, 12, 15, 19, 28,
|
112 |
-
9, 8, 10, 12, 16, 20, 27, 31,
|
113 |
-
11, 10, 12, 15, 20, 27, 31, 31,
|
114 |
-
12, 12, 14, 19, 27, 31, 31, 31,
|
115 |
-
16, 12, 19, 28, 31, 31, 31, 31],
|
116 |
-
[7, 7, 13, 24, 26, 31, 31, 31,
|
117 |
-
7, 12, 16, 21, 31, 31, 31, 31,
|
118 |
-
13, 16, 17, 31, 31, 31, 31, 31,
|
119 |
-
24, 21, 31, 31, 31, 31, 31, 31,
|
120 |
-
26, 31, 31, 31, 31, 31, 31, 31,
|
121 |
-
31, 31, 31, 31, 31, 31, 31, 31,
|
122 |
-
31, 31, 31, 31, 31, 31, 31, 31,
|
123 |
-
31, 31, 31, 31, 31, 31, 31, 31]
|
124 |
-
]},
|
125 |
-
'web_very_high': {'subsampling': 0, # "4:4:4"
|
126 |
-
'quantization': [
|
127 |
-
[2, 2, 2, 2, 3, 4, 5, 6,
|
128 |
-
2, 2, 2, 2, 3, 4, 5, 6,
|
129 |
-
2, 2, 2, 2, 4, 5, 7, 9,
|
130 |
-
2, 2, 2, 4, 5, 7, 9, 12,
|
131 |
-
3, 3, 4, 5, 8, 10, 12, 12,
|
132 |
-
4, 4, 5, 7, 10, 12, 12, 12,
|
133 |
-
5, 5, 7, 9, 12, 12, 12, 12,
|
134 |
-
6, 6, 9, 12, 12, 12, 12, 12],
|
135 |
-
[3, 3, 5, 9, 13, 15, 15, 15,
|
136 |
-
3, 4, 6, 11, 14, 12, 12, 12,
|
137 |
-
5, 6, 9, 14, 12, 12, 12, 12,
|
138 |
-
9, 11, 14, 12, 12, 12, 12, 12,
|
139 |
-
13, 14, 12, 12, 12, 12, 12, 12,
|
140 |
-
15, 12, 12, 12, 12, 12, 12, 12,
|
141 |
-
15, 12, 12, 12, 12, 12, 12, 12,
|
142 |
-
15, 12, 12, 12, 12, 12, 12, 12]
|
143 |
-
]},
|
144 |
-
'web_maximum': {'subsampling': 0, # "4:4:4"
|
145 |
-
'quantization': [
|
146 |
-
[1, 1, 1, 1, 1, 1, 1, 1,
|
147 |
-
1, 1, 1, 1, 1, 1, 1, 1,
|
148 |
-
1, 1, 1, 1, 1, 1, 1, 2,
|
149 |
-
1, 1, 1, 1, 1, 1, 2, 2,
|
150 |
-
1, 1, 1, 1, 1, 2, 2, 3,
|
151 |
-
1, 1, 1, 1, 2, 2, 3, 3,
|
152 |
-
1, 1, 1, 2, 2, 3, 3, 3,
|
153 |
-
1, 1, 2, 2, 3, 3, 3, 3],
|
154 |
-
[1, 1, 1, 2, 2, 3, 3, 3,
|
155 |
-
1, 1, 1, 2, 3, 3, 3, 3,
|
156 |
-
1, 1, 1, 3, 3, 3, 3, 3,
|
157 |
-
2, 2, 3, 3, 3, 3, 3, 3,
|
158 |
-
2, 3, 3, 3, 3, 3, 3, 3,
|
159 |
-
3, 3, 3, 3, 3, 3, 3, 3,
|
160 |
-
3, 3, 3, 3, 3, 3, 3, 3,
|
161 |
-
3, 3, 3, 3, 3, 3, 3, 3]
|
162 |
-
]},
|
163 |
-
'low': {'subsampling': 2, # "4:2:0"
|
164 |
-
'quantization': [
|
165 |
-
[18, 14, 14, 21, 30, 35, 34, 17,
|
166 |
-
14, 16, 16, 19, 26, 23, 12, 12,
|
167 |
-
14, 16, 17, 21, 23, 12, 12, 12,
|
168 |
-
21, 19, 21, 23, 12, 12, 12, 12,
|
169 |
-
30, 26, 23, 12, 12, 12, 12, 12,
|
170 |
-
35, 23, 12, 12, 12, 12, 12, 12,
|
171 |
-
34, 12, 12, 12, 12, 12, 12, 12,
|
172 |
-
17, 12, 12, 12, 12, 12, 12, 12],
|
173 |
-
[20, 19, 22, 27, 20, 20, 17, 17,
|
174 |
-
19, 25, 23, 14, 14, 12, 12, 12,
|
175 |
-
22, 23, 14, 14, 12, 12, 12, 12,
|
176 |
-
27, 14, 14, 12, 12, 12, 12, 12,
|
177 |
-
20, 14, 12, 12, 12, 12, 12, 12,
|
178 |
-
20, 12, 12, 12, 12, 12, 12, 12,
|
179 |
-
17, 12, 12, 12, 12, 12, 12, 12,
|
180 |
-
17, 12, 12, 12, 12, 12, 12, 12]
|
181 |
-
]},
|
182 |
-
'medium': {'subsampling': 2, # "4:2:0"
|
183 |
-
'quantization': [
|
184 |
-
[12, 8, 8, 12, 17, 21, 24, 17,
|
185 |
-
8, 9, 9, 11, 15, 19, 12, 12,
|
186 |
-
8, 9, 10, 12, 19, 12, 12, 12,
|
187 |
-
12, 11, 12, 21, 12, 12, 12, 12,
|
188 |
-
17, 15, 19, 12, 12, 12, 12, 12,
|
189 |
-
21, 19, 12, 12, 12, 12, 12, 12,
|
190 |
-
24, 12, 12, 12, 12, 12, 12, 12,
|
191 |
-
17, 12, 12, 12, 12, 12, 12, 12],
|
192 |
-
[13, 11, 13, 16, 20, 20, 17, 17,
|
193 |
-
11, 14, 14, 14, 14, 12, 12, 12,
|
194 |
-
13, 14, 14, 14, 12, 12, 12, 12,
|
195 |
-
16, 14, 14, 12, 12, 12, 12, 12,
|
196 |
-
20, 14, 12, 12, 12, 12, 12, 12,
|
197 |
-
20, 12, 12, 12, 12, 12, 12, 12,
|
198 |
-
17, 12, 12, 12, 12, 12, 12, 12,
|
199 |
-
17, 12, 12, 12, 12, 12, 12, 12]
|
200 |
-
]},
|
201 |
-
'high': {'subsampling': 0, # "4:4:4"
|
202 |
-
'quantization': [
|
203 |
-
[6, 4, 4, 6, 9, 11, 12, 16,
|
204 |
-
4, 5, 5, 6, 8, 10, 12, 12,
|
205 |
-
4, 5, 5, 6, 10, 12, 12, 12,
|
206 |
-
6, 6, 6, 11, 12, 12, 12, 12,
|
207 |
-
9, 8, 10, 12, 12, 12, 12, 12,
|
208 |
-
11, 10, 12, 12, 12, 12, 12, 12,
|
209 |
-
12, 12, 12, 12, 12, 12, 12, 12,
|
210 |
-
16, 12, 12, 12, 12, 12, 12, 12],
|
211 |
-
[7, 7, 13, 24, 20, 20, 17, 17,
|
212 |
-
7, 12, 16, 14, 14, 12, 12, 12,
|
213 |
-
13, 16, 14, 14, 12, 12, 12, 12,
|
214 |
-
24, 14, 14, 12, 12, 12, 12, 12,
|
215 |
-
20, 14, 12, 12, 12, 12, 12, 12,
|
216 |
-
20, 12, 12, 12, 12, 12, 12, 12,
|
217 |
-
17, 12, 12, 12, 12, 12, 12, 12,
|
218 |
-
17, 12, 12, 12, 12, 12, 12, 12]
|
219 |
-
]},
|
220 |
-
'maximum': {'subsampling': 0, # "4:4:4"
|
221 |
-
'quantization': [
|
222 |
-
[2, 2, 2, 2, 3, 4, 5, 6,
|
223 |
-
2, 2, 2, 2, 3, 4, 5, 6,
|
224 |
-
2, 2, 2, 2, 4, 5, 7, 9,
|
225 |
-
2, 2, 2, 4, 5, 7, 9, 12,
|
226 |
-
3, 3, 4, 5, 8, 10, 12, 12,
|
227 |
-
4, 4, 5, 7, 10, 12, 12, 12,
|
228 |
-
5, 5, 7, 9, 12, 12, 12, 12,
|
229 |
-
6, 6, 9, 12, 12, 12, 12, 12],
|
230 |
-
[3, 3, 5, 9, 13, 15, 15, 15,
|
231 |
-
3, 4, 6, 10, 14, 12, 12, 12,
|
232 |
-
5, 6, 9, 14, 12, 12, 12, 12,
|
233 |
-
9, 10, 14, 12, 12, 12, 12, 12,
|
234 |
-
13, 14, 12, 12, 12, 12, 12, 12,
|
235 |
-
15, 12, 12, 12, 12, 12, 12, 12,
|
236 |
-
15, 12, 12, 12, 12, 12, 12, 12,
|
237 |
-
15, 12, 12, 12, 12, 12, 12, 12]
|
238 |
-
]},
|
239 |
-
}
|
240 |
-
# fmt: on
|
|
|
|
|
|
|
|
|
|
|
|
|
|
|
|
|
|
|
|
|
|
|
|
|
|
|
|
|
|
|
|
|
|
|
|
|
|
|
|
|
|
|
|
|
|
|
|
|
|
|
|
|
|
|
|
|
|
|
|
|
|
|
|
|
|
|
|
|
|
|
|
|
|
|
|
|
|
|
|
|
|
|
|
|
|
|
|
|
|
|
|
|
|
|
|
|
|
|
|
|
|
|
|
|
|
|
|
|
|
|
|
|
|
|
|
|
|
|
|
|
|
|
|
|
|
|
|
|
|
|
|
|
|
|
|
|
|
|
|
|
|
|
|
|
|
|
|
|
|
|
|
|
|
|
|
|
|
|
|
|
|
|
|
|
|
|
|
|
|
|
|
|
|
|
|
|
|
|
|
|
|
|
|
|
|
|
|
|
|
|
|
|
|
|
|
|
|
|
|
|
|
|
|
|
|
|
|
|
|
|
|
|
|
|
|
|
|
|
|
|
|
|
|
|
|
|
|
|
|
|
|
|
|
|
|
|
|
|
|
|
|
|
|
|
|
|
|
|
|
|
|
|
|
|
|
|
|
|
|
|
|
|
|
|
|
|
|
|
|
|
|
|
|
|
|
|
|
|
|
|
|
|
|
|
|
|
|
|
|
|
|
|
|
|
|
|
|
|
|
|
|
|
|
|
|
|
|
|
|
|
|
|
|
|
|
|
|
|
|
|
|
|
|
|
|
|
|
|
|
|
|
|
|
|
|
|
|
|
|
|
|
|
|
|
|
|
|
|
|
|
|
|
|
|
|
|
|
|
|
|
|
|
|
|
|
|
|
|
|
|
|
|
|
|
|
|
|
|
|
|
|
|
|
|
|
|
|
|
|
|
|
|
|
|
|
|
|
|
|
|
|
|
|
|
|
|
|
|
|
|
|
|
|
|
|
|
|
|
|
|
|
|
|
|
|
|
|
|
|
|
|
|
|
|
|
|
|
|
|
|
|
|
|
|
|
|
|
|
|
|
|
|
|
|
|
|
|
|
|
|
|
|
|
|
|
|
|
|
|
|
|
|
|
|
|
|
|
|
|
|
|
spaces/DQChoi/gpt-demo/venv/lib/python3.11/site-packages/PIL/_util.py
DELETED
@@ -1,19 +0,0 @@
|
|
1 |
-
import os
|
2 |
-
from pathlib import Path
|
3 |
-
|
4 |
-
|
5 |
-
def is_path(f):
|
6 |
-
return isinstance(f, (bytes, str, Path))
|
7 |
-
|
8 |
-
|
9 |
-
def is_directory(f):
|
10 |
-
"""Checks if an object is a string, and that it points to a directory."""
|
11 |
-
return is_path(f) and os.path.isdir(f)
|
12 |
-
|
13 |
-
|
14 |
-
class DeferredError:
|
15 |
-
def __init__(self, ex):
|
16 |
-
self.ex = ex
|
17 |
-
|
18 |
-
def __getattr__(self, elt):
|
19 |
-
raise self.ex
|
|
|
|
|
|
|
|
|
|
|
|
|
|
|
|
|
|
|
|
|
|
|
|
|
|
|
|
|
|
|
|
|
|
|
|
|
|
|
|
spaces/DQChoi/gpt-demo/venv/lib/python3.11/site-packages/fontTools/ttLib/tables/G_S_U_B_.py
DELETED
@@ -1,5 +0,0 @@
|
|
1 |
-
from .otBase import BaseTTXConverter
|
2 |
-
|
3 |
-
|
4 |
-
class table_G_S_U_B_(BaseTTXConverter):
|
5 |
-
pass
|
|
|
|
|
|
|
|
|
|
|
|
spaces/DQChoi/gpt-demo/venv/lib/python3.11/site-packages/fontTools/varLib/instancer/names.py
DELETED
@@ -1,380 +0,0 @@
|
|
1 |
-
"""Helpers for instantiating name table records."""
|
2 |
-
|
3 |
-
from contextlib import contextmanager
|
4 |
-
from copy import deepcopy
|
5 |
-
from enum import IntEnum
|
6 |
-
import re
|
7 |
-
|
8 |
-
|
9 |
-
class NameID(IntEnum):
|
10 |
-
FAMILY_NAME = 1
|
11 |
-
SUBFAMILY_NAME = 2
|
12 |
-
UNIQUE_FONT_IDENTIFIER = 3
|
13 |
-
FULL_FONT_NAME = 4
|
14 |
-
VERSION_STRING = 5
|
15 |
-
POSTSCRIPT_NAME = 6
|
16 |
-
TYPOGRAPHIC_FAMILY_NAME = 16
|
17 |
-
TYPOGRAPHIC_SUBFAMILY_NAME = 17
|
18 |
-
VARIATIONS_POSTSCRIPT_NAME_PREFIX = 25
|
19 |
-
|
20 |
-
|
21 |
-
ELIDABLE_AXIS_VALUE_NAME = 2
|
22 |
-
|
23 |
-
|
24 |
-
def getVariationNameIDs(varfont):
|
25 |
-
used = []
|
26 |
-
if "fvar" in varfont:
|
27 |
-
fvar = varfont["fvar"]
|
28 |
-
for axis in fvar.axes:
|
29 |
-
used.append(axis.axisNameID)
|
30 |
-
for instance in fvar.instances:
|
31 |
-
used.append(instance.subfamilyNameID)
|
32 |
-
if instance.postscriptNameID != 0xFFFF:
|
33 |
-
used.append(instance.postscriptNameID)
|
34 |
-
if "STAT" in varfont:
|
35 |
-
stat = varfont["STAT"].table
|
36 |
-
for axis in stat.DesignAxisRecord.Axis if stat.DesignAxisRecord else ():
|
37 |
-
used.append(axis.AxisNameID)
|
38 |
-
for value in stat.AxisValueArray.AxisValue if stat.AxisValueArray else ():
|
39 |
-
used.append(value.ValueNameID)
|
40 |
-
elidedFallbackNameID = getattr(stat, "ElidedFallbackNameID", None)
|
41 |
-
if elidedFallbackNameID is not None:
|
42 |
-
used.append(elidedFallbackNameID)
|
43 |
-
# nameIDs <= 255 are reserved by OT spec so we don't touch them
|
44 |
-
return {nameID for nameID in used if nameID > 255}
|
45 |
-
|
46 |
-
|
47 |
-
@contextmanager
|
48 |
-
def pruningUnusedNames(varfont):
|
49 |
-
from . import log
|
50 |
-
|
51 |
-
origNameIDs = getVariationNameIDs(varfont)
|
52 |
-
|
53 |
-
yield
|
54 |
-
|
55 |
-
log.info("Pruning name table")
|
56 |
-
exclude = origNameIDs - getVariationNameIDs(varfont)
|
57 |
-
varfont["name"].names[:] = [
|
58 |
-
record for record in varfont["name"].names if record.nameID not in exclude
|
59 |
-
]
|
60 |
-
if "ltag" in varfont:
|
61 |
-
# Drop the whole 'ltag' table if all the language-dependent Unicode name
|
62 |
-
# records that reference it have been dropped.
|
63 |
-
# TODO: Only prune unused ltag tags, renumerating langIDs accordingly.
|
64 |
-
# Note ltag can also be used by feat or morx tables, so check those too.
|
65 |
-
if not any(
|
66 |
-
record
|
67 |
-
for record in varfont["name"].names
|
68 |
-
if record.platformID == 0 and record.langID != 0xFFFF
|
69 |
-
):
|
70 |
-
del varfont["ltag"]
|
71 |
-
|
72 |
-
|
73 |
-
def updateNameTable(varfont, axisLimits):
|
74 |
-
"""Update instatiated variable font's name table using STAT AxisValues.
|
75 |
-
|
76 |
-
Raises ValueError if the STAT table is missing or an Axis Value table is
|
77 |
-
missing for requested axis locations.
|
78 |
-
|
79 |
-
First, collect all STAT AxisValues that match the new default axis locations
|
80 |
-
(excluding "elided" ones); concatenate the strings in design axis order,
|
81 |
-
while giving priority to "synthetic" values (Format 4), to form the
|
82 |
-
typographic subfamily name associated with the new default instance.
|
83 |
-
Finally, update all related records in the name table, making sure that
|
84 |
-
legacy family/sub-family names conform to the the R/I/B/BI (Regular, Italic,
|
85 |
-
Bold, Bold Italic) naming model.
|
86 |
-
|
87 |
-
Example: Updating a partial variable font:
|
88 |
-
| >>> ttFont = TTFont("OpenSans[wdth,wght].ttf")
|
89 |
-
| >>> updateNameTable(ttFont, {"wght": (400, 900), "wdth": 75})
|
90 |
-
|
91 |
-
The name table records will be updated in the following manner:
|
92 |
-
NameID 1 familyName: "Open Sans" --> "Open Sans Condensed"
|
93 |
-
NameID 2 subFamilyName: "Regular" --> "Regular"
|
94 |
-
NameID 3 Unique font identifier: "3.000;GOOG;OpenSans-Regular" --> \
|
95 |
-
"3.000;GOOG;OpenSans-Condensed"
|
96 |
-
NameID 4 Full font name: "Open Sans Regular" --> "Open Sans Condensed"
|
97 |
-
NameID 6 PostScript name: "OpenSans-Regular" --> "OpenSans-Condensed"
|
98 |
-
NameID 16 Typographic Family name: None --> "Open Sans"
|
99 |
-
NameID 17 Typographic Subfamily name: None --> "Condensed"
|
100 |
-
|
101 |
-
References:
|
102 |
-
https://docs.microsoft.com/en-us/typography/opentype/spec/stat
|
103 |
-
https://docs.microsoft.com/en-us/typography/opentype/spec/name#name-ids
|
104 |
-
"""
|
105 |
-
from . import AxisLimits, axisValuesFromAxisLimits
|
106 |
-
|
107 |
-
if "STAT" not in varfont:
|
108 |
-
raise ValueError("Cannot update name table since there is no STAT table.")
|
109 |
-
stat = varfont["STAT"].table
|
110 |
-
if not stat.AxisValueArray:
|
111 |
-
raise ValueError("Cannot update name table since there are no STAT Axis Values")
|
112 |
-
fvar = varfont["fvar"]
|
113 |
-
|
114 |
-
# The updated name table will reflect the new 'zero origin' of the font.
|
115 |
-
# If we're instantiating a partial font, we will populate the unpinned
|
116 |
-
# axes with their default axis values from fvar.
|
117 |
-
axisLimits = AxisLimits(axisLimits).limitAxesAndPopulateDefaults(varfont)
|
118 |
-
partialDefaults = axisLimits.defaultLocation()
|
119 |
-
fvarDefaults = {a.axisTag: a.defaultValue for a in fvar.axes}
|
120 |
-
defaultAxisCoords = AxisLimits({**fvarDefaults, **partialDefaults})
|
121 |
-
assert all(v.minimum == v.maximum for v in defaultAxisCoords.values())
|
122 |
-
|
123 |
-
axisValueTables = axisValuesFromAxisLimits(stat, defaultAxisCoords)
|
124 |
-
checkAxisValuesExist(stat, axisValueTables, defaultAxisCoords.pinnedLocation())
|
125 |
-
|
126 |
-
# ignore "elidable" axis values, should be omitted in application font menus.
|
127 |
-
axisValueTables = [
|
128 |
-
v for v in axisValueTables if not v.Flags & ELIDABLE_AXIS_VALUE_NAME
|
129 |
-
]
|
130 |
-
axisValueTables = _sortAxisValues(axisValueTables)
|
131 |
-
_updateNameRecords(varfont, axisValueTables)
|
132 |
-
|
133 |
-
|
134 |
-
def checkAxisValuesExist(stat, axisValues, axisCoords):
|
135 |
-
seen = set()
|
136 |
-
designAxes = stat.DesignAxisRecord.Axis
|
137 |
-
for axisValueTable in axisValues:
|
138 |
-
axisValueFormat = axisValueTable.Format
|
139 |
-
if axisValueTable.Format in (1, 2, 3):
|
140 |
-
axisTag = designAxes[axisValueTable.AxisIndex].AxisTag
|
141 |
-
if axisValueFormat == 2:
|
142 |
-
axisValue = axisValueTable.NominalValue
|
143 |
-
else:
|
144 |
-
axisValue = axisValueTable.Value
|
145 |
-
if axisTag in axisCoords and axisValue == axisCoords[axisTag]:
|
146 |
-
seen.add(axisTag)
|
147 |
-
elif axisValueTable.Format == 4:
|
148 |
-
for rec in axisValueTable.AxisValueRecord:
|
149 |
-
axisTag = designAxes[rec.AxisIndex].AxisTag
|
150 |
-
if axisTag in axisCoords and rec.Value == axisCoords[axisTag]:
|
151 |
-
seen.add(axisTag)
|
152 |
-
|
153 |
-
missingAxes = set(axisCoords) - seen
|
154 |
-
if missingAxes:
|
155 |
-
missing = ", ".join(f"'{i}': {axisCoords[i]}" for i in missingAxes)
|
156 |
-
raise ValueError(f"Cannot find Axis Values {{{missing}}}")
|
157 |
-
|
158 |
-
|
159 |
-
def _sortAxisValues(axisValues):
|
160 |
-
# Sort by axis index, remove duplicates and ensure that format 4 AxisValues
|
161 |
-
# are dominant.
|
162 |
-
# The MS Spec states: "if a format 1, format 2 or format 3 table has a
|
163 |
-
# (nominal) value used in a format 4 table that also has values for
|
164 |
-
# other axes, the format 4 table, being the more specific match, is used",
|
165 |
-
# https://docs.microsoft.com/en-us/typography/opentype/spec/stat#axis-value-table-format-4
|
166 |
-
results = []
|
167 |
-
seenAxes = set()
|
168 |
-
# Sort format 4 axes so the tables with the most AxisValueRecords are first
|
169 |
-
format4 = sorted(
|
170 |
-
[v for v in axisValues if v.Format == 4],
|
171 |
-
key=lambda v: len(v.AxisValueRecord),
|
172 |
-
reverse=True,
|
173 |
-
)
|
174 |
-
|
175 |
-
for val in format4:
|
176 |
-
axisIndexes = set(r.AxisIndex for r in val.AxisValueRecord)
|
177 |
-
minIndex = min(axisIndexes)
|
178 |
-
if not seenAxes & axisIndexes:
|
179 |
-
seenAxes |= axisIndexes
|
180 |
-
results.append((minIndex, val))
|
181 |
-
|
182 |
-
for val in axisValues:
|
183 |
-
if val in format4:
|
184 |
-
continue
|
185 |
-
axisIndex = val.AxisIndex
|
186 |
-
if axisIndex not in seenAxes:
|
187 |
-
seenAxes.add(axisIndex)
|
188 |
-
results.append((axisIndex, val))
|
189 |
-
|
190 |
-
return [axisValue for _, axisValue in sorted(results)]
|
191 |
-
|
192 |
-
|
193 |
-
def _updateNameRecords(varfont, axisValues):
|
194 |
-
# Update nametable based on the axisValues using the R/I/B/BI model.
|
195 |
-
nametable = varfont["name"]
|
196 |
-
stat = varfont["STAT"].table
|
197 |
-
|
198 |
-
axisValueNameIDs = [a.ValueNameID for a in axisValues]
|
199 |
-
ribbiNameIDs = [n for n in axisValueNameIDs if _isRibbi(nametable, n)]
|
200 |
-
nonRibbiNameIDs = [n for n in axisValueNameIDs if n not in ribbiNameIDs]
|
201 |
-
elidedNameID = stat.ElidedFallbackNameID
|
202 |
-
elidedNameIsRibbi = _isRibbi(nametable, elidedNameID)
|
203 |
-
|
204 |
-
getName = nametable.getName
|
205 |
-
platforms = set((r.platformID, r.platEncID, r.langID) for r in nametable.names)
|
206 |
-
for platform in platforms:
|
207 |
-
if not all(getName(i, *platform) for i in (1, 2, elidedNameID)):
|
208 |
-
# Since no family name and subfamily name records were found,
|
209 |
-
# we cannot update this set of name Records.
|
210 |
-
continue
|
211 |
-
|
212 |
-
subFamilyName = " ".join(
|
213 |
-
getName(n, *platform).toUnicode() for n in ribbiNameIDs
|
214 |
-
)
|
215 |
-
if nonRibbiNameIDs:
|
216 |
-
typoSubFamilyName = " ".join(
|
217 |
-
getName(n, *platform).toUnicode() for n in axisValueNameIDs
|
218 |
-
)
|
219 |
-
else:
|
220 |
-
typoSubFamilyName = None
|
221 |
-
|
222 |
-
# If neither subFamilyName and typographic SubFamilyName exist,
|
223 |
-
# we will use the STAT's elidedFallbackName
|
224 |
-
if not typoSubFamilyName and not subFamilyName:
|
225 |
-
if elidedNameIsRibbi:
|
226 |
-
subFamilyName = getName(elidedNameID, *platform).toUnicode()
|
227 |
-
else:
|
228 |
-
typoSubFamilyName = getName(elidedNameID, *platform).toUnicode()
|
229 |
-
|
230 |
-
familyNameSuffix = " ".join(
|
231 |
-
getName(n, *platform).toUnicode() for n in nonRibbiNameIDs
|
232 |
-
)
|
233 |
-
|
234 |
-
_updateNameTableStyleRecords(
|
235 |
-
varfont,
|
236 |
-
familyNameSuffix,
|
237 |
-
subFamilyName,
|
238 |
-
typoSubFamilyName,
|
239 |
-
*platform,
|
240 |
-
)
|
241 |
-
|
242 |
-
|
243 |
-
def _isRibbi(nametable, nameID):
|
244 |
-
englishRecord = nametable.getName(nameID, 3, 1, 0x409)
|
245 |
-
return (
|
246 |
-
True
|
247 |
-
if englishRecord is not None
|
248 |
-
and englishRecord.toUnicode() in ("Regular", "Italic", "Bold", "Bold Italic")
|
249 |
-
else False
|
250 |
-
)
|
251 |
-
|
252 |
-
|
253 |
-
def _updateNameTableStyleRecords(
|
254 |
-
varfont,
|
255 |
-
familyNameSuffix,
|
256 |
-
subFamilyName,
|
257 |
-
typoSubFamilyName,
|
258 |
-
platformID=3,
|
259 |
-
platEncID=1,
|
260 |
-
langID=0x409,
|
261 |
-
):
|
262 |
-
# TODO (Marc F) It may be nice to make this part a standalone
|
263 |
-
# font renamer in the future.
|
264 |
-
nametable = varfont["name"]
|
265 |
-
platform = (platformID, platEncID, langID)
|
266 |
-
|
267 |
-
currentFamilyName = nametable.getName(
|
268 |
-
NameID.TYPOGRAPHIC_FAMILY_NAME, *platform
|
269 |
-
) or nametable.getName(NameID.FAMILY_NAME, *platform)
|
270 |
-
|
271 |
-
currentStyleName = nametable.getName(
|
272 |
-
NameID.TYPOGRAPHIC_SUBFAMILY_NAME, *platform
|
273 |
-
) or nametable.getName(NameID.SUBFAMILY_NAME, *platform)
|
274 |
-
|
275 |
-
if not all([currentFamilyName, currentStyleName]):
|
276 |
-
raise ValueError(f"Missing required NameIDs 1 and 2 for platform {platform}")
|
277 |
-
|
278 |
-
currentFamilyName = currentFamilyName.toUnicode()
|
279 |
-
currentStyleName = currentStyleName.toUnicode()
|
280 |
-
|
281 |
-
nameIDs = {
|
282 |
-
NameID.FAMILY_NAME: currentFamilyName,
|
283 |
-
NameID.SUBFAMILY_NAME: subFamilyName or "Regular",
|
284 |
-
}
|
285 |
-
if typoSubFamilyName:
|
286 |
-
nameIDs[NameID.FAMILY_NAME] = f"{currentFamilyName} {familyNameSuffix}".strip()
|
287 |
-
nameIDs[NameID.TYPOGRAPHIC_FAMILY_NAME] = currentFamilyName
|
288 |
-
nameIDs[NameID.TYPOGRAPHIC_SUBFAMILY_NAME] = typoSubFamilyName
|
289 |
-
else:
|
290 |
-
# Remove previous Typographic Family and SubFamily names since they're
|
291 |
-
# no longer required
|
292 |
-
for nameID in (
|
293 |
-
NameID.TYPOGRAPHIC_FAMILY_NAME,
|
294 |
-
NameID.TYPOGRAPHIC_SUBFAMILY_NAME,
|
295 |
-
):
|
296 |
-
nametable.removeNames(nameID=nameID)
|
297 |
-
|
298 |
-
newFamilyName = (
|
299 |
-
nameIDs.get(NameID.TYPOGRAPHIC_FAMILY_NAME) or nameIDs[NameID.FAMILY_NAME]
|
300 |
-
)
|
301 |
-
newStyleName = (
|
302 |
-
nameIDs.get(NameID.TYPOGRAPHIC_SUBFAMILY_NAME) or nameIDs[NameID.SUBFAMILY_NAME]
|
303 |
-
)
|
304 |
-
|
305 |
-
nameIDs[NameID.FULL_FONT_NAME] = f"{newFamilyName} {newStyleName}"
|
306 |
-
nameIDs[NameID.POSTSCRIPT_NAME] = _updatePSNameRecord(
|
307 |
-
varfont, newFamilyName, newStyleName, platform
|
308 |
-
)
|
309 |
-
|
310 |
-
uniqueID = _updateUniqueIdNameRecord(varfont, nameIDs, platform)
|
311 |
-
if uniqueID:
|
312 |
-
nameIDs[NameID.UNIQUE_FONT_IDENTIFIER] = uniqueID
|
313 |
-
|
314 |
-
for nameID, string in nameIDs.items():
|
315 |
-
assert string, nameID
|
316 |
-
nametable.setName(string, nameID, *platform)
|
317 |
-
|
318 |
-
if "fvar" not in varfont:
|
319 |
-
nametable.removeNames(NameID.VARIATIONS_POSTSCRIPT_NAME_PREFIX)
|
320 |
-
|
321 |
-
|
322 |
-
def _updatePSNameRecord(varfont, familyName, styleName, platform):
|
323 |
-
# Implementation based on Adobe Technical Note #5902 :
|
324 |
-
# https://wwwimages2.adobe.com/content/dam/acom/en/devnet/font/pdfs/5902.AdobePSNameGeneration.pdf
|
325 |
-
nametable = varfont["name"]
|
326 |
-
|
327 |
-
family_prefix = nametable.getName(
|
328 |
-
NameID.VARIATIONS_POSTSCRIPT_NAME_PREFIX, *platform
|
329 |
-
)
|
330 |
-
if family_prefix:
|
331 |
-
family_prefix = family_prefix.toUnicode()
|
332 |
-
else:
|
333 |
-
family_prefix = familyName
|
334 |
-
|
335 |
-
psName = f"{family_prefix}-{styleName}"
|
336 |
-
# Remove any characters other than uppercase Latin letters, lowercase
|
337 |
-
# Latin letters, digits and hyphens.
|
338 |
-
psName = re.sub(r"[^A-Za-z0-9-]", r"", psName)
|
339 |
-
|
340 |
-
if len(psName) > 127:
|
341 |
-
# Abbreviating the stylename so it fits within 127 characters whilst
|
342 |
-
# conforming to every vendor's specification is too complex. Instead
|
343 |
-
# we simply truncate the psname and add the required "..."
|
344 |
-
return f"{psName[:124]}..."
|
345 |
-
return psName
|
346 |
-
|
347 |
-
|
348 |
-
def _updateUniqueIdNameRecord(varfont, nameIDs, platform):
|
349 |
-
nametable = varfont["name"]
|
350 |
-
currentRecord = nametable.getName(NameID.UNIQUE_FONT_IDENTIFIER, *platform)
|
351 |
-
if not currentRecord:
|
352 |
-
return None
|
353 |
-
|
354 |
-
# Check if full name and postscript name are a substring of currentRecord
|
355 |
-
for nameID in (NameID.FULL_FONT_NAME, NameID.POSTSCRIPT_NAME):
|
356 |
-
nameRecord = nametable.getName(nameID, *platform)
|
357 |
-
if not nameRecord:
|
358 |
-
continue
|
359 |
-
if nameRecord.toUnicode() in currentRecord.toUnicode():
|
360 |
-
return currentRecord.toUnicode().replace(
|
361 |
-
nameRecord.toUnicode(), nameIDs[nameRecord.nameID]
|
362 |
-
)
|
363 |
-
|
364 |
-
# Create a new string since we couldn't find any substrings.
|
365 |
-
fontVersion = _fontVersion(varfont, platform)
|
366 |
-
achVendID = varfont["OS/2"].achVendID
|
367 |
-
# Remove non-ASCII characers and trailing spaces
|
368 |
-
vendor = re.sub(r"[^\x00-\x7F]", "", achVendID).strip()
|
369 |
-
psName = nameIDs[NameID.POSTSCRIPT_NAME]
|
370 |
-
return f"{fontVersion};{vendor};{psName}"
|
371 |
-
|
372 |
-
|
373 |
-
def _fontVersion(font, platform=(3, 1, 0x409)):
|
374 |
-
nameRecord = font["name"].getName(NameID.VERSION_STRING, *platform)
|
375 |
-
if nameRecord is None:
|
376 |
-
return f'{font["head"].fontRevision:.3f}'
|
377 |
-
# "Version 1.101; ttfautohint (v1.8.1.43-b0c9)" --> "1.101"
|
378 |
-
# Also works fine with inputs "Version 1.101" or "1.101" etc
|
379 |
-
versionNumber = nameRecord.toUnicode().split(";")[0]
|
380 |
-
return versionNumber.lstrip("Version ").strip()
|
|
|
|
|
|
|
|
|
|
|
|
|
|
|
|
|
|
|
|
|
|
|
|
|
|
|
|
|
|
|
|
|
|
|
|
|
|
|
|
|
|
|
|
|
|
|
|
|
|
|
|
|
|
|
|
|
|
|
|
|
|
|
|
|
|
|
|
|
|
|
|
|
|
|
|
|
|
|
|
|
|
|
|
|
|
|
|
|
|
|
|
|
|
|
|
|
|
|
|
|
|
|
|
|
|
|
|
|
|
|
|
|
|
|
|
|
|
|
|
|
|
|
|
|
|
|
|
|
|
|
|
|
|
|
|
|
|
|
|
|
|
|
|
|
|
|
|
|
|
|
|
|
|
|
|
|
|
|
|
|
|
|
|
|
|
|
|
|
|
|
|
|
|
|
|
|
|
|
|
|
|
|
|
|
|
|
|
|
|
|
|
|
|
|
|
|
|
|
|
|
|
|
|
|
|
|
|
|
|
|
|
|
|
|
|
|
|
|
|
|
|
|
|
|
|
|
|
|
|
|
|
|
|
|
|
|
|
|
|
|
|
|
|
|
|
|
|
|
|
|
|
|
|
|
|
|
|
|
|
|
|
|
|
|
|
|
|
|
|
|
|
|
|
|
|
|
|
|
|
|
|
|
|
|
|
|
|
|
|
|
|
|
|
|
|
|
|
|
|
|
|
|
|
|
|
|
|
|
|
|
|
|
|
|
|
|
|
|
|
|
|
|
|
|
|
|
|
|
|
|
|
|
|
|
|
|
|
|
|
|
|
|
|
|
|
|
|
|
|
|
|
|
|
|
|
|
|
|
|
|
|
|
|
|
|
|
|
|
|
|
|
|
|
|
|
|
|
|
|
|
|
|
|
|
|
|
|
|
|
|
|
|
|
|
|
|
|
|
|
|
|
|
|
|
|
|
|
|
|
|
|
|
|
|
|
|
|
|
|
|
|
|
|
|
|
|
|
|
|
|
|
|
|
|
|
|
|
|
|
|
|
|
|
|
|
|
|
|
|
|
|
|
|
|
|
|
|
|
|
|
|
|
|
|
|
|
|
|
|
|
|
|
|
|
|
|
|
|
|
|
|
|
|
|
|
|
|
|
|
|
|
|
|
|
|
|
|
|
|
|
|
|
|
|
|
|
|
|
|
|
|
|
|
|
|
|
|
|
|
|
|
|
|
|
|
|
|
|
|
|
|
|
|
|
|
|
|
|
|
|
|
|
|
|
|
|
|
|
|
|
|
|
|
|
|
|
|
|
|
|
|
|
|
|
|
|
|
|
|
|
|
|
|
|
|
|
|
|
|
|
|
|
|
|
|
|
|
|
|
|
|
|
|
|
|
|
|
|
|
|
|
|
|
|
|
|
|
|
|
|
|
|
|
|
|
|
|
|
|
|
|
|
|
|
|
|
|
|
|
|
|
|
|
|
|
|
|
|
|
|
|
|
|
|
|
|
|
|
|
|
|
|
|
|
|
|
|
|
|
|
|
|
|
|
|
|
|
|
|
|
|
|
|
|
|
|
|
|
|
|
|
|
|
|
|
|
|
|
|
|
|
|
|
|
|
|
|
|
|
|
|
|
|
|
|
|
|
|
|
|
|
|
|
|
|
|
|
|
|
|
|
|
|
|
|
|
|
|
|
|
|
|
|
|
|
|
|
|
|
|
|
|
|
|
|
|
|
|
|
|
|
|
|
|
|
|
|
|
|
|
|
spaces/Daniil-plotnikov/Daniil-plotnikov-russian-vision-v5-beta-3/README.md
DELETED
@@ -1,12 +0,0 @@
|
|
1 |
-
---
|
2 |
-
title: Daniil Plotnikov Russian Vision V5 Beta 3
|
3 |
-
emoji: ⚡
|
4 |
-
colorFrom: pink
|
5 |
-
colorTo: pink
|
6 |
-
sdk: gradio
|
7 |
-
sdk_version: 3.36.1
|
8 |
-
app_file: app.py
|
9 |
-
pinned: false
|
10 |
-
---
|
11 |
-
|
12 |
-
Check out the configuration reference at https://huggingface.co/docs/hub/spaces-config-reference
|
|
|
|
|
|
|
|
|
|
|
|
|
|
|
|
|
|
|
|
|
|
|
|
|
|
spaces/Datasculptor/StyleGAN-NADA/README.md
DELETED
@@ -1,14 +0,0 @@
|
|
1 |
-
---
|
2 |
-
title: StyleGAN NADA
|
3 |
-
emoji: 🌖
|
4 |
-
colorFrom: blue
|
5 |
-
colorTo: gray
|
6 |
-
sdk: gradio
|
7 |
-
sdk_version: 3.0.2
|
8 |
-
app_file: app.py
|
9 |
-
pinned: false
|
10 |
-
license: mit
|
11 |
-
duplicated_from: rinong/StyleGAN-NADA
|
12 |
-
---
|
13 |
-
|
14 |
-
Check out the configuration reference at https://huggingface.co/docs/hub/spaces#reference
|
|
|
|
|
|
|
|
|
|
|
|
|
|
|
|
|
|
|
|
|
|
|
|
|
|
|
|
|
|
spaces/Detomo/CuteRobot/README.md
DELETED
@@ -1,10 +0,0 @@
|
|
1 |
-
---
|
2 |
-
title: CuteRobot
|
3 |
-
emoji: 🤖
|
4 |
-
colorFrom: blue
|
5 |
-
colorTo: gray
|
6 |
-
sdk: static
|
7 |
-
pinned: false
|
8 |
-
---
|
9 |
-
|
10 |
-
Check out the configuration reference at https://huggingface.co/docs/hub/spaces-config-reference
|
|
|
|
|
|
|
|
|
|
|
|
|
|
|
|
|
|
|
|
|
|