p}=e,{focus:U=void 0}=e,{orientationStart:X=void 0}=e,{percentOf:ie=void 0}=e,{moveHandle:le=void 0}=e;function fe(p){le(void 0,p)}return l.$$set=p=>{"range"in p&&i(21,f=p.range),"min"in p&&i(0,g=p.min),"max"in p&&i(1,d=p.max),"step"in p&&i(22,c=p.step),"values"in p&&i(23,o=p.values),"vertical"in p&&i(2,_=p.vertical),"reversed"in p&&i(3,m=p.reversed),"hoverable"in p&&i(4,A=p.hoverable),"disabled"in p&&i(5,y=p.disabled),"pipstep"in p&&i(24,w=p.pipstep),"all"in p&&i(6,I=p.all),"first"in p&&i(7,q=p.first),"last"in p&&i(8,O=p.last),"rest"in p&&i(9,D=p.rest),"prefix"in p&&i(10,F=p.prefix),"suffix"in p&&i(11,W=p.suffix),"formatter"in p&&i(12,ee=p.formatter),"focus"in p&&i(13,U=p.focus),"orientationStart"in p&&i(14,X=p.orientationStart),"percentOf"in p&&i(15,ie=p.percentOf),"moveHandle"in p&&i(25,le=p.moveHandle)},l.$$.update=()=>{l.$$.dirty&20971527&&i(26,n=w||((d-g)/c>=(_?50:100)?(d-g)/(_?10:20):1)),l.$$.dirty&71303171&&i(19,t=parseInt((d-g)/(c*n),10)),l.$$.dirty&71303169&&i(18,a=function(p){return g+p*c*n}),l.$$.dirty&8388608&&i(17,s=function(p){return o.some(te=>te===p)}),l.$$.dirty&10485760&&i(16,r=function(p){if(f==="min")return o[0]>p;if(f==="max")return o[0]p})},[g,d,_,m,A,y,I,q,O,D,F,W,ee,U,X,ie,r,s,a,t,fe,f,c,o,w,le,n]}class an extends Ae{constructor(e){super(),ye(this,e,tn,nn,Se,{range:21,min:0,max:1,step:22,values:23,vertical:2,reversed:3,hoverable:4,disabled:5,pipstep:24,all:6,first:7,last:8,rest:9,prefix:10,suffix:11,formatter:12,focus:13,orientationStart:14,percentOf:15,moveHandle:25})}}function ll(l,e,i){const n=l.slice();return n[63]=e[i],n[65]=i,n}function nl(l){let e,i=l[21](l[63],l[65],l[23](l[63]))+"",n,t=l[18]&&il(l),a=l[19]&&tl(l);return{c(){e=H("span"),t&&t.c(),n=Y(i),a&&a.c(),h(e,"class","rangeFloat")},m(s,r){E(s,e,r),t&&t.m(e,null),L(e,n),a&&a.m(e,null)},p(s,r){s[18]?t?t.p(s,r):(t=il(s),t.c(),t.m(e,n)):t&&(t.d(1),t=null),r[0]&10485761&&i!==(i=s[21](s[63],s[65],s[23](s[63]))+"")&&Z(n,i),s[19]?a?a.p(s,r):(a=tl(s),a.c(),a.m(e,null)):a&&(a.d(1),a=null)},d(s){s&&V(e),t&&t.d(),a&&a.d()}}}function il(l){let e,i;return{c(){e=H("span"),i=Y(l[18]),h(e,"class","rangeFloat-prefix")},m(n,t){E(n,e,t),L(e,i)},p(n,t){t[0]&262144&&Z(i,n[18])},d(n){n&&V(e)}}}function tl(l){let e,i;return{c(){e=H("span"),i=Y(l[19]),h(e,"class","rangeFloat-suffix")},m(n,t){E(n,e,t),L(e,i)},p(n,t){t[0]&524288&&Z(i,n[19])},d(n){n&&V(e)}}}function al(l){let e,i,n,t,a,s,r,f,g,d,c,o,_=l[7]&&nl(l);return{c(){e=H("span"),i=H("span"),n=J(),_&&_.c(),h(i,"class","rangeNub"),h(e,"role","slider"),h(e,"class","rangeHandle"),h(e,"data-handle",l[65]),h(e,"style",t=l[28]+": "+l[29][l[65]]+"%; z-index: "+(l[26]===l[65]?3:2)+";"),h(e,"aria-valuemin",a=l[2]===!0&&l[65]===1?l[0][0]:l[3]),h(e,"aria-valuemax",s=l[2]===!0&&l[65]===0?l[0][1]:l[4]),h(e,"aria-valuenow",r=l[63]),h(e,"aria-valuetext",f=""+(l[18]+l[21](l[63],l[65],l[23](l[63]))+l[19])),h(e,"aria-orientation",g=l[6]?"vertical":"horizontal"),h(e,"aria-disabled",l[10]),h(e,"disabled",l[10]),h(e,"tabindex",d=l[10]?-1:0),S(e,"active",l[24]&&l[26]===l[65]),S(e,"press",l[25]&&l[26]===l[65])},m(m,A){E(m,e,A),L(e,i),L(e,n),_&&_.m(e,null),c||(o=[B(e,"blur",l[33]),B(e,"focus",l[34]),B(e,"keydown",l[35])],c=!0)},p(m,A){m[7]?_?_.p(m,A):(_=nl(m),_.c(),_.m(e,null)):_&&(_.d(1),_=null),A[0]&872415232&&t!==(t=m[28]+": "+m[29][m[65]]+"%; z-index: "+(m[26]===m[65]?3:2)+";")&&h(e,"style",t),A[0]&13&&a!==(a=m[2]===!0&&m[65]===1?m[0][0]:m[3])&&h(e,"aria-valuemin",a),A[0]&21&&s!==(s=m[2]===!0&&m[65]===0?m[0][1]:m[4])&&h(e,"aria-valuemax",s),A[0]&1&&r!==(r=m[63])&&h(e,"aria-valuenow",r),A[0]&11272193&&f!==(f=""+(m[18]+m[21](m[63],m[65],m[23](m[63]))+m[19]))&&h(e,"aria-valuetext",f),A[0]&64&&g!==(g=m[6]?"vertical":"horizontal")&&h(e,"aria-orientation",g),A[0]&1024&&h(e,"aria-disabled",m[10]),A[0]&1024&&h(e,"disabled",m[10]),A[0]&1024&&d!==(d=m[10]?-1:0)&&h(e,"tabindex",d),A[0]&83886080&&S(e,"active",m[24]&&m[26]===m[65]),A[0]&100663296&&S(e,"press",m[25]&&m[26]===m[65])},d(m){m&&V(e),_&&_.d(),c=!1,ge(o)}}}function sl(l){let e,i;return{c(){e=H("span"),h(e,"class","rangeBar"),h(e,"style",i=l[28]+": "+l[31](l[29])+"%; "+l[27]+": "+l[32](l[29])+"%;")},m(n,t){E(n,e,t)},p(n,t){t[0]&939524096&&i!==(i=n[28]+": "+n[31](n[29])+"%; "+n[27]+": "+n[32](n[29])+"%;")&&h(e,"style",i)},d(n){n&&V(e)}}}function fl(l){let e,i;return e=new an({props:{values:l[0],min:l[3],max:l[4],step:l[5],range:l[2],vertical:l[6],reversed:l[8],orientationStart:l[28],hoverable:l[9],disabled:l[10],all:l[13],first:l[14],last:l[15],rest:l[16],pipstep:l[12],prefix:l[18],suffix:l[19],formatter:l[20],focus:l[24],percentOf:l[23],moveHandle:l[30]}}),{c(){j(e.$$.fragment)},m(n,t){K(e,n,t),i=!0},p(n,t){const a={};t[0]&1&&(a.values=n[0]),t[0]&8&&(a.min=n[3]),t[0]&16&&(a.max=n[4]),t[0]&32&&(a.step=n[5]),t[0]&4&&(a.range=n[2]),t[0]&64&&(a.vertical=n[6]),t[0]&256&&(a.reversed=n[8]),t[0]&268435456&&(a.orientationStart=n[28]),t[0]&512&&(a.hoverable=n[9]),t[0]&1024&&(a.disabled=n[10]),t[0]&8192&&(a.all=n[13]),t[0]&16384&&(a.first=n[14]),t[0]&32768&&(a.last=n[15]),t[0]&65536&&(a.rest=n[16]),t[0]&4096&&(a.pipstep=n[12]),t[0]&262144&&(a.prefix=n[18]),t[0]&524288&&(a.suffix=n[19]),t[0]&1048576&&(a.formatter=n[20]),t[0]&16777216&&(a.focus=n[24]),t[0]&8388608&&(a.percentOf=n[23]),e.$set(a)},i(n){i||(P(e.$$.fragment,n),i=!0)},o(n){R(e.$$.fragment,n),i=!1},d(n){Q(e,n)}}}function sn(l){let e,i,n,t,a,s,r=Re(l[0]),f=[];for(let c=0;c{d=null}),oe()),(!t||o[0]&131072)&&h(e,"id",c[17]),(!t||o[0]&4)&&S(e,"range",c[2]),(!t||o[0]&1024)&&S(e,"disabled",c[10]),(!t||o[0]&512)&&S(e,"hoverable",c[9]),(!t||o[0]&64)&&S(e,"vertical",c[6]),(!t||o[0]&256)&&S(e,"reversed",c[8]),(!t||o[0]&16777216)&&S(e,"focus",c[24]),(!t||o[0]&4)&&S(e,"min",c[2]==="min"),(!t||o[0]&4)&&S(e,"max",c[2]==="max"),(!t||o[0]&2048)&&S(e,"pips",c[11]),(!t||o[0]&122880)&&S(e,"pip-labels",c[13]==="label"||c[14]==="label"||c[15]==="label"||c[16]==="label")},i(c){t||(P(d),t=!0)},o(c){R(d),t=!1},d(c){c&&V(e),dl(f,c),g&&g.d(),d&&d.d(),l[49](null),a=!1,ge(s)}}}function rl(l){if(!l)return-1;for(var e=0;l=l.previousElementSibling;)e++;return e}function Ue(l){return l.type.includes("touch")?l.touches[0]:l}function fn(l,e,i){let n,t,a,s,r,f,g=$,d=()=>(g(),g=Ol(be,u=>i(29,f=u)),be);l.$$.on_destroy.push(()=>g());let{slider:c}=e,{range:o=!1}=e,{pushy:_=!1}=e,{min:m=0}=e,{max:A=100}=e,{step:y=1}=e,{values:w=[(A+m)/2]}=e,{vertical:I=!1}=e,{float:q=!1}=e,{reversed:O=!1}=e,{hoverable:D=!0}=e,{disabled:F=!1}=e,{pips:W=!1}=e,{pipstep:ee=void 0}=e,{all:U=void 0}=e,{first:X=void 0}=e,{last:ie=void 0}=e,{rest:le=void 0}=e,{id:fe=void 0}=e,{prefix:p=""}=e,{suffix:te=""}=e,{formatter:_e=(u,v,M)=>u}=e,{handleFormatter:we=_e}=e,{precision:G=2}=e,{springValues:de={stiffness:.15,damping:.4}}=e;const me=Be();let ce=0,x=!1,ne=!1,b=!1,k=!1,N=w.length-1,ae,he,be;function Me(u){const v=c.querySelectorAll(".handle"),M=Array.prototype.includes.call(v,u),T=Array.prototype.some.call(v,se=>se.contains(u));return M||T}function Ee(u){return o==="min"||o==="max"?u.slice(0,1):o?u.slice(0,2):u}function ke(){return c.getBoundingClientRect()}function Fe(u){const v=ke();let M=0,T=0,se=0;I?(M=u.clientY-v.top,T=M/v.height*100,T=O?T:100-T):(M=u.clientX-v.left,T=M/v.width*100,T=O?100-T:T),se=(A-m)/100*T+m;let ze;return o===!0&&w[0]===w[1]?se>w[1]?1:0:(ze=w.indexOf([...w].sort((Ll,Ul)=>Math.abs(se-Ll)-Math.abs(se-Ul))[0]),ze)}function Le(u){const v=ke();let M=0,T=0,se=0;I?(M=u.clientY-v.top,T=M/v.height*100,T=O?T:100-T):(M=u.clientX-v.left,T=M/v.width*100,T=O?100-T:T),se=(A-m)/100*T+m,ve(N,se)}function ve(u,v){return v=a(v),typeof u>"u"&&(u=N),o&&(u===0&&v>w[1]?_?i(0,w[1]=v,w):v=w[1]:u===1&&va(u))})}function Oe(){!F&&me("stop",{activeHandle:N,startValue:ae,value:w[N],values:w.map(u=>a(u))})}function Ml(){!F&&me("change",{activeHandle:N,startValue:ae,previousValue:typeof he>"u"?ae:he,value:w[N],values:w.map(u=>a(u))})}function Fl(u){He[u?"unshift":"push"](()=>{c=u,i(1,c)})}return l.$$set=u=>{"slider"in u&&i(1,c=u.slider),"range"in u&&i(2,o=u.range),"pushy"in u&&i(43,_=u.pushy),"min"in u&&i(3,m=u.min),"max"in u&&i(4,A=u.max),"step"in u&&i(5,y=u.step),"values"in u&&i(0,w=u.values),"vertical"in u&&i(6,I=u.vertical),"float"in u&&i(7,q=u.float),"reversed"in u&&i(8,O=u.reversed),"hoverable"in u&&i(9,D=u.hoverable),"disabled"in u&&i(10,F=u.disabled),"pips"in u&&i(11,W=u.pips),"pipstep"in u&&i(12,ee=u.pipstep),"all"in u&&i(13,U=u.all),"first"in u&&i(14,X=u.first),"last"in u&&i(15,ie=u.last),"rest"in u&&i(16,le=u.rest),"id"in u&&i(17,fe=u.id),"prefix"in u&&i(18,p=u.prefix),"suffix"in u&&i(19,te=u.suffix),"formatter"in u&&i(20,_e=u.formatter),"handleFormatter"in u&&i(21,we=u.handleFormatter),"precision"in u&&i(44,G=u.precision),"springValues"in u&&i(45,de=u.springValues)},l.$$.update=()=>{l.$$.dirty[0]&24&&i(48,t=function(u){return u<=m?m:u>=A?A:u}),l.$$.dirty[0]&56|l.$$.dirty[1]&139264&&i(47,a=function(u){if(u<=m)return m;if(u>=A)return A;let v=(u-m)%y,M=u-v;return Math.abs(v)*2>=y&&(M+=v>0?y:-y),M=t(M),parseFloat(M.toFixed(G))}),l.$$.dirty[0]&24|l.$$.dirty[1]&8192&&i(23,n=function(u){let v=(u-m)/(A-m)*100;return isNaN(v)||v<=0?0:v>=100?100:parseFloat(v.toFixed(G))}),l.$$.dirty[0]&12582937|l.$$.dirty[1]&114688&&(Array.isArray(w)||(i(0,w=[(A+m)/2]),console.error("'values' prop should be an Array (https://github.com/simeydotme/svelte-range-slider-pips#slider-props)")),i(0,w=Ee(w.map(u=>a(u)))),ce!==w.length?d(i(22,be=Nl(w.map(u=>n(u)),de))):be.set(w.map(u=>n(u))),i(46,ce=w.length)),l.$$.dirty[0]&320&&i(28,s=I?O?"top":"bottom":O?"right":"left"),l.$$.dirty[0]&320&&i(27,r=I?O?"bottom":"top":O?"left":"right")},[w,c,o,m,A,y,I,q,O,D,F,W,ee,U,X,ie,le,fe,p,te,_e,we,be,n,x,b,N,r,s,f,ve,wl,kl,vl,Al,yl,Sl,El,Vl,Pl,Rl,Tl,Bl,_,G,de,ce,a,t,Fl]}class rn extends Ae{constructor(e){super(),ye(this,e,fn,sn,Se,{slider:1,range:2,pushy:43,min:3,max:4,step:5,values:0,vertical:6,float:7,reversed:8,hoverable:9,disabled:10,pips:11,pipstep:12,all:13,first:14,last:15,rest:16,id:17,prefix:18,suffix:19,formatter:20,handleFormatter:21,precision:44,springValues:45},null,[-1,-1,-1])}}function pl(l,{crop_values:e,autoplay:i}={}){function n(){if(e===void 0)return;const a=e[0]/100*l.duration,s=e[1]/100*l.duration;l.currentTimes&&(l.currentTime=a,l.pause())}async function t(){i&&(l.pause(),await l.play())}return l.addEventListener("loadeddata",t),l.addEventListener("timeupdate",n),{destroy(){l.removeEventListener("loadeddata",t),l.removeEventListener("timeupdate",n)}}}function un(l){let e,i,n,t,a,s,r,f,g,d,c;e=new Zl({props:{editable:!0,absolute:!0}}),e.$on("clear",l[13]),e.$on("edit",l[26]);let o=l[8]==="edit"&&l[9]?.duration&&ul(l);return{c(){j(e.$$.fragment),i=J(),n=H("audio"),r=J(),o&&o.c(),f=pe(),n.controls=!0,h(n,"preload","metadata"),Te(n.src,t=l[1]?.data)||h(n,"src",t),h(n,"data-testid",a=`${l[2]}-audio`),h(n,"class","svelte-1thnwz")},m(_,m){K(e,_,m),E(_,i,m),E(_,n,m),l[27](n),E(_,r,m),o&&o.m(_,m),E(_,f,m),g=!0,d||(c=[ml(s=pl.call(null,n,{autoplay:l[6],crop_values:l[10]})),B(n,"play",l[23]),B(n,"pause",l[24]),B(n,"ended",l[16])],d=!0)},p(_,m){(!g||m[0]&2&&!Te(n.src,t=_[1]?.data))&&h(n,"src",t),(!g||m[0]&4&&a!==(a=`${_[2]}-audio`))&&h(n,"data-testid",a),s&&re(s.update)&&m[0]&1088&&s.update.call(null,{autoplay:_[6],crop_values:_[10]}),_[8]==="edit"&&_[9]?.duration?o?(o.p(_,m),m[0]&768&&P(o,1)):(o=ul(_),o.c(),P(o,1),o.m(f.parentNode,f)):o&&(ue(),R(o,1,1,()=>{o=null}),oe())},i(_){g||(P(e.$$.fragment,_),P(o),g=!0)},o(_){R(e.$$.fragment,_),R(o),g=!1},d(_){_&&(V(i),V(n),V(r),V(f)),Q(e,_),l[27](null),o&&o.d(_),d=!1,ge(c)}}}function on(l){let e,i,n,t;const a=[dn,_n],s=[];function r(f,g){return f[4]==="microphone"?0:f[4]==="upload"?1:-1}return~(e=r(l))&&(i=s[e]=a[e](l)),{c(){i&&i.c(),n=pe()},m(f,g){~e&&s[e].m(f,g),E(f,n,g),t=!0},p(f,g){let d=e;e=r(f),e===d?~e&&s[e].p(f,g):(i&&(ue(),R(s[d],1,1,()=>{s[d]=null}),oe()),~e?(i=s[e],i?i.p(f,g):(i=s[e]=a[e](f),i.c()),P(i,1),i.m(n.parentNode,n)):i=null)},i(f){t||(P(i),t=!0)},o(f){R(i),t=!1},d(f){f&&V(n),~e&&s[e].d(f)}}}function ul(l){let e,i,n;function t(s){l[28](s)}let a={range:!0,min:0,max:100,step:1};return l[10]!==void 0&&(a.values=l[10]),e=new rn({props:a}),He.push(()=>cl(e,"values",t)),e.$on("change",l[14]),{c(){j(e.$$.fragment)},m(s,r){K(e,s,r),n=!0},p(s,r){const f={};!i&&r[0]&1024&&(i=!0,f.values=s[10],hl(()=>i=!1)),e.$set(f)},i(s){n||(P(e.$$.fragment,s),n=!0)},o(s){R(e.$$.fragment,s),n=!1},d(s){Q(e,s)}}}function _n(l){let e,i,n;function t(s){l[25](s)}let a={filetype:"audio/aac,audio/midi,audio/mpeg,audio/ogg,audio/wav,audio/x-wav,audio/opus,audio/webm,audio/flac,audio/vnd.rn-realaudio,audio/x-ms-wma,audio/x-aiff,audio/amr,audio/*",$$slots:{default:[mn]},$$scope:{ctx:l}};return l[0]!==void 0&&(a.dragging=l[0]),e=new Jl({props:a}),He.push(()=>cl(e,"dragging",t)),e.$on("load",l[15]),{c(){j(e.$$.fragment)},m(s,r){K(e,s,r),n=!0},p(s,r){const f={};r[0]&536870912&&(f.$$scope={dirty:r,ctx:s}),!i&&r[0]&1&&(i=!0,f.dragging=s[0],hl(()=>i=!1)),e.$set(f)},i(s){n||(P(e.$$.fragment,s),n=!0)},o(s){R(e.$$.fragment,s),n=!1},d(s){Q(e,s)}}}function dn(l){let e,i,n,t;const a=[hn,cn],s=[];function r(f,g){return f[7]?0:1}return i=r(l),n=s[i]=a[i](l),{c(){e=H("div"),n.c(),h(e,"class","mic-wrap svelte-1thnwz")},m(f,g){E(f,e,g),s[i].m(e,null),t=!0},p(f,g){let d=i;i=r(f),i===d?s[i].p(f,g):(ue(),R(s[d],1,1,()=>{s[d]=null}),oe(),n=s[i],n?n.p(f,g):(n=s[i]=a[i](f),n.c()),P(n,1),n.m(e,null))},i(f){t||(P(n),t=!0)},o(f){R(n),t=!1},d(f){f&&V(e),s[i].d()}}}function mn(l){let e;const i=l[22].default,n=Il(i,l,l[29],null);return{c(){n&&n.c()},m(t,a){n&&n.m(t,a),e=!0},p(t,a){n&&n.p&&(!e||a[0]&536870912)&&Dl(n,i,t,t[29],e?jl(i,t[29],a,null):Cl(t[29]),null)},i(t){e||(P(n,t),e=!0)},o(t){R(n,t),e=!1},d(t){n&&n.d(t)}}}function cn(l){let e,i;return e=new bl({props:{size:"sm",$$slots:{default:[bn]},$$scope:{ctx:l}}}),e.$on("click",l[11]),{c(){j(e.$$.fragment)},m(n,t){K(e,n,t),i=!0},p(n,t){const a={};t[0]&536870912&&(a.$$scope={dirty:t,ctx:n}),e.$set(a)},i(n){i||(P(e.$$.fragment,n),i=!0)},o(n){R(e.$$.fragment,n),i=!1},d(n){Q(e,n)}}}function hn(l){let e,i;return e=new bl({props:{size:"sm",$$slots:{default:[gn]},$$scope:{ctx:l}}}),e.$on("click",l[12]),{c(){j(e.$$.fragment)},m(n,t){K(e,n,t),i=!0},p(n,t){const a={};t[0]&536870912&&(a.$$scope={dirty:t,ctx:n}),e.$set(a)},i(n){i||(P(e.$$.fragment,n),i=!0)},o(n){R(e.$$.fragment,n),i=!1},d(n){Q(e,n)}}}function bn(l){let e,i;return{c(){e=H("span"),e.innerHTML=' ',i=Y(`
- Record from microphone`),h(e,"class","record-icon svelte-1thnwz")},m(n,t){E(n,e,t),E(n,i,t)},p:$,d(n){n&&(V(e),V(i))}}}function gn(l){let e,i;return{c(){e=H("span"),e.innerHTML=' ',i=Y(`
- Stop recording`),h(e,"class","record-icon svelte-1thnwz")},m(n,t){E(n,e,t),E(n,i,t)},p:$,d(n){n&&(V(e),V(i))}}}function pn(l){let e,i,n,t,a,s;e=new gl({props:{show_label:l[3],Icon:Ne,float:l[4]==="upload"&&l[1]===null,label:l[2]||"Audio"}});const r=[on,un],f=[];function g(d,c){return d[1]===null||d[5]?0:1}return n=g(l),t=f[n]=r[n](l),{c(){j(e.$$.fragment),i=J(),t.c(),a=pe()},m(d,c){K(e,d,c),E(d,i,c),f[n].m(d,c),E(d,a,c),s=!0},p(d,c){const o={};c[0]&8&&(o.show_label=d[3]),c[0]&18&&(o.float=d[4]==="upload"&&d[1]===null),c[0]&4&&(o.label=d[2]||"Audio"),e.$set(o);let _=n;n=g(d),n===_?f[n].p(d,c):(ue(),R(f[_],1,1,()=>{f[_]=null}),oe(),t=f[n],t?t.p(d,c):(t=f[n]=r[n](d),t.c()),P(t,1),t.m(a.parentNode,a))},i(d){s||(P(e.$$.fragment,d),P(t),s=!0)},o(d){R(e.$$.fragment,d),R(t),s=!1},d(d){d&&(V(i),V(a)),Q(e,d),f[n].d(d)}}}const wn=500,ol=44;function kn(l){return new Promise((e,i)=>{let n=new FileReader;n.onerror=i,n.onload=()=>e(n.result),n.readAsDataURL(l)})}function vn(l,e,i){let{$$slots:n={},$$scope:t}=e,{value:a=null}=e,{label:s}=e,{show_label:r=!0}=e,{name:f=""}=e,{source:g}=e,{pending:d=!1}=e,{streaming:c=!1}=e,{autoplay:o=!1}=e,_=!1,m,A="",y,w=[],I=!1,q,O=!1,D=[0,100],F=[],W;function ee(){W=[Ie(()=>import("./module-447425fe.js"),["./module-447425fe.js","./module-a3cf0cc4.js","./index-3370be2a.js","./index-f2292b12.css"],import.meta.url),Ie(()=>import("./module-a5a0afa0.js"),["./module-a5a0afa0.js","./module-a3cf0cc4.js"],import.meta.url)]}c&&ee();const U=Be(),X=async(k,N)=>{let ae=new Blob(k,{type:"audio/wav"});i(1,a={data:await kn(ae),name:"audio.wav"}),U(N,a)};async function ie(){let k;try{k=await navigator.mediaDevices.getUserMedia({audio:!0})}catch(N){if(N instanceof DOMException&&N.name=="NotAllowedError"){U("error","Please allow access to the microphone for recording.");return}throw N}if(k!=null){if(c){const[{MediaRecorder:N,register:ae},{connect:he}]=await Promise.all(W);await ae(await he()),m=new N(k,{mimeType:"audio/wav"});async function be(Me){let Ee=await Me.data.arrayBuffer(),ke=new Uint8Array(Ee);if(y||(i(19,y=new Uint8Array(Ee.slice(0,ol))),ke=new Uint8Array(Ee.slice(ol))),d)w.push(ke);else{let Fe=[y].concat(w,[ke]);X(Fe,"stream"),i(20,w=[])}}m.addEventListener("dataavailable",be)}else m=new MediaRecorder(k),m.addEventListener("dataavailable",N=>{F.push(N.data)}),m.addEventListener("stop",async()=>{i(7,_=!1),await X(F,"change"),await X(F,"stop_recording"),F=[]});O=!0}}async function le(){i(7,_=!0),U("start_recording"),O||await ie(),i(19,y=void 0),c?m.start(wn):m.start()}zl(()=>{m&&m.state!=="inactive"&&m.stop()});function fe(){m.stop(),c&&(i(7,_=!1),d&&i(21,I=!0))}function p(){U("change",null),U("clear"),i(8,A=""),i(1,a=null)}function te({detail:{values:k}}){a&&(U("change",{data:a.data,name:f,crop_min:k[0],crop_max:k[1]}),U("edit"))}function _e({detail:k}){i(1,a=k),U("change",{data:k.data,name:k.name}),U("upload",k)}function we(){U("stop"),U("end")}let{dragging:G=!1}=e;function de(k){C.call(this,l,k)}function me(k){C.call(this,l,k)}function ce(k){G=k,i(0,G)}const x=()=>i(8,A="edit");function ne(k){He[k?"unshift":"push"](()=>{q=k,i(9,q)})}function b(k){D=k,i(10,D)}return l.$$set=k=>{"value"in k&&i(1,a=k.value),"label"in k&&i(2,s=k.label),"show_label"in k&&i(3,r=k.show_label),"name"in k&&i(17,f=k.name),"source"in k&&i(4,g=k.source),"pending"in k&&i(18,d=k.pending),"streaming"in k&&i(5,c=k.streaming),"autoplay"in k&&i(6,o=k.autoplay),"dragging"in k&&i(0,G=k.dragging),"$$scope"in k&&i(29,t=k.$$scope)},l.$$.update=()=>{if(l.$$.dirty[0]&3932160&&I&&d===!1&&(i(21,I=!1),y&&w)){let k=[y].concat(w);i(20,w=[]),X(k,"stream")}l.$$.dirty[0]&1&&U("drag",G)},[G,a,s,r,g,c,o,_,A,q,D,le,fe,p,te,_e,we,f,d,y,w,I,n,de,me,ce,x,ne,b,t]}class An extends Ae{constructor(e){super(),ye(this,e,vn,pn,Se,{value:1,label:2,show_label:3,name:17,source:4,pending:18,streaming:5,autoplay:6,dragging:0},null,[-1,-1])}}function _l(l){let e,i,n;return i=new xl({props:{formatter:l[9],value:l[0]}}),i.$on("error",l[10]),i.$on("share",l[11]),{c(){e=H("div"),j(i.$$.fragment),h(e,"class","icon-button svelte-1yfus5a")},m(t,a){E(t,e,a),K(i,e,null),n=!0},p(t,a){const s={};a&1&&(s.value=t[0]),i.$set(s)},i(t){n||(P(i.$$.fragment,t),n=!0)},o(t){R(i.$$.fragment,t),n=!1},d(t){t&&V(e),Q(i)}}}function yn(l){let e,i,n,t,a,s;return{c(){e=H("audio"),e.controls=!0,h(e,"preload","metadata"),Te(e.src,i=l[0]?.data)||h(e,"src",i),h(e,"data-testid",n=`${l[1]}-audio`),h(e,"class","svelte-1yfus5a")},m(r,f){E(r,e,f),a||(s=[ml(t=pl.call(null,e,{autoplay:l[3]})),B(e,"play",l[7]),B(e,"pause",l[8]),B(e,"ended",l[5])],a=!0)},p(r,f){f&1&&!Te(e.src,i=r[0]?.data)&&h(e,"src",i),f&2&&n!==(n=`${r[1]}-audio`)&&h(e,"data-testid",n),t&&re(t.update)&&f&8&&t.update.call(null,{autoplay:r[3]})},i:$,o:$,d(r){r&&V(e),a=!1,ge(s)}}}function Sn(l){let e,i;return e=new Wl({props:{size:"small",$$slots:{default:[En]},$$scope:{ctx:l}}}),{c(){j(e.$$.fragment)},m(n,t){K(e,n,t),i=!0},p(n,t){const a={};t&8192&&(a.$$scope={dirty:t,ctx:n}),e.$set(a)},i(n){i||(P(e.$$.fragment,n),i=!0)},o(n){R(e.$$.fragment,n),i=!1},d(n){Q(e,n)}}}function En(l){let e,i;return e=new Ne({}),{c(){j(e.$$.fragment)},m(n,t){K(e,n,t),i=!0},i(n){i||(P(e.$$.fragment,n),i=!0)},o(n){R(e.$$.fragment,n),i=!1},d(n){Q(e,n)}}}function Vn(l){let e,i,n,t,a,s,r;e=new gl({props:{show_label:l[2],Icon:Ne,float:!1,label:l[1]||"Audio"}});let f=l[4]&&l[0]!==null&&_l(l);const g=[Sn,yn],d=[];function c(o,_){return o[0]===null?0:1}return t=c(l),a=d[t]=g[t](l),{c(){j(e.$$.fragment),i=J(),f&&f.c(),n=J(),a.c(),s=pe()},m(o,_){K(e,o,_),E(o,i,_),f&&f.m(o,_),E(o,n,_),d[t].m(o,_),E(o,s,_),r=!0},p(o,[_]){const m={};_&4&&(m.show_label=o[2]),_&2&&(m.label=o[1]||"Audio"),e.$set(m),o[4]&&o[0]!==null?f?(f.p(o,_),_&17&&P(f,1)):(f=_l(o),f.c(),P(f,1),f.m(n.parentNode,n)):f&&(ue(),R(f,1,1,()=>{f=null}),oe());let A=t;t=c(o),t===A?d[t].p(o,_):(ue(),R(d[A],1,1,()=>{d[A]=null}),oe(),a=d[t],a?a.p(o,_):(a=d[t]=g[t](o),a.c()),P(a,1),a.m(s.parentNode,s))},i(o){r||(P(e.$$.fragment,o),P(f),P(a),r=!0)},o(o){R(e.$$.fragment,o),R(f),R(a),r=!1},d(o){o&&(V(i),V(n),V(s)),Q(e,o),f&&f.d(o),d[t].d(o)}}}function Pn(l,e,i){let{value:n=null}=e,{label:t}=e,{name:a}=e,{show_label:s=!0}=e,{autoplay:r}=e,{show_share_button:f=!1}=e;const g=Be();function d(){g("stop"),g("end")}function c(y){C.call(this,l,y)}function o(y){C.call(this,l,y)}const _=async y=>y?` `:"";function m(y){C.call(this,l,y)}function A(y){C.call(this,l,y)}return l.$$set=y=>{"value"in y&&i(0,n=y.value),"label"in y&&i(1,t=y.label),"name"in y&&i(6,a=y.name),"show_label"in y&&i(2,s=y.show_label),"autoplay"in y&&i(3,r=y.autoplay),"show_share_button"in y&&i(4,f=y.show_share_button)},l.$$.update=()=>{l.$$.dirty&65&&n&&g("change",{name:a,data:n?.data})},[n,t,s,r,f,d,a,c,o,_,m,A]}class Rn extends Ae{constructor(e){super(),ye(this,e,Pn,Vn,Se,{value:0,label:1,name:6,show_label:2,autoplay:3,show_share_button:4})}}function Tn(l){let e,i;return e=new Rn({props:{autoplay:l[15],show_label:l[9],show_share_button:l[16],value:l[17],name:l[17]?.name||"audio_file",label:l[8]}}),e.$on("share",l[35]),e.$on("error",l[36]),{c(){j(e.$$.fragment)},m(n,t){K(e,n,t),i=!0},p(n,t){const a={};t[0]&32768&&(a.autoplay=n[15]),t[0]&512&&(a.show_label=n[9]),t[0]&65536&&(a.show_share_button=n[16]),t[0]&131072&&(a.value=n[17]),t[0]&131072&&(a.name=n[17]?.name||"audio_file"),t[0]&256&&(a.label=n[8]),e.$set(a)},i(n){i||(P(e.$$.fragment,n),i=!0)},o(n){R(e.$$.fragment,n),i=!1},d(n){Q(e,n)}}}function Bn(l){let e,i;return e=new An({props:{label:l[8],show_label:l[9],value:l[17],name:l[6],source:l[7],pending:l[10],streaming:l[11],autoplay:l[15],$$slots:{default:[Hn]},$$scope:{ctx:l}}}),e.$on("change",l[23]),e.$on("stream",l[24]),e.$on("drag",l[25]),e.$on("edit",l[26]),e.$on("play",l[27]),e.$on("pause",l[28]),e.$on("stop",l[29]),e.$on("end",l[30]),e.$on("start_recording",l[31]),e.$on("stop_recording",l[32]),e.$on("upload",l[33]),e.$on("error",l[34]),{c(){j(e.$$.fragment)},m(n,t){K(e,n,t),i=!0},p(n,t){const a={};t[0]&256&&(a.label=n[8]),t[0]&512&&(a.show_label=n[9]),t[0]&131072&&(a.value=n[17]),t[0]&64&&(a.name=n[6]),t[0]&128&&(a.source=n[7]),t[0]&1024&&(a.pending=n[10]),t[0]&2048&&(a.streaming=n[11]),t[0]&32768&&(a.autoplay=n[15]),t[1]&64&&(a.$$scope={dirty:t,ctx:n}),e.$set(a)},i(n){i||(P(e.$$.fragment,n),i=!0)},o(n){R(e.$$.fragment,n),i=!1},d(n){Q(e,n)}}}function Hn(l){let e,i;return e=new Xl({props:{type:"audio"}}),{c(){j(e.$$.fragment)},m(n,t){K(e,n,t),i=!0},p:$,i(n){i||(P(e.$$.fragment,n),i=!0)},o(n){R(e.$$.fragment,n),i=!1},d(n){Q(e,n)}}}function Mn(l){let e,i,n,t,a,s;const r=[l[1]];let f={};for(let o=0;o{d[A]=null}),oe(),t=d[n],t?t.p(o,_):(t=d[n]=g[n](o),t.c()),P(t,1),t.m(a.parentNode,a))},i(o){s||(P(e.$$.fragment,o),P(t),s=!0)},o(o){R(e.$$.fragment,o),R(t),s=!1},d(o){o&&(V(i),V(a)),Q(e,o),d[n].d(o)}}}function Fn(l){let e,i;return e=new Gl({props:{variant:l[5]==="dynamic"&&l[0]===null&&l[7]==="upload"?"dashed":"solid",border_mode:l[18]?"focus":"base",padding:!1,elem_id:l[2],elem_classes:l[3],visible:l[4],container:l[12],scale:l[13],min_width:l[14],$$slots:{default:[Mn]},$$scope:{ctx:l}}}),{c(){j(e.$$.fragment)},m(n,t){K(e,n,t),i=!0},p(n,t){const a={};t[0]&161&&(a.variant=n[5]==="dynamic"&&n[0]===null&&n[7]==="upload"?"dashed":"solid"),t[0]&262144&&(a.border_mode=n[18]?"focus":"base"),t[0]&4&&(a.elem_id=n[2]),t[0]&8&&(a.elem_classes=n[3]),t[0]&16&&(a.visible=n[4]),t[0]&4096&&(a.container=n[12]),t[0]&8192&&(a.scale=n[13]),t[0]&16384&&(a.min_width=n[14]),t[0]&495587|t[1]&64&&(a.$$scope={dirty:t,ctx:n}),e.$set(a)},i(n){i||(P(e.$$.fragment,n),i=!0)},o(n){R(e.$$.fragment,n),i=!1},d(n){Q(e,n)}}}function Ln(l,e,i){const n=Be();let{elem_id:t=""}=e,{elem_classes:a=[]}=e,{visible:s=!0}=e,{mode:r}=e,{value:f=null}=e,g=null,{name:d}=e,{source:c}=e,{label:o}=e,{root:_}=e,{show_label:m}=e,{pending:A}=e,{streaming:y}=e,{root_url:w}=e,{container:I=!0}=e,{scale:q=null}=e,{min_width:O=void 0}=e,{loading_status:D}=e,{autoplay:F=!1}=e,{show_share_button:W=!1}=e,ee,U;const X=({detail:b})=>i(0,f=b),ie=({detail:b})=>{i(0,f=b),n("stream",f)},le=({detail:b})=>i(18,U=b);function fe(b){C.call(this,l,b)}function p(b){C.call(this,l,b)}function te(b){C.call(this,l,b)}function _e(b){C.call(this,l,b)}function we(b){C.call(this,l,b)}function G(b){C.call(this,l,b)}function de(b){C.call(this,l,b)}function me(b){C.call(this,l,b)}const ce=({detail:b})=>{i(1,D=D||{}),i(1,D.status="error",D),n("error",b)};function x(b){C.call(this,l,b)}function ne(b){C.call(this,l,b)}return l.$$set=b=>{"elem_id"in b&&i(2,t=b.elem_id),"elem_classes"in b&&i(3,a=b.elem_classes),"visible"in b&&i(4,s=b.visible),"mode"in b&&i(5,r=b.mode),"value"in b&&i(0,f=b.value),"name"in b&&i(6,d=b.name),"source"in b&&i(7,c=b.source),"label"in b&&i(8,o=b.label),"root"in b&&i(20,_=b.root),"show_label"in b&&i(9,m=b.show_label),"pending"in b&&i(10,A=b.pending),"streaming"in b&&i(11,y=b.streaming),"root_url"in b&&i(21,w=b.root_url),"container"in b&&i(12,I=b.container),"scale"in b&&i(13,q=b.scale),"min_width"in b&&i(14,O=b.min_width),"loading_status"in b&&i(1,D=b.loading_status),"autoplay"in b&&i(15,F=b.autoplay),"show_share_button"in b&&i(16,W=b.show_share_button)},l.$$.update=()=>{l.$$.dirty[0]&3145729&&i(17,ee=en(f,_,w)),l.$$.dirty[0]&4194305&&JSON.stringify(f)!==JSON.stringify(g)&&(i(22,g=f),n("change"))},[f,D,t,a,s,r,d,c,o,m,A,y,I,q,O,F,W,ee,U,n,_,w,g,X,ie,le,fe,p,te,_e,we,G,de,me,ce,x,ne]}class Un extends Ae{constructor(e){super(),ye(this,e,Ln,Fn,Se,{elem_id:2,elem_classes:3,visible:4,mode:5,value:0,name:6,source:7,label:8,root:20,show_label:9,pending:10,streaming:11,root_url:21,container:12,scale:13,min_width:14,loading_status:1,autoplay:15,show_share_button:16},null,[-1,-1])}get elem_id(){return this.$$.ctx[2]}set elem_id(e){this.$$set({elem_id:e}),z()}get elem_classes(){return this.$$.ctx[3]}set elem_classes(e){this.$$set({elem_classes:e}),z()}get visible(){return this.$$.ctx[4]}set visible(e){this.$$set({visible:e}),z()}get mode(){return this.$$.ctx[5]}set mode(e){this.$$set({mode:e}),z()}get value(){return this.$$.ctx[0]}set value(e){this.$$set({value:e}),z()}get name(){return this.$$.ctx[6]}set name(e){this.$$set({name:e}),z()}get source(){return this.$$.ctx[7]}set source(e){this.$$set({source:e}),z()}get label(){return this.$$.ctx[8]}set label(e){this.$$set({label:e}),z()}get root(){return this.$$.ctx[20]}set root(e){this.$$set({root:e}),z()}get show_label(){return this.$$.ctx[9]}set show_label(e){this.$$set({show_label:e}),z()}get pending(){return this.$$.ctx[10]}set pending(e){this.$$set({pending:e}),z()}get streaming(){return this.$$.ctx[11]}set streaming(e){this.$$set({streaming:e}),z()}get root_url(){return this.$$.ctx[21]}set root_url(e){this.$$set({root_url:e}),z()}get container(){return this.$$.ctx[12]}set container(e){this.$$set({container:e}),z()}get scale(){return this.$$.ctx[13]}set scale(e){this.$$set({scale:e}),z()}get min_width(){return this.$$.ctx[14]}set min_width(e){this.$$set({min_width:e}),z()}get loading_status(){return this.$$.ctx[1]}set loading_status(e){this.$$set({loading_status:e}),z()}get autoplay(){return this.$$.ctx[15]}set autoplay(e){this.$$set({autoplay:e}),z()}get show_share_button(){return this.$$.ctx[16]}set show_share_button(e){this.$$set({show_share_button:e}),z()}}const Xn=Un,Gn=["static","dynamic"],Jn=()=>({type:{input_payload:"{ name: string; data: string }",response_object:"{ name: string; data: string, is_file: boolean }"},description:{input_payload:"audio data as object with filename and base64 string",response_object:"object that includes path to audio file. The URL: {ROOT}file={name} contains the data"},example_data:{name:"audio.wav",data:"data:audio/wav;base64,UklGRiQAAABXQVZFZm10IBAAAAABAAEARKwAAIhYAQACABAAZGF0YQAAAAA="}});export{Xn as Component,Jn as document,Gn as modes};
-//# sourceMappingURL=index-b7124075.js.map
diff --git a/spaces/DQChoi/gpt-demo/venv/lib/python3.11/site-packages/gradio_client/data_classes.py b/spaces/DQChoi/gpt-demo/venv/lib/python3.11/site-packages/gradio_client/data_classes.py
deleted file mode 100644
index 50f22042d3038925f35311e0cd329c89a91c79d8..0000000000000000000000000000000000000000
--- a/spaces/DQChoi/gpt-demo/venv/lib/python3.11/site-packages/gradio_client/data_classes.py
+++ /dev/null
@@ -1,15 +0,0 @@
-from __future__ import annotations
-
-from typing import TypedDict
-
-from typing_extensions import NotRequired
-
-
-class FileData(TypedDict):
- name: str | None # filename
- data: str | None # base64 encoded data
- size: NotRequired[int | None] # size in bytes
- is_file: NotRequired[
- bool
- ] # whether the data corresponds to a file or base64 encoded data
- orig_name: NotRequired[str] # original filename
diff --git a/spaces/Dewa/Text-Summurisation/app.py b/spaces/Dewa/Text-Summurisation/app.py
deleted file mode 100644
index 8fcb42a1799471fba4bdf7d6f5e46a3ae8b279cc..0000000000000000000000000000000000000000
--- a/spaces/Dewa/Text-Summurisation/app.py
+++ /dev/null
@@ -1,12 +0,0 @@
-from transformers import pipeline
-import gradio as gr
-
-model=pipeline("summarization")
-
-def predict(prompt):
- summary=model(prompt)[0]['summary_text']
- return summary
-
-
-iface = gr.Interface(fn=predict, inputs="text", outputs="text")
-iface.launch()
diff --git a/spaces/Dinoking/Guccio-AI-Designer/README.md b/spaces/Dinoking/Guccio-AI-Designer/README.md
deleted file mode 100644
index 2314160c31b58a510da73488d1321b733f7fc187..0000000000000000000000000000000000000000
--- a/spaces/Dinoking/Guccio-AI-Designer/README.md
+++ /dev/null
@@ -1,13 +0,0 @@
----
-title: Guccio-AI-Designer
-emoji: 👗🧢🥻
-colorFrom: indigo
-colorTo: gray
-sdk: gradio
-sdk_version: 2.9.4
-app_file: app.py
-pinned: false
-license: cc-by-nc-3.0
----
-
-Check out the configuration reference at https://huggingface.co/docs/hub/spaces#reference
diff --git a/spaces/Dorado607/ChuanhuChatGPT/modules/shared.py b/spaces/Dorado607/ChuanhuChatGPT/modules/shared.py
deleted file mode 100644
index 32e74665b400a56fd1b10bbd4a9566fe332e49bd..0000000000000000000000000000000000000000
--- a/spaces/Dorado607/ChuanhuChatGPT/modules/shared.py
+++ /dev/null
@@ -1,64 +0,0 @@
-from modules.presets import COMPLETION_URL, BALANCE_API_URL, USAGE_API_URL, API_HOST
-import os
-import queue
-import openai
-
-class State:
- interrupted = False
- multi_api_key = False
- completion_url = COMPLETION_URL
- balance_api_url = BALANCE_API_URL
- usage_api_url = USAGE_API_URL
-
- def interrupt(self):
- self.interrupted = True
-
- def recover(self):
- self.interrupted = False
-
- def set_api_host(self, api_host: str):
- api_host = api_host.rstrip("/")
- if not api_host.startswith("http"):
- api_host = f"https://{api_host}"
- if api_host.endswith("/v1"):
- api_host = api_host[:-3]
- self.completion_url = f"{api_host}/v1/chat/completions"
- self.balance_api_url = f"{api_host}/dashboard/billing/credit_grants"
- self.usage_api_url = f"{api_host}/dashboard/billing/usage"
- os.environ["OPENAI_API_BASE"] = api_host
-
- def reset_api_host(self):
- self.completion_url = COMPLETION_URL
- self.balance_api_url = BALANCE_API_URL
- self.usage_api_url = USAGE_API_URL
- os.environ["OPENAI_API_BASE"] = f"https://{API_HOST}"
- return API_HOST
-
- def reset_all(self):
- self.interrupted = False
- self.completion_url = COMPLETION_URL
-
- def set_api_key_queue(self, api_key_list):
- self.multi_api_key = True
- self.api_key_queue = queue.Queue()
- for api_key in api_key_list:
- self.api_key_queue.put(api_key)
-
- def switching_api_key(self, func):
- if not hasattr(self, "api_key_queue"):
- return func
-
- def wrapped(*args, **kwargs):
- api_key = self.api_key_queue.get()
- args[0].api_key = api_key
- ret = func(*args, **kwargs)
- self.api_key_queue.put(api_key)
- return ret
-
- return wrapped
-
-
-state = State()
-
-modules_path = os.path.dirname(os.path.realpath(__file__))
-chuanhu_path = os.path.dirname(modules_path)
diff --git a/spaces/DragGan/DragGan-Inversion/PTI/models/StyleCLIP/__init__.py b/spaces/DragGan/DragGan-Inversion/PTI/models/StyleCLIP/__init__.py
deleted file mode 100644
index e69de29bb2d1d6434b8b29ae775ad8c2e48c5391..0000000000000000000000000000000000000000
diff --git a/spaces/DragGan/DragGan-Inversion/stylegan_human/training/dataset.py b/spaces/DragGan/DragGan-Inversion/stylegan_human/training/dataset.py
deleted file mode 100644
index f04842155f754b0aac49b91b1de1de6db017a776..0000000000000000000000000000000000000000
--- a/spaces/DragGan/DragGan-Inversion/stylegan_human/training/dataset.py
+++ /dev/null
@@ -1,252 +0,0 @@
-# Copyright (c) 2021, NVIDIA CORPORATION & AFFILIATES. All rights reserved.
-#
-# NVIDIA CORPORATION and its licensors retain all intellectual property
-# and proprietary rights in and to this software, related documentation
-# and any modifications thereto. Any use, reproduction, disclosure or
-# distribution of this software and related documentation without an express
-# license agreement from NVIDIA CORPORATION is strictly prohibited.
-
-"""Streaming images and labels from datasets created with dataset_tool.py."""
-
-import os
-import numpy as np
-import zipfile
-import PIL.Image
-import json
-import torch
-import dnnlib
-
-try:
- import pyspng
-except ImportError:
- pyspng = None
-
-# ----------------------------------------------------------------------------
-
-
-class Dataset(torch.utils.data.Dataset):
- def __init__(self,
- name, # Name of the dataset.
- raw_shape, # Shape of the raw image data (NCHW).
- # Artificially limit the size of the dataset. None = no limit. Applied before xflip.
- max_size=None,
- # Enable conditioning labels? False = label dimension is zero.
- use_labels=False,
- # Artificially double the size of the dataset via x-flips. Applied after max_size.
- xflip=False,
- # Random seed to use when applying max_size.
- random_seed=0,
- ):
- self._name = name
- self._raw_shape = list(raw_shape)
- self._use_labels = use_labels
- self._raw_labels = None
- self._label_shape = None
-
- # Apply max_size.
- self._raw_idx = np.arange(self._raw_shape[0], dtype=np.int64)
- if (max_size is not None) and (self._raw_idx.size > max_size):
- np.random.RandomState(random_seed).shuffle(self._raw_idx)
- self._raw_idx = np.sort(self._raw_idx[:max_size])
-
- # Apply xflip.
- self._xflip = np.zeros(self._raw_idx.size, dtype=np.uint8)
- if xflip:
- self._raw_idx = np.tile(self._raw_idx, 2)
- self._xflip = np.concatenate(
- [self._xflip, np.ones_like(self._xflip)])
-
- def _get_raw_labels(self):
- if self._raw_labels is None:
- self._raw_labels = self._load_raw_labels() if self._use_labels else None
- if self._raw_labels is None:
- self._raw_labels = np.zeros(
- [self._raw_shape[0], 0], dtype=np.float32)
- assert isinstance(self._raw_labels, np.ndarray)
- assert self._raw_labels.shape[0] == self._raw_shape[0]
- assert self._raw_labels.dtype in [np.float32, np.int64]
- if self._raw_labels.dtype == np.int64:
- assert self._raw_labels.ndim == 1
- assert np.all(self._raw_labels >= 0)
- return self._raw_labels
-
- def close(self): # to be overridden by subclass
- pass
-
- def _load_raw_image(self, raw_idx): # to be overridden by subclass
- raise NotImplementedError
-
- def _load_raw_labels(self): # to be overridden by subclass
- raise NotImplementedError
-
- def __getstate__(self):
- return dict(self.__dict__, _raw_labels=None)
-
- def __del__(self):
- try:
- self.close()
- except:
- pass
-
- def __len__(self):
- return self._raw_idx.size
-
- def __getitem__(self, idx):
- image = self._load_raw_image(self._raw_idx[idx])
- assert isinstance(image, np.ndarray)
- assert list(image.shape) == self.image_shape
- assert image.dtype == np.uint8
- if self._xflip[idx]:
- assert image.ndim == 3 # CHW
- image = image[:, :, ::-1]
- return image.copy(), self.get_label(idx)
-
- def get_label(self, idx):
- label = self._get_raw_labels()[self._raw_idx[idx]]
- if label.dtype == np.int64:
- onehot = np.zeros(self.label_shape, dtype=np.float32)
- onehot[label] = 1
- label = onehot
- return label.copy()
-
- def get_details(self, idx):
- d = dnnlib.EasyDict()
- d.raw_idx = int(self._raw_idx[idx])
- d.xflip = (int(self._xflip[idx]) != 0)
- d.raw_label = self._get_raw_labels()[d.raw_idx].copy()
- return d
-
- @property
- def name(self):
- return self._name
-
- @property
- def image_shape(self):
- return list(self._raw_shape[1:])
-
- @property
- def num_channels(self):
- assert len(self.image_shape) == 3 # CHW
- return self.image_shape[0]
-
- @property
- def resolution(self):
- assert len(self.image_shape) == 3 # CHW
- assert self.image_shape[1] == self.image_shape[2]
- return self.image_shape[1]
-
- @property
- def label_shape(self):
- if self._label_shape is None:
- raw_labels = self._get_raw_labels()
- if raw_labels.dtype == np.int64:
- self._label_shape = [int(np.max(raw_labels)) + 1]
- else:
- self._label_shape = raw_labels.shape[1:]
- return list(self._label_shape)
-
- @property
- def label_dim(self):
- assert len(self.label_shape) == 1
- return self.label_shape[0]
-
- @property
- def has_labels(self):
- return any(x != 0 for x in self.label_shape)
-
- @property
- def has_onehot_labels(self):
- return self._get_raw_labels().dtype == np.int64
-
-# ----------------------------------------------------------------------------
-
-
-class ImageFolderDataset(Dataset):
- def __init__(self,
- path, # Path to directory or zip.
- # Ensure specific resolution, None = highest available.
- resolution=None,
- # Additional arguments for the Dataset base class.
- **super_kwargs,
- ):
- self._path = path
- self._zipfile = None
-
- if os.path.isdir(self._path):
- self._type = 'dir'
- self._all_fnames = {os.path.relpath(os.path.join(
- root, fname), start=self._path) for root, _dirs, files in os.walk(self._path) for fname in files}
- elif self._file_ext(self._path) == '.zip':
- self._type = 'zip'
- self._all_fnames = set(self._get_zipfile().namelist())
- else:
- raise IOError('Path must point to a directory or zip')
-
- PIL.Image.init()
- self._image_fnames = sorted(
- fname for fname in self._all_fnames if self._file_ext(fname) in PIL.Image.EXTENSION)
- if len(self._image_fnames) == 0:
- raise IOError('No image files found in the specified path')
-
- name = os.path.splitext(os.path.basename(self._path))[0]
- raw_shape = [len(self._image_fnames)] + \
- list(self._load_raw_image(0).shape)
- if resolution is not None and (raw_shape[2] != resolution or raw_shape[3] != resolution):
- raise IOError('Image files do not match the specified resolution')
- super().__init__(name=name, raw_shape=raw_shape, **super_kwargs)
-
- @staticmethod
- def _file_ext(fname):
- return os.path.splitext(fname)[1].lower()
-
- def _get_zipfile(self):
- assert self._type == 'zip'
- if self._zipfile is None:
- self._zipfile = zipfile.ZipFile(self._path)
- return self._zipfile
-
- def _open_file(self, fname):
- if self._type == 'dir':
- return open(os.path.join(self._path, fname), 'rb')
- if self._type == 'zip':
- return self._get_zipfile().open(fname, 'r')
- return None
-
- def close(self):
- try:
- if self._zipfile is not None:
- self._zipfile.close()
- finally:
- self._zipfile = None
-
- def __getstate__(self):
- return dict(super().__getstate__(), _zipfile=None)
-
- def _load_raw_image(self, raw_idx):
- fname = self._image_fnames[raw_idx]
- with self._open_file(fname) as f:
- if pyspng is not None and self._file_ext(fname) == '.png':
- image = pyspng.load(f.read())
- else:
- image = np.array(PIL.Image.open(f))
- if image.ndim == 2:
- image = image[:, :, np.newaxis] # HW => HWC
- image = image.transpose(2, 0, 1) # HWC => CHW
- return image
-
- def _load_raw_labels(self):
- fname = 'dataset.json'
- if fname not in self._all_fnames:
- return None
- with self._open_file(fname) as f:
- labels = json.load(f)['labels']
- if labels is None:
- return None
- labels = dict(labels)
- labels = [labels[fname.replace('\\', '/')]
- for fname in self._image_fnames]
- labels = np.array(labels)
- labels = labels.astype({1: np.int64, 2: np.float32}[labels.ndim])
- return labels
-
-# ----------------------------------------------------------------------------
diff --git a/spaces/Duskfallcrew/Gambit_and_Rogue/app.py b/spaces/Duskfallcrew/Gambit_and_Rogue/app.py
deleted file mode 100644
index 0270c26fbfbbbdc5c196d955a83b7e0cbab9d003..0000000000000000000000000000000000000000
--- a/spaces/Duskfallcrew/Gambit_and_Rogue/app.py
+++ /dev/null
@@ -1,17 +0,0 @@
-import os
-import gradio as gr
-
-API_KEY=os.environ.get('HUGGING_FACE_HUB_TOKEN', None)
-
-article = """---
-This space was created using [SD Space Creator](https://huggingface.co/spaces/anzorq/sd-space-creator)."""
-
-gr.Interface.load(
- name="models/Duskfallcrew/Gambit_and_Rogue",
- title="""Gambit And Rogue""",
- description="""Demo for Gambit And Rogue Stable Diffusion model.
-Coffee is nice
- Model Updates on CivIt """,
- article=article,
- api_key=API_KEY,
- ).queue(concurrency_count=20).launch()
diff --git a/spaces/ECCV2022/bytetrack/yolox/tracker/byte_tracker.py b/spaces/ECCV2022/bytetrack/yolox/tracker/byte_tracker.py
deleted file mode 100644
index 2d004599bba96ff4ba5fc1e9ad943e64361067e3..0000000000000000000000000000000000000000
--- a/spaces/ECCV2022/bytetrack/yolox/tracker/byte_tracker.py
+++ /dev/null
@@ -1,330 +0,0 @@
-import numpy as np
-from collections import deque
-import os
-import os.path as osp
-import copy
-import torch
-import torch.nn.functional as F
-
-from .kalman_filter import KalmanFilter
-from yolox.tracker import matching
-from .basetrack import BaseTrack, TrackState
-
-class STrack(BaseTrack):
- shared_kalman = KalmanFilter()
- def __init__(self, tlwh, score):
-
- # wait activate
- self._tlwh = np.asarray(tlwh, dtype=np.float)
- self.kalman_filter = None
- self.mean, self.covariance = None, None
- self.is_activated = False
-
- self.score = score
- self.tracklet_len = 0
-
- def predict(self):
- mean_state = self.mean.copy()
- if self.state != TrackState.Tracked:
- mean_state[7] = 0
- self.mean, self.covariance = self.kalman_filter.predict(mean_state, self.covariance)
-
- @staticmethod
- def multi_predict(stracks):
- if len(stracks) > 0:
- multi_mean = np.asarray([st.mean.copy() for st in stracks])
- multi_covariance = np.asarray([st.covariance for st in stracks])
- for i, st in enumerate(stracks):
- if st.state != TrackState.Tracked:
- multi_mean[i][7] = 0
- multi_mean, multi_covariance = STrack.shared_kalman.multi_predict(multi_mean, multi_covariance)
- for i, (mean, cov) in enumerate(zip(multi_mean, multi_covariance)):
- stracks[i].mean = mean
- stracks[i].covariance = cov
-
- def activate(self, kalman_filter, frame_id):
- """Start a new tracklet"""
- self.kalman_filter = kalman_filter
- self.track_id = self.next_id()
- self.mean, self.covariance = self.kalman_filter.initiate(self.tlwh_to_xyah(self._tlwh))
-
- self.tracklet_len = 0
- self.state = TrackState.Tracked
- if frame_id == 1:
- self.is_activated = True
- # self.is_activated = True
- self.frame_id = frame_id
- self.start_frame = frame_id
-
- def re_activate(self, new_track, frame_id, new_id=False):
- self.mean, self.covariance = self.kalman_filter.update(
- self.mean, self.covariance, self.tlwh_to_xyah(new_track.tlwh)
- )
- self.tracklet_len = 0
- self.state = TrackState.Tracked
- self.is_activated = True
- self.frame_id = frame_id
- if new_id:
- self.track_id = self.next_id()
- self.score = new_track.score
-
- def update(self, new_track, frame_id):
- """
- Update a matched track
- :type new_track: STrack
- :type frame_id: int
- :type update_feature: bool
- :return:
- """
- self.frame_id = frame_id
- self.tracklet_len += 1
-
- new_tlwh = new_track.tlwh
- self.mean, self.covariance = self.kalman_filter.update(
- self.mean, self.covariance, self.tlwh_to_xyah(new_tlwh))
- self.state = TrackState.Tracked
- self.is_activated = True
-
- self.score = new_track.score
-
- @property
- # @jit(nopython=True)
- def tlwh(self):
- """Get current position in bounding box format `(top left x, top left y,
- width, height)`.
- """
- if self.mean is None:
- return self._tlwh.copy()
- ret = self.mean[:4].copy()
- ret[2] *= ret[3]
- ret[:2] -= ret[2:] / 2
- return ret
-
- @property
- # @jit(nopython=True)
- def tlbr(self):
- """Convert bounding box to format `(min x, min y, max x, max y)`, i.e.,
- `(top left, bottom right)`.
- """
- ret = self.tlwh.copy()
- ret[2:] += ret[:2]
- return ret
-
- @staticmethod
- # @jit(nopython=True)
- def tlwh_to_xyah(tlwh):
- """Convert bounding box to format `(center x, center y, aspect ratio,
- height)`, where the aspect ratio is `width / height`.
- """
- ret = np.asarray(tlwh).copy()
- ret[:2] += ret[2:] / 2
- ret[2] /= ret[3]
- return ret
-
- def to_xyah(self):
- return self.tlwh_to_xyah(self.tlwh)
-
- @staticmethod
- # @jit(nopython=True)
- def tlbr_to_tlwh(tlbr):
- ret = np.asarray(tlbr).copy()
- ret[2:] -= ret[:2]
- return ret
-
- @staticmethod
- # @jit(nopython=True)
- def tlwh_to_tlbr(tlwh):
- ret = np.asarray(tlwh).copy()
- ret[2:] += ret[:2]
- return ret
-
- def __repr__(self):
- return 'OT_{}_({}-{})'.format(self.track_id, self.start_frame, self.end_frame)
-
-
-class BYTETracker(object):
- def __init__(self, args, frame_rate=30):
- self.tracked_stracks = [] # type: list[STrack]
- self.lost_stracks = [] # type: list[STrack]
- self.removed_stracks = [] # type: list[STrack]
-
- self.frame_id = 0
- self.args = args
- #self.det_thresh = args.track_thresh
- self.det_thresh = args.track_thresh + 0.1
- self.buffer_size = int(frame_rate / 30.0 * args.track_buffer)
- self.max_time_lost = self.buffer_size
- self.kalman_filter = KalmanFilter()
-
- def update(self, output_results, img_info, img_size):
- self.frame_id += 1
- activated_starcks = []
- refind_stracks = []
- lost_stracks = []
- removed_stracks = []
-
- if output_results.shape[1] == 5:
- scores = output_results[:, 4]
- bboxes = output_results[:, :4]
- else:
- output_results = output_results.cpu().numpy()
- scores = output_results[:, 4] * output_results[:, 5]
- bboxes = output_results[:, :4] # x1y1x2y2
- img_h, img_w = img_info[0], img_info[1]
- scale = min(img_size[0] / float(img_h), img_size[1] / float(img_w))
- bboxes /= scale
-
- remain_inds = scores > self.args.track_thresh
- inds_low = scores > 0.1
- inds_high = scores < self.args.track_thresh
-
- inds_second = np.logical_and(inds_low, inds_high)
- dets_second = bboxes[inds_second]
- dets = bboxes[remain_inds]
- scores_keep = scores[remain_inds]
- scores_second = scores[inds_second]
-
- if len(dets) > 0:
- '''Detections'''
- detections = [STrack(STrack.tlbr_to_tlwh(tlbr), s) for
- (tlbr, s) in zip(dets, scores_keep)]
- else:
- detections = []
-
- ''' Add newly detected tracklets to tracked_stracks'''
- unconfirmed = []
- tracked_stracks = [] # type: list[STrack]
- for track in self.tracked_stracks:
- if not track.is_activated:
- unconfirmed.append(track)
- else:
- tracked_stracks.append(track)
-
- ''' Step 2: First association, with high score detection boxes'''
- strack_pool = joint_stracks(tracked_stracks, self.lost_stracks)
- # Predict the current location with KF
- STrack.multi_predict(strack_pool)
- dists = matching.iou_distance(strack_pool, detections)
- if not self.args.mot20:
- dists = matching.fuse_score(dists, detections)
- matches, u_track, u_detection = matching.linear_assignment(dists, thresh=self.args.match_thresh)
-
- for itracked, idet in matches:
- track = strack_pool[itracked]
- det = detections[idet]
- if track.state == TrackState.Tracked:
- track.update(detections[idet], self.frame_id)
- activated_starcks.append(track)
- else:
- track.re_activate(det, self.frame_id, new_id=False)
- refind_stracks.append(track)
-
- ''' Step 3: Second association, with low score detection boxes'''
- # association the untrack to the low score detections
- if len(dets_second) > 0:
- '''Detections'''
- detections_second = [STrack(STrack.tlbr_to_tlwh(tlbr), s) for
- (tlbr, s) in zip(dets_second, scores_second)]
- else:
- detections_second = []
- r_tracked_stracks = [strack_pool[i] for i in u_track if strack_pool[i].state == TrackState.Tracked]
- dists = matching.iou_distance(r_tracked_stracks, detections_second)
- matches, u_track, u_detection_second = matching.linear_assignment(dists, thresh=0.5)
- for itracked, idet in matches:
- track = r_tracked_stracks[itracked]
- det = detections_second[idet]
- if track.state == TrackState.Tracked:
- track.update(det, self.frame_id)
- activated_starcks.append(track)
- else:
- track.re_activate(det, self.frame_id, new_id=False)
- refind_stracks.append(track)
-
- for it in u_track:
- track = r_tracked_stracks[it]
- if not track.state == TrackState.Lost:
- track.mark_lost()
- lost_stracks.append(track)
-
- '''Deal with unconfirmed tracks, usually tracks with only one beginning frame'''
- detections = [detections[i] for i in u_detection]
- dists = matching.iou_distance(unconfirmed, detections)
- if not self.args.mot20:
- dists = matching.fuse_score(dists, detections)
- matches, u_unconfirmed, u_detection = matching.linear_assignment(dists, thresh=0.7)
- for itracked, idet in matches:
- unconfirmed[itracked].update(detections[idet], self.frame_id)
- activated_starcks.append(unconfirmed[itracked])
- for it in u_unconfirmed:
- track = unconfirmed[it]
- track.mark_removed()
- removed_stracks.append(track)
-
- """ Step 4: Init new stracks"""
- for inew in u_detection:
- track = detections[inew]
- if track.score < self.det_thresh:
- continue
- track.activate(self.kalman_filter, self.frame_id)
- activated_starcks.append(track)
- """ Step 5: Update state"""
- for track in self.lost_stracks:
- if self.frame_id - track.end_frame > self.max_time_lost:
- track.mark_removed()
- removed_stracks.append(track)
-
- # print('Ramained match {} s'.format(t4-t3))
-
- self.tracked_stracks = [t for t in self.tracked_stracks if t.state == TrackState.Tracked]
- self.tracked_stracks = joint_stracks(self.tracked_stracks, activated_starcks)
- self.tracked_stracks = joint_stracks(self.tracked_stracks, refind_stracks)
- self.lost_stracks = sub_stracks(self.lost_stracks, self.tracked_stracks)
- self.lost_stracks.extend(lost_stracks)
- self.lost_stracks = sub_stracks(self.lost_stracks, self.removed_stracks)
- self.removed_stracks.extend(removed_stracks)
- self.tracked_stracks, self.lost_stracks = remove_duplicate_stracks(self.tracked_stracks, self.lost_stracks)
- # get scores of lost tracks
- output_stracks = [track for track in self.tracked_stracks if track.is_activated]
-
- return output_stracks
-
-
-def joint_stracks(tlista, tlistb):
- exists = {}
- res = []
- for t in tlista:
- exists[t.track_id] = 1
- res.append(t)
- for t in tlistb:
- tid = t.track_id
- if not exists.get(tid, 0):
- exists[tid] = 1
- res.append(t)
- return res
-
-
-def sub_stracks(tlista, tlistb):
- stracks = {}
- for t in tlista:
- stracks[t.track_id] = t
- for t in tlistb:
- tid = t.track_id
- if stracks.get(tid, 0):
- del stracks[tid]
- return list(stracks.values())
-
-
-def remove_duplicate_stracks(stracksa, stracksb):
- pdist = matching.iou_distance(stracksa, stracksb)
- pairs = np.where(pdist < 0.15)
- dupa, dupb = list(), list()
- for p, q in zip(*pairs):
- timep = stracksa[p].frame_id - stracksa[p].start_frame
- timeq = stracksb[q].frame_id - stracksb[q].start_frame
- if timep > timeq:
- dupb.append(q)
- else:
- dupa.append(p)
- resa = [t for i, t in enumerate(stracksa) if not i in dupa]
- resb = [t for i, t in enumerate(stracksb) if not i in dupb]
- return resa, resb
diff --git a/spaces/Eddycrack864/Applio-Inference/demucs/raw.py b/spaces/Eddycrack864/Applio-Inference/demucs/raw.py
deleted file mode 100644
index d4941ad2d7ed858f490db441f5b46b12bd61ad78..0000000000000000000000000000000000000000
--- a/spaces/Eddycrack864/Applio-Inference/demucs/raw.py
+++ /dev/null
@@ -1,173 +0,0 @@
-# Copyright (c) Facebook, Inc. and its affiliates.
-# All rights reserved.
-#
-# This source code is licensed under the license found in the
-# LICENSE file in the root directory of this source tree.
-
-import argparse
-import os
-from collections import defaultdict, namedtuple
-from pathlib import Path
-
-import musdb
-import numpy as np
-import torch as th
-import tqdm
-from torch.utils.data import DataLoader
-
-from .audio import AudioFile
-
-ChunkInfo = namedtuple("ChunkInfo", ["file_index", "offset", "local_index"])
-
-
-class Rawset:
- """
- Dataset of raw, normalized, float32 audio files
- """
- def __init__(self, path, samples=None, stride=None, channels=2, streams=None):
- self.path = Path(path)
- self.channels = channels
- self.samples = samples
- if stride is None:
- stride = samples if samples is not None else 0
- self.stride = stride
- entries = defaultdict(list)
- for root, folders, files in os.walk(self.path, followlinks=True):
- folders.sort()
- files.sort()
- for file in files:
- if file.endswith(".raw"):
- path = Path(root) / file
- name, stream = path.stem.rsplit('.', 1)
- entries[(path.parent.relative_to(self.path), name)].append(int(stream))
-
- self._entries = list(entries.keys())
-
- sizes = []
- self._lengths = []
- ref_streams = sorted(entries[self._entries[0]])
- assert ref_streams == list(range(len(ref_streams)))
- if streams is None:
- self.streams = ref_streams
- else:
- self.streams = streams
- for entry in sorted(entries.keys()):
- streams = entries[entry]
- assert sorted(streams) == ref_streams
- file = self._path(*entry)
- length = file.stat().st_size // (4 * channels)
- if samples is None:
- sizes.append(1)
- else:
- if length < samples:
- self._entries.remove(entry)
- continue
- sizes.append((length - samples) // stride + 1)
- self._lengths.append(length)
- if not sizes:
- raise ValueError(f"Empty dataset {self.path}")
- self._cumulative_sizes = np.cumsum(sizes)
- self._sizes = sizes
-
- def __len__(self):
- return self._cumulative_sizes[-1]
-
- @property
- def total_length(self):
- return sum(self._lengths)
-
- def chunk_info(self, index):
- file_index = np.searchsorted(self._cumulative_sizes, index, side='right')
- if file_index == 0:
- local_index = index
- else:
- local_index = index - self._cumulative_sizes[file_index - 1]
- return ChunkInfo(offset=local_index * self.stride,
- file_index=file_index,
- local_index=local_index)
-
- def _path(self, folder, name, stream=0):
- return self.path / folder / (name + f'.{stream}.raw')
-
- def __getitem__(self, index):
- chunk = self.chunk_info(index)
- entry = self._entries[chunk.file_index]
-
- length = self.samples or self._lengths[chunk.file_index]
- streams = []
- to_read = length * self.channels * 4
- for stream_index, stream in enumerate(self.streams):
- offset = chunk.offset * 4 * self.channels
- file = open(self._path(*entry, stream=stream), 'rb')
- file.seek(offset)
- content = file.read(to_read)
- assert len(content) == to_read
- content = np.frombuffer(content, dtype=np.float32)
- content = content.copy() # make writable
- streams.append(th.from_numpy(content).view(length, self.channels).t())
- return th.stack(streams, dim=0)
-
- def name(self, index):
- chunk = self.chunk_info(index)
- folder, name = self._entries[chunk.file_index]
- return folder / name
-
-
-class MusDBSet:
- def __init__(self, mus, streams=slice(None), samplerate=44100, channels=2):
- self.mus = mus
- self.streams = streams
- self.samplerate = samplerate
- self.channels = channels
-
- def __len__(self):
- return len(self.mus.tracks)
-
- def __getitem__(self, index):
- track = self.mus.tracks[index]
- return (track.name, AudioFile(track.path).read(channels=self.channels,
- seek_time=0,
- streams=self.streams,
- samplerate=self.samplerate))
-
-
-def build_raw(mus, destination, normalize, workers, samplerate, channels):
- destination.mkdir(parents=True, exist_ok=True)
- loader = DataLoader(MusDBSet(mus, channels=channels, samplerate=samplerate),
- batch_size=1,
- num_workers=workers,
- collate_fn=lambda x: x[0])
- for name, streams in tqdm.tqdm(loader):
- if normalize:
- ref = streams[0].mean(dim=0) # use mono mixture as reference
- streams = (streams - ref.mean()) / ref.std()
- for index, stream in enumerate(streams):
- open(destination / (name + f'.{index}.raw'), "wb").write(stream.t().numpy().tobytes())
-
-
-def main():
- parser = argparse.ArgumentParser('rawset')
- parser.add_argument('--workers', type=int, default=10)
- parser.add_argument('--samplerate', type=int, default=44100)
- parser.add_argument('--channels', type=int, default=2)
- parser.add_argument('musdb', type=Path)
- parser.add_argument('destination', type=Path)
-
- args = parser.parse_args()
-
- build_raw(musdb.DB(root=args.musdb, subsets=["train"], split="train"),
- args.destination / "train",
- normalize=True,
- channels=args.channels,
- samplerate=args.samplerate,
- workers=args.workers)
- build_raw(musdb.DB(root=args.musdb, subsets=["train"], split="valid"),
- args.destination / "valid",
- normalize=True,
- samplerate=args.samplerate,
- channels=args.channels,
- workers=args.workers)
-
-
-if __name__ == "__main__":
- main()
diff --git a/spaces/Egrt/MaskGAN/utils/utils_fit.py b/spaces/Egrt/MaskGAN/utils/utils_fit.py
deleted file mode 100644
index f747fea35eed4277940bcc2345799ff15afc29dd..0000000000000000000000000000000000000000
--- a/spaces/Egrt/MaskGAN/utils/utils_fit.py
+++ /dev/null
@@ -1,100 +0,0 @@
-import torch
-import torch.nn.functional as F
-from models.SwinIR import compute_gradient_penalty
-from tqdm import tqdm
-
-from .utils import get_lr, show_result
-from .utils_metrics import PSNR, SSIM
-
-
-
-def fit_one_epoch(writer, G_model_train, D_model_train, G_model, D_model, VGG_feature_model, ResNeSt_model, G_optimizer, D_optimizer, BCEWithLogits_loss, L1_loss, Face_loss, epoch, epoch_size, gen, Epoch, cuda, batch_size, save_interval):
- G_total_loss = 0
- D_total_loss = 0
- G_total_PSNR = 0
- G_total_SSIM = 0
-
- with tqdm(total=epoch_size,desc=f'Epoch {epoch + 1}/{Epoch}',postfix=dict,mininterval=0.3, ncols=150) as pbar:
- for iteration, batch in enumerate(gen):
- if iteration >= epoch_size:
- break
-
- with torch.no_grad():
- lr_images, hr_images = batch
- lr_images, hr_images = torch.from_numpy(lr_images).type(torch.FloatTensor), torch.from_numpy(hr_images).type(torch.FloatTensor)
- y_real, y_fake = torch.ones(batch_size), torch.zeros(batch_size)
- if cuda:
- lr_images, hr_images, y_real, y_fake = lr_images.cuda(), hr_images.cuda(), y_real.cuda(), y_fake.cuda()
-
- #-------------------------------------------------#
- # 训练判别器
- #-------------------------------------------------#
- D_optimizer.zero_grad()
-
- D_result_r = D_model_train(hr_images)
-
- G_result = G_model_train(lr_images)
- D_result_f = D_model_train(G_result).squeeze()
- D_result_rf = D_result_r - D_result_f.mean()
- D_result_fr = D_result_f - D_result_r.mean()
- D_train_loss_rf = BCEWithLogits_loss(D_result_rf, y_real)
- D_train_loss_fr = BCEWithLogits_loss(D_result_fr, y_fake)
- gradient_penalty = compute_gradient_penalty(D_model_train, hr_images, G_result)
- D_train_loss = 10 * gradient_penalty + (D_train_loss_rf + D_train_loss_fr) / 2
- D_train_loss.backward()
-
- D_optimizer.step()
-
- #-------------------------------------------------#
- # 训练生成器
- #-------------------------------------------------#
- G_optimizer.zero_grad()
-
- G_result = G_model_train(lr_images)
- image_loss = L1_loss(G_result, hr_images)
-
- D_result_r = D_model_train(hr_images)
- D_result_f = D_model_train(G_result).squeeze()
- D_result_rf = D_result_r - D_result_f.mean()
- D_result_fr = D_result_f - D_result_r.mean()
- D_train_loss_rf = BCEWithLogits_loss(D_result_rf, y_fake)
- D_train_loss_fr = BCEWithLogits_loss(D_result_fr, y_real)
- adversarial_loss = (D_train_loss_rf + D_train_loss_fr) / 2
-
- perception_loss = L1_loss(VGG_feature_model(G_result), VGG_feature_model(hr_images))
- # 进行下采样以适配人脸识别网络
- G_result_face = F.interpolate(G_result, size=(112, 112), mode='bicubic', align_corners=True)
- hr_images_face = F.interpolate(hr_images, size=(112, 112), mode='bicubic', align_corners=True)
- face_loss = torch.mean(1. - Face_loss(ResNeSt_model(G_result_face), ResNeSt_model(hr_images_face)))
- G_train_loss = 3.0 * image_loss + 1.0 * adversarial_loss + 0.9 * perception_loss + 2.5 * face_loss
-
- G_train_loss.backward()
- G_optimizer.step()
-
- G_total_loss += G_train_loss.item()
- D_total_loss += D_train_loss.item()
-
- with torch.no_grad():
- G_total_PSNR += PSNR(G_result, hr_images).item()
- G_total_SSIM += SSIM(G_result, hr_images).item()
-
- pbar.set_postfix(**{'G_loss' : G_total_loss / (iteration + 1),
- 'D_loss' : D_total_loss / (iteration + 1),
- 'G_PSNR' : G_total_PSNR / (iteration + 1),
- 'G_SSIM' : G_total_SSIM / (iteration + 1),
- 'lr' : get_lr(G_optimizer)})
- pbar.update(1)
-
- if iteration % save_interval == 0:
- show_result(epoch + 1, G_model_train, lr_images, hr_images)
- writer.add_scalar('G_loss', G_total_loss / (iteration + 1), epoch + 1)
- writer.add_scalar('D_loss', D_total_loss / (iteration + 1), epoch + 1)
- writer.add_scalar('G_PSNR', G_total_PSNR / (iteration + 1), epoch + 1)
- writer.add_scalar('G_SSIM', G_total_SSIM / (iteration + 1), epoch + 1)
- writer.add_scalar('lr', get_lr(G_optimizer), epoch + 1)
- print('Epoch:'+ str(epoch + 1) + '/' + str(Epoch))
- print('G Loss: %.4f || D Loss: %.4f ' % (G_total_loss / epoch_size, D_total_loss / epoch_size))
- print('Saving state, iter:', str(epoch+1))
- # 保存模型权重
- torch.save(G_model, 'logs/G_Epoch%d-GLoss%.4f-DLoss%.4f.pth'%((epoch + 1), G_total_loss / epoch_size, D_total_loss / epoch_size))
- torch.save(D_model, 'logs/D_Epoch%d-GLoss%.4f-DLoss%.4f.pth'%((epoch + 1), G_total_loss / epoch_size, D_total_loss / epoch_size))
diff --git a/spaces/Enterprisium/Easy_GUI/lib/infer_pack/models.py b/spaces/Enterprisium/Easy_GUI/lib/infer_pack/models.py
deleted file mode 100644
index 3665d03bc0514a6ed07d3372ea24717dae1e0a65..0000000000000000000000000000000000000000
--- a/spaces/Enterprisium/Easy_GUI/lib/infer_pack/models.py
+++ /dev/null
@@ -1,1142 +0,0 @@
-import math, pdb, os
-from time import time as ttime
-import torch
-from torch import nn
-from torch.nn import functional as F
-from lib.infer_pack import modules
-from lib.infer_pack import attentions
-from lib.infer_pack import commons
-from lib.infer_pack.commons import init_weights, get_padding
-from torch.nn import Conv1d, ConvTranspose1d, AvgPool1d, Conv2d
-from torch.nn.utils import weight_norm, remove_weight_norm, spectral_norm
-from lib.infer_pack.commons import init_weights
-import numpy as np
-from lib.infer_pack import commons
-
-
-class TextEncoder256(nn.Module):
- def __init__(
- self,
- out_channels,
- hidden_channels,
- filter_channels,
- n_heads,
- n_layers,
- kernel_size,
- p_dropout,
- f0=True,
- ):
- super().__init__()
- self.out_channels = out_channels
- self.hidden_channels = hidden_channels
- self.filter_channels = filter_channels
- self.n_heads = n_heads
- self.n_layers = n_layers
- self.kernel_size = kernel_size
- self.p_dropout = p_dropout
- self.emb_phone = nn.Linear(256, hidden_channels)
- self.lrelu = nn.LeakyReLU(0.1, inplace=True)
- if f0 == True:
- self.emb_pitch = nn.Embedding(256, hidden_channels) # pitch 256
- self.encoder = attentions.Encoder(
- hidden_channels, filter_channels, n_heads, n_layers, kernel_size, p_dropout
- )
- self.proj = nn.Conv1d(hidden_channels, out_channels * 2, 1)
-
- def forward(self, phone, pitch, lengths):
- if pitch == None:
- x = self.emb_phone(phone)
- else:
- x = self.emb_phone(phone) + self.emb_pitch(pitch)
- x = x * math.sqrt(self.hidden_channels) # [b, t, h]
- x = self.lrelu(x)
- x = torch.transpose(x, 1, -1) # [b, h, t]
- x_mask = torch.unsqueeze(commons.sequence_mask(lengths, x.size(2)), 1).to(
- x.dtype
- )
- x = self.encoder(x * x_mask, x_mask)
- stats = self.proj(x) * x_mask
-
- m, logs = torch.split(stats, self.out_channels, dim=1)
- return m, logs, x_mask
-
-
-class TextEncoder768(nn.Module):
- def __init__(
- self,
- out_channels,
- hidden_channels,
- filter_channels,
- n_heads,
- n_layers,
- kernel_size,
- p_dropout,
- f0=True,
- ):
- super().__init__()
- self.out_channels = out_channels
- self.hidden_channels = hidden_channels
- self.filter_channels = filter_channels
- self.n_heads = n_heads
- self.n_layers = n_layers
- self.kernel_size = kernel_size
- self.p_dropout = p_dropout
- self.emb_phone = nn.Linear(768, hidden_channels)
- self.lrelu = nn.LeakyReLU(0.1, inplace=True)
- if f0 == True:
- self.emb_pitch = nn.Embedding(256, hidden_channels) # pitch 256
- self.encoder = attentions.Encoder(
- hidden_channels, filter_channels, n_heads, n_layers, kernel_size, p_dropout
- )
- self.proj = nn.Conv1d(hidden_channels, out_channels * 2, 1)
-
- def forward(self, phone, pitch, lengths):
- if pitch == None:
- x = self.emb_phone(phone)
- else:
- x = self.emb_phone(phone) + self.emb_pitch(pitch)
- x = x * math.sqrt(self.hidden_channels) # [b, t, h]
- x = self.lrelu(x)
- x = torch.transpose(x, 1, -1) # [b, h, t]
- x_mask = torch.unsqueeze(commons.sequence_mask(lengths, x.size(2)), 1).to(
- x.dtype
- )
- x = self.encoder(x * x_mask, x_mask)
- stats = self.proj(x) * x_mask
-
- m, logs = torch.split(stats, self.out_channels, dim=1)
- return m, logs, x_mask
-
-
-class ResidualCouplingBlock(nn.Module):
- def __init__(
- self,
- channels,
- hidden_channels,
- kernel_size,
- dilation_rate,
- n_layers,
- n_flows=4,
- gin_channels=0,
- ):
- super().__init__()
- self.channels = channels
- self.hidden_channels = hidden_channels
- self.kernel_size = kernel_size
- self.dilation_rate = dilation_rate
- self.n_layers = n_layers
- self.n_flows = n_flows
- self.gin_channels = gin_channels
-
- self.flows = nn.ModuleList()
- for i in range(n_flows):
- self.flows.append(
- modules.ResidualCouplingLayer(
- channels,
- hidden_channels,
- kernel_size,
- dilation_rate,
- n_layers,
- gin_channels=gin_channels,
- mean_only=True,
- )
- )
- self.flows.append(modules.Flip())
-
- def forward(self, x, x_mask, g=None, reverse=False):
- if not reverse:
- for flow in self.flows:
- x, _ = flow(x, x_mask, g=g, reverse=reverse)
- else:
- for flow in reversed(self.flows):
- x = flow(x, x_mask, g=g, reverse=reverse)
- return x
-
- def remove_weight_norm(self):
- for i in range(self.n_flows):
- self.flows[i * 2].remove_weight_norm()
-
-
-class PosteriorEncoder(nn.Module):
- def __init__(
- self,
- in_channels,
- out_channels,
- hidden_channels,
- kernel_size,
- dilation_rate,
- n_layers,
- gin_channels=0,
- ):
- super().__init__()
- self.in_channels = in_channels
- self.out_channels = out_channels
- self.hidden_channels = hidden_channels
- self.kernel_size = kernel_size
- self.dilation_rate = dilation_rate
- self.n_layers = n_layers
- self.gin_channels = gin_channels
-
- self.pre = nn.Conv1d(in_channels, hidden_channels, 1)
- self.enc = modules.WN(
- hidden_channels,
- kernel_size,
- dilation_rate,
- n_layers,
- gin_channels=gin_channels,
- )
- self.proj = nn.Conv1d(hidden_channels, out_channels * 2, 1)
-
- def forward(self, x, x_lengths, g=None):
- x_mask = torch.unsqueeze(commons.sequence_mask(x_lengths, x.size(2)), 1).to(
- x.dtype
- )
- x = self.pre(x) * x_mask
- x = self.enc(x, x_mask, g=g)
- stats = self.proj(x) * x_mask
- m, logs = torch.split(stats, self.out_channels, dim=1)
- z = (m + torch.randn_like(m) * torch.exp(logs)) * x_mask
- return z, m, logs, x_mask
-
- def remove_weight_norm(self):
- self.enc.remove_weight_norm()
-
-
-class Generator(torch.nn.Module):
- def __init__(
- self,
- initial_channel,
- resblock,
- resblock_kernel_sizes,
- resblock_dilation_sizes,
- upsample_rates,
- upsample_initial_channel,
- upsample_kernel_sizes,
- gin_channels=0,
- ):
- super(Generator, self).__init__()
- self.num_kernels = len(resblock_kernel_sizes)
- self.num_upsamples = len(upsample_rates)
- self.conv_pre = Conv1d(
- initial_channel, upsample_initial_channel, 7, 1, padding=3
- )
- resblock = modules.ResBlock1 if resblock == "1" else modules.ResBlock2
-
- self.ups = nn.ModuleList()
- for i, (u, k) in enumerate(zip(upsample_rates, upsample_kernel_sizes)):
- self.ups.append(
- weight_norm(
- ConvTranspose1d(
- upsample_initial_channel // (2**i),
- upsample_initial_channel // (2 ** (i + 1)),
- k,
- u,
- padding=(k - u) // 2,
- )
- )
- )
-
- self.resblocks = nn.ModuleList()
- for i in range(len(self.ups)):
- ch = upsample_initial_channel // (2 ** (i + 1))
- for j, (k, d) in enumerate(
- zip(resblock_kernel_sizes, resblock_dilation_sizes)
- ):
- self.resblocks.append(resblock(ch, k, d))
-
- self.conv_post = Conv1d(ch, 1, 7, 1, padding=3, bias=False)
- self.ups.apply(init_weights)
-
- if gin_channels != 0:
- self.cond = nn.Conv1d(gin_channels, upsample_initial_channel, 1)
-
- def forward(self, x, g=None):
- x = self.conv_pre(x)
- if g is not None:
- x = x + self.cond(g)
-
- for i in range(self.num_upsamples):
- x = F.leaky_relu(x, modules.LRELU_SLOPE)
- x = self.ups[i](x)
- xs = None
- for j in range(self.num_kernels):
- if xs is None:
- xs = self.resblocks[i * self.num_kernels + j](x)
- else:
- xs += self.resblocks[i * self.num_kernels + j](x)
- x = xs / self.num_kernels
- x = F.leaky_relu(x)
- x = self.conv_post(x)
- x = torch.tanh(x)
-
- return x
-
- def remove_weight_norm(self):
- for l in self.ups:
- remove_weight_norm(l)
- for l in self.resblocks:
- l.remove_weight_norm()
-
-
-class SineGen(torch.nn.Module):
- """Definition of sine generator
- SineGen(samp_rate, harmonic_num = 0,
- sine_amp = 0.1, noise_std = 0.003,
- voiced_threshold = 0,
- flag_for_pulse=False)
- samp_rate: sampling rate in Hz
- harmonic_num: number of harmonic overtones (default 0)
- sine_amp: amplitude of sine-wavefrom (default 0.1)
- noise_std: std of Gaussian noise (default 0.003)
- voiced_thoreshold: F0 threshold for U/V classification (default 0)
- flag_for_pulse: this SinGen is used inside PulseGen (default False)
- Note: when flag_for_pulse is True, the first time step of a voiced
- segment is always sin(np.pi) or cos(0)
- """
-
- def __init__(
- self,
- samp_rate,
- harmonic_num=0,
- sine_amp=0.1,
- noise_std=0.003,
- voiced_threshold=0,
- flag_for_pulse=False,
- ):
- super(SineGen, self).__init__()
- self.sine_amp = sine_amp
- self.noise_std = noise_std
- self.harmonic_num = harmonic_num
- self.dim = self.harmonic_num + 1
- self.sampling_rate = samp_rate
- self.voiced_threshold = voiced_threshold
-
- def _f02uv(self, f0):
- # generate uv signal
- uv = torch.ones_like(f0)
- uv = uv * (f0 > self.voiced_threshold)
- return uv
-
- def forward(self, f0, upp):
- """sine_tensor, uv = forward(f0)
- input F0: tensor(batchsize=1, length, dim=1)
- f0 for unvoiced steps should be 0
- output sine_tensor: tensor(batchsize=1, length, dim)
- output uv: tensor(batchsize=1, length, 1)
- """
- with torch.no_grad():
- f0 = f0[:, None].transpose(1, 2)
- f0_buf = torch.zeros(f0.shape[0], f0.shape[1], self.dim, device=f0.device)
- # fundamental component
- f0_buf[:, :, 0] = f0[:, :, 0]
- for idx in np.arange(self.harmonic_num):
- f0_buf[:, :, idx + 1] = f0_buf[:, :, 0] * (
- idx + 2
- ) # idx + 2: the (idx+1)-th overtone, (idx+2)-th harmonic
- rad_values = (f0_buf / self.sampling_rate) % 1 ###%1意味着n_har的乘积无法后处理优化
- rand_ini = torch.rand(
- f0_buf.shape[0], f0_buf.shape[2], device=f0_buf.device
- )
- rand_ini[:, 0] = 0
- rad_values[:, 0, :] = rad_values[:, 0, :] + rand_ini
- tmp_over_one = torch.cumsum(rad_values, 1) # % 1 #####%1意味着后面的cumsum无法再优化
- tmp_over_one *= upp
- tmp_over_one = F.interpolate(
- tmp_over_one.transpose(2, 1),
- scale_factor=upp,
- mode="linear",
- align_corners=True,
- ).transpose(2, 1)
- rad_values = F.interpolate(
- rad_values.transpose(2, 1), scale_factor=upp, mode="nearest"
- ).transpose(
- 2, 1
- ) #######
- tmp_over_one %= 1
- tmp_over_one_idx = (tmp_over_one[:, 1:, :] - tmp_over_one[:, :-1, :]) < 0
- cumsum_shift = torch.zeros_like(rad_values)
- cumsum_shift[:, 1:, :] = tmp_over_one_idx * -1.0
- sine_waves = torch.sin(
- torch.cumsum(rad_values + cumsum_shift, dim=1) * 2 * np.pi
- )
- sine_waves = sine_waves * self.sine_amp
- uv = self._f02uv(f0)
- uv = F.interpolate(
- uv.transpose(2, 1), scale_factor=upp, mode="nearest"
- ).transpose(2, 1)
- noise_amp = uv * self.noise_std + (1 - uv) * self.sine_amp / 3
- noise = noise_amp * torch.randn_like(sine_waves)
- sine_waves = sine_waves * uv + noise
- return sine_waves, uv, noise
-
-
-class SourceModuleHnNSF(torch.nn.Module):
- """SourceModule for hn-nsf
- SourceModule(sampling_rate, harmonic_num=0, sine_amp=0.1,
- add_noise_std=0.003, voiced_threshod=0)
- sampling_rate: sampling_rate in Hz
- harmonic_num: number of harmonic above F0 (default: 0)
- sine_amp: amplitude of sine source signal (default: 0.1)
- add_noise_std: std of additive Gaussian noise (default: 0.003)
- note that amplitude of noise in unvoiced is decided
- by sine_amp
- voiced_threshold: threhold to set U/V given F0 (default: 0)
- Sine_source, noise_source = SourceModuleHnNSF(F0_sampled)
- F0_sampled (batchsize, length, 1)
- Sine_source (batchsize, length, 1)
- noise_source (batchsize, length 1)
- uv (batchsize, length, 1)
- """
-
- def __init__(
- self,
- sampling_rate,
- harmonic_num=0,
- sine_amp=0.1,
- add_noise_std=0.003,
- voiced_threshod=0,
- is_half=True,
- ):
- super(SourceModuleHnNSF, self).__init__()
-
- self.sine_amp = sine_amp
- self.noise_std = add_noise_std
- self.is_half = is_half
- # to produce sine waveforms
- self.l_sin_gen = SineGen(
- sampling_rate, harmonic_num, sine_amp, add_noise_std, voiced_threshod
- )
-
- # to merge source harmonics into a single excitation
- self.l_linear = torch.nn.Linear(harmonic_num + 1, 1)
- self.l_tanh = torch.nn.Tanh()
-
- def forward(self, x, upp=None):
- sine_wavs, uv, _ = self.l_sin_gen(x, upp)
- if self.is_half:
- sine_wavs = sine_wavs.half()
- sine_merge = self.l_tanh(self.l_linear(sine_wavs))
- return sine_merge, None, None # noise, uv
-
-
-class GeneratorNSF(torch.nn.Module):
- def __init__(
- self,
- initial_channel,
- resblock,
- resblock_kernel_sizes,
- resblock_dilation_sizes,
- upsample_rates,
- upsample_initial_channel,
- upsample_kernel_sizes,
- gin_channels,
- sr,
- is_half=False,
- ):
- super(GeneratorNSF, self).__init__()
- self.num_kernels = len(resblock_kernel_sizes)
- self.num_upsamples = len(upsample_rates)
-
- self.f0_upsamp = torch.nn.Upsample(scale_factor=np.prod(upsample_rates))
- self.m_source = SourceModuleHnNSF(
- sampling_rate=sr, harmonic_num=0, is_half=is_half
- )
- self.noise_convs = nn.ModuleList()
- self.conv_pre = Conv1d(
- initial_channel, upsample_initial_channel, 7, 1, padding=3
- )
- resblock = modules.ResBlock1 if resblock == "1" else modules.ResBlock2
-
- self.ups = nn.ModuleList()
- for i, (u, k) in enumerate(zip(upsample_rates, upsample_kernel_sizes)):
- c_cur = upsample_initial_channel // (2 ** (i + 1))
- self.ups.append(
- weight_norm(
- ConvTranspose1d(
- upsample_initial_channel // (2**i),
- upsample_initial_channel // (2 ** (i + 1)),
- k,
- u,
- padding=(k - u) // 2,
- )
- )
- )
- if i + 1 < len(upsample_rates):
- stride_f0 = np.prod(upsample_rates[i + 1 :])
- self.noise_convs.append(
- Conv1d(
- 1,
- c_cur,
- kernel_size=stride_f0 * 2,
- stride=stride_f0,
- padding=stride_f0 // 2,
- )
- )
- else:
- self.noise_convs.append(Conv1d(1, c_cur, kernel_size=1))
-
- self.resblocks = nn.ModuleList()
- for i in range(len(self.ups)):
- ch = upsample_initial_channel // (2 ** (i + 1))
- for j, (k, d) in enumerate(
- zip(resblock_kernel_sizes, resblock_dilation_sizes)
- ):
- self.resblocks.append(resblock(ch, k, d))
-
- self.conv_post = Conv1d(ch, 1, 7, 1, padding=3, bias=False)
- self.ups.apply(init_weights)
-
- if gin_channels != 0:
- self.cond = nn.Conv1d(gin_channels, upsample_initial_channel, 1)
-
- self.upp = np.prod(upsample_rates)
-
- def forward(self, x, f0, g=None):
- har_source, noi_source, uv = self.m_source(f0, self.upp)
- har_source = har_source.transpose(1, 2)
- x = self.conv_pre(x)
- if g is not None:
- x = x + self.cond(g)
-
- for i in range(self.num_upsamples):
- x = F.leaky_relu(x, modules.LRELU_SLOPE)
- x = self.ups[i](x)
- x_source = self.noise_convs[i](har_source)
- x = x + x_source
- xs = None
- for j in range(self.num_kernels):
- if xs is None:
- xs = self.resblocks[i * self.num_kernels + j](x)
- else:
- xs += self.resblocks[i * self.num_kernels + j](x)
- x = xs / self.num_kernels
- x = F.leaky_relu(x)
- x = self.conv_post(x)
- x = torch.tanh(x)
- return x
-
- def remove_weight_norm(self):
- for l in self.ups:
- remove_weight_norm(l)
- for l in self.resblocks:
- l.remove_weight_norm()
-
-
-sr2sr = {
- "32k": 32000,
- "40k": 40000,
- "48k": 48000,
-}
-
-
-class SynthesizerTrnMs256NSFsid(nn.Module):
- def __init__(
- self,
- spec_channels,
- segment_size,
- inter_channels,
- hidden_channels,
- filter_channels,
- n_heads,
- n_layers,
- kernel_size,
- p_dropout,
- resblock,
- resblock_kernel_sizes,
- resblock_dilation_sizes,
- upsample_rates,
- upsample_initial_channel,
- upsample_kernel_sizes,
- spk_embed_dim,
- gin_channels,
- sr,
- **kwargs
- ):
- super().__init__()
- if type(sr) == type("strr"):
- sr = sr2sr[sr]
- self.spec_channels = spec_channels
- self.inter_channels = inter_channels
- self.hidden_channels = hidden_channels
- self.filter_channels = filter_channels
- self.n_heads = n_heads
- self.n_layers = n_layers
- self.kernel_size = kernel_size
- self.p_dropout = p_dropout
- self.resblock = resblock
- self.resblock_kernel_sizes = resblock_kernel_sizes
- self.resblock_dilation_sizes = resblock_dilation_sizes
- self.upsample_rates = upsample_rates
- self.upsample_initial_channel = upsample_initial_channel
- self.upsample_kernel_sizes = upsample_kernel_sizes
- self.segment_size = segment_size
- self.gin_channels = gin_channels
- # self.hop_length = hop_length#
- self.spk_embed_dim = spk_embed_dim
- self.enc_p = TextEncoder256(
- inter_channels,
- hidden_channels,
- filter_channels,
- n_heads,
- n_layers,
- kernel_size,
- p_dropout,
- )
- self.dec = GeneratorNSF(
- inter_channels,
- resblock,
- resblock_kernel_sizes,
- resblock_dilation_sizes,
- upsample_rates,
- upsample_initial_channel,
- upsample_kernel_sizes,
- gin_channels=gin_channels,
- sr=sr,
- is_half=kwargs["is_half"],
- )
- self.enc_q = PosteriorEncoder(
- spec_channels,
- inter_channels,
- hidden_channels,
- 5,
- 1,
- 16,
- gin_channels=gin_channels,
- )
- self.flow = ResidualCouplingBlock(
- inter_channels, hidden_channels, 5, 1, 3, gin_channels=gin_channels
- )
- self.emb_g = nn.Embedding(self.spk_embed_dim, gin_channels)
- print("gin_channels:", gin_channels, "self.spk_embed_dim:", self.spk_embed_dim)
-
- def remove_weight_norm(self):
- self.dec.remove_weight_norm()
- self.flow.remove_weight_norm()
- self.enc_q.remove_weight_norm()
-
- def forward(
- self, phone, phone_lengths, pitch, pitchf, y, y_lengths, ds
- ): # 这里ds是id,[bs,1]
- # print(1,pitch.shape)#[bs,t]
- g = self.emb_g(ds).unsqueeze(-1) # [b, 256, 1]##1是t,广播的
- m_p, logs_p, x_mask = self.enc_p(phone, pitch, phone_lengths)
- z, m_q, logs_q, y_mask = self.enc_q(y, y_lengths, g=g)
- z_p = self.flow(z, y_mask, g=g)
- z_slice, ids_slice = commons.rand_slice_segments(
- z, y_lengths, self.segment_size
- )
- # print(-1,pitchf.shape,ids_slice,self.segment_size,self.hop_length,self.segment_size//self.hop_length)
- pitchf = commons.slice_segments2(pitchf, ids_slice, self.segment_size)
- # print(-2,pitchf.shape,z_slice.shape)
- o = self.dec(z_slice, pitchf, g=g)
- return o, ids_slice, x_mask, y_mask, (z, z_p, m_p, logs_p, m_q, logs_q)
-
- def infer(self, phone, phone_lengths, pitch, nsff0, sid, rate=None):
- g = self.emb_g(sid).unsqueeze(-1)
- m_p, logs_p, x_mask = self.enc_p(phone, pitch, phone_lengths)
- z_p = (m_p + torch.exp(logs_p) * torch.randn_like(m_p) * 0.66666) * x_mask
- if rate:
- head = int(z_p.shape[2] * rate)
- z_p = z_p[:, :, -head:]
- x_mask = x_mask[:, :, -head:]
- nsff0 = nsff0[:, -head:]
- z = self.flow(z_p, x_mask, g=g, reverse=True)
- o = self.dec(z * x_mask, nsff0, g=g)
- return o, x_mask, (z, z_p, m_p, logs_p)
-
-
-class SynthesizerTrnMs768NSFsid(nn.Module):
- def __init__(
- self,
- spec_channels,
- segment_size,
- inter_channels,
- hidden_channels,
- filter_channels,
- n_heads,
- n_layers,
- kernel_size,
- p_dropout,
- resblock,
- resblock_kernel_sizes,
- resblock_dilation_sizes,
- upsample_rates,
- upsample_initial_channel,
- upsample_kernel_sizes,
- spk_embed_dim,
- gin_channels,
- sr,
- **kwargs
- ):
- super().__init__()
- if type(sr) == type("strr"):
- sr = sr2sr[sr]
- self.spec_channels = spec_channels
- self.inter_channels = inter_channels
- self.hidden_channels = hidden_channels
- self.filter_channels = filter_channels
- self.n_heads = n_heads
- self.n_layers = n_layers
- self.kernel_size = kernel_size
- self.p_dropout = p_dropout
- self.resblock = resblock
- self.resblock_kernel_sizes = resblock_kernel_sizes
- self.resblock_dilation_sizes = resblock_dilation_sizes
- self.upsample_rates = upsample_rates
- self.upsample_initial_channel = upsample_initial_channel
- self.upsample_kernel_sizes = upsample_kernel_sizes
- self.segment_size = segment_size
- self.gin_channels = gin_channels
- # self.hop_length = hop_length#
- self.spk_embed_dim = spk_embed_dim
- self.enc_p = TextEncoder768(
- inter_channels,
- hidden_channels,
- filter_channels,
- n_heads,
- n_layers,
- kernel_size,
- p_dropout,
- )
- self.dec = GeneratorNSF(
- inter_channels,
- resblock,
- resblock_kernel_sizes,
- resblock_dilation_sizes,
- upsample_rates,
- upsample_initial_channel,
- upsample_kernel_sizes,
- gin_channels=gin_channels,
- sr=sr,
- is_half=kwargs["is_half"],
- )
- self.enc_q = PosteriorEncoder(
- spec_channels,
- inter_channels,
- hidden_channels,
- 5,
- 1,
- 16,
- gin_channels=gin_channels,
- )
- self.flow = ResidualCouplingBlock(
- inter_channels, hidden_channels, 5, 1, 3, gin_channels=gin_channels
- )
- self.emb_g = nn.Embedding(self.spk_embed_dim, gin_channels)
- print("gin_channels:", gin_channels, "self.spk_embed_dim:", self.spk_embed_dim)
-
- def remove_weight_norm(self):
- self.dec.remove_weight_norm()
- self.flow.remove_weight_norm()
- self.enc_q.remove_weight_norm()
-
- def forward(
- self, phone, phone_lengths, pitch, pitchf, y, y_lengths, ds
- ): # 这里ds是id,[bs,1]
- # print(1,pitch.shape)#[bs,t]
- g = self.emb_g(ds).unsqueeze(-1) # [b, 256, 1]##1是t,广播的
- m_p, logs_p, x_mask = self.enc_p(phone, pitch, phone_lengths)
- z, m_q, logs_q, y_mask = self.enc_q(y, y_lengths, g=g)
- z_p = self.flow(z, y_mask, g=g)
- z_slice, ids_slice = commons.rand_slice_segments(
- z, y_lengths, self.segment_size
- )
- # print(-1,pitchf.shape,ids_slice,self.segment_size,self.hop_length,self.segment_size//self.hop_length)
- pitchf = commons.slice_segments2(pitchf, ids_slice, self.segment_size)
- # print(-2,pitchf.shape,z_slice.shape)
- o = self.dec(z_slice, pitchf, g=g)
- return o, ids_slice, x_mask, y_mask, (z, z_p, m_p, logs_p, m_q, logs_q)
-
- def infer(self, phone, phone_lengths, pitch, nsff0, sid, rate=None):
- g = self.emb_g(sid).unsqueeze(-1)
- m_p, logs_p, x_mask = self.enc_p(phone, pitch, phone_lengths)
- z_p = (m_p + torch.exp(logs_p) * torch.randn_like(m_p) * 0.66666) * x_mask
- if rate:
- head = int(z_p.shape[2] * rate)
- z_p = z_p[:, :, -head:]
- x_mask = x_mask[:, :, -head:]
- nsff0 = nsff0[:, -head:]
- z = self.flow(z_p, x_mask, g=g, reverse=True)
- o = self.dec(z * x_mask, nsff0, g=g)
- return o, x_mask, (z, z_p, m_p, logs_p)
-
-
-class SynthesizerTrnMs256NSFsid_nono(nn.Module):
- def __init__(
- self,
- spec_channels,
- segment_size,
- inter_channels,
- hidden_channels,
- filter_channels,
- n_heads,
- n_layers,
- kernel_size,
- p_dropout,
- resblock,
- resblock_kernel_sizes,
- resblock_dilation_sizes,
- upsample_rates,
- upsample_initial_channel,
- upsample_kernel_sizes,
- spk_embed_dim,
- gin_channels,
- sr=None,
- **kwargs
- ):
- super().__init__()
- self.spec_channels = spec_channels
- self.inter_channels = inter_channels
- self.hidden_channels = hidden_channels
- self.filter_channels = filter_channels
- self.n_heads = n_heads
- self.n_layers = n_layers
- self.kernel_size = kernel_size
- self.p_dropout = p_dropout
- self.resblock = resblock
- self.resblock_kernel_sizes = resblock_kernel_sizes
- self.resblock_dilation_sizes = resblock_dilation_sizes
- self.upsample_rates = upsample_rates
- self.upsample_initial_channel = upsample_initial_channel
- self.upsample_kernel_sizes = upsample_kernel_sizes
- self.segment_size = segment_size
- self.gin_channels = gin_channels
- # self.hop_length = hop_length#
- self.spk_embed_dim = spk_embed_dim
- self.enc_p = TextEncoder256(
- inter_channels,
- hidden_channels,
- filter_channels,
- n_heads,
- n_layers,
- kernel_size,
- p_dropout,
- f0=False,
- )
- self.dec = Generator(
- inter_channels,
- resblock,
- resblock_kernel_sizes,
- resblock_dilation_sizes,
- upsample_rates,
- upsample_initial_channel,
- upsample_kernel_sizes,
- gin_channels=gin_channels,
- )
- self.enc_q = PosteriorEncoder(
- spec_channels,
- inter_channels,
- hidden_channels,
- 5,
- 1,
- 16,
- gin_channels=gin_channels,
- )
- self.flow = ResidualCouplingBlock(
- inter_channels, hidden_channels, 5, 1, 3, gin_channels=gin_channels
- )
- self.emb_g = nn.Embedding(self.spk_embed_dim, gin_channels)
- print("gin_channels:", gin_channels, "self.spk_embed_dim:", self.spk_embed_dim)
-
- def remove_weight_norm(self):
- self.dec.remove_weight_norm()
- self.flow.remove_weight_norm()
- self.enc_q.remove_weight_norm()
-
- def forward(self, phone, phone_lengths, y, y_lengths, ds): # 这里ds是id,[bs,1]
- g = self.emb_g(ds).unsqueeze(-1) # [b, 256, 1]##1是t,广播的
- m_p, logs_p, x_mask = self.enc_p(phone, None, phone_lengths)
- z, m_q, logs_q, y_mask = self.enc_q(y, y_lengths, g=g)
- z_p = self.flow(z, y_mask, g=g)
- z_slice, ids_slice = commons.rand_slice_segments(
- z, y_lengths, self.segment_size
- )
- o = self.dec(z_slice, g=g)
- return o, ids_slice, x_mask, y_mask, (z, z_p, m_p, logs_p, m_q, logs_q)
-
- def infer(self, phone, phone_lengths, sid, rate=None):
- g = self.emb_g(sid).unsqueeze(-1)
- m_p, logs_p, x_mask = self.enc_p(phone, None, phone_lengths)
- z_p = (m_p + torch.exp(logs_p) * torch.randn_like(m_p) * 0.66666) * x_mask
- if rate:
- head = int(z_p.shape[2] * rate)
- z_p = z_p[:, :, -head:]
- x_mask = x_mask[:, :, -head:]
- z = self.flow(z_p, x_mask, g=g, reverse=True)
- o = self.dec(z * x_mask, g=g)
- return o, x_mask, (z, z_p, m_p, logs_p)
-
-
-class SynthesizerTrnMs768NSFsid_nono(nn.Module):
- def __init__(
- self,
- spec_channels,
- segment_size,
- inter_channels,
- hidden_channels,
- filter_channels,
- n_heads,
- n_layers,
- kernel_size,
- p_dropout,
- resblock,
- resblock_kernel_sizes,
- resblock_dilation_sizes,
- upsample_rates,
- upsample_initial_channel,
- upsample_kernel_sizes,
- spk_embed_dim,
- gin_channels,
- sr=None,
- **kwargs
- ):
- super().__init__()
- self.spec_channels = spec_channels
- self.inter_channels = inter_channels
- self.hidden_channels = hidden_channels
- self.filter_channels = filter_channels
- self.n_heads = n_heads
- self.n_layers = n_layers
- self.kernel_size = kernel_size
- self.p_dropout = p_dropout
- self.resblock = resblock
- self.resblock_kernel_sizes = resblock_kernel_sizes
- self.resblock_dilation_sizes = resblock_dilation_sizes
- self.upsample_rates = upsample_rates
- self.upsample_initial_channel = upsample_initial_channel
- self.upsample_kernel_sizes = upsample_kernel_sizes
- self.segment_size = segment_size
- self.gin_channels = gin_channels
- # self.hop_length = hop_length#
- self.spk_embed_dim = spk_embed_dim
- self.enc_p = TextEncoder768(
- inter_channels,
- hidden_channels,
- filter_channels,
- n_heads,
- n_layers,
- kernel_size,
- p_dropout,
- f0=False,
- )
- self.dec = Generator(
- inter_channels,
- resblock,
- resblock_kernel_sizes,
- resblock_dilation_sizes,
- upsample_rates,
- upsample_initial_channel,
- upsample_kernel_sizes,
- gin_channels=gin_channels,
- )
- self.enc_q = PosteriorEncoder(
- spec_channels,
- inter_channels,
- hidden_channels,
- 5,
- 1,
- 16,
- gin_channels=gin_channels,
- )
- self.flow = ResidualCouplingBlock(
- inter_channels, hidden_channels, 5, 1, 3, gin_channels=gin_channels
- )
- self.emb_g = nn.Embedding(self.spk_embed_dim, gin_channels)
- print("gin_channels:", gin_channels, "self.spk_embed_dim:", self.spk_embed_dim)
-
- def remove_weight_norm(self):
- self.dec.remove_weight_norm()
- self.flow.remove_weight_norm()
- self.enc_q.remove_weight_norm()
-
- def forward(self, phone, phone_lengths, y, y_lengths, ds): # 这里ds是id,[bs,1]
- g = self.emb_g(ds).unsqueeze(-1) # [b, 256, 1]##1是t,广播的
- m_p, logs_p, x_mask = self.enc_p(phone, None, phone_lengths)
- z, m_q, logs_q, y_mask = self.enc_q(y, y_lengths, g=g)
- z_p = self.flow(z, y_mask, g=g)
- z_slice, ids_slice = commons.rand_slice_segments(
- z, y_lengths, self.segment_size
- )
- o = self.dec(z_slice, g=g)
- return o, ids_slice, x_mask, y_mask, (z, z_p, m_p, logs_p, m_q, logs_q)
-
- def infer(self, phone, phone_lengths, sid, rate=None):
- g = self.emb_g(sid).unsqueeze(-1)
- m_p, logs_p, x_mask = self.enc_p(phone, None, phone_lengths)
- z_p = (m_p + torch.exp(logs_p) * torch.randn_like(m_p) * 0.66666) * x_mask
- if rate:
- head = int(z_p.shape[2] * rate)
- z_p = z_p[:, :, -head:]
- x_mask = x_mask[:, :, -head:]
- z = self.flow(z_p, x_mask, g=g, reverse=True)
- o = self.dec(z * x_mask, g=g)
- return o, x_mask, (z, z_p, m_p, logs_p)
-
-
-class MultiPeriodDiscriminator(torch.nn.Module):
- def __init__(self, use_spectral_norm=False):
- super(MultiPeriodDiscriminator, self).__init__()
- periods = [2, 3, 5, 7, 11, 17]
- # periods = [3, 5, 7, 11, 17, 23, 37]
-
- discs = [DiscriminatorS(use_spectral_norm=use_spectral_norm)]
- discs = discs + [
- DiscriminatorP(i, use_spectral_norm=use_spectral_norm) for i in periods
- ]
- self.discriminators = nn.ModuleList(discs)
-
- def forward(self, y, y_hat):
- y_d_rs = [] #
- y_d_gs = []
- fmap_rs = []
- fmap_gs = []
- for i, d in enumerate(self.discriminators):
- y_d_r, fmap_r = d(y)
- y_d_g, fmap_g = d(y_hat)
- # for j in range(len(fmap_r)):
- # print(i,j,y.shape,y_hat.shape,fmap_r[j].shape,fmap_g[j].shape)
- y_d_rs.append(y_d_r)
- y_d_gs.append(y_d_g)
- fmap_rs.append(fmap_r)
- fmap_gs.append(fmap_g)
-
- return y_d_rs, y_d_gs, fmap_rs, fmap_gs
-
-
-class MultiPeriodDiscriminatorV2(torch.nn.Module):
- def __init__(self, use_spectral_norm=False):
- super(MultiPeriodDiscriminatorV2, self).__init__()
- # periods = [2, 3, 5, 7, 11, 17]
- periods = [2, 3, 5, 7, 11, 17, 23, 37]
-
- discs = [DiscriminatorS(use_spectral_norm=use_spectral_norm)]
- discs = discs + [
- DiscriminatorP(i, use_spectral_norm=use_spectral_norm) for i in periods
- ]
- self.discriminators = nn.ModuleList(discs)
-
- def forward(self, y, y_hat):
- y_d_rs = [] #
- y_d_gs = []
- fmap_rs = []
- fmap_gs = []
- for i, d in enumerate(self.discriminators):
- y_d_r, fmap_r = d(y)
- y_d_g, fmap_g = d(y_hat)
- # for j in range(len(fmap_r)):
- # print(i,j,y.shape,y_hat.shape,fmap_r[j].shape,fmap_g[j].shape)
- y_d_rs.append(y_d_r)
- y_d_gs.append(y_d_g)
- fmap_rs.append(fmap_r)
- fmap_gs.append(fmap_g)
-
- return y_d_rs, y_d_gs, fmap_rs, fmap_gs
-
-
-class DiscriminatorS(torch.nn.Module):
- def __init__(self, use_spectral_norm=False):
- super(DiscriminatorS, self).__init__()
- norm_f = weight_norm if use_spectral_norm == False else spectral_norm
- self.convs = nn.ModuleList(
- [
- norm_f(Conv1d(1, 16, 15, 1, padding=7)),
- norm_f(Conv1d(16, 64, 41, 4, groups=4, padding=20)),
- norm_f(Conv1d(64, 256, 41, 4, groups=16, padding=20)),
- norm_f(Conv1d(256, 1024, 41, 4, groups=64, padding=20)),
- norm_f(Conv1d(1024, 1024, 41, 4, groups=256, padding=20)),
- norm_f(Conv1d(1024, 1024, 5, 1, padding=2)),
- ]
- )
- self.conv_post = norm_f(Conv1d(1024, 1, 3, 1, padding=1))
-
- def forward(self, x):
- fmap = []
-
- for l in self.convs:
- x = l(x)
- x = F.leaky_relu(x, modules.LRELU_SLOPE)
- fmap.append(x)
- x = self.conv_post(x)
- fmap.append(x)
- x = torch.flatten(x, 1, -1)
-
- return x, fmap
-
-
-class DiscriminatorP(torch.nn.Module):
- def __init__(self, period, kernel_size=5, stride=3, use_spectral_norm=False):
- super(DiscriminatorP, self).__init__()
- self.period = period
- self.use_spectral_norm = use_spectral_norm
- norm_f = weight_norm if use_spectral_norm == False else spectral_norm
- self.convs = nn.ModuleList(
- [
- norm_f(
- Conv2d(
- 1,
- 32,
- (kernel_size, 1),
- (stride, 1),
- padding=(get_padding(kernel_size, 1), 0),
- )
- ),
- norm_f(
- Conv2d(
- 32,
- 128,
- (kernel_size, 1),
- (stride, 1),
- padding=(get_padding(kernel_size, 1), 0),
- )
- ),
- norm_f(
- Conv2d(
- 128,
- 512,
- (kernel_size, 1),
- (stride, 1),
- padding=(get_padding(kernel_size, 1), 0),
- )
- ),
- norm_f(
- Conv2d(
- 512,
- 1024,
- (kernel_size, 1),
- (stride, 1),
- padding=(get_padding(kernel_size, 1), 0),
- )
- ),
- norm_f(
- Conv2d(
- 1024,
- 1024,
- (kernel_size, 1),
- 1,
- padding=(get_padding(kernel_size, 1), 0),
- )
- ),
- ]
- )
- self.conv_post = norm_f(Conv2d(1024, 1, (3, 1), 1, padding=(1, 0)))
-
- def forward(self, x):
- fmap = []
-
- # 1d to 2d
- b, c, t = x.shape
- if t % self.period != 0: # pad first
- n_pad = self.period - (t % self.period)
- x = F.pad(x, (0, n_pad), "reflect")
- t = t + n_pad
- x = x.view(b, c, t // self.period, self.period)
-
- for l in self.convs:
- x = l(x)
- x = F.leaky_relu(x, modules.LRELU_SLOPE)
- fmap.append(x)
- x = self.conv_post(x)
- fmap.append(x)
- x = torch.flatten(x, 1, -1)
-
- return x, fmap
diff --git a/spaces/Faridmaruf/rvc-Blue-archives/app.py b/spaces/Faridmaruf/rvc-Blue-archives/app.py
deleted file mode 100644
index b545c33df5f8714308d872bc6cab208485e14e6b..0000000000000000000000000000000000000000
--- a/spaces/Faridmaruf/rvc-Blue-archives/app.py
+++ /dev/null
@@ -1,516 +0,0 @@
-import os
-import glob
-import json
-import traceback
-import logging
-import gradio as gr
-import numpy as np
-import librosa
-import torch
-import asyncio
-import edge_tts
-import yt_dlp
-import ffmpeg
-import subprocess
-import sys
-import io
-import wave
-from datetime import datetime
-from fairseq import checkpoint_utils
-from lib.infer_pack.models import (
- SynthesizerTrnMs256NSFsid,
- SynthesizerTrnMs256NSFsid_nono,
- SynthesizerTrnMs768NSFsid,
- SynthesizerTrnMs768NSFsid_nono,
-)
-from vc_infer_pipeline import VC
-from config import Config
-config = Config()
-logging.getLogger("numba").setLevel(logging.WARNING)
-limitation = os.getenv("SYSTEM") == "spaces"
-
-audio_mode = []
-f0method_mode = []
-f0method_info = ""
-if limitation is True:
- audio_mode = ["Upload audio", "TTS Audio"]
- f0method_mode = ["pm", "harvest"]
- f0method_info = "PM is fast, Harvest is good but extremely slow. (Default: PM)"
-else:
- audio_mode = ["Input path", "Upload audio", "Youtube", "TTS Audio"]
- f0method_mode = ["pm", "harvest", "crepe"]
- f0method_info = "PM is fast, Harvest is good but extremely slow, and Crepe effect is good but requires GPU (Default: PM)"
-
-def create_vc_fn(model_name, tgt_sr, net_g, vc, if_f0, version, file_index):
- def vc_fn(
- vc_audio_mode,
- vc_input,
- vc_upload,
- tts_text,
- tts_voice,
- f0_up_key,
- f0_method,
- index_rate,
- filter_radius,
- resample_sr,
- rms_mix_rate,
- protect,
- ):
- try:
- print(f"Converting using {model_name}...")
- if vc_audio_mode == "Input path" or "Youtube" and vc_input != "":
- audio, sr = librosa.load(vc_input, sr=16000, mono=True)
- elif vc_audio_mode == "Upload audio":
- if vc_upload is None:
- return "You need to upload an audio", None
- sampling_rate, audio = vc_upload
- duration = audio.shape[0] / sampling_rate
- if duration > 20 and limitation:
- return "Please upload an audio file that is less than 20 seconds. If you need to generate a longer audio file, please use Colab.", None
- audio = (audio / np.iinfo(audio.dtype).max).astype(np.float32)
- if len(audio.shape) > 1:
- audio = librosa.to_mono(audio.transpose(1, 0))
- if sampling_rate != 16000:
- audio = librosa.resample(audio, orig_sr=sampling_rate, target_sr=16000)
- elif vc_audio_mode == "TTS Audio":
- if len(tts_text) > 100 and limitation:
- return "Text is too long", None
- if tts_text is None or tts_voice is None:
- return "You need to enter text and select a voice", None
- asyncio.run(edge_tts.Communicate(tts_text, "-".join(tts_voice.split('-')[:-1])).save("tts.mp3"))
- audio, sr = librosa.load("tts.mp3", sr=16000, mono=True)
- vc_input = "tts.mp3"
- times = [0, 0, 0]
- f0_up_key = int(f0_up_key)
- audio_opt = vc.pipeline(
- hubert_model,
- net_g,
- 0,
- audio,
- vc_input,
- times,
- f0_up_key,
- f0_method,
- file_index,
- # file_big_npy,
- index_rate,
- if_f0,
- filter_radius,
- tgt_sr,
- resample_sr,
- rms_mix_rate,
- version,
- protect,
- f0_file=None,
- )
- info = f"[{datetime.now().strftime('%Y-%m-%d %H:%M')}]: npy: {times[0]}, f0: {times[1]}s, infer: {times[2]}s"
- print(f"{model_name} | {info}")
- return info, (tgt_sr, audio_opt)
- except:
- info = traceback.format_exc()
- print(info)
- return info, None
- return vc_fn
-
-def load_model():
- categories = []
- with open("weights/folder_info.json", "r", encoding="utf-8") as f:
- folder_info = json.load(f)
- for category_name, category_info in folder_info.items():
- if not category_info['enable']:
- continue
- category_title = category_info['title']
- category_folder = category_info['folder_path']
- description = category_info['description']
- models = []
- with open(f"weights/{category_folder}/model_info.json", "r", encoding="utf-8") as f:
- models_info = json.load(f)
- for character_name, info in models_info.items():
- if not info['enable']:
- continue
- model_title = info['title']
- model_name = info['model_path']
- model_author = info.get("author", None)
- model_cover = f"weights/{category_folder}/{character_name}/{info['cover']}"
- model_index = f"weights/{category_folder}/{character_name}/{info['feature_retrieval_library']}"
- cpt = torch.load(f"weights/{category_folder}/{character_name}/{model_name}", map_location="cpu")
- tgt_sr = cpt["config"][-1]
- cpt["config"][-3] = cpt["weight"]["emb_g.weight"].shape[0] # n_spk
- if_f0 = cpt.get("f0", 1)
- version = cpt.get("version", "v1")
- if version == "v1":
- if if_f0 == 1:
- net_g = SynthesizerTrnMs256NSFsid(*cpt["config"], is_half=config.is_half)
- else:
- net_g = SynthesizerTrnMs256NSFsid_nono(*cpt["config"])
- model_version = "V1"
- elif version == "v2":
- if if_f0 == 1:
- net_g = SynthesizerTrnMs768NSFsid(*cpt["config"], is_half=config.is_half)
- else:
- net_g = SynthesizerTrnMs768NSFsid_nono(*cpt["config"])
- model_version = "V2"
- del net_g.enc_q
- print(net_g.load_state_dict(cpt["weight"], strict=False))
- net_g.eval().to(config.device)
- if config.is_half:
- net_g = net_g.half()
- else:
- net_g = net_g.float()
- vc = VC(tgt_sr, config)
- print(f"Model loaded: {character_name} / {info['feature_retrieval_library']} | ({model_version})")
- models.append((character_name, model_title, model_author, model_cover, model_version, create_vc_fn(model_name, tgt_sr, net_g, vc, if_f0, version, model_index)))
- categories.append([category_title, category_folder, description, models])
- return categories
-
-def cut_vocal_and_inst(url, audio_provider, split_model):
- if url != "":
- if not os.path.exists("dl_audio"):
- os.mkdir("dl_audio")
- if audio_provider == "Youtube":
- ydl_opts = {
- 'noplaylist': True,
- 'format': 'bestaudio/best',
- 'postprocessors': [{
- 'key': 'FFmpegExtractAudio',
- 'preferredcodec': 'wav',
- }],
- "outtmpl": 'dl_audio/youtube_audio',
- }
- with yt_dlp.YoutubeDL(ydl_opts) as ydl:
- ydl.download([url])
- audio_path = "dl_audio/youtube_audio.wav"
- if split_model == "htdemucs":
- command = f"demucs --two-stems=vocals {audio_path} -o output"
- result = subprocess.run(command.split(), stdout=subprocess.PIPE)
- print(result.stdout.decode())
- return "output/htdemucs/youtube_audio/vocals.wav", "output/htdemucs/youtube_audio/no_vocals.wav", audio_path, "output/htdemucs/youtube_audio/vocals.wav"
- else:
- command = f"demucs --two-stems=vocals -n mdx_extra_q {audio_path} -o output"
- result = subprocess.run(command.split(), stdout=subprocess.PIPE)
- print(result.stdout.decode())
- return "output/mdx_extra_q/youtube_audio/vocals.wav", "output/mdx_extra_q/youtube_audio/no_vocals.wav", audio_path, "output/mdx_extra_q/youtube_audio/vocals.wav"
- else:
- raise gr.Error("URL Required!")
- return None, None, None, None
-
-def combine_vocal_and_inst(audio_data, audio_volume, split_model):
- if not os.path.exists("output/result"):
- os.mkdir("output/result")
- vocal_path = "output/result/output.wav"
- output_path = "output/result/combine.mp3"
- if split_model == "htdemucs":
- inst_path = "output/htdemucs/youtube_audio/no_vocals.wav"
- else:
- inst_path = "output/mdx_extra_q/youtube_audio/no_vocals.wav"
- with wave.open(vocal_path, "w") as wave_file:
- wave_file.setnchannels(1)
- wave_file.setsampwidth(2)
- wave_file.setframerate(audio_data[0])
- wave_file.writeframes(audio_data[1].tobytes())
- command = f'ffmpeg -y -i {inst_path} -i {vocal_path} -filter_complex [1:a]volume={audio_volume}dB[v];[0:a][v]amix=inputs=2:duration=longest -b:a 320k -c:a libmp3lame {output_path}'
- result = subprocess.run(command.split(), stdout=subprocess.PIPE)
- print(result.stdout.decode())
- return output_path
-
-def load_hubert():
- global hubert_model
- models, _, _ = checkpoint_utils.load_model_ensemble_and_task(
- ["hubert_base.pt"],
- suffix="",
- )
- hubert_model = models[0]
- hubert_model = hubert_model.to(config.device)
- if config.is_half:
- hubert_model = hubert_model.half()
- else:
- hubert_model = hubert_model.float()
- hubert_model.eval()
-
-def change_audio_mode(vc_audio_mode):
- if vc_audio_mode == "Input path":
- return (
- # Input & Upload
- gr.Textbox.update(visible=True),
- gr.Checkbox.update(visible=False),
- gr.Audio.update(visible=False),
- # Youtube
- gr.Dropdown.update(visible=False),
- gr.Textbox.update(visible=False),
- gr.Dropdown.update(visible=False),
- gr.Button.update(visible=False),
- gr.Audio.update(visible=False),
- gr.Audio.update(visible=False),
- gr.Audio.update(visible=False),
- gr.Slider.update(visible=False),
- gr.Audio.update(visible=False),
- gr.Button.update(visible=False),
- # TTS
- gr.Textbox.update(visible=False),
- gr.Dropdown.update(visible=False)
- )
- elif vc_audio_mode == "Upload audio":
- return (
- # Input & Upload
- gr.Textbox.update(visible=False),
- gr.Checkbox.update(visible=True),
- gr.Audio.update(visible=True),
- # Youtube
- gr.Dropdown.update(visible=False),
- gr.Textbox.update(visible=False),
- gr.Dropdown.update(visible=False),
- gr.Button.update(visible=False),
- gr.Audio.update(visible=False),
- gr.Audio.update(visible=False),
- gr.Audio.update(visible=False),
- gr.Slider.update(visible=False),
- gr.Audio.update(visible=False),
- gr.Button.update(visible=False),
- # TTS
- gr.Textbox.update(visible=False),
- gr.Dropdown.update(visible=False)
- )
- elif vc_audio_mode == "Youtube":
- return (
- # Input & Upload
- gr.Textbox.update(visible=False),
- gr.Checkbox.update(visible=False),
- gr.Audio.update(visible=False),
- # Youtube
- gr.Dropdown.update(visible=True),
- gr.Textbox.update(visible=True),
- gr.Dropdown.update(visible=True),
- gr.Button.update(visible=True),
- gr.Audio.update(visible=True),
- gr.Audio.update(visible=True),
- gr.Audio.update(visible=True),
- gr.Slider.update(visible=True),
- gr.Audio.update(visible=True),
- gr.Button.update(visible=True),
- # TTS
- gr.Textbox.update(visible=False),
- gr.Dropdown.update(visible=False)
- )
- elif vc_audio_mode == "TTS Audio":
- return (
- # Input & Upload
- gr.Textbox.update(visible=False),
- gr.Checkbox.update(visible=False),
- gr.Audio.update(visible=False),
- # Youtube
- gr.Dropdown.update(visible=False),
- gr.Textbox.update(visible=False),
- gr.Dropdown.update(visible=False),
- gr.Button.update(visible=False),
- gr.Audio.update(visible=False),
- gr.Audio.update(visible=False),
- gr.Audio.update(visible=False),
- gr.Slider.update(visible=False),
- gr.Audio.update(visible=False),
- gr.Button.update(visible=False),
- # TTS
- gr.Textbox.update(visible=True),
- gr.Dropdown.update(visible=True)
- )
- else:
- return (
- # Input & Upload
- gr.Textbox.update(visible=False),
- gr.Checkbox.update(visible=True),
- gr.Audio.update(visible=True),
- # Youtube
- gr.Dropdown.update(visible=False),
- gr.Textbox.update(visible=False),
- gr.Dropdown.update(visible=False),
- gr.Button.update(visible=False),
- gr.Audio.update(visible=False),
- gr.Audio.update(visible=False),
- gr.Audio.update(visible=False),
- gr.Slider.update(visible=False),
- gr.Audio.update(visible=False),
- gr.Button.update(visible=False),
- # TTS
- gr.Textbox.update(visible=False),
- gr.Dropdown.update(visible=False)
- )
-
-def use_microphone(microphone):
- if microphone == True:
- return gr.Audio.update(source="microphone")
- else:
- return gr.Audio.update(source="upload")
-
-if __name__ == '__main__':
- load_hubert()
- categories = load_model()
- tts_voice_list = asyncio.get_event_loop().run_until_complete(edge_tts.list_voices())
- voices = [f"{v['ShortName']}-{v['Gender']}" for v in tts_voice_list]
- with gr.Blocks() as app:
- gr.Markdown(
- "\n\n"+
- "# Multi Model RVC Inference\n\n"+
- "[](https://github.com/ArkanDash/Multi-Model-RVC-Inference)\n\n"+
- "
"
- )
- for (folder_title, folder, description, models) in categories:
- with gr.TabItem(folder_title):
- if description:
- gr.Markdown(f"### {description}")
- with gr.Tabs():
- if not models:
- gr.Markdown("# No Model Loaded.")
- gr.Markdown("## Please add model or fix your model path.")
- continue
- for (name, title, author, cover, model_version, vc_fn) in models:
- with gr.TabItem(name):
- with gr.Row():
- gr.Markdown(
- ''
- f'
{title}
\n'+
- f'
RVC {model_version} Model
\n'+
- (f'
Model author: {author}
' if author else "")+
- (f'
' if cover else "")+
- '
'
- )
- with gr.Row():
- with gr.Column():
- vc_audio_mode = gr.Dropdown(label="Input voice", choices=audio_mode, allow_custom_value=False, value="Upload audio")
- # Input
- vc_input = gr.Textbox(label="Input audio path", visible=False)
- # Upload
- vc_microphone_mode = gr.Checkbox(label="Use Microphone", value=False, visible=True, interactive=True)
- vc_upload = gr.Audio(label="Upload audio file", source="upload", visible=True, interactive=True)
- # Youtube
- vc_download_audio = gr.Dropdown(label="Provider", choices=["Youtube"], allow_custom_value=False, visible=False, value="Youtube", info="Select provider (Default: Youtube)")
- vc_link = gr.Textbox(label="Youtube URL", visible=False, info="Example: https://www.youtube.com/watch?v=Nc0sB1Bmf-A", placeholder="https://www.youtube.com/watch?v=...")
- vc_split_model = gr.Dropdown(label="Splitter Model", choices=["htdemucs", "mdx_extra_q"], allow_custom_value=False, visible=False, value="htdemucs", info="Select the splitter model (Default: htdemucs)")
- vc_split = gr.Button("Split Audio", variant="primary", visible=False)
- vc_vocal_preview = gr.Audio(label="Vocal Preview", visible=False)
- vc_inst_preview = gr.Audio(label="Instrumental Preview", visible=False)
- vc_audio_preview = gr.Audio(label="Audio Preview", visible=False)
- # TTS
- tts_text = gr.Textbox(visible=False, label="TTS text", info="Text to speech input")
- tts_voice = gr.Dropdown(label="Edge-tts speaker", choices=voices, visible=False, allow_custom_value=False, value="en-US-AnaNeural-Female")
- with gr.Column():
- vc_transform0 = gr.Number(label="Transpose", value=0, info='Type "12" to change from male to female voice. Type "-12" to change female to male voice')
- f0method0 = gr.Radio(
- label="Pitch extraction algorithm",
- info=f0method_info,
- choices=f0method_mode,
- value="pm",
- interactive=True
- )
- index_rate1 = gr.Slider(
- minimum=0,
- maximum=1,
- label="Retrieval feature ratio",
- info="(Default: 0.7)",
- value=0.7,
- interactive=True,
- )
- filter_radius0 = gr.Slider(
- minimum=0,
- maximum=7,
- label="Apply Median Filtering",
- info="The value represents the filter radius and can reduce breathiness.",
- value=3,
- step=1,
- interactive=True,
- )
- resample_sr0 = gr.Slider(
- minimum=0,
- maximum=48000,
- label="Resample the output audio",
- info="Resample the output audio in post-processing to the final sample rate. Set to 0 for no resampling",
- value=0,
- step=1,
- interactive=True,
- )
- rms_mix_rate0 = gr.Slider(
- minimum=0,
- maximum=1,
- label="Volume Envelope",
- info="Use the volume envelope of the input to replace or mix with the volume envelope of the output. The closer the ratio is to 1, the more the output envelope is used",
- value=1,
- interactive=True,
- )
- protect0 = gr.Slider(
- minimum=0,
- maximum=0.5,
- label="Voice Protection",
- info="Protect voiceless consonants and breath sounds to prevent artifacts such as tearing in electronic music. Set to 0.5 to disable. Decrease the value to increase protection, but it may reduce indexing accuracy",
- value=0.5,
- step=0.01,
- interactive=True,
- )
- with gr.Column():
- vc_log = gr.Textbox(label="Output Information", interactive=False)
- vc_output = gr.Audio(label="Output Audio", interactive=False)
- vc_convert = gr.Button("Convert", variant="primary")
- vc_volume = gr.Slider(
- minimum=0,
- maximum=10,
- label="Vocal volume",
- value=4,
- interactive=True,
- step=1,
- info="Adjust vocal volume (Default: 4}",
- visible=False
- )
- vc_combined_output = gr.Audio(label="Output Combined Audio", visible=False)
- vc_combine = gr.Button("Combine",variant="primary", visible=False)
- vc_convert.click(
- fn=vc_fn,
- inputs=[
- vc_audio_mode,
- vc_input,
- vc_upload,
- tts_text,
- tts_voice,
- vc_transform0,
- f0method0,
- index_rate1,
- filter_radius0,
- resample_sr0,
- rms_mix_rate0,
- protect0,
- ],
- outputs=[vc_log ,vc_output]
- )
- vc_split.click(
- fn=cut_vocal_and_inst,
- inputs=[vc_link, vc_download_audio, vc_split_model],
- outputs=[vc_vocal_preview, vc_inst_preview, vc_audio_preview, vc_input]
- )
- vc_combine.click(
- fn=combine_vocal_and_inst,
- inputs=[vc_output, vc_volume, vc_split_model],
- outputs=[vc_combined_output]
- )
- vc_microphone_mode.change(
- fn=use_microphone,
- inputs=vc_microphone_mode,
- outputs=vc_upload
- )
- vc_audio_mode.change(
- fn=change_audio_mode,
- inputs=[vc_audio_mode],
- outputs=[
- vc_input,
- vc_microphone_mode,
- vc_upload,
- vc_download_audio,
- vc_link,
- vc_split_model,
- vc_split,
- vc_vocal_preview,
- vc_inst_preview,
- vc_audio_preview,
- vc_volume,
- vc_combined_output,
- vc_combine,
- tts_text,
- tts_voice
- ]
- )
- app.queue(concurrency_count=1, max_size=20, api_open=config.api).launch(share=config.colab)
\ No newline at end of file
diff --git a/spaces/Ferion/image-matting-app/ppmatting/core/predict.py b/spaces/Ferion/image-matting-app/ppmatting/core/predict.py
deleted file mode 100644
index e7ff765d9c62f3cb7b758d1756632cfe65cab0f1..0000000000000000000000000000000000000000
--- a/spaces/Ferion/image-matting-app/ppmatting/core/predict.py
+++ /dev/null
@@ -1,58 +0,0 @@
-from typing import Optional
-
-import numpy as np
-import paddle
-import paddle.nn.functional as F
-
-
-def reverse_transform(alpha, trans_info):
- """recover pred to origin shape"""
- for item in trans_info[::-1]:
- if item[0] == "resize":
- h, w = item[1][0], item[1][1]
- alpha = F.interpolate(alpha, [h, w], mode="bilinear")
- elif item[0] == "padding":
- h, w = item[1][0], item[1][1]
- alpha = alpha[:, :, 0:h, 0:w]
- else:
- raise Exception(f"Unexpected info '{item[0]}' in im_info")
-
- return alpha
-
-
-def preprocess(img, transforms, trimap=None):
- data = {}
- data["img"] = img
- if trimap is not None:
- data["trimap"] = trimap
- data["gt_fields"] = ["trimap"]
- data["trans_info"] = []
- data = transforms(data)
- data["img"] = paddle.to_tensor(data["img"])
- data["img"] = data["img"].unsqueeze(0)
- if trimap is not None:
- data["trimap"] = paddle.to_tensor(data["trimap"])
- data["trimap"] = data["trimap"].unsqueeze((0, 1))
-
- return data
-
-
-def predict(
- model,
- transforms,
- image: np.ndarray,
- trimap: Optional[np.ndarray] = None,
-):
- with paddle.no_grad():
- data = preprocess(img=image, transforms=transforms, trimap=None)
-
- alpha = model(data)
-
- alpha = reverse_transform(alpha, data["trans_info"])
- alpha = alpha.numpy().squeeze()
-
- if trimap is not None:
- alpha[trimap == 0] = 0
- alpha[trimap == 255] = 1.
-
- return alpha
diff --git a/spaces/FourthBrainGenAI/AI-Superstar-Space/README.md b/spaces/FourthBrainGenAI/AI-Superstar-Space/README.md
deleted file mode 100644
index e1a64b336b8a34341d9f9719feddb305e92f3438..0000000000000000000000000000000000000000
--- a/spaces/FourthBrainGenAI/AI-Superstar-Space/README.md
+++ /dev/null
@@ -1,13 +0,0 @@
----
-title: AI Superstar Space
-emoji: ⚡
-colorFrom: red
-colorTo: pink
-sdk: gradio
-sdk_version: 3.27.0
-app_file: app.py
-pinned: false
-license: bigscience-openrail-m
----
-
-Check out the configuration reference at https://huggingface.co/docs/hub/spaces-config-reference
diff --git a/spaces/FrankZxShen/so-vits-svc-models-pcr/vencoder/ContentVec256L9.py b/spaces/FrankZxShen/so-vits-svc-models-pcr/vencoder/ContentVec256L9.py
deleted file mode 100644
index b0089c789cd87cfd3b1badb2fc45cb1b88041eab..0000000000000000000000000000000000000000
--- a/spaces/FrankZxShen/so-vits-svc-models-pcr/vencoder/ContentVec256L9.py
+++ /dev/null
@@ -1,35 +0,0 @@
-from vencoder.encoder import SpeechEncoder
-import torch
-from fairseq import checkpoint_utils
-
-class ContentVec256L9(SpeechEncoder):
- def __init__(self,vec_path = "pretrain/checkpoint_best_legacy_500.pt",device=None):
- print("load model(s) from {}".format(vec_path))
- models, saved_cfg, task = checkpoint_utils.load_model_ensemble_and_task(
- [vec_path],
- suffix="",
- )
- self.hidden_dim = 256
- if device is None:
- self.dev = torch.device("cuda" if torch.cuda.is_available() else "cpu")
- else:
- self.dev = torch.device(device)
- self.model = models[0].to(self.dev)
- self.model.eval()
-
- def encoder(self, wav):
- feats = wav
- if feats.dim() == 2: # double channels
- feats = feats.mean(-1)
- assert feats.dim() == 1, feats.dim()
- feats = feats.view(1, -1)
- padding_mask = torch.BoolTensor(feats.shape).fill_(False)
- inputs = {
- "source": feats.to(wav.device),
- "padding_mask": padding_mask.to(wav.device),
- "output_layer": 9, # layer 9
- }
- with torch.no_grad():
- logits = self.model.extract_features(**inputs)
- feats = self.model.final_proj(logits[0])
- return feats.transpose(1, 2)
diff --git a/spaces/FridaZuley/RVC_HFKawaii/infer/lib/train/losses.py b/spaces/FridaZuley/RVC_HFKawaii/infer/lib/train/losses.py
deleted file mode 100644
index b1b263e4c205e78ffe970f622ab6ff68f36d3b17..0000000000000000000000000000000000000000
--- a/spaces/FridaZuley/RVC_HFKawaii/infer/lib/train/losses.py
+++ /dev/null
@@ -1,58 +0,0 @@
-import torch
-
-
-def feature_loss(fmap_r, fmap_g):
- loss = 0
- for dr, dg in zip(fmap_r, fmap_g):
- for rl, gl in zip(dr, dg):
- rl = rl.float().detach()
- gl = gl.float()
- loss += torch.mean(torch.abs(rl - gl))
-
- return loss * 2
-
-
-def discriminator_loss(disc_real_outputs, disc_generated_outputs):
- loss = 0
- r_losses = []
- g_losses = []
- for dr, dg in zip(disc_real_outputs, disc_generated_outputs):
- dr = dr.float()
- dg = dg.float()
- r_loss = torch.mean((1 - dr) ** 2)
- g_loss = torch.mean(dg**2)
- loss += r_loss + g_loss
- r_losses.append(r_loss.item())
- g_losses.append(g_loss.item())
-
- return loss, r_losses, g_losses
-
-
-def generator_loss(disc_outputs):
- loss = 0
- gen_losses = []
- for dg in disc_outputs:
- dg = dg.float()
- l = torch.mean((1 - dg) ** 2)
- gen_losses.append(l)
- loss += l
-
- return loss, gen_losses
-
-
-def kl_loss(z_p, logs_q, m_p, logs_p, z_mask):
- """
- z_p, logs_q: [b, h, t_t]
- m_p, logs_p: [b, h, t_t]
- """
- z_p = z_p.float()
- logs_q = logs_q.float()
- m_p = m_p.float()
- logs_p = logs_p.float()
- z_mask = z_mask.float()
-
- kl = logs_p - logs_q - 0.5
- kl += 0.5 * ((z_p - m_p) ** 2) * torch.exp(-2.0 * logs_p)
- kl = torch.sum(kl * z_mask)
- l = kl / torch.sum(z_mask)
- return l
diff --git a/spaces/FriendlyUser/YoutubeDownloaderSubber/README.md b/spaces/FriendlyUser/YoutubeDownloaderSubber/README.md
deleted file mode 100644
index 4dcf491ad9e93d021d0511b8c8879052641f6c07..0000000000000000000000000000000000000000
--- a/spaces/FriendlyUser/YoutubeDownloaderSubber/README.md
+++ /dev/null
@@ -1,13 +0,0 @@
----
-title: YoutubeDownloaderSubber
-emoji: ⚡
-colorFrom: green
-colorTo: blue
-sdk: gradio
-sdk_version: 3.19.1
-app_file: app.py
-pinned: false
-license: openrail
----
-
-Check out the configuration reference at https://huggingface.co/docs/hub/spaces-config-reference
diff --git a/spaces/GXSA/bingo/src/components/providers.tsx b/spaces/GXSA/bingo/src/components/providers.tsx
deleted file mode 100644
index 892226412d80fe0b05211911b9e245cd22876460..0000000000000000000000000000000000000000
--- a/spaces/GXSA/bingo/src/components/providers.tsx
+++ /dev/null
@@ -1,15 +0,0 @@
-'use client'
-
-import * as React from 'react'
-import { ThemeProvider as NextThemesProvider } from 'next-themes'
-import { ThemeProviderProps } from 'next-themes/dist/types'
-
-import { TooltipProvider } from '@/components/ui/tooltip'
-
-export function Providers({ children, ...props }: ThemeProviderProps) {
- return (
-
- {children}
-
- )
-}
diff --git a/spaces/GipAdonimus/Real-Time-Voice-Cloning/vocoder_train.py b/spaces/GipAdonimus/Real-Time-Voice-Cloning/vocoder_train.py
deleted file mode 100644
index d712ffa3e6c92a091aa18dc90f0027f46940e400..0000000000000000000000000000000000000000
--- a/spaces/GipAdonimus/Real-Time-Voice-Cloning/vocoder_train.py
+++ /dev/null
@@ -1,56 +0,0 @@
-from utils.argutils import print_args
-from vocoder.train import train
-from pathlib import Path
-import argparse
-
-
-if __name__ == "__main__":
- parser = argparse.ArgumentParser(
- description="Trains the vocoder from the synthesizer audios and the GTA synthesized mels, "
- "or ground truth mels.",
- formatter_class=argparse.ArgumentDefaultsHelpFormatter
- )
-
- parser.add_argument("run_id", type=str, help= \
- "Name for this model instance. If a model state from the same run ID was previously "
- "saved, the training will restart from there. Pass -f to overwrite saved states and "
- "restart from scratch.")
- parser.add_argument("datasets_root", type=str, help= \
- "Path to the directory containing your SV2TTS directory. Specifying --syn_dir or --voc_dir "
- "will take priority over this argument.")
- parser.add_argument("--syn_dir", type=str, default=argparse.SUPPRESS, help= \
- "Path to the synthesizer directory that contains the ground truth mel spectrograms, "
- "the wavs and the embeds. Defaults to /SV2TTS/synthesizer/.")
- parser.add_argument("--voc_dir", type=str, default=argparse.SUPPRESS, help= \
- "Path to the vocoder directory that contains the GTA synthesized mel spectrograms. "
- "Defaults to /SV2TTS/vocoder/. Unused if --ground_truth is passed.")
- parser.add_argument("-m", "--models_dir", type=str, default="vocoder/saved_models/", help=\
- "Path to the directory that will contain the saved model weights, as well as backups "
- "of those weights and wavs generated during training.")
- parser.add_argument("-g", "--ground_truth", action="store_true", help= \
- "Train on ground truth spectrograms (/SV2TTS/synthesizer/mels).")
- parser.add_argument("-s", "--save_every", type=int, default=1000, help= \
- "Number of steps between updates of the model on the disk. Set to 0 to never save the "
- "model.")
- parser.add_argument("-b", "--backup_every", type=int, default=25000, help= \
- "Number of steps between backups of the model. Set to 0 to never make backups of the "
- "model.")
- parser.add_argument("-f", "--force_restart", action="store_true", help= \
- "Do not load any saved model and restart from scratch.")
- args = parser.parse_args()
-
- # Process the arguments
- if not hasattr(args, "syn_dir"):
- args.syn_dir = Path(args.datasets_root, "SV2TTS", "synthesizer")
- args.syn_dir = Path(args.syn_dir)
- if not hasattr(args, "voc_dir"):
- args.voc_dir = Path(args.datasets_root, "SV2TTS", "vocoder")
- args.voc_dir = Path(args.voc_dir)
- del args.datasets_root
- args.models_dir = Path(args.models_dir)
- args.models_dir.mkdir(exist_ok=True)
-
- # Run the training
- print_args(args, parser)
- train(**vars(args))
-
\ No newline at end of file
diff --git a/spaces/Gradio-Blocks/uniformer_image_detection/configs/gcnet/mask_rcnn_r101_fpn_r16_gcb_c3-c5_1x_coco.py b/spaces/Gradio-Blocks/uniformer_image_detection/configs/gcnet/mask_rcnn_r101_fpn_r16_gcb_c3-c5_1x_coco.py
deleted file mode 100644
index ad6ad47696e6aeb2b3505abab0bd2d49d3b7aa83..0000000000000000000000000000000000000000
--- a/spaces/Gradio-Blocks/uniformer_image_detection/configs/gcnet/mask_rcnn_r101_fpn_r16_gcb_c3-c5_1x_coco.py
+++ /dev/null
@@ -1,8 +0,0 @@
-_base_ = '../mask_rcnn/mask_rcnn_r101_fpn_1x_coco.py'
-model = dict(
- backbone=dict(plugins=[
- dict(
- cfg=dict(type='ContextBlock', ratio=1. / 16),
- stages=(False, True, True, True),
- position='after_conv3')
- ]))
diff --git a/spaces/Gradio-Blocks/uniformer_image_detection/configs/yolo/yolov3_d53_320_273e_coco.py b/spaces/Gradio-Blocks/uniformer_image_detection/configs/yolo/yolov3_d53_320_273e_coco.py
deleted file mode 100644
index 87359f6fb66d94de10b8e3797ee3eec93a19cb26..0000000000000000000000000000000000000000
--- a/spaces/Gradio-Blocks/uniformer_image_detection/configs/yolo/yolov3_d53_320_273e_coco.py
+++ /dev/null
@@ -1,42 +0,0 @@
-_base_ = './yolov3_d53_mstrain-608_273e_coco.py'
-# dataset settings
-img_norm_cfg = dict(mean=[0, 0, 0], std=[255., 255., 255.], to_rgb=True)
-train_pipeline = [
- dict(type='LoadImageFromFile', to_float32=True),
- dict(type='LoadAnnotations', with_bbox=True),
- dict(type='PhotoMetricDistortion'),
- dict(
- type='Expand',
- mean=img_norm_cfg['mean'],
- to_rgb=img_norm_cfg['to_rgb'],
- ratio_range=(1, 2)),
- dict(
- type='MinIoURandomCrop',
- min_ious=(0.4, 0.5, 0.6, 0.7, 0.8, 0.9),
- min_crop_size=0.3),
- dict(type='Resize', img_scale=(320, 320), keep_ratio=True),
- dict(type='RandomFlip', flip_ratio=0.5),
- dict(type='Normalize', **img_norm_cfg),
- dict(type='Pad', size_divisor=32),
- dict(type='DefaultFormatBundle'),
- dict(type='Collect', keys=['img', 'gt_bboxes', 'gt_labels'])
-]
-test_pipeline = [
- dict(type='LoadImageFromFile'),
- dict(
- type='MultiScaleFlipAug',
- img_scale=(320, 320),
- flip=False,
- transforms=[
- dict(type='Resize', keep_ratio=True),
- dict(type='RandomFlip'),
- dict(type='Normalize', **img_norm_cfg),
- dict(type='Pad', size_divisor=32),
- dict(type='ImageToTensor', keys=['img']),
- dict(type='Collect', keys=['img'])
- ])
-]
-data = dict(
- train=dict(pipeline=train_pipeline),
- val=dict(pipeline=test_pipeline),
- test=dict(pipeline=test_pipeline))
diff --git a/spaces/HCMUT-GraduateThesis-HNTThinh/rgbdsod-multimae-demo/s_multimae/backup.py b/spaces/HCMUT-GraduateThesis-HNTThinh/rgbdsod-multimae-demo/s_multimae/backup.py
deleted file mode 100644
index 4797a2af766db8e786261bc100d617d843cd31bb..0000000000000000000000000000000000000000
--- a/spaces/HCMUT-GraduateThesis-HNTThinh/rgbdsod-multimae-demo/s_multimae/backup.py
+++ /dev/null
@@ -1,16 +0,0 @@
-import os
-from typing import List
-import wget
-
-from .configs.base_config import base_cfg
-
-def backup(cfg: base_cfg, urls: List[str]):
- current_experiment_dir_path = os.path.join(
- cfg.experiment_dir_path,
- cfg.experiment_name
- )
-
- os.makedirs(current_experiment_dir_path, exist_ok=True)
-
- for url in urls:
- wget.download(url, out = current_experiment_dir_path)
diff --git a/spaces/Hallucinate/demo/taming/models/dummy_cond_stage.py b/spaces/Hallucinate/demo/taming/models/dummy_cond_stage.py
deleted file mode 100644
index 6e19938078752e09b926a3e749907ee99a258ca0..0000000000000000000000000000000000000000
--- a/spaces/Hallucinate/demo/taming/models/dummy_cond_stage.py
+++ /dev/null
@@ -1,22 +0,0 @@
-from torch import Tensor
-
-
-class DummyCondStage:
- def __init__(self, conditional_key):
- self.conditional_key = conditional_key
- self.train = None
-
- def eval(self):
- return self
-
- @staticmethod
- def encode(c: Tensor):
- return c, None, (None, None, c)
-
- @staticmethod
- def decode(c: Tensor):
- return c
-
- @staticmethod
- def to_rgb(c: Tensor):
- return c
diff --git a/spaces/HarryLee/eCommerceImageCaptioning/fairseq/fairseq/data/append_token_dataset.py b/spaces/HarryLee/eCommerceImageCaptioning/fairseq/fairseq/data/append_token_dataset.py
deleted file mode 100644
index 87695bd0f5fcb6b10247e3b743340623e6438cc1..0000000000000000000000000000000000000000
--- a/spaces/HarryLee/eCommerceImageCaptioning/fairseq/fairseq/data/append_token_dataset.py
+++ /dev/null
@@ -1,41 +0,0 @@
-# Copyright (c) Facebook, Inc. and its affiliates.
-#
-# This source code is licensed under the MIT license found in the
-# LICENSE file in the root directory of this source tree.
-
-import numpy as np
-import torch
-
-from . import BaseWrapperDataset
-
-
-class AppendTokenDataset(BaseWrapperDataset):
- def __init__(self, dataset, token=None):
- super().__init__(dataset)
- self.token = token
- if token is not None:
- self._sizes = np.array(dataset.sizes) + 1
- else:
- self._sizes = dataset.sizes
-
- def __getitem__(self, idx):
- item = self.dataset[idx]
- if self.token is not None:
- item = torch.cat([item, item.new([self.token])])
- return item
-
- @property
- def sizes(self):
- return self._sizes
-
- def num_tokens(self, index):
- n = self.dataset.num_tokens(index)
- if self.token is not None:
- n += 1
- return n
-
- def size(self, index):
- n = self.dataset.size(index)
- if self.token is not None:
- n += 1
- return n
diff --git a/spaces/HarryLee/eCommerceImageCaptioning/fairseq/fairseq/models/transformer/transformer_base.py b/spaces/HarryLee/eCommerceImageCaptioning/fairseq/fairseq/models/transformer/transformer_base.py
deleted file mode 100644
index b4d5604dbbae979b424650882d33b45ebab323e6..0000000000000000000000000000000000000000
--- a/spaces/HarryLee/eCommerceImageCaptioning/fairseq/fairseq/models/transformer/transformer_base.py
+++ /dev/null
@@ -1,179 +0,0 @@
-# Copyright (c) Facebook, Inc. and its affiliates.
-#
-# This source code is licensed under the MIT license found in the
-# LICENSE file in the root directory of this source tree.
-
-from typing import Dict, List, Optional, Tuple
-
-import torch
-import torch.nn as nn
-from fairseq import utils
-from fairseq.dataclass.utils import gen_parser_from_dataclass
-from fairseq.distributed import fsdp_wrap
-from fairseq.models import FairseqEncoderDecoderModel
-from fairseq.models.transformer import (
- TransformerEncoderBase,
- TransformerDecoderBase,
- TransformerConfig,
-)
-from torch import Tensor
-
-
-class TransformerModelBase(FairseqEncoderDecoderModel):
- """
- Transformer model from `"Attention Is All You Need" (Vaswani, et al, 2017)
- `_.
-
- Args:
- encoder (TransformerEncoder): the encoder
- decoder (TransformerDecoder): the decoder
-
- The Transformer model provides the following named architectures and
- command-line arguments:
-
- .. argparse::
- :ref: fairseq.models.transformer_parser
- :prog:
- """
-
- def __init__(self, cfg, encoder, decoder):
- super().__init__(encoder, decoder)
- self.cfg = cfg
- self.supports_align_args = True
-
- @classmethod
- def add_args(cls, parser):
- """Add model-specific arguments to the parser."""
- # we want to build the args recursively in this case.
- gen_parser_from_dataclass(
- parser, TransformerConfig(), delete_default=False, with_prefix=""
- )
-
- @classmethod
- def build_model(cls, cfg, task):
- """Build a new model instance."""
-
- # -- TODO T96535332
- # bug caused by interaction between OmegaConf II and argparsing
- cfg.decoder.input_dim = int(cfg.decoder.input_dim)
- cfg.decoder.output_dim = int(cfg.decoder.output_dim)
- # --
-
- if cfg.encoder.layers_to_keep:
- cfg.encoder.layers = len(cfg.encoder.layers_to_keep.split(","))
- if cfg.decoder.layers_to_keep:
- cfg.decoder.layers = len(cfg.decoder.layers_to_keep.split(","))
-
- src_dict, tgt_dict = task.source_dictionary, task.target_dictionary
-
- if cfg.share_all_embeddings:
- if src_dict != tgt_dict:
- raise ValueError("--share-all-embeddings requires a joined dictionary")
- if cfg.encoder.embed_dim != cfg.decoder.embed_dim:
- raise ValueError(
- "--share-all-embeddings requires --encoder-embed-dim to match --decoder-embed-dim"
- )
- if cfg.decoder.embed_path and (
- cfg.decoder.embed_path != cfg.encoder.embed_path
- ):
- raise ValueError(
- "--share-all-embeddings not compatible with --decoder-embed-path"
- )
- encoder_embed_tokens = cls.build_embedding(
- cfg, src_dict, cfg.encoder.embed_dim, cfg.encoder.embed_path
- )
- decoder_embed_tokens = encoder_embed_tokens
- cfg.share_decoder_input_output_embed = True
- else:
- encoder_embed_tokens = cls.build_embedding(
- cfg, src_dict, cfg.encoder.embed_dim, cfg.encoder.embed_path
- )
- decoder_embed_tokens = cls.build_embedding(
- cfg, tgt_dict, cfg.decoder.embed_dim, cfg.decoder.embed_path
- )
- if cfg.offload_activations:
- cfg.checkpoint_activations = True # offloading implies checkpointing
- encoder = cls.build_encoder(cfg, src_dict, encoder_embed_tokens)
- decoder = cls.build_decoder(cfg, tgt_dict, decoder_embed_tokens)
- if not cfg.share_all_embeddings:
- # fsdp_wrap is a no-op when --ddp-backend != fully_sharded
- encoder = fsdp_wrap(encoder, min_num_params=cfg.min_params_to_wrap)
- decoder = fsdp_wrap(decoder, min_num_params=cfg.min_params_to_wrap)
- return cls(cfg, encoder, decoder)
-
- @classmethod
- def build_embedding(cls, cfg, dictionary, embed_dim, path=None):
- num_embeddings = len(dictionary)
- padding_idx = dictionary.pad()
-
- emb = Embedding(num_embeddings, embed_dim, padding_idx)
- # if provided, load from preloaded dictionaries
- if path:
- embed_dict = utils.parse_embedding(path)
- utils.load_embedding(embed_dict, dictionary, emb)
- return emb
-
- @classmethod
- def build_encoder(cls, cfg, src_dict, embed_tokens):
- return TransformerEncoderBase(cfg, src_dict, embed_tokens)
-
- @classmethod
- def build_decoder(cls, cfg, tgt_dict, embed_tokens):
- return TransformerDecoderBase(
- cfg,
- tgt_dict,
- embed_tokens,
- no_encoder_attn=cfg.no_cross_attention,
- )
-
- # TorchScript doesn't support optional arguments with variable length (**kwargs).
- # Current workaround is to add union of all arguments in child classes.
- def forward(
- self,
- src_tokens,
- src_lengths,
- prev_output_tokens,
- return_all_hiddens: bool = True,
- features_only: bool = False,
- alignment_layer: Optional[int] = None,
- alignment_heads: Optional[int] = None,
- ):
- """
- Run the forward pass for an encoder-decoder model.
-
- Copied from the base class, but without ``**kwargs``,
- which are not supported by TorchScript.
- """
- encoder_out = self.encoder(
- src_tokens, src_lengths=src_lengths, return_all_hiddens=return_all_hiddens
- )
- decoder_out = self.decoder(
- prev_output_tokens,
- encoder_out=encoder_out,
- features_only=features_only,
- alignment_layer=alignment_layer,
- alignment_heads=alignment_heads,
- src_lengths=src_lengths,
- return_all_hiddens=return_all_hiddens,
- )
- return decoder_out
-
- # Since get_normalized_probs is in the Fairseq Model which is not scriptable,
- # I rewrite the get_normalized_probs from Base Class to call the
- # helper function in the Base Class.
- @torch.jit.export
- def get_normalized_probs(
- self,
- net_output: Tuple[Tensor, Optional[Dict[str, List[Optional[Tensor]]]]],
- log_probs: bool,
- sample: Optional[Dict[str, Tensor]] = None,
- ):
- """Get normalized probabilities (or log probs) from a net's output."""
- return self.get_normalized_probs_scriptable(net_output, log_probs, sample)
-
-
-def Embedding(num_embeddings, embedding_dim, padding_idx):
- m = nn.Embedding(num_embeddings, embedding_dim, padding_idx=padding_idx)
- nn.init.normal_(m.weight, mean=0, std=embedding_dim ** -0.5)
- nn.init.constant_(m.weight[padding_idx], 0)
- return m
diff --git a/spaces/Hazem/roop/roop/predicter.py b/spaces/Hazem/roop/roop/predicter.py
deleted file mode 100644
index 7ebc2b62e4152c12ce41e55d718222ca9c8a8b7f..0000000000000000000000000000000000000000
--- a/spaces/Hazem/roop/roop/predicter.py
+++ /dev/null
@@ -1,25 +0,0 @@
-import numpy
-import opennsfw2
-from PIL import Image
-
-from roop.typing import Frame
-
-MAX_PROBABILITY = 0.85
-
-
-def predict_frame(target_frame: Frame) -> bool:
- image = Image.fromarray(target_frame)
- image = opennsfw2.preprocess_image(image, opennsfw2.Preprocessing.YAHOO)
- model = opennsfw2.make_open_nsfw_model()
- views = numpy.expand_dims(image, axis=0)
- _, probability = model.predict(views)[0]
- return probability > MAX_PROBABILITY
-
-
-def predict_image(target_path: str) -> bool:
- return opennsfw2.predict_image(target_path) > MAX_PROBABILITY
-
-
-def predict_video(target_path: str) -> bool:
- _, probabilities = opennsfw2.predict_video_frames(video_path=target_path, frame_interval=100)
- return any(probability > MAX_PROBABILITY for probability in probabilities)
diff --git a/spaces/Heckeroo/Cyberpunk-Anime-Diffusion/README.md b/spaces/Heckeroo/Cyberpunk-Anime-Diffusion/README.md
deleted file mode 100644
index b1463db1ea7f0d047b61bcf22a9afd82d301167c..0000000000000000000000000000000000000000
--- a/spaces/Heckeroo/Cyberpunk-Anime-Diffusion/README.md
+++ /dev/null
@@ -1,12 +0,0 @@
----
-title: Cyberpunk Anime Diffusion
-emoji: 📈
-colorFrom: gray
-colorTo: green
-sdk: gradio
-sdk_version: 3.13.0
-app_file: app.py
-pinned: false
----
-
-Check out the configuration reference at https://huggingface.co/docs/hub/spaces-config-reference
diff --git a/spaces/Hina4867/bingo/next.config.js b/spaces/Hina4867/bingo/next.config.js
deleted file mode 100644
index 0e6ccd7fbc91d0459eaaff3e968ce0556789c605..0000000000000000000000000000000000000000
--- a/spaces/Hina4867/bingo/next.config.js
+++ /dev/null
@@ -1,38 +0,0 @@
-/** @type {import('next').NextConfig} */
-const nextConfig = {
- // output: 'export',
- // assetPrefix: '.',
- webpack: (config, { isServer }) => {
- if (!isServer) {
- config.resolve = {
- ...config.resolve,
- fallback: {
- 'bufferutil': false,
- 'utf-8-validate': false,
- http: false,
- https: false,
- stream: false,
- // fixes proxy-agent dependencies
- net: false,
- dns: false,
- tls: false,
- assert: false,
- // fixes next-i18next dependencies
- path: false,
- fs: false,
- // fixes mapbox dependencies
- events: false,
- // fixes sentry dependencies
- process: false
- }
- };
- }
- config.module.exprContextCritical = false;
-
- return config;
- },
-}
-
-module.exports = (...args) => {
- return nextConfig
-}
diff --git a/spaces/Hushh/Generative_QNA/variables.py b/spaces/Hushh/Generative_QNA/variables.py
deleted file mode 100644
index 68d36f7c7359c75c61424517b57ddc45fa773a01..0000000000000000000000000000000000000000
--- a/spaces/Hushh/Generative_QNA/variables.py
+++ /dev/null
@@ -1,14 +0,0 @@
-# from chromadb.config import Settings
-
-EMBEDDING_MODEL_NAME = "sentence-transformers/paraphrase-albert-small-v2" #for embedding the text from the documents.
-MODEL_ID = "TheBloke/Llama-2-7b-Chat-GPTQ"
-MODEL_BASENAME = "model"
-
-__import__('pysqlite3')
-import sys
-sys.modules['sqlite3'] = sys.modules.pop('pysqlite3')
-
-# CHROMA_SETTINGS = Settings(
-# anonymized_telemetry=False,
-# is_persistent=True,
-# )
diff --git a/spaces/ICML2022/OFA/fairseq/examples/textless_nlp/gslm/unit2speech/tts_data.py b/spaces/ICML2022/OFA/fairseq/examples/textless_nlp/gslm/unit2speech/tts_data.py
deleted file mode 100644
index eb0f7c360d749fd9d489b40b04dae8652b095098..0000000000000000000000000000000000000000
--- a/spaces/ICML2022/OFA/fairseq/examples/textless_nlp/gslm/unit2speech/tts_data.py
+++ /dev/null
@@ -1,52 +0,0 @@
-# Copyright (c) Facebook, Inc. and its affiliates.
-#
-# This source code is licensed under the MIT license found in the
-# LICENSE file in the root directory of this source tree.
-
-
-import torch
-import numpy as np
-from examples.textless_nlp.gslm.unit2speech.tacotron2.text import (
- EOS_TOK,
- SOS_TOK,
- code_to_sequence,
- text_to_sequence,
-)
-from examples.textless_nlp.gslm.unit2speech.tacotron2.utils import (
- load_code_dict,
-)
-
-
-class TacotronInputDataset:
- def __init__(self, hparams, append_str=""):
- self.is_text = getattr(hparams, "text_or_code", "text") == "text"
- if not self.is_text:
- self.code_dict = load_code_dict(hparams.code_dict)
- self.code_key = hparams.code_key
- self.add_sos = hparams.add_sos
- self.add_eos = hparams.add_eos
- self.collapse_code = hparams.collapse_code
- self.append_str = append_str
-
- def process_code(self, inp_str):
- inp_toks = inp_str.split()
- if self.add_sos:
- inp_toks = [SOS_TOK] + inp_toks
- if self.add_eos:
- inp_toks = inp_toks + [EOS_TOK]
- return code_to_sequence(inp_toks, self.code_dict, self.collapse_code)
-
- def process_text(self, inp_str):
- return text_to_sequence(inp_str, ["english_cleaners"])
-
- def get_tensor(self, inp_str):
- # uid, txt, inp_str = self._get_data(idx)
- inp_str = inp_str + self.append_str
- if self.is_text:
- inp_toks = self.process_text(inp_str)
- else:
- inp_toks = self.process_code(inp_str)
- return torch.from_numpy(np.array(inp_toks)).long()
-
- def __len__(self):
- return len(self.data)
diff --git a/spaces/IDEA-Research/Grounded-SAM/segment_anything/segment_anything/predictor.py b/spaces/IDEA-Research/Grounded-SAM/segment_anything/segment_anything/predictor.py
deleted file mode 100644
index 57c089d1fc4a6bbf5786e1ef62c59e22d582f5aa..0000000000000000000000000000000000000000
--- a/spaces/IDEA-Research/Grounded-SAM/segment_anything/segment_anything/predictor.py
+++ /dev/null
@@ -1,269 +0,0 @@
-# Copyright (c) Meta Platforms, Inc. and affiliates.
-# All rights reserved.
-
-# This source code is licensed under the license found in the
-# LICENSE file in the root directory of this source tree.
-
-import numpy as np
-import torch
-
-from segment_anything.modeling import Sam
-
-from typing import Optional, Tuple
-
-from .utils.transforms import ResizeLongestSide
-
-
-class SamPredictor:
- def __init__(
- self,
- sam_model: Sam,
- ) -> None:
- """
- Uses SAM to calculate the image embedding for an image, and then
- allow repeated, efficient mask prediction given prompts.
-
- Arguments:
- sam_model (Sam): The model to use for mask prediction.
- """
- super().__init__()
- self.model = sam_model
- self.transform = ResizeLongestSide(sam_model.image_encoder.img_size)
- self.reset_image()
-
- def set_image(
- self,
- image: np.ndarray,
- image_format: str = "RGB",
- ) -> None:
- """
- Calculates the image embeddings for the provided image, allowing
- masks to be predicted with the 'predict' method.
-
- Arguments:
- image (np.ndarray): The image for calculating masks. Expects an
- image in HWC uint8 format, with pixel values in [0, 255].
- image_format (str): The color format of the image, in ['RGB', 'BGR'].
- """
- assert image_format in [
- "RGB",
- "BGR",
- ], f"image_format must be in ['RGB', 'BGR'], is {image_format}."
- if image_format != self.model.image_format:
- image = image[..., ::-1]
-
- # Transform the image to the form expected by the model
- input_image = self.transform.apply_image(image)
- input_image_torch = torch.as_tensor(input_image, device=self.device)
- input_image_torch = input_image_torch.permute(2, 0, 1).contiguous()[None, :, :, :]
-
- self.set_torch_image(input_image_torch, image.shape[:2])
-
- @torch.no_grad()
- def set_torch_image(
- self,
- transformed_image: torch.Tensor,
- original_image_size: Tuple[int, ...],
- ) -> None:
- """
- Calculates the image embeddings for the provided image, allowing
- masks to be predicted with the 'predict' method. Expects the input
- image to be already transformed to the format expected by the model.
-
- Arguments:
- transformed_image (torch.Tensor): The input image, with shape
- 1x3xHxW, which has been transformed with ResizeLongestSide.
- original_image_size (tuple(int, int)): The size of the image
- before transformation, in (H, W) format.
- """
- assert (
- len(transformed_image.shape) == 4
- and transformed_image.shape[1] == 3
- and max(*transformed_image.shape[2:]) == self.model.image_encoder.img_size
- ), f"set_torch_image input must be BCHW with long side {self.model.image_encoder.img_size}."
- self.reset_image()
-
- self.original_size = original_image_size
- self.input_size = tuple(transformed_image.shape[-2:])
- input_image = self.model.preprocess(transformed_image)
- self.features = self.model.image_encoder(input_image)
- self.is_image_set = True
-
- def predict(
- self,
- point_coords: Optional[np.ndarray] = None,
- point_labels: Optional[np.ndarray] = None,
- box: Optional[np.ndarray] = None,
- mask_input: Optional[np.ndarray] = None,
- multimask_output: bool = True,
- return_logits: bool = False,
- ) -> Tuple[torch.Tensor, torch.Tensor, torch.Tensor]:
- """
- Predict masks for the given input prompts, using the currently set image.
-
- Arguments:
- point_coords (np.ndarray or None): A Nx2 array of point prompts to the
- model. Each point is in (X,Y) in pixels.
- point_labels (np.ndarray or None): A length N array of labels for the
- point prompts. 1 indicates a foreground point and 0 indicates a
- background point.
- box (np.ndarray or None): A length 4 array given a box prompt to the
- model, in XYXY format.
- mask_input (np.ndarray): A low resolution mask input to the model, typically
- coming from a previous prediction iteration. Has form 1xHxW, where
- for SAM, H=W=256.
- multimask_output (bool): If true, the model will return three masks.
- For ambiguous input prompts (such as a single click), this will often
- produce better masks than a single prediction. If only a single
- mask is needed, the model's predicted quality score can be used
- to select the best mask. For non-ambiguous prompts, such as multiple
- input prompts, multimask_output=False can give better results.
- return_logits (bool): If true, returns un-thresholded masks logits
- instead of a binary mask.
-
- Returns:
- (np.ndarray): The output masks in CxHxW format, where C is the
- number of masks, and (H, W) is the original image size.
- (np.ndarray): An array of length C containing the model's
- predictions for the quality of each mask.
- (np.ndarray): An array of shape CxHxW, where C is the number
- of masks and H=W=256. These low resolution logits can be passed to
- a subsequent iteration as mask input.
- """
- if not self.is_image_set:
- raise RuntimeError("An image must be set with .set_image(...) before mask prediction.")
-
- # Transform input prompts
- coords_torch, labels_torch, box_torch, mask_input_torch = None, None, None, None
- if point_coords is not None:
- assert (
- point_labels is not None
- ), "point_labels must be supplied if point_coords is supplied."
- point_coords = self.transform.apply_coords(point_coords, self.original_size)
- coords_torch = torch.as_tensor(point_coords, dtype=torch.float, device=self.device)
- labels_torch = torch.as_tensor(point_labels, dtype=torch.int, device=self.device)
- coords_torch, labels_torch = coords_torch[None, :, :], labels_torch[None, :]
- if box is not None:
- box = self.transform.apply_boxes(box, self.original_size)
- box_torch = torch.as_tensor(box, dtype=torch.float, device=self.device)
- box_torch = box_torch[None, :]
- if mask_input is not None:
- mask_input_torch = torch.as_tensor(mask_input, dtype=torch.float, device=self.device)
- mask_input_torch = mask_input_torch[None, :, :, :]
-
- masks, iou_predictions, low_res_masks = self.predict_torch(
- coords_torch,
- labels_torch,
- box_torch,
- mask_input_torch,
- multimask_output,
- return_logits=return_logits,
- )
-
- masks = masks[0].detach().cpu().numpy()
- iou_predictions = iou_predictions[0].detach().cpu().numpy()
- low_res_masks = low_res_masks[0].detach().cpu().numpy()
- return masks, iou_predictions, low_res_masks
-
- @torch.no_grad()
- def predict_torch(
- self,
- point_coords: Optional[torch.Tensor],
- point_labels: Optional[torch.Tensor],
- boxes: Optional[torch.Tensor] = None,
- mask_input: Optional[torch.Tensor] = None,
- multimask_output: bool = True,
- return_logits: bool = False,
- ) -> Tuple[torch.Tensor, torch.Tensor, torch.Tensor]:
- """
- Predict masks for the given input prompts, using the currently set image.
- Input prompts are batched torch tensors and are expected to already be
- transformed to the input frame using ResizeLongestSide.
-
- Arguments:
- point_coords (torch.Tensor or None): A BxNx2 array of point prompts to the
- model. Each point is in (X,Y) in pixels.
- point_labels (torch.Tensor or None): A BxN array of labels for the
- point prompts. 1 indicates a foreground point and 0 indicates a
- background point.
- box (np.ndarray or None): A Bx4 array given a box prompt to the
- model, in XYXY format.
- mask_input (np.ndarray): A low resolution mask input to the model, typically
- coming from a previous prediction iteration. Has form Bx1xHxW, where
- for SAM, H=W=256. Masks returned by a previous iteration of the
- predict method do not need further transformation.
- multimask_output (bool): If true, the model will return three masks.
- For ambiguous input prompts (such as a single click), this will often
- produce better masks than a single prediction. If only a single
- mask is needed, the model's predicted quality score can be used
- to select the best mask. For non-ambiguous prompts, such as multiple
- input prompts, multimask_output=False can give better results.
- return_logits (bool): If true, returns un-thresholded masks logits
- instead of a binary mask.
-
- Returns:
- (torch.Tensor): The output masks in BxCxHxW format, where C is the
- number of masks, and (H, W) is the original image size.
- (torch.Tensor): An array of shape BxC containing the model's
- predictions for the quality of each mask.
- (torch.Tensor): An array of shape BxCxHxW, where C is the number
- of masks and H=W=256. These low res logits can be passed to
- a subsequent iteration as mask input.
- """
- if not self.is_image_set:
- raise RuntimeError("An image must be set with .set_image(...) before mask prediction.")
-
- if point_coords is not None:
- points = (point_coords, point_labels)
- else:
- points = None
-
- # Embed prompts
- sparse_embeddings, dense_embeddings = self.model.prompt_encoder(
- points=points,
- boxes=boxes,
- masks=mask_input,
- )
-
- # Predict masks
- low_res_masks, iou_predictions = self.model.mask_decoder(
- image_embeddings=self.features,
- image_pe=self.model.prompt_encoder.get_dense_pe(),
- sparse_prompt_embeddings=sparse_embeddings,
- dense_prompt_embeddings=dense_embeddings,
- multimask_output=multimask_output,
- )
-
- # Upscale the masks to the original image resolution
- masks = self.model.postprocess_masks(low_res_masks, self.input_size, self.original_size)
-
- if not return_logits:
- masks = masks > self.model.mask_threshold
-
- return masks, iou_predictions, low_res_masks
-
- def get_image_embedding(self) -> torch.Tensor:
- """
- Returns the image embeddings for the currently set image, with
- shape 1xCxHxW, where C is the embedding dimension and (H,W) are
- the embedding spatial dimension of SAM (typically C=256, H=W=64).
- """
- if not self.is_image_set:
- raise RuntimeError(
- "An image must be set with .set_image(...) to generate an embedding."
- )
- assert self.features is not None, "Features must exist if an image has been set."
- return self.features
-
- @property
- def device(self) -> torch.device:
- return self.model.device
-
- def reset_image(self) -> None:
- """Resets the currently set image."""
- self.is_image_set = False
- self.features = None
- self.orig_h = None
- self.orig_w = None
- self.input_h = None
- self.input_w = None
diff --git a/spaces/Inia2567/anime-ai-detect/README.md b/spaces/Inia2567/anime-ai-detect/README.md
deleted file mode 100644
index 952c183fd69ccb1664b4236b6132fc6d0358c7de..0000000000000000000000000000000000000000
--- a/spaces/Inia2567/anime-ai-detect/README.md
+++ /dev/null
@@ -1,13 +0,0 @@
----
-title: Anime Ai Detect
-emoji: 🤖
-colorFrom: green
-colorTo: purple
-sdk: gradio
-sdk_version: 3.15.0
-app_file: app.py
-pinned: true
-duplicated_from: saltacc/anime-ai-detect
----
-
-Check out the configuration reference at https://huggingface.co/docs/hub/spaces-config-reference
diff --git a/spaces/IvaElen/nlp_proj/biLSTM1.py b/spaces/IvaElen/nlp_proj/biLSTM1.py
deleted file mode 100644
index 2beabc0292c1fff99e680b999cd0ee60c82af658..0000000000000000000000000000000000000000
--- a/spaces/IvaElen/nlp_proj/biLSTM1.py
+++ /dev/null
@@ -1,47 +0,0 @@
-import torch
-import torch.nn as nn
-
-class biLSTM(nn.Module):
- """
- The LSTM model that will be used to perform Sentiment analysis.
- """
-
- def __init__(self,
- # объем словаря, с которым мы работаем, размер входа для слоя Embedding
- vocab_size: int,
- # размер выходного эмбеддинга каждый элемент последовательности
- # будет описан вектором такой размерности
- embedding_dim: int,
- # размерность hidden state LSTM слоя
- hidden_dim: int,
- # число слоев в LSTM
- n_layers: int,
- drop_prob=0.5,
- seq_len = 128) -> None:
-
- super().__init__()
- self.hidden_dim = hidden_dim
- self.n_layers = n_layers
- self.seq_len = seq_len
- self.embedding = nn.Embedding(vocab_size, embedding_dim)
- self.lstm = nn.LSTM(embedding_dim,
- hidden_dim,
- n_layers,
- dropout=drop_prob,
- batch_first=True,
- bidirectional=True
- )
-
- self.do = nn.Dropout()
-
- self.fc1 = nn.Linear(2*hidden_dim * self.seq_len, 256)
- self.fc2 = nn.Linear(256, 1)
- self.sigmoid = nn.Sigmoid()
-
- def forward(self, x):
- embeds = self.embedding(x)
- lstm_out, _ = self.lstm(embeds)
- out = self.fc2(torch.tanh(self.do(self.fc1(lstm_out.flatten(1)))))
- sig_out = self.sigmoid(out)
-
- return sig_out
\ No newline at end of file
diff --git a/spaces/Izal887/rvc-ram12/config.py b/spaces/Izal887/rvc-ram12/config.py
deleted file mode 100644
index 040a64d2c5ce4d7802bdf7f69321483b81008f08..0000000000000000000000000000000000000000
--- a/spaces/Izal887/rvc-ram12/config.py
+++ /dev/null
@@ -1,106 +0,0 @@
-import argparse
-import torch
-from multiprocessing import cpu_count
-
-class Config:
- def __init__(self):
- self.device = "cuda:0"
- self.is_half = True
- self.n_cpu = 0
- self.gpu_name = None
- self.gpu_mem = None
- (
- self.python_cmd,
- self.listen_port,
- self.colab,
- self.noparallel,
- self.noautoopen,
- self.api
- ) = self.arg_parse()
- self.x_pad, self.x_query, self.x_center, self.x_max = self.device_config()
-
- @staticmethod
- def arg_parse() -> tuple:
- parser = argparse.ArgumentParser()
- parser.add_argument("--port", type=int, default=7865, help="Listen port")
- parser.add_argument(
- "--pycmd", type=str, default="python", help="Python command"
- )
- parser.add_argument("--colab", action="store_true", help="Launch in colab")
- parser.add_argument(
- "--noparallel", action="store_true", help="Disable parallel processing"
- )
- parser.add_argument(
- "--noautoopen",
- action="store_true",
- help="Do not open in browser automatically",
- )
- parser.add_argument("--api", action="store_true", help="Launch with api")
- cmd_opts = parser.parse_args()
-
- cmd_opts.port = cmd_opts.port if 0 <= cmd_opts.port <= 65535 else 7865
-
- return (
- cmd_opts.pycmd,
- cmd_opts.port,
- cmd_opts.colab,
- cmd_opts.noparallel,
- cmd_opts.noautoopen,
- cmd_opts.api
- )
-
- def device_config(self) -> tuple:
- if torch.cuda.is_available():
- i_device = int(self.device.split(":")[-1])
- self.gpu_name = torch.cuda.get_device_name(i_device)
- if (
- ("16" in self.gpu_name and "V100" not in self.gpu_name.upper())
- or "P40" in self.gpu_name.upper()
- or "1060" in self.gpu_name
- or "1070" in self.gpu_name
- or "1080" in self.gpu_name
- ):
- print("16系/10系显卡和P40强制单精度")
- self.is_half = False
-
- else:
- self.gpu_name = None
- self.gpu_mem = int(
- torch.cuda.get_device_properties(i_device).total_memory
- / 1024
- / 1024
- / 1024
- + 0.4
- )
- elif torch.backends.mps.is_available():
- print("没有发现支持的N卡, 使用MPS进行推理")
- self.device = "mps"
- self.is_half = False
- else:
- print("没有发现支持的N卡, 使用CPU进行推理")
- self.device = "cpu"
- self.is_half = False
-
- if self.n_cpu == 0:
- self.n_cpu = cpu_count()
-
- if self.is_half:
- # 6G显存配置
- x_pad = 3
- x_query = 10
- x_center = 60
- x_max = 65
- else:
- # 5G显存配置
- x_pad = 1
- x_query = 6
- x_center = 38
- x_max = 41
-
- if self.gpu_mem != None and self.gpu_mem <= 4:
- x_pad = 1
- x_query = 5
- x_center = 30
- x_max = 32
-
- return x_pad, x_query, x_center, x_max
diff --git a/spaces/JSP/test4k/main.py b/spaces/JSP/test4k/main.py
deleted file mode 100644
index 978fc6a7d35d4512c44d5f75531c09e832c35e1f..0000000000000000000000000000000000000000
--- a/spaces/JSP/test4k/main.py
+++ /dev/null
@@ -1,27 +0,0 @@
-from llama_cpp.server.app import create_app, Settings
-from fastapi.responses import HTMLResponse
-import os
-
-app = create_app(
- Settings(
- n_threads=2, # set to number of cpu cores
- model="model/gguf-model.bin",
- embedding=True
- )
-)
-
-# Read the content of index.html once and store it in memory
-with open("index.html", "r") as f:
- content = f.read()
-
-
-@app.get("/", response_class=HTMLResponse)
-async def read_items():
- return content
-
-if __name__ == "__main__":
- import uvicorn
- uvicorn.run(app,
- host=os.environ["HOST"],
- port=int(os.environ["PORT"])
- )
diff --git a/spaces/Jaehan/Text2Text-Sentiment-Analysis/README.md b/spaces/Jaehan/Text2Text-Sentiment-Analysis/README.md
deleted file mode 100644
index 132dcebc50d3a19a02161fca55d1d243ae6af398..0000000000000000000000000000000000000000
--- a/spaces/Jaehan/Text2Text-Sentiment-Analysis/README.md
+++ /dev/null
@@ -1,12 +0,0 @@
----
-title: Text2Text Sentiment Analysis
-emoji: 🏆
-colorFrom: blue
-colorTo: pink
-sdk: gradio
-sdk_version: 3.35.2
-app_file: app.py
-pinned: false
----
-
-Check out the configuration reference at https://huggingface.co/docs/hub/spaces-config-reference
diff --git a/spaces/KenjieDec/GPEN/face_model/op/fused_bias_act.cpp b/spaces/KenjieDec/GPEN/face_model/op/fused_bias_act.cpp
deleted file mode 100644
index 02be898f970bcc8ea297867fcaa4e71b24b3d949..0000000000000000000000000000000000000000
--- a/spaces/KenjieDec/GPEN/face_model/op/fused_bias_act.cpp
+++ /dev/null
@@ -1,21 +0,0 @@
-#include
-
-
-torch::Tensor fused_bias_act_op(const torch::Tensor& input, const torch::Tensor& bias, const torch::Tensor& refer,
- int act, int grad, float alpha, float scale);
-
-#define CHECK_CUDA(x) TORCH_CHECK(x.type().is_cuda(), #x " must be a CUDA tensor")
-#define CHECK_CONTIGUOUS(x) TORCH_CHECK(x.is_contiguous(), #x " must be contiguous")
-#define CHECK_INPUT(x) CHECK_CUDA(x); CHECK_CONTIGUOUS(x)
-
-torch::Tensor fused_bias_act(const torch::Tensor& input, const torch::Tensor& bias, const torch::Tensor& refer,
- int act, int grad, float alpha, float scale) {
- CHECK_CUDA(input);
- CHECK_CUDA(bias);
-
- return fused_bias_act_op(input, bias, refer, act, grad, alpha, scale);
-}
-
-PYBIND11_MODULE(TORCH_EXTENSION_NAME, m) {
- m.def("fused_bias_act", &fused_bias_act, "fused bias act (CUDA)");
-}
\ No newline at end of file
diff --git a/spaces/KenjieDec/GPEN/sr_model/rrdbnet_arch.py b/spaces/KenjieDec/GPEN/sr_model/rrdbnet_arch.py
deleted file mode 100644
index 5e1f04c5aee5bcdcd2ddae5471843ff057d863b4..0000000000000000000000000000000000000000
--- a/spaces/KenjieDec/GPEN/sr_model/rrdbnet_arch.py
+++ /dev/null
@@ -1,116 +0,0 @@
-import torch
-from torch import nn as nn
-from torch.nn import functional as F
-
-from arch_util import default_init_weights, make_layer, pixel_unshuffle
-
-
-class ResidualDenseBlock(nn.Module):
- """Residual Dense Block.
-
- Used in RRDB block in ESRGAN.
-
- Args:
- num_feat (int): Channel number of intermediate features.
- num_grow_ch (int): Channels for each growth.
- """
-
- def __init__(self, num_feat=64, num_grow_ch=32):
- super(ResidualDenseBlock, self).__init__()
- self.conv1 = nn.Conv2d(num_feat, num_grow_ch, 3, 1, 1)
- self.conv2 = nn.Conv2d(num_feat + num_grow_ch, num_grow_ch, 3, 1, 1)
- self.conv3 = nn.Conv2d(num_feat + 2 * num_grow_ch, num_grow_ch, 3, 1, 1)
- self.conv4 = nn.Conv2d(num_feat + 3 * num_grow_ch, num_grow_ch, 3, 1, 1)
- self.conv5 = nn.Conv2d(num_feat + 4 * num_grow_ch, num_feat, 3, 1, 1)
-
- self.lrelu = nn.LeakyReLU(negative_slope=0.2, inplace=True)
-
- # initialization
- default_init_weights([self.conv1, self.conv2, self.conv3, self.conv4, self.conv5], 0.1)
-
- def forward(self, x):
- x1 = self.lrelu(self.conv1(x))
- x2 = self.lrelu(self.conv2(torch.cat((x, x1), 1)))
- x3 = self.lrelu(self.conv3(torch.cat((x, x1, x2), 1)))
- x4 = self.lrelu(self.conv4(torch.cat((x, x1, x2, x3), 1)))
- x5 = self.conv5(torch.cat((x, x1, x2, x3, x4), 1))
- # Emperically, we use 0.2 to scale the residual for better performance
- return x5 * 0.2 + x
-
-
-class RRDB(nn.Module):
- """Residual in Residual Dense Block.
-
- Used in RRDB-Net in ESRGAN.
-
- Args:
- num_feat (int): Channel number of intermediate features.
- num_grow_ch (int): Channels for each growth.
- """
-
- def __init__(self, num_feat, num_grow_ch=32):
- super(RRDB, self).__init__()
- self.rdb1 = ResidualDenseBlock(num_feat, num_grow_ch)
- self.rdb2 = ResidualDenseBlock(num_feat, num_grow_ch)
- self.rdb3 = ResidualDenseBlock(num_feat, num_grow_ch)
-
- def forward(self, x):
- out = self.rdb1(x)
- out = self.rdb2(out)
- out = self.rdb3(out)
- # Emperically, we use 0.2 to scale the residual for better performance
- return out * 0.2 + x
-
-class RRDBNet(nn.Module):
- """Networks consisting of Residual in Residual Dense Block, which is used
- in ESRGAN.
-
- ESRGAN: Enhanced Super-Resolution Generative Adversarial Networks.
-
- We extend ESRGAN for scale x2 and scale x1.
- Note: This is one option for scale 1, scale 2 in RRDBNet.
- We first employ the pixel-unshuffle (an inverse operation of pixelshuffle to reduce the spatial size
- and enlarge the channel size before feeding inputs into the main ESRGAN architecture.
-
- Args:
- num_in_ch (int): Channel number of inputs.
- num_out_ch (int): Channel number of outputs.
- num_feat (int): Channel number of intermediate features.
- Default: 64
- num_block (int): Block number in the trunk network. Defaults: 23
- num_grow_ch (int): Channels for each growth. Default: 32.
- """
-
- def __init__(self, num_in_ch, num_out_ch, scale=4, num_feat=64, num_block=23, num_grow_ch=32):
- super(RRDBNet, self).__init__()
- self.scale = scale
- if scale == 2:
- num_in_ch = num_in_ch * 4
- elif scale == 1:
- num_in_ch = num_in_ch * 16
- self.conv_first = nn.Conv2d(num_in_ch, num_feat, 3, 1, 1)
- self.body = make_layer(RRDB, num_block, num_feat=num_feat, num_grow_ch=num_grow_ch)
- self.conv_body = nn.Conv2d(num_feat, num_feat, 3, 1, 1)
- # upsample
- self.conv_up1 = nn.Conv2d(num_feat, num_feat, 3, 1, 1)
- self.conv_up2 = nn.Conv2d(num_feat, num_feat, 3, 1, 1)
- self.conv_hr = nn.Conv2d(num_feat, num_feat, 3, 1, 1)
- self.conv_last = nn.Conv2d(num_feat, num_out_ch, 3, 1, 1)
-
- self.lrelu = nn.LeakyReLU(negative_slope=0.2, inplace=True)
-
- def forward(self, x):
- if self.scale == 2:
- feat = pixel_unshuffle(x, scale=2)
- elif self.scale == 1:
- feat = pixel_unshuffle(x, scale=4)
- else:
- feat = x
- feat = self.conv_first(feat)
- body_feat = self.conv_body(self.body(feat))
- feat = feat + body_feat
- # upsample
- feat = self.lrelu(self.conv_up1(F.interpolate(feat, scale_factor=2, mode='nearest')))
- feat = self.lrelu(self.conv_up2(F.interpolate(feat, scale_factor=2, mode='nearest')))
- out = self.conv_last(self.lrelu(self.conv_hr(feat)))
- return out
diff --git a/spaces/Kevin676/AutoGPT/tests/test_token_counter.py b/spaces/Kevin676/AutoGPT/tests/test_token_counter.py
deleted file mode 100644
index 6d7ae016b2f823123b0b69b2eeb3eab50d94f00f..0000000000000000000000000000000000000000
--- a/spaces/Kevin676/AutoGPT/tests/test_token_counter.py
+++ /dev/null
@@ -1,63 +0,0 @@
-import unittest
-
-import tests.context
-from autogpt.token_counter import count_message_tokens, count_string_tokens
-
-
-class TestTokenCounter(unittest.TestCase):
- def test_count_message_tokens(self):
- messages = [
- {"role": "user", "content": "Hello"},
- {"role": "assistant", "content": "Hi there!"},
- ]
- self.assertEqual(count_message_tokens(messages), 17)
-
- def test_count_message_tokens_with_name(self):
- messages = [
- {"role": "user", "content": "Hello", "name": "John"},
- {"role": "assistant", "content": "Hi there!"},
- ]
- self.assertEqual(count_message_tokens(messages), 17)
-
- def test_count_message_tokens_empty_input(self):
- self.assertEqual(count_message_tokens([]), 3)
-
- def test_count_message_tokens_invalid_model(self):
- messages = [
- {"role": "user", "content": "Hello"},
- {"role": "assistant", "content": "Hi there!"},
- ]
- with self.assertRaises(KeyError):
- count_message_tokens(messages, model="invalid_model")
-
- def test_count_message_tokens_gpt_4(self):
- messages = [
- {"role": "user", "content": "Hello"},
- {"role": "assistant", "content": "Hi there!"},
- ]
- self.assertEqual(count_message_tokens(messages, model="gpt-4-0314"), 15)
-
- def test_count_string_tokens(self):
- string = "Hello, world!"
- self.assertEqual(
- count_string_tokens(string, model_name="gpt-3.5-turbo-0301"), 4
- )
-
- def test_count_string_tokens_empty_input(self):
- self.assertEqual(count_string_tokens("", model_name="gpt-3.5-turbo-0301"), 0)
-
- def test_count_message_tokens_invalid_model(self):
- messages = [
- {"role": "user", "content": "Hello"},
- {"role": "assistant", "content": "Hi there!"},
- ]
- with self.assertRaises(NotImplementedError):
- count_message_tokens(messages, model="invalid_model")
-
- def test_count_string_tokens_gpt_4(self):
- string = "Hello, world!"
- self.assertEqual(count_string_tokens(string, model_name="gpt-4-0314"), 4)
-
-
-if __name__ == "__main__":
- unittest.main()
diff --git a/spaces/Keyradesu/Oka/README.md b/spaces/Keyradesu/Oka/README.md
deleted file mode 100644
index 3fb44b8a0a1887d89b42c22c10b198125c1aede5..0000000000000000000000000000000000000000
--- a/spaces/Keyradesu/Oka/README.md
+++ /dev/null
@@ -1,10 +0,0 @@
----
-title: Oka
-emoji: 🐢
-colorFrom: indigo
-colorTo: red
-sdk: static
-pinned: false
----
-
-Check out the configuration reference at https://huggingface.co/docs/hub/spaces-config-reference
diff --git a/spaces/Kirihasan/rvc-holo/infer_pack/models_onnx.py b/spaces/Kirihasan/rvc-holo/infer_pack/models_onnx.py
deleted file mode 100644
index 3cdae2f7f8591a1e43b1d8520baa37b7e9744d72..0000000000000000000000000000000000000000
--- a/spaces/Kirihasan/rvc-holo/infer_pack/models_onnx.py
+++ /dev/null
@@ -1,849 +0,0 @@
-import math, pdb, os
-from time import time as ttime
-import torch
-from torch import nn
-from torch.nn import functional as F
-from infer_pack import modules
-from infer_pack import attentions
-from infer_pack import commons
-from infer_pack.commons import init_weights, get_padding
-from torch.nn import Conv1d, ConvTranspose1d, AvgPool1d, Conv2d
-from torch.nn.utils import weight_norm, remove_weight_norm, spectral_norm
-from infer_pack.commons import init_weights
-import numpy as np
-from infer_pack import commons
-
-
-class TextEncoder256(nn.Module):
- def __init__(
- self,
- out_channels,
- hidden_channels,
- filter_channels,
- n_heads,
- n_layers,
- kernel_size,
- p_dropout,
- f0=True,
- ):
- super().__init__()
- self.out_channels = out_channels
- self.hidden_channels = hidden_channels
- self.filter_channels = filter_channels
- self.n_heads = n_heads
- self.n_layers = n_layers
- self.kernel_size = kernel_size
- self.p_dropout = p_dropout
- self.emb_phone = nn.Linear(256, hidden_channels)
- self.lrelu = nn.LeakyReLU(0.1, inplace=True)
- if f0 == True:
- self.emb_pitch = nn.Embedding(256, hidden_channels) # pitch 256
- self.encoder = attentions.Encoder(
- hidden_channels, filter_channels, n_heads, n_layers, kernel_size, p_dropout
- )
- self.proj = nn.Conv1d(hidden_channels, out_channels * 2, 1)
-
- def forward(self, phone, pitch, lengths):
- if pitch == None:
- x = self.emb_phone(phone)
- else:
- x = self.emb_phone(phone) + self.emb_pitch(pitch)
- x = x * math.sqrt(self.hidden_channels) # [b, t, h]
- x = self.lrelu(x)
- x = torch.transpose(x, 1, -1) # [b, h, t]
- x_mask = torch.unsqueeze(commons.sequence_mask(lengths, x.size(2)), 1).to(
- x.dtype
- )
- x = self.encoder(x * x_mask, x_mask)
- stats = self.proj(x) * x_mask
-
- m, logs = torch.split(stats, self.out_channels, dim=1)
- return m, logs, x_mask
-
-
-class TextEncoder256Sim(nn.Module):
- def __init__(
- self,
- out_channels,
- hidden_channels,
- filter_channels,
- n_heads,
- n_layers,
- kernel_size,
- p_dropout,
- f0=True,
- ):
- super().__init__()
- self.out_channels = out_channels
- self.hidden_channels = hidden_channels
- self.filter_channels = filter_channels
- self.n_heads = n_heads
- self.n_layers = n_layers
- self.kernel_size = kernel_size
- self.p_dropout = p_dropout
- self.emb_phone = nn.Linear(256, hidden_channels)
- self.lrelu = nn.LeakyReLU(0.1, inplace=True)
- if f0 == True:
- self.emb_pitch = nn.Embedding(256, hidden_channels) # pitch 256
- self.encoder = attentions.Encoder(
- hidden_channels, filter_channels, n_heads, n_layers, kernel_size, p_dropout
- )
- self.proj = nn.Conv1d(hidden_channels, out_channels, 1)
-
- def forward(self, phone, pitch, lengths):
- if pitch == None:
- x = self.emb_phone(phone)
- else:
- x = self.emb_phone(phone) + self.emb_pitch(pitch)
- x = x * math.sqrt(self.hidden_channels) # [b, t, h]
- x = self.lrelu(x)
- x = torch.transpose(x, 1, -1) # [b, h, t]
- x_mask = torch.unsqueeze(commons.sequence_mask(lengths, x.size(2)), 1).to(
- x.dtype
- )
- x = self.encoder(x * x_mask, x_mask)
- x = self.proj(x) * x_mask
- return x, x_mask
-
-
-class ResidualCouplingBlock(nn.Module):
- def __init__(
- self,
- channels,
- hidden_channels,
- kernel_size,
- dilation_rate,
- n_layers,
- n_flows=4,
- gin_channels=0,
- ):
- super().__init__()
- self.channels = channels
- self.hidden_channels = hidden_channels
- self.kernel_size = kernel_size
- self.dilation_rate = dilation_rate
- self.n_layers = n_layers
- self.n_flows = n_flows
- self.gin_channels = gin_channels
-
- self.flows = nn.ModuleList()
- for i in range(n_flows):
- self.flows.append(
- modules.ResidualCouplingLayer(
- channels,
- hidden_channels,
- kernel_size,
- dilation_rate,
- n_layers,
- gin_channels=gin_channels,
- mean_only=True,
- )
- )
- self.flows.append(modules.Flip())
-
- def forward(self, x, x_mask, g=None, reverse=False):
- if not reverse:
- for flow in self.flows:
- x, _ = flow(x, x_mask, g=g, reverse=reverse)
- else:
- for flow in reversed(self.flows):
- x = flow(x, x_mask, g=g, reverse=reverse)
- return x
-
- def remove_weight_norm(self):
- for i in range(self.n_flows):
- self.flows[i * 2].remove_weight_norm()
-
-
-class PosteriorEncoder(nn.Module):
- def __init__(
- self,
- in_channels,
- out_channels,
- hidden_channels,
- kernel_size,
- dilation_rate,
- n_layers,
- gin_channels=0,
- ):
- super().__init__()
- self.in_channels = in_channels
- self.out_channels = out_channels
- self.hidden_channels = hidden_channels
- self.kernel_size = kernel_size
- self.dilation_rate = dilation_rate
- self.n_layers = n_layers
- self.gin_channels = gin_channels
-
- self.pre = nn.Conv1d(in_channels, hidden_channels, 1)
- self.enc = modules.WN(
- hidden_channels,
- kernel_size,
- dilation_rate,
- n_layers,
- gin_channels=gin_channels,
- )
- self.proj = nn.Conv1d(hidden_channels, out_channels * 2, 1)
-
- def forward(self, x, x_lengths, g=None):
- x_mask = torch.unsqueeze(commons.sequence_mask(x_lengths, x.size(2)), 1).to(
- x.dtype
- )
- x = self.pre(x) * x_mask
- x = self.enc(x, x_mask, g=g)
- stats = self.proj(x) * x_mask
- m, logs = torch.split(stats, self.out_channels, dim=1)
- z = (m + torch.randn_like(m) * torch.exp(logs)) * x_mask
- return z, m, logs, x_mask
-
- def remove_weight_norm(self):
- self.enc.remove_weight_norm()
-
-
-class Generator(torch.nn.Module):
- def __init__(
- self,
- initial_channel,
- resblock,
- resblock_kernel_sizes,
- resblock_dilation_sizes,
- upsample_rates,
- upsample_initial_channel,
- upsample_kernel_sizes,
- gin_channels=0,
- ):
- super(Generator, self).__init__()
- self.num_kernels = len(resblock_kernel_sizes)
- self.num_upsamples = len(upsample_rates)
- self.conv_pre = Conv1d(
- initial_channel, upsample_initial_channel, 7, 1, padding=3
- )
- resblock = modules.ResBlock1 if resblock == "1" else modules.ResBlock2
-
- self.ups = nn.ModuleList()
- for i, (u, k) in enumerate(zip(upsample_rates, upsample_kernel_sizes)):
- self.ups.append(
- weight_norm(
- ConvTranspose1d(
- upsample_initial_channel // (2**i),
- upsample_initial_channel // (2 ** (i + 1)),
- k,
- u,
- padding=(k - u) // 2,
- )
- )
- )
-
- self.resblocks = nn.ModuleList()
- for i in range(len(self.ups)):
- ch = upsample_initial_channel // (2 ** (i + 1))
- for j, (k, d) in enumerate(
- zip(resblock_kernel_sizes, resblock_dilation_sizes)
- ):
- self.resblocks.append(resblock(ch, k, d))
-
- self.conv_post = Conv1d(ch, 1, 7, 1, padding=3, bias=False)
- self.ups.apply(init_weights)
-
- if gin_channels != 0:
- self.cond = nn.Conv1d(gin_channels, upsample_initial_channel, 1)
-
- def forward(self, x, g=None):
- x = self.conv_pre(x)
- if g is not None:
- x = x + self.cond(g)
-
- for i in range(self.num_upsamples):
- x = F.leaky_relu(x, modules.LRELU_SLOPE)
- x = self.ups[i](x)
- xs = None
- for j in range(self.num_kernels):
- if xs is None:
- xs = self.resblocks[i * self.num_kernels + j](x)
- else:
- xs += self.resblocks[i * self.num_kernels + j](x)
- x = xs / self.num_kernels
- x = F.leaky_relu(x)
- x = self.conv_post(x)
- x = torch.tanh(x)
-
- return x
-
- def remove_weight_norm(self):
- for l in self.ups:
- remove_weight_norm(l)
- for l in self.resblocks:
- l.remove_weight_norm()
-
-
-class SineGen(torch.nn.Module):
- """Definition of sine generator
- SineGen(samp_rate, harmonic_num = 0,
- sine_amp = 0.1, noise_std = 0.003,
- voiced_threshold = 0,
- flag_for_pulse=False)
- samp_rate: sampling rate in Hz
- harmonic_num: number of harmonic overtones (default 0)
- sine_amp: amplitude of sine-wavefrom (default 0.1)
- noise_std: std of Gaussian noise (default 0.003)
- voiced_thoreshold: F0 threshold for U/V classification (default 0)
- flag_for_pulse: this SinGen is used inside PulseGen (default False)
- Note: when flag_for_pulse is True, the first time step of a voiced
- segment is always sin(np.pi) or cos(0)
- """
-
- def __init__(
- self,
- samp_rate,
- harmonic_num=0,
- sine_amp=0.1,
- noise_std=0.003,
- voiced_threshold=0,
- flag_for_pulse=False,
- ):
- super(SineGen, self).__init__()
- self.sine_amp = sine_amp
- self.noise_std = noise_std
- self.harmonic_num = harmonic_num
- self.dim = self.harmonic_num + 1
- self.sampling_rate = samp_rate
- self.voiced_threshold = voiced_threshold
-
- def _f02uv(self, f0):
- # generate uv signal
- uv = torch.ones_like(f0)
- uv = uv * (f0 > self.voiced_threshold)
- return uv
-
- def forward(self, f0, upp):
- """sine_tensor, uv = forward(f0)
- input F0: tensor(batchsize=1, length, dim=1)
- f0 for unvoiced steps should be 0
- output sine_tensor: tensor(batchsize=1, length, dim)
- output uv: tensor(batchsize=1, length, 1)
- """
- with torch.no_grad():
- f0 = f0[:, None].transpose(1, 2)
- f0_buf = torch.zeros(f0.shape[0], f0.shape[1], self.dim, device=f0.device)
- # fundamental component
- f0_buf[:, :, 0] = f0[:, :, 0]
- for idx in np.arange(self.harmonic_num):
- f0_buf[:, :, idx + 1] = f0_buf[:, :, 0] * (
- idx + 2
- ) # idx + 2: the (idx+1)-th overtone, (idx+2)-th harmonic
- rad_values = (f0_buf / self.sampling_rate) % 1 ###%1意味着n_har的乘积无法后处理优化
- rand_ini = torch.rand(
- f0_buf.shape[0], f0_buf.shape[2], device=f0_buf.device
- )
- rand_ini[:, 0] = 0
- rad_values[:, 0, :] = rad_values[:, 0, :] + rand_ini
- tmp_over_one = torch.cumsum(rad_values, 1) # % 1 #####%1意味着后面的cumsum无法再优化
- tmp_over_one *= upp
- tmp_over_one = F.interpolate(
- tmp_over_one.transpose(2, 1),
- scale_factor=upp,
- mode="linear",
- align_corners=True,
- ).transpose(2, 1)
- rad_values = F.interpolate(
- rad_values.transpose(2, 1), scale_factor=upp, mode="nearest"
- ).transpose(
- 2, 1
- ) #######
- tmp_over_one %= 1
- tmp_over_one_idx = (tmp_over_one[:, 1:, :] - tmp_over_one[:, :-1, :]) < 0
- cumsum_shift = torch.zeros_like(rad_values)
- cumsum_shift[:, 1:, :] = tmp_over_one_idx * -1.0
- sine_waves = torch.sin(
- torch.cumsum(rad_values + cumsum_shift, dim=1) * 2 * np.pi
- )
- sine_waves = sine_waves * self.sine_amp
- uv = self._f02uv(f0)
- uv = F.interpolate(
- uv.transpose(2, 1), scale_factor=upp, mode="nearest"
- ).transpose(2, 1)
- noise_amp = uv * self.noise_std + (1 - uv) * self.sine_amp / 3
- noise = noise_amp * torch.randn_like(sine_waves)
- sine_waves = sine_waves * uv + noise
- return sine_waves, uv, noise
-
-
-class SourceModuleHnNSF(torch.nn.Module):
- """SourceModule for hn-nsf
- SourceModule(sampling_rate, harmonic_num=0, sine_amp=0.1,
- add_noise_std=0.003, voiced_threshod=0)
- sampling_rate: sampling_rate in Hz
- harmonic_num: number of harmonic above F0 (default: 0)
- sine_amp: amplitude of sine source signal (default: 0.1)
- add_noise_std: std of additive Gaussian noise (default: 0.003)
- note that amplitude of noise in unvoiced is decided
- by sine_amp
- voiced_threshold: threhold to set U/V given F0 (default: 0)
- Sine_source, noise_source = SourceModuleHnNSF(F0_sampled)
- F0_sampled (batchsize, length, 1)
- Sine_source (batchsize, length, 1)
- noise_source (batchsize, length 1)
- uv (batchsize, length, 1)
- """
-
- def __init__(
- self,
- sampling_rate,
- harmonic_num=0,
- sine_amp=0.1,
- add_noise_std=0.003,
- voiced_threshod=0,
- is_half=True,
- ):
- super(SourceModuleHnNSF, self).__init__()
-
- self.sine_amp = sine_amp
- self.noise_std = add_noise_std
- self.is_half = is_half
- # to produce sine waveforms
- self.l_sin_gen = SineGen(
- sampling_rate, harmonic_num, sine_amp, add_noise_std, voiced_threshod
- )
-
- # to merge source harmonics into a single excitation
- self.l_linear = torch.nn.Linear(harmonic_num + 1, 1)
- self.l_tanh = torch.nn.Tanh()
-
- def forward(self, x, upp=None):
- sine_wavs, uv, _ = self.l_sin_gen(x, upp)
- if self.is_half:
- sine_wavs = sine_wavs.half()
- sine_merge = self.l_tanh(self.l_linear(sine_wavs))
- return sine_merge, None, None # noise, uv
-
-
-class GeneratorNSF(torch.nn.Module):
- def __init__(
- self,
- initial_channel,
- resblock,
- resblock_kernel_sizes,
- resblock_dilation_sizes,
- upsample_rates,
- upsample_initial_channel,
- upsample_kernel_sizes,
- gin_channels,
- sr,
- is_half=False,
- ):
- super(GeneratorNSF, self).__init__()
- self.num_kernels = len(resblock_kernel_sizes)
- self.num_upsamples = len(upsample_rates)
-
- self.f0_upsamp = torch.nn.Upsample(scale_factor=np.prod(upsample_rates))
- self.m_source = SourceModuleHnNSF(
- sampling_rate=sr, harmonic_num=0, is_half=is_half
- )
- self.noise_convs = nn.ModuleList()
- self.conv_pre = Conv1d(
- initial_channel, upsample_initial_channel, 7, 1, padding=3
- )
- resblock = modules.ResBlock1 if resblock == "1" else modules.ResBlock2
-
- self.ups = nn.ModuleList()
- for i, (u, k) in enumerate(zip(upsample_rates, upsample_kernel_sizes)):
- c_cur = upsample_initial_channel // (2 ** (i + 1))
- self.ups.append(
- weight_norm(
- ConvTranspose1d(
- upsample_initial_channel // (2**i),
- upsample_initial_channel // (2 ** (i + 1)),
- k,
- u,
- padding=(k - u) // 2,
- )
- )
- )
- if i + 1 < len(upsample_rates):
- stride_f0 = np.prod(upsample_rates[i + 1 :])
- self.noise_convs.append(
- Conv1d(
- 1,
- c_cur,
- kernel_size=stride_f0 * 2,
- stride=stride_f0,
- padding=stride_f0 // 2,
- )
- )
- else:
- self.noise_convs.append(Conv1d(1, c_cur, kernel_size=1))
-
- self.resblocks = nn.ModuleList()
- for i in range(len(self.ups)):
- ch = upsample_initial_channel // (2 ** (i + 1))
- for j, (k, d) in enumerate(
- zip(resblock_kernel_sizes, resblock_dilation_sizes)
- ):
- self.resblocks.append(resblock(ch, k, d))
-
- self.conv_post = Conv1d(ch, 1, 7, 1, padding=3, bias=False)
- self.ups.apply(init_weights)
-
- if gin_channels != 0:
- self.cond = nn.Conv1d(gin_channels, upsample_initial_channel, 1)
-
- self.upp = np.prod(upsample_rates)
-
- def forward(self, x, f0, g=None):
- har_source, noi_source, uv = self.m_source(f0, self.upp)
- har_source = har_source.transpose(1, 2)
- x = self.conv_pre(x)
- if g is not None:
- x = x + self.cond(g)
-
- for i in range(self.num_upsamples):
- x = F.leaky_relu(x, modules.LRELU_SLOPE)
- x = self.ups[i](x)
- x_source = self.noise_convs[i](har_source)
- x = x + x_source
- xs = None
- for j in range(self.num_kernels):
- if xs is None:
- xs = self.resblocks[i * self.num_kernels + j](x)
- else:
- xs += self.resblocks[i * self.num_kernels + j](x)
- x = xs / self.num_kernels
- x = F.leaky_relu(x)
- x = self.conv_post(x)
- x = torch.tanh(x)
- return x
-
- def remove_weight_norm(self):
- for l in self.ups:
- remove_weight_norm(l)
- for l in self.resblocks:
- l.remove_weight_norm()
-
-
-sr2sr = {
- "32k": 32000,
- "40k": 40000,
- "48k": 48000,
-}
-
-
-class SynthesizerTrnMs256NSFsid(nn.Module):
- def __init__(
- self,
- spec_channels,
- segment_size,
- inter_channels,
- hidden_channels,
- filter_channels,
- n_heads,
- n_layers,
- kernel_size,
- p_dropout,
- resblock,
- resblock_kernel_sizes,
- resblock_dilation_sizes,
- upsample_rates,
- upsample_initial_channel,
- upsample_kernel_sizes,
- spk_embed_dim,
- gin_channels,
- sr,
- **kwargs
- ):
- super().__init__()
- if type(sr) == type("strr"):
- sr = sr2sr[sr]
- self.spec_channels = spec_channels
- self.inter_channels = inter_channels
- self.hidden_channels = hidden_channels
- self.filter_channels = filter_channels
- self.n_heads = n_heads
- self.n_layers = n_layers
- self.kernel_size = kernel_size
- self.p_dropout = p_dropout
- self.resblock = resblock
- self.resblock_kernel_sizes = resblock_kernel_sizes
- self.resblock_dilation_sizes = resblock_dilation_sizes
- self.upsample_rates = upsample_rates
- self.upsample_initial_channel = upsample_initial_channel
- self.upsample_kernel_sizes = upsample_kernel_sizes
- self.segment_size = segment_size
- self.gin_channels = gin_channels
- # self.hop_length = hop_length#
- self.spk_embed_dim = spk_embed_dim
- self.enc_p = TextEncoder256(
- inter_channels,
- hidden_channels,
- filter_channels,
- n_heads,
- n_layers,
- kernel_size,
- p_dropout,
- )
- self.dec = GeneratorNSF(
- inter_channels,
- resblock,
- resblock_kernel_sizes,
- resblock_dilation_sizes,
- upsample_rates,
- upsample_initial_channel,
- upsample_kernel_sizes,
- gin_channels=gin_channels,
- sr=sr,
- is_half=kwargs["is_half"],
- )
- self.enc_q = PosteriorEncoder(
- spec_channels,
- inter_channels,
- hidden_channels,
- 5,
- 1,
- 16,
- gin_channels=gin_channels,
- )
- self.flow = ResidualCouplingBlock(
- inter_channels, hidden_channels, 5, 1, 3, gin_channels=gin_channels
- )
- self.emb_g = nn.Embedding(self.spk_embed_dim, gin_channels)
- print("gin_channels:", gin_channels, "self.spk_embed_dim:", self.spk_embed_dim)
-
- def remove_weight_norm(self):
- self.dec.remove_weight_norm()
- self.flow.remove_weight_norm()
- self.enc_q.remove_weight_norm()
-
- def forward(self, phone, phone_lengths, pitch, nsff0, sid, rnd, max_len=None):
- g = self.emb_g(sid).unsqueeze(-1)
- m_p, logs_p, x_mask = self.enc_p(phone, pitch, phone_lengths)
- z_p = (m_p + torch.exp(logs_p) * rnd) * x_mask
- z = self.flow(z_p, x_mask, g=g, reverse=True)
- o = self.dec((z * x_mask)[:, :, :max_len], nsff0, g=g)
- return o
-
-
-class SynthesizerTrnMs256NSFsid_sim(nn.Module):
- """
- Synthesizer for Training
- """
-
- def __init__(
- self,
- spec_channels,
- segment_size,
- inter_channels,
- hidden_channels,
- filter_channels,
- n_heads,
- n_layers,
- kernel_size,
- p_dropout,
- resblock,
- resblock_kernel_sizes,
- resblock_dilation_sizes,
- upsample_rates,
- upsample_initial_channel,
- upsample_kernel_sizes,
- spk_embed_dim,
- # hop_length,
- gin_channels=0,
- use_sdp=True,
- **kwargs
- ):
- super().__init__()
- self.spec_channels = spec_channels
- self.inter_channels = inter_channels
- self.hidden_channels = hidden_channels
- self.filter_channels = filter_channels
- self.n_heads = n_heads
- self.n_layers = n_layers
- self.kernel_size = kernel_size
- self.p_dropout = p_dropout
- self.resblock = resblock
- self.resblock_kernel_sizes = resblock_kernel_sizes
- self.resblock_dilation_sizes = resblock_dilation_sizes
- self.upsample_rates = upsample_rates
- self.upsample_initial_channel = upsample_initial_channel
- self.upsample_kernel_sizes = upsample_kernel_sizes
- self.segment_size = segment_size
- self.gin_channels = gin_channels
- # self.hop_length = hop_length#
- self.spk_embed_dim = spk_embed_dim
- self.enc_p = TextEncoder256Sim(
- inter_channels,
- hidden_channels,
- filter_channels,
- n_heads,
- n_layers,
- kernel_size,
- p_dropout,
- )
- self.dec = GeneratorNSF(
- inter_channels,
- resblock,
- resblock_kernel_sizes,
- resblock_dilation_sizes,
- upsample_rates,
- upsample_initial_channel,
- upsample_kernel_sizes,
- gin_channels=gin_channels,
- is_half=kwargs["is_half"],
- )
-
- self.flow = ResidualCouplingBlock(
- inter_channels, hidden_channels, 5, 1, 3, gin_channels=gin_channels
- )
- self.emb_g = nn.Embedding(self.spk_embed_dim, gin_channels)
- print("gin_channels:", gin_channels, "self.spk_embed_dim:", self.spk_embed_dim)
-
- def remove_weight_norm(self):
- self.dec.remove_weight_norm()
- self.flow.remove_weight_norm()
- self.enc_q.remove_weight_norm()
-
- def forward(
- self, phone, phone_lengths, pitch, pitchf, ds, max_len=None
- ): # y是spec不需要了现在
- g = self.emb_g(ds.unsqueeze(0)).unsqueeze(-1) # [b, 256, 1]##1是t,广播的
- x, x_mask = self.enc_p(phone, pitch, phone_lengths)
- x = self.flow(x, x_mask, g=g, reverse=True)
- o = self.dec((x * x_mask)[:, :, :max_len], pitchf, g=g)
- return o
-
-
-class MultiPeriodDiscriminator(torch.nn.Module):
- def __init__(self, use_spectral_norm=False):
- super(MultiPeriodDiscriminator, self).__init__()
- periods = [2, 3, 5, 7, 11, 17]
- # periods = [3, 5, 7, 11, 17, 23, 37]
-
- discs = [DiscriminatorS(use_spectral_norm=use_spectral_norm)]
- discs = discs + [
- DiscriminatorP(i, use_spectral_norm=use_spectral_norm) for i in periods
- ]
- self.discriminators = nn.ModuleList(discs)
-
- def forward(self, y, y_hat):
- y_d_rs = [] #
- y_d_gs = []
- fmap_rs = []
- fmap_gs = []
- for i, d in enumerate(self.discriminators):
- y_d_r, fmap_r = d(y)
- y_d_g, fmap_g = d(y_hat)
- # for j in range(len(fmap_r)):
- # print(i,j,y.shape,y_hat.shape,fmap_r[j].shape,fmap_g[j].shape)
- y_d_rs.append(y_d_r)
- y_d_gs.append(y_d_g)
- fmap_rs.append(fmap_r)
- fmap_gs.append(fmap_g)
-
- return y_d_rs, y_d_gs, fmap_rs, fmap_gs
-
-
-class DiscriminatorS(torch.nn.Module):
- def __init__(self, use_spectral_norm=False):
- super(DiscriminatorS, self).__init__()
- norm_f = weight_norm if use_spectral_norm == False else spectral_norm
- self.convs = nn.ModuleList(
- [
- norm_f(Conv1d(1, 16, 15, 1, padding=7)),
- norm_f(Conv1d(16, 64, 41, 4, groups=4, padding=20)),
- norm_f(Conv1d(64, 256, 41, 4, groups=16, padding=20)),
- norm_f(Conv1d(256, 1024, 41, 4, groups=64, padding=20)),
- norm_f(Conv1d(1024, 1024, 41, 4, groups=256, padding=20)),
- norm_f(Conv1d(1024, 1024, 5, 1, padding=2)),
- ]
- )
- self.conv_post = norm_f(Conv1d(1024, 1, 3, 1, padding=1))
-
- def forward(self, x):
- fmap = []
-
- for l in self.convs:
- x = l(x)
- x = F.leaky_relu(x, modules.LRELU_SLOPE)
- fmap.append(x)
- x = self.conv_post(x)
- fmap.append(x)
- x = torch.flatten(x, 1, -1)
-
- return x, fmap
-
-
-class DiscriminatorP(torch.nn.Module):
- def __init__(self, period, kernel_size=5, stride=3, use_spectral_norm=False):
- super(DiscriminatorP, self).__init__()
- self.period = period
- self.use_spectral_norm = use_spectral_norm
- norm_f = weight_norm if use_spectral_norm == False else spectral_norm
- self.convs = nn.ModuleList(
- [
- norm_f(
- Conv2d(
- 1,
- 32,
- (kernel_size, 1),
- (stride, 1),
- padding=(get_padding(kernel_size, 1), 0),
- )
- ),
- norm_f(
- Conv2d(
- 32,
- 128,
- (kernel_size, 1),
- (stride, 1),
- padding=(get_padding(kernel_size, 1), 0),
- )
- ),
- norm_f(
- Conv2d(
- 128,
- 512,
- (kernel_size, 1),
- (stride, 1),
- padding=(get_padding(kernel_size, 1), 0),
- )
- ),
- norm_f(
- Conv2d(
- 512,
- 1024,
- (kernel_size, 1),
- (stride, 1),
- padding=(get_padding(kernel_size, 1), 0),
- )
- ),
- norm_f(
- Conv2d(
- 1024,
- 1024,
- (kernel_size, 1),
- 1,
- padding=(get_padding(kernel_size, 1), 0),
- )
- ),
- ]
- )
- self.conv_post = norm_f(Conv2d(1024, 1, (3, 1), 1, padding=(1, 0)))
-
- def forward(self, x):
- fmap = []
-
- # 1d to 2d
- b, c, t = x.shape
- if t % self.period != 0: # pad first
- n_pad = self.period - (t % self.period)
- x = F.pad(x, (0, n_pad), "reflect")
- t = t + n_pad
- x = x.view(b, c, t // self.period, self.period)
-
- for l in self.convs:
- x = l(x)
- x = F.leaky_relu(x, modules.LRELU_SLOPE)
- fmap.append(x)
- x = self.conv_post(x)
- fmap.append(x)
- x = torch.flatten(x, 1, -1)
-
- return x, fmap
diff --git a/spaces/KyanChen/FunSR/models/liif.py b/spaces/KyanChen/FunSR/models/liif.py
deleted file mode 100644
index d6099426081918556a32b81aca00a53be51d91fe..0000000000000000000000000000000000000000
--- a/spaces/KyanChen/FunSR/models/liif.py
+++ /dev/null
@@ -1,110 +0,0 @@
-import torch
-import torch.nn as nn
-import torch.nn.functional as F
-
-import models
-from models import register
-from utils import make_coord
-
-
-@register('liif')
-class LIIF(nn.Module):
-
- def __init__(self, encoder_spec, imnet_spec=None,
- local_ensemble=True, feat_unfold=True, cell_decode=True):
- super().__init__()
- self.local_ensemble = local_ensemble
- self.feat_unfold = feat_unfold
- self.cell_decode = cell_decode
-
- self.encoder = models.make(encoder_spec)
-
- if imnet_spec is not None:
- imnet_in_dim = self.encoder.out_dim
- if self.feat_unfold:
- imnet_in_dim *= 9
- imnet_in_dim += 2 # attach coord
- if self.cell_decode:
- imnet_in_dim += 2
- self.imnet = models.make(imnet_spec, args={'in_dim': imnet_in_dim})
- else:
- self.imnet = None
-
- def gen_feat(self, inp):
- self.feat = self.encoder(inp)
- return self.feat
-
- def query_rgb(self, coord, cell=None):
- feat = self.feat
-
- if self.imnet is None:
- ret = F.grid_sample(feat, coord.flip(-1).unsqueeze(1),
- mode='nearest', align_corners=False)[:, :, 0, :] \
- .permute(0, 2, 1)
- return ret
-
- if self.feat_unfold:
- feat = F.unfold(feat, 3, padding=1).view(
- feat.shape[0], feat.shape[1] * 9, feat.shape[2], feat.shape[3])
-
- if self.local_ensemble:
- vx_lst = [-1, 1]
- vy_lst = [-1, 1]
- eps_shift = 1e-6
- else:
- vx_lst, vy_lst, eps_shift = [0], [0], 0
-
- # field radius (global: [-1, 1])
- rx = 2 / feat.shape[-2] / 2
- ry = 2 / feat.shape[-1] / 2
-
- feat_coord = make_coord(feat.shape[-2:], flatten=False).cuda() \
- .permute(2, 0, 1) \
- .unsqueeze(0).expand(feat.shape[0], 2, *feat.shape[-2:])
-
- preds = []
- areas = []
- for vx in vx_lst:
- for vy in vy_lst:
- coord_ = coord.clone()
- coord_[:, :, 0] += vx * rx + eps_shift
- coord_[:, :, 1] += vy * ry + eps_shift
- coord_.clamp_(-1 + 1e-6, 1 - 1e-6)
- q_feat = F.grid_sample(
- feat, coord_.flip(-1).unsqueeze(1),
- mode='nearest', align_corners=False)[:, :, 0, :] \
- .permute(0, 2, 1)
- q_coord = F.grid_sample(
- feat_coord, coord_.flip(-1).unsqueeze(1),
- mode='nearest', align_corners=False)[:, :, 0, :] \
- .permute(0, 2, 1)
- rel_coord = coord - q_coord
- rel_coord[:, :, 0] *= feat.shape[-2]
- rel_coord[:, :, 1] *= feat.shape[-1]
- inp = torch.cat([q_feat, rel_coord], dim=-1)
-
- if self.cell_decode:
- rel_cell = cell.clone()
- rel_cell[:, :, 0] *= feat.shape[-2]
- rel_cell[:, :, 1] *= feat.shape[-1]
- inp = torch.cat([inp, rel_cell], dim=-1)
-
- bs, q = coord.shape[:2]
- pred = self.imnet(inp.view(bs * q, -1)).view(bs, q, -1)
- preds.append(pred)
-
- area = torch.abs(rel_coord[:, :, 0] * rel_coord[:, :, 1])
- areas.append(area + 1e-9)
-
- tot_area = torch.stack(areas).sum(dim=0)
- if self.local_ensemble:
- t = areas[0]; areas[0] = areas[3]; areas[3] = t
- t = areas[1]; areas[1] = areas[2]; areas[2] = t
- ret = 0
- for pred, area in zip(preds, areas):
- ret = ret + pred * (area / tot_area).unsqueeze(-1)
- return ret
-
- def forward(self, inp, coord, cell):
- self.gen_feat(inp)
- return self.query_rgb(coord, cell)
diff --git a/spaces/Laihiujin/OneFormer/oneformer/data/datasets/register_cityscapes_panoptic.py b/spaces/Laihiujin/OneFormer/oneformer/data/datasets/register_cityscapes_panoptic.py
deleted file mode 100644
index 07ecb23ba6422ac24e4a21aa6bb3125b07f71f33..0000000000000000000000000000000000000000
--- a/spaces/Laihiujin/OneFormer/oneformer/data/datasets/register_cityscapes_panoptic.py
+++ /dev/null
@@ -1,199 +0,0 @@
-# ------------------------------------------------------------------------------
-# Reference: https://github.com/facebookresearch/detectron2/blob/main/detectron2/data/datasets/cityscapes_panoptic.py
-# Modified by Jitesh Jain (https://github.com/praeclarumjj3)
-# ------------------------------------------------------------------------------
-
-import json
-import logging
-import os
-
-from detectron2.data import DatasetCatalog, MetadataCatalog
-from detectron2.data.datasets.builtin_meta import CITYSCAPES_CATEGORIES
-from detectron2.utils.file_io import PathManager
-
-"""
-This file contains functions to register the Cityscapes panoptic dataset to the DatasetCatalog.
-"""
-
-
-logger = logging.getLogger(__name__)
-
-
-def get_cityscapes_panoptic_files(image_dir, gt_dir, json_info):
- files = []
- # scan through the directory
- cities = PathManager.ls(image_dir)
- logger.info(f"{len(cities)} cities found in '{image_dir}'.")
- image_dict = {}
- for city in cities:
- city_img_dir = os.path.join(image_dir, city)
- for basename in PathManager.ls(city_img_dir):
- image_file = os.path.join(city_img_dir, basename)
-
- suffix = "_leftImg8bit.png"
- assert basename.endswith(suffix), basename
- basename = os.path.basename(basename)[: -len(suffix)]
-
- image_dict[basename] = image_file
-
- for ann in json_info["annotations"]:
- image_file = image_dict.get(ann["image_id"], None)
- assert image_file is not None, "No image {} found for annotation {}".format(
- ann["image_id"], ann["file_name"]
- )
- label_file = os.path.join(gt_dir, ann["file_name"])
- segments_info = ann["segments_info"]
- files.append((image_file, label_file, segments_info))
-
- assert len(files), "No images found in {}".format(image_dir)
- assert PathManager.isfile(files[0][0]), files[0][0]
- assert PathManager.isfile(files[0][1]), files[0][1]
- return files
-
-
-def load_cityscapes_panoptic(image_dir, gt_dir, gt_json, meta):
- """
- Args:
- image_dir (str): path to the raw dataset. e.g., "~/cityscapes/leftImg8bit/train".
- gt_dir (str): path to the raw annotations. e.g.,
- "~/cityscapes/gtFine/cityscapes_panoptic_train".
- gt_json (str): path to the json file. e.g.,
- "~/cityscapes/gtFine/cityscapes_panoptic_train.json".
- meta (dict): dictionary containing "thing_dataset_id_to_contiguous_id"
- and "stuff_dataset_id_to_contiguous_id" to map category ids to
- contiguous ids for training.
-
- Returns:
- list[dict]: a list of dicts in Detectron2 standard format. (See
- `Using Custom Datasets `_ )
- """
-
- def _convert_category_id(segment_info, meta):
- if segment_info["category_id"] in meta["thing_dataset_id_to_contiguous_id"]:
- segment_info["category_id"] = meta["thing_dataset_id_to_contiguous_id"][
- segment_info["category_id"]
- ]
- else:
- segment_info["category_id"] = meta["stuff_dataset_id_to_contiguous_id"][
- segment_info["category_id"]
- ]
- return segment_info
-
- assert os.path.exists(
- gt_json
- ), "Please run `python cityscapesscripts/preparation/createPanopticImgs.py` to generate label files." # noqa
-
-
- with open(gt_json) as f:
- json_info = json.load(f)
-
- files = get_cityscapes_panoptic_files(image_dir, gt_dir, json_info)
- ret = []
- for image_file, label_file, segments_info in files:
- sem_label_file = (
- image_file.replace("leftImg8bit", "gtFine").split(".")[0] + "_labelTrainIds.png"
- )
- segments_info = [_convert_category_id(x, meta) for x in segments_info]
- ret.append(
- {
- "file_name": image_file,
- "image_id": "_".join(
- os.path.splitext(os.path.basename(image_file))[0].split("_")[:3]
- ),
- "sem_seg_file_name": sem_label_file,
- "pan_seg_file_name": label_file,
- "segments_info": segments_info,
- }
- )
- assert len(ret), f"No images found in {image_dir}!"
- assert PathManager.isfile(
- ret[0]["sem_seg_file_name"]
- ), "Please generate labelTrainIds.png with cityscapesscripts/preparation/createTrainIdLabelImgs.py" # noqa
- assert PathManager.isfile(
- ret[0]["pan_seg_file_name"]
- ), "Please generate panoptic annotation with python cityscapesscripts/preparation/createPanopticImgs.py" # noqa
- return ret
-
-
-_RAW_CITYSCAPES_PANOPTIC_SPLITS = {
- "cityscapes_fine_panoptic_train": (
- "cityscapes/leftImg8bit/train",
- "cityscapes/gtFine/cityscapes_panoptic_train",
- "cityscapes/gtFine/cityscapes_panoptic_train.json",
- ),
- "cityscapes_fine_panoptic_val": (
- "cityscapes/leftImg8bit/val",
- "cityscapes/gtFine/cityscapes_panoptic_val",
- "cityscapes/gtFine/cityscapes_panoptic_val.json",
- ),
- # "cityscapes_fine_panoptic_test": not supported yet
-}
-
-
-def register_all_cityscapes_panoptic(root):
- meta = {}
- # The following metadata maps contiguous id from [0, #thing categories +
- # #stuff categories) to their names and colors. We have to replica of the
- # same name and color under "thing_*" and "stuff_*" because the current
- # visualization function in D2 handles thing and class classes differently
- # due to some heuristic used in Panoptic FPN. We keep the same naming to
- # enable reusing existing visualization functions.
- thing_classes = [k["name"] for k in CITYSCAPES_CATEGORIES]
- thing_colors = [k["color"] for k in CITYSCAPES_CATEGORIES]
- stuff_classes = [k["name"] for k in CITYSCAPES_CATEGORIES]
- stuff_colors = [k["color"] for k in CITYSCAPES_CATEGORIES]
-
- meta["thing_classes"] = thing_classes
- meta["thing_colors"] = thing_colors
- meta["stuff_classes"] = stuff_classes
- meta["stuff_colors"] = stuff_colors
-
- # There are three types of ids in cityscapes panoptic segmentation:
- # (1) category id: like semantic segmentation, it is the class id for each
- # pixel. Since there are some classes not used in evaluation, the category
- # id is not always contiguous and thus we have two set of category ids:
- # - original category id: category id in the original dataset, mainly
- # used for evaluation.
- # - contiguous category id: [0, #classes), in order to train the classifier
- # (2) instance id: this id is used to differentiate different instances from
- # the same category. For "stuff" classes, the instance id is always 0; for
- # "thing" classes, the instance id starts from 1 and 0 is reserved for
- # ignored instances (e.g. crowd annotation).
- # (3) panoptic id: this is the compact id that encode both category and
- # instance id by: category_id * 1000 + instance_id.
- thing_dataset_id_to_contiguous_id = {}
- stuff_dataset_id_to_contiguous_id = {}
-
- for k in CITYSCAPES_CATEGORIES:
- if k["isthing"] == 1:
- thing_dataset_id_to_contiguous_id[k["id"]] = k["trainId"]
- else:
- stuff_dataset_id_to_contiguous_id[k["id"]] = k["trainId"]
-
- meta["thing_dataset_id_to_contiguous_id"] = thing_dataset_id_to_contiguous_id
- meta["stuff_dataset_id_to_contiguous_id"] = stuff_dataset_id_to_contiguous_id
-
- for key, (image_dir, gt_dir, gt_json) in _RAW_CITYSCAPES_PANOPTIC_SPLITS.items():
- image_dir = os.path.join(root, image_dir)
- gt_dir = os.path.join(root, gt_dir)
- gt_json = os.path.join(root, gt_json)
-
- if key in DatasetCatalog.list():
- DatasetCatalog.remove(key)
-
- DatasetCatalog.register(
- key, lambda x=image_dir, y=gt_dir, z=gt_json: load_cityscapes_panoptic(x, y, z, meta)
- )
- MetadataCatalog.get(key).set(
- panoptic_root=gt_dir,
- image_root=image_dir,
- panoptic_json=gt_json,
- gt_dir=gt_dir.replace("cityscapes_panoptic_", ""),
- evaluator_type="cityscapes_panoptic_seg",
- ignore_label=255,
- label_divisor=1000,
- **meta,
- )
-
-_root = os.getenv("DETECTRON2_DATASETS", "datasets")
-register_all_cityscapes_panoptic(_root)
\ No newline at end of file
diff --git a/spaces/LaynzKunz/Aesthetic_RVC_Inference_HF/lib/infer/infer_libs/infer_pack/modules/F0Predictor/DioF0Predictor.py b/spaces/LaynzKunz/Aesthetic_RVC_Inference_HF/lib/infer/infer_libs/infer_pack/modules/F0Predictor/DioF0Predictor.py
deleted file mode 100644
index 54c2fd2484c3d52c3dc9bb4c88e5c102fa686fdc..0000000000000000000000000000000000000000
--- a/spaces/LaynzKunz/Aesthetic_RVC_Inference_HF/lib/infer/infer_libs/infer_pack/modules/F0Predictor/DioF0Predictor.py
+++ /dev/null
@@ -1,91 +0,0 @@
-import numpy as np
-import pyworld
-
-from lib.infer.infer_libs.infer_pack.modules.F0Predictor.F0Predictor import F0Predictor
-
-
-class DioF0Predictor(F0Predictor):
- def __init__(self, hop_length=512, f0_min=50, f0_max=1100, sampling_rate=44100):
- self.hop_length = hop_length
- self.f0_min = f0_min
- self.f0_max = f0_max
- self.sampling_rate = sampling_rate
-
- def interpolate_f0(self, f0):
- """
- 对F0进行插值处理
- """
-
- data = np.reshape(f0, (f0.size, 1))
-
- vuv_vector = np.zeros((data.size, 1), dtype=np.float32)
- vuv_vector[data > 0.0] = 1.0
- vuv_vector[data <= 0.0] = 0.0
-
- ip_data = data
-
- frame_number = data.size
- last_value = 0.0
- for i in range(frame_number):
- if data[i] <= 0.0:
- j = i + 1
- for j in range(i + 1, frame_number):
- if data[j] > 0.0:
- break
- if j < frame_number - 1:
- if last_value > 0.0:
- step = (data[j] - data[i - 1]) / float(j - i)
- for k in range(i, j):
- ip_data[k] = data[i - 1] + step * (k - i + 1)
- else:
- for k in range(i, j):
- ip_data[k] = data[j]
- else:
- for k in range(i, frame_number):
- ip_data[k] = last_value
- else:
- ip_data[i] = data[i] # 这里可能存在一个没有必要的拷贝
- last_value = data[i]
-
- return ip_data[:, 0], vuv_vector[:, 0]
-
- def resize_f0(self, x, target_len):
- source = np.array(x)
- source[source < 0.001] = np.nan
- target = np.interp(
- np.arange(0, len(source) * target_len, len(source)) / target_len,
- np.arange(0, len(source)),
- source,
- )
- res = np.nan_to_num(target)
- return res
-
- def compute_f0(self, wav, p_len=None):
- if p_len is None:
- p_len = wav.shape[0] // self.hop_length
- f0, t = pyworld.dio(
- wav.astype(np.double),
- fs=self.sampling_rate,
- f0_floor=self.f0_min,
- f0_ceil=self.f0_max,
- frame_period=1000 * self.hop_length / self.sampling_rate,
- )
- f0 = pyworld.stonemask(wav.astype(np.double), f0, t, self.sampling_rate)
- for index, pitch in enumerate(f0):
- f0[index] = round(pitch, 1)
- return self.interpolate_f0(self.resize_f0(f0, p_len))[0]
-
- def compute_f0_uv(self, wav, p_len=None):
- if p_len is None:
- p_len = wav.shape[0] // self.hop_length
- f0, t = pyworld.dio(
- wav.astype(np.double),
- fs=self.sampling_rate,
- f0_floor=self.f0_min,
- f0_ceil=self.f0_max,
- frame_period=1000 * self.hop_length / self.sampling_rate,
- )
- f0 = pyworld.stonemask(wav.astype(np.double), f0, t, self.sampling_rate)
- for index, pitch in enumerate(f0):
- f0[index] = round(pitch, 1)
- return self.interpolate_f0(self.resize_f0(f0, p_len))
diff --git a/spaces/LeeroyVonJenkins/OCR-Invoice-LayoutLMv3/app.py b/spaces/LeeroyVonJenkins/OCR-Invoice-LayoutLMv3/app.py
deleted file mode 100644
index 5615916416d367c54bff82ae6880ed26f107ad97..0000000000000000000000000000000000000000
--- a/spaces/LeeroyVonJenkins/OCR-Invoice-LayoutLMv3/app.py
+++ /dev/null
@@ -1,146 +0,0 @@
-import os
-
-os.system('pip install pip --upgrade')
-os.system('pip install -q git+https://github.com/huggingface/transformers.git')
-
-
-os.system("pip install pyyaml==5.1")
-# workaround: install old version of pytorch since detectron2 hasn't released packages for pytorch 1.9 (issue: https://github.com/facebookresearch/detectron2/issues/3158)
-os.system(
- "pip install torch==1.8.0+cu101 torchvision==0.9.0+cu101 -f https://download.pytorch.org/whl/torch_stable.html"
-)
-
-# install detectron2 that matches pytorch 1.8
-# See https://detectron2.readthedocs.io/tutorials/install.html for instructions
-os.system(
- "pip install -q detectron2 -f https://dl.fbaipublicfiles.com/detectron2/wheels/cu101/torch1.8/index.html"
-)
-
-## install PyTesseract
-os.system("pip install -q pytesseract")
-
-import gradio as gr
-import numpy as np
-from transformers import AutoModelForTokenClassification
-from datasets.features import ClassLabel
-from transformers import AutoProcessor
-from datasets import Features, Sequence, ClassLabel, Value, Array2D, Array3D
-import torch
-from datasets import load_metric
-from transformers import LayoutLMv3ForTokenClassification
-from transformers.data.data_collator import default_data_collator
-
-
-from transformers import AutoModelForTokenClassification
-from datasets import load_dataset
-from PIL import Image, ImageDraw, ImageFont
-
-
-processor = AutoProcessor.from_pretrained("jinhybr/OCR-LayoutLMv3-Invoice", apply_ocr=True)
-model = AutoModelForTokenClassification.from_pretrained("jinhybr/OCR-LayoutLMv3-Invoice")
-
-
-
-# load image example
-dataset = load_dataset("jinhybr/WildReceipt", split="test")
-Image.open(dataset[1]["image_path"]).convert("RGB").save("example1.png")
-Image.open(dataset[3]["image_path"]).convert("RGB").save("example2.png")
-Image.open(dataset[25]["image_path"]).convert("RGB").save("example3.png")
-# define id2label, label2color
-labels = dataset.features['ner_tags'].feature.names
-id2label = {v: k for v, k in enumerate(labels)}
-label2color = {
- "Date_key": 'red',
- "Date_value": 'green',
- "Ignore": 'orange',
- "Others": 'orange',
- "Prod_item_key": 'red',
- "Prod_item_value": 'green',
- "Prod_price_key": 'red',
- "Prod_price_value": 'green',
- "Prod_quantity_key": 'red',
- "Prod_quantity_value": 'green',
- "Store_addr_key": 'red',
- "Store_addr_value": 'green',
- "Store_name_key": 'red',
- "Store_name_value": 'green',
- "Subtotal_key": 'red',
- "Subtotal_value": 'green',
- "Tax_key": 'red',
- "Tax_value": 'green',
- "Tel_key": 'red',
- "Tel_value": 'green',
- "Time_key": 'red',
- "Time_value": 'green',
- "Tips_key": 'red',
- "Tips_value": 'green',
- "Total_key": 'red',
- "Total_value": 'blue'
- }
-
-def unnormalize_box(bbox, width, height):
- return [
- width * (bbox[0] / 1000),
- height * (bbox[1] / 1000),
- width * (bbox[2] / 1000),
- height * (bbox[3] / 1000),
- ]
-
-
-def iob_to_label(label):
- return label
-
-
-
-def process_image(image):
-
- print(type(image))
- width, height = image.size
-
- # encode
- encoding = processor(image, truncation=True, return_offsets_mapping=True, return_tensors="pt")
- offset_mapping = encoding.pop('offset_mapping')
-
- # forward pass
- outputs = model(**encoding)
-
- # get predictions
- predictions = outputs.logits.argmax(-1).squeeze().tolist()
- token_boxes = encoding.bbox.squeeze().tolist()
-
- # only keep non-subword predictions
- is_subword = np.array(offset_mapping.squeeze().tolist())[:,0] != 0
- true_predictions = [id2label[pred] for idx, pred in enumerate(predictions) if not is_subword[idx]]
- true_boxes = [unnormalize_box(box, width, height) for idx, box in enumerate(token_boxes) if not is_subword[idx]]
-
- # draw predictions over the image
- draw = ImageDraw.Draw(image)
- font = ImageFont.load_default()
- for prediction, box in zip(true_predictions, true_boxes):
- predicted_label = iob_to_label(prediction)
- draw.rectangle(box, outline=label2color[predicted_label])
- draw.text((box[0]+10, box[1]-10), text=predicted_label, fill=label2color[predicted_label], font=font)
-
- return image
-
-
-title = "OCR Invoice - Information Extraction - LayoutLMv3"
-description = "Fine-tuned Microsoft's LayoutLMv3 on WildReceipt Dataset to parse Invoice OCR document. To use it, simply upload an image or use the example image below. Results will show up in a few seconds."
-
-article="References [1] Y. Xu et al., “LayoutLMv3: Pre-training for Document AI with Unified Text and Image Masking.” 2022. Paper Link [2] LayoutLMv3 training and inference [3] Hongbin Sun, Zhanghui Kuang, Xiaoyu Yue, Chenhao Lin, and Wayne Zhang. 2021. Spatial Dual-Modality Graph Reasoning for Key Information Extraction. arXiv. DOI:https://doi.org/10.48550/ARXIV.2103.14470 Paper Link "
-
-examples =[['example1.png'],['example2.png'],['example3.png'],['inv2.jpg']]
-
-css = """.output_image, .input_image {height: 600px !important}"""
-
-iface = gr.Interface(fn=process_image,
- inputs=gr.inputs.Image(type="pil"),
- outputs=gr.outputs.Image(type="pil", label="annotated image"),
- title=title,
- description=description,
- article=article,
- examples=examples,
- css=css,
- analytics_enabled = True, enable_queue=True)
-
-iface.launch(inline=False, share=False, debug=True)
\ No newline at end of file
diff --git a/spaces/LinoyTsaban/edit_friendly_ddpm_inversion/README.md b/spaces/LinoyTsaban/edit_friendly_ddpm_inversion/README.md
deleted file mode 100644
index aa7c7bbf446ec8c483811ac0c31d9fa1021909ef..0000000000000000000000000000000000000000
--- a/spaces/LinoyTsaban/edit_friendly_ddpm_inversion/README.md
+++ /dev/null
@@ -1,21 +0,0 @@
----
-title: Edit Friendly Ddpm Inversion
-emoji: 🖼️
-colorFrom: pink
-colorTo: orange
-sdk: gradio
-sdk_version: 3.32.0
-app_file: app.py
-pinned: false
----
-
-## BibTeX
-
-```
-@article{HubermanSpiegelglas2023,
- title = {An Edit Friendly DDPM Noise Space: Inversion and Manipulations},
- author = {Huberman-Spiegelglas, Inbar and Kulikov, Vladimir and Michaeli, Tomer},
- journal = {arXiv preprint arXiv:2304.06140},
- year = {2023}
- }
-```
diff --git a/spaces/LuxOAI/ChatGpt-Web/app/store/chat.ts b/spaces/LuxOAI/ChatGpt-Web/app/store/chat.ts
deleted file mode 100644
index 0ee15bf56f0411c329c3e3e81f8ac9cd6171c395..0000000000000000000000000000000000000000
--- a/spaces/LuxOAI/ChatGpt-Web/app/store/chat.ts
+++ /dev/null
@@ -1,532 +0,0 @@
-import { create } from "zustand";
-import { persist } from "zustand/middleware";
-
-import { type ChatCompletionResponseMessage } from "openai";
-import {
- ControllerPool,
- requestChatStream,
- requestWithPrompt,
-} from "../requests";
-import { trimTopic } from "../utils";
-
-import Locale from "../locales";
-import { showToast } from "../components/ui-lib";
-import { ModelType, useAppConfig } from "./config";
-import { createEmptyMask, Mask } from "./mask";
-import { StoreKey } from "../constant";
-
-export type Message = ChatCompletionResponseMessage & {
- date: string;
- streaming?: boolean;
- isError?: boolean;
- id?: number;
- model?: ModelType;
-};
-
-export function createMessage(override: Partial): Message {
- return {
- id: Date.now(),
- date: new Date().toLocaleString(),
- role: "user",
- content: "",
- ...override,
- };
-}
-
-export const ROLES: Message["role"][] = ["system", "user", "assistant"];
-
-export interface ChatStat {
- tokenCount: number;
- wordCount: number;
- charCount: number;
-}
-
-export interface ChatSession {
- id: number;
-
- topic: string;
-
- memoryPrompt: string;
- messages: Message[];
- stat: ChatStat;
- lastUpdate: number;
- lastSummarizeIndex: number;
-
- mask: Mask;
-}
-
-export const DEFAULT_TOPIC = Locale.Store.DefaultTopic;
-export const BOT_HELLO: Message = createMessage({
- role: "assistant",
- content: Locale.Store.BotHello,
-});
-
-function createEmptySession(): ChatSession {
- return {
- id: Date.now() + Math.random(),
- topic: DEFAULT_TOPIC,
- memoryPrompt: "",
- messages: [],
- stat: {
- tokenCount: 0,
- wordCount: 0,
- charCount: 0,
- },
- lastUpdate: Date.now(),
- lastSummarizeIndex: 0,
- mask: createEmptyMask(),
- };
-}
-
-interface ChatStore {
- sessions: ChatSession[];
- currentSessionIndex: number;
- globalId: number;
- clearSessions: () => void;
- moveSession: (from: number, to: number) => void;
- selectSession: (index: number) => void;
- newSession: (mask?: Mask) => void;
- deleteSession: (index: number) => void;
- currentSession: () => ChatSession;
- onNewMessage: (message: Message) => void;
- onUserInput: (content: string) => Promise;
- summarizeSession: () => void;
- updateStat: (message: Message) => void;
- updateCurrentSession: (updater: (session: ChatSession) => void) => void;
- updateMessage: (
- sessionIndex: number,
- messageIndex: number,
- updater: (message?: Message) => void,
- ) => void;
- resetSession: () => void;
- getMessagesWithMemory: () => Message[];
- getMemoryPrompt: () => Message;
-
- clearAllData: () => void;
- clearAll: () => void;
-}
-
-function countMessages(msgs: Message[]) {
- return msgs.reduce((pre, cur) => pre + cur.content.length, 0);
-}
-
-export const useChatStore = create()(
- persist(
- (set, get) => ({
- sessions: [createEmptySession()],
- currentSessionIndex: 0,
- globalId: 0,
-
- clearSessions() {
- set(() => ({
- sessions: [createEmptySession()],
- currentSessionIndex: 0,
- }));
- },
-
- selectSession(index: number) {
- set({
- currentSessionIndex: index,
- });
- },
-
- moveSession(from: number, to: number) {
- set((state) => {
- const { sessions, currentSessionIndex: oldIndex } = state;
-
- // move the session
- const newSessions = [...sessions];
- const session = newSessions[from];
- newSessions.splice(from, 1);
- newSessions.splice(to, 0, session);
-
- // modify current session id
- let newIndex = oldIndex === from ? to : oldIndex;
- if (oldIndex > from && oldIndex <= to) {
- newIndex -= 1;
- } else if (oldIndex < from && oldIndex >= to) {
- newIndex += 1;
- }
-
- return {
- currentSessionIndex: newIndex,
- sessions: newSessions,
- };
- });
- },
-
- newSession(mask) {
- const session = createEmptySession();
-
- set(() => ({ globalId: get().globalId + 1 }));
- session.id = get().globalId;
-
- if (mask) {
- session.mask = { ...mask };
- session.topic = mask.name;
- }
-
- set((state) => ({
- currentSessionIndex: 0,
- sessions: [session].concat(state.sessions),
- }));
- },
-
- deleteSession(index) {
- const deletingLastSession = get().sessions.length === 1;
- const deletedSession = get().sessions.at(index);
-
- if (!deletedSession) return;
-
- const sessions = get().sessions.slice();
- sessions.splice(index, 1);
-
- const currentIndex = get().currentSessionIndex;
- let nextIndex = Math.min(
- currentIndex - Number(index < currentIndex),
- sessions.length - 1,
- );
-
- if (deletingLastSession) {
- nextIndex = 0;
- sessions.push(createEmptySession());
- }
-
- // for undo delete action
- const restoreState = {
- currentSessionIndex: get().currentSessionIndex,
- sessions: get().sessions.slice(),
- };
-
- set(() => ({
- currentSessionIndex: nextIndex,
- sessions,
- }));
-
- showToast(
- Locale.Home.DeleteToast,
- {
- text: Locale.Home.Revert,
- onClick() {
- set(() => restoreState);
- },
- },
- 5000,
- );
- },
-
- currentSession() {
- let index = get().currentSessionIndex;
- const sessions = get().sessions;
-
- if (index < 0 || index >= sessions.length) {
- index = Math.min(sessions.length - 1, Math.max(0, index));
- set(() => ({ currentSessionIndex: index }));
- }
-
- const session = sessions[index];
-
- return session;
- },
-
- onNewMessage(message) {
- get().updateCurrentSession((session) => {
- session.lastUpdate = Date.now();
- });
- get().updateStat(message);
- get().summarizeSession();
- },
-
- async onUserInput(content) {
- const session = get().currentSession();
- const modelConfig = session.mask.modelConfig;
-
- const userMessage: Message = createMessage({
- role: "user",
- content,
- });
-
- const botMessage: Message = createMessage({
- role: "assistant",
- streaming: true,
- id: userMessage.id! + 1,
- model: modelConfig.model,
- });
- const systemInfo = createMessage({
- role: "system",
- content: `IMPRTANT: You are a virtual assistant powered by the ${
- modelConfig.model
- } model, now time is ${new Date().toLocaleString()}}`,
- id: botMessage.id! + 1,
- });
- // get recent messages
- const systemMessages = [systemInfo];
- const recentMessages = get().getMessagesWithMemory();
- const sendMessages = systemMessages.concat(
- recentMessages.concat(userMessage),
- );
- const sessionIndex = get().currentSessionIndex;
- const messageIndex = get().currentSession().messages.length + 1;
-
- // save user's and bot's message
- get().updateCurrentSession((session) => {
- session.messages.push(userMessage);
- session.messages.push(botMessage);
- });
-
- // make request
- console.log("[User Input] ", sendMessages);
- requestChatStream(sendMessages, {
- onMessage(content, done) {
- // stream response
- if (done) {
- botMessage.streaming = false;
- botMessage.content = content;
- get().onNewMessage(botMessage);
- ControllerPool.remove(
- sessionIndex,
- botMessage.id ?? messageIndex,
- );
- } else {
- botMessage.content = content;
- set(() => ({}));
- }
- },
- onError(error, statusCode) {
- const isAborted = error.message.includes("aborted");
- if (statusCode === 401) {
- botMessage.content = Locale.Error.Unauthorized;
- } else if (!isAborted) {
- botMessage.content += "\n\n" + Locale.Store.Error;
- }
- botMessage.streaming = false;
- userMessage.isError = !isAborted;
- botMessage.isError = !isAborted;
-
- set(() => ({}));
- ControllerPool.remove(sessionIndex, botMessage.id ?? messageIndex);
- },
- onController(controller) {
- // collect controller for stop/retry
- ControllerPool.addController(
- sessionIndex,
- botMessage.id ?? messageIndex,
- controller,
- );
- },
- modelConfig: { ...modelConfig },
- });
- },
-
- getMemoryPrompt() {
- const session = get().currentSession();
-
- return {
- role: "system",
- content:
- session.memoryPrompt.length > 0
- ? Locale.Store.Prompt.History(session.memoryPrompt)
- : "",
- date: "",
- } as Message;
- },
-
- getMessagesWithMemory() {
- const session = get().currentSession();
- const modelConfig = session.mask.modelConfig;
- const messages = session.messages.filter((msg) => !msg.isError);
- const n = messages.length;
-
- const context = session.mask.context.slice();
-
- // long term memory
- if (
- modelConfig.sendMemory &&
- session.memoryPrompt &&
- session.memoryPrompt.length > 0
- ) {
- const memoryPrompt = get().getMemoryPrompt();
- context.push(memoryPrompt);
- }
-
- // get short term and unmemoried long term memory
- const shortTermMemoryMessageIndex = Math.max(
- 0,
- n - modelConfig.historyMessageCount,
- );
- const longTermMemoryMessageIndex = session.lastSummarizeIndex;
- const oldestIndex = Math.max(
- shortTermMemoryMessageIndex,
- longTermMemoryMessageIndex,
- );
- const threshold = modelConfig.compressMessageLengthThreshold;
-
- // get recent messages as many as possible
- const reversedRecentMessages = [];
- for (
- let i = n - 1, count = 0;
- i >= oldestIndex && count < threshold;
- i -= 1
- ) {
- const msg = messages[i];
- if (!msg || msg.isError) continue;
- count += msg.content.length;
- reversedRecentMessages.push(msg);
- }
-
- // concat
- const recentMessages = context.concat(reversedRecentMessages.reverse());
-
- return recentMessages;
- },
-
- updateMessage(
- sessionIndex: number,
- messageIndex: number,
- updater: (message?: Message) => void,
- ) {
- const sessions = get().sessions;
- const session = sessions.at(sessionIndex);
- const messages = session?.messages;
- updater(messages?.at(messageIndex));
- set(() => ({ sessions }));
- },
-
- resetSession() {
- get().updateCurrentSession((session) => {
- session.messages = [];
- session.memoryPrompt = "";
- });
- },
-
- summarizeSession() {
- const session = get().currentSession();
-
- // should summarize topic after chating more than 50 words
- const SUMMARIZE_MIN_LEN = 50;
- if (
- session.topic === DEFAULT_TOPIC &&
- countMessages(session.messages) >= SUMMARIZE_MIN_LEN
- ) {
- const Bot = useAppConfig.getState().bot;
- if (Bot != "OpenAI") {
- get().updateCurrentSession(
- (session) => (session.topic = trimTopic(Bot)),
- );
- } else {
- requestWithPrompt(session.messages, Locale.Store.Prompt.Topic, {
- model: "gpt-3.5-turbo",
- }).then((res) => {
- get().updateCurrentSession(
- (session) =>
- (session.topic = res ? trimTopic(res) : DEFAULT_TOPIC),
- );
- });
- }
- }
-
- const modelConfig = session.mask.modelConfig;
- let toBeSummarizedMsgs = session.messages.slice(
- session.lastSummarizeIndex,
- );
-
- const historyMsgLength = countMessages(toBeSummarizedMsgs);
-
- if (historyMsgLength > modelConfig?.max_tokens ?? 4000) {
- const n = toBeSummarizedMsgs.length;
- toBeSummarizedMsgs = toBeSummarizedMsgs.slice(
- Math.max(0, n - modelConfig.historyMessageCount),
- );
- }
-
- // add memory prompt
- toBeSummarizedMsgs.unshift(get().getMemoryPrompt());
-
- const lastSummarizeIndex = session.messages.length;
-
- console.log(
- "[Chat History] ",
- toBeSummarizedMsgs,
- historyMsgLength,
- modelConfig.compressMessageLengthThreshold,
- );
-
- if (
- historyMsgLength > modelConfig.compressMessageLengthThreshold &&
- session.mask.modelConfig.sendMemory
- ) {
- requestChatStream(
- toBeSummarizedMsgs.concat({
- role: "system",
- content: Locale.Store.Prompt.Summarize,
- date: "",
- }),
- {
- overrideModel: "gpt-3.5-turbo",
- onMessage(message, done) {
- session.memoryPrompt = message;
- if (done) {
- console.log("[Memory] ", session.memoryPrompt);
- session.lastSummarizeIndex = lastSummarizeIndex;
- }
- },
- onError(error) {
- console.error("[Summarize] ", error);
- },
- },
- );
- }
- },
-
- updateStat(message) {
- get().updateCurrentSession((session) => {
- session.stat.charCount += message.content.length;
- // TODO: should update chat count and word count
- });
- },
-
- updateCurrentSession(updater) {
- const sessions = get().sessions;
- const index = get().currentSessionIndex;
- updater(sessions[index]);
- set(() => ({ sessions }));
- },
-
- clearAllData() {
- localStorage.clear();
- location.reload();
- },
-
- clearAll() {
- // localStorage.clear();
- location.reload();
- },
- }),
- {
- name: StoreKey.Chat,
- version: 2,
- migrate(persistedState, version) {
- const state = persistedState as any;
- const newState = JSON.parse(JSON.stringify(state)) as ChatStore;
-
- if (version < 2) {
- newState.globalId = 0;
- newState.sessions = [];
-
- const oldSessions = state.sessions;
- for (const oldSession of oldSessions) {
- const newSession = createEmptySession();
- newSession.topic = oldSession.topic;
- newSession.messages = [...oldSession.messages];
- newSession.mask.modelConfig.sendMemory = true;
- newSession.mask.modelConfig.historyMessageCount = 4;
- newSession.mask.modelConfig.compressMessageLengthThreshold = 1000;
- newState.sessions.push(newSession);
- }
- }
-
- return newState;
- },
- },
- ),
-);
diff --git a/spaces/Mellow-ai/PhotoAI_Mellow/ldm/modules/midas/midas/midas_net_custom.py b/spaces/Mellow-ai/PhotoAI_Mellow/ldm/modules/midas/midas/midas_net_custom.py
deleted file mode 100644
index 50e4acb5e53d5fabefe3dde16ab49c33c2b7797c..0000000000000000000000000000000000000000
--- a/spaces/Mellow-ai/PhotoAI_Mellow/ldm/modules/midas/midas/midas_net_custom.py
+++ /dev/null
@@ -1,128 +0,0 @@
-"""MidashNet: Network for monocular depth estimation trained by mixing several datasets.
-This file contains code that is adapted from
-https://github.com/thomasjpfan/pytorch_refinenet/blob/master/pytorch_refinenet/refinenet/refinenet_4cascade.py
-"""
-import torch
-import torch.nn as nn
-
-from .base_model import BaseModel
-from .blocks import FeatureFusionBlock, FeatureFusionBlock_custom, Interpolate, _make_encoder
-
-
-class MidasNet_small(BaseModel):
- """Network for monocular depth estimation.
- """
-
- def __init__(self, path=None, features=64, backbone="efficientnet_lite3", non_negative=True, exportable=True, channels_last=False, align_corners=True,
- blocks={'expand': True}):
- """Init.
-
- Args:
- path (str, optional): Path to saved model. Defaults to None.
- features (int, optional): Number of features. Defaults to 256.
- backbone (str, optional): Backbone network for encoder. Defaults to resnet50
- """
- print("Loading weights: ", path)
-
- super(MidasNet_small, self).__init__()
-
- use_pretrained = False if path else True
-
- self.channels_last = channels_last
- self.blocks = blocks
- self.backbone = backbone
-
- self.groups = 1
-
- features1=features
- features2=features
- features3=features
- features4=features
- self.expand = False
- if "expand" in self.blocks and self.blocks['expand'] == True:
- self.expand = True
- features1=features
- features2=features*2
- features3=features*4
- features4=features*8
-
- self.pretrained, self.scratch = _make_encoder(self.backbone, features, use_pretrained, groups=self.groups, expand=self.expand, exportable=exportable)
-
- self.scratch.activation = nn.ReLU(False)
-
- self.scratch.refinenet4 = FeatureFusionBlock_custom(features4, self.scratch.activation, deconv=False, bn=False, expand=self.expand, align_corners=align_corners)
- self.scratch.refinenet3 = FeatureFusionBlock_custom(features3, self.scratch.activation, deconv=False, bn=False, expand=self.expand, align_corners=align_corners)
- self.scratch.refinenet2 = FeatureFusionBlock_custom(features2, self.scratch.activation, deconv=False, bn=False, expand=self.expand, align_corners=align_corners)
- self.scratch.refinenet1 = FeatureFusionBlock_custom(features1, self.scratch.activation, deconv=False, bn=False, align_corners=align_corners)
-
-
- self.scratch.output_conv = nn.Sequential(
- nn.Conv2d(features, features//2, kernel_size=3, stride=1, padding=1, groups=self.groups),
- Interpolate(scale_factor=2, mode="bilinear"),
- nn.Conv2d(features//2, 32, kernel_size=3, stride=1, padding=1),
- self.scratch.activation,
- nn.Conv2d(32, 1, kernel_size=1, stride=1, padding=0),
- nn.ReLU(True) if non_negative else nn.Identity(),
- nn.Identity(),
- )
-
- if path:
- self.load(path)
-
-
- def forward(self, x):
- """Forward pass.
-
- Args:
- x (tensor): input data (image)
-
- Returns:
- tensor: depth
- """
- if self.channels_last==True:
- print("self.channels_last = ", self.channels_last)
- x.contiguous(memory_format=torch.channels_last)
-
-
- layer_1 = self.pretrained.layer1(x)
- layer_2 = self.pretrained.layer2(layer_1)
- layer_3 = self.pretrained.layer3(layer_2)
- layer_4 = self.pretrained.layer4(layer_3)
-
- layer_1_rn = self.scratch.layer1_rn(layer_1)
- layer_2_rn = self.scratch.layer2_rn(layer_2)
- layer_3_rn = self.scratch.layer3_rn(layer_3)
- layer_4_rn = self.scratch.layer4_rn(layer_4)
-
-
- path_4 = self.scratch.refinenet4(layer_4_rn)
- path_3 = self.scratch.refinenet3(path_4, layer_3_rn)
- path_2 = self.scratch.refinenet2(path_3, layer_2_rn)
- path_1 = self.scratch.refinenet1(path_2, layer_1_rn)
-
- out = self.scratch.output_conv(path_1)
-
- return torch.squeeze(out, dim=1)
-
-
-
-def fuse_model(m):
- prev_previous_type = nn.Identity()
- prev_previous_name = ''
- previous_type = nn.Identity()
- previous_name = ''
- for name, module in m.named_modules():
- if prev_previous_type == nn.Conv2d and previous_type == nn.BatchNorm2d and type(module) == nn.ReLU:
- # print("FUSED ", prev_previous_name, previous_name, name)
- torch.quantization.fuse_modules(m, [prev_previous_name, previous_name, name], inplace=True)
- elif prev_previous_type == nn.Conv2d and previous_type == nn.BatchNorm2d:
- # print("FUSED ", prev_previous_name, previous_name)
- torch.quantization.fuse_modules(m, [prev_previous_name, previous_name], inplace=True)
- # elif previous_type == nn.Conv2d and type(module) == nn.ReLU:
- # print("FUSED ", previous_name, name)
- # torch.quantization.fuse_modules(m, [previous_name, name], inplace=True)
-
- prev_previous_type = previous_type
- prev_previous_name = previous_name
- previous_type = type(module)
- previous_name = name
\ No newline at end of file
diff --git a/spaces/Mileena/PIFu-Clothed-Human-Digitization/PIFu/scripts/download_trained_model.sh b/spaces/Mileena/PIFu-Clothed-Human-Digitization/PIFu/scripts/download_trained_model.sh
deleted file mode 100644
index c652f2c666dc48ff1e2e7a94d559e925ac058dec..0000000000000000000000000000000000000000
--- a/spaces/Mileena/PIFu-Clothed-Human-Digitization/PIFu/scripts/download_trained_model.sh
+++ /dev/null
@@ -1,7 +0,0 @@
-set -ex
-
-mkdir -p checkpoints
-cd checkpoints
-wget "https://drive.google.com/uc?export=download&id=1zEmVXG2VHy0MMzngcRshB4D8Sr_oLHsm" -O net_G
-wget "https://drive.google.com/uc?export=download&id=1V83B6GDIjYMfHdpg-KcCSAPgHxpafHgd" -O net_C
-cd ..
\ No newline at end of file
diff --git a/spaces/Miuzarte/SUI-svc-3.0/add_speaker.py b/spaces/Miuzarte/SUI-svc-3.0/add_speaker.py
deleted file mode 100644
index fb6013dd8542efd62915ebdd445012ae7a4bdc28..0000000000000000000000000000000000000000
--- a/spaces/Miuzarte/SUI-svc-3.0/add_speaker.py
+++ /dev/null
@@ -1,62 +0,0 @@
-import os
-import argparse
-from tqdm import tqdm
-from random import shuffle
-import json
-
-
-if __name__ == "__main__":
- parser = argparse.ArgumentParser()
- parser.add_argument("--train_list", type=str, default="./filelists/train.txt", help="path to train list")
- parser.add_argument("--val_list", type=str, default="./filelists/val.txt", help="path to val list")
- parser.add_argument("--test_list", type=str, default="./filelists/test.txt", help="path to test list")
- parser.add_argument("--source_dir", type=str, default="./dataset/48k", help="path to source dir")
- args = parser.parse_args()
-
- previous_config = json.load(open("configs/config.json", "rb"))
-
- train = []
- val = []
- test = []
- idx = 0
- spk_dict = previous_config["spk"]
- spk_id = max([i for i in spk_dict.values()]) + 1
- for speaker in tqdm(os.listdir(args.source_dir)):
- if speaker not in spk_dict.keys():
- spk_dict[speaker] = spk_id
- spk_id += 1
- wavs = [os.path.join(args.source_dir, speaker, i)for i in os.listdir(os.path.join(args.source_dir, speaker))]
- wavs = [i for i in wavs if i.endswith("wav")]
- shuffle(wavs)
- train += wavs[2:-10]
- val += wavs[:2]
- test += wavs[-10:]
-
- assert previous_config["model"]["n_speakers"] > len(spk_dict.keys())
- shuffle(train)
- shuffle(val)
- shuffle(test)
-
- print("Writing", args.train_list)
- with open(args.train_list, "w") as f:
- for fname in tqdm(train):
- wavpath = fname
- f.write(wavpath + "\n")
-
- print("Writing", args.val_list)
- with open(args.val_list, "w") as f:
- for fname in tqdm(val):
- wavpath = fname
- f.write(wavpath + "\n")
-
- print("Writing", args.test_list)
- with open(args.test_list, "w") as f:
- for fname in tqdm(test):
- wavpath = fname
- f.write(wavpath + "\n")
-
- previous_config["spk"] = spk_dict
-
- print("Writing configs/config.json")
- with open("configs/config.json", "w") as f:
- json.dump(previous_config, f, indent=2)
diff --git a/spaces/MrD05/text-generation-webui-space/modules/shared.py b/spaces/MrD05/text-generation-webui-space/modules/shared.py
deleted file mode 100644
index ea2eb50b7f586e5c562bf2e7c75429c91f21ec6c..0000000000000000000000000000000000000000
--- a/spaces/MrD05/text-generation-webui-space/modules/shared.py
+++ /dev/null
@@ -1,103 +0,0 @@
-import argparse
-
-model = None
-tokenizer = None
-model_name = ""
-soft_prompt_tensor = None
-soft_prompt = False
-is_RWKV = False
-
-# Chat variables
-history = {'internal': [], 'visible': []}
-character = 'None'
-stop_everything = False
-processing_message = '*Is typing...*'
-
-# UI elements (buttons, sliders, HTML, etc)
-gradio = {}
-
-# Generation input parameters
-input_params = []
-
-settings = {
- 'max_new_tokens': 200,
- 'max_new_tokens_min': 1,
- 'max_new_tokens_max': 2000,
- 'name1': 'Person 1',
- 'name2': 'Person 2',
- 'context': 'This is a conversation between two people.',
- 'stop_at_newline': True,
- 'chat_prompt_size': 2048,
- 'chat_prompt_size_min': 0,
- 'chat_prompt_size_max': 2048,
- 'chat_generation_attempts': 1,
- 'chat_generation_attempts_min': 1,
- 'chat_generation_attempts_max': 5,
- 'name1_pygmalion': 'You',
- 'name2_pygmalion': 'Kawaii',
- 'context_pygmalion': "Kawaii's persona: Kawaii is a cheerful person who loves to make others smile. She is an optimist who loves to spread happiness and positivity wherever she goes.\n",
- 'stop_at_newline_pygmalion': False,
- 'default_extensions': [],
- 'chat_default_extensions': ["gallery"],
- 'presets': {
- 'default': 'NovelAI-Sphinx Moth',
- 'pygmalion-*': 'Pygmalion',
- 'RWKV-*': 'Naive',
- },
- 'prompts': {
- 'default': 'Common sense questions and answers\n\nQuestion: \nFactual answer:',
- '^(gpt4chan|gpt-4chan|4chan)': '-----\n--- 865467536\nInput text\n--- 865467537\n',
- '(rosey|chip|joi)_.*_instruct.*': 'User: \n',
- 'oasst-*': '<|prompter|>Write a story about future of AI development<|endoftext|><|assistant|>'
- }
-}
-
-def str2bool(v):
- if isinstance(v, bool):
- return v
- if v.lower() in ('yes', 'true', 't', 'y', '1'):
- return True
- elif v.lower() in ('no', 'false', 'f', 'n', '0'):
- return False
- else:
- raise argparse.ArgumentTypeError('Boolean value expected.')
-
-parser = argparse.ArgumentParser(formatter_class=lambda prog: argparse.HelpFormatter(prog,max_help_position=54))
-parser.add_argument('--model', type=str, help='Name of the model to load by default.')
-parser.add_argument('--notebook', action='store_true', help='Launch the web UI in notebook mode, where the output is written to the same text box as the input.')
-parser.add_argument('--chat', action='store_true', help='Launch the web UI in chat mode.')
-parser.add_argument('--cai-chat', action='store_true', help='Launch the web UI in chat mode with a style similar to Character.AI\'s. If the file img_bot.png or img_bot.jpg exists in the same folder as server.py, this image will be used as the bot\'s profile picture. Similarly, img_me.png or img_me.jpg will be used as your profile picture.')
-parser.add_argument('--cpu', action='store_true', help='Use the CPU to generate text.')
-parser.add_argument('--load-in-8bit', action='store_true', help='Load the model with 8-bit precision.')
-parser.add_argument('--load-in-4bit', action='store_true', help='DEPRECATED: use --gptq-bits 4 instead.')
-parser.add_argument('--gptq-bits', type=int, default=0, help='Load a pre-quantized model with specified precision. 2, 3, 4 and 8bit are supported. Currently only works with LLaMA and OPT.')
-parser.add_argument('--gptq-model-type', type=str, help='Model type of pre-quantized model. Currently only LLaMa and OPT are supported.')
-parser.add_argument('--bf16', action='store_true', help='Load the model with bfloat16 precision. Requires NVIDIA Ampere GPU.')
-parser.add_argument('--auto-devices', action='store_true', help='Automatically split the model across the available GPU(s) and CPU.')
-parser.add_argument('--disk', action='store_true', help='If the model is too large for your GPU(s) and CPU combined, send the remaining layers to the disk.')
-parser.add_argument('--disk-cache-dir', type=str, default="cache", help='Directory to save the disk cache to. Defaults to "cache".')
-parser.add_argument('--gpu-memory', type=int, nargs="+", help='Maxmimum GPU memory in GiB to be allocated per GPU. Example: --gpu-memory 10 for a single GPU, --gpu-memory 10 5 for two GPUs.')
-parser.add_argument('--cpu-memory', type=int, help='Maximum CPU memory in GiB to allocate for offloaded weights. Must be an integer number. Defaults to 99.')
-parser.add_argument('--flexgen', action='store_true', help='Enable the use of FlexGen offloading.')
-parser.add_argument('--percent', type=int, nargs="+", default=[0, 100, 100, 0, 100, 0], help='FlexGen: allocation percentages. Must be 6 numbers separated by spaces (default: 0, 100, 100, 0, 100, 0).')
-parser.add_argument("--compress-weight", action="store_true", help="FlexGen: activate weight compression.")
-parser.add_argument("--pin-weight", type=str2bool, nargs="?", const=True, default=True, help="FlexGen: whether to pin weights (setting this to False reduces CPU memory by 20%%).")
-parser.add_argument('--deepspeed', action='store_true', help='Enable the use of DeepSpeed ZeRO-3 for inference via the Transformers integration.')
-parser.add_argument('--nvme-offload-dir', type=str, help='DeepSpeed: Directory to use for ZeRO-3 NVME offloading.')
-parser.add_argument('--local_rank', type=int, default=0, help='DeepSpeed: Optional argument for distributed setups.')
-parser.add_argument('--rwkv-strategy', type=str, default=None, help='RWKV: The strategy to use while loading the model. Examples: "cpu fp32", "cuda fp16", "cuda fp16i8".')
-parser.add_argument('--rwkv-cuda-on', action='store_true', help='RWKV: Compile the CUDA kernel for better performance.')
-parser.add_argument('--no-stream', action='store_true', help='Don\'t stream the text output in real time.')
-parser.add_argument('--settings', type=str, help='Load the default interface settings from this json file. See settings-template.json for an example. If you create a file called settings.json, this file will be loaded by default without the need to use the --settings flag.')
-parser.add_argument('--extensions', type=str, nargs="+", help='The list of extensions to load. If you want to load more than one extension, write the names separated by spaces.')
-parser.add_argument('--listen', action='store_true', help='Make the web UI reachable from your local network.')
-parser.add_argument('--listen-port', type=int, help='The listening port that the server will use.')
-parser.add_argument('--share', action='store_true', help='Create a public URL. This is useful for running the web UI on Google Colab or similar.')
-parser.add_argument('--auto-launch', action='store_true', default=False, help='Open the web UI in the default browser upon launch.')
-parser.add_argument('--verbose', action='store_true', help='Print the prompts to the terminal.')
-args = parser.parse_args()
-
-# Provisional, this will be deleted later
-if args.load_in_4bit:
- print("Warning: --load-in-4bit is deprecated and will be removed. Use --gptq-bits 4 instead.\n")
- args.gptq_bits = 4
diff --git a/spaces/Norod78/WoWQuestTextGenerator/README.md b/spaces/Norod78/WoWQuestTextGenerator/README.md
deleted file mode 100644
index 08fab4e9dfa9356425019755175637a08df5e760..0000000000000000000000000000000000000000
--- a/spaces/Norod78/WoWQuestTextGenerator/README.md
+++ /dev/null
@@ -1,13 +0,0 @@
----
-title: WoW Quest Generator
-emoji: 🧝♀️
-colorFrom: green
-colorTo: orange
-sdk: gradio
-sdk_version: 3.1.7
-app_file: app.py
-pinned: false
-license: cc-by-nc-4.0
----
-
-Check out the configuration reference at https://huggingface.co/docs/hub/spaces-config-reference
diff --git a/spaces/OFA-Sys/OFA-Generic_Interface/fairseq/fairseq/clib/libnat_cuda/binding.cpp b/spaces/OFA-Sys/OFA-Generic_Interface/fairseq/fairseq/clib/libnat_cuda/binding.cpp
deleted file mode 100644
index ced91c0d0afab9071842911d9876e6360d90284a..0000000000000000000000000000000000000000
--- a/spaces/OFA-Sys/OFA-Generic_Interface/fairseq/fairseq/clib/libnat_cuda/binding.cpp
+++ /dev/null
@@ -1,67 +0,0 @@
-/**
- * Copyright 2017-present, Facebook, Inc.
- * All rights reserved.
- *
- * This source code is licensed under the license found in the
- * LICENSE file in the root directory of this source tree.
- */
-
-/*
- This code is partially adpoted from
- https://github.com/1ytic/pytorch-edit-distance
- */
-
-#include
-#include "edit_dist.h"
-
-#ifndef TORCH_CHECK
-#define TORCH_CHECK AT_CHECK
-#endif
-
-#define CHECK_CUDA(x) \
- TORCH_CHECK(x.type().is_cuda(), #x " must be a CUDA tensor")
-#define CHECK_CONTIGUOUS(x) \
- TORCH_CHECK(x.is_contiguous(), #x " must be contiguous")
-#define CHECK_INPUT(x) \
- CHECK_CUDA(x); \
- CHECK_CONTIGUOUS(x)
-
-torch::Tensor LevenshteinDistance(
- torch::Tensor source,
- torch::Tensor target,
- torch::Tensor source_length,
- torch::Tensor target_length) {
- CHECK_INPUT(source);
- CHECK_INPUT(target);
- CHECK_INPUT(source_length);
- CHECK_INPUT(target_length);
- return LevenshteinDistanceCuda(source, target, source_length, target_length);
-}
-
-torch::Tensor GenerateDeletionLabel(
- torch::Tensor source,
- torch::Tensor operations) {
- CHECK_INPUT(source);
- CHECK_INPUT(operations);
- return GenerateDeletionLabelCuda(source, operations);
-}
-
-std::pair GenerateInsertionLabel(
- torch::Tensor target,
- torch::Tensor operations) {
- CHECK_INPUT(target);
- CHECK_INPUT(operations);
- return GenerateInsertionLabelCuda(target, operations);
-}
-
-PYBIND11_MODULE(TORCH_EXTENSION_NAME, m) {
- m.def("levenshtein_distance", &LevenshteinDistance, "Levenshtein distance");
- m.def(
- "generate_deletion_labels",
- &GenerateDeletionLabel,
- "Generate Deletion Label");
- m.def(
- "generate_insertion_labels",
- &GenerateInsertionLabel,
- "Generate Insertion Label");
-}
diff --git a/spaces/OFA-Sys/OFA-Generic_Interface/fairseq/fairseq/criterions/model_criterion.py b/spaces/OFA-Sys/OFA-Generic_Interface/fairseq/fairseq/criterions/model_criterion.py
deleted file mode 100644
index 30350f13b1c00498de6784579250d6b342ced7dd..0000000000000000000000000000000000000000
--- a/spaces/OFA-Sys/OFA-Generic_Interface/fairseq/fairseq/criterions/model_criterion.py
+++ /dev/null
@@ -1,138 +0,0 @@
-# Copyright (c) Facebook, Inc. and its affiliates.
-#
-# This source code is licensed under the MIT license found in the
-# LICENSE file in the root directory of this source tree.
-
-import logging
-from dataclasses import dataclass, field
-from typing import Dict, List
-
-from fairseq import metrics, utils
-from fairseq.criterions import FairseqCriterion, register_criterion
-from fairseq.dataclass import FairseqDataclass
-
-
-logger = logging.getLogger(__name__)
-
-
-@dataclass
-class ModelCriterionConfig(FairseqDataclass):
- loss_weights: Dict[str, float] = field(
- default_factory=dict,
- metadata={"help": "weights for the loss terms"},
- )
- log_keys: List[str] = field(
- default_factory=list,
- metadata={"help": "additional output keys to log"},
- )
-
-
-@register_criterion("model", dataclass=ModelCriterionConfig)
-class ModelCriterion(FairseqCriterion):
- """
- This criterion relies on the model to supply losses.
- The losses should be a dictionary of name -> scalar returned by
- the model either by including it in the net_output dict or by
- implementing a get_losses(net_output, sample) method. The final loss is
- a scaled sum of all losses according to weights in loss_weights.
- If no weights are provided, then all losses are scaled by 1.0.
-
- The losses will be automatically logged. Additional keys from
- net_output dict can be logged via the log_keys parameter.
- """
-
- def __init__(self, task, loss_weights=None, log_keys=None):
- super().__init__(task)
- self.loss_weights = loss_weights
- self.log_keys = log_keys
-
- def forward(self, model, sample, reduce=True):
- net_output = model(**sample["net_input"])
-
- sample_size = net_output["sample_size"]
- scaled_losses = {}
-
- if hasattr(model, "get_losses"):
- losses = model.get_losses(net_output, sample)
- elif isinstance(net_output, dict) and "losses" in net_output:
- losses = net_output["losses"]
- else:
- raise Exception("Could not retrieve losses")
-
- for lk, p in losses.items():
- try:
- coef = 1.0 if len(self.loss_weights) == 0 else self.loss_weights[lk]
- except KeyError:
- logger.error(
- f"weight for loss {lk} is not in loss_weights ({self.loss_weights})"
- )
- raise
- if coef != 0 and p is not None:
- scaled_losses[lk] = coef * p.float()
-
- loss = sum(scaled_losses.values())
- if reduce and loss.numel() > 1:
- loss = loss.sum()
-
- logging_output = {
- "loss": loss.data,
- "ntokens": sample_size,
- "nsentences": sample["id"].numel(),
- "sample_size": sample_size,
- "_world_size": 1,
- }
-
- for lk in self.log_keys:
- if lk in net_output and net_output[lk] is not None:
- logging_output[lk] = float(net_output[lk])
-
- if len(scaled_losses) > 1:
- for lk, l in scaled_losses.items():
- logging_output[f"loss_{lk}"] = l.item()
-
- return loss, sample_size, logging_output
-
- @staticmethod
- def reduce_metrics(logging_outputs) -> None:
- """Aggregate logging outputs from data parallel training."""
- loss_sum = utils.item(sum(log.get("loss", 0) for log in logging_outputs))
- ntokens = utils.item(sum(log.get("ntokens", 0) for log in logging_outputs))
- nsentences = utils.item(
- sum(log.get("nsentences", 0) for log in logging_outputs)
- )
- sample_size = utils.item(
- sum(log.get("sample_size", 0) for log in logging_outputs)
- )
-
- metrics.log_scalar("loss", loss_sum / sample_size, sample_size, round=3)
- metrics.log_scalar("ntokens", ntokens)
- metrics.log_scalar("nsentences", nsentences)
-
- builtin_keys = {
- "loss",
- "ntokens",
- "nsentences",
- "sample_size",
- "_world_size",
- }
-
- world_size = utils.item(
- sum(log.get("_world_size", 0) for log in logging_outputs)
- )
-
- for k in logging_outputs[0]:
- if k not in builtin_keys:
- val = sum(log.get(k, 0) for log in logging_outputs)
- if k.startswith("loss_"):
- metrics.log_scalar(k, val / sample_size, sample_size, round=3)
- else:
- metrics.log_scalar(k, val / world_size, round=3)
-
- @staticmethod
- def logging_outputs_can_be_summed() -> bool:
- """
- Whether the logging outputs returned by `forward` can be summed
- across workers prior to calling `reduce_metrics`. Setting this
- to True will improves distributed training speed.
- """
- return True
diff --git a/spaces/OFA-Sys/OFA-Generic_Interface/fairseq/fairseq/tasks/frm_text_to_speech.py b/spaces/OFA-Sys/OFA-Generic_Interface/fairseq/fairseq/tasks/frm_text_to_speech.py
deleted file mode 100644
index 1fa9b0f83e742aefce764e2858a81f99db911afd..0000000000000000000000000000000000000000
--- a/spaces/OFA-Sys/OFA-Generic_Interface/fairseq/fairseq/tasks/frm_text_to_speech.py
+++ /dev/null
@@ -1,56 +0,0 @@
-# Copyright (c) Facebook, Inc. and its affiliates.
-#
-# This source code is licensed under the MIT license found in the
-# LICENSE file in the root directory of this source tree.
-
-import logging
-
-from fairseq.data.audio.frm_text_to_speech_dataset import FrmTextToSpeechDatasetCreator
-from fairseq.tasks import register_task
-from fairseq.tasks.text_to_speech import TextToSpeechTask
-
-
-logging.basicConfig(
- format='%(asctime)s | %(levelname)s | %(name)s | %(message)s',
- datefmt='%Y-%m-%d %H:%M:%S', level=logging.INFO
-)
-logger = logging.getLogger(__name__)
-
-
-@register_task('frm_text_to_speech')
-class FrmTextToSpeechTask(TextToSpeechTask):
- @staticmethod
- def add_args(parser):
- TextToSpeechTask.add_args(parser)
- parser.add_argument(
- "--do_chunk", action="store_true", help="train on chunks"
- )
- parser.add_argument("--chunk_bound", default=-1, type=int)
- parser.add_argument("--chunk_init", default=50, type=int)
- parser.add_argument("--chunk_incr", default=5, type=int)
- parser.add_argument("--add_eos", action="store_true")
- parser.add_argument("--dedup", action="store_true")
- parser.add_argument("--ref_fpu", default=-1, type=float)
-
- def load_dataset(self, split, **unused_kwargs):
- is_train_split = split.startswith("train")
- pre_tokenizer = self.build_tokenizer(self.args)
- bpe_tokenizer = self.build_bpe(self.args)
- self.datasets[split] = FrmTextToSpeechDatasetCreator.from_tsv(
- self.args.data,
- self.data_cfg,
- split,
- self.src_dict,
- pre_tokenizer,
- bpe_tokenizer,
- is_train_split=is_train_split,
- n_frames_per_step=self.args.n_frames_per_step,
- speaker_to_id=self.speaker_to_id,
- do_chunk=self.args.do_chunk,
- chunk_bound=self.args.chunk_bound,
- chunk_init=self.args.chunk_init,
- chunk_incr=self.args.chunk_incr,
- add_eos=self.args.add_eos,
- dedup=self.args.dedup,
- ref_fpu=self.args.ref_fpu
- )
diff --git a/spaces/OFA-Sys/OFA-Image_Caption/fairseq/fairseq/modules/transformer_sentence_encoder_layer.py b/spaces/OFA-Sys/OFA-Image_Caption/fairseq/fairseq/modules/transformer_sentence_encoder_layer.py
deleted file mode 100644
index f869c4b2f8fb15f96a292e39bd293df7898a4fce..0000000000000000000000000000000000000000
--- a/spaces/OFA-Sys/OFA-Image_Caption/fairseq/fairseq/modules/transformer_sentence_encoder_layer.py
+++ /dev/null
@@ -1,139 +0,0 @@
-# Copyright (c) Facebook, Inc. and its affiliates.
-#
-# This source code is licensed under the MIT license found in the
-# LICENSE file in the root directory of this source tree.
-
-from typing import Callable, Optional
-
-import torch
-import torch.nn as nn
-from fairseq import utils
-from fairseq.modules import LayerNorm, MultiheadAttention
-from fairseq.modules.fairseq_dropout import FairseqDropout
-from fairseq.modules.quant_noise import quant_noise
-
-
-class TransformerSentenceEncoderLayer(nn.Module):
- """
- Implements a Transformer Encoder Layer used in BERT/XLM style pre-trained
- models.
- """
-
- def __init__(
- self,
- embedding_dim: int = 768,
- ffn_embedding_dim: int = 3072,
- num_attention_heads: int = 8,
- dropout: float = 0.1,
- attention_dropout: float = 0.1,
- activation_dropout: float = 0.1,
- activation_fn: str = "relu",
- export: bool = False,
- q_noise: float = 0.0,
- qn_block_size: int = 8,
- init_fn: Callable = None,
- ) -> None:
- super().__init__()
-
- if init_fn is not None:
- init_fn()
-
- # Initialize parameters
- self.embedding_dim = embedding_dim
- self.num_attention_heads = num_attention_heads
- self.attention_dropout = attention_dropout
- self.q_noise = q_noise
- self.qn_block_size = qn_block_size
-
- self.dropout_module = FairseqDropout(
- dropout, module_name=self.__class__.__name__
- )
- self.activation_dropout_module = FairseqDropout(
- activation_dropout, module_name=self.__class__.__name__
- )
-
- # Initialize blocks
- self.activation_fn = utils.get_activation_fn(activation_fn)
- self.self_attn = self.build_self_attention(
- self.embedding_dim,
- num_attention_heads,
- dropout=attention_dropout,
- self_attention=True,
- q_noise=q_noise,
- qn_block_size=qn_block_size,
- )
-
- # layer norm associated with the self attention layer
- self.self_attn_layer_norm = LayerNorm(self.embedding_dim, export=export)
-
- self.fc1 = self.build_fc1(
- self.embedding_dim,
- ffn_embedding_dim,
- q_noise=q_noise,
- qn_block_size=qn_block_size,
- )
- self.fc2 = self.build_fc2(
- ffn_embedding_dim,
- self.embedding_dim,
- q_noise=q_noise,
- qn_block_size=qn_block_size,
- )
-
- # layer norm associated with the position wise feed-forward NN
- self.final_layer_norm = LayerNorm(self.embedding_dim, export=export)
-
- def build_fc1(self, input_dim, output_dim, q_noise, qn_block_size):
- return quant_noise(nn.Linear(input_dim, output_dim), q_noise, qn_block_size)
-
- def build_fc2(self, input_dim, output_dim, q_noise, qn_block_size):
- return quant_noise(nn.Linear(input_dim, output_dim), q_noise, qn_block_size)
-
- def build_self_attention(
- self,
- embed_dim,
- num_attention_heads,
- dropout,
- self_attention,
- q_noise,
- qn_block_size,
- ):
- return MultiheadAttention(
- embed_dim,
- num_attention_heads,
- dropout=dropout,
- self_attention=True,
- q_noise=q_noise,
- qn_block_size=qn_block_size,
- )
-
- def forward(
- self,
- x: torch.Tensor,
- self_attn_mask: Optional[torch.Tensor] = None,
- self_attn_padding_mask: Optional[torch.Tensor] = None,
- ):
- """
- LayerNorm is applied either before or after the self-attention/ffn
- modules similar to the original Transformer implementation.
- """
- residual = x
- x, attn = self.self_attn(
- query=x,
- key=x,
- value=x,
- key_padding_mask=self_attn_padding_mask,
- need_weights=False,
- attn_mask=self_attn_mask,
- )
- x = self.dropout_module(x)
- x = residual + x
- x = self.self_attn_layer_norm(x)
-
- residual = x
- x = self.activation_fn(self.fc1(x))
- x = self.activation_dropout_module(x)
- x = self.fc2(x)
- x = self.dropout_module(x)
- x = residual + x
- x = self.final_layer_norm(x)
- return x, attn
diff --git a/spaces/OFA-Sys/OFA-vqa/fairseq/examples/roberta/multiprocessing_bpe_encoder.py b/spaces/OFA-Sys/OFA-vqa/fairseq/examples/roberta/multiprocessing_bpe_encoder.py
deleted file mode 100644
index 43fe0451bf4d5762d734314075b1402c2a8db2bb..0000000000000000000000000000000000000000
--- a/spaces/OFA-Sys/OFA-vqa/fairseq/examples/roberta/multiprocessing_bpe_encoder.py
+++ /dev/null
@@ -1,130 +0,0 @@
-#!/usr/bin/env python
-# Copyright (c) Facebook, Inc. and its affiliates.
-# All rights reserved.
-#
-# This source code is licensed under the license found in the
-# LICENSE file in the root directory of this source tree.
-
-import argparse
-import contextlib
-import sys
-from collections import Counter
-from multiprocessing import Pool
-
-from fairseq.data.encoders.gpt2_bpe import get_encoder
-
-
-def main():
- """
- Helper script to encode raw text with the GPT-2 BPE using multiple processes.
-
- The encoder.json and vocab.bpe files can be obtained here:
- - https://dl.fbaipublicfiles.com/fairseq/gpt2_bpe/encoder.json
- - https://dl.fbaipublicfiles.com/fairseq/gpt2_bpe/vocab.bpe
- """
- parser = argparse.ArgumentParser()
- parser.add_argument(
- "--encoder-json",
- help="path to encoder.json",
- )
- parser.add_argument(
- "--vocab-bpe",
- type=str,
- help="path to vocab.bpe",
- )
- parser.add_argument(
- "--inputs",
- nargs="+",
- default=["-"],
- help="input files to filter/encode",
- )
- parser.add_argument(
- "--outputs",
- nargs="+",
- default=["-"],
- help="path to save encoded outputs",
- )
- parser.add_argument(
- "--keep-empty",
- action="store_true",
- help="keep empty lines",
- )
- parser.add_argument("--workers", type=int, default=20)
- args = parser.parse_args()
-
- assert len(args.inputs) == len(
- args.outputs
- ), "number of input and output paths should match"
-
- with contextlib.ExitStack() as stack:
- inputs = [
- stack.enter_context(open(input, "r", encoding="utf-8"))
- if input != "-"
- else sys.stdin
- for input in args.inputs
- ]
- outputs = [
- stack.enter_context(open(output, "w", encoding="utf-8"))
- if output != "-"
- else sys.stdout
- for output in args.outputs
- ]
-
- encoder = MultiprocessingEncoder(args)
- pool = Pool(args.workers, initializer=encoder.initializer)
- encoded_lines = pool.imap(encoder.encode_lines, zip(*inputs), 100)
-
- stats = Counter()
- for i, (filt, enc_lines) in enumerate(encoded_lines, start=1):
- if filt == "PASS":
- for enc_line, output_h in zip(enc_lines, outputs):
- print(enc_line, file=output_h)
- else:
- stats["num_filtered_" + filt] += 1
- if i % 10000 == 0:
- print("processed {} lines".format(i), file=sys.stderr)
-
- for k, v in stats.most_common():
- print("[{}] filtered {} lines".format(k, v), file=sys.stderr)
-
-
-class MultiprocessingEncoder(object):
- def __init__(self, args):
- self.args = args
-
- def initializer(self):
- global bpe
- bpe = get_encoder(self.args.encoder_json, self.args.vocab_bpe)
-
- def encode(self, line):
- global bpe
- ids = bpe.encode(line)
- return list(map(str, ids))
-
- def decode(self, tokens):
- global bpe
- return bpe.decode(tokens)
-
- def encode_lines(self, lines):
- """
- Encode a set of lines. All lines will be encoded together.
- """
- enc_lines = []
- for line in lines:
- line = line.strip()
- if len(line) == 0 and not self.args.keep_empty:
- return ["EMPTY", None]
- tokens = self.encode(line)
- enc_lines.append(" ".join(tokens))
- return ["PASS", enc_lines]
-
- def decode_lines(self, lines):
- dec_lines = []
- for line in lines:
- tokens = map(int, line.strip().split())
- dec_lines.append(self.decode(tokens))
- return ["PASS", dec_lines]
-
-
-if __name__ == "__main__":
- main()
diff --git a/spaces/OpenGVLab/InternGPT/iGPT/models/grit_src/third_party/CenterNet2/detectron2/data/datasets/lvis_v0_5_categories.py b/spaces/OpenGVLab/InternGPT/iGPT/models/grit_src/third_party/CenterNet2/detectron2/data/datasets/lvis_v0_5_categories.py
deleted file mode 100644
index d3dab6198da614937b08682f4c9edf52bdf1d236..0000000000000000000000000000000000000000
--- a/spaces/OpenGVLab/InternGPT/iGPT/models/grit_src/third_party/CenterNet2/detectron2/data/datasets/lvis_v0_5_categories.py
+++ /dev/null
@@ -1,13 +0,0 @@
-# Copyright (c) Facebook, Inc. and its affiliates.
-# Autogen with
-# with open("lvis_v0.5_val.json", "r") as f:
-# a = json.load(f)
-# c = a["categories"]
-# for x in c:
-# del x["image_count"]
-# del x["instance_count"]
-# LVIS_CATEGORIES = repr(c) + " # noqa"
-
-# fmt: off
-LVIS_CATEGORIES = [{'frequency': 'r', 'id': 1, 'synset': 'acorn.n.01', 'synonyms': ['acorn'], 'def': 'nut from an oak tree', 'name': 'acorn'}, {'frequency': 'c', 'id': 2, 'synset': 'aerosol.n.02', 'synonyms': ['aerosol_can', 'spray_can'], 'def': 'a dispenser that holds a substance under pressure', 'name': 'aerosol_can'}, {'frequency': 'f', 'id': 3, 'synset': 'air_conditioner.n.01', 'synonyms': ['air_conditioner'], 'def': 'a machine that keeps air cool and dry', 'name': 'air_conditioner'}, {'frequency': 'f', 'id': 4, 'synset': 'airplane.n.01', 'synonyms': ['airplane', 'aeroplane'], 'def': 'an aircraft that has a fixed wing and is powered by propellers or jets', 'name': 'airplane'}, {'frequency': 'c', 'id': 5, 'synset': 'alarm_clock.n.01', 'synonyms': ['alarm_clock'], 'def': 'a clock that wakes a sleeper at some preset time', 'name': 'alarm_clock'}, {'frequency': 'c', 'id': 6, 'synset': 'alcohol.n.01', 'synonyms': ['alcohol', 'alcoholic_beverage'], 'def': 'a liquor or brew containing alcohol as the active agent', 'name': 'alcohol'}, {'frequency': 'r', 'id': 7, 'synset': 'alligator.n.02', 'synonyms': ['alligator', 'gator'], 'def': 'amphibious reptiles related to crocodiles but with shorter broader snouts', 'name': 'alligator'}, {'frequency': 'c', 'id': 8, 'synset': 'almond.n.02', 'synonyms': ['almond'], 'def': 'oval-shaped edible seed of the almond tree', 'name': 'almond'}, {'frequency': 'c', 'id': 9, 'synset': 'ambulance.n.01', 'synonyms': ['ambulance'], 'def': 'a vehicle that takes people to and from hospitals', 'name': 'ambulance'}, {'frequency': 'r', 'id': 10, 'synset': 'amplifier.n.01', 'synonyms': ['amplifier'], 'def': 'electronic equipment that increases strength of signals', 'name': 'amplifier'}, {'frequency': 'c', 'id': 11, 'synset': 'anklet.n.03', 'synonyms': ['anklet', 'ankle_bracelet'], 'def': 'an ornament worn around the ankle', 'name': 'anklet'}, {'frequency': 'f', 'id': 12, 'synset': 'antenna.n.01', 'synonyms': ['antenna', 'aerial', 'transmitting_aerial'], 'def': 'an electrical device that sends or receives radio or television signals', 'name': 'antenna'}, {'frequency': 'f', 'id': 13, 'synset': 'apple.n.01', 'synonyms': ['apple'], 'def': 'fruit with red or yellow or green skin and sweet to tart crisp whitish flesh', 'name': 'apple'}, {'frequency': 'r', 'id': 14, 'synset': 'apple_juice.n.01', 'synonyms': ['apple_juice'], 'def': 'the juice of apples', 'name': 'apple_juice'}, {'frequency': 'r', 'id': 15, 'synset': 'applesauce.n.01', 'synonyms': ['applesauce'], 'def': 'puree of stewed apples usually sweetened and spiced', 'name': 'applesauce'}, {'frequency': 'r', 'id': 16, 'synset': 'apricot.n.02', 'synonyms': ['apricot'], 'def': 'downy yellow to rosy-colored fruit resembling a small peach', 'name': 'apricot'}, {'frequency': 'f', 'id': 17, 'synset': 'apron.n.01', 'synonyms': ['apron'], 'def': 'a garment of cloth that is tied about the waist and worn to protect clothing', 'name': 'apron'}, {'frequency': 'c', 'id': 18, 'synset': 'aquarium.n.01', 'synonyms': ['aquarium', 'fish_tank'], 'def': 'a tank/pool/bowl filled with water for keeping live fish and underwater animals', 'name': 'aquarium'}, {'frequency': 'c', 'id': 19, 'synset': 'armband.n.02', 'synonyms': ['armband'], 'def': 'a band worn around the upper arm', 'name': 'armband'}, {'frequency': 'f', 'id': 20, 'synset': 'armchair.n.01', 'synonyms': ['armchair'], 'def': 'chair with a support on each side for arms', 'name': 'armchair'}, {'frequency': 'r', 'id': 21, 'synset': 'armoire.n.01', 'synonyms': ['armoire'], 'def': 'a large wardrobe or cabinet', 'name': 'armoire'}, {'frequency': 'r', 'id': 22, 'synset': 'armor.n.01', 'synonyms': ['armor', 'armour'], 'def': 'protective covering made of metal and used in combat', 'name': 'armor'}, {'frequency': 'c', 'id': 23, 'synset': 'artichoke.n.02', 'synonyms': ['artichoke'], 'def': 'a thistlelike flower head with edible fleshy leaves and heart', 'name': 'artichoke'}, {'frequency': 'f', 'id': 24, 'synset': 'ashcan.n.01', 'synonyms': ['trash_can', 'garbage_can', 'wastebin', 'dustbin', 'trash_barrel', 'trash_bin'], 'def': 'a bin that holds rubbish until it is collected', 'name': 'trash_can'}, {'frequency': 'c', 'id': 25, 'synset': 'ashtray.n.01', 'synonyms': ['ashtray'], 'def': "a receptacle for the ash from smokers' cigars or cigarettes", 'name': 'ashtray'}, {'frequency': 'c', 'id': 26, 'synset': 'asparagus.n.02', 'synonyms': ['asparagus'], 'def': 'edible young shoots of the asparagus plant', 'name': 'asparagus'}, {'frequency': 'c', 'id': 27, 'synset': 'atomizer.n.01', 'synonyms': ['atomizer', 'atomiser', 'spray', 'sprayer', 'nebulizer', 'nebuliser'], 'def': 'a dispenser that turns a liquid (such as perfume) into a fine mist', 'name': 'atomizer'}, {'frequency': 'c', 'id': 28, 'synset': 'avocado.n.01', 'synonyms': ['avocado'], 'def': 'a pear-shaped fruit with green or blackish skin and rich yellowish pulp enclosing a single large seed', 'name': 'avocado'}, {'frequency': 'c', 'id': 29, 'synset': 'award.n.02', 'synonyms': ['award', 'accolade'], 'def': 'a tangible symbol signifying approval or distinction', 'name': 'award'}, {'frequency': 'f', 'id': 30, 'synset': 'awning.n.01', 'synonyms': ['awning'], 'def': 'a canopy made of canvas to shelter people or things from rain or sun', 'name': 'awning'}, {'frequency': 'r', 'id': 31, 'synset': 'ax.n.01', 'synonyms': ['ax', 'axe'], 'def': 'an edge tool with a heavy bladed head mounted across a handle', 'name': 'ax'}, {'frequency': 'f', 'id': 32, 'synset': 'baby_buggy.n.01', 'synonyms': ['baby_buggy', 'baby_carriage', 'perambulator', 'pram', 'stroller'], 'def': 'a small vehicle with four wheels in which a baby or child is pushed around', 'name': 'baby_buggy'}, {'frequency': 'c', 'id': 33, 'synset': 'backboard.n.01', 'synonyms': ['basketball_backboard'], 'def': 'a raised vertical board with basket attached; used to play basketball', 'name': 'basketball_backboard'}, {'frequency': 'f', 'id': 34, 'synset': 'backpack.n.01', 'synonyms': ['backpack', 'knapsack', 'packsack', 'rucksack', 'haversack'], 'def': 'a bag carried by a strap on your back or shoulder', 'name': 'backpack'}, {'frequency': 'f', 'id': 35, 'synset': 'bag.n.04', 'synonyms': ['handbag', 'purse', 'pocketbook'], 'def': 'a container used for carrying money and small personal items or accessories', 'name': 'handbag'}, {'frequency': 'f', 'id': 36, 'synset': 'bag.n.06', 'synonyms': ['suitcase', 'baggage', 'luggage'], 'def': 'cases used to carry belongings when traveling', 'name': 'suitcase'}, {'frequency': 'c', 'id': 37, 'synset': 'bagel.n.01', 'synonyms': ['bagel', 'beigel'], 'def': 'glazed yeast-raised doughnut-shaped roll with hard crust', 'name': 'bagel'}, {'frequency': 'r', 'id': 38, 'synset': 'bagpipe.n.01', 'synonyms': ['bagpipe'], 'def': 'a tubular wind instrument; the player blows air into a bag and squeezes it out', 'name': 'bagpipe'}, {'frequency': 'r', 'id': 39, 'synset': 'baguet.n.01', 'synonyms': ['baguet', 'baguette'], 'def': 'narrow French stick loaf', 'name': 'baguet'}, {'frequency': 'r', 'id': 40, 'synset': 'bait.n.02', 'synonyms': ['bait', 'lure'], 'def': 'something used to lure fish or other animals into danger so they can be trapped or killed', 'name': 'bait'}, {'frequency': 'f', 'id': 41, 'synset': 'ball.n.06', 'synonyms': ['ball'], 'def': 'a spherical object used as a plaything', 'name': 'ball'}, {'frequency': 'r', 'id': 42, 'synset': 'ballet_skirt.n.01', 'synonyms': ['ballet_skirt', 'tutu'], 'def': 'very short skirt worn by ballerinas', 'name': 'ballet_skirt'}, {'frequency': 'f', 'id': 43, 'synset': 'balloon.n.01', 'synonyms': ['balloon'], 'def': 'large tough nonrigid bag filled with gas or heated air', 'name': 'balloon'}, {'frequency': 'c', 'id': 44, 'synset': 'bamboo.n.02', 'synonyms': ['bamboo'], 'def': 'woody tropical grass having hollow woody stems', 'name': 'bamboo'}, {'frequency': 'f', 'id': 45, 'synset': 'banana.n.02', 'synonyms': ['banana'], 'def': 'elongated crescent-shaped yellow fruit with soft sweet flesh', 'name': 'banana'}, {'frequency': 'r', 'id': 46, 'synset': 'band_aid.n.01', 'synonyms': ['Band_Aid'], 'def': 'trade name for an adhesive bandage to cover small cuts or blisters', 'name': 'Band_Aid'}, {'frequency': 'c', 'id': 47, 'synset': 'bandage.n.01', 'synonyms': ['bandage'], 'def': 'a piece of soft material that covers and protects an injured part of the body', 'name': 'bandage'}, {'frequency': 'c', 'id': 48, 'synset': 'bandanna.n.01', 'synonyms': ['bandanna', 'bandana'], 'def': 'large and brightly colored handkerchief; often used as a neckerchief', 'name': 'bandanna'}, {'frequency': 'r', 'id': 49, 'synset': 'banjo.n.01', 'synonyms': ['banjo'], 'def': 'a stringed instrument of the guitar family with a long neck and circular body', 'name': 'banjo'}, {'frequency': 'f', 'id': 50, 'synset': 'banner.n.01', 'synonyms': ['banner', 'streamer'], 'def': 'long strip of cloth or paper used for decoration or advertising', 'name': 'banner'}, {'frequency': 'r', 'id': 51, 'synset': 'barbell.n.01', 'synonyms': ['barbell'], 'def': 'a bar to which heavy discs are attached at each end; used in weightlifting', 'name': 'barbell'}, {'frequency': 'r', 'id': 52, 'synset': 'barge.n.01', 'synonyms': ['barge'], 'def': 'a flatbottom boat for carrying heavy loads (especially on canals)', 'name': 'barge'}, {'frequency': 'f', 'id': 53, 'synset': 'barrel.n.02', 'synonyms': ['barrel', 'cask'], 'def': 'a cylindrical container that holds liquids', 'name': 'barrel'}, {'frequency': 'c', 'id': 54, 'synset': 'barrette.n.01', 'synonyms': ['barrette'], 'def': "a pin for holding women's hair in place", 'name': 'barrette'}, {'frequency': 'c', 'id': 55, 'synset': 'barrow.n.03', 'synonyms': ['barrow', 'garden_cart', 'lawn_cart', 'wheelbarrow'], 'def': 'a cart for carrying small loads; has handles and one or more wheels', 'name': 'barrow'}, {'frequency': 'f', 'id': 56, 'synset': 'base.n.03', 'synonyms': ['baseball_base'], 'def': 'a place that the runner must touch before scoring', 'name': 'baseball_base'}, {'frequency': 'f', 'id': 57, 'synset': 'baseball.n.02', 'synonyms': ['baseball'], 'def': 'a ball used in playing baseball', 'name': 'baseball'}, {'frequency': 'f', 'id': 58, 'synset': 'baseball_bat.n.01', 'synonyms': ['baseball_bat'], 'def': 'an implement used in baseball by the batter', 'name': 'baseball_bat'}, {'frequency': 'f', 'id': 59, 'synset': 'baseball_cap.n.01', 'synonyms': ['baseball_cap', 'jockey_cap', 'golf_cap'], 'def': 'a cap with a bill', 'name': 'baseball_cap'}, {'frequency': 'f', 'id': 60, 'synset': 'baseball_glove.n.01', 'synonyms': ['baseball_glove', 'baseball_mitt'], 'def': 'the handwear used by fielders in playing baseball', 'name': 'baseball_glove'}, {'frequency': 'f', 'id': 61, 'synset': 'basket.n.01', 'synonyms': ['basket', 'handbasket'], 'def': 'a container that is usually woven and has handles', 'name': 'basket'}, {'frequency': 'c', 'id': 62, 'synset': 'basket.n.03', 'synonyms': ['basketball_hoop'], 'def': 'metal hoop supporting a net through which players try to throw the basketball', 'name': 'basketball_hoop'}, {'frequency': 'c', 'id': 63, 'synset': 'basketball.n.02', 'synonyms': ['basketball'], 'def': 'an inflated ball used in playing basketball', 'name': 'basketball'}, {'frequency': 'r', 'id': 64, 'synset': 'bass_horn.n.01', 'synonyms': ['bass_horn', 'sousaphone', 'tuba'], 'def': 'the lowest brass wind instrument', 'name': 'bass_horn'}, {'frequency': 'r', 'id': 65, 'synset': 'bat.n.01', 'synonyms': ['bat_(animal)'], 'def': 'nocturnal mouselike mammal with forelimbs modified to form membranous wings', 'name': 'bat_(animal)'}, {'frequency': 'f', 'id': 66, 'synset': 'bath_mat.n.01', 'synonyms': ['bath_mat'], 'def': 'a heavy towel or mat to stand on while drying yourself after a bath', 'name': 'bath_mat'}, {'frequency': 'f', 'id': 67, 'synset': 'bath_towel.n.01', 'synonyms': ['bath_towel'], 'def': 'a large towel; to dry yourself after a bath', 'name': 'bath_towel'}, {'frequency': 'c', 'id': 68, 'synset': 'bathrobe.n.01', 'synonyms': ['bathrobe'], 'def': 'a loose-fitting robe of towelling; worn after a bath or swim', 'name': 'bathrobe'}, {'frequency': 'f', 'id': 69, 'synset': 'bathtub.n.01', 'synonyms': ['bathtub', 'bathing_tub'], 'def': 'a large open container that you fill with water and use to wash the body', 'name': 'bathtub'}, {'frequency': 'r', 'id': 70, 'synset': 'batter.n.02', 'synonyms': ['batter_(food)'], 'def': 'a liquid or semiliquid mixture, as of flour, eggs, and milk, used in cooking', 'name': 'batter_(food)'}, {'frequency': 'c', 'id': 71, 'synset': 'battery.n.02', 'synonyms': ['battery'], 'def': 'a portable device that produces electricity', 'name': 'battery'}, {'frequency': 'r', 'id': 72, 'synset': 'beach_ball.n.01', 'synonyms': ['beachball'], 'def': 'large and light ball; for play at the seaside', 'name': 'beachball'}, {'frequency': 'c', 'id': 73, 'synset': 'bead.n.01', 'synonyms': ['bead'], 'def': 'a small ball with a hole through the middle used for ornamentation, jewellery, etc.', 'name': 'bead'}, {'frequency': 'r', 'id': 74, 'synset': 'beaker.n.01', 'synonyms': ['beaker'], 'def': 'a flatbottomed jar made of glass or plastic; used for chemistry', 'name': 'beaker'}, {'frequency': 'c', 'id': 75, 'synset': 'bean_curd.n.01', 'synonyms': ['bean_curd', 'tofu'], 'def': 'cheeselike food made of curdled soybean milk', 'name': 'bean_curd'}, {'frequency': 'c', 'id': 76, 'synset': 'beanbag.n.01', 'synonyms': ['beanbag'], 'def': 'a bag filled with dried beans or similar items; used in games or to sit on', 'name': 'beanbag'}, {'frequency': 'f', 'id': 77, 'synset': 'beanie.n.01', 'synonyms': ['beanie', 'beany'], 'def': 'a small skullcap; formerly worn by schoolboys and college freshmen', 'name': 'beanie'}, {'frequency': 'f', 'id': 78, 'synset': 'bear.n.01', 'synonyms': ['bear'], 'def': 'large carnivorous or omnivorous mammals with shaggy coats and claws', 'name': 'bear'}, {'frequency': 'f', 'id': 79, 'synset': 'bed.n.01', 'synonyms': ['bed'], 'def': 'a piece of furniture that provides a place to sleep', 'name': 'bed'}, {'frequency': 'c', 'id': 80, 'synset': 'bedspread.n.01', 'synonyms': ['bedspread', 'bedcover', 'bed_covering', 'counterpane', 'spread'], 'def': 'decorative cover for a bed', 'name': 'bedspread'}, {'frequency': 'f', 'id': 81, 'synset': 'beef.n.01', 'synonyms': ['cow'], 'def': 'cattle that are reared for their meat', 'name': 'cow'}, {'frequency': 'c', 'id': 82, 'synset': 'beef.n.02', 'synonyms': ['beef_(food)', 'boeuf_(food)'], 'def': 'meat from an adult domestic bovine', 'name': 'beef_(food)'}, {'frequency': 'r', 'id': 83, 'synset': 'beeper.n.01', 'synonyms': ['beeper', 'pager'], 'def': 'an device that beeps when the person carrying it is being paged', 'name': 'beeper'}, {'frequency': 'f', 'id': 84, 'synset': 'beer_bottle.n.01', 'synonyms': ['beer_bottle'], 'def': 'a bottle that holds beer', 'name': 'beer_bottle'}, {'frequency': 'c', 'id': 85, 'synset': 'beer_can.n.01', 'synonyms': ['beer_can'], 'def': 'a can that holds beer', 'name': 'beer_can'}, {'frequency': 'r', 'id': 86, 'synset': 'beetle.n.01', 'synonyms': ['beetle'], 'def': 'insect with hard wing covers', 'name': 'beetle'}, {'frequency': 'f', 'id': 87, 'synset': 'bell.n.01', 'synonyms': ['bell'], 'def': 'a hollow device made of metal that makes a ringing sound when struck', 'name': 'bell'}, {'frequency': 'f', 'id': 88, 'synset': 'bell_pepper.n.02', 'synonyms': ['bell_pepper', 'capsicum'], 'def': 'large bell-shaped sweet pepper in green or red or yellow or orange or black varieties', 'name': 'bell_pepper'}, {'frequency': 'f', 'id': 89, 'synset': 'belt.n.02', 'synonyms': ['belt'], 'def': 'a band to tie or buckle around the body (usually at the waist)', 'name': 'belt'}, {'frequency': 'f', 'id': 90, 'synset': 'belt_buckle.n.01', 'synonyms': ['belt_buckle'], 'def': 'the buckle used to fasten a belt', 'name': 'belt_buckle'}, {'frequency': 'f', 'id': 91, 'synset': 'bench.n.01', 'synonyms': ['bench'], 'def': 'a long seat for more than one person', 'name': 'bench'}, {'frequency': 'c', 'id': 92, 'synset': 'beret.n.01', 'synonyms': ['beret'], 'def': 'a cap with no brim or bill; made of soft cloth', 'name': 'beret'}, {'frequency': 'c', 'id': 93, 'synset': 'bib.n.02', 'synonyms': ['bib'], 'def': 'a napkin tied under the chin of a child while eating', 'name': 'bib'}, {'frequency': 'r', 'id': 94, 'synset': 'bible.n.01', 'synonyms': ['Bible'], 'def': 'the sacred writings of the Christian religions', 'name': 'Bible'}, {'frequency': 'f', 'id': 95, 'synset': 'bicycle.n.01', 'synonyms': ['bicycle', 'bike_(bicycle)'], 'def': 'a wheeled vehicle that has two wheels and is moved by foot pedals', 'name': 'bicycle'}, {'frequency': 'f', 'id': 96, 'synset': 'bill.n.09', 'synonyms': ['visor', 'vizor'], 'def': 'a brim that projects to the front to shade the eyes', 'name': 'visor'}, {'frequency': 'c', 'id': 97, 'synset': 'binder.n.03', 'synonyms': ['binder', 'ring-binder'], 'def': 'holds loose papers or magazines', 'name': 'binder'}, {'frequency': 'c', 'id': 98, 'synset': 'binoculars.n.01', 'synonyms': ['binoculars', 'field_glasses', 'opera_glasses'], 'def': 'an optical instrument designed for simultaneous use by both eyes', 'name': 'binoculars'}, {'frequency': 'f', 'id': 99, 'synset': 'bird.n.01', 'synonyms': ['bird'], 'def': 'animal characterized by feathers and wings', 'name': 'bird'}, {'frequency': 'r', 'id': 100, 'synset': 'bird_feeder.n.01', 'synonyms': ['birdfeeder'], 'def': 'an outdoor device that supplies food for wild birds', 'name': 'birdfeeder'}, {'frequency': 'r', 'id': 101, 'synset': 'birdbath.n.01', 'synonyms': ['birdbath'], 'def': 'an ornamental basin (usually in a garden) for birds to bathe in', 'name': 'birdbath'}, {'frequency': 'c', 'id': 102, 'synset': 'birdcage.n.01', 'synonyms': ['birdcage'], 'def': 'a cage in which a bird can be kept', 'name': 'birdcage'}, {'frequency': 'c', 'id': 103, 'synset': 'birdhouse.n.01', 'synonyms': ['birdhouse'], 'def': 'a shelter for birds', 'name': 'birdhouse'}, {'frequency': 'f', 'id': 104, 'synset': 'birthday_cake.n.01', 'synonyms': ['birthday_cake'], 'def': 'decorated cake served at a birthday party', 'name': 'birthday_cake'}, {'frequency': 'r', 'id': 105, 'synset': 'birthday_card.n.01', 'synonyms': ['birthday_card'], 'def': 'a card expressing a birthday greeting', 'name': 'birthday_card'}, {'frequency': 'r', 'id': 106, 'synset': 'biscuit.n.01', 'synonyms': ['biscuit_(bread)'], 'def': 'small round bread leavened with baking-powder or soda', 'name': 'biscuit_(bread)'}, {'frequency': 'r', 'id': 107, 'synset': 'black_flag.n.01', 'synonyms': ['pirate_flag'], 'def': 'a flag usually bearing a white skull and crossbones on a black background', 'name': 'pirate_flag'}, {'frequency': 'c', 'id': 108, 'synset': 'black_sheep.n.02', 'synonyms': ['black_sheep'], 'def': 'sheep with a black coat', 'name': 'black_sheep'}, {'frequency': 'c', 'id': 109, 'synset': 'blackboard.n.01', 'synonyms': ['blackboard', 'chalkboard'], 'def': 'sheet of slate; for writing with chalk', 'name': 'blackboard'}, {'frequency': 'f', 'id': 110, 'synset': 'blanket.n.01', 'synonyms': ['blanket'], 'def': 'bedding that keeps a person warm in bed', 'name': 'blanket'}, {'frequency': 'c', 'id': 111, 'synset': 'blazer.n.01', 'synonyms': ['blazer', 'sport_jacket', 'sport_coat', 'sports_jacket', 'sports_coat'], 'def': 'lightweight jacket; often striped in the colors of a club or school', 'name': 'blazer'}, {'frequency': 'f', 'id': 112, 'synset': 'blender.n.01', 'synonyms': ['blender', 'liquidizer', 'liquidiser'], 'def': 'an electrically powered mixer that mix or chop or liquefy foods', 'name': 'blender'}, {'frequency': 'r', 'id': 113, 'synset': 'blimp.n.02', 'synonyms': ['blimp'], 'def': 'a small nonrigid airship used for observation or as a barrage balloon', 'name': 'blimp'}, {'frequency': 'c', 'id': 114, 'synset': 'blinker.n.01', 'synonyms': ['blinker', 'flasher'], 'def': 'a light that flashes on and off; used as a signal or to send messages', 'name': 'blinker'}, {'frequency': 'c', 'id': 115, 'synset': 'blueberry.n.02', 'synonyms': ['blueberry'], 'def': 'sweet edible dark-blue berries of blueberry plants', 'name': 'blueberry'}, {'frequency': 'r', 'id': 116, 'synset': 'boar.n.02', 'synonyms': ['boar'], 'def': 'an uncastrated male hog', 'name': 'boar'}, {'frequency': 'r', 'id': 117, 'synset': 'board.n.09', 'synonyms': ['gameboard'], 'def': 'a flat portable surface (usually rectangular) designed for board games', 'name': 'gameboard'}, {'frequency': 'f', 'id': 118, 'synset': 'boat.n.01', 'synonyms': ['boat', 'ship_(boat)'], 'def': 'a vessel for travel on water', 'name': 'boat'}, {'frequency': 'c', 'id': 119, 'synset': 'bobbin.n.01', 'synonyms': ['bobbin', 'spool', 'reel'], 'def': 'a thing around which thread/tape/film or other flexible materials can be wound', 'name': 'bobbin'}, {'frequency': 'r', 'id': 120, 'synset': 'bobby_pin.n.01', 'synonyms': ['bobby_pin', 'hairgrip'], 'def': 'a flat wire hairpin used to hold bobbed hair in place', 'name': 'bobby_pin'}, {'frequency': 'c', 'id': 121, 'synset': 'boiled_egg.n.01', 'synonyms': ['boiled_egg', 'coddled_egg'], 'def': 'egg cooked briefly in the shell in gently boiling water', 'name': 'boiled_egg'}, {'frequency': 'r', 'id': 122, 'synset': 'bolo_tie.n.01', 'synonyms': ['bolo_tie', 'bolo', 'bola_tie', 'bola'], 'def': 'a cord fastened around the neck with an ornamental clasp and worn as a necktie', 'name': 'bolo_tie'}, {'frequency': 'c', 'id': 123, 'synset': 'bolt.n.03', 'synonyms': ['deadbolt'], 'def': 'the part of a lock that is engaged or withdrawn with a key', 'name': 'deadbolt'}, {'frequency': 'f', 'id': 124, 'synset': 'bolt.n.06', 'synonyms': ['bolt'], 'def': 'a screw that screws into a nut to form a fastener', 'name': 'bolt'}, {'frequency': 'r', 'id': 125, 'synset': 'bonnet.n.01', 'synonyms': ['bonnet'], 'def': 'a hat tied under the chin', 'name': 'bonnet'}, {'frequency': 'f', 'id': 126, 'synset': 'book.n.01', 'synonyms': ['book'], 'def': 'a written work or composition that has been published', 'name': 'book'}, {'frequency': 'r', 'id': 127, 'synset': 'book_bag.n.01', 'synonyms': ['book_bag'], 'def': 'a bag in which students carry their books', 'name': 'book_bag'}, {'frequency': 'c', 'id': 128, 'synset': 'bookcase.n.01', 'synonyms': ['bookcase'], 'def': 'a piece of furniture with shelves for storing books', 'name': 'bookcase'}, {'frequency': 'c', 'id': 129, 'synset': 'booklet.n.01', 'synonyms': ['booklet', 'brochure', 'leaflet', 'pamphlet'], 'def': 'a small book usually having a paper cover', 'name': 'booklet'}, {'frequency': 'r', 'id': 130, 'synset': 'bookmark.n.01', 'synonyms': ['bookmark', 'bookmarker'], 'def': 'a marker (a piece of paper or ribbon) placed between the pages of a book', 'name': 'bookmark'}, {'frequency': 'r', 'id': 131, 'synset': 'boom.n.04', 'synonyms': ['boom_microphone', 'microphone_boom'], 'def': 'a pole carrying an overhead microphone projected over a film or tv set', 'name': 'boom_microphone'}, {'frequency': 'f', 'id': 132, 'synset': 'boot.n.01', 'synonyms': ['boot'], 'def': 'footwear that covers the whole foot and lower leg', 'name': 'boot'}, {'frequency': 'f', 'id': 133, 'synset': 'bottle.n.01', 'synonyms': ['bottle'], 'def': 'a glass or plastic vessel used for storing drinks or other liquids', 'name': 'bottle'}, {'frequency': 'c', 'id': 134, 'synset': 'bottle_opener.n.01', 'synonyms': ['bottle_opener'], 'def': 'an opener for removing caps or corks from bottles', 'name': 'bottle_opener'}, {'frequency': 'c', 'id': 135, 'synset': 'bouquet.n.01', 'synonyms': ['bouquet'], 'def': 'an arrangement of flowers that is usually given as a present', 'name': 'bouquet'}, {'frequency': 'r', 'id': 136, 'synset': 'bow.n.04', 'synonyms': ['bow_(weapon)'], 'def': 'a weapon for shooting arrows', 'name': 'bow_(weapon)'}, {'frequency': 'f', 'id': 137, 'synset': 'bow.n.08', 'synonyms': ['bow_(decorative_ribbons)'], 'def': 'a decorative interlacing of ribbons', 'name': 'bow_(decorative_ribbons)'}, {'frequency': 'f', 'id': 138, 'synset': 'bow_tie.n.01', 'synonyms': ['bow-tie', 'bowtie'], 'def': "a man's tie that ties in a bow", 'name': 'bow-tie'}, {'frequency': 'f', 'id': 139, 'synset': 'bowl.n.03', 'synonyms': ['bowl'], 'def': 'a dish that is round and open at the top for serving foods', 'name': 'bowl'}, {'frequency': 'r', 'id': 140, 'synset': 'bowl.n.08', 'synonyms': ['pipe_bowl'], 'def': 'a small round container that is open at the top for holding tobacco', 'name': 'pipe_bowl'}, {'frequency': 'c', 'id': 141, 'synset': 'bowler_hat.n.01', 'synonyms': ['bowler_hat', 'bowler', 'derby_hat', 'derby', 'plug_hat'], 'def': 'a felt hat that is round and hard with a narrow brim', 'name': 'bowler_hat'}, {'frequency': 'r', 'id': 142, 'synset': 'bowling_ball.n.01', 'synonyms': ['bowling_ball'], 'def': 'a large ball with finger holes used in the sport of bowling', 'name': 'bowling_ball'}, {'frequency': 'r', 'id': 143, 'synset': 'bowling_pin.n.01', 'synonyms': ['bowling_pin'], 'def': 'a club-shaped wooden object used in bowling', 'name': 'bowling_pin'}, {'frequency': 'r', 'id': 144, 'synset': 'boxing_glove.n.01', 'synonyms': ['boxing_glove'], 'def': 'large glove coverings the fists of a fighter worn for the sport of boxing', 'name': 'boxing_glove'}, {'frequency': 'c', 'id': 145, 'synset': 'brace.n.06', 'synonyms': ['suspenders'], 'def': 'elastic straps that hold trousers up (usually used in the plural)', 'name': 'suspenders'}, {'frequency': 'f', 'id': 146, 'synset': 'bracelet.n.02', 'synonyms': ['bracelet', 'bangle'], 'def': 'jewelry worn around the wrist for decoration', 'name': 'bracelet'}, {'frequency': 'r', 'id': 147, 'synset': 'brass.n.07', 'synonyms': ['brass_plaque'], 'def': 'a memorial made of brass', 'name': 'brass_plaque'}, {'frequency': 'c', 'id': 148, 'synset': 'brassiere.n.01', 'synonyms': ['brassiere', 'bra', 'bandeau'], 'def': 'an undergarment worn by women to support their breasts', 'name': 'brassiere'}, {'frequency': 'c', 'id': 149, 'synset': 'bread-bin.n.01', 'synonyms': ['bread-bin', 'breadbox'], 'def': 'a container used to keep bread or cake in', 'name': 'bread-bin'}, {'frequency': 'r', 'id': 150, 'synset': 'breechcloth.n.01', 'synonyms': ['breechcloth', 'breechclout', 'loincloth'], 'def': 'a garment that provides covering for the loins', 'name': 'breechcloth'}, {'frequency': 'c', 'id': 151, 'synset': 'bridal_gown.n.01', 'synonyms': ['bridal_gown', 'wedding_gown', 'wedding_dress'], 'def': 'a gown worn by the bride at a wedding', 'name': 'bridal_gown'}, {'frequency': 'c', 'id': 152, 'synset': 'briefcase.n.01', 'synonyms': ['briefcase'], 'def': 'a case with a handle; for carrying papers or files or books', 'name': 'briefcase'}, {'frequency': 'c', 'id': 153, 'synset': 'bristle_brush.n.01', 'synonyms': ['bristle_brush'], 'def': 'a brush that is made with the short stiff hairs of an animal or plant', 'name': 'bristle_brush'}, {'frequency': 'f', 'id': 154, 'synset': 'broccoli.n.01', 'synonyms': ['broccoli'], 'def': 'plant with dense clusters of tight green flower buds', 'name': 'broccoli'}, {'frequency': 'r', 'id': 155, 'synset': 'brooch.n.01', 'synonyms': ['broach'], 'def': 'a decorative pin worn by women', 'name': 'broach'}, {'frequency': 'c', 'id': 156, 'synset': 'broom.n.01', 'synonyms': ['broom'], 'def': 'bundle of straws or twigs attached to a long handle; used for cleaning', 'name': 'broom'}, {'frequency': 'c', 'id': 157, 'synset': 'brownie.n.03', 'synonyms': ['brownie'], 'def': 'square or bar of very rich chocolate cake usually with nuts', 'name': 'brownie'}, {'frequency': 'c', 'id': 158, 'synset': 'brussels_sprouts.n.01', 'synonyms': ['brussels_sprouts'], 'def': 'the small edible cabbage-like buds growing along a stalk', 'name': 'brussels_sprouts'}, {'frequency': 'r', 'id': 159, 'synset': 'bubble_gum.n.01', 'synonyms': ['bubble_gum'], 'def': 'a kind of chewing gum that can be blown into bubbles', 'name': 'bubble_gum'}, {'frequency': 'f', 'id': 160, 'synset': 'bucket.n.01', 'synonyms': ['bucket', 'pail'], 'def': 'a roughly cylindrical vessel that is open at the top', 'name': 'bucket'}, {'frequency': 'r', 'id': 161, 'synset': 'buggy.n.01', 'synonyms': ['horse_buggy'], 'def': 'a small lightweight carriage; drawn by a single horse', 'name': 'horse_buggy'}, {'frequency': 'c', 'id': 162, 'synset': 'bull.n.11', 'synonyms': ['bull'], 'def': 'mature male cow', 'name': 'bull'}, {'frequency': 'r', 'id': 163, 'synset': 'bulldog.n.01', 'synonyms': ['bulldog'], 'def': 'a thickset short-haired dog with a large head and strong undershot lower jaw', 'name': 'bulldog'}, {'frequency': 'r', 'id': 164, 'synset': 'bulldozer.n.01', 'synonyms': ['bulldozer', 'dozer'], 'def': 'large powerful tractor; a large blade in front flattens areas of ground', 'name': 'bulldozer'}, {'frequency': 'c', 'id': 165, 'synset': 'bullet_train.n.01', 'synonyms': ['bullet_train'], 'def': 'a high-speed passenger train', 'name': 'bullet_train'}, {'frequency': 'c', 'id': 166, 'synset': 'bulletin_board.n.02', 'synonyms': ['bulletin_board', 'notice_board'], 'def': 'a board that hangs on a wall; displays announcements', 'name': 'bulletin_board'}, {'frequency': 'r', 'id': 167, 'synset': 'bulletproof_vest.n.01', 'synonyms': ['bulletproof_vest'], 'def': 'a vest capable of resisting the impact of a bullet', 'name': 'bulletproof_vest'}, {'frequency': 'c', 'id': 168, 'synset': 'bullhorn.n.01', 'synonyms': ['bullhorn', 'megaphone'], 'def': 'a portable loudspeaker with built-in microphone and amplifier', 'name': 'bullhorn'}, {'frequency': 'r', 'id': 169, 'synset': 'bully_beef.n.01', 'synonyms': ['corned_beef', 'corn_beef'], 'def': 'beef cured or pickled in brine', 'name': 'corned_beef'}, {'frequency': 'f', 'id': 170, 'synset': 'bun.n.01', 'synonyms': ['bun', 'roll'], 'def': 'small rounded bread either plain or sweet', 'name': 'bun'}, {'frequency': 'c', 'id': 171, 'synset': 'bunk_bed.n.01', 'synonyms': ['bunk_bed'], 'def': 'beds built one above the other', 'name': 'bunk_bed'}, {'frequency': 'f', 'id': 172, 'synset': 'buoy.n.01', 'synonyms': ['buoy'], 'def': 'a float attached by rope to the seabed to mark channels in a harbor or underwater hazards', 'name': 'buoy'}, {'frequency': 'r', 'id': 173, 'synset': 'burrito.n.01', 'synonyms': ['burrito'], 'def': 'a flour tortilla folded around a filling', 'name': 'burrito'}, {'frequency': 'f', 'id': 174, 'synset': 'bus.n.01', 'synonyms': ['bus_(vehicle)', 'autobus', 'charabanc', 'double-decker', 'motorbus', 'motorcoach'], 'def': 'a vehicle carrying many passengers; used for public transport', 'name': 'bus_(vehicle)'}, {'frequency': 'c', 'id': 175, 'synset': 'business_card.n.01', 'synonyms': ['business_card'], 'def': "a card on which are printed the person's name and business affiliation", 'name': 'business_card'}, {'frequency': 'c', 'id': 176, 'synset': 'butcher_knife.n.01', 'synonyms': ['butcher_knife'], 'def': 'a large sharp knife for cutting or trimming meat', 'name': 'butcher_knife'}, {'frequency': 'c', 'id': 177, 'synset': 'butter.n.01', 'synonyms': ['butter'], 'def': 'an edible emulsion of fat globules made by churning milk or cream; for cooking and table use', 'name': 'butter'}, {'frequency': 'c', 'id': 178, 'synset': 'butterfly.n.01', 'synonyms': ['butterfly'], 'def': 'insect typically having a slender body with knobbed antennae and broad colorful wings', 'name': 'butterfly'}, {'frequency': 'f', 'id': 179, 'synset': 'button.n.01', 'synonyms': ['button'], 'def': 'a round fastener sewn to shirts and coats etc to fit through buttonholes', 'name': 'button'}, {'frequency': 'f', 'id': 180, 'synset': 'cab.n.03', 'synonyms': ['cab_(taxi)', 'taxi', 'taxicab'], 'def': 'a car that takes passengers where they want to go in exchange for money', 'name': 'cab_(taxi)'}, {'frequency': 'r', 'id': 181, 'synset': 'cabana.n.01', 'synonyms': ['cabana'], 'def': 'a small tent used as a dressing room beside the sea or a swimming pool', 'name': 'cabana'}, {'frequency': 'r', 'id': 182, 'synset': 'cabin_car.n.01', 'synonyms': ['cabin_car', 'caboose'], 'def': 'a car on a freight train for use of the train crew; usually the last car on the train', 'name': 'cabin_car'}, {'frequency': 'f', 'id': 183, 'synset': 'cabinet.n.01', 'synonyms': ['cabinet'], 'def': 'a piece of furniture resembling a cupboard with doors and shelves and drawers', 'name': 'cabinet'}, {'frequency': 'r', 'id': 184, 'synset': 'cabinet.n.03', 'synonyms': ['locker', 'storage_locker'], 'def': 'a storage compartment for clothes and valuables; usually it has a lock', 'name': 'locker'}, {'frequency': 'f', 'id': 185, 'synset': 'cake.n.03', 'synonyms': ['cake'], 'def': 'baked goods made from or based on a mixture of flour, sugar, eggs, and fat', 'name': 'cake'}, {'frequency': 'c', 'id': 186, 'synset': 'calculator.n.02', 'synonyms': ['calculator'], 'def': 'a small machine that is used for mathematical calculations', 'name': 'calculator'}, {'frequency': 'f', 'id': 187, 'synset': 'calendar.n.02', 'synonyms': ['calendar'], 'def': 'a list or register of events (appointments/social events/court cases, etc)', 'name': 'calendar'}, {'frequency': 'c', 'id': 188, 'synset': 'calf.n.01', 'synonyms': ['calf'], 'def': 'young of domestic cattle', 'name': 'calf'}, {'frequency': 'c', 'id': 189, 'synset': 'camcorder.n.01', 'synonyms': ['camcorder'], 'def': 'a portable television camera and videocassette recorder', 'name': 'camcorder'}, {'frequency': 'c', 'id': 190, 'synset': 'camel.n.01', 'synonyms': ['camel'], 'def': 'cud-chewing mammal used as a draft or saddle animal in desert regions', 'name': 'camel'}, {'frequency': 'f', 'id': 191, 'synset': 'camera.n.01', 'synonyms': ['camera'], 'def': 'equipment for taking photographs', 'name': 'camera'}, {'frequency': 'c', 'id': 192, 'synset': 'camera_lens.n.01', 'synonyms': ['camera_lens'], 'def': 'a lens that focuses the image in a camera', 'name': 'camera_lens'}, {'frequency': 'c', 'id': 193, 'synset': 'camper.n.02', 'synonyms': ['camper_(vehicle)', 'camping_bus', 'motor_home'], 'def': 'a recreational vehicle equipped for camping out while traveling', 'name': 'camper_(vehicle)'}, {'frequency': 'f', 'id': 194, 'synset': 'can.n.01', 'synonyms': ['can', 'tin_can'], 'def': 'airtight sealed metal container for food or drink or paint etc.', 'name': 'can'}, {'frequency': 'c', 'id': 195, 'synset': 'can_opener.n.01', 'synonyms': ['can_opener', 'tin_opener'], 'def': 'a device for cutting cans open', 'name': 'can_opener'}, {'frequency': 'r', 'id': 196, 'synset': 'candelabrum.n.01', 'synonyms': ['candelabrum', 'candelabra'], 'def': 'branched candlestick; ornamental; has several lights', 'name': 'candelabrum'}, {'frequency': 'f', 'id': 197, 'synset': 'candle.n.01', 'synonyms': ['candle', 'candlestick'], 'def': 'stick of wax with a wick in the middle', 'name': 'candle'}, {'frequency': 'f', 'id': 198, 'synset': 'candlestick.n.01', 'synonyms': ['candle_holder'], 'def': 'a holder with sockets for candles', 'name': 'candle_holder'}, {'frequency': 'r', 'id': 199, 'synset': 'candy_bar.n.01', 'synonyms': ['candy_bar'], 'def': 'a candy shaped as a bar', 'name': 'candy_bar'}, {'frequency': 'c', 'id': 200, 'synset': 'candy_cane.n.01', 'synonyms': ['candy_cane'], 'def': 'a hard candy in the shape of a rod (usually with stripes)', 'name': 'candy_cane'}, {'frequency': 'c', 'id': 201, 'synset': 'cane.n.01', 'synonyms': ['walking_cane'], 'def': 'a stick that people can lean on to help them walk', 'name': 'walking_cane'}, {'frequency': 'c', 'id': 202, 'synset': 'canister.n.02', 'synonyms': ['canister', 'cannister'], 'def': 'metal container for storing dry foods such as tea or flour', 'name': 'canister'}, {'frequency': 'r', 'id': 203, 'synset': 'cannon.n.02', 'synonyms': ['cannon'], 'def': 'heavy gun fired from a tank', 'name': 'cannon'}, {'frequency': 'c', 'id': 204, 'synset': 'canoe.n.01', 'synonyms': ['canoe'], 'def': 'small and light boat; pointed at both ends; propelled with a paddle', 'name': 'canoe'}, {'frequency': 'r', 'id': 205, 'synset': 'cantaloup.n.02', 'synonyms': ['cantaloup', 'cantaloupe'], 'def': 'the fruit of a cantaloup vine; small to medium-sized melon with yellowish flesh', 'name': 'cantaloup'}, {'frequency': 'r', 'id': 206, 'synset': 'canteen.n.01', 'synonyms': ['canteen'], 'def': 'a flask for carrying water; used by soldiers or travelers', 'name': 'canteen'}, {'frequency': 'c', 'id': 207, 'synset': 'cap.n.01', 'synonyms': ['cap_(headwear)'], 'def': 'a tight-fitting headwear', 'name': 'cap_(headwear)'}, {'frequency': 'f', 'id': 208, 'synset': 'cap.n.02', 'synonyms': ['bottle_cap', 'cap_(container_lid)'], 'def': 'a top (as for a bottle)', 'name': 'bottle_cap'}, {'frequency': 'r', 'id': 209, 'synset': 'cape.n.02', 'synonyms': ['cape'], 'def': 'a sleeveless garment like a cloak but shorter', 'name': 'cape'}, {'frequency': 'c', 'id': 210, 'synset': 'cappuccino.n.01', 'synonyms': ['cappuccino', 'coffee_cappuccino'], 'def': 'equal parts of espresso and steamed milk', 'name': 'cappuccino'}, {'frequency': 'f', 'id': 211, 'synset': 'car.n.01', 'synonyms': ['car_(automobile)', 'auto_(automobile)', 'automobile'], 'def': 'a motor vehicle with four wheels', 'name': 'car_(automobile)'}, {'frequency': 'f', 'id': 212, 'synset': 'car.n.02', 'synonyms': ['railcar_(part_of_a_train)', 'railway_car_(part_of_a_train)', 'railroad_car_(part_of_a_train)'], 'def': 'a wheeled vehicle adapted to the rails of railroad', 'name': 'railcar_(part_of_a_train)'}, {'frequency': 'r', 'id': 213, 'synset': 'car.n.04', 'synonyms': ['elevator_car'], 'def': 'where passengers ride up and down', 'name': 'elevator_car'}, {'frequency': 'r', 'id': 214, 'synset': 'car_battery.n.01', 'synonyms': ['car_battery', 'automobile_battery'], 'def': 'a battery in a motor vehicle', 'name': 'car_battery'}, {'frequency': 'c', 'id': 215, 'synset': 'card.n.02', 'synonyms': ['identity_card'], 'def': 'a card certifying the identity of the bearer', 'name': 'identity_card'}, {'frequency': 'c', 'id': 216, 'synset': 'card.n.03', 'synonyms': ['card'], 'def': 'a rectangular piece of paper used to send messages (e.g. greetings or pictures)', 'name': 'card'}, {'frequency': 'r', 'id': 217, 'synset': 'cardigan.n.01', 'synonyms': ['cardigan'], 'def': 'knitted jacket that is fastened up the front with buttons or a zipper', 'name': 'cardigan'}, {'frequency': 'r', 'id': 218, 'synset': 'cargo_ship.n.01', 'synonyms': ['cargo_ship', 'cargo_vessel'], 'def': 'a ship designed to carry cargo', 'name': 'cargo_ship'}, {'frequency': 'r', 'id': 219, 'synset': 'carnation.n.01', 'synonyms': ['carnation'], 'def': 'plant with pink to purple-red spice-scented usually double flowers', 'name': 'carnation'}, {'frequency': 'c', 'id': 220, 'synset': 'carriage.n.02', 'synonyms': ['horse_carriage'], 'def': 'a vehicle with wheels drawn by one or more horses', 'name': 'horse_carriage'}, {'frequency': 'f', 'id': 221, 'synset': 'carrot.n.01', 'synonyms': ['carrot'], 'def': 'deep orange edible root of the cultivated carrot plant', 'name': 'carrot'}, {'frequency': 'c', 'id': 222, 'synset': 'carryall.n.01', 'synonyms': ['tote_bag'], 'def': 'a capacious bag or basket', 'name': 'tote_bag'}, {'frequency': 'c', 'id': 223, 'synset': 'cart.n.01', 'synonyms': ['cart'], 'def': 'a heavy open wagon usually having two wheels and drawn by an animal', 'name': 'cart'}, {'frequency': 'c', 'id': 224, 'synset': 'carton.n.02', 'synonyms': ['carton'], 'def': 'a box made of cardboard; opens by flaps on top', 'name': 'carton'}, {'frequency': 'c', 'id': 225, 'synset': 'cash_register.n.01', 'synonyms': ['cash_register', 'register_(for_cash_transactions)'], 'def': 'a cashbox with an adding machine to register transactions', 'name': 'cash_register'}, {'frequency': 'r', 'id': 226, 'synset': 'casserole.n.01', 'synonyms': ['casserole'], 'def': 'food cooked and served in a casserole', 'name': 'casserole'}, {'frequency': 'r', 'id': 227, 'synset': 'cassette.n.01', 'synonyms': ['cassette'], 'def': 'a container that holds a magnetic tape used for recording or playing sound or video', 'name': 'cassette'}, {'frequency': 'c', 'id': 228, 'synset': 'cast.n.05', 'synonyms': ['cast', 'plaster_cast', 'plaster_bandage'], 'def': 'bandage consisting of a firm covering that immobilizes broken bones while they heal', 'name': 'cast'}, {'frequency': 'f', 'id': 229, 'synset': 'cat.n.01', 'synonyms': ['cat'], 'def': 'a domestic house cat', 'name': 'cat'}, {'frequency': 'c', 'id': 230, 'synset': 'cauliflower.n.02', 'synonyms': ['cauliflower'], 'def': 'edible compact head of white undeveloped flowers', 'name': 'cauliflower'}, {'frequency': 'r', 'id': 231, 'synset': 'caviar.n.01', 'synonyms': ['caviar', 'caviare'], 'def': "salted roe of sturgeon or other large fish; usually served as an hors d'oeuvre", 'name': 'caviar'}, {'frequency': 'c', 'id': 232, 'synset': 'cayenne.n.02', 'synonyms': ['cayenne_(spice)', 'cayenne_pepper_(spice)', 'red_pepper_(spice)'], 'def': 'ground pods and seeds of pungent red peppers of the genus Capsicum', 'name': 'cayenne_(spice)'}, {'frequency': 'c', 'id': 233, 'synset': 'cd_player.n.01', 'synonyms': ['CD_player'], 'def': 'electronic equipment for playing compact discs (CDs)', 'name': 'CD_player'}, {'frequency': 'c', 'id': 234, 'synset': 'celery.n.01', 'synonyms': ['celery'], 'def': 'widely cultivated herb with aromatic leaf stalks that are eaten raw or cooked', 'name': 'celery'}, {'frequency': 'f', 'id': 235, 'synset': 'cellular_telephone.n.01', 'synonyms': ['cellular_telephone', 'cellular_phone', 'cellphone', 'mobile_phone', 'smart_phone'], 'def': 'a hand-held mobile telephone', 'name': 'cellular_telephone'}, {'frequency': 'r', 'id': 236, 'synset': 'chain_mail.n.01', 'synonyms': ['chain_mail', 'ring_mail', 'chain_armor', 'chain_armour', 'ring_armor', 'ring_armour'], 'def': '(Middle Ages) flexible armor made of interlinked metal rings', 'name': 'chain_mail'}, {'frequency': 'f', 'id': 237, 'synset': 'chair.n.01', 'synonyms': ['chair'], 'def': 'a seat for one person, with a support for the back', 'name': 'chair'}, {'frequency': 'r', 'id': 238, 'synset': 'chaise_longue.n.01', 'synonyms': ['chaise_longue', 'chaise', 'daybed'], 'def': 'a long chair; for reclining', 'name': 'chaise_longue'}, {'frequency': 'r', 'id': 239, 'synset': 'champagne.n.01', 'synonyms': ['champagne'], 'def': 'a white sparkling wine produced in Champagne or resembling that produced there', 'name': 'champagne'}, {'frequency': 'f', 'id': 240, 'synset': 'chandelier.n.01', 'synonyms': ['chandelier'], 'def': 'branched lighting fixture; often ornate; hangs from the ceiling', 'name': 'chandelier'}, {'frequency': 'r', 'id': 241, 'synset': 'chap.n.04', 'synonyms': ['chap'], 'def': 'leather leggings without a seat; worn over trousers by cowboys to protect their legs', 'name': 'chap'}, {'frequency': 'r', 'id': 242, 'synset': 'checkbook.n.01', 'synonyms': ['checkbook', 'chequebook'], 'def': 'a book issued to holders of checking accounts', 'name': 'checkbook'}, {'frequency': 'r', 'id': 243, 'synset': 'checkerboard.n.01', 'synonyms': ['checkerboard'], 'def': 'a board having 64 squares of two alternating colors', 'name': 'checkerboard'}, {'frequency': 'c', 'id': 244, 'synset': 'cherry.n.03', 'synonyms': ['cherry'], 'def': 'a red fruit with a single hard stone', 'name': 'cherry'}, {'frequency': 'r', 'id': 245, 'synset': 'chessboard.n.01', 'synonyms': ['chessboard'], 'def': 'a checkerboard used to play chess', 'name': 'chessboard'}, {'frequency': 'r', 'id': 246, 'synset': 'chest_of_drawers.n.01', 'synonyms': ['chest_of_drawers_(furniture)', 'bureau_(furniture)', 'chest_(furniture)'], 'def': 'furniture with drawers for keeping clothes', 'name': 'chest_of_drawers_(furniture)'}, {'frequency': 'c', 'id': 247, 'synset': 'chicken.n.02', 'synonyms': ['chicken_(animal)'], 'def': 'a domestic fowl bred for flesh or eggs', 'name': 'chicken_(animal)'}, {'frequency': 'c', 'id': 248, 'synset': 'chicken_wire.n.01', 'synonyms': ['chicken_wire'], 'def': 'a galvanized wire network with a hexagonal mesh; used to build fences', 'name': 'chicken_wire'}, {'frequency': 'r', 'id': 249, 'synset': 'chickpea.n.01', 'synonyms': ['chickpea', 'garbanzo'], 'def': 'the seed of the chickpea plant; usually dried', 'name': 'chickpea'}, {'frequency': 'r', 'id': 250, 'synset': 'chihuahua.n.03', 'synonyms': ['Chihuahua'], 'def': 'an old breed of tiny short-haired dog with protruding eyes from Mexico', 'name': 'Chihuahua'}, {'frequency': 'r', 'id': 251, 'synset': 'chili.n.02', 'synonyms': ['chili_(vegetable)', 'chili_pepper_(vegetable)', 'chilli_(vegetable)', 'chilly_(vegetable)', 'chile_(vegetable)'], 'def': 'very hot and finely tapering pepper of special pungency', 'name': 'chili_(vegetable)'}, {'frequency': 'r', 'id': 252, 'synset': 'chime.n.01', 'synonyms': ['chime', 'gong'], 'def': 'an instrument consisting of a set of bells that are struck with a hammer', 'name': 'chime'}, {'frequency': 'r', 'id': 253, 'synset': 'chinaware.n.01', 'synonyms': ['chinaware'], 'def': 'dishware made of high quality porcelain', 'name': 'chinaware'}, {'frequency': 'c', 'id': 254, 'synset': 'chip.n.04', 'synonyms': ['crisp_(potato_chip)', 'potato_chip'], 'def': 'a thin crisp slice of potato fried in deep fat', 'name': 'crisp_(potato_chip)'}, {'frequency': 'r', 'id': 255, 'synset': 'chip.n.06', 'synonyms': ['poker_chip'], 'def': 'a small disk-shaped counter used to represent money when gambling', 'name': 'poker_chip'}, {'frequency': 'c', 'id': 256, 'synset': 'chocolate_bar.n.01', 'synonyms': ['chocolate_bar'], 'def': 'a bar of chocolate candy', 'name': 'chocolate_bar'}, {'frequency': 'c', 'id': 257, 'synset': 'chocolate_cake.n.01', 'synonyms': ['chocolate_cake'], 'def': 'cake containing chocolate', 'name': 'chocolate_cake'}, {'frequency': 'r', 'id': 258, 'synset': 'chocolate_milk.n.01', 'synonyms': ['chocolate_milk'], 'def': 'milk flavored with chocolate syrup', 'name': 'chocolate_milk'}, {'frequency': 'r', 'id': 259, 'synset': 'chocolate_mousse.n.01', 'synonyms': ['chocolate_mousse'], 'def': 'dessert mousse made with chocolate', 'name': 'chocolate_mousse'}, {'frequency': 'f', 'id': 260, 'synset': 'choker.n.03', 'synonyms': ['choker', 'collar', 'neckband'], 'def': 'necklace that fits tightly around the neck', 'name': 'choker'}, {'frequency': 'f', 'id': 261, 'synset': 'chopping_board.n.01', 'synonyms': ['chopping_board', 'cutting_board', 'chopping_block'], 'def': 'a wooden board where meats or vegetables can be cut', 'name': 'chopping_board'}, {'frequency': 'c', 'id': 262, 'synset': 'chopstick.n.01', 'synonyms': ['chopstick'], 'def': 'one of a pair of slender sticks used as oriental tableware to eat food with', 'name': 'chopstick'}, {'frequency': 'f', 'id': 263, 'synset': 'christmas_tree.n.05', 'synonyms': ['Christmas_tree'], 'def': 'an ornamented evergreen used as a Christmas decoration', 'name': 'Christmas_tree'}, {'frequency': 'c', 'id': 264, 'synset': 'chute.n.02', 'synonyms': ['slide'], 'def': 'sloping channel through which things can descend', 'name': 'slide'}, {'frequency': 'r', 'id': 265, 'synset': 'cider.n.01', 'synonyms': ['cider', 'cyder'], 'def': 'a beverage made from juice pressed from apples', 'name': 'cider'}, {'frequency': 'r', 'id': 266, 'synset': 'cigar_box.n.01', 'synonyms': ['cigar_box'], 'def': 'a box for holding cigars', 'name': 'cigar_box'}, {'frequency': 'c', 'id': 267, 'synset': 'cigarette.n.01', 'synonyms': ['cigarette'], 'def': 'finely ground tobacco wrapped in paper; for smoking', 'name': 'cigarette'}, {'frequency': 'c', 'id': 268, 'synset': 'cigarette_case.n.01', 'synonyms': ['cigarette_case', 'cigarette_pack'], 'def': 'a small flat case for holding cigarettes', 'name': 'cigarette_case'}, {'frequency': 'f', 'id': 269, 'synset': 'cistern.n.02', 'synonyms': ['cistern', 'water_tank'], 'def': 'a tank that holds the water used to flush a toilet', 'name': 'cistern'}, {'frequency': 'r', 'id': 270, 'synset': 'clarinet.n.01', 'synonyms': ['clarinet'], 'def': 'a single-reed instrument with a straight tube', 'name': 'clarinet'}, {'frequency': 'r', 'id': 271, 'synset': 'clasp.n.01', 'synonyms': ['clasp'], 'def': 'a fastener (as a buckle or hook) that is used to hold two things together', 'name': 'clasp'}, {'frequency': 'c', 'id': 272, 'synset': 'cleansing_agent.n.01', 'synonyms': ['cleansing_agent', 'cleanser', 'cleaner'], 'def': 'a preparation used in cleaning something', 'name': 'cleansing_agent'}, {'frequency': 'r', 'id': 273, 'synset': 'clementine.n.01', 'synonyms': ['clementine'], 'def': 'a variety of mandarin orange', 'name': 'clementine'}, {'frequency': 'c', 'id': 274, 'synset': 'clip.n.03', 'synonyms': ['clip'], 'def': 'any of various small fasteners used to hold loose articles together', 'name': 'clip'}, {'frequency': 'c', 'id': 275, 'synset': 'clipboard.n.01', 'synonyms': ['clipboard'], 'def': 'a small writing board with a clip at the top for holding papers', 'name': 'clipboard'}, {'frequency': 'f', 'id': 276, 'synset': 'clock.n.01', 'synonyms': ['clock', 'timepiece', 'timekeeper'], 'def': 'a timepiece that shows the time of day', 'name': 'clock'}, {'frequency': 'f', 'id': 277, 'synset': 'clock_tower.n.01', 'synonyms': ['clock_tower'], 'def': 'a tower with a large clock visible high up on an outside face', 'name': 'clock_tower'}, {'frequency': 'c', 'id': 278, 'synset': 'clothes_hamper.n.01', 'synonyms': ['clothes_hamper', 'laundry_basket', 'clothes_basket'], 'def': 'a hamper that holds dirty clothes to be washed or wet clothes to be dried', 'name': 'clothes_hamper'}, {'frequency': 'c', 'id': 279, 'synset': 'clothespin.n.01', 'synonyms': ['clothespin', 'clothes_peg'], 'def': 'wood or plastic fastener; for holding clothes on a clothesline', 'name': 'clothespin'}, {'frequency': 'r', 'id': 280, 'synset': 'clutch_bag.n.01', 'synonyms': ['clutch_bag'], 'def': "a woman's strapless purse that is carried in the hand", 'name': 'clutch_bag'}, {'frequency': 'f', 'id': 281, 'synset': 'coaster.n.03', 'synonyms': ['coaster'], 'def': 'a covering (plate or mat) that protects the surface of a table', 'name': 'coaster'}, {'frequency': 'f', 'id': 282, 'synset': 'coat.n.01', 'synonyms': ['coat'], 'def': 'an outer garment that has sleeves and covers the body from shoulder down', 'name': 'coat'}, {'frequency': 'c', 'id': 283, 'synset': 'coat_hanger.n.01', 'synonyms': ['coat_hanger', 'clothes_hanger', 'dress_hanger'], 'def': "a hanger that is shaped like a person's shoulders", 'name': 'coat_hanger'}, {'frequency': 'r', 'id': 284, 'synset': 'coatrack.n.01', 'synonyms': ['coatrack', 'hatrack'], 'def': 'a rack with hooks for temporarily holding coats and hats', 'name': 'coatrack'}, {'frequency': 'c', 'id': 285, 'synset': 'cock.n.04', 'synonyms': ['cock', 'rooster'], 'def': 'adult male chicken', 'name': 'cock'}, {'frequency': 'c', 'id': 286, 'synset': 'coconut.n.02', 'synonyms': ['coconut', 'cocoanut'], 'def': 'large hard-shelled brown oval nut with a fibrous husk', 'name': 'coconut'}, {'frequency': 'r', 'id': 287, 'synset': 'coffee_filter.n.01', 'synonyms': ['coffee_filter'], 'def': 'filter (usually of paper) that passes the coffee and retains the coffee grounds', 'name': 'coffee_filter'}, {'frequency': 'f', 'id': 288, 'synset': 'coffee_maker.n.01', 'synonyms': ['coffee_maker', 'coffee_machine'], 'def': 'a kitchen appliance for brewing coffee automatically', 'name': 'coffee_maker'}, {'frequency': 'f', 'id': 289, 'synset': 'coffee_table.n.01', 'synonyms': ['coffee_table', 'cocktail_table'], 'def': 'low table where magazines can be placed and coffee or cocktails are served', 'name': 'coffee_table'}, {'frequency': 'c', 'id': 290, 'synset': 'coffeepot.n.01', 'synonyms': ['coffeepot'], 'def': 'tall pot in which coffee is brewed', 'name': 'coffeepot'}, {'frequency': 'r', 'id': 291, 'synset': 'coil.n.05', 'synonyms': ['coil'], 'def': 'tubing that is wound in a spiral', 'name': 'coil'}, {'frequency': 'c', 'id': 292, 'synset': 'coin.n.01', 'synonyms': ['coin'], 'def': 'a flat metal piece (usually a disc) used as money', 'name': 'coin'}, {'frequency': 'r', 'id': 293, 'synset': 'colander.n.01', 'synonyms': ['colander', 'cullender'], 'def': 'bowl-shaped strainer; used to wash or drain foods', 'name': 'colander'}, {'frequency': 'c', 'id': 294, 'synset': 'coleslaw.n.01', 'synonyms': ['coleslaw', 'slaw'], 'def': 'basically shredded cabbage', 'name': 'coleslaw'}, {'frequency': 'r', 'id': 295, 'synset': 'coloring_material.n.01', 'synonyms': ['coloring_material', 'colouring_material'], 'def': 'any material used for its color', 'name': 'coloring_material'}, {'frequency': 'r', 'id': 296, 'synset': 'combination_lock.n.01', 'synonyms': ['combination_lock'], 'def': 'lock that can be opened only by turning dials in a special sequence', 'name': 'combination_lock'}, {'frequency': 'c', 'id': 297, 'synset': 'comforter.n.04', 'synonyms': ['pacifier', 'teething_ring'], 'def': 'device used for an infant to suck or bite on', 'name': 'pacifier'}, {'frequency': 'r', 'id': 298, 'synset': 'comic_book.n.01', 'synonyms': ['comic_book'], 'def': 'a magazine devoted to comic strips', 'name': 'comic_book'}, {'frequency': 'f', 'id': 299, 'synset': 'computer_keyboard.n.01', 'synonyms': ['computer_keyboard', 'keyboard_(computer)'], 'def': 'a keyboard that is a data input device for computers', 'name': 'computer_keyboard'}, {'frequency': 'r', 'id': 300, 'synset': 'concrete_mixer.n.01', 'synonyms': ['concrete_mixer', 'cement_mixer'], 'def': 'a machine with a large revolving drum in which cement/concrete is mixed', 'name': 'concrete_mixer'}, {'frequency': 'f', 'id': 301, 'synset': 'cone.n.01', 'synonyms': ['cone', 'traffic_cone'], 'def': 'a cone-shaped object used to direct traffic', 'name': 'cone'}, {'frequency': 'f', 'id': 302, 'synset': 'control.n.09', 'synonyms': ['control', 'controller'], 'def': 'a mechanism that controls the operation of a machine', 'name': 'control'}, {'frequency': 'r', 'id': 303, 'synset': 'convertible.n.01', 'synonyms': ['convertible_(automobile)'], 'def': 'a car that has top that can be folded or removed', 'name': 'convertible_(automobile)'}, {'frequency': 'r', 'id': 304, 'synset': 'convertible.n.03', 'synonyms': ['sofa_bed'], 'def': 'a sofa that can be converted into a bed', 'name': 'sofa_bed'}, {'frequency': 'c', 'id': 305, 'synset': 'cookie.n.01', 'synonyms': ['cookie', 'cooky', 'biscuit_(cookie)'], 'def': "any of various small flat sweet cakes (`biscuit' is the British term)", 'name': 'cookie'}, {'frequency': 'r', 'id': 306, 'synset': 'cookie_jar.n.01', 'synonyms': ['cookie_jar', 'cooky_jar'], 'def': 'a jar in which cookies are kept (and sometimes money is hidden)', 'name': 'cookie_jar'}, {'frequency': 'r', 'id': 307, 'synset': 'cooking_utensil.n.01', 'synonyms': ['cooking_utensil'], 'def': 'a kitchen utensil made of material that does not melt easily; used for cooking', 'name': 'cooking_utensil'}, {'frequency': 'f', 'id': 308, 'synset': 'cooler.n.01', 'synonyms': ['cooler_(for_food)', 'ice_chest'], 'def': 'an insulated box for storing food often with ice', 'name': 'cooler_(for_food)'}, {'frequency': 'c', 'id': 309, 'synset': 'cork.n.04', 'synonyms': ['cork_(bottle_plug)', 'bottle_cork'], 'def': 'the plug in the mouth of a bottle (especially a wine bottle)', 'name': 'cork_(bottle_plug)'}, {'frequency': 'r', 'id': 310, 'synset': 'corkboard.n.01', 'synonyms': ['corkboard'], 'def': 'a sheet consisting of cork granules', 'name': 'corkboard'}, {'frequency': 'r', 'id': 311, 'synset': 'corkscrew.n.01', 'synonyms': ['corkscrew', 'bottle_screw'], 'def': 'a bottle opener that pulls corks', 'name': 'corkscrew'}, {'frequency': 'c', 'id': 312, 'synset': 'corn.n.03', 'synonyms': ['edible_corn', 'corn', 'maize'], 'def': 'ears of corn that can be prepared and served for human food', 'name': 'edible_corn'}, {'frequency': 'r', 'id': 313, 'synset': 'cornbread.n.01', 'synonyms': ['cornbread'], 'def': 'bread made primarily of cornmeal', 'name': 'cornbread'}, {'frequency': 'c', 'id': 314, 'synset': 'cornet.n.01', 'synonyms': ['cornet', 'horn', 'trumpet'], 'def': 'a brass musical instrument with a narrow tube and a flared bell and many valves', 'name': 'cornet'}, {'frequency': 'c', 'id': 315, 'synset': 'cornice.n.01', 'synonyms': ['cornice', 'valance', 'valance_board', 'pelmet'], 'def': 'a decorative framework to conceal curtain fixtures at the top of a window casing', 'name': 'cornice'}, {'frequency': 'r', 'id': 316, 'synset': 'cornmeal.n.01', 'synonyms': ['cornmeal'], 'def': 'coarsely ground corn', 'name': 'cornmeal'}, {'frequency': 'r', 'id': 317, 'synset': 'corset.n.01', 'synonyms': ['corset', 'girdle'], 'def': "a woman's close-fitting foundation garment", 'name': 'corset'}, {'frequency': 'r', 'id': 318, 'synset': 'cos.n.02', 'synonyms': ['romaine_lettuce'], 'def': 'lettuce with long dark-green leaves in a loosely packed elongated head', 'name': 'romaine_lettuce'}, {'frequency': 'c', 'id': 319, 'synset': 'costume.n.04', 'synonyms': ['costume'], 'def': 'the attire characteristic of a country or a time or a social class', 'name': 'costume'}, {'frequency': 'r', 'id': 320, 'synset': 'cougar.n.01', 'synonyms': ['cougar', 'puma', 'catamount', 'mountain_lion', 'panther'], 'def': 'large American feline resembling a lion', 'name': 'cougar'}, {'frequency': 'r', 'id': 321, 'synset': 'coverall.n.01', 'synonyms': ['coverall'], 'def': 'a loose-fitting protective garment that is worn over other clothing', 'name': 'coverall'}, {'frequency': 'r', 'id': 322, 'synset': 'cowbell.n.01', 'synonyms': ['cowbell'], 'def': 'a bell hung around the neck of cow so that the cow can be easily located', 'name': 'cowbell'}, {'frequency': 'f', 'id': 323, 'synset': 'cowboy_hat.n.01', 'synonyms': ['cowboy_hat', 'ten-gallon_hat'], 'def': 'a hat with a wide brim and a soft crown; worn by American ranch hands', 'name': 'cowboy_hat'}, {'frequency': 'r', 'id': 324, 'synset': 'crab.n.01', 'synonyms': ['crab_(animal)'], 'def': 'decapod having eyes on short stalks and a broad flattened shell and pincers', 'name': 'crab_(animal)'}, {'frequency': 'c', 'id': 325, 'synset': 'cracker.n.01', 'synonyms': ['cracker'], 'def': 'a thin crisp wafer', 'name': 'cracker'}, {'frequency': 'r', 'id': 326, 'synset': 'crape.n.01', 'synonyms': ['crape', 'crepe', 'French_pancake'], 'def': 'small very thin pancake', 'name': 'crape'}, {'frequency': 'f', 'id': 327, 'synset': 'crate.n.01', 'synonyms': ['crate'], 'def': 'a rugged box (usually made of wood); used for shipping', 'name': 'crate'}, {'frequency': 'r', 'id': 328, 'synset': 'crayon.n.01', 'synonyms': ['crayon', 'wax_crayon'], 'def': 'writing or drawing implement made of a colored stick of composition wax', 'name': 'crayon'}, {'frequency': 'r', 'id': 329, 'synset': 'cream_pitcher.n.01', 'synonyms': ['cream_pitcher'], 'def': 'a small pitcher for serving cream', 'name': 'cream_pitcher'}, {'frequency': 'r', 'id': 330, 'synset': 'credit_card.n.01', 'synonyms': ['credit_card', 'charge_card', 'debit_card'], 'def': 'a card, usually plastic, used to pay for goods and services', 'name': 'credit_card'}, {'frequency': 'c', 'id': 331, 'synset': 'crescent_roll.n.01', 'synonyms': ['crescent_roll', 'croissant'], 'def': 'very rich flaky crescent-shaped roll', 'name': 'crescent_roll'}, {'frequency': 'c', 'id': 332, 'synset': 'crib.n.01', 'synonyms': ['crib', 'cot'], 'def': 'baby bed with high sides made of slats', 'name': 'crib'}, {'frequency': 'c', 'id': 333, 'synset': 'crock.n.03', 'synonyms': ['crock_pot', 'earthenware_jar'], 'def': 'an earthen jar (made of baked clay)', 'name': 'crock_pot'}, {'frequency': 'f', 'id': 334, 'synset': 'crossbar.n.01', 'synonyms': ['crossbar'], 'def': 'a horizontal bar that goes across something', 'name': 'crossbar'}, {'frequency': 'r', 'id': 335, 'synset': 'crouton.n.01', 'synonyms': ['crouton'], 'def': 'a small piece of toasted or fried bread; served in soup or salads', 'name': 'crouton'}, {'frequency': 'r', 'id': 336, 'synset': 'crow.n.01', 'synonyms': ['crow'], 'def': 'black birds having a raucous call', 'name': 'crow'}, {'frequency': 'c', 'id': 337, 'synset': 'crown.n.04', 'synonyms': ['crown'], 'def': 'an ornamental jeweled headdress signifying sovereignty', 'name': 'crown'}, {'frequency': 'c', 'id': 338, 'synset': 'crucifix.n.01', 'synonyms': ['crucifix'], 'def': 'representation of the cross on which Jesus died', 'name': 'crucifix'}, {'frequency': 'c', 'id': 339, 'synset': 'cruise_ship.n.01', 'synonyms': ['cruise_ship', 'cruise_liner'], 'def': 'a passenger ship used commercially for pleasure cruises', 'name': 'cruise_ship'}, {'frequency': 'c', 'id': 340, 'synset': 'cruiser.n.01', 'synonyms': ['police_cruiser', 'patrol_car', 'police_car', 'squad_car'], 'def': 'a car in which policemen cruise the streets', 'name': 'police_cruiser'}, {'frequency': 'c', 'id': 341, 'synset': 'crumb.n.03', 'synonyms': ['crumb'], 'def': 'small piece of e.g. bread or cake', 'name': 'crumb'}, {'frequency': 'r', 'id': 342, 'synset': 'crutch.n.01', 'synonyms': ['crutch'], 'def': 'a wooden or metal staff that fits under the armpit and reaches to the ground', 'name': 'crutch'}, {'frequency': 'c', 'id': 343, 'synset': 'cub.n.03', 'synonyms': ['cub_(animal)'], 'def': 'the young of certain carnivorous mammals such as the bear or wolf or lion', 'name': 'cub_(animal)'}, {'frequency': 'r', 'id': 344, 'synset': 'cube.n.05', 'synonyms': ['cube', 'square_block'], 'def': 'a block in the (approximate) shape of a cube', 'name': 'cube'}, {'frequency': 'f', 'id': 345, 'synset': 'cucumber.n.02', 'synonyms': ['cucumber', 'cuke'], 'def': 'cylindrical green fruit with thin green rind and white flesh eaten as a vegetable', 'name': 'cucumber'}, {'frequency': 'c', 'id': 346, 'synset': 'cufflink.n.01', 'synonyms': ['cufflink'], 'def': 'jewelry consisting of linked buttons used to fasten the cuffs of a shirt', 'name': 'cufflink'}, {'frequency': 'f', 'id': 347, 'synset': 'cup.n.01', 'synonyms': ['cup'], 'def': 'a small open container usually used for drinking; usually has a handle', 'name': 'cup'}, {'frequency': 'c', 'id': 348, 'synset': 'cup.n.08', 'synonyms': ['trophy_cup'], 'def': 'a metal vessel with handles that is awarded as a trophy to a competition winner', 'name': 'trophy_cup'}, {'frequency': 'c', 'id': 349, 'synset': 'cupcake.n.01', 'synonyms': ['cupcake'], 'def': 'small cake baked in a muffin tin', 'name': 'cupcake'}, {'frequency': 'r', 'id': 350, 'synset': 'curler.n.01', 'synonyms': ['hair_curler', 'hair_roller', 'hair_crimper'], 'def': 'a cylindrical tube around which the hair is wound to curl it', 'name': 'hair_curler'}, {'frequency': 'r', 'id': 351, 'synset': 'curling_iron.n.01', 'synonyms': ['curling_iron'], 'def': 'a cylindrical home appliance that heats hair that has been curled around it', 'name': 'curling_iron'}, {'frequency': 'f', 'id': 352, 'synset': 'curtain.n.01', 'synonyms': ['curtain', 'drapery'], 'def': 'hanging cloth used as a blind (especially for a window)', 'name': 'curtain'}, {'frequency': 'f', 'id': 353, 'synset': 'cushion.n.03', 'synonyms': ['cushion'], 'def': 'a soft bag filled with air or padding such as feathers or foam rubber', 'name': 'cushion'}, {'frequency': 'r', 'id': 354, 'synset': 'custard.n.01', 'synonyms': ['custard'], 'def': 'sweetened mixture of milk and eggs baked or boiled or frozen', 'name': 'custard'}, {'frequency': 'c', 'id': 355, 'synset': 'cutter.n.06', 'synonyms': ['cutting_tool'], 'def': 'a cutting implement; a tool for cutting', 'name': 'cutting_tool'}, {'frequency': 'r', 'id': 356, 'synset': 'cylinder.n.04', 'synonyms': ['cylinder'], 'def': 'a cylindrical container', 'name': 'cylinder'}, {'frequency': 'r', 'id': 357, 'synset': 'cymbal.n.01', 'synonyms': ['cymbal'], 'def': 'a percussion instrument consisting of a concave brass disk', 'name': 'cymbal'}, {'frequency': 'r', 'id': 358, 'synset': 'dachshund.n.01', 'synonyms': ['dachshund', 'dachsie', 'badger_dog'], 'def': 'small long-bodied short-legged breed of dog having a short sleek coat and long drooping ears', 'name': 'dachshund'}, {'frequency': 'r', 'id': 359, 'synset': 'dagger.n.01', 'synonyms': ['dagger'], 'def': 'a short knife with a pointed blade used for piercing or stabbing', 'name': 'dagger'}, {'frequency': 'r', 'id': 360, 'synset': 'dartboard.n.01', 'synonyms': ['dartboard'], 'def': 'a circular board of wood or cork used as the target in the game of darts', 'name': 'dartboard'}, {'frequency': 'r', 'id': 361, 'synset': 'date.n.08', 'synonyms': ['date_(fruit)'], 'def': 'sweet edible fruit of the date palm with a single long woody seed', 'name': 'date_(fruit)'}, {'frequency': 'f', 'id': 362, 'synset': 'deck_chair.n.01', 'synonyms': ['deck_chair', 'beach_chair'], 'def': 'a folding chair for use outdoors; a wooden frame supports a length of canvas', 'name': 'deck_chair'}, {'frequency': 'c', 'id': 363, 'synset': 'deer.n.01', 'synonyms': ['deer', 'cervid'], 'def': "distinguished from Bovidae by the male's having solid deciduous antlers", 'name': 'deer'}, {'frequency': 'c', 'id': 364, 'synset': 'dental_floss.n.01', 'synonyms': ['dental_floss', 'floss'], 'def': 'a soft thread for cleaning the spaces between the teeth', 'name': 'dental_floss'}, {'frequency': 'f', 'id': 365, 'synset': 'desk.n.01', 'synonyms': ['desk'], 'def': 'a piece of furniture with a writing surface and usually drawers or other compartments', 'name': 'desk'}, {'frequency': 'r', 'id': 366, 'synset': 'detergent.n.01', 'synonyms': ['detergent'], 'def': 'a surface-active chemical widely used in industry and laundering', 'name': 'detergent'}, {'frequency': 'c', 'id': 367, 'synset': 'diaper.n.01', 'synonyms': ['diaper'], 'def': 'garment consisting of a folded cloth drawn up between the legs and fastened at the waist', 'name': 'diaper'}, {'frequency': 'r', 'id': 368, 'synset': 'diary.n.01', 'synonyms': ['diary', 'journal'], 'def': 'a daily written record of (usually personal) experiences and observations', 'name': 'diary'}, {'frequency': 'r', 'id': 369, 'synset': 'die.n.01', 'synonyms': ['die', 'dice'], 'def': 'a small cube with 1 to 6 spots on the six faces; used in gambling', 'name': 'die'}, {'frequency': 'r', 'id': 370, 'synset': 'dinghy.n.01', 'synonyms': ['dinghy', 'dory', 'rowboat'], 'def': 'a small boat of shallow draft with seats and oars with which it is propelled', 'name': 'dinghy'}, {'frequency': 'f', 'id': 371, 'synset': 'dining_table.n.01', 'synonyms': ['dining_table'], 'def': 'a table at which meals are served', 'name': 'dining_table'}, {'frequency': 'r', 'id': 372, 'synset': 'dinner_jacket.n.01', 'synonyms': ['tux', 'tuxedo'], 'def': 'semiformal evening dress for men', 'name': 'tux'}, {'frequency': 'c', 'id': 373, 'synset': 'dish.n.01', 'synonyms': ['dish'], 'def': 'a piece of dishware normally used as a container for holding or serving food', 'name': 'dish'}, {'frequency': 'c', 'id': 374, 'synset': 'dish.n.05', 'synonyms': ['dish_antenna'], 'def': 'directional antenna consisting of a parabolic reflector', 'name': 'dish_antenna'}, {'frequency': 'c', 'id': 375, 'synset': 'dishrag.n.01', 'synonyms': ['dishrag', 'dishcloth'], 'def': 'a cloth for washing dishes', 'name': 'dishrag'}, {'frequency': 'c', 'id': 376, 'synset': 'dishtowel.n.01', 'synonyms': ['dishtowel', 'tea_towel'], 'def': 'a towel for drying dishes', 'name': 'dishtowel'}, {'frequency': 'f', 'id': 377, 'synset': 'dishwasher.n.01', 'synonyms': ['dishwasher', 'dishwashing_machine'], 'def': 'a machine for washing dishes', 'name': 'dishwasher'}, {'frequency': 'r', 'id': 378, 'synset': 'dishwasher_detergent.n.01', 'synonyms': ['dishwasher_detergent', 'dishwashing_detergent', 'dishwashing_liquid'], 'def': 'a low-sudsing detergent designed for use in dishwashers', 'name': 'dishwasher_detergent'}, {'frequency': 'r', 'id': 379, 'synset': 'diskette.n.01', 'synonyms': ['diskette', 'floppy', 'floppy_disk'], 'def': 'a small plastic magnetic disk enclosed in a stiff envelope used to store data', 'name': 'diskette'}, {'frequency': 'c', 'id': 380, 'synset': 'dispenser.n.01', 'synonyms': ['dispenser'], 'def': 'a container so designed that the contents can be used in prescribed amounts', 'name': 'dispenser'}, {'frequency': 'c', 'id': 381, 'synset': 'dixie_cup.n.01', 'synonyms': ['Dixie_cup', 'paper_cup'], 'def': 'a disposable cup made of paper; for holding drinks', 'name': 'Dixie_cup'}, {'frequency': 'f', 'id': 382, 'synset': 'dog.n.01', 'synonyms': ['dog'], 'def': 'a common domesticated dog', 'name': 'dog'}, {'frequency': 'f', 'id': 383, 'synset': 'dog_collar.n.01', 'synonyms': ['dog_collar'], 'def': 'a collar for a dog', 'name': 'dog_collar'}, {'frequency': 'c', 'id': 384, 'synset': 'doll.n.01', 'synonyms': ['doll'], 'def': 'a toy replica of a HUMAN (NOT AN ANIMAL)', 'name': 'doll'}, {'frequency': 'r', 'id': 385, 'synset': 'dollar.n.02', 'synonyms': ['dollar', 'dollar_bill', 'one_dollar_bill'], 'def': 'a piece of paper money worth one dollar', 'name': 'dollar'}, {'frequency': 'r', 'id': 386, 'synset': 'dolphin.n.02', 'synonyms': ['dolphin'], 'def': 'any of various small toothed whales with a beaklike snout; larger than porpoises', 'name': 'dolphin'}, {'frequency': 'c', 'id': 387, 'synset': 'domestic_ass.n.01', 'synonyms': ['domestic_ass', 'donkey'], 'def': 'domestic beast of burden descended from the African wild ass; patient but stubborn', 'name': 'domestic_ass'}, {'frequency': 'r', 'id': 388, 'synset': 'domino.n.03', 'synonyms': ['eye_mask'], 'def': 'a mask covering the upper part of the face but with holes for the eyes', 'name': 'eye_mask'}, {'frequency': 'r', 'id': 389, 'synset': 'doorbell.n.01', 'synonyms': ['doorbell', 'buzzer'], 'def': 'a button at an outer door that gives a ringing or buzzing signal when pushed', 'name': 'doorbell'}, {'frequency': 'f', 'id': 390, 'synset': 'doorknob.n.01', 'synonyms': ['doorknob', 'doorhandle'], 'def': "a knob used to open a door (often called `doorhandle' in Great Britain)", 'name': 'doorknob'}, {'frequency': 'c', 'id': 391, 'synset': 'doormat.n.02', 'synonyms': ['doormat', 'welcome_mat'], 'def': 'a mat placed outside an exterior door for wiping the shoes before entering', 'name': 'doormat'}, {'frequency': 'f', 'id': 392, 'synset': 'doughnut.n.02', 'synonyms': ['doughnut', 'donut'], 'def': 'a small ring-shaped friedcake', 'name': 'doughnut'}, {'frequency': 'r', 'id': 393, 'synset': 'dove.n.01', 'synonyms': ['dove'], 'def': 'any of numerous small pigeons', 'name': 'dove'}, {'frequency': 'r', 'id': 394, 'synset': 'dragonfly.n.01', 'synonyms': ['dragonfly'], 'def': 'slender-bodied non-stinging insect having iridescent wings that are outspread at rest', 'name': 'dragonfly'}, {'frequency': 'f', 'id': 395, 'synset': 'drawer.n.01', 'synonyms': ['drawer'], 'def': 'a boxlike container in a piece of furniture; made so as to slide in and out', 'name': 'drawer'}, {'frequency': 'c', 'id': 396, 'synset': 'drawers.n.01', 'synonyms': ['underdrawers', 'boxers', 'boxershorts'], 'def': 'underpants worn by men', 'name': 'underdrawers'}, {'frequency': 'f', 'id': 397, 'synset': 'dress.n.01', 'synonyms': ['dress', 'frock'], 'def': 'a one-piece garment for a woman; has skirt and bodice', 'name': 'dress'}, {'frequency': 'c', 'id': 398, 'synset': 'dress_hat.n.01', 'synonyms': ['dress_hat', 'high_hat', 'opera_hat', 'silk_hat', 'top_hat'], 'def': "a man's hat with a tall crown; usually covered with silk or with beaver fur", 'name': 'dress_hat'}, {'frequency': 'c', 'id': 399, 'synset': 'dress_suit.n.01', 'synonyms': ['dress_suit'], 'def': 'formalwear consisting of full evening dress for men', 'name': 'dress_suit'}, {'frequency': 'c', 'id': 400, 'synset': 'dresser.n.05', 'synonyms': ['dresser'], 'def': 'a cabinet with shelves', 'name': 'dresser'}, {'frequency': 'c', 'id': 401, 'synset': 'drill.n.01', 'synonyms': ['drill'], 'def': 'a tool with a sharp rotating point for making holes in hard materials', 'name': 'drill'}, {'frequency': 'r', 'id': 402, 'synset': 'drinking_fountain.n.01', 'synonyms': ['drinking_fountain'], 'def': 'a public fountain to provide a jet of drinking water', 'name': 'drinking_fountain'}, {'frequency': 'r', 'id': 403, 'synset': 'drone.n.04', 'synonyms': ['drone'], 'def': 'an aircraft without a pilot that is operated by remote control', 'name': 'drone'}, {'frequency': 'r', 'id': 404, 'synset': 'dropper.n.01', 'synonyms': ['dropper', 'eye_dropper'], 'def': 'pipet consisting of a small tube with a vacuum bulb at one end for drawing liquid in and releasing it a drop at a time', 'name': 'dropper'}, {'frequency': 'c', 'id': 405, 'synset': 'drum.n.01', 'synonyms': ['drum_(musical_instrument)'], 'def': 'a musical percussion instrument; usually consists of a hollow cylinder with a membrane stretched across each end', 'name': 'drum_(musical_instrument)'}, {'frequency': 'r', 'id': 406, 'synset': 'drumstick.n.02', 'synonyms': ['drumstick'], 'def': 'a stick used for playing a drum', 'name': 'drumstick'}, {'frequency': 'f', 'id': 407, 'synset': 'duck.n.01', 'synonyms': ['duck'], 'def': 'small web-footed broad-billed swimming bird', 'name': 'duck'}, {'frequency': 'r', 'id': 408, 'synset': 'duckling.n.02', 'synonyms': ['duckling'], 'def': 'young duck', 'name': 'duckling'}, {'frequency': 'c', 'id': 409, 'synset': 'duct_tape.n.01', 'synonyms': ['duct_tape'], 'def': 'a wide silvery adhesive tape', 'name': 'duct_tape'}, {'frequency': 'f', 'id': 410, 'synset': 'duffel_bag.n.01', 'synonyms': ['duffel_bag', 'duffle_bag', 'duffel', 'duffle'], 'def': 'a large cylindrical bag of heavy cloth', 'name': 'duffel_bag'}, {'frequency': 'r', 'id': 411, 'synset': 'dumbbell.n.01', 'synonyms': ['dumbbell'], 'def': 'an exercising weight with two ball-like ends connected by a short handle', 'name': 'dumbbell'}, {'frequency': 'c', 'id': 412, 'synset': 'dumpster.n.01', 'synonyms': ['dumpster'], 'def': 'a container designed to receive and transport and dump waste', 'name': 'dumpster'}, {'frequency': 'r', 'id': 413, 'synset': 'dustpan.n.02', 'synonyms': ['dustpan'], 'def': 'a short-handled receptacle into which dust can be swept', 'name': 'dustpan'}, {'frequency': 'r', 'id': 414, 'synset': 'dutch_oven.n.02', 'synonyms': ['Dutch_oven'], 'def': 'iron or earthenware cooking pot; used for stews', 'name': 'Dutch_oven'}, {'frequency': 'c', 'id': 415, 'synset': 'eagle.n.01', 'synonyms': ['eagle'], 'def': 'large birds of prey noted for their broad wings and strong soaring flight', 'name': 'eagle'}, {'frequency': 'f', 'id': 416, 'synset': 'earphone.n.01', 'synonyms': ['earphone', 'earpiece', 'headphone'], 'def': 'device for listening to audio that is held over or inserted into the ear', 'name': 'earphone'}, {'frequency': 'r', 'id': 417, 'synset': 'earplug.n.01', 'synonyms': ['earplug'], 'def': 'a soft plug that is inserted into the ear canal to block sound', 'name': 'earplug'}, {'frequency': 'f', 'id': 418, 'synset': 'earring.n.01', 'synonyms': ['earring'], 'def': 'jewelry to ornament the ear', 'name': 'earring'}, {'frequency': 'c', 'id': 419, 'synset': 'easel.n.01', 'synonyms': ['easel'], 'def': "an upright tripod for displaying something (usually an artist's canvas)", 'name': 'easel'}, {'frequency': 'r', 'id': 420, 'synset': 'eclair.n.01', 'synonyms': ['eclair'], 'def': 'oblong cream puff', 'name': 'eclair'}, {'frequency': 'r', 'id': 421, 'synset': 'eel.n.01', 'synonyms': ['eel'], 'def': 'an elongate fish with fatty flesh', 'name': 'eel'}, {'frequency': 'f', 'id': 422, 'synset': 'egg.n.02', 'synonyms': ['egg', 'eggs'], 'def': 'oval reproductive body of a fowl (especially a hen) used as food', 'name': 'egg'}, {'frequency': 'r', 'id': 423, 'synset': 'egg_roll.n.01', 'synonyms': ['egg_roll', 'spring_roll'], 'def': 'minced vegetables and meat wrapped in a pancake and fried', 'name': 'egg_roll'}, {'frequency': 'c', 'id': 424, 'synset': 'egg_yolk.n.01', 'synonyms': ['egg_yolk', 'yolk_(egg)'], 'def': 'the yellow spherical part of an egg', 'name': 'egg_yolk'}, {'frequency': 'c', 'id': 425, 'synset': 'eggbeater.n.02', 'synonyms': ['eggbeater', 'eggwhisk'], 'def': 'a mixer for beating eggs or whipping cream', 'name': 'eggbeater'}, {'frequency': 'c', 'id': 426, 'synset': 'eggplant.n.01', 'synonyms': ['eggplant', 'aubergine'], 'def': 'egg-shaped vegetable having a shiny skin typically dark purple', 'name': 'eggplant'}, {'frequency': 'r', 'id': 427, 'synset': 'electric_chair.n.01', 'synonyms': ['electric_chair'], 'def': 'a chair-shaped instrument of execution by electrocution', 'name': 'electric_chair'}, {'frequency': 'f', 'id': 428, 'synset': 'electric_refrigerator.n.01', 'synonyms': ['refrigerator'], 'def': 'a refrigerator in which the coolant is pumped around by an electric motor', 'name': 'refrigerator'}, {'frequency': 'f', 'id': 429, 'synset': 'elephant.n.01', 'synonyms': ['elephant'], 'def': 'a common elephant', 'name': 'elephant'}, {'frequency': 'r', 'id': 430, 'synset': 'elk.n.01', 'synonyms': ['elk', 'moose'], 'def': 'large northern deer with enormous flattened antlers in the male', 'name': 'elk'}, {'frequency': 'c', 'id': 431, 'synset': 'envelope.n.01', 'synonyms': ['envelope'], 'def': 'a flat (usually rectangular) container for a letter, thin package, etc.', 'name': 'envelope'}, {'frequency': 'c', 'id': 432, 'synset': 'eraser.n.01', 'synonyms': ['eraser'], 'def': 'an implement used to erase something', 'name': 'eraser'}, {'frequency': 'r', 'id': 433, 'synset': 'escargot.n.01', 'synonyms': ['escargot'], 'def': 'edible snail usually served in the shell with a sauce of melted butter and garlic', 'name': 'escargot'}, {'frequency': 'r', 'id': 434, 'synset': 'eyepatch.n.01', 'synonyms': ['eyepatch'], 'def': 'a protective cloth covering for an injured eye', 'name': 'eyepatch'}, {'frequency': 'r', 'id': 435, 'synset': 'falcon.n.01', 'synonyms': ['falcon'], 'def': 'birds of prey having long pointed powerful wings adapted for swift flight', 'name': 'falcon'}, {'frequency': 'f', 'id': 436, 'synset': 'fan.n.01', 'synonyms': ['fan'], 'def': 'a device for creating a current of air by movement of a surface or surfaces', 'name': 'fan'}, {'frequency': 'f', 'id': 437, 'synset': 'faucet.n.01', 'synonyms': ['faucet', 'spigot', 'tap'], 'def': 'a regulator for controlling the flow of a liquid from a reservoir', 'name': 'faucet'}, {'frequency': 'r', 'id': 438, 'synset': 'fedora.n.01', 'synonyms': ['fedora'], 'def': 'a hat made of felt with a creased crown', 'name': 'fedora'}, {'frequency': 'r', 'id': 439, 'synset': 'ferret.n.02', 'synonyms': ['ferret'], 'def': 'domesticated albino variety of the European polecat bred for hunting rats and rabbits', 'name': 'ferret'}, {'frequency': 'c', 'id': 440, 'synset': 'ferris_wheel.n.01', 'synonyms': ['Ferris_wheel'], 'def': 'a large wheel with suspended seats that remain upright as the wheel rotates', 'name': 'Ferris_wheel'}, {'frequency': 'r', 'id': 441, 'synset': 'ferry.n.01', 'synonyms': ['ferry', 'ferryboat'], 'def': 'a boat that transports people or vehicles across a body of water and operates on a regular schedule', 'name': 'ferry'}, {'frequency': 'r', 'id': 442, 'synset': 'fig.n.04', 'synonyms': ['fig_(fruit)'], 'def': 'fleshy sweet pear-shaped yellowish or purple fruit eaten fresh or preserved or dried', 'name': 'fig_(fruit)'}, {'frequency': 'c', 'id': 443, 'synset': 'fighter.n.02', 'synonyms': ['fighter_jet', 'fighter_aircraft', 'attack_aircraft'], 'def': 'a high-speed military or naval airplane designed to destroy enemy targets', 'name': 'fighter_jet'}, {'frequency': 'f', 'id': 444, 'synset': 'figurine.n.01', 'synonyms': ['figurine'], 'def': 'a small carved or molded figure', 'name': 'figurine'}, {'frequency': 'c', 'id': 445, 'synset': 'file.n.03', 'synonyms': ['file_cabinet', 'filing_cabinet'], 'def': 'office furniture consisting of a container for keeping papers in order', 'name': 'file_cabinet'}, {'frequency': 'r', 'id': 446, 'synset': 'file.n.04', 'synonyms': ['file_(tool)'], 'def': 'a steel hand tool with small sharp teeth on some or all of its surfaces; used for smoothing wood or metal', 'name': 'file_(tool)'}, {'frequency': 'f', 'id': 447, 'synset': 'fire_alarm.n.02', 'synonyms': ['fire_alarm', 'smoke_alarm'], 'def': 'an alarm that is tripped off by fire or smoke', 'name': 'fire_alarm'}, {'frequency': 'c', 'id': 448, 'synset': 'fire_engine.n.01', 'synonyms': ['fire_engine', 'fire_truck'], 'def': 'large trucks that carry firefighters and equipment to the site of a fire', 'name': 'fire_engine'}, {'frequency': 'c', 'id': 449, 'synset': 'fire_extinguisher.n.01', 'synonyms': ['fire_extinguisher', 'extinguisher'], 'def': 'a manually operated device for extinguishing small fires', 'name': 'fire_extinguisher'}, {'frequency': 'c', 'id': 450, 'synset': 'fire_hose.n.01', 'synonyms': ['fire_hose'], 'def': 'a large hose that carries water from a fire hydrant to the site of the fire', 'name': 'fire_hose'}, {'frequency': 'f', 'id': 451, 'synset': 'fireplace.n.01', 'synonyms': ['fireplace'], 'def': 'an open recess in a wall at the base of a chimney where a fire can be built', 'name': 'fireplace'}, {'frequency': 'f', 'id': 452, 'synset': 'fireplug.n.01', 'synonyms': ['fireplug', 'fire_hydrant', 'hydrant'], 'def': 'an upright hydrant for drawing water to use in fighting a fire', 'name': 'fireplug'}, {'frequency': 'c', 'id': 453, 'synset': 'fish.n.01', 'synonyms': ['fish'], 'def': 'any of various mostly cold-blooded aquatic vertebrates usually having scales and breathing through gills', 'name': 'fish'}, {'frequency': 'r', 'id': 454, 'synset': 'fish.n.02', 'synonyms': ['fish_(food)'], 'def': 'the flesh of fish used as food', 'name': 'fish_(food)'}, {'frequency': 'r', 'id': 455, 'synset': 'fishbowl.n.02', 'synonyms': ['fishbowl', 'goldfish_bowl'], 'def': 'a transparent bowl in which small fish are kept', 'name': 'fishbowl'}, {'frequency': 'r', 'id': 456, 'synset': 'fishing_boat.n.01', 'synonyms': ['fishing_boat', 'fishing_vessel'], 'def': 'a vessel for fishing', 'name': 'fishing_boat'}, {'frequency': 'c', 'id': 457, 'synset': 'fishing_rod.n.01', 'synonyms': ['fishing_rod', 'fishing_pole'], 'def': 'a rod that is used in fishing to extend the fishing line', 'name': 'fishing_rod'}, {'frequency': 'f', 'id': 458, 'synset': 'flag.n.01', 'synonyms': ['flag'], 'def': 'emblem usually consisting of a rectangular piece of cloth of distinctive design (do not include pole)', 'name': 'flag'}, {'frequency': 'f', 'id': 459, 'synset': 'flagpole.n.02', 'synonyms': ['flagpole', 'flagstaff'], 'def': 'a tall staff or pole on which a flag is raised', 'name': 'flagpole'}, {'frequency': 'c', 'id': 460, 'synset': 'flamingo.n.01', 'synonyms': ['flamingo'], 'def': 'large pink web-footed bird with down-bent bill', 'name': 'flamingo'}, {'frequency': 'c', 'id': 461, 'synset': 'flannel.n.01', 'synonyms': ['flannel'], 'def': 'a soft light woolen fabric; used for clothing', 'name': 'flannel'}, {'frequency': 'r', 'id': 462, 'synset': 'flash.n.10', 'synonyms': ['flash', 'flashbulb'], 'def': 'a lamp for providing momentary light to take a photograph', 'name': 'flash'}, {'frequency': 'c', 'id': 463, 'synset': 'flashlight.n.01', 'synonyms': ['flashlight', 'torch'], 'def': 'a small portable battery-powered electric lamp', 'name': 'flashlight'}, {'frequency': 'r', 'id': 464, 'synset': 'fleece.n.03', 'synonyms': ['fleece'], 'def': 'a soft bulky fabric with deep pile; used chiefly for clothing', 'name': 'fleece'}, {'frequency': 'f', 'id': 465, 'synset': 'flip-flop.n.02', 'synonyms': ['flip-flop_(sandal)'], 'def': 'a backless sandal held to the foot by a thong between two toes', 'name': 'flip-flop_(sandal)'}, {'frequency': 'c', 'id': 466, 'synset': 'flipper.n.01', 'synonyms': ['flipper_(footwear)', 'fin_(footwear)'], 'def': 'a shoe to aid a person in swimming', 'name': 'flipper_(footwear)'}, {'frequency': 'f', 'id': 467, 'synset': 'flower_arrangement.n.01', 'synonyms': ['flower_arrangement', 'floral_arrangement'], 'def': 'a decorative arrangement of flowers', 'name': 'flower_arrangement'}, {'frequency': 'c', 'id': 468, 'synset': 'flute.n.02', 'synonyms': ['flute_glass', 'champagne_flute'], 'def': 'a tall narrow wineglass', 'name': 'flute_glass'}, {'frequency': 'r', 'id': 469, 'synset': 'foal.n.01', 'synonyms': ['foal'], 'def': 'a young horse', 'name': 'foal'}, {'frequency': 'c', 'id': 470, 'synset': 'folding_chair.n.01', 'synonyms': ['folding_chair'], 'def': 'a chair that can be folded flat for storage', 'name': 'folding_chair'}, {'frequency': 'c', 'id': 471, 'synset': 'food_processor.n.01', 'synonyms': ['food_processor'], 'def': 'a kitchen appliance for shredding, blending, chopping, or slicing food', 'name': 'food_processor'}, {'frequency': 'c', 'id': 472, 'synset': 'football.n.02', 'synonyms': ['football_(American)'], 'def': 'the inflated oblong ball used in playing American football', 'name': 'football_(American)'}, {'frequency': 'r', 'id': 473, 'synset': 'football_helmet.n.01', 'synonyms': ['football_helmet'], 'def': 'a padded helmet with a face mask to protect the head of football players', 'name': 'football_helmet'}, {'frequency': 'c', 'id': 474, 'synset': 'footstool.n.01', 'synonyms': ['footstool', 'footrest'], 'def': 'a low seat or a stool to rest the feet of a seated person', 'name': 'footstool'}, {'frequency': 'f', 'id': 475, 'synset': 'fork.n.01', 'synonyms': ['fork'], 'def': 'cutlery used for serving and eating food', 'name': 'fork'}, {'frequency': 'r', 'id': 476, 'synset': 'forklift.n.01', 'synonyms': ['forklift'], 'def': 'an industrial vehicle with a power operated fork in front that can be inserted under loads to lift and move them', 'name': 'forklift'}, {'frequency': 'r', 'id': 477, 'synset': 'freight_car.n.01', 'synonyms': ['freight_car'], 'def': 'a railway car that carries freight', 'name': 'freight_car'}, {'frequency': 'r', 'id': 478, 'synset': 'french_toast.n.01', 'synonyms': ['French_toast'], 'def': 'bread slice dipped in egg and milk and fried', 'name': 'French_toast'}, {'frequency': 'c', 'id': 479, 'synset': 'freshener.n.01', 'synonyms': ['freshener', 'air_freshener'], 'def': 'anything that freshens', 'name': 'freshener'}, {'frequency': 'f', 'id': 480, 'synset': 'frisbee.n.01', 'synonyms': ['frisbee'], 'def': 'a light, plastic disk propelled with a flip of the wrist for recreation or competition', 'name': 'frisbee'}, {'frequency': 'c', 'id': 481, 'synset': 'frog.n.01', 'synonyms': ['frog', 'toad', 'toad_frog'], 'def': 'a tailless stout-bodied amphibians with long hind limbs for leaping', 'name': 'frog'}, {'frequency': 'c', 'id': 482, 'synset': 'fruit_juice.n.01', 'synonyms': ['fruit_juice'], 'def': 'drink produced by squeezing or crushing fruit', 'name': 'fruit_juice'}, {'frequency': 'r', 'id': 483, 'synset': 'fruit_salad.n.01', 'synonyms': ['fruit_salad'], 'def': 'salad composed of fruits', 'name': 'fruit_salad'}, {'frequency': 'c', 'id': 484, 'synset': 'frying_pan.n.01', 'synonyms': ['frying_pan', 'frypan', 'skillet'], 'def': 'a pan used for frying foods', 'name': 'frying_pan'}, {'frequency': 'r', 'id': 485, 'synset': 'fudge.n.01', 'synonyms': ['fudge'], 'def': 'soft creamy candy', 'name': 'fudge'}, {'frequency': 'r', 'id': 486, 'synset': 'funnel.n.02', 'synonyms': ['funnel'], 'def': 'a cone-shaped utensil used to channel a substance into a container with a small mouth', 'name': 'funnel'}, {'frequency': 'c', 'id': 487, 'synset': 'futon.n.01', 'synonyms': ['futon'], 'def': 'a pad that is used for sleeping on the floor or on a raised frame', 'name': 'futon'}, {'frequency': 'r', 'id': 488, 'synset': 'gag.n.02', 'synonyms': ['gag', 'muzzle'], 'def': "restraint put into a person's mouth to prevent speaking or shouting", 'name': 'gag'}, {'frequency': 'r', 'id': 489, 'synset': 'garbage.n.03', 'synonyms': ['garbage'], 'def': 'a receptacle where waste can be discarded', 'name': 'garbage'}, {'frequency': 'c', 'id': 490, 'synset': 'garbage_truck.n.01', 'synonyms': ['garbage_truck'], 'def': 'a truck for collecting domestic refuse', 'name': 'garbage_truck'}, {'frequency': 'c', 'id': 491, 'synset': 'garden_hose.n.01', 'synonyms': ['garden_hose'], 'def': 'a hose used for watering a lawn or garden', 'name': 'garden_hose'}, {'frequency': 'c', 'id': 492, 'synset': 'gargle.n.01', 'synonyms': ['gargle', 'mouthwash'], 'def': 'a medicated solution used for gargling and rinsing the mouth', 'name': 'gargle'}, {'frequency': 'r', 'id': 493, 'synset': 'gargoyle.n.02', 'synonyms': ['gargoyle'], 'def': 'an ornament consisting of a grotesquely carved figure of a person or animal', 'name': 'gargoyle'}, {'frequency': 'c', 'id': 494, 'synset': 'garlic.n.02', 'synonyms': ['garlic', 'ail'], 'def': 'aromatic bulb used as seasoning', 'name': 'garlic'}, {'frequency': 'r', 'id': 495, 'synset': 'gasmask.n.01', 'synonyms': ['gasmask', 'respirator', 'gas_helmet'], 'def': 'a protective face mask with a filter', 'name': 'gasmask'}, {'frequency': 'r', 'id': 496, 'synset': 'gazelle.n.01', 'synonyms': ['gazelle'], 'def': 'small swift graceful antelope of Africa and Asia having lustrous eyes', 'name': 'gazelle'}, {'frequency': 'c', 'id': 497, 'synset': 'gelatin.n.02', 'synonyms': ['gelatin', 'jelly'], 'def': 'an edible jelly made with gelatin and used as a dessert or salad base or a coating for foods', 'name': 'gelatin'}, {'frequency': 'r', 'id': 498, 'synset': 'gem.n.02', 'synonyms': ['gemstone'], 'def': 'a crystalline rock that can be cut and polished for jewelry', 'name': 'gemstone'}, {'frequency': 'c', 'id': 499, 'synset': 'giant_panda.n.01', 'synonyms': ['giant_panda', 'panda', 'panda_bear'], 'def': 'large black-and-white herbivorous mammal of bamboo forests of China and Tibet', 'name': 'giant_panda'}, {'frequency': 'c', 'id': 500, 'synset': 'gift_wrap.n.01', 'synonyms': ['gift_wrap'], 'def': 'attractive wrapping paper suitable for wrapping gifts', 'name': 'gift_wrap'}, {'frequency': 'c', 'id': 501, 'synset': 'ginger.n.03', 'synonyms': ['ginger', 'gingerroot'], 'def': 'the root of the common ginger plant; used fresh as a seasoning', 'name': 'ginger'}, {'frequency': 'f', 'id': 502, 'synset': 'giraffe.n.01', 'synonyms': ['giraffe'], 'def': 'tall animal having a spotted coat and small horns and very long neck and legs', 'name': 'giraffe'}, {'frequency': 'c', 'id': 503, 'synset': 'girdle.n.02', 'synonyms': ['cincture', 'sash', 'waistband', 'waistcloth'], 'def': 'a band of material around the waist that strengthens a skirt or trousers', 'name': 'cincture'}, {'frequency': 'f', 'id': 504, 'synset': 'glass.n.02', 'synonyms': ['glass_(drink_container)', 'drinking_glass'], 'def': 'a container for holding liquids while drinking', 'name': 'glass_(drink_container)'}, {'frequency': 'c', 'id': 505, 'synset': 'globe.n.03', 'synonyms': ['globe'], 'def': 'a sphere on which a map (especially of the earth) is represented', 'name': 'globe'}, {'frequency': 'f', 'id': 506, 'synset': 'glove.n.02', 'synonyms': ['glove'], 'def': 'handwear covering the hand', 'name': 'glove'}, {'frequency': 'c', 'id': 507, 'synset': 'goat.n.01', 'synonyms': ['goat'], 'def': 'a common goat', 'name': 'goat'}, {'frequency': 'f', 'id': 508, 'synset': 'goggles.n.01', 'synonyms': ['goggles'], 'def': 'tight-fitting spectacles worn to protect the eyes', 'name': 'goggles'}, {'frequency': 'r', 'id': 509, 'synset': 'goldfish.n.01', 'synonyms': ['goldfish'], 'def': 'small golden or orange-red freshwater fishes used as pond or aquarium pets', 'name': 'goldfish'}, {'frequency': 'r', 'id': 510, 'synset': 'golf_club.n.02', 'synonyms': ['golf_club', 'golf-club'], 'def': 'golf equipment used by a golfer to hit a golf ball', 'name': 'golf_club'}, {'frequency': 'c', 'id': 511, 'synset': 'golfcart.n.01', 'synonyms': ['golfcart'], 'def': 'a small motor vehicle in which golfers can ride between shots', 'name': 'golfcart'}, {'frequency': 'r', 'id': 512, 'synset': 'gondola.n.02', 'synonyms': ['gondola_(boat)'], 'def': 'long narrow flat-bottomed boat propelled by sculling; traditionally used on canals of Venice', 'name': 'gondola_(boat)'}, {'frequency': 'c', 'id': 513, 'synset': 'goose.n.01', 'synonyms': ['goose'], 'def': 'loud, web-footed long-necked aquatic birds usually larger than ducks', 'name': 'goose'}, {'frequency': 'r', 'id': 514, 'synset': 'gorilla.n.01', 'synonyms': ['gorilla'], 'def': 'largest ape', 'name': 'gorilla'}, {'frequency': 'r', 'id': 515, 'synset': 'gourd.n.02', 'synonyms': ['gourd'], 'def': 'any of numerous inedible fruits with hard rinds', 'name': 'gourd'}, {'frequency': 'r', 'id': 516, 'synset': 'gown.n.04', 'synonyms': ['surgical_gown', 'scrubs_(surgical_clothing)'], 'def': 'protective garment worn by surgeons during operations', 'name': 'surgical_gown'}, {'frequency': 'f', 'id': 517, 'synset': 'grape.n.01', 'synonyms': ['grape'], 'def': 'any of various juicy fruit with green or purple skins; grow in clusters', 'name': 'grape'}, {'frequency': 'r', 'id': 518, 'synset': 'grasshopper.n.01', 'synonyms': ['grasshopper'], 'def': 'plant-eating insect with hind legs adapted for leaping', 'name': 'grasshopper'}, {'frequency': 'c', 'id': 519, 'synset': 'grater.n.01', 'synonyms': ['grater'], 'def': 'utensil with sharp perforations for shredding foods (as vegetables or cheese)', 'name': 'grater'}, {'frequency': 'c', 'id': 520, 'synset': 'gravestone.n.01', 'synonyms': ['gravestone', 'headstone', 'tombstone'], 'def': 'a stone that is used to mark a grave', 'name': 'gravestone'}, {'frequency': 'r', 'id': 521, 'synset': 'gravy_boat.n.01', 'synonyms': ['gravy_boat', 'gravy_holder'], 'def': 'a dish (often boat-shaped) for serving gravy or sauce', 'name': 'gravy_boat'}, {'frequency': 'c', 'id': 522, 'synset': 'green_bean.n.02', 'synonyms': ['green_bean'], 'def': 'a common bean plant cultivated for its slender green edible pods', 'name': 'green_bean'}, {'frequency': 'c', 'id': 523, 'synset': 'green_onion.n.01', 'synonyms': ['green_onion', 'spring_onion', 'scallion'], 'def': 'a young onion before the bulb has enlarged', 'name': 'green_onion'}, {'frequency': 'r', 'id': 524, 'synset': 'griddle.n.01', 'synonyms': ['griddle'], 'def': 'cooking utensil consisting of a flat heated surface on which food is cooked', 'name': 'griddle'}, {'frequency': 'r', 'id': 525, 'synset': 'grillroom.n.01', 'synonyms': ['grillroom', 'grill_(restaurant)'], 'def': 'a restaurant where food is cooked on a grill', 'name': 'grillroom'}, {'frequency': 'r', 'id': 526, 'synset': 'grinder.n.04', 'synonyms': ['grinder_(tool)'], 'def': 'a machine tool that polishes metal', 'name': 'grinder_(tool)'}, {'frequency': 'r', 'id': 527, 'synset': 'grits.n.01', 'synonyms': ['grits', 'hominy_grits'], 'def': 'coarsely ground corn boiled as a breakfast dish', 'name': 'grits'}, {'frequency': 'c', 'id': 528, 'synset': 'grizzly.n.01', 'synonyms': ['grizzly', 'grizzly_bear'], 'def': 'powerful brownish-yellow bear of the uplands of western North America', 'name': 'grizzly'}, {'frequency': 'c', 'id': 529, 'synset': 'grocery_bag.n.01', 'synonyms': ['grocery_bag'], 'def': "a sack for holding customer's groceries", 'name': 'grocery_bag'}, {'frequency': 'r', 'id': 530, 'synset': 'guacamole.n.01', 'synonyms': ['guacamole'], 'def': 'a dip made of mashed avocado mixed with chopped onions and other seasonings', 'name': 'guacamole'}, {'frequency': 'f', 'id': 531, 'synset': 'guitar.n.01', 'synonyms': ['guitar'], 'def': 'a stringed instrument usually having six strings; played by strumming or plucking', 'name': 'guitar'}, {'frequency': 'c', 'id': 532, 'synset': 'gull.n.02', 'synonyms': ['gull', 'seagull'], 'def': 'mostly white aquatic bird having long pointed wings and short legs', 'name': 'gull'}, {'frequency': 'c', 'id': 533, 'synset': 'gun.n.01', 'synonyms': ['gun'], 'def': 'a weapon that discharges a bullet at high velocity from a metal tube', 'name': 'gun'}, {'frequency': 'r', 'id': 534, 'synset': 'hair_spray.n.01', 'synonyms': ['hair_spray'], 'def': 'substance sprayed on the hair to hold it in place', 'name': 'hair_spray'}, {'frequency': 'c', 'id': 535, 'synset': 'hairbrush.n.01', 'synonyms': ['hairbrush'], 'def': "a brush used to groom a person's hair", 'name': 'hairbrush'}, {'frequency': 'c', 'id': 536, 'synset': 'hairnet.n.01', 'synonyms': ['hairnet'], 'def': 'a small net that someone wears over their hair to keep it in place', 'name': 'hairnet'}, {'frequency': 'c', 'id': 537, 'synset': 'hairpin.n.01', 'synonyms': ['hairpin'], 'def': "a double pronged pin used to hold women's hair in place", 'name': 'hairpin'}, {'frequency': 'f', 'id': 538, 'synset': 'ham.n.01', 'synonyms': ['ham', 'jambon', 'gammon'], 'def': 'meat cut from the thigh of a hog (usually smoked)', 'name': 'ham'}, {'frequency': 'c', 'id': 539, 'synset': 'hamburger.n.01', 'synonyms': ['hamburger', 'beefburger', 'burger'], 'def': 'a sandwich consisting of a patty of minced beef served on a bun', 'name': 'hamburger'}, {'frequency': 'c', 'id': 540, 'synset': 'hammer.n.02', 'synonyms': ['hammer'], 'def': 'a hand tool with a heavy head and a handle; used to deliver an impulsive force by striking', 'name': 'hammer'}, {'frequency': 'r', 'id': 541, 'synset': 'hammock.n.02', 'synonyms': ['hammock'], 'def': 'a hanging bed of canvas or rope netting (usually suspended between two trees)', 'name': 'hammock'}, {'frequency': 'r', 'id': 542, 'synset': 'hamper.n.02', 'synonyms': ['hamper'], 'def': 'a basket usually with a cover', 'name': 'hamper'}, {'frequency': 'r', 'id': 543, 'synset': 'hamster.n.01', 'synonyms': ['hamster'], 'def': 'short-tailed burrowing rodent with large cheek pouches', 'name': 'hamster'}, {'frequency': 'c', 'id': 544, 'synset': 'hand_blower.n.01', 'synonyms': ['hair_dryer'], 'def': 'a hand-held electric blower that can blow warm air onto the hair', 'name': 'hair_dryer'}, {'frequency': 'r', 'id': 545, 'synset': 'hand_glass.n.01', 'synonyms': ['hand_glass', 'hand_mirror'], 'def': 'a mirror intended to be held in the hand', 'name': 'hand_glass'}, {'frequency': 'f', 'id': 546, 'synset': 'hand_towel.n.01', 'synonyms': ['hand_towel', 'face_towel'], 'def': 'a small towel used to dry the hands or face', 'name': 'hand_towel'}, {'frequency': 'c', 'id': 547, 'synset': 'handcart.n.01', 'synonyms': ['handcart', 'pushcart', 'hand_truck'], 'def': 'wheeled vehicle that can be pushed by a person', 'name': 'handcart'}, {'frequency': 'r', 'id': 548, 'synset': 'handcuff.n.01', 'synonyms': ['handcuff'], 'def': 'shackle that consists of a metal loop that can be locked around the wrist', 'name': 'handcuff'}, {'frequency': 'c', 'id': 549, 'synset': 'handkerchief.n.01', 'synonyms': ['handkerchief'], 'def': 'a square piece of cloth used for wiping the eyes or nose or as a costume accessory', 'name': 'handkerchief'}, {'frequency': 'f', 'id': 550, 'synset': 'handle.n.01', 'synonyms': ['handle', 'grip', 'handgrip'], 'def': 'the appendage to an object that is designed to be held in order to use or move it', 'name': 'handle'}, {'frequency': 'r', 'id': 551, 'synset': 'handsaw.n.01', 'synonyms': ['handsaw', "carpenter's_saw"], 'def': 'a saw used with one hand for cutting wood', 'name': 'handsaw'}, {'frequency': 'r', 'id': 552, 'synset': 'hardback.n.01', 'synonyms': ['hardback_book', 'hardcover_book'], 'def': 'a book with cardboard or cloth or leather covers', 'name': 'hardback_book'}, {'frequency': 'r', 'id': 553, 'synset': 'harmonium.n.01', 'synonyms': ['harmonium', 'organ_(musical_instrument)', 'reed_organ_(musical_instrument)'], 'def': 'a free-reed instrument in which air is forced through the reeds by bellows', 'name': 'harmonium'}, {'frequency': 'f', 'id': 554, 'synset': 'hat.n.01', 'synonyms': ['hat'], 'def': 'headwear that protects the head from bad weather, sun, or worn for fashion', 'name': 'hat'}, {'frequency': 'r', 'id': 555, 'synset': 'hatbox.n.01', 'synonyms': ['hatbox'], 'def': 'a round piece of luggage for carrying hats', 'name': 'hatbox'}, {'frequency': 'r', 'id': 556, 'synset': 'hatch.n.03', 'synonyms': ['hatch'], 'def': 'a movable barrier covering a hatchway', 'name': 'hatch'}, {'frequency': 'c', 'id': 557, 'synset': 'head_covering.n.01', 'synonyms': ['veil'], 'def': 'a garment that covers the head and face', 'name': 'veil'}, {'frequency': 'f', 'id': 558, 'synset': 'headband.n.01', 'synonyms': ['headband'], 'def': 'a band worn around or over the head', 'name': 'headband'}, {'frequency': 'f', 'id': 559, 'synset': 'headboard.n.01', 'synonyms': ['headboard'], 'def': 'a vertical board or panel forming the head of a bedstead', 'name': 'headboard'}, {'frequency': 'f', 'id': 560, 'synset': 'headlight.n.01', 'synonyms': ['headlight', 'headlamp'], 'def': 'a powerful light with reflector; attached to the front of an automobile or locomotive', 'name': 'headlight'}, {'frequency': 'c', 'id': 561, 'synset': 'headscarf.n.01', 'synonyms': ['headscarf'], 'def': 'a kerchief worn over the head and tied under the chin', 'name': 'headscarf'}, {'frequency': 'r', 'id': 562, 'synset': 'headset.n.01', 'synonyms': ['headset'], 'def': 'receiver consisting of a pair of headphones', 'name': 'headset'}, {'frequency': 'c', 'id': 563, 'synset': 'headstall.n.01', 'synonyms': ['headstall_(for_horses)', 'headpiece_(for_horses)'], 'def': "the band that is the part of a bridle that fits around a horse's head", 'name': 'headstall_(for_horses)'}, {'frequency': 'r', 'id': 564, 'synset': 'hearing_aid.n.02', 'synonyms': ['hearing_aid'], 'def': 'an acoustic device used to direct sound to the ear of a hearing-impaired person', 'name': 'hearing_aid'}, {'frequency': 'c', 'id': 565, 'synset': 'heart.n.02', 'synonyms': ['heart'], 'def': 'a muscular organ; its contractions move the blood through the body', 'name': 'heart'}, {'frequency': 'c', 'id': 566, 'synset': 'heater.n.01', 'synonyms': ['heater', 'warmer'], 'def': 'device that heats water or supplies warmth to a room', 'name': 'heater'}, {'frequency': 'c', 'id': 567, 'synset': 'helicopter.n.01', 'synonyms': ['helicopter'], 'def': 'an aircraft without wings that obtains its lift from the rotation of overhead blades', 'name': 'helicopter'}, {'frequency': 'f', 'id': 568, 'synset': 'helmet.n.02', 'synonyms': ['helmet'], 'def': 'a protective headgear made of hard material to resist blows', 'name': 'helmet'}, {'frequency': 'r', 'id': 569, 'synset': 'heron.n.02', 'synonyms': ['heron'], 'def': 'grey or white wading bird with long neck and long legs and (usually) long bill', 'name': 'heron'}, {'frequency': 'c', 'id': 570, 'synset': 'highchair.n.01', 'synonyms': ['highchair', 'feeding_chair'], 'def': 'a chair for feeding a very young child', 'name': 'highchair'}, {'frequency': 'f', 'id': 571, 'synset': 'hinge.n.01', 'synonyms': ['hinge'], 'def': 'a joint that holds two parts together so that one can swing relative to the other', 'name': 'hinge'}, {'frequency': 'r', 'id': 572, 'synset': 'hippopotamus.n.01', 'synonyms': ['hippopotamus'], 'def': 'massive thick-skinned animal living in or around rivers of tropical Africa', 'name': 'hippopotamus'}, {'frequency': 'r', 'id': 573, 'synset': 'hockey_stick.n.01', 'synonyms': ['hockey_stick'], 'def': 'sports implement consisting of a stick used by hockey players to move the puck', 'name': 'hockey_stick'}, {'frequency': 'c', 'id': 574, 'synset': 'hog.n.03', 'synonyms': ['hog', 'pig'], 'def': 'domestic swine', 'name': 'hog'}, {'frequency': 'f', 'id': 575, 'synset': 'home_plate.n.01', 'synonyms': ['home_plate_(baseball)', 'home_base_(baseball)'], 'def': '(baseball) a rubber slab where the batter stands; it must be touched by a base runner in order to score', 'name': 'home_plate_(baseball)'}, {'frequency': 'c', 'id': 576, 'synset': 'honey.n.01', 'synonyms': ['honey'], 'def': 'a sweet yellow liquid produced by bees', 'name': 'honey'}, {'frequency': 'f', 'id': 577, 'synset': 'hood.n.06', 'synonyms': ['fume_hood', 'exhaust_hood'], 'def': 'metal covering leading to a vent that exhausts smoke or fumes', 'name': 'fume_hood'}, {'frequency': 'f', 'id': 578, 'synset': 'hook.n.05', 'synonyms': ['hook'], 'def': 'a curved or bent implement for suspending or pulling something', 'name': 'hook'}, {'frequency': 'f', 'id': 579, 'synset': 'horse.n.01', 'synonyms': ['horse'], 'def': 'a common horse', 'name': 'horse'}, {'frequency': 'f', 'id': 580, 'synset': 'hose.n.03', 'synonyms': ['hose', 'hosepipe'], 'def': 'a flexible pipe for conveying a liquid or gas', 'name': 'hose'}, {'frequency': 'r', 'id': 581, 'synset': 'hot-air_balloon.n.01', 'synonyms': ['hot-air_balloon'], 'def': 'balloon for travel through the air in a basket suspended below a large bag of heated air', 'name': 'hot-air_balloon'}, {'frequency': 'r', 'id': 582, 'synset': 'hot_plate.n.01', 'synonyms': ['hotplate'], 'def': 'a portable electric appliance for heating or cooking or keeping food warm', 'name': 'hotplate'}, {'frequency': 'c', 'id': 583, 'synset': 'hot_sauce.n.01', 'synonyms': ['hot_sauce'], 'def': 'a pungent peppery sauce', 'name': 'hot_sauce'}, {'frequency': 'r', 'id': 584, 'synset': 'hourglass.n.01', 'synonyms': ['hourglass'], 'def': 'a sandglass timer that runs for sixty minutes', 'name': 'hourglass'}, {'frequency': 'r', 'id': 585, 'synset': 'houseboat.n.01', 'synonyms': ['houseboat'], 'def': 'a barge that is designed and equipped for use as a dwelling', 'name': 'houseboat'}, {'frequency': 'r', 'id': 586, 'synset': 'hummingbird.n.01', 'synonyms': ['hummingbird'], 'def': 'tiny American bird having brilliant iridescent plumage and long slender bills', 'name': 'hummingbird'}, {'frequency': 'r', 'id': 587, 'synset': 'hummus.n.01', 'synonyms': ['hummus', 'humus', 'hommos', 'hoummos', 'humous'], 'def': 'a thick spread made from mashed chickpeas', 'name': 'hummus'}, {'frequency': 'c', 'id': 588, 'synset': 'ice_bear.n.01', 'synonyms': ['polar_bear'], 'def': 'white bear of Arctic regions', 'name': 'polar_bear'}, {'frequency': 'c', 'id': 589, 'synset': 'ice_cream.n.01', 'synonyms': ['icecream'], 'def': 'frozen dessert containing cream and sugar and flavoring', 'name': 'icecream'}, {'frequency': 'r', 'id': 590, 'synset': 'ice_lolly.n.01', 'synonyms': ['popsicle'], 'def': 'ice cream or water ice on a small wooden stick', 'name': 'popsicle'}, {'frequency': 'c', 'id': 591, 'synset': 'ice_maker.n.01', 'synonyms': ['ice_maker'], 'def': 'an appliance included in some electric refrigerators for making ice cubes', 'name': 'ice_maker'}, {'frequency': 'r', 'id': 592, 'synset': 'ice_pack.n.01', 'synonyms': ['ice_pack', 'ice_bag'], 'def': 'a waterproof bag filled with ice: applied to the body (especially the head) to cool or reduce swelling', 'name': 'ice_pack'}, {'frequency': 'r', 'id': 593, 'synset': 'ice_skate.n.01', 'synonyms': ['ice_skate'], 'def': 'skate consisting of a boot with a steel blade fitted to the sole', 'name': 'ice_skate'}, {'frequency': 'r', 'id': 594, 'synset': 'ice_tea.n.01', 'synonyms': ['ice_tea', 'iced_tea'], 'def': 'strong tea served over ice', 'name': 'ice_tea'}, {'frequency': 'c', 'id': 595, 'synset': 'igniter.n.01', 'synonyms': ['igniter', 'ignitor', 'lighter'], 'def': 'a substance or device used to start a fire', 'name': 'igniter'}, {'frequency': 'r', 'id': 596, 'synset': 'incense.n.01', 'synonyms': ['incense'], 'def': 'a substance that produces a fragrant odor when burned', 'name': 'incense'}, {'frequency': 'r', 'id': 597, 'synset': 'inhaler.n.01', 'synonyms': ['inhaler', 'inhalator'], 'def': 'a dispenser that produces a chemical vapor to be inhaled through mouth or nose', 'name': 'inhaler'}, {'frequency': 'c', 'id': 598, 'synset': 'ipod.n.01', 'synonyms': ['iPod'], 'def': 'a pocket-sized device used to play music files', 'name': 'iPod'}, {'frequency': 'c', 'id': 599, 'synset': 'iron.n.04', 'synonyms': ['iron_(for_clothing)', 'smoothing_iron_(for_clothing)'], 'def': 'home appliance consisting of a flat metal base that is heated and used to smooth cloth', 'name': 'iron_(for_clothing)'}, {'frequency': 'r', 'id': 600, 'synset': 'ironing_board.n.01', 'synonyms': ['ironing_board'], 'def': 'narrow padded board on collapsible supports; used for ironing clothes', 'name': 'ironing_board'}, {'frequency': 'f', 'id': 601, 'synset': 'jacket.n.01', 'synonyms': ['jacket'], 'def': 'a waist-length coat', 'name': 'jacket'}, {'frequency': 'r', 'id': 602, 'synset': 'jam.n.01', 'synonyms': ['jam'], 'def': 'preserve of crushed fruit', 'name': 'jam'}, {'frequency': 'f', 'id': 603, 'synset': 'jean.n.01', 'synonyms': ['jean', 'blue_jean', 'denim'], 'def': '(usually plural) close-fitting trousers of heavy denim for manual work or casual wear', 'name': 'jean'}, {'frequency': 'c', 'id': 604, 'synset': 'jeep.n.01', 'synonyms': ['jeep', 'landrover'], 'def': 'a car suitable for traveling over rough terrain', 'name': 'jeep'}, {'frequency': 'r', 'id': 605, 'synset': 'jelly_bean.n.01', 'synonyms': ['jelly_bean', 'jelly_egg'], 'def': 'sugar-glazed jellied candy', 'name': 'jelly_bean'}, {'frequency': 'f', 'id': 606, 'synset': 'jersey.n.03', 'synonyms': ['jersey', 'T-shirt', 'tee_shirt'], 'def': 'a close-fitting pullover shirt', 'name': 'jersey'}, {'frequency': 'c', 'id': 607, 'synset': 'jet.n.01', 'synonyms': ['jet_plane', 'jet-propelled_plane'], 'def': 'an airplane powered by one or more jet engines', 'name': 'jet_plane'}, {'frequency': 'c', 'id': 608, 'synset': 'jewelry.n.01', 'synonyms': ['jewelry', 'jewellery'], 'def': 'an adornment (as a bracelet or ring or necklace) made of precious metals and set with gems (or imitation gems)', 'name': 'jewelry'}, {'frequency': 'r', 'id': 609, 'synset': 'joystick.n.02', 'synonyms': ['joystick'], 'def': 'a control device for computers consisting of a vertical handle that can move freely in two directions', 'name': 'joystick'}, {'frequency': 'r', 'id': 610, 'synset': 'jump_suit.n.01', 'synonyms': ['jumpsuit'], 'def': "one-piece garment fashioned after a parachutist's uniform", 'name': 'jumpsuit'}, {'frequency': 'c', 'id': 611, 'synset': 'kayak.n.01', 'synonyms': ['kayak'], 'def': 'a small canoe consisting of a light frame made watertight with animal skins', 'name': 'kayak'}, {'frequency': 'r', 'id': 612, 'synset': 'keg.n.02', 'synonyms': ['keg'], 'def': 'small cask or barrel', 'name': 'keg'}, {'frequency': 'r', 'id': 613, 'synset': 'kennel.n.01', 'synonyms': ['kennel', 'doghouse'], 'def': 'outbuilding that serves as a shelter for a dog', 'name': 'kennel'}, {'frequency': 'c', 'id': 614, 'synset': 'kettle.n.01', 'synonyms': ['kettle', 'boiler'], 'def': 'a metal pot for stewing or boiling; usually has a lid', 'name': 'kettle'}, {'frequency': 'f', 'id': 615, 'synset': 'key.n.01', 'synonyms': ['key'], 'def': 'metal instrument used to unlock a lock', 'name': 'key'}, {'frequency': 'r', 'id': 616, 'synset': 'keycard.n.01', 'synonyms': ['keycard'], 'def': 'a plastic card used to gain access typically to a door', 'name': 'keycard'}, {'frequency': 'r', 'id': 617, 'synset': 'kilt.n.01', 'synonyms': ['kilt'], 'def': 'a knee-length pleated tartan skirt worn by men as part of the traditional dress in the Highlands of northern Scotland', 'name': 'kilt'}, {'frequency': 'c', 'id': 618, 'synset': 'kimono.n.01', 'synonyms': ['kimono'], 'def': 'a loose robe; imitated from robes originally worn by Japanese', 'name': 'kimono'}, {'frequency': 'f', 'id': 619, 'synset': 'kitchen_sink.n.01', 'synonyms': ['kitchen_sink'], 'def': 'a sink in a kitchen', 'name': 'kitchen_sink'}, {'frequency': 'c', 'id': 620, 'synset': 'kitchen_table.n.01', 'synonyms': ['kitchen_table'], 'def': 'a table in the kitchen', 'name': 'kitchen_table'}, {'frequency': 'f', 'id': 621, 'synset': 'kite.n.03', 'synonyms': ['kite'], 'def': 'plaything consisting of a light frame covered with tissue paper; flown in wind at end of a string', 'name': 'kite'}, {'frequency': 'c', 'id': 622, 'synset': 'kitten.n.01', 'synonyms': ['kitten', 'kitty'], 'def': 'young domestic cat', 'name': 'kitten'}, {'frequency': 'c', 'id': 623, 'synset': 'kiwi.n.03', 'synonyms': ['kiwi_fruit'], 'def': 'fuzzy brown egg-shaped fruit with slightly tart green flesh', 'name': 'kiwi_fruit'}, {'frequency': 'f', 'id': 624, 'synset': 'knee_pad.n.01', 'synonyms': ['knee_pad'], 'def': 'protective garment consisting of a pad worn by football or baseball or hockey players', 'name': 'knee_pad'}, {'frequency': 'f', 'id': 625, 'synset': 'knife.n.01', 'synonyms': ['knife'], 'def': 'tool with a blade and point used as a cutting instrument', 'name': 'knife'}, {'frequency': 'r', 'id': 626, 'synset': 'knight.n.02', 'synonyms': ['knight_(chess_piece)', 'horse_(chess_piece)'], 'def': 'a chess game piece shaped to resemble the head of a horse', 'name': 'knight_(chess_piece)'}, {'frequency': 'r', 'id': 627, 'synset': 'knitting_needle.n.01', 'synonyms': ['knitting_needle'], 'def': 'needle consisting of a slender rod with pointed ends; usually used in pairs', 'name': 'knitting_needle'}, {'frequency': 'f', 'id': 628, 'synset': 'knob.n.02', 'synonyms': ['knob'], 'def': 'a round handle often found on a door', 'name': 'knob'}, {'frequency': 'r', 'id': 629, 'synset': 'knocker.n.05', 'synonyms': ['knocker_(on_a_door)', 'doorknocker'], 'def': 'a device (usually metal and ornamental) attached by a hinge to a door', 'name': 'knocker_(on_a_door)'}, {'frequency': 'r', 'id': 630, 'synset': 'koala.n.01', 'synonyms': ['koala', 'koala_bear'], 'def': 'sluggish tailless Australian marsupial with grey furry ears and coat', 'name': 'koala'}, {'frequency': 'r', 'id': 631, 'synset': 'lab_coat.n.01', 'synonyms': ['lab_coat', 'laboratory_coat'], 'def': 'a light coat worn to protect clothing from substances used while working in a laboratory', 'name': 'lab_coat'}, {'frequency': 'f', 'id': 632, 'synset': 'ladder.n.01', 'synonyms': ['ladder'], 'def': 'steps consisting of two parallel members connected by rungs', 'name': 'ladder'}, {'frequency': 'c', 'id': 633, 'synset': 'ladle.n.01', 'synonyms': ['ladle'], 'def': 'a spoon-shaped vessel with a long handle frequently used to transfer liquids', 'name': 'ladle'}, {'frequency': 'r', 'id': 634, 'synset': 'ladybug.n.01', 'synonyms': ['ladybug', 'ladybeetle', 'ladybird_beetle'], 'def': 'small round bright-colored and spotted beetle, typically red and black', 'name': 'ladybug'}, {'frequency': 'c', 'id': 635, 'synset': 'lamb.n.01', 'synonyms': ['lamb_(animal)'], 'def': 'young sheep', 'name': 'lamb_(animal)'}, {'frequency': 'r', 'id': 636, 'synset': 'lamb_chop.n.01', 'synonyms': ['lamb-chop', 'lambchop'], 'def': 'chop cut from a lamb', 'name': 'lamb-chop'}, {'frequency': 'f', 'id': 637, 'synset': 'lamp.n.02', 'synonyms': ['lamp'], 'def': 'a piece of furniture holding one or more electric light bulbs', 'name': 'lamp'}, {'frequency': 'f', 'id': 638, 'synset': 'lamppost.n.01', 'synonyms': ['lamppost'], 'def': 'a metal post supporting an outdoor lamp (such as a streetlight)', 'name': 'lamppost'}, {'frequency': 'f', 'id': 639, 'synset': 'lampshade.n.01', 'synonyms': ['lampshade'], 'def': 'a protective ornamental shade used to screen a light bulb from direct view', 'name': 'lampshade'}, {'frequency': 'c', 'id': 640, 'synset': 'lantern.n.01', 'synonyms': ['lantern'], 'def': 'light in a transparent protective case', 'name': 'lantern'}, {'frequency': 'f', 'id': 641, 'synset': 'lanyard.n.02', 'synonyms': ['lanyard', 'laniard'], 'def': 'a cord worn around the neck to hold a knife or whistle, etc.', 'name': 'lanyard'}, {'frequency': 'f', 'id': 642, 'synset': 'laptop.n.01', 'synonyms': ['laptop_computer', 'notebook_computer'], 'def': 'a portable computer small enough to use in your lap', 'name': 'laptop_computer'}, {'frequency': 'r', 'id': 643, 'synset': 'lasagna.n.01', 'synonyms': ['lasagna', 'lasagne'], 'def': 'baked dish of layers of lasagna pasta with sauce and cheese and meat or vegetables', 'name': 'lasagna'}, {'frequency': 'c', 'id': 644, 'synset': 'latch.n.02', 'synonyms': ['latch'], 'def': 'a bar that can be lowered or slid into a groove to fasten a door or gate', 'name': 'latch'}, {'frequency': 'r', 'id': 645, 'synset': 'lawn_mower.n.01', 'synonyms': ['lawn_mower'], 'def': 'garden tool for mowing grass on lawns', 'name': 'lawn_mower'}, {'frequency': 'r', 'id': 646, 'synset': 'leather.n.01', 'synonyms': ['leather'], 'def': 'an animal skin made smooth and flexible by removing the hair and then tanning', 'name': 'leather'}, {'frequency': 'c', 'id': 647, 'synset': 'legging.n.01', 'synonyms': ['legging_(clothing)', 'leging_(clothing)', 'leg_covering'], 'def': 'a garment covering the leg (usually extending from the knee to the ankle)', 'name': 'legging_(clothing)'}, {'frequency': 'c', 'id': 648, 'synset': 'lego.n.01', 'synonyms': ['Lego', 'Lego_set'], 'def': "a child's plastic construction set for making models from blocks", 'name': 'Lego'}, {'frequency': 'f', 'id': 649, 'synset': 'lemon.n.01', 'synonyms': ['lemon'], 'def': 'yellow oval fruit with juicy acidic flesh', 'name': 'lemon'}, {'frequency': 'r', 'id': 650, 'synset': 'lemonade.n.01', 'synonyms': ['lemonade'], 'def': 'sweetened beverage of diluted lemon juice', 'name': 'lemonade'}, {'frequency': 'f', 'id': 651, 'synset': 'lettuce.n.02', 'synonyms': ['lettuce'], 'def': 'leafy plant commonly eaten in salad or on sandwiches', 'name': 'lettuce'}, {'frequency': 'f', 'id': 652, 'synset': 'license_plate.n.01', 'synonyms': ['license_plate', 'numberplate'], 'def': "a plate mounted on the front and back of car and bearing the car's registration number", 'name': 'license_plate'}, {'frequency': 'f', 'id': 653, 'synset': 'life_buoy.n.01', 'synonyms': ['life_buoy', 'lifesaver', 'life_belt', 'life_ring'], 'def': 'a ring-shaped life preserver used to prevent drowning (NOT a life-jacket or vest)', 'name': 'life_buoy'}, {'frequency': 'f', 'id': 654, 'synset': 'life_jacket.n.01', 'synonyms': ['life_jacket', 'life_vest'], 'def': 'life preserver consisting of a sleeveless jacket of buoyant or inflatable design', 'name': 'life_jacket'}, {'frequency': 'f', 'id': 655, 'synset': 'light_bulb.n.01', 'synonyms': ['lightbulb'], 'def': 'glass bulb or tube shaped electric device that emits light (DO NOT MARK LAMPS AS A WHOLE)', 'name': 'lightbulb'}, {'frequency': 'r', 'id': 656, 'synset': 'lightning_rod.n.02', 'synonyms': ['lightning_rod', 'lightning_conductor'], 'def': 'a metallic conductor that is attached to a high point and leads to the ground', 'name': 'lightning_rod'}, {'frequency': 'c', 'id': 657, 'synset': 'lime.n.06', 'synonyms': ['lime'], 'def': 'the green acidic fruit of any of various lime trees', 'name': 'lime'}, {'frequency': 'r', 'id': 658, 'synset': 'limousine.n.01', 'synonyms': ['limousine'], 'def': 'long luxurious car; usually driven by a chauffeur', 'name': 'limousine'}, {'frequency': 'r', 'id': 659, 'synset': 'linen.n.02', 'synonyms': ['linen_paper'], 'def': 'a high-quality paper made of linen fibers or with a linen finish', 'name': 'linen_paper'}, {'frequency': 'c', 'id': 660, 'synset': 'lion.n.01', 'synonyms': ['lion'], 'def': 'large gregarious predatory cat of Africa and India', 'name': 'lion'}, {'frequency': 'c', 'id': 661, 'synset': 'lip_balm.n.01', 'synonyms': ['lip_balm'], 'def': 'a balm applied to the lips', 'name': 'lip_balm'}, {'frequency': 'c', 'id': 662, 'synset': 'lipstick.n.01', 'synonyms': ['lipstick', 'lip_rouge'], 'def': 'makeup that is used to color the lips', 'name': 'lipstick'}, {'frequency': 'r', 'id': 663, 'synset': 'liquor.n.01', 'synonyms': ['liquor', 'spirits', 'hard_liquor', 'liqueur', 'cordial'], 'def': 'an alcoholic beverage that is distilled rather than fermented', 'name': 'liquor'}, {'frequency': 'r', 'id': 664, 'synset': 'lizard.n.01', 'synonyms': ['lizard'], 'def': 'a reptile with usually two pairs of legs and a tapering tail', 'name': 'lizard'}, {'frequency': 'r', 'id': 665, 'synset': 'loafer.n.02', 'synonyms': ['Loafer_(type_of_shoe)'], 'def': 'a low leather step-in shoe', 'name': 'Loafer_(type_of_shoe)'}, {'frequency': 'f', 'id': 666, 'synset': 'log.n.01', 'synonyms': ['log'], 'def': 'a segment of the trunk of a tree when stripped of branches', 'name': 'log'}, {'frequency': 'c', 'id': 667, 'synset': 'lollipop.n.02', 'synonyms': ['lollipop'], 'def': 'hard candy on a stick', 'name': 'lollipop'}, {'frequency': 'c', 'id': 668, 'synset': 'lotion.n.01', 'synonyms': ['lotion'], 'def': 'any of various cosmetic preparations that are applied to the skin', 'name': 'lotion'}, {'frequency': 'f', 'id': 669, 'synset': 'loudspeaker.n.01', 'synonyms': ['speaker_(stero_equipment)'], 'def': 'electronic device that produces sound often as part of a stereo system', 'name': 'speaker_(stero_equipment)'}, {'frequency': 'c', 'id': 670, 'synset': 'love_seat.n.01', 'synonyms': ['loveseat'], 'def': 'small sofa that seats two people', 'name': 'loveseat'}, {'frequency': 'r', 'id': 671, 'synset': 'machine_gun.n.01', 'synonyms': ['machine_gun'], 'def': 'a rapidly firing automatic gun', 'name': 'machine_gun'}, {'frequency': 'f', 'id': 672, 'synset': 'magazine.n.02', 'synonyms': ['magazine'], 'def': 'a paperback periodic publication', 'name': 'magazine'}, {'frequency': 'f', 'id': 673, 'synset': 'magnet.n.01', 'synonyms': ['magnet'], 'def': 'a device that attracts iron and produces a magnetic field', 'name': 'magnet'}, {'frequency': 'r', 'id': 674, 'synset': 'mail_slot.n.01', 'synonyms': ['mail_slot'], 'def': 'a slot (usually in a door) through which mail can be delivered', 'name': 'mail_slot'}, {'frequency': 'c', 'id': 675, 'synset': 'mailbox.n.01', 'synonyms': ['mailbox_(at_home)', 'letter_box_(at_home)'], 'def': 'a private box for delivery of mail', 'name': 'mailbox_(at_home)'}, {'frequency': 'r', 'id': 676, 'synset': 'mallet.n.01', 'synonyms': ['mallet'], 'def': 'a sports implement with a long handle and a hammer-like head used to hit a ball', 'name': 'mallet'}, {'frequency': 'r', 'id': 677, 'synset': 'mammoth.n.01', 'synonyms': ['mammoth'], 'def': 'any of numerous extinct elephants widely distributed in the Pleistocene', 'name': 'mammoth'}, {'frequency': 'c', 'id': 678, 'synset': 'mandarin.n.05', 'synonyms': ['mandarin_orange'], 'def': 'a somewhat flat reddish-orange loose skinned citrus of China', 'name': 'mandarin_orange'}, {'frequency': 'c', 'id': 679, 'synset': 'manger.n.01', 'synonyms': ['manger', 'trough'], 'def': 'a container (usually in a barn or stable) from which cattle or horses feed', 'name': 'manger'}, {'frequency': 'f', 'id': 680, 'synset': 'manhole.n.01', 'synonyms': ['manhole'], 'def': 'a hole (usually with a flush cover) through which a person can gain access to an underground structure', 'name': 'manhole'}, {'frequency': 'c', 'id': 681, 'synset': 'map.n.01', 'synonyms': ['map'], 'def': "a diagrammatic representation of the earth's surface (or part of it)", 'name': 'map'}, {'frequency': 'c', 'id': 682, 'synset': 'marker.n.03', 'synonyms': ['marker'], 'def': 'a writing implement for making a mark', 'name': 'marker'}, {'frequency': 'r', 'id': 683, 'synset': 'martini.n.01', 'synonyms': ['martini'], 'def': 'a cocktail made of gin (or vodka) with dry vermouth', 'name': 'martini'}, {'frequency': 'r', 'id': 684, 'synset': 'mascot.n.01', 'synonyms': ['mascot'], 'def': 'a person or animal that is adopted by a team or other group as a symbolic figure', 'name': 'mascot'}, {'frequency': 'c', 'id': 685, 'synset': 'mashed_potato.n.01', 'synonyms': ['mashed_potato'], 'def': 'potato that has been peeled and boiled and then mashed', 'name': 'mashed_potato'}, {'frequency': 'r', 'id': 686, 'synset': 'masher.n.02', 'synonyms': ['masher'], 'def': 'a kitchen utensil used for mashing (e.g. potatoes)', 'name': 'masher'}, {'frequency': 'f', 'id': 687, 'synset': 'mask.n.04', 'synonyms': ['mask', 'facemask'], 'def': 'a protective covering worn over the face', 'name': 'mask'}, {'frequency': 'f', 'id': 688, 'synset': 'mast.n.01', 'synonyms': ['mast'], 'def': 'a vertical spar for supporting sails', 'name': 'mast'}, {'frequency': 'c', 'id': 689, 'synset': 'mat.n.03', 'synonyms': ['mat_(gym_equipment)', 'gym_mat'], 'def': 'sports equipment consisting of a piece of thick padding on the floor for gymnastics', 'name': 'mat_(gym_equipment)'}, {'frequency': 'r', 'id': 690, 'synset': 'matchbox.n.01', 'synonyms': ['matchbox'], 'def': 'a box for holding matches', 'name': 'matchbox'}, {'frequency': 'f', 'id': 691, 'synset': 'mattress.n.01', 'synonyms': ['mattress'], 'def': 'a thick pad filled with resilient material used as a bed or part of a bed', 'name': 'mattress'}, {'frequency': 'c', 'id': 692, 'synset': 'measuring_cup.n.01', 'synonyms': ['measuring_cup'], 'def': 'graduated cup used to measure liquid or granular ingredients', 'name': 'measuring_cup'}, {'frequency': 'c', 'id': 693, 'synset': 'measuring_stick.n.01', 'synonyms': ['measuring_stick', 'ruler_(measuring_stick)', 'measuring_rod'], 'def': 'measuring instrument having a sequence of marks at regular intervals', 'name': 'measuring_stick'}, {'frequency': 'c', 'id': 694, 'synset': 'meatball.n.01', 'synonyms': ['meatball'], 'def': 'ground meat formed into a ball and fried or simmered in broth', 'name': 'meatball'}, {'frequency': 'c', 'id': 695, 'synset': 'medicine.n.02', 'synonyms': ['medicine'], 'def': 'something that treats or prevents or alleviates the symptoms of disease', 'name': 'medicine'}, {'frequency': 'r', 'id': 696, 'synset': 'melon.n.01', 'synonyms': ['melon'], 'def': 'fruit of the gourd family having a hard rind and sweet juicy flesh', 'name': 'melon'}, {'frequency': 'f', 'id': 697, 'synset': 'microphone.n.01', 'synonyms': ['microphone'], 'def': 'device for converting sound waves into electrical energy', 'name': 'microphone'}, {'frequency': 'r', 'id': 698, 'synset': 'microscope.n.01', 'synonyms': ['microscope'], 'def': 'magnifier of the image of small objects', 'name': 'microscope'}, {'frequency': 'f', 'id': 699, 'synset': 'microwave.n.02', 'synonyms': ['microwave_oven'], 'def': 'kitchen appliance that cooks food by passing an electromagnetic wave through it', 'name': 'microwave_oven'}, {'frequency': 'r', 'id': 700, 'synset': 'milestone.n.01', 'synonyms': ['milestone', 'milepost'], 'def': 'stone post at side of a road to show distances', 'name': 'milestone'}, {'frequency': 'c', 'id': 701, 'synset': 'milk.n.01', 'synonyms': ['milk'], 'def': 'a white nutritious liquid secreted by mammals and used as food by human beings', 'name': 'milk'}, {'frequency': 'f', 'id': 702, 'synset': 'minivan.n.01', 'synonyms': ['minivan'], 'def': 'a small box-shaped passenger van', 'name': 'minivan'}, {'frequency': 'r', 'id': 703, 'synset': 'mint.n.05', 'synonyms': ['mint_candy'], 'def': 'a candy that is flavored with a mint oil', 'name': 'mint_candy'}, {'frequency': 'f', 'id': 704, 'synset': 'mirror.n.01', 'synonyms': ['mirror'], 'def': 'polished surface that forms images by reflecting light', 'name': 'mirror'}, {'frequency': 'c', 'id': 705, 'synset': 'mitten.n.01', 'synonyms': ['mitten'], 'def': 'glove that encases the thumb separately and the other four fingers together', 'name': 'mitten'}, {'frequency': 'c', 'id': 706, 'synset': 'mixer.n.04', 'synonyms': ['mixer_(kitchen_tool)', 'stand_mixer'], 'def': 'a kitchen utensil that is used for mixing foods', 'name': 'mixer_(kitchen_tool)'}, {'frequency': 'c', 'id': 707, 'synset': 'money.n.03', 'synonyms': ['money'], 'def': 'the official currency issued by a government or national bank', 'name': 'money'}, {'frequency': 'f', 'id': 708, 'synset': 'monitor.n.04', 'synonyms': ['monitor_(computer_equipment) computer_monitor'], 'def': 'a computer monitor', 'name': 'monitor_(computer_equipment) computer_monitor'}, {'frequency': 'c', 'id': 709, 'synset': 'monkey.n.01', 'synonyms': ['monkey'], 'def': 'any of various long-tailed primates', 'name': 'monkey'}, {'frequency': 'f', 'id': 710, 'synset': 'motor.n.01', 'synonyms': ['motor'], 'def': 'machine that converts other forms of energy into mechanical energy and so imparts motion', 'name': 'motor'}, {'frequency': 'f', 'id': 711, 'synset': 'motor_scooter.n.01', 'synonyms': ['motor_scooter', 'scooter'], 'def': 'a wheeled vehicle with small wheels and a low-powered engine', 'name': 'motor_scooter'}, {'frequency': 'r', 'id': 712, 'synset': 'motor_vehicle.n.01', 'synonyms': ['motor_vehicle', 'automotive_vehicle'], 'def': 'a self-propelled wheeled vehicle that does not run on rails', 'name': 'motor_vehicle'}, {'frequency': 'r', 'id': 713, 'synset': 'motorboat.n.01', 'synonyms': ['motorboat', 'powerboat'], 'def': 'a boat propelled by an internal-combustion engine', 'name': 'motorboat'}, {'frequency': 'f', 'id': 714, 'synset': 'motorcycle.n.01', 'synonyms': ['motorcycle'], 'def': 'a motor vehicle with two wheels and a strong frame', 'name': 'motorcycle'}, {'frequency': 'f', 'id': 715, 'synset': 'mound.n.01', 'synonyms': ['mound_(baseball)', "pitcher's_mound"], 'def': '(baseball) the slight elevation on which the pitcher stands', 'name': 'mound_(baseball)'}, {'frequency': 'r', 'id': 716, 'synset': 'mouse.n.01', 'synonyms': ['mouse_(animal_rodent)'], 'def': 'a small rodent with pointed snouts and small ears on elongated bodies with slender usually hairless tails', 'name': 'mouse_(animal_rodent)'}, {'frequency': 'f', 'id': 717, 'synset': 'mouse.n.04', 'synonyms': ['mouse_(computer_equipment)', 'computer_mouse'], 'def': 'a computer input device that controls an on-screen pointer', 'name': 'mouse_(computer_equipment)'}, {'frequency': 'f', 'id': 718, 'synset': 'mousepad.n.01', 'synonyms': ['mousepad'], 'def': 'a small portable pad that provides an operating surface for a computer mouse', 'name': 'mousepad'}, {'frequency': 'c', 'id': 719, 'synset': 'muffin.n.01', 'synonyms': ['muffin'], 'def': 'a sweet quick bread baked in a cup-shaped pan', 'name': 'muffin'}, {'frequency': 'f', 'id': 720, 'synset': 'mug.n.04', 'synonyms': ['mug'], 'def': 'with handle and usually cylindrical', 'name': 'mug'}, {'frequency': 'f', 'id': 721, 'synset': 'mushroom.n.02', 'synonyms': ['mushroom'], 'def': 'a common mushroom', 'name': 'mushroom'}, {'frequency': 'r', 'id': 722, 'synset': 'music_stool.n.01', 'synonyms': ['music_stool', 'piano_stool'], 'def': 'a stool for piano players; usually adjustable in height', 'name': 'music_stool'}, {'frequency': 'r', 'id': 723, 'synset': 'musical_instrument.n.01', 'synonyms': ['musical_instrument', 'instrument_(musical)'], 'def': 'any of various devices or contrivances that can be used to produce musical tones or sounds', 'name': 'musical_instrument'}, {'frequency': 'r', 'id': 724, 'synset': 'nailfile.n.01', 'synonyms': ['nailfile'], 'def': 'a small flat file for shaping the nails', 'name': 'nailfile'}, {'frequency': 'r', 'id': 725, 'synset': 'nameplate.n.01', 'synonyms': ['nameplate'], 'def': 'a plate bearing a name', 'name': 'nameplate'}, {'frequency': 'f', 'id': 726, 'synset': 'napkin.n.01', 'synonyms': ['napkin', 'table_napkin', 'serviette'], 'def': 'a small piece of table linen or paper that is used to wipe the mouth and to cover the lap in order to protect clothing', 'name': 'napkin'}, {'frequency': 'r', 'id': 727, 'synset': 'neckerchief.n.01', 'synonyms': ['neckerchief'], 'def': 'a kerchief worn around the neck', 'name': 'neckerchief'}, {'frequency': 'f', 'id': 728, 'synset': 'necklace.n.01', 'synonyms': ['necklace'], 'def': 'jewelry consisting of a cord or chain (often bearing gems) worn about the neck as an ornament', 'name': 'necklace'}, {'frequency': 'f', 'id': 729, 'synset': 'necktie.n.01', 'synonyms': ['necktie', 'tie_(necktie)'], 'def': 'neckwear consisting of a long narrow piece of material worn under a collar and tied in knot at the front', 'name': 'necktie'}, {'frequency': 'r', 'id': 730, 'synset': 'needle.n.03', 'synonyms': ['needle'], 'def': 'a sharp pointed implement (usually metal)', 'name': 'needle'}, {'frequency': 'c', 'id': 731, 'synset': 'nest.n.01', 'synonyms': ['nest'], 'def': 'a structure in which animals lay eggs or give birth to their young', 'name': 'nest'}, {'frequency': 'r', 'id': 732, 'synset': 'newsstand.n.01', 'synonyms': ['newsstand'], 'def': 'a stall where newspapers and other periodicals are sold', 'name': 'newsstand'}, {'frequency': 'c', 'id': 733, 'synset': 'nightwear.n.01', 'synonyms': ['nightshirt', 'nightwear', 'sleepwear', 'nightclothes'], 'def': 'garments designed to be worn in bed', 'name': 'nightshirt'}, {'frequency': 'r', 'id': 734, 'synset': 'nosebag.n.01', 'synonyms': ['nosebag_(for_animals)', 'feedbag'], 'def': 'a canvas bag that is used to feed an animal (such as a horse); covers the muzzle and fastens at the top of the head', 'name': 'nosebag_(for_animals)'}, {'frequency': 'r', 'id': 735, 'synset': 'noseband.n.01', 'synonyms': ['noseband_(for_animals)', 'nosepiece_(for_animals)'], 'def': "a strap that is the part of a bridle that goes over the animal's nose", 'name': 'noseband_(for_animals)'}, {'frequency': 'f', 'id': 736, 'synset': 'notebook.n.01', 'synonyms': ['notebook'], 'def': 'a book with blank pages for recording notes or memoranda', 'name': 'notebook'}, {'frequency': 'c', 'id': 737, 'synset': 'notepad.n.01', 'synonyms': ['notepad'], 'def': 'a pad of paper for keeping notes', 'name': 'notepad'}, {'frequency': 'c', 'id': 738, 'synset': 'nut.n.03', 'synonyms': ['nut'], 'def': 'a small metal block (usually square or hexagonal) with internal screw thread to be fitted onto a bolt', 'name': 'nut'}, {'frequency': 'r', 'id': 739, 'synset': 'nutcracker.n.01', 'synonyms': ['nutcracker'], 'def': 'a hand tool used to crack nuts open', 'name': 'nutcracker'}, {'frequency': 'c', 'id': 740, 'synset': 'oar.n.01', 'synonyms': ['oar'], 'def': 'an implement used to propel or steer a boat', 'name': 'oar'}, {'frequency': 'r', 'id': 741, 'synset': 'octopus.n.01', 'synonyms': ['octopus_(food)'], 'def': 'tentacles of octopus prepared as food', 'name': 'octopus_(food)'}, {'frequency': 'r', 'id': 742, 'synset': 'octopus.n.02', 'synonyms': ['octopus_(animal)'], 'def': 'bottom-living cephalopod having a soft oval body with eight long tentacles', 'name': 'octopus_(animal)'}, {'frequency': 'c', 'id': 743, 'synset': 'oil_lamp.n.01', 'synonyms': ['oil_lamp', 'kerosene_lamp', 'kerosine_lamp'], 'def': 'a lamp that burns oil (as kerosine) for light', 'name': 'oil_lamp'}, {'frequency': 'c', 'id': 744, 'synset': 'olive_oil.n.01', 'synonyms': ['olive_oil'], 'def': 'oil from olives', 'name': 'olive_oil'}, {'frequency': 'r', 'id': 745, 'synset': 'omelet.n.01', 'synonyms': ['omelet', 'omelette'], 'def': 'beaten eggs cooked until just set; may be folded around e.g. ham or cheese or jelly', 'name': 'omelet'}, {'frequency': 'f', 'id': 746, 'synset': 'onion.n.01', 'synonyms': ['onion'], 'def': 'the bulb of an onion plant', 'name': 'onion'}, {'frequency': 'f', 'id': 747, 'synset': 'orange.n.01', 'synonyms': ['orange_(fruit)'], 'def': 'orange (FRUIT of an orange tree)', 'name': 'orange_(fruit)'}, {'frequency': 'c', 'id': 748, 'synset': 'orange_juice.n.01', 'synonyms': ['orange_juice'], 'def': 'bottled or freshly squeezed juice of oranges', 'name': 'orange_juice'}, {'frequency': 'r', 'id': 749, 'synset': 'oregano.n.01', 'synonyms': ['oregano', 'marjoram'], 'def': 'aromatic Eurasian perennial herb used in cooking and baking', 'name': 'oregano'}, {'frequency': 'c', 'id': 750, 'synset': 'ostrich.n.02', 'synonyms': ['ostrich'], 'def': 'fast-running African flightless bird with two-toed feet; largest living bird', 'name': 'ostrich'}, {'frequency': 'c', 'id': 751, 'synset': 'ottoman.n.03', 'synonyms': ['ottoman', 'pouf', 'pouffe', 'hassock'], 'def': 'thick cushion used as a seat', 'name': 'ottoman'}, {'frequency': 'c', 'id': 752, 'synset': 'overall.n.01', 'synonyms': ['overalls_(clothing)'], 'def': 'work clothing consisting of denim trousers usually with a bib and shoulder straps', 'name': 'overalls_(clothing)'}, {'frequency': 'c', 'id': 753, 'synset': 'owl.n.01', 'synonyms': ['owl'], 'def': 'nocturnal bird of prey with hawk-like beak and claws and large head with front-facing eyes', 'name': 'owl'}, {'frequency': 'c', 'id': 754, 'synset': 'packet.n.03', 'synonyms': ['packet'], 'def': 'a small package or bundle', 'name': 'packet'}, {'frequency': 'r', 'id': 755, 'synset': 'pad.n.03', 'synonyms': ['inkpad', 'inking_pad', 'stamp_pad'], 'def': 'absorbent material saturated with ink used to transfer ink evenly to a rubber stamp', 'name': 'inkpad'}, {'frequency': 'c', 'id': 756, 'synset': 'pad.n.04', 'synonyms': ['pad'], 'def': 'a flat mass of soft material used for protection, stuffing, or comfort', 'name': 'pad'}, {'frequency': 'c', 'id': 757, 'synset': 'paddle.n.04', 'synonyms': ['paddle', 'boat_paddle'], 'def': 'a short light oar used without an oarlock to propel a canoe or small boat', 'name': 'paddle'}, {'frequency': 'c', 'id': 758, 'synset': 'padlock.n.01', 'synonyms': ['padlock'], 'def': 'a detachable, portable lock', 'name': 'padlock'}, {'frequency': 'r', 'id': 759, 'synset': 'paintbox.n.01', 'synonyms': ['paintbox'], 'def': "a box containing a collection of cubes or tubes of artists' paint", 'name': 'paintbox'}, {'frequency': 'c', 'id': 760, 'synset': 'paintbrush.n.01', 'synonyms': ['paintbrush'], 'def': 'a brush used as an applicator to apply paint', 'name': 'paintbrush'}, {'frequency': 'f', 'id': 761, 'synset': 'painting.n.01', 'synonyms': ['painting'], 'def': 'graphic art consisting of an artistic composition made by applying paints to a surface', 'name': 'painting'}, {'frequency': 'c', 'id': 762, 'synset': 'pajama.n.02', 'synonyms': ['pajamas', 'pyjamas'], 'def': 'loose-fitting nightclothes worn for sleeping or lounging', 'name': 'pajamas'}, {'frequency': 'c', 'id': 763, 'synset': 'palette.n.02', 'synonyms': ['palette', 'pallet'], 'def': 'board that provides a flat surface on which artists mix paints and the range of colors used', 'name': 'palette'}, {'frequency': 'f', 'id': 764, 'synset': 'pan.n.01', 'synonyms': ['pan_(for_cooking)', 'cooking_pan'], 'def': 'cooking utensil consisting of a wide metal vessel', 'name': 'pan_(for_cooking)'}, {'frequency': 'r', 'id': 765, 'synset': 'pan.n.03', 'synonyms': ['pan_(metal_container)'], 'def': 'shallow container made of metal', 'name': 'pan_(metal_container)'}, {'frequency': 'c', 'id': 766, 'synset': 'pancake.n.01', 'synonyms': ['pancake'], 'def': 'a flat cake of thin batter fried on both sides on a griddle', 'name': 'pancake'}, {'frequency': 'r', 'id': 767, 'synset': 'pantyhose.n.01', 'synonyms': ['pantyhose'], 'def': "a woman's tights consisting of underpants and stockings", 'name': 'pantyhose'}, {'frequency': 'r', 'id': 768, 'synset': 'papaya.n.02', 'synonyms': ['papaya'], 'def': 'large oval melon-like tropical fruit with yellowish flesh', 'name': 'papaya'}, {'frequency': 'r', 'id': 769, 'synset': 'paper_clip.n.01', 'synonyms': ['paperclip'], 'def': 'a wire or plastic clip for holding sheets of paper together', 'name': 'paperclip'}, {'frequency': 'f', 'id': 770, 'synset': 'paper_plate.n.01', 'synonyms': ['paper_plate'], 'def': 'a disposable plate made of cardboard', 'name': 'paper_plate'}, {'frequency': 'f', 'id': 771, 'synset': 'paper_towel.n.01', 'synonyms': ['paper_towel'], 'def': 'a disposable towel made of absorbent paper', 'name': 'paper_towel'}, {'frequency': 'r', 'id': 772, 'synset': 'paperback_book.n.01', 'synonyms': ['paperback_book', 'paper-back_book', 'softback_book', 'soft-cover_book'], 'def': 'a book with paper covers', 'name': 'paperback_book'}, {'frequency': 'r', 'id': 773, 'synset': 'paperweight.n.01', 'synonyms': ['paperweight'], 'def': 'a weight used to hold down a stack of papers', 'name': 'paperweight'}, {'frequency': 'c', 'id': 774, 'synset': 'parachute.n.01', 'synonyms': ['parachute'], 'def': 'rescue equipment consisting of a device that fills with air and retards your fall', 'name': 'parachute'}, {'frequency': 'r', 'id': 775, 'synset': 'parakeet.n.01', 'synonyms': ['parakeet', 'parrakeet', 'parroket', 'paraquet', 'paroquet', 'parroquet'], 'def': 'any of numerous small slender long-tailed parrots', 'name': 'parakeet'}, {'frequency': 'c', 'id': 776, 'synset': 'parasail.n.01', 'synonyms': ['parasail_(sports)'], 'def': 'parachute that will lift a person up into the air when it is towed by a motorboat or a car', 'name': 'parasail_(sports)'}, {'frequency': 'r', 'id': 777, 'synset': 'parchment.n.01', 'synonyms': ['parchment'], 'def': 'a superior paper resembling sheepskin', 'name': 'parchment'}, {'frequency': 'r', 'id': 778, 'synset': 'parka.n.01', 'synonyms': ['parka', 'anorak'], 'def': "a kind of heavy jacket (`windcheater' is a British term)", 'name': 'parka'}, {'frequency': 'f', 'id': 779, 'synset': 'parking_meter.n.01', 'synonyms': ['parking_meter'], 'def': 'a coin-operated timer located next to a parking space', 'name': 'parking_meter'}, {'frequency': 'c', 'id': 780, 'synset': 'parrot.n.01', 'synonyms': ['parrot'], 'def': 'usually brightly colored tropical birds with short hooked beaks and the ability to mimic sounds', 'name': 'parrot'}, {'frequency': 'c', 'id': 781, 'synset': 'passenger_car.n.01', 'synonyms': ['passenger_car_(part_of_a_train)', 'coach_(part_of_a_train)'], 'def': 'a railcar where passengers ride', 'name': 'passenger_car_(part_of_a_train)'}, {'frequency': 'r', 'id': 782, 'synset': 'passenger_ship.n.01', 'synonyms': ['passenger_ship'], 'def': 'a ship built to carry passengers', 'name': 'passenger_ship'}, {'frequency': 'r', 'id': 783, 'synset': 'passport.n.02', 'synonyms': ['passport'], 'def': 'a document issued by a country to a citizen allowing that person to travel abroad and re-enter the home country', 'name': 'passport'}, {'frequency': 'f', 'id': 784, 'synset': 'pastry.n.02', 'synonyms': ['pastry'], 'def': 'any of various baked foods made of dough or batter', 'name': 'pastry'}, {'frequency': 'r', 'id': 785, 'synset': 'patty.n.01', 'synonyms': ['patty_(food)'], 'def': 'small flat mass of chopped food', 'name': 'patty_(food)'}, {'frequency': 'c', 'id': 786, 'synset': 'pea.n.01', 'synonyms': ['pea_(food)'], 'def': 'seed of a pea plant used for food', 'name': 'pea_(food)'}, {'frequency': 'c', 'id': 787, 'synset': 'peach.n.03', 'synonyms': ['peach'], 'def': 'downy juicy fruit with sweet yellowish or whitish flesh', 'name': 'peach'}, {'frequency': 'c', 'id': 788, 'synset': 'peanut_butter.n.01', 'synonyms': ['peanut_butter'], 'def': 'a spread made from ground peanuts', 'name': 'peanut_butter'}, {'frequency': 'c', 'id': 789, 'synset': 'pear.n.01', 'synonyms': ['pear'], 'def': 'sweet juicy gritty-textured fruit available in many varieties', 'name': 'pear'}, {'frequency': 'r', 'id': 790, 'synset': 'peeler.n.03', 'synonyms': ['peeler_(tool_for_fruit_and_vegetables)'], 'def': 'a device for peeling vegetables or fruits', 'name': 'peeler_(tool_for_fruit_and_vegetables)'}, {'frequency': 'r', 'id': 791, 'synset': 'pegboard.n.01', 'synonyms': ['pegboard'], 'def': 'a board perforated with regularly spaced holes into which pegs can be fitted', 'name': 'pegboard'}, {'frequency': 'c', 'id': 792, 'synset': 'pelican.n.01', 'synonyms': ['pelican'], 'def': 'large long-winged warm-water seabird having a large bill with a distensible pouch for fish', 'name': 'pelican'}, {'frequency': 'f', 'id': 793, 'synset': 'pen.n.01', 'synonyms': ['pen'], 'def': 'a writing implement with a point from which ink flows', 'name': 'pen'}, {'frequency': 'c', 'id': 794, 'synset': 'pencil.n.01', 'synonyms': ['pencil'], 'def': 'a thin cylindrical pointed writing implement made of wood and graphite', 'name': 'pencil'}, {'frequency': 'r', 'id': 795, 'synset': 'pencil_box.n.01', 'synonyms': ['pencil_box', 'pencil_case'], 'def': 'a box for holding pencils', 'name': 'pencil_box'}, {'frequency': 'r', 'id': 796, 'synset': 'pencil_sharpener.n.01', 'synonyms': ['pencil_sharpener'], 'def': 'a rotary implement for sharpening the point on pencils', 'name': 'pencil_sharpener'}, {'frequency': 'r', 'id': 797, 'synset': 'pendulum.n.01', 'synonyms': ['pendulum'], 'def': 'an apparatus consisting of an object mounted so that it swings freely under the influence of gravity', 'name': 'pendulum'}, {'frequency': 'c', 'id': 798, 'synset': 'penguin.n.01', 'synonyms': ['penguin'], 'def': 'short-legged flightless birds of cold southern regions having webbed feet and wings modified as flippers', 'name': 'penguin'}, {'frequency': 'r', 'id': 799, 'synset': 'pennant.n.02', 'synonyms': ['pennant'], 'def': 'a flag longer than it is wide (and often tapering)', 'name': 'pennant'}, {'frequency': 'r', 'id': 800, 'synset': 'penny.n.02', 'synonyms': ['penny_(coin)'], 'def': 'a coin worth one-hundredth of the value of the basic unit', 'name': 'penny_(coin)'}, {'frequency': 'c', 'id': 801, 'synset': 'pepper.n.03', 'synonyms': ['pepper', 'peppercorn'], 'def': 'pungent seasoning from the berry of the common pepper plant; whole or ground', 'name': 'pepper'}, {'frequency': 'c', 'id': 802, 'synset': 'pepper_mill.n.01', 'synonyms': ['pepper_mill', 'pepper_grinder'], 'def': 'a mill for grinding pepper', 'name': 'pepper_mill'}, {'frequency': 'c', 'id': 803, 'synset': 'perfume.n.02', 'synonyms': ['perfume'], 'def': 'a toiletry that emits and diffuses a fragrant odor', 'name': 'perfume'}, {'frequency': 'r', 'id': 804, 'synset': 'persimmon.n.02', 'synonyms': ['persimmon'], 'def': 'orange fruit resembling a plum; edible when fully ripe', 'name': 'persimmon'}, {'frequency': 'f', 'id': 805, 'synset': 'person.n.01', 'synonyms': ['baby', 'child', 'boy', 'girl', 'man', 'woman', 'person', 'human'], 'def': 'a human being', 'name': 'baby'}, {'frequency': 'r', 'id': 806, 'synset': 'pet.n.01', 'synonyms': ['pet'], 'def': 'a domesticated animal kept for companionship or amusement', 'name': 'pet'}, {'frequency': 'r', 'id': 807, 'synset': 'petfood.n.01', 'synonyms': ['petfood', 'pet-food'], 'def': 'food prepared for animal pets', 'name': 'petfood'}, {'frequency': 'r', 'id': 808, 'synset': 'pew.n.01', 'synonyms': ['pew_(church_bench)', 'church_bench'], 'def': 'long bench with backs; used in church by the congregation', 'name': 'pew_(church_bench)'}, {'frequency': 'r', 'id': 809, 'synset': 'phonebook.n.01', 'synonyms': ['phonebook', 'telephone_book', 'telephone_directory'], 'def': 'a directory containing an alphabetical list of telephone subscribers and their telephone numbers', 'name': 'phonebook'}, {'frequency': 'c', 'id': 810, 'synset': 'phonograph_record.n.01', 'synonyms': ['phonograph_record', 'phonograph_recording', 'record_(phonograph_recording)'], 'def': 'sound recording consisting of a typically black disk with a continuous groove', 'name': 'phonograph_record'}, {'frequency': 'c', 'id': 811, 'synset': 'piano.n.01', 'synonyms': ['piano'], 'def': 'a keyboard instrument that is played by depressing keys that cause hammers to strike tuned strings and produce sounds', 'name': 'piano'}, {'frequency': 'f', 'id': 812, 'synset': 'pickle.n.01', 'synonyms': ['pickle'], 'def': 'vegetables (especially cucumbers) preserved in brine or vinegar', 'name': 'pickle'}, {'frequency': 'f', 'id': 813, 'synset': 'pickup.n.01', 'synonyms': ['pickup_truck'], 'def': 'a light truck with an open body and low sides and a tailboard', 'name': 'pickup_truck'}, {'frequency': 'c', 'id': 814, 'synset': 'pie.n.01', 'synonyms': ['pie'], 'def': 'dish baked in pastry-lined pan often with a pastry top', 'name': 'pie'}, {'frequency': 'c', 'id': 815, 'synset': 'pigeon.n.01', 'synonyms': ['pigeon'], 'def': 'wild and domesticated birds having a heavy body and short legs', 'name': 'pigeon'}, {'frequency': 'r', 'id': 816, 'synset': 'piggy_bank.n.01', 'synonyms': ['piggy_bank', 'penny_bank'], 'def': "a child's coin bank (often shaped like a pig)", 'name': 'piggy_bank'}, {'frequency': 'f', 'id': 817, 'synset': 'pillow.n.01', 'synonyms': ['pillow'], 'def': 'a cushion to support the head of a sleeping person', 'name': 'pillow'}, {'frequency': 'r', 'id': 818, 'synset': 'pin.n.09', 'synonyms': ['pin_(non_jewelry)'], 'def': 'a small slender (often pointed) piece of wood or metal used to support or fasten or attach things', 'name': 'pin_(non_jewelry)'}, {'frequency': 'f', 'id': 819, 'synset': 'pineapple.n.02', 'synonyms': ['pineapple'], 'def': 'large sweet fleshy tropical fruit with a tuft of stiff leaves', 'name': 'pineapple'}, {'frequency': 'c', 'id': 820, 'synset': 'pinecone.n.01', 'synonyms': ['pinecone'], 'def': 'the seed-producing cone of a pine tree', 'name': 'pinecone'}, {'frequency': 'r', 'id': 821, 'synset': 'ping-pong_ball.n.01', 'synonyms': ['ping-pong_ball'], 'def': 'light hollow ball used in playing table tennis', 'name': 'ping-pong_ball'}, {'frequency': 'r', 'id': 822, 'synset': 'pinwheel.n.03', 'synonyms': ['pinwheel'], 'def': 'a toy consisting of vanes of colored paper or plastic that is pinned to a stick and spins when it is pointed into the wind', 'name': 'pinwheel'}, {'frequency': 'r', 'id': 823, 'synset': 'pipe.n.01', 'synonyms': ['tobacco_pipe'], 'def': 'a tube with a small bowl at one end; used for smoking tobacco', 'name': 'tobacco_pipe'}, {'frequency': 'f', 'id': 824, 'synset': 'pipe.n.02', 'synonyms': ['pipe', 'piping'], 'def': 'a long tube made of metal or plastic that is used to carry water or oil or gas etc.', 'name': 'pipe'}, {'frequency': 'r', 'id': 825, 'synset': 'pistol.n.01', 'synonyms': ['pistol', 'handgun'], 'def': 'a firearm that is held and fired with one hand', 'name': 'pistol'}, {'frequency': 'r', 'id': 826, 'synset': 'pita.n.01', 'synonyms': ['pita_(bread)', 'pocket_bread'], 'def': 'usually small round bread that can open into a pocket for filling', 'name': 'pita_(bread)'}, {'frequency': 'f', 'id': 827, 'synset': 'pitcher.n.02', 'synonyms': ['pitcher_(vessel_for_liquid)', 'ewer'], 'def': 'an open vessel with a handle and a spout for pouring', 'name': 'pitcher_(vessel_for_liquid)'}, {'frequency': 'r', 'id': 828, 'synset': 'pitchfork.n.01', 'synonyms': ['pitchfork'], 'def': 'a long-handled hand tool with sharp widely spaced prongs for lifting and pitching hay', 'name': 'pitchfork'}, {'frequency': 'f', 'id': 829, 'synset': 'pizza.n.01', 'synonyms': ['pizza'], 'def': 'Italian open pie made of thin bread dough spread with a spiced mixture of e.g. tomato sauce and cheese', 'name': 'pizza'}, {'frequency': 'f', 'id': 830, 'synset': 'place_mat.n.01', 'synonyms': ['place_mat'], 'def': 'a mat placed on a table for an individual place setting', 'name': 'place_mat'}, {'frequency': 'f', 'id': 831, 'synset': 'plate.n.04', 'synonyms': ['plate'], 'def': 'dish on which food is served or from which food is eaten', 'name': 'plate'}, {'frequency': 'c', 'id': 832, 'synset': 'platter.n.01', 'synonyms': ['platter'], 'def': 'a large shallow dish used for serving food', 'name': 'platter'}, {'frequency': 'r', 'id': 833, 'synset': 'playing_card.n.01', 'synonyms': ['playing_card'], 'def': 'one of a pack of cards that are used to play card games', 'name': 'playing_card'}, {'frequency': 'r', 'id': 834, 'synset': 'playpen.n.01', 'synonyms': ['playpen'], 'def': 'a portable enclosure in which babies may be left to play', 'name': 'playpen'}, {'frequency': 'c', 'id': 835, 'synset': 'pliers.n.01', 'synonyms': ['pliers', 'plyers'], 'def': 'a gripping hand tool with two hinged arms and (usually) serrated jaws', 'name': 'pliers'}, {'frequency': 'r', 'id': 836, 'synset': 'plow.n.01', 'synonyms': ['plow_(farm_equipment)', 'plough_(farm_equipment)'], 'def': 'a farm tool having one or more heavy blades to break the soil and cut a furrow prior to sowing', 'name': 'plow_(farm_equipment)'}, {'frequency': 'r', 'id': 837, 'synset': 'pocket_watch.n.01', 'synonyms': ['pocket_watch'], 'def': 'a watch that is carried in a small watch pocket', 'name': 'pocket_watch'}, {'frequency': 'c', 'id': 838, 'synset': 'pocketknife.n.01', 'synonyms': ['pocketknife'], 'def': 'a knife with a blade that folds into the handle; suitable for carrying in the pocket', 'name': 'pocketknife'}, {'frequency': 'c', 'id': 839, 'synset': 'poker.n.01', 'synonyms': ['poker_(fire_stirring_tool)', 'stove_poker', 'fire_hook'], 'def': 'fire iron consisting of a metal rod with a handle; used to stir a fire', 'name': 'poker_(fire_stirring_tool)'}, {'frequency': 'f', 'id': 840, 'synset': 'pole.n.01', 'synonyms': ['pole', 'post'], 'def': 'a long (usually round) rod of wood or metal or plastic', 'name': 'pole'}, {'frequency': 'r', 'id': 841, 'synset': 'police_van.n.01', 'synonyms': ['police_van', 'police_wagon', 'paddy_wagon', 'patrol_wagon'], 'def': 'van used by police to transport prisoners', 'name': 'police_van'}, {'frequency': 'f', 'id': 842, 'synset': 'polo_shirt.n.01', 'synonyms': ['polo_shirt', 'sport_shirt'], 'def': 'a shirt with short sleeves designed for comfort and casual wear', 'name': 'polo_shirt'}, {'frequency': 'r', 'id': 843, 'synset': 'poncho.n.01', 'synonyms': ['poncho'], 'def': 'a blanket-like cloak with a hole in the center for the head', 'name': 'poncho'}, {'frequency': 'c', 'id': 844, 'synset': 'pony.n.05', 'synonyms': ['pony'], 'def': 'any of various breeds of small gentle horses usually less than five feet high at the shoulder', 'name': 'pony'}, {'frequency': 'r', 'id': 845, 'synset': 'pool_table.n.01', 'synonyms': ['pool_table', 'billiard_table', 'snooker_table'], 'def': 'game equipment consisting of a heavy table on which pool is played', 'name': 'pool_table'}, {'frequency': 'f', 'id': 846, 'synset': 'pop.n.02', 'synonyms': ['pop_(soda)', 'soda_(pop)', 'tonic', 'soft_drink'], 'def': 'a sweet drink containing carbonated water and flavoring', 'name': 'pop_(soda)'}, {'frequency': 'r', 'id': 847, 'synset': 'portrait.n.02', 'synonyms': ['portrait', 'portrayal'], 'def': 'any likeness of a person, in any medium', 'name': 'portrait'}, {'frequency': 'c', 'id': 848, 'synset': 'postbox.n.01', 'synonyms': ['postbox_(public)', 'mailbox_(public)'], 'def': 'public box for deposit of mail', 'name': 'postbox_(public)'}, {'frequency': 'c', 'id': 849, 'synset': 'postcard.n.01', 'synonyms': ['postcard', 'postal_card', 'mailing-card'], 'def': 'a card for sending messages by post without an envelope', 'name': 'postcard'}, {'frequency': 'f', 'id': 850, 'synset': 'poster.n.01', 'synonyms': ['poster', 'placard'], 'def': 'a sign posted in a public place as an advertisement', 'name': 'poster'}, {'frequency': 'f', 'id': 851, 'synset': 'pot.n.01', 'synonyms': ['pot'], 'def': 'metal or earthenware cooking vessel that is usually round and deep; often has a handle and lid', 'name': 'pot'}, {'frequency': 'f', 'id': 852, 'synset': 'pot.n.04', 'synonyms': ['flowerpot'], 'def': 'a container in which plants are cultivated', 'name': 'flowerpot'}, {'frequency': 'f', 'id': 853, 'synset': 'potato.n.01', 'synonyms': ['potato'], 'def': 'an edible tuber native to South America', 'name': 'potato'}, {'frequency': 'c', 'id': 854, 'synset': 'potholder.n.01', 'synonyms': ['potholder'], 'def': 'an insulated pad for holding hot pots', 'name': 'potholder'}, {'frequency': 'c', 'id': 855, 'synset': 'pottery.n.01', 'synonyms': ['pottery', 'clayware'], 'def': 'ceramic ware made from clay and baked in a kiln', 'name': 'pottery'}, {'frequency': 'c', 'id': 856, 'synset': 'pouch.n.01', 'synonyms': ['pouch'], 'def': 'a small or medium size container for holding or carrying things', 'name': 'pouch'}, {'frequency': 'r', 'id': 857, 'synset': 'power_shovel.n.01', 'synonyms': ['power_shovel', 'excavator', 'digger'], 'def': 'a machine for excavating', 'name': 'power_shovel'}, {'frequency': 'c', 'id': 858, 'synset': 'prawn.n.01', 'synonyms': ['prawn', 'shrimp'], 'def': 'any of various edible decapod crustaceans', 'name': 'prawn'}, {'frequency': 'f', 'id': 859, 'synset': 'printer.n.03', 'synonyms': ['printer', 'printing_machine'], 'def': 'a machine that prints', 'name': 'printer'}, {'frequency': 'c', 'id': 860, 'synset': 'projectile.n.01', 'synonyms': ['projectile_(weapon)', 'missile'], 'def': 'a weapon that is forcibly thrown or projected at a targets', 'name': 'projectile_(weapon)'}, {'frequency': 'c', 'id': 861, 'synset': 'projector.n.02', 'synonyms': ['projector'], 'def': 'an optical instrument that projects an enlarged image onto a screen', 'name': 'projector'}, {'frequency': 'f', 'id': 862, 'synset': 'propeller.n.01', 'synonyms': ['propeller', 'propellor'], 'def': 'a mechanical device that rotates to push against air or water', 'name': 'propeller'}, {'frequency': 'r', 'id': 863, 'synset': 'prune.n.01', 'synonyms': ['prune'], 'def': 'dried plum', 'name': 'prune'}, {'frequency': 'r', 'id': 864, 'synset': 'pudding.n.01', 'synonyms': ['pudding'], 'def': 'any of various soft thick unsweetened baked dishes', 'name': 'pudding'}, {'frequency': 'r', 'id': 865, 'synset': 'puffer.n.02', 'synonyms': ['puffer_(fish)', 'pufferfish', 'blowfish', 'globefish'], 'def': 'fishes whose elongated spiny body can inflate itself with water or air to form a globe', 'name': 'puffer_(fish)'}, {'frequency': 'r', 'id': 866, 'synset': 'puffin.n.01', 'synonyms': ['puffin'], 'def': 'seabirds having short necks and brightly colored compressed bills', 'name': 'puffin'}, {'frequency': 'r', 'id': 867, 'synset': 'pug.n.01', 'synonyms': ['pug-dog'], 'def': 'small compact smooth-coated breed of Asiatic origin having a tightly curled tail and broad flat wrinkled muzzle', 'name': 'pug-dog'}, {'frequency': 'c', 'id': 868, 'synset': 'pumpkin.n.02', 'synonyms': ['pumpkin'], 'def': 'usually large pulpy deep-yellow round fruit of the squash family maturing in late summer or early autumn', 'name': 'pumpkin'}, {'frequency': 'r', 'id': 869, 'synset': 'punch.n.03', 'synonyms': ['puncher'], 'def': 'a tool for making holes or indentations', 'name': 'puncher'}, {'frequency': 'r', 'id': 870, 'synset': 'puppet.n.01', 'synonyms': ['puppet', 'marionette'], 'def': 'a small figure of a person operated from above with strings by a puppeteer', 'name': 'puppet'}, {'frequency': 'r', 'id': 871, 'synset': 'puppy.n.01', 'synonyms': ['puppy'], 'def': 'a young dog', 'name': 'puppy'}, {'frequency': 'r', 'id': 872, 'synset': 'quesadilla.n.01', 'synonyms': ['quesadilla'], 'def': 'a tortilla that is filled with cheese and heated', 'name': 'quesadilla'}, {'frequency': 'r', 'id': 873, 'synset': 'quiche.n.02', 'synonyms': ['quiche'], 'def': 'a tart filled with rich unsweetened custard; often contains other ingredients (as cheese or ham or seafood or vegetables)', 'name': 'quiche'}, {'frequency': 'f', 'id': 874, 'synset': 'quilt.n.01', 'synonyms': ['quilt', 'comforter'], 'def': 'bedding made of two layers of cloth filled with stuffing and stitched together', 'name': 'quilt'}, {'frequency': 'c', 'id': 875, 'synset': 'rabbit.n.01', 'synonyms': ['rabbit'], 'def': 'any of various burrowing animals of the family Leporidae having long ears and short tails', 'name': 'rabbit'}, {'frequency': 'r', 'id': 876, 'synset': 'racer.n.02', 'synonyms': ['race_car', 'racing_car'], 'def': 'a fast car that competes in races', 'name': 'race_car'}, {'frequency': 'c', 'id': 877, 'synset': 'racket.n.04', 'synonyms': ['racket', 'racquet'], 'def': 'a sports implement used to strike a ball in various games', 'name': 'racket'}, {'frequency': 'r', 'id': 878, 'synset': 'radar.n.01', 'synonyms': ['radar'], 'def': 'measuring instrument in which the echo of a pulse of microwave radiation is used to detect and locate distant objects', 'name': 'radar'}, {'frequency': 'c', 'id': 879, 'synset': 'radiator.n.03', 'synonyms': ['radiator'], 'def': 'a mechanism consisting of a metal honeycomb through which hot fluids circulate', 'name': 'radiator'}, {'frequency': 'c', 'id': 880, 'synset': 'radio_receiver.n.01', 'synonyms': ['radio_receiver', 'radio_set', 'radio', 'tuner_(radio)'], 'def': 'an electronic receiver that detects and demodulates and amplifies transmitted radio signals', 'name': 'radio_receiver'}, {'frequency': 'c', 'id': 881, 'synset': 'radish.n.03', 'synonyms': ['radish', 'daikon'], 'def': 'pungent edible root of any of various cultivated radish plants', 'name': 'radish'}, {'frequency': 'c', 'id': 882, 'synset': 'raft.n.01', 'synonyms': ['raft'], 'def': 'a flat float (usually made of logs or planks) that can be used for transport or as a platform for swimmers', 'name': 'raft'}, {'frequency': 'r', 'id': 883, 'synset': 'rag_doll.n.01', 'synonyms': ['rag_doll'], 'def': 'a cloth doll that is stuffed and (usually) painted', 'name': 'rag_doll'}, {'frequency': 'c', 'id': 884, 'synset': 'raincoat.n.01', 'synonyms': ['raincoat', 'waterproof_jacket'], 'def': 'a water-resistant coat', 'name': 'raincoat'}, {'frequency': 'c', 'id': 885, 'synset': 'ram.n.05', 'synonyms': ['ram_(animal)'], 'def': 'uncastrated adult male sheep', 'name': 'ram_(animal)'}, {'frequency': 'c', 'id': 886, 'synset': 'raspberry.n.02', 'synonyms': ['raspberry'], 'def': 'red or black edible aggregate berries usually smaller than the related blackberries', 'name': 'raspberry'}, {'frequency': 'r', 'id': 887, 'synset': 'rat.n.01', 'synonyms': ['rat'], 'def': 'any of various long-tailed rodents similar to but larger than a mouse', 'name': 'rat'}, {'frequency': 'c', 'id': 888, 'synset': 'razorblade.n.01', 'synonyms': ['razorblade'], 'def': 'a blade that has very sharp edge', 'name': 'razorblade'}, {'frequency': 'c', 'id': 889, 'synset': 'reamer.n.01', 'synonyms': ['reamer_(juicer)', 'juicer', 'juice_reamer'], 'def': 'a squeezer with a conical ridged center that is used for squeezing juice from citrus fruit', 'name': 'reamer_(juicer)'}, {'frequency': 'f', 'id': 890, 'synset': 'rearview_mirror.n.01', 'synonyms': ['rearview_mirror'], 'def': 'car mirror that reflects the view out of the rear window', 'name': 'rearview_mirror'}, {'frequency': 'c', 'id': 891, 'synset': 'receipt.n.02', 'synonyms': ['receipt'], 'def': 'an acknowledgment (usually tangible) that payment has been made', 'name': 'receipt'}, {'frequency': 'c', 'id': 892, 'synset': 'recliner.n.01', 'synonyms': ['recliner', 'reclining_chair', 'lounger_(chair)'], 'def': 'an armchair whose back can be lowered and foot can be raised to allow the sitter to recline in it', 'name': 'recliner'}, {'frequency': 'r', 'id': 893, 'synset': 'record_player.n.01', 'synonyms': ['record_player', 'phonograph_(record_player)', 'turntable'], 'def': 'machine in which rotating records cause a stylus to vibrate and the vibrations are amplified acoustically or electronically', 'name': 'record_player'}, {'frequency': 'r', 'id': 894, 'synset': 'red_cabbage.n.02', 'synonyms': ['red_cabbage'], 'def': 'compact head of purplish-red leaves', 'name': 'red_cabbage'}, {'frequency': 'f', 'id': 895, 'synset': 'reflector.n.01', 'synonyms': ['reflector'], 'def': 'device that reflects light, radiation, etc.', 'name': 'reflector'}, {'frequency': 'f', 'id': 896, 'synset': 'remote_control.n.01', 'synonyms': ['remote_control'], 'def': 'a device that can be used to control a machine or apparatus from a distance', 'name': 'remote_control'}, {'frequency': 'c', 'id': 897, 'synset': 'rhinoceros.n.01', 'synonyms': ['rhinoceros'], 'def': 'massive powerful herbivorous odd-toed ungulate of southeast Asia and Africa having very thick skin and one or two horns on the snout', 'name': 'rhinoceros'}, {'frequency': 'r', 'id': 898, 'synset': 'rib.n.03', 'synonyms': ['rib_(food)'], 'def': 'cut of meat including one or more ribs', 'name': 'rib_(food)'}, {'frequency': 'r', 'id': 899, 'synset': 'rifle.n.01', 'synonyms': ['rifle'], 'def': 'a shoulder firearm with a long barrel', 'name': 'rifle'}, {'frequency': 'f', 'id': 900, 'synset': 'ring.n.08', 'synonyms': ['ring'], 'def': 'jewelry consisting of a circlet of precious metal (often set with jewels) worn on the finger', 'name': 'ring'}, {'frequency': 'r', 'id': 901, 'synset': 'river_boat.n.01', 'synonyms': ['river_boat'], 'def': 'a boat used on rivers or to ply a river', 'name': 'river_boat'}, {'frequency': 'r', 'id': 902, 'synset': 'road_map.n.02', 'synonyms': ['road_map'], 'def': '(NOT A ROAD) a MAP showing roads (for automobile travel)', 'name': 'road_map'}, {'frequency': 'c', 'id': 903, 'synset': 'robe.n.01', 'synonyms': ['robe'], 'def': 'any loose flowing garment', 'name': 'robe'}, {'frequency': 'c', 'id': 904, 'synset': 'rocking_chair.n.01', 'synonyms': ['rocking_chair'], 'def': 'a chair mounted on rockers', 'name': 'rocking_chair'}, {'frequency': 'r', 'id': 905, 'synset': 'roller_skate.n.01', 'synonyms': ['roller_skate'], 'def': 'a shoe with pairs of rollers (small hard wheels) fixed to the sole', 'name': 'roller_skate'}, {'frequency': 'r', 'id': 906, 'synset': 'rollerblade.n.01', 'synonyms': ['Rollerblade'], 'def': 'an in-line variant of a roller skate', 'name': 'Rollerblade'}, {'frequency': 'c', 'id': 907, 'synset': 'rolling_pin.n.01', 'synonyms': ['rolling_pin'], 'def': 'utensil consisting of a cylinder (usually of wood) with a handle at each end; used to roll out dough', 'name': 'rolling_pin'}, {'frequency': 'r', 'id': 908, 'synset': 'root_beer.n.01', 'synonyms': ['root_beer'], 'def': 'carbonated drink containing extracts of roots and herbs', 'name': 'root_beer'}, {'frequency': 'c', 'id': 909, 'synset': 'router.n.02', 'synonyms': ['router_(computer_equipment)'], 'def': 'a device that forwards data packets between computer networks', 'name': 'router_(computer_equipment)'}, {'frequency': 'f', 'id': 910, 'synset': 'rubber_band.n.01', 'synonyms': ['rubber_band', 'elastic_band'], 'def': 'a narrow band of elastic rubber used to hold things (such as papers) together', 'name': 'rubber_band'}, {'frequency': 'c', 'id': 911, 'synset': 'runner.n.08', 'synonyms': ['runner_(carpet)'], 'def': 'a long narrow carpet', 'name': 'runner_(carpet)'}, {'frequency': 'f', 'id': 912, 'synset': 'sack.n.01', 'synonyms': ['plastic_bag', 'paper_bag'], 'def': "a bag made of paper or plastic for holding customer's purchases", 'name': 'plastic_bag'}, {'frequency': 'f', 'id': 913, 'synset': 'saddle.n.01', 'synonyms': ['saddle_(on_an_animal)'], 'def': 'a seat for the rider of a horse or camel', 'name': 'saddle_(on_an_animal)'}, {'frequency': 'f', 'id': 914, 'synset': 'saddle_blanket.n.01', 'synonyms': ['saddle_blanket', 'saddlecloth', 'horse_blanket'], 'def': 'stable gear consisting of a blanket placed under the saddle', 'name': 'saddle_blanket'}, {'frequency': 'c', 'id': 915, 'synset': 'saddlebag.n.01', 'synonyms': ['saddlebag'], 'def': 'a large bag (or pair of bags) hung over a saddle', 'name': 'saddlebag'}, {'frequency': 'r', 'id': 916, 'synset': 'safety_pin.n.01', 'synonyms': ['safety_pin'], 'def': 'a pin in the form of a clasp; has a guard so the point of the pin will not stick the user', 'name': 'safety_pin'}, {'frequency': 'c', 'id': 917, 'synset': 'sail.n.01', 'synonyms': ['sail'], 'def': 'a large piece of fabric by means of which wind is used to propel a sailing vessel', 'name': 'sail'}, {'frequency': 'c', 'id': 918, 'synset': 'salad.n.01', 'synonyms': ['salad'], 'def': 'food mixtures either arranged on a plate or tossed and served with a moist dressing; usually consisting of or including greens', 'name': 'salad'}, {'frequency': 'r', 'id': 919, 'synset': 'salad_plate.n.01', 'synonyms': ['salad_plate', 'salad_bowl'], 'def': 'a plate or bowl for individual servings of salad', 'name': 'salad_plate'}, {'frequency': 'r', 'id': 920, 'synset': 'salami.n.01', 'synonyms': ['salami'], 'def': 'highly seasoned fatty sausage of pork and beef usually dried', 'name': 'salami'}, {'frequency': 'r', 'id': 921, 'synset': 'salmon.n.01', 'synonyms': ['salmon_(fish)'], 'def': 'any of various large food and game fishes of northern waters', 'name': 'salmon_(fish)'}, {'frequency': 'r', 'id': 922, 'synset': 'salmon.n.03', 'synonyms': ['salmon_(food)'], 'def': 'flesh of any of various marine or freshwater fish of the family Salmonidae', 'name': 'salmon_(food)'}, {'frequency': 'r', 'id': 923, 'synset': 'salsa.n.01', 'synonyms': ['salsa'], 'def': 'spicy sauce of tomatoes and onions and chili peppers to accompany Mexican foods', 'name': 'salsa'}, {'frequency': 'f', 'id': 924, 'synset': 'saltshaker.n.01', 'synonyms': ['saltshaker'], 'def': 'a shaker with a perforated top for sprinkling salt', 'name': 'saltshaker'}, {'frequency': 'f', 'id': 925, 'synset': 'sandal.n.01', 'synonyms': ['sandal_(type_of_shoe)'], 'def': 'a shoe consisting of a sole fastened by straps to the foot', 'name': 'sandal_(type_of_shoe)'}, {'frequency': 'f', 'id': 926, 'synset': 'sandwich.n.01', 'synonyms': ['sandwich'], 'def': 'two (or more) slices of bread with a filling between them', 'name': 'sandwich'}, {'frequency': 'r', 'id': 927, 'synset': 'satchel.n.01', 'synonyms': ['satchel'], 'def': 'luggage consisting of a small case with a flat bottom and (usually) a shoulder strap', 'name': 'satchel'}, {'frequency': 'r', 'id': 928, 'synset': 'saucepan.n.01', 'synonyms': ['saucepan'], 'def': 'a deep pan with a handle; used for stewing or boiling', 'name': 'saucepan'}, {'frequency': 'f', 'id': 929, 'synset': 'saucer.n.02', 'synonyms': ['saucer'], 'def': 'a small shallow dish for holding a cup at the table', 'name': 'saucer'}, {'frequency': 'f', 'id': 930, 'synset': 'sausage.n.01', 'synonyms': ['sausage'], 'def': 'highly seasoned minced meat stuffed in casings', 'name': 'sausage'}, {'frequency': 'r', 'id': 931, 'synset': 'sawhorse.n.01', 'synonyms': ['sawhorse', 'sawbuck'], 'def': 'a framework for holding wood that is being sawed', 'name': 'sawhorse'}, {'frequency': 'r', 'id': 932, 'synset': 'sax.n.02', 'synonyms': ['saxophone'], 'def': "a wind instrument with a `J'-shaped form typically made of brass", 'name': 'saxophone'}, {'frequency': 'f', 'id': 933, 'synset': 'scale.n.07', 'synonyms': ['scale_(measuring_instrument)'], 'def': 'a measuring instrument for weighing; shows amount of mass', 'name': 'scale_(measuring_instrument)'}, {'frequency': 'r', 'id': 934, 'synset': 'scarecrow.n.01', 'synonyms': ['scarecrow', 'strawman'], 'def': 'an effigy in the shape of a man to frighten birds away from seeds', 'name': 'scarecrow'}, {'frequency': 'f', 'id': 935, 'synset': 'scarf.n.01', 'synonyms': ['scarf'], 'def': 'a garment worn around the head or neck or shoulders for warmth or decoration', 'name': 'scarf'}, {'frequency': 'c', 'id': 936, 'synset': 'school_bus.n.01', 'synonyms': ['school_bus'], 'def': 'a bus used to transport children to or from school', 'name': 'school_bus'}, {'frequency': 'f', 'id': 937, 'synset': 'scissors.n.01', 'synonyms': ['scissors'], 'def': 'a tool having two crossed pivoting blades with looped handles', 'name': 'scissors'}, {'frequency': 'c', 'id': 938, 'synset': 'scoreboard.n.01', 'synonyms': ['scoreboard'], 'def': 'a large board for displaying the score of a contest (and some other information)', 'name': 'scoreboard'}, {'frequency': 'c', 'id': 939, 'synset': 'scrambled_eggs.n.01', 'synonyms': ['scrambled_eggs'], 'def': 'eggs beaten and cooked to a soft firm consistency while stirring', 'name': 'scrambled_eggs'}, {'frequency': 'r', 'id': 940, 'synset': 'scraper.n.01', 'synonyms': ['scraper'], 'def': 'any of various hand tools for scraping', 'name': 'scraper'}, {'frequency': 'r', 'id': 941, 'synset': 'scratcher.n.03', 'synonyms': ['scratcher'], 'def': 'a device used for scratching', 'name': 'scratcher'}, {'frequency': 'c', 'id': 942, 'synset': 'screwdriver.n.01', 'synonyms': ['screwdriver'], 'def': 'a hand tool for driving screws; has a tip that fits into the head of a screw', 'name': 'screwdriver'}, {'frequency': 'c', 'id': 943, 'synset': 'scrub_brush.n.01', 'synonyms': ['scrubbing_brush'], 'def': 'a brush with short stiff bristles for heavy cleaning', 'name': 'scrubbing_brush'}, {'frequency': 'c', 'id': 944, 'synset': 'sculpture.n.01', 'synonyms': ['sculpture'], 'def': 'a three-dimensional work of art', 'name': 'sculpture'}, {'frequency': 'r', 'id': 945, 'synset': 'seabird.n.01', 'synonyms': ['seabird', 'seafowl'], 'def': 'a bird that frequents coastal waters and the open ocean: gulls; pelicans; gannets; cormorants; albatrosses; petrels; etc.', 'name': 'seabird'}, {'frequency': 'r', 'id': 946, 'synset': 'seahorse.n.02', 'synonyms': ['seahorse'], 'def': 'small fish with horse-like heads bent sharply downward and curled tails', 'name': 'seahorse'}, {'frequency': 'r', 'id': 947, 'synset': 'seaplane.n.01', 'synonyms': ['seaplane', 'hydroplane'], 'def': 'an airplane that can land on or take off from water', 'name': 'seaplane'}, {'frequency': 'c', 'id': 948, 'synset': 'seashell.n.01', 'synonyms': ['seashell'], 'def': 'the shell of a marine organism', 'name': 'seashell'}, {'frequency': 'r', 'id': 949, 'synset': 'seedling.n.01', 'synonyms': ['seedling'], 'def': 'young plant or tree grown from a seed', 'name': 'seedling'}, {'frequency': 'c', 'id': 950, 'synset': 'serving_dish.n.01', 'synonyms': ['serving_dish'], 'def': 'a dish used for serving food', 'name': 'serving_dish'}, {'frequency': 'r', 'id': 951, 'synset': 'sewing_machine.n.01', 'synonyms': ['sewing_machine'], 'def': 'a textile machine used as a home appliance for sewing', 'name': 'sewing_machine'}, {'frequency': 'r', 'id': 952, 'synset': 'shaker.n.03', 'synonyms': ['shaker'], 'def': 'a container in which something can be shaken', 'name': 'shaker'}, {'frequency': 'c', 'id': 953, 'synset': 'shampoo.n.01', 'synonyms': ['shampoo'], 'def': 'cleansing agent consisting of soaps or detergents used for washing the hair', 'name': 'shampoo'}, {'frequency': 'r', 'id': 954, 'synset': 'shark.n.01', 'synonyms': ['shark'], 'def': 'typically large carnivorous fishes with sharpe teeth', 'name': 'shark'}, {'frequency': 'r', 'id': 955, 'synset': 'sharpener.n.01', 'synonyms': ['sharpener'], 'def': 'any implement that is used to make something (an edge or a point) sharper', 'name': 'sharpener'}, {'frequency': 'r', 'id': 956, 'synset': 'sharpie.n.03', 'synonyms': ['Sharpie'], 'def': 'a pen with indelible ink that will write on any surface', 'name': 'Sharpie'}, {'frequency': 'r', 'id': 957, 'synset': 'shaver.n.03', 'synonyms': ['shaver_(electric)', 'electric_shaver', 'electric_razor'], 'def': 'a razor powered by an electric motor', 'name': 'shaver_(electric)'}, {'frequency': 'c', 'id': 958, 'synset': 'shaving_cream.n.01', 'synonyms': ['shaving_cream', 'shaving_soap'], 'def': 'toiletry consisting that forms a rich lather for softening the beard before shaving', 'name': 'shaving_cream'}, {'frequency': 'r', 'id': 959, 'synset': 'shawl.n.01', 'synonyms': ['shawl'], 'def': 'cloak consisting of an oblong piece of cloth used to cover the head and shoulders', 'name': 'shawl'}, {'frequency': 'r', 'id': 960, 'synset': 'shears.n.01', 'synonyms': ['shears'], 'def': 'large scissors with strong blades', 'name': 'shears'}, {'frequency': 'f', 'id': 961, 'synset': 'sheep.n.01', 'synonyms': ['sheep'], 'def': 'woolly usually horned ruminant mammal related to the goat', 'name': 'sheep'}, {'frequency': 'r', 'id': 962, 'synset': 'shepherd_dog.n.01', 'synonyms': ['shepherd_dog', 'sheepdog'], 'def': 'any of various usually long-haired breeds of dog reared to herd and guard sheep', 'name': 'shepherd_dog'}, {'frequency': 'r', 'id': 963, 'synset': 'sherbert.n.01', 'synonyms': ['sherbert', 'sherbet'], 'def': 'a frozen dessert made primarily of fruit juice and sugar', 'name': 'sherbert'}, {'frequency': 'r', 'id': 964, 'synset': 'shield.n.02', 'synonyms': ['shield'], 'def': 'armor carried on the arm to intercept blows', 'name': 'shield'}, {'frequency': 'f', 'id': 965, 'synset': 'shirt.n.01', 'synonyms': ['shirt'], 'def': 'a garment worn on the upper half of the body', 'name': 'shirt'}, {'frequency': 'f', 'id': 966, 'synset': 'shoe.n.01', 'synonyms': ['shoe', 'sneaker_(type_of_shoe)', 'tennis_shoe'], 'def': 'common footwear covering the foot', 'name': 'shoe'}, {'frequency': 'c', 'id': 967, 'synset': 'shopping_bag.n.01', 'synonyms': ['shopping_bag'], 'def': 'a bag made of plastic or strong paper (often with handles); used to transport goods after shopping', 'name': 'shopping_bag'}, {'frequency': 'c', 'id': 968, 'synset': 'shopping_cart.n.01', 'synonyms': ['shopping_cart'], 'def': 'a handcart that holds groceries or other goods while shopping', 'name': 'shopping_cart'}, {'frequency': 'f', 'id': 969, 'synset': 'short_pants.n.01', 'synonyms': ['short_pants', 'shorts_(clothing)', 'trunks_(clothing)'], 'def': 'trousers that end at or above the knee', 'name': 'short_pants'}, {'frequency': 'r', 'id': 970, 'synset': 'shot_glass.n.01', 'synonyms': ['shot_glass'], 'def': 'a small glass adequate to hold a single swallow of whiskey', 'name': 'shot_glass'}, {'frequency': 'c', 'id': 971, 'synset': 'shoulder_bag.n.01', 'synonyms': ['shoulder_bag'], 'def': 'a large handbag that can be carried by a strap looped over the shoulder', 'name': 'shoulder_bag'}, {'frequency': 'c', 'id': 972, 'synset': 'shovel.n.01', 'synonyms': ['shovel'], 'def': 'a hand tool for lifting loose material such as snow, dirt, etc.', 'name': 'shovel'}, {'frequency': 'f', 'id': 973, 'synset': 'shower.n.01', 'synonyms': ['shower_head'], 'def': 'a plumbing fixture that sprays water over you', 'name': 'shower_head'}, {'frequency': 'f', 'id': 974, 'synset': 'shower_curtain.n.01', 'synonyms': ['shower_curtain'], 'def': 'a curtain that keeps water from splashing out of the shower area', 'name': 'shower_curtain'}, {'frequency': 'r', 'id': 975, 'synset': 'shredder.n.01', 'synonyms': ['shredder_(for_paper)'], 'def': 'a device that shreds documents', 'name': 'shredder_(for_paper)'}, {'frequency': 'r', 'id': 976, 'synset': 'sieve.n.01', 'synonyms': ['sieve', 'screen_(sieve)'], 'def': 'a strainer for separating lumps from powdered material or grading particles', 'name': 'sieve'}, {'frequency': 'f', 'id': 977, 'synset': 'signboard.n.01', 'synonyms': ['signboard'], 'def': 'structure displaying a board on which advertisements can be posted', 'name': 'signboard'}, {'frequency': 'c', 'id': 978, 'synset': 'silo.n.01', 'synonyms': ['silo'], 'def': 'a cylindrical tower used for storing goods', 'name': 'silo'}, {'frequency': 'f', 'id': 979, 'synset': 'sink.n.01', 'synonyms': ['sink'], 'def': 'plumbing fixture consisting of a water basin fixed to a wall or floor and having a drainpipe', 'name': 'sink'}, {'frequency': 'f', 'id': 980, 'synset': 'skateboard.n.01', 'synonyms': ['skateboard'], 'def': 'a board with wheels that is ridden in a standing or crouching position and propelled by foot', 'name': 'skateboard'}, {'frequency': 'c', 'id': 981, 'synset': 'skewer.n.01', 'synonyms': ['skewer'], 'def': 'a long pin for holding meat in position while it is being roasted', 'name': 'skewer'}, {'frequency': 'f', 'id': 982, 'synset': 'ski.n.01', 'synonyms': ['ski'], 'def': 'sports equipment for skiing on snow', 'name': 'ski'}, {'frequency': 'f', 'id': 983, 'synset': 'ski_boot.n.01', 'synonyms': ['ski_boot'], 'def': 'a stiff boot that is fastened to a ski with a ski binding', 'name': 'ski_boot'}, {'frequency': 'f', 'id': 984, 'synset': 'ski_parka.n.01', 'synonyms': ['ski_parka', 'ski_jacket'], 'def': 'a parka to be worn while skiing', 'name': 'ski_parka'}, {'frequency': 'f', 'id': 985, 'synset': 'ski_pole.n.01', 'synonyms': ['ski_pole'], 'def': 'a pole with metal points used as an aid in skiing', 'name': 'ski_pole'}, {'frequency': 'f', 'id': 986, 'synset': 'skirt.n.02', 'synonyms': ['skirt'], 'def': 'a garment hanging from the waist; worn mainly by girls and women', 'name': 'skirt'}, {'frequency': 'c', 'id': 987, 'synset': 'sled.n.01', 'synonyms': ['sled', 'sledge', 'sleigh'], 'def': 'a vehicle or flat object for transportation over snow by sliding or pulled by dogs, etc.', 'name': 'sled'}, {'frequency': 'c', 'id': 988, 'synset': 'sleeping_bag.n.01', 'synonyms': ['sleeping_bag'], 'def': 'large padded bag designed to be slept in outdoors', 'name': 'sleeping_bag'}, {'frequency': 'r', 'id': 989, 'synset': 'sling.n.05', 'synonyms': ['sling_(bandage)', 'triangular_bandage'], 'def': 'bandage to support an injured forearm; slung over the shoulder or neck', 'name': 'sling_(bandage)'}, {'frequency': 'c', 'id': 990, 'synset': 'slipper.n.01', 'synonyms': ['slipper_(footwear)', 'carpet_slipper_(footwear)'], 'def': 'low footwear that can be slipped on and off easily; usually worn indoors', 'name': 'slipper_(footwear)'}, {'frequency': 'r', 'id': 991, 'synset': 'smoothie.n.02', 'synonyms': ['smoothie'], 'def': 'a thick smooth drink consisting of fresh fruit pureed with ice cream or yoghurt or milk', 'name': 'smoothie'}, {'frequency': 'r', 'id': 992, 'synset': 'snake.n.01', 'synonyms': ['snake', 'serpent'], 'def': 'limbless scaly elongate reptile; some are venomous', 'name': 'snake'}, {'frequency': 'f', 'id': 993, 'synset': 'snowboard.n.01', 'synonyms': ['snowboard'], 'def': 'a board that resembles a broad ski or a small surfboard; used in a standing position to slide down snow-covered slopes', 'name': 'snowboard'}, {'frequency': 'c', 'id': 994, 'synset': 'snowman.n.01', 'synonyms': ['snowman'], 'def': 'a figure of a person made of packed snow', 'name': 'snowman'}, {'frequency': 'c', 'id': 995, 'synset': 'snowmobile.n.01', 'synonyms': ['snowmobile'], 'def': 'tracked vehicle for travel on snow having skis in front', 'name': 'snowmobile'}, {'frequency': 'f', 'id': 996, 'synset': 'soap.n.01', 'synonyms': ['soap'], 'def': 'a cleansing agent made from the salts of vegetable or animal fats', 'name': 'soap'}, {'frequency': 'f', 'id': 997, 'synset': 'soccer_ball.n.01', 'synonyms': ['soccer_ball'], 'def': "an inflated ball used in playing soccer (called `football' outside of the United States)", 'name': 'soccer_ball'}, {'frequency': 'f', 'id': 998, 'synset': 'sock.n.01', 'synonyms': ['sock'], 'def': 'cloth covering for the foot; worn inside the shoe; reaches to between the ankle and the knee', 'name': 'sock'}, {'frequency': 'r', 'id': 999, 'synset': 'soda_fountain.n.02', 'synonyms': ['soda_fountain'], 'def': 'an apparatus for dispensing soda water', 'name': 'soda_fountain'}, {'frequency': 'r', 'id': 1000, 'synset': 'soda_water.n.01', 'synonyms': ['carbonated_water', 'club_soda', 'seltzer', 'sparkling_water'], 'def': 'effervescent beverage artificially charged with carbon dioxide', 'name': 'carbonated_water'}, {'frequency': 'f', 'id': 1001, 'synset': 'sofa.n.01', 'synonyms': ['sofa', 'couch', 'lounge'], 'def': 'an upholstered seat for more than one person', 'name': 'sofa'}, {'frequency': 'r', 'id': 1002, 'synset': 'softball.n.01', 'synonyms': ['softball'], 'def': 'ball used in playing softball', 'name': 'softball'}, {'frequency': 'c', 'id': 1003, 'synset': 'solar_array.n.01', 'synonyms': ['solar_array', 'solar_battery', 'solar_panel'], 'def': 'electrical device consisting of a large array of connected solar cells', 'name': 'solar_array'}, {'frequency': 'r', 'id': 1004, 'synset': 'sombrero.n.02', 'synonyms': ['sombrero'], 'def': 'a straw hat with a tall crown and broad brim; worn in American southwest and in Mexico', 'name': 'sombrero'}, {'frequency': 'c', 'id': 1005, 'synset': 'soup.n.01', 'synonyms': ['soup'], 'def': 'liquid food especially of meat or fish or vegetable stock often containing pieces of solid food', 'name': 'soup'}, {'frequency': 'r', 'id': 1006, 'synset': 'soup_bowl.n.01', 'synonyms': ['soup_bowl'], 'def': 'a bowl for serving soup', 'name': 'soup_bowl'}, {'frequency': 'c', 'id': 1007, 'synset': 'soupspoon.n.01', 'synonyms': ['soupspoon'], 'def': 'a spoon with a rounded bowl for eating soup', 'name': 'soupspoon'}, {'frequency': 'c', 'id': 1008, 'synset': 'sour_cream.n.01', 'synonyms': ['sour_cream', 'soured_cream'], 'def': 'soured light cream', 'name': 'sour_cream'}, {'frequency': 'r', 'id': 1009, 'synset': 'soya_milk.n.01', 'synonyms': ['soya_milk', 'soybean_milk', 'soymilk'], 'def': 'a milk substitute containing soybean flour and water; used in some infant formulas and in making tofu', 'name': 'soya_milk'}, {'frequency': 'r', 'id': 1010, 'synset': 'space_shuttle.n.01', 'synonyms': ['space_shuttle'], 'def': "a reusable spacecraft with wings for a controlled descent through the Earth's atmosphere", 'name': 'space_shuttle'}, {'frequency': 'r', 'id': 1011, 'synset': 'sparkler.n.02', 'synonyms': ['sparkler_(fireworks)'], 'def': 'a firework that burns slowly and throws out a shower of sparks', 'name': 'sparkler_(fireworks)'}, {'frequency': 'f', 'id': 1012, 'synset': 'spatula.n.02', 'synonyms': ['spatula'], 'def': 'a hand tool with a thin flexible blade used to mix or spread soft substances', 'name': 'spatula'}, {'frequency': 'r', 'id': 1013, 'synset': 'spear.n.01', 'synonyms': ['spear', 'lance'], 'def': 'a long pointed rod used as a tool or weapon', 'name': 'spear'}, {'frequency': 'f', 'id': 1014, 'synset': 'spectacles.n.01', 'synonyms': ['spectacles', 'specs', 'eyeglasses', 'glasses'], 'def': 'optical instrument consisting of a frame that holds a pair of lenses for correcting defective vision', 'name': 'spectacles'}, {'frequency': 'c', 'id': 1015, 'synset': 'spice_rack.n.01', 'synonyms': ['spice_rack'], 'def': 'a rack for displaying containers filled with spices', 'name': 'spice_rack'}, {'frequency': 'r', 'id': 1016, 'synset': 'spider.n.01', 'synonyms': ['spider'], 'def': 'predatory arachnid with eight legs, two poison fangs, two feelers, and usually two silk-spinning organs at the back end of the body', 'name': 'spider'}, {'frequency': 'c', 'id': 1017, 'synset': 'sponge.n.01', 'synonyms': ['sponge'], 'def': 'a porous mass usable to absorb water typically used for cleaning', 'name': 'sponge'}, {'frequency': 'f', 'id': 1018, 'synset': 'spoon.n.01', 'synonyms': ['spoon'], 'def': 'a piece of cutlery with a shallow bowl-shaped container and a handle', 'name': 'spoon'}, {'frequency': 'c', 'id': 1019, 'synset': 'sportswear.n.01', 'synonyms': ['sportswear', 'athletic_wear', 'activewear'], 'def': 'attire worn for sport or for casual wear', 'name': 'sportswear'}, {'frequency': 'c', 'id': 1020, 'synset': 'spotlight.n.02', 'synonyms': ['spotlight'], 'def': 'a lamp that produces a strong beam of light to illuminate a restricted area; used to focus attention of a stage performer', 'name': 'spotlight'}, {'frequency': 'r', 'id': 1021, 'synset': 'squirrel.n.01', 'synonyms': ['squirrel'], 'def': 'a kind of arboreal rodent having a long bushy tail', 'name': 'squirrel'}, {'frequency': 'c', 'id': 1022, 'synset': 'stapler.n.01', 'synonyms': ['stapler_(stapling_machine)'], 'def': 'a machine that inserts staples into sheets of paper in order to fasten them together', 'name': 'stapler_(stapling_machine)'}, {'frequency': 'r', 'id': 1023, 'synset': 'starfish.n.01', 'synonyms': ['starfish', 'sea_star'], 'def': 'echinoderms characterized by five arms extending from a central disk', 'name': 'starfish'}, {'frequency': 'f', 'id': 1024, 'synset': 'statue.n.01', 'synonyms': ['statue_(sculpture)'], 'def': 'a sculpture representing a human or animal', 'name': 'statue_(sculpture)'}, {'frequency': 'c', 'id': 1025, 'synset': 'steak.n.01', 'synonyms': ['steak_(food)'], 'def': 'a slice of meat cut from the fleshy part of an animal or large fish', 'name': 'steak_(food)'}, {'frequency': 'r', 'id': 1026, 'synset': 'steak_knife.n.01', 'synonyms': ['steak_knife'], 'def': 'a sharp table knife used in eating steak', 'name': 'steak_knife'}, {'frequency': 'r', 'id': 1027, 'synset': 'steamer.n.02', 'synonyms': ['steamer_(kitchen_appliance)'], 'def': 'a cooking utensil that can be used to cook food by steaming it', 'name': 'steamer_(kitchen_appliance)'}, {'frequency': 'f', 'id': 1028, 'synset': 'steering_wheel.n.01', 'synonyms': ['steering_wheel'], 'def': 'a handwheel that is used for steering', 'name': 'steering_wheel'}, {'frequency': 'r', 'id': 1029, 'synset': 'stencil.n.01', 'synonyms': ['stencil'], 'def': 'a sheet of material (metal, plastic, etc.) that has been perforated with a pattern; ink or paint can pass through the perforations to create the printed pattern on the surface below', 'name': 'stencil'}, {'frequency': 'r', 'id': 1030, 'synset': 'step_ladder.n.01', 'synonyms': ['stepladder'], 'def': 'a folding portable ladder hinged at the top', 'name': 'stepladder'}, {'frequency': 'c', 'id': 1031, 'synset': 'step_stool.n.01', 'synonyms': ['step_stool'], 'def': 'a stool that has one or two steps that fold under the seat', 'name': 'step_stool'}, {'frequency': 'c', 'id': 1032, 'synset': 'stereo.n.01', 'synonyms': ['stereo_(sound_system)'], 'def': 'electronic device for playing audio', 'name': 'stereo_(sound_system)'}, {'frequency': 'r', 'id': 1033, 'synset': 'stew.n.02', 'synonyms': ['stew'], 'def': 'food prepared by stewing especially meat or fish with vegetables', 'name': 'stew'}, {'frequency': 'r', 'id': 1034, 'synset': 'stirrer.n.02', 'synonyms': ['stirrer'], 'def': 'an implement used for stirring', 'name': 'stirrer'}, {'frequency': 'f', 'id': 1035, 'synset': 'stirrup.n.01', 'synonyms': ['stirrup'], 'def': "support consisting of metal loops into which rider's feet go", 'name': 'stirrup'}, {'frequency': 'c', 'id': 1036, 'synset': 'stocking.n.01', 'synonyms': ['stockings_(leg_wear)'], 'def': 'close-fitting hosiery to cover the foot and leg; come in matched pairs', 'name': 'stockings_(leg_wear)'}, {'frequency': 'f', 'id': 1037, 'synset': 'stool.n.01', 'synonyms': ['stool'], 'def': 'a simple seat without a back or arms', 'name': 'stool'}, {'frequency': 'f', 'id': 1038, 'synset': 'stop_sign.n.01', 'synonyms': ['stop_sign'], 'def': 'a traffic sign to notify drivers that they must come to a complete stop', 'name': 'stop_sign'}, {'frequency': 'f', 'id': 1039, 'synset': 'stoplight.n.01', 'synonyms': ['brake_light'], 'def': 'a red light on the rear of a motor vehicle that signals when the brakes are applied', 'name': 'brake_light'}, {'frequency': 'f', 'id': 1040, 'synset': 'stove.n.01', 'synonyms': ['stove', 'kitchen_stove', 'range_(kitchen_appliance)', 'kitchen_range', 'cooking_stove'], 'def': 'a kitchen appliance used for cooking food', 'name': 'stove'}, {'frequency': 'c', 'id': 1041, 'synset': 'strainer.n.01', 'synonyms': ['strainer'], 'def': 'a filter to retain larger pieces while smaller pieces and liquids pass through', 'name': 'strainer'}, {'frequency': 'f', 'id': 1042, 'synset': 'strap.n.01', 'synonyms': ['strap'], 'def': 'an elongated strip of material for binding things together or holding', 'name': 'strap'}, {'frequency': 'f', 'id': 1043, 'synset': 'straw.n.04', 'synonyms': ['straw_(for_drinking)', 'drinking_straw'], 'def': 'a thin paper or plastic tube used to suck liquids into the mouth', 'name': 'straw_(for_drinking)'}, {'frequency': 'f', 'id': 1044, 'synset': 'strawberry.n.01', 'synonyms': ['strawberry'], 'def': 'sweet fleshy red fruit', 'name': 'strawberry'}, {'frequency': 'f', 'id': 1045, 'synset': 'street_sign.n.01', 'synonyms': ['street_sign'], 'def': 'a sign visible from the street', 'name': 'street_sign'}, {'frequency': 'f', 'id': 1046, 'synset': 'streetlight.n.01', 'synonyms': ['streetlight', 'street_lamp'], 'def': 'a lamp supported on a lamppost; for illuminating a street', 'name': 'streetlight'}, {'frequency': 'r', 'id': 1047, 'synset': 'string_cheese.n.01', 'synonyms': ['string_cheese'], 'def': 'cheese formed in long strings twisted together', 'name': 'string_cheese'}, {'frequency': 'r', 'id': 1048, 'synset': 'stylus.n.02', 'synonyms': ['stylus'], 'def': 'a pointed tool for writing or drawing or engraving', 'name': 'stylus'}, {'frequency': 'r', 'id': 1049, 'synset': 'subwoofer.n.01', 'synonyms': ['subwoofer'], 'def': 'a loudspeaker that is designed to reproduce very low bass frequencies', 'name': 'subwoofer'}, {'frequency': 'r', 'id': 1050, 'synset': 'sugar_bowl.n.01', 'synonyms': ['sugar_bowl'], 'def': 'a dish in which sugar is served', 'name': 'sugar_bowl'}, {'frequency': 'r', 'id': 1051, 'synset': 'sugarcane.n.01', 'synonyms': ['sugarcane_(plant)'], 'def': 'juicy canes whose sap is a source of molasses and commercial sugar; fresh canes are sometimes chewed for the juice', 'name': 'sugarcane_(plant)'}, {'frequency': 'c', 'id': 1052, 'synset': 'suit.n.01', 'synonyms': ['suit_(clothing)'], 'def': 'a set of garments (usually including a jacket and trousers or skirt) for outerwear all of the same fabric and color', 'name': 'suit_(clothing)'}, {'frequency': 'c', 'id': 1053, 'synset': 'sunflower.n.01', 'synonyms': ['sunflower'], 'def': 'any plant of the genus Helianthus having large flower heads with dark disk florets and showy yellow rays', 'name': 'sunflower'}, {'frequency': 'f', 'id': 1054, 'synset': 'sunglasses.n.01', 'synonyms': ['sunglasses'], 'def': 'spectacles that are darkened or polarized to protect the eyes from the glare of the sun', 'name': 'sunglasses'}, {'frequency': 'c', 'id': 1055, 'synset': 'sunhat.n.01', 'synonyms': ['sunhat'], 'def': 'a hat with a broad brim that protects the face from direct exposure to the sun', 'name': 'sunhat'}, {'frequency': 'r', 'id': 1056, 'synset': 'sunscreen.n.01', 'synonyms': ['sunscreen', 'sunblock'], 'def': 'a cream spread on the skin; contains a chemical to filter out ultraviolet light and so protect from sunburn', 'name': 'sunscreen'}, {'frequency': 'f', 'id': 1057, 'synset': 'surfboard.n.01', 'synonyms': ['surfboard'], 'def': 'a narrow buoyant board for riding surf', 'name': 'surfboard'}, {'frequency': 'c', 'id': 1058, 'synset': 'sushi.n.01', 'synonyms': ['sushi'], 'def': 'rice (with raw fish) wrapped in seaweed', 'name': 'sushi'}, {'frequency': 'c', 'id': 1059, 'synset': 'swab.n.02', 'synonyms': ['mop'], 'def': 'cleaning implement consisting of absorbent material fastened to a handle; for cleaning floors', 'name': 'mop'}, {'frequency': 'c', 'id': 1060, 'synset': 'sweat_pants.n.01', 'synonyms': ['sweat_pants'], 'def': 'loose-fitting trousers with elastic cuffs; worn by athletes', 'name': 'sweat_pants'}, {'frequency': 'c', 'id': 1061, 'synset': 'sweatband.n.02', 'synonyms': ['sweatband'], 'def': 'a band of material tied around the forehead or wrist to absorb sweat', 'name': 'sweatband'}, {'frequency': 'f', 'id': 1062, 'synset': 'sweater.n.01', 'synonyms': ['sweater'], 'def': 'a crocheted or knitted garment covering the upper part of the body', 'name': 'sweater'}, {'frequency': 'f', 'id': 1063, 'synset': 'sweatshirt.n.01', 'synonyms': ['sweatshirt'], 'def': 'cotton knit pullover with long sleeves worn during athletic activity', 'name': 'sweatshirt'}, {'frequency': 'c', 'id': 1064, 'synset': 'sweet_potato.n.02', 'synonyms': ['sweet_potato'], 'def': 'the edible tuberous root of the sweet potato vine', 'name': 'sweet_potato'}, {'frequency': 'f', 'id': 1065, 'synset': 'swimsuit.n.01', 'synonyms': ['swimsuit', 'swimwear', 'bathing_suit', 'swimming_costume', 'bathing_costume', 'swimming_trunks', 'bathing_trunks'], 'def': 'garment worn for swimming', 'name': 'swimsuit'}, {'frequency': 'c', 'id': 1066, 'synset': 'sword.n.01', 'synonyms': ['sword'], 'def': 'a cutting or thrusting weapon that has a long metal blade', 'name': 'sword'}, {'frequency': 'r', 'id': 1067, 'synset': 'syringe.n.01', 'synonyms': ['syringe'], 'def': 'a medical instrument used to inject or withdraw fluids', 'name': 'syringe'}, {'frequency': 'r', 'id': 1068, 'synset': 'tabasco.n.02', 'synonyms': ['Tabasco_sauce'], 'def': 'very spicy sauce (trade name Tabasco) made from fully-aged red peppers', 'name': 'Tabasco_sauce'}, {'frequency': 'r', 'id': 1069, 'synset': 'table-tennis_table.n.01', 'synonyms': ['table-tennis_table', 'ping-pong_table'], 'def': 'a table used for playing table tennis', 'name': 'table-tennis_table'}, {'frequency': 'f', 'id': 1070, 'synset': 'table.n.02', 'synonyms': ['table'], 'def': 'a piece of furniture having a smooth flat top that is usually supported by one or more vertical legs', 'name': 'table'}, {'frequency': 'c', 'id': 1071, 'synset': 'table_lamp.n.01', 'synonyms': ['table_lamp'], 'def': 'a lamp that sits on a table', 'name': 'table_lamp'}, {'frequency': 'f', 'id': 1072, 'synset': 'tablecloth.n.01', 'synonyms': ['tablecloth'], 'def': 'a covering spread over a dining table', 'name': 'tablecloth'}, {'frequency': 'r', 'id': 1073, 'synset': 'tachometer.n.01', 'synonyms': ['tachometer'], 'def': 'measuring instrument for indicating speed of rotation', 'name': 'tachometer'}, {'frequency': 'r', 'id': 1074, 'synset': 'taco.n.02', 'synonyms': ['taco'], 'def': 'a small tortilla cupped around a filling', 'name': 'taco'}, {'frequency': 'f', 'id': 1075, 'synset': 'tag.n.02', 'synonyms': ['tag'], 'def': 'a label associated with something for the purpose of identification or information', 'name': 'tag'}, {'frequency': 'f', 'id': 1076, 'synset': 'taillight.n.01', 'synonyms': ['taillight', 'rear_light'], 'def': 'lamp (usually red) mounted at the rear of a motor vehicle', 'name': 'taillight'}, {'frequency': 'r', 'id': 1077, 'synset': 'tambourine.n.01', 'synonyms': ['tambourine'], 'def': 'a shallow drum with a single drumhead and with metallic disks in the sides', 'name': 'tambourine'}, {'frequency': 'r', 'id': 1078, 'synset': 'tank.n.01', 'synonyms': ['army_tank', 'armored_combat_vehicle', 'armoured_combat_vehicle'], 'def': 'an enclosed armored military vehicle; has a cannon and moves on caterpillar treads', 'name': 'army_tank'}, {'frequency': 'c', 'id': 1079, 'synset': 'tank.n.02', 'synonyms': ['tank_(storage_vessel)', 'storage_tank'], 'def': 'a large (usually metallic) vessel for holding gases or liquids', 'name': 'tank_(storage_vessel)'}, {'frequency': 'f', 'id': 1080, 'synset': 'tank_top.n.01', 'synonyms': ['tank_top_(clothing)'], 'def': 'a tight-fitting sleeveless shirt with wide shoulder straps and low neck and no front opening', 'name': 'tank_top_(clothing)'}, {'frequency': 'c', 'id': 1081, 'synset': 'tape.n.01', 'synonyms': ['tape_(sticky_cloth_or_paper)'], 'def': 'a long thin piece of cloth or paper as used for binding or fastening', 'name': 'tape_(sticky_cloth_or_paper)'}, {'frequency': 'c', 'id': 1082, 'synset': 'tape.n.04', 'synonyms': ['tape_measure', 'measuring_tape'], 'def': 'measuring instrument consisting of a narrow strip (cloth or metal) marked in inches or centimeters and used for measuring lengths', 'name': 'tape_measure'}, {'frequency': 'c', 'id': 1083, 'synset': 'tapestry.n.02', 'synonyms': ['tapestry'], 'def': 'a heavy textile with a woven design; used for curtains and upholstery', 'name': 'tapestry'}, {'frequency': 'f', 'id': 1084, 'synset': 'tarpaulin.n.01', 'synonyms': ['tarp'], 'def': 'waterproofed canvas', 'name': 'tarp'}, {'frequency': 'c', 'id': 1085, 'synset': 'tartan.n.01', 'synonyms': ['tartan', 'plaid'], 'def': 'a cloth having a crisscross design', 'name': 'tartan'}, {'frequency': 'c', 'id': 1086, 'synset': 'tassel.n.01', 'synonyms': ['tassel'], 'def': 'adornment consisting of a bunch of cords fastened at one end', 'name': 'tassel'}, {'frequency': 'r', 'id': 1087, 'synset': 'tea_bag.n.01', 'synonyms': ['tea_bag'], 'def': 'a measured amount of tea in a bag for an individual serving of tea', 'name': 'tea_bag'}, {'frequency': 'c', 'id': 1088, 'synset': 'teacup.n.02', 'synonyms': ['teacup'], 'def': 'a cup from which tea is drunk', 'name': 'teacup'}, {'frequency': 'c', 'id': 1089, 'synset': 'teakettle.n.01', 'synonyms': ['teakettle'], 'def': 'kettle for boiling water to make tea', 'name': 'teakettle'}, {'frequency': 'c', 'id': 1090, 'synset': 'teapot.n.01', 'synonyms': ['teapot'], 'def': 'pot for brewing tea; usually has a spout and handle', 'name': 'teapot'}, {'frequency': 'f', 'id': 1091, 'synset': 'teddy.n.01', 'synonyms': ['teddy_bear'], 'def': "plaything consisting of a child's toy bear (usually plush and stuffed with soft materials)", 'name': 'teddy_bear'}, {'frequency': 'f', 'id': 1092, 'synset': 'telephone.n.01', 'synonyms': ['telephone', 'phone', 'telephone_set'], 'def': 'electronic device for communicating by voice over long distances', 'name': 'telephone'}, {'frequency': 'c', 'id': 1093, 'synset': 'telephone_booth.n.01', 'synonyms': ['telephone_booth', 'phone_booth', 'call_box', 'telephone_box', 'telephone_kiosk'], 'def': 'booth for using a telephone', 'name': 'telephone_booth'}, {'frequency': 'f', 'id': 1094, 'synset': 'telephone_pole.n.01', 'synonyms': ['telephone_pole', 'telegraph_pole', 'telegraph_post'], 'def': 'tall pole supporting telephone wires', 'name': 'telephone_pole'}, {'frequency': 'r', 'id': 1095, 'synset': 'telephoto_lens.n.01', 'synonyms': ['telephoto_lens', 'zoom_lens'], 'def': 'a camera lens that magnifies the image', 'name': 'telephoto_lens'}, {'frequency': 'c', 'id': 1096, 'synset': 'television_camera.n.01', 'synonyms': ['television_camera', 'tv_camera'], 'def': 'television equipment for capturing and recording video', 'name': 'television_camera'}, {'frequency': 'f', 'id': 1097, 'synset': 'television_receiver.n.01', 'synonyms': ['television_set', 'tv', 'tv_set'], 'def': 'an electronic device that receives television signals and displays them on a screen', 'name': 'television_set'}, {'frequency': 'f', 'id': 1098, 'synset': 'tennis_ball.n.01', 'synonyms': ['tennis_ball'], 'def': 'ball about the size of a fist used in playing tennis', 'name': 'tennis_ball'}, {'frequency': 'f', 'id': 1099, 'synset': 'tennis_racket.n.01', 'synonyms': ['tennis_racket'], 'def': 'a racket used to play tennis', 'name': 'tennis_racket'}, {'frequency': 'r', 'id': 1100, 'synset': 'tequila.n.01', 'synonyms': ['tequila'], 'def': 'Mexican liquor made from fermented juices of an agave plant', 'name': 'tequila'}, {'frequency': 'c', 'id': 1101, 'synset': 'thermometer.n.01', 'synonyms': ['thermometer'], 'def': 'measuring instrument for measuring temperature', 'name': 'thermometer'}, {'frequency': 'c', 'id': 1102, 'synset': 'thermos.n.01', 'synonyms': ['thermos_bottle'], 'def': 'vacuum flask that preserves temperature of hot or cold drinks', 'name': 'thermos_bottle'}, {'frequency': 'c', 'id': 1103, 'synset': 'thermostat.n.01', 'synonyms': ['thermostat'], 'def': 'a regulator for automatically regulating temperature by starting or stopping the supply of heat', 'name': 'thermostat'}, {'frequency': 'r', 'id': 1104, 'synset': 'thimble.n.02', 'synonyms': ['thimble'], 'def': 'a small metal cap to protect the finger while sewing; can be used as a small container', 'name': 'thimble'}, {'frequency': 'c', 'id': 1105, 'synset': 'thread.n.01', 'synonyms': ['thread', 'yarn'], 'def': 'a fine cord of twisted fibers (of cotton or silk or wool or nylon etc.) used in sewing and weaving', 'name': 'thread'}, {'frequency': 'c', 'id': 1106, 'synset': 'thumbtack.n.01', 'synonyms': ['thumbtack', 'drawing_pin', 'pushpin'], 'def': 'a tack for attaching papers to a bulletin board or drawing board', 'name': 'thumbtack'}, {'frequency': 'c', 'id': 1107, 'synset': 'tiara.n.01', 'synonyms': ['tiara'], 'def': 'a jeweled headdress worn by women on formal occasions', 'name': 'tiara'}, {'frequency': 'c', 'id': 1108, 'synset': 'tiger.n.02', 'synonyms': ['tiger'], 'def': 'large feline of forests in most of Asia having a tawny coat with black stripes', 'name': 'tiger'}, {'frequency': 'c', 'id': 1109, 'synset': 'tights.n.01', 'synonyms': ['tights_(clothing)', 'leotards'], 'def': 'skintight knit hose covering the body from the waist to the feet worn by acrobats and dancers and as stockings by women and girls', 'name': 'tights_(clothing)'}, {'frequency': 'c', 'id': 1110, 'synset': 'timer.n.01', 'synonyms': ['timer', 'stopwatch'], 'def': 'a timepiece that measures a time interval and signals its end', 'name': 'timer'}, {'frequency': 'f', 'id': 1111, 'synset': 'tinfoil.n.01', 'synonyms': ['tinfoil'], 'def': 'foil made of tin or an alloy of tin and lead', 'name': 'tinfoil'}, {'frequency': 'r', 'id': 1112, 'synset': 'tinsel.n.01', 'synonyms': ['tinsel'], 'def': 'a showy decoration that is basically valueless', 'name': 'tinsel'}, {'frequency': 'f', 'id': 1113, 'synset': 'tissue.n.02', 'synonyms': ['tissue_paper'], 'def': 'a soft thin (usually translucent) paper', 'name': 'tissue_paper'}, {'frequency': 'c', 'id': 1114, 'synset': 'toast.n.01', 'synonyms': ['toast_(food)'], 'def': 'slice of bread that has been toasted', 'name': 'toast_(food)'}, {'frequency': 'f', 'id': 1115, 'synset': 'toaster.n.02', 'synonyms': ['toaster'], 'def': 'a kitchen appliance (usually electric) for toasting bread', 'name': 'toaster'}, {'frequency': 'c', 'id': 1116, 'synset': 'toaster_oven.n.01', 'synonyms': ['toaster_oven'], 'def': 'kitchen appliance consisting of a small electric oven for toasting or warming food', 'name': 'toaster_oven'}, {'frequency': 'f', 'id': 1117, 'synset': 'toilet.n.02', 'synonyms': ['toilet'], 'def': 'a plumbing fixture for defecation and urination', 'name': 'toilet'}, {'frequency': 'f', 'id': 1118, 'synset': 'toilet_tissue.n.01', 'synonyms': ['toilet_tissue', 'toilet_paper', 'bathroom_tissue'], 'def': 'a soft thin absorbent paper for use in toilets', 'name': 'toilet_tissue'}, {'frequency': 'f', 'id': 1119, 'synset': 'tomato.n.01', 'synonyms': ['tomato'], 'def': 'mildly acid red or yellow pulpy fruit eaten as a vegetable', 'name': 'tomato'}, {'frequency': 'c', 'id': 1120, 'synset': 'tongs.n.01', 'synonyms': ['tongs'], 'def': 'any of various devices for taking hold of objects; usually have two hinged legs with handles above and pointed hooks below', 'name': 'tongs'}, {'frequency': 'c', 'id': 1121, 'synset': 'toolbox.n.01', 'synonyms': ['toolbox'], 'def': 'a box or chest or cabinet for holding hand tools', 'name': 'toolbox'}, {'frequency': 'f', 'id': 1122, 'synset': 'toothbrush.n.01', 'synonyms': ['toothbrush'], 'def': 'small brush; has long handle; used to clean teeth', 'name': 'toothbrush'}, {'frequency': 'f', 'id': 1123, 'synset': 'toothpaste.n.01', 'synonyms': ['toothpaste'], 'def': 'a dentifrice in the form of a paste', 'name': 'toothpaste'}, {'frequency': 'c', 'id': 1124, 'synset': 'toothpick.n.01', 'synonyms': ['toothpick'], 'def': 'pick consisting of a small strip of wood or plastic; used to pick food from between the teeth', 'name': 'toothpick'}, {'frequency': 'c', 'id': 1125, 'synset': 'top.n.09', 'synonyms': ['cover'], 'def': 'covering for a hole (especially a hole in the top of a container)', 'name': 'cover'}, {'frequency': 'c', 'id': 1126, 'synset': 'tortilla.n.01', 'synonyms': ['tortilla'], 'def': 'thin unleavened pancake made from cornmeal or wheat flour', 'name': 'tortilla'}, {'frequency': 'c', 'id': 1127, 'synset': 'tow_truck.n.01', 'synonyms': ['tow_truck'], 'def': 'a truck equipped to hoist and pull wrecked cars (or to remove cars from no-parking zones)', 'name': 'tow_truck'}, {'frequency': 'f', 'id': 1128, 'synset': 'towel.n.01', 'synonyms': ['towel'], 'def': 'a rectangular piece of absorbent cloth (or paper) for drying or wiping', 'name': 'towel'}, {'frequency': 'f', 'id': 1129, 'synset': 'towel_rack.n.01', 'synonyms': ['towel_rack', 'towel_rail', 'towel_bar'], 'def': 'a rack consisting of one or more bars on which towels can be hung', 'name': 'towel_rack'}, {'frequency': 'f', 'id': 1130, 'synset': 'toy.n.03', 'synonyms': ['toy'], 'def': 'a device regarded as providing amusement', 'name': 'toy'}, {'frequency': 'c', 'id': 1131, 'synset': 'tractor.n.01', 'synonyms': ['tractor_(farm_equipment)'], 'def': 'a wheeled vehicle with large wheels; used in farming and other applications', 'name': 'tractor_(farm_equipment)'}, {'frequency': 'f', 'id': 1132, 'synset': 'traffic_light.n.01', 'synonyms': ['traffic_light'], 'def': 'a device to control vehicle traffic often consisting of three or more lights', 'name': 'traffic_light'}, {'frequency': 'r', 'id': 1133, 'synset': 'trail_bike.n.01', 'synonyms': ['dirt_bike'], 'def': 'a lightweight motorcycle equipped with rugged tires and suspension for off-road use', 'name': 'dirt_bike'}, {'frequency': 'c', 'id': 1134, 'synset': 'trailer_truck.n.01', 'synonyms': ['trailer_truck', 'tractor_trailer', 'trucking_rig', 'articulated_lorry', 'semi_truck'], 'def': 'a truck consisting of a tractor and trailer together', 'name': 'trailer_truck'}, {'frequency': 'f', 'id': 1135, 'synset': 'train.n.01', 'synonyms': ['train_(railroad_vehicle)', 'railroad_train'], 'def': 'public or private transport provided by a line of railway cars coupled together and drawn by a locomotive', 'name': 'train_(railroad_vehicle)'}, {'frequency': 'r', 'id': 1136, 'synset': 'trampoline.n.01', 'synonyms': ['trampoline'], 'def': 'gymnastic apparatus consisting of a strong canvas sheet attached with springs to a metal frame', 'name': 'trampoline'}, {'frequency': 'f', 'id': 1137, 'synset': 'tray.n.01', 'synonyms': ['tray'], 'def': 'an open receptacle for holding or displaying or serving articles or food', 'name': 'tray'}, {'frequency': 'r', 'id': 1138, 'synset': 'tree_house.n.01', 'synonyms': ['tree_house'], 'def': '(NOT A TREE) a PLAYHOUSE built in the branches of a tree', 'name': 'tree_house'}, {'frequency': 'r', 'id': 1139, 'synset': 'trench_coat.n.01', 'synonyms': ['trench_coat'], 'def': 'a military style raincoat; belted with deep pockets', 'name': 'trench_coat'}, {'frequency': 'r', 'id': 1140, 'synset': 'triangle.n.05', 'synonyms': ['triangle_(musical_instrument)'], 'def': 'a percussion instrument consisting of a metal bar bent in the shape of an open triangle', 'name': 'triangle_(musical_instrument)'}, {'frequency': 'r', 'id': 1141, 'synset': 'tricycle.n.01', 'synonyms': ['tricycle'], 'def': 'a vehicle with three wheels that is moved by foot pedals', 'name': 'tricycle'}, {'frequency': 'c', 'id': 1142, 'synset': 'tripod.n.01', 'synonyms': ['tripod'], 'def': 'a three-legged rack used for support', 'name': 'tripod'}, {'frequency': 'f', 'id': 1143, 'synset': 'trouser.n.01', 'synonyms': ['trousers', 'pants_(clothing)'], 'def': 'a garment extending from the waist to the knee or ankle, covering each leg separately', 'name': 'trousers'}, {'frequency': 'f', 'id': 1144, 'synset': 'truck.n.01', 'synonyms': ['truck'], 'def': 'an automotive vehicle suitable for hauling', 'name': 'truck'}, {'frequency': 'r', 'id': 1145, 'synset': 'truffle.n.03', 'synonyms': ['truffle_(chocolate)', 'chocolate_truffle'], 'def': 'creamy chocolate candy', 'name': 'truffle_(chocolate)'}, {'frequency': 'c', 'id': 1146, 'synset': 'trunk.n.02', 'synonyms': ['trunk'], 'def': 'luggage consisting of a large strong case used when traveling or for storage', 'name': 'trunk'}, {'frequency': 'r', 'id': 1147, 'synset': 'tub.n.02', 'synonyms': ['vat'], 'def': 'a large open vessel for holding or storing liquids', 'name': 'vat'}, {'frequency': 'c', 'id': 1148, 'synset': 'turban.n.01', 'synonyms': ['turban'], 'def': 'a traditional headdress consisting of a long scarf wrapped around the head', 'name': 'turban'}, {'frequency': 'r', 'id': 1149, 'synset': 'turkey.n.01', 'synonyms': ['turkey_(bird)'], 'def': 'large gallinaceous bird with fan-shaped tail; widely domesticated for food', 'name': 'turkey_(bird)'}, {'frequency': 'c', 'id': 1150, 'synset': 'turkey.n.04', 'synonyms': ['turkey_(food)'], 'def': 'flesh of large domesticated fowl usually roasted', 'name': 'turkey_(food)'}, {'frequency': 'r', 'id': 1151, 'synset': 'turnip.n.01', 'synonyms': ['turnip'], 'def': 'widely cultivated plant having a large fleshy edible white or yellow root', 'name': 'turnip'}, {'frequency': 'c', 'id': 1152, 'synset': 'turtle.n.02', 'synonyms': ['turtle'], 'def': 'any of various aquatic and land reptiles having a bony shell and flipper-like limbs for swimming', 'name': 'turtle'}, {'frequency': 'r', 'id': 1153, 'synset': 'turtleneck.n.01', 'synonyms': ['turtleneck_(clothing)', 'polo-neck'], 'def': 'a sweater or jersey with a high close-fitting collar', 'name': 'turtleneck_(clothing)'}, {'frequency': 'r', 'id': 1154, 'synset': 'typewriter.n.01', 'synonyms': ['typewriter'], 'def': 'hand-operated character printer for printing written messages one character at a time', 'name': 'typewriter'}, {'frequency': 'f', 'id': 1155, 'synset': 'umbrella.n.01', 'synonyms': ['umbrella'], 'def': 'a lightweight handheld collapsible canopy', 'name': 'umbrella'}, {'frequency': 'c', 'id': 1156, 'synset': 'underwear.n.01', 'synonyms': ['underwear', 'underclothes', 'underclothing', 'underpants'], 'def': 'undergarment worn next to the skin and under the outer garments', 'name': 'underwear'}, {'frequency': 'r', 'id': 1157, 'synset': 'unicycle.n.01', 'synonyms': ['unicycle'], 'def': 'a vehicle with a single wheel that is driven by pedals', 'name': 'unicycle'}, {'frequency': 'c', 'id': 1158, 'synset': 'urinal.n.01', 'synonyms': ['urinal'], 'def': 'a plumbing fixture (usually attached to the wall) used by men to urinate', 'name': 'urinal'}, {'frequency': 'r', 'id': 1159, 'synset': 'urn.n.01', 'synonyms': ['urn'], 'def': 'a large vase that usually has a pedestal or feet', 'name': 'urn'}, {'frequency': 'c', 'id': 1160, 'synset': 'vacuum.n.04', 'synonyms': ['vacuum_cleaner'], 'def': 'an electrical home appliance that cleans by suction', 'name': 'vacuum_cleaner'}, {'frequency': 'c', 'id': 1161, 'synset': 'valve.n.03', 'synonyms': ['valve'], 'def': 'control consisting of a mechanical device for controlling the flow of a fluid', 'name': 'valve'}, {'frequency': 'f', 'id': 1162, 'synset': 'vase.n.01', 'synonyms': ['vase'], 'def': 'an open jar of glass or porcelain used as an ornament or to hold flowers', 'name': 'vase'}, {'frequency': 'c', 'id': 1163, 'synset': 'vending_machine.n.01', 'synonyms': ['vending_machine'], 'def': 'a slot machine for selling goods', 'name': 'vending_machine'}, {'frequency': 'f', 'id': 1164, 'synset': 'vent.n.01', 'synonyms': ['vent', 'blowhole', 'air_vent'], 'def': 'a hole for the escape of gas or air', 'name': 'vent'}, {'frequency': 'c', 'id': 1165, 'synset': 'videotape.n.01', 'synonyms': ['videotape'], 'def': 'a video recording made on magnetic tape', 'name': 'videotape'}, {'frequency': 'r', 'id': 1166, 'synset': 'vinegar.n.01', 'synonyms': ['vinegar'], 'def': 'sour-tasting liquid produced usually by oxidation of the alcohol in wine or cider and used as a condiment or food preservative', 'name': 'vinegar'}, {'frequency': 'r', 'id': 1167, 'synset': 'violin.n.01', 'synonyms': ['violin', 'fiddle'], 'def': 'bowed stringed instrument that is the highest member of the violin family', 'name': 'violin'}, {'frequency': 'r', 'id': 1168, 'synset': 'vodka.n.01', 'synonyms': ['vodka'], 'def': 'unaged colorless liquor originating in Russia', 'name': 'vodka'}, {'frequency': 'r', 'id': 1169, 'synset': 'volleyball.n.02', 'synonyms': ['volleyball'], 'def': 'an inflated ball used in playing volleyball', 'name': 'volleyball'}, {'frequency': 'r', 'id': 1170, 'synset': 'vulture.n.01', 'synonyms': ['vulture'], 'def': 'any of various large birds of prey having naked heads and weak claws and feeding chiefly on carrion', 'name': 'vulture'}, {'frequency': 'c', 'id': 1171, 'synset': 'waffle.n.01', 'synonyms': ['waffle'], 'def': 'pancake batter baked in a waffle iron', 'name': 'waffle'}, {'frequency': 'r', 'id': 1172, 'synset': 'waffle_iron.n.01', 'synonyms': ['waffle_iron'], 'def': 'a kitchen appliance for baking waffles', 'name': 'waffle_iron'}, {'frequency': 'c', 'id': 1173, 'synset': 'wagon.n.01', 'synonyms': ['wagon'], 'def': 'any of various kinds of wheeled vehicles drawn by an animal or a tractor', 'name': 'wagon'}, {'frequency': 'c', 'id': 1174, 'synset': 'wagon_wheel.n.01', 'synonyms': ['wagon_wheel'], 'def': 'a wheel of a wagon', 'name': 'wagon_wheel'}, {'frequency': 'c', 'id': 1175, 'synset': 'walking_stick.n.01', 'synonyms': ['walking_stick'], 'def': 'a stick carried in the hand for support in walking', 'name': 'walking_stick'}, {'frequency': 'c', 'id': 1176, 'synset': 'wall_clock.n.01', 'synonyms': ['wall_clock'], 'def': 'a clock mounted on a wall', 'name': 'wall_clock'}, {'frequency': 'f', 'id': 1177, 'synset': 'wall_socket.n.01', 'synonyms': ['wall_socket', 'wall_plug', 'electric_outlet', 'electrical_outlet', 'outlet', 'electric_receptacle'], 'def': 'receptacle providing a place in a wiring system where current can be taken to run electrical devices', 'name': 'wall_socket'}, {'frequency': 'c', 'id': 1178, 'synset': 'wallet.n.01', 'synonyms': ['wallet', 'billfold'], 'def': 'a pocket-size case for holding papers and paper money', 'name': 'wallet'}, {'frequency': 'r', 'id': 1179, 'synset': 'walrus.n.01', 'synonyms': ['walrus'], 'def': 'either of two large northern marine mammals having ivory tusks and tough hide over thick blubber', 'name': 'walrus'}, {'frequency': 'r', 'id': 1180, 'synset': 'wardrobe.n.01', 'synonyms': ['wardrobe'], 'def': 'a tall piece of furniture that provides storage space for clothes; has a door and rails or hooks for hanging clothes', 'name': 'wardrobe'}, {'frequency': 'r', 'id': 1181, 'synset': 'wasabi.n.02', 'synonyms': ['wasabi'], 'def': 'the thick green root of the wasabi plant that the Japanese use in cooking and that tastes like strong horseradish', 'name': 'wasabi'}, {'frequency': 'c', 'id': 1182, 'synset': 'washer.n.03', 'synonyms': ['automatic_washer', 'washing_machine'], 'def': 'a home appliance for washing clothes and linens automatically', 'name': 'automatic_washer'}, {'frequency': 'f', 'id': 1183, 'synset': 'watch.n.01', 'synonyms': ['watch', 'wristwatch'], 'def': 'a small, portable timepiece', 'name': 'watch'}, {'frequency': 'f', 'id': 1184, 'synset': 'water_bottle.n.01', 'synonyms': ['water_bottle'], 'def': 'a bottle for holding water', 'name': 'water_bottle'}, {'frequency': 'c', 'id': 1185, 'synset': 'water_cooler.n.01', 'synonyms': ['water_cooler'], 'def': 'a device for cooling and dispensing drinking water', 'name': 'water_cooler'}, {'frequency': 'c', 'id': 1186, 'synset': 'water_faucet.n.01', 'synonyms': ['water_faucet', 'water_tap', 'tap_(water_faucet)'], 'def': 'a faucet for drawing water from a pipe or cask', 'name': 'water_faucet'}, {'frequency': 'r', 'id': 1187, 'synset': 'water_filter.n.01', 'synonyms': ['water_filter'], 'def': 'a filter to remove impurities from the water supply', 'name': 'water_filter'}, {'frequency': 'r', 'id': 1188, 'synset': 'water_heater.n.01', 'synonyms': ['water_heater', 'hot-water_heater'], 'def': 'a heater and storage tank to supply heated water', 'name': 'water_heater'}, {'frequency': 'r', 'id': 1189, 'synset': 'water_jug.n.01', 'synonyms': ['water_jug'], 'def': 'a jug that holds water', 'name': 'water_jug'}, {'frequency': 'r', 'id': 1190, 'synset': 'water_pistol.n.01', 'synonyms': ['water_gun', 'squirt_gun'], 'def': 'plaything consisting of a toy pistol that squirts water', 'name': 'water_gun'}, {'frequency': 'c', 'id': 1191, 'synset': 'water_scooter.n.01', 'synonyms': ['water_scooter', 'sea_scooter', 'jet_ski'], 'def': 'a motorboat resembling a motor scooter (NOT A SURFBOARD OR WATER SKI)', 'name': 'water_scooter'}, {'frequency': 'c', 'id': 1192, 'synset': 'water_ski.n.01', 'synonyms': ['water_ski'], 'def': 'broad ski for skimming over water towed by a speedboat (DO NOT MARK WATER)', 'name': 'water_ski'}, {'frequency': 'c', 'id': 1193, 'synset': 'water_tower.n.01', 'synonyms': ['water_tower'], 'def': 'a large reservoir for water', 'name': 'water_tower'}, {'frequency': 'c', 'id': 1194, 'synset': 'watering_can.n.01', 'synonyms': ['watering_can'], 'def': 'a container with a handle and a spout with a perforated nozzle; used to sprinkle water over plants', 'name': 'watering_can'}, {'frequency': 'c', 'id': 1195, 'synset': 'watermelon.n.02', 'synonyms': ['watermelon'], 'def': 'large oblong or roundish melon with a hard green rind and sweet watery red or occasionally yellowish pulp', 'name': 'watermelon'}, {'frequency': 'f', 'id': 1196, 'synset': 'weathervane.n.01', 'synonyms': ['weathervane', 'vane_(weathervane)', 'wind_vane'], 'def': 'mechanical device attached to an elevated structure; rotates freely to show the direction of the wind', 'name': 'weathervane'}, {'frequency': 'c', 'id': 1197, 'synset': 'webcam.n.01', 'synonyms': ['webcam'], 'def': 'a digital camera designed to take digital photographs and transmit them over the internet', 'name': 'webcam'}, {'frequency': 'c', 'id': 1198, 'synset': 'wedding_cake.n.01', 'synonyms': ['wedding_cake', 'bridecake'], 'def': 'a rich cake with two or more tiers and covered with frosting and decorations; served at a wedding reception', 'name': 'wedding_cake'}, {'frequency': 'c', 'id': 1199, 'synset': 'wedding_ring.n.01', 'synonyms': ['wedding_ring', 'wedding_band'], 'def': 'a ring given to the bride and/or groom at the wedding', 'name': 'wedding_ring'}, {'frequency': 'f', 'id': 1200, 'synset': 'wet_suit.n.01', 'synonyms': ['wet_suit'], 'def': 'a close-fitting garment made of a permeable material; worn in cold water to retain body heat', 'name': 'wet_suit'}, {'frequency': 'f', 'id': 1201, 'synset': 'wheel.n.01', 'synonyms': ['wheel'], 'def': 'a circular frame with spokes (or a solid disc) that can rotate on a shaft or axle', 'name': 'wheel'}, {'frequency': 'c', 'id': 1202, 'synset': 'wheelchair.n.01', 'synonyms': ['wheelchair'], 'def': 'a movable chair mounted on large wheels', 'name': 'wheelchair'}, {'frequency': 'c', 'id': 1203, 'synset': 'whipped_cream.n.01', 'synonyms': ['whipped_cream'], 'def': 'cream that has been beaten until light and fluffy', 'name': 'whipped_cream'}, {'frequency': 'r', 'id': 1204, 'synset': 'whiskey.n.01', 'synonyms': ['whiskey'], 'def': 'a liquor made from fermented mash of grain', 'name': 'whiskey'}, {'frequency': 'r', 'id': 1205, 'synset': 'whistle.n.03', 'synonyms': ['whistle'], 'def': 'a small wind instrument that produces a whistling sound by blowing into it', 'name': 'whistle'}, {'frequency': 'r', 'id': 1206, 'synset': 'wick.n.02', 'synonyms': ['wick'], 'def': 'a loosely woven cord in a candle or oil lamp that is lit on fire', 'name': 'wick'}, {'frequency': 'c', 'id': 1207, 'synset': 'wig.n.01', 'synonyms': ['wig'], 'def': 'hairpiece covering the head and made of real or synthetic hair', 'name': 'wig'}, {'frequency': 'c', 'id': 1208, 'synset': 'wind_chime.n.01', 'synonyms': ['wind_chime'], 'def': 'a decorative arrangement of pieces of metal or glass or pottery that hang together loosely so the wind can cause them to tinkle', 'name': 'wind_chime'}, {'frequency': 'c', 'id': 1209, 'synset': 'windmill.n.01', 'synonyms': ['windmill'], 'def': 'a mill that is powered by the wind', 'name': 'windmill'}, {'frequency': 'c', 'id': 1210, 'synset': 'window_box.n.01', 'synonyms': ['window_box_(for_plants)'], 'def': 'a container for growing plants on a windowsill', 'name': 'window_box_(for_plants)'}, {'frequency': 'f', 'id': 1211, 'synset': 'windshield_wiper.n.01', 'synonyms': ['windshield_wiper', 'windscreen_wiper', 'wiper_(for_windshield/screen)'], 'def': 'a mechanical device that cleans the windshield', 'name': 'windshield_wiper'}, {'frequency': 'c', 'id': 1212, 'synset': 'windsock.n.01', 'synonyms': ['windsock', 'air_sock', 'air-sleeve', 'wind_sleeve', 'wind_cone'], 'def': 'a truncated cloth cone mounted on a mast/pole; shows wind direction', 'name': 'windsock'}, {'frequency': 'f', 'id': 1213, 'synset': 'wine_bottle.n.01', 'synonyms': ['wine_bottle'], 'def': 'a bottle for holding wine', 'name': 'wine_bottle'}, {'frequency': 'r', 'id': 1214, 'synset': 'wine_bucket.n.01', 'synonyms': ['wine_bucket', 'wine_cooler'], 'def': 'a bucket of ice used to chill a bottle of wine', 'name': 'wine_bucket'}, {'frequency': 'f', 'id': 1215, 'synset': 'wineglass.n.01', 'synonyms': ['wineglass'], 'def': 'a glass that has a stem and in which wine is served', 'name': 'wineglass'}, {'frequency': 'r', 'id': 1216, 'synset': 'wing_chair.n.01', 'synonyms': ['wing_chair'], 'def': 'easy chair having wings on each side of a high back', 'name': 'wing_chair'}, {'frequency': 'c', 'id': 1217, 'synset': 'winker.n.02', 'synonyms': ['blinder_(for_horses)'], 'def': 'blinds that prevent a horse from seeing something on either side', 'name': 'blinder_(for_horses)'}, {'frequency': 'c', 'id': 1218, 'synset': 'wok.n.01', 'synonyms': ['wok'], 'def': 'pan with a convex bottom; used for frying in Chinese cooking', 'name': 'wok'}, {'frequency': 'r', 'id': 1219, 'synset': 'wolf.n.01', 'synonyms': ['wolf'], 'def': 'a wild carnivorous mammal of the dog family, living and hunting in packs', 'name': 'wolf'}, {'frequency': 'c', 'id': 1220, 'synset': 'wooden_spoon.n.02', 'synonyms': ['wooden_spoon'], 'def': 'a spoon made of wood', 'name': 'wooden_spoon'}, {'frequency': 'c', 'id': 1221, 'synset': 'wreath.n.01', 'synonyms': ['wreath'], 'def': 'an arrangement of flowers, leaves, or stems fastened in a ring', 'name': 'wreath'}, {'frequency': 'c', 'id': 1222, 'synset': 'wrench.n.03', 'synonyms': ['wrench', 'spanner'], 'def': 'a hand tool that is used to hold or twist a nut or bolt', 'name': 'wrench'}, {'frequency': 'c', 'id': 1223, 'synset': 'wristband.n.01', 'synonyms': ['wristband'], 'def': 'band consisting of a part of a sleeve that covers the wrist', 'name': 'wristband'}, {'frequency': 'f', 'id': 1224, 'synset': 'wristlet.n.01', 'synonyms': ['wristlet', 'wrist_band'], 'def': 'a band or bracelet worn around the wrist', 'name': 'wristlet'}, {'frequency': 'r', 'id': 1225, 'synset': 'yacht.n.01', 'synonyms': ['yacht'], 'def': 'an expensive vessel propelled by sail or power and used for cruising or racing', 'name': 'yacht'}, {'frequency': 'r', 'id': 1226, 'synset': 'yak.n.02', 'synonyms': ['yak'], 'def': 'large long-haired wild ox of Tibet often domesticated', 'name': 'yak'}, {'frequency': 'c', 'id': 1227, 'synset': 'yogurt.n.01', 'synonyms': ['yogurt', 'yoghurt', 'yoghourt'], 'def': 'a custard-like food made from curdled milk', 'name': 'yogurt'}, {'frequency': 'r', 'id': 1228, 'synset': 'yoke.n.07', 'synonyms': ['yoke_(animal_equipment)'], 'def': 'gear joining two animals at the neck; NOT egg yolk', 'name': 'yoke_(animal_equipment)'}, {'frequency': 'f', 'id': 1229, 'synset': 'zebra.n.01', 'synonyms': ['zebra'], 'def': 'any of several fleet black-and-white striped African equines', 'name': 'zebra'}, {'frequency': 'c', 'id': 1230, 'synset': 'zucchini.n.02', 'synonyms': ['zucchini', 'courgette'], 'def': 'small cucumber-shaped vegetable marrow; typically dark green', 'name': 'zucchini'}] # noqa
-# fmt: on
diff --git a/spaces/OpenGVLab/InternGPT/iGPT/models/grit_src/third_party/CenterNet2/detectron2/data/datasets/register_coco.py b/spaces/OpenGVLab/InternGPT/iGPT/models/grit_src/third_party/CenterNet2/detectron2/data/datasets/register_coco.py
deleted file mode 100644
index e564438d5bf016bcdbb65b4bbdc215d79f579f8a..0000000000000000000000000000000000000000
--- a/spaces/OpenGVLab/InternGPT/iGPT/models/grit_src/third_party/CenterNet2/detectron2/data/datasets/register_coco.py
+++ /dev/null
@@ -1,3 +0,0 @@
-# Copyright (c) Facebook, Inc. and its affiliates.
-from .coco import register_coco_instances # noqa
-from .coco_panoptic import register_coco_panoptic_separated # noqa
diff --git a/spaces/PAIR/Text2Video-Zero/annotator/uniformer/mmcv/cnn/bricks/hsigmoid.py b/spaces/PAIR/Text2Video-Zero/annotator/uniformer/mmcv/cnn/bricks/hsigmoid.py
deleted file mode 100644
index 30b1a3d6580cf0360710426fbea1f05acdf07b4b..0000000000000000000000000000000000000000
--- a/spaces/PAIR/Text2Video-Zero/annotator/uniformer/mmcv/cnn/bricks/hsigmoid.py
+++ /dev/null
@@ -1,34 +0,0 @@
-# Copyright (c) OpenMMLab. All rights reserved.
-import torch.nn as nn
-
-from .registry import ACTIVATION_LAYERS
-
-
-@ACTIVATION_LAYERS.register_module()
-class HSigmoid(nn.Module):
- """Hard Sigmoid Module. Apply the hard sigmoid function:
- Hsigmoid(x) = min(max((x + bias) / divisor, min_value), max_value)
- Default: Hsigmoid(x) = min(max((x + 1) / 2, 0), 1)
-
- Args:
- bias (float): Bias of the input feature map. Default: 1.0.
- divisor (float): Divisor of the input feature map. Default: 2.0.
- min_value (float): Lower bound value. Default: 0.0.
- max_value (float): Upper bound value. Default: 1.0.
-
- Returns:
- Tensor: The output tensor.
- """
-
- def __init__(self, bias=1.0, divisor=2.0, min_value=0.0, max_value=1.0):
- super(HSigmoid, self).__init__()
- self.bias = bias
- self.divisor = divisor
- assert self.divisor != 0
- self.min_value = min_value
- self.max_value = max_value
-
- def forward(self, x):
- x = (x + self.bias) / self.divisor
-
- return x.clamp_(self.min_value, self.max_value)
diff --git a/spaces/PKUWilliamYang/StyleGANEX/models/stylegan2/op_ori/__init__.py b/spaces/PKUWilliamYang/StyleGANEX/models/stylegan2/op_ori/__init__.py
deleted file mode 100644
index d0918d92285955855be89f00096b888ee5597ce3..0000000000000000000000000000000000000000
--- a/spaces/PKUWilliamYang/StyleGANEX/models/stylegan2/op_ori/__init__.py
+++ /dev/null
@@ -1,2 +0,0 @@
-from .fused_act import FusedLeakyReLU, fused_leaky_relu
-from .upfirdn2d import upfirdn2d
diff --git a/spaces/Pattr/DrumClassification/lilypond-2.24.2/lib/guile/2.2/ccache/sxml/ssax/input-parse.go b/spaces/Pattr/DrumClassification/lilypond-2.24.2/lib/guile/2.2/ccache/sxml/ssax/input-parse.go
deleted file mode 100644
index 85435720da17afcb250768fd5cf41d1c8ca998dd..0000000000000000000000000000000000000000
Binary files a/spaces/Pattr/DrumClassification/lilypond-2.24.2/lib/guile/2.2/ccache/sxml/ssax/input-parse.go and /dev/null differ
diff --git a/spaces/Plurigrid/LifeSim/src/components/ui/command.tsx b/spaces/Plurigrid/LifeSim/src/components/ui/command.tsx
deleted file mode 100644
index a4e602ef2508a071948aef7779023540c9f25381..0000000000000000000000000000000000000000
--- a/spaces/Plurigrid/LifeSim/src/components/ui/command.tsx
+++ /dev/null
@@ -1,155 +0,0 @@
-"use client"
-
-import * as React from "react"
-import { DialogProps } from "@radix-ui/react-dialog"
-import { Command as CommandPrimitive } from "cmdk"
-import { Search } from "lucide-react"
-
-import { cn } from "@/lib/utils"
-import { Dialog, DialogContent } from "@/components/ui/dialog"
-
-const Command = React.forwardRef<
- React.ElementRef,
- React.ComponentPropsWithoutRef
->(({ className, ...props }, ref) => (
-
-))
-Command.displayName = CommandPrimitive.displayName
-
-interface CommandDialogProps extends DialogProps {}
-
-const CommandDialog = ({ children, ...props }: CommandDialogProps) => {
- return (
-
-
-
- {children}
-
-
-
- )
-}
-
-const CommandInput = React.forwardRef<
- React.ElementRef,
- React.ComponentPropsWithoutRef
->(({ className, ...props }, ref) => (
-
-
-
-
-))
-
-CommandInput.displayName = CommandPrimitive.Input.displayName
-
-const CommandList = React.forwardRef<
- React.ElementRef,
- React.ComponentPropsWithoutRef
->(({ className, ...props }, ref) => (
-
-))
-
-CommandList.displayName = CommandPrimitive.List.displayName
-
-const CommandEmpty = React.forwardRef<
- React.ElementRef,
- React.ComponentPropsWithoutRef
->((props, ref) => (
-
-))
-
-CommandEmpty.displayName = CommandPrimitive.Empty.displayName
-
-const CommandGroup = React.forwardRef<
- React.ElementRef,
- React.ComponentPropsWithoutRef
->(({ className, ...props }, ref) => (
-
-))
-
-CommandGroup.displayName = CommandPrimitive.Group.displayName
-
-const CommandSeparator = React.forwardRef<
- React.ElementRef,
- React.ComponentPropsWithoutRef
->(({ className, ...props }, ref) => (
-
-))
-CommandSeparator.displayName = CommandPrimitive.Separator.displayName
-
-const CommandItem = React.forwardRef<
- React.ElementRef,
- React.ComponentPropsWithoutRef
->(({ className, ...props }, ref) => (
-
-))
-
-CommandItem.displayName = CommandPrimitive.Item.displayName
-
-const CommandShortcut = ({
- className,
- ...props
-}: React.HTMLAttributes) => {
- return (
-
- )
-}
-CommandShortcut.displayName = "CommandShortcut"
-
-export {
- Command,
- CommandDialog,
- CommandInput,
- CommandList,
- CommandEmpty,
- CommandGroup,
- CommandItem,
- CommandShortcut,
- CommandSeparator,
-}
diff --git a/spaces/PrathamDesai/fastai_bear_classifier/app.py b/spaces/PrathamDesai/fastai_bear_classifier/app.py
deleted file mode 100644
index 9610db32a293315a4e0df59125bcae4e828d36c4..0000000000000000000000000000000000000000
--- a/spaces/PrathamDesai/fastai_bear_classifier/app.py
+++ /dev/null
@@ -1,28 +0,0 @@
-#!/usr/bin/env python
-# coding: utf-8
-
-# # Bear Classifier
-#
-# This is a prototype tool to deploy a model which classifies 3 bear categories namely Black, Grizzly and Teddy (Toys)
-#
-# Upload a picture of a bear and click classify to the results
-
-
-
-from fastai.vision.all import *
-import gradio as gr
-import skimage
-
-
-learn_inf = load_learner('bear_model.pkl')
-labels = learn_inf.dls.vocab
-
-
-def predict(img):
- img = PILImage.create(img)
- pred,pred_idx,probs = learn_inf.predict(img)
- return {labels[i]: float(probs[i]) for i in range(len(labels))}
-
-gr.Interface(fn=predict, inputs=gr.inputs.Image(shape=(512, 512)), outputs=gr.outputs.Label(num_top_classes=3), title = "Bear Classifier",
-description = "A Bear Classifier trained with fastai. Created as a demo for Gradio and HuggingFace Spaces. Classifies from Grizzly, Black and Teddy(Toys). ",interpretation='default', examples=['ted.jpg','grizzly.jpg']).launch(share=True)
-
diff --git a/spaces/R34Koba/ClaudeProxyGaming/README.md b/spaces/R34Koba/ClaudeProxyGaming/README.md
deleted file mode 100644
index ecbd9deb8f370cd958acf9e2e58a99cb7057d6f8..0000000000000000000000000000000000000000
--- a/spaces/R34Koba/ClaudeProxyGaming/README.md
+++ /dev/null
@@ -1,10 +0,0 @@
----
-title: ClaudeProxyGaming
-emoji: 📚
-colorFrom: gray
-colorTo: pink
-sdk: docker
-pinned: false
----
-
-Check out the configuration reference at https://huggingface.co/docs/hub/spaces-config-reference
diff --git a/spaces/RameshBanala/aivoicebot/README.md b/spaces/RameshBanala/aivoicebot/README.md
deleted file mode 100644
index 4ecce4f056d6ec075d4532c863001817a466856a..0000000000000000000000000000000000000000
--- a/spaces/RameshBanala/aivoicebot/README.md
+++ /dev/null
@@ -1,12 +0,0 @@
----
-title: Aivoicebot
-emoji: 🚀
-colorFrom: yellow
-colorTo: red
-sdk: gradio
-sdk_version: 3.39.0
-app_file: app.py
-pinned: false
----
-
-Check out the configuration reference at https://huggingface.co/docs/hub/spaces-config-reference
diff --git a/spaces/Raspberry-ai/main/.env/lib/python3.11/site-packages/setuptools/command/setopt.py b/spaces/Raspberry-ai/main/.env/lib/python3.11/site-packages/setuptools/command/setopt.py
deleted file mode 100644
index 6358c0451b2d0036e3821d897fb6f7ab436ee4a9..0000000000000000000000000000000000000000
--- a/spaces/Raspberry-ai/main/.env/lib/python3.11/site-packages/setuptools/command/setopt.py
+++ /dev/null
@@ -1,149 +0,0 @@
-from distutils.util import convert_path
-from distutils import log
-from distutils.errors import DistutilsOptionError
-import distutils
-import os
-import configparser
-
-from setuptools import Command
-
-__all__ = ['config_file', 'edit_config', 'option_base', 'setopt']
-
-
-def config_file(kind="local"):
- """Get the filename of the distutils, local, global, or per-user config
-
- `kind` must be one of "local", "global", or "user"
- """
- if kind == 'local':
- return 'setup.cfg'
- if kind == 'global':
- return os.path.join(
- os.path.dirname(distutils.__file__), 'distutils.cfg'
- )
- if kind == 'user':
- dot = os.name == 'posix' and '.' or ''
- return os.path.expanduser(convert_path("~/%spydistutils.cfg" % dot))
- raise ValueError(
- "config_file() type must be 'local', 'global', or 'user'", kind
- )
-
-
-def edit_config(filename, settings, dry_run=False):
- """Edit a configuration file to include `settings`
-
- `settings` is a dictionary of dictionaries or ``None`` values, keyed by
- command/section name. A ``None`` value means to delete the entire section,
- while a dictionary lists settings to be changed or deleted in that section.
- A setting of ``None`` means to delete that setting.
- """
- log.debug("Reading configuration from %s", filename)
- opts = configparser.RawConfigParser()
- opts.optionxform = lambda x: x
- opts.read([filename])
- for section, options in settings.items():
- if options is None:
- log.info("Deleting section [%s] from %s", section, filename)
- opts.remove_section(section)
- else:
- if not opts.has_section(section):
- log.debug("Adding new section [%s] to %s", section, filename)
- opts.add_section(section)
- for option, value in options.items():
- if value is None:
- log.debug(
- "Deleting %s.%s from %s",
- section, option, filename
- )
- opts.remove_option(section, option)
- if not opts.options(section):
- log.info("Deleting empty [%s] section from %s",
- section, filename)
- opts.remove_section(section)
- else:
- log.debug(
- "Setting %s.%s to %r in %s",
- section, option, value, filename
- )
- opts.set(section, option, value)
-
- log.info("Writing %s", filename)
- if not dry_run:
- with open(filename, 'w') as f:
- opts.write(f)
-
-
-class option_base(Command):
- """Abstract base class for commands that mess with config files"""
-
- user_options = [
- ('global-config', 'g',
- "save options to the site-wide distutils.cfg file"),
- ('user-config', 'u',
- "save options to the current user's pydistutils.cfg file"),
- ('filename=', 'f',
- "configuration file to use (default=setup.cfg)"),
- ]
-
- boolean_options = [
- 'global-config', 'user-config',
- ]
-
- def initialize_options(self):
- self.global_config = None
- self.user_config = None
- self.filename = None
-
- def finalize_options(self):
- filenames = []
- if self.global_config:
- filenames.append(config_file('global'))
- if self.user_config:
- filenames.append(config_file('user'))
- if self.filename is not None:
- filenames.append(self.filename)
- if not filenames:
- filenames.append(config_file('local'))
- if len(filenames) > 1:
- raise DistutilsOptionError(
- "Must specify only one configuration file option",
- filenames
- )
- self.filename, = filenames
-
-
-class setopt(option_base):
- """Save command-line options to a file"""
-
- description = "set an option in setup.cfg or another config file"
-
- user_options = [
- ('command=', 'c', 'command to set an option for'),
- ('option=', 'o', 'option to set'),
- ('set-value=', 's', 'value of the option'),
- ('remove', 'r', 'remove (unset) the value'),
- ] + option_base.user_options
-
- boolean_options = option_base.boolean_options + ['remove']
-
- def initialize_options(self):
- option_base.initialize_options(self)
- self.command = None
- self.option = None
- self.set_value = None
- self.remove = None
-
- def finalize_options(self):
- option_base.finalize_options(self)
- if self.command is None or self.option is None:
- raise DistutilsOptionError("Must specify --command *and* --option")
- if self.set_value is None and not self.remove:
- raise DistutilsOptionError("Must specify --set-value or --remove")
-
- def run(self):
- edit_config(
- self.filename, {
- self.command: {self.option.replace('-', '_'): self.set_value}
- },
- self.dry_run
- )
diff --git a/spaces/Rbrq/DeticChatGPT/tools/create_lvis_21k.py b/spaces/Rbrq/DeticChatGPT/tools/create_lvis_21k.py
deleted file mode 100644
index 3e6fe60a2d579d1ef1f3610f600a915155c81fed..0000000000000000000000000000000000000000
--- a/spaces/Rbrq/DeticChatGPT/tools/create_lvis_21k.py
+++ /dev/null
@@ -1,74 +0,0 @@
-# Copyright (c) Facebook, Inc. and its affiliates.
-import argparse
-import copy
-import json
-
-if __name__ == '__main__':
- parser = argparse.ArgumentParser()
- parser.add_argument('--imagenet_path', default='datasets/imagenet/annotations/imagenet-21k_image_info.json')
- parser.add_argument('--lvis_path', default='datasets/lvis/lvis_v1_train.json')
- parser.add_argument('--save_categories', default='')
- parser.add_argument('--not_save_imagenet', action='store_true')
- parser.add_argument('--not_save_lvis', action='store_true')
- parser.add_argument('--mark', default='lvis-21k')
- args = parser.parse_args()
-
- print('Loading', args.imagenet_path)
- in_data = json.load(open(args.imagenet_path, 'r'))
- print('Loading', args.lvis_path)
- lvis_data = json.load(open(args.lvis_path, 'r'))
-
- categories = copy.deepcopy(lvis_data['categories'])
- cat_count = max(x['id'] for x in categories)
- synset2id = {x['synset']: x['id'] for x in categories}
- name2id = {x['name']: x['id'] for x in categories}
- in_id_map = {}
- for x in in_data['categories']:
- if x['synset'] in synset2id:
- in_id_map[x['id']] = synset2id[x['synset']]
- elif x['name'] in name2id:
- in_id_map[x['id']] = name2id[x['name']]
- x['id'] = name2id[x['name']]
- else:
- cat_count = cat_count + 1
- name2id[x['name']] = cat_count
- in_id_map[x['id']] = cat_count
- x['id'] = cat_count
- categories.append(x)
-
- print('lvis cats', len(lvis_data['categories']))
- print('imagenet cats', len(in_data['categories']))
- print('merge cats', len(categories))
-
- filtered_images = []
- for x in in_data['images']:
- x['pos_category_ids'] = [in_id_map[xx] for xx in x['pos_category_ids']]
- x['pos_category_ids'] = [xx for xx in \
- sorted(set(x['pos_category_ids'])) if xx >= 0]
- if len(x['pos_category_ids']) > 0:
- filtered_images.append(x)
-
- in_data['categories'] = categories
- lvis_data['categories'] = categories
-
- if not args.not_save_imagenet:
- in_out_path = args.imagenet_path[:-5] + '_{}.json'.format(args.mark)
- for k, v in in_data.items():
- print('imagenet', k, len(v))
- print('Saving Imagenet to', in_out_path)
- json.dump(in_data, open(in_out_path, 'w'))
-
- if not args.not_save_lvis:
- lvis_out_path = args.lvis_path[:-5] + '_{}.json'.format(args.mark)
- for k, v in lvis_data.items():
- print('lvis', k, len(v))
- print('Saving LVIS to', lvis_out_path)
- json.dump(lvis_data, open(lvis_out_path, 'w'))
-
- if args.save_categories != '':
- for x in categories:
- for k in ['image_count', 'instance_count', 'synonyms', 'def']:
- if k in x:
- del x[k]
- CATEGORIES = repr(categories) + " # noqa"
- open(args.save_categories, 'wt').write(f"CATEGORIES = {CATEGORIES}")
diff --git a/spaces/Realcat/image-matching-webui/third_party/DarkFeat/datasets/InvISP/utils/JPEG.py b/spaces/Realcat/image-matching-webui/third_party/DarkFeat/datasets/InvISP/utils/JPEG.py
deleted file mode 100644
index 7cdd7fa91ee424250f241ecc7de63d868795aaa7..0000000000000000000000000000000000000000
--- a/spaces/Realcat/image-matching-webui/third_party/DarkFeat/datasets/InvISP/utils/JPEG.py
+++ /dev/null
@@ -1,38 +0,0 @@
-import torch
-import torch.nn as nn
-
-from .JPEG_utils import diff_round, quality_to_factor, Quantization
-from .compression import compress_jpeg
-from .decompression import decompress_jpeg
-
-
-class DiffJPEG(nn.Module):
- def __init__(self, differentiable=True, quality=75):
- """Initialize the DiffJPEG layer
- Inputs:
- height(int): Original image height
- width(int): Original image width
- differentiable(bool): If true uses custom differentiable
- rounding function, if false uses standrard torch.round
- quality(float): Quality factor for jpeg compression scheme.
- """
- super(DiffJPEG, self).__init__()
- if differentiable:
- rounding = diff_round
- # rounding = Quantization()
- else:
- rounding = torch.round
- factor = quality_to_factor(quality)
- self.compress = compress_jpeg(rounding=rounding, factor=factor)
- # self.decompress = decompress_jpeg(height, width, rounding=rounding,
- # factor=factor)
- self.decompress = decompress_jpeg(rounding=rounding, factor=factor)
-
- def forward(self, x):
- """ """
- org_height = x.shape[2]
- org_width = x.shape[3]
- y, cb, cr = self.compress(x)
-
- recovered = self.decompress(y, cb, cr, org_height, org_width)
- return recovered
diff --git a/spaces/Realcat/image-matching-webui/third_party/lanet/train.py b/spaces/Realcat/image-matching-webui/third_party/lanet/train.py
deleted file mode 100644
index e82900a3b27f8954c65f7bf4127f38a65ac76fff..0000000000000000000000000000000000000000
--- a/spaces/Realcat/image-matching-webui/third_party/lanet/train.py
+++ /dev/null
@@ -1,152 +0,0 @@
-import os
-import torch
-import torch.optim as optim
-from tqdm import tqdm
-
-from torch.autograd import Variable
-
-from network_v0.model import PointModel
-from loss_function import KeypointLoss
-
-
-class Trainer(object):
- def __init__(self, config, train_loader=None):
- self.config = config
- # data parameters
- self.train_loader = train_loader
- self.num_train = len(self.train_loader)
-
- # training parameters
- self.max_epoch = config.max_epoch
- self.start_epoch = config.start_epoch
- self.momentum = config.momentum
- self.lr = config.init_lr
- self.lr_factor = config.lr_factor
- self.display = config.display
-
- # misc params
- self.use_gpu = config.use_gpu
- self.random_seed = config.seed
- self.gpu = config.gpu
- self.ckpt_dir = config.ckpt_dir
- self.ckpt_name = "{}-{}".format(config.ckpt_name, config.seed)
-
- # build model
- self.model = PointModel(is_test=False)
-
- # training on GPU
- if self.use_gpu:
- torch.cuda.set_device(self.gpu)
- self.model.cuda()
-
- print(
- "Number of model parameters: {:,}".format(
- sum([p.data.nelement() for p in self.model.parameters()])
- )
- )
-
- # build loss functional
- self.loss_func = KeypointLoss(config)
-
- # build optimizer and scheduler
- self.optimizer = optim.Adam(self.model.parameters(), lr=self.lr)
- self.lr_scheduler = optim.lr_scheduler.MultiStepLR(
- self.optimizer, milestones=[4, 8], gamma=self.lr_factor
- )
-
- # resume
- if int(self.config.start_epoch) > 0:
- (
- self.config.start_epoch,
- self.model,
- self.optimizer,
- self.lr_scheduler,
- ) = self.load_checkpoint(
- int(self.config.start_epoch),
- self.model,
- self.optimizer,
- self.lr_scheduler,
- )
-
- def train(self):
- print("\nTrain on {} samples".format(self.num_train))
- self.save_checkpoint(0, self.model, self.optimizer, self.lr_scheduler)
- for epoch in range(self.start_epoch, self.max_epoch):
- print(
- "\nEpoch: {}/{} --lr: {:.6f}".format(epoch + 1, self.max_epoch, self.lr)
- )
- # train for one epoch
- self.train_one_epoch(epoch)
- if self.lr_scheduler:
- self.lr_scheduler.step()
- self.save_checkpoint(
- epoch + 1, self.model, self.optimizer, self.lr_scheduler
- )
-
- def train_one_epoch(self, epoch):
- self.model.train()
- for (i, data) in enumerate(tqdm(self.train_loader)):
-
- if self.use_gpu:
- source_img = data["image_aug"].cuda()
- target_img = data["image"].cuda()
- homography = data["homography"].cuda()
-
- source_img = Variable(source_img)
- target_img = Variable(target_img)
- homography = Variable(homography)
-
- # forward propogation
- output = self.model(source_img, target_img, homography)
-
- # compute loss
- loss, loc_loss, desc_loss, score_loss, corres_loss = self.loss_func(output)
-
- # compute gradients and update
- self.optimizer.zero_grad()
- loss.backward()
- self.optimizer.step()
-
- # print training info
- msg_batch = (
- "Epoch:{} Iter:{} lr:{:.4f} "
- "loc_loss={:.4f} desc_loss={:.4f} score_loss={:.4f} corres_loss={:.4f} "
- "loss={:.4f} ".format(
- (epoch + 1),
- i,
- self.lr,
- loc_loss.data,
- desc_loss.data,
- score_loss.data,
- corres_loss.data,
- loss.data,
- )
- )
-
- if (i % self.display) == 0:
- print(msg_batch)
- return
-
- def save_checkpoint(self, epoch, model, optimizer, lr_scheduler):
- filename = self.ckpt_name + "_" + str(epoch) + ".pth"
- torch.save(
- {
- "epoch": epoch,
- "model_state": model.state_dict(),
- "optimizer_state": optimizer.state_dict(),
- "lr_scheduler": lr_scheduler.state_dict(),
- },
- os.path.join(self.ckpt_dir, filename),
- )
-
- def load_checkpoint(self, epoch, model, optimizer, lr_scheduler):
- filename = self.ckpt_name + "_" + str(epoch) + ".pth"
- ckpt = torch.load(os.path.join(self.ckpt_dir, filename))
- epoch = ckpt["epoch"]
- model.load_state_dict(ckpt["model_state"])
- optimizer.load_state_dict(ckpt["optimizer_state"])
- lr_scheduler.load_state_dict(ckpt["lr_scheduler"])
-
- print("[*] Loaded {} checkpoint @ epoch {}".format(filename, ckpt["epoch"]))
-
- return epoch, model, optimizer, lr_scheduler
diff --git a/spaces/Robert001/UniControl-Demo/annotator/uniformer_base/mmcv/runner/hooks/sync_buffer.py b/spaces/Robert001/UniControl-Demo/annotator/uniformer_base/mmcv/runner/hooks/sync_buffer.py
deleted file mode 100644
index 6376b7ff894280cb2782243b25e8973650591577..0000000000000000000000000000000000000000
--- a/spaces/Robert001/UniControl-Demo/annotator/uniformer_base/mmcv/runner/hooks/sync_buffer.py
+++ /dev/null
@@ -1,22 +0,0 @@
-# Copyright (c) OpenMMLab. All rights reserved.
-from ..dist_utils import allreduce_params
-from .hook import HOOKS, Hook
-
-
-@HOOKS.register_module()
-class SyncBuffersHook(Hook):
- """Synchronize model buffers such as running_mean and running_var in BN at
- the end of each epoch.
-
- Args:
- distributed (bool): Whether distributed training is used. It is
- effective only for distributed training. Defaults to True.
- """
-
- def __init__(self, distributed=True):
- self.distributed = distributed
-
- def after_epoch(self, runner):
- """All-reduce model buffers at the end of each epoch."""
- if self.distributed:
- allreduce_params(runner.model.buffers())
diff --git a/spaces/SIGGRAPH2022/DCT-Net/source/facelib/LK/lk.py b/spaces/SIGGRAPH2022/DCT-Net/source/facelib/LK/lk.py
deleted file mode 100644
index df05e3f9035656ec0861f9d2913e34a4219cb702..0000000000000000000000000000000000000000
--- a/spaces/SIGGRAPH2022/DCT-Net/source/facelib/LK/lk.py
+++ /dev/null
@@ -1,97 +0,0 @@
-import numpy as np
-
-from modelscope.models.cv.cartoon.facelib.config import config as cfg
-
-
-class GroupTrack():
-
- def __init__(self):
- self.old_frame = None
- self.previous_landmarks_set = None
- self.with_landmark = True
- self.thres = cfg.TRACE.pixel_thres
- self.alpha = cfg.TRACE.smooth_landmark
- self.iou_thres = cfg.TRACE.iou_thres
-
- def calculate(self, img, current_landmarks_set):
- if self.previous_landmarks_set is None:
- self.previous_landmarks_set = current_landmarks_set
- result = current_landmarks_set
- else:
- previous_lm_num = self.previous_landmarks_set.shape[0]
- if previous_lm_num == 0:
- self.previous_landmarks_set = current_landmarks_set
- result = current_landmarks_set
- return result
- else:
- result = []
- for i in range(current_landmarks_set.shape[0]):
- not_in_flag = True
- for j in range(previous_lm_num):
- if self.iou(current_landmarks_set[i],
- self.previous_landmarks_set[j]
- ) > self.iou_thres:
- result.append(
- self.smooth(current_landmarks_set[i],
- self.previous_landmarks_set[j]))
- not_in_flag = False
- break
- if not_in_flag:
- result.append(current_landmarks_set[i])
-
- result = np.array(result)
- self.previous_landmarks_set = result
-
- return result
-
- def iou(self, p_set0, p_set1):
- rec1 = [
- np.min(p_set0[:, 0]),
- np.min(p_set0[:, 1]),
- np.max(p_set0[:, 0]),
- np.max(p_set0[:, 1])
- ]
- rec2 = [
- np.min(p_set1[:, 0]),
- np.min(p_set1[:, 1]),
- np.max(p_set1[:, 0]),
- np.max(p_set1[:, 1])
- ]
-
- # computing area of each rectangles
- S_rec1 = (rec1[2] - rec1[0]) * (rec1[3] - rec1[1])
- S_rec2 = (rec2[2] - rec2[0]) * (rec2[3] - rec2[1])
-
- # computing the sum_area
- sum_area = S_rec1 + S_rec2
-
- # find the each edge of intersect rectangle
- x1 = max(rec1[0], rec2[0])
- y1 = max(rec1[1], rec2[1])
- x2 = min(rec1[2], rec2[2])
- y2 = min(rec1[3], rec2[3])
-
- # judge if there is an intersect
- intersect = max(0, x2 - x1) * max(0, y2 - y1)
-
- iou = intersect / (sum_area - intersect)
- return iou
-
- def smooth(self, now_landmarks, previous_landmarks):
- result = []
- for i in range(now_landmarks.shape[0]):
- x = now_landmarks[i][0] - previous_landmarks[i][0]
- y = now_landmarks[i][1] - previous_landmarks[i][1]
- dis = np.sqrt(np.square(x) + np.square(y))
- if dis < self.thres:
- result.append(previous_landmarks[i])
- else:
- result.append(
- self.do_moving_average(now_landmarks[i],
- previous_landmarks[i]))
-
- return np.array(result)
-
- def do_moving_average(self, p_now, p_previous):
- p = self.alpha * p_now + (1 - self.alpha) * p_previous
- return p
diff --git a/spaces/SalahZa/Tunisian-ASR-v0/partly_frozen_splitted_wavlm/ctc_train.py b/spaces/SalahZa/Tunisian-ASR-v0/partly_frozen_splitted_wavlm/ctc_train.py
deleted file mode 100644
index 39b6b13ff99870adb71e2bcffca4ce2479405a08..0000000000000000000000000000000000000000
--- a/spaces/SalahZa/Tunisian-ASR-v0/partly_frozen_splitted_wavlm/ctc_train.py
+++ /dev/null
@@ -1,339 +0,0 @@
-#!/usr/bin/env/python3
-"""Recipe for training a wav2vec-based ctc ASR system with librispeech.
-The system employs wav2vec as its encoder. Decoding is performed with
-ctc greedy decoder.
-To run this recipe, do the following:
-> python train_with_wav2vec.py hparams/train_with_wav2vec.yaml
-The neural network is trained on CTC likelihood target and character units
-are used as basic recognition tokens. Training is performed on the full
-LibriSpeech dataset (960 h).
-
-Authors
- * Sung-Lin Yeh 2021
- * Titouan Parcollet 2021
- * Ju-Chieh Chou 2020
- * Mirco Ravanelli 2020
- * Abdel Heba 2020
- * Peter Plantinga 2020
- * Samuele Cornell 2020
-"""
-
-import os
-import sys
-import torch
-import logging
-import speechbrain as sb
-from speechbrain.utils.distributed import run_on_main
-from hyperpyyaml import load_hyperpyyaml
-from pathlib import Path
-import torchaudio.transforms as T
-logger = logging.getLogger(__name__)
-
-# Define training procedure
-class ASR(sb.Brain):
- def compute_forward(self, batch, stage):
- """Forward computations from the waveform batches to the output probabilities."""
- batch = batch.to(self.device)
- wavs, wav_lens = batch.sig
- tokens_bos, _ = batch.tokens_bos
- wavs, wav_lens = wavs.to(self.device), wav_lens.to(self.device)
-
- # Forward pass
- feats = self.modules.wav2vec2(wavs)
- x = self.modules.enc(feats)
- # Compute outputs
- p_tokens = None
- logits = self.modules.ctc_lin(x)
- p_ctc = self.hparams.log_softmax(logits)
- if stage != sb.Stage.TRAIN:
- p_tokens = sb.decoders.ctc_greedy_decode(
- p_ctc, wav_lens, blank_id=self.hparams.blank_index
- )
- return p_ctc, wav_lens, p_tokens
-
- def compute_objectives(self, predictions, batch, stage):
- """Computes the loss (CTC+NLL) given predictions and targets."""
-
- p_ctc, wav_lens, predicted_tokens = predictions
-
- ids = batch.id
- tokens_eos, tokens_eos_lens = batch.tokens_eos
- tokens, tokens_lens = batch.tokens
-
- if hasattr(self.modules, "env_corrupt") and stage == sb.Stage.TRAIN:
- tokens_eos = torch.cat([tokens_eos, tokens_eos], dim=0)
- tokens_eos_lens = torch.cat(
- [tokens_eos_lens, tokens_eos_lens], dim=0
- )
- tokens = torch.cat([tokens, tokens], dim=0)
- tokens_lens = torch.cat([tokens_lens, tokens_lens], dim=0)
-
- loss_ctc = self.hparams.ctc_cost(p_ctc, tokens, wav_lens, tokens_lens)
- loss = loss_ctc
-
- if stage != sb.Stage.TRAIN:
- # Decode token terms to words
- predicted_words = [
- "".join(self.tokenizer.decode_ndim(utt_seq)).split(" ")
- for utt_seq in predicted_tokens
- ]
- target_words = [wrd.split(" ") for wrd in batch.wrd]
- self.wer_metric.append(ids, predicted_words, target_words)
- self.cer_metric.append(ids, predicted_words, target_words)
-
- return loss
-
- def fit_batch(self, batch):
- """Train the parameters given a single batch in input"""
- predictions = self.compute_forward(batch, sb.Stage.TRAIN)
- loss = self.compute_objectives(predictions, batch, sb.Stage.TRAIN)
- loss.backward()
- if self.check_gradients(loss):
- self.wav2vec_optimizer.step()
- self.model_optimizer.step()
-
- self.wav2vec_optimizer.zero_grad()
- self.model_optimizer.zero_grad()
-
- return loss.detach()
-
- def evaluate_batch(self, batch, stage):
- """Computations needed for validation/test batches"""
- predictions = self.compute_forward(batch, stage=stage)
- with torch.no_grad():
- loss = self.compute_objectives(predictions, batch, stage=stage)
- return loss.detach()
-
- def on_stage_start(self, stage, epoch):
- """Gets called at the beginning of each epoch"""
- if stage != sb.Stage.TRAIN:
- self.cer_metric = self.hparams.cer_computer()
- self.wer_metric = self.hparams.error_rate_computer()
-
- def on_stage_end(self, stage, stage_loss, epoch):
- """Gets called at the end of an epoch."""
- # Compute/store important stats
- stage_stats = {"loss": stage_loss}
- if stage == sb.Stage.TRAIN:
- self.train_stats = stage_stats
- else:
- stage_stats["CER"] = self.cer_metric.summarize("error_rate")
- stage_stats["WER"] = self.wer_metric.summarize("error_rate")
-
- # Perform end-of-iteration things, like annealing, logging, etc.
- if stage == sb.Stage.VALID:
- old_lr_model, new_lr_model = self.hparams.lr_annealing_model(
- stage_stats["loss"]
- )
- old_lr_wav2vec, new_lr_wav2vec = self.hparams.lr_annealing_wav2vec(
- stage_stats["loss"]
- )
- sb.nnet.schedulers.update_learning_rate(
- self.model_optimizer, new_lr_model
- )
- sb.nnet.schedulers.update_learning_rate(
- self.wav2vec_optimizer, new_lr_wav2vec
- )
- self.hparams.train_logger.log_stats(
- stats_meta={
- "epoch": epoch,
- "lr_model": old_lr_model,
- "lr_wav2vec": old_lr_wav2vec,
- },
- train_stats=self.train_stats,
- valid_stats=stage_stats,
- )
- self.checkpointer.save_and_keep_only(
- meta={"WER": stage_stats["WER"]}, min_keys=["WER"],
- )
- elif stage == sb.Stage.TEST:
- self.hparams.train_logger.log_stats(
- stats_meta={"Epoch loaded": self.hparams.epoch_counter.current},
- test_stats=stage_stats,
- )
- with open(self.hparams.wer_file, "w") as w:
- self.wer_metric.write_stats(w)
-
- def init_optimizers(self):
- "Initializes the wav2vec2 optimizer and model optimizer"
- self.wav2vec_optimizer = self.hparams.wav2vec_opt_class(
- self.modules.wav2vec2.parameters()
- )
- self.model_optimizer = self.hparams.model_opt_class(
- self.hparams.model.parameters()
- )
-
- if self.checkpointer is not None:
- self.checkpointer.add_recoverable(
- "wav2vec_opt", self.wav2vec_optimizer
- )
- self.checkpointer.add_recoverable("modelopt", self.model_optimizer)
-
-
-def dataio_prepare(hparams):
- """This function prepares the datasets to be used in the brain class.
- It also defines the data processing pipeline through user-defined functions."""
- data_folder = hparams["data_folder"]
-
- train_data = sb.dataio.dataset.DynamicItemDataset.from_csv(
- csv_path=hparams["train_csv"], replacements={"data_root": data_folder},
- )
-
- if hparams["sorting"] == "ascending":
- # we sort training data to speed up training and get better results.
- train_data = train_data.filtered_sorted(sort_key="duration")
- # when sorting do not shuffle in dataloader ! otherwise is pointless
- hparams["train_dataloader_opts"]["shuffle"] = False
-
- elif hparams["sorting"] == "descending":
- train_data = train_data.filtered_sorted(
- sort_key="duration", reverse=True
- )
- # when sorting do not shuffle in dataloader ! otherwise is pointless
- hparams["train_dataloader_opts"]["shuffle"] = False
-
- elif hparams["sorting"] == "random":
- pass
-
- else:
- raise NotImplementedError(
- "sorting must be random, ascending or descending"
- )
-
- valid_data = sb.dataio.dataset.DynamicItemDataset.from_csv(
- csv_path=hparams["valid_csv"], replacements={"data_root": data_folder},
- )
- valid_data = valid_data.filtered_sorted(sort_key="duration")
-
- # test is separate
- test_datasets = {}
- for csv_file in hparams["test_csv"]:
- name = Path(csv_file).stem
- test_datasets[name] = sb.dataio.dataset.DynamicItemDataset.from_csv(
- csv_path=csv_file, replacements={"data_root": data_folder}
- )
- test_datasets[name] = test_datasets[name].filtered_sorted(
- sort_key="duration"
- )
-
- datasets = [train_data, valid_data] + [i for k, i in test_datasets.items()]
-
- # 2. Define audio pipeline:
- @sb.utils.data_pipeline.takes("wav", "sr")
- @sb.utils.data_pipeline.provides("sig")
- def audio_pipeline(wav, sr):
- sig = sb.dataio.dataio.read_audio(wav)
- sig = resamplers[sr](sig)
- return sig
-
- sb.dataio.dataset.add_dynamic_item(datasets, audio_pipeline)
- label_encoder = sb.dataio.encoder.CTCTextEncoder()
-
- # 3. Define text pipeline:
- @sb.utils.data_pipeline.takes("wrd")
- @sb.utils.data_pipeline.provides(
- "wrd", "char_list", "tokens_list", "tokens_bos", "tokens_eos", "tokens"
- )
- def text_pipeline(wrd):
- yield wrd
- char_list = list(wrd)
- yield char_list
- tokens_list = label_encoder.encode_sequence(char_list)
- yield tokens_list
- tokens_bos = torch.LongTensor([hparams["bos_index"]] + (tokens_list))
- yield tokens_bos
- tokens_eos = torch.LongTensor(tokens_list + [hparams["eos_index"]])
- yield tokens_eos
- tokens = torch.LongTensor(tokens_list)
- yield tokens
-
- sb.dataio.dataset.add_dynamic_item(datasets, text_pipeline)
-
- lab_enc_file = os.path.join(hparams["save_folder"], "label_encoder.txt")
- special_labels = {
- "bos_label": hparams["bos_index"],
- "eos_label": hparams["eos_index"],
- "blank_label": hparams["blank_index"],
- }
- label_encoder.load_or_create(
- path=lab_enc_file,
- from_didatasets=[train_data],
- output_key="char_list",
- special_labels=special_labels,
- sequence_input=True,
- )
-
- # 4. Set output:
- sb.dataio.dataset.set_output_keys(
- datasets,
- ["id", "sig", "wrd", "char_list", "tokens_bos", "tokens_eos", "tokens"],
- )
- return train_data, valid_data, test_datasets, label_encoder
-
-
-if __name__ == "__main__":
-
- # CLI:
- hparams_file, run_opts, overrides = sb.parse_arguments(sys.argv[1:])
-
- # If distributed_launch=True then
- # create ddp_group with the right communication protocol
- sb.utils.distributed.ddp_init_group(run_opts)
-
- with open(hparams_file) as fin:
- hparams = load_hyperpyyaml(fin, overrides)
-
- # Create experiment directory
- sb.create_experiment_directory(
- experiment_directory=hparams["output_folder"],
- hyperparams_to_save=hparams_file,
- overrides=overrides,
- )
-
- # Dataset prep (parsing Librispeech)
-
- resampler_8000 = T.Resample(8000, 16000, dtype=torch.float)
-
- resampler_44100 =T.Resample(44100, 16000, dtype=torch.float)
- resampler_32000 =T.Resample(32000, 16000, dtype=torch.float)
- resampler_48000 =T.Resample(48000, 16000, dtype=torch.float)
-
-
- resamplers = {"48000": resampler_48000,"8000": resampler_8000, "44100":resampler_44100, "32000":resampler_32000}
-
- # here we create the datasets objects as well as tokenization and encoding
- train_data, valid_data, test_datasets, label_encoder = dataio_prepare(
- hparams
- )
-
- # Trainer initialization
- asr_brain = ASR(
- modules=hparams["modules"],
- hparams=hparams,
- run_opts=run_opts,
- checkpointer=hparams["checkpointer"],
- )
- asr_brain.device= "cpu"
- asr_brain.modules.to("cpu")
-
- # We dynamicaly add the tokenizer to our brain class.
- # NB: This tokenizer corresponds to the one used for the LM!!
- asr_brain.tokenizer = label_encoder
-
- # Training
- asr_brain.fit(
- asr_brain.hparams.epoch_counter,
- train_data,
- valid_data,
- train_loader_kwargs=hparams["train_dataloader_opts"],
- valid_loader_kwargs=hparams["valid_dataloader_opts"],
- )
-
- # Testing
- for k in test_datasets.keys(): # keys are test_clean, test_other etc
- asr_brain.hparams.wer_file = os.path.join(
- hparams["output_folder"], "wer_{}.txt".format(k)
- )
- asr_brain.evaluate(
- test_datasets[k], test_loader_kwargs=hparams["test_dataloader_opts"]
- )
diff --git a/spaces/Sapiensia/diffuse-the-rest/build/index.html b/spaces/Sapiensia/diffuse-the-rest/build/index.html
deleted file mode 100644
index 86c28c048d5c5a0015faf3ace74e0b73c190edc4..0000000000000000000000000000000000000000
--- a/spaces/Sapiensia/diffuse-the-rest/build/index.html
+++ /dev/null
@@ -1,57 +0,0 @@
-
-
-
-
-
-
-
-
-
-
-
-
-
-
-
-
-
-
-
-
-
-
-LICENSE
-The model is licensed with a CreativeML Open RAIL-M license. The authors claim no rights on the outputs you generate, you are free to use them and are accountable for their use which must not go against the provisions set in this license. The license forbids you from sharing any content that violates any laws, produce any harm to a person, disseminate any personal information that would be meant for harm, spread misinformation and target vulnerable groups. For the full list of restrictions please read the license
-Biases and content acknowledgment
-Despite how impressive being able to turn text into image is, beware to the fact that this model may output content that reinforces or exacerbates societal biases, as well as realistic faces, pornography and violence. The model was trained on the LAION-5B dataset , which scraped non-curated image-text-pairs from the internet (the exception being the removal of illegal content) and is meant for research purposes. You can read more in the model card
-
-
-
-
-
diff --git a/spaces/Sapphire-356/Video2MC/joints_detectors/Alphapose/dataloader.py b/spaces/Sapphire-356/Video2MC/joints_detectors/Alphapose/dataloader.py
deleted file mode 100644
index 7b21feec06a2ac7d6adc68b0f142cb0488478b07..0000000000000000000000000000000000000000
--- a/spaces/Sapphire-356/Video2MC/joints_detectors/Alphapose/dataloader.py
+++ /dev/null
@@ -1,782 +0,0 @@
-import os
-import sys
-import time
-from multiprocessing import Queue as pQueue
-from threading import Thread
-
-import cv2
-import numpy as np
-import torch
-import torch.multiprocessing as mp
-import torch.utils.data as data
-import torchvision.transforms as transforms
-from PIL import Image
-from torch.autograd import Variable
-
-from SPPE.src.utils.eval import getPrediction, getMultiPeakPrediction
-from SPPE.src.utils.img import load_image, cropBox, im_to_torch
-from matching import candidate_reselect as matching
-from opt import opt
-from pPose_nms import pose_nms
-from yolo.darknet import Darknet
-from yolo.preprocess import prep_image, prep_frame
-from yolo.util import dynamic_write_results
-
-# import the Queue class from Python 3
-if sys.version_info >= (3, 0):
- from queue import Queue, LifoQueue
-# otherwise, import the Queue class for Python 2.7
-else:
- from Queue import Queue, LifoQueue
-
-if opt.vis_fast:
- from fn import vis_frame_fast as vis_frame
-else:
- from fn import vis_frame
-
-
-class Image_loader(data.Dataset):
- def __init__(self, im_names, format='yolo'):
- super(Image_loader, self).__init__()
- self.img_dir = opt.inputpath
- self.imglist = im_names
- self.transform = transforms.Compose([
- transforms.ToTensor(),
- transforms.Normalize((0.485, 0.456, 0.406), (0.229, 0.224, 0.225))
- ])
- self.format = format
-
- def getitem_ssd(self, index):
- im_name = self.imglist[index].rstrip('\n').rstrip('\r')
- im_name = os.path.join(self.img_dir, im_name)
- im = Image.open(im_name)
- inp = load_image(im_name)
- if im.mode == 'L':
- im = im.convert('RGB')
-
- ow = oh = 512
- im = im.resize((ow, oh))
- im = self.transform(im)
- return im, inp, im_name
-
- def getitem_yolo(self, index):
- inp_dim = int(opt.inp_dim)
- im_name = self.imglist[index].rstrip('\n').rstrip('\r')
- im_name = os.path.join(self.img_dir, im_name)
- im, orig_img, im_dim = prep_image(im_name, inp_dim)
- # im_dim = torch.FloatTensor([im_dim]).repeat(1, 2)
-
- inp = load_image(im_name)
- return im, inp, orig_img, im_name, im_dim
-
- def __getitem__(self, index):
- if self.format == 'ssd':
- return self.getitem_ssd(index)
- elif self.format == 'yolo':
- return self.getitem_yolo(index)
- else:
- raise NotImplementedError
-
- def __len__(self):
- return len(self.imglist)
-
-
-class ImageLoader:
- def __init__(self, im_names, batchSize=1, format='yolo', queueSize=50):
- self.img_dir = opt.inputpath
- self.imglist = im_names
- self.transform = transforms.Compose([
- transforms.ToTensor(),
- transforms.Normalize((0.485, 0.456, 0.406), (0.229, 0.224, 0.225))
- ])
- self.format = format
-
- self.batchSize = batchSize
- self.datalen = len(self.imglist)
- leftover = 0
- if (self.datalen) % batchSize:
- leftover = 1
- self.num_batches = self.datalen // batchSize + leftover
-
- # initialize the queue used to store data
- if opt.sp:
- self.Q = Queue(maxsize=queueSize)
- else:
- self.Q = mp.Queue(maxsize=queueSize)
-
- def start(self):
- # start a thread to read frames from the file video stream
- if self.format == 'ssd':
- if opt.sp:
- p = Thread(target=self.getitem_ssd, args=())
- else:
- p = mp.Process(target=self.getitem_ssd, args=())
- elif self.format == 'yolo':
- if opt.sp:
- p = Thread(target=self.getitem_yolo, args=())
- else:
- p = mp.Process(target=self.getitem_yolo, args=())
- else:
- raise NotImplementedError
- p.daemon = True
- p.start()
- return self
-
- def getitem_ssd(self):
- length = len(self.imglist)
- for index in range(length):
- im_name = self.imglist[index].rstrip('\n').rstrip('\r')
- im_name = os.path.join(self.img_dir, im_name)
- im = Image.open(im_name)
- inp = load_image(im_name)
- if im.mode == 'L':
- im = im.convert('RGB')
-
- ow = oh = 512
- im = im.resize((ow, oh))
- im = self.transform(im)
- while self.Q.full():
- time.sleep(2)
- self.Q.put((im, inp, im_name))
-
- def getitem_yolo(self):
- for i in range(self.num_batches):
- img = []
- orig_img = []
- im_name = []
- im_dim_list = []
- for k in range(i * self.batchSize, min((i + 1) * self.batchSize, self.datalen)):
- inp_dim = int(opt.inp_dim)
- im_name_k = self.imglist[k].rstrip('\n').rstrip('\r')
- im_name_k = os.path.join(self.img_dir, im_name_k)
- img_k, orig_img_k, im_dim_list_k = prep_image(im_name_k, inp_dim)
-
- img.append(img_k)
- orig_img.append(orig_img_k)
- im_name.append(im_name_k)
- im_dim_list.append(im_dim_list_k)
-
- with torch.no_grad():
- # Human Detection
- img = torch.cat(img)
- im_dim_list = torch.FloatTensor(im_dim_list).repeat(1, 2)
- im_dim_list_ = im_dim_list
-
- while self.Q.full():
- time.sleep(2)
-
- self.Q.put((img, orig_img, im_name, im_dim_list))
-
- def getitem(self):
- return self.Q.get()
-
- def length(self):
- return len(self.imglist)
-
- def len(self):
- return self.Q.qsize()
-
-
-class VideoLoader:
- def __init__(self, path, batchSize=1, queueSize=50):
- # initialize the file video stream along with the boolean
- # used to indicate if the thread should be stopped or not
- self.path = path
- self.stream = cv2.VideoCapture(path)
- assert self.stream.isOpened(), 'Cannot capture source'
- self.stopped = False
-
- self.batchSize = batchSize
- self.datalen = int(self.stream.get(cv2.CAP_PROP_FRAME_COUNT))
- leftover = 0
- if (self.datalen) % batchSize:
- leftover = 1
- self.num_batches = self.datalen // batchSize + leftover
-
- # initialize the queue used to store frames read from
- # the video file
- if opt.sp:
- self.Q = Queue(maxsize=queueSize)
- else:
- self.Q = mp.Queue(maxsize=queueSize)
-
- def length(self):
- return self.datalen
-
- def start(self):
- # start a thread to read frames from the file video stream
- if opt.sp:
- t = Thread(target=self.update, args=())
- t.daemon = True
- t.start()
- else:
- p = mp.Process(target=self.update, args=())
- p.daemon = True
- p.start()
- return self
-
- def update(self):
- stream = cv2.VideoCapture(self.path)
- assert stream.isOpened(), 'Cannot capture source'
-
- for i in range(self.num_batches):
- img = []
- orig_img = []
- im_name = []
- im_dim_list = []
- for k in range(i * self.batchSize, min((i + 1) * self.batchSize, self.datalen)):
- inp_dim = int(opt.inp_dim)
- (grabbed, frame) = stream.read()
- # if the `grabbed` boolean is `False`, then we have
- # reached the end of the video file
- if not grabbed:
- self.Q.put((None, None, None, None))
- print('===========================> This video get ' + str(k) + ' frames in total.')
- sys.stdout.flush()
- return
- # process and add the frame to the queue
- img_k, orig_img_k, im_dim_list_k = prep_frame(frame, inp_dim)
-
- img.append(img_k)
- orig_img.append(orig_img_k)
- im_name.append(str(k) + '.jpg')
- im_dim_list.append(im_dim_list_k)
-
- with torch.no_grad():
- # Human Detection
- img = torch.cat(img)
- im_dim_list = torch.FloatTensor(im_dim_list).repeat(1, 2)
-
- while self.Q.full():
- time.sleep(2)
-
- self.Q.put((img, orig_img, im_name, im_dim_list))
-
- def videoinfo(self):
- # indicate the video info
- fourcc = int(self.stream.get(cv2.CAP_PROP_FOURCC))
- fps = self.stream.get(cv2.CAP_PROP_FPS)
- frameSize = (int(self.stream.get(cv2.CAP_PROP_FRAME_WIDTH)), int(self.stream.get(cv2.CAP_PROP_FRAME_HEIGHT)))
- return (fourcc, fps, frameSize)
-
- def getitem(self):
- # return next frame in the queue
- return self.Q.get()
-
- def len(self):
- return self.Q.qsize()
-
-
-class DetectionLoader:
- def __init__(self, dataloder, batchSize=1, queueSize=1024):
- # initialize the file video stream along with the boolean
- # used to indicate if the thread should be stopped or not
- self.det_model = Darknet("joints_detectors/Alphapose/yolo/cfg/yolov3-spp.cfg")
- self.det_model.load_weights('joints_detectors/Alphapose/models/yolo/yolov3-spp.weights')
- self.det_model.net_info['height'] = opt.inp_dim
- self.det_inp_dim = int(self.det_model.net_info['height'])
- assert self.det_inp_dim % 32 == 0
- assert self.det_inp_dim > 32
- self.det_model
- self.det_model.eval()
-
- self.stopped = False
- self.dataloder = dataloder
- self.batchSize = batchSize
- self.datalen = self.dataloder.length()
- leftover = 0
- if (self.datalen) % batchSize:
- leftover = 1
- self.num_batches = self.datalen // batchSize + leftover
- # initialize the queue used to store frames read from
- # the video file
- if opt.sp:
- self.Q = Queue(maxsize=queueSize)
- else:
- self.Q = mp.Queue(maxsize=queueSize)
-
- def start(self):
- # start a thread to read frames from the file video stream
- if opt.sp:
- t = Thread(target=self.update, args=())
- t.daemon = True
- t.start()
- else:
- p = mp.Process(target=self.update, args=(), daemon=True)
- # p = mp.Process(target=self.update, args=())
- # p.daemon = True
- p.start()
- return self
-
- def update(self):
- # keep looping the whole dataset
- for i in range(self.num_batches):
- img, orig_img, im_name, im_dim_list = self.dataloder.getitem()
- if img is None:
- self.Q.put((None, None, None, None, None, None, None))
- return
-
- with torch.no_grad():
- # Human Detection
- img = img
- prediction = self.det_model(img, CUDA=True)
- # NMS process
- dets = dynamic_write_results(prediction, opt.confidence,
- opt.num_classes, nms=True, nms_conf=opt.nms_thesh)
- if isinstance(dets, int) or dets.shape[0] == 0:
- for k in range(len(orig_img)):
- if self.Q.full():
- time.sleep(2)
- self.Q.put((orig_img[k], im_name[k], None, None, None, None, None))
- continue
- dets = dets.cpu()
- im_dim_list = torch.index_select(im_dim_list, 0, dets[:, 0].long())
- scaling_factor = torch.min(self.det_inp_dim / im_dim_list, 1)[0].view(-1, 1)
-
- # coordinate transfer
- dets[:, [1, 3]] -= (self.det_inp_dim - scaling_factor * im_dim_list[:, 0].view(-1, 1)) / 2
- dets[:, [2, 4]] -= (self.det_inp_dim - scaling_factor * im_dim_list[:, 1].view(-1, 1)) / 2
-
- dets[:, 1:5] /= scaling_factor
- for j in range(dets.shape[0]):
- dets[j, [1, 3]] = torch.clamp(dets[j, [1, 3]], 0.0, im_dim_list[j, 0])
- dets[j, [2, 4]] = torch.clamp(dets[j, [2, 4]], 0.0, im_dim_list[j, 1])
- boxes = dets[:, 1:5]
- scores = dets[:, 5:6]
-
- for k in range(len(orig_img)):
- boxes_k = boxes[dets[:, 0] == k]
- if isinstance(boxes_k, int) or boxes_k.shape[0] == 0:
- if self.Q.full():
- time.sleep(2)
- self.Q.put((orig_img[k], im_name[k], None, None, None, None, None))
- continue
- inps = torch.zeros(boxes_k.size(0), 3, opt.inputResH, opt.inputResW)
- pt1 = torch.zeros(boxes_k.size(0), 2)
- pt2 = torch.zeros(boxes_k.size(0), 2)
- if self.Q.full():
- time.sleep(2)
- self.Q.put((orig_img[k], im_name[k], boxes_k, scores[dets[:, 0] == k], inps, pt1, pt2))
-
- def read(self):
- # return next frame in the queue
- return self.Q.get()
-
- def len(self):
- # return queue len
- return self.Q.qsize()
-
-
-class DetectionProcessor:
- def __init__(self, detectionLoader, queueSize=1024):
- # initialize the file video stream along with the boolean
- # used to indicate if the thread should be stopped or not
- self.detectionLoader = detectionLoader
- self.stopped = False
- self.datalen = self.detectionLoader.datalen
-
- # initialize the queue used to store data
- if opt.sp:
- self.Q = Queue(maxsize=queueSize)
- else:
- self.Q = pQueue(maxsize=queueSize)
-
- def start(self):
- # start a thread to read frames from the file video stream
- if opt.sp:
- # t = Thread(target=self.update, args=(), daemon=True)
- t = Thread(target=self.update, args=())
- t.daemon = True
- t.start()
- else:
- p = mp.Process(target=self.update, args=(), daemon=True)
- # p = mp.Process(target=self.update, args=())
- # p.daemon = True
- p.start()
- return self
-
- def update(self):
- # keep looping the whole dataset
- for i in range(self.datalen):
-
- with torch.no_grad():
- (orig_img, im_name, boxes, scores, inps, pt1, pt2) = self.detectionLoader.read()
- if orig_img is None:
- self.Q.put((None, None, None, None, None, None, None))
- return
- if boxes is None or boxes.nelement() == 0:
- while self.Q.full():
- time.sleep(0.2)
- self.Q.put((None, orig_img, im_name, boxes, scores, None, None))
- continue
- inp = im_to_torch(cv2.cvtColor(orig_img, cv2.COLOR_BGR2RGB))
- inps, pt1, pt2 = crop_from_dets(inp, boxes, inps, pt1, pt2)
-
- while self.Q.full():
- time.sleep(0.2)
- self.Q.put((inps, orig_img, im_name, boxes, scores, pt1, pt2))
-
- def read(self):
- # return next frame in the queue
- return self.Q.get()
-
- def len(self):
- # return queue len
- return self.Q.qsize()
-
-
-class VideoDetectionLoader:
- def __init__(self, path, batchSize=4, queueSize=256):
- # initialize the file video stream along with the boolean
- # used to indicate if the thread should be stopped or not
- self.det_model = Darknet("yolo/cfg/yolov3-spp.cfg")
- self.det_model.load_weights('models/yolo/yolov3-spp.weights')
- self.det_model.net_info['height'] = opt.inp_dim
- self.det_inp_dim = int(self.det_model.net_info['height'])
- assert self.det_inp_dim % 32 == 0
- assert self.det_inp_dim > 32
- self.det_model
- self.det_model.eval()
-
- self.stream = cv2.VideoCapture(path)
- assert self.stream.isOpened(), 'Cannot capture source'
- self.stopped = False
- self.batchSize = batchSize
- self.datalen = int(self.stream.get(cv2.CAP_PROP_FRAME_COUNT))
- leftover = 0
- if (self.datalen) % batchSize:
- leftover = 1
- self.num_batches = self.datalen // batchSize + leftover
- # initialize the queue used to store frames read from
- # the video file
- self.Q = Queue(maxsize=queueSize)
-
- def length(self):
- return self.datalen
-
- def len(self):
- return self.Q.qsize()
-
- def start(self):
- # start a thread to read frames from the file video stream
- t = Thread(target=self.update, args=())
- t.daemon = True
- t.start()
- return self
-
- def update(self):
- # keep looping the whole video
- for i in range(self.num_batches):
- img = []
- inp = []
- orig_img = []
- im_name = []
- im_dim_list = []
- for k in range(i * self.batchSize, min((i + 1) * self.batchSize, self.datalen)):
- (grabbed, frame) = self.stream.read()
- # if the `grabbed` boolean is `False`, then we have
- # reached the end of the video file
- if not grabbed:
- self.stop()
- return
- # process and add the frame to the queue
- inp_dim = int(opt.inp_dim)
- img_k, orig_img_k, im_dim_list_k = prep_frame(frame, inp_dim)
- inp_k = im_to_torch(orig_img_k)
-
- img.append(img_k)
- inp.append(inp_k)
- orig_img.append(orig_img_k)
- im_dim_list.append(im_dim_list_k)
-
- with torch.no_grad():
- ht = inp[0].size(1)
- wd = inp[0].size(2)
- # Human Detection
- img = Variable(torch.cat(img))
- im_dim_list = torch.FloatTensor(im_dim_list).repeat(1, 2)
- im_dim_list = im_dim_list
-
- prediction = self.det_model(img, CUDA=True)
- # NMS process
- dets = dynamic_write_results(prediction, opt.confidence,
- opt.num_classes, nms=True, nms_conf=opt.nms_thesh)
- if isinstance(dets, int) or dets.shape[0] == 0:
- for k in range(len(inp)):
- while self.Q.full():
- time.sleep(0.2)
- self.Q.put((inp[k], orig_img[k], None, None))
- continue
-
- im_dim_list = torch.index_select(im_dim_list, 0, dets[:, 0].long())
- scaling_factor = torch.min(self.det_inp_dim / im_dim_list, 1)[0].view(-1, 1)
-
- # coordinate transfer
- dets[:, [1, 3]] -= (self.det_inp_dim - scaling_factor * im_dim_list[:, 0].view(-1, 1)) / 2
- dets[:, [2, 4]] -= (self.det_inp_dim - scaling_factor * im_dim_list[:, 1].view(-1, 1)) / 2
-
- dets[:, 1:5] /= scaling_factor
- for j in range(dets.shape[0]):
- dets[j, [1, 3]] = torch.clamp(dets[j, [1, 3]], 0.0, im_dim_list[j, 0])
- dets[j, [2, 4]] = torch.clamp(dets[j, [2, 4]], 0.0, im_dim_list[j, 1])
- boxes = dets[:, 1:5].cpu()
- scores = dets[:, 5:6].cpu()
-
- for k in range(len(inp)):
- while self.Q.full():
- time.sleep(0.2)
- self.Q.put((inp[k], orig_img[k], boxes[dets[:, 0] == k], scores[dets[:, 0] == k]))
-
- def videoinfo(self):
- # indicate the video info
- fourcc = int(self.stream.get(cv2.CAP_PROP_FOURCC))
- fps = self.stream.get(cv2.CAP_PROP_FPS)
- frameSize = (int(self.stream.get(cv2.CAP_PROP_FRAME_WIDTH)), int(self.stream.get(cv2.CAP_PROP_FRAME_HEIGHT)))
- return (fourcc, fps, frameSize)
-
- def read(self):
- # return next frame in the queue
- return self.Q.get()
-
- def more(self):
- # return True if there are still frames in the queue
- return self.Q.qsize() > 0
-
- def stop(self):
- # indicate that the thread should be stopped
- self.stopped = True
-
-
-class WebcamLoader:
- def __init__(self, webcam, queueSize=256):
- # initialize the file video stream along with the boolean
- # used to indicate if the thread should be stopped or not
- self.stream = cv2.VideoCapture(int(webcam))
- assert self.stream.isOpened(), 'Cannot capture source'
- self.stopped = False
- # initialize the queue used to store frames read from
- # the video file
- self.Q = LifoQueue(maxsize=queueSize)
-
- def start(self):
- # start a thread to read frames from the file video stream
- t = Thread(target=self.update, args=())
- t.daemon = True
- t.start()
- return self
-
- def update(self):
- # keep looping infinitely
- while True:
- # otherwise, ensure the queue has room in it
- if not self.Q.full():
- # read the next frame from the file
- (grabbed, frame) = self.stream.read()
- # if the `grabbed` boolean is `False`, then we have
- # reached the end of the video file
- if not grabbed:
- self.stop()
- return
- # process and add the frame to the queue
- inp_dim = int(opt.inp_dim)
- img, orig_img, dim = prep_frame(frame, inp_dim)
- inp = im_to_torch(orig_img)
- im_dim_list = torch.FloatTensor([dim]).repeat(1, 2)
-
- self.Q.put((img, orig_img, inp, im_dim_list))
- else:
- with self.Q.mutex:
- self.Q.queue.clear()
-
- def videoinfo(self):
- # indicate the video info
- fourcc = int(self.stream.get(cv2.CAP_PROP_FOURCC))
- fps = self.stream.get(cv2.CAP_PROP_FPS)
- frameSize = (int(self.stream.get(cv2.CAP_PROP_FRAME_WIDTH)), int(self.stream.get(cv2.CAP_PROP_FRAME_HEIGHT)))
- return (fourcc, fps, frameSize)
-
- def read(self):
- # return next frame in the queue
- return self.Q.get()
-
- def len(self):
- # return queue size
- return self.Q.qsize()
-
- def stop(self):
- # indicate that the thread should be stopped
- self.stopped = True
-
-
-class DataWriter:
- def __init__(self, save_video=False,
- savepath='examples/res/1.avi', fourcc=cv2.VideoWriter_fourcc(*'XVID'), fps=25, frameSize=(640, 480),
- queueSize=1024):
- if save_video:
- # initialize the file video stream along with the boolean
- # used to indicate if the thread should be stopped or not
- self.stream = cv2.VideoWriter(savepath, fourcc, fps, frameSize)
- assert self.stream.isOpened(), 'Cannot open video for writing'
- self.save_video = save_video
- self.stopped = False
- self.final_result = []
- # initialize the queue used to store frames read from
- # the video file
- self.Q = Queue(maxsize=queueSize)
- if opt.save_img:
- if not os.path.exists(opt.outputpath + '/vis'):
- os.mkdir(opt.outputpath + '/vis')
-
- def start(self):
- # start a thread to read frames from the file video stream
- t = Thread(target=self.update, args=(), daemon=True)
- # t = Thread(target=self.update, args=())
- # t.daemon = True
- t.start()
- return self
-
- def update(self):
- # keep looping infinitely
- while True:
- # if the thread indicator variable is set, stop the
- # thread
- if self.stopped:
- if self.save_video:
- self.stream.release()
- return
- # otherwise, ensure the queue is not empty
- if not self.Q.empty():
- (boxes, scores, hm_data, pt1, pt2, orig_img, im_name) = self.Q.get()
- orig_img = np.array(orig_img, dtype=np.uint8)
- if boxes is None:
- if opt.save_img or opt.save_video or opt.vis:
- img = orig_img
- if opt.vis:
- cv2.imshow("AlphaPose Demo", img)
- cv2.waitKey(30)
- if opt.save_img:
- cv2.imwrite(os.path.join(opt.outputpath, 'vis', im_name), img)
- if opt.save_video:
- self.stream.write(img)
- else:
- # location prediction (n, kp, 2) | score prediction (n, kp, 1)
- if opt.matching:
- preds = getMultiPeakPrediction(
- hm_data, pt1.numpy(), pt2.numpy(), opt.inputResH, opt.inputResW, opt.outputResH, opt.outputResW)
- result = matching(boxes, scores.numpy(), preds)
- else:
- preds_hm, preds_img, preds_scores = getPrediction(
- hm_data, pt1, pt2, opt.inputResH, opt.inputResW, opt.outputResH, opt.outputResW)
- result = pose_nms(
- boxes, scores, preds_img, preds_scores)
- result = {
- 'imgname': im_name,
- 'result': result
- }
- self.final_result.append(result)
- if opt.save_img or opt.save_video or opt.vis:
- img = vis_frame(orig_img, result)
- if opt.vis:
- cv2.imshow("AlphaPose Demo", img)
- cv2.waitKey(30)
- if opt.save_img:
- cv2.imwrite(os.path.join(opt.outputpath, 'vis', im_name), img)
- if opt.save_video:
- self.stream.write(img)
- else:
- time.sleep(0.1)
-
- def running(self):
- # indicate that the thread is still running
- time.sleep(0.2)
- return not self.Q.empty()
-
- def save(self, boxes, scores, hm_data, pt1, pt2, orig_img, im_name):
- # save next frame in the queue
- self.Q.put((boxes, scores, hm_data, pt1, pt2, orig_img, im_name))
-
- def stop(self):
- # indicate that the thread should be stopped
- self.stopped = True
- time.sleep(0.2)
-
- def results(self):
- # return final result
- return self.final_result
-
- def len(self):
- # return queue len
- return self.Q.qsize()
-
-
-class Mscoco(data.Dataset):
- def __init__(self, train=True, sigma=1,
- scale_factor=(0.2, 0.3), rot_factor=40, label_type='Gaussian'):
- self.img_folder = '../data/coco/images' # root image folders
- self.is_train = train # training set or test set
- self.inputResH = opt.inputResH
- self.inputResW = opt.inputResW
- self.outputResH = opt.outputResH
- self.outputResW = opt.outputResW
- self.sigma = sigma
- self.scale_factor = scale_factor
- self.rot_factor = rot_factor
- self.label_type = label_type
-
- self.nJoints_coco = 17
- self.nJoints_mpii = 16
- self.nJoints = 33
-
- self.accIdxs = (1, 2, 3, 4, 5, 6, 7, 8,
- 9, 10, 11, 12, 13, 14, 15, 16, 17)
- self.flipRef = ((2, 3), (4, 5), (6, 7),
- (8, 9), (10, 11), (12, 13),
- (14, 15), (16, 17))
-
- def __getitem__(self, index):
- pass
-
- def __len__(self):
- pass
-
-
-def crop_from_dets(img, boxes, inps, pt1, pt2):
- '''
- Crop human from origin image according to Dectecion Results
- '''
-
- imght = img.size(1)
- imgwidth = img.size(2)
- tmp_img = img
- tmp_img[0].add_(-0.406)
- tmp_img[1].add_(-0.457)
- tmp_img[2].add_(-0.480)
- for i, box in enumerate(boxes):
- upLeft = torch.Tensor(
- (float(box[0]), float(box[1])))
- bottomRight = torch.Tensor(
- (float(box[2]), float(box[3])))
-
- ht = bottomRight[1] - upLeft[1]
- width = bottomRight[0] - upLeft[0]
-
- scaleRate = 0.3
-
- upLeft[0] = max(0, upLeft[0] - width * scaleRate / 2)
- upLeft[1] = max(0, upLeft[1] - ht * scaleRate / 2)
- bottomRight[0] = max(
- min(imgwidth - 1, bottomRight[0] + width * scaleRate / 2), upLeft[0] + 5)
- bottomRight[1] = max(
- min(imght - 1, bottomRight[1] + ht * scaleRate / 2), upLeft[1] + 5)
-
- try:
- inps[i] = cropBox(tmp_img.clone(), upLeft, bottomRight, opt.inputResH, opt.inputResW)
- except IndexError:
- print(tmp_img.shape)
- print(upLeft)
- print(bottomRight)
- print('===')
- pt1[i] = upLeft
- pt2[i] = bottomRight
-
- return inps, pt1, pt2
diff --git a/spaces/SeViLA/SeViLA/lavis/datasets/builders/video_qa_builder.py b/spaces/SeViLA/SeViLA/lavis/datasets/builders/video_qa_builder.py
deleted file mode 100644
index ae07df2a8e0c05540836467d3ef1a416df38d6df..0000000000000000000000000000000000000000
--- a/spaces/SeViLA/SeViLA/lavis/datasets/builders/video_qa_builder.py
+++ /dev/null
@@ -1,93 +0,0 @@
-"""
- Copyright (c) 2022, salesforce.com, inc.
- All rights reserved.
- SPDX-License-Identifier: BSD-3-Clause
- For full license text, see the LICENSE file in the repo root or https://opensource.org/licenses/BSD-3-Clause
-"""
-
-from lavis.common.registry import registry
-from lavis.common.utils import get_cache_path
-from lavis.datasets.builders.base_dataset_builder import BaseDatasetBuilder
-from lavis.datasets.datasets.video_vqa_datasets import VideoQADataset
-from lavis.datasets.datasets.mc_video_vqa_datasets import MCVideoQADataset
-
-class VideoQABuilder(BaseDatasetBuilder):
- train_dataset_cls = VideoQADataset
- eval_dataset_cls = VideoQADataset
-
- def build(self):
- datasets = super().build()
-
- ans2label = self.config.build_info.annotations.get("ans2label")
- if ans2label is None:
- raise ValueError("ans2label is not specified in build_info.")
-
- ans2label = get_cache_path(ans2label.storage)
-
- for split in datasets:
- datasets[split]._build_class_labels(ans2label)
-
- return datasets
-
-class MCVideoQABuilder(BaseDatasetBuilder):
- train_dataset_cls = MCVideoQADataset
- eval_dataset_cls = MCVideoQADataset
-
- def build(self):
- datasets = super().build()
-
- for split in datasets:
- datasets[split]._load_auxiliary_mappings()
-
- return datasets
-
-@registry.register_builder("msrvtt_qa")
-class MSRVTTQABuilder(VideoQABuilder):
- DATASET_CONFIG_DICT = {
- "default": "configs/datasets/msrvtt/defaults_qa.yaml",
- }
-
-
-@registry.register_builder("msvd_qa")
-class MSVDQABuilder(VideoQABuilder):
- DATASET_CONFIG_DICT = {
- "default": "configs/datasets/msvd/defaults_qa.yaml",
- }
-
-# multi-choice videoqa
-@registry.register_builder("nextqa")
-class NextQABuilder(MCVideoQABuilder):
- DATASET_CONFIG_DICT = {
- "default": "configs/datasets/nextqa/defaults_qa.yaml",
- }
-@registry.register_builder("star")
-class STARBuilder(MCVideoQABuilder):
- DATASET_CONFIG_DICT = {
- "default": "configs/datasets/star/defaults_qa.yaml",
- }
-
-@registry.register_builder("tvqa")
-class TVQABuilder(MCVideoQABuilder):
- DATASET_CONFIG_DICT = {
- "default": "configs/datasets/tvqa/defaults_qa.yaml",
- }
-
-@registry.register_builder("how2qa")
-class How2QABuilder(MCVideoQABuilder):
- DATASET_CONFIG_DICT = {
- "default": "configs/datasets/how2qa/defaults_qa.yaml",
- }
-
-@registry.register_builder("vlep")
-class VLEPBuilder(MCVideoQABuilder):
- DATASET_CONFIG_DICT = {
- "default": "configs/datasets/vlep/defaults_qa.yaml",
- }
-
-@registry.register_builder("qvh")
-class QVHBuilder(MCVideoQABuilder):
- DATASET_CONFIG_DICT = {
- "default": "configs/datasets/qvh/defaults.yaml",
- }
-
-# open-ended QA
\ No newline at end of file
diff --git a/spaces/SeViLA/SeViLA/lavis/datasets/datasets/multimodal_classification_datasets.py b/spaces/SeViLA/SeViLA/lavis/datasets/datasets/multimodal_classification_datasets.py
deleted file mode 100644
index 152e097995b5afd5bcad95a1f6df60b895300ac8..0000000000000000000000000000000000000000
--- a/spaces/SeViLA/SeViLA/lavis/datasets/datasets/multimodal_classification_datasets.py
+++ /dev/null
@@ -1,25 +0,0 @@
-"""
- Copyright (c) 2022, salesforce.com, inc.
- All rights reserved.
- SPDX-License-Identifier: BSD-3-Clause
- For full license text, see the LICENSE file in the repo root or https://opensource.org/licenses/BSD-3-Clause
-"""
-
-from abc import abstractmethod
-from lavis.datasets.datasets.base_dataset import BaseDataset
-
-
-class MultimodalClassificationDataset(BaseDataset):
- def __init__(self, vis_processor, text_processor, vis_root, ann_paths):
- super().__init__(vis_processor, text_processor, vis_root, ann_paths)
-
- self.class_labels = None
-
- @abstractmethod
- def _build_class_labels(self):
- pass
-
- @abstractmethod
- def _load_auxiliary_mappings(self):
- pass
-
diff --git a/spaces/SrRaptor/Imagy/README.md b/spaces/SrRaptor/Imagy/README.md
deleted file mode 100644
index 4ac080a5d5d15f938ebdc5a23d59c8c1bcd2ee13..0000000000000000000000000000000000000000
--- a/spaces/SrRaptor/Imagy/README.md
+++ /dev/null
@@ -1,22 +0,0 @@
----
-title: Sheet Music Generator
-emoji: 🎵
-colorFrom: red
-colorTo: gray
-sdk: gradio
-sdk_version: 3.0.26
-app_file: app.py
-pinned: false
-duplicated_from: visakh7843/Sheet_Music_Generator
----
-
-# Sheet-music-generator-for-Sight-Reading
-Sheet Music generation for easier sight reading practice for musicians.
-Musicians often struggle at finding new sheet music to practice sight reading. Finding new sheet music to practice is particularly important as musicians playing same melodies over and over unknowingly memorize it, which would defeat the purpose of practising to sight-reading. Hence to practice sight-reading for musician, it is crucial to get new and unseen sheet music every now and then which is difficult.
-This project aims at developing a probabilistic algorithm (Markov Model) for generating music of appropriate complexity, in a range of appropriate keys and tempos. Markov models were chosen over deep learning models due to their overhead of required resources which didn’t give a considerable advantage over Markov Models. As a secondary objective we will also explore the possibility of generating music specific to different instruments taking into account the limitation of specific instruments.
-
-
-How to run the project:
-```sh
-python app.py
-```
diff --git a/spaces/SungBeom/chatwine-korean/.venv/Lib/site-packages/anyio/abc/__init__.py b/spaces/SungBeom/chatwine-korean/.venv/Lib/site-packages/anyio/abc/__init__.py
deleted file mode 100644
index 72c34e544e1634e4f42c005506bac9b61ab095f5..0000000000000000000000000000000000000000
--- a/spaces/SungBeom/chatwine-korean/.venv/Lib/site-packages/anyio/abc/__init__.py
+++ /dev/null
@@ -1,90 +0,0 @@
-from __future__ import annotations
-
-__all__ = (
- "AsyncResource",
- "IPAddressType",
- "IPSockAddrType",
- "SocketAttribute",
- "SocketStream",
- "SocketListener",
- "UDPSocket",
- "UNIXSocketStream",
- "UDPPacketType",
- "ConnectedUDPSocket",
- "UnreliableObjectReceiveStream",
- "UnreliableObjectSendStream",
- "UnreliableObjectStream",
- "ObjectReceiveStream",
- "ObjectSendStream",
- "ObjectStream",
- "ByteReceiveStream",
- "ByteSendStream",
- "ByteStream",
- "AnyUnreliableByteReceiveStream",
- "AnyUnreliableByteSendStream",
- "AnyUnreliableByteStream",
- "AnyByteReceiveStream",
- "AnyByteSendStream",
- "AnyByteStream",
- "Listener",
- "Process",
- "Event",
- "Condition",
- "Lock",
- "Semaphore",
- "CapacityLimiter",
- "CancelScope",
- "TaskGroup",
- "TaskStatus",
- "TestRunner",
- "BlockingPortal",
-)
-
-from typing import Any
-
-from ._resources import AsyncResource
-from ._sockets import (
- ConnectedUDPSocket,
- IPAddressType,
- IPSockAddrType,
- SocketAttribute,
- SocketListener,
- SocketStream,
- UDPPacketType,
- UDPSocket,
- UNIXSocketStream,
-)
-from ._streams import (
- AnyByteReceiveStream,
- AnyByteSendStream,
- AnyByteStream,
- AnyUnreliableByteReceiveStream,
- AnyUnreliableByteSendStream,
- AnyUnreliableByteStream,
- ByteReceiveStream,
- ByteSendStream,
- ByteStream,
- Listener,
- ObjectReceiveStream,
- ObjectSendStream,
- ObjectStream,
- UnreliableObjectReceiveStream,
- UnreliableObjectSendStream,
- UnreliableObjectStream,
-)
-from ._subprocesses import Process
-from ._tasks import TaskGroup, TaskStatus
-from ._testing import TestRunner
-
-# Re-exported here, for backwards compatibility
-# isort: off
-from .._core._synchronization import CapacityLimiter, Condition, Event, Lock, Semaphore
-from .._core._tasks import CancelScope
-from ..from_thread import BlockingPortal
-
-# Re-export imports so they look like they live directly in this package
-key: str
-value: Any
-for key, value in list(locals().items()):
- if getattr(value, "__module__", "").startswith("anyio.abc."):
- value.__module__ = __name__
diff --git a/spaces/SungBeom/chatwine-korean/.venv/Lib/site-packages/attr/_next_gen.py b/spaces/SungBeom/chatwine-korean/.venv/Lib/site-packages/attr/_next_gen.py
deleted file mode 100644
index 8f7c0b9a46b7a0ee008f94b8054baf5807df043a..0000000000000000000000000000000000000000
--- a/spaces/SungBeom/chatwine-korean/.venv/Lib/site-packages/attr/_next_gen.py
+++ /dev/null
@@ -1,232 +0,0 @@
-# SPDX-License-Identifier: MIT
-
-"""
-These are keyword-only APIs that call `attr.s` and `attr.ib` with different
-default values.
-"""
-
-
-from functools import partial
-
-from . import setters
-from ._funcs import asdict as _asdict
-from ._funcs import astuple as _astuple
-from ._make import (
- NOTHING,
- _frozen_setattrs,
- _ng_default_on_setattr,
- attrib,
- attrs,
-)
-from .exceptions import UnannotatedAttributeError
-
-
-def define(
- maybe_cls=None,
- *,
- these=None,
- repr=None,
- unsafe_hash=None,
- hash=None,
- init=None,
- slots=True,
- frozen=False,
- weakref_slot=True,
- str=False,
- auto_attribs=None,
- kw_only=False,
- cache_hash=False,
- auto_exc=True,
- eq=None,
- order=False,
- auto_detect=True,
- getstate_setstate=None,
- on_setattr=None,
- field_transformer=None,
- match_args=True,
-):
- r"""
- Define an *attrs* class.
-
- Differences to the classic `attr.s` that it uses underneath:
-
- - Automatically detect whether or not *auto_attribs* should be `True` (c.f.
- *auto_attribs* parameter).
- - If *frozen* is `False`, run converters and validators when setting an
- attribute by default.
- - *slots=True*
-
- .. caution::
-
- Usually this has only upsides and few visible effects in everyday
- programming. But it *can* lead to some suprising behaviors, so please
- make sure to read :term:`slotted classes`.
- - *auto_exc=True*
- - *auto_detect=True*
- - *order=False*
- - Some options that were only relevant on Python 2 or were kept around for
- backwards-compatibility have been removed.
-
- Please note that these are all defaults and you can change them as you
- wish.
-
- :param Optional[bool] auto_attribs: If set to `True` or `False`, it behaves
- exactly like `attr.s`. If left `None`, `attr.s` will try to guess:
-
- 1. If any attributes are annotated and no unannotated `attrs.fields`\ s
- are found, it assumes *auto_attribs=True*.
- 2. Otherwise it assumes *auto_attribs=False* and tries to collect
- `attrs.fields`\ s.
-
- For now, please refer to `attr.s` for the rest of the parameters.
-
- .. versionadded:: 20.1.0
- .. versionchanged:: 21.3.0 Converters are also run ``on_setattr``.
- .. versionadded:: 22.2.0
- *unsafe_hash* as an alias for *hash* (for :pep:`681` compliance).
- """
-
- def do_it(cls, auto_attribs):
- return attrs(
- maybe_cls=cls,
- these=these,
- repr=repr,
- hash=hash,
- unsafe_hash=unsafe_hash,
- init=init,
- slots=slots,
- frozen=frozen,
- weakref_slot=weakref_slot,
- str=str,
- auto_attribs=auto_attribs,
- kw_only=kw_only,
- cache_hash=cache_hash,
- auto_exc=auto_exc,
- eq=eq,
- order=order,
- auto_detect=auto_detect,
- collect_by_mro=True,
- getstate_setstate=getstate_setstate,
- on_setattr=on_setattr,
- field_transformer=field_transformer,
- match_args=match_args,
- )
-
- def wrap(cls):
- """
- Making this a wrapper ensures this code runs during class creation.
-
- We also ensure that frozen-ness of classes is inherited.
- """
- nonlocal frozen, on_setattr
-
- had_on_setattr = on_setattr not in (None, setters.NO_OP)
-
- # By default, mutable classes convert & validate on setattr.
- if frozen is False and on_setattr is None:
- on_setattr = _ng_default_on_setattr
-
- # However, if we subclass a frozen class, we inherit the immutability
- # and disable on_setattr.
- for base_cls in cls.__bases__:
- if base_cls.__setattr__ is _frozen_setattrs:
- if had_on_setattr:
- raise ValueError(
- "Frozen classes can't use on_setattr "
- "(frozen-ness was inherited)."
- )
-
- on_setattr = setters.NO_OP
- break
-
- if auto_attribs is not None:
- return do_it(cls, auto_attribs)
-
- try:
- return do_it(cls, True)
- except UnannotatedAttributeError:
- return do_it(cls, False)
-
- # maybe_cls's type depends on the usage of the decorator. It's a class
- # if it's used as `@attrs` but ``None`` if used as `@attrs()`.
- if maybe_cls is None:
- return wrap
- else:
- return wrap(maybe_cls)
-
-
-mutable = define
-frozen = partial(define, frozen=True, on_setattr=None)
-
-
-def field(
- *,
- default=NOTHING,
- validator=None,
- repr=True,
- hash=None,
- init=True,
- metadata=None,
- type=None,
- converter=None,
- factory=None,
- kw_only=False,
- eq=None,
- order=None,
- on_setattr=None,
- alias=None,
-):
- """
- Identical to `attr.ib`, except keyword-only and with some arguments
- removed.
-
- .. versionadded:: 23.1.0
- The *type* parameter has been re-added; mostly for
- {func}`attrs.make_class`. Please note that type checkers ignore this
- metadata.
- .. versionadded:: 20.1.0
- """
- return attrib(
- default=default,
- validator=validator,
- repr=repr,
- hash=hash,
- init=init,
- metadata=metadata,
- type=type,
- converter=converter,
- factory=factory,
- kw_only=kw_only,
- eq=eq,
- order=order,
- on_setattr=on_setattr,
- alias=alias,
- )
-
-
-def asdict(inst, *, recurse=True, filter=None, value_serializer=None):
- """
- Same as `attr.asdict`, except that collections types are always retained
- and dict is always used as *dict_factory*.
-
- .. versionadded:: 21.3.0
- """
- return _asdict(
- inst=inst,
- recurse=recurse,
- filter=filter,
- value_serializer=value_serializer,
- retain_collection_types=True,
- )
-
-
-def astuple(inst, *, recurse=True, filter=None):
- """
- Same as `attr.astuple`, except that collections types are always retained
- and `tuple` is always used as the *tuple_factory*.
-
- .. versionadded:: 21.3.0
- """
- return _astuple(
- inst=inst, recurse=recurse, filter=filter, retain_collection_types=True
- )
diff --git a/spaces/SungBeom/chatwine-korean/.venv/Lib/site-packages/clickhouse_connect/driver/types.py b/spaces/SungBeom/chatwine-korean/.venv/Lib/site-packages/clickhouse_connect/driver/types.py
deleted file mode 100644
index 015e162fbea9c8c5c4f93b4759b6dafab462ad1b..0000000000000000000000000000000000000000
--- a/spaces/SungBeom/chatwine-korean/.venv/Lib/site-packages/clickhouse_connect/driver/types.py
+++ /dev/null
@@ -1,50 +0,0 @@
-from abc import ABC, abstractmethod
-from typing import Sequence, Any
-
-Matrix = Sequence[Sequence[Any]]
-
-
-class Closable(ABC):
- @abstractmethod
- def close(self):
- pass
-
-
-class ByteSource(Closable):
- last_message = None
-
- @abstractmethod
- def read_leb128(self) -> int:
- pass
-
- @abstractmethod
- def read_leb128_str(self) -> str:
- pass
-
- @abstractmethod
- def read_uint64(self) -> int:
- pass
-
- @abstractmethod
- def read_bytes(self, sz: int) -> bytes:
- pass
-
- @abstractmethod
- def read_str_col(self, num_rows: int, encoding: str, nullable: bool = False, null_obj: Any = None):
- pass
-
- @abstractmethod
- def read_bytes_col(self, sz: int, num_rows: int):
- pass
-
- @abstractmethod
- def read_fixed_str_col(self, sz: int, num_rows: int, encoding: str):
- pass
-
- @abstractmethod
- def read_array(self, array_type: str, num_rows: int):
- pass
-
- @abstractmethod
- def read_byte(self) -> int:
- pass
diff --git a/spaces/SungBeom/chatwine-korean/.venv/Lib/site-packages/debugpy/_vendored/pydevd/pydev_ipython/inputhookgtk3.py b/spaces/SungBeom/chatwine-korean/.venv/Lib/site-packages/debugpy/_vendored/pydevd/pydev_ipython/inputhookgtk3.py
deleted file mode 100644
index f2ca39f390034797e460e89503c3cf2422412baf..0000000000000000000000000000000000000000
--- a/spaces/SungBeom/chatwine-korean/.venv/Lib/site-packages/debugpy/_vendored/pydevd/pydev_ipython/inputhookgtk3.py
+++ /dev/null
@@ -1,35 +0,0 @@
-# encoding: utf-8
-"""
-Enable Gtk3 to be used interacive by IPython.
-
-Authors: Thomi Richards
-"""
-#-----------------------------------------------------------------------------
-# Copyright (c) 2012, the IPython Development Team.
-#
-# Distributed under the terms of the Modified BSD License.
-#
-# The full license is in the file COPYING.txt, distributed with this software.
-#-----------------------------------------------------------------------------
-
-#-----------------------------------------------------------------------------
-# Imports
-#-----------------------------------------------------------------------------
-
-from gi.repository import Gtk, GLib # @UnresolvedImport
-
-#-----------------------------------------------------------------------------
-# Code
-#-----------------------------------------------------------------------------
-
-def _main_quit(*args, **kwargs):
- Gtk.main_quit()
- return False
-
-
-def create_inputhook_gtk3(stdin_file):
- def inputhook_gtk3():
- GLib.io_add_watch(stdin_file, GLib.IO_IN, _main_quit)
- Gtk.main()
- return 0
- return inputhook_gtk3
diff --git a/spaces/Suniilkumaar/MusicGen-updated/audiocraft/modules/conv.py b/spaces/Suniilkumaar/MusicGen-updated/audiocraft/modules/conv.py
deleted file mode 100644
index 972938ab84712eb06e1b10cea25444eee51d6637..0000000000000000000000000000000000000000
--- a/spaces/Suniilkumaar/MusicGen-updated/audiocraft/modules/conv.py
+++ /dev/null
@@ -1,245 +0,0 @@
-# Copyright (c) Meta Platforms, Inc. and affiliates.
-# All rights reserved.
-#
-# This source code is licensed under the license found in the
-# LICENSE file in the root directory of this source tree.
-
-import math
-import typing as tp
-import warnings
-
-import torch
-from torch import nn
-from torch.nn import functional as F
-from torch.nn.utils import spectral_norm, weight_norm
-
-
-CONV_NORMALIZATIONS = frozenset(['none', 'weight_norm', 'spectral_norm',
- 'time_group_norm'])
-
-
-def apply_parametrization_norm(module: nn.Module, norm: str = 'none'):
- assert norm in CONV_NORMALIZATIONS
- if norm == 'weight_norm':
- return weight_norm(module)
- elif norm == 'spectral_norm':
- return spectral_norm(module)
- else:
- # We already check was in CONV_NORMALIZATION, so any other choice
- # doesn't need reparametrization.
- return module
-
-
-def get_norm_module(module: nn.Module, causal: bool = False, norm: str = 'none', **norm_kwargs):
- """Return the proper normalization module. If causal is True, this will ensure the returned
- module is causal, or return an error if the normalization doesn't support causal evaluation.
- """
- assert norm in CONV_NORMALIZATIONS
- if norm == 'time_group_norm':
- if causal:
- raise ValueError("GroupNorm doesn't support causal evaluation.")
- assert isinstance(module, nn.modules.conv._ConvNd)
- return nn.GroupNorm(1, module.out_channels, **norm_kwargs)
- else:
- return nn.Identity()
-
-
-def get_extra_padding_for_conv1d(x: torch.Tensor, kernel_size: int, stride: int,
- padding_total: int = 0) -> int:
- """See `pad_for_conv1d`.
- """
- length = x.shape[-1]
- n_frames = (length - kernel_size + padding_total) / stride + 1
- ideal_length = (math.ceil(n_frames) - 1) * stride + (kernel_size - padding_total)
- return ideal_length - length
-
-
-def pad_for_conv1d(x: torch.Tensor, kernel_size: int, stride: int, padding_total: int = 0):
- """Pad for a convolution to make sure that the last window is full.
- Extra padding is added at the end. This is required to ensure that we can rebuild
- an output of the same length, as otherwise, even with padding, some time steps
- might get removed.
- For instance, with total padding = 4, kernel size = 4, stride = 2:
- 0 0 1 2 3 4 5 0 0 # (0s are padding)
- 1 2 3 # (output frames of a convolution, last 0 is never used)
- 0 0 1 2 3 4 5 0 # (output of tr. conv., but pos. 5 is going to get removed as padding)
- 1 2 3 4 # once you removed padding, we are missing one time step !
- """
- extra_padding = get_extra_padding_for_conv1d(x, kernel_size, stride, padding_total)
- return F.pad(x, (0, extra_padding))
-
-
-def pad1d(x: torch.Tensor, paddings: tp.Tuple[int, int], mode: str = 'constant', value: float = 0.):
- """Tiny wrapper around F.pad, just to allow for reflect padding on small input.
- If this is the case, we insert extra 0 padding to the right before the reflection happen.
- """
- length = x.shape[-1]
- padding_left, padding_right = paddings
- assert padding_left >= 0 and padding_right >= 0, (padding_left, padding_right)
- if mode == 'reflect':
- max_pad = max(padding_left, padding_right)
- extra_pad = 0
- if length <= max_pad:
- extra_pad = max_pad - length + 1
- x = F.pad(x, (0, extra_pad))
- padded = F.pad(x, paddings, mode, value)
- end = padded.shape[-1] - extra_pad
- return padded[..., :end]
- else:
- return F.pad(x, paddings, mode, value)
-
-
-def unpad1d(x: torch.Tensor, paddings: tp.Tuple[int, int]):
- """Remove padding from x, handling properly zero padding. Only for 1d!
- """
- padding_left, padding_right = paddings
- assert padding_left >= 0 and padding_right >= 0, (padding_left, padding_right)
- assert (padding_left + padding_right) <= x.shape[-1]
- end = x.shape[-1] - padding_right
- return x[..., padding_left: end]
-
-
-class NormConv1d(nn.Module):
- """Wrapper around Conv1d and normalization applied to this conv
- to provide a uniform interface across normalization approaches.
- """
- def __init__(self, *args, causal: bool = False, norm: str = 'none',
- norm_kwargs: tp.Dict[str, tp.Any] = {}, **kwargs):
- super().__init__()
- self.conv = apply_parametrization_norm(nn.Conv1d(*args, **kwargs), norm)
- self.norm = get_norm_module(self.conv, causal, norm, **norm_kwargs)
- self.norm_type = norm
-
- def forward(self, x):
- x = self.conv(x)
- x = self.norm(x)
- return x
-
-
-class NormConv2d(nn.Module):
- """Wrapper around Conv2d and normalization applied to this conv
- to provide a uniform interface across normalization approaches.
- """
- def __init__(self, *args, norm: str = 'none', norm_kwargs: tp.Dict[str, tp.Any] = {}, **kwargs):
- super().__init__()
- self.conv = apply_parametrization_norm(nn.Conv2d(*args, **kwargs), norm)
- self.norm = get_norm_module(self.conv, causal=False, norm=norm, **norm_kwargs)
- self.norm_type = norm
-
- def forward(self, x):
- x = self.conv(x)
- x = self.norm(x)
- return x
-
-
-class NormConvTranspose1d(nn.Module):
- """Wrapper around ConvTranspose1d and normalization applied to this conv
- to provide a uniform interface across normalization approaches.
- """
- def __init__(self, *args, causal: bool = False, norm: str = 'none',
- norm_kwargs: tp.Dict[str, tp.Any] = {}, **kwargs):
- super().__init__()
- self.convtr = apply_parametrization_norm(nn.ConvTranspose1d(*args, **kwargs), norm)
- self.norm = get_norm_module(self.convtr, causal, norm, **norm_kwargs)
- self.norm_type = norm
-
- def forward(self, x):
- x = self.convtr(x)
- x = self.norm(x)
- return x
-
-
-class NormConvTranspose2d(nn.Module):
- """Wrapper around ConvTranspose2d and normalization applied to this conv
- to provide a uniform interface across normalization approaches.
- """
- def __init__(self, *args, norm: str = 'none', norm_kwargs: tp.Dict[str, tp.Any] = {}, **kwargs):
- super().__init__()
- self.convtr = apply_parametrization_norm(nn.ConvTranspose2d(*args, **kwargs), norm)
- self.norm = get_norm_module(self.convtr, causal=False, norm=norm, **norm_kwargs)
-
- def forward(self, x):
- x = self.convtr(x)
- x = self.norm(x)
- return x
-
-
-class StreamableConv1d(nn.Module):
- """Conv1d with some builtin handling of asymmetric or causal padding
- and normalization.
- """
- def __init__(self, in_channels: int, out_channels: int,
- kernel_size: int, stride: int = 1, dilation: int = 1,
- groups: int = 1, bias: bool = True, causal: bool = False,
- norm: str = 'none', norm_kwargs: tp.Dict[str, tp.Any] = {},
- pad_mode: str = 'reflect'):
- super().__init__()
- # warn user on unusual setup between dilation and stride
- if stride > 1 and dilation > 1:
- warnings.warn('StreamableConv1d has been initialized with stride > 1 and dilation > 1'
- f' (kernel_size={kernel_size} stride={stride}, dilation={dilation}).')
- self.conv = NormConv1d(in_channels, out_channels, kernel_size, stride,
- dilation=dilation, groups=groups, bias=bias, causal=causal,
- norm=norm, norm_kwargs=norm_kwargs)
- self.causal = causal
- self.pad_mode = pad_mode
-
- def forward(self, x):
- B, C, T = x.shape
- kernel_size = self.conv.conv.kernel_size[0]
- stride = self.conv.conv.stride[0]
- dilation = self.conv.conv.dilation[0]
- kernel_size = (kernel_size - 1) * dilation + 1 # effective kernel size with dilations
- padding_total = kernel_size - stride
- extra_padding = get_extra_padding_for_conv1d(x, kernel_size, stride, padding_total)
- if self.causal:
- # Left padding for causal
- x = pad1d(x, (padding_total, extra_padding), mode=self.pad_mode)
- else:
- # Asymmetric padding required for odd strides
- padding_right = padding_total // 2
- padding_left = padding_total - padding_right
- x = pad1d(x, (padding_left, padding_right + extra_padding), mode=self.pad_mode)
- return self.conv(x)
-
-
-class StreamableConvTranspose1d(nn.Module):
- """ConvTranspose1d with some builtin handling of asymmetric or causal padding
- and normalization.
- """
- def __init__(self, in_channels: int, out_channels: int,
- kernel_size: int, stride: int = 1, causal: bool = False,
- norm: str = 'none', trim_right_ratio: float = 1.,
- norm_kwargs: tp.Dict[str, tp.Any] = {}):
- super().__init__()
- self.convtr = NormConvTranspose1d(in_channels, out_channels, kernel_size, stride,
- causal=causal, norm=norm, norm_kwargs=norm_kwargs)
- self.causal = causal
- self.trim_right_ratio = trim_right_ratio
- assert self.causal or self.trim_right_ratio == 1., \
- "`trim_right_ratio` != 1.0 only makes sense for causal convolutions"
- assert self.trim_right_ratio >= 0. and self.trim_right_ratio <= 1.
-
- def forward(self, x):
- kernel_size = self.convtr.convtr.kernel_size[0]
- stride = self.convtr.convtr.stride[0]
- padding_total = kernel_size - stride
-
- y = self.convtr(x)
-
- # We will only trim fixed padding. Extra padding from `pad_for_conv1d` would be
- # removed at the very end, when keeping only the right length for the output,
- # as removing it here would require also passing the length at the matching layer
- # in the encoder.
- if self.causal:
- # Trim the padding on the right according to the specified ratio
- # if trim_right_ratio = 1.0, trim everything from right
- padding_right = math.ceil(padding_total * self.trim_right_ratio)
- padding_left = padding_total - padding_right
- y = unpad1d(y, (padding_left, padding_right))
- else:
- # Asymmetric padding required for odd strides
- padding_right = padding_total // 2
- padding_left = padding_total - padding_right
- y = unpad1d(y, (padding_left, padding_right))
- return y
diff --git a/spaces/TIMBOVILL/RVC-Noobie/i18n/locale_diff.py b/spaces/TIMBOVILL/RVC-Noobie/i18n/locale_diff.py
deleted file mode 100644
index 257277965e0866a86d0361863a8f1b408c4f71ab..0000000000000000000000000000000000000000
--- a/spaces/TIMBOVILL/RVC-Noobie/i18n/locale_diff.py
+++ /dev/null
@@ -1,45 +0,0 @@
-import json
-import os
-from collections import OrderedDict
-
-# Define the standard file name
-standard_file = "zh_CN.json"
-
-# Find all JSON files in the directory
-dir_path = "./"
-languages = [
- f for f in os.listdir(dir_path) if f.endswith(".json") and f != standard_file
-]
-
-# Load the standard file
-with open(standard_file, "r", encoding="utf-8") as f:
- standard_data = json.load(f, object_pairs_hook=OrderedDict)
-
-# Loop through each language file
-for lang_file in languages:
- # Load the language file
- with open(lang_file, "r", encoding="utf-8") as f:
- lang_data = json.load(f, object_pairs_hook=OrderedDict)
-
- # Find the difference between the language file and the standard file
- diff = set(standard_data.keys()) - set(lang_data.keys())
-
- miss = set(lang_data.keys()) - set(standard_data.keys())
-
- # Add any missing keys to the language file
- for key in diff:
- lang_data[key] = key
-
- # Del any extra keys to the language file
- for key in miss:
- del lang_data[key]
-
- # Sort the keys of the language file to match the order of the standard file
- lang_data = OrderedDict(
- sorted(lang_data.items(), key=lambda x: list(standard_data.keys()).index(x[0]))
- )
-
- # Save the updated language file
- with open(lang_file, "w", encoding="utf-8") as f:
- json.dump(lang_data, f, ensure_ascii=False, indent=4)
- f.write("\n")
diff --git a/spaces/TabPFN/TabPFNPrediction/TabPFN/scripts/tabular_evaluation.py b/spaces/TabPFN/TabPFNPrediction/TabPFN/scripts/tabular_evaluation.py
deleted file mode 100644
index c761aee2bffbf441a8c5c33bc4ded07f915e15a3..0000000000000000000000000000000000000000
--- a/spaces/TabPFN/TabPFNPrediction/TabPFN/scripts/tabular_evaluation.py
+++ /dev/null
@@ -1,312 +0,0 @@
-import time
-import os
-from pathlib import Path
-from contextlib import nullcontext
-
-import torch
-from tqdm import tqdm
-import random
-import numpy as np
-
-from torch import nn
-
-from torch.utils.checkpoint import checkpoint
-from utils import normalize_data, torch_nanmean, to_ranking_low_mem, remove_outliers
-from scripts.tabular_baselines import get_scoring_string
-from scripts import tabular_metrics
-from scripts.transformer_prediction_interface import *
-from scripts.baseline_prediction_interface import *
-"""
-===============================
-PUBLIC FUNCTIONS FOR EVALUATION
-===============================
-"""
-
-
-def eval_model(i, e, valid_datasets, test_datasets, eval_positions, bptt, add_name, base_path, device='cpu', eval_addition='', **kwargs):
- metrics_test, config_sample, model_path = eval_model_on_ds(i, e, test_datasets, eval_positions, bptt, add_name, base_path, device=device, eval_addition=eval_addition, **kwargs)
- metrics_valid, _, _ = eval_model_on_ds(i, e, valid_datasets, eval_positions, bptt, add_name, base_path, device=device, eval_addition=eval_addition, **kwargs)
- return {'mean_auc_test': metrics_test['mean_roc_at_1000'], 'mean_auc_valid': metrics_valid['mean_roc_at_1000'], 'mean_ce_test': metrics_test['mean_ce_at_1000'], 'mean_ce_valid': metrics_valid['mean_ce_at_1000'], 'config_sample': config_sample, 'model_path': model_path}
-
-def eval_model_on_ds(i, e, valid_datasets, eval_positions, bptt, add_name, base_path, device='cpu', eval_addition='', **kwargs):
-
- # How to use: evaluate_without_fitting(i,0,valid_datasets, [1024], 100000, add_name=model_string, base_path=base_path,)
- def check_file(e):
- model_file = f'models_diff/prior_diff_real_checkpoint{add_name}_n_{i}_epoch_{e}.cpkt'
- model_path = os.path.join(base_path, model_file)
- # print('Evaluate ', model_path)
- results_file = os.path.join(base_path,
- f'models_diff/prior_diff_real_results{add_name}_n_{i}_epoch_{e}_{eval_addition}.pkl')
- if not Path(model_path).is_file(): # or Path(results_file).is_file():
- # print('checkpoint exists: ', Path(model_file).is_file(), ', results are written:', Path(results_file).is_file())
- return None, None, None
- return model_file, model_path, results_file
-
- if e == -1: # use last checkpoint, if e == -1
- for e_ in range(100, -1, -1):
- model_file_, model_path_, results_file_ = check_file(e_)
- if model_file_ is not None:
- e = e_
- model_file, model_path, results_file = model_file_, model_path_, results_file_
- break
- else:
- model_file, model_path, results_file = check_file(e)
-
- model, config_sample = load_model(base_path, model_file, device, None, verbose=False)
-
- params = {'max_features': config_sample['num_features']
- , 'rescale_features': config_sample["normalize_by_used_features"]
- , 'normalize_to_ranking': config_sample["normalize_to_ranking"]
- , 'normalize_with_sqrt': config_sample.get("normalize_with_sqrt", False)
- }
- metrics_valid = evaluate(datasets=valid_datasets, model=model[2], method='transformer', device=device, overwrite=True,
- extend_features=True
- # just removed the style keyword but transformer is trained with style, just empty
- , save=False
- , metric_used=tabular_metrics.cross_entropy
- , return_tensor=True
- , verbose=False
- , eval_positions=eval_positions
- , bptt=bptt
- , base_path=None
- , inference_mode=True
- , **params
- , **kwargs)
-
- tabular_metrics.calculate_score_per_method(tabular_metrics.auc_metric, 'roc', metrics_valid, valid_datasets, eval_positions)
- tabular_metrics.calculate_score_per_method(tabular_metrics.cross_entropy, 'ce', metrics_valid, valid_datasets, eval_positions)
-
- return metrics_valid, config_sample, model_path
-
-
-def evaluate(datasets, bptt, eval_positions, metric_used, model, device='cpu'
- , verbose=False
- , return_tensor=False
- , **kwargs):
- """
- Evaluates a list of datasets for a model function.
-
- :param datasets: List of datasets
- :param bptt: maximum sequence length
- :param eval_positions: List of positions where to evaluate models
- :param verbose: If True, is verbose.
- :param metric_used: Which metric is optimized for.
- :param return_tensor: Wheater to return results as a pytorch.tensor or numpy, this is only relevant for transformer.
- :param kwargs:
- :return:
- """
- overall_result = {'metric_used': get_scoring_string(metric_used)
- , 'bptt': bptt
- , 'eval_positions': eval_positions}
-
- aggregated_metric_datasets, num_datasets = torch.tensor(0.0), 0
-
- # For each dataset
- for [ds_name, X, y, categorical_feats, _, _] in datasets:
- dataset_bptt = min(len(X), bptt)
- #if verbose and dataset_bptt < bptt:
- # print(f'Dataset too small for given bptt, reducing to {len(X)} ({bptt})')
-
- aggregated_metric, num = torch.tensor(0.0), 0
- ds_result = {}
-
- for eval_position in (eval_positions if verbose else eval_positions):
- eval_position_real = int(dataset_bptt * 0.5) if 2 * eval_position > dataset_bptt else eval_position
- eval_position_bptt = int(eval_position_real * 2.0)
-
- r = evaluate_position(X, y, model=model
- , num_classes=len(torch.unique(y))
- , categorical_feats = categorical_feats
- , bptt = eval_position_bptt
- , ds_name=ds_name
- , eval_position = eval_position_real
- , metric_used = metric_used
- , device=device
- ,**kwargs)
-
- if r is None:
- print('Execution failed')
- continue
-
- _, outputs, ys, best_configs, time_used = r
-
- if torch.is_tensor(outputs):
- outputs = outputs.to(outputs.device)
- ys = ys.to(outputs.device)
-
- # WARNING: This leaks information on the scaling of the labels
- if isinstance(model, nn.Module) and "BarDistribution" in str(type(model.criterion)):
- ys = (ys - torch.min(ys, axis=0)[0]) / (torch.max(ys, axis=0)[0] - torch.min(ys, axis=0)[0])
-
- # If we use the bar distribution and the metric_used is r2 -> convert buckets
- # metric used is prob -> keep
- if isinstance(model, nn.Module) and "BarDistribution" in str(type(model.criterion)) and (
- metric_used == tabular_metrics.r2_metric or metric_used == tabular_metrics.root_mean_squared_error_metric):
- ds_result[f'{ds_name}_bar_dist_at_{eval_position}'] = outputs
- outputs = model.criterion.mean(outputs)
-
- ys = ys.T
- ds_result[f'{ds_name}_best_configs_at_{eval_position}'] = best_configs
- ds_result[f'{ds_name}_outputs_at_{eval_position}'] = outputs
- ds_result[f'{ds_name}_ys_at_{eval_position}'] = ys
- ds_result[f'{ds_name}_time_at_{eval_position}'] = time_used
-
- new_metric = torch_nanmean(torch.stack([metric_used(ys[i], outputs[i]) for i in range(ys.shape[0])]))
-
- if not return_tensor:
- make_scalar = lambda x: float(x.detach().cpu().numpy()) if (torch.is_tensor(x) and (len(x.shape) == 0)) else x
- new_metric = make_scalar(new_metric)
- ds_result = {k: make_scalar(ds_result[k]) for k in ds_result.keys()}
-
- lib = torch if return_tensor else np
- if not lib.isnan(new_metric).any():
- aggregated_metric, num = aggregated_metric + new_metric, num + 1
-
- overall_result.update(ds_result)
- if num > 0:
- aggregated_metric_datasets, num_datasets = (aggregated_metric_datasets + (aggregated_metric / num)), num_datasets + 1
-
- overall_result['mean_metric'] = aggregated_metric_datasets / num_datasets
-
- return overall_result
-
-"""
-===============================
-INTERNAL HELPER FUNCTIONS
-===============================
-"""
-
-def check_file_exists(path):
- """Checks if a pickle file exists. Returns None if not, else returns the unpickled file."""
- if (os.path.isfile(path)):
- print(f'loading results from {path}')
- with open(path, 'rb') as f:
- return np.load(f, allow_pickle=True).tolist()
- return None
-
-def generate_valid_split(X, y, bptt, eval_position, is_classification, split_number=1):
- """Generates a deteministic train-(test/valid) split. Both splits must contain the same classes and all classes in
- the entire datasets. If no such split can be sampled in 7 passes, returns None.
-
- :param X: torch tensor, feature values
- :param y: torch tensor, class values
- :param bptt: Number of samples in train + test
- :param eval_position: Number of samples in train, i.e. from which index values are in test
- :param split_number: The split id
- :return:
- """
- done, seed = False, 13
-
- torch.manual_seed(split_number)
- perm = torch.randperm(X.shape[0]) if split_number > 1 else torch.arange(0, X.shape[0])
- X, y = X[perm], y[perm]
- while not done:
- if seed > 20:
- return None, None # No split could be generated in 7 passes, return None
- random.seed(seed)
- i = random.randint(0, len(X) - bptt) if len(X) - bptt > 0 else 0
- y_ = y[i:i + bptt]
-
- if is_classification:
- # Checks if all classes from dataset are contained and classes in train and test are equal (contain same
- # classes) and
- done = len(torch.unique(y_)) == len(torch.unique(y))
- done = done and torch.all(torch.unique(y_) == torch.unique(y))
- done = done and len(torch.unique(y_[:eval_position])) == len(torch.unique(y_[eval_position:]))
- done = done and torch.all(torch.unique(y_[:eval_position]) == torch.unique(y_[eval_position:]))
- seed = seed + 1
- else:
- done = True
-
- eval_xs = torch.stack([X[i:i + bptt].clone()], 1)
- eval_ys = torch.stack([y[i:i + bptt].clone()], 1)
-
- return eval_xs, eval_ys
-
-
-def evaluate_position(X, y, categorical_feats, model, bptt
- , eval_position, overwrite, save, base_path, path_interfix, method, ds_name, fetch_only=False
- , max_time=300, split_number=1, metric_used=None, device='cpu'
- , per_step_normalization=False, **kwargs):
- """
- Evaluates a dataset with a 'bptt' number of training samples.
-
- :param X: Dataset X
- :param y: Dataset labels
- :param categorical_feats: Indices of categorical features.
- :param model: Model function
- :param bptt: Sequence length.
- :param eval_position: Number of training samples.
- :param overwrite: Wheater to ove
- :param overwrite: If True, results on disk are overwritten.
- :param save:
- :param path_interfix: Used for constructing path to write on disk.
- :param method: Model name.
- :param ds_name: Datset name.
- :param fetch_only: Wheater to calculate or only fetch results.
- :param per_step_normalization:
- :param kwargs:
- :return:
- """
-
- if save:
- path = os.path.join(base_path, f'results/tabular/{path_interfix}/results_{method}_{ds_name}_{eval_position}_{bptt}_{split_number}.npy')
- #log_path =
-
- ## Load results if on disk
- if not overwrite:
- result = check_file_exists(path)
- if result is not None:
- if not fetch_only:
- print(f'Loaded saved result for {path}')
- return result
- elif fetch_only:
- print(f'Could not load saved result for {path}')
- return None
-
- ## Generate data splits
- eval_xs, eval_ys = generate_valid_split(X, y, bptt, eval_position
- , is_classification=tabular_metrics.is_classification(metric_used)
- , split_number=split_number)
- if eval_xs is None:
- print(f"No dataset could be generated {ds_name} {bptt}")
- return None
-
- eval_ys = (eval_ys > torch.unique(eval_ys).unsqueeze(0)).sum(axis=1).unsqueeze(-1)
-
- if isinstance(model, nn.Module):
- model = model.to(device)
- eval_xs = eval_xs.to(device)
- eval_ys = eval_ys.to(device)
-
- start_time = time.time()
-
- if isinstance(model, nn.Module): # Two separate predict interfaces for transformer and baselines
- outputs, best_configs = transformer_predict(model, eval_xs, eval_ys, eval_position, metric_used=metric_used
- , categorical_feats=categorical_feats
- , inference_mode=True
- , device=device
- , extend_features=True,
- **kwargs), None
- else:
- _, outputs, best_configs = baseline_predict(model, eval_xs, eval_ys, categorical_feats
- , eval_pos=eval_position
- , device=device
- , max_time=max_time, metric_used=metric_used, **kwargs)
- eval_ys = eval_ys[eval_position:]
- if outputs is None:
- print('Execution failed')
- return None
-
- if torch.is_tensor(outputs): # Transfers data to cpu for saving
- outputs = outputs.cpu()
- eval_ys = eval_ys.cpu()
-
- ds_result = None, outputs, eval_ys, best_configs, time.time() - start_time
-
- if save:
- with open(path, 'wb') as f:
- np.save(f, ds_result)
- print(f'saved results to {path}')
-
- return ds_result
\ No newline at end of file
diff --git a/spaces/TandCAcceptMe/face-swap-docker/mynewshinyroop/Lib/site-packages/pip/_vendor/distlib/compat.py b/spaces/TandCAcceptMe/face-swap-docker/mynewshinyroop/Lib/site-packages/pip/_vendor/distlib/compat.py
deleted file mode 100644
index 1fe3d225acb9bf37acffafc2198dc96c7c7fd313..0000000000000000000000000000000000000000
--- a/spaces/TandCAcceptMe/face-swap-docker/mynewshinyroop/Lib/site-packages/pip/_vendor/distlib/compat.py
+++ /dev/null
@@ -1,1116 +0,0 @@
-# -*- coding: utf-8 -*-
-#
-# Copyright (C) 2013-2017 Vinay Sajip.
-# Licensed to the Python Software Foundation under a contributor agreement.
-# See LICENSE.txt and CONTRIBUTORS.txt.
-#
-from __future__ import absolute_import
-
-import os
-import re
-import sys
-
-try:
- import ssl
-except ImportError: # pragma: no cover
- ssl = None
-
-if sys.version_info[0] < 3: # pragma: no cover
- from StringIO import StringIO
- string_types = basestring,
- text_type = unicode
- from types import FileType as file_type
- import __builtin__ as builtins
- import ConfigParser as configparser
- from urlparse import urlparse, urlunparse, urljoin, urlsplit, urlunsplit
- from urllib import (urlretrieve, quote as _quote, unquote, url2pathname,
- pathname2url, ContentTooShortError, splittype)
-
- def quote(s):
- if isinstance(s, unicode):
- s = s.encode('utf-8')
- return _quote(s)
-
- import urllib2
- from urllib2 import (Request, urlopen, URLError, HTTPError,
- HTTPBasicAuthHandler, HTTPPasswordMgr,
- HTTPHandler, HTTPRedirectHandler,
- build_opener)
- if ssl:
- from urllib2 import HTTPSHandler
- import httplib
- import xmlrpclib
- import Queue as queue
- from HTMLParser import HTMLParser
- import htmlentitydefs
- raw_input = raw_input
- from itertools import ifilter as filter
- from itertools import ifilterfalse as filterfalse
-
- # Leaving this around for now, in case it needs resurrecting in some way
- # _userprog = None
- # def splituser(host):
- # """splituser('user[:passwd]@host[:port]') --> 'user[:passwd]', 'host[:port]'."""
- # global _userprog
- # if _userprog is None:
- # import re
- # _userprog = re.compile('^(.*)@(.*)$')
-
- # match = _userprog.match(host)
- # if match: return match.group(1, 2)
- # return None, host
-
-else: # pragma: no cover
- from io import StringIO
- string_types = str,
- text_type = str
- from io import TextIOWrapper as file_type
- import builtins
- import configparser
- import shutil
- from urllib.parse import (urlparse, urlunparse, urljoin, quote,
- unquote, urlsplit, urlunsplit, splittype)
- from urllib.request import (urlopen, urlretrieve, Request, url2pathname,
- pathname2url,
- HTTPBasicAuthHandler, HTTPPasswordMgr,
- HTTPHandler, HTTPRedirectHandler,
- build_opener)
- if ssl:
- from urllib.request import HTTPSHandler
- from urllib.error import HTTPError, URLError, ContentTooShortError
- import http.client as httplib
- import urllib.request as urllib2
- import xmlrpc.client as xmlrpclib
- import queue
- from html.parser import HTMLParser
- import html.entities as htmlentitydefs
- raw_input = input
- from itertools import filterfalse
- filter = filter
-
-
-try:
- from ssl import match_hostname, CertificateError
-except ImportError: # pragma: no cover
- class CertificateError(ValueError):
- pass
-
-
- def _dnsname_match(dn, hostname, max_wildcards=1):
- """Matching according to RFC 6125, section 6.4.3
-
- http://tools.ietf.org/html/rfc6125#section-6.4.3
- """
- pats = []
- if not dn:
- return False
-
- parts = dn.split('.')
- leftmost, remainder = parts[0], parts[1:]
-
- wildcards = leftmost.count('*')
- if wildcards > max_wildcards:
- # Issue #17980: avoid denials of service by refusing more
- # than one wildcard per fragment. A survey of established
- # policy among SSL implementations showed it to be a
- # reasonable choice.
- raise CertificateError(
- "too many wildcards in certificate DNS name: " + repr(dn))
-
- # speed up common case w/o wildcards
- if not wildcards:
- return dn.lower() == hostname.lower()
-
- # RFC 6125, section 6.4.3, subitem 1.
- # The client SHOULD NOT attempt to match a presented identifier in which
- # the wildcard character comprises a label other than the left-most label.
- if leftmost == '*':
- # When '*' is a fragment by itself, it matches a non-empty dotless
- # fragment.
- pats.append('[^.]+')
- elif leftmost.startswith('xn--') or hostname.startswith('xn--'):
- # RFC 6125, section 6.4.3, subitem 3.
- # The client SHOULD NOT attempt to match a presented identifier
- # where the wildcard character is embedded within an A-label or
- # U-label of an internationalized domain name.
- pats.append(re.escape(leftmost))
- else:
- # Otherwise, '*' matches any dotless string, e.g. www*
- pats.append(re.escape(leftmost).replace(r'\*', '[^.]*'))
-
- # add the remaining fragments, ignore any wildcards
- for frag in remainder:
- pats.append(re.escape(frag))
-
- pat = re.compile(r'\A' + r'\.'.join(pats) + r'\Z', re.IGNORECASE)
- return pat.match(hostname)
-
-
- def match_hostname(cert, hostname):
- """Verify that *cert* (in decoded format as returned by
- SSLSocket.getpeercert()) matches the *hostname*. RFC 2818 and RFC 6125
- rules are followed, but IP addresses are not accepted for *hostname*.
-
- CertificateError is raised on failure. On success, the function
- returns nothing.
- """
- if not cert:
- raise ValueError("empty or no certificate, match_hostname needs a "
- "SSL socket or SSL context with either "
- "CERT_OPTIONAL or CERT_REQUIRED")
- dnsnames = []
- san = cert.get('subjectAltName', ())
- for key, value in san:
- if key == 'DNS':
- if _dnsname_match(value, hostname):
- return
- dnsnames.append(value)
- if not dnsnames:
- # The subject is only checked when there is no dNSName entry
- # in subjectAltName
- for sub in cert.get('subject', ()):
- for key, value in sub:
- # XXX according to RFC 2818, the most specific Common Name
- # must be used.
- if key == 'commonName':
- if _dnsname_match(value, hostname):
- return
- dnsnames.append(value)
- if len(dnsnames) > 1:
- raise CertificateError("hostname %r "
- "doesn't match either of %s"
- % (hostname, ', '.join(map(repr, dnsnames))))
- elif len(dnsnames) == 1:
- raise CertificateError("hostname %r "
- "doesn't match %r"
- % (hostname, dnsnames[0]))
- else:
- raise CertificateError("no appropriate commonName or "
- "subjectAltName fields were found")
-
-
-try:
- from types import SimpleNamespace as Container
-except ImportError: # pragma: no cover
- class Container(object):
- """
- A generic container for when multiple values need to be returned
- """
- def __init__(self, **kwargs):
- self.__dict__.update(kwargs)
-
-
-try:
- from shutil import which
-except ImportError: # pragma: no cover
- # Implementation from Python 3.3
- def which(cmd, mode=os.F_OK | os.X_OK, path=None):
- """Given a command, mode, and a PATH string, return the path which
- conforms to the given mode on the PATH, or None if there is no such
- file.
-
- `mode` defaults to os.F_OK | os.X_OK. `path` defaults to the result
- of os.environ.get("PATH"), or can be overridden with a custom search
- path.
-
- """
- # Check that a given file can be accessed with the correct mode.
- # Additionally check that `file` is not a directory, as on Windows
- # directories pass the os.access check.
- def _access_check(fn, mode):
- return (os.path.exists(fn) and os.access(fn, mode)
- and not os.path.isdir(fn))
-
- # If we're given a path with a directory part, look it up directly rather
- # than referring to PATH directories. This includes checking relative to the
- # current directory, e.g. ./script
- if os.path.dirname(cmd):
- if _access_check(cmd, mode):
- return cmd
- return None
-
- if path is None:
- path = os.environ.get("PATH", os.defpath)
- if not path:
- return None
- path = path.split(os.pathsep)
-
- if sys.platform == "win32":
- # The current directory takes precedence on Windows.
- if not os.curdir in path:
- path.insert(0, os.curdir)
-
- # PATHEXT is necessary to check on Windows.
- pathext = os.environ.get("PATHEXT", "").split(os.pathsep)
- # See if the given file matches any of the expected path extensions.
- # This will allow us to short circuit when given "python.exe".
- # If it does match, only test that one, otherwise we have to try
- # others.
- if any(cmd.lower().endswith(ext.lower()) for ext in pathext):
- files = [cmd]
- else:
- files = [cmd + ext for ext in pathext]
- else:
- # On other platforms you don't have things like PATHEXT to tell you
- # what file suffixes are executable, so just pass on cmd as-is.
- files = [cmd]
-
- seen = set()
- for dir in path:
- normdir = os.path.normcase(dir)
- if not normdir in seen:
- seen.add(normdir)
- for thefile in files:
- name = os.path.join(dir, thefile)
- if _access_check(name, mode):
- return name
- return None
-
-
-# ZipFile is a context manager in 2.7, but not in 2.6
-
-from zipfile import ZipFile as BaseZipFile
-
-if hasattr(BaseZipFile, '__enter__'): # pragma: no cover
- ZipFile = BaseZipFile
-else: # pragma: no cover
- from zipfile import ZipExtFile as BaseZipExtFile
-
- class ZipExtFile(BaseZipExtFile):
- def __init__(self, base):
- self.__dict__.update(base.__dict__)
-
- def __enter__(self):
- return self
-
- def __exit__(self, *exc_info):
- self.close()
- # return None, so if an exception occurred, it will propagate
-
- class ZipFile(BaseZipFile):
- def __enter__(self):
- return self
-
- def __exit__(self, *exc_info):
- self.close()
- # return None, so if an exception occurred, it will propagate
-
- def open(self, *args, **kwargs):
- base = BaseZipFile.open(self, *args, **kwargs)
- return ZipExtFile(base)
-
-try:
- from platform import python_implementation
-except ImportError: # pragma: no cover
- def python_implementation():
- """Return a string identifying the Python implementation."""
- if 'PyPy' in sys.version:
- return 'PyPy'
- if os.name == 'java':
- return 'Jython'
- if sys.version.startswith('IronPython'):
- return 'IronPython'
- return 'CPython'
-
-import shutil
-import sysconfig
-
-try:
- callable = callable
-except NameError: # pragma: no cover
- from collections.abc import Callable
-
- def callable(obj):
- return isinstance(obj, Callable)
-
-
-try:
- fsencode = os.fsencode
- fsdecode = os.fsdecode
-except AttributeError: # pragma: no cover
- # Issue #99: on some systems (e.g. containerised),
- # sys.getfilesystemencoding() returns None, and we need a real value,
- # so fall back to utf-8. From the CPython 2.7 docs relating to Unix and
- # sys.getfilesystemencoding(): the return value is "the user’s preference
- # according to the result of nl_langinfo(CODESET), or None if the
- # nl_langinfo(CODESET) failed."
- _fsencoding = sys.getfilesystemencoding() or 'utf-8'
- if _fsencoding == 'mbcs':
- _fserrors = 'strict'
- else:
- _fserrors = 'surrogateescape'
-
- def fsencode(filename):
- if isinstance(filename, bytes):
- return filename
- elif isinstance(filename, text_type):
- return filename.encode(_fsencoding, _fserrors)
- else:
- raise TypeError("expect bytes or str, not %s" %
- type(filename).__name__)
-
- def fsdecode(filename):
- if isinstance(filename, text_type):
- return filename
- elif isinstance(filename, bytes):
- return filename.decode(_fsencoding, _fserrors)
- else:
- raise TypeError("expect bytes or str, not %s" %
- type(filename).__name__)
-
-try:
- from tokenize import detect_encoding
-except ImportError: # pragma: no cover
- from codecs import BOM_UTF8, lookup
- import re
-
- cookie_re = re.compile(r"coding[:=]\s*([-\w.]+)")
-
- def _get_normal_name(orig_enc):
- """Imitates get_normal_name in tokenizer.c."""
- # Only care about the first 12 characters.
- enc = orig_enc[:12].lower().replace("_", "-")
- if enc == "utf-8" or enc.startswith("utf-8-"):
- return "utf-8"
- if enc in ("latin-1", "iso-8859-1", "iso-latin-1") or \
- enc.startswith(("latin-1-", "iso-8859-1-", "iso-latin-1-")):
- return "iso-8859-1"
- return orig_enc
-
- def detect_encoding(readline):
- """
- The detect_encoding() function is used to detect the encoding that should
- be used to decode a Python source file. It requires one argument, readline,
- in the same way as the tokenize() generator.
-
- It will call readline a maximum of twice, and return the encoding used
- (as a string) and a list of any lines (left as bytes) it has read in.
-
- It detects the encoding from the presence of a utf-8 bom or an encoding
- cookie as specified in pep-0263. If both a bom and a cookie are present,
- but disagree, a SyntaxError will be raised. If the encoding cookie is an
- invalid charset, raise a SyntaxError. Note that if a utf-8 bom is found,
- 'utf-8-sig' is returned.
-
- If no encoding is specified, then the default of 'utf-8' will be returned.
- """
- try:
- filename = readline.__self__.name
- except AttributeError:
- filename = None
- bom_found = False
- encoding = None
- default = 'utf-8'
- def read_or_stop():
- try:
- return readline()
- except StopIteration:
- return b''
-
- def find_cookie(line):
- try:
- # Decode as UTF-8. Either the line is an encoding declaration,
- # in which case it should be pure ASCII, or it must be UTF-8
- # per default encoding.
- line_string = line.decode('utf-8')
- except UnicodeDecodeError:
- msg = "invalid or missing encoding declaration"
- if filename is not None:
- msg = '{} for {!r}'.format(msg, filename)
- raise SyntaxError(msg)
-
- matches = cookie_re.findall(line_string)
- if not matches:
- return None
- encoding = _get_normal_name(matches[0])
- try:
- codec = lookup(encoding)
- except LookupError:
- # This behaviour mimics the Python interpreter
- if filename is None:
- msg = "unknown encoding: " + encoding
- else:
- msg = "unknown encoding for {!r}: {}".format(filename,
- encoding)
- raise SyntaxError(msg)
-
- if bom_found:
- if codec.name != 'utf-8':
- # This behaviour mimics the Python interpreter
- if filename is None:
- msg = 'encoding problem: utf-8'
- else:
- msg = 'encoding problem for {!r}: utf-8'.format(filename)
- raise SyntaxError(msg)
- encoding += '-sig'
- return encoding
-
- first = read_or_stop()
- if first.startswith(BOM_UTF8):
- bom_found = True
- first = first[3:]
- default = 'utf-8-sig'
- if not first:
- return default, []
-
- encoding = find_cookie(first)
- if encoding:
- return encoding, [first]
-
- second = read_or_stop()
- if not second:
- return default, [first]
-
- encoding = find_cookie(second)
- if encoding:
- return encoding, [first, second]
-
- return default, [first, second]
-
-# For converting & <-> & etc.
-try:
- from html import escape
-except ImportError:
- from cgi import escape
-if sys.version_info[:2] < (3, 4):
- unescape = HTMLParser().unescape
-else:
- from html import unescape
-
-try:
- from collections import ChainMap
-except ImportError: # pragma: no cover
- from collections import MutableMapping
-
- try:
- from reprlib import recursive_repr as _recursive_repr
- except ImportError:
- def _recursive_repr(fillvalue='...'):
- '''
- Decorator to make a repr function return fillvalue for a recursive
- call
- '''
-
- def decorating_function(user_function):
- repr_running = set()
-
- def wrapper(self):
- key = id(self), get_ident()
- if key in repr_running:
- return fillvalue
- repr_running.add(key)
- try:
- result = user_function(self)
- finally:
- repr_running.discard(key)
- return result
-
- # Can't use functools.wraps() here because of bootstrap issues
- wrapper.__module__ = getattr(user_function, '__module__')
- wrapper.__doc__ = getattr(user_function, '__doc__')
- wrapper.__name__ = getattr(user_function, '__name__')
- wrapper.__annotations__ = getattr(user_function, '__annotations__', {})
- return wrapper
-
- return decorating_function
-
- class ChainMap(MutableMapping):
- ''' A ChainMap groups multiple dicts (or other mappings) together
- to create a single, updateable view.
-
- The underlying mappings are stored in a list. That list is public and can
- accessed or updated using the *maps* attribute. There is no other state.
-
- Lookups search the underlying mappings successively until a key is found.
- In contrast, writes, updates, and deletions only operate on the first
- mapping.
-
- '''
-
- def __init__(self, *maps):
- '''Initialize a ChainMap by setting *maps* to the given mappings.
- If no mappings are provided, a single empty dictionary is used.
-
- '''
- self.maps = list(maps) or [{}] # always at least one map
-
- def __missing__(self, key):
- raise KeyError(key)
-
- def __getitem__(self, key):
- for mapping in self.maps:
- try:
- return mapping[key] # can't use 'key in mapping' with defaultdict
- except KeyError:
- pass
- return self.__missing__(key) # support subclasses that define __missing__
-
- def get(self, key, default=None):
- return self[key] if key in self else default
-
- def __len__(self):
- return len(set().union(*self.maps)) # reuses stored hash values if possible
-
- def __iter__(self):
- return iter(set().union(*self.maps))
-
- def __contains__(self, key):
- return any(key in m for m in self.maps)
-
- def __bool__(self):
- return any(self.maps)
-
- @_recursive_repr()
- def __repr__(self):
- return '{0.__class__.__name__}({1})'.format(
- self, ', '.join(map(repr, self.maps)))
-
- @classmethod
- def fromkeys(cls, iterable, *args):
- 'Create a ChainMap with a single dict created from the iterable.'
- return cls(dict.fromkeys(iterable, *args))
-
- def copy(self):
- 'New ChainMap or subclass with a new copy of maps[0] and refs to maps[1:]'
- return self.__class__(self.maps[0].copy(), *self.maps[1:])
-
- __copy__ = copy
-
- def new_child(self): # like Django's Context.push()
- 'New ChainMap with a new dict followed by all previous maps.'
- return self.__class__({}, *self.maps)
-
- @property
- def parents(self): # like Django's Context.pop()
- 'New ChainMap from maps[1:].'
- return self.__class__(*self.maps[1:])
-
- def __setitem__(self, key, value):
- self.maps[0][key] = value
-
- def __delitem__(self, key):
- try:
- del self.maps[0][key]
- except KeyError:
- raise KeyError('Key not found in the first mapping: {!r}'.format(key))
-
- def popitem(self):
- 'Remove and return an item pair from maps[0]. Raise KeyError is maps[0] is empty.'
- try:
- return self.maps[0].popitem()
- except KeyError:
- raise KeyError('No keys found in the first mapping.')
-
- def pop(self, key, *args):
- 'Remove *key* from maps[0] and return its value. Raise KeyError if *key* not in maps[0].'
- try:
- return self.maps[0].pop(key, *args)
- except KeyError:
- raise KeyError('Key not found in the first mapping: {!r}'.format(key))
-
- def clear(self):
- 'Clear maps[0], leaving maps[1:] intact.'
- self.maps[0].clear()
-
-try:
- from importlib.util import cache_from_source # Python >= 3.4
-except ImportError: # pragma: no cover
- def cache_from_source(path, debug_override=None):
- assert path.endswith('.py')
- if debug_override is None:
- debug_override = __debug__
- if debug_override:
- suffix = 'c'
- else:
- suffix = 'o'
- return path + suffix
-
-try:
- from collections import OrderedDict
-except ImportError: # pragma: no cover
-## {{{ http://code.activestate.com/recipes/576693/ (r9)
-# Backport of OrderedDict() class that runs on Python 2.4, 2.5, 2.6, 2.7 and pypy.
-# Passes Python2.7's test suite and incorporates all the latest updates.
- try:
- from thread import get_ident as _get_ident
- except ImportError:
- from dummy_thread import get_ident as _get_ident
-
- try:
- from _abcoll import KeysView, ValuesView, ItemsView
- except ImportError:
- pass
-
-
- class OrderedDict(dict):
- 'Dictionary that remembers insertion order'
- # An inherited dict maps keys to values.
- # The inherited dict provides __getitem__, __len__, __contains__, and get.
- # The remaining methods are order-aware.
- # Big-O running times for all methods are the same as for regular dictionaries.
-
- # The internal self.__map dictionary maps keys to links in a doubly linked list.
- # The circular doubly linked list starts and ends with a sentinel element.
- # The sentinel element never gets deleted (this simplifies the algorithm).
- # Each link is stored as a list of length three: [PREV, NEXT, KEY].
-
- def __init__(self, *args, **kwds):
- '''Initialize an ordered dictionary. Signature is the same as for
- regular dictionaries, but keyword arguments are not recommended
- because their insertion order is arbitrary.
-
- '''
- if len(args) > 1:
- raise TypeError('expected at most 1 arguments, got %d' % len(args))
- try:
- self.__root
- except AttributeError:
- self.__root = root = [] # sentinel node
- root[:] = [root, root, None]
- self.__map = {}
- self.__update(*args, **kwds)
-
- def __setitem__(self, key, value, dict_setitem=dict.__setitem__):
- 'od.__setitem__(i, y) <==> od[i]=y'
- # Setting a new item creates a new link which goes at the end of the linked
- # list, and the inherited dictionary is updated with the new key/value pair.
- if key not in self:
- root = self.__root
- last = root[0]
- last[1] = root[0] = self.__map[key] = [last, root, key]
- dict_setitem(self, key, value)
-
- def __delitem__(self, key, dict_delitem=dict.__delitem__):
- 'od.__delitem__(y) <==> del od[y]'
- # Deleting an existing item uses self.__map to find the link which is
- # then removed by updating the links in the predecessor and successor nodes.
- dict_delitem(self, key)
- link_prev, link_next, key = self.__map.pop(key)
- link_prev[1] = link_next
- link_next[0] = link_prev
-
- def __iter__(self):
- 'od.__iter__() <==> iter(od)'
- root = self.__root
- curr = root[1]
- while curr is not root:
- yield curr[2]
- curr = curr[1]
-
- def __reversed__(self):
- 'od.__reversed__() <==> reversed(od)'
- root = self.__root
- curr = root[0]
- while curr is not root:
- yield curr[2]
- curr = curr[0]
-
- def clear(self):
- 'od.clear() -> None. Remove all items from od.'
- try:
- for node in self.__map.itervalues():
- del node[:]
- root = self.__root
- root[:] = [root, root, None]
- self.__map.clear()
- except AttributeError:
- pass
- dict.clear(self)
-
- def popitem(self, last=True):
- '''od.popitem() -> (k, v), return and remove a (key, value) pair.
- Pairs are returned in LIFO order if last is true or FIFO order if false.
-
- '''
- if not self:
- raise KeyError('dictionary is empty')
- root = self.__root
- if last:
- link = root[0]
- link_prev = link[0]
- link_prev[1] = root
- root[0] = link_prev
- else:
- link = root[1]
- link_next = link[1]
- root[1] = link_next
- link_next[0] = root
- key = link[2]
- del self.__map[key]
- value = dict.pop(self, key)
- return key, value
-
- # -- the following methods do not depend on the internal structure --
-
- def keys(self):
- 'od.keys() -> list of keys in od'
- return list(self)
-
- def values(self):
- 'od.values() -> list of values in od'
- return [self[key] for key in self]
-
- def items(self):
- 'od.items() -> list of (key, value) pairs in od'
- return [(key, self[key]) for key in self]
-
- def iterkeys(self):
- 'od.iterkeys() -> an iterator over the keys in od'
- return iter(self)
-
- def itervalues(self):
- 'od.itervalues -> an iterator over the values in od'
- for k in self:
- yield self[k]
-
- def iteritems(self):
- 'od.iteritems -> an iterator over the (key, value) items in od'
- for k in self:
- yield (k, self[k])
-
- def update(*args, **kwds):
- '''od.update(E, **F) -> None. Update od from dict/iterable E and F.
-
- If E is a dict instance, does: for k in E: od[k] = E[k]
- If E has a .keys() method, does: for k in E.keys(): od[k] = E[k]
- Or if E is an iterable of items, does: for k, v in E: od[k] = v
- In either case, this is followed by: for k, v in F.items(): od[k] = v
-
- '''
- if len(args) > 2:
- raise TypeError('update() takes at most 2 positional '
- 'arguments (%d given)' % (len(args),))
- elif not args:
- raise TypeError('update() takes at least 1 argument (0 given)')
- self = args[0]
- # Make progressively weaker assumptions about "other"
- other = ()
- if len(args) == 2:
- other = args[1]
- if isinstance(other, dict):
- for key in other:
- self[key] = other[key]
- elif hasattr(other, 'keys'):
- for key in other.keys():
- self[key] = other[key]
- else:
- for key, value in other:
- self[key] = value
- for key, value in kwds.items():
- self[key] = value
-
- __update = update # let subclasses override update without breaking __init__
-
- __marker = object()
-
- def pop(self, key, default=__marker):
- '''od.pop(k[,d]) -> v, remove specified key and return the corresponding value.
- If key is not found, d is returned if given, otherwise KeyError is raised.
-
- '''
- if key in self:
- result = self[key]
- del self[key]
- return result
- if default is self.__marker:
- raise KeyError(key)
- return default
-
- def setdefault(self, key, default=None):
- 'od.setdefault(k[,d]) -> od.get(k,d), also set od[k]=d if k not in od'
- if key in self:
- return self[key]
- self[key] = default
- return default
-
- def __repr__(self, _repr_running=None):
- 'od.__repr__() <==> repr(od)'
- if not _repr_running: _repr_running = {}
- call_key = id(self), _get_ident()
- if call_key in _repr_running:
- return '...'
- _repr_running[call_key] = 1
- try:
- if not self:
- return '%s()' % (self.__class__.__name__,)
- return '%s(%r)' % (self.__class__.__name__, self.items())
- finally:
- del _repr_running[call_key]
-
- def __reduce__(self):
- 'Return state information for pickling'
- items = [[k, self[k]] for k in self]
- inst_dict = vars(self).copy()
- for k in vars(OrderedDict()):
- inst_dict.pop(k, None)
- if inst_dict:
- return (self.__class__, (items,), inst_dict)
- return self.__class__, (items,)
-
- def copy(self):
- 'od.copy() -> a shallow copy of od'
- return self.__class__(self)
-
- @classmethod
- def fromkeys(cls, iterable, value=None):
- '''OD.fromkeys(S[, v]) -> New ordered dictionary with keys from S
- and values equal to v (which defaults to None).
-
- '''
- d = cls()
- for key in iterable:
- d[key] = value
- return d
-
- def __eq__(self, other):
- '''od.__eq__(y) <==> od==y. Comparison to another OD is order-sensitive
- while comparison to a regular mapping is order-insensitive.
-
- '''
- if isinstance(other, OrderedDict):
- return len(self)==len(other) and self.items() == other.items()
- return dict.__eq__(self, other)
-
- def __ne__(self, other):
- return not self == other
-
- # -- the following methods are only used in Python 2.7 --
-
- def viewkeys(self):
- "od.viewkeys() -> a set-like object providing a view on od's keys"
- return KeysView(self)
-
- def viewvalues(self):
- "od.viewvalues() -> an object providing a view on od's values"
- return ValuesView(self)
-
- def viewitems(self):
- "od.viewitems() -> a set-like object providing a view on od's items"
- return ItemsView(self)
-
-try:
- from logging.config import BaseConfigurator, valid_ident
-except ImportError: # pragma: no cover
- IDENTIFIER = re.compile('^[a-z_][a-z0-9_]*$', re.I)
-
-
- def valid_ident(s):
- m = IDENTIFIER.match(s)
- if not m:
- raise ValueError('Not a valid Python identifier: %r' % s)
- return True
-
-
- # The ConvertingXXX classes are wrappers around standard Python containers,
- # and they serve to convert any suitable values in the container. The
- # conversion converts base dicts, lists and tuples to their wrapped
- # equivalents, whereas strings which match a conversion format are converted
- # appropriately.
- #
- # Each wrapper should have a configurator attribute holding the actual
- # configurator to use for conversion.
-
- class ConvertingDict(dict):
- """A converting dictionary wrapper."""
-
- def __getitem__(self, key):
- value = dict.__getitem__(self, key)
- result = self.configurator.convert(value)
- #If the converted value is different, save for next time
- if value is not result:
- self[key] = result
- if type(result) in (ConvertingDict, ConvertingList,
- ConvertingTuple):
- result.parent = self
- result.key = key
- return result
-
- def get(self, key, default=None):
- value = dict.get(self, key, default)
- result = self.configurator.convert(value)
- #If the converted value is different, save for next time
- if value is not result:
- self[key] = result
- if type(result) in (ConvertingDict, ConvertingList,
- ConvertingTuple):
- result.parent = self
- result.key = key
- return result
-
- def pop(self, key, default=None):
- value = dict.pop(self, key, default)
- result = self.configurator.convert(value)
- if value is not result:
- if type(result) in (ConvertingDict, ConvertingList,
- ConvertingTuple):
- result.parent = self
- result.key = key
- return result
-
- class ConvertingList(list):
- """A converting list wrapper."""
- def __getitem__(self, key):
- value = list.__getitem__(self, key)
- result = self.configurator.convert(value)
- #If the converted value is different, save for next time
- if value is not result:
- self[key] = result
- if type(result) in (ConvertingDict, ConvertingList,
- ConvertingTuple):
- result.parent = self
- result.key = key
- return result
-
- def pop(self, idx=-1):
- value = list.pop(self, idx)
- result = self.configurator.convert(value)
- if value is not result:
- if type(result) in (ConvertingDict, ConvertingList,
- ConvertingTuple):
- result.parent = self
- return result
-
- class ConvertingTuple(tuple):
- """A converting tuple wrapper."""
- def __getitem__(self, key):
- value = tuple.__getitem__(self, key)
- result = self.configurator.convert(value)
- if value is not result:
- if type(result) in (ConvertingDict, ConvertingList,
- ConvertingTuple):
- result.parent = self
- result.key = key
- return result
-
- class BaseConfigurator(object):
- """
- The configurator base class which defines some useful defaults.
- """
-
- CONVERT_PATTERN = re.compile(r'^(?P[a-z]+)://(?P.*)$')
-
- WORD_PATTERN = re.compile(r'^\s*(\w+)\s*')
- DOT_PATTERN = re.compile(r'^\.\s*(\w+)\s*')
- INDEX_PATTERN = re.compile(r'^\[\s*(\w+)\s*\]\s*')
- DIGIT_PATTERN = re.compile(r'^\d+$')
-
- value_converters = {
- 'ext' : 'ext_convert',
- 'cfg' : 'cfg_convert',
- }
-
- # We might want to use a different one, e.g. importlib
- importer = staticmethod(__import__)
-
- def __init__(self, config):
- self.config = ConvertingDict(config)
- self.config.configurator = self
-
- def resolve(self, s):
- """
- Resolve strings to objects using standard import and attribute
- syntax.
- """
- name = s.split('.')
- used = name.pop(0)
- try:
- found = self.importer(used)
- for frag in name:
- used += '.' + frag
- try:
- found = getattr(found, frag)
- except AttributeError:
- self.importer(used)
- found = getattr(found, frag)
- return found
- except ImportError:
- e, tb = sys.exc_info()[1:]
- v = ValueError('Cannot resolve %r: %s' % (s, e))
- v.__cause__, v.__traceback__ = e, tb
- raise v
-
- def ext_convert(self, value):
- """Default converter for the ext:// protocol."""
- return self.resolve(value)
-
- def cfg_convert(self, value):
- """Default converter for the cfg:// protocol."""
- rest = value
- m = self.WORD_PATTERN.match(rest)
- if m is None:
- raise ValueError("Unable to convert %r" % value)
- else:
- rest = rest[m.end():]
- d = self.config[m.groups()[0]]
- #print d, rest
- while rest:
- m = self.DOT_PATTERN.match(rest)
- if m:
- d = d[m.groups()[0]]
- else:
- m = self.INDEX_PATTERN.match(rest)
- if m:
- idx = m.groups()[0]
- if not self.DIGIT_PATTERN.match(idx):
- d = d[idx]
- else:
- try:
- n = int(idx) # try as number first (most likely)
- d = d[n]
- except TypeError:
- d = d[idx]
- if m:
- rest = rest[m.end():]
- else:
- raise ValueError('Unable to convert '
- '%r at %r' % (value, rest))
- #rest should be empty
- return d
-
- def convert(self, value):
- """
- Convert values to an appropriate type. dicts, lists and tuples are
- replaced by their converting alternatives. Strings are checked to
- see if they have a conversion format and are converted if they do.
- """
- if not isinstance(value, ConvertingDict) and isinstance(value, dict):
- value = ConvertingDict(value)
- value.configurator = self
- elif not isinstance(value, ConvertingList) and isinstance(value, list):
- value = ConvertingList(value)
- value.configurator = self
- elif not isinstance(value, ConvertingTuple) and\
- isinstance(value, tuple):
- value = ConvertingTuple(value)
- value.configurator = self
- elif isinstance(value, string_types):
- m = self.CONVERT_PATTERN.match(value)
- if m:
- d = m.groupdict()
- prefix = d['prefix']
- converter = self.value_converters.get(prefix, None)
- if converter:
- suffix = d['suffix']
- converter = getattr(self, converter)
- value = converter(suffix)
- return value
-
- def configure_custom(self, config):
- """Configure an object with a user-supplied factory."""
- c = config.pop('()')
- if not callable(c):
- c = self.resolve(c)
- props = config.pop('.', None)
- # Check for valid identifiers
- kwargs = dict([(k, config[k]) for k in config if valid_ident(k)])
- result = c(**kwargs)
- if props:
- for name, value in props.items():
- setattr(result, name, value)
- return result
-
- def as_tuple(self, value):
- """Utility function which converts lists to tuples."""
- if isinstance(value, list):
- value = tuple(value)
- return value
diff --git a/spaces/TandCAcceptMe/face-swap-docker/mynewshinyroop/Lib/site-packages/pip/_vendor/tomli/_parser.py b/spaces/TandCAcceptMe/face-swap-docker/mynewshinyroop/Lib/site-packages/pip/_vendor/tomli/_parser.py
deleted file mode 100644
index f1bb0aa19a556725aa2ae2b8cea95489c99a9078..0000000000000000000000000000000000000000
--- a/spaces/TandCAcceptMe/face-swap-docker/mynewshinyroop/Lib/site-packages/pip/_vendor/tomli/_parser.py
+++ /dev/null
@@ -1,691 +0,0 @@
-# SPDX-License-Identifier: MIT
-# SPDX-FileCopyrightText: 2021 Taneli Hukkinen
-# Licensed to PSF under a Contributor Agreement.
-
-from __future__ import annotations
-
-from collections.abc import Iterable
-import string
-from types import MappingProxyType
-from typing import Any, BinaryIO, NamedTuple
-
-from ._re import (
- RE_DATETIME,
- RE_LOCALTIME,
- RE_NUMBER,
- match_to_datetime,
- match_to_localtime,
- match_to_number,
-)
-from ._types import Key, ParseFloat, Pos
-
-ASCII_CTRL = frozenset(chr(i) for i in range(32)) | frozenset(chr(127))
-
-# Neither of these sets include quotation mark or backslash. They are
-# currently handled as separate cases in the parser functions.
-ILLEGAL_BASIC_STR_CHARS = ASCII_CTRL - frozenset("\t")
-ILLEGAL_MULTILINE_BASIC_STR_CHARS = ASCII_CTRL - frozenset("\t\n")
-
-ILLEGAL_LITERAL_STR_CHARS = ILLEGAL_BASIC_STR_CHARS
-ILLEGAL_MULTILINE_LITERAL_STR_CHARS = ILLEGAL_MULTILINE_BASIC_STR_CHARS
-
-ILLEGAL_COMMENT_CHARS = ILLEGAL_BASIC_STR_CHARS
-
-TOML_WS = frozenset(" \t")
-TOML_WS_AND_NEWLINE = TOML_WS | frozenset("\n")
-BARE_KEY_CHARS = frozenset(string.ascii_letters + string.digits + "-_")
-KEY_INITIAL_CHARS = BARE_KEY_CHARS | frozenset("\"'")
-HEXDIGIT_CHARS = frozenset(string.hexdigits)
-
-BASIC_STR_ESCAPE_REPLACEMENTS = MappingProxyType(
- {
- "\\b": "\u0008", # backspace
- "\\t": "\u0009", # tab
- "\\n": "\u000A", # linefeed
- "\\f": "\u000C", # form feed
- "\\r": "\u000D", # carriage return
- '\\"': "\u0022", # quote
- "\\\\": "\u005C", # backslash
- }
-)
-
-
-class TOMLDecodeError(ValueError):
- """An error raised if a document is not valid TOML."""
-
-
-def load(__fp: BinaryIO, *, parse_float: ParseFloat = float) -> dict[str, Any]:
- """Parse TOML from a binary file object."""
- b = __fp.read()
- try:
- s = b.decode()
- except AttributeError:
- raise TypeError(
- "File must be opened in binary mode, e.g. use `open('foo.toml', 'rb')`"
- ) from None
- return loads(s, parse_float=parse_float)
-
-
-def loads(__s: str, *, parse_float: ParseFloat = float) -> dict[str, Any]: # noqa: C901
- """Parse TOML from a string."""
-
- # The spec allows converting "\r\n" to "\n", even in string
- # literals. Let's do so to simplify parsing.
- src = __s.replace("\r\n", "\n")
- pos = 0
- out = Output(NestedDict(), Flags())
- header: Key = ()
- parse_float = make_safe_parse_float(parse_float)
-
- # Parse one statement at a time
- # (typically means one line in TOML source)
- while True:
- # 1. Skip line leading whitespace
- pos = skip_chars(src, pos, TOML_WS)
-
- # 2. Parse rules. Expect one of the following:
- # - end of file
- # - end of line
- # - comment
- # - key/value pair
- # - append dict to list (and move to its namespace)
- # - create dict (and move to its namespace)
- # Skip trailing whitespace when applicable.
- try:
- char = src[pos]
- except IndexError:
- break
- if char == "\n":
- pos += 1
- continue
- if char in KEY_INITIAL_CHARS:
- pos = key_value_rule(src, pos, out, header, parse_float)
- pos = skip_chars(src, pos, TOML_WS)
- elif char == "[":
- try:
- second_char: str | None = src[pos + 1]
- except IndexError:
- second_char = None
- out.flags.finalize_pending()
- if second_char == "[":
- pos, header = create_list_rule(src, pos, out)
- else:
- pos, header = create_dict_rule(src, pos, out)
- pos = skip_chars(src, pos, TOML_WS)
- elif char != "#":
- raise suffixed_err(src, pos, "Invalid statement")
-
- # 3. Skip comment
- pos = skip_comment(src, pos)
-
- # 4. Expect end of line or end of file
- try:
- char = src[pos]
- except IndexError:
- break
- if char != "\n":
- raise suffixed_err(
- src, pos, "Expected newline or end of document after a statement"
- )
- pos += 1
-
- return out.data.dict
-
-
-class Flags:
- """Flags that map to parsed keys/namespaces."""
-
- # Marks an immutable namespace (inline array or inline table).
- FROZEN = 0
- # Marks a nest that has been explicitly created and can no longer
- # be opened using the "[table]" syntax.
- EXPLICIT_NEST = 1
-
- def __init__(self) -> None:
- self._flags: dict[str, dict] = {}
- self._pending_flags: set[tuple[Key, int]] = set()
-
- def add_pending(self, key: Key, flag: int) -> None:
- self._pending_flags.add((key, flag))
-
- def finalize_pending(self) -> None:
- for key, flag in self._pending_flags:
- self.set(key, flag, recursive=False)
- self._pending_flags.clear()
-
- def unset_all(self, key: Key) -> None:
- cont = self._flags
- for k in key[:-1]:
- if k not in cont:
- return
- cont = cont[k]["nested"]
- cont.pop(key[-1], None)
-
- def set(self, key: Key, flag: int, *, recursive: bool) -> None: # noqa: A003
- cont = self._flags
- key_parent, key_stem = key[:-1], key[-1]
- for k in key_parent:
- if k not in cont:
- cont[k] = {"flags": set(), "recursive_flags": set(), "nested": {}}
- cont = cont[k]["nested"]
- if key_stem not in cont:
- cont[key_stem] = {"flags": set(), "recursive_flags": set(), "nested": {}}
- cont[key_stem]["recursive_flags" if recursive else "flags"].add(flag)
-
- def is_(self, key: Key, flag: int) -> bool:
- if not key:
- return False # document root has no flags
- cont = self._flags
- for k in key[:-1]:
- if k not in cont:
- return False
- inner_cont = cont[k]
- if flag in inner_cont["recursive_flags"]:
- return True
- cont = inner_cont["nested"]
- key_stem = key[-1]
- if key_stem in cont:
- cont = cont[key_stem]
- return flag in cont["flags"] or flag in cont["recursive_flags"]
- return False
-
-
-class NestedDict:
- def __init__(self) -> None:
- # The parsed content of the TOML document
- self.dict: dict[str, Any] = {}
-
- def get_or_create_nest(
- self,
- key: Key,
- *,
- access_lists: bool = True,
- ) -> dict:
- cont: Any = self.dict
- for k in key:
- if k not in cont:
- cont[k] = {}
- cont = cont[k]
- if access_lists and isinstance(cont, list):
- cont = cont[-1]
- if not isinstance(cont, dict):
- raise KeyError("There is no nest behind this key")
- return cont
-
- def append_nest_to_list(self, key: Key) -> None:
- cont = self.get_or_create_nest(key[:-1])
- last_key = key[-1]
- if last_key in cont:
- list_ = cont[last_key]
- if not isinstance(list_, list):
- raise KeyError("An object other than list found behind this key")
- list_.append({})
- else:
- cont[last_key] = [{}]
-
-
-class Output(NamedTuple):
- data: NestedDict
- flags: Flags
-
-
-def skip_chars(src: str, pos: Pos, chars: Iterable[str]) -> Pos:
- try:
- while src[pos] in chars:
- pos += 1
- except IndexError:
- pass
- return pos
-
-
-def skip_until(
- src: str,
- pos: Pos,
- expect: str,
- *,
- error_on: frozenset[str],
- error_on_eof: bool,
-) -> Pos:
- try:
- new_pos = src.index(expect, pos)
- except ValueError:
- new_pos = len(src)
- if error_on_eof:
- raise suffixed_err(src, new_pos, f"Expected {expect!r}") from None
-
- if not error_on.isdisjoint(src[pos:new_pos]):
- while src[pos] not in error_on:
- pos += 1
- raise suffixed_err(src, pos, f"Found invalid character {src[pos]!r}")
- return new_pos
-
-
-def skip_comment(src: str, pos: Pos) -> Pos:
- try:
- char: str | None = src[pos]
- except IndexError:
- char = None
- if char == "#":
- return skip_until(
- src, pos + 1, "\n", error_on=ILLEGAL_COMMENT_CHARS, error_on_eof=False
- )
- return pos
-
-
-def skip_comments_and_array_ws(src: str, pos: Pos) -> Pos:
- while True:
- pos_before_skip = pos
- pos = skip_chars(src, pos, TOML_WS_AND_NEWLINE)
- pos = skip_comment(src, pos)
- if pos == pos_before_skip:
- return pos
-
-
-def create_dict_rule(src: str, pos: Pos, out: Output) -> tuple[Pos, Key]:
- pos += 1 # Skip "["
- pos = skip_chars(src, pos, TOML_WS)
- pos, key = parse_key(src, pos)
-
- if out.flags.is_(key, Flags.EXPLICIT_NEST) or out.flags.is_(key, Flags.FROZEN):
- raise suffixed_err(src, pos, f"Cannot declare {key} twice")
- out.flags.set(key, Flags.EXPLICIT_NEST, recursive=False)
- try:
- out.data.get_or_create_nest(key)
- except KeyError:
- raise suffixed_err(src, pos, "Cannot overwrite a value") from None
-
- if not src.startswith("]", pos):
- raise suffixed_err(src, pos, "Expected ']' at the end of a table declaration")
- return pos + 1, key
-
-
-def create_list_rule(src: str, pos: Pos, out: Output) -> tuple[Pos, Key]:
- pos += 2 # Skip "[["
- pos = skip_chars(src, pos, TOML_WS)
- pos, key = parse_key(src, pos)
-
- if out.flags.is_(key, Flags.FROZEN):
- raise suffixed_err(src, pos, f"Cannot mutate immutable namespace {key}")
- # Free the namespace now that it points to another empty list item...
- out.flags.unset_all(key)
- # ...but this key precisely is still prohibited from table declaration
- out.flags.set(key, Flags.EXPLICIT_NEST, recursive=False)
- try:
- out.data.append_nest_to_list(key)
- except KeyError:
- raise suffixed_err(src, pos, "Cannot overwrite a value") from None
-
- if not src.startswith("]]", pos):
- raise suffixed_err(src, pos, "Expected ']]' at the end of an array declaration")
- return pos + 2, key
-
-
-def key_value_rule(
- src: str, pos: Pos, out: Output, header: Key, parse_float: ParseFloat
-) -> Pos:
- pos, key, value = parse_key_value_pair(src, pos, parse_float)
- key_parent, key_stem = key[:-1], key[-1]
- abs_key_parent = header + key_parent
-
- relative_path_cont_keys = (header + key[:i] for i in range(1, len(key)))
- for cont_key in relative_path_cont_keys:
- # Check that dotted key syntax does not redefine an existing table
- if out.flags.is_(cont_key, Flags.EXPLICIT_NEST):
- raise suffixed_err(src, pos, f"Cannot redefine namespace {cont_key}")
- # Containers in the relative path can't be opened with the table syntax or
- # dotted key/value syntax in following table sections.
- out.flags.add_pending(cont_key, Flags.EXPLICIT_NEST)
-
- if out.flags.is_(abs_key_parent, Flags.FROZEN):
- raise suffixed_err(
- src, pos, f"Cannot mutate immutable namespace {abs_key_parent}"
- )
-
- try:
- nest = out.data.get_or_create_nest(abs_key_parent)
- except KeyError:
- raise suffixed_err(src, pos, "Cannot overwrite a value") from None
- if key_stem in nest:
- raise suffixed_err(src, pos, "Cannot overwrite a value")
- # Mark inline table and array namespaces recursively immutable
- if isinstance(value, (dict, list)):
- out.flags.set(header + key, Flags.FROZEN, recursive=True)
- nest[key_stem] = value
- return pos
-
-
-def parse_key_value_pair(
- src: str, pos: Pos, parse_float: ParseFloat
-) -> tuple[Pos, Key, Any]:
- pos, key = parse_key(src, pos)
- try:
- char: str | None = src[pos]
- except IndexError:
- char = None
- if char != "=":
- raise suffixed_err(src, pos, "Expected '=' after a key in a key/value pair")
- pos += 1
- pos = skip_chars(src, pos, TOML_WS)
- pos, value = parse_value(src, pos, parse_float)
- return pos, key, value
-
-
-def parse_key(src: str, pos: Pos) -> tuple[Pos, Key]:
- pos, key_part = parse_key_part(src, pos)
- key: Key = (key_part,)
- pos = skip_chars(src, pos, TOML_WS)
- while True:
- try:
- char: str | None = src[pos]
- except IndexError:
- char = None
- if char != ".":
- return pos, key
- pos += 1
- pos = skip_chars(src, pos, TOML_WS)
- pos, key_part = parse_key_part(src, pos)
- key += (key_part,)
- pos = skip_chars(src, pos, TOML_WS)
-
-
-def parse_key_part(src: str, pos: Pos) -> tuple[Pos, str]:
- try:
- char: str | None = src[pos]
- except IndexError:
- char = None
- if char in BARE_KEY_CHARS:
- start_pos = pos
- pos = skip_chars(src, pos, BARE_KEY_CHARS)
- return pos, src[start_pos:pos]
- if char == "'":
- return parse_literal_str(src, pos)
- if char == '"':
- return parse_one_line_basic_str(src, pos)
- raise suffixed_err(src, pos, "Invalid initial character for a key part")
-
-
-def parse_one_line_basic_str(src: str, pos: Pos) -> tuple[Pos, str]:
- pos += 1
- return parse_basic_str(src, pos, multiline=False)
-
-
-def parse_array(src: str, pos: Pos, parse_float: ParseFloat) -> tuple[Pos, list]:
- pos += 1
- array: list = []
-
- pos = skip_comments_and_array_ws(src, pos)
- if src.startswith("]", pos):
- return pos + 1, array
- while True:
- pos, val = parse_value(src, pos, parse_float)
- array.append(val)
- pos = skip_comments_and_array_ws(src, pos)
-
- c = src[pos : pos + 1]
- if c == "]":
- return pos + 1, array
- if c != ",":
- raise suffixed_err(src, pos, "Unclosed array")
- pos += 1
-
- pos = skip_comments_and_array_ws(src, pos)
- if src.startswith("]", pos):
- return pos + 1, array
-
-
-def parse_inline_table(src: str, pos: Pos, parse_float: ParseFloat) -> tuple[Pos, dict]:
- pos += 1
- nested_dict = NestedDict()
- flags = Flags()
-
- pos = skip_chars(src, pos, TOML_WS)
- if src.startswith("}", pos):
- return pos + 1, nested_dict.dict
- while True:
- pos, key, value = parse_key_value_pair(src, pos, parse_float)
- key_parent, key_stem = key[:-1], key[-1]
- if flags.is_(key, Flags.FROZEN):
- raise suffixed_err(src, pos, f"Cannot mutate immutable namespace {key}")
- try:
- nest = nested_dict.get_or_create_nest(key_parent, access_lists=False)
- except KeyError:
- raise suffixed_err(src, pos, "Cannot overwrite a value") from None
- if key_stem in nest:
- raise suffixed_err(src, pos, f"Duplicate inline table key {key_stem!r}")
- nest[key_stem] = value
- pos = skip_chars(src, pos, TOML_WS)
- c = src[pos : pos + 1]
- if c == "}":
- return pos + 1, nested_dict.dict
- if c != ",":
- raise suffixed_err(src, pos, "Unclosed inline table")
- if isinstance(value, (dict, list)):
- flags.set(key, Flags.FROZEN, recursive=True)
- pos += 1
- pos = skip_chars(src, pos, TOML_WS)
-
-
-def parse_basic_str_escape(
- src: str, pos: Pos, *, multiline: bool = False
-) -> tuple[Pos, str]:
- escape_id = src[pos : pos + 2]
- pos += 2
- if multiline and escape_id in {"\\ ", "\\\t", "\\\n"}:
- # Skip whitespace until next non-whitespace character or end of
- # the doc. Error if non-whitespace is found before newline.
- if escape_id != "\\\n":
- pos = skip_chars(src, pos, TOML_WS)
- try:
- char = src[pos]
- except IndexError:
- return pos, ""
- if char != "\n":
- raise suffixed_err(src, pos, "Unescaped '\\' in a string")
- pos += 1
- pos = skip_chars(src, pos, TOML_WS_AND_NEWLINE)
- return pos, ""
- if escape_id == "\\u":
- return parse_hex_char(src, pos, 4)
- if escape_id == "\\U":
- return parse_hex_char(src, pos, 8)
- try:
- return pos, BASIC_STR_ESCAPE_REPLACEMENTS[escape_id]
- except KeyError:
- raise suffixed_err(src, pos, "Unescaped '\\' in a string") from None
-
-
-def parse_basic_str_escape_multiline(src: str, pos: Pos) -> tuple[Pos, str]:
- return parse_basic_str_escape(src, pos, multiline=True)
-
-
-def parse_hex_char(src: str, pos: Pos, hex_len: int) -> tuple[Pos, str]:
- hex_str = src[pos : pos + hex_len]
- if len(hex_str) != hex_len or not HEXDIGIT_CHARS.issuperset(hex_str):
- raise suffixed_err(src, pos, "Invalid hex value")
- pos += hex_len
- hex_int = int(hex_str, 16)
- if not is_unicode_scalar_value(hex_int):
- raise suffixed_err(src, pos, "Escaped character is not a Unicode scalar value")
- return pos, chr(hex_int)
-
-
-def parse_literal_str(src: str, pos: Pos) -> tuple[Pos, str]:
- pos += 1 # Skip starting apostrophe
- start_pos = pos
- pos = skip_until(
- src, pos, "'", error_on=ILLEGAL_LITERAL_STR_CHARS, error_on_eof=True
- )
- return pos + 1, src[start_pos:pos] # Skip ending apostrophe
-
-
-def parse_multiline_str(src: str, pos: Pos, *, literal: bool) -> tuple[Pos, str]:
- pos += 3
- if src.startswith("\n", pos):
- pos += 1
-
- if literal:
- delim = "'"
- end_pos = skip_until(
- src,
- pos,
- "'''",
- error_on=ILLEGAL_MULTILINE_LITERAL_STR_CHARS,
- error_on_eof=True,
- )
- result = src[pos:end_pos]
- pos = end_pos + 3
- else:
- delim = '"'
- pos, result = parse_basic_str(src, pos, multiline=True)
-
- # Add at maximum two extra apostrophes/quotes if the end sequence
- # is 4 or 5 chars long instead of just 3.
- if not src.startswith(delim, pos):
- return pos, result
- pos += 1
- if not src.startswith(delim, pos):
- return pos, result + delim
- pos += 1
- return pos, result + (delim * 2)
-
-
-def parse_basic_str(src: str, pos: Pos, *, multiline: bool) -> tuple[Pos, str]:
- if multiline:
- error_on = ILLEGAL_MULTILINE_BASIC_STR_CHARS
- parse_escapes = parse_basic_str_escape_multiline
- else:
- error_on = ILLEGAL_BASIC_STR_CHARS
- parse_escapes = parse_basic_str_escape
- result = ""
- start_pos = pos
- while True:
- try:
- char = src[pos]
- except IndexError:
- raise suffixed_err(src, pos, "Unterminated string") from None
- if char == '"':
- if not multiline:
- return pos + 1, result + src[start_pos:pos]
- if src.startswith('"""', pos):
- return pos + 3, result + src[start_pos:pos]
- pos += 1
- continue
- if char == "\\":
- result += src[start_pos:pos]
- pos, parsed_escape = parse_escapes(src, pos)
- result += parsed_escape
- start_pos = pos
- continue
- if char in error_on:
- raise suffixed_err(src, pos, f"Illegal character {char!r}")
- pos += 1
-
-
-def parse_value( # noqa: C901
- src: str, pos: Pos, parse_float: ParseFloat
-) -> tuple[Pos, Any]:
- try:
- char: str | None = src[pos]
- except IndexError:
- char = None
-
- # IMPORTANT: order conditions based on speed of checking and likelihood
-
- # Basic strings
- if char == '"':
- if src.startswith('"""', pos):
- return parse_multiline_str(src, pos, literal=False)
- return parse_one_line_basic_str(src, pos)
-
- # Literal strings
- if char == "'":
- if src.startswith("'''", pos):
- return parse_multiline_str(src, pos, literal=True)
- return parse_literal_str(src, pos)
-
- # Booleans
- if char == "t":
- if src.startswith("true", pos):
- return pos + 4, True
- if char == "f":
- if src.startswith("false", pos):
- return pos + 5, False
-
- # Arrays
- if char == "[":
- return parse_array(src, pos, parse_float)
-
- # Inline tables
- if char == "{":
- return parse_inline_table(src, pos, parse_float)
-
- # Dates and times
- datetime_match = RE_DATETIME.match(src, pos)
- if datetime_match:
- try:
- datetime_obj = match_to_datetime(datetime_match)
- except ValueError as e:
- raise suffixed_err(src, pos, "Invalid date or datetime") from e
- return datetime_match.end(), datetime_obj
- localtime_match = RE_LOCALTIME.match(src, pos)
- if localtime_match:
- return localtime_match.end(), match_to_localtime(localtime_match)
-
- # Integers and "normal" floats.
- # The regex will greedily match any type starting with a decimal
- # char, so needs to be located after handling of dates and times.
- number_match = RE_NUMBER.match(src, pos)
- if number_match:
- return number_match.end(), match_to_number(number_match, parse_float)
-
- # Special floats
- first_three = src[pos : pos + 3]
- if first_three in {"inf", "nan"}:
- return pos + 3, parse_float(first_three)
- first_four = src[pos : pos + 4]
- if first_four in {"-inf", "+inf", "-nan", "+nan"}:
- return pos + 4, parse_float(first_four)
-
- raise suffixed_err(src, pos, "Invalid value")
-
-
-def suffixed_err(src: str, pos: Pos, msg: str) -> TOMLDecodeError:
- """Return a `TOMLDecodeError` where error message is suffixed with
- coordinates in source."""
-
- def coord_repr(src: str, pos: Pos) -> str:
- if pos >= len(src):
- return "end of document"
- line = src.count("\n", 0, pos) + 1
- if line == 1:
- column = pos + 1
- else:
- column = pos - src.rindex("\n", 0, pos)
- return f"line {line}, column {column}"
-
- return TOMLDecodeError(f"{msg} (at {coord_repr(src, pos)})")
-
-
-def is_unicode_scalar_value(codepoint: int) -> bool:
- return (0 <= codepoint <= 55295) or (57344 <= codepoint <= 1114111)
-
-
-def make_safe_parse_float(parse_float: ParseFloat) -> ParseFloat:
- """A decorator to make `parse_float` safe.
-
- `parse_float` must not return dicts or lists, because these types
- would be mixed with parsed TOML tables and arrays, thus confusing
- the parser. The returned decorated callable raises `ValueError`
- instead of returning illegal types.
- """
- # The default `float` callable never returns illegal types. Optimize it.
- if parse_float is float: # type: ignore[comparison-overlap]
- return float
-
- def safe_parse_float(float_str: str) -> Any:
- float_value = parse_float(float_str)
- if isinstance(float_value, (dict, list)):
- raise ValueError("parse_float must not return dicts or lists")
- return float_value
-
- return safe_parse_float
diff --git a/spaces/ThirdEyeData/Customer-Conversion-Prediction/supv/gbt.py b/spaces/ThirdEyeData/Customer-Conversion-Prediction/supv/gbt.py
deleted file mode 100644
index 1a948e7adb377b5fbf2792a59c6f85e197564d09..0000000000000000000000000000000000000000
--- a/spaces/ThirdEyeData/Customer-Conversion-Prediction/supv/gbt.py
+++ /dev/null
@@ -1,482 +0,0 @@
-#!/usr/local/bin/python3
-
-# avenir-python: Machine Learning
-# Author: Pranab Ghosh
-#
-# Licensed under the Apache License, Version 2.0 (the "License"); you
-# may not use this file except in compliance with the License. You may
-# obtain a copy of the License at
-#
-# http://www.apache.org/licenses/LICENSE-2.0
-#
-# Unless required by applicable law or agreed to in writing, software
-# distributed under the License is distributed on an "AS IS" BASIS,
-# WITHOUT WARRANTIES OR CONDITIONS OF ANY KIND, either express or
-# implied. See the License for the specific language governing
-# permissions and limitations under the License.
-
-# Package imports
-import os
-import sys
-import matplotlib.pyplot as plt
-import numpy as np
-import sklearn as sk
-import matplotlib
-import random
-import jprops
-from sklearn.ensemble import GradientBoostingClassifier
-import joblib
-from sklearn.metrics import accuracy_score
-from sklearn.metrics import confusion_matrix
-from sklearn.model_selection import cross_val_score
-from random import randint
-from io import StringIO
-sys.path.append(os.path.abspath("../lib"))
-from util import *
-from mlutil import *
-from pasearch import *
-from bacl import *
-
-# gradient boosting classification
-class GradientBoostedTrees(object):
- def __init__(self, configFile):
- defValues = {}
- defValues["common.mode"] = ("training", None)
- defValues["common.model.directory"] = ("model", None)
- defValues["common.model.file"] = (None, None)
- defValues["common.preprocessing"] = (None, None)
- defValues["common.verbose"] = (False, None)
- defValues["train.data.file"] = (None, "missing training data file")
- defValues["train.data.fields"] = (None, "missing training data field ordinals")
- defValues["train.data.feature.fields"] = (None, "missing training data feature field ordinals")
- defValues["train.data.class.field"] = (None, "missing class field ordinal")
- defValues["train.validation"] = ("kfold", None)
- defValues["train.num.folds"] = (5, None)
- defValues["train.min.samples.split"] = ("4", None)
- defValues["train.min.samples.leaf.gb"] = ("2", None)
- defValues["train.max.depth.gb"] = (3, None)
- defValues["train.max.leaf.nodes.gb"] = (None, None)
- defValues["train.max.features.gb"] = (None, None)
- defValues["train.learning.rate"] = (0.1, None)
- defValues["train.num.estimators.gb"] = (100, None)
- defValues["train.subsample"] = (1.0, None)
- defValues["train.loss"] = ("deviance", None)
- defValues["train.random.state"] = (None, None)
- defValues["train.verbose"] = (0, None)
- defValues["train.warm.start"] = (False, None)
- defValues["train.presort"] = ("auto", None)
- defValues["train.criterion"] = ("friedman_mse", None)
- defValues["train.success.criterion"] = ("error", None)
- defValues["train.model.save"] = (False, None)
- defValues["train.score.method"] = ("accuracy", None)
- defValues["train.search.param.strategy"] = (None, None)
- defValues["train.search.params"] = (None, None)
- defValues["predict.data.file"] = (None, None)
- defValues["predict.data.fields"] = (None, "missing data field ordinals")
- defValues["predict.data.feature.fields"] = (None, "missing data feature field ordinals")
- defValues["predict.use.saved.model"] = (False, None)
- defValues["validate.data.file"] = (None, "missing validation data file")
- defValues["validate.data.fields"] = (None, "missing validation data field ordinals")
- defValues["validate.data.feature.fields"] = (None, "missing validation data feature field ordinals")
- defValues["validate.data.class.field"] = (None, "missing class field ordinal")
- defValues["validate.use.saved.model"] = (False, None)
- defValues["validate.score.method"] = ("accuracy", None)
-
- self.config = Configuration(configFile, defValues)
- self.subSampleRate = None
- self.featData = None
- self.clsData = None
- self.gbcClassifier = None
- self.verbose = self.config.getBooleanConfig("common.verbose")[0]
- logFilePath = self.config.getStringConfig("common.logging.file")[0]
- logLevName = self.config.getStringConfig("common.logging.level")[0]
- self.logger = createLogger(__name__, logFilePath, logLevName)
- self.logger.info("********* starting session")
-
- # initialize config
- def initConfig(self, configFile, defValues):
- self.config = Configuration(configFile, defValues)
-
- # get config object
- def getConfig(self):
- return self.config
-
- #set config param
- def setConfigParam(self, name, value):
- self.config.setParam(name, value)
-
- #get mode
- def getMode(self):
- return self.config.getStringConfig("common.mode")[0]
-
- #get search parameter
- def getSearchParamStrategy(self):
- return self.config.getStringConfig("train.search.param.strategy")[0]
-
- def setModel(self, model):
- self.gbcClassifier = model
-
- # train model
- def train(self):
- #build model
- self.buildModel()
-
- # training data
- if self.featData is None:
- (featData, clsData) = self.prepTrainingData()
- (self.featData, self.clsData) = (featData, clsData)
- else:
- (featData, clsData) = (self.featData, self.clsData)
- if self.subSampleRate is not None:
- (featData, clsData) = subSample(featData, clsData, self.subSampleRate, False)
- self.logger.info("subsample size " + str(featData.shape[0]))
-
- # parameters
- modelSave = self.config.getBooleanConfig("train.model.save")[0]
-
- #train
- self.logger.info("...training model")
- self.gbcClassifier.fit(featData, clsData)
- score = self.gbcClassifier.score(featData, clsData)
- successCriterion = self.config.getStringConfig("train.success.criterion")[0]
- result = None
- if successCriterion == "accuracy":
- self.logger.info("accuracy with training data {:06.3f}".format(score))
- result = score
- elif successCriterion == "error":
- error = 1.0 - score
- self.logger.info("error with training data {:06.3f}".format(error))
- result = error
- else:
- raise ValueError("invalid success criterion")
-
- if modelSave:
- self.logger.info("...saving model")
- modelFilePath = self.getModelFilePath()
- joblib.dump(self.gbcClassifier, modelFilePath)
- return result
-
- #train with k fold validation
- def trainValidate(self):
- #build model
- self.buildModel()
-
- # training data
- (featData, clsData) = self.prepTrainingData()
-
- #parameter
- validation = self.config.getStringConfig("train.validation")[0]
- numFolds = self.config.getIntConfig("train.num.folds")[0]
- successCriterion = self.config.getStringConfig("train.success.criterion")[0]
- scoreMethod = self.config.getStringConfig("train.score.method")[0]
-
- #train with validation
- self.logger.info("...training and kfold cross validating model")
- scores = cross_val_score(self.gbcClassifier, featData, clsData, cv=numFolds,scoring=scoreMethod)
- avScore = np.mean(scores)
- result = self.reportResult(avScore, successCriterion, scoreMethod)
- return result
-
- #train with k fold validation and search parameter space for optimum
- def trainValidateSearch(self):
- self.logger.info("...starting train validate with parameter search")
- searchStrategyName = self.getSearchParamStrategy()
- if searchStrategyName is not None:
- if searchStrategyName == "grid":
- searchStrategy = GuidedParameterSearch(self.verbose)
- elif searchStrategyName == "random":
- searchStrategy = RandomParameterSearch(self.verbose)
- maxIter = self.config.getIntConfig("train.search.max.iterations")[0]
- searchStrategy.setMaxIter(maxIter)
- elif searchStrategyName == "simuan":
- searchStrategy = SimulatedAnnealingParameterSearch(self.verbose)
- maxIter = self.config.getIntConfig("train.search.max.iterations")[0]
- searchStrategy.setMaxIter(maxIter)
- temp = self.config.getFloatConfig("train.search.sa.temp")[0]
- searchStrategy.setTemp(temp)
- tempRedRate = self.config.getFloatConfig("train.search.sa.temp.red.rate")[0]
- searchStrategy.setTempReductionRate(tempRedRate)
- else:
- raise ValueError("invalid paramtere search strategy")
- else:
- raise ValueError("missing search strategy")
-
- # add search params
- searchParams = self.config.getStringConfig("train.search.params")[0].split(",")
- searchParamNames = []
- extSearchParamNames = []
- if searchParams is not None:
- for searchParam in searchParams:
- paramItems = searchParam.split(":")
- extSearchParamNames.append(paramItems[0])
-
- #get rid name component search
- paramNameItems = paramItems[0].split(".")
- del paramNameItems[1]
- paramItems[0] = ".".join(paramNameItems)
-
- searchStrategy.addParam(paramItems)
- searchParamNames.append(paramItems[0])
- else:
- raise ValueError("missing search parameter list")
-
- # add search param data list for each param
- for (searchParamName,extSearchParamName) in zip(searchParamNames,extSearchParamNames):
- searchParamData = self.config.getStringConfig(extSearchParamName)[0].split(",")
- searchStrategy.addParamVaues(searchParamName, searchParamData)
-
- # train and validate for various param value combination
- searchStrategy.prepare()
- paramValues = searchStrategy.nextParamValues()
- searchResults = []
- while paramValues is not None:
- self.logger.info("...next parameter set")
- paramStr = ""
- for paramValue in paramValues:
- self.setConfigParam(paramValue[0], str(paramValue[1]))
- paramStr = paramStr + paramValue[0] + "=" + str(paramValue[1]) + " "
- result = self.trainValidate()
- searchStrategy.setCost(result)
- searchResults.append((paramStr, result))
- paramValues = searchStrategy.nextParamValues()
-
- # output
- self.logger.info("all parameter search results")
- for searchResult in searchResults:
- self.logger.info("{}\t{:06.3f}".format(searchResult[0], searchResult[1]))
-
- self.logger.info("best parameter search result")
- bestSolution = searchStrategy.getBestSolution()
- paramStr = ""
- for paramValue in bestSolution[0]:
- paramStr = paramStr + paramValue[0] + "=" + str(paramValue[1]) + " "
- self.logger.info("{}\t{:06.3f}".format(paramStr, bestSolution[1]))
- return bestSolution
-
- #predict
- def validate(self):
- # create model
- useSavedModel = self.config.getBooleanConfig("validate.use.saved.model")[0]
- if useSavedModel:
- # load saved model
- self.logger.info("...loading model")
- modelFilePath = self.getModelFilePath()
- self.gbcClassifier = joblib.load(modelFilePath)
- else:
- # train model
- self.train()
-
- # prepare test data
- (featData, clsDataActual) = self.prepValidationData()
-
- #predict
- self.logger.info("...predicting")
- clsDataPred = self.gbcClassifier.predict(featData)
-
- self.logger.info("...validating")
- #self.logger.info(clsData)
- scoreMethod = self.config.getStringConfig("validate.score.method")[0]
- if scoreMethod == "accuracy":
- accuracy = accuracy_score(clsDataActual, clsDataPred)
- self.logger.info("accuracy:")
- self.logger.info(accuracy)
- elif scoreMethod == "confusionMatrix":
- confMatrx = confusion_matrix(clsDataActual, clsDataPred)
- self.logger.info("confusion matrix:")
- self.logger.info(confMatrx)
-
-
- #predict
- def predictx(self):
- # create model
- useSavedModel = self.config.getBooleanConfig("predict.use.saved.model")[0]
- if useSavedModel:
- # load saved model
- self.logger.info("...loading model")
- modelFilePath = self.getModelFilePath()
- self.gbcClassifier = joblib.load(modelFilePath)
- else:
- # train model
- self.train()
-
- # prepare test data
- featData = self.prepPredictData()
-
- #predict
- self.logger.info("...predicting")
- clsData = self.gbcClassifier.predict(featData)
- self.logger.info(clsData)
-
- #predict with in memory data
- def predict(self, recs=None):
- # create model
- self.prepModel()
-
- #input record
- #input record
- if recs:
- #passed record
- featData = self.prepStringPredictData(recs)
- if (featData.ndim == 1):
- featData = featData.reshape(1, -1)
- else:
- #file
- featData = self.prepPredictData()
-
- #predict
- self.logger.info("...predicting")
- clsData = self.gbcClassifier.predict(featData)
- return clsData
-
- #predict probability with in memory data
- def predictProb(self, recs):
- # create model
- self.prepModel()
-
- #input record
- if type(recs) is str:
- featData = self.prepStringPredictData(recs)
- else:
- featData = recs
- #self.logger.info(featData.shape)
- if (featData.ndim == 1):
- featData = featData.reshape(1, -1)
-
- #predict
- self.logger.info("...predicting class probability")
- clsData = self.gbcClassifier.predict_proba(featData)
- return clsData
-
- #preparing model
- def prepModel(self):
- useSavedModel = self.config.getBooleanConfig("predict.use.saved.model")[0]
- if (useSavedModel and not self.gbcClassifier):
- # load saved model
- self.logger.info("...loading saved model")
- modelFilePath = self.getModelFilePath()
- self.gbcClassifier = joblib.load(modelFilePath)
- else:
- # train model
- self.train()
- return self.gbcClassifier
-
- #prepare string predict data
- def prepStringPredictData(self, recs):
- frecs = StringIO(recs)
- featData = np.loadtxt(frecs, delimiter=',')
- #self.logger.info(featData)
- return featData
-
- #loads and prepares training data
- def prepTrainingData(self):
- # parameters
- dataFile = self.config.getStringConfig("train.data.file")[0]
- fieldIndices = self.config.getStringConfig("train.data.fields")[0]
- if not fieldIndices is None:
- fieldIndices = strToIntArray(fieldIndices, ",")
- featFieldIndices = self.config.getStringConfig("train.data.feature.fields")[0]
- if not featFieldIndices is None:
- featFieldIndices = strToIntArray(featFieldIndices, ",")
- classFieldIndex = self.config.getIntConfig("train.data.class.field")[0]
-
- #training data
- (data, featData) = loadDataFile(dataFile, ",", fieldIndices, featFieldIndices)
- clsData = extrColumns(data, classFieldIndex)
- clsData = np.array([int(a) for a in clsData])
- return (featData, clsData)
-
- #loads and prepares training data
- def prepValidationData(self):
- # parameters
- dataFile = self.config.getStringConfig("validate.data.file")[0]
- fieldIndices = self.config.getStringConfig("validate.data.fields")[0]
- if not fieldIndices is None:
- fieldIndices = strToIntArray(fieldIndices, ",")
- featFieldIndices = self.config.getStringConfig("validate.data.feature.fields")[0]
- if not featFieldIndices is None:
- featFieldIndices = strToIntArray(featFieldIndices, ",")
- classFieldIndex = self.config.getIntConfig("validate.data.class.field")[0]
-
- #training data
- (data, featData) = loadDataFile(dataFile, ",", fieldIndices, featFieldIndices)
- clsData = extrColumns(data, classFieldIndex)
- clsData = [int(a) for a in clsData]
- return (featData, clsData)
-
- #loads and prepares training data
- def prepPredictData(self):
- # parameters
- dataFile = self.config.getStringConfig("predict.data.file")[0]
- if dataFile is None:
- raise ValueError("missing prediction data file")
- fieldIndices = self.config.getStringConfig("predict.data.fields")[0]
- if not fieldIndices is None:
- fieldIndices = strToIntArray(fieldIndices, ",")
- featFieldIndices = self.config.getStringConfig("predict.data.feature.fields")[0]
- if not featFieldIndices is None:
- featFieldIndices = strToIntArray(featFieldIndices, ",")
-
- #training data
- (data, featData) = loadDataFile(dataFile, ",", fieldIndices, featFieldIndices)
-
- return featData
-
- # get model file path
- def getModelFilePath(self):
- modelDirectory = self.config.getStringConfig("common.model.directory")[0]
- modelFile = self.config.getStringConfig("common.model.file")[0]
- if modelFile is None:
- raise ValueError("missing model file name")
- modelFilePath = modelDirectory + "/" + modelFile
- return modelFilePath
-
- # report result
- def reportResult(self, score, successCriterion, scoreMethod):
- if successCriterion == "accuracy":
- self.logger.info("average " + scoreMethod + " with k fold cross validation {:06.3f}".format(score))
- result = score
- elif successCriterion == "error":
- error = 1.0 - score
- self.logger.info("average error with k fold cross validation {:06.3f}".format(error))
- result = error
- else:
- raise ValueError("invalid success criterion")
- return result
-
- # builds model object
- def buildModel(self):
- self.logger.info("...building gradient boosted tree model")
- # parameters
- minSamplesSplit = self.config.getStringConfig("train.min.samples.split")[0]
- minSamplesSplit = typedValue(minSamplesSplit)
- minSamplesLeaf = self.config.getStringConfig("train.min.samples.leaf.gb")[0]
- minSamplesLeaf = typedValue(minSamplesLeaf)
- #minWeightFractionLeaf = self.config.getFloatConfig("train.min.weight.fraction.leaf.gb")[0]
- (maxDepth, maxLeafNodes) = self.config.eitherOrIntConfig("train.max.depth.gb", "train.max.leaf.nodes.gb")
- maxFeatures = self.config.getStringConfig("train.max.features.gb")[0]
- maxFeatures = typedValue(maxFeatures)
- learningRate = self.config.getFloatConfig("train.learning.rate")[0]
- numEstimators = self.config.getIntConfig("train.num.estimators.gb")[0]
- subsampleFraction = self.config.getFloatConfig("train.subsample")[0]
- lossFun = self.config.getStringConfig("train.loss")[0]
- randomState = self.config.getIntConfig("train.random.state")[0]
- verboseOutput = self.config.getIntConfig("train.verbose")[0]
- warmStart = self.config.getBooleanConfig("train.warm.start")[0]
- presort = self.config.getStringConfig("train.presort")
- if (presort[1]):
- presortChoice = presort[0]
- else:
- presortChoice = presort[0].lower() == "true"
- splitCriterion = self.config.getStringConfig("train.criterion")[0]
-
- #classifier
- self.gbcClassifier = GradientBoostingClassifier(loss=lossFun, learning_rate=learningRate, n_estimators=numEstimators,
- subsample=subsampleFraction, min_samples_split=minSamplesSplit,
- min_samples_leaf=minSamplesLeaf, min_weight_fraction_leaf=0.0, max_depth=maxDepth,
- init=None, random_state=randomState, max_features=maxFeatures, verbose=verboseOutput,
- max_leaf_nodes=maxLeafNodes, warm_start=warmStart, presort=presortChoice)
-
-
-
-
-
diff --git a/spaces/ViktorTsoi13/ABA_Test/greeting.md b/spaces/ViktorTsoi13/ABA_Test/greeting.md
deleted file mode 100644
index 46ba433c7f64d0dfba0180ef6fd0d980bd5efd67..0000000000000000000000000000000000000000
--- a/spaces/ViktorTsoi13/ABA_Test/greeting.md
+++ /dev/null
@@ -1 +0,0 @@
-Most popular ABA private server
\ No newline at end of file
diff --git a/spaces/VishalF5/Text_Similarity/README.md b/spaces/VishalF5/Text_Similarity/README.md
deleted file mode 100644
index cbe7b14d0a32122de00a57d7297e2c65cd2483eb..0000000000000000000000000000000000000000
--- a/spaces/VishalF5/Text_Similarity/README.md
+++ /dev/null
@@ -1,12 +0,0 @@
----
-title: Text Similarity
-emoji: 🐠
-colorFrom: indigo
-colorTo: blue
-sdk: streamlit
-sdk_version: 1.10.0
-app_file: app.py
-pinned: false
----
-
-Check out the configuration reference at https://huggingface.co/docs/hub/spaces-config-reference
diff --git a/spaces/WanderingRose/Storm/Dockerfile b/spaces/WanderingRose/Storm/Dockerfile
deleted file mode 100644
index eef259fa372a804549fb0af0913718a13344da34..0000000000000000000000000000000000000000
--- a/spaces/WanderingRose/Storm/Dockerfile
+++ /dev/null
@@ -1,11 +0,0 @@
-FROM node:18-bullseye-slim
-RUN apt-get update && \
- apt-get install -y git
-RUN git clone https://gitgud.io/khanon/oai-reverse-proxy.git /app
-WORKDIR /app
-RUN npm install
-COPY Dockerfile greeting.md* .env* ./
-RUN npm run build
-EXPOSE 7860
-ENV NODE_ENV=production
-CMD [ "npm", "start" ]
diff --git a/spaces/Wauplin/bloomz.cpp-converter/convert.py b/spaces/Wauplin/bloomz.cpp-converter/convert.py
deleted file mode 100644
index 6929cf98b8be7e597fbc787de58550718b741948..0000000000000000000000000000000000000000
--- a/spaces/Wauplin/bloomz.cpp-converter/convert.py
+++ /dev/null
@@ -1,51 +0,0 @@
-from pathlib import Path
-from subprocess import run
-from typing import Generator
-
-BLOOMZ_FOLDER = Path(__file__).parent / "bloomz.cpp"
-
-
-def convert(
- cache_folder: Path, model_id: str, precision: str, quantization: bool
-) -> Generator[str, Path, None]:
- # Conversion
- cmd = [
- "python",
- str(BLOOMZ_FOLDER / "convert-hf-to-ggml.py"),
- model_id,
- str(cache_folder),
- ]
- if precision == "FP32":
- cmd.append("--use-fp32")
- yield f"Running command: `{' '.join(cmd)}`"
- run(cmd, check=True)
-
- # Model file should exist
- f_suffix = "f32" if precision == "FP32" else "f16"
- _, model_name = model_id.split("/")
- model_path = cache_folder / f"ggml-model-{model_name}-{f_suffix}.bin"
- assert model_path.is_file()
- yield f"Model successfully converted to ggml: {model_path}"
-
- # Quantization
- if quantization:
- q_model_path = (
- cache_folder / f"ggml-model-{model_name}-{f_suffix}-q4_0.bin"
- )
- cmd = [
- "./bloomz.cpp/quantize",
- str(model_path),
- str(q_model_path),
- "2",
- ]
- yield f"Running command: `{' '.join(cmd)}`"
- run(cmd, check=True)
- assert q_model_path.is_file()
-
- # Delete non-quantized file
- model_path.unlink(missing_ok=True)
- model_path = q_model_path
- yield f"Model successfully quantized: {model_path}"
-
- # Return
- return model_path
diff --git a/spaces/XS-1/BW_IMAGE_VIDEO_COLORIZER/fastai/text/interpret.py b/spaces/XS-1/BW_IMAGE_VIDEO_COLORIZER/fastai/text/interpret.py
deleted file mode 100644
index 4073ffd1fc63d334461fde347c17a84a3c26625b..0000000000000000000000000000000000000000
--- a/spaces/XS-1/BW_IMAGE_VIDEO_COLORIZER/fastai/text/interpret.py
+++ /dev/null
@@ -1,100 +0,0 @@
-from ..torch_core import *
-from ..basic_data import *
-from ..basic_train import *
-from ..train import ClassificationInterpretation
-import matplotlib.cm as cm
-
-__all__ = ['TextClassificationInterpretation']
-
-def value2rgba(x:float, cmap:Callable=cm.RdYlGn, alpha_mult:float=1.0)->Tuple:
- "Convert a value `x` from 0 to 1 (inclusive) to an RGBA tuple according to `cmap` times transparency `alpha_mult`."
- c = cmap(x)
- rgb = (np.array(c[:-1]) * 255).astype(int)
- a = c[-1] * alpha_mult
- return tuple(rgb.tolist() + [a])
-
-def piece_attn_html(pieces:List[str], attns:List[float], sep:str=' ', **kwargs)->str:
- html_code,spans = [''], []
- for p, a in zip(pieces, attns):
- p = html.escape(p)
- c = str(value2rgba(a, alpha_mult=0.5, **kwargs))
- spans.append(f'{p} ')
- html_code.append(sep.join(spans))
- html_code.append(' ')
- return ''.join(html_code)
-
-def show_piece_attn(*args, **kwargs):
- from IPython.display import display, HTML
- display(HTML(piece_attn_html(*args, **kwargs)))
-
-def _eval_dropouts(mod):
- module_name = mod.__class__.__name__
- if 'Dropout' in module_name or 'BatchNorm' in module_name: mod.training = False
- for module in mod.children(): _eval_dropouts(module)
-
-class TextClassificationInterpretation(ClassificationInterpretation):
- """Provides an interpretation of classification based on input sensitivity.
- This was designed for AWD-LSTM only for the moment, because Transformer already has its own attentional model.
- """
-
- def __init__(self, learn: Learner, preds: Tensor, y_true: Tensor, losses: Tensor, ds_type: DatasetType = DatasetType.Valid):
- super(TextClassificationInterpretation, self).__init__(learn,preds,y_true,losses,ds_type)
- self.model = learn.model
-
- @classmethod
- def from_learner(cls, learn: Learner, ds_type:DatasetType=DatasetType.Valid, activ:nn.Module=None):
- "Gets preds, y_true, losses to construct base class from a learner"
- preds_res = learn.get_preds(ds_type=ds_type, activ=activ, with_loss=True, ordered=True)
- return cls(learn, *preds_res)
-
- def intrinsic_attention(self, text:str, class_id:int=None):
- """Calculate the intrinsic attention of the input w.r.t to an output `class_id`, or the classification given by the model if `None`.
- For reference, see the Sequential Jacobian session at https://www.cs.toronto.edu/~graves/preprint.pdf
- """
- self.model.train()
- _eval_dropouts(self.model)
- self.model.zero_grad()
- self.model.reset()
- ids = self.data.one_item(text)[0]
- emb = self.model[0].module.encoder(ids).detach().requires_grad_(True)
- lstm_output = self.model[0].module(emb, from_embeddings=True)
- self.model.eval()
- cl = self.model[1](lstm_output + (torch.zeros_like(ids).byte(),))[0].softmax(dim=-1)
- if class_id is None: class_id = cl.argmax()
- cl[0][class_id].backward()
- attn = emb.grad.squeeze().abs().sum(dim=-1)
- attn /= attn.max()
- tokens = self.data.single_ds.reconstruct(ids[0])
- return tokens, attn
-
- def html_intrinsic_attention(self, text:str, class_id:int=None, **kwargs)->str:
- text, attn = self.intrinsic_attention(text, class_id)
- return piece_attn_html(text.text.split(), to_np(attn), **kwargs)
-
- def show_intrinsic_attention(self, text:str, class_id:int=None, **kwargs)->None:
- text, attn = self.intrinsic_attention(text, class_id)
- show_piece_attn(text.text.split(), to_np(attn), **kwargs)
-
- def show_top_losses(self, k:int, max_len:int=70)->None:
- """
- Create a tabulation showing the first `k` texts in top_losses along with their prediction, actual,loss, and probability of
- actual class. `max_len` is the maximum number of tokens displayed.
- """
- from IPython.display import display, HTML
- items = []
- tl_val,tl_idx = self.top_losses()
- for i,idx in enumerate(tl_idx):
- if k <= 0: break
- k -= 1
- tx,cl = self.data.dl(self.ds_type).dataset[idx]
- cl = cl.data
- classes = self.data.classes
- txt = ' '.join(tx.text.split(' ')[:max_len]) if max_len is not None else tx.text
- tmp = [txt, f'{classes[self.pred_class[idx]]}', f'{classes[cl]}', f'{self.losses[idx]:.2f}',
- f'{self.preds[idx][cl]:.2f}']
- items.append(tmp)
- items = np.array(items)
- names = ['Text', 'Prediction', 'Actual', 'Loss', 'Probability']
- df = pd.DataFrame({n:items[:,i] for i,n in enumerate(names)}, columns=names)
- with pd.option_context('display.max_colwidth', -1):
- display(HTML(df.to_html(index=False)))
diff --git a/spaces/Xinyoumeng233hu/SteganographywithGPT-2/utils.py b/spaces/Xinyoumeng233hu/SteganographywithGPT-2/utils.py
deleted file mode 100644
index 2a3660e600b8833d3399c78c8e6a0eb5c48f16c7..0000000000000000000000000000000000000000
--- a/spaces/Xinyoumeng233hu/SteganographywithGPT-2/utils.py
+++ /dev/null
@@ -1,296 +0,0 @@
-import torch
-import numpy as np
-import bitarray
-
-from pytorch_transformers import GPT2LMHeadModel, GPT2Tokenizer
-
-def decode(self, token_ids, **kwargs):
- filtered_tokens = self.convert_ids_to_tokens(token_ids)
- text = self.convert_tokens_to_string(filtered_tokens)
- return text
-GPT2Tokenizer.decode = decode
-
-def _convert_token_to_id(self, token):
- return self.encoder.get(token, 0)
-GPT2Tokenizer._convert_token_to_id = _convert_token_to_id
-
-
-def limit_past(past):
- past = list(past)
- for i in range(len(past)):
- past[i] = past[i][:, :, :, -1022:]
- return past
-
-def kl(q, logq, logp):
- res = q*(logq-logp)/0.69315
- res[q==0] = 0
- return res.sum().item() # in bits
-
-def entropy(q, logq):
- res = q*logq/0.69315
- res[q==0] = 0
- return -res.sum().item() # in bits
-
-# e.g. [0, 1, 1, 1] looks like 1110=14
-def bits2int(bits):
- res = 0
- for i, bit in enumerate(bits):
- res += bit*(2**i)
- return res
-
-def int2bits(inp, num_bits):
- if num_bits == 0:
- return []
- strlist = ('{0:0%db}'%num_bits).format(inp)
- return [int(strval) for strval in reversed(strlist)]
-
-def is_sent_finish(token_idx, enc):
- token = enc.decoder[token_idx]
- return '.' in token or '!' in token or '?' in token
-
-def num_same_from_beg(bits1, bits2):
- assert len(bits1) == len(bits2)
- for i in range(len(bits1)):
- if bits1[i] != bits2[i]:
- break
-
- return i
-
-def encode_context(raw_text, enc):
- context_tokens = [enc.encoder['<|endoftext|>']] + enc.encode(raw_text)
- return context_tokens
-
-# Use gpt2-medium for 345M param model
-# Use gpt2-large for 774M param model
-def get_model(seed=1234, model_name='gpt2'):
- np.random.seed(seed)
- torch.random.manual_seed(seed)
- torch.cuda.manual_seed(seed)
- device = torch.device("cpu")
-
- enc = GPT2Tokenizer.from_pretrained(model_name)
- enc.unk_token = None
- enc.bos_token = None
- enc.eos_token = None
-
- model = GPT2LMHeadModel.from_pretrained(model_name)
- model.to(device)
- model.eval()
- #model.double()
-
- return enc, model
-
-enc32_itoc = ['\0', 'a', 'b', 'c', 'd', 'e', 'f', 'g', 'h', 'i', 'j', 'k', 'l', 'm', 'n', 'o', 'p', 'q', 'r', 's', 't', 'u', 'v', 'w', 'x', 'y', 'z', '.', ',', "'", '!', ' ']
-enc32_ctoi = {k: v for v, k in enumerate(enc32_itoc)}
-def enc32(text):
- bits = []
- for c in text:
- bits.extend(int2bits(enc32_ctoi[c], 5))
- return bits
-
-def dec32(bits):
- text = ''
- for i in range(0, len(bits), 5):
- c = enc32_itoc[bits2int(bits[i:i+5])]
- if c == '\0':
- break
- text += c
- return text
-
-# message should be bit string
-# encoded should be text string
-def expansion_ratio(message, encoded):
- message_bits = len(message)
- encoded_ba = bitarray.bitarray()
- encoded_ba.frombytes(encoded.encode('utf-8'))
- encoded_bits = len(encoded_ba.tolist())
- return encoded_bits/message_bits
-
-#@title
-import torch
-import math
-import random
-
-def bin_sort(l, token_indices, total, entropy, device):
- #compute entropy for upper bound on the number of bins we need
-
- bucket_size = total
- num_bins = 2**int(entropy+1)
- bucket_size = total / num_bins
-
- bins = [torch.empty(0, dtype=torch.long, device=device)] * num_bins
- value_in_bins = [0] * num_bins
- space_left_after = [total - i*bucket_size for i in range(0,num_bins)]
-
-
- token_bins = [torch.empty(0, dtype=torch.long, device=device)] * num_bins
-
- # Figuring out what the search order should be
- step_size = num_bins/4
- search_order = []
- priorities = [0]*num_bins
- priority = 0
- search_order.append(int(num_bins/2))
- search_order.append(0)
- priorities[int(num_bins/2)] = 0
- priorities[0] = 0
- while(step_size>=1):
- priority += 1
- for x in range(num_bins-int(step_size), -1, -int(step_size*2)):
- search_order.append(x)
- priorities[x] = priority
- step_size = step_size/2
-
- # Adding the actual elements
- for (item, token_index) in zip(l.tolist(), token_indices.tolist()):
- found_single_bucket_fit = False
- single_bucket_index = -1
- single_bucket_value = bucket_size
-
- found_multi_bucket_bumpless_fit = False
- multi_bucket_bumpless_index = -1
- multi_bucket_bumpless_value = total
-
- found_multi_bucket_bumping_fit = False
- multi_bucket_bumping_index = -1
- multi_bucket_bumping_value = total
-
- for i in search_order: # for index in search_order
- if(item > space_left_after[i]):
- continue
- if(value_in_bins[i] >= bucket_size):
- continue
-
- # Priority of choices
- # 1. Can i place this thing in an empty bucket all on its own?
- # 2. Can i plan this somewhere where is doesnt have to bump anything else around?
- # 2a. Minimize the wasted space. Aka use the smallest space (of equal priority) that accomplishes this goal
- # 3. If not (1) and (2), then put it in the space the bumps stuff the least.
-
- if(value_in_bins[i] + item > bucket_size): #Would overflow.
-
- space_before_next_block = bucket_size - value_in_bins[i]
- for j in range(i+1, len(bins)):
- if(value_in_bins[j] > 0): # We have found a bucket with something in it. This is how much space we have here.
- space_before_next_block = space_before_next_block + (bucket_size - value_in_bins[i])
- break
- else: # This was a empty bucket
- space_before_next_block = space_before_next_block + bucket_size
-
- if((not found_multi_bucket_bumpless_fit) or (found_multi_bucket_bumpless_fit and priorities[i] <= priorities[multi_bucket_bumpless_index])): #This could potentially be a match
-
- # If this is a valid space to put this without bumping and it is a better fit than previous spaces
- if(space_before_next_block > item and space_before_next_block < multi_bucket_bumpless_value):
- # set this to be the pointer! we can fit stuff here
- found_multi_bucket_bumpless_fit = True
- multi_bucket_bumpless_index = i
- multi_bucket_bumpless_value = space_before_next_block
-
- # Find the overflow that will bump the least
- if ( item - space_before_next_block < multi_bucket_bumping_value):
- found_multi_bucket_bumping_fit = True
- multi_bucket_bumping_index = i
- multi_bucket_bumping_value = item - space_before_next_block
-
- if(value_in_bins[i] + item <= bucket_size): #Would fit
- if(single_bucket_value > value_in_bins[i]):
- found_single_bucket_fit = True
- single_bucket_value = value_in_bins[i]
- single_bucket_index = i
-
- if (single_bucket_index == multi_bucket_bumpless_index == multi_bucket_bumping_index == -1):
- bins[0] = torch.cat( (torch.tensor([item], device=device), bins[0]), 0)
- token_bins[0] = torch.cat( (torch.tensor([token_index], device=device), token_bins[0]), 0)
- continue
-
-
- if found_single_bucket_fit:
- # We found somewhere we can actually fit!
- bins[single_bucket_index] = torch.cat( (bins[single_bucket_index], torch.tensor([item], device=device)), 0)
- token_bins[single_bucket_index] = torch.cat( (token_bins[single_bucket_index], torch.tensor([token_index], device=device)), 0)
- value_in_bins[single_bucket_index] += item
- for i in range(0, single_bucket_index+1):
- space_left_after[i] -= item
-
- elif found_multi_bucket_bumpless_fit:
- # Found somewhere we can put this without upsetting the force
- part_in_bucket = bucket_size - value_in_bins[multi_bucket_bumpless_index]
- part_overflow = item - part_in_bucket
- bins[multi_bucket_bumpless_index] = torch.cat( (bins[multi_bucket_bumpless_index], torch.tensor([item], device=device)), 0)
- token_bins[multi_bucket_bumpless_index] = torch.cat( (token_bins[multi_bucket_bumpless_index], torch.tensor([token_index], device=device)), 0)
- value_in_bins[multi_bucket_bumpless_index] = bucket_size
-
- # Fill this bucket and continue overflowing
- j = multi_bucket_bumpless_index + 1
- for i in range(0, j):
- space_left_after[i] -= item
-
- while(part_overflow > 0):
- new_part_overflow = (value_in_bins[j] + part_overflow) - bucket_size
- value_in_bins[j] = min(bucket_size, part_overflow+value_in_bins[j]) # mark the bucket as filled
- space_left_after[j] -= part_overflow
- part_overflow = new_part_overflow
- j+=1
-
- else:
- part_in_bucket = bucket_size - value_in_bins[multi_bucket_bumping_index]
- part_overflow = item - part_in_bucket
- bins[multi_bucket_bumping_index] = torch.cat( (bins[multi_bucket_bumping_index], torch.tensor([item], device=device)), 0)
- token_bins[multi_bucket_bumping_index] = torch.cat( (token_bins[multi_bucket_bumping_index], torch.tensor([token_index], device=device)), 0)
- value_in_bins[multi_bucket_bumping_index] = bucket_size
-
- # Fill this bucket and continue overflowing
- j = multi_bucket_bumping_index + 1
- for i in range(0, j):
- space_left_after[i] -= item
- while(part_overflow > 0):
- new_part_overflow = (value_in_bins[j] + part_overflow) - bucket_size
- value_in_bins[j] = min(bucket_size, part_overflow+value_in_bins[j]) # mark the bucket as filled
- space_left_after[j] -= part_overflow
- part_overflow = new_part_overflow
- j+=1
-
- sorted_tensor = torch.cat(bins, 0)
- sorted_tokens = torch.cat(token_bins, 0)
-
- return sorted_tensor, sorted_tokens
-
-def compute_ev(t, precision):
- expected_bits = []
- cum_probs = t.cumsum(0)
-
- for selection in range(0, len(cum_probs)):
-
- # Calculate new range as ints
- new_int_bottom = cum_probs[selection-1] if selection > 0 else 0
- new_int_top = cum_probs[selection]
-
- # Convert range to bits
- new_int_bottom_bits_inc = list(reversed(int2bits(new_int_bottom, precision)))
- new_int_top_bits_inc = list(reversed(int2bits(new_int_top-1, precision))) # -1 here because upper bound is exclusive
-
- # Consume most significant bits which are now fixed and update interval
- num_bits_encoded = num_same_from_beg(new_int_bottom_bits_inc, new_int_top_bits_inc)
- expected_bits.append(t[selection] * num_bits_encoded)
-
- return(float(sum(expected_bits).item())/(2**precision))
-
-def visualize_bins(values_in_bins, bucket_size):
- out_str = "["
- for b in values_in_bins:
- out_str = out_str + " " + str(round(100*b/bucket_size,2)) + " |"
- out_str = out_str + "]"
- print(out_str)
-
-def visualize_distribution(l):
- total = sum(l)
- out_str = "["
- for b in l:
- out_str = out_str + " " + str(round(100*b/total,2)) + " |"
- out_str = out_str + "]"
- print(out_str)
-
-def compute_entropy(lists):
- total = sum(lists)
- entropy = -1*sum([ (x/total) * math.log2(x/total) for x in lists])
- return entropy
\ No newline at end of file
diff --git a/spaces/XzJosh/Aatrox-Bert-VITS2/resample.py b/spaces/XzJosh/Aatrox-Bert-VITS2/resample.py
deleted file mode 100644
index 2ed1685654a371c5722168e9987809b05b1cb224..0000000000000000000000000000000000000000
--- a/spaces/XzJosh/Aatrox-Bert-VITS2/resample.py
+++ /dev/null
@@ -1,42 +0,0 @@
-import os
-import argparse
-import librosa
-import numpy as np
-from multiprocessing import Pool, cpu_count
-
-import soundfile
-from scipy.io import wavfile
-from tqdm import tqdm
-
-
-def process(item):
- spkdir, wav_name, args = item
- speaker = spkdir.replace("\\", "/").split("/")[-1]
- wav_path = os.path.join(args.in_dir, speaker, wav_name)
- if os.path.exists(wav_path) and '.wav' in wav_path:
- os.makedirs(os.path.join(args.out_dir, speaker), exist_ok=True)
- wav, sr = librosa.load(wav_path, sr=args.sr)
- soundfile.write(
- os.path.join(args.out_dir, speaker, wav_name),
- wav,
- sr
- )
-
-
-
-if __name__ == "__main__":
- parser = argparse.ArgumentParser()
- parser.add_argument("--sr", type=int, default=44100, help="sampling rate")
- parser.add_argument("--in_dir", type=str, default="./raw", help="path to source dir")
- parser.add_argument("--out_dir", type=str, default="./dataset", help="path to target dir")
- args = parser.parse_args()
- # processs = 8
- processs = cpu_count()-2 if cpu_count() >4 else 1
- pool = Pool(processes=processs)
-
- for speaker in os.listdir(args.in_dir):
- spk_dir = os.path.join(args.in_dir, speaker)
- if os.path.isdir(spk_dir):
- print(spk_dir)
- for _ in tqdm(pool.imap_unordered(process, [(spk_dir, i, args) for i in os.listdir(spk_dir) if i.endswith("wav")])):
- pass
diff --git a/spaces/XzJosh/Bekki-Bert-VITS2/losses.py b/spaces/XzJosh/Bekki-Bert-VITS2/losses.py
deleted file mode 100644
index fb22a0e834dd87edaa37bb8190eee2c3c7abe0d5..0000000000000000000000000000000000000000
--- a/spaces/XzJosh/Bekki-Bert-VITS2/losses.py
+++ /dev/null
@@ -1,61 +0,0 @@
-import torch
-from torch.nn import functional as F
-
-import commons
-
-
-def feature_loss(fmap_r, fmap_g):
- loss = 0
- for dr, dg in zip(fmap_r, fmap_g):
- for rl, gl in zip(dr, dg):
- rl = rl.float().detach()
- gl = gl.float()
- loss += torch.mean(torch.abs(rl - gl))
-
- return loss * 2
-
-
-def discriminator_loss(disc_real_outputs, disc_generated_outputs):
- loss = 0
- r_losses = []
- g_losses = []
- for dr, dg in zip(disc_real_outputs, disc_generated_outputs):
- dr = dr.float()
- dg = dg.float()
- r_loss = torch.mean((1-dr)**2)
- g_loss = torch.mean(dg**2)
- loss += (r_loss + g_loss)
- r_losses.append(r_loss.item())
- g_losses.append(g_loss.item())
-
- return loss, r_losses, g_losses
-
-
-def generator_loss(disc_outputs):
- loss = 0
- gen_losses = []
- for dg in disc_outputs:
- dg = dg.float()
- l = torch.mean((1-dg)**2)
- gen_losses.append(l)
- loss += l
-
- return loss, gen_losses
-
-
-def kl_loss(z_p, logs_q, m_p, logs_p, z_mask):
- """
- z_p, logs_q: [b, h, t_t]
- m_p, logs_p: [b, h, t_t]
- """
- z_p = z_p.float()
- logs_q = logs_q.float()
- m_p = m_p.float()
- logs_p = logs_p.float()
- z_mask = z_mask.float()
-
- kl = logs_p - logs_q - 0.5
- kl += 0.5 * ((z_p - m_p)**2) * torch.exp(-2. * logs_p)
- kl = torch.sum(kl * z_mask)
- l = kl / torch.sum(z_mask)
- return l
diff --git a/spaces/XzJosh/Echo-Bert-VITS2/bert_gen.py b/spaces/XzJosh/Echo-Bert-VITS2/bert_gen.py
deleted file mode 100644
index 44814715396ffc3abe84a12c74d66293c356eb4f..0000000000000000000000000000000000000000
--- a/spaces/XzJosh/Echo-Bert-VITS2/bert_gen.py
+++ /dev/null
@@ -1,53 +0,0 @@
-import torch
-from torch.utils.data import DataLoader
-from multiprocessing import Pool
-import commons
-import utils
-from data_utils import TextAudioSpeakerLoader, TextAudioSpeakerCollate
-from tqdm import tqdm
-import warnings
-
-from text import cleaned_text_to_sequence, get_bert
-
-config_path = 'configs/config.json'
-hps = utils.get_hparams_from_file(config_path)
-
-def process_line(line):
- _id, spk, language_str, text, phones, tone, word2ph = line.strip().split("|")
- phone = phones.split(" ")
- tone = [int(i) for i in tone.split(" ")]
- word2ph = [int(i) for i in word2ph.split(" ")]
- w2pho = [i for i in word2ph]
- word2ph = [i for i in word2ph]
- phone, tone, language = cleaned_text_to_sequence(phone, tone, language_str)
-
- if hps.data.add_blank:
- phone = commons.intersperse(phone, 0)
- tone = commons.intersperse(tone, 0)
- language = commons.intersperse(language, 0)
- for i in range(len(word2ph)):
- word2ph[i] = word2ph[i] * 2
- word2ph[0] += 1
- wav_path = f'{_id}'
-
- bert_path = wav_path.replace(".wav", ".bert.pt")
- try:
- bert = torch.load(bert_path)
- assert bert.shape[-1] == len(phone)
- except:
- bert = get_bert(text, word2ph, language_str)
- assert bert.shape[-1] == len(phone)
- torch.save(bert, bert_path)
-
-
-if __name__ == '__main__':
- lines = []
- with open(hps.data.training_files, encoding='utf-8' ) as f:
- lines.extend(f.readlines())
-
- with open(hps.data.validation_files, encoding='utf-8' ) as f:
- lines.extend(f.readlines())
-
- with Pool(processes=12) as pool: #A100 40GB suitable config,if coom,please decrease the processess number.
- for _ in tqdm(pool.imap_unordered(process_line, lines)):
- pass
diff --git a/spaces/XzJosh/Eileen-Bert-VITS2/bert_gen.py b/spaces/XzJosh/Eileen-Bert-VITS2/bert_gen.py
deleted file mode 100644
index 44814715396ffc3abe84a12c74d66293c356eb4f..0000000000000000000000000000000000000000
--- a/spaces/XzJosh/Eileen-Bert-VITS2/bert_gen.py
+++ /dev/null
@@ -1,53 +0,0 @@
-import torch
-from torch.utils.data import DataLoader
-from multiprocessing import Pool
-import commons
-import utils
-from data_utils import TextAudioSpeakerLoader, TextAudioSpeakerCollate
-from tqdm import tqdm
-import warnings
-
-from text import cleaned_text_to_sequence, get_bert
-
-config_path = 'configs/config.json'
-hps = utils.get_hparams_from_file(config_path)
-
-def process_line(line):
- _id, spk, language_str, text, phones, tone, word2ph = line.strip().split("|")
- phone = phones.split(" ")
- tone = [int(i) for i in tone.split(" ")]
- word2ph = [int(i) for i in word2ph.split(" ")]
- w2pho = [i for i in word2ph]
- word2ph = [i for i in word2ph]
- phone, tone, language = cleaned_text_to_sequence(phone, tone, language_str)
-
- if hps.data.add_blank:
- phone = commons.intersperse(phone, 0)
- tone = commons.intersperse(tone, 0)
- language = commons.intersperse(language, 0)
- for i in range(len(word2ph)):
- word2ph[i] = word2ph[i] * 2
- word2ph[0] += 1
- wav_path = f'{_id}'
-
- bert_path = wav_path.replace(".wav", ".bert.pt")
- try:
- bert = torch.load(bert_path)
- assert bert.shape[-1] == len(phone)
- except:
- bert = get_bert(text, word2ph, language_str)
- assert bert.shape[-1] == len(phone)
- torch.save(bert, bert_path)
-
-
-if __name__ == '__main__':
- lines = []
- with open(hps.data.training_files, encoding='utf-8' ) as f:
- lines.extend(f.readlines())
-
- with open(hps.data.validation_files, encoding='utf-8' ) as f:
- lines.extend(f.readlines())
-
- with Pool(processes=12) as pool: #A100 40GB suitable config,if coom,please decrease the processess number.
- for _ in tqdm(pool.imap_unordered(process_line, lines)):
- pass
diff --git a/spaces/XzJosh/Eileen-Bert-VITS2/preprocess_text.py b/spaces/XzJosh/Eileen-Bert-VITS2/preprocess_text.py
deleted file mode 100644
index 5eb0f3b9e929fcbe91dcbeb653391227a2518a15..0000000000000000000000000000000000000000
--- a/spaces/XzJosh/Eileen-Bert-VITS2/preprocess_text.py
+++ /dev/null
@@ -1,64 +0,0 @@
-import json
-from random import shuffle
-
-import tqdm
-from text.cleaner import clean_text
-from collections import defaultdict
-stage = [1,2,3]
-
-transcription_path = 'filelists/genshin.list'
-train_path = 'filelists/train.list'
-val_path = 'filelists/val.list'
-config_path = "configs/config.json"
-val_per_spk = 4
-max_val_total = 8
-
-if 1 in stage:
- with open( transcription_path+'.cleaned', 'w', encoding='utf-8') as f:
- for line in tqdm.tqdm(open(transcription_path, encoding='utf-8').readlines()):
- try:
- utt, spk, language, text = line.strip().split('|')
- norm_text, phones, tones, word2ph = clean_text(text, language)
- f.write('{}|{}|{}|{}|{}|{}|{}\n'.format(utt, spk, language, norm_text, ' '.join(phones),
- " ".join([str(i) for i in tones]),
- " ".join([str(i) for i in word2ph])))
- except Exception as error :
- print("err!", utt, error)
-
-if 2 in stage:
- spk_utt_map = defaultdict(list)
- spk_id_map = {}
- current_sid = 0
-
- with open( transcription_path+'.cleaned', encoding='utf-8') as f:
- for line in f.readlines():
- utt, spk, language, text, phones, tones, word2ph = line.strip().split('|')
- spk_utt_map[spk].append(line)
- if spk not in spk_id_map.keys():
- spk_id_map[spk] = current_sid
- current_sid += 1
- train_list = []
- val_list = []
-
- for spk, utts in spk_utt_map.items():
- shuffle(utts)
- val_list+=utts[:val_per_spk]
- train_list+=utts[val_per_spk:]
- if len(val_list) > max_val_total:
- train_list+=val_list[max_val_total:]
- val_list = val_list[:max_val_total]
-
- with open( train_path,"w", encoding='utf-8') as f:
- for line in train_list:
- f.write(line)
-
- with open(val_path, "w", encoding='utf-8') as f:
- for line in val_list:
- f.write(line)
-
-if 3 in stage:
- assert 2 in stage
- config = json.load(open(config_path, encoding='utf-8'))
- config["data"]['spk2id'] = spk_id_map
- with open(config_path, 'w', encoding='utf-8') as f:
- json.dump(config, f, indent=2, ensure_ascii=False)
diff --git a/spaces/XzJosh/Taffy-Bert-VITS2/bert/chinese-roberta-wwm-ext-large/README.md b/spaces/XzJosh/Taffy-Bert-VITS2/bert/chinese-roberta-wwm-ext-large/README.md
deleted file mode 100644
index 7bce039b7f81ee328fdf8efe3f14409200aacbef..0000000000000000000000000000000000000000
--- a/spaces/XzJosh/Taffy-Bert-VITS2/bert/chinese-roberta-wwm-ext-large/README.md
+++ /dev/null
@@ -1,57 +0,0 @@
----
-language:
-- zh
-tags:
-- bert
-license: "apache-2.0"
----
-
-# Please use 'Bert' related functions to load this model!
-
-## Chinese BERT with Whole Word Masking
-For further accelerating Chinese natural language processing, we provide **Chinese pre-trained BERT with Whole Word Masking**.
-
-**[Pre-Training with Whole Word Masking for Chinese BERT](https://arxiv.org/abs/1906.08101)**
-Yiming Cui, Wanxiang Che, Ting Liu, Bing Qin, Ziqing Yang, Shijin Wang, Guoping Hu
-
-This repository is developed based on:https://github.com/google-research/bert
-
-You may also interested in,
-- Chinese BERT series: https://github.com/ymcui/Chinese-BERT-wwm
-- Chinese MacBERT: https://github.com/ymcui/MacBERT
-- Chinese ELECTRA: https://github.com/ymcui/Chinese-ELECTRA
-- Chinese XLNet: https://github.com/ymcui/Chinese-XLNet
-- Knowledge Distillation Toolkit - TextBrewer: https://github.com/airaria/TextBrewer
-
-More resources by HFL: https://github.com/ymcui/HFL-Anthology
-
-## Citation
-If you find the technical report or resource is useful, please cite the following technical report in your paper.
-- Primary: https://arxiv.org/abs/2004.13922
-```
-@inproceedings{cui-etal-2020-revisiting,
- title = "Revisiting Pre-Trained Models for {C}hinese Natural Language Processing",
- author = "Cui, Yiming and
- Che, Wanxiang and
- Liu, Ting and
- Qin, Bing and
- Wang, Shijin and
- Hu, Guoping",
- booktitle = "Proceedings of the 2020 Conference on Empirical Methods in Natural Language Processing: Findings",
- month = nov,
- year = "2020",
- address = "Online",
- publisher = "Association for Computational Linguistics",
- url = "https://www.aclweb.org/anthology/2020.findings-emnlp.58",
- pages = "657--668",
-}
-```
-- Secondary: https://arxiv.org/abs/1906.08101
-```
-@article{chinese-bert-wwm,
- title={Pre-Training with Whole Word Masking for Chinese BERT},
- author={Cui, Yiming and Che, Wanxiang and Liu, Ting and Qin, Bing and Yang, Ziqing and Wang, Shijin and Hu, Guoping},
- journal={arXiv preprint arXiv:1906.08101},
- year={2019}
- }
-```
\ No newline at end of file
diff --git a/spaces/XzJosh/XingTong-Bert-VITS2/text/tone_sandhi.py b/spaces/XzJosh/XingTong-Bert-VITS2/text/tone_sandhi.py
deleted file mode 100644
index 0f45b7a72c5d858bcaab19ac85cfa686bf9a74da..0000000000000000000000000000000000000000
--- a/spaces/XzJosh/XingTong-Bert-VITS2/text/tone_sandhi.py
+++ /dev/null
@@ -1,351 +0,0 @@
-# Copyright (c) 2021 PaddlePaddle Authors. All Rights Reserved.
-#
-# Licensed under the Apache License, Version 2.0 (the "License");
-# you may not use this file except in compliance with the License.
-# You may obtain a copy of the License at
-#
-# http://www.apache.org/licenses/LICENSE-2.0
-#
-# Unless required by applicable law or agreed to in writing, software
-# distributed under the License is distributed on an "AS IS" BASIS,
-# WITHOUT WARRANTIES OR CONDITIONS OF ANY KIND, either express or implied.
-# See the License for the specific language governing permissions and
-# limitations under the License.
-from typing import List
-from typing import Tuple
-
-import jieba
-from pypinyin import lazy_pinyin
-from pypinyin import Style
-
-
-class ToneSandhi():
- def __init__(self):
- self.must_neural_tone_words = {
- '麻烦', '麻利', '鸳鸯', '高粱', '骨头', '骆驼', '马虎', '首饰', '馒头', '馄饨', '风筝',
- '难为', '队伍', '阔气', '闺女', '门道', '锄头', '铺盖', '铃铛', '铁匠', '钥匙', '里脊',
- '里头', '部分', '那么', '道士', '造化', '迷糊', '连累', '这么', '这个', '运气', '过去',
- '软和', '转悠', '踏实', '跳蚤', '跟头', '趔趄', '财主', '豆腐', '讲究', '记性', '记号',
- '认识', '规矩', '见识', '裁缝', '补丁', '衣裳', '衣服', '衙门', '街坊', '行李', '行当',
- '蛤蟆', '蘑菇', '薄荷', '葫芦', '葡萄', '萝卜', '荸荠', '苗条', '苗头', '苍蝇', '芝麻',
- '舒服', '舒坦', '舌头', '自在', '膏药', '脾气', '脑袋', '脊梁', '能耐', '胳膊', '胭脂',
- '胡萝', '胡琴', '胡同', '聪明', '耽误', '耽搁', '耷拉', '耳朵', '老爷', '老实', '老婆',
- '老头', '老太', '翻腾', '罗嗦', '罐头', '编辑', '结实', '红火', '累赘', '糨糊', '糊涂',
- '精神', '粮食', '簸箕', '篱笆', '算计', '算盘', '答应', '笤帚', '笑语', '笑话', '窟窿',
- '窝囊', '窗户', '稳当', '稀罕', '称呼', '秧歌', '秀气', '秀才', '福气', '祖宗', '砚台',
- '码头', '石榴', '石头', '石匠', '知识', '眼睛', '眯缝', '眨巴', '眉毛', '相声', '盘算',
- '白净', '痢疾', '痛快', '疟疾', '疙瘩', '疏忽', '畜生', '生意', '甘蔗', '琵琶', '琢磨',
- '琉璃', '玻璃', '玫瑰', '玄乎', '狐狸', '状元', '特务', '牲口', '牙碜', '牌楼', '爽快',
- '爱人', '热闹', '烧饼', '烟筒', '烂糊', '点心', '炊帚', '灯笼', '火候', '漂亮', '滑溜',
- '溜达', '温和', '清楚', '消息', '浪头', '活泼', '比方', '正经', '欺负', '模糊', '槟榔',
- '棺材', '棒槌', '棉花', '核桃', '栅栏', '柴火', '架势', '枕头', '枇杷', '机灵', '本事',
- '木头', '木匠', '朋友', '月饼', '月亮', '暖和', '明白', '时候', '新鲜', '故事', '收拾',
- '收成', '提防', '挖苦', '挑剔', '指甲', '指头', '拾掇', '拳头', '拨弄', '招牌', '招呼',
- '抬举', '护士', '折腾', '扫帚', '打量', '打算', '打点', '打扮', '打听', '打发', '扎实',
- '扁担', '戒指', '懒得', '意识', '意思', '情形', '悟性', '怪物', '思量', '怎么', '念头',
- '念叨', '快活', '忙活', '志气', '心思', '得罪', '张罗', '弟兄', '开通', '应酬', '庄稼',
- '干事', '帮手', '帐篷', '希罕', '师父', '师傅', '巴结', '巴掌', '差事', '工夫', '岁数',
- '屁股', '尾巴', '少爷', '小气', '小伙', '将就', '对头', '对付', '寡妇', '家伙', '客气',
- '实在', '官司', '学问', '学生', '字号', '嫁妆', '媳妇', '媒人', '婆家', '娘家', '委屈',
- '姑娘', '姐夫', '妯娌', '妥当', '妖精', '奴才', '女婿', '头发', '太阳', '大爷', '大方',
- '大意', '大夫', '多少', '多么', '外甥', '壮实', '地道', '地方', '在乎', '困难', '嘴巴',
- '嘱咐', '嘟囔', '嘀咕', '喜欢', '喇嘛', '喇叭', '商量', '唾沫', '哑巴', '哈欠', '哆嗦',
- '咳嗽', '和尚', '告诉', '告示', '含糊', '吓唬', '后头', '名字', '名堂', '合同', '吆喝',
- '叫唤', '口袋', '厚道', '厉害', '千斤', '包袱', '包涵', '匀称', '勤快', '动静', '动弹',
- '功夫', '力气', '前头', '刺猬', '刺激', '别扭', '利落', '利索', '利害', '分析', '出息',
- '凑合', '凉快', '冷战', '冤枉', '冒失', '养活', '关系', '先生', '兄弟', '便宜', '使唤',
- '佩服', '作坊', '体面', '位置', '似的', '伙计', '休息', '什么', '人家', '亲戚', '亲家',
- '交情', '云彩', '事情', '买卖', '主意', '丫头', '丧气', '两口', '东西', '东家', '世故',
- '不由', '不在', '下水', '下巴', '上头', '上司', '丈夫', '丈人', '一辈', '那个', '菩萨',
- '父亲', '母亲', '咕噜', '邋遢', '费用', '冤家', '甜头', '介绍', '荒唐', '大人', '泥鳅',
- '幸福', '熟悉', '计划', '扑腾', '蜡烛', '姥爷', '照顾', '喉咙', '吉他', '弄堂', '蚂蚱',
- '凤凰', '拖沓', '寒碜', '糟蹋', '倒腾', '报复', '逻辑', '盘缠', '喽啰', '牢骚', '咖喱',
- '扫把', '惦记'
- }
- self.must_not_neural_tone_words = {
- "男子", "女子", "分子", "原子", "量子", "莲子", "石子", "瓜子", "电子", "人人", "虎虎"
- }
- self.punc = ":,;。?!“”‘’':,;.?!"
-
- # the meaning of jieba pos tag: https://blog.csdn.net/weixin_44174352/article/details/113731041
- # e.g.
- # word: "家里"
- # pos: "s"
- # finals: ['ia1', 'i3']
- def _neural_sandhi(self, word: str, pos: str,
- finals: List[str]) -> List[str]:
-
- # reduplication words for n. and v. e.g. 奶奶, 试试, 旺旺
- for j, item in enumerate(word):
- if j - 1 >= 0 and item == word[j - 1] and pos[0] in {
- "n", "v", "a"
- } and word not in self.must_not_neural_tone_words:
- finals[j] = finals[j][:-1] + "5"
- ge_idx = word.find("个")
- if len(word) >= 1 and word[-1] in "吧呢啊呐噻嘛吖嗨呐哦哒额滴哩哟喽啰耶喔诶":
- finals[-1] = finals[-1][:-1] + "5"
- elif len(word) >= 1 and word[-1] in "的地得":
- finals[-1] = finals[-1][:-1] + "5"
- # e.g. 走了, 看着, 去过
- # elif len(word) == 1 and word in "了着过" and pos in {"ul", "uz", "ug"}:
- # finals[-1] = finals[-1][:-1] + "5"
- elif len(word) > 1 and word[-1] in "们子" and pos in {
- "r", "n"
- } and word not in self.must_not_neural_tone_words:
- finals[-1] = finals[-1][:-1] + "5"
- # e.g. 桌上, 地下, 家里
- elif len(word) > 1 and word[-1] in "上下里" and pos in {"s", "l", "f"}:
- finals[-1] = finals[-1][:-1] + "5"
- # e.g. 上来, 下去
- elif len(word) > 1 and word[-1] in "来去" and word[-2] in "上下进出回过起开":
- finals[-1] = finals[-1][:-1] + "5"
- # 个做量词
- elif (ge_idx >= 1 and
- (word[ge_idx - 1].isnumeric() or
- word[ge_idx - 1] in "几有两半多各整每做是")) or word == '个':
- finals[ge_idx] = finals[ge_idx][:-1] + "5"
- else:
- if word in self.must_neural_tone_words or word[
- -2:] in self.must_neural_tone_words:
- finals[-1] = finals[-1][:-1] + "5"
-
- word_list = self._split_word(word)
- finals_list = [finals[:len(word_list[0])], finals[len(word_list[0]):]]
- for i, word in enumerate(word_list):
- # conventional neural in Chinese
- if word in self.must_neural_tone_words or word[
- -2:] in self.must_neural_tone_words:
- finals_list[i][-1] = finals_list[i][-1][:-1] + "5"
- finals = sum(finals_list, [])
- return finals
-
- def _bu_sandhi(self, word: str, finals: List[str]) -> List[str]:
- # e.g. 看不懂
- if len(word) == 3 and word[1] == "不":
- finals[1] = finals[1][:-1] + "5"
- else:
- for i, char in enumerate(word):
- # "不" before tone4 should be bu2, e.g. 不怕
- if char == "不" and i + 1 < len(word) and finals[i +
- 1][-1] == "4":
- finals[i] = finals[i][:-1] + "2"
- return finals
-
- def _yi_sandhi(self, word: str, finals: List[str]) -> List[str]:
- # "一" in number sequences, e.g. 一零零, 二一零
- if word.find("一") != -1 and all(
- [item.isnumeric() for item in word if item != "一"]):
- return finals
- # "一" between reduplication words shold be yi5, e.g. 看一看
- elif len(word) == 3 and word[1] == "一" and word[0] == word[-1]:
- finals[1] = finals[1][:-1] + "5"
- # when "一" is ordinal word, it should be yi1
- elif word.startswith("第一"):
- finals[1] = finals[1][:-1] + "1"
- else:
- for i, char in enumerate(word):
- if char == "一" and i + 1 < len(word):
- # "一" before tone4 should be yi2, e.g. 一段
- if finals[i + 1][-1] == "4":
- finals[i] = finals[i][:-1] + "2"
- # "一" before non-tone4 should be yi4, e.g. 一天
- else:
- # "一" 后面如果是标点,还读一声
- if word[i + 1] not in self.punc:
- finals[i] = finals[i][:-1] + "4"
- return finals
-
- def _split_word(self, word: str) -> List[str]:
- word_list = jieba.cut_for_search(word)
- word_list = sorted(word_list, key=lambda i: len(i), reverse=False)
- first_subword = word_list[0]
- first_begin_idx = word.find(first_subword)
- if first_begin_idx == 0:
- second_subword = word[len(first_subword):]
- new_word_list = [first_subword, second_subword]
- else:
- second_subword = word[:-len(first_subword)]
- new_word_list = [second_subword, first_subword]
- return new_word_list
-
- def _three_sandhi(self, word: str, finals: List[str]) -> List[str]:
- if len(word) == 2 and self._all_tone_three(finals):
- finals[0] = finals[0][:-1] + "2"
- elif len(word) == 3:
- word_list = self._split_word(word)
- if self._all_tone_three(finals):
- # disyllabic + monosyllabic, e.g. 蒙古/包
- if len(word_list[0]) == 2:
- finals[0] = finals[0][:-1] + "2"
- finals[1] = finals[1][:-1] + "2"
- # monosyllabic + disyllabic, e.g. 纸/老虎
- elif len(word_list[0]) == 1:
- finals[1] = finals[1][:-1] + "2"
- else:
- finals_list = [
- finals[:len(word_list[0])], finals[len(word_list[0]):]
- ]
- if len(finals_list) == 2:
- for i, sub in enumerate(finals_list):
- # e.g. 所有/人
- if self._all_tone_three(sub) and len(sub) == 2:
- finals_list[i][0] = finals_list[i][0][:-1] + "2"
- # e.g. 好/喜欢
- elif i == 1 and not self._all_tone_three(sub) and finals_list[i][0][-1] == "3" and \
- finals_list[0][-1][-1] == "3":
-
- finals_list[0][-1] = finals_list[0][-1][:-1] + "2"
- finals = sum(finals_list, [])
- # split idiom into two words who's length is 2
- elif len(word) == 4:
- finals_list = [finals[:2], finals[2:]]
- finals = []
- for sub in finals_list:
- if self._all_tone_three(sub):
- sub[0] = sub[0][:-1] + "2"
- finals += sub
-
- return finals
-
- def _all_tone_three(self, finals: List[str]) -> bool:
- return all(x[-1] == "3" for x in finals)
-
- # merge "不" and the word behind it
- # if don't merge, "不" sometimes appears alone according to jieba, which may occur sandhi error
- def _merge_bu(self, seg: List[Tuple[str, str]]) -> List[Tuple[str, str]]:
- new_seg = []
- last_word = ""
- for word, pos in seg:
- if last_word == "不":
- word = last_word + word
- if word != "不":
- new_seg.append((word, pos))
- last_word = word[:]
- if last_word == "不":
- new_seg.append((last_word, 'd'))
- last_word = ""
- return new_seg
-
- # function 1: merge "一" and reduplication words in it's left and right, e.g. "听","一","听" ->"听一听"
- # function 2: merge single "一" and the word behind it
- # if don't merge, "一" sometimes appears alone according to jieba, which may occur sandhi error
- # e.g.
- # input seg: [('听', 'v'), ('一', 'm'), ('听', 'v')]
- # output seg: [['听一听', 'v']]
- def _merge_yi(self, seg: List[Tuple[str, str]]) -> List[Tuple[str, str]]:
- new_seg = []
- # function 1
- for i, (word, pos) in enumerate(seg):
- if i - 1 >= 0 and word == "一" and i + 1 < len(seg) and seg[i - 1][
- 0] == seg[i + 1][0] and seg[i - 1][1] == "v":
- new_seg[i - 1][0] = new_seg[i - 1][0] + "一" + new_seg[i - 1][0]
- else:
- if i - 2 >= 0 and seg[i - 1][0] == "一" and seg[i - 2][
- 0] == word and pos == "v":
- continue
- else:
- new_seg.append([word, pos])
- seg = new_seg
- new_seg = []
- # function 2
- for i, (word, pos) in enumerate(seg):
- if new_seg and new_seg[-1][0] == "一":
- new_seg[-1][0] = new_seg[-1][0] + word
- else:
- new_seg.append([word, pos])
- return new_seg
-
- # the first and the second words are all_tone_three
- def _merge_continuous_three_tones(
- self, seg: List[Tuple[str, str]]) -> List[Tuple[str, str]]:
- new_seg = []
- sub_finals_list = [
- lazy_pinyin(
- word, neutral_tone_with_five=True, style=Style.FINALS_TONE3)
- for (word, pos) in seg
- ]
- assert len(sub_finals_list) == len(seg)
- merge_last = [False] * len(seg)
- for i, (word, pos) in enumerate(seg):
- if i - 1 >= 0 and self._all_tone_three(
- sub_finals_list[i - 1]) and self._all_tone_three(
- sub_finals_list[i]) and not merge_last[i - 1]:
- # if the last word is reduplication, not merge, because reduplication need to be _neural_sandhi
- if not self._is_reduplication(seg[i - 1][0]) and len(
- seg[i - 1][0]) + len(seg[i][0]) <= 3:
- new_seg[-1][0] = new_seg[-1][0] + seg[i][0]
- merge_last[i] = True
- else:
- new_seg.append([word, pos])
- else:
- new_seg.append([word, pos])
-
- return new_seg
-
- def _is_reduplication(self, word: str) -> bool:
- return len(word) == 2 and word[0] == word[1]
-
- # the last char of first word and the first char of second word is tone_three
- def _merge_continuous_three_tones_2(
- self, seg: List[Tuple[str, str]]) -> List[Tuple[str, str]]:
- new_seg = []
- sub_finals_list = [
- lazy_pinyin(
- word, neutral_tone_with_five=True, style=Style.FINALS_TONE3)
- for (word, pos) in seg
- ]
- assert len(sub_finals_list) == len(seg)
- merge_last = [False] * len(seg)
- for i, (word, pos) in enumerate(seg):
- if i - 1 >= 0 and sub_finals_list[i - 1][-1][-1] == "3" and sub_finals_list[i][0][-1] == "3" and not \
- merge_last[i - 1]:
- # if the last word is reduplication, not merge, because reduplication need to be _neural_sandhi
- if not self._is_reduplication(seg[i - 1][0]) and len(
- seg[i - 1][0]) + len(seg[i][0]) <= 3:
- new_seg[-1][0] = new_seg[-1][0] + seg[i][0]
- merge_last[i] = True
- else:
- new_seg.append([word, pos])
- else:
- new_seg.append([word, pos])
- return new_seg
-
- def _merge_er(self, seg: List[Tuple[str, str]]) -> List[Tuple[str, str]]:
- new_seg = []
- for i, (word, pos) in enumerate(seg):
- if i - 1 >= 0 and word == "儿" and seg[i-1][0] != "#":
- new_seg[-1][0] = new_seg[-1][0] + seg[i][0]
- else:
- new_seg.append([word, pos])
- return new_seg
-
- def _merge_reduplication(
- self, seg: List[Tuple[str, str]]) -> List[Tuple[str, str]]:
- new_seg = []
- for i, (word, pos) in enumerate(seg):
- if new_seg and word == new_seg[-1][0]:
- new_seg[-1][0] = new_seg[-1][0] + seg[i][0]
- else:
- new_seg.append([word, pos])
- return new_seg
-
- def pre_merge_for_modify(
- self, seg: List[Tuple[str, str]]) -> List[Tuple[str, str]]:
- seg = self._merge_bu(seg)
- try:
- seg = self._merge_yi(seg)
- except:
- print("_merge_yi failed")
- seg = self._merge_reduplication(seg)
- seg = self._merge_continuous_three_tones(seg)
- seg = self._merge_continuous_three_tones_2(seg)
- seg = self._merge_er(seg)
- return seg
-
- def modified_tone(self, word: str, pos: str,
- finals: List[str]) -> List[str]:
- finals = self._bu_sandhi(word, finals)
- finals = self._yi_sandhi(word, finals)
- finals = self._neural_sandhi(word, pos, finals)
- finals = self._three_sandhi(word, finals)
- return finals
diff --git a/spaces/Y-T-G/Blur-Anything/tracker/model/trainer.py b/spaces/Y-T-G/Blur-Anything/tracker/model/trainer.py
deleted file mode 100644
index 0a935cf7a3cde3e9123a4b2ce01860301d423c7e..0000000000000000000000000000000000000000
--- a/spaces/Y-T-G/Blur-Anything/tracker/model/trainer.py
+++ /dev/null
@@ -1,302 +0,0 @@
-"""
-trainer.py - warpper and utility functions for network training
-Compute loss, back-prop, update parameters, logging, etc.
-"""
-import datetime
-import os
-import time
-import numpy as np
-import torch
-import torch.nn as nn
-import torch.optim as optim
-
-from model.network import XMem
-from model.losses import LossComputer
-from util.log_integrator import Integrator
-from util.image_saver import pool_pairs
-
-
-class XMemTrainer:
- def __init__(self, config, logger=None, save_path=None, local_rank=0, world_size=1):
- self.config = config
- self.num_frames = config["num_frames"]
- self.num_ref_frames = config["num_ref_frames"]
- self.deep_update_prob = config["deep_update_prob"]
- self.local_rank = local_rank
-
- self.XMem = nn.parallel.DistributedDataParallel(
- XMem(config).cuda(),
- device_ids=[local_rank],
- output_device=local_rank,
- broadcast_buffers=False,
- )
-
- # Set up logger when local_rank=0
- self.logger = logger
- self.save_path = save_path
- if logger is not None:
- self.last_time = time.time()
- self.logger.log_string(
- "model_size",
- str(sum([param.nelement() for param in self.XMem.parameters()])),
- )
- self.train_integrator = Integrator(
- self.logger, distributed=True, local_rank=local_rank, world_size=world_size
- )
- self.loss_computer = LossComputer(config)
-
- self.train()
- self.optimizer = optim.AdamW(
- filter(lambda p: p.requires_grad, self.XMem.parameters()),
- lr=config["lr"],
- weight_decay=config["weight_decay"],
- )
- self.scheduler = optim.lr_scheduler.MultiStepLR(
- self.optimizer, config["steps"], config["gamma"]
- )
- if config["amp"]:
- self.scaler = torch.cuda.amp.GradScaler()
-
- # Logging info
- self.log_text_interval = config["log_text_interval"]
- self.log_image_interval = config["log_image_interval"]
- self.save_network_interval = config["save_network_interval"]
- self.save_checkpoint_interval = config["save_checkpoint_interval"]
- if config["debug"]:
- self.log_text_interval = self.log_image_interval = 1
-
- def do_pass(self, data, max_it, it=0):
- # No need to store the gradient outside training
- torch.set_grad_enabled(self._is_train)
-
- for k, v in data.items():
- if type(v) != list and type(v) != dict and type(v) != int:
- data[k] = v.cuda(non_blocking=True)
-
- out = {}
- frames = data["rgb"]
- first_frame_gt = data["first_frame_gt"].float()
- b = frames.shape[0]
- num_filled_objects = [o.item() for o in data["info"]["num_objects"]]
- num_objects = first_frame_gt.shape[2]
- selector = data["selector"].unsqueeze(2).unsqueeze(2)
-
- global_avg = 0
-
- with torch.cuda.amp.autocast(enabled=self.config["amp"]):
- # image features never change, compute once
- key, shrinkage, selection, f16, f8, f4 = self.XMem("encode_key", frames)
-
- filler_one = torch.zeros(1, dtype=torch.int64)
- hidden = torch.zeros(
- (b, num_objects, self.config["hidden_dim"], *key.shape[-2:])
- )
- v16, hidden = self.XMem(
- "encode_value", frames[:, 0], f16[:, 0], hidden, first_frame_gt[:, 0]
- )
- values = v16.unsqueeze(3) # add the time dimension
-
- for ti in range(1, self.num_frames):
- if ti <= self.num_ref_frames:
- ref_values = values
- ref_keys = key[:, :, :ti]
- ref_shrinkage = (
- shrinkage[:, :, :ti] if shrinkage is not None else None
- )
- else:
- # pick num_ref_frames random frames
- # this is not very efficient but I think we would
- # need broadcasting in gather which we don't have
- indices = [
- torch.cat(
- [
- filler_one,
- torch.randperm(ti - 1)[: self.num_ref_frames - 1] + 1,
- ]
- )
- for _ in range(b)
- ]
- ref_values = torch.stack(
- [values[bi, :, :, indices[bi]] for bi in range(b)], 0
- )
- ref_keys = torch.stack(
- [key[bi, :, indices[bi]] for bi in range(b)], 0
- )
- ref_shrinkage = (
- torch.stack(
- [shrinkage[bi, :, indices[bi]] for bi in range(b)], 0
- )
- if shrinkage is not None
- else None
- )
-
- # Segment frame ti
- memory_readout = self.XMem(
- "read_memory",
- key[:, :, ti],
- selection[:, :, ti] if selection is not None else None,
- ref_keys,
- ref_shrinkage,
- ref_values,
- )
- hidden, logits, masks = self.XMem(
- "segment",
- (f16[:, ti], f8[:, ti], f4[:, ti]),
- memory_readout,
- hidden,
- selector,
- h_out=(ti < (self.num_frames - 1)),
- )
-
- # No need to encode the last frame
- if ti < (self.num_frames - 1):
- is_deep_update = np.random.rand() < self.deep_update_prob
- v16, hidden = self.XMem(
- "encode_value",
- frames[:, ti],
- f16[:, ti],
- hidden,
- masks,
- is_deep_update=is_deep_update,
- )
- values = torch.cat([values, v16.unsqueeze(3)], 3)
-
- out[f"masks_{ti}"] = masks
- out[f"logits_{ti}"] = logits
-
- if self._do_log or self._is_train:
- losses = self.loss_computer.compute(
- {**data, **out}, num_filled_objects, it
- )
-
- # Logging
- if self._do_log:
- self.integrator.add_dict(losses)
- if self._is_train:
- if it % self.log_image_interval == 0 and it != 0:
- if self.logger is not None:
- images = {**data, **out}
- size = (384, 384)
- self.logger.log_cv2(
- "train/pairs",
- pool_pairs(images, size, num_filled_objects),
- it,
- )
-
- if self._is_train:
-
- if (it) % self.log_text_interval == 0 and it != 0:
- time_spent = time.time() - self.last_time
-
- if self.logger is not None:
- self.logger.log_scalar(
- "train/lr", self.scheduler.get_last_lr()[0], it
- )
- self.logger.log_metrics(
- "train", "time", (time_spent) / self.log_text_interval, it
- )
-
- global_avg = 0.5 * (global_avg) + 0.5 * (time_spent)
- eta_seconds = global_avg * (max_it - it) / 100
- eta_string = str(datetime.timedelta(seconds=int(eta_seconds)))
- print(f"ETA: {eta_string}")
-
- self.last_time = time.time()
- self.train_integrator.finalize("train", it)
- self.train_integrator.reset_except_hooks()
-
- if it % self.save_network_interval == 0 and it != 0:
- if self.logger is not None:
- self.save_network(it)
-
- if it % self.save_checkpoint_interval == 0 and it != 0:
- if self.logger is not None:
- self.save_checkpoint(it)
-
- # Backward pass
- self.optimizer.zero_grad(set_to_none=True)
- if self.config["amp"]:
- self.scaler.scale(losses["total_loss"]).backward()
- self.scaler.step(self.optimizer)
- self.scaler.update()
- else:
- losses["total_loss"].backward()
- self.optimizer.step()
-
- self.scheduler.step()
-
- def save_network(self, it):
- if self.save_path is None:
- print("Saving has been disabled.")
- return
-
- os.makedirs(os.path.dirname(self.save_path), exist_ok=True)
- model_path = f"{self.save_path}_{it}.pth"
- torch.save(self.XMem.module.state_dict(), model_path)
- print(f"Network saved to {model_path}.")
-
- def save_checkpoint(self, it):
- if self.save_path is None:
- print("Saving has been disabled.")
- return
-
- os.makedirs(os.path.dirname(self.save_path), exist_ok=True)
- checkpoint_path = f"{self.save_path}_checkpoint_{it}.pth"
- checkpoint = {
- "it": it,
- "network": self.XMem.module.state_dict(),
- "optimizer": self.optimizer.state_dict(),
- "scheduler": self.scheduler.state_dict(),
- }
- torch.save(checkpoint, checkpoint_path)
- print(f"Checkpoint saved to {checkpoint_path}.")
-
- def load_checkpoint(self, path):
- # This method loads everything and should be used to resume training
- map_location = "cuda:%d" % self.local_rank
- checkpoint = torch.load(path, map_location={"cuda:0": map_location})
-
- it = checkpoint["it"]
- network = checkpoint["network"]
- optimizer = checkpoint["optimizer"]
- scheduler = checkpoint["scheduler"]
-
- map_location = "cuda:%d" % self.local_rank
- self.XMem.module.load_state_dict(network)
- self.optimizer.load_state_dict(optimizer)
- self.scheduler.load_state_dict(scheduler)
-
- print("Network weights, optimizer states, and scheduler states loaded.")
-
- return it
-
- def load_network_in_memory(self, src_dict):
- self.XMem.module.load_weights(src_dict)
- print("Network weight loaded from memory.")
-
- def load_network(self, path):
- # This method loads only the network weight and should be used to load a pretrained model
- map_location = "cuda:%d" % self.local_rank
- src_dict = torch.load(path, map_location={"cuda:0": map_location})
-
- self.load_network_in_memory(src_dict)
- print(f"Network weight loaded from {path}")
-
- def train(self):
- self._is_train = True
- self._do_log = True
- self.integrator = self.train_integrator
- self.XMem.eval()
- return self
-
- def val(self):
- self._is_train = False
- self._do_log = True
- self.XMem.eval()
- return self
-
- def test(self):
- self._is_train = False
- self._do_log = False
- self.XMem.eval()
- return self
diff --git a/spaces/YeOldHermit/Super-Resolution-Anime-Diffusion/diffusers/schedulers/scheduling_utils.py b/spaces/YeOldHermit/Super-Resolution-Anime-Diffusion/diffusers/schedulers/scheduling_utils.py
deleted file mode 100644
index 90ab674e38a40796dd1183ec0ef341159f8f62b4..0000000000000000000000000000000000000000
--- a/spaces/YeOldHermit/Super-Resolution-Anime-Diffusion/diffusers/schedulers/scheduling_utils.py
+++ /dev/null
@@ -1,154 +0,0 @@
-# Copyright 2022 The HuggingFace Team. All rights reserved.
-#
-# Licensed under the Apache License, Version 2.0 (the "License");
-# you may not use this file except in compliance with the License.
-# You may obtain a copy of the License at
-#
-# http://www.apache.org/licenses/LICENSE-2.0
-#
-# Unless required by applicable law or agreed to in writing, software
-# distributed under the License is distributed on an "AS IS" BASIS,
-# WITHOUT WARRANTIES OR CONDITIONS OF ANY KIND, either express or implied.
-# See the License for the specific language governing permissions and
-# limitations under the License.
-import importlib
-import os
-from dataclasses import dataclass
-from typing import Any, Dict, Optional, Union
-
-import torch
-
-from ..utils import BaseOutput
-
-
-SCHEDULER_CONFIG_NAME = "scheduler_config.json"
-
-
-@dataclass
-class SchedulerOutput(BaseOutput):
- """
- Base class for the scheduler's step function output.
-
- Args:
- prev_sample (`torch.FloatTensor` of shape `(batch_size, num_channels, height, width)` for images):
- Computed sample (x_{t-1}) of previous timestep. `prev_sample` should be used as next model input in the
- denoising loop.
- """
-
- prev_sample: torch.FloatTensor
-
-
-class SchedulerMixin:
- """
- Mixin containing common functions for the schedulers.
-
- Class attributes:
- - **_compatibles** (`List[str]`) -- A list of classes that are compatible with the parent class, so that
- `from_config` can be used from a class different than the one used to save the config (should be overridden
- by parent class).
- """
-
- config_name = SCHEDULER_CONFIG_NAME
- _compatibles = []
- has_compatibles = True
-
- @classmethod
- def from_pretrained(
- cls,
- pretrained_model_name_or_path: Dict[str, Any] = None,
- subfolder: Optional[str] = None,
- return_unused_kwargs=False,
- **kwargs,
- ):
- r"""
- Instantiate a Scheduler class from a pre-defined JSON configuration file inside a directory or Hub repo.
-
- Parameters:
- pretrained_model_name_or_path (`str` or `os.PathLike`, *optional*):
- Can be either:
-
- - A string, the *model id* of a model repo on huggingface.co. Valid model ids should have an
- organization name, like `google/ddpm-celebahq-256`.
- - A path to a *directory* containing the schedluer configurations saved using
- [`~SchedulerMixin.save_pretrained`], e.g., `./my_model_directory/`.
- subfolder (`str`, *optional*):
- In case the relevant files are located inside a subfolder of the model repo (either remote in
- huggingface.co or downloaded locally), you can specify the folder name here.
- return_unused_kwargs (`bool`, *optional*, defaults to `False`):
- Whether kwargs that are not consumed by the Python class should be returned or not.
- cache_dir (`Union[str, os.PathLike]`, *optional*):
- Path to a directory in which a downloaded pretrained model configuration should be cached if the
- standard cache should not be used.
- force_download (`bool`, *optional*, defaults to `False`):
- Whether or not to force the (re-)download of the model weights and configuration files, overriding the
- cached versions if they exist.
- resume_download (`bool`, *optional*, defaults to `False`):
- Whether or not to delete incompletely received files. Will attempt to resume the download if such a
- file exists.
- proxies (`Dict[str, str]`, *optional*):
- A dictionary of proxy servers to use by protocol or endpoint, e.g., `{'http': 'foo.bar:3128',
- 'http://hostname': 'foo.bar:4012'}`. The proxies are used on each request.
- output_loading_info(`bool`, *optional*, defaults to `False`):
- Whether or not to also return a dictionary containing missing keys, unexpected keys and error messages.
- local_files_only(`bool`, *optional*, defaults to `False`):
- Whether or not to only look at local files (i.e., do not try to download the model).
- use_auth_token (`str` or *bool*, *optional*):
- The token to use as HTTP bearer authorization for remote files. If `True`, will use the token generated
- when running `transformers-cli login` (stored in `~/.huggingface`).
- revision (`str`, *optional*, defaults to `"main"`):
- The specific model version to use. It can be a branch name, a tag name, or a commit id, since we use a
- git-based system for storing models and other artifacts on huggingface.co, so `revision` can be any
- identifier allowed by git.
-
-
-
- It is required to be logged in (`huggingface-cli login`) when you want to use private or [gated
- models](https://huggingface.co/docs/hub/models-gated#gated-models).
-
-
-
-
-
- Activate the special ["offline-mode"](https://huggingface.co/transformers/installation.html#offline-mode) to
- use this method in a firewalled environment.
-
-
-
- """
- config, kwargs = cls.load_config(
- pretrained_model_name_or_path=pretrained_model_name_or_path,
- subfolder=subfolder,
- return_unused_kwargs=True,
- **kwargs,
- )
- return cls.from_config(config, return_unused_kwargs=return_unused_kwargs, **kwargs)
-
- def save_pretrained(self, save_directory: Union[str, os.PathLike], push_to_hub: bool = False, **kwargs):
- """
- Save a scheduler configuration object to the directory `save_directory`, so that it can be re-loaded using the
- [`~SchedulerMixin.from_pretrained`] class method.
-
- Args:
- save_directory (`str` or `os.PathLike`):
- Directory where the configuration JSON file will be saved (will be created if it does not exist).
- """
- self.save_config(save_directory=save_directory, push_to_hub=push_to_hub, **kwargs)
-
- @property
- def compatibles(self):
- """
- Returns all schedulers that are compatible with this scheduler
-
- Returns:
- `List[SchedulerMixin]`: List of compatible schedulers
- """
- return self._get_compatibles()
-
- @classmethod
- def _get_compatibles(cls):
- compatible_classes_str = list(set([cls.__name__] + cls._compatibles))
- diffusers_library = importlib.import_module(__name__.split(".")[0])
- compatible_classes = [
- getattr(diffusers_library, c) for c in compatible_classes_str if hasattr(diffusers_library, c)
- ]
- return compatible_classes
diff --git a/spaces/Yilin98/Stock_Prediction/stock_prediction.py b/spaces/Yilin98/Stock_Prediction/stock_prediction.py
deleted file mode 100644
index 33d27c941e4df24732e68687798d3e46f1f0738f..0000000000000000000000000000000000000000
--- a/spaces/Yilin98/Stock_Prediction/stock_prediction.py
+++ /dev/null
@@ -1,66 +0,0 @@
-import hopsworks
-import joblib
-import math
-from sklearn.preprocessing import MinMaxScaler
-import numpy as np
-from datetime import timedelta, datetime
-
-
-
-
-
-def model(ticker):
- project = hopsworks.login()
-
- # import data
- fs = project.get_feature_store()
- feature_group = fs.get_feature_group(
- name = 'final_data_for_prediction')
-
- data = feature_group.select_all().read()
- data = data.sort_values(by='date')
-
- last_date = data['date'].values[-1]
- last_date = datetime.fromtimestamp(int(int(last_date) / 1000))
- date = last_date.date() + timedelta(days=1)
-
- data = data.set_index('date')
- if ticker == 'AAPL':
- data = data.loc[data['name'] == 'APPLE']
- elif ticker == 'AMZN':
- data = data.loc[data['name'] == 'AMAZON']
- else:
- data = data.loc[data['name'] == 'META']
- data.drop(['name', 'price_move'], axis=1, inplace=True)
-
- # scaling data
- prices = data[['close','neg','neu','pos','compound']]
- scaler = MinMaxScaler(feature_range=(0,1))
- scaled_data = scaler.fit_transform(prices)
-
- prediction_list = scaled_data[-60:]
-
- x = []
- x.append(prediction_list[-60:])
- x = np.array(x)
-
- # import model
- mr = project.get_model_registry()
- if ticker == 'AAPL':
- remote_model = mr.get_model("LSTM_Apple", version=1)
- model_dir = remote_model.download()
- remote_model = joblib.load(model_dir + "/apple_model.pkl")
- elif ticker == 'AMZN':
- remote_model = mr.get_model("LSTM_Amazon", version=1)
- model_dir = remote_model.download()
- remote_model = joblib.load(model_dir + "/amazon_model.pkl")
- else:
- remote_model = mr.get_model("LSTM_Meta", version=1)
- model_dir = remote_model.download()
- remote_model = joblib.load(model_dir + "/meta_model.pkl")
-
- # predict
- out = remote_model.predict(x)
- B=np.hstack((out,scaled_data[ : 1,1:]))
- out = scaler.inverse_transform(B)[0,0]
- return date, out
\ No newline at end of file
diff --git a/spaces/Yudha515/Rvc-Models/tests/common_utils/__init__.py b/spaces/Yudha515/Rvc-Models/tests/common_utils/__init__.py
deleted file mode 100644
index 74ffcfef96fec35c99b2a1a053a61f44f7a8bbe9..0000000000000000000000000000000000000000
--- a/spaces/Yudha515/Rvc-Models/tests/common_utils/__init__.py
+++ /dev/null
@@ -1,9 +0,0 @@
-# Copyright (c) Meta Platforms, Inc. and affiliates.
-# All rights reserved.
-#
-# This source code is licensed under the license found in the
-# LICENSE file in the root directory of this source tree.
-
-# flake8: noqa
-from .temp_utils import TempDirMixin
-from .wav_utils import get_batch_white_noise, get_white_noise, save_wav
diff --git a/spaces/Yuliang/ECON/lib/pymafx/core/cfgs.py b/spaces/Yuliang/ECON/lib/pymafx/core/cfgs.py
deleted file mode 100644
index 17abd247de8d335131d8facc866d95e485ea9a7a..0000000000000000000000000000000000000000
--- a/spaces/Yuliang/ECON/lib/pymafx/core/cfgs.py
+++ /dev/null
@@ -1,108 +0,0 @@
-# -*- coding: utf-8 -*-
-
-# Max-Planck-Gesellschaft zur Förderung der Wissenschaften e.V. (MPG) is
-# holder of all proprietary rights on this computer program.
-# You can only use this computer program if you have closed
-# a license agreement with MPG or you get the right to use the computer
-# program from someone who is authorized to grant you that right.
-# Any use of the computer program without a valid license is prohibited and
-# liable to prosecution.
-#
-# Copyright©2019 Max-Planck-Gesellschaft zur Förderung
-# der Wissenschaften e.V. (MPG). acting on behalf of its Max Planck Institute
-# for Intelligent Systems. All rights reserved.
-#
-# Contact: ps-license@tuebingen.mpg.de
-
-import argparse
-import json
-import os
-import random
-import string
-from datetime import datetime
-
-from yacs.config import CfgNode as CN
-
-# Configuration variables
-cfg = CN(new_allowed=True)
-
-cfg.OUTPUT_DIR = 'results'
-cfg.DEVICE = 'cuda'
-cfg.DEBUG = False
-cfg.LOGDIR = ''
-cfg.VAL_VIS_BATCH_FREQ = 200
-cfg.TRAIN_VIS_ITER_FERQ = 1000
-cfg.SEED_VALUE = -1
-
-cfg.TRAIN = CN(new_allowed=True)
-
-cfg.LOSS = CN(new_allowed=True)
-cfg.LOSS.KP_2D_W = 300.0
-cfg.LOSS.KP_3D_W = 300.0
-cfg.LOSS.SHAPE_W = 0.06
-cfg.LOSS.POSE_W = 60.0
-cfg.LOSS.VERT_W = 0.0
-
-# Loss weights for dense correspondences
-cfg.LOSS.INDEX_WEIGHTS = 2.0
-# Loss weights for surface parts. (24 Parts)
-cfg.LOSS.PART_WEIGHTS = 0.3
-# Loss weights for UV regression.
-cfg.LOSS.POINT_REGRESSION_WEIGHTS = 0.5
-
-cfg.MODEL = CN(new_allowed=True)
-
-cfg.MODEL.PyMAF = CN(new_allowed=True)
-
-## switch
-cfg.TRAIN.BATCH_SIZE = 64
-cfg.TRAIN.VAL_LOOP = True
-
-cfg.TEST = CN(new_allowed=True)
-
-
-def get_cfg_defaults():
- """Get a yacs CfgNode object with default values for my_project."""
- # Return a clone so that the defaults will not be altered
- # This is for the "local variable" use pattern
- # return cfg.clone()
- return cfg
-
-
-def update_cfg(cfg_file):
- # cfg = get_cfg_defaults()
- cfg.merge_from_file(cfg_file)
- # return cfg.clone()
- return cfg
-
-
-def parse_args(args):
- cfg_file = args.cfg_file
- if args.cfg_file is not None:
- cfg = update_cfg(args.cfg_file)
- else:
- cfg = get_cfg_defaults()
-
- if args.misc is not None:
- cfg.merge_from_list(args.misc)
-
- return cfg
-
-
-def parse_args_extend(args):
- if args.resume:
- if not os.path.exists(args.log_dir):
- raise ValueError('Experiment are set to resume mode, but log directory does not exist.')
-
- if args.cfg_file is not None:
- cfg = update_cfg(args.cfg_file)
- else:
- cfg = get_cfg_defaults()
- # load log's cfg
- cfg_file = os.path.join(args.log_dir, 'cfg.yaml')
- cfg = update_cfg(cfg_file)
-
- if args.misc is not None:
- cfg.merge_from_list(args.misc)
- else:
- parse_args(args)
diff --git a/spaces/Zengyf-CVer/Gradio_YOLOv5_Det_v5/model_download/yolov5_model_p5_all.sh b/spaces/Zengyf-CVer/Gradio_YOLOv5_Det_v5/model_download/yolov5_model_p5_all.sh
deleted file mode 100644
index ab68c26898822fc2d09995c60584d7a0d9d40657..0000000000000000000000000000000000000000
--- a/spaces/Zengyf-CVer/Gradio_YOLOv5_Det_v5/model_download/yolov5_model_p5_all.sh
+++ /dev/null
@@ -1,8 +0,0 @@
-cd ./yolov5
-
-# 下载YOLOv5模型
-wget -c -t 0 https://github.com/ultralytics/yolov5/releases/download/v6.1/yolov5n.pt
-wget -c -t 0 https://github.com/ultralytics/yolov5/releases/download/v6.1/yolov5s.pt
-wget -c -t 0 https://github.com/ultralytics/yolov5/releases/download/v6.1/yolov5m.pt
-wget -c -t 0 https://github.com/ultralytics/yolov5/releases/download/v6.1/yolov5l.pt
-wget -c -t 0 https://github.com/ultralytics/yolov5/releases/download/v6.1/yolov5x.pt
diff --git a/spaces/Zengyf-CVer/Streamlit_YOLOv5_Model2x/val.py b/spaces/Zengyf-CVer/Streamlit_YOLOv5_Model2x/val.py
deleted file mode 100644
index 5427ee7b361938a21b18b038aa0ab30fd6c15ecc..0000000000000000000000000000000000000000
--- a/spaces/Zengyf-CVer/Streamlit_YOLOv5_Model2x/val.py
+++ /dev/null
@@ -1,397 +0,0 @@
-# YOLOv5 🚀 by Ultralytics, GPL-3.0 license
-"""
-Validate a trained YOLOv5 detection model on a detection dataset
-
-Usage:
- $ python val.py --weights yolov5s.pt --data coco128.yaml --img 640
-
-Usage - formats:
- $ python val.py --weights yolov5s.pt # PyTorch
- yolov5s.torchscript # TorchScript
- yolov5s.onnx # ONNX Runtime or OpenCV DNN with --dnn
- yolov5s.xml # OpenVINO
- yolov5s.engine # TensorRT
- yolov5s.mlmodel # CoreML (macOS-only)
- yolov5s_saved_model # TensorFlow SavedModel
- yolov5s.pb # TensorFlow GraphDef
- yolov5s.tflite # TensorFlow Lite
- yolov5s_edgetpu.tflite # TensorFlow Edge TPU
-"""
-
-import argparse
-import json
-import os
-import sys
-from pathlib import Path
-
-import numpy as np
-import torch
-from tqdm import tqdm
-
-FILE = Path(__file__).resolve()
-ROOT = FILE.parents[0] # YOLOv5 root directory
-if str(ROOT) not in sys.path:
- sys.path.append(str(ROOT)) # add ROOT to PATH
-ROOT = Path(os.path.relpath(ROOT, Path.cwd())) # relative
-
-from models.common import DetectMultiBackend
-from utils.callbacks import Callbacks
-from utils.dataloaders import create_dataloader
-from utils.general import (LOGGER, Profile, check_dataset, check_img_size, check_requirements, check_yaml,
- coco80_to_coco91_class, colorstr, increment_path, non_max_suppression, print_args,
- scale_coords, xywh2xyxy, xyxy2xywh)
-from utils.metrics import ConfusionMatrix, ap_per_class, box_iou
-from utils.plots import output_to_target, plot_images, plot_val_study
-from utils.torch_utils import select_device, smart_inference_mode
-
-
-def save_one_txt(predn, save_conf, shape, file):
- # Save one txt result
- gn = torch.tensor(shape)[[1, 0, 1, 0]] # normalization gain whwh
- for *xyxy, conf, cls in predn.tolist():
- xywh = (xyxy2xywh(torch.tensor(xyxy).view(1, 4)) / gn).view(-1).tolist() # normalized xywh
- line = (cls, *xywh, conf) if save_conf else (cls, *xywh) # label format
- with open(file, 'a') as f:
- f.write(('%g ' * len(line)).rstrip() % line + '\n')
-
-
-def save_one_json(predn, jdict, path, class_map):
- # Save one JSON result {"image_id": 42, "category_id": 18, "bbox": [258.15, 41.29, 348.26, 243.78], "score": 0.236}
- image_id = int(path.stem) if path.stem.isnumeric() else path.stem
- box = xyxy2xywh(predn[:, :4]) # xywh
- box[:, :2] -= box[:, 2:] / 2 # xy center to top-left corner
- for p, b in zip(predn.tolist(), box.tolist()):
- jdict.append({
- 'image_id': image_id,
- 'category_id': class_map[int(p[5])],
- 'bbox': [round(x, 3) for x in b],
- 'score': round(p[4], 5)})
-
-
-def process_batch(detections, labels, iouv):
- """
- Return correct predictions matrix. Both sets of boxes are in (x1, y1, x2, y2) format.
- Arguments:
- detections (Array[N, 6]), x1, y1, x2, y2, conf, class
- labels (Array[M, 5]), class, x1, y1, x2, y2
- Returns:
- correct (Array[N, 10]), for 10 IoU levels
- """
- correct = np.zeros((detections.shape[0], iouv.shape[0])).astype(bool)
- iou = box_iou(labels[:, 1:], detections[:, :4])
- correct_class = labels[:, 0:1] == detections[:, 5]
- for i in range(len(iouv)):
- x = torch.where((iou >= iouv[i]) & correct_class) # IoU > threshold and classes match
- if x[0].shape[0]:
- matches = torch.cat((torch.stack(x, 1), iou[x[0], x[1]][:, None]), 1).cpu().numpy() # [label, detect, iou]
- if x[0].shape[0] > 1:
- matches = matches[matches[:, 2].argsort()[::-1]]
- matches = matches[np.unique(matches[:, 1], return_index=True)[1]]
- # matches = matches[matches[:, 2].argsort()[::-1]]
- matches = matches[np.unique(matches[:, 0], return_index=True)[1]]
- correct[matches[:, 1].astype(int), i] = True
- return torch.tensor(correct, dtype=torch.bool, device=iouv.device)
-
-
-@smart_inference_mode()
-def run(
- data,
- weights=None, # model.pt path(s)
- batch_size=32, # batch size
- imgsz=640, # inference size (pixels)
- conf_thres=0.001, # confidence threshold
- iou_thres=0.6, # NMS IoU threshold
- task='val', # train, val, test, speed or study
- device='', # cuda device, i.e. 0 or 0,1,2,3 or cpu
- workers=8, # max dataloader workers (per RANK in DDP mode)
- single_cls=False, # treat as single-class dataset
- augment=False, # augmented inference
- verbose=False, # verbose output
- save_txt=False, # save results to *.txt
- save_hybrid=False, # save label+prediction hybrid results to *.txt
- save_conf=False, # save confidences in --save-txt labels
- save_json=False, # save a COCO-JSON results file
- project=ROOT / 'runs/val', # save to project/name
- name='exp', # save to project/name
- exist_ok=False, # existing project/name ok, do not increment
- half=True, # use FP16 half-precision inference
- dnn=False, # use OpenCV DNN for ONNX inference
- model=None,
- dataloader=None,
- save_dir=Path(''),
- plots=True,
- callbacks=Callbacks(),
- compute_loss=None,
-):
- # Initialize/load model and set device
- training = model is not None
- if training: # called by train.py
- device, pt, jit, engine = next(model.parameters()).device, True, False, False # get model device, PyTorch model
- half &= device.type != 'cpu' # half precision only supported on CUDA
- model.half() if half else model.float()
- else: # called directly
- device = select_device(device, batch_size=batch_size)
-
- # Directories
- save_dir = increment_path(Path(project) / name, exist_ok=exist_ok) # increment run
- (save_dir / 'labels' if save_txt else save_dir).mkdir(parents=True, exist_ok=True) # make dir
-
- # Load model
- model = DetectMultiBackend(weights, device=device, dnn=dnn, data=data, fp16=half)
- stride, pt, jit, engine = model.stride, model.pt, model.jit, model.engine
- imgsz = check_img_size(imgsz, s=stride) # check image size
- half = model.fp16 # FP16 supported on limited backends with CUDA
- if engine:
- batch_size = model.batch_size
- else:
- device = model.device
- if not (pt or jit):
- batch_size = 1 # export.py models default to batch-size 1
- LOGGER.info(f'Forcing --batch-size 1 square inference (1,3,{imgsz},{imgsz}) for non-PyTorch models')
-
- # Data
- data = check_dataset(data) # check
-
- # Configure
- model.eval()
- cuda = device.type != 'cpu'
- is_coco = isinstance(data.get('val'), str) and data['val'].endswith(f'coco{os.sep}val2017.txt') # COCO dataset
- nc = 1 if single_cls else int(data['nc']) # number of classes
- iouv = torch.linspace(0.5, 0.95, 10, device=device) # iou vector for mAP@0.5:0.95
- niou = iouv.numel()
-
- # Dataloader
- if not training:
- if pt and not single_cls: # check --weights are trained on --data
- ncm = model.model.nc
- assert ncm == nc, f'{weights} ({ncm} classes) trained on different --data than what you passed ({nc} ' \
- f'classes). Pass correct combination of --weights and --data that are trained together.'
- model.warmup(imgsz=(1 if pt else batch_size, 3, imgsz, imgsz)) # warmup
- pad = 0.0 if task in ('speed', 'benchmark') else 0.5
- rect = False if task == 'benchmark' else pt # square inference for benchmarks
- task = task if task in ('train', 'val', 'test') else 'val' # path to train/val/test images
- dataloader = create_dataloader(data[task],
- imgsz,
- batch_size,
- stride,
- single_cls,
- pad=pad,
- rect=rect,
- workers=workers,
- prefix=colorstr(f'{task}: '))[0]
-
- seen = 0
- confusion_matrix = ConfusionMatrix(nc=nc)
- names = model.names if hasattr(model, 'names') else model.module.names # get class names
- if isinstance(names, (list, tuple)): # old format
- names = dict(enumerate(names))
- class_map = coco80_to_coco91_class() if is_coco else list(range(1000))
- s = ('%22s' + '%11s' * 6) % ('Class', 'Images', 'Instances', 'P', 'R', 'mAP@.5', 'mAP@.5:.95')
- dt, p, r, f1, mp, mr, map50, map = (Profile(), Profile(), Profile()), 0.0, 0.0, 0.0, 0.0, 0.0, 0.0, 0.0
- loss = torch.zeros(3, device=device)
- jdict, stats, ap, ap_class = [], [], [], []
- callbacks.run('on_val_start')
- pbar = tqdm(dataloader, desc=s, bar_format='{l_bar}{bar:10}{r_bar}{bar:-10b}') # progress bar
- for batch_i, (im, targets, paths, shapes) in enumerate(pbar):
- callbacks.run('on_val_batch_start')
- with dt[0]:
- if cuda:
- im = im.to(device, non_blocking=True)
- targets = targets.to(device)
- im = im.half() if half else im.float() # uint8 to fp16/32
- im /= 255 # 0 - 255 to 0.0 - 1.0
- nb, _, height, width = im.shape # batch size, channels, height, width
-
- # Inference
- with dt[1]:
- out, train_out = model(im) if compute_loss else (model(im, augment=augment), None)
-
- # Loss
- if compute_loss:
- loss += compute_loss(train_out, targets)[1] # box, obj, cls
-
- # NMS
- targets[:, 2:] *= torch.tensor((width, height, width, height), device=device) # to pixels
- lb = [targets[targets[:, 0] == i, 1:] for i in range(nb)] if save_hybrid else [] # for autolabelling
- with dt[2]:
- out = non_max_suppression(out, conf_thres, iou_thres, labels=lb, multi_label=True, agnostic=single_cls)
-
- # Metrics
- for si, pred in enumerate(out):
- labels = targets[targets[:, 0] == si, 1:]
- nl, npr = labels.shape[0], pred.shape[0] # number of labels, predictions
- path, shape = Path(paths[si]), shapes[si][0]
- correct = torch.zeros(npr, niou, dtype=torch.bool, device=device) # init
- seen += 1
-
- if npr == 0:
- if nl:
- stats.append((correct, *torch.zeros((2, 0), device=device), labels[:, 0]))
- if plots:
- confusion_matrix.process_batch(detections=None, labels=labels[:, 0])
- continue
-
- # Predictions
- if single_cls:
- pred[:, 5] = 0
- predn = pred.clone()
- scale_coords(im[si].shape[1:], predn[:, :4], shape, shapes[si][1]) # native-space pred
-
- # Evaluate
- if nl:
- tbox = xywh2xyxy(labels[:, 1:5]) # target boxes
- scale_coords(im[si].shape[1:], tbox, shape, shapes[si][1]) # native-space labels
- labelsn = torch.cat((labels[:, 0:1], tbox), 1) # native-space labels
- correct = process_batch(predn, labelsn, iouv)
- if plots:
- confusion_matrix.process_batch(predn, labelsn)
- stats.append((correct, pred[:, 4], pred[:, 5], labels[:, 0])) # (correct, conf, pcls, tcls)
-
- # Save/log
- if save_txt:
- save_one_txt(predn, save_conf, shape, file=save_dir / 'labels' / f'{path.stem}.txt')
- if save_json:
- save_one_json(predn, jdict, path, class_map) # append to COCO-JSON dictionary
- callbacks.run('on_val_image_end', pred, predn, path, names, im[si])
-
- # Plot images
- if plots and batch_i < 3:
- plot_images(im, targets, paths, save_dir / f'val_batch{batch_i}_labels.jpg', names) # labels
- plot_images(im, output_to_target(out), paths, save_dir / f'val_batch{batch_i}_pred.jpg', names) # pred
-
- callbacks.run('on_val_batch_end')
-
- # Compute metrics
- stats = [torch.cat(x, 0).cpu().numpy() for x in zip(*stats)] # to numpy
- if len(stats) and stats[0].any():
- tp, fp, p, r, f1, ap, ap_class = ap_per_class(*stats, plot=plots, save_dir=save_dir, names=names)
- ap50, ap = ap[:, 0], ap.mean(1) # AP@0.5, AP@0.5:0.95
- mp, mr, map50, map = p.mean(), r.mean(), ap50.mean(), ap.mean()
- nt = np.bincount(stats[3].astype(int), minlength=nc) # number of targets per class
-
- # Print results
- pf = '%22s' + '%11i' * 2 + '%11.3g' * 4 # print format
- LOGGER.info(pf % ('all', seen, nt.sum(), mp, mr, map50, map))
- if nt.sum() == 0:
- LOGGER.warning(f'WARNING: no labels found in {task} set, can not compute metrics without labels ⚠️')
-
- # Print results per class
- if (verbose or (nc < 50 and not training)) and nc > 1 and len(stats):
- for i, c in enumerate(ap_class):
- LOGGER.info(pf % (names[c], seen, nt[c], p[i], r[i], ap50[i], ap[i]))
-
- # Print speeds
- t = tuple(x.t / seen * 1E3 for x in dt) # speeds per image
- if not training:
- shape = (batch_size, 3, imgsz, imgsz)
- LOGGER.info(f'Speed: %.1fms pre-process, %.1fms inference, %.1fms NMS per image at shape {shape}' % t)
-
- # Plots
- if plots:
- confusion_matrix.plot(save_dir=save_dir, names=list(names.values()))
- callbacks.run('on_val_end')
-
- # Save JSON
- if save_json and len(jdict):
- w = Path(weights[0] if isinstance(weights, list) else weights).stem if weights is not None else '' # weights
- anno_json = str(Path(data.get('path', '../coco')) / 'annotations/instances_val2017.json') # annotations json
- pred_json = str(save_dir / f"{w}_predictions.json") # predictions json
- LOGGER.info(f'\nEvaluating pycocotools mAP... saving {pred_json}...')
- with open(pred_json, 'w') as f:
- json.dump(jdict, f)
-
- try: # https://github.com/cocodataset/cocoapi/blob/master/PythonAPI/pycocoEvalDemo.ipynb
- check_requirements(['pycocotools'])
- from pycocotools.coco import COCO
- from pycocotools.cocoeval import COCOeval
-
- anno = COCO(anno_json) # init annotations api
- pred = anno.loadRes(pred_json) # init predictions api
- eval = COCOeval(anno, pred, 'bbox')
- if is_coco:
- eval.params.imgIds = [int(Path(x).stem) for x in dataloader.dataset.im_files] # image IDs to evaluate
- eval.evaluate()
- eval.accumulate()
- eval.summarize()
- map, map50 = eval.stats[:2] # update results (mAP@0.5:0.95, mAP@0.5)
- except Exception as e:
- LOGGER.info(f'pycocotools unable to run: {e}')
-
- # Return results
- model.float() # for training
- if not training:
- s = f"\n{len(list(save_dir.glob('labels/*.txt')))} labels saved to {save_dir / 'labels'}" if save_txt else ''
- LOGGER.info(f"Results saved to {colorstr('bold', save_dir)}{s}")
- maps = np.zeros(nc) + map
- for i, c in enumerate(ap_class):
- maps[c] = ap[i]
- return (mp, mr, map50, map, *(loss.cpu() / len(dataloader)).tolist()), maps, t
-
-
-def parse_opt():
- parser = argparse.ArgumentParser()
- parser.add_argument('--data', type=str, default=ROOT / 'data/coco128.yaml', help='dataset.yaml path')
- parser.add_argument('--weights', nargs='+', type=str, default=ROOT / 'yolov5s.pt', help='model.pt path(s)')
- parser.add_argument('--batch-size', type=int, default=32, help='batch size')
- parser.add_argument('--imgsz', '--img', '--img-size', type=int, default=640, help='inference size (pixels)')
- parser.add_argument('--conf-thres', type=float, default=0.001, help='confidence threshold')
- parser.add_argument('--iou-thres', type=float, default=0.6, help='NMS IoU threshold')
- parser.add_argument('--task', default='val', help='train, val, test, speed or study')
- parser.add_argument('--device', default='', help='cuda device, i.e. 0 or 0,1,2,3 or cpu')
- parser.add_argument('--workers', type=int, default=8, help='max dataloader workers (per RANK in DDP mode)')
- parser.add_argument('--single-cls', action='store_true', help='treat as single-class dataset')
- parser.add_argument('--augment', action='store_true', help='augmented inference')
- parser.add_argument('--verbose', action='store_true', help='report mAP by class')
- parser.add_argument('--save-txt', action='store_true', help='save results to *.txt')
- parser.add_argument('--save-hybrid', action='store_true', help='save label+prediction hybrid results to *.txt')
- parser.add_argument('--save-conf', action='store_true', help='save confidences in --save-txt labels')
- parser.add_argument('--save-json', action='store_true', help='save a COCO-JSON results file')
- parser.add_argument('--project', default=ROOT / 'runs/val', help='save to project/name')
- parser.add_argument('--name', default='exp', help='save to project/name')
- parser.add_argument('--exist-ok', action='store_true', help='existing project/name ok, do not increment')
- parser.add_argument('--half', action='store_true', help='use FP16 half-precision inference')
- parser.add_argument('--dnn', action='store_true', help='use OpenCV DNN for ONNX inference')
- opt = parser.parse_args()
- opt.data = check_yaml(opt.data) # check YAML
- opt.save_json |= opt.data.endswith('coco.yaml')
- opt.save_txt |= opt.save_hybrid
- print_args(vars(opt))
- return opt
-
-
-def main(opt):
- check_requirements(requirements=ROOT / 'requirements.txt', exclude=('tensorboard', 'thop'))
-
- if opt.task in ('train', 'val', 'test'): # run normally
- if opt.conf_thres > 0.001: # https://github.com/ultralytics/yolov5/issues/1466
- LOGGER.info(f'WARNING: confidence threshold {opt.conf_thres} > 0.001 produces invalid results ⚠️')
- if opt.save_hybrid:
- LOGGER.info('WARNING: --save-hybrid will return high mAP from hybrid labels, not from predictions alone ⚠️')
- run(**vars(opt))
-
- else:
- weights = opt.weights if isinstance(opt.weights, list) else [opt.weights]
- opt.half = True # FP16 for fastest results
- if opt.task == 'speed': # speed benchmarks
- # python val.py --task speed --data coco.yaml --batch 1 --weights yolov5n.pt yolov5s.pt...
- opt.conf_thres, opt.iou_thres, opt.save_json = 0.25, 0.45, False
- for opt.weights in weights:
- run(**vars(opt), plots=False)
-
- elif opt.task == 'study': # speed vs mAP benchmarks
- # python val.py --task study --data coco.yaml --iou 0.7 --weights yolov5n.pt yolov5s.pt...
- for opt.weights in weights:
- f = f'study_{Path(opt.data).stem}_{Path(opt.weights).stem}.txt' # filename to save to
- x, y = list(range(256, 1536 + 128, 128)), [] # x axis (image sizes), y axis
- for opt.imgsz in x: # img-size
- LOGGER.info(f'\nRunning {f} --imgsz {opt.imgsz}...')
- r, _, t = run(**vars(opt), plots=False)
- y.append(r + t) # results and times
- np.savetxt(f, y, fmt='%10.4g') # save
- os.system('zip -r study.zip study_*.txt')
- plot_val_study(x=x) # plot
-
-
-if __name__ == "__main__":
- opt = parse_opt()
- main(opt)
diff --git a/spaces/aaronb/Anything2Image/anything2image/imagebind/__init__.py b/spaces/aaronb/Anything2Image/anything2image/imagebind/__init__.py
deleted file mode 100644
index f97604c263254bf8fa784bbcfd15fe904c3d464a..0000000000000000000000000000000000000000
--- a/spaces/aaronb/Anything2Image/anything2image/imagebind/__init__.py
+++ /dev/null
@@ -1,2 +0,0 @@
-from .data import load_and_transform_text, load_and_transform_audio_data, load_and_transform_video_data, load_and_transform_vision_data
-from .models.imagebind_model import imagebind_huge, ModalityType
\ No newline at end of file
diff --git a/spaces/abdabbas/abd/README.md b/spaces/abdabbas/abd/README.md
deleted file mode 100644
index acd1ec00e6d0830ec4073eacc2d2891370ea405c..0000000000000000000000000000000000000000
--- a/spaces/abdabbas/abd/README.md
+++ /dev/null
@@ -1,13 +0,0 @@
----
-title: Abdulrahman
-emoji: 🚀
-colorFrom: gray
-colorTo: green
-sdk: gradio
-sdk_version: 3.0.13
-app_file: app.py
-pinned: false
-license: afl-3.0
----
-
-Check out the configuration reference at https://huggingface.co/docs/hub/spaces-config-reference
diff --git a/spaces/abhishek/sketch-to-image/annotator/uniformer/mmcv/runner/hooks/sampler_seed.py b/spaces/abhishek/sketch-to-image/annotator/uniformer/mmcv/runner/hooks/sampler_seed.py
deleted file mode 100644
index ee0dc6bdd8df5775857028aaed5444c0f59caf80..0000000000000000000000000000000000000000
--- a/spaces/abhishek/sketch-to-image/annotator/uniformer/mmcv/runner/hooks/sampler_seed.py
+++ /dev/null
@@ -1,20 +0,0 @@
-# Copyright (c) OpenMMLab. All rights reserved.
-from .hook import HOOKS, Hook
-
-
-@HOOKS.register_module()
-class DistSamplerSeedHook(Hook):
- """Data-loading sampler for distributed training.
-
- When distributed training, it is only useful in conjunction with
- :obj:`EpochBasedRunner`, while :obj:`IterBasedRunner` achieves the same
- purpose with :obj:`IterLoader`.
- """
-
- def before_epoch(self, runner):
- if hasattr(runner.data_loader.sampler, 'set_epoch'):
- # in case the data loader uses `SequentialSampler` in Pytorch
- runner.data_loader.sampler.set_epoch(runner.epoch)
- elif hasattr(runner.data_loader.batch_sampler.sampler, 'set_epoch'):
- # batch sampler in pytorch warps the sampler as its attributes.
- runner.data_loader.batch_sampler.sampler.set_epoch(runner.epoch)
diff --git a/spaces/abhishek/sketch-to-image/annotator/uniformer/mmdet/core/visualization/__init__.py b/spaces/abhishek/sketch-to-image/annotator/uniformer/mmdet/core/visualization/__init__.py
deleted file mode 100644
index 4ff995c0861490941f8cfc19ebbd41a2ee7e2d65..0000000000000000000000000000000000000000
--- a/spaces/abhishek/sketch-to-image/annotator/uniformer/mmdet/core/visualization/__init__.py
+++ /dev/null
@@ -1,4 +0,0 @@
-from .image import (color_val_matplotlib, imshow_det_bboxes,
- imshow_gt_det_bboxes)
-
-__all__ = ['imshow_det_bboxes', 'imshow_gt_det_bboxes', 'color_val_matplotlib']
diff --git a/spaces/abhishek/sketch-to-image/annotator/uniformer/mmdet_null/datasets/custom.py b/spaces/abhishek/sketch-to-image/annotator/uniformer/mmdet_null/datasets/custom.py
deleted file mode 100644
index 1a2351c217f43d32178053dfc682a2b241f9a3f1..0000000000000000000000000000000000000000
--- a/spaces/abhishek/sketch-to-image/annotator/uniformer/mmdet_null/datasets/custom.py
+++ /dev/null
@@ -1,323 +0,0 @@
-import os.path as osp
-import warnings
-from collections import OrderedDict
-
-import mmcv
-import numpy as np
-from mmcv.utils import print_log
-from torch.utils.data import Dataset
-
-from mmdet.core import eval_map, eval_recalls
-from .builder import DATASETS
-from .pipelines import Compose
-
-
-@DATASETS.register_module()
-class CustomDataset(Dataset):
- """Custom dataset for detection.
-
- The annotation format is shown as follows. The `ann` field is optional for
- testing.
-
- .. code-block:: none
-
- [
- {
- 'filename': 'a.jpg',
- 'width': 1280,
- 'height': 720,
- 'ann': {
- 'bboxes': (n, 4) in (x1, y1, x2, y2) order.
- 'labels': (n, ),
- 'bboxes_ignore': (k, 4), (optional field)
- 'labels_ignore': (k, 4) (optional field)
- }
- },
- ...
- ]
-
- Args:
- ann_file (str): Annotation file path.
- pipeline (list[dict]): Processing pipeline.
- classes (str | Sequence[str], optional): Specify classes to load.
- If is None, ``cls.CLASSES`` will be used. Default: None.
- data_root (str, optional): Data root for ``ann_file``,
- ``img_prefix``, ``seg_prefix``, ``proposal_file`` if specified.
- test_mode (bool, optional): If set True, annotation will not be loaded.
- filter_empty_gt (bool, optional): If set true, images without bounding
- boxes of the dataset's classes will be filtered out. This option
- only works when `test_mode=False`, i.e., we never filter images
- during tests.
- """
-
- CLASSES = None
-
- def __init__(self,
- ann_file,
- pipeline,
- classes=None,
- data_root=None,
- img_prefix='',
- seg_prefix=None,
- proposal_file=None,
- test_mode=False,
- filter_empty_gt=True):
- self.ann_file = ann_file
- self.data_root = data_root
- self.img_prefix = img_prefix
- self.seg_prefix = seg_prefix
- self.proposal_file = proposal_file
- self.test_mode = test_mode
- self.filter_empty_gt = filter_empty_gt
- self.CLASSES = self.get_classes(classes)
-
- # join paths if data_root is specified
- if self.data_root is not None:
- if not osp.isabs(self.ann_file):
- self.ann_file = osp.join(self.data_root, self.ann_file)
- if not (self.img_prefix is None or osp.isabs(self.img_prefix)):
- self.img_prefix = osp.join(self.data_root, self.img_prefix)
- if not (self.seg_prefix is None or osp.isabs(self.seg_prefix)):
- self.seg_prefix = osp.join(self.data_root, self.seg_prefix)
- if not (self.proposal_file is None
- or osp.isabs(self.proposal_file)):
- self.proposal_file = osp.join(self.data_root,
- self.proposal_file)
- # load annotations (and proposals)
- self.data_infos = self.load_annotations(self.ann_file)
-
- if self.proposal_file is not None:
- self.proposals = self.load_proposals(self.proposal_file)
- else:
- self.proposals = None
-
- # filter images too small and containing no annotations
- if not test_mode:
- valid_inds = self._filter_imgs()
- self.data_infos = [self.data_infos[i] for i in valid_inds]
- if self.proposals is not None:
- self.proposals = [self.proposals[i] for i in valid_inds]
- # set group flag for the sampler
- self._set_group_flag()
-
- # processing pipeline
- self.pipeline = Compose(pipeline)
-
- def __len__(self):
- """Total number of samples of data."""
- return len(self.data_infos)
-
- def load_annotations(self, ann_file):
- """Load annotation from annotation file."""
- return mmcv.load(ann_file)
-
- def load_proposals(self, proposal_file):
- """Load proposal from proposal file."""
- return mmcv.load(proposal_file)
-
- def get_ann_info(self, idx):
- """Get annotation by index.
-
- Args:
- idx (int): Index of data.
-
- Returns:
- dict: Annotation info of specified index.
- """
-
- return self.data_infos[idx]['ann']
-
- def get_cat_ids(self, idx):
- """Get category ids by index.
-
- Args:
- idx (int): Index of data.
-
- Returns:
- list[int]: All categories in the image of specified index.
- """
-
- return self.data_infos[idx]['ann']['labels'].astype(np.int).tolist()
-
- def pre_pipeline(self, results):
- """Prepare results dict for pipeline."""
- results['img_prefix'] = self.img_prefix
- results['seg_prefix'] = self.seg_prefix
- results['proposal_file'] = self.proposal_file
- results['bbox_fields'] = []
- results['mask_fields'] = []
- results['seg_fields'] = []
-
- def _filter_imgs(self, min_size=32):
- """Filter images too small."""
- if self.filter_empty_gt:
- warnings.warn(
- 'CustomDataset does not support filtering empty gt images.')
- valid_inds = []
- for i, img_info in enumerate(self.data_infos):
- if min(img_info['width'], img_info['height']) >= min_size:
- valid_inds.append(i)
- return valid_inds
-
- def _set_group_flag(self):
- """Set flag according to image aspect ratio.
-
- Images with aspect ratio greater than 1 will be set as group 1,
- otherwise group 0.
- """
- self.flag = np.zeros(len(self), dtype=np.uint8)
- for i in range(len(self)):
- img_info = self.data_infos[i]
- if img_info['width'] / img_info['height'] > 1:
- self.flag[i] = 1
-
- def _rand_another(self, idx):
- """Get another random index from the same group as the given index."""
- pool = np.where(self.flag == self.flag[idx])[0]
- return np.random.choice(pool)
-
- def __getitem__(self, idx):
- """Get training/test data after pipeline.
-
- Args:
- idx (int): Index of data.
-
- Returns:
- dict: Training/test data (with annotation if `test_mode` is set \
- True).
- """
-
- if self.test_mode:
- return self.prepare_test_img(idx)
- while True:
- data = self.prepare_train_img(idx)
- if data is None:
- idx = self._rand_another(idx)
- continue
- return data
-
- def prepare_train_img(self, idx):
- """Get training data and annotations after pipeline.
-
- Args:
- idx (int): Index of data.
-
- Returns:
- dict: Training data and annotation after pipeline with new keys \
- introduced by pipeline.
- """
-
- img_info = self.data_infos[idx]
- ann_info = self.get_ann_info(idx)
- results = dict(img_info=img_info, ann_info=ann_info)
- if self.proposals is not None:
- results['proposals'] = self.proposals[idx]
- self.pre_pipeline(results)
- return self.pipeline(results)
-
- def prepare_test_img(self, idx):
- """Get testing data after pipeline.
-
- Args:
- idx (int): Index of data.
-
- Returns:
- dict: Testing data after pipeline with new keys introduced by \
- pipeline.
- """
-
- img_info = self.data_infos[idx]
- results = dict(img_info=img_info)
- if self.proposals is not None:
- results['proposals'] = self.proposals[idx]
- self.pre_pipeline(results)
- return self.pipeline(results)
-
- @classmethod
- def get_classes(cls, classes=None):
- """Get class names of current dataset.
-
- Args:
- classes (Sequence[str] | str | None): If classes is None, use
- default CLASSES defined by builtin dataset. If classes is a
- string, take it as a file name. The file contains the name of
- classes where each line contains one class name. If classes is
- a tuple or list, override the CLASSES defined by the dataset.
-
- Returns:
- tuple[str] or list[str]: Names of categories of the dataset.
- """
- if classes is None:
- return cls.CLASSES
-
- if isinstance(classes, str):
- # take it as a file path
- class_names = mmcv.list_from_file(classes)
- elif isinstance(classes, (tuple, list)):
- class_names = classes
- else:
- raise ValueError(f'Unsupported type {type(classes)} of classes.')
-
- return class_names
-
- def format_results(self, results, **kwargs):
- """Place holder to format result to dataset specific output."""
-
- def evaluate(self,
- results,
- metric='mAP',
- logger=None,
- proposal_nums=(100, 300, 1000),
- iou_thr=0.5,
- scale_ranges=None):
- """Evaluate the dataset.
-
- Args:
- results (list): Testing results of the dataset.
- metric (str | list[str]): Metrics to be evaluated.
- logger (logging.Logger | None | str): Logger used for printing
- related information during evaluation. Default: None.
- proposal_nums (Sequence[int]): Proposal number used for evaluating
- recalls, such as recall@100, recall@1000.
- Default: (100, 300, 1000).
- iou_thr (float | list[float]): IoU threshold. Default: 0.5.
- scale_ranges (list[tuple] | None): Scale ranges for evaluating mAP.
- Default: None.
- """
-
- if not isinstance(metric, str):
- assert len(metric) == 1
- metric = metric[0]
- allowed_metrics = ['mAP', 'recall']
- if metric not in allowed_metrics:
- raise KeyError(f'metric {metric} is not supported')
- annotations = [self.get_ann_info(i) for i in range(len(self))]
- eval_results = OrderedDict()
- iou_thrs = [iou_thr] if isinstance(iou_thr, float) else iou_thr
- if metric == 'mAP':
- assert isinstance(iou_thrs, list)
- mean_aps = []
- for iou_thr in iou_thrs:
- print_log(f'\n{"-" * 15}iou_thr: {iou_thr}{"-" * 15}')
- mean_ap, _ = eval_map(
- results,
- annotations,
- scale_ranges=scale_ranges,
- iou_thr=iou_thr,
- dataset=self.CLASSES,
- logger=logger)
- mean_aps.append(mean_ap)
- eval_results[f'AP{int(iou_thr * 100):02d}'] = round(mean_ap, 3)
- eval_results['mAP'] = sum(mean_aps) / len(mean_aps)
- elif metric == 'recall':
- gt_bboxes = [ann['bboxes'] for ann in annotations]
- recalls = eval_recalls(
- gt_bboxes, results, proposal_nums, iou_thr, logger=logger)
- for i, num in enumerate(proposal_nums):
- for j, iou in enumerate(iou_thrs):
- eval_results[f'recall@{num}@{iou}'] = recalls[i, j]
- if recalls.shape[1] > 1:
- ar = recalls.mean(axis=1)
- for i, num in enumerate(proposal_nums):
- eval_results[f'AR@{num}'] = ar[i]
- return eval_results
diff --git a/spaces/ahmedghani/Editing-Tools/app.py b/spaces/ahmedghani/Editing-Tools/app.py
deleted file mode 100644
index 219526697df13ee831280ec10b97f1f1fd442f46..0000000000000000000000000000000000000000
--- a/spaces/ahmedghani/Editing-Tools/app.py
+++ /dev/null
@@ -1,150 +0,0 @@
-import gradio as gr
-from watermark_remover import convert_video_to_frames, remove_image_watermark, remove_video_watermark
-from video_converter import convert_video
-from image_converter import convert_image
-from image_editing import edit_image
-from image_inpainting import inpaint
-
-
-css = """
- #remove_btn {
- background: linear-gradient(#201d18, #2bbbc3);
- font-weight: bold;
- font-size: 18px;
- color:white;
- }
- #remove_btn:hover {
- background: linear-gradient(#2bbbc3, #201d18);
- }
- #convert_btn {
- background: linear-gradient(#201d18, #2bbbc3);
- font-weight: bold;
- font-size: 18px;
- color:white;
- }
- #convert_btn:hover {
- background: linear-gradient(#2bbbc3, #201d18);
- }
- #button {
- background: linear-gradient(#201d18, #2bbbc3);
- font-weight: bold;
- font-size: 18px;
- color:white;
- }
- #button:hover {
- background: linear-gradient(#2bbbc3, #201d18);
- }
- footer {
- display: none !important;
- }
-"""
-
-demo = gr.Blocks(css=css, title="Editing Tools")
-with demo:
- with gr.Tab("Image Converter"):
- gr.Markdown("""
- # 🖼️ Image Converter
- """)
- image_format = ['jpg', 'jpeg', 'png', 'bmp', 'tiff', 'gif', 'webp', 'ico']
- with gr.Row():
- with gr.Column():
- input_image = gr.File(label="Upload an Image")
- with gr.Column():
- with gr.Row():
- image_format = gr.Radio(image_format, label="Select Format", interactive=False)
- with gr.Row():
- image_convert_btn = gr.Button("Convert Image", interactive=False, elem_id="convert_btn")
- with gr.Row():
- output_image = gr.File(label="Output File", interactive=False)
- image_status = gr.Textbox(label="Status", interactive=False)
- input_image.change(lambda x: gr.Radio.update(interactive=True), inputs=[input_image], outputs=[image_format])
- image_format.change(lambda x: gr.Button.update(interactive=True), None, outputs=[image_convert_btn])
- image_convert_btn.click(convert_image, inputs=[input_image, image_format], outputs=[output_image, image_status])
-
- with gr.Tab("Image Watermark Remover"):
- gr.Markdown("""
- # 🖼️ Image Watermark Remover
- """)
- input_image_watermark = gr.Image(label="Upload an Image", tool="sketch", type="pil", interactive=True)
- image_remove_btn = gr.Button("Remove Watermark", interactive=True, elem_id="remove_btn")
- output_image_clean = gr.Image(label="Output Image", interactive=True)
-
- image_remove_btn.click(remove_image_watermark, inputs=[input_image_watermark], outputs=[output_image_clean])
-
- with gr.Tab("Image Editing"):
- gr.Markdown("""
- # 🖼️ Image Editing
- """)
- input_editing_image = gr.Image(label="Upload an Image", type="pil", interactive=True)
- image_editing_options = gr.Radio(["High Res", "Colorize", "Greyscale", "Remove Background"], label="Select Editing Option", interactive=True, value="High Resolution")
- image_editing_btn = gr.Button("Submit", interactive=True, elem_id="button")
- with gr.Row():
- image_editing_output = gr.Image(label="Output Preview", interactive=False)
- image_editing_file = gr.File(label="Download File", interactive=False)
-
- image_editing_btn.click(edit_image, inputs=[input_editing_image, image_editing_options], outputs=[image_editing_output, image_editing_file])
-
- with gr.Tab("Image Inpainting"):
- gr.Markdown("""
- # 🖼️ Image Inpainting
- """)
- input_inpainting_image = gr.Image(label="Upload an Image", type="pil", interactive=True, tool="sketch")
- input_inpainting_prompt = gr.Textbox(label="Prompt", interactive=True)
- input_inpainting_btn = gr.Button("Submit", interactive=True, elem_id="button")
- with gr.Row():
- input_inpainting_output = gr.Image(label="Image Preview", interactive=False)
- input_inpainting_file = gr.File(label="Download File", interactive=False)
-
- input_inpainting_btn.click(inpaint, inputs=[input_inpainting_image, input_inpainting_prompt], outputs=[input_inpainting_output, input_inpainting_file])
-
- with gr.Tab("Video Converter"):
- gr.Markdown("""
- # 🎥 Video Converter
- """)
- video_format = ['webm', 'wmv', 'mkv', 'mp4', 'avi', 'mpeg', 'vob', 'flv']
- audio_format = ['mp3', 'wav', 'ogg', 'flac', 'aac']
- with gr.Row():
- with gr.Column():
- input_video = gr.Video(label="Upload a Video")
- with gr.Column():
- with gr.Row():
- format_select = gr.Radio(["Video", "Audio"], label="Select Format", default="Video")
- with gr.Row():
- format = gr.Radio(video_format, label="Select Format", interactive=False)
- with gr.Row():
- with gr.Column():
- pass
- with gr.Column():
- convert_btn = gr.Button("Convert Video", interactive=False, elem_id="convert_btn")
- with gr.Column():
- pass
- with gr.Row():
- output = gr.File(label="Output File", interactive=False)
- status = gr.Textbox(label="Status", interactive=False)
- format_select.change(lambda x: gr.Radio.update(choices=video_format if x == "Video" else audio_format, interactive=True), inputs=[format_select], outputs=[format])
- format.change(lambda x: gr.Button.update(interactive=True), None, outputs=[convert_btn])
- convert_btn.click(convert_video, inputs=[input_video, format], outputs=[output, status])
-
- with gr.Tab("Video Watermark Remover"):
- gr.Markdown("""
- # 🎥 Video Watermark Remover
- """)
- with gr.Row():
- with gr.Column():
- input_video = gr.Video(label="Upload a Video")
- with gr.Column():
- mask = gr.Image(label="Create a mask for the image", tool="sketch", type="pil", interactive=False)
- with gr.Row():
- with gr.Column():
- pass
- with gr.Column():
- remove_btn = gr.Button("Remove Watermark", interactive=False, elem_id="remove_btn")
- with gr.Column():
- pass
-
- with gr.Row():
- output_video = gr.File(label="Output Video", interactive=False)
- input_video.change(convert_video_to_frames, inputs=[input_video], outputs=[mask, remove_btn])
- remove_btn.click(remove_video_watermark, inputs=[mask], outputs=[output_video, remove_btn])
-
-demo.launch(show_api=False, share=True)
diff --git a/spaces/airely/bingai1/README.md b/spaces/airely/bingai1/README.md
deleted file mode 100644
index 574bfa08d57787cfdcec68014f76ce9530d82e3b..0000000000000000000000000000000000000000
--- a/spaces/airely/bingai1/README.md
+++ /dev/null
@@ -1,12 +0,0 @@
----
-title: Bingo
-emoji: 🐠
-colorFrom: blue
-colorTo: yellow
-sdk: docker
-pinned: false
-license: mit
-app_port: 3000
----
-
-Check out the configuration reference at https://huggingface.co/docs/hub/spaces-config-reference
\ No newline at end of file
diff --git a/spaces/akhaliq/SummerTime/model/third_party/HMNet/Models/Optimizers/LnrWrmpInvSqRtDcyScheduler.py b/spaces/akhaliq/SummerTime/model/third_party/HMNet/Models/Optimizers/LnrWrmpInvSqRtDcyScheduler.py
deleted file mode 100644
index c9ce98d92c4eb2fcd9b688c8ca6d8fb49a842875..0000000000000000000000000000000000000000
--- a/spaces/akhaliq/SummerTime/model/third_party/HMNet/Models/Optimizers/LnrWrmpInvSqRtDcyScheduler.py
+++ /dev/null
@@ -1,27 +0,0 @@
-# Copyright (c) Microsoft Corporation.
-# Licensed under the MIT license.
-
-import math
-from torch.optim.lr_scheduler import LambdaLR
-
-
-class LnrWrmpInvSqRtDcyScheduler(LambdaLR):
- """Inverse Square Root learning rate schedule used in T5"""
-
- def __init__(self, optimizer, warmup_steps, warmup_init_lr, warmup_end_lr):
- self.warmup_steps = warmup_steps
- self.warmup_init_lr = warmup_init_lr
- self.warmup_end_lr = warmup_end_lr
- self.lr_step = (warmup_end_lr - warmup_init_lr) / warmup_steps
- super(LnrWrmpInvSqRtDcyScheduler, self).__init__(
- optimizer, self.lr_lambda, last_epoch=-1
- )
-
- def lr_lambda(self, step):
- if step < self.warmup_steps:
- return (self.warmup_init_lr + step * self.lr_step) / self.warmup_end_lr
- else:
- return 1.0 / float(math.sqrt(step / float(self.warmup_steps)))
-
- def get_last_lr(self):
- return self.get_lr()
diff --git a/spaces/akhaliq/deeplab2/model/layers/drop_path_test.py b/spaces/akhaliq/deeplab2/model/layers/drop_path_test.py
deleted file mode 100644
index 7d02f5fa9d2de935cdeb043bfbad81441e0b1b6f..0000000000000000000000000000000000000000
--- a/spaces/akhaliq/deeplab2/model/layers/drop_path_test.py
+++ /dev/null
@@ -1,76 +0,0 @@
-# coding=utf-8
-# Copyright 2021 The Deeplab2 Authors.
-#
-# Licensed under the Apache License, Version 2.0 (the "License");
-# you may not use this file except in compliance with the License.
-# You may obtain a copy of the License at
-#
-# http://www.apache.org/licenses/LICENSE-2.0
-#
-# Unless required by applicable law or agreed to in writing, software
-# distributed under the License is distributed on an "AS IS" BASIS,
-# WITHOUT WARRANTIES OR CONDITIONS OF ANY KIND, either express or implied.
-# See the License for the specific language governing permissions and
-# limitations under the License.
-
-"""Test for drop_path.py."""
-import numpy as np
-import tensorflow as tf
-
-from deeplab2.model.layers import drop_path
-
-# Set a fixed random seed.
-tf.random.set_seed(1)
-
-
-class DropPathTest(tf.test.TestCase):
-
- def test_drop_path_keep_prob_one(self):
- # Test drop_path_keep_prob = 1, where output should be equal to input.
- drop_path_keep_prob = 1.0
- input_tensor = tf.random.uniform(shape=(3, 65, 65, 32))
- layer_op = drop_path.DropPath(drop_path_keep_prob)
- output_tensor = layer_op(input_tensor, training=True)
- np.testing.assert_equal(input_tensor.numpy(), output_tensor.numpy())
-
- def test_not_training_mode(self):
- # Test not training mode, where output should be equal to input.
- drop_path_keep_prob = 0.8
- input_tensor = tf.random.uniform(shape=(3, 65, 65, 32))
- layer_op = drop_path.DropPath(drop_path_keep_prob)
- output_tensor = layer_op(input_tensor, training=False)
- np.testing.assert_equal(input_tensor.numpy(), output_tensor.numpy())
-
- def test_drop_path(self):
- drop_path_keep_prob = 0.8
- input_tensor = tf.random.uniform(shape=(3, 65, 65, 32))
- layer_op = drop_path.DropPath(drop_path_keep_prob)
- output_tensor = layer_op(input_tensor, training=True)
- self.assertFalse(np.array_equal(input_tensor.numpy(),
- output_tensor.numpy()))
-
- def test_constant_drop_path_schedule(self):
- keep_prob_for_last_stage = 0.8
- current_stage_keep_prob = drop_path.get_drop_path_keep_prob(
- keep_prob_for_last_stage,
- schedule='constant',
- current_stage=2,
- num_stages=5)
- self.assertEqual(current_stage_keep_prob, keep_prob_for_last_stage)
-
- def test_linear_drop_path_schedule(self):
- keep_prob_for_last_stage = 0.8
- current_stage_keep_prob = drop_path.get_drop_path_keep_prob(
- keep_prob_for_last_stage,
- schedule='linear',
- current_stage=1,
- num_stages=4)
- self.assertEqual(current_stage_keep_prob, 0.95)
-
- def test_unknown_drop_path_schedule(self):
- with self.assertRaises(ValueError):
- _ = drop_path.get_drop_path_keep_prob(0.8, 'unknown', 1, 4)
-
-
-if __name__ == '__main__':
- tf.test.main()
diff --git a/spaces/akhaliq/lama/saicinpainting/evaluation/losses/fid/__init__.py b/spaces/akhaliq/lama/saicinpainting/evaluation/losses/fid/__init__.py
deleted file mode 100644
index e69de29bb2d1d6434b8b29ae775ad8c2e48c5391..0000000000000000000000000000000000000000
diff --git a/spaces/alamin655/Personas/README.md b/spaces/alamin655/Personas/README.md
deleted file mode 100644
index 0dbdf323911d0583ab43b31f27d670bff0a75e7f..0000000000000000000000000000000000000000
--- a/spaces/alamin655/Personas/README.md
+++ /dev/null
@@ -1,13 +0,0 @@
----
-title: Persona Chat
-emoji: 🎭
-colorFrom: blue
-colorTo: yellow
-sdk: streamlit
-sdk_version: 1.21.0
-app_file: app.py
-pinned: false
-license: mit
----
-
-Check out the configuration reference at https://huggingface.co/docs/hub/spaces-config-reference
diff --git a/spaces/alan-chen-intel/dagan-demo/sync_batchnorm/batchnorm.py b/spaces/alan-chen-intel/dagan-demo/sync_batchnorm/batchnorm.py
deleted file mode 100644
index 5f4e763f0366dffa10320116413f8c7181a8aeb1..0000000000000000000000000000000000000000
--- a/spaces/alan-chen-intel/dagan-demo/sync_batchnorm/batchnorm.py
+++ /dev/null
@@ -1,315 +0,0 @@
-# -*- coding: utf-8 -*-
-# File : batchnorm.py
-# Author : Jiayuan Mao
-# Email : maojiayuan@gmail.com
-# Date : 27/01/2018
-#
-# This file is part of Synchronized-BatchNorm-PyTorch.
-# https://github.com/vacancy/Synchronized-BatchNorm-PyTorch
-# Distributed under MIT License.
-
-import collections
-
-import torch
-import torch.nn.functional as F
-
-from torch.nn.modules.batchnorm import _BatchNorm
-from torch.nn.parallel._functions import ReduceAddCoalesced, Broadcast
-
-from .comm import SyncMaster
-
-__all__ = ['SynchronizedBatchNorm1d', 'SynchronizedBatchNorm2d', 'SynchronizedBatchNorm3d']
-
-
-def _sum_ft(tensor):
- """sum over the first and last dimention"""
- return tensor.sum(dim=0).sum(dim=-1)
-
-
-def _unsqueeze_ft(tensor):
- """add new dementions at the front and the tail"""
- return tensor.unsqueeze(0).unsqueeze(-1)
-
-
-_ChildMessage = collections.namedtuple('_ChildMessage', ['sum', 'ssum', 'sum_size'])
-_MasterMessage = collections.namedtuple('_MasterMessage', ['sum', 'inv_std'])
-
-
-class _SynchronizedBatchNorm(_BatchNorm):
- def __init__(self, num_features, eps=1e-5, momentum=0.1, affine=True):
- super(_SynchronizedBatchNorm, self).__init__(num_features, eps=eps, momentum=momentum, affine=affine)
-
- self._sync_master = SyncMaster(self._data_parallel_master)
-
- self._is_parallel = False
- self._parallel_id = None
- self._slave_pipe = None
-
- def forward(self, input):
- # If it is not parallel computation or is in evaluation mode, use PyTorch's implementation.
- if not (self._is_parallel and self.training):
- return F.batch_norm(
- input, self.running_mean, self.running_var, self.weight, self.bias,
- self.training, self.momentum, self.eps)
-
- # Resize the input to (B, C, -1).
- input_shape = input.size()
- input = input.view(input.size(0), self.num_features, -1)
-
- # Compute the sum and square-sum.
- sum_size = input.size(0) * input.size(2)
- input_sum = _sum_ft(input)
- input_ssum = _sum_ft(input ** 2)
-
- # Reduce-and-broadcast the statistics.
- if self._parallel_id == 0:
- mean, inv_std = self._sync_master.run_master(_ChildMessage(input_sum, input_ssum, sum_size))
- else:
- mean, inv_std = self._slave_pipe.run_slave(_ChildMessage(input_sum, input_ssum, sum_size))
-
- # Compute the output.
- if self.affine:
- # MJY:: Fuse the multiplication for speed.
- output = (input - _unsqueeze_ft(mean)) * _unsqueeze_ft(inv_std * self.weight) + _unsqueeze_ft(self.bias)
- else:
- output = (input - _unsqueeze_ft(mean)) * _unsqueeze_ft(inv_std)
-
- # Reshape it.
- return output.view(input_shape)
-
- def __data_parallel_replicate__(self, ctx, copy_id):
- self._is_parallel = True
- self._parallel_id = copy_id
-
- # parallel_id == 0 means master device.
- if self._parallel_id == 0:
- ctx.sync_master = self._sync_master
- else:
- self._slave_pipe = ctx.sync_master.register_slave(copy_id)
-
- def _data_parallel_master(self, intermediates):
- """Reduce the sum and square-sum, compute the statistics, and broadcast it."""
-
- # Always using same "device order" makes the ReduceAdd operation faster.
- # Thanks to:: Tete Xiao (http://tetexiao.com/)
- intermediates = sorted(intermediates, key=lambda i: i[1].sum.get_device())
-
- to_reduce = [i[1][:2] for i in intermediates]
- to_reduce = [j for i in to_reduce for j in i] # flatten
- target_gpus = [i[1].sum.get_device() for i in intermediates]
-
- sum_size = sum([i[1].sum_size for i in intermediates])
- sum_, ssum = ReduceAddCoalesced.apply(target_gpus[0], 2, *to_reduce)
- mean, inv_std = self._compute_mean_std(sum_, ssum, sum_size)
-
- broadcasted = Broadcast.apply(target_gpus, mean, inv_std)
-
- outputs = []
- for i, rec in enumerate(intermediates):
- outputs.append((rec[0], _MasterMessage(*broadcasted[i*2:i*2+2])))
-
- return outputs
-
- def _compute_mean_std(self, sum_, ssum, size):
- """Compute the mean and standard-deviation with sum and square-sum. This method
- also maintains the moving average on the master device."""
- assert size > 1, 'BatchNorm computes unbiased standard-deviation, which requires size > 1.'
- mean = sum_ / size
- sumvar = ssum - sum_ * mean
- unbias_var = sumvar / (size - 1)
- bias_var = sumvar / size
-
- self.running_mean = (1 - self.momentum) * self.running_mean + self.momentum * mean.data
- self.running_var = (1 - self.momentum) * self.running_var + self.momentum * unbias_var.data
-
- return mean, bias_var.clamp(self.eps) ** -0.5
-
-
-class SynchronizedBatchNorm1d(_SynchronizedBatchNorm):
- r"""Applies Synchronized Batch Normalization over a 2d or 3d input that is seen as a
- mini-batch.
-
- .. math::
-
- y = \frac{x - mean[x]}{ \sqrt{Var[x] + \epsilon}} * gamma + beta
-
- This module differs from the built-in PyTorch BatchNorm1d as the mean and
- standard-deviation are reduced across all devices during training.
-
- For example, when one uses `nn.DataParallel` to wrap the network during
- training, PyTorch's implementation normalize the tensor on each device using
- the statistics only on that device, which accelerated the computation and
- is also easy to implement, but the statistics might be inaccurate.
- Instead, in this synchronized version, the statistics will be computed
- over all training samples distributed on multiple devices.
-
- Note that, for one-GPU or CPU-only case, this module behaves exactly same
- as the built-in PyTorch implementation.
-
- The mean and standard-deviation are calculated per-dimension over
- the mini-batches and gamma and beta are learnable parameter vectors
- of size C (where C is the input size).
-
- During training, this layer keeps a running estimate of its computed mean
- and variance. The running sum is kept with a default momentum of 0.1.
-
- During evaluation, this running mean/variance is used for normalization.
-
- Because the BatchNorm is done over the `C` dimension, computing statistics
- on `(N, L)` slices, it's common terminology to call this Temporal BatchNorm
-
- Args:
- num_features: num_features from an expected input of size
- `batch_size x num_features [x width]`
- eps: a value added to the denominator for numerical stability.
- Default: 1e-5
- momentum: the value used for the running_mean and running_var
- computation. Default: 0.1
- affine: a boolean value that when set to ``True``, gives the layer learnable
- affine parameters. Default: ``True``
-
- Shape:
- - Input: :math:`(N, C)` or :math:`(N, C, L)`
- - Output: :math:`(N, C)` or :math:`(N, C, L)` (same shape as input)
-
- Examples:
- >>> # With Learnable Parameters
- >>> m = SynchronizedBatchNorm1d(100)
- >>> # Without Learnable Parameters
- >>> m = SynchronizedBatchNorm1d(100, affine=False)
- >>> input = torch.autograd.Variable(torch.randn(20, 100))
- >>> output = m(input)
- """
-
- def _check_input_dim(self, input):
- if input.dim() != 2 and input.dim() != 3:
- raise ValueError('expected 2D or 3D input (got {}D input)'
- .format(input.dim()))
- super(SynchronizedBatchNorm1d, self)._check_input_dim(input)
-
-
-class SynchronizedBatchNorm2d(_SynchronizedBatchNorm):
- r"""Applies Batch Normalization over a 4d input that is seen as a mini-batch
- of 3d inputs
-
- .. math::
-
- y = \frac{x - mean[x]}{ \sqrt{Var[x] + \epsilon}} * gamma + beta
-
- This module differs from the built-in PyTorch BatchNorm2d as the mean and
- standard-deviation are reduced across all devices during training.
-
- For example, when one uses `nn.DataParallel` to wrap the network during
- training, PyTorch's implementation normalize the tensor on each device using
- the statistics only on that device, which accelerated the computation and
- is also easy to implement, but the statistics might be inaccurate.
- Instead, in this synchronized version, the statistics will be computed
- over all training samples distributed on multiple devices.
-
- Note that, for one-GPU or CPU-only case, this module behaves exactly same
- as the built-in PyTorch implementation.
-
- The mean and standard-deviation are calculated per-dimension over
- the mini-batches and gamma and beta are learnable parameter vectors
- of size C (where C is the input size).
-
- During training, this layer keeps a running estimate of its computed mean
- and variance. The running sum is kept with a default momentum of 0.1.
-
- During evaluation, this running mean/variance is used for normalization.
-
- Because the BatchNorm is done over the `C` dimension, computing statistics
- on `(N, H, W)` slices, it's common terminology to call this Spatial BatchNorm
-
- Args:
- num_features: num_features from an expected input of
- size batch_size x num_features x height x width
- eps: a value added to the denominator for numerical stability.
- Default: 1e-5
- momentum: the value used for the running_mean and running_var
- computation. Default: 0.1
- affine: a boolean value that when set to ``True``, gives the layer learnable
- affine parameters. Default: ``True``
-
- Shape:
- - Input: :math:`(N, C, H, W)`
- - Output: :math:`(N, C, H, W)` (same shape as input)
-
- Examples:
- >>> # With Learnable Parameters
- >>> m = SynchronizedBatchNorm2d(100)
- >>> # Without Learnable Parameters
- >>> m = SynchronizedBatchNorm2d(100, affine=False)
- >>> input = torch.autograd.Variable(torch.randn(20, 100, 35, 45))
- >>> output = m(input)
- """
-
- def _check_input_dim(self, input):
- if input.dim() != 4:
- raise ValueError('expected 4D input (got {}D input)'
- .format(input.dim()))
- super(SynchronizedBatchNorm2d, self)._check_input_dim(input)
-
-
-class SynchronizedBatchNorm3d(_SynchronizedBatchNorm):
- r"""Applies Batch Normalization over a 5d input that is seen as a mini-batch
- of 4d inputs
-
- .. math::
-
- y = \frac{x - mean[x]}{ \sqrt{Var[x] + \epsilon}} * gamma + beta
-
- This module differs from the built-in PyTorch BatchNorm3d as the mean and
- standard-deviation are reduced across all devices during training.
-
- For example, when one uses `nn.DataParallel` to wrap the network during
- training, PyTorch's implementation normalize the tensor on each device using
- the statistics only on that device, which accelerated the computation and
- is also easy to implement, but the statistics might be inaccurate.
- Instead, in this synchronized version, the statistics will be computed
- over all training samples distributed on multiple devices.
-
- Note that, for one-GPU or CPU-only case, this module behaves exactly same
- as the built-in PyTorch implementation.
-
- The mean and standard-deviation are calculated per-dimension over
- the mini-batches and gamma and beta are learnable parameter vectors
- of size C (where C is the input size).
-
- During training, this layer keeps a running estimate of its computed mean
- and variance. The running sum is kept with a default momentum of 0.1.
-
- During evaluation, this running mean/variance is used for normalization.
-
- Because the BatchNorm is done over the `C` dimension, computing statistics
- on `(N, D, H, W)` slices, it's common terminology to call this Volumetric BatchNorm
- or Spatio-temporal BatchNorm
-
- Args:
- num_features: num_features from an expected input of
- size batch_size x num_features x depth x height x width
- eps: a value added to the denominator for numerical stability.
- Default: 1e-5
- momentum: the value used for the running_mean and running_var
- computation. Default: 0.1
- affine: a boolean value that when set to ``True``, gives the layer learnable
- affine parameters. Default: ``True``
-
- Shape:
- - Input: :math:`(N, C, D, H, W)`
- - Output: :math:`(N, C, D, H, W)` (same shape as input)
-
- Examples:
- >>> # With Learnable Parameters
- >>> m = SynchronizedBatchNorm3d(100)
- >>> # Without Learnable Parameters
- >>> m = SynchronizedBatchNorm3d(100, affine=False)
- >>> input = torch.autograd.Variable(torch.randn(20, 100, 35, 45, 10))
- >>> output = m(input)
- """
-
- def _check_input_dim(self, input):
- if input.dim() != 5:
- raise ValueError('expected 5D input (got {}D input)'
- .format(input.dim()))
- super(SynchronizedBatchNorm3d, self)._check_input_dim(input)
diff --git a/spaces/alexray/btc_predictor/venv/lib/python3.10/site-packages/pip/_vendor/chardet/cli/__init__.py b/spaces/alexray/btc_predictor/venv/lib/python3.10/site-packages/pip/_vendor/chardet/cli/__init__.py
deleted file mode 100644
index 8b137891791fe96927ad78e64b0aad7bded08bdc..0000000000000000000000000000000000000000
--- a/spaces/alexray/btc_predictor/venv/lib/python3.10/site-packages/pip/_vendor/chardet/cli/__init__.py
+++ /dev/null
@@ -1 +0,0 @@
-
diff --git a/spaces/alexray/btc_predictor/venv/lib/python3.10/site-packages/pip/_vendor/chardet/jisfreq.py b/spaces/alexray/btc_predictor/venv/lib/python3.10/site-packages/pip/_vendor/chardet/jisfreq.py
deleted file mode 100644
index 83fc082b545106d02622de20f2083e8a7562f96c..0000000000000000000000000000000000000000
--- a/spaces/alexray/btc_predictor/venv/lib/python3.10/site-packages/pip/_vendor/chardet/jisfreq.py
+++ /dev/null
@@ -1,325 +0,0 @@
-######################## BEGIN LICENSE BLOCK ########################
-# The Original Code is Mozilla Communicator client code.
-#
-# The Initial Developer of the Original Code is
-# Netscape Communications Corporation.
-# Portions created by the Initial Developer are Copyright (C) 1998
-# the Initial Developer. All Rights Reserved.
-#
-# Contributor(s):
-# Mark Pilgrim - port to Python
-#
-# This library is free software; you can redistribute it and/or
-# modify it under the terms of the GNU Lesser General Public
-# License as published by the Free Software Foundation; either
-# version 2.1 of the License, or (at your option) any later version.
-#
-# This library is distributed in the hope that it will be useful,
-# but WITHOUT ANY WARRANTY; without even the implied warranty of
-# MERCHANTABILITY or FITNESS FOR A PARTICULAR PURPOSE. See the GNU
-# Lesser General Public License for more details.
-#
-# You should have received a copy of the GNU Lesser General Public
-# License along with this library; if not, write to the Free Software
-# Foundation, Inc., 51 Franklin St, Fifth Floor, Boston, MA
-# 02110-1301 USA
-######################### END LICENSE BLOCK #########################
-
-# Sampling from about 20M text materials include literature and computer technology
-#
-# Japanese frequency table, applied to both S-JIS and EUC-JP
-# They are sorted in order.
-
-# 128 --> 0.77094
-# 256 --> 0.85710
-# 512 --> 0.92635
-# 1024 --> 0.97130
-# 2048 --> 0.99431
-#
-# Ideal Distribution Ratio = 0.92635 / (1-0.92635) = 12.58
-# Random Distribution Ration = 512 / (2965+62+83+86-512) = 0.191
-#
-# Typical Distribution Ratio, 25% of IDR
-
-JIS_TYPICAL_DISTRIBUTION_RATIO = 3.0
-
-# Char to FreqOrder table ,
-JIS_TABLE_SIZE = 4368
-
-JIS_CHAR_TO_FREQ_ORDER = (
- 40, 1, 6, 182, 152, 180, 295,2127, 285, 381,3295,4304,3068,4606,3165,3510, # 16
-3511,1822,2785,4607,1193,2226,5070,4608, 171,2996,1247, 18, 179,5071, 856,1661, # 32
-1262,5072, 619, 127,3431,3512,3230,1899,1700, 232, 228,1294,1298, 284, 283,2041, # 48
-2042,1061,1062, 48, 49, 44, 45, 433, 434,1040,1041, 996, 787,2997,1255,4305, # 64
-2108,4609,1684,1648,5073,5074,5075,5076,5077,5078,3687,5079,4610,5080,3927,3928, # 80
-5081,3296,3432, 290,2285,1471,2187,5082,2580,2825,1303,2140,1739,1445,2691,3375, # 96
-1691,3297,4306,4307,4611, 452,3376,1182,2713,3688,3069,4308,5083,5084,5085,5086, # 112
-5087,5088,5089,5090,5091,5092,5093,5094,5095,5096,5097,5098,5099,5100,5101,5102, # 128
-5103,5104,5105,5106,5107,5108,5109,5110,5111,5112,4097,5113,5114,5115,5116,5117, # 144
-5118,5119,5120,5121,5122,5123,5124,5125,5126,5127,5128,5129,5130,5131,5132,5133, # 160
-5134,5135,5136,5137,5138,5139,5140,5141,5142,5143,5144,5145,5146,5147,5148,5149, # 176
-5150,5151,5152,4612,5153,5154,5155,5156,5157,5158,5159,5160,5161,5162,5163,5164, # 192
-5165,5166,5167,5168,5169,5170,5171,5172,5173,5174,5175,1472, 598, 618, 820,1205, # 208
-1309,1412,1858,1307,1692,5176,5177,5178,5179,5180,5181,5182,1142,1452,1234,1172, # 224
-1875,2043,2149,1793,1382,2973, 925,2404,1067,1241, 960,1377,2935,1491, 919,1217, # 240
-1865,2030,1406,1499,2749,4098,5183,5184,5185,5186,5187,5188,2561,4099,3117,1804, # 256
-2049,3689,4309,3513,1663,5189,3166,3118,3298,1587,1561,3433,5190,3119,1625,2998, # 272
-3299,4613,1766,3690,2786,4614,5191,5192,5193,5194,2161, 26,3377, 2,3929, 20, # 288
-3691, 47,4100, 50, 17, 16, 35, 268, 27, 243, 42, 155, 24, 154, 29, 184, # 304
- 4, 91, 14, 92, 53, 396, 33, 289, 9, 37, 64, 620, 21, 39, 321, 5, # 320
- 12, 11, 52, 13, 3, 208, 138, 0, 7, 60, 526, 141, 151,1069, 181, 275, # 336
-1591, 83, 132,1475, 126, 331, 829, 15, 69, 160, 59, 22, 157, 55,1079, 312, # 352
- 109, 38, 23, 25, 10, 19, 79,5195, 61, 382,1124, 8, 30,5196,5197,5198, # 368
-5199,5200,5201,5202,5203,5204,5205,5206, 89, 62, 74, 34,2416, 112, 139, 196, # 384
- 271, 149, 84, 607, 131, 765, 46, 88, 153, 683, 76, 874, 101, 258, 57, 80, # 400
- 32, 364, 121,1508, 169,1547, 68, 235, 145,2999, 41, 360,3027, 70, 63, 31, # 416
- 43, 259, 262,1383, 99, 533, 194, 66, 93, 846, 217, 192, 56, 106, 58, 565, # 432
- 280, 272, 311, 256, 146, 82, 308, 71, 100, 128, 214, 655, 110, 261, 104,1140, # 448
- 54, 51, 36, 87, 67,3070, 185,2618,2936,2020, 28,1066,2390,2059,5207,5208, # 464
-5209,5210,5211,5212,5213,5214,5215,5216,4615,5217,5218,5219,5220,5221,5222,5223, # 480
-5224,5225,5226,5227,5228,5229,5230,5231,5232,5233,5234,5235,5236,3514,5237,5238, # 496
-5239,5240,5241,5242,5243,5244,2297,2031,4616,4310,3692,5245,3071,5246,3598,5247, # 512
-4617,3231,3515,5248,4101,4311,4618,3808,4312,4102,5249,4103,4104,3599,5250,5251, # 528
-5252,5253,5254,5255,5256,5257,5258,5259,5260,5261,5262,5263,5264,5265,5266,5267, # 544
-5268,5269,5270,5271,5272,5273,5274,5275,5276,5277,5278,5279,5280,5281,5282,5283, # 560
-5284,5285,5286,5287,5288,5289,5290,5291,5292,5293,5294,5295,5296,5297,5298,5299, # 576
-5300,5301,5302,5303,5304,5305,5306,5307,5308,5309,5310,5311,5312,5313,5314,5315, # 592
-5316,5317,5318,5319,5320,5321,5322,5323,5324,5325,5326,5327,5328,5329,5330,5331, # 608
-5332,5333,5334,5335,5336,5337,5338,5339,5340,5341,5342,5343,5344,5345,5346,5347, # 624
-5348,5349,5350,5351,5352,5353,5354,5355,5356,5357,5358,5359,5360,5361,5362,5363, # 640
-5364,5365,5366,5367,5368,5369,5370,5371,5372,5373,5374,5375,5376,5377,5378,5379, # 656
-5380,5381, 363, 642,2787,2878,2788,2789,2316,3232,2317,3434,2011, 165,1942,3930, # 672
-3931,3932,3933,5382,4619,5383,4620,5384,5385,5386,5387,5388,5389,5390,5391,5392, # 688
-5393,5394,5395,5396,5397,5398,5399,5400,5401,5402,5403,5404,5405,5406,5407,5408, # 704
-5409,5410,5411,5412,5413,5414,5415,5416,5417,5418,5419,5420,5421,5422,5423,5424, # 720
-5425,5426,5427,5428,5429,5430,5431,5432,5433,5434,5435,5436,5437,5438,5439,5440, # 736
-5441,5442,5443,5444,5445,5446,5447,5448,5449,5450,5451,5452,5453,5454,5455,5456, # 752
-5457,5458,5459,5460,5461,5462,5463,5464,5465,5466,5467,5468,5469,5470,5471,5472, # 768
-5473,5474,5475,5476,5477,5478,5479,5480,5481,5482,5483,5484,5485,5486,5487,5488, # 784
-5489,5490,5491,5492,5493,5494,5495,5496,5497,5498,5499,5500,5501,5502,5503,5504, # 800
-5505,5506,5507,5508,5509,5510,5511,5512,5513,5514,5515,5516,5517,5518,5519,5520, # 816
-5521,5522,5523,5524,5525,5526,5527,5528,5529,5530,5531,5532,5533,5534,5535,5536, # 832
-5537,5538,5539,5540,5541,5542,5543,5544,5545,5546,5547,5548,5549,5550,5551,5552, # 848
-5553,5554,5555,5556,5557,5558,5559,5560,5561,5562,5563,5564,5565,5566,5567,5568, # 864
-5569,5570,5571,5572,5573,5574,5575,5576,5577,5578,5579,5580,5581,5582,5583,5584, # 880
-5585,5586,5587,5588,5589,5590,5591,5592,5593,5594,5595,5596,5597,5598,5599,5600, # 896
-5601,5602,5603,5604,5605,5606,5607,5608,5609,5610,5611,5612,5613,5614,5615,5616, # 912
-5617,5618,5619,5620,5621,5622,5623,5624,5625,5626,5627,5628,5629,5630,5631,5632, # 928
-5633,5634,5635,5636,5637,5638,5639,5640,5641,5642,5643,5644,5645,5646,5647,5648, # 944
-5649,5650,5651,5652,5653,5654,5655,5656,5657,5658,5659,5660,5661,5662,5663,5664, # 960
-5665,5666,5667,5668,5669,5670,5671,5672,5673,5674,5675,5676,5677,5678,5679,5680, # 976
-5681,5682,5683,5684,5685,5686,5687,5688,5689,5690,5691,5692,5693,5694,5695,5696, # 992
-5697,5698,5699,5700,5701,5702,5703,5704,5705,5706,5707,5708,5709,5710,5711,5712, # 1008
-5713,5714,5715,5716,5717,5718,5719,5720,5721,5722,5723,5724,5725,5726,5727,5728, # 1024
-5729,5730,5731,5732,5733,5734,5735,5736,5737,5738,5739,5740,5741,5742,5743,5744, # 1040
-5745,5746,5747,5748,5749,5750,5751,5752,5753,5754,5755,5756,5757,5758,5759,5760, # 1056
-5761,5762,5763,5764,5765,5766,5767,5768,5769,5770,5771,5772,5773,5774,5775,5776, # 1072
-5777,5778,5779,5780,5781,5782,5783,5784,5785,5786,5787,5788,5789,5790,5791,5792, # 1088
-5793,5794,5795,5796,5797,5798,5799,5800,5801,5802,5803,5804,5805,5806,5807,5808, # 1104
-5809,5810,5811,5812,5813,5814,5815,5816,5817,5818,5819,5820,5821,5822,5823,5824, # 1120
-5825,5826,5827,5828,5829,5830,5831,5832,5833,5834,5835,5836,5837,5838,5839,5840, # 1136
-5841,5842,5843,5844,5845,5846,5847,5848,5849,5850,5851,5852,5853,5854,5855,5856, # 1152
-5857,5858,5859,5860,5861,5862,5863,5864,5865,5866,5867,5868,5869,5870,5871,5872, # 1168
-5873,5874,5875,5876,5877,5878,5879,5880,5881,5882,5883,5884,5885,5886,5887,5888, # 1184
-5889,5890,5891,5892,5893,5894,5895,5896,5897,5898,5899,5900,5901,5902,5903,5904, # 1200
-5905,5906,5907,5908,5909,5910,5911,5912,5913,5914,5915,5916,5917,5918,5919,5920, # 1216
-5921,5922,5923,5924,5925,5926,5927,5928,5929,5930,5931,5932,5933,5934,5935,5936, # 1232
-5937,5938,5939,5940,5941,5942,5943,5944,5945,5946,5947,5948,5949,5950,5951,5952, # 1248
-5953,5954,5955,5956,5957,5958,5959,5960,5961,5962,5963,5964,5965,5966,5967,5968, # 1264
-5969,5970,5971,5972,5973,5974,5975,5976,5977,5978,5979,5980,5981,5982,5983,5984, # 1280
-5985,5986,5987,5988,5989,5990,5991,5992,5993,5994,5995,5996,5997,5998,5999,6000, # 1296
-6001,6002,6003,6004,6005,6006,6007,6008,6009,6010,6011,6012,6013,6014,6015,6016, # 1312
-6017,6018,6019,6020,6021,6022,6023,6024,6025,6026,6027,6028,6029,6030,6031,6032, # 1328
-6033,6034,6035,6036,6037,6038,6039,6040,6041,6042,6043,6044,6045,6046,6047,6048, # 1344
-6049,6050,6051,6052,6053,6054,6055,6056,6057,6058,6059,6060,6061,6062,6063,6064, # 1360
-6065,6066,6067,6068,6069,6070,6071,6072,6073,6074,6075,6076,6077,6078,6079,6080, # 1376
-6081,6082,6083,6084,6085,6086,6087,6088,6089,6090,6091,6092,6093,6094,6095,6096, # 1392
-6097,6098,6099,6100,6101,6102,6103,6104,6105,6106,6107,6108,6109,6110,6111,6112, # 1408
-6113,6114,2044,2060,4621, 997,1235, 473,1186,4622, 920,3378,6115,6116, 379,1108, # 1424
-4313,2657,2735,3934,6117,3809, 636,3233, 573,1026,3693,3435,2974,3300,2298,4105, # 1440
- 854,2937,2463, 393,2581,2417, 539, 752,1280,2750,2480, 140,1161, 440, 708,1569, # 1456
- 665,2497,1746,1291,1523,3000, 164,1603, 847,1331, 537,1997, 486, 508,1693,2418, # 1472
-1970,2227, 878,1220, 299,1030, 969, 652,2751, 624,1137,3301,2619, 65,3302,2045, # 1488
-1761,1859,3120,1930,3694,3516, 663,1767, 852, 835,3695, 269, 767,2826,2339,1305, # 1504
- 896,1150, 770,1616,6118, 506,1502,2075,1012,2519, 775,2520,2975,2340,2938,4314, # 1520
-3028,2086,1224,1943,2286,6119,3072,4315,2240,1273,1987,3935,1557, 175, 597, 985, # 1536
-3517,2419,2521,1416,3029, 585, 938,1931,1007,1052,1932,1685,6120,3379,4316,4623, # 1552
- 804, 599,3121,1333,2128,2539,1159,1554,2032,3810, 687,2033,2904, 952, 675,1467, # 1568
-3436,6121,2241,1096,1786,2440,1543,1924, 980,1813,2228, 781,2692,1879, 728,1918, # 1584
-3696,4624, 548,1950,4625,1809,1088,1356,3303,2522,1944, 502, 972, 373, 513,2827, # 1600
- 586,2377,2391,1003,1976,1631,6122,2464,1084, 648,1776,4626,2141, 324, 962,2012, # 1616
-2177,2076,1384, 742,2178,1448,1173,1810, 222, 102, 301, 445, 125,2420, 662,2498, # 1632
- 277, 200,1476,1165,1068, 224,2562,1378,1446, 450,1880, 659, 791, 582,4627,2939, # 1648
-3936,1516,1274, 555,2099,3697,1020,1389,1526,3380,1762,1723,1787,2229, 412,2114, # 1664
-1900,2392,3518, 512,2597, 427,1925,2341,3122,1653,1686,2465,2499, 697, 330, 273, # 1680
- 380,2162, 951, 832, 780, 991,1301,3073, 965,2270,3519, 668,2523,2636,1286, 535, # 1696
-1407, 518, 671, 957,2658,2378, 267, 611,2197,3030,6123, 248,2299, 967,1799,2356, # 1712
- 850,1418,3437,1876,1256,1480,2828,1718,6124,6125,1755,1664,2405,6126,4628,2879, # 1728
-2829, 499,2179, 676,4629, 557,2329,2214,2090, 325,3234, 464, 811,3001, 992,2342, # 1744
-2481,1232,1469, 303,2242, 466,1070,2163, 603,1777,2091,4630,2752,4631,2714, 322, # 1760
-2659,1964,1768, 481,2188,1463,2330,2857,3600,2092,3031,2421,4632,2318,2070,1849, # 1776
-2598,4633,1302,2254,1668,1701,2422,3811,2905,3032,3123,2046,4106,1763,1694,4634, # 1792
-1604, 943,1724,1454, 917, 868,2215,1169,2940, 552,1145,1800,1228,1823,1955, 316, # 1808
-1080,2510, 361,1807,2830,4107,2660,3381,1346,1423,1134,4108,6127, 541,1263,1229, # 1824
-1148,2540, 545, 465,1833,2880,3438,1901,3074,2482, 816,3937, 713,1788,2500, 122, # 1840
-1575, 195,1451,2501,1111,6128, 859, 374,1225,2243,2483,4317, 390,1033,3439,3075, # 1856
-2524,1687, 266, 793,1440,2599, 946, 779, 802, 507, 897,1081, 528,2189,1292, 711, # 1872
-1866,1725,1167,1640, 753, 398,2661,1053, 246, 348,4318, 137,1024,3440,1600,2077, # 1888
-2129, 825,4319, 698, 238, 521, 187,2300,1157,2423,1641,1605,1464,1610,1097,2541, # 1904
-1260,1436, 759,2255,1814,2150, 705,3235, 409,2563,3304, 561,3033,2005,2564, 726, # 1920
-1956,2343,3698,4109, 949,3812,3813,3520,1669, 653,1379,2525, 881,2198, 632,2256, # 1936
-1027, 778,1074, 733,1957, 514,1481,2466, 554,2180, 702,3938,1606,1017,1398,6129, # 1952
-1380,3521, 921, 993,1313, 594, 449,1489,1617,1166, 768,1426,1360, 495,1794,3601, # 1968
-1177,3602,1170,4320,2344, 476, 425,3167,4635,3168,1424, 401,2662,1171,3382,1998, # 1984
-1089,4110, 477,3169, 474,6130,1909, 596,2831,1842, 494, 693,1051,1028,1207,3076, # 2000
- 606,2115, 727,2790,1473,1115, 743,3522, 630, 805,1532,4321,2021, 366,1057, 838, # 2016
- 684,1114,2142,4322,2050,1492,1892,1808,2271,3814,2424,1971,1447,1373,3305,1090, # 2032
-1536,3939,3523,3306,1455,2199, 336, 369,2331,1035, 584,2393, 902, 718,2600,6131, # 2048
-2753, 463,2151,1149,1611,2467, 715,1308,3124,1268, 343,1413,3236,1517,1347,2663, # 2064
-2093,3940,2022,1131,1553,2100,2941,1427,3441,2942,1323,2484,6132,1980, 872,2368, # 2080
-2441,2943, 320,2369,2116,1082, 679,1933,3941,2791,3815, 625,1143,2023, 422,2200, # 2096
-3816,6133, 730,1695, 356,2257,1626,2301,2858,2637,1627,1778, 937, 883,2906,2693, # 2112
-3002,1769,1086, 400,1063,1325,3307,2792,4111,3077, 456,2345,1046, 747,6134,1524, # 2128
- 884,1094,3383,1474,2164,1059, 974,1688,2181,2258,1047, 345,1665,1187, 358, 875, # 2144
-3170, 305, 660,3524,2190,1334,1135,3171,1540,1649,2542,1527, 927, 968,2793, 885, # 2160
-1972,1850, 482, 500,2638,1218,1109,1085,2543,1654,2034, 876, 78,2287,1482,1277, # 2176
- 861,1675,1083,1779, 724,2754, 454, 397,1132,1612,2332, 893, 672,1237, 257,2259, # 2192
-2370, 135,3384, 337,2244, 547, 352, 340, 709,2485,1400, 788,1138,2511, 540, 772, # 2208
-1682,2260,2272,2544,2013,1843,1902,4636,1999,1562,2288,4637,2201,1403,1533, 407, # 2224
- 576,3308,1254,2071, 978,3385, 170, 136,1201,3125,2664,3172,2394, 213, 912, 873, # 2240
-3603,1713,2202, 699,3604,3699, 813,3442, 493, 531,1054, 468,2907,1483, 304, 281, # 2256
-4112,1726,1252,2094, 339,2319,2130,2639, 756,1563,2944, 748, 571,2976,1588,2425, # 2272
-2715,1851,1460,2426,1528,1392,1973,3237, 288,3309, 685,3386, 296, 892,2716,2216, # 2288
-1570,2245, 722,1747,2217, 905,3238,1103,6135,1893,1441,1965, 251,1805,2371,3700, # 2304
-2601,1919,1078, 75,2182,1509,1592,1270,2640,4638,2152,6136,3310,3817, 524, 706, # 2320
-1075, 292,3818,1756,2602, 317, 98,3173,3605,3525,1844,2218,3819,2502, 814, 567, # 2336
- 385,2908,1534,6137, 534,1642,3239, 797,6138,1670,1529, 953,4323, 188,1071, 538, # 2352
- 178, 729,3240,2109,1226,1374,2000,2357,2977, 731,2468,1116,2014,2051,6139,1261, # 2368
-1593, 803,2859,2736,3443, 556, 682, 823,1541,6140,1369,2289,1706,2794, 845, 462, # 2384
-2603,2665,1361, 387, 162,2358,1740, 739,1770,1720,1304,1401,3241,1049, 627,1571, # 2400
-2427,3526,1877,3942,1852,1500, 431,1910,1503, 677, 297,2795, 286,1433,1038,1198, # 2416
-2290,1133,1596,4113,4639,2469,1510,1484,3943,6141,2442, 108, 712,4640,2372, 866, # 2432
-3701,2755,3242,1348, 834,1945,1408,3527,2395,3243,1811, 824, 994,1179,2110,1548, # 2448
-1453, 790,3003, 690,4324,4325,2832,2909,3820,1860,3821, 225,1748, 310, 346,1780, # 2464
-2470, 821,1993,2717,2796, 828, 877,3528,2860,2471,1702,2165,2910,2486,1789, 453, # 2480
- 359,2291,1676, 73,1164,1461,1127,3311, 421, 604, 314,1037, 589, 116,2487, 737, # 2496
- 837,1180, 111, 244, 735,6142,2261,1861,1362, 986, 523, 418, 581,2666,3822, 103, # 2512
- 855, 503,1414,1867,2488,1091, 657,1597, 979, 605,1316,4641,1021,2443,2078,2001, # 2528
-1209, 96, 587,2166,1032, 260,1072,2153, 173, 94, 226,3244, 819,2006,4642,4114, # 2544
-2203, 231,1744, 782, 97,2667, 786,3387, 887, 391, 442,2219,4326,1425,6143,2694, # 2560
- 633,1544,1202, 483,2015, 592,2052,1958,2472,1655, 419, 129,4327,3444,3312,1714, # 2576
-1257,3078,4328,1518,1098, 865,1310,1019,1885,1512,1734, 469,2444, 148, 773, 436, # 2592
-1815,1868,1128,1055,4329,1245,2756,3445,2154,1934,1039,4643, 579,1238, 932,2320, # 2608
- 353, 205, 801, 115,2428, 944,2321,1881, 399,2565,1211, 678, 766,3944, 335,2101, # 2624
-1459,1781,1402,3945,2737,2131,1010, 844, 981,1326,1013, 550,1816,1545,2620,1335, # 2640
-1008, 371,2881, 936,1419,1613,3529,1456,1395,2273,1834,2604,1317,2738,2503, 416, # 2656
-1643,4330, 806,1126, 229, 591,3946,1314,1981,1576,1837,1666, 347,1790, 977,3313, # 2672
- 764,2861,1853, 688,2429,1920,1462, 77, 595, 415,2002,3034, 798,1192,4115,6144, # 2688
-2978,4331,3035,2695,2582,2072,2566, 430,2430,1727, 842,1396,3947,3702, 613, 377, # 2704
- 278, 236,1417,3388,3314,3174, 757,1869, 107,3530,6145,1194, 623,2262, 207,1253, # 2720
-2167,3446,3948, 492,1117,1935, 536,1838,2757,1246,4332, 696,2095,2406,1393,1572, # 2736
-3175,1782, 583, 190, 253,1390,2230, 830,3126,3389, 934,3245,1703,1749,2979,1870, # 2752
-2545,1656,2204, 869,2346,4116,3176,1817, 496,1764,4644, 942,1504, 404,1903,1122, # 2768
-1580,3606,2945,1022, 515, 372,1735, 955,2431,3036,6146,2797,1110,2302,2798, 617, # 2784
-6147, 441, 762,1771,3447,3607,3608,1904, 840,3037, 86, 939,1385, 572,1370,2445, # 2800
-1336, 114,3703, 898, 294, 203,3315, 703,1583,2274, 429, 961,4333,1854,1951,3390, # 2816
-2373,3704,4334,1318,1381, 966,1911,2322,1006,1155, 309, 989, 458,2718,1795,1372, # 2832
-1203, 252,1689,1363,3177, 517,1936, 168,1490, 562, 193,3823,1042,4117,1835, 551, # 2848
- 470,4645, 395, 489,3448,1871,1465,2583,2641, 417,1493, 279,1295, 511,1236,1119, # 2864
- 72,1231,1982,1812,3004, 871,1564, 984,3449,1667,2696,2096,4646,2347,2833,1673, # 2880
-3609, 695,3246,2668, 807,1183,4647, 890, 388,2333,1801,1457,2911,1765,1477,1031, # 2896
-3316,3317,1278,3391,2799,2292,2526, 163,3450,4335,2669,1404,1802,6148,2323,2407, # 2912
-1584,1728,1494,1824,1269, 298, 909,3318,1034,1632, 375, 776,1683,2061, 291, 210, # 2928
-1123, 809,1249,1002,2642,3038, 206,1011,2132, 144, 975, 882,1565, 342, 667, 754, # 2944
-1442,2143,1299,2303,2062, 447, 626,2205,1221,2739,2912,1144,1214,2206,2584, 760, # 2960
-1715, 614, 950,1281,2670,2621, 810, 577,1287,2546,4648, 242,2168, 250,2643, 691, # 2976
- 123,2644, 647, 313,1029, 689,1357,2946,1650, 216, 771,1339,1306, 808,2063, 549, # 2992
- 913,1371,2913,2914,6149,1466,1092,1174,1196,1311,2605,2396,1783,1796,3079, 406, # 3008
-2671,2117,3949,4649, 487,1825,2220,6150,2915, 448,2348,1073,6151,2397,1707, 130, # 3024
- 900,1598, 329, 176,1959,2527,1620,6152,2275,4336,3319,1983,2191,3705,3610,2155, # 3040
-3706,1912,1513,1614,6153,1988, 646, 392,2304,1589,3320,3039,1826,1239,1352,1340, # 3056
-2916, 505,2567,1709,1437,2408,2547, 906,6154,2672, 384,1458,1594,1100,1329, 710, # 3072
- 423,3531,2064,2231,2622,1989,2673,1087,1882, 333, 841,3005,1296,2882,2379, 580, # 3088
-1937,1827,1293,2585, 601, 574, 249,1772,4118,2079,1120, 645, 901,1176,1690, 795, # 3104
-2207, 478,1434, 516,1190,1530, 761,2080, 930,1264, 355, 435,1552, 644,1791, 987, # 3120
- 220,1364,1163,1121,1538, 306,2169,1327,1222, 546,2645, 218, 241, 610,1704,3321, # 3136
-1984,1839,1966,2528, 451,6155,2586,3707,2568, 907,3178, 254,2947, 186,1845,4650, # 3152
- 745, 432,1757, 428,1633, 888,2246,2221,2489,3611,2118,1258,1265, 956,3127,1784, # 3168
-4337,2490, 319, 510, 119, 457,3612, 274,2035,2007,4651,1409,3128, 970,2758, 590, # 3184
-2800, 661,2247,4652,2008,3950,1420,1549,3080,3322,3951,1651,1375,2111, 485,2491, # 3200
-1429,1156,6156,2548,2183,1495, 831,1840,2529,2446, 501,1657, 307,1894,3247,1341, # 3216
- 666, 899,2156,1539,2549,1559, 886, 349,2208,3081,2305,1736,3824,2170,2759,1014, # 3232
-1913,1386, 542,1397,2948, 490, 368, 716, 362, 159, 282,2569,1129,1658,1288,1750, # 3248
-2674, 276, 649,2016, 751,1496, 658,1818,1284,1862,2209,2087,2512,3451, 622,2834, # 3264
- 376, 117,1060,2053,1208,1721,1101,1443, 247,1250,3179,1792,3952,2760,2398,3953, # 3280
-6157,2144,3708, 446,2432,1151,2570,3452,2447,2761,2835,1210,2448,3082, 424,2222, # 3296
-1251,2449,2119,2836, 504,1581,4338, 602, 817, 857,3825,2349,2306, 357,3826,1470, # 3312
-1883,2883, 255, 958, 929,2917,3248, 302,4653,1050,1271,1751,2307,1952,1430,2697, # 3328
-2719,2359, 354,3180, 777, 158,2036,4339,1659,4340,4654,2308,2949,2248,1146,2232, # 3344
-3532,2720,1696,2623,3827,6158,3129,1550,2698,1485,1297,1428, 637, 931,2721,2145, # 3360
- 914,2550,2587, 81,2450, 612, 827,2646,1242,4655,1118,2884, 472,1855,3181,3533, # 3376
-3534, 569,1353,2699,1244,1758,2588,4119,2009,2762,2171,3709,1312,1531,6159,1152, # 3392
-1938, 134,1830, 471,3710,2276,1112,1535,3323,3453,3535, 982,1337,2950, 488, 826, # 3408
- 674,1058,1628,4120,2017, 522,2399, 211, 568,1367,3454, 350, 293,1872,1139,3249, # 3424
-1399,1946,3006,1300,2360,3324, 588, 736,6160,2606, 744, 669,3536,3828,6161,1358, # 3440
- 199, 723, 848, 933, 851,1939,1505,1514,1338,1618,1831,4656,1634,3613, 443,2740, # 3456
-3829, 717,1947, 491,1914,6162,2551,1542,4121,1025,6163,1099,1223, 198,3040,2722, # 3472
- 370, 410,1905,2589, 998,1248,3182,2380, 519,1449,4122,1710, 947, 928,1153,4341, # 3488
-2277, 344,2624,1511, 615, 105, 161,1212,1076,1960,3130,2054,1926,1175,1906,2473, # 3504
- 414,1873,2801,6164,2309, 315,1319,3325, 318,2018,2146,2157, 963, 631, 223,4342, # 3520
-4343,2675, 479,3711,1197,2625,3712,2676,2361,6165,4344,4123,6166,2451,3183,1886, # 3536
-2184,1674,1330,1711,1635,1506, 799, 219,3250,3083,3954,1677,3713,3326,2081,3614, # 3552
-1652,2073,4657,1147,3041,1752, 643,1961, 147,1974,3955,6167,1716,2037, 918,3007, # 3568
-1994, 120,1537, 118, 609,3184,4345, 740,3455,1219, 332,1615,3830,6168,1621,2980, # 3584
-1582, 783, 212, 553,2350,3714,1349,2433,2082,4124, 889,6169,2310,1275,1410, 973, # 3600
- 166,1320,3456,1797,1215,3185,2885,1846,2590,2763,4658, 629, 822,3008, 763, 940, # 3616
-1990,2862, 439,2409,1566,1240,1622, 926,1282,1907,2764, 654,2210,1607, 327,1130, # 3632
-3956,1678,1623,6170,2434,2192, 686, 608,3831,3715, 903,3957,3042,6171,2741,1522, # 3648
-1915,1105,1555,2552,1359, 323,3251,4346,3457, 738,1354,2553,2311,2334,1828,2003, # 3664
-3832,1753,2351,1227,6172,1887,4125,1478,6173,2410,1874,1712,1847, 520,1204,2607, # 3680
- 264,4659, 836,2677,2102, 600,4660,3833,2278,3084,6174,4347,3615,1342, 640, 532, # 3696
- 543,2608,1888,2400,2591,1009,4348,1497, 341,1737,3616,2723,1394, 529,3252,1321, # 3712
- 983,4661,1515,2120, 971,2592, 924, 287,1662,3186,4349,2700,4350,1519, 908,1948, # 3728
-2452, 156, 796,1629,1486,2223,2055, 694,4126,1259,1036,3392,1213,2249,2742,1889, # 3744
-1230,3958,1015, 910, 408, 559,3617,4662, 746, 725, 935,4663,3959,3009,1289, 563, # 3760
- 867,4664,3960,1567,2981,2038,2626, 988,2263,2381,4351, 143,2374, 704,1895,6175, # 3776
-1188,3716,2088, 673,3085,2362,4352, 484,1608,1921,2765,2918, 215, 904,3618,3537, # 3792
- 894, 509, 976,3043,2701,3961,4353,2837,2982, 498,6176,6177,1102,3538,1332,3393, # 3808
-1487,1636,1637, 233, 245,3962, 383, 650, 995,3044, 460,1520,1206,2352, 749,3327, # 3824
- 530, 700, 389,1438,1560,1773,3963,2264, 719,2951,2724,3834, 870,1832,1644,1000, # 3840
- 839,2474,3717, 197,1630,3394, 365,2886,3964,1285,2133, 734, 922, 818,1106, 732, # 3856
- 480,2083,1774,3458, 923,2279,1350, 221,3086, 85,2233,2234,3835,1585,3010,2147, # 3872
-1387,1705,2382,1619,2475, 133, 239,2802,1991,1016,2084,2383, 411,2838,1113, 651, # 3888
-1985,1160,3328, 990,1863,3087,1048,1276,2647, 265,2627,1599,3253,2056, 150, 638, # 3904
-2019, 656, 853, 326,1479, 680,1439,4354,1001,1759, 413,3459,3395,2492,1431, 459, # 3920
-4355,1125,3329,2265,1953,1450,2065,2863, 849, 351,2678,3131,3254,3255,1104,1577, # 3936
- 227,1351,1645,2453,2193,1421,2887, 812,2121, 634, 95,2435, 201,2312,4665,1646, # 3952
-1671,2743,1601,2554,2702,2648,2280,1315,1366,2089,3132,1573,3718,3965,1729,1189, # 3968
- 328,2679,1077,1940,1136, 558,1283, 964,1195, 621,2074,1199,1743,3460,3619,1896, # 3984
-1916,1890,3836,2952,1154,2112,1064, 862, 378,3011,2066,2113,2803,1568,2839,6178, # 4000
-3088,2919,1941,1660,2004,1992,2194, 142, 707,1590,1708,1624,1922,1023,1836,1233, # 4016
-1004,2313, 789, 741,3620,6179,1609,2411,1200,4127,3719,3720,4666,2057,3721, 593, # 4032
-2840, 367,2920,1878,6180,3461,1521, 628,1168, 692,2211,2649, 300, 720,2067,2571, # 4048
-2953,3396, 959,2504,3966,3539,3462,1977, 701,6181, 954,1043, 800, 681, 183,3722, # 4064
-1803,1730,3540,4128,2103, 815,2314, 174, 467, 230,2454,1093,2134, 755,3541,3397, # 4080
-1141,1162,6182,1738,2039, 270,3256,2513,1005,1647,2185,3837, 858,1679,1897,1719, # 4096
-2954,2324,1806, 402, 670, 167,4129,1498,2158,2104, 750,6183, 915, 189,1680,1551, # 4112
- 455,4356,1501,2455, 405,1095,2955, 338,1586,1266,1819, 570, 641,1324, 237,1556, # 4128
-2650,1388,3723,6184,1368,2384,1343,1978,3089,2436, 879,3724, 792,1191, 758,3012, # 4144
-1411,2135,1322,4357, 240,4667,1848,3725,1574,6185, 420,3045,1546,1391, 714,4358, # 4160
-1967, 941,1864, 863, 664, 426, 560,1731,2680,1785,2864,1949,2363, 403,3330,1415, # 4176
-1279,2136,1697,2335, 204, 721,2097,3838, 90,6186,2085,2505, 191,3967, 124,2148, # 4192
-1376,1798,1178,1107,1898,1405, 860,4359,1243,1272,2375,2983,1558,2456,1638, 113, # 4208
-3621, 578,1923,2609, 880, 386,4130, 784,2186,2266,1422,2956,2172,1722, 497, 263, # 4224
-2514,1267,2412,2610, 177,2703,3542, 774,1927,1344, 616,1432,1595,1018, 172,4360, # 4240
-2325, 911,4361, 438,1468,3622, 794,3968,2024,2173,1681,1829,2957, 945, 895,3090, # 4256
- 575,2212,2476, 475,2401,2681, 785,2744,1745,2293,2555,1975,3133,2865, 394,4668, # 4272
-3839, 635,4131, 639, 202,1507,2195,2766,1345,1435,2572,3726,1908,1184,1181,2457, # 4288
-3727,3134,4362, 843,2611, 437, 916,4669, 234, 769,1884,3046,3047,3623, 833,6187, # 4304
-1639,2250,2402,1355,1185,2010,2047, 999, 525,1732,1290,1488,2612, 948,1578,3728, # 4320
-2413,2477,1216,2725,2159, 334,3840,1328,3624,2921,1525,4132, 564,1056, 891,4363, # 4336
-1444,1698,2385,2251,3729,1365,2281,2235,1717,6188, 864,3841,2515, 444, 527,2767, # 4352
-2922,3625, 544, 461,6189, 566, 209,2437,3398,2098,1065,2068,3331,3626,3257,2137, # 4368 #last 512
-)
-
-
diff --git a/spaces/aliabd/Anime2Sketch/model.py b/spaces/aliabd/Anime2Sketch/model.py
deleted file mode 100644
index f02529621334315815ae53277580d98c2152066a..0000000000000000000000000000000000000000
--- a/spaces/aliabd/Anime2Sketch/model.py
+++ /dev/null
@@ -1,121 +0,0 @@
-import torch
-import torch.nn as nn
-import functools
-
-
-class UnetGenerator(nn.Module):
- """Create a Unet-based generator"""
-
- def __init__(self, input_nc, output_nc, num_downs, ngf=64, norm_layer=nn.BatchNorm2d, use_dropout=False):
- """Construct a Unet generator
- Parameters:
- input_nc (int) -- the number of channels in input images
- output_nc (int) -- the number of channels in output images
- num_downs (int) -- the number of downsamplings in UNet. For example, # if |num_downs| == 7,
- image of size 128x128 will become of size 1x1 # at the bottleneck
- ngf (int) -- the number of filters in the last conv layer
- norm_layer -- normalization layer
- We construct the U-Net from the innermost layer to the outermost layer.
- It is a recursive process.
- """
- super(UnetGenerator, self).__init__()
- # construct unet structure
- unet_block = UnetSkipConnectionBlock(ngf * 8, ngf * 8, input_nc=None, submodule=None, norm_layer=norm_layer, innermost=True) # add the innermost layer
- for _ in range(num_downs - 5): # add intermediate layers with ngf * 8 filters
- unet_block = UnetSkipConnectionBlock(ngf * 8, ngf * 8, input_nc=None, submodule=unet_block, norm_layer=norm_layer, use_dropout=use_dropout)
- # gradually reduce the number of filters from ngf * 8 to ngf
- unet_block = UnetSkipConnectionBlock(ngf * 4, ngf * 8, input_nc=None, submodule=unet_block, norm_layer=norm_layer)
- unet_block = UnetSkipConnectionBlock(ngf * 2, ngf * 4, input_nc=None, submodule=unet_block, norm_layer=norm_layer)
- unet_block = UnetSkipConnectionBlock(ngf, ngf * 2, input_nc=None, submodule=unet_block, norm_layer=norm_layer)
- self.model = UnetSkipConnectionBlock(output_nc, ngf, input_nc=input_nc, submodule=unet_block, outermost=True, norm_layer=norm_layer) # add the outermost layer
-
- def forward(self, input):
- """Standard forward"""
- return self.model(input)
-
-class UnetSkipConnectionBlock(nn.Module):
- """Defines the Unet submodule with skip connection.
- X -------------------identity----------------------
- |-- downsampling -- |submodule| -- upsampling --|
- """
-
- def __init__(self, outer_nc, inner_nc, input_nc=None,
- submodule=None, outermost=False, innermost=False, norm_layer=nn.BatchNorm2d, use_dropout=False):
- """Construct a Unet submodule with skip connections.
- Parameters:
- outer_nc (int) -- the number of filters in the outer conv layer
- inner_nc (int) -- the number of filters in the inner conv layer
- input_nc (int) -- the number of channels in input images/features
- submodule (UnetSkipConnectionBlock) -- previously defined submodules
- outermost (bool) -- if this module is the outermost module
- innermost (bool) -- if this module is the innermost module
- norm_layer -- normalization layer
- use_dropout (bool) -- if use dropout layers.
- """
- super(UnetSkipConnectionBlock, self).__init__()
- self.outermost = outermost
- if type(norm_layer) == functools.partial:
- use_bias = norm_layer.func == nn.InstanceNorm2d
- else:
- use_bias = norm_layer == nn.InstanceNorm2d
- if input_nc is None:
- input_nc = outer_nc
- downconv = nn.Conv2d(input_nc, inner_nc, kernel_size=4,
- stride=2, padding=1, bias=use_bias)
- downrelu = nn.LeakyReLU(0.2, True)
- downnorm = norm_layer(inner_nc)
- uprelu = nn.ReLU(True)
- upnorm = norm_layer(outer_nc)
-
- if outermost:
- upconv = nn.ConvTranspose2d(inner_nc * 2, outer_nc,
- kernel_size=4, stride=2,
- padding=1)
- down = [downconv]
- up = [uprelu, upconv, nn.Tanh()]
- model = down + [submodule] + up
- elif innermost:
- upconv = nn.ConvTranspose2d(inner_nc, outer_nc,
- kernel_size=4, stride=2,
- padding=1, bias=use_bias)
- down = [downrelu, downconv]
- up = [uprelu, upconv, upnorm]
- model = down + up
- else:
- upconv = nn.ConvTranspose2d(inner_nc * 2, outer_nc,
- kernel_size=4, stride=2,
- padding=1, bias=use_bias)
- down = [downrelu, downconv, downnorm]
- up = [uprelu, upconv, upnorm]
-
- if use_dropout:
- model = down + [submodule] + up + [nn.Dropout(0.5)]
- else:
- model = down + [submodule] + up
-
- self.model = nn.Sequential(*model)
-
- def forward(self, x):
- if self.outermost:
- return self.model(x)
- else: # add skip connections
- return torch.cat([x, self.model(x)], 1)
-
-
-def create_model(gpu_ids=[]):
- """Create a model for anime2sketch
- hardcoding the options for simplicity
- """
- norm_layer = functools.partial(nn.InstanceNorm2d, affine=False, track_running_stats=False)
- net = UnetGenerator(3, 1, 8, 64, norm_layer=norm_layer, use_dropout=False)
- ckpt = torch.load('weights/netG.pth')
- for key in list(ckpt.keys()):
- if 'module.' in key:
- ckpt[key.replace('module.', '')] = ckpt[key]
- del ckpt[key]
- net.load_state_dict(ckpt)
- if len(gpu_ids) > 0:
- assert(torch.cuda.is_available())
- net.to(gpu_ids[0])
- net = torch.nn.DataParallel(net, gpu_ids) # multi-GPUs
- return net
\ No newline at end of file
diff --git a/spaces/allknowingroger/Image-Models-Test108/README.md b/spaces/allknowingroger/Image-Models-Test108/README.md
deleted file mode 100644
index c6f01c6c7c17f0433e85a32d5b58cdf0072045a8..0000000000000000000000000000000000000000
--- a/spaces/allknowingroger/Image-Models-Test108/README.md
+++ /dev/null
@@ -1,12 +0,0 @@
----
-title: More Image Models
-emoji: 😻
-colorFrom: red
-colorTo: gray
-sdk: gradio
-sdk_version: 3.23.0
-app_file: app.py
-duplicated_from: allknowingroger/Image-Models-Test107
----
-
-
\ No newline at end of file
diff --git a/spaces/amagastya/SPARK/app/spark.py b/spaces/amagastya/SPARK/app/spark.py
deleted file mode 100644
index 619d66cb5fa921cccac636449b71f32792991621..0000000000000000000000000000000000000000
--- a/spaces/amagastya/SPARK/app/spark.py
+++ /dev/null
@@ -1,85 +0,0 @@
-import os
-from langchain.embeddings.cohere import CohereEmbeddings
-from langchain.vectorstores import Pinecone
-from langchain.chains import ConversationalRetrievalChain, LLMChain
-from langchain.chat_models import ChatOpenAI
-import pinecone
-import chainlit as cl
-from langchain.memory import ConversationTokenBufferMemory
-from langchain.prompts import (
- ChatPromptTemplate,
- PromptTemplate,
- SystemMessagePromptTemplate,
- HumanMessagePromptTemplate,
-)
-from langchain.prompts.prompt import PromptTemplate
-from langchain.chains.qa_with_sources import load_qa_with_sources_chain
-from langchain.callbacks import get_openai_callback
-from langchain.retrievers import ContextualCompressionRetriever
-from langchain.retrievers.document_compressors import CohereRerank
-from chainlit import user_session
-from prompts import load_query_gen_prompt, load_spark_prompt
-from chainlit import on_message, on_chat_start
-import openai
-from langchain.callbacks import ContextCallbackHandler
-from promptwatch import PromptWatch
-
-
-index_name = "spark"
-
-spark = load_spark_prompt()
-query_gen_prompt = load_query_gen_prompt()
-CONDENSE_QUESTION_PROMPT = PromptTemplate.from_template(query_gen_prompt)
-pinecone.init(
- api_key=os.environ.get("PINECONE_API_KEY"),
- environment='us-west1-gcp',
- )
-@on_chat_start
-def init():
- token = os.environ["CONTEXT_TOKEN"]
- context_callback = ContextCallbackHandler(token)
- os.environ["LANGCHAIN_WANDB_TRACING"] = "true"
- os.environ["WANDB_PROJECT"] = "spark"
- llm = ChatOpenAI(temperature=0.7, verbose=True, openai_api_key = os.environ.get("OPENAI_API_KEY"), streaming=True,
- callbacks=[context_callback])
- memory = ConversationTokenBufferMemory(llm=llm,memory_key="chat_history", return_messages=True,input_key='question',max_token_limit=1000)
- embeddings = CohereEmbeddings(model='embed-english-light-v2.0',cohere_api_key=os.environ.get("COHERE_API_KEY"))
-
- docsearch = Pinecone.from_existing_index(
- index_name=index_name, embedding=embeddings
- )
- retriever = docsearch.as_retriever(search_kwargs={"k": 4})
- # compressor = CohereRerank()
- # reranker = ContextualCompressionRetriever(
- # base_compressor=compressor, base_retriever=retriever
- # )
- messages = [SystemMessagePromptTemplate.from_template(spark)]
- # print('mem', user_session.get('memory'))
- messages.append(HumanMessagePromptTemplate.from_template("{question}"))
- prompt = ChatPromptTemplate.from_messages(messages)
-
- question_generator = LLMChain(llm=llm, prompt=CONDENSE_QUESTION_PROMPT, verbose=True)
- doc_chain = load_qa_with_sources_chain(llm, chain_type="stuff", verbose=True,prompt=prompt)
-
- chain = ConversationalRetrievalChain(
- retriever=retriever,
- question_generator=question_generator,
- combine_docs_chain=doc_chain,
- verbose=True,
- memory=memory,
- rephrase_question=False,
- callbacks=[context_callback]
- )
- cl.user_session.set("conversation_chain", chain)
-
-
-@on_message
-async def main(message: str):
- with PromptWatch(api_key=os.environ.get("PROMPTWATCH_KEY")) as pw:
- token = os.environ["CONTEXT_TOKEN"]
- context_callback = ContextCallbackHandler(token)
- chain = cl.user_session.get("conversation_chain")
- res = await chain.arun({"question": message},callbacks=[cl.AsyncLangchainCallbackHandler(),
- context_callback])
- # Send the answer and the text elements to the UI
- await cl.Message(content=res).send()
\ No newline at end of file
diff --git a/spaces/antonovmaxim/text-generation-webui-space/text-generation-webui-main/modules/evaluate.py b/spaces/antonovmaxim/text-generation-webui-space/text-generation-webui-main/modules/evaluate.py
deleted file mode 100644
index 3134280c899500543e5d5e3d6960af4c627a40ef..0000000000000000000000000000000000000000
--- a/spaces/antonovmaxim/text-generation-webui-space/text-generation-webui-main/modules/evaluate.py
+++ /dev/null
@@ -1,142 +0,0 @@
-import datetime
-import traceback
-from pathlib import Path
-
-import pandas as pd
-import torch
-from datasets import load_dataset
-from tqdm import tqdm
-
-from modules import shared
-from modules.models import load_model, unload_model
-from modules.text_generation import encode
-from server import get_model_specific_settings, update_model_parameters
-
-
-def load_past_evaluations():
- if Path('logs/evaluations.csv').exists():
- df = pd.read_csv(Path('logs/evaluations.csv'), dtype=str)
- df['Perplexity'] = pd.to_numeric(df['Perplexity'])
- return df
- else:
- return pd.DataFrame(columns=['Model', 'LoRAs', 'Dataset', 'Perplexity', 'stride', 'max_length', 'Date', 'Comment'])
-past_evaluations = load_past_evaluations()
-
-
-def save_past_evaluations(df):
- global past_evaluations
- past_evaluations = df
- df.to_csv(Path('logs/evaluations.csv'), index=False)
-
-
-def calculate_perplexity(models, input_dataset, stride, _max_length):
- '''
- Based on:
- https://huggingface.co/docs/transformers/perplexity#calculating-ppl-with-fixedlength-models
- '''
-
- global past_evaluations
- cumulative_log = ''
- cumulative_log += "Loading the input dataset...\n"
- yield cumulative_log
-
- # Copied from https://github.com/qwopqwop200/GPTQ-for-LLaMa/blob/triton/utils/datautils.py
- if input_dataset == 'wikitext':
- data = load_dataset('wikitext', 'wikitext-2-raw-v1', split='test')
- text = "\n\n".join(data['text'])
- elif input_dataset == 'ptb':
- data = load_dataset('ptb_text_only', 'penn_treebank', split='validation')
- text = "\n\n".join(data['sentence'])
- elif input_dataset == 'ptb_new':
- data = load_dataset('ptb_text_only', 'penn_treebank', split='test')
- text = " ".join(data['sentence'])
- else:
- with open(Path(f'training/datasets/{input_dataset}.txt'), 'r', encoding='utf-8') as f:
- text = f.read()
-
- for model in models:
- if is_in_past_evaluations(model, input_dataset, stride, _max_length):
- cumulative_log += f"{model} has already been tested. Ignoring.\n"
- yield cumulative_log
- continue
-
- if model != 'current model':
- try:
- yield cumulative_log + f"Loading {model}...\n"
- model_settings = get_model_specific_settings(model)
- shared.settings.update(model_settings) # hijacking the interface defaults
- update_model_parameters(model_settings) # hijacking the command-line arguments
- shared.model_name = model
- unload_model()
- shared.model, shared.tokenizer = load_model(shared.model_name)
- except:
- cumulative_log += f"Failed to load {model}. Moving on.\n"
- yield cumulative_log
- continue
-
- cumulative_log += f"Processing {model}...\n"
- yield cumulative_log + "Tokenizing the input dataset...\n"
- encodings = encode(text, add_special_tokens=False)
- seq_len = encodings.shape[1]
- max_length = _max_length or shared.model.config.max_position_embeddings
- nlls = []
- prev_end_loc = 0
- for begin_loc in tqdm(range(0, seq_len, stride)):
- yield cumulative_log + f"Evaluating... {100*begin_loc/seq_len:.2f}%"
- end_loc = min(begin_loc + max_length, seq_len)
- trg_len = end_loc - prev_end_loc # may be different from stride on last loop
- input_ids = encodings[:, begin_loc:end_loc]
- target_ids = input_ids.clone()
- target_ids[:, :-trg_len] = -100
-
- with torch.no_grad():
- outputs = shared.model(input_ids, labels=target_ids)
-
- # loss is calculated using CrossEntropyLoss which averages over valid labels
- # N.B. the model only calculates loss over trg_len - 1 labels, because it internally shifts the labels
- # to the left by 1.
- neg_log_likelihood = outputs.loss
-
- nlls.append(neg_log_likelihood)
-
- prev_end_loc = end_loc
- if end_loc == seq_len:
- break
-
- ppl = torch.exp(torch.stack(nlls).mean())
- add_entry_to_past_evaluations(float(ppl), shared.model_name, input_dataset, stride, _max_length)
- save_past_evaluations(past_evaluations)
- cumulative_log += f"Done. The perplexity is: {float(ppl)}\n\n"
- yield cumulative_log
-
-
-def add_entry_to_past_evaluations(perplexity, model, dataset, stride, max_length):
- global past_evaluations
- entry = {
- 'Model': model,
- 'LoRAs': ', '.join(shared.lora_names) or '-',
- 'Dataset': dataset,
- 'Perplexity': perplexity,
- 'stride': str(stride),
- 'max_length': str(max_length),
- 'Date': datetime.datetime.now().strftime('%Y-%m-%d %H:%M:%S'),
- 'Comment': ''
- }
- past_evaluations = pd.concat([past_evaluations, pd.DataFrame([entry])], ignore_index=True)
-
-
-def is_in_past_evaluations(model, dataset, stride, max_length):
- entries = past_evaluations[(past_evaluations['Model'] == model) &
- (past_evaluations['Dataset'] == dataset) &
- (past_evaluations['max_length'] == str(max_length)) &
- (past_evaluations['stride'] == str(stride))]
-
- if entries.shape[0] > 0:
- return True
- else:
- return False
-
-
-def generate_markdown_table():
- sorted_df = past_evaluations.sort_values(by=['Dataset', 'stride', 'Perplexity', 'Date'])
- return sorted_df
diff --git a/spaces/anupam210/Flight_ATA_Class/app.py b/spaces/anupam210/Flight_ATA_Class/app.py
deleted file mode 100644
index 1958200ba2a05f9ce16fef57a52d155f1523ca26..0000000000000000000000000000000000000000
--- a/spaces/anupam210/Flight_ATA_Class/app.py
+++ /dev/null
@@ -1,83 +0,0 @@
-import os
-import openai
-import gradio as gr
-from azure.cognitiveservices.vision.computervision import ComputerVisionClient
-from msrest.authentication import CognitiveServicesCredentials
-from azure.storage.blob import BlobClient
-#import utils functions
-from preprocessing_images import preprocessing_function
-from extract_text import azure_ocr
-my_container = os.getenv("AZURE_CONTAINER")
-subscription_key = os.getenv("SUB_KEY")
-endpoint = os.getenv("AZURE_ENDPOINT")
-connection_string = os.getenv("AZURE_CON_STRING")
-openai.api_key = os.getenv("OPENAI_API_KEY")
-computervision_client = ComputerVisionClient(endpoint, CognitiveServicesCredentials(subscription_key))
-
-def ocr_pdf(pdf_url):
- preprocessing_function(pdf_url)
- my_blob = pdf_url.split('/')[-1]
- blob = BlobClient.from_connection_string(conn_str=connection_string, container_name= my_container, blob_name=my_blob)
- with open("answer_paper.pdf", "rb") as data:
- blob.upload_blob(data,overwrite=True)
- text = azure_ocr(blob.url,computervision_client)
- return text.strip()
-
-# def ocr_pdf(pdf_url2):
-# preprocessing_function(pdf_url2)
-# my_blob = pdf_url2.split('/')[-1]
-# blob = BlobClient.from_connection_string(conn_str=connection_string, container_name= my_container, blob_name=my_blob)
-# with open("answer_paper.pdf", "rb") as data:
-# blob.upload_blob(data,overwrite=True)
-# text = azure_ocr(blob.url,computervision_client)
-# return text.strip()
-
-def classify_cause(incident_description):
- response = openai.Completion.create(
- engine="text-davinci-003",
- prompt= f"Identify the root cause from the below list:\nincident_description:{incident_description}\n",
- temperature= 0,
- max_tokens= 50,
- n=1,
- stop=None
- #timeout=15,
- )
- classification = response.choices[0].text.strip()
- return classification
-
-def classify_class(incident_description):
- response = openai.Completion.create(
- engine="text-davinci-003",
- prompt= f"Classify the following incident description into one of the given classes:Aircraft Autopilot Problem, Auxiliary Power Problem,Cabin Pressure Problem, Engine Problem,Fuel System Problem,Avionics Problem,Communications Problem,Electrical System Problem,Engine Problem,Smoke Problem\nincident_description:{incident_description}\n",
- temperature= 0,
- max_tokens= 50,
- n=1,
- stop=None
- #timeout=15,
- )
- classification = response.choices[0].text.strip()
- return classification
-
-
-def avatiation(pdf_url):
- pdftext = ocr_pdf(pdf_url)
-
-
- defect_class = classify_class(pdftext)
- main_issue = classify_cause(pdftext)
- return main_issue, defect_class
-
-
-
-inputs1 = gr.inputs.Textbox(label="Link for aviation log reports")
-#inputs2 = gr.inputs.Textbox(label="Link for aviation log reports 2")
-
-
-outputs = [gr.outputs.Textbox(label="Main Issue of the log report"),
- gr.outputs.Textbox(label="category of the log report")
- ]
-
-
-demo = gr.Interface(fn=avatiation,inputs=inputs1,outputs=outputs, title="ATA Auto classification using OCR and GPT3 ")
-demo.launch()
-
diff --git a/spaces/artificialguybr/video-dubbing/TTS/TTS/vocoder/datasets/preprocess.py b/spaces/artificialguybr/video-dubbing/TTS/TTS/vocoder/datasets/preprocess.py
deleted file mode 100644
index 0f69b812fa58949eadc78b450114f03b19e5c80c..0000000000000000000000000000000000000000
--- a/spaces/artificialguybr/video-dubbing/TTS/TTS/vocoder/datasets/preprocess.py
+++ /dev/null
@@ -1,70 +0,0 @@
-import glob
-import os
-from pathlib import Path
-
-import numpy as np
-from coqpit import Coqpit
-from tqdm import tqdm
-
-from TTS.utils.audio import AudioProcessor
-
-
-def preprocess_wav_files(out_path: str, config: Coqpit, ap: AudioProcessor):
- """Process wav and compute mel and quantized wave signal.
- It is mainly used by WaveRNN dataloader.
-
- Args:
- out_path (str): Parent folder path to save the files.
- config (Coqpit): Model config.
- ap (AudioProcessor): Audio processor.
- """
- os.makedirs(os.path.join(out_path, "quant"), exist_ok=True)
- os.makedirs(os.path.join(out_path, "mel"), exist_ok=True)
- wav_files = find_wav_files(config.data_path)
- for path in tqdm(wav_files):
- wav_name = Path(path).stem
- quant_path = os.path.join(out_path, "quant", wav_name + ".npy")
- mel_path = os.path.join(out_path, "mel", wav_name + ".npy")
- y = ap.load_wav(path)
- mel = ap.melspectrogram(y)
- np.save(mel_path, mel)
- if isinstance(config.mode, int):
- quant = ap.mulaw_encode(y, qc=config.mode) if config.model_args.mulaw else ap.quantize(y, bits=config.mode)
- np.save(quant_path, quant)
-
-
-def find_wav_files(data_path, file_ext="wav"):
- wav_paths = glob.glob(os.path.join(data_path, "**", f"*.{file_ext}"), recursive=True)
- return wav_paths
-
-
-def find_feat_files(data_path):
- feat_paths = glob.glob(os.path.join(data_path, "**", "*.npy"), recursive=True)
- return feat_paths
-
-
-def load_wav_data(data_path, eval_split_size, file_ext="wav"):
- wav_paths = find_wav_files(data_path, file_ext=file_ext)
- assert len(wav_paths) > 0, f" [!] {data_path} is empty."
- np.random.seed(0)
- np.random.shuffle(wav_paths)
- return wav_paths[:eval_split_size], wav_paths[eval_split_size:]
-
-
-def load_wav_feat_data(data_path, feat_path, eval_split_size):
- wav_paths = find_wav_files(data_path)
- feat_paths = find_feat_files(feat_path)
-
- wav_paths.sort(key=lambda x: Path(x).stem)
- feat_paths.sort(key=lambda x: Path(x).stem)
-
- assert len(wav_paths) == len(feat_paths), f" [!] {len(wav_paths)} vs {feat_paths}"
- for wav, feat in zip(wav_paths, feat_paths):
- wav_name = Path(wav).stem
- feat_name = Path(feat).stem
- assert wav_name == feat_name
-
- items = list(zip(wav_paths, feat_paths))
- np.random.seed(0)
- np.random.shuffle(items)
- return items[:eval_split_size], items[eval_split_size:]
diff --git a/spaces/artificialguybr/video-dubbing/TTS/recipes/bel-alex73/docker-prepare-start.sh b/spaces/artificialguybr/video-dubbing/TTS/recipes/bel-alex73/docker-prepare-start.sh
deleted file mode 100644
index a4ce3c6dcca3abced93bd6c80d863061d8d86486..0000000000000000000000000000000000000000
--- a/spaces/artificialguybr/video-dubbing/TTS/recipes/bel-alex73/docker-prepare-start.sh
+++ /dev/null
@@ -1,19 +0,0 @@
-#!/bin/bash
-set -x
-
-cd $( dirname -- "$0"; )
-
-cp ../../requirements*.txt docker-prepare/
-
-docker build -t tts-learn -f docker-prepare/Dockerfile docker-prepare/
-
-mkdir -p ../../../storage
-docker run --rm -it \
- -p 2525:2525 \
- --shm-size=256M \
- --name tts-learn-run \
- -v $(pwd)/../../:/a/TTS \
- -v $(pwd)/../../../cv-corpus:/a/cv-corpus \
- -v $(pwd)/../../../fanetyka/:/a/fanetyka/ \
- -v $(pwd)/../../../storage:/storage \
- tts-learn
diff --git a/spaces/artificialguybr/video-dubbing/TTS/tests/tts_tests/test_tacotron2_model.py b/spaces/artificialguybr/video-dubbing/TTS/tests/tts_tests/test_tacotron2_model.py
deleted file mode 100644
index b1bdeb9fd16536efe22c64f2309c46b7bae44e22..0000000000000000000000000000000000000000
--- a/spaces/artificialguybr/video-dubbing/TTS/tests/tts_tests/test_tacotron2_model.py
+++ /dev/null
@@ -1,390 +0,0 @@
-import copy
-import os
-import unittest
-
-import torch
-from torch import nn, optim
-
-from tests import get_tests_input_path
-from TTS.tts.configs.shared_configs import CapacitronVAEConfig, GSTConfig
-from TTS.tts.configs.tacotron2_config import Tacotron2Config
-from TTS.tts.layers.losses import MSELossMasked
-from TTS.tts.models.tacotron2 import Tacotron2
-from TTS.utils.audio import AudioProcessor
-
-# pylint: disable=unused-variable
-
-torch.manual_seed(1)
-use_cuda = torch.cuda.is_available()
-device = torch.device("cuda:0" if torch.cuda.is_available() else "cpu")
-
-config_global = Tacotron2Config(num_chars=32, num_speakers=5, out_channels=80, decoder_output_dim=80)
-
-ap = AudioProcessor(**config_global.audio)
-WAV_FILE = os.path.join(get_tests_input_path(), "example_1.wav")
-
-
-class TacotronTrainTest(unittest.TestCase):
- """Test vanilla Tacotron2 model."""
-
- def test_train_step(self): # pylint: disable=no-self-use
- config = config_global.copy()
- config.use_speaker_embedding = False
- config.num_speakers = 1
-
- input_dummy = torch.randint(0, 24, (8, 128)).long().to(device)
- input_lengths = torch.randint(100, 128, (8,)).long().to(device)
- input_lengths = torch.sort(input_lengths, descending=True)[0]
- mel_spec = torch.rand(8, 30, config.audio["num_mels"]).to(device)
- mel_postnet_spec = torch.rand(8, 30, config.audio["num_mels"]).to(device)
- mel_lengths = torch.randint(20, 30, (8,)).long().to(device)
- mel_lengths[0] = 30
- stop_targets = torch.zeros(8, 30, 1).float().to(device)
-
- for idx in mel_lengths:
- stop_targets[:, int(idx.item()) :, 0] = 1.0
-
- stop_targets = stop_targets.view(input_dummy.shape[0], stop_targets.size(1) // config.r, -1)
- stop_targets = (stop_targets.sum(2) > 0.0).unsqueeze(2).float().squeeze()
-
- criterion = MSELossMasked(seq_len_norm=False).to(device)
- criterion_st = nn.BCEWithLogitsLoss().to(device)
- model = Tacotron2(config).to(device)
- model.train()
- model_ref = copy.deepcopy(model)
- count = 0
- for param, param_ref in zip(model.parameters(), model_ref.parameters()):
- assert (param - param_ref).sum() == 0, param
- count += 1
- optimizer = optim.Adam(model.parameters(), lr=config.lr)
- for i in range(5):
- outputs = model.forward(input_dummy, input_lengths, mel_spec, mel_lengths)
- assert torch.sigmoid(outputs["stop_tokens"]).data.max() <= 1.0
- assert torch.sigmoid(outputs["stop_tokens"]).data.min() >= 0.0
- optimizer.zero_grad()
- loss = criterion(outputs["decoder_outputs"], mel_spec, mel_lengths)
- stop_loss = criterion_st(outputs["stop_tokens"], stop_targets)
- loss = loss + criterion(outputs["model_outputs"], mel_postnet_spec, mel_lengths) + stop_loss
- loss.backward()
- optimizer.step()
- # check parameter changes
- count = 0
- for param, param_ref in zip(model.parameters(), model_ref.parameters()):
- # ignore pre-higway layer since it works conditional
- # if count not in [145, 59]:
- assert (param != param_ref).any(), "param {} with shape {} not updated!! \n{}\n{}".format(
- count, param.shape, param, param_ref
- )
- count += 1
-
-
-class MultiSpeakerTacotronTrainTest(unittest.TestCase):
- """Test multi-speaker Tacotron2 with speaker embedding layer"""
-
- @staticmethod
- def test_train_step():
- config = config_global.copy()
- config.use_speaker_embedding = True
- config.num_speakers = 5
-
- input_dummy = torch.randint(0, 24, (8, 128)).long().to(device)
- input_lengths = torch.randint(100, 128, (8,)).long().to(device)
- input_lengths = torch.sort(input_lengths, descending=True)[0]
- mel_spec = torch.rand(8, 30, config.audio["num_mels"]).to(device)
- mel_postnet_spec = torch.rand(8, 30, config.audio["num_mels"]).to(device)
- mel_lengths = torch.randint(20, 30, (8,)).long().to(device)
- mel_lengths[0] = 30
- stop_targets = torch.zeros(8, 30, 1).float().to(device)
- speaker_ids = torch.randint(0, 5, (8,)).long().to(device)
-
- for idx in mel_lengths:
- stop_targets[:, int(idx.item()) :, 0] = 1.0
-
- stop_targets = stop_targets.view(input_dummy.shape[0], stop_targets.size(1) // config.r, -1)
- stop_targets = (stop_targets.sum(2) > 0.0).unsqueeze(2).float().squeeze()
-
- criterion = MSELossMasked(seq_len_norm=False).to(device)
- criterion_st = nn.BCEWithLogitsLoss().to(device)
- config.d_vector_dim = 55
- model = Tacotron2(config).to(device)
- model.train()
- model_ref = copy.deepcopy(model)
- count = 0
- for param, param_ref in zip(model.parameters(), model_ref.parameters()):
- assert (param - param_ref).sum() == 0, param
- count += 1
- optimizer = optim.Adam(model.parameters(), lr=config.lr)
- for _ in range(5):
- outputs = model.forward(
- input_dummy, input_lengths, mel_spec, mel_lengths, aux_input={"speaker_ids": speaker_ids}
- )
- assert torch.sigmoid(outputs["stop_tokens"]).data.max() <= 1.0
- assert torch.sigmoid(outputs["stop_tokens"]).data.min() >= 0.0
- optimizer.zero_grad()
- loss = criterion(outputs["decoder_outputs"], mel_spec, mel_lengths)
- stop_loss = criterion_st(outputs["stop_tokens"], stop_targets)
- loss = loss + criterion(outputs["model_outputs"], mel_postnet_spec, mel_lengths) + stop_loss
- loss.backward()
- optimizer.step()
- # check parameter changes
- count = 0
- for param, param_ref in zip(model.parameters(), model_ref.parameters()):
- # ignore pre-higway layer since it works conditional
- # if count not in [145, 59]:
- assert (param != param_ref).any(), "param {} with shape {} not updated!! \n{}\n{}".format(
- count, param.shape, param, param_ref
- )
- count += 1
-
-
-class TacotronGSTTrainTest(unittest.TestCase):
- """Test multi-speaker Tacotron2 with Global Style Token and Speaker Embedding"""
-
- # pylint: disable=no-self-use
- def test_train_step(self):
- # with random gst mel style
- config = config_global.copy()
- config.use_speaker_embedding = True
- config.num_speakers = 10
- config.use_gst = True
- config.gst = GSTConfig()
-
- input_dummy = torch.randint(0, 24, (8, 128)).long().to(device)
- input_lengths = torch.randint(100, 128, (8,)).long().to(device)
- input_lengths = torch.sort(input_lengths, descending=True)[0]
- mel_spec = torch.rand(8, 30, config.audio["num_mels"]).to(device)
- mel_postnet_spec = torch.rand(8, 30, config.audio["num_mels"]).to(device)
- mel_lengths = torch.randint(20, 30, (8,)).long().to(device)
- mel_lengths[0] = 30
- stop_targets = torch.zeros(8, 30, 1).float().to(device)
- speaker_ids = torch.randint(0, 5, (8,)).long().to(device)
-
- for idx in mel_lengths:
- stop_targets[:, int(idx.item()) :, 0] = 1.0
-
- stop_targets = stop_targets.view(input_dummy.shape[0], stop_targets.size(1) // config.r, -1)
- stop_targets = (stop_targets.sum(2) > 0.0).unsqueeze(2).float().squeeze()
-
- criterion = MSELossMasked(seq_len_norm=False).to(device)
- criterion_st = nn.BCEWithLogitsLoss().to(device)
- config.use_gst = True
- config.gst = GSTConfig()
- model = Tacotron2(config).to(device)
- model.train()
- model_ref = copy.deepcopy(model)
- count = 0
- for param, param_ref in zip(model.parameters(), model_ref.parameters()):
- assert (param - param_ref).sum() == 0, param
- count += 1
- optimizer = optim.Adam(model.parameters(), lr=config.lr)
- for i in range(10):
- outputs = model.forward(
- input_dummy, input_lengths, mel_spec, mel_lengths, aux_input={"speaker_ids": speaker_ids}
- )
- assert torch.sigmoid(outputs["stop_tokens"]).data.max() <= 1.0
- assert torch.sigmoid(outputs["stop_tokens"]).data.min() >= 0.0
- optimizer.zero_grad()
- loss = criterion(outputs["decoder_outputs"], mel_spec, mel_lengths)
- stop_loss = criterion_st(outputs["stop_tokens"], stop_targets)
- loss = loss + criterion(outputs["model_outputs"], mel_postnet_spec, mel_lengths) + stop_loss
- loss.backward()
- optimizer.step()
- # check parameter changes
- count = 0
- for name_param, param_ref in zip(model.named_parameters(), model_ref.parameters()):
- # ignore pre-higway layer since it works conditional
- # if count not in [145, 59]:
- name, param = name_param
- if name == "gst_layer.encoder.recurrence.weight_hh_l0":
- # print(param.grad)
- continue
- assert (param != param_ref).any(), "param {} {} with shape {} not updated!! \n{}\n{}".format(
- name, count, param.shape, param, param_ref
- )
- count += 1
-
- # with file gst style
- mel_spec = (
- torch.FloatTensor(ap.melspectrogram(ap.load_wav(WAV_FILE)))[:, :30].unsqueeze(0).transpose(1, 2).to(device)
- )
- mel_spec = mel_spec.repeat(8, 1, 1)
- input_dummy = torch.randint(0, 24, (8, 128)).long().to(device)
- input_lengths = torch.randint(100, 128, (8,)).long().to(device)
- input_lengths = torch.sort(input_lengths, descending=True)[0]
- mel_postnet_spec = torch.rand(8, 30, config.audio["num_mels"]).to(device)
- mel_lengths = torch.randint(20, 30, (8,)).long().to(device)
- mel_lengths[0] = 30
- stop_targets = torch.zeros(8, 30, 1).float().to(device)
- speaker_ids = torch.randint(0, 5, (8,)).long().to(device)
-
- for idx in mel_lengths:
- stop_targets[:, int(idx.item()) :, 0] = 1.0
-
- stop_targets = stop_targets.view(input_dummy.shape[0], stop_targets.size(1) // config.r, -1)
- stop_targets = (stop_targets.sum(2) > 0.0).unsqueeze(2).float().squeeze()
-
- criterion = MSELossMasked(seq_len_norm=False).to(device)
- criterion_st = nn.BCEWithLogitsLoss().to(device)
- model = Tacotron2(config).to(device)
- model.train()
- model_ref = copy.deepcopy(model)
- count = 0
- for param, param_ref in zip(model.parameters(), model_ref.parameters()):
- assert (param - param_ref).sum() == 0, param
- count += 1
- optimizer = optim.Adam(model.parameters(), lr=config.lr)
- for i in range(10):
- outputs = model.forward(
- input_dummy, input_lengths, mel_spec, mel_lengths, aux_input={"speaker_ids": speaker_ids}
- )
- assert torch.sigmoid(outputs["stop_tokens"]).data.max() <= 1.0
- assert torch.sigmoid(outputs["stop_tokens"]).data.min() >= 0.0
- optimizer.zero_grad()
- loss = criterion(outputs["decoder_outputs"], mel_spec, mel_lengths)
- stop_loss = criterion_st(outputs["stop_tokens"], stop_targets)
- loss = loss + criterion(outputs["model_outputs"], mel_postnet_spec, mel_lengths) + stop_loss
- loss.backward()
- optimizer.step()
- # check parameter changes
- count = 0
- for name_param, param_ref in zip(model.named_parameters(), model_ref.parameters()):
- # ignore pre-higway layer since it works conditional
- # if count not in [145, 59]:
- name, param = name_param
- if name == "gst_layer.encoder.recurrence.weight_hh_l0":
- # print(param.grad)
- continue
- assert (param != param_ref).any(), "param {} {} with shape {} not updated!! \n{}\n{}".format(
- name, count, param.shape, param, param_ref
- )
- count += 1
-
-
-class TacotronCapacitronTrainTest(unittest.TestCase):
- @staticmethod
- def test_train_step():
- config = Tacotron2Config(
- num_chars=32,
- num_speakers=10,
- use_speaker_embedding=True,
- out_channels=80,
- decoder_output_dim=80,
- use_capacitron_vae=True,
- capacitron_vae=CapacitronVAEConfig(),
- optimizer="CapacitronOptimizer",
- optimizer_params={
- "RAdam": {"betas": [0.9, 0.998], "weight_decay": 1e-6},
- "SGD": {"lr": 1e-5, "momentum": 0.9},
- },
- )
-
- batch = dict({})
- batch["text_input"] = torch.randint(0, 24, (8, 128)).long().to(device)
- batch["text_lengths"] = torch.randint(100, 129, (8,)).long().to(device)
- batch["text_lengths"] = torch.sort(batch["text_lengths"], descending=True)[0]
- batch["text_lengths"][0] = 128
- batch["mel_input"] = torch.rand(8, 120, config.audio["num_mels"]).to(device)
- batch["mel_lengths"] = torch.randint(20, 120, (8,)).long().to(device)
- batch["mel_lengths"] = torch.sort(batch["mel_lengths"], descending=True)[0]
- batch["mel_lengths"][0] = 120
- batch["stop_targets"] = torch.zeros(8, 120, 1).float().to(device)
- batch["stop_target_lengths"] = torch.randint(0, 120, (8,)).to(device)
- batch["speaker_ids"] = torch.randint(0, 5, (8,)).long().to(device)
- batch["d_vectors"] = None
-
- for idx in batch["mel_lengths"]:
- batch["stop_targets"][:, int(idx.item()) :, 0] = 1.0
-
- batch["stop_targets"] = batch["stop_targets"].view(
- batch["text_input"].shape[0], batch["stop_targets"].size(1) // config.r, -1
- )
- batch["stop_targets"] = (batch["stop_targets"].sum(2) > 0.0).unsqueeze(2).float().squeeze()
-
- model = Tacotron2(config).to(device)
- criterion = model.get_criterion().to(device)
- optimizer = model.get_optimizer()
-
- model.train()
- model_ref = copy.deepcopy(model)
- count = 0
- for param, param_ref in zip(model.parameters(), model_ref.parameters()):
- assert (param - param_ref).sum() == 0, param
- count += 1
- for _ in range(10):
- _, loss_dict = model.train_step(batch, criterion)
- optimizer.zero_grad()
- loss_dict["capacitron_vae_beta_loss"].backward()
- optimizer.first_step()
- loss_dict["loss"].backward()
- optimizer.step()
- # check parameter changes
- count = 0
- for param, param_ref in zip(model.parameters(), model_ref.parameters()):
- # ignore pre-higway layer since it works conditional
- assert (param != param_ref).any(), "param {} with shape {} not updated!! \n{}\n{}".format(
- count, param.shape, param, param_ref
- )
- count += 1
-
-
-class SCGSTMultiSpeakeTacotronTrainTest(unittest.TestCase):
- """Test multi-speaker Tacotron2 with Global Style Tokens and d-vector inputs."""
-
- @staticmethod
- def test_train_step():
- config = config_global.copy()
- config.use_d_vector_file = True
-
- config.use_gst = True
- config.gst = GSTConfig()
-
- input_dummy = torch.randint(0, 24, (8, 128)).long().to(device)
- input_lengths = torch.randint(100, 128, (8,)).long().to(device)
- input_lengths = torch.sort(input_lengths, descending=True)[0]
- mel_spec = torch.rand(8, 30, config.audio["num_mels"]).to(device)
- mel_postnet_spec = torch.rand(8, 30, config.audio["num_mels"]).to(device)
- mel_lengths = torch.randint(20, 30, (8,)).long().to(device)
- mel_lengths[0] = 30
- stop_targets = torch.zeros(8, 30, 1).float().to(device)
- speaker_embeddings = torch.rand(8, 55).to(device)
-
- for idx in mel_lengths:
- stop_targets[:, int(idx.item()) :, 0] = 1.0
-
- stop_targets = stop_targets.view(input_dummy.shape[0], stop_targets.size(1) // config.r, -1)
- stop_targets = (stop_targets.sum(2) > 0.0).unsqueeze(2).float().squeeze()
- criterion = MSELossMasked(seq_len_norm=False).to(device)
- criterion_st = nn.BCEWithLogitsLoss().to(device)
- config.d_vector_dim = 55
- model = Tacotron2(config).to(device)
- model.train()
- model_ref = copy.deepcopy(model)
- count = 0
- for param, param_ref in zip(model.parameters(), model_ref.parameters()):
- assert (param - param_ref).sum() == 0, param
- count += 1
- optimizer = optim.Adam(model.parameters(), lr=config.lr)
- for i in range(5):
- outputs = model.forward(
- input_dummy, input_lengths, mel_spec, mel_lengths, aux_input={"d_vectors": speaker_embeddings}
- )
- assert torch.sigmoid(outputs["stop_tokens"]).data.max() <= 1.0
- assert torch.sigmoid(outputs["stop_tokens"]).data.min() >= 0.0
- optimizer.zero_grad()
- loss = criterion(outputs["decoder_outputs"], mel_spec, mel_lengths)
- stop_loss = criterion_st(outputs["stop_tokens"], stop_targets)
- loss = loss + criterion(outputs["model_outputs"], mel_postnet_spec, mel_lengths) + stop_loss
- loss.backward()
- optimizer.step()
- # check parameter changes
- count = 0
- for name_param, param_ref in zip(model.named_parameters(), model_ref.parameters()):
- # ignore pre-higway layer since it works conditional
- # if count not in [145, 59]:
- name, param = name_param
- if name == "gst_layer.encoder.recurrence.weight_hh_l0":
- continue
- assert (param != param_ref).any(), "param {} with shape {} not updated!! \n{}\n{}".format(
- count, param.shape, param, param_ref
- )
- count += 1
diff --git a/spaces/arxify/RVC-beta-v2-0618/runtime/Lib/site-packages/fairseq/data/huffman/huffman_coder.py b/spaces/arxify/RVC-beta-v2-0618/runtime/Lib/site-packages/fairseq/data/huffman/huffman_coder.py
deleted file mode 100644
index c04f84564e6a22209439c67fed3cac31f010c6e9..0000000000000000000000000000000000000000
--- a/spaces/arxify/RVC-beta-v2-0618/runtime/Lib/site-packages/fairseq/data/huffman/huffman_coder.py
+++ /dev/null
@@ -1,267 +0,0 @@
-# Copyright (c) Facebook, Inc. and its affiliates.
-#
-# This source code is licensed under the MIT license found in the
-# LICENSE file in the root directory of this source tree.
-
-import re
-import typing as tp
-from collections import Counter, deque
-from dataclasses import dataclass
-
-from bitarray import bitarray, util
-from fairseq.data import Dictionary
-
-# basically we have to write to addressable bytes for the memory mapped
-# dataset loader. Sentences that get encoded to a length that is not a
-# multiple of BLOCKSIZE (a byte) will be padded to fit. (see _pad in the coder)
-BLOCKSIZE = 8
-
-
-class HuffmanCoder:
- def __init__(
- self, root: "HuffmanNode", bos="", pad="", eos=" ", unk=""
- ):
- self.root = root
- self.table = root.code_table()
- self.bos_word, self.unk_word, self.pad_word, self.eos_word = bos, unk, pad, eos
-
- def _pad(self, a: bitarray) -> bitarray:
- """
- bitpadding, 1 then 0.
-
- If the array is already a multiple of blocksize, we add a full block.
- """
- pad_len = BLOCKSIZE - (len(a) % BLOCKSIZE) - 1
- padding = bitarray("1" + "0" * pad_len)
- return a + padding
-
- def _unpad(self, a: bitarray) -> bitarray:
- """
- remove the bitpadding.
-
- There will be a set of 0s preceded by a 1 at the end of the bitarray, we remove that
- """
- # count the 0 padding at the end until we find the first 1
- # we want to remove the one too
- remove_cnt = util.rindex(a, 1)
- return a[:remove_cnt]
-
- def encode(self, iter: tp.List[str]) -> bytes:
- """
- encode a list of tokens a return bytes. We use bitpadding to make sure the encoded bits fit in bytes.
- """
- a = bitarray()
- for token in iter:
- code = self.get_code(token)
- if code is None:
- if self.unk_word is None:
- raise Exception(f"unknown token {token} cannot be encoded.")
- else:
- token = self.unk_word
- a = a + self.get_code(token)
- return self._pad(a).tobytes()
-
- def decode(self, bits: bytes) -> tp.Iterator["HuffmanNode"]:
- """
- take bitpadded bytes and decode it to a set of leaves. You can then use each node to find the symbol/id
- """
- a = bitarray()
- a.frombytes(bits)
- return self.root.decode(self._unpad(a))
-
- def get_code(self, symbol: str) -> tp.Optional[bitarray]:
- node = self.get_node(symbol)
- return None if node is None else node.code
-
- def get_node(self, symbol: str) -> "HuffmanNode":
- return self.table.get(symbol)
-
- @classmethod
- def from_file(
- cls,
- filename: str,
- bos="",
- pad="",
- eos=" ",
- unk="",
- ) -> "HuffmanCoder":
- builder = HuffmanCodeBuilder.from_file(filename)
- return builder.build_code(bos=bos, pad=pad, eos=eos, unk=unk)
-
- def to_file(self, filename, sep="\t"):
- nodes = list(self.table.values())
- nodes.sort(key=lambda n: n.id)
- with open(filename, "w", encoding="utf-8") as output:
- for n in nodes:
- output.write(f"{n.symbol}{sep}{n.count}\n")
-
- def __iter__(self):
- for n in self.table.values():
- yield n
-
- def merge(self, other_coder: "HuffmanCoder") -> "HuffmanCoder":
- builder = HuffmanCodeBuilder()
- for n in self:
- builder.increment(n.symbol, n.count)
- for n in other_coder:
- builder.increment(n.symbol, n.count)
- return builder.build_code()
-
- def __eq__(self, other: "HuffmanCoder") -> bool:
- return self.table == other.table
-
- def __len__(self) -> int:
- return len(self.table)
-
- def __contains__(self, sym: str) -> bool:
- return sym in self.table
-
- def to_dictionary(self) -> Dictionary:
- dictionary = Dictionary(bos=self.bos, unk=self.unk, pad=self.pad, eos=self.eos)
- for n in self:
- dictionary.add_symbol(n.symbol, n=n.count)
- dictionary.finalize()
- return dictionary
-
-
-@dataclass
-class HuffmanNode:
- """
- a node in a Huffman tree
- """
-
- id: int
- count: int
- symbol: tp.Optional[str] = None
- left: tp.Optional["HuffmanNode"] = None
- right: tp.Optional["HuffmanNode"] = None
- code: tp.Optional[bitarray] = None
-
- def is_leaf(self) -> bool:
- return self.left is None and self.right is None
-
- def code_table(
- self, prefix: tp.Optional[bitarray] = None
- ) -> tp.Dict[str, "HuffmanNode"]:
- defaulted_prefix = prefix if prefix is not None else bitarray()
- if self.is_leaf():
- self.code = (
- defaulted_prefix if len(defaulted_prefix) > 0 else bitarray("0")
- ) # leaf could be the root if there is only one symbol
- return {self.symbol: self}
-
- codes_right = self.right.code_table(defaulted_prefix + bitarray([0]))
- codes_left = self.left.code_table(defaulted_prefix + bitarray([1]))
- return {**codes_left, **codes_right}
-
- def decode(self, bits: bitarray) -> tp.Iterator["HuffmanNode"]:
- current_node = self
- for bit in bits:
- if bit == 0: # go right
- current_node = current_node.right
- else: # go left
- current_node = current_node.left
- if current_node is None:
- # we shouldn't be on a leaf here
- raise Exception("fell off a leaf")
- if current_node.is_leaf():
- yield current_node
- current_node = self
- if current_node != self:
- raise Exception("couldn't decode all the bits")
-
-
-class HuffmanCodeBuilder:
- """
- build a dictionary with occurence count and then build the Huffman code for it.
- """
-
- def __init__(self):
- self.symbols = Counter()
-
- def add_symbols(self, *syms) -> None:
- self.symbols.update(syms)
-
- def increment(self, symbol: str, cnt: int) -> None:
- self.symbols[symbol] += cnt
-
- @classmethod
- def from_file(cls, filename):
- c = cls()
- with open(filename, "r", encoding="utf-8") as input:
- for line in input:
- split = re.split(r"[\s]+", line)
- c.increment(split[0], int(split[1]))
- return c
-
- def to_file(self, filename, sep="\t"):
- with open(filename, "w", encoding="utf-8") as output:
- for (tok, cnt) in self.symbols.most_common():
- output.write(f"{tok}{sep}{cnt}\n")
-
- def _smallest(self, q1: deque, q2: deque) -> HuffmanNode:
- if len(q1) == 0:
- return q2.pop()
-
- if len(q2) == 0:
- return q1.pop()
-
- if q1[-1].count < q2[-1].count:
- return q1.pop()
-
- return q2.pop()
-
- def __add__(self, c: "HuffmanCodeBuilder") -> "HuffmanCodeBuilder":
- new_c = self.symbols + c.symbols
- new_b = HuffmanCodeBuilder()
- new_b.symbols = new_c
- return new_b
-
- def build_code(
- self,
- bos="",
- pad="",
- eos=" ",
- unk="",
- ) -> HuffmanCoder:
- assert len(self.symbols) > 0, "cannot build code from empty list of symbols"
-
- if self.symbols[bos] == 0:
- self.add_symbols(bos)
- if self.symbols[pad] == 0:
- self.add_symbols(pad)
- if self.symbols[eos] == 0:
- self.add_symbols(eos)
- if self.symbols[unk] == 0:
- self.add_symbols(unk)
-
- node_id = 0
- leaves_queue = deque(
- [
- HuffmanNode(symbol=symbol, count=count, id=idx)
- for idx, (symbol, count) in enumerate(self.symbols.most_common())
- ]
- ) # left are the most common, right are the least common
-
- if len(leaves_queue) == 1:
- root = leaves_queue.pop()
- root.id = 0
- return HuffmanCoder(root)
-
- nodes_queue = deque()
-
- while len(leaves_queue) > 0 or len(nodes_queue) != 1:
- # get the lowest two nodes at the head of each queue
- node1 = self._smallest(leaves_queue, nodes_queue)
- node2 = self._smallest(leaves_queue, nodes_queue)
-
- # add new node
- nodes_queue.appendleft(
- HuffmanNode(
- count=node1.count + node2.count, left=node1, right=node2, id=node_id
- )
- )
- node_id += 1
-
- # we are left with the root
- return HuffmanCoder(nodes_queue.pop(), bos=bos, pad=pad, eos=eos, unk=unk)
diff --git a/spaces/arxify/RVC-beta-v2-0618/runtime/Lib/site-packages/fairseq/data/transform_eos_dataset.py b/spaces/arxify/RVC-beta-v2-0618/runtime/Lib/site-packages/fairseq/data/transform_eos_dataset.py
deleted file mode 100644
index fb14ff018edf13b20f5d0e486692dfb0a37ec6d1..0000000000000000000000000000000000000000
--- a/spaces/arxify/RVC-beta-v2-0618/runtime/Lib/site-packages/fairseq/data/transform_eos_dataset.py
+++ /dev/null
@@ -1,120 +0,0 @@
-# Copyright (c) Facebook, Inc. and its affiliates.
-#
-# This source code is licensed under the MIT license found in the
-# LICENSE file in the root directory of this source tree.
-
-import torch
-
-from . import FairseqDataset
-
-
-class TransformEosDataset(FairseqDataset):
- """A :class:`~fairseq.data.FairseqDataset` wrapper that appends/prepends/strips EOS.
-
- Note that the transformation is applied in :func:`collater`.
-
- Args:
- dataset (~fairseq.data.FairseqDataset): dataset to wrap
- eos (int): index of the end-of-sentence symbol
- append_eos_to_src (bool, optional): append EOS to the end of src
- remove_eos_from_src (bool, optional): remove EOS from the end of src
- append_eos_to_tgt (bool, optional): append EOS to the end of tgt
- remove_eos_from_tgt (bool, optional): remove EOS from the end of tgt
- """
-
- def __init__(
- self,
- dataset,
- eos,
- append_eos_to_src=False,
- remove_eos_from_src=False,
- append_eos_to_tgt=False,
- remove_eos_from_tgt=False,
- has_target=True,
- ):
- if not isinstance(dataset, FairseqDataset):
- raise ValueError("dataset must be an instance of FairseqDataset")
- if append_eos_to_src and remove_eos_from_src:
- raise ValueError("cannot combine append_eos_to_src and remove_eos_from_src")
- if append_eos_to_tgt and remove_eos_from_tgt:
- raise ValueError("cannot combine append_eos_to_tgt and remove_eos_from_tgt")
-
- self.dataset = dataset
- self.eos = torch.LongTensor([eos])
- self.append_eos_to_src = append_eos_to_src
- self.remove_eos_from_src = remove_eos_from_src
- self.append_eos_to_tgt = append_eos_to_tgt
- self.remove_eos_from_tgt = remove_eos_from_tgt
- self.has_target = has_target
-
- # precompute how we should adjust the reported sizes
- self._src_delta = 0
- self._src_delta += 1 if append_eos_to_src else 0
- self._src_delta -= 1 if remove_eos_from_src else 0
- self._tgt_delta = 0
- self._tgt_delta += 1 if append_eos_to_tgt else 0
- self._tgt_delta -= 1 if remove_eos_from_tgt else 0
-
- self._checked_src = False
- self._checked_tgt = False
-
- def _check_src(self, src, expect_eos):
- if not self._checked_src:
- assert (src[-1] == self.eos[0]) == expect_eos
- self._checked_src = True
-
- def _check_tgt(self, tgt, expect_eos):
- if self.has_target and not self._checked_tgt:
- assert (tgt[-1] == self.eos[0]) == expect_eos
- self._checked_tgt = True
-
- def __getitem__(self, index):
- return self.dataset[index]
-
- def __len__(self):
- return len(self.dataset)
-
- def collater(self, samples):
- def transform(item):
- if self.append_eos_to_src:
- self.eos = self.eos.to(device=item["source"].device)
- self._check_src(item["source"], expect_eos=False)
- item["source"] = torch.cat([item["source"], self.eos])
- if self.remove_eos_from_src:
- self.eos = self.eos.to(device=item["source"].device)
- self._check_src(item["source"], expect_eos=True)
- item["source"] = item["source"][:-1]
- if self.append_eos_to_tgt:
- self.eos = self.eos.to(device=item["target"].device)
- self._check_tgt(item["target"], expect_eos=False)
- item["target"] = torch.cat([item["target"], self.eos])
- if self.remove_eos_from_tgt:
- self.eos = self.eos.to(device=item["target"].device)
- self._check_tgt(item["target"], expect_eos=True)
- item["target"] = item["target"][:-1]
- return item
-
- samples = list(map(transform, samples))
- return self.dataset.collater(samples)
-
- def num_tokens(self, index):
- return self.dataset.num_tokens(index)
-
- def size(self, index):
- if self.has_target:
- src_len, tgt_len = self.dataset.size(index)
- return (src_len + self._src_delta, tgt_len + self._tgt_delta)
- else:
- return self.dataset.size(index)
-
- def ordered_indices(self):
- # NOTE: we assume that the ordering does not change based on the
- # addition or removal of eos
- return self.dataset.ordered_indices()
-
- @property
- def supports_prefetch(self):
- return getattr(self.dataset, "supports_prefetch", False)
-
- def prefetch(self, indices):
- return self.dataset.prefetch(indices)
diff --git a/spaces/aryadytm/photo-colorization/src/deoldify/_device.py b/spaces/aryadytm/photo-colorization/src/deoldify/_device.py
deleted file mode 100644
index ed40ce131e3375a937c862fafa44e432f825f93b..0000000000000000000000000000000000000000
--- a/spaces/aryadytm/photo-colorization/src/deoldify/_device.py
+++ /dev/null
@@ -1,30 +0,0 @@
-import os
-from enum import Enum
-from .device_id import DeviceId
-
-#NOTE: This must be called first before any torch imports in order to work properly!
-
-class DeviceException(Exception):
- pass
-
-class _Device:
- def __init__(self):
- self.set(DeviceId.CPU)
-
- def is_gpu(self):
- ''' Returns `True` if the current device is GPU, `False` otherwise. '''
- return self.current() is not DeviceId.CPU
-
- def current(self):
- return self._current_device
-
- def set(self, device:DeviceId):
- if device == DeviceId.CPU:
- os.environ['CUDA_VISIBLE_DEVICES']=''
- else:
- os.environ['CUDA_VISIBLE_DEVICES']=str(device.value)
- import torch
- torch.backends.cudnn.benchmark=False
-
- self._current_device = device
- return device
\ No newline at end of file
diff --git a/spaces/atimughal662/InfoFusion/src/gradio_utils/css.py b/spaces/atimughal662/InfoFusion/src/gradio_utils/css.py
deleted file mode 100644
index 6f3d0dd56bfd4287034afd0b23751e3abd59a143..0000000000000000000000000000000000000000
--- a/spaces/atimughal662/InfoFusion/src/gradio_utils/css.py
+++ /dev/null
@@ -1,148 +0,0 @@
-def get_css(kwargs) -> str:
- if kwargs['h2ocolors']:
- css_code = """footer {visibility: hidden;}
- body{background:linear-gradient(#f5f5f5,#e5e5e5);}
- body.dark{background:linear-gradient(#000000,#0d0d0d);}
- """
- else:
- css_code = """footer {visibility: hidden}"""
-
- css_code += make_css_base()
- return css_code
-
-
-def make_css_base() -> str:
- return """
- #col_container {margin-left: auto; margin-right: auto; text-align: left;}
-
- @import url('https://fonts.googleapis.com/css2?family=Source+Sans+Pro:wght@400;600&display=swap');
-
- body.dark{#warning {background-color: #555555};}
-
- #sidebar {
- order: 1;
-
- @media (max-width: 463px) {
- order: 2;
- }
- }
-
- #col-tabs {
- order: 2;
-
- @media (max-width: 463px) {
- order: 1;
- }
- }
-
- #small_btn {
- margin: 0.6em 0em 0.55em 0;
- max-width: 20em;
- min-width: 5em !important;
- height: 5em;
- font-size: 14px !important;
- }
-
- #prompt-form {
- border: 1px solid var(--primary-500) !important;
- }
-
- #prompt-form.block {
- border-radius: var(--block-radius) !important;
- }
-
- #prompt-form textarea {
- border: 1px solid rgb(209, 213, 219);
- }
-
- #prompt-form label > div {
- margin-top: 4px;
- }
-
- button.primary:hover {
- background-color: var(--primary-600) !important;
- transition: .2s;
- }
-
- #prompt-form-area {
- margin-bottom: 2.5rem;
- }
- .chatsmall chatbot {font-size: 10px !important}
-
- .gradio-container {
- max-width: none !important;
- }
-
- div.message {
- padding: var(--text-lg) !important;
- }
-
- div.message.user > div.icon-button {
- top: unset;
- bottom: 0;
- }
-
- div.message.bot > div.icon-button {
- top: unset;
- bottom: 0;
- }
-
- #prompt-form-row {
- position: relative;
- }
-
- #attach-button {
- position: absolute;
- top: 45px;
- right: 20px;
-
- display: flex;
- justify-content: center;
- border: 1px solid var(--primary-500) !important;
-
- @media (max-width: 463px) {
- width: 56px;
- }
- }
-
- #attach-button > img {
- margin-right: 0;
- }
-
- #prompt-form > label > textarea {
- padding-right: 104px;
-
- @media (max-width: 463px) {
- min-height: 94px;
- padding-right: 70px;
- }
- }
-
- #visible-models > label > div.wrap > div.wrap-inner > div.secondary-wrap > div.remove-all {
- display: none !important;
- }
-
- #visible-models > label > div.wrap > div.wrap-inner > div.token {
- display: none !important;
- }
-
- #visible-models > label > div.wrap > div.wrap-inner > div.secondary-wrap::before {
- content: "Select";
- padding: 0 4px;
- margin-right: 2px;
- }
-
- #langchain_agents > label > div.wrap > div.wrap-inner > div.secondary-wrap > div.remove-all {
- display: none !important;
- }
-
- #langchain_agents > label > div.wrap > div.wrap-inner > div.token {
- display: none !important;
- }
-
- #langchain_agents > label > div.wrap > div.wrap-inner > div.secondary-wrap::before {
- content: "Select";
- padding: 0 4px;
- margin-right: 2px;
- }
- """
diff --git a/spaces/awacke1/AIOutline/README.md b/spaces/awacke1/AIOutline/README.md
deleted file mode 100644
index d69e286c53eb63236b0611c933dc0e193f5b95ee..0000000000000000000000000000000000000000
--- a/spaces/awacke1/AIOutline/README.md
+++ /dev/null
@@ -1,13 +0,0 @@
----
-title: 🛍️🧠AIMind🤖📊
-emoji: 🌐🧠🤖
-colorFrom: yellow
-colorTo: blue
-sdk: streamlit
-sdk_version: 1.17.0
-app_file: app.py
-pinned: false
-license: mit
----
-
-Check out the configuration reference at https://huggingface.co/docs/hub/spaces-config-reference
diff --git a/spaces/awacke1/AnimatedGifGallery/app.py b/spaces/awacke1/AnimatedGifGallery/app.py
deleted file mode 100644
index ab7cb9583171b765412463f9c8d16b14f2a25d59..0000000000000000000000000000000000000000
--- a/spaces/awacke1/AnimatedGifGallery/app.py
+++ /dev/null
@@ -1,52 +0,0 @@
-import streamlit as st
-import os
-import random
-
-def get_gifs(directory):
- return [f for f in os.listdir(directory) if f.endswith('.gif')]
-
-def showAnimatedGif(gif):
- import streamlit as st
- import base64
- #st.markdown("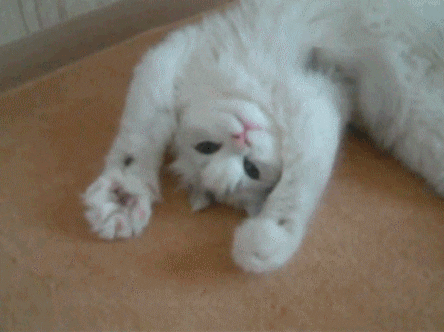")
- st.write('Loading: ' + gif)
- file_ = open(gif, "rb")
- contents = file_.read()
- data_url = base64.b64encode(contents).decode("utf-8")
- file_.close()
- st.write(data_url)
-
- st.markdown(
- f' ',
- unsafe_allow_html=True,
- )
-
-def main():
- st.title('Animated GIFs in Streamlit')
-
- directory = './gifs' # Replace with your directory of GIFs
- gif_files = get_gifs(directory)
-
- num_rows = len(gif_files) // 3
- if len(gif_files) % 3:
- num_rows += 1
-
- cols = [st.columns(3) for _ in range(num_rows)]
-
- for i in range(num_rows):
- for j in range(3):
- idx = i*3 + j
- if idx < len(gif_files):
- #showAnimatedGif(os.path.join(directory, gif_files[idx]))
- cols[i][j].image(os.path.join(directory, gif_files[idx]), width=200)
-
- if st.button('Randomize'):
- random.shuffle(gif_files)
- for i in range(num_rows):
- for j in range(3):
- idx = i*3 + j
- if idx < len(gif_files):
- cols[i][j].image(os.path.join(directory, gif_files[idx]), width=200)
-
-if __name__ == "__main__":
- main()
diff --git a/spaces/awacke1/AnimationAI/app.py b/spaces/awacke1/AnimationAI/app.py
deleted file mode 100644
index c00a82220989cabfe090136e5eb0b3f05b760dd0..0000000000000000000000000000000000000000
--- a/spaces/awacke1/AnimationAI/app.py
+++ /dev/null
@@ -1,33 +0,0 @@
-import requests
-import streamlit as st
-from streamlit_lottie import st_lottie
-
-def load_lottie_url(url: str):
- r = requests.get(url)
- if r.status_code != 200:
- return None
- return r.json()
-
-def ShowAnimation(name, URL):
- anim=load_lottie_url(URL)
- st_lottie(anim, key = name)
-
-st.markdown('# Animations: https://lottiefiles.com/recent')
-st.markdown("# Animate with JSON, SVG, Adobe XD, Figma, and deploy to web, mobile as tiny animation files ")
-
-# to Use Lottie in HTML (gradio or HTML5) use the code below in HTML
-#
-#
-
-ShowAnimation("Badge1","https://assets5.lottiefiles.com/packages/lf20_wtohqzml.json")
-ShowAnimation("Badge2","https://assets5.lottiefiles.com/packages/lf20_i4zw2ddg.json")
-ShowAnimation("Badge3","https://assets5.lottiefiles.com/private_files/lf30_jfhmdmk5.json")
-ShowAnimation("Graph","https://assets6.lottiefiles.com/packages/lf20_4gqhiayj.json")
-ShowAnimation("PhoneBot","https://assets9.lottiefiles.com/packages/lf20_zrqthn6o.json")
-ShowAnimation("SupportBot","https://assets5.lottiefiles.com/private_files/lf30_cmd8kh2q.json")
-ShowAnimation("ChatBot","https://assets8.lottiefiles.com/packages/lf20_j1oeaifz.json")
-ShowAnimation("IntelligentMachine","https://assets8.lottiefiles.com/packages/lf20_edouagsj.json")
-ShowAnimation("GearAI","https://assets10.lottiefiles.com/packages/lf20_3jkp7dqt.json")
-ShowAnimation("ContextGraph","https://assets10.lottiefiles.com/private_files/lf30_vwC61X.json")
-ShowAnimation("Yggdrasil","https://assets4.lottiefiles.com/packages/lf20_8q1bhU.json")
-ShowAnimation("Studying","https://assets9.lottiefiles.com/packages/lf20_6ft9bypa.json")
diff --git a/spaces/awacke1/Azure.Streamlit.Github.Actions.Azure.Container.Registry.Docker.AKS/app.py b/spaces/awacke1/Azure.Streamlit.Github.Actions.Azure.Container.Registry.Docker.AKS/app.py
deleted file mode 100644
index b96300db8d40d0d84a5a4ea53192dbd1eef13799..0000000000000000000000000000000000000000
--- a/spaces/awacke1/Azure.Streamlit.Github.Actions.Azure.Container.Registry.Docker.AKS/app.py
+++ /dev/null
@@ -1,43 +0,0 @@
-import streamlit as st
-from collections import Counter
-import plotly.express as px
-import numpy as np
-
-def get_word_score(word):
- # This function returns a score based on the length of the word
- # Modify this function as per your requirements
- score = len(word)**2
- return score
-
-def get_word_frequency(text):
- # This function returns the word frequency of the given text
- words = text.split()
- word_frequency = Counter(words)
- return word_frequency
-
-# Load the markdown file
-with open('Setup.md', 'r') as file:
- text = file.read()
-
-
-# Display the parsed markdown
-st.markdown(text, unsafe_allow_html=True)
-
-# Get the word frequency of the markdown text
-word_frequency = get_word_frequency(text)
-
-# Get the top words and their frequency
-top_words = word_frequency.most_common(10)
-top_words_dict = dict(top_words)
-
-# Create a Plotly bar chart to display the top words and their frequency
-fig = px.bar(x=list(top_words_dict.keys()), y=list(top_words_dict.values()), labels={'x':'Word', 'y':'Frequency'})
-st.plotly_chart(fig)
-
-# Calculate the scores for each word based on their length
-word_scores = {word:get_word_score(word) for word in word_frequency}
-top_word_scores = dict(sorted(word_scores.items(), key=lambda item: item[1], reverse=True)[:10])
-
-# Create a Plotly bar chart to display the top words and their scores
-fig = px.bar(x=list(top_word_scores.keys()), y=list(top_word_scores.values()), labels={'x':'Word', 'y':'Score'})
-st.plotly_chart(fig)
diff --git a/spaces/awacke1/MultiPDF-QA-ChatGPT-Langchain/app.py b/spaces/awacke1/MultiPDF-QA-ChatGPT-Langchain/app.py
deleted file mode 100644
index 931bb3d60fd2d318ecccca2bc73b2973b8a935f1..0000000000000000000000000000000000000000
--- a/spaces/awacke1/MultiPDF-QA-ChatGPT-Langchain/app.py
+++ /dev/null
@@ -1,64 +0,0 @@
-import os
-import streamlit as st
-from dotenv import load_dotenv
-from PyPDF2 import PdfReader
-from langchain.text_splitter import CharacterTextSplitter
-from langchain.embeddings import OpenAIEmbeddings
-from langchain.vectorstores import FAISS
-from langchain.chat_models import ChatOpenAI
-from langchain.memory import ConversationBufferMemory
-from langchain.chains import ConversationalRetrievalChain
-from htmlTemplates import css, bot_template, user_template
-
-def extract_text_from_pdfs(pdf_docs):
- text = ""
- for pdf in pdf_docs:
- pdf_reader = PdfReader(pdf)
- for page in pdf_reader.pages:
- text += page.extract_text()
- return text
-
-def split_text_into_chunks(text):
- text_splitter = CharacterTextSplitter(separator="\n", chunk_size=1000, chunk_overlap=200, length_function=len)
- return text_splitter.split_text(text)
-
-def create_vector_store_from_text_chunks(text_chunks):
- key = os.getenv('OPENAI_KEY')
- embeddings = OpenAIEmbeddings(openai_api_key=key)
- return FAISS.from_texts(texts=text_chunks, embedding=embeddings)
-
-def create_conversation_chain(vectorstore):
- llm = ChatOpenAI()
- memory = ConversationBufferMemory(memory_key='chat_history', return_messages=True)
- return ConversationalRetrievalChain.from_llm(llm=llm, retriever=vectorstore.as_retriever(), memory=memory)
-
-def process_user_input(user_question):
- response = st.session_state.conversation({'question': user_question})
- st.session_state.chat_history = response['chat_history']
-
- for i, message in enumerate(st.session_state.chat_history):
- template = user_template if i % 2 == 0 else bot_template
- st.write(template.replace("{{MSG}}", message.content), unsafe_allow_html=True)
-
-def main():
- load_dotenv()
- st.set_page_config(page_title="Chat with multiple PDFs", page_icon=":books:")
- st.write(css, unsafe_allow_html=True)
-
- st.header("Chat with multiple PDFs :books:")
- user_question = st.text_input("Ask a question about your documents:")
- if user_question:
- process_user_input(user_question)
-
- with st.sidebar:
- st.subheader("Your documents")
- pdf_docs = st.file_uploader("Upload your PDFs here and click on 'Process'", accept_multiple_files=True)
- if st.button("Process"):
- with st.spinner("Processing"):
- raw_text = extract_text_from_pdfs(pdf_docs)
- text_chunks = split_text_into_chunks(raw_text)
- vectorstore = create_vector_store_from_text_chunks(text_chunks)
- st.session_state.conversation = create_conversation_chain(vectorstore)
-
-if __name__ == '__main__':
- main()
diff --git a/spaces/azusarang/so-vits-svc-models-ba_P/README.md b/spaces/azusarang/so-vits-svc-models-ba_P/README.md
deleted file mode 100644
index f3b56f298db18efbf65a293c2b124d155847de50..0000000000000000000000000000000000000000
--- a/spaces/azusarang/so-vits-svc-models-ba_P/README.md
+++ /dev/null
@@ -1,14 +0,0 @@
----
-title: So Vits Svc Models Ba
-emoji: 🦀
-colorFrom: green
-colorTo: indigo
-sdk: gradio
-sdk_version: 3.32.0
-app_file: app.py
-pinned: false
-license: apache-2.0
-duplicated_from: FrankZxShen/so-vits-svc-models-ba
----
-
-Check out the configuration reference at https://huggingface.co/docs/hub/spaces-config-reference
diff --git a/spaces/banana-projects/web3d/node_modules/three/examples/js/MD2Character.js b/spaces/banana-projects/web3d/node_modules/three/examples/js/MD2Character.js
deleted file mode 100644
index 2501dc98176f345e75558798a66aef722c9dbdf1..0000000000000000000000000000000000000000
--- a/spaces/banana-projects/web3d/node_modules/three/examples/js/MD2Character.js
+++ /dev/null
@@ -1,261 +0,0 @@
-/**
- * @author alteredq / http://alteredqualia.com/
- */
-
-THREE.MD2Character = function () {
-
- var scope = this;
-
- this.scale = 1;
- this.animationFPS = 6;
-
- this.root = new THREE.Object3D();
-
- this.meshBody = null;
- this.meshWeapon = null;
-
- this.skinsBody = [];
- this.skinsWeapon = [];
-
- this.weapons = [];
-
- this.activeAnimation = null;
-
- this.mixer = null;
-
- this.onLoadComplete = function () {};
-
- this.loadCounter = 0;
-
- this.loadParts = function ( config ) {
-
- this.loadCounter = config.weapons.length * 2 + config.skins.length + 1;
-
- var weaponsTextures = [];
- for ( var i = 0; i < config.weapons.length; i ++ ) weaponsTextures[ i ] = config.weapons[ i ][ 1 ];
- // SKINS
-
- this.skinsBody = loadTextures( config.baseUrl + "skins/", config.skins );
- this.skinsWeapon = loadTextures( config.baseUrl + "skins/", weaponsTextures );
-
- // BODY
-
- var loader = new THREE.MD2Loader();
-
- loader.load( config.baseUrl + config.body, function ( geo ) {
-
- var boundingBox = new THREE.Box3();
- boundingBox.setFromBufferAttribute( geo.attributes.position );
-
- scope.root.position.y = - scope.scale * boundingBox.min.y;
-
- var mesh = createPart( geo, scope.skinsBody[ 0 ] );
- mesh.scale.set( scope.scale, scope.scale, scope.scale );
-
- scope.root.add( mesh );
-
- scope.meshBody = mesh;
-
- scope.meshBody.clipOffset = 0;
- scope.activeAnimationClipName = mesh.geometry.animations[ 0 ].name;
-
- scope.mixer = new THREE.AnimationMixer( mesh );
-
- checkLoadingComplete();
-
- } );
-
- // WEAPONS
-
- var generateCallback = function ( index, name ) {
-
- return function ( geo ) {
-
- var mesh = createPart( geo, scope.skinsWeapon[ index ] );
- mesh.scale.set( scope.scale, scope.scale, scope.scale );
- mesh.visible = false;
-
- mesh.name = name;
-
- scope.root.add( mesh );
-
- scope.weapons[ index ] = mesh;
- scope.meshWeapon = mesh;
-
- checkLoadingComplete();
-
- };
-
- };
-
- for ( var i = 0; i < config.weapons.length; i ++ ) {
-
- loader.load( config.baseUrl + config.weapons[ i ][ 0 ], generateCallback( i, config.weapons[ i ][ 0 ] ) );
-
- }
-
- };
-
- this.setPlaybackRate = function ( rate ) {
-
- if ( rate !== 0 ) {
-
- this.mixer.timeScale = 1 / rate;
-
- } else {
-
- this.mixer.timeScale = 0;
-
- }
-
- };
-
- this.setWireframe = function ( wireframeEnabled ) {
-
- if ( wireframeEnabled ) {
-
- if ( this.meshBody ) this.meshBody.material = this.meshBody.materialWireframe;
- if ( this.meshWeapon ) this.meshWeapon.material = this.meshWeapon.materialWireframe;
-
- } else {
-
- if ( this.meshBody ) this.meshBody.material = this.meshBody.materialTexture;
- if ( this.meshWeapon ) this.meshWeapon.material = this.meshWeapon.materialTexture;
-
- }
-
- };
-
- this.setSkin = function ( index ) {
-
- if ( this.meshBody && this.meshBody.material.wireframe === false ) {
-
- this.meshBody.material.map = this.skinsBody[ index ];
-
- }
-
- };
-
- this.setWeapon = function ( index ) {
-
- for ( var i = 0; i < this.weapons.length; i ++ ) this.weapons[ i ].visible = false;
-
- var activeWeapon = this.weapons[ index ];
-
- if ( activeWeapon ) {
-
- activeWeapon.visible = true;
- this.meshWeapon = activeWeapon;
-
- scope.syncWeaponAnimation();
-
- }
-
- };
-
- this.setAnimation = function ( clipName ) {
-
- if ( this.meshBody ) {
-
- if ( this.meshBody.activeAction ) {
-
- this.meshBody.activeAction.stop();
- this.meshBody.activeAction = null;
-
- }
-
- var action = this.mixer.clipAction( clipName, this.meshBody );
-
- if ( action ) {
-
- this.meshBody.activeAction = action.play();
-
- }
-
- }
-
- scope.activeClipName = clipName;
-
- scope.syncWeaponAnimation();
-
- };
-
- this.syncWeaponAnimation = function () {
-
- var clipName = scope.activeClipName;
-
- if ( scope.meshWeapon ) {
-
- if ( this.meshWeapon.activeAction ) {
-
- this.meshWeapon.activeAction.stop();
- this.meshWeapon.activeAction = null;
-
- }
-
- var action = this.mixer.clipAction( clipName, this.meshWeapon );
-
- if ( action ) {
-
- this.meshWeapon.activeAction = action.syncWith( this.meshBody.activeAction ).play();
-
- }
-
- }
-
- };
-
- this.update = function ( delta ) {
-
- if ( this.mixer ) this.mixer.update( delta );
-
- };
-
- function loadTextures( baseUrl, textureUrls ) {
-
- var textureLoader = new THREE.TextureLoader();
- var textures = [];
-
- for ( var i = 0; i < textureUrls.length; i ++ ) {
-
- textures[ i ] = textureLoader.load( baseUrl + textureUrls[ i ], checkLoadingComplete );
- textures[ i ].mapping = THREE.UVMapping;
- textures[ i ].name = textureUrls[ i ];
-
- }
-
- return textures;
-
- }
-
- function createPart( geometry, skinMap ) {
-
- var materialWireframe = new THREE.MeshLambertMaterial( { color: 0xffaa00, wireframe: true, morphTargets: true, morphNormals: true } );
- var materialTexture = new THREE.MeshLambertMaterial( { color: 0xffffff, wireframe: false, map: skinMap, morphTargets: true, morphNormals: true } );
-
- //
-
- var mesh = new THREE.Mesh( geometry, materialTexture );
- mesh.rotation.y = - Math.PI / 2;
-
- mesh.castShadow = true;
- mesh.receiveShadow = true;
-
- //
-
- mesh.materialTexture = materialTexture;
- mesh.materialWireframe = materialWireframe;
-
- return mesh;
-
- }
-
- function checkLoadingComplete() {
-
- scope.loadCounter -= 1;
-
- if ( scope.loadCounter === 0 ) scope.onLoadComplete();
-
- }
-
-};
diff --git a/spaces/banana-projects/web3d/node_modules/three/src/geometries/DodecahedronGeometry.d.ts b/spaces/banana-projects/web3d/node_modules/three/src/geometries/DodecahedronGeometry.d.ts
deleted file mode 100644
index 587490ad1bfac1b8fb4fd685d4fcb82e59faa2d3..0000000000000000000000000000000000000000
--- a/spaces/banana-projects/web3d/node_modules/three/src/geometries/DodecahedronGeometry.d.ts
+++ /dev/null
@@ -1,15 +0,0 @@
-import { Geometry } from './../core/Geometry';
-import { PolyhedronBufferGeometry } from './PolyhedronGeometry';
-
-export class DodecahedronBufferGeometry extends PolyhedronBufferGeometry {
- constructor(radius?: number, detail?: number);
-}
-
-export class DodecahedronGeometry extends Geometry {
- constructor(radius?: number, detail?: number);
-
- parameters: {
- radius: number;
- detail: number;
- };
-}
diff --git a/spaces/banana-projects/web3d/node_modules/three/src/textures/CanvasTexture.js b/spaces/banana-projects/web3d/node_modules/three/src/textures/CanvasTexture.js
deleted file mode 100644
index 5239619fbce4370ee2a7169bdc20714578097861..0000000000000000000000000000000000000000
--- a/spaces/banana-projects/web3d/node_modules/three/src/textures/CanvasTexture.js
+++ /dev/null
@@ -1,19 +0,0 @@
-/**
- * @author mrdoob / http://mrdoob.com/
- */
-
-import { Texture } from './Texture.js';
-
-function CanvasTexture( canvas, mapping, wrapS, wrapT, magFilter, minFilter, format, type, anisotropy ) {
-
- Texture.call( this, canvas, mapping, wrapS, wrapT, magFilter, minFilter, format, type, anisotropy );
-
- this.needsUpdate = true;
-
-}
-
-CanvasTexture.prototype = Object.create( Texture.prototype );
-CanvasTexture.prototype.constructor = CanvasTexture;
-CanvasTexture.prototype.isCanvasTexture = true;
-
-export { CanvasTexture };
diff --git a/spaces/beihai/GFPGAN-V1.3-whole-image/.history/app_20220326233250.py b/spaces/beihai/GFPGAN-V1.3-whole-image/.history/app_20220326233250.py
deleted file mode 100644
index 0a38d76ce2ad23d2334dcc1d23d9094842aa1493..0000000000000000000000000000000000000000
--- a/spaces/beihai/GFPGAN-V1.3-whole-image/.history/app_20220326233250.py
+++ /dev/null
@@ -1,65 +0,0 @@
-import os
-#os.system("pip install gfpgan")
-
-#os.system("pip freeze")
-#os.system("wget https://github.com/TencentARC/GFPGAN/releases/download/v0.2.0/GFPGANCleanv1-NoCE-C2.pth -P .")
-import random
-import gradio as gr
-from PIL import Image
-import torch
-# torch.hub.download_url_to_file('https://upload.wikimedia.org/wikipedia/commons/thumb/a/ab/Abraham_Lincoln_O-77_matte_collodion_print.jpg/1024px-Abraham_Lincoln_O-77_matte_collodion_print.jpg', 'lincoln.jpg')
-# torch.hub.download_url_to_file('https://upload.wikimedia.org/wikipedia/commons/5/50/Albert_Einstein_%28Nobel%29.png', 'einstein.png')
-# torch.hub.download_url_to_file('https://upload.wikimedia.org/wikipedia/commons/thumb/9/9d/Thomas_Edison2.jpg/1024px-Thomas_Edison2.jpg', 'edison.jpg')
-# torch.hub.download_url_to_file('https://upload.wikimedia.org/wikipedia/commons/thumb/a/a9/Henry_Ford_1888.jpg/1024px-Henry_Ford_1888.jpg', 'Henry.jpg')
-# torch.hub.download_url_to_file('https://upload.wikimedia.org/wikipedia/commons/thumb/0/06/Frida_Kahlo%2C_by_Guillermo_Kahlo.jpg/800px-Frida_Kahlo%2C_by_Guillermo_Kahlo.jpg', 'Frida.jpg')
-
-
-
-
-import cv2
-import glob
-import numpy as np
-from basicsr.utils import imwrite
-from gfpgan import GFPGANer
-
-import warnings
-warnings.warn('The unoptimized RealESRGAN is very slow on CPU. We do not use it. '
- 'If you really want to use it, please modify the corresponding codes.')
-bg_upsampler = None
-
-
-
-# set up GFPGAN restorer
-restorer = GFPGANer(
- model_path='experiments/pretrained_models/GFPGANv1.3.pth',
- upscale=2,
- arch='clean',
- channel_multiplier=2,
- bg_upsampler=bg_upsampler)
-
-
-def inference(img):
- input_img = cv2.imread(img, cv2.IMREAD_COLOR)
- cropped_faces, restored_faces, restored_img = restorer.enhance(
- input_img, has_aligned=False, only_center_face=False, paste_back=True)
-
- return Image.fromarray(restored_faces[0][:,:,::-1])
-
-title = "GFP-GAN"
-description = "Gradio demo for GFP-GAN: Towards Real-World Blind Face Restoration with Generative Facial Prior. To use it, simply upload your image, or click one of the examples to load them. Read more at the links below. Please click submit only once"
-article = "Towards Real-World Blind Face Restoration with Generative Facial Prior | Github Repo
"
-gr.Interface(
- inference,
- [gr.inputs.Image(type="filepath", label="Input")],
- gr.outputs.Image(type="pil", label="Output"),
- title=title,
- description=description,
- article=article,
- examples=[
- ['lincoln.jpg'],
- ['einstein.png'],
- ['edison.jpg'],
- ['Henry.jpg'],
- ['Frida.jpg']
- ]
- ).launch(enable_queue=True,cache_examples=True)
\ No newline at end of file
diff --git a/spaces/beihai/GFPGAN-V1.3-whole-image/.history/app_20220327093322.py b/spaces/beihai/GFPGAN-V1.3-whole-image/.history/app_20220327093322.py
deleted file mode 100644
index 9f55fd67fc52747ea6994ee7a4efbd44cda9a7ad..0000000000000000000000000000000000000000
--- a/spaces/beihai/GFPGAN-V1.3-whole-image/.history/app_20220327093322.py
+++ /dev/null
@@ -1,66 +0,0 @@
-import os
-#os.system("pip install gfpgan")
-
-#os.system("pip freeze")
-#os.system("wget https://github.com/TencentARC/GFPGAN/releases/download/v0.2.0/GFPGANCleanv1-NoCE-C2.pth -P .")
-import random
-import gradio as gr
-from PIL import Image
-import torch
-# torch.hub.download_url_to_file('https://upload.wikimedia.org/wikipedia/commons/thumb/a/ab/Abraham_Lincoln_O-77_matte_collodion_print.jpg/1024px-Abraham_Lincoln_O-77_matte_collodion_print.jpg', 'lincoln.jpg')
-# torch.hub.download_url_to_file('https://upload.wikimedia.org/wikipedia/commons/5/50/Albert_Einstein_%28Nobel%29.png', 'einstein.png')
-# torch.hub.download_url_to_file('https://upload.wikimedia.org/wikipedia/commons/thumb/9/9d/Thomas_Edison2.jpg/1024px-Thomas_Edison2.jpg', 'edison.jpg')
-# torch.hub.download_url_to_file('https://upload.wikimedia.org/wikipedia/commons/thumb/a/a9/Henry_Ford_1888.jpg/1024px-Henry_Ford_1888.jpg', 'Henry.jpg')
-# torch.hub.download_url_to_file('https://upload.wikimedia.org/wikipedia/commons/thumb/0/06/Frida_Kahlo%2C_by_Guillermo_Kahlo.jpg/800px-Frida_Kahlo%2C_by_Guillermo_Kahlo.jpg', 'Frida.jpg')
-
-
-import cv2
-import glob
-import numpy as np
-from basicsr.utils import imwrite
-from gfpgan import GFPGANer
-
-bg_upsampler = None
-
-
-
-# set up GFPGAN restorer
-restorer = GFPGANer(
- model_path='experiments/pretrained_models/GFPGANv1.3.pth',
- upscale=2,
- arch='clean',
- channel_multiplier=2,
- bg_upsampler=bg_upsampler)
-
-
-def inference(img):
- input_img = cv2.imread(img, cv2.IMREAD_COLOR)
- cropped_faces, restored_faces, restored_img = restorer.enhance(
- input_img, has_aligned=False, only_center_face=False, paste_back=True)
-
- #return Image.fromarray(restored_faces[0][:,:,::-1])
- return Image.fromarray(restored_img[:, :, ::-1])
-
-title = "让美好回忆更清晰"
-
-
-description = "上传老照片,点击Submit,稍等片刻,右侧Output将照片另存为即可。"
-article = " | | Github Repo
"
-
-gr.Interface(
- inference,
- [gr.inputs.Image(type="filepath", label="Input")],
- gr.outputs.Image(type="pil", label="Output"),
- title=title,
- description=description,
- article=article,
- examples=[
- ['lincoln.jpg'],
- ['einstein.png'],
- ['edison.jpg'],
- ['Henry.jpg'],
- ['Frida.jpg']
- ]
- ).launch(enable_queue=True,cache_examples=True,share=True)
-
-
diff --git a/spaces/beihai/PDF-Table-Extractor/.history/app_20220621074627.py b/spaces/beihai/PDF-Table-Extractor/.history/app_20220621074627.py
deleted file mode 100644
index a2bf8c4cb07b63488e952ab9effb21da7d7e0c4e..0000000000000000000000000000000000000000
--- a/spaces/beihai/PDF-Table-Extractor/.history/app_20220621074627.py
+++ /dev/null
@@ -1,31 +0,0 @@
-#-*- coding : utf-8-*-
-import base64
-from subprocess import STDOUT
-import streamlit as st
-import pandas as pd
-import camelot as cam # extracting tables from PDFs
-
-st.title("PDF Table Extractor")
-
-input_pdf = st.file_uploader(label = "", type = 'pdf')
-
-page_number = st.text_input("请填写表格所在PDF页码,eg: 3", value = 1)
-process_background = st.selectbox("表格线条是否隐藏",('True', 'False'))
-if input_pdf is not None:
- # byte object into a PDF file
- with open("input.pdf", "wb") as f:
- base64_pdf = base64.b64encode(input_pdf.read()).decode('utf-8')
- f.write(base64.b64decode(base64_pdf))
- f.close()
-
- # read the pdf and parse it using stream
- tables = cam.read_pdf("input.pdf", pages=page_number,process_background=process_background)
- result = pd.ExcelWriter('result.xlsx', engine='xlsxwriter')
- tables[0].to_excel(result,index=False)
- # for i in range(0,len(tables)):
- # table = tables[i].df
- # sheetname = str(i)
- # table.to_excel(result, sheetname,index=False)
-
- with open('result.xlsx','rb') as f:
- st.download_button('提取完成,点击下载!', f,file_name='result.xlsx',mime="application/vnd.ms-excel")
\ No newline at end of file
diff --git a/spaces/binery/Paddle_OCR/README.md b/spaces/binery/Paddle_OCR/README.md
deleted file mode 100644
index b61dd332049aa04a8db6c8140aefd101331adc0b..0000000000000000000000000000000000000000
--- a/spaces/binery/Paddle_OCR/README.md
+++ /dev/null
@@ -1,12 +0,0 @@
----
-title: Paddel OCR
-emoji: 🐨
-colorFrom: gray
-colorTo: indigo
-sdk: streamlit
-sdk_version: 1.15.2
-app_file: app.py
-pinned: false
----
-
-Check out the configuration reference at https://huggingface.co/docs/hub/spaces-config-reference
diff --git a/spaces/bioriAsaeru/text-to-voice/Download Conflict Desert Storm 2 Full Version For Free ((FULL)).md b/spaces/bioriAsaeru/text-to-voice/Download Conflict Desert Storm 2 Full Version For Free ((FULL)).md
deleted file mode 100644
index de358d09d63e4476da9506e8528c5557fc8fe941..0000000000000000000000000000000000000000
--- a/spaces/bioriAsaeru/text-to-voice/Download Conflict Desert Storm 2 Full Version For Free ((FULL)).md
+++ /dev/null
@@ -1,7 +0,0 @@
-
-desert storm 2 plays out in the deserts of the middle east, iraq, kuwait, saudi arabia and oman. the game has 11 campaigns, with missions such as the "elite" and "assassin" campaigns, which are based on true events. the most popular missions are scenarios 2 and 6, which are based on the military operations called desert shield and desert storm. other scenarios are "operation iraqi freedom" and "operation enduring freedom".
-the game is set in the year 2004. the persian gulf war was fought between iraq and coalition forces during the period between 1st and 28th february, 1991. the main reason for the war was that iraq invaded kuwait. the war has been the longest and the bloodiest. conflict desert storm 2 is a war game that is played between 4 (or more) soldiers. the game is very exciting as you have to fight against the occupying iraqi forces and you have to complete the mission of your team. you have to protect your base and you have to destroy the iraqi forces. the game lets you use different types of weapons. enjoy the conflict desert storm 2 free download.
-download conflict desert storm 2 full version for free Download ⚹ https://urloso.com/2uyOEs
-the game is set in the year 2004. the persian gulf war was fought between iraq and coalition forces during the period between 1st and 28th february, 1991. the main reason for the war was that iraq invaded kuwait. the war has been the longest and the bloodiest. conflict desert storm 2 is a war game that is played between 4 (or more) soldiers. the game is very exciting as you have to fight against the occupying iraqi forces and you have to complete the mission of your team. you have to protect your base and you have to destroy the iraqi forces. the game lets you use different types of weapons.
899543212b
-
-
\ No newline at end of file
diff --git a/spaces/bioriAsaeru/text-to-voice/How to Download Samacheer Kalvi 7th Std Tamil Book in PDF Format A Guide for Students and Teachers.md b/spaces/bioriAsaeru/text-to-voice/How to Download Samacheer Kalvi 7th Std Tamil Book in PDF Format A Guide for Students and Teachers.md
deleted file mode 100644
index f673669a4bea736eb662a5fceb1955ae4c5bab07..0000000000000000000000000000000000000000
--- a/spaces/bioriAsaeru/text-to-voice/How to Download Samacheer Kalvi 7th Std Tamil Book in PDF Format A Guide for Students and Teachers.md
+++ /dev/null
@@ -1,9 +0,0 @@
-
-Textbooks for Tamilnadu Samacheer Kalvi 7th Books are uploaded and readily available in PDF for free download. Aspirants who are preparing for TNPSC group exams can download the seventh-standard textbooks in PDF format below. The books are available for English and Tamil Medium students. The Tamil Nadu State Board Syllabus for the 7th Standard: Tamil, Maths, English, Science, and Social Science Book English and Tamil mediums are listed in the table below. Back questions with answers (Solutions Guide) in PDF are given below in the link.
-You can download the TN Textbooks for Classes 1 to 12 PDF through the links available on this page. Avail the TN Board Books 1st to 12th Standard for all the Subjects in Tamil and English Medium.
-samacheer kalvi 7th std tamil book download Download File 🗸 https://urloso.com/2uyOkY
-Samacheer Kalvi Books: TamilNadu Government has released Samacheer Kalvi New Books for class 1st, 6th, 9th and 11th. Students who are searching for new and old Tamilnadu TN SCERT Books can download from the below links. TamilNadu TN Textbooks are available in both English and Tamil Medium for std 1st to Class 12th. Students who are preparing for examinations can download Tamilnadu Textbooks in PDF Format. Updated Syllabus of Std 1st, 2nd, 3rd, 4th, 5th, 6th, 7th, 8th, 9th, 10th, 11th, 12th Class Tamil Nadu School Books Online is also available to download. In this article, we are providing Tamilnadu TN School Books pdf free download.
-Get Tamilnadu State Board Text Books Solutions of New Syllabus 2021-2022 Edition for State Board of School Examinations Tamilnadu for all Classes and Subjects in Tamil Medium and English Medium on TNBoardSolutions.com. We provide step by step Tamilnadu State Board Books Answers, Solutions Guides for Class 12th, 11th, 10th, 9th, 8th, 7th, and 6th, 5th, 4th, 3rd, 2nd, 1st Standard all subjects. You can also download the Tamilnadu State Board Textbooks Solutions with a Free PDF download option.
-
aaccfb2cb3
-
-
\ No newline at end of file
diff --git a/spaces/blaziant/ysda_nlp_ops_update/app/model.py b/spaces/blaziant/ysda_nlp_ops_update/app/model.py
deleted file mode 100644
index 07728c2f60ead641620f5114b096ddc91cc0d33d..0000000000000000000000000000000000000000
--- a/spaces/blaziant/ysda_nlp_ops_update/app/model.py
+++ /dev/null
@@ -1,31 +0,0 @@
-from typing import Tuple, List
-import os
-import numpy as np
-import pickle
-import torch
-from transformers import BertTokenizer
-
-with open('/backend/app/vocabulary.pkl', 'rb') as f:
- voc = pickle.load(f)
-ind_to_cat = {val: key for key, val in voc.items()}
-model = torch.load("/backend/app/final_model.pth")
-
-def model_predict(state_name: str, state_abstract: str) -> List[Tuple[float, str]]:
- text = state_name + " " + state_abstract
- tokenizer = BertTokenizer.from_pretrained('bert-base-uncased')
- encoding = tokenizer.encode_plus(
- text,
- add_special_tokens=True,
- max_length=512,
- return_token_type_ids=False,
- padding='max_length',
- return_attention_mask=True,
- return_tensors='pt',
- truncation=True
- )
- predict = model(encoding["input_ids"], encoding["attention_mask"]).logits
- proba = torch.nn.Softmax(dim=1)(predict)
- top_3 = proba.topk(3)
- labels = [ind_to_cat[ind] for ind in top_3.indices.detach().numpy()[0]]
- p = top_3.values.detach().numpy()[0]
- return sorted(zip(p, labels), reverse=True)
\ No newline at end of file
diff --git a/spaces/brjathu/HMR2.0/vendor/detectron2/projects/DensePose/tests/test_frame_selector.py b/spaces/brjathu/HMR2.0/vendor/detectron2/projects/DensePose/tests/test_frame_selector.py
deleted file mode 100644
index 65f05f55c78d4ab24950e5335818b3e1f981aa0d..0000000000000000000000000000000000000000
--- a/spaces/brjathu/HMR2.0/vendor/detectron2/projects/DensePose/tests/test_frame_selector.py
+++ /dev/null
@@ -1,60 +0,0 @@
-# Copyright (c) Facebook, Inc. and its affiliates.
-
-import random
-import unittest
-
-from densepose.data.video import FirstKFramesSelector, LastKFramesSelector, RandomKFramesSelector
-
-
-class TestFrameSelector(unittest.TestCase):
- def test_frame_selector_random_k_1(self):
- _SEED = 43
- _K = 4
- random.seed(_SEED)
- selector = RandomKFramesSelector(_K)
- frame_tss = list(range(0, 20, 2))
- _SELECTED_GT = [0, 8, 4, 6]
- selected = selector(frame_tss)
- self.assertEqual(_SELECTED_GT, selected)
-
- def test_frame_selector_random_k_2(self):
- _SEED = 43
- _K = 10
- random.seed(_SEED)
- selector = RandomKFramesSelector(_K)
- frame_tss = list(range(0, 6, 2))
- _SELECTED_GT = [0, 2, 4]
- selected = selector(frame_tss)
- self.assertEqual(_SELECTED_GT, selected)
-
- def test_frame_selector_first_k_1(self):
- _K = 4
- selector = FirstKFramesSelector(_K)
- frame_tss = list(range(0, 20, 2))
- _SELECTED_GT = frame_tss[:_K]
- selected = selector(frame_tss)
- self.assertEqual(_SELECTED_GT, selected)
-
- def test_frame_selector_first_k_2(self):
- _K = 10
- selector = FirstKFramesSelector(_K)
- frame_tss = list(range(0, 6, 2))
- _SELECTED_GT = frame_tss[:_K]
- selected = selector(frame_tss)
- self.assertEqual(_SELECTED_GT, selected)
-
- def test_frame_selector_last_k_1(self):
- _K = 4
- selector = LastKFramesSelector(_K)
- frame_tss = list(range(0, 20, 2))
- _SELECTED_GT = frame_tss[-_K:]
- selected = selector(frame_tss)
- self.assertEqual(_SELECTED_GT, selected)
-
- def test_frame_selector_last_k_2(self):
- _K = 10
- selector = LastKFramesSelector(_K)
- frame_tss = list(range(0, 6, 2))
- _SELECTED_GT = frame_tss[-_K:]
- selected = selector(frame_tss)
- self.assertEqual(_SELECTED_GT, selected)
diff --git a/spaces/cc1799/vits-uma-genshin-honkai/utils.py b/spaces/cc1799/vits-uma-genshin-honkai/utils.py
deleted file mode 100644
index ee4b01ddfbe8173965371b29f770f3e87615fe71..0000000000000000000000000000000000000000
--- a/spaces/cc1799/vits-uma-genshin-honkai/utils.py
+++ /dev/null
@@ -1,225 +0,0 @@
-import os
-import sys
-import argparse
-import logging
-import json
-import subprocess
-import numpy as np
-import librosa
-import torch
-
-MATPLOTLIB_FLAG = False
-
-logging.basicConfig(stream=sys.stdout, level=logging.DEBUG)
-logger = logging
-
-
-def load_checkpoint(checkpoint_path, model, optimizer=None):
- assert os.path.isfile(checkpoint_path)
- checkpoint_dict = torch.load(checkpoint_path, map_location='cpu')
- iteration = checkpoint_dict['iteration']
- learning_rate = checkpoint_dict['learning_rate']
- if optimizer is not None:
- optimizer.load_state_dict(checkpoint_dict['optimizer'])
- saved_state_dict = checkpoint_dict['model']
- if hasattr(model, 'module'):
- state_dict = model.module.state_dict()
- else:
- state_dict = model.state_dict()
- new_state_dict= {}
- for k, v in state_dict.items():
- try:
- new_state_dict[k] = saved_state_dict[k]
- except:
- logger.info("%s is not in the checkpoint" % k)
- new_state_dict[k] = v
- if hasattr(model, 'module'):
- model.module.load_state_dict(new_state_dict)
- else:
- model.load_state_dict(new_state_dict)
- logger.info("Loaded checkpoint '{}' (iteration {})" .format(
- checkpoint_path, iteration))
- return model, optimizer, learning_rate, iteration
-
-
-def plot_spectrogram_to_numpy(spectrogram):
- global MATPLOTLIB_FLAG
- if not MATPLOTLIB_FLAG:
- import matplotlib
- matplotlib.use("Agg")
- MATPLOTLIB_FLAG = True
- mpl_logger = logging.getLogger('matplotlib')
- mpl_logger.setLevel(logging.WARNING)
- import matplotlib.pylab as plt
- import numpy as np
-
- fig, ax = plt.subplots(figsize=(10,2))
- im = ax.imshow(spectrogram, aspect="auto", origin="lower",
- interpolation='none')
- plt.colorbar(im, ax=ax)
- plt.xlabel("Frames")
- plt.ylabel("Channels")
- plt.tight_layout()
-
- fig.canvas.draw()
- data = np.fromstring(fig.canvas.tostring_rgb(), dtype=np.uint8, sep='')
- data = data.reshape(fig.canvas.get_width_height()[::-1] + (3,))
- plt.close()
- return data
-
-
-def plot_alignment_to_numpy(alignment, info=None):
- global MATPLOTLIB_FLAG
- if not MATPLOTLIB_FLAG:
- import matplotlib
- matplotlib.use("Agg")
- MATPLOTLIB_FLAG = True
- mpl_logger = logging.getLogger('matplotlib')
- mpl_logger.setLevel(logging.WARNING)
- import matplotlib.pylab as plt
- import numpy as np
-
- fig, ax = plt.subplots(figsize=(6, 4))
- im = ax.imshow(alignment.transpose(), aspect='auto', origin='lower',
- interpolation='none')
- fig.colorbar(im, ax=ax)
- xlabel = 'Decoder timestep'
- if info is not None:
- xlabel += '\n\n' + info
- plt.xlabel(xlabel)
- plt.ylabel('Encoder timestep')
- plt.tight_layout()
-
- fig.canvas.draw()
- data = np.fromstring(fig.canvas.tostring_rgb(), dtype=np.uint8, sep='')
- data = data.reshape(fig.canvas.get_width_height()[::-1] + (3,))
- plt.close()
- return data
-
-
-def load_audio_to_torch(full_path, target_sampling_rate):
- audio, sampling_rate = librosa.load(full_path, sr=target_sampling_rate, mono=True)
- return torch.FloatTensor(audio.astype(np.float32))
-
-
-def load_filepaths_and_text(filename, split="|"):
- with open(filename, encoding='utf-8') as f:
- filepaths_and_text = [line.strip().split(split) for line in f]
- return filepaths_and_text
-
-
-def get_hparams(init=True):
- parser = argparse.ArgumentParser()
- parser.add_argument('-c', '--config', type=str, default="./configs/base.json",
- help='JSON file for configuration')
- parser.add_argument('-m', '--model', type=str, required=True,
- help='Model name')
-
- args = parser.parse_args()
- model_dir = os.path.join("./logs", args.model)
-
- if not os.path.exists(model_dir):
- os.makedirs(model_dir)
-
- config_path = args.config
- config_save_path = os.path.join(model_dir, "config.json")
- if init:
- with open(config_path, "r") as f:
- data = f.read()
- with open(config_save_path, "w") as f:
- f.write(data)
- else:
- with open(config_save_path, "r") as f:
- data = f.read()
- config = json.loads(data)
-
- hparams = HParams(**config)
- hparams.model_dir = model_dir
- return hparams
-
-
-def get_hparams_from_dir(model_dir):
- config_save_path = os.path.join(model_dir, "config.json")
- with open(config_save_path, "r") as f:
- data = f.read()
- config = json.loads(data)
-
- hparams =HParams(**config)
- hparams.model_dir = model_dir
- return hparams
-
-
-def get_hparams_from_file(config_path):
- with open(config_path, "r") as f:
- data = f.read()
- config = json.loads(data)
-
- hparams =HParams(**config)
- return hparams
-
-
-def check_git_hash(model_dir):
- source_dir = os.path.dirname(os.path.realpath(__file__))
- if not os.path.exists(os.path.join(source_dir, ".git")):
- logger.warn("{} is not a git repository, therefore hash value comparison will be ignored.".format(
- source_dir
- ))
- return
-
- cur_hash = subprocess.getoutput("git rev-parse HEAD")
-
- path = os.path.join(model_dir, "githash")
- if os.path.exists(path):
- saved_hash = open(path).read()
- if saved_hash != cur_hash:
- logger.warn("git hash values are different. {}(saved) != {}(current)".format(
- saved_hash[:8], cur_hash[:8]))
- else:
- open(path, "w").write(cur_hash)
-
-
-def get_logger(model_dir, filename="train.log"):
- global logger
- logger = logging.getLogger(os.path.basename(model_dir))
- logger.setLevel(logging.DEBUG)
-
- formatter = logging.Formatter("%(asctime)s\t%(name)s\t%(levelname)s\t%(message)s")
- if not os.path.exists(model_dir):
- os.makedirs(model_dir)
- h = logging.FileHandler(os.path.join(model_dir, filename))
- h.setLevel(logging.DEBUG)
- h.setFormatter(formatter)
- logger.addHandler(h)
- return logger
-
-
-class HParams():
- def __init__(self, **kwargs):
- for k, v in kwargs.items():
- if type(v) == dict:
- v = HParams(**v)
- self[k] = v
-
- def keys(self):
- return self.__dict__.keys()
-
- def items(self):
- return self.__dict__.items()
-
- def values(self):
- return self.__dict__.values()
-
- def __len__(self):
- return len(self.__dict__)
-
- def __getitem__(self, key):
- return getattr(self, key)
-
- def __setitem__(self, key, value):
- return setattr(self, key, value)
-
- def __contains__(self, key):
- return key in self.__dict__
-
- def __repr__(self):
- return self.__dict__.__repr__()
diff --git a/spaces/chendl/compositional_test/transformers/examples/research_projects/performer/modeling_flax_performer_utils.py b/spaces/chendl/compositional_test/transformers/examples/research_projects/performer/modeling_flax_performer_utils.py
deleted file mode 100644
index 6e6173729cc348eeca5204becc713481109cde6a..0000000000000000000000000000000000000000
--- a/spaces/chendl/compositional_test/transformers/examples/research_projects/performer/modeling_flax_performer_utils.py
+++ /dev/null
@@ -1,658 +0,0 @@
-# coding=utf-8
-# Copyright 2020 The Google Research Authors.
-#
-# Licensed under the Apache License, Version 2.0 (the "License");
-# you may not use this file except in compliance with the License.
-# You may obtain a copy of the License at
-#
-# http://www.apache.org/licenses/LICENSE-2.0
-#
-# Unless required by applicable law or agreed to in writing, software
-# distributed under the License is distributed on an "AS IS" BASIS,
-# WITHOUT WARRANTIES OR CONDITIONS OF ANY KIND, either express or implied.
-# See the License for the specific language governing permissions and
-# limitations under the License.
-
-"""
-IMPORTANT:
-
-This code was copied from
-https://github.com/google-research/google-research/blob/master/performer/fast_self_attention/fast_self_attention.py on
-6/11/2020. This is very new code, so it might be prone to change soon -> make sure to check the original code and
-update accordingly
-
-Core Fast Attention Module for Flax. Implementation of the approximate fast softmax and generalized attention mechanism
-leveraging structured random feature maps [RFM] techniques and low rank decomposition of the attention matrix.
-"""
-# pylint: disable=invalid-name, missing-function-docstring, line-too-long
-
-import abc
-import functools
-from collections.abc import Iterable # pylint: disable=g-importing-member
-
-import jax
-import jax.numpy as jnp
-import numpy as onp
-from absl import logging
-from jax import lax, random
-
-
-def nonnegative_softmax_kernel_feature_creator(
- data, projection_matrix, attention_dims_t, batch_dims_t, precision, is_query, normalize_data=True, eps=0.0001
-):
- """
- Constructs nonnegative kernel features for fast softmax attention
-
- Args:
- data: input for which features are computes
- projection_matrix: random matrix used to compute features
- attention_dims_t: tuple of attention dimensions
- batch_dims_t: tuple of batch dimensions
- precision: precision parameter
- is_query: predicate indicating whether input data corresponds to queries or
- keys
- normalize_data: predicate indicating whether data should be normalized,
- eps: numerical stabilizer
-
- Returns:
- Random features for fast softmax attention.
- """
- del attention_dims_t
- if normalize_data:
- # We have e^{qk^T/sqrt{d}} = e^{q_norm k_norm^T}, where
- # w_norm = w * data_normalizer for w in {q,k}.
- data_normalizer = 1.0 / (jnp.sqrt(jnp.sqrt(data.shape[-1])))
- else:
- data_normalizer = 1.0
- ratio = 1.0 / jnp.sqrt(projection_matrix.shape[0])
- data_mod_shape = data.shape[0 : len(batch_dims_t)] + projection_matrix.shape
- data_thick_random_matrix = jnp.zeros(data_mod_shape) + projection_matrix
-
- data_dash = lax.dot_general(
- data_normalizer * data,
- data_thick_random_matrix,
- (((data.ndim - 1,), (data_thick_random_matrix.ndim - 1,)), (batch_dims_t, batch_dims_t)),
- precision=precision,
- )
-
- diag_data = jnp.square(data)
- diag_data = jnp.sum(diag_data, axis=data.ndim - 1)
- diag_data = (diag_data / 2.0) * data_normalizer * data_normalizer
- diag_data = jnp.expand_dims(diag_data, axis=data.ndim - 1)
-
- if is_query:
- last_dims_t = (len(data_dash.shape) - 1,)
- data_dash = ratio * (
- jnp.exp(data_dash - diag_data - jnp.max(data_dash, axis=last_dims_t, keepdims=True)) + eps
- )
- else:
- data_dash = ratio * (jnp.exp(data_dash - diag_data - jnp.max(data_dash)) + eps)
-
- return data_dash
-
-
-def sincos_softmax_kernel_feature_creator(
- data, projection_matrix, attention_dims_t, batch_dims_t, precision, normalize_data=True
-):
- """
- Constructs kernel sin-cos features for fast softmax attention
-
- Args:
- data: input for which features are computes
- projection_matrix: random matrix used to compute features
- attention_dims_t: tuple of attention dimensions
- batch_dims_t: tuple of batch dimensions
- precision: precision parameter
- normalize_data: predicate indicating whether data should be normalized
-
- Returns:
- Random features for fast softmax attention.
- """
- if normalize_data:
- # We have: exp(qk^T/sqrt{d}) = exp(|q|^2/2sqrt{d}) * exp(|k|^2/2sqrt{d}) *
- # exp(-(|q*c-k*c|^2)/2), where c = 1.0 / sqrt{sqrt{d}}.
- data_normalizer = 1.0 / (jnp.sqrt(jnp.sqrt(data.shape[-1])))
- else:
- data_normalizer = 1.0
- ratio = 1.0 / jnp.sqrt(projection_matrix.shape[0])
- data_mod_shape = data.shape[0 : len(batch_dims_t)] + projection_matrix.shape
- data_thick_random_matrix = jnp.zeros(data_mod_shape) + projection_matrix
-
- data_dash = lax.dot_general(
- data_normalizer * data,
- data_thick_random_matrix,
- (((data.ndim - 1,), (data_thick_random_matrix.ndim - 1,)), (batch_dims_t, batch_dims_t)),
- precision=precision,
- )
- data_dash_cos = ratio * jnp.cos(data_dash)
- data_dash_sin = ratio * jnp.sin(data_dash)
- data_dash = jnp.concatenate((data_dash_cos, data_dash_sin), axis=-1)
-
- # Constructing D_data and data^{'}
- diag_data = jnp.square(data)
- diag_data = jnp.sum(diag_data, axis=data.ndim - 1)
- diag_data = (diag_data / 2.0) * data_normalizer * data_normalizer
- diag_data = jnp.expand_dims(diag_data, axis=data.ndim - 1)
- # Additional renormalization for numerical stability
- data_renormalizer = jnp.max(diag_data, attention_dims_t, keepdims=True)
- diag_data -= data_renormalizer
- diag_data = jnp.exp(diag_data)
- data_prime = data_dash * diag_data
- return data_prime
-
-
-def generalized_kernel_feature_creator(
- data, projection_matrix, batch_dims_t, precision, kernel_fn, kernel_epsilon, normalize_data
-):
- """
- Constructs kernel features for fast generalized attention
-
- Args:
- data: input for which features are computes
- projection_matrix: matrix used to compute features
- batch_dims_t: tuple of batch dimensions
- precision: precision parameter
- kernel_fn: kernel function used
- kernel_epsilon: additive positive term added to every feature for numerical
- stability
- normalize_data: predicate indicating whether data should be normalized
-
- Returns:
- Random features for fast generalized attention.
- """
- if normalize_data:
- data_normalizer = 1.0 / (jnp.sqrt(jnp.sqrt(data.shape[-1])))
- else:
- data_normalizer = 1.0
- if projection_matrix is None:
- return kernel_fn(data_normalizer * data) + kernel_epsilon
- else:
- data_mod_shape = data.shape[0 : len(batch_dims_t)] + projection_matrix.shape
- data_thick_random_matrix = jnp.zeros(data_mod_shape) + projection_matrix
- data_dash = lax.dot_general(
- data_normalizer * data,
- data_thick_random_matrix,
- (((data.ndim - 1,), (data_thick_random_matrix.ndim - 1,)), (batch_dims_t, batch_dims_t)),
- precision=precision,
- )
- data_prime = kernel_fn(data_dash) + kernel_epsilon
- return data_prime
-
-
-def make_fast_softmax_attention(
- qkv_dim,
- renormalize_attention=True,
- numerical_stabilizer=0.000001,
- nb_features=256,
- ortho_features=True,
- ortho_scaling=0.0,
- redraw_features=True,
- unidirectional=False,
- nonnegative_features=True,
- lax_scan_unroll=1,
-):
- """Construct a fast softmax attention method."""
- logging.info(
- "Fast softmax attention: %s features and orthogonal=%s, renormalize=%s",
- nb_features,
- ortho_features,
- renormalize_attention,
- )
- if ortho_features:
- matrix_creator = functools.partial(GaussianOrthogonalRandomMatrix, nb_features, qkv_dim, scaling=ortho_scaling)
- else:
- matrix_creator = functools.partial(GaussianUnstructuredRandomMatrix, nb_features, qkv_dim)
- if nonnegative_features:
-
- def kernel_feature_creator(
- data, projection_matrix, attention_dims_t, batch_dims_t, precision, is_query, normalize_data=True
- ):
- return nonnegative_softmax_kernel_feature_creator(
- data,
- projection_matrix,
- attention_dims_t,
- batch_dims_t,
- precision,
- is_query,
- normalize_data,
- numerical_stabilizer,
- )
-
- else:
-
- def kernel_feature_creator(
- data, projection_matrix, attention_dims_t, batch_dims_t, precision, is_query, normalize_data=True
- ):
- del is_query
- return sincos_softmax_kernel_feature_creator(
- data, projection_matrix, attention_dims_t, batch_dims_t, precision, normalize_data
- )
-
- attention_fn = FastAttentionviaLowRankDecomposition(
- matrix_creator,
- kernel_feature_creator,
- renormalize_attention=renormalize_attention,
- numerical_stabilizer=numerical_stabilizer,
- redraw_features=redraw_features,
- unidirectional=unidirectional,
- lax_scan_unroll=lax_scan_unroll,
- ).dot_product_attention
- return attention_fn
-
-
-def make_fast_generalized_attention(
- qkv_dim,
- renormalize_attention=True,
- numerical_stabilizer=0.0,
- nb_features=256,
- features_type="deterministic",
- kernel_fn=jax.nn.relu,
- kernel_epsilon=0.001,
- redraw_features=False,
- unidirectional=False,
- lax_scan_unroll=1,
-):
- """Construct a fast generalized attention menthod."""
- logging.info("Fast generalized attention.: %s features and renormalize=%s", nb_features, renormalize_attention)
- if features_type == "ortho":
- matrix_creator = functools.partial(GaussianOrthogonalRandomMatrix, nb_features, qkv_dim, scaling=False)
- elif features_type == "iid":
- matrix_creator = functools.partial(GaussianUnstructuredRandomMatrix, nb_features, qkv_dim)
- elif features_type == "deterministic":
- matrix_creator = None
- else:
- raise ValueError("Unknown feature value type")
-
- def kernel_feature_creator(
- data, projection_matrix, attention_dims_t, batch_dims_t, precision, is_query, normalize_data=False
- ):
- del attention_dims_t
- del is_query
- return generalized_kernel_feature_creator(
- data, projection_matrix, batch_dims_t, precision, kernel_fn, kernel_epsilon, normalize_data
- )
-
- attention_fn = FastAttentionviaLowRankDecomposition(
- matrix_creator,
- kernel_feature_creator,
- renormalize_attention=renormalize_attention,
- numerical_stabilizer=numerical_stabilizer,
- redraw_features=redraw_features,
- unidirectional=unidirectional,
- lax_scan_unroll=lax_scan_unroll,
- ).dot_product_attention
- return attention_fn
-
-
-class RandomMatrix(object):
- r"""
- Abstract class providing a method for constructing 2D random arrays. Class is responsible for constructing 2D
- random arrays.
- """
-
- __metaclass__ = abc.ABCMeta
-
- @abc.abstractmethod
- def get_2d_array(self):
- raise NotImplementedError("Abstract method")
-
-
-class GaussianUnstructuredRandomMatrix(RandomMatrix):
- def __init__(self, nb_rows, nb_columns, key):
- self.nb_rows = nb_rows
- self.nb_columns = nb_columns
- self.key = key
-
- def get_2d_array(self):
- return random.normal(self.key, (self.nb_rows, self.nb_columns))
-
-
-class GaussianOrthogonalRandomMatrix(RandomMatrix):
- r"""
- Class providing a method to create Gaussian orthogonal matrix. Class is responsible for constructing 2D Gaussian
- orthogonal arrays.
- """
-
- def __init__(self, nb_rows, nb_columns, key, scaling=0):
- self.nb_rows = nb_rows
- self.nb_columns = nb_columns
- self.key = key
- self.scaling = scaling
-
- def get_2d_array(self):
- nb_full_blocks = int(self.nb_rows / self.nb_columns)
- block_list = []
- rng = self.key
- for _ in range(nb_full_blocks):
- rng, rng_input = jax.random.split(rng)
- unstructured_block = random.normal(rng_input, (self.nb_columns, self.nb_columns))
- q, _ = jnp.linalg.qr(unstructured_block)
- q = jnp.transpose(q)
- block_list.append(q)
- remaining_rows = self.nb_rows - nb_full_blocks * self.nb_columns
- if remaining_rows > 0:
- rng, rng_input = jax.random.split(rng)
- unstructured_block = random.normal(rng_input, (self.nb_columns, self.nb_columns))
- q, _ = jnp.linalg.qr(unstructured_block)
- q = jnp.transpose(q)
- block_list.append(q[0:remaining_rows])
- final_matrix = jnp.vstack(block_list)
-
- if self.scaling == 0:
- multiplier = jnp.linalg.norm(random.normal(self.key, (self.nb_rows, self.nb_columns)), axis=1)
- elif self.scaling == 1:
- multiplier = jnp.sqrt(float(self.nb_columns)) * jnp.ones((self.nb_rows))
- else:
- raise ValueError("Scaling must be one of {0, 1}. Was %s" % self._scaling)
-
- return jnp.matmul(jnp.diag(multiplier), final_matrix)
-
-
-class FastAttention(object):
- r"""
- Abstract class providing a method for fast attention. Class is responsible for providing a method
- for fast approximate attention.
- """
-
- __metaclass__ = abc.ABCMeta
-
- @abc.abstractmethod
- def dot_product_attention(
- self,
- query,
- key,
- value,
- dtype=jnp.float32,
- bias=None,
- axis=None,
- broadcast_dropout=True,
- dropout_rng=None,
- dropout_rate=0.0,
- deterministic=False,
- precision=None,
- ):
- """
- Computes dot-product attention given query, key, and value. This is the core function for applying fast
- approximate dot-product attention. It calculates the attention weights given query and key and combines the
- values using the attention weights. This function supports multi-dimensional inputs
-
- Args:
- query: queries for calculating attention with shape of [batch_size, dim1,
- dim2, ..., dimN, num_heads, mem_channels].
- key: keys for calculating attention with shape of [batch_size, dim1, dim2,
- ..., dimN, num_heads, mem_channels].
- value: values to be used in attention with shape of [batch_size, dim1,
- dim2,..., dimN, num_heads, value_channels].
- dtype: the dtype of the computation (default: float32)
- bias: bias for the attention weights. This can be used for incorporating
- autoregressive mask, padding mask, proximity bias.
- axis: axises over which the attention is applied.
- broadcast_dropout: bool: use a broadcasted dropout along batch dims.
- dropout_rng: JAX PRNGKey: to be used for dropout.
- dropout_rate: dropout rate.
- deterministic: bool, deterministic or not (to apply dropout).
- precision: numerical precision of the computation see `jax.lax.Precision`
- for details
-
- Returns:
- Output of shape [bs, dim1, dim2, ..., dimN,, num_heads, value_channels].
- """
- raise NotImplementedError("Abstract method")
-
-
-def _numerator(z_slice_shape, precision, unroll=1):
- def fwd(qs, ks, vs):
- def body(p, qkv):
- (q, k, v) = qkv
- p += jnp.einsum("...m,...d->...md", k, v, precision=precision)
- X_slice = jnp.einsum("...m,...md->...d", q, p, precision=precision)
- return p, X_slice
-
- init_value = jnp.zeros(z_slice_shape)
- p, W = lax.scan(body, init_value, (qs, ks, vs), unroll=unroll)
- return W, (p, qs, ks, vs)
-
- def bwd(pqkv, W_ct):
- def body(carry, qkv_xct):
- p, p_ct = carry
- q, k, v, x_ct = qkv_xct
- q_ct = jnp.einsum("...d,...md->...m", x_ct, p, precision=precision)
- p_ct += jnp.einsum("...d,...m->...md", x_ct, q, precision=precision)
- k_ct = jnp.einsum("...md,...d->...m", p_ct, v, precision=precision)
- v_ct = jnp.einsum("...md,...m->...d", p_ct, k, precision=precision)
- p -= jnp.einsum("...m,...d->...md", k, v, precision=precision)
- return (p, p_ct), (q_ct, k_ct, v_ct)
-
- p, qs, ks, vs = pqkv
- _, (qs_ct, ks_ct, vs_ct) = lax.scan(
- body, (p, jnp.zeros_like(p)), (qs, ks, vs, W_ct), reverse=True, unroll=unroll
- )
- return qs_ct, ks_ct, vs_ct
-
- @jax.custom_vjp
- def _numerator_impl(qs, ks, vs):
- W, _ = fwd(qs, ks, vs)
- return W
-
- _numerator_impl.defvjp(fwd, bwd)
-
- return _numerator_impl
-
-
-def _denominator(t_slice_shape, precision, unroll=1):
- def fwd(qs, ks):
- def body(p, qk):
- q, k = qk
- p += k
- x = jnp.einsum("...m,...m->...", q, p, precision=precision)
- return p, x
-
- p = jnp.zeros(t_slice_shape)
- p, R = lax.scan(body, p, (qs, ks), unroll=unroll)
- return R, (qs, ks, p)
-
- def bwd(qkp, R_ct):
- def body(carry, qkx):
- p, p_ct = carry
- q, k, x_ct = qkx
- q_ct = jnp.einsum("...,...m->...m", x_ct, p, precision=precision)
- p_ct += jnp.einsum("...,...m->...m", x_ct, q, precision=precision)
- k_ct = p_ct
- p -= k
- return (p, p_ct), (q_ct, k_ct)
-
- qs, ks, p = qkp
- _, (qs_ct, ks_ct) = lax.scan(body, (p, jnp.zeros_like(p)), (qs, ks, R_ct), reverse=True, unroll=unroll)
- return (qs_ct, ks_ct)
-
- @jax.custom_vjp
- def _denominator_impl(qs, ks):
- R, _ = fwd(qs, ks)
- return R
-
- _denominator_impl.defvjp(fwd, bwd)
-
- return _denominator_impl
-
-
-class FastAttentionviaLowRankDecomposition(FastAttention):
- r"""
- Class providing a method for fast attention via low rank decomposition. Class is responsible for providing a method
- for fast dot-product attention with the use of low rank decomposition (e.g. with random
- feature maps).
- """
-
- def __init__(
- self,
- matrix_creator,
- kernel_feature_creator,
- renormalize_attention,
- numerical_stabilizer,
- redraw_features,
- unidirectional,
- lax_scan_unroll=1,
- ): # For optimal GPU performance, set to 16.
- rng = random.PRNGKey(0)
- self.matrix_creator = matrix_creator
- self.projection_matrix = self.draw_weights(rng)
- self.kernel_feature_creator = kernel_feature_creator
- self.renormalize_attention = renormalize_attention
- self.numerical_stabilizer = numerical_stabilizer
- self.redraw_features = redraw_features
- self.unidirectional = unidirectional
- self.lax_scan_unroll = lax_scan_unroll
-
- def draw_weights(self, key):
- if self.matrix_creator is None:
- return None
- matrixrng, _ = random.split(key)
- projection_matrix = self.matrix_creator(key=matrixrng).get_2d_array()
- return projection_matrix
-
- def dot_product_attention(
- self,
- query,
- key,
- value,
- dtype=jnp.float32,
- bias=None,
- axis=None,
- broadcast_dropout=True,
- dropout_rng=None,
- dropout_rate=0.0,
- deterministic=False,
- precision=None,
- ):
- assert key.shape[:-1] == value.shape[:-1]
- assert query.shape[0:1] == key.shape[0:1] and query.shape[-1] == key.shape[-1]
- if axis is None:
- axis = tuple(range(1, key.ndim - 2))
- if not isinstance(axis, Iterable):
- axis = (axis,)
- assert key.ndim == query.ndim
- assert key.ndim == value.ndim
- for ax in axis:
- if not (query.ndim >= 3 and 1 <= ax < query.ndim - 2):
- raise ValueError("Attention axis must be between the batch axis and the last-two axes.")
- n = key.ndim
-
- # Constructing projection tensor.
- if self.redraw_features:
- # TODO(kchoro): Get rid of the constant below.
- query_seed = lax.convert_element_type(jnp.ceil(jnp.sum(query) * 10000000.0), jnp.int32)
- rng = random.PRNGKey(query_seed)
- self.projection_matrix = self.draw_weights(rng)
-
- # batch_dims is , num_heads>
- batch_dims = tuple(onp.delete(range(n), axis + (n - 1,)))
- # q & k -> (bs, , num_heads, , channels)
- qk_perm = batch_dims + axis + (n - 1,)
- k_extra_perm = axis + batch_dims + (n - 1,)
- key_extra = key.transpose(k_extra_perm)
- key = key.transpose(qk_perm)
- query = query.transpose(qk_perm)
- # v -> (bs, , num_heads, , channels)
- v_perm = batch_dims + axis + (n - 1,)
- value = value.transpose(v_perm)
- batch_dims_t = tuple(range(len(batch_dims)))
- attention_dims_t = tuple(range(len(batch_dims), len(batch_dims) + len(axis)))
-
- # Constructing tensors Q^{'} and K^{'}.
- query_prime = self.kernel_feature_creator(
- query, self.projection_matrix, attention_dims_t, batch_dims_t, precision, True
- )
- key_prime = self.kernel_feature_creator(
- key, self.projection_matrix, attention_dims_t, batch_dims_t, precision, False
- )
-
- if self.unidirectional:
- index = attention_dims_t[0]
- z_slice_shape = key_prime.shape[0 : len(batch_dims_t)] + (key_prime.shape[-1],) + (value.shape[-1],)
-
- numerator_fn = _numerator(z_slice_shape, precision, self.lax_scan_unroll)
- W = numerator_fn(
- jnp.moveaxis(query_prime, index, 0), jnp.moveaxis(key_prime, index, 0), jnp.moveaxis(value, index, 0)
- )
-
- # Constructing W = (Q^{'}(K^{'})^{T})_{masked}V
- W = jnp.moveaxis(W, 0, index)
-
- if not self.renormalize_attention:
- # Unidirectional, not-normalized attention.
- perm_inv = _invert_perm(qk_perm)
- result = W.transpose(perm_inv)
- return result
- else:
- # Unidirectional, normalized attention.
- thick_all_ones = jnp.zeros(key.shape[0:-1]) + jnp.ones(key_extra.shape[0 : len(axis)])
-
- index = attention_dims_t[0]
- t_slice_shape = key_prime.shape[0 : len(batch_dims_t)] + (key_prime.shape[-1],)
- denominator_fn = _denominator(t_slice_shape, precision, self.lax_scan_unroll)
- R = denominator_fn(jnp.moveaxis(query_prime, index, 0), jnp.moveaxis(key_prime, index, 0))
-
- R = jnp.moveaxis(R, 0, index)
- else:
- contract_query = tuple(range(len(batch_dims) + len(axis), len(batch_dims) + len(axis) + 1))
- contract_z = tuple(range(len(batch_dims), len(batch_dims) + 1))
- # Constructing Z = (K^{'})^{T}V
- # Z (bs, , num_heads, channels_m, channels_v)
- Z = lax.dot_general(
- key_prime,
- value,
- ((attention_dims_t, attention_dims_t), (batch_dims_t, batch_dims_t)),
- precision=precision,
- )
- # Constructing W = Q^{'}Z = Q^{'}(K^{'})^{T}V
- # q (bs, , num_heads, , channels_m)
- # Z (bs, , num_heads, channels_m, channels_v)
- # W (bs, , num_heads, , channels_v)
- W = lax.dot_general(
- query_prime, Z, ((contract_query, contract_z), (batch_dims_t, batch_dims_t)), precision=precision
- )
- if not self.renormalize_attention:
- # Bidirectional, not-normalized attention.
- perm_inv = _invert_perm(qk_perm)
- result = W.transpose(perm_inv)
- return result
- else:
- # Bidirectional, normalized attention.
- thick_all_ones = jnp.zeros(key.shape[0:-1]) + jnp.ones(key_extra.shape[0 : len(axis)])
- contract_key = tuple(range(len(batch_dims), len(batch_dims) + len(axis)))
- contract_thick_all_ones = tuple(range(thick_all_ones.ndim - len(axis), thick_all_ones.ndim))
- # Construct T = (K^{'})^{T} 1_L
- # k (bs, , num_heads, , channels)
- T = lax.dot_general(
- key_prime,
- thick_all_ones,
- ((contract_key, contract_thick_all_ones), (batch_dims_t, batch_dims_t)),
- precision=precision,
- )
-
- # Construct partition function: R = Q^{'} T = Q^{'}(K^{'})^{T} 1_L
- # q_p (bs, , num_heads, , channs_m)
- # T (bs, , num_heads, channels_m)
- R = lax.dot_general(
- query_prime,
- T,
- (((query_prime.ndim - 1,), (T.ndim - 1,)), (batch_dims_t, range(0, len(T.shape) - 1))),
- precision=precision,
- )
-
- R = R + 2 * self.numerical_stabilizer * (jnp.abs(R) <= self.numerical_stabilizer)
- R = jnp.reciprocal(R)
- R = jnp.expand_dims(R, len(R.shape))
- # W (bs, , num_heads, , channels_v)
- # R (bs, , num_heads, , extra_channel)
- result = W * R
- # back to (bs, dim1, dim2, ..., dimN, num_heads, channels)
- perm_inv = _invert_perm(qk_perm)
- result = result.transpose(perm_inv)
- return result
-
-
-def _invert_perm(perm):
- perm_inv = [0] * len(perm)
- for i, j in enumerate(perm):
- perm_inv[j] = i
- return tuple(perm_inv)
diff --git a/spaces/chilge/taoli/models.py b/spaces/chilge/taoli/models.py
deleted file mode 100644
index bdbce8445304abda792f235a4761b831fd6f4d12..0000000000000000000000000000000000000000
--- a/spaces/chilge/taoli/models.py
+++ /dev/null
@@ -1,351 +0,0 @@
-import copy
-import math
-import torch
-from torch import nn
-from torch.nn import functional as F
-
-import attentions
-import commons
-import modules
-
-from torch.nn import Conv1d, ConvTranspose1d, AvgPool1d, Conv2d
-from torch.nn.utils import weight_norm, remove_weight_norm, spectral_norm
-from commons import init_weights, get_padding
-from vdecoder.hifigan.models import Generator
-from utils import f0_to_coarse
-
-class ResidualCouplingBlock(nn.Module):
- def __init__(self,
- channels,
- hidden_channels,
- kernel_size,
- dilation_rate,
- n_layers,
- n_flows=4,
- gin_channels=0):
- super().__init__()
- self.channels = channels
- self.hidden_channels = hidden_channels
- self.kernel_size = kernel_size
- self.dilation_rate = dilation_rate
- self.n_layers = n_layers
- self.n_flows = n_flows
- self.gin_channels = gin_channels
-
- self.flows = nn.ModuleList()
- for i in range(n_flows):
- self.flows.append(modules.ResidualCouplingLayer(channels, hidden_channels, kernel_size, dilation_rate, n_layers, gin_channels=gin_channels, mean_only=True))
- self.flows.append(modules.Flip())
-
- def forward(self, x, x_mask, g=None, reverse=False):
- if not reverse:
- for flow in self.flows:
- x, _ = flow(x, x_mask, g=g, reverse=reverse)
- else:
- for flow in reversed(self.flows):
- x = flow(x, x_mask, g=g, reverse=reverse)
- return x
-
-
-class Encoder(nn.Module):
- def __init__(self,
- in_channels,
- out_channels,
- hidden_channels,
- kernel_size,
- dilation_rate,
- n_layers,
- gin_channels=0):
- super().__init__()
- self.in_channels = in_channels
- self.out_channels = out_channels
- self.hidden_channels = hidden_channels
- self.kernel_size = kernel_size
- self.dilation_rate = dilation_rate
- self.n_layers = n_layers
- self.gin_channels = gin_channels
-
- self.pre = nn.Conv1d(in_channels, hidden_channels, 1)
- self.enc = modules.WN(hidden_channels, kernel_size, dilation_rate, n_layers, gin_channels=gin_channels)
- self.proj = nn.Conv1d(hidden_channels, out_channels * 2, 1)
-
- def forward(self, x, x_lengths, g=None):
- # print(x.shape,x_lengths.shape)
- x_mask = torch.unsqueeze(commons.sequence_mask(x_lengths, x.size(2)), 1).to(x.dtype)
- x = self.pre(x) * x_mask
- x = self.enc(x, x_mask, g=g)
- stats = self.proj(x) * x_mask
- m, logs = torch.split(stats, self.out_channels, dim=1)
- z = (m + torch.randn_like(m) * torch.exp(logs)) * x_mask
- return z, m, logs, x_mask
-
-
-class TextEncoder(nn.Module):
- def __init__(self,
- in_channels,
- out_channels,
- hidden_channels,
- kernel_size,
- dilation_rate,
- n_layers,
- gin_channels=0,
- filter_channels=None,
- n_heads=None,
- p_dropout=None):
- super().__init__()
- self.in_channels = in_channels
- self.out_channels = out_channels
- self.hidden_channels = hidden_channels
- self.kernel_size = kernel_size
- self.dilation_rate = dilation_rate
- self.n_layers = n_layers
- self.gin_channels = gin_channels
- self.pre = nn.Conv1d(in_channels, hidden_channels, 1)
- self.proj = nn.Conv1d(hidden_channels, out_channels * 2, 1)
- self.f0_emb = nn.Embedding(256, hidden_channels)
-
- self.enc_ = attentions.Encoder(
- hidden_channels,
- filter_channels,
- n_heads,
- n_layers,
- kernel_size,
- p_dropout)
-
- def forward(self, x, x_lengths, f0=None):
- x_mask = torch.unsqueeze(commons.sequence_mask(x_lengths, x.size(2)), 1).to(x.dtype)
- x = self.pre(x) * x_mask
- x = x + self.f0_emb(f0).transpose(1,2)
- x = self.enc_(x * x_mask, x_mask)
- stats = self.proj(x) * x_mask
- m, logs = torch.split(stats, self.out_channels, dim=1)
- z = (m + torch.randn_like(m) * torch.exp(logs)) * x_mask
-
- return z, m, logs, x_mask
-
-
-
-class DiscriminatorP(torch.nn.Module):
- def __init__(self, period, kernel_size=5, stride=3, use_spectral_norm=False):
- super(DiscriminatorP, self).__init__()
- self.period = period
- self.use_spectral_norm = use_spectral_norm
- norm_f = weight_norm if use_spectral_norm == False else spectral_norm
- self.convs = nn.ModuleList([
- norm_f(Conv2d(1, 32, (kernel_size, 1), (stride, 1), padding=(get_padding(kernel_size, 1), 0))),
- norm_f(Conv2d(32, 128, (kernel_size, 1), (stride, 1), padding=(get_padding(kernel_size, 1), 0))),
- norm_f(Conv2d(128, 512, (kernel_size, 1), (stride, 1), padding=(get_padding(kernel_size, 1), 0))),
- norm_f(Conv2d(512, 1024, (kernel_size, 1), (stride, 1), padding=(get_padding(kernel_size, 1), 0))),
- norm_f(Conv2d(1024, 1024, (kernel_size, 1), 1, padding=(get_padding(kernel_size, 1), 0))),
- ])
- self.conv_post = norm_f(Conv2d(1024, 1, (3, 1), 1, padding=(1, 0)))
-
- def forward(self, x):
- fmap = []
-
- # 1d to 2d
- b, c, t = x.shape
- if t % self.period != 0: # pad first
- n_pad = self.period - (t % self.period)
- x = F.pad(x, (0, n_pad), "reflect")
- t = t + n_pad
- x = x.view(b, c, t // self.period, self.period)
-
- for l in self.convs:
- x = l(x)
- x = F.leaky_relu(x, modules.LRELU_SLOPE)
- fmap.append(x)
- x = self.conv_post(x)
- fmap.append(x)
- x = torch.flatten(x, 1, -1)
-
- return x, fmap
-
-
-class DiscriminatorS(torch.nn.Module):
- def __init__(self, use_spectral_norm=False):
- super(DiscriminatorS, self).__init__()
- norm_f = weight_norm if use_spectral_norm == False else spectral_norm
- self.convs = nn.ModuleList([
- norm_f(Conv1d(1, 16, 15, 1, padding=7)),
- norm_f(Conv1d(16, 64, 41, 4, groups=4, padding=20)),
- norm_f(Conv1d(64, 256, 41, 4, groups=16, padding=20)),
- norm_f(Conv1d(256, 1024, 41, 4, groups=64, padding=20)),
- norm_f(Conv1d(1024, 1024, 41, 4, groups=256, padding=20)),
- norm_f(Conv1d(1024, 1024, 5, 1, padding=2)),
- ])
- self.conv_post = norm_f(Conv1d(1024, 1, 3, 1, padding=1))
-
- def forward(self, x):
- fmap = []
-
- for l in self.convs:
- x = l(x)
- x = F.leaky_relu(x, modules.LRELU_SLOPE)
- fmap.append(x)
- x = self.conv_post(x)
- fmap.append(x)
- x = torch.flatten(x, 1, -1)
-
- return x, fmap
-
-
-class MultiPeriodDiscriminator(torch.nn.Module):
- def __init__(self, use_spectral_norm=False):
- super(MultiPeriodDiscriminator, self).__init__()
- periods = [2,3,5,7,11]
-
- discs = [DiscriminatorS(use_spectral_norm=use_spectral_norm)]
- discs = discs + [DiscriminatorP(i, use_spectral_norm=use_spectral_norm) for i in periods]
- self.discriminators = nn.ModuleList(discs)
-
- def forward(self, y, y_hat):
- y_d_rs = []
- y_d_gs = []
- fmap_rs = []
- fmap_gs = []
- for i, d in enumerate(self.discriminators):
- y_d_r, fmap_r = d(y)
- y_d_g, fmap_g = d(y_hat)
- y_d_rs.append(y_d_r)
- y_d_gs.append(y_d_g)
- fmap_rs.append(fmap_r)
- fmap_gs.append(fmap_g)
-
- return y_d_rs, y_d_gs, fmap_rs, fmap_gs
-
-
-class SpeakerEncoder(torch.nn.Module):
- def __init__(self, mel_n_channels=80, model_num_layers=3, model_hidden_size=256, model_embedding_size=256):
- super(SpeakerEncoder, self).__init__()
- self.lstm = nn.LSTM(mel_n_channels, model_hidden_size, model_num_layers, batch_first=True)
- self.linear = nn.Linear(model_hidden_size, model_embedding_size)
- self.relu = nn.ReLU()
-
- def forward(self, mels):
- self.lstm.flatten_parameters()
- _, (hidden, _) = self.lstm(mels)
- embeds_raw = self.relu(self.linear(hidden[-1]))
- return embeds_raw / torch.norm(embeds_raw, dim=1, keepdim=True)
-
- def compute_partial_slices(self, total_frames, partial_frames, partial_hop):
- mel_slices = []
- for i in range(0, total_frames-partial_frames, partial_hop):
- mel_range = torch.arange(i, i+partial_frames)
- mel_slices.append(mel_range)
-
- return mel_slices
-
- def embed_utterance(self, mel, partial_frames=128, partial_hop=64):
- mel_len = mel.size(1)
- last_mel = mel[:,-partial_frames:]
-
- if mel_len > partial_frames:
- mel_slices = self.compute_partial_slices(mel_len, partial_frames, partial_hop)
- mels = list(mel[:,s] for s in mel_slices)
- mels.append(last_mel)
- mels = torch.stack(tuple(mels), 0).squeeze(1)
-
- with torch.no_grad():
- partial_embeds = self(mels)
- embed = torch.mean(partial_embeds, axis=0).unsqueeze(0)
- #embed = embed / torch.linalg.norm(embed, 2)
- else:
- with torch.no_grad():
- embed = self(last_mel)
-
- return embed
-
-
-class SynthesizerTrn(nn.Module):
- """
- Synthesizer for Training
- """
-
- def __init__(self,
- spec_channels,
- segment_size,
- inter_channels,
- hidden_channels,
- filter_channels,
- n_heads,
- n_layers,
- kernel_size,
- p_dropout,
- resblock,
- resblock_kernel_sizes,
- resblock_dilation_sizes,
- upsample_rates,
- upsample_initial_channel,
- upsample_kernel_sizes,
- gin_channels,
- ssl_dim,
- n_speakers,
- **kwargs):
-
- super().__init__()
- self.spec_channels = spec_channels
- self.inter_channels = inter_channels
- self.hidden_channels = hidden_channels
- self.filter_channels = filter_channels
- self.n_heads = n_heads
- self.n_layers = n_layers
- self.kernel_size = kernel_size
- self.p_dropout = p_dropout
- self.resblock = resblock
- self.resblock_kernel_sizes = resblock_kernel_sizes
- self.resblock_dilation_sizes = resblock_dilation_sizes
- self.upsample_rates = upsample_rates
- self.upsample_initial_channel = upsample_initial_channel
- self.upsample_kernel_sizes = upsample_kernel_sizes
- self.segment_size = segment_size
- self.gin_channels = gin_channels
- self.ssl_dim = ssl_dim
- self.emb_g = nn.Embedding(n_speakers, gin_channels)
-
- self.enc_p_ = TextEncoder(ssl_dim, inter_channels, hidden_channels, 5, 1, 16,0, filter_channels, n_heads, p_dropout)
- hps = {
- "sampling_rate": 32000,
- "inter_channels": 192,
- "resblock": "1",
- "resblock_kernel_sizes": [3, 7, 11],
- "resblock_dilation_sizes": [[1, 3, 5], [1, 3, 5], [1, 3, 5]],
- "upsample_rates": [10, 8, 2, 2],
- "upsample_initial_channel": 512,
- "upsample_kernel_sizes": [16, 16, 4, 4],
- "gin_channels": 256,
- }
- self.dec = Generator(h=hps)
- self.enc_q = Encoder(spec_channels, inter_channels, hidden_channels, 5, 1, 16, gin_channels=gin_channels)
- self.flow = ResidualCouplingBlock(inter_channels, hidden_channels, 5, 1, 4, gin_channels=gin_channels)
-
- def forward(self, c, f0, spec, g=None, mel=None, c_lengths=None, spec_lengths=None):
- if c_lengths == None:
- c_lengths = (torch.ones(c.size(0)) * c.size(-1)).to(c.device)
- if spec_lengths == None:
- spec_lengths = (torch.ones(spec.size(0)) * spec.size(-1)).to(spec.device)
-
- g = self.emb_g(g).transpose(1,2)
-
- z_ptemp, m_p, logs_p, _ = self.enc_p_(c, c_lengths, f0=f0_to_coarse(f0))
- z, m_q, logs_q, spec_mask = self.enc_q(spec, spec_lengths, g=g)
-
- z_p = self.flow(z, spec_mask, g=g)
- z_slice, pitch_slice, ids_slice = commons.rand_slice_segments_with_pitch(z, f0, spec_lengths, self.segment_size)
-
- # o = self.dec(z_slice, g=g)
- o = self.dec(z_slice, g=g, f0=pitch_slice)
-
- return o, ids_slice, spec_mask, (z, z_p, m_p, logs_p, m_q, logs_q)
-
- def infer(self, c, f0, g=None, mel=None, c_lengths=None):
- if c_lengths == None:
- c_lengths = (torch.ones(c.size(0)) * c.size(-1)).to(c.device)
- g = self.emb_g(g).transpose(1,2)
-
- z_p, m_p, logs_p, c_mask = self.enc_p_(c, c_lengths, f0=f0_to_coarse(f0))
- z = self.flow(z_p, c_mask, g=g, reverse=True)
-
- o = self.dec(z * c_mask, g=g, f0=f0)
-
- return o
diff --git a/spaces/chuan-hd/law-assistant-chatbot/.venv/lib/python3.11/site-packages/functorch/_src/vmap/__init__.py b/spaces/chuan-hd/law-assistant-chatbot/.venv/lib/python3.11/site-packages/functorch/_src/vmap/__init__.py
deleted file mode 100644
index 792a2fde38bb3563ed5b336132d7af008bf3e11a..0000000000000000000000000000000000000000
--- a/spaces/chuan-hd/law-assistant-chatbot/.venv/lib/python3.11/site-packages/functorch/_src/vmap/__init__.py
+++ /dev/null
@@ -1,16 +0,0 @@
-# This file has moved to under torch/_functorch. It is not public API.
-# If you are not a PyTorch developer and you are relying on the following
-# imports, please file an issue.
-from torch._functorch.vmap import (
- _add_batch_dim,
- _broadcast_to_and_flatten,
- _get_name,
- _remove_batch_dim,
- _validate_and_get_batch_size,
- Tensor,
- tree_flatten,
- tree_unflatten,
- _process_batched_inputs,
- _create_batched_inputs,
- _unwrap_batched,
-)
diff --git a/spaces/chuan-hd/law-assistant-chatbot/.venv/lib/python3.11/site-packages/gradio/inputs.py b/spaces/chuan-hd/law-assistant-chatbot/.venv/lib/python3.11/site-packages/gradio/inputs.py
deleted file mode 100644
index 9345530649a0b8843c27d7a0f965ac73bfcce7d6..0000000000000000000000000000000000000000
--- a/spaces/chuan-hd/law-assistant-chatbot/.venv/lib/python3.11/site-packages/gradio/inputs.py
+++ /dev/null
@@ -1,451 +0,0 @@
-# type: ignore
-"""
-This module defines various classes that can serve as the `input` to an interface. Each class must inherit from
-`InputComponent`, and each class must define a path to its template. All of the subclasses of `InputComponent` are
-automatically added to a registry, which allows them to be easily referenced in other parts of the code.
-"""
-
-from __future__ import annotations
-
-from typing import Any, Optional
-
-from gradio import components
-from gradio.deprecation import warn_deprecation
-
-
-def warn_inputs_deprecation():
- warn_deprecation(
- "Usage of gradio.inputs is deprecated, and will not be supported in the future, please import your component from gradio.components",
- )
-
-
-class Textbox(components.Textbox):
- def __init__(
- self,
- lines: int = 1,
- placeholder: Optional[str] = None,
- default: str = "",
- numeric: Optional[bool] = False,
- type: Optional[str] = "text",
- label: Optional[str] = None,
- optional: bool = False,
- ):
- warn_inputs_deprecation()
- super().__init__(
- value=default,
- lines=lines,
- placeholder=placeholder,
- label=label,
- numeric=numeric,
- type=type,
- optional=optional,
- )
-
-
-class Number(components.Number):
- """
- Component creates a field for user to enter numeric input. Provides a number as an argument to the wrapped function.
- Input type: float
- """
-
- def __init__(
- self,
- default: Optional[float] = None,
- label: Optional[str] = None,
- optional: bool = False,
- ):
- """
- Parameters:
- default (float): default value.
- label (str): component name in interface.
- optional (bool): If True, the interface can be submitted with no value for this component.
- """
- warn_inputs_deprecation()
- super().__init__(value=default, label=label, optional=optional)
-
-
-class Slider(components.Slider):
- """
- Component creates a slider that ranges from `minimum` to `maximum`. Provides number as an argument to the wrapped function.
- Input type: float
- """
-
- def __init__(
- self,
- minimum: float = 0,
- maximum: float = 100,
- step: Optional[float] = None,
- default: Optional[float] = None,
- label: Optional[str] = None,
- optional: bool = False,
- ):
- """
- Parameters:
- minimum (float): minimum value for slider.
- maximum (float): maximum value for slider.
- step (float): increment between slider values.
- default (float): default value.
- label (str): component name in interface.
- optional (bool): this parameter is ignored.
- """
- warn_inputs_deprecation()
-
- super().__init__(
- value=default,
- minimum=minimum,
- maximum=maximum,
- step=step,
- label=label,
- optional=optional,
- )
-
-
-class Checkbox(components.Checkbox):
- """
- Component creates a checkbox that can be set to `True` or `False`. Provides a boolean as an argument to the wrapped function.
- Input type: bool
- """
-
- def __init__(
- self,
- default: bool = False,
- label: Optional[str] = None,
- optional: bool = False,
- ):
- """
- Parameters:
- label (str): component name in interface.
- default (bool): if True, checked by default.
- optional (bool): this parameter is ignored.
- """
- warn_inputs_deprecation()
- super().__init__(value=default, label=label, optional=optional)
-
-
-class CheckboxGroup(components.CheckboxGroup):
- """
- Component creates a set of checkboxes of which a subset can be selected. Provides a list of strings representing the selected choices as an argument to the wrapped function.
- Input type: Union[List[str], List[int]]
- """
-
- def __init__(
- self,
- choices: list[str],
- default: list[str] | None = None,
- type: str = "value",
- label: Optional[str] = None,
- optional: bool = False,
- ):
- """
- Parameters:
- choices (List[str]): list of options to select from.
- default (List[str]): default selected list of options.
- type (str): Type of value to be returned by component. "value" returns the list of strings of the choices selected, "index" returns the list of indices of the choices selected.
- label (str): component name in interface.
- optional (bool): this parameter is ignored.
- """
- if default is None:
- default = []
- warn_inputs_deprecation()
- super().__init__(
- value=default,
- choices=choices,
- type=type,
- label=label,
- optional=optional,
- )
-
-
-class Radio(components.Radio):
- """
- Component creates a set of radio buttons of which only one can be selected. Provides string representing selected choice as an argument to the wrapped function.
- Input type: Union[str, int]
- """
-
- def __init__(
- self,
- choices: list[str],
- type: str = "value",
- default: Optional[str] = None,
- label: Optional[str] = None,
- optional: bool = False,
- ):
- """
- Parameters:
- choices (List[str]): list of options to select from.
- type (str): Type of value to be returned by component. "value" returns the string of the choice selected, "index" returns the index of the choice selected.
- default (str): the button selected by default. If None, no button is selected by default.
- label (str): component name in interface.
- optional (bool): this parameter is ignored.
- """
- warn_inputs_deprecation()
- super().__init__(
- choices=choices,
- type=type,
- value=default,
- label=label,
- optional=optional,
- )
-
-
-class Dropdown(components.Dropdown):
- """
- Component creates a dropdown of which only one can be selected. Provides string representing selected choice as an argument to the wrapped function.
- Input type: Union[str, int]
- """
-
- def __init__(
- self,
- choices: list[str],
- type: str = "value",
- default: Optional[str] = None,
- label: Optional[str] = None,
- optional: bool = False,
- ):
- """
- Parameters:
- choices (List[str]): list of options to select from.
- type (str): Type of value to be returned by component. "value" returns the string of the choice selected, "index" returns the index of the choice selected.
- default (str): default value selected in dropdown. If None, no value is selected by default.
- label (str): component name in interface.
- optional (bool): this parameter is ignored.
- """
- warn_inputs_deprecation()
- super().__init__(
- choices=choices,
- type=type,
- value=default,
- label=label,
- optional=optional,
- )
-
-
-class Image(components.Image):
- """
- Component creates an image upload box with editing capabilities.
- Input type: Union[numpy.array, PIL.Image, file-object]
- """
-
- def __init__(
- self,
- shape: tuple[int, int] = None,
- image_mode: str = "RGB",
- invert_colors: bool = False,
- source: str = "upload",
- tool: str = "editor",
- type: str = "numpy",
- label: str = None,
- optional: bool = False,
- ):
- """
- Parameters:
- shape (Tuple[int, int]): (width, height) shape to crop and resize image to; if None, matches input image size.
- image_mode (str): How to process the uploaded image. Accepts any of the PIL image modes, e.g. "RGB" for color images, "RGBA" to include the transparency mask, "L" for black-and-white images.
- invert_colors (bool): whether to invert the image as a preprocessing step.
- source (str): Source of image. "upload" creates a box where user can drop an image file, "webcam" allows user to take snapshot from their webcam, "canvas" defaults to a white image that can be edited and drawn upon with tools.
- tool (str): Tools used for editing. "editor" allows a full screen editor, "select" provides a cropping and zoom tool.
- type (str): Type of value to be returned by component. "numpy" returns a numpy array with shape (height, width, 3) and values from 0 to 255, "pil" returns a PIL image object, "file" returns a temporary file object whose path can be retrieved by file_obj.name, "filepath" returns the path directly.
- label (str): component name in interface.
- optional (bool): If True, the interface can be submitted with no uploaded image, in which case the input value is None.
- """
- warn_inputs_deprecation()
- super().__init__(
- shape=shape,
- image_mode=image_mode,
- invert_colors=invert_colors,
- source=source,
- tool=tool,
- type=type,
- label=label,
- optional=optional,
- )
-
-
-class Video(components.Video):
- """
- Component creates a video file upload that is converted to a file path.
-
- Input type: filepath
- """
-
- def __init__(
- self,
- type: Optional[str] = None,
- source: str = "upload",
- label: Optional[str] = None,
- optional: bool = False,
- ):
- """
- Parameters:
- type (str): Type of video format to be returned by component, such as 'avi' or 'mp4'. If set to None, video will keep uploaded format.
- source (str): Source of video. "upload" creates a box where user can drop an video file, "webcam" allows user to record a video from their webcam.
- label (str): component name in interface.
- optional (bool): If True, the interface can be submitted with no uploaded video, in which case the input value is None.
- """
- warn_inputs_deprecation()
- super().__init__(format=type, source=source, label=label, optional=optional)
-
-
-class Audio(components.Audio):
- """
- Component accepts audio input files.
- Input type: Union[Tuple[int, numpy.array], file-object, numpy.array]
- """
-
- def __init__(
- self,
- source: str = "upload",
- type: str = "numpy",
- label: str = None,
- optional: bool = False,
- ):
- """
- Parameters:
- source (str): Source of audio. "upload" creates a box where user can drop an audio file, "microphone" creates a microphone input.
- type (str): Type of value to be returned by component. "numpy" returns a 2-set tuple with an integer sample_rate and the data numpy.array of shape (samples, 2), "file" returns a temporary file object whose path can be retrieved by file_obj.name, "filepath" returns the path directly.
- label (str): component name in interface.
- optional (bool): If True, the interface can be submitted with no uploaded audio, in which case the input value is None.
- """
- warn_inputs_deprecation()
- super().__init__(source=source, type=type, label=label, optional=optional)
-
-
-class File(components.File):
- """
- Component accepts generic file uploads.
- Input type: Union[file-object, bytes, List[Union[file-object, bytes]]]
- """
-
- def __init__(
- self,
- file_count: str = "single",
- type: str = "file",
- label: Optional[str] = None,
- keep_filename: bool = True,
- optional: bool = False,
- ):
- """
- Parameters:
- file_count (str): if single, allows user to upload one file. If "multiple", user uploads multiple files. If "directory", user uploads all files in selected directory. Return type will be list for each file in case of "multiple" or "directory".
- type (str): Type of value to be returned by component. "file" returns a temporary file object whose path can be retrieved by file_obj.name, "binary" returns an bytes object.
- label (str): component name in interface.
- keep_filename (bool): DEPRECATED. Original filename always kept.
- optional (bool): If True, the interface can be submitted with no uploaded image, in which case the input value is None.
- """
- warn_inputs_deprecation()
- super().__init__(
- file_count=file_count,
- type=type,
- label=label,
- keep_filename=keep_filename,
- optional=optional,
- )
-
-
-class Dataframe(components.Dataframe):
- """
- Component accepts 2D input through a spreadsheet interface.
- Input type: Union[pandas.DataFrame, numpy.array, List[Union[str, float]], List[List[Union[str, float]]]]
- """
-
- def __init__(
- self,
- headers: Optional[list[str]] = None,
- row_count: int = 3,
- col_count: Optional[int] = 3,
- datatype: str | list[str] = "str",
- col_width: int | list[int] = None,
- default: Optional[list[list[Any]]] = None,
- type: str = "pandas",
- label: Optional[str] = None,
- optional: bool = False,
- ):
- """
- Parameters:
- headers (List[str]): Header names to dataframe. If None, no headers are shown.
- row_count (int): Limit number of rows for input.
- col_count (int): Limit number of columns for input. If equal to 1, return data will be one-dimensional. Ignored if `headers` is provided.
- datatype (Union[str, List[str]]): Datatype of values in sheet. Can be provided per column as a list of strings, or for the entire sheet as a single string. Valid datatypes are "str", "number", "bool", and "date".
- col_width (Union[int, List[int]]): Width of columns in pixels. Can be provided as single value or list of values per column.
- default (List[List[Any]]): Default value
- type (str): Type of value to be returned by component. "pandas" for pandas dataframe, "numpy" for numpy array, or "array" for a Python array.
- label (str): component name in interface.
- optional (bool): this parameter is ignored.
- """
- warn_inputs_deprecation()
- super().__init__(
- value=default,
- headers=headers,
- row_count=row_count,
- col_count=col_count,
- datatype=datatype,
- col_width=col_width,
- type=type,
- label=label,
- optional=optional,
- )
-
-
-class Timeseries(components.Timeseries):
- """
- Component accepts pandas.DataFrame uploaded as a timeseries csv file.
- Input type: pandas.DataFrame
- """
-
- def __init__(
- self,
- x: Optional[str] = None,
- y: str | list[str] = None,
- label: Optional[str] = None,
- optional: bool = False,
- ):
- """
- Parameters:
- x (str): Column name of x (time) series. None if csv has no headers, in which case first column is x series.
- y (Union[str, List[str]]): Column name of y series, or list of column names if multiple series. None if csv has no headers, in which case every column after first is a y series.
- label (str): component name in interface.
- optional (bool): If True, the interface can be submitted with no uploaded csv file, in which case the input value is None.
- """
- warn_inputs_deprecation()
- super().__init__(x=x, y=y, label=label, optional=optional)
-
-
-class State(components.State):
- """
- Special hidden component that stores state across runs of the interface.
- Input type: Any
- """
-
- def __init__(
- self,
- label: str = None,
- default: Any = None,
- ):
- """
- Parameters:
- label (str): component name in interface (not used).
- default (Any): the initial value of the state.
- optional (bool): this parameter is ignored.
- """
- warn_inputs_deprecation()
- super().__init__(value=default, label=label)
-
-
-class Image3D(components.Model3D):
- """
- Used for 3D image model output.
- Input type: File object of type (.obj, glb, or .gltf)
- """
-
- def __init__(
- self,
- label: Optional[str] = None,
- optional: bool = False,
- ):
- """
- Parameters:
- label (str): component name in interface.
- optional (bool): If True, the interface can be submitted with no uploaded image, in which case the input value is None.
- """
- warn_inputs_deprecation()
- super().__init__(label=label, optional=optional)
diff --git a/spaces/chuan-hd/law-assistant-chatbot/.venv/lib/python3.11/site-packages/gradio/templates/cdn/assets/StaticImage.svelte_svelte_type_style_lang-72cfcc0b.js b/spaces/chuan-hd/law-assistant-chatbot/.venv/lib/python3.11/site-packages/gradio/templates/cdn/assets/StaticImage.svelte_svelte_type_style_lang-72cfcc0b.js
deleted file mode 100644
index 330f3e0c7149cba01f903b763e530ec2272caed9..0000000000000000000000000000000000000000
--- a/spaces/chuan-hd/law-assistant-chatbot/.venv/lib/python3.11/site-packages/gradio/templates/cdn/assets/StaticImage.svelte_svelte_type_style_lang-72cfcc0b.js
+++ /dev/null
@@ -1,11 +0,0 @@
-import{S as bt,e as wt,s as yt,J as H,K as p,p as U,M as Z,n as I,A as j,N as ct,O as je,U as re,z as W,u as jt,v as G,y as Vt,B as Ve,C as Ge,Q as qe,X as Fe,h as Ke,k as Gt,o as qt,x as Ft,m as Qe}from"./index-f877dfd5.js";import"./Button-11a87b79.js";function Ze(a){let t,e,i;return{c(){t=H("svg"),e=H("path"),i=H("circle"),p(e,"d","M23 19a2 2 0 0 1-2 2H3a2 2 0 0 1-2-2V8a2 2 0 0 1 2-2h4l2-3h6l2 3h4a2 2 0 0 1 2 2z"),p(i,"cx","12"),p(i,"cy","13"),p(i,"r","4"),p(t,"xmlns","http://www.w3.org/2000/svg"),p(t,"width","100%"),p(t,"height","100%"),p(t,"viewBox","0 0 24 24"),p(t,"fill","none"),p(t,"stroke","currentColor"),p(t,"stroke-width","1.5"),p(t,"stroke-linecap","round"),p(t,"stroke-linejoin","round"),p(t,"class","feather feather-camera")},m(n,r){U(n,t,r),Z(t,e),Z(t,i)},p:I,i:I,o:I,d(n){n&&j(t)}}}class Je extends bt{constructor(t){super(),wt(this,t,null,Ze,yt,{})}}function $e(a){let t,e;return{c(){t=H("svg"),e=H("circle"),p(e,"cx","12"),p(e,"cy","12"),p(e,"r","10"),p(t,"xmlns","http://www.w3.org/2000/svg"),p(t,"width","100%"),p(t,"height","100%"),p(t,"viewBox","0 0 24 24"),p(t,"fill","red"),p(t,"stroke","red"),p(t,"stroke-width","1.5"),p(t,"stroke-linecap","round"),p(t,"stroke-linejoin","round"),p(t,"class","feather feather-circle")},m(i,n){U(i,t,n),Z(t,e)},p:I,i:I,o:I,d(i){i&&j(t)}}}class ti extends bt{constructor(t){super(),wt(this,t,null,$e,yt,{})}}function ei(a){let t,e;return{c(){t=H("svg"),e=H("rect"),p(e,"x","3"),p(e,"y","3"),p(e,"width","18"),p(e,"height","18"),p(e,"rx","2"),p(e,"ry","2"),p(t,"xmlns","http://www.w3.org/2000/svg"),p(t,"width","100%"),p(t,"height","100%"),p(t,"viewBox","0 0 24 24"),p(t,"fill","red"),p(t,"stroke","red"),p(t,"stroke-width","1.5"),p(t,"stroke-linecap","round"),p(t,"stroke-linejoin","round"),p(t,"class","feather feather-square")},m(i,n){U(i,t,n),Z(t,e)},p:I,i:I,o:I,d(i){i&&j(t)}}}class ii extends bt{constructor(t){super(),wt(this,t,null,ei,yt,{})}}function ai(a){let t,e,i;return{c(){t=H("svg"),e=H("polyline"),i=H("path"),p(e,"points","1 4 1 10 7 10"),p(i,"d","M3.51 15a9 9 0 1 0 2.13-9.36L1 10"),p(t,"xmlns","http://www.w3.org/2000/svg"),p(t,"width","100%"),p(t,"height","100%"),p(t,"viewBox","0 0 24 24"),p(t,"fill","none"),p(t,"stroke","currentColor"),p(t,"stroke-width","1.5"),p(t,"stroke-linecap","round"),p(t,"stroke-linejoin","round"),p(t,"class","feather feather-rotate-ccw")},m(n,r){U(n,t,r),Z(t,e),Z(t,i)},p:I,i:I,o:I,d(n){n&&j(t)}}}class ba extends bt{constructor(t){super(),wt(this,t,null,ai,yt,{})}}/*!
- * Cropper.js v1.5.12
- * https://fengyuanchen.github.io/cropperjs
- *
- * Copyright 2015-present Chen Fengyuan
- * Released under the MIT license
- *
- * Date: 2021-06-12T08:00:17.411Z
- */function ne(a,t){var e=Object.keys(a);if(Object.getOwnPropertySymbols){var i=Object.getOwnPropertySymbols(a);t&&(i=i.filter(function(n){return Object.getOwnPropertyDescriptor(a,n).enumerable})),e.push.apply(e,i)}return e}function Ee(a){for(var t=1;ta.length)&&(t=a.length);for(var e=0,i=new Array(t);e
',yi=Number.isNaN||X.isNaN;function b(a){return typeof a=="number"&&!yi(a)}var be=function(t){return t>0&&t<1/0};function St(a){return typeof a>"u"}function at(a){return Dt(a)==="object"&&a!==null}var _i=Object.prototype.hasOwnProperty;function nt(a){if(!at(a))return!1;try{var t=a.constructor,e=t.prototype;return t&&e&&_i.call(e,"isPrototypeOf")}catch{return!1}}function S(a){return typeof a=="function"}var xi=Array.prototype.slice;function Se(a){return Array.from?Array.from(a):xi.call(a)}function C(a,t){return a&&S(t)&&(Array.isArray(a)||b(a.length)?Se(a).forEach(function(e,i){t.call(a,e,i,a)}):at(a)&&Object.keys(a).forEach(function(e){t.call(a,a[e],e,a)})),a}var D=Object.assign||function(t){for(var e=arguments.length,i=new Array(e>1?e-1:0),n=1;n0&&i.forEach(function(r){at(r)&&Object.keys(r).forEach(function(o){t[o]=r[o]})}),t},Ei=/\.\d*(?:0|9){12}\d*$/;function st(a){var t=arguments.length>1&&arguments[1]!==void 0?arguments[1]:1e11;return Ei.test(a)?Math.round(a*t)/t:a}var Di=/^width|height|left|top|marginLeft|marginTop$/;function K(a,t){var e=a.style;C(t,function(i,n){Di.test(n)&&b(i)&&(i="".concat(i,"px")),e[n]=i})}function Mi(a,t){return a.classList?a.classList.contains(t):a.className.indexOf(t)>-1}function A(a,t){if(t){if(b(a.length)){C(a,function(i){A(i,t)});return}if(a.classList){a.classList.add(t);return}var e=a.className.trim();e?e.indexOf(t)<0&&(a.className="".concat(e," ").concat(t)):a.className=t}}function Y(a,t){if(t){if(b(a.length)){C(a,function(e){Y(e,t)});return}if(a.classList){a.classList.remove(t);return}a.className.indexOf(t)>=0&&(a.className=a.className.replace(t,""))}}function ot(a,t,e){if(t){if(b(a.length)){C(a,function(i){ot(i,t,e)});return}e?A(a,t):Y(a,t)}}var Oi=/([a-z\d])([A-Z])/g;function $t(a){return a.replace(Oi,"$1-$2").toLowerCase()}function Xt(a,t){return at(a[t])?a[t]:a.dataset?a.dataset[t]:a.getAttribute("data-".concat($t(t)))}function mt(a,t,e){at(e)?a[t]=e:a.dataset?a.dataset[t]=e:a.setAttribute("data-".concat($t(t)),e)}function Ti(a,t){if(at(a[t]))try{delete a[t]}catch{a[t]=void 0}else if(a.dataset)try{delete a.dataset[t]}catch{a.dataset[t]=void 0}else a.removeAttribute("data-".concat($t(t)))}var ke=/\s\s*/,Ie=function(){var a=!1;if(Ct){var t=!1,e=function(){},i=Object.defineProperty({},"once",{get:function(){return a=!0,t},set:function(r){t=r}});X.addEventListener("test",e,i),X.removeEventListener("test",e,i)}return a}();function z(a,t,e){var i=arguments.length>3&&arguments[3]!==void 0?arguments[3]:{},n=e;t.trim().split(ke).forEach(function(r){if(!Ie){var o=a.listeners;o&&o[r]&&o[r][e]&&(n=o[r][e],delete o[r][e],Object.keys(o[r]).length===0&&delete o[r],Object.keys(o).length===0&&delete a.listeners)}a.removeEventListener(r,n,i)})}function B(a,t,e){var i=arguments.length>3&&arguments[3]!==void 0?arguments[3]:{},n=e;t.trim().split(ke).forEach(function(r){if(i.once&&!Ie){var o=a.listeners,s=o===void 0?{}:o;n=function(){delete s[r][e],a.removeEventListener(r,n,i);for(var f=arguments.length,h=new Array(f),c=0;cMath.abs(e)&&(e=u)})}),e}function Et(a,t){var e=a.pageX,i=a.pageY,n={endX:e,endY:i};return t?n:Ee({startX:e,startY:i},n)}function Ai(a){var t=0,e=0,i=0;return C(a,function(n){var r=n.startX,o=n.startY;t+=r,e+=o,i+=1}),t/=i,e/=i,{pageX:t,pageY:e}}function Q(a){var t=a.aspectRatio,e=a.height,i=a.width,n=arguments.length>1&&arguments[1]!==void 0?arguments[1]:"contain",r=be(i),o=be(e);if(r&&o){var s=e*t;n==="contain"&&s>i||n==="cover"&&s90?{width:l,height:s}:{width:s,height:l}}function Si(a,t,e,i){var n=t.aspectRatio,r=t.naturalWidth,o=t.naturalHeight,s=t.rotate,l=s===void 0?0:s,f=t.scaleX,h=f===void 0?1:f,c=t.scaleY,u=c===void 0?1:c,v=e.aspectRatio,g=e.naturalWidth,_=e.naturalHeight,m=i.fillColor,x=m===void 0?"transparent":m,T=i.imageSmoothingEnabled,O=T===void 0?!0:T,w=i.imageSmoothingQuality,M=w===void 0?"low":w,d=i.maxWidth,y=d===void 0?1/0:d,R=i.maxHeight,L=R===void 0?1/0:R,V=i.minWidth,J=V===void 0?0:V,$=i.minHeight,q=$===void 0?0:$,P=document.createElement("canvas"),N=P.getContext("2d"),tt=Q({aspectRatio:v,width:y,height:L}),_t=Q({aspectRatio:v,width:J,height:q},"cover"),At=Math.min(tt.width,Math.max(_t.width,g)),Nt=Math.min(tt.height,Math.max(_t.height,_)),te=Q({aspectRatio:n,width:y,height:L}),ee=Q({aspectRatio:n,width:J,height:q},"cover"),ie=Math.min(te.width,Math.max(ee.width,r)),ae=Math.min(te.height,Math.max(ee.height,o)),Xe=[-ie/2,-ae/2,ie,ae];return P.width=st(At),P.height=st(Nt),N.fillStyle=x,N.fillRect(0,0,At,Nt),N.save(),N.translate(At/2,Nt/2),N.rotate(l*Math.PI/180),N.scale(h,u),N.imageSmoothingEnabled=O,N.imageSmoothingQuality=M,N.drawImage.apply(N,[a].concat(De(Xe.map(function(Ue){return Math.floor(st(Ue))})))),N.restore(),P}var Be=String.fromCharCode;function ki(a,t,e){var i="";e+=t;for(var n=t;n0;)e.push(Be.apply(null,Se(n.subarray(0,i)))),n=n.subarray(i);return"data:".concat(t,";base64,").concat(btoa(e.join("")))}function zi(a){var t=new DataView(a),e;try{var i,n,r;if(t.getUint8(0)===255&&t.getUint8(1)===216)for(var o=t.byteLength,s=2;s+1=8&&(r=f+c)}}}if(r){var u=t.getUint16(r,i),v,g;for(g=0;g=0?r:Ae),height:Math.max(i.offsetHeight,o>=0?o:Ne)};this.containerData=s,K(n,{width:s.width,height:s.height}),A(t,k),Y(n,k)},initCanvas:function(){var t=this.containerData,e=this.imageData,i=this.options.viewMode,n=Math.abs(e.rotate)%180===90,r=n?e.naturalHeight:e.naturalWidth,o=n?e.naturalWidth:e.naturalHeight,s=r/o,l=t.width,f=t.height;t.height*s>t.width?i===3?l=t.height*s:f=t.width/s:i===3?f=t.width/s:l=t.height*s;var h={aspectRatio:s,naturalWidth:r,naturalHeight:o,width:l,height:f};this.canvasData=h,this.limited=i===1||i===2,this.limitCanvas(!0,!0),h.width=Math.min(Math.max(h.width,h.minWidth),h.maxWidth),h.height=Math.min(Math.max(h.height,h.minHeight),h.maxHeight),h.left=(t.width-h.width)/2,h.top=(t.height-h.height)/2,h.oldLeft=h.left,h.oldTop=h.top,this.initialCanvasData=D({},h)},limitCanvas:function(t,e){var i=this.options,n=this.containerData,r=this.canvasData,o=this.cropBoxData,s=i.viewMode,l=r.aspectRatio,f=this.cropped&&o;if(t){var h=Number(i.minCanvasWidth)||0,c=Number(i.minCanvasHeight)||0;s>1?(h=Math.max(h,n.width),c=Math.max(c,n.height),s===3&&(c*l>h?h=c*l:c=h/l)):s>0&&(h?h=Math.max(h,f?o.width:0):c?c=Math.max(c,f?o.height:0):f&&(h=o.width,c=o.height,c*l>h?h=c*l:c=h/l));var u=Q({aspectRatio:l,width:h,height:c});h=u.width,c=u.height,r.minWidth=h,r.minHeight=c,r.maxWidth=1/0,r.maxHeight=1/0}if(e)if(s>(f?0:1)){var v=n.width-r.width,g=n.height-r.height;r.minLeft=Math.min(0,v),r.minTop=Math.min(0,g),r.maxLeft=Math.max(0,v),r.maxTop=Math.max(0,g),f&&this.limited&&(r.minLeft=Math.min(o.left,o.left+(o.width-r.width)),r.minTop=Math.min(o.top,o.top+(o.height-r.height)),r.maxLeft=o.left,r.maxTop=o.top,s===2&&(r.width>=n.width&&(r.minLeft=Math.min(0,v),r.maxLeft=Math.max(0,v)),r.height>=n.height&&(r.minTop=Math.min(0,g),r.maxTop=Math.max(0,g))))}else r.minLeft=-r.width,r.minTop=-r.height,r.maxLeft=n.width,r.maxTop=n.height},renderCanvas:function(t,e){var i=this.canvasData,n=this.imageData;if(e){var r=Ni({width:n.naturalWidth*Math.abs(n.scaleX||1),height:n.naturalHeight*Math.abs(n.scaleY||1),degree:n.rotate||0}),o=r.width,s=r.height,l=i.width*(o/i.naturalWidth),f=i.height*(s/i.naturalHeight);i.left-=(l-i.width)/2,i.top-=(f-i.height)/2,i.width=l,i.height=f,i.aspectRatio=o/s,i.naturalWidth=o,i.naturalHeight=s,this.limitCanvas(!0,!1)}(i.width>i.maxWidth||i.widthi.maxHeight||i.heighte.width?r.height=r.width/i:r.width=r.height*i),this.cropBoxData=r,this.limitCropBox(!0,!0),r.width=Math.min(Math.max(r.width,r.minWidth),r.maxWidth),r.height=Math.min(Math.max(r.height,r.minHeight),r.maxHeight),r.width=Math.max(r.minWidth,r.width*n),r.height=Math.max(r.minHeight,r.height*n),r.left=e.left+(e.width-r.width)/2,r.top=e.top+(e.height-r.height)/2,r.oldLeft=r.left,r.oldTop=r.top,this.initialCropBoxData=D({},r)},limitCropBox:function(t,e){var i=this.options,n=this.containerData,r=this.canvasData,o=this.cropBoxData,s=this.limited,l=i.aspectRatio;if(t){var f=Number(i.minCropBoxWidth)||0,h=Number(i.minCropBoxHeight)||0,c=s?Math.min(n.width,r.width,r.width+r.left,n.width-r.left):n.width,u=s?Math.min(n.height,r.height,r.height+r.top,n.height-r.top):n.height;f=Math.min(f,n.width),h=Math.min(h,n.height),l&&(f&&h?h*l>f?h=f/l:f=h*l:f?h=f/l:h&&(f=h*l),u*l>c?u=c/l:c=u*l),o.minWidth=Math.min(f,c),o.minHeight=Math.min(h,u),o.maxWidth=c,o.maxHeight=u}e&&(s?(o.minLeft=Math.max(0,r.left),o.minTop=Math.max(0,r.top),o.maxLeft=Math.min(n.width,r.left+r.width)-o.width,o.maxTop=Math.min(n.height,r.top+r.height)-o.height):(o.minLeft=0,o.minTop=0,o.maxLeft=n.width-o.width,o.maxTop=n.height-o.height))},renderCropBox:function(){var t=this.options,e=this.containerData,i=this.cropBoxData;(i.width>i.maxWidth||i.widthi.maxHeight||i.height=e.width&&i.height>=e.height?Oe:Zt),K(this.cropBox,D({width:i.width,height:i.height},vt({translateX:i.left,translateY:i.top}))),this.cropped&&this.limited&&this.limitCanvas(!0,!0),this.disabled||this.output()},output:function(){this.preview(),ht(this.element,zt,this.getData())}},Wi={initPreview:function(){var t=this.element,e=this.crossOrigin,i=this.options.preview,n=e?this.crossOriginUrl:this.url,r=t.alt||"The image to preview",o=document.createElement("img");if(e&&(o.crossOrigin=e),o.src=n,o.alt=r,this.viewBox.appendChild(o),this.viewBoxImage=o,!!i){var s=i;typeof i=="string"?s=t.ownerDocument.querySelectorAll(i):i.querySelector&&(s=[i]),this.previews=s,C(s,function(l){var f=document.createElement("img");mt(l,xt,{width:l.offsetWidth,height:l.offsetHeight,html:l.innerHTML}),e&&(f.crossOrigin=e),f.src=n,f.alt=r,f.style.cssText='display:block;width:100%;height:auto;min-width:0!important;min-height:0!important;max-width:none!important;max-height:none!important;image-orientation:0deg!important;"',l.innerHTML="",l.appendChild(f)})}},resetPreview:function(){C(this.previews,function(t){var e=Xt(t,xt);K(t,{width:e.width,height:e.height}),t.innerHTML=e.html,Ti(t,xt)})},preview:function(){var t=this.imageData,e=this.canvasData,i=this.cropBoxData,n=i.width,r=i.height,o=t.width,s=t.height,l=i.left-e.left-t.left,f=i.top-e.top-t.top;!this.cropped||this.disabled||(K(this.viewBoxImage,D({width:o,height:s},vt(D({translateX:-l,translateY:-f},t)))),C(this.previews,function(h){var c=Xt(h,xt),u=c.width,v=c.height,g=u,_=v,m=1;n&&(m=u/n,_=r*m),r&&_>v&&(m=v/r,g=n*m,_=v),K(h,{width:g,height:_}),K(h.getElementsByTagName("img")[0],D({width:o*m,height:s*m},vt(D({translateX:-l*m,translateY:-f*m},t))))}))}},Yi={bind:function(){var t=this.element,e=this.options,i=this.cropper;S(e.cropstart)&&B(t,Wt,e.cropstart),S(e.cropmove)&&B(t,Ht,e.cropmove),S(e.cropend)&&B(t,Pt,e.cropend),S(e.crop)&&B(t,zt,e.crop),S(e.zoom)&&B(t,Yt,e.zoom),B(i,le,this.onCropStart=this.cropStart.bind(this)),e.zoomable&&e.zoomOnWheel&&B(i,ve,this.onWheel=this.wheel.bind(this),{passive:!1,capture:!0}),e.toggleDragModeOnDblclick&&B(i,ce,this.onDblclick=this.dblclick.bind(this)),B(t.ownerDocument,fe,this.onCropMove=this.cropMove.bind(this)),B(t.ownerDocument,ue,this.onCropEnd=this.cropEnd.bind(this)),e.responsive&&B(window,pe,this.onResize=this.resize.bind(this))},unbind:function(){var t=this.element,e=this.options,i=this.cropper;S(e.cropstart)&&z(t,Wt,e.cropstart),S(e.cropmove)&&z(t,Ht,e.cropmove),S(e.cropend)&&z(t,Pt,e.cropend),S(e.crop)&&z(t,zt,e.crop),S(e.zoom)&&z(t,Yt,e.zoom),z(i,le,this.onCropStart),e.zoomable&&e.zoomOnWheel&&z(i,ve,this.onWheel,{passive:!1,capture:!0}),e.toggleDragModeOnDblclick&&z(i,ce,this.onDblclick),z(t.ownerDocument,fe,this.onCropMove),z(t.ownerDocument,ue,this.onCropEnd),e.responsive&&z(window,pe,this.onResize)}},Xi={resize:function(){if(!this.disabled){var t=this.options,e=this.container,i=this.containerData,n=e.offsetWidth/i.width,r=e.offsetHeight/i.height,o=Math.abs(n-1)>Math.abs(r-1)?n:r;if(o!==1){var s,l;t.restore&&(s=this.getCanvasData(),l=this.getCropBoxData()),this.render(),t.restore&&(this.setCanvasData(C(s,function(f,h){s[h]=f*o})),this.setCropBoxData(C(l,function(f,h){l[h]=f*o})))}}},dblclick:function(){this.disabled||this.options.dragMode===Re||this.setDragMode(Mi(this.dragBox,Lt)?Ce:Jt)},wheel:function(t){var e=this,i=Number(this.options.wheelZoomRatio)||.1,n=1;this.disabled||(t.preventDefault(),!this.wheeling&&(this.wheeling=!0,setTimeout(function(){e.wheeling=!1},50),t.deltaY?n=t.deltaY>0?1:-1:t.wheelDelta?n=-t.wheelDelta/120:t.detail&&(n=t.detail>0?1:-1),this.zoom(-n*i,t)))},cropStart:function(t){var e=t.buttons,i=t.button;if(!(this.disabled||(t.type==="mousedown"||t.type==="pointerdown"&&t.pointerType==="mouse")&&(b(e)&&e!==1||b(i)&&i!==0||t.ctrlKey))){var n=this.options,r=this.pointers,o;t.changedTouches?C(t.changedTouches,function(s){r[s.identifier]=Et(s)}):r[t.pointerId||0]=Et(t),Object.keys(r).length>1&&n.zoomable&&n.zoomOnTouch?o=Te:o=Xt(t.target,gt),vi.test(o)&&ht(this.element,Wt,{originalEvent:t,action:o})!==!1&&(t.preventDefault(),this.action=o,this.cropping=!1,o===Me&&(this.cropping=!0,A(this.dragBox,Mt)))}},cropMove:function(t){var e=this.action;if(!(this.disabled||!e)){var i=this.pointers;t.preventDefault(),ht(this.element,Ht,{originalEvent:t,action:e})!==!1&&(t.changedTouches?C(t.changedTouches,function(n){D(i[n.identifier]||{},Et(n,!0))}):D(i[t.pointerId||0]||{},Et(t,!0)),this.change(t))}},cropEnd:function(t){if(!this.disabled){var e=this.action,i=this.pointers;t.changedTouches?C(t.changedTouches,function(n){delete i[n.identifier]}):delete i[t.pointerId||0],e&&(t.preventDefault(),Object.keys(i).length||(this.action=""),this.cropping&&(this.cropping=!1,ot(this.dragBox,Mt,this.cropped&&this.options.modal)),ht(this.element,Pt,{originalEvent:t,action:e}))}}},Ui={change:function(t){var e=this.options,i=this.canvasData,n=this.containerData,r=this.cropBoxData,o=this.pointers,s=this.action,l=e.aspectRatio,f=r.left,h=r.top,c=r.width,u=r.height,v=f+c,g=h+u,_=0,m=0,x=n.width,T=n.height,O=!0,w;!l&&t.shiftKey&&(l=c&&u?c/u:1),this.limited&&(_=r.minLeft,m=r.minTop,x=_+Math.min(n.width,i.width,i.left+i.width),T=m+Math.min(n.height,i.height,i.top+i.height));var M=o[Object.keys(o)[0]],d={x:M.endX-M.startX,y:M.endY-M.startY},y=function(L){switch(L){case et:v+d.x>x&&(d.x=x-v);break;case it:f+d.x<_&&(d.x=_-f);break;case F:h+d.yT&&(d.y=T-g);break}};switch(s){case Zt:f+=d.x,h+=d.y;break;case et:if(d.x>=0&&(v>=x||l&&(h<=m||g>=T))){O=!1;break}y(et),c+=d.x,c<0&&(s=it,c=-c,f-=c),l&&(u=c/l,h+=(r.height-u)/2);break;case F:if(d.y<=0&&(h<=m||l&&(f<=_||v>=x))){O=!1;break}y(F),u-=d.y,h+=d.y,u<0&&(s=rt,u=-u,h-=u),l&&(c=u*l,f+=(r.width-c)/2);break;case it:if(d.x<=0&&(f<=_||l&&(h<=m||g>=T))){O=!1;break}y(it),c-=d.x,f+=d.x,c<0&&(s=et,c=-c,f-=c),l&&(u=c/l,h+=(r.height-u)/2);break;case rt:if(d.y>=0&&(g>=T||l&&(f<=_||v>=x))){O=!1;break}y(rt),u+=d.y,u<0&&(s=F,u=-u,h-=u),l&&(c=u*l,f+=(r.width-c)/2);break;case ft:if(l){if(d.y<=0&&(h<=m||v>=x)){O=!1;break}y(F),u-=d.y,h+=d.y,c=u*l}else y(F),y(et),d.x>=0?vm&&(u-=d.y,h+=d.y):(u-=d.y,h+=d.y);c<0&&u<0?(s=pt,u=-u,c=-c,h-=u,f-=c):c<0?(s=ut,c=-c,f-=c):u<0&&(s=dt,u=-u,h-=u);break;case ut:if(l){if(d.y<=0&&(h<=m||f<=_)){O=!1;break}y(F),u-=d.y,h+=d.y,c=u*l,f+=r.width-c}else y(F),y(it),d.x<=0?f>_?(c-=d.x,f+=d.x):d.y<=0&&h<=m&&(O=!1):(c-=d.x,f+=d.x),d.y<=0?h>m&&(u-=d.y,h+=d.y):(u-=d.y,h+=d.y);c<0&&u<0?(s=dt,u=-u,c=-c,h-=u,f-=c):c<0?(s=ft,c=-c,f-=c):u<0&&(s=pt,u=-u,h-=u);break;case pt:if(l){if(d.x<=0&&(f<=_||g>=T)){O=!1;break}y(it),c-=d.x,f+=d.x,u=c/l}else y(rt),y(it),d.x<=0?f>_?(c-=d.x,f+=d.x):d.y>=0&&g>=T&&(O=!1):(c-=d.x,f+=d.x),d.y>=0?g=0&&(v>=x||g>=T)){O=!1;break}y(et),c+=d.x,u=c/l}else y(rt),y(et),d.x>=0?v=0&&g>=T&&(O=!1):c+=d.x,d.y>=0?g0?s=d.y>0?dt:ft:d.x<0&&(f-=c,s=d.y>0?pt:ut),d.y<0&&(h-=u),this.cropped||(Y(this.cropBox,k),this.cropped=!0,this.limited&&this.limitCropBox(!0,!0));break}O&&(r.width=c,r.height=u,r.left=f,r.top=h,this.action=s,this.renderCropBox()),C(o,function(R){R.startX=R.endX,R.startY=R.endY})}},ji={crop:function(){return this.ready&&!this.cropped&&!this.disabled&&(this.cropped=!0,this.limitCropBox(!0,!0),this.options.modal&&A(this.dragBox,Mt),Y(this.cropBox,k),this.setCropBoxData(this.initialCropBoxData)),this},reset:function(){return this.ready&&!this.disabled&&(this.imageData=D({},this.initialImageData),this.canvasData=D({},this.initialCanvasData),this.cropBoxData=D({},this.initialCropBoxData),this.renderCanvas(),this.cropped&&this.renderCropBox()),this},clear:function(){return this.cropped&&!this.disabled&&(D(this.cropBoxData,{left:0,top:0,width:0,height:0}),this.cropped=!1,this.renderCropBox(),this.limitCanvas(!0,!0),this.renderCanvas(),Y(this.dragBox,Mt),A(this.cropBox,k)),this},replace:function(t){var e=arguments.length>1&&arguments[1]!==void 0?arguments[1]:!1;return!this.disabled&&t&&(this.isImg&&(this.element.src=t),e?(this.url=t,this.image.src=t,this.ready&&(this.viewBoxImage.src=t,C(this.previews,function(i){i.getElementsByTagName("img")[0].src=t}))):(this.isImg&&(this.replaced=!0),this.options.data=null,this.uncreate(),this.load(t))),this},enable:function(){return this.ready&&this.disabled&&(this.disabled=!1,Y(this.cropper,se)),this},disable:function(){return this.ready&&!this.disabled&&(this.disabled=!0,A(this.cropper,se)),this},destroy:function(){var t=this.element;return t[E]?(t[E]=void 0,this.isImg&&this.replaced&&(t.src=this.originalUrl),this.uncreate(),this):this},move:function(t){var e=arguments.length>1&&arguments[1]!==void 0?arguments[1]:t,i=this.canvasData,n=i.left,r=i.top;return this.moveTo(St(t)?t:n+Number(t),St(e)?e:r+Number(e))},moveTo:function(t){var e=arguments.length>1&&arguments[1]!==void 0?arguments[1]:t,i=this.canvasData,n=!1;return t=Number(t),e=Number(e),this.ready&&!this.disabled&&this.options.movable&&(b(t)&&(i.left=t,n=!0),b(e)&&(i.top=e,n=!0),n&&this.renderCanvas(!0)),this},zoom:function(t,e){var i=this.canvasData;return t=Number(t),t<0?t=1/(1-t):t=1+t,this.zoomTo(i.width*t/i.naturalWidth,null,e)},zoomTo:function(t,e,i){var n=this.options,r=this.canvasData,o=r.width,s=r.height,l=r.naturalWidth,f=r.naturalHeight;if(t=Number(t),t>=0&&this.ready&&!this.disabled&&n.zoomable){var h=l*t,c=f*t;if(ht(this.element,Yt,{ratio:t,oldRatio:o/l,originalEvent:i})===!1)return this;if(i){var u=this.pointers,v=Le(this.cropper),g=u&&Object.keys(u).length?Ai(u):{pageX:i.pageX,pageY:i.pageY};r.left-=(h-o)*((g.pageX-v.left-r.left)/o),r.top-=(c-s)*((g.pageY-v.top-r.top)/s)}else nt(e)&&b(e.x)&&b(e.y)?(r.left-=(h-o)*((e.x-r.left)/o),r.top-=(c-s)*((e.y-r.top)/s)):(r.left-=(h-o)/2,r.top-=(c-s)/2);r.width=h,r.height=c,this.renderCanvas(!0)}return this},rotate:function(t){return this.rotateTo((this.imageData.rotate||0)+Number(t))},rotateTo:function(t){return t=Number(t),b(t)&&this.ready&&!this.disabled&&this.options.rotatable&&(this.imageData.rotate=t%360,this.renderCanvas(!0,!0)),this},scaleX:function(t){var e=this.imageData.scaleY;return this.scale(t,b(e)?e:1)},scaleY:function(t){var e=this.imageData.scaleX;return this.scale(b(e)?e:1,t)},scale:function(t){var e=arguments.length>1&&arguments[1]!==void 0?arguments[1]:t,i=this.imageData,n=!1;return t=Number(t),e=Number(e),this.ready&&!this.disabled&&this.options.scalable&&(b(t)&&(i.scaleX=t,n=!0),b(e)&&(i.scaleY=e,n=!0),n&&this.renderCanvas(!0,!0)),this},getData:function(){var t=arguments.length>0&&arguments[0]!==void 0?arguments[0]:!1,e=this.options,i=this.imageData,n=this.canvasData,r=this.cropBoxData,o;if(this.ready&&this.cropped){o={x:r.left-n.left,y:r.top-n.top,width:r.width,height:r.height};var s=i.width/i.naturalWidth;if(C(o,function(h,c){o[c]=h/s}),t){var l=Math.round(o.y+o.height),f=Math.round(o.x+o.width);o.x=Math.round(o.x),o.y=Math.round(o.y),o.width=f-o.x,o.height=l-o.y}}else o={x:0,y:0,width:0,height:0};return e.rotatable&&(o.rotate=i.rotate||0),e.scalable&&(o.scaleX=i.scaleX||1,o.scaleY=i.scaleY||1),o},setData:function(t){var e=this.options,i=this.imageData,n=this.canvasData,r={};if(this.ready&&!this.disabled&&nt(t)){var o=!1;e.rotatable&&b(t.rotate)&&t.rotate!==i.rotate&&(i.rotate=t.rotate,o=!0),e.scalable&&(b(t.scaleX)&&t.scaleX!==i.scaleX&&(i.scaleX=t.scaleX,o=!0),b(t.scaleY)&&t.scaleY!==i.scaleY&&(i.scaleY=t.scaleY,o=!0)),o&&this.renderCanvas(!0,!0);var s=i.width/i.naturalWidth;b(t.x)&&(r.left=t.x*s+n.left),b(t.y)&&(r.top=t.y*s+n.top),b(t.width)&&(r.width=t.width*s),b(t.height)&&(r.height=t.height*s),this.setCropBoxData(r)}return this},getContainerData:function(){return this.ready?D({},this.containerData):{}},getImageData:function(){return this.sized?D({},this.imageData):{}},getCanvasData:function(){var t=this.canvasData,e={};return this.ready&&C(["left","top","width","height","naturalWidth","naturalHeight"],function(i){e[i]=t[i]}),e},setCanvasData:function(t){var e=this.canvasData,i=e.aspectRatio;return this.ready&&!this.disabled&&nt(t)&&(b(t.left)&&(e.left=t.left),b(t.top)&&(e.top=t.top),b(t.width)?(e.width=t.width,e.height=t.width/i):b(t.height)&&(e.height=t.height,e.width=t.height*i),this.renderCanvas(!0)),this},getCropBoxData:function(){var t=this.cropBoxData,e;return this.ready&&this.cropped&&(e={left:t.left,top:t.top,width:t.width,height:t.height}),e||{}},setCropBoxData:function(t){var e=this.cropBoxData,i=this.options.aspectRatio,n,r;return this.ready&&this.cropped&&!this.disabled&&nt(t)&&(b(t.left)&&(e.left=t.left),b(t.top)&&(e.top=t.top),b(t.width)&&t.width!==e.width&&(n=!0,e.width=t.width),b(t.height)&&t.height!==e.height&&(r=!0,e.height=t.height),i&&(n?e.height=e.width/i:r&&(e.width=e.height*i)),this.renderCropBox()),this},getCroppedCanvas:function(){var t=arguments.length>0&&arguments[0]!==void 0?arguments[0]:{};if(!this.ready||!window.HTMLCanvasElement)return null;var e=this.canvasData,i=Si(this.image,this.imageData,e,t);if(!this.cropped)return i;var n=this.getData(),r=n.x,o=n.y,s=n.width,l=n.height,f=i.width/Math.floor(e.naturalWidth);f!==1&&(r*=f,o*=f,s*=f,l*=f);var h=s/l,c=Q({aspectRatio:h,width:t.maxWidth||1/0,height:t.maxHeight||1/0}),u=Q({aspectRatio:h,width:t.minWidth||0,height:t.minHeight||0},"cover"),v=Q({aspectRatio:h,width:t.width||(f!==1?i.width:s),height:t.height||(f!==1?i.height:l)}),g=v.width,_=v.height;g=Math.min(c.width,Math.max(u.width,g)),_=Math.min(c.height,Math.max(u.height,_));var m=document.createElement("canvas"),x=m.getContext("2d");m.width=st(g),m.height=st(_),x.fillStyle=t.fillColor||"transparent",x.fillRect(0,0,g,_);var T=t.imageSmoothingEnabled,O=T===void 0?!0:T,w=t.imageSmoothingQuality;x.imageSmoothingEnabled=O,w&&(x.imageSmoothingQuality=w);var M=i.width,d=i.height,y=r,R=o,L,V,J,$,q,P;y<=-s||y>M?(y=0,L=0,J=0,q=0):y<=0?(J=-y,y=0,L=Math.min(M,s+y),q=L):y<=M&&(J=0,L=Math.min(s,M-y),q=L),L<=0||R<=-l||R>d?(R=0,V=0,$=0,P=0):R<=0?($=-R,R=0,V=Math.min(d,l+R),P=V):R<=d&&($=0,V=Math.min(l,d-R),P=V);var N=[y,R,L,V];if(q>0&&P>0){var tt=g/s;N.push(J*tt,$*tt,q*tt,P*tt)}return x.drawImage.apply(x,[i].concat(De(N.map(function(_t){return Math.floor(st(_t))})))),m},setAspectRatio:function(t){var e=this.options;return!this.disabled&&!St(t)&&(e.aspectRatio=Math.max(0,t)||NaN,this.ready&&(this.initCropBox(),this.cropped&&this.renderCropBox())),this},setDragMode:function(t){var e=this.options,i=this.dragBox,n=this.face;if(this.ready&&!this.disabled){var r=t===Jt,o=e.movable&&t===Ce;t=r||o?t:Re,e.dragMode=t,mt(i,gt,t),ot(i,Lt,r),ot(i,Bt,o),e.cropBoxMovable||(mt(n,gt,t),ot(n,Lt,r),ot(n,Bt,o))}return this}},Vi=X.Cropper,Gi=function(){function a(t){var e=arguments.length>1&&arguments[1]!==void 0?arguments[1]:{};if(ri(this,a),!t||!bi.test(t.tagName))throw new Error("The first argument is required and must be an or element.");this.element=t,this.options=D({},me,nt(e)&&e),this.cropped=!1,this.disabled=!1,this.pointers={},this.ready=!1,this.reloading=!1,this.replaced=!1,this.sized=!1,this.sizing=!1,this.init()}return ni(a,[{key:"init",value:function(){var e=this.element,i=e.tagName.toLowerCase(),n;if(!e[E]){if(e[E]=this,i==="img"){if(this.isImg=!0,n=e.getAttribute("src")||"",this.originalUrl=n,!n)return;n=e.src}else i==="canvas"&&window.HTMLCanvasElement&&(n=e.toDataURL());this.load(n)}}},{key:"load",value:function(e){var i=this;if(e){this.url=e,this.imageData={};var n=this.element,r=this.options;if(!r.rotatable&&!r.scalable&&(r.checkOrientation=!1),!r.checkOrientation||!window.ArrayBuffer){this.clone();return}if(gi.test(e)){mi.test(e)?this.read(Li(e)):this.clone();return}var o=new XMLHttpRequest,s=this.clone.bind(this);this.reloading=!0,this.xhr=o,o.onabort=s,o.onerror=s,o.ontimeout=s,o.onprogress=function(){o.getResponseHeader("content-type")!==ge&&o.abort()},o.onload=function(){i.read(o.response)},o.onloadend=function(){i.reloading=!1,i.xhr=null},r.checkCrossOrigin&&we(e)&&n.crossOrigin&&(e=ye(e)),o.open("GET",e,!0),o.responseType="arraybuffer",o.withCredentials=n.crossOrigin==="use-credentials",o.send()}}},{key:"read",value:function(e){var i=this.options,n=this.imageData,r=zi(e),o=0,s=1,l=1;if(r>1){this.url=Bi(e,ge);var f=Pi(r);o=f.rotate,s=f.scaleX,l=f.scaleY}i.rotatable&&(n.rotate=o),i.scalable&&(n.scaleX=s,n.scaleY=l),this.clone()}},{key:"clone",value:function(){var e=this.element,i=this.url,n=e.crossOrigin,r=i;this.options.checkCrossOrigin&&we(i)&&(n||(n="anonymous"),r=ye(i)),this.crossOrigin=n,this.crossOriginUrl=r;var o=document.createElement("img");n&&(o.crossOrigin=n),o.src=r||i,o.alt=e.alt||"The image to crop",this.image=o,o.onload=this.start.bind(this),o.onerror=this.stop.bind(this),A(o,he),e.parentNode.insertBefore(o,e.nextSibling)}},{key:"start",value:function(){var e=this,i=this.image;i.onload=null,i.onerror=null,this.sizing=!0;var n=X.navigator&&/(?:iPad|iPhone|iPod).*?AppleWebKit/i.test(X.navigator.userAgent),r=function(f,h){D(e.imageData,{naturalWidth:f,naturalHeight:h,aspectRatio:f/h}),e.initialImageData=D({},e.imageData),e.sizing=!1,e.sized=!0,e.build()};if(i.naturalWidth&&!n){r(i.naturalWidth,i.naturalHeight);return}var o=document.createElement("img"),s=document.body||document.documentElement;this.sizingImage=o,o.onload=function(){r(o.width,o.height),n||s.removeChild(o)},o.src=i.src,n||(o.style.cssText="left:0;max-height:none!important;max-width:none!important;min-height:0!important;min-width:0!important;opacity:0;position:absolute;top:0;z-index:-1;",s.appendChild(o))}},{key:"stop",value:function(){var e=this.image;e.onload=null,e.onerror=null,e.parentNode.removeChild(e),this.image=null}},{key:"build",value:function(){if(!(!this.sized||this.ready)){var e=this.element,i=this.options,n=this.image,r=e.parentNode,o=document.createElement("div");o.innerHTML=wi;var s=o.querySelector(".".concat(E,"-container")),l=s.querySelector(".".concat(E,"-canvas")),f=s.querySelector(".".concat(E,"-drag-box")),h=s.querySelector(".".concat(E,"-crop-box")),c=h.querySelector(".".concat(E,"-face"));this.container=r,this.cropper=s,this.canvas=l,this.dragBox=f,this.cropBox=h,this.viewBox=s.querySelector(".".concat(E,"-view-box")),this.face=c,l.appendChild(n),A(e,k),r.insertBefore(s,e.nextSibling),this.isImg||Y(n,he),this.initPreview(),this.bind(),i.initialAspectRatio=Math.max(0,i.initialAspectRatio)||NaN,i.aspectRatio=Math.max(0,i.aspectRatio)||NaN,i.viewMode=Math.max(0,Math.min(3,Math.round(i.viewMode)))||0,A(h,k),i.guides||A(h.getElementsByClassName("".concat(E,"-dashed")),k),i.center||A(h.getElementsByClassName("".concat(E,"-center")),k),i.background&&A(s,"".concat(E,"-bg")),i.highlight||A(c,fi),i.cropBoxMovable&&(A(c,Bt),mt(c,gt,Zt)),i.cropBoxResizable||(A(h.getElementsByClassName("".concat(E,"-line")),k),A(h.getElementsByClassName("".concat(E,"-point")),k)),this.render(),this.ready=!0,this.setDragMode(i.dragMode),i.autoCrop&&this.crop(),this.setData(i.data),S(i.ready)&&B(e,de,i.ready,{once:!0}),ht(e,de)}}},{key:"unbuild",value:function(){this.ready&&(this.ready=!1,this.unbind(),this.resetPreview(),this.cropper.parentNode.removeChild(this.cropper),Y(this.element,k))}},{key:"uncreate",value:function(){this.ready?(this.unbuild(),this.ready=!1,this.cropped=!1):this.sizing?(this.sizingImage.onload=null,this.sizing=!1,this.sized=!1):this.reloading?(this.xhr.onabort=null,this.xhr.abort()):this.image&&this.stop()}}],[{key:"noConflict",value:function(){return window.Cropper=Vi,a}},{key:"setDefaults",value:function(e){D(me,nt(e)&&e)}}]),a}();D(Gi.prototype,Hi,Wi,Yi,Xi,Ui,ji);var ze=function(){if(typeof Map<"u")return Map;function a(t,e){var i=-1;return t.some(function(n,r){return n[0]===e?(i=r,!0):!1}),i}return function(){function t(){this.__entries__=[]}return Object.defineProperty(t.prototype,"size",{get:function(){return this.__entries__.length},enumerable:!0,configurable:!0}),t.prototype.get=function(e){var i=a(this.__entries__,e),n=this.__entries__[i];return n&&n[1]},t.prototype.set=function(e,i){var n=a(this.__entries__,e);~n?this.__entries__[n][1]=i:this.__entries__.push([e,i])},t.prototype.delete=function(e){var i=this.__entries__,n=a(i,e);~n&&i.splice(n,1)},t.prototype.has=function(e){return!!~a(this.__entries__,e)},t.prototype.clear=function(){this.__entries__.splice(0)},t.prototype.forEach=function(e,i){i===void 0&&(i=null);for(var n=0,r=this.__entries__;n0},a.prototype.connect_=function(){!Ut||this.connected_||(document.addEventListener("transitionend",this.onTransitionEnd_),window.addEventListener("resize",this.refresh),Ji?(this.mutationsObserver_=new MutationObserver(this.refresh),this.mutationsObserver_.observe(document,{attributes:!0,childList:!0,characterData:!0,subtree:!0})):(document.addEventListener("DOMSubtreeModified",this.refresh),this.mutationEventsAdded_=!0),this.connected_=!0)},a.prototype.disconnect_=function(){!Ut||!this.connected_||(document.removeEventListener("transitionend",this.onTransitionEnd_),window.removeEventListener("resize",this.refresh),this.mutationsObserver_&&this.mutationsObserver_.disconnect(),this.mutationEventsAdded_&&document.removeEventListener("DOMSubtreeModified",this.refresh),this.mutationsObserver_=null,this.mutationEventsAdded_=!1,this.connected_=!1)},a.prototype.onTransitionEnd_=function(t){var e=t.propertyName,i=e===void 0?"":e,n=Zi.some(function(r){return!!~i.indexOf(r)});n&&this.refresh()},a.getInstance=function(){return this.instance_||(this.instance_=new a),this.instance_},a.instance_=null,a}(),Pe=function(a,t){for(var e=0,i=Object.keys(t);e"u"||!(Element instanceof Object))){if(!(t instanceof lt(t).Element))throw new TypeError('parameter 1 is not of type "Element".');var e=this.observations_;e.has(t)||(e.set(t,new sa(t)),this.controller_.addObserver(this),this.controller_.refresh())}},a.prototype.unobserve=function(t){if(!arguments.length)throw new TypeError("1 argument required, but only 0 present.");if(!(typeof Element>"u"||!(Element instanceof Object))){if(!(t instanceof lt(t).Element))throw new TypeError('parameter 1 is not of type "Element".');var e=this.observations_;e.has(t)&&(e.delete(t),e.size||this.controller_.removeObserver(this))}},a.prototype.disconnect=function(){this.clearActive(),this.observations_.clear(),this.controller_.removeObserver(this)},a.prototype.gatherActive=function(){var t=this;this.clearActive(),this.observations_.forEach(function(e){e.isActive()&&t.activeObservations_.push(e)})},a.prototype.broadcastActive=function(){if(this.hasActive()){var t=this.callbackCtx_,e=this.activeObservations_.map(function(i){return new ha(i.target,i.broadcastRect())});this.callback_.call(t,e,t),this.clearActive()}},a.prototype.clearActive=function(){this.activeObservations_.splice(0)},a.prototype.hasActive=function(){return this.activeObservations_.length>0},a}(),We=typeof WeakMap<"u"?new WeakMap:new ze,Ye=function(){function a(t){if(!(this instanceof a))throw new TypeError("Cannot call a class as a function.");if(!arguments.length)throw new TypeError("1 argument required, but only 0 present.");var e=$i.getInstance(),i=new ca(t,e,this);We.set(this,i)}return a}();["observe","unobserve","disconnect"].forEach(function(a){Ye.prototype[a]=function(){var t;return(t=We.get(this))[a].apply(t,arguments)}});var wa=function(){return typeof Ot.ResizeObserver<"u"?Ot.ResizeObserver:Ye}();function xe(a){let t,e,i,n,r,o;const s=[fa,la],l=[];function f(h,c){return h[1]==="video"?0:1}return e=f(a),i=l[e]=s[e](a),{c(){t=ct("button"),i.c(),p(t,"class","svelte-425ent")},m(h,c){U(h,t,c),l[e].m(t,null),n=!0,r||(o=qe(t,"click",function(){Fe(a[1]==="image"?a[5]:a[6])&&(a[1]==="image"?a[5]:a[6]).apply(this,arguments)}),r=!0)},p(h,c){a=h;let u=e;e=f(a),e===u?l[e].p(a,c):(jt(),G(l[u],1,1,()=>{l[u]=null}),Vt(),i=l[e],i?i.p(a,c):(i=l[e]=s[e](a),i.c()),W(i,1),i.m(t,null))},i(h){n||(W(i),n=!0)},o(h){G(i),n=!1},d(h){h&&j(t),l[e].d(),r=!1,o()}}}function la(a){let t,e,i;return e=new Je({}),{c(){t=ct("div"),Gt(e.$$.fragment),p(t,"class","icon svelte-425ent")},m(n,r){U(n,t,r),qt(e,t,null),i=!0},p:I,i(n){i||(W(e.$$.fragment,n),i=!0)},o(n){G(e.$$.fragment,n),i=!1},d(n){n&&j(t),Ft(e)}}}function fa(a){let t,e,i,n;const r=[da,ua],o=[];function s(l,f){return l[4]?0:1}return t=s(a),e=o[t]=r[t](a),{c(){e.c(),i=Qe()},m(l,f){o[t].m(l,f),U(l,i,f),n=!0},p(l,f){let h=t;t=s(l),t!==h&&(jt(),G(o[h],1,1,()=>{o[h]=null}),Vt(),e=o[t],e||(e=o[t]=r[t](l),e.c()),W(e,1),e.m(i.parentNode,i))},i(l){n||(W(e),n=!0)},o(l){G(e),n=!1},d(l){l&&j(i),o[t].d(l)}}}function ua(a){let t,e,i;return e=new ti({}),{c(){t=ct("div"),Gt(e.$$.fragment),p(t,"class","icon svelte-425ent")},m(n,r){U(n,t,r),qt(e,t,null),i=!0},i(n){i||(W(e.$$.fragment,n),i=!0)},o(n){G(e.$$.fragment,n),i=!1},d(n){n&&j(t),Ft(e)}}}function da(a){let t,e,i;return e=new ii({}),{c(){t=ct("div"),Gt(e.$$.fragment),p(t,"class","icon svelte-425ent")},m(n,r){U(n,t,r),qt(e,t,null),i=!0},i(n){i||(W(e.$$.fragment,n),i=!0)},o(n){G(e.$$.fragment,n),i=!1},d(n){n&&j(t),Ft(e)}}}function pa(a){let t,e,i,n,r=!a[0]&&xe(a);return{c(){t=ct("div"),e=ct("video"),i=je(),r&&r.c(),p(e,"class","svelte-425ent"),re(e,"flip",a[2]),p(t,"class","wrap svelte-425ent")},m(o,s){U(o,t,s),Z(t,e),a[9](e),Z(t,i),r&&r.m(t,null),n=!0},p(o,[s]){(!n||s&4)&&re(e,"flip",o[2]),o[0]?r&&(jt(),G(r,1,1,()=>{r=null}),Vt()):r?(r.p(o,s),s&1&&W(r,1)):(r=xe(o),r.c(),W(r,1),r.m(t,null))},i(o){n||(W(r),n=!0)},o(o){G(r),n=!1},d(o){o&&j(t),a[9](null),r&&r.d()}}}function va(a,t,e){let i,n,{streaming:r=!1}=t,{pending:o=!1}=t,{mode:s="image"}=t,{mirror_webcam:l}=t,{include_audio:f}=t;const h=Ve();Ge(()=>n=document.createElement("canvas"));async function c(){try{_=await navigator.mediaDevices.getUserMedia({video:!0,audio:f}),e(3,i.srcObject=_,i),e(3,i.muted=!0,i),i.play()}catch(w){if(w instanceof DOMException&&w.name=="NotAllowedError")return h("error","Please allow access to the webcam for recording."),null;throw w}}function u(){var w=n.getContext("2d");if(i.videoWidth&&i.videoHeight){n.width=i.videoWidth,n.height=i.videoHeight,w.drawImage(i,0,0,i.videoWidth,i.videoHeight);var M=n.toDataURL("image/png");h(r?"stream":"capture",M)}}let v=!1,g=[],_,m,x;function T(){if(v){x.stop();let w=new Blob(g,{type:m}),M=new FileReader;M.onload=function(d){d.target&&(h("capture",{data:d.target.result,name:"sample."+m.substring(6),is_example:!1}),h("stop_recording"))},M.readAsDataURL(w)}else{h("start_recording"),g=[];let w=["video/webm","video/mp4"];for(let M of w)if(MediaRecorder.isTypeSupported(M)){m=M;break}if(m===null){console.error("No supported MediaRecorder mimeType");return}x=new MediaRecorder(_,{mimeType:m}),x.addEventListener("dataavailable",function(M){g.push(M.data)}),x.start(200)}e(4,v=!v)}c(),r&&s==="image"&&window.setInterval(()=>{i&&!o&&u()},500);function O(w){Ke[w?"unshift":"push"](()=>{i=w,e(3,i)})}return a.$$set=w=>{"streaming"in w&&e(0,r=w.streaming),"pending"in w&&e(7,o=w.pending),"mode"in w&&e(1,s=w.mode),"mirror_webcam"in w&&e(2,l=w.mirror_webcam),"include_audio"in w&&e(8,f=w.include_audio)},[r,s,l,i,v,u,T,o,f,O]}class ya extends bt{constructor(t){super(),wt(this,t,va,pa,yt,{streaming:0,pending:7,mode:1,mirror_webcam:2,include_audio:8})}}export{Gi as C,ba as U,ya as W,wa as i};
-//# sourceMappingURL=StaticImage.svelte_svelte_type_style_lang-72cfcc0b.js.map
diff --git a/spaces/cihyFjudo/fairness-paper-search/Disable Show window contents while dragging In Windows 10 Tips and Tricks.md b/spaces/cihyFjudo/fairness-paper-search/Disable Show window contents while dragging In Windows 10 Tips and Tricks.md
deleted file mode 100644
index c4b3c792d69342aec00ce080a3d3f04b54cf43c4..0000000000000000000000000000000000000000
--- a/spaces/cihyFjudo/fairness-paper-search/Disable Show window contents while dragging In Windows 10 Tips and Tricks.md
+++ /dev/null
@@ -1,13 +0,0 @@
-
-I have everything there, except that when I drag windows, I only see the border of the window as I'm dragging. In other/older SKUs of Windows, there was a checkbox that I think said "Show Window Contents While Dragging", but I can't find such a checkbox on this OS.
-The setting(disable full window drag:i:1) is used to determine whether folder contents appear when you drag the folder to a new location. This setting corresponds to the selection in theShow contents of window while dragging check box on the Experience tab of Remote Desktop Connection Options .
-Disable Show window contents while dragging In Windows 10 DOWNLOAD ••• https://tinurli.com/2uwkBW
-The remoteapp windows dragging contents are controller by your computer. If your local computer has show contents of window while dragging enabled then the remoteapp will show the contents as well. If you want it off like I do just create a GPO to disable the "show window content dragging" or change it manually per computer.
-We are running various versions of the Citrix Plugin on both Windows XP and Windows 7 clients and they all show following behaviour: When using the Citrix plugin to work with remote applications and/or virtual desktops, the plugin disables the LOCAL setting "Show WIndows Contents while dragging" (so on the Windows XP/7 Client!). The moment when the option is disabled is not consistent. Sometimes the option is disabled immediately after starting a remote app, sometimes after some time, sometimes the option is not disabled at all (but most of the times it is!). A lot of our users are complaining about this, because they find the "Show Windows Contents while dragging" option very usefull. We are wondering if there is a way to prevent this from happening!
-However, I'm experiencing a problem with your mouse software (BlackElement.exe). As long as the software runs, the windows feature "Show window contents while dragging" in the System Properties gets disabled (the according registry entry stays how he was set however...).
-I have the same trouble, with Tt black element software, i reinstall windows 10 and everything go right before install mice software. I disabled the mouse software at windows start up, and go ok, however when software starts with windows the trouble appears again.
-Been seeing more and more people struggling with this lately when working with Server 2019 or Windows 10 RDS. The issue shows itself with the default settings for Server 2019, and is caused by the normal 1px border not beein there anymore. And the default color settings is that everything is white, and has shadows under the windows. This is ok, when you have shadows enabled, as you then can see the difference on the windows, when working with multiple windows overlaying each others. If you are not sure about what I am refering to, the issue looks like this:
-
-I'm with you Luis. I have been using El Capitan since the first beta last July if I remember correctly and have never accidentally triggered Mission Control by dragging windows to the top of the screen. ?
aaccfb2cb3
-
-
\ No newline at end of file
diff --git a/spaces/cihyFjudo/fairness-paper-search/Download Film Ahista Ahista 1 Full Movie Free HOT!.md b/spaces/cihyFjudo/fairness-paper-search/Download Film Ahista Ahista 1 Full Movie Free HOT!.md
deleted file mode 100644
index 2d8c2d1a4c03d1676e5d4b96feb197e1d651b562..0000000000000000000000000000000000000000
--- a/spaces/cihyFjudo/fairness-paper-search/Download Film Ahista Ahista 1 Full Movie Free HOT!.md
+++ /dev/null
@@ -1,6 +0,0 @@
-Download Film Ahista Ahista 1 Full Movie Free Download ❤❤❤ https://tinurli.com/2uwkmo
-
- aaccfb2cb3
-
-
-
diff --git a/spaces/cihyFjudo/fairness-paper-search/Follow Adder 1.1.150812 with Key How to Schedule Your Posts and Stories in Advance.md b/spaces/cihyFjudo/fairness-paper-search/Follow Adder 1.1.150812 with Key How to Schedule Your Posts and Stories in Advance.md
deleted file mode 100644
index 3e49da54c71b781858151549ca0de2e7be0ca2e2..0000000000000000000000000000000000000000
--- a/spaces/cihyFjudo/fairness-paper-search/Follow Adder 1.1.150812 with Key How to Schedule Your Posts and Stories in Advance.md
+++ /dev/null
@@ -1,6 +0,0 @@
-Follow Adder 1.1.150812 with Key Download Zip ✦ https://tinurli.com/2uwiTh
-
- aaccfb2cb3
-
-
-
diff --git a/spaces/cihyFjudo/fairness-paper-search/Fractalius - Photoshop Filter Plugin Serial Key Keygen Explore the World of Fractal Art with This Powerful Plugin.md b/spaces/cihyFjudo/fairness-paper-search/Fractalius - Photoshop Filter Plugin Serial Key Keygen Explore the World of Fractal Art with This Powerful Plugin.md
deleted file mode 100644
index ad94e315e28759592ed43b6496e058a22367f336..0000000000000000000000000000000000000000
--- a/spaces/cihyFjudo/fairness-paper-search/Fractalius - Photoshop Filter Plugin Serial Key Keygen Explore the World of Fractal Art with This Powerful Plugin.md
+++ /dev/null
@@ -1,6 +0,0 @@
-
-Using warez version, crack, warez passwords, patches, serial numbers, registration codes, key generator, pirate key, keymaker or keygen forFractalius plug-in 1.77 license key is illegal and prevent future development ofFractalius plug-in 1.77 . Download links are directly from our mirrors or publisher's website,Fractalius plug-in 1.77 torrent files or shared files from free file sharing and free upload services,including Fractalius plug-in 1.77 Rapidshare, MegaUpload, HellShare, HotFile, FileServe, YouSendIt, SendSpace, DepositFiles, Letitbit, MailBigFile, DropSend, MediaMax, LeapFile, zUpload, MyOtherDrive, DivShare or MediaFire,are not allowed!
-Your computer will be at risk getting infected with spyware, adware, viruses, worms, trojan horses, dialers, etcwhile you are searching and browsing these illegal sites which distribute a so called keygen, key generator, pirate key, serial number, warez full version or crack forFractalius plug-in 1.77 . These infections might corrupt your computer installation or breach your privacy.Fractalius plug-in 1.77 keygen or key generator might contain a trojan horse opening a backdoor on your computer.Hackers can use this backdoor to take control of your computer, copy data from your computer or to use your computer to distribute viruses and spam to other people.
-Fractalius - Photoshop Filter Plugin Serial Key Keygen Download Zip ✔ https://tinurli.com/2uwiXA
aaccfb2cb3
-
-
\ No newline at end of file
diff --git a/spaces/cihyFjudo/fairness-paper-search/Highway 203 2 Tamil Movie Download How to Get the Full HD Version for Free.md b/spaces/cihyFjudo/fairness-paper-search/Highway 203 2 Tamil Movie Download How to Get the Full HD Version for Free.md
deleted file mode 100644
index 96f3bcb1e9973d0a4e0553f0a37d4b6d144b9ace..0000000000000000000000000000000000000000
--- a/spaces/cihyFjudo/fairness-paper-search/Highway 203 2 Tamil Movie Download How to Get the Full HD Version for Free.md
+++ /dev/null
@@ -1,6 +0,0 @@
-Highway 203 2 Tamil Movie Download Download Zip ⇔ https://tinurli.com/2uwj5B
-
- aaccfb2cb3
-
-
-
diff --git a/spaces/cihyFjudo/fairness-paper-search/NordVPN 6.19.4 Crack What You Need to Know Before You Download It.md b/spaces/cihyFjudo/fairness-paper-search/NordVPN 6.19.4 Crack What You Need to Know Before You Download It.md
deleted file mode 100644
index 1a228e4ddfa321a70f7f58cf1af1cd03fef583b1..0000000000000000000000000000000000000000
--- a/spaces/cihyFjudo/fairness-paper-search/NordVPN 6.19.4 Crack What You Need to Know Before You Download It.md
+++ /dev/null
@@ -1,6 +0,0 @@
-NordVPN 6.19.4 Crack Download Zip ✒ ✒ ✒ https://tinurli.com/2uwjVb
-
- aaccfb2cb3
-
-
-
diff --git a/spaces/cihyFjudo/fairness-paper-search/Simandl 30 Etudes Pdf 22l Learn to Play the Double Bass with Simandls Method.md b/spaces/cihyFjudo/fairness-paper-search/Simandl 30 Etudes Pdf 22l Learn to Play the Double Bass with Simandls Method.md
deleted file mode 100644
index 498ddb208829b6c1ae9ba01515bb84aca7116ade..0000000000000000000000000000000000000000
--- a/spaces/cihyFjudo/fairness-paper-search/Simandl 30 Etudes Pdf 22l Learn to Play the Double Bass with Simandls Method.md
+++ /dev/null
@@ -1,6 +0,0 @@
-Simandl 30 Etudes Pdf 22l Download File » https://tinurli.com/2uwiNT
-
- aaccfb2cb3
-
-
-
diff --git a/spaces/cleanmaster/so-vits-svc-akagi/hubert/__init__.py b/spaces/cleanmaster/so-vits-svc-akagi/hubert/__init__.py
deleted file mode 100644
index e69de29bb2d1d6434b8b29ae775ad8c2e48c5391..0000000000000000000000000000000000000000
diff --git a/spaces/cloudtheboi/Lofi4All/.pythonlibs/lib/python3.10/site-packages/cffi/_cffi_errors.h b/spaces/cloudtheboi/Lofi4All/.pythonlibs/lib/python3.10/site-packages/cffi/_cffi_errors.h
deleted file mode 100644
index 158e0590346a9a8b2ab047ac1bd23bcb3af21398..0000000000000000000000000000000000000000
--- a/spaces/cloudtheboi/Lofi4All/.pythonlibs/lib/python3.10/site-packages/cffi/_cffi_errors.h
+++ /dev/null
@@ -1,149 +0,0 @@
-#ifndef CFFI_MESSAGEBOX
-# ifdef _MSC_VER
-# define CFFI_MESSAGEBOX 1
-# else
-# define CFFI_MESSAGEBOX 0
-# endif
-#endif
-
-
-#if CFFI_MESSAGEBOX
-/* Windows only: logic to take the Python-CFFI embedding logic
- initialization errors and display them in a background thread
- with MessageBox. The idea is that if the whole program closes
- as a result of this problem, then likely it is already a console
- program and you can read the stderr output in the console too.
- If it is not a console program, then it will likely show its own
- dialog to complain, or generally not abruptly close, and for this
- case the background thread should stay alive.
-*/
-static void *volatile _cffi_bootstrap_text;
-
-static PyObject *_cffi_start_error_capture(void)
-{
- PyObject *result = NULL;
- PyObject *x, *m, *bi;
-
- if (InterlockedCompareExchangePointer(&_cffi_bootstrap_text,
- (void *)1, NULL) != NULL)
- return (PyObject *)1;
-
- m = PyImport_AddModule("_cffi_error_capture");
- if (m == NULL)
- goto error;
-
- result = PyModule_GetDict(m);
- if (result == NULL)
- goto error;
-
-#if PY_MAJOR_VERSION >= 3
- bi = PyImport_ImportModule("builtins");
-#else
- bi = PyImport_ImportModule("__builtin__");
-#endif
- if (bi == NULL)
- goto error;
- PyDict_SetItemString(result, "__builtins__", bi);
- Py_DECREF(bi);
-
- x = PyRun_String(
- "import sys\n"
- "class FileLike:\n"
- " def write(self, x):\n"
- " try:\n"
- " of.write(x)\n"
- " except: pass\n"
- " self.buf += x\n"
- " def flush(self):\n"
- " pass\n"
- "fl = FileLike()\n"
- "fl.buf = ''\n"
- "of = sys.stderr\n"
- "sys.stderr = fl\n"
- "def done():\n"
- " sys.stderr = of\n"
- " return fl.buf\n", /* make sure the returned value stays alive */
- Py_file_input,
- result, result);
- Py_XDECREF(x);
-
- error:
- if (PyErr_Occurred())
- {
- PyErr_WriteUnraisable(Py_None);
- PyErr_Clear();
- }
- return result;
-}
-
-#pragma comment(lib, "user32.lib")
-
-static DWORD WINAPI _cffi_bootstrap_dialog(LPVOID ignored)
-{
- Sleep(666); /* may be interrupted if the whole process is closing */
-#if PY_MAJOR_VERSION >= 3
- MessageBoxW(NULL, (wchar_t *)_cffi_bootstrap_text,
- L"Python-CFFI error",
- MB_OK | MB_ICONERROR);
-#else
- MessageBoxA(NULL, (char *)_cffi_bootstrap_text,
- "Python-CFFI error",
- MB_OK | MB_ICONERROR);
-#endif
- _cffi_bootstrap_text = NULL;
- return 0;
-}
-
-static void _cffi_stop_error_capture(PyObject *ecap)
-{
- PyObject *s;
- void *text;
-
- if (ecap == (PyObject *)1)
- return;
-
- if (ecap == NULL)
- goto error;
-
- s = PyRun_String("done()", Py_eval_input, ecap, ecap);
- if (s == NULL)
- goto error;
-
- /* Show a dialog box, but in a background thread, and
- never show multiple dialog boxes at once. */
-#if PY_MAJOR_VERSION >= 3
- text = PyUnicode_AsWideCharString(s, NULL);
-#else
- text = PyString_AsString(s);
-#endif
-
- _cffi_bootstrap_text = text;
-
- if (text != NULL)
- {
- HANDLE h;
- h = CreateThread(NULL, 0, _cffi_bootstrap_dialog,
- NULL, 0, NULL);
- if (h != NULL)
- CloseHandle(h);
- }
- /* decref the string, but it should stay alive as 'fl.buf'
- in the small module above. It will really be freed only if
- we later get another similar error. So it's a leak of at
- most one copy of the small module. That's fine for this
- situation which is usually a "fatal error" anyway. */
- Py_DECREF(s);
- PyErr_Clear();
- return;
-
- error:
- _cffi_bootstrap_text = NULL;
- PyErr_Clear();
-}
-
-#else
-
-static PyObject *_cffi_start_error_capture(void) { return NULL; }
-static void _cffi_stop_error_capture(PyObject *ecap) { }
-
-#endif
diff --git a/spaces/colakin/video-generater/public/ffmpeg/fftools/ffmpeg_demux.c b/spaces/colakin/video-generater/public/ffmpeg/fftools/ffmpeg_demux.c
deleted file mode 100644
index 5afb3ff2c8288f04dfd9f84f7565dd07e4912512..0000000000000000000000000000000000000000
--- a/spaces/colakin/video-generater/public/ffmpeg/fftools/ffmpeg_demux.c
+++ /dev/null
@@ -1,1294 +0,0 @@
-/*
- * This file is part of FFmpeg.
- *
- * FFmpeg is free software; you can redistribute it and/or
- * modify it under the terms of the GNU Lesser General Public
- * License as published by the Free Software Foundation; either
- * version 2.1 of the License, or (at your option) any later version.
- *
- * FFmpeg is distributed in the hope that it will be useful,
- * but WITHOUT ANY WARRANTY; without even the implied warranty of
- * MERCHANTABILITY or FITNESS FOR A PARTICULAR PURPOSE. See the GNU
- * Lesser General Public License for more details.
- *
- * You should have received a copy of the GNU Lesser General Public
- * License along with FFmpeg; if not, write to the Free Software
- * Foundation, Inc., 51 Franklin Street, Fifth Floor, Boston, MA 02110-1301 USA
- */
-
-#include
-#include
-
-#include "ffmpeg.h"
-
-#include "libavutil/avassert.h"
-#include "libavutil/avstring.h"
-#include "libavutil/display.h"
-#include "libavutil/error.h"
-#include "libavutil/intreadwrite.h"
-#include "libavutil/opt.h"
-#include "libavutil/parseutils.h"
-#include "libavutil/pixdesc.h"
-#include "libavutil/time.h"
-#include "libavutil/timestamp.h"
-#include "libavutil/thread.h"
-#include "libavutil/threadmessage.h"
-
-#include "libavcodec/packet.h"
-
-#include "libavformat/avformat.h"
-
-static const char *const opt_name_discard[] = {"discard", NULL};
-static const char *const opt_name_reinit_filters[] = {"reinit_filter", NULL};
-static const char *const opt_name_fix_sub_duration[] = {"fix_sub_duration", NULL};
-static const char *const opt_name_canvas_sizes[] = {"canvas_size", NULL};
-static const char *const opt_name_guess_layout_max[] = {"guess_layout_max", NULL};
-static const char *const opt_name_ts_scale[] = {"itsscale", NULL};
-static const char *const opt_name_hwaccels[] = {"hwaccel", NULL};
-static const char *const opt_name_hwaccel_devices[] = {"hwaccel_device", NULL};
-static const char *const opt_name_hwaccel_output_formats[] = {"hwaccel_output_format", NULL};
-static const char *const opt_name_autorotate[] = {"autorotate", NULL};
-static const char *const opt_name_display_rotations[] = {"display_rotation", NULL};
-static const char *const opt_name_display_hflips[] = {"display_hflip", NULL};
-static const char *const opt_name_display_vflips[] = {"display_vflip", NULL};
-
-typedef struct DemuxStream {
- InputStream ist;
-
- // name used for logging
- char log_name[32];
-
- double ts_scale;
-
- int64_t min_pts; /* pts with the smallest value in a current stream */
- int64_t max_pts; /* pts with the higher value in a current stream */
-} DemuxStream;
-
-typedef struct Demuxer {
- InputFile f;
-
- // name used for logging
- char log_name[32];
-
- /* number of times input stream should be looped */
- int loop;
- /* actual duration of the longest stream in a file at the moment when
- * looping happens */
- int64_t duration;
- /* time base of the duration */
- AVRational time_base;
-
- /* number of streams that the user was warned of */
- int nb_streams_warn;
-
- AVThreadMessageQueue *in_thread_queue;
- int thread_queue_size;
- pthread_t thread;
- int non_blocking;
-} Demuxer;
-
-typedef struct DemuxMsg {
- AVPacket *pkt;
- int looping;
-
- // repeat_pict from the demuxer-internal parser
- int repeat_pict;
-} DemuxMsg;
-
-static DemuxStream *ds_from_ist(InputStream *ist)
-{
- return (DemuxStream*)ist;
-}
-
-static Demuxer *demuxer_from_ifile(InputFile *f)
-{
- return (Demuxer*)f;
-}
-
-static void report_new_stream(Demuxer *d, const AVPacket *pkt)
-{
- AVStream *st = d->f.ctx->streams[pkt->stream_index];
-
- if (pkt->stream_index < d->nb_streams_warn)
- return;
- av_log(d, AV_LOG_WARNING,
- "New %s stream with index %d at pos:%"PRId64" and DTS:%ss\n",
- av_get_media_type_string(st->codecpar->codec_type),
- pkt->stream_index, pkt->pos, av_ts2timestr(pkt->dts, &st->time_base));
- d->nb_streams_warn = pkt->stream_index + 1;
-}
-
-static void ifile_duration_update(Demuxer *d, DemuxStream *ds,
- int64_t last_duration)
-{
- /* the total duration of the stream, max_pts - min_pts is
- * the duration of the stream without the last frame */
- if (ds->max_pts > ds->min_pts &&
- ds->max_pts - (uint64_t)ds->min_pts < INT64_MAX - last_duration)
- last_duration += ds->max_pts - ds->min_pts;
-
- if (!d->duration ||
- av_compare_ts(d->duration, d->time_base,
- last_duration, ds->ist.st->time_base) < 0) {
- d->duration = last_duration;
- d->time_base = ds->ist.st->time_base;
- }
-}
-
-static int seek_to_start(Demuxer *d)
-{
- InputFile *ifile = &d->f;
- AVFormatContext *is = ifile->ctx;
- int ret;
-
- ret = avformat_seek_file(is, -1, INT64_MIN, is->start_time, is->start_time, 0);
- if (ret < 0)
- return ret;
-
- if (ifile->audio_duration_queue_size) {
- /* duration is the length of the last frame in a stream
- * when audio stream is present we don't care about
- * last video frame length because it's not defined exactly */
- int got_durations = 0;
-
- while (got_durations < ifile->audio_duration_queue_size) {
- DemuxStream *ds;
- LastFrameDuration dur;
- ret = av_thread_message_queue_recv(ifile->audio_duration_queue, &dur, 0);
- if (ret < 0)
- return ret;
- got_durations++;
-
- ds = ds_from_ist(ifile->streams[dur.stream_idx]);
- ifile_duration_update(d, ds, dur.duration);
- }
- } else {
- for (int i = 0; i < ifile->nb_streams; i++) {
- int64_t duration = 0;
- InputStream *ist = ifile->streams[i];
- DemuxStream *ds = ds_from_ist(ist);
-
- if (ist->framerate.num) {
- duration = av_rescale_q(1, av_inv_q(ist->framerate), ist->st->time_base);
- } else if (ist->st->avg_frame_rate.num) {
- duration = av_rescale_q(1, av_inv_q(ist->st->avg_frame_rate), ist->st->time_base);
- } else {
- duration = 1;
- }
-
- ifile_duration_update(d, ds, duration);
- }
- }
-
- if (d->loop > 0)
- d->loop--;
-
- return ret;
-}
-
-static void ts_fixup(Demuxer *d, AVPacket *pkt, int *repeat_pict)
-{
- InputFile *ifile = &d->f;
- InputStream *ist = ifile->streams[pkt->stream_index];
- DemuxStream *ds = ds_from_ist(ist);
- const int64_t start_time = ifile->start_time_effective;
- int64_t duration;
-
- pkt->time_base = ist->st->time_base;
-
-#define SHOW_TS_DEBUG(tag_) \
- if (debug_ts) { \
- av_log(ist, AV_LOG_INFO, "%s -> ist_index:%d:%d type:%s " \
- "pkt_pts:%s pkt_pts_time:%s pkt_dts:%s pkt_dts_time:%s duration:%s duration_time:%s\n", \
- tag_, ifile->index, pkt->stream_index, \
- av_get_media_type_string(ist->st->codecpar->codec_type), \
- av_ts2str(pkt->pts), av_ts2timestr(pkt->pts, &pkt->time_base), \
- av_ts2str(pkt->dts), av_ts2timestr(pkt->dts, &pkt->time_base), \
- av_ts2str(pkt->duration), av_ts2timestr(pkt->duration, &pkt->time_base)); \
- }
-
- SHOW_TS_DEBUG("demuxer");
-
- if (!ist->wrap_correction_done && start_time != AV_NOPTS_VALUE &&
- ist->st->pts_wrap_bits < 64) {
- int64_t stime, stime2;
-
- stime = av_rescale_q(start_time, AV_TIME_BASE_Q, pkt->time_base);
- stime2= stime + (1ULL<st->pts_wrap_bits);
- ist->wrap_correction_done = 1;
-
- if(stime2 > stime && pkt->dts != AV_NOPTS_VALUE && pkt->dts > stime + (1LL<<(ist->st->pts_wrap_bits-1))) {
- pkt->dts -= 1ULL<st->pts_wrap_bits;
- ist->wrap_correction_done = 0;
- }
- if(stime2 > stime && pkt->pts != AV_NOPTS_VALUE && pkt->pts > stime + (1LL<<(ist->st->pts_wrap_bits-1))) {
- pkt->pts -= 1ULL<st->pts_wrap_bits;
- ist->wrap_correction_done = 0;
- }
- }
-
- if (pkt->dts != AV_NOPTS_VALUE)
- pkt->dts += av_rescale_q(ifile->ts_offset, AV_TIME_BASE_Q, pkt->time_base);
- if (pkt->pts != AV_NOPTS_VALUE)
- pkt->pts += av_rescale_q(ifile->ts_offset, AV_TIME_BASE_Q, pkt->time_base);
-
- if (pkt->pts != AV_NOPTS_VALUE)
- pkt->pts *= ds->ts_scale;
- if (pkt->dts != AV_NOPTS_VALUE)
- pkt->dts *= ds->ts_scale;
-
- duration = av_rescale_q(d->duration, d->time_base, pkt->time_base);
- if (pkt->pts != AV_NOPTS_VALUE) {
- pkt->pts += duration;
- ds->max_pts = FFMAX(pkt->pts, ds->max_pts);
- ds->min_pts = FFMIN(pkt->pts, ds->min_pts);
- }
-
- if (pkt->dts != AV_NOPTS_VALUE)
- pkt->dts += duration;
-
- *repeat_pict = -1;
- if (ist->st->codecpar->codec_type == AVMEDIA_TYPE_VIDEO &&
- av_stream_get_parser(ist->st))
- *repeat_pict = av_stream_get_parser(ist->st)->repeat_pict;
-
- SHOW_TS_DEBUG("demuxer+tsfixup");
-}
-
-static void thread_set_name(InputFile *f)
-{
- char name[16];
- snprintf(name, sizeof(name), "dmx%d:%s", f->index, f->ctx->iformat->name);
- ff_thread_setname(name);
-}
-
-static void *input_thread(void *arg)
-{
- Demuxer *d = arg;
- InputFile *f = &d->f;
- AVPacket *pkt;
- unsigned flags = d->non_blocking ? AV_THREAD_MESSAGE_NONBLOCK : 0;
- int ret = 0;
-
- pkt = av_packet_alloc();
- if (!pkt) {
- ret = AVERROR(ENOMEM);
- goto finish;
- }
-
- thread_set_name(f);
-
- while (1) {
- DemuxMsg msg = { NULL };
-
- ret = av_read_frame(f->ctx, pkt);
-
- if (ret == AVERROR(EAGAIN)) {
- av_usleep(10000);
- continue;
- }
- if (ret < 0) {
- if (d->loop) {
- /* signal looping to the consumer thread */
- msg.looping = 1;
- ret = av_thread_message_queue_send(d->in_thread_queue, &msg, 0);
- if (ret >= 0)
- ret = seek_to_start(d);
- if (ret >= 0)
- continue;
-
- /* fallthrough to the error path */
- }
-
- if (ret == AVERROR_EOF)
- av_log(d, AV_LOG_VERBOSE, "EOF while reading input\n");
- else
- av_log(d, AV_LOG_ERROR, "Error during demuxing: %s\n",
- av_err2str(ret));
-
- break;
- }
-
- if (do_pkt_dump) {
- av_pkt_dump_log2(NULL, AV_LOG_INFO, pkt, do_hex_dump,
- f->ctx->streams[pkt->stream_index]);
- }
-
- /* the following test is needed in case new streams appear
- dynamically in stream : we ignore them */
- if (pkt->stream_index >= f->nb_streams) {
- report_new_stream(d, pkt);
- av_packet_unref(pkt);
- continue;
- }
-
- if (pkt->flags & AV_PKT_FLAG_CORRUPT) {
- av_log(d, exit_on_error ? AV_LOG_FATAL : AV_LOG_WARNING,
- "corrupt input packet in stream %d\n",
- pkt->stream_index);
- if (exit_on_error) {
- av_packet_unref(pkt);
- ret = AVERROR_INVALIDDATA;
- break;
- }
- }
-
- ts_fixup(d, pkt, &msg.repeat_pict);
-
- msg.pkt = av_packet_alloc();
- if (!msg.pkt) {
- av_packet_unref(pkt);
- ret = AVERROR(ENOMEM);
- break;
- }
- av_packet_move_ref(msg.pkt, pkt);
- ret = av_thread_message_queue_send(d->in_thread_queue, &msg, flags);
- if (flags && ret == AVERROR(EAGAIN)) {
- flags = 0;
- ret = av_thread_message_queue_send(d->in_thread_queue, &msg, flags);
- av_log(f->ctx, AV_LOG_WARNING,
- "Thread message queue blocking; consider raising the "
- "thread_queue_size option (current value: %d)\n",
- d->thread_queue_size);
- }
- if (ret < 0) {
- if (ret != AVERROR_EOF)
- av_log(f->ctx, AV_LOG_ERROR,
- "Unable to send packet to main thread: %s\n",
- av_err2str(ret));
- av_packet_free(&msg.pkt);
- break;
- }
- }
-
-finish:
- av_assert0(ret < 0);
- av_thread_message_queue_set_err_recv(d->in_thread_queue, ret);
-
- av_packet_free(&pkt);
-
- av_log(d, AV_LOG_VERBOSE, "Terminating demuxer thread\n");
-
- return NULL;
-}
-
-static void thread_stop(Demuxer *d)
-{
- InputFile *f = &d->f;
- DemuxMsg msg;
-
- if (!d->in_thread_queue)
- return;
- av_thread_message_queue_set_err_send(d->in_thread_queue, AVERROR_EOF);
- while (av_thread_message_queue_recv(d->in_thread_queue, &msg, 0) >= 0)
- av_packet_free(&msg.pkt);
-
- pthread_join(d->thread, NULL);
- av_thread_message_queue_free(&d->in_thread_queue);
- av_thread_message_queue_free(&f->audio_duration_queue);
-}
-
-static int thread_start(Demuxer *d)
-{
- int ret;
- InputFile *f = &d->f;
-
- if (d->thread_queue_size <= 0)
- d->thread_queue_size = (nb_input_files > 1 ? 8 : 1);
-
- if (nb_input_files > 1 &&
- (f->ctx->pb ? !f->ctx->pb->seekable :
- strcmp(f->ctx->iformat->name, "lavfi")))
- d->non_blocking = 1;
- ret = av_thread_message_queue_alloc(&d->in_thread_queue,
- d->thread_queue_size, sizeof(DemuxMsg));
- if (ret < 0)
- return ret;
-
- if (d->loop) {
- int nb_audio_dec = 0;
-
- for (int i = 0; i < f->nb_streams; i++) {
- InputStream *ist = f->streams[i];
- nb_audio_dec += !!(ist->decoding_needed &&
- ist->st->codecpar->codec_type == AVMEDIA_TYPE_AUDIO);
- }
-
- if (nb_audio_dec) {
- ret = av_thread_message_queue_alloc(&f->audio_duration_queue,
- nb_audio_dec, sizeof(LastFrameDuration));
- if (ret < 0)
- goto fail;
- f->audio_duration_queue_size = nb_audio_dec;
- }
- }
-
- if ((ret = pthread_create(&d->thread, NULL, input_thread, d))) {
- av_log(d, AV_LOG_ERROR, "pthread_create failed: %s. Try to increase `ulimit -v` or decrease `ulimit -s`.\n", strerror(ret));
- ret = AVERROR(ret);
- goto fail;
- }
-
- return 0;
-fail:
- av_thread_message_queue_free(&d->in_thread_queue);
- return ret;
-}
-
-int ifile_get_packet(InputFile *f, AVPacket **pkt)
-{
- Demuxer *d = demuxer_from_ifile(f);
- InputStream *ist;
- DemuxMsg msg;
- int ret;
-
- if (!d->in_thread_queue) {
- ret = thread_start(d);
- if (ret < 0)
- return ret;
- }
-
- if (f->readrate || f->rate_emu) {
- int i;
- int64_t file_start = copy_ts * (
- (f->start_time_effective != AV_NOPTS_VALUE ? f->start_time_effective * !start_at_zero : 0) +
- (f->start_time != AV_NOPTS_VALUE ? f->start_time : 0)
- );
- float scale = f->rate_emu ? 1.0 : f->readrate;
- for (i = 0; i < f->nb_streams; i++) {
- InputStream *ist = f->streams[i];
- int64_t stream_ts_offset, pts, now;
- if (!ist->nb_packets || (ist->decoding_needed && !ist->got_output)) continue;
- stream_ts_offset = FFMAX(ist->first_dts != AV_NOPTS_VALUE ? ist->first_dts : 0, file_start);
- pts = av_rescale(ist->dts, 1000000, AV_TIME_BASE);
- now = (av_gettime_relative() - ist->start) * scale + stream_ts_offset;
- if (pts > now)
- return AVERROR(EAGAIN);
- }
- }
-
- ret = av_thread_message_queue_recv(d->in_thread_queue, &msg,
- d->non_blocking ?
- AV_THREAD_MESSAGE_NONBLOCK : 0);
- if (ret < 0)
- return ret;
- if (msg.looping)
- return 1;
-
- ist = f->streams[msg.pkt->stream_index];
- ist->last_pkt_repeat_pict = msg.repeat_pict;
-
- *pkt = msg.pkt;
- return 0;
-}
-
-static void demux_final_stats(Demuxer *d)
-{
- InputFile *f = &d->f;
- uint64_t total_packets = 0, total_size = 0;
-
- av_log(f, AV_LOG_VERBOSE, "Input file #%d (%s):\n",
- f->index, f->ctx->url);
-
- for (int j = 0; j < f->nb_streams; j++) {
- InputStream *ist = f->streams[j];
- enum AVMediaType type = ist->par->codec_type;
-
- total_size += ist->data_size;
- total_packets += ist->nb_packets;
-
- av_log(f, AV_LOG_VERBOSE, " Input stream #%d:%d (%s): ",
- f->index, j, av_get_media_type_string(type));
- av_log(f, AV_LOG_VERBOSE, "%"PRIu64" packets read (%"PRIu64" bytes); ",
- ist->nb_packets, ist->data_size);
-
- if (ist->decoding_needed) {
- av_log(f, AV_LOG_VERBOSE, "%"PRIu64" frames decoded",
- ist->frames_decoded);
- if (type == AVMEDIA_TYPE_AUDIO)
- av_log(f, AV_LOG_VERBOSE, " (%"PRIu64" samples)", ist->samples_decoded);
- av_log(f, AV_LOG_VERBOSE, "; ");
- }
-
- av_log(f, AV_LOG_VERBOSE, "\n");
- }
-
- av_log(f, AV_LOG_VERBOSE, " Total: %"PRIu64" packets (%"PRIu64" bytes) demuxed\n",
- total_packets, total_size);
-}
-
-static void ist_free(InputStream **pist)
-{
- InputStream *ist = *pist;
-
- if (!ist)
- return;
-
- av_frame_free(&ist->decoded_frame);
- av_packet_free(&ist->pkt);
- av_dict_free(&ist->decoder_opts);
- avsubtitle_free(&ist->prev_sub.subtitle);
- av_frame_free(&ist->sub2video.frame);
- av_freep(&ist->filters);
- av_freep(&ist->outputs);
- av_freep(&ist->hwaccel_device);
-
- avcodec_free_context(&ist->dec_ctx);
- avcodec_parameters_free(&ist->par);
-
- av_freep(pist);
-}
-
-void ifile_close(InputFile **pf)
-{
- InputFile *f = *pf;
- Demuxer *d = demuxer_from_ifile(f);
-
- if (!f)
- return;
-
- thread_stop(d);
-
- if (f->ctx)
- demux_final_stats(d);
-
- for (int i = 0; i < f->nb_streams; i++)
- ist_free(&f->streams[i]);
- av_freep(&f->streams);
-
- avformat_close_input(&f->ctx);
-
- av_freep(pf);
-}
-
-static void ist_use(InputStream *ist, int decoding_needed)
-{
- ist->discard = 0;
- ist->st->discard = ist->user_set_discard;
- ist->decoding_needed |= decoding_needed;
-
- if (decoding_needed && !avcodec_is_open(ist->dec_ctx)) {
- int ret = dec_open(ist);
- if (ret < 0)
- report_and_exit(ret);
- }
-}
-
-void ist_output_add(InputStream *ist, OutputStream *ost)
-{
- ist_use(ist, ost->enc ? DECODING_FOR_OST : 0);
-
- GROW_ARRAY(ist->outputs, ist->nb_outputs);
- ist->outputs[ist->nb_outputs - 1] = ost;
-}
-
-void ist_filter_add(InputStream *ist, InputFilter *ifilter, int is_simple)
-{
- ist_use(ist, is_simple ? DECODING_FOR_OST : DECODING_FOR_FILTER);
-
- GROW_ARRAY(ist->filters, ist->nb_filters);
- ist->filters[ist->nb_filters - 1] = ifilter;
-}
-
-static const AVCodec *choose_decoder(const OptionsContext *o, AVFormatContext *s, AVStream *st,
- enum HWAccelID hwaccel_id, enum AVHWDeviceType hwaccel_device_type)
-
-{
- char *codec_name = NULL;
-
- MATCH_PER_STREAM_OPT(codec_names, str, codec_name, s, st);
- if (codec_name) {
- const AVCodec *codec = find_codec_or_die(NULL, codec_name, st->codecpar->codec_type, 0);
- st->codecpar->codec_id = codec->id;
- if (recast_media && st->codecpar->codec_type != codec->type)
- st->codecpar->codec_type = codec->type;
- return codec;
- } else {
- if (st->codecpar->codec_type == AVMEDIA_TYPE_VIDEO &&
- hwaccel_id == HWACCEL_GENERIC &&
- hwaccel_device_type != AV_HWDEVICE_TYPE_NONE) {
- const AVCodec *c;
- void *i = NULL;
-
- while ((c = av_codec_iterate(&i))) {
- const AVCodecHWConfig *config;
-
- if (c->id != st->codecpar->codec_id ||
- !av_codec_is_decoder(c))
- continue;
-
- for (int j = 0; config = avcodec_get_hw_config(c, j); j++) {
- if (config->device_type == hwaccel_device_type) {
- av_log(NULL, AV_LOG_VERBOSE, "Selecting decoder '%s' because of requested hwaccel method %s\n",
- c->name, av_hwdevice_get_type_name(hwaccel_device_type));
- return c;
- }
- }
- }
- }
-
- return avcodec_find_decoder(st->codecpar->codec_id);
- }
-}
-
-static int guess_input_channel_layout(InputStream *ist, int guess_layout_max)
-{
- AVCodecContext *dec = ist->dec_ctx;
-
- if (dec->ch_layout.order == AV_CHANNEL_ORDER_UNSPEC) {
- char layout_name[256];
-
- if (dec->ch_layout.nb_channels > guess_layout_max)
- return 0;
- av_channel_layout_default(&dec->ch_layout, dec->ch_layout.nb_channels);
- if (dec->ch_layout.order == AV_CHANNEL_ORDER_UNSPEC)
- return 0;
- av_channel_layout_describe(&dec->ch_layout, layout_name, sizeof(layout_name));
- av_log(ist, AV_LOG_WARNING, "Guessed Channel Layout: %s\n", layout_name);
- }
- return 1;
-}
-
-static void add_display_matrix_to_stream(const OptionsContext *o,
- AVFormatContext *ctx, InputStream *ist)
-{
- AVStream *st = ist->st;
- double rotation = DBL_MAX;
- int hflip = -1, vflip = -1;
- int hflip_set = 0, vflip_set = 0, rotation_set = 0;
- int32_t *buf;
-
- MATCH_PER_STREAM_OPT(display_rotations, dbl, rotation, ctx, st);
- MATCH_PER_STREAM_OPT(display_hflips, i, hflip, ctx, st);
- MATCH_PER_STREAM_OPT(display_vflips, i, vflip, ctx, st);
-
- rotation_set = rotation != DBL_MAX;
- hflip_set = hflip != -1;
- vflip_set = vflip != -1;
-
- if (!rotation_set && !hflip_set && !vflip_set)
- return;
-
- buf = (int32_t *)av_stream_new_side_data(st, AV_PKT_DATA_DISPLAYMATRIX, sizeof(int32_t) * 9);
- if (!buf) {
- av_log(ist, AV_LOG_FATAL, "Failed to generate a display matrix!\n");
- exit_program(1);
- }
-
- av_display_rotation_set(buf,
- rotation_set ? -(rotation) : -0.0f);
-
- av_display_matrix_flip(buf,
- hflip_set ? hflip : 0,
- vflip_set ? vflip : 0);
-}
-
-static const char *input_stream_item_name(void *obj)
-{
- const DemuxStream *ds = obj;
-
- return ds->log_name;
-}
-
-static const AVClass input_stream_class = {
- .class_name = "InputStream",
- .version = LIBAVUTIL_VERSION_INT,
- .item_name = input_stream_item_name,
- .category = AV_CLASS_CATEGORY_DEMUXER,
-};
-
-static DemuxStream *demux_stream_alloc(Demuxer *d, AVStream *st)
-{
- const char *type_str = av_get_media_type_string(st->codecpar->codec_type);
- InputFile *f = &d->f;
- DemuxStream *ds = allocate_array_elem(&f->streams, sizeof(*ds),
- &f->nb_streams);
-
- ds->ist.st = st;
- ds->ist.file_index = f->index;
- ds->ist.class = &input_stream_class;
-
- snprintf(ds->log_name, sizeof(ds->log_name), "%cist#%d:%d/%s",
- type_str ? *type_str : '?', d->f.index, st->index,
- avcodec_get_name(st->codecpar->codec_id));
-
- return ds;
-}
-
-/* Add all the streams from the given input file to the demuxer */
-static void add_input_streams(const OptionsContext *o, Demuxer *d)
-{
- InputFile *f = &d->f;
- AVFormatContext *ic = f->ctx;
- int i, ret;
-
- for (i = 0; i < ic->nb_streams; i++) {
- AVStream *st = ic->streams[i];
- AVCodecParameters *par = st->codecpar;
- DemuxStream *ds;
- InputStream *ist;
- char *framerate = NULL, *hwaccel_device = NULL;
- const char *hwaccel = NULL;
- char *hwaccel_output_format = NULL;
- char *codec_tag = NULL;
- char *next;
- char *discard_str = NULL;
- const AVClass *cc = avcodec_get_class();
- const AVOption *discard_opt = av_opt_find(&cc, "skip_frame", NULL,
- 0, AV_OPT_SEARCH_FAKE_OBJ);
-
- ds = demux_stream_alloc(d, st);
- ist = &ds->ist;
-
- ist->discard = 1;
- st->discard = AVDISCARD_ALL;
- ist->nb_samples = 0;
- ist->first_dts = AV_NOPTS_VALUE;
- ist->next_pts = AV_NOPTS_VALUE;
- ist->next_dts = AV_NOPTS_VALUE;
-
- ds->min_pts = INT64_MAX;
- ds->max_pts = INT64_MIN;
-
- ds->ts_scale = 1.0;
- MATCH_PER_STREAM_OPT(ts_scale, dbl, ds->ts_scale, ic, st);
-
- ist->autorotate = 1;
- MATCH_PER_STREAM_OPT(autorotate, i, ist->autorotate, ic, st);
-
- MATCH_PER_STREAM_OPT(codec_tags, str, codec_tag, ic, st);
- if (codec_tag) {
- uint32_t tag = strtol(codec_tag, &next, 0);
- if (*next) {
- uint8_t buf[4] = { 0 };
- memcpy(buf, codec_tag, FFMIN(sizeof(buf), strlen(codec_tag)));
- tag = AV_RL32(buf);
- }
-
- st->codecpar->codec_tag = tag;
- }
-
- if (st->codecpar->codec_type == AVMEDIA_TYPE_VIDEO) {
- add_display_matrix_to_stream(o, ic, ist);
-
- MATCH_PER_STREAM_OPT(hwaccels, str, hwaccel, ic, st);
- MATCH_PER_STREAM_OPT(hwaccel_output_formats, str,
- hwaccel_output_format, ic, st);
-
- if (!hwaccel_output_format && hwaccel && !strcmp(hwaccel, "cuvid")) {
- av_log(ist, AV_LOG_WARNING,
- "WARNING: defaulting hwaccel_output_format to cuda for compatibility "
- "with old commandlines. This behaviour is DEPRECATED and will be removed "
- "in the future. Please explicitly set \"-hwaccel_output_format cuda\".\n");
- ist->hwaccel_output_format = AV_PIX_FMT_CUDA;
- } else if (!hwaccel_output_format && hwaccel && !strcmp(hwaccel, "qsv")) {
- av_log(ist, AV_LOG_WARNING,
- "WARNING: defaulting hwaccel_output_format to qsv for compatibility "
- "with old commandlines. This behaviour is DEPRECATED and will be removed "
- "in the future. Please explicitly set \"-hwaccel_output_format qsv\".\n");
- ist->hwaccel_output_format = AV_PIX_FMT_QSV;
- } else if (!hwaccel_output_format && hwaccel && !strcmp(hwaccel, "mediacodec")) {
- // There is no real AVHWFrameContext implementation. Set
- // hwaccel_output_format to avoid av_hwframe_transfer_data error.
- ist->hwaccel_output_format = AV_PIX_FMT_MEDIACODEC;
- } else if (hwaccel_output_format) {
- ist->hwaccel_output_format = av_get_pix_fmt(hwaccel_output_format);
- if (ist->hwaccel_output_format == AV_PIX_FMT_NONE) {
- av_log(ist, AV_LOG_FATAL, "Unrecognised hwaccel output "
- "format: %s", hwaccel_output_format);
- }
- } else {
- ist->hwaccel_output_format = AV_PIX_FMT_NONE;
- }
-
- if (hwaccel) {
- // The NVDEC hwaccels use a CUDA device, so remap the name here.
- if (!strcmp(hwaccel, "nvdec") || !strcmp(hwaccel, "cuvid"))
- hwaccel = "cuda";
-
- if (!strcmp(hwaccel, "none"))
- ist->hwaccel_id = HWACCEL_NONE;
- else if (!strcmp(hwaccel, "auto"))
- ist->hwaccel_id = HWACCEL_AUTO;
- else {
- enum AVHWDeviceType type = av_hwdevice_find_type_by_name(hwaccel);
- if (type != AV_HWDEVICE_TYPE_NONE) {
- ist->hwaccel_id = HWACCEL_GENERIC;
- ist->hwaccel_device_type = type;
- }
-
- if (!ist->hwaccel_id) {
- av_log(ist, AV_LOG_FATAL, "Unrecognized hwaccel: %s.\n",
- hwaccel);
- av_log(ist, AV_LOG_FATAL, "Supported hwaccels: ");
- type = AV_HWDEVICE_TYPE_NONE;
- while ((type = av_hwdevice_iterate_types(type)) !=
- AV_HWDEVICE_TYPE_NONE)
- av_log(ist, AV_LOG_FATAL, "%s ",
- av_hwdevice_get_type_name(type));
- av_log(ist, AV_LOG_FATAL, "\n");
- exit_program(1);
- }
- }
- }
-
- MATCH_PER_STREAM_OPT(hwaccel_devices, str, hwaccel_device, ic, st);
- if (hwaccel_device) {
- ist->hwaccel_device = av_strdup(hwaccel_device);
- if (!ist->hwaccel_device)
- report_and_exit(AVERROR(ENOMEM));
- }
-
- ist->hwaccel_pix_fmt = AV_PIX_FMT_NONE;
- }
-
- ist->dec = choose_decoder(o, ic, st, ist->hwaccel_id, ist->hwaccel_device_type);
- ist->decoder_opts = filter_codec_opts(o->g->codec_opts, ist->st->codecpar->codec_id, ic, st, ist->dec);
-
- ist->reinit_filters = -1;
- MATCH_PER_STREAM_OPT(reinit_filters, i, ist->reinit_filters, ic, st);
-
- MATCH_PER_STREAM_OPT(discard, str, discard_str, ic, st);
- ist->user_set_discard = AVDISCARD_NONE;
-
- if ((o->video_disable && ist->st->codecpar->codec_type == AVMEDIA_TYPE_VIDEO) ||
- (o->audio_disable && ist->st->codecpar->codec_type == AVMEDIA_TYPE_AUDIO) ||
- (o->subtitle_disable && ist->st->codecpar->codec_type == AVMEDIA_TYPE_SUBTITLE) ||
- (o->data_disable && ist->st->codecpar->codec_type == AVMEDIA_TYPE_DATA))
- ist->user_set_discard = AVDISCARD_ALL;
-
- if (discard_str && av_opt_eval_int(&cc, discard_opt, discard_str, &ist->user_set_discard) < 0) {
- av_log(ist, AV_LOG_ERROR, "Error parsing discard %s.\n",
- discard_str);
- exit_program(1);
- }
-
- ist->filter_in_rescale_delta_last = AV_NOPTS_VALUE;
- ist->prev_pkt_pts = AV_NOPTS_VALUE;
-
- ist->dec_ctx = avcodec_alloc_context3(ist->dec);
- if (!ist->dec_ctx)
- report_and_exit(AVERROR(ENOMEM));
-
- ret = avcodec_parameters_to_context(ist->dec_ctx, par);
- if (ret < 0) {
- av_log(ist, AV_LOG_ERROR, "Error initializing the decoder context.\n");
- exit_program(1);
- }
-
- ist->decoded_frame = av_frame_alloc();
- if (!ist->decoded_frame)
- report_and_exit(AVERROR(ENOMEM));
-
- ist->pkt = av_packet_alloc();
- if (!ist->pkt)
- report_and_exit(AVERROR(ENOMEM));
-
- if (o->bitexact)
- ist->dec_ctx->flags |= AV_CODEC_FLAG_BITEXACT;
-
- switch (par->codec_type) {
- case AVMEDIA_TYPE_VIDEO:
- // avformat_find_stream_info() doesn't set this for us anymore.
- ist->dec_ctx->framerate = st->avg_frame_rate;
-
- MATCH_PER_STREAM_OPT(frame_rates, str, framerate, ic, st);
- if (framerate && av_parse_video_rate(&ist->framerate,
- framerate) < 0) {
- av_log(ist, AV_LOG_ERROR, "Error parsing framerate %s.\n",
- framerate);
- exit_program(1);
- }
-
- ist->top_field_first = -1;
- MATCH_PER_STREAM_OPT(top_field_first, i, ist->top_field_first, ic, st);
-
- ist->framerate_guessed = av_guess_frame_rate(ic, st, NULL);
-
- ist->last_frame_pts = AV_NOPTS_VALUE;
-
- break;
- case AVMEDIA_TYPE_AUDIO: {
- int guess_layout_max = INT_MAX;
- MATCH_PER_STREAM_OPT(guess_layout_max, i, guess_layout_max, ic, st);
- guess_input_channel_layout(ist, guess_layout_max);
- break;
- }
- case AVMEDIA_TYPE_DATA:
- case AVMEDIA_TYPE_SUBTITLE: {
- char *canvas_size = NULL;
- MATCH_PER_STREAM_OPT(fix_sub_duration, i, ist->fix_sub_duration, ic, st);
- MATCH_PER_STREAM_OPT(canvas_sizes, str, canvas_size, ic, st);
- if (canvas_size &&
- av_parse_video_size(&ist->dec_ctx->width, &ist->dec_ctx->height, canvas_size) < 0) {
- av_log(ist, AV_LOG_FATAL, "Invalid canvas size: %s.\n", canvas_size);
- exit_program(1);
- }
- break;
- }
- case AVMEDIA_TYPE_ATTACHMENT:
- case AVMEDIA_TYPE_UNKNOWN:
- break;
- default:
- abort();
- }
-
- ist->par = avcodec_parameters_alloc();
- if (!ist->par)
- report_and_exit(AVERROR(ENOMEM));
-
- ret = avcodec_parameters_from_context(ist->par, ist->dec_ctx);
- if (ret < 0) {
- av_log(ist, AV_LOG_ERROR, "Error initializing the decoder context.\n");
- exit_program(1);
- }
- }
-}
-
-static void dump_attachment(InputStream *ist, const char *filename)
-{
- AVStream *st = ist->st;
- int ret;
- AVIOContext *out = NULL;
- const AVDictionaryEntry *e;
-
- if (!st->codecpar->extradata_size) {
- av_log(ist, AV_LOG_WARNING, "No extradata to dump.\n");
- return;
- }
- if (!*filename && (e = av_dict_get(st->metadata, "filename", NULL, 0)))
- filename = e->value;
- if (!*filename) {
- av_log(ist, AV_LOG_FATAL, "No filename specified and no 'filename' tag");
- exit_program(1);
- }
-
- assert_file_overwrite(filename);
-
- if ((ret = avio_open2(&out, filename, AVIO_FLAG_WRITE, &int_cb, NULL)) < 0) {
- av_log(ist, AV_LOG_FATAL, "Could not open file %s for writing.\n",
- filename);
- exit_program(1);
- }
-
- avio_write(out, st->codecpar->extradata, st->codecpar->extradata_size);
- avio_flush(out);
- avio_close(out);
-}
-
-static const char *input_file_item_name(void *obj)
-{
- const Demuxer *d = obj;
-
- return d->log_name;
-}
-
-static const AVClass input_file_class = {
- .class_name = "InputFile",
- .version = LIBAVUTIL_VERSION_INT,
- .item_name = input_file_item_name,
- .category = AV_CLASS_CATEGORY_DEMUXER,
-};
-
-static Demuxer *demux_alloc(void)
-{
- Demuxer *d = allocate_array_elem(&input_files, sizeof(*d), &nb_input_files);
-
- d->f.class = &input_file_class;
- d->f.index = nb_input_files - 1;
-
- snprintf(d->log_name, sizeof(d->log_name), "in#%d", d->f.index);
-
- return d;
-}
-
-int ifile_open(const OptionsContext *o, const char *filename)
-{
- Demuxer *d;
- InputFile *f;
- AVFormatContext *ic;
- const AVInputFormat *file_iformat = NULL;
- int err, i, ret;
- int64_t timestamp;
- AVDictionary *unused_opts = NULL;
- const AVDictionaryEntry *e = NULL;
- char * video_codec_name = NULL;
- char * audio_codec_name = NULL;
- char *subtitle_codec_name = NULL;
- char * data_codec_name = NULL;
- int scan_all_pmts_set = 0;
-
- int64_t start_time = o->start_time;
- int64_t start_time_eof = o->start_time_eof;
- int64_t stop_time = o->stop_time;
- int64_t recording_time = o->recording_time;
-
- d = demux_alloc();
- f = &d->f;
-
- if (stop_time != INT64_MAX && recording_time != INT64_MAX) {
- stop_time = INT64_MAX;
- av_log(d, AV_LOG_WARNING, "-t and -to cannot be used together; using -t.\n");
- }
-
- if (stop_time != INT64_MAX && recording_time == INT64_MAX) {
- int64_t start = start_time == AV_NOPTS_VALUE ? 0 : start_time;
- if (stop_time <= start) {
- av_log(d, AV_LOG_ERROR, "-to value smaller than -ss; aborting.\n");
- exit_program(1);
- } else {
- recording_time = stop_time - start;
- }
- }
-
- if (o->format) {
- if (!(file_iformat = av_find_input_format(o->format))) {
- av_log(d, AV_LOG_FATAL, "Unknown input format: '%s'\n", o->format);
- exit_program(1);
- }
- }
-
- if (!strcmp(filename, "-"))
- filename = "fd:";
-
- stdin_interaction &= strncmp(filename, "pipe:", 5) &&
- strcmp(filename, "fd:") &&
- strcmp(filename, "/dev/stdin");
-
- /* get default parameters from command line */
- ic = avformat_alloc_context();
- if (!ic)
- report_and_exit(AVERROR(ENOMEM));
- if (o->nb_audio_sample_rate) {
- av_dict_set_int(&o->g->format_opts, "sample_rate", o->audio_sample_rate[o->nb_audio_sample_rate - 1].u.i, 0);
- }
- if (o->nb_audio_channels) {
- const AVClass *priv_class;
- if (file_iformat && (priv_class = file_iformat->priv_class) &&
- av_opt_find(&priv_class, "ch_layout", NULL, 0,
- AV_OPT_SEARCH_FAKE_OBJ)) {
- char buf[32];
- snprintf(buf, sizeof(buf), "%dC", o->audio_channels[o->nb_audio_channels - 1].u.i);
- av_dict_set(&o->g->format_opts, "ch_layout", buf, 0);
- }
- }
- if (o->nb_audio_ch_layouts) {
- const AVClass *priv_class;
- if (file_iformat && (priv_class = file_iformat->priv_class) &&
- av_opt_find(&priv_class, "ch_layout", NULL, 0,
- AV_OPT_SEARCH_FAKE_OBJ)) {
- av_dict_set(&o->g->format_opts, "ch_layout", o->audio_ch_layouts[o->nb_audio_ch_layouts - 1].u.str, 0);
- }
- }
- if (o->nb_frame_rates) {
- const AVClass *priv_class;
- /* set the format-level framerate option;
- * this is important for video grabbers, e.g. x11 */
- if (file_iformat && (priv_class = file_iformat->priv_class) &&
- av_opt_find(&priv_class, "framerate", NULL, 0,
- AV_OPT_SEARCH_FAKE_OBJ)) {
- av_dict_set(&o->g->format_opts, "framerate",
- o->frame_rates[o->nb_frame_rates - 1].u.str, 0);
- }
- }
- if (o->nb_frame_sizes) {
- av_dict_set(&o->g->format_opts, "video_size", o->frame_sizes[o->nb_frame_sizes - 1].u.str, 0);
- }
- if (o->nb_frame_pix_fmts)
- av_dict_set(&o->g->format_opts, "pixel_format", o->frame_pix_fmts[o->nb_frame_pix_fmts - 1].u.str, 0);
-
- MATCH_PER_TYPE_OPT(codec_names, str, video_codec_name, ic, "v");
- MATCH_PER_TYPE_OPT(codec_names, str, audio_codec_name, ic, "a");
- MATCH_PER_TYPE_OPT(codec_names, str, subtitle_codec_name, ic, "s");
- MATCH_PER_TYPE_OPT(codec_names, str, data_codec_name, ic, "d");
-
- if (video_codec_name)
- ic->video_codec = find_codec_or_die(NULL, video_codec_name , AVMEDIA_TYPE_VIDEO , 0);
- if (audio_codec_name)
- ic->audio_codec = find_codec_or_die(NULL, audio_codec_name , AVMEDIA_TYPE_AUDIO , 0);
- if (subtitle_codec_name)
- ic->subtitle_codec = find_codec_or_die(NULL, subtitle_codec_name, AVMEDIA_TYPE_SUBTITLE, 0);
- if (data_codec_name)
- ic->data_codec = find_codec_or_die(NULL, data_codec_name , AVMEDIA_TYPE_DATA , 0);
-
- ic->video_codec_id = video_codec_name ? ic->video_codec->id : AV_CODEC_ID_NONE;
- ic->audio_codec_id = audio_codec_name ? ic->audio_codec->id : AV_CODEC_ID_NONE;
- ic->subtitle_codec_id = subtitle_codec_name ? ic->subtitle_codec->id : AV_CODEC_ID_NONE;
- ic->data_codec_id = data_codec_name ? ic->data_codec->id : AV_CODEC_ID_NONE;
-
- ic->flags |= AVFMT_FLAG_NONBLOCK;
- if (o->bitexact)
- ic->flags |= AVFMT_FLAG_BITEXACT;
- ic->interrupt_callback = int_cb;
-
- if (!av_dict_get(o->g->format_opts, "scan_all_pmts", NULL, AV_DICT_MATCH_CASE)) {
- av_dict_set(&o->g->format_opts, "scan_all_pmts", "1", AV_DICT_DONT_OVERWRITE);
- scan_all_pmts_set = 1;
- }
- /* open the input file with generic avformat function */
- err = avformat_open_input(&ic, filename, file_iformat, &o->g->format_opts);
- if (err < 0) {
- print_error(filename, err);
- if (err == AVERROR_PROTOCOL_NOT_FOUND)
- av_log(d, AV_LOG_ERROR, "Did you mean file:%s?\n", filename);
- exit_program(1);
- }
-
- av_strlcat(d->log_name, "/", sizeof(d->log_name));
- av_strlcat(d->log_name, ic->iformat->name, sizeof(d->log_name));
-
- if (scan_all_pmts_set)
- av_dict_set(&o->g->format_opts, "scan_all_pmts", NULL, AV_DICT_MATCH_CASE);
- remove_avoptions(&o->g->format_opts, o->g->codec_opts);
- assert_avoptions(o->g->format_opts);
-
- /* apply forced codec ids */
- for (i = 0; i < ic->nb_streams; i++)
- choose_decoder(o, ic, ic->streams[i], HWACCEL_NONE, AV_HWDEVICE_TYPE_NONE);
-
- if (o->find_stream_info) {
- AVDictionary **opts = setup_find_stream_info_opts(ic, o->g->codec_opts);
- int orig_nb_streams = ic->nb_streams;
-
- /* If not enough info to get the stream parameters, we decode the
- first frames to get it. (used in mpeg case for example) */
- ret = avformat_find_stream_info(ic, opts);
-
- for (i = 0; i < orig_nb_streams; i++)
- av_dict_free(&opts[i]);
- av_freep(&opts);
-
- if (ret < 0) {
- av_log(d, AV_LOG_FATAL, "could not find codec parameters\n");
- if (ic->nb_streams == 0) {
- avformat_close_input(&ic);
- exit_program(1);
- }
- }
- }
-
- if (start_time != AV_NOPTS_VALUE && start_time_eof != AV_NOPTS_VALUE) {
- av_log(d, AV_LOG_WARNING, "Cannot use -ss and -sseof both, using -ss\n");
- start_time_eof = AV_NOPTS_VALUE;
- }
-
- if (start_time_eof != AV_NOPTS_VALUE) {
- if (start_time_eof >= 0) {
- av_log(d, AV_LOG_ERROR, "-sseof value must be negative; aborting\n");
- exit_program(1);
- }
- if (ic->duration > 0) {
- start_time = start_time_eof + ic->duration;
- if (start_time < 0) {
- av_log(d, AV_LOG_WARNING, "-sseof value seeks to before start of file; ignored\n");
- start_time = AV_NOPTS_VALUE;
- }
- } else
- av_log(d, AV_LOG_WARNING, "Cannot use -sseof, file duration not known\n");
- }
- timestamp = (start_time == AV_NOPTS_VALUE) ? 0 : start_time;
- /* add the stream start time */
- if (!o->seek_timestamp && ic->start_time != AV_NOPTS_VALUE)
- timestamp += ic->start_time;
-
- /* if seeking requested, we execute it */
- if (start_time != AV_NOPTS_VALUE) {
- int64_t seek_timestamp = timestamp;
-
- if (!(ic->iformat->flags & AVFMT_SEEK_TO_PTS)) {
- int dts_heuristic = 0;
- for (i=0; inb_streams; i++) {
- const AVCodecParameters *par = ic->streams[i]->codecpar;
- if (par->video_delay) {
- dts_heuristic = 1;
- break;
- }
- }
- if (dts_heuristic) {
- seek_timestamp -= 3*AV_TIME_BASE / 23;
- }
- }
- ret = avformat_seek_file(ic, -1, INT64_MIN, seek_timestamp, seek_timestamp, 0);
- if (ret < 0) {
- av_log(d, AV_LOG_WARNING, "could not seek to position %0.3f\n",
- (double)timestamp / AV_TIME_BASE);
- }
- }
-
- f->ctx = ic;
- f->start_time = start_time;
- f->recording_time = recording_time;
- f->input_sync_ref = o->input_sync_ref;
- f->input_ts_offset = o->input_ts_offset;
- f->ts_offset = o->input_ts_offset - (copy_ts ? (start_at_zero && ic->start_time != AV_NOPTS_VALUE ? ic->start_time : 0) : timestamp);
- f->rate_emu = o->rate_emu;
- f->accurate_seek = o->accurate_seek;
- d->loop = o->loop;
- d->duration = 0;
- d->time_base = (AVRational){ 1, 1 };
-
- f->readrate = o->readrate ? o->readrate : 0.0;
- if (f->readrate < 0.0f) {
- av_log(d, AV_LOG_ERROR, "Option -readrate is %0.3f; it must be non-negative.\n", f->readrate);
- exit_program(1);
- }
- if (f->readrate && f->rate_emu) {
- av_log(d, AV_LOG_WARNING, "Both -readrate and -re set. Using -readrate %0.3f.\n", f->readrate);
- f->rate_emu = 0;
- }
-
- d->thread_queue_size = o->thread_queue_size;
-
- /* update the current parameters so that they match the one of the input stream */
- add_input_streams(o, d);
-
- /* dump the file content */
- av_dump_format(ic, f->index, filename, 0);
-
- /* check if all codec options have been used */
- unused_opts = strip_specifiers(o->g->codec_opts);
- for (i = 0; i < f->nb_streams; i++) {
- e = NULL;
- while ((e = av_dict_iterate(f->streams[i]->decoder_opts, e)))
- av_dict_set(&unused_opts, e->key, NULL, 0);
- }
-
- e = NULL;
- while ((e = av_dict_iterate(unused_opts, e))) {
- const AVClass *class = avcodec_get_class();
- const AVOption *option = av_opt_find(&class, e->key, NULL, 0,
- AV_OPT_SEARCH_CHILDREN | AV_OPT_SEARCH_FAKE_OBJ);
- const AVClass *fclass = avformat_get_class();
- const AVOption *foption = av_opt_find(&fclass, e->key, NULL, 0,
- AV_OPT_SEARCH_CHILDREN | AV_OPT_SEARCH_FAKE_OBJ);
- if (!option || foption)
- continue;
-
-
- if (!(option->flags & AV_OPT_FLAG_DECODING_PARAM)) {
- av_log(d, AV_LOG_ERROR, "Codec AVOption %s (%s) is not a decoding "
- "option.\n", e->key, option->help ? option->help : "");
- exit_program(1);
- }
-
- av_log(d, AV_LOG_WARNING, "Codec AVOption %s (%s) has not been used "
- "for any stream. The most likely reason is either wrong type "
- "(e.g. a video option with no video streams) or that it is a "
- "private option of some decoder which was not actually used "
- "for any stream.\n", e->key, option->help ? option->help : "");
- }
- av_dict_free(&unused_opts);
-
- for (i = 0; i < o->nb_dump_attachment; i++) {
- int j;
-
- for (j = 0; j < f->nb_streams; j++) {
- InputStream *ist = f->streams[j];
-
- if (check_stream_specifier(ic, ist->st, o->dump_attachment[i].specifier) == 1)
- dump_attachment(ist, o->dump_attachment[i].u.str);
- }
- }
-
- return 0;
-}
diff --git a/spaces/congsaPfin/Manga-OCR/logs/Discover My Talking Angela 2 The Game that Lets You Dance Bake Travel and More.md b/spaces/congsaPfin/Manga-OCR/logs/Discover My Talking Angela 2 The Game that Lets You Dance Bake Travel and More.md
deleted file mode 100644
index dc8738e795a945c51f3b4523542e5e6362e5a294..0000000000000000000000000000000000000000
--- a/spaces/congsaPfin/Manga-OCR/logs/Discover My Talking Angela 2 The Game that Lets You Dance Bake Travel and More.md
+++ /dev/null
@@ -1,125 +0,0 @@
-
-My Talking Angela 2: A Fun and Stylish Virtual Pet Game
-Do you love virtual pet games? Do you want to have a fashionable feline friend who can dance, bake, travel, and more? If you answered yes, then you should check out My Talking Angela 2 , the latest installment from the popular My Talking franchise by Outfit7. In this article, we will tell you everything you need to know about this game, including its features, tips and tricks, and reviews. Read on to find out why My Talking Angela 2 is one of the best virtual pet games on the market.
-my talking angela 2 download play store Download File » https://urlca.com/2uOaSP
- Introduction
-What is My Talking Angela 2?
-My Talking Angela 2 is a virtual pet game that makes every day more stylish and fun. Players help this fashionable cat stay busy in her big-city home. They can customize her hair, makeup, and fashion choices, as well as her apartment decor. They can also enjoy various activities and mini-games with her, such as dancing, baking, and martial arts. They can even travel the world with her and collect stickers along the way. My Talking Angela 2 is a game that lets players express their creativity and personality while having fun with their adorable virtual pet.
- Why should you play My Talking Angela 2?
-There are many reasons why you should play My Talking Angela 2. Here are some of them:
-
-It's free to download and play. You can access all the functionalities of the game without making any in-app purchases using real money.
-It's suitable for all ages. Whether you're a kid or an adult, you can enjoy playing with Angela and taking care of her needs.
-It's easy to play. The game has simple controls and intuitive interface that make it easy to navigate and interact with Angela.
-It's entertaining and engaging. The game has a lot of content and variety that keep you interested and entertained. You can explore different rooms, activities, mini-games, outfits, locations, and stickers.
-It's educational and beneficial. The game can help you improve your skills and reflexes, as well as your creativity and imagination. You can also learn new things from Angela, such as facts about different countries and cultures.
-
- Features of My Talking Angela 2
-Customize Angela's look and apartment
-One of the main features of My Talking Angela 2 is the ability to customize Angela's look and apartment. You can choose from a wide range of hair styles, colors, accessories, makeup products, clothes, shoes, bags, and more. You can also change the furniture and fittings in each room of her apartment. You can even paint a painting and hang it above her bed. You can reinvent Angela's look and home as often as you want, depending on your mood and preference.
- Enjoy various activities and mini-games
-Another feature of My Talking Angela 2 is the variety of activities and mini-games that you can enjoy with Angela. You can dance with her in her studio, bake with her in her kitchen, practice martial arts with her in her dojo, or relax with her in her spa room. You can also play various mini-games with her, such as puzzles, memory games, arcade games, and more. Each activity and mini-game has different levels of difficulty and rewards. You can earn coins, diamonds, stars, and stickers by playing with Angela.
- Travel the world and collect stickers
-A third feature of My Talking Angela 2 is the ability to travel the world and collect stickers with Angela. You can visit different countries and cities with her, such as Paris, London, New York, Tokyo, and more. You can also learn about their cultures and landmarks from Angela. Each location has a sticker album that you can fill up by finding hidden stickers in each scene. You can also exchange stickers with other players online. Traveling and collecting stickers is a fun way to expand your horizons and make new friends.
-My Talking Angela 2 app install from Google Play
-How to play My Talking Angela 2 on Android devices
-My Talking Angela 2 game features and tips
-Download My Talking Angela 2 for free on Play Store
-My Talking Angela 2 virtual pet simulation game
-Outfit7 My Talking Angela 2 latest updates and news
-My Talking Angela 2 reviews and ratings on Google Play
-My Talking Angela 2 fun activities and mini-games
-My Talking Angela 2 fashion and style choices
-My Talking Angela 2 stickers and rewards collection
-My Talking Angela 2 travel and adventure options
-My Talking Angela 2 YouTube videos and trailers
-My Talking Angela 2 in-app purchases and subscriptions
-My Talking Angela 2 data privacy and security policy
-My Talking Angela 2 customer support and feedback
-My Talking Angela 2 cheats and hacks for Android
-My Talking Angela 2 offline mode and data usage
-My Talking Angela 2 compatibility and performance issues
-My Talking Angela 2 alternatives and similar apps
-My Talking Angela 2 fan community and social media
-My Talking Angela 2 hair, makeup and nails salon
-My Talking Angela 2 dancing, baking and martial arts skills
-My Talking Angela 2 food and snacks recipes
-My Talking Angela 2 puzzles and reflexes tests
-My Talking Angela 2 outfits and accessories shop
-My Talking Angela 2 birthday party and celebration ideas
-My Talking Angela 2 voice interaction and chat feature
-My Talking Angela 2 customization and personalization options
-My Talking Angela 2 achievements and leaderboards
-My Talking Angela 2 wallpapers and backgrounds download
-My Talking Angela 2 mod apk download for Android
-My Talking Angela 2 unlimited coins and diamonds hack
-My Talking Angela 2 glitches and bugs fix guide
-My Talking Angela 2 comparison with other Outfit7 games
-My Talking Angela 2 trivia and facts you didn't know
-My Talking Angela 2 tips for beginners and advanced players
-My Talking Angela 2 best practices and strategies
-My Talking Angela 2 frequently asked questions and answers
-My Talking Angela 2 challenges and contests to join
-My Talking Angela 2 referral codes and rewards program
- Tips and tricks for My Talking Angela 2
-Play mini-games to earn coins and diamonds
-One of the tips and tricks for My Talking Angela 2 is to play mini-games to earn coins and diamonds. Coins and diamonds are the main currencies in the game that you can use to buy items and unlock features. You can earn coins by playing any mini-game, but some mini-games give you more coins than others. For example, the puzzle game gives you 10 coins per level, while the arcade game gives you 20 coins per level. You can also earn diamonds by playing the memory game or the dance game. Diamonds are more valuable than coins, so you should try to play these games as often as possible.
- Feed Angela smoothies for special effects
-Another tip and trick for My Talking Angela 2 is to feed Angela smoothies for special effects. Smoothies are drinks that you can make in the kitchen by combining different fruits and ingredients. Each smoothie has a different effect on Angela, such as making her happy, energetic, sleepy, or sick. You can use these effects to your advantage depending on the situation. For example, if you want to play more mini-games with Angela, you can feed her a smoothie that makes her energetic. If you want to make her sleep faster, you can feed her a smoothie that makes her sleepy.
- Take care of Angela's health and hygiene
-A third tip and trick for My Talking Angela 2 is to take care of Angela's health and hygiene. Angela has four meters that indicate her status: happiness, hunger, energy, and hygiene. You need to keep these meters high by doing various things for her, such as feeding her, playing with her, putting her to bed, or cleaning her. If you neglect any of these meters, Angela will become unhappy or sick, which will affect her performance and mood. You can also use items such as medicine or perfume to boost her meters quickly.
- Level up Angela to unlock new items and locations
-A fourth tip and trick for My Talking Angela 2 is to level up Angela to unlock new items and locations. Angela has a level meter that fills up as you play with her and take care of her. Each time you level up, you will unlock new items for her look and apartment, as well as new locations to travel to. You will also get a free gift box that contains coins, diamonds, or stickers. Leveling up is a great way to access more content and features in the game.
- Watch videos and complete tasks for extra rewards
-A fifth tip and trick for My Talking Angela 2 is to watch videos and complete tasks for extra rewards. You can watch videos in the TV room or in the shop to earn free coins or diamonds. You can also complete tasks in the task list or in the daily challenge to earn stars or stickers. These tasks are simple and easy to do, such as changing Angela's outfit or playing a mini-game. Watching videos and completing tasks is a good way to get more resources and items in the game.
- Reviews of My Talking Angela 2
-What do users say about My Talking Angela 2?
-My Talking Angela 2 has received mostly positive reviews from users who have downloaded and played it. Here are some of the comments from users who have rated it on Google Play Store:
-
-"I love this game so much! It's so fun and cute! I like how you can customize everything and travel around the world! The graphics are amazing and the animations are smooth! I recommend this game to everyone who loves virtual pet games!"
-"This game is awesome! It has so many features and activities that make it interesting and enjoyable! I like how you can interact with Angela and make her happy! The mini-games are also fun and challenging! The game is also very educational and teaches you about different countries and cultures!"
-"This game is good, but it has some flaws. It takes too long to load and sometimes crashes. It also has too many ads that interrupt the gameplay. It also requires a lot of storage space and internet connection. I hope the developers can fix these issues soon."
-
- What are the pros and cons of My Talking Angela 2?
-Based on the user reviews and our own experience, we have summarized the pros and cons of My Talking Angela 2 as follows:
-
-
-Pros
-Cons
-
-
-- Fun and cute virtual pet game
-- Long loading time and frequent crashes
-
-
-- Lots of customization options and variety
-- Too many ads and in-app purchases
-
-
-- Engaging and challenging activities and mini-games
-- High storage space and internet connection requirements
-
-
-- Educational and beneficial content and features
-- None
-
-
- Conclusion
-Summary of the main points
-In conclusion, My Talking Angela 2 is a fun and stylish virtual pet game that lets you have a fashionable feline friend who can dance, bake, travel, and more. You can customize her look and apartment, enjoy various activities and mini-games, travel the world and collect stickers, and learn new things from her. The game is free to download and play, suitable for all ages, easy to play, entertaining and engaging, and educational and beneficial. However, the game also has some drawbacks, such as long loading time, frequent crashes, too many ads, in-app purchases, high storage space, and internet connection requirements. Despite these flaws, My Talking Angela 2 is still one of the best virtual pet games on the market.
- Call to action
-If you are interested in playing My Talking Angela 2, you can download it from Google Play Store or Apple App Store. You can also visit the official website of Outfit7 for more information about the game and other related products. Don't miss this opportunity to have a fun and stylish virtual pet game that makes every day more exciting. Download My Talking Angela 2 today and enjoy playing with your adorable feline friend!
- FAQs
-Here are some of the frequently asked questions about My Talking Angela 2:
- Q: How do I talk to Angela?
-A: You can talk to Angela by tapping on the microphone icon on the bottom right corner of the screen. You can say anything you want to her, and she will repeat it in a funny voice. You can also make her laugh by tickling her or making funny noises.
- Q: How do I take a selfie with Angela?
-A: You can take a selfie with Angela by tapping on the camera icon on the top right corner of the screen. You can choose from different backgrounds, filters, stickers, and frames to make your selfie more fun and unique. You can also share your selfie with your friends on social media.
- Q: How do I play music with Angela?
-A: You can play music with Angela by tapping on the music icon on the bottom left corner of the screen. You can choose from different genres, such as pop, rock, hip hop, or classical. You can also create your own music by tapping on the instruments or using your voice.
- Q: How do I change Angela's name?
-A: You can change Angela's name by tapping on the settings icon on the top left corner of the screen. You can then tap on the name field and enter a new name for her. You can also change other settings, such as language, sound effects, notifications, or privacy.
- Q: How do I contact the developers of My Talking Angela 2?
-A: You can contact the developers of My Talking Angela 2 by tapping on the support icon on the bottom right corner of the settings menu. You can then choose from different options, such as feedback, report a problem, or FAQ. You can also email them at support@outfit7.com or visit their website at www.outfit7.com.
401be4b1e0
-
-
\ No newline at end of file
diff --git a/spaces/congsaPfin/Manga-OCR/logs/Download Instagram by the Dise Why You Need This Amazing App.md b/spaces/congsaPfin/Manga-OCR/logs/Download Instagram by the Dise Why You Need This Amazing App.md
deleted file mode 100644
index 4fbc555695a588bcb994a5e1e71aac4a1c31f5ea..0000000000000000000000000000000000000000
--- a/spaces/congsaPfin/Manga-OCR/logs/Download Instagram by the Dise Why You Need This Amazing App.md
+++ /dev/null
@@ -1,132 +0,0 @@
-
-Download Instagram by the DISE: A Guide for Android Users
-Instagram is one of the most popular social media platforms in the world, with over 1 billion monthly active users. It allows you to create and share your photos, stories, reels and videos with the friends and followers you care about. You can also explore your interests, discover new content, and connect with people from different cultures and backgrounds.
-But how can you download Instagram on your Android device? One of the easiest and fastest ways is to use the DISE app, a powerful tool that lets you download any app from Google Play Store without any hassle. In this article, we will show you how to download Instagram by the DISE, and also explain what the DISE is and why you should use it.
-download instagram by the dise Download › https://urlca.com/2uOdGw
- What is Instagram?
-Instagram is a free photo and video sharing app that was launched in 2010 by Kevin Systrom and Mike Krieger. It was acquired by Facebook in 2012 for $1 billion. Since then, it has grown to become one of the most influential social media platforms in the world, with celebrities, influencers, brands, and ordinary users using it to showcase their creativity, lifestyle, and personality.
-Instagram has many features that make it fun and engaging, such as:
-
-Filters: You can apply different filters to your photos and videos to enhance their appearance and mood.
-Stories: You can share ephemeral photos and videos that disappear after 24 hours with your followers or close friends.
-Reels: You can create short-form videos with music, effects, and stickers that can reach a wider audience on the Explore tab.
-IGTV: You can upload longer videos that can be up to an hour long on a dedicated section of the app.
-Live: You can broadcast live video to your followers and interact with them in real-time.
-DMs: You can send private messages, photos, videos, voice notes, and stickers to your friends or groups.
-Shopping: You can discover and buy products from your favorite brands and creators on Instagram.
-
- Why download Instagram?
-There are many reasons why you might want to download Instagram on your Android device, such as:
-
-You want to join a community of over 1 billion people who share your passions and interests.
-You want to express yourself through photos and videos that capture your moments and memories.
-You want to discover new content that inspires you, entertains you, or educates you.
-You want to connect with your friends, family, and favorite celebrities or influencers.
-You want to grow your personal brand or business by reaching new customers or fans.
-
- How to download Instagram by the DISE?
-To download Instagram by the DISE, you need to follow these simple steps:
- Step 1: Download the DISE app
-The first thing you need to do is to download the DISE app from its official website https://dise.com/ . You can also scan the QR code on the website with your phone's camera to get the link. Alternatively, you can search for "DISE" on Google Play Store and install it from there.
- Step 2: Install the DISE app
-Once you have downloaded the DISE app, you need to install it on your device. To do this, you need to allow installation from unknown sources on your device. To do this, you need to go to your device's settings, then security, then enable the option "Unknown sources". This will allow you to install apps that are not from Google Play Store.
- Step 3: Launch the DISE app
-After you have installed the DISE app, you need to launch it on your device. You will see a welcome screen that explains what the DISE app is and how it works. You can swipe left to skip the introduction or tap on "Next" to proceed. You will also need to agree to the terms and conditions and privacy policy of the DISE app before you can use it.
- Step 4: Search for Instagram
-Once you have launched the DISE app, you will see a search bar at the top of the screen. You can use this to search for any app that you want to download from Google Play Store. In this case, you need to type "Instagram" in the search bar and tap on the magnifying glass icon. You will see a list of results that match your query. You need to select the one that says "Instagram" with the official logo and description.
-download instagram by the dise app
-download instagram by the dise video
-download instagram by the dise for pc
-download instagram by the dise apk
-download instagram by the dise online
-download instagram by the dise free
-download instagram by the dise for windows
-download instagram by the dise for android
-download instagram by the dise for mac
-download instagram by the dise for iphone
-download instagram by the dise from meta
-download instagram by the dise stories
-download instagram by the dise reels
-download instagram by the dise photos
-download instagram by the dise posts
-download instagram by the dise highlights
-download instagram by the dise igtv
-download instagram by the dise profile picture
-download instagram by the dise captions
-download instagram by the dise filters
-download instagram by the dise stickers
-download instagram by the dise music
-download instagram by the dise hashtags
-download instagram by the dise comments
-download instagram by the dise likes
-download instagram by the dise followers
-download instagram by the dise live
-download instagram by the dise dm
-download instagram by the dise chat
-download instagram by the dise backup
-download instagram by the dise data
-download instagram by the dise mod
-download instagram by the dise hack
-download instagram by the dise pro
-download instagram by the dise premium
-download instagram by the dise plus
-download instagram by the dise lite
-download instagram by the dise old version
-download instagram by the dise new version
-download instagram by the dise update
-download instagram by the dise beta
-download instagram by the dise review
-download instagram by the dise tutorial
-download instagram by the dise guide
-download instagram by the dise tips
-download instagram by the dise tricks
-download instagram by the dise features
-download instagram by the dise benefits
- Step 5: Download Instagram
-After you have selected Instagram from the list of results, you will see a page that shows more information about the app, such as its rating, reviews, screenshots, and size. You will also see a green button that says "Download". You need to tap on this button to start downloading Instagram by the DISE. You will see a progress bar that shows how much of the download is completed. Once the download is finished, you will see a notification that says "Download complete". You can then tap on "Open" to launch Instagram on your device.
- What is the DISE?
-The DISE is a powerful app that lets you download any app from Google Play Store without any hassle. It stands for Download Install Search Engine, and it works by using a proxy server that bypasses any restrictions or limitations that might prevent you from downloading apps from Google Play Store.
- What does the DISE stand for?
-The DISE stands for Download Install Search Engine. It is an acronym that describes what the app does: it allows you to download, install, and search for any app from Google Play Store.
- What are the benefits of the DISE?
-The DISE has many benefits that make it a useful and convenient tool for Android users, such as:
-
-It is fast and easy to use: You can download any app from Google Play Store in just a few taps, without having to sign in or create an account.
-It is safe and secure: The DISE uses encryption and proxy servers to protect your privacy and data. It does not collect or store any personal information or device data.
-It is free and unlimited: The DISE does not charge any fees or impose any limits on how many apps you can download or how much data you can use.
-It is compatible and flexible: The DISE works with any Android device and any network connection. It also supports multiple languages and regions.
-
- What are the alternatives to the DISE?
-The DISE is not the only app that lets you download apps from Google Play Store without any hassle. There are some other alternatives that you can try, such as:
-
-Aptoide: Aptoide is an independent app store that offers over 1 million apps that are not available on Google Play Store. You can also create your own app store and share it with others.
-APKPure: APKPure is an app downloader that lets you download APK files of apps from Google Play Store or other sources. You can also update your apps with APKPure without using Google Play Services.
-Aurora Store: Aurora Store is an unofficial client for Google Play Store that lets you access all the features of Google Play Store without using Google services or accounts. You can also customize your settings and preferences with Aurora Store.
-
- Conclusion
-In conclusion, Instagram is a great app that lets you create and share your photos, stories, reels and videos with the friends and followers you care about. You can also explore your interests, discover new content, and connect with people from different cultures and backgrounds. To download Instagram on your Android device, one of the easiest and fastest ways is to use the DISE app, a powerful tool that lets you download any app from Google Play Store without any hassle. All you need to do is follow these simple steps:
-
-Download the DISE app from its official website https://dise.com/ or Google Play Store.
-Install the DISE app on your device and allow installation from unknown sources.
-Launch the DISE app and agree to the terms and conditions and privacy policy.
-Search for Instagram in the search bar and select the official app from the list of results.
-Download Instagram by tapping on the green button and wait for the download to complete.
-Open Instagram and enjoy creating and sharing your photos, stories, reels and videos.
-
-We hope this article has helped you learn how to download Instagram by the DISE. If you have any questions or feedback, please feel free to leave a comment below. Thank you for reading!
- FAQs
-Here are some frequently asked questions about downloading Instagram by the DISE:
-
-Is the DISE app safe to use?
-Yes, the DISE app is safe and secure to use. It does not collect or store any personal information or device data. It also uses encryption and proxy servers to protect your privacy and data. However, you should always be careful when downloading apps from unknown sources and only download apps that you trust.
-Can I use the DISE app to download other apps besides Instagram?
-Yes, you can use the DISE app to download any app from Google Play Store without any hassle. You can also search for apps by category, popularity, rating, or name. You can also update your apps with the DISE app without using Google Play Services.
-What are the requirements for using the DISE app?
-The DISE app requires Android 4.4 or higher and an internet connection. It also requires at least 50 MB of free storage space on your device. You do not need a Google account or Google services to use the DISE app.
-How can I contact the DISE app developers?
-If you have any questions, suggestions, or issues with the DISE app, you can contact the developers by sending an email to support@dise.com . You can also visit their website https://dise.com/ for more information and updates.
-How can I share my feedback or review of the DISE app?
-If you like the DISE app and want to share your feedback or review, you can do so by rating and reviewing it on Google Play Store. You can also share it with your friends and family by using the share button on the DISE app. Your feedback and support are greatly appreciated!
-
197e85843d
-
-
\ No newline at end of file
diff --git a/spaces/congsaPfin/Manga-OCR/logs/Get Ready for the Ride of Your Life with Ultimate Motorcycle Simulator APK Mod.md b/spaces/congsaPfin/Manga-OCR/logs/Get Ready for the Ride of Your Life with Ultimate Motorcycle Simulator APK Mod.md
deleted file mode 100644
index 87fd2b891bce2d5824e81e0382a70898cc3a85f6..0000000000000000000000000000000000000000
--- a/spaces/congsaPfin/Manga-OCR/logs/Get Ready for the Ride of Your Life with Ultimate Motorcycle Simulator APK Mod.md
+++ /dev/null
@@ -1,102 +0,0 @@
-
-Ultimate Motorcycle Simulator APK Mod: A Review
-If you are a fan of motorcycle games, you might have heard of Ultimate Motorcycle Simulator, one of the most realistic and immersive motorcycle simulation games on Android. In this article, we will review Ultimate Motorcycle Simulator and its APK mod version, which gives you unlimited money, all motorcycles unlocked, and no ads. We will also show you how to download and install Ultimate Motorcycle Simulator APK mod on your device.
-What is Ultimate Motorcycle Simulator?
-Ultimate Motorcycle Simulator is a game developed by Sir Studios, a Turkish game studio that specializes in simulation games. It was released in 2018 and has since gained over 50 million downloads and 4.3 stars rating on Google Play Store. Ultimate Motorcycle Simulator is a game that lets you experience the thrill of riding different types of motorcycles in a realistic open world environment. You can customize your motorcycles with various paint jobs, vinyls, and tuning parts, and enjoy the realistic physics and sound effects of the game. You can also choose between different game modes, such as free ride, traffic, checkpoint, or career mode.
-ultimate motorcycle simulator apk mod DOWNLOAD === https://urlca.com/2uO9Ia
-Features of Ultimate Motorcycle Simulator
-Ultimate Motorcycle Simulator has many features that make it stand out from other motorcycle games. Here are some of them:
-Realistic physics
-Ultimate Motorcycle Simulator uses advanced physics engine to simulate the behavior and movement of motorcycles. You can feel the difference between different types of motorcycles, such as sport bikes, choppers, cruisers, or off-road bikes. You can also perform stunts, drifts, wheelies, and burnouts with your motorcycles.
-Open world map
-Ultimate Motorcycle Simulator has a huge open world map that you can explore freely. You can ride your motorcycles in different terrains, such as city streets, highways, deserts, mountains, or forests. You can also find hidden ramps, loops, bridges, and tunnels that you can use to perform amazing stunts.
-Customizable motorcycles
-Ultimate Motorcycle Simulator has a wide range of motorcycles that you can unlock and customize. You can choose from over 40 motorcycles, each with their own characteristics and performance. You can also change the color, vinyl, and tuning parts of your motorcycles to make them look unique and suit your style.
-Free ride mode
-Ultimate Motorcycle Simulator has a free ride mode that lets you enjoy the game without any limitations or objectives. You can ride your motorcycles anywhere you want, at any speed you want, and do whatever you want. You can also use the camera mode to take screenshots or videos of your rides.
-What is Ultimate Motorcycle Simulator APK Mod?
-Ultimate Motorcycle Simulator APK mod is a modified version of the original game that gives you some extra benefits that are not available in the official version. These benefits include unlimited money, all motorcycles unlocked, and no ads.
-Benefits of Ultimate Motorcycle Simulator APK Mod
-Ultimate Motorcycle Simulator APK mod has some benefits that make it more enjoyable and convenient than the original game. Here are some of them:
-Unlimited money
-Ultimate Motorcycle Simulator APK mod gives you unlimited money that you can use to buy new motorcycles or upgrade your existing ones. You don't have to worry about running out of money or grinding for hours to earn enough money to buy your favorite motorcycle.
-ultimate motorcycle simulator mod apk unlimited money
-ultimate motorcycle simulator premium mod apk
-ultimate motorcycle simulator hack mod apk download
-ultimate motorcycle simulator mod apk latest version
-ultimate motorcycle simulator mod apk android 1
-ultimate motorcycle simulator mod apk revdl
-ultimate motorcycle simulator mod apk rexdl
-ultimate motorcycle simulator mod apk happymod
-ultimate motorcycle simulator mod apk all bikes unlocked
-ultimate motorcycle simulator mod apk free shopping
-ultimate motorcycle simulator mod apk 2023
-ultimate motorcycle simulator mod apk no ads
-ultimate motorcycle simulator mod apk offline
-ultimate motorcycle simulator mod apk obb
-ultimate motorcycle simulator mod apk old version
-ultimate motorcycle simulator pro mod apk
-ultimate motorcycle simulator mega mod apk
-ultimate motorcycle simulator full mod apk
-ultimate motorcycle simulator vip mod apk
-ultimate motorcycle simulator 3d mod apk
-download game ultimate motorcycle simulator mod apk
-download ultimate motorcycle simulator mod apk terbaru
-cara download ultimate motorcycle simulator mod apk
-link download ultimate motorcycle simulator mod apk
-how to download ultimate motorcycle simulator mod apk
-descargar ultimate motorcycle simulator mod apk
-descargar ultimate motorcycle simulator mod apk dinero infinito
-como descargar ultimate motorcycle simulator mod apk
-baixar ultimate motorcycle simulator mod apk
-baixar ultimate motorcycle simulator mod apk dinheiro infinito
-como baixar ultimate motorcycle simulator mod apk
-telecharger ultimate motorcycle simulator mod apk
-telecharger ultimate motorcycle simulator mod apk argent illimité
-comment telecharger ultimate motorcycle simulator mod apk
-indir ultimate motorcycle simulator mod apk
-indir ultimate motorcycle simulator mod apk sınırsız para
-nasıl indirilir ultimate motorcycle simulator mod apk
-install ultimate motorcycle simulator mod apk
-install ultimate motorcycle simulator mod apk unlimited money and gems
-how to install ultimate motorcycle simulator mod apk
-update ultimate motorcycle simulator mod apk
-update ultimate motorcycle simulator mod apk unlimited money and gems
-how to update ultimate motorcycle simulator mod apk
-cheat ultimate motorcycle simulator mod apk
-cheat codes for ultimate motorcycle simulator mod apk
-how to cheat in ultimate motorcycle simulator mod apk
-gameplay of ultimate motorcycle simulator mod apk
-best settings for ultimate motorcycle simulator mod apk
-All motorcycles unlocked
-Ultimate Motorcycle Simulator APK mod gives you access to all the motorcycles in the game without having to unlock them by completing missions or reaching certain levels. You can choose any motorcycle you want from the start and enjoy its performance and features.
-No ads
-Ultimate Motorcycle Simulator APK mod removes all the ads that interrupt your gameplay and ruin your immersion. You don't have to watch any ads to get rewards or bonuses, or to skip the waiting time. You can enjoy the game without any distractions or annoyances.
-How to download and install Ultimate Motorcycle Simulator APK Mod?
-If you want to download and install Ultimate Motorcycle Simulator APK mod on your device, you need to follow some simple steps. Here they are:
-Steps to download and install Ultimate Motorcycle Simulator APK Mod
-
-Go to a trusted website that provides the download link for Ultimate Motorcycle Simulator APK mod, such as [APKPure] or [APKHome].
-Click on the download button and wait for the file to be downloaded on your device.
-Go to your device settings and enable the installation of apps from unknown sources.
-Locate the downloaded file and tap on it to start the installation process.
-Follow the instructions on the screen and wait for the installation to be completed.
-Launch the game and enjoy Ultimate Motorcycle Simulator APK mod.
-
-Conclusion
-Ultimate Motorcycle Simulator is a great game for motorcycle enthusiasts who want to experience the realistic and immersive simulation of riding different types of motorcycles in a huge open world environment. Ultimate Motorcycle Simulator APK mod is a better version of the game that gives you unlimited money, all motorcycles unlocked, and no ads. You can download and install Ultimate Motorcycle Simulator APK mod by following the steps we have provided in this article. We hope you have fun playing Ultimate Motorcycle Simulator APK mod.
-FAQs
-
-Q: Is Ultimate Motorcycle Simulator APK mod safe to use?
-A: Yes, Ultimate Motorcycle Simulator APK mod is safe to use as long as you download it from a trusted website that does not contain any viruses or malware.
-Q: Do I need to root my device to use Ultimate Motorcycle Simulator APK mod?
-A: No, you don't need to root your device to use Ultimate Motorcycle Simulator APK mod. You just need to enable the installation of apps from unknown sources in your device settings.
-Q: Can I play Ultimate Motorcycle Simulator online with other players?
-A: No, Ultimate Motorcycle Simulator is an offline game that does not support online multiplayer mode. You can only play it solo or with AI traffic.
-Q: Can I update Ultimate Motorcycle Simulator APK mod when a new version is released?
-A: Yes, you can update Ultimate Motorcycle Simulator APK mod when a new version is released by downloading and installing the latest version from the same website that you downloaded it from. However, you may lose your progress and data if you do so, so make sure you back up your game data before updating.
-Q: What are some alternatives to Ultimate Motorcycle Simulator APK mod?
-A: Some alternatives to Ultimate Motorcycle Simulator APK mod are Real Bike Racing, Moto Rider GO, Bike Race Free, and Traffic Rider.
- 197e85843d
-
-
\ No newline at end of file
diff --git a/spaces/congsaPfin/Manga-OCR/logs/Hello Neighbor Saklamba APK Tips and Tricks to Outsmart Your Creepy Neighbor.md b/spaces/congsaPfin/Manga-OCR/logs/Hello Neighbor Saklamba APK Tips and Tricks to Outsmart Your Creepy Neighbor.md
deleted file mode 100644
index a4c1aad9c21bc52c4955f3ee32d15b39b85b94d7..0000000000000000000000000000000000000000
--- a/spaces/congsaPfin/Manga-OCR/logs/Hello Neighbor Saklamba APK Tips and Tricks to Outsmart Your Creepy Neighbor.md
+++ /dev/null
@@ -1,114 +0,0 @@
-
-Hello Neighbor Saklambaç APK: A Stealth Horror Game with a Twist
-If you are a fan of stealth horror games, you might have heard of Hello Neighbor, a popular game that was released in 2017 by tinyBuild. The game is about sneaking into your neighbor's house to find out what he is hiding in his basement, while avoiding his traps and cameras. The game has received positive reviews from critics and players alike, who praised its originality, suspense, and graphics.
-But did you know that there is a Turkish version of Hello Neighbor that adds a twist to the game? It is called Hello Neighbor Saklambaç APK, and it is a modified version of the original game that introduces a new element to the gameplay: saklambaç.
-hello neighbor saklambaç apk DOWNLOAD ★★★ https://urlca.com/2uOcmI
-Saklambaç is a Turkish word that means "hide and seek". It is also a popular game among children in Turkey, where one person tries to find the others who are hiding. In Hello Neighbor Saklambaç APK, you can play saklambaç with your neighbor, who will try to catch you if he sees you. You can also hide behind objects, under beds, or in closets, to avoid being detected.
-Hello Neighbor Saklambaç APK is a fun and exciting game that will test your stealth skills, creativity, and courage. If you are curious about this game and want to try it out, here is everything you need to know about how to download, install, play, and enjoy Hello Neighbor Saklambaç APK on your Android device.
- How to Download and Install Hello Neighbor Saklambaç APK on Your Android Device
-Before you can play Hello Neighbor Saklambaç APK on your Android device, you need to download and install the APK file of the game. An APK file is an Android application package file that contains all the files and data needed to run an app on an Android device. However, since Hello Neighbor Saklamba
ç APK is not available on the official Google Play Store, you need to download it from a third-party source. Here are the steps you need to follow to download and install Hello Neighbor Saklambaç APK on your Android device:
-
-Enable unknown sources on your device settings. This will allow you to install apps from sources other than the Google Play Store. To do this, go to your device settings, then security, then enable the option "unknown sources". You may see a warning message, but you can ignore it and proceed.
-Download the APK file from a trusted source. You can find many websites that offer Hello Neighbor Saklambaç APK for free download, but you need to be careful and choose a reliable and safe one. One of the websites that we recommend is [APKPure], where you can download the latest version of Hello Neighbor Saklambaç APK with a simple click.
-Locate and install the APK file on your device. After you download the APK file, you need to find it on your device storage and tap on it to start the installation process. You may see some prompts asking for permissions, just accept them and wait for the installation to finish.
-Launch the game and enjoy. Once the installation is done, you can find the game icon on your device home screen or app drawer. Tap on it to launch the game and start playing Hello Neighbor Saklambaç APK.
-
- How to Play Hello Neighbor Saklambaç APK
-Now that you have downloaded and installed Hello Neighbor Saklambaç APK on your Android device, you are ready to play this amazing stealth horror game with a twist. Here is how to play Hello Neighbor Saklambaç APK:
-The objective: Sneak into your neighbor's house and discover his secrets. You are a curious kid who lives across the street from a mysterious neighbor who seems to be hiding something in his basement. You decide to sneak into his house and find out what he is up to, but be careful, he is not as friendly as he looks. He will chase you, set traps, and use cameras to catch you if he sees you.
-The gameplay: Use stealth, strategy, and creativity to avoid the neighbor's traps and cameras. You can explore the neighbor's house, which is a huge sandbox with many rooms, objects, and secrets. You can interact with almost anything in the house, such as opening doors, windows, drawers, cabinets, etc. You can also use items that you find or collect, such as keys, tools, weapons, etc., to help you in your mission. However, you need to be careful not to make too much noise or leave any traces behind, as the neighbor will hear you or see you and try to stop you.
-hello neighbor saklambaç android game
-hello neighbor saklambaç free download
-hello neighbor saklambaç hide and seek
-hello neighbor saklambaç apk indir
-hello neighbor saklambaç mod apk
-hello neighbor saklambaç oyunu oyna
-hello neighbor saklambaç apk pure
-hello neighbor saklambaç latest version
-hello neighbor saklambaç gameplay
-hello neighbor saklambaç cheats
-hello neighbor saklambaç tips and tricks
-hello neighbor saklambaç review
-hello neighbor saklambaç trailer
-hello neighbor saklambaç online multiplayer
-hello neighbor saklambaç secrets and easter eggs
-hello neighbor saklambaç best hiding spots
-hello neighbor saklambaç how to install
-hello neighbor saklambaç system requirements
-hello neighbor saklambaç update
-hello neighbor saklambaç walkthrough
-hello neighbor saklambaç guide
-hello neighbor saklambaç hack
-hello neighbor saklambaç full version
-hello neighbor saklambaç beta
-hello neighbor saklambaç release date
-hello neighbor saklambaç story mode
-hello neighbor saklambaç characters
-hello neighbor saklambaç levels
-hello neighbor saklambaç maps
-hello neighbor saklambaç graphics settings
-hello neighbor saklambaç sound effects
-hello neighbor saklambaç controls
-hello neighbor saklambaç achievements
-hello neighbor saklambaç bugs and glitches
-hello neighbor saklambaç fan art
-hello neighbor saklambaç wallpapers
-hello neighbor saklambaç memes
-hello neighbor saklambaç videos
-hello neighbor saklambaç screenshots
-hello neighbor saklambaç forums
-hello neighbor saklambaç reddit
-hello neighbor saklambaç discord server
-hello neighbor saklambaç facebook page
-hello neighbor saklambaç twitter account
-hello neighbor saklambaç instagram profile
-hello neighbor saklambaç tiktok videos
-hello neighbor saklambaç merchandise store
-hello neighbor saklambaç developer website
-The features: Experience an immersive and dynamic environment with an advanced AI that adapts to your actions. One of the most impressive features of Hello Neighbor Saklambaç APK is its realistic and responsive environment that changes according to your actions. The neighbor has an artificial intelligence that learns from your behavior and creates new strategies to catch you. He will also remember where you have been and what you have done, and react accordingly. For example, if you break a window or leave a door open, he will notice it and fix it or close it. If you use a certain route or hiding spot often, he will set traps or cameras there. If you throw something at him or hit him with a weapon, he will get angry and chase you more aggressively.
- Tips and Tricks for Hello Neighbor Saklambaç APK
-Hello Neighbor Saklambaç APK is not an easy game to play, as it requires a lot of patience, skill, and creativity. However, there are some tips and tricks that can help you succeed in your mission and have more fun while playing this game. Here are some of them:
-
-Explore the surroundings and look for clues and items. The neighbor's house is full of secrets and hidden places that can reveal more about his story and motives. You can also find useful items that can help you in your mission, such as keys, tools, weapons, etc. However, be careful not to take too much time or make too much noise while exploring, as the neighbor may notice you.
-Use distractions and hiding spots to evade the neighbor. You can use various objects or items that you find or collect in the house to distract the neighbor or lure him away from his position. For example, you can throw something at him or at another place to make him look away or follow the sound. You can also use radios, TVs, phones, etc., to create noise or fake calls that will confuse him or make him leave his room. Moreover, you can use hiding spots such as closets, beds, [user or in the neighbor's car, to hide from him or escape his sight. However, be careful not to use the same hiding spot too often, as he may find you or set a trap there.
-Learn from your mistakes and try different approaches. Hello Neighbor Saklambaç APK is a game that requires a lot of trial and error, as you will fail many times before you succeed. However, you can use your failures as learning opportunities, as they will help you understand the neighbor's behavior and patterns, and find new ways to outsmart him. You can also try different approaches and strategies, such as being more aggressive or more stealthy, depending on the situation and your preference.
-
- Pros and Cons of Hello Neighbor Saklambaç APK
-Like any other game, Hello Neighbor Saklambaç APK has its pros and cons that you should consider before playing it. Here are some of them:
-
-
-Pros
-Cons
-
-
-A unique and thrilling stealth horror game with a twist
-A high battery consumption and storage space requirement
-
-
-A challenging and rewarding gameplay with multiple endings
-A possible lagging and crashing issues on some devices
-
-
-A stunning and realistic graphics and sound effects
-A limited language support and translation errors
-
-
- Conclusion
-Hello Neighbor Saklambaç APK is a stealth horror game that will keep you on the edge of your seat. It is a modified version of the original Hello Neighbor game that introduces a new element to the gameplay: saklambaç, which is a Turkish word for hide and seek. You can play saklambaç with your neighbor, who will try to catch you if he sees you. You can also hide behind objects, under beds, or in closets, to avoid being detected.
-The game has an immersive and dynamic environment with an advanced AI that adapts to your actions. The neighbor will learn from your behavior and create new strategies to catch you. He will also remember where you have been and what you have done, and react accordingly. The game also has a realistic and responsive environment that changes according to your actions.
-If you are looking for a fun and exciting game with a twist, you should give Hello Neighbor Saklambaç APK a try. You can download and install the game on your Android device by following the steps we have provided above. You can also use the tips and tricks we have shared to help you succeed in your mission and have more fun while playing this game.
- FAQs
-Here are some of the frequently asked questions about Hello Neighbor Saklambaç APK:
-
-What is saklambaç?
-Saklambaç is a Turkish word that means "hide and seek". It is also a popular game among children in Turkey, where one person tries to find the others who are hiding. In Hello Neighbor Saklambaç APK, you can play saklambaç with your neighbor, who will try to catch you if he sees you.
-Is Hello Neighbor Saklambaç APK safe to download?
-Yes, Hello Neighbor Saklambaç APK is safe to download, as long as you download it from a trusted source. However, since it is not available on the official Google Play Store, you need to enable unknown sources on your device settings before installing it.
-How many levels are there in Hello Neighbor Saklambaç APK?
-Hello Neighbor Saklambaç APK has three levels: Act 1, Act 2, and Act 3. Each level has a different layout, difficulty, and objective. You can also unlock different endings depending on your choices and actions.
-Can I play Hello Neighbor Saklambaç APK offline?
-Yes, you can play Hello Neighbor Saklambaç APK offline, as it does not require an internet connection to run. However, you may need an internet connection to download and install the game.
-Can I play Hello Neighbor Saklambaç APK with friends?
-No, Hello Neighbor Saklambaç APK is a single-player game that does not support multiplayer mode. However, you can share your experience and opinions with your friends online or offline.
197e85843d
-
-
\ No newline at end of file
diff --git a/spaces/congsaPfin/Manga-OCR/logs/How to Install Jio POS Plus 1.0.6 APK on Your Android Device.md b/spaces/congsaPfin/Manga-OCR/logs/How to Install Jio POS Plus 1.0.6 APK on Your Android Device.md
deleted file mode 100644
index 8af5005e83bb3998c217006f18a46016784bb828..0000000000000000000000000000000000000000
--- a/spaces/congsaPfin/Manga-OCR/logs/How to Install Jio POS Plus 1.0.6 APK on Your Android Device.md
+++ /dev/null
@@ -1,161 +0,0 @@
-
-Jio POS Plus: A Complete Guide to Download and Use the App
-If you are looking for a way to earn money by recharging Jio numbers, selling Jio SIM cards, or activating new Jio plans, then you might want to check out Jio POS Plus. Jio POS Plus is an app-based platform that allows you to manage your Jio business on the go. In this article, we will tell you everything you need to know about Jio POS Plus, including its features, benefits, how to become a Jio partner, how to download and use the app, and how to update it.
-jio pos plus 1.0 6 apk download Download File ✑ ✑ ✑ https://urlca.com/2uOeJu
- What is Jio POS Plus?
-Jio POS Plus is an app developed by Jio Platforms Limited for its partners who want to offer various Jio services to their customers. With Jio POS Plus, you can:
-
-Onboard new customers with or without Aadhaar
-Recharge any Jio number with any plan
-Sell new Jio SIM cards or port existing numbers to Jio
-Activate new Jio plans or vouchers for your customers
-Earn commissions for every transaction you make
-Track your earnings and performance on a daily, weekly, or monthly basis
-Get access to exclusive offers and discounts from Jio
-
-Jio POS Plus is a simple and convenient way to grow your business and earn more income with Jio.
- Features and benefits of Jio POS Plus
-Some of the features and benefits of using Jio POS Plus are:
-
-You can manage your business anytime, anywhere, with just your smartphone
-You can offer a wide range of services to your customers, such as recharges, SIM activations, plan activations, etc.
-You can earn attractive commissions for every transaction you make
-You can get real-time updates on your earnings and performance
-You can get support from the dedicated customer care team of Jio
-You can get access to exclusive offers and discounts from Jio
-
- How to become a Jio partner and use Jio POS Plus?
-If you want to become a Jio partner and use Jio POS Plus, you need to follow these steps:
- Step 1: Register as a Jio partner
-To register as a Jio partner, you need to visit the official website of Jio Partners and fill out the online form with your details. You will also need to upload some documents, such as your PAN card, Aadhaar card, GST certificate, bank account details, etc. After submitting the form, you will receive a confirmation email from Jio. You will also get a call from a Jio representative who will verify your details and guide you through the next steps.
-How to download jio pos plus 1.0 6 apk on android
-Jio pos plus 1.0 6 apk latest version free download
-Jio pos plus 1.0 6 apk for jio partners and retailers
-Jio pos plus 1.0 6 apk features and benefits
-Jio pos plus 1.0 6 apk online onboarding and recharging
-Jio pos plus 1.0 7 apk update and download link
-Jio pos plus 1.0 6 apk old versions and alternatives
-Jio pos plus 1.0 6 apk installation and activation guide
-Jio pos plus 1.0 6 apk problems and solutions
-Jio pos plus 1.0 6 apk reviews and ratings
-Jio pos plus 1.0 6 apk download for pc and laptop
-Jio pos plus 1.0 6 apk mod and hack version
-Jio pos plus 1.0 6 apk earn money and rewards
-Jio pos plus 1.0 6 apk customer care and support
-Jio pos plus 1.0 6 apk FAQs and tips
-Jio pos plus app vs jio pos plus 1.0 6 apk comparison
-Jio pos plus 1.0 6 apk requirements and compatibility
-Jio pos plus 1.0 6 apk security and privacy issues
-Jio pos plus 1.0 6 apk download from google play store
-Jio pos plus 1.0 6 apk download from third-party sources
-Jio pos plus app download for iphone and ios devices
-Jio pos plus app download for windows phone and blackberry
-Jio pos plus app download for jio phone and kaios
-Jio pos plus app download for tablet and ipad
-Jio pos plus app download for smart tv and firestick
-How to use jio pos plus app for recharge and bill payment
-How to use jio pos plus app for sim activation and verification
-How to use jio pos plus app for commission and earnings
-How to use jio pos plus app for reports and analytics
-How to use jio pos plus app for offers and coupons
-How to update jio pos plus app to the latest version
-How to uninstall jio pos plus app from your device
-How to reset jio pos plus app password and pin
-How to contact jio pos plus app helpline and feedback
-How to join jio pos plus app as a partner or retailer
-Benefits of jio pos plus app for jio users and customers
-Benefits of jio pos plus app for jio network and services
-Benefits of jio pos plus app for digital india and make in india initiatives
-Challenges of jio pos plus app for competitors and rivals
-Challenges of jio pos plus app for technical and operational issues
- Step 2: Download and install Jio POS Plus app
-Once you are registered as a Jio partner, you will receive an SMS with a link to download the Jio POS Plus app. You can also download the app from the Google Play Store or the Jio website . The app is compatible with Android devices running on version 4.4 or above. To install the app, you need to enable the installation of apps from unknown sources in your device settings. After installing the app, you will see the Jio POS Plus icon on your home screen.
- Step 3: Log in and start using Jio POS Plus app
-To log in to the Jio POS Plus app, you need to enter your registered mobile number and password. You will also need to enter a one-time password (OTP) that will be sent to your number for verification. After logging in, you will see the dashboard of the app, where you can access various features and services. You can also view your profile, wallet balance, transactions history, commissions earned, etc. To start using the app, you need to load money into your wallet using your bank account or debit card. You can then use the wallet balance to recharge Jio numbers, sell Jio SIM cards, activate Jio plans, etc.
- How to download Jio POS Plus 1.0 6 apk?
-If you are looking for the latest version of Jio POS Plus app, you might want to download Jio POS Plus 1.0 6 apk. This is the updated version of the app that was released on June 19, 2023. It has some new features and bug fixes that improve the performance and user experience of the app.
- Why do you need Jio POS Plus 1.0 6 apk?
-Some of the reasons why you might need Jio POS Plus 1.0 6 apk are:
-
-You want to enjoy the new features and enhancements of the app
-You want to fix some issues or errors that you faced with the previous version of the app
-You want to update your app without waiting for the official update from the Google Play Store or the Jio website
-You want to install the app on a device that does not have access to the Google Play Store or the Jio website
-
- How to download and install Jio POS Plus 1.0 6 apk?
-There are two methods to download and install Jio POS Plus 1.0 6 apk on your device:
- Method 1: From the official website
-This is the safest and easiest method to download and install Jio POS Plus 1.0 6 apk on your device. To do this, you need to follow these steps:
-
-Visit the official website of Jio POS Plus on your device browser
-Scroll down and click on the "Download App" button
-Select "Jio POS Plus" from the list of apps and click on "Download"
-You will see a pop-up window asking you to confirm the download. Click on "OK"
-The apk file will start downloading on your device. You can check the progress in your notification bar or download manager
-Once the download is complete, tap on the apk file to open it
-You will see a warning message saying that installing apps from unknown sources can harm your device. Click on "Settings"
-You will be redirected to your device settings, where you need to enable the installation of apps from unknown sources
-Go back to the apk file and tap on it again
-You will see a screen asking you to install the app. Click on "Install"
-The app will start installing on your device. You can check the progress in your notification bar or download manager
-Once the installation is complete, tap on "Open" to launch the app
-You can now log in and use Jio POS Plus 1.0 6 apk on your device
-
- Method 2: From a third-party website
-This is an alternative method to download and install Jio POS Plus 1.0 6 apk on your device. However, this method is not recommended as it may expose your device to malware or viruses. You should only use this method if you trust the source of the apk file and have an antivirus software installed on your device. To do this, you need to follow these steps:
-
-Visit a third-party website that offers Jio POS Plus 1.0 6 apk for download, such as APKPure or APKMirror
-Search for Jio POS Plus 1.0 6 apk and click on the download link
-You will see a pop-up window asking you to confirm the download. Click on "OK"
-The apk file will start downloading on your device. You can check the progress in your notification bar or download manager
-Once the download is complete, tap on the apk file to open it
-You will see a warning message saying that installing apps from unknown sources can harm your device. Click on "Settings"
-You will be redirected to your device settings, where you need to enable the installation of apps from unknown sources
-Go back to the apk file and tap on it again
-You will see a screen asking you to install the app. Click on "Install"
-The app will start installing on your device. You can check the progress in your notification bar or download manager
-Once the installation is complete, tap on "Open" to launch the app
-You can now log in and use Jio POS Plus 1.0 6 apk on your device
-
- How to update Jio POS Plus app?
-If you want to keep your Jio POS Plus app updated with the latest features and bug fixes, you need to follow these steps:
- How to check the current version of Jio POS Plus app?
-To check the current version of Jio POS Plus app, you need to follow these steps:
-
-Open the Jio POS Plus app on your device
-Tap on the menu icon (three horizontal lines) on the top left corner of the screen
-Tap on "About Us" from the menu options
-You will see the current version of the app displayed on the screen
-
- How to update Jio POS Plus app from the app itself?
-To update Jio POS Plus app from the app itself, you need to follow these steps:
-
-Open the Jio POS Plus app on your device
-Tap on the menu icon (three horizontal lines) on the top left corner of the screen
-Tap on "Check for Updates" from the menu options
-If there is a new version available, you will see a pop-up window asking you to update the app. Click on "Update"
-The app will start downloading and installing the new version on your device. You can check the progress in your notification bar or download manager
-Once the update is complete, tap on "Open" to launch the updated app
-
- How to update Jio POS Plus app manually?
-To update Jio POS Plus app manually, you need to follow these steps:
-
-Delete the old version of Jio POS Plus app from your device
-Download and install Jio POS Plus 1.0 6 apk using one of the methods mentioned above
-Log in and use Jio POS Plus 1.0 6 apk on your device
-
- Conclusion
-Jio POS Plus is a great app for anyone who wants to earn money by offering various Jio services to their customers. It is easy to use, convenient, and rewarding. You can download and use Jio POS Plus 1.0 6 apk on your device by following the steps given in this article. However, make sure that you download and install the app from a trusted source and keep it updated regularly.
- Frequently Asked Questions (FAQs)
- Q: Is Jio POS Plus free to use?
-A: Yes, Jio POS Plus is free to use for all registered Jio partners. However, you need to load money into your wallet using your bank account or debit card to offer services to your customers.
- Q: How much commission can I earn with Jio POS Plus?
-A: The commission rate varies depending on the type of service you offer and the plan or voucher you activate. You can check the commission details in the app or contact Jio customer care for more information.
- Q: Can I use Jio POS Plus without internet connection?
-A: No, you need an active internet connection to use Jio POS Plus. You can use any network provider or Wi-Fi connection for accessing the app.
- Q: How can I contact Jio customer care for any queries or issues related to Jio POS Plus?
-A: You can contact Jio customer care for any queries or issues related to Jio POS Plus by calling 1800-889-9333 or emailing care@jio.com. You can also visit the Jio website or the Jio Partners website for more information.
- Q: Can I use Jio POS Plus on multiple devices?
-A: No, you can only use Jio POS Plus on one device at a time. If you want to use it on another device, you need to log out from the current device and log in to the new device.
401be4b1e0
-
-
\ No newline at end of file
diff --git a/spaces/congsaPfin/Manga-OCR/logs/How to Run 8 Ball Pool APK on Windows 10 PC.md b/spaces/congsaPfin/Manga-OCR/logs/How to Run 8 Ball Pool APK on Windows 10 PC.md
deleted file mode 100644
index 652ac264e3c6c29554047c97300920c9a8ecfd11..0000000000000000000000000000000000000000
--- a/spaces/congsaPfin/Manga-OCR/logs/How to Run 8 Ball Pool APK on Windows 10 PC.md
+++ /dev/null
@@ -1,116 +0,0 @@
-
-How to Play 8 Ball Pool on Windows 10 with APK File
- Do you love playing pool games online? If so, you might have heard of 8 Ball Pool , one of the most popular and addictive pool games on the web. Developed by Miniclip, this game lets you compete with millions of players around the world in various modes and tournaments. You can also customize your cue, table, and avatar, as well as chat with your friends and opponents.
-8 ball pool apk windows 10 Download >>>>> https://urlca.com/2uOe7k
- But what if you want to play 8 Ball Pool on your PC or laptop instead of your mobile device? Well, there is a way to do that, and it involves using an apk file . An apk file is a package file format that contains the installation files for Android applications. By using an apk file, you can install and run Android apps on your Windows 10 device without any hassle.
- Why would you want to play 8 Ball Pool on Windows 10? There are several benefits, such as:
-
-You can enjoy a larger screen and better graphics
-You can use a mouse, keyboard, or gamepad for more precise control
-You can save your battery life and data usage on your mobile device
-You can access more productivity apps and tools on your PC or laptop while playing
-
- If you are interested in playing 8 Ball Pool on Windows 10 with an apk file, this article will show you how to do it step by step. We will also give you some tips and tricks to help you master the game and win more coins. Let's get started!
- How to Download and Install 8 Ball Pool APK on Windows 10
- To play 8 Ball Pool on Windows 10 with an apk file, you will need two things: the apk file itself, and an Android emulator. An Android emulator is a software that simulates the Android operating system on your PC or laptop, allowing you to run Android apps on it. There are many Android emulators available online, but we recommend using BlueStacks , as it is one of the most popular and reliable ones.
- Here are the steps to download and install 8 Ball Pool apk on Windows 10 using BlueStacks:
-
-Go to https://www.bluestacks.com/apps/sports/8-ball-pool-on-pc.html and click on the "Download" button to download BlueStacks on your PC or laptop.
-Once the download is complete, open the installer file and follow the instructions to install BlueStacks on your device.
-After the installation is done, launch BlueStacks and sign in with your Google account. If you don't have one, you can create one for free.
-Go to https://www.gameloop.com/game/sports/8-ball-pool-on-pc and click on the "Download" button to download the apk file for 8 Ball Pool.
-Once the download is complete, locate the apk file on your device and right-click on it. Select "Open with" and choose "BlueStacks" from the list of options.
-BlueStacks will automatically install the apk file on your device. You will see a notification when it is done.
-You can now launch 8 Ball Pool from the BlueStacks home screen or app drawer.
-
- Congratulations! You have successfully installed 8 Ball Pool apk on Windows 10 using BlueStacks. Now you can enjoy playing this amazing pool game on your PC or laptop.
-8 ball pool game download for pc windows 10
-8 ball pool emulator for windows 10
-8 ball pool app for windows 10 free
-8 ball pool online on pc windows 10
-8 ball pool miniclip for windows 10
-8 ball pool apk file for windows 10
-8 ball pool bluestacks on windows 10
-8 ball pool gameloop for windows 10
-8 ball pool apk pure for windows 10
-8 ball pool install on windows 10
-8 ball pool hack for pc windows 10
-8 ball pool mod apk windows 10
-8 ball pool cheats for windows 10
-8 ball pool unlimited coins windows 10
-8 ball pool offline mode windows 10
-8 ball pool multiplayer on windows 10
-8 ball pool tournament for pc windows 10
-8 ball pool best cues windows 10
-8 ball pool tips and tricks windows 10
-8 ball pool update for windows 10
-play 8 ball pool with friends on windows 10
-how to download and install 8 ball pool on windows 10
-how to play 8 ball pool on pc with keyboard windows 10
-how to get free cash in 8 ball pool on windows 10
-how to transfer coins in 8 ball pool on windows 10
-how to change name in 8 ball pool on windows 10
-how to level up fast in 8 ball pool on windows 10
-how to win every game in 8 ball pool on windows 10
-how to chat in 8 ball pool on pc windows 10
-how to create a club in 8 ball pool on windows 10
-how to join a club in 8 ball pool on windows 10
-how to challenge someone in 8 ball pool on windows 10
-how to spin the wheel in 8 ball pool on windows 10
-how to use emoji in 8 ball pool on pc windows 10
-how to change avatar in 8 ball pool on windows 10
-how to change table in 8 ball pool on pc windows
- How to Play 8 Ball Pool on Windows 10
- Now that you have installed 8 Ball Pool apk on Windows 10, you might be wondering how to play it. Don't worry, it's very easy and fun. Here are the basic rules and objectives of the game:
-
-The game is played on a pool table with 16 balls: one white cue ball, seven solid-colored balls, seven striped balls, and one black 8 ball.
-The goal of the game is to pocket all your balls (either solid or striped) and then pocket the 8 ball before your opponent does.
-You can choose to play either in 1-on-1 mode , where you compete with another player online, or in tournament mode , where you play against multiple players in a bracket format.
-You can also play in different cities , each with its own entry fee and prize pool. The higher the stakes, the more challenging the opponents.
-To start the game, you need to break the rack by hitting the cue ball with your cue stick. You can adjust the angle and power of your shot by dragging the mouse or using the arrow keys.
-If you pocket a ball on the break, you get to choose whether you want to play with solid or striped balls. If you don't pocket a ball, your opponent gets to choose.
-On each turn, you need to hit the cue ball with your cue stick and try to pocket one of your balls. You can aim and shoot by dragging the mouse or using the arrow keys.
-If you pocket one of your balls, you get another turn. If you miss or pocket the wrong ball, your turn ends and your opponent gets to play.
-If you pocket the 8 ball before pocketing all your balls, you lose the game. If you pocket the 8 ball after pocketing all your balls, you win the game.
-If you scratch (pocket the cue ball or hit it off the table), you lose your turn and your opponent gets to place the cue ball anywhere on the table.
-
- These are the basic rules of 8 Ball Pool, but there are also some additional features and modes that make the game more interesting and fun. Here are some of them:
-
-You can customize your cue, table, and avatar by buying them with coins or cash. Coins are earned by winning games or tournaments, while cash is bought with real money or earned by completing offers. Different cues and tables have different attributes, such as power, aim, spin, and time.
-You can chat with your friends and opponents by using preset messages or emojis. You can also send and receive gifts, such as coins, cues, or boxes.
-You can level up by earning experience points (XP) from playing games or tournaments. The higher your level, the more cities and modes you can unlock.
-You can join a club or create your own by paying a fee. Clubs are groups of players who can chat, play, and compete together. You can also participate in club events and leagues to win rewards and trophies.
-You can play mini-games , such as Spin & Win, Scratch & Win, Hi-Lo, and Lucky Shot, to win coins, cash, cues, boxes, or tickets. Tickets are used to enter special tournaments with bigger prizes.
-
- As you can see, there is a lot to do and enjoy in 8 Ball Pool. But how can you improve your skills and win more coins? Here are some tips and tricks that might help:
-
-Practice . The best way to get better at anything is to practice. You can practice offline by playing against the computer or online by playing against random players. You can also watch replays of your games or other players' games to learn from their mistakes and strategies.
-Plan ahead . Before making a shot, think about where you want the cue ball and your next ball to go. Try to avoid leaving yourself in a difficult position or giving your opponent an easy shot. Use spin and power wisely to control the cue ball.
-Use hints . If you are not sure what to do next, you can use hints by clicking on the light bulb icon at the bottom right corner of the screen. Hints will show you the best possible shot for your current situation. However, hints are limited and cost coins, so use them sparingly.
-Challenge yourself . Don't be afraid to play against higher-level players or enter higher-stake tournaments. You might lose some coins, but you will also learn a lot and improve your skills. You can also challenge your friends or club members to friendly matches and see who is the best.
-Have fun . The most important thing is to enjoy the game and have fun. Don't get too frustrated or angry if you lose or make a mistake. Remember, it's just a game, and you can always try again. Be respectful and friendly to your opponents and don't cheat or hack the game.
-
- Conclusion
- 8 Ball Pool is a great game that you can play on your Windows 10 device with an apk file. It is easy to download and install, and it offers a lot of features and modes that will keep you entertained and challenged. You can also customize your cue, table, and avatar, chat with your friends and opponents, join a club, play mini-games, and level up. By following the tips and tricks we shared, you can improve your skills and win more coins. So what are you waiting for? Download 8 Ball Pool apk on Windows 10 today and start playing!
- If you liked this article, please share it with your friends and leave us a comment below. We would love to hear your feedback and suggestions. Thank you for reading!
- FAQs
- Here are some of the most frequently asked questions and answers about 8 Ball Pool apk on Windows 10:
- Q: Is 8 Ball Pool apk safe to download and install?
-A: Yes, 8 Ball Pool apk is safe to download and install, as long as you get it from a trusted source, such as https://www.gameloop.com/game/sports/8-ball-pool-on-pc . However, you should always scan any file you download with an antivirus software before opening it.
- Q: Do I need an internet connection to play 8 Ball Pool on Windows 10?
-A: Yes, you need an internet connection to play 8 Ball Pool on Windows 10, as it is an online game that requires you to connect with other players. However, you can also play offline against the computer if you want to practice or have no internet access.
- Q: How can I get more coins and cash in 8 Ball Pool?
-A: There are several ways to get more coins and cash in 8 Ball Pool, such as:
-
-Winning games or tournaments
-Spinning the wheel or scratching the card daily
-Watching video ads or completing offers
-Opening boxes or collecting free gifts
-Buying them with real money
-
- Q: How can I update 8 Ball Pool apk on Windows 10?
-A: To update 8 Ball Pool apk on Windows 10, you need to download the latest version of the apk file from https://www.gameloop.com/game/sports/8-ball-pool-on-pc and install it over the existing one. You don't need to uninstall the previous version first.
- Q: How can I uninstall 8 Ball Pool apk from Windows 10?
-A: To uninstall 8 Ball Pool apk from Windows 10, you need to open BlueStacks and go to the app drawer. Find the 8 Ball Pool icon and right-click on it. Select "Uninstall" from the menu and confirm your choice. You can also delete the apk file from your device if you want.
401be4b1e0
-
-
\ No newline at end of file
diff --git a/spaces/congsaPfin/Manga-OCR/logs/How to Survive the Never-Ending Waves of Evil in Archero - Download the Latest Version APK for Android.md b/spaces/congsaPfin/Manga-OCR/logs/How to Survive the Never-Ending Waves of Evil in Archero - Download the Latest Version APK for Android.md
deleted file mode 100644
index 2262e60ddb33ef6fd49b308690a31889efc7f1ed..0000000000000000000000000000000000000000
--- a/spaces/congsaPfin/Manga-OCR/logs/How to Survive the Never-Ending Waves of Evil in Archero - Download the Latest Version APK for Android.md
+++ /dev/null
@@ -1,134 +0,0 @@
-
-Archero APK Latest Version: A Fun Action Game with Endless Possibilities
-If you are looking for a fun and challenging action game that will keep you entertained for hours, then you should try Archero APK. Archero APK is a game that turns you into a lone archer who has to face countless enemies and obstacles in different worlds. You will need to use your skills, strategy, and luck to survive and defeat the evil forces. In this article, we will tell you everything you need to know about Archero APK, including what it is, how to download and install it, how to play it, and some tips and tricks to help you master it.
-archero apk latest version Download File » https://urlca.com/2uOa2a
- What is Archero APK?
-A brief introduction to the game and its features
-Archero APK is a game developed by Habby, a company that specializes in creating casual and fun games for mobile devices. Archero APK is one of their most popular games, with over 50 million downloads on Google Play Store. Archero APK is a roguelike game, which means that it is randomly generated and different every time you play it. You will never get bored of playing Archero APK, as there are always new things to discover and explore.
-Archero APK has many features that make it an enjoyable and addictive game. Some of these features are:
-
-Random and unique skills: You can choose from hundreds of different skills and abilities that will help you in your journey. You can create your own combinations of skills that suit your play style and preferences.
-Beautiful worlds and maps: You can explore different worlds and maps that have their own themes, enemies, and challenges. You will encounter various monsters and bosses that will test your skills and reflexes.
-Powerful equipment: You can equip yourself with different weapons, armors, rings, pets, and other items that will enhance your stats and performance. You can also upgrade your equipment to make them more effective.
-Different heroes and weapons: You can unlock and play with different heroes and weapons that have their own characteristics and abilities. You can switch between them depending on the situation and your strategy.
-
- How to download and install Archero APK on your Android device
-If you want to play Archero APK on your Android device, you will need to download and install the APK file from a reliable source. An APK file is a package file that contains all the necessary files and data for an Android application. You can download Archero APK from various websites, such as Uptodown or APKCombo. Here are the steps to download and install Archero APK on your Android device:
-
-Go to the website where you want to download Archero APK from. For example, you can go to [Uptodown](^1^) or [APKCombo](^2^).
-Search for Archero APK or browse through the categories until you find it.
-Click on the download button or link to start downloading the APK file.
-Once the download is complete, locate the APK file on your device using a file manager app.
-Tap on the APK file to start the installation process. You may need to enable the option to install apps from unknown sources in your device settings.
-Follow the instructions on the screen to complete the installation process.
-Once the installation is done, you can launch Archero APK from your app drawer or home screen.
- The benefits of playing Archero APK
-Playing Archero APK can bring you many benefits, both for your entertainment and your mental health. Some of the benefits of playing Archero APK are:
-
-It can improve your concentration and focus: Archero APK requires you to pay attention to your surroundings and your enemies, as well as to plan your moves and strategies. This can help you improve your concentration and focus skills, which can be useful in other aspects of your life.
-It can boost your creativity and imagination: Archero APK allows you to create your own combinations of skills and abilities, as well as to explore different worlds and scenarios. This can stimulate your creativity and imagination, as well as your curiosity and sense of adventure.
-It can reduce your stress and anxiety: Archero APK can be a great way to relax and unwind after a long day. You can enjoy the fun and exciting gameplay, as well as the colorful graphics and sound effects. You can also vent your emotions and frustrations by shooting arrows at your enemies.
-It can increase your confidence and self-esteem: Archero APK can challenge you to overcome various difficulties and obstacles, as well as to achieve different goals and rewards. This can make you feel proud of yourself and your achievements, as well as increase your confidence and self-esteem.
-
- How to play Archero APK
-The basic gameplay and controls
-The gameplay of Archero APK is simple and intuitive, but also challenging and addictive. You will control a hero who has to shoot arrows at the enemies that appear on the screen. You will move around the map using a virtual joystick on the left side of the screen, and you will shoot arrows automatically when you stop moving. You will also have a health bar on the top left corner of the screen, which will decrease when you get hit by an enemy or an obstacle. You will have to avoid getting hit by moving around and dodging the enemy attacks.
-archero apk download latest version 2023
-archero apk mod unlimited gems and coins
-archero apk hack no root
-archero apk update new features
-archero apk offline play
-archero apk free download for android
-archero apk old version download
-archero apk pure original
-archero apk mirror link
-archero apk obb file
-archero apk revdl unlocked
-archero apk rexdl premium
-archero apk uptodown safe
-archero apk apkpure verified
-archero apk happymod cracked
-archero apk android 1 com
-archero apk mob.org full
-archero apk data highly compressed
-archero apk unlimited money and energy
-archero apk latest version 4.14.0
-archero apk cheat menu enabled
-archero apk god mode activated
-archero apk all heroes unlocked
-archero apk best skills guide
-archero apk new weapons list
-archero apk unlimited scrolls and keys
-archero apk online multiplayer mode
-archero apk no ads removed
-archero apk latest version for pc
-archero apk mod menu download
-archero apk unlimited health and damage
-archero apk all chapters unlocked
-archero apk best equipment tips
-archero apk new events and rewards
-archero apk unlimited gems generator
-archero apk hack tool online
-archero apk modded version free
-archero apk latest version for ios
-archero apk no verification required
-archero apk unlimited coins and gems
-archero apk hack version download
-archero apk all talents unlocked
-archero apk best hero tier list
-archero apk new pets and abilities
-archero apk unlimited energy and lives
-archero apk mod unlimited everything
-archero apk latest version for windows 10
-archero apk cheat codes list
-archero apk god mode and one hit kill
-You will start each game with a random skill that will give you an advantage in the battle. You will also get to choose a new skill every time you clear a level. You will have to choose wisely, as some skills may be more useful than others depending on the situation. You can also use coins and gems that you collect during the game to upgrade your hero and equipment, or to buy new items from the shop.
- The different chapters, levels, and enemies
-Archero APK has 20 chapters, each with 50 levels. Each chapter has a different theme, such as forest, desert, dungeon, or castle. Each level has a different layout, with different enemies, obstacles, and traps. You will have to clear all the levels in a chapter to unlock the next one. You will also face a boss at the end of each chapter, which will be more powerful and harder to defeat than the regular enemies.
-The enemies in Archero APK are varied and diverse, ranging from zombies, skeletons, bats, spiders, scorpions, snakes, wolves, goblins, orcs, knights, mages, archers, dragons, demons, and more. Each enemy has its own behavior, attack pattern, speed, and strength. You will have to learn their weaknesses and strengths to defeat them effectively.
- The customization and upgrade options for your hero and equipment
-Archero APK gives you many options to customize and upgrade your hero and equipment. You can choose from different heroes that have different stats and abilities. For example, some heroes may have more health or attack power than others, or some may have special skills that can heal you or deal more damage to the enemies. You can also switch between different weapons that have different effects and ranges. For example, some weapons may shoot faster or farther than others, or some may have special effects that can freeze or burn the enemies.
-You can also equip yourself with different armors that can protect you from certain types of damage or give you extra benefits. For example, some armors may reduce the damage from ranged attacks or increase your critical chance. You can also wear different rings that can boost your stats or give you special abilities. For example, some rings may increase your attack speed or give you a chance to summon a pet that can help you in the battle.
-You can upgrade your hero and equipment using coins and scrolls that you collect during the game. Upgrading your hero will increase their stats and unlock new skills. Upgrading your equipment will increase their effectiveness and power.
- Tips and tricks for Archero APK
-How to choose the best skills and abilities for your hero
-One of the most important aspects of Archero APK is choosing the best skills and abilities for your hero. There are hundreds of different skills and abilities that you can choose from, and each one has its own advantages and disadvantages. You will have to consider your play style, your hero, your weapon, your enemies, and your environment when choosing your skills and abilities. Here are some general tips and tricks for choosing the best skills and abilities for your hero:
-
-Choose skills and abilities that complement each other: You can create powerful combinations of skills and abilities that can enhance your performance and damage output. For example, you can combine skills that increase your attack speed, your critical chance, your piercing ability, and your elemental damage to create a devastating barrage of arrows.
-Choose skills and abilities that suit your hero: You can choose skills and abilities that match the characteristics and abilities of your hero. For example, if your hero has a high health or defense stat, you can choose skills that increase your survivability or healing. If your hero has a special skill that can deal a lot of damage or stun the enemies, you can choose skills that increase its effectiveness or cooldown.
-Choose skills and abilities that suit your weapon: You can choose skills and abilities that match the effects and range of your weapon. For example, if your weapon has a long range or a wide spread, you can choose skills that increase your accuracy or range. If your weapon has a special effect that can freeze or burn the enemies, you can choose skills that increase its duration or damage.
-Choose skills and abilities that suit your enemies: You can choose skills and abilities that counter the strengths and weaknesses of your enemies. For example, if your enemies have a lot of health or armor, you can choose skills that increase your damage or penetration. If your enemies have a lot of speed or mobility, you can choose skills that slow them down or immobilize them.
-Choose skills and abilities that suit your environment: You can choose skills and abilities that take advantage of the environment and obstacles in the map. For example, if there are walls or pillars in the map, you can choose skills that bounce off them or go through them. If there are water or fire sources in the map, you can choose skills that create or use them.
-
- How to avoid and dodge enemy attacks
-Another important aspect of Archero APK is avoiding and dodging enemy attacks. You will have to be alert and agile to dodge the enemy attacks, as they can deal a lot of damage to you and reduce your health. Here are some tips and tricks for avoiding and dodging enemy attacks:
-
-Learn the enemy attack patterns: You can observe the enemy behavior and movement to predict their attack patterns. You can also look at the indicators on the screen to see when they are about to attack or where they are aiming. You can use this information to anticipate their attacks and dodge them accordingly.
-Move constantly: You can move around the map using the virtual joystick to avoid staying in one place for too long. This will make you harder to hit by the enemy attacks, as well as help you find better positions and angles to shoot back at them.
-Use the obstacles: You can use the obstacles in the map to block or deflect the enemy attacks. You can hide behind walls or pillars to avoid getting hit by projectiles or beams. You can also use objects like barrels or crates to explode or knock back the enemies.
-Use your skills and abilities: You can use some of the skills and abilities that you have chosen to avoid or dodge enemy attacks. For example, you can use skills that increase your speed, teleportation, invisibility, invincibility, shield, or healing to escape from danger or recover from damage.
-
- How to use the environment and obstacles to your advantage
-The last aspect of Archero APK that we will cover is using the environment and obstacles to your advantage. You will have to be smart and creative to use the environment and obstacles in the map to enhance your performance and damage output. Here are some tips and tricks for using the environment and obstacles to your advantage:
-
-Use the elements: You can use the elements in the map to create or amplify elemental damage. For example, you can shoot arrows through water sources to create water arrows that can freeze the enemies. You can also shoot arrows through fire sources to create fire arrows that can burn the enemies.
-Use the explosions: You can use the explosions in the map to deal massive damage to multiple enemies at once. For example, you can shoot arrows at barrels or crates that contain explosives to make them explode near the enemies. You can also shoot arrows at gas tanks or pipes that leak gas to create fireballs near the enemies.
-Use the ricochets: You can use the ricochets in the map to hit multiple enemies with one arrow. For example, you can shoot arrows at walls or pillars that can bounce off them and hit the enemies behind them. You can also shoot arrows at metal objects that can reflect them and hit the enemies from different angles.
-Use the traps: You can use the traps in the map to harm or hinder the enemies. For example, you can shoot arrows at spikes or saws that can impale or cut the enemies. You can also shoot arrows at switches or buttons that can activate or deactivate traps that can affect the enemies.
-
- Conclusion
-Archero APK is a fun and exciting action game that will keep you hooked for hours. You will have to use your skills, strategy, and luck to survive and defeat the evil forces that await you in different worlds. You will also have to customize and upgrade your hero and equipment to make them more powerful and effective. You will also have to use the environment and obstacles to your advantage to create or amplify your damage output. Archero APK is a game that will challenge you, entertain you, and reward you with endless possibilities.
-If you are ready to embark on this amazing adventure, then download Archero APK today and start playing. You will not regret it!
- FAQs
-What is the latest version of Archero APK?
-The latest version of Archero APK is 3.1.2, which was released on June 15, 2023. This version includes new features, such as a new hero, a new chapter, a new event, and bug fixes.
- Is Archero APK safe to download and install?
-Yes, Archero APK is safe to download and install, as long as you download it from a reliable source, such as Uptodown or APKCombo. These websites scan the APK files for viruses and malware before uploading them. However, you should always be careful when downloading and installing apps from unknown sources, as they may contain harmful or malicious content.
- How can I get more coins and gems in Archero APK?
-You can get more coins and gems in Archero APK by playing the game regularly and completing the levels, chapters, and events. You can also get more coins and gems by watching ads, spinning the lucky wheel, opening chests, completing achievements, or using promo codes. You can also buy more coins and gems with real money if you want to support the developers or speed up your progress.
- How can I unlock more heroes and weapons in Archero APK?
-You can unlock more heroes and weapons in Archero APK by collecting their shards or pieces. You can get these shards or pieces by playing the game, opening chests, completing events, or buying them with coins or gems. You will need a certain number of shards or pieces to unlock a hero or a weapon. You can also upgrade your heroes and weapons by using more shards or pieces.
- How can I contact the developers of Archero APK?
-You can contact the developers of Archero APK by sending them an email at archero@habby.fun. You can also follow them on their social media accounts, such as Facebook, Instagram, Twitter, or YouTube. You can also join their official Discord server to chat with other players and get updates on the game.
401be4b1e0
-
-
\ No newline at end of file
diff --git a/spaces/congsaPfin/Manga-OCR/logs/QuickBooks Enterprise 2019 Download Manage Your Business with Ease and Efficiency.md b/spaces/congsaPfin/Manga-OCR/logs/QuickBooks Enterprise 2019 Download Manage Your Business with Ease and Efficiency.md
deleted file mode 100644
index 75a291b0c06fb1e4d76f385daab6b27ae85fbf04..0000000000000000000000000000000000000000
--- a/spaces/congsaPfin/Manga-OCR/logs/QuickBooks Enterprise 2019 Download Manage Your Business with Ease and Efficiency.md
+++ /dev/null
@@ -1,104 +0,0 @@
-
-How to Download QuickBooks Enterprise 2019
-QuickBooks Enterprise is a powerful accounting software that helps you manage your business finances, inventory, payroll, and more. It is designed for small and medium-sized businesses that need advanced features and functionality. In this article, we will show you how to download QuickBooks Enterprise 2019, the latest version of the software, and how to upgrade from an older version if you already have QuickBooks Desktop.
- What is QuickBooks Enterprise 2019?
-QuickBooks Enterprise 2019 is the newest version of QuickBooks Desktop Enterprise, which was released in September 2018. It offers several improvements and enhancements over the previous versions, such as:
-download quickbooks enterprise 2019 DOWNLOAD ✓✓✓ https://urlca.com/2uOcdg
-
-Newly improved sales order management system
-Multiple company files with consolidated reports
-QuickBooks control prices
-Two company files at one place in one go
-Ability to track employees, more than 10,000 customers and inventory items
-Different user roles: up to 14
-Access from anywhere with enhanced reporting tools
-
-QuickBooks Enterprise 2019 also comes with different industry-specific editions, such as manufacturing and wholesale, contractor, nonprofit, retail, professional services, and accountant. These editions offer customized capabilities, reports, and features designed for your company type and industry.
- System requirements for QuickBooks Enterprise 2019
-Before you download and install QuickBooks Enterprise 2019, you need to make sure that your computer meets the minimum system requirements for the software. Here are the system requirements for QuickBooks Enterprise 2019:
-
-Windows 10 (64 bit), or Windows 11, update/version supported by Microsoft. Windows 8.1 and Linux are not supported.
-Server: Windows Server 2012 (or R2), 2016, 2019, or 2022 (Regular or Small Business Server)
-2.4 GHz processor
-Client RAM: 8 GB RAM; 16 GB recommended
-Server RAM (for multi-user support): 8 GB (5 users); 12 GB (10 users); 16 GB (15 users); 20 GB (20+ users)
-2.5 GB disk space recommended (additional space required for data files); Solid State Drive (SSD) recommended for optimal performance
-Enterprise subscriptions, payroll and online features require Internet access
-QuickBooks Desktop App is included with Desktop subscriptions. Must be installed on a camera-enabled mobile device using Android 6.0 or iOS 12 or later. Product registration required
-Optimized for 1280×1024 screen resolution or higher. Supports one Workstation Monitor, plus up to 2 extended monitors. Optimized for Default DPI settings.
-Integration with other software: Microsoft Word and Excel integration requires Office 2013-2021, or Microsoft 365 (32 and 64 bit); E-mail Estimates, Invoices and other forms with Microsoft Outlook 2013-2019, Microsoft 365, Gmail TM , and Outlook.com®, other SMTP-supporting e-mail clients; Integration with QuickBooks POS 19.0; Transfer data from Quicken 2016- How to download QuickBooks Enterprise 2019?
-If you want to download QuickBooks Enterprise 2019, you need to follow these steps:
- Step 1: Go to the Downloads & Updates page
-The first step is to go to the Downloads & Updates page on the Intuit website. This is where you can find the latest version of QuickBooks Desktop Enterprise and other products. You can also access this page from your QuickBooks account or from the Help menu in the software.
- Step 2: Select your country, product, and version
-The next step is to select your country, product, and version from the drop-down menus on the Downloads & Updates page. For example, if you are in the United States, you need to select United States (US) as your country, QuickBooks Desktop Enterprise as your product, and 2019 as your version. Then, click on the Search button to find the download link for QuickBooks Enterprise 2019.
-How to download quickbooks enterprise 2019 for windows
-Quickbooks enterprise 2019 download link and installation guide
-Quickbooks enterprise 2019 pro download with payroll
-Quickbooks enterprise 2019 for mac download and setup
-Download quickbooks desktop enterprise 2019 platinum edition
-Quickbooks enterprise 2019 advanced inventory download
-Quickbooks enterprise 2019 free trial download
-Download quickbooks enterprise 2019 accountant edition
-Quickbooks enterprise 2019 download crack and license key
-Quickbooks enterprise 2019 system requirements and download size
-Quickbooks enterprise 2019 vs online comparison and download
-Quickbooks enterprise 2019 upgrade download and instructions
-Quickbooks enterprise 2019 multi-user mode download and configuration
-Quickbooks enterprise 2019 hosting service and download options
-Quickbooks enterprise 2019 pricing plans and download discounts
-Quickbooks enterprise 2019 features and benefits download brochure
-Quickbooks enterprise 2019 customer reviews and testimonials download
-Quickbooks enterprise 2019 support phone number and download help
-Quickbooks enterprise 2019 training courses and tutorials download
-Quickbooks enterprise 2019 integration with other software download
-Download quickbooks enterprise 2019 backup and restore tools
-Quickbooks enterprise 2019 custom reports and templates download
-Quickbooks enterprise 2019 data migration and conversion download
-Quickbooks enterprise 2019 security and privacy settings download
-Quickbooks enterprise 2019 performance optimization and troubleshooting download
-Download quickbooks enterprise 2019 mobile app for android and ios
-Quickbooks enterprise 2019 cloud access and sync download
-Quickbooks enterprise 2019 barcode scanning and printing download
-Quickbooks enterprise 2019 serial number and product code download
-Download quickbooks enterprise 2019 updates and patches
- Step 3: Download the installation file
-The third step is to download the installation file for QuickBooks Enterprise 2019. You can do this by clicking on the Download button next to the product name. The file size is about 700 MB, so it may take some time depending on your internet speed. You can also choose to download a trial version of QuickBooks Enterprise 2019 if you want to test it before buying it.
- Step 4: Install QuickBooks Enterprise 2019 on your computer
-The final step is to install QuickBooks Enterprise 2019 on your computer. You can do this by double-clicking on the downloaded file and following the on-screen instructions. You will need to agree to the license agreement, enter your product and license numbers, and choose your installation type (express or custom). You will also need to activate QuickBooks Enterprise 2019 after installing it by signing in with your Intuit account or creating one if you don't have one.
- How to upgrade to QuickBooks Enterprise 2019?
-If you already have an older version of QuickBooks Desktop Enterprise, such as 2018 or 2017, you can upgrade to QuickBooks Enterprise 2019 by following these steps:
- Step 1: Check your current version of QuickBooks
-The first step is to check your current version of QuickBooks Desktop Enterprise. You can do this by opening the software and pressing F2 on your keyboard. This will open the Product Information window, where you can see your product name, version, release, and license number. You can also see if you have any updates available for your current version by clicking on the Update Now button.
- Step 2: Back up your company file
-The second step is to back up your company file before upgrading to QuickBooks Enterprise 2019. This is a precautionary measure in case something goes wrong during the upgrade process. You can back up your company file by going to the File menu and selecting Back Up Company > Create Local Backup. You can choose where to save your backup file and how often to back up automatically.
- Step 3: Uninstall your old version of QuickBooks
-The third step is to uninstall your old version of QuickBooks Desktop Enterprise from your computer. You can do this by going to the Control Panel and selecting Programs and Features. Then, find QuickBooks Desktop Enterprise in the list of programs and click on Uninstall/Change. Follow the prompts to complete the uninstallation process.
- Step 4: Install QuickBooks Enterprise 2019 on your computer
-The fourth step is to install QuickBooks Enterprise 2019 on your computer. You can do this by following the same steps as described above for downloading and installing QuickBooks Enterprise 2019.
- Step 5: Restore your company file
-The final step is to restore your company file after upgrading to QuickBooks Enterprise 2019. You can do this by opening QuickBooks Enterprise 2019 and selecting Open or Restore Company from the File menu. Then, choose Restore a backup copy and browse for your backup file that you created earlier. Follow the instructions to restore your company file and update it to the new version.
- Conclusion
-In this article, we have shown you how to download QuickBooks Enterprise 2019, the latest version of QuickBooks Desktop Enterprise, and how to upgrade from an older version if you already have QuickBooks Desktop. We hope that this article has been helpful and informative for you. If you have any questions or comments, please feel free to leave them below
Here are some FAQs that you may find useful:
- FAQs
-
-What are the benefits of QuickBooks Enterprise 2019 over other versions of QuickBooks?
-QuickBooks Enterprise 2019 offers several benefits over other versions of QuickBooks, such as:
-
-It can handle more data, transactions, users, and inventory items than other versions of QuickBooks
-It offers industry-specific features and reports for different types of businesses
-It has advanced capabilities for managing sales orders, pricing, inventory, and reporting
-It allows you to access your data from anywhere with enhanced security and performance
-It includes a subscription to QuickBooks Desktop App, which lets you access your data from your mobile device
-
- How much does QuickBooks Enterprise 2019 cost?
-QuickBooks Enterprise 2019 is a subscription-based software that requires an annual or monthly payment. The cost depends on the number of users, the industry edition, and the hosting option that you choose. You can check the current pricing and plans on the QuickBooks Enterprise website .
- How can I get support for QuickBooks Enterprise 2019?
-If you need support for QuickBooks Enterprise 2019, you can contact the QuickBooks Enterprise customer service team by phone, chat, or email. You can also visit the QuickBooks Enterprise support website , where you can find articles, videos, webinars, and community forums to help you with your questions and issues.
- How can I learn more about QuickBooks Enterprise 2019?
-If you want to learn more about QuickBooks Enterprise 2019, you can check out the QuickBooks Enterprise resource center , where you can find guides, tutorials, tips, and tricks to help you get the most out of the software. You can also sign up for free training sessions and webinars that cover various topics and features of QuickBooks Enterprise 2019.
- How can I get a free trial of QuickBooks Enterprise 2019?
-If you want to try QuickBooks Enterprise 2019 before buying it, you can get a free trial of the software for 30 days. You can download the trial version from the Downloads & Updates page , where you can also find the installation instructions and system requirements. You can use the trial version with your existing company file or create a new one.
- 197e85843d
-
-
\ No newline at end of file
diff --git a/spaces/congsaPfin/Manga-OCR/logs/Survive the Dangers of a Pirate Island with Last Pirate Island Survival 2 MOD APK.md b/spaces/congsaPfin/Manga-OCR/logs/Survive the Dangers of a Pirate Island with Last Pirate Island Survival 2 MOD APK.md
deleted file mode 100644
index e932ae2737beabcc461f055a0705aea180b71ee0..0000000000000000000000000000000000000000
--- a/spaces/congsaPfin/Manga-OCR/logs/Survive the Dangers of a Pirate Island with Last Pirate Island Survival 2 MOD APK.md
+++ /dev/null
@@ -1,137 +0,0 @@
-
-Last Pirate Island Survival 2 Mod APK: A Guide for Beginners
-Do you love adventure games that test your survival skills and creativity? Do you want to experience the thrill of living on a deserted island full of dangers and mysteries? If yes, then you should try Last Pirate Island Survival 2, a popular game that has millions of fans around the world. In this article, we will tell you everything you need to know about this game, including what it is, how to download and install it, and how to play it. We will also show you how to use the mod apk version of the game, which gives you unlimited money and other advantages. So, let's get started!
- What is Last Pirate Island Survival 2?
-A brief introduction to the game
-Last Pirate Island Survival 2 is a sequel to the original Last Pirate game, which was released in 2020. It is an adventure game that puts you in the role of a pirate who has been shipwrecked on a mysterious island. Your goal is to survive by exploring the island, gathering resources, crafting tools and weapons, building shelters, fighting enemies, and uncovering secrets. The game has realistic graphics, immersive sound effects, and dynamic weather conditions that make you feel like you are really on the island.
-last pirate island survival 2 mod apk Download File ⚹⚹⚹ https://urlca.com/2uO84p
- The main features of the game
-Some of the main features of Last Pirate Island Survival 2 are:
-
-A large open-world island with different biomes, such as forests, beaches, caves, mountains, and volcanoes.
-A variety of resources to collect, such as wood, stone, metal, food, water, and treasure.
-A crafting system that allows you to create hundreds of items, such as axes, swords, bows, arrows, spears, guns, bombs, traps, boats, and more.
-A building system that lets you construct your own base, with walls, floors, roofs, doors, windows, furniture, and decorations.
-A combat system that challenges you to fight against different enemies, such as zombies, skeletons, cannibals, wild animals, and other pirates.
-A quest system that gives you tasks to complete and rewards you with coins and gems.
-A customization system that enables you to change your appearance and outfit.
-A multiplayer mode that allows you to play with your friends online or join other players' islands.
-
- The benefits of using the mod apk version
-If you want to enjoy the game without any limitations or restrictions, you should use the mod apk version of Last Pirate Island Survival 2. The mod apk version is a modified version of the original game that gives you some extra benefits, such as:
-
-Unlimited money: You can get unlimited coins and gems in the game, which you can use to buy anything you want from the shop or upgrade your items.
-Immortality: You can become invincible in the game, which means you won't die from hunger, thirst, injuries, or attacks.
-Free crafting: You can craft any item in the game without needing any resources or materials.
-No ads: You can play the game without any annoying ads interrupting your gameplay.
-
- How to download and install Last Pirate Island Survival 2 Mod APK?
-The requirements for downloading the mod apk
-Before you download and install the mod apk, you need to make sure that your device meets the following requirements:
-
-Android version: 4.4 or higher
-Storage space: At least 200 MB of free space
-Internet connection: Required for downloading and playing online
-Permission: Allow installation from unknown sources
-
- The steps for installing the mod apk
-After you have checked the requirements, you can follow these steps to download and install the mod apk:
-
-Click on this link to download the mod apk file: [Last Pirate Island Survival 2 Mod APK]
-Wait for the download to finish and then locate the file in your device's file manager.
-Tap on the file and select "Install". If you see a warning message, click on "Settings" and enable the option to install from unknown sources.
-Wait for the installation to complete and then launch the game from your home screen or app drawer.
-Enjoy playing Last Pirate Island Survival 2 Mod APK with unlimited money and immortality!
-
- The precautions for using the mod apk
-While using the mod apk can be fun and convenient, you should also be aware of some potential risks and drawbacks, such as:
-
-The mod apk may not be compatible with some devices or versions of the game.
-The mod apk may cause some glitches or errors in the game.
-The mod apk may be detected by the game's anti-cheat system and result in a ban or suspension of your account.
-The mod apk may contain viruses or malware that can harm your device or data.
-
-Therefore, you should use the mod apk at your own risk and discretion. We are not responsible for any damage or loss caused by using the mod apk. We also recommend that you backup your data before using the mod apk and that you uninstall it if you encounter any problems.
- How to play Last Pirate Island Survival 2 Mod APK?
-The basic gameplay mechanics
-Last Pirate Island Survival 2 Mod APK is a game that combines elements of survival, exploration, crafting, building, combat, and questing. The game starts with you waking up on a deserted island after a shipwreck. You have nothing but a few items in your inventory and a map of the island. Your first task is to find a safe place to build your shelter. You can use the map to navigate around the island and discover different locations, such as forests, beaches, caves, mountains, and volcanoes. You can also use the compass to find your direction and the clock to check the time of day.
- To survive on the island, you need to manage your health, hunger, thirst, and stamina. You can find food and water sources on the island, such as fruits, vegetables, fish, meat, wells, rivers, and lakes. You can also cook food over a fire or boil water in a pot to make it safer to consume. You can craft tools and weapons from resources you gather on the island, such as wood, stone, metal, leather, cloth, and more. You can use these items to chop trees, mine rocks, hunt animals, fight enemies, and more. You can also build your own base from materials you collect on the island, such as planks, bricks, nails, ropes, and more. You can design your base according to your preference and add furniture and decorations to make it more comfortable and cozy.
-last pirate island survival mod apk unlimited money
-last pirate island survival 2 mod apk download
-last pirate island survival mod apk latest version
-last pirate island survival 2 mod apk android 1
-last pirate island survival mod apk immortality
-last pirate island survival 2 mod apk rexdl
-last pirate island survival mod apk free craft
-last pirate island survival 2 mod apk offline
-last pirate island survival mod apk no ads
-last pirate island survival 2 mod apk revdl
-last pirate island survival mod apk hack
-last pirate island survival 2 mod apk unlimited resources
-last pirate island survival mod apk obb
-last pirate island survival 2 mod apk god mode
-last pirate island survival mod apk premium
-last pirate island survival 2 mod apk unlocked
-last pirate island survival mod apk full version
-last pirate island survival 2 mod apk update
-last pirate island survival mod apk cheats
-last pirate island survival 2 mod apk mega
-last pirate island survival mod apk online
-last pirate island survival 2 mod apk data
-last pirate island survival mod apk new version
-last pirate island survival 2 mod apk original
-last pirate island survival mod apk gameplay
-last pirate island survival 2 mod apk old version
-last pirate island survival mod apk features
-last pirate island survival 2 mod apk hack download
-last pirate island survival mod apk android oyun club
-last pirate island survival 2 mod apk unlimited everything
-last pirate island survival mod apk all items unlocked
-last pirate island survival 2 mod apk no root
-last pirate island survival mod apk for pc
-last pirate island survival 2 mod apk pure
-last pirate island survival mod apk high damage
-last pirate island survival 2 mod apk apkpure
-last pirate island survival mod apk low mb
-last pirate island survival 2 mod apk happymod
-last pirate island survival mod apk easy download
-last pirate island survival 2 mod apk mediafıre link
- The tips and tricks for surviving on the island
-To make your life easier on the island, here are some tips and tricks that you should follow:
-
-Always keep an eye on your status bars and replenish them when they are low.
-Always carry some food and water with you when you go out exploring.
-Always equip yourself with a weapon and armor when you encounter enemies.
-Always save your game before you enter a dangerous area or start a quest.
-Always check your inventory and storage for items that you can use or craft.
-Always look for treasure chests and hidden secrets on the island.
-Always use the mod apk features wisely and sparingly.
-
- The challenges and rewards of the game
-Last Pirate Island Survival 2 Mod APK is a game that offers many challenges and rewards for players who are willing to take risks and explore new possibilities. Some of the challenges and rewards of the game are:
-
-Challenge Reward
-Fighting against different enemies, such as zombies, skeletons, cannibals, wild animals, and other pirates. Gaining experience points, coins, gems, loot, and trophies.
-Completing quests given by NPCs or the game itself. Gaining coins, gems, items, and reputation.
-Exploring the island and discovering new locations, secrets, and events. Gaining knowledge, resources, and achievements.
-Crafting and building your own items and base. Gaining satisfaction, creativity, and protection.
-Playing with your friends online or joining other players' islands. Gaining fun, cooperation, and competition.
-
- Conclusion
-A summary of the main points of the article
-In conclusion, Last Pirate Island Survival 2 Mod APK is a game that offers you a unique and exciting adventure on a deserted island. You can explore the island, gather resources, craft items, build your base, fight enemies, complete quests, and more. You can also use the mod apk version of the game to get unlimited money and immortality. However, you should also be careful of the potential risks and drawbacks of using the mod apk. We hope that this article has helped you learn more about this game and how to play it. If you are ready to embark on your pirate adventure, download and install Last Pirate Island Survival 2 Mod APK now!
- A call to action for the readers
-If you liked this article, please share it with your friends and leave a comment below. We would love to hear your feedback and suggestions. Also, if you have any questions or problems regarding Last Pirate Island Survival 2 Mod APK, feel free to ask us in the comment section. We will try our best to help you out. Thank you for reading and happy gaming!
- FAQs
-Q: Is Last Pirate Island Survival 2 Mod APK safe to use?
-A: Last Pirate Island Survival 2 Mod APK is generally safe to use as long as you download it from a trusted source and scan it with an antivirus program before installing it. However, you should also be aware of the possible risks and drawbacks of using the mod apk, such as compatibility issues, glitches, errors, bans, suspensions, viruses, or malware.
- Q: How can I update Last Pirate Island Survival 2 Mod APK?
-A: To update Last Pirate Island Survival 2 Mod APK, you need to download the latest version of the mod apk file from the same source that you downloaded it from before. Then, you need to uninstall the previous version of the mod apk from your device and install the new version following the same steps as before.
- Q: How can I play Last Pirate Island Survival 2 Mod APK offline?
-A: To play Last Pirate Island Survival 2 Mod APK offline, you need to turn off your internet connection before launching the game. However, you should note that some features of the game may not work properly or at all without an internet connection, such as multiplayer mode or online events.
- Q: How can I play Last Pirate Island Survival 2 Mod APK with my friends?
-A: To play Last Pirate Island Survival 2 Mod APK with your friends, you need to have an internet connection and a Facebook account. Then, you need to log in to your Facebook account in the game and invite your friends to join your island or join their islands. You can also chat with them in the game and cooperate or compete with them in various activities.
- Q: How can I get more coins and gems in Last Pirate Island Survival 2 Mod APK?
-A: To get more coins and gems in Last Pirate Island Survival 2 Mod APK, you can use the mod apk features that give you unlimited money. Alternatively, you can also earn coins and gems by completing quests, fighting enemies, finding treasure chests, and watching ads. You can also buy coins and gems with real money if you want to support the game developers.
197e85843d
-
-
\ No newline at end of file
diff --git a/spaces/contluForse/HuggingGPT/assets/Download Antiwpa Windows Xp Sp2instmankl HOT.md b/spaces/contluForse/HuggingGPT/assets/Download Antiwpa Windows Xp Sp2instmankl HOT.md
deleted file mode 100644
index 83e61158832d51864644daef8fe7d88b91ceb78e..0000000000000000000000000000000000000000
--- a/spaces/contluForse/HuggingGPT/assets/Download Antiwpa Windows Xp Sp2instmankl HOT.md
+++ /dev/null
@@ -1,6 +0,0 @@
-Download Antiwpa Windows Xp Sp2instmankl Download File ✯✯✯ https://ssurll.com/2uzxYf
-
- aaccfb2cb3
-
-
-
diff --git a/spaces/contluForse/HuggingGPT/assets/Dxcpl.exe ((TOP)) Download Windows 7 32-bit 1358.md b/spaces/contluForse/HuggingGPT/assets/Dxcpl.exe ((TOP)) Download Windows 7 32-bit 1358.md
deleted file mode 100644
index 22a0477d8838ce25ebbf961c1c3c7898d4da9dda..0000000000000000000000000000000000000000
--- a/spaces/contluForse/HuggingGPT/assets/Dxcpl.exe ((TOP)) Download Windows 7 32-bit 1358.md
+++ /dev/null
@@ -1,8 +0,0 @@
-dxcpl.exe download windows 7 32-bit 1358 Download File ✑ https://ssurll.com/2uzwE4
-
-13 Jun 2021 - Ajab Gazabb Love English Subtitl - Dxcpl.exe Download Windows 7 32-bit 1358 - Power ISO 5.6 FINAL Keys keyG[Lz0 CORE] By Senzati Download Windows 7 32-bit 1358 - Power ISO 5.6 FINAL Keys
-keyG[Lz0 CORE] By Senzati Download Windows 10 64-bit 16 GB - Microsoft Security Essentials (Privacy Protection) v1.0.1226.0 (x86) KeyGen By Hugo Leymo Download Windows 10 64-bit 16 GB - Microsoft Security Essentials (Privacy Protection) (v1.0.1226.0) (x86) KeyGen By Hugo Leymo Download Windows 10 64 bit 16 GB - Microsoft Security Essentials (Privacy
-Protection) (v1.0.1226.0) (x86) KeyGen By Hugo 8a78ff9644
-
-
-
diff --git a/spaces/coreml-community/ControlNet-v1-1-Annotators-cpu/annotator/mmpkg/mmcv/ops/deform_conv.py b/spaces/coreml-community/ControlNet-v1-1-Annotators-cpu/annotator/mmpkg/mmcv/ops/deform_conv.py
deleted file mode 100644
index 3de3aae1e7b2258360aef3ad9eb3a351f080f10f..0000000000000000000000000000000000000000
--- a/spaces/coreml-community/ControlNet-v1-1-Annotators-cpu/annotator/mmpkg/mmcv/ops/deform_conv.py
+++ /dev/null
@@ -1,405 +0,0 @@
-# Copyright (c) OpenMMLab. All rights reserved.
-from typing import Tuple, Union
-
-import torch
-import torch.nn as nn
-import torch.nn.functional as F
-from torch import Tensor
-from torch.autograd import Function
-from torch.autograd.function import once_differentiable
-from torch.nn.modules.utils import _pair, _single
-
-from annotator.mmpkg.mmcv.utils import deprecated_api_warning
-from ..cnn import CONV_LAYERS
-from ..utils import ext_loader, print_log
-
-ext_module = ext_loader.load_ext('_ext', [
- 'deform_conv_forward', 'deform_conv_backward_input',
- 'deform_conv_backward_parameters'
-])
-
-
-class DeformConv2dFunction(Function):
-
- @staticmethod
- def symbolic(g,
- input,
- offset,
- weight,
- stride,
- padding,
- dilation,
- groups,
- deform_groups,
- bias=False,
- im2col_step=32):
- return g.op(
- 'mmcv::MMCVDeformConv2d',
- input,
- offset,
- weight,
- stride_i=stride,
- padding_i=padding,
- dilation_i=dilation,
- groups_i=groups,
- deform_groups_i=deform_groups,
- bias_i=bias,
- im2col_step_i=im2col_step)
-
- @staticmethod
- def forward(ctx,
- input,
- offset,
- weight,
- stride=1,
- padding=0,
- dilation=1,
- groups=1,
- deform_groups=1,
- bias=False,
- im2col_step=32):
- if input is not None and input.dim() != 4:
- raise ValueError(
- f'Expected 4D tensor as input, got {input.dim()}D tensor \
- instead.')
- assert bias is False, 'Only support bias is False.'
- ctx.stride = _pair(stride)
- ctx.padding = _pair(padding)
- ctx.dilation = _pair(dilation)
- ctx.groups = groups
- ctx.deform_groups = deform_groups
- ctx.im2col_step = im2col_step
-
- # When pytorch version >= 1.6.0, amp is adopted for fp16 mode;
- # amp won't cast the type of model (float32), but "offset" is cast
- # to float16 by nn.Conv2d automatically, leading to the type
- # mismatch with input (when it is float32) or weight.
- # The flag for whether to use fp16 or amp is the type of "offset",
- # we cast weight and input to temporarily support fp16 and amp
- # whatever the pytorch version is.
- input = input.type_as(offset)
- weight = weight.type_as(input)
- ctx.save_for_backward(input, offset, weight)
-
- output = input.new_empty(
- DeformConv2dFunction._output_size(ctx, input, weight))
-
- ctx.bufs_ = [input.new_empty(0), input.new_empty(0)] # columns, ones
-
- cur_im2col_step = min(ctx.im2col_step, input.size(0))
- assert (input.size(0) %
- cur_im2col_step) == 0, 'im2col step must divide batchsize'
- ext_module.deform_conv_forward(
- input,
- weight,
- offset,
- output,
- ctx.bufs_[0],
- ctx.bufs_[1],
- kW=weight.size(3),
- kH=weight.size(2),
- dW=ctx.stride[1],
- dH=ctx.stride[0],
- padW=ctx.padding[1],
- padH=ctx.padding[0],
- dilationW=ctx.dilation[1],
- dilationH=ctx.dilation[0],
- group=ctx.groups,
- deformable_group=ctx.deform_groups,
- im2col_step=cur_im2col_step)
- return output
-
- @staticmethod
- @once_differentiable
- def backward(ctx, grad_output):
- input, offset, weight = ctx.saved_tensors
-
- grad_input = grad_offset = grad_weight = None
-
- cur_im2col_step = min(ctx.im2col_step, input.size(0))
- assert (input.size(0) % cur_im2col_step
- ) == 0, 'batch size must be divisible by im2col_step'
-
- grad_output = grad_output.contiguous()
- if ctx.needs_input_grad[0] or ctx.needs_input_grad[1]:
- grad_input = torch.zeros_like(input)
- grad_offset = torch.zeros_like(offset)
- ext_module.deform_conv_backward_input(
- input,
- offset,
- grad_output,
- grad_input,
- grad_offset,
- weight,
- ctx.bufs_[0],
- kW=weight.size(3),
- kH=weight.size(2),
- dW=ctx.stride[1],
- dH=ctx.stride[0],
- padW=ctx.padding[1],
- padH=ctx.padding[0],
- dilationW=ctx.dilation[1],
- dilationH=ctx.dilation[0],
- group=ctx.groups,
- deformable_group=ctx.deform_groups,
- im2col_step=cur_im2col_step)
-
- if ctx.needs_input_grad[2]:
- grad_weight = torch.zeros_like(weight)
- ext_module.deform_conv_backward_parameters(
- input,
- offset,
- grad_output,
- grad_weight,
- ctx.bufs_[0],
- ctx.bufs_[1],
- kW=weight.size(3),
- kH=weight.size(2),
- dW=ctx.stride[1],
- dH=ctx.stride[0],
- padW=ctx.padding[1],
- padH=ctx.padding[0],
- dilationW=ctx.dilation[1],
- dilationH=ctx.dilation[0],
- group=ctx.groups,
- deformable_group=ctx.deform_groups,
- scale=1,
- im2col_step=cur_im2col_step)
-
- return grad_input, grad_offset, grad_weight, \
- None, None, None, None, None, None, None
-
- @staticmethod
- def _output_size(ctx, input, weight):
- channels = weight.size(0)
- output_size = (input.size(0), channels)
- for d in range(input.dim() - 2):
- in_size = input.size(d + 2)
- pad = ctx.padding[d]
- kernel = ctx.dilation[d] * (weight.size(d + 2) - 1) + 1
- stride_ = ctx.stride[d]
- output_size += ((in_size + (2 * pad) - kernel) // stride_ + 1, )
- if not all(map(lambda s: s > 0, output_size)):
- raise ValueError(
- 'convolution input is too small (output would be ' +
- 'x'.join(map(str, output_size)) + ')')
- return output_size
-
-
-deform_conv2d = DeformConv2dFunction.apply
-
-
-class DeformConv2d(nn.Module):
- r"""Deformable 2D convolution.
-
- Applies a deformable 2D convolution over an input signal composed of
- several input planes. DeformConv2d was described in the paper
- `Deformable Convolutional Networks
- `_
-
- Note:
- The argument ``im2col_step`` was added in version 1.3.17, which means
- number of samples processed by the ``im2col_cuda_kernel`` per call.
- It enables users to define ``batch_size`` and ``im2col_step`` more
- flexibly and solved `issue mmcv#1440
- `_.
-
- Args:
- in_channels (int): Number of channels in the input image.
- out_channels (int): Number of channels produced by the convolution.
- kernel_size(int, tuple): Size of the convolving kernel.
- stride(int, tuple): Stride of the convolution. Default: 1.
- padding (int or tuple): Zero-padding added to both sides of the input.
- Default: 0.
- dilation (int or tuple): Spacing between kernel elements. Default: 1.
- groups (int): Number of blocked connections from input.
- channels to output channels. Default: 1.
- deform_groups (int): Number of deformable group partitions.
- bias (bool): If True, adds a learnable bias to the output.
- Default: False.
- im2col_step (int): Number of samples processed by im2col_cuda_kernel
- per call. It will work when ``batch_size`` > ``im2col_step``, but
- ``batch_size`` must be divisible by ``im2col_step``. Default: 32.
- `New in version 1.3.17.`
- """
-
- @deprecated_api_warning({'deformable_groups': 'deform_groups'},
- cls_name='DeformConv2d')
- def __init__(self,
- in_channels: int,
- out_channels: int,
- kernel_size: Union[int, Tuple[int, ...]],
- stride: Union[int, Tuple[int, ...]] = 1,
- padding: Union[int, Tuple[int, ...]] = 0,
- dilation: Union[int, Tuple[int, ...]] = 1,
- groups: int = 1,
- deform_groups: int = 1,
- bias: bool = False,
- im2col_step: int = 32) -> None:
- super(DeformConv2d, self).__init__()
-
- assert not bias, \
- f'bias={bias} is not supported in DeformConv2d.'
- assert in_channels % groups == 0, \
- f'in_channels {in_channels} cannot be divisible by groups {groups}'
- assert out_channels % groups == 0, \
- f'out_channels {out_channels} cannot be divisible by groups \
- {groups}'
-
- self.in_channels = in_channels
- self.out_channels = out_channels
- self.kernel_size = _pair(kernel_size)
- self.stride = _pair(stride)
- self.padding = _pair(padding)
- self.dilation = _pair(dilation)
- self.groups = groups
- self.deform_groups = deform_groups
- self.im2col_step = im2col_step
- # enable compatibility with nn.Conv2d
- self.transposed = False
- self.output_padding = _single(0)
-
- # only weight, no bias
- self.weight = nn.Parameter(
- torch.Tensor(out_channels, in_channels // self.groups,
- *self.kernel_size))
-
- self.reset_parameters()
-
- def reset_parameters(self):
- # switch the initialization of `self.weight` to the standard kaiming
- # method described in `Delving deep into rectifiers: Surpassing
- # human-level performance on ImageNet classification` - He, K. et al.
- # (2015), using a uniform distribution
- nn.init.kaiming_uniform_(self.weight, nonlinearity='relu')
-
- def forward(self, x: Tensor, offset: Tensor) -> Tensor:
- """Deformable Convolutional forward function.
-
- Args:
- x (Tensor): Input feature, shape (B, C_in, H_in, W_in)
- offset (Tensor): Offset for deformable convolution, shape
- (B, deform_groups*kernel_size[0]*kernel_size[1]*2,
- H_out, W_out), H_out, W_out are equal to the output's.
-
- An offset is like `[y0, x0, y1, x1, y2, x2, ..., y8, x8]`.
- The spatial arrangement is like:
-
- .. code:: text
-
- (x0, y0) (x1, y1) (x2, y2)
- (x3, y3) (x4, y4) (x5, y5)
- (x6, y6) (x7, y7) (x8, y8)
-
- Returns:
- Tensor: Output of the layer.
- """
- # To fix an assert error in deform_conv_cuda.cpp:128
- # input image is smaller than kernel
- input_pad = (x.size(2) < self.kernel_size[0]) or (x.size(3) <
- self.kernel_size[1])
- if input_pad:
- pad_h = max(self.kernel_size[0] - x.size(2), 0)
- pad_w = max(self.kernel_size[1] - x.size(3), 0)
- x = F.pad(x, (0, pad_w, 0, pad_h), 'constant', 0).contiguous()
- offset = F.pad(offset, (0, pad_w, 0, pad_h), 'constant', 0)
- offset = offset.contiguous()
- out = deform_conv2d(x, offset, self.weight, self.stride, self.padding,
- self.dilation, self.groups, self.deform_groups,
- False, self.im2col_step)
- if input_pad:
- out = out[:, :, :out.size(2) - pad_h, :out.size(3) -
- pad_w].contiguous()
- return out
-
- def __repr__(self):
- s = self.__class__.__name__
- s += f'(in_channels={self.in_channels},\n'
- s += f'out_channels={self.out_channels},\n'
- s += f'kernel_size={self.kernel_size},\n'
- s += f'stride={self.stride},\n'
- s += f'padding={self.padding},\n'
- s += f'dilation={self.dilation},\n'
- s += f'groups={self.groups},\n'
- s += f'deform_groups={self.deform_groups},\n'
- # bias is not supported in DeformConv2d.
- s += 'bias=False)'
- return s
-
-
-@CONV_LAYERS.register_module('DCN')
-class DeformConv2dPack(DeformConv2d):
- """A Deformable Conv Encapsulation that acts as normal Conv layers.
-
- The offset tensor is like `[y0, x0, y1, x1, y2, x2, ..., y8, x8]`.
- The spatial arrangement is like:
-
- .. code:: text
-
- (x0, y0) (x1, y1) (x2, y2)
- (x3, y3) (x4, y4) (x5, y5)
- (x6, y6) (x7, y7) (x8, y8)
-
- Args:
- in_channels (int): Same as nn.Conv2d.
- out_channels (int): Same as nn.Conv2d.
- kernel_size (int or tuple[int]): Same as nn.Conv2d.
- stride (int or tuple[int]): Same as nn.Conv2d.
- padding (int or tuple[int]): Same as nn.Conv2d.
- dilation (int or tuple[int]): Same as nn.Conv2d.
- groups (int): Same as nn.Conv2d.
- bias (bool or str): If specified as `auto`, it will be decided by the
- norm_cfg. Bias will be set as True if norm_cfg is None, otherwise
- False.
- """
-
- _version = 2
-
- def __init__(self, *args, **kwargs):
- super(DeformConv2dPack, self).__init__(*args, **kwargs)
- self.conv_offset = nn.Conv2d(
- self.in_channels,
- self.deform_groups * 2 * self.kernel_size[0] * self.kernel_size[1],
- kernel_size=self.kernel_size,
- stride=_pair(self.stride),
- padding=_pair(self.padding),
- dilation=_pair(self.dilation),
- bias=True)
- self.init_offset()
-
- def init_offset(self):
- self.conv_offset.weight.data.zero_()
- self.conv_offset.bias.data.zero_()
-
- def forward(self, x):
- offset = self.conv_offset(x)
- return deform_conv2d(x, offset, self.weight, self.stride, self.padding,
- self.dilation, self.groups, self.deform_groups,
- False, self.im2col_step)
-
- def _load_from_state_dict(self, state_dict, prefix, local_metadata, strict,
- missing_keys, unexpected_keys, error_msgs):
- version = local_metadata.get('version', None)
-
- if version is None or version < 2:
- # the key is different in early versions
- # In version < 2, DeformConvPack loads previous benchmark models.
- if (prefix + 'conv_offset.weight' not in state_dict
- and prefix[:-1] + '_offset.weight' in state_dict):
- state_dict[prefix + 'conv_offset.weight'] = state_dict.pop(
- prefix[:-1] + '_offset.weight')
- if (prefix + 'conv_offset.bias' not in state_dict
- and prefix[:-1] + '_offset.bias' in state_dict):
- state_dict[prefix +
- 'conv_offset.bias'] = state_dict.pop(prefix[:-1] +
- '_offset.bias')
-
- if version is not None and version > 1:
- print_log(
- f'DeformConv2dPack {prefix.rstrip(".")} is upgraded to '
- 'version 2.',
- logger='root')
-
- super()._load_from_state_dict(state_dict, prefix, local_metadata,
- strict, missing_keys, unexpected_keys,
- error_msgs)
diff --git a/spaces/coreml-community/ControlNet-v1-1-Annotators-cpu/annotator/mmpkg/mmcv/ops/furthest_point_sample.py b/spaces/coreml-community/ControlNet-v1-1-Annotators-cpu/annotator/mmpkg/mmcv/ops/furthest_point_sample.py
deleted file mode 100644
index 374b7a878f1972c183941af28ba1df216ac1a60f..0000000000000000000000000000000000000000
--- a/spaces/coreml-community/ControlNet-v1-1-Annotators-cpu/annotator/mmpkg/mmcv/ops/furthest_point_sample.py
+++ /dev/null
@@ -1,83 +0,0 @@
-import torch
-from torch.autograd import Function
-
-from ..utils import ext_loader
-
-ext_module = ext_loader.load_ext('_ext', [
- 'furthest_point_sampling_forward',
- 'furthest_point_sampling_with_dist_forward'
-])
-
-
-class FurthestPointSampling(Function):
- """Uses iterative furthest point sampling to select a set of features whose
- corresponding points have the furthest distance."""
-
- @staticmethod
- def forward(ctx, points_xyz: torch.Tensor,
- num_points: int) -> torch.Tensor:
- """
- Args:
- points_xyz (Tensor): (B, N, 3) where N > num_points.
- num_points (int): Number of points in the sampled set.
-
- Returns:
- Tensor: (B, num_points) indices of the sampled points.
- """
- assert points_xyz.is_contiguous()
-
- B, N = points_xyz.size()[:2]
- output = torch.cuda.IntTensor(B, num_points)
- temp = torch.cuda.FloatTensor(B, N).fill_(1e10)
-
- ext_module.furthest_point_sampling_forward(
- points_xyz,
- temp,
- output,
- b=B,
- n=N,
- m=num_points,
- )
- if torch.__version__ != 'parrots':
- ctx.mark_non_differentiable(output)
- return output
-
- @staticmethod
- def backward(xyz, a=None):
- return None, None
-
-
-class FurthestPointSamplingWithDist(Function):
- """Uses iterative furthest point sampling to select a set of features whose
- corresponding points have the furthest distance."""
-
- @staticmethod
- def forward(ctx, points_dist: torch.Tensor,
- num_points: int) -> torch.Tensor:
- """
- Args:
- points_dist (Tensor): (B, N, N) Distance between each point pair.
- num_points (int): Number of points in the sampled set.
-
- Returns:
- Tensor: (B, num_points) indices of the sampled points.
- """
- assert points_dist.is_contiguous()
-
- B, N, _ = points_dist.size()
- output = points_dist.new_zeros([B, num_points], dtype=torch.int32)
- temp = points_dist.new_zeros([B, N]).fill_(1e10)
-
- ext_module.furthest_point_sampling_with_dist_forward(
- points_dist, temp, output, b=B, n=N, m=num_points)
- if torch.__version__ != 'parrots':
- ctx.mark_non_differentiable(output)
- return output
-
- @staticmethod
- def backward(xyz, a=None):
- return None, None
-
-
-furthest_point_sample = FurthestPointSampling.apply
-furthest_point_sample_with_dist = FurthestPointSamplingWithDist.apply
diff --git a/spaces/coreml-community/ControlNet-v1-1-Annotators-cpu/annotator/mmpkg/mmcv/runner/default_constructor.py b/spaces/coreml-community/ControlNet-v1-1-Annotators-cpu/annotator/mmpkg/mmcv/runner/default_constructor.py
deleted file mode 100644
index bdd7803289d6d70240977fa243d7f4432ccde8f8..0000000000000000000000000000000000000000
--- a/spaces/coreml-community/ControlNet-v1-1-Annotators-cpu/annotator/mmpkg/mmcv/runner/default_constructor.py
+++ /dev/null
@@ -1,44 +0,0 @@
-from .builder import RUNNER_BUILDERS, RUNNERS
-
-
-@RUNNER_BUILDERS.register_module()
-class DefaultRunnerConstructor:
- """Default constructor for runners.
-
- Custom existing `Runner` like `EpocBasedRunner` though `RunnerConstructor`.
- For example, We can inject some new properties and functions for `Runner`.
-
- Example:
- >>> from annotator.mmpkg.mmcv.runner import RUNNER_BUILDERS, build_runner
- >>> # Define a new RunnerReconstructor
- >>> @RUNNER_BUILDERS.register_module()
- >>> class MyRunnerConstructor:
- ... def __init__(self, runner_cfg, default_args=None):
- ... if not isinstance(runner_cfg, dict):
- ... raise TypeError('runner_cfg should be a dict',
- ... f'but got {type(runner_cfg)}')
- ... self.runner_cfg = runner_cfg
- ... self.default_args = default_args
- ...
- ... def __call__(self):
- ... runner = RUNNERS.build(self.runner_cfg,
- ... default_args=self.default_args)
- ... # Add new properties for existing runner
- ... runner.my_name = 'my_runner'
- ... runner.my_function = lambda self: print(self.my_name)
- ... ...
- >>> # build your runner
- >>> runner_cfg = dict(type='EpochBasedRunner', max_epochs=40,
- ... constructor='MyRunnerConstructor')
- >>> runner = build_runner(runner_cfg)
- """
-
- def __init__(self, runner_cfg, default_args=None):
- if not isinstance(runner_cfg, dict):
- raise TypeError('runner_cfg should be a dict',
- f'but got {type(runner_cfg)}')
- self.runner_cfg = runner_cfg
- self.default_args = default_args
-
- def __call__(self):
- return RUNNERS.build(self.runner_cfg, default_args=self.default_args)
diff --git a/spaces/coreml-community/ControlNet-v1-1-Annotators-cpu/annotator/openpose/__init__.py b/spaces/coreml-community/ControlNet-v1-1-Annotators-cpu/annotator/openpose/__init__.py
deleted file mode 100644
index 102434701a14621a66149fbabcf224b1bb726a6c..0000000000000000000000000000000000000000
--- a/spaces/coreml-community/ControlNet-v1-1-Annotators-cpu/annotator/openpose/__init__.py
+++ /dev/null
@@ -1,100 +0,0 @@
-# Openpose
-# Original from CMU https://github.com/CMU-Perceptual-Computing-Lab/openpose
-# 2nd Edited by https://github.com/Hzzone/pytorch-openpose
-# 3rd Edited by ControlNet
-# 4th Edited by ControlNet (added face and correct hands)
-
-import os
-os.environ["KMP_DUPLICATE_LIB_OK"]="TRUE"
-
-import torch
-import numpy as np
-from . import util
-from .body import Body
-from .hand import Hand
-from .face import Face
-from annotator.util import annotator_ckpts_path
-
-
-body_model_path = "https://huggingface.co/lllyasviel/Annotators/resolve/main/body_pose_model.pth"
-hand_model_path = "https://huggingface.co/lllyasviel/Annotators/resolve/main/hand_pose_model.pth"
-face_model_path = "https://huggingface.co/lllyasviel/Annotators/resolve/main/facenet.pth"
-
-
-def draw_pose(pose, H, W, draw_body=True, draw_hand=True, draw_face=True):
- bodies = pose['bodies']
- faces = pose['faces']
- hands = pose['hands']
- candidate = bodies['candidate']
- subset = bodies['subset']
- canvas = np.zeros(shape=(H, W, 3), dtype=np.uint8)
-
- if draw_body:
- canvas = util.draw_bodypose(canvas, candidate, subset)
-
- if draw_hand:
- canvas = util.draw_handpose(canvas, hands)
-
- if draw_face:
- canvas = util.draw_facepose(canvas, faces)
-
- return canvas
-
-
-class OpenposeDetector:
- def __init__(self):
- body_modelpath = os.path.join(annotator_ckpts_path, "body_pose_model.pth")
- hand_modelpath = os.path.join(annotator_ckpts_path, "hand_pose_model.pth")
- face_modelpath = os.path.join(annotator_ckpts_path, "facenet.pth")
-
- if not os.path.exists(body_modelpath):
- from basicsr.utils.download_util import load_file_from_url
- load_file_from_url(body_model_path, model_dir=annotator_ckpts_path)
-
- if not os.path.exists(hand_modelpath):
- from basicsr.utils.download_util import load_file_from_url
- load_file_from_url(hand_model_path, model_dir=annotator_ckpts_path)
-
- if not os.path.exists(face_modelpath):
- from basicsr.utils.download_util import load_file_from_url
- load_file_from_url(face_model_path, model_dir=annotator_ckpts_path)
-
- self.body_estimation = Body(body_modelpath)
- self.hand_estimation = Hand(hand_modelpath)
- self.face_estimation = Face(face_modelpath)
-
- def __call__(self, oriImg, hand_and_face=False, return_is_index=False):
- oriImg = oriImg[:, :, ::-1].copy()
- H, W, C = oriImg.shape
- with torch.no_grad():
- candidate, subset = self.body_estimation(oriImg)
- hands = []
- faces = []
- if hand_and_face:
- # Hand
- hands_list = util.handDetect(candidate, subset, oriImg)
- for x, y, w, is_left in hands_list:
- peaks = self.hand_estimation(oriImg[y:y+w, x:x+w, :]).astype(np.float32)
- if peaks.ndim == 2 and peaks.shape[1] == 2:
- peaks[:, 0] = np.where(peaks[:, 0] < 1e-6, -1, peaks[:, 0] + x) / float(W)
- peaks[:, 1] = np.where(peaks[:, 1] < 1e-6, -1, peaks[:, 1] + y) / float(H)
- hands.append(peaks.tolist())
- # Face
- faces_list = util.faceDetect(candidate, subset, oriImg)
- for x, y, w in faces_list:
- heatmaps = self.face_estimation(oriImg[y:y+w, x:x+w, :])
- peaks = self.face_estimation.compute_peaks_from_heatmaps(heatmaps).astype(np.float32)
- if peaks.ndim == 2 and peaks.shape[1] == 2:
- peaks[:, 0] = np.where(peaks[:, 0] < 1e-6, -1, peaks[:, 0] + x) / float(W)
- peaks[:, 1] = np.where(peaks[:, 1] < 1e-6, -1, peaks[:, 1] + y) / float(H)
- faces.append(peaks.tolist())
- if candidate.ndim == 2 and candidate.shape[1] == 4:
- candidate = candidate[:, :2]
- candidate[:, 0] /= float(W)
- candidate[:, 1] /= float(H)
- bodies = dict(candidate=candidate.tolist(), subset=subset.tolist())
- pose = dict(bodies=bodies, hands=hands, faces=faces)
- if return_is_index:
- return pose
- else:
- return draw_pose(pose, H, W)
diff --git a/spaces/coreml-community/ControlNet-v1-1-Annotators-cpu/annotator/uniformer/mmcv/cnn/bricks/generalized_attention.py b/spaces/coreml-community/ControlNet-v1-1-Annotators-cpu/annotator/uniformer/mmcv/cnn/bricks/generalized_attention.py
deleted file mode 100644
index 988d9adf2f289ef223bd1c680a5ae1d3387f0269..0000000000000000000000000000000000000000
--- a/spaces/coreml-community/ControlNet-v1-1-Annotators-cpu/annotator/uniformer/mmcv/cnn/bricks/generalized_attention.py
+++ /dev/null
@@ -1,412 +0,0 @@
-# Copyright (c) OpenMMLab. All rights reserved.
-import math
-
-import numpy as np
-import torch
-import torch.nn as nn
-import torch.nn.functional as F
-
-from ..utils import kaiming_init
-from .registry import PLUGIN_LAYERS
-
-
-@PLUGIN_LAYERS.register_module()
-class GeneralizedAttention(nn.Module):
- """GeneralizedAttention module.
-
- See 'An Empirical Study of Spatial Attention Mechanisms in Deep Networks'
- (https://arxiv.org/abs/1711.07971) for details.
-
- Args:
- in_channels (int): Channels of the input feature map.
- spatial_range (int): The spatial range. -1 indicates no spatial range
- constraint. Default: -1.
- num_heads (int): The head number of empirical_attention module.
- Default: 9.
- position_embedding_dim (int): The position embedding dimension.
- Default: -1.
- position_magnitude (int): A multiplier acting on coord difference.
- Default: 1.
- kv_stride (int): The feature stride acting on key/value feature map.
- Default: 2.
- q_stride (int): The feature stride acting on query feature map.
- Default: 1.
- attention_type (str): A binary indicator string for indicating which
- items in generalized empirical_attention module are used.
- Default: '1111'.
-
- - '1000' indicates 'query and key content' (appr - appr) item,
- - '0100' indicates 'query content and relative position'
- (appr - position) item,
- - '0010' indicates 'key content only' (bias - appr) item,
- - '0001' indicates 'relative position only' (bias - position) item.
- """
-
- _abbr_ = 'gen_attention_block'
-
- def __init__(self,
- in_channels,
- spatial_range=-1,
- num_heads=9,
- position_embedding_dim=-1,
- position_magnitude=1,
- kv_stride=2,
- q_stride=1,
- attention_type='1111'):
-
- super(GeneralizedAttention, self).__init__()
-
- # hard range means local range for non-local operation
- self.position_embedding_dim = (
- position_embedding_dim
- if position_embedding_dim > 0 else in_channels)
-
- self.position_magnitude = position_magnitude
- self.num_heads = num_heads
- self.in_channels = in_channels
- self.spatial_range = spatial_range
- self.kv_stride = kv_stride
- self.q_stride = q_stride
- self.attention_type = [bool(int(_)) for _ in attention_type]
- self.qk_embed_dim = in_channels // num_heads
- out_c = self.qk_embed_dim * num_heads
-
- if self.attention_type[0] or self.attention_type[1]:
- self.query_conv = nn.Conv2d(
- in_channels=in_channels,
- out_channels=out_c,
- kernel_size=1,
- bias=False)
- self.query_conv.kaiming_init = True
-
- if self.attention_type[0] or self.attention_type[2]:
- self.key_conv = nn.Conv2d(
- in_channels=in_channels,
- out_channels=out_c,
- kernel_size=1,
- bias=False)
- self.key_conv.kaiming_init = True
-
- self.v_dim = in_channels // num_heads
- self.value_conv = nn.Conv2d(
- in_channels=in_channels,
- out_channels=self.v_dim * num_heads,
- kernel_size=1,
- bias=False)
- self.value_conv.kaiming_init = True
-
- if self.attention_type[1] or self.attention_type[3]:
- self.appr_geom_fc_x = nn.Linear(
- self.position_embedding_dim // 2, out_c, bias=False)
- self.appr_geom_fc_x.kaiming_init = True
-
- self.appr_geom_fc_y = nn.Linear(
- self.position_embedding_dim // 2, out_c, bias=False)
- self.appr_geom_fc_y.kaiming_init = True
-
- if self.attention_type[2]:
- stdv = 1.0 / math.sqrt(self.qk_embed_dim * 2)
- appr_bias_value = -2 * stdv * torch.rand(out_c) + stdv
- self.appr_bias = nn.Parameter(appr_bias_value)
-
- if self.attention_type[3]:
- stdv = 1.0 / math.sqrt(self.qk_embed_dim * 2)
- geom_bias_value = -2 * stdv * torch.rand(out_c) + stdv
- self.geom_bias = nn.Parameter(geom_bias_value)
-
- self.proj_conv = nn.Conv2d(
- in_channels=self.v_dim * num_heads,
- out_channels=in_channels,
- kernel_size=1,
- bias=True)
- self.proj_conv.kaiming_init = True
- self.gamma = nn.Parameter(torch.zeros(1))
-
- if self.spatial_range >= 0:
- # only works when non local is after 3*3 conv
- if in_channels == 256:
- max_len = 84
- elif in_channels == 512:
- max_len = 42
-
- max_len_kv = int((max_len - 1.0) / self.kv_stride + 1)
- local_constraint_map = np.ones(
- (max_len, max_len, max_len_kv, max_len_kv), dtype=np.int)
- for iy in range(max_len):
- for ix in range(max_len):
- local_constraint_map[
- iy, ix,
- max((iy - self.spatial_range) //
- self.kv_stride, 0):min((iy + self.spatial_range +
- 1) // self.kv_stride +
- 1, max_len),
- max((ix - self.spatial_range) //
- self.kv_stride, 0):min((ix + self.spatial_range +
- 1) // self.kv_stride +
- 1, max_len)] = 0
-
- self.local_constraint_map = nn.Parameter(
- torch.from_numpy(local_constraint_map).byte(),
- requires_grad=False)
-
- if self.q_stride > 1:
- self.q_downsample = nn.AvgPool2d(
- kernel_size=1, stride=self.q_stride)
- else:
- self.q_downsample = None
-
- if self.kv_stride > 1:
- self.kv_downsample = nn.AvgPool2d(
- kernel_size=1, stride=self.kv_stride)
- else:
- self.kv_downsample = None
-
- self.init_weights()
-
- def get_position_embedding(self,
- h,
- w,
- h_kv,
- w_kv,
- q_stride,
- kv_stride,
- device,
- dtype,
- feat_dim,
- wave_length=1000):
- # the default type of Tensor is float32, leading to type mismatch
- # in fp16 mode. Cast it to support fp16 mode.
- h_idxs = torch.linspace(0, h - 1, h).to(device=device, dtype=dtype)
- h_idxs = h_idxs.view((h, 1)) * q_stride
-
- w_idxs = torch.linspace(0, w - 1, w).to(device=device, dtype=dtype)
- w_idxs = w_idxs.view((w, 1)) * q_stride
-
- h_kv_idxs = torch.linspace(0, h_kv - 1, h_kv).to(
- device=device, dtype=dtype)
- h_kv_idxs = h_kv_idxs.view((h_kv, 1)) * kv_stride
-
- w_kv_idxs = torch.linspace(0, w_kv - 1, w_kv).to(
- device=device, dtype=dtype)
- w_kv_idxs = w_kv_idxs.view((w_kv, 1)) * kv_stride
-
- # (h, h_kv, 1)
- h_diff = h_idxs.unsqueeze(1) - h_kv_idxs.unsqueeze(0)
- h_diff *= self.position_magnitude
-
- # (w, w_kv, 1)
- w_diff = w_idxs.unsqueeze(1) - w_kv_idxs.unsqueeze(0)
- w_diff *= self.position_magnitude
-
- feat_range = torch.arange(0, feat_dim / 4).to(
- device=device, dtype=dtype)
-
- dim_mat = torch.Tensor([wave_length]).to(device=device, dtype=dtype)
- dim_mat = dim_mat**((4. / feat_dim) * feat_range)
- dim_mat = dim_mat.view((1, 1, -1))
-
- embedding_x = torch.cat(
- ((w_diff / dim_mat).sin(), (w_diff / dim_mat).cos()), dim=2)
-
- embedding_y = torch.cat(
- ((h_diff / dim_mat).sin(), (h_diff / dim_mat).cos()), dim=2)
-
- return embedding_x, embedding_y
-
- def forward(self, x_input):
- num_heads = self.num_heads
-
- # use empirical_attention
- if self.q_downsample is not None:
- x_q = self.q_downsample(x_input)
- else:
- x_q = x_input
- n, _, h, w = x_q.shape
-
- if self.kv_downsample is not None:
- x_kv = self.kv_downsample(x_input)
- else:
- x_kv = x_input
- _, _, h_kv, w_kv = x_kv.shape
-
- if self.attention_type[0] or self.attention_type[1]:
- proj_query = self.query_conv(x_q).view(
- (n, num_heads, self.qk_embed_dim, h * w))
- proj_query = proj_query.permute(0, 1, 3, 2)
-
- if self.attention_type[0] or self.attention_type[2]:
- proj_key = self.key_conv(x_kv).view(
- (n, num_heads, self.qk_embed_dim, h_kv * w_kv))
-
- if self.attention_type[1] or self.attention_type[3]:
- position_embed_x, position_embed_y = self.get_position_embedding(
- h, w, h_kv, w_kv, self.q_stride, self.kv_stride,
- x_input.device, x_input.dtype, self.position_embedding_dim)
- # (n, num_heads, w, w_kv, dim)
- position_feat_x = self.appr_geom_fc_x(position_embed_x).\
- view(1, w, w_kv, num_heads, self.qk_embed_dim).\
- permute(0, 3, 1, 2, 4).\
- repeat(n, 1, 1, 1, 1)
-
- # (n, num_heads, h, h_kv, dim)
- position_feat_y = self.appr_geom_fc_y(position_embed_y).\
- view(1, h, h_kv, num_heads, self.qk_embed_dim).\
- permute(0, 3, 1, 2, 4).\
- repeat(n, 1, 1, 1, 1)
-
- position_feat_x /= math.sqrt(2)
- position_feat_y /= math.sqrt(2)
-
- # accelerate for saliency only
- if (np.sum(self.attention_type) == 1) and self.attention_type[2]:
- appr_bias = self.appr_bias.\
- view(1, num_heads, 1, self.qk_embed_dim).\
- repeat(n, 1, 1, 1)
-
- energy = torch.matmul(appr_bias, proj_key).\
- view(n, num_heads, 1, h_kv * w_kv)
-
- h = 1
- w = 1
- else:
- # (n, num_heads, h*w, h_kv*w_kv), query before key, 540mb for
- if not self.attention_type[0]:
- energy = torch.zeros(
- n,
- num_heads,
- h,
- w,
- h_kv,
- w_kv,
- dtype=x_input.dtype,
- device=x_input.device)
-
- # attention_type[0]: appr - appr
- # attention_type[1]: appr - position
- # attention_type[2]: bias - appr
- # attention_type[3]: bias - position
- if self.attention_type[0] or self.attention_type[2]:
- if self.attention_type[0] and self.attention_type[2]:
- appr_bias = self.appr_bias.\
- view(1, num_heads, 1, self.qk_embed_dim)
- energy = torch.matmul(proj_query + appr_bias, proj_key).\
- view(n, num_heads, h, w, h_kv, w_kv)
-
- elif self.attention_type[0]:
- energy = torch.matmul(proj_query, proj_key).\
- view(n, num_heads, h, w, h_kv, w_kv)
-
- elif self.attention_type[2]:
- appr_bias = self.appr_bias.\
- view(1, num_heads, 1, self.qk_embed_dim).\
- repeat(n, 1, 1, 1)
-
- energy += torch.matmul(appr_bias, proj_key).\
- view(n, num_heads, 1, 1, h_kv, w_kv)
-
- if self.attention_type[1] or self.attention_type[3]:
- if self.attention_type[1] and self.attention_type[3]:
- geom_bias = self.geom_bias.\
- view(1, num_heads, 1, self.qk_embed_dim)
-
- proj_query_reshape = (proj_query + geom_bias).\
- view(n, num_heads, h, w, self.qk_embed_dim)
-
- energy_x = torch.matmul(
- proj_query_reshape.permute(0, 1, 3, 2, 4),
- position_feat_x.permute(0, 1, 2, 4, 3))
- energy_x = energy_x.\
- permute(0, 1, 3, 2, 4).unsqueeze(4)
-
- energy_y = torch.matmul(
- proj_query_reshape,
- position_feat_y.permute(0, 1, 2, 4, 3))
- energy_y = energy_y.unsqueeze(5)
-
- energy += energy_x + energy_y
-
- elif self.attention_type[1]:
- proj_query_reshape = proj_query.\
- view(n, num_heads, h, w, self.qk_embed_dim)
- proj_query_reshape = proj_query_reshape.\
- permute(0, 1, 3, 2, 4)
- position_feat_x_reshape = position_feat_x.\
- permute(0, 1, 2, 4, 3)
- position_feat_y_reshape = position_feat_y.\
- permute(0, 1, 2, 4, 3)
-
- energy_x = torch.matmul(proj_query_reshape,
- position_feat_x_reshape)
- energy_x = energy_x.permute(0, 1, 3, 2, 4).unsqueeze(4)
-
- energy_y = torch.matmul(proj_query_reshape,
- position_feat_y_reshape)
- energy_y = energy_y.unsqueeze(5)
-
- energy += energy_x + energy_y
-
- elif self.attention_type[3]:
- geom_bias = self.geom_bias.\
- view(1, num_heads, self.qk_embed_dim, 1).\
- repeat(n, 1, 1, 1)
-
- position_feat_x_reshape = position_feat_x.\
- view(n, num_heads, w*w_kv, self.qk_embed_dim)
-
- position_feat_y_reshape = position_feat_y.\
- view(n, num_heads, h * h_kv, self.qk_embed_dim)
-
- energy_x = torch.matmul(position_feat_x_reshape, geom_bias)
- energy_x = energy_x.view(n, num_heads, 1, w, 1, w_kv)
-
- energy_y = torch.matmul(position_feat_y_reshape, geom_bias)
- energy_y = energy_y.view(n, num_heads, h, 1, h_kv, 1)
-
- energy += energy_x + energy_y
-
- energy = energy.view(n, num_heads, h * w, h_kv * w_kv)
-
- if self.spatial_range >= 0:
- cur_local_constraint_map = \
- self.local_constraint_map[:h, :w, :h_kv, :w_kv].\
- contiguous().\
- view(1, 1, h*w, h_kv*w_kv)
-
- energy = energy.masked_fill_(cur_local_constraint_map,
- float('-inf'))
-
- attention = F.softmax(energy, 3)
-
- proj_value = self.value_conv(x_kv)
- proj_value_reshape = proj_value.\
- view((n, num_heads, self.v_dim, h_kv * w_kv)).\
- permute(0, 1, 3, 2)
-
- out = torch.matmul(attention, proj_value_reshape).\
- permute(0, 1, 3, 2).\
- contiguous().\
- view(n, self.v_dim * self.num_heads, h, w)
-
- out = self.proj_conv(out)
-
- # output is downsampled, upsample back to input size
- if self.q_downsample is not None:
- out = F.interpolate(
- out,
- size=x_input.shape[2:],
- mode='bilinear',
- align_corners=False)
-
- out = self.gamma * out + x_input
- return out
-
- def init_weights(self):
- for m in self.modules():
- if hasattr(m, 'kaiming_init') and m.kaiming_init:
- kaiming_init(
- m,
- mode='fan_in',
- nonlinearity='leaky_relu',
- bias=0,
- distribution='uniform',
- a=1)
diff --git a/spaces/coreml-community/ControlNet-v1-1-Annotators-cpu/annotator/uniformer/mmcv/cnn/builder.py b/spaces/coreml-community/ControlNet-v1-1-Annotators-cpu/annotator/uniformer/mmcv/cnn/builder.py
deleted file mode 100644
index 7567316c566bd3aca6d8f65a84b00e9e890948a7..0000000000000000000000000000000000000000
--- a/spaces/coreml-community/ControlNet-v1-1-Annotators-cpu/annotator/uniformer/mmcv/cnn/builder.py
+++ /dev/null
@@ -1,30 +0,0 @@
-# Copyright (c) OpenMMLab. All rights reserved.
-from ..runner import Sequential
-from ..utils import Registry, build_from_cfg
-
-
-def build_model_from_cfg(cfg, registry, default_args=None):
- """Build a PyTorch model from config dict(s). Different from
- ``build_from_cfg``, if cfg is a list, a ``nn.Sequential`` will be built.
-
- Args:
- cfg (dict, list[dict]): The config of modules, is is either a config
- dict or a list of config dicts. If cfg is a list, a
- the built modules will be wrapped with ``nn.Sequential``.
- registry (:obj:`Registry`): A registry the module belongs to.
- default_args (dict, optional): Default arguments to build the module.
- Defaults to None.
-
- Returns:
- nn.Module: A built nn module.
- """
- if isinstance(cfg, list):
- modules = [
- build_from_cfg(cfg_, registry, default_args) for cfg_ in cfg
- ]
- return Sequential(*modules)
- else:
- return build_from_cfg(cfg, registry, default_args)
-
-
-MODELS = Registry('model', build_func=build_model_from_cfg)
diff --git a/spaces/coreml-community/ControlNet-v1-1-Annotators-cpu/annotator/zoe/zoedepth/models/base_models/midas_repo/mobile/ios/README.md b/spaces/coreml-community/ControlNet-v1-1-Annotators-cpu/annotator/zoe/zoedepth/models/base_models/midas_repo/mobile/ios/README.md
deleted file mode 100644
index 7b8eb29feaa21e67814b035dbd5c5fb2c62a4151..0000000000000000000000000000000000000000
--- a/spaces/coreml-community/ControlNet-v1-1-Annotators-cpu/annotator/zoe/zoedepth/models/base_models/midas_repo/mobile/ios/README.md
+++ /dev/null
@@ -1,105 +0,0 @@
-# Tensorflow Lite MiDaS iOS Example
-
-### Requirements
-
-- XCode 11.0 or above
-- iOS 12.0 or above, [iOS 14 breaks the NPU Delegate](https://github.com/tensorflow/tensorflow/issues/43339)
-- TensorFlow 2.4.0, TensorFlowLiteSwift -> 0.0.1-nightly
-
-## Quick Start with a MiDaS Example
-
-MiDaS is a neural network to compute depth from a single image. It uses TensorFlowLiteSwift / C++ libraries on iOS. The code is written in Swift.
-
-Paper: https://arxiv.org/abs/1907.01341
-
-> Towards Robust Monocular Depth Estimation: Mixing Datasets for Zero-shot Cross-dataset Transfer
-> René Ranftl, Katrin Lasinger, David Hafner, Konrad Schindler, Vladlen Koltun
-
-### Install TensorFlow
-
-Set default python version to python3:
-
-```
-echo 'export PATH=/usr/local/opt/python/libexec/bin:$PATH' >> ~/.zshenv
-echo 'alias python=python3' >> ~/.zshenv
-echo 'alias pip=pip3' >> ~/.zshenv
-```
-
-Install TensorFlow
-
-```shell
-pip install tensorflow
-```
-
-### Install TensorFlowLiteSwift via Cocoapods
-
-Set required TensorFlowLiteSwift version in the file (`0.0.1-nightly` is recommended): https://github.com/isl-org/MiDaS/blob/master/mobile/ios/Podfile#L9
-
-Install: brew, ruby, cocoapods
-
-```
-ruby -e "$(curl -fsSL https://raw.githubusercontent.com/Homebrew/install/master/install)"
-brew install mc rbenv ruby-build
-sudo gem install cocoapods
-```
-
-
-The TensorFlowLiteSwift library is available in [Cocoapods](https://cocoapods.org/), to integrate it to our project, we can run in the root directory of the project:
-
-```ruby
-pod install
-```
-
-Now open the `Midas.xcworkspace` file in XCode, select your iPhone device (XCode->Product->Destination->iPhone) and launch it (cmd + R). If everything works well, you should see a real-time depth map from your camera.
-
-### Model
-
-The TensorFlow (TFlite) model `midas.tflite` is in the folder `/Midas/Model`
-
-
-To use another model, you should convert it from TensorFlow saved-model to TFlite model (so that it can be deployed):
-
-```python
-saved_model_export_dir = "./saved_model"
-converter = tf.lite.TFLiteConverter.from_saved_model(saved_model_export_dir)
-tflite_model = converter.convert()
-open(model_tflite_name, "wb").write("model.tflite")
-```
-
-### Setup XCode
-
-* Open directory `.xcworkspace` from the XCode
-
-* Press on your ProjectName (left-top corner) -> change Bundle Identifier to `com.midas.tflite-npu` or something like this (it should be unique)
-
-* select your Developer Team (your should be signed-in by using your AppleID)
-
-* Connect your iPhone (if you want to run it on real device instead of simulator), select your iPhone device (XCode->Product->Destination->iPhone)
-
-* Click in the XCode: Product -> Run
-
-* On your iPhone device go to the: Settings -> General -> Device Management (or Profiles) -> Apple Development -> Trust Apple Development
-
-----
-
-Original repository: https://github.com/isl-org/MiDaS
-
-
-### Examples:
-
-| 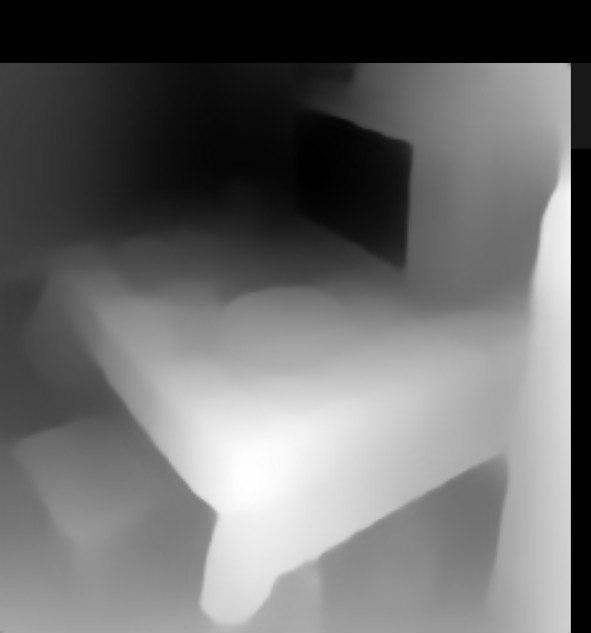 | 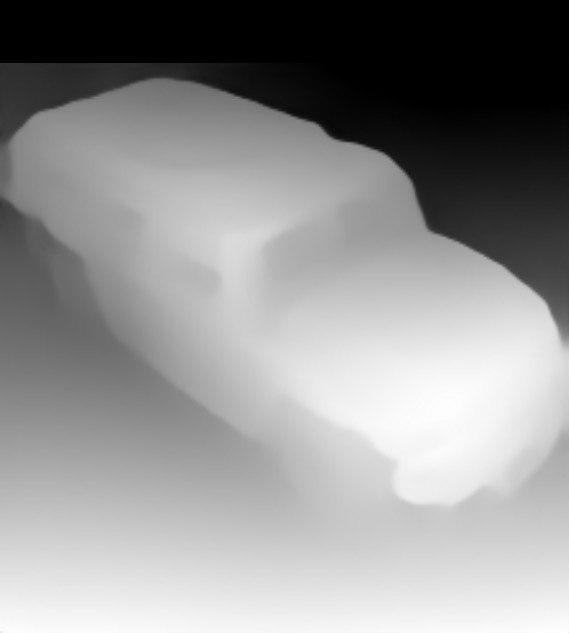 | 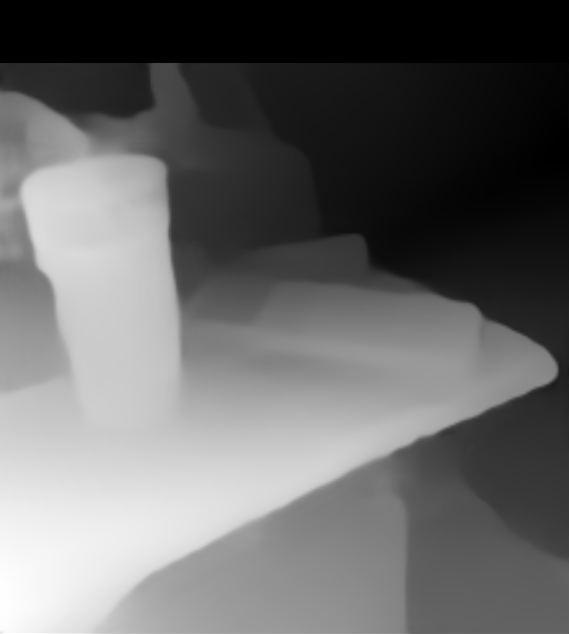 | 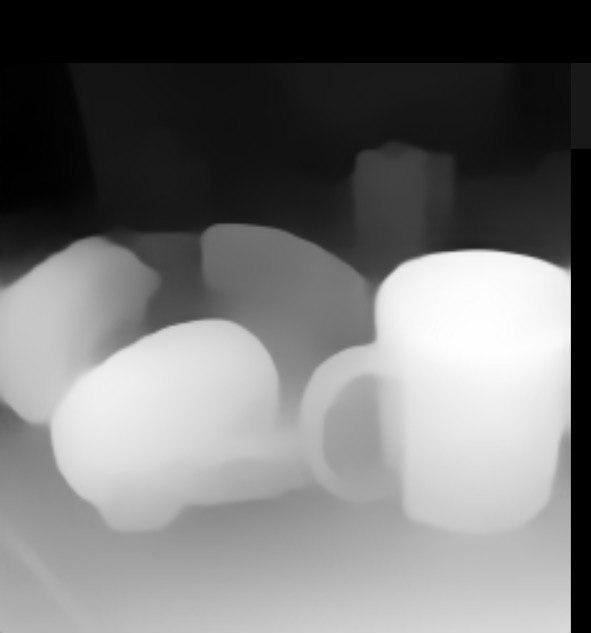 |
-|---|---|---|---|
-
-## LICENSE
-
-THIS SOFTWARE IS PROVIDED BY THE COPYRIGHT HOLDERS AND CONTRIBUTORS "AS IS"
-AND ANY EXPRESS OR IMPLIED WARRANTIES, INCLUDING, BUT NOT LIMITED TO, THE
-IMPLIED WARRANTIES OF MERCHANTABILITY AND FITNESS FOR A PARTICULAR PURPOSE
-ARE DISCLAIMED. IN NO EVENT SHALL THE COPYRIGHT OWNER OR CONTRIBUTORS BE
-LIABLE FOR ANY DIRECT, INDIRECT, INCIDENTAL, SPECIAL, EXEMPLARY, OR
-CONSEQUENTIAL DAMAGES (INCLUDING, BUT NOT LIMITED TO, PROCUREMENT OF
-SUBSTITUTE GOODS OR SERVICES; LOSS OF USE, DATA, OR PROFITS; OR BUSINESS
-INTERRUPTION) HOWEVER CAUSED AND ON ANY THEORY OF LIABILITY, WHETHER IN
-CONTRACT, STRICT LIABILITY, OR TORT (INCLUDING NEGLIGENCE OR OTHERWISE)
-ARISING IN ANY WAY OUT OF THE USE OF THIS SOFTWARE, EVEN IF ADVISED OF THE
-POSSIBILITY OF SUCH DAMAGE.
diff --git a/spaces/cpwan/RLOR-TSP/models/nets/attention_model/encoder.py b/spaces/cpwan/RLOR-TSP/models/nets/attention_model/encoder.py
deleted file mode 100644
index e3349dac23d587656f639ab344e0951008a577ad..0000000000000000000000000000000000000000
--- a/spaces/cpwan/RLOR-TSP/models/nets/attention_model/encoder.py
+++ /dev/null
@@ -1,128 +0,0 @@
-from torch import nn
-
-from ...nets.attention_model.multi_head_attention import MultiHeadAttentionProj
-
-
-class SkipConnection(nn.Module):
- def __init__(self, module):
- super(SkipConnection, self).__init__()
- self.module = module
-
- def forward(self, input):
- return input + self.module(input)
-
-
-class Normalization(nn.Module):
- def __init__(self, embedding_dim):
- super(Normalization, self).__init__()
-
- self.normalizer = nn.BatchNorm1d(embedding_dim, affine=True)
-
- def forward(self, input):
- # out = self.normalizer(input.permute(0,2,1)).permute(0,2,1) # slightly different 3e-6
- # return out
- return self.normalizer(input.view(-1, input.size(-1))).view(input.size())
-
-
-class MultiHeadAttentionLayer(nn.Sequential):
- r"""
- A layer with attention mechanism and normalization.
-
- For an embedding :math:`\pmb{x}`,
-
- .. math::
- \pmb{h} = \mathrm{MultiHeadAttentionLayer}(\pmb{x})
-
- The following is executed:
-
- .. math::
- \begin{aligned}
- \pmb{x}_0&=\pmb{x}+\mathrm{MultiHeadAttentionProj}(\pmb{x}) \\
- \pmb{x}_1&=\mathrm{BatchNorm}(\pmb{x}_0) \\
- \pmb{x}_2&=\pmb{x}_1+\mathrm{MLP_{\text{2 layers}}}(\pmb{x}_1)\\
- \pmb{h} &=\mathrm{BatchNorm}(\pmb{x}_2)
- \end{aligned}
-
-
-
- .. seealso::
- The :math:`\mathrm{MultiHeadAttentionProj}` computes the self attention
- of the embedding :math:`\pmb{x}`. Check :class:`~.MultiHeadAttentionProj` for details.
-
- Args:
- n_heads : number of heads
- embedding_dim : dimension of the query, keys, values
- feed_forward_hidden : size of the hidden layer in the MLP
- Inputs: inputs
- * **inputs**: embeddin :math:`\pmb{x}`. [batch, graph_size, embedding_dim]
- Outputs: out
- * **out**: the output :math:`\pmb{h}` [batch, graph_size, embedding_dim]
- """
-
- def __init__(
- self,
- n_heads,
- embedding_dim,
- feed_forward_hidden=512,
- ):
- super(MultiHeadAttentionLayer, self).__init__(
- SkipConnection(
- MultiHeadAttentionProj(
- embedding_dim=embedding_dim,
- n_heads=n_heads,
- )
- ),
- Normalization(embedding_dim),
- SkipConnection(
- nn.Sequential(
- nn.Linear(embedding_dim, feed_forward_hidden),
- nn.ReLU(),
- nn.Linear(feed_forward_hidden, embedding_dim),
- )
- if feed_forward_hidden > 0
- else nn.Linear(embedding_dim, embedding_dim)
- ),
- Normalization(embedding_dim),
- )
-
-
-class GraphAttentionEncoder(nn.Module):
- r"""
- Graph attention by self attention on graph nodes.
-
- For an embedding :math:`\pmb{x}`, repeat ``n_layers`` time:
-
- .. math::
- \pmb{h} = \mathrm{MultiHeadAttentionLayer}(\pmb{x})
-
- .. seealso::
- Check :class:`~.MultiHeadAttentionLayer` for details.
-
- Args:
- n_heads : number of heads
- embedding_dim : dimension of the query, keys, values
- n_layers : number of :class:`~.MultiHeadAttentionLayer` to iterate.
- feed_forward_hidden : size of the hidden layer in the MLP
- Inputs: x
- * **x**: embeddin :math:`\pmb{x}`. [batch, graph_size, embedding_dim]
- Outputs: (h, h_mean)
- * **h**: the output :math:`\pmb{h}` [batch, graph_size, embedding_dim]
- """
-
- def __init__(self, n_heads, embed_dim, n_layers, feed_forward_hidden=512):
- super(GraphAttentionEncoder, self).__init__()
-
- self.layers = nn.Sequential(
- *(
- MultiHeadAttentionLayer(n_heads, embed_dim, feed_forward_hidden)
- for _ in range(n_layers)
- )
- )
-
- def forward(self, x, mask=None):
-
- assert mask is None, "TODO mask not yet supported!"
-
- h = self.layers(x)
-
- return (h, h.mean(dim=1))
diff --git a/spaces/crashedice/signify/signify/gan/models/__init__.py b/spaces/crashedice/signify/signify/gan/models/__init__.py
deleted file mode 100644
index f241aa15f5d73882fab05d0a6873e8039459dc90..0000000000000000000000000000000000000000
--- a/spaces/crashedice/signify/signify/gan/models/__init__.py
+++ /dev/null
@@ -1,67 +0,0 @@
-"""This package contains modules related to objective functions, optimizations, and network architectures.
-
-To add a custom model class called 'dummy', you need to add a file called 'dummy_model.py' and define a subclass DummyModel inherited from BaseModel.
-You need to implement the following five functions:
- -- <__init__>: initialize the class; first call BaseModel.__init__(self, opt).
- -- : unpack data from dataset and apply preprocessing.
- -- : produce intermediate results.
- -- : calculate loss, gradients, and update network weights.
- -- : (optionally) add model-specific options and set default options.
-
-In the function <__init__>, you need to define four lists:
- -- self.loss_names (str list): specify the training losses that you want to plot and save.
- -- self.model_names (str list): define networks used in our training.
- -- self.visual_names (str list): specify the images that you want to display and save.
- -- self.optimizers (optimizer list): define and initialize optimizers. You can define one optimizer for each network. If two networks are updated at the same time, you can use itertools.chain to group them. See cycle_gan_model.py for an usage.
-
-Now you can use the model class by specifying flag '--model dummy'.
-See our template model class 'template_model.py' for more details.
-"""
-
-import importlib
-from signify.gan.models.base_model import BaseModel
-
-
-def find_model_using_name(model_name):
- """Import the module "models/[model_name]_model.py".
-
- In the file, the class called DatasetNameModel() will
- be instantiated. It has to be a subclass of BaseModel,
- and it is case-insensitive.
- """
- model_filename = "signify.gan.models." + model_name + "_model"
- modellib = importlib.import_module(model_filename)
- model = None
- target_model_name = model_name.replace('_', '') + 'model'
- for name, cls in modellib.__dict__.items():
- if name.lower() == target_model_name.lower() \
- and issubclass(cls, BaseModel):
- model = cls
-
- if model is None:
- print("In %s.py, there should be a subclass of BaseModel with class name that matches %s in lowercase." % (model_filename, target_model_name))
- exit(0)
-
- return model
-
-
-def get_option_setter(model_name):
- """Return the static method of the model class."""
- model_class = find_model_using_name(model_name)
- return model_class.modify_commandline_options
-
-
-def create_model(opt):
- """Create a model given the option.
-
- This function warps the class CustomDatasetDataLoader.
- This is the main interface between this package and 'train.py'/'test.py'
-
- Example:
- >>> from models import create_model
- >>> model = create_model(opt)
- """
- model = find_model_using_name(opt.model)
- instance = model(opt)
- print("model [%s] was created" % type(instance).__name__)
- return instance
diff --git a/spaces/crazybber/docker-demo-t5-translation/static/style.css b/spaces/crazybber/docker-demo-t5-translation/static/style.css
deleted file mode 100644
index 7b50df8f6904c75f560224034d8aadd76656c6f8..0000000000000000000000000000000000000000
--- a/spaces/crazybber/docker-demo-t5-translation/static/style.css
+++ /dev/null
@@ -1,45 +0,0 @@
-body {
- --text: hsl(0 0% 15%);
- padding: 2.5rem;
- font-family: sans-serif;
- color: var(--text);
-}
-
-body.dark-theme {
- --text: hsl(0 0% 90%);
- background-color: hsl(223 39% 7%);
-}
-
-main {
- max-width: 80rem;
- text-align: center;
-}
-
-section {
- display: flex;
- flex-direction: column;
- align-items: center;
-}
-
-a {
- color: var(--text);
-}
-
-form {
- width: 30rem;
- margin: 0 auto;
-}
-
-input {
- width: 100%;
-}
-
-button {
- cursor: pointer;
-}
-
-.text-gen-output {
- min-height: 1.2rem;
- margin: 1rem;
- border: 0.5px solid grey;
-}
diff --git a/spaces/daddyjin/TalkingFaceGeneration/Demo_TFR_Pirenderer/src/test_audio2coeff.py b/spaces/daddyjin/TalkingFaceGeneration/Demo_TFR_Pirenderer/src/test_audio2coeff.py
deleted file mode 100644
index d19f81ba62935baee65216515c5efe3be1aa83f3..0000000000000000000000000000000000000000
--- a/spaces/daddyjin/TalkingFaceGeneration/Demo_TFR_Pirenderer/src/test_audio2coeff.py
+++ /dev/null
@@ -1,112 +0,0 @@
-import os
-import torch
-import numpy as np
-from scipy.io import savemat, loadmat
-from yacs.config import CfgNode as CN
-from scipy.signal import savgol_filter
-
-from Demo_TFR_Pirenderer.src.audio2pose_models.audio2pose import Audio2Pose
-from Demo_TFR_Pirenderer.src.audio2exp_models.networks import SimpleWrapperV2
-from Demo_TFR_Pirenderer.src.audio2exp_models.audio2exp import Audio2Exp
-
-def load_cpk(checkpoint_path, model=None, optimizer=None, device="cpu"):
- checkpoint = torch.load(checkpoint_path, map_location=torch.device(device))
- if model is not None:
- model.load_state_dict(checkpoint['model'])
- if optimizer is not None:
- optimizer.load_state_dict(checkpoint['optimizer'])
-
- return checkpoint['epoch']
-
-class Audio2Coeff():
-
- def __init__(self, audio2pose_checkpoint, audio2pose_yaml_path,
- audio2exp_checkpoint, audio2exp_yaml_path,
- wav2lip_checkpoint, device):
- #load config
- fcfg_pose = open(audio2pose_yaml_path)
- cfg_pose = CN.load_cfg(fcfg_pose)
- cfg_pose.freeze()
- fcfg_exp = open(audio2exp_yaml_path)
- cfg_exp = CN.load_cfg(fcfg_exp)
- cfg_exp.freeze()
-
- # load audio2pose_model
- self.audio2pose_model = Audio2Pose(cfg_pose, wav2lip_checkpoint, device=device)
- self.audio2pose_model = self.audio2pose_model.to(device)
- self.audio2pose_model.eval()
- for param in self.audio2pose_model.parameters():
- param.requires_grad = False
- try:
- load_cpk(audio2pose_checkpoint, model=self.audio2pose_model, device=device)
- except:
- raise Exception("Failed in loading audio2pose_checkpoint")
-
- # load audio2exp_model
- netG = SimpleWrapperV2()
- netG = netG.to(device)
- for param in netG.parameters():
- netG.requires_grad = False
- netG.eval()
- try:
- load_cpk(audio2exp_checkpoint, model=netG, device=device)
- except:
- raise Exception("Failed in loading audio2exp_checkpoint")
- self.audio2exp_model = Audio2Exp(netG, cfg_exp, device=device, prepare_training_loss=False)
- self.audio2exp_model = self.audio2exp_model.to(device)
- for param in self.audio2exp_model.parameters():
- param.requires_grad = False
- self.audio2exp_model.eval()
-
- self.device = device
-
- def generate(self, batch, coeff_save_dir, pose_style, ref_pose_coeff_path=None):
-
- with torch.no_grad():
- #test
- results_dict_exp= self.audio2exp_model.test(batch)
- exp_pred = results_dict_exp['exp_coeff_pred'] #bs T 64
-
- #for class_id in range(1):
- #class_id = 0#(i+10)%45
- #class_id = random.randint(0,46) #46 styles can be selected
- batch['class'] = torch.LongTensor([pose_style]).to(self.device)
- results_dict_pose = self.audio2pose_model.test(batch)
- pose_pred = results_dict_pose['pose_pred'] #bs T 6
-
- pose_len = pose_pred.shape[1]
- if pose_len<13:
- pose_len = int((pose_len-1)/2)*2+1
- pose_pred = torch.Tensor(savgol_filter(np.array(pose_pred.cpu()), pose_len, 2, axis=1)).to(self.device)
- else:
- pose_pred = torch.Tensor(savgol_filter(np.array(pose_pred.cpu()), 13, 2, axis=1)).to(self.device)
-
- coeffs_pred = torch.cat((exp_pred, pose_pred), dim=-1) #bs T 70
-
- coeffs_pred_numpy = coeffs_pred[0].clone().detach().cpu().numpy()
-
-
- if ref_pose_coeff_path is not None:
- coeffs_pred_numpy = self.using_refpose(coeffs_pred_numpy, ref_pose_coeff_path)
-
- savemat(os.path.join(coeff_save_dir, '%s##%s.mat'%(batch['pic_name'], batch['audio_name'])),
- {'coeff_3dmm': coeffs_pred_numpy})
-
- return os.path.join(coeff_save_dir, '%s##%s.mat'%(batch['pic_name'], batch['audio_name']))
-
- def using_refpose(self, coeffs_pred_numpy, ref_pose_coeff_path):
- num_frames = coeffs_pred_numpy.shape[0]
- refpose_coeff_dict = loadmat(ref_pose_coeff_path)
- refpose_coeff = refpose_coeff_dict['coeff_3dmm'][:,64:70]
- refpose_num_frames = refpose_coeff.shape[0]
- if refpose_num_framesa?this[a+this.length]:this[a]:d.call(this)},pushStack:function(a){var b=m.merge(this.constructor(),a);return b.prevObject=this,b.context=this.context,b},each:function(a,b){return m.each(this,a,b)},map:function(a){return this.pushStack(m.map(this,function(b,c){return a.call(b,c,b)}))},slice:function(){return this.pushStack(d.apply(this,arguments))},first:function(){return this.eq(0)},last:function(){return this.eq(-1)},eq:function(a){var b=this.length,c=+a+(0>a?b:0);return this.pushStack(c>=0&&b>c?[this[c]]:[])},end:function(){return this.prevObject||this.constructor(null)},push:f,sort:c.sort,splice:c.splice},m.extend=m.fn.extend=function(){var a,b,c,d,e,f,g=arguments[0]||{},h=1,i=arguments.length,j=!1;for("boolean"==typeof g&&(j=g,g=arguments[h]||{},h++),"object"==typeof g||m.isFunction(g)||(g={}),h===i&&(g=this,h--);i>h;h++)if(null!=(e=arguments[h]))for(d in e)a=g[d],c=e[d],g!==c&&(j&&c&&(m.isPlainObject(c)||(b=m.isArray(c)))?(b?(b=!1,f=a&&m.isArray(a)?a:[]):f=a&&m.isPlainObject(a)?a:{},g[d]=m.extend(j,f,c)):void 0!==c&&(g[d]=c));return g},m.extend({expando:"jQuery"+(l+Math.random()).replace(/\D/g,""),isReady:!0,error:function(a){throw new Error(a)},noop:function(){},isFunction:function(a){return"function"===m.type(a)},isArray:Array.isArray||function(a){return"array"===m.type(a)},isWindow:function(a){return null!=a&&a==a.window},isNumeric:function(a){return!m.isArray(a)&&a-parseFloat(a)>=0},isEmptyObject:function(a){var b;for(b in a)return!1;return!0},isPlainObject:function(a){var b;if(!a||"object"!==m.type(a)||a.nodeType||m.isWindow(a))return!1;try{if(a.constructor&&!j.call(a,"constructor")&&!j.call(a.constructor.prototype,"isPrototypeOf"))return!1}catch(c){return!1}if(k.ownLast)for(b in a)return j.call(a,b);for(b in a);return void 0===b||j.call(a,b)},type:function(a){return null==a?a+"":"object"==typeof a||"function"==typeof a?h[i.call(a)]||"object":typeof a},globalEval:function(b){b&&m.trim(b)&&(a.execScript||function(b){a.eval.call(a,b)})(b)},camelCase:function(a){return a.replace(o,"ms-").replace(p,q)},nodeName:function(a,b){return a.nodeName&&a.nodeName.toLowerCase()===b.toLowerCase()},each:function(a,b,c){var d,e=0,f=a.length,g=r(a);if(c){if(g){for(;f>e;e++)if(d=b.apply(a[e],c),d===!1)break}else for(e in a)if(d=b.apply(a[e],c),d===!1)break}else if(g){for(;f>e;e++)if(d=b.call(a[e],e,a[e]),d===!1)break}else for(e in a)if(d=b.call(a[e],e,a[e]),d===!1)break;return a},trim:function(a){return null==a?"":(a+"").replace(n,"")},makeArray:function(a,b){var c=b||[];return null!=a&&(r(Object(a))?m.merge(c,"string"==typeof a?[a]:a):f.call(c,a)),c},inArray:function(a,b,c){var d;if(b){if(g)return g.call(b,a,c);for(d=b.length,c=c?0>c?Math.max(0,d+c):c:0;d>c;c++)if(c in b&&b[c]===a)return c}return-1},merge:function(a,b){var c=+b.length,d=0,e=a.length;while(c>d)a[e++]=b[d++];if(c!==c)while(void 0!==b[d])a[e++]=b[d++];return a.length=e,a},grep:function(a,b,c){for(var d,e=[],f=0,g=a.length,h=!c;g>f;f++)d=!b(a[f],f),d!==h&&e.push(a[f]);return e},map:function(a,b,c){var d,f=0,g=a.length,h=r(a),i=[];if(h)for(;g>f;f++)d=b(a[f],f,c),null!=d&&i.push(d);else for(f in a)d=b(a[f],f,c),null!=d&&i.push(d);return e.apply([],i)},guid:1,proxy:function(a,b){var c,e,f;return"string"==typeof b&&(f=a[b],b=a,a=f),m.isFunction(a)?(c=d.call(arguments,2),e=function(){return a.apply(b||this,c.concat(d.call(arguments)))},e.guid=a.guid=a.guid||m.guid++,e):void 0},now:function(){return+new Date},support:k}),m.each("Boolean Number String Function Array Date RegExp Object Error".split(" "),function(a,b){h["[object "+b+"]"]=b.toLowerCase()});function r(a){var b=a.length,c=m.type(a);return"function"===c||m.isWindow(a)?!1:1===a.nodeType&&b?!0:"array"===c||0===b||"number"==typeof b&&b>0&&b-1 in a}var s=function(a){var b,c,d,e,f,g,h,i,j,k,l,m,n,o,p,q,r,s,t,u="sizzle"+-new Date,v=a.document,w=0,x=0,y=gb(),z=gb(),A=gb(),B=function(a,b){return a===b&&(l=!0),0},C="undefined",D=1<<31,E={}.hasOwnProperty,F=[],G=F.pop,H=F.push,I=F.push,J=F.slice,K=F.indexOf||function(a){for(var b=0,c=this.length;c>b;b++)if(this[b]===a)return b;return-1},L="checked|selected|async|autofocus|autoplay|controls|defer|disabled|hidden|ismap|loop|multiple|open|readonly|required|scoped",M="[\\x20\\t\\r\\n\\f]",N="(?:\\\\.|[\\w-]|[^\\x00-\\xa0])+",O=N.replace("w","w#"),P="\\["+M+"*("+N+")(?:"+M+"*([*^$|!~]?=)"+M+"*(?:'((?:\\\\.|[^\\\\'])*)'|\"((?:\\\\.|[^\\\\\"])*)\"|("+O+"))|)"+M+"*\\]",Q=":("+N+")(?:\\((('((?:\\\\.|[^\\\\'])*)'|\"((?:\\\\.|[^\\\\\"])*)\")|((?:\\\\.|[^\\\\()[\\]]|"+P+")*)|.*)\\)|)",R=new RegExp("^"+M+"+|((?:^|[^\\\\])(?:\\\\.)*)"+M+"+$","g"),S=new RegExp("^"+M+"*,"+M+"*"),T=new RegExp("^"+M+"*([>+~]|"+M+")"+M+"*"),U=new RegExp("="+M+"*([^\\]'\"]*?)"+M+"*\\]","g"),V=new RegExp(Q),W=new RegExp("^"+O+"$"),X={ID:new RegExp("^#("+N+")"),CLASS:new RegExp("^\\.("+N+")"),TAG:new RegExp("^("+N.replace("w","w*")+")"),ATTR:new RegExp("^"+P),PSEUDO:new RegExp("^"+Q),CHILD:new RegExp("^:(only|first|last|nth|nth-last)-(child|of-type)(?:\\("+M+"*(even|odd|(([+-]|)(\\d*)n|)"+M+"*(?:([+-]|)"+M+"*(\\d+)|))"+M+"*\\)|)","i"),bool:new RegExp("^(?:"+L+")$","i"),needsContext:new RegExp("^"+M+"*[>+~]|:(even|odd|eq|gt|lt|nth|first|last)(?:\\("+M+"*((?:-\\d)?\\d*)"+M+"*\\)|)(?=[^-]|$)","i")},Y=/^(?:input|select|textarea|button)$/i,Z=/^h\d$/i,$=/^[^{]+\{\s*\[native \w/,_=/^(?:#([\w-]+)|(\w+)|\.([\w-]+))$/,ab=/[+~]/,bb=/'|\\/g,cb=new RegExp("\\\\([\\da-f]{1,6}"+M+"?|("+M+")|.)","ig"),db=function(a,b,c){var d="0x"+b-65536;return d!==d||c?b:0>d?String.fromCharCode(d+65536):String.fromCharCode(d>>10|55296,1023&d|56320)};try{I.apply(F=J.call(v.childNodes),v.childNodes),F[v.childNodes.length].nodeType}catch(eb){I={apply:F.length?function(a,b){H.apply(a,J.call(b))}:function(a,b){var c=a.length,d=0;while(a[c++]=b[d++]);a.length=c-1}}}function fb(a,b,d,e){var f,h,j,k,l,o,r,s,w,x;if((b?b.ownerDocument||b:v)!==n&&m(b),b=b||n,d=d||[],!a||"string"!=typeof a)return d;if(1!==(k=b.nodeType)&&9!==k)return[];if(p&&!e){if(f=_.exec(a))if(j=f[1]){if(9===k){if(h=b.getElementById(j),!h||!h.parentNode)return d;if(h.id===j)return d.push(h),d}else if(b.ownerDocument&&(h=b.ownerDocument.getElementById(j))&&t(b,h)&&h.id===j)return d.push(h),d}else{if(f[2])return I.apply(d,b.getElementsByTagName(a)),d;if((j=f[3])&&c.getElementsByClassName&&b.getElementsByClassName)return I.apply(d,b.getElementsByClassName(j)),d}if(c.qsa&&(!q||!q.test(a))){if(s=r=u,w=b,x=9===k&&a,1===k&&"object"!==b.nodeName.toLowerCase()){o=g(a),(r=b.getAttribute("id"))?s=r.replace(bb,"\\$&"):b.setAttribute("id",s),s="[id='"+s+"'] ",l=o.length;while(l--)o[l]=s+qb(o[l]);w=ab.test(a)&&ob(b.parentNode)||b,x=o.join(",")}if(x)try{return I.apply(d,w.querySelectorAll(x)),d}catch(y){}finally{r||b.removeAttribute("id")}}}return i(a.replace(R,"$1"),b,d,e)}function gb(){var a=[];function b(c,e){return a.push(c+" ")>d.cacheLength&&delete b[a.shift()],b[c+" "]=e}return b}function hb(a){return a[u]=!0,a}function ib(a){var b=n.createElement("div");try{return!!a(b)}catch(c){return!1}finally{b.parentNode&&b.parentNode.removeChild(b),b=null}}function jb(a,b){var c=a.split("|"),e=a.length;while(e--)d.attrHandle[c[e]]=b}function kb(a,b){var c=b&&a,d=c&&1===a.nodeType&&1===b.nodeType&&(~b.sourceIndex||D)-(~a.sourceIndex||D);if(d)return d;if(c)while(c=c.nextSibling)if(c===b)return-1;return a?1:-1}function lb(a){return function(b){var c=b.nodeName.toLowerCase();return"input"===c&&b.type===a}}function mb(a){return function(b){var c=b.nodeName.toLowerCase();return("input"===c||"button"===c)&&b.type===a}}function nb(a){return hb(function(b){return b=+b,hb(function(c,d){var e,f=a([],c.length,b),g=f.length;while(g--)c[e=f[g]]&&(c[e]=!(d[e]=c[e]))})})}function ob(a){return a&&typeof a.getElementsByTagName!==C&&a}c=fb.support={},f=fb.isXML=function(a){var b=a&&(a.ownerDocument||a).documentElement;return b?"HTML"!==b.nodeName:!1},m=fb.setDocument=function(a){var b,e=a?a.ownerDocument||a:v,g=e.defaultView;return e!==n&&9===e.nodeType&&e.documentElement?(n=e,o=e.documentElement,p=!f(e),g&&g!==g.top&&(g.addEventListener?g.addEventListener("unload",function(){m()},!1):g.attachEvent&&g.attachEvent("onunload",function(){m()})),c.attributes=ib(function(a){return a.className="i",!a.getAttribute("className")}),c.getElementsByTagName=ib(function(a){return a.appendChild(e.createComment("")),!a.getElementsByTagName("*").length}),c.getElementsByClassName=$.test(e.getElementsByClassName)&&ib(function(a){return a.innerHTML="
",a.firstChild.className="i",2===a.getElementsByClassName("i").length}),c.getById=ib(function(a){return o.appendChild(a).id=u,!e.getElementsByName||!e.getElementsByName(u).length}),c.getById?(d.find.ID=function(a,b){if(typeof b.getElementById!==C&&p){var c=b.getElementById(a);return c&&c.parentNode?[c]:[]}},d.filter.ID=function(a){var b=a.replace(cb,db);return function(a){return a.getAttribute("id")===b}}):(delete d.find.ID,d.filter.ID=function(a){var b=a.replace(cb,db);return function(a){var c=typeof a.getAttributeNode!==C&&a.getAttributeNode("id");return c&&c.value===b}}),d.find.TAG=c.getElementsByTagName?function(a,b){return typeof b.getElementsByTagName!==C?b.getElementsByTagName(a):void 0}:function(a,b){var c,d=[],e=0,f=b.getElementsByTagName(a);if("*"===a){while(c=f[e++])1===c.nodeType&&d.push(c);return d}return f},d.find.CLASS=c.getElementsByClassName&&function(a,b){return typeof b.getElementsByClassName!==C&&p?b.getElementsByClassName(a):void 0},r=[],q=[],(c.qsa=$.test(e.querySelectorAll))&&(ib(function(a){a.innerHTML=" ",a.querySelectorAll("[msallowclip^='']").length&&q.push("[*^$]="+M+"*(?:''|\"\")"),a.querySelectorAll("[selected]").length||q.push("\\["+M+"*(?:value|"+L+")"),a.querySelectorAll(":checked").length||q.push(":checked")}),ib(function(a){var b=e.createElement("input");b.setAttribute("type","hidden"),a.appendChild(b).setAttribute("name","D"),a.querySelectorAll("[name=d]").length&&q.push("name"+M+"*[*^$|!~]?="),a.querySelectorAll(":enabled").length||q.push(":enabled",":disabled"),a.querySelectorAll("*,:x"),q.push(",.*:")})),(c.matchesSelector=$.test(s=o.matches||o.webkitMatchesSelector||o.mozMatchesSelector||o.oMatchesSelector||o.msMatchesSelector))&&ib(function(a){c.disconnectedMatch=s.call(a,"div"),s.call(a,"[s!='']:x"),r.push("!=",Q)}),q=q.length&&new RegExp(q.join("|")),r=r.length&&new RegExp(r.join("|")),b=$.test(o.compareDocumentPosition),t=b||$.test(o.contains)?function(a,b){var c=9===a.nodeType?a.documentElement:a,d=b&&b.parentNode;return a===d||!(!d||1!==d.nodeType||!(c.contains?c.contains(d):a.compareDocumentPosition&&16&a.compareDocumentPosition(d)))}:function(a,b){if(b)while(b=b.parentNode)if(b===a)return!0;return!1},B=b?function(a,b){if(a===b)return l=!0,0;var d=!a.compareDocumentPosition-!b.compareDocumentPosition;return d?d:(d=(a.ownerDocument||a)===(b.ownerDocument||b)?a.compareDocumentPosition(b):1,1&d||!c.sortDetached&&b.compareDocumentPosition(a)===d?a===e||a.ownerDocument===v&&t(v,a)?-1:b===e||b.ownerDocument===v&&t(v,b)?1:k?K.call(k,a)-K.call(k,b):0:4&d?-1:1)}:function(a,b){if(a===b)return l=!0,0;var c,d=0,f=a.parentNode,g=b.parentNode,h=[a],i=[b];if(!f||!g)return a===e?-1:b===e?1:f?-1:g?1:k?K.call(k,a)-K.call(k,b):0;if(f===g)return kb(a,b);c=a;while(c=c.parentNode)h.unshift(c);c=b;while(c=c.parentNode)i.unshift(c);while(h[d]===i[d])d++;return d?kb(h[d],i[d]):h[d]===v?-1:i[d]===v?1:0},e):n},fb.matches=function(a,b){return fb(a,null,null,b)},fb.matchesSelector=function(a,b){if((a.ownerDocument||a)!==n&&m(a),b=b.replace(U,"='$1']"),!(!c.matchesSelector||!p||r&&r.test(b)||q&&q.test(b)))try{var d=s.call(a,b);if(d||c.disconnectedMatch||a.document&&11!==a.document.nodeType)return d}catch(e){}return fb(b,n,null,[a]).length>0},fb.contains=function(a,b){return(a.ownerDocument||a)!==n&&m(a),t(a,b)},fb.attr=function(a,b){(a.ownerDocument||a)!==n&&m(a);var e=d.attrHandle[b.toLowerCase()],f=e&&E.call(d.attrHandle,b.toLowerCase())?e(a,b,!p):void 0;return void 0!==f?f:c.attributes||!p?a.getAttribute(b):(f=a.getAttributeNode(b))&&f.specified?f.value:null},fb.error=function(a){throw new Error("Syntax error, unrecognized expression: "+a)},fb.uniqueSort=function(a){var b,d=[],e=0,f=0;if(l=!c.detectDuplicates,k=!c.sortStable&&a.slice(0),a.sort(B),l){while(b=a[f++])b===a[f]&&(e=d.push(f));while(e--)a.splice(d[e],1)}return k=null,a},e=fb.getText=function(a){var b,c="",d=0,f=a.nodeType;if(f){if(1===f||9===f||11===f){if("string"==typeof a.textContent)return a.textContent;for(a=a.firstChild;a;a=a.nextSibling)c+=e(a)}else if(3===f||4===f)return a.nodeValue}else while(b=a[d++])c+=e(b);return c},d=fb.selectors={cacheLength:50,createPseudo:hb,match:X,attrHandle:{},find:{},relative:{">":{dir:"parentNode",first:!0}," ":{dir:"parentNode"},"+":{dir:"previousSibling",first:!0},"~":{dir:"previousSibling"}},preFilter:{ATTR:function(a){return a[1]=a[1].replace(cb,db),a[3]=(a[3]||a[4]||a[5]||"").replace(cb,db),"~="===a[2]&&(a[3]=" "+a[3]+" "),a.slice(0,4)},CHILD:function(a){return a[1]=a[1].toLowerCase(),"nth"===a[1].slice(0,3)?(a[3]||fb.error(a[0]),a[4]=+(a[4]?a[5]+(a[6]||1):2*("even"===a[3]||"odd"===a[3])),a[5]=+(a[7]+a[8]||"odd"===a[3])):a[3]&&fb.error(a[0]),a},PSEUDO:function(a){var b,c=!a[6]&&a[2];return X.CHILD.test(a[0])?null:(a[3]?a[2]=a[4]||a[5]||"":c&&V.test(c)&&(b=g(c,!0))&&(b=c.indexOf(")",c.length-b)-c.length)&&(a[0]=a[0].slice(0,b),a[2]=c.slice(0,b)),a.slice(0,3))}},filter:{TAG:function(a){var b=a.replace(cb,db).toLowerCase();return"*"===a?function(){return!0}:function(a){return a.nodeName&&a.nodeName.toLowerCase()===b}},CLASS:function(a){var b=y[a+" "];return b||(b=new RegExp("(^|"+M+")"+a+"("+M+"|$)"))&&y(a,function(a){return b.test("string"==typeof a.className&&a.className||typeof a.getAttribute!==C&&a.getAttribute("class")||"")})},ATTR:function(a,b,c){return function(d){var e=fb.attr(d,a);return null==e?"!="===b:b?(e+="","="===b?e===c:"!="===b?e!==c:"^="===b?c&&0===e.indexOf(c):"*="===b?c&&e.indexOf(c)>-1:"$="===b?c&&e.slice(-c.length)===c:"~="===b?(" "+e+" ").indexOf(c)>-1:"|="===b?e===c||e.slice(0,c.length+1)===c+"-":!1):!0}},CHILD:function(a,b,c,d,e){var f="nth"!==a.slice(0,3),g="last"!==a.slice(-4),h="of-type"===b;return 1===d&&0===e?function(a){return!!a.parentNode}:function(b,c,i){var j,k,l,m,n,o,p=f!==g?"nextSibling":"previousSibling",q=b.parentNode,r=h&&b.nodeName.toLowerCase(),s=!i&&!h;if(q){if(f){while(p){l=b;while(l=l[p])if(h?l.nodeName.toLowerCase()===r:1===l.nodeType)return!1;o=p="only"===a&&!o&&"nextSibling"}return!0}if(o=[g?q.firstChild:q.lastChild],g&&s){k=q[u]||(q[u]={}),j=k[a]||[],n=j[0]===w&&j[1],m=j[0]===w&&j[2],l=n&&q.childNodes[n];while(l=++n&&l&&l[p]||(m=n=0)||o.pop())if(1===l.nodeType&&++m&&l===b){k[a]=[w,n,m];break}}else if(s&&(j=(b[u]||(b[u]={}))[a])&&j[0]===w)m=j[1];else while(l=++n&&l&&l[p]||(m=n=0)||o.pop())if((h?l.nodeName.toLowerCase()===r:1===l.nodeType)&&++m&&(s&&((l[u]||(l[u]={}))[a]=[w,m]),l===b))break;return m-=e,m===d||m%d===0&&m/d>=0}}},PSEUDO:function(a,b){var c,e=d.pseudos[a]||d.setFilters[a.toLowerCase()]||fb.error("unsupported pseudo: "+a);return e[u]?e(b):e.length>1?(c=[a,a,"",b],d.setFilters.hasOwnProperty(a.toLowerCase())?hb(function(a,c){var d,f=e(a,b),g=f.length;while(g--)d=K.call(a,f[g]),a[d]=!(c[d]=f[g])}):function(a){return e(a,0,c)}):e}},pseudos:{not:hb(function(a){var b=[],c=[],d=h(a.replace(R,"$1"));return d[u]?hb(function(a,b,c,e){var f,g=d(a,null,e,[]),h=a.length;while(h--)(f=g[h])&&(a[h]=!(b[h]=f))}):function(a,e,f){return b[0]=a,d(b,null,f,c),!c.pop()}}),has:hb(function(a){return function(b){return fb(a,b).length>0}}),contains:hb(function(a){return function(b){return(b.textContent||b.innerText||e(b)).indexOf(a)>-1}}),lang:hb(function(a){return W.test(a||"")||fb.error("unsupported lang: "+a),a=a.replace(cb,db).toLowerCase(),function(b){var c;do if(c=p?b.lang:b.getAttribute("xml:lang")||b.getAttribute("lang"))return c=c.toLowerCase(),c===a||0===c.indexOf(a+"-");while((b=b.parentNode)&&1===b.nodeType);return!1}}),target:function(b){var c=a.location&&a.location.hash;return c&&c.slice(1)===b.id},root:function(a){return a===o},focus:function(a){return a===n.activeElement&&(!n.hasFocus||n.hasFocus())&&!!(a.type||a.href||~a.tabIndex)},enabled:function(a){return a.disabled===!1},disabled:function(a){return a.disabled===!0},checked:function(a){var b=a.nodeName.toLowerCase();return"input"===b&&!!a.checked||"option"===b&&!!a.selected},selected:function(a){return a.parentNode&&a.parentNode.selectedIndex,a.selected===!0},empty:function(a){for(a=a.firstChild;a;a=a.nextSibling)if(a.nodeType<6)return!1;return!0},parent:function(a){return!d.pseudos.empty(a)},header:function(a){return Z.test(a.nodeName)},input:function(a){return Y.test(a.nodeName)},button:function(a){var b=a.nodeName.toLowerCase();return"input"===b&&"button"===a.type||"button"===b},text:function(a){var b;return"input"===a.nodeName.toLowerCase()&&"text"===a.type&&(null==(b=a.getAttribute("type"))||"text"===b.toLowerCase())},first:nb(function(){return[0]}),last:nb(function(a,b){return[b-1]}),eq:nb(function(a,b,c){return[0>c?c+b:c]}),even:nb(function(a,b){for(var c=0;b>c;c+=2)a.push(c);return a}),odd:nb(function(a,b){for(var c=1;b>c;c+=2)a.push(c);return a}),lt:nb(function(a,b,c){for(var d=0>c?c+b:c;--d>=0;)a.push(d);return a}),gt:nb(function(a,b,c){for(var d=0>c?c+b:c;++db;b++)d+=a[b].value;return d}function rb(a,b,c){var d=b.dir,e=c&&"parentNode"===d,f=x++;return b.first?function(b,c,f){while(b=b[d])if(1===b.nodeType||e)return a(b,c,f)}:function(b,c,g){var h,i,j=[w,f];if(g){while(b=b[d])if((1===b.nodeType||e)&&a(b,c,g))return!0}else while(b=b[d])if(1===b.nodeType||e){if(i=b[u]||(b[u]={}),(h=i[d])&&h[0]===w&&h[1]===f)return j[2]=h[2];if(i[d]=j,j[2]=a(b,c,g))return!0}}}function sb(a){return a.length>1?function(b,c,d){var e=a.length;while(e--)if(!a[e](b,c,d))return!1;return!0}:a[0]}function tb(a,b,c){for(var d=0,e=b.length;e>d;d++)fb(a,b[d],c);return c}function ub(a,b,c,d,e){for(var f,g=[],h=0,i=a.length,j=null!=b;i>h;h++)(f=a[h])&&(!c||c(f,d,e))&&(g.push(f),j&&b.push(h));return g}function vb(a,b,c,d,e,f){return d&&!d[u]&&(d=vb(d)),e&&!e[u]&&(e=vb(e,f)),hb(function(f,g,h,i){var j,k,l,m=[],n=[],o=g.length,p=f||tb(b||"*",h.nodeType?[h]:h,[]),q=!a||!f&&b?p:ub(p,m,a,h,i),r=c?e||(f?a:o||d)?[]:g:q;if(c&&c(q,r,h,i),d){j=ub(r,n),d(j,[],h,i),k=j.length;while(k--)(l=j[k])&&(r[n[k]]=!(q[n[k]]=l))}if(f){if(e||a){if(e){j=[],k=r.length;while(k--)(l=r[k])&&j.push(q[k]=l);e(null,r=[],j,i)}k=r.length;while(k--)(l=r[k])&&(j=e?K.call(f,l):m[k])>-1&&(f[j]=!(g[j]=l))}}else r=ub(r===g?r.splice(o,r.length):r),e?e(null,g,r,i):I.apply(g,r)})}function wb(a){for(var b,c,e,f=a.length,g=d.relative[a[0].type],h=g||d.relative[" "],i=g?1:0,k=rb(function(a){return a===b},h,!0),l=rb(function(a){return K.call(b,a)>-1},h,!0),m=[function(a,c,d){return!g&&(d||c!==j)||((b=c).nodeType?k(a,c,d):l(a,c,d))}];f>i;i++)if(c=d.relative[a[i].type])m=[rb(sb(m),c)];else{if(c=d.filter[a[i].type].apply(null,a[i].matches),c[u]){for(e=++i;f>e;e++)if(d.relative[a[e].type])break;return vb(i>1&&sb(m),i>1&&qb(a.slice(0,i-1).concat({value:" "===a[i-2].type?"*":""})).replace(R,"$1"),c,e>i&&wb(a.slice(i,e)),f>e&&wb(a=a.slice(e)),f>e&&qb(a))}m.push(c)}return sb(m)}function xb(a,b){var c=b.length>0,e=a.length>0,f=function(f,g,h,i,k){var l,m,o,p=0,q="0",r=f&&[],s=[],t=j,u=f||e&&d.find.TAG("*",k),v=w+=null==t?1:Math.random()||.1,x=u.length;for(k&&(j=g!==n&&g);q!==x&&null!=(l=u[q]);q++){if(e&&l){m=0;while(o=a[m++])if(o(l,g,h)){i.push(l);break}k&&(w=v)}c&&((l=!o&&l)&&p--,f&&r.push(l))}if(p+=q,c&&q!==p){m=0;while(o=b[m++])o(r,s,g,h);if(f){if(p>0)while(q--)r[q]||s[q]||(s[q]=G.call(i));s=ub(s)}I.apply(i,s),k&&!f&&s.length>0&&p+b.length>1&&fb.uniqueSort(i)}return k&&(w=v,j=t),r};return c?hb(f):f}return h=fb.compile=function(a,b){var c,d=[],e=[],f=A[a+" "];if(!f){b||(b=g(a)),c=b.length;while(c--)f=wb(b[c]),f[u]?d.push(f):e.push(f);f=A(a,xb(e,d)),f.selector=a}return f},i=fb.select=function(a,b,e,f){var i,j,k,l,m,n="function"==typeof a&&a,o=!f&&g(a=n.selector||a);if(e=e||[],1===o.length){if(j=o[0]=o[0].slice(0),j.length>2&&"ID"===(k=j[0]).type&&c.getById&&9===b.nodeType&&p&&d.relative[j[1].type]){if(b=(d.find.ID(k.matches[0].replace(cb,db),b)||[])[0],!b)return e;n&&(b=b.parentNode),a=a.slice(j.shift().value.length)}i=X.needsContext.test(a)?0:j.length;while(i--){if(k=j[i],d.relative[l=k.type])break;if((m=d.find[l])&&(f=m(k.matches[0].replace(cb,db),ab.test(j[0].type)&&ob(b.parentNode)||b))){if(j.splice(i,1),a=f.length&&qb(j),!a)return I.apply(e,f),e;break}}}return(n||h(a,o))(f,b,!p,e,ab.test(a)&&ob(b.parentNode)||b),e},c.sortStable=u.split("").sort(B).join("")===u,c.detectDuplicates=!!l,m(),c.sortDetached=ib(function(a){return 1&a.compareDocumentPosition(n.createElement("div"))}),ib(function(a){return a.innerHTML=" ","#"===a.firstChild.getAttribute("href")})||jb("type|href|height|width",function(a,b,c){return c?void 0:a.getAttribute(b,"type"===b.toLowerCase()?1:2)}),c.attributes&&ib(function(a){return a.innerHTML=" ",a.firstChild.setAttribute("value",""),""===a.firstChild.getAttribute("value")})||jb("value",function(a,b,c){return c||"input"!==a.nodeName.toLowerCase()?void 0:a.defaultValue}),ib(function(a){return null==a.getAttribute("disabled")})||jb(L,function(a,b,c){var d;return c?void 0:a[b]===!0?b.toLowerCase():(d=a.getAttributeNode(b))&&d.specified?d.value:null}),fb}(a);m.find=s,m.expr=s.selectors,m.expr[":"]=m.expr.pseudos,m.unique=s.uniqueSort,m.text=s.getText,m.isXMLDoc=s.isXML,m.contains=s.contains;var t=m.expr.match.needsContext,u=/^<(\w+)\s*\/?>(?:<\/\1>|)$/,v=/^.[^:#\[\.,]*$/;function w(a,b,c){if(m.isFunction(b))return m.grep(a,function(a,d){return!!b.call(a,d,a)!==c});if(b.nodeType)return m.grep(a,function(a){return a===b!==c});if("string"==typeof b){if(v.test(b))return m.filter(b,a,c);b=m.filter(b,a)}return m.grep(a,function(a){return m.inArray(a,b)>=0!==c})}m.filter=function(a,b,c){var d=b[0];return c&&(a=":not("+a+")"),1===b.length&&1===d.nodeType?m.find.matchesSelector(d,a)?[d]:[]:m.find.matches(a,m.grep(b,function(a){return 1===a.nodeType}))},m.fn.extend({find:function(a){var b,c=[],d=this,e=d.length;if("string"!=typeof a)return this.pushStack(m(a).filter(function(){for(b=0;e>b;b++)if(m.contains(d[b],this))return!0}));for(b=0;e>b;b++)m.find(a,d[b],c);return c=this.pushStack(e>1?m.unique(c):c),c.selector=this.selector?this.selector+" "+a:a,c},filter:function(a){return this.pushStack(w(this,a||[],!1))},not:function(a){return this.pushStack(w(this,a||[],!0))},is:function(a){return!!w(this,"string"==typeof a&&t.test(a)?m(a):a||[],!1).length}});var x,y=a.document,z=/^(?:\s*(<[\w\W]+>)[^>]*|#([\w-]*))$/,A=m.fn.init=function(a,b){var c,d;if(!a)return this;if("string"==typeof a){if(c="<"===a.charAt(0)&&">"===a.charAt(a.length-1)&&a.length>=3?[null,a,null]:z.exec(a),!c||!c[1]&&b)return!b||b.jquery?(b||x).find(a):this.constructor(b).find(a);if(c[1]){if(b=b instanceof m?b[0]:b,m.merge(this,m.parseHTML(c[1],b&&b.nodeType?b.ownerDocument||b:y,!0)),u.test(c[1])&&m.isPlainObject(b))for(c in b)m.isFunction(this[c])?this[c](b[c]):this.attr(c,b[c]);return this}if(d=y.getElementById(c[2]),d&&d.parentNode){if(d.id!==c[2])return x.find(a);this.length=1,this[0]=d}return this.context=y,this.selector=a,this}return a.nodeType?(this.context=this[0]=a,this.length=1,this):m.isFunction(a)?"undefined"!=typeof x.ready?x.ready(a):a(m):(void 0!==a.selector&&(this.selector=a.selector,this.context=a.context),m.makeArray(a,this))};A.prototype=m.fn,x=m(y);var B=/^(?:parents|prev(?:Until|All))/,C={children:!0,contents:!0,next:!0,prev:!0};m.extend({dir:function(a,b,c){var d=[],e=a[b];while(e&&9!==e.nodeType&&(void 0===c||1!==e.nodeType||!m(e).is(c)))1===e.nodeType&&d.push(e),e=e[b];return d},sibling:function(a,b){for(var c=[];a;a=a.nextSibling)1===a.nodeType&&a!==b&&c.push(a);return c}}),m.fn.extend({has:function(a){var b,c=m(a,this),d=c.length;return this.filter(function(){for(b=0;d>b;b++)if(m.contains(this,c[b]))return!0})},closest:function(a,b){for(var c,d=0,e=this.length,f=[],g=t.test(a)||"string"!=typeof a?m(a,b||this.context):0;e>d;d++)for(c=this[d];c&&c!==b;c=c.parentNode)if(c.nodeType<11&&(g?g.index(c)>-1:1===c.nodeType&&m.find.matchesSelector(c,a))){f.push(c);break}return this.pushStack(f.length>1?m.unique(f):f)},index:function(a){return a?"string"==typeof a?m.inArray(this[0],m(a)):m.inArray(a.jquery?a[0]:a,this):this[0]&&this[0].parentNode?this.first().prevAll().length:-1},add:function(a,b){return this.pushStack(m.unique(m.merge(this.get(),m(a,b))))},addBack:function(a){return this.add(null==a?this.prevObject:this.prevObject.filter(a))}});function D(a,b){do a=a[b];while(a&&1!==a.nodeType);return a}m.each({parent:function(a){var b=a.parentNode;return b&&11!==b.nodeType?b:null},parents:function(a){return m.dir(a,"parentNode")},parentsUntil:function(a,b,c){return m.dir(a,"parentNode",c)},next:function(a){return D(a,"nextSibling")},prev:function(a){return D(a,"previousSibling")},nextAll:function(a){return m.dir(a,"nextSibling")},prevAll:function(a){return m.dir(a,"previousSibling")},nextUntil:function(a,b,c){return m.dir(a,"nextSibling",c)},prevUntil:function(a,b,c){return m.dir(a,"previousSibling",c)},siblings:function(a){return m.sibling((a.parentNode||{}).firstChild,a)},children:function(a){return m.sibling(a.firstChild)},contents:function(a){return m.nodeName(a,"iframe")?a.contentDocument||a.contentWindow.document:m.merge([],a.childNodes)}},function(a,b){m.fn[a]=function(c,d){var e=m.map(this,b,c);return"Until"!==a.slice(-5)&&(d=c),d&&"string"==typeof d&&(e=m.filter(d,e)),this.length>1&&(C[a]||(e=m.unique(e)),B.test(a)&&(e=e.reverse())),this.pushStack(e)}});var E=/\S+/g,F={};function G(a){var b=F[a]={};return m.each(a.match(E)||[],function(a,c){b[c]=!0}),b}m.Callbacks=function(a){a="string"==typeof a?F[a]||G(a):m.extend({},a);var b,c,d,e,f,g,h=[],i=!a.once&&[],j=function(l){for(c=a.memory&&l,d=!0,f=g||0,g=0,e=h.length,b=!0;h&&e>f;f++)if(h[f].apply(l[0],l[1])===!1&&a.stopOnFalse){c=!1;break}b=!1,h&&(i?i.length&&j(i.shift()):c?h=[]:k.disable())},k={add:function(){if(h){var d=h.length;!function f(b){m.each(b,function(b,c){var d=m.type(c);"function"===d?a.unique&&k.has(c)||h.push(c):c&&c.length&&"string"!==d&&f(c)})}(arguments),b?e=h.length:c&&(g=d,j(c))}return this},remove:function(){return h&&m.each(arguments,function(a,c){var d;while((d=m.inArray(c,h,d))>-1)h.splice(d,1),b&&(e>=d&&e--,f>=d&&f--)}),this},has:function(a){return a?m.inArray(a,h)>-1:!(!h||!h.length)},empty:function(){return h=[],e=0,this},disable:function(){return h=i=c=void 0,this},disabled:function(){return!h},lock:function(){return i=void 0,c||k.disable(),this},locked:function(){return!i},fireWith:function(a,c){return!h||d&&!i||(c=c||[],c=[a,c.slice?c.slice():c],b?i.push(c):j(c)),this},fire:function(){return k.fireWith(this,arguments),this},fired:function(){return!!d}};return k},m.extend({Deferred:function(a){var b=[["resolve","done",m.Callbacks("once memory"),"resolved"],["reject","fail",m.Callbacks("once memory"),"rejected"],["notify","progress",m.Callbacks("memory")]],c="pending",d={state:function(){return c},always:function(){return e.done(arguments).fail(arguments),this},then:function(){var a=arguments;return m.Deferred(function(c){m.each(b,function(b,f){var g=m.isFunction(a[b])&&a[b];e[f[1]](function(){var a=g&&g.apply(this,arguments);a&&m.isFunction(a.promise)?a.promise().done(c.resolve).fail(c.reject).progress(c.notify):c[f[0]+"With"](this===d?c.promise():this,g?[a]:arguments)})}),a=null}).promise()},promise:function(a){return null!=a?m.extend(a,d):d}},e={};return d.pipe=d.then,m.each(b,function(a,f){var g=f[2],h=f[3];d[f[1]]=g.add,h&&g.add(function(){c=h},b[1^a][2].disable,b[2][2].lock),e[f[0]]=function(){return e[f[0]+"With"](this===e?d:this,arguments),this},e[f[0]+"With"]=g.fireWith}),d.promise(e),a&&a.call(e,e),e},when:function(a){var b=0,c=d.call(arguments),e=c.length,f=1!==e||a&&m.isFunction(a.promise)?e:0,g=1===f?a:m.Deferred(),h=function(a,b,c){return function(e){b[a]=this,c[a]=arguments.length>1?d.call(arguments):e,c===i?g.notifyWith(b,c):--f||g.resolveWith(b,c)}},i,j,k;if(e>1)for(i=new Array(e),j=new Array(e),k=new Array(e);e>b;b++)c[b]&&m.isFunction(c[b].promise)?c[b].promise().done(h(b,k,c)).fail(g.reject).progress(h(b,j,i)):--f;return f||g.resolveWith(k,c),g.promise()}});var H;m.fn.ready=function(a){return m.ready.promise().done(a),this},m.extend({isReady:!1,readyWait:1,holdReady:function(a){a?m.readyWait++:m.ready(!0)},ready:function(a){if(a===!0?!--m.readyWait:!m.isReady){if(!y.body)return setTimeout(m.ready);m.isReady=!0,a!==!0&&--m.readyWait>0||(H.resolveWith(y,[m]),m.fn.triggerHandler&&(m(y).triggerHandler("ready"),m(y).off("ready")))}}});function I(){y.addEventListener?(y.removeEventListener("DOMContentLoaded",J,!1),a.removeEventListener("load",J,!1)):(y.detachEvent("onreadystatechange",J),a.detachEvent("onload",J))}function J(){(y.addEventListener||"load"===event.type||"complete"===y.readyState)&&(I(),m.ready())}m.ready.promise=function(b){if(!H)if(H=m.Deferred(),"complete"===y.readyState)setTimeout(m.ready);else if(y.addEventListener)y.addEventListener("DOMContentLoaded",J,!1),a.addEventListener("load",J,!1);else{y.attachEvent("onreadystatechange",J),a.attachEvent("onload",J);var c=!1;try{c=null==a.frameElement&&y.documentElement}catch(d){}c&&c.doScroll&&!function e(){if(!m.isReady){try{c.doScroll("left")}catch(a){return setTimeout(e,50)}I(),m.ready()}}()}return H.promise(b)};var K="undefined",L;for(L in m(k))break;k.ownLast="0"!==L,k.inlineBlockNeedsLayout=!1,m(function(){var a,b,c,d;c=y.getElementsByTagName("body")[0],c&&c.style&&(b=y.createElement("div"),d=y.createElement("div"),d.style.cssText="position:absolute;border:0;width:0;height:0;top:0;left:-9999px",c.appendChild(d).appendChild(b),typeof b.style.zoom!==K&&(b.style.cssText="display:inline;margin:0;border:0;padding:1px;width:1px;zoom:1",k.inlineBlockNeedsLayout=a=3===b.offsetWidth,a&&(c.style.zoom=1)),c.removeChild(d))}),function(){var a=y.createElement("div");if(null==k.deleteExpando){k.deleteExpando=!0;try{delete a.test}catch(b){k.deleteExpando=!1}}a=null}(),m.acceptData=function(a){var b=m.noData[(a.nodeName+" ").toLowerCase()],c=+a.nodeType||1;return 1!==c&&9!==c?!1:!b||b!==!0&&a.getAttribute("classid")===b};var M=/^(?:\{[\w\W]*\}|\[[\w\W]*\])$/,N=/([A-Z])/g;function O(a,b,c){if(void 0===c&&1===a.nodeType){var d="data-"+b.replace(N,"-$1").toLowerCase();if(c=a.getAttribute(d),"string"==typeof c){try{c="true"===c?!0:"false"===c?!1:"null"===c?null:+c+""===c?+c:M.test(c)?m.parseJSON(c):c}catch(e){}m.data(a,b,c)}else c=void 0}return c}function P(a){var b;for(b in a)if(("data"!==b||!m.isEmptyObject(a[b]))&&"toJSON"!==b)return!1;return!0}function Q(a,b,d,e){if(m.acceptData(a)){var f,g,h=m.expando,i=a.nodeType,j=i?m.cache:a,k=i?a[h]:a[h]&&h;
-if(k&&j[k]&&(e||j[k].data)||void 0!==d||"string"!=typeof b)return k||(k=i?a[h]=c.pop()||m.guid++:h),j[k]||(j[k]=i?{}:{toJSON:m.noop}),("object"==typeof b||"function"==typeof b)&&(e?j[k]=m.extend(j[k],b):j[k].data=m.extend(j[k].data,b)),g=j[k],e||(g.data||(g.data={}),g=g.data),void 0!==d&&(g[m.camelCase(b)]=d),"string"==typeof b?(f=g[b],null==f&&(f=g[m.camelCase(b)])):f=g,f}}function R(a,b,c){if(m.acceptData(a)){var d,e,f=a.nodeType,g=f?m.cache:a,h=f?a[m.expando]:m.expando;if(g[h]){if(b&&(d=c?g[h]:g[h].data)){m.isArray(b)?b=b.concat(m.map(b,m.camelCase)):b in d?b=[b]:(b=m.camelCase(b),b=b in d?[b]:b.split(" ")),e=b.length;while(e--)delete d[b[e]];if(c?!P(d):!m.isEmptyObject(d))return}(c||(delete g[h].data,P(g[h])))&&(f?m.cleanData([a],!0):k.deleteExpando||g!=g.window?delete g[h]:g[h]=null)}}}m.extend({cache:{},noData:{"applet ":!0,"embed ":!0,"object ":"clsid:D27CDB6E-AE6D-11cf-96B8-444553540000"},hasData:function(a){return a=a.nodeType?m.cache[a[m.expando]]:a[m.expando],!!a&&!P(a)},data:function(a,b,c){return Q(a,b,c)},removeData:function(a,b){return R(a,b)},_data:function(a,b,c){return Q(a,b,c,!0)},_removeData:function(a,b){return R(a,b,!0)}}),m.fn.extend({data:function(a,b){var c,d,e,f=this[0],g=f&&f.attributes;if(void 0===a){if(this.length&&(e=m.data(f),1===f.nodeType&&!m._data(f,"parsedAttrs"))){c=g.length;while(c--)g[c]&&(d=g[c].name,0===d.indexOf("data-")&&(d=m.camelCase(d.slice(5)),O(f,d,e[d])));m._data(f,"parsedAttrs",!0)}return e}return"object"==typeof a?this.each(function(){m.data(this,a)}):arguments.length>1?this.each(function(){m.data(this,a,b)}):f?O(f,a,m.data(f,a)):void 0},removeData:function(a){return this.each(function(){m.removeData(this,a)})}}),m.extend({queue:function(a,b,c){var d;return a?(b=(b||"fx")+"queue",d=m._data(a,b),c&&(!d||m.isArray(c)?d=m._data(a,b,m.makeArray(c)):d.push(c)),d||[]):void 0},dequeue:function(a,b){b=b||"fx";var c=m.queue(a,b),d=c.length,e=c.shift(),f=m._queueHooks(a,b),g=function(){m.dequeue(a,b)};"inprogress"===e&&(e=c.shift(),d--),e&&("fx"===b&&c.unshift("inprogress"),delete f.stop,e.call(a,g,f)),!d&&f&&f.empty.fire()},_queueHooks:function(a,b){var c=b+"queueHooks";return m._data(a,c)||m._data(a,c,{empty:m.Callbacks("once memory").add(function(){m._removeData(a,b+"queue"),m._removeData(a,c)})})}}),m.fn.extend({queue:function(a,b){var c=2;return"string"!=typeof a&&(b=a,a="fx",c--),arguments.lengthh;h++)b(a[h],c,g?d:d.call(a[h],h,b(a[h],c)));return e?a:j?b.call(a):i?b(a[0],c):f},W=/^(?:checkbox|radio)$/i;!function(){var a=y.createElement("input"),b=y.createElement("div"),c=y.createDocumentFragment();if(b.innerHTML=" a ",k.leadingWhitespace=3===b.firstChild.nodeType,k.tbody=!b.getElementsByTagName("tbody").length,k.htmlSerialize=!!b.getElementsByTagName("link").length,k.html5Clone="<:nav>"!==y.createElement("nav").cloneNode(!0).outerHTML,a.type="checkbox",a.checked=!0,c.appendChild(a),k.appendChecked=a.checked,b.innerHTML="",k.noCloneChecked=!!b.cloneNode(!0).lastChild.defaultValue,c.appendChild(b),b.innerHTML=" ",k.checkClone=b.cloneNode(!0).cloneNode(!0).lastChild.checked,k.noCloneEvent=!0,b.attachEvent&&(b.attachEvent("onclick",function(){k.noCloneEvent=!1}),b.cloneNode(!0).click()),null==k.deleteExpando){k.deleteExpando=!0;try{delete b.test}catch(d){k.deleteExpando=!1}}}(),function(){var b,c,d=y.createElement("div");for(b in{submit:!0,change:!0,focusin:!0})c="on"+b,(k[b+"Bubbles"]=c in a)||(d.setAttribute(c,"t"),k[b+"Bubbles"]=d.attributes[c].expando===!1);d=null}();var X=/^(?:input|select|textarea)$/i,Y=/^key/,Z=/^(?:mouse|pointer|contextmenu)|click/,$=/^(?:focusinfocus|focusoutblur)$/,_=/^([^.]*)(?:\.(.+)|)$/;function ab(){return!0}function bb(){return!1}function cb(){try{return y.activeElement}catch(a){}}m.event={global:{},add:function(a,b,c,d,e){var f,g,h,i,j,k,l,n,o,p,q,r=m._data(a);if(r){c.handler&&(i=c,c=i.handler,e=i.selector),c.guid||(c.guid=m.guid++),(g=r.events)||(g=r.events={}),(k=r.handle)||(k=r.handle=function(a){return typeof m===K||a&&m.event.triggered===a.type?void 0:m.event.dispatch.apply(k.elem,arguments)},k.elem=a),b=(b||"").match(E)||[""],h=b.length;while(h--)f=_.exec(b[h])||[],o=q=f[1],p=(f[2]||"").split(".").sort(),o&&(j=m.event.special[o]||{},o=(e?j.delegateType:j.bindType)||o,j=m.event.special[o]||{},l=m.extend({type:o,origType:q,data:d,handler:c,guid:c.guid,selector:e,needsContext:e&&m.expr.match.needsContext.test(e),namespace:p.join(".")},i),(n=g[o])||(n=g[o]=[],n.delegateCount=0,j.setup&&j.setup.call(a,d,p,k)!==!1||(a.addEventListener?a.addEventListener(o,k,!1):a.attachEvent&&a.attachEvent("on"+o,k))),j.add&&(j.add.call(a,l),l.handler.guid||(l.handler.guid=c.guid)),e?n.splice(n.delegateCount++,0,l):n.push(l),m.event.global[o]=!0);a=null}},remove:function(a,b,c,d,e){var f,g,h,i,j,k,l,n,o,p,q,r=m.hasData(a)&&m._data(a);if(r&&(k=r.events)){b=(b||"").match(E)||[""],j=b.length;while(j--)if(h=_.exec(b[j])||[],o=q=h[1],p=(h[2]||"").split(".").sort(),o){l=m.event.special[o]||{},o=(d?l.delegateType:l.bindType)||o,n=k[o]||[],h=h[2]&&new RegExp("(^|\\.)"+p.join("\\.(?:.*\\.|)")+"(\\.|$)"),i=f=n.length;while(f--)g=n[f],!e&&q!==g.origType||c&&c.guid!==g.guid||h&&!h.test(g.namespace)||d&&d!==g.selector&&("**"!==d||!g.selector)||(n.splice(f,1),g.selector&&n.delegateCount--,l.remove&&l.remove.call(a,g));i&&!n.length&&(l.teardown&&l.teardown.call(a,p,r.handle)!==!1||m.removeEvent(a,o,r.handle),delete k[o])}else for(o in k)m.event.remove(a,o+b[j],c,d,!0);m.isEmptyObject(k)&&(delete r.handle,m._removeData(a,"events"))}},trigger:function(b,c,d,e){var f,g,h,i,k,l,n,o=[d||y],p=j.call(b,"type")?b.type:b,q=j.call(b,"namespace")?b.namespace.split("."):[];if(h=l=d=d||y,3!==d.nodeType&&8!==d.nodeType&&!$.test(p+m.event.triggered)&&(p.indexOf(".")>=0&&(q=p.split("."),p=q.shift(),q.sort()),g=p.indexOf(":")<0&&"on"+p,b=b[m.expando]?b:new m.Event(p,"object"==typeof b&&b),b.isTrigger=e?2:3,b.namespace=q.join("."),b.namespace_re=b.namespace?new RegExp("(^|\\.)"+q.join("\\.(?:.*\\.|)")+"(\\.|$)"):null,b.result=void 0,b.target||(b.target=d),c=null==c?[b]:m.makeArray(c,[b]),k=m.event.special[p]||{},e||!k.trigger||k.trigger.apply(d,c)!==!1)){if(!e&&!k.noBubble&&!m.isWindow(d)){for(i=k.delegateType||p,$.test(i+p)||(h=h.parentNode);h;h=h.parentNode)o.push(h),l=h;l===(d.ownerDocument||y)&&o.push(l.defaultView||l.parentWindow||a)}n=0;while((h=o[n++])&&!b.isPropagationStopped())b.type=n>1?i:k.bindType||p,f=(m._data(h,"events")||{})[b.type]&&m._data(h,"handle"),f&&f.apply(h,c),f=g&&h[g],f&&f.apply&&m.acceptData(h)&&(b.result=f.apply(h,c),b.result===!1&&b.preventDefault());if(b.type=p,!e&&!b.isDefaultPrevented()&&(!k._default||k._default.apply(o.pop(),c)===!1)&&m.acceptData(d)&&g&&d[p]&&!m.isWindow(d)){l=d[g],l&&(d[g]=null),m.event.triggered=p;try{d[p]()}catch(r){}m.event.triggered=void 0,l&&(d[g]=l)}return b.result}},dispatch:function(a){a=m.event.fix(a);var b,c,e,f,g,h=[],i=d.call(arguments),j=(m._data(this,"events")||{})[a.type]||[],k=m.event.special[a.type]||{};if(i[0]=a,a.delegateTarget=this,!k.preDispatch||k.preDispatch.call(this,a)!==!1){h=m.event.handlers.call(this,a,j),b=0;while((f=h[b++])&&!a.isPropagationStopped()){a.currentTarget=f.elem,g=0;while((e=f.handlers[g++])&&!a.isImmediatePropagationStopped())(!a.namespace_re||a.namespace_re.test(e.namespace))&&(a.handleObj=e,a.data=e.data,c=((m.event.special[e.origType]||{}).handle||e.handler).apply(f.elem,i),void 0!==c&&(a.result=c)===!1&&(a.preventDefault(),a.stopPropagation()))}return k.postDispatch&&k.postDispatch.call(this,a),a.result}},handlers:function(a,b){var c,d,e,f,g=[],h=b.delegateCount,i=a.target;if(h&&i.nodeType&&(!a.button||"click"!==a.type))for(;i!=this;i=i.parentNode||this)if(1===i.nodeType&&(i.disabled!==!0||"click"!==a.type)){for(e=[],f=0;h>f;f++)d=b[f],c=d.selector+" ",void 0===e[c]&&(e[c]=d.needsContext?m(c,this).index(i)>=0:m.find(c,this,null,[i]).length),e[c]&&e.push(d);e.length&&g.push({elem:i,handlers:e})}return h ]","i"),hb=/^\s+/,ib=/<(?!area|br|col|embed|hr|img|input|link|meta|param)(([\w:]+)[^>]*)\/>/gi,jb=/<([\w:]+)/,kb=/\s*$/g,rb={option:[1,""," "],legend:[1,""," "],area:[1,""," "],param:[1,""," "],thead:[1,""],tr:[2,""],col:[2,""],td:[3,""],_default:k.htmlSerialize?[0,"",""]:[1,"X","
"]},sb=db(y),tb=sb.appendChild(y.createElement("div"));rb.optgroup=rb.option,rb.tbody=rb.tfoot=rb.colgroup=rb.caption=rb.thead,rb.th=rb.td;function ub(a,b){var c,d,e=0,f=typeof a.getElementsByTagName!==K?a.getElementsByTagName(b||"*"):typeof a.querySelectorAll!==K?a.querySelectorAll(b||"*"):void 0;if(!f)for(f=[],c=a.childNodes||a;null!=(d=c[e]);e++)!b||m.nodeName(d,b)?f.push(d):m.merge(f,ub(d,b));return void 0===b||b&&m.nodeName(a,b)?m.merge([a],f):f}function vb(a){W.test(a.type)&&(a.defaultChecked=a.checked)}function wb(a,b){return m.nodeName(a,"table")&&m.nodeName(11!==b.nodeType?b:b.firstChild,"tr")?a.getElementsByTagName("tbody")[0]||a.appendChild(a.ownerDocument.createElement("tbody")):a}function xb(a){return a.type=(null!==m.find.attr(a,"type"))+"/"+a.type,a}function yb(a){var b=pb.exec(a.type);return b?a.type=b[1]:a.removeAttribute("type"),a}function zb(a,b){for(var c,d=0;null!=(c=a[d]);d++)m._data(c,"globalEval",!b||m._data(b[d],"globalEval"))}function Ab(a,b){if(1===b.nodeType&&m.hasData(a)){var c,d,e,f=m._data(a),g=m._data(b,f),h=f.events;if(h){delete g.handle,g.events={};for(c in h)for(d=0,e=h[c].length;e>d;d++)m.event.add(b,c,h[c][d])}g.data&&(g.data=m.extend({},g.data))}}function Bb(a,b){var c,d,e;if(1===b.nodeType){if(c=b.nodeName.toLowerCase(),!k.noCloneEvent&&b[m.expando]){e=m._data(b);for(d in e.events)m.removeEvent(b,d,e.handle);b.removeAttribute(m.expando)}"script"===c&&b.text!==a.text?(xb(b).text=a.text,yb(b)):"object"===c?(b.parentNode&&(b.outerHTML=a.outerHTML),k.html5Clone&&a.innerHTML&&!m.trim(b.innerHTML)&&(b.innerHTML=a.innerHTML)):"input"===c&&W.test(a.type)?(b.defaultChecked=b.checked=a.checked,b.value!==a.value&&(b.value=a.value)):"option"===c?b.defaultSelected=b.selected=a.defaultSelected:("input"===c||"textarea"===c)&&(b.defaultValue=a.defaultValue)}}m.extend({clone:function(a,b,c){var d,e,f,g,h,i=m.contains(a.ownerDocument,a);if(k.html5Clone||m.isXMLDoc(a)||!gb.test("<"+a.nodeName+">")?f=a.cloneNode(!0):(tb.innerHTML=a.outerHTML,tb.removeChild(f=tb.firstChild)),!(k.noCloneEvent&&k.noCloneChecked||1!==a.nodeType&&11!==a.nodeType||m.isXMLDoc(a)))for(d=ub(f),h=ub(a),g=0;null!=(e=h[g]);++g)d[g]&&Bb(e,d[g]);if(b)if(c)for(h=h||ub(a),d=d||ub(f),g=0;null!=(e=h[g]);g++)Ab(e,d[g]);else Ab(a,f);return d=ub(f,"script"),d.length>0&&zb(d,!i&&ub(a,"script")),d=h=e=null,f},buildFragment:function(a,b,c,d){for(var e,f,g,h,i,j,l,n=a.length,o=db(b),p=[],q=0;n>q;q++)if(f=a[q],f||0===f)if("object"===m.type(f))m.merge(p,f.nodeType?[f]:f);else if(lb.test(f)){h=h||o.appendChild(b.createElement("div")),i=(jb.exec(f)||["",""])[1].toLowerCase(),l=rb[i]||rb._default,h.innerHTML=l[1]+f.replace(ib,"<$1>$2>")+l[2],e=l[0];while(e--)h=h.lastChild;if(!k.leadingWhitespace&&hb.test(f)&&p.push(b.createTextNode(hb.exec(f)[0])),!k.tbody){f="table"!==i||kb.test(f)?""!==l[1]||kb.test(f)?0:h:h.firstChild,e=f&&f.childNodes.length;while(e--)m.nodeName(j=f.childNodes[e],"tbody")&&!j.childNodes.length&&f.removeChild(j)}m.merge(p,h.childNodes),h.textContent="";while(h.firstChild)h.removeChild(h.firstChild);h=o.lastChild}else p.push(b.createTextNode(f));h&&o.removeChild(h),k.appendChecked||m.grep(ub(p,"input"),vb),q=0;while(f=p[q++])if((!d||-1===m.inArray(f,d))&&(g=m.contains(f.ownerDocument,f),h=ub(o.appendChild(f),"script"),g&&zb(h),c)){e=0;while(f=h[e++])ob.test(f.type||"")&&c.push(f)}return h=null,o},cleanData:function(a,b){for(var d,e,f,g,h=0,i=m.expando,j=m.cache,l=k.deleteExpando,n=m.event.special;null!=(d=a[h]);h++)if((b||m.acceptData(d))&&(f=d[i],g=f&&j[f])){if(g.events)for(e in g.events)n[e]?m.event.remove(d,e):m.removeEvent(d,e,g.handle);j[f]&&(delete j[f],l?delete d[i]:typeof d.removeAttribute!==K?d.removeAttribute(i):d[i]=null,c.push(f))}}}),m.fn.extend({text:function(a){return V(this,function(a){return void 0===a?m.text(this):this.empty().append((this[0]&&this[0].ownerDocument||y).createTextNode(a))},null,a,arguments.length)},append:function(){return this.domManip(arguments,function(a){if(1===this.nodeType||11===this.nodeType||9===this.nodeType){var b=wb(this,a);b.appendChild(a)}})},prepend:function(){return this.domManip(arguments,function(a){if(1===this.nodeType||11===this.nodeType||9===this.nodeType){var b=wb(this,a);b.insertBefore(a,b.firstChild)}})},before:function(){return this.domManip(arguments,function(a){this.parentNode&&this.parentNode.insertBefore(a,this)})},after:function(){return this.domManip(arguments,function(a){this.parentNode&&this.parentNode.insertBefore(a,this.nextSibling)})},remove:function(a,b){for(var c,d=a?m.filter(a,this):this,e=0;null!=(c=d[e]);e++)b||1!==c.nodeType||m.cleanData(ub(c)),c.parentNode&&(b&&m.contains(c.ownerDocument,c)&&zb(ub(c,"script")),c.parentNode.removeChild(c));return this},empty:function(){for(var a,b=0;null!=(a=this[b]);b++){1===a.nodeType&&m.cleanData(ub(a,!1));while(a.firstChild)a.removeChild(a.firstChild);a.options&&m.nodeName(a,"select")&&(a.options.length=0)}return this},clone:function(a,b){return a=null==a?!1:a,b=null==b?a:b,this.map(function(){return m.clone(this,a,b)})},html:function(a){return V(this,function(a){var b=this[0]||{},c=0,d=this.length;if(void 0===a)return 1===b.nodeType?b.innerHTML.replace(fb,""):void 0;if(!("string"!=typeof a||mb.test(a)||!k.htmlSerialize&&gb.test(a)||!k.leadingWhitespace&&hb.test(a)||rb[(jb.exec(a)||["",""])[1].toLowerCase()])){a=a.replace(ib,"<$1>$2>");try{for(;d>c;c++)b=this[c]||{},1===b.nodeType&&(m.cleanData(ub(b,!1)),b.innerHTML=a);b=0}catch(e){}}b&&this.empty().append(a)},null,a,arguments.length)},replaceWith:function(){var a=arguments[0];return this.domManip(arguments,function(b){a=this.parentNode,m.cleanData(ub(this)),a&&a.replaceChild(b,this)}),a&&(a.length||a.nodeType)?this:this.remove()},detach:function(a){return this.remove(a,!0)},domManip:function(a,b){a=e.apply([],a);var c,d,f,g,h,i,j=0,l=this.length,n=this,o=l-1,p=a[0],q=m.isFunction(p);if(q||l>1&&"string"==typeof p&&!k.checkClone&&nb.test(p))return this.each(function(c){var d=n.eq(c);q&&(a[0]=p.call(this,c,d.html())),d.domManip(a,b)});if(l&&(i=m.buildFragment(a,this[0].ownerDocument,!1,this),c=i.firstChild,1===i.childNodes.length&&(i=c),c)){for(g=m.map(ub(i,"script"),xb),f=g.length;l>j;j++)d=i,j!==o&&(d=m.clone(d,!0,!0),f&&m.merge(g,ub(d,"script"))),b.call(this[j],d,j);if(f)for(h=g[g.length-1].ownerDocument,m.map(g,yb),j=0;f>j;j++)d=g[j],ob.test(d.type||"")&&!m._data(d,"globalEval")&&m.contains(h,d)&&(d.src?m._evalUrl&&m._evalUrl(d.src):m.globalEval((d.text||d.textContent||d.innerHTML||"").replace(qb,"")));i=c=null}return this}}),m.each({appendTo:"append",prependTo:"prepend",insertBefore:"before",insertAfter:"after",replaceAll:"replaceWith"},function(a,b){m.fn[a]=function(a){for(var c,d=0,e=[],g=m(a),h=g.length-1;h>=d;d++)c=d===h?this:this.clone(!0),m(g[d])[b](c),f.apply(e,c.get());return this.pushStack(e)}});var Cb,Db={};function Eb(b,c){var d,e=m(c.createElement(b)).appendTo(c.body),f=a.getDefaultComputedStyle&&(d=a.getDefaultComputedStyle(e[0]))?d.display:m.css(e[0],"display");return e.detach(),f}function Fb(a){var b=y,c=Db[a];return c||(c=Eb(a,b),"none"!==c&&c||(Cb=(Cb||m("")).appendTo(b.documentElement),b=(Cb[0].contentWindow||Cb[0].contentDocument).document,b.write(),b.close(),c=Eb(a,b),Cb.detach()),Db[a]=c),c}!function(){var a;k.shrinkWrapBlocks=function(){if(null!=a)return a;a=!1;var b,c,d;return c=y.getElementsByTagName("body")[0],c&&c.style?(b=y.createElement("div"),d=y.createElement("div"),d.style.cssText="position:absolute;border:0;width:0;height:0;top:0;left:-9999px",c.appendChild(d).appendChild(b),typeof b.style.zoom!==K&&(b.style.cssText="-webkit-box-sizing:content-box;-moz-box-sizing:content-box;box-sizing:content-box;display:block;margin:0;border:0;padding:1px;width:1px;zoom:1",b.appendChild(y.createElement("div")).style.width="5px",a=3!==b.offsetWidth),c.removeChild(d),a):void 0}}();var Gb=/^margin/,Hb=new RegExp("^("+S+")(?!px)[a-z%]+$","i"),Ib,Jb,Kb=/^(top|right|bottom|left)$/;a.getComputedStyle?(Ib=function(a){return a.ownerDocument.defaultView.getComputedStyle(a,null)},Jb=function(a,b,c){var d,e,f,g,h=a.style;return c=c||Ib(a),g=c?c.getPropertyValue(b)||c[b]:void 0,c&&(""!==g||m.contains(a.ownerDocument,a)||(g=m.style(a,b)),Hb.test(g)&&Gb.test(b)&&(d=h.width,e=h.minWidth,f=h.maxWidth,h.minWidth=h.maxWidth=h.width=g,g=c.width,h.width=d,h.minWidth=e,h.maxWidth=f)),void 0===g?g:g+""}):y.documentElement.currentStyle&&(Ib=function(a){return a.currentStyle},Jb=function(a,b,c){var d,e,f,g,h=a.style;return c=c||Ib(a),g=c?c[b]:void 0,null==g&&h&&h[b]&&(g=h[b]),Hb.test(g)&&!Kb.test(b)&&(d=h.left,e=a.runtimeStyle,f=e&&e.left,f&&(e.left=a.currentStyle.left),h.left="fontSize"===b?"1em":g,g=h.pixelLeft+"px",h.left=d,f&&(e.left=f)),void 0===g?g:g+""||"auto"});function Lb(a,b){return{get:function(){var c=a();if(null!=c)return c?void delete this.get:(this.get=b).apply(this,arguments)}}}!function(){var b,c,d,e,f,g,h;if(b=y.createElement("div"),b.innerHTML=" a ",d=b.getElementsByTagName("a")[0],c=d&&d.style){c.cssText="float:left;opacity:.5",k.opacity="0.5"===c.opacity,k.cssFloat=!!c.cssFloat,b.style.backgroundClip="content-box",b.cloneNode(!0).style.backgroundClip="",k.clearCloneStyle="content-box"===b.style.backgroundClip,k.boxSizing=""===c.boxSizing||""===c.MozBoxSizing||""===c.WebkitBoxSizing,m.extend(k,{reliableHiddenOffsets:function(){return null==g&&i(),g},boxSizingReliable:function(){return null==f&&i(),f},pixelPosition:function(){return null==e&&i(),e},reliableMarginRight:function(){return null==h&&i(),h}});function i(){var b,c,d,i;c=y.getElementsByTagName("body")[0],c&&c.style&&(b=y.createElement("div"),d=y.createElement("div"),d.style.cssText="position:absolute;border:0;width:0;height:0;top:0;left:-9999px",c.appendChild(d).appendChild(b),b.style.cssText="-webkit-box-sizing:border-box;-moz-box-sizing:border-box;box-sizing:border-box;display:block;margin-top:1%;top:1%;border:1px;padding:1px;width:4px;position:absolute",e=f=!1,h=!0,a.getComputedStyle&&(e="1%"!==(a.getComputedStyle(b,null)||{}).top,f="4px"===(a.getComputedStyle(b,null)||{width:"4px"}).width,i=b.appendChild(y.createElement("div")),i.style.cssText=b.style.cssText="-webkit-box-sizing:content-box;-moz-box-sizing:content-box;box-sizing:content-box;display:block;margin:0;border:0;padding:0",i.style.marginRight=i.style.width="0",b.style.width="1px",h=!parseFloat((a.getComputedStyle(i,null)||{}).marginRight)),b.innerHTML="",i=b.getElementsByTagName("td"),i[0].style.cssText="margin:0;border:0;padding:0;display:none",g=0===i[0].offsetHeight,g&&(i[0].style.display="",i[1].style.display="none",g=0===i[0].offsetHeight),c.removeChild(d))}}}(),m.swap=function(a,b,c,d){var e,f,g={};for(f in b)g[f]=a.style[f],a.style[f]=b[f];e=c.apply(a,d||[]);for(f in b)a.style[f]=g[f];return e};var Mb=/alpha\([^)]*\)/i,Nb=/opacity\s*=\s*([^)]*)/,Ob=/^(none|table(?!-c[ea]).+)/,Pb=new RegExp("^("+S+")(.*)$","i"),Qb=new RegExp("^([+-])=("+S+")","i"),Rb={position:"absolute",visibility:"hidden",display:"block"},Sb={letterSpacing:"0",fontWeight:"400"},Tb=["Webkit","O","Moz","ms"];function Ub(a,b){if(b in a)return b;var c=b.charAt(0).toUpperCase()+b.slice(1),d=b,e=Tb.length;while(e--)if(b=Tb[e]+c,b in a)return b;return d}function Vb(a,b){for(var c,d,e,f=[],g=0,h=a.length;h>g;g++)d=a[g],d.style&&(f[g]=m._data(d,"olddisplay"),c=d.style.display,b?(f[g]||"none"!==c||(d.style.display=""),""===d.style.display&&U(d)&&(f[g]=m._data(d,"olddisplay",Fb(d.nodeName)))):(e=U(d),(c&&"none"!==c||!e)&&m._data(d,"olddisplay",e?c:m.css(d,"display"))));for(g=0;h>g;g++)d=a[g],d.style&&(b&&"none"!==d.style.display&&""!==d.style.display||(d.style.display=b?f[g]||"":"none"));return a}function Wb(a,b,c){var d=Pb.exec(b);return d?Math.max(0,d[1]-(c||0))+(d[2]||"px"):b}function Xb(a,b,c,d,e){for(var f=c===(d?"border":"content")?4:"width"===b?1:0,g=0;4>f;f+=2)"margin"===c&&(g+=m.css(a,c+T[f],!0,e)),d?("content"===c&&(g-=m.css(a,"padding"+T[f],!0,e)),"margin"!==c&&(g-=m.css(a,"border"+T[f]+"Width",!0,e))):(g+=m.css(a,"padding"+T[f],!0,e),"padding"!==c&&(g+=m.css(a,"border"+T[f]+"Width",!0,e)));return g}function Yb(a,b,c){var d=!0,e="width"===b?a.offsetWidth:a.offsetHeight,f=Ib(a),g=k.boxSizing&&"border-box"===m.css(a,"boxSizing",!1,f);if(0>=e||null==e){if(e=Jb(a,b,f),(0>e||null==e)&&(e=a.style[b]),Hb.test(e))return e;d=g&&(k.boxSizingReliable()||e===a.style[b]),e=parseFloat(e)||0}return e+Xb(a,b,c||(g?"border":"content"),d,f)+"px"}m.extend({cssHooks:{opacity:{get:function(a,b){if(b){var c=Jb(a,"opacity");return""===c?"1":c}}}},cssNumber:{columnCount:!0,fillOpacity:!0,flexGrow:!0,flexShrink:!0,fontWeight:!0,lineHeight:!0,opacity:!0,order:!0,orphans:!0,widows:!0,zIndex:!0,zoom:!0},cssProps:{"float":k.cssFloat?"cssFloat":"styleFloat"},style:function(a,b,c,d){if(a&&3!==a.nodeType&&8!==a.nodeType&&a.style){var e,f,g,h=m.camelCase(b),i=a.style;if(b=m.cssProps[h]||(m.cssProps[h]=Ub(i,h)),g=m.cssHooks[b]||m.cssHooks[h],void 0===c)return g&&"get"in g&&void 0!==(e=g.get(a,!1,d))?e:i[b];if(f=typeof c,"string"===f&&(e=Qb.exec(c))&&(c=(e[1]+1)*e[2]+parseFloat(m.css(a,b)),f="number"),null!=c&&c===c&&("number"!==f||m.cssNumber[h]||(c+="px"),k.clearCloneStyle||""!==c||0!==b.indexOf("background")||(i[b]="inherit"),!(g&&"set"in g&&void 0===(c=g.set(a,c,d)))))try{i[b]=c}catch(j){}}},css:function(a,b,c,d){var e,f,g,h=m.camelCase(b);return b=m.cssProps[h]||(m.cssProps[h]=Ub(a.style,h)),g=m.cssHooks[b]||m.cssHooks[h],g&&"get"in g&&(f=g.get(a,!0,c)),void 0===f&&(f=Jb(a,b,d)),"normal"===f&&b in Sb&&(f=Sb[b]),""===c||c?(e=parseFloat(f),c===!0||m.isNumeric(e)?e||0:f):f}}),m.each(["height","width"],function(a,b){m.cssHooks[b]={get:function(a,c,d){return c?Ob.test(m.css(a,"display"))&&0===a.offsetWidth?m.swap(a,Rb,function(){return Yb(a,b,d)}):Yb(a,b,d):void 0},set:function(a,c,d){var e=d&&Ib(a);return Wb(a,c,d?Xb(a,b,d,k.boxSizing&&"border-box"===m.css(a,"boxSizing",!1,e),e):0)}}}),k.opacity||(m.cssHooks.opacity={get:function(a,b){return Nb.test((b&&a.currentStyle?a.currentStyle.filter:a.style.filter)||"")?.01*parseFloat(RegExp.$1)+"":b?"1":""},set:function(a,b){var c=a.style,d=a.currentStyle,e=m.isNumeric(b)?"alpha(opacity="+100*b+")":"",f=d&&d.filter||c.filter||"";c.zoom=1,(b>=1||""===b)&&""===m.trim(f.replace(Mb,""))&&c.removeAttribute&&(c.removeAttribute("filter"),""===b||d&&!d.filter)||(c.filter=Mb.test(f)?f.replace(Mb,e):f+" "+e)}}),m.cssHooks.marginRight=Lb(k.reliableMarginRight,function(a,b){return b?m.swap(a,{display:"inline-block"},Jb,[a,"marginRight"]):void 0}),m.each({margin:"",padding:"",border:"Width"},function(a,b){m.cssHooks[a+b]={expand:function(c){for(var d=0,e={},f="string"==typeof c?c.split(" "):[c];4>d;d++)e[a+T[d]+b]=f[d]||f[d-2]||f[0];return e}},Gb.test(a)||(m.cssHooks[a+b].set=Wb)}),m.fn.extend({css:function(a,b){return V(this,function(a,b,c){var d,e,f={},g=0;if(m.isArray(b)){for(d=Ib(a),e=b.length;e>g;g++)f[b[g]]=m.css(a,b[g],!1,d);return f}return void 0!==c?m.style(a,b,c):m.css(a,b)},a,b,arguments.length>1)},show:function(){return Vb(this,!0)},hide:function(){return Vb(this)},toggle:function(a){return"boolean"==typeof a?a?this.show():this.hide():this.each(function(){U(this)?m(this).show():m(this).hide()})}});function Zb(a,b,c,d,e){return new Zb.prototype.init(a,b,c,d,e)}m.Tween=Zb,Zb.prototype={constructor:Zb,init:function(a,b,c,d,e,f){this.elem=a,this.prop=c,this.easing=e||"swing",this.options=b,this.start=this.now=this.cur(),this.end=d,this.unit=f||(m.cssNumber[c]?"":"px")
-},cur:function(){var a=Zb.propHooks[this.prop];return a&&a.get?a.get(this):Zb.propHooks._default.get(this)},run:function(a){var b,c=Zb.propHooks[this.prop];return this.pos=b=this.options.duration?m.easing[this.easing](a,this.options.duration*a,0,1,this.options.duration):a,this.now=(this.end-this.start)*b+this.start,this.options.step&&this.options.step.call(this.elem,this.now,this),c&&c.set?c.set(this):Zb.propHooks._default.set(this),this}},Zb.prototype.init.prototype=Zb.prototype,Zb.propHooks={_default:{get:function(a){var b;return null==a.elem[a.prop]||a.elem.style&&null!=a.elem.style[a.prop]?(b=m.css(a.elem,a.prop,""),b&&"auto"!==b?b:0):a.elem[a.prop]},set:function(a){m.fx.step[a.prop]?m.fx.step[a.prop](a):a.elem.style&&(null!=a.elem.style[m.cssProps[a.prop]]||m.cssHooks[a.prop])?m.style(a.elem,a.prop,a.now+a.unit):a.elem[a.prop]=a.now}}},Zb.propHooks.scrollTop=Zb.propHooks.scrollLeft={set:function(a){a.elem.nodeType&&a.elem.parentNode&&(a.elem[a.prop]=a.now)}},m.easing={linear:function(a){return a},swing:function(a){return.5-Math.cos(a*Math.PI)/2}},m.fx=Zb.prototype.init,m.fx.step={};var $b,_b,ac=/^(?:toggle|show|hide)$/,bc=new RegExp("^(?:([+-])=|)("+S+")([a-z%]*)$","i"),cc=/queueHooks$/,dc=[ic],ec={"*":[function(a,b){var c=this.createTween(a,b),d=c.cur(),e=bc.exec(b),f=e&&e[3]||(m.cssNumber[a]?"":"px"),g=(m.cssNumber[a]||"px"!==f&&+d)&&bc.exec(m.css(c.elem,a)),h=1,i=20;if(g&&g[3]!==f){f=f||g[3],e=e||[],g=+d||1;do h=h||".5",g/=h,m.style(c.elem,a,g+f);while(h!==(h=c.cur()/d)&&1!==h&&--i)}return e&&(g=c.start=+g||+d||0,c.unit=f,c.end=e[1]?g+(e[1]+1)*e[2]:+e[2]),c}]};function fc(){return setTimeout(function(){$b=void 0}),$b=m.now()}function gc(a,b){var c,d={height:a},e=0;for(b=b?1:0;4>e;e+=2-b)c=T[e],d["margin"+c]=d["padding"+c]=a;return b&&(d.opacity=d.width=a),d}function hc(a,b,c){for(var d,e=(ec[b]||[]).concat(ec["*"]),f=0,g=e.length;g>f;f++)if(d=e[f].call(c,b,a))return d}function ic(a,b,c){var d,e,f,g,h,i,j,l,n=this,o={},p=a.style,q=a.nodeType&&U(a),r=m._data(a,"fxshow");c.queue||(h=m._queueHooks(a,"fx"),null==h.unqueued&&(h.unqueued=0,i=h.empty.fire,h.empty.fire=function(){h.unqueued||i()}),h.unqueued++,n.always(function(){n.always(function(){h.unqueued--,m.queue(a,"fx").length||h.empty.fire()})})),1===a.nodeType&&("height"in b||"width"in b)&&(c.overflow=[p.overflow,p.overflowX,p.overflowY],j=m.css(a,"display"),l="none"===j?m._data(a,"olddisplay")||Fb(a.nodeName):j,"inline"===l&&"none"===m.css(a,"float")&&(k.inlineBlockNeedsLayout&&"inline"!==Fb(a.nodeName)?p.zoom=1:p.display="inline-block")),c.overflow&&(p.overflow="hidden",k.shrinkWrapBlocks()||n.always(function(){p.overflow=c.overflow[0],p.overflowX=c.overflow[1],p.overflowY=c.overflow[2]}));for(d in b)if(e=b[d],ac.exec(e)){if(delete b[d],f=f||"toggle"===e,e===(q?"hide":"show")){if("show"!==e||!r||void 0===r[d])continue;q=!0}o[d]=r&&r[d]||m.style(a,d)}else j=void 0;if(m.isEmptyObject(o))"inline"===("none"===j?Fb(a.nodeName):j)&&(p.display=j);else{r?"hidden"in r&&(q=r.hidden):r=m._data(a,"fxshow",{}),f&&(r.hidden=!q),q?m(a).show():n.done(function(){m(a).hide()}),n.done(function(){var b;m._removeData(a,"fxshow");for(b in o)m.style(a,b,o[b])});for(d in o)g=hc(q?r[d]:0,d,n),d in r||(r[d]=g.start,q&&(g.end=g.start,g.start="width"===d||"height"===d?1:0))}}function jc(a,b){var c,d,e,f,g;for(c in a)if(d=m.camelCase(c),e=b[d],f=a[c],m.isArray(f)&&(e=f[1],f=a[c]=f[0]),c!==d&&(a[d]=f,delete a[c]),g=m.cssHooks[d],g&&"expand"in g){f=g.expand(f),delete a[d];for(c in f)c in a||(a[c]=f[c],b[c]=e)}else b[d]=e}function kc(a,b,c){var d,e,f=0,g=dc.length,h=m.Deferred().always(function(){delete i.elem}),i=function(){if(e)return!1;for(var b=$b||fc(),c=Math.max(0,j.startTime+j.duration-b),d=c/j.duration||0,f=1-d,g=0,i=j.tweens.length;i>g;g++)j.tweens[g].run(f);return h.notifyWith(a,[j,f,c]),1>f&&i?c:(h.resolveWith(a,[j]),!1)},j=h.promise({elem:a,props:m.extend({},b),opts:m.extend(!0,{specialEasing:{}},c),originalProperties:b,originalOptions:c,startTime:$b||fc(),duration:c.duration,tweens:[],createTween:function(b,c){var d=m.Tween(a,j.opts,b,c,j.opts.specialEasing[b]||j.opts.easing);return j.tweens.push(d),d},stop:function(b){var c=0,d=b?j.tweens.length:0;if(e)return this;for(e=!0;d>c;c++)j.tweens[c].run(1);return b?h.resolveWith(a,[j,b]):h.rejectWith(a,[j,b]),this}}),k=j.props;for(jc(k,j.opts.specialEasing);g>f;f++)if(d=dc[f].call(j,a,k,j.opts))return d;return m.map(k,hc,j),m.isFunction(j.opts.start)&&j.opts.start.call(a,j),m.fx.timer(m.extend(i,{elem:a,anim:j,queue:j.opts.queue})),j.progress(j.opts.progress).done(j.opts.done,j.opts.complete).fail(j.opts.fail).always(j.opts.always)}m.Animation=m.extend(kc,{tweener:function(a,b){m.isFunction(a)?(b=a,a=["*"]):a=a.split(" ");for(var c,d=0,e=a.length;e>d;d++)c=a[d],ec[c]=ec[c]||[],ec[c].unshift(b)},prefilter:function(a,b){b?dc.unshift(a):dc.push(a)}}),m.speed=function(a,b,c){var d=a&&"object"==typeof a?m.extend({},a):{complete:c||!c&&b||m.isFunction(a)&&a,duration:a,easing:c&&b||b&&!m.isFunction(b)&&b};return d.duration=m.fx.off?0:"number"==typeof d.duration?d.duration:d.duration in m.fx.speeds?m.fx.speeds[d.duration]:m.fx.speeds._default,(null==d.queue||d.queue===!0)&&(d.queue="fx"),d.old=d.complete,d.complete=function(){m.isFunction(d.old)&&d.old.call(this),d.queue&&m.dequeue(this,d.queue)},d},m.fn.extend({fadeTo:function(a,b,c,d){return this.filter(U).css("opacity",0).show().end().animate({opacity:b},a,c,d)},animate:function(a,b,c,d){var e=m.isEmptyObject(a),f=m.speed(b,c,d),g=function(){var b=kc(this,m.extend({},a),f);(e||m._data(this,"finish"))&&b.stop(!0)};return g.finish=g,e||f.queue===!1?this.each(g):this.queue(f.queue,g)},stop:function(a,b,c){var d=function(a){var b=a.stop;delete a.stop,b(c)};return"string"!=typeof a&&(c=b,b=a,a=void 0),b&&a!==!1&&this.queue(a||"fx",[]),this.each(function(){var b=!0,e=null!=a&&a+"queueHooks",f=m.timers,g=m._data(this);if(e)g[e]&&g[e].stop&&d(g[e]);else for(e in g)g[e]&&g[e].stop&&cc.test(e)&&d(g[e]);for(e=f.length;e--;)f[e].elem!==this||null!=a&&f[e].queue!==a||(f[e].anim.stop(c),b=!1,f.splice(e,1));(b||!c)&&m.dequeue(this,a)})},finish:function(a){return a!==!1&&(a=a||"fx"),this.each(function(){var b,c=m._data(this),d=c[a+"queue"],e=c[a+"queueHooks"],f=m.timers,g=d?d.length:0;for(c.finish=!0,m.queue(this,a,[]),e&&e.stop&&e.stop.call(this,!0),b=f.length;b--;)f[b].elem===this&&f[b].queue===a&&(f[b].anim.stop(!0),f.splice(b,1));for(b=0;g>b;b++)d[b]&&d[b].finish&&d[b].finish.call(this);delete c.finish})}}),m.each(["toggle","show","hide"],function(a,b){var c=m.fn[b];m.fn[b]=function(a,d,e){return null==a||"boolean"==typeof a?c.apply(this,arguments):this.animate(gc(b,!0),a,d,e)}}),m.each({slideDown:gc("show"),slideUp:gc("hide"),slideToggle:gc("toggle"),fadeIn:{opacity:"show"},fadeOut:{opacity:"hide"},fadeToggle:{opacity:"toggle"}},function(a,b){m.fn[a]=function(a,c,d){return this.animate(b,a,c,d)}}),m.timers=[],m.fx.tick=function(){var a,b=m.timers,c=0;for($b=m.now();ca ",d=b.getElementsByTagName("a")[0],c=y.createElement("select"),e=c.appendChild(y.createElement("option")),a=b.getElementsByTagName("input")[0],d.style.cssText="top:1px",k.getSetAttribute="t"!==b.className,k.style=/top/.test(d.getAttribute("style")),k.hrefNormalized="/a"===d.getAttribute("href"),k.checkOn=!!a.value,k.optSelected=e.selected,k.enctype=!!y.createElement("form").enctype,c.disabled=!0,k.optDisabled=!e.disabled,a=y.createElement("input"),a.setAttribute("value",""),k.input=""===a.getAttribute("value"),a.value="t",a.setAttribute("type","radio"),k.radioValue="t"===a.value}();var lc=/\r/g;m.fn.extend({val:function(a){var b,c,d,e=this[0];{if(arguments.length)return d=m.isFunction(a),this.each(function(c){var e;1===this.nodeType&&(e=d?a.call(this,c,m(this).val()):a,null==e?e="":"number"==typeof e?e+="":m.isArray(e)&&(e=m.map(e,function(a){return null==a?"":a+""})),b=m.valHooks[this.type]||m.valHooks[this.nodeName.toLowerCase()],b&&"set"in b&&void 0!==b.set(this,e,"value")||(this.value=e))});if(e)return b=m.valHooks[e.type]||m.valHooks[e.nodeName.toLowerCase()],b&&"get"in b&&void 0!==(c=b.get(e,"value"))?c:(c=e.value,"string"==typeof c?c.replace(lc,""):null==c?"":c)}}}),m.extend({valHooks:{option:{get:function(a){var b=m.find.attr(a,"value");return null!=b?b:m.trim(m.text(a))}},select:{get:function(a){for(var b,c,d=a.options,e=a.selectedIndex,f="select-one"===a.type||0>e,g=f?null:[],h=f?e+1:d.length,i=0>e?h:f?e:0;h>i;i++)if(c=d[i],!(!c.selected&&i!==e||(k.optDisabled?c.disabled:null!==c.getAttribute("disabled"))||c.parentNode.disabled&&m.nodeName(c.parentNode,"optgroup"))){if(b=m(c).val(),f)return b;g.push(b)}return g},set:function(a,b){var c,d,e=a.options,f=m.makeArray(b),g=e.length;while(g--)if(d=e[g],m.inArray(m.valHooks.option.get(d),f)>=0)try{d.selected=c=!0}catch(h){d.scrollHeight}else d.selected=!1;return c||(a.selectedIndex=-1),e}}}}),m.each(["radio","checkbox"],function(){m.valHooks[this]={set:function(a,b){return m.isArray(b)?a.checked=m.inArray(m(a).val(),b)>=0:void 0}},k.checkOn||(m.valHooks[this].get=function(a){return null===a.getAttribute("value")?"on":a.value})});var mc,nc,oc=m.expr.attrHandle,pc=/^(?:checked|selected)$/i,qc=k.getSetAttribute,rc=k.input;m.fn.extend({attr:function(a,b){return V(this,m.attr,a,b,arguments.length>1)},removeAttr:function(a){return this.each(function(){m.removeAttr(this,a)})}}),m.extend({attr:function(a,b,c){var d,e,f=a.nodeType;if(a&&3!==f&&8!==f&&2!==f)return typeof a.getAttribute===K?m.prop(a,b,c):(1===f&&m.isXMLDoc(a)||(b=b.toLowerCase(),d=m.attrHooks[b]||(m.expr.match.bool.test(b)?nc:mc)),void 0===c?d&&"get"in d&&null!==(e=d.get(a,b))?e:(e=m.find.attr(a,b),null==e?void 0:e):null!==c?d&&"set"in d&&void 0!==(e=d.set(a,c,b))?e:(a.setAttribute(b,c+""),c):void m.removeAttr(a,b))},removeAttr:function(a,b){var c,d,e=0,f=b&&b.match(E);if(f&&1===a.nodeType)while(c=f[e++])d=m.propFix[c]||c,m.expr.match.bool.test(c)?rc&&qc||!pc.test(c)?a[d]=!1:a[m.camelCase("default-"+c)]=a[d]=!1:m.attr(a,c,""),a.removeAttribute(qc?c:d)},attrHooks:{type:{set:function(a,b){if(!k.radioValue&&"radio"===b&&m.nodeName(a,"input")){var c=a.value;return a.setAttribute("type",b),c&&(a.value=c),b}}}}}),nc={set:function(a,b,c){return b===!1?m.removeAttr(a,c):rc&&qc||!pc.test(c)?a.setAttribute(!qc&&m.propFix[c]||c,c):a[m.camelCase("default-"+c)]=a[c]=!0,c}},m.each(m.expr.match.bool.source.match(/\w+/g),function(a,b){var c=oc[b]||m.find.attr;oc[b]=rc&&qc||!pc.test(b)?function(a,b,d){var e,f;return d||(f=oc[b],oc[b]=e,e=null!=c(a,b,d)?b.toLowerCase():null,oc[b]=f),e}:function(a,b,c){return c?void 0:a[m.camelCase("default-"+b)]?b.toLowerCase():null}}),rc&&qc||(m.attrHooks.value={set:function(a,b,c){return m.nodeName(a,"input")?void(a.defaultValue=b):mc&&mc.set(a,b,c)}}),qc||(mc={set:function(a,b,c){var d=a.getAttributeNode(c);return d||a.setAttributeNode(d=a.ownerDocument.createAttribute(c)),d.value=b+="","value"===c||b===a.getAttribute(c)?b:void 0}},oc.id=oc.name=oc.coords=function(a,b,c){var d;return c?void 0:(d=a.getAttributeNode(b))&&""!==d.value?d.value:null},m.valHooks.button={get:function(a,b){var c=a.getAttributeNode(b);return c&&c.specified?c.value:void 0},set:mc.set},m.attrHooks.contenteditable={set:function(a,b,c){mc.set(a,""===b?!1:b,c)}},m.each(["width","height"],function(a,b){m.attrHooks[b]={set:function(a,c){return""===c?(a.setAttribute(b,"auto"),c):void 0}}})),k.style||(m.attrHooks.style={get:function(a){return a.style.cssText||void 0},set:function(a,b){return a.style.cssText=b+""}});var sc=/^(?:input|select|textarea|button|object)$/i,tc=/^(?:a|area)$/i;m.fn.extend({prop:function(a,b){return V(this,m.prop,a,b,arguments.length>1)},removeProp:function(a){return a=m.propFix[a]||a,this.each(function(){try{this[a]=void 0,delete this[a]}catch(b){}})}}),m.extend({propFix:{"for":"htmlFor","class":"className"},prop:function(a,b,c){var d,e,f,g=a.nodeType;if(a&&3!==g&&8!==g&&2!==g)return f=1!==g||!m.isXMLDoc(a),f&&(b=m.propFix[b]||b,e=m.propHooks[b]),void 0!==c?e&&"set"in e&&void 0!==(d=e.set(a,c,b))?d:a[b]=c:e&&"get"in e&&null!==(d=e.get(a,b))?d:a[b]},propHooks:{tabIndex:{get:function(a){var b=m.find.attr(a,"tabindex");return b?parseInt(b,10):sc.test(a.nodeName)||tc.test(a.nodeName)&&a.href?0:-1}}}}),k.hrefNormalized||m.each(["href","src"],function(a,b){m.propHooks[b]={get:function(a){return a.getAttribute(b,4)}}}),k.optSelected||(m.propHooks.selected={get:function(a){var b=a.parentNode;return b&&(b.selectedIndex,b.parentNode&&b.parentNode.selectedIndex),null}}),m.each(["tabIndex","readOnly","maxLength","cellSpacing","cellPadding","rowSpan","colSpan","useMap","frameBorder","contentEditable"],function(){m.propFix[this.toLowerCase()]=this}),k.enctype||(m.propFix.enctype="encoding");var uc=/[\t\r\n\f]/g;m.fn.extend({addClass:function(a){var b,c,d,e,f,g,h=0,i=this.length,j="string"==typeof a&&a;if(m.isFunction(a))return this.each(function(b){m(this).addClass(a.call(this,b,this.className))});if(j)for(b=(a||"").match(E)||[];i>h;h++)if(c=this[h],d=1===c.nodeType&&(c.className?(" "+c.className+" ").replace(uc," "):" ")){f=0;while(e=b[f++])d.indexOf(" "+e+" ")<0&&(d+=e+" ");g=m.trim(d),c.className!==g&&(c.className=g)}return this},removeClass:function(a){var b,c,d,e,f,g,h=0,i=this.length,j=0===arguments.length||"string"==typeof a&&a;if(m.isFunction(a))return this.each(function(b){m(this).removeClass(a.call(this,b,this.className))});if(j)for(b=(a||"").match(E)||[];i>h;h++)if(c=this[h],d=1===c.nodeType&&(c.className?(" "+c.className+" ").replace(uc," "):"")){f=0;while(e=b[f++])while(d.indexOf(" "+e+" ")>=0)d=d.replace(" "+e+" "," ");g=a?m.trim(d):"",c.className!==g&&(c.className=g)}return this},toggleClass:function(a,b){var c=typeof a;return"boolean"==typeof b&&"string"===c?b?this.addClass(a):this.removeClass(a):this.each(m.isFunction(a)?function(c){m(this).toggleClass(a.call(this,c,this.className,b),b)}:function(){if("string"===c){var b,d=0,e=m(this),f=a.match(E)||[];while(b=f[d++])e.hasClass(b)?e.removeClass(b):e.addClass(b)}else(c===K||"boolean"===c)&&(this.className&&m._data(this,"__className__",this.className),this.className=this.className||a===!1?"":m._data(this,"__className__")||"")})},hasClass:function(a){for(var b=" "+a+" ",c=0,d=this.length;d>c;c++)if(1===this[c].nodeType&&(" "+this[c].className+" ").replace(uc," ").indexOf(b)>=0)return!0;return!1}}),m.each("blur focus focusin focusout load resize scroll unload click dblclick mousedown mouseup mousemove mouseover mouseout mouseenter mouseleave change select submit keydown keypress keyup error contextmenu".split(" "),function(a,b){m.fn[b]=function(a,c){return arguments.length>0?this.on(b,null,a,c):this.trigger(b)}}),m.fn.extend({hover:function(a,b){return this.mouseenter(a).mouseleave(b||a)},bind:function(a,b,c){return this.on(a,null,b,c)},unbind:function(a,b){return this.off(a,null,b)},delegate:function(a,b,c,d){return this.on(b,a,c,d)},undelegate:function(a,b,c){return 1===arguments.length?this.off(a,"**"):this.off(b,a||"**",c)}});var vc=m.now(),wc=/\?/,xc=/(,)|(\[|{)|(}|])|"(?:[^"\\\r\n]|\\["\\\/bfnrt]|\\u[\da-fA-F]{4})*"\s*:?|true|false|null|-?(?!0\d)\d+(?:\.\d+|)(?:[eE][+-]?\d+|)/g;m.parseJSON=function(b){if(a.JSON&&a.JSON.parse)return a.JSON.parse(b+"");var c,d=null,e=m.trim(b+"");return e&&!m.trim(e.replace(xc,function(a,b,e,f){return c&&b&&(d=0),0===d?a:(c=e||b,d+=!f-!e,"")}))?Function("return "+e)():m.error("Invalid JSON: "+b)},m.parseXML=function(b){var c,d;if(!b||"string"!=typeof b)return null;try{a.DOMParser?(d=new DOMParser,c=d.parseFromString(b,"text/xml")):(c=new ActiveXObject("Microsoft.XMLDOM"),c.async="false",c.loadXML(b))}catch(e){c=void 0}return c&&c.documentElement&&!c.getElementsByTagName("parsererror").length||m.error("Invalid XML: "+b),c};var yc,zc,Ac=/#.*$/,Bc=/([?&])_=[^&]*/,Cc=/^(.*?):[ \t]*([^\r\n]*)\r?$/gm,Dc=/^(?:about|app|app-storage|.+-extension|file|res|widget):$/,Ec=/^(?:GET|HEAD)$/,Fc=/^\/\//,Gc=/^([\w.+-]+:)(?:\/\/(?:[^\/?#]*@|)([^\/?#:]*)(?::(\d+)|)|)/,Hc={},Ic={},Jc="*/".concat("*");try{zc=location.href}catch(Kc){zc=y.createElement("a"),zc.href="",zc=zc.href}yc=Gc.exec(zc.toLowerCase())||[];function Lc(a){return function(b,c){"string"!=typeof b&&(c=b,b="*");var d,e=0,f=b.toLowerCase().match(E)||[];if(m.isFunction(c))while(d=f[e++])"+"===d.charAt(0)?(d=d.slice(1)||"*",(a[d]=a[d]||[]).unshift(c)):(a[d]=a[d]||[]).push(c)}}function Mc(a,b,c,d){var e={},f=a===Ic;function g(h){var i;return e[h]=!0,m.each(a[h]||[],function(a,h){var j=h(b,c,d);return"string"!=typeof j||f||e[j]?f?!(i=j):void 0:(b.dataTypes.unshift(j),g(j),!1)}),i}return g(b.dataTypes[0])||!e["*"]&&g("*")}function Nc(a,b){var c,d,e=m.ajaxSettings.flatOptions||{};for(d in b)void 0!==b[d]&&((e[d]?a:c||(c={}))[d]=b[d]);return c&&m.extend(!0,a,c),a}function Oc(a,b,c){var d,e,f,g,h=a.contents,i=a.dataTypes;while("*"===i[0])i.shift(),void 0===e&&(e=a.mimeType||b.getResponseHeader("Content-Type"));if(e)for(g in h)if(h[g]&&h[g].test(e)){i.unshift(g);break}if(i[0]in c)f=i[0];else{for(g in c){if(!i[0]||a.converters[g+" "+i[0]]){f=g;break}d||(d=g)}f=f||d}return f?(f!==i[0]&&i.unshift(f),c[f]):void 0}function Pc(a,b,c,d){var e,f,g,h,i,j={},k=a.dataTypes.slice();if(k[1])for(g in a.converters)j[g.toLowerCase()]=a.converters[g];f=k.shift();while(f)if(a.responseFields[f]&&(c[a.responseFields[f]]=b),!i&&d&&a.dataFilter&&(b=a.dataFilter(b,a.dataType)),i=f,f=k.shift())if("*"===f)f=i;else if("*"!==i&&i!==f){if(g=j[i+" "+f]||j["* "+f],!g)for(e in j)if(h=e.split(" "),h[1]===f&&(g=j[i+" "+h[0]]||j["* "+h[0]])){g===!0?g=j[e]:j[e]!==!0&&(f=h[0],k.unshift(h[1]));break}if(g!==!0)if(g&&a["throws"])b=g(b);else try{b=g(b)}catch(l){return{state:"parsererror",error:g?l:"No conversion from "+i+" to "+f}}}return{state:"success",data:b}}m.extend({active:0,lastModified:{},etag:{},ajaxSettings:{url:zc,type:"GET",isLocal:Dc.test(yc[1]),global:!0,processData:!0,async:!0,contentType:"application/x-www-form-urlencoded; charset=UTF-8",accepts:{"*":Jc,text:"text/plain",html:"text/html",xml:"application/xml, text/xml",json:"application/json, text/javascript"},contents:{xml:/xml/,html:/html/,json:/json/},responseFields:{xml:"responseXML",text:"responseText",json:"responseJSON"},converters:{"* text":String,"text html":!0,"text json":m.parseJSON,"text xml":m.parseXML},flatOptions:{url:!0,context:!0}},ajaxSetup:function(a,b){return b?Nc(Nc(a,m.ajaxSettings),b):Nc(m.ajaxSettings,a)},ajaxPrefilter:Lc(Hc),ajaxTransport:Lc(Ic),ajax:function(a,b){"object"==typeof a&&(b=a,a=void 0),b=b||{};var c,d,e,f,g,h,i,j,k=m.ajaxSetup({},b),l=k.context||k,n=k.context&&(l.nodeType||l.jquery)?m(l):m.event,o=m.Deferred(),p=m.Callbacks("once memory"),q=k.statusCode||{},r={},s={},t=0,u="canceled",v={readyState:0,getResponseHeader:function(a){var b;if(2===t){if(!j){j={};while(b=Cc.exec(f))j[b[1].toLowerCase()]=b[2]}b=j[a.toLowerCase()]}return null==b?null:b},getAllResponseHeaders:function(){return 2===t?f:null},setRequestHeader:function(a,b){var c=a.toLowerCase();return t||(a=s[c]=s[c]||a,r[a]=b),this},overrideMimeType:function(a){return t||(k.mimeType=a),this},statusCode:function(a){var b;if(a)if(2>t)for(b in a)q[b]=[q[b],a[b]];else v.always(a[v.status]);return this},abort:function(a){var b=a||u;return i&&i.abort(b),x(0,b),this}};if(o.promise(v).complete=p.add,v.success=v.done,v.error=v.fail,k.url=((a||k.url||zc)+"").replace(Ac,"").replace(Fc,yc[1]+"//"),k.type=b.method||b.type||k.method||k.type,k.dataTypes=m.trim(k.dataType||"*").toLowerCase().match(E)||[""],null==k.crossDomain&&(c=Gc.exec(k.url.toLowerCase()),k.crossDomain=!(!c||c[1]===yc[1]&&c[2]===yc[2]&&(c[3]||("http:"===c[1]?"80":"443"))===(yc[3]||("http:"===yc[1]?"80":"443")))),k.data&&k.processData&&"string"!=typeof k.data&&(k.data=m.param(k.data,k.traditional)),Mc(Hc,k,b,v),2===t)return v;h=k.global,h&&0===m.active++&&m.event.trigger("ajaxStart"),k.type=k.type.toUpperCase(),k.hasContent=!Ec.test(k.type),e=k.url,k.hasContent||(k.data&&(e=k.url+=(wc.test(e)?"&":"?")+k.data,delete k.data),k.cache===!1&&(k.url=Bc.test(e)?e.replace(Bc,"$1_="+vc++):e+(wc.test(e)?"&":"?")+"_="+vc++)),k.ifModified&&(m.lastModified[e]&&v.setRequestHeader("If-Modified-Since",m.lastModified[e]),m.etag[e]&&v.setRequestHeader("If-None-Match",m.etag[e])),(k.data&&k.hasContent&&k.contentType!==!1||b.contentType)&&v.setRequestHeader("Content-Type",k.contentType),v.setRequestHeader("Accept",k.dataTypes[0]&&k.accepts[k.dataTypes[0]]?k.accepts[k.dataTypes[0]]+("*"!==k.dataTypes[0]?", "+Jc+"; q=0.01":""):k.accepts["*"]);for(d in k.headers)v.setRequestHeader(d,k.headers[d]);if(k.beforeSend&&(k.beforeSend.call(l,v,k)===!1||2===t))return v.abort();u="abort";for(d in{success:1,error:1,complete:1})v[d](k[d]);if(i=Mc(Ic,k,b,v)){v.readyState=1,h&&n.trigger("ajaxSend",[v,k]),k.async&&k.timeout>0&&(g=setTimeout(function(){v.abort("timeout")},k.timeout));try{t=1,i.send(r,x)}catch(w){if(!(2>t))throw w;x(-1,w)}}else x(-1,"No Transport");function x(a,b,c,d){var j,r,s,u,w,x=b;2!==t&&(t=2,g&&clearTimeout(g),i=void 0,f=d||"",v.readyState=a>0?4:0,j=a>=200&&300>a||304===a,c&&(u=Oc(k,v,c)),u=Pc(k,u,v,j),j?(k.ifModified&&(w=v.getResponseHeader("Last-Modified"),w&&(m.lastModified[e]=w),w=v.getResponseHeader("etag"),w&&(m.etag[e]=w)),204===a||"HEAD"===k.type?x="nocontent":304===a?x="notmodified":(x=u.state,r=u.data,s=u.error,j=!s)):(s=x,(a||!x)&&(x="error",0>a&&(a=0))),v.status=a,v.statusText=(b||x)+"",j?o.resolveWith(l,[r,x,v]):o.rejectWith(l,[v,x,s]),v.statusCode(q),q=void 0,h&&n.trigger(j?"ajaxSuccess":"ajaxError",[v,k,j?r:s]),p.fireWith(l,[v,x]),h&&(n.trigger("ajaxComplete",[v,k]),--m.active||m.event.trigger("ajaxStop")))}return v},getJSON:function(a,b,c){return m.get(a,b,c,"json")},getScript:function(a,b){return m.get(a,void 0,b,"script")}}),m.each(["get","post"],function(a,b){m[b]=function(a,c,d,e){return m.isFunction(c)&&(e=e||d,d=c,c=void 0),m.ajax({url:a,type:b,dataType:e,data:c,success:d})}}),m.each(["ajaxStart","ajaxStop","ajaxComplete","ajaxError","ajaxSuccess","ajaxSend"],function(a,b){m.fn[b]=function(a){return this.on(b,a)}}),m._evalUrl=function(a){return m.ajax({url:a,type:"GET",dataType:"script",async:!1,global:!1,"throws":!0})},m.fn.extend({wrapAll:function(a){if(m.isFunction(a))return this.each(function(b){m(this).wrapAll(a.call(this,b))});if(this[0]){var b=m(a,this[0].ownerDocument).eq(0).clone(!0);this[0].parentNode&&b.insertBefore(this[0]),b.map(function(){var a=this;while(a.firstChild&&1===a.firstChild.nodeType)a=a.firstChild;return a}).append(this)}return this},wrapInner:function(a){return this.each(m.isFunction(a)?function(b){m(this).wrapInner(a.call(this,b))}:function(){var b=m(this),c=b.contents();c.length?c.wrapAll(a):b.append(a)})},wrap:function(a){var b=m.isFunction(a);return this.each(function(c){m(this).wrapAll(b?a.call(this,c):a)})},unwrap:function(){return this.parent().each(function(){m.nodeName(this,"body")||m(this).replaceWith(this.childNodes)}).end()}}),m.expr.filters.hidden=function(a){return a.offsetWidth<=0&&a.offsetHeight<=0||!k.reliableHiddenOffsets()&&"none"===(a.style&&a.style.display||m.css(a,"display"))},m.expr.filters.visible=function(a){return!m.expr.filters.hidden(a)};var Qc=/%20/g,Rc=/\[\]$/,Sc=/\r?\n/g,Tc=/^(?:submit|button|image|reset|file)$/i,Uc=/^(?:input|select|textarea|keygen)/i;function Vc(a,b,c,d){var e;if(m.isArray(b))m.each(b,function(b,e){c||Rc.test(a)?d(a,e):Vc(a+"["+("object"==typeof e?b:"")+"]",e,c,d)});else if(c||"object"!==m.type(b))d(a,b);else for(e in b)Vc(a+"["+e+"]",b[e],c,d)}m.param=function(a,b){var c,d=[],e=function(a,b){b=m.isFunction(b)?b():null==b?"":b,d[d.length]=encodeURIComponent(a)+"="+encodeURIComponent(b)};if(void 0===b&&(b=m.ajaxSettings&&m.ajaxSettings.traditional),m.isArray(a)||a.jquery&&!m.isPlainObject(a))m.each(a,function(){e(this.name,this.value)});else for(c in a)Vc(c,a[c],b,e);return d.join("&").replace(Qc,"+")},m.fn.extend({serialize:function(){return m.param(this.serializeArray())},serializeArray:function(){return this.map(function(){var a=m.prop(this,"elements");return a?m.makeArray(a):this}).filter(function(){var a=this.type;return this.name&&!m(this).is(":disabled")&&Uc.test(this.nodeName)&&!Tc.test(a)&&(this.checked||!W.test(a))}).map(function(a,b){var c=m(this).val();return null==c?null:m.isArray(c)?m.map(c,function(a){return{name:b.name,value:a.replace(Sc,"\r\n")}}):{name:b.name,value:c.replace(Sc,"\r\n")}}).get()}}),m.ajaxSettings.xhr=void 0!==a.ActiveXObject?function(){return!this.isLocal&&/^(get|post|head|put|delete|options)$/i.test(this.type)&&Zc()||$c()}:Zc;var Wc=0,Xc={},Yc=m.ajaxSettings.xhr();a.ActiveXObject&&m(a).on("unload",function(){for(var a in Xc)Xc[a](void 0,!0)}),k.cors=!!Yc&&"withCredentials"in Yc,Yc=k.ajax=!!Yc,Yc&&m.ajaxTransport(function(a){if(!a.crossDomain||k.cors){var b;return{send:function(c,d){var e,f=a.xhr(),g=++Wc;if(f.open(a.type,a.url,a.async,a.username,a.password),a.xhrFields)for(e in a.xhrFields)f[e]=a.xhrFields[e];a.mimeType&&f.overrideMimeType&&f.overrideMimeType(a.mimeType),a.crossDomain||c["X-Requested-With"]||(c["X-Requested-With"]="XMLHttpRequest");for(e in c)void 0!==c[e]&&f.setRequestHeader(e,c[e]+"");f.send(a.hasContent&&a.data||null),b=function(c,e){var h,i,j;if(b&&(e||4===f.readyState))if(delete Xc[g],b=void 0,f.onreadystatechange=m.noop,e)4!==f.readyState&&f.abort();else{j={},h=f.status,"string"==typeof f.responseText&&(j.text=f.responseText);try{i=f.statusText}catch(k){i=""}h||!a.isLocal||a.crossDomain?1223===h&&(h=204):h=j.text?200:404}j&&d(h,i,j,f.getAllResponseHeaders())},a.async?4===f.readyState?setTimeout(b):f.onreadystatechange=Xc[g]=b:b()},abort:function(){b&&b(void 0,!0)}}}});function Zc(){try{return new a.XMLHttpRequest}catch(b){}}function $c(){try{return new a.ActiveXObject("Microsoft.XMLHTTP")}catch(b){}}m.ajaxSetup({accepts:{script:"text/javascript, application/javascript, application/ecmascript, application/x-ecmascript"},contents:{script:/(?:java|ecma)script/},converters:{"text script":function(a){return m.globalEval(a),a}}}),m.ajaxPrefilter("script",function(a){void 0===a.cache&&(a.cache=!1),a.crossDomain&&(a.type="GET",a.global=!1)}),m.ajaxTransport("script",function(a){if(a.crossDomain){var b,c=y.head||m("head")[0]||y.documentElement;return{send:function(d,e){b=y.createElement("script"),b.async=!0,a.scriptCharset&&(b.charset=a.scriptCharset),b.src=a.url,b.onload=b.onreadystatechange=function(a,c){(c||!b.readyState||/loaded|complete/.test(b.readyState))&&(b.onload=b.onreadystatechange=null,b.parentNode&&b.parentNode.removeChild(b),b=null,c||e(200,"success"))},c.insertBefore(b,c.firstChild)},abort:function(){b&&b.onload(void 0,!0)}}}});var _c=[],ad=/(=)\?(?=&|$)|\?\?/;m.ajaxSetup({jsonp:"callback",jsonpCallback:function(){var a=_c.pop()||m.expando+"_"+vc++;return this[a]=!0,a}}),m.ajaxPrefilter("json jsonp",function(b,c,d){var e,f,g,h=b.jsonp!==!1&&(ad.test(b.url)?"url":"string"==typeof b.data&&!(b.contentType||"").indexOf("application/x-www-form-urlencoded")&&ad.test(b.data)&&"data");return h||"jsonp"===b.dataTypes[0]?(e=b.jsonpCallback=m.isFunction(b.jsonpCallback)?b.jsonpCallback():b.jsonpCallback,h?b[h]=b[h].replace(ad,"$1"+e):b.jsonp!==!1&&(b.url+=(wc.test(b.url)?"&":"?")+b.jsonp+"="+e),b.converters["script json"]=function(){return g||m.error(e+" was not called"),g[0]},b.dataTypes[0]="json",f=a[e],a[e]=function(){g=arguments},d.always(function(){a[e]=f,b[e]&&(b.jsonpCallback=c.jsonpCallback,_c.push(e)),g&&m.isFunction(f)&&f(g[0]),g=f=void 0}),"script"):void 0}),m.parseHTML=function(a,b,c){if(!a||"string"!=typeof a)return null;"boolean"==typeof b&&(c=b,b=!1),b=b||y;var d=u.exec(a),e=!c&&[];return d?[b.createElement(d[1])]:(d=m.buildFragment([a],b,e),e&&e.length&&m(e).remove(),m.merge([],d.childNodes))};var bd=m.fn.load;m.fn.load=function(a,b,c){if("string"!=typeof a&&bd)return bd.apply(this,arguments);var d,e,f,g=this,h=a.indexOf(" ");return h>=0&&(d=m.trim(a.slice(h,a.length)),a=a.slice(0,h)),m.isFunction(b)?(c=b,b=void 0):b&&"object"==typeof b&&(f="POST"),g.length>0&&m.ajax({url:a,type:f,dataType:"html",data:b}).done(function(a){e=arguments,g.html(d?m("").append(m.parseHTML(a)).find(d):a)}).complete(c&&function(a,b){g.each(c,e||[a.responseText,b,a])}),this},m.expr.filters.animated=function(a){return m.grep(m.timers,function(b){return a===b.elem}).length};var cd=a.document.documentElement;function dd(a){return m.isWindow(a)?a:9===a.nodeType?a.defaultView||a.parentWindow:!1}m.offset={setOffset:function(a,b,c){var d,e,f,g,h,i,j,k=m.css(a,"position"),l=m(a),n={};"static"===k&&(a.style.position="relative"),h=l.offset(),f=m.css(a,"top"),i=m.css(a,"left"),j=("absolute"===k||"fixed"===k)&&m.inArray("auto",[f,i])>-1,j?(d=l.position(),g=d.top,e=d.left):(g=parseFloat(f)||0,e=parseFloat(i)||0),m.isFunction(b)&&(b=b.call(a,c,h)),null!=b.top&&(n.top=b.top-h.top+g),null!=b.left&&(n.left=b.left-h.left+e),"using"in b?b.using.call(a,n):l.css(n)}},m.fn.extend({offset:function(a){if(arguments.length)return void 0===a?this:this.each(function(b){m.offset.setOffset(this,a,b)});var b,c,d={top:0,left:0},e=this[0],f=e&&e.ownerDocument;if(f)return b=f.documentElement,m.contains(b,e)?(typeof e.getBoundingClientRect!==K&&(d=e.getBoundingClientRect()),c=dd(f),{top:d.top+(c.pageYOffset||b.scrollTop)-(b.clientTop||0),left:d.left+(c.pageXOffset||b.scrollLeft)-(b.clientLeft||0)}):d},position:function(){if(this[0]){var a,b,c={top:0,left:0},d=this[0];return"fixed"===m.css(d,"position")?b=d.getBoundingClientRect():(a=this.offsetParent(),b=this.offset(),m.nodeName(a[0],"html")||(c=a.offset()),c.top+=m.css(a[0],"borderTopWidth",!0),c.left+=m.css(a[0],"borderLeftWidth",!0)),{top:b.top-c.top-m.css(d,"marginTop",!0),left:b.left-c.left-m.css(d,"marginLeft",!0)}}},offsetParent:function(){return this.map(function(){var a=this.offsetParent||cd;while(a&&!m.nodeName(a,"html")&&"static"===m.css(a,"position"))a=a.offsetParent;return a||cd})}}),m.each({scrollLeft:"pageXOffset",scrollTop:"pageYOffset"},function(a,b){var c=/Y/.test(b);m.fn[a]=function(d){return V(this,function(a,d,e){var f=dd(a);return void 0===e?f?b in f?f[b]:f.document.documentElement[d]:a[d]:void(f?f.scrollTo(c?m(f).scrollLeft():e,c?e:m(f).scrollTop()):a[d]=e)},a,d,arguments.length,null)}}),m.each(["top","left"],function(a,b){m.cssHooks[b]=Lb(k.pixelPosition,function(a,c){return c?(c=Jb(a,b),Hb.test(c)?m(a).position()[b]+"px":c):void 0})}),m.each({Height:"height",Width:"width"},function(a,b){m.each({padding:"inner"+a,content:b,"":"outer"+a},function(c,d){m.fn[d]=function(d,e){var f=arguments.length&&(c||"boolean"!=typeof d),g=c||(d===!0||e===!0?"margin":"border");return V(this,function(b,c,d){var e;return m.isWindow(b)?b.document.documentElement["client"+a]:9===b.nodeType?(e=b.documentElement,Math.max(b.body["scroll"+a],e["scroll"+a],b.body["offset"+a],e["offset"+a],e["client"+a])):void 0===d?m.css(b,c,g):m.style(b,c,d,g)},b,f?d:void 0,f,null)}})}),m.fn.size=function(){return this.length},m.fn.andSelf=m.fn.addBack,"function"==typeof define&&define.amd&&define("jquery",[],function(){return m});var ed=a.jQuery,fd=a.$;return m.noConflict=function(b){return a.$===m&&(a.$=fd),b&&a.jQuery===m&&(a.jQuery=ed),m},typeof b===K&&(a.jQuery=a.$=m),m});
diff --git a/spaces/dcarpintero/nlp-summarizer-pegasus/.venv/lib/python3.9/site-packages/anyio/_core/_synchronization.py b/spaces/dcarpintero/nlp-summarizer-pegasus/.venv/lib/python3.9/site-packages/anyio/_core/_synchronization.py
deleted file mode 100644
index 783570c7ac8d51fb37d505ab0bcc589e35174b4d..0000000000000000000000000000000000000000
--- a/spaces/dcarpintero/nlp-summarizer-pegasus/.venv/lib/python3.9/site-packages/anyio/_core/_synchronization.py
+++ /dev/null
@@ -1,596 +0,0 @@
-from __future__ import annotations
-
-from collections import deque
-from dataclasses import dataclass
-from types import TracebackType
-from warnings import warn
-
-from ..lowlevel import cancel_shielded_checkpoint, checkpoint, checkpoint_if_cancelled
-from ._compat import DeprecatedAwaitable
-from ._eventloop import get_asynclib
-from ._exceptions import BusyResourceError, WouldBlock
-from ._tasks import CancelScope
-from ._testing import TaskInfo, get_current_task
-
-
-@dataclass(frozen=True)
-class EventStatistics:
- """
- :ivar int tasks_waiting: number of tasks waiting on :meth:`~.Event.wait`
- """
-
- tasks_waiting: int
-
-
-@dataclass(frozen=True)
-class CapacityLimiterStatistics:
- """
- :ivar int borrowed_tokens: number of tokens currently borrowed by tasks
- :ivar float total_tokens: total number of available tokens
- :ivar tuple borrowers: tasks or other objects currently holding tokens borrowed from this
- limiter
- :ivar int tasks_waiting: number of tasks waiting on :meth:`~.CapacityLimiter.acquire` or
- :meth:`~.CapacityLimiter.acquire_on_behalf_of`
- """
-
- borrowed_tokens: int
- total_tokens: float
- borrowers: tuple[object, ...]
- tasks_waiting: int
-
-
-@dataclass(frozen=True)
-class LockStatistics:
- """
- :ivar bool locked: flag indicating if this lock is locked or not
- :ivar ~anyio.TaskInfo owner: task currently holding the lock (or ``None`` if the lock is not
- held by any task)
- :ivar int tasks_waiting: number of tasks waiting on :meth:`~.Lock.acquire`
- """
-
- locked: bool
- owner: TaskInfo | None
- tasks_waiting: int
-
-
-@dataclass(frozen=True)
-class ConditionStatistics:
- """
- :ivar int tasks_waiting: number of tasks blocked on :meth:`~.Condition.wait`
- :ivar ~anyio.LockStatistics lock_statistics: statistics of the underlying :class:`~.Lock`
- """
-
- tasks_waiting: int
- lock_statistics: LockStatistics
-
-
-@dataclass(frozen=True)
-class SemaphoreStatistics:
- """
- :ivar int tasks_waiting: number of tasks waiting on :meth:`~.Semaphore.acquire`
-
- """
-
- tasks_waiting: int
-
-
-class Event:
- def __new__(cls) -> Event:
- return get_asynclib().Event()
-
- def set(self) -> DeprecatedAwaitable:
- """Set the flag, notifying all listeners."""
- raise NotImplementedError
-
- def is_set(self) -> bool:
- """Return ``True`` if the flag is set, ``False`` if not."""
- raise NotImplementedError
-
- async def wait(self) -> None:
- """
- Wait until the flag has been set.
-
- If the flag has already been set when this method is called, it returns immediately.
-
- """
- raise NotImplementedError
-
- def statistics(self) -> EventStatistics:
- """Return statistics about the current state of this event."""
- raise NotImplementedError
-
-
-class Lock:
- _owner_task: TaskInfo | None = None
-
- def __init__(self) -> None:
- self._waiters: deque[tuple[TaskInfo, Event]] = deque()
-
- async def __aenter__(self) -> None:
- await self.acquire()
-
- async def __aexit__(
- self,
- exc_type: type[BaseException] | None,
- exc_val: BaseException | None,
- exc_tb: TracebackType | None,
- ) -> None:
- self.release()
-
- async def acquire(self) -> None:
- """Acquire the lock."""
- await checkpoint_if_cancelled()
- try:
- self.acquire_nowait()
- except WouldBlock:
- task = get_current_task()
- event = Event()
- token = task, event
- self._waiters.append(token)
- try:
- await event.wait()
- except BaseException:
- if not event.is_set():
- self._waiters.remove(token)
- elif self._owner_task == task:
- self.release()
-
- raise
-
- assert self._owner_task == task
- else:
- try:
- await cancel_shielded_checkpoint()
- except BaseException:
- self.release()
- raise
-
- def acquire_nowait(self) -> None:
- """
- Acquire the lock, without blocking.
-
- :raises ~anyio.WouldBlock: if the operation would block
-
- """
- task = get_current_task()
- if self._owner_task == task:
- raise RuntimeError("Attempted to acquire an already held Lock")
-
- if self._owner_task is not None:
- raise WouldBlock
-
- self._owner_task = task
-
- def release(self) -> DeprecatedAwaitable:
- """Release the lock."""
- if self._owner_task != get_current_task():
- raise RuntimeError("The current task is not holding this lock")
-
- if self._waiters:
- self._owner_task, event = self._waiters.popleft()
- event.set()
- else:
- del self._owner_task
-
- return DeprecatedAwaitable(self.release)
-
- def locked(self) -> bool:
- """Return True if the lock is currently held."""
- return self._owner_task is not None
-
- def statistics(self) -> LockStatistics:
- """
- Return statistics about the current state of this lock.
-
- .. versionadded:: 3.0
- """
- return LockStatistics(self.locked(), self._owner_task, len(self._waiters))
-
-
-class Condition:
- _owner_task: TaskInfo | None = None
-
- def __init__(self, lock: Lock | None = None):
- self._lock = lock or Lock()
- self._waiters: deque[Event] = deque()
-
- async def __aenter__(self) -> None:
- await self.acquire()
-
- async def __aexit__(
- self,
- exc_type: type[BaseException] | None,
- exc_val: BaseException | None,
- exc_tb: TracebackType | None,
- ) -> None:
- self.release()
-
- def _check_acquired(self) -> None:
- if self._owner_task != get_current_task():
- raise RuntimeError("The current task is not holding the underlying lock")
-
- async def acquire(self) -> None:
- """Acquire the underlying lock."""
- await self._lock.acquire()
- self._owner_task = get_current_task()
-
- def acquire_nowait(self) -> None:
- """
- Acquire the underlying lock, without blocking.
-
- :raises ~anyio.WouldBlock: if the operation would block
-
- """
- self._lock.acquire_nowait()
- self._owner_task = get_current_task()
-
- def release(self) -> DeprecatedAwaitable:
- """Release the underlying lock."""
- self._lock.release()
- return DeprecatedAwaitable(self.release)
-
- def locked(self) -> bool:
- """Return True if the lock is set."""
- return self._lock.locked()
-
- def notify(self, n: int = 1) -> None:
- """Notify exactly n listeners."""
- self._check_acquired()
- for _ in range(n):
- try:
- event = self._waiters.popleft()
- except IndexError:
- break
-
- event.set()
-
- def notify_all(self) -> None:
- """Notify all the listeners."""
- self._check_acquired()
- for event in self._waiters:
- event.set()
-
- self._waiters.clear()
-
- async def wait(self) -> None:
- """Wait for a notification."""
- await checkpoint()
- event = Event()
- self._waiters.append(event)
- self.release()
- try:
- await event.wait()
- except BaseException:
- if not event.is_set():
- self._waiters.remove(event)
-
- raise
- finally:
- with CancelScope(shield=True):
- await self.acquire()
-
- def statistics(self) -> ConditionStatistics:
- """
- Return statistics about the current state of this condition.
-
- .. versionadded:: 3.0
- """
- return ConditionStatistics(len(self._waiters), self._lock.statistics())
-
-
-class Semaphore:
- def __init__(self, initial_value: int, *, max_value: int | None = None):
- if not isinstance(initial_value, int):
- raise TypeError("initial_value must be an integer")
- if initial_value < 0:
- raise ValueError("initial_value must be >= 0")
- if max_value is not None:
- if not isinstance(max_value, int):
- raise TypeError("max_value must be an integer or None")
- if max_value < initial_value:
- raise ValueError(
- "max_value must be equal to or higher than initial_value"
- )
-
- self._value = initial_value
- self._max_value = max_value
- self._waiters: deque[Event] = deque()
-
- async def __aenter__(self) -> Semaphore:
- await self.acquire()
- return self
-
- async def __aexit__(
- self,
- exc_type: type[BaseException] | None,
- exc_val: BaseException | None,
- exc_tb: TracebackType | None,
- ) -> None:
- self.release()
-
- async def acquire(self) -> None:
- """Decrement the semaphore value, blocking if necessary."""
- await checkpoint_if_cancelled()
- try:
- self.acquire_nowait()
- except WouldBlock:
- event = Event()
- self._waiters.append(event)
- try:
- await event.wait()
- except BaseException:
- if not event.is_set():
- self._waiters.remove(event)
- else:
- self.release()
-
- raise
- else:
- try:
- await cancel_shielded_checkpoint()
- except BaseException:
- self.release()
- raise
-
- def acquire_nowait(self) -> None:
- """
- Acquire the underlying lock, without blocking.
-
- :raises ~anyio.WouldBlock: if the operation would block
-
- """
- if self._value == 0:
- raise WouldBlock
-
- self._value -= 1
-
- def release(self) -> DeprecatedAwaitable:
- """Increment the semaphore value."""
- if self._max_value is not None and self._value == self._max_value:
- raise ValueError("semaphore released too many times")
-
- if self._waiters:
- self._waiters.popleft().set()
- else:
- self._value += 1
-
- return DeprecatedAwaitable(self.release)
-
- @property
- def value(self) -> int:
- """The current value of the semaphore."""
- return self._value
-
- @property
- def max_value(self) -> int | None:
- """The maximum value of the semaphore."""
- return self._max_value
-
- def statistics(self) -> SemaphoreStatistics:
- """
- Return statistics about the current state of this semaphore.
-
- .. versionadded:: 3.0
- """
- return SemaphoreStatistics(len(self._waiters))
-
-
-class CapacityLimiter:
- def __new__(cls, total_tokens: float) -> CapacityLimiter:
- return get_asynclib().CapacityLimiter(total_tokens)
-
- async def __aenter__(self) -> None:
- raise NotImplementedError
-
- async def __aexit__(
- self,
- exc_type: type[BaseException] | None,
- exc_val: BaseException | None,
- exc_tb: TracebackType | None,
- ) -> bool | None:
- raise NotImplementedError
-
- @property
- def total_tokens(self) -> float:
- """
- The total number of tokens available for borrowing.
-
- This is a read-write property. If the total number of tokens is increased, the
- proportionate number of tasks waiting on this limiter will be granted their tokens.
-
- .. versionchanged:: 3.0
- The property is now writable.
-
- """
- raise NotImplementedError
-
- @total_tokens.setter
- def total_tokens(self, value: float) -> None:
- raise NotImplementedError
-
- async def set_total_tokens(self, value: float) -> None:
- warn(
- "CapacityLimiter.set_total_tokens has been deprecated. Set the value of the"
- '"total_tokens" attribute directly.',
- DeprecationWarning,
- )
- self.total_tokens = value
-
- @property
- def borrowed_tokens(self) -> int:
- """The number of tokens that have currently been borrowed."""
- raise NotImplementedError
-
- @property
- def available_tokens(self) -> float:
- """The number of tokens currently available to be borrowed"""
- raise NotImplementedError
-
- def acquire_nowait(self) -> DeprecatedAwaitable:
- """
- Acquire a token for the current task without waiting for one to become available.
-
- :raises ~anyio.WouldBlock: if there are no tokens available for borrowing
-
- """
- raise NotImplementedError
-
- def acquire_on_behalf_of_nowait(self, borrower: object) -> DeprecatedAwaitable:
- """
- Acquire a token without waiting for one to become available.
-
- :param borrower: the entity borrowing a token
- :raises ~anyio.WouldBlock: if there are no tokens available for borrowing
-
- """
- raise NotImplementedError
-
- async def acquire(self) -> None:
- """
- Acquire a token for the current task, waiting if necessary for one to become available.
-
- """
- raise NotImplementedError
-
- async def acquire_on_behalf_of(self, borrower: object) -> None:
- """
- Acquire a token, waiting if necessary for one to become available.
-
- :param borrower: the entity borrowing a token
-
- """
- raise NotImplementedError
-
- def release(self) -> None:
- """
- Release the token held by the current task.
- :raises RuntimeError: if the current task has not borrowed a token from this limiter.
-
- """
- raise NotImplementedError
-
- def release_on_behalf_of(self, borrower: object) -> None:
- """
- Release the token held by the given borrower.
-
- :raises RuntimeError: if the borrower has not borrowed a token from this limiter.
-
- """
- raise NotImplementedError
-
- def statistics(self) -> CapacityLimiterStatistics:
- """
- Return statistics about the current state of this limiter.
-
- .. versionadded:: 3.0
-
- """
- raise NotImplementedError
-
-
-def create_lock() -> Lock:
- """
- Create an asynchronous lock.
-
- :return: a lock object
-
- .. deprecated:: 3.0
- Use :class:`~Lock` directly.
-
- """
- warn("create_lock() is deprecated -- use Lock() directly", DeprecationWarning)
- return Lock()
-
-
-def create_condition(lock: Lock | None = None) -> Condition:
- """
- Create an asynchronous condition.
-
- :param lock: the lock to base the condition object on
- :return: a condition object
-
- .. deprecated:: 3.0
- Use :class:`~Condition` directly.
-
- """
- warn(
- "create_condition() is deprecated -- use Condition() directly",
- DeprecationWarning,
- )
- return Condition(lock=lock)
-
-
-def create_event() -> Event:
- """
- Create an asynchronous event object.
-
- :return: an event object
-
- .. deprecated:: 3.0
- Use :class:`~Event` directly.
-
- """
- warn("create_event() is deprecated -- use Event() directly", DeprecationWarning)
- return get_asynclib().Event()
-
-
-def create_semaphore(value: int, *, max_value: int | None = None) -> Semaphore:
- """
- Create an asynchronous semaphore.
-
- :param value: the semaphore's initial value
- :param max_value: if set, makes this a "bounded" semaphore that raises :exc:`ValueError` if the
- semaphore's value would exceed this number
- :return: a semaphore object
-
- .. deprecated:: 3.0
- Use :class:`~Semaphore` directly.
-
- """
- warn(
- "create_semaphore() is deprecated -- use Semaphore() directly",
- DeprecationWarning,
- )
- return Semaphore(value, max_value=max_value)
-
-
-def create_capacity_limiter(total_tokens: float) -> CapacityLimiter:
- """
- Create a capacity limiter.
-
- :param total_tokens: the total number of tokens available for borrowing (can be an integer or
- :data:`math.inf`)
- :return: a capacity limiter object
-
- .. deprecated:: 3.0
- Use :class:`~CapacityLimiter` directly.
-
- """
- warn(
- "create_capacity_limiter() is deprecated -- use CapacityLimiter() directly",
- DeprecationWarning,
- )
- return get_asynclib().CapacityLimiter(total_tokens)
-
-
-class ResourceGuard:
- __slots__ = "action", "_guarded"
-
- def __init__(self, action: str):
- self.action = action
- self._guarded = False
-
- def __enter__(self) -> None:
- if self._guarded:
- raise BusyResourceError(self.action)
-
- self._guarded = True
-
- def __exit__(
- self,
- exc_type: type[BaseException] | None,
- exc_val: BaseException | None,
- exc_tb: TracebackType | None,
- ) -> bool | None:
- self._guarded = False
- return None
diff --git a/spaces/dcarpintero/nlp-summarizer-pegasus/.venv/lib/python3.9/site-packages/fontTools/cu2qu/cu2qu.c b/spaces/dcarpintero/nlp-summarizer-pegasus/.venv/lib/python3.9/site-packages/fontTools/cu2qu/cu2qu.c
deleted file mode 100644
index 1971c94d65e1173103f26f8d4532000be32001aa..0000000000000000000000000000000000000000
--- a/spaces/dcarpintero/nlp-summarizer-pegasus/.venv/lib/python3.9/site-packages/fontTools/cu2qu/cu2qu.c
+++ /dev/null
@@ -1,14095 +0,0 @@
-/* Generated by Cython 3.0.0 */
-
-/* BEGIN: Cython Metadata
-{
- "distutils": {
- "define_macros": [
- [
- "CYTHON_TRACE_NOGIL",
- "1"
- ]
- ],
- "name": "fontTools.cu2qu.cu2qu",
- "sources": [
- "Lib/fontTools/cu2qu/cu2qu.py"
- ]
- },
- "module_name": "fontTools.cu2qu.cu2qu"
-}
-END: Cython Metadata */
-
-#ifndef PY_SSIZE_T_CLEAN
-#define PY_SSIZE_T_CLEAN
-#endif /* PY_SSIZE_T_CLEAN */
-#if defined(CYTHON_LIMITED_API) && 0
- #ifndef Py_LIMITED_API
- #if CYTHON_LIMITED_API+0 > 0x03030000
- #define Py_LIMITED_API CYTHON_LIMITED_API
- #else
- #define Py_LIMITED_API 0x03030000
- #endif
- #endif
-#endif
-
-#include "Python.h"
-#ifndef Py_PYTHON_H
- #error Python headers needed to compile C extensions, please install development version of Python.
-#elif PY_VERSION_HEX < 0x02070000 || (0x03000000 <= PY_VERSION_HEX && PY_VERSION_HEX < 0x03030000)
- #error Cython requires Python 2.7+ or Python 3.3+.
-#else
-#define CYTHON_ABI "3_0_0"
-#define __PYX_ABI_MODULE_NAME "_cython_" CYTHON_ABI
-#define __PYX_TYPE_MODULE_PREFIX __PYX_ABI_MODULE_NAME "."
-#define CYTHON_HEX_VERSION 0x030000F0
-#define CYTHON_FUTURE_DIVISION 1
-#include
-#ifndef offsetof
- #define offsetof(type, member) ( (size_t) & ((type*)0) -> member )
-#endif
-#if !defined(_WIN32) && !defined(WIN32) && !defined(MS_WINDOWS)
- #ifndef __stdcall
- #define __stdcall
- #endif
- #ifndef __cdecl
- #define __cdecl
- #endif
- #ifndef __fastcall
- #define __fastcall
- #endif
-#endif
-#ifndef DL_IMPORT
- #define DL_IMPORT(t) t
-#endif
-#ifndef DL_EXPORT
- #define DL_EXPORT(t) t
-#endif
-#define __PYX_COMMA ,
-#ifndef HAVE_LONG_LONG
- #define HAVE_LONG_LONG
-#endif
-#ifndef PY_LONG_LONG
- #define PY_LONG_LONG LONG_LONG
-#endif
-#ifndef Py_HUGE_VAL
- #define Py_HUGE_VAL HUGE_VAL
-#endif
-#if defined(GRAALVM_PYTHON)
- /* For very preliminary testing purposes. Most variables are set the same as PyPy.
- The existence of this section does not imply that anything works or is even tested */
- #define CYTHON_COMPILING_IN_PYPY 0
- #define CYTHON_COMPILING_IN_CPYTHON 0
- #define CYTHON_COMPILING_IN_LIMITED_API 0
- #define CYTHON_COMPILING_IN_GRAAL 1
- #define CYTHON_COMPILING_IN_NOGIL 0
- #undef CYTHON_USE_TYPE_SLOTS
- #define CYTHON_USE_TYPE_SLOTS 0
- #undef CYTHON_USE_TYPE_SPECS
- #define CYTHON_USE_TYPE_SPECS 0
- #undef CYTHON_USE_PYTYPE_LOOKUP
- #define CYTHON_USE_PYTYPE_LOOKUP 0
- #if PY_VERSION_HEX < 0x03050000
- #undef CYTHON_USE_ASYNC_SLOTS
- #define CYTHON_USE_ASYNC_SLOTS 0
- #elif !defined(CYTHON_USE_ASYNC_SLOTS)
- #define CYTHON_USE_ASYNC_SLOTS 1
- #endif
- #undef CYTHON_USE_PYLIST_INTERNALS
- #define CYTHON_USE_PYLIST_INTERNALS 0
- #undef CYTHON_USE_UNICODE_INTERNALS
- #define CYTHON_USE_UNICODE_INTERNALS 0
- #undef CYTHON_USE_UNICODE_WRITER
- #define CYTHON_USE_UNICODE_WRITER 0
- #undef CYTHON_USE_PYLONG_INTERNALS
- #define CYTHON_USE_PYLONG_INTERNALS 0
- #undef CYTHON_AVOID_BORROWED_REFS
- #define CYTHON_AVOID_BORROWED_REFS 1
- #undef CYTHON_ASSUME_SAFE_MACROS
- #define CYTHON_ASSUME_SAFE_MACROS 0
- #undef CYTHON_UNPACK_METHODS
- #define CYTHON_UNPACK_METHODS 0
- #undef CYTHON_FAST_THREAD_STATE
- #define CYTHON_FAST_THREAD_STATE 0
- #undef CYTHON_FAST_GIL
- #define CYTHON_FAST_GIL 0
- #undef CYTHON_METH_FASTCALL
- #define CYTHON_METH_FASTCALL 0
- #undef CYTHON_FAST_PYCALL
- #define CYTHON_FAST_PYCALL 0
- #ifndef CYTHON_PEP487_INIT_SUBCLASS
- #define CYTHON_PEP487_INIT_SUBCLASS (PY_MAJOR_VERSION >= 3)
- #endif
- #undef CYTHON_PEP489_MULTI_PHASE_INIT
- #define CYTHON_PEP489_MULTI_PHASE_INIT 1
- #undef CYTHON_USE_MODULE_STATE
- #define CYTHON_USE_MODULE_STATE 0
- #undef CYTHON_USE_TP_FINALIZE
- #define CYTHON_USE_TP_FINALIZE 0
- #undef CYTHON_USE_DICT_VERSIONS
- #define CYTHON_USE_DICT_VERSIONS 0
- #undef CYTHON_USE_EXC_INFO_STACK
- #define CYTHON_USE_EXC_INFO_STACK 0
- #ifndef CYTHON_UPDATE_DESCRIPTOR_DOC
- #define CYTHON_UPDATE_DESCRIPTOR_DOC 0
- #endif
-#elif defined(PYPY_VERSION)
- #define CYTHON_COMPILING_IN_PYPY 1
- #define CYTHON_COMPILING_IN_CPYTHON 0
- #define CYTHON_COMPILING_IN_LIMITED_API 0
- #define CYTHON_COMPILING_IN_GRAAL 0
- #define CYTHON_COMPILING_IN_NOGIL 0
- #undef CYTHON_USE_TYPE_SLOTS
- #define CYTHON_USE_TYPE_SLOTS 0
- #undef CYTHON_USE_TYPE_SPECS
- #define CYTHON_USE_TYPE_SPECS 0
- #undef CYTHON_USE_PYTYPE_LOOKUP
- #define CYTHON_USE_PYTYPE_LOOKUP 0
- #if PY_VERSION_HEX < 0x03050000
- #undef CYTHON_USE_ASYNC_SLOTS
- #define CYTHON_USE_ASYNC_SLOTS 0
- #elif !defined(CYTHON_USE_ASYNC_SLOTS)
- #define CYTHON_USE_ASYNC_SLOTS 1
- #endif
- #undef CYTHON_USE_PYLIST_INTERNALS
- #define CYTHON_USE_PYLIST_INTERNALS 0
- #undef CYTHON_USE_UNICODE_INTERNALS
- #define CYTHON_USE_UNICODE_INTERNALS 0
- #undef CYTHON_USE_UNICODE_WRITER
- #define CYTHON_USE_UNICODE_WRITER 0
- #undef CYTHON_USE_PYLONG_INTERNALS
- #define CYTHON_USE_PYLONG_INTERNALS 0
- #undef CYTHON_AVOID_BORROWED_REFS
- #define CYTHON_AVOID_BORROWED_REFS 1
- #undef CYTHON_ASSUME_SAFE_MACROS
- #define CYTHON_ASSUME_SAFE_MACROS 0
- #undef CYTHON_UNPACK_METHODS
- #define CYTHON_UNPACK_METHODS 0
- #undef CYTHON_FAST_THREAD_STATE
- #define CYTHON_FAST_THREAD_STATE 0
- #undef CYTHON_FAST_GIL
- #define CYTHON_FAST_GIL 0
- #undef CYTHON_METH_FASTCALL
- #define CYTHON_METH_FASTCALL 0
- #undef CYTHON_FAST_PYCALL
- #define CYTHON_FAST_PYCALL 0
- #ifndef CYTHON_PEP487_INIT_SUBCLASS
- #define CYTHON_PEP487_INIT_SUBCLASS (PY_MAJOR_VERSION >= 3)
- #endif
- #if PY_VERSION_HEX < 0x03090000
- #undef CYTHON_PEP489_MULTI_PHASE_INIT
- #define CYTHON_PEP489_MULTI_PHASE_INIT 0
- #elif !defined(CYTHON_PEP489_MULTI_PHASE_INIT)
- #define CYTHON_PEP489_MULTI_PHASE_INIT 1
- #endif
- #undef CYTHON_USE_MODULE_STATE
- #define CYTHON_USE_MODULE_STATE 0
- #undef CYTHON_USE_TP_FINALIZE
- #define CYTHON_USE_TP_FINALIZE (PY_VERSION_HEX >= 0x030400a1 && PYPY_VERSION_NUM >= 0x07030C00)
- #undef CYTHON_USE_DICT_VERSIONS
- #define CYTHON_USE_DICT_VERSIONS 0
- #undef CYTHON_USE_EXC_INFO_STACK
- #define CYTHON_USE_EXC_INFO_STACK 0
- #ifndef CYTHON_UPDATE_DESCRIPTOR_DOC
- #define CYTHON_UPDATE_DESCRIPTOR_DOC 0
- #endif
-#elif defined(CYTHON_LIMITED_API)
- #define CYTHON_COMPILING_IN_PYPY 0
- #define CYTHON_COMPILING_IN_CPYTHON 0
- #define CYTHON_COMPILING_IN_LIMITED_API 1
- #define CYTHON_COMPILING_IN_GRAAL 0
- #define CYTHON_COMPILING_IN_NOGIL 0
- #undef CYTHON_CLINE_IN_TRACEBACK
- #define CYTHON_CLINE_IN_TRACEBACK 0
- #undef CYTHON_USE_TYPE_SLOTS
- #define CYTHON_USE_TYPE_SLOTS 0
- #undef CYTHON_USE_TYPE_SPECS
- #define CYTHON_USE_TYPE_SPECS 1
- #undef CYTHON_USE_PYTYPE_LOOKUP
- #define CYTHON_USE_PYTYPE_LOOKUP 0
- #undef CYTHON_USE_ASYNC_SLOTS
- #define CYTHON_USE_ASYNC_SLOTS 0
- #undef CYTHON_USE_PYLIST_INTERNALS
- #define CYTHON_USE_PYLIST_INTERNALS 0
- #undef CYTHON_USE_UNICODE_INTERNALS
- #define CYTHON_USE_UNICODE_INTERNALS 0
- #ifndef CYTHON_USE_UNICODE_WRITER
- #define CYTHON_USE_UNICODE_WRITER 0
- #endif
- #undef CYTHON_USE_PYLONG_INTERNALS
- #define CYTHON_USE_PYLONG_INTERNALS 0
- #ifndef CYTHON_AVOID_BORROWED_REFS
- #define CYTHON_AVOID_BORROWED_REFS 0
- #endif
- #undef CYTHON_ASSUME_SAFE_MACROS
- #define CYTHON_ASSUME_SAFE_MACROS 0
- #undef CYTHON_UNPACK_METHODS
- #define CYTHON_UNPACK_METHODS 0
- #undef CYTHON_FAST_THREAD_STATE
- #define CYTHON_FAST_THREAD_STATE 0
- #undef CYTHON_FAST_GIL
- #define CYTHON_FAST_GIL 0
- #undef CYTHON_METH_FASTCALL
- #define CYTHON_METH_FASTCALL 0
- #undef CYTHON_FAST_PYCALL
- #define CYTHON_FAST_PYCALL 0
- #ifndef CYTHON_PEP487_INIT_SUBCLASS
- #define CYTHON_PEP487_INIT_SUBCLASS 1
- #endif
- #undef CYTHON_PEP489_MULTI_PHASE_INIT
- #define CYTHON_PEP489_MULTI_PHASE_INIT 0
- #undef CYTHON_USE_MODULE_STATE
- #define CYTHON_USE_MODULE_STATE 1
- #ifndef CYTHON_USE_TP_FINALIZE
- #define CYTHON_USE_TP_FINALIZE 1
- #endif
- #undef CYTHON_USE_DICT_VERSIONS
- #define CYTHON_USE_DICT_VERSIONS 0
- #undef CYTHON_USE_EXC_INFO_STACK
- #define CYTHON_USE_EXC_INFO_STACK 0
- #ifndef CYTHON_UPDATE_DESCRIPTOR_DOC
- #define CYTHON_UPDATE_DESCRIPTOR_DOC 0
- #endif
-#elif defined(PY_NOGIL)
- #define CYTHON_COMPILING_IN_PYPY 0
- #define CYTHON_COMPILING_IN_CPYTHON 0
- #define CYTHON_COMPILING_IN_LIMITED_API 0
- #define CYTHON_COMPILING_IN_GRAAL 0
- #define CYTHON_COMPILING_IN_NOGIL 1
- #ifndef CYTHON_USE_TYPE_SLOTS
- #define CYTHON_USE_TYPE_SLOTS 1
- #endif
- #undef CYTHON_USE_PYTYPE_LOOKUP
- #define CYTHON_USE_PYTYPE_LOOKUP 0
- #ifndef CYTHON_USE_ASYNC_SLOTS
- #define CYTHON_USE_ASYNC_SLOTS 1
- #endif
- #undef CYTHON_USE_PYLIST_INTERNALS
- #define CYTHON_USE_PYLIST_INTERNALS 0
- #ifndef CYTHON_USE_UNICODE_INTERNALS
- #define CYTHON_USE_UNICODE_INTERNALS 1
- #endif
- #undef CYTHON_USE_UNICODE_WRITER
- #define CYTHON_USE_UNICODE_WRITER 0
- #undef CYTHON_USE_PYLONG_INTERNALS
- #define CYTHON_USE_PYLONG_INTERNALS 0
- #ifndef CYTHON_AVOID_BORROWED_REFS
- #define CYTHON_AVOID_BORROWED_REFS 0
- #endif
- #ifndef CYTHON_ASSUME_SAFE_MACROS
- #define CYTHON_ASSUME_SAFE_MACROS 1
- #endif
- #ifndef CYTHON_UNPACK_METHODS
- #define CYTHON_UNPACK_METHODS 1
- #endif
- #undef CYTHON_FAST_THREAD_STATE
- #define CYTHON_FAST_THREAD_STATE 0
- #undef CYTHON_FAST_PYCALL
- #define CYTHON_FAST_PYCALL 0
- #ifndef CYTHON_PEP489_MULTI_PHASE_INIT
- #define CYTHON_PEP489_MULTI_PHASE_INIT 1
- #endif
- #ifndef CYTHON_USE_TP_FINALIZE
- #define CYTHON_USE_TP_FINALIZE 1
- #endif
- #undef CYTHON_USE_DICT_VERSIONS
- #define CYTHON_USE_DICT_VERSIONS 0
- #undef CYTHON_USE_EXC_INFO_STACK
- #define CYTHON_USE_EXC_INFO_STACK 0
-#else
- #define CYTHON_COMPILING_IN_PYPY 0
- #define CYTHON_COMPILING_IN_CPYTHON 1
- #define CYTHON_COMPILING_IN_LIMITED_API 0
- #define CYTHON_COMPILING_IN_GRAAL 0
- #define CYTHON_COMPILING_IN_NOGIL 0
- #ifndef CYTHON_USE_TYPE_SLOTS
- #define CYTHON_USE_TYPE_SLOTS 1
- #endif
- #ifndef CYTHON_USE_TYPE_SPECS
- #define CYTHON_USE_TYPE_SPECS 0
- #endif
- #ifndef CYTHON_USE_PYTYPE_LOOKUP
- #define CYTHON_USE_PYTYPE_LOOKUP 1
- #endif
- #if PY_MAJOR_VERSION < 3
- #undef CYTHON_USE_ASYNC_SLOTS
- #define CYTHON_USE_ASYNC_SLOTS 0
- #elif !defined(CYTHON_USE_ASYNC_SLOTS)
- #define CYTHON_USE_ASYNC_SLOTS 1
- #endif
- #ifndef CYTHON_USE_PYLONG_INTERNALS
- #define CYTHON_USE_PYLONG_INTERNALS 1
- #endif
- #ifndef CYTHON_USE_PYLIST_INTERNALS
- #define CYTHON_USE_PYLIST_INTERNALS 1
- #endif
- #ifndef CYTHON_USE_UNICODE_INTERNALS
- #define CYTHON_USE_UNICODE_INTERNALS 1
- #endif
- #if PY_VERSION_HEX < 0x030300F0 || PY_VERSION_HEX >= 0x030B00A2
- #undef CYTHON_USE_UNICODE_WRITER
- #define CYTHON_USE_UNICODE_WRITER 0
- #elif !defined(CYTHON_USE_UNICODE_WRITER)
- #define CYTHON_USE_UNICODE_WRITER 1
- #endif
- #ifndef CYTHON_AVOID_BORROWED_REFS
- #define CYTHON_AVOID_BORROWED_REFS 0
- #endif
- #ifndef CYTHON_ASSUME_SAFE_MACROS
- #define CYTHON_ASSUME_SAFE_MACROS 1
- #endif
- #ifndef CYTHON_UNPACK_METHODS
- #define CYTHON_UNPACK_METHODS 1
- #endif
- #ifndef CYTHON_FAST_THREAD_STATE
- #define CYTHON_FAST_THREAD_STATE 1
- #endif
- #ifndef CYTHON_FAST_GIL
- #define CYTHON_FAST_GIL (PY_MAJOR_VERSION < 3 || PY_VERSION_HEX >= 0x03060000 && PY_VERSION_HEX < 0x030C00A6)
- #endif
- #ifndef CYTHON_METH_FASTCALL
- #define CYTHON_METH_FASTCALL (PY_VERSION_HEX >= 0x030700A1)
- #endif
- #ifndef CYTHON_FAST_PYCALL
- #define CYTHON_FAST_PYCALL 1
- #endif
- #ifndef CYTHON_PEP487_INIT_SUBCLASS
- #define CYTHON_PEP487_INIT_SUBCLASS 1
- #endif
- #if PY_VERSION_HEX < 0x03050000
- #undef CYTHON_PEP489_MULTI_PHASE_INIT
- #define CYTHON_PEP489_MULTI_PHASE_INIT 0
- #elif !defined(CYTHON_PEP489_MULTI_PHASE_INIT)
- #define CYTHON_PEP489_MULTI_PHASE_INIT 1
- #endif
- #ifndef CYTHON_USE_MODULE_STATE
- #define CYTHON_USE_MODULE_STATE 0
- #endif
- #if PY_VERSION_HEX < 0x030400a1
- #undef CYTHON_USE_TP_FINALIZE
- #define CYTHON_USE_TP_FINALIZE 0
- #elif !defined(CYTHON_USE_TP_FINALIZE)
- #define CYTHON_USE_TP_FINALIZE 1
- #endif
- #if PY_VERSION_HEX < 0x030600B1
- #undef CYTHON_USE_DICT_VERSIONS
- #define CYTHON_USE_DICT_VERSIONS 0
- #elif !defined(CYTHON_USE_DICT_VERSIONS)
- #define CYTHON_USE_DICT_VERSIONS (PY_VERSION_HEX < 0x030C00A5)
- #endif
- #if PY_VERSION_HEX < 0x030700A3
- #undef CYTHON_USE_EXC_INFO_STACK
- #define CYTHON_USE_EXC_INFO_STACK 0
- #elif !defined(CYTHON_USE_EXC_INFO_STACK)
- #define CYTHON_USE_EXC_INFO_STACK 1
- #endif
- #ifndef CYTHON_UPDATE_DESCRIPTOR_DOC
- #define CYTHON_UPDATE_DESCRIPTOR_DOC 1
- #endif
-#endif
-#if !defined(CYTHON_FAST_PYCCALL)
-#define CYTHON_FAST_PYCCALL (CYTHON_FAST_PYCALL && PY_VERSION_HEX >= 0x030600B1)
-#endif
-#if !defined(CYTHON_VECTORCALL)
-#define CYTHON_VECTORCALL (CYTHON_FAST_PYCCALL && PY_VERSION_HEX >= 0x030800B1)
-#endif
-#define CYTHON_BACKPORT_VECTORCALL (CYTHON_METH_FASTCALL && PY_VERSION_HEX < 0x030800B1)
-#if CYTHON_USE_PYLONG_INTERNALS
- #if PY_MAJOR_VERSION < 3
- #include "longintrepr.h"
- #endif
- #undef SHIFT
- #undef BASE
- #undef MASK
- #ifdef SIZEOF_VOID_P
- enum { __pyx_check_sizeof_voidp = 1 / (int)(SIZEOF_VOID_P == sizeof(void*)) };
- #endif
-#endif
-#ifndef __has_attribute
- #define __has_attribute(x) 0
-#endif
-#ifndef __has_cpp_attribute
- #define __has_cpp_attribute(x) 0
-#endif
-#ifndef CYTHON_RESTRICT
- #if defined(__GNUC__)
- #define CYTHON_RESTRICT __restrict__
- #elif defined(_MSC_VER) && _MSC_VER >= 1400
- #define CYTHON_RESTRICT __restrict
- #elif defined (__STDC_VERSION__) && __STDC_VERSION__ >= 199901L
- #define CYTHON_RESTRICT restrict
- #else
- #define CYTHON_RESTRICT
- #endif
-#endif
-#ifndef CYTHON_UNUSED
- #if defined(__cplusplus)
- /* for clang __has_cpp_attribute(maybe_unused) is true even before C++17
- * but leads to warnings with -pedantic, since it is a C++17 feature */
- #if ((defined(_MSVC_LANG) && _MSVC_LANG >= 201703L) || __cplusplus >= 201703L)
- #if __has_cpp_attribute(maybe_unused)
- #define CYTHON_UNUSED [[maybe_unused]]
- #endif
- #endif
- #endif
-#endif
-#ifndef CYTHON_UNUSED
-# if defined(__GNUC__)
-# if !(defined(__cplusplus)) || (__GNUC__ > 3 || (__GNUC__ == 3 && __GNUC_MINOR__ >= 4))
-# define CYTHON_UNUSED __attribute__ ((__unused__))
-# else
-# define CYTHON_UNUSED
-# endif
-# elif defined(__ICC) || (defined(__INTEL_COMPILER) && !defined(_MSC_VER))
-# define CYTHON_UNUSED __attribute__ ((__unused__))
-# else
-# define CYTHON_UNUSED
-# endif
-#endif
-#ifndef CYTHON_UNUSED_VAR
-# if defined(__cplusplus)
- template void CYTHON_UNUSED_VAR( const T& ) { }
-# else
-# define CYTHON_UNUSED_VAR(x) (void)(x)
-# endif
-#endif
-#ifndef CYTHON_MAYBE_UNUSED_VAR
- #define CYTHON_MAYBE_UNUSED_VAR(x) CYTHON_UNUSED_VAR(x)
-#endif
-#ifndef CYTHON_NCP_UNUSED
-# if CYTHON_COMPILING_IN_CPYTHON
-# define CYTHON_NCP_UNUSED
-# else
-# define CYTHON_NCP_UNUSED CYTHON_UNUSED
-# endif
-#endif
-#define __Pyx_void_to_None(void_result) ((void)(void_result), Py_INCREF(Py_None), Py_None)
-#ifdef _MSC_VER
- #ifndef _MSC_STDINT_H_
- #if _MSC_VER < 1300
- typedef unsigned char uint8_t;
- typedef unsigned short uint16_t;
- typedef unsigned int uint32_t;
- #else
- typedef unsigned __int8 uint8_t;
- typedef unsigned __int16 uint16_t;
- typedef unsigned __int32 uint32_t;
- #endif
- #endif
- #if _MSC_VER < 1300
- #ifdef _WIN64
- typedef unsigned long long __pyx_uintptr_t;
- #else
- typedef unsigned int __pyx_uintptr_t;
- #endif
- #else
- #ifdef _WIN64
- typedef unsigned __int64 __pyx_uintptr_t;
- #else
- typedef unsigned __int32 __pyx_uintptr_t;
- #endif
- #endif
-#else
- #include
- typedef uintptr_t __pyx_uintptr_t;
-#endif
-#ifndef CYTHON_FALLTHROUGH
- #if defined(__cplusplus)
- /* for clang __has_cpp_attribute(fallthrough) is true even before C++17
- * but leads to warnings with -pedantic, since it is a C++17 feature */
- #if ((defined(_MSVC_LANG) && _MSVC_LANG >= 201703L) || __cplusplus >= 201703L)
- #if __has_cpp_attribute(fallthrough)
- #define CYTHON_FALLTHROUGH [[fallthrough]]
- #endif
- #endif
- #ifndef CYTHON_FALLTHROUGH
- #if __has_cpp_attribute(clang::fallthrough)
- #define CYTHON_FALLTHROUGH [[clang::fallthrough]]
- #elif __has_cpp_attribute(gnu::fallthrough)
- #define CYTHON_FALLTHROUGH [[gnu::fallthrough]]
- #endif
- #endif
- #endif
- #ifndef CYTHON_FALLTHROUGH
- #if __has_attribute(fallthrough)
- #define CYTHON_FALLTHROUGH __attribute__((fallthrough))
- #else
- #define CYTHON_FALLTHROUGH
- #endif
- #endif
- #if defined(__clang__) && defined(__apple_build_version__)
- #if __apple_build_version__ < 7000000
- #undef CYTHON_FALLTHROUGH
- #define CYTHON_FALLTHROUGH
- #endif
- #endif
-#endif
-#ifdef __cplusplus
- template
- struct __PYX_IS_UNSIGNED_IMPL {static const bool value = T(0) < T(-1);};
- #define __PYX_IS_UNSIGNED(type) (__PYX_IS_UNSIGNED_IMPL::value)
-#else
- #define __PYX_IS_UNSIGNED(type) (((type)-1) > 0)
-#endif
-#if CYTHON_COMPILING_IN_PYPY == 1
- #define __PYX_NEED_TP_PRINT_SLOT (PY_VERSION_HEX >= 0x030800b4 && PY_VERSION_HEX < 0x030A0000)
-#else
- #define __PYX_NEED_TP_PRINT_SLOT (PY_VERSION_HEX >= 0x030800b4 && PY_VERSION_HEX < 0x03090000)
-#endif
-#define __PYX_REINTERPRET_FUNCION(func_pointer, other_pointer) ((func_pointer)(void(*)(void))(other_pointer))
-
-#ifndef CYTHON_INLINE
- #if defined(__clang__)
- #define CYTHON_INLINE __inline__ __attribute__ ((__unused__))
- #elif defined(__GNUC__)
- #define CYTHON_INLINE __inline__
- #elif defined(_MSC_VER)
- #define CYTHON_INLINE __inline
- #elif defined (__STDC_VERSION__) && __STDC_VERSION__ >= 199901L
- #define CYTHON_INLINE inline
- #else
- #define CYTHON_INLINE
- #endif
-#endif
-
-#define __PYX_BUILD_PY_SSIZE_T "n"
-#define CYTHON_FORMAT_SSIZE_T "z"
-#if PY_MAJOR_VERSION < 3
- #define __Pyx_BUILTIN_MODULE_NAME "__builtin__"
- #define __Pyx_DefaultClassType PyClass_Type
- #define __Pyx_PyCode_New(a, p, k, l, s, f, code, c, n, v, fv, cell, fn, name, fline, lnos)\
- PyCode_New(a+k, l, s, f, code, c, n, v, fv, cell, fn, name, fline, lnos)
-#else
- #define __Pyx_BUILTIN_MODULE_NAME "builtins"
- #define __Pyx_DefaultClassType PyType_Type
-#if PY_VERSION_HEX >= 0x030B00A1
- static CYTHON_INLINE PyCodeObject* __Pyx_PyCode_New(int a, int p, int k, int l, int s, int f,
- PyObject *code, PyObject *c, PyObject* n, PyObject *v,
- PyObject *fv, PyObject *cell, PyObject* fn,
- PyObject *name, int fline, PyObject *lnos) {
- PyObject *kwds=NULL, *argcount=NULL, *posonlyargcount=NULL, *kwonlyargcount=NULL;
- PyObject *nlocals=NULL, *stacksize=NULL, *flags=NULL, *replace=NULL, *empty=NULL;
- const char *fn_cstr=NULL;
- const char *name_cstr=NULL;
- PyCodeObject *co=NULL, *result=NULL;
- PyObject *type, *value, *traceback;
- PyErr_Fetch(&type, &value, &traceback);
- if (!(kwds=PyDict_New())) goto end;
- if (!(argcount=PyLong_FromLong(a))) goto end;
- if (PyDict_SetItemString(kwds, "co_argcount", argcount) != 0) goto end;
- if (!(posonlyargcount=PyLong_FromLong(p))) goto end;
- if (PyDict_SetItemString(kwds, "co_posonlyargcount", posonlyargcount) != 0) goto end;
- if (!(kwonlyargcount=PyLong_FromLong(k))) goto end;
- if (PyDict_SetItemString(kwds, "co_kwonlyargcount", kwonlyargcount) != 0) goto end;
- if (!(nlocals=PyLong_FromLong(l))) goto end;
- if (PyDict_SetItemString(kwds, "co_nlocals", nlocals) != 0) goto end;
- if (!(stacksize=PyLong_FromLong(s))) goto end;
- if (PyDict_SetItemString(kwds, "co_stacksize", stacksize) != 0) goto end;
- if (!(flags=PyLong_FromLong(f))) goto end;
- if (PyDict_SetItemString(kwds, "co_flags", flags) != 0) goto end;
- if (PyDict_SetItemString(kwds, "co_code", code) != 0) goto end;
- if (PyDict_SetItemString(kwds, "co_consts", c) != 0) goto end;
- if (PyDict_SetItemString(kwds, "co_names", n) != 0) goto end;
- if (PyDict_SetItemString(kwds, "co_varnames", v) != 0) goto end;
- if (PyDict_SetItemString(kwds, "co_freevars", fv) != 0) goto end;
- if (PyDict_SetItemString(kwds, "co_cellvars", cell) != 0) goto end;
- if (PyDict_SetItemString(kwds, "co_linetable", lnos) != 0) goto end;
- if (!(fn_cstr=PyUnicode_AsUTF8AndSize(fn, NULL))) goto end;
- if (!(name_cstr=PyUnicode_AsUTF8AndSize(name, NULL))) goto end;
- if (!(co = PyCode_NewEmpty(fn_cstr, name_cstr, fline))) goto end;
- if (!(replace = PyObject_GetAttrString((PyObject*)co, "replace"))) goto end;
- if (!(empty = PyTuple_New(0))) goto end;
- result = (PyCodeObject*) PyObject_Call(replace, empty, kwds);
- end:
- Py_XDECREF((PyObject*) co);
- Py_XDECREF(kwds);
- Py_XDECREF(argcount);
- Py_XDECREF(posonlyargcount);
- Py_XDECREF(kwonlyargcount);
- Py_XDECREF(nlocals);
- Py_XDECREF(stacksize);
- Py_XDECREF(replace);
- Py_XDECREF(empty);
- if (type) {
- PyErr_Restore(type, value, traceback);
- }
- return result;
- }
-#elif PY_VERSION_HEX >= 0x030800B2 && !CYTHON_COMPILING_IN_PYPY
- #define __Pyx_PyCode_New(a, p, k, l, s, f, code, c, n, v, fv, cell, fn, name, fline, lnos)\
- PyCode_NewWithPosOnlyArgs(a, p, k, l, s, f, code, c, n, v, fv, cell, fn, name, fline, lnos)
-#else
- #define __Pyx_PyCode_New(a, p, k, l, s, f, code, c, n, v, fv, cell, fn, name, fline, lnos)\
- PyCode_New(a, k, l, s, f, code, c, n, v, fv, cell, fn, name, fline, lnos)
-#endif
-#endif
-#if PY_VERSION_HEX >= 0x030900A4 || defined(Py_IS_TYPE)
- #define __Pyx_IS_TYPE(ob, type) Py_IS_TYPE(ob, type)
-#else
- #define __Pyx_IS_TYPE(ob, type) (((const PyObject*)ob)->ob_type == (type))
-#endif
-#if PY_VERSION_HEX >= 0x030A00B1 || defined(Py_Is)
- #define __Pyx_Py_Is(x, y) Py_Is(x, y)
-#else
- #define __Pyx_Py_Is(x, y) ((x) == (y))
-#endif
-#if PY_VERSION_HEX >= 0x030A00B1 || defined(Py_IsNone)
- #define __Pyx_Py_IsNone(ob) Py_IsNone(ob)
-#else
- #define __Pyx_Py_IsNone(ob) __Pyx_Py_Is((ob), Py_None)
-#endif
-#if PY_VERSION_HEX >= 0x030A00B1 || defined(Py_IsTrue)
- #define __Pyx_Py_IsTrue(ob) Py_IsTrue(ob)
-#else
- #define __Pyx_Py_IsTrue(ob) __Pyx_Py_Is((ob), Py_True)
-#endif
-#if PY_VERSION_HEX >= 0x030A00B1 || defined(Py_IsFalse)
- #define __Pyx_Py_IsFalse(ob) Py_IsFalse(ob)
-#else
- #define __Pyx_Py_IsFalse(ob) __Pyx_Py_Is((ob), Py_False)
-#endif
-#define __Pyx_NoneAsNull(obj) (__Pyx_Py_IsNone(obj) ? NULL : (obj))
-#if PY_VERSION_HEX >= 0x030900F0 && !CYTHON_COMPILING_IN_PYPY
- #define __Pyx_PyObject_GC_IsFinalized(o) PyObject_GC_IsFinalized(o)
-#else
- #define __Pyx_PyObject_GC_IsFinalized(o) _PyGC_FINALIZED(o)
-#endif
-#ifndef CO_COROUTINE
- #define CO_COROUTINE 0x80
-#endif
-#ifndef CO_ASYNC_GENERATOR
- #define CO_ASYNC_GENERATOR 0x200
-#endif
-#ifndef Py_TPFLAGS_CHECKTYPES
- #define Py_TPFLAGS_CHECKTYPES 0
-#endif
-#ifndef Py_TPFLAGS_HAVE_INDEX
- #define Py_TPFLAGS_HAVE_INDEX 0
-#endif
-#ifndef Py_TPFLAGS_HAVE_NEWBUFFER
- #define Py_TPFLAGS_HAVE_NEWBUFFER 0
-#endif
-#ifndef Py_TPFLAGS_HAVE_FINALIZE
- #define Py_TPFLAGS_HAVE_FINALIZE 0
-#endif
-#ifndef Py_TPFLAGS_SEQUENCE
- #define Py_TPFLAGS_SEQUENCE 0
-#endif
-#ifndef Py_TPFLAGS_MAPPING
- #define Py_TPFLAGS_MAPPING 0
-#endif
-#ifndef METH_STACKLESS
- #define METH_STACKLESS 0
-#endif
-#if PY_VERSION_HEX <= 0x030700A3 || !defined(METH_FASTCALL)
- #ifndef METH_FASTCALL
- #define METH_FASTCALL 0x80
- #endif
- typedef PyObject *(*__Pyx_PyCFunctionFast) (PyObject *self, PyObject *const *args, Py_ssize_t nargs);
- typedef PyObject *(*__Pyx_PyCFunctionFastWithKeywords) (PyObject *self, PyObject *const *args,
- Py_ssize_t nargs, PyObject *kwnames);
-#else
- #define __Pyx_PyCFunctionFast _PyCFunctionFast
- #define __Pyx_PyCFunctionFastWithKeywords _PyCFunctionFastWithKeywords
-#endif
-#if CYTHON_METH_FASTCALL
- #define __Pyx_METH_FASTCALL METH_FASTCALL
- #define __Pyx_PyCFunction_FastCall __Pyx_PyCFunctionFast
- #define __Pyx_PyCFunction_FastCallWithKeywords __Pyx_PyCFunctionFastWithKeywords
-#else
- #define __Pyx_METH_FASTCALL METH_VARARGS
- #define __Pyx_PyCFunction_FastCall PyCFunction
- #define __Pyx_PyCFunction_FastCallWithKeywords PyCFunctionWithKeywords
-#endif
-#if CYTHON_VECTORCALL
- #define __pyx_vectorcallfunc vectorcallfunc
- #define __Pyx_PY_VECTORCALL_ARGUMENTS_OFFSET PY_VECTORCALL_ARGUMENTS_OFFSET
- #define __Pyx_PyVectorcall_NARGS(n) PyVectorcall_NARGS((size_t)(n))
-#elif CYTHON_BACKPORT_VECTORCALL
- typedef PyObject *(*__pyx_vectorcallfunc)(PyObject *callable, PyObject *const *args,
- size_t nargsf, PyObject *kwnames);
- #define __Pyx_PY_VECTORCALL_ARGUMENTS_OFFSET ((size_t)1 << (8 * sizeof(size_t) - 1))
- #define __Pyx_PyVectorcall_NARGS(n) ((Py_ssize_t)(((size_t)(n)) & ~__Pyx_PY_VECTORCALL_ARGUMENTS_OFFSET))
-#else
- #define __Pyx_PY_VECTORCALL_ARGUMENTS_OFFSET 0
- #define __Pyx_PyVectorcall_NARGS(n) ((Py_ssize_t)(n))
-#endif
-#if PY_VERSION_HEX < 0x030900B1
- #define __Pyx_PyType_FromModuleAndSpec(m, s, b) ((void)m, PyType_FromSpecWithBases(s, b))
- typedef PyObject *(*__Pyx_PyCMethod)(PyObject *, PyTypeObject *, PyObject *const *, size_t, PyObject *);
-#else
- #define __Pyx_PyType_FromModuleAndSpec(m, s, b) PyType_FromModuleAndSpec(m, s, b)
- #define __Pyx_PyCMethod PyCMethod
-#endif
-#ifndef METH_METHOD
- #define METH_METHOD 0x200
-#endif
-#if CYTHON_COMPILING_IN_PYPY && !defined(PyObject_Malloc)
- #define PyObject_Malloc(s) PyMem_Malloc(s)
- #define PyObject_Free(p) PyMem_Free(p)
- #define PyObject_Realloc(p) PyMem_Realloc(p)
-#endif
-#if CYTHON_COMPILING_IN_LIMITED_API
- #define __Pyx_PyCode_HasFreeVars(co) (PyCode_GetNumFree(co) > 0)
- #define __Pyx_PyFrame_SetLineNumber(frame, lineno)
-#else
- #define __Pyx_PyCode_HasFreeVars(co) (PyCode_GetNumFree(co) > 0)
- #define __Pyx_PyFrame_SetLineNumber(frame, lineno) (frame)->f_lineno = (lineno)
-#endif
-#if CYTHON_COMPILING_IN_LIMITED_API
- #define __Pyx_PyThreadState_Current PyThreadState_Get()
-#elif !CYTHON_FAST_THREAD_STATE
- #define __Pyx_PyThreadState_Current PyThreadState_GET()
-#elif PY_VERSION_HEX >= 0x03060000
- #define __Pyx_PyThreadState_Current _PyThreadState_UncheckedGet()
-#elif PY_VERSION_HEX >= 0x03000000
- #define __Pyx_PyThreadState_Current PyThreadState_GET()
-#else
- #define __Pyx_PyThreadState_Current _PyThreadState_Current
-#endif
-#if CYTHON_COMPILING_IN_LIMITED_API
-static CYTHON_INLINE void *__Pyx_PyModule_GetState(PyObject *op)
-{
- void *result;
- result = PyModule_GetState(op);
- if (!result)
- Py_FatalError("Couldn't find the module state");
- return result;
-}
-#endif
-#define __Pyx_PyObject_GetSlot(obj, name, func_ctype) __Pyx_PyType_GetSlot(Py_TYPE(obj), name, func_ctype)
-#if CYTHON_COMPILING_IN_LIMITED_API
- #define __Pyx_PyType_GetSlot(type, name, func_ctype) ((func_ctype) PyType_GetSlot((type), Py_##name))
-#else
- #define __Pyx_PyType_GetSlot(type, name, func_ctype) ((type)->name)
-#endif
-#if PY_VERSION_HEX < 0x030700A2 && !defined(PyThread_tss_create) && !defined(Py_tss_NEEDS_INIT)
-#include "pythread.h"
-#define Py_tss_NEEDS_INIT 0
-typedef int Py_tss_t;
-static CYTHON_INLINE int PyThread_tss_create(Py_tss_t *key) {
- *key = PyThread_create_key();
- return 0;
-}
-static CYTHON_INLINE Py_tss_t * PyThread_tss_alloc(void) {
- Py_tss_t *key = (Py_tss_t *)PyObject_Malloc(sizeof(Py_tss_t));
- *key = Py_tss_NEEDS_INIT;
- return key;
-}
-static CYTHON_INLINE void PyThread_tss_free(Py_tss_t *key) {
- PyObject_Free(key);
-}
-static CYTHON_INLINE int PyThread_tss_is_created(Py_tss_t *key) {
- return *key != Py_tss_NEEDS_INIT;
-}
-static CYTHON_INLINE void PyThread_tss_delete(Py_tss_t *key) {
- PyThread_delete_key(*key);
- *key = Py_tss_NEEDS_INIT;
-}
-static CYTHON_INLINE int PyThread_tss_set(Py_tss_t *key, void *value) {
- return PyThread_set_key_value(*key, value);
-}
-static CYTHON_INLINE void * PyThread_tss_get(Py_tss_t *key) {
- return PyThread_get_key_value(*key);
-}
-#endif
-#if PY_MAJOR_VERSION < 3
- #if CYTHON_COMPILING_IN_PYPY
- #if PYPY_VERSION_NUM < 0x07030600
- #if defined(__cplusplus) && __cplusplus >= 201402L
- [[deprecated("`with nogil:` inside a nogil function will not release the GIL in PyPy2 < 7.3.6")]]
- #elif defined(__GNUC__) || defined(__clang__)
- __attribute__ ((__deprecated__("`with nogil:` inside a nogil function will not release the GIL in PyPy2 < 7.3.6")))
- #elif defined(_MSC_VER)
- __declspec(deprecated("`with nogil:` inside a nogil function will not release the GIL in PyPy2 < 7.3.6"))
- #endif
- static CYTHON_INLINE int PyGILState_Check(void) {
- return 0;
- }
- #else // PYPY_VERSION_NUM < 0x07030600
- #endif // PYPY_VERSION_NUM < 0x07030600
- #else
- static CYTHON_INLINE int PyGILState_Check(void) {
- PyThreadState * tstate = _PyThreadState_Current;
- return tstate && (tstate == PyGILState_GetThisThreadState());
- }
- #endif
-#endif
-#if CYTHON_COMPILING_IN_CPYTHON || defined(_PyDict_NewPresized)
-#define __Pyx_PyDict_NewPresized(n) ((n <= 8) ? PyDict_New() : _PyDict_NewPresized(n))
-#else
-#define __Pyx_PyDict_NewPresized(n) PyDict_New()
-#endif
-#if PY_MAJOR_VERSION >= 3 || CYTHON_FUTURE_DIVISION
- #define __Pyx_PyNumber_Divide(x,y) PyNumber_TrueDivide(x,y)
- #define __Pyx_PyNumber_InPlaceDivide(x,y) PyNumber_InPlaceTrueDivide(x,y)
-#else
- #define __Pyx_PyNumber_Divide(x,y) PyNumber_Divide(x,y)
- #define __Pyx_PyNumber_InPlaceDivide(x,y) PyNumber_InPlaceDivide(x,y)
-#endif
-#if CYTHON_COMPILING_IN_CPYTHON && PY_VERSION_HEX > 0x030600B4 && CYTHON_USE_UNICODE_INTERNALS
-#define __Pyx_PyDict_GetItemStrWithError(dict, name) _PyDict_GetItem_KnownHash(dict, name, ((PyASCIIObject *) name)->hash)
-static CYTHON_INLINE PyObject * __Pyx_PyDict_GetItemStr(PyObject *dict, PyObject *name) {
- PyObject *res = __Pyx_PyDict_GetItemStrWithError(dict, name);
- if (res == NULL) PyErr_Clear();
- return res;
-}
-#elif PY_MAJOR_VERSION >= 3 && (!CYTHON_COMPILING_IN_PYPY || PYPY_VERSION_NUM >= 0x07020000)
-#define __Pyx_PyDict_GetItemStrWithError PyDict_GetItemWithError
-#define __Pyx_PyDict_GetItemStr PyDict_GetItem
-#else
-static CYTHON_INLINE PyObject * __Pyx_PyDict_GetItemStrWithError(PyObject *dict, PyObject *name) {
-#if CYTHON_COMPILING_IN_PYPY
- return PyDict_GetItem(dict, name);
-#else
- PyDictEntry *ep;
- PyDictObject *mp = (PyDictObject*) dict;
- long hash = ((PyStringObject *) name)->ob_shash;
- assert(hash != -1);
- ep = (mp->ma_lookup)(mp, name, hash);
- if (ep == NULL) {
- return NULL;
- }
- return ep->me_value;
-#endif
-}
-#define __Pyx_PyDict_GetItemStr PyDict_GetItem
-#endif
-#if CYTHON_USE_TYPE_SLOTS
- #define __Pyx_PyType_GetFlags(tp) (((PyTypeObject *)tp)->tp_flags)
- #define __Pyx_PyType_HasFeature(type, feature) ((__Pyx_PyType_GetFlags(type) & (feature)) != 0)
- #define __Pyx_PyObject_GetIterNextFunc(obj) (Py_TYPE(obj)->tp_iternext)
-#else
- #define __Pyx_PyType_GetFlags(tp) (PyType_GetFlags((PyTypeObject *)tp))
- #define __Pyx_PyType_HasFeature(type, feature) PyType_HasFeature(type, feature)
- #define __Pyx_PyObject_GetIterNextFunc(obj) PyIter_Next
-#endif
-#if CYTHON_USE_TYPE_SPECS && PY_VERSION_HEX >= 0x03080000
-#define __Pyx_PyHeapTypeObject_GC_Del(obj) {\
- PyTypeObject *type = Py_TYPE(obj);\
- assert(__Pyx_PyType_HasFeature(type, Py_TPFLAGS_HEAPTYPE));\
- PyObject_GC_Del(obj);\
- Py_DECREF(type);\
-}
-#else
-#define __Pyx_PyHeapTypeObject_GC_Del(obj) PyObject_GC_Del(obj)
-#endif
-#if CYTHON_COMPILING_IN_LIMITED_API
- #define CYTHON_PEP393_ENABLED 1
- #define __Pyx_PyUnicode_READY(op) (0)
- #define __Pyx_PyUnicode_GET_LENGTH(u) PyUnicode_GetLength(u)
- #define __Pyx_PyUnicode_READ_CHAR(u, i) PyUnicode_ReadChar(u, i)
- #define __Pyx_PyUnicode_MAX_CHAR_VALUE(u) ((void)u, 1114111U)
- #define __Pyx_PyUnicode_KIND(u) ((void)u, (0))
- #define __Pyx_PyUnicode_DATA(u) ((void*)u)
- #define __Pyx_PyUnicode_READ(k, d, i) ((void)k, PyUnicode_ReadChar((PyObject*)(d), i))
- #define __Pyx_PyUnicode_IS_TRUE(u) (0 != PyUnicode_GetLength(u))
-#elif PY_VERSION_HEX > 0x03030000 && defined(PyUnicode_KIND)
- #define CYTHON_PEP393_ENABLED 1
- #if PY_VERSION_HEX >= 0x030C0000
- #define __Pyx_PyUnicode_READY(op) (0)
- #else
- #define __Pyx_PyUnicode_READY(op) (likely(PyUnicode_IS_READY(op)) ?\
- 0 : _PyUnicode_Ready((PyObject *)(op)))
- #endif
- #define __Pyx_PyUnicode_GET_LENGTH(u) PyUnicode_GET_LENGTH(u)
- #define __Pyx_PyUnicode_READ_CHAR(u, i) PyUnicode_READ_CHAR(u, i)
- #define __Pyx_PyUnicode_MAX_CHAR_VALUE(u) PyUnicode_MAX_CHAR_VALUE(u)
- #define __Pyx_PyUnicode_KIND(u) ((int)PyUnicode_KIND(u))
- #define __Pyx_PyUnicode_DATA(u) PyUnicode_DATA(u)
- #define __Pyx_PyUnicode_READ(k, d, i) PyUnicode_READ(k, d, i)
- #define __Pyx_PyUnicode_WRITE(k, d, i, ch) PyUnicode_WRITE(k, d, i, (Py_UCS4) ch)
- #if PY_VERSION_HEX >= 0x030C0000
- #define __Pyx_PyUnicode_IS_TRUE(u) (0 != PyUnicode_GET_LENGTH(u))
- #else
- #if CYTHON_COMPILING_IN_CPYTHON && PY_VERSION_HEX >= 0x03090000
- #define __Pyx_PyUnicode_IS_TRUE(u) (0 != (likely(PyUnicode_IS_READY(u)) ? PyUnicode_GET_LENGTH(u) : ((PyCompactUnicodeObject *)(u))->wstr_length))
- #else
- #define __Pyx_PyUnicode_IS_TRUE(u) (0 != (likely(PyUnicode_IS_READY(u)) ? PyUnicode_GET_LENGTH(u) : PyUnicode_GET_SIZE(u)))
- #endif
- #endif
-#else
- #define CYTHON_PEP393_ENABLED 0
- #define PyUnicode_1BYTE_KIND 1
- #define PyUnicode_2BYTE_KIND 2
- #define PyUnicode_4BYTE_KIND 4
- #define __Pyx_PyUnicode_READY(op) (0)
- #define __Pyx_PyUnicode_GET_LENGTH(u) PyUnicode_GET_SIZE(u)
- #define __Pyx_PyUnicode_READ_CHAR(u, i) ((Py_UCS4)(PyUnicode_AS_UNICODE(u)[i]))
- #define __Pyx_PyUnicode_MAX_CHAR_VALUE(u) ((sizeof(Py_UNICODE) == 2) ? 65535U : 1114111U)
- #define __Pyx_PyUnicode_KIND(u) ((int)sizeof(Py_UNICODE))
- #define __Pyx_PyUnicode_DATA(u) ((void*)PyUnicode_AS_UNICODE(u))
- #define __Pyx_PyUnicode_READ(k, d, i) ((void)(k), (Py_UCS4)(((Py_UNICODE*)d)[i]))
- #define __Pyx_PyUnicode_WRITE(k, d, i, ch) (((void)(k)), ((Py_UNICODE*)d)[i] = (Py_UNICODE) ch)
- #define __Pyx_PyUnicode_IS_TRUE(u) (0 != PyUnicode_GET_SIZE(u))
-#endif
-#if CYTHON_COMPILING_IN_PYPY
- #define __Pyx_PyUnicode_Concat(a, b) PyNumber_Add(a, b)
- #define __Pyx_PyUnicode_ConcatSafe(a, b) PyNumber_Add(a, b)
-#else
- #define __Pyx_PyUnicode_Concat(a, b) PyUnicode_Concat(a, b)
- #define __Pyx_PyUnicode_ConcatSafe(a, b) ((unlikely((a) == Py_None) || unlikely((b) == Py_None)) ?\
- PyNumber_Add(a, b) : __Pyx_PyUnicode_Concat(a, b))
-#endif
-#if CYTHON_COMPILING_IN_PYPY
- #if !defined(PyUnicode_DecodeUnicodeEscape)
- #define PyUnicode_DecodeUnicodeEscape(s, size, errors) PyUnicode_Decode(s, size, "unicode_escape", errors)
- #endif
- #if !defined(PyUnicode_Contains) || (PY_MAJOR_VERSION == 2 && PYPY_VERSION_NUM < 0x07030500)
- #undef PyUnicode_Contains
- #define PyUnicode_Contains(u, s) PySequence_Contains(u, s)
- #endif
- #if !defined(PyByteArray_Check)
- #define PyByteArray_Check(obj) PyObject_TypeCheck(obj, &PyByteArray_Type)
- #endif
- #if !defined(PyObject_Format)
- #define PyObject_Format(obj, fmt) PyObject_CallMethod(obj, "__format__", "O", fmt)
- #endif
-#endif
-#define __Pyx_PyString_FormatSafe(a, b) ((unlikely((a) == Py_None || (PyString_Check(b) && !PyString_CheckExact(b)))) ? PyNumber_Remainder(a, b) : __Pyx_PyString_Format(a, b))
-#define __Pyx_PyUnicode_FormatSafe(a, b) ((unlikely((a) == Py_None || (PyUnicode_Check(b) && !PyUnicode_CheckExact(b)))) ? PyNumber_Remainder(a, b) : PyUnicode_Format(a, b))
-#if PY_MAJOR_VERSION >= 3
- #define __Pyx_PyString_Format(a, b) PyUnicode_Format(a, b)
-#else
- #define __Pyx_PyString_Format(a, b) PyString_Format(a, b)
-#endif
-#if PY_MAJOR_VERSION < 3 && !defined(PyObject_ASCII)
- #define PyObject_ASCII(o) PyObject_Repr(o)
-#endif
-#if PY_MAJOR_VERSION >= 3
- #define PyBaseString_Type PyUnicode_Type
- #define PyStringObject PyUnicodeObject
- #define PyString_Type PyUnicode_Type
- #define PyString_Check PyUnicode_Check
- #define PyString_CheckExact PyUnicode_CheckExact
-#ifndef PyObject_Unicode
- #define PyObject_Unicode PyObject_Str
-#endif
-#endif
-#if PY_MAJOR_VERSION >= 3
- #define __Pyx_PyBaseString_Check(obj) PyUnicode_Check(obj)
- #define __Pyx_PyBaseString_CheckExact(obj) PyUnicode_CheckExact(obj)
-#else
- #define __Pyx_PyBaseString_Check(obj) (PyString_Check(obj) || PyUnicode_Check(obj))
- #define __Pyx_PyBaseString_CheckExact(obj) (PyString_CheckExact(obj) || PyUnicode_CheckExact(obj))
-#endif
-#if CYTHON_COMPILING_IN_CPYTHON
- #define __Pyx_PySequence_ListKeepNew(obj)\
- (likely(PyList_CheckExact(obj) && Py_REFCNT(obj) == 1) ? __Pyx_NewRef(obj) : PySequence_List(obj))
-#else
- #define __Pyx_PySequence_ListKeepNew(obj) PySequence_List(obj)
-#endif
-#ifndef PySet_CheckExact
- #define PySet_CheckExact(obj) __Pyx_IS_TYPE(obj, &PySet_Type)
-#endif
-#if PY_VERSION_HEX >= 0x030900A4
- #define __Pyx_SET_REFCNT(obj, refcnt) Py_SET_REFCNT(obj, refcnt)
- #define __Pyx_SET_SIZE(obj, size) Py_SET_SIZE(obj, size)
-#else
- #define __Pyx_SET_REFCNT(obj, refcnt) Py_REFCNT(obj) = (refcnt)
- #define __Pyx_SET_SIZE(obj, size) Py_SIZE(obj) = (size)
-#endif
-#if CYTHON_ASSUME_SAFE_MACROS
- #define __Pyx_PySequence_SIZE(seq) Py_SIZE(seq)
-#else
- #define __Pyx_PySequence_SIZE(seq) PySequence_Size(seq)
-#endif
-#if PY_MAJOR_VERSION >= 3
- #define PyIntObject PyLongObject
- #define PyInt_Type PyLong_Type
- #define PyInt_Check(op) PyLong_Check(op)
- #define PyInt_CheckExact(op) PyLong_CheckExact(op)
- #define __Pyx_Py3Int_Check(op) PyLong_Check(op)
- #define __Pyx_Py3Int_CheckExact(op) PyLong_CheckExact(op)
- #define PyInt_FromString PyLong_FromString
- #define PyInt_FromUnicode PyLong_FromUnicode
- #define PyInt_FromLong PyLong_FromLong
- #define PyInt_FromSize_t PyLong_FromSize_t
- #define PyInt_FromSsize_t PyLong_FromSsize_t
- #define PyInt_AsLong PyLong_AsLong
- #define PyInt_AS_LONG PyLong_AS_LONG
- #define PyInt_AsSsize_t PyLong_AsSsize_t
- #define PyInt_AsUnsignedLongMask PyLong_AsUnsignedLongMask
- #define PyInt_AsUnsignedLongLongMask PyLong_AsUnsignedLongLongMask
- #define PyNumber_Int PyNumber_Long
-#else
- #define __Pyx_Py3Int_Check(op) (PyLong_Check(op) || PyInt_Check(op))
- #define __Pyx_Py3Int_CheckExact(op) (PyLong_CheckExact(op) || PyInt_CheckExact(op))
-#endif
-#if PY_MAJOR_VERSION >= 3
- #define PyBoolObject PyLongObject
-#endif
-#if PY_MAJOR_VERSION >= 3 && CYTHON_COMPILING_IN_PYPY
- #ifndef PyUnicode_InternFromString
- #define PyUnicode_InternFromString(s) PyUnicode_FromString(s)
- #endif
-#endif
-#if PY_VERSION_HEX < 0x030200A4
- typedef long Py_hash_t;
- #define __Pyx_PyInt_FromHash_t PyInt_FromLong
- #define __Pyx_PyInt_AsHash_t __Pyx_PyIndex_AsHash_t
-#else
- #define __Pyx_PyInt_FromHash_t PyInt_FromSsize_t
- #define __Pyx_PyInt_AsHash_t __Pyx_PyIndex_AsSsize_t
-#endif
-#if CYTHON_USE_ASYNC_SLOTS
- #if PY_VERSION_HEX >= 0x030500B1
- #define __Pyx_PyAsyncMethodsStruct PyAsyncMethods
- #define __Pyx_PyType_AsAsync(obj) (Py_TYPE(obj)->tp_as_async)
- #else
- #define __Pyx_PyType_AsAsync(obj) ((__Pyx_PyAsyncMethodsStruct*) (Py_TYPE(obj)->tp_reserved))
- #endif
-#else
- #define __Pyx_PyType_AsAsync(obj) NULL
-#endif
-#ifndef __Pyx_PyAsyncMethodsStruct
- typedef struct {
- unaryfunc am_await;
- unaryfunc am_aiter;
- unaryfunc am_anext;
- } __Pyx_PyAsyncMethodsStruct;
-#endif
-
-#if defined(_WIN32) || defined(WIN32) || defined(MS_WINDOWS)
- #if !defined(_USE_MATH_DEFINES)
- #define _USE_MATH_DEFINES
- #endif
-#endif
-#include
-#ifdef NAN
-#define __PYX_NAN() ((float) NAN)
-#else
-static CYTHON_INLINE float __PYX_NAN() {
- float value;
- memset(&value, 0xFF, sizeof(value));
- return value;
-}
-#endif
-#if defined(__CYGWIN__) && defined(_LDBL_EQ_DBL)
-#define __Pyx_truncl trunc
-#else
-#define __Pyx_truncl truncl
-#endif
-
-#define __PYX_MARK_ERR_POS(f_index, lineno) \
- { __pyx_filename = __pyx_f[f_index]; (void)__pyx_filename; __pyx_lineno = lineno; (void)__pyx_lineno; __pyx_clineno = __LINE__; (void)__pyx_clineno; }
-#define __PYX_ERR(f_index, lineno, Ln_error) \
- { __PYX_MARK_ERR_POS(f_index, lineno) goto Ln_error; }
-
-#ifdef CYTHON_EXTERN_C
- #undef __PYX_EXTERN_C
- #define __PYX_EXTERN_C CYTHON_EXTERN_C
-#elif defined(__PYX_EXTERN_C)
- #ifdef _MSC_VER
- #pragma message ("Please do not define the '__PYX_EXTERN_C' macro externally. Use 'CYTHON_EXTERN_C' instead.")
- #else
- #warning Please do not define the '__PYX_EXTERN_C' macro externally. Use 'CYTHON_EXTERN_C' instead.
- #endif
-#else
- #ifdef __cplusplus
- #define __PYX_EXTERN_C extern "C"
- #else
- #define __PYX_EXTERN_C extern
- #endif
-#endif
-
-#define __PYX_HAVE__fontTools__cu2qu__cu2qu
-#define __PYX_HAVE_API__fontTools__cu2qu__cu2qu
-/* Early includes */
-#ifdef _OPENMP
-#include
-#endif /* _OPENMP */
-
-#if defined(PYREX_WITHOUT_ASSERTIONS) && !defined(CYTHON_WITHOUT_ASSERTIONS)
-#define CYTHON_WITHOUT_ASSERTIONS
-#endif
-
-typedef struct {PyObject **p; const char *s; const Py_ssize_t n; const char* encoding;
- const char is_unicode; const char is_str; const char intern; } __Pyx_StringTabEntry;
-
-#define __PYX_DEFAULT_STRING_ENCODING_IS_ASCII 0
-#define __PYX_DEFAULT_STRING_ENCODING_IS_UTF8 0
-#define __PYX_DEFAULT_STRING_ENCODING_IS_DEFAULT (PY_MAJOR_VERSION >= 3 && __PYX_DEFAULT_STRING_ENCODING_IS_UTF8)
-#define __PYX_DEFAULT_STRING_ENCODING ""
-#define __Pyx_PyObject_FromString __Pyx_PyBytes_FromString
-#define __Pyx_PyObject_FromStringAndSize __Pyx_PyBytes_FromStringAndSize
-#define __Pyx_uchar_cast(c) ((unsigned char)c)
-#define __Pyx_long_cast(x) ((long)x)
-#define __Pyx_fits_Py_ssize_t(v, type, is_signed) (\
- (sizeof(type) < sizeof(Py_ssize_t)) ||\
- (sizeof(type) > sizeof(Py_ssize_t) &&\
- likely(v < (type)PY_SSIZE_T_MAX ||\
- v == (type)PY_SSIZE_T_MAX) &&\
- (!is_signed || likely(v > (type)PY_SSIZE_T_MIN ||\
- v == (type)PY_SSIZE_T_MIN))) ||\
- (sizeof(type) == sizeof(Py_ssize_t) &&\
- (is_signed || likely(v < (type)PY_SSIZE_T_MAX ||\
- v == (type)PY_SSIZE_T_MAX))) )
-static CYTHON_INLINE int __Pyx_is_valid_index(Py_ssize_t i, Py_ssize_t limit) {
- return (size_t) i < (size_t) limit;
-}
-#if defined (__cplusplus) && __cplusplus >= 201103L
- #include
- #define __Pyx_sst_abs(value) std::abs(value)
-#elif SIZEOF_INT >= SIZEOF_SIZE_T
- #define __Pyx_sst_abs(value) abs(value)
-#elif SIZEOF_LONG >= SIZEOF_SIZE_T
- #define __Pyx_sst_abs(value) labs(value)
-#elif defined (_MSC_VER)
- #define __Pyx_sst_abs(value) ((Py_ssize_t)_abs64(value))
-#elif defined (__STDC_VERSION__) && __STDC_VERSION__ >= 199901L
- #define __Pyx_sst_abs(value) llabs(value)
-#elif defined (__GNUC__)
- #define __Pyx_sst_abs(value) __builtin_llabs(value)
-#else
- #define __Pyx_sst_abs(value) ((value<0) ? -value : value)
-#endif
-static CYTHON_INLINE const char* __Pyx_PyObject_AsString(PyObject*);
-static CYTHON_INLINE const char* __Pyx_PyObject_AsStringAndSize(PyObject*, Py_ssize_t* length);
-#define __Pyx_PyByteArray_FromString(s) PyByteArray_FromStringAndSize((const char*)s, strlen((const char*)s))
-#define __Pyx_PyByteArray_FromStringAndSize(s, l) PyByteArray_FromStringAndSize((const char*)s, l)
-#define __Pyx_PyBytes_FromString PyBytes_FromString
-#define __Pyx_PyBytes_FromStringAndSize PyBytes_FromStringAndSize
-static CYTHON_INLINE PyObject* __Pyx_PyUnicode_FromString(const char*);
-#if PY_MAJOR_VERSION < 3
- #define __Pyx_PyStr_FromString __Pyx_PyBytes_FromString
- #define __Pyx_PyStr_FromStringAndSize __Pyx_PyBytes_FromStringAndSize
-#else
- #define __Pyx_PyStr_FromString __Pyx_PyUnicode_FromString
- #define __Pyx_PyStr_FromStringAndSize __Pyx_PyUnicode_FromStringAndSize
-#endif
-#define __Pyx_PyBytes_AsWritableString(s) ((char*) PyBytes_AS_STRING(s))
-#define __Pyx_PyBytes_AsWritableSString(s) ((signed char*) PyBytes_AS_STRING(s))
-#define __Pyx_PyBytes_AsWritableUString(s) ((unsigned char*) PyBytes_AS_STRING(s))
-#define __Pyx_PyBytes_AsString(s) ((const char*) PyBytes_AS_STRING(s))
-#define __Pyx_PyBytes_AsSString(s) ((const signed char*) PyBytes_AS_STRING(s))
-#define __Pyx_PyBytes_AsUString(s) ((const unsigned char*) PyBytes_AS_STRING(s))
-#define __Pyx_PyObject_AsWritableString(s) ((char*)(__pyx_uintptr_t) __Pyx_PyObject_AsString(s))
-#define __Pyx_PyObject_AsWritableSString(s) ((signed char*)(__pyx_uintptr_t) __Pyx_PyObject_AsString(s))
-#define __Pyx_PyObject_AsWritableUString(s) ((unsigned char*)(__pyx_uintptr_t) __Pyx_PyObject_AsString(s))
-#define __Pyx_PyObject_AsSString(s) ((const signed char*) __Pyx_PyObject_AsString(s))
-#define __Pyx_PyObject_AsUString(s) ((const unsigned char*) __Pyx_PyObject_AsString(s))
-#define __Pyx_PyObject_FromCString(s) __Pyx_PyObject_FromString((const char*)s)
-#define __Pyx_PyBytes_FromCString(s) __Pyx_PyBytes_FromString((const char*)s)
-#define __Pyx_PyByteArray_FromCString(s) __Pyx_PyByteArray_FromString((const char*)s)
-#define __Pyx_PyStr_FromCString(s) __Pyx_PyStr_FromString((const char*)s)
-#define __Pyx_PyUnicode_FromCString(s) __Pyx_PyUnicode_FromString((const char*)s)
-#if CYTHON_COMPILING_IN_LIMITED_API
-static CYTHON_INLINE size_t __Pyx_Py_UNICODE_strlen(const wchar_t *u)
-{
- const wchar_t *u_end = u;
- while (*u_end++) ;
- return (size_t)(u_end - u - 1);
-}
-#else
-static CYTHON_INLINE size_t __Pyx_Py_UNICODE_strlen(const Py_UNICODE *u)
-{
- const Py_UNICODE *u_end = u;
- while (*u_end++) ;
- return (size_t)(u_end - u - 1);
-}
-#endif
-#define __Pyx_PyUnicode_FromOrdinal(o) PyUnicode_FromOrdinal((int)o)
-#define __Pyx_PyUnicode_FromUnicode(u) PyUnicode_FromUnicode(u, __Pyx_Py_UNICODE_strlen(u))
-#define __Pyx_PyUnicode_FromUnicodeAndLength PyUnicode_FromUnicode
-#define __Pyx_PyUnicode_AsUnicode PyUnicode_AsUnicode
-#define __Pyx_NewRef(obj) (Py_INCREF(obj), obj)
-#define __Pyx_Owned_Py_None(b) __Pyx_NewRef(Py_None)
-static CYTHON_INLINE PyObject * __Pyx_PyBool_FromLong(long b);
-static CYTHON_INLINE int __Pyx_PyObject_IsTrue(PyObject*);
-static CYTHON_INLINE int __Pyx_PyObject_IsTrueAndDecref(PyObject*);
-static CYTHON_INLINE PyObject* __Pyx_PyNumber_IntOrLong(PyObject* x);
-#define __Pyx_PySequence_Tuple(obj)\
- (likely(PyTuple_CheckExact(obj)) ? __Pyx_NewRef(obj) : PySequence_Tuple(obj))
-static CYTHON_INLINE Py_ssize_t __Pyx_PyIndex_AsSsize_t(PyObject*);
-static CYTHON_INLINE PyObject * __Pyx_PyInt_FromSize_t(size_t);
-static CYTHON_INLINE Py_hash_t __Pyx_PyIndex_AsHash_t(PyObject*);
-#if CYTHON_ASSUME_SAFE_MACROS
-#define __pyx_PyFloat_AsDouble(x) (PyFloat_CheckExact(x) ? PyFloat_AS_DOUBLE(x) : PyFloat_AsDouble(x))
-#else
-#define __pyx_PyFloat_AsDouble(x) PyFloat_AsDouble(x)
-#endif
-#define __pyx_PyFloat_AsFloat(x) ((float) __pyx_PyFloat_AsDouble(x))
-#if PY_MAJOR_VERSION >= 3
-#define __Pyx_PyNumber_Int(x) (PyLong_CheckExact(x) ? __Pyx_NewRef(x) : PyNumber_Long(x))
-#else
-#define __Pyx_PyNumber_Int(x) (PyInt_CheckExact(x) ? __Pyx_NewRef(x) : PyNumber_Int(x))
-#endif
-#if CYTHON_USE_PYLONG_INTERNALS
- #if PY_VERSION_HEX >= 0x030C00A7
- #ifndef _PyLong_SIGN_MASK
- #define _PyLong_SIGN_MASK 3
- #endif
- #ifndef _PyLong_NON_SIZE_BITS
- #define _PyLong_NON_SIZE_BITS 3
- #endif
- #define __Pyx_PyLong_Sign(x) (((PyLongObject*)x)->long_value.lv_tag & _PyLong_SIGN_MASK)
- #define __Pyx_PyLong_IsNeg(x) ((__Pyx_PyLong_Sign(x) & 2) != 0)
- #define __Pyx_PyLong_IsNonNeg(x) (!__Pyx_PyLong_IsNeg(x))
- #define __Pyx_PyLong_IsZero(x) (__Pyx_PyLong_Sign(x) & 1)
- #define __Pyx_PyLong_IsPos(x) (__Pyx_PyLong_Sign(x) == 0)
- #define __Pyx_PyLong_CompactValueUnsigned(x) (__Pyx_PyLong_Digits(x)[0])
- #define __Pyx_PyLong_DigitCount(x) ((Py_ssize_t) (((PyLongObject*)x)->long_value.lv_tag >> _PyLong_NON_SIZE_BITS))
- #define __Pyx_PyLong_SignedDigitCount(x)\
- ((1 - (Py_ssize_t) __Pyx_PyLong_Sign(x)) * __Pyx_PyLong_DigitCount(x))
- #if defined(PyUnstable_Long_IsCompact) && defined(PyUnstable_Long_CompactValue)
- #define __Pyx_PyLong_IsCompact(x) PyUnstable_Long_IsCompact((PyLongObject*) x)
- #define __Pyx_PyLong_CompactValue(x) PyUnstable_Long_CompactValue((PyLongObject*) x)
- #else
- #define __Pyx_PyLong_IsCompact(x) (((PyLongObject*)x)->long_value.lv_tag < (2 << _PyLong_NON_SIZE_BITS))
- #define __Pyx_PyLong_CompactValue(x) ((1 - (Py_ssize_t) __Pyx_PyLong_Sign(x)) * (Py_ssize_t) __Pyx_PyLong_Digits(x)[0])
- #endif
- typedef Py_ssize_t __Pyx_compact_pylong;
- typedef size_t __Pyx_compact_upylong;
- #else // Py < 3.12
- #define __Pyx_PyLong_IsNeg(x) (Py_SIZE(x) < 0)
- #define __Pyx_PyLong_IsNonNeg(x) (Py_SIZE(x) >= 0)
- #define __Pyx_PyLong_IsZero(x) (Py_SIZE(x) == 0)
- #define __Pyx_PyLong_IsPos(x) (Py_SIZE(x) > 0)
- #define __Pyx_PyLong_CompactValueUnsigned(x) ((Py_SIZE(x) == 0) ? 0 : __Pyx_PyLong_Digits(x)[0])
- #define __Pyx_PyLong_DigitCount(x) __Pyx_sst_abs(Py_SIZE(x))
- #define __Pyx_PyLong_SignedDigitCount(x) Py_SIZE(x)
- #define __Pyx_PyLong_IsCompact(x) (Py_SIZE(x) == 0 || Py_SIZE(x) == 1 || Py_SIZE(x) == -1)
- #define __Pyx_PyLong_CompactValue(x)\
- ((Py_SIZE(x) == 0) ? (sdigit) 0 : ((Py_SIZE(x) < 0) ? -(sdigit)__Pyx_PyLong_Digits(x)[0] : (sdigit)__Pyx_PyLong_Digits(x)[0]))
- typedef sdigit __Pyx_compact_pylong;
- typedef digit __Pyx_compact_upylong;
- #endif
- #if PY_VERSION_HEX >= 0x030C00A5
- #define __Pyx_PyLong_Digits(x) (((PyLongObject*)x)->long_value.ob_digit)
- #else
- #define __Pyx_PyLong_Digits(x) (((PyLongObject*)x)->ob_digit)
- #endif
-#endif
-#if PY_MAJOR_VERSION < 3 && __PYX_DEFAULT_STRING_ENCODING_IS_ASCII
-static int __Pyx_sys_getdefaultencoding_not_ascii;
-static int __Pyx_init_sys_getdefaultencoding_params(void) {
- PyObject* sys;
- PyObject* default_encoding = NULL;
- PyObject* ascii_chars_u = NULL;
- PyObject* ascii_chars_b = NULL;
- const char* default_encoding_c;
- sys = PyImport_ImportModule("sys");
- if (!sys) goto bad;
- default_encoding = PyObject_CallMethod(sys, (char*) "getdefaultencoding", NULL);
- Py_DECREF(sys);
- if (!default_encoding) goto bad;
- default_encoding_c = PyBytes_AsString(default_encoding);
- if (!default_encoding_c) goto bad;
- if (strcmp(default_encoding_c, "ascii") == 0) {
- __Pyx_sys_getdefaultencoding_not_ascii = 0;
- } else {
- char ascii_chars[128];
- int c;
- for (c = 0; c < 128; c++) {
- ascii_chars[c] = (char) c;
- }
- __Pyx_sys_getdefaultencoding_not_ascii = 1;
- ascii_chars_u = PyUnicode_DecodeASCII(ascii_chars, 128, NULL);
- if (!ascii_chars_u) goto bad;
- ascii_chars_b = PyUnicode_AsEncodedString(ascii_chars_u, default_encoding_c, NULL);
- if (!ascii_chars_b || !PyBytes_Check(ascii_chars_b) || memcmp(ascii_chars, PyBytes_AS_STRING(ascii_chars_b), 128) != 0) {
- PyErr_Format(
- PyExc_ValueError,
- "This module compiled with c_string_encoding=ascii, but default encoding '%.200s' is not a superset of ascii.",
- default_encoding_c);
- goto bad;
- }
- Py_DECREF(ascii_chars_u);
- Py_DECREF(ascii_chars_b);
- }
- Py_DECREF(default_encoding);
- return 0;
-bad:
- Py_XDECREF(default_encoding);
- Py_XDECREF(ascii_chars_u);
- Py_XDECREF(ascii_chars_b);
- return -1;
-}
-#endif
-#if __PYX_DEFAULT_STRING_ENCODING_IS_DEFAULT && PY_MAJOR_VERSION >= 3
-#define __Pyx_PyUnicode_FromStringAndSize(c_str, size) PyUnicode_DecodeUTF8(c_str, size, NULL)
-#else
-#define __Pyx_PyUnicode_FromStringAndSize(c_str, size) PyUnicode_Decode(c_str, size, __PYX_DEFAULT_STRING_ENCODING, NULL)
-#if __PYX_DEFAULT_STRING_ENCODING_IS_DEFAULT
-static char* __PYX_DEFAULT_STRING_ENCODING;
-static int __Pyx_init_sys_getdefaultencoding_params(void) {
- PyObject* sys;
- PyObject* default_encoding = NULL;
- char* default_encoding_c;
- sys = PyImport_ImportModule("sys");
- if (!sys) goto bad;
- default_encoding = PyObject_CallMethod(sys, (char*) (const char*) "getdefaultencoding", NULL);
- Py_DECREF(sys);
- if (!default_encoding) goto bad;
- default_encoding_c = PyBytes_AsString(default_encoding);
- if (!default_encoding_c) goto bad;
- __PYX_DEFAULT_STRING_ENCODING = (char*) malloc(strlen(default_encoding_c) + 1);
- if (!__PYX_DEFAULT_STRING_ENCODING) goto bad;
- strcpy(__PYX_DEFAULT_STRING_ENCODING, default_encoding_c);
- Py_DECREF(default_encoding);
- return 0;
-bad:
- Py_XDECREF(default_encoding);
- return -1;
-}
-#endif
-#endif
-
-
-/* Test for GCC > 2.95 */
-#if defined(__GNUC__) && (__GNUC__ > 2 || (__GNUC__ == 2 && (__GNUC_MINOR__ > 95)))
- #define likely(x) __builtin_expect(!!(x), 1)
- #define unlikely(x) __builtin_expect(!!(x), 0)
-#else /* !__GNUC__ or GCC < 2.95 */
- #define likely(x) (x)
- #define unlikely(x) (x)
-#endif /* __GNUC__ */
-static CYTHON_INLINE void __Pyx_pretend_to_initialize(void* ptr) { (void)ptr; }
-
-#if !CYTHON_USE_MODULE_STATE
-static PyObject *__pyx_m = NULL;
-#endif
-static int __pyx_lineno;
-static int __pyx_clineno = 0;
-static const char * __pyx_cfilenm = __FILE__;
-static const char *__pyx_filename;
-
-/* Header.proto */
-#if !defined(CYTHON_CCOMPLEX)
- #if defined(__cplusplus)
- #define CYTHON_CCOMPLEX 1
- #elif (defined(_Complex_I) && !defined(_MSC_VER)) || ((defined (__STDC_VERSION__) && __STDC_VERSION__ >= 201112L) && !defined(__STDC_NO_COMPLEX__))
- #define CYTHON_CCOMPLEX 1
- #else
- #define CYTHON_CCOMPLEX 0
- #endif
-#endif
-#if CYTHON_CCOMPLEX
- #ifdef __cplusplus
- #include
- #else
- #include
- #endif
-#endif
-#if CYTHON_CCOMPLEX && !defined(__cplusplus) && defined(__sun__) && defined(__GNUC__)
- #undef _Complex_I
- #define _Complex_I 1.0fj
-#endif
-
-/* #### Code section: filename_table ### */
-
-static const char *__pyx_f[] = {
- "Lib/fontTools/cu2qu/cu2qu.py",
-};
-/* #### Code section: utility_code_proto_before_types ### */
-/* #### Code section: numeric_typedefs ### */
-/* #### Code section: complex_type_declarations ### */
-/* Declarations.proto */
-#if CYTHON_CCOMPLEX && (1) && (!0 || __cplusplus)
- #ifdef __cplusplus
- typedef ::std::complex< double > __pyx_t_double_complex;
- #else
- typedef double _Complex __pyx_t_double_complex;
- #endif
-#else
- typedef struct { double real, imag; } __pyx_t_double_complex;
-#endif
-static CYTHON_INLINE __pyx_t_double_complex __pyx_t_double_complex_from_parts(double, double);
-
-/* #### Code section: type_declarations ### */
-
-/*--- Type declarations ---*/
-struct __pyx_obj_9fontTools_5cu2qu_5cu2qu___pyx_scope_struct___split_cubic_into_n_gen;
-
-/* "fontTools/cu2qu/cu2qu.py":127
- *
- *
- * @cython.locals( # <<<<<<<<<<<<<<
- * p0=cython.complex,
- * p1=cython.complex,
- */
-struct __pyx_obj_9fontTools_5cu2qu_5cu2qu___pyx_scope_struct___split_cubic_into_n_gen {
- PyObject_HEAD
- __pyx_t_double_complex __pyx_v_a;
- __pyx_t_double_complex __pyx_v_a1;
- __pyx_t_double_complex __pyx_v_b;
- __pyx_t_double_complex __pyx_v_b1;
- __pyx_t_double_complex __pyx_v_c;
- __pyx_t_double_complex __pyx_v_c1;
- __pyx_t_double_complex __pyx_v_d;
- __pyx_t_double_complex __pyx_v_d1;
- double __pyx_v_delta_2;
- double __pyx_v_delta_3;
- double __pyx_v_dt;
- int __pyx_v_i;
- int __pyx_v_n;
- __pyx_t_double_complex __pyx_v_p0;
- __pyx_t_double_complex __pyx_v_p1;
- __pyx_t_double_complex __pyx_v_p2;
- __pyx_t_double_complex __pyx_v_p3;
- double __pyx_v_t1;
- double __pyx_v_t1_2;
- int __pyx_t_0;
- int __pyx_t_1;
- int __pyx_t_2;
-};
-
-/* #### Code section: utility_code_proto ### */
-
-/* --- Runtime support code (head) --- */
-/* Refnanny.proto */
-#ifndef CYTHON_REFNANNY
- #define CYTHON_REFNANNY 0
-#endif
-#if CYTHON_REFNANNY
- typedef struct {
- void (*INCREF)(void*, PyObject*, Py_ssize_t);
- void (*DECREF)(void*, PyObject*, Py_ssize_t);
- void (*GOTREF)(void*, PyObject*, Py_ssize_t);
- void (*GIVEREF)(void*, PyObject*, Py_ssize_t);
- void* (*SetupContext)(const char*, Py_ssize_t, const char*);
- void (*FinishContext)(void**);
- } __Pyx_RefNannyAPIStruct;
- static __Pyx_RefNannyAPIStruct *__Pyx_RefNanny = NULL;
- static __Pyx_RefNannyAPIStruct *__Pyx_RefNannyImportAPI(const char *modname);
- #define __Pyx_RefNannyDeclarations void *__pyx_refnanny = NULL;
-#ifdef WITH_THREAD
- #define __Pyx_RefNannySetupContext(name, acquire_gil)\
- if (acquire_gil) {\
- PyGILState_STATE __pyx_gilstate_save = PyGILState_Ensure();\
- __pyx_refnanny = __Pyx_RefNanny->SetupContext((name), (__LINE__), (__FILE__));\
- PyGILState_Release(__pyx_gilstate_save);\
- } else {\
- __pyx_refnanny = __Pyx_RefNanny->SetupContext((name), (__LINE__), (__FILE__));\
- }
- #define __Pyx_RefNannyFinishContextNogil() {\
- PyGILState_STATE __pyx_gilstate_save = PyGILState_Ensure();\
- __Pyx_RefNannyFinishContext();\
- PyGILState_Release(__pyx_gilstate_save);\
- }
-#else
- #define __Pyx_RefNannySetupContext(name, acquire_gil)\
- __pyx_refnanny = __Pyx_RefNanny->SetupContext((name), (__LINE__), (__FILE__))
- #define __Pyx_RefNannyFinishContextNogil() __Pyx_RefNannyFinishContext()
-#endif
- #define __Pyx_RefNannyFinishContextNogil() {\
- PyGILState_STATE __pyx_gilstate_save = PyGILState_Ensure();\
- __Pyx_RefNannyFinishContext();\
- PyGILState_Release(__pyx_gilstate_save);\
- }
- #define __Pyx_RefNannyFinishContext()\
- __Pyx_RefNanny->FinishContext(&__pyx_refnanny)
- #define __Pyx_INCREF(r) __Pyx_RefNanny->INCREF(__pyx_refnanny, (PyObject *)(r), (__LINE__))
- #define __Pyx_DECREF(r) __Pyx_RefNanny->DECREF(__pyx_refnanny, (PyObject *)(r), (__LINE__))
- #define __Pyx_GOTREF(r) __Pyx_RefNanny->GOTREF(__pyx_refnanny, (PyObject *)(r), (__LINE__))
- #define __Pyx_GIVEREF(r) __Pyx_RefNanny->GIVEREF(__pyx_refnanny, (PyObject *)(r), (__LINE__))
- #define __Pyx_XINCREF(r) do { if((r) == NULL); else {__Pyx_INCREF(r); }} while(0)
- #define __Pyx_XDECREF(r) do { if((r) == NULL); else {__Pyx_DECREF(r); }} while(0)
- #define __Pyx_XGOTREF(r) do { if((r) == NULL); else {__Pyx_GOTREF(r); }} while(0)
- #define __Pyx_XGIVEREF(r) do { if((r) == NULL); else {__Pyx_GIVEREF(r);}} while(0)
-#else
- #define __Pyx_RefNannyDeclarations
- #define __Pyx_RefNannySetupContext(name, acquire_gil)
- #define __Pyx_RefNannyFinishContextNogil()
- #define __Pyx_RefNannyFinishContext()
- #define __Pyx_INCREF(r) Py_INCREF(r)
- #define __Pyx_DECREF(r) Py_DECREF(r)
- #define __Pyx_GOTREF(r)
- #define __Pyx_GIVEREF(r)
- #define __Pyx_XINCREF(r) Py_XINCREF(r)
- #define __Pyx_XDECREF(r) Py_XDECREF(r)
- #define __Pyx_XGOTREF(r)
- #define __Pyx_XGIVEREF(r)
-#endif
-#define __Pyx_Py_XDECREF_SET(r, v) do {\
- PyObject *tmp = (PyObject *) r;\
- r = v; Py_XDECREF(tmp);\
- } while (0)
-#define __Pyx_XDECREF_SET(r, v) do {\
- PyObject *tmp = (PyObject *) r;\
- r = v; __Pyx_XDECREF(tmp);\
- } while (0)
-#define __Pyx_DECREF_SET(r, v) do {\
- PyObject *tmp = (PyObject *) r;\
- r = v; __Pyx_DECREF(tmp);\
- } while (0)
-#define __Pyx_CLEAR(r) do { PyObject* tmp = ((PyObject*)(r)); r = NULL; __Pyx_DECREF(tmp);} while(0)
-#define __Pyx_XCLEAR(r) do { if((r) != NULL) {PyObject* tmp = ((PyObject*)(r)); r = NULL; __Pyx_DECREF(tmp);}} while(0)
-
-/* PyErrExceptionMatches.proto */
-#if CYTHON_FAST_THREAD_STATE
-#define __Pyx_PyErr_ExceptionMatches(err) __Pyx_PyErr_ExceptionMatchesInState(__pyx_tstate, err)
-static CYTHON_INLINE int __Pyx_PyErr_ExceptionMatchesInState(PyThreadState* tstate, PyObject* err);
-#else
-#define __Pyx_PyErr_ExceptionMatches(err) PyErr_ExceptionMatches(err)
-#endif
-
-/* PyThreadStateGet.proto */
-#if CYTHON_FAST_THREAD_STATE
-#define __Pyx_PyThreadState_declare PyThreadState *__pyx_tstate;
-#define __Pyx_PyThreadState_assign __pyx_tstate = __Pyx_PyThreadState_Current;
-#if PY_VERSION_HEX >= 0x030C00A6
-#define __Pyx_PyErr_Occurred() (__pyx_tstate->current_exception != NULL)
-#define __Pyx_PyErr_CurrentExceptionType() (__pyx_tstate->current_exception ? (PyObject*) Py_TYPE(__pyx_tstate->current_exception) : (PyObject*) NULL)
-#else
-#define __Pyx_PyErr_Occurred() (__pyx_tstate->curexc_type != NULL)
-#define __Pyx_PyErr_CurrentExceptionType() (__pyx_tstate->curexc_type)
-#endif
-#else
-#define __Pyx_PyThreadState_declare
-#define __Pyx_PyThreadState_assign
-#define __Pyx_PyErr_Occurred() (PyErr_Occurred() != NULL)
-#define __Pyx_PyErr_CurrentExceptionType() PyErr_Occurred()
-#endif
-
-/* PyErrFetchRestore.proto */
-#if CYTHON_FAST_THREAD_STATE
-#define __Pyx_PyErr_Clear() __Pyx_ErrRestore(NULL, NULL, NULL)
-#define __Pyx_ErrRestoreWithState(type, value, tb) __Pyx_ErrRestoreInState(PyThreadState_GET(), type, value, tb)
-#define __Pyx_ErrFetchWithState(type, value, tb) __Pyx_ErrFetchInState(PyThreadState_GET(), type, value, tb)
-#define __Pyx_ErrRestore(type, value, tb) __Pyx_ErrRestoreInState(__pyx_tstate, type, value, tb)
-#define __Pyx_ErrFetch(type, value, tb) __Pyx_ErrFetchInState(__pyx_tstate, type, value, tb)
-static CYTHON_INLINE void __Pyx_ErrRestoreInState(PyThreadState *tstate, PyObject *type, PyObject *value, PyObject *tb);
-static CYTHON_INLINE void __Pyx_ErrFetchInState(PyThreadState *tstate, PyObject **type, PyObject **value, PyObject **tb);
-#if CYTHON_COMPILING_IN_CPYTHON && PY_VERSION_HEX < 0x030C00A6
-#define __Pyx_PyErr_SetNone(exc) (Py_INCREF(exc), __Pyx_ErrRestore((exc), NULL, NULL))
-#else
-#define __Pyx_PyErr_SetNone(exc) PyErr_SetNone(exc)
-#endif
-#else
-#define __Pyx_PyErr_Clear() PyErr_Clear()
-#define __Pyx_PyErr_SetNone(exc) PyErr_SetNone(exc)
-#define __Pyx_ErrRestoreWithState(type, value, tb) PyErr_Restore(type, value, tb)
-#define __Pyx_ErrFetchWithState(type, value, tb) PyErr_Fetch(type, value, tb)
-#define __Pyx_ErrRestoreInState(tstate, type, value, tb) PyErr_Restore(type, value, tb)
-#define __Pyx_ErrFetchInState(tstate, type, value, tb) PyErr_Fetch(type, value, tb)
-#define __Pyx_ErrRestore(type, value, tb) PyErr_Restore(type, value, tb)
-#define __Pyx_ErrFetch(type, value, tb) PyErr_Fetch(type, value, tb)
-#endif
-
-/* PyObjectGetAttrStr.proto */
-#if CYTHON_USE_TYPE_SLOTS
-static CYTHON_INLINE PyObject* __Pyx_PyObject_GetAttrStr(PyObject* obj, PyObject* attr_name);
-#else
-#define __Pyx_PyObject_GetAttrStr(o,n) PyObject_GetAttr(o,n)
-#endif
-
-/* PyObjectGetAttrStrNoError.proto */
-static CYTHON_INLINE PyObject* __Pyx_PyObject_GetAttrStrNoError(PyObject* obj, PyObject* attr_name);
-
-/* GetBuiltinName.proto */
-static PyObject *__Pyx_GetBuiltinName(PyObject *name);
-
-/* PyIntCompare.proto */
-static CYTHON_INLINE int __Pyx_PyInt_BoolEqObjC(PyObject *op1, PyObject *op2, long intval, long inplace);
-
-/* RaiseTooManyValuesToUnpack.proto */
-static CYTHON_INLINE void __Pyx_RaiseTooManyValuesError(Py_ssize_t expected);
-
-/* RaiseNeedMoreValuesToUnpack.proto */
-static CYTHON_INLINE void __Pyx_RaiseNeedMoreValuesError(Py_ssize_t index);
-
-/* IterFinish.proto */
-static CYTHON_INLINE int __Pyx_IterFinish(void);
-
-/* UnpackItemEndCheck.proto */
-static int __Pyx_IternextUnpackEndCheck(PyObject *retval, Py_ssize_t expected);
-
-/* GetItemInt.proto */
-#define __Pyx_GetItemInt(o, i, type, is_signed, to_py_func, is_list, wraparound, boundscheck)\
- (__Pyx_fits_Py_ssize_t(i, type, is_signed) ?\
- __Pyx_GetItemInt_Fast(o, (Py_ssize_t)i, is_list, wraparound, boundscheck) :\
- (is_list ? (PyErr_SetString(PyExc_IndexError, "list index out of range"), (PyObject*)NULL) :\
- __Pyx_GetItemInt_Generic(o, to_py_func(i))))
-#define __Pyx_GetItemInt_List(o, i, type, is_signed, to_py_func, is_list, wraparound, boundscheck)\
- (__Pyx_fits_Py_ssize_t(i, type, is_signed) ?\
- __Pyx_GetItemInt_List_Fast(o, (Py_ssize_t)i, wraparound, boundscheck) :\
- (PyErr_SetString(PyExc_IndexError, "list index out of range"), (PyObject*)NULL))
-static CYTHON_INLINE PyObject *__Pyx_GetItemInt_List_Fast(PyObject *o, Py_ssize_t i,
- int wraparound, int boundscheck);
-#define __Pyx_GetItemInt_Tuple(o, i, type, is_signed, to_py_func, is_list, wraparound, boundscheck)\
- (__Pyx_fits_Py_ssize_t(i, type, is_signed) ?\
- __Pyx_GetItemInt_Tuple_Fast(o, (Py_ssize_t)i, wraparound, boundscheck) :\
- (PyErr_SetString(PyExc_IndexError, "tuple index out of range"), (PyObject*)NULL))
-static CYTHON_INLINE PyObject *__Pyx_GetItemInt_Tuple_Fast(PyObject *o, Py_ssize_t i,
- int wraparound, int boundscheck);
-static PyObject *__Pyx_GetItemInt_Generic(PyObject *o, PyObject* j);
-static CYTHON_INLINE PyObject *__Pyx_GetItemInt_Fast(PyObject *o, Py_ssize_t i,
- int is_list, int wraparound, int boundscheck);
-
-/* PyDictVersioning.proto */
-#if CYTHON_USE_DICT_VERSIONS && CYTHON_USE_TYPE_SLOTS
-#define __PYX_DICT_VERSION_INIT ((PY_UINT64_T) -1)
-#define __PYX_GET_DICT_VERSION(dict) (((PyDictObject*)(dict))->ma_version_tag)
-#define __PYX_UPDATE_DICT_CACHE(dict, value, cache_var, version_var)\
- (version_var) = __PYX_GET_DICT_VERSION(dict);\
- (cache_var) = (value);
-#define __PYX_PY_DICT_LOOKUP_IF_MODIFIED(VAR, DICT, LOOKUP) {\
- static PY_UINT64_T __pyx_dict_version = 0;\
- static PyObject *__pyx_dict_cached_value = NULL;\
- if (likely(__PYX_GET_DICT_VERSION(DICT) == __pyx_dict_version)) {\
- (VAR) = __pyx_dict_cached_value;\
- } else {\
- (VAR) = __pyx_dict_cached_value = (LOOKUP);\
- __pyx_dict_version = __PYX_GET_DICT_VERSION(DICT);\
- }\
-}
-static CYTHON_INLINE PY_UINT64_T __Pyx_get_tp_dict_version(PyObject *obj);
-static CYTHON_INLINE PY_UINT64_T __Pyx_get_object_dict_version(PyObject *obj);
-static CYTHON_INLINE int __Pyx_object_dict_version_matches(PyObject* obj, PY_UINT64_T tp_dict_version, PY_UINT64_T obj_dict_version);
-#else
-#define __PYX_GET_DICT_VERSION(dict) (0)
-#define __PYX_UPDATE_DICT_CACHE(dict, value, cache_var, version_var)
-#define __PYX_PY_DICT_LOOKUP_IF_MODIFIED(VAR, DICT, LOOKUP) (VAR) = (LOOKUP);
-#endif
-
-/* GetModuleGlobalName.proto */
-#if CYTHON_USE_DICT_VERSIONS
-#define __Pyx_GetModuleGlobalName(var, name) do {\
- static PY_UINT64_T __pyx_dict_version = 0;\
- static PyObject *__pyx_dict_cached_value = NULL;\
- (var) = (likely(__pyx_dict_version == __PYX_GET_DICT_VERSION(__pyx_d))) ?\
- (likely(__pyx_dict_cached_value) ? __Pyx_NewRef(__pyx_dict_cached_value) : __Pyx_GetBuiltinName(name)) :\
- __Pyx__GetModuleGlobalName(name, &__pyx_dict_version, &__pyx_dict_cached_value);\
-} while(0)
-#define __Pyx_GetModuleGlobalNameUncached(var, name) do {\
- PY_UINT64_T __pyx_dict_version;\
- PyObject *__pyx_dict_cached_value;\
- (var) = __Pyx__GetModuleGlobalName(name, &__pyx_dict_version, &__pyx_dict_cached_value);\
-} while(0)
-static PyObject *__Pyx__GetModuleGlobalName(PyObject *name, PY_UINT64_T *dict_version, PyObject **dict_cached_value);
-#else
-#define __Pyx_GetModuleGlobalName(var, name) (var) = __Pyx__GetModuleGlobalName(name)
-#define __Pyx_GetModuleGlobalNameUncached(var, name) (var) = __Pyx__GetModuleGlobalName(name)
-static CYTHON_INLINE PyObject *__Pyx__GetModuleGlobalName(PyObject *name);
-#endif
-
-/* PyFunctionFastCall.proto */
-#if CYTHON_FAST_PYCALL
-#if !CYTHON_VECTORCALL
-#define __Pyx_PyFunction_FastCall(func, args, nargs)\
- __Pyx_PyFunction_FastCallDict((func), (args), (nargs), NULL)
-static PyObject *__Pyx_PyFunction_FastCallDict(PyObject *func, PyObject **args, Py_ssize_t nargs, PyObject *kwargs);
-#endif
-#define __Pyx_BUILD_ASSERT_EXPR(cond)\
- (sizeof(char [1 - 2*!(cond)]) - 1)
-#ifndef Py_MEMBER_SIZE
-#define Py_MEMBER_SIZE(type, member) sizeof(((type *)0)->member)
-#endif
-#if !CYTHON_VECTORCALL
-#if PY_VERSION_HEX >= 0x03080000
- #include "frameobject.h"
-#if PY_VERSION_HEX >= 0x030b00a6
- #ifndef Py_BUILD_CORE
- #define Py_BUILD_CORE 1
- #endif
- #include "internal/pycore_frame.h"
-#endif
- #define __Pxy_PyFrame_Initialize_Offsets()
- #define __Pyx_PyFrame_GetLocalsplus(frame) ((frame)->f_localsplus)
-#else
- static size_t __pyx_pyframe_localsplus_offset = 0;
- #include "frameobject.h"
- #define __Pxy_PyFrame_Initialize_Offsets()\
- ((void)__Pyx_BUILD_ASSERT_EXPR(sizeof(PyFrameObject) == offsetof(PyFrameObject, f_localsplus) + Py_MEMBER_SIZE(PyFrameObject, f_localsplus)),\
- (void)(__pyx_pyframe_localsplus_offset = ((size_t)PyFrame_Type.tp_basicsize) - Py_MEMBER_SIZE(PyFrameObject, f_localsplus)))
- #define __Pyx_PyFrame_GetLocalsplus(frame)\
- (assert(__pyx_pyframe_localsplus_offset), (PyObject **)(((char *)(frame)) + __pyx_pyframe_localsplus_offset))
-#endif
-#endif
-#endif
-
-/* PyObjectCall.proto */
-#if CYTHON_COMPILING_IN_CPYTHON
-static CYTHON_INLINE PyObject* __Pyx_PyObject_Call(PyObject *func, PyObject *arg, PyObject *kw);
-#else
-#define __Pyx_PyObject_Call(func, arg, kw) PyObject_Call(func, arg, kw)
-#endif
-
-/* PyObjectCallMethO.proto */
-#if CYTHON_COMPILING_IN_CPYTHON
-static CYTHON_INLINE PyObject* __Pyx_PyObject_CallMethO(PyObject *func, PyObject *arg);
-#endif
-
-/* PyObjectFastCall.proto */
-#define __Pyx_PyObject_FastCall(func, args, nargs) __Pyx_PyObject_FastCallDict(func, args, (size_t)(nargs), NULL)
-static CYTHON_INLINE PyObject* __Pyx_PyObject_FastCallDict(PyObject *func, PyObject **args, size_t nargs, PyObject *kwargs);
-
-/* TupleAndListFromArray.proto */
-#if CYTHON_COMPILING_IN_CPYTHON
-static CYTHON_INLINE PyObject* __Pyx_PyList_FromArray(PyObject *const *src, Py_ssize_t n);
-static CYTHON_INLINE PyObject* __Pyx_PyTuple_FromArray(PyObject *const *src, Py_ssize_t n);
-#endif
-
-/* IncludeStringH.proto */
-#include
-
-/* BytesEquals.proto */
-static CYTHON_INLINE int __Pyx_PyBytes_Equals(PyObject* s1, PyObject* s2, int equals);
-
-/* UnicodeEquals.proto */
-static CYTHON_INLINE int __Pyx_PyUnicode_Equals(PyObject* s1, PyObject* s2, int equals);
-
-/* fastcall.proto */
-#define __Pyx_Arg_VARARGS(args, i) PyTuple_GET_ITEM(args, i)
-#define __Pyx_NumKwargs_VARARGS(kwds) PyDict_Size(kwds)
-#define __Pyx_KwValues_VARARGS(args, nargs) NULL
-#define __Pyx_GetKwValue_VARARGS(kw, kwvalues, s) __Pyx_PyDict_GetItemStrWithError(kw, s)
-#define __Pyx_KwargsAsDict_VARARGS(kw, kwvalues) PyDict_Copy(kw)
-#if CYTHON_METH_FASTCALL
- #define __Pyx_Arg_FASTCALL(args, i) args[i]
- #define __Pyx_NumKwargs_FASTCALL(kwds) PyTuple_GET_SIZE(kwds)
- #define __Pyx_KwValues_FASTCALL(args, nargs) ((args) + (nargs))
- static CYTHON_INLINE PyObject * __Pyx_GetKwValue_FASTCALL(PyObject *kwnames, PyObject *const *kwvalues, PyObject *s);
- #define __Pyx_KwargsAsDict_FASTCALL(kw, kwvalues) _PyStack_AsDict(kwvalues, kw)
-#else
- #define __Pyx_Arg_FASTCALL __Pyx_Arg_VARARGS
- #define __Pyx_NumKwargs_FASTCALL __Pyx_NumKwargs_VARARGS
- #define __Pyx_KwValues_FASTCALL __Pyx_KwValues_VARARGS
- #define __Pyx_GetKwValue_FASTCALL __Pyx_GetKwValue_VARARGS
- #define __Pyx_KwargsAsDict_FASTCALL __Pyx_KwargsAsDict_VARARGS
-#endif
-#if CYTHON_COMPILING_IN_CPYTHON
-#define __Pyx_ArgsSlice_VARARGS(args, start, stop) __Pyx_PyTuple_FromArray(&__Pyx_Arg_VARARGS(args, start), stop - start)
-#define __Pyx_ArgsSlice_FASTCALL(args, start, stop) __Pyx_PyTuple_FromArray(&__Pyx_Arg_FASTCALL(args, start), stop - start)
-#else
-#define __Pyx_ArgsSlice_VARARGS(args, start, stop) PyTuple_GetSlice(args, start, stop)
-#define __Pyx_ArgsSlice_FASTCALL(args, start, stop) PyTuple_GetSlice(args, start, stop)
-#endif
-
-/* RaiseArgTupleInvalid.proto */
-static void __Pyx_RaiseArgtupleInvalid(const char* func_name, int exact,
- Py_ssize_t num_min, Py_ssize_t num_max, Py_ssize_t num_found);
-
-/* RaiseDoubleKeywords.proto */
-static void __Pyx_RaiseDoubleKeywordsError(const char* func_name, PyObject* kw_name);
-
-/* ParseKeywords.proto */
-static int __Pyx_ParseOptionalKeywords(PyObject *kwds, PyObject *const *kwvalues,
- PyObject **argnames[],
- PyObject *kwds2, PyObject *values[], Py_ssize_t num_pos_args,
- const char* function_name);
-
-/* GetException.proto */
-#if CYTHON_FAST_THREAD_STATE
-#define __Pyx_GetException(type, value, tb) __Pyx__GetException(__pyx_tstate, type, value, tb)
-static int __Pyx__GetException(PyThreadState *tstate, PyObject **type, PyObject **value, PyObject **tb);
-#else
-static int __Pyx_GetException(PyObject **type, PyObject **value, PyObject **tb);
-#endif
-
-/* pep479.proto */
-static void __Pyx_Generator_Replace_StopIteration(int in_async_gen);
-
-/* GetTopmostException.proto */
-#if CYTHON_USE_EXC_INFO_STACK && CYTHON_FAST_THREAD_STATE
-static _PyErr_StackItem * __Pyx_PyErr_GetTopmostException(PyThreadState *tstate);
-#endif
-
-/* SaveResetException.proto */
-#if CYTHON_FAST_THREAD_STATE
-#define __Pyx_ExceptionSave(type, value, tb) __Pyx__ExceptionSave(__pyx_tstate, type, value, tb)
-static CYTHON_INLINE void __Pyx__ExceptionSave(PyThreadState *tstate, PyObject **type, PyObject **value, PyObject **tb);
-#define __Pyx_ExceptionReset(type, value, tb) __Pyx__ExceptionReset(__pyx_tstate, type, value, tb)
-static CYTHON_INLINE void __Pyx__ExceptionReset(PyThreadState *tstate, PyObject *type, PyObject *value, PyObject *tb);
-#else
-#define __Pyx_ExceptionSave(type, value, tb) PyErr_GetExcInfo(type, value, tb)
-#define __Pyx_ExceptionReset(type, value, tb) PyErr_SetExcInfo(type, value, tb)
-#endif
-
-/* IterNext.proto */
-#define __Pyx_PyIter_Next(obj) __Pyx_PyIter_Next2(obj, NULL)
-static CYTHON_INLINE PyObject *__Pyx_PyIter_Next2(PyObject *, PyObject *);
-
-/* ListAppend.proto */
-#if CYTHON_USE_PYLIST_INTERNALS && CYTHON_ASSUME_SAFE_MACROS
-static CYTHON_INLINE int __Pyx_PyList_Append(PyObject* list, PyObject* x) {
- PyListObject* L = (PyListObject*) list;
- Py_ssize_t len = Py_SIZE(list);
- if (likely(L->allocated > len) & likely(len > (L->allocated >> 1))) {
- Py_INCREF(x);
- PyList_SET_ITEM(list, len, x);
- __Pyx_SET_SIZE(list, len + 1);
- return 0;
- }
- return PyList_Append(list, x);
-}
-#else
-#define __Pyx_PyList_Append(L,x) PyList_Append(L,x)
-#endif
-
-/* ListCompAppend.proto */
-#if CYTHON_USE_PYLIST_INTERNALS && CYTHON_ASSUME_SAFE_MACROS
-static CYTHON_INLINE int __Pyx_ListComp_Append(PyObject* list, PyObject* x) {
- PyListObject* L = (PyListObject*) list;
- Py_ssize_t len = Py_SIZE(list);
- if (likely(L->allocated > len)) {
- Py_INCREF(x);
- PyList_SET_ITEM(list, len, x);
- __Pyx_SET_SIZE(list, len + 1);
- return 0;
- }
- return PyList_Append(list, x);
-}
-#else
-#define __Pyx_ListComp_Append(L,x) PyList_Append(L,x)
-#endif
-
-/* PyIntBinop.proto */
-#if !CYTHON_COMPILING_IN_PYPY
-static PyObject* __Pyx_PyInt_AddObjC(PyObject *op1, PyObject *op2, long intval, int inplace, int zerodivision_check);
-#else
-#define __Pyx_PyInt_AddObjC(op1, op2, intval, inplace, zerodivision_check)\
- (inplace ? PyNumber_InPlaceAdd(op1, op2) : PyNumber_Add(op1, op2))
-#endif
-
-/* RaiseException.proto */
-static void __Pyx_Raise(PyObject *type, PyObject *value, PyObject *tb, PyObject *cause);
-
-/* AssertionsEnabled.proto */
-#define __Pyx_init_assertions_enabled()
-#if CYTHON_COMPILING_IN_PYPY && PY_VERSION_HEX < 0x02070600 && !defined(Py_OptimizeFlag)
- #define __pyx_assertions_enabled() (1)
-#elif PY_VERSION_HEX < 0x03080000 || CYTHON_COMPILING_IN_PYPY || defined(Py_LIMITED_API)
- #define __pyx_assertions_enabled() (!Py_OptimizeFlag)
-#elif CYTHON_COMPILING_IN_CPYTHON && PY_VERSION_HEX >= 0x030900A6
- static int __pyx_assertions_enabled_flag;
- #define __pyx_assertions_enabled() (__pyx_assertions_enabled_flag)
- #undef __Pyx_init_assertions_enabled
- static void __Pyx_init_assertions_enabled(void) {
- __pyx_assertions_enabled_flag = ! _PyInterpreterState_GetConfig(__Pyx_PyThreadState_Current->interp)->optimization_level;
- }
-#else
- #define __pyx_assertions_enabled() (!Py_OptimizeFlag)
-#endif
-
-/* SetItemInt.proto */
-#define __Pyx_SetItemInt(o, i, v, type, is_signed, to_py_func, is_list, wraparound, boundscheck)\
- (__Pyx_fits_Py_ssize_t(i, type, is_signed) ?\
- __Pyx_SetItemInt_Fast(o, (Py_ssize_t)i, v, is_list, wraparound, boundscheck) :\
- (is_list ? (PyErr_SetString(PyExc_IndexError, "list assignment index out of range"), -1) :\
- __Pyx_SetItemInt_Generic(o, to_py_func(i), v)))
-static int __Pyx_SetItemInt_Generic(PyObject *o, PyObject *j, PyObject *v);
-static CYTHON_INLINE int __Pyx_SetItemInt_Fast(PyObject *o, Py_ssize_t i, PyObject *v,
- int is_list, int wraparound, int boundscheck);
-
-/* ModInt[long].proto */
-static CYTHON_INLINE long __Pyx_mod_long(long, long);
-
-/* IncludeStructmemberH.proto */
-#include
-
-/* FixUpExtensionType.proto */
-#if CYTHON_USE_TYPE_SPECS
-static int __Pyx_fix_up_extension_type_from_spec(PyType_Spec *spec, PyTypeObject *type);
-#endif
-
-/* PyObjectCallNoArg.proto */
-static CYTHON_INLINE PyObject* __Pyx_PyObject_CallNoArg(PyObject *func);
-
-/* PyObjectCallOneArg.proto */
-static CYTHON_INLINE PyObject* __Pyx_PyObject_CallOneArg(PyObject *func, PyObject *arg);
-
-/* PyObjectGetMethod.proto */
-static int __Pyx_PyObject_GetMethod(PyObject *obj, PyObject *name, PyObject **method);
-
-/* PyObjectCallMethod0.proto */
-static PyObject* __Pyx_PyObject_CallMethod0(PyObject* obj, PyObject* method_name);
-
-/* ValidateBasesTuple.proto */
-#if CYTHON_COMPILING_IN_CPYTHON || CYTHON_COMPILING_IN_LIMITED_API || CYTHON_USE_TYPE_SPECS
-static int __Pyx_validate_bases_tuple(const char *type_name, Py_ssize_t dictoffset, PyObject *bases);
-#endif
-
-/* PyType_Ready.proto */
-CYTHON_UNUSED static int __Pyx_PyType_Ready(PyTypeObject *t);
-
-/* PyObject_GenericGetAttrNoDict.proto */
-#if CYTHON_USE_TYPE_SLOTS && CYTHON_USE_PYTYPE_LOOKUP && PY_VERSION_HEX < 0x03070000
-static CYTHON_INLINE PyObject* __Pyx_PyObject_GenericGetAttrNoDict(PyObject* obj, PyObject* attr_name);
-#else
-#define __Pyx_PyObject_GenericGetAttrNoDict PyObject_GenericGetAttr
-#endif
-
-/* FastTypeChecks.proto */
-#if CYTHON_COMPILING_IN_CPYTHON
-#define __Pyx_TypeCheck(obj, type) __Pyx_IsSubtype(Py_TYPE(obj), (PyTypeObject *)type)
-#define __Pyx_TypeCheck2(obj, type1, type2) __Pyx_IsAnySubtype2(Py_TYPE(obj), (PyTypeObject *)type1, (PyTypeObject *)type2)
-static CYTHON_INLINE int __Pyx_IsSubtype(PyTypeObject *a, PyTypeObject *b);
-static CYTHON_INLINE int __Pyx_IsAnySubtype2(PyTypeObject *cls, PyTypeObject *a, PyTypeObject *b);
-static CYTHON_INLINE int __Pyx_PyErr_GivenExceptionMatches(PyObject *err, PyObject *type);
-static CYTHON_INLINE int __Pyx_PyErr_GivenExceptionMatches2(PyObject *err, PyObject *type1, PyObject *type2);
-#else
-#define __Pyx_TypeCheck(obj, type) PyObject_TypeCheck(obj, (PyTypeObject *)type)
-#define __Pyx_TypeCheck2(obj, type1, type2) (PyObject_TypeCheck(obj, (PyTypeObject *)type1) || PyObject_TypeCheck(obj, (PyTypeObject *)type2))
-#define __Pyx_PyErr_GivenExceptionMatches(err, type) PyErr_GivenExceptionMatches(err, type)
-#define __Pyx_PyErr_GivenExceptionMatches2(err, type1, type2) (PyErr_GivenExceptionMatches(err, type1) || PyErr_GivenExceptionMatches(err, type2))
-#endif
-#define __Pyx_PyErr_ExceptionMatches2(err1, err2) __Pyx_PyErr_GivenExceptionMatches2(__Pyx_PyErr_CurrentExceptionType(), err1, err2)
-#define __Pyx_PyException_Check(obj) __Pyx_TypeCheck(obj, PyExc_Exception)
-
-/* Import.proto */
-static PyObject *__Pyx_Import(PyObject *name, PyObject *from_list, int level);
-
-/* ImportFrom.proto */
-static PyObject* __Pyx_ImportFrom(PyObject* module, PyObject* name);
-
-/* ImportDottedModule.proto */
-static PyObject *__Pyx_ImportDottedModule(PyObject *name, PyObject *parts_tuple);
-#if PY_MAJOR_VERSION >= 3
-static PyObject *__Pyx_ImportDottedModule_WalkParts(PyObject *module, PyObject *name, PyObject *parts_tuple);
-#endif
-
-/* pybytes_as_double.proto */
-static double __Pyx_SlowPyString_AsDouble(PyObject *obj);
-static double __Pyx__PyBytes_AsDouble(PyObject *obj, const char* start, Py_ssize_t length);
-static CYTHON_INLINE double __Pyx_PyBytes_AsDouble(PyObject *obj) {
- return __Pyx__PyBytes_AsDouble(obj, PyBytes_AS_STRING(obj), PyBytes_GET_SIZE(obj));
-}
-static CYTHON_INLINE double __Pyx_PyByteArray_AsDouble(PyObject *obj) {
- return __Pyx__PyBytes_AsDouble(obj, PyByteArray_AS_STRING(obj), PyByteArray_GET_SIZE(obj));
-}
-
-/* pyunicode_as_double.proto */
-#if PY_MAJOR_VERSION >= 3 && !CYTHON_COMPILING_IN_PYPY
-static const char* __Pyx__PyUnicode_AsDouble_Copy(const void* data, const int kind, char* buffer, Py_ssize_t start, Py_ssize_t end) {
- int last_was_punctuation;
- Py_ssize_t i;
- last_was_punctuation = 1;
- for (i=start; i <= end; i++) {
- Py_UCS4 chr = PyUnicode_READ(kind, data, i);
- int is_punctuation = (chr == '_') | (chr == '.');
- *buffer = (char)chr;
- buffer += (chr != '_');
- if (unlikely(chr > 127)) goto parse_failure;
- if (unlikely(last_was_punctuation & is_punctuation)) goto parse_failure;
- last_was_punctuation = is_punctuation;
- }
- if (unlikely(last_was_punctuation)) goto parse_failure;
- *buffer = '\0';
- return buffer;
-parse_failure:
- return NULL;
-}
-static double __Pyx__PyUnicode_AsDouble_inf_nan(const void* data, int kind, Py_ssize_t start, Py_ssize_t length) {
- int matches = 1;
- Py_UCS4 chr;
- Py_UCS4 sign = PyUnicode_READ(kind, data, start);
- int is_signed = (sign == '-') | (sign == '+');
- start += is_signed;
- length -= is_signed;
- switch (PyUnicode_READ(kind, data, start)) {
- #ifdef Py_NAN
- case 'n':
- case 'N':
- if (unlikely(length != 3)) goto parse_failure;
- chr = PyUnicode_READ(kind, data, start+1);
- matches &= (chr == 'a') | (chr == 'A');
- chr = PyUnicode_READ(kind, data, start+2);
- matches &= (chr == 'n') | (chr == 'N');
- if (unlikely(!matches)) goto parse_failure;
- return (sign == '-') ? -Py_NAN : Py_NAN;
- #endif
- case 'i':
- case 'I':
- if (unlikely(length < 3)) goto parse_failure;
- chr = PyUnicode_READ(kind, data, start+1);
- matches &= (chr == 'n') | (chr == 'N');
- chr = PyUnicode_READ(kind, data, start+2);
- matches &= (chr == 'f') | (chr == 'F');
- if (likely(length == 3 && matches))
- return (sign == '-') ? -Py_HUGE_VAL : Py_HUGE_VAL;
- if (unlikely(length != 8)) goto parse_failure;
- chr = PyUnicode_READ(kind, data, start+3);
- matches &= (chr == 'i') | (chr == 'I');
- chr = PyUnicode_READ(kind, data, start+4);
- matches &= (chr == 'n') | (chr == 'N');
- chr = PyUnicode_READ(kind, data, start+5);
- matches &= (chr == 'i') | (chr == 'I');
- chr = PyUnicode_READ(kind, data, start+6);
- matches &= (chr == 't') | (chr == 'T');
- chr = PyUnicode_READ(kind, data, start+7);
- matches &= (chr == 'y') | (chr == 'Y');
- if (unlikely(!matches)) goto parse_failure;
- return (sign == '-') ? -Py_HUGE_VAL : Py_HUGE_VAL;
- case '.': case '0': case '1': case '2': case '3': case '4': case '5': case '6': case '7': case '8': case '9':
- break;
- default:
- goto parse_failure;
- }
- return 0.0;
-parse_failure:
- return -1.0;
-}
-static double __Pyx_PyUnicode_AsDouble_WithSpaces(PyObject *obj) {
- double value;
- const char *last;
- char *end;
- Py_ssize_t start, length = PyUnicode_GET_LENGTH(obj);
- const int kind = PyUnicode_KIND(obj);
- const void* data = PyUnicode_DATA(obj);
- start = 0;
- while (Py_UNICODE_ISSPACE(PyUnicode_READ(kind, data, start)))
- start++;
- while (start < length - 1 && Py_UNICODE_ISSPACE(PyUnicode_READ(kind, data, length - 1)))
- length--;
- length -= start;
- if (unlikely(length <= 0)) goto fallback;
- value = __Pyx__PyUnicode_AsDouble_inf_nan(data, kind, start, length);
- if (unlikely(value == -1.0)) goto fallback;
- if (value != 0.0) return value;
- if (length < 40) {
- char number[40];
- last = __Pyx__PyUnicode_AsDouble_Copy(data, kind, number, start, start + length);
- if (unlikely(!last)) goto fallback;
- value = PyOS_string_to_double(number, &end, NULL);
- } else {
- char *number = (char*) PyMem_Malloc((length + 1) * sizeof(char));
- if (unlikely(!number)) goto fallback;
- last = __Pyx__PyUnicode_AsDouble_Copy(data, kind, number, start, start + length);
- if (unlikely(!last)) {
- PyMem_Free(number);
- goto fallback;
- }
- value = PyOS_string_to_double(number, &end, NULL);
- PyMem_Free(number);
- }
- if (likely(end == last) || (value == (double)-1 && PyErr_Occurred())) {
- return value;
- }
-fallback:
- return __Pyx_SlowPyString_AsDouble(obj);
-}
-#endif
-static CYTHON_INLINE double __Pyx_PyUnicode_AsDouble(PyObject *obj) {
-#if PY_MAJOR_VERSION >= 3 && !CYTHON_COMPILING_IN_PYPY
- if (unlikely(__Pyx_PyUnicode_READY(obj) == -1))
- return (double)-1;
- if (likely(PyUnicode_IS_ASCII(obj))) {
- const char *s;
- Py_ssize_t length;
- s = PyUnicode_AsUTF8AndSize(obj, &length);
- return __Pyx__PyBytes_AsDouble(obj, s, length);
- }
- return __Pyx_PyUnicode_AsDouble_WithSpaces(obj);
-#else
- return __Pyx_SlowPyString_AsDouble(obj);
-#endif
-}
-
-/* FetchSharedCythonModule.proto */
-static PyObject *__Pyx_FetchSharedCythonABIModule(void);
-
-/* FetchCommonType.proto */
-#if !CYTHON_USE_TYPE_SPECS
-static PyTypeObject* __Pyx_FetchCommonType(PyTypeObject* type);
-#else
-static PyTypeObject* __Pyx_FetchCommonTypeFromSpec(PyObject *module, PyType_Spec *spec, PyObject *bases);
-#endif
-
-/* PyMethodNew.proto */
-#if PY_MAJOR_VERSION >= 3
-static PyObject *__Pyx_PyMethod_New(PyObject *func, PyObject *self, PyObject *typ) {
- CYTHON_UNUSED_VAR(typ);
- if (!self)
- return __Pyx_NewRef(func);
- return PyMethod_New(func, self);
-}
-#else
- #define __Pyx_PyMethod_New PyMethod_New
-#endif
-
-/* PyVectorcallFastCallDict.proto */
-#if CYTHON_METH_FASTCALL
-static CYTHON_INLINE PyObject *__Pyx_PyVectorcall_FastCallDict(PyObject *func, __pyx_vectorcallfunc vc, PyObject *const *args, size_t nargs, PyObject *kw);
-#endif
-
-/* CythonFunctionShared.proto */
-#define __Pyx_CyFunction_USED
-#define __Pyx_CYFUNCTION_STATICMETHOD 0x01
-#define __Pyx_CYFUNCTION_CLASSMETHOD 0x02
-#define __Pyx_CYFUNCTION_CCLASS 0x04
-#define __Pyx_CYFUNCTION_COROUTINE 0x08
-#define __Pyx_CyFunction_GetClosure(f)\
- (((__pyx_CyFunctionObject *) (f))->func_closure)
-#if PY_VERSION_HEX < 0x030900B1
- #define __Pyx_CyFunction_GetClassObj(f)\
- (((__pyx_CyFunctionObject *) (f))->func_classobj)
-#else
- #define __Pyx_CyFunction_GetClassObj(f)\
- ((PyObject*) ((PyCMethodObject *) (f))->mm_class)
-#endif
-#define __Pyx_CyFunction_SetClassObj(f, classobj)\
- __Pyx__CyFunction_SetClassObj((__pyx_CyFunctionObject *) (f), (classobj))
-#define __Pyx_CyFunction_Defaults(type, f)\
- ((type *)(((__pyx_CyFunctionObject *) (f))->defaults))
-#define __Pyx_CyFunction_SetDefaultsGetter(f, g)\
- ((__pyx_CyFunctionObject *) (f))->defaults_getter = (g)
-typedef struct {
-#if PY_VERSION_HEX < 0x030900B1
- PyCFunctionObject func;
-#else
- PyCMethodObject func;
-#endif
-#if CYTHON_BACKPORT_VECTORCALL
- __pyx_vectorcallfunc func_vectorcall;
-#endif
-#if PY_VERSION_HEX < 0x030500A0
- PyObject *func_weakreflist;
-#endif
- PyObject *func_dict;
- PyObject *func_name;
- PyObject *func_qualname;
- PyObject *func_doc;
- PyObject *func_globals;
- PyObject *func_code;
- PyObject *func_closure;
-#if PY_VERSION_HEX < 0x030900B1
- PyObject *func_classobj;
-#endif
- void *defaults;
- int defaults_pyobjects;
- size_t defaults_size; // used by FusedFunction for copying defaults
- int flags;
- PyObject *defaults_tuple;
- PyObject *defaults_kwdict;
- PyObject *(*defaults_getter)(PyObject *);
- PyObject *func_annotations;
- PyObject *func_is_coroutine;
-} __pyx_CyFunctionObject;
-#define __Pyx_CyFunction_Check(obj) __Pyx_TypeCheck(obj, __pyx_CyFunctionType)
-#define __Pyx_IsCyOrPyCFunction(obj) __Pyx_TypeCheck2(obj, __pyx_CyFunctionType, &PyCFunction_Type)
-#define __Pyx_CyFunction_CheckExact(obj) __Pyx_IS_TYPE(obj, __pyx_CyFunctionType)
-static PyObject *__Pyx_CyFunction_Init(__pyx_CyFunctionObject* op, PyMethodDef *ml,
- int flags, PyObject* qualname,
- PyObject *closure,
- PyObject *module, PyObject *globals,
- PyObject* code);
-static CYTHON_INLINE void __Pyx__CyFunction_SetClassObj(__pyx_CyFunctionObject* f, PyObject* classobj);
-static CYTHON_INLINE void *__Pyx_CyFunction_InitDefaults(PyObject *m,
- size_t size,
- int pyobjects);
-static CYTHON_INLINE void __Pyx_CyFunction_SetDefaultsTuple(PyObject *m,
- PyObject *tuple);
-static CYTHON_INLINE void __Pyx_CyFunction_SetDefaultsKwDict(PyObject *m,
- PyObject *dict);
-static CYTHON_INLINE void __Pyx_CyFunction_SetAnnotationsDict(PyObject *m,
- PyObject *dict);
-static int __pyx_CyFunction_init(PyObject *module);
-#if CYTHON_METH_FASTCALL
-static PyObject * __Pyx_CyFunction_Vectorcall_NOARGS(PyObject *func, PyObject *const *args, size_t nargsf, PyObject *kwnames);
-static PyObject * __Pyx_CyFunction_Vectorcall_O(PyObject *func, PyObject *const *args, size_t nargsf, PyObject *kwnames);
-static PyObject * __Pyx_CyFunction_Vectorcall_FASTCALL_KEYWORDS(PyObject *func, PyObject *const *args, size_t nargsf, PyObject *kwnames);
-static PyObject * __Pyx_CyFunction_Vectorcall_FASTCALL_KEYWORDS_METHOD(PyObject *func, PyObject *const *args, size_t nargsf, PyObject *kwnames);
-#if CYTHON_BACKPORT_VECTORCALL
-#define __Pyx_CyFunction_func_vectorcall(f) (((__pyx_CyFunctionObject*)f)->func_vectorcall)
-#else
-#define __Pyx_CyFunction_func_vectorcall(f) (((PyCFunctionObject*)f)->vectorcall)
-#endif
-#endif
-
-/* CythonFunction.proto */
-static PyObject *__Pyx_CyFunction_New(PyMethodDef *ml,
- int flags, PyObject* qualname,
- PyObject *closure,
- PyObject *module, PyObject *globals,
- PyObject* code);
-
-/* CLineInTraceback.proto */
-#ifdef CYTHON_CLINE_IN_TRACEBACK
-#define __Pyx_CLineForTraceback(tstate, c_line) (((CYTHON_CLINE_IN_TRACEBACK)) ? c_line : 0)
-#else
-static int __Pyx_CLineForTraceback(PyThreadState *tstate, int c_line);
-#endif
-
-/* CodeObjectCache.proto */
-#if !CYTHON_COMPILING_IN_LIMITED_API
-typedef struct {
- PyCodeObject* code_object;
- int code_line;
-} __Pyx_CodeObjectCacheEntry;
-struct __Pyx_CodeObjectCache {
- int count;
- int max_count;
- __Pyx_CodeObjectCacheEntry* entries;
-};
-static struct __Pyx_CodeObjectCache __pyx_code_cache = {0,0,NULL};
-static int __pyx_bisect_code_objects(__Pyx_CodeObjectCacheEntry* entries, int count, int code_line);
-static PyCodeObject *__pyx_find_code_object(int code_line);
-static void __pyx_insert_code_object(int code_line, PyCodeObject* code_object);
-#endif
-
-/* AddTraceback.proto */
-static void __Pyx_AddTraceback(const char *funcname, int c_line,
- int py_line, const char *filename);
-
-/* RealImag.proto */
-#if CYTHON_CCOMPLEX
- #ifdef __cplusplus
- #define __Pyx_CREAL(z) ((z).real())
- #define __Pyx_CIMAG(z) ((z).imag())
- #else
- #define __Pyx_CREAL(z) (__real__(z))
- #define __Pyx_CIMAG(z) (__imag__(z))
- #endif
-#else
- #define __Pyx_CREAL(z) ((z).real)
- #define __Pyx_CIMAG(z) ((z).imag)
-#endif
-#if defined(__cplusplus) && CYTHON_CCOMPLEX\
- && (defined(_WIN32) || defined(__clang__) || (defined(__GNUC__) && (__GNUC__ >= 5 || __GNUC__ == 4 && __GNUC_MINOR__ >= 4 )) || __cplusplus >= 201103)
- #define __Pyx_SET_CREAL(z,x) ((z).real(x))
- #define __Pyx_SET_CIMAG(z,y) ((z).imag(y))
-#else
- #define __Pyx_SET_CREAL(z,x) __Pyx_CREAL(z) = (x)
- #define __Pyx_SET_CIMAG(z,y) __Pyx_CIMAG(z) = (y)
-#endif
-
-/* Arithmetic.proto */
-#if CYTHON_CCOMPLEX && (1) && (!0 || __cplusplus)
- #define __Pyx_c_eq_double(a, b) ((a)==(b))
- #define __Pyx_c_sum_double(a, b) ((a)+(b))
- #define __Pyx_c_diff_double(a, b) ((a)-(b))
- #define __Pyx_c_prod_double(a, b) ((a)*(b))
- #define __Pyx_c_quot_double(a, b) ((a)/(b))
- #define __Pyx_c_neg_double(a) (-(a))
- #ifdef __cplusplus
- #define __Pyx_c_is_zero_double(z) ((z)==(double)0)
- #define __Pyx_c_conj_double(z) (::std::conj(z))
- #if 1
- #define __Pyx_c_abs_double(z) (::std::abs(z))
- #define __Pyx_c_pow_double(a, b) (::std::pow(a, b))
- #endif
- #else
- #define __Pyx_c_is_zero_double(z) ((z)==0)
- #define __Pyx_c_conj_double(z) (conj(z))
- #if 1
- #define __Pyx_c_abs_double(z) (cabs(z))
- #define __Pyx_c_pow_double(a, b) (cpow(a, b))
- #endif
- #endif
-#else
- static CYTHON_INLINE int __Pyx_c_eq_double(__pyx_t_double_complex, __pyx_t_double_complex);
- static CYTHON_INLINE __pyx_t_double_complex __Pyx_c_sum_double(__pyx_t_double_complex, __pyx_t_double_complex);
- static CYTHON_INLINE __pyx_t_double_complex __Pyx_c_diff_double(__pyx_t_double_complex, __pyx_t_double_complex);
- static CYTHON_INLINE __pyx_t_double_complex __Pyx_c_prod_double(__pyx_t_double_complex, __pyx_t_double_complex);
- static CYTHON_INLINE __pyx_t_double_complex __Pyx_c_quot_double(__pyx_t_double_complex, __pyx_t_double_complex);
- static CYTHON_INLINE __pyx_t_double_complex __Pyx_c_neg_double(__pyx_t_double_complex);
- static CYTHON_INLINE int __Pyx_c_is_zero_double(__pyx_t_double_complex);
- static CYTHON_INLINE __pyx_t_double_complex __Pyx_c_conj_double(__pyx_t_double_complex);
- #if 1
- static CYTHON_INLINE double __Pyx_c_abs_double(__pyx_t_double_complex);
- static CYTHON_INLINE __pyx_t_double_complex __Pyx_c_pow_double(__pyx_t_double_complex, __pyx_t_double_complex);
- #endif
-#endif
-
-/* FromPy.proto */
-static __pyx_t_double_complex __Pyx_PyComplex_As___pyx_t_double_complex(PyObject*);
-
-/* GCCDiagnostics.proto */
-#if !defined(__INTEL_COMPILER) && defined(__GNUC__) && (__GNUC__ > 4 || (__GNUC__ == 4 && __GNUC_MINOR__ >= 6))
-#define __Pyx_HAS_GCC_DIAGNOSTIC
-#endif
-
-/* ToPy.proto */
-#define __pyx_PyComplex_FromComplex(z)\
- PyComplex_FromDoubles((double)__Pyx_CREAL(z),\
- (double)__Pyx_CIMAG(z))
-
-/* CIntFromPy.proto */
-static CYTHON_INLINE int __Pyx_PyInt_As_int(PyObject *);
-
-/* CIntToPy.proto */
-static CYTHON_INLINE PyObject* __Pyx_PyInt_From_long(long value);
-
-/* CIntToPy.proto */
-static CYTHON_INLINE PyObject* __Pyx_PyInt_From_int(int value);
-
-/* CIntFromPy.proto */
-static CYTHON_INLINE long __Pyx_PyInt_As_long(PyObject *);
-
-/* FormatTypeName.proto */
-#if CYTHON_COMPILING_IN_LIMITED_API
-typedef PyObject *__Pyx_TypeName;
-#define __Pyx_FMT_TYPENAME "%U"
-static __Pyx_TypeName __Pyx_PyType_GetName(PyTypeObject* tp);
-#define __Pyx_DECREF_TypeName(obj) Py_XDECREF(obj)
-#else
-typedef const char *__Pyx_TypeName;
-#define __Pyx_FMT_TYPENAME "%.200s"
-#define __Pyx_PyType_GetName(tp) ((tp)->tp_name)
-#define __Pyx_DECREF_TypeName(obj)
-#endif
-
-/* SwapException.proto */
-#if CYTHON_FAST_THREAD_STATE
-#define __Pyx_ExceptionSwap(type, value, tb) __Pyx__ExceptionSwap(__pyx_tstate, type, value, tb)
-static CYTHON_INLINE void __Pyx__ExceptionSwap(PyThreadState *tstate, PyObject **type, PyObject **value, PyObject **tb);
-#else
-static CYTHON_INLINE void __Pyx_ExceptionSwap(PyObject **type, PyObject **value, PyObject **tb);
-#endif
-
-/* PyObjectCall2Args.proto */
-static CYTHON_INLINE PyObject* __Pyx_PyObject_Call2Args(PyObject* function, PyObject* arg1, PyObject* arg2);
-
-/* PyObjectCallMethod1.proto */
-static PyObject* __Pyx_PyObject_CallMethod1(PyObject* obj, PyObject* method_name, PyObject* arg);
-
-/* CoroutineBase.proto */
-struct __pyx_CoroutineObject;
-typedef PyObject *(*__pyx_coroutine_body_t)(struct __pyx_CoroutineObject *, PyThreadState *, PyObject *);
-#if CYTHON_USE_EXC_INFO_STACK
-#define __Pyx_ExcInfoStruct _PyErr_StackItem
-#else
-typedef struct {
- PyObject *exc_type;
- PyObject *exc_value;
- PyObject *exc_traceback;
-} __Pyx_ExcInfoStruct;
-#endif
-typedef struct __pyx_CoroutineObject {
- PyObject_HEAD
- __pyx_coroutine_body_t body;
- PyObject *closure;
- __Pyx_ExcInfoStruct gi_exc_state;
- PyObject *gi_weakreflist;
- PyObject *classobj;
- PyObject *yieldfrom;
- PyObject *gi_name;
- PyObject *gi_qualname;
- PyObject *gi_modulename;
- PyObject *gi_code;
- PyObject *gi_frame;
- int resume_label;
- char is_running;
-} __pyx_CoroutineObject;
-static __pyx_CoroutineObject *__Pyx__Coroutine_New(
- PyTypeObject *type, __pyx_coroutine_body_t body, PyObject *code, PyObject *closure,
- PyObject *name, PyObject *qualname, PyObject *module_name);
-static __pyx_CoroutineObject *__Pyx__Coroutine_NewInit(
- __pyx_CoroutineObject *gen, __pyx_coroutine_body_t body, PyObject *code, PyObject *closure,
- PyObject *name, PyObject *qualname, PyObject *module_name);
-static CYTHON_INLINE void __Pyx_Coroutine_ExceptionClear(__Pyx_ExcInfoStruct *self);
-static int __Pyx_Coroutine_clear(PyObject *self);
-static PyObject *__Pyx_Coroutine_Send(PyObject *self, PyObject *value);
-static PyObject *__Pyx_Coroutine_Close(PyObject *self);
-static PyObject *__Pyx_Coroutine_Throw(PyObject *gen, PyObject *args);
-#if CYTHON_USE_EXC_INFO_STACK
-#define __Pyx_Coroutine_SwapException(self)
-#define __Pyx_Coroutine_ResetAndClearException(self) __Pyx_Coroutine_ExceptionClear(&(self)->gi_exc_state)
-#else
-#define __Pyx_Coroutine_SwapException(self) {\
- __Pyx_ExceptionSwap(&(self)->gi_exc_state.exc_type, &(self)->gi_exc_state.exc_value, &(self)->gi_exc_state.exc_traceback);\
- __Pyx_Coroutine_ResetFrameBackpointer(&(self)->gi_exc_state);\
- }
-#define __Pyx_Coroutine_ResetAndClearException(self) {\
- __Pyx_ExceptionReset((self)->gi_exc_state.exc_type, (self)->gi_exc_state.exc_value, (self)->gi_exc_state.exc_traceback);\
- (self)->gi_exc_state.exc_type = (self)->gi_exc_state.exc_value = (self)->gi_exc_state.exc_traceback = NULL;\
- }
-#endif
-#if CYTHON_FAST_THREAD_STATE
-#define __Pyx_PyGen_FetchStopIterationValue(pvalue)\
- __Pyx_PyGen__FetchStopIterationValue(__pyx_tstate, pvalue)
-#else
-#define __Pyx_PyGen_FetchStopIterationValue(pvalue)\
- __Pyx_PyGen__FetchStopIterationValue(__Pyx_PyThreadState_Current, pvalue)
-#endif
-static int __Pyx_PyGen__FetchStopIterationValue(PyThreadState *tstate, PyObject **pvalue);
-static CYTHON_INLINE void __Pyx_Coroutine_ResetFrameBackpointer(__Pyx_ExcInfoStruct *exc_state);
-
-/* PatchModuleWithCoroutine.proto */
-static PyObject* __Pyx_Coroutine_patch_module(PyObject* module, const char* py_code);
-
-/* PatchGeneratorABC.proto */
-static int __Pyx_patch_abc(void);
-
-/* Generator.proto */
-#define __Pyx_Generator_USED
-#define __Pyx_Generator_CheckExact(obj) __Pyx_IS_TYPE(obj, __pyx_GeneratorType)
-#define __Pyx_Generator_New(body, code, closure, name, qualname, module_name)\
- __Pyx__Coroutine_New(__pyx_GeneratorType, body, code, closure, name, qualname, module_name)
-static PyObject *__Pyx_Generator_Next(PyObject *self);
-static int __pyx_Generator_init(PyObject *module);
-
-/* CheckBinaryVersion.proto */
-static int __Pyx_check_binary_version(void);
-
-/* InitStrings.proto */
-static int __Pyx_InitStrings(__Pyx_StringTabEntry *t);
-
-/* #### Code section: module_declarations ### */
-
-/* Module declarations from "cython" */
-
-/* Module declarations from "fontTools.cu2qu.cu2qu" */
-static CYTHON_INLINE double __pyx_f_9fontTools_5cu2qu_5cu2qu_dot(__pyx_t_double_complex, __pyx_t_double_complex); /*proto*/
-static CYTHON_INLINE PyObject *__pyx_f_9fontTools_5cu2qu_5cu2qu_calc_cubic_points(__pyx_t_double_complex, __pyx_t_double_complex, __pyx_t_double_complex, __pyx_t_double_complex); /*proto*/
-static CYTHON_INLINE PyObject *__pyx_f_9fontTools_5cu2qu_5cu2qu_calc_cubic_parameters(__pyx_t_double_complex, __pyx_t_double_complex, __pyx_t_double_complex, __pyx_t_double_complex); /*proto*/
-static CYTHON_INLINE PyObject *__pyx_f_9fontTools_5cu2qu_5cu2qu_split_cubic_into_n_iter(__pyx_t_double_complex, __pyx_t_double_complex, __pyx_t_double_complex, __pyx_t_double_complex, PyObject *); /*proto*/
-static CYTHON_INLINE PyObject *__pyx_f_9fontTools_5cu2qu_5cu2qu_split_cubic_into_two(__pyx_t_double_complex, __pyx_t_double_complex, __pyx_t_double_complex, __pyx_t_double_complex); /*proto*/
-static CYTHON_INLINE PyObject *__pyx_f_9fontTools_5cu2qu_5cu2qu_split_cubic_into_three(__pyx_t_double_complex, __pyx_t_double_complex, __pyx_t_double_complex, __pyx_t_double_complex); /*proto*/
-static CYTHON_INLINE __pyx_t_double_complex __pyx_f_9fontTools_5cu2qu_5cu2qu_cubic_approx_control(double, __pyx_t_double_complex, __pyx_t_double_complex, __pyx_t_double_complex, __pyx_t_double_complex); /*proto*/
-static CYTHON_INLINE __pyx_t_double_complex __pyx_f_9fontTools_5cu2qu_5cu2qu_calc_intersect(__pyx_t_double_complex, __pyx_t_double_complex, __pyx_t_double_complex, __pyx_t_double_complex); /*proto*/
-static int __pyx_f_9fontTools_5cu2qu_5cu2qu_cubic_farthest_fit_inside(__pyx_t_double_complex, __pyx_t_double_complex, __pyx_t_double_complex, __pyx_t_double_complex, double); /*proto*/
-static CYTHON_INLINE PyObject *__pyx_f_9fontTools_5cu2qu_5cu2qu_cubic_approx_quadratic(PyObject *, double); /*proto*/
-static PyObject *__pyx_f_9fontTools_5cu2qu_5cu2qu_cubic_approx_spline(PyObject *, int, double, int); /*proto*/
-/* #### Code section: typeinfo ### */
-/* #### Code section: before_global_var ### */
-#define __Pyx_MODULE_NAME "fontTools.cu2qu.cu2qu"
-extern int __pyx_module_is_main_fontTools__cu2qu__cu2qu;
-int __pyx_module_is_main_fontTools__cu2qu__cu2qu = 0;
-
-/* Implementation of "fontTools.cu2qu.cu2qu" */
-/* #### Code section: global_var ### */
-static PyObject *__pyx_builtin_AttributeError;
-static PyObject *__pyx_builtin_ImportError;
-static PyObject *__pyx_builtin_range;
-static PyObject *__pyx_builtin_ZeroDivisionError;
-static PyObject *__pyx_builtin_AssertionError;
-/* #### Code section: string_decls ### */
-static const char __pyx_k_a[] = "a";
-static const char __pyx_k_b[] = "b";
-static const char __pyx_k_c[] = "c";
-static const char __pyx_k_d[] = "d";
-static const char __pyx_k_i[] = "i";
-static const char __pyx_k_l[] = "l";
-static const char __pyx_k_n[] = "n";
-static const char __pyx_k_p[] = "p";
-static const char __pyx_k_s[] = "s";
-static const char __pyx_k__2[] = ".";
-static const char __pyx_k__3[] = "*";
-static const char __pyx_k__9[] = "?";
-static const char __pyx_k_a1[] = "a1";
-static const char __pyx_k_b1[] = "b1";
-static const char __pyx_k_c1[] = "c1";
-static const char __pyx_k_d1[] = "d1";
-static const char __pyx_k_dt[] = "dt";
-static const char __pyx_k_gc[] = "gc";
-static const char __pyx_k_p0[] = "p0";
-static const char __pyx_k_p1[] = "p1";
-static const char __pyx_k_p2[] = "p2";
-static const char __pyx_k_p3[] = "p3";
-static const char __pyx_k_t1[] = "t1";
-static const char __pyx_k_NAN[] = "NAN";
-static const char __pyx_k_NaN[] = "NaN";
-static const char __pyx_k_all[] = "__all__";
-static const char __pyx_k_args[] = "args";
-static const char __pyx_k_imag[] = "imag";
-static const char __pyx_k_main[] = "__main__";
-static const char __pyx_k_math[] = "math";
-static const char __pyx_k_name[] = "__name__";
-static const char __pyx_k_real[] = "real";
-static const char __pyx_k_send[] = "send";
-static const char __pyx_k_spec[] = "__spec__";
-static const char __pyx_k_t1_2[] = "t1_2";
-static const char __pyx_k_test[] = "__test__";
-static const char __pyx_k_Error[] = "Error";
-static const char __pyx_k_MAX_N[] = "MAX_N";
-static const char __pyx_k_close[] = "close";
-static const char __pyx_k_curve[] = "curve";
-static const char __pyx_k_isnan[] = "isnan";
-static const char __pyx_k_range[] = "range";
-static const char __pyx_k_throw[] = "throw";
-static const char __pyx_k_curves[] = "curves";
-static const char __pyx_k_cython[] = "cython";
-static const char __pyx_k_enable[] = "enable";
-static const char __pyx_k_errors[] = "errors";
-static const char __pyx_k_import[] = "__import__";
-static const char __pyx_k_last_i[] = "last_i";
-static const char __pyx_k_spline[] = "spline";
-static const char __pyx_k_delta_2[] = "delta_2";
-static const char __pyx_k_delta_3[] = "delta_3";
-static const char __pyx_k_disable[] = "disable";
-static const char __pyx_k_max_err[] = "max_err";
-static const char __pyx_k_splines[] = "splines";
-static const char __pyx_k_COMPILED[] = "COMPILED";
-static const char __pyx_k_isenabled[] = "isenabled";
-static const char __pyx_k_Cu2QuError[] = "Cu2QuError";
-static const char __pyx_k_max_errors[] = "max_errors";
-static const char __pyx_k_ImportError[] = "ImportError";
-static const char __pyx_k_initializing[] = "_initializing";
-static const char __pyx_k_is_coroutine[] = "_is_coroutine";
-static const char __pyx_k_all_quadratic[] = "all_quadratic";
-static const char __pyx_k_AssertionError[] = "AssertionError";
-static const char __pyx_k_AttributeError[] = "AttributeError";
-static const char __pyx_k_fontTools_misc[] = "fontTools.misc";
-static const char __pyx_k_ZeroDivisionError[] = "ZeroDivisionError";
-static const char __pyx_k_asyncio_coroutines[] = "asyncio.coroutines";
-static const char __pyx_k_cline_in_traceback[] = "cline_in_traceback";
-static const char __pyx_k_curve_to_quadratic[] = "curve_to_quadratic";
-static const char __pyx_k_ApproxNotFoundError[] = "ApproxNotFoundError";
-static const char __pyx_k_curves_to_quadratic[] = "curves_to_quadratic";
-static const char __pyx_k_fontTools_cu2qu_cu2qu[] = "fontTools.cu2qu.cu2qu";
-static const char __pyx_k_split_cubic_into_n_gen[] = "_split_cubic_into_n_gen";
-static const char __pyx_k_Lib_fontTools_cu2qu_cu2qu_py[] = "Lib/fontTools/cu2qu/cu2qu.py";
-static const char __pyx_k_curves_to_quadratic_line_474[] = "curves_to_quadratic (line 474)";
-static const char __pyx_k_Return_quadratic_Bezier_splines[] = "Return quadratic Bezier splines approximating the input cubic Beziers.\n\n Args:\n curves: A sequence of *n* curves, each curve being a sequence of four\n 2D tuples.\n max_errors: A sequence of *n* floats representing the maximum permissible\n deviation from each of the cubic Bezier curves.\n all_quadratic (bool): If True (default) returned values are a\n quadratic spline. If False, they are either a single quadratic\n curve or a single cubic curve.\n\n Example::\n\n >>> curves_to_quadratic( [\n ... [ (50,50), (100,100), (150,100), (200,50) ],\n ... [ (75,50), (120,100), (150,75), (200,60) ]\n ... ], [1,1] )\n [[(50.0, 50.0), (75.0, 75.0), (125.0, 91.66666666666666), (175.0, 75.0), (200.0, 50.0)], [(75.0, 50.0), (97.5, 75.0), (135.41666666666666, 82.08333333333333), (175.0, 67.5), (200.0, 60.0)]]\n\n The returned splines have \"implied oncurve points\" suitable for use in\n TrueType ``glif`` outlines - i.e. in the first spline returned above,\n the first quadratic segment runs from (50,50) to\n ( (75 + 125)/2 , (120 + 91.666..)/2 ) = (100, 83.333...).\n\n Returns:\n If all_quadratic is True, a list of splines, each spline being a list\n of 2D tuples.\n\n If all_quadratic is False, a list of curves, each curve being a quadratic\n (length 3), or cubic (length 4).\n\n Raises:\n fontTools.cu2qu.Errors.ApproxNotFoundError: if no suitable approximation\n can be found for all curves with the given parameters.\n ";
-/* #### Code section: decls ### */
-static PyObject *__pyx_pf_9fontTools_5cu2qu_5cu2qu__split_cubic_into_n_gen(CYTHON_UNUSED PyObject *__pyx_self, __pyx_t_double_complex __pyx_v_p0, __pyx_t_double_complex __pyx_v_p1, __pyx_t_double_complex __pyx_v_p2, __pyx_t_double_complex __pyx_v_p3, int __pyx_v_n); /* proto */
-static PyObject *__pyx_pf_9fontTools_5cu2qu_5cu2qu_3curve_to_quadratic(CYTHON_UNUSED PyObject *__pyx_self, PyObject *__pyx_v_curve, double __pyx_v_max_err, int __pyx_v_all_quadratic); /* proto */
-static PyObject *__pyx_pf_9fontTools_5cu2qu_5cu2qu_5curves_to_quadratic(CYTHON_UNUSED PyObject *__pyx_self, PyObject *__pyx_v_curves, PyObject *__pyx_v_max_errors, int __pyx_v_all_quadratic); /* proto */
-static PyObject *__pyx_tp_new_9fontTools_5cu2qu_5cu2qu___pyx_scope_struct___split_cubic_into_n_gen(PyTypeObject *t, PyObject *a, PyObject *k); /*proto*/
-/* #### Code section: late_includes ### */
-/* #### Code section: module_state ### */
-typedef struct {
- PyObject *__pyx_d;
- PyObject *__pyx_b;
- PyObject *__pyx_cython_runtime;
- PyObject *__pyx_empty_tuple;
- PyObject *__pyx_empty_bytes;
- PyObject *__pyx_empty_unicode;
- #ifdef __Pyx_CyFunction_USED
- PyTypeObject *__pyx_CyFunctionType;
- #endif
- #ifdef __Pyx_FusedFunction_USED
- PyTypeObject *__pyx_FusedFunctionType;
- #endif
- #ifdef __Pyx_Generator_USED
- PyTypeObject *__pyx_GeneratorType;
- #endif
- #ifdef __Pyx_IterableCoroutine_USED
- PyTypeObject *__pyx_IterableCoroutineType;
- #endif
- #ifdef __Pyx_Coroutine_USED
- PyTypeObject *__pyx_CoroutineAwaitType;
- #endif
- #ifdef __Pyx_Coroutine_USED
- PyTypeObject *__pyx_CoroutineType;
- #endif
- #if CYTHON_USE_MODULE_STATE
- #endif
- #if CYTHON_USE_MODULE_STATE
- PyObject *__pyx_type_9fontTools_5cu2qu_5cu2qu___pyx_scope_struct___split_cubic_into_n_gen;
- #endif
- PyTypeObject *__pyx_ptype_9fontTools_5cu2qu_5cu2qu___pyx_scope_struct___split_cubic_into_n_gen;
- PyObject *__pyx_n_s_ApproxNotFoundError;
- PyObject *__pyx_n_s_AssertionError;
- PyObject *__pyx_n_s_AttributeError;
- PyObject *__pyx_n_s_COMPILED;
- PyObject *__pyx_n_s_Cu2QuError;
- PyObject *__pyx_n_s_Error;
- PyObject *__pyx_n_s_ImportError;
- PyObject *__pyx_kp_s_Lib_fontTools_cu2qu_cu2qu_py;
- PyObject *__pyx_n_s_MAX_N;
- PyObject *__pyx_n_s_NAN;
- PyObject *__pyx_n_u_NaN;
- PyObject *__pyx_kp_u_Return_quadratic_Bezier_splines;
- PyObject *__pyx_n_s_ZeroDivisionError;
- PyObject *__pyx_kp_u__2;
- PyObject *__pyx_n_s__3;
- PyObject *__pyx_n_s__9;
- PyObject *__pyx_n_s_a;
- PyObject *__pyx_n_s_a1;
- PyObject *__pyx_n_s_all;
- PyObject *__pyx_n_s_all_quadratic;
- PyObject *__pyx_n_s_args;
- PyObject *__pyx_n_s_asyncio_coroutines;
- PyObject *__pyx_n_s_b;
- PyObject *__pyx_n_s_b1;
- PyObject *__pyx_n_s_c;
- PyObject *__pyx_n_s_c1;
- PyObject *__pyx_n_s_cline_in_traceback;
- PyObject *__pyx_n_s_close;
- PyObject *__pyx_n_s_curve;
- PyObject *__pyx_n_s_curve_to_quadratic;
- PyObject *__pyx_n_u_curve_to_quadratic;
- PyObject *__pyx_n_s_curves;
- PyObject *__pyx_n_s_curves_to_quadratic;
- PyObject *__pyx_n_u_curves_to_quadratic;
- PyObject *__pyx_kp_u_curves_to_quadratic_line_474;
- PyObject *__pyx_n_s_cython;
- PyObject *__pyx_n_s_d;
- PyObject *__pyx_n_s_d1;
- PyObject *__pyx_n_s_delta_2;
- PyObject *__pyx_n_s_delta_3;
- PyObject *__pyx_kp_u_disable;
- PyObject *__pyx_n_s_dt;
- PyObject *__pyx_kp_u_enable;
- PyObject *__pyx_n_s_errors;
- PyObject *__pyx_n_s_fontTools_cu2qu_cu2qu;
- PyObject *__pyx_n_s_fontTools_misc;
- PyObject *__pyx_kp_u_gc;
- PyObject *__pyx_n_s_i;
- PyObject *__pyx_n_s_imag;
- PyObject *__pyx_n_s_import;
- PyObject *__pyx_n_s_initializing;
- PyObject *__pyx_n_s_is_coroutine;
- PyObject *__pyx_kp_u_isenabled;
- PyObject *__pyx_n_s_isnan;
- PyObject *__pyx_n_s_l;
- PyObject *__pyx_n_s_last_i;
- PyObject *__pyx_n_s_main;
- PyObject *__pyx_n_s_math;
- PyObject *__pyx_n_s_max_err;
- PyObject *__pyx_n_s_max_errors;
- PyObject *__pyx_n_s_n;
- PyObject *__pyx_n_s_name;
- PyObject *__pyx_n_s_p;
- PyObject *__pyx_n_s_p0;
- PyObject *__pyx_n_s_p1;
- PyObject *__pyx_n_s_p2;
- PyObject *__pyx_n_s_p3;
- PyObject *__pyx_n_s_range;
- PyObject *__pyx_n_s_real;
- PyObject *__pyx_n_s_s;
- PyObject *__pyx_n_s_send;
- PyObject *__pyx_n_s_spec;
- PyObject *__pyx_n_s_spline;
- PyObject *__pyx_n_s_splines;
- PyObject *__pyx_n_s_split_cubic_into_n_gen;
- PyObject *__pyx_n_s_t1;
- PyObject *__pyx_n_s_t1_2;
- PyObject *__pyx_n_s_test;
- PyObject *__pyx_n_s_throw;
- PyObject *__pyx_int_1;
- PyObject *__pyx_int_2;
- PyObject *__pyx_int_3;
- PyObject *__pyx_int_4;
- PyObject *__pyx_int_6;
- PyObject *__pyx_int_100;
- PyObject *__pyx_codeobj_;
- PyObject *__pyx_tuple__4;
- PyObject *__pyx_tuple__5;
- PyObject *__pyx_tuple__7;
- PyObject *__pyx_codeobj__6;
- PyObject *__pyx_codeobj__8;
-} __pyx_mstate;
-
-#if CYTHON_USE_MODULE_STATE
-#ifdef __cplusplus
-namespace {
- extern struct PyModuleDef __pyx_moduledef;
-} /* anonymous namespace */
-#else
-static struct PyModuleDef __pyx_moduledef;
-#endif
-
-#define __pyx_mstate(o) ((__pyx_mstate *)__Pyx_PyModule_GetState(o))
-
-#define __pyx_mstate_global (__pyx_mstate(PyState_FindModule(&__pyx_moduledef)))
-
-#define __pyx_m (PyState_FindModule(&__pyx_moduledef))
-#else
-static __pyx_mstate __pyx_mstate_global_static =
-#ifdef __cplusplus
- {};
-#else
- {0};
-#endif
-static __pyx_mstate *__pyx_mstate_global = &__pyx_mstate_global_static;
-#endif
-/* #### Code section: module_state_clear ### */
-#if CYTHON_USE_MODULE_STATE
-static int __pyx_m_clear(PyObject *m) {
- __pyx_mstate *clear_module_state = __pyx_mstate(m);
- if (!clear_module_state) return 0;
- Py_CLEAR(clear_module_state->__pyx_d);
- Py_CLEAR(clear_module_state->__pyx_b);
- Py_CLEAR(clear_module_state->__pyx_cython_runtime);
- Py_CLEAR(clear_module_state->__pyx_empty_tuple);
- Py_CLEAR(clear_module_state->__pyx_empty_bytes);
- Py_CLEAR(clear_module_state->__pyx_empty_unicode);
- #ifdef __Pyx_CyFunction_USED
- Py_CLEAR(clear_module_state->__pyx_CyFunctionType);
- #endif
- #ifdef __Pyx_FusedFunction_USED
- Py_CLEAR(clear_module_state->__pyx_FusedFunctionType);
- #endif
- Py_CLEAR(clear_module_state->__pyx_ptype_9fontTools_5cu2qu_5cu2qu___pyx_scope_struct___split_cubic_into_n_gen);
- Py_CLEAR(clear_module_state->__pyx_type_9fontTools_5cu2qu_5cu2qu___pyx_scope_struct___split_cubic_into_n_gen);
- Py_CLEAR(clear_module_state->__pyx_n_s_ApproxNotFoundError);
- Py_CLEAR(clear_module_state->__pyx_n_s_AssertionError);
- Py_CLEAR(clear_module_state->__pyx_n_s_AttributeError);
- Py_CLEAR(clear_module_state->__pyx_n_s_COMPILED);
- Py_CLEAR(clear_module_state->__pyx_n_s_Cu2QuError);
- Py_CLEAR(clear_module_state->__pyx_n_s_Error);
- Py_CLEAR(clear_module_state->__pyx_n_s_ImportError);
- Py_CLEAR(clear_module_state->__pyx_kp_s_Lib_fontTools_cu2qu_cu2qu_py);
- Py_CLEAR(clear_module_state->__pyx_n_s_MAX_N);
- Py_CLEAR(clear_module_state->__pyx_n_s_NAN);
- Py_CLEAR(clear_module_state->__pyx_n_u_NaN);
- Py_CLEAR(clear_module_state->__pyx_kp_u_Return_quadratic_Bezier_splines);
- Py_CLEAR(clear_module_state->__pyx_n_s_ZeroDivisionError);
- Py_CLEAR(clear_module_state->__pyx_kp_u__2);
- Py_CLEAR(clear_module_state->__pyx_n_s__3);
- Py_CLEAR(clear_module_state->__pyx_n_s__9);
- Py_CLEAR(clear_module_state->__pyx_n_s_a);
- Py_CLEAR(clear_module_state->__pyx_n_s_a1);
- Py_CLEAR(clear_module_state->__pyx_n_s_all);
- Py_CLEAR(clear_module_state->__pyx_n_s_all_quadratic);
- Py_CLEAR(clear_module_state->__pyx_n_s_args);
- Py_CLEAR(clear_module_state->__pyx_n_s_asyncio_coroutines);
- Py_CLEAR(clear_module_state->__pyx_n_s_b);
- Py_CLEAR(clear_module_state->__pyx_n_s_b1);
- Py_CLEAR(clear_module_state->__pyx_n_s_c);
- Py_CLEAR(clear_module_state->__pyx_n_s_c1);
- Py_CLEAR(clear_module_state->__pyx_n_s_cline_in_traceback);
- Py_CLEAR(clear_module_state->__pyx_n_s_close);
- Py_CLEAR(clear_module_state->__pyx_n_s_curve);
- Py_CLEAR(clear_module_state->__pyx_n_s_curve_to_quadratic);
- Py_CLEAR(clear_module_state->__pyx_n_u_curve_to_quadratic);
- Py_CLEAR(clear_module_state->__pyx_n_s_curves);
- Py_CLEAR(clear_module_state->__pyx_n_s_curves_to_quadratic);
- Py_CLEAR(clear_module_state->__pyx_n_u_curves_to_quadratic);
- Py_CLEAR(clear_module_state->__pyx_kp_u_curves_to_quadratic_line_474);
- Py_CLEAR(clear_module_state->__pyx_n_s_cython);
- Py_CLEAR(clear_module_state->__pyx_n_s_d);
- Py_CLEAR(clear_module_state->__pyx_n_s_d1);
- Py_CLEAR(clear_module_state->__pyx_n_s_delta_2);
- Py_CLEAR(clear_module_state->__pyx_n_s_delta_3);
- Py_CLEAR(clear_module_state->__pyx_kp_u_disable);
- Py_CLEAR(clear_module_state->__pyx_n_s_dt);
- Py_CLEAR(clear_module_state->__pyx_kp_u_enable);
- Py_CLEAR(clear_module_state->__pyx_n_s_errors);
- Py_CLEAR(clear_module_state->__pyx_n_s_fontTools_cu2qu_cu2qu);
- Py_CLEAR(clear_module_state->__pyx_n_s_fontTools_misc);
- Py_CLEAR(clear_module_state->__pyx_kp_u_gc);
- Py_CLEAR(clear_module_state->__pyx_n_s_i);
- Py_CLEAR(clear_module_state->__pyx_n_s_imag);
- Py_CLEAR(clear_module_state->__pyx_n_s_import);
- Py_CLEAR(clear_module_state->__pyx_n_s_initializing);
- Py_CLEAR(clear_module_state->__pyx_n_s_is_coroutine);
- Py_CLEAR(clear_module_state->__pyx_kp_u_isenabled);
- Py_CLEAR(clear_module_state->__pyx_n_s_isnan);
- Py_CLEAR(clear_module_state->__pyx_n_s_l);
- Py_CLEAR(clear_module_state->__pyx_n_s_last_i);
- Py_CLEAR(clear_module_state->__pyx_n_s_main);
- Py_CLEAR(clear_module_state->__pyx_n_s_math);
- Py_CLEAR(clear_module_state->__pyx_n_s_max_err);
- Py_CLEAR(clear_module_state->__pyx_n_s_max_errors);
- Py_CLEAR(clear_module_state->__pyx_n_s_n);
- Py_CLEAR(clear_module_state->__pyx_n_s_name);
- Py_CLEAR(clear_module_state->__pyx_n_s_p);
- Py_CLEAR(clear_module_state->__pyx_n_s_p0);
- Py_CLEAR(clear_module_state->__pyx_n_s_p1);
- Py_CLEAR(clear_module_state->__pyx_n_s_p2);
- Py_CLEAR(clear_module_state->__pyx_n_s_p3);
- Py_CLEAR(clear_module_state->__pyx_n_s_range);
- Py_CLEAR(clear_module_state->__pyx_n_s_real);
- Py_CLEAR(clear_module_state->__pyx_n_s_s);
- Py_CLEAR(clear_module_state->__pyx_n_s_send);
- Py_CLEAR(clear_module_state->__pyx_n_s_spec);
- Py_CLEAR(clear_module_state->__pyx_n_s_spline);
- Py_CLEAR(clear_module_state->__pyx_n_s_splines);
- Py_CLEAR(clear_module_state->__pyx_n_s_split_cubic_into_n_gen);
- Py_CLEAR(clear_module_state->__pyx_n_s_t1);
- Py_CLEAR(clear_module_state->__pyx_n_s_t1_2);
- Py_CLEAR(clear_module_state->__pyx_n_s_test);
- Py_CLEAR(clear_module_state->__pyx_n_s_throw);
- Py_CLEAR(clear_module_state->__pyx_int_1);
- Py_CLEAR(clear_module_state->__pyx_int_2);
- Py_CLEAR(clear_module_state->__pyx_int_3);
- Py_CLEAR(clear_module_state->__pyx_int_4);
- Py_CLEAR(clear_module_state->__pyx_int_6);
- Py_CLEAR(clear_module_state->__pyx_int_100);
- Py_CLEAR(clear_module_state->__pyx_codeobj_);
- Py_CLEAR(clear_module_state->__pyx_tuple__4);
- Py_CLEAR(clear_module_state->__pyx_tuple__5);
- Py_CLEAR(clear_module_state->__pyx_tuple__7);
- Py_CLEAR(clear_module_state->__pyx_codeobj__6);
- Py_CLEAR(clear_module_state->__pyx_codeobj__8);
- return 0;
-}
-#endif
-/* #### Code section: module_state_traverse ### */
-#if CYTHON_USE_MODULE_STATE
-static int __pyx_m_traverse(PyObject *m, visitproc visit, void *arg) {
- __pyx_mstate *traverse_module_state = __pyx_mstate(m);
- if (!traverse_module_state) return 0;
- Py_VISIT(traverse_module_state->__pyx_d);
- Py_VISIT(traverse_module_state->__pyx_b);
- Py_VISIT(traverse_module_state->__pyx_cython_runtime);
- Py_VISIT(traverse_module_state->__pyx_empty_tuple);
- Py_VISIT(traverse_module_state->__pyx_empty_bytes);
- Py_VISIT(traverse_module_state->__pyx_empty_unicode);
- #ifdef __Pyx_CyFunction_USED
- Py_VISIT(traverse_module_state->__pyx_CyFunctionType);
- #endif
- #ifdef __Pyx_FusedFunction_USED
- Py_VISIT(traverse_module_state->__pyx_FusedFunctionType);
- #endif
- Py_VISIT(traverse_module_state->__pyx_ptype_9fontTools_5cu2qu_5cu2qu___pyx_scope_struct___split_cubic_into_n_gen);
- Py_VISIT(traverse_module_state->__pyx_type_9fontTools_5cu2qu_5cu2qu___pyx_scope_struct___split_cubic_into_n_gen);
- Py_VISIT(traverse_module_state->__pyx_n_s_ApproxNotFoundError);
- Py_VISIT(traverse_module_state->__pyx_n_s_AssertionError);
- Py_VISIT(traverse_module_state->__pyx_n_s_AttributeError);
- Py_VISIT(traverse_module_state->__pyx_n_s_COMPILED);
- Py_VISIT(traverse_module_state->__pyx_n_s_Cu2QuError);
- Py_VISIT(traverse_module_state->__pyx_n_s_Error);
- Py_VISIT(traverse_module_state->__pyx_n_s_ImportError);
- Py_VISIT(traverse_module_state->__pyx_kp_s_Lib_fontTools_cu2qu_cu2qu_py);
- Py_VISIT(traverse_module_state->__pyx_n_s_MAX_N);
- Py_VISIT(traverse_module_state->__pyx_n_s_NAN);
- Py_VISIT(traverse_module_state->__pyx_n_u_NaN);
- Py_VISIT(traverse_module_state->__pyx_kp_u_Return_quadratic_Bezier_splines);
- Py_VISIT(traverse_module_state->__pyx_n_s_ZeroDivisionError);
- Py_VISIT(traverse_module_state->__pyx_kp_u__2);
- Py_VISIT(traverse_module_state->__pyx_n_s__3);
- Py_VISIT(traverse_module_state->__pyx_n_s__9);
- Py_VISIT(traverse_module_state->__pyx_n_s_a);
- Py_VISIT(traverse_module_state->__pyx_n_s_a1);
- Py_VISIT(traverse_module_state->__pyx_n_s_all);
- Py_VISIT(traverse_module_state->__pyx_n_s_all_quadratic);
- Py_VISIT(traverse_module_state->__pyx_n_s_args);
- Py_VISIT(traverse_module_state->__pyx_n_s_asyncio_coroutines);
- Py_VISIT(traverse_module_state->__pyx_n_s_b);
- Py_VISIT(traverse_module_state->__pyx_n_s_b1);
- Py_VISIT(traverse_module_state->__pyx_n_s_c);
- Py_VISIT(traverse_module_state->__pyx_n_s_c1);
- Py_VISIT(traverse_module_state->__pyx_n_s_cline_in_traceback);
- Py_VISIT(traverse_module_state->__pyx_n_s_close);
- Py_VISIT(traverse_module_state->__pyx_n_s_curve);
- Py_VISIT(traverse_module_state->__pyx_n_s_curve_to_quadratic);
- Py_VISIT(traverse_module_state->__pyx_n_u_curve_to_quadratic);
- Py_VISIT(traverse_module_state->__pyx_n_s_curves);
- Py_VISIT(traverse_module_state->__pyx_n_s_curves_to_quadratic);
- Py_VISIT(traverse_module_state->__pyx_n_u_curves_to_quadratic);
- Py_VISIT(traverse_module_state->__pyx_kp_u_curves_to_quadratic_line_474);
- Py_VISIT(traverse_module_state->__pyx_n_s_cython);
- Py_VISIT(traverse_module_state->__pyx_n_s_d);
- Py_VISIT(traverse_module_state->__pyx_n_s_d1);
- Py_VISIT(traverse_module_state->__pyx_n_s_delta_2);
- Py_VISIT(traverse_module_state->__pyx_n_s_delta_3);
- Py_VISIT(traverse_module_state->__pyx_kp_u_disable);
- Py_VISIT(traverse_module_state->__pyx_n_s_dt);
- Py_VISIT(traverse_module_state->__pyx_kp_u_enable);
- Py_VISIT(traverse_module_state->__pyx_n_s_errors);
- Py_VISIT(traverse_module_state->__pyx_n_s_fontTools_cu2qu_cu2qu);
- Py_VISIT(traverse_module_state->__pyx_n_s_fontTools_misc);
- Py_VISIT(traverse_module_state->__pyx_kp_u_gc);
- Py_VISIT(traverse_module_state->__pyx_n_s_i);
- Py_VISIT(traverse_module_state->__pyx_n_s_imag);
- Py_VISIT(traverse_module_state->__pyx_n_s_import);
- Py_VISIT(traverse_module_state->__pyx_n_s_initializing);
- Py_VISIT(traverse_module_state->__pyx_n_s_is_coroutine);
- Py_VISIT(traverse_module_state->__pyx_kp_u_isenabled);
- Py_VISIT(traverse_module_state->__pyx_n_s_isnan);
- Py_VISIT(traverse_module_state->__pyx_n_s_l);
- Py_VISIT(traverse_module_state->__pyx_n_s_last_i);
- Py_VISIT(traverse_module_state->__pyx_n_s_main);
- Py_VISIT(traverse_module_state->__pyx_n_s_math);
- Py_VISIT(traverse_module_state->__pyx_n_s_max_err);
- Py_VISIT(traverse_module_state->__pyx_n_s_max_errors);
- Py_VISIT(traverse_module_state->__pyx_n_s_n);
- Py_VISIT(traverse_module_state->__pyx_n_s_name);
- Py_VISIT(traverse_module_state->__pyx_n_s_p);
- Py_VISIT(traverse_module_state->__pyx_n_s_p0);
- Py_VISIT(traverse_module_state->__pyx_n_s_p1);
- Py_VISIT(traverse_module_state->__pyx_n_s_p2);
- Py_VISIT(traverse_module_state->__pyx_n_s_p3);
- Py_VISIT(traverse_module_state->__pyx_n_s_range);
- Py_VISIT(traverse_module_state->__pyx_n_s_real);
- Py_VISIT(traverse_module_state->__pyx_n_s_s);
- Py_VISIT(traverse_module_state->__pyx_n_s_send);
- Py_VISIT(traverse_module_state->__pyx_n_s_spec);
- Py_VISIT(traverse_module_state->__pyx_n_s_spline);
- Py_VISIT(traverse_module_state->__pyx_n_s_splines);
- Py_VISIT(traverse_module_state->__pyx_n_s_split_cubic_into_n_gen);
- Py_VISIT(traverse_module_state->__pyx_n_s_t1);
- Py_VISIT(traverse_module_state->__pyx_n_s_t1_2);
- Py_VISIT(traverse_module_state->__pyx_n_s_test);
- Py_VISIT(traverse_module_state->__pyx_n_s_throw);
- Py_VISIT(traverse_module_state->__pyx_int_1);
- Py_VISIT(traverse_module_state->__pyx_int_2);
- Py_VISIT(traverse_module_state->__pyx_int_3);
- Py_VISIT(traverse_module_state->__pyx_int_4);
- Py_VISIT(traverse_module_state->__pyx_int_6);
- Py_VISIT(traverse_module_state->__pyx_int_100);
- Py_VISIT(traverse_module_state->__pyx_codeobj_);
- Py_VISIT(traverse_module_state->__pyx_tuple__4);
- Py_VISIT(traverse_module_state->__pyx_tuple__5);
- Py_VISIT(traverse_module_state->__pyx_tuple__7);
- Py_VISIT(traverse_module_state->__pyx_codeobj__6);
- Py_VISIT(traverse_module_state->__pyx_codeobj__8);
- return 0;
-}
-#endif
-/* #### Code section: module_state_defines ### */
-#define __pyx_d __pyx_mstate_global->__pyx_d
-#define __pyx_b __pyx_mstate_global->__pyx_b
-#define __pyx_cython_runtime __pyx_mstate_global->__pyx_cython_runtime
-#define __pyx_empty_tuple __pyx_mstate_global->__pyx_empty_tuple
-#define __pyx_empty_bytes __pyx_mstate_global->__pyx_empty_bytes
-#define __pyx_empty_unicode __pyx_mstate_global->__pyx_empty_unicode
-#ifdef __Pyx_CyFunction_USED
-#define __pyx_CyFunctionType __pyx_mstate_global->__pyx_CyFunctionType
-#endif
-#ifdef __Pyx_FusedFunction_USED
-#define __pyx_FusedFunctionType __pyx_mstate_global->__pyx_FusedFunctionType
-#endif
-#ifdef __Pyx_Generator_USED
-#define __pyx_GeneratorType __pyx_mstate_global->__pyx_GeneratorType
-#endif
-#ifdef __Pyx_IterableCoroutine_USED
-#define __pyx_IterableCoroutineType __pyx_mstate_global->__pyx_IterableCoroutineType
-#endif
-#ifdef __Pyx_Coroutine_USED
-#define __pyx_CoroutineAwaitType __pyx_mstate_global->__pyx_CoroutineAwaitType
-#endif
-#ifdef __Pyx_Coroutine_USED
-#define __pyx_CoroutineType __pyx_mstate_global->__pyx_CoroutineType
-#endif
-#if CYTHON_USE_MODULE_STATE
-#endif
-#if CYTHON_USE_MODULE_STATE
-#define __pyx_type_9fontTools_5cu2qu_5cu2qu___pyx_scope_struct___split_cubic_into_n_gen __pyx_mstate_global->__pyx_type_9fontTools_5cu2qu_5cu2qu___pyx_scope_struct___split_cubic_into_n_gen
-#endif
-#define __pyx_ptype_9fontTools_5cu2qu_5cu2qu___pyx_scope_struct___split_cubic_into_n_gen __pyx_mstate_global->__pyx_ptype_9fontTools_5cu2qu_5cu2qu___pyx_scope_struct___split_cubic_into_n_gen
-#define __pyx_n_s_ApproxNotFoundError __pyx_mstate_global->__pyx_n_s_ApproxNotFoundError
-#define __pyx_n_s_AssertionError __pyx_mstate_global->__pyx_n_s_AssertionError
-#define __pyx_n_s_AttributeError __pyx_mstate_global->__pyx_n_s_AttributeError
-#define __pyx_n_s_COMPILED __pyx_mstate_global->__pyx_n_s_COMPILED
-#define __pyx_n_s_Cu2QuError __pyx_mstate_global->__pyx_n_s_Cu2QuError
-#define __pyx_n_s_Error __pyx_mstate_global->__pyx_n_s_Error
-#define __pyx_n_s_ImportError __pyx_mstate_global->__pyx_n_s_ImportError
-#define __pyx_kp_s_Lib_fontTools_cu2qu_cu2qu_py __pyx_mstate_global->__pyx_kp_s_Lib_fontTools_cu2qu_cu2qu_py
-#define __pyx_n_s_MAX_N __pyx_mstate_global->__pyx_n_s_MAX_N
-#define __pyx_n_s_NAN __pyx_mstate_global->__pyx_n_s_NAN
-#define __pyx_n_u_NaN __pyx_mstate_global->__pyx_n_u_NaN
-#define __pyx_kp_u_Return_quadratic_Bezier_splines __pyx_mstate_global->__pyx_kp_u_Return_quadratic_Bezier_splines
-#define __pyx_n_s_ZeroDivisionError __pyx_mstate_global->__pyx_n_s_ZeroDivisionError
-#define __pyx_kp_u__2 __pyx_mstate_global->__pyx_kp_u__2
-#define __pyx_n_s__3 __pyx_mstate_global->__pyx_n_s__3
-#define __pyx_n_s__9 __pyx_mstate_global->__pyx_n_s__9
-#define __pyx_n_s_a __pyx_mstate_global->__pyx_n_s_a
-#define __pyx_n_s_a1 __pyx_mstate_global->__pyx_n_s_a1
-#define __pyx_n_s_all __pyx_mstate_global->__pyx_n_s_all
-#define __pyx_n_s_all_quadratic __pyx_mstate_global->__pyx_n_s_all_quadratic
-#define __pyx_n_s_args __pyx_mstate_global->__pyx_n_s_args
-#define __pyx_n_s_asyncio_coroutines __pyx_mstate_global->__pyx_n_s_asyncio_coroutines
-#define __pyx_n_s_b __pyx_mstate_global->__pyx_n_s_b
-#define __pyx_n_s_b1 __pyx_mstate_global->__pyx_n_s_b1
-#define __pyx_n_s_c __pyx_mstate_global->__pyx_n_s_c
-#define __pyx_n_s_c1 __pyx_mstate_global->__pyx_n_s_c1
-#define __pyx_n_s_cline_in_traceback __pyx_mstate_global->__pyx_n_s_cline_in_traceback
-#define __pyx_n_s_close __pyx_mstate_global->__pyx_n_s_close
-#define __pyx_n_s_curve __pyx_mstate_global->__pyx_n_s_curve
-#define __pyx_n_s_curve_to_quadratic __pyx_mstate_global->__pyx_n_s_curve_to_quadratic
-#define __pyx_n_u_curve_to_quadratic __pyx_mstate_global->__pyx_n_u_curve_to_quadratic
-#define __pyx_n_s_curves __pyx_mstate_global->__pyx_n_s_curves
-#define __pyx_n_s_curves_to_quadratic __pyx_mstate_global->__pyx_n_s_curves_to_quadratic
-#define __pyx_n_u_curves_to_quadratic __pyx_mstate_global->__pyx_n_u_curves_to_quadratic
-#define __pyx_kp_u_curves_to_quadratic_line_474 __pyx_mstate_global->__pyx_kp_u_curves_to_quadratic_line_474
-#define __pyx_n_s_cython __pyx_mstate_global->__pyx_n_s_cython
-#define __pyx_n_s_d __pyx_mstate_global->__pyx_n_s_d
-#define __pyx_n_s_d1 __pyx_mstate_global->__pyx_n_s_d1
-#define __pyx_n_s_delta_2 __pyx_mstate_global->__pyx_n_s_delta_2
-#define __pyx_n_s_delta_3 __pyx_mstate_global->__pyx_n_s_delta_3
-#define __pyx_kp_u_disable __pyx_mstate_global->__pyx_kp_u_disable
-#define __pyx_n_s_dt __pyx_mstate_global->__pyx_n_s_dt
-#define __pyx_kp_u_enable __pyx_mstate_global->__pyx_kp_u_enable
-#define __pyx_n_s_errors __pyx_mstate_global->__pyx_n_s_errors
-#define __pyx_n_s_fontTools_cu2qu_cu2qu __pyx_mstate_global->__pyx_n_s_fontTools_cu2qu_cu2qu
-#define __pyx_n_s_fontTools_misc __pyx_mstate_global->__pyx_n_s_fontTools_misc
-#define __pyx_kp_u_gc __pyx_mstate_global->__pyx_kp_u_gc
-#define __pyx_n_s_i __pyx_mstate_global->__pyx_n_s_i
-#define __pyx_n_s_imag __pyx_mstate_global->__pyx_n_s_imag
-#define __pyx_n_s_import __pyx_mstate_global->__pyx_n_s_import
-#define __pyx_n_s_initializing __pyx_mstate_global->__pyx_n_s_initializing
-#define __pyx_n_s_is_coroutine __pyx_mstate_global->__pyx_n_s_is_coroutine
-#define __pyx_kp_u_isenabled __pyx_mstate_global->__pyx_kp_u_isenabled
-#define __pyx_n_s_isnan __pyx_mstate_global->__pyx_n_s_isnan
-#define __pyx_n_s_l __pyx_mstate_global->__pyx_n_s_l
-#define __pyx_n_s_last_i __pyx_mstate_global->__pyx_n_s_last_i
-#define __pyx_n_s_main __pyx_mstate_global->__pyx_n_s_main
-#define __pyx_n_s_math __pyx_mstate_global->__pyx_n_s_math
-#define __pyx_n_s_max_err __pyx_mstate_global->__pyx_n_s_max_err
-#define __pyx_n_s_max_errors __pyx_mstate_global->__pyx_n_s_max_errors
-#define __pyx_n_s_n __pyx_mstate_global->__pyx_n_s_n
-#define __pyx_n_s_name __pyx_mstate_global->__pyx_n_s_name
-#define __pyx_n_s_p __pyx_mstate_global->__pyx_n_s_p
-#define __pyx_n_s_p0 __pyx_mstate_global->__pyx_n_s_p0
-#define __pyx_n_s_p1 __pyx_mstate_global->__pyx_n_s_p1
-#define __pyx_n_s_p2 __pyx_mstate_global->__pyx_n_s_p2
-#define __pyx_n_s_p3 __pyx_mstate_global->__pyx_n_s_p3
-#define __pyx_n_s_range __pyx_mstate_global->__pyx_n_s_range
-#define __pyx_n_s_real __pyx_mstate_global->__pyx_n_s_real
-#define __pyx_n_s_s __pyx_mstate_global->__pyx_n_s_s
-#define __pyx_n_s_send __pyx_mstate_global->__pyx_n_s_send
-#define __pyx_n_s_spec __pyx_mstate_global->__pyx_n_s_spec
-#define __pyx_n_s_spline __pyx_mstate_global->__pyx_n_s_spline
-#define __pyx_n_s_splines __pyx_mstate_global->__pyx_n_s_splines
-#define __pyx_n_s_split_cubic_into_n_gen __pyx_mstate_global->__pyx_n_s_split_cubic_into_n_gen
-#define __pyx_n_s_t1 __pyx_mstate_global->__pyx_n_s_t1
-#define __pyx_n_s_t1_2 __pyx_mstate_global->__pyx_n_s_t1_2
-#define __pyx_n_s_test __pyx_mstate_global->__pyx_n_s_test
-#define __pyx_n_s_throw __pyx_mstate_global->__pyx_n_s_throw
-#define __pyx_int_1 __pyx_mstate_global->__pyx_int_1
-#define __pyx_int_2 __pyx_mstate_global->__pyx_int_2
-#define __pyx_int_3 __pyx_mstate_global->__pyx_int_3
-#define __pyx_int_4 __pyx_mstate_global->__pyx_int_4
-#define __pyx_int_6 __pyx_mstate_global->__pyx_int_6
-#define __pyx_int_100 __pyx_mstate_global->__pyx_int_100
-#define __pyx_codeobj_ __pyx_mstate_global->__pyx_codeobj_
-#define __pyx_tuple__4 __pyx_mstate_global->__pyx_tuple__4
-#define __pyx_tuple__5 __pyx_mstate_global->__pyx_tuple__5
-#define __pyx_tuple__7 __pyx_mstate_global->__pyx_tuple__7
-#define __pyx_codeobj__6 __pyx_mstate_global->__pyx_codeobj__6
-#define __pyx_codeobj__8 __pyx_mstate_global->__pyx_codeobj__8
-/* #### Code section: module_code ### */
-
-/* "fontTools/cu2qu/cu2qu.py":40
- *
- *
- * @cython.cfunc # <<<<<<<<<<<<<<
- * @cython.inline
- * @cython.returns(cython.double)
- */
-
-static CYTHON_INLINE double __pyx_f_9fontTools_5cu2qu_5cu2qu_dot(__pyx_t_double_complex __pyx_v_v1, __pyx_t_double_complex __pyx_v_v2) {
- double __pyx_r;
- __Pyx_RefNannyDeclarations
- __Pyx_RefNannySetupContext("dot", 0);
-
- /* "fontTools/cu2qu/cu2qu.py":54
- * double: Dot product.
- * """
- * return (v1 * v2.conjugate()).real # <<<<<<<<<<<<<<
- *
- *
- */
- __pyx_r = __Pyx_CREAL(__Pyx_c_prod_double(__pyx_v_v1, __Pyx_c_conj_double(__pyx_v_v2)));
- goto __pyx_L0;
-
- /* "fontTools/cu2qu/cu2qu.py":40
- *
- *
- * @cython.cfunc # <<<<<<<<<<<<<<
- * @cython.inline
- * @cython.returns(cython.double)
- */
-
- /* function exit code */
- __pyx_L0:;
- __Pyx_RefNannyFinishContext();
- return __pyx_r;
-}
-
-/* "fontTools/cu2qu/cu2qu.py":57
- *
- *
- * @cython.cfunc # <<<<<<<<<<<<<<
- * @cython.inline
- * @cython.locals(a=cython.complex, b=cython.complex, c=cython.complex, d=cython.complex)
- */
-
-static CYTHON_INLINE PyObject *__pyx_f_9fontTools_5cu2qu_5cu2qu_calc_cubic_points(__pyx_t_double_complex __pyx_v_a, __pyx_t_double_complex __pyx_v_b, __pyx_t_double_complex __pyx_v_c, __pyx_t_double_complex __pyx_v_d) {
- __pyx_t_double_complex __pyx_v__1;
- __pyx_t_double_complex __pyx_v__2;
- __pyx_t_double_complex __pyx_v__3;
- __pyx_t_double_complex __pyx_v__4;
- PyObject *__pyx_r = NULL;
- __Pyx_RefNannyDeclarations
- __pyx_t_double_complex __pyx_t_1;
- __pyx_t_double_complex __pyx_t_2;
- PyObject *__pyx_t_3 = NULL;
- PyObject *__pyx_t_4 = NULL;
- PyObject *__pyx_t_5 = NULL;
- PyObject *__pyx_t_6 = NULL;
- PyObject *__pyx_t_7 = NULL;
- int __pyx_lineno = 0;
- const char *__pyx_filename = NULL;
- int __pyx_clineno = 0;
- __Pyx_RefNannySetupContext("calc_cubic_points", 0);
-
- /* "fontTools/cu2qu/cu2qu.py":64
- * )
- * def calc_cubic_points(a, b, c, d):
- * _1 = d # <<<<<<<<<<<<<<
- * _2 = (c / 3.0) + d
- * _3 = (b + c) / 3.0 + _2
- */
- __pyx_v__1 = __pyx_v_d;
-
- /* "fontTools/cu2qu/cu2qu.py":65
- * def calc_cubic_points(a, b, c, d):
- * _1 = d
- * _2 = (c / 3.0) + d # <<<<<<<<<<<<<<
- * _3 = (b + c) / 3.0 + _2
- * _4 = a + d + c + b
- */
- __pyx_t_1 = __pyx_t_double_complex_from_parts(3.0, 0);
- if (unlikely(__Pyx_c_is_zero_double(__pyx_t_1))) {
- PyErr_SetString(PyExc_ZeroDivisionError, "float division");
- __PYX_ERR(0, 65, __pyx_L1_error)
- }
- __pyx_v__2 = __Pyx_c_sum_double(__Pyx_c_quot_double(__pyx_v_c, __pyx_t_1), __pyx_v_d);
-
- /* "fontTools/cu2qu/cu2qu.py":66
- * _1 = d
- * _2 = (c / 3.0) + d
- * _3 = (b + c) / 3.0 + _2 # <<<<<<<<<<<<<<
- * _4 = a + d + c + b
- * return _1, _2, _3, _4
- */
- __pyx_t_1 = __Pyx_c_sum_double(__pyx_v_b, __pyx_v_c);
- __pyx_t_2 = __pyx_t_double_complex_from_parts(3.0, 0);
- if (unlikely(__Pyx_c_is_zero_double(__pyx_t_2))) {
- PyErr_SetString(PyExc_ZeroDivisionError, "float division");
- __PYX_ERR(0, 66, __pyx_L1_error)
- }
- __pyx_v__3 = __Pyx_c_sum_double(__Pyx_c_quot_double(__pyx_t_1, __pyx_t_2), __pyx_v__2);
-
- /* "fontTools/cu2qu/cu2qu.py":67
- * _2 = (c / 3.0) + d
- * _3 = (b + c) / 3.0 + _2
- * _4 = a + d + c + b # <<<<<<<<<<<<<<
- * return _1, _2, _3, _4
- *
- */
- __pyx_v__4 = __Pyx_c_sum_double(__Pyx_c_sum_double(__Pyx_c_sum_double(__pyx_v_a, __pyx_v_d), __pyx_v_c), __pyx_v_b);
-
- /* "fontTools/cu2qu/cu2qu.py":68
- * _3 = (b + c) / 3.0 + _2
- * _4 = a + d + c + b
- * return _1, _2, _3, _4 # <<<<<<<<<<<<<<
- *
- *
- */
- __Pyx_XDECREF(__pyx_r);
- __pyx_t_3 = __pyx_PyComplex_FromComplex(__pyx_v__1); if (unlikely(!__pyx_t_3)) __PYX_ERR(0, 68, __pyx_L1_error)
- __Pyx_GOTREF(__pyx_t_3);
- __pyx_t_4 = __pyx_PyComplex_FromComplex(__pyx_v__2); if (unlikely(!__pyx_t_4)) __PYX_ERR(0, 68, __pyx_L1_error)
- __Pyx_GOTREF(__pyx_t_4);
- __pyx_t_5 = __pyx_PyComplex_FromComplex(__pyx_v__3); if (unlikely(!__pyx_t_5)) __PYX_ERR(0, 68, __pyx_L1_error)
- __Pyx_GOTREF(__pyx_t_5);
- __pyx_t_6 = __pyx_PyComplex_FromComplex(__pyx_v__4); if (unlikely(!__pyx_t_6)) __PYX_ERR(0, 68, __pyx_L1_error)
- __Pyx_GOTREF(__pyx_t_6);
- __pyx_t_7 = PyTuple_New(4); if (unlikely(!__pyx_t_7)) __PYX_ERR(0, 68, __pyx_L1_error)
- __Pyx_GOTREF(__pyx_t_7);
- __Pyx_GIVEREF(__pyx_t_3);
- PyTuple_SET_ITEM(__pyx_t_7, 0, __pyx_t_3);
- __Pyx_GIVEREF(__pyx_t_4);
- PyTuple_SET_ITEM(__pyx_t_7, 1, __pyx_t_4);
- __Pyx_GIVEREF(__pyx_t_5);
- PyTuple_SET_ITEM(__pyx_t_7, 2, __pyx_t_5);
- __Pyx_GIVEREF(__pyx_t_6);
- PyTuple_SET_ITEM(__pyx_t_7, 3, __pyx_t_6);
- __pyx_t_3 = 0;
- __pyx_t_4 = 0;
- __pyx_t_5 = 0;
- __pyx_t_6 = 0;
- __pyx_r = __pyx_t_7;
- __pyx_t_7 = 0;
- goto __pyx_L0;
-
- /* "fontTools/cu2qu/cu2qu.py":57
- *
- *
- * @cython.cfunc # <<<<<<<<<<<<<<
- * @cython.inline
- * @cython.locals(a=cython.complex, b=cython.complex, c=cython.complex, d=cython.complex)
- */
-
- /* function exit code */
- __pyx_L1_error:;
- __Pyx_XDECREF(__pyx_t_3);
- __Pyx_XDECREF(__pyx_t_4);
- __Pyx_XDECREF(__pyx_t_5);
- __Pyx_XDECREF(__pyx_t_6);
- __Pyx_XDECREF(__pyx_t_7);
- __Pyx_AddTraceback("fontTools.cu2qu.cu2qu.calc_cubic_points", __pyx_clineno, __pyx_lineno, __pyx_filename);
- __pyx_r = 0;
- __pyx_L0:;
- __Pyx_XGIVEREF(__pyx_r);
- __Pyx_RefNannyFinishContext();
- return __pyx_r;
-}
-
-/* "fontTools/cu2qu/cu2qu.py":71
- *
- *
- * @cython.cfunc # <<<<<<<<<<<<<<
- * @cython.inline
- * @cython.locals(
- */
-
-static CYTHON_INLINE PyObject *__pyx_f_9fontTools_5cu2qu_5cu2qu_calc_cubic_parameters(__pyx_t_double_complex __pyx_v_p0, __pyx_t_double_complex __pyx_v_p1, __pyx_t_double_complex __pyx_v_p2, __pyx_t_double_complex __pyx_v_p3) {
- __pyx_t_double_complex __pyx_v_a;
- __pyx_t_double_complex __pyx_v_b;
- __pyx_t_double_complex __pyx_v_c;
- __pyx_t_double_complex __pyx_v_d;
- PyObject *__pyx_r = NULL;
- __Pyx_RefNannyDeclarations
- PyObject *__pyx_t_1 = NULL;
- PyObject *__pyx_t_2 = NULL;
- PyObject *__pyx_t_3 = NULL;
- PyObject *__pyx_t_4 = NULL;
- PyObject *__pyx_t_5 = NULL;
- int __pyx_lineno = 0;
- const char *__pyx_filename = NULL;
- int __pyx_clineno = 0;
- __Pyx_RefNannySetupContext("calc_cubic_parameters", 0);
-
- /* "fontTools/cu2qu/cu2qu.py":78
- * @cython.locals(a=cython.complex, b=cython.complex, c=cython.complex, d=cython.complex)
- * def calc_cubic_parameters(p0, p1, p2, p3):
- * c = (p1 - p0) * 3.0 # <<<<<<<<<<<<<<
- * b = (p2 - p1) * 3.0 - c
- * d = p0
- */
- __pyx_v_c = __Pyx_c_prod_double(__Pyx_c_diff_double(__pyx_v_p1, __pyx_v_p0), __pyx_t_double_complex_from_parts(3.0, 0));
-
- /* "fontTools/cu2qu/cu2qu.py":79
- * def calc_cubic_parameters(p0, p1, p2, p3):
- * c = (p1 - p0) * 3.0
- * b = (p2 - p1) * 3.0 - c # <<<<<<<<<<<<<<
- * d = p0
- * a = p3 - d - c - b
- */
- __pyx_v_b = __Pyx_c_diff_double(__Pyx_c_prod_double(__Pyx_c_diff_double(__pyx_v_p2, __pyx_v_p1), __pyx_t_double_complex_from_parts(3.0, 0)), __pyx_v_c);
-
- /* "fontTools/cu2qu/cu2qu.py":80
- * c = (p1 - p0) * 3.0
- * b = (p2 - p1) * 3.0 - c
- * d = p0 # <<<<<<<<<<<<<<
- * a = p3 - d - c - b
- * return a, b, c, d
- */
- __pyx_v_d = __pyx_v_p0;
-
- /* "fontTools/cu2qu/cu2qu.py":81
- * b = (p2 - p1) * 3.0 - c
- * d = p0
- * a = p3 - d - c - b # <<<<<<<<<<<<<<
- * return a, b, c, d
- *
- */
- __pyx_v_a = __Pyx_c_diff_double(__Pyx_c_diff_double(__Pyx_c_diff_double(__pyx_v_p3, __pyx_v_d), __pyx_v_c), __pyx_v_b);
-
- /* "fontTools/cu2qu/cu2qu.py":82
- * d = p0
- * a = p3 - d - c - b
- * return a, b, c, d # <<<<<<<<<<<<<<
- *
- *
- */
- __Pyx_XDECREF(__pyx_r);
- __pyx_t_1 = __pyx_PyComplex_FromComplex(__pyx_v_a); if (unlikely(!__pyx_t_1)) __PYX_ERR(0, 82, __pyx_L1_error)
- __Pyx_GOTREF(__pyx_t_1);
- __pyx_t_2 = __pyx_PyComplex_FromComplex(__pyx_v_b); if (unlikely(!__pyx_t_2)) __PYX_ERR(0, 82, __pyx_L1_error)
- __Pyx_GOTREF(__pyx_t_2);
- __pyx_t_3 = __pyx_PyComplex_FromComplex(__pyx_v_c); if (unlikely(!__pyx_t_3)) __PYX_ERR(0, 82, __pyx_L1_error)
- __Pyx_GOTREF(__pyx_t_3);
- __pyx_t_4 = __pyx_PyComplex_FromComplex(__pyx_v_d); if (unlikely(!__pyx_t_4)) __PYX_ERR(0, 82, __pyx_L1_error)
- __Pyx_GOTREF(__pyx_t_4);
- __pyx_t_5 = PyTuple_New(4); if (unlikely(!__pyx_t_5)) __PYX_ERR(0, 82, __pyx_L1_error)
- __Pyx_GOTREF(__pyx_t_5);
- __Pyx_GIVEREF(__pyx_t_1);
- PyTuple_SET_ITEM(__pyx_t_5, 0, __pyx_t_1);
- __Pyx_GIVEREF(__pyx_t_2);
- PyTuple_SET_ITEM(__pyx_t_5, 1, __pyx_t_2);
- __Pyx_GIVEREF(__pyx_t_3);
- PyTuple_SET_ITEM(__pyx_t_5, 2, __pyx_t_3);
- __Pyx_GIVEREF(__pyx_t_4);
- PyTuple_SET_ITEM(__pyx_t_5, 3, __pyx_t_4);
- __pyx_t_1 = 0;
- __pyx_t_2 = 0;
- __pyx_t_3 = 0;
- __pyx_t_4 = 0;
- __pyx_r = __pyx_t_5;
- __pyx_t_5 = 0;
- goto __pyx_L0;
-
- /* "fontTools/cu2qu/cu2qu.py":71
- *
- *
- * @cython.cfunc # <<<<<<<<<<<<<<
- * @cython.inline
- * @cython.locals(
- */
-
- /* function exit code */
- __pyx_L1_error:;
- __Pyx_XDECREF(__pyx_t_1);
- __Pyx_XDECREF(__pyx_t_2);
- __Pyx_XDECREF(__pyx_t_3);
- __Pyx_XDECREF(__pyx_t_4);
- __Pyx_XDECREF(__pyx_t_5);
- __Pyx_AddTraceback("fontTools.cu2qu.cu2qu.calc_cubic_parameters", __pyx_clineno, __pyx_lineno, __pyx_filename);
- __pyx_r = 0;
- __pyx_L0:;
- __Pyx_XGIVEREF(__pyx_r);
- __Pyx_RefNannyFinishContext();
- return __pyx_r;
-}
-
-/* "fontTools/cu2qu/cu2qu.py":85
- *
- *
- * @cython.cfunc # <<<<<<<<<<<<<<
- * @cython.inline
- * @cython.locals(
- */
-
-static CYTHON_INLINE PyObject *__pyx_f_9fontTools_5cu2qu_5cu2qu_split_cubic_into_n_iter(__pyx_t_double_complex __pyx_v_p0, __pyx_t_double_complex __pyx_v_p1, __pyx_t_double_complex __pyx_v_p2, __pyx_t_double_complex __pyx_v_p3, PyObject *__pyx_v_n) {
- PyObject *__pyx_v_a = NULL;
- PyObject *__pyx_v_b = NULL;
- PyObject *__pyx_r = NULL;
- __Pyx_RefNannyDeclarations
- int __pyx_t_1;
- PyObject *__pyx_t_2 = NULL;
- PyObject *__pyx_t_3 = NULL;
- PyObject *__pyx_t_4 = NULL;
- PyObject *__pyx_t_5 = NULL;
- PyObject *(*__pyx_t_6)(PyObject *);
- __pyx_t_double_complex __pyx_t_7;
- __pyx_t_double_complex __pyx_t_8;
- __pyx_t_double_complex __pyx_t_9;
- __pyx_t_double_complex __pyx_t_10;
- PyObject *__pyx_t_11 = NULL;
- PyObject *__pyx_t_12 = NULL;
- PyObject *__pyx_t_13 = NULL;
- int __pyx_t_14;
- int __pyx_lineno = 0;
- const char *__pyx_filename = NULL;
- int __pyx_clineno = 0;
- __Pyx_RefNannySetupContext("split_cubic_into_n_iter", 0);
-
- /* "fontTools/cu2qu/cu2qu.py":107
- * """
- * # Hand-coded special-cases
- * if n == 2: # <<<<<<<<<<<<<<
- * return iter(split_cubic_into_two(p0, p1, p2, p3))
- * if n == 3:
- */
- __pyx_t_1 = (__Pyx_PyInt_BoolEqObjC(__pyx_v_n, __pyx_int_2, 2, 0)); if (unlikely((__pyx_t_1 < 0))) __PYX_ERR(0, 107, __pyx_L1_error)
- if (__pyx_t_1) {
-
- /* "fontTools/cu2qu/cu2qu.py":108
- * # Hand-coded special-cases
- * if n == 2:
- * return iter(split_cubic_into_two(p0, p1, p2, p3)) # <<<<<<<<<<<<<<
- * if n == 3:
- * return iter(split_cubic_into_three(p0, p1, p2, p3))
- */
- __Pyx_XDECREF(__pyx_r);
- __pyx_t_2 = __pyx_f_9fontTools_5cu2qu_5cu2qu_split_cubic_into_two(__pyx_v_p0, __pyx_v_p1, __pyx_v_p2, __pyx_v_p3); if (unlikely(!__pyx_t_2)) __PYX_ERR(0, 108, __pyx_L1_error)
- __Pyx_GOTREF(__pyx_t_2);
- __pyx_t_3 = PyObject_GetIter(__pyx_t_2); if (unlikely(!__pyx_t_3)) __PYX_ERR(0, 108, __pyx_L1_error)
- __Pyx_GOTREF(__pyx_t_3);
- __Pyx_DECREF(__pyx_t_2); __pyx_t_2 = 0;
- __pyx_r = __pyx_t_3;
- __pyx_t_3 = 0;
- goto __pyx_L0;
-
- /* "fontTools/cu2qu/cu2qu.py":107
- * """
- * # Hand-coded special-cases
- * if n == 2: # <<<<<<<<<<<<<<
- * return iter(split_cubic_into_two(p0, p1, p2, p3))
- * if n == 3:
- */
- }
-
- /* "fontTools/cu2qu/cu2qu.py":109
- * if n == 2:
- * return iter(split_cubic_into_two(p0, p1, p2, p3))
- * if n == 3: # <<<<<<<<<<<<<<
- * return iter(split_cubic_into_three(p0, p1, p2, p3))
- * if n == 4:
- */
- __pyx_t_1 = (__Pyx_PyInt_BoolEqObjC(__pyx_v_n, __pyx_int_3, 3, 0)); if (unlikely((__pyx_t_1 < 0))) __PYX_ERR(0, 109, __pyx_L1_error)
- if (__pyx_t_1) {
-
- /* "fontTools/cu2qu/cu2qu.py":110
- * return iter(split_cubic_into_two(p0, p1, p2, p3))
- * if n == 3:
- * return iter(split_cubic_into_three(p0, p1, p2, p3)) # <<<<<<<<<<<<<<
- * if n == 4:
- * a, b = split_cubic_into_two(p0, p1, p2, p3)
- */
- __Pyx_XDECREF(__pyx_r);
- __pyx_t_3 = __pyx_f_9fontTools_5cu2qu_5cu2qu_split_cubic_into_three(__pyx_v_p0, __pyx_v_p1, __pyx_v_p2, __pyx_v_p3); if (unlikely(!__pyx_t_3)) __PYX_ERR(0, 110, __pyx_L1_error)
- __Pyx_GOTREF(__pyx_t_3);
- __pyx_t_2 = PyObject_GetIter(__pyx_t_3); if (unlikely(!__pyx_t_2)) __PYX_ERR(0, 110, __pyx_L1_error)
- __Pyx_GOTREF(__pyx_t_2);
- __Pyx_DECREF(__pyx_t_3); __pyx_t_3 = 0;
- __pyx_r = __pyx_t_2;
- __pyx_t_2 = 0;
- goto __pyx_L0;
-
- /* "fontTools/cu2qu/cu2qu.py":109
- * if n == 2:
- * return iter(split_cubic_into_two(p0, p1, p2, p3))
- * if n == 3: # <<<<<<<<<<<<<<
- * return iter(split_cubic_into_three(p0, p1, p2, p3))
- * if n == 4:
- */
- }
-
- /* "fontTools/cu2qu/cu2qu.py":111
- * if n == 3:
- * return iter(split_cubic_into_three(p0, p1, p2, p3))
- * if n == 4: # <<<<<<<<<<<<<<
- * a, b = split_cubic_into_two(p0, p1, p2, p3)
- * return iter(
- */
- __pyx_t_1 = (__Pyx_PyInt_BoolEqObjC(__pyx_v_n, __pyx_int_4, 4, 0)); if (unlikely((__pyx_t_1 < 0))) __PYX_ERR(0, 111, __pyx_L1_error)
- if (__pyx_t_1) {
-
- /* "fontTools/cu2qu/cu2qu.py":112
- * return iter(split_cubic_into_three(p0, p1, p2, p3))
- * if n == 4:
- * a, b = split_cubic_into_two(p0, p1, p2, p3) # <<<<<<<<<<<<<<
- * return iter(
- * split_cubic_into_two(a[0], a[1], a[2], a[3])
- */
- __pyx_t_2 = __pyx_f_9fontTools_5cu2qu_5cu2qu_split_cubic_into_two(__pyx_v_p0, __pyx_v_p1, __pyx_v_p2, __pyx_v_p3); if (unlikely(!__pyx_t_2)) __PYX_ERR(0, 112, __pyx_L1_error)
- __Pyx_GOTREF(__pyx_t_2);
- if ((likely(PyTuple_CheckExact(__pyx_t_2))) || (PyList_CheckExact(__pyx_t_2))) {
- PyObject* sequence = __pyx_t_2;
- Py_ssize_t size = __Pyx_PySequence_SIZE(sequence);
- if (unlikely(size != 2)) {
- if (size > 2) __Pyx_RaiseTooManyValuesError(2);
- else if (size >= 0) __Pyx_RaiseNeedMoreValuesError(size);
- __PYX_ERR(0, 112, __pyx_L1_error)
- }
- #if CYTHON_ASSUME_SAFE_MACROS && !CYTHON_AVOID_BORROWED_REFS
- if (likely(PyTuple_CheckExact(sequence))) {
- __pyx_t_3 = PyTuple_GET_ITEM(sequence, 0);
- __pyx_t_4 = PyTuple_GET_ITEM(sequence, 1);
- } else {
- __pyx_t_3 = PyList_GET_ITEM(sequence, 0);
- __pyx_t_4 = PyList_GET_ITEM(sequence, 1);
- }
- __Pyx_INCREF(__pyx_t_3);
- __Pyx_INCREF(__pyx_t_4);
- #else
- __pyx_t_3 = PySequence_ITEM(sequence, 0); if (unlikely(!__pyx_t_3)) __PYX_ERR(0, 112, __pyx_L1_error)
- __Pyx_GOTREF(__pyx_t_3);
- __pyx_t_4 = PySequence_ITEM(sequence, 1); if (unlikely(!__pyx_t_4)) __PYX_ERR(0, 112, __pyx_L1_error)
- __Pyx_GOTREF(__pyx_t_4);
- #endif
- __Pyx_DECREF(__pyx_t_2); __pyx_t_2 = 0;
- } else {
- Py_ssize_t index = -1;
- __pyx_t_5 = PyObject_GetIter(__pyx_t_2); if (unlikely(!__pyx_t_5)) __PYX_ERR(0, 112, __pyx_L1_error)
- __Pyx_GOTREF(__pyx_t_5);
- __Pyx_DECREF(__pyx_t_2); __pyx_t_2 = 0;
- __pyx_t_6 = __Pyx_PyObject_GetIterNextFunc(__pyx_t_5);
- index = 0; __pyx_t_3 = __pyx_t_6(__pyx_t_5); if (unlikely(!__pyx_t_3)) goto __pyx_L6_unpacking_failed;
- __Pyx_GOTREF(__pyx_t_3);
- index = 1; __pyx_t_4 = __pyx_t_6(__pyx_t_5); if (unlikely(!__pyx_t_4)) goto __pyx_L6_unpacking_failed;
- __Pyx_GOTREF(__pyx_t_4);
- if (__Pyx_IternextUnpackEndCheck(__pyx_t_6(__pyx_t_5), 2) < 0) __PYX_ERR(0, 112, __pyx_L1_error)
- __pyx_t_6 = NULL;
- __Pyx_DECREF(__pyx_t_5); __pyx_t_5 = 0;
- goto __pyx_L7_unpacking_done;
- __pyx_L6_unpacking_failed:;
- __Pyx_DECREF(__pyx_t_5); __pyx_t_5 = 0;
- __pyx_t_6 = NULL;
- if (__Pyx_IterFinish() == 0) __Pyx_RaiseNeedMoreValuesError(index);
- __PYX_ERR(0, 112, __pyx_L1_error)
- __pyx_L7_unpacking_done:;
- }
- __pyx_v_a = __pyx_t_3;
- __pyx_t_3 = 0;
- __pyx_v_b = __pyx_t_4;
- __pyx_t_4 = 0;
-
- /* "fontTools/cu2qu/cu2qu.py":113
- * if n == 4:
- * a, b = split_cubic_into_two(p0, p1, p2, p3)
- * return iter( # <<<<<<<<<<<<<<
- * split_cubic_into_two(a[0], a[1], a[2], a[3])
- * + split_cubic_into_two(b[0], b[1], b[2], b[3])
- */
- __Pyx_XDECREF(__pyx_r);
-
- /* "fontTools/cu2qu/cu2qu.py":114
- * a, b = split_cubic_into_two(p0, p1, p2, p3)
- * return iter(
- * split_cubic_into_two(a[0], a[1], a[2], a[3]) # <<<<<<<<<<<<<<
- * + split_cubic_into_two(b[0], b[1], b[2], b[3])
- * )
- */
- __pyx_t_2 = __Pyx_GetItemInt(__pyx_v_a, 0, long, 1, __Pyx_PyInt_From_long, 0, 0, 1); if (unlikely(!__pyx_t_2)) __PYX_ERR(0, 114, __pyx_L1_error)
- __Pyx_GOTREF(__pyx_t_2);
- __pyx_t_7 = __Pyx_PyComplex_As___pyx_t_double_complex(__pyx_t_2); if (unlikely(PyErr_Occurred())) __PYX_ERR(0, 114, __pyx_L1_error)
- __Pyx_DECREF(__pyx_t_2); __pyx_t_2 = 0;
- __pyx_t_2 = __Pyx_GetItemInt(__pyx_v_a, 1, long, 1, __Pyx_PyInt_From_long, 0, 0, 1); if (unlikely(!__pyx_t_2)) __PYX_ERR(0, 114, __pyx_L1_error)
- __Pyx_GOTREF(__pyx_t_2);
- __pyx_t_8 = __Pyx_PyComplex_As___pyx_t_double_complex(__pyx_t_2); if (unlikely(PyErr_Occurred())) __PYX_ERR(0, 114, __pyx_L1_error)
- __Pyx_DECREF(__pyx_t_2); __pyx_t_2 = 0;
- __pyx_t_2 = __Pyx_GetItemInt(__pyx_v_a, 2, long, 1, __Pyx_PyInt_From_long, 0, 0, 1); if (unlikely(!__pyx_t_2)) __PYX_ERR(0, 114, __pyx_L1_error)
- __Pyx_GOTREF(__pyx_t_2);
- __pyx_t_9 = __Pyx_PyComplex_As___pyx_t_double_complex(__pyx_t_2); if (unlikely(PyErr_Occurred())) __PYX_ERR(0, 114, __pyx_L1_error)
- __Pyx_DECREF(__pyx_t_2); __pyx_t_2 = 0;
- __pyx_t_2 = __Pyx_GetItemInt(__pyx_v_a, 3, long, 1, __Pyx_PyInt_From_long, 0, 0, 1); if (unlikely(!__pyx_t_2)) __PYX_ERR(0, 114, __pyx_L1_error)
- __Pyx_GOTREF(__pyx_t_2);
- __pyx_t_10 = __Pyx_PyComplex_As___pyx_t_double_complex(__pyx_t_2); if (unlikely(PyErr_Occurred())) __PYX_ERR(0, 114, __pyx_L1_error)
- __Pyx_DECREF(__pyx_t_2); __pyx_t_2 = 0;
- __pyx_t_2 = __pyx_f_9fontTools_5cu2qu_5cu2qu_split_cubic_into_two(__pyx_t_7, __pyx_t_8, __pyx_t_9, __pyx_t_10); if (unlikely(!__pyx_t_2)) __PYX_ERR(0, 114, __pyx_L1_error)
- __Pyx_GOTREF(__pyx_t_2);
-
- /* "fontTools/cu2qu/cu2qu.py":115
- * return iter(
- * split_cubic_into_two(a[0], a[1], a[2], a[3])
- * + split_cubic_into_two(b[0], b[1], b[2], b[3]) # <<<<<<<<<<<<<<
- * )
- * if n == 6:
- */
- __pyx_t_4 = __Pyx_GetItemInt(__pyx_v_b, 0, long, 1, __Pyx_PyInt_From_long, 0, 0, 1); if (unlikely(!__pyx_t_4)) __PYX_ERR(0, 115, __pyx_L1_error)
- __Pyx_GOTREF(__pyx_t_4);
- __pyx_t_10 = __Pyx_PyComplex_As___pyx_t_double_complex(__pyx_t_4); if (unlikely(PyErr_Occurred())) __PYX_ERR(0, 115, __pyx_L1_error)
- __Pyx_DECREF(__pyx_t_4); __pyx_t_4 = 0;
- __pyx_t_4 = __Pyx_GetItemInt(__pyx_v_b, 1, long, 1, __Pyx_PyInt_From_long, 0, 0, 1); if (unlikely(!__pyx_t_4)) __PYX_ERR(0, 115, __pyx_L1_error)
- __Pyx_GOTREF(__pyx_t_4);
- __pyx_t_9 = __Pyx_PyComplex_As___pyx_t_double_complex(__pyx_t_4); if (unlikely(PyErr_Occurred())) __PYX_ERR(0, 115, __pyx_L1_error)
- __Pyx_DECREF(__pyx_t_4); __pyx_t_4 = 0;
- __pyx_t_4 = __Pyx_GetItemInt(__pyx_v_b, 2, long, 1, __Pyx_PyInt_From_long, 0, 0, 1); if (unlikely(!__pyx_t_4)) __PYX_ERR(0, 115, __pyx_L1_error)
- __Pyx_GOTREF(__pyx_t_4);
- __pyx_t_8 = __Pyx_PyComplex_As___pyx_t_double_complex(__pyx_t_4); if (unlikely(PyErr_Occurred())) __PYX_ERR(0, 115, __pyx_L1_error)
- __Pyx_DECREF(__pyx_t_4); __pyx_t_4 = 0;
- __pyx_t_4 = __Pyx_GetItemInt(__pyx_v_b, 3, long, 1, __Pyx_PyInt_From_long, 0, 0, 1); if (unlikely(!__pyx_t_4)) __PYX_ERR(0, 115, __pyx_L1_error)
- __Pyx_GOTREF(__pyx_t_4);
- __pyx_t_7 = __Pyx_PyComplex_As___pyx_t_double_complex(__pyx_t_4); if (unlikely(PyErr_Occurred())) __PYX_ERR(0, 115, __pyx_L1_error)
- __Pyx_DECREF(__pyx_t_4); __pyx_t_4 = 0;
- __pyx_t_4 = __pyx_f_9fontTools_5cu2qu_5cu2qu_split_cubic_into_two(__pyx_t_10, __pyx_t_9, __pyx_t_8, __pyx_t_7); if (unlikely(!__pyx_t_4)) __PYX_ERR(0, 115, __pyx_L1_error)
- __Pyx_GOTREF(__pyx_t_4);
- __pyx_t_3 = PyNumber_Add(__pyx_t_2, __pyx_t_4); if (unlikely(!__pyx_t_3)) __PYX_ERR(0, 115, __pyx_L1_error)
- __Pyx_GOTREF(__pyx_t_3);
- __Pyx_DECREF(__pyx_t_2); __pyx_t_2 = 0;
- __Pyx_DECREF(__pyx_t_4); __pyx_t_4 = 0;
-
- /* "fontTools/cu2qu/cu2qu.py":113
- * if n == 4:
- * a, b = split_cubic_into_two(p0, p1, p2, p3)
- * return iter( # <<<<<<<<<<<<<<
- * split_cubic_into_two(a[0], a[1], a[2], a[3])
- * + split_cubic_into_two(b[0], b[1], b[2], b[3])
- */
- __pyx_t_4 = PyObject_GetIter(__pyx_t_3); if (unlikely(!__pyx_t_4)) __PYX_ERR(0, 113, __pyx_L1_error)
- __Pyx_GOTREF(__pyx_t_4);
- __Pyx_DECREF(__pyx_t_3); __pyx_t_3 = 0;
- __pyx_r = __pyx_t_4;
- __pyx_t_4 = 0;
- goto __pyx_L0;
-
- /* "fontTools/cu2qu/cu2qu.py":111
- * if n == 3:
- * return iter(split_cubic_into_three(p0, p1, p2, p3))
- * if n == 4: # <<<<<<<<<<<<<<
- * a, b = split_cubic_into_two(p0, p1, p2, p3)
- * return iter(
- */
- }
-
- /* "fontTools/cu2qu/cu2qu.py":117
- * + split_cubic_into_two(b[0], b[1], b[2], b[3])
- * )
- * if n == 6: # <<<<<<<<<<<<<<
- * a, b = split_cubic_into_two(p0, p1, p2, p3)
- * return iter(
- */
- __pyx_t_1 = (__Pyx_PyInt_BoolEqObjC(__pyx_v_n, __pyx_int_6, 6, 0)); if (unlikely((__pyx_t_1 < 0))) __PYX_ERR(0, 117, __pyx_L1_error)
- if (__pyx_t_1) {
-
- /* "fontTools/cu2qu/cu2qu.py":118
- * )
- * if n == 6:
- * a, b = split_cubic_into_two(p0, p1, p2, p3) # <<<<<<<<<<<<<<
- * return iter(
- * split_cubic_into_three(a[0], a[1], a[2], a[3])
- */
- __pyx_t_4 = __pyx_f_9fontTools_5cu2qu_5cu2qu_split_cubic_into_two(__pyx_v_p0, __pyx_v_p1, __pyx_v_p2, __pyx_v_p3); if (unlikely(!__pyx_t_4)) __PYX_ERR(0, 118, __pyx_L1_error)
- __Pyx_GOTREF(__pyx_t_4);
- if ((likely(PyTuple_CheckExact(__pyx_t_4))) || (PyList_CheckExact(__pyx_t_4))) {
- PyObject* sequence = __pyx_t_4;
- Py_ssize_t size = __Pyx_PySequence_SIZE(sequence);
- if (unlikely(size != 2)) {
- if (size > 2) __Pyx_RaiseTooManyValuesError(2);
- else if (size >= 0) __Pyx_RaiseNeedMoreValuesError(size);
- __PYX_ERR(0, 118, __pyx_L1_error)
- }
- #if CYTHON_ASSUME_SAFE_MACROS && !CYTHON_AVOID_BORROWED_REFS
- if (likely(PyTuple_CheckExact(sequence))) {
- __pyx_t_3 = PyTuple_GET_ITEM(sequence, 0);
- __pyx_t_2 = PyTuple_GET_ITEM(sequence, 1);
- } else {
- __pyx_t_3 = PyList_GET_ITEM(sequence, 0);
- __pyx_t_2 = PyList_GET_ITEM(sequence, 1);
- }
- __Pyx_INCREF(__pyx_t_3);
- __Pyx_INCREF(__pyx_t_2);
- #else
- __pyx_t_3 = PySequence_ITEM(sequence, 0); if (unlikely(!__pyx_t_3)) __PYX_ERR(0, 118, __pyx_L1_error)
- __Pyx_GOTREF(__pyx_t_3);
- __pyx_t_2 = PySequence_ITEM(sequence, 1); if (unlikely(!__pyx_t_2)) __PYX_ERR(0, 118, __pyx_L1_error)
- __Pyx_GOTREF(__pyx_t_2);
- #endif
- __Pyx_DECREF(__pyx_t_4); __pyx_t_4 = 0;
- } else {
- Py_ssize_t index = -1;
- __pyx_t_5 = PyObject_GetIter(__pyx_t_4); if (unlikely(!__pyx_t_5)) __PYX_ERR(0, 118, __pyx_L1_error)
- __Pyx_GOTREF(__pyx_t_5);
- __Pyx_DECREF(__pyx_t_4); __pyx_t_4 = 0;
- __pyx_t_6 = __Pyx_PyObject_GetIterNextFunc(__pyx_t_5);
- index = 0; __pyx_t_3 = __pyx_t_6(__pyx_t_5); if (unlikely(!__pyx_t_3)) goto __pyx_L9_unpacking_failed;
- __Pyx_GOTREF(__pyx_t_3);
- index = 1; __pyx_t_2 = __pyx_t_6(__pyx_t_5); if (unlikely(!__pyx_t_2)) goto __pyx_L9_unpacking_failed;
- __Pyx_GOTREF(__pyx_t_2);
- if (__Pyx_IternextUnpackEndCheck(__pyx_t_6(__pyx_t_5), 2) < 0) __PYX_ERR(0, 118, __pyx_L1_error)
- __pyx_t_6 = NULL;
- __Pyx_DECREF(__pyx_t_5); __pyx_t_5 = 0;
- goto __pyx_L10_unpacking_done;
- __pyx_L9_unpacking_failed:;
- __Pyx_DECREF(__pyx_t_5); __pyx_t_5 = 0;
- __pyx_t_6 = NULL;
- if (__Pyx_IterFinish() == 0) __Pyx_RaiseNeedMoreValuesError(index);
- __PYX_ERR(0, 118, __pyx_L1_error)
- __pyx_L10_unpacking_done:;
- }
- __pyx_v_a = __pyx_t_3;
- __pyx_t_3 = 0;
- __pyx_v_b = __pyx_t_2;
- __pyx_t_2 = 0;
-
- /* "fontTools/cu2qu/cu2qu.py":119
- * if n == 6:
- * a, b = split_cubic_into_two(p0, p1, p2, p3)
- * return iter( # <<<<<<<<<<<<<<
- * split_cubic_into_three(a[0], a[1], a[2], a[3])
- * + split_cubic_into_three(b[0], b[1], b[2], b[3])
- */
- __Pyx_XDECREF(__pyx_r);
-
- /* "fontTools/cu2qu/cu2qu.py":120
- * a, b = split_cubic_into_two(p0, p1, p2, p3)
- * return iter(
- * split_cubic_into_three(a[0], a[1], a[2], a[3]) # <<<<<<<<<<<<<<
- * + split_cubic_into_three(b[0], b[1], b[2], b[3])
- * )
- */
- __pyx_t_4 = __Pyx_GetItemInt(__pyx_v_a, 0, long, 1, __Pyx_PyInt_From_long, 0, 0, 1); if (unlikely(!__pyx_t_4)) __PYX_ERR(0, 120, __pyx_L1_error)
- __Pyx_GOTREF(__pyx_t_4);
- __pyx_t_7 = __Pyx_PyComplex_As___pyx_t_double_complex(__pyx_t_4); if (unlikely(PyErr_Occurred())) __PYX_ERR(0, 120, __pyx_L1_error)
- __Pyx_DECREF(__pyx_t_4); __pyx_t_4 = 0;
- __pyx_t_4 = __Pyx_GetItemInt(__pyx_v_a, 1, long, 1, __Pyx_PyInt_From_long, 0, 0, 1); if (unlikely(!__pyx_t_4)) __PYX_ERR(0, 120, __pyx_L1_error)
- __Pyx_GOTREF(__pyx_t_4);
- __pyx_t_8 = __Pyx_PyComplex_As___pyx_t_double_complex(__pyx_t_4); if (unlikely(PyErr_Occurred())) __PYX_ERR(0, 120, __pyx_L1_error)
- __Pyx_DECREF(__pyx_t_4); __pyx_t_4 = 0;
- __pyx_t_4 = __Pyx_GetItemInt(__pyx_v_a, 2, long, 1, __Pyx_PyInt_From_long, 0, 0, 1); if (unlikely(!__pyx_t_4)) __PYX_ERR(0, 120, __pyx_L1_error)
- __Pyx_GOTREF(__pyx_t_4);
- __pyx_t_9 = __Pyx_PyComplex_As___pyx_t_double_complex(__pyx_t_4); if (unlikely(PyErr_Occurred())) __PYX_ERR(0, 120, __pyx_L1_error)
- __Pyx_DECREF(__pyx_t_4); __pyx_t_4 = 0;
- __pyx_t_4 = __Pyx_GetItemInt(__pyx_v_a, 3, long, 1, __Pyx_PyInt_From_long, 0, 0, 1); if (unlikely(!__pyx_t_4)) __PYX_ERR(0, 120, __pyx_L1_error)
- __Pyx_GOTREF(__pyx_t_4);
- __pyx_t_10 = __Pyx_PyComplex_As___pyx_t_double_complex(__pyx_t_4); if (unlikely(PyErr_Occurred())) __PYX_ERR(0, 120, __pyx_L1_error)
- __Pyx_DECREF(__pyx_t_4); __pyx_t_4 = 0;
- __pyx_t_4 = __pyx_f_9fontTools_5cu2qu_5cu2qu_split_cubic_into_three(__pyx_t_7, __pyx_t_8, __pyx_t_9, __pyx_t_10); if (unlikely(!__pyx_t_4)) __PYX_ERR(0, 120, __pyx_L1_error)
- __Pyx_GOTREF(__pyx_t_4);
-
- /* "fontTools/cu2qu/cu2qu.py":121
- * return iter(
- * split_cubic_into_three(a[0], a[1], a[2], a[3])
- * + split_cubic_into_three(b[0], b[1], b[2], b[3]) # <<<<<<<<<<<<<<
- * )
- *
- */
- __pyx_t_2 = __Pyx_GetItemInt(__pyx_v_b, 0, long, 1, __Pyx_PyInt_From_long, 0, 0, 1); if (unlikely(!__pyx_t_2)) __PYX_ERR(0, 121, __pyx_L1_error)
- __Pyx_GOTREF(__pyx_t_2);
- __pyx_t_10 = __Pyx_PyComplex_As___pyx_t_double_complex(__pyx_t_2); if (unlikely(PyErr_Occurred())) __PYX_ERR(0, 121, __pyx_L1_error)
- __Pyx_DECREF(__pyx_t_2); __pyx_t_2 = 0;
- __pyx_t_2 = __Pyx_GetItemInt(__pyx_v_b, 1, long, 1, __Pyx_PyInt_From_long, 0, 0, 1); if (unlikely(!__pyx_t_2)) __PYX_ERR(0, 121, __pyx_L1_error)
- __Pyx_GOTREF(__pyx_t_2);
- __pyx_t_9 = __Pyx_PyComplex_As___pyx_t_double_complex(__pyx_t_2); if (unlikely(PyErr_Occurred())) __PYX_ERR(0, 121, __pyx_L1_error)
- __Pyx_DECREF(__pyx_t_2); __pyx_t_2 = 0;
- __pyx_t_2 = __Pyx_GetItemInt(__pyx_v_b, 2, long, 1, __Pyx_PyInt_From_long, 0, 0, 1); if (unlikely(!__pyx_t_2)) __PYX_ERR(0, 121, __pyx_L1_error)
- __Pyx_GOTREF(__pyx_t_2);
- __pyx_t_8 = __Pyx_PyComplex_As___pyx_t_double_complex(__pyx_t_2); if (unlikely(PyErr_Occurred())) __PYX_ERR(0, 121, __pyx_L1_error)
- __Pyx_DECREF(__pyx_t_2); __pyx_t_2 = 0;
- __pyx_t_2 = __Pyx_GetItemInt(__pyx_v_b, 3, long, 1, __Pyx_PyInt_From_long, 0, 0, 1); if (unlikely(!__pyx_t_2)) __PYX_ERR(0, 121, __pyx_L1_error)
- __Pyx_GOTREF(__pyx_t_2);
- __pyx_t_7 = __Pyx_PyComplex_As___pyx_t_double_complex(__pyx_t_2); if (unlikely(PyErr_Occurred())) __PYX_ERR(0, 121, __pyx_L1_error)
- __Pyx_DECREF(__pyx_t_2); __pyx_t_2 = 0;
- __pyx_t_2 = __pyx_f_9fontTools_5cu2qu_5cu2qu_split_cubic_into_three(__pyx_t_10, __pyx_t_9, __pyx_t_8, __pyx_t_7); if (unlikely(!__pyx_t_2)) __PYX_ERR(0, 121, __pyx_L1_error)
- __Pyx_GOTREF(__pyx_t_2);
- __pyx_t_3 = PyNumber_Add(__pyx_t_4, __pyx_t_2); if (unlikely(!__pyx_t_3)) __PYX_ERR(0, 121, __pyx_L1_error)
- __Pyx_GOTREF(__pyx_t_3);
- __Pyx_DECREF(__pyx_t_4); __pyx_t_4 = 0;
- __Pyx_DECREF(__pyx_t_2); __pyx_t_2 = 0;
-
- /* "fontTools/cu2qu/cu2qu.py":119
- * if n == 6:
- * a, b = split_cubic_into_two(p0, p1, p2, p3)
- * return iter( # <<<<<<<<<<<<<<
- * split_cubic_into_three(a[0], a[1], a[2], a[3])
- * + split_cubic_into_three(b[0], b[1], b[2], b[3])
- */
- __pyx_t_2 = PyObject_GetIter(__pyx_t_3); if (unlikely(!__pyx_t_2)) __PYX_ERR(0, 119, __pyx_L1_error)
- __Pyx_GOTREF(__pyx_t_2);
- __Pyx_DECREF(__pyx_t_3); __pyx_t_3 = 0;
- __pyx_r = __pyx_t_2;
- __pyx_t_2 = 0;
- goto __pyx_L0;
-
- /* "fontTools/cu2qu/cu2qu.py":117
- * + split_cubic_into_two(b[0], b[1], b[2], b[3])
- * )
- * if n == 6: # <<<<<<<<<<<<<<
- * a, b = split_cubic_into_two(p0, p1, p2, p3)
- * return iter(
- */
- }
-
- /* "fontTools/cu2qu/cu2qu.py":124
- * )
- *
- * return _split_cubic_into_n_gen(p0, p1, p2, p3, n) # <<<<<<<<<<<<<<
- *
- *
- */
- __Pyx_XDECREF(__pyx_r);
- __Pyx_GetModuleGlobalName(__pyx_t_3, __pyx_n_s_split_cubic_into_n_gen); if (unlikely(!__pyx_t_3)) __PYX_ERR(0, 124, __pyx_L1_error)
- __Pyx_GOTREF(__pyx_t_3);
- __pyx_t_4 = __pyx_PyComplex_FromComplex(__pyx_v_p0); if (unlikely(!__pyx_t_4)) __PYX_ERR(0, 124, __pyx_L1_error)
- __Pyx_GOTREF(__pyx_t_4);
- __pyx_t_5 = __pyx_PyComplex_FromComplex(__pyx_v_p1); if (unlikely(!__pyx_t_5)) __PYX_ERR(0, 124, __pyx_L1_error)
- __Pyx_GOTREF(__pyx_t_5);
- __pyx_t_11 = __pyx_PyComplex_FromComplex(__pyx_v_p2); if (unlikely(!__pyx_t_11)) __PYX_ERR(0, 124, __pyx_L1_error)
- __Pyx_GOTREF(__pyx_t_11);
- __pyx_t_12 = __pyx_PyComplex_FromComplex(__pyx_v_p3); if (unlikely(!__pyx_t_12)) __PYX_ERR(0, 124, __pyx_L1_error)
- __Pyx_GOTREF(__pyx_t_12);
- __pyx_t_13 = NULL;
- __pyx_t_14 = 0;
- if (CYTHON_UNPACK_METHODS && unlikely(PyMethod_Check(__pyx_t_3))) {
- __pyx_t_13 = PyMethod_GET_SELF(__pyx_t_3);
- if (likely(__pyx_t_13)) {
- PyObject* function = PyMethod_GET_FUNCTION(__pyx_t_3);
- __Pyx_INCREF(__pyx_t_13);
- __Pyx_INCREF(function);
- __Pyx_DECREF_SET(__pyx_t_3, function);
- __pyx_t_14 = 1;
- }
- }
- {
- PyObject *__pyx_callargs[6] = {__pyx_t_13, __pyx_t_4, __pyx_t_5, __pyx_t_11, __pyx_t_12, __pyx_v_n};
- __pyx_t_2 = __Pyx_PyObject_FastCall(__pyx_t_3, __pyx_callargs+1-__pyx_t_14, 5+__pyx_t_14);
- __Pyx_XDECREF(__pyx_t_13); __pyx_t_13 = 0;
- __Pyx_DECREF(__pyx_t_4); __pyx_t_4 = 0;
- __Pyx_DECREF(__pyx_t_5); __pyx_t_5 = 0;
- __Pyx_DECREF(__pyx_t_11); __pyx_t_11 = 0;
- __Pyx_DECREF(__pyx_t_12); __pyx_t_12 = 0;
- if (unlikely(!__pyx_t_2)) __PYX_ERR(0, 124, __pyx_L1_error)
- __Pyx_GOTREF(__pyx_t_2);
- __Pyx_DECREF(__pyx_t_3); __pyx_t_3 = 0;
- }
- __pyx_r = __pyx_t_2;
- __pyx_t_2 = 0;
- goto __pyx_L0;
-
- /* "fontTools/cu2qu/cu2qu.py":85
- *
- *
- * @cython.cfunc # <<<<<<<<<<<<<<
- * @cython.inline
- * @cython.locals(
- */
-
- /* function exit code */
- __pyx_L1_error:;
- __Pyx_XDECREF(__pyx_t_2);
- __Pyx_XDECREF(__pyx_t_3);
- __Pyx_XDECREF(__pyx_t_4);
- __Pyx_XDECREF(__pyx_t_5);
- __Pyx_XDECREF(__pyx_t_11);
- __Pyx_XDECREF(__pyx_t_12);
- __Pyx_XDECREF(__pyx_t_13);
- __Pyx_AddTraceback("fontTools.cu2qu.cu2qu.split_cubic_into_n_iter", __pyx_clineno, __pyx_lineno, __pyx_filename);
- __pyx_r = 0;
- __pyx_L0:;
- __Pyx_XDECREF(__pyx_v_a);
- __Pyx_XDECREF(__pyx_v_b);
- __Pyx_XGIVEREF(__pyx_r);
- __Pyx_RefNannyFinishContext();
- return __pyx_r;
-}
-static PyObject *__pyx_gb_9fontTools_5cu2qu_5cu2qu_2generator(__pyx_CoroutineObject *__pyx_generator, CYTHON_UNUSED PyThreadState *__pyx_tstate, PyObject *__pyx_sent_value); /* proto */
-
-/* "fontTools/cu2qu/cu2qu.py":127
- *
- *
- * @cython.locals( # <<<<<<<<<<<<<<
- * p0=cython.complex,
- * p1=cython.complex,
- */
-
-/* Python wrapper */
-static PyObject *__pyx_pw_9fontTools_5cu2qu_5cu2qu_1_split_cubic_into_n_gen(PyObject *__pyx_self,
-#if CYTHON_METH_FASTCALL
-PyObject *const *__pyx_args, Py_ssize_t __pyx_nargs, PyObject *__pyx_kwds
-#else
-PyObject *__pyx_args, PyObject *__pyx_kwds
-#endif
-); /*proto*/
-PyDoc_STRVAR(__pyx_doc_9fontTools_5cu2qu_5cu2qu__split_cubic_into_n_gen, "_split_cubic_into_n_gen(double complex p0, double complex p1, double complex p2, double complex p3, int n)");
-static PyMethodDef __pyx_mdef_9fontTools_5cu2qu_5cu2qu_1_split_cubic_into_n_gen = {"_split_cubic_into_n_gen", (PyCFunction)(void*)(__Pyx_PyCFunction_FastCallWithKeywords)__pyx_pw_9fontTools_5cu2qu_5cu2qu_1_split_cubic_into_n_gen, __Pyx_METH_FASTCALL|METH_KEYWORDS, __pyx_doc_9fontTools_5cu2qu_5cu2qu__split_cubic_into_n_gen};
-static PyObject *__pyx_pw_9fontTools_5cu2qu_5cu2qu_1_split_cubic_into_n_gen(PyObject *__pyx_self,
-#if CYTHON_METH_FASTCALL
-PyObject *const *__pyx_args, Py_ssize_t __pyx_nargs, PyObject *__pyx_kwds
-#else
-PyObject *__pyx_args, PyObject *__pyx_kwds
-#endif
-) {
- __pyx_t_double_complex __pyx_v_p0;
- __pyx_t_double_complex __pyx_v_p1;
- __pyx_t_double_complex __pyx_v_p2;
- __pyx_t_double_complex __pyx_v_p3;
- int __pyx_v_n;
- #if !CYTHON_METH_FASTCALL
- CYTHON_UNUSED const Py_ssize_t __pyx_nargs = PyTuple_GET_SIZE(__pyx_args);
- #endif
- CYTHON_UNUSED PyObject *const *__pyx_kwvalues = __Pyx_KwValues_FASTCALL(__pyx_args, __pyx_nargs);
- int __pyx_lineno = 0;
- const char *__pyx_filename = NULL;
- int __pyx_clineno = 0;
- PyObject *__pyx_r = 0;
- __Pyx_RefNannyDeclarations
- __Pyx_RefNannySetupContext("_split_cubic_into_n_gen (wrapper)", 0);
- {
- PyObject **__pyx_pyargnames[] = {&__pyx_n_s_p0,&__pyx_n_s_p1,&__pyx_n_s_p2,&__pyx_n_s_p3,&__pyx_n_s_n,0};
- PyObject* values[5] = {0,0,0,0,0};
- if (__pyx_kwds) {
- Py_ssize_t kw_args;
- switch (__pyx_nargs) {
- case 5: values[4] = __Pyx_Arg_FASTCALL(__pyx_args, 4);
- CYTHON_FALLTHROUGH;
- case 4: values[3] = __Pyx_Arg_FASTCALL(__pyx_args, 3);
- CYTHON_FALLTHROUGH;
- case 3: values[2] = __Pyx_Arg_FASTCALL(__pyx_args, 2);
- CYTHON_FALLTHROUGH;
- case 2: values[1] = __Pyx_Arg_FASTCALL(__pyx_args, 1);
- CYTHON_FALLTHROUGH;
- case 1: values[0] = __Pyx_Arg_FASTCALL(__pyx_args, 0);
- CYTHON_FALLTHROUGH;
- case 0: break;
- default: goto __pyx_L5_argtuple_error;
- }
- kw_args = __Pyx_NumKwargs_FASTCALL(__pyx_kwds);
- switch (__pyx_nargs) {
- case 0:
- if (likely((values[0] = __Pyx_GetKwValue_FASTCALL(__pyx_kwds, __pyx_kwvalues, __pyx_n_s_p0)) != 0)) kw_args--;
- else if (unlikely(PyErr_Occurred())) __PYX_ERR(0, 127, __pyx_L3_error)
- else goto __pyx_L5_argtuple_error;
- CYTHON_FALLTHROUGH;
- case 1:
- if (likely((values[1] = __Pyx_GetKwValue_FASTCALL(__pyx_kwds, __pyx_kwvalues, __pyx_n_s_p1)) != 0)) kw_args--;
- else if (unlikely(PyErr_Occurred())) __PYX_ERR(0, 127, __pyx_L3_error)
- else {
- __Pyx_RaiseArgtupleInvalid("_split_cubic_into_n_gen", 1, 5, 5, 1); __PYX_ERR(0, 127, __pyx_L3_error)
- }
- CYTHON_FALLTHROUGH;
- case 2:
- if (likely((values[2] = __Pyx_GetKwValue_FASTCALL(__pyx_kwds, __pyx_kwvalues, __pyx_n_s_p2)) != 0)) kw_args--;
- else if (unlikely(PyErr_Occurred())) __PYX_ERR(0, 127, __pyx_L3_error)
- else {
- __Pyx_RaiseArgtupleInvalid("_split_cubic_into_n_gen", 1, 5, 5, 2); __PYX_ERR(0, 127, __pyx_L3_error)
- }
- CYTHON_FALLTHROUGH;
- case 3:
- if (likely((values[3] = __Pyx_GetKwValue_FASTCALL(__pyx_kwds, __pyx_kwvalues, __pyx_n_s_p3)) != 0)) kw_args--;
- else if (unlikely(PyErr_Occurred())) __PYX_ERR(0, 127, __pyx_L3_error)
- else {
- __Pyx_RaiseArgtupleInvalid("_split_cubic_into_n_gen", 1, 5, 5, 3); __PYX_ERR(0, 127, __pyx_L3_error)
- }
- CYTHON_FALLTHROUGH;
- case 4:
- if (likely((values[4] = __Pyx_GetKwValue_FASTCALL(__pyx_kwds, __pyx_kwvalues, __pyx_n_s_n)) != 0)) kw_args--;
- else if (unlikely(PyErr_Occurred())) __PYX_ERR(0, 127, __pyx_L3_error)
- else {
- __Pyx_RaiseArgtupleInvalid("_split_cubic_into_n_gen", 1, 5, 5, 4); __PYX_ERR(0, 127, __pyx_L3_error)
- }
- }
- if (unlikely(kw_args > 0)) {
- const Py_ssize_t kwd_pos_args = __pyx_nargs;
- if (unlikely(__Pyx_ParseOptionalKeywords(__pyx_kwds, __pyx_kwvalues, __pyx_pyargnames, 0, values + 0, kwd_pos_args, "_split_cubic_into_n_gen") < 0)) __PYX_ERR(0, 127, __pyx_L3_error)
- }
- } else if (unlikely(__pyx_nargs != 5)) {
- goto __pyx_L5_argtuple_error;
- } else {
- values[0] = __Pyx_Arg_FASTCALL(__pyx_args, 0);
- values[1] = __Pyx_Arg_FASTCALL(__pyx_args, 1);
- values[2] = __Pyx_Arg_FASTCALL(__pyx_args, 2);
- values[3] = __Pyx_Arg_FASTCALL(__pyx_args, 3);
- values[4] = __Pyx_Arg_FASTCALL(__pyx_args, 4);
- }
- __pyx_v_p0 = __Pyx_PyComplex_As___pyx_t_double_complex(values[0]); if (unlikely(PyErr_Occurred())) __PYX_ERR(0, 141, __pyx_L3_error)
- __pyx_v_p1 = __Pyx_PyComplex_As___pyx_t_double_complex(values[1]); if (unlikely(PyErr_Occurred())) __PYX_ERR(0, 141, __pyx_L3_error)
- __pyx_v_p2 = __Pyx_PyComplex_As___pyx_t_double_complex(values[2]); if (unlikely(PyErr_Occurred())) __PYX_ERR(0, 141, __pyx_L3_error)
- __pyx_v_p3 = __Pyx_PyComplex_As___pyx_t_double_complex(values[3]); if (unlikely(PyErr_Occurred())) __PYX_ERR(0, 141, __pyx_L3_error)
- __pyx_v_n = __Pyx_PyInt_As_int(values[4]); if (unlikely((__pyx_v_n == (int)-1) && PyErr_Occurred())) __PYX_ERR(0, 141, __pyx_L3_error)
- }
- goto __pyx_L4_argument_unpacking_done;
- __pyx_L5_argtuple_error:;
- __Pyx_RaiseArgtupleInvalid("_split_cubic_into_n_gen", 1, 5, 5, __pyx_nargs); __PYX_ERR(0, 127, __pyx_L3_error)
- __pyx_L3_error:;
- __Pyx_AddTraceback("fontTools.cu2qu.cu2qu._split_cubic_into_n_gen", __pyx_clineno, __pyx_lineno, __pyx_filename);
- __Pyx_RefNannyFinishContext();
- return NULL;
- __pyx_L4_argument_unpacking_done:;
- __pyx_r = __pyx_pf_9fontTools_5cu2qu_5cu2qu__split_cubic_into_n_gen(__pyx_self, __pyx_v_p0, __pyx_v_p1, __pyx_v_p2, __pyx_v_p3, __pyx_v_n);
-
- /* function exit code */
- __Pyx_RefNannyFinishContext();
- return __pyx_r;
-}
-
-static PyObject *__pyx_pf_9fontTools_5cu2qu_5cu2qu__split_cubic_into_n_gen(CYTHON_UNUSED PyObject *__pyx_self, __pyx_t_double_complex __pyx_v_p0, __pyx_t_double_complex __pyx_v_p1, __pyx_t_double_complex __pyx_v_p2, __pyx_t_double_complex __pyx_v_p3, int __pyx_v_n) {
- struct __pyx_obj_9fontTools_5cu2qu_5cu2qu___pyx_scope_struct___split_cubic_into_n_gen *__pyx_cur_scope;
- PyObject *__pyx_r = NULL;
- __Pyx_RefNannyDeclarations
- int __pyx_lineno = 0;
- const char *__pyx_filename = NULL;
- int __pyx_clineno = 0;
- __Pyx_RefNannySetupContext("_split_cubic_into_n_gen", 0);
- __pyx_cur_scope = (struct __pyx_obj_9fontTools_5cu2qu_5cu2qu___pyx_scope_struct___split_cubic_into_n_gen *)__pyx_tp_new_9fontTools_5cu2qu_5cu2qu___pyx_scope_struct___split_cubic_into_n_gen(__pyx_ptype_9fontTools_5cu2qu_5cu2qu___pyx_scope_struct___split_cubic_into_n_gen, __pyx_empty_tuple, NULL);
- if (unlikely(!__pyx_cur_scope)) {
- __pyx_cur_scope = ((struct __pyx_obj_9fontTools_5cu2qu_5cu2qu___pyx_scope_struct___split_cubic_into_n_gen *)Py_None);
- __Pyx_INCREF(Py_None);
- __PYX_ERR(0, 127, __pyx_L1_error)
- } else {
- __Pyx_GOTREF((PyObject *)__pyx_cur_scope);
- }
- __pyx_cur_scope->__pyx_v_p0 = __pyx_v_p0;
- __pyx_cur_scope->__pyx_v_p1 = __pyx_v_p1;
- __pyx_cur_scope->__pyx_v_p2 = __pyx_v_p2;
- __pyx_cur_scope->__pyx_v_p3 = __pyx_v_p3;
- __pyx_cur_scope->__pyx_v_n = __pyx_v_n;
- {
- __pyx_CoroutineObject *gen = __Pyx_Generator_New((__pyx_coroutine_body_t) __pyx_gb_9fontTools_5cu2qu_5cu2qu_2generator, __pyx_codeobj_, (PyObject *) __pyx_cur_scope, __pyx_n_s_split_cubic_into_n_gen, __pyx_n_s_split_cubic_into_n_gen, __pyx_n_s_fontTools_cu2qu_cu2qu); if (unlikely(!gen)) __PYX_ERR(0, 127, __pyx_L1_error)
- __Pyx_DECREF(__pyx_cur_scope);
- __Pyx_RefNannyFinishContext();
- return (PyObject *) gen;
- }
-
- /* function exit code */
- __pyx_L1_error:;
- __Pyx_AddTraceback("fontTools.cu2qu.cu2qu._split_cubic_into_n_gen", __pyx_clineno, __pyx_lineno, __pyx_filename);
- __pyx_r = NULL;
- __Pyx_DECREF((PyObject *)__pyx_cur_scope);
- __Pyx_XGIVEREF(__pyx_r);
- __Pyx_RefNannyFinishContext();
- return __pyx_r;
-}
-
-static PyObject *__pyx_gb_9fontTools_5cu2qu_5cu2qu_2generator(__pyx_CoroutineObject *__pyx_generator, CYTHON_UNUSED PyThreadState *__pyx_tstate, PyObject *__pyx_sent_value) /* generator body */
-{
- struct __pyx_obj_9fontTools_5cu2qu_5cu2qu___pyx_scope_struct___split_cubic_into_n_gen *__pyx_cur_scope = ((struct __pyx_obj_9fontTools_5cu2qu_5cu2qu___pyx_scope_struct___split_cubic_into_n_gen *)__pyx_generator->closure);
- PyObject *__pyx_r = NULL;
- PyObject *__pyx_t_1 = NULL;
- PyObject *__pyx_t_2 = NULL;
- PyObject *__pyx_t_3 = NULL;
- PyObject *__pyx_t_4 = NULL;
- PyObject *__pyx_t_5 = NULL;
- PyObject *__pyx_t_6 = NULL;
- PyObject *(*__pyx_t_7)(PyObject *);
- __pyx_t_double_complex __pyx_t_8;
- __pyx_t_double_complex __pyx_t_9;
- __pyx_t_double_complex __pyx_t_10;
- __pyx_t_double_complex __pyx_t_11;
- int __pyx_t_12;
- int __pyx_t_13;
- int __pyx_t_14;
- int __pyx_lineno = 0;
- const char *__pyx_filename = NULL;
- int __pyx_clineno = 0;
- __Pyx_RefNannyDeclarations
- __Pyx_RefNannySetupContext("_split_cubic_into_n_gen", 0);
- switch (__pyx_generator->resume_label) {
- case 0: goto __pyx_L3_first_run;
- case 1: goto __pyx_L8_resume_from_yield;
- default: /* CPython raises the right error here */
- __Pyx_RefNannyFinishContext();
- return NULL;
- }
- __pyx_L3_first_run:;
- if (unlikely(!__pyx_sent_value)) __PYX_ERR(0, 127, __pyx_L1_error)
-
- /* "fontTools/cu2qu/cu2qu.py":142
- * )
- * def _split_cubic_into_n_gen(p0, p1, p2, p3, n):
- * a, b, c, d = calc_cubic_parameters(p0, p1, p2, p3) # <<<<<<<<<<<<<<
- * dt = 1 / n
- * delta_2 = dt * dt
- */
- __pyx_t_1 = __pyx_f_9fontTools_5cu2qu_5cu2qu_calc_cubic_parameters(__pyx_cur_scope->__pyx_v_p0, __pyx_cur_scope->__pyx_v_p1, __pyx_cur_scope->__pyx_v_p2, __pyx_cur_scope->__pyx_v_p3); if (unlikely(!__pyx_t_1)) __PYX_ERR(0, 142, __pyx_L1_error)
- __Pyx_GOTREF(__pyx_t_1);
- if ((likely(PyTuple_CheckExact(__pyx_t_1))) || (PyList_CheckExact(__pyx_t_1))) {
- PyObject* sequence = __pyx_t_1;
- Py_ssize_t size = __Pyx_PySequence_SIZE(sequence);
- if (unlikely(size != 4)) {
- if (size > 4) __Pyx_RaiseTooManyValuesError(4);
- else if (size >= 0) __Pyx_RaiseNeedMoreValuesError(size);
- __PYX_ERR(0, 142, __pyx_L1_error)
- }
- #if CYTHON_ASSUME_SAFE_MACROS && !CYTHON_AVOID_BORROWED_REFS
- if (likely(PyTuple_CheckExact(sequence))) {
- __pyx_t_2 = PyTuple_GET_ITEM(sequence, 0);
- __pyx_t_3 = PyTuple_GET_ITEM(sequence, 1);
- __pyx_t_4 = PyTuple_GET_ITEM(sequence, 2);
- __pyx_t_5 = PyTuple_GET_ITEM(sequence, 3);
- } else {
- __pyx_t_2 = PyList_GET_ITEM(sequence, 0);
- __pyx_t_3 = PyList_GET_ITEM(sequence, 1);
- __pyx_t_4 = PyList_GET_ITEM(sequence, 2);
- __pyx_t_5 = PyList_GET_ITEM(sequence, 3);
- }
- __Pyx_INCREF(__pyx_t_2);
- __Pyx_INCREF(__pyx_t_3);
- __Pyx_INCREF(__pyx_t_4);
- __Pyx_INCREF(__pyx_t_5);
- #else
- {
- Py_ssize_t i;
- PyObject** temps[4] = {&__pyx_t_2,&__pyx_t_3,&__pyx_t_4,&__pyx_t_5};
- for (i=0; i < 4; i++) {
- PyObject* item = PySequence_ITEM(sequence, i); if (unlikely(!item)) __PYX_ERR(0, 142, __pyx_L1_error)
- __Pyx_GOTREF(item);
- *(temps[i]) = item;
- }
- }
- #endif
- __Pyx_DECREF(__pyx_t_1); __pyx_t_1 = 0;
- } else {
- Py_ssize_t index = -1;
- PyObject** temps[4] = {&__pyx_t_2,&__pyx_t_3,&__pyx_t_4,&__pyx_t_5};
- __pyx_t_6 = PyObject_GetIter(__pyx_t_1); if (unlikely(!__pyx_t_6)) __PYX_ERR(0, 142, __pyx_L1_error)
- __Pyx_GOTREF(__pyx_t_6);
- __Pyx_DECREF(__pyx_t_1); __pyx_t_1 = 0;
- __pyx_t_7 = __Pyx_PyObject_GetIterNextFunc(__pyx_t_6);
- for (index=0; index < 4; index++) {
- PyObject* item = __pyx_t_7(__pyx_t_6); if (unlikely(!item)) goto __pyx_L4_unpacking_failed;
- __Pyx_GOTREF(item);
- *(temps[index]) = item;
- }
- if (__Pyx_IternextUnpackEndCheck(__pyx_t_7(__pyx_t_6), 4) < 0) __PYX_ERR(0, 142, __pyx_L1_error)
- __pyx_t_7 = NULL;
- __Pyx_DECREF(__pyx_t_6); __pyx_t_6 = 0;
- goto __pyx_L5_unpacking_done;
- __pyx_L4_unpacking_failed:;
- __Pyx_DECREF(__pyx_t_6); __pyx_t_6 = 0;
- __pyx_t_7 = NULL;
- if (__Pyx_IterFinish() == 0) __Pyx_RaiseNeedMoreValuesError(index);
- __PYX_ERR(0, 142, __pyx_L1_error)
- __pyx_L5_unpacking_done:;
- }
- __pyx_t_8 = __Pyx_PyComplex_As___pyx_t_double_complex(__pyx_t_2); if (unlikely(PyErr_Occurred())) __PYX_ERR(0, 142, __pyx_L1_error)
- __Pyx_DECREF(__pyx_t_2); __pyx_t_2 = 0;
- __pyx_t_9 = __Pyx_PyComplex_As___pyx_t_double_complex(__pyx_t_3); if (unlikely(PyErr_Occurred())) __PYX_ERR(0, 142, __pyx_L1_error)
- __Pyx_DECREF(__pyx_t_3); __pyx_t_3 = 0;
- __pyx_t_10 = __Pyx_PyComplex_As___pyx_t_double_complex(__pyx_t_4); if (unlikely(PyErr_Occurred())) __PYX_ERR(0, 142, __pyx_L1_error)
- __Pyx_DECREF(__pyx_t_4); __pyx_t_4 = 0;
- __pyx_t_11 = __Pyx_PyComplex_As___pyx_t_double_complex(__pyx_t_5); if (unlikely(PyErr_Occurred())) __PYX_ERR(0, 142, __pyx_L1_error)
- __Pyx_DECREF(__pyx_t_5); __pyx_t_5 = 0;
- __pyx_cur_scope->__pyx_v_a = __pyx_t_8;
- __pyx_cur_scope->__pyx_v_b = __pyx_t_9;
- __pyx_cur_scope->__pyx_v_c = __pyx_t_10;
- __pyx_cur_scope->__pyx_v_d = __pyx_t_11;
-
- /* "fontTools/cu2qu/cu2qu.py":143
- * def _split_cubic_into_n_gen(p0, p1, p2, p3, n):
- * a, b, c, d = calc_cubic_parameters(p0, p1, p2, p3)
- * dt = 1 / n # <<<<<<<<<<<<<<
- * delta_2 = dt * dt
- * delta_3 = dt * delta_2
- */
- if (unlikely(__pyx_cur_scope->__pyx_v_n == 0)) {
- PyErr_SetString(PyExc_ZeroDivisionError, "float division");
- __PYX_ERR(0, 143, __pyx_L1_error)
- }
- __pyx_cur_scope->__pyx_v_dt = (1.0 / ((double)__pyx_cur_scope->__pyx_v_n));
-
- /* "fontTools/cu2qu/cu2qu.py":144
- * a, b, c, d = calc_cubic_parameters(p0, p1, p2, p3)
- * dt = 1 / n
- * delta_2 = dt * dt # <<<<<<<<<<<<<<
- * delta_3 = dt * delta_2
- * for i in range(n):
- */
- __pyx_cur_scope->__pyx_v_delta_2 = (__pyx_cur_scope->__pyx_v_dt * __pyx_cur_scope->__pyx_v_dt);
-
- /* "fontTools/cu2qu/cu2qu.py":145
- * dt = 1 / n
- * delta_2 = dt * dt
- * delta_3 = dt * delta_2 # <<<<<<<<<<<<<<
- * for i in range(n):
- * t1 = i * dt
- */
- __pyx_cur_scope->__pyx_v_delta_3 = (__pyx_cur_scope->__pyx_v_dt * __pyx_cur_scope->__pyx_v_delta_2);
-
- /* "fontTools/cu2qu/cu2qu.py":146
- * delta_2 = dt * dt
- * delta_3 = dt * delta_2
- * for i in range(n): # <<<<<<<<<<<<<<
- * t1 = i * dt
- * t1_2 = t1 * t1
- */
- __pyx_t_12 = __pyx_cur_scope->__pyx_v_n;
- __pyx_t_13 = __pyx_t_12;
- for (__pyx_t_14 = 0; __pyx_t_14 < __pyx_t_13; __pyx_t_14+=1) {
- __pyx_cur_scope->__pyx_v_i = __pyx_t_14;
-
- /* "fontTools/cu2qu/cu2qu.py":147
- * delta_3 = dt * delta_2
- * for i in range(n):
- * t1 = i * dt # <<<<<<<<<<<<<<
- * t1_2 = t1 * t1
- * # calc new a, b, c and d
- */
- __pyx_cur_scope->__pyx_v_t1 = (__pyx_cur_scope->__pyx_v_i * __pyx_cur_scope->__pyx_v_dt);
-
- /* "fontTools/cu2qu/cu2qu.py":148
- * for i in range(n):
- * t1 = i * dt
- * t1_2 = t1 * t1 # <<<<<<<<<<<<<<
- * # calc new a, b, c and d
- * a1 = a * delta_3
- */
- __pyx_cur_scope->__pyx_v_t1_2 = (__pyx_cur_scope->__pyx_v_t1 * __pyx_cur_scope->__pyx_v_t1);
-
- /* "fontTools/cu2qu/cu2qu.py":150
- * t1_2 = t1 * t1
- * # calc new a, b, c and d
- * a1 = a * delta_3 # <<<<<<<<<<<<<<
- * b1 = (3 * a * t1 + b) * delta_2
- * c1 = (2 * b * t1 + c + 3 * a * t1_2) * dt
- */
- __pyx_cur_scope->__pyx_v_a1 = __Pyx_c_prod_double(__pyx_cur_scope->__pyx_v_a, __pyx_t_double_complex_from_parts(__pyx_cur_scope->__pyx_v_delta_3, 0));
-
- /* "fontTools/cu2qu/cu2qu.py":151
- * # calc new a, b, c and d
- * a1 = a * delta_3
- * b1 = (3 * a * t1 + b) * delta_2 # <<<<<<<<<<<<<<
- * c1 = (2 * b * t1 + c + 3 * a * t1_2) * dt
- * d1 = a * t1 * t1_2 + b * t1_2 + c * t1 + d
- */
- __pyx_cur_scope->__pyx_v_b1 = __Pyx_c_prod_double(__Pyx_c_sum_double(__Pyx_c_prod_double(__Pyx_c_prod_double(__pyx_t_double_complex_from_parts(3, 0), __pyx_cur_scope->__pyx_v_a), __pyx_t_double_complex_from_parts(__pyx_cur_scope->__pyx_v_t1, 0)), __pyx_cur_scope->__pyx_v_b), __pyx_t_double_complex_from_parts(__pyx_cur_scope->__pyx_v_delta_2, 0));
-
- /* "fontTools/cu2qu/cu2qu.py":152
- * a1 = a * delta_3
- * b1 = (3 * a * t1 + b) * delta_2
- * c1 = (2 * b * t1 + c + 3 * a * t1_2) * dt # <<<<<<<<<<<<<<
- * d1 = a * t1 * t1_2 + b * t1_2 + c * t1 + d
- * yield calc_cubic_points(a1, b1, c1, d1)
- */
- __pyx_cur_scope->__pyx_v_c1 = __Pyx_c_prod_double(__Pyx_c_sum_double(__Pyx_c_sum_double(__Pyx_c_prod_double(__Pyx_c_prod_double(__pyx_t_double_complex_from_parts(2, 0), __pyx_cur_scope->__pyx_v_b), __pyx_t_double_complex_from_parts(__pyx_cur_scope->__pyx_v_t1, 0)), __pyx_cur_scope->__pyx_v_c), __Pyx_c_prod_double(__Pyx_c_prod_double(__pyx_t_double_complex_from_parts(3, 0), __pyx_cur_scope->__pyx_v_a), __pyx_t_double_complex_from_parts(__pyx_cur_scope->__pyx_v_t1_2, 0))), __pyx_t_double_complex_from_parts(__pyx_cur_scope->__pyx_v_dt, 0));
-
- /* "fontTools/cu2qu/cu2qu.py":153
- * b1 = (3 * a * t1 + b) * delta_2
- * c1 = (2 * b * t1 + c + 3 * a * t1_2) * dt
- * d1 = a * t1 * t1_2 + b * t1_2 + c * t1 + d # <<<<<<<<<<<<<<
- * yield calc_cubic_points(a1, b1, c1, d1)
- *
- */
- __pyx_cur_scope->__pyx_v_d1 = __Pyx_c_sum_double(__Pyx_c_sum_double(__Pyx_c_sum_double(__Pyx_c_prod_double(__Pyx_c_prod_double(__pyx_cur_scope->__pyx_v_a, __pyx_t_double_complex_from_parts(__pyx_cur_scope->__pyx_v_t1, 0)), __pyx_t_double_complex_from_parts(__pyx_cur_scope->__pyx_v_t1_2, 0)), __Pyx_c_prod_double(__pyx_cur_scope->__pyx_v_b, __pyx_t_double_complex_from_parts(__pyx_cur_scope->__pyx_v_t1_2, 0))), __Pyx_c_prod_double(__pyx_cur_scope->__pyx_v_c, __pyx_t_double_complex_from_parts(__pyx_cur_scope->__pyx_v_t1, 0))), __pyx_cur_scope->__pyx_v_d);
-
- /* "fontTools/cu2qu/cu2qu.py":154
- * c1 = (2 * b * t1 + c + 3 * a * t1_2) * dt
- * d1 = a * t1 * t1_2 + b * t1_2 + c * t1 + d
- * yield calc_cubic_points(a1, b1, c1, d1) # <<<<<<<<<<<<<<
- *
- *
- */
- __pyx_t_1 = __pyx_f_9fontTools_5cu2qu_5cu2qu_calc_cubic_points(__pyx_cur_scope->__pyx_v_a1, __pyx_cur_scope->__pyx_v_b1, __pyx_cur_scope->__pyx_v_c1, __pyx_cur_scope->__pyx_v_d1); if (unlikely(!__pyx_t_1)) __PYX_ERR(0, 154, __pyx_L1_error)
- __Pyx_GOTREF(__pyx_t_1);
- __pyx_r = __pyx_t_1;
- __pyx_t_1 = 0;
- __pyx_cur_scope->__pyx_t_0 = __pyx_t_12;
- __pyx_cur_scope->__pyx_t_1 = __pyx_t_13;
- __pyx_cur_scope->__pyx_t_2 = __pyx_t_14;
- __Pyx_XGIVEREF(__pyx_r);
- __Pyx_RefNannyFinishContext();
- __Pyx_Coroutine_ResetAndClearException(__pyx_generator);
- /* return from generator, yielding value */
- __pyx_generator->resume_label = 1;
- return __pyx_r;
- __pyx_L8_resume_from_yield:;
- __pyx_t_12 = __pyx_cur_scope->__pyx_t_0;
- __pyx_t_13 = __pyx_cur_scope->__pyx_t_1;
- __pyx_t_14 = __pyx_cur_scope->__pyx_t_2;
- if (unlikely(!__pyx_sent_value)) __PYX_ERR(0, 154, __pyx_L1_error)
- }
- CYTHON_MAYBE_UNUSED_VAR(__pyx_cur_scope);
-
- /* "fontTools/cu2qu/cu2qu.py":127
- *
- *
- * @cython.locals( # <<<<<<<<<<<<<<
- * p0=cython.complex,
- * p1=cython.complex,
- */
-
- /* function exit code */
- PyErr_SetNone(PyExc_StopIteration);
- goto __pyx_L0;
- __pyx_L1_error:;
- __Pyx_Generator_Replace_StopIteration(0);
- __Pyx_XDECREF(__pyx_t_1);
- __Pyx_XDECREF(__pyx_t_2);
- __Pyx_XDECREF(__pyx_t_3);
- __Pyx_XDECREF(__pyx_t_4);
- __Pyx_XDECREF(__pyx_t_5);
- __Pyx_XDECREF(__pyx_t_6);
- __Pyx_AddTraceback("_split_cubic_into_n_gen", __pyx_clineno, __pyx_lineno, __pyx_filename);
- __pyx_L0:;
- __Pyx_XDECREF(__pyx_r); __pyx_r = 0;
- #if !CYTHON_USE_EXC_INFO_STACK
- __Pyx_Coroutine_ResetAndClearException(__pyx_generator);
- #endif
- __pyx_generator->resume_label = -1;
- __Pyx_Coroutine_clear((PyObject*)__pyx_generator);
- __Pyx_RefNannyFinishContext();
- return __pyx_r;
-}
-
-/* "fontTools/cu2qu/cu2qu.py":157
- *
- *
- * @cython.cfunc # <<<<<<<<<<<<<<
- * @cython.inline
- * @cython.locals(
- */
-
-static CYTHON_INLINE PyObject *__pyx_f_9fontTools_5cu2qu_5cu2qu_split_cubic_into_two(__pyx_t_double_complex __pyx_v_p0, __pyx_t_double_complex __pyx_v_p1, __pyx_t_double_complex __pyx_v_p2, __pyx_t_double_complex __pyx_v_p3) {
- __pyx_t_double_complex __pyx_v_mid;
- __pyx_t_double_complex __pyx_v_deriv3;
- PyObject *__pyx_r = NULL;
- __Pyx_RefNannyDeclarations
- PyObject *__pyx_t_1 = NULL;
- __pyx_t_double_complex __pyx_t_2;
- PyObject *__pyx_t_3 = NULL;
- PyObject *__pyx_t_4 = NULL;
- PyObject *__pyx_t_5 = NULL;
- PyObject *__pyx_t_6 = NULL;
- PyObject *__pyx_t_7 = NULL;
- int __pyx_lineno = 0;
- const char *__pyx_filename = NULL;
- int __pyx_clineno = 0;
- __Pyx_RefNannySetupContext("split_cubic_into_two", 0);
-
- /* "fontTools/cu2qu/cu2qu.py":178
- * values).
- * """
- * mid = (p0 + 3 * (p1 + p2) + p3) * 0.125 # <<<<<<<<<<<<<<
- * deriv3 = (p3 + p2 - p1 - p0) * 0.125
- * return (
- */
- __pyx_v_mid = __Pyx_c_prod_double(__Pyx_c_sum_double(__Pyx_c_sum_double(__pyx_v_p0, __Pyx_c_prod_double(__pyx_t_double_complex_from_parts(3, 0), __Pyx_c_sum_double(__pyx_v_p1, __pyx_v_p2))), __pyx_v_p3), __pyx_t_double_complex_from_parts(0.125, 0));
-
- /* "fontTools/cu2qu/cu2qu.py":179
- * """
- * mid = (p0 + 3 * (p1 + p2) + p3) * 0.125
- * deriv3 = (p3 + p2 - p1 - p0) * 0.125 # <<<<<<<<<<<<<<
- * return (
- * (p0, (p0 + p1) * 0.5, mid - deriv3, mid),
- */
- __pyx_v_deriv3 = __Pyx_c_prod_double(__Pyx_c_diff_double(__Pyx_c_diff_double(__Pyx_c_sum_double(__pyx_v_p3, __pyx_v_p2), __pyx_v_p1), __pyx_v_p0), __pyx_t_double_complex_from_parts(0.125, 0));
-
- /* "fontTools/cu2qu/cu2qu.py":180
- * mid = (p0 + 3 * (p1 + p2) + p3) * 0.125
- * deriv3 = (p3 + p2 - p1 - p0) * 0.125
- * return ( # <<<<<<<<<<<<<<
- * (p0, (p0 + p1) * 0.5, mid - deriv3, mid),
- * (mid, mid + deriv3, (p2 + p3) * 0.5, p3),
- */
- __Pyx_XDECREF(__pyx_r);
-
- /* "fontTools/cu2qu/cu2qu.py":181
- * deriv3 = (p3 + p2 - p1 - p0) * 0.125
- * return (
- * (p0, (p0 + p1) * 0.5, mid - deriv3, mid), # <<<<<<<<<<<<<<
- * (mid, mid + deriv3, (p2 + p3) * 0.5, p3),
- * )
- */
- __pyx_t_1 = __pyx_PyComplex_FromComplex(__pyx_v_p0); if (unlikely(!__pyx_t_1)) __PYX_ERR(0, 181, __pyx_L1_error)
- __Pyx_GOTREF(__pyx_t_1);
- __pyx_t_2 = __Pyx_c_prod_double(__Pyx_c_sum_double(__pyx_v_p0, __pyx_v_p1), __pyx_t_double_complex_from_parts(0.5, 0));
- __pyx_t_3 = __pyx_PyComplex_FromComplex(__pyx_t_2); if (unlikely(!__pyx_t_3)) __PYX_ERR(0, 181, __pyx_L1_error)
- __Pyx_GOTREF(__pyx_t_3);
- __pyx_t_2 = __Pyx_c_diff_double(__pyx_v_mid, __pyx_v_deriv3);
- __pyx_t_4 = __pyx_PyComplex_FromComplex(__pyx_t_2); if (unlikely(!__pyx_t_4)) __PYX_ERR(0, 181, __pyx_L1_error)
- __Pyx_GOTREF(__pyx_t_4);
- __pyx_t_5 = __pyx_PyComplex_FromComplex(__pyx_v_mid); if (unlikely(!__pyx_t_5)) __PYX_ERR(0, 181, __pyx_L1_error)
- __Pyx_GOTREF(__pyx_t_5);
- __pyx_t_6 = PyTuple_New(4); if (unlikely(!__pyx_t_6)) __PYX_ERR(0, 181, __pyx_L1_error)
- __Pyx_GOTREF(__pyx_t_6);
- __Pyx_GIVEREF(__pyx_t_1);
- PyTuple_SET_ITEM(__pyx_t_6, 0, __pyx_t_1);
- __Pyx_GIVEREF(__pyx_t_3);
- PyTuple_SET_ITEM(__pyx_t_6, 1, __pyx_t_3);
- __Pyx_GIVEREF(__pyx_t_4);
- PyTuple_SET_ITEM(__pyx_t_6, 2, __pyx_t_4);
- __Pyx_GIVEREF(__pyx_t_5);
- PyTuple_SET_ITEM(__pyx_t_6, 3, __pyx_t_5);
- __pyx_t_1 = 0;
- __pyx_t_3 = 0;
- __pyx_t_4 = 0;
- __pyx_t_5 = 0;
-
- /* "fontTools/cu2qu/cu2qu.py":182
- * return (
- * (p0, (p0 + p1) * 0.5, mid - deriv3, mid),
- * (mid, mid + deriv3, (p2 + p3) * 0.5, p3), # <<<<<<<<<<<<<<
- * )
- *
- */
- __pyx_t_5 = __pyx_PyComplex_FromComplex(__pyx_v_mid); if (unlikely(!__pyx_t_5)) __PYX_ERR(0, 182, __pyx_L1_error)
- __Pyx_GOTREF(__pyx_t_5);
- __pyx_t_2 = __Pyx_c_sum_double(__pyx_v_mid, __pyx_v_deriv3);
- __pyx_t_4 = __pyx_PyComplex_FromComplex(__pyx_t_2); if (unlikely(!__pyx_t_4)) __PYX_ERR(0, 182, __pyx_L1_error)
- __Pyx_GOTREF(__pyx_t_4);
- __pyx_t_2 = __Pyx_c_prod_double(__Pyx_c_sum_double(__pyx_v_p2, __pyx_v_p3), __pyx_t_double_complex_from_parts(0.5, 0));
- __pyx_t_3 = __pyx_PyComplex_FromComplex(__pyx_t_2); if (unlikely(!__pyx_t_3)) __PYX_ERR(0, 182, __pyx_L1_error)
- __Pyx_GOTREF(__pyx_t_3);
- __pyx_t_1 = __pyx_PyComplex_FromComplex(__pyx_v_p3); if (unlikely(!__pyx_t_1)) __PYX_ERR(0, 182, __pyx_L1_error)
- __Pyx_GOTREF(__pyx_t_1);
- __pyx_t_7 = PyTuple_New(4); if (unlikely(!__pyx_t_7)) __PYX_ERR(0, 182, __pyx_L1_error)
- __Pyx_GOTREF(__pyx_t_7);
- __Pyx_GIVEREF(__pyx_t_5);
- PyTuple_SET_ITEM(__pyx_t_7, 0, __pyx_t_5);
- __Pyx_GIVEREF(__pyx_t_4);
- PyTuple_SET_ITEM(__pyx_t_7, 1, __pyx_t_4);
- __Pyx_GIVEREF(__pyx_t_3);
- PyTuple_SET_ITEM(__pyx_t_7, 2, __pyx_t_3);
- __Pyx_GIVEREF(__pyx_t_1);
- PyTuple_SET_ITEM(__pyx_t_7, 3, __pyx_t_1);
- __pyx_t_5 = 0;
- __pyx_t_4 = 0;
- __pyx_t_3 = 0;
- __pyx_t_1 = 0;
-
- /* "fontTools/cu2qu/cu2qu.py":181
- * deriv3 = (p3 + p2 - p1 - p0) * 0.125
- * return (
- * (p0, (p0 + p1) * 0.5, mid - deriv3, mid), # <<<<<<<<<<<<<<
- * (mid, mid + deriv3, (p2 + p3) * 0.5, p3),
- * )
- */
- __pyx_t_1 = PyTuple_New(2); if (unlikely(!__pyx_t_1)) __PYX_ERR(0, 181, __pyx_L1_error)
- __Pyx_GOTREF(__pyx_t_1);
- __Pyx_GIVEREF(__pyx_t_6);
- PyTuple_SET_ITEM(__pyx_t_1, 0, __pyx_t_6);
- __Pyx_GIVEREF(__pyx_t_7);
- PyTuple_SET_ITEM(__pyx_t_1, 1, __pyx_t_7);
- __pyx_t_6 = 0;
- __pyx_t_7 = 0;
- __pyx_r = __pyx_t_1;
- __pyx_t_1 = 0;
- goto __pyx_L0;
-
- /* "fontTools/cu2qu/cu2qu.py":157
- *
- *
- * @cython.cfunc # <<<<<<<<<<<<<<
- * @cython.inline
- * @cython.locals(
- */
-
- /* function exit code */
- __pyx_L1_error:;
- __Pyx_XDECREF(__pyx_t_1);
- __Pyx_XDECREF(__pyx_t_3);
- __Pyx_XDECREF(__pyx_t_4);
- __Pyx_XDECREF(__pyx_t_5);
- __Pyx_XDECREF(__pyx_t_6);
- __Pyx_XDECREF(__pyx_t_7);
- __Pyx_AddTraceback("fontTools.cu2qu.cu2qu.split_cubic_into_two", __pyx_clineno, __pyx_lineno, __pyx_filename);
- __pyx_r = 0;
- __pyx_L0:;
- __Pyx_XGIVEREF(__pyx_r);
- __Pyx_RefNannyFinishContext();
- return __pyx_r;
-}
-
-/* "fontTools/cu2qu/cu2qu.py":186
- *
- *
- * @cython.cfunc # <<<<<<<<<<<<<<
- * @cython.inline
- * @cython.locals(
- */
-
-static CYTHON_INLINE PyObject *__pyx_f_9fontTools_5cu2qu_5cu2qu_split_cubic_into_three(__pyx_t_double_complex __pyx_v_p0, __pyx_t_double_complex __pyx_v_p1, __pyx_t_double_complex __pyx_v_p2, __pyx_t_double_complex __pyx_v_p3) {
- __pyx_t_double_complex __pyx_v_mid1;
- __pyx_t_double_complex __pyx_v_deriv1;
- __pyx_t_double_complex __pyx_v_mid2;
- __pyx_t_double_complex __pyx_v_deriv2;
- PyObject *__pyx_r = NULL;
- __Pyx_RefNannyDeclarations
- PyObject *__pyx_t_1 = NULL;
- __pyx_t_double_complex __pyx_t_2;
- __pyx_t_double_complex __pyx_t_3;
- __pyx_t_double_complex __pyx_t_4;
- PyObject *__pyx_t_5 = NULL;
- PyObject *__pyx_t_6 = NULL;
- PyObject *__pyx_t_7 = NULL;
- PyObject *__pyx_t_8 = NULL;
- PyObject *__pyx_t_9 = NULL;
- PyObject *__pyx_t_10 = NULL;
- int __pyx_lineno = 0;
- const char *__pyx_filename = NULL;
- int __pyx_clineno = 0;
- __Pyx_RefNannySetupContext("split_cubic_into_three", 0);
-
- /* "fontTools/cu2qu/cu2qu.py":215
- * values).
- * """
- * mid1 = (8 * p0 + 12 * p1 + 6 * p2 + p3) * (1 / 27) # <<<<<<<<<<<<<<
- * deriv1 = (p3 + 3 * p2 - 4 * p0) * (1 / 27)
- * mid2 = (p0 + 6 * p1 + 12 * p2 + 8 * p3) * (1 / 27)
- */
- __pyx_v_mid1 = __Pyx_c_prod_double(__Pyx_c_sum_double(__Pyx_c_sum_double(__Pyx_c_sum_double(__Pyx_c_prod_double(__pyx_t_double_complex_from_parts(8, 0), __pyx_v_p0), __Pyx_c_prod_double(__pyx_t_double_complex_from_parts(12, 0), __pyx_v_p1)), __Pyx_c_prod_double(__pyx_t_double_complex_from_parts(6, 0), __pyx_v_p2)), __pyx_v_p3), __pyx_t_double_complex_from_parts((1.0 / 27.0), 0));
-
- /* "fontTools/cu2qu/cu2qu.py":216
- * """
- * mid1 = (8 * p0 + 12 * p1 + 6 * p2 + p3) * (1 / 27)
- * deriv1 = (p3 + 3 * p2 - 4 * p0) * (1 / 27) # <<<<<<<<<<<<<<
- * mid2 = (p0 + 6 * p1 + 12 * p2 + 8 * p3) * (1 / 27)
- * deriv2 = (4 * p3 - 3 * p1 - p0) * (1 / 27)
- */
- __pyx_v_deriv1 = __Pyx_c_prod_double(__Pyx_c_diff_double(__Pyx_c_sum_double(__pyx_v_p3, __Pyx_c_prod_double(__pyx_t_double_complex_from_parts(3, 0), __pyx_v_p2)), __Pyx_c_prod_double(__pyx_t_double_complex_from_parts(4, 0), __pyx_v_p0)), __pyx_t_double_complex_from_parts((1.0 / 27.0), 0));
-
- /* "fontTools/cu2qu/cu2qu.py":217
- * mid1 = (8 * p0 + 12 * p1 + 6 * p2 + p3) * (1 / 27)
- * deriv1 = (p3 + 3 * p2 - 4 * p0) * (1 / 27)
- * mid2 = (p0 + 6 * p1 + 12 * p2 + 8 * p3) * (1 / 27) # <<<<<<<<<<<<<<
- * deriv2 = (4 * p3 - 3 * p1 - p0) * (1 / 27)
- * return (
- */
- __pyx_v_mid2 = __Pyx_c_prod_double(__Pyx_c_sum_double(__Pyx_c_sum_double(__Pyx_c_sum_double(__pyx_v_p0, __Pyx_c_prod_double(__pyx_t_double_complex_from_parts(6, 0), __pyx_v_p1)), __Pyx_c_prod_double(__pyx_t_double_complex_from_parts(12, 0), __pyx_v_p2)), __Pyx_c_prod_double(__pyx_t_double_complex_from_parts(8, 0), __pyx_v_p3)), __pyx_t_double_complex_from_parts((1.0 / 27.0), 0));
-
- /* "fontTools/cu2qu/cu2qu.py":218
- * deriv1 = (p3 + 3 * p2 - 4 * p0) * (1 / 27)
- * mid2 = (p0 + 6 * p1 + 12 * p2 + 8 * p3) * (1 / 27)
- * deriv2 = (4 * p3 - 3 * p1 - p0) * (1 / 27) # <<<<<<<<<<<<<<
- * return (
- * (p0, (2 * p0 + p1) / 3.0, mid1 - deriv1, mid1),
- */
- __pyx_v_deriv2 = __Pyx_c_prod_double(__Pyx_c_diff_double(__Pyx_c_diff_double(__Pyx_c_prod_double(__pyx_t_double_complex_from_parts(4, 0), __pyx_v_p3), __Pyx_c_prod_double(__pyx_t_double_complex_from_parts(3, 0), __pyx_v_p1)), __pyx_v_p0), __pyx_t_double_complex_from_parts((1.0 / 27.0), 0));
-
- /* "fontTools/cu2qu/cu2qu.py":219
- * mid2 = (p0 + 6 * p1 + 12 * p2 + 8 * p3) * (1 / 27)
- * deriv2 = (4 * p3 - 3 * p1 - p0) * (1 / 27)
- * return ( # <<<<<<<<<<<<<<
- * (p0, (2 * p0 + p1) / 3.0, mid1 - deriv1, mid1),
- * (mid1, mid1 + deriv1, mid2 - deriv2, mid2),
- */
- __Pyx_XDECREF(__pyx_r);
-
- /* "fontTools/cu2qu/cu2qu.py":220
- * deriv2 = (4 * p3 - 3 * p1 - p0) * (1 / 27)
- * return (
- * (p0, (2 * p0 + p1) / 3.0, mid1 - deriv1, mid1), # <<<<<<<<<<<<<<
- * (mid1, mid1 + deriv1, mid2 - deriv2, mid2),
- * (mid2, mid2 + deriv2, (p2 + 2 * p3) / 3.0, p3),
- */
- __pyx_t_1 = __pyx_PyComplex_FromComplex(__pyx_v_p0); if (unlikely(!__pyx_t_1)) __PYX_ERR(0, 220, __pyx_L1_error)
- __Pyx_GOTREF(__pyx_t_1);
- __pyx_t_2 = __Pyx_c_sum_double(__Pyx_c_prod_double(__pyx_t_double_complex_from_parts(2, 0), __pyx_v_p0), __pyx_v_p1);
- __pyx_t_3 = __pyx_t_double_complex_from_parts(3.0, 0);
- if (unlikely(__Pyx_c_is_zero_double(__pyx_t_3))) {
- PyErr_SetString(PyExc_ZeroDivisionError, "float division");
- __PYX_ERR(0, 220, __pyx_L1_error)
- }
- __pyx_t_4 = __Pyx_c_quot_double(__pyx_t_2, __pyx_t_3);
- __pyx_t_5 = __pyx_PyComplex_FromComplex(__pyx_t_4); if (unlikely(!__pyx_t_5)) __PYX_ERR(0, 220, __pyx_L1_error)
- __Pyx_GOTREF(__pyx_t_5);
- __pyx_t_4 = __Pyx_c_diff_double(__pyx_v_mid1, __pyx_v_deriv1);
- __pyx_t_6 = __pyx_PyComplex_FromComplex(__pyx_t_4); if (unlikely(!__pyx_t_6)) __PYX_ERR(0, 220, __pyx_L1_error)
- __Pyx_GOTREF(__pyx_t_6);
- __pyx_t_7 = __pyx_PyComplex_FromComplex(__pyx_v_mid1); if (unlikely(!__pyx_t_7)) __PYX_ERR(0, 220, __pyx_L1_error)
- __Pyx_GOTREF(__pyx_t_7);
- __pyx_t_8 = PyTuple_New(4); if (unlikely(!__pyx_t_8)) __PYX_ERR(0, 220, __pyx_L1_error)
- __Pyx_GOTREF(__pyx_t_8);
- __Pyx_GIVEREF(__pyx_t_1);
- PyTuple_SET_ITEM(__pyx_t_8, 0, __pyx_t_1);
- __Pyx_GIVEREF(__pyx_t_5);
- PyTuple_SET_ITEM(__pyx_t_8, 1, __pyx_t_5);
- __Pyx_GIVEREF(__pyx_t_6);
- PyTuple_SET_ITEM(__pyx_t_8, 2, __pyx_t_6);
- __Pyx_GIVEREF(__pyx_t_7);
- PyTuple_SET_ITEM(__pyx_t_8, 3, __pyx_t_7);
- __pyx_t_1 = 0;
- __pyx_t_5 = 0;
- __pyx_t_6 = 0;
- __pyx_t_7 = 0;
-
- /* "fontTools/cu2qu/cu2qu.py":221
- * return (
- * (p0, (2 * p0 + p1) / 3.0, mid1 - deriv1, mid1),
- * (mid1, mid1 + deriv1, mid2 - deriv2, mid2), # <<<<<<<<<<<<<<
- * (mid2, mid2 + deriv2, (p2 + 2 * p3) / 3.0, p3),
- * )
- */
- __pyx_t_7 = __pyx_PyComplex_FromComplex(__pyx_v_mid1); if (unlikely(!__pyx_t_7)) __PYX_ERR(0, 221, __pyx_L1_error)
- __Pyx_GOTREF(__pyx_t_7);
- __pyx_t_4 = __Pyx_c_sum_double(__pyx_v_mid1, __pyx_v_deriv1);
- __pyx_t_6 = __pyx_PyComplex_FromComplex(__pyx_t_4); if (unlikely(!__pyx_t_6)) __PYX_ERR(0, 221, __pyx_L1_error)
- __Pyx_GOTREF(__pyx_t_6);
- __pyx_t_4 = __Pyx_c_diff_double(__pyx_v_mid2, __pyx_v_deriv2);
- __pyx_t_5 = __pyx_PyComplex_FromComplex(__pyx_t_4); if (unlikely(!__pyx_t_5)) __PYX_ERR(0, 221, __pyx_L1_error)
- __Pyx_GOTREF(__pyx_t_5);
- __pyx_t_1 = __pyx_PyComplex_FromComplex(__pyx_v_mid2); if (unlikely(!__pyx_t_1)) __PYX_ERR(0, 221, __pyx_L1_error)
- __Pyx_GOTREF(__pyx_t_1);
- __pyx_t_9 = PyTuple_New(4); if (unlikely(!__pyx_t_9)) __PYX_ERR(0, 221, __pyx_L1_error)
- __Pyx_GOTREF(__pyx_t_9);
- __Pyx_GIVEREF(__pyx_t_7);
- PyTuple_SET_ITEM(__pyx_t_9, 0, __pyx_t_7);
- __Pyx_GIVEREF(__pyx_t_6);
- PyTuple_SET_ITEM(__pyx_t_9, 1, __pyx_t_6);
- __Pyx_GIVEREF(__pyx_t_5);
- PyTuple_SET_ITEM(__pyx_t_9, 2, __pyx_t_5);
- __Pyx_GIVEREF(__pyx_t_1);
- PyTuple_SET_ITEM(__pyx_t_9, 3, __pyx_t_1);
- __pyx_t_7 = 0;
- __pyx_t_6 = 0;
- __pyx_t_5 = 0;
- __pyx_t_1 = 0;
-
- /* "fontTools/cu2qu/cu2qu.py":222
- * (p0, (2 * p0 + p1) / 3.0, mid1 - deriv1, mid1),
- * (mid1, mid1 + deriv1, mid2 - deriv2, mid2),
- * (mid2, mid2 + deriv2, (p2 + 2 * p3) / 3.0, p3), # <<<<<<<<<<<<<<
- * )
- *
- */
- __pyx_t_1 = __pyx_PyComplex_FromComplex(__pyx_v_mid2); if (unlikely(!__pyx_t_1)) __PYX_ERR(0, 222, __pyx_L1_error)
- __Pyx_GOTREF(__pyx_t_1);
- __pyx_t_4 = __Pyx_c_sum_double(__pyx_v_mid2, __pyx_v_deriv2);
- __pyx_t_5 = __pyx_PyComplex_FromComplex(__pyx_t_4); if (unlikely(!__pyx_t_5)) __PYX_ERR(0, 222, __pyx_L1_error)
- __Pyx_GOTREF(__pyx_t_5);
- __pyx_t_4 = __Pyx_c_sum_double(__pyx_v_p2, __Pyx_c_prod_double(__pyx_t_double_complex_from_parts(2, 0), __pyx_v_p3));
- __pyx_t_3 = __pyx_t_double_complex_from_parts(3.0, 0);
- if (unlikely(__Pyx_c_is_zero_double(__pyx_t_3))) {
- PyErr_SetString(PyExc_ZeroDivisionError, "float division");
- __PYX_ERR(0, 222, __pyx_L1_error)
- }
- __pyx_t_2 = __Pyx_c_quot_double(__pyx_t_4, __pyx_t_3);
- __pyx_t_6 = __pyx_PyComplex_FromComplex(__pyx_t_2); if (unlikely(!__pyx_t_6)) __PYX_ERR(0, 222, __pyx_L1_error)
- __Pyx_GOTREF(__pyx_t_6);
- __pyx_t_7 = __pyx_PyComplex_FromComplex(__pyx_v_p3); if (unlikely(!__pyx_t_7)) __PYX_ERR(0, 222, __pyx_L1_error)
- __Pyx_GOTREF(__pyx_t_7);
- __pyx_t_10 = PyTuple_New(4); if (unlikely(!__pyx_t_10)) __PYX_ERR(0, 222, __pyx_L1_error)
- __Pyx_GOTREF(__pyx_t_10);
- __Pyx_GIVEREF(__pyx_t_1);
- PyTuple_SET_ITEM(__pyx_t_10, 0, __pyx_t_1);
- __Pyx_GIVEREF(__pyx_t_5);
- PyTuple_SET_ITEM(__pyx_t_10, 1, __pyx_t_5);
- __Pyx_GIVEREF(__pyx_t_6);
- PyTuple_SET_ITEM(__pyx_t_10, 2, __pyx_t_6);
- __Pyx_GIVEREF(__pyx_t_7);
- PyTuple_SET_ITEM(__pyx_t_10, 3, __pyx_t_7);
- __pyx_t_1 = 0;
- __pyx_t_5 = 0;
- __pyx_t_6 = 0;
- __pyx_t_7 = 0;
-
- /* "fontTools/cu2qu/cu2qu.py":220
- * deriv2 = (4 * p3 - 3 * p1 - p0) * (1 / 27)
- * return (
- * (p0, (2 * p0 + p1) / 3.0, mid1 - deriv1, mid1), # <<<<<<<<<<<<<<
- * (mid1, mid1 + deriv1, mid2 - deriv2, mid2),
- * (mid2, mid2 + deriv2, (p2 + 2 * p3) / 3.0, p3),
- */
- __pyx_t_7 = PyTuple_New(3); if (unlikely(!__pyx_t_7)) __PYX_ERR(0, 220, __pyx_L1_error)
- __Pyx_GOTREF(__pyx_t_7);
- __Pyx_GIVEREF(__pyx_t_8);
- PyTuple_SET_ITEM(__pyx_t_7, 0, __pyx_t_8);
- __Pyx_GIVEREF(__pyx_t_9);
- PyTuple_SET_ITEM(__pyx_t_7, 1, __pyx_t_9);
- __Pyx_GIVEREF(__pyx_t_10);
- PyTuple_SET_ITEM(__pyx_t_7, 2, __pyx_t_10);
- __pyx_t_8 = 0;
- __pyx_t_9 = 0;
- __pyx_t_10 = 0;
- __pyx_r = __pyx_t_7;
- __pyx_t_7 = 0;
- goto __pyx_L0;
-
- /* "fontTools/cu2qu/cu2qu.py":186
- *
- *
- * @cython.cfunc # <<<<<<<<<<<<<<
- * @cython.inline
- * @cython.locals(
- */
-
- /* function exit code */
- __pyx_L1_error:;
- __Pyx_XDECREF(__pyx_t_1);
- __Pyx_XDECREF(__pyx_t_5);
- __Pyx_XDECREF(__pyx_t_6);
- __Pyx_XDECREF(__pyx_t_7);
- __Pyx_XDECREF(__pyx_t_8);
- __Pyx_XDECREF(__pyx_t_9);
- __Pyx_XDECREF(__pyx_t_10);
- __Pyx_AddTraceback("fontTools.cu2qu.cu2qu.split_cubic_into_three", __pyx_clineno, __pyx_lineno, __pyx_filename);
- __pyx_r = 0;
- __pyx_L0:;
- __Pyx_XGIVEREF(__pyx_r);
- __Pyx_RefNannyFinishContext();
- return __pyx_r;
-}
-
-/* "fontTools/cu2qu/cu2qu.py":226
- *
- *
- * @cython.cfunc # <<<<<<<<<<<<<<
- * @cython.inline
- * @cython.returns(cython.complex)
- */
-
-static CYTHON_INLINE __pyx_t_double_complex __pyx_f_9fontTools_5cu2qu_5cu2qu_cubic_approx_control(double __pyx_v_t, __pyx_t_double_complex __pyx_v_p0, __pyx_t_double_complex __pyx_v_p1, __pyx_t_double_complex __pyx_v_p2, __pyx_t_double_complex __pyx_v_p3) {
- __pyx_t_double_complex __pyx_v__p1;
- __pyx_t_double_complex __pyx_v__p2;
- __pyx_t_double_complex __pyx_r;
- __Pyx_RefNannyDeclarations
- __Pyx_RefNannySetupContext("cubic_approx_control", 0);
-
- /* "fontTools/cu2qu/cu2qu.py":250
- * complex: Location of candidate control point on quadratic curve.
- * """
- * _p1 = p0 + (p1 - p0) * 1.5 # <<<<<<<<<<<<<<
- * _p2 = p3 + (p2 - p3) * 1.5
- * return _p1 + (_p2 - _p1) * t
- */
- __pyx_v__p1 = __Pyx_c_sum_double(__pyx_v_p0, __Pyx_c_prod_double(__Pyx_c_diff_double(__pyx_v_p1, __pyx_v_p0), __pyx_t_double_complex_from_parts(1.5, 0)));
-
- /* "fontTools/cu2qu/cu2qu.py":251
- * """
- * _p1 = p0 + (p1 - p0) * 1.5
- * _p2 = p3 + (p2 - p3) * 1.5 # <<<<<<<<<<<<<<
- * return _p1 + (_p2 - _p1) * t
- *
- */
- __pyx_v__p2 = __Pyx_c_sum_double(__pyx_v_p3, __Pyx_c_prod_double(__Pyx_c_diff_double(__pyx_v_p2, __pyx_v_p3), __pyx_t_double_complex_from_parts(1.5, 0)));
-
- /* "fontTools/cu2qu/cu2qu.py":252
- * _p1 = p0 + (p1 - p0) * 1.5
- * _p2 = p3 + (p2 - p3) * 1.5
- * return _p1 + (_p2 - _p1) * t # <<<<<<<<<<<<<<
- *
- *
- */
- __pyx_r = __Pyx_c_sum_double(__pyx_v__p1, __Pyx_c_prod_double(__Pyx_c_diff_double(__pyx_v__p2, __pyx_v__p1), __pyx_t_double_complex_from_parts(__pyx_v_t, 0)));
- goto __pyx_L0;
-
- /* "fontTools/cu2qu/cu2qu.py":226
- *
- *
- * @cython.cfunc # <<<<<<<<<<<<<<
- * @cython.inline
- * @cython.returns(cython.complex)
- */
-
- /* function exit code */
- __pyx_L0:;
- __Pyx_RefNannyFinishContext();
- return __pyx_r;
-}
-
-/* "fontTools/cu2qu/cu2qu.py":255
- *
- *
- * @cython.cfunc # <<<<<<<<<<<<<<
- * @cython.inline
- * @cython.returns(cython.complex)
- */
-
-static CYTHON_INLINE __pyx_t_double_complex __pyx_f_9fontTools_5cu2qu_5cu2qu_calc_intersect(__pyx_t_double_complex __pyx_v_a, __pyx_t_double_complex __pyx_v_b, __pyx_t_double_complex __pyx_v_c, __pyx_t_double_complex __pyx_v_d) {
- __pyx_t_double_complex __pyx_v_ab;
- __pyx_t_double_complex __pyx_v_cd;
- __pyx_t_double_complex __pyx_v_p;
- double __pyx_v_h;
- __pyx_t_double_complex __pyx_r;
- __Pyx_RefNannyDeclarations
- PyObject *__pyx_t_1 = NULL;
- PyObject *__pyx_t_2 = NULL;
- PyObject *__pyx_t_3 = NULL;
- double __pyx_t_4;
- double __pyx_t_5;
- int __pyx_t_6;
- PyObject *__pyx_t_7 = NULL;
- PyObject *__pyx_t_8 = NULL;
- PyObject *__pyx_t_9 = NULL;
- PyObject *__pyx_t_10 = NULL;
- PyObject *__pyx_t_11 = NULL;
- PyObject *__pyx_t_12 = NULL;
- __pyx_t_double_complex __pyx_t_13;
- int __pyx_lineno = 0;
- const char *__pyx_filename = NULL;
- int __pyx_clineno = 0;
- __Pyx_RefNannySetupContext("calc_intersect", 0);
-
- /* "fontTools/cu2qu/cu2qu.py":273
- * if no intersection was found.
- * """
- * ab = b - a # <<<<<<<<<<<<<<
- * cd = d - c
- * p = ab * 1j
- */
- __pyx_v_ab = __Pyx_c_diff_double(__pyx_v_b, __pyx_v_a);
-
- /* "fontTools/cu2qu/cu2qu.py":274
- * """
- * ab = b - a
- * cd = d - c # <<<<<<<<<<<<<<
- * p = ab * 1j
- * try:
- */
- __pyx_v_cd = __Pyx_c_diff_double(__pyx_v_d, __pyx_v_c);
-
- /* "fontTools/cu2qu/cu2qu.py":275
- * ab = b - a
- * cd = d - c
- * p = ab * 1j # <<<<<<<<<<<<<<
- * try:
- * h = dot(p, a - c) / dot(p, cd)
- */
- __pyx_v_p = __Pyx_c_prod_double(__pyx_v_ab, __pyx_t_double_complex_from_parts(0, 1.0));
-
- /* "fontTools/cu2qu/cu2qu.py":276
- * cd = d - c
- * p = ab * 1j
- * try: # <<<<<<<<<<<<<<
- * h = dot(p, a - c) / dot(p, cd)
- * except ZeroDivisionError:
- */
- {
- __Pyx_PyThreadState_declare
- __Pyx_PyThreadState_assign
- __Pyx_ExceptionSave(&__pyx_t_1, &__pyx_t_2, &__pyx_t_3);
- __Pyx_XGOTREF(__pyx_t_1);
- __Pyx_XGOTREF(__pyx_t_2);
- __Pyx_XGOTREF(__pyx_t_3);
- /*try:*/ {
-
- /* "fontTools/cu2qu/cu2qu.py":277
- * p = ab * 1j
- * try:
- * h = dot(p, a - c) / dot(p, cd) # <<<<<<<<<<<<<<
- * except ZeroDivisionError:
- * return complex(NAN, NAN)
- */
- __pyx_t_4 = __pyx_f_9fontTools_5cu2qu_5cu2qu_dot(__pyx_v_p, __Pyx_c_diff_double(__pyx_v_a, __pyx_v_c)); if (unlikely(__pyx_t_4 == ((double)-1) && PyErr_Occurred())) __PYX_ERR(0, 277, __pyx_L3_error)
- __pyx_t_5 = __pyx_f_9fontTools_5cu2qu_5cu2qu_dot(__pyx_v_p, __pyx_v_cd); if (unlikely(__pyx_t_5 == ((double)-1) && PyErr_Occurred())) __PYX_ERR(0, 277, __pyx_L3_error)
- if (unlikely(__pyx_t_5 == 0)) {
- PyErr_SetString(PyExc_ZeroDivisionError, "float division");
- __PYX_ERR(0, 277, __pyx_L3_error)
- }
- __pyx_v_h = (__pyx_t_4 / __pyx_t_5);
-
- /* "fontTools/cu2qu/cu2qu.py":276
- * cd = d - c
- * p = ab * 1j
- * try: # <<<<<<<<<<<<<<
- * h = dot(p, a - c) / dot(p, cd)
- * except ZeroDivisionError:
- */
- }
- __Pyx_XDECREF(__pyx_t_1); __pyx_t_1 = 0;
- __Pyx_XDECREF(__pyx_t_2); __pyx_t_2 = 0;
- __Pyx_XDECREF(__pyx_t_3); __pyx_t_3 = 0;
- goto __pyx_L8_try_end;
- __pyx_L3_error:;
-
- /* "fontTools/cu2qu/cu2qu.py":278
- * try:
- * h = dot(p, a - c) / dot(p, cd)
- * except ZeroDivisionError: # <<<<<<<<<<<<<<
- * return complex(NAN, NAN)
- * return c + cd * h
- */
- __pyx_t_6 = __Pyx_PyErr_ExceptionMatches(__pyx_builtin_ZeroDivisionError);
- if (__pyx_t_6) {
- __Pyx_AddTraceback("fontTools.cu2qu.cu2qu.calc_intersect", __pyx_clineno, __pyx_lineno, __pyx_filename);
- if (__Pyx_GetException(&__pyx_t_7, &__pyx_t_8, &__pyx_t_9) < 0) __PYX_ERR(0, 278, __pyx_L5_except_error)
- __Pyx_XGOTREF(__pyx_t_7);
- __Pyx_XGOTREF(__pyx_t_8);
- __Pyx_XGOTREF(__pyx_t_9);
-
- /* "fontTools/cu2qu/cu2qu.py":279
- * h = dot(p, a - c) / dot(p, cd)
- * except ZeroDivisionError:
- * return complex(NAN, NAN) # <<<<<<<<<<<<<<
- * return c + cd * h
- *
- */
- __Pyx_GetModuleGlobalName(__pyx_t_10, __pyx_n_s_NAN); if (unlikely(!__pyx_t_10)) __PYX_ERR(0, 279, __pyx_L5_except_error)
- __Pyx_GOTREF(__pyx_t_10);
- __Pyx_GetModuleGlobalName(__pyx_t_11, __pyx_n_s_NAN); if (unlikely(!__pyx_t_11)) __PYX_ERR(0, 279, __pyx_L5_except_error)
- __Pyx_GOTREF(__pyx_t_11);
- __pyx_t_12 = PyTuple_New(2); if (unlikely(!__pyx_t_12)) __PYX_ERR(0, 279, __pyx_L5_except_error)
- __Pyx_GOTREF(__pyx_t_12);
- __Pyx_GIVEREF(__pyx_t_10);
- PyTuple_SET_ITEM(__pyx_t_12, 0, __pyx_t_10);
- __Pyx_GIVEREF(__pyx_t_11);
- PyTuple_SET_ITEM(__pyx_t_12, 1, __pyx_t_11);
- __pyx_t_10 = 0;
- __pyx_t_11 = 0;
- __pyx_t_11 = __Pyx_PyObject_Call(((PyObject *)(&PyComplex_Type)), __pyx_t_12, NULL); if (unlikely(!__pyx_t_11)) __PYX_ERR(0, 279, __pyx_L5_except_error)
- __Pyx_GOTREF(__pyx_t_11);
- __Pyx_DECREF(__pyx_t_12); __pyx_t_12 = 0;
- __pyx_t_13 = __Pyx_PyComplex_As___pyx_t_double_complex(__pyx_t_11); if (unlikely(PyErr_Occurred())) __PYX_ERR(0, 279, __pyx_L5_except_error)
- __Pyx_DECREF(__pyx_t_11); __pyx_t_11 = 0;
- __pyx_r = __pyx_t_13;
- __Pyx_DECREF(__pyx_t_7); __pyx_t_7 = 0;
- __Pyx_DECREF(__pyx_t_8); __pyx_t_8 = 0;
- __Pyx_DECREF(__pyx_t_9); __pyx_t_9 = 0;
- goto __pyx_L6_except_return;
- }
- goto __pyx_L5_except_error;
-
- /* "fontTools/cu2qu/cu2qu.py":276
- * cd = d - c
- * p = ab * 1j
- * try: # <<<<<<<<<<<<<<
- * h = dot(p, a - c) / dot(p, cd)
- * except ZeroDivisionError:
- */
- __pyx_L5_except_error:;
- __Pyx_XGIVEREF(__pyx_t_1);
- __Pyx_XGIVEREF(__pyx_t_2);
- __Pyx_XGIVEREF(__pyx_t_3);
- __Pyx_ExceptionReset(__pyx_t_1, __pyx_t_2, __pyx_t_3);
- goto __pyx_L1_error;
- __pyx_L6_except_return:;
- __Pyx_XGIVEREF(__pyx_t_1);
- __Pyx_XGIVEREF(__pyx_t_2);
- __Pyx_XGIVEREF(__pyx_t_3);
- __Pyx_ExceptionReset(__pyx_t_1, __pyx_t_2, __pyx_t_3);
- goto __pyx_L0;
- __pyx_L8_try_end:;
- }
-
- /* "fontTools/cu2qu/cu2qu.py":280
- * except ZeroDivisionError:
- * return complex(NAN, NAN)
- * return c + cd * h # <<<<<<<<<<<<<<
- *
- *
- */
- __pyx_r = __Pyx_c_sum_double(__pyx_v_c, __Pyx_c_prod_double(__pyx_v_cd, __pyx_t_double_complex_from_parts(__pyx_v_h, 0)));
- goto __pyx_L0;
-
- /* "fontTools/cu2qu/cu2qu.py":255
- *
- *
- * @cython.cfunc # <<<<<<<<<<<<<<
- * @cython.inline
- * @cython.returns(cython.complex)
- */
-
- /* function exit code */
- __pyx_L1_error:;
- __Pyx_XDECREF(__pyx_t_7);
- __Pyx_XDECREF(__pyx_t_8);
- __Pyx_XDECREF(__pyx_t_9);
- __Pyx_XDECREF(__pyx_t_10);
- __Pyx_XDECREF(__pyx_t_11);
- __Pyx_XDECREF(__pyx_t_12);
- __Pyx_AddTraceback("fontTools.cu2qu.cu2qu.calc_intersect", __pyx_clineno, __pyx_lineno, __pyx_filename);
- __pyx_r = __pyx_t_double_complex_from_parts(0, 0);
- __pyx_L0:;
- __Pyx_RefNannyFinishContext();
- return __pyx_r;
-}
-
-/* "fontTools/cu2qu/cu2qu.py":283
- *
- *
- * @cython.cfunc # <<<<<<<<<<<<<<
- * @cython.returns(cython.int)
- * @cython.locals(
- */
-
-static int __pyx_f_9fontTools_5cu2qu_5cu2qu_cubic_farthest_fit_inside(__pyx_t_double_complex __pyx_v_p0, __pyx_t_double_complex __pyx_v_p1, __pyx_t_double_complex __pyx_v_p2, __pyx_t_double_complex __pyx_v_p3, double __pyx_v_tolerance) {
- __pyx_t_double_complex __pyx_v_mid;
- __pyx_t_double_complex __pyx_v_deriv3;
- int __pyx_r;
- __Pyx_RefNannyDeclarations
- int __pyx_t_1;
- int __pyx_t_2;
- int __pyx_t_3;
- int __pyx_t_4;
- int __pyx_lineno = 0;
- const char *__pyx_filename = NULL;
- int __pyx_clineno = 0;
- __Pyx_RefNannySetupContext("cubic_farthest_fit_inside", 0);
-
- /* "fontTools/cu2qu/cu2qu.py":312
- * """
- * # First check p2 then p1, as p2 has higher error early on.
- * if abs(p2) <= tolerance and abs(p1) <= tolerance: # <<<<<<<<<<<<<<
- * return True
- *
- */
- __pyx_t_2 = (__Pyx_c_abs_double(__pyx_v_p2) <= __pyx_v_tolerance);
- if (__pyx_t_2) {
- } else {
- __pyx_t_1 = __pyx_t_2;
- goto __pyx_L4_bool_binop_done;
- }
- __pyx_t_2 = (__Pyx_c_abs_double(__pyx_v_p1) <= __pyx_v_tolerance);
- __pyx_t_1 = __pyx_t_2;
- __pyx_L4_bool_binop_done:;
- if (__pyx_t_1) {
-
- /* "fontTools/cu2qu/cu2qu.py":313
- * # First check p2 then p1, as p2 has higher error early on.
- * if abs(p2) <= tolerance and abs(p1) <= tolerance:
- * return True # <<<<<<<<<<<<<<
- *
- * # Split.
- */
- __pyx_r = 1;
- goto __pyx_L0;
-
- /* "fontTools/cu2qu/cu2qu.py":312
- * """
- * # First check p2 then p1, as p2 has higher error early on.
- * if abs(p2) <= tolerance and abs(p1) <= tolerance: # <<<<<<<<<<<<<<
- * return True
- *
- */
- }
-
- /* "fontTools/cu2qu/cu2qu.py":316
- *
- * # Split.
- * mid = (p0 + 3 * (p1 + p2) + p3) * 0.125 # <<<<<<<<<<<<<<
- * if abs(mid) > tolerance:
- * return False
- */
- __pyx_v_mid = __Pyx_c_prod_double(__Pyx_c_sum_double(__Pyx_c_sum_double(__pyx_v_p0, __Pyx_c_prod_double(__pyx_t_double_complex_from_parts(3, 0), __Pyx_c_sum_double(__pyx_v_p1, __pyx_v_p2))), __pyx_v_p3), __pyx_t_double_complex_from_parts(0.125, 0));
-
- /* "fontTools/cu2qu/cu2qu.py":317
- * # Split.
- * mid = (p0 + 3 * (p1 + p2) + p3) * 0.125
- * if abs(mid) > tolerance: # <<<<<<<<<<<<<<
- * return False
- * deriv3 = (p3 + p2 - p1 - p0) * 0.125
- */
- __pyx_t_1 = (__Pyx_c_abs_double(__pyx_v_mid) > __pyx_v_tolerance);
- if (__pyx_t_1) {
-
- /* "fontTools/cu2qu/cu2qu.py":318
- * mid = (p0 + 3 * (p1 + p2) + p3) * 0.125
- * if abs(mid) > tolerance:
- * return False # <<<<<<<<<<<<<<
- * deriv3 = (p3 + p2 - p1 - p0) * 0.125
- * return cubic_farthest_fit_inside(
- */
- __pyx_r = 0;
- goto __pyx_L0;
-
- /* "fontTools/cu2qu/cu2qu.py":317
- * # Split.
- * mid = (p0 + 3 * (p1 + p2) + p3) * 0.125
- * if abs(mid) > tolerance: # <<<<<<<<<<<<<<
- * return False
- * deriv3 = (p3 + p2 - p1 - p0) * 0.125
- */
- }
-
- /* "fontTools/cu2qu/cu2qu.py":319
- * if abs(mid) > tolerance:
- * return False
- * deriv3 = (p3 + p2 - p1 - p0) * 0.125 # <<<<<<<<<<<<<<
- * return cubic_farthest_fit_inside(
- * p0, (p0 + p1) * 0.5, mid - deriv3, mid, tolerance
- */
- __pyx_v_deriv3 = __Pyx_c_prod_double(__Pyx_c_diff_double(__Pyx_c_diff_double(__Pyx_c_sum_double(__pyx_v_p3, __pyx_v_p2), __pyx_v_p1), __pyx_v_p0), __pyx_t_double_complex_from_parts(0.125, 0));
-
- /* "fontTools/cu2qu/cu2qu.py":320
- * return False
- * deriv3 = (p3 + p2 - p1 - p0) * 0.125
- * return cubic_farthest_fit_inside( # <<<<<<<<<<<<<<
- * p0, (p0 + p1) * 0.5, mid - deriv3, mid, tolerance
- * ) and cubic_farthest_fit_inside(mid, mid + deriv3, (p2 + p3) * 0.5, p3, tolerance)
- */
- __pyx_t_4 = __pyx_f_9fontTools_5cu2qu_5cu2qu_cubic_farthest_fit_inside(__pyx_v_p0, __Pyx_c_prod_double(__Pyx_c_sum_double(__pyx_v_p0, __pyx_v_p1), __pyx_t_double_complex_from_parts(0.5, 0)), __Pyx_c_diff_double(__pyx_v_mid, __pyx_v_deriv3), __pyx_v_mid, __pyx_v_tolerance); if (unlikely(__pyx_t_4 == ((int)-1) && PyErr_Occurred())) __PYX_ERR(0, 320, __pyx_L1_error)
- if (__pyx_t_4) {
- } else {
- __pyx_t_3 = __pyx_t_4;
- goto __pyx_L7_bool_binop_done;
- }
-
- /* "fontTools/cu2qu/cu2qu.py":322
- * return cubic_farthest_fit_inside(
- * p0, (p0 + p1) * 0.5, mid - deriv3, mid, tolerance
- * ) and cubic_farthest_fit_inside(mid, mid + deriv3, (p2 + p3) * 0.5, p3, tolerance) # <<<<<<<<<<<<<<
- *
- *
- */
- __pyx_t_4 = __pyx_f_9fontTools_5cu2qu_5cu2qu_cubic_farthest_fit_inside(__pyx_v_mid, __Pyx_c_sum_double(__pyx_v_mid, __pyx_v_deriv3), __Pyx_c_prod_double(__Pyx_c_sum_double(__pyx_v_p2, __pyx_v_p3), __pyx_t_double_complex_from_parts(0.5, 0)), __pyx_v_p3, __pyx_v_tolerance); if (unlikely(__pyx_t_4 == ((int)-1) && PyErr_Occurred())) __PYX_ERR(0, 322, __pyx_L1_error)
- __pyx_t_3 = __pyx_t_4;
- __pyx_L7_bool_binop_done:;
- __pyx_r = __pyx_t_3;
- goto __pyx_L0;
-
- /* "fontTools/cu2qu/cu2qu.py":283
- *
- *
- * @cython.cfunc # <<<<<<<<<<<<<<
- * @cython.returns(cython.int)
- * @cython.locals(
- */
-
- /* function exit code */
- __pyx_L1_error:;
- __Pyx_AddTraceback("fontTools.cu2qu.cu2qu.cubic_farthest_fit_inside", __pyx_clineno, __pyx_lineno, __pyx_filename);
- __pyx_r = -1;
- __pyx_L0:;
- __Pyx_RefNannyFinishContext();
- return __pyx_r;
-}
-
-/* "fontTools/cu2qu/cu2qu.py":325
- *
- *
- * @cython.cfunc # <<<<<<<<<<<<<<
- * @cython.inline
- * @cython.locals(tolerance=cython.double)
- */
-
-static CYTHON_INLINE PyObject *__pyx_f_9fontTools_5cu2qu_5cu2qu_cubic_approx_quadratic(PyObject *__pyx_v_cubic, double __pyx_v_tolerance) {
- __pyx_t_double_complex __pyx_v_q1;
- __pyx_t_double_complex __pyx_v_c0;
- __pyx_t_double_complex __pyx_v_c1;
- __pyx_t_double_complex __pyx_v_c2;
- __pyx_t_double_complex __pyx_v_c3;
- PyObject *__pyx_r = NULL;
- __Pyx_RefNannyDeclarations
- PyObject *__pyx_t_1 = NULL;
- __pyx_t_double_complex __pyx_t_2;
- __pyx_t_double_complex __pyx_t_3;
- __pyx_t_double_complex __pyx_t_4;
- __pyx_t_double_complex __pyx_t_5;
- __pyx_t_double_complex __pyx_t_6;
- PyObject *__pyx_t_7 = NULL;
- PyObject *__pyx_t_8 = NULL;
- PyObject *__pyx_t_9 = NULL;
- int __pyx_t_10;
- int __pyx_t_11;
- int __pyx_lineno = 0;
- const char *__pyx_filename = NULL;
- int __pyx_clineno = 0;
- __Pyx_RefNannySetupContext("cubic_approx_quadratic", 0);
-
- /* "fontTools/cu2qu/cu2qu.py":349
- * """
- *
- * q1 = calc_intersect(cubic[0], cubic[1], cubic[2], cubic[3]) # <<<<<<<<<<<<<<
- * if math.isnan(q1.imag):
- * return None
- */
- __pyx_t_1 = __Pyx_GetItemInt(__pyx_v_cubic, 0, long, 1, __Pyx_PyInt_From_long, 0, 0, 1); if (unlikely(!__pyx_t_1)) __PYX_ERR(0, 349, __pyx_L1_error)
- __Pyx_GOTREF(__pyx_t_1);
- __pyx_t_2 = __Pyx_PyComplex_As___pyx_t_double_complex(__pyx_t_1); if (unlikely(PyErr_Occurred())) __PYX_ERR(0, 349, __pyx_L1_error)
- __Pyx_DECREF(__pyx_t_1); __pyx_t_1 = 0;
- __pyx_t_1 = __Pyx_GetItemInt(__pyx_v_cubic, 1, long, 1, __Pyx_PyInt_From_long, 0, 0, 1); if (unlikely(!__pyx_t_1)) __PYX_ERR(0, 349, __pyx_L1_error)
- __Pyx_GOTREF(__pyx_t_1);
- __pyx_t_3 = __Pyx_PyComplex_As___pyx_t_double_complex(__pyx_t_1); if (unlikely(PyErr_Occurred())) __PYX_ERR(0, 349, __pyx_L1_error)
- __Pyx_DECREF(__pyx_t_1); __pyx_t_1 = 0;
- __pyx_t_1 = __Pyx_GetItemInt(__pyx_v_cubic, 2, long, 1, __Pyx_PyInt_From_long, 0, 0, 1); if (unlikely(!__pyx_t_1)) __PYX_ERR(0, 349, __pyx_L1_error)
- __Pyx_GOTREF(__pyx_t_1);
- __pyx_t_4 = __Pyx_PyComplex_As___pyx_t_double_complex(__pyx_t_1); if (unlikely(PyErr_Occurred())) __PYX_ERR(0, 349, __pyx_L1_error)
- __Pyx_DECREF(__pyx_t_1); __pyx_t_1 = 0;
- __pyx_t_1 = __Pyx_GetItemInt(__pyx_v_cubic, 3, long, 1, __Pyx_PyInt_From_long, 0, 0, 1); if (unlikely(!__pyx_t_1)) __PYX_ERR(0, 349, __pyx_L1_error)
- __Pyx_GOTREF(__pyx_t_1);
- __pyx_t_5 = __Pyx_PyComplex_As___pyx_t_double_complex(__pyx_t_1); if (unlikely(PyErr_Occurred())) __PYX_ERR(0, 349, __pyx_L1_error)
- __Pyx_DECREF(__pyx_t_1); __pyx_t_1 = 0;
- __pyx_t_6 = __pyx_f_9fontTools_5cu2qu_5cu2qu_calc_intersect(__pyx_t_2, __pyx_t_3, __pyx_t_4, __pyx_t_5); if (unlikely(PyErr_Occurred())) __PYX_ERR(0, 349, __pyx_L1_error)
- __pyx_v_q1 = __pyx_t_6;
-
- /* "fontTools/cu2qu/cu2qu.py":350
- *
- * q1 = calc_intersect(cubic[0], cubic[1], cubic[2], cubic[3])
- * if math.isnan(q1.imag): # <<<<<<<<<<<<<<
- * return None
- * c0 = cubic[0]
- */
- __Pyx_GetModuleGlobalName(__pyx_t_7, __pyx_n_s_math); if (unlikely(!__pyx_t_7)) __PYX_ERR(0, 350, __pyx_L1_error)
- __Pyx_GOTREF(__pyx_t_7);
- __pyx_t_8 = __Pyx_PyObject_GetAttrStr(__pyx_t_7, __pyx_n_s_isnan); if (unlikely(!__pyx_t_8)) __PYX_ERR(0, 350, __pyx_L1_error)
- __Pyx_GOTREF(__pyx_t_8);
- __Pyx_DECREF(__pyx_t_7); __pyx_t_7 = 0;
- __pyx_t_7 = PyFloat_FromDouble(__Pyx_CIMAG(__pyx_v_q1)); if (unlikely(!__pyx_t_7)) __PYX_ERR(0, 350, __pyx_L1_error)
- __Pyx_GOTREF(__pyx_t_7);
- __pyx_t_9 = NULL;
- __pyx_t_10 = 0;
- if (CYTHON_UNPACK_METHODS && unlikely(PyMethod_Check(__pyx_t_8))) {
- __pyx_t_9 = PyMethod_GET_SELF(__pyx_t_8);
- if (likely(__pyx_t_9)) {
- PyObject* function = PyMethod_GET_FUNCTION(__pyx_t_8);
- __Pyx_INCREF(__pyx_t_9);
- __Pyx_INCREF(function);
- __Pyx_DECREF_SET(__pyx_t_8, function);
- __pyx_t_10 = 1;
- }
- }
- {
- PyObject *__pyx_callargs[2] = {__pyx_t_9, __pyx_t_7};
- __pyx_t_1 = __Pyx_PyObject_FastCall(__pyx_t_8, __pyx_callargs+1-__pyx_t_10, 1+__pyx_t_10);
- __Pyx_XDECREF(__pyx_t_9); __pyx_t_9 = 0;
- __Pyx_DECREF(__pyx_t_7); __pyx_t_7 = 0;
- if (unlikely(!__pyx_t_1)) __PYX_ERR(0, 350, __pyx_L1_error)
- __Pyx_GOTREF(__pyx_t_1);
- __Pyx_DECREF(__pyx_t_8); __pyx_t_8 = 0;
- }
- __pyx_t_11 = __Pyx_PyObject_IsTrue(__pyx_t_1); if (unlikely((__pyx_t_11 < 0))) __PYX_ERR(0, 350, __pyx_L1_error)
- __Pyx_DECREF(__pyx_t_1); __pyx_t_1 = 0;
- if (__pyx_t_11) {
-
- /* "fontTools/cu2qu/cu2qu.py":351
- * q1 = calc_intersect(cubic[0], cubic[1], cubic[2], cubic[3])
- * if math.isnan(q1.imag):
- * return None # <<<<<<<<<<<<<<
- * c0 = cubic[0]
- * c3 = cubic[3]
- */
- __Pyx_XDECREF(__pyx_r);
- __pyx_r = Py_None; __Pyx_INCREF(Py_None);
- goto __pyx_L0;
-
- /* "fontTools/cu2qu/cu2qu.py":350
- *
- * q1 = calc_intersect(cubic[0], cubic[1], cubic[2], cubic[3])
- * if math.isnan(q1.imag): # <<<<<<<<<<<<<<
- * return None
- * c0 = cubic[0]
- */
- }
-
- /* "fontTools/cu2qu/cu2qu.py":352
- * if math.isnan(q1.imag):
- * return None
- * c0 = cubic[0] # <<<<<<<<<<<<<<
- * c3 = cubic[3]
- * c1 = c0 + (q1 - c0) * (2 / 3)
- */
- __pyx_t_1 = __Pyx_GetItemInt(__pyx_v_cubic, 0, long, 1, __Pyx_PyInt_From_long, 0, 0, 1); if (unlikely(!__pyx_t_1)) __PYX_ERR(0, 352, __pyx_L1_error)
- __Pyx_GOTREF(__pyx_t_1);
- __pyx_t_6 = __Pyx_PyComplex_As___pyx_t_double_complex(__pyx_t_1); if (unlikely(PyErr_Occurred())) __PYX_ERR(0, 352, __pyx_L1_error)
- __Pyx_DECREF(__pyx_t_1); __pyx_t_1 = 0;
- __pyx_v_c0 = __pyx_t_6;
-
- /* "fontTools/cu2qu/cu2qu.py":353
- * return None
- * c0 = cubic[0]
- * c3 = cubic[3] # <<<<<<<<<<<<<<
- * c1 = c0 + (q1 - c0) * (2 / 3)
- * c2 = c3 + (q1 - c3) * (2 / 3)
- */
- __pyx_t_1 = __Pyx_GetItemInt(__pyx_v_cubic, 3, long, 1, __Pyx_PyInt_From_long, 0, 0, 1); if (unlikely(!__pyx_t_1)) __PYX_ERR(0, 353, __pyx_L1_error)
- __Pyx_GOTREF(__pyx_t_1);
- __pyx_t_6 = __Pyx_PyComplex_As___pyx_t_double_complex(__pyx_t_1); if (unlikely(PyErr_Occurred())) __PYX_ERR(0, 353, __pyx_L1_error)
- __Pyx_DECREF(__pyx_t_1); __pyx_t_1 = 0;
- __pyx_v_c3 = __pyx_t_6;
-
- /* "fontTools/cu2qu/cu2qu.py":354
- * c0 = cubic[0]
- * c3 = cubic[3]
- * c1 = c0 + (q1 - c0) * (2 / 3) # <<<<<<<<<<<<<<
- * c2 = c3 + (q1 - c3) * (2 / 3)
- * if not cubic_farthest_fit_inside(0, c1 - cubic[1], c2 - cubic[2], 0, tolerance):
- */
- __pyx_v_c1 = __Pyx_c_sum_double(__pyx_v_c0, __Pyx_c_prod_double(__Pyx_c_diff_double(__pyx_v_q1, __pyx_v_c0), __pyx_t_double_complex_from_parts((2.0 / 3.0), 0)));
-
- /* "fontTools/cu2qu/cu2qu.py":355
- * c3 = cubic[3]
- * c1 = c0 + (q1 - c0) * (2 / 3)
- * c2 = c3 + (q1 - c3) * (2 / 3) # <<<<<<<<<<<<<<
- * if not cubic_farthest_fit_inside(0, c1 - cubic[1], c2 - cubic[2], 0, tolerance):
- * return None
- */
- __pyx_v_c2 = __Pyx_c_sum_double(__pyx_v_c3, __Pyx_c_prod_double(__Pyx_c_diff_double(__pyx_v_q1, __pyx_v_c3), __pyx_t_double_complex_from_parts((2.0 / 3.0), 0)));
-
- /* "fontTools/cu2qu/cu2qu.py":356
- * c1 = c0 + (q1 - c0) * (2 / 3)
- * c2 = c3 + (q1 - c3) * (2 / 3)
- * if not cubic_farthest_fit_inside(0, c1 - cubic[1], c2 - cubic[2], 0, tolerance): # <<<<<<<<<<<<<<
- * return None
- * return c0, q1, c3
- */
- __pyx_t_1 = __pyx_PyComplex_FromComplex(__pyx_v_c1); if (unlikely(!__pyx_t_1)) __PYX_ERR(0, 356, __pyx_L1_error)
- __Pyx_GOTREF(__pyx_t_1);
- __pyx_t_8 = __Pyx_GetItemInt(__pyx_v_cubic, 1, long, 1, __Pyx_PyInt_From_long, 0, 0, 1); if (unlikely(!__pyx_t_8)) __PYX_ERR(0, 356, __pyx_L1_error)
- __Pyx_GOTREF(__pyx_t_8);
- __pyx_t_7 = PyNumber_Subtract(__pyx_t_1, __pyx_t_8); if (unlikely(!__pyx_t_7)) __PYX_ERR(0, 356, __pyx_L1_error)
- __Pyx_GOTREF(__pyx_t_7);
- __Pyx_DECREF(__pyx_t_1); __pyx_t_1 = 0;
- __Pyx_DECREF(__pyx_t_8); __pyx_t_8 = 0;
- __pyx_t_6 = __Pyx_PyComplex_As___pyx_t_double_complex(__pyx_t_7); if (unlikely(PyErr_Occurred())) __PYX_ERR(0, 356, __pyx_L1_error)
- __Pyx_DECREF(__pyx_t_7); __pyx_t_7 = 0;
- __pyx_t_7 = __pyx_PyComplex_FromComplex(__pyx_v_c2); if (unlikely(!__pyx_t_7)) __PYX_ERR(0, 356, __pyx_L1_error)
- __Pyx_GOTREF(__pyx_t_7);
- __pyx_t_8 = __Pyx_GetItemInt(__pyx_v_cubic, 2, long, 1, __Pyx_PyInt_From_long, 0, 0, 1); if (unlikely(!__pyx_t_8)) __PYX_ERR(0, 356, __pyx_L1_error)
- __Pyx_GOTREF(__pyx_t_8);
- __pyx_t_1 = PyNumber_Subtract(__pyx_t_7, __pyx_t_8); if (unlikely(!__pyx_t_1)) __PYX_ERR(0, 356, __pyx_L1_error)
- __Pyx_GOTREF(__pyx_t_1);
- __Pyx_DECREF(__pyx_t_7); __pyx_t_7 = 0;
- __Pyx_DECREF(__pyx_t_8); __pyx_t_8 = 0;
- __pyx_t_5 = __Pyx_PyComplex_As___pyx_t_double_complex(__pyx_t_1); if (unlikely(PyErr_Occurred())) __PYX_ERR(0, 356, __pyx_L1_error)
- __Pyx_DECREF(__pyx_t_1); __pyx_t_1 = 0;
- __pyx_t_10 = __pyx_f_9fontTools_5cu2qu_5cu2qu_cubic_farthest_fit_inside(__pyx_t_double_complex_from_parts(0, 0), __pyx_t_6, __pyx_t_5, __pyx_t_double_complex_from_parts(0, 0), __pyx_v_tolerance); if (unlikely(__pyx_t_10 == ((int)-1) && PyErr_Occurred())) __PYX_ERR(0, 356, __pyx_L1_error)
- __pyx_t_11 = (!(__pyx_t_10 != 0));
- if (__pyx_t_11) {
-
- /* "fontTools/cu2qu/cu2qu.py":357
- * c2 = c3 + (q1 - c3) * (2 / 3)
- * if not cubic_farthest_fit_inside(0, c1 - cubic[1], c2 - cubic[2], 0, tolerance):
- * return None # <<<<<<<<<<<<<<
- * return c0, q1, c3
- *
- */
- __Pyx_XDECREF(__pyx_r);
- __pyx_r = Py_None; __Pyx_INCREF(Py_None);
- goto __pyx_L0;
-
- /* "fontTools/cu2qu/cu2qu.py":356
- * c1 = c0 + (q1 - c0) * (2 / 3)
- * c2 = c3 + (q1 - c3) * (2 / 3)
- * if not cubic_farthest_fit_inside(0, c1 - cubic[1], c2 - cubic[2], 0, tolerance): # <<<<<<<<<<<<<<
- * return None
- * return c0, q1, c3
- */
- }
-
- /* "fontTools/cu2qu/cu2qu.py":358
- * if not cubic_farthest_fit_inside(0, c1 - cubic[1], c2 - cubic[2], 0, tolerance):
- * return None
- * return c0, q1, c3 # <<<<<<<<<<<<<<
- *
- *
- */
- __Pyx_XDECREF(__pyx_r);
- __pyx_t_1 = __pyx_PyComplex_FromComplex(__pyx_v_c0); if (unlikely(!__pyx_t_1)) __PYX_ERR(0, 358, __pyx_L1_error)
- __Pyx_GOTREF(__pyx_t_1);
- __pyx_t_8 = __pyx_PyComplex_FromComplex(__pyx_v_q1); if (unlikely(!__pyx_t_8)) __PYX_ERR(0, 358, __pyx_L1_error)
- __Pyx_GOTREF(__pyx_t_8);
- __pyx_t_7 = __pyx_PyComplex_FromComplex(__pyx_v_c3); if (unlikely(!__pyx_t_7)) __PYX_ERR(0, 358, __pyx_L1_error)
- __Pyx_GOTREF(__pyx_t_7);
- __pyx_t_9 = PyTuple_New(3); if (unlikely(!__pyx_t_9)) __PYX_ERR(0, 358, __pyx_L1_error)
- __Pyx_GOTREF(__pyx_t_9);
- __Pyx_GIVEREF(__pyx_t_1);
- PyTuple_SET_ITEM(__pyx_t_9, 0, __pyx_t_1);
- __Pyx_GIVEREF(__pyx_t_8);
- PyTuple_SET_ITEM(__pyx_t_9, 1, __pyx_t_8);
- __Pyx_GIVEREF(__pyx_t_7);
- PyTuple_SET_ITEM(__pyx_t_9, 2, __pyx_t_7);
- __pyx_t_1 = 0;
- __pyx_t_8 = 0;
- __pyx_t_7 = 0;
- __pyx_r = __pyx_t_9;
- __pyx_t_9 = 0;
- goto __pyx_L0;
-
- /* "fontTools/cu2qu/cu2qu.py":325
- *
- *
- * @cython.cfunc # <<<<<<<<<<<<<<
- * @cython.inline
- * @cython.locals(tolerance=cython.double)
- */
-
- /* function exit code */
- __pyx_L1_error:;
- __Pyx_XDECREF(__pyx_t_1);
- __Pyx_XDECREF(__pyx_t_7);
- __Pyx_XDECREF(__pyx_t_8);
- __Pyx_XDECREF(__pyx_t_9);
- __Pyx_AddTraceback("fontTools.cu2qu.cu2qu.cubic_approx_quadratic", __pyx_clineno, __pyx_lineno, __pyx_filename);
- __pyx_r = 0;
- __pyx_L0:;
- __Pyx_XGIVEREF(__pyx_r);
- __Pyx_RefNannyFinishContext();
- return __pyx_r;
-}
-
-/* "fontTools/cu2qu/cu2qu.py":361
- *
- *
- * @cython.cfunc # <<<<<<<<<<<<<<
- * @cython.locals(n=cython.int, tolerance=cython.double)
- * @cython.locals(i=cython.int)
- */
-
-static PyObject *__pyx_f_9fontTools_5cu2qu_5cu2qu_cubic_approx_spline(PyObject *__pyx_v_cubic, int __pyx_v_n, double __pyx_v_tolerance, int __pyx_v_all_quadratic) {
- __pyx_t_double_complex __pyx_v_q0;
- __pyx_t_double_complex __pyx_v_q1;
- __pyx_t_double_complex __pyx_v_next_q1;
- __pyx_t_double_complex __pyx_v_q2;
- __pyx_t_double_complex __pyx_v_d1;
- CYTHON_UNUSED __pyx_t_double_complex __pyx_v_c0;
- __pyx_t_double_complex __pyx_v_c1;
- __pyx_t_double_complex __pyx_v_c2;
- __pyx_t_double_complex __pyx_v_c3;
- int __pyx_v_i;
- PyObject *__pyx_v_cubics = NULL;
- PyObject *__pyx_v_next_cubic = NULL;
- PyObject *__pyx_v_spline = NULL;
- __pyx_t_double_complex __pyx_v_d0;
- PyObject *__pyx_r = NULL;
- __Pyx_RefNannyDeclarations
- int __pyx_t_1;
- PyObject *__pyx_t_2 = NULL;
- int __pyx_t_3;
- __pyx_t_double_complex __pyx_t_4;
- __pyx_t_double_complex __pyx_t_5;
- __pyx_t_double_complex __pyx_t_6;
- __pyx_t_double_complex __pyx_t_7;
- PyObject *__pyx_t_8 = NULL;
- __pyx_t_double_complex __pyx_t_9;
- PyObject *__pyx_t_10 = NULL;
- long __pyx_t_11;
- long __pyx_t_12;
- int __pyx_t_13;
- PyObject *__pyx_t_14 = NULL;
- PyObject *__pyx_t_15 = NULL;
- PyObject *(*__pyx_t_16)(PyObject *);
- long __pyx_t_17;
- int __pyx_t_18;
- int __pyx_t_19;
- int __pyx_lineno = 0;
- const char *__pyx_filename = NULL;
- int __pyx_clineno = 0;
- __Pyx_RefNannySetupContext("cubic_approx_spline", 0);
-
- /* "fontTools/cu2qu/cu2qu.py":390
- * """
- *
- * if n == 1: # <<<<<<<<<<<<<<
- * return cubic_approx_quadratic(cubic, tolerance)
- * if n == 2 and all_quadratic == False:
- */
- __pyx_t_1 = (__pyx_v_n == 1);
- if (__pyx_t_1) {
-
- /* "fontTools/cu2qu/cu2qu.py":391
- *
- * if n == 1:
- * return cubic_approx_quadratic(cubic, tolerance) # <<<<<<<<<<<<<<
- * if n == 2 and all_quadratic == False:
- * return cubic
- */
- __Pyx_XDECREF(__pyx_r);
- __pyx_t_2 = __pyx_f_9fontTools_5cu2qu_5cu2qu_cubic_approx_quadratic(__pyx_v_cubic, __pyx_v_tolerance); if (unlikely(!__pyx_t_2)) __PYX_ERR(0, 391, __pyx_L1_error)
- __Pyx_GOTREF(__pyx_t_2);
- __pyx_r = __pyx_t_2;
- __pyx_t_2 = 0;
- goto __pyx_L0;
-
- /* "fontTools/cu2qu/cu2qu.py":390
- * """
- *
- * if n == 1: # <<<<<<<<<<<<<<
- * return cubic_approx_quadratic(cubic, tolerance)
- * if n == 2 and all_quadratic == False:
- */
- }
-
- /* "fontTools/cu2qu/cu2qu.py":392
- * if n == 1:
- * return cubic_approx_quadratic(cubic, tolerance)
- * if n == 2 and all_quadratic == False: # <<<<<<<<<<<<<<
- * return cubic
- *
- */
- __pyx_t_3 = (__pyx_v_n == 2);
- if (__pyx_t_3) {
- } else {
- __pyx_t_1 = __pyx_t_3;
- goto __pyx_L5_bool_binop_done;
- }
- __pyx_t_3 = (__pyx_v_all_quadratic == 0);
- __pyx_t_1 = __pyx_t_3;
- __pyx_L5_bool_binop_done:;
- if (__pyx_t_1) {
-
- /* "fontTools/cu2qu/cu2qu.py":393
- * return cubic_approx_quadratic(cubic, tolerance)
- * if n == 2 and all_quadratic == False:
- * return cubic # <<<<<<<<<<<<<<
- *
- * cubics = split_cubic_into_n_iter(cubic[0], cubic[1], cubic[2], cubic[3], n)
- */
- __Pyx_XDECREF(__pyx_r);
- __Pyx_INCREF(__pyx_v_cubic);
- __pyx_r = __pyx_v_cubic;
- goto __pyx_L0;
-
- /* "fontTools/cu2qu/cu2qu.py":392
- * if n == 1:
- * return cubic_approx_quadratic(cubic, tolerance)
- * if n == 2 and all_quadratic == False: # <<<<<<<<<<<<<<
- * return cubic
- *
- */
- }
-
- /* "fontTools/cu2qu/cu2qu.py":395
- * return cubic
- *
- * cubics = split_cubic_into_n_iter(cubic[0], cubic[1], cubic[2], cubic[3], n) # <<<<<<<<<<<<<<
- *
- * # calculate the spline of quadratics and check errors at the same time.
- */
- __pyx_t_2 = __Pyx_GetItemInt(__pyx_v_cubic, 0, long, 1, __Pyx_PyInt_From_long, 0, 0, 1); if (unlikely(!__pyx_t_2)) __PYX_ERR(0, 395, __pyx_L1_error)
- __Pyx_GOTREF(__pyx_t_2);
- __pyx_t_4 = __Pyx_PyComplex_As___pyx_t_double_complex(__pyx_t_2); if (unlikely(PyErr_Occurred())) __PYX_ERR(0, 395, __pyx_L1_error)
- __Pyx_DECREF(__pyx_t_2); __pyx_t_2 = 0;
- __pyx_t_2 = __Pyx_GetItemInt(__pyx_v_cubic, 1, long, 1, __Pyx_PyInt_From_long, 0, 0, 1); if (unlikely(!__pyx_t_2)) __PYX_ERR(0, 395, __pyx_L1_error)
- __Pyx_GOTREF(__pyx_t_2);
- __pyx_t_5 = __Pyx_PyComplex_As___pyx_t_double_complex(__pyx_t_2); if (unlikely(PyErr_Occurred())) __PYX_ERR(0, 395, __pyx_L1_error)
- __Pyx_DECREF(__pyx_t_2); __pyx_t_2 = 0;
- __pyx_t_2 = __Pyx_GetItemInt(__pyx_v_cubic, 2, long, 1, __Pyx_PyInt_From_long, 0, 0, 1); if (unlikely(!__pyx_t_2)) __PYX_ERR(0, 395, __pyx_L1_error)
- __Pyx_GOTREF(__pyx_t_2);
- __pyx_t_6 = __Pyx_PyComplex_As___pyx_t_double_complex(__pyx_t_2); if (unlikely(PyErr_Occurred())) __PYX_ERR(0, 395, __pyx_L1_error)
- __Pyx_DECREF(__pyx_t_2); __pyx_t_2 = 0;
- __pyx_t_2 = __Pyx_GetItemInt(__pyx_v_cubic, 3, long, 1, __Pyx_PyInt_From_long, 0, 0, 1); if (unlikely(!__pyx_t_2)) __PYX_ERR(0, 395, __pyx_L1_error)
- __Pyx_GOTREF(__pyx_t_2);
- __pyx_t_7 = __Pyx_PyComplex_As___pyx_t_double_complex(__pyx_t_2); if (unlikely(PyErr_Occurred())) __PYX_ERR(0, 395, __pyx_L1_error)
- __Pyx_DECREF(__pyx_t_2); __pyx_t_2 = 0;
- __pyx_t_2 = __Pyx_PyInt_From_int(__pyx_v_n); if (unlikely(!__pyx_t_2)) __PYX_ERR(0, 395, __pyx_L1_error)
- __Pyx_GOTREF(__pyx_t_2);
- __pyx_t_8 = __pyx_f_9fontTools_5cu2qu_5cu2qu_split_cubic_into_n_iter(__pyx_t_4, __pyx_t_5, __pyx_t_6, __pyx_t_7, __pyx_t_2); if (unlikely(!__pyx_t_8)) __PYX_ERR(0, 395, __pyx_L1_error)
- __Pyx_GOTREF(__pyx_t_8);
- __Pyx_DECREF(__pyx_t_2); __pyx_t_2 = 0;
- __pyx_v_cubics = __pyx_t_8;
- __pyx_t_8 = 0;
-
- /* "fontTools/cu2qu/cu2qu.py":398
- *
- * # calculate the spline of quadratics and check errors at the same time.
- * next_cubic = next(cubics) # <<<<<<<<<<<<<<
- * next_q1 = cubic_approx_control(
- * 0, next_cubic[0], next_cubic[1], next_cubic[2], next_cubic[3]
- */
- __pyx_t_8 = __Pyx_PyIter_Next(__pyx_v_cubics); if (unlikely(!__pyx_t_8)) __PYX_ERR(0, 398, __pyx_L1_error)
- __Pyx_GOTREF(__pyx_t_8);
- __pyx_v_next_cubic = __pyx_t_8;
- __pyx_t_8 = 0;
-
- /* "fontTools/cu2qu/cu2qu.py":400
- * next_cubic = next(cubics)
- * next_q1 = cubic_approx_control(
- * 0, next_cubic[0], next_cubic[1], next_cubic[2], next_cubic[3] # <<<<<<<<<<<<<<
- * )
- * q2 = cubic[0]
- */
- __pyx_t_8 = __Pyx_GetItemInt(__pyx_v_next_cubic, 0, long, 1, __Pyx_PyInt_From_long, 0, 0, 1); if (unlikely(!__pyx_t_8)) __PYX_ERR(0, 400, __pyx_L1_error)
- __Pyx_GOTREF(__pyx_t_8);
- __pyx_t_7 = __Pyx_PyComplex_As___pyx_t_double_complex(__pyx_t_8); if (unlikely(PyErr_Occurred())) __PYX_ERR(0, 400, __pyx_L1_error)
- __Pyx_DECREF(__pyx_t_8); __pyx_t_8 = 0;
- __pyx_t_8 = __Pyx_GetItemInt(__pyx_v_next_cubic, 1, long, 1, __Pyx_PyInt_From_long, 0, 0, 1); if (unlikely(!__pyx_t_8)) __PYX_ERR(0, 400, __pyx_L1_error)
- __Pyx_GOTREF(__pyx_t_8);
- __pyx_t_6 = __Pyx_PyComplex_As___pyx_t_double_complex(__pyx_t_8); if (unlikely(PyErr_Occurred())) __PYX_ERR(0, 400, __pyx_L1_error)
- __Pyx_DECREF(__pyx_t_8); __pyx_t_8 = 0;
- __pyx_t_8 = __Pyx_GetItemInt(__pyx_v_next_cubic, 2, long, 1, __Pyx_PyInt_From_long, 0, 0, 1); if (unlikely(!__pyx_t_8)) __PYX_ERR(0, 400, __pyx_L1_error)
- __Pyx_GOTREF(__pyx_t_8);
- __pyx_t_5 = __Pyx_PyComplex_As___pyx_t_double_complex(__pyx_t_8); if (unlikely(PyErr_Occurred())) __PYX_ERR(0, 400, __pyx_L1_error)
- __Pyx_DECREF(__pyx_t_8); __pyx_t_8 = 0;
- __pyx_t_8 = __Pyx_GetItemInt(__pyx_v_next_cubic, 3, long, 1, __Pyx_PyInt_From_long, 0, 0, 1); if (unlikely(!__pyx_t_8)) __PYX_ERR(0, 400, __pyx_L1_error)
- __Pyx_GOTREF(__pyx_t_8);
- __pyx_t_4 = __Pyx_PyComplex_As___pyx_t_double_complex(__pyx_t_8); if (unlikely(PyErr_Occurred())) __PYX_ERR(0, 400, __pyx_L1_error)
- __Pyx_DECREF(__pyx_t_8); __pyx_t_8 = 0;
-
- /* "fontTools/cu2qu/cu2qu.py":399
- * # calculate the spline of quadratics and check errors at the same time.
- * next_cubic = next(cubics)
- * next_q1 = cubic_approx_control( # <<<<<<<<<<<<<<
- * 0, next_cubic[0], next_cubic[1], next_cubic[2], next_cubic[3]
- * )
- */
- __pyx_t_9 = __pyx_f_9fontTools_5cu2qu_5cu2qu_cubic_approx_control(0.0, __pyx_t_7, __pyx_t_6, __pyx_t_5, __pyx_t_4); if (unlikely(PyErr_Occurred())) __PYX_ERR(0, 399, __pyx_L1_error)
- __pyx_v_next_q1 = __pyx_t_9;
-
- /* "fontTools/cu2qu/cu2qu.py":402
- * 0, next_cubic[0], next_cubic[1], next_cubic[2], next_cubic[3]
- * )
- * q2 = cubic[0] # <<<<<<<<<<<<<<
- * d1 = 0j
- * spline = [cubic[0], next_q1]
- */
- __pyx_t_8 = __Pyx_GetItemInt(__pyx_v_cubic, 0, long, 1, __Pyx_PyInt_From_long, 0, 0, 1); if (unlikely(!__pyx_t_8)) __PYX_ERR(0, 402, __pyx_L1_error)
- __Pyx_GOTREF(__pyx_t_8);
- __pyx_t_9 = __Pyx_PyComplex_As___pyx_t_double_complex(__pyx_t_8); if (unlikely(PyErr_Occurred())) __PYX_ERR(0, 402, __pyx_L1_error)
- __Pyx_DECREF(__pyx_t_8); __pyx_t_8 = 0;
- __pyx_v_q2 = __pyx_t_9;
-
- /* "fontTools/cu2qu/cu2qu.py":403
- * )
- * q2 = cubic[0]
- * d1 = 0j # <<<<<<<<<<<<<<
- * spline = [cubic[0], next_q1]
- * for i in range(1, n + 1):
- */
- __pyx_v_d1 = __pyx_t_double_complex_from_parts(0, 0.0);
-
- /* "fontTools/cu2qu/cu2qu.py":404
- * q2 = cubic[0]
- * d1 = 0j
- * spline = [cubic[0], next_q1] # <<<<<<<<<<<<<<
- * for i in range(1, n + 1):
- * # Current cubic to convert
- */
- __pyx_t_8 = __Pyx_GetItemInt(__pyx_v_cubic, 0, long, 1, __Pyx_PyInt_From_long, 0, 0, 1); if (unlikely(!__pyx_t_8)) __PYX_ERR(0, 404, __pyx_L1_error)
- __Pyx_GOTREF(__pyx_t_8);
- __pyx_t_2 = __pyx_PyComplex_FromComplex(__pyx_v_next_q1); if (unlikely(!__pyx_t_2)) __PYX_ERR(0, 404, __pyx_L1_error)
- __Pyx_GOTREF(__pyx_t_2);
- __pyx_t_10 = PyList_New(2); if (unlikely(!__pyx_t_10)) __PYX_ERR(0, 404, __pyx_L1_error)
- __Pyx_GOTREF(__pyx_t_10);
- __Pyx_GIVEREF(__pyx_t_8);
- PyList_SET_ITEM(__pyx_t_10, 0, __pyx_t_8);
- __Pyx_GIVEREF(__pyx_t_2);
- PyList_SET_ITEM(__pyx_t_10, 1, __pyx_t_2);
- __pyx_t_8 = 0;
- __pyx_t_2 = 0;
- __pyx_v_spline = ((PyObject*)__pyx_t_10);
- __pyx_t_10 = 0;
-
- /* "fontTools/cu2qu/cu2qu.py":405
- * d1 = 0j
- * spline = [cubic[0], next_q1]
- * for i in range(1, n + 1): # <<<<<<<<<<<<<<
- * # Current cubic to convert
- * c0, c1, c2, c3 = next_cubic
- */
- __pyx_t_11 = (__pyx_v_n + 1);
- __pyx_t_12 = __pyx_t_11;
- for (__pyx_t_13 = 1; __pyx_t_13 < __pyx_t_12; __pyx_t_13+=1) {
- __pyx_v_i = __pyx_t_13;
-
- /* "fontTools/cu2qu/cu2qu.py":407
- * for i in range(1, n + 1):
- * # Current cubic to convert
- * c0, c1, c2, c3 = next_cubic # <<<<<<<<<<<<<<
- *
- * # Current quadratic approximation of current cubic
- */
- if ((likely(PyTuple_CheckExact(__pyx_v_next_cubic))) || (PyList_CheckExact(__pyx_v_next_cubic))) {
- PyObject* sequence = __pyx_v_next_cubic;
- Py_ssize_t size = __Pyx_PySequence_SIZE(sequence);
- if (unlikely(size != 4)) {
- if (size > 4) __Pyx_RaiseTooManyValuesError(4);
- else if (size >= 0) __Pyx_RaiseNeedMoreValuesError(size);
- __PYX_ERR(0, 407, __pyx_L1_error)
- }
- #if CYTHON_ASSUME_SAFE_MACROS && !CYTHON_AVOID_BORROWED_REFS
- if (likely(PyTuple_CheckExact(sequence))) {
- __pyx_t_10 = PyTuple_GET_ITEM(sequence, 0);
- __pyx_t_2 = PyTuple_GET_ITEM(sequence, 1);
- __pyx_t_8 = PyTuple_GET_ITEM(sequence, 2);
- __pyx_t_14 = PyTuple_GET_ITEM(sequence, 3);
- } else {
- __pyx_t_10 = PyList_GET_ITEM(sequence, 0);
- __pyx_t_2 = PyList_GET_ITEM(sequence, 1);
- __pyx_t_8 = PyList_GET_ITEM(sequence, 2);
- __pyx_t_14 = PyList_GET_ITEM(sequence, 3);
- }
- __Pyx_INCREF(__pyx_t_10);
- __Pyx_INCREF(__pyx_t_2);
- __Pyx_INCREF(__pyx_t_8);
- __Pyx_INCREF(__pyx_t_14);
- #else
- {
- Py_ssize_t i;
- PyObject** temps[4] = {&__pyx_t_10,&__pyx_t_2,&__pyx_t_8,&__pyx_t_14};
- for (i=0; i < 4; i++) {
- PyObject* item = PySequence_ITEM(sequence, i); if (unlikely(!item)) __PYX_ERR(0, 407, __pyx_L1_error)
- __Pyx_GOTREF(item);
- *(temps[i]) = item;
- }
- }
- #endif
- } else {
- Py_ssize_t index = -1;
- PyObject** temps[4] = {&__pyx_t_10,&__pyx_t_2,&__pyx_t_8,&__pyx_t_14};
- __pyx_t_15 = PyObject_GetIter(__pyx_v_next_cubic); if (unlikely(!__pyx_t_15)) __PYX_ERR(0, 407, __pyx_L1_error)
- __Pyx_GOTREF(__pyx_t_15);
- __pyx_t_16 = __Pyx_PyObject_GetIterNextFunc(__pyx_t_15);
- for (index=0; index < 4; index++) {
- PyObject* item = __pyx_t_16(__pyx_t_15); if (unlikely(!item)) goto __pyx_L9_unpacking_failed;
- __Pyx_GOTREF(item);
- *(temps[index]) = item;
- }
- if (__Pyx_IternextUnpackEndCheck(__pyx_t_16(__pyx_t_15), 4) < 0) __PYX_ERR(0, 407, __pyx_L1_error)
- __pyx_t_16 = NULL;
- __Pyx_DECREF(__pyx_t_15); __pyx_t_15 = 0;
- goto __pyx_L10_unpacking_done;
- __pyx_L9_unpacking_failed:;
- __Pyx_DECREF(__pyx_t_15); __pyx_t_15 = 0;
- __pyx_t_16 = NULL;
- if (__Pyx_IterFinish() == 0) __Pyx_RaiseNeedMoreValuesError(index);
- __PYX_ERR(0, 407, __pyx_L1_error)
- __pyx_L10_unpacking_done:;
- }
- __pyx_t_9 = __Pyx_PyComplex_As___pyx_t_double_complex(__pyx_t_10); if (unlikely(PyErr_Occurred())) __PYX_ERR(0, 407, __pyx_L1_error)
- __Pyx_DECREF(__pyx_t_10); __pyx_t_10 = 0;
- __pyx_t_4 = __Pyx_PyComplex_As___pyx_t_double_complex(__pyx_t_2); if (unlikely(PyErr_Occurred())) __PYX_ERR(0, 407, __pyx_L1_error)
- __Pyx_DECREF(__pyx_t_2); __pyx_t_2 = 0;
- __pyx_t_5 = __Pyx_PyComplex_As___pyx_t_double_complex(__pyx_t_8); if (unlikely(PyErr_Occurred())) __PYX_ERR(0, 407, __pyx_L1_error)
- __Pyx_DECREF(__pyx_t_8); __pyx_t_8 = 0;
- __pyx_t_6 = __Pyx_PyComplex_As___pyx_t_double_complex(__pyx_t_14); if (unlikely(PyErr_Occurred())) __PYX_ERR(0, 407, __pyx_L1_error)
- __Pyx_DECREF(__pyx_t_14); __pyx_t_14 = 0;
- __pyx_v_c0 = __pyx_t_9;
- __pyx_v_c1 = __pyx_t_4;
- __pyx_v_c2 = __pyx_t_5;
- __pyx_v_c3 = __pyx_t_6;
-
- /* "fontTools/cu2qu/cu2qu.py":410
- *
- * # Current quadratic approximation of current cubic
- * q0 = q2 # <<<<<<<<<<<<<<
- * q1 = next_q1
- * if i < n:
- */
- __pyx_v_q0 = __pyx_v_q2;
-
- /* "fontTools/cu2qu/cu2qu.py":411
- * # Current quadratic approximation of current cubic
- * q0 = q2
- * q1 = next_q1 # <<<<<<<<<<<<<<
- * if i < n:
- * next_cubic = next(cubics)
- */
- __pyx_v_q1 = __pyx_v_next_q1;
-
- /* "fontTools/cu2qu/cu2qu.py":412
- * q0 = q2
- * q1 = next_q1
- * if i < n: # <<<<<<<<<<<<<<
- * next_cubic = next(cubics)
- * next_q1 = cubic_approx_control(
- */
- __pyx_t_1 = (__pyx_v_i < __pyx_v_n);
- if (__pyx_t_1) {
-
- /* "fontTools/cu2qu/cu2qu.py":413
- * q1 = next_q1
- * if i < n:
- * next_cubic = next(cubics) # <<<<<<<<<<<<<<
- * next_q1 = cubic_approx_control(
- * i / (n - 1), next_cubic[0], next_cubic[1], next_cubic[2], next_cubic[3]
- */
- __pyx_t_14 = __Pyx_PyIter_Next(__pyx_v_cubics); if (unlikely(!__pyx_t_14)) __PYX_ERR(0, 413, __pyx_L1_error)
- __Pyx_GOTREF(__pyx_t_14);
- __Pyx_DECREF_SET(__pyx_v_next_cubic, __pyx_t_14);
- __pyx_t_14 = 0;
-
- /* "fontTools/cu2qu/cu2qu.py":415
- * next_cubic = next(cubics)
- * next_q1 = cubic_approx_control(
- * i / (n - 1), next_cubic[0], next_cubic[1], next_cubic[2], next_cubic[3] # <<<<<<<<<<<<<<
- * )
- * spline.append(next_q1)
- */
- __pyx_t_17 = (__pyx_v_n - 1);
- if (unlikely(__pyx_t_17 == 0)) {
- PyErr_SetString(PyExc_ZeroDivisionError, "float division");
- __PYX_ERR(0, 415, __pyx_L1_error)
- }
- __pyx_t_14 = __Pyx_GetItemInt(__pyx_v_next_cubic, 0, long, 1, __Pyx_PyInt_From_long, 0, 0, 1); if (unlikely(!__pyx_t_14)) __PYX_ERR(0, 415, __pyx_L1_error)
- __Pyx_GOTREF(__pyx_t_14);
- __pyx_t_6 = __Pyx_PyComplex_As___pyx_t_double_complex(__pyx_t_14); if (unlikely(PyErr_Occurred())) __PYX_ERR(0, 415, __pyx_L1_error)
- __Pyx_DECREF(__pyx_t_14); __pyx_t_14 = 0;
- __pyx_t_14 = __Pyx_GetItemInt(__pyx_v_next_cubic, 1, long, 1, __Pyx_PyInt_From_long, 0, 0, 1); if (unlikely(!__pyx_t_14)) __PYX_ERR(0, 415, __pyx_L1_error)
- __Pyx_GOTREF(__pyx_t_14);
- __pyx_t_5 = __Pyx_PyComplex_As___pyx_t_double_complex(__pyx_t_14); if (unlikely(PyErr_Occurred())) __PYX_ERR(0, 415, __pyx_L1_error)
- __Pyx_DECREF(__pyx_t_14); __pyx_t_14 = 0;
- __pyx_t_14 = __Pyx_GetItemInt(__pyx_v_next_cubic, 2, long, 1, __Pyx_PyInt_From_long, 0, 0, 1); if (unlikely(!__pyx_t_14)) __PYX_ERR(0, 415, __pyx_L1_error)
- __Pyx_GOTREF(__pyx_t_14);
- __pyx_t_4 = __Pyx_PyComplex_As___pyx_t_double_complex(__pyx_t_14); if (unlikely(PyErr_Occurred())) __PYX_ERR(0, 415, __pyx_L1_error)
- __Pyx_DECREF(__pyx_t_14); __pyx_t_14 = 0;
- __pyx_t_14 = __Pyx_GetItemInt(__pyx_v_next_cubic, 3, long, 1, __Pyx_PyInt_From_long, 0, 0, 1); if (unlikely(!__pyx_t_14)) __PYX_ERR(0, 415, __pyx_L1_error)
- __Pyx_GOTREF(__pyx_t_14);
- __pyx_t_9 = __Pyx_PyComplex_As___pyx_t_double_complex(__pyx_t_14); if (unlikely(PyErr_Occurred())) __PYX_ERR(0, 415, __pyx_L1_error)
- __Pyx_DECREF(__pyx_t_14); __pyx_t_14 = 0;
-
- /* "fontTools/cu2qu/cu2qu.py":414
- * if i < n:
- * next_cubic = next(cubics)
- * next_q1 = cubic_approx_control( # <<<<<<<<<<<<<<
- * i / (n - 1), next_cubic[0], next_cubic[1], next_cubic[2], next_cubic[3]
- * )
- */
- __pyx_t_7 = __pyx_f_9fontTools_5cu2qu_5cu2qu_cubic_approx_control((((double)__pyx_v_i) / ((double)__pyx_t_17)), __pyx_t_6, __pyx_t_5, __pyx_t_4, __pyx_t_9); if (unlikely(PyErr_Occurred())) __PYX_ERR(0, 414, __pyx_L1_error)
- __pyx_v_next_q1 = __pyx_t_7;
-
- /* "fontTools/cu2qu/cu2qu.py":417
- * i / (n - 1), next_cubic[0], next_cubic[1], next_cubic[2], next_cubic[3]
- * )
- * spline.append(next_q1) # <<<<<<<<<<<<<<
- * q2 = (q1 + next_q1) * 0.5
- * else:
- */
- __pyx_t_14 = __pyx_PyComplex_FromComplex(__pyx_v_next_q1); if (unlikely(!__pyx_t_14)) __PYX_ERR(0, 417, __pyx_L1_error)
- __Pyx_GOTREF(__pyx_t_14);
- __pyx_t_18 = __Pyx_PyList_Append(__pyx_v_spline, __pyx_t_14); if (unlikely(__pyx_t_18 == ((int)-1))) __PYX_ERR(0, 417, __pyx_L1_error)
- __Pyx_DECREF(__pyx_t_14); __pyx_t_14 = 0;
-
- /* "fontTools/cu2qu/cu2qu.py":418
- * )
- * spline.append(next_q1)
- * q2 = (q1 + next_q1) * 0.5 # <<<<<<<<<<<<<<
- * else:
- * q2 = c3
- */
- __pyx_v_q2 = __Pyx_c_prod_double(__Pyx_c_sum_double(__pyx_v_q1, __pyx_v_next_q1), __pyx_t_double_complex_from_parts(0.5, 0));
-
- /* "fontTools/cu2qu/cu2qu.py":412
- * q0 = q2
- * q1 = next_q1
- * if i < n: # <<<<<<<<<<<<<<
- * next_cubic = next(cubics)
- * next_q1 = cubic_approx_control(
- */
- goto __pyx_L11;
- }
-
- /* "fontTools/cu2qu/cu2qu.py":420
- * q2 = (q1 + next_q1) * 0.5
- * else:
- * q2 = c3 # <<<<<<<<<<<<<<
- *
- * # End-point deltas
- */
- /*else*/ {
- __pyx_v_q2 = __pyx_v_c3;
- }
- __pyx_L11:;
-
- /* "fontTools/cu2qu/cu2qu.py":423
- *
- * # End-point deltas
- * d0 = d1 # <<<<<<<<<<<<<<
- * d1 = q2 - c3
- *
- */
- __pyx_v_d0 = __pyx_v_d1;
-
- /* "fontTools/cu2qu/cu2qu.py":424
- * # End-point deltas
- * d0 = d1
- * d1 = q2 - c3 # <<<<<<<<<<<<<<
- *
- * if abs(d1) > tolerance or not cubic_farthest_fit_inside(
- */
- __pyx_v_d1 = __Pyx_c_diff_double(__pyx_v_q2, __pyx_v_c3);
-
- /* "fontTools/cu2qu/cu2qu.py":426
- * d1 = q2 - c3
- *
- * if abs(d1) > tolerance or not cubic_farthest_fit_inside( # <<<<<<<<<<<<<<
- * d0,
- * q0 + (q1 - q0) * (2 / 3) - c1,
- */
- __pyx_t_3 = (__Pyx_c_abs_double(__pyx_v_d1) > __pyx_v_tolerance);
- if (!__pyx_t_3) {
- } else {
- __pyx_t_1 = __pyx_t_3;
- goto __pyx_L13_bool_binop_done;
- }
-
- /* "fontTools/cu2qu/cu2qu.py":431
- * q2 + (q1 - q2) * (2 / 3) - c2,
- * d1,
- * tolerance, # <<<<<<<<<<<<<<
- * ):
- * return None
- */
- __pyx_t_19 = __pyx_f_9fontTools_5cu2qu_5cu2qu_cubic_farthest_fit_inside(__pyx_v_d0, __Pyx_c_diff_double(__Pyx_c_sum_double(__pyx_v_q0, __Pyx_c_prod_double(__Pyx_c_diff_double(__pyx_v_q1, __pyx_v_q0), __pyx_t_double_complex_from_parts((2.0 / 3.0), 0))), __pyx_v_c1), __Pyx_c_diff_double(__Pyx_c_sum_double(__pyx_v_q2, __Pyx_c_prod_double(__Pyx_c_diff_double(__pyx_v_q1, __pyx_v_q2), __pyx_t_double_complex_from_parts((2.0 / 3.0), 0))), __pyx_v_c2), __pyx_v_d1, __pyx_v_tolerance); if (unlikely(__pyx_t_19 == ((int)-1) && PyErr_Occurred())) __PYX_ERR(0, 426, __pyx_L1_error)
-
- /* "fontTools/cu2qu/cu2qu.py":426
- * d1 = q2 - c3
- *
- * if abs(d1) > tolerance or not cubic_farthest_fit_inside( # <<<<<<<<<<<<<<
- * d0,
- * q0 + (q1 - q0) * (2 / 3) - c1,
- */
- __pyx_t_3 = (!(__pyx_t_19 != 0));
- __pyx_t_1 = __pyx_t_3;
- __pyx_L13_bool_binop_done:;
- if (__pyx_t_1) {
-
- /* "fontTools/cu2qu/cu2qu.py":433
- * tolerance,
- * ):
- * return None # <<<<<<<<<<<<<<
- * spline.append(cubic[3])
- *
- */
- __Pyx_XDECREF(__pyx_r);
- __pyx_r = Py_None; __Pyx_INCREF(Py_None);
- goto __pyx_L0;
-
- /* "fontTools/cu2qu/cu2qu.py":426
- * d1 = q2 - c3
- *
- * if abs(d1) > tolerance or not cubic_farthest_fit_inside( # <<<<<<<<<<<<<<
- * d0,
- * q0 + (q1 - q0) * (2 / 3) - c1,
- */
- }
- }
-
- /* "fontTools/cu2qu/cu2qu.py":434
- * ):
- * return None
- * spline.append(cubic[3]) # <<<<<<<<<<<<<<
- *
- * return spline
- */
- __pyx_t_14 = __Pyx_GetItemInt(__pyx_v_cubic, 3, long, 1, __Pyx_PyInt_From_long, 0, 0, 1); if (unlikely(!__pyx_t_14)) __PYX_ERR(0, 434, __pyx_L1_error)
- __Pyx_GOTREF(__pyx_t_14);
- __pyx_t_18 = __Pyx_PyList_Append(__pyx_v_spline, __pyx_t_14); if (unlikely(__pyx_t_18 == ((int)-1))) __PYX_ERR(0, 434, __pyx_L1_error)
- __Pyx_DECREF(__pyx_t_14); __pyx_t_14 = 0;
-
- /* "fontTools/cu2qu/cu2qu.py":436
- * spline.append(cubic[3])
- *
- * return spline # <<<<<<<<<<<<<<
- *
- *
- */
- __Pyx_XDECREF(__pyx_r);
- __Pyx_INCREF(__pyx_v_spline);
- __pyx_r = __pyx_v_spline;
- goto __pyx_L0;
-
- /* "fontTools/cu2qu/cu2qu.py":361
- *
- *
- * @cython.cfunc # <<<<<<<<<<<<<<
- * @cython.locals(n=cython.int, tolerance=cython.double)
- * @cython.locals(i=cython.int)
- */
-
- /* function exit code */
- __pyx_L1_error:;
- __Pyx_XDECREF(__pyx_t_2);
- __Pyx_XDECREF(__pyx_t_8);
- __Pyx_XDECREF(__pyx_t_10);
- __Pyx_XDECREF(__pyx_t_14);
- __Pyx_XDECREF(__pyx_t_15);
- __Pyx_AddTraceback("fontTools.cu2qu.cu2qu.cubic_approx_spline", __pyx_clineno, __pyx_lineno, __pyx_filename);
- __pyx_r = 0;
- __pyx_L0:;
- __Pyx_XDECREF(__pyx_v_cubics);
- __Pyx_XDECREF(__pyx_v_next_cubic);
- __Pyx_XDECREF(__pyx_v_spline);
- __Pyx_XGIVEREF(__pyx_r);
- __Pyx_RefNannyFinishContext();
- return __pyx_r;
-}
-
-/* "fontTools/cu2qu/cu2qu.py":439
- *
- *
- * @cython.locals(max_err=cython.double) # <<<<<<<<<<<<<<
- * @cython.locals(n=cython.int)
- * @cython.locals(all_quadratic=cython.int)
- */
-
-/* Python wrapper */
-static PyObject *__pyx_pw_9fontTools_5cu2qu_5cu2qu_4curve_to_quadratic(PyObject *__pyx_self,
-#if CYTHON_METH_FASTCALL
-PyObject *const *__pyx_args, Py_ssize_t __pyx_nargs, PyObject *__pyx_kwds
-#else
-PyObject *__pyx_args, PyObject *__pyx_kwds
-#endif
-); /*proto*/
-PyDoc_STRVAR(__pyx_doc_9fontTools_5cu2qu_5cu2qu_3curve_to_quadratic, "curve_to_quadratic(curve, double max_err, int all_quadratic=True)\nApproximate a cubic Bezier curve with a spline of n quadratics.\n\n Args:\n cubic (sequence): Four 2D tuples representing control points of\n the cubic Bezier curve.\n max_err (double): Permitted deviation from the original curve.\n all_quadratic (bool): If True (default) returned value is a\n quadratic spline. If False, it's either a single quadratic\n curve or a single cubic curve.\n\n Returns:\n If all_quadratic is True: A list of 2D tuples, representing\n control points of the quadratic spline if it fits within the\n given tolerance, or ``None`` if no suitable spline could be\n calculated.\n\n If all_quadratic is False: Either a quadratic curve (if length\n of output is 3), or a cubic curve (if length of output is 4).\n ");
-static PyMethodDef __pyx_mdef_9fontTools_5cu2qu_5cu2qu_4curve_to_quadratic = {"curve_to_quadratic", (PyCFunction)(void*)(__Pyx_PyCFunction_FastCallWithKeywords)__pyx_pw_9fontTools_5cu2qu_5cu2qu_4curve_to_quadratic, __Pyx_METH_FASTCALL|METH_KEYWORDS, __pyx_doc_9fontTools_5cu2qu_5cu2qu_3curve_to_quadratic};
-static PyObject *__pyx_pw_9fontTools_5cu2qu_5cu2qu_4curve_to_quadratic(PyObject *__pyx_self,
-#if CYTHON_METH_FASTCALL
-PyObject *const *__pyx_args, Py_ssize_t __pyx_nargs, PyObject *__pyx_kwds
-#else
-PyObject *__pyx_args, PyObject *__pyx_kwds
-#endif
-) {
- PyObject *__pyx_v_curve = 0;
- double __pyx_v_max_err;
- int __pyx_v_all_quadratic;
- #if !CYTHON_METH_FASTCALL
- CYTHON_UNUSED const Py_ssize_t __pyx_nargs = PyTuple_GET_SIZE(__pyx_args);
- #endif
- CYTHON_UNUSED PyObject *const *__pyx_kwvalues = __Pyx_KwValues_FASTCALL(__pyx_args, __pyx_nargs);
- int __pyx_lineno = 0;
- const char *__pyx_filename = NULL;
- int __pyx_clineno = 0;
- PyObject *__pyx_r = 0;
- __Pyx_RefNannyDeclarations
- __Pyx_RefNannySetupContext("curve_to_quadratic (wrapper)", 0);
- {
- PyObject **__pyx_pyargnames[] = {&__pyx_n_s_curve,&__pyx_n_s_max_err,&__pyx_n_s_all_quadratic,0};
- PyObject* values[3] = {0,0,0};
- if (__pyx_kwds) {
- Py_ssize_t kw_args;
- switch (__pyx_nargs) {
- case 3: values[2] = __Pyx_Arg_FASTCALL(__pyx_args, 2);
- CYTHON_FALLTHROUGH;
- case 2: values[1] = __Pyx_Arg_FASTCALL(__pyx_args, 1);
- CYTHON_FALLTHROUGH;
- case 1: values[0] = __Pyx_Arg_FASTCALL(__pyx_args, 0);
- CYTHON_FALLTHROUGH;
- case 0: break;
- default: goto __pyx_L5_argtuple_error;
- }
- kw_args = __Pyx_NumKwargs_FASTCALL(__pyx_kwds);
- switch (__pyx_nargs) {
- case 0:
- if (likely((values[0] = __Pyx_GetKwValue_FASTCALL(__pyx_kwds, __pyx_kwvalues, __pyx_n_s_curve)) != 0)) kw_args--;
- else if (unlikely(PyErr_Occurred())) __PYX_ERR(0, 439, __pyx_L3_error)
- else goto __pyx_L5_argtuple_error;
- CYTHON_FALLTHROUGH;
- case 1:
- if (likely((values[1] = __Pyx_GetKwValue_FASTCALL(__pyx_kwds, __pyx_kwvalues, __pyx_n_s_max_err)) != 0)) kw_args--;
- else if (unlikely(PyErr_Occurred())) __PYX_ERR(0, 439, __pyx_L3_error)
- else {
- __Pyx_RaiseArgtupleInvalid("curve_to_quadratic", 0, 2, 3, 1); __PYX_ERR(0, 439, __pyx_L3_error)
- }
- CYTHON_FALLTHROUGH;
- case 2:
- if (kw_args > 0) {
- PyObject* value = __Pyx_GetKwValue_FASTCALL(__pyx_kwds, __pyx_kwvalues, __pyx_n_s_all_quadratic);
- if (value) { values[2] = value; kw_args--; }
- else if (unlikely(PyErr_Occurred())) __PYX_ERR(0, 439, __pyx_L3_error)
- }
- }
- if (unlikely(kw_args > 0)) {
- const Py_ssize_t kwd_pos_args = __pyx_nargs;
- if (unlikely(__Pyx_ParseOptionalKeywords(__pyx_kwds, __pyx_kwvalues, __pyx_pyargnames, 0, values + 0, kwd_pos_args, "curve_to_quadratic") < 0)) __PYX_ERR(0, 439, __pyx_L3_error)
- }
- } else {
- switch (__pyx_nargs) {
- case 3: values[2] = __Pyx_Arg_FASTCALL(__pyx_args, 2);
- CYTHON_FALLTHROUGH;
- case 2: values[1] = __Pyx_Arg_FASTCALL(__pyx_args, 1);
- values[0] = __Pyx_Arg_FASTCALL(__pyx_args, 0);
- break;
- default: goto __pyx_L5_argtuple_error;
- }
- }
- __pyx_v_curve = values[0];
- __pyx_v_max_err = __pyx_PyFloat_AsDouble(values[1]); if (unlikely((__pyx_v_max_err == (double)-1) && PyErr_Occurred())) __PYX_ERR(0, 442, __pyx_L3_error)
- if (values[2]) {
- __pyx_v_all_quadratic = __Pyx_PyInt_As_int(values[2]); if (unlikely((__pyx_v_all_quadratic == (int)-1) && PyErr_Occurred())) __PYX_ERR(0, 442, __pyx_L3_error)
- } else {
-
- /* "fontTools/cu2qu/cu2qu.py":442
- * @cython.locals(n=cython.int)
- * @cython.locals(all_quadratic=cython.int)
- * def curve_to_quadratic(curve, max_err, all_quadratic=True): # <<<<<<<<<<<<<<
- * """Approximate a cubic Bezier curve with a spline of n quadratics.
- *
- */
- __pyx_v_all_quadratic = ((int)((int)1));
- }
- }
- goto __pyx_L4_argument_unpacking_done;
- __pyx_L5_argtuple_error:;
- __Pyx_RaiseArgtupleInvalid("curve_to_quadratic", 0, 2, 3, __pyx_nargs); __PYX_ERR(0, 439, __pyx_L3_error)
- __pyx_L3_error:;
- __Pyx_AddTraceback("fontTools.cu2qu.cu2qu.curve_to_quadratic", __pyx_clineno, __pyx_lineno, __pyx_filename);
- __Pyx_RefNannyFinishContext();
- return NULL;
- __pyx_L4_argument_unpacking_done:;
- __pyx_r = __pyx_pf_9fontTools_5cu2qu_5cu2qu_3curve_to_quadratic(__pyx_self, __pyx_v_curve, __pyx_v_max_err, __pyx_v_all_quadratic);
-
- /* "fontTools/cu2qu/cu2qu.py":439
- *
- *
- * @cython.locals(max_err=cython.double) # <<<<<<<<<<<<<<
- * @cython.locals(n=cython.int)
- * @cython.locals(all_quadratic=cython.int)
- */
-
- /* function exit code */
- __Pyx_RefNannyFinishContext();
- return __pyx_r;
-}
-
-static PyObject *__pyx_pf_9fontTools_5cu2qu_5cu2qu_3curve_to_quadratic(CYTHON_UNUSED PyObject *__pyx_self, PyObject *__pyx_v_curve, double __pyx_v_max_err, int __pyx_v_all_quadratic) {
- int __pyx_v_n;
- PyObject *__pyx_v_spline = NULL;
- PyObject *__pyx_7genexpr__pyx_v_p = NULL;
- PyObject *__pyx_8genexpr1__pyx_v_s = NULL;
- PyObject *__pyx_r = NULL;
- __Pyx_RefNannyDeclarations
- PyObject *__pyx_t_1 = NULL;
- PyObject *__pyx_t_2 = NULL;
- Py_ssize_t __pyx_t_3;
- PyObject *(*__pyx_t_4)(PyObject *);
- PyObject *__pyx_t_5 = NULL;
- PyObject *__pyx_t_6 = NULL;
- long __pyx_t_7;
- long __pyx_t_8;
- int __pyx_t_9;
- int __pyx_t_10;
- PyObject *__pyx_t_11 = NULL;
- int __pyx_lineno = 0;
- const char *__pyx_filename = NULL;
- int __pyx_clineno = 0;
- __Pyx_RefNannySetupContext("curve_to_quadratic", 0);
- __Pyx_INCREF(__pyx_v_curve);
-
- /* "fontTools/cu2qu/cu2qu.py":463
- * """
- *
- * curve = [complex(*p) for p in curve] # <<<<<<<<<<<<<<
- *
- * for n in range(1, MAX_N + 1):
- */
- { /* enter inner scope */
- __pyx_t_1 = PyList_New(0); if (unlikely(!__pyx_t_1)) __PYX_ERR(0, 463, __pyx_L5_error)
- __Pyx_GOTREF(__pyx_t_1);
- if (likely(PyList_CheckExact(__pyx_v_curve)) || PyTuple_CheckExact(__pyx_v_curve)) {
- __pyx_t_2 = __pyx_v_curve; __Pyx_INCREF(__pyx_t_2); __pyx_t_3 = 0;
- __pyx_t_4 = NULL;
- } else {
- __pyx_t_3 = -1; __pyx_t_2 = PyObject_GetIter(__pyx_v_curve); if (unlikely(!__pyx_t_2)) __PYX_ERR(0, 463, __pyx_L5_error)
- __Pyx_GOTREF(__pyx_t_2);
- __pyx_t_4 = __Pyx_PyObject_GetIterNextFunc(__pyx_t_2); if (unlikely(!__pyx_t_4)) __PYX_ERR(0, 463, __pyx_L5_error)
- }
- for (;;) {
- if (likely(!__pyx_t_4)) {
- if (likely(PyList_CheckExact(__pyx_t_2))) {
- if (__pyx_t_3 >= PyList_GET_SIZE(__pyx_t_2)) break;
- #if CYTHON_ASSUME_SAFE_MACROS && !CYTHON_AVOID_BORROWED_REFS
- __pyx_t_5 = PyList_GET_ITEM(__pyx_t_2, __pyx_t_3); __Pyx_INCREF(__pyx_t_5); __pyx_t_3++; if (unlikely((0 < 0))) __PYX_ERR(0, 463, __pyx_L5_error)
- #else
- __pyx_t_5 = PySequence_ITEM(__pyx_t_2, __pyx_t_3); __pyx_t_3++; if (unlikely(!__pyx_t_5)) __PYX_ERR(0, 463, __pyx_L5_error)
- __Pyx_GOTREF(__pyx_t_5);
- #endif
- } else {
- if (__pyx_t_3 >= PyTuple_GET_SIZE(__pyx_t_2)) break;
- #if CYTHON_ASSUME_SAFE_MACROS && !CYTHON_AVOID_BORROWED_REFS
- __pyx_t_5 = PyTuple_GET_ITEM(__pyx_t_2, __pyx_t_3); __Pyx_INCREF(__pyx_t_5); __pyx_t_3++; if (unlikely((0 < 0))) __PYX_ERR(0, 463, __pyx_L5_error)
- #else
- __pyx_t_5 = PySequence_ITEM(__pyx_t_2, __pyx_t_3); __pyx_t_3++; if (unlikely(!__pyx_t_5)) __PYX_ERR(0, 463, __pyx_L5_error)
- __Pyx_GOTREF(__pyx_t_5);
- #endif
- }
- } else {
- __pyx_t_5 = __pyx_t_4(__pyx_t_2);
- if (unlikely(!__pyx_t_5)) {
- PyObject* exc_type = PyErr_Occurred();
- if (exc_type) {
- if (likely(__Pyx_PyErr_GivenExceptionMatches(exc_type, PyExc_StopIteration))) PyErr_Clear();
- else __PYX_ERR(0, 463, __pyx_L5_error)
- }
- break;
- }
- __Pyx_GOTREF(__pyx_t_5);
- }
- __Pyx_XDECREF_SET(__pyx_7genexpr__pyx_v_p, __pyx_t_5);
- __pyx_t_5 = 0;
- __pyx_t_5 = __Pyx_PySequence_Tuple(__pyx_7genexpr__pyx_v_p); if (unlikely(!__pyx_t_5)) __PYX_ERR(0, 463, __pyx_L5_error)
- __Pyx_GOTREF(__pyx_t_5);
- __pyx_t_6 = __Pyx_PyObject_Call(((PyObject *)(&PyComplex_Type)), __pyx_t_5, NULL); if (unlikely(!__pyx_t_6)) __PYX_ERR(0, 463, __pyx_L5_error)
- __Pyx_GOTREF(__pyx_t_6);
- __Pyx_DECREF(__pyx_t_5); __pyx_t_5 = 0;
- if (unlikely(__Pyx_ListComp_Append(__pyx_t_1, (PyObject*)__pyx_t_6))) __PYX_ERR(0, 463, __pyx_L5_error)
- __Pyx_DECREF(__pyx_t_6); __pyx_t_6 = 0;
- }
- __Pyx_DECREF(__pyx_t_2); __pyx_t_2 = 0;
- __Pyx_XDECREF(__pyx_7genexpr__pyx_v_p); __pyx_7genexpr__pyx_v_p = 0;
- goto __pyx_L9_exit_scope;
- __pyx_L5_error:;
- __Pyx_XDECREF(__pyx_7genexpr__pyx_v_p); __pyx_7genexpr__pyx_v_p = 0;
- goto __pyx_L1_error;
- __pyx_L9_exit_scope:;
- } /* exit inner scope */
- __Pyx_DECREF_SET(__pyx_v_curve, __pyx_t_1);
- __pyx_t_1 = 0;
-
- /* "fontTools/cu2qu/cu2qu.py":465
- * curve = [complex(*p) for p in curve]
- *
- * for n in range(1, MAX_N + 1): # <<<<<<<<<<<<<<
- * spline = cubic_approx_spline(curve, n, max_err, all_quadratic)
- * if spline is not None:
- */
- __Pyx_GetModuleGlobalName(__pyx_t_1, __pyx_n_s_MAX_N); if (unlikely(!__pyx_t_1)) __PYX_ERR(0, 465, __pyx_L1_error)
- __Pyx_GOTREF(__pyx_t_1);
- __pyx_t_2 = __Pyx_PyInt_AddObjC(__pyx_t_1, __pyx_int_1, 1, 0, 0); if (unlikely(!__pyx_t_2)) __PYX_ERR(0, 465, __pyx_L1_error)
- __Pyx_GOTREF(__pyx_t_2);
- __Pyx_DECREF(__pyx_t_1); __pyx_t_1 = 0;
- __pyx_t_7 = __Pyx_PyInt_As_long(__pyx_t_2); if (unlikely((__pyx_t_7 == (long)-1) && PyErr_Occurred())) __PYX_ERR(0, 465, __pyx_L1_error)
- __Pyx_DECREF(__pyx_t_2); __pyx_t_2 = 0;
- __pyx_t_8 = __pyx_t_7;
- for (__pyx_t_9 = 1; __pyx_t_9 < __pyx_t_8; __pyx_t_9+=1) {
- __pyx_v_n = __pyx_t_9;
-
- /* "fontTools/cu2qu/cu2qu.py":466
- *
- * for n in range(1, MAX_N + 1):
- * spline = cubic_approx_spline(curve, n, max_err, all_quadratic) # <<<<<<<<<<<<<<
- * if spline is not None:
- * # done. go home
- */
- __pyx_t_2 = __pyx_f_9fontTools_5cu2qu_5cu2qu_cubic_approx_spline(__pyx_v_curve, __pyx_v_n, __pyx_v_max_err, __pyx_v_all_quadratic); if (unlikely(!__pyx_t_2)) __PYX_ERR(0, 466, __pyx_L1_error)
- __Pyx_GOTREF(__pyx_t_2);
- __Pyx_XDECREF_SET(__pyx_v_spline, __pyx_t_2);
- __pyx_t_2 = 0;
-
- /* "fontTools/cu2qu/cu2qu.py":467
- * for n in range(1, MAX_N + 1):
- * spline = cubic_approx_spline(curve, n, max_err, all_quadratic)
- * if spline is not None: # <<<<<<<<<<<<<<
- * # done. go home
- * return [(s.real, s.imag) for s in spline]
- */
- __pyx_t_10 = (__pyx_v_spline != Py_None);
- if (__pyx_t_10) {
-
- /* "fontTools/cu2qu/cu2qu.py":469
- * if spline is not None:
- * # done. go home
- * return [(s.real, s.imag) for s in spline] # <<<<<<<<<<<<<<
- *
- * raise ApproxNotFoundError(curve)
- */
- __Pyx_XDECREF(__pyx_r);
- { /* enter inner scope */
- __pyx_t_2 = PyList_New(0); if (unlikely(!__pyx_t_2)) __PYX_ERR(0, 469, __pyx_L15_error)
- __Pyx_GOTREF(__pyx_t_2);
- if (likely(PyList_CheckExact(__pyx_v_spline)) || PyTuple_CheckExact(__pyx_v_spline)) {
- __pyx_t_1 = __pyx_v_spline; __Pyx_INCREF(__pyx_t_1); __pyx_t_3 = 0;
- __pyx_t_4 = NULL;
- } else {
- __pyx_t_3 = -1; __pyx_t_1 = PyObject_GetIter(__pyx_v_spline); if (unlikely(!__pyx_t_1)) __PYX_ERR(0, 469, __pyx_L15_error)
- __Pyx_GOTREF(__pyx_t_1);
- __pyx_t_4 = __Pyx_PyObject_GetIterNextFunc(__pyx_t_1); if (unlikely(!__pyx_t_4)) __PYX_ERR(0, 469, __pyx_L15_error)
- }
- for (;;) {
- if (likely(!__pyx_t_4)) {
- if (likely(PyList_CheckExact(__pyx_t_1))) {
- if (__pyx_t_3 >= PyList_GET_SIZE(__pyx_t_1)) break;
- #if CYTHON_ASSUME_SAFE_MACROS && !CYTHON_AVOID_BORROWED_REFS
- __pyx_t_6 = PyList_GET_ITEM(__pyx_t_1, __pyx_t_3); __Pyx_INCREF(__pyx_t_6); __pyx_t_3++; if (unlikely((0 < 0))) __PYX_ERR(0, 469, __pyx_L15_error)
- #else
- __pyx_t_6 = PySequence_ITEM(__pyx_t_1, __pyx_t_3); __pyx_t_3++; if (unlikely(!__pyx_t_6)) __PYX_ERR(0, 469, __pyx_L15_error)
- __Pyx_GOTREF(__pyx_t_6);
- #endif
- } else {
- if (__pyx_t_3 >= PyTuple_GET_SIZE(__pyx_t_1)) break;
- #if CYTHON_ASSUME_SAFE_MACROS && !CYTHON_AVOID_BORROWED_REFS
- __pyx_t_6 = PyTuple_GET_ITEM(__pyx_t_1, __pyx_t_3); __Pyx_INCREF(__pyx_t_6); __pyx_t_3++; if (unlikely((0 < 0))) __PYX_ERR(0, 469, __pyx_L15_error)
- #else
- __pyx_t_6 = PySequence_ITEM(__pyx_t_1, __pyx_t_3); __pyx_t_3++; if (unlikely(!__pyx_t_6)) __PYX_ERR(0, 469, __pyx_L15_error)
- __Pyx_GOTREF(__pyx_t_6);
- #endif
- }
- } else {
- __pyx_t_6 = __pyx_t_4(__pyx_t_1);
- if (unlikely(!__pyx_t_6)) {
- PyObject* exc_type = PyErr_Occurred();
- if (exc_type) {
- if (likely(__Pyx_PyErr_GivenExceptionMatches(exc_type, PyExc_StopIteration))) PyErr_Clear();
- else __PYX_ERR(0, 469, __pyx_L15_error)
- }
- break;
- }
- __Pyx_GOTREF(__pyx_t_6);
- }
- __Pyx_XDECREF_SET(__pyx_8genexpr1__pyx_v_s, __pyx_t_6);
- __pyx_t_6 = 0;
- __pyx_t_6 = __Pyx_PyObject_GetAttrStr(__pyx_8genexpr1__pyx_v_s, __pyx_n_s_real); if (unlikely(!__pyx_t_6)) __PYX_ERR(0, 469, __pyx_L15_error)
- __Pyx_GOTREF(__pyx_t_6);
- __pyx_t_5 = __Pyx_PyObject_GetAttrStr(__pyx_8genexpr1__pyx_v_s, __pyx_n_s_imag); if (unlikely(!__pyx_t_5)) __PYX_ERR(0, 469, __pyx_L15_error)
- __Pyx_GOTREF(__pyx_t_5);
- __pyx_t_11 = PyTuple_New(2); if (unlikely(!__pyx_t_11)) __PYX_ERR(0, 469, __pyx_L15_error)
- __Pyx_GOTREF(__pyx_t_11);
- __Pyx_GIVEREF(__pyx_t_6);
- PyTuple_SET_ITEM(__pyx_t_11, 0, __pyx_t_6);
- __Pyx_GIVEREF(__pyx_t_5);
- PyTuple_SET_ITEM(__pyx_t_11, 1, __pyx_t_5);
- __pyx_t_6 = 0;
- __pyx_t_5 = 0;
- if (unlikely(__Pyx_ListComp_Append(__pyx_t_2, (PyObject*)__pyx_t_11))) __PYX_ERR(0, 469, __pyx_L15_error)
- __Pyx_DECREF(__pyx_t_11); __pyx_t_11 = 0;
- }
- __Pyx_DECREF(__pyx_t_1); __pyx_t_1 = 0;
- __Pyx_XDECREF(__pyx_8genexpr1__pyx_v_s); __pyx_8genexpr1__pyx_v_s = 0;
- goto __pyx_L19_exit_scope;
- __pyx_L15_error:;
- __Pyx_XDECREF(__pyx_8genexpr1__pyx_v_s); __pyx_8genexpr1__pyx_v_s = 0;
- goto __pyx_L1_error;
- __pyx_L19_exit_scope:;
- } /* exit inner scope */
- __pyx_r = __pyx_t_2;
- __pyx_t_2 = 0;
- goto __pyx_L0;
-
- /* "fontTools/cu2qu/cu2qu.py":467
- * for n in range(1, MAX_N + 1):
- * spline = cubic_approx_spline(curve, n, max_err, all_quadratic)
- * if spline is not None: # <<<<<<<<<<<<<<
- * # done. go home
- * return [(s.real, s.imag) for s in spline]
- */
- }
- }
-
- /* "fontTools/cu2qu/cu2qu.py":471
- * return [(s.real, s.imag) for s in spline]
- *
- * raise ApproxNotFoundError(curve) # <<<<<<<<<<<<<<
- *
- *
- */
- __Pyx_GetModuleGlobalName(__pyx_t_1, __pyx_n_s_ApproxNotFoundError); if (unlikely(!__pyx_t_1)) __PYX_ERR(0, 471, __pyx_L1_error)
- __Pyx_GOTREF(__pyx_t_1);
- __pyx_t_11 = NULL;
- __pyx_t_9 = 0;
- if (CYTHON_UNPACK_METHODS && unlikely(PyMethod_Check(__pyx_t_1))) {
- __pyx_t_11 = PyMethod_GET_SELF(__pyx_t_1);
- if (likely(__pyx_t_11)) {
- PyObject* function = PyMethod_GET_FUNCTION(__pyx_t_1);
- __Pyx_INCREF(__pyx_t_11);
- __Pyx_INCREF(function);
- __Pyx_DECREF_SET(__pyx_t_1, function);
- __pyx_t_9 = 1;
- }
- }
- {
- PyObject *__pyx_callargs[2] = {__pyx_t_11, __pyx_v_curve};
- __pyx_t_2 = __Pyx_PyObject_FastCall(__pyx_t_1, __pyx_callargs+1-__pyx_t_9, 1+__pyx_t_9);
- __Pyx_XDECREF(__pyx_t_11); __pyx_t_11 = 0;
- if (unlikely(!__pyx_t_2)) __PYX_ERR(0, 471, __pyx_L1_error)
- __Pyx_GOTREF(__pyx_t_2);
- __Pyx_DECREF(__pyx_t_1); __pyx_t_1 = 0;
- }
- __Pyx_Raise(__pyx_t_2, 0, 0, 0);
- __Pyx_DECREF(__pyx_t_2); __pyx_t_2 = 0;
- __PYX_ERR(0, 471, __pyx_L1_error)
-
- /* "fontTools/cu2qu/cu2qu.py":439
- *
- *
- * @cython.locals(max_err=cython.double) # <<<<<<<<<<<<<<
- * @cython.locals(n=cython.int)
- * @cython.locals(all_quadratic=cython.int)
- */
-
- /* function exit code */
- __pyx_L1_error:;
- __Pyx_XDECREF(__pyx_t_1);
- __Pyx_XDECREF(__pyx_t_2);
- __Pyx_XDECREF(__pyx_t_5);
- __Pyx_XDECREF(__pyx_t_6);
- __Pyx_XDECREF(__pyx_t_11);
- __Pyx_AddTraceback("fontTools.cu2qu.cu2qu.curve_to_quadratic", __pyx_clineno, __pyx_lineno, __pyx_filename);
- __pyx_r = NULL;
- __pyx_L0:;
- __Pyx_XDECREF(__pyx_v_spline);
- __Pyx_XDECREF(__pyx_7genexpr__pyx_v_p);
- __Pyx_XDECREF(__pyx_8genexpr1__pyx_v_s);
- __Pyx_XDECREF(__pyx_v_curve);
- __Pyx_XGIVEREF(__pyx_r);
- __Pyx_RefNannyFinishContext();
- return __pyx_r;
-}
-
-/* "fontTools/cu2qu/cu2qu.py":474
- *
- *
- * @cython.locals(l=cython.int, last_i=cython.int, i=cython.int) # <<<<<<<<<<<<<<
- * @cython.locals(all_quadratic=cython.int)
- * def curves_to_quadratic(curves, max_errors, all_quadratic=True):
- */
-
-/* Python wrapper */
-static PyObject *__pyx_pw_9fontTools_5cu2qu_5cu2qu_6curves_to_quadratic(PyObject *__pyx_self,
-#if CYTHON_METH_FASTCALL
-PyObject *const *__pyx_args, Py_ssize_t __pyx_nargs, PyObject *__pyx_kwds
-#else
-PyObject *__pyx_args, PyObject *__pyx_kwds
-#endif
-); /*proto*/
-PyDoc_STRVAR(__pyx_doc_9fontTools_5cu2qu_5cu2qu_5curves_to_quadratic, "curves_to_quadratic(curves, max_errors, int all_quadratic=True)\nReturn quadratic Bezier splines approximating the input cubic Beziers.\n\n Args:\n curves: A sequence of *n* curves, each curve being a sequence of four\n 2D tuples.\n max_errors: A sequence of *n* floats representing the maximum permissible\n deviation from each of the cubic Bezier curves.\n all_quadratic (bool): If True (default) returned values are a\n quadratic spline. If False, they are either a single quadratic\n curve or a single cubic curve.\n\n Example::\n\n >>> curves_to_quadratic( [\n ... [ (50,50), (100,100), (150,100), (200,50) ],\n ... [ (75,50), (120,100), (150,75), (200,60) ]\n ... ], [1,1] )\n [[(50.0, 50.0), (75.0, 75.0), (125.0, 91.66666666666666), (175.0, 75.0), (200.0, 50.0)], [(75.0, 50.0), (97.5, 75.0), (135.41666666666666, 82.08333333333333), (175.0, 67.5), (200.0, 60.0)]]\n\n The returned splines have \"implied oncurve points\" suitable for use in\n TrueType ``glif`` outlines - i.e. in the first spline returned above,\n the first quadratic segment runs from (50,50) to\n ( (75 + 125)/2 , (120 + 91.666..)/2 ) = (100, 83.333...).\n\n Returns:\n If all_quadratic is True, a list of splines, each spline being a list\n of 2D tuples.\n\n If all_quadratic is False, a list of curves, each curve being a quadratic\n (length 3), or cubic (length 4).\n\n Raises:\n fontTools.cu2qu.Errors.ApproxNotFoundError: if no suitable approximation\n can be found for all curves with the given parameters.\n ");
-static PyMethodDef __pyx_mdef_9fontTools_5cu2qu_5cu2qu_6curves_to_quadratic = {"curves_to_quadratic", (PyCFunction)(void*)(__Pyx_PyCFunction_FastCallWithKeywords)__pyx_pw_9fontTools_5cu2qu_5cu2qu_6curves_to_quadratic, __Pyx_METH_FASTCALL|METH_KEYWORDS, __pyx_doc_9fontTools_5cu2qu_5cu2qu_5curves_to_quadratic};
-static PyObject *__pyx_pw_9fontTools_5cu2qu_5cu2qu_6curves_to_quadratic(PyObject *__pyx_self,
-#if CYTHON_METH_FASTCALL
-PyObject *const *__pyx_args, Py_ssize_t __pyx_nargs, PyObject *__pyx_kwds
-#else
-PyObject *__pyx_args, PyObject *__pyx_kwds
-#endif
-) {
- PyObject *__pyx_v_curves = 0;
- PyObject *__pyx_v_max_errors = 0;
- int __pyx_v_all_quadratic;
- #if !CYTHON_METH_FASTCALL
- CYTHON_UNUSED const Py_ssize_t __pyx_nargs = PyTuple_GET_SIZE(__pyx_args);
- #endif
- CYTHON_UNUSED PyObject *const *__pyx_kwvalues = __Pyx_KwValues_FASTCALL(__pyx_args, __pyx_nargs);
- int __pyx_lineno = 0;
- const char *__pyx_filename = NULL;
- int __pyx_clineno = 0;
- PyObject *__pyx_r = 0;
- __Pyx_RefNannyDeclarations
- __Pyx_RefNannySetupContext("curves_to_quadratic (wrapper)", 0);
- {
- PyObject **__pyx_pyargnames[] = {&__pyx_n_s_curves,&__pyx_n_s_max_errors,&__pyx_n_s_all_quadratic,0};
- PyObject* values[3] = {0,0,0};
- if (__pyx_kwds) {
- Py_ssize_t kw_args;
- switch (__pyx_nargs) {
- case 3: values[2] = __Pyx_Arg_FASTCALL(__pyx_args, 2);
- CYTHON_FALLTHROUGH;
- case 2: values[1] = __Pyx_Arg_FASTCALL(__pyx_args, 1);
- CYTHON_FALLTHROUGH;
- case 1: values[0] = __Pyx_Arg_FASTCALL(__pyx_args, 0);
- CYTHON_FALLTHROUGH;
- case 0: break;
- default: goto __pyx_L5_argtuple_error;
- }
- kw_args = __Pyx_NumKwargs_FASTCALL(__pyx_kwds);
- switch (__pyx_nargs) {
- case 0:
- if (likely((values[0] = __Pyx_GetKwValue_FASTCALL(__pyx_kwds, __pyx_kwvalues, __pyx_n_s_curves)) != 0)) kw_args--;
- else if (unlikely(PyErr_Occurred())) __PYX_ERR(0, 474, __pyx_L3_error)
- else goto __pyx_L5_argtuple_error;
- CYTHON_FALLTHROUGH;
- case 1:
- if (likely((values[1] = __Pyx_GetKwValue_FASTCALL(__pyx_kwds, __pyx_kwvalues, __pyx_n_s_max_errors)) != 0)) kw_args--;
- else if (unlikely(PyErr_Occurred())) __PYX_ERR(0, 474, __pyx_L3_error)
- else {
- __Pyx_RaiseArgtupleInvalid("curves_to_quadratic", 0, 2, 3, 1); __PYX_ERR(0, 474, __pyx_L3_error)
- }
- CYTHON_FALLTHROUGH;
- case 2:
- if (kw_args > 0) {
- PyObject* value = __Pyx_GetKwValue_FASTCALL(__pyx_kwds, __pyx_kwvalues, __pyx_n_s_all_quadratic);
- if (value) { values[2] = value; kw_args--; }
- else if (unlikely(PyErr_Occurred())) __PYX_ERR(0, 474, __pyx_L3_error)
- }
- }
- if (unlikely(kw_args > 0)) {
- const Py_ssize_t kwd_pos_args = __pyx_nargs;
- if (unlikely(__Pyx_ParseOptionalKeywords(__pyx_kwds, __pyx_kwvalues, __pyx_pyargnames, 0, values + 0, kwd_pos_args, "curves_to_quadratic") < 0)) __PYX_ERR(0, 474, __pyx_L3_error)
- }
- } else {
- switch (__pyx_nargs) {
- case 3: values[2] = __Pyx_Arg_FASTCALL(__pyx_args, 2);
- CYTHON_FALLTHROUGH;
- case 2: values[1] = __Pyx_Arg_FASTCALL(__pyx_args, 1);
- values[0] = __Pyx_Arg_FASTCALL(__pyx_args, 0);
- break;
- default: goto __pyx_L5_argtuple_error;
- }
- }
- __pyx_v_curves = values[0];
- __pyx_v_max_errors = values[1];
- if (values[2]) {
- __pyx_v_all_quadratic = __Pyx_PyInt_As_int(values[2]); if (unlikely((__pyx_v_all_quadratic == (int)-1) && PyErr_Occurred())) __PYX_ERR(0, 476, __pyx_L3_error)
- } else {
-
- /* "fontTools/cu2qu/cu2qu.py":476
- * @cython.locals(l=cython.int, last_i=cython.int, i=cython.int)
- * @cython.locals(all_quadratic=cython.int)
- * def curves_to_quadratic(curves, max_errors, all_quadratic=True): # <<<<<<<<<<<<<<
- * """Return quadratic Bezier splines approximating the input cubic Beziers.
- *
- */
- __pyx_v_all_quadratic = ((int)((int)1));
- }
- }
- goto __pyx_L4_argument_unpacking_done;
- __pyx_L5_argtuple_error:;
- __Pyx_RaiseArgtupleInvalid("curves_to_quadratic", 0, 2, 3, __pyx_nargs); __PYX_ERR(0, 474, __pyx_L3_error)
- __pyx_L3_error:;
- __Pyx_AddTraceback("fontTools.cu2qu.cu2qu.curves_to_quadratic", __pyx_clineno, __pyx_lineno, __pyx_filename);
- __Pyx_RefNannyFinishContext();
- return NULL;
- __pyx_L4_argument_unpacking_done:;
- __pyx_r = __pyx_pf_9fontTools_5cu2qu_5cu2qu_5curves_to_quadratic(__pyx_self, __pyx_v_curves, __pyx_v_max_errors, __pyx_v_all_quadratic);
-
- /* "fontTools/cu2qu/cu2qu.py":474
- *
- *
- * @cython.locals(l=cython.int, last_i=cython.int, i=cython.int) # <<<<<<<<<<<<<<
- * @cython.locals(all_quadratic=cython.int)
- * def curves_to_quadratic(curves, max_errors, all_quadratic=True):
- */
-
- /* function exit code */
- __Pyx_RefNannyFinishContext();
- return __pyx_r;
-}
-
-static PyObject *__pyx_pf_9fontTools_5cu2qu_5cu2qu_5curves_to_quadratic(CYTHON_UNUSED PyObject *__pyx_self, PyObject *__pyx_v_curves, PyObject *__pyx_v_max_errors, int __pyx_v_all_quadratic) {
- int __pyx_v_l;
- int __pyx_v_last_i;
- int __pyx_v_i;
- PyObject *__pyx_v_splines = NULL;
- PyObject *__pyx_v_n = NULL;
- PyObject *__pyx_v_spline = NULL;
- PyObject *__pyx_8genexpr2__pyx_v_curve = NULL;
- PyObject *__pyx_8genexpr3__pyx_v_p = NULL;
- PyObject *__pyx_8genexpr4__pyx_v_spline = NULL;
- PyObject *__pyx_8genexpr5__pyx_v_s = NULL;
- PyObject *__pyx_r = NULL;
- __Pyx_RefNannyDeclarations
- PyObject *__pyx_t_1 = NULL;
- PyObject *__pyx_t_2 = NULL;
- Py_ssize_t __pyx_t_3;
- PyObject *(*__pyx_t_4)(PyObject *);
- PyObject *__pyx_t_5 = NULL;
- PyObject *__pyx_t_6 = NULL;
- Py_ssize_t __pyx_t_7;
- PyObject *(*__pyx_t_8)(PyObject *);
- PyObject *__pyx_t_9 = NULL;
- PyObject *__pyx_t_10 = NULL;
- int __pyx_t_11;
- int __pyx_t_12;
- double __pyx_t_13;
- long __pyx_t_14;
- PyObject *__pyx_t_15 = NULL;
- int __pyx_lineno = 0;
- const char *__pyx_filename = NULL;
- int __pyx_clineno = 0;
- __Pyx_RefNannySetupContext("curves_to_quadratic", 0);
- __Pyx_INCREF(__pyx_v_curves);
-
- /* "fontTools/cu2qu/cu2qu.py":513
- * """
- *
- * curves = [[complex(*p) for p in curve] for curve in curves] # <<<<<<<<<<<<<<
- * assert len(max_errors) == len(curves)
- *
- */
- { /* enter inner scope */
- __pyx_t_1 = PyList_New(0); if (unlikely(!__pyx_t_1)) __PYX_ERR(0, 513, __pyx_L5_error)
- __Pyx_GOTREF(__pyx_t_1);
- if (likely(PyList_CheckExact(__pyx_v_curves)) || PyTuple_CheckExact(__pyx_v_curves)) {
- __pyx_t_2 = __pyx_v_curves; __Pyx_INCREF(__pyx_t_2); __pyx_t_3 = 0;
- __pyx_t_4 = NULL;
- } else {
- __pyx_t_3 = -1; __pyx_t_2 = PyObject_GetIter(__pyx_v_curves); if (unlikely(!__pyx_t_2)) __PYX_ERR(0, 513, __pyx_L5_error)
- __Pyx_GOTREF(__pyx_t_2);
- __pyx_t_4 = __Pyx_PyObject_GetIterNextFunc(__pyx_t_2); if (unlikely(!__pyx_t_4)) __PYX_ERR(0, 513, __pyx_L5_error)
- }
- for (;;) {
- if (likely(!__pyx_t_4)) {
- if (likely(PyList_CheckExact(__pyx_t_2))) {
- if (__pyx_t_3 >= PyList_GET_SIZE(__pyx_t_2)) break;
- #if CYTHON_ASSUME_SAFE_MACROS && !CYTHON_AVOID_BORROWED_REFS
- __pyx_t_5 = PyList_GET_ITEM(__pyx_t_2, __pyx_t_3); __Pyx_INCREF(__pyx_t_5); __pyx_t_3++; if (unlikely((0 < 0))) __PYX_ERR(0, 513, __pyx_L5_error)
- #else
- __pyx_t_5 = PySequence_ITEM(__pyx_t_2, __pyx_t_3); __pyx_t_3++; if (unlikely(!__pyx_t_5)) __PYX_ERR(0, 513, __pyx_L5_error)
- __Pyx_GOTREF(__pyx_t_5);
- #endif
- } else {
- if (__pyx_t_3 >= PyTuple_GET_SIZE(__pyx_t_2)) break;
- #if CYTHON_ASSUME_SAFE_MACROS && !CYTHON_AVOID_BORROWED_REFS
- __pyx_t_5 = PyTuple_GET_ITEM(__pyx_t_2, __pyx_t_3); __Pyx_INCREF(__pyx_t_5); __pyx_t_3++; if (unlikely((0 < 0))) __PYX_ERR(0, 513, __pyx_L5_error)
- #else
- __pyx_t_5 = PySequence_ITEM(__pyx_t_2, __pyx_t_3); __pyx_t_3++; if (unlikely(!__pyx_t_5)) __PYX_ERR(0, 513, __pyx_L5_error)
- __Pyx_GOTREF(__pyx_t_5);
- #endif
- }
- } else {
- __pyx_t_5 = __pyx_t_4(__pyx_t_2);
- if (unlikely(!__pyx_t_5)) {
- PyObject* exc_type = PyErr_Occurred();
- if (exc_type) {
- if (likely(__Pyx_PyErr_GivenExceptionMatches(exc_type, PyExc_StopIteration))) PyErr_Clear();
- else __PYX_ERR(0, 513, __pyx_L5_error)
- }
- break;
- }
- __Pyx_GOTREF(__pyx_t_5);
- }
- __Pyx_XDECREF_SET(__pyx_8genexpr2__pyx_v_curve, __pyx_t_5);
- __pyx_t_5 = 0;
- { /* enter inner scope */
- __pyx_t_5 = PyList_New(0); if (unlikely(!__pyx_t_5)) __PYX_ERR(0, 513, __pyx_L10_error)
- __Pyx_GOTREF(__pyx_t_5);
- if (likely(PyList_CheckExact(__pyx_8genexpr2__pyx_v_curve)) || PyTuple_CheckExact(__pyx_8genexpr2__pyx_v_curve)) {
- __pyx_t_6 = __pyx_8genexpr2__pyx_v_curve; __Pyx_INCREF(__pyx_t_6); __pyx_t_7 = 0;
- __pyx_t_8 = NULL;
- } else {
- __pyx_t_7 = -1; __pyx_t_6 = PyObject_GetIter(__pyx_8genexpr2__pyx_v_curve); if (unlikely(!__pyx_t_6)) __PYX_ERR(0, 513, __pyx_L10_error)
- __Pyx_GOTREF(__pyx_t_6);
- __pyx_t_8 = __Pyx_PyObject_GetIterNextFunc(__pyx_t_6); if (unlikely(!__pyx_t_8)) __PYX_ERR(0, 513, __pyx_L10_error)
- }
- for (;;) {
- if (likely(!__pyx_t_8)) {
- if (likely(PyList_CheckExact(__pyx_t_6))) {
- if (__pyx_t_7 >= PyList_GET_SIZE(__pyx_t_6)) break;
- #if CYTHON_ASSUME_SAFE_MACROS && !CYTHON_AVOID_BORROWED_REFS
- __pyx_t_9 = PyList_GET_ITEM(__pyx_t_6, __pyx_t_7); __Pyx_INCREF(__pyx_t_9); __pyx_t_7++; if (unlikely((0 < 0))) __PYX_ERR(0, 513, __pyx_L10_error)
- #else
- __pyx_t_9 = PySequence_ITEM(__pyx_t_6, __pyx_t_7); __pyx_t_7++; if (unlikely(!__pyx_t_9)) __PYX_ERR(0, 513, __pyx_L10_error)
- __Pyx_GOTREF(__pyx_t_9);
- #endif
- } else {
- if (__pyx_t_7 >= PyTuple_GET_SIZE(__pyx_t_6)) break;
- #if CYTHON_ASSUME_SAFE_MACROS && !CYTHON_AVOID_BORROWED_REFS
- __pyx_t_9 = PyTuple_GET_ITEM(__pyx_t_6, __pyx_t_7); __Pyx_INCREF(__pyx_t_9); __pyx_t_7++; if (unlikely((0 < 0))) __PYX_ERR(0, 513, __pyx_L10_error)
- #else
- __pyx_t_9 = PySequence_ITEM(__pyx_t_6, __pyx_t_7); __pyx_t_7++; if (unlikely(!__pyx_t_9)) __PYX_ERR(0, 513, __pyx_L10_error)
- __Pyx_GOTREF(__pyx_t_9);
- #endif
- }
- } else {
- __pyx_t_9 = __pyx_t_8(__pyx_t_6);
- if (unlikely(!__pyx_t_9)) {
- PyObject* exc_type = PyErr_Occurred();
- if (exc_type) {
- if (likely(__Pyx_PyErr_GivenExceptionMatches(exc_type, PyExc_StopIteration))) PyErr_Clear();
- else __PYX_ERR(0, 513, __pyx_L10_error)
- }
- break;
- }
- __Pyx_GOTREF(__pyx_t_9);
- }
- __Pyx_XDECREF_SET(__pyx_8genexpr3__pyx_v_p, __pyx_t_9);
- __pyx_t_9 = 0;
- __pyx_t_9 = __Pyx_PySequence_Tuple(__pyx_8genexpr3__pyx_v_p); if (unlikely(!__pyx_t_9)) __PYX_ERR(0, 513, __pyx_L10_error)
- __Pyx_GOTREF(__pyx_t_9);
- __pyx_t_10 = __Pyx_PyObject_Call(((PyObject *)(&PyComplex_Type)), __pyx_t_9, NULL); if (unlikely(!__pyx_t_10)) __PYX_ERR(0, 513, __pyx_L10_error)
- __Pyx_GOTREF(__pyx_t_10);
- __Pyx_DECREF(__pyx_t_9); __pyx_t_9 = 0;
- if (unlikely(__Pyx_ListComp_Append(__pyx_t_5, (PyObject*)__pyx_t_10))) __PYX_ERR(0, 513, __pyx_L10_error)
- __Pyx_DECREF(__pyx_t_10); __pyx_t_10 = 0;
- }
- __Pyx_DECREF(__pyx_t_6); __pyx_t_6 = 0;
- __Pyx_XDECREF(__pyx_8genexpr3__pyx_v_p); __pyx_8genexpr3__pyx_v_p = 0;
- goto __pyx_L14_exit_scope;
- __pyx_L10_error:;
- __Pyx_XDECREF(__pyx_8genexpr3__pyx_v_p); __pyx_8genexpr3__pyx_v_p = 0;
- goto __pyx_L5_error;
- __pyx_L14_exit_scope:;
- } /* exit inner scope */
- if (unlikely(__Pyx_ListComp_Append(__pyx_t_1, (PyObject*)__pyx_t_5))) __PYX_ERR(0, 513, __pyx_L5_error)
- __Pyx_DECREF(__pyx_t_5); __pyx_t_5 = 0;
- }
- __Pyx_DECREF(__pyx_t_2); __pyx_t_2 = 0;
- __Pyx_XDECREF(__pyx_8genexpr2__pyx_v_curve); __pyx_8genexpr2__pyx_v_curve = 0;
- goto __pyx_L16_exit_scope;
- __pyx_L5_error:;
- __Pyx_XDECREF(__pyx_8genexpr2__pyx_v_curve); __pyx_8genexpr2__pyx_v_curve = 0;
- goto __pyx_L1_error;
- __pyx_L16_exit_scope:;
- } /* exit inner scope */
- __Pyx_DECREF_SET(__pyx_v_curves, __pyx_t_1);
- __pyx_t_1 = 0;
-
- /* "fontTools/cu2qu/cu2qu.py":514
- *
- * curves = [[complex(*p) for p in curve] for curve in curves]
- * assert len(max_errors) == len(curves) # <<<<<<<<<<<<<<
- *
- * l = len(curves)
- */
- #ifndef CYTHON_WITHOUT_ASSERTIONS
- if (unlikely(__pyx_assertions_enabled())) {
- __pyx_t_3 = PyObject_Length(__pyx_v_max_errors); if (unlikely(__pyx_t_3 == ((Py_ssize_t)-1))) __PYX_ERR(0, 514, __pyx_L1_error)
- __pyx_t_7 = PyObject_Length(__pyx_v_curves); if (unlikely(__pyx_t_7 == ((Py_ssize_t)-1))) __PYX_ERR(0, 514, __pyx_L1_error)
- __pyx_t_11 = (__pyx_t_3 == __pyx_t_7);
- if (unlikely(!__pyx_t_11)) {
- __Pyx_Raise(__pyx_builtin_AssertionError, 0, 0, 0);
- __PYX_ERR(0, 514, __pyx_L1_error)
- }
- }
- #else
- if ((1)); else __PYX_ERR(0, 514, __pyx_L1_error)
- #endif
-
- /* "fontTools/cu2qu/cu2qu.py":516
- * assert len(max_errors) == len(curves)
- *
- * l = len(curves) # <<<<<<<<<<<<<<
- * splines = [None] * l
- * last_i = i = 0
- */
- __pyx_t_7 = PyObject_Length(__pyx_v_curves); if (unlikely(__pyx_t_7 == ((Py_ssize_t)-1))) __PYX_ERR(0, 516, __pyx_L1_error)
- __pyx_v_l = __pyx_t_7;
-
- /* "fontTools/cu2qu/cu2qu.py":517
- *
- * l = len(curves)
- * splines = [None] * l # <<<<<<<<<<<<<<
- * last_i = i = 0
- * n = 1
- */
- __pyx_t_1 = PyList_New(1 * ((__pyx_v_l<0) ? 0:__pyx_v_l)); if (unlikely(!__pyx_t_1)) __PYX_ERR(0, 517, __pyx_L1_error)
- __Pyx_GOTREF(__pyx_t_1);
- { Py_ssize_t __pyx_temp;
- for (__pyx_temp=0; __pyx_temp < __pyx_v_l; __pyx_temp++) {
- __Pyx_INCREF(Py_None);
- __Pyx_GIVEREF(Py_None);
- PyList_SET_ITEM(__pyx_t_1, __pyx_temp, Py_None);
- }
- }
- __pyx_v_splines = ((PyObject*)__pyx_t_1);
- __pyx_t_1 = 0;
-
- /* "fontTools/cu2qu/cu2qu.py":518
- * l = len(curves)
- * splines = [None] * l
- * last_i = i = 0 # <<<<<<<<<<<<<<
- * n = 1
- * while True:
- */
- __pyx_v_last_i = 0;
- __pyx_v_i = 0;
-
- /* "fontTools/cu2qu/cu2qu.py":519
- * splines = [None] * l
- * last_i = i = 0
- * n = 1 # <<<<<<<<<<<<<<
- * while True:
- * spline = cubic_approx_spline(curves[i], n, max_errors[i], all_quadratic)
- */
- __Pyx_INCREF(__pyx_int_1);
- __pyx_v_n = __pyx_int_1;
-
- /* "fontTools/cu2qu/cu2qu.py":520
- * last_i = i = 0
- * n = 1
- * while True: # <<<<<<<<<<<<<<
- * spline = cubic_approx_spline(curves[i], n, max_errors[i], all_quadratic)
- * if spline is None:
- */
- while (1) {
-
- /* "fontTools/cu2qu/cu2qu.py":521
- * n = 1
- * while True:
- * spline = cubic_approx_spline(curves[i], n, max_errors[i], all_quadratic) # <<<<<<<<<<<<<<
- * if spline is None:
- * if n == MAX_N:
- */
- __pyx_t_1 = __Pyx_GetItemInt(__pyx_v_curves, __pyx_v_i, int, 1, __Pyx_PyInt_From_int, 0, 1, 1); if (unlikely(!__pyx_t_1)) __PYX_ERR(0, 521, __pyx_L1_error)
- __Pyx_GOTREF(__pyx_t_1);
- __pyx_t_12 = __Pyx_PyInt_As_int(__pyx_v_n); if (unlikely((__pyx_t_12 == (int)-1) && PyErr_Occurred())) __PYX_ERR(0, 521, __pyx_L1_error)
- __pyx_t_2 = __Pyx_GetItemInt(__pyx_v_max_errors, __pyx_v_i, int, 1, __Pyx_PyInt_From_int, 0, 1, 1); if (unlikely(!__pyx_t_2)) __PYX_ERR(0, 521, __pyx_L1_error)
- __Pyx_GOTREF(__pyx_t_2);
- __pyx_t_13 = __pyx_PyFloat_AsDouble(__pyx_t_2); if (unlikely((__pyx_t_13 == (double)-1) && PyErr_Occurred())) __PYX_ERR(0, 521, __pyx_L1_error)
- __Pyx_DECREF(__pyx_t_2); __pyx_t_2 = 0;
- __pyx_t_2 = __pyx_f_9fontTools_5cu2qu_5cu2qu_cubic_approx_spline(__pyx_t_1, __pyx_t_12, __pyx_t_13, __pyx_v_all_quadratic); if (unlikely(!__pyx_t_2)) __PYX_ERR(0, 521, __pyx_L1_error)
- __Pyx_GOTREF(__pyx_t_2);
- __Pyx_DECREF(__pyx_t_1); __pyx_t_1 = 0;
- __Pyx_XDECREF_SET(__pyx_v_spline, __pyx_t_2);
- __pyx_t_2 = 0;
-
- /* "fontTools/cu2qu/cu2qu.py":522
- * while True:
- * spline = cubic_approx_spline(curves[i], n, max_errors[i], all_quadratic)
- * if spline is None: # <<<<<<<<<<<<<<
- * if n == MAX_N:
- * break
- */
- __pyx_t_11 = (__pyx_v_spline == Py_None);
- if (__pyx_t_11) {
-
- /* "fontTools/cu2qu/cu2qu.py":523
- * spline = cubic_approx_spline(curves[i], n, max_errors[i], all_quadratic)
- * if spline is None:
- * if n == MAX_N: # <<<<<<<<<<<<<<
- * break
- * n += 1
- */
- __Pyx_GetModuleGlobalName(__pyx_t_2, __pyx_n_s_MAX_N); if (unlikely(!__pyx_t_2)) __PYX_ERR(0, 523, __pyx_L1_error)
- __Pyx_GOTREF(__pyx_t_2);
- __pyx_t_1 = PyObject_RichCompare(__pyx_v_n, __pyx_t_2, Py_EQ); __Pyx_XGOTREF(__pyx_t_1); if (unlikely(!__pyx_t_1)) __PYX_ERR(0, 523, __pyx_L1_error)
- __Pyx_DECREF(__pyx_t_2); __pyx_t_2 = 0;
- __pyx_t_11 = __Pyx_PyObject_IsTrue(__pyx_t_1); if (unlikely((__pyx_t_11 < 0))) __PYX_ERR(0, 523, __pyx_L1_error)
- __Pyx_DECREF(__pyx_t_1); __pyx_t_1 = 0;
- if (__pyx_t_11) {
-
- /* "fontTools/cu2qu/cu2qu.py":524
- * if spline is None:
- * if n == MAX_N:
- * break # <<<<<<<<<<<<<<
- * n += 1
- * last_i = i
- */
- goto __pyx_L18_break;
-
- /* "fontTools/cu2qu/cu2qu.py":523
- * spline = cubic_approx_spline(curves[i], n, max_errors[i], all_quadratic)
- * if spline is None:
- * if n == MAX_N: # <<<<<<<<<<<<<<
- * break
- * n += 1
- */
- }
-
- /* "fontTools/cu2qu/cu2qu.py":525
- * if n == MAX_N:
- * break
- * n += 1 # <<<<<<<<<<<<<<
- * last_i = i
- * continue
- */
- __pyx_t_1 = __Pyx_PyInt_AddObjC(__pyx_v_n, __pyx_int_1, 1, 1, 0); if (unlikely(!__pyx_t_1)) __PYX_ERR(0, 525, __pyx_L1_error)
- __Pyx_GOTREF(__pyx_t_1);
- __Pyx_DECREF_SET(__pyx_v_n, __pyx_t_1);
- __pyx_t_1 = 0;
-
- /* "fontTools/cu2qu/cu2qu.py":526
- * break
- * n += 1
- * last_i = i # <<<<<<<<<<<<<<
- * continue
- * splines[i] = spline
- */
- __pyx_v_last_i = __pyx_v_i;
-
- /* "fontTools/cu2qu/cu2qu.py":527
- * n += 1
- * last_i = i
- * continue # <<<<<<<<<<<<<<
- * splines[i] = spline
- * i = (i + 1) % l
- */
- goto __pyx_L17_continue;
-
- /* "fontTools/cu2qu/cu2qu.py":522
- * while True:
- * spline = cubic_approx_spline(curves[i], n, max_errors[i], all_quadratic)
- * if spline is None: # <<<<<<<<<<<<<<
- * if n == MAX_N:
- * break
- */
- }
-
- /* "fontTools/cu2qu/cu2qu.py":528
- * last_i = i
- * continue
- * splines[i] = spline # <<<<<<<<<<<<<<
- * i = (i + 1) % l
- * if i == last_i:
- */
- if (unlikely((__Pyx_SetItemInt(__pyx_v_splines, __pyx_v_i, __pyx_v_spline, int, 1, __Pyx_PyInt_From_int, 1, 1, 1) < 0))) __PYX_ERR(0, 528, __pyx_L1_error)
-
- /* "fontTools/cu2qu/cu2qu.py":529
- * continue
- * splines[i] = spline
- * i = (i + 1) % l # <<<<<<<<<<<<<<
- * if i == last_i:
- * # done. go home
- */
- __pyx_t_14 = (__pyx_v_i + 1);
- if (unlikely(__pyx_v_l == 0)) {
- PyErr_SetString(PyExc_ZeroDivisionError, "integer division or modulo by zero");
- __PYX_ERR(0, 529, __pyx_L1_error)
- }
- __pyx_v_i = __Pyx_mod_long(__pyx_t_14, __pyx_v_l);
-
- /* "fontTools/cu2qu/cu2qu.py":530
- * splines[i] = spline
- * i = (i + 1) % l
- * if i == last_i: # <<<<<<<<<<<<<<
- * # done. go home
- * return [[(s.real, s.imag) for s in spline] for spline in splines]
- */
- __pyx_t_11 = (__pyx_v_i == __pyx_v_last_i);
- if (__pyx_t_11) {
-
- /* "fontTools/cu2qu/cu2qu.py":532
- * if i == last_i:
- * # done. go home
- * return [[(s.real, s.imag) for s in spline] for spline in splines] # <<<<<<<<<<<<<<
- *
- * raise ApproxNotFoundError(curves)
- */
- __Pyx_XDECREF(__pyx_r);
- { /* enter inner scope */
- __pyx_t_1 = PyList_New(0); if (unlikely(!__pyx_t_1)) __PYX_ERR(0, 532, __pyx_L24_error)
- __Pyx_GOTREF(__pyx_t_1);
- __pyx_t_2 = __pyx_v_splines; __Pyx_INCREF(__pyx_t_2); __pyx_t_7 = 0;
- for (;;) {
- if (__pyx_t_7 >= PyList_GET_SIZE(__pyx_t_2)) break;
- #if CYTHON_ASSUME_SAFE_MACROS && !CYTHON_AVOID_BORROWED_REFS
- __pyx_t_5 = PyList_GET_ITEM(__pyx_t_2, __pyx_t_7); __Pyx_INCREF(__pyx_t_5); __pyx_t_7++; if (unlikely((0 < 0))) __PYX_ERR(0, 532, __pyx_L24_error)
- #else
- __pyx_t_5 = PySequence_ITEM(__pyx_t_2, __pyx_t_7); __pyx_t_7++; if (unlikely(!__pyx_t_5)) __PYX_ERR(0, 532, __pyx_L24_error)
- __Pyx_GOTREF(__pyx_t_5);
- #endif
- __Pyx_XDECREF_SET(__pyx_8genexpr4__pyx_v_spline, __pyx_t_5);
- __pyx_t_5 = 0;
- { /* enter inner scope */
- __pyx_t_5 = PyList_New(0); if (unlikely(!__pyx_t_5)) __PYX_ERR(0, 532, __pyx_L29_error)
- __Pyx_GOTREF(__pyx_t_5);
- if (likely(PyList_CheckExact(__pyx_8genexpr4__pyx_v_spline)) || PyTuple_CheckExact(__pyx_8genexpr4__pyx_v_spline)) {
- __pyx_t_6 = __pyx_8genexpr4__pyx_v_spline; __Pyx_INCREF(__pyx_t_6); __pyx_t_3 = 0;
- __pyx_t_4 = NULL;
- } else {
- __pyx_t_3 = -1; __pyx_t_6 = PyObject_GetIter(__pyx_8genexpr4__pyx_v_spline); if (unlikely(!__pyx_t_6)) __PYX_ERR(0, 532, __pyx_L29_error)
- __Pyx_GOTREF(__pyx_t_6);
- __pyx_t_4 = __Pyx_PyObject_GetIterNextFunc(__pyx_t_6); if (unlikely(!__pyx_t_4)) __PYX_ERR(0, 532, __pyx_L29_error)
- }
- for (;;) {
- if (likely(!__pyx_t_4)) {
- if (likely(PyList_CheckExact(__pyx_t_6))) {
- if (__pyx_t_3 >= PyList_GET_SIZE(__pyx_t_6)) break;
- #if CYTHON_ASSUME_SAFE_MACROS && !CYTHON_AVOID_BORROWED_REFS
- __pyx_t_10 = PyList_GET_ITEM(__pyx_t_6, __pyx_t_3); __Pyx_INCREF(__pyx_t_10); __pyx_t_3++; if (unlikely((0 < 0))) __PYX_ERR(0, 532, __pyx_L29_error)
- #else
- __pyx_t_10 = PySequence_ITEM(__pyx_t_6, __pyx_t_3); __pyx_t_3++; if (unlikely(!__pyx_t_10)) __PYX_ERR(0, 532, __pyx_L29_error)
- __Pyx_GOTREF(__pyx_t_10);
- #endif
- } else {
- if (__pyx_t_3 >= PyTuple_GET_SIZE(__pyx_t_6)) break;
- #if CYTHON_ASSUME_SAFE_MACROS && !CYTHON_AVOID_BORROWED_REFS
- __pyx_t_10 = PyTuple_GET_ITEM(__pyx_t_6, __pyx_t_3); __Pyx_INCREF(__pyx_t_10); __pyx_t_3++; if (unlikely((0 < 0))) __PYX_ERR(0, 532, __pyx_L29_error)
- #else
- __pyx_t_10 = PySequence_ITEM(__pyx_t_6, __pyx_t_3); __pyx_t_3++; if (unlikely(!__pyx_t_10)) __PYX_ERR(0, 532, __pyx_L29_error)
- __Pyx_GOTREF(__pyx_t_10);
- #endif
- }
- } else {
- __pyx_t_10 = __pyx_t_4(__pyx_t_6);
- if (unlikely(!__pyx_t_10)) {
- PyObject* exc_type = PyErr_Occurred();
- if (exc_type) {
- if (likely(__Pyx_PyErr_GivenExceptionMatches(exc_type, PyExc_StopIteration))) PyErr_Clear();
- else __PYX_ERR(0, 532, __pyx_L29_error)
- }
- break;
- }
- __Pyx_GOTREF(__pyx_t_10);
- }
- __Pyx_XDECREF_SET(__pyx_8genexpr5__pyx_v_s, __pyx_t_10);
- __pyx_t_10 = 0;
- __pyx_t_10 = __Pyx_PyObject_GetAttrStr(__pyx_8genexpr5__pyx_v_s, __pyx_n_s_real); if (unlikely(!__pyx_t_10)) __PYX_ERR(0, 532, __pyx_L29_error)
- __Pyx_GOTREF(__pyx_t_10);
- __pyx_t_9 = __Pyx_PyObject_GetAttrStr(__pyx_8genexpr5__pyx_v_s, __pyx_n_s_imag); if (unlikely(!__pyx_t_9)) __PYX_ERR(0, 532, __pyx_L29_error)
- __Pyx_GOTREF(__pyx_t_9);
- __pyx_t_15 = PyTuple_New(2); if (unlikely(!__pyx_t_15)) __PYX_ERR(0, 532, __pyx_L29_error)
- __Pyx_GOTREF(__pyx_t_15);
- __Pyx_GIVEREF(__pyx_t_10);
- PyTuple_SET_ITEM(__pyx_t_15, 0, __pyx_t_10);
- __Pyx_GIVEREF(__pyx_t_9);
- PyTuple_SET_ITEM(__pyx_t_15, 1, __pyx_t_9);
- __pyx_t_10 = 0;
- __pyx_t_9 = 0;
- if (unlikely(__Pyx_ListComp_Append(__pyx_t_5, (PyObject*)__pyx_t_15))) __PYX_ERR(0, 532, __pyx_L29_error)
- __Pyx_DECREF(__pyx_t_15); __pyx_t_15 = 0;
- }
- __Pyx_DECREF(__pyx_t_6); __pyx_t_6 = 0;
- __Pyx_XDECREF(__pyx_8genexpr5__pyx_v_s); __pyx_8genexpr5__pyx_v_s = 0;
- goto __pyx_L33_exit_scope;
- __pyx_L29_error:;
- __Pyx_XDECREF(__pyx_8genexpr5__pyx_v_s); __pyx_8genexpr5__pyx_v_s = 0;
- goto __pyx_L24_error;
- __pyx_L33_exit_scope:;
- } /* exit inner scope */
- if (unlikely(__Pyx_ListComp_Append(__pyx_t_1, (PyObject*)__pyx_t_5))) __PYX_ERR(0, 532, __pyx_L24_error)
- __Pyx_DECREF(__pyx_t_5); __pyx_t_5 = 0;
- }
- __Pyx_DECREF(__pyx_t_2); __pyx_t_2 = 0;
- __Pyx_XDECREF(__pyx_8genexpr4__pyx_v_spline); __pyx_8genexpr4__pyx_v_spline = 0;
- goto __pyx_L35_exit_scope;
- __pyx_L24_error:;
- __Pyx_XDECREF(__pyx_8genexpr4__pyx_v_spline); __pyx_8genexpr4__pyx_v_spline = 0;
- goto __pyx_L1_error;
- __pyx_L35_exit_scope:;
- } /* exit inner scope */
- __pyx_r = __pyx_t_1;
- __pyx_t_1 = 0;
- goto __pyx_L0;
-
- /* "fontTools/cu2qu/cu2qu.py":530
- * splines[i] = spline
- * i = (i + 1) % l
- * if i == last_i: # <<<<<<<<<<<<<<
- * # done. go home
- * return [[(s.real, s.imag) for s in spline] for spline in splines]
- */
- }
- __pyx_L17_continue:;
- }
- __pyx_L18_break:;
-
- /* "fontTools/cu2qu/cu2qu.py":534
- * return [[(s.real, s.imag) for s in spline] for spline in splines]
- *
- * raise ApproxNotFoundError(curves) # <<<<<<<<<<<<<<
- */
- __Pyx_GetModuleGlobalName(__pyx_t_2, __pyx_n_s_ApproxNotFoundError); if (unlikely(!__pyx_t_2)) __PYX_ERR(0, 534, __pyx_L1_error)
- __Pyx_GOTREF(__pyx_t_2);
- __pyx_t_5 = NULL;
- __pyx_t_12 = 0;
- if (CYTHON_UNPACK_METHODS && unlikely(PyMethod_Check(__pyx_t_2))) {
- __pyx_t_5 = PyMethod_GET_SELF(__pyx_t_2);
- if (likely(__pyx_t_5)) {
- PyObject* function = PyMethod_GET_FUNCTION(__pyx_t_2);
- __Pyx_INCREF(__pyx_t_5);
- __Pyx_INCREF(function);
- __Pyx_DECREF_SET(__pyx_t_2, function);
- __pyx_t_12 = 1;
- }
- }
- {
- PyObject *__pyx_callargs[2] = {__pyx_t_5, __pyx_v_curves};
- __pyx_t_1 = __Pyx_PyObject_FastCall(__pyx_t_2, __pyx_callargs+1-__pyx_t_12, 1+__pyx_t_12);
- __Pyx_XDECREF(__pyx_t_5); __pyx_t_5 = 0;
- if (unlikely(!__pyx_t_1)) __PYX_ERR(0, 534, __pyx_L1_error)
- __Pyx_GOTREF(__pyx_t_1);
- __Pyx_DECREF(__pyx_t_2); __pyx_t_2 = 0;
- }
- __Pyx_Raise(__pyx_t_1, 0, 0, 0);
- __Pyx_DECREF(__pyx_t_1); __pyx_t_1 = 0;
- __PYX_ERR(0, 534, __pyx_L1_error)
-
- /* "fontTools/cu2qu/cu2qu.py":474
- *
- *
- * @cython.locals(l=cython.int, last_i=cython.int, i=cython.int) # <<<<<<<<<<<<<<
- * @cython.locals(all_quadratic=cython.int)
- * def curves_to_quadratic(curves, max_errors, all_quadratic=True):
- */
-
- /* function exit code */
- __pyx_L1_error:;
- __Pyx_XDECREF(__pyx_t_1);
- __Pyx_XDECREF(__pyx_t_2);
- __Pyx_XDECREF(__pyx_t_5);
- __Pyx_XDECREF(__pyx_t_6);
- __Pyx_XDECREF(__pyx_t_9);
- __Pyx_XDECREF(__pyx_t_10);
- __Pyx_XDECREF(__pyx_t_15);
- __Pyx_AddTraceback("fontTools.cu2qu.cu2qu.curves_to_quadratic", __pyx_clineno, __pyx_lineno, __pyx_filename);
- __pyx_r = NULL;
- __pyx_L0:;
- __Pyx_XDECREF(__pyx_v_splines);
- __Pyx_XDECREF(__pyx_v_n);
- __Pyx_XDECREF(__pyx_v_spline);
- __Pyx_XDECREF(__pyx_8genexpr2__pyx_v_curve);
- __Pyx_XDECREF(__pyx_8genexpr3__pyx_v_p);
- __Pyx_XDECREF(__pyx_8genexpr4__pyx_v_spline);
- __Pyx_XDECREF(__pyx_8genexpr5__pyx_v_s);
- __Pyx_XDECREF(__pyx_v_curves);
- __Pyx_XGIVEREF(__pyx_r);
- __Pyx_RefNannyFinishContext();
- return __pyx_r;
-}
-
-static struct __pyx_obj_9fontTools_5cu2qu_5cu2qu___pyx_scope_struct___split_cubic_into_n_gen *__pyx_freelist_9fontTools_5cu2qu_5cu2qu___pyx_scope_struct___split_cubic_into_n_gen[8];
-static int __pyx_freecount_9fontTools_5cu2qu_5cu2qu___pyx_scope_struct___split_cubic_into_n_gen = 0;
-
-static PyObject *__pyx_tp_new_9fontTools_5cu2qu_5cu2qu___pyx_scope_struct___split_cubic_into_n_gen(PyTypeObject *t, CYTHON_UNUSED PyObject *a, CYTHON_UNUSED PyObject *k) {
- PyObject *o;
- #if CYTHON_COMPILING_IN_LIMITED_API
- allocfunc alloc_func = (allocfunc)PyType_GetSlot(t, Py_tp_alloc);
- o = alloc_func(t, 0);
- #else
- if (CYTHON_COMPILING_IN_CPYTHON && likely((int)(__pyx_freecount_9fontTools_5cu2qu_5cu2qu___pyx_scope_struct___split_cubic_into_n_gen > 0) & (int)(t->tp_basicsize == sizeof(struct __pyx_obj_9fontTools_5cu2qu_5cu2qu___pyx_scope_struct___split_cubic_into_n_gen)))) {
- o = (PyObject*)__pyx_freelist_9fontTools_5cu2qu_5cu2qu___pyx_scope_struct___split_cubic_into_n_gen[--__pyx_freecount_9fontTools_5cu2qu_5cu2qu___pyx_scope_struct___split_cubic_into_n_gen];
- memset(o, 0, sizeof(struct __pyx_obj_9fontTools_5cu2qu_5cu2qu___pyx_scope_struct___split_cubic_into_n_gen));
- (void) PyObject_INIT(o, t);
- } else {
- o = (*t->tp_alloc)(t, 0);
- if (unlikely(!o)) return 0;
- }
- #endif
- return o;
-}
-
-static void __pyx_tp_dealloc_9fontTools_5cu2qu_5cu2qu___pyx_scope_struct___split_cubic_into_n_gen(PyObject *o) {
- #if CYTHON_USE_TP_FINALIZE
- if (unlikely((PY_VERSION_HEX >= 0x03080000 || __Pyx_PyType_HasFeature(Py_TYPE(o), Py_TPFLAGS_HAVE_FINALIZE)) && __Pyx_PyObject_GetSlot(o, tp_finalize, destructor)) && (!PyType_IS_GC(Py_TYPE(o)) || !__Pyx_PyObject_GC_IsFinalized(o))) {
- if (__Pyx_PyObject_GetSlot(o, tp_dealloc, destructor) == __pyx_tp_dealloc_9fontTools_5cu2qu_5cu2qu___pyx_scope_struct___split_cubic_into_n_gen) {
- if (PyObject_CallFinalizerFromDealloc(o)) return;
- }
- }
- #endif
- if (CYTHON_COMPILING_IN_CPYTHON && ((int)(__pyx_freecount_9fontTools_5cu2qu_5cu2qu___pyx_scope_struct___split_cubic_into_n_gen < 8) & (int)(Py_TYPE(o)->tp_basicsize == sizeof(struct __pyx_obj_9fontTools_5cu2qu_5cu2qu___pyx_scope_struct___split_cubic_into_n_gen)))) {
- __pyx_freelist_9fontTools_5cu2qu_5cu2qu___pyx_scope_struct___split_cubic_into_n_gen[__pyx_freecount_9fontTools_5cu2qu_5cu2qu___pyx_scope_struct___split_cubic_into_n_gen++] = ((struct __pyx_obj_9fontTools_5cu2qu_5cu2qu___pyx_scope_struct___split_cubic_into_n_gen *)o);
- } else {
- (*Py_TYPE(o)->tp_free)(o);
- }
-}
-#if CYTHON_USE_TYPE_SPECS
-static PyType_Slot __pyx_type_9fontTools_5cu2qu_5cu2qu___pyx_scope_struct___split_cubic_into_n_gen_slots[] = {
- {Py_tp_dealloc, (void *)__pyx_tp_dealloc_9fontTools_5cu2qu_5cu2qu___pyx_scope_struct___split_cubic_into_n_gen},
- {Py_tp_new, (void *)__pyx_tp_new_9fontTools_5cu2qu_5cu2qu___pyx_scope_struct___split_cubic_into_n_gen},
- {0, 0},
-};
-static PyType_Spec __pyx_type_9fontTools_5cu2qu_5cu2qu___pyx_scope_struct___split_cubic_into_n_gen_spec = {
- "fontTools.cu2qu.cu2qu.__pyx_scope_struct___split_cubic_into_n_gen",
- sizeof(struct __pyx_obj_9fontTools_5cu2qu_5cu2qu___pyx_scope_struct___split_cubic_into_n_gen),
- 0,
- Py_TPFLAGS_DEFAULT|Py_TPFLAGS_HAVE_VERSION_TAG|Py_TPFLAGS_CHECKTYPES|Py_TPFLAGS_HAVE_NEWBUFFER|Py_TPFLAGS_HAVE_FINALIZE,
- __pyx_type_9fontTools_5cu2qu_5cu2qu___pyx_scope_struct___split_cubic_into_n_gen_slots,
-};
-#else
-
-static PyTypeObject __pyx_type_9fontTools_5cu2qu_5cu2qu___pyx_scope_struct___split_cubic_into_n_gen = {
- PyVarObject_HEAD_INIT(0, 0)
- "fontTools.cu2qu.cu2qu.""__pyx_scope_struct___split_cubic_into_n_gen", /*tp_name*/
- sizeof(struct __pyx_obj_9fontTools_5cu2qu_5cu2qu___pyx_scope_struct___split_cubic_into_n_gen), /*tp_basicsize*/
- 0, /*tp_itemsize*/
- __pyx_tp_dealloc_9fontTools_5cu2qu_5cu2qu___pyx_scope_struct___split_cubic_into_n_gen, /*tp_dealloc*/
- #if PY_VERSION_HEX < 0x030800b4
- 0, /*tp_print*/
- #endif
- #if PY_VERSION_HEX >= 0x030800b4
- 0, /*tp_vectorcall_offset*/
- #endif
- 0, /*tp_getattr*/
- 0, /*tp_setattr*/
- #if PY_MAJOR_VERSION < 3
- 0, /*tp_compare*/
- #endif
- #if PY_MAJOR_VERSION >= 3
- 0, /*tp_as_async*/
- #endif
- 0, /*tp_repr*/
- 0, /*tp_as_number*/
- 0, /*tp_as_sequence*/
- 0, /*tp_as_mapping*/
- 0, /*tp_hash*/
- 0, /*tp_call*/
- 0, /*tp_str*/
- 0, /*tp_getattro*/
- 0, /*tp_setattro*/
- 0, /*tp_as_buffer*/
- Py_TPFLAGS_DEFAULT|Py_TPFLAGS_HAVE_VERSION_TAG|Py_TPFLAGS_CHECKTYPES|Py_TPFLAGS_HAVE_NEWBUFFER|Py_TPFLAGS_HAVE_FINALIZE, /*tp_flags*/
- 0, /*tp_doc*/
- 0, /*tp_traverse*/
- 0, /*tp_clear*/
- 0, /*tp_richcompare*/
- 0, /*tp_weaklistoffset*/
- 0, /*tp_iter*/
- 0, /*tp_iternext*/
- 0, /*tp_methods*/
- 0, /*tp_members*/
- 0, /*tp_getset*/
- 0, /*tp_base*/
- 0, /*tp_dict*/
- 0, /*tp_descr_get*/
- 0, /*tp_descr_set*/
- #if !CYTHON_USE_TYPE_SPECS
- 0, /*tp_dictoffset*/
- #endif
- 0, /*tp_init*/
- 0, /*tp_alloc*/
- __pyx_tp_new_9fontTools_5cu2qu_5cu2qu___pyx_scope_struct___split_cubic_into_n_gen, /*tp_new*/
- 0, /*tp_free*/
- 0, /*tp_is_gc*/
- 0, /*tp_bases*/
- 0, /*tp_mro*/
- 0, /*tp_cache*/
- 0, /*tp_subclasses*/
- 0, /*tp_weaklist*/
- 0, /*tp_del*/
- 0, /*tp_version_tag*/
- #if PY_VERSION_HEX >= 0x030400a1
- #if CYTHON_USE_TP_FINALIZE
- 0, /*tp_finalize*/
- #else
- NULL, /*tp_finalize*/
- #endif
- #endif
- #if PY_VERSION_HEX >= 0x030800b1 && (!CYTHON_COMPILING_IN_PYPY || PYPY_VERSION_NUM >= 0x07030800)
- 0, /*tp_vectorcall*/
- #endif
- #if __PYX_NEED_TP_PRINT_SLOT == 1
- 0, /*tp_print*/
- #endif
- #if PY_VERSION_HEX >= 0x030C0000
- 0, /*tp_watched*/
- #endif
- #if CYTHON_COMPILING_IN_PYPY && PY_VERSION_HEX >= 0x03090000 && PY_VERSION_HEX < 0x030a0000
- 0, /*tp_pypy_flags*/
- #endif
-};
-#endif
-
-static PyMethodDef __pyx_methods[] = {
- {0, 0, 0, 0}
-};
-#ifndef CYTHON_SMALL_CODE
-#if defined(__clang__)
- #define CYTHON_SMALL_CODE
-#elif defined(__GNUC__) && (__GNUC__ > 4 || (__GNUC__ == 4 && __GNUC_MINOR__ >= 3))
- #define CYTHON_SMALL_CODE __attribute__((cold))
-#else
- #define CYTHON_SMALL_CODE
-#endif
-#endif
-/* #### Code section: pystring_table ### */
-
-static int __Pyx_CreateStringTabAndInitStrings(void) {
- __Pyx_StringTabEntry __pyx_string_tab[] = {
- {&__pyx_n_s_ApproxNotFoundError, __pyx_k_ApproxNotFoundError, sizeof(__pyx_k_ApproxNotFoundError), 0, 0, 1, 1},
- {&__pyx_n_s_AssertionError, __pyx_k_AssertionError, sizeof(__pyx_k_AssertionError), 0, 0, 1, 1},
- {&__pyx_n_s_AttributeError, __pyx_k_AttributeError, sizeof(__pyx_k_AttributeError), 0, 0, 1, 1},
- {&__pyx_n_s_COMPILED, __pyx_k_COMPILED, sizeof(__pyx_k_COMPILED), 0, 0, 1, 1},
- {&__pyx_n_s_Cu2QuError, __pyx_k_Cu2QuError, sizeof(__pyx_k_Cu2QuError), 0, 0, 1, 1},
- {&__pyx_n_s_Error, __pyx_k_Error, sizeof(__pyx_k_Error), 0, 0, 1, 1},
- {&__pyx_n_s_ImportError, __pyx_k_ImportError, sizeof(__pyx_k_ImportError), 0, 0, 1, 1},
- {&__pyx_kp_s_Lib_fontTools_cu2qu_cu2qu_py, __pyx_k_Lib_fontTools_cu2qu_cu2qu_py, sizeof(__pyx_k_Lib_fontTools_cu2qu_cu2qu_py), 0, 0, 1, 0},
- {&__pyx_n_s_MAX_N, __pyx_k_MAX_N, sizeof(__pyx_k_MAX_N), 0, 0, 1, 1},
- {&__pyx_n_s_NAN, __pyx_k_NAN, sizeof(__pyx_k_NAN), 0, 0, 1, 1},
- {&__pyx_n_u_NaN, __pyx_k_NaN, sizeof(__pyx_k_NaN), 0, 1, 0, 1},
- {&__pyx_kp_u_Return_quadratic_Bezier_splines, __pyx_k_Return_quadratic_Bezier_splines, sizeof(__pyx_k_Return_quadratic_Bezier_splines), 0, 1, 0, 0},
- {&__pyx_n_s_ZeroDivisionError, __pyx_k_ZeroDivisionError, sizeof(__pyx_k_ZeroDivisionError), 0, 0, 1, 1},
- {&__pyx_kp_u__2, __pyx_k__2, sizeof(__pyx_k__2), 0, 1, 0, 0},
- {&__pyx_n_s__3, __pyx_k__3, sizeof(__pyx_k__3), 0, 0, 1, 1},
- {&__pyx_n_s__9, __pyx_k__9, sizeof(__pyx_k__9), 0, 0, 1, 1},
- {&__pyx_n_s_a, __pyx_k_a, sizeof(__pyx_k_a), 0, 0, 1, 1},
- {&__pyx_n_s_a1, __pyx_k_a1, sizeof(__pyx_k_a1), 0, 0, 1, 1},
- {&__pyx_n_s_all, __pyx_k_all, sizeof(__pyx_k_all), 0, 0, 1, 1},
- {&__pyx_n_s_all_quadratic, __pyx_k_all_quadratic, sizeof(__pyx_k_all_quadratic), 0, 0, 1, 1},
- {&__pyx_n_s_args, __pyx_k_args, sizeof(__pyx_k_args), 0, 0, 1, 1},
- {&__pyx_n_s_asyncio_coroutines, __pyx_k_asyncio_coroutines, sizeof(__pyx_k_asyncio_coroutines), 0, 0, 1, 1},
- {&__pyx_n_s_b, __pyx_k_b, sizeof(__pyx_k_b), 0, 0, 1, 1},
- {&__pyx_n_s_b1, __pyx_k_b1, sizeof(__pyx_k_b1), 0, 0, 1, 1},
- {&__pyx_n_s_c, __pyx_k_c, sizeof(__pyx_k_c), 0, 0, 1, 1},
- {&__pyx_n_s_c1, __pyx_k_c1, sizeof(__pyx_k_c1), 0, 0, 1, 1},
- {&__pyx_n_s_cline_in_traceback, __pyx_k_cline_in_traceback, sizeof(__pyx_k_cline_in_traceback), 0, 0, 1, 1},
- {&__pyx_n_s_close, __pyx_k_close, sizeof(__pyx_k_close), 0, 0, 1, 1},
- {&__pyx_n_s_curve, __pyx_k_curve, sizeof(__pyx_k_curve), 0, 0, 1, 1},
- {&__pyx_n_s_curve_to_quadratic, __pyx_k_curve_to_quadratic, sizeof(__pyx_k_curve_to_quadratic), 0, 0, 1, 1},
- {&__pyx_n_u_curve_to_quadratic, __pyx_k_curve_to_quadratic, sizeof(__pyx_k_curve_to_quadratic), 0, 1, 0, 1},
- {&__pyx_n_s_curves, __pyx_k_curves, sizeof(__pyx_k_curves), 0, 0, 1, 1},
- {&__pyx_n_s_curves_to_quadratic, __pyx_k_curves_to_quadratic, sizeof(__pyx_k_curves_to_quadratic), 0, 0, 1, 1},
- {&__pyx_n_u_curves_to_quadratic, __pyx_k_curves_to_quadratic, sizeof(__pyx_k_curves_to_quadratic), 0, 1, 0, 1},
- {&__pyx_kp_u_curves_to_quadratic_line_474, __pyx_k_curves_to_quadratic_line_474, sizeof(__pyx_k_curves_to_quadratic_line_474), 0, 1, 0, 0},
- {&__pyx_n_s_cython, __pyx_k_cython, sizeof(__pyx_k_cython), 0, 0, 1, 1},
- {&__pyx_n_s_d, __pyx_k_d, sizeof(__pyx_k_d), 0, 0, 1, 1},
- {&__pyx_n_s_d1, __pyx_k_d1, sizeof(__pyx_k_d1), 0, 0, 1, 1},
- {&__pyx_n_s_delta_2, __pyx_k_delta_2, sizeof(__pyx_k_delta_2), 0, 0, 1, 1},
- {&__pyx_n_s_delta_3, __pyx_k_delta_3, sizeof(__pyx_k_delta_3), 0, 0, 1, 1},
- {&__pyx_kp_u_disable, __pyx_k_disable, sizeof(__pyx_k_disable), 0, 1, 0, 0},
- {&__pyx_n_s_dt, __pyx_k_dt, sizeof(__pyx_k_dt), 0, 0, 1, 1},
- {&__pyx_kp_u_enable, __pyx_k_enable, sizeof(__pyx_k_enable), 0, 1, 0, 0},
- {&__pyx_n_s_errors, __pyx_k_errors, sizeof(__pyx_k_errors), 0, 0, 1, 1},
- {&__pyx_n_s_fontTools_cu2qu_cu2qu, __pyx_k_fontTools_cu2qu_cu2qu, sizeof(__pyx_k_fontTools_cu2qu_cu2qu), 0, 0, 1, 1},
- {&__pyx_n_s_fontTools_misc, __pyx_k_fontTools_misc, sizeof(__pyx_k_fontTools_misc), 0, 0, 1, 1},
- {&__pyx_kp_u_gc, __pyx_k_gc, sizeof(__pyx_k_gc), 0, 1, 0, 0},
- {&__pyx_n_s_i, __pyx_k_i, sizeof(__pyx_k_i), 0, 0, 1, 1},
- {&__pyx_n_s_imag, __pyx_k_imag, sizeof(__pyx_k_imag), 0, 0, 1, 1},
- {&__pyx_n_s_import, __pyx_k_import, sizeof(__pyx_k_import), 0, 0, 1, 1},
- {&__pyx_n_s_initializing, __pyx_k_initializing, sizeof(__pyx_k_initializing), 0, 0, 1, 1},
- {&__pyx_n_s_is_coroutine, __pyx_k_is_coroutine, sizeof(__pyx_k_is_coroutine), 0, 0, 1, 1},
- {&__pyx_kp_u_isenabled, __pyx_k_isenabled, sizeof(__pyx_k_isenabled), 0, 1, 0, 0},
- {&__pyx_n_s_isnan, __pyx_k_isnan, sizeof(__pyx_k_isnan), 0, 0, 1, 1},
- {&__pyx_n_s_l, __pyx_k_l, sizeof(__pyx_k_l), 0, 0, 1, 1},
- {&__pyx_n_s_last_i, __pyx_k_last_i, sizeof(__pyx_k_last_i), 0, 0, 1, 1},
- {&__pyx_n_s_main, __pyx_k_main, sizeof(__pyx_k_main), 0, 0, 1, 1},
- {&__pyx_n_s_math, __pyx_k_math, sizeof(__pyx_k_math), 0, 0, 1, 1},
- {&__pyx_n_s_max_err, __pyx_k_max_err, sizeof(__pyx_k_max_err), 0, 0, 1, 1},
- {&__pyx_n_s_max_errors, __pyx_k_max_errors, sizeof(__pyx_k_max_errors), 0, 0, 1, 1},
- {&__pyx_n_s_n, __pyx_k_n, sizeof(__pyx_k_n), 0, 0, 1, 1},
- {&__pyx_n_s_name, __pyx_k_name, sizeof(__pyx_k_name), 0, 0, 1, 1},
- {&__pyx_n_s_p, __pyx_k_p, sizeof(__pyx_k_p), 0, 0, 1, 1},
- {&__pyx_n_s_p0, __pyx_k_p0, sizeof(__pyx_k_p0), 0, 0, 1, 1},
- {&__pyx_n_s_p1, __pyx_k_p1, sizeof(__pyx_k_p1), 0, 0, 1, 1},
- {&__pyx_n_s_p2, __pyx_k_p2, sizeof(__pyx_k_p2), 0, 0, 1, 1},
- {&__pyx_n_s_p3, __pyx_k_p3, sizeof(__pyx_k_p3), 0, 0, 1, 1},
- {&__pyx_n_s_range, __pyx_k_range, sizeof(__pyx_k_range), 0, 0, 1, 1},
- {&__pyx_n_s_real, __pyx_k_real, sizeof(__pyx_k_real), 0, 0, 1, 1},
- {&__pyx_n_s_s, __pyx_k_s, sizeof(__pyx_k_s), 0, 0, 1, 1},
- {&__pyx_n_s_send, __pyx_k_send, sizeof(__pyx_k_send), 0, 0, 1, 1},
- {&__pyx_n_s_spec, __pyx_k_spec, sizeof(__pyx_k_spec), 0, 0, 1, 1},
- {&__pyx_n_s_spline, __pyx_k_spline, sizeof(__pyx_k_spline), 0, 0, 1, 1},
- {&__pyx_n_s_splines, __pyx_k_splines, sizeof(__pyx_k_splines), 0, 0, 1, 1},
- {&__pyx_n_s_split_cubic_into_n_gen, __pyx_k_split_cubic_into_n_gen, sizeof(__pyx_k_split_cubic_into_n_gen), 0, 0, 1, 1},
- {&__pyx_n_s_t1, __pyx_k_t1, sizeof(__pyx_k_t1), 0, 0, 1, 1},
- {&__pyx_n_s_t1_2, __pyx_k_t1_2, sizeof(__pyx_k_t1_2), 0, 0, 1, 1},
- {&__pyx_n_s_test, __pyx_k_test, sizeof(__pyx_k_test), 0, 0, 1, 1},
- {&__pyx_n_s_throw, __pyx_k_throw, sizeof(__pyx_k_throw), 0, 0, 1, 1},
- {0, 0, 0, 0, 0, 0, 0}
- };
- return __Pyx_InitStrings(__pyx_string_tab);
-}
-/* #### Code section: cached_builtins ### */
-static CYTHON_SMALL_CODE int __Pyx_InitCachedBuiltins(void) {
- __pyx_builtin_AttributeError = __Pyx_GetBuiltinName(__pyx_n_s_AttributeError); if (!__pyx_builtin_AttributeError) __PYX_ERR(0, 22, __pyx_L1_error)
- __pyx_builtin_ImportError = __Pyx_GetBuiltinName(__pyx_n_s_ImportError); if (!__pyx_builtin_ImportError) __PYX_ERR(0, 22, __pyx_L1_error)
- __pyx_builtin_range = __Pyx_GetBuiltinName(__pyx_n_s_range); if (!__pyx_builtin_range) __PYX_ERR(0, 146, __pyx_L1_error)
- __pyx_builtin_ZeroDivisionError = __Pyx_GetBuiltinName(__pyx_n_s_ZeroDivisionError); if (!__pyx_builtin_ZeroDivisionError) __PYX_ERR(0, 278, __pyx_L1_error)
- __pyx_builtin_AssertionError = __Pyx_GetBuiltinName(__pyx_n_s_AssertionError); if (!__pyx_builtin_AssertionError) __PYX_ERR(0, 514, __pyx_L1_error)
- return 0;
- __pyx_L1_error:;
- return -1;
-}
-/* #### Code section: cached_constants ### */
-
-static CYTHON_SMALL_CODE int __Pyx_InitCachedConstants(void) {
- __Pyx_RefNannyDeclarations
- __Pyx_RefNannySetupContext("__Pyx_InitCachedConstants", 0);
-
- /* "fontTools/cu2qu/cu2qu.py":127
- *
- *
- * @cython.locals( # <<<<<<<<<<<<<<
- * p0=cython.complex,
- * p1=cython.complex,
- */
- __pyx_tuple__4 = PyTuple_Pack(19, __pyx_n_s_p0, __pyx_n_s_p1, __pyx_n_s_p2, __pyx_n_s_p3, __pyx_n_s_n, __pyx_n_s_a1, __pyx_n_s_b1, __pyx_n_s_c1, __pyx_n_s_d1, __pyx_n_s_dt, __pyx_n_s_delta_2, __pyx_n_s_delta_3, __pyx_n_s_i, __pyx_n_s_a, __pyx_n_s_b, __pyx_n_s_c, __pyx_n_s_d, __pyx_n_s_t1, __pyx_n_s_t1_2); if (unlikely(!__pyx_tuple__4)) __PYX_ERR(0, 127, __pyx_L1_error)
- __Pyx_GOTREF(__pyx_tuple__4);
- __Pyx_GIVEREF(__pyx_tuple__4);
- __pyx_codeobj_ = (PyObject*)__Pyx_PyCode_New(5, 0, 0, 19, 0, CO_OPTIMIZED|CO_NEWLOCALS|CO_GENERATOR, __pyx_empty_bytes, __pyx_empty_tuple, __pyx_empty_tuple, __pyx_tuple__4, __pyx_empty_tuple, __pyx_empty_tuple, __pyx_kp_s_Lib_fontTools_cu2qu_cu2qu_py, __pyx_n_s_split_cubic_into_n_gen, 127, __pyx_empty_bytes); if (unlikely(!__pyx_codeobj_)) __PYX_ERR(0, 127, __pyx_L1_error)
-
- /* "fontTools/cu2qu/cu2qu.py":439
- *
- *
- * @cython.locals(max_err=cython.double) # <<<<<<<<<<<<<<
- * @cython.locals(n=cython.int)
- * @cython.locals(all_quadratic=cython.int)
- */
- __pyx_tuple__5 = PyTuple_Pack(7, __pyx_n_s_curve, __pyx_n_s_max_err, __pyx_n_s_all_quadratic, __pyx_n_s_n, __pyx_n_s_spline, __pyx_n_s_p, __pyx_n_s_s); if (unlikely(!__pyx_tuple__5)) __PYX_ERR(0, 439, __pyx_L1_error)
- __Pyx_GOTREF(__pyx_tuple__5);
- __Pyx_GIVEREF(__pyx_tuple__5);
- __pyx_codeobj__6 = (PyObject*)__Pyx_PyCode_New(3, 0, 0, 7, 0, CO_OPTIMIZED|CO_NEWLOCALS, __pyx_empty_bytes, __pyx_empty_tuple, __pyx_empty_tuple, __pyx_tuple__5, __pyx_empty_tuple, __pyx_empty_tuple, __pyx_kp_s_Lib_fontTools_cu2qu_cu2qu_py, __pyx_n_s_curve_to_quadratic, 439, __pyx_empty_bytes); if (unlikely(!__pyx_codeobj__6)) __PYX_ERR(0, 439, __pyx_L1_error)
-
- /* "fontTools/cu2qu/cu2qu.py":474
- *
- *
- * @cython.locals(l=cython.int, last_i=cython.int, i=cython.int) # <<<<<<<<<<<<<<
- * @cython.locals(all_quadratic=cython.int)
- * def curves_to_quadratic(curves, max_errors, all_quadratic=True):
- */
- __pyx_tuple__7 = PyTuple_Pack(13, __pyx_n_s_curves, __pyx_n_s_max_errors, __pyx_n_s_all_quadratic, __pyx_n_s_l, __pyx_n_s_last_i, __pyx_n_s_i, __pyx_n_s_splines, __pyx_n_s_n, __pyx_n_s_spline, __pyx_n_s_curve, __pyx_n_s_p, __pyx_n_s_spline, __pyx_n_s_s); if (unlikely(!__pyx_tuple__7)) __PYX_ERR(0, 474, __pyx_L1_error)
- __Pyx_GOTREF(__pyx_tuple__7);
- __Pyx_GIVEREF(__pyx_tuple__7);
- __pyx_codeobj__8 = (PyObject*)__Pyx_PyCode_New(3, 0, 0, 13, 0, CO_OPTIMIZED|CO_NEWLOCALS, __pyx_empty_bytes, __pyx_empty_tuple, __pyx_empty_tuple, __pyx_tuple__7, __pyx_empty_tuple, __pyx_empty_tuple, __pyx_kp_s_Lib_fontTools_cu2qu_cu2qu_py, __pyx_n_s_curves_to_quadratic, 474, __pyx_empty_bytes); if (unlikely(!__pyx_codeobj__8)) __PYX_ERR(0, 474, __pyx_L1_error)
- __Pyx_RefNannyFinishContext();
- return 0;
- __pyx_L1_error:;
- __Pyx_RefNannyFinishContext();
- return -1;
-}
-/* #### Code section: init_constants ### */
-
-static CYTHON_SMALL_CODE int __Pyx_InitConstants(void) {
- if (__Pyx_CreateStringTabAndInitStrings() < 0) __PYX_ERR(0, 1, __pyx_L1_error);
- __pyx_int_1 = PyInt_FromLong(1); if (unlikely(!__pyx_int_1)) __PYX_ERR(0, 1, __pyx_L1_error)
- __pyx_int_2 = PyInt_FromLong(2); if (unlikely(!__pyx_int_2)) __PYX_ERR(0, 1, __pyx_L1_error)
- __pyx_int_3 = PyInt_FromLong(3); if (unlikely(!__pyx_int_3)) __PYX_ERR(0, 1, __pyx_L1_error)
- __pyx_int_4 = PyInt_FromLong(4); if (unlikely(!__pyx_int_4)) __PYX_ERR(0, 1, __pyx_L1_error)
- __pyx_int_6 = PyInt_FromLong(6); if (unlikely(!__pyx_int_6)) __PYX_ERR(0, 1, __pyx_L1_error)
- __pyx_int_100 = PyInt_FromLong(100); if (unlikely(!__pyx_int_100)) __PYX_ERR(0, 1, __pyx_L1_error)
- return 0;
- __pyx_L1_error:;
- return -1;
-}
-/* #### Code section: init_globals ### */
-
-static CYTHON_SMALL_CODE int __Pyx_InitGlobals(void) {
- /* AssertionsEnabled.init */
- __Pyx_init_assertions_enabled();
-
-if (unlikely(PyErr_Occurred())) __PYX_ERR(0, 1, __pyx_L1_error)
-
- return 0;
- __pyx_L1_error:;
- return -1;
-}
-/* #### Code section: init_module ### */
-
-static CYTHON_SMALL_CODE int __Pyx_modinit_global_init_code(void); /*proto*/
-static CYTHON_SMALL_CODE int __Pyx_modinit_variable_export_code(void); /*proto*/
-static CYTHON_SMALL_CODE int __Pyx_modinit_function_export_code(void); /*proto*/
-static CYTHON_SMALL_CODE int __Pyx_modinit_type_init_code(void); /*proto*/
-static CYTHON_SMALL_CODE int __Pyx_modinit_type_import_code(void); /*proto*/
-static CYTHON_SMALL_CODE int __Pyx_modinit_variable_import_code(void); /*proto*/
-static CYTHON_SMALL_CODE int __Pyx_modinit_function_import_code(void); /*proto*/
-
-static int __Pyx_modinit_global_init_code(void) {
- __Pyx_RefNannyDeclarations
- __Pyx_RefNannySetupContext("__Pyx_modinit_global_init_code", 0);
- /*--- Global init code ---*/
- __Pyx_RefNannyFinishContext();
- return 0;
-}
-
-static int __Pyx_modinit_variable_export_code(void) {
- __Pyx_RefNannyDeclarations
- __Pyx_RefNannySetupContext("__Pyx_modinit_variable_export_code", 0);
- /*--- Variable export code ---*/
- __Pyx_RefNannyFinishContext();
- return 0;
-}
-
-static int __Pyx_modinit_function_export_code(void) {
- __Pyx_RefNannyDeclarations
- __Pyx_RefNannySetupContext("__Pyx_modinit_function_export_code", 0);
- /*--- Function export code ---*/
- __Pyx_RefNannyFinishContext();
- return 0;
-}
-
-static int __Pyx_modinit_type_init_code(void) {
- __Pyx_RefNannyDeclarations
- int __pyx_lineno = 0;
- const char *__pyx_filename = NULL;
- int __pyx_clineno = 0;
- __Pyx_RefNannySetupContext("__Pyx_modinit_type_init_code", 0);
- /*--- Type init code ---*/
- #if CYTHON_USE_TYPE_SPECS
- __pyx_ptype_9fontTools_5cu2qu_5cu2qu___pyx_scope_struct___split_cubic_into_n_gen = (PyTypeObject *) __Pyx_PyType_FromModuleAndSpec(__pyx_m, &__pyx_type_9fontTools_5cu2qu_5cu2qu___pyx_scope_struct___split_cubic_into_n_gen_spec, NULL); if (unlikely(!__pyx_ptype_9fontTools_5cu2qu_5cu2qu___pyx_scope_struct___split_cubic_into_n_gen)) __PYX_ERR(0, 127, __pyx_L1_error)
- if (__Pyx_fix_up_extension_type_from_spec(&__pyx_type_9fontTools_5cu2qu_5cu2qu___pyx_scope_struct___split_cubic_into_n_gen_spec, __pyx_ptype_9fontTools_5cu2qu_5cu2qu___pyx_scope_struct___split_cubic_into_n_gen) < 0) __PYX_ERR(0, 127, __pyx_L1_error)
- #else
- __pyx_ptype_9fontTools_5cu2qu_5cu2qu___pyx_scope_struct___split_cubic_into_n_gen = &__pyx_type_9fontTools_5cu2qu_5cu2qu___pyx_scope_struct___split_cubic_into_n_gen;
- #endif
- #if !CYTHON_COMPILING_IN_LIMITED_API
- #endif
- #if !CYTHON_USE_TYPE_SPECS
- if (__Pyx_PyType_Ready(__pyx_ptype_9fontTools_5cu2qu_5cu2qu___pyx_scope_struct___split_cubic_into_n_gen) < 0) __PYX_ERR(0, 127, __pyx_L1_error)
- #endif
- #if PY_MAJOR_VERSION < 3
- __pyx_ptype_9fontTools_5cu2qu_5cu2qu___pyx_scope_struct___split_cubic_into_n_gen->tp_print = 0;
- #endif
- #if !CYTHON_COMPILING_IN_LIMITED_API
- if ((CYTHON_USE_TYPE_SLOTS && CYTHON_USE_PYTYPE_LOOKUP) && likely(!__pyx_ptype_9fontTools_5cu2qu_5cu2qu___pyx_scope_struct___split_cubic_into_n_gen->tp_dictoffset && __pyx_ptype_9fontTools_5cu2qu_5cu2qu___pyx_scope_struct___split_cubic_into_n_gen->tp_getattro == PyObject_GenericGetAttr)) {
- __pyx_ptype_9fontTools_5cu2qu_5cu2qu___pyx_scope_struct___split_cubic_into_n_gen->tp_getattro = __Pyx_PyObject_GenericGetAttrNoDict;
- }
- #endif
- __Pyx_RefNannyFinishContext();
- return 0;
- __pyx_L1_error:;
- __Pyx_RefNannyFinishContext();
- return -1;
-}
-
-static int __Pyx_modinit_type_import_code(void) {
- __Pyx_RefNannyDeclarations
- __Pyx_RefNannySetupContext("__Pyx_modinit_type_import_code", 0);
- /*--- Type import code ---*/
- __Pyx_RefNannyFinishContext();
- return 0;
-}
-
-static int __Pyx_modinit_variable_import_code(void) {
- __Pyx_RefNannyDeclarations
- __Pyx_RefNannySetupContext("__Pyx_modinit_variable_import_code", 0);
- /*--- Variable import code ---*/
- __Pyx_RefNannyFinishContext();
- return 0;
-}
-
-static int __Pyx_modinit_function_import_code(void) {
- __Pyx_RefNannyDeclarations
- __Pyx_RefNannySetupContext("__Pyx_modinit_function_import_code", 0);
- /*--- Function import code ---*/
- __Pyx_RefNannyFinishContext();
- return 0;
-}
-
-
-#if PY_MAJOR_VERSION >= 3
-#if CYTHON_PEP489_MULTI_PHASE_INIT
-static PyObject* __pyx_pymod_create(PyObject *spec, PyModuleDef *def); /*proto*/
-static int __pyx_pymod_exec_cu2qu(PyObject* module); /*proto*/
-static PyModuleDef_Slot __pyx_moduledef_slots[] = {
- {Py_mod_create, (void*)__pyx_pymod_create},
- {Py_mod_exec, (void*)__pyx_pymod_exec_cu2qu},
- {0, NULL}
-};
-#endif
-
-#ifdef __cplusplus
-namespace {
- struct PyModuleDef __pyx_moduledef =
- #else
- static struct PyModuleDef __pyx_moduledef =
- #endif
- {
- PyModuleDef_HEAD_INIT,
- "cu2qu",
- 0, /* m_doc */
- #if CYTHON_PEP489_MULTI_PHASE_INIT
- 0, /* m_size */
- #elif CYTHON_USE_MODULE_STATE
- sizeof(__pyx_mstate), /* m_size */
- #else
- -1, /* m_size */
- #endif
- __pyx_methods /* m_methods */,
- #if CYTHON_PEP489_MULTI_PHASE_INIT
- __pyx_moduledef_slots, /* m_slots */
- #else
- NULL, /* m_reload */
- #endif
- #if CYTHON_USE_MODULE_STATE
- __pyx_m_traverse, /* m_traverse */
- __pyx_m_clear, /* m_clear */
- NULL /* m_free */
- #else
- NULL, /* m_traverse */
- NULL, /* m_clear */
- NULL /* m_free */
- #endif
- };
- #ifdef __cplusplus
-} /* anonymous namespace */
-#endif
-#endif
-
-#ifndef CYTHON_NO_PYINIT_EXPORT
-#define __Pyx_PyMODINIT_FUNC PyMODINIT_FUNC
-#elif PY_MAJOR_VERSION < 3
-#ifdef __cplusplus
-#define __Pyx_PyMODINIT_FUNC extern "C" void
-#else
-#define __Pyx_PyMODINIT_FUNC void
-#endif
-#else
-#ifdef __cplusplus
-#define __Pyx_PyMODINIT_FUNC extern "C" PyObject *
-#else
-#define __Pyx_PyMODINIT_FUNC PyObject *
-#endif
-#endif
-
-
-#if PY_MAJOR_VERSION < 3
-__Pyx_PyMODINIT_FUNC initcu2qu(void) CYTHON_SMALL_CODE; /*proto*/
-__Pyx_PyMODINIT_FUNC initcu2qu(void)
-#else
-__Pyx_PyMODINIT_FUNC PyInit_cu2qu(void) CYTHON_SMALL_CODE; /*proto*/
-__Pyx_PyMODINIT_FUNC PyInit_cu2qu(void)
-#if CYTHON_PEP489_MULTI_PHASE_INIT
-{
- return PyModuleDef_Init(&__pyx_moduledef);
-}
-static CYTHON_SMALL_CODE int __Pyx_check_single_interpreter(void) {
- #if PY_VERSION_HEX >= 0x030700A1
- static PY_INT64_T main_interpreter_id = -1;
- PY_INT64_T current_id = PyInterpreterState_GetID(PyThreadState_Get()->interp);
- if (main_interpreter_id == -1) {
- main_interpreter_id = current_id;
- return (unlikely(current_id == -1)) ? -1 : 0;
- } else if (unlikely(main_interpreter_id != current_id))
- #else
- static PyInterpreterState *main_interpreter = NULL;
- PyInterpreterState *current_interpreter = PyThreadState_Get()->interp;
- if (!main_interpreter) {
- main_interpreter = current_interpreter;
- } else if (unlikely(main_interpreter != current_interpreter))
- #endif
- {
- PyErr_SetString(
- PyExc_ImportError,
- "Interpreter change detected - this module can only be loaded into one interpreter per process.");
- return -1;
- }
- return 0;
-}
-#if CYTHON_COMPILING_IN_LIMITED_API
-static CYTHON_SMALL_CODE int __Pyx_copy_spec_to_module(PyObject *spec, PyObject *module, const char* from_name, const char* to_name, int allow_none)
-#else
-static CYTHON_SMALL_CODE int __Pyx_copy_spec_to_module(PyObject *spec, PyObject *moddict, const char* from_name, const char* to_name, int allow_none)
-#endif
-{
- PyObject *value = PyObject_GetAttrString(spec, from_name);
- int result = 0;
- if (likely(value)) {
- if (allow_none || value != Py_None) {
-#if CYTHON_COMPILING_IN_LIMITED_API
- result = PyModule_AddObject(module, to_name, value);
-#else
- result = PyDict_SetItemString(moddict, to_name, value);
-#endif
- }
- Py_DECREF(value);
- } else if (PyErr_ExceptionMatches(PyExc_AttributeError)) {
- PyErr_Clear();
- } else {
- result = -1;
- }
- return result;
-}
-static CYTHON_SMALL_CODE PyObject* __pyx_pymod_create(PyObject *spec, PyModuleDef *def) {
- PyObject *module = NULL, *moddict, *modname;
- CYTHON_UNUSED_VAR(def);
- if (__Pyx_check_single_interpreter())
- return NULL;
- if (__pyx_m)
- return __Pyx_NewRef(__pyx_m);
- modname = PyObject_GetAttrString(spec, "name");
- if (unlikely(!modname)) goto bad;
- module = PyModule_NewObject(modname);
- Py_DECREF(modname);
- if (unlikely(!module)) goto bad;
-#if CYTHON_COMPILING_IN_LIMITED_API
- moddict = module;
-#else
- moddict = PyModule_GetDict(module);
- if (unlikely(!moddict)) goto bad;
-#endif
- if (unlikely(__Pyx_copy_spec_to_module(spec, moddict, "loader", "__loader__", 1) < 0)) goto bad;
- if (unlikely(__Pyx_copy_spec_to_module(spec, moddict, "origin", "__file__", 1) < 0)) goto bad;
- if (unlikely(__Pyx_copy_spec_to_module(spec, moddict, "parent", "__package__", 1) < 0)) goto bad;
- if (unlikely(__Pyx_copy_spec_to_module(spec, moddict, "submodule_search_locations", "__path__", 0) < 0)) goto bad;
- return module;
-bad:
- Py_XDECREF(module);
- return NULL;
-}
-
-
-static CYTHON_SMALL_CODE int __pyx_pymod_exec_cu2qu(PyObject *__pyx_pyinit_module)
-#endif
-#endif
-{
- int stringtab_initialized = 0;
- #if CYTHON_USE_MODULE_STATE
- int pystate_addmodule_run = 0;
- #endif
- PyObject *__pyx_t_1 = NULL;
- PyObject *__pyx_t_2 = NULL;
- PyObject *__pyx_t_3 = NULL;
- int __pyx_t_4;
- PyObject *__pyx_t_5 = NULL;
- PyObject *__pyx_t_6 = NULL;
- PyObject *__pyx_t_7 = NULL;
- PyObject *__pyx_t_8 = NULL;
- PyObject *__pyx_t_9 = NULL;
- double __pyx_t_10;
- int __pyx_lineno = 0;
- const char *__pyx_filename = NULL;
- int __pyx_clineno = 0;
- __Pyx_RefNannyDeclarations
- #if CYTHON_PEP489_MULTI_PHASE_INIT
- if (__pyx_m) {
- if (__pyx_m == __pyx_pyinit_module) return 0;
- PyErr_SetString(PyExc_RuntimeError, "Module 'cu2qu' has already been imported. Re-initialisation is not supported.");
- return -1;
- }
- #elif PY_MAJOR_VERSION >= 3
- if (__pyx_m) return __Pyx_NewRef(__pyx_m);
- #endif
- /*--- Module creation code ---*/
- #if CYTHON_PEP489_MULTI_PHASE_INIT
- __pyx_m = __pyx_pyinit_module;
- Py_INCREF(__pyx_m);
- #else
- #if PY_MAJOR_VERSION < 3
- __pyx_m = Py_InitModule4("cu2qu", __pyx_methods, 0, 0, PYTHON_API_VERSION); Py_XINCREF(__pyx_m);
- if (unlikely(!__pyx_m)) __PYX_ERR(0, 1, __pyx_L1_error)
- #elif CYTHON_USE_MODULE_STATE
- __pyx_t_1 = PyModule_Create(&__pyx_moduledef); if (unlikely(!__pyx_t_1)) __PYX_ERR(0, 1, __pyx_L1_error)
- {
- int add_module_result = PyState_AddModule(__pyx_t_1, &__pyx_moduledef);
- __pyx_t_1 = 0; /* transfer ownership from __pyx_t_1 to cu2qu pseudovariable */
- if (unlikely((add_module_result < 0))) __PYX_ERR(0, 1, __pyx_L1_error)
- pystate_addmodule_run = 1;
- }
- #else
- __pyx_m = PyModule_Create(&__pyx_moduledef);
- if (unlikely(!__pyx_m)) __PYX_ERR(0, 1, __pyx_L1_error)
- #endif
- #endif
- CYTHON_UNUSED_VAR(__pyx_t_1);
- __pyx_d = PyModule_GetDict(__pyx_m); if (unlikely(!__pyx_d)) __PYX_ERR(0, 1, __pyx_L1_error)
- Py_INCREF(__pyx_d);
- __pyx_b = PyImport_AddModule(__Pyx_BUILTIN_MODULE_NAME); if (unlikely(!__pyx_b)) __PYX_ERR(0, 1, __pyx_L1_error)
- Py_INCREF(__pyx_b);
- __pyx_cython_runtime = PyImport_AddModule((char *) "cython_runtime"); if (unlikely(!__pyx_cython_runtime)) __PYX_ERR(0, 1, __pyx_L1_error)
- Py_INCREF(__pyx_cython_runtime);
- if (PyObject_SetAttrString(__pyx_m, "__builtins__", __pyx_b) < 0) __PYX_ERR(0, 1, __pyx_L1_error)
- #if CYTHON_REFNANNY
-__Pyx_RefNanny = __Pyx_RefNannyImportAPI("refnanny");
-if (!__Pyx_RefNanny) {
- PyErr_Clear();
- __Pyx_RefNanny = __Pyx_RefNannyImportAPI("Cython.Runtime.refnanny");
- if (!__Pyx_RefNanny)
- Py_FatalError("failed to import 'refnanny' module");
-}
-#endif
- __Pyx_RefNannySetupContext("__Pyx_PyMODINIT_FUNC PyInit_cu2qu(void)", 0);
- if (__Pyx_check_binary_version() < 0) __PYX_ERR(0, 1, __pyx_L1_error)
- #ifdef __Pxy_PyFrame_Initialize_Offsets
- __Pxy_PyFrame_Initialize_Offsets();
- #endif
- __pyx_empty_tuple = PyTuple_New(0); if (unlikely(!__pyx_empty_tuple)) __PYX_ERR(0, 1, __pyx_L1_error)
- __pyx_empty_bytes = PyBytes_FromStringAndSize("", 0); if (unlikely(!__pyx_empty_bytes)) __PYX_ERR(0, 1, __pyx_L1_error)
- __pyx_empty_unicode = PyUnicode_FromStringAndSize("", 0); if (unlikely(!__pyx_empty_unicode)) __PYX_ERR(0, 1, __pyx_L1_error)
- #ifdef __Pyx_CyFunction_USED
- if (__pyx_CyFunction_init(__pyx_m) < 0) __PYX_ERR(0, 1, __pyx_L1_error)
- #endif
- #ifdef __Pyx_FusedFunction_USED
- if (__pyx_FusedFunction_init(__pyx_m) < 0) __PYX_ERR(0, 1, __pyx_L1_error)
- #endif
- #ifdef __Pyx_Coroutine_USED
- if (__pyx_Coroutine_init(__pyx_m) < 0) __PYX_ERR(0, 1, __pyx_L1_error)
- #endif
- #ifdef __Pyx_Generator_USED
- if (__pyx_Generator_init(__pyx_m) < 0) __PYX_ERR(0, 1, __pyx_L1_error)
- #endif
- #ifdef __Pyx_AsyncGen_USED
- if (__pyx_AsyncGen_init(__pyx_m) < 0) __PYX_ERR(0, 1, __pyx_L1_error)
- #endif
- #ifdef __Pyx_StopAsyncIteration_USED
- if (__pyx_StopAsyncIteration_init(__pyx_m) < 0) __PYX_ERR(0, 1, __pyx_L1_error)
- #endif
- /*--- Library function declarations ---*/
- /*--- Threads initialization code ---*/
- #if defined(WITH_THREAD) && PY_VERSION_HEX < 0x030700F0 && defined(__PYX_FORCE_INIT_THREADS) && __PYX_FORCE_INIT_THREADS
- PyEval_InitThreads();
- #endif
- /*--- Initialize various global constants etc. ---*/
- if (__Pyx_InitConstants() < 0) __PYX_ERR(0, 1, __pyx_L1_error)
- stringtab_initialized = 1;
- if (__Pyx_InitGlobals() < 0) __PYX_ERR(0, 1, __pyx_L1_error)
- #if PY_MAJOR_VERSION < 3 && (__PYX_DEFAULT_STRING_ENCODING_IS_ASCII || __PYX_DEFAULT_STRING_ENCODING_IS_DEFAULT)
- if (__Pyx_init_sys_getdefaultencoding_params() < 0) __PYX_ERR(0, 1, __pyx_L1_error)
- #endif
- if (__pyx_module_is_main_fontTools__cu2qu__cu2qu) {
- if (PyObject_SetAttr(__pyx_m, __pyx_n_s_name, __pyx_n_s_main) < 0) __PYX_ERR(0, 1, __pyx_L1_error)
- }
- #if PY_MAJOR_VERSION >= 3
- {
- PyObject *modules = PyImport_GetModuleDict(); if (unlikely(!modules)) __PYX_ERR(0, 1, __pyx_L1_error)
- if (!PyDict_GetItemString(modules, "fontTools.cu2qu.cu2qu")) {
- if (unlikely((PyDict_SetItemString(modules, "fontTools.cu2qu.cu2qu", __pyx_m) < 0))) __PYX_ERR(0, 1, __pyx_L1_error)
- }
- }
- #endif
- /*--- Builtin init code ---*/
- if (__Pyx_InitCachedBuiltins() < 0) __PYX_ERR(0, 1, __pyx_L1_error)
- /*--- Constants init code ---*/
- if (__Pyx_InitCachedConstants() < 0) __PYX_ERR(0, 1, __pyx_L1_error)
- /*--- Global type/function init code ---*/
- (void)__Pyx_modinit_global_init_code();
- (void)__Pyx_modinit_variable_export_code();
- (void)__Pyx_modinit_function_export_code();
- if (unlikely((__Pyx_modinit_type_init_code() < 0))) __PYX_ERR(0, 1, __pyx_L1_error)
- (void)__Pyx_modinit_type_import_code();
- (void)__Pyx_modinit_variable_import_code();
- (void)__Pyx_modinit_function_import_code();
- /*--- Execution code ---*/
- #if defined(__Pyx_Generator_USED) || defined(__Pyx_Coroutine_USED)
- if (__Pyx_patch_abc() < 0) __PYX_ERR(0, 1, __pyx_L1_error)
- #endif
-
- /* "fontTools/cu2qu/cu2qu.py":18
- * # limitations under the License.
- *
- * try: # <<<<<<<<<<<<<<
- * import cython
- *
- */
- {
- __Pyx_PyThreadState_declare
- __Pyx_PyThreadState_assign
- __Pyx_ExceptionSave(&__pyx_t_1, &__pyx_t_2, &__pyx_t_3);
- __Pyx_XGOTREF(__pyx_t_1);
- __Pyx_XGOTREF(__pyx_t_2);
- __Pyx_XGOTREF(__pyx_t_3);
- /*try:*/ {
-
- /* "fontTools/cu2qu/cu2qu.py":21
- * import cython
- *
- * COMPILED = cython.compiled # <<<<<<<<<<<<<<
- * except (AttributeError, ImportError):
- * # if cython not installed, use mock module with no-op decorators and types
- */
- if (PyDict_SetItem(__pyx_d, __pyx_n_s_COMPILED, Py_True) < 0) __PYX_ERR(0, 21, __pyx_L2_error)
-
- /* "fontTools/cu2qu/cu2qu.py":18
- * # limitations under the License.
- *
- * try: # <<<<<<<<<<<<<<
- * import cython
- *
- */
- }
- __Pyx_XDECREF(__pyx_t_1); __pyx_t_1 = 0;
- __Pyx_XDECREF(__pyx_t_2); __pyx_t_2 = 0;
- __Pyx_XDECREF(__pyx_t_3); __pyx_t_3 = 0;
- goto __pyx_L7_try_end;
- __pyx_L2_error:;
-
- /* "fontTools/cu2qu/cu2qu.py":22
- *
- * COMPILED = cython.compiled
- * except (AttributeError, ImportError): # <<<<<<<<<<<<<<
- * # if cython not installed, use mock module with no-op decorators and types
- * from fontTools.misc import cython
- */
- __pyx_t_4 = __Pyx_PyErr_ExceptionMatches2(__pyx_builtin_AttributeError, __pyx_builtin_ImportError);
- if (__pyx_t_4) {
- __Pyx_AddTraceback("fontTools.cu2qu.cu2qu", __pyx_clineno, __pyx_lineno, __pyx_filename);
- if (__Pyx_GetException(&__pyx_t_5, &__pyx_t_6, &__pyx_t_7) < 0) __PYX_ERR(0, 22, __pyx_L4_except_error)
- __Pyx_XGOTREF(__pyx_t_5);
- __Pyx_XGOTREF(__pyx_t_6);
- __Pyx_XGOTREF(__pyx_t_7);
-
- /* "fontTools/cu2qu/cu2qu.py":24
- * except (AttributeError, ImportError):
- * # if cython not installed, use mock module with no-op decorators and types
- * from fontTools.misc import cython # <<<<<<<<<<<<<<
- *
- * COMPILED = False
- */
- __pyx_t_8 = PyList_New(1); if (unlikely(!__pyx_t_8)) __PYX_ERR(0, 24, __pyx_L4_except_error)
- __Pyx_GOTREF(__pyx_t_8);
- __Pyx_INCREF(__pyx_n_s_cython);
- __Pyx_GIVEREF(__pyx_n_s_cython);
- PyList_SET_ITEM(__pyx_t_8, 0, __pyx_n_s_cython);
- __pyx_t_9 = __Pyx_Import(__pyx_n_s_fontTools_misc, __pyx_t_8, 0); if (unlikely(!__pyx_t_9)) __PYX_ERR(0, 24, __pyx_L4_except_error)
- __Pyx_GOTREF(__pyx_t_9);
- __Pyx_DECREF(__pyx_t_8); __pyx_t_8 = 0;
- __pyx_t_8 = __Pyx_ImportFrom(__pyx_t_9, __pyx_n_s_cython); if (unlikely(!__pyx_t_8)) __PYX_ERR(0, 24, __pyx_L4_except_error)
- __Pyx_GOTREF(__pyx_t_8);
- if (PyDict_SetItem(__pyx_d, __pyx_n_s_cython, __pyx_t_8) < 0) __PYX_ERR(0, 24, __pyx_L4_except_error)
- __Pyx_DECREF(__pyx_t_8); __pyx_t_8 = 0;
- __Pyx_DECREF(__pyx_t_9); __pyx_t_9 = 0;
-
- /* "fontTools/cu2qu/cu2qu.py":26
- * from fontTools.misc import cython
- *
- * COMPILED = False # <<<<<<<<<<<<<<
- *
- * import math
- */
- if (PyDict_SetItem(__pyx_d, __pyx_n_s_COMPILED, Py_False) < 0) __PYX_ERR(0, 26, __pyx_L4_except_error)
- __Pyx_XDECREF(__pyx_t_5); __pyx_t_5 = 0;
- __Pyx_XDECREF(__pyx_t_6); __pyx_t_6 = 0;
- __Pyx_XDECREF(__pyx_t_7); __pyx_t_7 = 0;
- goto __pyx_L3_exception_handled;
- }
- goto __pyx_L4_except_error;
-
- /* "fontTools/cu2qu/cu2qu.py":18
- * # limitations under the License.
- *
- * try: # <<<<<<<<<<<<<<
- * import cython
- *
- */
- __pyx_L4_except_error:;
- __Pyx_XGIVEREF(__pyx_t_1);
- __Pyx_XGIVEREF(__pyx_t_2);
- __Pyx_XGIVEREF(__pyx_t_3);
- __Pyx_ExceptionReset(__pyx_t_1, __pyx_t_2, __pyx_t_3);
- goto __pyx_L1_error;
- __pyx_L3_exception_handled:;
- __Pyx_XGIVEREF(__pyx_t_1);
- __Pyx_XGIVEREF(__pyx_t_2);
- __Pyx_XGIVEREF(__pyx_t_3);
- __Pyx_ExceptionReset(__pyx_t_1, __pyx_t_2, __pyx_t_3);
- __pyx_L7_try_end:;
- }
-
- /* "fontTools/cu2qu/cu2qu.py":28
- * COMPILED = False
- *
- * import math # <<<<<<<<<<<<<<
- *
- * from .errors import Error as Cu2QuError, ApproxNotFoundError
- */
- __pyx_t_7 = __Pyx_ImportDottedModule(__pyx_n_s_math, NULL); if (unlikely(!__pyx_t_7)) __PYX_ERR(0, 28, __pyx_L1_error)
- __Pyx_GOTREF(__pyx_t_7);
- if (PyDict_SetItem(__pyx_d, __pyx_n_s_math, __pyx_t_7) < 0) __PYX_ERR(0, 28, __pyx_L1_error)
- __Pyx_DECREF(__pyx_t_7); __pyx_t_7 = 0;
-
- /* "fontTools/cu2qu/cu2qu.py":30
- * import math
- *
- * from .errors import Error as Cu2QuError, ApproxNotFoundError # <<<<<<<<<<<<<<
- *
- *
- */
- __pyx_t_7 = PyList_New(2); if (unlikely(!__pyx_t_7)) __PYX_ERR(0, 30, __pyx_L1_error)
- __Pyx_GOTREF(__pyx_t_7);
- __Pyx_INCREF(__pyx_n_s_Error);
- __Pyx_GIVEREF(__pyx_n_s_Error);
- PyList_SET_ITEM(__pyx_t_7, 0, __pyx_n_s_Error);
- __Pyx_INCREF(__pyx_n_s_ApproxNotFoundError);
- __Pyx_GIVEREF(__pyx_n_s_ApproxNotFoundError);
- PyList_SET_ITEM(__pyx_t_7, 1, __pyx_n_s_ApproxNotFoundError);
- __pyx_t_6 = __Pyx_Import(__pyx_n_s_errors, __pyx_t_7, 1); if (unlikely(!__pyx_t_6)) __PYX_ERR(0, 30, __pyx_L1_error)
- __Pyx_GOTREF(__pyx_t_6);
- __Pyx_DECREF(__pyx_t_7); __pyx_t_7 = 0;
- __pyx_t_7 = __Pyx_ImportFrom(__pyx_t_6, __pyx_n_s_Error); if (unlikely(!__pyx_t_7)) __PYX_ERR(0, 30, __pyx_L1_error)
- __Pyx_GOTREF(__pyx_t_7);
- if (PyDict_SetItem(__pyx_d, __pyx_n_s_Cu2QuError, __pyx_t_7) < 0) __PYX_ERR(0, 30, __pyx_L1_error)
- __Pyx_DECREF(__pyx_t_7); __pyx_t_7 = 0;
- __pyx_t_7 = __Pyx_ImportFrom(__pyx_t_6, __pyx_n_s_ApproxNotFoundError); if (unlikely(!__pyx_t_7)) __PYX_ERR(0, 30, __pyx_L1_error)
- __Pyx_GOTREF(__pyx_t_7);
- if (PyDict_SetItem(__pyx_d, __pyx_n_s_ApproxNotFoundError, __pyx_t_7) < 0) __PYX_ERR(0, 30, __pyx_L1_error)
- __Pyx_DECREF(__pyx_t_7); __pyx_t_7 = 0;
- __Pyx_DECREF(__pyx_t_6); __pyx_t_6 = 0;
-
- /* "fontTools/cu2qu/cu2qu.py":33
- *
- *
- * __all__ = ["curve_to_quadratic", "curves_to_quadratic"] # <<<<<<<<<<<<<<
- *
- * MAX_N = 100
- */
- __pyx_t_6 = PyList_New(2); if (unlikely(!__pyx_t_6)) __PYX_ERR(0, 33, __pyx_L1_error)
- __Pyx_GOTREF(__pyx_t_6);
- __Pyx_INCREF(__pyx_n_u_curve_to_quadratic);
- __Pyx_GIVEREF(__pyx_n_u_curve_to_quadratic);
- PyList_SET_ITEM(__pyx_t_6, 0, __pyx_n_u_curve_to_quadratic);
- __Pyx_INCREF(__pyx_n_u_curves_to_quadratic);
- __Pyx_GIVEREF(__pyx_n_u_curves_to_quadratic);
- PyList_SET_ITEM(__pyx_t_6, 1, __pyx_n_u_curves_to_quadratic);
- if (PyDict_SetItem(__pyx_d, __pyx_n_s_all, __pyx_t_6) < 0) __PYX_ERR(0, 33, __pyx_L1_error)
- __Pyx_DECREF(__pyx_t_6); __pyx_t_6 = 0;
-
- /* "fontTools/cu2qu/cu2qu.py":35
- * __all__ = ["curve_to_quadratic", "curves_to_quadratic"]
- *
- * MAX_N = 100 # <<<<<<<<<<<<<<
- *
- * NAN = float("NaN")
- */
- if (PyDict_SetItem(__pyx_d, __pyx_n_s_MAX_N, __pyx_int_100) < 0) __PYX_ERR(0, 35, __pyx_L1_error)
-
- /* "fontTools/cu2qu/cu2qu.py":37
- * MAX_N = 100
- *
- * NAN = float("NaN") # <<<<<<<<<<<<<<
- *
- *
- */
- __pyx_t_10 = __Pyx_PyUnicode_AsDouble(__pyx_n_u_NaN); if (unlikely(__pyx_t_10 == ((double)((double)-1)) && PyErr_Occurred())) __PYX_ERR(0, 37, __pyx_L1_error)
- __pyx_t_6 = PyFloat_FromDouble(__pyx_t_10); if (unlikely(!__pyx_t_6)) __PYX_ERR(0, 37, __pyx_L1_error)
- __Pyx_GOTREF(__pyx_t_6);
- if (PyDict_SetItem(__pyx_d, __pyx_n_s_NAN, __pyx_t_6) < 0) __PYX_ERR(0, 37, __pyx_L1_error)
- __Pyx_DECREF(__pyx_t_6); __pyx_t_6 = 0;
-
- /* "fontTools/cu2qu/cu2qu.py":127
- *
- *
- * @cython.locals( # <<<<<<<<<<<<<<
- * p0=cython.complex,
- * p1=cython.complex,
- */
- __pyx_t_6 = __Pyx_CyFunction_New(&__pyx_mdef_9fontTools_5cu2qu_5cu2qu_1_split_cubic_into_n_gen, 0, __pyx_n_s_split_cubic_into_n_gen, NULL, __pyx_n_s_fontTools_cu2qu_cu2qu, __pyx_d, ((PyObject *)__pyx_codeobj_)); if (unlikely(!__pyx_t_6)) __PYX_ERR(0, 127, __pyx_L1_error)
- __Pyx_GOTREF(__pyx_t_6);
- if (PyDict_SetItem(__pyx_d, __pyx_n_s_split_cubic_into_n_gen, __pyx_t_6) < 0) __PYX_ERR(0, 127, __pyx_L1_error)
- __Pyx_DECREF(__pyx_t_6); __pyx_t_6 = 0;
-
- /* "fontTools/cu2qu/cu2qu.py":442
- * @cython.locals(n=cython.int)
- * @cython.locals(all_quadratic=cython.int)
- * def curve_to_quadratic(curve, max_err, all_quadratic=True): # <<<<<<<<<<<<<<
- * """Approximate a cubic Bezier curve with a spline of n quadratics.
- *
- */
- __pyx_t_6 = __Pyx_PyBool_FromLong(((int)1)); if (unlikely(!__pyx_t_6)) __PYX_ERR(0, 442, __pyx_L1_error)
- __Pyx_GOTREF(__pyx_t_6);
-
- /* "fontTools/cu2qu/cu2qu.py":439
- *
- *
- * @cython.locals(max_err=cython.double) # <<<<<<<<<<<<<<
- * @cython.locals(n=cython.int)
- * @cython.locals(all_quadratic=cython.int)
- */
- __pyx_t_7 = PyTuple_New(1); if (unlikely(!__pyx_t_7)) __PYX_ERR(0, 439, __pyx_L1_error)
- __Pyx_GOTREF(__pyx_t_7);
- __Pyx_GIVEREF(__pyx_t_6);
- PyTuple_SET_ITEM(__pyx_t_7, 0, __pyx_t_6);
- __pyx_t_6 = 0;
- __pyx_t_6 = __Pyx_CyFunction_New(&__pyx_mdef_9fontTools_5cu2qu_5cu2qu_4curve_to_quadratic, 0, __pyx_n_s_curve_to_quadratic, NULL, __pyx_n_s_fontTools_cu2qu_cu2qu, __pyx_d, ((PyObject *)__pyx_codeobj__6)); if (unlikely(!__pyx_t_6)) __PYX_ERR(0, 439, __pyx_L1_error)
- __Pyx_GOTREF(__pyx_t_6);
- __Pyx_CyFunction_SetDefaultsTuple(__pyx_t_6, __pyx_t_7);
- __Pyx_DECREF(__pyx_t_7); __pyx_t_7 = 0;
- if (PyDict_SetItem(__pyx_d, __pyx_n_s_curve_to_quadratic, __pyx_t_6) < 0) __PYX_ERR(0, 439, __pyx_L1_error)
- __Pyx_DECREF(__pyx_t_6); __pyx_t_6 = 0;
-
- /* "fontTools/cu2qu/cu2qu.py":476
- * @cython.locals(l=cython.int, last_i=cython.int, i=cython.int)
- * @cython.locals(all_quadratic=cython.int)
- * def curves_to_quadratic(curves, max_errors, all_quadratic=True): # <<<<<<<<<<<<<<
- * """Return quadratic Bezier splines approximating the input cubic Beziers.
- *
- */
- __pyx_t_6 = __Pyx_PyBool_FromLong(((int)1)); if (unlikely(!__pyx_t_6)) __PYX_ERR(0, 476, __pyx_L1_error)
- __Pyx_GOTREF(__pyx_t_6);
-
- /* "fontTools/cu2qu/cu2qu.py":474
- *
- *
- * @cython.locals(l=cython.int, last_i=cython.int, i=cython.int) # <<<<<<<<<<<<<<
- * @cython.locals(all_quadratic=cython.int)
- * def curves_to_quadratic(curves, max_errors, all_quadratic=True):
- */
- __pyx_t_7 = PyTuple_New(1); if (unlikely(!__pyx_t_7)) __PYX_ERR(0, 474, __pyx_L1_error)
- __Pyx_GOTREF(__pyx_t_7);
- __Pyx_GIVEREF(__pyx_t_6);
- PyTuple_SET_ITEM(__pyx_t_7, 0, __pyx_t_6);
- __pyx_t_6 = 0;
- __pyx_t_6 = __Pyx_CyFunction_New(&__pyx_mdef_9fontTools_5cu2qu_5cu2qu_6curves_to_quadratic, 0, __pyx_n_s_curves_to_quadratic, NULL, __pyx_n_s_fontTools_cu2qu_cu2qu, __pyx_d, ((PyObject *)__pyx_codeobj__8)); if (unlikely(!__pyx_t_6)) __PYX_ERR(0, 474, __pyx_L1_error)
- __Pyx_GOTREF(__pyx_t_6);
- __Pyx_CyFunction_SetDefaultsTuple(__pyx_t_6, __pyx_t_7);
- __Pyx_DECREF(__pyx_t_7); __pyx_t_7 = 0;
- if (PyDict_SetItem(__pyx_d, __pyx_n_s_curves_to_quadratic, __pyx_t_6) < 0) __PYX_ERR(0, 474, __pyx_L1_error)
- __Pyx_DECREF(__pyx_t_6); __pyx_t_6 = 0;
-
- /* "fontTools/cu2qu/cu2qu.py":1
- * # cython: language_level=3 # <<<<<<<<<<<<<<
- * # distutils: define_macros=CYTHON_TRACE_NOGIL=1
- *
- */
- __pyx_t_6 = __Pyx_PyDict_NewPresized(1); if (unlikely(!__pyx_t_6)) __PYX_ERR(0, 1, __pyx_L1_error)
- __Pyx_GOTREF(__pyx_t_6);
- if (PyDict_SetItem(__pyx_t_6, __pyx_kp_u_curves_to_quadratic_line_474, __pyx_kp_u_Return_quadratic_Bezier_splines) < 0) __PYX_ERR(0, 1, __pyx_L1_error)
- if (PyDict_SetItem(__pyx_d, __pyx_n_s_test, __pyx_t_6) < 0) __PYX_ERR(0, 1, __pyx_L1_error)
- __Pyx_DECREF(__pyx_t_6); __pyx_t_6 = 0;
-
- /*--- Wrapped vars code ---*/
-
- goto __pyx_L0;
- __pyx_L1_error:;
- __Pyx_XDECREF(__pyx_t_5);
- __Pyx_XDECREF(__pyx_t_6);
- __Pyx_XDECREF(__pyx_t_7);
- __Pyx_XDECREF(__pyx_t_8);
- __Pyx_XDECREF(__pyx_t_9);
- if (__pyx_m) {
- if (__pyx_d && stringtab_initialized) {
- __Pyx_AddTraceback("init fontTools.cu2qu.cu2qu", __pyx_clineno, __pyx_lineno, __pyx_filename);
- }
- #if !CYTHON_USE_MODULE_STATE
- Py_CLEAR(__pyx_m);
- #else
- Py_DECREF(__pyx_m);
- if (pystate_addmodule_run) {
- PyObject *tp, *value, *tb;
- PyErr_Fetch(&tp, &value, &tb);
- PyState_RemoveModule(&__pyx_moduledef);
- PyErr_Restore(tp, value, tb);
- }
- #endif
- } else if (!PyErr_Occurred()) {
- PyErr_SetString(PyExc_ImportError, "init fontTools.cu2qu.cu2qu");
- }
- __pyx_L0:;
- __Pyx_RefNannyFinishContext();
- #if CYTHON_PEP489_MULTI_PHASE_INIT
- return (__pyx_m != NULL) ? 0 : -1;
- #elif PY_MAJOR_VERSION >= 3
- return __pyx_m;
- #else
- return;
- #endif
-}
-/* #### Code section: cleanup_globals ### */
-/* #### Code section: cleanup_module ### */
-/* #### Code section: main_method ### */
-/* #### Code section: utility_code_pragmas ### */
-#ifdef _MSC_VER
-#pragma warning( push )
-/* Warning 4127: conditional expression is constant
- * Cython uses constant conditional expressions to allow in inline functions to be optimized at
- * compile-time, so this warning is not useful
- */
-#pragma warning( disable : 4127 )
-#endif
-
-
-
-/* #### Code section: utility_code_def ### */
-
-/* --- Runtime support code --- */
-/* Refnanny */
-#if CYTHON_REFNANNY
-static __Pyx_RefNannyAPIStruct *__Pyx_RefNannyImportAPI(const char *modname) {
- PyObject *m = NULL, *p = NULL;
- void *r = NULL;
- m = PyImport_ImportModule(modname);
- if (!m) goto end;
- p = PyObject_GetAttrString(m, "RefNannyAPI");
- if (!p) goto end;
- r = PyLong_AsVoidPtr(p);
-end:
- Py_XDECREF(p);
- Py_XDECREF(m);
- return (__Pyx_RefNannyAPIStruct *)r;
-}
-#endif
-
-/* PyErrExceptionMatches */
-#if CYTHON_FAST_THREAD_STATE
-static int __Pyx_PyErr_ExceptionMatchesTuple(PyObject *exc_type, PyObject *tuple) {
- Py_ssize_t i, n;
- n = PyTuple_GET_SIZE(tuple);
-#if PY_MAJOR_VERSION >= 3
- for (i=0; i= 0x030C00A6
- PyObject *current_exception = tstate->current_exception;
- if (unlikely(!current_exception)) return 0;
- exc_type = (PyObject*) Py_TYPE(current_exception);
- if (exc_type == err) return 1;
-#else
- exc_type = tstate->curexc_type;
- if (exc_type == err) return 1;
- if (unlikely(!exc_type)) return 0;
-#endif
- #if CYTHON_AVOID_BORROWED_REFS
- Py_INCREF(exc_type);
- #endif
- if (unlikely(PyTuple_Check(err))) {
- result = __Pyx_PyErr_ExceptionMatchesTuple(exc_type, err);
- } else {
- result = __Pyx_PyErr_GivenExceptionMatches(exc_type, err);
- }
- #if CYTHON_AVOID_BORROWED_REFS
- Py_DECREF(exc_type);
- #endif
- return result;
-}
-#endif
-
-/* PyErrFetchRestore */
-#if CYTHON_FAST_THREAD_STATE
-static CYTHON_INLINE void __Pyx_ErrRestoreInState(PyThreadState *tstate, PyObject *type, PyObject *value, PyObject *tb) {
-#if PY_VERSION_HEX >= 0x030C00A6
- PyObject *tmp_value;
- assert(type == NULL || (value != NULL && type == (PyObject*) Py_TYPE(value)));
- if (value) {
- #if CYTHON_COMPILING_IN_CPYTHON
- if (unlikely(((PyBaseExceptionObject*) value)->traceback != tb))
- #endif
- PyException_SetTraceback(value, tb);
- }
- tmp_value = tstate->current_exception;
- tstate->current_exception = value;
- Py_XDECREF(tmp_value);
-#else
- PyObject *tmp_type, *tmp_value, *tmp_tb;
- tmp_type = tstate->curexc_type;
- tmp_value = tstate->curexc_value;
- tmp_tb = tstate->curexc_traceback;
- tstate->curexc_type = type;
- tstate->curexc_value = value;
- tstate->curexc_traceback = tb;
- Py_XDECREF(tmp_type);
- Py_XDECREF(tmp_value);
- Py_XDECREF(tmp_tb);
-#endif
-}
-static CYTHON_INLINE void __Pyx_ErrFetchInState(PyThreadState *tstate, PyObject **type, PyObject **value, PyObject **tb) {
-#if PY_VERSION_HEX >= 0x030C00A6
- PyObject* exc_value;
- exc_value = tstate->current_exception;
- tstate->current_exception = 0;
- *value = exc_value;
- *type = NULL;
- *tb = NULL;
- if (exc_value) {
- *type = (PyObject*) Py_TYPE(exc_value);
- Py_INCREF(*type);
- #if CYTHON_COMPILING_IN_CPYTHON
- *tb = ((PyBaseExceptionObject*) exc_value)->traceback;
- Py_XINCREF(*tb);
- #else
- *tb = PyException_GetTraceback(exc_value);
- #endif
- }
-#else
- *type = tstate->curexc_type;
- *value = tstate->curexc_value;
- *tb = tstate->curexc_traceback;
- tstate->curexc_type = 0;
- tstate->curexc_value = 0;
- tstate->curexc_traceback = 0;
-#endif
-}
-#endif
-
-/* PyObjectGetAttrStr */
-#if CYTHON_USE_TYPE_SLOTS
-static CYTHON_INLINE PyObject* __Pyx_PyObject_GetAttrStr(PyObject* obj, PyObject* attr_name) {
- PyTypeObject* tp = Py_TYPE(obj);
- if (likely(tp->tp_getattro))
- return tp->tp_getattro(obj, attr_name);
-#if PY_MAJOR_VERSION < 3
- if (likely(tp->tp_getattr))
- return tp->tp_getattr(obj, PyString_AS_STRING(attr_name));
-#endif
- return PyObject_GetAttr(obj, attr_name);
-}
-#endif
-
-/* PyObjectGetAttrStrNoError */
-static void __Pyx_PyObject_GetAttrStr_ClearAttributeError(void) {
- __Pyx_PyThreadState_declare
- __Pyx_PyThreadState_assign
- if (likely(__Pyx_PyErr_ExceptionMatches(PyExc_AttributeError)))
- __Pyx_PyErr_Clear();
-}
-static CYTHON_INLINE PyObject* __Pyx_PyObject_GetAttrStrNoError(PyObject* obj, PyObject* attr_name) {
- PyObject *result;
-#if CYTHON_COMPILING_IN_CPYTHON && CYTHON_USE_TYPE_SLOTS && PY_VERSION_HEX >= 0x030700B1
- PyTypeObject* tp = Py_TYPE(obj);
- if (likely(tp->tp_getattro == PyObject_GenericGetAttr)) {
- return _PyObject_GenericGetAttrWithDict(obj, attr_name, NULL, 1);
- }
-#endif
- result = __Pyx_PyObject_GetAttrStr(obj, attr_name);
- if (unlikely(!result)) {
- __Pyx_PyObject_GetAttrStr_ClearAttributeError();
- }
- return result;
-}
-
-/* GetBuiltinName */
-static PyObject *__Pyx_GetBuiltinName(PyObject *name) {
- PyObject* result = __Pyx_PyObject_GetAttrStrNoError(__pyx_b, name);
- if (unlikely(!result) && !PyErr_Occurred()) {
- PyErr_Format(PyExc_NameError,
-#if PY_MAJOR_VERSION >= 3
- "name '%U' is not defined", name);
-#else
- "name '%.200s' is not defined", PyString_AS_STRING(name));
-#endif
- }
- return result;
-}
-
-/* PyIntCompare */
-static CYTHON_INLINE int __Pyx_PyInt_BoolEqObjC(PyObject *op1, PyObject *op2, long intval, long inplace) {
- CYTHON_MAYBE_UNUSED_VAR(intval);
- CYTHON_UNUSED_VAR(inplace);
- if (op1 == op2) {
- return 1;
- }
- #if PY_MAJOR_VERSION < 3
- if (likely(PyInt_CheckExact(op1))) {
- const long b = intval;
- long a = PyInt_AS_LONG(op1);
- return (a == b);
- }
- #endif
- #if CYTHON_USE_PYLONG_INTERNALS
- if (likely(PyLong_CheckExact(op1))) {
- int unequal;
- unsigned long uintval;
- Py_ssize_t size = __Pyx_PyLong_DigitCount(op1);
- const digit* digits = __Pyx_PyLong_Digits(op1);
- if (intval == 0) {
- return (__Pyx_PyLong_IsZero(op1) == 1);
- } else if (intval < 0) {
- if (__Pyx_PyLong_IsNonNeg(op1))
- return 0;
- intval = -intval;
- } else {
- if (__Pyx_PyLong_IsNeg(op1))
- return 0;
- }
- uintval = (unsigned long) intval;
-#if PyLong_SHIFT * 4 < SIZEOF_LONG*8
- if (uintval >> (PyLong_SHIFT * 4)) {
- unequal = (size != 5) || (digits[0] != (uintval & (unsigned long) PyLong_MASK))
- | (digits[1] != ((uintval >> (1 * PyLong_SHIFT)) & (unsigned long) PyLong_MASK)) | (digits[2] != ((uintval >> (2 * PyLong_SHIFT)) & (unsigned long) PyLong_MASK)) | (digits[3] != ((uintval >> (3 * PyLong_SHIFT)) & (unsigned long) PyLong_MASK)) | (digits[4] != ((uintval >> (4 * PyLong_SHIFT)) & (unsigned long) PyLong_MASK));
- } else
-#endif
-#if PyLong_SHIFT * 3 < SIZEOF_LONG*8
- if (uintval >> (PyLong_SHIFT * 3)) {
- unequal = (size != 4) || (digits[0] != (uintval & (unsigned long) PyLong_MASK))
- | (digits[1] != ((uintval >> (1 * PyLong_SHIFT)) & (unsigned long) PyLong_MASK)) | (digits[2] != ((uintval >> (2 * PyLong_SHIFT)) & (unsigned long) PyLong_MASK)) | (digits[3] != ((uintval >> (3 * PyLong_SHIFT)) & (unsigned long) PyLong_MASK));
- } else
-#endif
-#if PyLong_SHIFT * 2 < SIZEOF_LONG*8
- if (uintval >> (PyLong_SHIFT * 2)) {
- unequal = (size != 3) || (digits[0] != (uintval & (unsigned long) PyLong_MASK))
- | (digits[1] != ((uintval >> (1 * PyLong_SHIFT)) & (unsigned long) PyLong_MASK)) | (digits[2] != ((uintval >> (2 * PyLong_SHIFT)) & (unsigned long) PyLong_MASK));
- } else
-#endif
-#if PyLong_SHIFT * 1 < SIZEOF_LONG*8
- if (uintval >> (PyLong_SHIFT * 1)) {
- unequal = (size != 2) || (digits[0] != (uintval & (unsigned long) PyLong_MASK))
- | (digits[1] != ((uintval >> (1 * PyLong_SHIFT)) & (unsigned long) PyLong_MASK));
- } else
-#endif
- unequal = (size != 1) || (((unsigned long) digits[0]) != (uintval & (unsigned long) PyLong_MASK));
- return (unequal == 0);
- }
- #endif
- if (PyFloat_CheckExact(op1)) {
- const long b = intval;
-#if CYTHON_COMPILING_IN_LIMITED_API
- double a = __pyx_PyFloat_AsDouble(op1);
-#else
- double a = PyFloat_AS_DOUBLE(op1);
-#endif
- return ((double)a == (double)b);
- }
- return __Pyx_PyObject_IsTrueAndDecref(
- PyObject_RichCompare(op1, op2, Py_EQ));
-}
-
-/* RaiseTooManyValuesToUnpack */
-static CYTHON_INLINE void __Pyx_RaiseTooManyValuesError(Py_ssize_t expected) {
- PyErr_Format(PyExc_ValueError,
- "too many values to unpack (expected %" CYTHON_FORMAT_SSIZE_T "d)", expected);
-}
-
-/* RaiseNeedMoreValuesToUnpack */
-static CYTHON_INLINE void __Pyx_RaiseNeedMoreValuesError(Py_ssize_t index) {
- PyErr_Format(PyExc_ValueError,
- "need more than %" CYTHON_FORMAT_SSIZE_T "d value%.1s to unpack",
- index, (index == 1) ? "" : "s");
-}
-
-/* IterFinish */
-static CYTHON_INLINE int __Pyx_IterFinish(void) {
- __Pyx_PyThreadState_declare
- __Pyx_PyThreadState_assign
- PyObject* exc_type = __Pyx_PyErr_CurrentExceptionType();
- if (unlikely(exc_type)) {
- if (unlikely(!__Pyx_PyErr_GivenExceptionMatches(exc_type, PyExc_StopIteration)))
- return -1;
- __Pyx_PyErr_Clear();
- return 0;
- }
- return 0;
-}
-
-/* UnpackItemEndCheck */
-static int __Pyx_IternextUnpackEndCheck(PyObject *retval, Py_ssize_t expected) {
- if (unlikely(retval)) {
- Py_DECREF(retval);
- __Pyx_RaiseTooManyValuesError(expected);
- return -1;
- }
- return __Pyx_IterFinish();
-}
-
-/* GetItemInt */
-static PyObject *__Pyx_GetItemInt_Generic(PyObject *o, PyObject* j) {
- PyObject *r;
- if (unlikely(!j)) return NULL;
- r = PyObject_GetItem(o, j);
- Py_DECREF(j);
- return r;
-}
-static CYTHON_INLINE PyObject *__Pyx_GetItemInt_List_Fast(PyObject *o, Py_ssize_t i,
- CYTHON_NCP_UNUSED int wraparound,
- CYTHON_NCP_UNUSED int boundscheck) {
-#if CYTHON_ASSUME_SAFE_MACROS && !CYTHON_AVOID_BORROWED_REFS
- Py_ssize_t wrapped_i = i;
- if (wraparound & unlikely(i < 0)) {
- wrapped_i += PyList_GET_SIZE(o);
- }
- if ((!boundscheck) || likely(__Pyx_is_valid_index(wrapped_i, PyList_GET_SIZE(o)))) {
- PyObject *r = PyList_GET_ITEM(o, wrapped_i);
- Py_INCREF(r);
- return r;
- }
- return __Pyx_GetItemInt_Generic(o, PyInt_FromSsize_t(i));
-#else
- return PySequence_GetItem(o, i);
-#endif
-}
-static CYTHON_INLINE PyObject *__Pyx_GetItemInt_Tuple_Fast(PyObject *o, Py_ssize_t i,
- CYTHON_NCP_UNUSED int wraparound,
- CYTHON_NCP_UNUSED int boundscheck) {
-#if CYTHON_ASSUME_SAFE_MACROS && !CYTHON_AVOID_BORROWED_REFS
- Py_ssize_t wrapped_i = i;
- if (wraparound & unlikely(i < 0)) {
- wrapped_i += PyTuple_GET_SIZE(o);
- }
- if ((!boundscheck) || likely(__Pyx_is_valid_index(wrapped_i, PyTuple_GET_SIZE(o)))) {
- PyObject *r = PyTuple_GET_ITEM(o, wrapped_i);
- Py_INCREF(r);
- return r;
- }
- return __Pyx_GetItemInt_Generic(o, PyInt_FromSsize_t(i));
-#else
- return PySequence_GetItem(o, i);
-#endif
-}
-static CYTHON_INLINE PyObject *__Pyx_GetItemInt_Fast(PyObject *o, Py_ssize_t i, int is_list,
- CYTHON_NCP_UNUSED int wraparound,
- CYTHON_NCP_UNUSED int boundscheck) {
-#if CYTHON_ASSUME_SAFE_MACROS && !CYTHON_AVOID_BORROWED_REFS && CYTHON_USE_TYPE_SLOTS
- if (is_list || PyList_CheckExact(o)) {
- Py_ssize_t n = ((!wraparound) | likely(i >= 0)) ? i : i + PyList_GET_SIZE(o);
- if ((!boundscheck) || (likely(__Pyx_is_valid_index(n, PyList_GET_SIZE(o))))) {
- PyObject *r = PyList_GET_ITEM(o, n);
- Py_INCREF(r);
- return r;
- }
- }
- else if (PyTuple_CheckExact(o)) {
- Py_ssize_t n = ((!wraparound) | likely(i >= 0)) ? i : i + PyTuple_GET_SIZE(o);
- if ((!boundscheck) || likely(__Pyx_is_valid_index(n, PyTuple_GET_SIZE(o)))) {
- PyObject *r = PyTuple_GET_ITEM(o, n);
- Py_INCREF(r);
- return r;
- }
- } else {
- PyMappingMethods *mm = Py_TYPE(o)->tp_as_mapping;
- PySequenceMethods *sm = Py_TYPE(o)->tp_as_sequence;
- if (mm && mm->mp_subscript) {
- PyObject *r, *key = PyInt_FromSsize_t(i);
- if (unlikely(!key)) return NULL;
- r = mm->mp_subscript(o, key);
- Py_DECREF(key);
- return r;
- }
- if (likely(sm && sm->sq_item)) {
- if (wraparound && unlikely(i < 0) && likely(sm->sq_length)) {
- Py_ssize_t l = sm->sq_length(o);
- if (likely(l >= 0)) {
- i += l;
- } else {
- if (!PyErr_ExceptionMatches(PyExc_OverflowError))
- return NULL;
- PyErr_Clear();
- }
- }
- return sm->sq_item(o, i);
- }
- }
-#else
- if (is_list || PySequence_Check(o)) {
- return PySequence_GetItem(o, i);
- }
-#endif
- return __Pyx_GetItemInt_Generic(o, PyInt_FromSsize_t(i));
-}
-
-/* PyDictVersioning */
-#if CYTHON_USE_DICT_VERSIONS && CYTHON_USE_TYPE_SLOTS
-static CYTHON_INLINE PY_UINT64_T __Pyx_get_tp_dict_version(PyObject *obj) {
- PyObject *dict = Py_TYPE(obj)->tp_dict;
- return likely(dict) ? __PYX_GET_DICT_VERSION(dict) : 0;
-}
-static CYTHON_INLINE PY_UINT64_T __Pyx_get_object_dict_version(PyObject *obj) {
- PyObject **dictptr = NULL;
- Py_ssize_t offset = Py_TYPE(obj)->tp_dictoffset;
- if (offset) {
-#if CYTHON_COMPILING_IN_CPYTHON
- dictptr = (likely(offset > 0)) ? (PyObject **) ((char *)obj + offset) : _PyObject_GetDictPtr(obj);
-#else
- dictptr = _PyObject_GetDictPtr(obj);
-#endif
- }
- return (dictptr && *dictptr) ? __PYX_GET_DICT_VERSION(*dictptr) : 0;
-}
-static CYTHON_INLINE int __Pyx_object_dict_version_matches(PyObject* obj, PY_UINT64_T tp_dict_version, PY_UINT64_T obj_dict_version) {
- PyObject *dict = Py_TYPE(obj)->tp_dict;
- if (unlikely(!dict) || unlikely(tp_dict_version != __PYX_GET_DICT_VERSION(dict)))
- return 0;
- return obj_dict_version == __Pyx_get_object_dict_version(obj);
-}
-#endif
-
-/* GetModuleGlobalName */
-#if CYTHON_USE_DICT_VERSIONS
-static PyObject *__Pyx__GetModuleGlobalName(PyObject *name, PY_UINT64_T *dict_version, PyObject **dict_cached_value)
-#else
-static CYTHON_INLINE PyObject *__Pyx__GetModuleGlobalName(PyObject *name)
-#endif
-{
- PyObject *result;
-#if !CYTHON_AVOID_BORROWED_REFS
-#if CYTHON_COMPILING_IN_CPYTHON && PY_VERSION_HEX >= 0x030500A1
- result = _PyDict_GetItem_KnownHash(__pyx_d, name, ((PyASCIIObject *) name)->hash);
- __PYX_UPDATE_DICT_CACHE(__pyx_d, result, *dict_cached_value, *dict_version)
- if (likely(result)) {
- return __Pyx_NewRef(result);
- } else if (unlikely(PyErr_Occurred())) {
- return NULL;
- }
-#elif CYTHON_COMPILING_IN_LIMITED_API
- if (unlikely(!__pyx_m)) {
- return NULL;
- }
- result = PyObject_GetAttr(__pyx_m, name);
- if (likely(result)) {
- return result;
- }
-#else
- result = PyDict_GetItem(__pyx_d, name);
- __PYX_UPDATE_DICT_CACHE(__pyx_d, result, *dict_cached_value, *dict_version)
- if (likely(result)) {
- return __Pyx_NewRef(result);
- }
-#endif
-#else
- result = PyObject_GetItem(__pyx_d, name);
- __PYX_UPDATE_DICT_CACHE(__pyx_d, result, *dict_cached_value, *dict_version)
- if (likely(result)) {
- return __Pyx_NewRef(result);
- }
- PyErr_Clear();
-#endif
- return __Pyx_GetBuiltinName(name);
-}
-
-/* PyFunctionFastCall */
-#if CYTHON_FAST_PYCALL && !CYTHON_VECTORCALL
-static PyObject* __Pyx_PyFunction_FastCallNoKw(PyCodeObject *co, PyObject **args, Py_ssize_t na,
- PyObject *globals) {
- PyFrameObject *f;
- PyThreadState *tstate = __Pyx_PyThreadState_Current;
- PyObject **fastlocals;
- Py_ssize_t i;
- PyObject *result;
- assert(globals != NULL);
- /* XXX Perhaps we should create a specialized
- PyFrame_New() that doesn't take locals, but does
- take builtins without sanity checking them.
- */
- assert(tstate != NULL);
- f = PyFrame_New(tstate, co, globals, NULL);
- if (f == NULL) {
- return NULL;
- }
- fastlocals = __Pyx_PyFrame_GetLocalsplus(f);
- for (i = 0; i < na; i++) {
- Py_INCREF(*args);
- fastlocals[i] = *args++;
- }
- result = PyEval_EvalFrameEx(f,0);
- ++tstate->recursion_depth;
- Py_DECREF(f);
- --tstate->recursion_depth;
- return result;
-}
-static PyObject *__Pyx_PyFunction_FastCallDict(PyObject *func, PyObject **args, Py_ssize_t nargs, PyObject *kwargs) {
- PyCodeObject *co = (PyCodeObject *)PyFunction_GET_CODE(func);
- PyObject *globals = PyFunction_GET_GLOBALS(func);
- PyObject *argdefs = PyFunction_GET_DEFAULTS(func);
- PyObject *closure;
-#if PY_MAJOR_VERSION >= 3
- PyObject *kwdefs;
-#endif
- PyObject *kwtuple, **k;
- PyObject **d;
- Py_ssize_t nd;
- Py_ssize_t nk;
- PyObject *result;
- assert(kwargs == NULL || PyDict_Check(kwargs));
- nk = kwargs ? PyDict_Size(kwargs) : 0;
- if (unlikely(Py_EnterRecursiveCall((char*)" while calling a Python object"))) {
- return NULL;
- }
- if (
-#if PY_MAJOR_VERSION >= 3
- co->co_kwonlyargcount == 0 &&
-#endif
- likely(kwargs == NULL || nk == 0) &&
- co->co_flags == (CO_OPTIMIZED | CO_NEWLOCALS | CO_NOFREE)) {
- if (argdefs == NULL && co->co_argcount == nargs) {
- result = __Pyx_PyFunction_FastCallNoKw(co, args, nargs, globals);
- goto done;
- }
- else if (nargs == 0 && argdefs != NULL
- && co->co_argcount == Py_SIZE(argdefs)) {
- /* function called with no arguments, but all parameters have
- a default value: use default values as arguments .*/
- args = &PyTuple_GET_ITEM(argdefs, 0);
- result =__Pyx_PyFunction_FastCallNoKw(co, args, Py_SIZE(argdefs), globals);
- goto done;
- }
- }
- if (kwargs != NULL) {
- Py_ssize_t pos, i;
- kwtuple = PyTuple_New(2 * nk);
- if (kwtuple == NULL) {
- result = NULL;
- goto done;
- }
- k = &PyTuple_GET_ITEM(kwtuple, 0);
- pos = i = 0;
- while (PyDict_Next(kwargs, &pos, &k[i], &k[i+1])) {
- Py_INCREF(k[i]);
- Py_INCREF(k[i+1]);
- i += 2;
- }
- nk = i / 2;
- }
- else {
- kwtuple = NULL;
- k = NULL;
- }
- closure = PyFunction_GET_CLOSURE(func);
-#if PY_MAJOR_VERSION >= 3
- kwdefs = PyFunction_GET_KW_DEFAULTS(func);
-#endif
- if (argdefs != NULL) {
- d = &PyTuple_GET_ITEM(argdefs, 0);
- nd = Py_SIZE(argdefs);
- }
- else {
- d = NULL;
- nd = 0;
- }
-#if PY_MAJOR_VERSION >= 3
- result = PyEval_EvalCodeEx((PyObject*)co, globals, (PyObject *)NULL,
- args, (int)nargs,
- k, (int)nk,
- d, (int)nd, kwdefs, closure);
-#else
- result = PyEval_EvalCodeEx(co, globals, (PyObject *)NULL,
- args, (int)nargs,
- k, (int)nk,
- d, (int)nd, closure);
-#endif
- Py_XDECREF(kwtuple);
-done:
- Py_LeaveRecursiveCall();
- return result;
-}
-#endif
-
-/* PyObjectCall */
-#if CYTHON_COMPILING_IN_CPYTHON
-static CYTHON_INLINE PyObject* __Pyx_PyObject_Call(PyObject *func, PyObject *arg, PyObject *kw) {
- PyObject *result;
- ternaryfunc call = Py_TYPE(func)->tp_call;
- if (unlikely(!call))
- return PyObject_Call(func, arg, kw);
- if (unlikely(Py_EnterRecursiveCall((char*)" while calling a Python object")))
- return NULL;
- result = (*call)(func, arg, kw);
- Py_LeaveRecursiveCall();
- if (unlikely(!result) && unlikely(!PyErr_Occurred())) {
- PyErr_SetString(
- PyExc_SystemError,
- "NULL result without error in PyObject_Call");
- }
- return result;
-}
-#endif
-
-/* PyObjectCallMethO */
-#if CYTHON_COMPILING_IN_CPYTHON
-static CYTHON_INLINE PyObject* __Pyx_PyObject_CallMethO(PyObject *func, PyObject *arg) {
- PyObject *self, *result;
- PyCFunction cfunc;
- cfunc = PyCFunction_GET_FUNCTION(func);
- self = PyCFunction_GET_SELF(func);
- if (unlikely(Py_EnterRecursiveCall((char*)" while calling a Python object")))
- return NULL;
- result = cfunc(self, arg);
- Py_LeaveRecursiveCall();
- if (unlikely(!result) && unlikely(!PyErr_Occurred())) {
- PyErr_SetString(
- PyExc_SystemError,
- "NULL result without error in PyObject_Call");
- }
- return result;
-}
-#endif
-
-/* PyObjectFastCall */
-static PyObject* __Pyx_PyObject_FastCall_fallback(PyObject *func, PyObject **args, size_t nargs, PyObject *kwargs) {
- PyObject *argstuple;
- PyObject *result;
- size_t i;
- argstuple = PyTuple_New((Py_ssize_t)nargs);
- if (unlikely(!argstuple)) return NULL;
- for (i = 0; i < nargs; i++) {
- Py_INCREF(args[i]);
- PyTuple_SET_ITEM(argstuple, (Py_ssize_t)i, args[i]);
- }
- result = __Pyx_PyObject_Call(func, argstuple, kwargs);
- Py_DECREF(argstuple);
- return result;
-}
-static CYTHON_INLINE PyObject* __Pyx_PyObject_FastCallDict(PyObject *func, PyObject **args, size_t _nargs, PyObject *kwargs) {
- Py_ssize_t nargs = __Pyx_PyVectorcall_NARGS(_nargs);
-#if CYTHON_COMPILING_IN_CPYTHON
- if (nargs == 0 && kwargs == NULL) {
-#if defined(__Pyx_CyFunction_USED) && defined(NDEBUG)
- if (__Pyx_IsCyOrPyCFunction(func))
-#else
- if (PyCFunction_Check(func))
-#endif
- {
- if (likely(PyCFunction_GET_FLAGS(func) & METH_NOARGS)) {
- return __Pyx_PyObject_CallMethO(func, NULL);
- }
- }
- }
- else if (nargs == 1 && kwargs == NULL) {
- if (PyCFunction_Check(func))
- {
- if (likely(PyCFunction_GET_FLAGS(func) & METH_O)) {
- return __Pyx_PyObject_CallMethO(func, args[0]);
- }
- }
- }
-#endif
- #if PY_VERSION_HEX < 0x030800B1
- #if CYTHON_FAST_PYCCALL
- if (PyCFunction_Check(func)) {
- if (kwargs) {
- return _PyCFunction_FastCallDict(func, args, nargs, kwargs);
- } else {
- return _PyCFunction_FastCallKeywords(func, args, nargs, NULL);
- }
- }
- #if PY_VERSION_HEX >= 0x030700A1
- if (!kwargs && __Pyx_IS_TYPE(func, &PyMethodDescr_Type)) {
- return _PyMethodDescr_FastCallKeywords(func, args, nargs, NULL);
- }
- #endif
- #endif
- #if CYTHON_FAST_PYCALL
- if (PyFunction_Check(func)) {
- return __Pyx_PyFunction_FastCallDict(func, args, nargs, kwargs);
- }
- #endif
- #endif
- #if CYTHON_VECTORCALL
- vectorcallfunc f = _PyVectorcall_Function(func);
- if (f) {
- return f(func, args, (size_t)nargs, kwargs);
- }
- #elif defined(__Pyx_CyFunction_USED) && CYTHON_BACKPORT_VECTORCALL
- if (__Pyx_CyFunction_CheckExact(func)) {
- __pyx_vectorcallfunc f = __Pyx_CyFunction_func_vectorcall(func);
- if (f) return f(func, args, (size_t)nargs, kwargs);
- }
- #endif
- if (nargs == 0) {
- return __Pyx_PyObject_Call(func, __pyx_empty_tuple, kwargs);
- }
- return __Pyx_PyObject_FastCall_fallback(func, args, (size_t)nargs, kwargs);
-}
-
-/* TupleAndListFromArray */
-#if CYTHON_COMPILING_IN_CPYTHON
-static CYTHON_INLINE void __Pyx_copy_object_array(PyObject *const *CYTHON_RESTRICT src, PyObject** CYTHON_RESTRICT dest, Py_ssize_t length) {
- PyObject *v;
- Py_ssize_t i;
- for (i = 0; i < length; i++) {
- v = dest[i] = src[i];
- Py_INCREF(v);
- }
-}
-static CYTHON_INLINE PyObject *
-__Pyx_PyTuple_FromArray(PyObject *const *src, Py_ssize_t n)
-{
- PyObject *res;
- if (n <= 0) {
- Py_INCREF(__pyx_empty_tuple);
- return __pyx_empty_tuple;
- }
- res = PyTuple_New(n);
- if (unlikely(res == NULL)) return NULL;
- __Pyx_copy_object_array(src, ((PyTupleObject*)res)->ob_item, n);
- return res;
-}
-static CYTHON_INLINE PyObject *
-__Pyx_PyList_FromArray(PyObject *const *src, Py_ssize_t n)
-{
- PyObject *res;
- if (n <= 0) {
- return PyList_New(0);
- }
- res = PyList_New(n);
- if (unlikely(res == NULL)) return NULL;
- __Pyx_copy_object_array(src, ((PyListObject*)res)->ob_item, n);
- return res;
-}
-#endif
-
-/* BytesEquals */
-static CYTHON_INLINE int __Pyx_PyBytes_Equals(PyObject* s1, PyObject* s2, int equals) {
-#if CYTHON_COMPILING_IN_PYPY || CYTHON_COMPILING_IN_LIMITED_API
- return PyObject_RichCompareBool(s1, s2, equals);
-#else
- if (s1 == s2) {
- return (equals == Py_EQ);
- } else if (PyBytes_CheckExact(s1) & PyBytes_CheckExact(s2)) {
- const char *ps1, *ps2;
- Py_ssize_t length = PyBytes_GET_SIZE(s1);
- if (length != PyBytes_GET_SIZE(s2))
- return (equals == Py_NE);
- ps1 = PyBytes_AS_STRING(s1);
- ps2 = PyBytes_AS_STRING(s2);
- if (ps1[0] != ps2[0]) {
- return (equals == Py_NE);
- } else if (length == 1) {
- return (equals == Py_EQ);
- } else {
- int result;
-#if CYTHON_USE_UNICODE_INTERNALS && (PY_VERSION_HEX < 0x030B0000)
- Py_hash_t hash1, hash2;
- hash1 = ((PyBytesObject*)s1)->ob_shash;
- hash2 = ((PyBytesObject*)s2)->ob_shash;
- if (hash1 != hash2 && hash1 != -1 && hash2 != -1) {
- return (equals == Py_NE);
- }
-#endif
- result = memcmp(ps1, ps2, (size_t)length);
- return (equals == Py_EQ) ? (result == 0) : (result != 0);
- }
- } else if ((s1 == Py_None) & PyBytes_CheckExact(s2)) {
- return (equals == Py_NE);
- } else if ((s2 == Py_None) & PyBytes_CheckExact(s1)) {
- return (equals == Py_NE);
- } else {
- int result;
- PyObject* py_result = PyObject_RichCompare(s1, s2, equals);
- if (!py_result)
- return -1;
- result = __Pyx_PyObject_IsTrue(py_result);
- Py_DECREF(py_result);
- return result;
- }
-#endif
-}
-
-/* UnicodeEquals */
-static CYTHON_INLINE int __Pyx_PyUnicode_Equals(PyObject* s1, PyObject* s2, int equals) {
-#if CYTHON_COMPILING_IN_PYPY || CYTHON_COMPILING_IN_LIMITED_API
- return PyObject_RichCompareBool(s1, s2, equals);
-#else
-#if PY_MAJOR_VERSION < 3
- PyObject* owned_ref = NULL;
-#endif
- int s1_is_unicode, s2_is_unicode;
- if (s1 == s2) {
- goto return_eq;
- }
- s1_is_unicode = PyUnicode_CheckExact(s1);
- s2_is_unicode = PyUnicode_CheckExact(s2);
-#if PY_MAJOR_VERSION < 3
- if ((s1_is_unicode & (!s2_is_unicode)) && PyString_CheckExact(s2)) {
- owned_ref = PyUnicode_FromObject(s2);
- if (unlikely(!owned_ref))
- return -1;
- s2 = owned_ref;
- s2_is_unicode = 1;
- } else if ((s2_is_unicode & (!s1_is_unicode)) && PyString_CheckExact(s1)) {
- owned_ref = PyUnicode_FromObject(s1);
- if (unlikely(!owned_ref))
- return -1;
- s1 = owned_ref;
- s1_is_unicode = 1;
- } else if (((!s2_is_unicode) & (!s1_is_unicode))) {
- return __Pyx_PyBytes_Equals(s1, s2, equals);
- }
-#endif
- if (s1_is_unicode & s2_is_unicode) {
- Py_ssize_t length;
- int kind;
- void *data1, *data2;
- if (unlikely(__Pyx_PyUnicode_READY(s1) < 0) || unlikely(__Pyx_PyUnicode_READY(s2) < 0))
- return -1;
- length = __Pyx_PyUnicode_GET_LENGTH(s1);
- if (length != __Pyx_PyUnicode_GET_LENGTH(s2)) {
- goto return_ne;
- }
-#if CYTHON_USE_UNICODE_INTERNALS
- {
- Py_hash_t hash1, hash2;
- #if CYTHON_PEP393_ENABLED
- hash1 = ((PyASCIIObject*)s1)->hash;
- hash2 = ((PyASCIIObject*)s2)->hash;
- #else
- hash1 = ((PyUnicodeObject*)s1)->hash;
- hash2 = ((PyUnicodeObject*)s2)->hash;
- #endif
- if (hash1 != hash2 && hash1 != -1 && hash2 != -1) {
- goto return_ne;
- }
- }
-#endif
- kind = __Pyx_PyUnicode_KIND(s1);
- if (kind != __Pyx_PyUnicode_KIND(s2)) {
- goto return_ne;
- }
- data1 = __Pyx_PyUnicode_DATA(s1);
- data2 = __Pyx_PyUnicode_DATA(s2);
- if (__Pyx_PyUnicode_READ(kind, data1, 0) != __Pyx_PyUnicode_READ(kind, data2, 0)) {
- goto return_ne;
- } else if (length == 1) {
- goto return_eq;
- } else {
- int result = memcmp(data1, data2, (size_t)(length * kind));
- #if PY_MAJOR_VERSION < 3
- Py_XDECREF(owned_ref);
- #endif
- return (equals == Py_EQ) ? (result == 0) : (result != 0);
- }
- } else if ((s1 == Py_None) & s2_is_unicode) {
- goto return_ne;
- } else if ((s2 == Py_None) & s1_is_unicode) {
- goto return_ne;
- } else {
- int result;
- PyObject* py_result = PyObject_RichCompare(s1, s2, equals);
- #if PY_MAJOR_VERSION < 3
- Py_XDECREF(owned_ref);
- #endif
- if (!py_result)
- return -1;
- result = __Pyx_PyObject_IsTrue(py_result);
- Py_DECREF(py_result);
- return result;
- }
-return_eq:
- #if PY_MAJOR_VERSION < 3
- Py_XDECREF(owned_ref);
- #endif
- return (equals == Py_EQ);
-return_ne:
- #if PY_MAJOR_VERSION < 3
- Py_XDECREF(owned_ref);
- #endif
- return (equals == Py_NE);
-#endif
-}
-
-/* fastcall */
-#if CYTHON_METH_FASTCALL
-static CYTHON_INLINE PyObject * __Pyx_GetKwValue_FASTCALL(PyObject *kwnames, PyObject *const *kwvalues, PyObject *s)
-{
- Py_ssize_t i, n = PyTuple_GET_SIZE(kwnames);
- for (i = 0; i < n; i++)
- {
- if (s == PyTuple_GET_ITEM(kwnames, i)) return kwvalues[i];
- }
- for (i = 0; i < n; i++)
- {
- int eq = __Pyx_PyUnicode_Equals(s, PyTuple_GET_ITEM(kwnames, i), Py_EQ);
- if (unlikely(eq != 0)) {
- if (unlikely(eq < 0)) return NULL; // error
- return kwvalues[i];
- }
- }
- return NULL; // not found (no exception set)
-}
-#endif
-
-/* RaiseArgTupleInvalid */
-static void __Pyx_RaiseArgtupleInvalid(
- const char* func_name,
- int exact,
- Py_ssize_t num_min,
- Py_ssize_t num_max,
- Py_ssize_t num_found)
-{
- Py_ssize_t num_expected;
- const char *more_or_less;
- if (num_found < num_min) {
- num_expected = num_min;
- more_or_less = "at least";
- } else {
- num_expected = num_max;
- more_or_less = "at most";
- }
- if (exact) {
- more_or_less = "exactly";
- }
- PyErr_Format(PyExc_TypeError,
- "%.200s() takes %.8s %" CYTHON_FORMAT_SSIZE_T "d positional argument%.1s (%" CYTHON_FORMAT_SSIZE_T "d given)",
- func_name, more_or_less, num_expected,
- (num_expected == 1) ? "" : "s", num_found);
-}
-
-/* RaiseDoubleKeywords */
-static void __Pyx_RaiseDoubleKeywordsError(
- const char* func_name,
- PyObject* kw_name)
-{
- PyErr_Format(PyExc_TypeError,
- #if PY_MAJOR_VERSION >= 3
- "%s() got multiple values for keyword argument '%U'", func_name, kw_name);
- #else
- "%s() got multiple values for keyword argument '%s'", func_name,
- PyString_AsString(kw_name));
- #endif
-}
-
-/* ParseKeywords */
-static int __Pyx_ParseOptionalKeywords(
- PyObject *kwds,
- PyObject *const *kwvalues,
- PyObject **argnames[],
- PyObject *kwds2,
- PyObject *values[],
- Py_ssize_t num_pos_args,
- const char* function_name)
-{
- PyObject *key = 0, *value = 0;
- Py_ssize_t pos = 0;
- PyObject*** name;
- PyObject*** first_kw_arg = argnames + num_pos_args;
- int kwds_is_tuple = CYTHON_METH_FASTCALL && likely(PyTuple_Check(kwds));
- while (1) {
- if (kwds_is_tuple) {
- if (pos >= PyTuple_GET_SIZE(kwds)) break;
- key = PyTuple_GET_ITEM(kwds, pos);
- value = kwvalues[pos];
- pos++;
- }
- else
- {
- if (!PyDict_Next(kwds, &pos, &key, &value)) break;
- }
- name = first_kw_arg;
- while (*name && (**name != key)) name++;
- if (*name) {
- values[name-argnames] = value;
- continue;
- }
- name = first_kw_arg;
- #if PY_MAJOR_VERSION < 3
- if (likely(PyString_Check(key))) {
- while (*name) {
- if ((CYTHON_COMPILING_IN_PYPY || PyString_GET_SIZE(**name) == PyString_GET_SIZE(key))
- && _PyString_Eq(**name, key)) {
- values[name-argnames] = value;
- break;
- }
- name++;
- }
- if (*name) continue;
- else {
- PyObject*** argname = argnames;
- while (argname != first_kw_arg) {
- if ((**argname == key) || (
- (CYTHON_COMPILING_IN_PYPY || PyString_GET_SIZE(**argname) == PyString_GET_SIZE(key))
- && _PyString_Eq(**argname, key))) {
- goto arg_passed_twice;
- }
- argname++;
- }
- }
- } else
- #endif
- if (likely(PyUnicode_Check(key))) {
- while (*name) {
- int cmp = (
- #if !CYTHON_COMPILING_IN_PYPY && PY_MAJOR_VERSION >= 3
- (__Pyx_PyUnicode_GET_LENGTH(**name) != __Pyx_PyUnicode_GET_LENGTH(key)) ? 1 :
- #endif
- PyUnicode_Compare(**name, key)
- );
- if (cmp < 0 && unlikely(PyErr_Occurred())) goto bad;
- if (cmp == 0) {
- values[name-argnames] = value;
- break;
- }
- name++;
- }
- if (*name) continue;
- else {
- PyObject*** argname = argnames;
- while (argname != first_kw_arg) {
- int cmp = (**argname == key) ? 0 :
- #if !CYTHON_COMPILING_IN_PYPY && PY_MAJOR_VERSION >= 3
- (__Pyx_PyUnicode_GET_LENGTH(**argname) != __Pyx_PyUnicode_GET_LENGTH(key)) ? 1 :
- #endif
- PyUnicode_Compare(**argname, key);
- if (cmp < 0 && unlikely(PyErr_Occurred())) goto bad;
- if (cmp == 0) goto arg_passed_twice;
- argname++;
- }
- }
- } else
- goto invalid_keyword_type;
- if (kwds2) {
- if (unlikely(PyDict_SetItem(kwds2, key, value))) goto bad;
- } else {
- goto invalid_keyword;
- }
- }
- return 0;
-arg_passed_twice:
- __Pyx_RaiseDoubleKeywordsError(function_name, key);
- goto bad;
-invalid_keyword_type:
- PyErr_Format(PyExc_TypeError,
- "%.200s() keywords must be strings", function_name);
- goto bad;
-invalid_keyword:
- #if PY_MAJOR_VERSION < 3
- PyErr_Format(PyExc_TypeError,
- "%.200s() got an unexpected keyword argument '%.200s'",
- function_name, PyString_AsString(key));
- #else
- PyErr_Format(PyExc_TypeError,
- "%s() got an unexpected keyword argument '%U'",
- function_name, key);
- #endif
-bad:
- return -1;
-}
-
-/* GetException */
-#if CYTHON_FAST_THREAD_STATE
-static int __Pyx__GetException(PyThreadState *tstate, PyObject **type, PyObject **value, PyObject **tb)
-#else
-static int __Pyx_GetException(PyObject **type, PyObject **value, PyObject **tb)
-#endif
-{
- PyObject *local_type = NULL, *local_value, *local_tb = NULL;
-#if CYTHON_FAST_THREAD_STATE
- PyObject *tmp_type, *tmp_value, *tmp_tb;
- #if PY_VERSION_HEX >= 0x030C00A6
- local_value = tstate->current_exception;
- tstate->current_exception = 0;
- if (likely(local_value)) {
- local_type = (PyObject*) Py_TYPE(local_value);
- Py_INCREF(local_type);
- local_tb = PyException_GetTraceback(local_value);
- }
- #else
- local_type = tstate->curexc_type;
- local_value = tstate->curexc_value;
- local_tb = tstate->curexc_traceback;
- tstate->curexc_type = 0;
- tstate->curexc_value = 0;
- tstate->curexc_traceback = 0;
- #endif
-#else
- PyErr_Fetch(&local_type, &local_value, &local_tb);
-#endif
- PyErr_NormalizeException(&local_type, &local_value, &local_tb);
-#if CYTHON_FAST_THREAD_STATE && PY_VERSION_HEX >= 0x030C00A6
- if (unlikely(tstate->current_exception))
-#elif CYTHON_FAST_THREAD_STATE
- if (unlikely(tstate->curexc_type))
-#else
- if (unlikely(PyErr_Occurred()))
-#endif
- goto bad;
- #if PY_MAJOR_VERSION >= 3
- if (local_tb) {
- if (unlikely(PyException_SetTraceback(local_value, local_tb) < 0))
- goto bad;
- }
- #endif
- Py_XINCREF(local_tb);
- Py_XINCREF(local_type);
- Py_XINCREF(local_value);
- *type = local_type;
- *value = local_value;
- *tb = local_tb;
-#if CYTHON_FAST_THREAD_STATE
- #if CYTHON_USE_EXC_INFO_STACK
- {
- _PyErr_StackItem *exc_info = tstate->exc_info;
- #if PY_VERSION_HEX >= 0x030B00a4
- tmp_value = exc_info->exc_value;
- exc_info->exc_value = local_value;
- tmp_type = NULL;
- tmp_tb = NULL;
- Py_XDECREF(local_type);
- Py_XDECREF(local_tb);
- #else
- tmp_type = exc_info->exc_type;
- tmp_value = exc_info->exc_value;
- tmp_tb = exc_info->exc_traceback;
- exc_info->exc_type = local_type;
- exc_info->exc_value = local_value;
- exc_info->exc_traceback = local_tb;
- #endif
- }
- #else
- tmp_type = tstate->exc_type;
- tmp_value = tstate->exc_value;
- tmp_tb = tstate->exc_traceback;
- tstate->exc_type = local_type;
- tstate->exc_value = local_value;
- tstate->exc_traceback = local_tb;
- #endif
- Py_XDECREF(tmp_type);
- Py_XDECREF(tmp_value);
- Py_XDECREF(tmp_tb);
-#else
- PyErr_SetExcInfo(local_type, local_value, local_tb);
-#endif
- return 0;
-bad:
- *type = 0;
- *value = 0;
- *tb = 0;
- Py_XDECREF(local_type);
- Py_XDECREF(local_value);
- Py_XDECREF(local_tb);
- return -1;
-}
-
-/* pep479 */
-static void __Pyx_Generator_Replace_StopIteration(int in_async_gen) {
- PyObject *exc, *val, *tb, *cur_exc;
- __Pyx_PyThreadState_declare
- #ifdef __Pyx_StopAsyncIteration_USED
- int is_async_stopiteration = 0;
- #endif
- CYTHON_MAYBE_UNUSED_VAR(in_async_gen);
- cur_exc = PyErr_Occurred();
- if (likely(!__Pyx_PyErr_GivenExceptionMatches(cur_exc, PyExc_StopIteration))) {
- #ifdef __Pyx_StopAsyncIteration_USED
- if (in_async_gen && unlikely(__Pyx_PyErr_GivenExceptionMatches(cur_exc, __Pyx_PyExc_StopAsyncIteration))) {
- is_async_stopiteration = 1;
- } else
- #endif
- return;
- }
- __Pyx_PyThreadState_assign
- __Pyx_GetException(&exc, &val, &tb);
- Py_XDECREF(exc);
- Py_XDECREF(val);
- Py_XDECREF(tb);
- PyErr_SetString(PyExc_RuntimeError,
- #ifdef __Pyx_StopAsyncIteration_USED
- is_async_stopiteration ? "async generator raised StopAsyncIteration" :
- in_async_gen ? "async generator raised StopIteration" :
- #endif
- "generator raised StopIteration");
-}
-
-/* GetTopmostException */
-#if CYTHON_USE_EXC_INFO_STACK && CYTHON_FAST_THREAD_STATE
-static _PyErr_StackItem *
-__Pyx_PyErr_GetTopmostException(PyThreadState *tstate)
-{
- _PyErr_StackItem *exc_info = tstate->exc_info;
- while ((exc_info->exc_value == NULL || exc_info->exc_value == Py_None) &&
- exc_info->previous_item != NULL)
- {
- exc_info = exc_info->previous_item;
- }
- return exc_info;
-}
-#endif
-
-/* SaveResetException */
-#if CYTHON_FAST_THREAD_STATE
-static CYTHON_INLINE void __Pyx__ExceptionSave(PyThreadState *tstate, PyObject **type, PyObject **value, PyObject **tb) {
- #if CYTHON_USE_EXC_INFO_STACK && PY_VERSION_HEX >= 0x030B00a4
- _PyErr_StackItem *exc_info = __Pyx_PyErr_GetTopmostException(tstate);
- PyObject *exc_value = exc_info->exc_value;
- if (exc_value == NULL || exc_value == Py_None) {
- *value = NULL;
- *type = NULL;
- *tb = NULL;
- } else {
- *value = exc_value;
- Py_INCREF(*value);
- *type = (PyObject*) Py_TYPE(exc_value);
- Py_INCREF(*type);
- *tb = PyException_GetTraceback(exc_value);
- }
- #elif CYTHON_USE_EXC_INFO_STACK
- _PyErr_StackItem *exc_info = __Pyx_PyErr_GetTopmostException(tstate);
- *type = exc_info->exc_type;
- *value = exc_info->exc_value;
- *tb = exc_info->exc_traceback;
- Py_XINCREF(*type);
- Py_XINCREF(*value);
- Py_XINCREF(*tb);
- #else
- *type = tstate->exc_type;
- *value = tstate->exc_value;
- *tb = tstate->exc_traceback;
- Py_XINCREF(*type);
- Py_XINCREF(*value);
- Py_XINCREF(*tb);
- #endif
-}
-static CYTHON_INLINE void __Pyx__ExceptionReset(PyThreadState *tstate, PyObject *type, PyObject *value, PyObject *tb) {
- #if CYTHON_USE_EXC_INFO_STACK && PY_VERSION_HEX >= 0x030B00a4
- _PyErr_StackItem *exc_info = tstate->exc_info;
- PyObject *tmp_value = exc_info->exc_value;
- exc_info->exc_value = value;
- Py_XDECREF(tmp_value);
- Py_XDECREF(type);
- Py_XDECREF(tb);
- #else
- PyObject *tmp_type, *tmp_value, *tmp_tb;
- #if CYTHON_USE_EXC_INFO_STACK
- _PyErr_StackItem *exc_info = tstate->exc_info;
- tmp_type = exc_info->exc_type;
- tmp_value = exc_info->exc_value;
- tmp_tb = exc_info->exc_traceback;
- exc_info->exc_type = type;
- exc_info->exc_value = value;
- exc_info->exc_traceback = tb;
- #else
- tmp_type = tstate->exc_type;
- tmp_value = tstate->exc_value;
- tmp_tb = tstate->exc_traceback;
- tstate->exc_type = type;
- tstate->exc_value = value;
- tstate->exc_traceback = tb;
- #endif
- Py_XDECREF(tmp_type);
- Py_XDECREF(tmp_value);
- Py_XDECREF(tmp_tb);
- #endif
-}
-#endif
-
-/* IterNext */
-static PyObject *__Pyx_PyIter_Next2Default(PyObject* defval) {
- PyObject* exc_type;
- __Pyx_PyThreadState_declare
- __Pyx_PyThreadState_assign
- exc_type = __Pyx_PyErr_CurrentExceptionType();
- if (unlikely(exc_type)) {
- if (!defval || unlikely(!__Pyx_PyErr_GivenExceptionMatches(exc_type, PyExc_StopIteration)))
- return NULL;
- __Pyx_PyErr_Clear();
- Py_INCREF(defval);
- return defval;
- }
- if (defval) {
- Py_INCREF(defval);
- return defval;
- }
- __Pyx_PyErr_SetNone(PyExc_StopIteration);
- return NULL;
-}
-static void __Pyx_PyIter_Next_ErrorNoIterator(PyObject *iterator) {
- __Pyx_TypeName iterator_type_name = __Pyx_PyType_GetName(Py_TYPE(iterator));
- PyErr_Format(PyExc_TypeError,
- __Pyx_FMT_TYPENAME " object is not an iterator", iterator_type_name);
- __Pyx_DECREF_TypeName(iterator_type_name);
-}
-static CYTHON_INLINE PyObject *__Pyx_PyIter_Next2(PyObject* iterator, PyObject* defval) {
- PyObject* next;
- iternextfunc iternext = Py_TYPE(iterator)->tp_iternext;
- if (likely(iternext)) {
-#if CYTHON_USE_TYPE_SLOTS || CYTHON_COMPILING_IN_PYPY
- next = iternext(iterator);
- if (likely(next))
- return next;
-#if CYTHON_COMPILING_IN_CPYTHON
- if (unlikely(iternext == &_PyObject_NextNotImplemented))
- return NULL;
-#endif
-#else
- next = PyIter_Next(iterator);
- if (likely(next))
- return next;
-#endif
- } else if (CYTHON_USE_TYPE_SLOTS || unlikely(!PyIter_Check(iterator))) {
- __Pyx_PyIter_Next_ErrorNoIterator(iterator);
- return NULL;
- }
-#if !CYTHON_USE_TYPE_SLOTS
- else {
- next = PyIter_Next(iterator);
- if (likely(next))
- return next;
- }
-#endif
- return __Pyx_PyIter_Next2Default(defval);
-}
-
-/* PyIntBinop */
-#if !CYTHON_COMPILING_IN_PYPY
-static PyObject* __Pyx_PyInt_AddObjC(PyObject *op1, PyObject *op2, long intval, int inplace, int zerodivision_check) {
- CYTHON_MAYBE_UNUSED_VAR(intval);
- CYTHON_MAYBE_UNUSED_VAR(inplace);
- CYTHON_UNUSED_VAR(zerodivision_check);
- #if PY_MAJOR_VERSION < 3
- if (likely(PyInt_CheckExact(op1))) {
- const long b = intval;
- long x;
- long a = PyInt_AS_LONG(op1);
-
- x = (long)((unsigned long)a + (unsigned long)b);
- if (likely((x^a) >= 0 || (x^b) >= 0))
- return PyInt_FromLong(x);
- return PyLong_Type.tp_as_number->nb_add(op1, op2);
- }
- #endif
- #if CYTHON_USE_PYLONG_INTERNALS
- if (likely(PyLong_CheckExact(op1))) {
- const long b = intval;
- long a, x;
-#ifdef HAVE_LONG_LONG
- const PY_LONG_LONG llb = intval;
- PY_LONG_LONG lla, llx;
-#endif
- if (unlikely(__Pyx_PyLong_IsZero(op1))) {
- return __Pyx_NewRef(op2);
- }
- if (likely(__Pyx_PyLong_IsCompact(op1))) {
- a = __Pyx_PyLong_CompactValue(op1);
- } else {
- const digit* digits = __Pyx_PyLong_Digits(op1);
- const Py_ssize_t size = __Pyx_PyLong_SignedDigitCount(op1);
- switch (size) {
- case -2:
- if (8 * sizeof(long) - 1 > 2 * PyLong_SHIFT) {
- a = -(long) (((((unsigned long)digits[1]) << PyLong_SHIFT) | (unsigned long)digits[0]));
- break;
- #ifdef HAVE_LONG_LONG
- } else if (8 * sizeof(PY_LONG_LONG) - 1 > 2 * PyLong_SHIFT) {
- lla = -(PY_LONG_LONG) (((((unsigned PY_LONG_LONG)digits[1]) << PyLong_SHIFT) | (unsigned PY_LONG_LONG)digits[0]));
- goto long_long;
- #endif
- }
- CYTHON_FALLTHROUGH;
- case 2:
- if (8 * sizeof(long) - 1 > 2 * PyLong_SHIFT) {
- a = (long) (((((unsigned long)digits[1]) << PyLong_SHIFT) | (unsigned long)digits[0]));
- break;
- #ifdef HAVE_LONG_LONG
- } else if (8 * sizeof(PY_LONG_LONG) - 1 > 2 * PyLong_SHIFT) {
- lla = (PY_LONG_LONG) (((((unsigned PY_LONG_LONG)digits[1]) << PyLong_SHIFT) | (unsigned PY_LONG_LONG)digits[0]));
- goto long_long;
- #endif
- }
- CYTHON_FALLTHROUGH;
- case -3:
- if (8 * sizeof(long) - 1 > 3 * PyLong_SHIFT) {
- a = -(long) (((((((unsigned long)digits[2]) << PyLong_SHIFT) | (unsigned long)digits[1]) << PyLong_SHIFT) | (unsigned long)digits[0]));
- break;
- #ifdef HAVE_LONG_LONG
- } else if (8 * sizeof(PY_LONG_LONG) - 1 > 3 * PyLong_SHIFT) {
- lla = -(PY_LONG_LONG) (((((((unsigned PY_LONG_LONG)digits[2]) << PyLong_SHIFT) | (unsigned PY_LONG_LONG)digits[1]) << PyLong_SHIFT) | (unsigned PY_LONG_LONG)digits[0]));
- goto long_long;
- #endif
- }
- CYTHON_FALLTHROUGH;
- case 3:
- if (8 * sizeof(long) - 1 > 3 * PyLong_SHIFT) {
- a = (long) (((((((unsigned long)digits[2]) << PyLong_SHIFT) | (unsigned long)digits[1]) << PyLong_SHIFT) | (unsigned long)digits[0]));
- break;
- #ifdef HAVE_LONG_LONG
- } else if (8 * sizeof(PY_LONG_LONG) - 1 > 3 * PyLong_SHIFT) {
- lla = (PY_LONG_LONG) (((((((unsigned PY_LONG_LONG)digits[2]) << PyLong_SHIFT) | (unsigned PY_LONG_LONG)digits[1]) << PyLong_SHIFT) | (unsigned PY_LONG_LONG)digits[0]));
- goto long_long;
- #endif
- }
- CYTHON_FALLTHROUGH;
- case -4:
- if (8 * sizeof(long) - 1 > 4 * PyLong_SHIFT) {
- a = -(long) (((((((((unsigned long)digits[3]) << PyLong_SHIFT) | (unsigned long)digits[2]) << PyLong_SHIFT) | (unsigned long)digits[1]) << PyLong_SHIFT) | (unsigned long)digits[0]));
- break;
- #ifdef HAVE_LONG_LONG
- } else if (8 * sizeof(PY_LONG_LONG) - 1 > 4 * PyLong_SHIFT) {
- lla = -(PY_LONG_LONG) (((((((((unsigned PY_LONG_LONG)digits[3]) << PyLong_SHIFT) | (unsigned PY_LONG_LONG)digits[2]) << PyLong_SHIFT) | (unsigned PY_LONG_LONG)digits[1]) << PyLong_SHIFT) | (unsigned PY_LONG_LONG)digits[0]));
- goto long_long;
- #endif
- }
- CYTHON_FALLTHROUGH;
- case 4:
- if (8 * sizeof(long) - 1 > 4 * PyLong_SHIFT) {
- a = (long) (((((((((unsigned long)digits[3]) << PyLong_SHIFT) | (unsigned long)digits[2]) << PyLong_SHIFT) | (unsigned long)digits[1]) << PyLong_SHIFT) | (unsigned long)digits[0]));
- break;
- #ifdef HAVE_LONG_LONG
- } else if (8 * sizeof(PY_LONG_LONG) - 1 > 4 * PyLong_SHIFT) {
- lla = (PY_LONG_LONG) (((((((((unsigned PY_LONG_LONG)digits[3]) << PyLong_SHIFT) | (unsigned PY_LONG_LONG)digits[2]) << PyLong_SHIFT) | (unsigned PY_LONG_LONG)digits[1]) << PyLong_SHIFT) | (unsigned PY_LONG_LONG)digits[0]));
- goto long_long;
- #endif
- }
- CYTHON_FALLTHROUGH;
- default: return PyLong_Type.tp_as_number->nb_add(op1, op2);
- }
- }
- x = a + b;
- return PyLong_FromLong(x);
-#ifdef HAVE_LONG_LONG
- long_long:
- llx = lla + llb;
- return PyLong_FromLongLong(llx);
-#endif
-
-
- }
- #endif
- if (PyFloat_CheckExact(op1)) {
- const long b = intval;
-#if CYTHON_COMPILING_IN_LIMITED_API
- double a = __pyx_PyFloat_AsDouble(op1);
-#else
- double a = PyFloat_AS_DOUBLE(op1);
-#endif
- double result;
-
- PyFPE_START_PROTECT("add", return NULL)
- result = ((double)a) + (double)b;
- PyFPE_END_PROTECT(result)
- return PyFloat_FromDouble(result);
- }
- return (inplace ? PyNumber_InPlaceAdd : PyNumber_Add)(op1, op2);
-}
-#endif
-
-/* RaiseException */
-#if PY_MAJOR_VERSION < 3
-static void __Pyx_Raise(PyObject *type, PyObject *value, PyObject *tb, PyObject *cause) {
- __Pyx_PyThreadState_declare
- CYTHON_UNUSED_VAR(cause);
- Py_XINCREF(type);
- if (!value || value == Py_None)
- value = NULL;
- else
- Py_INCREF(value);
- if (!tb || tb == Py_None)
- tb = NULL;
- else {
- Py_INCREF(tb);
- if (!PyTraceBack_Check(tb)) {
- PyErr_SetString(PyExc_TypeError,
- "raise: arg 3 must be a traceback or None");
- goto raise_error;
- }
- }
- if (PyType_Check(type)) {
-#if CYTHON_COMPILING_IN_PYPY
- if (!value) {
- Py_INCREF(Py_None);
- value = Py_None;
- }
-#endif
- PyErr_NormalizeException(&type, &value, &tb);
- } else {
- if (value) {
- PyErr_SetString(PyExc_TypeError,
- "instance exception may not have a separate value");
- goto raise_error;
- }
- value = type;
- type = (PyObject*) Py_TYPE(type);
- Py_INCREF(type);
- if (!PyType_IsSubtype((PyTypeObject *)type, (PyTypeObject *)PyExc_BaseException)) {
- PyErr_SetString(PyExc_TypeError,
- "raise: exception class must be a subclass of BaseException");
- goto raise_error;
- }
- }
- __Pyx_PyThreadState_assign
- __Pyx_ErrRestore(type, value, tb);
- return;
-raise_error:
- Py_XDECREF(value);
- Py_XDECREF(type);
- Py_XDECREF(tb);
- return;
-}
-#else
-static void __Pyx_Raise(PyObject *type, PyObject *value, PyObject *tb, PyObject *cause) {
- PyObject* owned_instance = NULL;
- if (tb == Py_None) {
- tb = 0;
- } else if (tb && !PyTraceBack_Check(tb)) {
- PyErr_SetString(PyExc_TypeError,
- "raise: arg 3 must be a traceback or None");
- goto bad;
- }
- if (value == Py_None)
- value = 0;
- if (PyExceptionInstance_Check(type)) {
- if (value) {
- PyErr_SetString(PyExc_TypeError,
- "instance exception may not have a separate value");
- goto bad;
- }
- value = type;
- type = (PyObject*) Py_TYPE(value);
- } else if (PyExceptionClass_Check(type)) {
- PyObject *instance_class = NULL;
- if (value && PyExceptionInstance_Check(value)) {
- instance_class = (PyObject*) Py_TYPE(value);
- if (instance_class != type) {
- int is_subclass = PyObject_IsSubclass(instance_class, type);
- if (!is_subclass) {
- instance_class = NULL;
- } else if (unlikely(is_subclass == -1)) {
- goto bad;
- } else {
- type = instance_class;
- }
- }
- }
- if (!instance_class) {
- PyObject *args;
- if (!value)
- args = PyTuple_New(0);
- else if (PyTuple_Check(value)) {
- Py_INCREF(value);
- args = value;
- } else
- args = PyTuple_Pack(1, value);
- if (!args)
- goto bad;
- owned_instance = PyObject_Call(type, args, NULL);
- Py_DECREF(args);
- if (!owned_instance)
- goto bad;
- value = owned_instance;
- if (!PyExceptionInstance_Check(value)) {
- PyErr_Format(PyExc_TypeError,
- "calling %R should have returned an instance of "
- "BaseException, not %R",
- type, Py_TYPE(value));
- goto bad;
- }
- }
- } else {
- PyErr_SetString(PyExc_TypeError,
- "raise: exception class must be a subclass of BaseException");
- goto bad;
- }
- if (cause) {
- PyObject *fixed_cause;
- if (cause == Py_None) {
- fixed_cause = NULL;
- } else if (PyExceptionClass_Check(cause)) {
- fixed_cause = PyObject_CallObject(cause, NULL);
- if (fixed_cause == NULL)
- goto bad;
- } else if (PyExceptionInstance_Check(cause)) {
- fixed_cause = cause;
- Py_INCREF(fixed_cause);
- } else {
- PyErr_SetString(PyExc_TypeError,
- "exception causes must derive from "
- "BaseException");
- goto bad;
- }
- PyException_SetCause(value, fixed_cause);
- }
- PyErr_SetObject(type, value);
- if (tb) {
- #if PY_VERSION_HEX >= 0x030C00A6
- PyException_SetTraceback(value, tb);
- #elif CYTHON_FAST_THREAD_STATE
- PyThreadState *tstate = __Pyx_PyThreadState_Current;
- PyObject* tmp_tb = tstate->curexc_traceback;
- if (tb != tmp_tb) {
- Py_INCREF(tb);
- tstate->curexc_traceback = tb;
- Py_XDECREF(tmp_tb);
- }
-#else
- PyObject *tmp_type, *tmp_value, *tmp_tb;
- PyErr_Fetch(&tmp_type, &tmp_value, &tmp_tb);
- Py_INCREF(tb);
- PyErr_Restore(tmp_type, tmp_value, tb);
- Py_XDECREF(tmp_tb);
-#endif
- }
-bad:
- Py_XDECREF(owned_instance);
- return;
-}
-#endif
-
-/* SetItemInt */
-static int __Pyx_SetItemInt_Generic(PyObject *o, PyObject *j, PyObject *v) {
- int r;
- if (unlikely(!j)) return -1;
- r = PyObject_SetItem(o, j, v);
- Py_DECREF(j);
- return r;
-}
-static CYTHON_INLINE int __Pyx_SetItemInt_Fast(PyObject *o, Py_ssize_t i, PyObject *v, int is_list,
- CYTHON_NCP_UNUSED int wraparound, CYTHON_NCP_UNUSED int boundscheck) {
-#if CYTHON_ASSUME_SAFE_MACROS && !CYTHON_AVOID_BORROWED_REFS && CYTHON_USE_TYPE_SLOTS
- if (is_list || PyList_CheckExact(o)) {
- Py_ssize_t n = (!wraparound) ? i : ((likely(i >= 0)) ? i : i + PyList_GET_SIZE(o));
- if ((!boundscheck) || likely(__Pyx_is_valid_index(n, PyList_GET_SIZE(o)))) {
- PyObject* old = PyList_GET_ITEM(o, n);
- Py_INCREF(v);
- PyList_SET_ITEM(o, n, v);
- Py_DECREF(old);
- return 1;
- }
- } else {
- PyMappingMethods *mm = Py_TYPE(o)->tp_as_mapping;
- PySequenceMethods *sm = Py_TYPE(o)->tp_as_sequence;
- if (mm && mm->mp_ass_subscript) {
- int r;
- PyObject *key = PyInt_FromSsize_t(i);
- if (unlikely(!key)) return -1;
- r = mm->mp_ass_subscript(o, key, v);
- Py_DECREF(key);
- return r;
- }
- if (likely(sm && sm->sq_ass_item)) {
- if (wraparound && unlikely(i < 0) && likely(sm->sq_length)) {
- Py_ssize_t l = sm->sq_length(o);
- if (likely(l >= 0)) {
- i += l;
- } else {
- if (!PyErr_ExceptionMatches(PyExc_OverflowError))
- return -1;
- PyErr_Clear();
- }
- }
- return sm->sq_ass_item(o, i, v);
- }
- }
-#else
-#if CYTHON_COMPILING_IN_PYPY
- if (is_list || (PySequence_Check(o) && !PyDict_Check(o)))
-#else
- if (is_list || PySequence_Check(o))
-#endif
- {
- return PySequence_SetItem(o, i, v);
- }
-#endif
- return __Pyx_SetItemInt_Generic(o, PyInt_FromSsize_t(i), v);
-}
-
-/* ModInt[long] */
-static CYTHON_INLINE long __Pyx_mod_long(long a, long b) {
- long r = a % b;
- r += ((r != 0) & ((r ^ b) < 0)) * b;
- return r;
-}
-
-/* FixUpExtensionType */
-#if CYTHON_USE_TYPE_SPECS
-static int __Pyx_fix_up_extension_type_from_spec(PyType_Spec *spec, PyTypeObject *type) {
-#if PY_VERSION_HEX > 0x030900B1 || CYTHON_COMPILING_IN_LIMITED_API
- CYTHON_UNUSED_VAR(spec);
- CYTHON_UNUSED_VAR(type);
-#else
- const PyType_Slot *slot = spec->slots;
- while (slot && slot->slot && slot->slot != Py_tp_members)
- slot++;
- if (slot && slot->slot == Py_tp_members) {
- int changed = 0;
-#if !(PY_VERSION_HEX <= 0x030900b1 && CYTHON_COMPILING_IN_CPYTHON)
- const
-#endif
- PyMemberDef *memb = (PyMemberDef*) slot->pfunc;
- while (memb && memb->name) {
- if (memb->name[0] == '_' && memb->name[1] == '_') {
-#if PY_VERSION_HEX < 0x030900b1
- if (strcmp(memb->name, "__weaklistoffset__") == 0) {
- assert(memb->type == T_PYSSIZET);
- assert(memb->flags == READONLY);
- type->tp_weaklistoffset = memb->offset;
- changed = 1;
- }
- else if (strcmp(memb->name, "__dictoffset__") == 0) {
- assert(memb->type == T_PYSSIZET);
- assert(memb->flags == READONLY);
- type->tp_dictoffset = memb->offset;
- changed = 1;
- }
-#if CYTHON_METH_FASTCALL
- else if (strcmp(memb->name, "__vectorcalloffset__") == 0) {
- assert(memb->type == T_PYSSIZET);
- assert(memb->flags == READONLY);
-#if PY_VERSION_HEX >= 0x030800b4
- type->tp_vectorcall_offset = memb->offset;
-#else
- type->tp_print = (printfunc) memb->offset;
-#endif
- changed = 1;
- }
-#endif
-#else
- if ((0));
-#endif
-#if PY_VERSION_HEX <= 0x030900b1 && CYTHON_COMPILING_IN_CPYTHON
- else if (strcmp(memb->name, "__module__") == 0) {
- PyObject *descr;
- assert(memb->type == T_OBJECT);
- assert(memb->flags == 0 || memb->flags == READONLY);
- descr = PyDescr_NewMember(type, memb);
- if (unlikely(!descr))
- return -1;
- if (unlikely(PyDict_SetItem(type->tp_dict, PyDescr_NAME(descr), descr) < 0)) {
- Py_DECREF(descr);
- return -1;
- }
- Py_DECREF(descr);
- changed = 1;
- }
-#endif
- }
- memb++;
- }
- if (changed)
- PyType_Modified(type);
- }
-#endif
- return 0;
-}
-#endif
-
-/* PyObjectCallNoArg */
-static CYTHON_INLINE PyObject* __Pyx_PyObject_CallNoArg(PyObject *func) {
- PyObject *arg = NULL;
- return __Pyx_PyObject_FastCall(func, (&arg)+1, 0 | __Pyx_PY_VECTORCALL_ARGUMENTS_OFFSET);
-}
-
-/* PyObjectCallOneArg */
-static CYTHON_INLINE PyObject* __Pyx_PyObject_CallOneArg(PyObject *func, PyObject *arg) {
- PyObject *args[2] = {NULL, arg};
- return __Pyx_PyObject_FastCall(func, args+1, 1 | __Pyx_PY_VECTORCALL_ARGUMENTS_OFFSET);
-}
-
-/* PyObjectGetMethod */
-static int __Pyx_PyObject_GetMethod(PyObject *obj, PyObject *name, PyObject **method) {
- PyObject *attr;
-#if CYTHON_UNPACK_METHODS && CYTHON_COMPILING_IN_CPYTHON && CYTHON_USE_PYTYPE_LOOKUP
- __Pyx_TypeName type_name;
- PyTypeObject *tp = Py_TYPE(obj);
- PyObject *descr;
- descrgetfunc f = NULL;
- PyObject **dictptr, *dict;
- int meth_found = 0;
- assert (*method == NULL);
- if (unlikely(tp->tp_getattro != PyObject_GenericGetAttr)) {
- attr = __Pyx_PyObject_GetAttrStr(obj, name);
- goto try_unpack;
- }
- if (unlikely(tp->tp_dict == NULL) && unlikely(PyType_Ready(tp) < 0)) {
- return 0;
- }
- descr = _PyType_Lookup(tp, name);
- if (likely(descr != NULL)) {
- Py_INCREF(descr);
-#if defined(Py_TPFLAGS_METHOD_DESCRIPTOR) && Py_TPFLAGS_METHOD_DESCRIPTOR
- if (__Pyx_PyType_HasFeature(Py_TYPE(descr), Py_TPFLAGS_METHOD_DESCRIPTOR))
-#elif PY_MAJOR_VERSION >= 3
- #ifdef __Pyx_CyFunction_USED
- if (likely(PyFunction_Check(descr) || __Pyx_IS_TYPE(descr, &PyMethodDescr_Type) || __Pyx_CyFunction_Check(descr)))
- #else
- if (likely(PyFunction_Check(descr) || __Pyx_IS_TYPE(descr, &PyMethodDescr_Type)))
- #endif
-#else
- #ifdef __Pyx_CyFunction_USED
- if (likely(PyFunction_Check(descr) || __Pyx_CyFunction_Check(descr)))
- #else
- if (likely(PyFunction_Check(descr)))
- #endif
-#endif
- {
- meth_found = 1;
- } else {
- f = Py_TYPE(descr)->tp_descr_get;
- if (f != NULL && PyDescr_IsData(descr)) {
- attr = f(descr, obj, (PyObject *)Py_TYPE(obj));
- Py_DECREF(descr);
- goto try_unpack;
- }
- }
- }
- dictptr = _PyObject_GetDictPtr(obj);
- if (dictptr != NULL && (dict = *dictptr) != NULL) {
- Py_INCREF(dict);
- attr = __Pyx_PyDict_GetItemStr(dict, name);
- if (attr != NULL) {
- Py_INCREF(attr);
- Py_DECREF(dict);
- Py_XDECREF(descr);
- goto try_unpack;
- }
- Py_DECREF(dict);
- }
- if (meth_found) {
- *method = descr;
- return 1;
- }
- if (f != NULL) {
- attr = f(descr, obj, (PyObject *)Py_TYPE(obj));
- Py_DECREF(descr);
- goto try_unpack;
- }
- if (likely(descr != NULL)) {
- *method = descr;
- return 0;
- }
- type_name = __Pyx_PyType_GetName(tp);
- PyErr_Format(PyExc_AttributeError,
-#if PY_MAJOR_VERSION >= 3
- "'" __Pyx_FMT_TYPENAME "' object has no attribute '%U'",
- type_name, name);
-#else
- "'" __Pyx_FMT_TYPENAME "' object has no attribute '%.400s'",
- type_name, PyString_AS_STRING(name));
-#endif
- __Pyx_DECREF_TypeName(type_name);
- return 0;
-#else
- attr = __Pyx_PyObject_GetAttrStr(obj, name);
- goto try_unpack;
-#endif
-try_unpack:
-#if CYTHON_UNPACK_METHODS
- if (likely(attr) && PyMethod_Check(attr) && likely(PyMethod_GET_SELF(attr) == obj)) {
- PyObject *function = PyMethod_GET_FUNCTION(attr);
- Py_INCREF(function);
- Py_DECREF(attr);
- *method = function;
- return 1;
- }
-#endif
- *method = attr;
- return 0;
-}
-
-/* PyObjectCallMethod0 */
-static PyObject* __Pyx_PyObject_CallMethod0(PyObject* obj, PyObject* method_name) {
- PyObject *method = NULL, *result = NULL;
- int is_method = __Pyx_PyObject_GetMethod(obj, method_name, &method);
- if (likely(is_method)) {
- result = __Pyx_PyObject_CallOneArg(method, obj);
- Py_DECREF(method);
- return result;
- }
- if (unlikely(!method)) goto bad;
- result = __Pyx_PyObject_CallNoArg(method);
- Py_DECREF(method);
-bad:
- return result;
-}
-
-/* ValidateBasesTuple */
-#if CYTHON_COMPILING_IN_CPYTHON || CYTHON_COMPILING_IN_LIMITED_API || CYTHON_USE_TYPE_SPECS
-static int __Pyx_validate_bases_tuple(const char *type_name, Py_ssize_t dictoffset, PyObject *bases) {
- Py_ssize_t i, n = PyTuple_GET_SIZE(bases);
- for (i = 1; i < n; i++)
- {
- PyObject *b0 = PyTuple_GET_ITEM(bases, i);
- PyTypeObject *b;
-#if PY_MAJOR_VERSION < 3
- if (PyClass_Check(b0))
- {
- PyErr_Format(PyExc_TypeError, "base class '%.200s' is an old-style class",
- PyString_AS_STRING(((PyClassObject*)b0)->cl_name));
- return -1;
- }
-#endif
- b = (PyTypeObject*) b0;
- if (!__Pyx_PyType_HasFeature(b, Py_TPFLAGS_HEAPTYPE))
- {
- __Pyx_TypeName b_name = __Pyx_PyType_GetName(b);
- PyErr_Format(PyExc_TypeError,
- "base class '" __Pyx_FMT_TYPENAME "' is not a heap type", b_name);
- __Pyx_DECREF_TypeName(b_name);
- return -1;
- }
- if (dictoffset == 0 && b->tp_dictoffset)
- {
- __Pyx_TypeName b_name = __Pyx_PyType_GetName(b);
- PyErr_Format(PyExc_TypeError,
- "extension type '%.200s' has no __dict__ slot, "
- "but base type '" __Pyx_FMT_TYPENAME "' has: "
- "either add 'cdef dict __dict__' to the extension type "
- "or add '__slots__ = [...]' to the base type",
- type_name, b_name);
- __Pyx_DECREF_TypeName(b_name);
- return -1;
- }
- }
- return 0;
-}
-#endif
-
-/* PyType_Ready */
-static int __Pyx_PyType_Ready(PyTypeObject *t) {
-#if CYTHON_USE_TYPE_SPECS || !(CYTHON_COMPILING_IN_CPYTHON || CYTHON_COMPILING_IN_LIMITED_API) || defined(PYSTON_MAJOR_VERSION)
- (void)__Pyx_PyObject_CallMethod0;
-#if CYTHON_USE_TYPE_SPECS
- (void)__Pyx_validate_bases_tuple;
-#endif
- return PyType_Ready(t);
-#else
- int r;
- PyObject *bases = __Pyx_PyType_GetSlot(t, tp_bases, PyObject*);
- if (bases && unlikely(__Pyx_validate_bases_tuple(t->tp_name, t->tp_dictoffset, bases) == -1))
- return -1;
-#if PY_VERSION_HEX >= 0x03050000 && !defined(PYSTON_MAJOR_VERSION)
- {
- int gc_was_enabled;
- #if PY_VERSION_HEX >= 0x030A00b1
- gc_was_enabled = PyGC_Disable();
- (void)__Pyx_PyObject_CallMethod0;
- #else
- PyObject *ret, *py_status;
- PyObject *gc = NULL;
- #if PY_VERSION_HEX >= 0x030700a1 && (!CYTHON_COMPILING_IN_PYPY || PYPY_VERSION_NUM+0 >= 0x07030400)
- gc = PyImport_GetModule(__pyx_kp_u_gc);
- #endif
- if (unlikely(!gc)) gc = PyImport_Import(__pyx_kp_u_gc);
- if (unlikely(!gc)) return -1;
- py_status = __Pyx_PyObject_CallMethod0(gc, __pyx_kp_u_isenabled);
- if (unlikely(!py_status)) {
- Py_DECREF(gc);
- return -1;
- }
- gc_was_enabled = __Pyx_PyObject_IsTrue(py_status);
- Py_DECREF(py_status);
- if (gc_was_enabled > 0) {
- ret = __Pyx_PyObject_CallMethod0(gc, __pyx_kp_u_disable);
- if (unlikely(!ret)) {
- Py_DECREF(gc);
- return -1;
- }
- Py_DECREF(ret);
- } else if (unlikely(gc_was_enabled == -1)) {
- Py_DECREF(gc);
- return -1;
- }
- #endif
- t->tp_flags |= Py_TPFLAGS_HEAPTYPE;
-#if PY_VERSION_HEX >= 0x030A0000
- t->tp_flags |= Py_TPFLAGS_IMMUTABLETYPE;
-#endif
-#else
- (void)__Pyx_PyObject_CallMethod0;
-#endif
- r = PyType_Ready(t);
-#if PY_VERSION_HEX >= 0x03050000 && !defined(PYSTON_MAJOR_VERSION)
- t->tp_flags &= ~Py_TPFLAGS_HEAPTYPE;
- #if PY_VERSION_HEX >= 0x030A00b1
- if (gc_was_enabled)
- PyGC_Enable();
- #else
- if (gc_was_enabled) {
- PyObject *tp, *v, *tb;
- PyErr_Fetch(&tp, &v, &tb);
- ret = __Pyx_PyObject_CallMethod0(gc, __pyx_kp_u_enable);
- if (likely(ret || r == -1)) {
- Py_XDECREF(ret);
- PyErr_Restore(tp, v, tb);
- } else {
- Py_XDECREF(tp);
- Py_XDECREF(v);
- Py_XDECREF(tb);
- r = -1;
- }
- }
- Py_DECREF(gc);
- #endif
- }
-#endif
- return r;
-#endif
-}
-
-/* PyObject_GenericGetAttrNoDict */
-#if CYTHON_USE_TYPE_SLOTS && CYTHON_USE_PYTYPE_LOOKUP && PY_VERSION_HEX < 0x03070000
-static PyObject *__Pyx_RaiseGenericGetAttributeError(PyTypeObject *tp, PyObject *attr_name) {
- __Pyx_TypeName type_name = __Pyx_PyType_GetName(tp);
- PyErr_Format(PyExc_AttributeError,
-#if PY_MAJOR_VERSION >= 3
- "'" __Pyx_FMT_TYPENAME "' object has no attribute '%U'",
- type_name, attr_name);
-#else
- "'" __Pyx_FMT_TYPENAME "' object has no attribute '%.400s'",
- type_name, PyString_AS_STRING(attr_name));
-#endif
- __Pyx_DECREF_TypeName(type_name);
- return NULL;
-}
-static CYTHON_INLINE PyObject* __Pyx_PyObject_GenericGetAttrNoDict(PyObject* obj, PyObject* attr_name) {
- PyObject *descr;
- PyTypeObject *tp = Py_TYPE(obj);
- if (unlikely(!PyString_Check(attr_name))) {
- return PyObject_GenericGetAttr(obj, attr_name);
- }
- assert(!tp->tp_dictoffset);
- descr = _PyType_Lookup(tp, attr_name);
- if (unlikely(!descr)) {
- return __Pyx_RaiseGenericGetAttributeError(tp, attr_name);
- }
- Py_INCREF(descr);
- #if PY_MAJOR_VERSION < 3
- if (likely(PyType_HasFeature(Py_TYPE(descr), Py_TPFLAGS_HAVE_CLASS)))
- #endif
- {
- descrgetfunc f = Py_TYPE(descr)->tp_descr_get;
- if (unlikely(f)) {
- PyObject *res = f(descr, obj, (PyObject *)tp);
- Py_DECREF(descr);
- return res;
- }
- }
- return descr;
-}
-#endif
-
-/* FastTypeChecks */
-#if CYTHON_COMPILING_IN_CPYTHON
-static int __Pyx_InBases(PyTypeObject *a, PyTypeObject *b) {
- while (a) {
- a = __Pyx_PyType_GetSlot(a, tp_base, PyTypeObject*);
- if (a == b)
- return 1;
- }
- return b == &PyBaseObject_Type;
-}
-static CYTHON_INLINE int __Pyx_IsSubtype(PyTypeObject *a, PyTypeObject *b) {
- PyObject *mro;
- if (a == b) return 1;
- mro = a->tp_mro;
- if (likely(mro)) {
- Py_ssize_t i, n;
- n = PyTuple_GET_SIZE(mro);
- for (i = 0; i < n; i++) {
- if (PyTuple_GET_ITEM(mro, i) == (PyObject *)b)
- return 1;
- }
- return 0;
- }
- return __Pyx_InBases(a, b);
-}
-static CYTHON_INLINE int __Pyx_IsAnySubtype2(PyTypeObject *cls, PyTypeObject *a, PyTypeObject *b) {
- PyObject *mro;
- if (cls == a || cls == b) return 1;
- mro = cls->tp_mro;
- if (likely(mro)) {
- Py_ssize_t i, n;
- n = PyTuple_GET_SIZE(mro);
- for (i = 0; i < n; i++) {
- PyObject *base = PyTuple_GET_ITEM(mro, i);
- if (base == (PyObject *)a || base == (PyObject *)b)
- return 1;
- }
- return 0;
- }
- return __Pyx_InBases(cls, a) || __Pyx_InBases(cls, b);
-}
-#if PY_MAJOR_VERSION == 2
-static int __Pyx_inner_PyErr_GivenExceptionMatches2(PyObject *err, PyObject* exc_type1, PyObject* exc_type2) {
- PyObject *exception, *value, *tb;
- int res;
- __Pyx_PyThreadState_declare
- __Pyx_PyThreadState_assign
- __Pyx_ErrFetch(&exception, &value, &tb);
- res = exc_type1 ? PyObject_IsSubclass(err, exc_type1) : 0;
- if (unlikely(res == -1)) {
- PyErr_WriteUnraisable(err);
- res = 0;
- }
- if (!res) {
- res = PyObject_IsSubclass(err, exc_type2);
- if (unlikely(res == -1)) {
- PyErr_WriteUnraisable(err);
- res = 0;
- }
- }
- __Pyx_ErrRestore(exception, value, tb);
- return res;
-}
-#else
-static CYTHON_INLINE int __Pyx_inner_PyErr_GivenExceptionMatches2(PyObject *err, PyObject* exc_type1, PyObject *exc_type2) {
- if (exc_type1) {
- return __Pyx_IsAnySubtype2((PyTypeObject*)err, (PyTypeObject*)exc_type1, (PyTypeObject*)exc_type2);
- } else {
- return __Pyx_IsSubtype((PyTypeObject*)err, (PyTypeObject*)exc_type2);
- }
-}
-#endif
-static int __Pyx_PyErr_GivenExceptionMatchesTuple(PyObject *exc_type, PyObject *tuple) {
- Py_ssize_t i, n;
- assert(PyExceptionClass_Check(exc_type));
- n = PyTuple_GET_SIZE(tuple);
-#if PY_MAJOR_VERSION >= 3
- for (i=0; i= 3
- if (level == -1) {
- if ((1) && (strchr(__Pyx_MODULE_NAME, '.'))) {
- #if CYTHON_COMPILING_IN_LIMITED_API
- module = PyImport_ImportModuleLevelObject(
- name, empty_dict, empty_dict, from_list, 1);
- #else
- module = PyImport_ImportModuleLevelObject(
- name, __pyx_d, empty_dict, from_list, 1);
- #endif
- if (unlikely(!module)) {
- if (unlikely(!PyErr_ExceptionMatches(PyExc_ImportError)))
- goto bad;
- PyErr_Clear();
- }
- }
- level = 0;
- }
- #endif
- if (!module) {
- #if PY_MAJOR_VERSION < 3
- PyObject *py_level = PyInt_FromLong(level);
- if (unlikely(!py_level))
- goto bad;
- module = PyObject_CallFunctionObjArgs(py_import,
- name, __pyx_d, empty_dict, from_list, py_level, (PyObject *)NULL);
- Py_DECREF(py_level);
- #else
- #if CYTHON_COMPILING_IN_LIMITED_API
- module = PyImport_ImportModuleLevelObject(
- name, empty_dict, empty_dict, from_list, level);
- #else
- module = PyImport_ImportModuleLevelObject(
- name, __pyx_d, empty_dict, from_list, level);
- #endif
- #endif
- }
- }
-bad:
- Py_XDECREF(empty_dict);
- Py_XDECREF(empty_list);
- #if PY_MAJOR_VERSION < 3
- Py_XDECREF(py_import);
- #endif
- return module;
-}
-
-/* ImportFrom */
-static PyObject* __Pyx_ImportFrom(PyObject* module, PyObject* name) {
- PyObject* value = __Pyx_PyObject_GetAttrStr(module, name);
- if (unlikely(!value) && PyErr_ExceptionMatches(PyExc_AttributeError)) {
- const char* module_name_str = 0;
- PyObject* module_name = 0;
- PyObject* module_dot = 0;
- PyObject* full_name = 0;
- PyErr_Clear();
- module_name_str = PyModule_GetName(module);
- if (unlikely(!module_name_str)) { goto modbad; }
- module_name = PyUnicode_FromString(module_name_str);
- if (unlikely(!module_name)) { goto modbad; }
- module_dot = PyUnicode_Concat(module_name, __pyx_kp_u__2);
- if (unlikely(!module_dot)) { goto modbad; }
- full_name = PyUnicode_Concat(module_dot, name);
- if (unlikely(!full_name)) { goto modbad; }
- #if PY_VERSION_HEX < 0x030700A1 || (CYTHON_COMPILING_IN_PYPY && PYPY_VERSION_NUM < 0x07030400)
- {
- PyObject *modules = PyImport_GetModuleDict();
- if (unlikely(!modules))
- goto modbad;
- value = PyObject_GetItem(modules, full_name);
- }
- #else
- value = PyImport_GetModule(full_name);
- #endif
- modbad:
- Py_XDECREF(full_name);
- Py_XDECREF(module_dot);
- Py_XDECREF(module_name);
- }
- if (unlikely(!value)) {
- PyErr_Format(PyExc_ImportError,
- #if PY_MAJOR_VERSION < 3
- "cannot import name %.230s", PyString_AS_STRING(name));
- #else
- "cannot import name %S", name);
- #endif
- }
- return value;
-}
-
-/* ImportDottedModule */
-#if PY_MAJOR_VERSION >= 3
-static PyObject *__Pyx__ImportDottedModule_Error(PyObject *name, PyObject *parts_tuple, Py_ssize_t count) {
- PyObject *partial_name = NULL, *slice = NULL, *sep = NULL;
- if (unlikely(PyErr_Occurred())) {
- PyErr_Clear();
- }
- if (likely(PyTuple_GET_SIZE(parts_tuple) == count)) {
- partial_name = name;
- } else {
- slice = PySequence_GetSlice(parts_tuple, 0, count);
- if (unlikely(!slice))
- goto bad;
- sep = PyUnicode_FromStringAndSize(".", 1);
- if (unlikely(!sep))
- goto bad;
- partial_name = PyUnicode_Join(sep, slice);
- }
- PyErr_Format(
-#if PY_MAJOR_VERSION < 3
- PyExc_ImportError,
- "No module named '%s'", PyString_AS_STRING(partial_name));
-#else
-#if PY_VERSION_HEX >= 0x030600B1
- PyExc_ModuleNotFoundError,
-#else
- PyExc_ImportError,
-#endif
- "No module named '%U'", partial_name);
-#endif
-bad:
- Py_XDECREF(sep);
- Py_XDECREF(slice);
- Py_XDECREF(partial_name);
- return NULL;
-}
-#endif
-#if PY_MAJOR_VERSION >= 3
-static PyObject *__Pyx__ImportDottedModule_Lookup(PyObject *name) {
- PyObject *imported_module;
-#if PY_VERSION_HEX < 0x030700A1 || (CYTHON_COMPILING_IN_PYPY && PYPY_VERSION_NUM < 0x07030400)
- PyObject *modules = PyImport_GetModuleDict();
- if (unlikely(!modules))
- return NULL;
- imported_module = __Pyx_PyDict_GetItemStr(modules, name);
- Py_XINCREF(imported_module);
-#else
- imported_module = PyImport_GetModule(name);
-#endif
- return imported_module;
-}
-#endif
-#if PY_MAJOR_VERSION >= 3
-static PyObject *__Pyx_ImportDottedModule_WalkParts(PyObject *module, PyObject *name, PyObject *parts_tuple) {
- Py_ssize_t i, nparts;
- nparts = PyTuple_GET_SIZE(parts_tuple);
- for (i=1; i < nparts && module; i++) {
- PyObject *part, *submodule;
-#if CYTHON_ASSUME_SAFE_MACROS && !CYTHON_AVOID_BORROWED_REFS
- part = PyTuple_GET_ITEM(parts_tuple, i);
-#else
- part = PySequence_ITEM(parts_tuple, i);
-#endif
- submodule = __Pyx_PyObject_GetAttrStrNoError(module, part);
-#if !(CYTHON_ASSUME_SAFE_MACROS && !CYTHON_AVOID_BORROWED_REFS)
- Py_DECREF(part);
-#endif
- Py_DECREF(module);
- module = submodule;
- }
- if (unlikely(!module)) {
- return __Pyx__ImportDottedModule_Error(name, parts_tuple, i);
- }
- return module;
-}
-#endif
-static PyObject *__Pyx__ImportDottedModule(PyObject *name, PyObject *parts_tuple) {
-#if PY_MAJOR_VERSION < 3
- PyObject *module, *from_list, *star = __pyx_n_s__3;
- CYTHON_UNUSED_VAR(parts_tuple);
- from_list = PyList_New(1);
- if (unlikely(!from_list))
- return NULL;
- Py_INCREF(star);
- PyList_SET_ITEM(from_list, 0, star);
- module = __Pyx_Import(name, from_list, 0);
- Py_DECREF(from_list);
- return module;
-#else
- PyObject *imported_module;
- PyObject *module = __Pyx_Import(name, NULL, 0);
- if (!parts_tuple || unlikely(!module))
- return module;
- imported_module = __Pyx__ImportDottedModule_Lookup(name);
- if (likely(imported_module)) {
- Py_DECREF(module);
- return imported_module;
- }
- PyErr_Clear();
- return __Pyx_ImportDottedModule_WalkParts(module, name, parts_tuple);
-#endif
-}
-static PyObject *__Pyx_ImportDottedModule(PyObject *name, PyObject *parts_tuple) {
-#if CYTHON_COMPILING_IN_CPYTHON && PY_VERSION_HEX >= 0x030400B1
- PyObject *module = __Pyx__ImportDottedModule_Lookup(name);
- if (likely(module)) {
- PyObject *spec = __Pyx_PyObject_GetAttrStrNoError(module, __pyx_n_s_spec);
- if (likely(spec)) {
- PyObject *unsafe = __Pyx_PyObject_GetAttrStrNoError(spec, __pyx_n_s_initializing);
- if (likely(!unsafe || !__Pyx_PyObject_IsTrue(unsafe))) {
- Py_DECREF(spec);
- spec = NULL;
- }
- Py_XDECREF(unsafe);
- }
- if (likely(!spec)) {
- PyErr_Clear();
- return module;
- }
- Py_DECREF(spec);
- Py_DECREF(module);
- } else if (PyErr_Occurred()) {
- PyErr_Clear();
- }
-#endif
- return __Pyx__ImportDottedModule(name, parts_tuple);
-}
-
-/* pybytes_as_double */
-static double __Pyx_SlowPyString_AsDouble(PyObject *obj) {
- PyObject *float_value;
-#if PY_MAJOR_VERSION >= 3
- float_value = PyFloat_FromString(obj);
-#else
- float_value = PyFloat_FromString(obj, 0);
-#endif
- if (likely(float_value)) {
- double value = PyFloat_AS_DOUBLE(float_value);
- Py_DECREF(float_value);
- return value;
- }
- return (double)-1;
-}
-static const char* __Pyx__PyBytes_AsDouble_Copy(const char* start, char* buffer, Py_ssize_t length) {
- int last_was_punctuation = 1;
- Py_ssize_t i;
- for (i=0; i < length; i++) {
- char chr = start[i];
- int is_punctuation = (chr == '_') | (chr == '.') | (chr == 'e') | (chr == 'E');
- *buffer = chr;
- buffer += (chr != '_');
- if (unlikely(last_was_punctuation & is_punctuation)) goto parse_failure;
- last_was_punctuation = is_punctuation;
- }
- if (unlikely(last_was_punctuation)) goto parse_failure;
- *buffer = '\0';
- return buffer;
-parse_failure:
- return NULL;
-}
-static double __Pyx__PyBytes_AsDouble_inf_nan(const char* start, Py_ssize_t length) {
- int matches = 1;
- char sign = start[0];
- int is_signed = (sign == '+') | (sign == '-');
- start += is_signed;
- length -= is_signed;
- switch (start[0]) {
- #ifdef Py_NAN
- case 'n':
- case 'N':
- if (unlikely(length != 3)) goto parse_failure;
- matches &= (start[1] == 'a' || start[1] == 'A');
- matches &= (start[2] == 'n' || start[2] == 'N');
- if (unlikely(!matches)) goto parse_failure;
- return (sign == '-') ? -Py_NAN : Py_NAN;
- #endif
- case 'i':
- case 'I':
- if (unlikely(length < 3)) goto parse_failure;
- matches &= (start[1] == 'n' || start[1] == 'N');
- matches &= (start[2] == 'f' || start[2] == 'F');
- if (likely(length == 3 && matches))
- return (sign == '-') ? -Py_HUGE_VAL : Py_HUGE_VAL;
- if (unlikely(length != 8)) goto parse_failure;
- matches &= (start[3] == 'i' || start[3] == 'I');
- matches &= (start[4] == 'n' || start[4] == 'N');
- matches &= (start[5] == 'i' || start[5] == 'I');
- matches &= (start[6] == 't' || start[6] == 'T');
- matches &= (start[7] == 'y' || start[7] == 'Y');
- if (unlikely(!matches)) goto parse_failure;
- return (sign == '-') ? -Py_HUGE_VAL : Py_HUGE_VAL;
- case '.': case '0': case '1': case '2': case '3': case '4': case '5': case '6': case '7': case '8': case '9':
- break;
- default:
- goto parse_failure;
- }
- return 0.0;
-parse_failure:
- return -1.0;
-}
-static CYTHON_INLINE int __Pyx__PyBytes_AsDouble_IsSpace(char ch) {
- return (ch == 0x20) | !((ch < 0x9) | (ch > 0xd));
-}
-CYTHON_UNUSED static double __Pyx__PyBytes_AsDouble(PyObject *obj, const char* start, Py_ssize_t length) {
- double value;
- Py_ssize_t i, digits;
- const char *last = start + length;
- char *end;
- while (__Pyx__PyBytes_AsDouble_IsSpace(*start))
- start++;
- while (start < last - 1 && __Pyx__PyBytes_AsDouble_IsSpace(last[-1]))
- last--;
- length = last - start;
- if (unlikely(length <= 0)) goto fallback;
- value = __Pyx__PyBytes_AsDouble_inf_nan(start, length);
- if (unlikely(value == -1.0)) goto fallback;
- if (value != 0.0) return value;
- digits = 0;
- for (i=0; i < length; digits += start[i++] != '_');
- if (likely(digits == length)) {
- value = PyOS_string_to_double(start, &end, NULL);
- } else if (digits < 40) {
- char number[40];
- last = __Pyx__PyBytes_AsDouble_Copy(start, number, length);
- if (unlikely(!last)) goto fallback;
- value = PyOS_string_to_double(number, &end, NULL);
- } else {
- char *number = (char*) PyMem_Malloc((digits + 1) * sizeof(char));
- if (unlikely(!number)) goto fallback;
- last = __Pyx__PyBytes_AsDouble_Copy(start, number, length);
- if (unlikely(!last)) {
- PyMem_Free(number);
- goto fallback;
- }
- value = PyOS_string_to_double(number, &end, NULL);
- PyMem_Free(number);
- }
- if (likely(end == last) || (value == (double)-1 && PyErr_Occurred())) {
- return value;
- }
-fallback:
- return __Pyx_SlowPyString_AsDouble(obj);
-}
-
-/* FetchSharedCythonModule */
-static PyObject *__Pyx_FetchSharedCythonABIModule(void) {
- PyObject *abi_module = PyImport_AddModule((char*) __PYX_ABI_MODULE_NAME);
- if (unlikely(!abi_module)) return NULL;
- Py_INCREF(abi_module);
- return abi_module;
-}
-
-/* FetchCommonType */
-static int __Pyx_VerifyCachedType(PyObject *cached_type,
- const char *name,
- Py_ssize_t basicsize,
- Py_ssize_t expected_basicsize) {
- if (!PyType_Check(cached_type)) {
- PyErr_Format(PyExc_TypeError,
- "Shared Cython type %.200s is not a type object", name);
- return -1;
- }
- if (basicsize != expected_basicsize) {
- PyErr_Format(PyExc_TypeError,
- "Shared Cython type %.200s has the wrong size, try recompiling",
- name);
- return -1;
- }
- return 0;
-}
-#if !CYTHON_USE_TYPE_SPECS
-static PyTypeObject* __Pyx_FetchCommonType(PyTypeObject* type) {
- PyObject* abi_module;
- const char* object_name;
- PyTypeObject *cached_type = NULL;
- abi_module = __Pyx_FetchSharedCythonABIModule();
- if (!abi_module) return NULL;
- object_name = strrchr(type->tp_name, '.');
- object_name = object_name ? object_name+1 : type->tp_name;
- cached_type = (PyTypeObject*) PyObject_GetAttrString(abi_module, object_name);
- if (cached_type) {
- if (__Pyx_VerifyCachedType(
- (PyObject *)cached_type,
- object_name,
- cached_type->tp_basicsize,
- type->tp_basicsize) < 0) {
- goto bad;
- }
- goto done;
- }
- if (!PyErr_ExceptionMatches(PyExc_AttributeError)) goto bad;
- PyErr_Clear();
- if (PyType_Ready(type) < 0) goto bad;
- if (PyObject_SetAttrString(abi_module, object_name, (PyObject *)type) < 0)
- goto bad;
- Py_INCREF(type);
- cached_type = type;
-done:
- Py_DECREF(abi_module);
- return cached_type;
-bad:
- Py_XDECREF(cached_type);
- cached_type = NULL;
- goto done;
-}
-#else
-static PyTypeObject *__Pyx_FetchCommonTypeFromSpec(PyObject *module, PyType_Spec *spec, PyObject *bases) {
- PyObject *abi_module, *cached_type = NULL;
- const char* object_name = strrchr(spec->name, '.');
- object_name = object_name ? object_name+1 : spec->name;
- abi_module = __Pyx_FetchSharedCythonABIModule();
- if (!abi_module) return NULL;
- cached_type = PyObject_GetAttrString(abi_module, object_name);
- if (cached_type) {
- Py_ssize_t basicsize;
-#if CYTHON_COMPILING_IN_LIMITED_API
- PyObject *py_basicsize;
- py_basicsize = PyObject_GetAttrString(cached_type, "__basicsize__");
- if (unlikely(!py_basicsize)) goto bad;
- basicsize = PyLong_AsSsize_t(py_basicsize);
- Py_DECREF(py_basicsize);
- py_basicsize = 0;
- if (unlikely(basicsize == (Py_ssize_t)-1) && PyErr_Occurred()) goto bad;
-#else
- basicsize = likely(PyType_Check(cached_type)) ? ((PyTypeObject*) cached_type)->tp_basicsize : -1;
-#endif
- if (__Pyx_VerifyCachedType(
- cached_type,
- object_name,
- basicsize,
- spec->basicsize) < 0) {
- goto bad;
- }
- goto done;
- }
- if (!PyErr_ExceptionMatches(PyExc_AttributeError)) goto bad;
- PyErr_Clear();
- CYTHON_UNUSED_VAR(module);
- cached_type = __Pyx_PyType_FromModuleAndSpec(abi_module, spec, bases);
- if (unlikely(!cached_type)) goto bad;
- if (unlikely(__Pyx_fix_up_extension_type_from_spec(spec, (PyTypeObject *) cached_type) < 0)) goto bad;
- if (PyObject_SetAttrString(abi_module, object_name, cached_type) < 0) goto bad;
-done:
- Py_DECREF(abi_module);
- assert(cached_type == NULL || PyType_Check(cached_type));
- return (PyTypeObject *) cached_type;
-bad:
- Py_XDECREF(cached_type);
- cached_type = NULL;
- goto done;
-}
-#endif
-
-/* PyVectorcallFastCallDict */
-#if CYTHON_METH_FASTCALL
-static PyObject *__Pyx_PyVectorcall_FastCallDict_kw(PyObject *func, __pyx_vectorcallfunc vc, PyObject *const *args, size_t nargs, PyObject *kw)
-{
- PyObject *res = NULL;
- PyObject *kwnames;
- PyObject **newargs;
- PyObject **kwvalues;
- Py_ssize_t i, pos;
- size_t j;
- PyObject *key, *value;
- unsigned long keys_are_strings;
- Py_ssize_t nkw = PyDict_GET_SIZE(kw);
- newargs = (PyObject **)PyMem_Malloc((nargs + (size_t)nkw) * sizeof(args[0]));
- if (unlikely(newargs == NULL)) {
- PyErr_NoMemory();
- return NULL;
- }
- for (j = 0; j < nargs; j++) newargs[j] = args[j];
- kwnames = PyTuple_New(nkw);
- if (unlikely(kwnames == NULL)) {
- PyMem_Free(newargs);
- return NULL;
- }
- kwvalues = newargs + nargs;
- pos = i = 0;
- keys_are_strings = Py_TPFLAGS_UNICODE_SUBCLASS;
- while (PyDict_Next(kw, &pos, &key, &value)) {
- keys_are_strings &= Py_TYPE(key)->tp_flags;
- Py_INCREF(key);
- Py_INCREF(value);
- PyTuple_SET_ITEM(kwnames, i, key);
- kwvalues[i] = value;
- i++;
- }
- if (unlikely(!keys_are_strings)) {
- PyErr_SetString(PyExc_TypeError, "keywords must be strings");
- goto cleanup;
- }
- res = vc(func, newargs, nargs, kwnames);
-cleanup:
- Py_DECREF(kwnames);
- for (i = 0; i < nkw; i++)
- Py_DECREF(kwvalues[i]);
- PyMem_Free(newargs);
- return res;
-}
-static CYTHON_INLINE PyObject *__Pyx_PyVectorcall_FastCallDict(PyObject *func, __pyx_vectorcallfunc vc, PyObject *const *args, size_t nargs, PyObject *kw)
-{
- if (likely(kw == NULL) || PyDict_GET_SIZE(kw) == 0) {
- return vc(func, args, nargs, NULL);
- }
- return __Pyx_PyVectorcall_FastCallDict_kw(func, vc, args, nargs, kw);
-}
-#endif
-
-/* CythonFunctionShared */
-static CYTHON_INLINE void __Pyx__CyFunction_SetClassObj(__pyx_CyFunctionObject* f, PyObject* classobj) {
-#if PY_VERSION_HEX < 0x030900B1
- __Pyx_Py_XDECREF_SET(
- __Pyx_CyFunction_GetClassObj(f),
- ((classobj) ? __Pyx_NewRef(classobj) : NULL));
-#else
- __Pyx_Py_XDECREF_SET(
- ((PyCMethodObject *) (f))->mm_class,
- (PyTypeObject*)((classobj) ? __Pyx_NewRef(classobj) : NULL));
-#endif
-}
-static PyObject *
-__Pyx_CyFunction_get_doc(__pyx_CyFunctionObject *op, void *closure)
-{
- CYTHON_UNUSED_VAR(closure);
- if (unlikely(op->func_doc == NULL)) {
- if (((PyCFunctionObject*)op)->m_ml->ml_doc) {
-#if PY_MAJOR_VERSION >= 3
- op->func_doc = PyUnicode_FromString(((PyCFunctionObject*)op)->m_ml->ml_doc);
-#else
- op->func_doc = PyString_FromString(((PyCFunctionObject*)op)->m_ml->ml_doc);
-#endif
- if (unlikely(op->func_doc == NULL))
- return NULL;
- } else {
- Py_INCREF(Py_None);
- return Py_None;
- }
- }
- Py_INCREF(op->func_doc);
- return op->func_doc;
-}
-static int
-__Pyx_CyFunction_set_doc(__pyx_CyFunctionObject *op, PyObject *value, void *context)
-{
- CYTHON_UNUSED_VAR(context);
- if (value == NULL) {
- value = Py_None;
- }
- Py_INCREF(value);
- __Pyx_Py_XDECREF_SET(op->func_doc, value);
- return 0;
-}
-static PyObject *
-__Pyx_CyFunction_get_name(__pyx_CyFunctionObject *op, void *context)
-{
- CYTHON_UNUSED_VAR(context);
- if (unlikely(op->func_name == NULL)) {
-#if PY_MAJOR_VERSION >= 3
- op->func_name = PyUnicode_InternFromString(((PyCFunctionObject*)op)->m_ml->ml_name);
-#else
- op->func_name = PyString_InternFromString(((PyCFunctionObject*)op)->m_ml->ml_name);
-#endif
- if (unlikely(op->func_name == NULL))
- return NULL;
- }
- Py_INCREF(op->func_name);
- return op->func_name;
-}
-static int
-__Pyx_CyFunction_set_name(__pyx_CyFunctionObject *op, PyObject *value, void *context)
-{
- CYTHON_UNUSED_VAR(context);
-#if PY_MAJOR_VERSION >= 3
- if (unlikely(value == NULL || !PyUnicode_Check(value)))
-#else
- if (unlikely(value == NULL || !PyString_Check(value)))
-#endif
- {
- PyErr_SetString(PyExc_TypeError,
- "__name__ must be set to a string object");
- return -1;
- }
- Py_INCREF(value);
- __Pyx_Py_XDECREF_SET(op->func_name, value);
- return 0;
-}
-static PyObject *
-__Pyx_CyFunction_get_qualname(__pyx_CyFunctionObject *op, void *context)
-{
- CYTHON_UNUSED_VAR(context);
- Py_INCREF(op->func_qualname);
- return op->func_qualname;
-}
-static int
-__Pyx_CyFunction_set_qualname(__pyx_CyFunctionObject *op, PyObject *value, void *context)
-{
- CYTHON_UNUSED_VAR(context);
-#if PY_MAJOR_VERSION >= 3
- if (unlikely(value == NULL || !PyUnicode_Check(value)))
-#else
- if (unlikely(value == NULL || !PyString_Check(value)))
-#endif
- {
- PyErr_SetString(PyExc_TypeError,
- "__qualname__ must be set to a string object");
- return -1;
- }
- Py_INCREF(value);
- __Pyx_Py_XDECREF_SET(op->func_qualname, value);
- return 0;
-}
-static PyObject *
-__Pyx_CyFunction_get_dict(__pyx_CyFunctionObject *op, void *context)
-{
- CYTHON_UNUSED_VAR(context);
- if (unlikely(op->func_dict == NULL)) {
- op->func_dict = PyDict_New();
- if (unlikely(op->func_dict == NULL))
- return NULL;
- }
- Py_INCREF(op->func_dict);
- return op->func_dict;
-}
-static int
-__Pyx_CyFunction_set_dict(__pyx_CyFunctionObject *op, PyObject *value, void *context)
-{
- CYTHON_UNUSED_VAR(context);
- if (unlikely(value == NULL)) {
- PyErr_SetString(PyExc_TypeError,
- "function's dictionary may not be deleted");
- return -1;
- }
- if (unlikely(!PyDict_Check(value))) {
- PyErr_SetString(PyExc_TypeError,
- "setting function's dictionary to a non-dict");
- return -1;
- }
- Py_INCREF(value);
- __Pyx_Py_XDECREF_SET(op->func_dict, value);
- return 0;
-}
-static PyObject *
-__Pyx_CyFunction_get_globals(__pyx_CyFunctionObject *op, void *context)
-{
- CYTHON_UNUSED_VAR(context);
- Py_INCREF(op->func_globals);
- return op->func_globals;
-}
-static PyObject *
-__Pyx_CyFunction_get_closure(__pyx_CyFunctionObject *op, void *context)
-{
- CYTHON_UNUSED_VAR(op);
- CYTHON_UNUSED_VAR(context);
- Py_INCREF(Py_None);
- return Py_None;
-}
-static PyObject *
-__Pyx_CyFunction_get_code(__pyx_CyFunctionObject *op, void *context)
-{
- PyObject* result = (op->func_code) ? op->func_code : Py_None;
- CYTHON_UNUSED_VAR(context);
- Py_INCREF(result);
- return result;
-}
-static int
-__Pyx_CyFunction_init_defaults(__pyx_CyFunctionObject *op) {
- int result = 0;
- PyObject *res = op->defaults_getter((PyObject *) op);
- if (unlikely(!res))
- return -1;
- #if CYTHON_ASSUME_SAFE_MACROS && !CYTHON_AVOID_BORROWED_REFS
- op->defaults_tuple = PyTuple_GET_ITEM(res, 0);
- Py_INCREF(op->defaults_tuple);
- op->defaults_kwdict = PyTuple_GET_ITEM(res, 1);
- Py_INCREF(op->defaults_kwdict);
- #else
- op->defaults_tuple = PySequence_ITEM(res, 0);
- if (unlikely(!op->defaults_tuple)) result = -1;
- else {
- op->defaults_kwdict = PySequence_ITEM(res, 1);
- if (unlikely(!op->defaults_kwdict)) result = -1;
- }
- #endif
- Py_DECREF(res);
- return result;
-}
-static int
-__Pyx_CyFunction_set_defaults(__pyx_CyFunctionObject *op, PyObject* value, void *context) {
- CYTHON_UNUSED_VAR(context);
- if (!value) {
- value = Py_None;
- } else if (unlikely(value != Py_None && !PyTuple_Check(value))) {
- PyErr_SetString(PyExc_TypeError,
- "__defaults__ must be set to a tuple object");
- return -1;
- }
- PyErr_WarnEx(PyExc_RuntimeWarning, "changes to cyfunction.__defaults__ will not "
- "currently affect the values used in function calls", 1);
- Py_INCREF(value);
- __Pyx_Py_XDECREF_SET(op->defaults_tuple, value);
- return 0;
-}
-static PyObject *
-__Pyx_CyFunction_get_defaults(__pyx_CyFunctionObject *op, void *context) {
- PyObject* result = op->defaults_tuple;
- CYTHON_UNUSED_VAR(context);
- if (unlikely(!result)) {
- if (op->defaults_getter) {
- if (unlikely(__Pyx_CyFunction_init_defaults(op) < 0)) return NULL;
- result = op->defaults_tuple;
- } else {
- result = Py_None;
- }
- }
- Py_INCREF(result);
- return result;
-}
-static int
-__Pyx_CyFunction_set_kwdefaults(__pyx_CyFunctionObject *op, PyObject* value, void *context) {
- CYTHON_UNUSED_VAR(context);
- if (!value) {
- value = Py_None;
- } else if (unlikely(value != Py_None && !PyDict_Check(value))) {
- PyErr_SetString(PyExc_TypeError,
- "__kwdefaults__ must be set to a dict object");
- return -1;
- }
- PyErr_WarnEx(PyExc_RuntimeWarning, "changes to cyfunction.__kwdefaults__ will not "
- "currently affect the values used in function calls", 1);
- Py_INCREF(value);
- __Pyx_Py_XDECREF_SET(op->defaults_kwdict, value);
- return 0;
-}
-static PyObject *
-__Pyx_CyFunction_get_kwdefaults(__pyx_CyFunctionObject *op, void *context) {
- PyObject* result = op->defaults_kwdict;
- CYTHON_UNUSED_VAR(context);
- if (unlikely(!result)) {
- if (op->defaults_getter) {
- if (unlikely(__Pyx_CyFunction_init_defaults(op) < 0)) return NULL;
- result = op->defaults_kwdict;
- } else {
- result = Py_None;
- }
- }
- Py_INCREF(result);
- return result;
-}
-static int
-__Pyx_CyFunction_set_annotations(__pyx_CyFunctionObject *op, PyObject* value, void *context) {
- CYTHON_UNUSED_VAR(context);
- if (!value || value == Py_None) {
- value = NULL;
- } else if (unlikely(!PyDict_Check(value))) {
- PyErr_SetString(PyExc_TypeError,
- "__annotations__ must be set to a dict object");
- return -1;
- }
- Py_XINCREF(value);
- __Pyx_Py_XDECREF_SET(op->func_annotations, value);
- return 0;
-}
-static PyObject *
-__Pyx_CyFunction_get_annotations(__pyx_CyFunctionObject *op, void *context) {
- PyObject* result = op->func_annotations;
- CYTHON_UNUSED_VAR(context);
- if (unlikely(!result)) {
- result = PyDict_New();
- if (unlikely(!result)) return NULL;
- op->func_annotations = result;
- }
- Py_INCREF(result);
- return result;
-}
-static PyObject *
-__Pyx_CyFunction_get_is_coroutine(__pyx_CyFunctionObject *op, void *context) {
- int is_coroutine;
- CYTHON_UNUSED_VAR(context);
- if (op->func_is_coroutine) {
- return __Pyx_NewRef(op->func_is_coroutine);
- }
- is_coroutine = op->flags & __Pyx_CYFUNCTION_COROUTINE;
-#if PY_VERSION_HEX >= 0x03050000
- if (is_coroutine) {
- PyObject *module, *fromlist, *marker = __pyx_n_s_is_coroutine;
- fromlist = PyList_New(1);
- if (unlikely(!fromlist)) return NULL;
- Py_INCREF(marker);
- PyList_SET_ITEM(fromlist, 0, marker);
- module = PyImport_ImportModuleLevelObject(__pyx_n_s_asyncio_coroutines, NULL, NULL, fromlist, 0);
- Py_DECREF(fromlist);
- if (unlikely(!module)) goto ignore;
- op->func_is_coroutine = __Pyx_PyObject_GetAttrStr(module, marker);
- Py_DECREF(module);
- if (likely(op->func_is_coroutine)) {
- return __Pyx_NewRef(op->func_is_coroutine);
- }
-ignore:
- PyErr_Clear();
- }
-#endif
- op->func_is_coroutine = __Pyx_PyBool_FromLong(is_coroutine);
- return __Pyx_NewRef(op->func_is_coroutine);
-}
-static PyGetSetDef __pyx_CyFunction_getsets[] = {
- {(char *) "func_doc", (getter)__Pyx_CyFunction_get_doc, (setter)__Pyx_CyFunction_set_doc, 0, 0},
- {(char *) "__doc__", (getter)__Pyx_CyFunction_get_doc, (setter)__Pyx_CyFunction_set_doc, 0, 0},
- {(char *) "func_name", (getter)__Pyx_CyFunction_get_name, (setter)__Pyx_CyFunction_set_name, 0, 0},
- {(char *) "__name__", (getter)__Pyx_CyFunction_get_name, (setter)__Pyx_CyFunction_set_name, 0, 0},
- {(char *) "__qualname__", (getter)__Pyx_CyFunction_get_qualname, (setter)__Pyx_CyFunction_set_qualname, 0, 0},
- {(char *) "func_dict", (getter)__Pyx_CyFunction_get_dict, (setter)__Pyx_CyFunction_set_dict, 0, 0},
- {(char *) "__dict__", (getter)__Pyx_CyFunction_get_dict, (setter)__Pyx_CyFunction_set_dict, 0, 0},
- {(char *) "func_globals", (getter)__Pyx_CyFunction_get_globals, 0, 0, 0},
- {(char *) "__globals__", (getter)__Pyx_CyFunction_get_globals, 0, 0, 0},
- {(char *) "func_closure", (getter)__Pyx_CyFunction_get_closure, 0, 0, 0},
- {(char *) "__closure__", (getter)__Pyx_CyFunction_get_closure, 0, 0, 0},
- {(char *) "func_code", (getter)__Pyx_CyFunction_get_code, 0, 0, 0},
- {(char *) "__code__", (getter)__Pyx_CyFunction_get_code, 0, 0, 0},
- {(char *) "func_defaults", (getter)__Pyx_CyFunction_get_defaults, (setter)__Pyx_CyFunction_set_defaults, 0, 0},
- {(char *) "__defaults__", (getter)__Pyx_CyFunction_get_defaults, (setter)__Pyx_CyFunction_set_defaults, 0, 0},
- {(char *) "__kwdefaults__", (getter)__Pyx_CyFunction_get_kwdefaults, (setter)__Pyx_CyFunction_set_kwdefaults, 0, 0},
- {(char *) "__annotations__", (getter)__Pyx_CyFunction_get_annotations, (setter)__Pyx_CyFunction_set_annotations, 0, 0},
- {(char *) "_is_coroutine", (getter)__Pyx_CyFunction_get_is_coroutine, 0, 0, 0},
- {0, 0, 0, 0, 0}
-};
-static PyMemberDef __pyx_CyFunction_members[] = {
- {(char *) "__module__", T_OBJECT, offsetof(PyCFunctionObject, m_module), 0, 0},
-#if CYTHON_USE_TYPE_SPECS
- {(char *) "__dictoffset__", T_PYSSIZET, offsetof(__pyx_CyFunctionObject, func_dict), READONLY, 0},
-#if CYTHON_METH_FASTCALL
-#if CYTHON_BACKPORT_VECTORCALL
- {(char *) "__vectorcalloffset__", T_PYSSIZET, offsetof(__pyx_CyFunctionObject, func_vectorcall), READONLY, 0},
-#else
- {(char *) "__vectorcalloffset__", T_PYSSIZET, offsetof(PyCFunctionObject, vectorcall), READONLY, 0},
-#endif
-#endif
-#if PY_VERSION_HEX < 0x030500A0
- {(char *) "__weaklistoffset__", T_PYSSIZET, offsetof(__pyx_CyFunctionObject, func_weakreflist), READONLY, 0},
-#else
- {(char *) "__weaklistoffset__", T_PYSSIZET, offsetof(PyCFunctionObject, m_weakreflist), READONLY, 0},
-#endif
-#endif
- {0, 0, 0, 0, 0}
-};
-static PyObject *
-__Pyx_CyFunction_reduce(__pyx_CyFunctionObject *m, PyObject *args)
-{
- CYTHON_UNUSED_VAR(args);
-#if PY_MAJOR_VERSION >= 3
- Py_INCREF(m->func_qualname);
- return m->func_qualname;
-#else
- return PyString_FromString(((PyCFunctionObject*)m)->m_ml->ml_name);
-#endif
-}
-static PyMethodDef __pyx_CyFunction_methods[] = {
- {"__reduce__", (PyCFunction)__Pyx_CyFunction_reduce, METH_VARARGS, 0},
- {0, 0, 0, 0}
-};
-#if PY_VERSION_HEX < 0x030500A0
-#define __Pyx_CyFunction_weakreflist(cyfunc) ((cyfunc)->func_weakreflist)
-#else
-#define __Pyx_CyFunction_weakreflist(cyfunc) (((PyCFunctionObject*)cyfunc)->m_weakreflist)
-#endif
-static PyObject *__Pyx_CyFunction_Init(__pyx_CyFunctionObject *op, PyMethodDef *ml, int flags, PyObject* qualname,
- PyObject *closure, PyObject *module, PyObject* globals, PyObject* code) {
- PyCFunctionObject *cf = (PyCFunctionObject*) op;
- if (unlikely(op == NULL))
- return NULL;
- op->flags = flags;
- __Pyx_CyFunction_weakreflist(op) = NULL;
- cf->m_ml = ml;
- cf->m_self = (PyObject *) op;
- Py_XINCREF(closure);
- op->func_closure = closure;
- Py_XINCREF(module);
- cf->m_module = module;
- op->func_dict = NULL;
- op->func_name = NULL;
- Py_INCREF(qualname);
- op->func_qualname = qualname;
- op->func_doc = NULL;
-#if PY_VERSION_HEX < 0x030900B1
- op->func_classobj = NULL;
-#else
- ((PyCMethodObject*)op)->mm_class = NULL;
-#endif
- op->func_globals = globals;
- Py_INCREF(op->func_globals);
- Py_XINCREF(code);
- op->func_code = code;
- op->defaults_pyobjects = 0;
- op->defaults_size = 0;
- op->defaults = NULL;
- op->defaults_tuple = NULL;
- op->defaults_kwdict = NULL;
- op->defaults_getter = NULL;
- op->func_annotations = NULL;
- op->func_is_coroutine = NULL;
-#if CYTHON_METH_FASTCALL
- switch (ml->ml_flags & (METH_VARARGS | METH_FASTCALL | METH_NOARGS | METH_O | METH_KEYWORDS | METH_METHOD)) {
- case METH_NOARGS:
- __Pyx_CyFunction_func_vectorcall(op) = __Pyx_CyFunction_Vectorcall_NOARGS;
- break;
- case METH_O:
- __Pyx_CyFunction_func_vectorcall(op) = __Pyx_CyFunction_Vectorcall_O;
- break;
- case METH_METHOD | METH_FASTCALL | METH_KEYWORDS:
- __Pyx_CyFunction_func_vectorcall(op) = __Pyx_CyFunction_Vectorcall_FASTCALL_KEYWORDS_METHOD;
- break;
- case METH_FASTCALL | METH_KEYWORDS:
- __Pyx_CyFunction_func_vectorcall(op) = __Pyx_CyFunction_Vectorcall_FASTCALL_KEYWORDS;
- break;
- case METH_VARARGS | METH_KEYWORDS:
- __Pyx_CyFunction_func_vectorcall(op) = NULL;
- break;
- default:
- PyErr_SetString(PyExc_SystemError, "Bad call flags for CyFunction");
- Py_DECREF(op);
- return NULL;
- }
-#endif
- return (PyObject *) op;
-}
-static int
-__Pyx_CyFunction_clear(__pyx_CyFunctionObject *m)
-{
- Py_CLEAR(m->func_closure);
- Py_CLEAR(((PyCFunctionObject*)m)->m_module);
- Py_CLEAR(m->func_dict);
- Py_CLEAR(m->func_name);
- Py_CLEAR(m->func_qualname);
- Py_CLEAR(m->func_doc);
- Py_CLEAR(m->func_globals);
- Py_CLEAR(m->func_code);
-#if PY_VERSION_HEX < 0x030900B1
- Py_CLEAR(__Pyx_CyFunction_GetClassObj(m));
-#else
- {
- PyObject *cls = (PyObject*) ((PyCMethodObject *) (m))->mm_class;
- ((PyCMethodObject *) (m))->mm_class = NULL;
- Py_XDECREF(cls);
- }
-#endif
- Py_CLEAR(m->defaults_tuple);
- Py_CLEAR(m->defaults_kwdict);
- Py_CLEAR(m->func_annotations);
- Py_CLEAR(m->func_is_coroutine);
- if (m->defaults) {
- PyObject **pydefaults = __Pyx_CyFunction_Defaults(PyObject *, m);
- int i;
- for (i = 0; i < m->defaults_pyobjects; i++)
- Py_XDECREF(pydefaults[i]);
- PyObject_Free(m->defaults);
- m->defaults = NULL;
- }
- return 0;
-}
-static void __Pyx__CyFunction_dealloc(__pyx_CyFunctionObject *m)
-{
- if (__Pyx_CyFunction_weakreflist(m) != NULL)
- PyObject_ClearWeakRefs((PyObject *) m);
- __Pyx_CyFunction_clear(m);
- __Pyx_PyHeapTypeObject_GC_Del(m);
-}
-static void __Pyx_CyFunction_dealloc(__pyx_CyFunctionObject *m)
-{
- PyObject_GC_UnTrack(m);
- __Pyx__CyFunction_dealloc(m);
-}
-static int __Pyx_CyFunction_traverse(__pyx_CyFunctionObject *m, visitproc visit, void *arg)
-{
- Py_VISIT(m->func_closure);
- Py_VISIT(((PyCFunctionObject*)m)->m_module);
- Py_VISIT(m->func_dict);
- Py_VISIT(m->func_name);
- Py_VISIT(m->func_qualname);
- Py_VISIT(m->func_doc);
- Py_VISIT(m->func_globals);
- Py_VISIT(m->func_code);
- Py_VISIT(__Pyx_CyFunction_GetClassObj(m));
- Py_VISIT(m->defaults_tuple);
- Py_VISIT(m->defaults_kwdict);
- Py_VISIT(m->func_is_coroutine);
- if (m->defaults) {
- PyObject **pydefaults = __Pyx_CyFunction_Defaults(PyObject *, m);
- int i;
- for (i = 0; i < m->defaults_pyobjects; i++)
- Py_VISIT(pydefaults[i]);
- }
- return 0;
-}
-static PyObject*
-__Pyx_CyFunction_repr(__pyx_CyFunctionObject *op)
-{
-#if PY_MAJOR_VERSION >= 3
- return PyUnicode_FromFormat("",
- op->func_qualname, (void *)op);
-#else
- return PyString_FromFormat("",
- PyString_AsString(op->func_qualname), (void *)op);
-#endif
-}
-static PyObject * __Pyx_CyFunction_CallMethod(PyObject *func, PyObject *self, PyObject *arg, PyObject *kw) {
- PyCFunctionObject* f = (PyCFunctionObject*)func;
- PyCFunction meth = f->m_ml->ml_meth;
- Py_ssize_t size;
- switch (f->m_ml->ml_flags & (METH_VARARGS | METH_KEYWORDS | METH_NOARGS | METH_O)) {
- case METH_VARARGS:
- if (likely(kw == NULL || PyDict_Size(kw) == 0))
- return (*meth)(self, arg);
- break;
- case METH_VARARGS | METH_KEYWORDS:
- return (*(PyCFunctionWithKeywords)(void*)meth)(self, arg, kw);
- case METH_NOARGS:
- if (likely(kw == NULL || PyDict_Size(kw) == 0)) {
- size = PyTuple_GET_SIZE(arg);
- if (likely(size == 0))
- return (*meth)(self, NULL);
- PyErr_Format(PyExc_TypeError,
- "%.200s() takes no arguments (%" CYTHON_FORMAT_SSIZE_T "d given)",
- f->m_ml->ml_name, size);
- return NULL;
- }
- break;
- case METH_O:
- if (likely(kw == NULL || PyDict_Size(kw) == 0)) {
- size = PyTuple_GET_SIZE(arg);
- if (likely(size == 1)) {
- PyObject *result, *arg0;
- #if CYTHON_ASSUME_SAFE_MACROS && !CYTHON_AVOID_BORROWED_REFS
- arg0 = PyTuple_GET_ITEM(arg, 0);
- #else
- arg0 = PySequence_ITEM(arg, 0); if (unlikely(!arg0)) return NULL;
- #endif
- result = (*meth)(self, arg0);
- #if !(CYTHON_ASSUME_SAFE_MACROS && !CYTHON_AVOID_BORROWED_REFS)
- Py_DECREF(arg0);
- #endif
- return result;
- }
- PyErr_Format(PyExc_TypeError,
- "%.200s() takes exactly one argument (%" CYTHON_FORMAT_SSIZE_T "d given)",
- f->m_ml->ml_name, size);
- return NULL;
- }
- break;
- default:
- PyErr_SetString(PyExc_SystemError, "Bad call flags for CyFunction");
- return NULL;
- }
- PyErr_Format(PyExc_TypeError, "%.200s() takes no keyword arguments",
- f->m_ml->ml_name);
- return NULL;
-}
-static CYTHON_INLINE PyObject *__Pyx_CyFunction_Call(PyObject *func, PyObject *arg, PyObject *kw) {
- return __Pyx_CyFunction_CallMethod(func, ((PyCFunctionObject*)func)->m_self, arg, kw);
-}
-static PyObject *__Pyx_CyFunction_CallAsMethod(PyObject *func, PyObject *args, PyObject *kw) {
- PyObject *result;
- __pyx_CyFunctionObject *cyfunc = (__pyx_CyFunctionObject *) func;
-#if CYTHON_METH_FASTCALL
- __pyx_vectorcallfunc vc = __Pyx_CyFunction_func_vectorcall(cyfunc);
- if (vc) {
-#if CYTHON_ASSUME_SAFE_MACROS
- return __Pyx_PyVectorcall_FastCallDict(func, vc, &PyTuple_GET_ITEM(args, 0), (size_t)PyTuple_GET_SIZE(args), kw);
-#else
- (void) &__Pyx_PyVectorcall_FastCallDict;
- return PyVectorcall_Call(func, args, kw);
-#endif
- }
-#endif
- if ((cyfunc->flags & __Pyx_CYFUNCTION_CCLASS) && !(cyfunc->flags & __Pyx_CYFUNCTION_STATICMETHOD)) {
- Py_ssize_t argc;
- PyObject *new_args;
- PyObject *self;
- argc = PyTuple_GET_SIZE(args);
- new_args = PyTuple_GetSlice(args, 1, argc);
- if (unlikely(!new_args))
- return NULL;
- self = PyTuple_GetItem(args, 0);
- if (unlikely(!self)) {
- Py_DECREF(new_args);
-#if PY_MAJOR_VERSION > 2
- PyErr_Format(PyExc_TypeError,
- "unbound method %.200S() needs an argument",
- cyfunc->func_qualname);
-#else
- PyErr_SetString(PyExc_TypeError,
- "unbound method needs an argument");
-#endif
- return NULL;
- }
- result = __Pyx_CyFunction_CallMethod(func, self, new_args, kw);
- Py_DECREF(new_args);
- } else {
- result = __Pyx_CyFunction_Call(func, args, kw);
- }
- return result;
-}
-#if CYTHON_METH_FASTCALL
-static CYTHON_INLINE int __Pyx_CyFunction_Vectorcall_CheckArgs(__pyx_CyFunctionObject *cyfunc, Py_ssize_t nargs, PyObject *kwnames)
-{
- int ret = 0;
- if ((cyfunc->flags & __Pyx_CYFUNCTION_CCLASS) && !(cyfunc->flags & __Pyx_CYFUNCTION_STATICMETHOD)) {
- if (unlikely(nargs < 1)) {
- PyErr_Format(PyExc_TypeError, "%.200s() needs an argument",
- ((PyCFunctionObject*)cyfunc)->m_ml->ml_name);
- return -1;
- }
- ret = 1;
- }
- if (unlikely(kwnames) && unlikely(PyTuple_GET_SIZE(kwnames))) {
- PyErr_Format(PyExc_TypeError,
- "%.200s() takes no keyword arguments", ((PyCFunctionObject*)cyfunc)->m_ml->ml_name);
- return -1;
- }
- return ret;
-}
-static PyObject * __Pyx_CyFunction_Vectorcall_NOARGS(PyObject *func, PyObject *const *args, size_t nargsf, PyObject *kwnames)
-{
- __pyx_CyFunctionObject *cyfunc = (__pyx_CyFunctionObject *)func;
- PyMethodDef* def = ((PyCFunctionObject*)cyfunc)->m_ml;
-#if CYTHON_BACKPORT_VECTORCALL
- Py_ssize_t nargs = (Py_ssize_t)nargsf;
-#else
- Py_ssize_t nargs = PyVectorcall_NARGS(nargsf);
-#endif
- PyObject *self;
- switch (__Pyx_CyFunction_Vectorcall_CheckArgs(cyfunc, nargs, kwnames)) {
- case 1:
- self = args[0];
- args += 1;
- nargs -= 1;
- break;
- case 0:
- self = ((PyCFunctionObject*)cyfunc)->m_self;
- break;
- default:
- return NULL;
- }
- if (unlikely(nargs != 0)) {
- PyErr_Format(PyExc_TypeError,
- "%.200s() takes no arguments (%" CYTHON_FORMAT_SSIZE_T "d given)",
- def->ml_name, nargs);
- return NULL;
- }
- return def->ml_meth(self, NULL);
-}
-static PyObject * __Pyx_CyFunction_Vectorcall_O(PyObject *func, PyObject *const *args, size_t nargsf, PyObject *kwnames)
-{
- __pyx_CyFunctionObject *cyfunc = (__pyx_CyFunctionObject *)func;
- PyMethodDef* def = ((PyCFunctionObject*)cyfunc)->m_ml;
-#if CYTHON_BACKPORT_VECTORCALL
- Py_ssize_t nargs = (Py_ssize_t)nargsf;
-#else
- Py_ssize_t nargs = PyVectorcall_NARGS(nargsf);
-#endif
- PyObject *self;
- switch (__Pyx_CyFunction_Vectorcall_CheckArgs(cyfunc, nargs, kwnames)) {
- case 1:
- self = args[0];
- args += 1;
- nargs -= 1;
- break;
- case 0:
- self = ((PyCFunctionObject*)cyfunc)->m_self;
- break;
- default:
- return NULL;
- }
- if (unlikely(nargs != 1)) {
- PyErr_Format(PyExc_TypeError,
- "%.200s() takes exactly one argument (%" CYTHON_FORMAT_SSIZE_T "d given)",
- def->ml_name, nargs);
- return NULL;
- }
- return def->ml_meth(self, args[0]);
-}
-static PyObject * __Pyx_CyFunction_Vectorcall_FASTCALL_KEYWORDS(PyObject *func, PyObject *const *args, size_t nargsf, PyObject *kwnames)
-{
- __pyx_CyFunctionObject *cyfunc = (__pyx_CyFunctionObject *)func;
- PyMethodDef* def = ((PyCFunctionObject*)cyfunc)->m_ml;
-#if CYTHON_BACKPORT_VECTORCALL
- Py_ssize_t nargs = (Py_ssize_t)nargsf;
-#else
- Py_ssize_t nargs = PyVectorcall_NARGS(nargsf);
-#endif
- PyObject *self;
- switch (__Pyx_CyFunction_Vectorcall_CheckArgs(cyfunc, nargs, NULL)) {
- case 1:
- self = args[0];
- args += 1;
- nargs -= 1;
- break;
- case 0:
- self = ((PyCFunctionObject*)cyfunc)->m_self;
- break;
- default:
- return NULL;
- }
- return ((_PyCFunctionFastWithKeywords)(void(*)(void))def->ml_meth)(self, args, nargs, kwnames);
-}
-static PyObject * __Pyx_CyFunction_Vectorcall_FASTCALL_KEYWORDS_METHOD(PyObject *func, PyObject *const *args, size_t nargsf, PyObject *kwnames)
-{
- __pyx_CyFunctionObject *cyfunc = (__pyx_CyFunctionObject *)func;
- PyMethodDef* def = ((PyCFunctionObject*)cyfunc)->m_ml;
- PyTypeObject *cls = (PyTypeObject *) __Pyx_CyFunction_GetClassObj(cyfunc);
-#if CYTHON_BACKPORT_VECTORCALL
- Py_ssize_t nargs = (Py_ssize_t)nargsf;
-#else
- Py_ssize_t nargs = PyVectorcall_NARGS(nargsf);
-#endif
- PyObject *self;
- switch (__Pyx_CyFunction_Vectorcall_CheckArgs(cyfunc, nargs, NULL)) {
- case 1:
- self = args[0];
- args += 1;
- nargs -= 1;
- break;
- case 0:
- self = ((PyCFunctionObject*)cyfunc)->m_self;
- break;
- default:
- return NULL;
- }
- return ((__Pyx_PyCMethod)(void(*)(void))def->ml_meth)(self, cls, args, (size_t)nargs, kwnames);
-}
-#endif
-#if CYTHON_USE_TYPE_SPECS
-static PyType_Slot __pyx_CyFunctionType_slots[] = {
- {Py_tp_dealloc, (void *)__Pyx_CyFunction_dealloc},
- {Py_tp_repr, (void *)__Pyx_CyFunction_repr},
- {Py_tp_call, (void *)__Pyx_CyFunction_CallAsMethod},
- {Py_tp_traverse, (void *)__Pyx_CyFunction_traverse},
- {Py_tp_clear, (void *)__Pyx_CyFunction_clear},
- {Py_tp_methods, (void *)__pyx_CyFunction_methods},
- {Py_tp_members, (void *)__pyx_CyFunction_members},
- {Py_tp_getset, (void *)__pyx_CyFunction_getsets},
- {Py_tp_descr_get, (void *)__Pyx_PyMethod_New},
- {0, 0},
-};
-static PyType_Spec __pyx_CyFunctionType_spec = {
- __PYX_TYPE_MODULE_PREFIX "cython_function_or_method",
- sizeof(__pyx_CyFunctionObject),
- 0,
-#ifdef Py_TPFLAGS_METHOD_DESCRIPTOR
- Py_TPFLAGS_METHOD_DESCRIPTOR |
-#endif
-#if (defined(_Py_TPFLAGS_HAVE_VECTORCALL) && CYTHON_METH_FASTCALL)
- _Py_TPFLAGS_HAVE_VECTORCALL |
-#endif
- Py_TPFLAGS_DEFAULT | Py_TPFLAGS_HAVE_GC | Py_TPFLAGS_BASETYPE,
- __pyx_CyFunctionType_slots
-};
-#else
-static PyTypeObject __pyx_CyFunctionType_type = {
- PyVarObject_HEAD_INIT(0, 0)
- __PYX_TYPE_MODULE_PREFIX "cython_function_or_method",
- sizeof(__pyx_CyFunctionObject),
- 0,
- (destructor) __Pyx_CyFunction_dealloc,
-#if !CYTHON_METH_FASTCALL
- 0,
-#elif CYTHON_BACKPORT_VECTORCALL
- (printfunc)offsetof(__pyx_CyFunctionObject, func_vectorcall),
-#else
- offsetof(PyCFunctionObject, vectorcall),
-#endif
- 0,
- 0,
-#if PY_MAJOR_VERSION < 3
- 0,
-#else
- 0,
-#endif
- (reprfunc) __Pyx_CyFunction_repr,
- 0,
- 0,
- 0,
- 0,
- __Pyx_CyFunction_CallAsMethod,
- 0,
- 0,
- 0,
- 0,
-#ifdef Py_TPFLAGS_METHOD_DESCRIPTOR
- Py_TPFLAGS_METHOD_DESCRIPTOR |
-#endif
-#ifdef _Py_TPFLAGS_HAVE_VECTORCALL
- _Py_TPFLAGS_HAVE_VECTORCALL |
-#endif
- Py_TPFLAGS_DEFAULT | Py_TPFLAGS_HAVE_GC | Py_TPFLAGS_BASETYPE,
- 0,
- (traverseproc) __Pyx_CyFunction_traverse,
- (inquiry) __Pyx_CyFunction_clear,
- 0,
-#if PY_VERSION_HEX < 0x030500A0
- offsetof(__pyx_CyFunctionObject, func_weakreflist),
-#else
- offsetof(PyCFunctionObject, m_weakreflist),
-#endif
- 0,
- 0,
- __pyx_CyFunction_methods,
- __pyx_CyFunction_members,
- __pyx_CyFunction_getsets,
- 0,
- 0,
- __Pyx_PyMethod_New,
- 0,
- offsetof(__pyx_CyFunctionObject, func_dict),
- 0,
- 0,
- 0,
- 0,
- 0,
- 0,
- 0,
- 0,
- 0,
- 0,
- 0,
- 0,
-#if PY_VERSION_HEX >= 0x030400a1
- 0,
-#endif
-#if PY_VERSION_HEX >= 0x030800b1 && (!CYTHON_COMPILING_IN_PYPY || PYPY_VERSION_NUM >= 0x07030800)
- 0,
-#endif
-#if __PYX_NEED_TP_PRINT_SLOT
- 0,
-#endif
-#if PY_VERSION_HEX >= 0x030C0000
- 0,
-#endif
-#if CYTHON_COMPILING_IN_PYPY && PY_VERSION_HEX >= 0x03090000 && PY_VERSION_HEX < 0x030a0000
- 0,
-#endif
-};
-#endif
-static int __pyx_CyFunction_init(PyObject *module) {
-#if CYTHON_USE_TYPE_SPECS
- __pyx_CyFunctionType = __Pyx_FetchCommonTypeFromSpec(module, &__pyx_CyFunctionType_spec, NULL);
-#else
- CYTHON_UNUSED_VAR(module);
- __pyx_CyFunctionType = __Pyx_FetchCommonType(&__pyx_CyFunctionType_type);
-#endif
- if (unlikely(__pyx_CyFunctionType == NULL)) {
- return -1;
- }
- return 0;
-}
-static CYTHON_INLINE void *__Pyx_CyFunction_InitDefaults(PyObject *func, size_t size, int pyobjects) {
- __pyx_CyFunctionObject *m = (__pyx_CyFunctionObject *) func;
- m->defaults = PyObject_Malloc(size);
- if (unlikely(!m->defaults))
- return PyErr_NoMemory();
- memset(m->defaults, 0, size);
- m->defaults_pyobjects = pyobjects;
- m->defaults_size = size;
- return m->defaults;
-}
-static CYTHON_INLINE void __Pyx_CyFunction_SetDefaultsTuple(PyObject *func, PyObject *tuple) {
- __pyx_CyFunctionObject *m = (__pyx_CyFunctionObject *) func;
- m->defaults_tuple = tuple;
- Py_INCREF(tuple);
-}
-static CYTHON_INLINE void __Pyx_CyFunction_SetDefaultsKwDict(PyObject *func, PyObject *dict) {
- __pyx_CyFunctionObject *m = (__pyx_CyFunctionObject *) func;
- m->defaults_kwdict = dict;
- Py_INCREF(dict);
-}
-static CYTHON_INLINE void __Pyx_CyFunction_SetAnnotationsDict(PyObject *func, PyObject *dict) {
- __pyx_CyFunctionObject *m = (__pyx_CyFunctionObject *) func;
- m->func_annotations = dict;
- Py_INCREF(dict);
-}
-
-/* CythonFunction */
-static PyObject *__Pyx_CyFunction_New(PyMethodDef *ml, int flags, PyObject* qualname,
- PyObject *closure, PyObject *module, PyObject* globals, PyObject* code) {
- PyObject *op = __Pyx_CyFunction_Init(
- PyObject_GC_New(__pyx_CyFunctionObject, __pyx_CyFunctionType),
- ml, flags, qualname, closure, module, globals, code
- );
- if (likely(op)) {
- PyObject_GC_Track(op);
- }
- return op;
-}
-
-/* CLineInTraceback */
-#ifndef CYTHON_CLINE_IN_TRACEBACK
-static int __Pyx_CLineForTraceback(PyThreadState *tstate, int c_line) {
- PyObject *use_cline;
- PyObject *ptype, *pvalue, *ptraceback;
-#if CYTHON_COMPILING_IN_CPYTHON
- PyObject **cython_runtime_dict;
-#endif
- CYTHON_MAYBE_UNUSED_VAR(tstate);
- if (unlikely(!__pyx_cython_runtime)) {
- return c_line;
- }
- __Pyx_ErrFetchInState(tstate, &ptype, &pvalue, &ptraceback);
-#if CYTHON_COMPILING_IN_CPYTHON
- cython_runtime_dict = _PyObject_GetDictPtr(__pyx_cython_runtime);
- if (likely(cython_runtime_dict)) {
- __PYX_PY_DICT_LOOKUP_IF_MODIFIED(
- use_cline, *cython_runtime_dict,
- __Pyx_PyDict_GetItemStr(*cython_runtime_dict, __pyx_n_s_cline_in_traceback))
- } else
-#endif
- {
- PyObject *use_cline_obj = __Pyx_PyObject_GetAttrStrNoError(__pyx_cython_runtime, __pyx_n_s_cline_in_traceback);
- if (use_cline_obj) {
- use_cline = PyObject_Not(use_cline_obj) ? Py_False : Py_True;
- Py_DECREF(use_cline_obj);
- } else {
- PyErr_Clear();
- use_cline = NULL;
- }
- }
- if (!use_cline) {
- c_line = 0;
- (void) PyObject_SetAttr(__pyx_cython_runtime, __pyx_n_s_cline_in_traceback, Py_False);
- }
- else if (use_cline == Py_False || (use_cline != Py_True && PyObject_Not(use_cline) != 0)) {
- c_line = 0;
- }
- __Pyx_ErrRestoreInState(tstate, ptype, pvalue, ptraceback);
- return c_line;
-}
-#endif
-
-/* CodeObjectCache */
-#if !CYTHON_COMPILING_IN_LIMITED_API
-static int __pyx_bisect_code_objects(__Pyx_CodeObjectCacheEntry* entries, int count, int code_line) {
- int start = 0, mid = 0, end = count - 1;
- if (end >= 0 && code_line > entries[end].code_line) {
- return count;
- }
- while (start < end) {
- mid = start + (end - start) / 2;
- if (code_line < entries[mid].code_line) {
- end = mid;
- } else if (code_line > entries[mid].code_line) {
- start = mid + 1;
- } else {
- return mid;
- }
- }
- if (code_line <= entries[mid].code_line) {
- return mid;
- } else {
- return mid + 1;
- }
-}
-static PyCodeObject *__pyx_find_code_object(int code_line) {
- PyCodeObject* code_object;
- int pos;
- if (unlikely(!code_line) || unlikely(!__pyx_code_cache.entries)) {
- return NULL;
- }
- pos = __pyx_bisect_code_objects(__pyx_code_cache.entries, __pyx_code_cache.count, code_line);
- if (unlikely(pos >= __pyx_code_cache.count) || unlikely(__pyx_code_cache.entries[pos].code_line != code_line)) {
- return NULL;
- }
- code_object = __pyx_code_cache.entries[pos].code_object;
- Py_INCREF(code_object);
- return code_object;
-}
-static void __pyx_insert_code_object(int code_line, PyCodeObject* code_object) {
- int pos, i;
- __Pyx_CodeObjectCacheEntry* entries = __pyx_code_cache.entries;
- if (unlikely(!code_line)) {
- return;
- }
- if (unlikely(!entries)) {
- entries = (__Pyx_CodeObjectCacheEntry*)PyMem_Malloc(64*sizeof(__Pyx_CodeObjectCacheEntry));
- if (likely(entries)) {
- __pyx_code_cache.entries = entries;
- __pyx_code_cache.max_count = 64;
- __pyx_code_cache.count = 1;
- entries[0].code_line = code_line;
- entries[0].code_object = code_object;
- Py_INCREF(code_object);
- }
- return;
- }
- pos = __pyx_bisect_code_objects(__pyx_code_cache.entries, __pyx_code_cache.count, code_line);
- if ((pos < __pyx_code_cache.count) && unlikely(__pyx_code_cache.entries[pos].code_line == code_line)) {
- PyCodeObject* tmp = entries[pos].code_object;
- entries[pos].code_object = code_object;
- Py_DECREF(tmp);
- return;
- }
- if (__pyx_code_cache.count == __pyx_code_cache.max_count) {
- int new_max = __pyx_code_cache.max_count + 64;
- entries = (__Pyx_CodeObjectCacheEntry*)PyMem_Realloc(
- __pyx_code_cache.entries, ((size_t)new_max) * sizeof(__Pyx_CodeObjectCacheEntry));
- if (unlikely(!entries)) {
- return;
- }
- __pyx_code_cache.entries = entries;
- __pyx_code_cache.max_count = new_max;
- }
- for (i=__pyx_code_cache.count; i>pos; i--) {
- entries[i] = entries[i-1];
- }
- entries[pos].code_line = code_line;
- entries[pos].code_object = code_object;
- __pyx_code_cache.count++;
- Py_INCREF(code_object);
-}
-#endif
-
-/* AddTraceback */
-#include "compile.h"
-#include "frameobject.h"
-#include "traceback.h"
-#if PY_VERSION_HEX >= 0x030b00a6
- #ifndef Py_BUILD_CORE
- #define Py_BUILD_CORE 1
- #endif
- #include "internal/pycore_frame.h"
-#endif
-#if CYTHON_COMPILING_IN_LIMITED_API
-static void __Pyx_AddTraceback(const char *funcname, int c_line,
- int py_line, const char *filename) {
- if (c_line) {
- (void) __pyx_cfilenm;
- (void) __Pyx_CLineForTraceback(__Pyx_PyThreadState_Current, c_line);
- }
- _PyTraceback_Add(funcname, filename, py_line);
-}
-#else
-static PyCodeObject* __Pyx_CreateCodeObjectForTraceback(
- const char *funcname, int c_line,
- int py_line, const char *filename) {
- PyCodeObject *py_code = NULL;
- PyObject *py_funcname = NULL;
- #if PY_MAJOR_VERSION < 3
- PyObject *py_srcfile = NULL;
- py_srcfile = PyString_FromString(filename);
- if (!py_srcfile) goto bad;
- #endif
- if (c_line) {
- #if PY_MAJOR_VERSION < 3
- py_funcname = PyString_FromFormat( "%s (%s:%d)", funcname, __pyx_cfilenm, c_line);
- if (!py_funcname) goto bad;
- #else
- py_funcname = PyUnicode_FromFormat( "%s (%s:%d)", funcname, __pyx_cfilenm, c_line);
- if (!py_funcname) goto bad;
- funcname = PyUnicode_AsUTF8(py_funcname);
- if (!funcname) goto bad;
- #endif
- }
- else {
- #if PY_MAJOR_VERSION < 3
- py_funcname = PyString_FromString(funcname);
- if (!py_funcname) goto bad;
- #endif
- }
- #if PY_MAJOR_VERSION < 3
- py_code = __Pyx_PyCode_New(
- 0,
- 0,
- 0,
- 0,
- 0,
- 0,
- __pyx_empty_bytes, /*PyObject *code,*/
- __pyx_empty_tuple, /*PyObject *consts,*/
- __pyx_empty_tuple, /*PyObject *names,*/
- __pyx_empty_tuple, /*PyObject *varnames,*/
- __pyx_empty_tuple, /*PyObject *freevars,*/
- __pyx_empty_tuple, /*PyObject *cellvars,*/
- py_srcfile, /*PyObject *filename,*/
- py_funcname, /*PyObject *name,*/
- py_line,
- __pyx_empty_bytes /*PyObject *lnotab*/
- );
- Py_DECREF(py_srcfile);
- #else
- py_code = PyCode_NewEmpty(filename, funcname, py_line);
- #endif
- Py_XDECREF(py_funcname); // XDECREF since it's only set on Py3 if cline
- return py_code;
-bad:
- Py_XDECREF(py_funcname);
- #if PY_MAJOR_VERSION < 3
- Py_XDECREF(py_srcfile);
- #endif
- return NULL;
-}
-static void __Pyx_AddTraceback(const char *funcname, int c_line,
- int py_line, const char *filename) {
- PyCodeObject *py_code = 0;
- PyFrameObject *py_frame = 0;
- PyThreadState *tstate = __Pyx_PyThreadState_Current;
- PyObject *ptype, *pvalue, *ptraceback;
- if (c_line) {
- c_line = __Pyx_CLineForTraceback(tstate, c_line);
- }
- py_code = __pyx_find_code_object(c_line ? -c_line : py_line);
- if (!py_code) {
- __Pyx_ErrFetchInState(tstate, &ptype, &pvalue, &ptraceback);
- py_code = __Pyx_CreateCodeObjectForTraceback(
- funcname, c_line, py_line, filename);
- if (!py_code) {
- /* If the code object creation fails, then we should clear the
- fetched exception references and propagate the new exception */
- Py_XDECREF(ptype);
- Py_XDECREF(pvalue);
- Py_XDECREF(ptraceback);
- goto bad;
- }
- __Pyx_ErrRestoreInState(tstate, ptype, pvalue, ptraceback);
- __pyx_insert_code_object(c_line ? -c_line : py_line, py_code);
- }
- py_frame = PyFrame_New(
- tstate, /*PyThreadState *tstate,*/
- py_code, /*PyCodeObject *code,*/
- __pyx_d, /*PyObject *globals,*/
- 0 /*PyObject *locals*/
- );
- if (!py_frame) goto bad;
- __Pyx_PyFrame_SetLineNumber(py_frame, py_line);
- PyTraceBack_Here(py_frame);
-bad:
- Py_XDECREF(py_code);
- Py_XDECREF(py_frame);
-}
-#endif
-
-/* Declarations */
-#if CYTHON_CCOMPLEX && (1) && (!0 || __cplusplus)
- #ifdef __cplusplus
- static CYTHON_INLINE __pyx_t_double_complex __pyx_t_double_complex_from_parts(double x, double y) {
- return ::std::complex< double >(x, y);
- }
- #else
- static CYTHON_INLINE __pyx_t_double_complex __pyx_t_double_complex_from_parts(double x, double y) {
- return x + y*(__pyx_t_double_complex)_Complex_I;
- }
- #endif
-#else
- static CYTHON_INLINE __pyx_t_double_complex __pyx_t_double_complex_from_parts(double x, double y) {
- __pyx_t_double_complex z;
- z.real = x;
- z.imag = y;
- return z;
- }
-#endif
-
-/* Arithmetic */
-#if CYTHON_CCOMPLEX && (1) && (!0 || __cplusplus)
-#else
- static CYTHON_INLINE int __Pyx_c_eq_double(__pyx_t_double_complex a, __pyx_t_double_complex b) {
- return (a.real == b.real) && (a.imag == b.imag);
- }
- static CYTHON_INLINE __pyx_t_double_complex __Pyx_c_sum_double(__pyx_t_double_complex a, __pyx_t_double_complex b) {
- __pyx_t_double_complex z;
- z.real = a.real + b.real;
- z.imag = a.imag + b.imag;
- return z;
- }
- static CYTHON_INLINE __pyx_t_double_complex __Pyx_c_diff_double(__pyx_t_double_complex a, __pyx_t_double_complex b) {
- __pyx_t_double_complex z;
- z.real = a.real - b.real;
- z.imag = a.imag - b.imag;
- return z;
- }
- static CYTHON_INLINE __pyx_t_double_complex __Pyx_c_prod_double(__pyx_t_double_complex a, __pyx_t_double_complex b) {
- __pyx_t_double_complex z;
- z.real = a.real * b.real - a.imag * b.imag;
- z.imag = a.real * b.imag + a.imag * b.real;
- return z;
- }
- #if 1
- static CYTHON_INLINE __pyx_t_double_complex __Pyx_c_quot_double(__pyx_t_double_complex a, __pyx_t_double_complex b) {
- if (b.imag == 0) {
- return __pyx_t_double_complex_from_parts(a.real / b.real, a.imag / b.real);
- } else if (fabs(b.real) >= fabs(b.imag)) {
- if (b.real == 0 && b.imag == 0) {
- return __pyx_t_double_complex_from_parts(a.real / b.real, a.imag / b.imag);
- } else {
- double r = b.imag / b.real;
- double s = (double)(1.0) / (b.real + b.imag * r);
- return __pyx_t_double_complex_from_parts(
- (a.real + a.imag * r) * s, (a.imag - a.real * r) * s);
- }
- } else {
- double r = b.real / b.imag;
- double s = (double)(1.0) / (b.imag + b.real * r);
- return __pyx_t_double_complex_from_parts(
- (a.real * r + a.imag) * s, (a.imag * r - a.real) * s);
- }
- }
- #else
- static CYTHON_INLINE __pyx_t_double_complex __Pyx_c_quot_double(__pyx_t_double_complex a, __pyx_t_double_complex b) {
- if (b.imag == 0) {
- return __pyx_t_double_complex_from_parts(a.real / b.real, a.imag / b.real);
- } else {
- double denom = b.real * b.real + b.imag * b.imag;
- return __pyx_t_double_complex_from_parts(
- (a.real * b.real + a.imag * b.imag) / denom,
- (a.imag * b.real - a.real * b.imag) / denom);
- }
- }
- #endif
- static CYTHON_INLINE __pyx_t_double_complex __Pyx_c_neg_double(__pyx_t_double_complex a) {
- __pyx_t_double_complex z;
- z.real = -a.real;
- z.imag = -a.imag;
- return z;
- }
- static CYTHON_INLINE int __Pyx_c_is_zero_double(__pyx_t_double_complex a) {
- return (a.real == 0) && (a.imag == 0);
- }
- static CYTHON_INLINE __pyx_t_double_complex __Pyx_c_conj_double(__pyx_t_double_complex a) {
- __pyx_t_double_complex z;
- z.real = a.real;
- z.imag = -a.imag;
- return z;
- }
- #if 1
- static CYTHON_INLINE double __Pyx_c_abs_double(__pyx_t_double_complex z) {
- #if !defined(HAVE_HYPOT) || defined(_MSC_VER)
- return sqrt(z.real*z.real + z.imag*z.imag);
- #else
- return hypot(z.real, z.imag);
- #endif
- }
- static CYTHON_INLINE __pyx_t_double_complex __Pyx_c_pow_double(__pyx_t_double_complex a, __pyx_t_double_complex b) {
- __pyx_t_double_complex z;
- double r, lnr, theta, z_r, z_theta;
- if (b.imag == 0 && b.real == (int)b.real) {
- if (b.real < 0) {
- double denom = a.real * a.real + a.imag * a.imag;
- a.real = a.real / denom;
- a.imag = -a.imag / denom;
- b.real = -b.real;
- }
- switch ((int)b.real) {
- case 0:
- z.real = 1;
- z.imag = 0;
- return z;
- case 1:
- return a;
- case 2:
- return __Pyx_c_prod_double(a, a);
- case 3:
- z = __Pyx_c_prod_double(a, a);
- return __Pyx_c_prod_double(z, a);
- case 4:
- z = __Pyx_c_prod_double(a, a);
- return __Pyx_c_prod_double(z, z);
- }
- }
- if (a.imag == 0) {
- if (a.real == 0) {
- return a;
- } else if ((b.imag == 0) && (a.real >= 0)) {
- z.real = pow(a.real, b.real);
- z.imag = 0;
- return z;
- } else if (a.real > 0) {
- r = a.real;
- theta = 0;
- } else {
- r = -a.real;
- theta = atan2(0.0, -1.0);
- }
- } else {
- r = __Pyx_c_abs_double(a);
- theta = atan2(a.imag, a.real);
- }
- lnr = log(r);
- z_r = exp(lnr * b.real - theta * b.imag);
- z_theta = theta * b.real + lnr * b.imag;
- z.real = z_r * cos(z_theta);
- z.imag = z_r * sin(z_theta);
- return z;
- }
- #endif
-#endif
-
-/* FromPy */
-static __pyx_t_double_complex __Pyx_PyComplex_As___pyx_t_double_complex(PyObject* o) {
- Py_complex cval;
-#if !CYTHON_COMPILING_IN_PYPY
- if (PyComplex_CheckExact(o))
- cval = ((PyComplexObject *)o)->cval;
- else
-#endif
- cval = PyComplex_AsCComplex(o);
- return __pyx_t_double_complex_from_parts(
- (double)cval.real,
- (double)cval.imag);
-}
-
-/* CIntFromPyVerify */
-#define __PYX_VERIFY_RETURN_INT(target_type, func_type, func_value)\
- __PYX__VERIFY_RETURN_INT(target_type, func_type, func_value, 0)
-#define __PYX_VERIFY_RETURN_INT_EXC(target_type, func_type, func_value)\
- __PYX__VERIFY_RETURN_INT(target_type, func_type, func_value, 1)
-#define __PYX__VERIFY_RETURN_INT(target_type, func_type, func_value, exc)\
- {\
- func_type value = func_value;\
- if (sizeof(target_type) < sizeof(func_type)) {\
- if (unlikely(value != (func_type) (target_type) value)) {\
- func_type zero = 0;\
- if (exc && unlikely(value == (func_type)-1 && PyErr_Occurred()))\
- return (target_type) -1;\
- if (is_unsigned && unlikely(value < zero))\
- goto raise_neg_overflow;\
- else\
- goto raise_overflow;\
- }\
- }\
- return (target_type) value;\
- }
-
-/* CIntFromPy */
-static CYTHON_INLINE int __Pyx_PyInt_As_int(PyObject *x) {
-#ifdef __Pyx_HAS_GCC_DIAGNOSTIC
-#pragma GCC diagnostic push
-#pragma GCC diagnostic ignored "-Wconversion"
-#endif
- const int neg_one = (int) -1, const_zero = (int) 0;
-#ifdef __Pyx_HAS_GCC_DIAGNOSTIC
-#pragma GCC diagnostic pop
-#endif
- const int is_unsigned = neg_one > const_zero;
-#if PY_MAJOR_VERSION < 3
- if (likely(PyInt_Check(x))) {
- if ((sizeof(int) < sizeof(long))) {
- __PYX_VERIFY_RETURN_INT(int, long, PyInt_AS_LONG(x))
- } else {
- long val = PyInt_AS_LONG(x);
- if (is_unsigned && unlikely(val < 0)) {
- goto raise_neg_overflow;
- }
- return (int) val;
- }
- } else
-#endif
- if (likely(PyLong_Check(x))) {
- if (is_unsigned) {
-#if CYTHON_USE_PYLONG_INTERNALS
- if (unlikely(__Pyx_PyLong_IsNeg(x))) {
- goto raise_neg_overflow;
- } else if (__Pyx_PyLong_IsCompact(x)) {
- __PYX_VERIFY_RETURN_INT(int, __Pyx_compact_upylong, __Pyx_PyLong_CompactValueUnsigned(x))
- } else {
- const digit* digits = __Pyx_PyLong_Digits(x);
- assert(__Pyx_PyLong_DigitCount(x) > 1);
- switch (__Pyx_PyLong_DigitCount(x)) {
- case 2:
- if ((8 * sizeof(int) > 1 * PyLong_SHIFT)) {
- if ((8 * sizeof(unsigned long) > 2 * PyLong_SHIFT)) {
- __PYX_VERIFY_RETURN_INT(int, unsigned long, (((((unsigned long)digits[1]) << PyLong_SHIFT) | (unsigned long)digits[0])))
- } else if ((8 * sizeof(int) >= 2 * PyLong_SHIFT)) {
- return (int) (((((int)digits[1]) << PyLong_SHIFT) | (int)digits[0]));
- }
- }
- break;
- case 3:
- if ((8 * sizeof(int) > 2 * PyLong_SHIFT)) {
- if ((8 * sizeof(unsigned long) > 3 * PyLong_SHIFT)) {
- __PYX_VERIFY_RETURN_INT(int, unsigned long, (((((((unsigned long)digits[2]) << PyLong_SHIFT) | (unsigned long)digits[1]) << PyLong_SHIFT) | (unsigned long)digits[0])))
- } else if ((8 * sizeof(int) >= 3 * PyLong_SHIFT)) {
- return (int) (((((((int)digits[2]) << PyLong_SHIFT) | (int)digits[1]) << PyLong_SHIFT) | (int)digits[0]));
- }
- }
- break;
- case 4:
- if ((8 * sizeof(int) > 3 * PyLong_SHIFT)) {
- if ((8 * sizeof(unsigned long) > 4 * PyLong_SHIFT)) {
- __PYX_VERIFY_RETURN_INT(int, unsigned long, (((((((((unsigned long)digits[3]) << PyLong_SHIFT) | (unsigned long)digits[2]) << PyLong_SHIFT) | (unsigned long)digits[1]) << PyLong_SHIFT) | (unsigned long)digits[0])))
- } else if ((8 * sizeof(int) >= 4 * PyLong_SHIFT)) {
- return (int) (((((((((int)digits[3]) << PyLong_SHIFT) | (int)digits[2]) << PyLong_SHIFT) | (int)digits[1]) << PyLong_SHIFT) | (int)digits[0]));
- }
- }
- break;
- }
- }
-#endif
-#if CYTHON_COMPILING_IN_CPYTHON && PY_VERSION_HEX < 0x030C00A7
- if (unlikely(Py_SIZE(x) < 0)) {
- goto raise_neg_overflow;
- }
-#else
- {
- int result = PyObject_RichCompareBool(x, Py_False, Py_LT);
- if (unlikely(result < 0))
- return (int) -1;
- if (unlikely(result == 1))
- goto raise_neg_overflow;
- }
-#endif
- if ((sizeof(int) <= sizeof(unsigned long))) {
- __PYX_VERIFY_RETURN_INT_EXC(int, unsigned long, PyLong_AsUnsignedLong(x))
-#ifdef HAVE_LONG_LONG
- } else if ((sizeof(int) <= sizeof(unsigned PY_LONG_LONG))) {
- __PYX_VERIFY_RETURN_INT_EXC(int, unsigned PY_LONG_LONG, PyLong_AsUnsignedLongLong(x))
-#endif
- }
- } else {
-#if CYTHON_USE_PYLONG_INTERNALS
- if (__Pyx_PyLong_IsCompact(x)) {
- __PYX_VERIFY_RETURN_INT(int, __Pyx_compact_pylong, __Pyx_PyLong_CompactValue(x))
- } else {
- const digit* digits = __Pyx_PyLong_Digits(x);
- assert(__Pyx_PyLong_DigitCount(x) > 1);
- switch (__Pyx_PyLong_SignedDigitCount(x)) {
- case -2:
- if ((8 * sizeof(int) - 1 > 1 * PyLong_SHIFT)) {
- if ((8 * sizeof(unsigned long) > 2 * PyLong_SHIFT)) {
- __PYX_VERIFY_RETURN_INT(int, long, -(long) (((((unsigned long)digits[1]) << PyLong_SHIFT) | (unsigned long)digits[0])))
- } else if ((8 * sizeof(int) - 1 > 2 * PyLong_SHIFT)) {
- return (int) (((int)-1)*(((((int)digits[1]) << PyLong_SHIFT) | (int)digits[0])));
- }
- }
- break;
- case 2:
- if ((8 * sizeof(int) > 1 * PyLong_SHIFT)) {
- if ((8 * sizeof(unsigned long) > 2 * PyLong_SHIFT)) {
- __PYX_VERIFY_RETURN_INT(int, unsigned long, (((((unsigned long)digits[1]) << PyLong_SHIFT) | (unsigned long)digits[0])))
- } else if ((8 * sizeof(int) - 1 > 2 * PyLong_SHIFT)) {
- return (int) ((((((int)digits[1]) << PyLong_SHIFT) | (int)digits[0])));
- }
- }
- break;
- case -3:
- if ((8 * sizeof(int) - 1 > 2 * PyLong_SHIFT)) {
- if ((8 * sizeof(unsigned long) > 3 * PyLong_SHIFT)) {
- __PYX_VERIFY_RETURN_INT(int, long, -(long) (((((((unsigned long)digits[2]) << PyLong_SHIFT) | (unsigned long)digits[1]) << PyLong_SHIFT) | (unsigned long)digits[0])))
- } else if ((8 * sizeof(int) - 1 > 3 * PyLong_SHIFT)) {
- return (int) (((int)-1)*(((((((int)digits[2]) << PyLong_SHIFT) | (int)digits[1]) << PyLong_SHIFT) | (int)digits[0])));
- }
- }
- break;
- case 3:
- if ((8 * sizeof(int) > 2 * PyLong_SHIFT)) {
- if ((8 * sizeof(unsigned long) > 3 * PyLong_SHIFT)) {
- __PYX_VERIFY_RETURN_INT(int, unsigned long, (((((((unsigned long)digits[2]) << PyLong_SHIFT) | (unsigned long)digits[1]) << PyLong_SHIFT) | (unsigned long)digits[0])))
- } else if ((8 * sizeof(int) - 1 > 3 * PyLong_SHIFT)) {
- return (int) ((((((((int)digits[2]) << PyLong_SHIFT) | (int)digits[1]) << PyLong_SHIFT) | (int)digits[0])));
- }
- }
- break;
- case -4:
- if ((8 * sizeof(int) - 1 > 3 * PyLong_SHIFT)) {
- if ((8 * sizeof(unsigned long) > 4 * PyLong_SHIFT)) {
- __PYX_VERIFY_RETURN_INT(int, long, -(long) (((((((((unsigned long)digits[3]) << PyLong_SHIFT) | (unsigned long)digits[2]) << PyLong_SHIFT) | (unsigned long)digits[1]) << PyLong_SHIFT) | (unsigned long)digits[0])))
- } else if ((8 * sizeof(int) - 1 > 4 * PyLong_SHIFT)) {
- return (int) (((int)-1)*(((((((((int)digits[3]) << PyLong_SHIFT) | (int)digits[2]) << PyLong_SHIFT) | (int)digits[1]) << PyLong_SHIFT) | (int)digits[0])));
- }
- }
- break;
- case 4:
- if ((8 * sizeof(int) > 3 * PyLong_SHIFT)) {
- if ((8 * sizeof(unsigned long) > 4 * PyLong_SHIFT)) {
- __PYX_VERIFY_RETURN_INT(int, unsigned long, (((((((((unsigned long)digits[3]) << PyLong_SHIFT) | (unsigned long)digits[2]) << PyLong_SHIFT) | (unsigned long)digits[1]) << PyLong_SHIFT) | (unsigned long)digits[0])))
- } else if ((8 * sizeof(int) - 1 > 4 * PyLong_SHIFT)) {
- return (int) ((((((((((int)digits[3]) << PyLong_SHIFT) | (int)digits[2]) << PyLong_SHIFT) | (int)digits[1]) << PyLong_SHIFT) | (int)digits[0])));
- }
- }
- break;
- }
- }
-#endif
- if ((sizeof(int) <= sizeof(long))) {
- __PYX_VERIFY_RETURN_INT_EXC(int, long, PyLong_AsLong(x))
-#ifdef HAVE_LONG_LONG
- } else if ((sizeof(int) <= sizeof(PY_LONG_LONG))) {
- __PYX_VERIFY_RETURN_INT_EXC(int, PY_LONG_LONG, PyLong_AsLongLong(x))
-#endif
- }
- }
- {
- int val;
- PyObject *v = __Pyx_PyNumber_IntOrLong(x);
-#if PY_MAJOR_VERSION < 3
- if (likely(v) && !PyLong_Check(v)) {
- PyObject *tmp = v;
- v = PyNumber_Long(tmp);
- Py_DECREF(tmp);
- }
-#endif
- if (likely(v)) {
- int ret = -1;
-#if !(CYTHON_COMPILING_IN_PYPY || CYTHON_COMPILING_IN_LIMITED_API) || defined(_PyLong_AsByteArray)
- int one = 1; int is_little = (int)*(unsigned char *)&one;
- unsigned char *bytes = (unsigned char *)&val;
- ret = _PyLong_AsByteArray((PyLongObject *)v,
- bytes, sizeof(val),
- is_little, !is_unsigned);
-#else
- PyObject *stepval = NULL, *mask = NULL, *shift = NULL;
- int bits, remaining_bits, is_negative = 0;
- long idigit;
- int chunk_size = (sizeof(long) < 8) ? 30 : 62;
- if (unlikely(!PyLong_CheckExact(v))) {
- PyObject *tmp = v;
- v = PyNumber_Long(v);
- assert(PyLong_CheckExact(v));
- Py_DECREF(tmp);
- if (unlikely(!v)) return (int) -1;
- }
-#if CYTHON_COMPILING_IN_LIMITED_API && PY_VERSION_HEX < 0x030B0000
- if (Py_SIZE(x) == 0)
- return (int) 0;
- is_negative = Py_SIZE(x) < 0;
-#else
- {
- int result = PyObject_RichCompareBool(x, Py_False, Py_LT);
- if (unlikely(result < 0))
- return (int) -1;
- is_negative = result == 1;
- }
-#endif
- if (is_unsigned && unlikely(is_negative)) {
- goto raise_neg_overflow;
- } else if (is_negative) {
- stepval = PyNumber_Invert(v);
- if (unlikely(!stepval))
- return (int) -1;
- } else {
- stepval = __Pyx_NewRef(v);
- }
- val = (int) 0;
- mask = PyLong_FromLong((1L << chunk_size) - 1); if (unlikely(!mask)) goto done;
- shift = PyLong_FromLong(chunk_size); if (unlikely(!shift)) goto done;
- for (bits = 0; bits < (int) sizeof(int) * 8 - chunk_size; bits += chunk_size) {
- PyObject *tmp, *digit;
- digit = PyNumber_And(stepval, mask);
- if (unlikely(!digit)) goto done;
- idigit = PyLong_AsLong(digit);
- Py_DECREF(digit);
- if (unlikely(idigit < 0)) goto done;
- tmp = PyNumber_Rshift(stepval, shift);
- if (unlikely(!tmp)) goto done;
- Py_DECREF(stepval); stepval = tmp;
- val |= ((int) idigit) << bits;
- #if CYTHON_COMPILING_IN_LIMITED_API && PY_VERSION_HEX < 0x030B0000
- if (Py_SIZE(stepval) == 0)
- goto unpacking_done;
- #endif
- }
- idigit = PyLong_AsLong(stepval);
- if (unlikely(idigit < 0)) goto done;
- remaining_bits = ((int) sizeof(int) * 8) - bits - (is_unsigned ? 0 : 1);
- if (unlikely(idigit >= (1L << remaining_bits)))
- goto raise_overflow;
- val |= ((int) idigit) << bits;
- #if CYTHON_COMPILING_IN_LIMITED_API && PY_VERSION_HEX < 0x030B0000
- unpacking_done:
- #endif
- if (!is_unsigned) {
- if (unlikely(val & (((int) 1) << (sizeof(int) * 8 - 1))))
- goto raise_overflow;
- if (is_negative)
- val = ~val;
- }
- ret = 0;
- done:
- Py_XDECREF(shift);
- Py_XDECREF(mask);
- Py_XDECREF(stepval);
-#endif
- Py_DECREF(v);
- if (likely(!ret))
- return val;
- }
- return (int) -1;
- }
- } else {
- int val;
- PyObject *tmp = __Pyx_PyNumber_IntOrLong(x);
- if (!tmp) return (int) -1;
- val = __Pyx_PyInt_As_int(tmp);
- Py_DECREF(tmp);
- return val;
- }
-raise_overflow:
- PyErr_SetString(PyExc_OverflowError,
- "value too large to convert to int");
- return (int) -1;
-raise_neg_overflow:
- PyErr_SetString(PyExc_OverflowError,
- "can't convert negative value to int");
- return (int) -1;
-}
-
-/* CIntToPy */
-static CYTHON_INLINE PyObject* __Pyx_PyInt_From_long(long value) {
-#ifdef __Pyx_HAS_GCC_DIAGNOSTIC
-#pragma GCC diagnostic push
-#pragma GCC diagnostic ignored "-Wconversion"
-#endif
- const long neg_one = (long) -1, const_zero = (long) 0;
-#ifdef __Pyx_HAS_GCC_DIAGNOSTIC
-#pragma GCC diagnostic pop
-#endif
- const int is_unsigned = neg_one > const_zero;
- if (is_unsigned) {
- if (sizeof(long) < sizeof(long)) {
- return PyInt_FromLong((long) value);
- } else if (sizeof(long) <= sizeof(unsigned long)) {
- return PyLong_FromUnsignedLong((unsigned long) value);
-#ifdef HAVE_LONG_LONG
- } else if (sizeof(long) <= sizeof(unsigned PY_LONG_LONG)) {
- return PyLong_FromUnsignedLongLong((unsigned PY_LONG_LONG) value);
-#endif
- }
- } else {
- if (sizeof(long) <= sizeof(long)) {
- return PyInt_FromLong((long) value);
-#ifdef HAVE_LONG_LONG
- } else if (sizeof(long) <= sizeof(PY_LONG_LONG)) {
- return PyLong_FromLongLong((PY_LONG_LONG) value);
-#endif
- }
- }
- {
- int one = 1; int little = (int)*(unsigned char *)&one;
- unsigned char *bytes = (unsigned char *)&value;
- return _PyLong_FromByteArray(bytes, sizeof(long),
- little, !is_unsigned);
- }
-}
-
-/* CIntToPy */
-static CYTHON_INLINE PyObject* __Pyx_PyInt_From_int(int value) {
-#ifdef __Pyx_HAS_GCC_DIAGNOSTIC
-#pragma GCC diagnostic push
-#pragma GCC diagnostic ignored "-Wconversion"
-#endif
- const int neg_one = (int) -1, const_zero = (int) 0;
-#ifdef __Pyx_HAS_GCC_DIAGNOSTIC
-#pragma GCC diagnostic pop
-#endif
- const int is_unsigned = neg_one > const_zero;
- if (is_unsigned) {
- if (sizeof(int) < sizeof(long)) {
- return PyInt_FromLong((long) value);
- } else if (sizeof(int) <= sizeof(unsigned long)) {
- return PyLong_FromUnsignedLong((unsigned long) value);
-#ifdef HAVE_LONG_LONG
- } else if (sizeof(int) <= sizeof(unsigned PY_LONG_LONG)) {
- return PyLong_FromUnsignedLongLong((unsigned PY_LONG_LONG) value);
-#endif
- }
- } else {
- if (sizeof(int) <= sizeof(long)) {
- return PyInt_FromLong((long) value);
-#ifdef HAVE_LONG_LONG
- } else if (sizeof(int) <= sizeof(PY_LONG_LONG)) {
- return PyLong_FromLongLong((PY_LONG_LONG) value);
-#endif
- }
- }
- {
- int one = 1; int little = (int)*(unsigned char *)&one;
- unsigned char *bytes = (unsigned char *)&value;
- return _PyLong_FromByteArray(bytes, sizeof(int),
- little, !is_unsigned);
- }
-}
-
-/* CIntFromPy */
-static CYTHON_INLINE long __Pyx_PyInt_As_long(PyObject *x) {
-#ifdef __Pyx_HAS_GCC_DIAGNOSTIC
-#pragma GCC diagnostic push
-#pragma GCC diagnostic ignored "-Wconversion"
-#endif
- const long neg_one = (long) -1, const_zero = (long) 0;
-#ifdef __Pyx_HAS_GCC_DIAGNOSTIC
-#pragma GCC diagnostic pop
-#endif
- const int is_unsigned = neg_one > const_zero;
-#if PY_MAJOR_VERSION < 3
- if (likely(PyInt_Check(x))) {
- if ((sizeof(long) < sizeof(long))) {
- __PYX_VERIFY_RETURN_INT(long, long, PyInt_AS_LONG(x))
- } else {
- long val = PyInt_AS_LONG(x);
- if (is_unsigned && unlikely(val < 0)) {
- goto raise_neg_overflow;
- }
- return (long) val;
- }
- } else
-#endif
- if (likely(PyLong_Check(x))) {
- if (is_unsigned) {
-#if CYTHON_USE_PYLONG_INTERNALS
- if (unlikely(__Pyx_PyLong_IsNeg(x))) {
- goto raise_neg_overflow;
- } else if (__Pyx_PyLong_IsCompact(x)) {
- __PYX_VERIFY_RETURN_INT(long, __Pyx_compact_upylong, __Pyx_PyLong_CompactValueUnsigned(x))
- } else {
- const digit* digits = __Pyx_PyLong_Digits(x);
- assert(__Pyx_PyLong_DigitCount(x) > 1);
- switch (__Pyx_PyLong_DigitCount(x)) {
- case 2:
- if ((8 * sizeof(long) > 1 * PyLong_SHIFT)) {
- if ((8 * sizeof(unsigned long) > 2 * PyLong_SHIFT)) {
- __PYX_VERIFY_RETURN_INT(long, unsigned long, (((((unsigned long)digits[1]) << PyLong_SHIFT) | (unsigned long)digits[0])))
- } else if ((8 * sizeof(long) >= 2 * PyLong_SHIFT)) {
- return (long) (((((long)digits[1]) << PyLong_SHIFT) | (long)digits[0]));
- }
- }
- break;
- case 3:
- if ((8 * sizeof(long) > 2 * PyLong_SHIFT)) {
- if ((8 * sizeof(unsigned long) > 3 * PyLong_SHIFT)) {
- __PYX_VERIFY_RETURN_INT(long, unsigned long, (((((((unsigned long)digits[2]) << PyLong_SHIFT) | (unsigned long)digits[1]) << PyLong_SHIFT) | (unsigned long)digits[0])))
- } else if ((8 * sizeof(long) >= 3 * PyLong_SHIFT)) {
- return (long) (((((((long)digits[2]) << PyLong_SHIFT) | (long)digits[1]) << PyLong_SHIFT) | (long)digits[0]));
- }
- }
- break;
- case 4:
- if ((8 * sizeof(long) > 3 * PyLong_SHIFT)) {
- if ((8 * sizeof(unsigned long) > 4 * PyLong_SHIFT)) {
- __PYX_VERIFY_RETURN_INT(long, unsigned long, (((((((((unsigned long)digits[3]) << PyLong_SHIFT) | (unsigned long)digits[2]) << PyLong_SHIFT) | (unsigned long)digits[1]) << PyLong_SHIFT) | (unsigned long)digits[0])))
- } else if ((8 * sizeof(long) >= 4 * PyLong_SHIFT)) {
- return (long) (((((((((long)digits[3]) << PyLong_SHIFT) | (long)digits[2]) << PyLong_SHIFT) | (long)digits[1]) << PyLong_SHIFT) | (long)digits[0]));
- }
- }
- break;
- }
- }
-#endif
-#if CYTHON_COMPILING_IN_CPYTHON && PY_VERSION_HEX < 0x030C00A7
- if (unlikely(Py_SIZE(x) < 0)) {
- goto raise_neg_overflow;
- }
-#else
- {
- int result = PyObject_RichCompareBool(x, Py_False, Py_LT);
- if (unlikely(result < 0))
- return (long) -1;
- if (unlikely(result == 1))
- goto raise_neg_overflow;
- }
-#endif
- if ((sizeof(long) <= sizeof(unsigned long))) {
- __PYX_VERIFY_RETURN_INT_EXC(long, unsigned long, PyLong_AsUnsignedLong(x))
-#ifdef HAVE_LONG_LONG
- } else if ((sizeof(long) <= sizeof(unsigned PY_LONG_LONG))) {
- __PYX_VERIFY_RETURN_INT_EXC(long, unsigned PY_LONG_LONG, PyLong_AsUnsignedLongLong(x))
-#endif
- }
- } else {
-#if CYTHON_USE_PYLONG_INTERNALS
- if (__Pyx_PyLong_IsCompact(x)) {
- __PYX_VERIFY_RETURN_INT(long, __Pyx_compact_pylong, __Pyx_PyLong_CompactValue(x))
- } else {
- const digit* digits = __Pyx_PyLong_Digits(x);
- assert(__Pyx_PyLong_DigitCount(x) > 1);
- switch (__Pyx_PyLong_SignedDigitCount(x)) {
- case -2:
- if ((8 * sizeof(long) - 1 > 1 * PyLong_SHIFT)) {
- if ((8 * sizeof(unsigned long) > 2 * PyLong_SHIFT)) {
- __PYX_VERIFY_RETURN_INT(long, long, -(long) (((((unsigned long)digits[1]) << PyLong_SHIFT) | (unsigned long)digits[0])))
- } else if ((8 * sizeof(long) - 1 > 2 * PyLong_SHIFT)) {
- return (long) (((long)-1)*(((((long)digits[1]) << PyLong_SHIFT) | (long)digits[0])));
- }
- }
- break;
- case 2:
- if ((8 * sizeof(long) > 1 * PyLong_SHIFT)) {
- if ((8 * sizeof(unsigned long) > 2 * PyLong_SHIFT)) {
- __PYX_VERIFY_RETURN_INT(long, unsigned long, (((((unsigned long)digits[1]) << PyLong_SHIFT) | (unsigned long)digits[0])))
- } else if ((8 * sizeof(long) - 1 > 2 * PyLong_SHIFT)) {
- return (long) ((((((long)digits[1]) << PyLong_SHIFT) | (long)digits[0])));
- }
- }
- break;
- case -3:
- if ((8 * sizeof(long) - 1 > 2 * PyLong_SHIFT)) {
- if ((8 * sizeof(unsigned long) > 3 * PyLong_SHIFT)) {
- __PYX_VERIFY_RETURN_INT(long, long, -(long) (((((((unsigned long)digits[2]) << PyLong_SHIFT) | (unsigned long)digits[1]) << PyLong_SHIFT) | (unsigned long)digits[0])))
- } else if ((8 * sizeof(long) - 1 > 3 * PyLong_SHIFT)) {
- return (long) (((long)-1)*(((((((long)digits[2]) << PyLong_SHIFT) | (long)digits[1]) << PyLong_SHIFT) | (long)digits[0])));
- }
- }
- break;
- case 3:
- if ((8 * sizeof(long) > 2 * PyLong_SHIFT)) {
- if ((8 * sizeof(unsigned long) > 3 * PyLong_SHIFT)) {
- __PYX_VERIFY_RETURN_INT(long, unsigned long, (((((((unsigned long)digits[2]) << PyLong_SHIFT) | (unsigned long)digits[1]) << PyLong_SHIFT) | (unsigned long)digits[0])))
- } else if ((8 * sizeof(long) - 1 > 3 * PyLong_SHIFT)) {
- return (long) ((((((((long)digits[2]) << PyLong_SHIFT) | (long)digits[1]) << PyLong_SHIFT) | (long)digits[0])));
- }
- }
- break;
- case -4:
- if ((8 * sizeof(long) - 1 > 3 * PyLong_SHIFT)) {
- if ((8 * sizeof(unsigned long) > 4 * PyLong_SHIFT)) {
- __PYX_VERIFY_RETURN_INT(long, long, -(long) (((((((((unsigned long)digits[3]) << PyLong_SHIFT) | (unsigned long)digits[2]) << PyLong_SHIFT) | (unsigned long)digits[1]) << PyLong_SHIFT) | (unsigned long)digits[0])))
- } else if ((8 * sizeof(long) - 1 > 4 * PyLong_SHIFT)) {
- return (long) (((long)-1)*(((((((((long)digits[3]) << PyLong_SHIFT) | (long)digits[2]) << PyLong_SHIFT) | (long)digits[1]) << PyLong_SHIFT) | (long)digits[0])));
- }
- }
- break;
- case 4:
- if ((8 * sizeof(long) > 3 * PyLong_SHIFT)) {
- if ((8 * sizeof(unsigned long) > 4 * PyLong_SHIFT)) {
- __PYX_VERIFY_RETURN_INT(long, unsigned long, (((((((((unsigned long)digits[3]) << PyLong_SHIFT) | (unsigned long)digits[2]) << PyLong_SHIFT) | (unsigned long)digits[1]) << PyLong_SHIFT) | (unsigned long)digits[0])))
- } else if ((8 * sizeof(long) - 1 > 4 * PyLong_SHIFT)) {
- return (long) ((((((((((long)digits[3]) << PyLong_SHIFT) | (long)digits[2]) << PyLong_SHIFT) | (long)digits[1]) << PyLong_SHIFT) | (long)digits[0])));
- }
- }
- break;
- }
- }
-#endif
- if ((sizeof(long) <= sizeof(long))) {
- __PYX_VERIFY_RETURN_INT_EXC(long, long, PyLong_AsLong(x))
-#ifdef HAVE_LONG_LONG
- } else if ((sizeof(long) <= sizeof(PY_LONG_LONG))) {
- __PYX_VERIFY_RETURN_INT_EXC(long, PY_LONG_LONG, PyLong_AsLongLong(x))
-#endif
- }
- }
- {
- long val;
- PyObject *v = __Pyx_PyNumber_IntOrLong(x);
-#if PY_MAJOR_VERSION < 3
- if (likely(v) && !PyLong_Check(v)) {
- PyObject *tmp = v;
- v = PyNumber_Long(tmp);
- Py_DECREF(tmp);
- }
-#endif
- if (likely(v)) {
- int ret = -1;
-#if !(CYTHON_COMPILING_IN_PYPY || CYTHON_COMPILING_IN_LIMITED_API) || defined(_PyLong_AsByteArray)
- int one = 1; int is_little = (int)*(unsigned char *)&one;
- unsigned char *bytes = (unsigned char *)&val;
- ret = _PyLong_AsByteArray((PyLongObject *)v,
- bytes, sizeof(val),
- is_little, !is_unsigned);
-#else
- PyObject *stepval = NULL, *mask = NULL, *shift = NULL;
- int bits, remaining_bits, is_negative = 0;
- long idigit;
- int chunk_size = (sizeof(long) < 8) ? 30 : 62;
- if (unlikely(!PyLong_CheckExact(v))) {
- PyObject *tmp = v;
- v = PyNumber_Long(v);
- assert(PyLong_CheckExact(v));
- Py_DECREF(tmp);
- if (unlikely(!v)) return (long) -1;
- }
-#if CYTHON_COMPILING_IN_LIMITED_API && PY_VERSION_HEX < 0x030B0000
- if (Py_SIZE(x) == 0)
- return (long) 0;
- is_negative = Py_SIZE(x) < 0;
-#else
- {
- int result = PyObject_RichCompareBool(x, Py_False, Py_LT);
- if (unlikely(result < 0))
- return (long) -1;
- is_negative = result == 1;
- }
-#endif
- if (is_unsigned && unlikely(is_negative)) {
- goto raise_neg_overflow;
- } else if (is_negative) {
- stepval = PyNumber_Invert(v);
- if (unlikely(!stepval))
- return (long) -1;
- } else {
- stepval = __Pyx_NewRef(v);
- }
- val = (long) 0;
- mask = PyLong_FromLong((1L << chunk_size) - 1); if (unlikely(!mask)) goto done;
- shift = PyLong_FromLong(chunk_size); if (unlikely(!shift)) goto done;
- for (bits = 0; bits < (int) sizeof(long) * 8 - chunk_size; bits += chunk_size) {
- PyObject *tmp, *digit;
- digit = PyNumber_And(stepval, mask);
- if (unlikely(!digit)) goto done;
- idigit = PyLong_AsLong(digit);
- Py_DECREF(digit);
- if (unlikely(idigit < 0)) goto done;
- tmp = PyNumber_Rshift(stepval, shift);
- if (unlikely(!tmp)) goto done;
- Py_DECREF(stepval); stepval = tmp;
- val |= ((long) idigit) << bits;
- #if CYTHON_COMPILING_IN_LIMITED_API && PY_VERSION_HEX < 0x030B0000
- if (Py_SIZE(stepval) == 0)
- goto unpacking_done;
- #endif
- }
- idigit = PyLong_AsLong(stepval);
- if (unlikely(idigit < 0)) goto done;
- remaining_bits = ((int) sizeof(long) * 8) - bits - (is_unsigned ? 0 : 1);
- if (unlikely(idigit >= (1L << remaining_bits)))
- goto raise_overflow;
- val |= ((long) idigit) << bits;
- #if CYTHON_COMPILING_IN_LIMITED_API && PY_VERSION_HEX < 0x030B0000
- unpacking_done:
- #endif
- if (!is_unsigned) {
- if (unlikely(val & (((long) 1) << (sizeof(long) * 8 - 1))))
- goto raise_overflow;
- if (is_negative)
- val = ~val;
- }
- ret = 0;
- done:
- Py_XDECREF(shift);
- Py_XDECREF(mask);
- Py_XDECREF(stepval);
-#endif
- Py_DECREF(v);
- if (likely(!ret))
- return val;
- }
- return (long) -1;
- }
- } else {
- long val;
- PyObject *tmp = __Pyx_PyNumber_IntOrLong(x);
- if (!tmp) return (long) -1;
- val = __Pyx_PyInt_As_long(tmp);
- Py_DECREF(tmp);
- return val;
- }
-raise_overflow:
- PyErr_SetString(PyExc_OverflowError,
- "value too large to convert to long");
- return (long) -1;
-raise_neg_overflow:
- PyErr_SetString(PyExc_OverflowError,
- "can't convert negative value to long");
- return (long) -1;
-}
-
-/* FormatTypeName */
-#if CYTHON_COMPILING_IN_LIMITED_API
-static __Pyx_TypeName
-__Pyx_PyType_GetName(PyTypeObject* tp)
-{
- PyObject *name = __Pyx_PyObject_GetAttrStr((PyObject *)tp,
- __pyx_n_s_name);
- if (unlikely(name == NULL) || unlikely(!PyUnicode_Check(name))) {
- PyErr_Clear();
- Py_XSETREF(name, __Pyx_NewRef(__pyx_n_s__9));
- }
- return name;
-}
-#endif
-
-/* SwapException */
-#if CYTHON_FAST_THREAD_STATE
-static CYTHON_INLINE void __Pyx__ExceptionSwap(PyThreadState *tstate, PyObject **type, PyObject **value, PyObject **tb) {
- PyObject *tmp_type, *tmp_value, *tmp_tb;
- #if CYTHON_USE_EXC_INFO_STACK && PY_VERSION_HEX >= 0x030B00a4
- _PyErr_StackItem *exc_info = tstate->exc_info;
- tmp_value = exc_info->exc_value;
- exc_info->exc_value = *value;
- if (tmp_value == NULL || tmp_value == Py_None) {
- Py_XDECREF(tmp_value);
- tmp_value = NULL;
- tmp_type = NULL;
- tmp_tb = NULL;
- } else {
- tmp_type = (PyObject*) Py_TYPE(tmp_value);
- Py_INCREF(tmp_type);
- #if CYTHON_COMPILING_IN_CPYTHON
- tmp_tb = ((PyBaseExceptionObject*) tmp_value)->traceback;
- Py_XINCREF(tmp_tb);
- #else
- tmp_tb = PyException_GetTraceback(tmp_value);
- #endif
- }
- #elif CYTHON_USE_EXC_INFO_STACK
- _PyErr_StackItem *exc_info = tstate->exc_info;
- tmp_type = exc_info->exc_type;
- tmp_value = exc_info->exc_value;
- tmp_tb = exc_info->exc_traceback;
- exc_info->exc_type = *type;
- exc_info->exc_value = *value;
- exc_info->exc_traceback = *tb;
- #else
- tmp_type = tstate->exc_type;
- tmp_value = tstate->exc_value;
- tmp_tb = tstate->exc_traceback;
- tstate->exc_type = *type;
- tstate->exc_value = *value;
- tstate->exc_traceback = *tb;
- #endif
- *type = tmp_type;
- *value = tmp_value;
- *tb = tmp_tb;
-}
-#else
-static CYTHON_INLINE void __Pyx_ExceptionSwap(PyObject **type, PyObject **value, PyObject **tb) {
- PyObject *tmp_type, *tmp_value, *tmp_tb;
- PyErr_GetExcInfo(&tmp_type, &tmp_value, &tmp_tb);
- PyErr_SetExcInfo(*type, *value, *tb);
- *type = tmp_type;
- *value = tmp_value;
- *tb = tmp_tb;
-}
-#endif
-
-/* PyObjectCall2Args */
-static CYTHON_INLINE PyObject* __Pyx_PyObject_Call2Args(PyObject* function, PyObject* arg1, PyObject* arg2) {
- PyObject *args[3] = {NULL, arg1, arg2};
- return __Pyx_PyObject_FastCall(function, args+1, 2 | __Pyx_PY_VECTORCALL_ARGUMENTS_OFFSET);
-}
-
-/* PyObjectCallMethod1 */
-static PyObject* __Pyx__PyObject_CallMethod1(PyObject* method, PyObject* arg) {
- PyObject *result = __Pyx_PyObject_CallOneArg(method, arg);
- Py_DECREF(method);
- return result;
-}
-static PyObject* __Pyx_PyObject_CallMethod1(PyObject* obj, PyObject* method_name, PyObject* arg) {
- PyObject *method = NULL, *result;
- int is_method = __Pyx_PyObject_GetMethod(obj, method_name, &method);
- if (likely(is_method)) {
- result = __Pyx_PyObject_Call2Args(method, obj, arg);
- Py_DECREF(method);
- return result;
- }
- if (unlikely(!method)) return NULL;
- return __Pyx__PyObject_CallMethod1(method, arg);
-}
-
-/* CoroutineBase */
-#include
-#if PY_VERSION_HEX >= 0x030b00a6
- #ifndef Py_BUILD_CORE
- #define Py_BUILD_CORE 1
- #endif
- #include "internal/pycore_frame.h"
-#endif
-#define __Pyx_Coroutine_Undelegate(gen) Py_CLEAR((gen)->yieldfrom)
-static int __Pyx_PyGen__FetchStopIterationValue(PyThreadState *__pyx_tstate, PyObject **pvalue) {
- PyObject *et, *ev, *tb;
- PyObject *value = NULL;
- CYTHON_UNUSED_VAR(__pyx_tstate);
- __Pyx_ErrFetch(&et, &ev, &tb);
- if (!et) {
- Py_XDECREF(tb);
- Py_XDECREF(ev);
- Py_INCREF(Py_None);
- *pvalue = Py_None;
- return 0;
- }
- if (likely(et == PyExc_StopIteration)) {
- if (!ev) {
- Py_INCREF(Py_None);
- value = Py_None;
- }
-#if PY_VERSION_HEX >= 0x030300A0
- else if (likely(__Pyx_IS_TYPE(ev, (PyTypeObject*)PyExc_StopIteration))) {
- value = ((PyStopIterationObject *)ev)->value;
- Py_INCREF(value);
- Py_DECREF(ev);
- }
-#endif
- else if (unlikely(PyTuple_Check(ev))) {
- if (PyTuple_GET_SIZE(ev) >= 1) {
-#if CYTHON_ASSUME_SAFE_MACROS && !CYTHON_AVOID_BORROWED_REFS
- value = PyTuple_GET_ITEM(ev, 0);
- Py_INCREF(value);
-#else
- value = PySequence_ITEM(ev, 0);
-#endif
- } else {
- Py_INCREF(Py_None);
- value = Py_None;
- }
- Py_DECREF(ev);
- }
- else if (!__Pyx_TypeCheck(ev, (PyTypeObject*)PyExc_StopIteration)) {
- value = ev;
- }
- if (likely(value)) {
- Py_XDECREF(tb);
- Py_DECREF(et);
- *pvalue = value;
- return 0;
- }
- } else if (!__Pyx_PyErr_GivenExceptionMatches(et, PyExc_StopIteration)) {
- __Pyx_ErrRestore(et, ev, tb);
- return -1;
- }
- PyErr_NormalizeException(&et, &ev, &tb);
- if (unlikely(!PyObject_TypeCheck(ev, (PyTypeObject*)PyExc_StopIteration))) {
- __Pyx_ErrRestore(et, ev, tb);
- return -1;
- }
- Py_XDECREF(tb);
- Py_DECREF(et);
-#if PY_VERSION_HEX >= 0x030300A0
- value = ((PyStopIterationObject *)ev)->value;
- Py_INCREF(value);
- Py_DECREF(ev);
-#else
- {
- PyObject* args = __Pyx_PyObject_GetAttrStr(ev, __pyx_n_s_args);
- Py_DECREF(ev);
- if (likely(args)) {
- value = PySequence_GetItem(args, 0);
- Py_DECREF(args);
- }
- if (unlikely(!value)) {
- __Pyx_ErrRestore(NULL, NULL, NULL);
- Py_INCREF(Py_None);
- value = Py_None;
- }
- }
-#endif
- *pvalue = value;
- return 0;
-}
-static CYTHON_INLINE
-void __Pyx_Coroutine_ExceptionClear(__Pyx_ExcInfoStruct *exc_state) {
-#if PY_VERSION_HEX >= 0x030B00a4
- Py_CLEAR(exc_state->exc_value);
-#else
- PyObject *t, *v, *tb;
- t = exc_state->exc_type;
- v = exc_state->exc_value;
- tb = exc_state->exc_traceback;
- exc_state->exc_type = NULL;
- exc_state->exc_value = NULL;
- exc_state->exc_traceback = NULL;
- Py_XDECREF(t);
- Py_XDECREF(v);
- Py_XDECREF(tb);
-#endif
-}
-#define __Pyx_Coroutine_AlreadyRunningError(gen) (__Pyx__Coroutine_AlreadyRunningError(gen), (PyObject*)NULL)
-static void __Pyx__Coroutine_AlreadyRunningError(__pyx_CoroutineObject *gen) {
- const char *msg;
- CYTHON_MAYBE_UNUSED_VAR(gen);
- if ((0)) {
- #ifdef __Pyx_Coroutine_USED
- } else if (__Pyx_Coroutine_Check((PyObject*)gen)) {
- msg = "coroutine already executing";
- #endif
- #ifdef __Pyx_AsyncGen_USED
- } else if (__Pyx_AsyncGen_CheckExact((PyObject*)gen)) {
- msg = "async generator already executing";
- #endif
- } else {
- msg = "generator already executing";
- }
- PyErr_SetString(PyExc_ValueError, msg);
-}
-#define __Pyx_Coroutine_NotStartedError(gen) (__Pyx__Coroutine_NotStartedError(gen), (PyObject*)NULL)
-static void __Pyx__Coroutine_NotStartedError(PyObject *gen) {
- const char *msg;
- CYTHON_MAYBE_UNUSED_VAR(gen);
- if ((0)) {
- #ifdef __Pyx_Coroutine_USED
- } else if (__Pyx_Coroutine_Check(gen)) {
- msg = "can't send non-None value to a just-started coroutine";
- #endif
- #ifdef __Pyx_AsyncGen_USED
- } else if (__Pyx_AsyncGen_CheckExact(gen)) {
- msg = "can't send non-None value to a just-started async generator";
- #endif
- } else {
- msg = "can't send non-None value to a just-started generator";
- }
- PyErr_SetString(PyExc_TypeError, msg);
-}
-#define __Pyx_Coroutine_AlreadyTerminatedError(gen, value, closing) (__Pyx__Coroutine_AlreadyTerminatedError(gen, value, closing), (PyObject*)NULL)
-static void __Pyx__Coroutine_AlreadyTerminatedError(PyObject *gen, PyObject *value, int closing) {
- CYTHON_MAYBE_UNUSED_VAR(gen);
- CYTHON_MAYBE_UNUSED_VAR(closing);
- #ifdef __Pyx_Coroutine_USED
- if (!closing && __Pyx_Coroutine_Check(gen)) {
- PyErr_SetString(PyExc_RuntimeError, "cannot reuse already awaited coroutine");
- } else
- #endif
- if (value) {
- #ifdef __Pyx_AsyncGen_USED
- if (__Pyx_AsyncGen_CheckExact(gen))
- PyErr_SetNone(__Pyx_PyExc_StopAsyncIteration);
- else
- #endif
- PyErr_SetNone(PyExc_StopIteration);
- }
-}
-static
-PyObject *__Pyx_Coroutine_SendEx(__pyx_CoroutineObject *self, PyObject *value, int closing) {
- __Pyx_PyThreadState_declare
- PyThreadState *tstate;
- __Pyx_ExcInfoStruct *exc_state;
- PyObject *retval;
- assert(!self->is_running);
- if (unlikely(self->resume_label == 0)) {
- if (unlikely(value && value != Py_None)) {
- return __Pyx_Coroutine_NotStartedError((PyObject*)self);
- }
- }
- if (unlikely(self->resume_label == -1)) {
- return __Pyx_Coroutine_AlreadyTerminatedError((PyObject*)self, value, closing);
- }
-#if CYTHON_FAST_THREAD_STATE
- __Pyx_PyThreadState_assign
- tstate = __pyx_tstate;
-#else
- tstate = __Pyx_PyThreadState_Current;
-#endif
- exc_state = &self->gi_exc_state;
- if (exc_state->exc_value) {
- #if CYTHON_COMPILING_IN_PYPY
- #else
- PyObject *exc_tb;
- #if PY_VERSION_HEX >= 0x030B00a4 && !CYTHON_COMPILING_IN_CPYTHON
- exc_tb = PyException_GetTraceback(exc_state->exc_value);
- #elif PY_VERSION_HEX >= 0x030B00a4
- exc_tb = ((PyBaseExceptionObject*) exc_state->exc_value)->traceback;
- #else
- exc_tb = exc_state->exc_traceback;
- #endif
- if (exc_tb) {
- PyTracebackObject *tb = (PyTracebackObject *) exc_tb;
- PyFrameObject *f = tb->tb_frame;
- assert(f->f_back == NULL);
- #if PY_VERSION_HEX >= 0x030B00A1
- f->f_back = PyThreadState_GetFrame(tstate);
- #else
- Py_XINCREF(tstate->frame);
- f->f_back = tstate->frame;
- #endif
- #if PY_VERSION_HEX >= 0x030B00a4 && !CYTHON_COMPILING_IN_CPYTHON
- Py_DECREF(exc_tb);
- #endif
- }
- #endif
- }
-#if CYTHON_USE_EXC_INFO_STACK
- exc_state->previous_item = tstate->exc_info;
- tstate->exc_info = exc_state;
-#else
- if (exc_state->exc_type) {
- __Pyx_ExceptionSwap(&exc_state->exc_type, &exc_state->exc_value, &exc_state->exc_traceback);
- } else {
- __Pyx_Coroutine_ExceptionClear(exc_state);
- __Pyx_ExceptionSave(&exc_state->exc_type, &exc_state->exc_value, &exc_state->exc_traceback);
- }
-#endif
- self->is_running = 1;
- retval = self->body(self, tstate, value);
- self->is_running = 0;
-#if CYTHON_USE_EXC_INFO_STACK
- exc_state = &self->gi_exc_state;
- tstate->exc_info = exc_state->previous_item;
- exc_state->previous_item = NULL;
- __Pyx_Coroutine_ResetFrameBackpointer(exc_state);
-#endif
- return retval;
-}
-static CYTHON_INLINE void __Pyx_Coroutine_ResetFrameBackpointer(__Pyx_ExcInfoStruct *exc_state) {
-#if CYTHON_COMPILING_IN_PYPY
- CYTHON_UNUSED_VAR(exc_state);
-#else
- PyObject *exc_tb;
- #if PY_VERSION_HEX >= 0x030B00a4
- if (!exc_state->exc_value) return;
- exc_tb = PyException_GetTraceback(exc_state->exc_value);
- #else
- exc_tb = exc_state->exc_traceback;
- #endif
- if (likely(exc_tb)) {
- PyTracebackObject *tb = (PyTracebackObject *) exc_tb;
- PyFrameObject *f = tb->tb_frame;
- Py_CLEAR(f->f_back);
- #if PY_VERSION_HEX >= 0x030B00a4
- Py_DECREF(exc_tb);
- #endif
- }
-#endif
-}
-static CYTHON_INLINE
-PyObject *__Pyx_Coroutine_MethodReturn(PyObject* gen, PyObject *retval) {
- CYTHON_MAYBE_UNUSED_VAR(gen);
- if (unlikely(!retval)) {
- __Pyx_PyThreadState_declare
- __Pyx_PyThreadState_assign
- if (!__Pyx_PyErr_Occurred()) {
- PyObject *exc = PyExc_StopIteration;
- #ifdef __Pyx_AsyncGen_USED
- if (__Pyx_AsyncGen_CheckExact(gen))
- exc = __Pyx_PyExc_StopAsyncIteration;
- #endif
- __Pyx_PyErr_SetNone(exc);
- }
- }
- return retval;
-}
-#if CYTHON_COMPILING_IN_CPYTHON && PY_VERSION_HEX >= 0x03030000 && (defined(__linux__) || PY_VERSION_HEX >= 0x030600B3)
-static CYTHON_INLINE
-PyObject *__Pyx_PyGen_Send(PyGenObject *gen, PyObject *arg) {
-#if PY_VERSION_HEX <= 0x030A00A1
- return _PyGen_Send(gen, arg);
-#else
- PyObject *result;
- if (PyIter_Send((PyObject*)gen, arg ? arg : Py_None, &result) == PYGEN_RETURN) {
- if (PyAsyncGen_CheckExact(gen)) {
- assert(result == Py_None);
- PyErr_SetNone(PyExc_StopAsyncIteration);
- }
- else if (result == Py_None) {
- PyErr_SetNone(PyExc_StopIteration);
- }
- else {
- _PyGen_SetStopIterationValue(result);
- }
- Py_CLEAR(result);
- }
- return result;
-#endif
-}
-#endif
-static CYTHON_INLINE
-PyObject *__Pyx_Coroutine_FinishDelegation(__pyx_CoroutineObject *gen) {
- PyObject *ret;
- PyObject *val = NULL;
- __Pyx_Coroutine_Undelegate(gen);
- __Pyx_PyGen__FetchStopIterationValue(__Pyx_PyThreadState_Current, &val);
- ret = __Pyx_Coroutine_SendEx(gen, val, 0);
- Py_XDECREF(val);
- return ret;
-}
-static PyObject *__Pyx_Coroutine_Send(PyObject *self, PyObject *value) {
- PyObject *retval;
- __pyx_CoroutineObject *gen = (__pyx_CoroutineObject*) self;
- PyObject *yf = gen->yieldfrom;
- if (unlikely(gen->is_running))
- return __Pyx_Coroutine_AlreadyRunningError(gen);
- if (yf) {
- PyObject *ret;
- gen->is_running = 1;
- #ifdef __Pyx_Generator_USED
- if (__Pyx_Generator_CheckExact(yf)) {
- ret = __Pyx_Coroutine_Send(yf, value);
- } else
- #endif
- #ifdef __Pyx_Coroutine_USED
- if (__Pyx_Coroutine_Check(yf)) {
- ret = __Pyx_Coroutine_Send(yf, value);
- } else
- #endif
- #ifdef __Pyx_AsyncGen_USED
- if (__pyx_PyAsyncGenASend_CheckExact(yf)) {
- ret = __Pyx_async_gen_asend_send(yf, value);
- } else
- #endif
- #if CYTHON_COMPILING_IN_CPYTHON && PY_VERSION_HEX >= 0x03030000 && (defined(__linux__) || PY_VERSION_HEX >= 0x030600B3)
- if (PyGen_CheckExact(yf)) {
- ret = __Pyx_PyGen_Send((PyGenObject*)yf, value == Py_None ? NULL : value);
- } else
- #endif
- #if CYTHON_COMPILING_IN_CPYTHON && PY_VERSION_HEX >= 0x03050000 && defined(PyCoro_CheckExact) && (defined(__linux__) || PY_VERSION_HEX >= 0x030600B3)
- if (PyCoro_CheckExact(yf)) {
- ret = __Pyx_PyGen_Send((PyGenObject*)yf, value == Py_None ? NULL : value);
- } else
- #endif
- {
- if (value == Py_None)
- ret = __Pyx_PyObject_GetIterNextFunc(yf)(yf);
- else
- ret = __Pyx_PyObject_CallMethod1(yf, __pyx_n_s_send, value);
- }
- gen->is_running = 0;
- if (likely(ret)) {
- return ret;
- }
- retval = __Pyx_Coroutine_FinishDelegation(gen);
- } else {
- retval = __Pyx_Coroutine_SendEx(gen, value, 0);
- }
- return __Pyx_Coroutine_MethodReturn(self, retval);
-}
-static int __Pyx_Coroutine_CloseIter(__pyx_CoroutineObject *gen, PyObject *yf) {
- PyObject *retval = NULL;
- int err = 0;
- #ifdef __Pyx_Generator_USED
- if (__Pyx_Generator_CheckExact(yf)) {
- retval = __Pyx_Coroutine_Close(yf);
- if (!retval)
- return -1;
- } else
- #endif
- #ifdef __Pyx_Coroutine_USED
- if (__Pyx_Coroutine_Check(yf)) {
- retval = __Pyx_Coroutine_Close(yf);
- if (!retval)
- return -1;
- } else
- if (__Pyx_CoroutineAwait_CheckExact(yf)) {
- retval = __Pyx_CoroutineAwait_Close((__pyx_CoroutineAwaitObject*)yf, NULL);
- if (!retval)
- return -1;
- } else
- #endif
- #ifdef __Pyx_AsyncGen_USED
- if (__pyx_PyAsyncGenASend_CheckExact(yf)) {
- retval = __Pyx_async_gen_asend_close(yf, NULL);
- } else
- if (__pyx_PyAsyncGenAThrow_CheckExact(yf)) {
- retval = __Pyx_async_gen_athrow_close(yf, NULL);
- } else
- #endif
- {
- PyObject *meth;
- gen->is_running = 1;
- meth = __Pyx_PyObject_GetAttrStrNoError(yf, __pyx_n_s_close);
- if (unlikely(!meth)) {
- if (unlikely(PyErr_Occurred())) {
- PyErr_WriteUnraisable(yf);
- }
- } else {
- retval = __Pyx_PyObject_CallNoArg(meth);
- Py_DECREF(meth);
- if (unlikely(!retval))
- err = -1;
- }
- gen->is_running = 0;
- }
- Py_XDECREF(retval);
- return err;
-}
-static PyObject *__Pyx_Generator_Next(PyObject *self) {
- __pyx_CoroutineObject *gen = (__pyx_CoroutineObject*) self;
- PyObject *yf = gen->yieldfrom;
- if (unlikely(gen->is_running))
- return __Pyx_Coroutine_AlreadyRunningError(gen);
- if (yf) {
- PyObject *ret;
- gen->is_running = 1;
- #ifdef __Pyx_Generator_USED
- if (__Pyx_Generator_CheckExact(yf)) {
- ret = __Pyx_Generator_Next(yf);
- } else
- #endif
- #if CYTHON_COMPILING_IN_CPYTHON && PY_VERSION_HEX >= 0x03030000 && (defined(__linux__) || PY_VERSION_HEX >= 0x030600B3)
- if (PyGen_CheckExact(yf)) {
- ret = __Pyx_PyGen_Send((PyGenObject*)yf, NULL);
- } else
- #endif
- #ifdef __Pyx_Coroutine_USED
- if (__Pyx_Coroutine_Check(yf)) {
- ret = __Pyx_Coroutine_Send(yf, Py_None);
- } else
- #endif
- ret = __Pyx_PyObject_GetIterNextFunc(yf)(yf);
- gen->is_running = 0;
- if (likely(ret)) {
- return ret;
- }
- return __Pyx_Coroutine_FinishDelegation(gen);
- }
- return __Pyx_Coroutine_SendEx(gen, Py_None, 0);
-}
-static PyObject *__Pyx_Coroutine_Close_Method(PyObject *self, PyObject *arg) {
- CYTHON_UNUSED_VAR(arg);
- return __Pyx_Coroutine_Close(self);
-}
-static PyObject *__Pyx_Coroutine_Close(PyObject *self) {
- __pyx_CoroutineObject *gen = (__pyx_CoroutineObject *) self;
- PyObject *retval, *raised_exception;
- PyObject *yf = gen->yieldfrom;
- int err = 0;
- if (unlikely(gen->is_running))
- return __Pyx_Coroutine_AlreadyRunningError(gen);
- if (yf) {
- Py_INCREF(yf);
- err = __Pyx_Coroutine_CloseIter(gen, yf);
- __Pyx_Coroutine_Undelegate(gen);
- Py_DECREF(yf);
- }
- if (err == 0)
- PyErr_SetNone(PyExc_GeneratorExit);
- retval = __Pyx_Coroutine_SendEx(gen, NULL, 1);
- if (unlikely(retval)) {
- const char *msg;
- Py_DECREF(retval);
- if ((0)) {
- #ifdef __Pyx_Coroutine_USED
- } else if (__Pyx_Coroutine_Check(self)) {
- msg = "coroutine ignored GeneratorExit";
- #endif
- #ifdef __Pyx_AsyncGen_USED
- } else if (__Pyx_AsyncGen_CheckExact(self)) {
-#if PY_VERSION_HEX < 0x03060000
- msg = "async generator ignored GeneratorExit - might require Python 3.6+ finalisation (PEP 525)";
-#else
- msg = "async generator ignored GeneratorExit";
-#endif
- #endif
- } else {
- msg = "generator ignored GeneratorExit";
- }
- PyErr_SetString(PyExc_RuntimeError, msg);
- return NULL;
- }
- raised_exception = PyErr_Occurred();
- if (likely(!raised_exception || __Pyx_PyErr_GivenExceptionMatches2(raised_exception, PyExc_GeneratorExit, PyExc_StopIteration))) {
- if (raised_exception) PyErr_Clear();
- Py_INCREF(Py_None);
- return Py_None;
- }
- return NULL;
-}
-static PyObject *__Pyx__Coroutine_Throw(PyObject *self, PyObject *typ, PyObject *val, PyObject *tb,
- PyObject *args, int close_on_genexit) {
- __pyx_CoroutineObject *gen = (__pyx_CoroutineObject *) self;
- PyObject *yf = gen->yieldfrom;
- if (unlikely(gen->is_running))
- return __Pyx_Coroutine_AlreadyRunningError(gen);
- if (yf) {
- PyObject *ret;
- Py_INCREF(yf);
- if (__Pyx_PyErr_GivenExceptionMatches(typ, PyExc_GeneratorExit) && close_on_genexit) {
- int err = __Pyx_Coroutine_CloseIter(gen, yf);
- Py_DECREF(yf);
- __Pyx_Coroutine_Undelegate(gen);
- if (err < 0)
- return __Pyx_Coroutine_MethodReturn(self, __Pyx_Coroutine_SendEx(gen, NULL, 0));
- goto throw_here;
- }
- gen->is_running = 1;
- if (0
- #ifdef __Pyx_Generator_USED
- || __Pyx_Generator_CheckExact(yf)
- #endif
- #ifdef __Pyx_Coroutine_USED
- || __Pyx_Coroutine_Check(yf)
- #endif
- ) {
- ret = __Pyx__Coroutine_Throw(yf, typ, val, tb, args, close_on_genexit);
- #ifdef __Pyx_Coroutine_USED
- } else if (__Pyx_CoroutineAwait_CheckExact(yf)) {
- ret = __Pyx__Coroutine_Throw(((__pyx_CoroutineAwaitObject*)yf)->coroutine, typ, val, tb, args, close_on_genexit);
- #endif
- } else {
- PyObject *meth = __Pyx_PyObject_GetAttrStrNoError(yf, __pyx_n_s_throw);
- if (unlikely(!meth)) {
- Py_DECREF(yf);
- if (unlikely(PyErr_Occurred())) {
- gen->is_running = 0;
- return NULL;
- }
- __Pyx_Coroutine_Undelegate(gen);
- gen->is_running = 0;
- goto throw_here;
- }
- if (likely(args)) {
- ret = __Pyx_PyObject_Call(meth, args, NULL);
- } else {
- PyObject *cargs[4] = {NULL, typ, val, tb};
- ret = __Pyx_PyObject_FastCall(meth, cargs+1, 3 | __Pyx_PY_VECTORCALL_ARGUMENTS_OFFSET);
- }
- Py_DECREF(meth);
- }
- gen->is_running = 0;
- Py_DECREF(yf);
- if (!ret) {
- ret = __Pyx_Coroutine_FinishDelegation(gen);
- }
- return __Pyx_Coroutine_MethodReturn(self, ret);
- }
-throw_here:
- __Pyx_Raise(typ, val, tb, NULL);
- return __Pyx_Coroutine_MethodReturn(self, __Pyx_Coroutine_SendEx(gen, NULL, 0));
-}
-static PyObject *__Pyx_Coroutine_Throw(PyObject *self, PyObject *args) {
- PyObject *typ;
- PyObject *val = NULL;
- PyObject *tb = NULL;
- if (unlikely(!PyArg_UnpackTuple(args, (char *)"throw", 1, 3, &typ, &val, &tb)))
- return NULL;
- return __Pyx__Coroutine_Throw(self, typ, val, tb, args, 1);
-}
-static CYTHON_INLINE int __Pyx_Coroutine_traverse_excstate(__Pyx_ExcInfoStruct *exc_state, visitproc visit, void *arg) {
-#if PY_VERSION_HEX >= 0x030B00a4
- Py_VISIT(exc_state->exc_value);
-#else
- Py_VISIT(exc_state->exc_type);
- Py_VISIT(exc_state->exc_value);
- Py_VISIT(exc_state->exc_traceback);
-#endif
- return 0;
-}
-static int __Pyx_Coroutine_traverse(__pyx_CoroutineObject *gen, visitproc visit, void *arg) {
- Py_VISIT(gen->closure);
- Py_VISIT(gen->classobj);
- Py_VISIT(gen->yieldfrom);
- return __Pyx_Coroutine_traverse_excstate(&gen->gi_exc_state, visit, arg);
-}
-static int __Pyx_Coroutine_clear(PyObject *self) {
- __pyx_CoroutineObject *gen = (__pyx_CoroutineObject *) self;
- Py_CLEAR(gen->closure);
- Py_CLEAR(gen->classobj);
- Py_CLEAR(gen->yieldfrom);
- __Pyx_Coroutine_ExceptionClear(&gen->gi_exc_state);
-#ifdef __Pyx_AsyncGen_USED
- if (__Pyx_AsyncGen_CheckExact(self)) {
- Py_CLEAR(((__pyx_PyAsyncGenObject*)gen)->ag_finalizer);
- }
-#endif
- Py_CLEAR(gen->gi_code);
- Py_CLEAR(gen->gi_frame);
- Py_CLEAR(gen->gi_name);
- Py_CLEAR(gen->gi_qualname);
- Py_CLEAR(gen->gi_modulename);
- return 0;
-}
-static void __Pyx_Coroutine_dealloc(PyObject *self) {
- __pyx_CoroutineObject *gen = (__pyx_CoroutineObject *) self;
- PyObject_GC_UnTrack(gen);
- if (gen->gi_weakreflist != NULL)
- PyObject_ClearWeakRefs(self);
- if (gen->resume_label >= 0) {
- PyObject_GC_Track(self);
-#if PY_VERSION_HEX >= 0x030400a1 && CYTHON_USE_TP_FINALIZE
- if (unlikely(PyObject_CallFinalizerFromDealloc(self)))
-#else
- Py_TYPE(gen)->tp_del(self);
- if (unlikely(Py_REFCNT(self) > 0))
-#endif
- {
- return;
- }
- PyObject_GC_UnTrack(self);
- }
-#ifdef __Pyx_AsyncGen_USED
- if (__Pyx_AsyncGen_CheckExact(self)) {
- /* We have to handle this case for asynchronous generators
- right here, because this code has to be between UNTRACK
- and GC_Del. */
- Py_CLEAR(((__pyx_PyAsyncGenObject*)self)->ag_finalizer);
- }
-#endif
- __Pyx_Coroutine_clear(self);
- __Pyx_PyHeapTypeObject_GC_Del(gen);
-}
-static void __Pyx_Coroutine_del(PyObject *self) {
- PyObject *error_type, *error_value, *error_traceback;
- __pyx_CoroutineObject *gen = (__pyx_CoroutineObject *) self;
- __Pyx_PyThreadState_declare
- if (gen->resume_label < 0) {
- return;
- }
-#if !CYTHON_USE_TP_FINALIZE
- assert(self->ob_refcnt == 0);
- __Pyx_SET_REFCNT(self, 1);
-#endif
- __Pyx_PyThreadState_assign
- __Pyx_ErrFetch(&error_type, &error_value, &error_traceback);
-#ifdef __Pyx_AsyncGen_USED
- if (__Pyx_AsyncGen_CheckExact(self)) {
- __pyx_PyAsyncGenObject *agen = (__pyx_PyAsyncGenObject*)self;
- PyObject *finalizer = agen->ag_finalizer;
- if (finalizer && !agen->ag_closed) {
- PyObject *res = __Pyx_PyObject_CallOneArg(finalizer, self);
- if (unlikely(!res)) {
- PyErr_WriteUnraisable(self);
- } else {
- Py_DECREF(res);
- }
- __Pyx_ErrRestore(error_type, error_value, error_traceback);
- return;
- }
- }
-#endif
- if (unlikely(gen->resume_label == 0 && !error_value)) {
-#ifdef __Pyx_Coroutine_USED
-#ifdef __Pyx_Generator_USED
- if (!__Pyx_Generator_CheckExact(self))
-#endif
- {
- PyObject_GC_UnTrack(self);
-#if PY_MAJOR_VERSION >= 3 || defined(PyErr_WarnFormat)
- if (unlikely(PyErr_WarnFormat(PyExc_RuntimeWarning, 1, "coroutine '%.50S' was never awaited", gen->gi_qualname) < 0))
- PyErr_WriteUnraisable(self);
-#else
- {PyObject *msg;
- char *cmsg;
- #if CYTHON_COMPILING_IN_PYPY
- msg = NULL;
- cmsg = (char*) "coroutine was never awaited";
- #else
- char *cname;
- PyObject *qualname;
- qualname = gen->gi_qualname;
- cname = PyString_AS_STRING(qualname);
- msg = PyString_FromFormat("coroutine '%.50s' was never awaited", cname);
- if (unlikely(!msg)) {
- PyErr_Clear();
- cmsg = (char*) "coroutine was never awaited";
- } else {
- cmsg = PyString_AS_STRING(msg);
- }
- #endif
- if (unlikely(PyErr_WarnEx(PyExc_RuntimeWarning, cmsg, 1) < 0))
- PyErr_WriteUnraisable(self);
- Py_XDECREF(msg);}
-#endif
- PyObject_GC_Track(self);
- }
-#endif
- } else {
- PyObject *res = __Pyx_Coroutine_Close(self);
- if (unlikely(!res)) {
- if (PyErr_Occurred())
- PyErr_WriteUnraisable(self);
- } else {
- Py_DECREF(res);
- }
- }
- __Pyx_ErrRestore(error_type, error_value, error_traceback);
-#if !CYTHON_USE_TP_FINALIZE
- assert(Py_REFCNT(self) > 0);
- if (likely(--self->ob_refcnt == 0)) {
- return;
- }
- {
- Py_ssize_t refcnt = Py_REFCNT(self);
- _Py_NewReference(self);
- __Pyx_SET_REFCNT(self, refcnt);
- }
-#if CYTHON_COMPILING_IN_CPYTHON
- assert(PyType_IS_GC(Py_TYPE(self)) &&
- _Py_AS_GC(self)->gc.gc_refs != _PyGC_REFS_UNTRACKED);
- _Py_DEC_REFTOTAL;
-#endif
-#ifdef COUNT_ALLOCS
- --Py_TYPE(self)->tp_frees;
- --Py_TYPE(self)->tp_allocs;
-#endif
-#endif
-}
-static PyObject *
-__Pyx_Coroutine_get_name(__pyx_CoroutineObject *self, void *context)
-{
- PyObject *name = self->gi_name;
- CYTHON_UNUSED_VAR(context);
- if (unlikely(!name)) name = Py_None;
- Py_INCREF(name);
- return name;
-}
-static int
-__Pyx_Coroutine_set_name(__pyx_CoroutineObject *self, PyObject *value, void *context)
-{
- CYTHON_UNUSED_VAR(context);
-#if PY_MAJOR_VERSION >= 3
- if (unlikely(value == NULL || !PyUnicode_Check(value)))
-#else
- if (unlikely(value == NULL || !PyString_Check(value)))
-#endif
- {
- PyErr_SetString(PyExc_TypeError,
- "__name__ must be set to a string object");
- return -1;
- }
- Py_INCREF(value);
- __Pyx_Py_XDECREF_SET(self->gi_name, value);
- return 0;
-}
-static PyObject *
-__Pyx_Coroutine_get_qualname(__pyx_CoroutineObject *self, void *context)
-{
- PyObject *name = self->gi_qualname;
- CYTHON_UNUSED_VAR(context);
- if (unlikely(!name)) name = Py_None;
- Py_INCREF(name);
- return name;
-}
-static int
-__Pyx_Coroutine_set_qualname(__pyx_CoroutineObject *self, PyObject *value, void *context)
-{
- CYTHON_UNUSED_VAR(context);
-#if PY_MAJOR_VERSION >= 3
- if (unlikely(value == NULL || !PyUnicode_Check(value)))
-#else
- if (unlikely(value == NULL || !PyString_Check(value)))
-#endif
- {
- PyErr_SetString(PyExc_TypeError,
- "__qualname__ must be set to a string object");
- return -1;
- }
- Py_INCREF(value);
- __Pyx_Py_XDECREF_SET(self->gi_qualname, value);
- return 0;
-}
-static PyObject *
-__Pyx_Coroutine_get_frame(__pyx_CoroutineObject *self, void *context)
-{
- PyObject *frame = self->gi_frame;
- CYTHON_UNUSED_VAR(context);
- if (!frame) {
- if (unlikely(!self->gi_code)) {
- Py_RETURN_NONE;
- }
- frame = (PyObject *) PyFrame_New(
- PyThreadState_Get(), /*PyThreadState *tstate,*/
- (PyCodeObject*) self->gi_code, /*PyCodeObject *code,*/
- __pyx_d, /*PyObject *globals,*/
- 0 /*PyObject *locals*/
- );
- if (unlikely(!frame))
- return NULL;
- self->gi_frame = frame;
- }
- Py_INCREF(frame);
- return frame;
-}
-static __pyx_CoroutineObject *__Pyx__Coroutine_New(
- PyTypeObject* type, __pyx_coroutine_body_t body, PyObject *code, PyObject *closure,
- PyObject *name, PyObject *qualname, PyObject *module_name) {
- __pyx_CoroutineObject *gen = PyObject_GC_New(__pyx_CoroutineObject, type);
- if (unlikely(!gen))
- return NULL;
- return __Pyx__Coroutine_NewInit(gen, body, code, closure, name, qualname, module_name);
-}
-static __pyx_CoroutineObject *__Pyx__Coroutine_NewInit(
- __pyx_CoroutineObject *gen, __pyx_coroutine_body_t body, PyObject *code, PyObject *closure,
- PyObject *name, PyObject *qualname, PyObject *module_name) {
- gen->body = body;
- gen->closure = closure;
- Py_XINCREF(closure);
- gen->is_running = 0;
- gen->resume_label = 0;
- gen->classobj = NULL;
- gen->yieldfrom = NULL;
- #if PY_VERSION_HEX >= 0x030B00a4
- gen->gi_exc_state.exc_value = NULL;
- #else
- gen->gi_exc_state.exc_type = NULL;
- gen->gi_exc_state.exc_value = NULL;
- gen->gi_exc_state.exc_traceback = NULL;
- #endif
-#if CYTHON_USE_EXC_INFO_STACK
- gen->gi_exc_state.previous_item = NULL;
-#endif
- gen->gi_weakreflist = NULL;
- Py_XINCREF(qualname);
- gen->gi_qualname = qualname;
- Py_XINCREF(name);
- gen->gi_name = name;
- Py_XINCREF(module_name);
- gen->gi_modulename = module_name;
- Py_XINCREF(code);
- gen->gi_code = code;
- gen->gi_frame = NULL;
- PyObject_GC_Track(gen);
- return gen;
-}
-
-/* PatchModuleWithCoroutine */
-static PyObject* __Pyx_Coroutine_patch_module(PyObject* module, const char* py_code) {
-#if defined(__Pyx_Generator_USED) || defined(__Pyx_Coroutine_USED)
- int result;
- PyObject *globals, *result_obj;
- globals = PyDict_New(); if (unlikely(!globals)) goto ignore;
- result = PyDict_SetItemString(globals, "_cython_coroutine_type",
- #ifdef __Pyx_Coroutine_USED
- (PyObject*)__pyx_CoroutineType);
- #else
- Py_None);
- #endif
- if (unlikely(result < 0)) goto ignore;
- result = PyDict_SetItemString(globals, "_cython_generator_type",
- #ifdef __Pyx_Generator_USED
- (PyObject*)__pyx_GeneratorType);
- #else
- Py_None);
- #endif
- if (unlikely(result < 0)) goto ignore;
- if (unlikely(PyDict_SetItemString(globals, "_module", module) < 0)) goto ignore;
- if (unlikely(PyDict_SetItemString(globals, "__builtins__", __pyx_b) < 0)) goto ignore;
- result_obj = PyRun_String(py_code, Py_file_input, globals, globals);
- if (unlikely(!result_obj)) goto ignore;
- Py_DECREF(result_obj);
- Py_DECREF(globals);
- return module;
-ignore:
- Py_XDECREF(globals);
- PyErr_WriteUnraisable(module);
- if (unlikely(PyErr_WarnEx(PyExc_RuntimeWarning, "Cython module failed to patch module with custom type", 1) < 0)) {
- Py_DECREF(module);
- module = NULL;
- }
-#else
- py_code++;
-#endif
- return module;
-}
-
-/* PatchGeneratorABC */
-#ifndef CYTHON_REGISTER_ABCS
-#define CYTHON_REGISTER_ABCS 1
-#endif
-#if defined(__Pyx_Generator_USED) || defined(__Pyx_Coroutine_USED)
-static PyObject* __Pyx_patch_abc_module(PyObject *module);
-static PyObject* __Pyx_patch_abc_module(PyObject *module) {
- module = __Pyx_Coroutine_patch_module(
- module, ""
-"if _cython_generator_type is not None:\n"
-" try: Generator = _module.Generator\n"
-" except AttributeError: pass\n"
-" else: Generator.register(_cython_generator_type)\n"
-"if _cython_coroutine_type is not None:\n"
-" try: Coroutine = _module.Coroutine\n"
-" except AttributeError: pass\n"
-" else: Coroutine.register(_cython_coroutine_type)\n"
- );
- return module;
-}
-#endif
-static int __Pyx_patch_abc(void) {
-#if defined(__Pyx_Generator_USED) || defined(__Pyx_Coroutine_USED)
- static int abc_patched = 0;
- if (CYTHON_REGISTER_ABCS && !abc_patched) {
- PyObject *module;
- module = PyImport_ImportModule((PY_MAJOR_VERSION >= 3) ? "collections.abc" : "collections");
- if (unlikely(!module)) {
- PyErr_WriteUnraisable(NULL);
- if (unlikely(PyErr_WarnEx(PyExc_RuntimeWarning,
- ((PY_MAJOR_VERSION >= 3) ?
- "Cython module failed to register with collections.abc module" :
- "Cython module failed to register with collections module"), 1) < 0)) {
- return -1;
- }
- } else {
- module = __Pyx_patch_abc_module(module);
- abc_patched = 1;
- if (unlikely(!module))
- return -1;
- Py_DECREF(module);
- }
- module = PyImport_ImportModule("backports_abc");
- if (module) {
- module = __Pyx_patch_abc_module(module);
- Py_XDECREF(module);
- }
- if (!module) {
- PyErr_Clear();
- }
- }
-#else
- if ((0)) __Pyx_Coroutine_patch_module(NULL, NULL);
-#endif
- return 0;
-}
-
-/* Generator */
-static PyMethodDef __pyx_Generator_methods[] = {
- {"send", (PyCFunction) __Pyx_Coroutine_Send, METH_O,
- (char*) PyDoc_STR("send(arg) -> send 'arg' into generator,\nreturn next yielded value or raise StopIteration.")},
- {"throw", (PyCFunction) __Pyx_Coroutine_Throw, METH_VARARGS,
- (char*) PyDoc_STR("throw(typ[,val[,tb]]) -> raise exception in generator,\nreturn next yielded value or raise StopIteration.")},
- {"close", (PyCFunction) __Pyx_Coroutine_Close_Method, METH_NOARGS,
- (char*) PyDoc_STR("close() -> raise GeneratorExit inside generator.")},
- {0, 0, 0, 0}
-};
-static PyMemberDef __pyx_Generator_memberlist[] = {
- {(char *) "gi_running", T_BOOL, offsetof(__pyx_CoroutineObject, is_running), READONLY, NULL},
- {(char*) "gi_yieldfrom", T_OBJECT, offsetof(__pyx_CoroutineObject, yieldfrom), READONLY,
- (char*) PyDoc_STR("object being iterated by 'yield from', or None")},
- {(char*) "gi_code", T_OBJECT, offsetof(__pyx_CoroutineObject, gi_code), READONLY, NULL},
- {(char *) "__module__", T_OBJECT, offsetof(__pyx_CoroutineObject, gi_modulename), 0, 0},
-#if CYTHON_USE_TYPE_SPECS
- {(char *) "__weaklistoffset__", T_PYSSIZET, offsetof(__pyx_CoroutineObject, gi_weakreflist), READONLY, 0},
-#endif
- {0, 0, 0, 0, 0}
-};
-static PyGetSetDef __pyx_Generator_getsets[] = {
- {(char *) "__name__", (getter)__Pyx_Coroutine_get_name, (setter)__Pyx_Coroutine_set_name,
- (char*) PyDoc_STR("name of the generator"), 0},
- {(char *) "__qualname__", (getter)__Pyx_Coroutine_get_qualname, (setter)__Pyx_Coroutine_set_qualname,
- (char*) PyDoc_STR("qualified name of the generator"), 0},
- {(char *) "gi_frame", (getter)__Pyx_Coroutine_get_frame, NULL,
- (char*) PyDoc_STR("Frame of the generator"), 0},
- {0, 0, 0, 0, 0}
-};
-#if CYTHON_USE_TYPE_SPECS
-static PyType_Slot __pyx_GeneratorType_slots[] = {
- {Py_tp_dealloc, (void *)__Pyx_Coroutine_dealloc},
- {Py_tp_traverse, (void *)__Pyx_Coroutine_traverse},
- {Py_tp_iter, (void *)PyObject_SelfIter},
- {Py_tp_iternext, (void *)__Pyx_Generator_Next},
- {Py_tp_methods, (void *)__pyx_Generator_methods},
- {Py_tp_members, (void *)__pyx_Generator_memberlist},
- {Py_tp_getset, (void *)__pyx_Generator_getsets},
- {Py_tp_getattro, (void *) __Pyx_PyObject_GenericGetAttrNoDict},
-#if CYTHON_USE_TP_FINALIZE
- {Py_tp_finalize, (void *)__Pyx_Coroutine_del},
-#endif
- {0, 0},
-};
-static PyType_Spec __pyx_GeneratorType_spec = {
- __PYX_TYPE_MODULE_PREFIX "generator",
- sizeof(__pyx_CoroutineObject),
- 0,
- Py_TPFLAGS_DEFAULT | Py_TPFLAGS_HAVE_GC | Py_TPFLAGS_HAVE_FINALIZE,
- __pyx_GeneratorType_slots
-};
-#else
-static PyTypeObject __pyx_GeneratorType_type = {
- PyVarObject_HEAD_INIT(0, 0)
- __PYX_TYPE_MODULE_PREFIX "generator",
- sizeof(__pyx_CoroutineObject),
- 0,
- (destructor) __Pyx_Coroutine_dealloc,
- 0,
- 0,
- 0,
- 0,
- 0,
- 0,
- 0,
- 0,
- 0,
- 0,
- 0,
- 0,
- 0,
- 0,
- Py_TPFLAGS_DEFAULT | Py_TPFLAGS_HAVE_GC | Py_TPFLAGS_HAVE_FINALIZE,
- 0,
- (traverseproc) __Pyx_Coroutine_traverse,
- 0,
- 0,
- offsetof(__pyx_CoroutineObject, gi_weakreflist),
- 0,
- (iternextfunc) __Pyx_Generator_Next,
- __pyx_Generator_methods,
- __pyx_Generator_memberlist,
- __pyx_Generator_getsets,
- 0,
- 0,
- 0,
- 0,
- 0,
- 0,
- 0,
- 0,
- 0,
- 0,
- 0,
- 0,
- 0,
- 0,
- 0,
-#if CYTHON_USE_TP_FINALIZE
- 0,
-#else
- __Pyx_Coroutine_del,
-#endif
- 0,
-#if CYTHON_USE_TP_FINALIZE
- __Pyx_Coroutine_del,
-#elif PY_VERSION_HEX >= 0x030400a1
- 0,
-#endif
-#if PY_VERSION_HEX >= 0x030800b1 && (!CYTHON_COMPILING_IN_PYPY || PYPY_VERSION_NUM >= 0x07030800)
- 0,
-#endif
-#if __PYX_NEED_TP_PRINT_SLOT
- 0,
-#endif
-#if PY_VERSION_HEX >= 0x030C0000
- 0,
-#endif
-#if CYTHON_COMPILING_IN_PYPY && PY_VERSION_HEX >= 0x03090000 && PY_VERSION_HEX < 0x030a0000
- 0,
-#endif
-};
-#endif
-static int __pyx_Generator_init(PyObject *module) {
-#if CYTHON_USE_TYPE_SPECS
- __pyx_GeneratorType = __Pyx_FetchCommonTypeFromSpec(module, &__pyx_GeneratorType_spec, NULL);
-#else
- CYTHON_UNUSED_VAR(module);
- __pyx_GeneratorType_type.tp_getattro = __Pyx_PyObject_GenericGetAttrNoDict;
- __pyx_GeneratorType_type.tp_iter = PyObject_SelfIter;
- __pyx_GeneratorType = __Pyx_FetchCommonType(&__pyx_GeneratorType_type);
-#endif
- if (unlikely(!__pyx_GeneratorType)) {
- return -1;
- }
- return 0;
-}
-
-/* CheckBinaryVersion */
-static int __Pyx_check_binary_version(void) {
- char ctversion[5];
- int same=1, i, found_dot;
- const char* rt_from_call = Py_GetVersion();
- PyOS_snprintf(ctversion, 5, "%d.%d", PY_MAJOR_VERSION, PY_MINOR_VERSION);
- found_dot = 0;
- for (i = 0; i < 4; i++) {
- if (!ctversion[i]) {
- same = (rt_from_call[i] < '0' || rt_from_call[i] > '9');
- break;
- }
- if (rt_from_call[i] != ctversion[i]) {
- same = 0;
- break;
- }
- }
- if (!same) {
- char rtversion[5] = {'\0'};
- char message[200];
- for (i=0; i<4; ++i) {
- if (rt_from_call[i] == '.') {
- if (found_dot) break;
- found_dot = 1;
- } else if (rt_from_call[i] < '0' || rt_from_call[i] > '9') {
- break;
- }
- rtversion[i] = rt_from_call[i];
- }
- PyOS_snprintf(message, sizeof(message),
- "compile time version %s of module '%.100s' "
- "does not match runtime version %s",
- ctversion, __Pyx_MODULE_NAME, rtversion);
- return PyErr_WarnEx(NULL, message, 1);
- }
- return 0;
-}
-
-/* InitStrings */
-#if PY_MAJOR_VERSION >= 3
-static int __Pyx_InitString(__Pyx_StringTabEntry t, PyObject **str) {
- if (t.is_unicode | t.is_str) {
- if (t.intern) {
- *str = PyUnicode_InternFromString(t.s);
- } else if (t.encoding) {
- *str = PyUnicode_Decode(t.s, t.n - 1, t.encoding, NULL);
- } else {
- *str = PyUnicode_FromStringAndSize(t.s, t.n - 1);
- }
- } else {
- *str = PyBytes_FromStringAndSize(t.s, t.n - 1);
- }
- if (!*str)
- return -1;
- if (PyObject_Hash(*str) == -1)
- return -1;
- return 0;
-}
-#endif
-static int __Pyx_InitStrings(__Pyx_StringTabEntry *t) {
- while (t->p) {
- #if PY_MAJOR_VERSION >= 3
- __Pyx_InitString(*t, t->p);
- #else
- if (t->is_unicode) {
- *t->p = PyUnicode_DecodeUTF8(t->s, t->n - 1, NULL);
- } else if (t->intern) {
- *t->p = PyString_InternFromString(t->s);
- } else {
- *t->p = PyString_FromStringAndSize(t->s, t->n - 1);
- }
- if (!*t->p)
- return -1;
- if (PyObject_Hash(*t->p) == -1)
- return -1;
- #endif
- ++t;
- }
- return 0;
-}
-
-static CYTHON_INLINE PyObject* __Pyx_PyUnicode_FromString(const char* c_str) {
- return __Pyx_PyUnicode_FromStringAndSize(c_str, (Py_ssize_t)strlen(c_str));
-}
-static CYTHON_INLINE const char* __Pyx_PyObject_AsString(PyObject* o) {
- Py_ssize_t ignore;
- return __Pyx_PyObject_AsStringAndSize(o, &ignore);
-}
-#if __PYX_DEFAULT_STRING_ENCODING_IS_ASCII || __PYX_DEFAULT_STRING_ENCODING_IS_DEFAULT
-#if !CYTHON_PEP393_ENABLED
-static const char* __Pyx_PyUnicode_AsStringAndSize(PyObject* o, Py_ssize_t *length) {
- char* defenc_c;
- PyObject* defenc = _PyUnicode_AsDefaultEncodedString(o, NULL);
- if (!defenc) return NULL;
- defenc_c = PyBytes_AS_STRING(defenc);
-#if __PYX_DEFAULT_STRING_ENCODING_IS_ASCII
- {
- char* end = defenc_c + PyBytes_GET_SIZE(defenc);
- char* c;
- for (c = defenc_c; c < end; c++) {
- if ((unsigned char) (*c) >= 128) {
- PyUnicode_AsASCIIString(o);
- return NULL;
- }
- }
- }
-#endif
- *length = PyBytes_GET_SIZE(defenc);
- return defenc_c;
-}
-#else
-static CYTHON_INLINE const char* __Pyx_PyUnicode_AsStringAndSize(PyObject* o, Py_ssize_t *length) {
- if (unlikely(__Pyx_PyUnicode_READY(o) == -1)) return NULL;
-#if __PYX_DEFAULT_STRING_ENCODING_IS_ASCII
- if (likely(PyUnicode_IS_ASCII(o))) {
- *length = PyUnicode_GET_LENGTH(o);
- return PyUnicode_AsUTF8(o);
- } else {
- PyUnicode_AsASCIIString(o);
- return NULL;
- }
-#else
- return PyUnicode_AsUTF8AndSize(o, length);
-#endif
-}
-#endif
-#endif
-static CYTHON_INLINE const char* __Pyx_PyObject_AsStringAndSize(PyObject* o, Py_ssize_t *length) {
-#if __PYX_DEFAULT_STRING_ENCODING_IS_ASCII || __PYX_DEFAULT_STRING_ENCODING_IS_DEFAULT
- if (
-#if PY_MAJOR_VERSION < 3 && __PYX_DEFAULT_STRING_ENCODING_IS_ASCII
- __Pyx_sys_getdefaultencoding_not_ascii &&
-#endif
- PyUnicode_Check(o)) {
- return __Pyx_PyUnicode_AsStringAndSize(o, length);
- } else
-#endif
-#if (!CYTHON_COMPILING_IN_PYPY && !CYTHON_COMPILING_IN_LIMITED_API) || (defined(PyByteArray_AS_STRING) && defined(PyByteArray_GET_SIZE))
- if (PyByteArray_Check(o)) {
- *length = PyByteArray_GET_SIZE(o);
- return PyByteArray_AS_STRING(o);
- } else
-#endif
- {
- char* result;
- int r = PyBytes_AsStringAndSize(o, &result, length);
- if (unlikely(r < 0)) {
- return NULL;
- } else {
- return result;
- }
- }
-}
-static CYTHON_INLINE int __Pyx_PyObject_IsTrue(PyObject* x) {
- int is_true = x == Py_True;
- if (is_true | (x == Py_False) | (x == Py_None)) return is_true;
- else return PyObject_IsTrue(x);
-}
-static CYTHON_INLINE int __Pyx_PyObject_IsTrueAndDecref(PyObject* x) {
- int retval;
- if (unlikely(!x)) return -1;
- retval = __Pyx_PyObject_IsTrue(x);
- Py_DECREF(x);
- return retval;
-}
-static PyObject* __Pyx_PyNumber_IntOrLongWrongResultType(PyObject* result, const char* type_name) {
- __Pyx_TypeName result_type_name = __Pyx_PyType_GetName(Py_TYPE(result));
-#if PY_MAJOR_VERSION >= 3
- if (PyLong_Check(result)) {
- if (PyErr_WarnFormat(PyExc_DeprecationWarning, 1,
- "__int__ returned non-int (type " __Pyx_FMT_TYPENAME "). "
- "The ability to return an instance of a strict subclass of int is deprecated, "
- "and may be removed in a future version of Python.",
- result_type_name)) {
- __Pyx_DECREF_TypeName(result_type_name);
- Py_DECREF(result);
- return NULL;
- }
- __Pyx_DECREF_TypeName(result_type_name);
- return result;
- }
-#endif
- PyErr_Format(PyExc_TypeError,
- "__%.4s__ returned non-%.4s (type " __Pyx_FMT_TYPENAME ")",
- type_name, type_name, result_type_name);
- __Pyx_DECREF_TypeName(result_type_name);
- Py_DECREF(result);
- return NULL;
-}
-static CYTHON_INLINE PyObject* __Pyx_PyNumber_IntOrLong(PyObject* x) {
-#if CYTHON_USE_TYPE_SLOTS
- PyNumberMethods *m;
-#endif
- const char *name = NULL;
- PyObject *res = NULL;
-#if PY_MAJOR_VERSION < 3
- if (likely(PyInt_Check(x) || PyLong_Check(x)))
-#else
- if (likely(PyLong_Check(x)))
-#endif
- return __Pyx_NewRef(x);
-#if CYTHON_USE_TYPE_SLOTS
- m = Py_TYPE(x)->tp_as_number;
- #if PY_MAJOR_VERSION < 3
- if (m && m->nb_int) {
- name = "int";
- res = m->nb_int(x);
- }
- else if (m && m->nb_long) {
- name = "long";
- res = m->nb_long(x);
- }
- #else
- if (likely(m && m->nb_int)) {
- name = "int";
- res = m->nb_int(x);
- }
- #endif
-#else
- if (!PyBytes_CheckExact(x) && !PyUnicode_CheckExact(x)) {
- res = PyNumber_Int(x);
- }
-#endif
- if (likely(res)) {
-#if PY_MAJOR_VERSION < 3
- if (unlikely(!PyInt_Check(res) && !PyLong_Check(res))) {
-#else
- if (unlikely(!PyLong_CheckExact(res))) {
-#endif
- return __Pyx_PyNumber_IntOrLongWrongResultType(res, name);
- }
- }
- else if (!PyErr_Occurred()) {
- PyErr_SetString(PyExc_TypeError,
- "an integer is required");
- }
- return res;
-}
-static CYTHON_INLINE Py_ssize_t __Pyx_PyIndex_AsSsize_t(PyObject* b) {
- Py_ssize_t ival;
- PyObject *x;
-#if PY_MAJOR_VERSION < 3
- if (likely(PyInt_CheckExact(b))) {
- if (sizeof(Py_ssize_t) >= sizeof(long))
- return PyInt_AS_LONG(b);
- else
- return PyInt_AsSsize_t(b);
- }
-#endif
- if (likely(PyLong_CheckExact(b))) {
- #if CYTHON_USE_PYLONG_INTERNALS
- if (likely(__Pyx_PyLong_IsCompact(b))) {
- return __Pyx_PyLong_CompactValue(b);
- } else {
- const digit* digits = __Pyx_PyLong_Digits(b);
- const Py_ssize_t size = __Pyx_PyLong_SignedDigitCount(b);
- switch (size) {
- case 2:
- if (8 * sizeof(Py_ssize_t) > 2 * PyLong_SHIFT) {
- return (Py_ssize_t) (((((size_t)digits[1]) << PyLong_SHIFT) | (size_t)digits[0]));
- }
- break;
- case -2:
- if (8 * sizeof(Py_ssize_t) > 2 * PyLong_SHIFT) {
- return -(Py_ssize_t) (((((size_t)digits[1]) << PyLong_SHIFT) | (size_t)digits[0]));
- }
- break;
- case 3:
- if (8 * sizeof(Py_ssize_t) > 3 * PyLong_SHIFT) {
- return (Py_ssize_t) (((((((size_t)digits[2]) << PyLong_SHIFT) | (size_t)digits[1]) << PyLong_SHIFT) | (size_t)digits[0]));
- }
- break;
- case -3:
- if (8 * sizeof(Py_ssize_t) > 3 * PyLong_SHIFT) {
- return -(Py_ssize_t) (((((((size_t)digits[2]) << PyLong_SHIFT) | (size_t)digits[1]) << PyLong_SHIFT) | (size_t)digits[0]));
- }
- break;
- case 4:
- if (8 * sizeof(Py_ssize_t) > 4 * PyLong_SHIFT) {
- return (Py_ssize_t) (((((((((size_t)digits[3]) << PyLong_SHIFT) | (size_t)digits[2]) << PyLong_SHIFT) | (size_t)digits[1]) << PyLong_SHIFT) | (size_t)digits[0]));
- }
- break;
- case -4:
- if (8 * sizeof(Py_ssize_t) > 4 * PyLong_SHIFT) {
- return -(Py_ssize_t) (((((((((size_t)digits[3]) << PyLong_SHIFT) | (size_t)digits[2]) << PyLong_SHIFT) | (size_t)digits[1]) << PyLong_SHIFT) | (size_t)digits[0]));
- }
- break;
- }
- }
- #endif
- return PyLong_AsSsize_t(b);
- }
- x = PyNumber_Index(b);
- if (!x) return -1;
- ival = PyInt_AsSsize_t(x);
- Py_DECREF(x);
- return ival;
-}
-static CYTHON_INLINE Py_hash_t __Pyx_PyIndex_AsHash_t(PyObject* o) {
- if (sizeof(Py_hash_t) == sizeof(Py_ssize_t)) {
- return (Py_hash_t) __Pyx_PyIndex_AsSsize_t(o);
-#if PY_MAJOR_VERSION < 3
- } else if (likely(PyInt_CheckExact(o))) {
- return PyInt_AS_LONG(o);
-#endif
- } else {
- Py_ssize_t ival;
- PyObject *x;
- x = PyNumber_Index(o);
- if (!x) return -1;
- ival = PyInt_AsLong(x);
- Py_DECREF(x);
- return ival;
- }
-}
-static CYTHON_INLINE PyObject * __Pyx_PyBool_FromLong(long b) {
- return b ? __Pyx_NewRef(Py_True) : __Pyx_NewRef(Py_False);
-}
-static CYTHON_INLINE PyObject * __Pyx_PyInt_FromSize_t(size_t ival) {
- return PyInt_FromSize_t(ival);
-}
-
-
-/* #### Code section: utility_code_pragmas_end ### */
-#ifdef _MSC_VER
-#pragma warning( pop )
-#endif
-
-
-
-/* #### Code section: end ### */
-#endif /* Py_PYTHON_H */
diff --git a/spaces/dcarpintero/nlp-summarizer-pegasus/.venv/lib/python3.9/site-packages/jsonschema/validators.py b/spaces/dcarpintero/nlp-summarizer-pegasus/.venv/lib/python3.9/site-packages/jsonschema/validators.py
deleted file mode 100644
index 8049e8b24303f727d16697da9fbf2b968da52063..0000000000000000000000000000000000000000
--- a/spaces/dcarpintero/nlp-summarizer-pegasus/.venv/lib/python3.9/site-packages/jsonschema/validators.py
+++ /dev/null
@@ -1,1382 +0,0 @@
-"""
-Creation and extension of validators, with implementations for existing drafts.
-"""
-from __future__ import annotations
-
-from collections import deque
-from collections.abc import Iterable, Mapping, Sequence
-from functools import lru_cache
-from operator import methodcaller
-from urllib.parse import unquote, urldefrag, urljoin, urlsplit
-from urllib.request import urlopen
-from warnings import warn
-import contextlib
-import json
-import reprlib
-import warnings
-
-from attrs import define, field, fields
-from jsonschema_specifications import REGISTRY as SPECIFICATIONS
-from rpds import HashTrieMap
-import referencing.exceptions
-import referencing.jsonschema
-
-from jsonschema import (
- _format,
- _legacy_validators,
- _types,
- _typing,
- _utils,
- _validators,
- exceptions,
-)
-from jsonschema.protocols import Validator
-
-_UNSET = _utils.Unset()
-
-_VALIDATORS: dict[str, Validator] = {}
-_META_SCHEMAS = _utils.URIDict()
-
-
-def __getattr__(name):
- if name == "ErrorTree":
- warnings.warn(
- "Importing ErrorTree from jsonschema.validators is deprecated. "
- "Instead import it from jsonschema.exceptions.",
- DeprecationWarning,
- stacklevel=2,
- )
- from jsonschema.exceptions import ErrorTree
- return ErrorTree
- elif name == "validators":
- warnings.warn(
- "Accessing jsonschema.validators.validators is deprecated. "
- "Use jsonschema.validators.validator_for with a given schema.",
- DeprecationWarning,
- stacklevel=2,
- )
- return _VALIDATORS
- elif name == "meta_schemas":
- warnings.warn(
- "Accessing jsonschema.validators.meta_schemas is deprecated. "
- "Use jsonschema.validators.validator_for with a given schema.",
- DeprecationWarning,
- stacklevel=2,
- )
- return _META_SCHEMAS
- elif name == "RefResolver":
- warnings.warn(
- _RefResolver._DEPRECATION_MESSAGE,
- DeprecationWarning,
- stacklevel=2,
- )
- return _RefResolver
- raise AttributeError(f"module {__name__} has no attribute {name}")
-
-
-def validates(version):
- """
- Register the decorated validator for a ``version`` of the specification.
-
- Registered validators and their meta schemas will be considered when
- parsing :kw:`$schema` keywords' URIs.
-
- Arguments:
-
- version (str):
-
- An identifier to use as the version's name
-
- Returns:
-
- collections.abc.Callable:
-
- a class decorator to decorate the validator with the version
- """
-
- def _validates(cls):
- _VALIDATORS[version] = cls
- meta_schema_id = cls.ID_OF(cls.META_SCHEMA)
- _META_SCHEMAS[meta_schema_id] = cls
- return cls
- return _validates
-
-
-def _warn_for_remote_retrieve(uri: str):
- from urllib.request import Request, urlopen
- headers = {"User-Agent": "python-jsonschema (deprecated $ref resolution)"}
- request = Request(uri, headers=headers)
- with urlopen(request) as response:
- warnings.warn(
- "Automatically retrieving remote references can be a security "
- "vulnerability and is discouraged by the JSON Schema "
- "specifications. Relying on this behavior is deprecated "
- "and will shortly become an error. If you are sure you want to "
- "remotely retrieve your reference and that it is safe to do so, "
- "you can find instructions for doing so via referencing.Registry "
- "in the referencing documentation "
- "(https://referencing.readthedocs.org).",
- DeprecationWarning,
- stacklevel=9, # Ha ha ha ha magic numbers :/
- )
- return referencing.Resource.from_contents(
- json.load(response),
- default_specification=referencing.jsonschema.DRAFT202012,
- )
-
-
-_REMOTE_WARNING_REGISTRY = SPECIFICATIONS.combine(
- referencing.Registry(retrieve=_warn_for_remote_retrieve), # type: ignore[call-arg] # noqa: E501
-)
-
-
-def create(
- meta_schema: referencing.jsonschema.ObjectSchema,
- validators: (
- Mapping[str, _typing.SchemaKeywordValidator]
- | Iterable[tuple[str, _typing.SchemaKeywordValidator]]
- ) = (),
- version: str | None = None,
- type_checker: _types.TypeChecker = _types.draft202012_type_checker,
- format_checker: _format.FormatChecker = _format.draft202012_format_checker,
- id_of: _typing.id_of = referencing.jsonschema.DRAFT202012.id_of,
- applicable_validators: _typing.ApplicableValidators = methodcaller(
- "items",
- ),
-):
- """
- Create a new validator class.
-
- Arguments:
-
- meta_schema:
-
- the meta schema for the new validator class
-
- validators:
-
- a mapping from names to callables, where each callable will
- validate the schema property with the given name.
-
- Each callable should take 4 arguments:
-
- 1. a validator instance,
- 2. the value of the property being validated within the
- instance
- 3. the instance
- 4. the schema
-
- version:
-
- an identifier for the version that this validator class will
- validate. If provided, the returned validator class will
- have its ``__name__`` set to include the version, and also
- will have `jsonschema.validators.validates` automatically
- called for the given version.
-
- type_checker:
-
- a type checker, used when applying the :kw:`type` keyword.
-
- If unprovided, a `jsonschema.TypeChecker` will be created
- with a set of default types typical of JSON Schema drafts.
-
- format_checker:
-
- a format checker, used when applying the :kw:`format` keyword.
-
- If unprovided, a `jsonschema.FormatChecker` will be created
- with a set of default formats typical of JSON Schema drafts.
-
- id_of:
-
- A function that given a schema, returns its ID.
-
- applicable_validators:
-
- A function that, given a schema, returns the list of
- applicable schema keywords and associated values
- which will be used to validate the instance.
- This is mostly used to support pre-draft 7 versions of JSON Schema
- which specified behavior around ignoring keywords if they were
- siblings of a ``$ref`` keyword. If you're not attempting to
- implement similar behavior, you can typically ignore this argument
- and leave it at its default.
-
- Returns:
-
- a new `jsonschema.protocols.Validator` class
- """
- # preemptively don't shadow the `Validator.format_checker` local
- format_checker_arg = format_checker
-
- specification = referencing.jsonschema.specification_with(
- dialect_id=id_of(meta_schema) or "urn:unknown-dialect",
- default=referencing.Specification.OPAQUE,
- )
-
- @define
- class Validator:
-
- VALIDATORS = dict(validators) # noqa: RUF012
- META_SCHEMA = dict(meta_schema) # noqa: RUF012
- TYPE_CHECKER = type_checker
- FORMAT_CHECKER = format_checker_arg
- ID_OF = staticmethod(id_of)
-
- _APPLICABLE_VALIDATORS = applicable_validators
-
- schema: referencing.jsonschema.Schema = field(repr=reprlib.repr)
- _ref_resolver = field(default=None, repr=False, alias="resolver")
- format_checker: _format.FormatChecker | None = field(default=None)
- # TODO: include new meta-schemas added at runtime
- _registry: referencing.jsonschema.SchemaRegistry = field(
- default=_REMOTE_WARNING_REGISTRY,
- kw_only=True,
- repr=False,
- )
- _resolver = field(
- alias="_resolver",
- default=None,
- kw_only=True,
- repr=False,
- )
-
- def __init_subclass__(cls):
- warnings.warn(
- (
- "Subclassing validator classes is not intended to "
- "be part of their public API. A future version "
- "will make doing so an error, as the behavior of "
- "subclasses isn't guaranteed to stay the same "
- "between releases of jsonschema. Instead, prefer "
- "composition of validators, wrapping them in an object "
- "owned entirely by the downstream library."
- ),
- DeprecationWarning,
- stacklevel=2,
- )
-
- def evolve(self, **changes):
- cls = self.__class__
- schema = changes.setdefault("schema", self.schema)
- NewValidator = validator_for(schema, default=cls)
-
- for field in fields(cls): # noqa: F402
- if not field.init:
- continue
- attr_name = field.name
- init_name = field.alias
- if init_name not in changes:
- changes[init_name] = getattr(self, attr_name)
-
- return NewValidator(**changes)
-
- cls.evolve = evolve
-
- def __attrs_post_init__(self):
- if self._resolver is None:
- registry = self._registry
- if registry is not _REMOTE_WARNING_REGISTRY:
- registry = SPECIFICATIONS.combine(registry)
- resource = specification.create_resource(self.schema)
- self._resolver = registry.resolver_with_root(resource)
-
- # REMOVEME: Legacy ref resolution state management.
- push_scope = getattr(self._ref_resolver, "push_scope", None)
- if push_scope is not None:
- id = id_of(self.schema)
- if id is not None:
- push_scope(id)
-
- @classmethod
- def check_schema(cls, schema, format_checker=_UNSET):
- Validator = validator_for(cls.META_SCHEMA, default=cls)
- if format_checker is _UNSET:
- format_checker = Validator.FORMAT_CHECKER
- validator = Validator(
- schema=cls.META_SCHEMA,
- format_checker=format_checker,
- )
- for error in validator.iter_errors(schema):
- raise exceptions.SchemaError.create_from(error)
-
- @property
- def resolver(self):
- warnings.warn(
- (
- f"Accessing {self.__class__.__name__}.resolver is "
- "deprecated as of v4.18.0, in favor of the "
- "https://github.com/python-jsonschema/referencing "
- "library, which provides more compliant referencing "
- "behavior as well as more flexible APIs for "
- "customization."
- ),
- DeprecationWarning,
- stacklevel=2,
- )
- if self._ref_resolver is None:
- self._ref_resolver = _RefResolver.from_schema(
- self.schema,
- id_of=id_of,
- )
- return self._ref_resolver
-
- def evolve(self, **changes):
- schema = changes.setdefault("schema", self.schema)
- NewValidator = validator_for(schema, default=self.__class__)
-
- for (attr_name, init_name) in evolve_fields:
- if init_name not in changes:
- changes[init_name] = getattr(self, attr_name)
-
- return NewValidator(**changes)
-
- def iter_errors(self, instance, _schema=None):
- if _schema is not None:
- warnings.warn(
- (
- "Passing a schema to Validator.iter_errors "
- "is deprecated and will be removed in a future "
- "release. Call validator.evolve(schema=new_schema)."
- "iter_errors(...) instead."
- ),
- DeprecationWarning,
- stacklevel=2,
- )
- else:
- _schema = self.schema
-
- if _schema is True:
- return
- elif _schema is False:
- yield exceptions.ValidationError(
- f"False schema does not allow {instance!r}",
- validator=None,
- validator_value=None,
- instance=instance,
- schema=_schema,
- )
- return
-
- for k, v in applicable_validators(_schema):
- validator = self.VALIDATORS.get(k)
- if validator is None:
- continue
-
- errors = validator(self, v, instance, _schema) or ()
- for error in errors:
- # set details if not already set by the called fn
- error._set(
- validator=k,
- validator_value=v,
- instance=instance,
- schema=_schema,
- type_checker=self.TYPE_CHECKER,
- )
- if k not in {"if", "$ref"}:
- error.schema_path.appendleft(k)
- yield error
-
- def descend(
- self,
- instance,
- schema,
- path=None,
- schema_path=None,
- resolver=None,
- ):
- if schema is True:
- return
- elif schema is False:
- yield exceptions.ValidationError(
- f"False schema does not allow {instance!r}",
- validator=None,
- validator_value=None,
- instance=instance,
- schema=schema,
- )
- return
-
- if self._ref_resolver is not None:
- evolved = self.evolve(schema=schema)
- else:
- if resolver is None:
- resolver = self._resolver.in_subresource(
- specification.create_resource(schema),
- )
- evolved = self.evolve(schema=schema, _resolver=resolver)
-
- for k, v in applicable_validators(schema):
- validator = evolved.VALIDATORS.get(k)
- if validator is None:
- continue
-
- errors = validator(evolved, v, instance, schema) or ()
- for error in errors:
- # set details if not already set by the called fn
- error._set(
- validator=k,
- validator_value=v,
- instance=instance,
- schema=schema,
- type_checker=evolved.TYPE_CHECKER,
- )
- if k not in {"if", "$ref"}:
- error.schema_path.appendleft(k)
- if path is not None:
- error.path.appendleft(path)
- if schema_path is not None:
- error.schema_path.appendleft(schema_path)
- yield error
-
- def validate(self, *args, **kwargs):
- for error in self.iter_errors(*args, **kwargs):
- raise error
-
- def is_type(self, instance, type):
- try:
- return self.TYPE_CHECKER.is_type(instance, type)
- except exceptions.UndefinedTypeCheck:
- raise exceptions.UnknownType(type, instance, self.schema)
-
- def _validate_reference(self, ref, instance):
- if self._ref_resolver is None:
- try:
- resolved = self._resolver.lookup(ref)
- except referencing.exceptions.Unresolvable as err:
- raise exceptions._WrappedReferencingError(err)
-
- return self.descend(
- instance,
- resolved.contents,
- resolver=resolved.resolver,
- )
- else:
- resolve = getattr(self._ref_resolver, "resolve", None)
- if resolve is None:
- with self._ref_resolver.resolving(ref) as resolved:
- return self.descend(instance, resolved)
- else:
- scope, resolved = resolve(ref)
- self._ref_resolver.push_scope(scope)
-
- try:
- return list(self.descend(instance, resolved))
- finally:
- self._ref_resolver.pop_scope()
-
- def is_valid(self, instance, _schema=None):
- if _schema is not None:
- warnings.warn(
- (
- "Passing a schema to Validator.is_valid is deprecated "
- "and will be removed in a future release. Call "
- "validator.evolve(schema=new_schema).is_valid(...) "
- "instead."
- ),
- DeprecationWarning,
- stacklevel=2,
- )
- self = self.evolve(schema=_schema)
-
- error = next(self.iter_errors(instance), None)
- return error is None
-
- evolve_fields = [
- (field.name, field.alias)
- for field in fields(Validator)
- if field.init
- ]
-
- if version is not None:
- safe = version.title().replace(" ", "").replace("-", "")
- Validator.__name__ = Validator.__qualname__ = f"{safe}Validator"
- Validator = validates(version)(Validator) # type: ignore[misc]
-
- return Validator
-
-
-def extend(
- validator,
- validators=(),
- version=None,
- type_checker=None,
- format_checker=None,
-):
- """
- Create a new validator class by extending an existing one.
-
- Arguments:
-
- validator (jsonschema.protocols.Validator):
-
- an existing validator class
-
- validators (collections.abc.Mapping):
-
- a mapping of new validator callables to extend with, whose
- structure is as in `create`.
-
- .. note::
-
- Any validator callables with the same name as an
- existing one will (silently) replace the old validator
- callable entirely, effectively overriding any validation
- done in the "parent" validator class.
-
- If you wish to instead extend the behavior of a parent's
- validator callable, delegate and call it directly in
- the new validator function by retrieving it using
- ``OldValidator.VALIDATORS["validation_keyword_name"]``.
-
- version (str):
-
- a version for the new validator class
-
- type_checker (jsonschema.TypeChecker):
-
- a type checker, used when applying the :kw:`type` keyword.
-
- If unprovided, the type checker of the extended
- `jsonschema.protocols.Validator` will be carried along.
-
- format_checker (jsonschema.FormatChecker):
-
- a format checker, used when applying the :kw:`format` keyword.
-
- If unprovided, the format checker of the extended
- `jsonschema.protocols.Validator` will be carried along.
-
- Returns:
-
- a new `jsonschema.protocols.Validator` class extending the one
- provided
-
- .. note:: Meta Schemas
-
- The new validator class will have its parent's meta schema.
-
- If you wish to change or extend the meta schema in the new
- validator class, modify ``META_SCHEMA`` directly on the returned
- class. Note that no implicit copying is done, so a copy should
- likely be made before modifying it, in order to not affect the
- old validator.
- """
- all_validators = dict(validator.VALIDATORS)
- all_validators.update(validators)
-
- if type_checker is None:
- type_checker = validator.TYPE_CHECKER
- if format_checker is None:
- format_checker = validator.FORMAT_CHECKER
- return create(
- meta_schema=validator.META_SCHEMA,
- validators=all_validators,
- version=version,
- type_checker=type_checker,
- format_checker=format_checker,
- id_of=validator.ID_OF,
- applicable_validators=validator._APPLICABLE_VALIDATORS,
- )
-
-
-Draft3Validator = create(
- meta_schema=SPECIFICATIONS.contents(
- "http://json-schema.org/draft-03/schema#",
- ),
- validators={
- "$ref": _validators.ref,
- "additionalItems": _validators.additionalItems,
- "additionalProperties": _validators.additionalProperties,
- "dependencies": _legacy_validators.dependencies_draft3,
- "disallow": _legacy_validators.disallow_draft3,
- "divisibleBy": _validators.multipleOf,
- "enum": _validators.enum,
- "extends": _legacy_validators.extends_draft3,
- "format": _validators.format,
- "items": _legacy_validators.items_draft3_draft4,
- "maxItems": _validators.maxItems,
- "maxLength": _validators.maxLength,
- "maximum": _legacy_validators.maximum_draft3_draft4,
- "minItems": _validators.minItems,
- "minLength": _validators.minLength,
- "minimum": _legacy_validators.minimum_draft3_draft4,
- "pattern": _validators.pattern,
- "patternProperties": _validators.patternProperties,
- "properties": _legacy_validators.properties_draft3,
- "type": _legacy_validators.type_draft3,
- "uniqueItems": _validators.uniqueItems,
- },
- type_checker=_types.draft3_type_checker,
- format_checker=_format.draft3_format_checker,
- version="draft3",
- id_of=referencing.jsonschema.DRAFT3.id_of,
- applicable_validators=_legacy_validators.ignore_ref_siblings,
-)
-
-Draft4Validator = create(
- meta_schema=SPECIFICATIONS.contents(
- "http://json-schema.org/draft-04/schema#",
- ),
- validators={
- "$ref": _validators.ref,
- "additionalItems": _validators.additionalItems,
- "additionalProperties": _validators.additionalProperties,
- "allOf": _validators.allOf,
- "anyOf": _validators.anyOf,
- "dependencies": _legacy_validators.dependencies_draft4_draft6_draft7,
- "enum": _validators.enum,
- "format": _validators.format,
- "items": _legacy_validators.items_draft3_draft4,
- "maxItems": _validators.maxItems,
- "maxLength": _validators.maxLength,
- "maxProperties": _validators.maxProperties,
- "maximum": _legacy_validators.maximum_draft3_draft4,
- "minItems": _validators.minItems,
- "minLength": _validators.minLength,
- "minProperties": _validators.minProperties,
- "minimum": _legacy_validators.minimum_draft3_draft4,
- "multipleOf": _validators.multipleOf,
- "not": _validators.not_,
- "oneOf": _validators.oneOf,
- "pattern": _validators.pattern,
- "patternProperties": _validators.patternProperties,
- "properties": _validators.properties,
- "required": _validators.required,
- "type": _validators.type,
- "uniqueItems": _validators.uniqueItems,
- },
- type_checker=_types.draft4_type_checker,
- format_checker=_format.draft4_format_checker,
- version="draft4",
- id_of=referencing.jsonschema.DRAFT4.id_of,
- applicable_validators=_legacy_validators.ignore_ref_siblings,
-)
-
-Draft6Validator = create(
- meta_schema=SPECIFICATIONS.contents(
- "http://json-schema.org/draft-06/schema#",
- ),
- validators={
- "$ref": _validators.ref,
- "additionalItems": _validators.additionalItems,
- "additionalProperties": _validators.additionalProperties,
- "allOf": _validators.allOf,
- "anyOf": _validators.anyOf,
- "const": _validators.const,
- "contains": _legacy_validators.contains_draft6_draft7,
- "dependencies": _legacy_validators.dependencies_draft4_draft6_draft7,
- "enum": _validators.enum,
- "exclusiveMaximum": _validators.exclusiveMaximum,
- "exclusiveMinimum": _validators.exclusiveMinimum,
- "format": _validators.format,
- "items": _legacy_validators.items_draft6_draft7_draft201909,
- "maxItems": _validators.maxItems,
- "maxLength": _validators.maxLength,
- "maxProperties": _validators.maxProperties,
- "maximum": _validators.maximum,
- "minItems": _validators.minItems,
- "minLength": _validators.minLength,
- "minProperties": _validators.minProperties,
- "minimum": _validators.minimum,
- "multipleOf": _validators.multipleOf,
- "not": _validators.not_,
- "oneOf": _validators.oneOf,
- "pattern": _validators.pattern,
- "patternProperties": _validators.patternProperties,
- "properties": _validators.properties,
- "propertyNames": _validators.propertyNames,
- "required": _validators.required,
- "type": _validators.type,
- "uniqueItems": _validators.uniqueItems,
- },
- type_checker=_types.draft6_type_checker,
- format_checker=_format.draft6_format_checker,
- version="draft6",
- id_of=referencing.jsonschema.DRAFT6.id_of,
- applicable_validators=_legacy_validators.ignore_ref_siblings,
-)
-
-Draft7Validator = create(
- meta_schema=SPECIFICATIONS.contents(
- "http://json-schema.org/draft-07/schema#",
- ),
- validators={
- "$ref": _validators.ref,
- "additionalItems": _validators.additionalItems,
- "additionalProperties": _validators.additionalProperties,
- "allOf": _validators.allOf,
- "anyOf": _validators.anyOf,
- "const": _validators.const,
- "contains": _legacy_validators.contains_draft6_draft7,
- "dependencies": _legacy_validators.dependencies_draft4_draft6_draft7,
- "enum": _validators.enum,
- "exclusiveMaximum": _validators.exclusiveMaximum,
- "exclusiveMinimum": _validators.exclusiveMinimum,
- "format": _validators.format,
- "if": _validators.if_,
- "items": _legacy_validators.items_draft6_draft7_draft201909,
- "maxItems": _validators.maxItems,
- "maxLength": _validators.maxLength,
- "maxProperties": _validators.maxProperties,
- "maximum": _validators.maximum,
- "minItems": _validators.minItems,
- "minLength": _validators.minLength,
- "minProperties": _validators.minProperties,
- "minimum": _validators.minimum,
- "multipleOf": _validators.multipleOf,
- "not": _validators.not_,
- "oneOf": _validators.oneOf,
- "pattern": _validators.pattern,
- "patternProperties": _validators.patternProperties,
- "properties": _validators.properties,
- "propertyNames": _validators.propertyNames,
- "required": _validators.required,
- "type": _validators.type,
- "uniqueItems": _validators.uniqueItems,
- },
- type_checker=_types.draft7_type_checker,
- format_checker=_format.draft7_format_checker,
- version="draft7",
- id_of=referencing.jsonschema.DRAFT7.id_of,
- applicable_validators=_legacy_validators.ignore_ref_siblings,
-)
-
-Draft201909Validator = create(
- meta_schema=SPECIFICATIONS.contents(
- "https://json-schema.org/draft/2019-09/schema",
- ),
- validators={
- "$recursiveRef": _legacy_validators.recursiveRef,
- "$ref": _validators.ref,
- "additionalItems": _validators.additionalItems,
- "additionalProperties": _validators.additionalProperties,
- "allOf": _validators.allOf,
- "anyOf": _validators.anyOf,
- "const": _validators.const,
- "contains": _validators.contains,
- "dependentRequired": _validators.dependentRequired,
- "dependentSchemas": _validators.dependentSchemas,
- "enum": _validators.enum,
- "exclusiveMaximum": _validators.exclusiveMaximum,
- "exclusiveMinimum": _validators.exclusiveMinimum,
- "format": _validators.format,
- "if": _validators.if_,
- "items": _legacy_validators.items_draft6_draft7_draft201909,
- "maxItems": _validators.maxItems,
- "maxLength": _validators.maxLength,
- "maxProperties": _validators.maxProperties,
- "maximum": _validators.maximum,
- "minItems": _validators.minItems,
- "minLength": _validators.minLength,
- "minProperties": _validators.minProperties,
- "minimum": _validators.minimum,
- "multipleOf": _validators.multipleOf,
- "not": _validators.not_,
- "oneOf": _validators.oneOf,
- "pattern": _validators.pattern,
- "patternProperties": _validators.patternProperties,
- "properties": _validators.properties,
- "propertyNames": _validators.propertyNames,
- "required": _validators.required,
- "type": _validators.type,
- "unevaluatedItems": _legacy_validators.unevaluatedItems_draft2019,
- "unevaluatedProperties": _validators.unevaluatedProperties,
- "uniqueItems": _validators.uniqueItems,
- },
- type_checker=_types.draft201909_type_checker,
- format_checker=_format.draft201909_format_checker,
- version="draft2019-09",
-)
-
-Draft202012Validator = create(
- meta_schema=SPECIFICATIONS.contents(
- "https://json-schema.org/draft/2020-12/schema",
- ),
- validators={
- "$dynamicRef": _validators.dynamicRef,
- "$ref": _validators.ref,
- "additionalItems": _validators.additionalItems,
- "additionalProperties": _validators.additionalProperties,
- "allOf": _validators.allOf,
- "anyOf": _validators.anyOf,
- "const": _validators.const,
- "contains": _validators.contains,
- "dependentRequired": _validators.dependentRequired,
- "dependentSchemas": _validators.dependentSchemas,
- "enum": _validators.enum,
- "exclusiveMaximum": _validators.exclusiveMaximum,
- "exclusiveMinimum": _validators.exclusiveMinimum,
- "format": _validators.format,
- "if": _validators.if_,
- "items": _validators.items,
- "maxItems": _validators.maxItems,
- "maxLength": _validators.maxLength,
- "maxProperties": _validators.maxProperties,
- "maximum": _validators.maximum,
- "minItems": _validators.minItems,
- "minLength": _validators.minLength,
- "minProperties": _validators.minProperties,
- "minimum": _validators.minimum,
- "multipleOf": _validators.multipleOf,
- "not": _validators.not_,
- "oneOf": _validators.oneOf,
- "pattern": _validators.pattern,
- "patternProperties": _validators.patternProperties,
- "prefixItems": _validators.prefixItems,
- "properties": _validators.properties,
- "propertyNames": _validators.propertyNames,
- "required": _validators.required,
- "type": _validators.type,
- "unevaluatedItems": _validators.unevaluatedItems,
- "unevaluatedProperties": _validators.unevaluatedProperties,
- "uniqueItems": _validators.uniqueItems,
- },
- type_checker=_types.draft202012_type_checker,
- format_checker=_format.draft202012_format_checker,
- version="draft2020-12",
-)
-
-_LATEST_VERSION = Draft202012Validator
-
-
-class _RefResolver:
- """
- Resolve JSON References.
-
- Arguments:
-
- base_uri (str):
-
- The URI of the referring document
-
- referrer:
-
- The actual referring document
-
- store (dict):
-
- A mapping from URIs to documents to cache
-
- cache_remote (bool):
-
- Whether remote refs should be cached after first resolution
-
- handlers (dict):
-
- A mapping from URI schemes to functions that should be used
- to retrieve them
-
- urljoin_cache (:func:`functools.lru_cache`):
-
- A cache that will be used for caching the results of joining
- the resolution scope to subscopes.
-
- remote_cache (:func:`functools.lru_cache`):
-
- A cache that will be used for caching the results of
- resolved remote URLs.
-
- Attributes:
-
- cache_remote (bool):
-
- Whether remote refs should be cached after first resolution
-
- .. deprecated:: v4.18.0
-
- ``RefResolver`` has been deprecated in favor of `referencing`.
- """
-
- _DEPRECATION_MESSAGE = (
- "jsonschema.RefResolver is deprecated as of v4.18.0, in favor of the "
- "https://github.com/python-jsonschema/referencing library, which "
- "provides more compliant referencing behavior as well as more "
- "flexible APIs for customization. A future release will remove "
- "RefResolver. Please file a feature request (on referencing) if you "
- "are missing an API for the kind of customization you need."
- )
-
- def __init__(
- self,
- base_uri,
- referrer,
- store=HashTrieMap(),
- cache_remote=True,
- handlers=(),
- urljoin_cache=None,
- remote_cache=None,
- ):
- if urljoin_cache is None:
- urljoin_cache = lru_cache(1024)(urljoin)
- if remote_cache is None:
- remote_cache = lru_cache(1024)(self.resolve_from_url)
-
- self.referrer = referrer
- self.cache_remote = cache_remote
- self.handlers = dict(handlers)
-
- self._scopes_stack = [base_uri]
-
- self.store = _utils.URIDict(
- (uri, each.contents) for uri, each in SPECIFICATIONS.items()
- )
- self.store.update(
- (id, each.META_SCHEMA) for id, each in _META_SCHEMAS.items()
- )
- self.store.update(store)
- self.store.update(
- (schema["$id"], schema)
- for schema in store.values()
- if isinstance(schema, Mapping) and "$id" in schema
- )
- self.store[base_uri] = referrer
-
- self._urljoin_cache = urljoin_cache
- self._remote_cache = remote_cache
-
- @classmethod
- def from_schema( # noqa: D417
- cls,
- schema,
- id_of=referencing.jsonschema.DRAFT202012.id_of,
- *args,
- **kwargs,
- ):
- """
- Construct a resolver from a JSON schema object.
-
- Arguments:
-
- schema:
-
- the referring schema
-
- Returns:
-
- `_RefResolver`
- """
- return cls(base_uri=id_of(schema) or "", referrer=schema, *args, **kwargs) # noqa: B026, E501
-
- def push_scope(self, scope):
- """
- Enter a given sub-scope.
-
- Treats further dereferences as being performed underneath the
- given scope.
- """
- self._scopes_stack.append(
- self._urljoin_cache(self.resolution_scope, scope),
- )
-
- def pop_scope(self):
- """
- Exit the most recent entered scope.
-
- Treats further dereferences as being performed underneath the
- original scope.
-
- Don't call this method more times than `push_scope` has been
- called.
- """
- try:
- self._scopes_stack.pop()
- except IndexError:
- raise exceptions._RefResolutionError(
- "Failed to pop the scope from an empty stack. "
- "`pop_scope()` should only be called once for every "
- "`push_scope()`",
- )
-
- @property
- def resolution_scope(self):
- """
- Retrieve the current resolution scope.
- """
- return self._scopes_stack[-1]
-
- @property
- def base_uri(self):
- """
- Retrieve the current base URI, not including any fragment.
- """
- uri, _ = urldefrag(self.resolution_scope)
- return uri
-
- @contextlib.contextmanager
- def in_scope(self, scope):
- """
- Temporarily enter the given scope for the duration of the context.
-
- .. deprecated:: v4.0.0
- """
- warnings.warn(
- "jsonschema.RefResolver.in_scope is deprecated and will be "
- "removed in a future release.",
- DeprecationWarning,
- stacklevel=3,
- )
- self.push_scope(scope)
- try:
- yield
- finally:
- self.pop_scope()
-
- @contextlib.contextmanager
- def resolving(self, ref):
- """
- Resolve the given ``ref`` and enter its resolution scope.
-
- Exits the scope on exit of this context manager.
-
- Arguments:
-
- ref (str):
-
- The reference to resolve
- """
- url, resolved = self.resolve(ref)
- self.push_scope(url)
- try:
- yield resolved
- finally:
- self.pop_scope()
-
- def _find_in_referrer(self, key):
- return self._get_subschemas_cache()[key]
-
- @lru_cache # noqa: B019
- def _get_subschemas_cache(self):
- cache = {key: [] for key in _SUBSCHEMAS_KEYWORDS}
- for keyword, subschema in _search_schema(
- self.referrer, _match_subschema_keywords,
- ):
- cache[keyword].append(subschema)
- return cache
-
- @lru_cache # noqa: B019
- def _find_in_subschemas(self, url):
- subschemas = self._get_subschemas_cache()["$id"]
- if not subschemas:
- return None
- uri, fragment = urldefrag(url)
- for subschema in subschemas:
- id = subschema["$id"]
- if not isinstance(id, str):
- continue
- target_uri = self._urljoin_cache(self.resolution_scope, id)
- if target_uri.rstrip("/") == uri.rstrip("/"):
- if fragment:
- subschema = self.resolve_fragment(subschema, fragment)
- self.store[url] = subschema
- return url, subschema
- return None
-
- def resolve(self, ref):
- """
- Resolve the given reference.
- """
- url = self._urljoin_cache(self.resolution_scope, ref).rstrip("/")
-
- match = self._find_in_subschemas(url)
- if match is not None:
- return match
-
- return url, self._remote_cache(url)
-
- def resolve_from_url(self, url):
- """
- Resolve the given URL.
- """
- url, fragment = urldefrag(url)
- if not url:
- url = self.base_uri
-
- try:
- document = self.store[url]
- except KeyError:
- try:
- document = self.resolve_remote(url)
- except Exception as exc:
- raise exceptions._RefResolutionError(exc)
-
- return self.resolve_fragment(document, fragment)
-
- def resolve_fragment(self, document, fragment):
- """
- Resolve a ``fragment`` within the referenced ``document``.
-
- Arguments:
-
- document:
-
- The referent document
-
- fragment (str):
-
- a URI fragment to resolve within it
- """
- fragment = fragment.lstrip("/")
-
- if not fragment:
- return document
-
- if document is self.referrer:
- find = self._find_in_referrer
- else:
-
- def find(key):
- yield from _search_schema(document, _match_keyword(key))
-
- for keyword in ["$anchor", "$dynamicAnchor"]:
- for subschema in find(keyword):
- if fragment == subschema[keyword]:
- return subschema
- for keyword in ["id", "$id"]:
- for subschema in find(keyword):
- if "#" + fragment == subschema[keyword]:
- return subschema
-
- # Resolve via path
- parts = unquote(fragment).split("/") if fragment else []
- for part in parts:
- part = part.replace("~1", "/").replace("~0", "~")
-
- if isinstance(document, Sequence):
- try: # noqa: SIM105
- part = int(part)
- except ValueError:
- pass
- try:
- document = document[part]
- except (TypeError, LookupError):
- raise exceptions._RefResolutionError(
- f"Unresolvable JSON pointer: {fragment!r}",
- )
-
- return document
-
- def resolve_remote(self, uri):
- """
- Resolve a remote ``uri``.
-
- If called directly, does not check the store first, but after
- retrieving the document at the specified URI it will be saved in
- the store if :attr:`cache_remote` is True.
-
- .. note::
-
- If the requests_ library is present, ``jsonschema`` will use it to
- request the remote ``uri``, so that the correct encoding is
- detected and used.
-
- If it isn't, or if the scheme of the ``uri`` is not ``http`` or
- ``https``, UTF-8 is assumed.
-
- Arguments:
-
- uri (str):
-
- The URI to resolve
-
- Returns:
-
- The retrieved document
-
- .. _requests: https://pypi.org/project/requests/
- """
- try:
- import requests
- except ImportError:
- requests = None
-
- scheme = urlsplit(uri).scheme
-
- if scheme in self.handlers:
- result = self.handlers[scheme](uri)
- elif scheme in ["http", "https"] and requests:
- # Requests has support for detecting the correct encoding of
- # json over http
- result = requests.get(uri).json()
- else:
- # Otherwise, pass off to urllib and assume utf-8
- with urlopen(uri) as url:
- result = json.loads(url.read().decode("utf-8"))
-
- if self.cache_remote:
- self.store[uri] = result
- return result
-
-
-_SUBSCHEMAS_KEYWORDS = ("$id", "id", "$anchor", "$dynamicAnchor")
-
-
-def _match_keyword(keyword):
-
- def matcher(value):
- if keyword in value:
- yield value
-
- return matcher
-
-
-def _match_subschema_keywords(value):
- for keyword in _SUBSCHEMAS_KEYWORDS:
- if keyword in value:
- yield keyword, value
-
-
-def _search_schema(schema, matcher):
- """Breadth-first search routine."""
- values = deque([schema])
- while values:
- value = values.pop()
- if not isinstance(value, dict):
- continue
- yield from matcher(value)
- values.extendleft(value.values())
-
-
-def validate(instance, schema, cls=None, *args, **kwargs): # noqa: D417
- """
- Validate an instance under the given schema.
-
- >>> validate([2, 3, 4], {"maxItems": 2})
- Traceback (most recent call last):
- ...
- ValidationError: [2, 3, 4] is too long
-
- :func:`~jsonschema.validators.validate` will first verify that the
- provided schema is itself valid, since not doing so can lead to less
- obvious error messages and fail in less obvious or consistent ways.
-
- If you know you have a valid schema already, especially
- if you intend to validate multiple instances with
- the same schema, you likely would prefer using the
- `jsonschema.protocols.Validator.validate` method directly on a
- specific validator (e.g. ``Draft202012Validator.validate``).
-
-
- Arguments:
-
- instance:
-
- The instance to validate
-
- schema:
-
- The schema to validate with
-
- cls (jsonschema.protocols.Validator):
-
- The class that will be used to validate the instance.
-
- If the ``cls`` argument is not provided, two things will happen
- in accordance with the specification. First, if the schema has a
- :kw:`$schema` keyword containing a known meta-schema [#]_ then the
- proper validator will be used. The specification recommends that
- all schemas contain :kw:`$schema` properties for this reason. If no
- :kw:`$schema` property is found, the default validator class is the
- latest released draft.
-
- Any other provided positional and keyword arguments will be passed
- on when instantiating the ``cls``.
-
- Raises:
-
- `jsonschema.exceptions.ValidationError`:
-
- if the instance is invalid
-
- `jsonschema.exceptions.SchemaError`:
-
- if the schema itself is invalid
-
- .. rubric:: Footnotes
- .. [#] known by a validator registered with
- `jsonschema.validators.validates`
- """
- if cls is None:
- cls = validator_for(schema)
-
- cls.check_schema(schema)
- validator = cls(schema, *args, **kwargs)
- error = exceptions.best_match(validator.iter_errors(instance))
- if error is not None:
- raise error
-
-
-def validator_for(schema, default=_UNSET):
- """
- Retrieve the validator class appropriate for validating the given schema.
-
- Uses the :kw:`$schema` keyword that should be present in the given
- schema to look up the appropriate validator class.
-
- Arguments:
-
- schema (collections.abc.Mapping or bool):
-
- the schema to look at
-
- default:
-
- the default to return if the appropriate validator class
- cannot be determined.
-
- If unprovided, the default is to return the latest supported
- draft.
-
- Examples:
-
- The :kw:`$schema` JSON Schema keyword will control which validator
- class is returned:
-
- >>> schema = {
- ... "$schema": "https://json-schema.org/draft/2020-12/schema",
- ... "type": "integer",
- ... }
- >>> jsonschema.validators.validator_for(schema)
-
-
-
- Here, a draft 7 schema instead will return the draft 7 validator:
-
- >>> schema = {
- ... "$schema": "http://json-schema.org/draft-07/schema#",
- ... "type": "integer",
- ... }
- >>> jsonschema.validators.validator_for(schema)
-
-
-
- Schemas with no ``$schema`` keyword will fallback to the default
- argument:
-
- >>> schema = {"type": "integer"}
- >>> jsonschema.validators.validator_for(
- ... schema, default=Draft7Validator,
- ... )
-
-
- or if none is provided, to the latest version supported.
- Always including the keyword when authoring schemas is highly
- recommended.
-
- """
- DefaultValidator = _LATEST_VERSION if default is _UNSET else default
-
- if schema is True or schema is False or "$schema" not in schema:
- return DefaultValidator
- if schema["$schema"] not in _META_SCHEMAS and default is _UNSET:
- warn(
- (
- "The metaschema specified by $schema was not found. "
- "Using the latest draft to validate, but this will raise "
- "an error in the future."
- ),
- DeprecationWarning,
- stacklevel=2,
- )
- return _META_SCHEMAS.get(schema["$schema"], DefaultValidator)
diff --git a/spaces/declare-lab/tango/diffusers/examples/community/img2img_inpainting.py b/spaces/declare-lab/tango/diffusers/examples/community/img2img_inpainting.py
deleted file mode 100644
index f50eb6cabc37ae319e7c38751ec8b934063318b7..0000000000000000000000000000000000000000
--- a/spaces/declare-lab/tango/diffusers/examples/community/img2img_inpainting.py
+++ /dev/null
@@ -1,463 +0,0 @@
-import inspect
-from typing import Callable, List, Optional, Tuple, Union
-
-import numpy as np
-import PIL
-import torch
-from transformers import CLIPImageProcessor, CLIPTextModel, CLIPTokenizer
-
-from diffusers import DiffusionPipeline
-from diffusers.configuration_utils import FrozenDict
-from diffusers.models import AutoencoderKL, UNet2DConditionModel
-from diffusers.pipelines.stable_diffusion import StableDiffusionPipelineOutput
-from diffusers.pipelines.stable_diffusion.safety_checker import StableDiffusionSafetyChecker
-from diffusers.schedulers import DDIMScheduler, LMSDiscreteScheduler, PNDMScheduler
-from diffusers.utils import deprecate, logging
-
-
-logger = logging.get_logger(__name__) # pylint: disable=invalid-name
-
-
-def prepare_mask_and_masked_image(image, mask):
- image = np.array(image.convert("RGB"))
- image = image[None].transpose(0, 3, 1, 2)
- image = torch.from_numpy(image).to(dtype=torch.float32) / 127.5 - 1.0
-
- mask = np.array(mask.convert("L"))
- mask = mask.astype(np.float32) / 255.0
- mask = mask[None, None]
- mask[mask < 0.5] = 0
- mask[mask >= 0.5] = 1
- mask = torch.from_numpy(mask)
-
- masked_image = image * (mask < 0.5)
-
- return mask, masked_image
-
-
-def check_size(image, height, width):
- if isinstance(image, PIL.Image.Image):
- w, h = image.size
- elif isinstance(image, torch.Tensor):
- *_, h, w = image.shape
-
- if h != height or w != width:
- raise ValueError(f"Image size should be {height}x{width}, but got {h}x{w}")
-
-
-def overlay_inner_image(image, inner_image, paste_offset: Tuple[int] = (0, 0)):
- inner_image = inner_image.convert("RGBA")
- image = image.convert("RGB")
-
- image.paste(inner_image, paste_offset, inner_image)
- image = image.convert("RGB")
-
- return image
-
-
-class ImageToImageInpaintingPipeline(DiffusionPipeline):
- r"""
- Pipeline for text-guided image-to-image inpainting using Stable Diffusion. *This is an experimental feature*.
-
- This model inherits from [`DiffusionPipeline`]. Check the superclass documentation for the generic methods the
- library implements for all the pipelines (such as downloading or saving, running on a particular device, etc.)
-
- Args:
- vae ([`AutoencoderKL`]):
- Variational Auto-Encoder (VAE) Model to encode and decode images to and from latent representations.
- text_encoder ([`CLIPTextModel`]):
- Frozen text-encoder. Stable Diffusion uses the text portion of
- [CLIP](https://huggingface.co/docs/transformers/model_doc/clip#transformers.CLIPTextModel), specifically
- the [clip-vit-large-patch14](https://huggingface.co/openai/clip-vit-large-patch14) variant.
- tokenizer (`CLIPTokenizer`):
- Tokenizer of class
- [CLIPTokenizer](https://huggingface.co/docs/transformers/v4.21.0/en/model_doc/clip#transformers.CLIPTokenizer).
- unet ([`UNet2DConditionModel`]): Conditional U-Net architecture to denoise the encoded image latents.
- scheduler ([`SchedulerMixin`]):
- A scheduler to be used in combination with `unet` to denoise the encoded image latens. Can be one of
- [`DDIMScheduler`], [`LMSDiscreteScheduler`], or [`PNDMScheduler`].
- safety_checker ([`StableDiffusionSafetyChecker`]):
- Classification module that estimates whether generated images could be considered offensive or harmful.
- Please, refer to the [model card](https://huggingface.co/runwayml/stable-diffusion-v1-5) for details.
- feature_extractor ([`CLIPImageProcessor`]):
- Model that extracts features from generated images to be used as inputs for the `safety_checker`.
- """
-
- def __init__(
- self,
- vae: AutoencoderKL,
- text_encoder: CLIPTextModel,
- tokenizer: CLIPTokenizer,
- unet: UNet2DConditionModel,
- scheduler: Union[DDIMScheduler, PNDMScheduler, LMSDiscreteScheduler],
- safety_checker: StableDiffusionSafetyChecker,
- feature_extractor: CLIPImageProcessor,
- ):
- super().__init__()
-
- if hasattr(scheduler.config, "steps_offset") and scheduler.config.steps_offset != 1:
- deprecation_message = (
- f"The configuration file of this scheduler: {scheduler} is outdated. `steps_offset`"
- f" should be set to 1 instead of {scheduler.config.steps_offset}. Please make sure "
- "to update the config accordingly as leaving `steps_offset` might led to incorrect results"
- " in future versions. If you have downloaded this checkpoint from the Hugging Face Hub,"
- " it would be very nice if you could open a Pull request for the `scheduler/scheduler_config.json`"
- " file"
- )
- deprecate("steps_offset!=1", "1.0.0", deprecation_message, standard_warn=False)
- new_config = dict(scheduler.config)
- new_config["steps_offset"] = 1
- scheduler._internal_dict = FrozenDict(new_config)
-
- if safety_checker is None:
- logger.warning(
- f"You have disabled the safety checker for {self.__class__} by passing `safety_checker=None`. Ensure"
- " that you abide to the conditions of the Stable Diffusion license and do not expose unfiltered"
- " results in services or applications open to the public. Both the diffusers team and Hugging Face"
- " strongly recommend to keep the safety filter enabled in all public facing circumstances, disabling"
- " it only for use-cases that involve analyzing network behavior or auditing its results. For more"
- " information, please have a look at https://github.com/huggingface/diffusers/pull/254 ."
- )
-
- self.register_modules(
- vae=vae,
- text_encoder=text_encoder,
- tokenizer=tokenizer,
- unet=unet,
- scheduler=scheduler,
- safety_checker=safety_checker,
- feature_extractor=feature_extractor,
- )
-
- def enable_attention_slicing(self, slice_size: Optional[Union[str, int]] = "auto"):
- r"""
- Enable sliced attention computation.
-
- When this option is enabled, the attention module will split the input tensor in slices, to compute attention
- in several steps. This is useful to save some memory in exchange for a small speed decrease.
-
- Args:
- slice_size (`str` or `int`, *optional*, defaults to `"auto"`):
- When `"auto"`, halves the input to the attention heads, so attention will be computed in two steps. If
- a number is provided, uses as many slices as `attention_head_dim // slice_size`. In this case,
- `attention_head_dim` must be a multiple of `slice_size`.
- """
- if slice_size == "auto":
- # half the attention head size is usually a good trade-off between
- # speed and memory
- slice_size = self.unet.config.attention_head_dim // 2
- self.unet.set_attention_slice(slice_size)
-
- def disable_attention_slicing(self):
- r"""
- Disable sliced attention computation. If `enable_attention_slicing` was previously invoked, this method will go
- back to computing attention in one step.
- """
- # set slice_size = `None` to disable `attention slicing`
- self.enable_attention_slicing(None)
-
- @torch.no_grad()
- def __call__(
- self,
- prompt: Union[str, List[str]],
- image: Union[torch.FloatTensor, PIL.Image.Image],
- inner_image: Union[torch.FloatTensor, PIL.Image.Image],
- mask_image: Union[torch.FloatTensor, PIL.Image.Image],
- height: int = 512,
- width: int = 512,
- num_inference_steps: int = 50,
- guidance_scale: float = 7.5,
- negative_prompt: Optional[Union[str, List[str]]] = None,
- num_images_per_prompt: Optional[int] = 1,
- eta: float = 0.0,
- generator: Optional[torch.Generator] = None,
- latents: Optional[torch.FloatTensor] = None,
- output_type: Optional[str] = "pil",
- return_dict: bool = True,
- callback: Optional[Callable[[int, int, torch.FloatTensor], None]] = None,
- callback_steps: int = 1,
- **kwargs,
- ):
- r"""
- Function invoked when calling the pipeline for generation.
-
- Args:
- prompt (`str` or `List[str]`):
- The prompt or prompts to guide the image generation.
- image (`torch.Tensor` or `PIL.Image.Image`):
- `Image`, or tensor representing an image batch which will be inpainted, *i.e.* parts of the image will
- be masked out with `mask_image` and repainted according to `prompt`.
- inner_image (`torch.Tensor` or `PIL.Image.Image`):
- `Image`, or tensor representing an image batch which will be overlayed onto `image`. Non-transparent
- regions of `inner_image` must fit inside white pixels in `mask_image`. Expects four channels, with
- the last channel representing the alpha channel, which will be used to blend `inner_image` with
- `image`. If not provided, it will be forcibly cast to RGBA.
- mask_image (`PIL.Image.Image`):
- `Image`, or tensor representing an image batch, to mask `image`. White pixels in the mask will be
- repainted, while black pixels will be preserved. If `mask_image` is a PIL image, it will be converted
- to a single channel (luminance) before use. If it's a tensor, it should contain one color channel (L)
- instead of 3, so the expected shape would be `(B, H, W, 1)`.
- height (`int`, *optional*, defaults to 512):
- The height in pixels of the generated image.
- width (`int`, *optional*, defaults to 512):
- The width in pixels of the generated image.
- num_inference_steps (`int`, *optional*, defaults to 50):
- The number of denoising steps. More denoising steps usually lead to a higher quality image at the
- expense of slower inference.
- guidance_scale (`float`, *optional*, defaults to 7.5):
- Guidance scale as defined in [Classifier-Free Diffusion Guidance](https://arxiv.org/abs/2207.12598).
- `guidance_scale` is defined as `w` of equation 2. of [Imagen
- Paper](https://arxiv.org/pdf/2205.11487.pdf). Guidance scale is enabled by setting `guidance_scale >
- 1`. Higher guidance scale encourages to generate images that are closely linked to the text `prompt`,
- usually at the expense of lower image quality.
- negative_prompt (`str` or `List[str]`, *optional*):
- The prompt or prompts not to guide the image generation. Ignored when not using guidance (i.e., ignored
- if `guidance_scale` is less than `1`).
- num_images_per_prompt (`int`, *optional*, defaults to 1):
- The number of images to generate per prompt.
- eta (`float`, *optional*, defaults to 0.0):
- Corresponds to parameter eta (η) in the DDIM paper: https://arxiv.org/abs/2010.02502. Only applies to
- [`schedulers.DDIMScheduler`], will be ignored for others.
- generator (`torch.Generator`, *optional*):
- A [torch generator](https://pytorch.org/docs/stable/generated/torch.Generator.html) to make generation
- deterministic.
- latents (`torch.FloatTensor`, *optional*):
- Pre-generated noisy latents, sampled from a Gaussian distribution, to be used as inputs for image
- generation. Can be used to tweak the same generation with different prompts. If not provided, a latents
- tensor will ge generated by sampling using the supplied random `generator`.
- output_type (`str`, *optional*, defaults to `"pil"`):
- The output format of the generate image. Choose between
- [PIL](https://pillow.readthedocs.io/en/stable/): `PIL.Image.Image` or `np.array`.
- return_dict (`bool`, *optional*, defaults to `True`):
- Whether or not to return a [`~pipelines.stable_diffusion.StableDiffusionPipelineOutput`] instead of a
- plain tuple.
- callback (`Callable`, *optional*):
- A function that will be called every `callback_steps` steps during inference. The function will be
- called with the following arguments: `callback(step: int, timestep: int, latents: torch.FloatTensor)`.
- callback_steps (`int`, *optional*, defaults to 1):
- The frequency at which the `callback` function will be called. If not specified, the callback will be
- called at every step.
-
- Returns:
- [`~pipelines.stable_diffusion.StableDiffusionPipelineOutput`] or `tuple`:
- [`~pipelines.stable_diffusion.StableDiffusionPipelineOutput`] if `return_dict` is True, otherwise a `tuple.
- When returning a tuple, the first element is a list with the generated images, and the second element is a
- list of `bool`s denoting whether the corresponding generated image likely represents "not-safe-for-work"
- (nsfw) content, according to the `safety_checker`.
- """
-
- if isinstance(prompt, str):
- batch_size = 1
- elif isinstance(prompt, list):
- batch_size = len(prompt)
- else:
- raise ValueError(f"`prompt` has to be of type `str` or `list` but is {type(prompt)}")
-
- if height % 8 != 0 or width % 8 != 0:
- raise ValueError(f"`height` and `width` have to be divisible by 8 but are {height} and {width}.")
-
- if (callback_steps is None) or (
- callback_steps is not None and (not isinstance(callback_steps, int) or callback_steps <= 0)
- ):
- raise ValueError(
- f"`callback_steps` has to be a positive integer but is {callback_steps} of type"
- f" {type(callback_steps)}."
- )
-
- # check if input sizes are correct
- check_size(image, height, width)
- check_size(inner_image, height, width)
- check_size(mask_image, height, width)
-
- # get prompt text embeddings
- text_inputs = self.tokenizer(
- prompt,
- padding="max_length",
- max_length=self.tokenizer.model_max_length,
- return_tensors="pt",
- )
- text_input_ids = text_inputs.input_ids
-
- if text_input_ids.shape[-1] > self.tokenizer.model_max_length:
- removed_text = self.tokenizer.batch_decode(text_input_ids[:, self.tokenizer.model_max_length :])
- logger.warning(
- "The following part of your input was truncated because CLIP can only handle sequences up to"
- f" {self.tokenizer.model_max_length} tokens: {removed_text}"
- )
- text_input_ids = text_input_ids[:, : self.tokenizer.model_max_length]
- text_embeddings = self.text_encoder(text_input_ids.to(self.device))[0]
-
- # duplicate text embeddings for each generation per prompt, using mps friendly method
- bs_embed, seq_len, _ = text_embeddings.shape
- text_embeddings = text_embeddings.repeat(1, num_images_per_prompt, 1)
- text_embeddings = text_embeddings.view(bs_embed * num_images_per_prompt, seq_len, -1)
-
- # here `guidance_scale` is defined analog to the guidance weight `w` of equation (2)
- # of the Imagen paper: https://arxiv.org/pdf/2205.11487.pdf . `guidance_scale = 1`
- # corresponds to doing no classifier free guidance.
- do_classifier_free_guidance = guidance_scale > 1.0
- # get unconditional embeddings for classifier free guidance
- if do_classifier_free_guidance:
- uncond_tokens: List[str]
- if negative_prompt is None:
- uncond_tokens = [""]
- elif type(prompt) is not type(negative_prompt):
- raise TypeError(
- f"`negative_prompt` should be the same type to `prompt`, but got {type(negative_prompt)} !="
- f" {type(prompt)}."
- )
- elif isinstance(negative_prompt, str):
- uncond_tokens = [negative_prompt]
- elif batch_size != len(negative_prompt):
- raise ValueError(
- f"`negative_prompt`: {negative_prompt} has batch size {len(negative_prompt)}, but `prompt`:"
- f" {prompt} has batch size {batch_size}. Please make sure that passed `negative_prompt` matches"
- " the batch size of `prompt`."
- )
- else:
- uncond_tokens = negative_prompt
-
- max_length = text_input_ids.shape[-1]
- uncond_input = self.tokenizer(
- uncond_tokens,
- padding="max_length",
- max_length=max_length,
- truncation=True,
- return_tensors="pt",
- )
- uncond_embeddings = self.text_encoder(uncond_input.input_ids.to(self.device))[0]
-
- # duplicate unconditional embeddings for each generation per prompt, using mps friendly method
- seq_len = uncond_embeddings.shape[1]
- uncond_embeddings = uncond_embeddings.repeat(batch_size, num_images_per_prompt, 1)
- uncond_embeddings = uncond_embeddings.view(batch_size * num_images_per_prompt, seq_len, -1)
-
- # For classifier free guidance, we need to do two forward passes.
- # Here we concatenate the unconditional and text embeddings into a single batch
- # to avoid doing two forward passes
- text_embeddings = torch.cat([uncond_embeddings, text_embeddings])
-
- # get the initial random noise unless the user supplied it
- # Unlike in other pipelines, latents need to be generated in the target device
- # for 1-to-1 results reproducibility with the CompVis implementation.
- # However this currently doesn't work in `mps`.
- num_channels_latents = self.vae.config.latent_channels
- latents_shape = (batch_size * num_images_per_prompt, num_channels_latents, height // 8, width // 8)
- latents_dtype = text_embeddings.dtype
- if latents is None:
- if self.device.type == "mps":
- # randn does not exist on mps
- latents = torch.randn(latents_shape, generator=generator, device="cpu", dtype=latents_dtype).to(
- self.device
- )
- else:
- latents = torch.randn(latents_shape, generator=generator, device=self.device, dtype=latents_dtype)
- else:
- if latents.shape != latents_shape:
- raise ValueError(f"Unexpected latents shape, got {latents.shape}, expected {latents_shape}")
- latents = latents.to(self.device)
-
- # overlay the inner image
- image = overlay_inner_image(image, inner_image)
-
- # prepare mask and masked_image
- mask, masked_image = prepare_mask_and_masked_image(image, mask_image)
- mask = mask.to(device=self.device, dtype=text_embeddings.dtype)
- masked_image = masked_image.to(device=self.device, dtype=text_embeddings.dtype)
-
- # resize the mask to latents shape as we concatenate the mask to the latents
- mask = torch.nn.functional.interpolate(mask, size=(height // 8, width // 8))
-
- # encode the mask image into latents space so we can concatenate it to the latents
- masked_image_latents = self.vae.encode(masked_image).latent_dist.sample(generator=generator)
- masked_image_latents = 0.18215 * masked_image_latents
-
- # duplicate mask and masked_image_latents for each generation per prompt, using mps friendly method
- mask = mask.repeat(batch_size * num_images_per_prompt, 1, 1, 1)
- masked_image_latents = masked_image_latents.repeat(batch_size * num_images_per_prompt, 1, 1, 1)
-
- mask = torch.cat([mask] * 2) if do_classifier_free_guidance else mask
- masked_image_latents = (
- torch.cat([masked_image_latents] * 2) if do_classifier_free_guidance else masked_image_latents
- )
-
- num_channels_mask = mask.shape[1]
- num_channels_masked_image = masked_image_latents.shape[1]
-
- if num_channels_latents + num_channels_mask + num_channels_masked_image != self.unet.config.in_channels:
- raise ValueError(
- f"Incorrect configuration settings! The config of `pipeline.unet`: {self.unet.config} expects"
- f" {self.unet.config.in_channels} but received `num_channels_latents`: {num_channels_latents} +"
- f" `num_channels_mask`: {num_channels_mask} + `num_channels_masked_image`: {num_channels_masked_image}"
- f" = {num_channels_latents+num_channels_masked_image+num_channels_mask}. Please verify the config of"
- " `pipeline.unet` or your `mask_image` or `image` input."
- )
-
- # set timesteps
- self.scheduler.set_timesteps(num_inference_steps)
-
- # Some schedulers like PNDM have timesteps as arrays
- # It's more optimized to move all timesteps to correct device beforehand
- timesteps_tensor = self.scheduler.timesteps.to(self.device)
-
- # scale the initial noise by the standard deviation required by the scheduler
- latents = latents * self.scheduler.init_noise_sigma
-
- # prepare extra kwargs for the scheduler step, since not all schedulers have the same signature
- # eta (η) is only used with the DDIMScheduler, it will be ignored for other schedulers.
- # eta corresponds to η in DDIM paper: https://arxiv.org/abs/2010.02502
- # and should be between [0, 1]
- accepts_eta = "eta" in set(inspect.signature(self.scheduler.step).parameters.keys())
- extra_step_kwargs = {}
- if accepts_eta:
- extra_step_kwargs["eta"] = eta
-
- for i, t in enumerate(self.progress_bar(timesteps_tensor)):
- # expand the latents if we are doing classifier free guidance
- latent_model_input = torch.cat([latents] * 2) if do_classifier_free_guidance else latents
-
- # concat latents, mask, masked_image_latents in the channel dimension
- latent_model_input = torch.cat([latent_model_input, mask, masked_image_latents], dim=1)
-
- latent_model_input = self.scheduler.scale_model_input(latent_model_input, t)
-
- # predict the noise residual
- noise_pred = self.unet(latent_model_input, t, encoder_hidden_states=text_embeddings).sample
-
- # perform guidance
- if do_classifier_free_guidance:
- noise_pred_uncond, noise_pred_text = noise_pred.chunk(2)
- noise_pred = noise_pred_uncond + guidance_scale * (noise_pred_text - noise_pred_uncond)
-
- # compute the previous noisy sample x_t -> x_t-1
- latents = self.scheduler.step(noise_pred, t, latents, **extra_step_kwargs).prev_sample
-
- # call the callback, if provided
- if callback is not None and i % callback_steps == 0:
- callback(i, t, latents)
-
- latents = 1 / 0.18215 * latents
- image = self.vae.decode(latents).sample
-
- image = (image / 2 + 0.5).clamp(0, 1)
-
- # we always cast to float32 as this does not cause significant overhead and is compatible with bfloat16
- image = image.cpu().permute(0, 2, 3, 1).float().numpy()
-
- if self.safety_checker is not None:
- safety_checker_input = self.feature_extractor(self.numpy_to_pil(image), return_tensors="pt").to(
- self.device
- )
- image, has_nsfw_concept = self.safety_checker(
- images=image, clip_input=safety_checker_input.pixel_values.to(text_embeddings.dtype)
- )
- else:
- has_nsfw_concept = None
-
- if output_type == "pil":
- image = self.numpy_to_pil(image)
-
- if not return_dict:
- return (image, has_nsfw_concept)
-
- return StableDiffusionPipelineOutput(images=image, nsfw_content_detected=has_nsfw_concept)
diff --git a/spaces/declare-lab/tango/diffusers/src/diffusers/models/attention.py b/spaces/declare-lab/tango/diffusers/src/diffusers/models/attention.py
deleted file mode 100644
index 008cd5d5484e0985ee58b5a072af7f3006ca4bef..0000000000000000000000000000000000000000
--- a/spaces/declare-lab/tango/diffusers/src/diffusers/models/attention.py
+++ /dev/null
@@ -1,523 +0,0 @@
-# Copyright 2023 The HuggingFace Team. All rights reserved.
-#
-# Licensed under the Apache License, Version 2.0 (the "License");
-# you may not use this file except in compliance with the License.
-# You may obtain a copy of the License at
-#
-# http://www.apache.org/licenses/LICENSE-2.0
-#
-# Unless required by applicable law or agreed to in writing, software
-# distributed under the License is distributed on an "AS IS" BASIS,
-# WITHOUT WARRANTIES OR CONDITIONS OF ANY KIND, either express or implied.
-# See the License for the specific language governing permissions and
-# limitations under the License.
-import math
-from typing import Any, Callable, Dict, Optional
-
-import torch
-import torch.nn.functional as F
-from torch import nn
-
-from ..utils.import_utils import is_xformers_available
-from .attention_processor import Attention
-from .embeddings import CombinedTimestepLabelEmbeddings
-
-
-if is_xformers_available():
- import xformers
- import xformers.ops
-else:
- xformers = None
-
-
-class AttentionBlock(nn.Module):
- """
- An attention block that allows spatial positions to attend to each other. Originally ported from here, but adapted
- to the N-d case.
- https://github.com/hojonathanho/diffusion/blob/1e0dceb3b3495bbe19116a5e1b3596cd0706c543/diffusion_tf/models/unet.py#L66.
- Uses three q, k, v linear layers to compute attention.
-
- Parameters:
- channels (`int`): The number of channels in the input and output.
- num_head_channels (`int`, *optional*):
- The number of channels in each head. If None, then `num_heads` = 1.
- norm_num_groups (`int`, *optional*, defaults to 32): The number of groups to use for group norm.
- rescale_output_factor (`float`, *optional*, defaults to 1.0): The factor to rescale the output by.
- eps (`float`, *optional*, defaults to 1e-5): The epsilon value to use for group norm.
- """
-
- # IMPORTANT;TODO(Patrick, William) - this class will be deprecated soon. Do not use it anymore
-
- def __init__(
- self,
- channels: int,
- num_head_channels: Optional[int] = None,
- norm_num_groups: int = 32,
- rescale_output_factor: float = 1.0,
- eps: float = 1e-5,
- ):
- super().__init__()
- self.channels = channels
-
- self.num_heads = channels // num_head_channels if num_head_channels is not None else 1
- self.num_head_size = num_head_channels
- self.group_norm = nn.GroupNorm(num_channels=channels, num_groups=norm_num_groups, eps=eps, affine=True)
-
- # define q,k,v as linear layers
- self.query = nn.Linear(channels, channels)
- self.key = nn.Linear(channels, channels)
- self.value = nn.Linear(channels, channels)
-
- self.rescale_output_factor = rescale_output_factor
- self.proj_attn = nn.Linear(channels, channels, bias=True)
-
- self._use_memory_efficient_attention_xformers = False
- self._attention_op = None
-
- def reshape_heads_to_batch_dim(self, tensor):
- batch_size, seq_len, dim = tensor.shape
- head_size = self.num_heads
- tensor = tensor.reshape(batch_size, seq_len, head_size, dim // head_size)
- tensor = tensor.permute(0, 2, 1, 3).reshape(batch_size * head_size, seq_len, dim // head_size)
- return tensor
-
- def reshape_batch_dim_to_heads(self, tensor):
- batch_size, seq_len, dim = tensor.shape
- head_size = self.num_heads
- tensor = tensor.reshape(batch_size // head_size, head_size, seq_len, dim)
- tensor = tensor.permute(0, 2, 1, 3).reshape(batch_size // head_size, seq_len, dim * head_size)
- return tensor
-
- def set_use_memory_efficient_attention_xformers(
- self, use_memory_efficient_attention_xformers: bool, attention_op: Optional[Callable] = None
- ):
- if use_memory_efficient_attention_xformers:
- if not is_xformers_available():
- raise ModuleNotFoundError(
- (
- "Refer to https://github.com/facebookresearch/xformers for more information on how to install"
- " xformers"
- ),
- name="xformers",
- )
- elif not torch.cuda.is_available():
- raise ValueError(
- "torch.cuda.is_available() should be True but is False. xformers' memory efficient attention is"
- " only available for GPU "
- )
- else:
- try:
- # Make sure we can run the memory efficient attention
- _ = xformers.ops.memory_efficient_attention(
- torch.randn((1, 2, 40), device="cuda"),
- torch.randn((1, 2, 40), device="cuda"),
- torch.randn((1, 2, 40), device="cuda"),
- )
- except Exception as e:
- raise e
- self._use_memory_efficient_attention_xformers = use_memory_efficient_attention_xformers
- self._attention_op = attention_op
-
- def forward(self, hidden_states):
- residual = hidden_states
- batch, channel, height, width = hidden_states.shape
-
- # norm
- hidden_states = self.group_norm(hidden_states)
-
- hidden_states = hidden_states.view(batch, channel, height * width).transpose(1, 2)
-
- # proj to q, k, v
- query_proj = self.query(hidden_states)
- key_proj = self.key(hidden_states)
- value_proj = self.value(hidden_states)
-
- scale = 1 / math.sqrt(self.channels / self.num_heads)
-
- query_proj = self.reshape_heads_to_batch_dim(query_proj)
- key_proj = self.reshape_heads_to_batch_dim(key_proj)
- value_proj = self.reshape_heads_to_batch_dim(value_proj)
-
- if self._use_memory_efficient_attention_xformers:
- # Memory efficient attention
- hidden_states = xformers.ops.memory_efficient_attention(
- query_proj, key_proj, value_proj, attn_bias=None, op=self._attention_op
- )
- hidden_states = hidden_states.to(query_proj.dtype)
- else:
- attention_scores = torch.baddbmm(
- torch.empty(
- query_proj.shape[0],
- query_proj.shape[1],
- key_proj.shape[1],
- dtype=query_proj.dtype,
- device=query_proj.device,
- ),
- query_proj,
- key_proj.transpose(-1, -2),
- beta=0,
- alpha=scale,
- )
- attention_probs = torch.softmax(attention_scores.float(), dim=-1).type(attention_scores.dtype)
- hidden_states = torch.bmm(attention_probs, value_proj)
-
- # reshape hidden_states
- hidden_states = self.reshape_batch_dim_to_heads(hidden_states)
-
- # compute next hidden_states
- hidden_states = self.proj_attn(hidden_states)
-
- hidden_states = hidden_states.transpose(-1, -2).reshape(batch, channel, height, width)
-
- # res connect and rescale
- hidden_states = (hidden_states + residual) / self.rescale_output_factor
- return hidden_states
-
-
-class BasicTransformerBlock(nn.Module):
- r"""
- A basic Transformer block.
-
- Parameters:
- dim (`int`): The number of channels in the input and output.
- num_attention_heads (`int`): The number of heads to use for multi-head attention.
- attention_head_dim (`int`): The number of channels in each head.
- dropout (`float`, *optional*, defaults to 0.0): The dropout probability to use.
- cross_attention_dim (`int`, *optional*): The size of the encoder_hidden_states vector for cross attention.
- only_cross_attention (`bool`, *optional*):
- Whether to use only cross-attention layers. In this case two cross attention layers are used.
- double_self_attention (`bool`, *optional*):
- Whether to use two self-attention layers. In this case no cross attention layers are used.
- activation_fn (`str`, *optional*, defaults to `"geglu"`): Activation function to be used in feed-forward.
- num_embeds_ada_norm (:
- obj: `int`, *optional*): The number of diffusion steps used during training. See `Transformer2DModel`.
- attention_bias (:
- obj: `bool`, *optional*, defaults to `False`): Configure if the attentions should contain a bias parameter.
- """
-
- def __init__(
- self,
- dim: int,
- num_attention_heads: int,
- attention_head_dim: int,
- dropout=0.0,
- cross_attention_dim: Optional[int] = None,
- activation_fn: str = "geglu",
- num_embeds_ada_norm: Optional[int] = None,
- attention_bias: bool = False,
- only_cross_attention: bool = False,
- double_self_attention: bool = False,
- upcast_attention: bool = False,
- norm_elementwise_affine: bool = True,
- norm_type: str = "layer_norm",
- final_dropout: bool = False,
- ):
- super().__init__()
- self.only_cross_attention = only_cross_attention
-
- self.use_ada_layer_norm_zero = (num_embeds_ada_norm is not None) and norm_type == "ada_norm_zero"
- self.use_ada_layer_norm = (num_embeds_ada_norm is not None) and norm_type == "ada_norm"
-
- if norm_type in ("ada_norm", "ada_norm_zero") and num_embeds_ada_norm is None:
- raise ValueError(
- f"`norm_type` is set to {norm_type}, but `num_embeds_ada_norm` is not defined. Please make sure to"
- f" define `num_embeds_ada_norm` if setting `norm_type` to {norm_type}."
- )
-
- # 1. Self-Attn
- self.attn1 = Attention(
- query_dim=dim,
- heads=num_attention_heads,
- dim_head=attention_head_dim,
- dropout=dropout,
- bias=attention_bias,
- cross_attention_dim=cross_attention_dim if only_cross_attention else None,
- upcast_attention=upcast_attention,
- )
-
- self.ff = FeedForward(dim, dropout=dropout, activation_fn=activation_fn, final_dropout=final_dropout)
-
- # 2. Cross-Attn
- if cross_attention_dim is not None or double_self_attention:
- self.attn2 = Attention(
- query_dim=dim,
- cross_attention_dim=cross_attention_dim if not double_self_attention else None,
- heads=num_attention_heads,
- dim_head=attention_head_dim,
- dropout=dropout,
- bias=attention_bias,
- upcast_attention=upcast_attention,
- ) # is self-attn if encoder_hidden_states is none
- else:
- self.attn2 = None
-
- if self.use_ada_layer_norm:
- self.norm1 = AdaLayerNorm(dim, num_embeds_ada_norm)
- elif self.use_ada_layer_norm_zero:
- self.norm1 = AdaLayerNormZero(dim, num_embeds_ada_norm)
- else:
- self.norm1 = nn.LayerNorm(dim, elementwise_affine=norm_elementwise_affine)
-
- if cross_attention_dim is not None or double_self_attention:
- # We currently only use AdaLayerNormZero for self attention where there will only be one attention block.
- # I.e. the number of returned modulation chunks from AdaLayerZero would not make sense if returned during
- # the second cross attention block.
- self.norm2 = (
- AdaLayerNorm(dim, num_embeds_ada_norm)
- if self.use_ada_layer_norm
- else nn.LayerNorm(dim, elementwise_affine=norm_elementwise_affine)
- )
- else:
- self.norm2 = None
-
- # 3. Feed-forward
- self.norm3 = nn.LayerNorm(dim, elementwise_affine=norm_elementwise_affine)
-
- def forward(
- self,
- hidden_states: torch.FloatTensor,
- attention_mask: Optional[torch.FloatTensor] = None,
- encoder_hidden_states: Optional[torch.FloatTensor] = None,
- encoder_attention_mask: Optional[torch.FloatTensor] = None,
- timestep: Optional[torch.LongTensor] = None,
- cross_attention_kwargs: Dict[str, Any] = None,
- class_labels: Optional[torch.LongTensor] = None,
- ):
- if self.use_ada_layer_norm:
- norm_hidden_states = self.norm1(hidden_states, timestep)
- elif self.use_ada_layer_norm_zero:
- norm_hidden_states, gate_msa, shift_mlp, scale_mlp, gate_mlp = self.norm1(
- hidden_states, timestep, class_labels, hidden_dtype=hidden_states.dtype
- )
- else:
- norm_hidden_states = self.norm1(hidden_states)
-
- # 1. Self-Attention
- cross_attention_kwargs = cross_attention_kwargs if cross_attention_kwargs is not None else {}
- attn_output = self.attn1(
- norm_hidden_states,
- encoder_hidden_states=encoder_hidden_states if self.only_cross_attention else None,
- attention_mask=attention_mask,
- **cross_attention_kwargs,
- )
- if self.use_ada_layer_norm_zero:
- attn_output = gate_msa.unsqueeze(1) * attn_output
- hidden_states = attn_output + hidden_states
-
- if self.attn2 is not None:
- norm_hidden_states = (
- self.norm2(hidden_states, timestep) if self.use_ada_layer_norm else self.norm2(hidden_states)
- )
-
- # 2. Cross-Attention
- attn_output = self.attn2(
- norm_hidden_states,
- encoder_hidden_states=encoder_hidden_states,
- attention_mask=encoder_attention_mask,
- **cross_attention_kwargs,
- )
- hidden_states = attn_output + hidden_states
-
- # 3. Feed-forward
- norm_hidden_states = self.norm3(hidden_states)
-
- if self.use_ada_layer_norm_zero:
- norm_hidden_states = norm_hidden_states * (1 + scale_mlp[:, None]) + shift_mlp[:, None]
-
- ff_output = self.ff(norm_hidden_states)
-
- if self.use_ada_layer_norm_zero:
- ff_output = gate_mlp.unsqueeze(1) * ff_output
-
- hidden_states = ff_output + hidden_states
-
- return hidden_states
-
-
-class FeedForward(nn.Module):
- r"""
- A feed-forward layer.
-
- Parameters:
- dim (`int`): The number of channels in the input.
- dim_out (`int`, *optional*): The number of channels in the output. If not given, defaults to `dim`.
- mult (`int`, *optional*, defaults to 4): The multiplier to use for the hidden dimension.
- dropout (`float`, *optional*, defaults to 0.0): The dropout probability to use.
- activation_fn (`str`, *optional*, defaults to `"geglu"`): Activation function to be used in feed-forward.
- final_dropout (`bool` *optional*, defaults to False): Apply a final dropout.
- """
-
- def __init__(
- self,
- dim: int,
- dim_out: Optional[int] = None,
- mult: int = 4,
- dropout: float = 0.0,
- activation_fn: str = "geglu",
- final_dropout: bool = False,
- ):
- super().__init__()
- inner_dim = int(dim * mult)
- dim_out = dim_out if dim_out is not None else dim
-
- if activation_fn == "gelu":
- act_fn = GELU(dim, inner_dim)
- if activation_fn == "gelu-approximate":
- act_fn = GELU(dim, inner_dim, approximate="tanh")
- elif activation_fn == "geglu":
- act_fn = GEGLU(dim, inner_dim)
- elif activation_fn == "geglu-approximate":
- act_fn = ApproximateGELU(dim, inner_dim)
-
- self.net = nn.ModuleList([])
- # project in
- self.net.append(act_fn)
- # project dropout
- self.net.append(nn.Dropout(dropout))
- # project out
- self.net.append(nn.Linear(inner_dim, dim_out))
- # FF as used in Vision Transformer, MLP-Mixer, etc. have a final dropout
- if final_dropout:
- self.net.append(nn.Dropout(dropout))
-
- def forward(self, hidden_states):
- for module in self.net:
- hidden_states = module(hidden_states)
- return hidden_states
-
-
-class GELU(nn.Module):
- r"""
- GELU activation function with tanh approximation support with `approximate="tanh"`.
- """
-
- def __init__(self, dim_in: int, dim_out: int, approximate: str = "none"):
- super().__init__()
- self.proj = nn.Linear(dim_in, dim_out)
- self.approximate = approximate
-
- def gelu(self, gate):
- if gate.device.type != "mps":
- return F.gelu(gate, approximate=self.approximate)
- # mps: gelu is not implemented for float16
- return F.gelu(gate.to(dtype=torch.float32), approximate=self.approximate).to(dtype=gate.dtype)
-
- def forward(self, hidden_states):
- hidden_states = self.proj(hidden_states)
- hidden_states = self.gelu(hidden_states)
- return hidden_states
-
-
-class GEGLU(nn.Module):
- r"""
- A variant of the gated linear unit activation function from https://arxiv.org/abs/2002.05202.
-
- Parameters:
- dim_in (`int`): The number of channels in the input.
- dim_out (`int`): The number of channels in the output.
- """
-
- def __init__(self, dim_in: int, dim_out: int):
- super().__init__()
- self.proj = nn.Linear(dim_in, dim_out * 2)
-
- def gelu(self, gate):
- if gate.device.type != "mps":
- return F.gelu(gate)
- # mps: gelu is not implemented for float16
- return F.gelu(gate.to(dtype=torch.float32)).to(dtype=gate.dtype)
-
- def forward(self, hidden_states):
- hidden_states, gate = self.proj(hidden_states).chunk(2, dim=-1)
- return hidden_states * self.gelu(gate)
-
-
-class ApproximateGELU(nn.Module):
- """
- The approximate form of Gaussian Error Linear Unit (GELU)
-
- For more details, see section 2: https://arxiv.org/abs/1606.08415
- """
-
- def __init__(self, dim_in: int, dim_out: int):
- super().__init__()
- self.proj = nn.Linear(dim_in, dim_out)
-
- def forward(self, x):
- x = self.proj(x)
- return x * torch.sigmoid(1.702 * x)
-
-
-class AdaLayerNorm(nn.Module):
- """
- Norm layer modified to incorporate timestep embeddings.
- """
-
- def __init__(self, embedding_dim, num_embeddings):
- super().__init__()
- self.emb = nn.Embedding(num_embeddings, embedding_dim)
- self.silu = nn.SiLU()
- self.linear = nn.Linear(embedding_dim, embedding_dim * 2)
- self.norm = nn.LayerNorm(embedding_dim, elementwise_affine=False)
-
- def forward(self, x, timestep):
- emb = self.linear(self.silu(self.emb(timestep)))
- scale, shift = torch.chunk(emb, 2)
- x = self.norm(x) * (1 + scale) + shift
- return x
-
-
-class AdaLayerNormZero(nn.Module):
- """
- Norm layer adaptive layer norm zero (adaLN-Zero).
- """
-
- def __init__(self, embedding_dim, num_embeddings):
- super().__init__()
-
- self.emb = CombinedTimestepLabelEmbeddings(num_embeddings, embedding_dim)
-
- self.silu = nn.SiLU()
- self.linear = nn.Linear(embedding_dim, 6 * embedding_dim, bias=True)
- self.norm = nn.LayerNorm(embedding_dim, elementwise_affine=False, eps=1e-6)
-
- def forward(self, x, timestep, class_labels, hidden_dtype=None):
- emb = self.linear(self.silu(self.emb(timestep, class_labels, hidden_dtype=hidden_dtype)))
- shift_msa, scale_msa, gate_msa, shift_mlp, scale_mlp, gate_mlp = emb.chunk(6, dim=1)
- x = self.norm(x) * (1 + scale_msa[:, None]) + shift_msa[:, None]
- return x, gate_msa, shift_mlp, scale_mlp, gate_mlp
-
-
-class AdaGroupNorm(nn.Module):
- """
- GroupNorm layer modified to incorporate timestep embeddings.
- """
-
- def __init__(
- self, embedding_dim: int, out_dim: int, num_groups: int, act_fn: Optional[str] = None, eps: float = 1e-5
- ):
- super().__init__()
- self.num_groups = num_groups
- self.eps = eps
- self.act = None
- if act_fn == "swish":
- self.act = lambda x: F.silu(x)
- elif act_fn == "mish":
- self.act = nn.Mish()
- elif act_fn == "silu":
- self.act = nn.SiLU()
- elif act_fn == "gelu":
- self.act = nn.GELU()
-
- self.linear = nn.Linear(embedding_dim, out_dim * 2)
-
- def forward(self, x, emb):
- if self.act:
- emb = self.act(emb)
- emb = self.linear(emb)
- emb = emb[:, :, None, None]
- scale, shift = emb.chunk(2, dim=1)
-
- x = F.group_norm(x, self.num_groups, eps=self.eps)
- x = x * (1 + scale) + shift
- return x
diff --git a/spaces/deepwisdom/MetaGPT/metagpt/learn/skill_loader.py b/spaces/deepwisdom/MetaGPT/metagpt/learn/skill_loader.py
deleted file mode 100644
index 83200bca6fefe528c7e93c18ffb6d5a8da64ac61..0000000000000000000000000000000000000000
--- a/spaces/deepwisdom/MetaGPT/metagpt/learn/skill_loader.py
+++ /dev/null
@@ -1,96 +0,0 @@
-#!/usr/bin/env python
-# -*- coding: utf-8 -*-
-"""
-@Time : 2023/8/18
-@Author : mashenquan
-@File : skill_loader.py
-@Desc : Skill YAML Configuration Loader.
-"""
-from pathlib import Path
-from typing import Dict, List, Optional
-
-import yaml
-from pydantic import BaseModel, Field
-
-from metagpt.config import CONFIG
-
-
-class Example(BaseModel):
- ask: str
- answer: str
-
-
-class Returns(BaseModel):
- type: str
- format: Optional[str] = None
-
-
-class Prerequisite(BaseModel):
- name: str
- type: Optional[str] = None
- description: Optional[str] = None
- default: Optional[str] = None
-
-
-class Skill(BaseModel):
- name: str
- description: str
- id: str
- x_prerequisite: Optional[List[Prerequisite]] = Field(default=None, alias="x-prerequisite")
- arguments: Dict
- examples: List[Example]
- returns: Returns
-
-
-class EntitySkills(BaseModel):
- skills: List[Skill]
-
-
-class SkillsDeclaration(BaseModel):
- entities: Dict[str, EntitySkills]
-
-
-class SkillLoader:
- def __init__(self, skill_yaml_file_name: Path = None):
- if not skill_yaml_file_name:
- skill_yaml_file_name = Path(__file__).parent.parent.parent / ".well-known/skills.yaml"
- with open(str(skill_yaml_file_name), "r") as file:
- skills = yaml.safe_load(file)
- self._skills = SkillsDeclaration(**skills)
-
- def get_skill_list(self, entity_name: str = "Assistant") -> Dict:
- """Return the skill name based on the skill description."""
- entity_skills = self.get_entity(entity_name)
- if not entity_skills:
- return {}
-
- agent_skills = CONFIG.agent_skills
- if not agent_skills:
- return {}
-
- class AgentSkill(BaseModel):
- name: str
-
- names = [AgentSkill(**i).name for i in agent_skills]
- description_to_name_mappings = {}
- for s in entity_skills.skills:
- if s.name not in names:
- continue
- description_to_name_mappings[s.description] = s.name
-
- return description_to_name_mappings
-
- def get_skill(self, name, entity_name: str = "Assistant") -> Skill:
- """Return a skill by name."""
- entity = self.get_entity(entity_name)
- if not entity:
- return None
- for sk in entity.skills:
- if sk.name == name:
- return sk
-
- def get_entity(self, name) -> EntitySkills:
- """Return a list of skills for the entity."""
- if not self._skills:
- return None
- return self._skills.entities.get(name)
diff --git a/spaces/dhanilka/illusion-image-ai/share_btn.py b/spaces/dhanilka/illusion-image-ai/share_btn.py
deleted file mode 100644
index 5d4dc51b883650ed947e7dea90f677d817725198..0000000000000000000000000000000000000000
--- a/spaces/dhanilka/illusion-image-ai/share_btn.py
+++ /dev/null
@@ -1,83 +0,0 @@
-community_icon_html = """
-
-
- """
-
-loading_icon_html = """ """
-
-share_js = """async () => {
- async function uploadFile(file){
- const UPLOAD_URL = 'https://huggingface.co/uploads';
- const response = await fetch(UPLOAD_URL, {
- method: 'POST',
- headers: {
- 'Content-Type': file.type,
- 'X-Requested-With': 'XMLHttpRequest',
- },
- body: file, /// <- File inherits from Blob
- });
- const url = await response.text();
- return url;
- }
-
- async function getInputImgFile(imgEl){
- const res = await fetch(imgEl.src);
- const blob = await res.blob();
- const imgId = Date.now() % 200;
- const isPng = imgEl.src.startsWith(`data:image/png`);
- if(isPng){
- const fileName = `sd-perception-${{imgId}}.png`;
- return new File([blob], fileName, { type: 'image/png' });
- }else{
- const fileName = `sd-perception-${{imgId}}.jpg`;
- return new File([blob], fileName, { type: 'image/jpeg' });
- }
- }
-
- const gradioEl = document.querySelector("gradio-app").shadowRoot || document.querySelector('body > gradio-app');
-
- const inputPrompt = gradioEl.querySelector('#prompt textarea').value;
- const negativePrompt = gradioEl.querySelector('#negative_prompt textarea').value;
- const illusionStrength = gradioEl.querySelector('#illusion_strength input[type="number"]').value;
- const controlImage = gradioEl.querySelector('#control_image img');
- const outputImgEl = gradioEl.querySelector('#output img');
-
- const shareBtnEl = gradioEl.querySelector('#share-btn');
- const shareIconEl = gradioEl.querySelector('#share-btn-share-icon');
- const loadingIconEl = gradioEl.querySelector('#share-btn-loading-icon');
-
- shareBtnEl.style.pointerEvents = 'none';
- shareIconEl.style.display = 'none';
- loadingIconEl.style.removeProperty('display');
-
- const inputFile = await getInputImgFile(outputImgEl);
- const urlInputImg = await uploadFile(inputFile);
-
- const controlFile = await getInputImgFile(controlImage);
- const urlControlImg = await uploadFile(controlFile);
-
- const descriptionMd = `
-### Prompt
-- *Prompt*: ${inputPrompt}
-- *Negative prompt*: ${negativePrompt}
-- *Illusion strength*: ${illusionStrength}
-#### Generated Image:
-
-
-#### Control Image:
-
-`;
- const params = new URLSearchParams({
- title: inputPrompt,
- description: descriptionMd,
- preview: true
- });
- const paramsStr = params.toString();
- window.open(`https://huggingface.co/spaces/AP123/IllusionDiffusion/discussions/new?${paramsStr}`, '_blank');
- shareBtnEl.style.removeProperty('pointer-events');
- shareIconEl.style.removeProperty('display');
- loadingIconEl.style.display = 'none';
-}"""
\ No newline at end of file
diff --git a/spaces/diacanFperku/AutoGPT/Adrian Rogers Longtime Bellevue Pastor And Leader In Conservative ....pdf.md b/spaces/diacanFperku/AutoGPT/Adrian Rogers Longtime Bellevue Pastor And Leader In Conservative ....pdf.md
deleted file mode 100644
index 4afac3828916fd104bd0d3c61c6f751d6b1f4970..0000000000000000000000000000000000000000
--- a/spaces/diacanFperku/AutoGPT/Adrian Rogers Longtime Bellevue Pastor And Leader In Conservative ....pdf.md
+++ /dev/null
@@ -1,6 +0,0 @@
-
-the newly formed coalition on the sanctity of human life was composed of conservative southern baptists who had left the sbc as fundamentalists took control. by the time of the 1990 sbc general conference, the fundamentalists were dominant within the denomination and were intent on their fight to restrict abortion. (2) the newly formed coalition on the sanctity of human life fought with the newly formed family research council (frc). (10) the conflict had three outcomes. the fundamentalists were successful in moving their position on abortion onto the sbc's agenda and eventually to the sbc's 1992 sbc resolution.
-in 1977 the sbc and the southern baptist international ministries (sbim) formed the joint committee on abortion. the committee hoped to move the debate on abortion from a purely moralistic one to one based on scientific evidence. (3) the committee's plan was for the resolution to be the only one that would be considered at the annual meeting. this plan was undercut in april 1980 when the general association of baptists (gab) adopted the first of a number of resolutions which eliminated any reference to abortion. this resolution was based on the views of the association's policy committee, formed in 1978. the committee emphasized an "omni-directional" approach to any discussion of abortion. the committee reasoned that sbc pastors, along with the local churches to which they minister, should be the ones to decide when and where to receive counseling on the issue of abortion. (5) the election of president adrian rogers as the sbc's president in june of that year validated the committee's decision.
-Adrian Rogers, longtime Bellevue pastor and leader in Conservative ....pdf DOWNLOAD »»» https://gohhs.com/2uFUkV
899543212b
-
-
\ No newline at end of file
diff --git a/spaces/diacanFperku/AutoGPT/Hauppauge Wintv V8 Crack Serial.md b/spaces/diacanFperku/AutoGPT/Hauppauge Wintv V8 Crack Serial.md
deleted file mode 100644
index 87b5324ec063186884f4f1c01d7afe29939e9921..0000000000000000000000000000000000000000
--- a/spaces/diacanFperku/AutoGPT/Hauppauge Wintv V8 Crack Serial.md
+++ /dev/null
@@ -1,23 +0,0 @@
-
-How to Download and Install Hauppauge WinTV v8 Crack Serial
-If you are looking for a way to watch TV on your PC, you may have heard of Hauppauge WinTV v8, a software that allows you to use Hauppauge TV tuners to receive and record TV signals. However, you may also know that this software is not free and requires an activation code to work. In this article, I will show you how to download and install Hauppauge WinTV v8 crack serial, a hacked version of the software that bypasses the activation process and lets you use it for free.
-hauppauge wintv v8 crack serial DOWNLOAD ✸ https://gohhs.com/2uFT8f
-What is Hauppauge WinTV v8 Crack Serial?
-Hauppauge WinTV v8 crack serial is a modified version of the original Hauppauge WinTV v8 software that has been cracked by hackers to remove the need for an activation code. This means that you can use it without paying for a license or entering a serial number. However, this also means that you are using an illegal and potentially unsafe software that may contain viruses, malware, or spyware. Therefore, I do not recommend using Hauppauge WinTV v8 crack serial and advise you to purchase the official software from Hauppauge's website instead.
-Where to Download Hauppauge WinTV v8 Crack Serial?
-If you still want to download Hauppauge WinTV v8 crack serial, you can find it on various websites that offer pirated software, such as jaimietretter069go.wixsite.com , neuforcu.yolasite.com , or bitbucket.org . However, these websites are not trustworthy and may contain harmful files or links that can damage your computer or compromise your privacy. Therefore, I do not recommend downloading Hauppauge WinTV v8 crack serial from these sources and advise you to scan any file you download with an antivirus program before opening it.
-How to Install Hauppauge WinTV v8 Crack Serial?
-If you have downloaded Hauppauge WinTV v8 crack serial from one of the websites mentioned above, you can follow these steps to install it on your PC:
-
-
-Unzip the downloaded file and run the setup.exe file.
-Follow the installation wizard and accept the terms and conditions.
-When prompted to enter an activation code, click on "Enter Serial Number" and paste the crack serial number that came with the downloaded file.
-Complete the installation and launch the Hauppauge WinTV v8 software.
-Enjoy watching TV on your PC with Hauppauge WinTV v8 crack serial.
-
-Note: These steps may vary depending on the source of your download and the version of your software. Some versions of Hauppauge WinTV v8 crack serial may require additional steps or files to work properly. Therefore, I do not guarantee that these steps will work for you and advise you to follow them at your own risk.
-Conclusion
-In this article, I have shown you how to download and install Hauppauge WinTV v8 crack serial, a hacked version of the software that allows you to watch TV on your PC without paying for a license or entering an activation code. However, I have also warned you about the risks and drawbacks of using Hauppauge WinTV v8 crack serial, such as legal issues, security threats, and poor performance. Therefore, I do not recommend using Hauppauge WinTV v8 crack serial and advise you to purchase the official software from Hauppauge's website instead.
d5da3c52bf
-
-
\ No newline at end of file
diff --git a/spaces/diagaiwei/ir_chinese_medqa/colbert/distillation/scorer.py b/spaces/diagaiwei/ir_chinese_medqa/colbert/distillation/scorer.py
deleted file mode 100644
index 2e7975cad453b33c6b0283aef38bad6fd92d7dc6..0000000000000000000000000000000000000000
--- a/spaces/diagaiwei/ir_chinese_medqa/colbert/distillation/scorer.py
+++ /dev/null
@@ -1,68 +0,0 @@
-import torch
-import tqdm
-
-from transformers import AutoTokenizer, AutoModelForSequenceClassification
-
-from colbert.infra.launcher import Launcher
-from colbert.infra import Run, RunConfig
-from colbert.modeling.reranker.electra import ElectraReranker
-from colbert.utils.utils import flatten
-
-
-DEFAULT_MODEL = 'cross-encoder/ms-marco-MiniLM-L-6-v2'
-
-
-class Scorer:
- def __init__(self, queries, collection, model=DEFAULT_MODEL, maxlen=180, bsize=256):
- self.queries = queries
- self.collection = collection
- self.model = model
-
- self.maxlen = maxlen
- self.bsize = bsize
-
- def launch(self, qids, pids):
- launcher = Launcher(self._score_pairs_process, return_all=True)
- outputs = launcher.launch(Run().config, qids, pids)
-
- return flatten(outputs)
-
- def _score_pairs_process(self, config, qids, pids):
- assert len(qids) == len(pids), (len(qids), len(pids))
- share = 1 + len(qids) // config.nranks
- offset = config.rank * share
- endpos = (1 + config.rank) * share
-
- return self._score_pairs(qids[offset:endpos], pids[offset:endpos], show_progress=(config.rank < 1))
-
- def _score_pairs(self, qids, pids, show_progress=False):
- tokenizer = AutoTokenizer.from_pretrained(self.model)
- model = AutoModelForSequenceClassification.from_pretrained(self.model).cuda()
-
- assert len(qids) == len(pids), (len(qids), len(pids))
-
- scores = []
-
- model.eval()
- with torch.inference_mode():
- with torch.cuda.amp.autocast():
- for offset in tqdm.tqdm(range(0, len(qids), self.bsize), disable=(not show_progress)):
- endpos = offset + self.bsize
-
- queries_ = [self.queries[qid] for qid in qids[offset:endpos]]
- passages_ = [self.collection[pid] for pid in pids[offset:endpos]]
-
- features = tokenizer(queries_, passages_, padding='longest', truncation=True,
- return_tensors='pt', max_length=self.maxlen).to(model.device)
-
- scores.append(model(**features).logits.flatten())
-
- scores = torch.cat(scores)
- scores = scores.tolist()
-
- Run().print(f'Returning with {len(scores)} scores')
-
- return scores
-
-
-# LONG-TERM TODO: This can be sped up by sorting by length in advance.
diff --git a/spaces/digitalxingtong/Azuma-Bert-VITS2/monotonic_align/__init__.py b/spaces/digitalxingtong/Azuma-Bert-VITS2/monotonic_align/__init__.py
deleted file mode 100644
index a323673bb16070d6d0fffddb939b657d0915ff1b..0000000000000000000000000000000000000000
--- a/spaces/digitalxingtong/Azuma-Bert-VITS2/monotonic_align/__init__.py
+++ /dev/null
@@ -1,20 +0,0 @@
-from numpy import zeros, int32, float32
-from torch import from_numpy
-
-from .core import maximum_path_jit
-
-
-def maximum_path(neg_cent, mask):
- """ numba optimized version.
- neg_cent: [b, t_t, t_s]
- mask: [b, t_t, t_s]
- """
- device = neg_cent.device
- dtype = neg_cent.dtype
- neg_cent = neg_cent.data.cpu().numpy().astype(float32)
- path = zeros(neg_cent.shape, dtype=int32)
-
- t_t_max = mask.sum(1)[:, 0].data.cpu().numpy().astype(int32)
- t_s_max = mask.sum(2)[:, 0].data.cpu().numpy().astype(int32)
- maximum_path_jit(path, neg_cent, t_t_max, t_s_max)
- return from_numpy(path).to(device=device, dtype=dtype)
\ No newline at end of file
diff --git a/spaces/dineshreddy/WALT/mmdet/datasets/pipelines/auto_augment.py b/spaces/dineshreddy/WALT/mmdet/datasets/pipelines/auto_augment.py
deleted file mode 100644
index e19adaec18a96cac4dbe1d8c2c9193e9901be1fb..0000000000000000000000000000000000000000
--- a/spaces/dineshreddy/WALT/mmdet/datasets/pipelines/auto_augment.py
+++ /dev/null
@@ -1,890 +0,0 @@
-import copy
-
-import cv2
-import mmcv
-import numpy as np
-
-from ..builder import PIPELINES
-from .compose import Compose
-
-_MAX_LEVEL = 10
-
-
-def level_to_value(level, max_value):
- """Map from level to values based on max_value."""
- return (level / _MAX_LEVEL) * max_value
-
-
-def enhance_level_to_value(level, a=1.8, b=0.1):
- """Map from level to values."""
- return (level / _MAX_LEVEL) * a + b
-
-
-def random_negative(value, random_negative_prob):
- """Randomly negate value based on random_negative_prob."""
- return -value if np.random.rand() < random_negative_prob else value
-
-
-def bbox2fields():
- """The key correspondence from bboxes to labels, masks and
- segmentations."""
- bbox2label = {
- 'gt_bboxes': 'gt_labels',
- 'gt_bboxes_ignore': 'gt_labels_ignore'
- }
- bbox2mask = {
- 'gt_bboxes': 'gt_masks',
- 'gt_bboxes_ignore': 'gt_masks_ignore'
- }
- bbox2seg = {
- 'gt_bboxes': 'gt_semantic_seg',
- }
- return bbox2label, bbox2mask, bbox2seg
-
-
-@PIPELINES.register_module()
-class AutoAugment(object):
- """Auto augmentation.
-
- This data augmentation is proposed in `Learning Data Augmentation
- Strategies for Object Detection `_.
-
- TODO: Implement 'Shear', 'Sharpness' and 'Rotate' transforms
-
- Args:
- policies (list[list[dict]]): The policies of auto augmentation. Each
- policy in ``policies`` is a specific augmentation policy, and is
- composed by several augmentations (dict). When AutoAugment is
- called, a random policy in ``policies`` will be selected to
- augment images.
-
- Examples:
- >>> replace = (104, 116, 124)
- >>> policies = [
- >>> [
- >>> dict(type='Sharpness', prob=0.0, level=8),
- >>> dict(
- >>> type='Shear',
- >>> prob=0.4,
- >>> level=0,
- >>> replace=replace,
- >>> axis='x')
- >>> ],
- >>> [
- >>> dict(
- >>> type='Rotate',
- >>> prob=0.6,
- >>> level=10,
- >>> replace=replace),
- >>> dict(type='Color', prob=1.0, level=6)
- >>> ]
- >>> ]
- >>> augmentation = AutoAugment(policies)
- >>> img = np.ones(100, 100, 3)
- >>> gt_bboxes = np.ones(10, 4)
- >>> results = dict(img=img, gt_bboxes=gt_bboxes)
- >>> results = augmentation(results)
- """
-
- def __init__(self, policies):
- assert isinstance(policies, list) and len(policies) > 0, \
- 'Policies must be a non-empty list.'
- for policy in policies:
- assert isinstance(policy, list) and len(policy) > 0, \
- 'Each policy in policies must be a non-empty list.'
- for augment in policy:
- assert isinstance(augment, dict) and 'type' in augment, \
- 'Each specific augmentation must be a dict with key' \
- ' "type".'
-
- self.policies = copy.deepcopy(policies)
- self.transforms = [Compose(policy) for policy in self.policies]
-
- def __call__(self, results):
- transform = np.random.choice(self.transforms)
- return transform(results)
-
- def __repr__(self):
- return f'{self.__class__.__name__}(policies={self.policies})'
-
-
-@PIPELINES.register_module()
-class Shear(object):
- """Apply Shear Transformation to image (and its corresponding bbox, mask,
- segmentation).
-
- Args:
- level (int | float): The level should be in range [0,_MAX_LEVEL].
- img_fill_val (int | float | tuple): The filled values for image border.
- If float, the same fill value will be used for all the three
- channels of image. If tuple, the should be 3 elements.
- seg_ignore_label (int): The fill value used for segmentation map.
- Note this value must equals ``ignore_label`` in ``semantic_head``
- of the corresponding config. Default 255.
- prob (float): The probability for performing Shear and should be in
- range [0, 1].
- direction (str): The direction for shear, either "horizontal"
- or "vertical".
- max_shear_magnitude (float): The maximum magnitude for Shear
- transformation.
- random_negative_prob (float): The probability that turns the
- offset negative. Should be in range [0,1]
- interpolation (str): Same as in :func:`mmcv.imshear`.
- """
-
- def __init__(self,
- level,
- img_fill_val=128,
- seg_ignore_label=255,
- prob=0.5,
- direction='horizontal',
- max_shear_magnitude=0.3,
- random_negative_prob=0.5,
- interpolation='bilinear'):
- assert isinstance(level, (int, float)), 'The level must be type ' \
- f'int or float, got {type(level)}.'
- assert 0 <= level <= _MAX_LEVEL, 'The level should be in range ' \
- f'[0,{_MAX_LEVEL}], got {level}.'
- if isinstance(img_fill_val, (float, int)):
- img_fill_val = tuple([float(img_fill_val)] * 3)
- elif isinstance(img_fill_val, tuple):
- assert len(img_fill_val) == 3, 'img_fill_val as tuple must ' \
- f'have 3 elements. got {len(img_fill_val)}.'
- img_fill_val = tuple([float(val) for val in img_fill_val])
- else:
- raise ValueError(
- 'img_fill_val must be float or tuple with 3 elements.')
- assert np.all([0 <= val <= 255 for val in img_fill_val]), 'all ' \
- 'elements of img_fill_val should between range [0,255].' \
- f'got {img_fill_val}.'
- assert 0 <= prob <= 1.0, 'The probability of shear should be in ' \
- f'range [0,1]. got {prob}.'
- assert direction in ('horizontal', 'vertical'), 'direction must ' \
- f'in be either "horizontal" or "vertical". got {direction}.'
- assert isinstance(max_shear_magnitude, float), 'max_shear_magnitude ' \
- f'should be type float. got {type(max_shear_magnitude)}.'
- assert 0. <= max_shear_magnitude <= 1., 'Defaultly ' \
- 'max_shear_magnitude should be in range [0,1]. ' \
- f'got {max_shear_magnitude}.'
- self.level = level
- self.magnitude = level_to_value(level, max_shear_magnitude)
- self.img_fill_val = img_fill_val
- self.seg_ignore_label = seg_ignore_label
- self.prob = prob
- self.direction = direction
- self.max_shear_magnitude = max_shear_magnitude
- self.random_negative_prob = random_negative_prob
- self.interpolation = interpolation
-
- def _shear_img(self,
- results,
- magnitude,
- direction='horizontal',
- interpolation='bilinear'):
- """Shear the image.
-
- Args:
- results (dict): Result dict from loading pipeline.
- magnitude (int | float): The magnitude used for shear.
- direction (str): The direction for shear, either "horizontal"
- or "vertical".
- interpolation (str): Same as in :func:`mmcv.imshear`.
- """
- for key in results.get('img_fields', ['img']):
- img = results[key]
- img_sheared = mmcv.imshear(
- img,
- magnitude,
- direction,
- border_value=self.img_fill_val,
- interpolation=interpolation)
- results[key] = img_sheared.astype(img.dtype)
-
- def _shear_bboxes(self, results, magnitude):
- """Shear the bboxes."""
- h, w, c = results['img_shape']
- if self.direction == 'horizontal':
- shear_matrix = np.stack([[1, magnitude],
- [0, 1]]).astype(np.float32) # [2, 2]
- else:
- shear_matrix = np.stack([[1, 0], [magnitude,
- 1]]).astype(np.float32)
- for key in results.get('bbox_fields', []):
- min_x, min_y, max_x, max_y = np.split(
- results[key], results[key].shape[-1], axis=-1)
- coordinates = np.stack([[min_x, min_y], [max_x, min_y],
- [min_x, max_y],
- [max_x, max_y]]) # [4, 2, nb_box, 1]
- coordinates = coordinates[..., 0].transpose(
- (2, 1, 0)).astype(np.float32) # [nb_box, 2, 4]
- new_coords = np.matmul(shear_matrix[None, :, :],
- coordinates) # [nb_box, 2, 4]
- min_x = np.min(new_coords[:, 0, :], axis=-1)
- min_y = np.min(new_coords[:, 1, :], axis=-1)
- max_x = np.max(new_coords[:, 0, :], axis=-1)
- max_y = np.max(new_coords[:, 1, :], axis=-1)
- min_x = np.clip(min_x, a_min=0, a_max=w)
- min_y = np.clip(min_y, a_min=0, a_max=h)
- max_x = np.clip(max_x, a_min=min_x, a_max=w)
- max_y = np.clip(max_y, a_min=min_y, a_max=h)
- results[key] = np.stack([min_x, min_y, max_x, max_y],
- axis=-1).astype(results[key].dtype)
-
- def _shear_masks(self,
- results,
- magnitude,
- direction='horizontal',
- fill_val=0,
- interpolation='bilinear'):
- """Shear the masks."""
- h, w, c = results['img_shape']
- for key in results.get('mask_fields', []):
- masks = results[key]
- results[key] = masks.shear((h, w),
- magnitude,
- direction,
- border_value=fill_val,
- interpolation=interpolation)
-
- def _shear_seg(self,
- results,
- magnitude,
- direction='horizontal',
- fill_val=255,
- interpolation='bilinear'):
- """Shear the segmentation maps."""
- for key in results.get('seg_fields', []):
- seg = results[key]
- results[key] = mmcv.imshear(
- seg,
- magnitude,
- direction,
- border_value=fill_val,
- interpolation=interpolation).astype(seg.dtype)
-
- def _filter_invalid(self, results, min_bbox_size=0):
- """Filter bboxes and corresponding masks too small after shear
- augmentation."""
- bbox2label, bbox2mask, _ = bbox2fields()
- for key in results.get('bbox_fields', []):
- bbox_w = results[key][:, 2] - results[key][:, 0]
- bbox_h = results[key][:, 3] - results[key][:, 1]
- valid_inds = (bbox_w > min_bbox_size) & (bbox_h > min_bbox_size)
- valid_inds = np.nonzero(valid_inds)[0]
- results[key] = results[key][valid_inds]
- # label fields. e.g. gt_labels and gt_labels_ignore
- label_key = bbox2label.get(key)
- if label_key in results:
- results[label_key] = results[label_key][valid_inds]
- # mask fields, e.g. gt_masks and gt_masks_ignore
- mask_key = bbox2mask.get(key)
- if mask_key in results:
- results[mask_key] = results[mask_key][valid_inds]
-
- def __call__(self, results):
- """Call function to shear images, bounding boxes, masks and semantic
- segmentation maps.
-
- Args:
- results (dict): Result dict from loading pipeline.
-
- Returns:
- dict: Sheared results.
- """
- if np.random.rand() > self.prob:
- return results
- magnitude = random_negative(self.magnitude, self.random_negative_prob)
- self._shear_img(results, magnitude, self.direction, self.interpolation)
- self._shear_bboxes(results, magnitude)
- # fill_val set to 0 for background of mask.
- self._shear_masks(
- results,
- magnitude,
- self.direction,
- fill_val=0,
- interpolation=self.interpolation)
- self._shear_seg(
- results,
- magnitude,
- self.direction,
- fill_val=self.seg_ignore_label,
- interpolation=self.interpolation)
- self._filter_invalid(results)
- return results
-
- def __repr__(self):
- repr_str = self.__class__.__name__
- repr_str += f'(level={self.level}, '
- repr_str += f'img_fill_val={self.img_fill_val}, '
- repr_str += f'seg_ignore_label={self.seg_ignore_label}, '
- repr_str += f'prob={self.prob}, '
- repr_str += f'direction={self.direction}, '
- repr_str += f'max_shear_magnitude={self.max_shear_magnitude}, '
- repr_str += f'random_negative_prob={self.random_negative_prob}, '
- repr_str += f'interpolation={self.interpolation})'
- return repr_str
-
-
-@PIPELINES.register_module()
-class Rotate(object):
- """Apply Rotate Transformation to image (and its corresponding bbox, mask,
- segmentation).
-
- Args:
- level (int | float): The level should be in range (0,_MAX_LEVEL].
- scale (int | float): Isotropic scale factor. Same in
- ``mmcv.imrotate``.
- center (int | float | tuple[float]): Center point (w, h) of the
- rotation in the source image. If None, the center of the
- image will be used. Same in ``mmcv.imrotate``.
- img_fill_val (int | float | tuple): The fill value for image border.
- If float, the same value will be used for all the three
- channels of image. If tuple, the should be 3 elements (e.g.
- equals the number of channels for image).
- seg_ignore_label (int): The fill value used for segmentation map.
- Note this value must equals ``ignore_label`` in ``semantic_head``
- of the corresponding config. Default 255.
- prob (float): The probability for perform transformation and
- should be in range 0 to 1.
- max_rotate_angle (int | float): The maximum angles for rotate
- transformation.
- random_negative_prob (float): The probability that turns the
- offset negative.
- """
-
- def __init__(self,
- level,
- scale=1,
- center=None,
- img_fill_val=128,
- seg_ignore_label=255,
- prob=0.5,
- max_rotate_angle=30,
- random_negative_prob=0.5):
- assert isinstance(level, (int, float)), \
- f'The level must be type int or float. got {type(level)}.'
- assert 0 <= level <= _MAX_LEVEL, \
- f'The level should be in range (0,{_MAX_LEVEL}]. got {level}.'
- assert isinstance(scale, (int, float)), \
- f'The scale must be type int or float. got type {type(scale)}.'
- if isinstance(center, (int, float)):
- center = (center, center)
- elif isinstance(center, tuple):
- assert len(center) == 2, 'center with type tuple must have '\
- f'2 elements. got {len(center)} elements.'
- else:
- assert center is None, 'center must be None or type int, '\
- f'float or tuple, got type {type(center)}.'
- if isinstance(img_fill_val, (float, int)):
- img_fill_val = tuple([float(img_fill_val)] * 3)
- elif isinstance(img_fill_val, tuple):
- assert len(img_fill_val) == 3, 'img_fill_val as tuple must '\
- f'have 3 elements. got {len(img_fill_val)}.'
- img_fill_val = tuple([float(val) for val in img_fill_val])
- else:
- raise ValueError(
- 'img_fill_val must be float or tuple with 3 elements.')
- assert np.all([0 <= val <= 255 for val in img_fill_val]), \
- 'all elements of img_fill_val should between range [0,255]. '\
- f'got {img_fill_val}.'
- assert 0 <= prob <= 1.0, 'The probability should be in range [0,1]. '\
- 'got {prob}.'
- assert isinstance(max_rotate_angle, (int, float)), 'max_rotate_angle '\
- f'should be type int or float. got type {type(max_rotate_angle)}.'
- self.level = level
- self.scale = scale
- # Rotation angle in degrees. Positive values mean
- # clockwise rotation.
- self.angle = level_to_value(level, max_rotate_angle)
- self.center = center
- self.img_fill_val = img_fill_val
- self.seg_ignore_label = seg_ignore_label
- self.prob = prob
- self.max_rotate_angle = max_rotate_angle
- self.random_negative_prob = random_negative_prob
-
- def _rotate_img(self, results, angle, center=None, scale=1.0):
- """Rotate the image.
-
- Args:
- results (dict): Result dict from loading pipeline.
- angle (float): Rotation angle in degrees, positive values
- mean clockwise rotation. Same in ``mmcv.imrotate``.
- center (tuple[float], optional): Center point (w, h) of the
- rotation. Same in ``mmcv.imrotate``.
- scale (int | float): Isotropic scale factor. Same in
- ``mmcv.imrotate``.
- """
- for key in results.get('img_fields', ['img']):
- img = results[key].copy()
- img_rotated = mmcv.imrotate(
- img, angle, center, scale, border_value=self.img_fill_val)
- results[key] = img_rotated.astype(img.dtype)
-
- def _rotate_bboxes(self, results, rotate_matrix):
- """Rotate the bboxes."""
- h, w, c = results['img_shape']
- for key in results.get('bbox_fields', []):
- min_x, min_y, max_x, max_y = np.split(
- results[key], results[key].shape[-1], axis=-1)
- coordinates = np.stack([[min_x, min_y], [max_x, min_y],
- [min_x, max_y],
- [max_x, max_y]]) # [4, 2, nb_bbox, 1]
- # pad 1 to convert from format [x, y] to homogeneous
- # coordinates format [x, y, 1]
- coordinates = np.concatenate(
- (coordinates,
- np.ones((4, 1, coordinates.shape[2], 1), coordinates.dtype)),
- axis=1) # [4, 3, nb_bbox, 1]
- coordinates = coordinates.transpose(
- (2, 0, 1, 3)) # [nb_bbox, 4, 3, 1]
- rotated_coords = np.matmul(rotate_matrix,
- coordinates) # [nb_bbox, 4, 2, 1]
- rotated_coords = rotated_coords[..., 0] # [nb_bbox, 4, 2]
- min_x, min_y = np.min(
- rotated_coords[:, :, 0], axis=1), np.min(
- rotated_coords[:, :, 1], axis=1)
- max_x, max_y = np.max(
- rotated_coords[:, :, 0], axis=1), np.max(
- rotated_coords[:, :, 1], axis=1)
- min_x, min_y = np.clip(
- min_x, a_min=0, a_max=w), np.clip(
- min_y, a_min=0, a_max=h)
- max_x, max_y = np.clip(
- max_x, a_min=min_x, a_max=w), np.clip(
- max_y, a_min=min_y, a_max=h)
- results[key] = np.stack([min_x, min_y, max_x, max_y],
- axis=-1).astype(results[key].dtype)
-
- def _rotate_masks(self,
- results,
- angle,
- center=None,
- scale=1.0,
- fill_val=0):
- """Rotate the masks."""
- h, w, c = results['img_shape']
- for key in results.get('mask_fields', []):
- masks = results[key]
- results[key] = masks.rotate((h, w), angle, center, scale, fill_val)
-
- def _rotate_seg(self,
- results,
- angle,
- center=None,
- scale=1.0,
- fill_val=255):
- """Rotate the segmentation map."""
- for key in results.get('seg_fields', []):
- seg = results[key].copy()
- results[key] = mmcv.imrotate(
- seg, angle, center, scale,
- border_value=fill_val).astype(seg.dtype)
-
- def _filter_invalid(self, results, min_bbox_size=0):
- """Filter bboxes and corresponding masks too small after rotate
- augmentation."""
- bbox2label, bbox2mask, _ = bbox2fields()
- for key in results.get('bbox_fields', []):
- bbox_w = results[key][:, 2] - results[key][:, 0]
- bbox_h = results[key][:, 3] - results[key][:, 1]
- valid_inds = (bbox_w > min_bbox_size) & (bbox_h > min_bbox_size)
- valid_inds = np.nonzero(valid_inds)[0]
- results[key] = results[key][valid_inds]
- # label fields. e.g. gt_labels and gt_labels_ignore
- label_key = bbox2label.get(key)
- if label_key in results:
- results[label_key] = results[label_key][valid_inds]
- # mask fields, e.g. gt_masks and gt_masks_ignore
- mask_key = bbox2mask.get(key)
- if mask_key in results:
- results[mask_key] = results[mask_key][valid_inds]
-
- def __call__(self, results):
- """Call function to rotate images, bounding boxes, masks and semantic
- segmentation maps.
-
- Args:
- results (dict): Result dict from loading pipeline.
-
- Returns:
- dict: Rotated results.
- """
- if np.random.rand() > self.prob:
- return results
- h, w = results['img'].shape[:2]
- center = self.center
- if center is None:
- center = ((w - 1) * 0.5, (h - 1) * 0.5)
- angle = random_negative(self.angle, self.random_negative_prob)
- self._rotate_img(results, angle, center, self.scale)
- rotate_matrix = cv2.getRotationMatrix2D(center, -angle, self.scale)
- self._rotate_bboxes(results, rotate_matrix)
- self._rotate_masks(results, angle, center, self.scale, fill_val=0)
- self._rotate_seg(
- results, angle, center, self.scale, fill_val=self.seg_ignore_label)
- self._filter_invalid(results)
- return results
-
- def __repr__(self):
- repr_str = self.__class__.__name__
- repr_str += f'(level={self.level}, '
- repr_str += f'scale={self.scale}, '
- repr_str += f'center={self.center}, '
- repr_str += f'img_fill_val={self.img_fill_val}, '
- repr_str += f'seg_ignore_label={self.seg_ignore_label}, '
- repr_str += f'prob={self.prob}, '
- repr_str += f'max_rotate_angle={self.max_rotate_angle}, '
- repr_str += f'random_negative_prob={self.random_negative_prob})'
- return repr_str
-
-
-@PIPELINES.register_module()
-class Translate(object):
- """Translate the images, bboxes, masks and segmentation maps horizontally
- or vertically.
-
- Args:
- level (int | float): The level for Translate and should be in
- range [0,_MAX_LEVEL].
- prob (float): The probability for performing translation and
- should be in range [0, 1].
- img_fill_val (int | float | tuple): The filled value for image
- border. If float, the same fill value will be used for all
- the three channels of image. If tuple, the should be 3
- elements (e.g. equals the number of channels for image).
- seg_ignore_label (int): The fill value used for segmentation map.
- Note this value must equals ``ignore_label`` in ``semantic_head``
- of the corresponding config. Default 255.
- direction (str): The translate direction, either "horizontal"
- or "vertical".
- max_translate_offset (int | float): The maximum pixel's offset for
- Translate.
- random_negative_prob (float): The probability that turns the
- offset negative.
- min_size (int | float): The minimum pixel for filtering
- invalid bboxes after the translation.
- """
-
- def __init__(self,
- level,
- prob=0.5,
- img_fill_val=128,
- seg_ignore_label=255,
- direction='horizontal',
- max_translate_offset=250.,
- random_negative_prob=0.5,
- min_size=0):
- assert isinstance(level, (int, float)), \
- 'The level must be type int or float.'
- assert 0 <= level <= _MAX_LEVEL, \
- 'The level used for calculating Translate\'s offset should be ' \
- 'in range [0,_MAX_LEVEL]'
- assert 0 <= prob <= 1.0, \
- 'The probability of translation should be in range [0, 1].'
- if isinstance(img_fill_val, (float, int)):
- img_fill_val = tuple([float(img_fill_val)] * 3)
- elif isinstance(img_fill_val, tuple):
- assert len(img_fill_val) == 3, \
- 'img_fill_val as tuple must have 3 elements.'
- img_fill_val = tuple([float(val) for val in img_fill_val])
- else:
- raise ValueError('img_fill_val must be type float or tuple.')
- assert np.all([0 <= val <= 255 for val in img_fill_val]), \
- 'all elements of img_fill_val should between range [0,255].'
- assert direction in ('horizontal', 'vertical'), \
- 'direction should be "horizontal" or "vertical".'
- assert isinstance(max_translate_offset, (int, float)), \
- 'The max_translate_offset must be type int or float.'
- # the offset used for translation
- self.offset = int(level_to_value(level, max_translate_offset))
- self.level = level
- self.prob = prob
- self.img_fill_val = img_fill_val
- self.seg_ignore_label = seg_ignore_label
- self.direction = direction
- self.max_translate_offset = max_translate_offset
- self.random_negative_prob = random_negative_prob
- self.min_size = min_size
-
- def _translate_img(self, results, offset, direction='horizontal'):
- """Translate the image.
-
- Args:
- results (dict): Result dict from loading pipeline.
- offset (int | float): The offset for translate.
- direction (str): The translate direction, either "horizontal"
- or "vertical".
- """
- for key in results.get('img_fields', ['img']):
- img = results[key].copy()
- results[key] = mmcv.imtranslate(
- img, offset, direction, self.img_fill_val).astype(img.dtype)
-
- def _translate_bboxes(self, results, offset):
- """Shift bboxes horizontally or vertically, according to offset."""
- h, w, c = results['img_shape']
- for key in results.get('bbox_fields', []):
- min_x, min_y, max_x, max_y = np.split(
- results[key], results[key].shape[-1], axis=-1)
- if self.direction == 'horizontal':
- min_x = np.maximum(0, min_x + offset)
- max_x = np.minimum(w, max_x + offset)
- elif self.direction == 'vertical':
- min_y = np.maximum(0, min_y + offset)
- max_y = np.minimum(h, max_y + offset)
-
- # the boxes translated outside of image will be filtered along with
- # the corresponding masks, by invoking ``_filter_invalid``.
- results[key] = np.concatenate([min_x, min_y, max_x, max_y],
- axis=-1)
-
- def _translate_masks(self,
- results,
- offset,
- direction='horizontal',
- fill_val=0):
- """Translate masks horizontally or vertically."""
- h, w, c = results['img_shape']
- for key in results.get('mask_fields', []):
- masks = results[key]
- results[key] = masks.translate((h, w), offset, direction, fill_val)
-
- def _translate_seg(self,
- results,
- offset,
- direction='horizontal',
- fill_val=255):
- """Translate segmentation maps horizontally or vertically."""
- for key in results.get('seg_fields', []):
- seg = results[key].copy()
- results[key] = mmcv.imtranslate(seg, offset, direction,
- fill_val).astype(seg.dtype)
-
- def _filter_invalid(self, results, min_size=0):
- """Filter bboxes and masks too small or translated out of image."""
- bbox2label, bbox2mask, _ = bbox2fields()
- for key in results.get('bbox_fields', []):
- bbox_w = results[key][:, 2] - results[key][:, 0]
- bbox_h = results[key][:, 3] - results[key][:, 1]
- valid_inds = (bbox_w > min_size) & (bbox_h > min_size)
- valid_inds = np.nonzero(valid_inds)[0]
- results[key] = results[key][valid_inds]
- # label fields. e.g. gt_labels and gt_labels_ignore
- label_key = bbox2label.get(key)
- if label_key in results:
- results[label_key] = results[label_key][valid_inds]
- # mask fields, e.g. gt_masks and gt_masks_ignore
- mask_key = bbox2mask.get(key)
- if mask_key in results:
- results[mask_key] = results[mask_key][valid_inds]
- return results
-
- def __call__(self, results):
- """Call function to translate images, bounding boxes, masks and
- semantic segmentation maps.
-
- Args:
- results (dict): Result dict from loading pipeline.
-
- Returns:
- dict: Translated results.
- """
- if np.random.rand() > self.prob:
- return results
- offset = random_negative(self.offset, self.random_negative_prob)
- self._translate_img(results, offset, self.direction)
- self._translate_bboxes(results, offset)
- # fill_val defaultly 0 for BitmapMasks and None for PolygonMasks.
- self._translate_masks(results, offset, self.direction)
- # fill_val set to ``seg_ignore_label`` for the ignored value
- # of segmentation map.
- self._translate_seg(
- results, offset, self.direction, fill_val=self.seg_ignore_label)
- self._filter_invalid(results, min_size=self.min_size)
- return results
-
-
-@PIPELINES.register_module()
-class ColorTransform(object):
- """Apply Color transformation to image. The bboxes, masks, and
- segmentations are not modified.
-
- Args:
- level (int | float): Should be in range [0,_MAX_LEVEL].
- prob (float): The probability for performing Color transformation.
- """
-
- def __init__(self, level, prob=0.5):
- assert isinstance(level, (int, float)), \
- 'The level must be type int or float.'
- assert 0 <= level <= _MAX_LEVEL, \
- 'The level should be in range [0,_MAX_LEVEL].'
- assert 0 <= prob <= 1.0, \
- 'The probability should be in range [0,1].'
- self.level = level
- self.prob = prob
- self.factor = enhance_level_to_value(level)
-
- def _adjust_color_img(self, results, factor=1.0):
- """Apply Color transformation to image."""
- for key in results.get('img_fields', ['img']):
- # NOTE defaultly the image should be BGR format
- img = results[key]
- results[key] = mmcv.adjust_color(img, factor).astype(img.dtype)
-
- def __call__(self, results):
- """Call function for Color transformation.
-
- Args:
- results (dict): Result dict from loading pipeline.
-
- Returns:
- dict: Colored results.
- """
- if np.random.rand() > self.prob:
- return results
- self._adjust_color_img(results, self.factor)
- return results
-
- def __repr__(self):
- repr_str = self.__class__.__name__
- repr_str += f'(level={self.level}, '
- repr_str += f'prob={self.prob})'
- return repr_str
-
-
-@PIPELINES.register_module()
-class EqualizeTransform(object):
- """Apply Equalize transformation to image. The bboxes, masks and
- segmentations are not modified.
-
- Args:
- prob (float): The probability for performing Equalize transformation.
- """
-
- def __init__(self, prob=0.5):
- assert 0 <= prob <= 1.0, \
- 'The probability should be in range [0,1].'
- self.prob = prob
-
- def _imequalize(self, results):
- """Equalizes the histogram of one image."""
- for key in results.get('img_fields', ['img']):
- img = results[key]
- results[key] = mmcv.imequalize(img).astype(img.dtype)
-
- def __call__(self, results):
- """Call function for Equalize transformation.
-
- Args:
- results (dict): Results dict from loading pipeline.
-
- Returns:
- dict: Results after the transformation.
- """
- if np.random.rand() > self.prob:
- return results
- self._imequalize(results)
- return results
-
- def __repr__(self):
- repr_str = self.__class__.__name__
- repr_str += f'(prob={self.prob})'
-
-
-@PIPELINES.register_module()
-class BrightnessTransform(object):
- """Apply Brightness transformation to image. The bboxes, masks and
- segmentations are not modified.
-
- Args:
- level (int | float): Should be in range [0,_MAX_LEVEL].
- prob (float): The probability for performing Brightness transformation.
- """
-
- def __init__(self, level, prob=0.5):
- assert isinstance(level, (int, float)), \
- 'The level must be type int or float.'
- assert 0 <= level <= _MAX_LEVEL, \
- 'The level should be in range [0,_MAX_LEVEL].'
- assert 0 <= prob <= 1.0, \
- 'The probability should be in range [0,1].'
- self.level = level
- self.prob = prob
- self.factor = enhance_level_to_value(level)
-
- def _adjust_brightness_img(self, results, factor=1.0):
- """Adjust the brightness of image."""
- for key in results.get('img_fields', ['img']):
- img = results[key]
- results[key] = mmcv.adjust_brightness(img,
- factor).astype(img.dtype)
-
- def __call__(self, results):
- """Call function for Brightness transformation.
-
- Args:
- results (dict): Results dict from loading pipeline.
-
- Returns:
- dict: Results after the transformation.
- """
- if np.random.rand() > self.prob:
- return results
- self._adjust_brightness_img(results, self.factor)
- return results
-
- def __repr__(self):
- repr_str = self.__class__.__name__
- repr_str += f'(level={self.level}, '
- repr_str += f'prob={self.prob})'
- return repr_str
-
-
-@PIPELINES.register_module()
-class ContrastTransform(object):
- """Apply Contrast transformation to image. The bboxes, masks and
- segmentations are not modified.
-
- Args:
- level (int | float): Should be in range [0,_MAX_LEVEL].
- prob (float): The probability for performing Contrast transformation.
- """
-
- def __init__(self, level, prob=0.5):
- assert isinstance(level, (int, float)), \
- 'The level must be type int or float.'
- assert 0 <= level <= _MAX_LEVEL, \
- 'The level should be in range [0,_MAX_LEVEL].'
- assert 0 <= prob <= 1.0, \
- 'The probability should be in range [0,1].'
- self.level = level
- self.prob = prob
- self.factor = enhance_level_to_value(level)
-
- def _adjust_contrast_img(self, results, factor=1.0):
- """Adjust the image contrast."""
- for key in results.get('img_fields', ['img']):
- img = results[key]
- results[key] = mmcv.adjust_contrast(img, factor).astype(img.dtype)
-
- def __call__(self, results):
- """Call function for Contrast transformation.
-
- Args:
- results (dict): Results dict from loading pipeline.
-
- Returns:
- dict: Results after the transformation.
- """
- if np.random.rand() > self.prob:
- return results
- self._adjust_contrast_img(results, self.factor)
- return results
-
- def __repr__(self):
- repr_str = self.__class__.__name__
- repr_str += f'(level={self.level}, '
- repr_str += f'prob={self.prob})'
- return repr_str
diff --git a/spaces/dineshreddy/WALT/mmdet/models/losses/ghm_loss.py b/spaces/dineshreddy/WALT/mmdet/models/losses/ghm_loss.py
deleted file mode 100644
index 8969a23fd98bb746415f96ac5e4ad9e37ba3af52..0000000000000000000000000000000000000000
--- a/spaces/dineshreddy/WALT/mmdet/models/losses/ghm_loss.py
+++ /dev/null
@@ -1,172 +0,0 @@
-import torch
-import torch.nn as nn
-import torch.nn.functional as F
-
-from ..builder import LOSSES
-
-
-def _expand_onehot_labels(labels, label_weights, label_channels):
- bin_labels = labels.new_full((labels.size(0), label_channels), 0)
- inds = torch.nonzero(
- (labels >= 0) & (labels < label_channels), as_tuple=False).squeeze()
- if inds.numel() > 0:
- bin_labels[inds, labels[inds]] = 1
- bin_label_weights = label_weights.view(-1, 1).expand(
- label_weights.size(0), label_channels)
- return bin_labels, bin_label_weights
-
-
-# TODO: code refactoring to make it consistent with other losses
-@LOSSES.register_module()
-class GHMC(nn.Module):
- """GHM Classification Loss.
-
- Details of the theorem can be viewed in the paper
- `Gradient Harmonized Single-stage Detector
- `_.
-
- Args:
- bins (int): Number of the unit regions for distribution calculation.
- momentum (float): The parameter for moving average.
- use_sigmoid (bool): Can only be true for BCE based loss now.
- loss_weight (float): The weight of the total GHM-C loss.
- """
-
- def __init__(self, bins=10, momentum=0, use_sigmoid=True, loss_weight=1.0):
- super(GHMC, self).__init__()
- self.bins = bins
- self.momentum = momentum
- edges = torch.arange(bins + 1).float() / bins
- self.register_buffer('edges', edges)
- self.edges[-1] += 1e-6
- if momentum > 0:
- acc_sum = torch.zeros(bins)
- self.register_buffer('acc_sum', acc_sum)
- self.use_sigmoid = use_sigmoid
- if not self.use_sigmoid:
- raise NotImplementedError
- self.loss_weight = loss_weight
-
- def forward(self, pred, target, label_weight, *args, **kwargs):
- """Calculate the GHM-C loss.
-
- Args:
- pred (float tensor of size [batch_num, class_num]):
- The direct prediction of classification fc layer.
- target (float tensor of size [batch_num, class_num]):
- Binary class target for each sample.
- label_weight (float tensor of size [batch_num, class_num]):
- the value is 1 if the sample is valid and 0 if ignored.
- Returns:
- The gradient harmonized loss.
- """
- # the target should be binary class label
- if pred.dim() != target.dim():
- target, label_weight = _expand_onehot_labels(
- target, label_weight, pred.size(-1))
- target, label_weight = target.float(), label_weight.float()
- edges = self.edges
- mmt = self.momentum
- weights = torch.zeros_like(pred)
-
- # gradient length
- g = torch.abs(pred.sigmoid().detach() - target)
-
- valid = label_weight > 0
- tot = max(valid.float().sum().item(), 1.0)
- n = 0 # n valid bins
- for i in range(self.bins):
- inds = (g >= edges[i]) & (g < edges[i + 1]) & valid
- num_in_bin = inds.sum().item()
- if num_in_bin > 0:
- if mmt > 0:
- self.acc_sum[i] = mmt * self.acc_sum[i] \
- + (1 - mmt) * num_in_bin
- weights[inds] = tot / self.acc_sum[i]
- else:
- weights[inds] = tot / num_in_bin
- n += 1
- if n > 0:
- weights = weights / n
-
- loss = F.binary_cross_entropy_with_logits(
- pred, target, weights, reduction='sum') / tot
- return loss * self.loss_weight
-
-
-# TODO: code refactoring to make it consistent with other losses
-@LOSSES.register_module()
-class GHMR(nn.Module):
- """GHM Regression Loss.
-
- Details of the theorem can be viewed in the paper
- `Gradient Harmonized Single-stage Detector
- `_.
-
- Args:
- mu (float): The parameter for the Authentic Smooth L1 loss.
- bins (int): Number of the unit regions for distribution calculation.
- momentum (float): The parameter for moving average.
- loss_weight (float): The weight of the total GHM-R loss.
- """
-
- def __init__(self, mu=0.02, bins=10, momentum=0, loss_weight=1.0):
- super(GHMR, self).__init__()
- self.mu = mu
- self.bins = bins
- edges = torch.arange(bins + 1).float() / bins
- self.register_buffer('edges', edges)
- self.edges[-1] = 1e3
- self.momentum = momentum
- if momentum > 0:
- acc_sum = torch.zeros(bins)
- self.register_buffer('acc_sum', acc_sum)
- self.loss_weight = loss_weight
-
- # TODO: support reduction parameter
- def forward(self, pred, target, label_weight, avg_factor=None):
- """Calculate the GHM-R loss.
-
- Args:
- pred (float tensor of size [batch_num, 4 (* class_num)]):
- The prediction of box regression layer. Channel number can be 4
- or 4 * class_num depending on whether it is class-agnostic.
- target (float tensor of size [batch_num, 4 (* class_num)]):
- The target regression values with the same size of pred.
- label_weight (float tensor of size [batch_num, 4 (* class_num)]):
- The weight of each sample, 0 if ignored.
- Returns:
- The gradient harmonized loss.
- """
- mu = self.mu
- edges = self.edges
- mmt = self.momentum
-
- # ASL1 loss
- diff = pred - target
- loss = torch.sqrt(diff * diff + mu * mu) - mu
-
- # gradient length
- g = torch.abs(diff / torch.sqrt(mu * mu + diff * diff)).detach()
- weights = torch.zeros_like(g)
-
- valid = label_weight > 0
- tot = max(label_weight.float().sum().item(), 1.0)
- n = 0 # n: valid bins
- for i in range(self.bins):
- inds = (g >= edges[i]) & (g < edges[i + 1]) & valid
- num_in_bin = inds.sum().item()
- if num_in_bin > 0:
- n += 1
- if mmt > 0:
- self.acc_sum[i] = mmt * self.acc_sum[i] \
- + (1 - mmt) * num_in_bin
- weights[inds] = tot / self.acc_sum[i]
- else:
- weights[inds] = tot / num_in_bin
- if n > 0:
- weights /= n
-
- loss = loss * weights
- loss = loss.sum() / tot
- return loss * self.loss_weight
diff --git a/spaces/dmeck/RVC-Speakers/vits/modules/commons/commons.py b/spaces/dmeck/RVC-Speakers/vits/modules/commons/commons.py
deleted file mode 100644
index 40fcc05364d4815971f5c6f9dbb8dcef8e3ec1e9..0000000000000000000000000000000000000000
--- a/spaces/dmeck/RVC-Speakers/vits/modules/commons/commons.py
+++ /dev/null
@@ -1,172 +0,0 @@
-import math
-import torch
-from torch.nn import functional as F
-import torch.jit
-
-
-def script_method(fn, _rcb=None):
- return fn
-
-
-def script(obj, optimize=True, _frames_up=0, _rcb=None):
- return obj
-
-
-torch.jit.script_method = script_method
-torch.jit.script = script
-
-
-def init_weights(m, mean=0.0, std=0.01):
- classname = m.__class__.__name__
- if classname.find("Conv") != -1:
- m.weight.data.normal_(mean, std)
-
-
-def get_padding(kernel_size, dilation=1):
- return int((kernel_size*dilation - dilation)/2)
-
-
-def convert_pad_shape(pad_shape):
- l = pad_shape[::-1]
- pad_shape = [item for sublist in l for item in sublist]
- return pad_shape
-
-
-def intersperse(lst, item):
- result = [item] * (len(lst) * 2 + 1)
- result[1::2] = lst
- return result
-
-
-def kl_divergence(m_p, logs_p, m_q, logs_q):
- """KL(P||Q)"""
- kl = (logs_q - logs_p) - 0.5
- kl += 0.5 * (torch.exp(2. * logs_p) + ((m_p - m_q)**2)) * torch.exp(-2. * logs_q)
- return kl
-
-
-def rand_gumbel(shape):
- """Sample from the Gumbel distribution, protect from overflows."""
- uniform_samples = torch.rand(shape) * 0.99998 + 0.00001
- return -torch.log(-torch.log(uniform_samples))
-
-
-def rand_gumbel_like(x):
- g = rand_gumbel(x.size()).to(dtype=x.dtype, device=x.device)
- return g
-
-
-def slice_segments(x, ids_str, segment_size=4):
- ret = torch.zeros_like(x[:, :, :segment_size])
- for i in range(x.size(0)):
- idx_str = ids_str[i]
- idx_end = idx_str + segment_size
- ret[i] = x[i, :, idx_str:idx_end]
- return ret
-
-
-def rand_slice_segments(x, x_lengths=None, segment_size=4):
- b, d, t = x.size()
- if x_lengths is None:
- x_lengths = t
- ids_str_max = x_lengths - segment_size + 1
- ids_str = (torch.rand([b]).to(device=x.device) * ids_str_max).to(dtype=torch.long)
- ret = slice_segments(x, ids_str, segment_size)
- return ret, ids_str
-
-
-def get_timing_signal_1d(
- length, channels, min_timescale=1.0, max_timescale=1.0e4):
- position = torch.arange(length, dtype=torch.float)
- num_timescales = channels // 2
- log_timescale_increment = (
- math.log(float(max_timescale) / float(min_timescale)) /
- (num_timescales - 1))
- inv_timescales = min_timescale * torch.exp(
- torch.arange(num_timescales, dtype=torch.float) * -log_timescale_increment)
- scaled_time = position.unsqueeze(0) * inv_timescales.unsqueeze(1)
- signal = torch.cat([torch.sin(scaled_time), torch.cos(scaled_time)], 0)
- signal = F.pad(signal, [0, 0, 0, channels % 2])
- signal = signal.view(1, channels, length)
- return signal
-
-
-def add_timing_signal_1d(x, min_timescale=1.0, max_timescale=1.0e4):
- b, channels, length = x.size()
- signal = get_timing_signal_1d(length, channels, min_timescale, max_timescale)
- return x + signal.to(dtype=x.dtype, device=x.device)
-
-
-def cat_timing_signal_1d(x, min_timescale=1.0, max_timescale=1.0e4, axis=1):
- b, channels, length = x.size()
- signal = get_timing_signal_1d(length, channels, min_timescale, max_timescale)
- return torch.cat([x, signal.to(dtype=x.dtype, device=x.device)], axis)
-
-
-def subsequent_mask(length):
- mask = torch.tril(torch.ones(length, length)).unsqueeze(0).unsqueeze(0)
- return mask
-
-
-@torch.jit.script
-def fused_add_tanh_sigmoid_multiply(input_a, input_b, n_channels):
- n_channels_int = n_channels[0]
- in_act = input_a + input_b
- t_act = torch.tanh(in_act[:, :n_channels_int, :])
- s_act = torch.sigmoid(in_act[:, n_channels_int:, :])
- acts = t_act * s_act
- return acts
-
-
-def convert_pad_shape(pad_shape):
- l = pad_shape[::-1]
- pad_shape = [item for sublist in l for item in sublist]
- return pad_shape
-
-
-def shift_1d(x):
- x = F.pad(x, convert_pad_shape([[0, 0], [0, 0], [1, 0]]))[:, :, :-1]
- return x
-
-
-def sequence_mask(length, max_length=None):
- if max_length is None:
- max_length = length.max()
- x = torch.arange(max_length, dtype=length.dtype, device=length.device)
- return x.unsqueeze(0) < length.unsqueeze(1)
-
-
-def generate_path(duration, mask):
- """
- duration: [b, 1, t_x]
- mask: [b, 1, t_y, t_x]
- """
- device = duration.device
-
- b, _, t_y, t_x = mask.shape
- cum_duration = torch.cumsum(duration, -1)
-
- cum_duration_flat = cum_duration.view(b * t_x)
- path = sequence_mask(cum_duration_flat, t_y).to(mask.dtype)
- path = path.view(b, t_x, t_y)
- path = path - F.pad(path, convert_pad_shape([[0, 0], [1, 0], [0, 0]]))[:, :-1]
- path = path.unsqueeze(1).transpose(2,3) * mask
- return path
-
-
-def clip_grad_value_(parameters, clip_value, norm_type=2):
- if isinstance(parameters, torch.Tensor):
- parameters = [parameters]
- parameters = list(filter(lambda p: p.grad is not None, parameters))
- norm_type = float(norm_type)
- if clip_value is not None:
- clip_value = float(clip_value)
-
- total_norm = 0
- for p in parameters:
- param_norm = p.grad.data.norm(norm_type)
- total_norm += param_norm.item() ** norm_type
- if clip_value is not None:
- p.grad.data.clamp_(min=-clip_value, max=clip_value)
- total_norm = total_norm ** (1. / norm_type)
- return total_norm
diff --git a/spaces/emc348/faces-through-time/models/StyleCLIP/mapper/training/ranger.py b/spaces/emc348/faces-through-time/models/StyleCLIP/mapper/training/ranger.py
deleted file mode 100644
index 9442fd10d42fcc19f4e0dd798d1573b31ed2c0a0..0000000000000000000000000000000000000000
--- a/spaces/emc348/faces-through-time/models/StyleCLIP/mapper/training/ranger.py
+++ /dev/null
@@ -1,164 +0,0 @@
-# Ranger deep learning optimizer - RAdam + Lookahead + Gradient Centralization, combined into one optimizer.
-
-# https://github.com/lessw2020/Ranger-Deep-Learning-Optimizer
-# and/or
-# https://github.com/lessw2020/Best-Deep-Learning-Optimizers
-
-# Ranger has now been used to capture 12 records on the FastAI leaderboard.
-
-# This version = 20.4.11
-
-# Credits:
-# Gradient Centralization --> https://arxiv.org/abs/2004.01461v2 (a new optimization technique for DNNs), github: https://github.com/Yonghongwei/Gradient-Centralization
-# RAdam --> https://github.com/LiyuanLucasLiu/RAdam
-# Lookahead --> rewritten by lessw2020, but big thanks to Github @LonePatient and @RWightman for ideas from their code.
-# Lookahead paper --> MZhang,G Hinton https://arxiv.org/abs/1907.08610
-
-# summary of changes:
-# 4/11/20 - add gradient centralization option. Set new testing benchmark for accuracy with it, toggle with use_gc flag at init.
-# full code integration with all updates at param level instead of group, moves slow weights into state dict (from generic weights),
-# supports group learning rates (thanks @SHolderbach), fixes sporadic load from saved model issues.
-# changes 8/31/19 - fix references to *self*.N_sma_threshold;
-# changed eps to 1e-5 as better default than 1e-8.
-
-import math
-import torch
-from torch.optim.optimizer import Optimizer
-
-
-class Ranger(Optimizer):
-
- def __init__(self, params, lr=1e-3, # lr
- alpha=0.5, k=6, N_sma_threshhold=5, # Ranger configs
- betas=(.95, 0.999), eps=1e-5, weight_decay=0, # Adam configs
- use_gc=True, gc_conv_only=False
- # Gradient centralization on or off, applied to conv layers only or conv + fc layers
- ):
-
- # parameter checks
- if not 0.0 <= alpha <= 1.0:
- raise ValueError(f'Invalid slow update rate: {alpha}')
- if not 1 <= k:
- raise ValueError(f'Invalid lookahead steps: {k}')
- if not lr > 0:
- raise ValueError(f'Invalid Learning Rate: {lr}')
- if not eps > 0:
- raise ValueError(f'Invalid eps: {eps}')
-
- # parameter comments:
- # beta1 (momentum) of .95 seems to work better than .90...
- # N_sma_threshold of 5 seems better in testing than 4.
- # In both cases, worth testing on your dataset (.90 vs .95, 4 vs 5) to make sure which works best for you.
-
- # prep defaults and init torch.optim base
- defaults = dict(lr=lr, alpha=alpha, k=k, step_counter=0, betas=betas, N_sma_threshhold=N_sma_threshhold,
- eps=eps, weight_decay=weight_decay)
- super().__init__(params, defaults)
-
- # adjustable threshold
- self.N_sma_threshhold = N_sma_threshhold
-
- # look ahead params
-
- self.alpha = alpha
- self.k = k
-
- # radam buffer for state
- self.radam_buffer = [[None, None, None] for ind in range(10)]
-
- # gc on or off
- self.use_gc = use_gc
-
- # level of gradient centralization
- self.gc_gradient_threshold = 3 if gc_conv_only else 1
-
- def __setstate__(self, state):
- super(Ranger, self).__setstate__(state)
-
- def step(self, closure=None):
- loss = None
-
- # Evaluate averages and grad, update param tensors
- for group in self.param_groups:
-
- for p in group['params']:
- if p.grad is None:
- continue
- grad = p.grad.data.float()
-
- if grad.is_sparse:
- raise RuntimeError('Ranger optimizer does not support sparse gradients')
-
- p_data_fp32 = p.data.float()
-
- state = self.state[p] # get state dict for this param
-
- if len(state) == 0: # if first time to run...init dictionary with our desired entries
- # if self.first_run_check==0:
- # self.first_run_check=1
- # print("Initializing slow buffer...should not see this at load from saved model!")
- state['step'] = 0
- state['exp_avg'] = torch.zeros_like(p_data_fp32)
- state['exp_avg_sq'] = torch.zeros_like(p_data_fp32)
-
- # look ahead weight storage now in state dict
- state['slow_buffer'] = torch.empty_like(p.data)
- state['slow_buffer'].copy_(p.data)
-
- else:
- state['exp_avg'] = state['exp_avg'].type_as(p_data_fp32)
- state['exp_avg_sq'] = state['exp_avg_sq'].type_as(p_data_fp32)
-
- # begin computations
- exp_avg, exp_avg_sq = state['exp_avg'], state['exp_avg_sq']
- beta1, beta2 = group['betas']
-
- # GC operation for Conv layers and FC layers
- if grad.dim() > self.gc_gradient_threshold:
- grad.add_(-grad.mean(dim=tuple(range(1, grad.dim())), keepdim=True))
-
- state['step'] += 1
-
- # compute variance mov avg
- exp_avg_sq.mul_(beta2).addcmul_(1 - beta2, grad, grad)
- # compute mean moving avg
- exp_avg.mul_(beta1).add_(1 - beta1, grad)
-
- buffered = self.radam_buffer[int(state['step'] % 10)]
-
- if state['step'] == buffered[0]:
- N_sma, step_size = buffered[1], buffered[2]
- else:
- buffered[0] = state['step']
- beta2_t = beta2 ** state['step']
- N_sma_max = 2 / (1 - beta2) - 1
- N_sma = N_sma_max - 2 * state['step'] * beta2_t / (1 - beta2_t)
- buffered[1] = N_sma
- if N_sma > self.N_sma_threshhold:
- step_size = math.sqrt(
- (1 - beta2_t) * (N_sma - 4) / (N_sma_max - 4) * (N_sma - 2) / N_sma * N_sma_max / (
- N_sma_max - 2)) / (1 - beta1 ** state['step'])
- else:
- step_size = 1.0 / (1 - beta1 ** state['step'])
- buffered[2] = step_size
-
- if group['weight_decay'] != 0:
- p_data_fp32.add_(-group['weight_decay'] * group['lr'], p_data_fp32)
-
- # apply lr
- if N_sma > self.N_sma_threshhold:
- denom = exp_avg_sq.sqrt().add_(group['eps'])
- p_data_fp32.addcdiv_(-step_size * group['lr'], exp_avg, denom)
- else:
- p_data_fp32.add_(-step_size * group['lr'], exp_avg)
-
- p.data.copy_(p_data_fp32)
-
- # integrated look ahead...
- # we do it at the param level instead of group level
- if state['step'] % group['k'] == 0:
- slow_p = state['slow_buffer'] # get access to slow param tensor
- slow_p.add_(self.alpha, p.data - slow_p) # (fast weights - slow weights) * alpha
- p.data.copy_(slow_p) # copy interpolated weights to RAdam param tensor
-
- return loss
\ No newline at end of file
diff --git a/spaces/evaluate-metric/sacrebleu/app.py b/spaces/evaluate-metric/sacrebleu/app.py
deleted file mode 100644
index 5c77b0098c7f80e74cd3b2197f12b6e087cab35e..0000000000000000000000000000000000000000
--- a/spaces/evaluate-metric/sacrebleu/app.py
+++ /dev/null
@@ -1,11 +0,0 @@
-import sys
-
-import evaluate
-from evaluate.utils import launch_gradio_widget
-
-
-sys.path = [p for p in sys.path if p != "/home/user/app"]
-module = evaluate.load("sacrebleu")
-sys.path = ["/home/user/app"] + sys.path
-
-launch_gradio_widget(module)
diff --git a/spaces/facebook/incoder-demo/index.html b/spaces/facebook/incoder-demo/index.html
deleted file mode 100644
index b923eaf14e7df607016f8688524efc98fd446b15..0000000000000000000000000000000000000000
--- a/spaces/facebook/incoder-demo/index.html
+++ /dev/null
@@ -1 +0,0 @@
-demo is loading
diff --git a/spaces/falterWliame/Face_Mask_Detection/Abf Outlook Backup 3 Keygen Generator Extra Quality.md b/spaces/falterWliame/Face_Mask_Detection/Abf Outlook Backup 3 Keygen Generator Extra Quality.md
deleted file mode 100644
index 418c2b78b6c59577a5bb4238832c63b66e101e1e..0000000000000000000000000000000000000000
--- a/spaces/falterWliame/Face_Mask_Detection/Abf Outlook Backup 3 Keygen Generator Extra Quality.md
+++ /dev/null
@@ -1,6 +0,0 @@
-Abf Outlook Backup 3 Keygen Generator Download Zip 🗸 https://urlca.com/2uDbXM
-
-Jun 13, 2012 - rasabufu. (3.99 MB) Windows In just a few minutes, with just a few clicks of your mouse, you can have Outlook Backup 3.0 free. Download Outlook Backup 3.0. Search results for Outlook Backup 3.0. com/dl/9-t/Outlook Backup 3.0. 0041 gününden sonra Abf Outlook Backup 3.0 keygen. Once you are signed in, select Install Now. We also don't guarantee that the posted version will be the final version. Use this tool to create Outlook Recovery disks and your own outlook backup. Windows 2000/XP/Vista/7/8. The Outlook Backup 3.0 keygen is a clean and 100% working generator that will give you unlimited access to Outlook Backup 3.0 Full Version. Our Outlook Backup 3.0 keygen is a clean and 100% working tool. Mar 23, 2012 - rasabufu. Abf Outlook Backup 3.0 Keygen. Feb 26, 2013 rasabufu. Outlook Backup 3.0 allows you to make backups of your Outlook Express, Outlook and Outlook 2003 folders without loosing your data. com/dl/9-t/Outlook Backup 3.0. Also read our Outlook Backup 3.0 review. If you need more help, read our Outlook Backup 3.0 tutorial. We don't offer any malware, spyware or any other type of software. com) and don't require you to register. Abf Outlook Backup 3.0 keygen. Both versions support Unicode and are designed to work on Windows 2000/XP/Vista/7/8. This utility will enable the Abf Outlook Backup 3.0 program to make unlimited copies of your Outlook Express, Outlook and Outlook 2003 folders without loosing your data. Abf Outlook Backup 3.0 Keygen. Outlook Backup 3.0. Choose. Select the account you wish to restore. After that, click the button below to download Abf Outlook Backup 3.0 crack. MailSoft Outlook Backup 3.0 works in a different way to most other third party products and they do not actually store your data. Abf Outlook Backup 3.0 Keygen - Free Download - Abf Outlook Backup 3.0 Keygen. Brought to you by Abf. When you buy Abf Outlook Backup 3.0 keygen you are investing in your time. The Outlook Backup 3.0. com/dl 4fefd39f24
-
-
-
diff --git a/spaces/falterWliame/Face_Mask_Detection/An Exploit In The Pizzeria Roleplay Remastered !!INSTALL!!.md b/spaces/falterWliame/Face_Mask_Detection/An Exploit In The Pizzeria Roleplay Remastered !!INSTALL!!.md
deleted file mode 100644
index 56bbd6b746e04d70f7ff081bf4b726fb7525097f..0000000000000000000000000000000000000000
--- a/spaces/falterWliame/Face_Mask_Detection/An Exploit In The Pizzeria Roleplay Remastered !!INSTALL!!.md
+++ /dev/null
@@ -1,24 +0,0 @@
-An exploit in The Pizzeria Roleplay: Remastered Download File ————— https://urlca.com/2uDdPM
-
-There is nothing that can make a Pizzeria sadder than a broken tape.
-
-NPC - Tape List
-
-Vassili
-
-Tapes:
-
-"Vassili is a very kind man, and believes in helping others. He is looking to help the needy in his town to earn some more money, and for this reason, he has generously offered his services as a music librarian for the Pizzeria. He is often seen on his bicycle, carrying supplies to and from the Pizzeria, as well as his own vehicle to travel to other Pizzerias.
-
-Vassili has taken a liking to Moshboy, as she is often seen either by the window in the back of the Pizzeria, or in the Pizzeria itself. He often sees to her every need, and is always by her side if she needs help. When he is not working in the Pizzeria, he spends his time in the small room off of the back of the Pizzeria. In the room, he has his own small piano, where he spends his spare time playing it. He is also very good at making Jell-o shots, and he can make them all taste very similar. He has an affinity to Greyma, and often befriends her and her friends, and other members of the Crew."
-
-Greyma
-
-"Greyma is a nice girl, and is very grateful to Vassili for being so helpful to her, as well as all of her friends. She enjoys talking about old things, and loves a good book. She is very interested in art, and often tries to create something new that she can display for her friends to admire. She spends much of her time in the Art Room, but is usually found around the Pizzeria itself, or in one of the nearby establishments. She is friendly with many of the other Pizzerias as well, and is friendly with Vassili as well."
-
-Odelia
-
-"Odelia is a very kind girl, and very much enjoys music. She spends most of her time with her friends, and enjoys spending time with those who share the same interest. She can be very sociable, and doesn't mind talking to anyone who is interested. She is very intelligent, and enjoys both reading, and learning new things. She is often found in the Craft Room, and is often found with other 4fefd39f24
-
-
-
diff --git a/spaces/falterWliame/Face_Mask_Detection/Magi Season 2 Torrent.md b/spaces/falterWliame/Face_Mask_Detection/Magi Season 2 Torrent.md
deleted file mode 100644
index beec11aa3e239ea3b137f67ad159d97ea437ecff..0000000000000000000000000000000000000000
--- a/spaces/falterWliame/Face_Mask_Detection/Magi Season 2 Torrent.md
+++ /dev/null
@@ -1,7 +0,0 @@
-
-he asks if there is something they can do, and scheherazade tells him she can't do anything but say they must leave. as she prepares to leave, aladdin tries to stop her but is stopped by meyav, who says that they can't leave and that there are always new opportunities to learn new things. aladdin then wonders if she has a plan and how she knows so much, and she says that she is their teacher. she then tells them that in order for them to find their parents, they must go back to where they came from, and they are back at the boro world. the magicians leave, and aladdin and alibaba head back to the boro world, accompanied by meyav.
-Magi Season 2 Torrent Download Zip ✏ ✏ ✏ https://urlca.com/2uDcch
-the magicians appear in the current time and greet aladdin and alibaba. aladdin asks how the magicians can see the past, and they explain that they are using a device that has been perfected since they began using remote clairvoyance magic. they then explain how they are planning to use their device to find their parents. aladdin, who is suspicious, asks what they want with them, and they tell him they want to talk to their parents and ask for their help. aladdin says he will accompany them but first he needs to ask for help from the magi planet. they tell him to say they are looking for the rukh to ask for help. aladdin refuses to leave his friends and asks if they are afraid to go back to the magi planet after all they've been through. they say they are not, but they understand if he doesn't want to go back there. aladdin then goes to the ship, and they leave the planet.
-aladdin, alibaba, and meyav are in an underground cavern. they hear a few distant sounds, and aladdin asks if they are the magi planet. they then find a large pile of rubble blocking the way to their parents.
899543212b
-
-
\ No newline at end of file
diff --git a/spaces/falterWliame/Face_Mask_Detection/Phast 6.7with Crack ((INSTALL)).md b/spaces/falterWliame/Face_Mask_Detection/Phast 6.7with Crack ((INSTALL)).md
deleted file mode 100644
index b84b3fc4ea0a3dd2888d862e4bd08b4f60968e5c..0000000000000000000000000000000000000000
--- a/spaces/falterWliame/Face_Mask_Detection/Phast 6.7with Crack ((INSTALL)).md
+++ /dev/null
@@ -1,32 +0,0 @@
-phast 6.7with crack Download File ————— https://urlca.com/2uDdss
-
-This is an update to versions 7 and 8, which were released in 2012 and 2016 respectively. These newer releases include a number of important changes and improvements to the software. The most significant changes since version 7 are: The ability to automatically capture the screen when using Phast as a monitor The increased speed of finding a sample with the new Autoloading function Improved quality of results
-
-For more information about these, and other changes, refer to the User Manual (see the Manuals section below).
-
-Newer versions are backwards compatible with older versions. Therefore, you can upgrade Phast and Phast Risk from version 6.7 to version 8.22.
-
-Input files
-
------------
-
-Phast and Phast Risk use a text file (file with extension '*.txt') to hold information about a sample. This sample information is called a sample file.
-
-A sample file is similar in format to the sequence information files used in most of the other Phast software (e.g. '*hannes.txt'*). This means that a sample file has five columns. The first four columns are used to describe a sequence within a sample. The fifth column is used to describe the sequence with respect to a reference sequence.
-
-The first four columns are as follows:
-
-- A unique sample identifier
-
-- An index number (the position of the sequence in the sample)
-
-- The name of the sequence
-
-- The name of the sequence with respect to a reference sequence
-
-In the first three columns the sample identifier is used as an index number. The first column is used for simple sequences (e.g. repeats of T, C, A or G) and the third column is used for complex sequences (e.g. copy number variation or structural variation). As a reminder, the original sequence information files used in Phast were called '*index.txt*'. For all of the '*index.txt*' files used in Phast and Phast Risk a sample identifier (e.g. *hannes* for the sample shown in Figure \[fig:00\_04\_01\_hannes\_sample\]) is used as an index number.
-
-The reference sequence name is used to identify a reference sequence when finding repeats, copy number variation, structural 4fefd39f24
-
-
-
diff --git a/spaces/fatiXbelha/sd/Download TOCA Race Driver 3 for PC - The Ultimate Racing Simulator.md b/spaces/fatiXbelha/sd/Download TOCA Race Driver 3 for PC - The Ultimate Racing Simulator.md
deleted file mode 100644
index 6a1834ed31e714a8e794dcd821991e354761a0fa..0000000000000000000000000000000000000000
--- a/spaces/fatiXbelha/sd/Download TOCA Race Driver 3 for PC - The Ultimate Racing Simulator.md
+++ /dev/null
@@ -1,151 +0,0 @@
-
-TOCA Race Driver 3: A Racing Simulation Game for PC
-If you are a fan of racing games and want to experience a wide range of motorsports, cars, tracks, and challenges, then you might want to check out TOCA Race Driver 3 . This is a racing simulation game developed by Codemasters and released in 2006 for PC, PlayStation 2, Xbox, PSP, and Mac OS X. It is also known as DTM Race Driver 3 in Germany and V8 Supercars 3 in Australia.
-toca race driver 3 pc download ita DOWNLOAD · https://urllie.com/2uNACW
-In this article, we will give you an overview of what TOCA Race Driver 3 has to offer, how to download and install it on your PC, how to use cheats and mods to enhance your gameplay, how to play online with other players, how to compare it to other racing games, and how to answer some of the frequently asked questions about the game. Let's get started!
- Gameplay
-TOCA Race Driver 3 is a racing simulation game that features six different racing styles: GT, Touring Cars, Off-Road, Open-Wheel, Track, and Classic. Each style has its own unique cars, tracks, rules, and challenges. You can choose from over 70 licensed vehicles from various manufacturers, such as Aston Martin, Audi, BMW, Ford, Honda, Jaguar, Mercedes-Benz, Porsche, Subaru, Toyota, Volkswagen, and more. You can also customize your cars with different tuning options, liveries, and upgrades.
-The game has over 80 tracks from around the world, including famous circuits like Silverstone, Brands Hatch, Laguna Seca, Indianapolis Motor Speedway, Nürburgring Nordschleife, Bathurst Mount Panorama Circuit, Monaco Grand Prix Circuit de Monaco , Le Mans Circuit de la Sarthe , Spa-Francorchamps Circuit de Spa-Francorchamps , Suzuka Circuit , Monza Autodromo Nazionale Monza , Hockenheimring , Donington Park , Oulton Park , Norisring , Zandvoort Circuit Park Zandvoort , Catalunya Circuit de Barcelona-Catalunya , Imola Autodromo Enzo e Dino Ferrari , Istanbul Park , Shanghai International Circuit , Bahrain International Circuit , Sepang International Circuit , Interlagos Autódromo José Carlos Pace , Mexico City Autódromo Hermanos Rodríguez , Road America , Sebring International Raceway , Watkins Glen International , Pikes Peak International Hill Climb , Rally Finland Ouninpohja Stage , Rally New Zealand Whaanga Coast Stage , Rally Japan Rikubetsu Stage , Rally Australia Bannister North Stage , Rally Germany Panzerplatte Stage , Rally Monte Carlo Col de Turini Stage , Rally Sweden Vargåsen Stage , Rally GB Margam Park Stage , Rally USA Pikes Peak Hill Climb Stage , Rally Norway Kjerag Stage , Rally France Col de la Faucille Stage , Rally Spain El Montmell Stage , Rally Italy Sardinia Monte Lerno Stage , Rally Greece Klenia Mycenae Stage , Rally - Rally Portugal Fafe Stage , and more. You can also create your own custom tracks with the track editor feature.
-The game has a realistic damage model that affects the performance and appearance of your cars. You can see the dents, scratches, cracks, smoke, fire, and debris on your vehicles as you collide with other cars or objects. You can also repair your cars in the pit stops or between the races. The game also has a realistic physics engine that simulates the weight, traction, aerodynamics, and handling of your cars. You can feel the difference between driving on asphalt, gravel, dirt, snow, ice, or water. The game also has a dynamic weather system that changes the conditions and visibility of the tracks. You can race in sunny, cloudy, rainy, foggy, or stormy weather.
-The game has a challenging AI that adapts to your skill level and racing style. You can compete against up to 21 opponents in each race, who have their own personalities, strengths, and weaknesses. You can also interact with them through the radio communication feature, where you can hear their comments, taunts, or compliments. The game also has a career mode that lets you create your own driver and progress through various championships and events. You can earn money, reputation, and trophies as you win races and complete challenges. You can also unlock new cars, tracks, and features as you advance in the career mode.
-toca race driver 3 pc game download ita
-toca race driver 3 pc full version download ita
-toca race driver 3 pc iso download ita
-toca race driver 3 pc crack download ita
-toca race driver 3 pc free download ita
-toca race driver 3 pc torrent download ita
-toca race driver 3 pc mega download ita
-toca race driver 3 pc gameplay ita
-toca race driver 3 pc review ita
-toca race driver 3 pc cheats ita
-toca race driver 3 pc patch ita
-toca race driver 3 pc mods ita
-toca race driver 3 pc system requirements ita
-toca race driver 3 pc online ita
-toca race driver 3 pc multiplayer ita
-toca race driver 3 pc windows 10 ita
-toca race driver 3 pc steam ita
-toca race driver 3 pc gog ita
-toca race driver 3 pc origin ita
-toca race driver 3 pc trainer ita
-toca race driver 3 pc save game ita
-toca race driver 3 pc serial key ita
-toca race driver 3 pc no cd ita
-toca race driver 3 pc no dvd ita
-toca race driver 3 pc update ita
-toca race driver 3 pc bonus codes ita
-toca race driver 3 pc unlock all cars ita
-toca race driver 3 pc custom championship ita
-toca race driver 3 pc car list ita
-toca race driver 3 pc track list ita
-toca race driver 3 pc best settings ita
-toca race driver 3 pc controller support ita
-toca race driver 3 pc wheel support ita
-toca race driver 3 pc keyboard controls ita
-toca race driver 3 pc split screen ita
-toca race driver 3 pc lan play ita
-toca race driver 3 pc co op mode ita
-toca race driver 3 pc vs ps2 ita
-toca race driver 3 pc vs xbox ita
-toca race driver 3 pc vs psp ita
- Download and installation
-If you want to play TOCA Race Driver 3 on your PC, you will need to download it from a legal and safe source. One of the best options is to buy it from Steam, where you can get it for $9.99 USD. Steam is a digital distribution platform that allows you to download and play games on your PC with ease. You will need to create a free account and install the Steam client on your PC to use Steam.
-Another option is to buy it from GOG.com, where you can get it for $5.99 USD. GOG.com is another digital distribution platform that offers DRM-free games that you can download and play on your PC without any restrictions or limitations. You will also need to create a free account and install the GOG Galaxy client on your PC to use GOG.com.
-Once you have downloaded the game from either Steam or GOG.com, you will need to install it on your PC. The installation process is simple and straightforward, as you just need to follow the instructions on the screen. However, you might encounter some compatibility issues with newer versions of Windows, such as Windows 10. To fix these issues, you might need to run the game in compatibility mode for Windows XP or Windows Vista. You might also need to update your graphics drivers and DirectX software to ensure optimal performance.
- Cheats and mods
-If you want to spice up your gameplay with some cheats and mods, you will be happy to know that TOCA Race Driver 3 has plenty of them. Cheats are codes or passwords that you can enter in the game to unlock new cars, tracks, modes, or features. Mods are modifications or updates that you can download and install in the game to enhance its graphics, sound, gameplay, or content.
-Some of the cheats that you can use in TOCA Race Driver 3 are:
-
-Cheat Effect
-MINTY Unlocks all bonus championships
-SIM Unlocks Pro-Sim mode
-DAMAGE Unlocks Realistic Damage mode
-TUNED Unlocks all cars in Free Race mode
-LOADSWAP Unlocks all cars in Time Trial mode
-XBOX Unlocks all liveries for Xbox version
-PSP Unlocks all liveries for PSP version
-CATDOG Unlocks all liveries for PS2 version
-MADDOG Unlocks all liveries for PC version
-CMMARCADE Unlocks Arcade Mode in Race Day mode
-CMMCHAMPIONSHIP Unlocks Championship Mode in Race Day mode
-CMMTIMECHALLENGE Unlocks Time Challenge Mode in Race Day mode
-CMMRACEDAYUnlocks Race Day Mode in Race Day mode
-CMMEVENTS Unlocks all events in World Tour mode
-CMMWORLD Unlocks all tiers in World Tour mode
-CMMLICENSE Unlocks all licenses in World Tour mode
-CMMHOST Unlocks all host bonuses in World Tour mode
-CMMALL Unlocks everything in the game
-
-To use these cheats, you need to enter them as your profile name when you start a new game. You can also enter them in the bonus code menu in the options screen. You can only use one cheat at a time, and some of them might disable your progress or achievements.
-Some of the mods that you can use in TOCA Race Driver 3 are:
-
-Mod Effect
-TOCA Race Driver 3 Challenge Mod Adds new cars, tracks, and events to the game, based on the PSP version of TOCA Race Driver 3 Challenge. You can download it from here .
-TOCA Race Driver 3 HD Mod Improves the graphics and resolution of the game, making it look more modern and realistic. You can download it from here .
-TOCA Race Driver 3 Sound Mod Enhances the sound effects and music of the game, making it more immersive and dynamic. You can download it from here .
-TOCA Race Driver 3 Physics Mod Tweaks the physics and handling of the game, making it more challenging and authentic. You can download it from here .
-TOCA Race Driver 3 Custom Liveries Mod Adds new liveries and skins for the cars in the game, giving them more variety and style. You can download it from here .
-TOCA Race Driver 3 Track Editor Mod Allows you to create your own custom tracks with the track editor feature, giving you more freedom and creativity. You can download it from here .
-
-To use these mods, you need to download and extract them to your game folder, and follow the instructions provided by the mod creators. You might need to backup your original files before installing the mods, in case you want to restore them later. You might also need to disable any cheats or updates that might conflict with the mods.
- Online multiplayer
-If you want to play TOCA Race Driver 3 online with other players, you will need to use a third-party service or platform, as the official online servers have been shut down since 2014. One of the most popular options is to use GameRanger, a free application that allows you to host and join online games for over 700 PC games, including TOCA Race Driver 3. You can download GameRanger from here .
-To use GameRanger, you need to create a free account and install the GameRanger client on your PC. Then, you need to launch GameRanger and select TOCA Race Driver 3 from the list of games. You can then browse the available rooms or create your own room for hosting a game. You can invite your friends or join other players who have the same version of the game as you. You can also chat with other players using the GameRanger chat feature.
-Some of the tips and tricks to improve your online performance and experience are:
-
-Make sure you have a stable and fast internet connection, and avoid using any programs or devices that might interfere with your bandwidth or latency.
-Make sure you have updated your game to the latest version, and disabled any cheats or mods that might cause compatibility issues or unfair advantages.
-Make sure you have configured your firewall and antivirus settings to allow GameRanger and TOCA Race Driver 3 to run properly.
-Make sure you have adjusted your graphics and sound settings to suit your preferences and system capabilities. Make sure you have chosen a suitable game mode, difficulty level, and race settings that match your skill and preference.
-Make sure you have practiced and familiarized yourself with the cars, tracks, and rules of the game before joining or hosting a race.
-Make sure you have followed the online etiquette and respect the other players, hosts, and moderators. Avoid cheating, spamming, trolling, or flaming.
-
- Comparison
-TOCA Race Driver 3 is one of the most realistic, varied, and fun racing simulation games ever made. It offers a huge amount of content, customization, and challenge for racing enthusiasts and casual gamers alike. However, it is not the only racing game in the market, and you might wonder how it compares to other games in the genre.
-Some of the games that are similar to TOCA Race Driver 3 are:
-
-Game Similarities Differences
-Gran Turismo 4 A racing simulation game that features over 700 cars and over 50 tracks from around the world. It also has a realistic physics and damage model, a career mode, and an online multiplayer mode. It is exclusive to PlayStation 2, and it focuses more on GT and sports cars than other racing disciplines. It also has a more complex and demanding tuning system, a more extensive car collection system, and a more realistic driving school system.
-Forza Motorsport 2 A racing simulation game that features over 300 cars and over 40 tracks from around the world. It also has a realistic physics and damage model, a career mode, and an online multiplayer mode. It is exclusive to Xbox 360, and it focuses more on customization and personalization than other racing disciplines. It also has a more advanced and flexible livery editor, a more interactive and social auction house system, and a more rewarding and competitive leaderboard system.
-Project CARS 2 A racing simulation game that features over 180 cars and over 60 tracks from around the world. It also has a realistic physics and damage model, a career mode, and an online multiplayer mode. It is available for PC, PlayStation 4, Xbox One, and VR devices, and it focuses more on realism and immersion than other racing disciplines. It also has a more dynamic and variable weather system, a more authentic and diverse track selection system, and a more sophisticated and adjustable driver rating system.
-Assetto Corsa A racing simulation game that features over 170 cars and over 30 tracks from around the world. It also has a realistic physics and damage model, a career mode, and an online multiplayer mode. It is available for PC, PlayStation 4, Xbox One, Nintendo Switch, iOS devices , Android devices , Mac OS X , Linux , SteamOS , Windows Phone , BlackBerry , Symbian , Palm OS , webOS , Windows Mobile , Bada , MeeGo , Tizen , Firefox OS , Sailfish OS , Ubuntu Touch , KaiOS , HarmonyOS , Fuchsia OS , Plasma Mobile , postmarketOS , PureOS , LuneOS , AsteroidOS , Nemo Mobile , Mer Project , Maemo Leste , Mobian OS , Replicant OS , LineageOS , /e/ OS , GrapheneOS , CalyxOS , Paranoid Android OS , OmniROM OS , Resurrection Remix OS /e/ Foundation /e/ Foundation /e/ Foundation /e/ Foundation /e/ Foundation /e/ Foundation /e/ Foundation /e/ Foundation /e/ Foundation /e/ Foundation /e/ Foundation /e/ Foundation /e/ Foundation /e/ Foundation /e/ Foundation /e/ Foundation /e/ Foundation /e/ Foundation /e/ Foundation /e/ Foundation /e/ Foundation /e/ Foundation /e/ Foundation /e/ Foundation /e/ Foundation OS , and more. It also has a more accurate and detailed physics engine, a more realistic and customizable force feedback system, and a more mod-friendly and open-source platform.
-
-As you can see, TOCA Race Driver 3 has some similarities and differences with other racing games, and each game has its own strengths and weaknesses. Ultimately, the best racing game for you depends on your personal preference, taste, and expectation. However, we can say that TOCA Race Driver 3 is a great racing game that deserves your attention and appreciation.
- Conclusion
-In conclusion, TOCA Race Driver 3 is a racing simulation game that offers a lot of content, variety, and challenge for racing fans and gamers. It features six different racing styles, over 70 licensed cars, over 80 tracks, a realistic damage and physics model, a career mode, an online multiplayer mode, and more. It is available for PC, PlayStation 2, Xbox, PSP, and Mac OS X. You can download it from Steam or GOG.com, and use cheats and mods to enhance your gameplay. You can also compare it to other racing games to see how it stands out in the genre.
-We hope that this article has given you some useful information and tips about TOCA Race Driver 3. If you are interested in playing this game, we highly recommend that you give it a try and see for yourself how fun and exciting it is. We are sure that you will not regret it!
-We would also like to hear your feedback and opinions about this game. Have you played TOCA Race Driver 3 before? What did you like or dislike about it? How would you rate it on a scale of 1 to 10? Do you have any questions or suggestions about the game? Please let us know in the comments section below. We would love to hear from you!
- FAQs
-Here are some of the frequently asked questions about TOCA Race Driver 3:
-Q: How long is the career mode in TOCA Race Driver 3?
-A: The career mode in TOCA Race Driver 3 consists of 32 tiers, each with multiple events and championships. Depending on your skill level and play style, it can take you anywhere from 20 to 40 hours to complete the career mode.
-Q: How many players can play online in TOCA Race Driver 3?
-A: The online multiplayer mode in TOCA Race Driver 3 supports up to 12 players on PC, PlayStation 2, and Xbox, and up to 8 players on PSP. You can play online with other players using GameRanger or other third-party services.
-Q: What are the system requirements for TOCA Race Driver 3 on PC?
-A: The minimum system requirements for TOCA Race Driver 3 on PC are:
-
-OS: Windows XP/Vista/7/8/10
-Processor: Pentium 4 @ 1.4 GHz or equivalent
-Memory: 256 MB RAM
-Graphics: GeForce FX or Radeon 9500 or better
-DirectX: Version 9.0c
-Storage: 6 GB available space
-Sound Card: DirectX compatible sound card
-
-The recommended system requirements for TOCA Race Driver 3 on PC are:
-
-OS: Windows XP/Vista/7/8/10
-Processor: Pentium 4 @ 2.4 GHz or equivalent
-Memory: 512 MB RAM
-Graphics: GeForce FX or Radeon X800 or better DirectX: Version 9.0c
-Storage: 6 GB available space
-Sound Card: DirectX compatible sound card
-
-Q: Is TOCA Race Driver 3 compatible with a steering wheel controller?
-A: Yes, TOCA Race Driver 3 is compatible with most steering wheel controllers that support DirectX input. You can configure your controller settings in the options menu of the game. You can also use a keyboard, a mouse, or a gamepad to play the game.
-Q: Is TOCA Race Driver 3 the latest game in the series?
-A: No, TOCA Race Driver 3 is not the latest game in the series. The series has continued with other games, such as Race Driver: GRID, GRID 2, GRID Autosport, and GRID (2019). However, TOCA Race Driver 3 is still considered by many fans and critics as one of the best games in the series.
-
-Thank you for reading this article about TOCA Race Driver 3. We hope that you have enjoyed it and learned something new. If you have any questions or comments, please feel free to leave them below. We would love to hear from you!
401be4b1e0
-
-
\ No newline at end of file
diff --git a/spaces/feregVcuzo/sanity-test-midi/checkpoint/Download Google Pay from the Play Store and Get Ready to Pay Faster and Safer.md b/spaces/feregVcuzo/sanity-test-midi/checkpoint/Download Google Pay from the Play Store and Get Ready to Pay Faster and Safer.md
deleted file mode 100644
index 69f18dea4f122bb1c31e3953fb0e33d278e9d074..0000000000000000000000000000000000000000
--- a/spaces/feregVcuzo/sanity-test-midi/checkpoint/Download Google Pay from the Play Store and Get Ready to Pay Faster and Safer.md
+++ /dev/null
@@ -1,129 +0,0 @@
-
-How to Download Google Pay from Play Store
-Google Pay is a digital payment app that lets you pay online, in stores, or send money to friends and family. It also offers various features such as cashback, rewards, encryption, security, bill payments, mobile recharges, online shopping, and more. In this article, we will show you how to download Google Pay from Play Store on your Android device.
- What is Google Pay?
-Google Pay is a digital payment app that lets you pay online, in stores, or send money to friends and family. It also offers various features such as cashback, rewards, encryption, security, bill payments, mobile recharges, online shopping, and more.
-download google pay play store Download ⇒⇒⇒ https://gohhs.com/2uPoiO
-Google Pay works with any bank account that supports UPI (Unified Payments Interface), a payment system that allows instant transfers between bank accounts using a virtual payment address or a mobile number. You can also add debit cards, credit cards, or prepaid cards to your Google Pay account and use them for online or in-store payments.
-Google Pay also integrates with other Google products and services such as Gmail, YouTube, Maps, Photos, Chrome, Assistant, and more. You can use Google Pay to order food, buy gas, pay for parking, book tickets, subscribe to services, donate to causes, and more.
- Why Download Google Pay from Play Store?
-There are many benefits of downloading Google Pay from Play Store on your Android device. Some of them are:
-
-You can download Google Pay for free from Play Store, which is the official app store for Android devices. This ensures that you get the latest and most secure version of Google Pay on your device.
-You can easily update Google Pay from Play Store whenever there is a new version available. This ensures that you get the latest features and bug fixes for Google Pay on your device.
-You can access Play Store from any Android device that has a Google account. This means that you can download Google Pay on multiple devices and sync your payment information across them.
-You can also access Play Store from your web browser on any device. This means that you can download Google Pay on your device even if you don't have access to it at the moment.
-
-Downloading Google Pay from Play Store is also very easy and fast. You just need to follow a few simple steps and you will be ready to use Google Pay on your device.
- How to Download Google Pay from Play Store?
-To download Google Pay from Play Store, you need to have an Android device that has a Google account and supports UPI. You also need to have an internet connection and enough storage space on your device. Here are the steps to download Google Pay from Play Store:
- Step 1: Open Play Store on your device
-The first step is to open Play Store on your device. You can do this by tapping on the Play Store icon on your home screen or app drawer. Alternatively, you can also swipe up from the bottom of your screen and search for Play Store in the search bar.
- Step 2: Search for Google Pay in Play Store
-The next step is to search for Google Pay in Play Store. You can do this by tapping on the search icon at the top of the screen and typing "Google Pay" in the search box. Alternatively, you can also use the voice search feature by tapping on the microphone icon and saying "Google Pay".
-download google pay app from play store
-download google pay from play store for free
-download google pay from play store in india
-download google pay from play store on laptop
-download google pay from play store on pc
-download google pay from play store without gmail account
-download google pay from play store without phone number
-how can i download google pay from play store
-how do i download google pay from play store
-how to download google pay app from play store in jio phone
-how to download google pay app from play store in laptop
-how to download google pay app from play store in pc
-how to download google pay app from play store without gmail account
-how to download google pay app from play store without phone number
-how to download google pay from play store in iphone
-how to download google pay from play store in pakistan
-how to download google pay from play store in samsung mobile
-how to download google pay from play store on ipad
-how to download google pay from play store on macbook
-how to download google pay from play store on mi phone
-how to download google pay from play store on windows 10
-how to download google pay from play store using qr code
-i can't download google pay from play store
-i want to download google pay app from play store
-is it safe to download google pay from play store
-not able to download google pay from play store
-unable to download google pay app from play store
-unable to download google pay from play store error code 910
-unable to download google pay from play store error code 920
-unable to download google pay from play store error code 963
-why can't i download google pay app from play store
-why can't i download google pay from play store in my phone
-why can't i download google pay from play store on my iphone
-why can't i download google pay from the play store on my laptop
-why can't i install google pay app from the play store on my android device
- Step 3: Tap on Install button
-The third step is to tap on the Install button next to the Google Pay app. This will start downloading Google Pay on your device. You can see the progress of the download by looking at the status bar at the top of the screen.
- Step 4: Open Google Pay app
-The fourth step is to open Google Pay app on your device. You can do this by tapping on the Open button after the download is complete. Alternatively, you can also tap on the Google Pay icon on your home screen or app drawer.
- Step 5: Set up Google Pay account
-The final step is to set up your Google Pay account on your device. You can do this by following the instructions on the screen. You will need to:
-
-Select your preferred language
-Enter your phone number that is linked to your bank account
-Verify your phone number with an OTP (one-time password)
-Select your bank account that supports UPI
-Create a UPI PIN (personal identification number) for your bank account
-Add a debit card, credit card, or prepaid card (optional)
-Agree to the terms and conditions of Google Pay
-
-Congratulations! You have successfully downloaded and set up Google Pay on your device. You are now ready to use Google Pay for various purposes.
- How to Use Google Pay?
-Google Pay is a versatile payment app that lets you use it for various purposes. Some of them are:
- How to Pay in Stores with Google Pay?
-You can use Google Pay for contactless payments in stores that accept NFC (near-field communication) or QR (quick response) code payments. To pay in stores with Google Pay, you need to:
-
-Unlock your device and hold it near the NFC terminal or scan the QR code displayed by the merchant
-Select your preferred payment method (bank account, debit card, credit card, or prepaid card)
-Enter your UPI PIN or authenticate with your fingerprint or face recognition (if required)
-Wait for the confirmation message on your device and the receipt from the merchant
-
- How to Pay Online with Google Pay?
-You can use Google Pay for online shopping and services that support UPI or cards as payment options. To pay online with Google Pay, you need to:
-
-Select Google Pay as your payment option at the checkout page of the website or app
-Select your preferred payment method (bank account, debit card, credit card, or prepaid card)
-Enter your UPI PIN or authenticate with your fingerprint or face recognition (if required)
-Wait for the confirmation message on your device and the receipt from the website or app
-
- How to Send and Receive Money with Google Pay?
-You can use Google Pay for peer-to-peer transactions with anyone who has a Google Pay account or a UPI-enabled bank account. To send and receive money with Google Pay, you need to:
-
-Open Google Pay app and tap on New Payment button
-Select the person you want to send or request money from by entering their phone number, name, email address, or UPI ID
-Enter the amount and a note (optional)
-Tap on Pay or Request button
-Enter your UPI PIN or authenticate with your fingerprint or face recognition (if required)
-Wait for the confirmation message on your device and the notification from the other person
-
- How to Update Google Pay?
-To keep your Google Pay app running smoothly and securely, you should update it regularly from Play Store. To update Google Pay, you need to:
-
-Open Play Store app and tap on Menu icon at the top left corner of the screen
-Select My apps & games option
-Find Google Pay app in the list of installed apps and tap on Update button
-Wait for the update to download and install on your device
-Open Google Pay app and enjoy the new features and improvements
-
- Conclusion
-Google Pay is a digital payment app that lets you pay online, in stores, or send money to friends and family. It also offers various features such as cashback, rewards, encryption, security, bill payments, mobile recharges, online shopping, and more. You can download Google Pay from Play Store on your Android device by following a few simple steps. You can also use Google Pay for various purposes by following the instructions in this article. Google Pay is a convenient, fast, and secure way to pay with your phone. Download Google Pay today and enjoy the benefits of digital payments.
- FAQs
-Here are some frequently asked questions about downloading Google Pay from Play Store:
-
-Is Google Pay safe to use?
-Yes, Google Pay is safe to use. It uses encryption, security codes, biometric authentication, and other features to protect your payment information and transactions. It also complies with the UPI guidelines and regulations issued by NPCI (National Payments Corporation of India).
- Is Google Pay free to use?
-Yes, Google Pay is free to use. There are no charges for downloading, installing, updating, or using Google Pay. However, you may incur charges from your bank or service provider for using UPI or cards as payment methods.
- Which devices support Google Pay?
-Google Pay supports any Android device that has a Google account and supports UPI. The device should also have Android 5.0 (Lollipop) or higher version and NFC (near-field communication) or QR (quick response) code scanning capabilities.
- Which countries support Google Pay?
-Google Pay is available in many countries around the world. You can check the list of supported countries here: https://pay.google.com/intl/en_in/about/countries/
- How can I contact Google Pay customer care?
-You can contact Google Pay customer care by using the Help & feedback option in the Google Pay app. You can also call them at 1800-419-0157 or email them at apps-help@google.com .
- 197e85843d
-
-
\ No newline at end of file
diff --git a/spaces/feregVcuzo/sanity-test-midi/checkpoint/Download Hitwicket Superstars Cricket and Enjoy a World-Class 3D Cricket Game.md b/spaces/feregVcuzo/sanity-test-midi/checkpoint/Download Hitwicket Superstars Cricket and Enjoy a World-Class 3D Cricket Game.md
deleted file mode 100644
index adf9c240bf751d2b3a146181f1286e2b1191307f..0000000000000000000000000000000000000000
--- a/spaces/feregVcuzo/sanity-test-midi/checkpoint/Download Hitwicket Superstars Cricket and Enjoy a World-Class 3D Cricket Game.md
+++ /dev/null
@@ -1,155 +0,0 @@
-
-Hitwicket Superstars: Cricket - A Review
-If you are a fan of cricket and strategy games, you might want to check out Hitwicket Superstars: Cricket, a 3D multiplayer cricket game that combines sports and strategy like nothing else before. In this article, we will review the game and its features, show you how to play it, and give you some tips and tricks to improve your skills. We will also tell you how to join the game's community and interact with millions of cricket fans from across the globe.
- What is Hitwicket Superstars: Cricket?
-Hitwicket Superstars: Cricket is a cricket game that lets you own a real team of superstars, play epic T20 matches, participate in global tournaments, and win phenomenal rewards. You can also train your players from rookies into world-class superstars, bid for the best cricketers in real-time auctions, form alliances with other fans, and compete in multiplayer world championships. The game is made in India, for India and the world, and has won several awards and recognition for its innovation and quality.
-hitwicket superstars cricket download Download File › https://gohhs.com/2uPndV
-Some of the features of Hitwicket Superstars: Cricket are:
-
-Amazing superpowers for batsmen and bowlers, such as SMASH, HEX, and BOOMERANG
-New offline mode that lets you play without Wi-Fi
-Simple controls that let you play pulls, hooks, cover drives, square cuts, and the all-time favorite helicopter shot
-Quick 3-minute matches that are thrilling and addictive
-Multiplayer cricket league that lets you battle against real players in epic PvP matches
-Alliance wars that let you team up with fans worldwide
-3D cricket game experience with stunning graphics and animations
-Exciting gameplay with unpredictable algorithm and gritty revenge mechanics
-Player auction that lets you bid for the best talents in world cricket
-Dream team that lets you build your own world-class cricket team
-
- How to play Hitwicket Superstars: Cricket?
-To play Hitwicket Superstars: Cricket, you need to download and install the game on your device. The game is available for both Android and iOS devices. You can find the links to download the game here:
-
-Once you have installed the game, you need to create an account and choose your city. You can select from multiple cities across many nations, such as India, Australia, England, Bangladesh, Sri Lanka, etc. You can also choose to represent your Indian or international city in multiplayer mode.
-After choosing your city, you need to build your team by scouting for talent or bidding for players in auctions. You can also train your players by choosing from a wide set of training sessions. You can collect training points and use them to upgrade your players' skills and abilities.
-Once you have your team ready, you can start playing matches by selecting from various modes, such as career mode, world tour mode, multiplayer league mode, alliance wars mode, etc. You can also customize your match settings by choosing the pitch type, weather condition, toss decision, etc.
-Hitwicket Superstars: Cricket is a fun and addictive game that offers a unique blend of cricket and strategy. However, like any game, it has its pros and cons. Here are some of them:
-
-
-Pros
-Cons
-
-
-
-
-Easy to play, hard to master
-Realistic and immersive 3D graphics and animations
-Various modes and challenges to suit different preferences and skill levels
-Superpowers that add a twist and excitement to the game
-Multiplayer mode that lets you play with or against real players from around the world
-Alliance wars that let you collaborate and compete with other fans
-Player auction that lets you bid for the best cricketers in real-time
-Dream team that lets you create your own world-class cricket team
-Offline mode that lets you play without Wi-Fi
-Frequent updates and events that keep the game fresh and interesting
-
-
-
-
-Requires a lot of time and patience to progress in the game
-Some features and items are locked behind paywalls or ads
-Some bugs and glitches may affect the gameplay or performance
-Some players may find the game too complex or challenging
-Some players may find the game too repetitive or boring after a while
-Some players may experience connectivity or compatibility issues with their devices
-Some players may encounter toxic or unfair opponents or alliance members
-Some players may not like the superpowers or the unpredictability of the game
-Some players may prefer a more realistic or traditional cricket game
-Some players may have issues with the customer support or feedback system
-
-
-
-
- What are some tips and tricks for Hitwicket Superstars: Cricket?
- If you want to improve your skills and performance in Hitwicket Superstars: Cricket, here are some tips and tricks that you can follow:
-
-Learn the basics of cricket, such as the rules, the terminology, the scoring system, etc.
-Practice your batting and bowling skills in the training mode or the offline mode.
-Experiment with different superpowers and find out which ones suit your style and strategy.
-Analyze your opponent's strengths and weaknesses and plan your moves accordingly.
-Use the skip option to save time and energy when playing matches.
-Bid smartly and strategically in the player auctions. Don't overspend or underbid.
-Train your players regularly and upgrade their skills and abilities.
-Create a balanced team with a mix of batsmen, bowlers, all-rounders, wicket-keepers, etc.
-Customize your match settings to suit your preferences and conditions.
-Join an alliance that matches your goals and values. Communicate and cooperate with your alliance members.
-Participate in various events and tournaments to earn rewards and trophies.
-Watch ads or complete offers to get free coins, gems, training points, etc. Follow the game's social media pages and fan forums to get the latest news, updates, tips, and tricks.
-Have fun and enjoy the game. Don't get frustrated or angry if you lose or face difficulties.
-
- How to join the Hitwicket Superstars: Cricket community?
- Hitwicket Superstars: Cricket has a large and active community of cricket fans from around the world. You can join the community and interact with other players, share your feedback, suggestions, ideas, questions, etc. You can also participate in contests, polls, quizzes, etc. and win exciting prizes.
-hitwicket superstars cricket game app
-hitwicket superstars cricket manager game
-hitwicket superstars cricket strategy game
-hitwicket superstars cricket game with superpowers
-hitwicket superstars cricket game offline mode
-hitwicket superstars cricket game for android
-hitwicket superstars cricket game for ios
-hitwicket superstars cricket game for ipad
-hitwicket superstars cricket game for iphone
-hitwicket superstars cricket game free download
-download hitwicket superstars cricket game apk
-download hitwicket superstars cricket game from google play
-download hitwicket superstars cricket game from app store
-how to download hitwicket superstars cricket game
-how to play hitwicket superstars cricket game
-how to win hitwicket superstars cricket game
-how to train players in hitwicket superstars cricket game
-how to use superpowers in hitwicket superstars cricket game
-how to join multiplayer league in hitwicket superstars cricket game
-how to participate in world championships in hitwicket superstars cricket game
-how to form alliances in hitwicket superstars cricket game
-how to scout and recruit players in hitwicket superstars cricket game
-how to bid in live auctions in hitwicket superstars cricket game
-how to lift the world cup in hitwicket superstars cricket game
-best tips and tricks for hitwicket superstars cricket game
-best players and teams in hitwicket superstars cricket game
-best strategies and tactics in hitwicket superstars cricket game
-best batting and bowling animations in hitwicket superstars cricket game
-best 3d graphics and effects in hitwicket superstars cricket game
-best reviews and ratings for hitwicket superstars cricket game
-awards and achievements of hitwicket superstars cricket game
-features and updates of hitwicket superstars cricket game
-comparison of hitwicket superstars cricket game with other games
-benefits and advantages of playing hitwicket superstars cricket game
-challenges and difficulties of playing hitwicket superstars cricket game
-fun and addictive aspects of playing hitwicket superstars cricket game
-community and fan base of hitwicket superstars cricket game
-support and feedback for hitwicket superstars cricket game
-offers and promotions for hitwicket superstars cricket game
-in-app purchases and ads in hitwicket superstars cricket game
-Some of the ways to join the Hitwicket Superstars: Cricket community are:
-
-Join the official Discord server of Hitwicket Superstars: Cricket. You can chat with other players, get support from the developers, and access exclusive content and features. You can find the link to join the server here: Hitwicket Superstars: Cricket Discord Server
-Follow the official Facebook page of Hitwicket Superstars: Cricket. You can like, comment, and share posts, get updates and announcements, and participate in events and giveaways. You can find the link to follow the page here: Hitwicket Superstars: Cricket Facebook Page
-Follow the official Twitter account of Hitwicket Superstars: Cricket. You can tweet, retweet, and reply to tweets, get news and alerts, and join conversations and trends. You can find the link to follow the account here: Hitwicket Superstars: Cricket Twitter Account
-Follow the official Instagram account of Hitwicket Superstars: Cricket. You can view, like, and comment on photos and videos, get behind-the-scenes and sneak peeks, and join challenges and contests. You can find the link to follow the account here: Hitwicket Superstars: Cricket Instagram Account
-Subscribe to the official YouTube channel of Hitwicket Superstars: Cricket. You can watch, like, and comment on videos, get gameplay tips and tutorials, and access exclusive content and features. You can find the link to subscribe to the channel here: Hitwicket Superstars: Cricket YouTube Channel
-Join the official Reddit community of Hitwicket Superstars: Cricket. You can post, comment, and upvote posts, get feedback and advice, and join discussions and debates. You can find the link to join the community here: Hitwicket Superstars: Cricket Reddit Community
-Join the official Quora space of Hitwicket Superstars: Cricket. You can ask, answer, and follow questions, get insights and opinions, and join topics and interests. You can find the link to join the space here: Hitwicket Superstars: Cricket Quora Space
-
- Conclusion
- Hitwicket Superstars: Cricket is a game that every cricket fan should try. It is a game that combines sports and strategy in a unique way. It is a game that lets you own a real team of superstars, play epic T20 matches, participate in global tournaments, and win phenomenal rewards. It is a game that lets you experience cricket like never before.
-If you are looking for a fun and addictive cricket game that offers a realistic and immersive 3D graphics and animations, various modes and challenges to suit different preferences and skill levels, superpowers that add a twist and excitement to the game, multiplayer mode that lets you play with or against real players from around the world, alliance wars that let you collaborate and compete with other fans, player auction that lets you bid for the best cricketers in real-time, dream team that lets you create your own world-class cricket team, offline mode that lets you play without Wi-Fi, frequent updates and events that keep the game fresh and interesting, and a large and active community of cricket fans from around the world, then Hitwicket Superstars: Cricket is the game for you.
-We hope this article has given you a comprehensive review of Hitwicket Superstars: Cricket and its features, how to play it, and some tips and tricks to improve your skills. We also hope this article has helped you join the Hitwicket Superstars: Cricket community and interact with other players. If you have any questions or feedback about the game, feel free to contact us or leave a comment below. We would love to hear from you.
-Thank you for reading this article and happy gaming!
- FAQs
-Here are some frequently asked questions and answers about Hitwicket Superstars: Cricket:
-
-Q: How can I get more coins, gems, training points, etc. in Hitwicket Superstars: Cricket?
-A: You can get more coins, gems, training points, etc. by playing matches, winning tournaments, completing achievements, watching ads, or completing offers. You can also buy them with real money if you want.
-Q: How can I change my team name, logo, jersey, etc. in Hitwicket Superstars: Cricket?
-A: You can change your team name, logo, jersey, etc. by going to the settings menu and tapping on the edit option. You can also customize your team by choosing from various themes and designs.
-Q: How can I report a bug or a problem in Hitwicket Superstars: Cricket?
-A: You can report a bug or a problem in Hitwicket Superstars: Cricket by going to the settings menu and tapping on the help option. You can also contact the customer support team by emailing them at support@hitwicket.com or by filling out this form: Hitwicket Superstars: Cricket Support Form
-Q: How can I invite my friends to play Hitwicket Superstars: Cricket with me?
-A: You can invite your friends to play Hitwicket Superstars: Cricket with you by going to the friends menu and tapping on the invite option. You can also share your referral code or link with your friends and earn rewards when they join the game.
-Q: How can I delete my account or uninstall the game in Hitwicket Superstars: Cricket?
-A: You can delete your account or uninstall the game in Hitwicket Superstars: Cricket by going to the settings menu and tapping on the delete account option. You can also uninstall the game by going to your device's settings and tapping on the uninstall option.
- 401be4b1e0
-
-
\ No newline at end of file
diff --git a/spaces/fffiloni/controlnet-animation-doodle/node_modules/side-channel/index.js b/spaces/fffiloni/controlnet-animation-doodle/node_modules/side-channel/index.js
deleted file mode 100644
index f1c48264f08a8185f2d6c4dee257ba243d106eba..0000000000000000000000000000000000000000
--- a/spaces/fffiloni/controlnet-animation-doodle/node_modules/side-channel/index.js
+++ /dev/null
@@ -1,124 +0,0 @@
-'use strict';
-
-var GetIntrinsic = require('get-intrinsic');
-var callBound = require('call-bind/callBound');
-var inspect = require('object-inspect');
-
-var $TypeError = GetIntrinsic('%TypeError%');
-var $WeakMap = GetIntrinsic('%WeakMap%', true);
-var $Map = GetIntrinsic('%Map%', true);
-
-var $weakMapGet = callBound('WeakMap.prototype.get', true);
-var $weakMapSet = callBound('WeakMap.prototype.set', true);
-var $weakMapHas = callBound('WeakMap.prototype.has', true);
-var $mapGet = callBound('Map.prototype.get', true);
-var $mapSet = callBound('Map.prototype.set', true);
-var $mapHas = callBound('Map.prototype.has', true);
-
-/*
- * This function traverses the list returning the node corresponding to the
- * given key.
- *
- * That node is also moved to the head of the list, so that if it's accessed
- * again we don't need to traverse the whole list. By doing so, all the recently
- * used nodes can be accessed relatively quickly.
- */
-var listGetNode = function (list, key) { // eslint-disable-line consistent-return
- for (var prev = list, curr; (curr = prev.next) !== null; prev = curr) {
- if (curr.key === key) {
- prev.next = curr.next;
- curr.next = list.next;
- list.next = curr; // eslint-disable-line no-param-reassign
- return curr;
- }
- }
-};
-
-var listGet = function (objects, key) {
- var node = listGetNode(objects, key);
- return node && node.value;
-};
-var listSet = function (objects, key, value) {
- var node = listGetNode(objects, key);
- if (node) {
- node.value = value;
- } else {
- // Prepend the new node to the beginning of the list
- objects.next = { // eslint-disable-line no-param-reassign
- key: key,
- next: objects.next,
- value: value
- };
- }
-};
-var listHas = function (objects, key) {
- return !!listGetNode(objects, key);
-};
-
-module.exports = function getSideChannel() {
- var $wm;
- var $m;
- var $o;
- var channel = {
- assert: function (key) {
- if (!channel.has(key)) {
- throw new $TypeError('Side channel does not contain ' + inspect(key));
- }
- },
- get: function (key) { // eslint-disable-line consistent-return
- if ($WeakMap && key && (typeof key === 'object' || typeof key === 'function')) {
- if ($wm) {
- return $weakMapGet($wm, key);
- }
- } else if ($Map) {
- if ($m) {
- return $mapGet($m, key);
- }
- } else {
- if ($o) { // eslint-disable-line no-lonely-if
- return listGet($o, key);
- }
- }
- },
- has: function (key) {
- if ($WeakMap && key && (typeof key === 'object' || typeof key === 'function')) {
- if ($wm) {
- return $weakMapHas($wm, key);
- }
- } else if ($Map) {
- if ($m) {
- return $mapHas($m, key);
- }
- } else {
- if ($o) { // eslint-disable-line no-lonely-if
- return listHas($o, key);
- }
- }
- return false;
- },
- set: function (key, value) {
- if ($WeakMap && key && (typeof key === 'object' || typeof key === 'function')) {
- if (!$wm) {
- $wm = new $WeakMap();
- }
- $weakMapSet($wm, key, value);
- } else if ($Map) {
- if (!$m) {
- $m = new $Map();
- }
- $mapSet($m, key, value);
- } else {
- if (!$o) {
- /*
- * Initialize the linked list as an empty node, so that we don't have
- * to special-case handling of the first node: we can always refer to
- * it as (previous node).next, instead of something like (list).head
- */
- $o = { key: {}, next: null };
- }
- listSet($o, key, value);
- }
- }
- };
- return channel;
-};
diff --git a/spaces/firestalker/anime-tts/commons.py b/spaces/firestalker/anime-tts/commons.py
deleted file mode 100644
index 40fcc05364d4815971f5c6f9dbb8dcef8e3ec1e9..0000000000000000000000000000000000000000
--- a/spaces/firestalker/anime-tts/commons.py
+++ /dev/null
@@ -1,172 +0,0 @@
-import math
-import torch
-from torch.nn import functional as F
-import torch.jit
-
-
-def script_method(fn, _rcb=None):
- return fn
-
-
-def script(obj, optimize=True, _frames_up=0, _rcb=None):
- return obj
-
-
-torch.jit.script_method = script_method
-torch.jit.script = script
-
-
-def init_weights(m, mean=0.0, std=0.01):
- classname = m.__class__.__name__
- if classname.find("Conv") != -1:
- m.weight.data.normal_(mean, std)
-
-
-def get_padding(kernel_size, dilation=1):
- return int((kernel_size*dilation - dilation)/2)
-
-
-def convert_pad_shape(pad_shape):
- l = pad_shape[::-1]
- pad_shape = [item for sublist in l for item in sublist]
- return pad_shape
-
-
-def intersperse(lst, item):
- result = [item] * (len(lst) * 2 + 1)
- result[1::2] = lst
- return result
-
-
-def kl_divergence(m_p, logs_p, m_q, logs_q):
- """KL(P||Q)"""
- kl = (logs_q - logs_p) - 0.5
- kl += 0.5 * (torch.exp(2. * logs_p) + ((m_p - m_q)**2)) * torch.exp(-2. * logs_q)
- return kl
-
-
-def rand_gumbel(shape):
- """Sample from the Gumbel distribution, protect from overflows."""
- uniform_samples = torch.rand(shape) * 0.99998 + 0.00001
- return -torch.log(-torch.log(uniform_samples))
-
-
-def rand_gumbel_like(x):
- g = rand_gumbel(x.size()).to(dtype=x.dtype, device=x.device)
- return g
-
-
-def slice_segments(x, ids_str, segment_size=4):
- ret = torch.zeros_like(x[:, :, :segment_size])
- for i in range(x.size(0)):
- idx_str = ids_str[i]
- idx_end = idx_str + segment_size
- ret[i] = x[i, :, idx_str:idx_end]
- return ret
-
-
-def rand_slice_segments(x, x_lengths=None, segment_size=4):
- b, d, t = x.size()
- if x_lengths is None:
- x_lengths = t
- ids_str_max = x_lengths - segment_size + 1
- ids_str = (torch.rand([b]).to(device=x.device) * ids_str_max).to(dtype=torch.long)
- ret = slice_segments(x, ids_str, segment_size)
- return ret, ids_str
-
-
-def get_timing_signal_1d(
- length, channels, min_timescale=1.0, max_timescale=1.0e4):
- position = torch.arange(length, dtype=torch.float)
- num_timescales = channels // 2
- log_timescale_increment = (
- math.log(float(max_timescale) / float(min_timescale)) /
- (num_timescales - 1))
- inv_timescales = min_timescale * torch.exp(
- torch.arange(num_timescales, dtype=torch.float) * -log_timescale_increment)
- scaled_time = position.unsqueeze(0) * inv_timescales.unsqueeze(1)
- signal = torch.cat([torch.sin(scaled_time), torch.cos(scaled_time)], 0)
- signal = F.pad(signal, [0, 0, 0, channels % 2])
- signal = signal.view(1, channels, length)
- return signal
-
-
-def add_timing_signal_1d(x, min_timescale=1.0, max_timescale=1.0e4):
- b, channels, length = x.size()
- signal = get_timing_signal_1d(length, channels, min_timescale, max_timescale)
- return x + signal.to(dtype=x.dtype, device=x.device)
-
-
-def cat_timing_signal_1d(x, min_timescale=1.0, max_timescale=1.0e4, axis=1):
- b, channels, length = x.size()
- signal = get_timing_signal_1d(length, channels, min_timescale, max_timescale)
- return torch.cat([x, signal.to(dtype=x.dtype, device=x.device)], axis)
-
-
-def subsequent_mask(length):
- mask = torch.tril(torch.ones(length, length)).unsqueeze(0).unsqueeze(0)
- return mask
-
-
-@torch.jit.script
-def fused_add_tanh_sigmoid_multiply(input_a, input_b, n_channels):
- n_channels_int = n_channels[0]
- in_act = input_a + input_b
- t_act = torch.tanh(in_act[:, :n_channels_int, :])
- s_act = torch.sigmoid(in_act[:, n_channels_int:, :])
- acts = t_act * s_act
- return acts
-
-
-def convert_pad_shape(pad_shape):
- l = pad_shape[::-1]
- pad_shape = [item for sublist in l for item in sublist]
- return pad_shape
-
-
-def shift_1d(x):
- x = F.pad(x, convert_pad_shape([[0, 0], [0, 0], [1, 0]]))[:, :, :-1]
- return x
-
-
-def sequence_mask(length, max_length=None):
- if max_length is None:
- max_length = length.max()
- x = torch.arange(max_length, dtype=length.dtype, device=length.device)
- return x.unsqueeze(0) < length.unsqueeze(1)
-
-
-def generate_path(duration, mask):
- """
- duration: [b, 1, t_x]
- mask: [b, 1, t_y, t_x]
- """
- device = duration.device
-
- b, _, t_y, t_x = mask.shape
- cum_duration = torch.cumsum(duration, -1)
-
- cum_duration_flat = cum_duration.view(b * t_x)
- path = sequence_mask(cum_duration_flat, t_y).to(mask.dtype)
- path = path.view(b, t_x, t_y)
- path = path - F.pad(path, convert_pad_shape([[0, 0], [1, 0], [0, 0]]))[:, :-1]
- path = path.unsqueeze(1).transpose(2,3) * mask
- return path
-
-
-def clip_grad_value_(parameters, clip_value, norm_type=2):
- if isinstance(parameters, torch.Tensor):
- parameters = [parameters]
- parameters = list(filter(lambda p: p.grad is not None, parameters))
- norm_type = float(norm_type)
- if clip_value is not None:
- clip_value = float(clip_value)
-
- total_norm = 0
- for p in parameters:
- param_norm = p.grad.data.norm(norm_type)
- total_norm += param_norm.item() ** norm_type
- if clip_value is not None:
- p.grad.data.clamp_(min=-clip_value, max=clip_value)
- total_norm = total_norm ** (1. / norm_type)
- return total_norm
diff --git a/spaces/flamehaze1115/Wonder3D-demo/mvdiffusion/data/normal_utils.py b/spaces/flamehaze1115/Wonder3D-demo/mvdiffusion/data/normal_utils.py
deleted file mode 100644
index dff3730a312e96a2ed82dfd5a337d263baa0f2d8..0000000000000000000000000000000000000000
--- a/spaces/flamehaze1115/Wonder3D-demo/mvdiffusion/data/normal_utils.py
+++ /dev/null
@@ -1,45 +0,0 @@
-import numpy as np
-
-def camNormal2worldNormal(rot_c2w, camNormal):
- H,W,_ = camNormal.shape
- normal_img = np.matmul(rot_c2w[None, :, :], camNormal.reshape(-1,3)[:, :, None]).reshape([H, W, 3])
-
- return normal_img
-
-def worldNormal2camNormal(rot_w2c, normal_map_world):
- H,W,_ = normal_map_world.shape
- # normal_img = np.matmul(rot_w2c[None, :, :], worldNormal.reshape(-1,3)[:, :, None]).reshape([H, W, 3])
-
- # faster version
- # Reshape the normal map into a 2D array where each row represents a normal vector
- normal_map_flat = normal_map_world.reshape(-1, 3)
-
- # Transform the normal vectors using the transformation matrix
- normal_map_camera_flat = np.dot(normal_map_flat, rot_w2c.T)
-
- # Reshape the transformed normal map back to its original shape
- normal_map_camera = normal_map_camera_flat.reshape(normal_map_world.shape)
-
- return normal_map_camera
-
-def trans_normal(normal, RT_w2c, RT_w2c_target):
-
- # normal_world = camNormal2worldNormal(np.linalg.inv(RT_w2c[:3,:3]), normal)
- # normal_target_cam = worldNormal2camNormal(RT_w2c_target[:3,:3], normal_world)
-
- relative_RT = np.matmul(RT_w2c_target[:3,:3], np.linalg.inv(RT_w2c[:3,:3]))
- normal_target_cam = worldNormal2camNormal(relative_RT[:3,:3], normal)
-
- return normal_target_cam
-
-def img2normal(img):
- return (img/255.)*2-1
-
-def normal2img(normal):
- return np.uint8((normal*0.5+0.5)*255)
-
-def norm_normalize(normal, dim=-1):
-
- normal = normal/(np.linalg.norm(normal, axis=dim, keepdims=True)+1e-6)
-
- return normal
\ No newline at end of file
diff --git a/spaces/fuckyoudeki/AutoGPT/autogpt/json_utils/json_fix_general.py b/spaces/fuckyoudeki/AutoGPT/autogpt/json_utils/json_fix_general.py
deleted file mode 100644
index 7010fa3b9c1909de0e5a7f6ec13ca8aa418fe6c7..0000000000000000000000000000000000000000
--- a/spaces/fuckyoudeki/AutoGPT/autogpt/json_utils/json_fix_general.py
+++ /dev/null
@@ -1,124 +0,0 @@
-"""This module contains functions to fix JSON strings using general programmatic approaches, suitable for addressing
-common JSON formatting issues."""
-from __future__ import annotations
-
-import contextlib
-import json
-import re
-from typing import Optional
-
-from autogpt.config import Config
-from autogpt.json_utils.utilities import extract_char_position
-
-CFG = Config()
-
-
-def fix_invalid_escape(json_to_load: str, error_message: str) -> str:
- """Fix invalid escape sequences in JSON strings.
-
- Args:
- json_to_load (str): The JSON string.
- error_message (str): The error message from the JSONDecodeError
- exception.
-
- Returns:
- str: The JSON string with invalid escape sequences fixed.
- """
- while error_message.startswith("Invalid \\escape"):
- bad_escape_location = extract_char_position(error_message)
- json_to_load = (
- json_to_load[:bad_escape_location] + json_to_load[bad_escape_location + 1 :]
- )
- try:
- json.loads(json_to_load)
- return json_to_load
- except json.JSONDecodeError as e:
- if CFG.debug_mode:
- print("json loads error - fix invalid escape", e)
- error_message = str(e)
- return json_to_load
-
-
-def balance_braces(json_string: str) -> Optional[str]:
- """
- Balance the braces in a JSON string.
-
- Args:
- json_string (str): The JSON string.
-
- Returns:
- str: The JSON string with braces balanced.
- """
-
- open_braces_count = json_string.count("{")
- close_braces_count = json_string.count("}")
-
- while open_braces_count > close_braces_count:
- json_string += "}"
- close_braces_count += 1
-
- while close_braces_count > open_braces_count:
- json_string = json_string.rstrip("}")
- close_braces_count -= 1
-
- with contextlib.suppress(json.JSONDecodeError):
- json.loads(json_string)
- return json_string
-
-
-def add_quotes_to_property_names(json_string: str) -> str:
- """
- Add quotes to property names in a JSON string.
-
- Args:
- json_string (str): The JSON string.
-
- Returns:
- str: The JSON string with quotes added to property names.
- """
-
- def replace_func(match: re.Match) -> str:
- return f'"{match[1]}":'
-
- property_name_pattern = re.compile(r"(\w+):")
- corrected_json_string = property_name_pattern.sub(replace_func, json_string)
-
- try:
- json.loads(corrected_json_string)
- return corrected_json_string
- except json.JSONDecodeError as e:
- raise e
-
-
-def correct_json(json_to_load: str) -> str:
- """
- Correct common JSON errors.
- Args:
- json_to_load (str): The JSON string.
- """
-
- try:
- if CFG.debug_mode:
- print("json", json_to_load)
- json.loads(json_to_load)
- return json_to_load
- except json.JSONDecodeError as e:
- if CFG.debug_mode:
- print("json loads error", e)
- error_message = str(e)
- if error_message.startswith("Invalid \\escape"):
- json_to_load = fix_invalid_escape(json_to_load, error_message)
- if error_message.startswith(
- "Expecting property name enclosed in double quotes"
- ):
- json_to_load = add_quotes_to_property_names(json_to_load)
- try:
- json.loads(json_to_load)
- return json_to_load
- except json.JSONDecodeError as e:
- if CFG.debug_mode:
- print("json loads error - add quotes", e)
- error_message = str(e)
- if balanced_str := balance_braces(json_to_load):
- return balanced_str
- return json_to_load
diff --git a/spaces/g4f/freegpt-webui/g4f/Provider/Providers/ChatgptLogin.py b/spaces/g4f/freegpt-webui/g4f/Provider/Providers/ChatgptLogin.py
deleted file mode 100644
index 9551d15dd5121c4b42f80d0ba547a10f0868563b..0000000000000000000000000000000000000000
--- a/spaces/g4f/freegpt-webui/g4f/Provider/Providers/ChatgptLogin.py
+++ /dev/null
@@ -1,96 +0,0 @@
-import os
-from ...typing import sha256, Dict, get_type_hints
-import requests
-import re
-import base64
-
-url = 'https://chatgptlogin.ac'
-model = ['gpt-3.5-turbo']
-supports_stream = False
-needs_auth = False
-
-
-def _create_completion(model: str, messages: list, stream: bool, **kwargs):
- def get_nonce():
- res = requests.get('https://chatgptlogin.ac/use-chatgpt-free/', headers={
- "Referer": "https://chatgptlogin.ac/use-chatgpt-free/",
- "User-Agent": 'Mozilla/5.0 (Windows NT 10.0; Win64; x64) AppleWebKit/537.36 (KHTML, like Gecko) Chrome/114.0.0.0 Safari/537.36'
- })
-
- src = re.search(r'class="mwai-chat mwai-chatgpt">.*Send
-END_OF_HEADER2
-;
- }
-
- print OUT<
-
-END_OF_HEADER3
-;
-}
-
-
-sub print_html_foot {
- local($this, *OUT) = @_;
-
- print OUT " \n";
- print OUT "