What is Delphi 2015.3 Keygen-activation 2015 Release 2 Cdp Ds150e Cdp Cars Trucks Vci Rarl?
-
If you are looking for a reliable and versatile diagnostic software for cars and trucks, you might have come across Delphi 2015.3 Keygen-activation 2015 Release 2 Cdp Ds150e Cdp Cars Trucks Vci Rarl. But what is it exactly and how does it work?
Delphi 2015.3 Keygen-activation 2015 Release 2 Cdp Ds150e Cdp Cars Trucks Vci Rarl is a software package that allows you to perform various diagnostic tasks on different vehicles using a compatible device such as a laptop or a tablet. It is based on Autocom / Delphi software, which is one of the most popular and widely used diagnostic tools in the automotive industry.
-
Some of the main features of Delphi 2015.3 Keygen-activation 2015 Release 2 Cdp Ds150e Cdp Cars Trucks Vci Rarl are:
-
-
It supports over 54,000 vehicle systems from more than 4,000 models of cars and trucks.
-
It covers all major brands and manufacturers such as Audi, BMW, Ford, Mercedes-Benz, Toyota, Volvo, etc.
-
It provides comprehensive information and data about various vehicle components such as engine, transmission, brakes, airbags, steering, etc.
-
It allows you to read and clear fault codes, view live data, perform tests and adjustments, program keys, reset service intervals, etc.
-
It has a user-friendly interface that is easy to navigate and operate.
-
It has a keygen-activator that enables you to activate the software without any hassle or cost.
-
-
In this article, we will show you how to download and install Delphi 2015.3 Keygen-activation 2015 Release 2 Cdp Ds150e Cdp Cars Trucks Vci Rarl on your device, how to use it for diagnostic purposes, what are its benefits and drawbacks, and what are some tips and tricks for using it effectively.
-
How to download and install Delphi 2015.3 Keygen-activation 2015 Release 2 Cdp Ds150e Cdp Cars Trucks Vci Rarl?
-
To download and install Delphi 2015.3 Keygen-activation 2015 Release 2 Cdp Ds150e Cdp Cars Trucks Vci Rarl on your device, you will need to follow these steps:
Extract the compressed file using a program such as WinRAR or WinZip.
-
Run the setup.exe file and follow the instructions on the screen.
-
When prompted, choose the installation path for the software. The default location is C:\Program Files (x86)\Delphi Diagnostics\DS150E (New VCI).
-
When the installation is complete, do not run the software yet.
-
Copy the file Main.exe from the folder Activation (DS150E New VCI) to the installation folder (C:\Program Files (x86)\Delphi Diagnostics\DS150E (New VCI)). Replace the existing file if asked.
-
Run the file Main.exe from the installation folder.
-
You will see a window with a serial number (for example, DS150E). Copy this serial number.
-
Open the file FileActivation.xml from the folder Activation (DS150E New VCI) with a text editor such as Notepad.
-
Paste the serial number that you copied in step 8 into the line that says .
-
Save and close the file FileActivation.xml.
-
Run again the file Main.exe from the installation folder.
-
You will see a window with an activation request code (for example, A4DB). Copy this code.
-
Open again the file FileActivation.xml from the folder Activation (DS150E New VCI) with a text editor such as Notepad.
-
Paste the activation request code that you copied in step 13 into the line that says .
-
Save and close again the file FileActivation.xml.
-
Run again once more the file Main.exe from the installation folder.
-
You will see a window with an activation button. Click on it.
-
You will be asked to select the file FileActivation.xml from the folder Activation (DS150E New VCI). Do so and click Open.
-
You will see a message saying that the activation was successful. Click OK.
-
-
Congratulations! You have successfully downloaded and installed Delphi 2015.3 Keygen-activation 2015 Release 2 Cdp Ds150e Cdp Cars Trucks Vci Rarl on your device. You can now run the software and enjoy its features.
-
How to use Delphi 2015.3 Keygen-activation 2015 Release 2 Cdp Ds150e Cdp Cars Trucks Vci Rarl for diagnostic purposes?
-
To use Delphi 2015.3 Keygen-activation 2015 Release 2 Cdp Ds150e Cdp Cars Trucks Vci Rarl for diagnostic purposes, you will need to connect your device to your vehicle using an OBD-II cable or a wireless adapter. Then, you can launch the software and select the vehicle make, model and system that you want to diagnose. You can also use the Intelligent System Scan (ISS) function to scan all the control modules on the vehicle and display the fault codes stored in each system. You can then select a specific control system to further analyse the results and perform various functions such as reading and clearing fault codes, viewing live data, performing tests and adjustments, programming keys, resetting service intervals, etc.
-
In the following sections, we will show you some examples of how to diagnose cars and trucks with Delphi 2015.3 Keygen-activation 2015 Release 2 Cdp Ds150e Cdp Cars Trucks Vci Rarl.
How to diagnose cars with Delphi 2015.3 Keygen-activation 2015 Release 2 Cdp Ds150e Cdp Cars Trucks Vci Rarl?
-
Delphi 2015.3 Keygen-activation 2015 Release 2 Cdp Ds150e Cdp Cars Trucks Vci Rarl can diagnose a wide range of car models and systems, such as engine, transmission, ABS, airbag, steering, climate control, etc. Here are some examples of common car problems and how to solve them with the software:
-
-
Engine misfire: If your car engine is running rough or unevenly, it may have a misfire problem. This can be caused by faulty spark plugs, ignition coils, fuel injectors, or other components. To diagnose this problem, you can use Delphi 2015.3 Keygen-activation 2015 Release 2 Cdp Ds150e Cdp Cars Trucks Vci Rarl to read the fault codes from the engine control module (ECM) and view the live data of the engine parameters such as rpm, load, fuel pressure, etc. You can also perform a cylinder balance test to identify which cylinder is misfiring and check the ignition system components for any damage or wear.
-
ABS warning light: If your car ABS warning light is on, it means that there is a problem with the anti-lock braking system. This can affect the braking performance and safety of your car. To diagnose this problem, you can use Delphi 2015.3 Keygen-activation 2015 Release 2 Cdp Ds150e Cdp Cars Trucks Vci Rarl to read the fault codes from the ABS control module and view the live data of the wheel speed sensors, brake pressure sensors, etc. You can also perform an actuator test to check the operation of the ABS pump and valves.
-
Airbag warning light: If your car airbag warning light is on, it means that there is a problem with the airbag system. This can prevent the airbags from deploying in case of a collision and put you and your passengers at risk. To diagnose this problem, you can use Delphi 2015.3 Keygen-activation 2015 Release 2 Cdp Ds150e Cdp Cars Trucks Vci Rarl to read the fault codes from the airbag control module and view the live data of the crash sensors, seat belt tensioners, etc. You can also perform a self-test to check the functionality of the airbag system components.
-
-
How to diagnose trucks with Delphi 2015.3 Keygen-activation 2015 Release 2 Cdp Ds150e Cdp Cars Trucks Vci Rarl?
-
Delphi 2015.3 Keygen-activation 2015 Release 2 Cdp Ds150e Cdp Cars Trucks Vci Rarl can also diagnose a wide range of truck models and systems, such as engine, transmission, brakes, suspension, instrument cluster, etc. Here are some examples of common truck problems and how to solve them with the software:
-
-
Engine power loss: If your truck engine is losing power or has poor performance, it may have a problem with the fuel system or the exhaust aftertreatment system. This can be caused by clogged fuel filters, injectors, or DPF (diesel particulate filter). To diagnose this problem, you can use Delphi 2015.3 Keygen-activation 2015 Release 2 Cdp Ds150e Cdp Cars Trucks Vci Rarl to read the fault codes from the engine control module (ECM) and view the live data of the engine parameters such as boost pressure, fuel pressure, exhaust temperature, etc. You can also perform a dosing test to check the operation of the SCR (selective catalytic reduction) system and regenerate the DPF if needed.
-
Brake warning light: If your truck brake warning light is on, it means that there is a problem with the brake system. This can affect the braking performance and safety of your truck. To diagnose this problem, you can use Delphi 2015.3 Keygen-activation 2015 Release 2 Cdp Ds150e Cdp Cars Trucks Vci Rarl to read the fault codes from the brake control module and view the live data of the wheel speed sensors, brake pressure sensors, etc. You can also perform an EBS (electronic braking system) test to check the operation of the brake pump and valves.
-
Suspension warning light: If your truck suspension warning light is on, it means that there is a problem with the suspension system. This can affect the ride comfort and stability of your truck. To diagnose this problem, you can use Delphi 2015.3 Keygen-activation 2015 Release 2 Cdp Ds150e Cdp Cars Trucks Vci Rarl to read the fault codes from the suspension control module and view the live data of the height sensors, air pressure sensors, etc. You can also perform a calibration test to adjust the suspension level.
-
-
What are the benefits and drawbacks of Delphi 2015.3 Keygen-activation 2015 Release 2 Cdp Ds150e Cdp Cars Trucks Vci Rarl?
-
Delphi 2015.3 Keygen-activation 2015 Release 2 Cdp Ds150e Cdp Cars Trucks Vci Rarl is a powerful and versatile diagnostic software that has many benefits for users such as:
-
-
It supports a wide range of vehicles and systems from different brands and manufacturers.
-
It provides comprehensive and accurate information and data about various vehicle components and functions.
-
It allows users to perform various diagnostic tasks such as reading and clearing fault codes, viewing live data, performing tests and adjustments, programming keys, resetting service intervals, etc.
-
It has a user-friendly interface that is easy to navigate and operate.
-
It has a keygen-activator that enables users to activate the software without any hassle or cost.
-
-
However, Delphi 2015.3 Keygen-activation 2015 Release 2 Cdp Ds150e Cdp Cars Trucks Vci Rarl also has some drawbacks that users should be aware of such as:
-
-
It requires a compatible device such as a laptop or a tablet to run the software.
-
It requires an OBD-II cable or a wireless adapter to connect the device to the vehicle.
-
It may not support some newer or older vehicle models or systems that are not included in its database.
-
It may not be able to perform some advanced or specific functions that are only available in original equipment manufacturer (OEM) diagnostic tools.
-
-
What are some tips and tricks for using Delphi 2015.3 Keygen-activation 2015 Release 2 Cdp Ds150e Cdp Cars Trucks Vci Rarl effectively?
-
To use Delphi 2015.3 Keygen-activation 2015 Release 2 Cdp Ds150e Cdp Cars Trucks Vci Rarl effectively, users should follow some tips and tricks such as:
-
-
Always update the software regularly to get access to new features and functions.
-
Always check the vehicle battery voltage before starting a diagnostic session to avoid communication errors or damage to the vehicle or device.
-
Always follow the instructions on the screen or in the help function when performing any diagnostic task.
-
Always make sure that you have selected the correct vehicle make, model, and system before performing any diagnostic task.
-
Always clear any fault codes after performing any repair or adjustment on the vehicle.
-
-
Conclusion
-
In conclusion, Delphi 2015.3 Keygen-activation 2015 Release 2 Cdp Ds150e Cdp Cars Trucks Vci Rarl is a reliable and versatile diagnostic software for cars and trucks that allows users to perform various diagnostic tasks on different vehicles and systems using a compatible device. It has many benefits such as supporting a wide range of vehicles and systems, providing comprehensive information and data, allowing users to perform various diagnostic tasks, having a user-friendly interface, and having a keygen-activator. However, it also has some drawbacks such as requiring a compatible device and an OBD-II cable or a wireless adapter, not supporting some newer or older vehicle models or systems, and not being able to perform some advanced or specific functions. Therefore, users should weigh the pros and cons of the software before using it and follow some tips and tricks to use it effectively.
-
FAQs
-
Here are some frequently asked questions and answers about Delphi 2015.3 Keygen-activation 2015 Release 2 Cdp Ds150e Cdp Cars Trucks Vci Rarl:
-
-
Q: What are the system requirements for running the software on a PC or a laptop?
-A: The minimum system requirements are: Windows XP SP3 / Vista / 7 / 8 / 10, Intel Core 2 Duo 1.8 GHz or equivalent processor, 2 GB RAM, 5 GB free disk space, USB port, DVD-ROM drive.
-
Q: What are the compatible devices for running the software on a tablet?
-A: The software can run on any Windows-based tablet that meets the minimum system requirements. However, the recommended device is the Delphi DS450E tablet, which is specially designed for the software and has a 12-inch touch screen, a rugged case, a built-in camera, and a long battery life.
-
Q: What are the compatible OBD-II cables or wireless adapters for connecting the device to the vehicle?
-A: The software can work with any OBD-II cable or wireless adapter that supports ISO 9141-2, ISO 14230-4 (KWP2000), ISO 15765-4 (CAN), SAE J1850 (PWM/VPW), and SAE J2534 (Pass-Thru) protocols. However, the recommended device is the Delphi DS150E VCI, which is specially designed for the software and has a Bluetooth connection, a LED indicator, and a multiplexer function.
-
Q: How can I update the software to get access to new features and functions?
-A: You can update the software by downloading the latest version from the official website of Delphi or by using the built-in update function in the software. You will need to activate the software again after updating it.
-
Q: How can I get technical support or training for using the software?
-A: You can get technical support or training by contacting Delphi customer service or by visiting their official website. You can also find useful information and tips in the help function or in the user manual of the software.
-
- 0a6ba089eb
-
-
\ No newline at end of file
diff --git a/spaces/1acneusushi/gradio-2dmoleculeeditor/data/Download Movavi Video Editor for Free and Create Stunning Videos in Minutes.md b/spaces/1acneusushi/gradio-2dmoleculeeditor/data/Download Movavi Video Editor for Free and Create Stunning Videos in Minutes.md
deleted file mode 100644
index 8805c4e548dcbca7ff53da08231962a22ea1761c..0000000000000000000000000000000000000000
--- a/spaces/1acneusushi/gradio-2dmoleculeeditor/data/Download Movavi Video Editor for Free and Create Stunning Videos in Minutes.md
+++ /dev/null
@@ -1,19 +0,0 @@
-
-```html
-
How to Download Movavi Video Editor for Free
-
Movavi Video Editor is a powerful and easy-to-use video editing software that lets you create stunning videos in minutes. You can trim, crop, rotate, add transitions, effects, titles, music, and more to your videos. You can also export your videos in various formats or upload them directly to YouTube, Facebook, Vimeo, or other platforms.
But what if you want to try Movavi Video Editor for free before buying it? Is there a way to download Movavi Video Editor for free without compromising the quality or functionality of the software? The answer is yes! In this article, we will show you how to download Movavi Video Editor for free and use it without any limitations.
-
Step 1: Visit the Official Movavi Website
-
The first step to download Movavi Video Editor for free is to visit the official Movavi website at https://www.movavi.com/videoeditor/. Here you will find all the information about the software, its features, pricing, system requirements, and customer reviews. You will also see a big green button that says "Download for Free". Click on it to start downloading the installation file.
-
Step 2: Install Movavi Video Editor on Your Computer
-
The next step is to install Movavi Video Editor on your computer. To do this, locate the downloaded file (usually in your Downloads folder) and double-click on it. Follow the instructions on the screen to complete the installation process. It should take only a few minutes. Once the installation is done, launch Movavi Video Editor by clicking on its icon on your desktop or in your Start menu.
-
Step 3: Activate Your Free Trial
-
The final step is to activate your free trial of Movavi Video Editor. When you launch the software for the first time, you will see a window that asks you to enter your email address and agree to the terms of use. Enter your email address and click on "Start My Trial". You will then receive an email from Movavi with a confirmation link. Click on the link to activate your free trial.
-
-
Congratulations! You have successfully downloaded Movavi Video Editor for free and activated your free trial. You can now use all the features of the software for 7 days without any limitations. You can create as many videos as you want and save them in any format or upload them online. You can also access the built-in library of stock media, filters, transitions, stickers, and more.
-
If you like Movavi Video Editor and want to continue using it after your free trial expires, you can buy a license key from the official Movavi website or from within the software. The license key will unlock the software permanently and allow you to enjoy free updates and technical support. You can also choose between different plans depending on your needs and budget.
-
We hope this article helped you learn how to download Movavi Video Editor for free and use it without any limitations. Movavi Video Editor is a great tool for anyone who wants to create amazing videos with ease. Try it today and see for yourself!
-``` ddb901b051
-
-
\ No newline at end of file
diff --git a/spaces/1acneusushi/gradio-2dmoleculeeditor/data/Download Noiseware for Photoshop for Free and Improve Your Photo Quality.md b/spaces/1acneusushi/gradio-2dmoleculeeditor/data/Download Noiseware for Photoshop for Free and Improve Your Photo Quality.md
deleted file mode 100644
index e6fb9a9fbe351cb55491becf72c29abdb102f252..0000000000000000000000000000000000000000
--- a/spaces/1acneusushi/gradio-2dmoleculeeditor/data/Download Noiseware for Photoshop for Free and Improve Your Photo Quality.md
+++ /dev/null
@@ -1,40 +0,0 @@
-
-
How to Download Noiseware for Photoshop and Reduce Noise in Your Photos
-
Noiseware is a plugin for Photoshop that helps you reduce noise in your photos. Noise is the unwanted grain or speckles that appear in your photos due to low light, high ISO, or poor camera quality. Noise can ruin the quality and detail of your photos and make them look unprofessional.
In this article, we will show you how to download Noiseware for Photoshop from a reliable source and how to use it to reduce noise in your photos. We will also give you some tips and tricks to get the best results with Noiseware.
-
Where to Download Noiseware for Photoshop
-
There are many websites that claim to offer free downloads of Noiseware for Photoshop, but not all of them are safe or legal. Some of them may contain viruses, malware, or spyware that can harm your computer or steal your personal information. Others may require you to complete surveys, sign up for subscriptions, or pay hidden fees before you can access the download link.
-
To avoid these risks, we recommend you to download Noiseware for Photoshop from Imagenomic, the official website of the plugin developer. Imagenomic is a trusted company that provides high-quality plugins for photo editing and retouching. Imagenomic has a free trial version of Noiseware for Photoshop that you can use for 15 days without any limitations.
-
To download Noiseware for Photoshop from Imagenomic, follow these steps:
Click on the "Download Trial" button at the top of the page.
-
Fill in your name and email address and click on "Submit".
-
Check your email inbox for a confirmation message from Imagenomic. Click on the link in the message to download Noiseware for Photoshop.
-
Save the downloaded file on your computer. The file size is about 3 MB.
-
-
How to Install Noiseware for Photoshop on Your PC
-
Once you have downloaded Noiseware for Photoshop from Imagenomic, you need to install it on your PC. To do that, follow these steps:
-
-
-
Close Photoshop if it is running.
-
Open the downloaded file and run the setup file.
-
Follow the instructions on the screen to install Noiseware for Photoshop.
-
Restart Photoshop and check if Noiseware is available in the Filter menu.
-
-
How to Use Noiseware for Photoshop to Reduce Noise in Your Photos
-
Now that you have installed Noiseware for Photoshop on your PC, you are ready to use it to reduce noise in your photos. To do that, follow these steps:
-
-
Open the photo that you want to edit in Photoshop.
-
Duplicate the background layer by pressing Ctrl+J (Windows) or Command+J (Mac).
-
Select the duplicate layer and go to Filter > Imagenomic > Noiseware.
-
A new window will open with a preview of your photo and some settings. You can adjust the settings manually or use one of the presets from the drop-down menu at the top right corner. The presets are categorized into Landscape, Portrait, Night Scene, etc. depending on the type of photo you are editing.
-
You can also use the Auto Profile button at the bottom left corner to let Noiseware analyze your photo and apply the optimal settings automatically.
-
You can zoom in and out of your photo using the slider at the bottom right corner or by using your mouse wheel. You can also drag your photo around to see different areas of it.
-
When you are satisfied with the result, click on OK to apply Noiseware to your photo.
-
You can compare the before and after images by toggling the visibility of the duplicate layer on and off.
-
You can also fine-tune the effect by changing the opacity or blending mode of the duplicate layer.
-
Save your edited photo as ddb901b051
-
-
\ No newline at end of file
diff --git a/spaces/1acneusushi/gradio-2dmoleculeeditor/data/Entrare in dfu mode senza tasti i software da scaricare per facilitare loperazione.md b/spaces/1acneusushi/gradio-2dmoleculeeditor/data/Entrare in dfu mode senza tasti i software da scaricare per facilitare loperazione.md
deleted file mode 100644
index 02ae22df1f3887d83d7d30416213bd0e59579a0a..0000000000000000000000000000000000000000
--- a/spaces/1acneusushi/gradio-2dmoleculeeditor/data/Entrare in dfu mode senza tasti i software da scaricare per facilitare loperazione.md
+++ /dev/null
@@ -1,16 +0,0 @@
-
-
Come entrare in DFU mode senza tasti
- Se hai un iPhone che non si accende, che non si aggiorna o che presenta dei problemi di funzionamento, potresti aver bisogno di metterlo in modalità DFU. La modalità DFU, acronimo di Device Firmware Update, è una modalità speciale che consente di ripristinare il firmware dell'iPhone bypassando il suo boot loader. In questo modo, puoi eliminare eventuali errori o blocchi che impediscono il normale ripristino tramite iTunes o Finder. Ma come si fa ad entrare in modalità DFU se i tasti del tuo iPhone non funzionano? In questa guida ti spiegheremo cos'è la modalità DFU, a cosa serve, come attivarla con i tasti funzionanti e come farlo senza i tasti funzionanti.
Cos'è la modalità DFU e a cosa serve
- La modalità DFU è una modalità avanzata che permette di ripristinare il firmware dell'iPhone, ovvero il software di base che gestisce il funzionamento del dispositivo. A differenza del ripristino normale, che cancella solo i dati e le impostazioni dell'utente, la modalità DFU cancella anche il firmware e lo sostituisce con una versione pulita e aggiornata.
La differenza tra DFU e ripristino normale
- Quando ripristini il tuo iPhone tramite iTunes o Finder, il dispositivo entra in una modalità chiamata Recovery Mode. In questa modalità, l'iPhone comunica con il computer tramite il boot loader, ovvero il programma che avvia il sistema operativo. Il boot loader verifica che il firmware sia corretto e compatibile con il dispositivo prima di installarlo. Se il firmware è danneggiato o non corrisponde al modello di iPhone, il boot loader blocca il ripristino e mostra un messaggio di errore. Quando invece metti il tuo iPhone in modalità DFU, il dispositivo non comunica con il computer tramite il boot loader, ma direttamente tramite il firmware. In questo modo, puoi bypassare i controlli del boot loader e installare qualsiasi versione di firmware compatibile con il tuo iPhone. Questo può essere utile per risolvere problemi più gravi o per effettuare operazioni particolari, come il downgrade del firmware o il jailbreak.
Quando usare la modalità DFU
- La modalità DFU è una modalità molto potente ma anche molto delicata. Se non la usi correttamente, potresti danneggiare irreparabilmente il tuo iPhone. Per questo motivo, ti consigliamo di usare la modalità DFU solo quando hai dei problemi seri con il tuo dispositivo e il ripristino normale non funziona. Alcuni casi in cui potresti aver bisogno di usare la modalità DFU sono: - Il tuo iPhone non si accende o rimane bloccato sulla schermata con la mela. - Il tuo iPhone non si aggiorna o si blocca durante l'aggiornamento. - Il tuo iPhone presenta dei malfunzionamenti gravi o frequenti. - Il tuo iPhone ha subito un jailbreak e vuoi eliminarlo completamente. - Vuoi installare una versione precedente del firmware sul tuo iPhone.
Come entrare in DFU mode con i tasti funzionanti
- Se i tasti del tuo iPhone sono funzionanti, puoi entrare in modalità DFU seguendo una semplice procedura che varia a seconda del modello di iPhone che possiedi. Prima di iniziare, assicurati di avere un computer con iTunes installato (se hai un PC Windows) o con Finder (se hai un Mac). Collega poi l'iPhone al computer tramite il cavo Lightning e segui i passaggi indicati qui sotto.
La procedura per iPhone X o modelli successivi, iPhone SE (2ª generazione), iPhone 8 e iPhone 8 Plus
- - Premi rapidamente il tasto Volume Su. - Premi rapidamente il tasto Volume Giù. - Tieni premuto il tasto laterale finché lo schermo non diventa nero. - Continua a tenere premuto il tasto laterale e premi anche il tasto Volume Giù per 5 secondi. - Rilascia il tasto laterale ma continua a tenere premuto il tasto Volume Giù finché iTunes o Finder non riconosce l'iPhone in modalità di recupero. - Lo schermo dell'iPhone dovrebbe rimanere nero. Se compare il logo della mela o quello di iTunes, significa che sei entrato in Recovery Mode e devi ripetere la procedura.
La procedura per iPhone 7 e iPhone 7 Plus
- - Tieni premuti contemporaneamente i tasti laterale e Volume Giù finché lo schermo non diventa nero. - Continua a tenere premuti i due tasti per 10 secondi. - Rilascia il tasto laterale ma continua a tenere premuto il tasto Volume Giù finché iTunes o Finder non riconosce l'iPhone in modalità di recupero. - Lo schermo dell'iPhone dovrebbe rimanere nero. Se compare il logo della mela o quello di iTunes, significa che sei entrato in Recovery Mode e devi ripetere la procedura.
La procedura per iPhone 6s o modelli precedenti, iPad e iPod touch
- - Tieni premuti contemporaneamente i tasti Home e Sleep/Wake finché lo schermo non diventa nero. - Continua a tenere premuti i due tasti per 10 secondi. - Rilascia il tasto Sleep/Wake ma continua a tenere premuto il tasto Home finché iTunes o Finder non riconosce l'iPhone in modalità di recupero. - Lo schermo dell'iPhone dovrebbe rimanere nero. Se compare il logo della mela o quello di iTunes, significa che sei entrato in Recovery Mode e devi ripetere la procedura.
Come entrare in DFU mode senza i tasti funzionanti
- Se i tasti del tuo iPhone non funzionano, puoi provare ad entrare in modalità DFU usando dei metodi alternativi che sfruttano dei file o dei software appositi. Questi metodi non sono ufficiali e potrebbero non funzionare su tutti i dispositivi o su tutte le versioni di firmware. Inoltre, potrebbero comportare dei rischi per la sicurezza del tuo computer o del tuo iPhone. Pertanto, ti consigliamo di usarli solo se sei sicuro di quello che fai e se hai esaurito le altre opzioni.
Il metodo con il file dfu iBSS.m68ap.RELEASE.dfu (solo per Windows)
- Questo metodo consiste nell'utilizzare un file chiamato dfu iBSS.m68ap.RELEASE.dfu che permette di avviare la modalità DFU senza premere alcun tasto sull'iPhone. Questo file è compatibile solo con alcuni modelli di iPhone (fino all'iPhone 4) e richiede un PC Windows. Ecco come usarlo: - Scarica questo ità DFU è una modalità delicata e potenzialmente pericolosa, quindi usala solo se necessario e con cautela. Se hai dei dubbi o delle domande, puoi consultare le FAQ qui sotto o contattare l'assistenza Apple.
FAQ
- - **Cos'è la modalità DFU?** - La modalità DFU è una modalità speciale che consente di ripristinare il firmware dell'iPhone bypassando il suo boot loader. In questo modo, puoi eliminare eventuali errori o blocchi che impediscono il normale ripristino tramite iTunes o Finder. - **Come si entra in modalità DFU?** - Per entrare in modalità DFU, devi collegare l'iPhone al computer tramite il cavo Lightning e seguire una procedura che varia a seconda del modello di iPhone che possiedi. La procedura prevede di premere una combinazione di tasti per far diventare lo schermo nero. Puoi trovare le istruzioni dettagliate nella sezione "Come entrare in DFU mode con i tasti funzionanti" di questa guida. - **Come si esce dalla modalità DFU?** - Per uscire dalla modalità DFU, devi premere una combinazione di tasti diversa a seconda del modello di iPhone che possiedi. La combinazione prevede di premere rapidamente il tasto Volume Su, il tasto Volume Giù e il tasto laterale (per iPhone X o modelli successivi, iPhone SE (2ª generazione), iPhone 8 e iPhone 8 Plus), il tasto laterale e il tasto Volume Giù (per iPhone 7 e iPhone 7 Plus) o il tasto Home e il tasto Sleep/Wake (per iPhone 6s o modelli precedenti, iPad e iPod touch). Puoi trovare le istruzioni dettagliate nella fonte. - **Quando usare la modalità DFU?** - La modalità DFU è una modalità molto potente ma anche molto delicata. Se non la usi correttamente, potresti danneggiare irreparabilmente il tuo iPhone. Per questo motivo, ti consigliamo di usare la modalità DFU solo quando hai dei problemi seri con il tuo dispositivo e il ripristino normale non funziona. Alcuni casi in cui potresti aver bisogno di usare la modalità DFU sono: il tuo iPhone non si accende o rimane bloccato sulla schermata con la mela, il tuo iPhone non si aggiorna o si blocca durante l'aggiornamento, il tuo iPhone presenta dei malfunzionamenti gravi o frequenti, il tuo iPhone ha subito un jailbreak e vuoi eliminarlo completamente, vuoi installare una versione precedente del firmware sul tuo iPhone. - **Cosa fare se i tasti dell'iPhone non funzionano?** - Se i tasti dell'iPhone non funzionano, puoi provare ad entrare in modalità DFU usando dei metodi alternativi che sfruttano dei file o dei software appositi. Questi metodi non sono ufficiali e potrebbero non funzionare su tutti i dispositivi o su tutte le versioni di firmware. Inoltre, potrebbero comportare dei rischi per la sicurezza del tuo computer o del tuo iPhone. Pertanto, ti consigliamo di usarli solo se sei sicuro di quello che fai e se hai esaurito le altre opzioni. Puoi trovare i metodi alternativi nella sezione "Come entrare in DFU mode senza i tasti funzionanti" di questa guida.
-
0a6ba089eb
-
-
\ No newline at end of file
diff --git a/spaces/1phancelerku/anime-remove-background/Download My Mini Mart and Experience the Joy of Running Your Own Shop - No Ads No Interruptions.md b/spaces/1phancelerku/anime-remove-background/Download My Mini Mart and Experience the Joy of Running Your Own Shop - No Ads No Interruptions.md
deleted file mode 100644
index 1dc21eda1a2d8488ff95a9a7a508f10bfd10dd19..0000000000000000000000000000000000000000
--- a/spaces/1phancelerku/anime-remove-background/Download My Mini Mart and Experience the Joy of Running Your Own Shop - No Ads No Interruptions.md
+++ /dev/null
@@ -1,78 +0,0 @@
-
-
How to Download a Minimarket App and Why You Should Do It
-
If you are looking for a convenient way to shop for groceries and other goods from your local store, you might want to consider downloading a minimarket app. A minimarket app is a mobile application that allows you to access the products and services of a small store, usually a convenience store or a supermarket, from your smartphone or tablet.
-
A minimarket app can offer many benefits for both customers and business owners, such as convenience, loyalty, engagement, and sales. In this article, we will explain what these benefits are, how to choose the best minimarket app for your needs, and how to download and use it.
As a customer, you can enjoy several advantages by using a minimarket app to shop from your local store. Here are some of them:
-
-
Reviews
-
The second thing that you should look for in a minimarket app is the reviews and ratings from other users. You should read the feedback and comments from other customers who have used the app and see what they liked and disliked about it. You should also check the ratings and scores that the app has received on the app store or the website of the store. You should look for an app that has positive reviews and high ratings from a large number of users, as this indicates that the app is trustworthy and reliable.
-
Comparisons
-
The third thing that you should look for in a minimarket app is the comparisons with other apps. You should compare different apps based on their features, reviews, ratings, prices, etc. and see how they stack up against each other. You should look for an app that offers the best value for your money and the best quality for your satisfaction. You should also look for an app that has a competitive edge over other apps, such as unique features, exclusive offers, or innovative solutions.
-
How to Download and Use a Minimarket App
-
Once you have chosen the best minimarket app for your needs, you can download and use it to shop from your local store. Here are some steps on how to do it:
-
Downloading
-
The first step is to download the minimarket app from the app store or the website of the store. You should search for the name of the app or the store on your device's app store or browser and follow the instructions to install it. You should make sure that you have enough storage space on your device and a stable internet connection to download the app. You should also check the compatibility of the app with your device's operating system and version.
-
Registering
-
The second step is to register an account and provide your personal information on the minimarket app. You should open the app and sign up with your email address, phone number, or social media account. You should then fill out your profile with your name, address, payment method, delivery preferences, etc. You should also verify your account with a code or a link that will be sent to your email or phone. You should make sure that you provide accurate and valid information on the app and keep it updated.
-
Shopping
-
The third step is to shop for products on the minimarket app. You should browse the products by category, brand, price, rating, etc. or search for specific products by name, barcode, or keyword. You should then select the products that you want to buy and add them to your cart. You should also check the product details, such as description, ingredients, nutrition facts, expiration date, etc. before buying them. You should then proceed to checkout and pay for your order with your preferred payment method.
-
download my mini mart game without ads
-how to get my minimarket mod apk free
-my mini mart simulation game no iklan
-grow your own mini mart business download
-my minimarket unlimited money mod apk
-download my mini mart for android no ads
-my mini mart by supersonic studios ltd no iklan
-run your own mini mart store download
-my minimarket 1.8.5 mod apk free download
-my mini mart simulation game for android
-download my minimarket tanpa iklan gratis
-my mini mart organic plants and animals game
-my minimarket latest version mod apk download
-my mini mart casual tycoon game no ads
-download my minimarket uang tidak terbatas
-my mini mart management simulation game download
-my minimarket apk for android no iklan
-my mini mart fun and relaxing game download
-my minimarket mod apk unlimited everything
-download my mini mart without ads for free
-my mini mart grow a pretend business game
-my minimarket 1.8.5 apk no iklan download
-my mini mart simulation game with tons of activities
-download my minimarket mod apk terbaru
-my mini mart expand your marts game no ads
-download my mini mart simulation game offline
-my minimarket free simulation game no iklan
-my mini mart challenge of running a small store game
-my minimarket mod apk tanpa iklan download
-my mini mart simulation game with cute graphics
-download my minimarket mod apk offline mode
-my mini mart hire and build your marts game no ads
-my minimarket simulation game by supersonic studios ltd
-my mini mart become a successful business tycoon game download
-download my minimarket mod apk unlimited coins and gems
-my mini mart simulation game with in-app purchases no ads
-my minimarket 1.8.5 mod apk no ads free download
-my mini mart simulation game with rolling offer and daily spin
-download my minimarket mod apk latest version 2023
-my mini mart simulation game with 14 different marts no ads
-
Delivery
-
The fourth step is to choose your delivery option and track your order on the minimarket app. You should choose whether you want to pick up your order from the store or have it delivered to your address. You should also choose when you want to receive your order, such as same-day delivery, next-day delivery, or scheduled delivery. You should then confirm your order details and wait for a confirmation message from the store. You should also track your order status and progress on the app or contact customer service if you have any issues or questions.
-
Conclusion
-
In conclusion, downloading a minimarket app can be a great way to shop for groceries and other goods from your local store with convenience, loyalty, engagement, and sales benefits. To choose the best minimarket app for your needs, you should consider its features, reviews reviews, and comparisons. To download and use a minimarket app, you should follow the steps of downloading, registering, shopping, and delivery. We hope that this article has helped you understand how to download a minimarket app and why you should do it. If you have any questions or comments, please feel free to contact us. Thank you for reading and happy shopping!
-
FAQs
-
Here are some frequently asked questions related to downloading a minimarket app:
-
How do I contact customer service on the minimarket app?
-
Most minimarket apps have a customer service feature that allows you to chat, call, or email the store staff if you have any issues or questions. You can usually find this feature on the app's menu, settings, or help section. You can also check the app's website or social media pages for more contact information.
-
How do I update my payment information on the minimarket app?
-
To update your payment information on the minimarket app, you should go to your profile or account section and select the payment option. You can then add, edit, or delete your payment methods, such as credit card, debit card, PayPal, etc. You should make sure that your payment information is correct and secure before making any transactions.
-
How do I cancel or return my order on the minimarket app?
-
To cancel or return your order on the minimarket app, you should check the store's cancellation and return policy first. Some stores may allow you to cancel or return your order within a certain period of time or under certain conditions. You can then contact the store or use the app's order management feature to request a cancellation or return. You may need to provide your order number, reason, and proof of purchase. You may also need to pay for the shipping or restocking fees.
-
How do I share my feedback or review on the minimarket app?
-
To share your feedback or review on the minimarket app, you should go to the product page or the app's review section and rate and write your opinion about the product or the app. You can also upload photos or videos to show your experience. You should be honest, respectful, and constructive when sharing your feedback or review. You should also avoid spamming, trolling, or abusing other users or the store.
-
How do I find the best deals and offers on the minimarket app?
-
To find the best deals and offers on the minimarket app, you should check the app's homepage, banner, or notification section for any special events, promotions, or discounts that are available. You can also use the app's search filter, sorting, or recommendation feature to find the products that suit your budget and preferences. You can also join the app's loyalty program or newsletter to get exclusive deals and offers.
401be4b1e0
-
-
\ No newline at end of file
diff --git a/spaces/1phancelerku/anime-remove-background/Download Voyage 4 MOD APK (v2.54) and Experience the Relaxation of Driving - Unlimited Money Included.md b/spaces/1phancelerku/anime-remove-background/Download Voyage 4 MOD APK (v2.54) and Experience the Relaxation of Driving - Unlimited Money Included.md
deleted file mode 100644
index df4d6046e36f8b84bbe4d0c530f4342ae4121238..0000000000000000000000000000000000000000
--- a/spaces/1phancelerku/anime-remove-background/Download Voyage 4 MOD APK (v2.54) and Experience the Relaxation of Driving - Unlimited Money Included.md
+++ /dev/null
@@ -1,106 +0,0 @@
-
-
Voyage 4 Mod APK Son Sürüm: A Guide to the Ultimate Road Trip Game
-
Do you love driving games that let you explore realistic and diverse landscapes? Do you want to experience a cinematic adventure game that captures the essence of shared exploration? Do you want to play a game that is simple, non-violent, and beautiful? If you answered yes to any of these questions, then you should try Voyage 4 Mod APK Son Sürüm, a game that will take you on a memorable road trip across Russia.
-
What is Voyage 4 and why should you play it?
-
Voyage 4 is a realistic driving simulator game that lets you travel on Russian roads with various cars. You can choose from over 50 vehicles, ranging from sedans and SUVs to trucks and buses. You can also customize your car with tuning parts, visual effects, and sounds.
The game features a large and detailed map of Russia, with over 1000 cities and towns, as well as different regions, weather conditions, and historical landmarks. You can drive on highways, country roads, dirt roads, and even off-road. You can also encounter traffic, police, accidents, and other events that make the game more realistic and challenging.
-
Voyage 4 is not just a driving game, but also an adventure game that tells a story through its environment and gameplay. You can discover secrets, mysteries, and surprises along the way. You can also interact with other drivers and passengers, who have their own personalities and stories. You can even play with a friend in co-op mode, where you can share the same car or drive separately.
-
What is Voyage 4 Mod APK Son Sürüm and how to download it?
-
Voyage 4 Mod APK Son Sürüm is a modified version of the game that gives you unlimited money, unlocked cars, and no ads. This means that you can enjoy the game without any limitations or interruptions. You can access all the cars and tuning parts without spending real money. You can also have more fun and challenge with the game's realistic physics and graphics.
-
To download Voyage 4 Mod APK Son Sürüm, you need to follow these simple steps:
-
-
Go to a reliable source like APKCombo, which offers safe and fast downloads of APK files.
-
Search for Voyage 4 Mod APK Son Sürüm in the search bar or browse the categories.
-
Click on the download button and wait for the file to be downloaded.
-
Enable unknown sources in your device settings by going to Settings > Security > Unknown Sources.
-
Install the APK file by tapping on it and following the instructions.
-
Launch the game and enjoy!
-
-
What are the features and benefits of Voyage 4 Mod APK Son Sürüm?
-
Voyage 4 Mod APK Son Sürüm has many features and benefits that make it a great choice for anyone who loves driving games. Here are some of them:
-
-
You can enjoy the game without any limitations or interruptions. You don't have to worry about running out of money, unlocking cars, or watching ads.
-
You can access all the cars and tuning parts without spending real money. You can choose from over 50 vehicles, each with its own characteristics and performance. You can also customize your car with tuning parts, visual effects, and sounds.
-
You can have more fun and challenge with the game's realistic physics and graphics. The game uses advanced physics engine that simulates the behavior of real cars on different surfaces and conditions. The game also has stunning graphics that create a realistic and immersive atmosphere.
-
-
What are some tips and tricks to play Voyage 4 Mod APK Son Sürüm?
-
Voyage 4 Mod APK Son Sürüm is a game that requires skill, patience, and attention. Here are some tips and tricks to help you play better:
-
-
Use the triangle button to get directions if you are lost or stuck. The game will show you the nearest city or town where you can find gas stations, repair shops, hotels, or other facilities.
-
Use the console to adjust the settings and optimize the game performance. You can change the graphics quality, sound volume, control sensitivity, camera angle, language, and other options.
-
Try different cars and routes to discover new places and secrets. The game has a lot of variety and diversity in its map, cars, events, and stories. You can drive on different roads, explore different regions, encounter different situations, and uncover different secrets.
-
-
What are some reviews and ratings of Voyage 4 Mod APK Son Sürüm?
-
Voyage 4 Mod APK Son Sürüm has a high rating and positive feedback from users who downloaded it from APKCombo. Here are some of their reviews:
-
voyage 4 mod apk son sürüm indir
-voyage 4 mod apk son sürüm hileli
-voyage 4 mod apk son sürüm android oyun club
-voyage 4 mod apk son sürüm güncel
-voyage 4 mod apk son sürüm para hilesi
-voyage 4 mod apk son sürüm mega hile
-voyage 4 mod apk son sürüm türkçe
-voyage 4 mod apk son sürüm ücretsiz
-voyage 4 mod apk son sürüm full
-voyage 4 mod apk son sürüm kurulumu
-voyage 4 mod apk son sürüm nasıl indirilir
-voyage 4 mod apk son sürüm nasıl yüklenir
-voyage 4 mod apk son sürüm nasıl oynanır
-voyage 4 mod apk son sürüm oyun indir club
-voyage 4 mod apk son sürüm oyunu indir
-voyage 4 mod apk son sürüm oyunu oyna
-voyage 4 mod apk son sürüm oyunu hakkında
-voyage 4 mod apk son sürüm oyunu inceleme
-voyage 4 mod apk son sürüm oyunu yorumlar
-voyage 4 mod apk son sürüm oyunu özellikleri
-voyage 4 mod apk son sürüm oyunu sistem gereksinimleri
-voyage 4 mod apk son sürüm oyunu videoları
-voyage 4 mod apk son sürüm oyunu resimleri
-voyage 4 mod apk son sürüm oyunu hileleri
-voyage 4 mod apk son sürüm oyunu ipuçları
-voyage 4 mod apk son sürüm oyunu rehberi
-voyage 4 mod apk son sürüm oyunu haritası
-voyage 4 mod apk son sürüm oyunu araçları
-voyage 4 mod apk son sürüm oyunu görevleri
-voyage 4 mod apk son sürüm oyunu müzikleri
-voyage 4 mod apk son sürüm download
-voyage 4 mod apk son sürüm free download
-voyage 4 mod apk son sürüm latest version download
-voyage 4 mod apk son sürüm updated version download
-voyage 4 mod apk son sürüm offline download
-voyage 4 mod apk son sürüm online download
-voyage 4 mod apk son sürüm direct download link
-voyage 4 mod apk son sürüm mediafire download link
-voyage 4 mod apk son sürüm google drive download link
-voyage 4 mod apk son sürüm mega download link
-voyage 4 mod apk son sürüm unlimited money download
-voyage 4 mod apk son sürüm unlimited coins download
-voyage 4 mod apk son sürüm unlimited gems download
-voyage 4 mod apk son sürüm unlimited fuel download
-voyage 4 mod apk son sürüm unlocked all cars download
-voyage 4 mod apk son sürüm unlocked all maps download
-voyage 4 mod apk son sürüm unlocked all features download
-voyage 4 mod apk son sürüm no ads download
-voyage 4 mod apk son sürüm no root download
-voyage 4 mod apk son sürüm no virus download
-
-
User
Rating
Review
-
Mehmet
5 stars
Very good game, I like the graphics and the physics. The mod apk is also very good, it gives me unlimited money and unlocked cars. I recommend it to everyone who likes driving games.
-
Ayşe
4 stars
I enjoy playing this game, it is very relaxing and fun. The mod apk is also very helpful, it removes the ads and gives me more options to customize my car. The only thing I don't like is that the game sometimes crashes or freezes.
-
Ali
5 stars
This game is amazing, it is like a real road trip across Russia. The mod apk is also amazing, it gives me everything I need to play the game without any problems. I love the game and the mod apk.
-
-
Conclusion
-
Voyage 4 Mod APK Son Sürüm is a great way to experience the game's amazing features and benefits. You can download it easily and safely from APKCombo and enjoy a short and simple game with a beautiful aesthetic. You can also share your adventure with a friend or play solo with an AI companion. Voyage 4 Mod APK Son Sürüm is a game that will make you feel the joy of exploration and discovery.
-
FAQs
-
Q1. Is Voyage 4 Mod APK Son Sürüm safe to download and install?
-
A1. Yes, as long as you download it from a trusted source like APKCombo, which scans all the APK files for viruses and malware.
-
Q2. How long is Voyage 4 Mod APK Son Sürüm?
-
A2. The game's runtime is under 2 hours, but you can replay it with different cars and routes to see more of the world.
-
Q3. Can I play Voyage 4 Mod APK Son Sürüm without internet or Google Play service?
-
A3. Yes, you can play offline, but you will not be able to save your results or see other players' results in Google Play.
-
Q4. Does Voyage 4 Mod APK Son Sürüm have any dialogue or text?
-
A4. No, the game does not use any dialogue or text to tell its story. It relies on visuals, sounds, and gestures instead.
-
Q5. What are some other games similar to Voyage 4 Mod APK Son Sürüm?
-
A5. Some other games that have a similar style and theme are Journey, Limbo, Inside, Brothers: A Tale of Two Sons, and Unravel.
197e85843d
-
-
\ No newline at end of file
diff --git a/spaces/1phancelerku/anime-remove-background/Evertale 2.0.64 Mod Apk Free Shopping and Unlimited Money.md b/spaces/1phancelerku/anime-remove-background/Evertale 2.0.64 Mod Apk Free Shopping and Unlimited Money.md
deleted file mode 100644
index 9a2bfa97b473f3d777d609ad4779576df08b6a10..0000000000000000000000000000000000000000
--- a/spaces/1phancelerku/anime-remove-background/Evertale 2.0.64 Mod Apk Free Shopping and Unlimited Money.md
+++ /dev/null
@@ -1,113 +0,0 @@
-
-
Evertale 2.0.64 Mod Apk: A Guide for Beginners
-
If you are a fan of fantasy RPGs with monster-catching and battling elements, you might have heard of Evertale, a popular game by ZigZaGame Inc. that has been compared to Pokémon and other similar games. But did you know that there is a modified version of the game that gives you access to some developer functions that can make your gameplay easier and more fun? In this article, we will tell you everything you need to know about Evertale 2.0.64 Mod Apk, including what it is, how to download and install it, and how to play it.
-
What is Evertale?
-
Before we dive into the details of the mod apk, let's first review what Evertale is and why it is worth playing.
Evertale is a game that takes you to the fantasy world of Erden, where you can catch, train, and evolve over 180 monsters and heroes across an impressive story-driven adventure. You can explore sprawling landscapes, bustling cities, and mythical dungeons in this expansive open-world RPG. You can also join a band of unlikely heroes and free the world of Erden from the deadly Pandemonium, an ancient curse that descends once every 100 years.
-
A rich story mode and online features
-
Evertale has a lot to offer for both offline and online players. You can immerse yourself in the engaging single-player story mode that has a lot of quests, characters, secrets, and rewards to discover. You can also jump online to compete in real-time PvP leagues and form guilds with other players to unlock limited-edition gear, power-ups, and more. You can also participate in weekly online events that offer exclusive unlockables and limited characters to add to your collection.
-
A game with positive reviews and high ratings
-
Evertale has been well-received by strategy RPG enthusiasts and beginners alike, with over 5 million downloads from the Google Play Store alone. It has also received positive reviews from critics and players who praised its solid writing, lovely art style, strategic combat, and variety of content. It has been rated 4.5 out of 5 stars on both Android and iOS platforms.
-
What is Evertale 2.0.64 Mod Apk?
-
Now that you have an idea of what Evertale is, let's talk about what Evertale 2.0.64 Mod Apk is and how it differs from the original game.
-
evertale 2.0.64 mod apk unlimited money
-evertale 2.0.64 mod apk free download
-evertale 2.0.64 mod apk latest version
-evertale 2.0.64 mod apk android 1
-evertale 2.0.64 mod apk offline
-evertale 2.0.64 mod apk unlimited soul stones
-evertale 2.0.64 mod apk no root
-evertale 2.0.64 mod apk obb
-evertale 2.0.64 mod apk rexdl
-evertale 2.0.64 mod apk revdl
-evertale 2.0.64 mod apk hack
-evertale 2.0.64 mod apk god mode
-evertale 2.0.64 mod apk unlimited everything
-evertale 2.0.64 mod apk mega
-evertale 2.0.64 mod apk data
-evertale 2.0.64 mod apk full version
-evertale 2.0.64 mod apk premium
-evertale 2.0.64 mod apk unlocked
-evertale 2.0.64 mod apk vip
-evertale 2.0.64 mod apk pro
-evertale 2.0.64 mod apk all characters
-evertale 2.0.64 mod apk high damage
-evertale 2.0.64 mod apk one hit kill
-evertale 2.0.64 mod apk unlimited gems
-evertale 2.0.64 mod apk unlimited coins
-evertale 2.0.64 mod apk unlimited capture stones
-evertale 2.0.64 mod apk unlimited mana
-evertale 2.0.64 mod apk unlimited gold
-evertale 2.0.64 mod apk unlimited silver
-evertale 2.0.64 mod apk unlimited tickets
-evertale 2.0.64 mod apk unlimited keys
-evertale 2.0.64 mod apk unlimited chests
-evertale 2.0.64 mod apk unlimited weapons
-evertale 2.0.64 mod apk unlimited items
-evertale 2.0.64 mod apk unlimited resources
-evertale 2.0.64 mod apk unlimited skills
-evertale 2.0.64 mod apk unlimited levels
-evertale 2.0.64 mod apk unlimited stars
-evertale 2.0.64 mod apk unlimited quests
-evertale 2.0.64 mod apk unlimited events
-
A modified version of the game with developer functions
-
Evertale 2.0.64 Mod Apk is a modified version of the game that gives you access to some developer functions that are not available in the original game. These functions include:
-
-
Characters can't die
-
100% catch rate
-
Free shopping
-
Unlimited soul stones
-
No ads
-
-
These functions can make your gameplay more convenient and enjoyable, as you can easily catch and upgrade your monsters, buy anything you want from the shop, and avoid annoying ads. However, they also come with some drawbacks that you should be aware of.
-
The benefits and risks of using the mod apk
-
Using the mod apk can have some benefits, such as:
-
-
You can save time and money by not having to grind for resources or spend real money on in-app purchases.
-
You can experiment with different combinations of monsters and heroes without worrying about losing battles or wasting soul stones.
-
You can experience the full story mode without any interruptions or difficulties.
-
-
However, using the mod apk can also have some risks, such as:
-
-
You might lose the thrill and challenge of the game, as you can easily breeze through any obstacle or enemy.
-
You might get bored of the game sooner, as you have nothing to strive for or achieve.
-
You might face legal issues or bans from the game developers, as using the mod apk is against their terms of service and can be considered as cheating or hacking.
-
-
Therefore, you should weigh the pros and cons of using the mod apk before deciding to download and install it. You should also be careful about where you download it from, as some sources might contain viruses or malware that can harm your device or steal your personal information.
-
How to download and install the mod apk
-
If you have decided to use the mod apk, here are the steps you need to follow to download and install it:
-
-
Uninstall the original Evertale game from your device if you have it installed.
-
Go to a trusted website that provides the Evertale 2.0.64 Mod Apk file, such as [APKPure] or [APKDone].
-
Download the mod apk file to your device.
-
Enable the installation of apps from unknown sources in your device settings.
-
Locate and tap on the mod apk file to start the installation process.
-
Follow the instructions on the screen to complete the installation.
-
Launch the game and enjoy!
-
-
How to play Evertale 2.0.64 Mod Apk?
-
Now that you have successfully installed the mod apk, you might be wondering how to play it. Here are some tips and tricks that can help you get started and master the game.
-
The basics of the battle system
-
Evertale has a turn-based battle system that allows you to control up to four characters at a time. Each character has a unique set of skills and abilities that can be activated by spending mana points (MP). You can also switch between different characters during battle by tapping on their icons at the bottom of the screen. You can win battles by defeating all enemies or by capturing them with soul stones.
-
The tips and tricks for catching and training monsters
-
Evertale has over 180 monsters that you can catch and train to become your allies. You can catch monsters by using soul stones during battle, which have a 100% success rate with the mod apk. You can also find monsters in chests, events, or by exploring the world map. You can train your monsters by leveling them up, evolving them, equipping them with gear, and teaching them new skills. You can also customize your monsters' names, appearances, and personalities.
-
The best strategies for progressing in the story mode and online events
-
Evertale has a captivating story mode that spans over six chapters, each with its own plot, characters, and locations. You can progress in the story mode by completing quests, solving puzzles, fighting enemies, and collecting items. You can also unlock new areas and secrets by revisiting previous locations with different characters or abilities. You can also participate in online events that offer exclusive rewards and challenges. You can compete in PvP leagues, join guilds, cooperate with other players, and more.
-
Conclusion
-
you can follow the steps we have provided to download and install it. You can also use our tips and tricks to play it and enjoy its features. Evertale is a game that can offer you hours of fun and entertainment, whether you play it with or without the mod apk. We hope you found this article helpful and informative. Happy gaming!
-
FAQs
-
Here are some frequently asked questions about Evertale 2.0.64 Mod Apk that you might find useful.
-
Q: Is Evertale 2.0.64 Mod Apk safe to use?
-
A: Evertale 2.0.64 Mod Apk is not an official version of the game, so it might not be safe to use. It might contain viruses or malware that can harm your device or steal your personal information. It might also cause legal issues or bans from the game developers, as using it is against their terms of service and can be considered as cheating or hacking. Therefore, you should use it at your own risk and discretion.
-
Q: Can I play Evertale 2.0.64 Mod Apk offline?
-
A: Yes, you can play Evertale 2.0.64 Mod Apk offline, as it does not require an internet connection to run. However, you will not be able to access some online features, such as PvP leagues, guilds, events, and more.
-
Q: Can I update Evertale 2.0.64 Mod Apk to the latest version?
-
A: No, you cannot update Evertale 2.0.64 Mod Apk to the latest version, as it is not compatible with the original game. If you want to update the game, you will have to uninstall the mod apk and install the original game from the official sources.
-
Q: Can I transfer my progress from Evertale 2.0.64 Mod Apk to the original game?
-
A: No, you cannot transfer your progress from Evertale 2.0.64 Mod Apk to the original game, as they are not compatible with each other. If you want to play the original game, you will have to start from scratch.
-
Q: Can I use Evertale 2.0.64 Mod Apk with other mods or hacks?
-
A: No, you cannot use Evertale 2.0.64 Mod Apk with other mods or hacks, as they might cause conflicts or errors in the game. You should only use one mod or hack at a time.
401be4b1e0
-
-
\ No newline at end of file
diff --git a/spaces/2023Liu2023/bingo/README.md b/spaces/2023Liu2023/bingo/README.md
deleted file mode 100644
index 218767d1d7debd26932ffddca2ec0f421c0171a9..0000000000000000000000000000000000000000
--- a/spaces/2023Liu2023/bingo/README.md
+++ /dev/null
@@ -1,195 +0,0 @@
----
-title: bingo
-emoji: 📉
-colorFrom: red
-colorTo: red
-sdk: docker
-pinned: true
-license: mit
-duplicated_from: hf4all/bingo
----
-
-
-
-# Bingo
-
-Bingo,一个让你呼吸顺畅 New Bing。
-
-高度还原 New Bing 网页版的主要操作,国内可用,兼容绝大多数微软 Bing AI 的功能,可自行部署使用。
-
-
-
-[](https://hub.docker.com/repository/docker/weaigc/bingo/)
-[](https://hub.docker.com/repository/docker/weaigc/bingo/)
-[](https://github.com/weaigc/bingo/blob/main/license)
-
-
- Demo for 22h Diffusion v0-2 Stable Diffusion model.
- {"Add the following tokens to your prompts for the model to work properly: estilovintedois" if prefix else ""}
-
- Running on {"GPU 🔥" if torch.cuda.is_available() else f"CPU 🥶. For faster inference it is recommended to upgrade to GPU in Settings"}
')
- tts_text = gr.Textbox(label="TTS text (100 words limitation)")
- audio_input = gr.Audio(label = 'Please upload audio file that less than 30 seconds', visible = False)
- tts_voice = gr.Dropdown(choices= tts_get_voices_list())
- predict_f0 = gr.Checkbox(label = 'Auto predict F0', value = False)
- audio_mode = gr.Checkbox(label = 'Upload audio instead', value = False)
- audio_output = gr.Audio(label="Output Audio")
- btn_submit = gr.Button("Generate")
-
- btn_submit.click(infer, [tts_text, tts_voice, audio_input, predict_f0, audio_mode], [audio_output])
- audio_mode.change(change_to_audio_mode, audio_mode, [audio_input, tts_text, tts_voice])
-
- app.queue(concurrency_count=1, api_open=args.api).launch(share=args.share)
diff --git a/spaces/AlekseyKorshuk/instagram-filter-removal/configs/default.py b/spaces/AlekseyKorshuk/instagram-filter-removal/configs/default.py
deleted file mode 100644
index b46610ba35392fdbab20e208d28b5d93d1dcf547..0000000000000000000000000000000000000000
--- a/spaces/AlekseyKorshuk/instagram-filter-removal/configs/default.py
+++ /dev/null
@@ -1,88 +0,0 @@
-from yacs.config import CfgNode as CN
-
-_C = CN()
-
-_C.SYSTEM = CN()
-_C.SYSTEM.NUM_GPU = 2
-_C.SYSTEM.NUM_WORKERS = 4
-
-_C.WANDB = CN()
-_C.WANDB.PROJECT_NAME = "instagram-filter-removal"
-_C.WANDB.ENTITY = "vvgl-ozu"
-_C.WANDB.RUN = 12
-_C.WANDB.LOG_DIR = ""
-_C.WANDB.NUM_ROW = 0
-
-_C.TRAIN = CN()
-_C.TRAIN.NUM_TOTAL_STEP = 120000
-_C.TRAIN.START_STEP = 0
-_C.TRAIN.BATCH_SIZE = 8
-_C.TRAIN.SHUFFLE = True
-_C.TRAIN.LOG_INTERVAL = 100
-_C.TRAIN.SAVE_INTERVAL = 5000
-_C.TRAIN.SAVE_DIR = "./weights"
-_C.TRAIN.RESUME = True
-_C.TRAIN.VISUALIZE_INTERVAL = 100
-_C.TRAIN.TUNE = False
-
-_C.MODEL = CN()
-_C.MODEL.NAME = "ifr-no-aux"
-_C.MODEL.IS_TRAIN = True
-_C.MODEL.NUM_CLASS = 17
-_C.MODEL.CKPT = ""
-
-_C.MODEL.IFR = CN()
-_C.MODEL.IFR.NAME = "InstaFilterRemovalNetwork"
-_C.MODEL.IFR.NUM_CHANNELS = 32
-_C.MODEL.IFR.DESTYLER_CHANNELS = 32
-_C.MODEL.IFR.SOLVER = CN()
-_C.MODEL.IFR.SOLVER.LR = 2e-4
-_C.MODEL.IFR.SOLVER.BETAS = (0.5, 0.9)
-_C.MODEL.IFR.SOLVER.SCHEDULER = []
-_C.MODEL.IFR.SOLVER.DECAY_RATE = 0.
-
-_C.MODEL.D = CN()
-_C.MODEL.D.NAME = "1-ChOutputDiscriminator"
-_C.MODEL.D.NUM_CHANNELS = 32
-_C.MODEL.D.NUM_CRITICS = 5
-_C.MODEL.D.SOLVER = CN()
-_C.MODEL.D.SOLVER.LR = 1e-3
-_C.MODEL.D.SOLVER.BETAS = (0.5, 0.9)
-_C.MODEL.D.SOLVER.SCHEDULER = []
-_C.MODEL.D.SOLVER.DECAY_RATE = 0.5
-
-_C.OPTIM = CN()
-_C.OPTIM.GP = 10
-_C.OPTIM.MASK = 1
-_C.OPTIM.RECON = 1.4
-_C.OPTIM.SEMANTIC = 1e-4
-_C.OPTIM.TEXTURE = 1e-3
-_C.OPTIM.ADVERSARIAL = 1e-3
-_C.OPTIM.AUX = 0.5
-
-_C.DATASET = CN()
-_C.DATASET.NAME = "IFFI" # "IFFI" # "DIV2K?" #
-_C.DATASET.ROOT = "../../Datasets/IFFI-dataset/train" # "../../Datasets/IFFI-dataset" # "/media/birdortyedi/e5042b8f-ca5e-4a22-ac68-7e69ff648bc4/IFFI-dataset"
-_C.DATASET.TEST_ROOT = "../../Datasets/IFFI-dataset"
-_C.DATASET.SIZE = 256
-_C.DATASET.CROP_SIZE = 512
-_C.DATASET.MEAN = [0.5, 0.5, 0.5]
-_C.DATASET.STD = [0.5, 0.5, 0.5]
-
-_C.TEST = CN()
-_C.TEST.OUTPUT_DIR = "./outputs"
-_C.TEST.ABLATION = False
-_C.TEST.WEIGHTS = ""
-_C.TEST.BATCH_SIZE = 64
-_C.TEST.IMG_ID = 52
-
-
-def get_cfg_defaults():
- """Get a yacs CfgNode object with default values for my_project."""
- # Return a clone so that the defaults will not be altered
- # This is for the "local variable" use pattern
- return _C.clone()
-
-
-# provide a way to import the defaults as a global singleton:
-cfg = _C # users can `from config import cfg`
diff --git a/spaces/Andy1621/uniformer_image_detection/configs/guided_anchoring/ga_retinanet_x101_64x4d_fpn_1x_coco.py b/spaces/Andy1621/uniformer_image_detection/configs/guided_anchoring/ga_retinanet_x101_64x4d_fpn_1x_coco.py
deleted file mode 100644
index 1b18c2ba41d1493380bab3515be8e29547988ebf..0000000000000000000000000000000000000000
--- a/spaces/Andy1621/uniformer_image_detection/configs/guided_anchoring/ga_retinanet_x101_64x4d_fpn_1x_coco.py
+++ /dev/null
@@ -1,13 +0,0 @@
-_base_ = './ga_retinanet_r50_fpn_1x_coco.py'
-model = dict(
- pretrained='open-mmlab://resnext101_64x4d',
- backbone=dict(
- type='ResNeXt',
- depth=101,
- groups=64,
- base_width=4,
- num_stages=4,
- out_indices=(0, 1, 2, 3),
- frozen_stages=1,
- norm_cfg=dict(type='BN', requires_grad=True),
- style='pytorch'))
diff --git a/spaces/Andy1621/uniformer_image_detection/mmdet/core/evaluation/recall.py b/spaces/Andy1621/uniformer_image_detection/mmdet/core/evaluation/recall.py
deleted file mode 100644
index 23ec744f552db1a4a76bfa63b7cc8b357deb3140..0000000000000000000000000000000000000000
--- a/spaces/Andy1621/uniformer_image_detection/mmdet/core/evaluation/recall.py
+++ /dev/null
@@ -1,189 +0,0 @@
-from collections.abc import Sequence
-
-import numpy as np
-from mmcv.utils import print_log
-from terminaltables import AsciiTable
-
-from .bbox_overlaps import bbox_overlaps
-
-
-def _recalls(all_ious, proposal_nums, thrs):
-
- img_num = all_ious.shape[0]
- total_gt_num = sum([ious.shape[0] for ious in all_ious])
-
- _ious = np.zeros((proposal_nums.size, total_gt_num), dtype=np.float32)
- for k, proposal_num in enumerate(proposal_nums):
- tmp_ious = np.zeros(0)
- for i in range(img_num):
- ious = all_ious[i][:, :proposal_num].copy()
- gt_ious = np.zeros((ious.shape[0]))
- if ious.size == 0:
- tmp_ious = np.hstack((tmp_ious, gt_ious))
- continue
- for j in range(ious.shape[0]):
- gt_max_overlaps = ious.argmax(axis=1)
- max_ious = ious[np.arange(0, ious.shape[0]), gt_max_overlaps]
- gt_idx = max_ious.argmax()
- gt_ious[j] = max_ious[gt_idx]
- box_idx = gt_max_overlaps[gt_idx]
- ious[gt_idx, :] = -1
- ious[:, box_idx] = -1
- tmp_ious = np.hstack((tmp_ious, gt_ious))
- _ious[k, :] = tmp_ious
-
- _ious = np.fliplr(np.sort(_ious, axis=1))
- recalls = np.zeros((proposal_nums.size, thrs.size))
- for i, thr in enumerate(thrs):
- recalls[:, i] = (_ious >= thr).sum(axis=1) / float(total_gt_num)
-
- return recalls
-
-
-def set_recall_param(proposal_nums, iou_thrs):
- """Check proposal_nums and iou_thrs and set correct format."""
- if isinstance(proposal_nums, Sequence):
- _proposal_nums = np.array(proposal_nums)
- elif isinstance(proposal_nums, int):
- _proposal_nums = np.array([proposal_nums])
- else:
- _proposal_nums = proposal_nums
-
- if iou_thrs is None:
- _iou_thrs = np.array([0.5])
- elif isinstance(iou_thrs, Sequence):
- _iou_thrs = np.array(iou_thrs)
- elif isinstance(iou_thrs, float):
- _iou_thrs = np.array([iou_thrs])
- else:
- _iou_thrs = iou_thrs
-
- return _proposal_nums, _iou_thrs
-
-
-def eval_recalls(gts,
- proposals,
- proposal_nums=None,
- iou_thrs=0.5,
- logger=None):
- """Calculate recalls.
-
- Args:
- gts (list[ndarray]): a list of arrays of shape (n, 4)
- proposals (list[ndarray]): a list of arrays of shape (k, 4) or (k, 5)
- proposal_nums (int | Sequence[int]): Top N proposals to be evaluated.
- iou_thrs (float | Sequence[float]): IoU thresholds. Default: 0.5.
- logger (logging.Logger | str | None): The way to print the recall
- summary. See `mmcv.utils.print_log()` for details. Default: None.
-
- Returns:
- ndarray: recalls of different ious and proposal nums
- """
-
- img_num = len(gts)
- assert img_num == len(proposals)
-
- proposal_nums, iou_thrs = set_recall_param(proposal_nums, iou_thrs)
-
- all_ious = []
- for i in range(img_num):
- if proposals[i].ndim == 2 and proposals[i].shape[1] == 5:
- scores = proposals[i][:, 4]
- sort_idx = np.argsort(scores)[::-1]
- img_proposal = proposals[i][sort_idx, :]
- else:
- img_proposal = proposals[i]
- prop_num = min(img_proposal.shape[0], proposal_nums[-1])
- if gts[i] is None or gts[i].shape[0] == 0:
- ious = np.zeros((0, img_proposal.shape[0]), dtype=np.float32)
- else:
- ious = bbox_overlaps(gts[i], img_proposal[:prop_num, :4])
- all_ious.append(ious)
- all_ious = np.array(all_ious)
- recalls = _recalls(all_ious, proposal_nums, iou_thrs)
-
- print_recall_summary(recalls, proposal_nums, iou_thrs, logger=logger)
- return recalls
-
-
-def print_recall_summary(recalls,
- proposal_nums,
- iou_thrs,
- row_idxs=None,
- col_idxs=None,
- logger=None):
- """Print recalls in a table.
-
- Args:
- recalls (ndarray): calculated from `bbox_recalls`
- proposal_nums (ndarray or list): top N proposals
- iou_thrs (ndarray or list): iou thresholds
- row_idxs (ndarray): which rows(proposal nums) to print
- col_idxs (ndarray): which cols(iou thresholds) to print
- logger (logging.Logger | str | None): The way to print the recall
- summary. See `mmcv.utils.print_log()` for details. Default: None.
- """
- proposal_nums = np.array(proposal_nums, dtype=np.int32)
- iou_thrs = np.array(iou_thrs)
- if row_idxs is None:
- row_idxs = np.arange(proposal_nums.size)
- if col_idxs is None:
- col_idxs = np.arange(iou_thrs.size)
- row_header = [''] + iou_thrs[col_idxs].tolist()
- table_data = [row_header]
- for i, num in enumerate(proposal_nums[row_idxs]):
- row = [f'{val:.3f}' for val in recalls[row_idxs[i], col_idxs].tolist()]
- row.insert(0, num)
- table_data.append(row)
- table = AsciiTable(table_data)
- print_log('\n' + table.table, logger=logger)
-
-
-def plot_num_recall(recalls, proposal_nums):
- """Plot Proposal_num-Recalls curve.
-
- Args:
- recalls(ndarray or list): shape (k,)
- proposal_nums(ndarray or list): same shape as `recalls`
- """
- if isinstance(proposal_nums, np.ndarray):
- _proposal_nums = proposal_nums.tolist()
- else:
- _proposal_nums = proposal_nums
- if isinstance(recalls, np.ndarray):
- _recalls = recalls.tolist()
- else:
- _recalls = recalls
-
- import matplotlib.pyplot as plt
- f = plt.figure()
- plt.plot([0] + _proposal_nums, [0] + _recalls)
- plt.xlabel('Proposal num')
- plt.ylabel('Recall')
- plt.axis([0, proposal_nums.max(), 0, 1])
- f.show()
-
-
-def plot_iou_recall(recalls, iou_thrs):
- """Plot IoU-Recalls curve.
-
- Args:
- recalls(ndarray or list): shape (k,)
- iou_thrs(ndarray or list): same shape as `recalls`
- """
- if isinstance(iou_thrs, np.ndarray):
- _iou_thrs = iou_thrs.tolist()
- else:
- _iou_thrs = iou_thrs
- if isinstance(recalls, np.ndarray):
- _recalls = recalls.tolist()
- else:
- _recalls = recalls
-
- import matplotlib.pyplot as plt
- f = plt.figure()
- plt.plot(_iou_thrs + [1.0], _recalls + [0.])
- plt.xlabel('IoU')
- plt.ylabel('Recall')
- plt.axis([iou_thrs.min(), 1, 0, 1])
- f.show()
diff --git a/spaces/Anonymous-123/ImageNet-Editing/object_removal/TFill/scripts/test.sh b/spaces/Anonymous-123/ImageNet-Editing/object_removal/TFill/scripts/test.sh
deleted file mode 100644
index b70d6e93277362e285e86d65e7fdf066f3cb88a2..0000000000000000000000000000000000000000
--- a/spaces/Anonymous-123/ImageNet-Editing/object_removal/TFill/scripts/test.sh
+++ /dev/null
@@ -1,15 +0,0 @@
-python test.py \
---name celeba \
---img_file ./examples/imagenet/img/ \
---mask_file ./examples/imagenet/mask/ \
---results_dir ./results \
---model tc \
---coarse_or_refine refine \
---gpu_id 0 \
---no_shuffle \
---batch_size 1 \
---preprocess scale_shortside \
---mask_type 3 \
---load_size 512 \
---attn_G \
---add_noise
diff --git a/spaces/Anonymous-sub/Rerender/ControlNet/annotator/uniformer/configs/_base_/datasets/cityscapes.py b/spaces/Anonymous-sub/Rerender/ControlNet/annotator/uniformer/configs/_base_/datasets/cityscapes.py
deleted file mode 100644
index f21867c63e1835f6fceb61f066e802fd8fd2a735..0000000000000000000000000000000000000000
--- a/spaces/Anonymous-sub/Rerender/ControlNet/annotator/uniformer/configs/_base_/datasets/cityscapes.py
+++ /dev/null
@@ -1,54 +0,0 @@
-# dataset settings
-dataset_type = 'CityscapesDataset'
-data_root = 'data/cityscapes/'
-img_norm_cfg = dict(
- mean=[123.675, 116.28, 103.53], std=[58.395, 57.12, 57.375], to_rgb=True)
-crop_size = (512, 1024)
-train_pipeline = [
- dict(type='LoadImageFromFile'),
- dict(type='LoadAnnotations'),
- dict(type='Resize', img_scale=(2048, 1024), ratio_range=(0.5, 2.0)),
- dict(type='RandomCrop', crop_size=crop_size, cat_max_ratio=0.75),
- dict(type='RandomFlip', prob=0.5),
- dict(type='PhotoMetricDistortion'),
- dict(type='Normalize', **img_norm_cfg),
- dict(type='Pad', size=crop_size, pad_val=0, seg_pad_val=255),
- dict(type='DefaultFormatBundle'),
- dict(type='Collect', keys=['img', 'gt_semantic_seg']),
-]
-test_pipeline = [
- dict(type='LoadImageFromFile'),
- dict(
- type='MultiScaleFlipAug',
- img_scale=(2048, 1024),
- # img_ratios=[0.5, 0.75, 1.0, 1.25, 1.5, 1.75],
- flip=False,
- transforms=[
- dict(type='Resize', keep_ratio=True),
- dict(type='RandomFlip'),
- dict(type='Normalize', **img_norm_cfg),
- dict(type='ImageToTensor', keys=['img']),
- dict(type='Collect', keys=['img']),
- ])
-]
-data = dict(
- samples_per_gpu=2,
- workers_per_gpu=2,
- train=dict(
- type=dataset_type,
- data_root=data_root,
- img_dir='leftImg8bit/train',
- ann_dir='gtFine/train',
- pipeline=train_pipeline),
- val=dict(
- type=dataset_type,
- data_root=data_root,
- img_dir='leftImg8bit/val',
- ann_dir='gtFine/val',
- pipeline=test_pipeline),
- test=dict(
- type=dataset_type,
- data_root=data_root,
- img_dir='leftImg8bit/val',
- ann_dir='gtFine/val',
- pipeline=test_pipeline))
diff --git a/spaces/Anonymous-sub/Rerender/ControlNet/annotator/uniformer/mmcv/parallel/__init__.py b/spaces/Anonymous-sub/Rerender/ControlNet/annotator/uniformer/mmcv/parallel/__init__.py
deleted file mode 100644
index 2ed2c17ad357742e423beeaf4d35db03fe9af469..0000000000000000000000000000000000000000
--- a/spaces/Anonymous-sub/Rerender/ControlNet/annotator/uniformer/mmcv/parallel/__init__.py
+++ /dev/null
@@ -1,13 +0,0 @@
-# Copyright (c) OpenMMLab. All rights reserved.
-from .collate import collate
-from .data_container import DataContainer
-from .data_parallel import MMDataParallel
-from .distributed import MMDistributedDataParallel
-from .registry import MODULE_WRAPPERS
-from .scatter_gather import scatter, scatter_kwargs
-from .utils import is_module_wrapper
-
-__all__ = [
- 'collate', 'DataContainer', 'MMDataParallel', 'MMDistributedDataParallel',
- 'scatter', 'scatter_kwargs', 'is_module_wrapper', 'MODULE_WRAPPERS'
-]
diff --git a/spaces/Armandoliv/whisper-biomedical-ner/app.py b/spaces/Armandoliv/whisper-biomedical-ner/app.py
deleted file mode 100644
index 29a9c5a91da4c3ae1080ea62664e8ea1f41e9deb..0000000000000000000000000000000000000000
--- a/spaces/Armandoliv/whisper-biomedical-ner/app.py
+++ /dev/null
@@ -1,56 +0,0 @@
-import gradio as gr
-import torch
-import spacy
-import os
-import whisper
-
-os.system('pip install https://huggingface.co/Armandoliv/es_pipeline/resolve/main/es_pipeline-any-py3-none-any.whl')
-device = torch.device("cuda:0" if torch.cuda.is_available() else "cpu")
-model_whisper = whisper.load_model("small")
-nlp_ner = spacy.load("es_pipeline")
-
-def main_generator(youtube_id:str):
- YouTubeID = youtube_id.split("https://www.youtube.com/watch?v=") #
- if len(YouTubeID)>1:
- YouTubeID = YouTubeID[1]
- else:
- YouTubeID ='XfyGv-xwjlI'
-
- OutputFile = f'test_audio_youtube_{YouTubeID}.m4a'
-
- os.system(f"youtube-dl -o {OutputFile} {YouTubeID} --extract-audio --restrict-filenames -f 'bestaudio[ext=m4a]'")
-
- result = model_whisper.transcribe(OutputFile)
- text = result['text']
- doc = nlp_ner(text)
-
- output_list = []
- for ent in doc.ents:
- result_dict = {
- 'entity': ent.label_,
- 'word': ent.text,
- 'start':ent.start_char,
- 'end': ent.end_char
- }
- output_list.append(result_dict)
-
- return {"text": text, "entities": output_list}
-inputs = [gr.Textbox(lines=1, placeholder="Link of youtube video here...", label="Input")]
-outputs = gr.HighlightedText()
-title="ASR FOR SPANISH MEDICAL RECORDS"
-description = "This demo uses AI Models to create an AUDIO ANNOTATION FOR MEDICAL RECORDS"
-examples = ['https://www.youtube.com/watch?v=xOZM-1p-jAk']
-
-io = gr.Interface(fn=main_generator, inputs=inputs, outputs=outputs, title=title, description = description, examples = examples,
-
- css= """.gr-button-primary { background: -webkit-linear-gradient(
- 90deg, #355764 0%, #55a8a1 100% ) !important; background: #355764;
- background: linear-gradient(
- 90deg, #355764 0%, #55a8a1 100% ) !important;
- background: -moz-linear-gradient( 90deg, #355764 0%, #55a8a1 100% ) !important;
- background: -webkit-linear-gradient(
- 90deg, #355764 0%, #55a8a1 100% ) !important;
- color:white !important}"""
- )
-
-io.launch()
\ No newline at end of file
diff --git a/spaces/Ataturk-Chatbot/HuggingFaceChat/venv/lib/python3.11/site-packages/pip/_internal/utils/_log.py b/spaces/Ataturk-Chatbot/HuggingFaceChat/venv/lib/python3.11/site-packages/pip/_internal/utils/_log.py
deleted file mode 100644
index 92c4c6a193873ce09629f6cfaa2dabc4f14ecb03..0000000000000000000000000000000000000000
--- a/spaces/Ataturk-Chatbot/HuggingFaceChat/venv/lib/python3.11/site-packages/pip/_internal/utils/_log.py
+++ /dev/null
@@ -1,38 +0,0 @@
-"""Customize logging
-
-Defines custom logger class for the `logger.verbose(...)` method.
-
-init_logging() must be called before any other modules that call logging.getLogger.
-"""
-
-import logging
-from typing import Any, cast
-
-# custom log level for `--verbose` output
-# between DEBUG and INFO
-VERBOSE = 15
-
-
-class VerboseLogger(logging.Logger):
- """Custom Logger, defining a verbose log-level
-
- VERBOSE is between INFO and DEBUG.
- """
-
- def verbose(self, msg: str, *args: Any, **kwargs: Any) -> None:
- return self.log(VERBOSE, msg, *args, **kwargs)
-
-
-def getLogger(name: str) -> VerboseLogger:
- """logging.getLogger, but ensures our VerboseLogger class is returned"""
- return cast(VerboseLogger, logging.getLogger(name))
-
-
-def init_logging() -> None:
- """Register our VerboseLogger and VERBOSE log level.
-
- Should be called before any calls to getLogger(),
- i.e. in pip._internal.__init__
- """
- logging.setLoggerClass(VerboseLogger)
- logging.addLevelName(VERBOSE, "VERBOSE")
diff --git a/spaces/Ataturk-Chatbot/HuggingFaceChat/venv/lib/python3.11/site-packages/pip/_vendor/urllib3/util/response.py b/spaces/Ataturk-Chatbot/HuggingFaceChat/venv/lib/python3.11/site-packages/pip/_vendor/urllib3/util/response.py
deleted file mode 100644
index 5ea609ccedf18eb4ab70f8fc6990448eb6407237..0000000000000000000000000000000000000000
--- a/spaces/Ataturk-Chatbot/HuggingFaceChat/venv/lib/python3.11/site-packages/pip/_vendor/urllib3/util/response.py
+++ /dev/null
@@ -1,107 +0,0 @@
-from __future__ import absolute_import
-
-from email.errors import MultipartInvariantViolationDefect, StartBoundaryNotFoundDefect
-
-from ..exceptions import HeaderParsingError
-from ..packages.six.moves import http_client as httplib
-
-
-def is_fp_closed(obj):
- """
- Checks whether a given file-like object is closed.
-
- :param obj:
- The file-like object to check.
- """
-
- try:
- # Check `isclosed()` first, in case Python3 doesn't set `closed`.
- # GH Issue #928
- return obj.isclosed()
- except AttributeError:
- pass
-
- try:
- # Check via the official file-like-object way.
- return obj.closed
- except AttributeError:
- pass
-
- try:
- # Check if the object is a container for another file-like object that
- # gets released on exhaustion (e.g. HTTPResponse).
- return obj.fp is None
- except AttributeError:
- pass
-
- raise ValueError("Unable to determine whether fp is closed.")
-
-
-def assert_header_parsing(headers):
- """
- Asserts whether all headers have been successfully parsed.
- Extracts encountered errors from the result of parsing headers.
-
- Only works on Python 3.
-
- :param http.client.HTTPMessage headers: Headers to verify.
-
- :raises urllib3.exceptions.HeaderParsingError:
- If parsing errors are found.
- """
-
- # This will fail silently if we pass in the wrong kind of parameter.
- # To make debugging easier add an explicit check.
- if not isinstance(headers, httplib.HTTPMessage):
- raise TypeError("expected httplib.Message, got {0}.".format(type(headers)))
-
- defects = getattr(headers, "defects", None)
- get_payload = getattr(headers, "get_payload", None)
-
- unparsed_data = None
- if get_payload:
- # get_payload is actually email.message.Message.get_payload;
- # we're only interested in the result if it's not a multipart message
- if not headers.is_multipart():
- payload = get_payload()
-
- if isinstance(payload, (bytes, str)):
- unparsed_data = payload
- if defects:
- # httplib is assuming a response body is available
- # when parsing headers even when httplib only sends
- # header data to parse_headers() This results in
- # defects on multipart responses in particular.
- # See: https://github.com/urllib3/urllib3/issues/800
-
- # So we ignore the following defects:
- # - StartBoundaryNotFoundDefect:
- # The claimed start boundary was never found.
- # - MultipartInvariantViolationDefect:
- # A message claimed to be a multipart but no subparts were found.
- defects = [
- defect
- for defect in defects
- if not isinstance(
- defect, (StartBoundaryNotFoundDefect, MultipartInvariantViolationDefect)
- )
- ]
-
- if defects or unparsed_data:
- raise HeaderParsingError(defects=defects, unparsed_data=unparsed_data)
-
-
-def is_response_to_head(response):
- """
- Checks whether the request of a response has been a HEAD-request.
- Handles the quirks of AppEngine.
-
- :param http.client.HTTPResponse response:
- Response to check if the originating request
- used 'HEAD' as a method.
- """
- # FIXME: Can we do this somehow without accessing private httplib _method?
- method = response._method
- if isinstance(method, int): # Platform-specific: Appengine
- return method == 3
- return method.upper() == "HEAD"
diff --git a/spaces/AtheneaEdu/README/README.md b/spaces/AtheneaEdu/README/README.md
deleted file mode 100644
index bbdb386d2b61d51e0b9e2041fc9e026a2916149b..0000000000000000000000000000000000000000
--- a/spaces/AtheneaEdu/README/README.md
+++ /dev/null
@@ -1,16 +0,0 @@
----
-title: README
-emoji: 🦀
-colorFrom: pink
-colorTo: blue
-sdk: static
-pinned: false
----
-
-👩🎓 Athenea helps educators to teach better, delivering relevant learnings to students and custom feedback.
-
-👩💻 We build custom LLM to help teachers and students become exceptional.
-
-
-
-
diff --git a/spaces/AutoGeneralAI/voice-assistant/app.py b/spaces/AutoGeneralAI/voice-assistant/app.py
deleted file mode 100644
index c7b3db5073e9d4666cb944c4a9e200e6f1ab1e25..0000000000000000000000000000000000000000
--- a/spaces/AutoGeneralAI/voice-assistant/app.py
+++ /dev/null
@@ -1,46 +0,0 @@
-import gradio as gr
-import openai, subprocess
-import os
-# import config
-# openai.api_key = config.OPENAI_API_KEY
-
-messages = [{"role": "system", "content": 'You are a therapist. Respond to all input in 25 words or less.'}]
-
-def transcribe(key, audio):
- openai.api_key = key
- global messages
-
- audio_filename_with_extension = audio + '.wav'
- os.rename(audio, audio_filename_with_extension)
-
- audio_file = open(audio_filename_with_extension, "rb")
- transcript = openai.Audio.transcribe("whisper-1", audio_file)
-
- messages.append({"role": "user", "content": transcript["text"]})
-
- response = openai.ChatCompletion.create(model="gpt-3.5-turbo", messages=messages)
-
- system_message = response["choices"][0]["message"]
- messages.append(system_message)
-
- #subprocess.call(["say", system_message['content']])
- print("output: " + system_message['content'] + "\n")
-
- chat_transcript = ""
- for message in messages:
- if message['role'] != 'system':
- chat_transcript += message['role'] + ": " + message['content'] + "\n\n"
-
- return chat_transcript
-
-# ui = gr.Interface(fn=transcribe, inputs=["text", gr.Audio(source="microphone", type="filepath")], outputs="text").launch()
-keyTxt = gr.Textbox(
- show_label=True,
- placeholder=f"Your API-key...",
- type="password",
- visible=True,
- label="API-Key",
- )
-ui = gr.Interface(fn=transcribe, inputs=[keyTxt, gr.Audio(source="microphone", type="filepath")], outputs="text").launch()
-
-ui.launch()
diff --git a/spaces/Awiny/Image2Paragraph/models/grit_src/grit/data/custom_build_augmentation.py b/spaces/Awiny/Image2Paragraph/models/grit_src/grit/data/custom_build_augmentation.py
deleted file mode 100644
index 49a52d011c09dbe027d41ee7e50127c392a8bf33..0000000000000000000000000000000000000000
--- a/spaces/Awiny/Image2Paragraph/models/grit_src/grit/data/custom_build_augmentation.py
+++ /dev/null
@@ -1,44 +0,0 @@
-# Copyright (c) Facebook, Inc. and its affiliates.
-from detectron2.data import transforms as T
-from .transforms.custom_augmentation_impl import EfficientDetResizeCrop
-
-
-def build_custom_augmentation(cfg, is_train, scale=None, size=None, \
- min_size=None, max_size=None):
- """
- Create a list of default :class:`Augmentation` from config.
- Now it includes resizing and flipping.
-
- Returns:
- list[Augmentation]
- """
- if cfg.INPUT.CUSTOM_AUG == 'ResizeShortestEdge':
- if is_train:
- min_size = cfg.INPUT.MIN_SIZE_TRAIN if min_size is None else min_size
- max_size = cfg.INPUT.MAX_SIZE_TRAIN if max_size is None else max_size
- sample_style = cfg.INPUT.MIN_SIZE_TRAIN_SAMPLING
- else:
- min_size = cfg.INPUT.MIN_SIZE_TEST
- max_size = cfg.INPUT.MAX_SIZE_TEST
- sample_style = "choice"
- augmentation = [T.ResizeShortestEdge(min_size, max_size, sample_style)]
- elif cfg.INPUT.CUSTOM_AUG == 'EfficientDetResizeCrop':
- if is_train:
- scale = cfg.INPUT.SCALE_RANGE if scale is None else scale
- size = cfg.INPUT.TRAIN_SIZE if size is None else size
- else:
- scale = (1, 1)
- size = cfg.INPUT.TEST_SIZE
- augmentation = [EfficientDetResizeCrop(size, scale)]
- else:
- assert 0, cfg.INPUT.CUSTOM_AUG
-
- if is_train:
- augmentation.append(T.RandomFlip())
- return augmentation
-
-
-build_custom_transform_gen = build_custom_augmentation
-"""
-Alias for backward-compatibility.
-"""
\ No newline at end of file
diff --git a/spaces/Awiny/Image2Paragraph/models/grit_src/third_party/CenterNet2/configs/common/models/keypoint_rcnn_fpn.py b/spaces/Awiny/Image2Paragraph/models/grit_src/third_party/CenterNet2/configs/common/models/keypoint_rcnn_fpn.py
deleted file mode 100644
index 56b3994df249884d4816fc9a5c7f553a9ab6f400..0000000000000000000000000000000000000000
--- a/spaces/Awiny/Image2Paragraph/models/grit_src/third_party/CenterNet2/configs/common/models/keypoint_rcnn_fpn.py
+++ /dev/null
@@ -1,33 +0,0 @@
-from detectron2.config import LazyCall as L
-from detectron2.layers import ShapeSpec
-from detectron2.modeling.poolers import ROIPooler
-from detectron2.modeling.roi_heads import KRCNNConvDeconvUpsampleHead
-
-from .mask_rcnn_fpn import model
-
-[model.roi_heads.pop(x) for x in ["mask_in_features", "mask_pooler", "mask_head"]]
-
-model.roi_heads.update(
- num_classes=1,
- keypoint_in_features=["p2", "p3", "p4", "p5"],
- keypoint_pooler=L(ROIPooler)(
- output_size=14,
- scales=(1.0 / 4, 1.0 / 8, 1.0 / 16, 1.0 / 32),
- sampling_ratio=0,
- pooler_type="ROIAlignV2",
- ),
- keypoint_head=L(KRCNNConvDeconvUpsampleHead)(
- input_shape=ShapeSpec(channels=256, width=14, height=14),
- num_keypoints=17,
- conv_dims=[512] * 8,
- loss_normalizer="visible",
- ),
-)
-
-# Detectron1 uses 2000 proposals per-batch, but this option is per-image in detectron2.
-# 1000 proposals per-image is found to hurt box AP.
-# Therefore we increase it to 1500 per-image.
-model.proposal_generator.post_nms_topk = (1500, 1000)
-
-# Keypoint AP degrades (though box AP improves) when using plain L1 loss
-model.roi_heads.box_predictor.smooth_l1_beta = 0.5
diff --git a/spaces/BMukhtar/BookRecognitionKz/upload_image.py b/spaces/BMukhtar/BookRecognitionKz/upload_image.py
deleted file mode 100644
index d49c5e80803a461b149743c9fa9beb1afc4520b2..0000000000000000000000000000000000000000
--- a/spaces/BMukhtar/BookRecognitionKz/upload_image.py
+++ /dev/null
@@ -1,25 +0,0 @@
-import pandas as pd
-import numpy as np
-import streamlit as st
-import easyocr
-import PIL
-from PIL import Image, ImageDraw
-from matplotlib import pyplot as plt
-
-
-# main title
-st.set_page_config(layout="wide")
-st.title("Get text from image with EasyOCR")
-# subtitle
-st.markdown("## EasyOCRR with Streamlit")
-col1, col2 = st.columns(2)
-uploaded_file = col1.file_uploader("Upload your file here ",type=['png','jpeg','jpg'])
-if uploaded_file is not None:
- col1.image(uploaded_file) #display
- #print("GOGO ",type(uploaded_file))
- image = Image.open(uploaded_file)
- reader = easyocr.Reader(['tr','en'], gpu=False)
- result = reader.readtext(np.array(image),paragraph=True) # turn image to numpy array
- #print(len(result))
- result_text = "\n\n".join([item[1] for item in result])
- col2.markdown(result_text)
\ No newline at end of file
diff --git a/spaces/Bart92/RVC_HF/infer/lib/infer_pack/modules/F0Predictor/PMF0Predictor.py b/spaces/Bart92/RVC_HF/infer/lib/infer_pack/modules/F0Predictor/PMF0Predictor.py
deleted file mode 100644
index 06f2b79f5e5c6f2049bf8220c29ae20c3f82d524..0000000000000000000000000000000000000000
--- a/spaces/Bart92/RVC_HF/infer/lib/infer_pack/modules/F0Predictor/PMF0Predictor.py
+++ /dev/null
@@ -1,98 +0,0 @@
-import numpy as np
-import parselmouth
-
-from infer.lib.infer_pack.modules.F0Predictor.F0Predictor import F0Predictor
-
-
-class PMF0Predictor(F0Predictor):
- def __init__(self, hop_length=512, f0_min=50, f0_max=1100, sampling_rate=44100):
- self.hop_length = hop_length
- self.f0_min = f0_min
- self.f0_max = f0_max
- self.sampling_rate = sampling_rate
-
- def interpolate_f0(self, f0):
- """
- 对F0进行插值处理
- """
-
- data = np.reshape(f0, (f0.size, 1))
-
- vuv_vector = np.zeros((data.size, 1), dtype=np.float32)
- vuv_vector[data > 0.0] = 1.0
- vuv_vector[data <= 0.0] = 0.0
-
- ip_data = data
-
- frame_number = data.size
- last_value = 0.0
- for i in range(frame_number):
- if data[i] <= 0.0:
- j = i + 1
- for j in range(i + 1, frame_number):
- if data[j] > 0.0:
- break
- if j < frame_number - 1:
- if last_value > 0.0:
- step = (data[j] - data[i - 1]) / float(j - i)
- for k in range(i, j):
- ip_data[k] = data[i - 1] + step * (k - i + 1)
- else:
- for k in range(i, j):
- ip_data[k] = data[j]
- else:
- for k in range(i, frame_number):
- ip_data[k] = last_value
- else:
- ip_data[i] = data[i] # 这里可能存在一个没有必要的拷贝
- last_value = data[i]
-
- return ip_data[:, 0], vuv_vector[:, 0]
-
- def compute_f0(self, wav, p_len=None):
- x = wav
- if p_len is None:
- p_len = x.shape[0] // self.hop_length
- else:
- assert abs(p_len - x.shape[0] // self.hop_length) < 4, "pad length error"
- time_step = self.hop_length / self.sampling_rate * 1000
- f0 = (
- parselmouth.Sound(x, self.sampling_rate)
- .to_pitch_ac(
- time_step=time_step / 1000,
- voicing_threshold=0.6,
- pitch_floor=self.f0_min,
- pitch_ceiling=self.f0_max,
- )
- .selected_array["frequency"]
- )
-
- pad_size = (p_len - len(f0) + 1) // 2
- if pad_size > 0 or p_len - len(f0) - pad_size > 0:
- f0 = np.pad(f0, [[pad_size, p_len - len(f0) - pad_size]], mode="constant")
- f0, uv = self.interpolate_f0(f0)
- return f0
-
- def compute_f0_uv(self, wav, p_len=None):
- x = wav
- if p_len is None:
- p_len = x.shape[0] // self.hop_length
- else:
- assert abs(p_len - x.shape[0] // self.hop_length) < 4, "pad length error"
- time_step = self.hop_length / self.sampling_rate * 1000
- f0 = (
- parselmouth.Sound(x, self.sampling_rate)
- .to_pitch_ac(
- time_step=time_step / 1000,
- voicing_threshold=0.6,
- pitch_floor=self.f0_min,
- pitch_ceiling=self.f0_max,
- )
- .selected_array["frequency"]
- )
-
- pad_size = (p_len - len(f0) + 1) // 2
- if pad_size > 0 or p_len - len(f0) - pad_size > 0:
- f0 = np.pad(f0, [[pad_size, p_len - len(f0) - pad_size]], mode="constant")
- f0, uv = self.interpolate_f0(f0)
- return f0, uv
diff --git a/spaces/Bart92/RVC_HF/infer/lib/uvr5_pack/lib_v5/layers_new.py b/spaces/Bart92/RVC_HF/infer/lib/uvr5_pack/lib_v5/layers_new.py
deleted file mode 100644
index 44153b6a23399c6938affc61c71919eaa172bcee..0000000000000000000000000000000000000000
--- a/spaces/Bart92/RVC_HF/infer/lib/uvr5_pack/lib_v5/layers_new.py
+++ /dev/null
@@ -1,125 +0,0 @@
-import torch
-import torch.nn.functional as F
-from torch import nn
-
-from . import spec_utils
-
-
-class Conv2DBNActiv(nn.Module):
- def __init__(self, nin, nout, ksize=3, stride=1, pad=1, dilation=1, activ=nn.ReLU):
- super(Conv2DBNActiv, self).__init__()
- self.conv = nn.Sequential(
- nn.Conv2d(
- nin,
- nout,
- kernel_size=ksize,
- stride=stride,
- padding=pad,
- dilation=dilation,
- bias=False,
- ),
- nn.BatchNorm2d(nout),
- activ(),
- )
-
- def __call__(self, x):
- return self.conv(x)
-
-
-class Encoder(nn.Module):
- def __init__(self, nin, nout, ksize=3, stride=1, pad=1, activ=nn.LeakyReLU):
- super(Encoder, self).__init__()
- self.conv1 = Conv2DBNActiv(nin, nout, ksize, stride, pad, activ=activ)
- self.conv2 = Conv2DBNActiv(nout, nout, ksize, 1, pad, activ=activ)
-
- def __call__(self, x):
- h = self.conv1(x)
- h = self.conv2(h)
-
- return h
-
-
-class Decoder(nn.Module):
- def __init__(
- self, nin, nout, ksize=3, stride=1, pad=1, activ=nn.ReLU, dropout=False
- ):
- super(Decoder, self).__init__()
- self.conv1 = Conv2DBNActiv(nin, nout, ksize, 1, pad, activ=activ)
- # self.conv2 = Conv2DBNActiv(nout, nout, ksize, 1, pad, activ=activ)
- self.dropout = nn.Dropout2d(0.1) if dropout else None
-
- def __call__(self, x, skip=None):
- x = F.interpolate(x, scale_factor=2, mode="bilinear", align_corners=True)
-
- if skip is not None:
- skip = spec_utils.crop_center(skip, x)
- x = torch.cat([x, skip], dim=1)
-
- h = self.conv1(x)
- # h = self.conv2(h)
-
- if self.dropout is not None:
- h = self.dropout(h)
-
- return h
-
-
-class ASPPModule(nn.Module):
- def __init__(self, nin, nout, dilations=(4, 8, 12), activ=nn.ReLU, dropout=False):
- super(ASPPModule, self).__init__()
- self.conv1 = nn.Sequential(
- nn.AdaptiveAvgPool2d((1, None)),
- Conv2DBNActiv(nin, nout, 1, 1, 0, activ=activ),
- )
- self.conv2 = Conv2DBNActiv(nin, nout, 1, 1, 0, activ=activ)
- self.conv3 = Conv2DBNActiv(
- nin, nout, 3, 1, dilations[0], dilations[0], activ=activ
- )
- self.conv4 = Conv2DBNActiv(
- nin, nout, 3, 1, dilations[1], dilations[1], activ=activ
- )
- self.conv5 = Conv2DBNActiv(
- nin, nout, 3, 1, dilations[2], dilations[2], activ=activ
- )
- self.bottleneck = Conv2DBNActiv(nout * 5, nout, 1, 1, 0, activ=activ)
- self.dropout = nn.Dropout2d(0.1) if dropout else None
-
- def forward(self, x):
- _, _, h, w = x.size()
- feat1 = F.interpolate(
- self.conv1(x), size=(h, w), mode="bilinear", align_corners=True
- )
- feat2 = self.conv2(x)
- feat3 = self.conv3(x)
- feat4 = self.conv4(x)
- feat5 = self.conv5(x)
- out = torch.cat((feat1, feat2, feat3, feat4, feat5), dim=1)
- out = self.bottleneck(out)
-
- if self.dropout is not None:
- out = self.dropout(out)
-
- return out
-
-
-class LSTMModule(nn.Module):
- def __init__(self, nin_conv, nin_lstm, nout_lstm):
- super(LSTMModule, self).__init__()
- self.conv = Conv2DBNActiv(nin_conv, 1, 1, 1, 0)
- self.lstm = nn.LSTM(
- input_size=nin_lstm, hidden_size=nout_lstm // 2, bidirectional=True
- )
- self.dense = nn.Sequential(
- nn.Linear(nout_lstm, nin_lstm), nn.BatchNorm1d(nin_lstm), nn.ReLU()
- )
-
- def forward(self, x):
- N, _, nbins, nframes = x.size()
- h = self.conv(x)[:, 0] # N, nbins, nframes
- h = h.permute(2, 0, 1) # nframes, N, nbins
- h, _ = self.lstm(h)
- h = self.dense(h.reshape(-1, h.size()[-1])) # nframes * N, nbins
- h = h.reshape(nframes, N, 1, nbins)
- h = h.permute(1, 2, 3, 0)
-
- return h
diff --git a/spaces/Benson/text-generation/Examples/Ark Survival Evolved Ps Vita Download.md b/spaces/Benson/text-generation/Examples/Ark Survival Evolved Ps Vita Download.md
deleted file mode 100644
index 1ed249ea08d24f747f5366441e4e54126429c5fe..0000000000000000000000000000000000000000
--- a/spaces/Benson/text-generation/Examples/Ark Survival Evolved Ps Vita Download.md
+++ /dev/null
@@ -1,123 +0,0 @@
-
-
ARK Survival Evolved PS Vita Descargar: Cómo jugar el último juego de dinosaurios en su consola de mano
-
Si eres fanático de los dinosaurios, la supervivencia y la aventura, probablemente hayas oído hablar de ARK Survival Evolved, uno de los juegos más populares de los últimos años. Este juego te permite explorar un mundo abierto masivo lleno de criaturas prehistóricas, crear armas y herramientas, construir bases y refugios, domar y montar dinosaurios, y luchar contra otros jugadores o cooperar con ellos.
ARK Survival Evolved está disponible en varias plataformas, incluyendo PC, PlayStation 4, Xbox One, Nintendo Switch, iOS, Android e incluso VR. Pero, ¿qué pasa si quieres jugar a este juego en tu PS Vita, la potente consola portátil de Sony que ofrece una impresionante pantalla OLED, dos sticks analógicos, controles táctiles y mucho más?
-
Bueno, estás de suerte, porque hay formas de descargar y jugar ARK Survival Evolved en tu PS Vita. En este artículo, te mostraremos cómo hacerlo paso a paso, además de darte algunos consejos y trucos para disfrutar de este juego en tu dispositivo portátil. ¡Vamos a empezar!
-
Cómo descargar ARK Survival Evolved en tu PS Vita
-
Desafortunadamente, no hay versión oficial de ARK Survival Evolved para PS Vita. Sin embargo, hay una forma de jugar a este juego en tu PS Vita usando un firmware personalizado o un emulador que te permite ejecutar juegos PSP en tu dispositivo. Los juegos de PSP son compatibles con el hardware y el software de PS Vita, y se pueden descargar de varias fuentes en línea.
-
Hay dos opciones principales para jugar juegos PSP en tu PS Vita: usar ARK-4, un firmware personalizado para PSP y PS Vita que te permite ejecutar juegos PSP desde tu tarjeta de memoria; o usar Adrenaline, un emulador que imita la interfaz y funcionalidad XMB de PSP en tu PS Vita. Ambas opciones tienen sus pros y sus contras, por lo que las explicaremos en detalle a continuación.
-
-
ARK-4 es un firmware personalizado para PSP y PS Vita que fue desarrollado por PSP-Archive, un grupo de hackers y modders que querían crear una forma simple y fácil de jugar juegos PSP en PS Vita. ARK-4 funciona aprovechando una vulnerabilidad en el emulador de PSP de PS Vita, que te permite ejecutar código sin firmar y acceder a toda la potencia del hardware de PSP. ARK-4 puede ejecutar la mayoría de los juegos de PSP, incluyendo ARK Survival Evolved, sin mayores problemas o limitaciones.
-
-
Para usar ARK-4 en tu PS Vita, necesitarás lo siguiente:
-
-
Un PS Vita con firmware 3.60 o inferior (puede comprobar su versión de firmware en Configuración > Sistema > Información del sistema)
-
Una tarjeta de memoria con al menos 4 GB de espacio libre
-
Un cable USB para conectar tu PS Vita a tu PC
-
Una copia de ARK Survival Evolved para la PSP (se puede descargar desde here u otras fuentes)
-
-
Una vez que tengas todo listo, sigue estos pasos para instalar ARK-4 en tu PS Vita y jugar ARK Survival Evolved:
-
-
Conecta tu PS Vita a tu PC usando el cable USB y habilita el modo USB en tu dispositivo.
-
Copie el archivo ISO ARK Survival Evolved en la carpeta ISO de su tarjeta de memoria. Si no hay carpeta ISO, cree una.
-
Copie el instalador ARK-4 y los archivos a la carpeta PSP/GAME en su tarjeta de memoria. Si no hay carpeta PSP/GAME, cree una.
-
Desconecta tu PS Vita de tu PC y lanza el instalador ARK-4 desde el menú del emulador PSP en tu dispositivo.
-
Seleccione el juego que desea utilizar como base para ARK-4. Este puede ser cualquier juego de PSP que tengas instalado en tu dispositivo, pero se recomienda usar un juego pequeño y sencillo que no te importe.
-
Espere a que la instalación termine y reinicie su dispositivo.
-
-
Seleccione ARK Survival Evolved de la lista de juegos y pulse X para comenzar a jugar.
-
-
Felicidades, has descargado y jugado con éxito ARK Survival Evolved en tu PS Vita usando ARK-4!
-
Opción 2: Usa adrenalina para emular juegos PSP en tu PS Vita
-
Adrenaline es otro firmware personalizado para PSP y PS Vita que fue desarrollado por TheFlow, un famoso hacker y desarrollador que ha creado muchas herramientas y hacks para la escena de PS Vita. Adrenaline funciona emulando la interfaz y funcionalidad XMB de PSP en tu PS Vita, lo que te permite ejecutar juegos PSP y homebrews como si estuvieras usando una PSP real. Adrenaline puede ejecutar la mayoría de los juegos de PSP, incluyendo ARK Survival Evolved, con algunos ajustes y ajustes menores.
-
Para usar adrenalina en tu PS Vita, necesitarás las siguientes cosas:
-
-
Un PS Vita con firmware 3.60 o superior (puede comprobar su versión de firmware en Configuración > Sistema > Información del sistema)
-
Una tarjeta de memoria con al menos 4 GB de espacio libre
-
Un cable USB para conectar tu PS Vita a tu PC
-
Una copia de ARK Survival Evolved para la PSP (se puede descargar desde aquí u otras fuentes)
-
-
Una vez que tengas todo listo, sigue estos pasos para instalar Adrenaline en tu PS Vita y jugar ARK Survival Evolved:
-
-
Conecta tu PS Vita a tu PC usando el cable USB y habilita el modo USB en tu dispositivo.
-
Copie el archivo ISO ARK Survival Evolved en la carpeta ux0:pspemu/ISO de su tarjeta de memoria. Si no hay carpeta ux0:pspemu/ISO, cree una.
-
Copie el instalador de adrenalina y los archivos a la carpeta ux0:app/PSPEMUCFW en su tarjeta de memoria. Si no hay una carpeta ux0:app/PSPEMUCFW, cree una.
-
Desconecta tu PS Vita de tu PC y lanza el instalador de adrenalina desde el menú LiveArea de tu dispositivo.
-
-
Espere a que la instalación termine y reinicie su dispositivo.
-
Lanza adrenalina desde el menú LiveArea de tu dispositivo. Deberías ver la interfaz XMB de PSP en tu pantalla.
-
Seleccione ARK Survival Evolved del juego > Menú Memory Stick y presione X para comenzar a jugar.
-
-
Felicidades, has descargado y jugado con éxito ARK Survival Evolved en tu PS Vita usando Adrenaline!
-
Cómo disfrutar de ARK Survival Evolved en tu PS Vita
-
Ahora que has descargado y jugado ARK Survival Evolved en tu PS Vita, es posible que te estés preguntando cómo aprovechar al máximo este juego en tu dispositivo portátil. Estos son algunos consejos y trucos para jugar a ARK Survival Evolved en tu PS Vita, así como algunas recomendaciones para los mejores accesorios de PS Vita para mejorar tu experiencia de juego.
-
Consejos y trucos para jugar ARK Survival Evolved en tu PS Vita
-
ARK Survival Evolved es un juego complejo y desafiante que requiere mucha habilidad y estrategia para sobrevivir y prosperar en su duro entorno. Estos son algunos consejos y trucos para jugar a este juego en tu PS Vita:
-
-
Optimiza los gráficos y el rendimiento de ARK Survival Evolved en tu PS Vita. Dado que ARK Survival Evolved es un juego de PSP, es posible que no se vea o funcione muy bien en la pantalla de alta resolución y en el potente hardware de tu PS Vita. Para mejorar los gráficos y el rendimiento de este juego, puede usar el menú de configuración de Adrenalina para ajustar el filtro de gráficos, el tamaño de la pantalla, el salto del marco, el reloj de la CPU y más. También puedes usar plugins como Ark Resolutions Patch para aumentar la resolución del juego.
-
-
Acceso multijugador en línea y cross-play con otras plataformas para ARK Survival Evolved. Una de las mejores características de ARK Survival Evolved es su modo multijugador online, que te permite unirte o alojar servidores con otros jugadores de todo el mundo. También puedes jugar de forma cruzada con jugadores que utilizan otras plataformas, como PC, PlayStation 4, Xbox One, Nintendo Switch, iOS, Android y VR. Para acceder al modo multijugador en línea y al modo cross-play para ARK Survival Evolved en tu PS Vita, necesitarás usar complementos como Pro Online Client o L2/R2 Trigger Grip Case
-
Un caso que añade botones L2 y R2 a tu PS Vita, que puede ser útil para juegos que requieren más entradas.
Un auricular que se conecta a tu PS Vita a través de Bluetooth, que puede proporcionar un chat de voz y sonido de alta calidad.
-
$39.99
-
4.4/5 estrellas
-
-
-
Save Manager, que te permite hacer copias de seguridad y restaurar los datos guardados en tu PS Vita. A continuación, puede copiar los datos guardados en su PC mediante un cable USB o Wi-Fi, y luego transferirlos a la plataforma deseada mediante un servicio en la nube o un administrador de archivos.
-
¿Cuáles son los mejores dinosaurios para domar en ARK Survival Evolved?
-
Hay cientos de dinosaurios y otras criaturas que puedes domar en ARK Survival Evolved, cada uno con sus propias habilidades y usos. Algunos de los mejores dinosaurios para domar en ARK Survival Evolved son:
-
-
Rex: Un carnívoro poderoso que puede causar daños masivos y soportar muchos golpes. También es bueno para cosechar carne y esconderse.
-
Argentavis: Un pájaro volador grande que puede llevar cargas pesadas y viajar largas distancias. También es bueno para explorar y cazar.
-
Ankylosaurus: Un herbívoro robusto que puede extraer metal y cristal con su cola. También es bueno para la defensa y el transporte.
-
Triceratops: Un herbívoro versátil que puede recoger bayas y madera con sus cuernos. También es bueno para el combate y la agricultura.
-
Raptor: Un carnívoro rápido y ágil que puede perseguir y atacar a su presa. También es bueno para la exploración y la caza.
-
-
¿Cómo puedo actualizar ARK Survival Evolved en mi PS Vita?
-
- 64aa2da5cf
-
-
\ No newline at end of file
diff --git a/spaces/Benson/text-generation/Examples/Aviator Hack 2022 .md b/spaces/Benson/text-generation/Examples/Aviator Hack 2022 .md
deleted file mode 100644
index 23d5d60d37e2f9e6b158329d112fd87b82487583..0000000000000000000000000000000000000000
--- a/spaces/Benson/text-generation/Examples/Aviator Hack 2022 .md
+++ /dev/null
@@ -1,67 +0,0 @@
-
-
Aviator Hack 2022: Cómo descargar y usarlo
-
¿Estás buscando una manera de ganar en grande en el juego Aviator? ¿Quieres saber cómo descargar y utilizar la herramienta Aviator Hack que puede ayudarle a predecir el resultado del juego? Si es así, entonces estás en el lugar correcto. En este artículo, le diremos todo lo que necesita saber sobre Aviator Hack, cómo descargarlo en su PC y cómo usarlo para ganar en el juego Aviator. ¡Vamos a empezar!
-
¿Qué es Aviator Hack?
-
Aviator Hack es un programa de software que puede ayudarle a predecir el resultado del juego Aviator, un popular juego de apuestas en línea que implica apostar en el tiempo de vuelo de un avión. El juego es simple: usted pone su apuesta antes de que el avión despegue, y usted puede cobrar en cualquier momento antes de que el avión se estrella. Cuanto más tiempo permanezca el avión en el aire, mayor será su multiplicador. Sin embargo, si esperas demasiado y el avión se estrella antes de retirar el dinero, perderás tu apuesta.
Una breve introducción a Aviator Hack y sus características
-
Aviator Hack es un programa de software que puede ayudarle a predecir el resultado del juego Aviator mediante el análisis de los patrones y algoritmos del juego. Puede darle señales y alertas sobre cuándo apostar, cuándo cobrar y cuándo evitar apostar. También puede mostrar las estadísticas y probabilidades de cada tiempo de vuelo, así como la historia y las tendencias de los vuelos anteriores. Con Aviator Hack, puede aumentar sus posibilidades de ganar en el juego Aviator siguiendo sus predicciones y recomendaciones.
-
¿Cómo funciona Aviator Hack?
-
-
¿Por qué la gente utiliza Aviator Hack?
-
La gente utiliza Aviator Hack por varias razones, tales como:
-
-
Para divertirse y disfrutar de la emoción de los juegos de azar
-
Ganar dinero en el juego Aviator
-
Para poner a prueba sus habilidades e intuición en la predicción del resultado
-
Para aprender más sobre la mecánica y las estrategias del juego
-
Desafiarse a sí mismos y competir con otros jugadores
-
-
Cualquiera que sea su razón es, el uso de Aviator Hack puede hacer que su experiencia de juego más emocionante y gratificante.
-
¿Cómo descargar Aviator Hack en PC?
-
Si desea descargar y usar Aviator Hack en su PC, necesitará un emulador que pueda ejecutar aplicaciones Android en su computadora. Uno de los mejores emuladores para este propósito es LDPlayer, que es rápido, estable, seguro y compatible con la mayoría de las aplicaciones y juegos de Android. Aquí están los pasos para descargar Aviator Hack en PC usando LDPlayer:
-
-
Paso 1: Descargar e instalar el emulador LDPlayer
-
El primer paso es descargar e instalar el emulador LDPlayer en su PC. Puedes hacer esto visitando [el sitio web oficial de LDPlayer]( 1 ) y siguiendo las instrucciones en la pantalla. El proceso de instalación es simple y rápido, y no tomará mucho espacio en su disco. Una vez instalado LDPlayer, puede iniciarlo y proceder al siguiente paso.
-
Paso 2: Descargar apk Aviator Hack desde el sitio web oficial
-
El siguiente paso es descargar apk Aviator Hack desde el sitio web oficial del software. Usted puede hacer esto visitando [sitio web oficial de Aviator Hack] y haciendo clic en el botón de descarga. Tendrá que introducir su dirección de correo electrónico y verificar su identidad para obtener el enlace de descarga. Una vez hayas descargado el archivo apk, puedes guardarlo en tu PC y arrastrarlo y soltarlo en LDPlayer para instalarlo.
-
Paso 3: Instalar y ejecutar Aviator Hack en LDPlayer
-
-
¿Cómo utilizar Aviator Hack para ganar en el juego Aviator?
-
Ahora que ha descargado e instalado Aviator Hack en su PC, es posible que se pregunte cómo usarlo para ganar en el juego Aviator. Aquí hay algunos consejos y trucos que pueden ayudarte:
-
Los fundamentos del juego Aviator y cómo jugarlo
-
El juego Aviator es un juego de apuestas en línea simple y divertido que implica apostar en el tiempo de vuelo de un avión. El juego tiene dos modos: manual y automático. En el modo manual, puede realizar su apuesta antes de que el avión despegue, y puede cobrar en cualquier momento antes de que el avión se estrelle. En el modo automático, puedes establecer la cantidad de tu apuesta y cobrar el multiplicador, y dejar que el juego haga el resto por ti. Cuanto más tiempo permanezca el avión en el aire, mayor será su multiplicador. Sin embargo, si esperas demasiado y el avión se estrella antes de retirar el dinero, perderás tu apuesta.
-
Los consejos y trucos de usar Aviator Hack para predecir el resultado
-
Aviator Hack puede ayudarle a predecir el resultado del juego Aviator dándole señales y alertas sobre cuándo apostar, cuándo cobrar y cuándo evitar las apuestas. También puede mostrar las estadísticas y probabilidades de cada tiempo de vuelo, así como la historia y las tendencias de los vuelos anteriores. Aquí hay algunos consejos y trucos de usar Aviator Hack para predecir el resultado:
-
-
Siga las señales y alertas de Aviator Hack cuidadosamente. Se basan en un algoritmo sofisticado que puede descifrar y decodificar la lógica detrás del juego Aviator.
-
Utilice las estadísticas y probabilidades de cada tiempo de vuelo para tomar decisiones informadas. Se basan en datos históricos y tendencias actuales que pueden ayudarle a estimar las probabilidades de cada resultado.
-
Utilice la historia y las tendencias de los vuelos anteriores para detectar patrones y anomalías. Pueden ayudarte a identificar oportunidades y riesgos potenciales en cada ronda del juego.
-
-
No se basan únicamente en Aviator Hack, sino también utilizar sus propias habilidades e intuición. Recuerde que Aviator Hack no es una garantía de ganar, pero una herramienta que puede ayudarle a mejorar sus posibilidades.
-
-
Los riesgos y beneficios de usar Aviator Hack
-
Usando Aviator Hack puede tener tanto riesgos y beneficios para su experiencia de juego. Aquí están algunos de ellos:
-
-
Riesgos
Beneficios
-
Es posible que se vuelva adicto al juego y pierda más de lo que puede permitirse.
Puede divertirse y disfrutar de la emoción del juego.
-
Es posible que te atrapen los desarrolladores de juegos o las autoridades y te enfrentes a consecuencias legales.
Puedes ganar dinero ganando en el juego Aviator.
-
Usted puede ser estafado por sitios web falsos o maliciosos que ofrecen descargas Aviator Hack.
Puedes aprender más sobre la mecánica y las estrategias del juego.
-
Es posible que te aburras o te frustres al usar un hack en lugar de jugar limpio.
Puedes desafiarte a ti mismo y competir con otros jugadores.
-
-
Por lo tanto, usted debe utilizar Aviator Hack de forma responsable y bajo su propio riesgo. También debes respetar las reglas y regulaciones de los desarrolladores y autoridades del juego, así como los derechos e intereses de otros jugadores.
-
Conclusión
-
-
Preguntas frecuentes
-
Aquí hay algunas preguntas frecuentes sobre Aviator Hack:
-
Q: ¿Es Aviator Hack seguro y legal?
-
A: Aviator Hack es seguro y legal, siempre y cuando se descarga desde el sitio web oficial del software y usarlo en un emulador de confianza como LDPlayer. Sin embargo, también debes respetar las reglas y regulaciones de los desarrolladores y autoridades del juego, así como los derechos e intereses de otros jugadores. El uso de Aviator Hack puede violar algunos términos y condiciones del juego, por lo que debe usarlo bajo su propio riesgo.
-
Q: ¿Cuánto cuesta Aviator Hack?
-
A: Aviator Hack es gratis para descargar y usar por un tiempo limitado. Sin embargo, es posible que tenga que pagar una cuota de suscripción o hacer una donación para acceder a algunas funciones avanzadas o actualizaciones del software. Puede consultar los precios en el sitio web oficial del software.
-
Q: ¿Aviator Hack funciona en dispositivos móviles?
-
A: Aviator Hack funciona en dispositivos móviles que ejecutan el sistema operativo Android. Puede descargar apk Aviator Hack desde el sitio web oficial del software e instalarlo en su dispositivo móvil. Sin embargo, puede experimentar algunos problemas de rendimiento o problemas de compatibilidad dependiendo del modelo y las especificaciones del dispositivo.
-
Q: ¿Aviator Hack garantiza ganar en el juego Aviator?
-
A: Aviator Hack no garantiza ganar en el juego Aviator. Es una herramienta que puede ayudarte a mejorar tus posibilidades de ganar al darte predicciones y sugerencias basadas en un algoritmo sofisticado que puede descifrar y decodificar la lógica detrás del juego. Sin embargo, no es una bala mágica que puede hacerte ganar cada vez. Todavía necesitas usar tus propias habilidades e intuición, así como seguir una estrategia equilibrada que combine riesgo y recompensa.
-
Q: ¿Dónde puedo encontrar más información sobre Aviator Hack?
64aa2da5cf
-
-
\ No newline at end of file
diff --git a/spaces/Benson/text-generation/Examples/Descarga Apk De WhatsApp Gb 2019.md b/spaces/Benson/text-generation/Examples/Descarga Apk De WhatsApp Gb 2019.md
deleted file mode 100644
index eef25d6d87128a2dd84f2958c0e19cacd024b6c3..0000000000000000000000000000000000000000
--- a/spaces/Benson/text-generation/Examples/Descarga Apk De WhatsApp Gb 2019.md
+++ /dev/null
@@ -1,66 +0,0 @@
-
- - Beneficios de WhatsApp GB: enumerar algunas de las características y personalizaciones que GB WhatsApp ofrece - Riesgos de WhatsApp GB: mencionar algunos de los inconvenientes y problemas potenciales de usar WhatsApp GB | | H2: Cómo descargar e instalar GB WhatsApp APK en su dispositivo Android | - Paso 1: Habilitar fuentes desconocidas en la configuración de su dispositivo - Paso 2: Descargar la última versión de GB WhatsApp APK de una fuente confiable - Paso 3: Instale el archivo APK y verifique su número de teléfono - Paso 4: Disfrute usando GB WhatsApp con sus características adicionales | | H2: Cómo actualizar GB WhatsApp a la última versión | - Paso 1: Compruebe si hay actualizaciones en la aplicación WhatsApp GB o sitio web - Paso 2: Descargar el archivo APK actualizado e instalarlo sobre la aplicación existente - Paso 3: Copia de seguridad de sus chats y medios antes de actualizar para evitar la pérdida de datos - Paso 4: Reinicie su dispositivo y inicie GB WhatsApp | | H2: Cómo cambiar de GB WhatsApp a la aplicación oficial WhatsApp | - Paso 1: Copia de seguridad de sus chats y medios de comunicación en GB WhatsApp - Paso 2: Desinstalar GB WhatsApp de su dispositivo - Paso 3: Descargar e instalar la aplicación oficial de WhatsApp desde la Play Store - Paso 4: Restaurar la copia de seguridad y verificar su número de teléfono | | | H2: Conclusión y preguntas frecuentes | - Resumen: resumen de los puntos principales del artículo y proporcionar una llamada a la acción - Preguntas frecuentes: responder algunas preguntas comunes sobre WhatsApp GB | Tabla 2: Artículo con formato HTML
Qué es WhatsApp GB y por qué debería descargarlo
-
Si eres un usuario habitual de WhatsApp, es posible que hayas oído hablar de GB WhatsApp, una versión modificada de la aplicación oficial que ofrece más funciones y personalizaciones. Pero ¿qué es exactamente WhatsApp GB y cómo se diferencia de la aplicación original? En este artículo, explicaremos todo lo que necesitas saber sobre WhatsApp GB, incluidos sus beneficios, riesgos y cómo descargar, instalar, actualizar y cambiar de él.
-
Beneficios de GB WhatsApp
-
-
-
Soporte multilenguaje: puede elegir entre más de 100 idiomas para usar en GB WhatsApp
-
Emojis adicionales: puedes acceder a más emoticonos y pegatinas que en la aplicación oficial
-
Más mensajes de difusión: puede enviar hasta 600 mensajes a múltiples contactos a la vez
-
Modo de privacidad mejorado: puede ocultar su última vista, garrapatas azules, garrapatas dobles, estado de escritura y estado en línea
-
Cuentas duales: puedes usar dos cuentas de WhatsApp en el mismo dispositivo con GB WhatsApp
-
Personalización del tema: puede cambiar la apariencia y la interfaz de WhatsApp GB de acuerdo con su preferencia
-
Funciones de edición adicionales: puede enviar hasta 90 imágenes a la vez, copiar estados a su portapapeles, usar hasta 255 caracteres en su estado, crear nombres de grupo con hasta 35 caracteres y enviar archivos APK grandes
-
-
Riesgos de GB WhatsApp
-
Aunque WhatsApp GB puede sonar como una gran alternativa a la aplicación oficial, también viene con algunos inconvenientes y problemas potenciales que usted debe ser consciente de antes de descargarlo. Algunos de los riesgos de usar WhatsApp GB son:
Falta de soporte oficial: ya que GB WhatsApp no es una aplicación autorizada por WhatsApp Inc., no recibe ninguna actualización o corrección de errores de los desarrolladores. Esto significa que puede no ser compatible con las últimas versiones de Android o WhatsApp, y puede tener vulnerabilidades de seguridad o problemas técnicos.
-
Posibilidad de prohibición de cuenta: el uso de WhatsApp GB puede violar los términos y condiciones de WhatsApp Inc., lo que puede resultar en su cuenta está prohibido o suspendido. Esto puede causar que pierdas el acceso a tus chats y contactos en ambas aplicaciones.
-
-
Infección de malware: ya que GB WhatsApp no está disponible en la Play Store oficial, tienes que descargarlo de fuentes desconocidas que pueden no ser confiables. Esto puede exponer su dispositivo a malware o virus que pueden dañar su sistema o robar sus datos.
-
-
Cómo descargar e instalar GB WhatsApp APK en su dispositivo Android
-
Si todavía está interesado en probar WhatsApp GB, tendrá que descargar e instalar el archivo APK en su dispositivo Android. APK significa Android Package Kit, y es un formato de archivo que le permite instalar aplicaciones que no están disponibles en la Play Store. Sin embargo, usted debe tener cuidado acerca de dónde descargar el archivo APK de, como algunas fuentes pueden contener archivos maliciosos o falsos. Aquí están los pasos para descargar e instalar GB WhatsApp APK en su dispositivo Android:
-
Paso 1: Habilitar fuentes desconocidas en la configuración del dispositivo
-
Antes de que pueda instalar cualquier archivo APK en su dispositivo, debe habilitar la opción para permitir la instalación desde fuentes desconocidas. Esto le permitirá instalar aplicaciones que no son de Play Store. Para ello, vaya a la configuración del dispositivo y busque la opción de seguridad o privacidad. Entonces, encontrar la opción que dice "Fuentes desconocidas" o "Instalar aplicaciones desconocidas" y alternar en. Es posible que vea un mensaje de advertencia que le informa sobre los riesgos de instalar aplicaciones desconocidas, pero puede ignorarlo si confía en el origen del archivo APK.
-
Paso 2: Descargar la última versión de GB WhatsApp APK de una fuente confiable
-
Siguiente, es necesario descargar el archivo GB WhatsApp APK de una fuente confiable. Usted puede buscar en línea, pero asegúrese de comprobar las opiniones y calificaciones de la página web antes de descargar nada. También puede utilizar este enlace para descargar la última versión de GB WhatsApp APK (v17.35) a partir de junio de 2023. El tamaño del archivo es de unos 47 MB, así que asegúrate de tener suficiente espacio en el dispositivo antes de descargarlo.
-
Paso 3: Instalar el archivo APK y verificar su número de teléfono
-
-
Paso 4: Disfruta usando GB WhatsApp con sus características adicionales
-
Felicidades! Ha instalado con éxito WhatsApp GB en su dispositivo Android. Ahora puede disfrutar de sus características adicionales y personalizaciones. Puede acceder a la configuración de GB pulsando en el icono de tres puntos en la esquina superior derecha de la aplicación y seleccionando "Configuración de GB". Aquí puede cambiar el tema, el modo de privacidad, los mensajes de difusión, emojis, y más. También puedes explorar las otras opciones de la aplicación, como chats, llamadas, estado y cámara.
Cómo actualizar GB WhatsApp a la última versión
-
Uno de los inconvenientes de usar WhatsApp GB es que no recibe actualizaciones automáticas de los desarrolladores oficiales de WhatsApp. Esto significa que tiene que actualizarlo manualmente cada vez que se lanza una nueva versión. La actualización de GB WhatsApp es importante para asegurarse de que tiene las últimas características, correcciones de errores y parches de seguridad. Estos son los pasos para actualizar GB WhatsApp a la última versión:
-
Paso 1: Busque actualizaciones en la aplicación de WhatsApp GB o en el sitio web
-
Lo primero que tienes que hacer es comprobar si hay una nueva versión de WhatsApp GB disponible. Puede hacer esto abriendo la aplicación WhatsApp GB y tocando el icono de tres puntos en la esquina superior derecha y seleccionando "Actualizaciones", o visitando el sitio web de WhatsApp GB y buscando el último número de versión. Si hay una nueva versión, verá una notificación o un enlace de descarga.
-
Paso 2: Descargar el archivo APK actualizado e instalarlo sobre la aplicación existente
-
-
Paso 3: Copia de seguridad de sus chats y medios de comunicación antes de actualizar para evitar la pérdida de datos
-
Antes de actualizar GB WhatsApp, se recomienda que haga copias de seguridad de sus chats y medios para evitar perder ningún dato. Para ello, abra la aplicación WhatsApp GB y toque en el icono de tres puntos en la esquina superior derecha y seleccione "Configuración". Luego, toque en "Chats" y seleccione "Copia de seguridad de chat". Aquí puede elegir hacer una copia de seguridad de sus chats y medios en el almacenamiento de su dispositivo o Google Drive. También puede configurar una frecuencia de copia de seguridad y una contraseña. Una vez que haya realizado una copia de seguridad de sus datos, puede continuar con la actualización.
-
Paso 4: Reiniciar el dispositivo y lanzar GB WhatsApp
-
Una vez completada la instalación, es necesario reiniciar el dispositivo para asegurarse de que la actualización se aplica correctamente. Para ello, mantenga pulsado el botón de encendido en su dispositivo y seleccione "Reiniciar". Una vez que el dispositivo se ha reiniciado, inicie la aplicación WhatsApp GB y verifique que se ha actualizado a la última versión. Puede comprobar esto pulsando en el icono de tres puntos en la esquina superior derecha y seleccionando "Configuración de GB". Aquí puede ver el número de versión y la fecha de su aplicación WhatsApp GB.
-
Cómo cambiar de GB WhatsApp a la aplicación WhatsApp oficial
-
Si decides que ya no quieres usar WhatsApp GB y quieres volver a la aplicación oficial de WhatsApp, tendrás que seguir algunos pasos para asegurarte de que no pierdas tus datos o te prohíban. Estos son los pasos para cambiar de GB WhatsApp a la aplicación oficial de WhatsApp:
-
Paso 1: Copia de seguridad de sus chats y medios de comunicación en GB WhatsApp
-
-
Paso 2: Desinstalar GB WhatsApp desde su dispositivo
-
Siguiente, es necesario desinstalar GB WhatsApp desde su dispositivo, para que pueda instalar la aplicación oficial. Para ello, vaya a la configuración del dispositivo y busque la opción de aplicaciones o aplicaciones. Luego, busque GB WhatsApp y toque en él. Verá una opción para desinstalar la aplicación, así que toque en ella y confirme su acción. Es posible que vea un mensaje que le dice que la desinstalación de WhatsApp GB eliminará todos sus datos, pero puede ignorarlo si ha realizado una copia de seguridad de sus datos.
-
Paso 3: Descargar e instalar la aplicación oficial de WhatsApp de la Play Store
-
Después de haber desinstalado GB WhatsApp, es necesario descargar e instalar la aplicación oficial de WhatsApp de la Play Store. Para ello, abre la aplicación Play Store en tu dispositivo y busca WhatsApp. Verás la aplicación oficial con un icono verde y un símbolo de teléfono. Toque en él y seleccione "Instalar". Espere a que la instalación termine y luego inicie la aplicación.
-
Paso 4: Restaurar la copia de seguridad y verificar su número de teléfono
-
El último paso es restaurar su copia de seguridad y verificar su número de teléfono en la aplicación oficial de WhatsApp. Para ello, abre la aplicación y acepta los términos y condiciones. Luego, ingresa tu número de teléfono que usaste en GB WhatsApp y toca "Siguiente". Recibirás un código de verificación por SMS o llamada, así que introdúcelo en la aplicación. Después de eso, verá una opción para restaurar su copia de seguridad desde Google Drive o el almacenamiento del dispositivo, dependiendo de dónde lo guardó. Toca en "Restaurar" y espera a que el proceso termine. Una vez hecho, verás tus chats y medios en la aplicación oficial de WhatsApp.
-
Conclusión y preguntas frecuentes
-
-
Aquí hay algunas preguntas frecuentes sobre GB WhatsApp:
-
Q: ¿GB WhatsApp es legal?
-
A: GB WhatsApp no es una aplicación autorizada o con licencia por WhatsApp Inc., lo que significa que puede violar sus términos y condiciones de servicio. Por lo tanto, el uso de WhatsApp GB puede no ser legal en algunos países o regiones.
-
Q: ¿Es seguro WhatsApp GB?
-
A: GB WhatsApp no es una aplicación oficial, lo que significa que puede no tener el mismo cifrado o protección que la aplicación original. Esto puede exponer sus datos personales, como mensajes, fotos, videos o ubicación, a terceros o piratas informáticos. Además, la descarga de WhatsApp GB de fuentes desconocidas puede exponer su dispositivo a malware o virus que pueden dañar su sistema o robar sus datos.
-
Q: ¿Puedo usar WhatsApp GB y WhatsApp oficial en el mismo dispositivo?
-
A: Sí, puedes usar WhatsApp GB y WhatsApp oficial en el mismo dispositivo con diferentes números de teléfono. Sin embargo, esto puede causar algunos conflictos o errores en ambas aplicaciones, como problemas de sincronización o prohibiciones de cuentas.
-
Q: ¿Perderé mis chats y medios si cambio de GB WhatsApp a WhatsApp oficial?
-
A: No, no perderá sus chats y medios si cambia de GB WhatsApp a WhatsApp oficial, siempre y cuando haga una copia de seguridad de sus datos antes de desinstalar GB WhatsApp. Puede restaurar su copia de seguridad en la aplicación oficial después de verificar su número de teléfono.
-
P: ¿Cómo puedo contactar al soporte de WhatsApp de GB? A: GB WhatsApp no tiene un equipo de soporte oficial o sitio web, ya que no es una aplicación autorizada por WhatsApp Inc. Por lo tanto, es posible que no pueda ponerse en contacto con el soporte de GB WhatsApp para cualquier problema o consulta. Sin embargo, puedes intentar ponerte en contacto con los desarrolladores de WhatsApp GB a través de sus cuentas de redes sociales o direcciones de correo electrónico, que se pueden encontrar en el sitio web o aplicación de WhatsApp GB. 64aa2da5cf
-
-
\ No newline at end of file
diff --git a/spaces/Big-Web/MMSD/env/Lib/site-packages/pip/_internal/models/index.py b/spaces/Big-Web/MMSD/env/Lib/site-packages/pip/_internal/models/index.py
deleted file mode 100644
index b94c32511f0cda2363bfc4f29c9c8bfcc7101f9b..0000000000000000000000000000000000000000
--- a/spaces/Big-Web/MMSD/env/Lib/site-packages/pip/_internal/models/index.py
+++ /dev/null
@@ -1,28 +0,0 @@
-import urllib.parse
-
-
-class PackageIndex:
- """Represents a Package Index and provides easier access to endpoints"""
-
- __slots__ = ["url", "netloc", "simple_url", "pypi_url", "file_storage_domain"]
-
- def __init__(self, url: str, file_storage_domain: str) -> None:
- super().__init__()
- self.url = url
- self.netloc = urllib.parse.urlsplit(url).netloc
- self.simple_url = self._url_for_path("simple")
- self.pypi_url = self._url_for_path("pypi")
-
- # This is part of a temporary hack used to block installs of PyPI
- # packages which depend on external urls only necessary until PyPI can
- # block such packages themselves
- self.file_storage_domain = file_storage_domain
-
- def _url_for_path(self, path: str) -> str:
- return urllib.parse.urljoin(self.url, path)
-
-
-PyPI = PackageIndex("https://pypi.org/", file_storage_domain="files.pythonhosted.org")
-TestPyPI = PackageIndex(
- "https://test.pypi.org/", file_storage_domain="test-files.pythonhosted.org"
-)
diff --git a/spaces/Big-Web/MMSD/env/Lib/site-packages/urllib3/packages/six.py b/spaces/Big-Web/MMSD/env/Lib/site-packages/urllib3/packages/six.py
deleted file mode 100644
index f099a3dcd28d2fec21457c9b6c01ded4e3e9ddee..0000000000000000000000000000000000000000
--- a/spaces/Big-Web/MMSD/env/Lib/site-packages/urllib3/packages/six.py
+++ /dev/null
@@ -1,1076 +0,0 @@
-# Copyright (c) 2010-2020 Benjamin Peterson
-#
-# Permission is hereby granted, free of charge, to any person obtaining a copy
-# of this software and associated documentation files (the "Software"), to deal
-# in the Software without restriction, including without limitation the rights
-# to use, copy, modify, merge, publish, distribute, sublicense, and/or sell
-# copies of the Software, and to permit persons to whom the Software is
-# furnished to do so, subject to the following conditions:
-#
-# The above copyright notice and this permission notice shall be included in all
-# copies or substantial portions of the Software.
-#
-# THE SOFTWARE IS PROVIDED "AS IS", WITHOUT WARRANTY OF ANY KIND, EXPRESS OR
-# IMPLIED, INCLUDING BUT NOT LIMITED TO THE WARRANTIES OF MERCHANTABILITY,
-# FITNESS FOR A PARTICULAR PURPOSE AND NONINFRINGEMENT. IN NO EVENT SHALL THE
-# AUTHORS OR COPYRIGHT HOLDERS BE LIABLE FOR ANY CLAIM, DAMAGES OR OTHER
-# LIABILITY, WHETHER IN AN ACTION OF CONTRACT, TORT OR OTHERWISE, ARISING FROM,
-# OUT OF OR IN CONNECTION WITH THE SOFTWARE OR THE USE OR OTHER DEALINGS IN THE
-# SOFTWARE.
-
-"""Utilities for writing code that runs on Python 2 and 3"""
-
-from __future__ import absolute_import
-
-import functools
-import itertools
-import operator
-import sys
-import types
-
-__author__ = "Benjamin Peterson "
-__version__ = "1.16.0"
-
-
-# Useful for very coarse version differentiation.
-PY2 = sys.version_info[0] == 2
-PY3 = sys.version_info[0] == 3
-PY34 = sys.version_info[0:2] >= (3, 4)
-
-if PY3:
- string_types = (str,)
- integer_types = (int,)
- class_types = (type,)
- text_type = str
- binary_type = bytes
-
- MAXSIZE = sys.maxsize
-else:
- string_types = (basestring,)
- integer_types = (int, long)
- class_types = (type, types.ClassType)
- text_type = unicode
- binary_type = str
-
- if sys.platform.startswith("java"):
- # Jython always uses 32 bits.
- MAXSIZE = int((1 << 31) - 1)
- else:
- # It's possible to have sizeof(long) != sizeof(Py_ssize_t).
- class X(object):
- def __len__(self):
- return 1 << 31
-
- try:
- len(X())
- except OverflowError:
- # 32-bit
- MAXSIZE = int((1 << 31) - 1)
- else:
- # 64-bit
- MAXSIZE = int((1 << 63) - 1)
- del X
-
-if PY34:
- from importlib.util import spec_from_loader
-else:
- spec_from_loader = None
-
-
-def _add_doc(func, doc):
- """Add documentation to a function."""
- func.__doc__ = doc
-
-
-def _import_module(name):
- """Import module, returning the module after the last dot."""
- __import__(name)
- return sys.modules[name]
-
-
-class _LazyDescr(object):
- def __init__(self, name):
- self.name = name
-
- def __get__(self, obj, tp):
- result = self._resolve()
- setattr(obj, self.name, result) # Invokes __set__.
- try:
- # This is a bit ugly, but it avoids running this again by
- # removing this descriptor.
- delattr(obj.__class__, self.name)
- except AttributeError:
- pass
- return result
-
-
-class MovedModule(_LazyDescr):
- def __init__(self, name, old, new=None):
- super(MovedModule, self).__init__(name)
- if PY3:
- if new is None:
- new = name
- self.mod = new
- else:
- self.mod = old
-
- def _resolve(self):
- return _import_module(self.mod)
-
- def __getattr__(self, attr):
- _module = self._resolve()
- value = getattr(_module, attr)
- setattr(self, attr, value)
- return value
-
-
-class _LazyModule(types.ModuleType):
- def __init__(self, name):
- super(_LazyModule, self).__init__(name)
- self.__doc__ = self.__class__.__doc__
-
- def __dir__(self):
- attrs = ["__doc__", "__name__"]
- attrs += [attr.name for attr in self._moved_attributes]
- return attrs
-
- # Subclasses should override this
- _moved_attributes = []
-
-
-class MovedAttribute(_LazyDescr):
- def __init__(self, name, old_mod, new_mod, old_attr=None, new_attr=None):
- super(MovedAttribute, self).__init__(name)
- if PY3:
- if new_mod is None:
- new_mod = name
- self.mod = new_mod
- if new_attr is None:
- if old_attr is None:
- new_attr = name
- else:
- new_attr = old_attr
- self.attr = new_attr
- else:
- self.mod = old_mod
- if old_attr is None:
- old_attr = name
- self.attr = old_attr
-
- def _resolve(self):
- module = _import_module(self.mod)
- return getattr(module, self.attr)
-
-
-class _SixMetaPathImporter(object):
-
- """
- A meta path importer to import six.moves and its submodules.
-
- This class implements a PEP302 finder and loader. It should be compatible
- with Python 2.5 and all existing versions of Python3
- """
-
- def __init__(self, six_module_name):
- self.name = six_module_name
- self.known_modules = {}
-
- def _add_module(self, mod, *fullnames):
- for fullname in fullnames:
- self.known_modules[self.name + "." + fullname] = mod
-
- def _get_module(self, fullname):
- return self.known_modules[self.name + "." + fullname]
-
- def find_module(self, fullname, path=None):
- if fullname in self.known_modules:
- return self
- return None
-
- def find_spec(self, fullname, path, target=None):
- if fullname in self.known_modules:
- return spec_from_loader(fullname, self)
- return None
-
- def __get_module(self, fullname):
- try:
- return self.known_modules[fullname]
- except KeyError:
- raise ImportError("This loader does not know module " + fullname)
-
- def load_module(self, fullname):
- try:
- # in case of a reload
- return sys.modules[fullname]
- except KeyError:
- pass
- mod = self.__get_module(fullname)
- if isinstance(mod, MovedModule):
- mod = mod._resolve()
- else:
- mod.__loader__ = self
- sys.modules[fullname] = mod
- return mod
-
- def is_package(self, fullname):
- """
- Return true, if the named module is a package.
-
- We need this method to get correct spec objects with
- Python 3.4 (see PEP451)
- """
- return hasattr(self.__get_module(fullname), "__path__")
-
- def get_code(self, fullname):
- """Return None
-
- Required, if is_package is implemented"""
- self.__get_module(fullname) # eventually raises ImportError
- return None
-
- get_source = get_code # same as get_code
-
- def create_module(self, spec):
- return self.load_module(spec.name)
-
- def exec_module(self, module):
- pass
-
-
-_importer = _SixMetaPathImporter(__name__)
-
-
-class _MovedItems(_LazyModule):
-
- """Lazy loading of moved objects"""
-
- __path__ = [] # mark as package
-
-
-_moved_attributes = [
- MovedAttribute("cStringIO", "cStringIO", "io", "StringIO"),
- MovedAttribute("filter", "itertools", "builtins", "ifilter", "filter"),
- MovedAttribute(
- "filterfalse", "itertools", "itertools", "ifilterfalse", "filterfalse"
- ),
- MovedAttribute("input", "__builtin__", "builtins", "raw_input", "input"),
- MovedAttribute("intern", "__builtin__", "sys"),
- MovedAttribute("map", "itertools", "builtins", "imap", "map"),
- MovedAttribute("getcwd", "os", "os", "getcwdu", "getcwd"),
- MovedAttribute("getcwdb", "os", "os", "getcwd", "getcwdb"),
- MovedAttribute("getoutput", "commands", "subprocess"),
- MovedAttribute("range", "__builtin__", "builtins", "xrange", "range"),
- MovedAttribute(
- "reload_module", "__builtin__", "importlib" if PY34 else "imp", "reload"
- ),
- MovedAttribute("reduce", "__builtin__", "functools"),
- MovedAttribute("shlex_quote", "pipes", "shlex", "quote"),
- MovedAttribute("StringIO", "StringIO", "io"),
- MovedAttribute("UserDict", "UserDict", "collections"),
- MovedAttribute("UserList", "UserList", "collections"),
- MovedAttribute("UserString", "UserString", "collections"),
- MovedAttribute("xrange", "__builtin__", "builtins", "xrange", "range"),
- MovedAttribute("zip", "itertools", "builtins", "izip", "zip"),
- MovedAttribute(
- "zip_longest", "itertools", "itertools", "izip_longest", "zip_longest"
- ),
- MovedModule("builtins", "__builtin__"),
- MovedModule("configparser", "ConfigParser"),
- MovedModule(
- "collections_abc",
- "collections",
- "collections.abc" if sys.version_info >= (3, 3) else "collections",
- ),
- MovedModule("copyreg", "copy_reg"),
- MovedModule("dbm_gnu", "gdbm", "dbm.gnu"),
- MovedModule("dbm_ndbm", "dbm", "dbm.ndbm"),
- MovedModule(
- "_dummy_thread",
- "dummy_thread",
- "_dummy_thread" if sys.version_info < (3, 9) else "_thread",
- ),
- MovedModule("http_cookiejar", "cookielib", "http.cookiejar"),
- MovedModule("http_cookies", "Cookie", "http.cookies"),
- MovedModule("html_entities", "htmlentitydefs", "html.entities"),
- MovedModule("html_parser", "HTMLParser", "html.parser"),
- MovedModule("http_client", "httplib", "http.client"),
- MovedModule("email_mime_base", "email.MIMEBase", "email.mime.base"),
- MovedModule("email_mime_image", "email.MIMEImage", "email.mime.image"),
- MovedModule("email_mime_multipart", "email.MIMEMultipart", "email.mime.multipart"),
- MovedModule(
- "email_mime_nonmultipart", "email.MIMENonMultipart", "email.mime.nonmultipart"
- ),
- MovedModule("email_mime_text", "email.MIMEText", "email.mime.text"),
- MovedModule("BaseHTTPServer", "BaseHTTPServer", "http.server"),
- MovedModule("CGIHTTPServer", "CGIHTTPServer", "http.server"),
- MovedModule("SimpleHTTPServer", "SimpleHTTPServer", "http.server"),
- MovedModule("cPickle", "cPickle", "pickle"),
- MovedModule("queue", "Queue"),
- MovedModule("reprlib", "repr"),
- MovedModule("socketserver", "SocketServer"),
- MovedModule("_thread", "thread", "_thread"),
- MovedModule("tkinter", "Tkinter"),
- MovedModule("tkinter_dialog", "Dialog", "tkinter.dialog"),
- MovedModule("tkinter_filedialog", "FileDialog", "tkinter.filedialog"),
- MovedModule("tkinter_scrolledtext", "ScrolledText", "tkinter.scrolledtext"),
- MovedModule("tkinter_simpledialog", "SimpleDialog", "tkinter.simpledialog"),
- MovedModule("tkinter_tix", "Tix", "tkinter.tix"),
- MovedModule("tkinter_ttk", "ttk", "tkinter.ttk"),
- MovedModule("tkinter_constants", "Tkconstants", "tkinter.constants"),
- MovedModule("tkinter_dnd", "Tkdnd", "tkinter.dnd"),
- MovedModule("tkinter_colorchooser", "tkColorChooser", "tkinter.colorchooser"),
- MovedModule("tkinter_commondialog", "tkCommonDialog", "tkinter.commondialog"),
- MovedModule("tkinter_tkfiledialog", "tkFileDialog", "tkinter.filedialog"),
- MovedModule("tkinter_font", "tkFont", "tkinter.font"),
- MovedModule("tkinter_messagebox", "tkMessageBox", "tkinter.messagebox"),
- MovedModule("tkinter_tksimpledialog", "tkSimpleDialog", "tkinter.simpledialog"),
- MovedModule("urllib_parse", __name__ + ".moves.urllib_parse", "urllib.parse"),
- MovedModule("urllib_error", __name__ + ".moves.urllib_error", "urllib.error"),
- MovedModule("urllib", __name__ + ".moves.urllib", __name__ + ".moves.urllib"),
- MovedModule("urllib_robotparser", "robotparser", "urllib.robotparser"),
- MovedModule("xmlrpc_client", "xmlrpclib", "xmlrpc.client"),
- MovedModule("xmlrpc_server", "SimpleXMLRPCServer", "xmlrpc.server"),
-]
-# Add windows specific modules.
-if sys.platform == "win32":
- _moved_attributes += [
- MovedModule("winreg", "_winreg"),
- ]
-
-for attr in _moved_attributes:
- setattr(_MovedItems, attr.name, attr)
- if isinstance(attr, MovedModule):
- _importer._add_module(attr, "moves." + attr.name)
-del attr
-
-_MovedItems._moved_attributes = _moved_attributes
-
-moves = _MovedItems(__name__ + ".moves")
-_importer._add_module(moves, "moves")
-
-
-class Module_six_moves_urllib_parse(_LazyModule):
-
- """Lazy loading of moved objects in six.moves.urllib_parse"""
-
-
-_urllib_parse_moved_attributes = [
- MovedAttribute("ParseResult", "urlparse", "urllib.parse"),
- MovedAttribute("SplitResult", "urlparse", "urllib.parse"),
- MovedAttribute("parse_qs", "urlparse", "urllib.parse"),
- MovedAttribute("parse_qsl", "urlparse", "urllib.parse"),
- MovedAttribute("urldefrag", "urlparse", "urllib.parse"),
- MovedAttribute("urljoin", "urlparse", "urllib.parse"),
- MovedAttribute("urlparse", "urlparse", "urllib.parse"),
- MovedAttribute("urlsplit", "urlparse", "urllib.parse"),
- MovedAttribute("urlunparse", "urlparse", "urllib.parse"),
- MovedAttribute("urlunsplit", "urlparse", "urllib.parse"),
- MovedAttribute("quote", "urllib", "urllib.parse"),
- MovedAttribute("quote_plus", "urllib", "urllib.parse"),
- MovedAttribute("unquote", "urllib", "urllib.parse"),
- MovedAttribute("unquote_plus", "urllib", "urllib.parse"),
- MovedAttribute(
- "unquote_to_bytes", "urllib", "urllib.parse", "unquote", "unquote_to_bytes"
- ),
- MovedAttribute("urlencode", "urllib", "urllib.parse"),
- MovedAttribute("splitquery", "urllib", "urllib.parse"),
- MovedAttribute("splittag", "urllib", "urllib.parse"),
- MovedAttribute("splituser", "urllib", "urllib.parse"),
- MovedAttribute("splitvalue", "urllib", "urllib.parse"),
- MovedAttribute("uses_fragment", "urlparse", "urllib.parse"),
- MovedAttribute("uses_netloc", "urlparse", "urllib.parse"),
- MovedAttribute("uses_params", "urlparse", "urllib.parse"),
- MovedAttribute("uses_query", "urlparse", "urllib.parse"),
- MovedAttribute("uses_relative", "urlparse", "urllib.parse"),
-]
-for attr in _urllib_parse_moved_attributes:
- setattr(Module_six_moves_urllib_parse, attr.name, attr)
-del attr
-
-Module_six_moves_urllib_parse._moved_attributes = _urllib_parse_moved_attributes
-
-_importer._add_module(
- Module_six_moves_urllib_parse(__name__ + ".moves.urllib_parse"),
- "moves.urllib_parse",
- "moves.urllib.parse",
-)
-
-
-class Module_six_moves_urllib_error(_LazyModule):
-
- """Lazy loading of moved objects in six.moves.urllib_error"""
-
-
-_urllib_error_moved_attributes = [
- MovedAttribute("URLError", "urllib2", "urllib.error"),
- MovedAttribute("HTTPError", "urllib2", "urllib.error"),
- MovedAttribute("ContentTooShortError", "urllib", "urllib.error"),
-]
-for attr in _urllib_error_moved_attributes:
- setattr(Module_six_moves_urllib_error, attr.name, attr)
-del attr
-
-Module_six_moves_urllib_error._moved_attributes = _urllib_error_moved_attributes
-
-_importer._add_module(
- Module_six_moves_urllib_error(__name__ + ".moves.urllib.error"),
- "moves.urllib_error",
- "moves.urllib.error",
-)
-
-
-class Module_six_moves_urllib_request(_LazyModule):
-
- """Lazy loading of moved objects in six.moves.urllib_request"""
-
-
-_urllib_request_moved_attributes = [
- MovedAttribute("urlopen", "urllib2", "urllib.request"),
- MovedAttribute("install_opener", "urllib2", "urllib.request"),
- MovedAttribute("build_opener", "urllib2", "urllib.request"),
- MovedAttribute("pathname2url", "urllib", "urllib.request"),
- MovedAttribute("url2pathname", "urllib", "urllib.request"),
- MovedAttribute("getproxies", "urllib", "urllib.request"),
- MovedAttribute("Request", "urllib2", "urllib.request"),
- MovedAttribute("OpenerDirector", "urllib2", "urllib.request"),
- MovedAttribute("HTTPDefaultErrorHandler", "urllib2", "urllib.request"),
- MovedAttribute("HTTPRedirectHandler", "urllib2", "urllib.request"),
- MovedAttribute("HTTPCookieProcessor", "urllib2", "urllib.request"),
- MovedAttribute("ProxyHandler", "urllib2", "urllib.request"),
- MovedAttribute("BaseHandler", "urllib2", "urllib.request"),
- MovedAttribute("HTTPPasswordMgr", "urllib2", "urllib.request"),
- MovedAttribute("HTTPPasswordMgrWithDefaultRealm", "urllib2", "urllib.request"),
- MovedAttribute("AbstractBasicAuthHandler", "urllib2", "urllib.request"),
- MovedAttribute("HTTPBasicAuthHandler", "urllib2", "urllib.request"),
- MovedAttribute("ProxyBasicAuthHandler", "urllib2", "urllib.request"),
- MovedAttribute("AbstractDigestAuthHandler", "urllib2", "urllib.request"),
- MovedAttribute("HTTPDigestAuthHandler", "urllib2", "urllib.request"),
- MovedAttribute("ProxyDigestAuthHandler", "urllib2", "urllib.request"),
- MovedAttribute("HTTPHandler", "urllib2", "urllib.request"),
- MovedAttribute("HTTPSHandler", "urllib2", "urllib.request"),
- MovedAttribute("FileHandler", "urllib2", "urllib.request"),
- MovedAttribute("FTPHandler", "urllib2", "urllib.request"),
- MovedAttribute("CacheFTPHandler", "urllib2", "urllib.request"),
- MovedAttribute("UnknownHandler", "urllib2", "urllib.request"),
- MovedAttribute("HTTPErrorProcessor", "urllib2", "urllib.request"),
- MovedAttribute("urlretrieve", "urllib", "urllib.request"),
- MovedAttribute("urlcleanup", "urllib", "urllib.request"),
- MovedAttribute("URLopener", "urllib", "urllib.request"),
- MovedAttribute("FancyURLopener", "urllib", "urllib.request"),
- MovedAttribute("proxy_bypass", "urllib", "urllib.request"),
- MovedAttribute("parse_http_list", "urllib2", "urllib.request"),
- MovedAttribute("parse_keqv_list", "urllib2", "urllib.request"),
-]
-for attr in _urllib_request_moved_attributes:
- setattr(Module_six_moves_urllib_request, attr.name, attr)
-del attr
-
-Module_six_moves_urllib_request._moved_attributes = _urllib_request_moved_attributes
-
-_importer._add_module(
- Module_six_moves_urllib_request(__name__ + ".moves.urllib.request"),
- "moves.urllib_request",
- "moves.urllib.request",
-)
-
-
-class Module_six_moves_urllib_response(_LazyModule):
-
- """Lazy loading of moved objects in six.moves.urllib_response"""
-
-
-_urllib_response_moved_attributes = [
- MovedAttribute("addbase", "urllib", "urllib.response"),
- MovedAttribute("addclosehook", "urllib", "urllib.response"),
- MovedAttribute("addinfo", "urllib", "urllib.response"),
- MovedAttribute("addinfourl", "urllib", "urllib.response"),
-]
-for attr in _urllib_response_moved_attributes:
- setattr(Module_six_moves_urllib_response, attr.name, attr)
-del attr
-
-Module_six_moves_urllib_response._moved_attributes = _urllib_response_moved_attributes
-
-_importer._add_module(
- Module_six_moves_urllib_response(__name__ + ".moves.urllib.response"),
- "moves.urllib_response",
- "moves.urllib.response",
-)
-
-
-class Module_six_moves_urllib_robotparser(_LazyModule):
-
- """Lazy loading of moved objects in six.moves.urllib_robotparser"""
-
-
-_urllib_robotparser_moved_attributes = [
- MovedAttribute("RobotFileParser", "robotparser", "urllib.robotparser"),
-]
-for attr in _urllib_robotparser_moved_attributes:
- setattr(Module_six_moves_urllib_robotparser, attr.name, attr)
-del attr
-
-Module_six_moves_urllib_robotparser._moved_attributes = (
- _urllib_robotparser_moved_attributes
-)
-
-_importer._add_module(
- Module_six_moves_urllib_robotparser(__name__ + ".moves.urllib.robotparser"),
- "moves.urllib_robotparser",
- "moves.urllib.robotparser",
-)
-
-
-class Module_six_moves_urllib(types.ModuleType):
-
- """Create a six.moves.urllib namespace that resembles the Python 3 namespace"""
-
- __path__ = [] # mark as package
- parse = _importer._get_module("moves.urllib_parse")
- error = _importer._get_module("moves.urllib_error")
- request = _importer._get_module("moves.urllib_request")
- response = _importer._get_module("moves.urllib_response")
- robotparser = _importer._get_module("moves.urllib_robotparser")
-
- def __dir__(self):
- return ["parse", "error", "request", "response", "robotparser"]
-
-
-_importer._add_module(
- Module_six_moves_urllib(__name__ + ".moves.urllib"), "moves.urllib"
-)
-
-
-def add_move(move):
- """Add an item to six.moves."""
- setattr(_MovedItems, move.name, move)
-
-
-def remove_move(name):
- """Remove item from six.moves."""
- try:
- delattr(_MovedItems, name)
- except AttributeError:
- try:
- del moves.__dict__[name]
- except KeyError:
- raise AttributeError("no such move, %r" % (name,))
-
-
-if PY3:
- _meth_func = "__func__"
- _meth_self = "__self__"
-
- _func_closure = "__closure__"
- _func_code = "__code__"
- _func_defaults = "__defaults__"
- _func_globals = "__globals__"
-else:
- _meth_func = "im_func"
- _meth_self = "im_self"
-
- _func_closure = "func_closure"
- _func_code = "func_code"
- _func_defaults = "func_defaults"
- _func_globals = "func_globals"
-
-
-try:
- advance_iterator = next
-except NameError:
-
- def advance_iterator(it):
- return it.next()
-
-
-next = advance_iterator
-
-
-try:
- callable = callable
-except NameError:
-
- def callable(obj):
- return any("__call__" in klass.__dict__ for klass in type(obj).__mro__)
-
-
-if PY3:
-
- def get_unbound_function(unbound):
- return unbound
-
- create_bound_method = types.MethodType
-
- def create_unbound_method(func, cls):
- return func
-
- Iterator = object
-else:
-
- def get_unbound_function(unbound):
- return unbound.im_func
-
- def create_bound_method(func, obj):
- return types.MethodType(func, obj, obj.__class__)
-
- def create_unbound_method(func, cls):
- return types.MethodType(func, None, cls)
-
- class Iterator(object):
- def next(self):
- return type(self).__next__(self)
-
- callable = callable
-_add_doc(
- get_unbound_function, """Get the function out of a possibly unbound function"""
-)
-
-
-get_method_function = operator.attrgetter(_meth_func)
-get_method_self = operator.attrgetter(_meth_self)
-get_function_closure = operator.attrgetter(_func_closure)
-get_function_code = operator.attrgetter(_func_code)
-get_function_defaults = operator.attrgetter(_func_defaults)
-get_function_globals = operator.attrgetter(_func_globals)
-
-
-if PY3:
-
- def iterkeys(d, **kw):
- return iter(d.keys(**kw))
-
- def itervalues(d, **kw):
- return iter(d.values(**kw))
-
- def iteritems(d, **kw):
- return iter(d.items(**kw))
-
- def iterlists(d, **kw):
- return iter(d.lists(**kw))
-
- viewkeys = operator.methodcaller("keys")
-
- viewvalues = operator.methodcaller("values")
-
- viewitems = operator.methodcaller("items")
-else:
-
- def iterkeys(d, **kw):
- return d.iterkeys(**kw)
-
- def itervalues(d, **kw):
- return d.itervalues(**kw)
-
- def iteritems(d, **kw):
- return d.iteritems(**kw)
-
- def iterlists(d, **kw):
- return d.iterlists(**kw)
-
- viewkeys = operator.methodcaller("viewkeys")
-
- viewvalues = operator.methodcaller("viewvalues")
-
- viewitems = operator.methodcaller("viewitems")
-
-_add_doc(iterkeys, "Return an iterator over the keys of a dictionary.")
-_add_doc(itervalues, "Return an iterator over the values of a dictionary.")
-_add_doc(iteritems, "Return an iterator over the (key, value) pairs of a dictionary.")
-_add_doc(
- iterlists, "Return an iterator over the (key, [values]) pairs of a dictionary."
-)
-
-
-if PY3:
-
- def b(s):
- return s.encode("latin-1")
-
- def u(s):
- return s
-
- unichr = chr
- import struct
-
- int2byte = struct.Struct(">B").pack
- del struct
- byte2int = operator.itemgetter(0)
- indexbytes = operator.getitem
- iterbytes = iter
- import io
-
- StringIO = io.StringIO
- BytesIO = io.BytesIO
- del io
- _assertCountEqual = "assertCountEqual"
- if sys.version_info[1] <= 1:
- _assertRaisesRegex = "assertRaisesRegexp"
- _assertRegex = "assertRegexpMatches"
- _assertNotRegex = "assertNotRegexpMatches"
- else:
- _assertRaisesRegex = "assertRaisesRegex"
- _assertRegex = "assertRegex"
- _assertNotRegex = "assertNotRegex"
-else:
-
- def b(s):
- return s
-
- # Workaround for standalone backslash
-
- def u(s):
- return unicode(s.replace(r"\\", r"\\\\"), "unicode_escape")
-
- unichr = unichr
- int2byte = chr
-
- def byte2int(bs):
- return ord(bs[0])
-
- def indexbytes(buf, i):
- return ord(buf[i])
-
- iterbytes = functools.partial(itertools.imap, ord)
- import StringIO
-
- StringIO = BytesIO = StringIO.StringIO
- _assertCountEqual = "assertItemsEqual"
- _assertRaisesRegex = "assertRaisesRegexp"
- _assertRegex = "assertRegexpMatches"
- _assertNotRegex = "assertNotRegexpMatches"
-_add_doc(b, """Byte literal""")
-_add_doc(u, """Text literal""")
-
-
-def assertCountEqual(self, *args, **kwargs):
- return getattr(self, _assertCountEqual)(*args, **kwargs)
-
-
-def assertRaisesRegex(self, *args, **kwargs):
- return getattr(self, _assertRaisesRegex)(*args, **kwargs)
-
-
-def assertRegex(self, *args, **kwargs):
- return getattr(self, _assertRegex)(*args, **kwargs)
-
-
-def assertNotRegex(self, *args, **kwargs):
- return getattr(self, _assertNotRegex)(*args, **kwargs)
-
-
-if PY3:
- exec_ = getattr(moves.builtins, "exec")
-
- def reraise(tp, value, tb=None):
- try:
- if value is None:
- value = tp()
- if value.__traceback__ is not tb:
- raise value.with_traceback(tb)
- raise value
- finally:
- value = None
- tb = None
-
-else:
-
- def exec_(_code_, _globs_=None, _locs_=None):
- """Execute code in a namespace."""
- if _globs_ is None:
- frame = sys._getframe(1)
- _globs_ = frame.f_globals
- if _locs_ is None:
- _locs_ = frame.f_locals
- del frame
- elif _locs_ is None:
- _locs_ = _globs_
- exec ("""exec _code_ in _globs_, _locs_""")
-
- exec_(
- """def reraise(tp, value, tb=None):
- try:
- raise tp, value, tb
- finally:
- tb = None
-"""
- )
-
-
-if sys.version_info[:2] > (3,):
- exec_(
- """def raise_from(value, from_value):
- try:
- raise value from from_value
- finally:
- value = None
-"""
- )
-else:
-
- def raise_from(value, from_value):
- raise value
-
-
-print_ = getattr(moves.builtins, "print", None)
-if print_ is None:
-
- def print_(*args, **kwargs):
- """The new-style print function for Python 2.4 and 2.5."""
- fp = kwargs.pop("file", sys.stdout)
- if fp is None:
- return
-
- def write(data):
- if not isinstance(data, basestring):
- data = str(data)
- # If the file has an encoding, encode unicode with it.
- if (
- isinstance(fp, file)
- and isinstance(data, unicode)
- and fp.encoding is not None
- ):
- errors = getattr(fp, "errors", None)
- if errors is None:
- errors = "strict"
- data = data.encode(fp.encoding, errors)
- fp.write(data)
-
- want_unicode = False
- sep = kwargs.pop("sep", None)
- if sep is not None:
- if isinstance(sep, unicode):
- want_unicode = True
- elif not isinstance(sep, str):
- raise TypeError("sep must be None or a string")
- end = kwargs.pop("end", None)
- if end is not None:
- if isinstance(end, unicode):
- want_unicode = True
- elif not isinstance(end, str):
- raise TypeError("end must be None or a string")
- if kwargs:
- raise TypeError("invalid keyword arguments to print()")
- if not want_unicode:
- for arg in args:
- if isinstance(arg, unicode):
- want_unicode = True
- break
- if want_unicode:
- newline = unicode("\n")
- space = unicode(" ")
- else:
- newline = "\n"
- space = " "
- if sep is None:
- sep = space
- if end is None:
- end = newline
- for i, arg in enumerate(args):
- if i:
- write(sep)
- write(arg)
- write(end)
-
-
-if sys.version_info[:2] < (3, 3):
- _print = print_
-
- def print_(*args, **kwargs):
- fp = kwargs.get("file", sys.stdout)
- flush = kwargs.pop("flush", False)
- _print(*args, **kwargs)
- if flush and fp is not None:
- fp.flush()
-
-
-_add_doc(reraise, """Reraise an exception.""")
-
-if sys.version_info[0:2] < (3, 4):
- # This does exactly the same what the :func:`py3:functools.update_wrapper`
- # function does on Python versions after 3.2. It sets the ``__wrapped__``
- # attribute on ``wrapper`` object and it doesn't raise an error if any of
- # the attributes mentioned in ``assigned`` and ``updated`` are missing on
- # ``wrapped`` object.
- def _update_wrapper(
- wrapper,
- wrapped,
- assigned=functools.WRAPPER_ASSIGNMENTS,
- updated=functools.WRAPPER_UPDATES,
- ):
- for attr in assigned:
- try:
- value = getattr(wrapped, attr)
- except AttributeError:
- continue
- else:
- setattr(wrapper, attr, value)
- for attr in updated:
- getattr(wrapper, attr).update(getattr(wrapped, attr, {}))
- wrapper.__wrapped__ = wrapped
- return wrapper
-
- _update_wrapper.__doc__ = functools.update_wrapper.__doc__
-
- def wraps(
- wrapped,
- assigned=functools.WRAPPER_ASSIGNMENTS,
- updated=functools.WRAPPER_UPDATES,
- ):
- return functools.partial(
- _update_wrapper, wrapped=wrapped, assigned=assigned, updated=updated
- )
-
- wraps.__doc__ = functools.wraps.__doc__
-
-else:
- wraps = functools.wraps
-
-
-def with_metaclass(meta, *bases):
- """Create a base class with a metaclass."""
- # This requires a bit of explanation: the basic idea is to make a dummy
- # metaclass for one level of class instantiation that replaces itself with
- # the actual metaclass.
- class metaclass(type):
- def __new__(cls, name, this_bases, d):
- if sys.version_info[:2] >= (3, 7):
- # This version introduced PEP 560 that requires a bit
- # of extra care (we mimic what is done by __build_class__).
- resolved_bases = types.resolve_bases(bases)
- if resolved_bases is not bases:
- d["__orig_bases__"] = bases
- else:
- resolved_bases = bases
- return meta(name, resolved_bases, d)
-
- @classmethod
- def __prepare__(cls, name, this_bases):
- return meta.__prepare__(name, bases)
-
- return type.__new__(metaclass, "temporary_class", (), {})
-
-
-def add_metaclass(metaclass):
- """Class decorator for creating a class with a metaclass."""
-
- def wrapper(cls):
- orig_vars = cls.__dict__.copy()
- slots = orig_vars.get("__slots__")
- if slots is not None:
- if isinstance(slots, str):
- slots = [slots]
- for slots_var in slots:
- orig_vars.pop(slots_var)
- orig_vars.pop("__dict__", None)
- orig_vars.pop("__weakref__", None)
- if hasattr(cls, "__qualname__"):
- orig_vars["__qualname__"] = cls.__qualname__
- return metaclass(cls.__name__, cls.__bases__, orig_vars)
-
- return wrapper
-
-
-def ensure_binary(s, encoding="utf-8", errors="strict"):
- """Coerce **s** to six.binary_type.
-
- For Python 2:
- - `unicode` -> encoded to `str`
- - `str` -> `str`
-
- For Python 3:
- - `str` -> encoded to `bytes`
- - `bytes` -> `bytes`
- """
- if isinstance(s, binary_type):
- return s
- if isinstance(s, text_type):
- return s.encode(encoding, errors)
- raise TypeError("not expecting type '%s'" % type(s))
-
-
-def ensure_str(s, encoding="utf-8", errors="strict"):
- """Coerce *s* to `str`.
-
- For Python 2:
- - `unicode` -> encoded to `str`
- - `str` -> `str`
-
- For Python 3:
- - `str` -> `str`
- - `bytes` -> decoded to `str`
- """
- # Optimization: Fast return for the common case.
- if type(s) is str:
- return s
- if PY2 and isinstance(s, text_type):
- return s.encode(encoding, errors)
- elif PY3 and isinstance(s, binary_type):
- return s.decode(encoding, errors)
- elif not isinstance(s, (text_type, binary_type)):
- raise TypeError("not expecting type '%s'" % type(s))
- return s
-
-
-def ensure_text(s, encoding="utf-8", errors="strict"):
- """Coerce *s* to six.text_type.
-
- For Python 2:
- - `unicode` -> `unicode`
- - `str` -> `unicode`
-
- For Python 3:
- - `str` -> `str`
- - `bytes` -> decoded to `str`
- """
- if isinstance(s, binary_type):
- return s.decode(encoding, errors)
- elif isinstance(s, text_type):
- return s
- else:
- raise TypeError("not expecting type '%s'" % type(s))
-
-
-def python_2_unicode_compatible(klass):
- """
- A class decorator that defines __unicode__ and __str__ methods under Python 2.
- Under Python 3 it does nothing.
-
- To support Python 2 and 3 with a single code base, define a __str__ method
- returning text and apply this decorator to the class.
- """
- if PY2:
- if "__str__" not in klass.__dict__:
- raise ValueError(
- "@python_2_unicode_compatible cannot be applied "
- "to %s because it doesn't define __str__()." % klass.__name__
- )
- klass.__unicode__ = klass.__str__
- klass.__str__ = lambda self: self.__unicode__().encode("utf-8")
- return klass
-
-
-# Complete the moves implementation.
-# This code is at the end of this module to speed up module loading.
-# Turn this module into a package.
-__path__ = [] # required for PEP 302 and PEP 451
-__package__ = __name__ # see PEP 366 @ReservedAssignment
-if globals().get("__spec__") is not None:
- __spec__.submodule_search_locations = [] # PEP 451 @UndefinedVariable
-# Remove other six meta path importers, since they cause problems. This can
-# happen if six is removed from sys.modules and then reloaded. (Setuptools does
-# this for some reason.)
-if sys.meta_path:
- for i, importer in enumerate(sys.meta_path):
- # Here's some real nastiness: Another "instance" of the six module might
- # be floating around. Therefore, we can't use isinstance() to check for
- # the six meta path importer, since the other six instance will have
- # inserted an importer with different class.
- if (
- type(importer).__name__ == "_SixMetaPathImporter"
- and importer.name == __name__
- ):
- del sys.meta_path[i]
- break
- del i, importer
-# Finally, add the importer to the meta path import hook.
-sys.meta_path.append(_importer)
diff --git a/spaces/CC123123/blip2_t/utils.py b/spaces/CC123123/blip2_t/utils.py
deleted file mode 100644
index a5a67d654a67ee37847d428c94524c7cabee3e1d..0000000000000000000000000000000000000000
--- a/spaces/CC123123/blip2_t/utils.py
+++ /dev/null
@@ -1,27 +0,0 @@
-import os
-
-
-class Endpoint:
- def __init__(self):
- self._url = None
-
- @property
- def url(self):
- if self._url is None:
- self._url = self.get_url()
-
- return self._url
-
- def get_url(self):
- endpoint = os.environ.get("endpoint")
-
- return endpoint
-
-
-def get_token():
- token = os.environ.get("auth_token")
-
- if token is None:
- raise ValueError("auth-token not found in environment variables")
-
- return token
diff --git a/spaces/CVPR/Dual-Key_Backdoor_Attacks/datagen/detectron2/detectron2/utils/serialize.py b/spaces/CVPR/Dual-Key_Backdoor_Attacks/datagen/detectron2/detectron2/utils/serialize.py
deleted file mode 100644
index 734a62c2c4ecfd520eb9e8b941857b6f7e17d4c8..0000000000000000000000000000000000000000
--- a/spaces/CVPR/Dual-Key_Backdoor_Attacks/datagen/detectron2/detectron2/utils/serialize.py
+++ /dev/null
@@ -1,29 +0,0 @@
-# Copyright (c) Facebook, Inc. and its affiliates. All Rights Reserved
-import cloudpickle
-
-
-class PicklableWrapper(object):
- """
- Wrap an object to make it more picklable, note that it uses
- heavy weight serialization libraries that are slower than pickle.
- It's best to use it only on closures (which are usually not picklable).
-
- This is a simplified version of
- https://github.com/joblib/joblib/blob/master/joblib/externals/loky/cloudpickle_wrapper.py
- """
-
- def __init__(self, obj):
- self._obj = obj
-
- def __reduce__(self):
- s = cloudpickle.dumps(self._obj)
- return cloudpickle.loads, (s,)
-
- def __call__(self, *args, **kwargs):
- return self._obj(*args, **kwargs)
-
- def __getattr__(self, attr):
- # Ensure that the wrapped object can be used seamlessly as the previous object.
- if attr not in ["_obj"]:
- return getattr(self._obj, attr)
- return getattr(self, attr)
diff --git a/spaces/CVPR/LIVE/pybind11/include/pybind11/detail/internals.h b/spaces/CVPR/LIVE/pybind11/include/pybind11/detail/internals.h
deleted file mode 100644
index cf40e9fe995cd952e0dec8378b44b3ac8477f235..0000000000000000000000000000000000000000
--- a/spaces/CVPR/LIVE/pybind11/include/pybind11/detail/internals.h
+++ /dev/null
@@ -1,352 +0,0 @@
-/*
- pybind11/detail/internals.h: Internal data structure and related functions
-
- Copyright (c) 2017 Wenzel Jakob
-
- All rights reserved. Use of this source code is governed by a
- BSD-style license that can be found in the LICENSE file.
-*/
-
-#pragma once
-
-#include "../pytypes.h"
-
-PYBIND11_NAMESPACE_BEGIN(PYBIND11_NAMESPACE)
-PYBIND11_NAMESPACE_BEGIN(detail)
-// Forward declarations
-inline PyTypeObject *make_static_property_type();
-inline PyTypeObject *make_default_metaclass();
-inline PyObject *make_object_base_type(PyTypeObject *metaclass);
-
-// The old Python Thread Local Storage (TLS) API is deprecated in Python 3.7 in favor of the new
-// Thread Specific Storage (TSS) API.
-#if PY_VERSION_HEX >= 0x03070000
-# define PYBIND11_TLS_KEY_INIT(var) Py_tss_t *var = nullptr
-# define PYBIND11_TLS_GET_VALUE(key) PyThread_tss_get((key))
-# define PYBIND11_TLS_REPLACE_VALUE(key, value) PyThread_tss_set((key), (value))
-# define PYBIND11_TLS_DELETE_VALUE(key) PyThread_tss_set((key), nullptr)
-# define PYBIND11_TLS_FREE(key) PyThread_tss_free(key)
-#else
- // Usually an int but a long on Cygwin64 with Python 3.x
-# define PYBIND11_TLS_KEY_INIT(var) decltype(PyThread_create_key()) var = 0
-# define PYBIND11_TLS_GET_VALUE(key) PyThread_get_key_value((key))
-# if PY_MAJOR_VERSION < 3
-# define PYBIND11_TLS_DELETE_VALUE(key) \
- PyThread_delete_key_value(key)
-# define PYBIND11_TLS_REPLACE_VALUE(key, value) \
- do { \
- PyThread_delete_key_value((key)); \
- PyThread_set_key_value((key), (value)); \
- } while (false)
-# else
-# define PYBIND11_TLS_DELETE_VALUE(key) \
- PyThread_set_key_value((key), nullptr)
-# define PYBIND11_TLS_REPLACE_VALUE(key, value) \
- PyThread_set_key_value((key), (value))
-# endif
-# define PYBIND11_TLS_FREE(key) (void)key
-#endif
-
-// Python loads modules by default with dlopen with the RTLD_LOCAL flag; under libc++ and possibly
-// other STLs, this means `typeid(A)` from one module won't equal `typeid(A)` from another module
-// even when `A` is the same, non-hidden-visibility type (e.g. from a common include). Under
-// libstdc++, this doesn't happen: equality and the type_index hash are based on the type name,
-// which works. If not under a known-good stl, provide our own name-based hash and equality
-// functions that use the type name.
-#if defined(__GLIBCXX__)
-inline bool same_type(const std::type_info &lhs, const std::type_info &rhs) { return lhs == rhs; }
-using type_hash = std::hash;
-using type_equal_to = std::equal_to;
-#else
-inline bool same_type(const std::type_info &lhs, const std::type_info &rhs) {
- return lhs.name() == rhs.name() || std::strcmp(lhs.name(), rhs.name()) == 0;
-}
-
-struct type_hash {
- size_t operator()(const std::type_index &t) const {
- size_t hash = 5381;
- const char *ptr = t.name();
- while (auto c = static_cast(*ptr++))
- hash = (hash * 33) ^ c;
- return hash;
- }
-};
-
-struct type_equal_to {
- bool operator()(const std::type_index &lhs, const std::type_index &rhs) const {
- return lhs.name() == rhs.name() || std::strcmp(lhs.name(), rhs.name()) == 0;
- }
-};
-#endif
-
-template
-using type_map = std::unordered_map;
-
-struct overload_hash {
- inline size_t operator()(const std::pair& v) const {
- size_t value = std::hash()(v.first);
- value ^= std::hash()(v.second) + 0x9e3779b9 + (value<<6) + (value>>2);
- return value;
- }
-};
-
-/// Internal data structure used to track registered instances and types.
-/// Whenever binary incompatible changes are made to this structure,
-/// `PYBIND11_INTERNALS_VERSION` must be incremented.
-struct internals {
- type_map registered_types_cpp; // std::type_index -> pybind11's type information
- std::unordered_map> registered_types_py; // PyTypeObject* -> base type_info(s)
- std::unordered_multimap registered_instances; // void * -> instance*
- std::unordered_set, overload_hash> inactive_overload_cache;
- type_map> direct_conversions;
- std::unordered_map> patients;
- std::forward_list registered_exception_translators;
- std::unordered_map shared_data; // Custom data to be shared across extensions
- std::vector loader_patient_stack; // Used by `loader_life_support`
- std::forward_list static_strings; // Stores the std::strings backing detail::c_str()
- PyTypeObject *static_property_type;
- PyTypeObject *default_metaclass;
- PyObject *instance_base;
-#if defined(WITH_THREAD)
- PYBIND11_TLS_KEY_INIT(tstate);
- PyInterpreterState *istate = nullptr;
- ~internals() {
- // This destructor is called *after* Py_Finalize() in finalize_interpreter().
- // That *SHOULD BE* fine. The following details what happens whe PyThread_tss_free is called.
- // PYBIND11_TLS_FREE is PyThread_tss_free on python 3.7+. On older python, it does nothing.
- // PyThread_tss_free calls PyThread_tss_delete and PyMem_RawFree.
- // PyThread_tss_delete just calls TlsFree (on Windows) or pthread_key_delete (on *NIX). Neither
- // of those have anything to do with CPython internals.
- // PyMem_RawFree *requires* that the `tstate` be allocated with the CPython allocator.
- PYBIND11_TLS_FREE(tstate);
- }
-#endif
-};
-
-/// Additional type information which does not fit into the PyTypeObject.
-/// Changes to this struct also require bumping `PYBIND11_INTERNALS_VERSION`.
-struct type_info {
- PyTypeObject *type;
- const std::type_info *cpptype;
- size_t type_size, type_align, holder_size_in_ptrs;
- void *(*operator_new)(size_t);
- void (*init_instance)(instance *, const void *);
- void (*dealloc)(value_and_holder &v_h);
- std::vector implicit_conversions;
- std::vector> implicit_casts;
- std::vector *direct_conversions;
- buffer_info *(*get_buffer)(PyObject *, void *) = nullptr;
- void *get_buffer_data = nullptr;
- void *(*module_local_load)(PyObject *, const type_info *) = nullptr;
- /* A simple type never occurs as a (direct or indirect) parent
- * of a class that makes use of multiple inheritance */
- bool simple_type : 1;
- /* True if there is no multiple inheritance in this type's inheritance tree */
- bool simple_ancestors : 1;
- /* for base vs derived holder_type checks */
- bool default_holder : 1;
- /* true if this is a type registered with py::module_local */
- bool module_local : 1;
-};
-
-/// Tracks the `internals` and `type_info` ABI version independent of the main library version
-#define PYBIND11_INTERNALS_VERSION 4
-
-/// On MSVC, debug and release builds are not ABI-compatible!
-#if defined(_MSC_VER) && defined(_DEBUG)
-# define PYBIND11_BUILD_TYPE "_debug"
-#else
-# define PYBIND11_BUILD_TYPE ""
-#endif
-
-/// Let's assume that different compilers are ABI-incompatible.
-#if defined(_MSC_VER)
-# define PYBIND11_COMPILER_TYPE "_msvc"
-#elif defined(__INTEL_COMPILER)
-# define PYBIND11_COMPILER_TYPE "_icc"
-#elif defined(__clang__)
-# define PYBIND11_COMPILER_TYPE "_clang"
-#elif defined(__PGI)
-# define PYBIND11_COMPILER_TYPE "_pgi"
-#elif defined(__MINGW32__)
-# define PYBIND11_COMPILER_TYPE "_mingw"
-#elif defined(__CYGWIN__)
-# define PYBIND11_COMPILER_TYPE "_gcc_cygwin"
-#elif defined(__GNUC__)
-# define PYBIND11_COMPILER_TYPE "_gcc"
-#else
-# define PYBIND11_COMPILER_TYPE "_unknown"
-#endif
-
-#if defined(_LIBCPP_VERSION)
-# define PYBIND11_STDLIB "_libcpp"
-#elif defined(__GLIBCXX__) || defined(__GLIBCPP__)
-# define PYBIND11_STDLIB "_libstdcpp"
-#else
-# define PYBIND11_STDLIB ""
-#endif
-
-/// On Linux/OSX, changes in __GXX_ABI_VERSION__ indicate ABI incompatibility.
-#if defined(__GXX_ABI_VERSION)
-# define PYBIND11_BUILD_ABI "_cxxabi" PYBIND11_TOSTRING(__GXX_ABI_VERSION)
-#else
-# define PYBIND11_BUILD_ABI ""
-#endif
-
-#if defined(WITH_THREAD)
-# define PYBIND11_INTERNALS_KIND ""
-#else
-# define PYBIND11_INTERNALS_KIND "_without_thread"
-#endif
-
-#define PYBIND11_INTERNALS_ID "__pybind11_internals_v" \
- PYBIND11_TOSTRING(PYBIND11_INTERNALS_VERSION) PYBIND11_INTERNALS_KIND PYBIND11_COMPILER_TYPE PYBIND11_STDLIB PYBIND11_BUILD_ABI PYBIND11_BUILD_TYPE "__"
-
-#define PYBIND11_MODULE_LOCAL_ID "__pybind11_module_local_v" \
- PYBIND11_TOSTRING(PYBIND11_INTERNALS_VERSION) PYBIND11_INTERNALS_KIND PYBIND11_COMPILER_TYPE PYBIND11_STDLIB PYBIND11_BUILD_ABI PYBIND11_BUILD_TYPE "__"
-
-/// Each module locally stores a pointer to the `internals` data. The data
-/// itself is shared among modules with the same `PYBIND11_INTERNALS_ID`.
-inline internals **&get_internals_pp() {
- static internals **internals_pp = nullptr;
- return internals_pp;
-}
-
-inline void translate_exception(std::exception_ptr p) {
- try {
- if (p) std::rethrow_exception(p);
- } catch (error_already_set &e) { e.restore(); return;
- } catch (const builtin_exception &e) { e.set_error(); return;
- } catch (const std::bad_alloc &e) { PyErr_SetString(PyExc_MemoryError, e.what()); return;
- } catch (const std::domain_error &e) { PyErr_SetString(PyExc_ValueError, e.what()); return;
- } catch (const std::invalid_argument &e) { PyErr_SetString(PyExc_ValueError, e.what()); return;
- } catch (const std::length_error &e) { PyErr_SetString(PyExc_ValueError, e.what()); return;
- } catch (const std::out_of_range &e) { PyErr_SetString(PyExc_IndexError, e.what()); return;
- } catch (const std::range_error &e) { PyErr_SetString(PyExc_ValueError, e.what()); return;
- } catch (const std::overflow_error &e) { PyErr_SetString(PyExc_OverflowError, e.what()); return;
- } catch (const std::exception &e) { PyErr_SetString(PyExc_RuntimeError, e.what()); return;
- } catch (...) {
- PyErr_SetString(PyExc_RuntimeError, "Caught an unknown exception!");
- return;
- }
-}
-
-#if !defined(__GLIBCXX__)
-inline void translate_local_exception(std::exception_ptr p) {
- try {
- if (p) std::rethrow_exception(p);
- } catch (error_already_set &e) { e.restore(); return;
- } catch (const builtin_exception &e) { e.set_error(); return;
- }
-}
-#endif
-
-/// Return a reference to the current `internals` data
-PYBIND11_NOINLINE inline internals &get_internals() {
- auto **&internals_pp = get_internals_pp();
- if (internals_pp && *internals_pp)
- return **internals_pp;
-
- // Ensure that the GIL is held since we will need to make Python calls.
- // Cannot use py::gil_scoped_acquire here since that constructor calls get_internals.
- struct gil_scoped_acquire_local {
- gil_scoped_acquire_local() : state (PyGILState_Ensure()) {}
- ~gil_scoped_acquire_local() { PyGILState_Release(state); }
- const PyGILState_STATE state;
- } gil;
-
- constexpr auto *id = PYBIND11_INTERNALS_ID;
- auto builtins = handle(PyEval_GetBuiltins());
- if (builtins.contains(id) && isinstance(builtins[id])) {
- internals_pp = static_cast(capsule(builtins[id]));
-
- // We loaded builtins through python's builtins, which means that our `error_already_set`
- // and `builtin_exception` may be different local classes than the ones set up in the
- // initial exception translator, below, so add another for our local exception classes.
- //
- // libstdc++ doesn't require this (types there are identified only by name)
-#if !defined(__GLIBCXX__)
- (*internals_pp)->registered_exception_translators.push_front(&translate_local_exception);
-#endif
- } else {
- if (!internals_pp) internals_pp = new internals*();
- auto *&internals_ptr = *internals_pp;
- internals_ptr = new internals();
-#if defined(WITH_THREAD)
-
- #if PY_VERSION_HEX < 0x03090000
- PyEval_InitThreads();
- #endif
- PyThreadState *tstate = PyThreadState_Get();
- #if PY_VERSION_HEX >= 0x03070000
- internals_ptr->tstate = PyThread_tss_alloc();
- if (!internals_ptr->tstate || PyThread_tss_create(internals_ptr->tstate))
- pybind11_fail("get_internals: could not successfully initialize the TSS key!");
- PyThread_tss_set(internals_ptr->tstate, tstate);
- #else
- internals_ptr->tstate = PyThread_create_key();
- if (internals_ptr->tstate == -1)
- pybind11_fail("get_internals: could not successfully initialize the TLS key!");
- PyThread_set_key_value(internals_ptr->tstate, tstate);
- #endif
- internals_ptr->istate = tstate->interp;
-#endif
- builtins[id] = capsule(internals_pp);
- internals_ptr->registered_exception_translators.push_front(&translate_exception);
- internals_ptr->static_property_type = make_static_property_type();
- internals_ptr->default_metaclass = make_default_metaclass();
- internals_ptr->instance_base = make_object_base_type(internals_ptr->default_metaclass);
- }
- return **internals_pp;
-}
-
-/// Works like `internals.registered_types_cpp`, but for module-local registered types:
-inline type_map ®istered_local_types_cpp() {
- static type_map locals{};
- return locals;
-}
-
-/// Constructs a std::string with the given arguments, stores it in `internals`, and returns its
-/// `c_str()`. Such strings objects have a long storage duration -- the internal strings are only
-/// cleared when the program exits or after interpreter shutdown (when embedding), and so are
-/// suitable for c-style strings needed by Python internals (such as PyTypeObject's tp_name).
-template
-const char *c_str(Args &&...args) {
- auto &strings = get_internals().static_strings;
- strings.emplace_front(std::forward(args)...);
- return strings.front().c_str();
-}
-
-PYBIND11_NAMESPACE_END(detail)
-
-/// Returns a named pointer that is shared among all extension modules (using the same
-/// pybind11 version) running in the current interpreter. Names starting with underscores
-/// are reserved for internal usage. Returns `nullptr` if no matching entry was found.
-inline PYBIND11_NOINLINE void *get_shared_data(const std::string &name) {
- auto &internals = detail::get_internals();
- auto it = internals.shared_data.find(name);
- return it != internals.shared_data.end() ? it->second : nullptr;
-}
-
-/// Set the shared data that can be later recovered by `get_shared_data()`.
-inline PYBIND11_NOINLINE void *set_shared_data(const std::string &name, void *data) {
- detail::get_internals().shared_data[name] = data;
- return data;
-}
-
-/// Returns a typed reference to a shared data entry (by using `get_shared_data()`) if
-/// such entry exists. Otherwise, a new object of default-constructible type `T` is
-/// added to the shared data under the given name and a reference to it is returned.
-template
-T &get_or_create_shared_data(const std::string &name) {
- auto &internals = detail::get_internals();
- auto it = internals.shared_data.find(name);
- T *ptr = (T *) (it != internals.shared_data.end() ? it->second : nullptr);
- if (!ptr) {
- ptr = new T();
- internals.shared_data[name] = ptr;
- }
- return *ptr;
-}
-
-PYBIND11_NAMESPACE_END(PYBIND11_NAMESPACE)
diff --git a/spaces/CVPR/LIVE/thrust/thrust/system/detail/adl/async/transform.h b/spaces/CVPR/LIVE/thrust/thrust/system/detail/adl/async/transform.h
deleted file mode 100644
index abb2163ead0654a805deb3b31ca29f8c576ac9e9..0000000000000000000000000000000000000000
--- a/spaces/CVPR/LIVE/thrust/thrust/system/detail/adl/async/transform.h
+++ /dev/null
@@ -1,34 +0,0 @@
-/*
- * Copyright 2008-2018 NVIDIA Corporation
- *
- * Licensed under the Apache License, Version 2.0 (the "License");
- * you may not use this file except in compliance with the License.
- * You may obtain a transform of the License at
- *
- * http://www.apache.org/licenses/LICENSE-2.0
- *
- * Unless required by applicable law or agreed to in writing, software
- * distributed under the License is distributed on an "AS IS" BASIS,
- * WITHOUT WARRANTIES OR CONDITIONS OF ANY KIND, either express or implied.
- * See the License for the specific language governing permissions and
- * limitations under the License.
- */
-
-// The purpose of this header is to #include the async/transform.h header of the
-// sequential, host, and device systems. It should be #included in any code
-// which uses ADL to dispatch async transform.
-
-#pragma once
-
-#include
-
-//#include
-
-//#define __THRUST_HOST_SYSTEM_ASYNC_TRANSFORM_HEADER <__THRUST_HOST_SYSTEM_ROOT/detail/async/transform.h>
-//#include __THRUST_HOST_SYSTEM_ASYNC_TRANSFORM_HEADER
-//#undef __THRUST_HOST_SYSTEM_ASYNC_TRANSFORM_HEADER
-
-#define __THRUST_DEVICE_SYSTEM_ASYNC_TRANSFORM_HEADER <__THRUST_DEVICE_SYSTEM_ROOT/detail/async/transform.h>
-#include __THRUST_DEVICE_SYSTEM_ASYNC_TRANSFORM_HEADER
-#undef __THRUST_DEVICE_SYSTEM_ASYNC_TRANSFORM_HEADER
-
diff --git a/spaces/CVPR/lama-example/saicinpainting/utils.py b/spaces/CVPR/lama-example/saicinpainting/utils.py
deleted file mode 100644
index d0914320eab96e197ae379b94ea7eeb2fe5dfd79..0000000000000000000000000000000000000000
--- a/spaces/CVPR/lama-example/saicinpainting/utils.py
+++ /dev/null
@@ -1,174 +0,0 @@
-import bisect
-import functools
-import logging
-import numbers
-import os
-import signal
-import sys
-import traceback
-import warnings
-
-import torch
-from pytorch_lightning import seed_everything
-
-LOGGER = logging.getLogger(__name__)
-
-
-def check_and_warn_input_range(tensor, min_value, max_value, name):
- actual_min = tensor.min()
- actual_max = tensor.max()
- if actual_min < min_value or actual_max > max_value:
- warnings.warn(f"{name} must be in {min_value}..{max_value} range, but it ranges {actual_min}..{actual_max}")
-
-
-def sum_dict_with_prefix(target, cur_dict, prefix, default=0):
- for k, v in cur_dict.items():
- target_key = prefix + k
- target[target_key] = target.get(target_key, default) + v
-
-
-def average_dicts(dict_list):
- result = {}
- norm = 1e-3
- for dct in dict_list:
- sum_dict_with_prefix(result, dct, '')
- norm += 1
- for k in list(result):
- result[k] /= norm
- return result
-
-
-def add_prefix_to_keys(dct, prefix):
- return {prefix + k: v for k, v in dct.items()}
-
-
-def set_requires_grad(module, value):
- for param in module.parameters():
- param.requires_grad = value
-
-
-def flatten_dict(dct):
- result = {}
- for k, v in dct.items():
- if isinstance(k, tuple):
- k = '_'.join(k)
- if isinstance(v, dict):
- for sub_k, sub_v in flatten_dict(v).items():
- result[f'{k}_{sub_k}'] = sub_v
- else:
- result[k] = v
- return result
-
-
-class LinearRamp:
- def __init__(self, start_value=0, end_value=1, start_iter=-1, end_iter=0):
- self.start_value = start_value
- self.end_value = end_value
- self.start_iter = start_iter
- self.end_iter = end_iter
-
- def __call__(self, i):
- if i < self.start_iter:
- return self.start_value
- if i >= self.end_iter:
- return self.end_value
- part = (i - self.start_iter) / (self.end_iter - self.start_iter)
- return self.start_value * (1 - part) + self.end_value * part
-
-
-class LadderRamp:
- def __init__(self, start_iters, values):
- self.start_iters = start_iters
- self.values = values
- assert len(values) == len(start_iters) + 1, (len(values), len(start_iters))
-
- def __call__(self, i):
- segment_i = bisect.bisect_right(self.start_iters, i)
- return self.values[segment_i]
-
-
-def get_ramp(kind='ladder', **kwargs):
- if kind == 'linear':
- return LinearRamp(**kwargs)
- if kind == 'ladder':
- return LadderRamp(**kwargs)
- raise ValueError(f'Unexpected ramp kind: {kind}')
-
-
-def print_traceback_handler(sig, frame):
- LOGGER.warning(f'Received signal {sig}')
- bt = ''.join(traceback.format_stack())
- LOGGER.warning(f'Requested stack trace:\n{bt}')
-
-
-def register_debug_signal_handlers(sig=signal.SIGUSR1, handler=print_traceback_handler):
- LOGGER.warning(f'Setting signal {sig} handler {handler}')
- signal.signal(sig, handler)
-
-
-def handle_deterministic_config(config):
- seed = dict(config).get('seed', None)
- if seed is None:
- return False
-
- seed_everything(seed)
- return True
-
-
-def get_shape(t):
- if torch.is_tensor(t):
- return tuple(t.shape)
- elif isinstance(t, dict):
- return {n: get_shape(q) for n, q in t.items()}
- elif isinstance(t, (list, tuple)):
- return [get_shape(q) for q in t]
- elif isinstance(t, numbers.Number):
- return type(t)
- else:
- raise ValueError('unexpected type {}'.format(type(t)))
-
-
-def get_has_ddp_rank():
- master_port = os.environ.get('MASTER_PORT', None)
- node_rank = os.environ.get('NODE_RANK', None)
- local_rank = os.environ.get('LOCAL_RANK', None)
- world_size = os.environ.get('WORLD_SIZE', None)
- has_rank = master_port is not None or node_rank is not None or local_rank is not None or world_size is not None
- return has_rank
-
-
-def handle_ddp_subprocess():
- def main_decorator(main_func):
- @functools.wraps(main_func)
- def new_main(*args, **kwargs):
- # Trainer sets MASTER_PORT, NODE_RANK, LOCAL_RANK, WORLD_SIZE
- parent_cwd = os.environ.get('TRAINING_PARENT_WORK_DIR', None)
- has_parent = parent_cwd is not None
- has_rank = get_has_ddp_rank()
- assert has_parent == has_rank, f'Inconsistent state: has_parent={has_parent}, has_rank={has_rank}'
-
- if has_parent:
- # we are in the worker
- sys.argv.extend([
- f'hydra.run.dir={parent_cwd}',
- # 'hydra/hydra_logging=disabled',
- # 'hydra/job_logging=disabled'
- ])
- # do nothing if this is a top-level process
- # TRAINING_PARENT_WORK_DIR is set in handle_ddp_parent_process after hydra initialization
-
- main_func(*args, **kwargs)
- return new_main
- return main_decorator
-
-
-def handle_ddp_parent_process():
- parent_cwd = os.environ.get('TRAINING_PARENT_WORK_DIR', None)
- has_parent = parent_cwd is not None
- has_rank = get_has_ddp_rank()
- assert has_parent == has_rank, f'Inconsistent state: has_parent={has_parent}, has_rank={has_rank}'
-
- if parent_cwd is None:
- os.environ['TRAINING_PARENT_WORK_DIR'] = os.getcwd()
-
- return has_parent
diff --git a/spaces/CVPR/monoscene_lite/monoscene/unet3d_nyu.py b/spaces/CVPR/monoscene_lite/monoscene/unet3d_nyu.py
deleted file mode 100644
index e9e3b3718999248efa1b2925658465ba59801b13..0000000000000000000000000000000000000000
--- a/spaces/CVPR/monoscene_lite/monoscene/unet3d_nyu.py
+++ /dev/null
@@ -1,90 +0,0 @@
-# encoding: utf-8
-import torch
-import torch.nn as nn
-import torch.nn.functional as F
-import numpy as np
-from monoscene.CRP3D import CPMegaVoxels
-from monoscene.modules import (
- Process,
- Upsample,
- Downsample,
- SegmentationHead,
- ASPP,
-)
-
-
-class UNet3D(nn.Module):
- def __init__(
- self,
- class_num,
- norm_layer,
- feature,
- full_scene_size,
- n_relations=4,
- project_res=[],
- context_prior=True,
- bn_momentum=0.1,
- ):
- super(UNet3D, self).__init__()
- self.business_layer = []
- self.project_res = project_res
-
- self.feature_1_4 = feature
- self.feature_1_8 = feature * 2
- self.feature_1_16 = feature * 4
-
- self.feature_1_16_dec = self.feature_1_16
- self.feature_1_8_dec = self.feature_1_8
- self.feature_1_4_dec = self.feature_1_4
-
- self.process_1_4 = nn.Sequential(
- Process(self.feature_1_4, norm_layer, bn_momentum, dilations=[1, 2, 3]),
- Downsample(self.feature_1_4, norm_layer, bn_momentum),
- )
- self.process_1_8 = nn.Sequential(
- Process(self.feature_1_8, norm_layer, bn_momentum, dilations=[1, 2, 3]),
- Downsample(self.feature_1_8, norm_layer, bn_momentum),
- )
- self.up_1_16_1_8 = Upsample(
- self.feature_1_16_dec, self.feature_1_8_dec, norm_layer, bn_momentum
- )
- self.up_1_8_1_4 = Upsample(
- self.feature_1_8_dec, self.feature_1_4_dec, norm_layer, bn_momentum
- )
- self.ssc_head_1_4 = SegmentationHead(
- self.feature_1_4_dec, self.feature_1_4_dec, class_num, [1, 2, 3]
- )
-
- self.context_prior = context_prior
- size_1_16 = tuple(np.ceil(i / 4).astype(int) for i in full_scene_size)
-
- if context_prior:
- self.CP_mega_voxels = CPMegaVoxels(
- self.feature_1_16,
- size_1_16,
- n_relations=n_relations,
- bn_momentum=bn_momentum,
- )
-
- #
- def forward(self, input_dict):
- res = {}
-
- x3d_1_4 = input_dict["x3d"]
- x3d_1_8 = self.process_1_4(x3d_1_4)
- x3d_1_16 = self.process_1_8(x3d_1_8)
-
- if self.context_prior:
- ret = self.CP_mega_voxels(x3d_1_16)
- x3d_1_16 = ret["x"]
- for k in ret.keys():
- res[k] = ret[k]
-
- x3d_up_1_8 = self.up_1_16_1_8(x3d_1_16) + x3d_1_8
- x3d_up_1_4 = self.up_1_8_1_4(x3d_up_1_8) + x3d_1_4
-
- ssc_logit_1_4 = self.ssc_head_1_4(x3d_up_1_4)
-
- res["ssc_logit"] = ssc_logit_1_4
-
- return res
diff --git a/spaces/ChandraMohanNayal/AutoGPT/benchmark/__init__.py b/spaces/ChandraMohanNayal/AutoGPT/benchmark/__init__.py
deleted file mode 100644
index e69de29bb2d1d6434b8b29ae775ad8c2e48c5391..0000000000000000000000000000000000000000
diff --git a/spaces/ChandraMohanNayal/AutoGPT/tests/test_prompt_generator.py b/spaces/ChandraMohanNayal/AutoGPT/tests/test_prompt_generator.py
deleted file mode 100644
index 6a0bfd6c7bbdbfaa3750e9dee621bd25e17a448b..0000000000000000000000000000000000000000
--- a/spaces/ChandraMohanNayal/AutoGPT/tests/test_prompt_generator.py
+++ /dev/null
@@ -1,114 +0,0 @@
-from unittest import TestCase
-
-from autogpt.promptgenerator import PromptGenerator
-
-
-class TestPromptGenerator(TestCase):
- """
- Test cases for the PromptGenerator class, which is responsible for generating
- prompts for the AI with constraints, commands, resources, and performance evaluations.
- """
-
- @classmethod
- def setUpClass(cls):
- """
- Set up the initial state for each test method by creating an instance of PromptGenerator.
- """
- cls.generator = PromptGenerator()
-
- # Test whether the add_constraint() method adds a constraint to the generator's constraints list
- def test_add_constraint(self):
- """
- Test if the add_constraint() method adds a constraint to the generator's constraints list.
- """
- constraint = "Constraint1"
- self.generator.add_constraint(constraint)
- self.assertIn(constraint, self.generator.constraints)
-
- # Test whether the add_command() method adds a command to the generator's commands list
- def test_add_command(self):
- """
- Test if the add_command() method adds a command to the generator's commands list.
- """
- command_label = "Command Label"
- command_name = "command_name"
- args = {"arg1": "value1", "arg2": "value2"}
- self.generator.add_command(command_label, command_name, args)
- command = {
- "label": command_label,
- "name": command_name,
- "args": args,
- }
- self.assertIn(command, self.generator.commands)
-
- def test_add_resource(self):
- """
- Test if the add_resource() method adds a resource to the generator's resources list.
- """
- resource = "Resource1"
- self.generator.add_resource(resource)
- self.assertIn(resource, self.generator.resources)
-
- def test_add_performance_evaluation(self):
- """
- Test if the add_performance_evaluation() method adds an evaluation to the generator's
- performance_evaluation list.
- """
- evaluation = "Evaluation1"
- self.generator.add_performance_evaluation(evaluation)
- self.assertIn(evaluation, self.generator.performance_evaluation)
-
- def test_generate_prompt_string(self):
- """
- Test if the generate_prompt_string() method generates a prompt string with all the added
- constraints, commands, resources, and evaluations.
- """
- # Define the test data
- constraints = ["Constraint1", "Constraint2"]
- commands = [
- {
- "label": "Command1",
- "name": "command_name1",
- "args": {"arg1": "value1"},
- },
- {
- "label": "Command2",
- "name": "command_name2",
- "args": {},
- },
- ]
- resources = ["Resource1", "Resource2"]
- evaluations = ["Evaluation1", "Evaluation2"]
-
- # Add test data to the generator
- for constraint in constraints:
- self.generator.add_constraint(constraint)
- for command in commands:
- self.generator.add_command(
- command["label"], command["name"], command["args"]
- )
- for resource in resources:
- self.generator.add_resource(resource)
- for evaluation in evaluations:
- self.generator.add_performance_evaluation(evaluation)
-
- # Generate the prompt string and verify its correctness
- prompt_string = self.generator.generate_prompt_string()
- self.assertIsNotNone(prompt_string)
-
- # Check if all constraints, commands, resources, and evaluations are present in the prompt string
- for constraint in constraints:
- self.assertIn(constraint, prompt_string)
- for command in commands:
- self.assertIn(command["name"], prompt_string)
- for key, value in command["args"].items():
- self.assertIn(f'"{key}": "{value}"', prompt_string)
- for resource in resources:
- self.assertIn(resource, prompt_string)
- for evaluation in evaluations:
- self.assertIn(evaluation, prompt_string)
-
- self.assertIn("constraints", prompt_string.lower())
- self.assertIn("commands", prompt_string.lower())
- self.assertIn("resources", prompt_string.lower())
- self.assertIn("performance evaluation", prompt_string.lower())
diff --git a/spaces/Chomkwoy/Nilkessye/cpool_new/src/bottom_pool.cpp b/spaces/Chomkwoy/Nilkessye/cpool_new/src/bottom_pool.cpp
deleted file mode 100644
index 607d6366b063f4e59195085be5718cf6e22974c4..0000000000000000000000000000000000000000
--- a/spaces/Chomkwoy/Nilkessye/cpool_new/src/bottom_pool.cpp
+++ /dev/null
@@ -1,90 +0,0 @@
-#include
-
-#include
-
-std::vector pool_forward(
- torch::Tensor input
-) {
- // Initialize output
- torch::Tensor output = torch::zeros_like(input);
-
- // Get height
- int64_t height = input.size(2);
-
- // Copy the last column
- torch::Tensor input_temp = input.select(2, 0);
- torch::Tensor output_temp = output.select(2, 0);
- output_temp.copy_(input_temp);
-
- torch::Tensor max_temp;
- for (int64_t ind = 0; ind < height - 1; ++ind) {
- input_temp = input.select(2, ind + 1);
- output_temp = output.select(2, ind);
- max_temp = output.select(2, ind + 1);
-
- torch::max_out(max_temp, input_temp, output_temp);
- }
-
- return {
- output
- };
-}
-
-std::vector pool_backward(
- torch::Tensor input,
- torch::Tensor grad_output
-) {
- auto output = torch::zeros_like(input);
-
- int32_t batch = input.size(0);
- int32_t channel = input.size(1);
- int32_t height = input.size(2);
- int32_t width = input.size(3);
-
- // auto max_val = torch::zeros(torch::CUDA(torch::kFloat), {batch, channel, width});
- // auto max_ind = torch::zeros(torch::CUDA(torch::kLong), {batch, channel, width});
- auto max_val = torch::zeros({batch, channel, width}, torch::TensorOptions().dtype(torch::kFloat).device(torch::kCUDA));
- auto max_ind = torch::zeros({batch, channel, width}, torch::TensorOptions().dtype(torch::kLong).device(torch::kCUDA));
-
- auto input_temp = input.select(2, 0);
- max_val.copy_(input_temp);
-
- max_ind.fill_(0);
-
- auto output_temp = output.select(2, 0);
- auto grad_output_temp = grad_output.select(2, 0);
- output_temp.copy_(grad_output_temp);
-
- auto un_max_ind = max_ind.unsqueeze(2);
- // auto gt_mask = torch::zeros(torch::CUDA(torch::kByte), {batch, channel, width});
- // auto max_temp = torch::zeros(torch::CUDA(torch::kFloat), {batch, channel, width});
- auto gt_mask = torch::zeros({batch, channel, width}, torch::TensorOptions().dtype(torch::kByte).device(torch::kCUDA));
- auto max_temp = torch::zeros({batch, channel, width}, torch::TensorOptions().dtype(torch::kFloat).device(torch::kCUDA));
-
- for (int32_t ind = 0; ind < height - 1; ++ind) {
- input_temp = input.select(2, ind + 1);
- torch::gt_out(gt_mask, input_temp, max_val);
-
- torch::masked_select_out(max_temp, input_temp, gt_mask);
- max_val.masked_scatter_(gt_mask, max_temp);
- max_ind.masked_fill_(gt_mask, ind + 1);
-
- grad_output_temp = grad_output.select(2, ind + 1).unsqueeze(2);
- output.scatter_add_(2, un_max_ind, grad_output_temp);
- }
-
- return {
- output
- };
-}
-
-PYBIND11_MODULE(TORCH_EXTENSION_NAME, m) {
- m.def(
- "forward", &pool_forward, "Bottom Pool Forward",
- py::call_guard()
- );
- m.def(
- "backward", &pool_backward, "Bottom Pool Backward",
- py::call_guard()
- );
-}
diff --git a/spaces/CikeyQI/Yunzai/Yunzai/plugins/ws-plugin/CHANGELOG.md b/spaces/CikeyQI/Yunzai/Yunzai/plugins/ws-plugin/CHANGELOG.md
deleted file mode 100644
index 4fbfdfef0354dc77f132f7224be3b60b2f8facae..0000000000000000000000000000000000000000
--- a/spaces/CikeyQI/Yunzai/Yunzai/plugins/ws-plugin/CHANGELOG.md
+++ /dev/null
@@ -1,295 +0,0 @@
-# 0.5.15
-
-* RedProtocol 尝试适配喵崽
-
-# 0.5.14
-
-* `#ws重新连接` 改为指定
-
-# 0.5.13
-
-* red 非win系统可以使用`#ws设置red转发2`使发送伪造消息为直接发送
-
-# 0.5.12
-
-* 修改red连接过程
-* onebot增加快速操作api
-* 尝试存储trss icqq插件发送的消息
-
-# 0.5.11
-
-* Chronocat 0.0.48 支持私聊导入抽卡记录等
-
-# 0.5.10
-
-* Chronocat响应群临时消息
-
-# 0.5.9
-
-* 将消息存储到数据库,默认存储7天
- * 仅支持 Miao-Yunzai & TRSS-Yunzai, Yunzai-Bot还是存储在redis
-* Chronocat 0.0.47之前的版本获取群历史消息没有user_id,可能会导致获取失败,建议更新至0.0.47
-
-# 0.5.8
-
-* 增加一个设置项`#ws设置禁言拦截 开启/关闭`,详细请查看`#ws设置`
-
-# 0.5.7
-
-* 支持正向http 反向http
-* 部分细节优化
-
-# 0.5.6
-
-* 正向ws连接支持/api /event接口
-
-# 0.5.5
-
-* QQNT 转发消息发送者改为固定
-* 可能适配了ReadStream的file
-
-# 0.5.4
-
-* Chronocat 0.0.43 发送转发消息有message_id返回了
-
-# 0.5.3
-
-* 支持了TRSS-Yunzai 的 ICQQ-Plugin
- * 目前 TRSS-Yunzai 的可用协议为
- * GenshinUID Core 全部协议均可使用
- * onebot 仅支持 QQNT 以及 ICQQ
-
-# 0.5.2
-
-* QQNT 拦截配置项中没有uin的连接
- * uin为需要连接的账号
-* 优化添加连接之后的提示词
-
-# 0.5.1
-
-* QQNT 将嵌套转发消息改成不嵌套
- * 暂时不支持嵌套转发
-
-# 0.5.0
-
-* 重构一下QQNT相关的代码
-* 优化一下onebotApi相关的代码
- * 之后可能会用得上
-* QQNT支持正向和反向ws连接,需要对QQNT连接的账号发送#ws添加连接
-* QQNT增加群禁言,全体禁言,踢群
- * 如果QQNT不在前台且焦点不在指定的群,获取的群成员列表可能为空
- * 如果为空的话则只会获取启动机器人之后在群内发送过消息的人
-* QQNT修复使用Chronocat0.0.42时的某些bug
-* QQNT增加请求Api超时输出日志
-
-# 0.4.20
-
-* QQNT 简单支持一下文字的转发消息,(图片会把消息发给自己然后撤回取直链)
- * 需要更新Chronocat 0.0.40
- * 需要64位QQNT,如果不是64位不能伪造转发消息
- * 机器人所在的QQNT看不了发送的转发消息
- * 发送转发消息之前至少要有一条消息
- * 转发消息目前不能主动撤回
-
-# 0.4.19
-
-* QQNT 增加获取群历史消息
- * 因为回复消息没有msgId,所以将seq存在redis获取对应的msgId,默认存储10分钟
-* QQNT 优化使用TRSS脚本自动获取Token
-
-# 0.4.18
-
-* QQNT 增加发送视频 需要ffmpeg
-
-# 0.4.17
-
-* QQNT 增加发送文件
-
-# 0.4.16
-
-* QQNT 发送语音修改为使用ffmpeg,请自行配置ffmpeg,否则可能无法发送语音
- * 更新后需要重新安装依赖 pnpm install --filter=ws-plugin
-
-# 0.4.15
-
-* QQNT 优化进群通知,增加禁言通知
-
-# 0.4.14
-
-* 增加 TRSS-Yunzai 连接 gsuid_core
-* 优化CQ:node
-
-# 0.4.13
-
-* QQNT 增加发送回复消息
- * 回复会默认带一个 `@`
-
-# 0.4.12
-
-* QQNT 若不填Token则自动获取Token
-
-# 0.4.11
-
-* QQNT 增加发送语音,小表情
- * 语音只有手机上能听
-
-# 0.4.10
-
-* QQNT 增加入群通知
-
-# 0.4.9
-
-* QQNT 增加主动撤回消息
-
-# 0.4.8
-
-* 优化`#ws添加连接`
-
-# 0.4.7
-
-* 增加 TRSS-Yunzai 连接 QQNT
-
-# 0.4.6
-
-* 增加白名单
-
-# 0.4.5
-
-* 优化代码
-
-# 0.4.4
-
-* 增加api
- * get_essence_msg_list 获取精华消息列表
- * _get_group_notice 获取群公告
-
-# 0.4.3
-
-* 增加匿名以及群匿名用户禁言API
-
-# 0.4.2
-
-* 增加设置项`#ws设置全部 开启/关闭` 一键操作所有设置项
-
-# 0.4.1
-
-* 优化存储消息,其他插件使用e.reply时也能存储,防止get_msg报错
-
-# 0.4.0
-
-* 适配锅巴
-* 增加大部分api
-* 增加请求上报
-* 增加`#ws更新日志`
-
-# 0.3.12
-
-* 修复更换为Bot.on之后仅at不生效的问题
-* 增加可单独禁用群聊
- * `#ws禁用群123456` 不带群号则默认为当前群
- * `#ws启用群123456` 不带群号则默认为当前群
- * `#ws查看禁用群`
- * 详细可查看`#ws帮助`
-
-# 0.3.11
-
-* 新增CQ码[CQ:music] 音乐自定义分享 Copyright xiaofei-plugin
-
-# 0.3.10
-
-* 修改message_id存储于redis
-* 增加设置项`#ws设置存储600`
-* 增加对message_id为null的判断
-* 获取消息仅针对`用户发送`以及`ws-plugin插件发送`,如果是其他插件发送的消息会获取不到
-
-# 0.3.9
-
-* 修改为Bot.on
-* 删除设置项`#ws设置优先级`
-
-# 0.3.8
-
-* 修复前缀判断时错误的匹配e.msg的问题
-* 反向ws连接添加缺少的请求头X-Client-Role
-
-# 0.3.7
-
-* 增加api
- * get_group_root_files 获取群根目录文件列表
- * get_group_files_by_folder 获取群子目录文件列表
- * get_group_file_url 获取群文件资源链接
-
-# 0.3.6
-
-* 增加CQ码[CQ:record] 语音
-
-# 0.3.5
-
-* 增加发送消息成功后的日志输出
-* 适配根据歌曲id分享音乐
-
-# 0.3.4
-
-* 可能修复了连接失败时关闭和删除连接无效的bug
-* 增加一个设置项`#ws设置优先级1` 设置完重启后生效
-* 增加了几个bug
-
-# 0.3.3
-
-* 增加CQ码[CQ:face] QQ表情
-
-# 0.3.2
-
-* 重新开放一下正向ws连接
-
-# 0.3.1
-
-* 增加指令`#ws帮助` Copyright miao-plugin
-
-# 0.3.0
-
-* 增加指令`#ws关闭连接``#ws打开连接``#ws查看连接`
- *`#ws关闭连接` 不会删除已有连接,同时不进行连接
- *`#ws打开连接` 打开已关闭的连接
- *`#ws查看连接` 查看已有的所有连接名字和状态
- *`#ws添加连接` 添加一个新的连接
- *`#ws删除连接` 删除一个已有的连接
- *`#ws重新连接` 强制断开已有的所有连接并重新连接
-* 暂时关闭正向ws连接
-
-# 0.2.0
-
-* 增加通知事件上报,默认关闭,需要可自行使用`#ws设置`进行开启
- * 增加以下通知事件
- * 群管理员变动,群成员减少,群成员增加
- * 群禁言,好友添加,群消息撤回
- * 好友消息撤回,群内戳一戳
-
-# 0.1.0
-
-* 增加指令`#ws版本``#ws设置` Copyright miao-plugin
-
-# 0.0.5
-
-* 增加指令`#ws重新连接`
-* 增加首次连接时将结果通知主人设置
-
-# 0.0.4
-
-* 增加断线自动重新连接
-* 增加断线和重连通知主人设置
-
-# 0.0.3
-
-* 适配gsuid群聊导出抽卡记录和私聊发送抽卡记录json文件
-
-# 0.0.2
-
-* 增加指令`#ws添加连接``#ws删除连接`
-
-# 0.0.1
-
-* 初始化插件
-* 可连接支持onebotv11协议的bot以及gsuid_core
-* 适配了部分onebot api
-
diff --git a/spaces/CofAI/chat.b4/client/css/main.css b/spaces/CofAI/chat.b4/client/css/main.css
deleted file mode 100644
index ec1f1dd80247747912e1976413a1e3897f1308db..0000000000000000000000000000000000000000
--- a/spaces/CofAI/chat.b4/client/css/main.css
+++ /dev/null
@@ -1,14 +0,0 @@
-.main-container {
- display: flex;
- padding: var(--section-gap);
- height: 100vh;
- justify-content: center;
- box-sizing: border-box;
-}
-
-@media screen and (max-width: 360px) {
- .main-container {
- padding: 0px;
- height: 90vh;
- }
-}
\ No newline at end of file
diff --git a/spaces/Coweed/BadTrip/README.md b/spaces/Coweed/BadTrip/README.md
deleted file mode 100644
index 6cf0b15ad1a17a3bae11beefcea69b4a42eedaa7..0000000000000000000000000000000000000000
--- a/spaces/Coweed/BadTrip/README.md
+++ /dev/null
@@ -1,10 +0,0 @@
----
-title: BadTrip
-emoji: 👁
-colorFrom: purple
-colorTo: green
-sdk: docker
-pinned: false
----
-
-Check out the configuration reference at https://huggingface.co/docs/hub/spaces-config-reference
diff --git a/spaces/DQChoi/gpt-demo/venv/lib/python3.11/site-packages/attr/exceptions.py b/spaces/DQChoi/gpt-demo/venv/lib/python3.11/site-packages/attr/exceptions.py
deleted file mode 100644
index 2883493085143c64c95d60a249e5444f8840cbc6..0000000000000000000000000000000000000000
--- a/spaces/DQChoi/gpt-demo/venv/lib/python3.11/site-packages/attr/exceptions.py
+++ /dev/null
@@ -1,91 +0,0 @@
-# SPDX-License-Identifier: MIT
-
-
-class FrozenError(AttributeError):
- """
- A frozen/immutable instance or attribute have been attempted to be
- modified.
-
- It mirrors the behavior of ``namedtuples`` by using the same error message
- and subclassing `AttributeError`.
-
- .. versionadded:: 20.1.0
- """
-
- msg = "can't set attribute"
- args = [msg]
-
-
-class FrozenInstanceError(FrozenError):
- """
- A frozen instance has been attempted to be modified.
-
- .. versionadded:: 16.1.0
- """
-
-
-class FrozenAttributeError(FrozenError):
- """
- A frozen attribute has been attempted to be modified.
-
- .. versionadded:: 20.1.0
- """
-
-
-class AttrsAttributeNotFoundError(ValueError):
- """
- An *attrs* function couldn't find an attribute that the user asked for.
-
- .. versionadded:: 16.2.0
- """
-
-
-class NotAnAttrsClassError(ValueError):
- """
- A non-*attrs* class has been passed into an *attrs* function.
-
- .. versionadded:: 16.2.0
- """
-
-
-class DefaultAlreadySetError(RuntimeError):
- """
- A default has been set when defining the field and is attempted to be reset
- using the decorator.
-
- .. versionadded:: 17.1.0
- """
-
-
-class UnannotatedAttributeError(RuntimeError):
- """
- A class with ``auto_attribs=True`` has a field without a type annotation.
-
- .. versionadded:: 17.3.0
- """
-
-
-class PythonTooOldError(RuntimeError):
- """
- It was attempted to use an *attrs* feature that requires a newer Python
- version.
-
- .. versionadded:: 18.2.0
- """
-
-
-class NotCallableError(TypeError):
- """
- A field requiring a callable has been set with a value that is not
- callable.
-
- .. versionadded:: 19.2.0
- """
-
- def __init__(self, msg, value):
- super(TypeError, self).__init__(msg, value)
- self.msg = msg
- self.value = value
-
- def __str__(self):
- return str(self.msg)
diff --git a/spaces/DQChoi/gpt-demo/venv/lib/python3.11/site-packages/fontTools/cu2qu/cu2qu.py b/spaces/DQChoi/gpt-demo/venv/lib/python3.11/site-packages/fontTools/cu2qu/cu2qu.py
deleted file mode 100644
index e620b48a55bd0ce720a34c309d295839edabe5aa..0000000000000000000000000000000000000000
--- a/spaces/DQChoi/gpt-demo/venv/lib/python3.11/site-packages/fontTools/cu2qu/cu2qu.py
+++ /dev/null
@@ -1,534 +0,0 @@
-# cython: language_level=3
-# distutils: define_macros=CYTHON_TRACE_NOGIL=1
-
-# Copyright 2015 Google Inc. All Rights Reserved.
-#
-# Licensed under the Apache License, Version 2.0 (the "License");
-# you may not use this file except in compliance with the License.
-# You may obtain a copy of the License at
-#
-# http://www.apache.org/licenses/LICENSE-2.0
-#
-# Unless required by applicable law or agreed to in writing, software
-# distributed under the License is distributed on an "AS IS" BASIS,
-# WITHOUT WARRANTIES OR CONDITIONS OF ANY KIND, either express or implied.
-# See the License for the specific language governing permissions and
-# limitations under the License.
-
-try:
- import cython
-
- COMPILED = cython.compiled
-except (AttributeError, ImportError):
- # if cython not installed, use mock module with no-op decorators and types
- from fontTools.misc import cython
-
- COMPILED = False
-
-import math
-
-from .errors import Error as Cu2QuError, ApproxNotFoundError
-
-
-__all__ = ["curve_to_quadratic", "curves_to_quadratic"]
-
-MAX_N = 100
-
-NAN = float("NaN")
-
-
-@cython.cfunc
-@cython.inline
-@cython.returns(cython.double)
-@cython.locals(v1=cython.complex, v2=cython.complex)
-def dot(v1, v2):
- """Return the dot product of two vectors.
-
- Args:
- v1 (complex): First vector.
- v2 (complex): Second vector.
-
- Returns:
- double: Dot product.
- """
- return (v1 * v2.conjugate()).real
-
-
-@cython.cfunc
-@cython.inline
-@cython.locals(a=cython.complex, b=cython.complex, c=cython.complex, d=cython.complex)
-@cython.locals(
- _1=cython.complex, _2=cython.complex, _3=cython.complex, _4=cython.complex
-)
-def calc_cubic_points(a, b, c, d):
- _1 = d
- _2 = (c / 3.0) + d
- _3 = (b + c) / 3.0 + _2
- _4 = a + d + c + b
- return _1, _2, _3, _4
-
-
-@cython.cfunc
-@cython.inline
-@cython.locals(
- p0=cython.complex, p1=cython.complex, p2=cython.complex, p3=cython.complex
-)
-@cython.locals(a=cython.complex, b=cython.complex, c=cython.complex, d=cython.complex)
-def calc_cubic_parameters(p0, p1, p2, p3):
- c = (p1 - p0) * 3.0
- b = (p2 - p1) * 3.0 - c
- d = p0
- a = p3 - d - c - b
- return a, b, c, d
-
-
-@cython.cfunc
-@cython.inline
-@cython.locals(
- p0=cython.complex, p1=cython.complex, p2=cython.complex, p3=cython.complex
-)
-def split_cubic_into_n_iter(p0, p1, p2, p3, n):
- """Split a cubic Bezier into n equal parts.
-
- Splits the curve into `n` equal parts by curve time.
- (t=0..1/n, t=1/n..2/n, ...)
-
- Args:
- p0 (complex): Start point of curve.
- p1 (complex): First handle of curve.
- p2 (complex): Second handle of curve.
- p3 (complex): End point of curve.
-
- Returns:
- An iterator yielding the control points (four complex values) of the
- subcurves.
- """
- # Hand-coded special-cases
- if n == 2:
- return iter(split_cubic_into_two(p0, p1, p2, p3))
- if n == 3:
- return iter(split_cubic_into_three(p0, p1, p2, p3))
- if n == 4:
- a, b = split_cubic_into_two(p0, p1, p2, p3)
- return iter(
- split_cubic_into_two(a[0], a[1], a[2], a[3])
- + split_cubic_into_two(b[0], b[1], b[2], b[3])
- )
- if n == 6:
- a, b = split_cubic_into_two(p0, p1, p2, p3)
- return iter(
- split_cubic_into_three(a[0], a[1], a[2], a[3])
- + split_cubic_into_three(b[0], b[1], b[2], b[3])
- )
-
- return _split_cubic_into_n_gen(p0, p1, p2, p3, n)
-
-
-@cython.locals(
- p0=cython.complex,
- p1=cython.complex,
- p2=cython.complex,
- p3=cython.complex,
- n=cython.int,
-)
-@cython.locals(a=cython.complex, b=cython.complex, c=cython.complex, d=cython.complex)
-@cython.locals(
- dt=cython.double, delta_2=cython.double, delta_3=cython.double, i=cython.int
-)
-@cython.locals(
- a1=cython.complex, b1=cython.complex, c1=cython.complex, d1=cython.complex
-)
-def _split_cubic_into_n_gen(p0, p1, p2, p3, n):
- a, b, c, d = calc_cubic_parameters(p0, p1, p2, p3)
- dt = 1 / n
- delta_2 = dt * dt
- delta_3 = dt * delta_2
- for i in range(n):
- t1 = i * dt
- t1_2 = t1 * t1
- # calc new a, b, c and d
- a1 = a * delta_3
- b1 = (3 * a * t1 + b) * delta_2
- c1 = (2 * b * t1 + c + 3 * a * t1_2) * dt
- d1 = a * t1 * t1_2 + b * t1_2 + c * t1 + d
- yield calc_cubic_points(a1, b1, c1, d1)
-
-
-@cython.cfunc
-@cython.inline
-@cython.locals(
- p0=cython.complex, p1=cython.complex, p2=cython.complex, p3=cython.complex
-)
-@cython.locals(mid=cython.complex, deriv3=cython.complex)
-def split_cubic_into_two(p0, p1, p2, p3):
- """Split a cubic Bezier into two equal parts.
-
- Splits the curve into two equal parts at t = 0.5
-
- Args:
- p0 (complex): Start point of curve.
- p1 (complex): First handle of curve.
- p2 (complex): Second handle of curve.
- p3 (complex): End point of curve.
-
- Returns:
- tuple: Two cubic Beziers (each expressed as a tuple of four complex
- values).
- """
- mid = (p0 + 3 * (p1 + p2) + p3) * 0.125
- deriv3 = (p3 + p2 - p1 - p0) * 0.125
- return (
- (p0, (p0 + p1) * 0.5, mid - deriv3, mid),
- (mid, mid + deriv3, (p2 + p3) * 0.5, p3),
- )
-
-
-@cython.cfunc
-@cython.inline
-@cython.locals(
- p0=cython.complex,
- p1=cython.complex,
- p2=cython.complex,
- p3=cython.complex,
-)
-@cython.locals(
- mid1=cython.complex,
- deriv1=cython.complex,
- mid2=cython.complex,
- deriv2=cython.complex,
-)
-def split_cubic_into_three(p0, p1, p2, p3):
- """Split a cubic Bezier into three equal parts.
-
- Splits the curve into three equal parts at t = 1/3 and t = 2/3
-
- Args:
- p0 (complex): Start point of curve.
- p1 (complex): First handle of curve.
- p2 (complex): Second handle of curve.
- p3 (complex): End point of curve.
-
- Returns:
- tuple: Three cubic Beziers (each expressed as a tuple of four complex
- values).
- """
- mid1 = (8 * p0 + 12 * p1 + 6 * p2 + p3) * (1 / 27)
- deriv1 = (p3 + 3 * p2 - 4 * p0) * (1 / 27)
- mid2 = (p0 + 6 * p1 + 12 * p2 + 8 * p3) * (1 / 27)
- deriv2 = (4 * p3 - 3 * p1 - p0) * (1 / 27)
- return (
- (p0, (2 * p0 + p1) / 3.0, mid1 - deriv1, mid1),
- (mid1, mid1 + deriv1, mid2 - deriv2, mid2),
- (mid2, mid2 + deriv2, (p2 + 2 * p3) / 3.0, p3),
- )
-
-
-@cython.cfunc
-@cython.inline
-@cython.returns(cython.complex)
-@cython.locals(
- t=cython.double,
- p0=cython.complex,
- p1=cython.complex,
- p2=cython.complex,
- p3=cython.complex,
-)
-@cython.locals(_p1=cython.complex, _p2=cython.complex)
-def cubic_approx_control(t, p0, p1, p2, p3):
- """Approximate a cubic Bezier using a quadratic one.
-
- Args:
- t (double): Position of control point.
- p0 (complex): Start point of curve.
- p1 (complex): First handle of curve.
- p2 (complex): Second handle of curve.
- p3 (complex): End point of curve.
-
- Returns:
- complex: Location of candidate control point on quadratic curve.
- """
- _p1 = p0 + (p1 - p0) * 1.5
- _p2 = p3 + (p2 - p3) * 1.5
- return _p1 + (_p2 - _p1) * t
-
-
-@cython.cfunc
-@cython.inline
-@cython.returns(cython.complex)
-@cython.locals(a=cython.complex, b=cython.complex, c=cython.complex, d=cython.complex)
-@cython.locals(ab=cython.complex, cd=cython.complex, p=cython.complex, h=cython.double)
-def calc_intersect(a, b, c, d):
- """Calculate the intersection of two lines.
-
- Args:
- a (complex): Start point of first line.
- b (complex): End point of first line.
- c (complex): Start point of second line.
- d (complex): End point of second line.
-
- Returns:
- complex: Location of intersection if one present, ``complex(NaN,NaN)``
- if no intersection was found.
- """
- ab = b - a
- cd = d - c
- p = ab * 1j
- try:
- h = dot(p, a - c) / dot(p, cd)
- except ZeroDivisionError:
- return complex(NAN, NAN)
- return c + cd * h
-
-
-@cython.cfunc
-@cython.returns(cython.int)
-@cython.locals(
- tolerance=cython.double,
- p0=cython.complex,
- p1=cython.complex,
- p2=cython.complex,
- p3=cython.complex,
-)
-@cython.locals(mid=cython.complex, deriv3=cython.complex)
-def cubic_farthest_fit_inside(p0, p1, p2, p3, tolerance):
- """Check if a cubic Bezier lies within a given distance of the origin.
-
- "Origin" means *the* origin (0,0), not the start of the curve. Note that no
- checks are made on the start and end positions of the curve; this function
- only checks the inside of the curve.
-
- Args:
- p0 (complex): Start point of curve.
- p1 (complex): First handle of curve.
- p2 (complex): Second handle of curve.
- p3 (complex): End point of curve.
- tolerance (double): Distance from origin.
-
- Returns:
- bool: True if the cubic Bezier ``p`` entirely lies within a distance
- ``tolerance`` of the origin, False otherwise.
- """
- # First check p2 then p1, as p2 has higher error early on.
- if abs(p2) <= tolerance and abs(p1) <= tolerance:
- return True
-
- # Split.
- mid = (p0 + 3 * (p1 + p2) + p3) * 0.125
- if abs(mid) > tolerance:
- return False
- deriv3 = (p3 + p2 - p1 - p0) * 0.125
- return cubic_farthest_fit_inside(
- p0, (p0 + p1) * 0.5, mid - deriv3, mid, tolerance
- ) and cubic_farthest_fit_inside(mid, mid + deriv3, (p2 + p3) * 0.5, p3, tolerance)
-
-
-@cython.cfunc
-@cython.inline
-@cython.locals(tolerance=cython.double)
-@cython.locals(
- q1=cython.complex,
- c0=cython.complex,
- c1=cython.complex,
- c2=cython.complex,
- c3=cython.complex,
-)
-def cubic_approx_quadratic(cubic, tolerance):
- """Approximate a cubic Bezier with a single quadratic within a given tolerance.
-
- Args:
- cubic (sequence): Four complex numbers representing control points of
- the cubic Bezier curve.
- tolerance (double): Permitted deviation from the original curve.
-
- Returns:
- Three complex numbers representing control points of the quadratic
- curve if it fits within the given tolerance, or ``None`` if no suitable
- curve could be calculated.
- """
-
- q1 = calc_intersect(cubic[0], cubic[1], cubic[2], cubic[3])
- if math.isnan(q1.imag):
- return None
- c0 = cubic[0]
- c3 = cubic[3]
- c1 = c0 + (q1 - c0) * (2 / 3)
- c2 = c3 + (q1 - c3) * (2 / 3)
- if not cubic_farthest_fit_inside(0, c1 - cubic[1], c2 - cubic[2], 0, tolerance):
- return None
- return c0, q1, c3
-
-
-@cython.cfunc
-@cython.locals(n=cython.int, tolerance=cython.double)
-@cython.locals(i=cython.int)
-@cython.locals(all_quadratic=cython.int)
-@cython.locals(
- c0=cython.complex, c1=cython.complex, c2=cython.complex, c3=cython.complex
-)
-@cython.locals(
- q0=cython.complex,
- q1=cython.complex,
- next_q1=cython.complex,
- q2=cython.complex,
- d1=cython.complex,
-)
-def cubic_approx_spline(cubic, n, tolerance, all_quadratic):
- """Approximate a cubic Bezier curve with a spline of n quadratics.
-
- Args:
- cubic (sequence): Four complex numbers representing control points of
- the cubic Bezier curve.
- n (int): Number of quadratic Bezier curves in the spline.
- tolerance (double): Permitted deviation from the original curve.
-
- Returns:
- A list of ``n+2`` complex numbers, representing control points of the
- quadratic spline if it fits within the given tolerance, or ``None`` if
- no suitable spline could be calculated.
- """
-
- if n == 1:
- return cubic_approx_quadratic(cubic, tolerance)
- if n == 2 and all_quadratic == False:
- return cubic
-
- cubics = split_cubic_into_n_iter(cubic[0], cubic[1], cubic[2], cubic[3], n)
-
- # calculate the spline of quadratics and check errors at the same time.
- next_cubic = next(cubics)
- next_q1 = cubic_approx_control(
- 0, next_cubic[0], next_cubic[1], next_cubic[2], next_cubic[3]
- )
- q2 = cubic[0]
- d1 = 0j
- spline = [cubic[0], next_q1]
- for i in range(1, n + 1):
- # Current cubic to convert
- c0, c1, c2, c3 = next_cubic
-
- # Current quadratic approximation of current cubic
- q0 = q2
- q1 = next_q1
- if i < n:
- next_cubic = next(cubics)
- next_q1 = cubic_approx_control(
- i / (n - 1), next_cubic[0], next_cubic[1], next_cubic[2], next_cubic[3]
- )
- spline.append(next_q1)
- q2 = (q1 + next_q1) * 0.5
- else:
- q2 = c3
-
- # End-point deltas
- d0 = d1
- d1 = q2 - c3
-
- if abs(d1) > tolerance or not cubic_farthest_fit_inside(
- d0,
- q0 + (q1 - q0) * (2 / 3) - c1,
- q2 + (q1 - q2) * (2 / 3) - c2,
- d1,
- tolerance,
- ):
- return None
- spline.append(cubic[3])
-
- return spline
-
-
-@cython.locals(max_err=cython.double)
-@cython.locals(n=cython.int)
-@cython.locals(all_quadratic=cython.int)
-def curve_to_quadratic(curve, max_err, all_quadratic=True):
- """Approximate a cubic Bezier curve with a spline of n quadratics.
-
- Args:
- cubic (sequence): Four 2D tuples representing control points of
- the cubic Bezier curve.
- max_err (double): Permitted deviation from the original curve.
- all_quadratic (bool): If True (default) returned value is a
- quadratic spline. If False, it's either a single quadratic
- curve or a single cubic curve.
-
- Returns:
- If all_quadratic is True: A list of 2D tuples, representing
- control points of the quadratic spline if it fits within the
- given tolerance, or ``None`` if no suitable spline could be
- calculated.
-
- If all_quadratic is False: Either a quadratic curve (if length
- of output is 3), or a cubic curve (if length of output is 4).
- """
-
- curve = [complex(*p) for p in curve]
-
- for n in range(1, MAX_N + 1):
- spline = cubic_approx_spline(curve, n, max_err, all_quadratic)
- if spline is not None:
- # done. go home
- return [(s.real, s.imag) for s in spline]
-
- raise ApproxNotFoundError(curve)
-
-
-@cython.locals(l=cython.int, last_i=cython.int, i=cython.int)
-@cython.locals(all_quadratic=cython.int)
-def curves_to_quadratic(curves, max_errors, all_quadratic=True):
- """Return quadratic Bezier splines approximating the input cubic Beziers.
-
- Args:
- curves: A sequence of *n* curves, each curve being a sequence of four
- 2D tuples.
- max_errors: A sequence of *n* floats representing the maximum permissible
- deviation from each of the cubic Bezier curves.
- all_quadratic (bool): If True (default) returned values are a
- quadratic spline. If False, they are either a single quadratic
- curve or a single cubic curve.
-
- Example::
-
- >>> curves_to_quadratic( [
- ... [ (50,50), (100,100), (150,100), (200,50) ],
- ... [ (75,50), (120,100), (150,75), (200,60) ]
- ... ], [1,1] )
- [[(50.0, 50.0), (75.0, 75.0), (125.0, 91.66666666666666), (175.0, 75.0), (200.0, 50.0)], [(75.0, 50.0), (97.5, 75.0), (135.41666666666666, 82.08333333333333), (175.0, 67.5), (200.0, 60.0)]]
-
- The returned splines have "implied oncurve points" suitable for use in
- TrueType ``glif`` outlines - i.e. in the first spline returned above,
- the first quadratic segment runs from (50,50) to
- ( (75 + 125)/2 , (120 + 91.666..)/2 ) = (100, 83.333...).
-
- Returns:
- If all_quadratic is True, a list of splines, each spline being a list
- of 2D tuples.
-
- If all_quadratic is False, a list of curves, each curve being a quadratic
- (length 3), or cubic (length 4).
-
- Raises:
- fontTools.cu2qu.Errors.ApproxNotFoundError: if no suitable approximation
- can be found for all curves with the given parameters.
- """
-
- curves = [[complex(*p) for p in curve] for curve in curves]
- assert len(max_errors) == len(curves)
-
- l = len(curves)
- splines = [None] * l
- last_i = i = 0
- n = 1
- while True:
- spline = cubic_approx_spline(curves[i], n, max_errors[i], all_quadratic)
- if spline is None:
- if n == MAX_N:
- break
- n += 1
- last_i = i
- continue
- splines[i] = spline
- i = (i + 1) % l
- if i == last_i:
- # done. go home
- return [[(s.real, s.imag) for s in spline] for spline in splines]
-
- raise ApproxNotFoundError(curves)
diff --git a/spaces/DQChoi/gpt-demo/venv/lib/python3.11/site-packages/fontTools/ttLib/tables/G__l_o_c.py b/spaces/DQChoi/gpt-demo/venv/lib/python3.11/site-packages/fontTools/ttLib/tables/G__l_o_c.py
deleted file mode 100644
index 7973b9be911d450f2504e83704705c9bb8e4b810..0000000000000000000000000000000000000000
--- a/spaces/DQChoi/gpt-demo/venv/lib/python3.11/site-packages/fontTools/ttLib/tables/G__l_o_c.py
+++ /dev/null
@@ -1,84 +0,0 @@
-from fontTools.misc import sstruct
-from fontTools.misc.textTools import safeEval
-from . import DefaultTable
-import array
-import sys
-
-
-Gloc_header = """
- > # big endian
- version: 16.16F # Table version
- flags: H # bit 0: 1=long format, 0=short format
- # bit 1: 1=attribute names, 0=no names
- numAttribs: H # NUmber of attributes
-"""
-
-
-class table_G__l_o_c(DefaultTable.DefaultTable):
- """
- Support Graphite Gloc tables
- """
-
- dependencies = ["Glat"]
-
- def __init__(self, tag=None):
- DefaultTable.DefaultTable.__init__(self, tag)
- self.attribIds = None
- self.numAttribs = 0
-
- def decompile(self, data, ttFont):
- _, data = sstruct.unpack2(Gloc_header, data, self)
- flags = self.flags
- del self.flags
- self.locations = array.array("I" if flags & 1 else "H")
- self.locations.frombytes(data[: len(data) - self.numAttribs * (flags & 2)])
- if sys.byteorder != "big":
- self.locations.byteswap()
- self.attribIds = array.array("H")
- if flags & 2:
- self.attribIds.frombytes(data[-self.numAttribs * 2 :])
- if sys.byteorder != "big":
- self.attribIds.byteswap()
-
- def compile(self, ttFont):
- data = sstruct.pack(
- Gloc_header,
- dict(
- version=1.0,
- flags=(bool(self.attribIds) << 1) + (self.locations.typecode == "I"),
- numAttribs=self.numAttribs,
- ),
- )
- if sys.byteorder != "big":
- self.locations.byteswap()
- data += self.locations.tobytes()
- if sys.byteorder != "big":
- self.locations.byteswap()
- if self.attribIds:
- if sys.byteorder != "big":
- self.attribIds.byteswap()
- data += self.attribIds.tobytes()
- if sys.byteorder != "big":
- self.attribIds.byteswap()
- return data
-
- def set(self, locations):
- long_format = max(locations) >= 65536
- self.locations = array.array("I" if long_format else "H", locations)
-
- def toXML(self, writer, ttFont):
- writer.simpletag("attributes", number=self.numAttribs)
- writer.newline()
-
- def fromXML(self, name, attrs, content, ttFont):
- if name == "attributes":
- self.numAttribs = int(safeEval(attrs["number"]))
-
- def __getitem__(self, index):
- return self.locations[index]
-
- def __len__(self):
- return len(self.locations)
-
- def __iter__(self):
- return iter(self.locations)
diff --git a/spaces/DQChoi/gpt-demo/venv/lib/python3.11/site-packages/gradio/templates/frontend/assets/index-8dee978a.js b/spaces/DQChoi/gpt-demo/venv/lib/python3.11/site-packages/gradio/templates/frontend/assets/index-8dee978a.js
deleted file mode 100644
index 8678b06f01a5441601d37b931c6d04dc10386908..0000000000000000000000000000000000000000
--- a/spaces/DQChoi/gpt-demo/venv/lib/python3.11/site-packages/gradio/templates/frontend/assets/index-8dee978a.js
+++ /dev/null
@@ -1,2 +0,0 @@
-import{S as c,e as m,s as v,a9 as b,N as f,K as r,L as o,U as d,p as g,M as p,ab as h,ac as w,ad as y,z as G,v as j,A as k}from"./index-3370be2a.js";function C(n){let s,l,u,i;const _=n[4].default,a=b(_,n,n[3],null);return{c(){s=f("div"),l=f("div"),a&&a.c(),r(l,"class","styler svelte-iyf88w"),o(l,"--block-radius","0px"),o(l,"--block-border-width","0px"),o(l,"--layout-gap","1px"),o(l,"--form-gap-width","1px"),o(l,"--button-border-width","0px"),o(l,"--button-large-radius","0px"),o(l,"--button-small-radius","0px"),r(s,"id",n[0]),r(s,"class",u="gr-group "+n[1].join(" ")+" svelte-iyf88w"),d(s,"hide",!n[2])},m(e,t){g(e,s,t),p(s,l),a&&a.m(l,null),i=!0},p(e,[t]){a&&a.p&&(!i||t&8)&&h(a,_,e,e[3],i?y(_,e[3],t,null):w(e[3]),null),(!i||t&1)&&r(s,"id",e[0]),(!i||t&2&&u!==(u="gr-group "+e[1].join(" ")+" svelte-iyf88w"))&&r(s,"class",u),(!i||t&6)&&d(s,"hide",!e[2])},i(e){i||(G(a,e),i=!0)},o(e){j(a,e),i=!1},d(e){e&&k(s),a&&a.d(e)}}}function S(n,s,l){let{$$slots:u={},$$scope:i}=s,{elem_id:_=""}=s,{elem_classes:a=[]}=s,{visible:e=!0}=s;return n.$$set=t=>{"elem_id"in t&&l(0,_=t.elem_id),"elem_classes"in t&&l(1,a=t.elem_classes),"visible"in t&&l(2,e=t.visible),"$$scope"in t&&l(3,i=t.$$scope)},[_,a,e,i,u]}class q extends c{constructor(s){super(),m(this,s,S,C,v,{elem_id:0,elem_classes:1,visible:2})}}const A=q,K=["static"];export{A as Component,K as modes};
-//# sourceMappingURL=index-8dee978a.js.map
diff --git a/spaces/DaleChen/AutoGPT/autogpt/cli.py b/spaces/DaleChen/AutoGPT/autogpt/cli.py
deleted file mode 100644
index a2e99cb421cad005528cb160e948ce59ccfcdb66..0000000000000000000000000000000000000000
--- a/spaces/DaleChen/AutoGPT/autogpt/cli.py
+++ /dev/null
@@ -1,145 +0,0 @@
-"""Main script for the autogpt package."""
-import click
-
-
-@click.group(invoke_without_command=True)
-@click.option("-c", "--continuous", is_flag=True, help="Enable Continuous Mode")
-@click.option(
- "--skip-reprompt",
- "-y",
- is_flag=True,
- help="Skips the re-prompting messages at the beginning of the script",
-)
-@click.option(
- "--ai-settings",
- "-C",
- help="Specifies which ai_settings.yaml file to use, will also automatically skip the re-prompt.",
-)
-@click.option(
- "-l",
- "--continuous-limit",
- type=int,
- help="Defines the number of times to run in continuous mode",
-)
-@click.option("--speak", is_flag=True, help="Enable Speak Mode")
-@click.option("--debug", is_flag=True, help="Enable Debug Mode")
-@click.option("--gpt3only", is_flag=True, help="Enable GPT3.5 Only Mode")
-@click.option("--gpt4only", is_flag=True, help="Enable GPT4 Only Mode")
-@click.option(
- "--use-memory",
- "-m",
- "memory_type",
- type=str,
- help="Defines which Memory backend to use",
-)
-@click.option(
- "-b",
- "--browser-name",
- help="Specifies which web-browser to use when using selenium to scrape the web.",
-)
-@click.option(
- "--allow-downloads",
- is_flag=True,
- help="Dangerous: Allows Auto-GPT to download files natively.",
-)
-@click.option(
- "--skip-news",
- is_flag=True,
- help="Specifies whether to suppress the output of latest news on startup.",
-)
-@click.pass_context
-def main(
- ctx: click.Context,
- continuous: bool,
- continuous_limit: int,
- ai_settings: str,
- skip_reprompt: bool,
- speak: bool,
- debug: bool,
- gpt3only: bool,
- gpt4only: bool,
- memory_type: str,
- browser_name: str,
- allow_downloads: bool,
- skip_news: bool,
-) -> None:
- """
- Welcome to AutoGPT an experimental open-source application showcasing the capabilities of the GPT-4 pushing the boundaries of AI.
-
- Start an Auto-GPT assistant.
- """
- # Put imports inside function to avoid importing everything when starting the CLI
- import logging
-
- from colorama import Fore
-
- from autogpt.agent.agent import Agent
- from autogpt.config import Config, check_openai_api_key
- from autogpt.configurator import create_config
- from autogpt.logs import logger
- from autogpt.memory import get_memory
- from autogpt.prompt import construct_prompt
- from autogpt.utils import get_current_git_branch, get_latest_bulletin
-
- if ctx.invoked_subcommand is None:
- cfg = Config()
- # TODO: fill in llm values here
- check_openai_api_key()
- create_config(
- continuous,
- continuous_limit,
- ai_settings,
- skip_reprompt,
- speak,
- debug,
- gpt3only,
- gpt4only,
- memory_type,
- browser_name,
- allow_downloads,
- skip_news,
- )
- logger.set_level(logging.DEBUG if cfg.debug_mode else logging.INFO)
- ai_name = ""
- if not cfg.skip_news:
- motd = get_latest_bulletin()
- if motd:
- logger.typewriter_log("NEWS: ", Fore.GREEN, motd)
- git_branch = get_current_git_branch()
- if git_branch and git_branch != "stable":
- logger.typewriter_log(
- "WARNING: ",
- Fore.RED,
- f"You are running on `{git_branch}` branch "
- "- this is not a supported branch.",
- )
- system_prompt = construct_prompt()
- # print(prompt)
- # Initialize variables
- full_message_history = []
- next_action_count = 0
- # Make a constant:
- triggering_prompt = (
- "Determine which next command to use, and respond using the"
- " format specified above:"
- )
- # Initialize memory and make sure it is empty.
- # this is particularly important for indexing and referencing pinecone memory
- memory = get_memory(cfg, init=True)
- logger.typewriter_log(
- "Using memory of type:", Fore.GREEN, f"{memory.__class__.__name__}"
- )
- logger.typewriter_log("Using Browser:", Fore.GREEN, cfg.selenium_web_browser)
- agent = Agent(
- ai_name=ai_name,
- memory=memory,
- full_message_history=full_message_history,
- next_action_count=next_action_count,
- system_prompt=system_prompt,
- triggering_prompt=triggering_prompt,
- )
- agent.start_interaction_loop()
-
-
-if __name__ == "__main__":
- main()
diff --git a/spaces/DamarJati/DamarJati-NSFW-filter-DecentScan/README.md b/spaces/DamarJati/DamarJati-NSFW-filter-DecentScan/README.md
deleted file mode 100644
index 4625ca074aa5cfac803813452d565cd75f751cac..0000000000000000000000000000000000000000
--- a/spaces/DamarJati/DamarJati-NSFW-filter-DecentScan/README.md
+++ /dev/null
@@ -1,12 +0,0 @@
----
-title: NSFW Filter DecentScan
-emoji: 👀
-colorFrom: yellow
-colorTo: pink
-sdk: gradio
-sdk_version: 3.45.2
-app_file: app.py
-pinned: false
----
-
-Check out the configuration reference at https://huggingface.co/docs/hub/spaces-config-reference
\ No newline at end of file
diff --git a/spaces/DeeeTeeee01/VODAFONE-CUSTOMER-CHURN-PREDICTION-APP/app.py b/spaces/DeeeTeeee01/VODAFONE-CUSTOMER-CHURN-PREDICTION-APP/app.py
deleted file mode 100644
index 3cdac0af665e66c74140f5059c0e17fedd297e13..0000000000000000000000000000000000000000
--- a/spaces/DeeeTeeee01/VODAFONE-CUSTOMER-CHURN-PREDICTION-APP/app.py
+++ /dev/null
@@ -1,139 +0,0 @@
-#Importing the libraries
-import gradio as gr
-import pickle
-import pandas as pd
-import numpy as np
-import joblib
-from PIL import Image
-
-#using joblib to load the model:
-num_imputer = joblib.load('num_imputer.joblib') # loading the imputer
-cat_imputer = joblib.load('cat_imputer.joblib') # loading the imputer
-encoder = joblib.load('encoder.joblib') # loading the encoder
-scaler = joblib.load('scaler.joblib') # loading the scaler
-model = joblib.load('ml.joblib') # loading the model
-
-
-# Create a function that applies the ML pipeline and makes predictions
-def predict(gender,SeniorCitizen,Partner,Dependents, tenure, PhoneService,MultipleLines,
- InternetService,OnlineSecurity,OnlineBackup,DeviceProtection,TechSupport,StreamingTV,StreamingMovies,
- Contract,PaperlessBilling,PaymentMethod,MonthlyCharges,TotalCharges):
-
-
-
- # Create a dataframe with the input data
- input_df = pd.DataFrame({
- 'gender': [gender],
- 'SeniorCitizen': [SeniorCitizen],
- 'Partner': [Partner],
- 'Dependents': [Dependents],
- 'tenure': [tenure],
- 'PhoneService': [PhoneService],
- 'MultipleLines': [MultipleLines],
- 'InternetService': [InternetService],
- 'OnlineSecurity': [OnlineSecurity],
- 'OnlineBackup': [OnlineBackup],
- 'DeviceProtection': [DeviceProtection],
- 'TechSupport': [TechSupport],
- 'StreamingTV': [StreamingTV],
- 'StreamingMovies': [StreamingMovies],
- 'Contract': [Contract],
- 'PaperlessBilling': [PaperlessBilling],
- 'PaymentMethod': [PaymentMethod],
- 'MonthlyCharges': [MonthlyCharges],
- 'TotalCharges': [TotalCharges]
-
- })
-
-# Create a list with the categorical and numerical columns
- cat_columns = [col for col in input_df.columns if input_df[col].dtype == 'object']
- num_columns = [col for col in input_df.columns if input_df[col].dtype != 'object']
-
- # Impute the missing values
- input_df_imputed_cat = cat_imputer.transform(input_df[cat_columns])
- input_df_imputed_num = num_imputer.transform(input_df[num_columns])
-
- # Encode the categorical columns
- input_encoded_df = pd.DataFrame(encoder.transform(input_df_imputed_cat).toarray(),
- columns=encoder.get_feature_names_out(cat_columns))
-
- # Scale the numerical columns
- input_df_scaled = scaler.transform(input_df_imputed_num)
- input_scaled_df = pd.DataFrame(input_df_scaled , columns = num_columns)
-
-
- #joining the cat encoded and num scaled
- final_df = pd.concat([input_encoded_df, input_scaled_df], axis=1)
-
- final_df = final_df.reindex(columns=['SeniorCitizen','tenure','MonthlyCharges','TotalCharges',
- 'gender_Female','gender_Male','Partner_No','Partner_Yes','Dependents_No','Dependents_Yes','PhoneService_No',
- 'PhoneService_Yes','MultipleLines_No','MultipleLines_Yes','InternetService_DSL','InternetService_Fiber optic',
- 'InternetService_No','OnlineSecurity_No','OnlineSecurity_Yes','OnlineBackup_No','OnlineBackup_Yes','DeviceProtection_No',
- 'DeviceProtection_Yes','TechSupport_No','TechSupport_Yes','StreamingTV_No','StreamingTV_Yes','StreamingMovies_No',
- 'StreamingMovies_Yes','Contract_Month-to-month','Contract_One year','Contract_Two year','PaperlessBilling_No',
- 'PaperlessBilling_Yes','PaymentMethod_Bank transfer (automatic)','PaymentMethod_Credit card (automatic)','PaymentMethod_Electronic check',
- 'PaymentMethod_Mailed check'])
-
- # Make predictions using the model
- predict = model.predict(final_df)
-
-
- prediction_label = "THIS CUSTOMER WILL CHURN" if predict.item() == "Yes" else "THIS CUSTOMER WILL NOT CHURN"
-
-
- return prediction_label
-
- #return predictions
-
-#define the input interface
-
-
-input_interface = []
-
-with gr.Blocks(css=".gradio-container {background-color:silver}") as app:
- title = gr.Label('VODAFONE CUSTOMER CHURN PREDICTION')
- img = gr.Image("VODA.png").style(height= 210 , width= 1250)
-
-
- with gr.Row():
- gr.Markdown("This application provides predictions on whether a customer will churn or remain with the Company. Please enter the customer's information below and click PREDICT to view the prediction outcome.")
-
- with gr.Row():
- with gr.Column(scale=3.5, min_width=500):
- input_interface = [
- gr.components.Radio(['male', 'female'], label='What is your Gender?'),
- gr.components.Number(label="Are you a Seniorcitizen? (No=0 and Yes=1), 55years and above"),
- gr.components.Radio(['Yes', 'No'], label='Do you have a Partner?'),
- gr.components.Dropdown(['No', 'Yes'], label='Do you have any Dependents?'),
- gr.components.Number(label='Length of Tenure (No. of months with Vodafone)'),
- gr.components.Radio(['No', 'Yes'], label='Do you use Phone Service?'),
- gr.components.Radio(['No', 'Yes'], label='Do you use Multiple Lines?'),
- gr.components.Radio(['DSL', 'Fiber optic', 'No'], label='Do you use Internet Service?'),
- gr.components.Radio(['No', 'Yes'], label='Do you use Online Security?'),
- gr.components.Radio(['No', 'Yes'], label='Do you use Online Backup?'),
- gr.components.Radio(['No', 'Yes'], label='Do you use Device Protection?'),
- gr.components.Radio(['No', 'Yes'], label='Do you use the Tech Support?'),
- gr.components.Radio(['No', 'Yes'], label='Do you Streaming TV?'),
- gr.components.Radio(['No', 'Yes'], label='Do you Streaming Movies?'),
- gr.components.Dropdown(['Month-to-month', 'One year', 'Two year'], label='Please what Contract Type do you Subscribe to?'),
- gr.components.Radio(['Yes', 'No'], label='Do you use Paperless Billing?'),
- gr.components.Dropdown(['Electronic check', 'Mailed check', 'Bank transfer (automatic)',
- 'Credit card (automatic)'], label='What type of Payment Method do you use please?'),
- gr.components.Number(label="How much is you Monthly Charges?"),
- gr.components.Number(label="How much is your Total Charges?")
- ]
-
- with gr.Row():
- predict_btn = gr.Button('Predict')
-
-
-
-# Define the output interfaces
- output_interface = gr.Label(label="churn")
-
- predict_btn.click(fn=predict, inputs=input_interface, outputs=output_interface)
-
-
- app.launch(share=False)
-
-
diff --git a/spaces/DragGan/DragGan-Inversion/PTI/torch_utils/training_stats.py b/spaces/DragGan/DragGan-Inversion/PTI/torch_utils/training_stats.py
deleted file mode 100644
index 26f467f9eaa074ee13de1cf2625cd7da44880847..0000000000000000000000000000000000000000
--- a/spaces/DragGan/DragGan-Inversion/PTI/torch_utils/training_stats.py
+++ /dev/null
@@ -1,268 +0,0 @@
-# Copyright (c) 2021, NVIDIA CORPORATION. All rights reserved.
-#
-# NVIDIA CORPORATION and its licensors retain all intellectual property
-# and proprietary rights in and to this software, related documentation
-# and any modifications thereto. Any use, reproduction, disclosure or
-# distribution of this software and related documentation without an express
-# license agreement from NVIDIA CORPORATION is strictly prohibited.
-
-"""Facilities for reporting and collecting training statistics across
-multiple processes and devices. The interface is designed to minimize
-synchronization overhead as well as the amount of boilerplate in user
-code."""
-
-import re
-import numpy as np
-import torch
-import dnnlib
-
-from . import misc
-
-#----------------------------------------------------------------------------
-
-_num_moments = 3 # [num_scalars, sum_of_scalars, sum_of_squares]
-_reduce_dtype = torch.float32 # Data type to use for initial per-tensor reduction.
-_counter_dtype = torch.float64 # Data type to use for the internal counters.
-_rank = 0 # Rank of the current process.
-_sync_device = None # Device to use for multiprocess communication. None = single-process.
-_sync_called = False # Has _sync() been called yet?
-_counters = dict() # Running counters on each device, updated by report(): name => device => torch.Tensor
-_cumulative = dict() # Cumulative counters on the CPU, updated by _sync(): name => torch.Tensor
-
-#----------------------------------------------------------------------------
-
-def init_multiprocessing(rank, sync_device):
- r"""Initializes `torch_utils.training_stats` for collecting statistics
- across multiple processes.
-
- This function must be called after
- `torch.distributed.init_process_group()` and before `Collector.update()`.
- The call is not necessary if multi-process collection is not needed.
-
- Args:
- rank: Rank of the current process.
- sync_device: PyTorch device to use for inter-process
- communication, or None to disable multi-process
- collection. Typically `torch.device('cuda', rank)`.
- """
- global _rank, _sync_device
- assert not _sync_called
- _rank = rank
- _sync_device = sync_device
-
-#----------------------------------------------------------------------------
-
-@misc.profiled_function
-def report(name, value):
- r"""Broadcasts the given set of scalars to all interested instances of
- `Collector`, across device and process boundaries.
-
- This function is expected to be extremely cheap and can be safely
- called from anywhere in the training loop, loss function, or inside a
- `torch.nn.Module`.
-
- Warning: The current implementation expects the set of unique names to
- be consistent across processes. Please make sure that `report()` is
- called at least once for each unique name by each process, and in the
- same order. If a given process has no scalars to broadcast, it can do
- `report(name, [])` (empty list).
-
- Args:
- name: Arbitrary string specifying the name of the statistic.
- Averages are accumulated separately for each unique name.
- value: Arbitrary set of scalars. Can be a list, tuple,
- NumPy array, PyTorch tensor, or Python scalar.
-
- Returns:
- The same `value` that was passed in.
- """
- if name not in _counters:
- _counters[name] = dict()
-
- elems = torch.as_tensor(value)
- if elems.numel() == 0:
- return value
-
- elems = elems.detach().flatten().to(_reduce_dtype)
- moments = torch.stack([
- torch.ones_like(elems).sum(),
- elems.sum(),
- elems.square().sum(),
- ])
- assert moments.ndim == 1 and moments.shape[0] == _num_moments
- moments = moments.to(_counter_dtype)
-
- device = moments.device
- if device not in _counters[name]:
- _counters[name][device] = torch.zeros_like(moments)
- _counters[name][device].add_(moments)
- return value
-
-#----------------------------------------------------------------------------
-
-def report0(name, value):
- r"""Broadcasts the given set of scalars by the first process (`rank = 0`),
- but ignores any scalars provided by the other processes.
- See `report()` for further details.
- """
- report(name, value if _rank == 0 else [])
- return value
-
-#----------------------------------------------------------------------------
-
-class Collector:
- r"""Collects the scalars broadcasted by `report()` and `report0()` and
- computes their long-term averages (mean and standard deviation) over
- user-defined periods of time.
-
- The averages are first collected into internal counters that are not
- directly visible to the user. They are then copied to the user-visible
- state as a result of calling `update()` and can then be queried using
- `mean()`, `std()`, `as_dict()`, etc. Calling `update()` also resets the
- internal counters for the next round, so that the user-visible state
- effectively reflects averages collected between the last two calls to
- `update()`.
-
- Args:
- regex: Regular expression defining which statistics to
- collect. The default is to collect everything.
- keep_previous: Whether to retain the previous averages if no
- scalars were collected on a given round
- (default: True).
- """
- def __init__(self, regex='.*', keep_previous=True):
- self._regex = re.compile(regex)
- self._keep_previous = keep_previous
- self._cumulative = dict()
- self._moments = dict()
- self.update()
- self._moments.clear()
-
- def names(self):
- r"""Returns the names of all statistics broadcasted so far that
- match the regular expression specified at construction time.
- """
- return [name for name in _counters if self._regex.fullmatch(name)]
-
- def update(self):
- r"""Copies current values of the internal counters to the
- user-visible state and resets them for the next round.
-
- If `keep_previous=True` was specified at construction time, the
- operation is skipped for statistics that have received no scalars
- since the last update, retaining their previous averages.
-
- This method performs a number of GPU-to-CPU transfers and one
- `torch.distributed.all_reduce()`. It is intended to be called
- periodically in the main training loop, typically once every
- N training steps.
- """
- if not self._keep_previous:
- self._moments.clear()
- for name, cumulative in _sync(self.names()):
- if name not in self._cumulative:
- self._cumulative[name] = torch.zeros([_num_moments], dtype=_counter_dtype)
- delta = cumulative - self._cumulative[name]
- self._cumulative[name].copy_(cumulative)
- if float(delta[0]) != 0:
- self._moments[name] = delta
-
- def _get_delta(self, name):
- r"""Returns the raw moments that were accumulated for the given
- statistic between the last two calls to `update()`, or zero if
- no scalars were collected.
- """
- assert self._regex.fullmatch(name)
- if name not in self._moments:
- self._moments[name] = torch.zeros([_num_moments], dtype=_counter_dtype)
- return self._moments[name]
-
- def num(self, name):
- r"""Returns the number of scalars that were accumulated for the given
- statistic between the last two calls to `update()`, or zero if
- no scalars were collected.
- """
- delta = self._get_delta(name)
- return int(delta[0])
-
- def mean(self, name):
- r"""Returns the mean of the scalars that were accumulated for the
- given statistic between the last two calls to `update()`, or NaN if
- no scalars were collected.
- """
- delta = self._get_delta(name)
- if int(delta[0]) == 0:
- return float('nan')
- return float(delta[1] / delta[0])
-
- def std(self, name):
- r"""Returns the standard deviation of the scalars that were
- accumulated for the given statistic between the last two calls to
- `update()`, or NaN if no scalars were collected.
- """
- delta = self._get_delta(name)
- if int(delta[0]) == 0 or not np.isfinite(float(delta[1])):
- return float('nan')
- if int(delta[0]) == 1:
- return float(0)
- mean = float(delta[1] / delta[0])
- raw_var = float(delta[2] / delta[0])
- return np.sqrt(max(raw_var - np.square(mean), 0))
-
- def as_dict(self):
- r"""Returns the averages accumulated between the last two calls to
- `update()` as an `dnnlib.EasyDict`. The contents are as follows:
-
- dnnlib.EasyDict(
- NAME = dnnlib.EasyDict(num=FLOAT, mean=FLOAT, std=FLOAT),
- ...
- )
- """
- stats = dnnlib.EasyDict()
- for name in self.names():
- stats[name] = dnnlib.EasyDict(num=self.num(name), mean=self.mean(name), std=self.std(name))
- return stats
-
- def __getitem__(self, name):
- r"""Convenience getter.
- `collector[name]` is a synonym for `collector.mean(name)`.
- """
- return self.mean(name)
-
-#----------------------------------------------------------------------------
-
-def _sync(names):
- r"""Synchronize the global cumulative counters across devices and
- processes. Called internally by `Collector.update()`.
- """
- if len(names) == 0:
- return []
- global _sync_called
- _sync_called = True
-
- # Collect deltas within current rank.
- deltas = []
- device = _sync_device if _sync_device is not None else torch.device('cpu')
- for name in names:
- delta = torch.zeros([_num_moments], dtype=_counter_dtype, device=device)
- for counter in _counters[name].values():
- delta.add_(counter.to(device))
- counter.copy_(torch.zeros_like(counter))
- deltas.append(delta)
- deltas = torch.stack(deltas)
-
- # Sum deltas across ranks.
- if _sync_device is not None:
- torch.distributed.all_reduce(deltas)
-
- # Update cumulative values.
- deltas = deltas.cpu()
- for idx, name in enumerate(names):
- if name not in _cumulative:
- _cumulative[name] = torch.zeros([_num_moments], dtype=_counter_dtype)
- _cumulative[name].add_(deltas[idx])
-
- # Return name-value pairs.
- return [(name, _cumulative[name]) for name in names]
-
-#----------------------------------------------------------------------------
diff --git a/spaces/ECCV2022/bytetrack/yolox/utils/metric.py b/spaces/ECCV2022/bytetrack/yolox/utils/metric.py
deleted file mode 100644
index 4840b8dd0e97d26891fb8c515b6999cf35bd9544..0000000000000000000000000000000000000000
--- a/spaces/ECCV2022/bytetrack/yolox/utils/metric.py
+++ /dev/null
@@ -1,123 +0,0 @@
-#!/usr/bin/env python3
-# -*- coding: utf-8 -*-
-# Copyright (c) 2014-2021 Megvii Inc. All rights reserved.
-import numpy as np
-
-import torch
-
-import functools
-import os
-import time
-from collections import defaultdict, deque
-
-__all__ = [
- "AverageMeter",
- "MeterBuffer",
- "get_total_and_free_memory_in_Mb",
- "occupy_mem",
- "gpu_mem_usage",
-]
-
-
-def get_total_and_free_memory_in_Mb(cuda_device):
- devices_info_str = os.popen(
- "nvidia-smi --query-gpu=memory.total,memory.used --format=csv,nounits,noheader"
- )
- devices_info = devices_info_str.read().strip().split("\n")
- total, used = devices_info[int(cuda_device)].split(",")
- return int(total), int(used)
-
-
-def occupy_mem(cuda_device, mem_ratio=0.95):
- """
- pre-allocate gpu memory for training to avoid memory Fragmentation.
- """
- total, used = get_total_and_free_memory_in_Mb(cuda_device)
- max_mem = int(total * mem_ratio)
- block_mem = max_mem - used
- x = torch.cuda.FloatTensor(256, 1024, block_mem)
- del x
- time.sleep(5)
-
-
-def gpu_mem_usage():
- """
- Compute the GPU memory usage for the current device (MB).
- """
- mem_usage_bytes = torch.cuda.max_memory_allocated()
- return mem_usage_bytes / (1024 * 1024)
-
-
-class AverageMeter:
- """Track a series of values and provide access to smoothed values over a
- window or the global series average.
- """
-
- def __init__(self, window_size=50):
- self._deque = deque(maxlen=window_size)
- self._total = 0.0
- self._count = 0
-
- def update(self, value):
- self._deque.append(value)
- self._count += 1
- self._total += value
-
- @property
- def median(self):
- d = np.array(list(self._deque))
- return np.median(d)
-
- @property
- def avg(self):
- # if deque is empty, nan will be returned.
- d = np.array(list(self._deque))
- return d.mean()
-
- @property
- def global_avg(self):
- return self._total / max(self._count, 1e-5)
-
- @property
- def latest(self):
- return self._deque[-1] if len(self._deque) > 0 else None
-
- @property
- def total(self):
- return self._total
-
- def reset(self):
- self._deque.clear()
- self._total = 0.0
- self._count = 0
-
- def clear(self):
- self._deque.clear()
-
-
-class MeterBuffer(defaultdict):
- """Computes and stores the average and current value"""
-
- def __init__(self, window_size=20):
- factory = functools.partial(AverageMeter, window_size=window_size)
- super().__init__(factory)
-
- def reset(self):
- for v in self.values():
- v.reset()
-
- def get_filtered_meter(self, filter_key="time"):
- return {k: v for k, v in self.items() if filter_key in k}
-
- def update(self, values=None, **kwargs):
- if values is None:
- values = {}
- values.update(kwargs)
- for k, v in values.items():
- if isinstance(v, torch.Tensor):
- v = v.detach()
- self[k].update(v)
-
- def clear_meters(self):
- for v in self.values():
- v.clear()
diff --git a/spaces/EronSamez/RVC_HFmeu/train/data_utils.py b/spaces/EronSamez/RVC_HFmeu/train/data_utils.py
deleted file mode 100644
index 71c0eff1815469a52399dc90a093a2f8a29223eb..0000000000000000000000000000000000000000
--- a/spaces/EronSamez/RVC_HFmeu/train/data_utils.py
+++ /dev/null
@@ -1,512 +0,0 @@
-import os, traceback
-import numpy as np
-import torch
-import torch.utils.data
-
-from mel_processing import spectrogram_torch
-from utils import load_wav_to_torch, load_filepaths_and_text
-
-
-class TextAudioLoaderMultiNSFsid(torch.utils.data.Dataset):
- """
- 1) loads audio, text pairs
- 2) normalizes text and converts them to sequences of integers
- 3) computes spectrograms from audio files.
- """
-
- def __init__(self, audiopaths_and_text, hparams):
- self.audiopaths_and_text = load_filepaths_and_text(audiopaths_and_text)
- self.max_wav_value = hparams.max_wav_value
- self.sampling_rate = hparams.sampling_rate
- self.filter_length = hparams.filter_length
- self.hop_length = hparams.hop_length
- self.win_length = hparams.win_length
- self.sampling_rate = hparams.sampling_rate
- self.min_text_len = getattr(hparams, "min_text_len", 1)
- self.max_text_len = getattr(hparams, "max_text_len", 5000)
- self._filter()
-
- def _filter(self):
- """
- Filter text & store spec lengths
- """
- # Store spectrogram lengths for Bucketing
- # wav_length ~= file_size / (wav_channels * Bytes per dim) = file_size / (1 * 2)
- # spec_length = wav_length // hop_length
- audiopaths_and_text_new = []
- lengths = []
- for audiopath, text, pitch, pitchf, dv in self.audiopaths_and_text:
- if self.min_text_len <= len(text) and len(text) <= self.max_text_len:
- audiopaths_and_text_new.append([audiopath, text, pitch, pitchf, dv])
- lengths.append(os.path.getsize(audiopath) // (3 * self.hop_length))
- self.audiopaths_and_text = audiopaths_and_text_new
- self.lengths = lengths
-
- def get_sid(self, sid):
- sid = torch.LongTensor([int(sid)])
- return sid
-
- def get_audio_text_pair(self, audiopath_and_text):
- # separate filename and text
- file = audiopath_and_text[0]
- phone = audiopath_and_text[1]
- pitch = audiopath_and_text[2]
- pitchf = audiopath_and_text[3]
- dv = audiopath_and_text[4]
-
- phone, pitch, pitchf = self.get_labels(phone, pitch, pitchf)
- spec, wav = self.get_audio(file)
- dv = self.get_sid(dv)
-
- len_phone = phone.size()[0]
- len_spec = spec.size()[-1]
- # print(123,phone.shape,pitch.shape,spec.shape)
- if len_phone != len_spec:
- len_min = min(len_phone, len_spec)
- # amor
- len_wav = len_min * self.hop_length
-
- spec = spec[:, :len_min]
- wav = wav[:, :len_wav]
-
- phone = phone[:len_min, :]
- pitch = pitch[:len_min]
- pitchf = pitchf[:len_min]
-
- return (spec, wav, phone, pitch, pitchf, dv)
-
- def get_labels(self, phone, pitch, pitchf):
- phone = np.load(phone)
- phone = np.repeat(phone, 2, axis=0)
- pitch = np.load(pitch)
- pitchf = np.load(pitchf)
- n_num = min(phone.shape[0], 900) # DistributedBucketSampler
- # print(234,phone.shape,pitch.shape)
- phone = phone[:n_num, :]
- pitch = pitch[:n_num]
- pitchf = pitchf[:n_num]
- phone = torch.FloatTensor(phone)
- pitch = torch.LongTensor(pitch)
- pitchf = torch.FloatTensor(pitchf)
- return phone, pitch, pitchf
-
- def get_audio(self, filename):
- audio, sampling_rate = load_wav_to_torch(filename)
- if sampling_rate != self.sampling_rate:
- raise ValueError(
- "{} SR doesn't match target {} SR".format(
- sampling_rate, self.sampling_rate
- )
- )
- audio_norm = audio
- # audio_norm = audio / self.max_wav_value
- # audio_norm = audio / np.abs(audio).max()
-
- audio_norm = audio_norm.unsqueeze(0)
- spec_filename = filename.replace(".wav", ".spec.pt")
- if os.path.exists(spec_filename):
- try:
- spec = torch.load(spec_filename)
- except:
- print(spec_filename, traceback.format_exc())
- spec = spectrogram_torch(
- audio_norm,
- self.filter_length,
- self.sampling_rate,
- self.hop_length,
- self.win_length,
- center=False,
- )
- spec = torch.squeeze(spec, 0)
- torch.save(spec, spec_filename, _use_new_zipfile_serialization=False)
- else:
- spec = spectrogram_torch(
- audio_norm,
- self.filter_length,
- self.sampling_rate,
- self.hop_length,
- self.win_length,
- center=False,
- )
- spec = torch.squeeze(spec, 0)
- torch.save(spec, spec_filename, _use_new_zipfile_serialization=False)
- return spec, audio_norm
-
- def __getitem__(self, index):
- return self.get_audio_text_pair(self.audiopaths_and_text[index])
-
- def __len__(self):
- return len(self.audiopaths_and_text)
-
-
-class TextAudioCollateMultiNSFsid:
- """Zero-pads model inputs and targets"""
-
- def __init__(self, return_ids=False):
- self.return_ids = return_ids
-
- def __call__(self, batch):
- """Collate's training batch from normalized text and aduio
- PARAMS
- ------
- batch: [text_normalized, spec_normalized, wav_normalized]
- """
- # Right zero-pad all one-hot text sequences to max input length
- _, ids_sorted_decreasing = torch.sort(
- torch.LongTensor([x[0].size(1) for x in batch]), dim=0, descending=True
- )
-
- max_spec_len = max([x[0].size(1) for x in batch])
- max_wave_len = max([x[1].size(1) for x in batch])
- spec_lengths = torch.LongTensor(len(batch))
- wave_lengths = torch.LongTensor(len(batch))
- spec_padded = torch.FloatTensor(len(batch), batch[0][0].size(0), max_spec_len)
- wave_padded = torch.FloatTensor(len(batch), 1, max_wave_len)
- spec_padded.zero_()
- wave_padded.zero_()
-
- max_phone_len = max([x[2].size(0) for x in batch])
- phone_lengths = torch.LongTensor(len(batch))
- phone_padded = torch.FloatTensor(
- len(batch), max_phone_len, batch[0][2].shape[1]
- ) # (spec, wav, phone, pitch)
- pitch_padded = torch.LongTensor(len(batch), max_phone_len)
- pitchf_padded = torch.FloatTensor(len(batch), max_phone_len)
- phone_padded.zero_()
- pitch_padded.zero_()
- pitchf_padded.zero_()
- # dv = torch.FloatTensor(len(batch), 256)#gin=256
- sid = torch.LongTensor(len(batch))
-
- for i in range(len(ids_sorted_decreasing)):
- row = batch[ids_sorted_decreasing[i]]
-
- spec = row[0]
- spec_padded[i, :, : spec.size(1)] = spec
- spec_lengths[i] = spec.size(1)
-
- wave = row[1]
- wave_padded[i, :, : wave.size(1)] = wave
- wave_lengths[i] = wave.size(1)
-
- phone = row[2]
- phone_padded[i, : phone.size(0), :] = phone
- phone_lengths[i] = phone.size(0)
-
- pitch = row[3]
- pitch_padded[i, : pitch.size(0)] = pitch
- pitchf = row[4]
- pitchf_padded[i, : pitchf.size(0)] = pitchf
-
- # dv[i] = row[5]
- sid[i] = row[5]
-
- return (
- phone_padded,
- phone_lengths,
- pitch_padded,
- pitchf_padded,
- spec_padded,
- spec_lengths,
- wave_padded,
- wave_lengths,
- # dv
- sid,
- )
-
-
-class TextAudioLoader(torch.utils.data.Dataset):
- """
- 1) loads audio, text pairs
- 2) normalizes text and converts them to sequences of integers
- 3) computes spectrograms from audio files.
- """
-
- def __init__(self, audiopaths_and_text, hparams):
- self.audiopaths_and_text = load_filepaths_and_text(audiopaths_and_text)
- self.max_wav_value = hparams.max_wav_value
- self.sampling_rate = hparams.sampling_rate
- self.filter_length = hparams.filter_length
- self.hop_length = hparams.hop_length
- self.win_length = hparams.win_length
- self.sampling_rate = hparams.sampling_rate
- self.min_text_len = getattr(hparams, "min_text_len", 1)
- self.max_text_len = getattr(hparams, "max_text_len", 5000)
- self._filter()
-
- def _filter(self):
- """
- Filter text & store spec lengths
- """
- # Store spectrogram lengths for Bucketing
- # wav_length ~= file_size / (wav_channels * Bytes per dim) = file_size / (1 * 2)
- # spec_length = wav_length // hop_length
- audiopaths_and_text_new = []
- lengths = []
- for audiopath, text, dv in self.audiopaths_and_text:
- if self.min_text_len <= len(text) and len(text) <= self.max_text_len:
- audiopaths_and_text_new.append([audiopath, text, dv])
- lengths.append(os.path.getsize(audiopath) // (3 * self.hop_length))
- self.audiopaths_and_text = audiopaths_and_text_new
- self.lengths = lengths
-
- def get_sid(self, sid):
- sid = torch.LongTensor([int(sid)])
- return sid
-
- def get_audio_text_pair(self, audiopath_and_text):
- # separate filename and text
- file = audiopath_and_text[0]
- phone = audiopath_and_text[1]
- dv = audiopath_and_text[2]
-
- phone = self.get_labels(phone)
- spec, wav = self.get_audio(file)
- dv = self.get_sid(dv)
-
- len_phone = phone.size()[0]
- len_spec = spec.size()[-1]
- if len_phone != len_spec:
- len_min = min(len_phone, len_spec)
- len_wav = len_min * self.hop_length
- spec = spec[:, :len_min]
- wav = wav[:, :len_wav]
- phone = phone[:len_min, :]
- return (spec, wav, phone, dv)
-
- def get_labels(self, phone):
- phone = np.load(phone)
- phone = np.repeat(phone, 2, axis=0)
- n_num = min(phone.shape[0], 900) # DistributedBucketSampler
- phone = phone[:n_num, :]
- phone = torch.FloatTensor(phone)
- return phone
-
- def get_audio(self, filename):
- audio, sampling_rate = load_wav_to_torch(filename)
- if sampling_rate != self.sampling_rate:
- raise ValueError(
- "{} SR doesn't match target {} SR".format(
- sampling_rate, self.sampling_rate
- )
- )
- audio_norm = audio
- # audio_norm = audio / self.max_wav_value
- # audio_norm = audio / np.abs(audio).max()
-
- audio_norm = audio_norm.unsqueeze(0)
- spec_filename = filename.replace(".wav", ".spec.pt")
- if os.path.exists(spec_filename):
- try:
- spec = torch.load(spec_filename)
- except:
- print(spec_filename, traceback.format_exc())
- spec = spectrogram_torch(
- audio_norm,
- self.filter_length,
- self.sampling_rate,
- self.hop_length,
- self.win_length,
- center=False,
- )
- spec = torch.squeeze(spec, 0)
- torch.save(spec, spec_filename, _use_new_zipfile_serialization=False)
- else:
- spec = spectrogram_torch(
- audio_norm,
- self.filter_length,
- self.sampling_rate,
- self.hop_length,
- self.win_length,
- center=False,
- )
- spec = torch.squeeze(spec, 0)
- torch.save(spec, spec_filename, _use_new_zipfile_serialization=False)
- return spec, audio_norm
-
- def __getitem__(self, index):
- return self.get_audio_text_pair(self.audiopaths_and_text[index])
-
- def __len__(self):
- return len(self.audiopaths_and_text)
-
-
-class TextAudioCollate:
- """Zero-pads model inputs and targets"""
-
- def __init__(self, return_ids=False):
- self.return_ids = return_ids
-
- def __call__(self, batch):
- """Collate's training batch from normalized text and aduio
- PARAMS
- ------
- batch: [text_normalized, spec_normalized, wav_normalized]
- """
- # Right zero-pad all one-hot text sequences to max input length
- _, ids_sorted_decreasing = torch.sort(
- torch.LongTensor([x[0].size(1) for x in batch]), dim=0, descending=True
- )
-
- max_spec_len = max([x[0].size(1) for x in batch])
- max_wave_len = max([x[1].size(1) for x in batch])
- spec_lengths = torch.LongTensor(len(batch))
- wave_lengths = torch.LongTensor(len(batch))
- spec_padded = torch.FloatTensor(len(batch), batch[0][0].size(0), max_spec_len)
- wave_padded = torch.FloatTensor(len(batch), 1, max_wave_len)
- spec_padded.zero_()
- wave_padded.zero_()
-
- max_phone_len = max([x[2].size(0) for x in batch])
- phone_lengths = torch.LongTensor(len(batch))
- phone_padded = torch.FloatTensor(
- len(batch), max_phone_len, batch[0][2].shape[1]
- )
- phone_padded.zero_()
- sid = torch.LongTensor(len(batch))
-
- for i in range(len(ids_sorted_decreasing)):
- row = batch[ids_sorted_decreasing[i]]
-
- spec = row[0]
- spec_padded[i, :, : spec.size(1)] = spec
- spec_lengths[i] = spec.size(1)
-
- wave = row[1]
- wave_padded[i, :, : wave.size(1)] = wave
- wave_lengths[i] = wave.size(1)
-
- phone = row[2]
- phone_padded[i, : phone.size(0), :] = phone
- phone_lengths[i] = phone.size(0)
-
- sid[i] = row[3]
-
- return (
- phone_padded,
- phone_lengths,
- spec_padded,
- spec_lengths,
- wave_padded,
- wave_lengths,
- sid,
- )
-
-
-class DistributedBucketSampler(torch.utils.data.distributed.DistributedSampler):
- """
- Maintain similar input lengths in a batch.
- Length groups are specified by boundaries.
- Ex) boundaries = [b1, b2, b3] -> any batch is included either {x | b1 < length(x) <=b2} or {x | b2 < length(x) <= b3}.
-
- It removes samples which are not included in the boundaries.
- Ex) boundaries = [b1, b2, b3] -> any x s.t. length(x) <= b1 or length(x) > b3 are discarded.
- """
-
- def __init__(
- self,
- dataset,
- batch_size,
- boundaries,
- num_replicas=None,
- rank=None,
- shuffle=True,
- ):
- super().__init__(dataset, num_replicas=num_replicas, rank=rank, shuffle=shuffle)
- self.lengths = dataset.lengths
- self.batch_size = batch_size
- self.boundaries = boundaries
-
- self.buckets, self.num_samples_per_bucket = self._create_buckets()
- self.total_size = sum(self.num_samples_per_bucket)
- self.num_samples = self.total_size // self.num_replicas
-
- def _create_buckets(self):
- buckets = [[] for _ in range(len(self.boundaries) - 1)]
- for i in range(len(self.lengths)):
- length = self.lengths[i]
- idx_bucket = self._bisect(length)
- if idx_bucket != -1:
- buckets[idx_bucket].append(i)
-
- for i in range(len(buckets) - 1, -1, -1): #
- if len(buckets[i]) == 0:
- buckets.pop(i)
- self.boundaries.pop(i + 1)
-
- num_samples_per_bucket = []
- for i in range(len(buckets)):
- len_bucket = len(buckets[i])
- total_batch_size = self.num_replicas * self.batch_size
- rem = (
- total_batch_size - (len_bucket % total_batch_size)
- ) % total_batch_size
- num_samples_per_bucket.append(len_bucket + rem)
- return buckets, num_samples_per_bucket
-
- def __iter__(self):
- # deterministically shuffle based on epoch
- g = torch.Generator()
- g.manual_seed(self.epoch)
-
- indices = []
- if self.shuffle:
- for bucket in self.buckets:
- indices.append(torch.randperm(len(bucket), generator=g).tolist())
- else:
- for bucket in self.buckets:
- indices.append(list(range(len(bucket))))
-
- batches = []
- for i in range(len(self.buckets)):
- bucket = self.buckets[i]
- len_bucket = len(bucket)
- ids_bucket = indices[i]
- num_samples_bucket = self.num_samples_per_bucket[i]
-
- # add extra samples to make it evenly divisible
- rem = num_samples_bucket - len_bucket
- ids_bucket = (
- ids_bucket
- + ids_bucket * (rem // len_bucket)
- + ids_bucket[: (rem % len_bucket)]
- )
-
- # subsample
- ids_bucket = ids_bucket[self.rank :: self.num_replicas]
-
- # batching
- for j in range(len(ids_bucket) // self.batch_size):
- batch = [
- bucket[idx]
- for idx in ids_bucket[
- j * self.batch_size : (j + 1) * self.batch_size
- ]
- ]
- batches.append(batch)
-
- if self.shuffle:
- batch_ids = torch.randperm(len(batches), generator=g).tolist()
- batches = [batches[i] for i in batch_ids]
- self.batches = batches
-
- assert len(self.batches) * self.batch_size == self.num_samples
- return iter(self.batches)
-
- def _bisect(self, x, lo=0, hi=None):
- if hi is None:
- hi = len(self.boundaries) - 1
-
- if hi > lo:
- mid = (hi + lo) // 2
- if self.boundaries[mid] < x and x <= self.boundaries[mid + 1]:
- return mid
- elif x <= self.boundaries[mid]:
- return self._bisect(x, lo, mid)
- else:
- return self._bisect(x, mid + 1, hi)
- else:
- return -1
-
- def __len__(self):
- return self.num_samples // self.batch_size
diff --git a/spaces/FFZG-cleopatra/latvian-twitter-sentiment-classifier/app.py b/spaces/FFZG-cleopatra/latvian-twitter-sentiment-classifier/app.py
deleted file mode 100644
index 1df372cac0b7ca30b6962d63231ef6a82cfa66ca..0000000000000000000000000000000000000000
--- a/spaces/FFZG-cleopatra/latvian-twitter-sentiment-classifier/app.py
+++ /dev/null
@@ -1,113 +0,0 @@
-import torch
-from utils import label_full_decoder
-import sys
-import dataset
-import engine
-from model import BERTBaseUncased
-
-import config
-from transformers import pipeline, AutoTokenizer, AutoModel
-import gradio as gr
-
-from ekphrasis.classes.preprocessor import TextPreProcessor
-from ekphrasis.classes.tokenizer import SocialTokenizer
-from ekphrasis.dicts.emoticons import emoticons
-
-device = config.device
-model = BERTBaseUncased()
-model.load_state_dict(torch.load(config.MODEL_PATH, map_location=torch.device(device)),strict=False)
-model.to(device)
-
-# T = tokenizer.TweetTokenizer(
-# preserve_handles=True, preserve_hashes=True, preserve_case=False, preserve_url=False)
-
-# text_processor = TextPreProcessor(
-# # terms that will be normalized
-# normalize=['url', 'email', 'percent', 'money', 'phone', 'user'],
-# # terms that will be annotated
-# annotate={},
-# fix_html=True, # fix HTML tokens
-
-# # corpus from which the word statistics are going to be used
-# # for word segmentation
-# segmenter="twitter",
-
-# # corpus from which the word statistics are going to be used
-# # for spell correction
-# corrector="twitter",
-
-# unpack_hashtags=False, # perform word segmentation on hashtags
-# unpack_contractions=False, # Unpack contractions (can't -> can not)
-# spell_correct_elong=False, # spell correction for elongated words
-
-# # select a tokenizer. You can use SocialTokenizer, or pass your own
-# # the tokenizer, should take as input a string and return a list of tokens
-# tokenizer=SocialTokenizer(lowercase=True).tokenize,
-
-# # list of dictionaries, for replacing tokens extracted from the text,
-# # with other expressions. You can pass more than one dictionaries.
-# dicts=[]
-# )
-
-
-social_tokenizer=SocialTokenizer(lowercase=True).tokenize
-
-def preprocess(text):
- # tokens = T.tokenize(text)
- # tokens = text_processor.pre_process_docs(text)
-
- tokens = social_tokenizer(text)
- print(tokens, file=sys.stderr)
- ptokens = []
- for index, token in enumerate(tokens):
- if "@" in token:
- if index > 0:
- # check if previous token was mention
- if "@" in tokens[index-1]:
- pass
- else:
- ptokens.append("mention_0")
- else:
- ptokens.append("mention_0")
- else:
- ptokens.append(token)
-
- print(ptokens, file=sys.stderr)
- return " ".join(ptokens)
-
-
-def predict_sentiment(sentence = ""):
- sentence = preprocess(sentence)
-
- model_path = config.MODEL_PATH
-
- test_dataset = dataset.BERTDataset(
- review=[sentence],
- target=[0]
- )
-
- test_data_loader = torch.utils.data.DataLoader(
- test_dataset,
- batch_size=config.VALID_BATCH_SIZE,
- num_workers=2
- )
-
- outputs, [] = engine.predict_fn(test_data_loader, model, device)
-
- print(outputs)
- return label_full_decoder(outputs[0]) #{"label":outputs[0]}
-
-
-
-
-interface = gr.Interface(
- fn=predict_sentiment,
- inputs='text',
- outputs=['label'],
- title='Latvian Twitter Sentiment Analysis',
- examples= ["Es mīlu Tevi","Es ienīstu kafiju"],
- description='Get the positive/neutral/negative sentiment for the given input.'
-)
-
-interface.launch(inline = False)
-
diff --git a/spaces/FelixLuoX/codeformer/CodeFormer/basicsr/ops/dcn/deform_conv.py b/spaces/FelixLuoX/codeformer/CodeFormer/basicsr/ops/dcn/deform_conv.py
deleted file mode 100644
index 734154f9ed9447d585eae7df6886acb136f8a3cf..0000000000000000000000000000000000000000
--- a/spaces/FelixLuoX/codeformer/CodeFormer/basicsr/ops/dcn/deform_conv.py
+++ /dev/null
@@ -1,377 +0,0 @@
-import math
-import torch
-from torch import nn as nn
-from torch.autograd import Function
-from torch.autograd.function import once_differentiable
-from torch.nn import functional as F
-from torch.nn.modules.utils import _pair, _single
-
-try:
- from . import deform_conv_ext
-except ImportError:
- import os
- BASICSR_JIT = os.getenv('BASICSR_JIT')
- if BASICSR_JIT == 'True':
- from torch.utils.cpp_extension import load
- module_path = os.path.dirname(__file__)
- deform_conv_ext = load(
- 'deform_conv',
- sources=[
- os.path.join(module_path, 'src', 'deform_conv_ext.cpp'),
- os.path.join(module_path, 'src', 'deform_conv_cuda.cpp'),
- os.path.join(module_path, 'src', 'deform_conv_cuda_kernel.cu'),
- ],
- )
-
-
-class DeformConvFunction(Function):
-
- @staticmethod
- def forward(ctx,
- input,
- offset,
- weight,
- stride=1,
- padding=0,
- dilation=1,
- groups=1,
- deformable_groups=1,
- im2col_step=64):
- if input is not None and input.dim() != 4:
- raise ValueError(f'Expected 4D tensor as input, got {input.dim()}' 'D tensor instead.')
- ctx.stride = _pair(stride)
- ctx.padding = _pair(padding)
- ctx.dilation = _pair(dilation)
- ctx.groups = groups
- ctx.deformable_groups = deformable_groups
- ctx.im2col_step = im2col_step
-
- ctx.save_for_backward(input, offset, weight)
-
- output = input.new_empty(DeformConvFunction._output_size(input, weight, ctx.padding, ctx.dilation, ctx.stride))
-
- ctx.bufs_ = [input.new_empty(0), input.new_empty(0)] # columns, ones
-
- if not input.is_cuda:
- raise NotImplementedError
- else:
- cur_im2col_step = min(ctx.im2col_step, input.shape[0])
- assert (input.shape[0] % cur_im2col_step) == 0, 'im2col step must divide batchsize'
- deform_conv_ext.deform_conv_forward(input, weight,
- offset, output, ctx.bufs_[0], ctx.bufs_[1], weight.size(3),
- weight.size(2), ctx.stride[1], ctx.stride[0], ctx.padding[1],
- ctx.padding[0], ctx.dilation[1], ctx.dilation[0], ctx.groups,
- ctx.deformable_groups, cur_im2col_step)
- return output
-
- @staticmethod
- @once_differentiable
- def backward(ctx, grad_output):
- input, offset, weight = ctx.saved_tensors
-
- grad_input = grad_offset = grad_weight = None
-
- if not grad_output.is_cuda:
- raise NotImplementedError
- else:
- cur_im2col_step = min(ctx.im2col_step, input.shape[0])
- assert (input.shape[0] % cur_im2col_step) == 0, 'im2col step must divide batchsize'
-
- if ctx.needs_input_grad[0] or ctx.needs_input_grad[1]:
- grad_input = torch.zeros_like(input)
- grad_offset = torch.zeros_like(offset)
- deform_conv_ext.deform_conv_backward_input(input, offset, grad_output, grad_input,
- grad_offset, weight, ctx.bufs_[0], weight.size(3),
- weight.size(2), ctx.stride[1], ctx.stride[0], ctx.padding[1],
- ctx.padding[0], ctx.dilation[1], ctx.dilation[0], ctx.groups,
- ctx.deformable_groups, cur_im2col_step)
-
- if ctx.needs_input_grad[2]:
- grad_weight = torch.zeros_like(weight)
- deform_conv_ext.deform_conv_backward_parameters(input, offset, grad_output, grad_weight,
- ctx.bufs_[0], ctx.bufs_[1], weight.size(3),
- weight.size(2), ctx.stride[1], ctx.stride[0],
- ctx.padding[1], ctx.padding[0], ctx.dilation[1],
- ctx.dilation[0], ctx.groups, ctx.deformable_groups, 1,
- cur_im2col_step)
-
- return (grad_input, grad_offset, grad_weight, None, None, None, None, None)
-
- @staticmethod
- def _output_size(input, weight, padding, dilation, stride):
- channels = weight.size(0)
- output_size = (input.size(0), channels)
- for d in range(input.dim() - 2):
- in_size = input.size(d + 2)
- pad = padding[d]
- kernel = dilation[d] * (weight.size(d + 2) - 1) + 1
- stride_ = stride[d]
- output_size += ((in_size + (2 * pad) - kernel) // stride_ + 1, )
- if not all(map(lambda s: s > 0, output_size)):
- raise ValueError('convolution input is too small (output would be ' f'{"x".join(map(str, output_size))})')
- return output_size
-
-
-class ModulatedDeformConvFunction(Function):
-
- @staticmethod
- def forward(ctx,
- input,
- offset,
- mask,
- weight,
- bias=None,
- stride=1,
- padding=0,
- dilation=1,
- groups=1,
- deformable_groups=1):
- ctx.stride = stride
- ctx.padding = padding
- ctx.dilation = dilation
- ctx.groups = groups
- ctx.deformable_groups = deformable_groups
- ctx.with_bias = bias is not None
- if not ctx.with_bias:
- bias = input.new_empty(1) # fake tensor
- if not input.is_cuda:
- raise NotImplementedError
- if weight.requires_grad or mask.requires_grad or offset.requires_grad \
- or input.requires_grad:
- ctx.save_for_backward(input, offset, mask, weight, bias)
- output = input.new_empty(ModulatedDeformConvFunction._infer_shape(ctx, input, weight))
- ctx._bufs = [input.new_empty(0), input.new_empty(0)]
- deform_conv_ext.modulated_deform_conv_forward(input, weight, bias, ctx._bufs[0], offset, mask, output,
- ctx._bufs[1], weight.shape[2], weight.shape[3], ctx.stride,
- ctx.stride, ctx.padding, ctx.padding, ctx.dilation, ctx.dilation,
- ctx.groups, ctx.deformable_groups, ctx.with_bias)
- return output
-
- @staticmethod
- @once_differentiable
- def backward(ctx, grad_output):
- if not grad_output.is_cuda:
- raise NotImplementedError
- input, offset, mask, weight, bias = ctx.saved_tensors
- grad_input = torch.zeros_like(input)
- grad_offset = torch.zeros_like(offset)
- grad_mask = torch.zeros_like(mask)
- grad_weight = torch.zeros_like(weight)
- grad_bias = torch.zeros_like(bias)
- deform_conv_ext.modulated_deform_conv_backward(input, weight, bias, ctx._bufs[0], offset, mask, ctx._bufs[1],
- grad_input, grad_weight, grad_bias, grad_offset, grad_mask,
- grad_output, weight.shape[2], weight.shape[3], ctx.stride,
- ctx.stride, ctx.padding, ctx.padding, ctx.dilation, ctx.dilation,
- ctx.groups, ctx.deformable_groups, ctx.with_bias)
- if not ctx.with_bias:
- grad_bias = None
-
- return (grad_input, grad_offset, grad_mask, grad_weight, grad_bias, None, None, None, None, None)
-
- @staticmethod
- def _infer_shape(ctx, input, weight):
- n = input.size(0)
- channels_out = weight.size(0)
- height, width = input.shape[2:4]
- kernel_h, kernel_w = weight.shape[2:4]
- height_out = (height + 2 * ctx.padding - (ctx.dilation * (kernel_h - 1) + 1)) // ctx.stride + 1
- width_out = (width + 2 * ctx.padding - (ctx.dilation * (kernel_w - 1) + 1)) // ctx.stride + 1
- return n, channels_out, height_out, width_out
-
-
-deform_conv = DeformConvFunction.apply
-modulated_deform_conv = ModulatedDeformConvFunction.apply
-
-
-class DeformConv(nn.Module):
-
- def __init__(self,
- in_channels,
- out_channels,
- kernel_size,
- stride=1,
- padding=0,
- dilation=1,
- groups=1,
- deformable_groups=1,
- bias=False):
- super(DeformConv, self).__init__()
-
- assert not bias
- assert in_channels % groups == 0, \
- f'in_channels {in_channels} is not divisible by groups {groups}'
- assert out_channels % groups == 0, \
- f'out_channels {out_channels} is not divisible ' \
- f'by groups {groups}'
-
- self.in_channels = in_channels
- self.out_channels = out_channels
- self.kernel_size = _pair(kernel_size)
- self.stride = _pair(stride)
- self.padding = _pair(padding)
- self.dilation = _pair(dilation)
- self.groups = groups
- self.deformable_groups = deformable_groups
- # enable compatibility with nn.Conv2d
- self.transposed = False
- self.output_padding = _single(0)
-
- self.weight = nn.Parameter(torch.Tensor(out_channels, in_channels // self.groups, *self.kernel_size))
-
- self.reset_parameters()
-
- def reset_parameters(self):
- n = self.in_channels
- for k in self.kernel_size:
- n *= k
- stdv = 1. / math.sqrt(n)
- self.weight.data.uniform_(-stdv, stdv)
-
- def forward(self, x, offset):
- # To fix an assert error in deform_conv_cuda.cpp:128
- # input image is smaller than kernel
- input_pad = (x.size(2) < self.kernel_size[0] or x.size(3) < self.kernel_size[1])
- if input_pad:
- pad_h = max(self.kernel_size[0] - x.size(2), 0)
- pad_w = max(self.kernel_size[1] - x.size(3), 0)
- x = F.pad(x, (0, pad_w, 0, pad_h), 'constant', 0).contiguous()
- offset = F.pad(offset, (0, pad_w, 0, pad_h), 'constant', 0).contiguous()
- out = deform_conv(x, offset, self.weight, self.stride, self.padding, self.dilation, self.groups,
- self.deformable_groups)
- if input_pad:
- out = out[:, :, :out.size(2) - pad_h, :out.size(3) - pad_w].contiguous()
- return out
-
-
-class DeformConvPack(DeformConv):
- """A Deformable Conv Encapsulation that acts as normal Conv layers.
-
- Args:
- in_channels (int): Same as nn.Conv2d.
- out_channels (int): Same as nn.Conv2d.
- kernel_size (int or tuple[int]): Same as nn.Conv2d.
- stride (int or tuple[int]): Same as nn.Conv2d.
- padding (int or tuple[int]): Same as nn.Conv2d.
- dilation (int or tuple[int]): Same as nn.Conv2d.
- groups (int): Same as nn.Conv2d.
- bias (bool or str): If specified as `auto`, it will be decided by the
- norm_cfg. Bias will be set as True if norm_cfg is None, otherwise
- False.
- """
-
- _version = 2
-
- def __init__(self, *args, **kwargs):
- super(DeformConvPack, self).__init__(*args, **kwargs)
-
- self.conv_offset = nn.Conv2d(
- self.in_channels,
- self.deformable_groups * 2 * self.kernel_size[0] * self.kernel_size[1],
- kernel_size=self.kernel_size,
- stride=_pair(self.stride),
- padding=_pair(self.padding),
- dilation=_pair(self.dilation),
- bias=True)
- self.init_offset()
-
- def init_offset(self):
- self.conv_offset.weight.data.zero_()
- self.conv_offset.bias.data.zero_()
-
- def forward(self, x):
- offset = self.conv_offset(x)
- return deform_conv(x, offset, self.weight, self.stride, self.padding, self.dilation, self.groups,
- self.deformable_groups)
-
-
-class ModulatedDeformConv(nn.Module):
-
- def __init__(self,
- in_channels,
- out_channels,
- kernel_size,
- stride=1,
- padding=0,
- dilation=1,
- groups=1,
- deformable_groups=1,
- bias=True):
- super(ModulatedDeformConv, self).__init__()
- self.in_channels = in_channels
- self.out_channels = out_channels
- self.kernel_size = _pair(kernel_size)
- self.stride = stride
- self.padding = padding
- self.dilation = dilation
- self.groups = groups
- self.deformable_groups = deformable_groups
- self.with_bias = bias
- # enable compatibility with nn.Conv2d
- self.transposed = False
- self.output_padding = _single(0)
-
- self.weight = nn.Parameter(torch.Tensor(out_channels, in_channels // groups, *self.kernel_size))
- if bias:
- self.bias = nn.Parameter(torch.Tensor(out_channels))
- else:
- self.register_parameter('bias', None)
- self.init_weights()
-
- def init_weights(self):
- n = self.in_channels
- for k in self.kernel_size:
- n *= k
- stdv = 1. / math.sqrt(n)
- self.weight.data.uniform_(-stdv, stdv)
- if self.bias is not None:
- self.bias.data.zero_()
-
- def forward(self, x, offset, mask):
- return modulated_deform_conv(x, offset, mask, self.weight, self.bias, self.stride, self.padding, self.dilation,
- self.groups, self.deformable_groups)
-
-
-class ModulatedDeformConvPack(ModulatedDeformConv):
- """A ModulatedDeformable Conv Encapsulation that acts as normal Conv layers.
-
- Args:
- in_channels (int): Same as nn.Conv2d.
- out_channels (int): Same as nn.Conv2d.
- kernel_size (int or tuple[int]): Same as nn.Conv2d.
- stride (int or tuple[int]): Same as nn.Conv2d.
- padding (int or tuple[int]): Same as nn.Conv2d.
- dilation (int or tuple[int]): Same as nn.Conv2d.
- groups (int): Same as nn.Conv2d.
- bias (bool or str): If specified as `auto`, it will be decided by the
- norm_cfg. Bias will be set as True if norm_cfg is None, otherwise
- False.
- """
-
- _version = 2
-
- def __init__(self, *args, **kwargs):
- super(ModulatedDeformConvPack, self).__init__(*args, **kwargs)
-
- self.conv_offset = nn.Conv2d(
- self.in_channels,
- self.deformable_groups * 3 * self.kernel_size[0] * self.kernel_size[1],
- kernel_size=self.kernel_size,
- stride=_pair(self.stride),
- padding=_pair(self.padding),
- dilation=_pair(self.dilation),
- bias=True)
- self.init_weights()
-
- def init_weights(self):
- super(ModulatedDeformConvPack, self).init_weights()
- if hasattr(self, 'conv_offset'):
- self.conv_offset.weight.data.zero_()
- self.conv_offset.bias.data.zero_()
-
- def forward(self, x):
- out = self.conv_offset(x)
- o1, o2, mask = torch.chunk(out, 3, dim=1)
- offset = torch.cat((o1, o2), dim=1)
- mask = torch.sigmoid(mask)
- return modulated_deform_conv(x, offset, mask, self.weight, self.bias, self.stride, self.padding, self.dilation,
- self.groups, self.deformable_groups)
diff --git a/spaces/Fernando22/freegpt-webui/g4f/Provider/Provider.py b/spaces/Fernando22/freegpt-webui/g4f/Provider/Provider.py
deleted file mode 100644
index d24df76b6a6ccfc9b244f13a51bfc124b398a271..0000000000000000000000000000000000000000
--- a/spaces/Fernando22/freegpt-webui/g4f/Provider/Provider.py
+++ /dev/null
@@ -1,16 +0,0 @@
-import os
-from ..typing import sha256, Dict, get_type_hints
-
-url = None
-model = None
-supports_stream = False
-needs_auth = False
-
-
-def _create_completion(model: str, messages: list, stream: bool, **kwargs):
- return
-
-
-params = f'g4f.Providers.{os.path.basename(__file__)[:-3]} supports: ' + \
- '(%s)' % ', '.join(
- [f"{name}: {get_type_hints(_create_completion)[name].__name__}" for name in _create_completion.__code__.co_varnames[:_create_completion.__code__.co_argcount]])
diff --git a/spaces/FrankZxShen/vits-fast-finetuning-umamusume/text/korean.py b/spaces/FrankZxShen/vits-fast-finetuning-umamusume/text/korean.py
deleted file mode 100644
index edee07429a450c55e3d8e246997faaa1e0b89cc9..0000000000000000000000000000000000000000
--- a/spaces/FrankZxShen/vits-fast-finetuning-umamusume/text/korean.py
+++ /dev/null
@@ -1,210 +0,0 @@
-import re
-from jamo import h2j, j2hcj
-import ko_pron
-
-
-# This is a list of Korean classifiers preceded by pure Korean numerals.
-_korean_classifiers = '군데 권 개 그루 닢 대 두 마리 모 모금 뭇 발 발짝 방 번 벌 보루 살 수 술 시 쌈 움큼 정 짝 채 척 첩 축 켤레 톨 통'
-
-# List of (hangul, hangul divided) pairs:
-_hangul_divided = [(re.compile('%s' % x[0]), x[1]) for x in [
- ('ㄳ', 'ㄱㅅ'),
- ('ㄵ', 'ㄴㅈ'),
- ('ㄶ', 'ㄴㅎ'),
- ('ㄺ', 'ㄹㄱ'),
- ('ㄻ', 'ㄹㅁ'),
- ('ㄼ', 'ㄹㅂ'),
- ('ㄽ', 'ㄹㅅ'),
- ('ㄾ', 'ㄹㅌ'),
- ('ㄿ', 'ㄹㅍ'),
- ('ㅀ', 'ㄹㅎ'),
- ('ㅄ', 'ㅂㅅ'),
- ('ㅘ', 'ㅗㅏ'),
- ('ㅙ', 'ㅗㅐ'),
- ('ㅚ', 'ㅗㅣ'),
- ('ㅝ', 'ㅜㅓ'),
- ('ㅞ', 'ㅜㅔ'),
- ('ㅟ', 'ㅜㅣ'),
- ('ㅢ', 'ㅡㅣ'),
- ('ㅑ', 'ㅣㅏ'),
- ('ㅒ', 'ㅣㅐ'),
- ('ㅕ', 'ㅣㅓ'),
- ('ㅖ', 'ㅣㅔ'),
- ('ㅛ', 'ㅣㅗ'),
- ('ㅠ', 'ㅣㅜ')
-]]
-
-# List of (Latin alphabet, hangul) pairs:
-_latin_to_hangul = [(re.compile('%s' % x[0], re.IGNORECASE), x[1]) for x in [
- ('a', '에이'),
- ('b', '비'),
- ('c', '시'),
- ('d', '디'),
- ('e', '이'),
- ('f', '에프'),
- ('g', '지'),
- ('h', '에이치'),
- ('i', '아이'),
- ('j', '제이'),
- ('k', '케이'),
- ('l', '엘'),
- ('m', '엠'),
- ('n', '엔'),
- ('o', '오'),
- ('p', '피'),
- ('q', '큐'),
- ('r', '아르'),
- ('s', '에스'),
- ('t', '티'),
- ('u', '유'),
- ('v', '브이'),
- ('w', '더블유'),
- ('x', '엑스'),
- ('y', '와이'),
- ('z', '제트')
-]]
-
-# List of (ipa, lazy ipa) pairs:
-_ipa_to_lazy_ipa = [(re.compile('%s' % x[0], re.IGNORECASE), x[1]) for x in [
- ('t͡ɕ','ʧ'),
- ('d͡ʑ','ʥ'),
- ('ɲ','n^'),
- ('ɕ','ʃ'),
- ('ʷ','w'),
- ('ɭ','l`'),
- ('ʎ','ɾ'),
- ('ɣ','ŋ'),
- ('ɰ','ɯ'),
- ('ʝ','j'),
- ('ʌ','ə'),
- ('ɡ','g'),
- ('\u031a','#'),
- ('\u0348','='),
- ('\u031e',''),
- ('\u0320',''),
- ('\u0339','')
-]]
-
-
-def latin_to_hangul(text):
- for regex, replacement in _latin_to_hangul:
- text = re.sub(regex, replacement, text)
- return text
-
-
-def divide_hangul(text):
- text = j2hcj(h2j(text))
- for regex, replacement in _hangul_divided:
- text = re.sub(regex, replacement, text)
- return text
-
-
-def hangul_number(num, sino=True):
- '''Reference https://github.com/Kyubyong/g2pK'''
- num = re.sub(',', '', num)
-
- if num == '0':
- return '영'
- if not sino and num == '20':
- return '스무'
-
- digits = '123456789'
- names = '일이삼사오육칠팔구'
- digit2name = {d: n for d, n in zip(digits, names)}
-
- modifiers = '한 두 세 네 다섯 여섯 일곱 여덟 아홉'
- decimals = '열 스물 서른 마흔 쉰 예순 일흔 여든 아흔'
- digit2mod = {d: mod for d, mod in zip(digits, modifiers.split())}
- digit2dec = {d: dec for d, dec in zip(digits, decimals.split())}
-
- spelledout = []
- for i, digit in enumerate(num):
- i = len(num) - i - 1
- if sino:
- if i == 0:
- name = digit2name.get(digit, '')
- elif i == 1:
- name = digit2name.get(digit, '') + '십'
- name = name.replace('일십', '십')
- else:
- if i == 0:
- name = digit2mod.get(digit, '')
- elif i == 1:
- name = digit2dec.get(digit, '')
- if digit == '0':
- if i % 4 == 0:
- last_three = spelledout[-min(3, len(spelledout)):]
- if ''.join(last_three) == '':
- spelledout.append('')
- continue
- else:
- spelledout.append('')
- continue
- if i == 2:
- name = digit2name.get(digit, '') + '백'
- name = name.replace('일백', '백')
- elif i == 3:
- name = digit2name.get(digit, '') + '천'
- name = name.replace('일천', '천')
- elif i == 4:
- name = digit2name.get(digit, '') + '만'
- name = name.replace('일만', '만')
- elif i == 5:
- name = digit2name.get(digit, '') + '십'
- name = name.replace('일십', '십')
- elif i == 6:
- name = digit2name.get(digit, '') + '백'
- name = name.replace('일백', '백')
- elif i == 7:
- name = digit2name.get(digit, '') + '천'
- name = name.replace('일천', '천')
- elif i == 8:
- name = digit2name.get(digit, '') + '억'
- elif i == 9:
- name = digit2name.get(digit, '') + '십'
- elif i == 10:
- name = digit2name.get(digit, '') + '백'
- elif i == 11:
- name = digit2name.get(digit, '') + '천'
- elif i == 12:
- name = digit2name.get(digit, '') + '조'
- elif i == 13:
- name = digit2name.get(digit, '') + '십'
- elif i == 14:
- name = digit2name.get(digit, '') + '백'
- elif i == 15:
- name = digit2name.get(digit, '') + '천'
- spelledout.append(name)
- return ''.join(elem for elem in spelledout)
-
-
-def number_to_hangul(text):
- '''Reference https://github.com/Kyubyong/g2pK'''
- tokens = set(re.findall(r'(\d[\d,]*)([\uac00-\ud71f]+)', text))
- for token in tokens:
- num, classifier = token
- if classifier[:2] in _korean_classifiers or classifier[0] in _korean_classifiers:
- spelledout = hangul_number(num, sino=False)
- else:
- spelledout = hangul_number(num, sino=True)
- text = text.replace(f'{num}{classifier}', f'{spelledout}{classifier}')
- # digit by digit for remaining digits
- digits = '0123456789'
- names = '영일이삼사오육칠팔구'
- for d, n in zip(digits, names):
- text = text.replace(d, n)
- return text
-
-
-def korean_to_lazy_ipa(text):
- text = latin_to_hangul(text)
- text = number_to_hangul(text)
- text=re.sub('[\uac00-\ud7af]+',lambda x:ko_pron.romanise(x.group(0),'ipa').split('] ~ [')[0],text)
- for regex, replacement in _ipa_to_lazy_ipa:
- text = re.sub(regex, replacement, text)
- return text
-
-
-def korean_to_ipa(text):
- text = korean_to_lazy_ipa(text)
- return text.replace('ʧ','tʃ').replace('ʥ','dʑ')
diff --git a/spaces/Gradio-Blocks/uniformer_image_detection/mmdet/models/detectors/two_stage.py b/spaces/Gradio-Blocks/uniformer_image_detection/mmdet/models/detectors/two_stage.py
deleted file mode 100644
index ba5bdde980dc0cd76375455c9c7ffaae4b25531e..0000000000000000000000000000000000000000
--- a/spaces/Gradio-Blocks/uniformer_image_detection/mmdet/models/detectors/two_stage.py
+++ /dev/null
@@ -1,215 +0,0 @@
-import torch
-import torch.nn as nn
-
-# from mmdet.core import bbox2result, bbox2roi, build_assigner, build_sampler
-from ..builder import DETECTORS, build_backbone, build_head, build_neck
-from .base import BaseDetector
-
-
-@DETECTORS.register_module()
-class TwoStageDetector(BaseDetector):
- """Base class for two-stage detectors.
-
- Two-stage detectors typically consisting of a region proposal network and a
- task-specific regression head.
- """
-
- def __init__(self,
- backbone,
- neck=None,
- rpn_head=None,
- roi_head=None,
- train_cfg=None,
- test_cfg=None,
- pretrained=None):
- super(TwoStageDetector, self).__init__()
- self.backbone = build_backbone(backbone)
-
- if neck is not None:
- self.neck = build_neck(neck)
-
- if rpn_head is not None:
- rpn_train_cfg = train_cfg.rpn if train_cfg is not None else None
- rpn_head_ = rpn_head.copy()
- rpn_head_.update(train_cfg=rpn_train_cfg, test_cfg=test_cfg.rpn)
- self.rpn_head = build_head(rpn_head_)
-
- if roi_head is not None:
- # update train and test cfg here for now
- # TODO: refactor assigner & sampler
- rcnn_train_cfg = train_cfg.rcnn if train_cfg is not None else None
- roi_head.update(train_cfg=rcnn_train_cfg)
- roi_head.update(test_cfg=test_cfg.rcnn)
- self.roi_head = build_head(roi_head)
-
- self.train_cfg = train_cfg
- self.test_cfg = test_cfg
-
- self.init_weights(pretrained=pretrained)
-
- @property
- def with_rpn(self):
- """bool: whether the detector has RPN"""
- return hasattr(self, 'rpn_head') and self.rpn_head is not None
-
- @property
- def with_roi_head(self):
- """bool: whether the detector has a RoI head"""
- return hasattr(self, 'roi_head') and self.roi_head is not None
-
- def init_weights(self, pretrained=None):
- """Initialize the weights in detector.
-
- Args:
- pretrained (str, optional): Path to pre-trained weights.
- Defaults to None.
- """
- super(TwoStageDetector, self).init_weights(pretrained)
- self.backbone.init_weights(pretrained=pretrained)
- if self.with_neck:
- if isinstance(self.neck, nn.Sequential):
- for m in self.neck:
- m.init_weights()
- else:
- self.neck.init_weights()
- if self.with_rpn:
- self.rpn_head.init_weights()
- if self.with_roi_head:
- self.roi_head.init_weights(pretrained)
-
- def extract_feat(self, img):
- """Directly extract features from the backbone+neck."""
- x = self.backbone(img)
- if self.with_neck:
- x = self.neck(x)
- return x
-
- def forward_dummy(self, img):
- """Used for computing network flops.
-
- See `mmdetection/tools/analysis_tools/get_flops.py`
- """
- outs = ()
- # backbone
- x = self.extract_feat(img)
- # rpn
- if self.with_rpn:
- rpn_outs = self.rpn_head(x)
- outs = outs + (rpn_outs, )
- proposals = torch.randn(1000, 4).to(img.device)
- # roi_head
- roi_outs = self.roi_head.forward_dummy(x, proposals)
- outs = outs + (roi_outs, )
- return outs
-
- def forward_train(self,
- img,
- img_metas,
- gt_bboxes,
- gt_labels,
- gt_bboxes_ignore=None,
- gt_masks=None,
- proposals=None,
- **kwargs):
- """
- Args:
- img (Tensor): of shape (N, C, H, W) encoding input images.
- Typically these should be mean centered and std scaled.
-
- img_metas (list[dict]): list of image info dict where each dict
- has: 'img_shape', 'scale_factor', 'flip', and may also contain
- 'filename', 'ori_shape', 'pad_shape', and 'img_norm_cfg'.
- For details on the values of these keys see
- `mmdet/datasets/pipelines/formatting.py:Collect`.
-
- gt_bboxes (list[Tensor]): Ground truth bboxes for each image with
- shape (num_gts, 4) in [tl_x, tl_y, br_x, br_y] format.
-
- gt_labels (list[Tensor]): class indices corresponding to each box
-
- gt_bboxes_ignore (None | list[Tensor]): specify which bounding
- boxes can be ignored when computing the loss.
-
- gt_masks (None | Tensor) : true segmentation masks for each box
- used if the architecture supports a segmentation task.
-
- proposals : override rpn proposals with custom proposals. Use when
- `with_rpn` is False.
-
- Returns:
- dict[str, Tensor]: a dictionary of loss components
- """
- x = self.extract_feat(img)
-
- losses = dict()
-
- # RPN forward and loss
- if self.with_rpn:
- proposal_cfg = self.train_cfg.get('rpn_proposal',
- self.test_cfg.rpn)
- rpn_losses, proposal_list = self.rpn_head.forward_train(
- x,
- img_metas,
- gt_bboxes,
- gt_labels=None,
- gt_bboxes_ignore=gt_bboxes_ignore,
- proposal_cfg=proposal_cfg)
- losses.update(rpn_losses)
- else:
- proposal_list = proposals
-
- roi_losses = self.roi_head.forward_train(x, img_metas, proposal_list,
- gt_bboxes, gt_labels,
- gt_bboxes_ignore, gt_masks,
- **kwargs)
- losses.update(roi_losses)
-
- return losses
-
- async def async_simple_test(self,
- img,
- img_meta,
- proposals=None,
- rescale=False):
- """Async test without augmentation."""
- assert self.with_bbox, 'Bbox head must be implemented.'
- x = self.extract_feat(img)
-
- if proposals is None:
- proposal_list = await self.rpn_head.async_simple_test_rpn(
- x, img_meta)
- else:
- proposal_list = proposals
-
- return await self.roi_head.async_simple_test(
- x, proposal_list, img_meta, rescale=rescale)
-
- def simple_test(self, img, img_metas, proposals=None, rescale=False):
- """Test without augmentation."""
- assert self.with_bbox, 'Bbox head must be implemented.'
-
- x = self.extract_feat(img)
-
- # get origin input shape to onnx dynamic input shape
- if torch.onnx.is_in_onnx_export():
- img_shape = torch._shape_as_tensor(img)[2:]
- img_metas[0]['img_shape_for_onnx'] = img_shape
-
- if proposals is None:
- proposal_list = self.rpn_head.simple_test_rpn(x, img_metas)
- else:
- proposal_list = proposals
-
- return self.roi_head.simple_test(
- x, proposal_list, img_metas, rescale=rescale)
-
- def aug_test(self, imgs, img_metas, rescale=False):
- """Test with augmentations.
-
- If rescale is False, then returned bboxes and masks will fit the scale
- of imgs[0].
- """
- x = self.extract_feats(imgs)
- proposal_list = self.rpn_head.aug_test_rpn(x, img_metas)
- return self.roi_head.aug_test(
- x, proposal_list, img_metas, rescale=rescale)
diff --git a/spaces/Gradio-Blocks/uniformer_image_segmentation/mmseg/models/decode_heads/dnl_head.py b/spaces/Gradio-Blocks/uniformer_image_segmentation/mmseg/models/decode_heads/dnl_head.py
deleted file mode 100644
index 52a662ccb6ae8ff00930eb54ed71113724b6494e..0000000000000000000000000000000000000000
--- a/spaces/Gradio-Blocks/uniformer_image_segmentation/mmseg/models/decode_heads/dnl_head.py
+++ /dev/null
@@ -1,131 +0,0 @@
-import torch
-from mmcv.cnn import NonLocal2d
-from torch import nn
-
-from ..builder import HEADS
-from .fcn_head import FCNHead
-
-
-class DisentangledNonLocal2d(NonLocal2d):
- """Disentangled Non-Local Blocks.
-
- Args:
- temperature (float): Temperature to adjust attention. Default: 0.05
- """
-
- def __init__(self, *arg, temperature, **kwargs):
- super().__init__(*arg, **kwargs)
- self.temperature = temperature
- self.conv_mask = nn.Conv2d(self.in_channels, 1, kernel_size=1)
-
- def embedded_gaussian(self, theta_x, phi_x):
- """Embedded gaussian with temperature."""
-
- # NonLocal2d pairwise_weight: [N, HxW, HxW]
- pairwise_weight = torch.matmul(theta_x, phi_x)
- if self.use_scale:
- # theta_x.shape[-1] is `self.inter_channels`
- pairwise_weight /= theta_x.shape[-1]**0.5
- pairwise_weight /= self.temperature
- pairwise_weight = pairwise_weight.softmax(dim=-1)
- return pairwise_weight
-
- def forward(self, x):
- # x: [N, C, H, W]
- n = x.size(0)
-
- # g_x: [N, HxW, C]
- g_x = self.g(x).view(n, self.inter_channels, -1)
- g_x = g_x.permute(0, 2, 1)
-
- # theta_x: [N, HxW, C], phi_x: [N, C, HxW]
- if self.mode == 'gaussian':
- theta_x = x.view(n, self.in_channels, -1)
- theta_x = theta_x.permute(0, 2, 1)
- if self.sub_sample:
- phi_x = self.phi(x).view(n, self.in_channels, -1)
- else:
- phi_x = x.view(n, self.in_channels, -1)
- elif self.mode == 'concatenation':
- theta_x = self.theta(x).view(n, self.inter_channels, -1, 1)
- phi_x = self.phi(x).view(n, self.inter_channels, 1, -1)
- else:
- theta_x = self.theta(x).view(n, self.inter_channels, -1)
- theta_x = theta_x.permute(0, 2, 1)
- phi_x = self.phi(x).view(n, self.inter_channels, -1)
-
- # subtract mean
- theta_x -= theta_x.mean(dim=-2, keepdim=True)
- phi_x -= phi_x.mean(dim=-1, keepdim=True)
-
- pairwise_func = getattr(self, self.mode)
- # pairwise_weight: [N, HxW, HxW]
- pairwise_weight = pairwise_func(theta_x, phi_x)
-
- # y: [N, HxW, C]
- y = torch.matmul(pairwise_weight, g_x)
- # y: [N, C, H, W]
- y = y.permute(0, 2, 1).contiguous().reshape(n, self.inter_channels,
- *x.size()[2:])
-
- # unary_mask: [N, 1, HxW]
- unary_mask = self.conv_mask(x)
- unary_mask = unary_mask.view(n, 1, -1)
- unary_mask = unary_mask.softmax(dim=-1)
- # unary_x: [N, 1, C]
- unary_x = torch.matmul(unary_mask, g_x)
- # unary_x: [N, C, 1, 1]
- unary_x = unary_x.permute(0, 2, 1).contiguous().reshape(
- n, self.inter_channels, 1, 1)
-
- output = x + self.conv_out(y + unary_x)
-
- return output
-
-
-@HEADS.register_module()
-class DNLHead(FCNHead):
- """Disentangled Non-Local Neural Networks.
-
- This head is the implementation of `DNLNet
- `_.
-
- Args:
- reduction (int): Reduction factor of projection transform. Default: 2.
- use_scale (bool): Whether to scale pairwise_weight by
- sqrt(1/inter_channels). Default: False.
- mode (str): The nonlocal mode. Options are 'embedded_gaussian',
- 'dot_product'. Default: 'embedded_gaussian.'.
- temperature (float): Temperature to adjust attention. Default: 0.05
- """
-
- def __init__(self,
- reduction=2,
- use_scale=True,
- mode='embedded_gaussian',
- temperature=0.05,
- **kwargs):
- super(DNLHead, self).__init__(num_convs=2, **kwargs)
- self.reduction = reduction
- self.use_scale = use_scale
- self.mode = mode
- self.temperature = temperature
- self.dnl_block = DisentangledNonLocal2d(
- in_channels=self.channels,
- reduction=self.reduction,
- use_scale=self.use_scale,
- conv_cfg=self.conv_cfg,
- norm_cfg=self.norm_cfg,
- mode=self.mode,
- temperature=self.temperature)
-
- def forward(self, inputs):
- """Forward function."""
- x = self._transform_inputs(inputs)
- output = self.convs[0](x)
- output = self.dnl_block(output)
- output = self.convs[1](output)
- if self.concat_input:
- output = self.conv_cat(torch.cat([x, output], dim=1))
- output = self.cls_seg(output)
- return output
diff --git a/spaces/GrandaddyShmax/AudioCraft_Plus/audiocraft/adversarial/discriminators/__init__.py b/spaces/GrandaddyShmax/AudioCraft_Plus/audiocraft/adversarial/discriminators/__init__.py
deleted file mode 100644
index f9e5ff59950ee0b1d1a67c9b3831d67d08048148..0000000000000000000000000000000000000000
--- a/spaces/GrandaddyShmax/AudioCraft_Plus/audiocraft/adversarial/discriminators/__init__.py
+++ /dev/null
@@ -1,10 +0,0 @@
-# Copyright (c) Meta Platforms, Inc. and affiliates.
-# All rights reserved.
-#
-# This source code is licensed under the license found in the
-# LICENSE file in the root directory of this source tree.
-
-# flake8: noqa
-from .mpd import MultiPeriodDiscriminator
-from .msd import MultiScaleDiscriminator
-from .msstftd import MultiScaleSTFTDiscriminator
diff --git a/spaces/GrandaddyShmax/AudioCraft_Plus/audiocraft/metrics/__init__.py b/spaces/GrandaddyShmax/AudioCraft_Plus/audiocraft/metrics/__init__.py
deleted file mode 100644
index 3474bdc4f1c88b21904d2a21ba077c93a8a70c8b..0000000000000000000000000000000000000000
--- a/spaces/GrandaddyShmax/AudioCraft_Plus/audiocraft/metrics/__init__.py
+++ /dev/null
@@ -1,14 +0,0 @@
-# Copyright (c) Meta Platforms, Inc. and affiliates.
-# All rights reserved.
-#
-# This source code is licensed under the license found in the
-# LICENSE file in the root directory of this source tree.
-"""Metrics like CLAP score, FAD, KLD, Visqol, Chroma similarity, etc.
-"""
-# flake8: noqa
-from .clap_consistency import CLAPTextConsistencyMetric, TextConsistencyMetric
-from .chroma_cosinesim import ChromaCosineSimilarityMetric
-from .fad import FrechetAudioDistanceMetric
-from .kld import KLDivergenceMetric, PasstKLDivergenceMetric
-from .rvm import RelativeVolumeMel
-from .visqol import ViSQOL
diff --git a/spaces/GrandaddyShmax/AudioCraft_Plus/scripts/mos.py b/spaces/GrandaddyShmax/AudioCraft_Plus/scripts/mos.py
deleted file mode 100644
index a711c9ece23e72ed3a07032c7834ef7c56ab4f11..0000000000000000000000000000000000000000
--- a/spaces/GrandaddyShmax/AudioCraft_Plus/scripts/mos.py
+++ /dev/null
@@ -1,286 +0,0 @@
-# Copyright (c) Meta Platforms, Inc. and affiliates.
-# All rights reserved.
-#
-# This source code is licensed under the license found in the
-# LICENSE file in the root directory of this source tree.
-
-
-"""
-To run this script, from the root of the repo. Make sure to have Flask installed
-
- FLASK_DEBUG=1 FLASK_APP=scripts.mos flask run -p 4567
- # or if you have gunicorn
- gunicorn -w 4 -b 127.0.0.1:8895 -t 120 'scripts.mos:app' --access-logfile -
-
-"""
-from collections import defaultdict
-from functools import wraps
-from hashlib import sha1
-import json
-import math
-from pathlib import Path
-import random
-import typing as tp
-
-from flask import Flask, redirect, render_template, request, session, url_for
-
-from audiocraft import train
-from audiocraft.utils.samples.manager import get_samples_for_xps
-
-
-SAMPLES_PER_PAGE = 8
-MAX_RATING = 5
-storage = Path(train.main.dora.dir / 'mos_storage')
-storage.mkdir(exist_ok=True)
-surveys = storage / 'surveys'
-surveys.mkdir(exist_ok=True)
-magma_root = Path(train.__file__).parent.parent
-app = Flask('mos', static_folder=str(magma_root / 'scripts/static'),
- template_folder=str(magma_root / 'scripts/templates'))
-app.secret_key = b'audiocraft makes the best songs'
-
-
-def normalize_path(path: Path):
- """Just to make path a bit nicer, make them relative to the Dora root dir.
- """
- path = path.resolve()
- dora_dir = train.main.dora.dir.resolve() / 'xps'
- return path.relative_to(dora_dir)
-
-
-def get_full_path(normalized_path: Path):
- """Revert `normalize_path`.
- """
- return train.main.dora.dir.resolve() / 'xps' / normalized_path
-
-
-def get_signature(xps: tp.List[str]):
- """Return a signature for a list of XP signatures.
- """
- return sha1(json.dumps(xps).encode()).hexdigest()[:10]
-
-
-def ensure_logged(func):
- """Ensure user is logged in.
- """
- @wraps(func)
- def _wrapped(*args, **kwargs):
- user = session.get('user')
- if user is None:
- return redirect(url_for('login', redirect_to=request.url))
- return func(*args, **kwargs)
- return _wrapped
-
-
-@app.route('/login', methods=['GET', 'POST'])
-def login():
- """Login user if not already, then redirect.
- """
- user = session.get('user')
- if user is None:
- error = None
- if request.method == 'POST':
- user = request.form['user']
- if not user:
- error = 'User cannot be empty'
- if user is None or error:
- return render_template('login.html', error=error)
- assert user
- session['user'] = user
- redirect_to = request.args.get('redirect_to')
- if redirect_to is None:
- redirect_to = url_for('index')
- return redirect(redirect_to)
-
-
-@app.route('/', methods=['GET', 'POST'])
-@ensure_logged
-def index():
- """Offer to create a new study.
- """
- errors = []
- if request.method == 'POST':
- xps_or_grids = [part.strip() for part in request.form['xps'].split()]
- xps = set()
- for xp_or_grid in xps_or_grids:
- xp_path = train.main.dora.dir / 'xps' / xp_or_grid
- if xp_path.exists():
- xps.add(xp_or_grid)
- continue
- grid_path = train.main.dora.dir / 'grids' / xp_or_grid
- if grid_path.exists():
- for child in grid_path.iterdir():
- if child.is_symlink():
- xps.add(child.name)
- continue
- errors.append(f'{xp_or_grid} is neither an XP nor a grid!')
- assert xps or errors
- blind = 'true' if request.form.get('blind') == 'on' else 'false'
- xps = list(xps)
- if not errors:
- signature = get_signature(xps)
- manifest = {
- 'xps': xps,
- }
- survey_path = surveys / signature
- survey_path.mkdir(exist_ok=True)
- with open(survey_path / 'manifest.json', 'w') as f:
- json.dump(manifest, f, indent=2)
- return redirect(url_for('survey', blind=blind, signature=signature))
- return render_template('index.html', errors=errors)
-
-
-@app.route('/survey/', methods=['GET', 'POST'])
-@ensure_logged
-def survey(signature):
- success = request.args.get('success', False)
- seed = int(request.args.get('seed', 4321))
- blind = request.args.get('blind', 'false') in ['true', 'on', 'True']
- exclude_prompted = request.args.get('exclude_prompted', 'false') in ['true', 'on', 'True']
- exclude_unprompted = request.args.get('exclude_unprompted', 'false') in ['true', 'on', 'True']
- max_epoch = int(request.args.get('max_epoch', '-1'))
- survey_path = surveys / signature
- assert survey_path.exists(), survey_path
-
- user = session['user']
- result_folder = survey_path / 'results'
- result_folder.mkdir(exist_ok=True)
- result_file = result_folder / f'{user}_{seed}.json'
-
- with open(survey_path / 'manifest.json') as f:
- manifest = json.load(f)
-
- xps = [train.main.get_xp_from_sig(xp) for xp in manifest['xps']]
- names, ref_name = train.main.get_names(xps)
-
- samples_kwargs = {
- 'exclude_prompted': exclude_prompted,
- 'exclude_unprompted': exclude_unprompted,
- 'max_epoch': max_epoch,
- }
- matched_samples = get_samples_for_xps(xps, epoch=-1, **samples_kwargs) # fetch latest epoch
- models_by_id = {
- id: [{
- 'xp': xps[idx],
- 'xp_name': names[idx],
- 'model_id': f'{xps[idx].sig}-{sample.id}',
- 'sample': sample,
- 'is_prompted': sample.prompt is not None,
- 'errors': [],
- } for idx, sample in enumerate(samples)]
- for id, samples in matched_samples.items()
- }
- experiments = [
- {'xp': xp, 'name': names[idx], 'epoch': list(matched_samples.values())[0][idx].epoch}
- for idx, xp in enumerate(xps)
- ]
-
- keys = list(matched_samples.keys())
- keys.sort()
- rng = random.Random(seed)
- rng.shuffle(keys)
- model_ids = keys[:SAMPLES_PER_PAGE]
-
- if blind:
- for key in model_ids:
- rng.shuffle(models_by_id[key])
-
- ok = True
- if request.method == 'POST':
- all_samples_results = []
- for id in model_ids:
- models = models_by_id[id]
- result = {
- 'id': id,
- 'is_prompted': models[0]['is_prompted'],
- 'models': {}
- }
- all_samples_results.append(result)
- for model in models:
- rating = request.form[model['model_id']]
- if rating:
- rating = int(rating)
- assert rating <= MAX_RATING and rating >= 1
- result['models'][model['xp'].sig] = rating
- model['rating'] = rating
- else:
- ok = False
- model['errors'].append('Please rate this model.')
- if ok:
- result = {
- 'results': all_samples_results,
- 'seed': seed,
- 'user': user,
- 'blind': blind,
- 'exclude_prompted': exclude_prompted,
- 'exclude_unprompted': exclude_unprompted,
- }
- print(result)
- with open(result_file, 'w') as f:
- json.dump(result, f)
- seed = seed + 1
- return redirect(url_for(
- 'survey', signature=signature, blind=blind, seed=seed,
- exclude_prompted=exclude_prompted, exclude_unprompted=exclude_unprompted,
- max_epoch=max_epoch, success=True))
-
- ratings = list(range(1, MAX_RATING + 1))
- return render_template(
- 'survey.html', ratings=ratings, blind=blind, seed=seed, signature=signature, success=success,
- exclude_prompted=exclude_prompted, exclude_unprompted=exclude_unprompted, max_epoch=max_epoch,
- experiments=experiments, models_by_id=models_by_id, model_ids=model_ids, errors=[],
- ref_name=ref_name, already_filled=result_file.exists())
-
-
-@app.route('/audio/')
-def audio(path: str):
- full_path = Path('/') / path
- assert full_path.suffix in [".mp3", ".wav"]
- return full_path.read_bytes(), {'Content-Type': 'audio/mpeg'}
-
-
-def mean(x):
- return sum(x) / len(x)
-
-
-def std(x):
- m = mean(x)
- return math.sqrt(sum((i - m)**2 for i in x) / len(x))
-
-
-@app.route('/results/')
-@ensure_logged
-def results(signature):
-
- survey_path = surveys / signature
- assert survey_path.exists(), survey_path
- result_folder = survey_path / 'results'
- result_folder.mkdir(exist_ok=True)
-
- # ratings per model, then per user.
- ratings_per_model = defaultdict(list)
- users = []
- for result_file in result_folder.iterdir():
- if result_file.suffix != '.json':
- continue
- with open(result_file) as f:
- results = json.load(f)
- users.append(results['user'])
- for result in results['results']:
- for sig, rating in result['models'].items():
- ratings_per_model[sig].append(rating)
-
- fmt = '{:.2f}'
- models = []
- for model in sorted(ratings_per_model.keys()):
- ratings = ratings_per_model[model]
-
- models.append({
- 'sig': model,
- 'samples': len(ratings),
- 'mean_rating': fmt.format(mean(ratings)),
- # the value 1.96 was probably chosen to achieve some
- # confidence interval assuming gaussianity.
- 'std_rating': fmt.format(1.96 * std(ratings) / len(ratings)**0.5),
- })
- return render_template('results.html', signature=signature, models=models, users=users)
diff --git a/spaces/Grezz/generate_human_motion/VQ-Trans/models/pos_encoding.py b/spaces/Grezz/generate_human_motion/VQ-Trans/models/pos_encoding.py
deleted file mode 100644
index 066be3e1f8a1636f7eaabd1c534b9c618ee3e9f8..0000000000000000000000000000000000000000
--- a/spaces/Grezz/generate_human_motion/VQ-Trans/models/pos_encoding.py
+++ /dev/null
@@ -1,43 +0,0 @@
-"""
-Various positional encodings for the transformer.
-"""
-import math
-import torch
-from torch import nn
-
-def PE1d_sincos(seq_length, dim):
- """
- :param d_model: dimension of the model
- :param length: length of positions
- :return: length*d_model position matrix
- """
- if dim % 2 != 0:
- raise ValueError("Cannot use sin/cos positional encoding with "
- "odd dim (got dim={:d})".format(dim))
- pe = torch.zeros(seq_length, dim)
- position = torch.arange(0, seq_length).unsqueeze(1)
- div_term = torch.exp((torch.arange(0, dim, 2, dtype=torch.float) *
- -(math.log(10000.0) / dim)))
- pe[:, 0::2] = torch.sin(position.float() * div_term)
- pe[:, 1::2] = torch.cos(position.float() * div_term)
-
- return pe.unsqueeze(1)
-
-
-class PositionEmbedding(nn.Module):
- """
- Absolute pos embedding (standard), learned.
- """
- def __init__(self, seq_length, dim, dropout, grad=False):
- super().__init__()
- self.embed = nn.Parameter(data=PE1d_sincos(seq_length, dim), requires_grad=grad)
- self.dropout = nn.Dropout(p=dropout)
-
- def forward(self, x):
- # x.shape: bs, seq_len, feat_dim
- l = x.shape[1]
- x = x.permute(1, 0, 2) + self.embed[:l].expand(x.permute(1, 0, 2).shape)
- x = self.dropout(x.permute(1, 0, 2))
- return x
-
-
\ No newline at end of file
diff --git a/spaces/HCMUT-GraduateThesis-HNTThinh/rgbdsod-multimae-demo/s_multimae/generate_saliency_maps.py b/spaces/HCMUT-GraduateThesis-HNTThinh/rgbdsod-multimae-demo/s_multimae/generate_saliency_maps.py
deleted file mode 100644
index a1cbee97c5d425c44d07a8f944208e7c62acc100..0000000000000000000000000000000000000000
--- a/spaces/HCMUT-GraduateThesis-HNTThinh/rgbdsod-multimae-demo/s_multimae/generate_saliency_maps.py
+++ /dev/null
@@ -1,113 +0,0 @@
-from typing import List
-import torch, os
-from tqdm import tqdm
-from torch.utils.data import DataLoader
-from torch import nn, Tensor
-import cv2
-import numpy as np
-import torch.nn.functional as F
-
-from .rgbd_model import RGBDModel
-from .configs.base_config import base_cfg
-from .checkpoint import load_checkpoint
-from .utils import clean_dir
-from .dataset_fn import TestDataset
-from .device import device
-
-@torch.no_grad()
-def generate_saliency_maps_per_dataloader(
- cfg: base_cfg,
- dataloader: DataLoader,
- model: RGBDModel,
- save_dataset_dir: str,
- is_padding: bool = True, is_fp16: bool = False
-) -> None:
- os.makedirs(save_dataset_dir, exist_ok=True)
- for i_batch, (images, depths, gts, indices, image_sizes, image_names) in tqdm(
- enumerate(dataloader), total=len(dataloader.dataset) // dataloader.batch_size
- ):
- gpu_images: Tensor = images.to(device)
- gpu_depths: Tensor = depths.to(device)
- gpu_gts: Tensor = depths.to(device)
- with torch.cuda.amp.autocast(enabled=is_fp16):
- preds_no_sigmoid: Tensor = model.inference(gpu_images, gpu_depths)
-
- for pred_no_sigmoid, image_name, image_size in zip(preds_no_sigmoid, image_names, image_sizes):
- if is_padding:
- w, h = image_size.numpy()
- k = max(w, h)
- res: Tensor = F.interpolate(
- pred_no_sigmoid.unsqueeze(0), size=(k, k),
- mode='bilinear', align_corners=False
- )
- res = res[:, :, int((k-h)/2.): int((k+h)/2.), int((k-w)/2.): int((k+w)/2.)]
- else:
- res: Tensor = F.interpolate(
- pred_no_sigmoid.unsqueeze(0), size=(image_size[1], image_size[0]),
- mode='bilinear', align_corners=False
- )
- res = res.sigmoid().data.cpu().numpy().squeeze()
- res = (res - res.min()) / (res.max() - res.min() + 1e-8)
-
- if is_fp16:
- res = np.float32(res)
- cv2.imwrite(os.path.join(save_dataset_dir, image_name),res*255)
-
- del gpu_images, gpu_depths, gpu_gts, preds_no_sigmoid
-
-def get_experiment_saliency_maps_working_dir(cfg: base_cfg, epoch: int) -> str:
- rs = f'{cfg.experiment_name}_epoch{epoch}{"_padding" if cfg.is_padding_for_test else ""}'
- if cfg.is_inference_with_no_depth:
- rs += '_nodepth'
- return rs
-
-@torch.no_grad()
-def generate_saliency_maps(
- cfg: base_cfg, model: nn.Module,
- epochs_lst: List[int], # List of epochs [400, 500, ...]
- data_augmentation_version: int,
- set_version: int = 1, # Set version 1, 2
-) -> List[str]:
- experiment_names: List[str] = []
-
- test_dataset_names = cfg.test_dataset_names
-
- for epoch in epochs_lst:
- ckpt_path = os.path.join(cfg.experiment_dir_path, cfg.experiment_name, f'checkpoint_{epoch}.pt')
- load_checkpoint(model, None, None, None, ckpt_path)
- experiment_name = get_experiment_saliency_maps_working_dir(cfg, epoch)
- experiment_names.append(experiment_name)
- experiment_saliency_maps_working_dir = os.path.join(
- cfg.sotas_working_dir, experiment_name
- )
- clean_dir(experiment_saliency_maps_working_dir)
- print(f'Output to directory {experiment_saliency_maps_working_dir}')
-
- model.to(device)
- model.eval()
-
- batch_size = cfg.test_batch_size
-
- dataset_working_dir_paths: List[str] = [
- os.path.join(cfg.test_datasets_working_dir_path, dataset_name) \
- for dataset_name in test_dataset_names
- ] # Colab
-
- for dataset_name in test_dataset_names:
- print(f'Dataset {dataset_name}')
- dataset_working_dir_path = os.path.join(
- cfg.test_datasets_working_dir_path, dataset_name
- )
- dataset = TestDataset(cfg, dataset_working_dir_path)
- dataloader = DataLoader(
- dataset, batch_size=batch_size,
- shuffle=False, num_workers=cfg.num_workers
- )
- generate_saliency_maps_per_dataloader(
- cfg, dataloader, model,
- os.path.join(experiment_saliency_maps_working_dir, dataset_name),
- is_fp16 = cfg.is_fp16,
- is_padding = cfg.is_padding_for_test
- )
- return experiment_names
-
\ No newline at end of file
diff --git a/spaces/HaloMaster/chinesesummary/fengshen/data/hubert/hubert_dataset.py b/spaces/HaloMaster/chinesesummary/fengshen/data/hubert/hubert_dataset.py
deleted file mode 100644
index d8eaa25a5238740cc86a05af257aa3e0996f1499..0000000000000000000000000000000000000000
--- a/spaces/HaloMaster/chinesesummary/fengshen/data/hubert/hubert_dataset.py
+++ /dev/null
@@ -1,361 +0,0 @@
-# Copyright (c) Facebook, Inc. and its affiliates.
-#
-# This source code is licensed under the MIT license found in the
-# LICENSE file in the root directory of this source tree.
-
-import itertools
-import logging
-import os
-import sys
-from typing import Any, List, Optional, Union
-
-import numpy as np
-
-import torch
-import torch.nn.functional as F
-from fairseq.data import data_utils
-from fairseq.data.fairseq_dataset import FairseqDataset
-
-logger = logging.getLogger(__name__)
-
-
-def add_data_specific_args(parent_args):
- parser = parent_args.add_argument_group('Hubert Dataset')
- parser.add_argument('--data', type=str)
- parser.add_argument('--sample_rate', type=float, default=16000)
- parser.add_argument('--label_dir', type=str)
- parser.add_argument('--labels', type=str, nargs='+')
- parser.add_argument('--label_rate', type=float)
- parser.add_argument('--max_keep_size', type=int, default=None)
- parser.add_argument('--min_sample_size', type=int)
- parser.add_argument('--max_sample_size', type=int)
- parser.add_argument('--pad_audio', type=bool)
- parser.add_argument('--normalize', type=bool)
- parser.add_argument('--random_crop', type=bool)
- parser.add_argument('--single_target', type=bool, default=False)
- return parent_args
-
-
-def load_audio(manifest_path, max_keep, min_keep):
- n_long, n_short = 0, 0
- names, inds, sizes = [], [], []
- with open(manifest_path) as f:
- root = f.readline().strip()
- for ind, line in enumerate(f):
- items = line.strip().split("\t")
- assert len(items) == 2, line
- sz = int(items[1])
- if min_keep is not None and sz < min_keep:
- n_short += 1
- elif max_keep is not None and sz > max_keep:
- n_long += 1
- else:
- names.append(items[0])
- inds.append(ind)
- sizes.append(sz)
- tot = ind + 1
- logger.info(
- (
- f"max_keep={max_keep}, min_keep={min_keep}, "
- f"loaded {len(names)}, skipped {n_short} short and {n_long} long, "
- f"longest-loaded={max(sizes)}, shortest-loaded={min(sizes)}"
- )
- )
- return root, names, inds, tot, sizes
-
-
-def load_label(label_path, inds, tot):
- with open(label_path) as f:
- labels = [line.rstrip() for line in f]
- assert (
- len(labels) == tot
- ), f"number of labels does not match ({len(labels)} != {tot})"
- labels = [labels[i] for i in inds]
- return labels
-
-
-def load_label_offset(label_path, inds, tot):
- with open(label_path) as f:
- code_lengths = [len(line.encode("utf-8")) for line in f]
- assert (
- len(code_lengths) == tot
- ), f"number of labels does not match ({len(code_lengths)} != {tot})"
- offsets = list(itertools.accumulate([0] + code_lengths))
- offsets = [(offsets[i], offsets[i + 1]) for i in inds]
- return offsets
-
-
-def verify_label_lengths(
- audio_sizes,
- audio_rate,
- label_path,
- label_rate,
- inds,
- tot,
- tol=0.1, # tolerance in seconds
-):
- if label_rate < 0:
- logger.info(f"{label_path} is sequence label. skipped")
- return
-
- with open(label_path) as f:
- lengths = [len(line.rstrip().split()) for line in f]
- assert len(lengths) == tot
- lengths = [lengths[i] for i in inds]
- num_invalid = 0
- for i, ind in enumerate(inds):
- dur_from_audio = audio_sizes[i] / audio_rate
- dur_from_label = lengths[i] / label_rate
- if abs(dur_from_audio - dur_from_label) > tol:
- logger.warning(
- (
- f"audio and label duration differ too much "
- f"(|{dur_from_audio} - {dur_from_label}| > {tol}) "
- f"in line {ind+1} of {label_path}. Check if `label_rate` "
- f"is correctly set (currently {label_rate}). "
- f"num. of samples = {audio_sizes[i]}; "
- f"label length = {lengths[i]}"
- )
- )
- num_invalid += 1
- if num_invalid > 0:
- logger.warning(
- f"total {num_invalid} (audio, label) pairs with mismatched lengths"
- )
-
-
-class HubertDataset(FairseqDataset):
- def __init__(
- self,
- manifest_path: str,
- sample_rate: float,
- label_paths: List[str],
- label_rates: Union[List[float], float], # -1 for sequence labels
- pad_list: List[str],
- eos_list: List[str],
- label_processors: Optional[List[Any]] = None,
- max_keep_sample_size: Optional[int] = None,
- min_keep_sample_size: Optional[int] = None,
- max_sample_size: Optional[int] = None,
- shuffle: bool = True,
- pad_audio: bool = False,
- normalize: bool = False,
- store_labels: bool = True,
- random_crop: bool = False,
- single_target: bool = False,
- ):
- self.audio_root, self.audio_names, inds, tot, self.sizes = load_audio(
- manifest_path, max_keep_sample_size, min_keep_sample_size
- )
- self.sample_rate = sample_rate
- self.shuffle = shuffle
- self.random_crop = random_crop
-
- self.num_labels = len(label_paths)
- self.pad_list = pad_list
- self.eos_list = eos_list
- self.label_processors = label_processors
- self.single_target = single_target
- self.label_rates = (
- [label_rates for _ in range(len(label_paths))]
- if isinstance(label_rates, float)
- else label_rates
- )
- self.store_labels = store_labels
- if store_labels:
- self.label_list = [load_label(p, inds, tot) for p in label_paths]
- else:
- self.label_paths = label_paths
- self.label_offsets_list = [
- load_label_offset(p, inds, tot) for p in label_paths
- ]
- assert label_processors is None or len(label_processors) == self.num_labels
- for label_path, label_rate in zip(label_paths, self.label_rates):
- verify_label_lengths(
- self.sizes, sample_rate, label_path, label_rate, inds, tot
- )
-
- self.max_sample_size = (
- max_sample_size if max_sample_size is not None else sys.maxsize
- )
- self.pad_audio = pad_audio
- self.normalize = normalize
- logger.info(
- f"pad_audio={pad_audio}, random_crop={random_crop}, "
- f"normalize={normalize}, max_sample_size={self.max_sample_size}"
- )
-
- def get_audio(self, index):
- import soundfile as sf
-
- wav_path = os.path.join(self.audio_root, self.audio_names[index])
- wav, cur_sample_rate = sf.read(wav_path)
- wav = torch.from_numpy(wav).float()
- wav = self.postprocess(wav, cur_sample_rate)
- return wav
-
- def get_label(self, index, label_idx):
- if self.store_labels:
- label = self.label_list[label_idx][index]
- else:
- with open(self.label_paths[label_idx]) as f:
- offset_s, offset_e = self.label_offsets_list[label_idx][index]
- f.seek(offset_s)
- label = f.read(offset_e - offset_s)
-
- if self.label_processors is not None:
- label = self.label_processors[label_idx](label)
- return label
-
- def get_labels(self, index):
- return [self.get_label(index, i) for i in range(self.num_labels)]
-
- def __getitem__(self, index):
- wav = self.get_audio(index)
- labels = self.get_labels(index)
- return {"id": index, "source": wav, "label_list": labels}
-
- def __len__(self):
- return len(self.sizes)
-
- def crop_to_max_size(self, wav, target_size):
- size = len(wav)
- diff = size - target_size
- if diff <= 0:
- return wav, 0
-
- start, end = 0, target_size
- if self.random_crop:
- start = np.random.randint(0, diff + 1)
- end = size - diff + start
- return wav[start:end], start
-
- def collater(self, samples):
- # target = max(sizes) -> random_crop not used
- # target = max_sample_size -> random_crop used for long
- samples = [s for s in samples if s["source"] is not None]
- if len(samples) == 0:
- return {}
-
- audios = [s["source"] for s in samples]
- audio_sizes = [len(s) for s in audios]
- if self.pad_audio:
- audio_size = min(max(audio_sizes), self.max_sample_size)
- else:
- audio_size = min(min(audio_sizes), self.max_sample_size)
- collated_audios, padding_mask, audio_starts = self.collater_audio(
- audios, audio_size
- )
-
- targets_by_label = [
- [s["label_list"][i] for s in samples] for i in range(self.num_labels)
- ]
- targets_list, lengths_list, ntokens_list = self.collater_label(
- targets_by_label, audio_size, audio_starts
- )
-
- net_input = {"source": collated_audios, "padding_mask": padding_mask}
- batch = {
- "id": torch.LongTensor([s["id"] for s in samples]),
- "net_input": net_input,
- }
-
- if self.single_target:
- batch["target_lengths"] = lengths_list[0]
- batch["ntokens"] = ntokens_list[0]
- batch["target"] = targets_list[0]
- else:
- batch["target_lengths_list"] = lengths_list
- batch["ntokens_list"] = ntokens_list
- batch["target_list"] = targets_list
- return batch
-
- def collater_audio(self, audios, audio_size):
- collated_audios = audios[0].new_zeros(len(audios), audio_size)
- padding_mask = (
- torch.BoolTensor(collated_audios.shape).fill_(False)
- # if self.pad_audio else None
- )
- audio_starts = [0 for _ in audios]
- for i, audio in enumerate(audios):
- diff = len(audio) - audio_size
- if diff == 0:
- collated_audios[i] = audio
- elif diff < 0:
- assert self.pad_audio
- collated_audios[i] = torch.cat([audio, audio.new_full((-diff,), 0.0)])
- padding_mask[i, diff:] = True
- else:
- collated_audios[i], audio_starts[i] = self.crop_to_max_size(
- audio, audio_size
- )
- return collated_audios, padding_mask, audio_starts
-
- def collater_frm_label(self, targets, audio_size, audio_starts, label_rate, pad):
- assert label_rate > 0
- s2f = label_rate / self.sample_rate
- frm_starts = [int(round(s * s2f)) for s in audio_starts]
- frm_size = int(round(audio_size * s2f))
- if not self.pad_audio:
- rem_size = [len(t) - s for t, s in zip(targets, frm_starts)]
- frm_size = min(frm_size, *rem_size)
- targets = [t[s: s + frm_size] for t, s in zip(targets, frm_starts)]
- logger.debug(f"audio_starts={audio_starts}")
- logger.debug(f"frame_starts={frm_starts}")
- logger.debug(f"frame_size={frm_size}")
-
- lengths = torch.LongTensor([len(t) for t in targets])
- ntokens = lengths.sum().item()
- targets = data_utils.collate_tokens(targets, pad_idx=pad, left_pad=False)
- return targets, lengths, ntokens
-
- def collater_seq_label(self, targets, pad):
- lengths = torch.LongTensor([len(t) for t in targets])
- ntokens = lengths.sum().item()
- targets = data_utils.collate_tokens(targets, pad_idx=pad, left_pad=False)
- return targets, lengths, ntokens
-
- def collater_label(self, targets_by_label, audio_size, audio_starts):
- targets_list, lengths_list, ntokens_list = [], [], []
- itr = zip(targets_by_label, self.label_rates, self.pad_list)
- for targets, label_rate, pad in itr:
- if label_rate == -1.0:
- targets, lengths, ntokens = self.collater_seq_label(targets, pad)
- else:
- targets, lengths, ntokens = self.collater_frm_label(
- targets, audio_size, audio_starts, label_rate, pad
- )
- targets_list.append(targets)
- lengths_list.append(lengths)
- ntokens_list.append(ntokens)
- return targets_list, lengths_list, ntokens_list
-
- def num_tokens(self, index):
- return self.size(index)
-
- def size(self, index):
- if self.pad_audio:
- return self.sizes[index]
- return min(self.sizes[index], self.max_sample_size)
-
- def ordered_indices(self):
- if self.shuffle:
- order = [np.random.permutation(len(self))]
- else:
- order = [np.arange(len(self))]
-
- order.append(self.sizes)
- return np.lexsort(order)[::-1]
-
- def postprocess(self, wav, cur_sample_rate):
- if wav.dim() == 2:
- wav = wav.mean(-1)
- assert wav.dim() == 1, wav.dim()
-
- if cur_sample_rate != self.sample_rate:
- raise Exception(f"sr {cur_sample_rate} != {self.sample_rate}")
-
- if self.normalize:
- with torch.no_grad():
- wav = F.layer_norm(wav, wav.shape)
- return wav
diff --git a/spaces/HarryLee/eCommerceImageCaptioning/fairseq/examples/criss/README.md b/spaces/HarryLee/eCommerceImageCaptioning/fairseq/examples/criss/README.md
deleted file mode 100644
index 4689ed7c10497a5100b28fe6d6801a7c089da569..0000000000000000000000000000000000000000
--- a/spaces/HarryLee/eCommerceImageCaptioning/fairseq/examples/criss/README.md
+++ /dev/null
@@ -1,61 +0,0 @@
-# Cross-lingual Retrieval for Iterative Self-Supervised Training
-
-https://arxiv.org/pdf/2006.09526.pdf
-
-## Introduction
-
-CRISS is a multilingual sequence-to-sequnce pretraining method where mining and training processes are applied iteratively, improving cross-lingual alignment and translation ability at the same time.
-
-## Requirements:
-
-* faiss: https://github.com/facebookresearch/faiss
-* mosesdecoder: https://github.com/moses-smt/mosesdecoder
-* flores: https://github.com/facebookresearch/flores
-* LASER: https://github.com/facebookresearch/LASER
-
-## Unsupervised Machine Translation
-##### 1. Download and decompress CRISS checkpoints
-```
-cd examples/criss
-wget https://dl.fbaipublicfiles.com/criss/criss_3rd_checkpoints.tar.gz
-tar -xf criss_checkpoints.tar.gz
-```
-##### 2. Download and preprocess Flores test dataset
-Make sure to run all scripts from examples/criss directory
-```
-bash download_and_preprocess_flores_test.sh
-```
-
-##### 3. Run Evaluation on Sinhala-English
-```
-bash unsupervised_mt/eval.sh
-```
-
-## Sentence Retrieval
-##### 1. Download and preprocess Tatoeba dataset
-```
-bash download_and_preprocess_tatoeba.sh
-```
-
-##### 2. Run Sentence Retrieval on Tatoeba Kazakh-English
-```
-bash sentence_retrieval/sentence_retrieval_tatoeba.sh
-```
-
-## Mining
-##### 1. Install faiss
-Follow instructions on https://github.com/facebookresearch/faiss/blob/master/INSTALL.md
-##### 2. Mine pseudo-parallel data between Kazakh and English
-```
-bash mining/mine_example.sh
-```
-
-## Citation
-```bibtex
-@article{tran2020cross,
- title={Cross-lingual retrieval for iterative self-supervised training},
- author={Tran, Chau and Tang, Yuqing and Li, Xian and Gu, Jiatao},
- journal={arXiv preprint arXiv:2006.09526},
- year={2020}
-}
-```
diff --git a/spaces/HarryLee/eCommerceImageCaptioning/fairseq/examples/wav2vec/unsupervised/w2vu_generate.py b/spaces/HarryLee/eCommerceImageCaptioning/fairseq/examples/wav2vec/unsupervised/w2vu_generate.py
deleted file mode 100644
index 6177239dc75f6937d036462a5a2379aaee202e7d..0000000000000000000000000000000000000000
--- a/spaces/HarryLee/eCommerceImageCaptioning/fairseq/examples/wav2vec/unsupervised/w2vu_generate.py
+++ /dev/null
@@ -1,707 +0,0 @@
-#!/usr/bin/env python3 -u
-# Copyright (c) Facebook, Inc. and its affiliates.
-#
-# This source code is licensed under the MIT license found in the
-# LICENSE file in the root directory of this source tree.
-
-"""
-Run inference for pre-processed data with a trained model.
-"""
-
-import ast
-from collections import namedtuple
-from dataclasses import dataclass, field
-from enum import Enum, auto
-import hydra
-from hydra.core.config_store import ConfigStore
-import logging
-import math
-import os
-from omegaconf import OmegaConf
-from typing import Optional
-import sys
-
-import editdistance
-import torch
-
-from hydra.core.hydra_config import HydraConfig
-
-from fairseq import checkpoint_utils, progress_bar, tasks, utils
-from fairseq.data.data_utils import post_process
-from fairseq.dataclass.configs import FairseqDataclass, FairseqConfig
-from fairseq.logging.meters import StopwatchMeter
-from omegaconf import open_dict
-
-from examples.speech_recognition.kaldi.kaldi_decoder import KaldiDecoderConfig
-
-logging.root.setLevel(logging.INFO)
-logging.basicConfig(stream=sys.stdout, level=logging.INFO)
-logger = logging.getLogger(__name__)
-
-
-class DecoderType(Enum):
- VITERBI = auto()
- KENLM = auto()
- FAIRSEQ = auto()
- KALDI = auto()
-
-
-@dataclass
-class UnsupGenerateConfig(FairseqDataclass):
- fairseq: FairseqConfig = FairseqConfig()
- lm_weight: float = field(
- default=2.0,
- metadata={"help": "language model weight"},
- )
- w2l_decoder: DecoderType = field(
- default=DecoderType.VITERBI,
- metadata={"help": "type of decoder to use"},
- )
- kaldi_decoder_config: Optional[KaldiDecoderConfig] = None
- lexicon: Optional[str] = field(
- default=None,
- metadata={
- "help": "path to lexicon. This is also used to 'phonemize' for unsupvised param tuning"
- },
- )
- lm_model: Optional[str] = field(
- default=None,
- metadata={"help": "path to language model (kenlm or fairseq)"},
- )
- unit_lm: bool = field(
- default=False,
- metadata={"help": "whether to use unit lm"},
- )
- beam_threshold: float = field(
- default=50.0,
- metadata={"help": "beam score threshold"},
- )
- beam_size_token: float = field(
- default=100.0,
- metadata={"help": "max tokens per beam"},
- )
- beam: int = field(
- default=5,
- metadata={"help": "decoder beam size"},
- )
- nbest: int = field(
- default=1,
- metadata={"help": "number of results to return"},
- )
- word_score: float = field(
- default=1.0,
- metadata={"help": "word score to add at end of word"},
- )
- unk_weight: float = field(
- default=-math.inf,
- metadata={"help": "unknown token weight"},
- )
- sil_weight: float = field(
- default=0.0,
- metadata={"help": "silence token weight"},
- )
- targets: Optional[str] = field(
- default=None,
- metadata={"help": "extension of ground truth labels to compute UER"},
- )
- results_path: Optional[str] = field(
- default=None,
- metadata={"help": "where to store results"},
- )
- post_process: Optional[str] = field(
- default=None,
- metadata={"help": "how to post process results"},
- )
- vocab_usage_power: float = field(
- default=2,
- metadata={"help": "for unsupervised param tuning"},
- )
-
- viterbi_transcript: Optional[str] = field(
- default=None,
- metadata={"help": "for unsupervised param tuning"},
- )
- min_lm_ppl: float = field(
- default=0,
- metadata={"help": "for unsupervised param tuning"},
- )
- min_vt_uer: float = field(
- default=0,
- metadata={"help": "for unsupervised param tuning"},
- )
-
- blank_weight: float = field(
- default=0,
- metadata={"help": "value to add or set for blank emission"},
- )
- blank_mode: str = field(
- default="set",
- metadata={
- "help": "can be add or set, how to modify blank emission with blank weight"
- },
- )
- sil_is_blank: bool = field(
- default=False,
- metadata={"help": "if true, token is same as blank token"},
- )
-
- unsupervised_tuning: bool = field(
- default=False,
- metadata={
- "help": "if true, returns a score based on unsupervised param selection metric instead of UER"
- },
- )
- is_ax: bool = field(
- default=False,
- metadata={
- "help": "if true, assumes we are using ax for tuning and returns a tuple for ax to consume"
- },
- )
-
-
-def get_dataset_itr(cfg, task):
- return task.get_batch_iterator(
- dataset=task.dataset(cfg.fairseq.dataset.gen_subset),
- max_tokens=cfg.fairseq.dataset.max_tokens,
- max_sentences=cfg.fairseq.dataset.batch_size,
- max_positions=(sys.maxsize, sys.maxsize),
- ignore_invalid_inputs=cfg.fairseq.dataset.skip_invalid_size_inputs_valid_test,
- required_batch_size_multiple=cfg.fairseq.dataset.required_batch_size_multiple,
- num_shards=cfg.fairseq.dataset.num_shards,
- shard_id=cfg.fairseq.dataset.shard_id,
- num_workers=cfg.fairseq.dataset.num_workers,
- data_buffer_size=cfg.fairseq.dataset.data_buffer_size,
- ).next_epoch_itr(shuffle=False)
-
-
-def process_predictions(
- cfg: UnsupGenerateConfig,
- hypos,
- tgt_dict,
- target_tokens,
- res_files,
-):
- retval = []
- word_preds = []
- transcriptions = []
- dec_scores = []
-
- for i, hypo in enumerate(hypos[: min(len(hypos), cfg.nbest)]):
- if torch.is_tensor(hypo["tokens"]):
- tokens = hypo["tokens"].int().cpu()
- tokens = tokens[tokens >= tgt_dict.nspecial]
- hyp_pieces = tgt_dict.string(tokens)
- else:
- hyp_pieces = " ".join(hypo["tokens"])
-
- if "words" in hypo and len(hypo["words"]) > 0:
- hyp_words = " ".join(hypo["words"])
- else:
- hyp_words = post_process(hyp_pieces, cfg.post_process)
-
- to_write = {}
- if res_files is not None:
- to_write[res_files["hypo.units"]] = hyp_pieces
- to_write[res_files["hypo.words"]] = hyp_words
-
- tgt_words = ""
- if target_tokens is not None:
- if isinstance(target_tokens, str):
- tgt_pieces = tgt_words = target_tokens
- else:
- tgt_pieces = tgt_dict.string(target_tokens)
- tgt_words = post_process(tgt_pieces, cfg.post_process)
-
- if res_files is not None:
- to_write[res_files["ref.units"]] = tgt_pieces
- to_write[res_files["ref.words"]] = tgt_words
-
- if not cfg.fairseq.common_eval.quiet:
- logger.info(f"HYPO {i}:" + hyp_words)
- if tgt_words:
- logger.info("TARGET:" + tgt_words)
-
- if "am_score" in hypo and "lm_score" in hypo:
- logger.info(
- f"DECODER AM SCORE: {hypo['am_score']}, DECODER LM SCORE: {hypo['lm_score']}, DECODER SCORE: {hypo['score']}"
- )
- elif "score" in hypo:
- logger.info(f"DECODER SCORE: {hypo['score']}")
-
- logger.info("___________________")
-
- hyp_words_arr = hyp_words.split()
- tgt_words_arr = tgt_words.split()
-
- retval.append(
- (
- editdistance.eval(hyp_words_arr, tgt_words_arr),
- len(hyp_words_arr),
- len(tgt_words_arr),
- hyp_pieces,
- hyp_words,
- )
- )
- word_preds.append(hyp_words_arr)
- transcriptions.append(to_write)
- dec_scores.append(-hypo.get("score", 0)) # negate cuz kaldi returns NLL
-
- if len(retval) > 1:
- best = None
- for r, t in zip(retval, transcriptions):
- if best is None or r[0] < best[0][0]:
- best = r, t
- for dest, tran in best[1].items():
- print(tran, file=dest)
- dest.flush()
- return best[0]
-
- assert len(transcriptions) == 1
- for dest, tran in transcriptions[0].items():
- print(tran, file=dest)
-
- return retval[0]
-
-
-def prepare_result_files(cfg: UnsupGenerateConfig):
- def get_res_file(file_prefix):
- if cfg.fairseq.dataset.num_shards > 1:
- file_prefix = f"{cfg.fairseq.dataset.shard_id}_{file_prefix}"
- path = os.path.join(
- cfg.results_path,
- "{}{}.txt".format(
- cfg.fairseq.dataset.gen_subset,
- file_prefix,
- ),
- )
- return open(path, "w", buffering=1)
-
- if not cfg.results_path:
- return None
-
- return {
- "hypo.words": get_res_file(""),
- "hypo.units": get_res_file("_units"),
- "ref.words": get_res_file("_ref"),
- "ref.units": get_res_file("_ref_units"),
- "hypo.nbest.words": get_res_file("_nbest_words"),
- }
-
-
-def optimize_models(cfg: UnsupGenerateConfig, use_cuda, models):
- """Optimize ensemble for generation"""
- for model in models:
- model.eval()
- if cfg.fairseq.common.fp16:
- model.half()
- if use_cuda:
- model.cuda()
-
-
-GenResult = namedtuple(
- "GenResult",
- [
- "count",
- "errs_t",
- "gen_timer",
- "lengths_hyp_unit_t",
- "lengths_hyp_t",
- "lengths_t",
- "lm_score_t",
- "num_feats",
- "num_sentences",
- "num_symbols",
- "vt_err_t",
- "vt_length_t",
- ],
-)
-
-
-def generate(cfg: UnsupGenerateConfig, models, saved_cfg, use_cuda):
- task = tasks.setup_task(cfg.fairseq.task)
- saved_cfg.task.labels = cfg.fairseq.task.labels
- task.load_dataset(cfg.fairseq.dataset.gen_subset, task_cfg=saved_cfg.task)
- # Set dictionary
- tgt_dict = task.target_dictionary
- logger.info(
- "| {} {} {} examples".format(
- cfg.fairseq.task.data,
- cfg.fairseq.dataset.gen_subset,
- len(task.dataset(cfg.fairseq.dataset.gen_subset)),
- )
- )
- # Load dataset (possibly sharded)
- itr = get_dataset_itr(cfg, task)
- # Initialize generator
- gen_timer = StopwatchMeter()
-
- def build_generator(cfg: UnsupGenerateConfig):
- w2l_decoder = cfg.w2l_decoder
- if w2l_decoder == DecoderType.VITERBI:
- from examples.speech_recognition.w2l_decoder import W2lViterbiDecoder
-
- return W2lViterbiDecoder(cfg, task.target_dictionary)
- elif w2l_decoder == DecoderType.KENLM:
- from examples.speech_recognition.w2l_decoder import W2lKenLMDecoder
-
- return W2lKenLMDecoder(cfg, task.target_dictionary)
- elif w2l_decoder == DecoderType.FAIRSEQ:
- from examples.speech_recognition.w2l_decoder import W2lFairseqLMDecoder
-
- return W2lFairseqLMDecoder(cfg, task.target_dictionary)
- elif w2l_decoder == DecoderType.KALDI:
- from examples.speech_recognition.kaldi.kaldi_decoder import KaldiDecoder
-
- assert cfg.kaldi_decoder_config is not None
-
- return KaldiDecoder(
- cfg.kaldi_decoder_config,
- cfg.beam,
- )
- else:
- raise NotImplementedError(
- "only wav2letter decoders with (viterbi, kenlm, fairseqlm) options are supported at the moment but found "
- + str(w2l_decoder)
- )
-
- generator = build_generator(cfg)
-
- kenlm = None
- fairseq_lm = None
- if cfg.lm_model is not None:
- import kenlm
-
- kenlm = kenlm.Model(cfg.lm_model)
-
- num_sentences = 0
- if cfg.results_path is not None and not os.path.exists(cfg.results_path):
- os.makedirs(cfg.results_path)
-
- res_files = prepare_result_files(cfg)
- errs_t = 0
- lengths_hyp_t = 0
- lengths_hyp_unit_t = 0
- lengths_t = 0
- count = 0
- num_feats = 0
- all_hyp_pieces = []
- all_hyp_words = []
-
- num_symbols = (
- len([s for s in tgt_dict.symbols if not s.startswith("madeup")])
- - tgt_dict.nspecial
- )
- targets = None
- if cfg.targets is not None:
- tgt_path = os.path.join(
- cfg.fairseq.task.data, cfg.fairseq.dataset.gen_subset + "." + cfg.targets
- )
- if os.path.exists(tgt_path):
- with open(tgt_path, "r") as f:
- targets = f.read().splitlines()
- viterbi_transcript = None
- if cfg.viterbi_transcript is not None and len(cfg.viterbi_transcript) > 0:
- logger.info(f"loading viterbi transcript from {cfg.viterbi_transcript}")
- with open(cfg.viterbi_transcript, "r") as vf:
- viterbi_transcript = vf.readlines()
- viterbi_transcript = [v.rstrip().split() for v in viterbi_transcript]
-
- gen_timer.start()
-
- start = 0
- end = len(itr)
-
- hypo_futures = None
- if cfg.w2l_decoder == DecoderType.KALDI:
- logger.info("Extracting features")
- hypo_futures = []
- samples = []
- with progress_bar.build_progress_bar(cfg.fairseq.common, itr) as t:
- for i, sample in enumerate(t):
- if "net_input" not in sample or i < start or i >= end:
- continue
- if "padding_mask" not in sample["net_input"]:
- sample["net_input"]["padding_mask"] = None
-
- hypos, num_feats = gen_hypos(
- generator, models, num_feats, sample, task, use_cuda
- )
- hypo_futures.append(hypos)
- samples.append(sample)
- itr = list(zip(hypo_futures, samples))
- start = 0
- end = len(itr)
- logger.info("Finished extracting features")
-
- with progress_bar.build_progress_bar(cfg.fairseq.common, itr) as t:
- for i, sample in enumerate(t):
- if i < start or i >= end:
- continue
-
- if hypo_futures is not None:
- hypos, sample = sample
- hypos = [h.result() for h in hypos]
- else:
- if "net_input" not in sample:
- continue
-
- hypos, num_feats = gen_hypos(
- generator, models, num_feats, sample, task, use_cuda
- )
-
- for i, sample_id in enumerate(sample["id"].tolist()):
- if targets is not None:
- target_tokens = targets[sample_id]
- elif "target" in sample or "target_label" in sample:
- toks = (
- sample["target"][i, :]
- if "target_label" not in sample
- else sample["target_label"][i, :]
- )
-
- target_tokens = utils.strip_pad(toks, tgt_dict.pad()).int().cpu()
- else:
- target_tokens = None
-
- # Process top predictions
- (
- errs,
- length_hyp,
- length,
- hyp_pieces,
- hyp_words,
- ) = process_predictions(
- cfg,
- hypos[i],
- tgt_dict,
- target_tokens,
- res_files,
- )
- errs_t += errs
- lengths_hyp_t += length_hyp
- lengths_hyp_unit_t += (
- len(hyp_pieces) if len(hyp_pieces) > 0 else len(hyp_words)
- )
- lengths_t += length
- count += 1
- all_hyp_pieces.append(hyp_pieces)
- all_hyp_words.append(hyp_words)
-
- num_sentences += (
- sample["nsentences"] if "nsentences" in sample else sample["id"].numel()
- )
-
- lm_score_sum = 0
- if kenlm is not None:
-
- if cfg.unit_lm:
- lm_score_sum = sum(kenlm.score(w) for w in all_hyp_pieces)
- else:
- lm_score_sum = sum(kenlm.score(w) for w in all_hyp_words)
- elif fairseq_lm is not None:
- lm_score_sum = sum(fairseq_lm.score([h.split() for h in all_hyp_words])[0])
-
- vt_err_t = 0
- vt_length_t = 0
- if viterbi_transcript is not None:
- unit_hyps = []
- if cfg.targets is not None and cfg.lexicon is not None:
- lex = {}
- with open(cfg.lexicon, "r") as lf:
- for line in lf:
- items = line.rstrip().split()
- lex[items[0]] = items[1:]
- for h in all_hyp_pieces:
- hyp_ws = []
- for w in h.split():
- assert w in lex, w
- hyp_ws.extend(lex[w])
- unit_hyps.append(hyp_ws)
-
- else:
- unit_hyps.extend([h.split() for h in all_hyp_words])
-
- vt_err_t = sum(
- editdistance.eval(vt, h) for vt, h in zip(viterbi_transcript, unit_hyps)
- )
-
- vt_length_t = sum(len(h) for h in viterbi_transcript)
-
- if res_files is not None:
- for r in res_files.values():
- r.close()
-
- gen_timer.stop(lengths_hyp_t)
-
- return GenResult(
- count,
- errs_t,
- gen_timer,
- lengths_hyp_unit_t,
- lengths_hyp_t,
- lengths_t,
- lm_score_sum,
- num_feats,
- num_sentences,
- num_symbols,
- vt_err_t,
- vt_length_t,
- )
-
-
-def gen_hypos(generator, models, num_feats, sample, task, use_cuda):
- sample = utils.move_to_cuda(sample) if use_cuda else sample
-
- if "features" in sample["net_input"]:
- sample["net_input"]["dense_x_only"] = True
- num_feats += (
- sample["net_input"]["features"].shape[0]
- * sample["net_input"]["features"].shape[1]
- )
- hypos = task.inference_step(generator, models, sample, None)
- return hypos, num_feats
-
-
-def main(cfg: UnsupGenerateConfig, model=None):
- if (
- cfg.fairseq.dataset.max_tokens is None
- and cfg.fairseq.dataset.batch_size is None
- ):
- cfg.fairseq.dataset.max_tokens = 1024000
-
- use_cuda = torch.cuda.is_available() and not cfg.fairseq.common.cpu
-
- task = tasks.setup_task(cfg.fairseq.task)
-
- overrides = ast.literal_eval(cfg.fairseq.common_eval.model_overrides)
-
- if cfg.fairseq.task._name == "unpaired_audio_text":
- overrides["model"] = {
- "blank_weight": cfg.blank_weight,
- "blank_mode": cfg.blank_mode,
- "blank_is_sil": cfg.sil_is_blank,
- "no_softmax": True,
- "segmentation": {
- "type": "NONE",
- },
- }
- else:
- overrides["model"] = {
- "blank_weight": cfg.blank_weight,
- "blank_mode": cfg.blank_mode,
- }
-
- if model is None:
- # Load ensemble
- logger.info("| loading model(s) from {}".format(cfg.fairseq.common_eval.path))
- models, saved_cfg = checkpoint_utils.load_model_ensemble(
- cfg.fairseq.common_eval.path.split("\\"),
- arg_overrides=overrides,
- task=task,
- suffix=cfg.fairseq.checkpoint.checkpoint_suffix,
- strict=(cfg.fairseq.checkpoint.checkpoint_shard_count == 1),
- num_shards=cfg.fairseq.checkpoint.checkpoint_shard_count,
- )
- optimize_models(cfg, use_cuda, models)
- else:
- models = [model]
- saved_cfg = cfg.fairseq
-
- with open_dict(saved_cfg.task):
- saved_cfg.task.shuffle = False
- saved_cfg.task.sort_by_length = False
-
- gen_result = generate(cfg, models, saved_cfg, use_cuda)
-
- wer = None
- if gen_result.lengths_t > 0:
- wer = gen_result.errs_t * 100.0 / gen_result.lengths_t
- logger.info(f"WER: {wer}")
-
- lm_ppl = float("inf")
-
- if gen_result.lm_score_t != 0 and gen_result.lengths_hyp_t > 0:
- hyp_len = gen_result.lengths_hyp_t
- lm_ppl = math.pow(
- 10, -gen_result.lm_score_t / (hyp_len + gen_result.num_sentences)
- )
- logger.info(f"LM PPL: {lm_ppl}")
-
- logger.info(
- "| Processed {} sentences ({} tokens) in {:.1f}s ({:.2f}"
- " sentences/s, {:.2f} tokens/s)".format(
- gen_result.num_sentences,
- gen_result.gen_timer.n,
- gen_result.gen_timer.sum,
- gen_result.num_sentences / gen_result.gen_timer.sum,
- 1.0 / gen_result.gen_timer.avg,
- )
- )
-
- vt_diff = None
- if gen_result.vt_length_t > 0:
- vt_diff = gen_result.vt_err_t / gen_result.vt_length_t
- vt_diff = max(cfg.min_vt_uer, vt_diff)
-
- lm_ppl = max(cfg.min_lm_ppl, lm_ppl)
-
- if not cfg.unsupervised_tuning == 0:
- weighted_score = wer
- else:
- weighted_score = math.log(lm_ppl) * (vt_diff or 1.0)
-
- res = (
- f"| Generate {cfg.fairseq.dataset.gen_subset} with beam={cfg.beam}, "
- f"lm_weight={cfg.kaldi_decoder_config.acoustic_scale if cfg.kaldi_decoder_config else cfg.lm_weight}, "
- f"word_score={cfg.word_score}, sil_weight={cfg.sil_weight}, blank_weight={cfg.blank_weight}, "
- f"WER: {wer}, LM_PPL: {lm_ppl}, num feats: {gen_result.num_feats}, "
- f"length: {gen_result.lengths_hyp_t}, UER to viterbi: {(vt_diff or 0) * 100}, score: {weighted_score}"
- )
-
- logger.info(res)
- # print(res)
-
- return task, weighted_score
-
-
-@hydra.main(
- config_path=os.path.join("../../..", "fairseq", "config"), config_name="config"
-)
-def hydra_main(cfg):
- with open_dict(cfg):
- # make hydra logging work with ddp (see # see https://github.com/facebookresearch/hydra/issues/1126)
- cfg.job_logging_cfg = OmegaConf.to_container(
- HydraConfig.get().job_logging, resolve=True
- )
-
- cfg = OmegaConf.create(
- OmegaConf.to_container(cfg, resolve=False, enum_to_str=False)
- )
- OmegaConf.set_struct(cfg, True)
- logger.info(cfg)
-
- utils.import_user_module(cfg.fairseq.common)
-
- _, score = main(cfg)
-
- if cfg.is_ax:
- return score, None
- return score
-
-
-def cli_main():
- try:
- from hydra._internal.utils import get_args
-
- cfg_name = get_args().config_name or "config"
- except:
- logger.warning("Failed to get config name from hydra args")
- cfg_name = "config"
-
- cs = ConfigStore.instance()
- cs.store(name=cfg_name, node=UnsupGenerateConfig)
- hydra_main()
-
-
-if __name__ == "__main__":
- cli_main()
diff --git a/spaces/Hazem/Pub_face/README.md b/spaces/Hazem/Pub_face/README.md
deleted file mode 100644
index fcc481fcbcc054b0f552030fc6efd94791b86910..0000000000000000000000000000000000000000
--- a/spaces/Hazem/Pub_face/README.md
+++ /dev/null
@@ -1,14 +0,0 @@
----
-title: Image Face Upscale Restoration-GFPGAN
-emoji: 📈
-colorFrom: blue
-colorTo: gray
-sdk: gradio
-sdk_version: 3.1.7
-app_file: app.py
-pinned: false
-license: apache-2.0
-duplicated_from: nightfury/Image_Face_Upscale_Restoration-GFPGAN
----
-
-Check out the configuration reference at https://huggingface.co/docs/hub/spaces-config-reference
diff --git a/spaces/Himanshusingh/KernAI-stock-news-distilbert/app.py b/spaces/Himanshusingh/KernAI-stock-news-distilbert/app.py
deleted file mode 100644
index 3ea07041ef10da45cf98369b0aa1c9f219f547b2..0000000000000000000000000000000000000000
--- a/spaces/Himanshusingh/KernAI-stock-news-distilbert/app.py
+++ /dev/null
@@ -1,3 +0,0 @@
-import gradio as gr
-
-gr.Interface.load("models/KernAI/stock-news-distilbert").launch()
\ No newline at end of file
diff --git a/spaces/HuangLab/CELL-E_2-Sequence_Prediction/celle/transformer.py b/spaces/HuangLab/CELL-E_2-Sequence_Prediction/celle/transformer.py
deleted file mode 100644
index 9186ab4772261591cbe58c9db5882f14cf3bd66a..0000000000000000000000000000000000000000
--- a/spaces/HuangLab/CELL-E_2-Sequence_Prediction/celle/transformer.py
+++ /dev/null
@@ -1,213 +0,0 @@
-from functools import partial
-
-import torch
-from torch import nn
-import torch.nn.functional as F
-from einops import rearrange
-
-from celle.reversible import SequentialSequence
-from celle.attention import Attention
-
-from rotary_embedding_torch import RotaryEmbedding, broadcat
-from celle.utils import exists, default, cast_tuple
-
-# https://arxiv.org/abs/2103.17239
-class LayerScale(nn.Module):
- def __init__(self, dim, depth, fn):
- super().__init__()
- if depth <= 18:
- init_eps = 0.1
- elif depth > 18 and depth <= 24:
- init_eps = 1e-5
- else:
- init_eps = 1e-6
-
- scale = torch.zeros(1, 1, dim).fill_(init_eps)
- self.scale = nn.Parameter(scale)
- self.fn = fn
-
- def forward(self, x, **kwargs):
- return self.fn(x, **kwargs) * self.scale
-
-
-# layer norm
-class PreNorm(nn.Module):
- def __init__(self, dim, fn):
- super().__init__()
- self.norm = nn.LayerNorm(dim)
- self.norm_out = nn.Identity()
- self.fn = fn
-
- def forward(self, x, **kwargs):
- x = self.norm(x)
- x = self.fn(x, **kwargs)
- return self.norm_out(x)
-
-
-# feed forward
-
-
-class GEGLU(nn.Module):
- def forward(self, x):
- x, gates = x.chunk(2, dim=-1)
- return x * F.gelu(gates)
-
-
-class FeedForward(nn.Module):
- def __init__(self, dim, dropout=0.0, mult=4.0):
- super().__init__()
- self.net = nn.Sequential(
- nn.Linear(dim, dim * mult * 2),
- GEGLU(),
- nn.Dropout(dropout),
- nn.Linear(dim * mult, dim),
- )
-
- def forward(self, x):
- return self.net(x)
-
-
-# main transformer class
-class Transformer(nn.Module):
- def __init__(
- self,
- *,
- dim,
- depth,
- seq_len,
- causal=True,
- heads=8,
- dim_head=64,
- ff_mult=4,
- attn_dropout=0.0,
- ff_dropout=0.0,
- image_fmap_size=None,
- num_images=None,
- stable=False,
- rotary_emb=True,
- ):
- super().__init__()
- layers = nn.ModuleList([])
-
- self.seq_len = seq_len
- self.image_fmap_size = image_fmap_size
-
- for ind in range(depth):
-
- attn_class = partial(Attention, stable=stable)
-
- attn = attn_class(
- dim,
- causal=causal,
- seq_len=seq_len,
- heads=heads,
- dim_head=dim_head,
- dropout=attn_dropout,
- )
-
- ff = FeedForward(dim, mult=ff_mult, dropout=ff_dropout)
-
- layers.append(
- nn.ModuleList(
- [
- LayerScale(
- dim, ind + 1, PreNorm(dim, attn)
- ),
- LayerScale(
- dim, ind + 1, PreNorm(dim, ff)
- ),
- ]
- )
- )
-
- # pairs arguments with attention layer
- route_attn = ((True, False),) * depth
- attn_route_map = {
- "mask": route_attn,
- "rotary_pos_emb": route_attn,
- }
-
- self.layers = SequentialSequence(layers, args_route=attn_route_map)
-
- # generate positional embeddings for rotary
-
- pos_emb = None
- if rotary_emb:
- rot_dim = dim_head // 3
- img_seq_len = ((image_fmap_size // num_images) ** 2) * num_images
-
- text_len = seq_len - img_seq_len + 1
-
- text_pos_emb = RotaryEmbedding(dim=rot_dim)
-
- img_axial_pos_emb = RotaryEmbedding(dim=rot_dim, freqs_for="pixel")
-
- text_freqs = text_pos_emb(torch.arange(text_len))
-
- img_to_text_freqs = text_pos_emb(
- torch.full((img_seq_len,), 8192)
- ) # image is given a position far away from text
-
- text_freqs = torch.cat((text_freqs, img_to_text_freqs), dim=0)
-
- img_freqs_axial = img_axial_pos_emb(
- torch.linspace(-1, 1, steps=image_fmap_size)
- )
-
- if num_images > 1:
- split_img_freqs_axial = torch.split(
- img_freqs_axial, image_fmap_size // num_images, dim=0
- )
-
- split_img_freqs = [
- broadcat(
- (
- rearrange(img_freqs_axial_per_image, "i d -> i () d"),
- rearrange(img_freqs_axial_per_image, "j d -> () j d"),
- ),
- dim=-1,
- )
- for img_freqs_axial_per_image in split_img_freqs_axial
- ]
-
- split_img_freqs = [
- rearrange(img_freqs_per_image, "h w d -> (h w) d")
- for img_freqs_per_image in split_img_freqs
- ]
-
- # concat per image-image_freqs
-
- img_freqs = torch.cat(split_img_freqs, dim=0)
-
- elif num_images == 1:
- img_freqs = broadcat(
- (
- rearrange(img_freqs_axial, "i d -> i () d"),
- rearrange(img_freqs_axial, "j d -> () j d"),
- ),
- dim=-1,
- )
-
- img_freqs = rearrange(img_freqs, "h w d -> (h w) d")
-
- else:
- assert False, "num_images must be int greater than 0"
- self.img_axial_pos_emb = img_axial_pos_emb
- self.text_pos_emb = text_pos_emb
-
- text_axial_freqs = img_axial_pos_emb(
- torch.full((text_len,), -10.0)
- ) # text is given a position of -10 apart from the image axial positions, which is from range [-1, 1]
-
- text_axial_freqs = torch.cat((text_axial_freqs, text_axial_freqs), dim=-1)
-
- img_freqs = torch.cat((text_axial_freqs, img_freqs), dim=0)
-
- pos_emb = torch.cat((text_freqs, img_freqs), dim=-1)
-
- pos_emb = rearrange(pos_emb, "n d -> () n d")
-
- self.register_buffer("pos_emb", pos_emb)
-
- def forward(self, x, **kwargs):
- return self.layers(x, rotary_pos_emb=self.pos_emb, **kwargs)
\ No newline at end of file
diff --git a/spaces/ICML2022/resefa/utils/visualizers/grid_visualizer.py b/spaces/ICML2022/resefa/utils/visualizers/grid_visualizer.py
deleted file mode 100644
index 291e5fee45816a9775242c3a138ebd0f55f1df20..0000000000000000000000000000000000000000
--- a/spaces/ICML2022/resefa/utils/visualizers/grid_visualizer.py
+++ /dev/null
@@ -1,232 +0,0 @@
-# python3.7
-"""Contains the visualizer to visualize images by composing them as a gird."""
-
-from ..image_utils import get_blank_image
-from ..image_utils import get_grid_shape
-from ..image_utils import parse_image_size
-from ..image_utils import load_image
-from ..image_utils import save_image
-from ..image_utils import resize_image
-from ..image_utils import list_images_from_dir
-
-__all__ = ['GridVisualizer']
-
-
-class GridVisualizer(object):
- """Defines the visualizer that visualizes images as a grid.
-
- Basically, given a collection of images, this visualizer stitches them one
- by one. Notably, this class also supports adding spaces between images,
- adding borders around images, and using white/black background.
-
- Example:
-
- grid = GridVisualizer(num_rows, num_cols)
- for i in range(num_rows):
- for j in range(num_cols):
- grid.add(i, j, image)
- grid.save('visualize.jpg')
- """
-
- def __init__(self,
- grid_size=0,
- num_rows=0,
- num_cols=0,
- is_portrait=False,
- image_size=None,
- image_channels=0,
- row_spacing=0,
- col_spacing=0,
- border_left=0,
- border_right=0,
- border_top=0,
- border_bottom=0,
- use_black_background=True):
- """Initializes the grid visualizer.
-
- Args:
- grid_size: Total number of cells, i.e., height * width. (default: 0)
- num_rows: Number of rows. (default: 0)
- num_cols: Number of columns. (default: 0)
- is_portrait: Whether the grid should be portrait or landscape.
- This is only used when it requires to compute `num_rows` and
- `num_cols` automatically. See function `get_grid_shape()` in
- file `./image_utils.py` for details. (default: False)
- image_size: Size to visualize each image. (default: 0)
- image_channels: Number of image channels. (default: 0)
- row_spacing: Spacing between rows. (default: 0)
- col_spacing: Spacing between columns. (default: 0)
- border_left: Width of left border. (default: 0)
- border_right: Width of right border. (default: 0)
- border_top: Width of top border. (default: 0)
- border_bottom: Width of bottom border. (default: 0)
- use_black_background: Whether to use black background.
- (default: True)
- """
- self.reset(grid_size, num_rows, num_cols, is_portrait)
- self.set_image_size(image_size)
- self.set_image_channels(image_channels)
- self.set_row_spacing(row_spacing)
- self.set_col_spacing(col_spacing)
- self.set_border_left(border_left)
- self.set_border_right(border_right)
- self.set_border_top(border_top)
- self.set_border_bottom(border_bottom)
- self.set_background(use_black_background)
- self.grid = None
-
- def reset(self,
- grid_size=0,
- num_rows=0,
- num_cols=0,
- is_portrait=False):
- """Resets the grid shape, i.e., number of rows/columns."""
- if grid_size > 0:
- num_rows, num_cols = get_grid_shape(grid_size,
- height=num_rows,
- width=num_cols,
- is_portrait=is_portrait)
- self.grid_size = num_rows * num_cols
- self.num_rows = num_rows
- self.num_cols = num_cols
- self.grid = None
-
- def set_image_size(self, image_size=None):
- """Sets the image size of each cell in the grid."""
- height, width = parse_image_size(image_size)
- self.image_height = height
- self.image_width = width
-
- def set_image_channels(self, image_channels=0):
- """Sets the number of channels of the grid."""
- self.image_channels = image_channels
-
- def set_row_spacing(self, row_spacing=0):
- """Sets the spacing between grid rows."""
- self.row_spacing = row_spacing
-
- def set_col_spacing(self, col_spacing=0):
- """Sets the spacing between grid columns."""
- self.col_spacing = col_spacing
-
- def set_border_left(self, border_left=0):
- """Sets the width of the left border of the grid."""
- self.border_left = border_left
-
- def set_border_right(self, border_right=0):
- """Sets the width of the right border of the grid."""
- self.border_right = border_right
-
- def set_border_top(self, border_top=0):
- """Sets the width of the top border of the grid."""
- self.border_top = border_top
-
- def set_border_bottom(self, border_bottom=0):
- """Sets the width of the bottom border of the grid."""
- self.border_bottom = border_bottom
-
- def set_background(self, use_black=True):
- """Sets the grid background."""
- self.use_black_background = use_black
-
- def init_grid(self):
- """Initializes the grid with a blank image."""
- assert self.num_rows > 0
- assert self.num_cols > 0
- assert self.image_height > 0
- assert self.image_width > 0
- assert self.image_channels > 0
- grid_height = (self.image_height * self.num_rows +
- self.row_spacing * (self.num_rows - 1) +
- self.border_top + self.border_bottom)
- grid_width = (self.image_width * self.num_cols +
- self.col_spacing * (self.num_cols - 1) +
- self.border_left + self.border_right)
- self.grid = get_blank_image(grid_height, grid_width,
- channels=self.image_channels,
- use_black=self.use_black_background)
-
- def add(self, i, j, image):
- """Adds an image into the grid.
-
- NOTE: The input image is assumed to be with `RGB` channel order.
- """
- if self.grid is None:
- height, width = image.shape[0:2]
- channels = 1 if image.ndim == 2 else image.shape[2]
- height = self.image_height or height
- width = self.image_width or width
- channels = self.image_channels or channels
- self.set_image_size((height, width))
- self.set_image_channels(channels)
- self.init_grid()
- if image.shape[0:2] != (self.image_height, self.image_width):
- image = resize_image(image, (self.image_width, self.image_height))
- y = self.border_top + i * (self.image_height + self.row_spacing)
- x = self.border_left + j * (self.image_width + self.col_spacing)
- self.grid[y:y + self.image_height, x:x + self.image_width] = image
-
- def visualize_collection(self,
- images,
- save_path=None,
- num_rows=0,
- num_cols=0,
- is_portrait=False,
- is_row_major=True):
- """Visualizes a collection of images one by one."""
- self.grid = None
- self.reset(grid_size=len(images),
- num_rows=num_rows,
- num_cols=num_cols,
- is_portrait=is_portrait)
- for idx, image in enumerate(images):
- if is_row_major:
- row_idx, col_idx = divmod(idx, self.num_cols)
- else:
- col_idx, row_idx = divmod(idx, self.num_rows)
- self.add(row_idx, col_idx, image)
- if save_path:
- self.save(save_path)
-
- def visualize_list(self,
- image_list,
- save_path=None,
- num_rows=0,
- num_cols=0,
- is_portrait=False,
- is_row_major=True):
- """Visualizes a list of image files."""
- self.grid = None
- self.reset(grid_size=len(image_list),
- num_rows=num_rows,
- num_cols=num_cols,
- is_portrait=is_portrait)
- for idx, filename in enumerate(image_list):
- image = load_image(filename)
- if is_row_major:
- row_idx, col_idx = divmod(idx, self.num_cols)
- else:
- col_idx, row_idx = divmod(idx, self.num_rows)
- self.add(row_idx, col_idx, image)
- if save_path:
- self.save(save_path)
-
- def visualize_directory(self,
- directory,
- save_path=None,
- num_rows=0,
- num_cols=0,
- is_portrait=False,
- is_row_major=True):
- """Visualizes all images under a directory."""
- image_list = list_images_from_dir(directory)
- self.visualize_list(image_list=image_list,
- save_path=save_path,
- num_rows=num_rows,
- num_cols=num_cols,
- is_portrait=is_portrait,
- is_row_major=is_row_major)
-
- def save(self, path):
- """Saves the grid."""
- save_image(path, self.grid)
diff --git a/spaces/IDEA-Research/Grounded-SAM/GroundingDINO/groundingdino/models/__init__.py b/spaces/IDEA-Research/Grounded-SAM/GroundingDINO/groundingdino/models/__init__.py
deleted file mode 100644
index e3413961d1d184b99835eb1e919b052d70298bc6..0000000000000000000000000000000000000000
--- a/spaces/IDEA-Research/Grounded-SAM/GroundingDINO/groundingdino/models/__init__.py
+++ /dev/null
@@ -1,18 +0,0 @@
-# ------------------------------------------------------------------------
-# Grounding DINO
-# url: https://github.com/IDEA-Research/GroundingDINO
-# Copyright (c) 2023 IDEA. All Rights Reserved.
-# Licensed under the Apache License, Version 2.0 [see LICENSE for details]
-# ------------------------------------------------------------------------
-# Copyright (c) Facebook, Inc. and its affiliates. All Rights Reserved
-from .GroundingDINO import build_groundingdino
-
-
-def build_model(args):
- # we use register to maintain models from catdet6 on.
- from .registry import MODULE_BUILD_FUNCS
-
- assert args.modelname in MODULE_BUILD_FUNCS._module_dict
- build_func = MODULE_BUILD_FUNCS.get(args.modelname)
- model = build_func(args)
- return model
diff --git a/spaces/IXIAOHEII/NB/README.md b/spaces/IXIAOHEII/NB/README.md
deleted file mode 100644
index afc64c1a83b524f02feac26a499f0d5089476943..0000000000000000000000000000000000000000
--- a/spaces/IXIAOHEII/NB/README.md
+++ /dev/null
@@ -1,11 +0,0 @@
----
-title: NB
-emoji: 🔥
-colorFrom: blue
-colorTo: yellow
-sdk: docker
-pinned: false
-license: mit
----
-
-Check out the configuration reference at https://huggingface.co/docs/hub/spaces-config-reference
diff --git a/spaces/InpaintAI/Inpaint-Anything/third_party/lama/models/ade20k/segm_lib/utils/__init__.py b/spaces/InpaintAI/Inpaint-Anything/third_party/lama/models/ade20k/segm_lib/utils/__init__.py
deleted file mode 100644
index abe3cbe49477fe37d4fc16249de8a10f4fb4a013..0000000000000000000000000000000000000000
--- a/spaces/InpaintAI/Inpaint-Anything/third_party/lama/models/ade20k/segm_lib/utils/__init__.py
+++ /dev/null
@@ -1 +0,0 @@
-from .th import *
diff --git a/spaces/InpaintAI/Inpaint-Anything/third_party/segment-anything/demo/src/components/helpers/onnxModelAPI.tsx b/spaces/InpaintAI/Inpaint-Anything/third_party/segment-anything/demo/src/components/helpers/onnxModelAPI.tsx
deleted file mode 100644
index 2e006c95b407ff4a7c0c071badf6a9cf2fe34ef0..0000000000000000000000000000000000000000
--- a/spaces/InpaintAI/Inpaint-Anything/third_party/segment-anything/demo/src/components/helpers/onnxModelAPI.tsx
+++ /dev/null
@@ -1,71 +0,0 @@
-// Copyright (c) Meta Platforms, Inc. and affiliates.
-// All rights reserved.
-
-// This source code is licensed under the license found in the
-// LICENSE file in the root directory of this source tree.
-
-import { Tensor } from "onnxruntime-web";
-import { modeDataProps } from "./Interfaces";
-
-const modelData = ({ clicks, tensor, modelScale }: modeDataProps) => {
- const imageEmbedding = tensor;
- let pointCoords;
- let pointLabels;
- let pointCoordsTensor;
- let pointLabelsTensor;
-
- // Check there are input click prompts
- if (clicks) {
- let n = clicks.length;
-
- // If there is no box input, a single padding point with
- // label -1 and coordinates (0.0, 0.0) should be concatenated
- // so initialize the array to support (n + 1) points.
- pointCoords = new Float32Array(2 * (n + 1));
- pointLabels = new Float32Array(n + 1);
-
- // Add clicks and scale to what SAM expects
- for (let i = 0; i < n; i++) {
- pointCoords[2 * i] = clicks[i].x * modelScale.samScale;
- pointCoords[2 * i + 1] = clicks[i].y * modelScale.samScale;
- pointLabels[i] = clicks[i].clickType;
- }
-
- // Add in the extra point/label when only clicks and no box
- // The extra point is at (0, 0) with label -1
- pointCoords[2 * n] = 0.0;
- pointCoords[2 * n + 1] = 0.0;
- pointLabels[n] = -1.0;
-
- // Create the tensor
- pointCoordsTensor = new Tensor("float32", pointCoords, [1, n + 1, 2]);
- pointLabelsTensor = new Tensor("float32", pointLabels, [1, n + 1]);
- }
- const imageSizeTensor = new Tensor("float32", [
- modelScale.height,
- modelScale.width,
- ]);
-
- if (pointCoordsTensor === undefined || pointLabelsTensor === undefined)
- return;
-
- // There is no previous mask, so default to an empty tensor
- const maskInput = new Tensor(
- "float32",
- new Float32Array(256 * 256),
- [1, 1, 256, 256]
- );
- // There is no previous mask, so default to 0
- const hasMaskInput = new Tensor("float32", [0]);
-
- return {
- image_embeddings: imageEmbedding,
- point_coords: pointCoordsTensor,
- point_labels: pointLabelsTensor,
- orig_im_size: imageSizeTensor,
- mask_input: maskInput,
- has_mask_input: hasMaskInput,
- };
-};
-
-export { modelData };
diff --git a/spaces/JadAssaf/STPIzeimer/app.py b/spaces/JadAssaf/STPIzeimer/app.py
deleted file mode 100644
index e083af925b92f96e68f54d5066ea91c38ba06017..0000000000000000000000000000000000000000
--- a/spaces/JadAssaf/STPIzeimer/app.py
+++ /dev/null
@@ -1,62 +0,0 @@
-# %%
-import gradio as gr
-import joblib
-loaded_rf_2way = joblib.load("STPI_2WAY_RandomForest.joblib")
-loaded_rf_3way = joblib.load("STPI_3WAY_RandomForest.joblib")
-
-
-def STPI(t_0_5_MaxValue,t_1_0_MaxValue,t_2_0_MaxValue,
-# Acc_0_5__1_0_MaxValue,
-Abs_Diff_t_0_5_MaxValue,Abs_Diff_t_1_0_MaxValue,Abs_Diff_t_2_0_MaxValue):
- print('------------------')
-
- X = [t_0_5_MaxValue,t_1_0_MaxValue,t_2_0_MaxValue,
- # Acc_0_5__1_0_MaxValue,
- Abs_Diff_t_0_5_MaxValue,Abs_Diff_t_1_0_MaxValue,Abs_Diff_t_2_0_MaxValue]
- print(X)
- outcome_decoded = ['Normal','Keratoconic','Suspect']
- file_object = open('stpi_data.txt', 'a')
- file_object.write(str(t_0_5_MaxValue))
- file_object.write(';')
- file_object.write(str(t_1_0_MaxValue))
- file_object.write(';')
- file_object.write(str(t_2_0_MaxValue))
- file_object.write(';')
- # file_object.write(str(Acc_0_5__1_0_MaxValue))
- # file_object.write(';')
- file_object.write(str(Abs_Diff_t_0_5_MaxValue))
- file_object.write(';')
- file_object.write(str(Abs_Diff_t_1_0_MaxValue))
- file_object.write(';')
- file_object.write(str(Abs_Diff_t_2_0_MaxValue))
- file_object.write(';')
- file_object.write('\n')
- file_object.close()
-
- result_2way = loaded_rf_2way.predict([X])
- print('The patient is ', outcome_decoded[int(result_2way)], ' through the 2way method')
-
- result_3way = loaded_rf_3way.predict([X])
- if result_2way == 0:
- print('The patient is ', outcome_decoded[int(result_3way)], 'through the 3way method')
- # result = 'The 3-way classification resulted in a ', outcome_decoded[int(result_3way)] + ' patient.'
- # further_analysis = 'Futher analysis using the 2-way classification resulted in a ' + outcome_decoded[int(result_2way)] + ' label.'
- return 'The patient is ' + outcome_decoded[int(result_3way)] + '.'
-
- # result = 'The 2-way classification resulted in a ', outcome_decoded[int(result_2way)] + ' patient.'
- # further_analysis = 'Futher analysis using the 3-way classification resulted in a ' + outcome_decoded[int(result_3way)] + ' label.'
-
- return 'The patient is ' + outcome_decoded[int(result_2way)] + '.'
-
-iface = gr.Interface(
- fn=STPI,
- title='TSPI Calculator',
- description='Calculates the Thickness Speed Progression Index (TSPI) through summarized tomographic parameters. Beta version made for Zeimer by Prof. Shady Awwad and Jad Assaf MD.',
- inputs=["number", "number","number",
- # "number",
- "number", "number","number"],
- outputs="text")
-iface.launch(
- # share=True
- )
-# %%
diff --git a/spaces/Jean-Baptiste/email_parser/README.md b/spaces/Jean-Baptiste/email_parser/README.md
deleted file mode 100644
index 4c1fe5b42995d26ce750bfa089a24c1ff7f475e0..0000000000000000000000000000000000000000
--- a/spaces/Jean-Baptiste/email_parser/README.md
+++ /dev/null
@@ -1,37 +0,0 @@
----
-title: Email_parser
-emoji: 🌖
-colorFrom: yellow
-colorTo: red
-sdk: gradio
-app_file: app.py
-pinned: false
----
-
-# Configuration
-
-`title`: _string_
-Display title for the Space
-
-`emoji`: _string_
-Space emoji (emoji-only character allowed)
-
-`colorFrom`: _string_
-Color for Thumbnail gradient (red, yellow, green, blue, indigo, purple, pink, gray)
-
-`colorTo`: _string_
-Color for Thumbnail gradient (red, yellow, green, blue, indigo, purple, pink, gray)
-
-`sdk`: _string_
-Can be either `gradio` or `streamlit`
-
-`sdk_version` : _string_
-Only applicable for `streamlit` SDK.
-See [doc](https://hf.co/docs/hub/spaces) for more info on supported versions.
-
-`app_file`: _string_
-Path to your main application file (which contains either `gradio` or `streamlit` Python code).
-Path is relative to the root of the repository.
-
-`pinned`: _boolean_
-Whether the Space stays on top of your list.
diff --git a/spaces/JeffJing/ZookChatBot/steamship/invocable/invocable.py b/spaces/JeffJing/ZookChatBot/steamship/invocable/invocable.py
deleted file mode 100644
index 757ea1c0a19b4a5c50c238f53e0a467d055c5f92..0000000000000000000000000000000000000000
--- a/spaces/JeffJing/ZookChatBot/steamship/invocable/invocable.py
+++ /dev/null
@@ -1,264 +0,0 @@
-"""Please see https://docs.steamship.com/ for information about building a Steamship Package"""
-import inspect
-import logging
-import pathlib
-import time
-from abc import ABC
-from collections import defaultdict
-from functools import wraps
-from http import HTTPStatus
-from typing import Any, Dict, Optional, Type, Union
-
-import toml
-
-from steamship.base.package_spec import MethodSpec, PackageSpec
-from steamship.client.steamship import Steamship
-from steamship.invocable import Config
-from steamship.invocable.config import ConfigParameter
-from steamship.invocable.invocable_request import InvocableRequest, InvocationContext
-from steamship.invocable.invocable_response import InvocableResponse
-from steamship.utils.url import Verb
-
-
-def make_registering_decorator(decorator):
- """
- Returns a copy of foreignDecorator, which is identical in every
- way(*), except also appends a .decorator property to the callable it
- spits out.
-
- (*)We can be somewhat "hygienic", but newDecorator still isn't signature-preserving,
- i.e. you will not be able to get a runtime list of parameters.
- For that, you need hackish libraries...but in this case, the only argument is func, so it's not a big issue
- """
-
- def new_decorator(func):
- # Call to newDecorator(method)
- # Exactly like old decorator, but output keeps track of what decorated it
- output = decorator(
- func
- ) # apply foreignDecorator, like call to foreignDecorator(method) would have done
- output.decorator = new_decorator # keep track of decorator
- # R.original = func # might as well keep track of everything!
- return output
-
- new_decorator.__name__ = decorator.__name__
- new_decorator.__doc__ = decorator.__doc__
- new_decorator.__is_endpoint__ = True
- return new_decorator
-
-
-# https://stackoverflow.com/questions/2366713/can-a-decorator-of-an-instance-method-access-the-class
-# noinspection PyUnusedLocal
-def endpoint(verb: str = None, path: str = None, **kwargs):
- """By using ``kwargs`` we can tag the function with Any parameters.""" # noqa: RST210
-
- def decorator(function):
- # This is used in conjunction with the __init_subclass__ code!
- # Otherwise the __name__ won't be correct in maybeDecorated.__name__!
- # noinspection PyShadowingNames
- @wraps(function)
- def wrap(self, *args, **kwargs):
- return function(self, *args, **kwargs)
-
- # Build a dictionary of String->Primitive Types to pass back with endpoint
- # This enables the Engine to add support for features like public=True, etc, without the Client changing.
- config: Dict[str, Union[str, bool, int, float]] = {}
- for key, val in kwargs.items():
- if isinstance(val, (str, bool, int, float)):
- config[key] = val
-
- wrap.__path__ = path
- wrap.__verb__ = verb
- wrap.__endpoint_config__ = config
-
- return wrap
-
- decorator = make_registering_decorator(decorator)
- return decorator
-
-
-def get(path: str, **kwargs):
- return endpoint(verb=Verb.GET, path=path, **kwargs)
-
-
-def post(path: str, **kwargs):
- return endpoint(verb=Verb.POST, path=path, **kwargs)
-
-
-class Invocable(ABC):
- """A Steamship microservice.
-
- This model.py class:
-
- 1. Provide a pre-authenticated instance of the Steamship client
- 2. Provides a Lambda handler that routes to registered functions
- 3. Provides useful methods connecting functions to the router.
- """
-
- _method_mappings = defaultdict(dict)
- _package_spec: PackageSpec
- config: Config
- context: InvocationContext
-
- def __init__(
- self,
- client: Steamship = None,
- config: Dict[str, Any] = None,
- context: InvocationContext = None,
- ):
- self.context = context
-
- try:
- secret_kwargs = toml.load(".steamship/secrets.toml")
- except FileNotFoundError: # Support local secret loading
- try:
- local_secrets_file = (
- pathlib.Path(inspect.getfile(type(self))).parent / ".steamship" / "secrets.toml"
- )
- secret_kwargs = toml.load(str(local_secrets_file))
- except (TypeError, FileNotFoundError):
- secret_kwargs = {}
-
- # The configuration for the Invocable is the union of:
- #
- # 1) The `secret_kwargs` dict, read in from .steamship/secrets.toml, if it exists, and
- # 2) The `config` dict, provided upon instantiation.
- #
- # When invoked from within Steamship, the `config` dict is frozen, at the instance level, upon instance
- # creation. All subsequent method invocations reuse that frozen config.
- config = {
- **secret_kwargs,
- **{k: v for k, v in (config or {}).items() if v != ""},
- }
-
- # Finally, we set the config object to an instance of the class returned by `self.config_cls`
- if config:
- self.config = self.config_cls()(**config)
- else:
- self.config = self.config_cls()()
-
- self.client = client
-
- def __init_subclass__(cls, **kwargs):
- super().__init_subclass__(**kwargs)
-
- start_time = time.time()
- cls._package_spec = PackageSpec(name=cls.__name__, doc=cls.__doc__, methods=[])
- cls._method_mappings = defaultdict(dict)
- base_fn_list = [
- may_be_decorated
- for base_cls in cls.__bases__
- for may_be_decorated in base_cls.__dict__.values()
- ]
- for attribute in base_fn_list + list(cls.__dict__.values()):
- decorator = getattr(attribute, "decorator", None)
- if decorator:
- if getattr(decorator, "__is_endpoint__", False):
- path = getattr(attribute, "__path__", None)
- verb = getattr(attribute, "__verb__", None)
- config = getattr(attribute, "__endpoint_config__", {})
- method_spec = cls._register_mapping(
- name=attribute.__name__, verb=verb, path=path, config=config
- )
- cls._package_spec.methods.append(method_spec)
-
- # Add the HTTP GET /__dir__ method which returns a serialization of the PackageSpec.
- # Wired up to both GET and POST for convenience (since POST is the default from the Python client, but
- # GET is the default if using from a browser).
- cls._register_mapping(name="__steamship_dir__", verb=Verb.GET, path="/__dir__")
- cls._register_mapping(name="__steamship_dir__", verb=Verb.POST, path="/__dir__")
- end_time = time.time()
- logging.info(f"Registered package functions in {end_time - start_time} seconds.")
-
- def __steamship_dir__(self) -> dict:
- """Return this Invocable's PackageSpec for remote inspection -- e.g. documentation or OpenAPI generation."""
- return self._package_spec.dict()
-
- @classmethod
- def config_cls(cls) -> Type[Config]:
- """Returns the configuration object for the Invocable.
-
- By default, Steamship packages and plugins will not take any configuration. Steamship packages and plugins may
- declare a configuration object which extends from Config, if needed, as follows:
-
- class MyPackageOrPlugin:
- class MyConfig(Config):
- ...
-
- @classmethod
- def config_cls(cls):
- return MyPackageOrPlugin.MyConfig
- """ # noqa: RST301
- return Config
-
- @classmethod
- def _register_mapping(
- cls,
- name: str,
- verb: Optional[Verb] = None,
- path: str = "",
- config: Dict[str, Union[int, float, bool, str]] = None,
- ) -> MethodSpec:
- """Registering a mapping permits the method to be invoked via HTTP."""
- method_spec = MethodSpec(cls, name, path=path, verb=verb, config=config)
- # It's important to use method_spec.path below since that's the CLEANED path.
- cls._method_mappings[verb][method_spec.path] = name
- logging.info(f"[{cls.__name__}] {verb} {path} => {name}")
- return method_spec
-
- def __call__(self, request: InvocableRequest, context: Any = None) -> InvocableResponse:
- """Invokes a method call if it is registered."""
- if not hasattr(self.__class__, "_method_mappings"):
- logging.error("__call__: No mappings available on invocable.")
- return InvocableResponse.error(
- code=HTTPStatus.NOT_FOUND, message="No mappings available for invocable."
- )
-
- if request.invocation is None:
- logging.error("__call__: No invocation on request.")
- return InvocableResponse.error(
- code=HTTPStatus.NOT_FOUND, message="No invocation was found."
- )
-
- verb = Verb(request.invocation.http_verb.strip().upper())
- path = request.invocation.invocation_path
-
- path = MethodSpec.clean_path(path)
-
- logging.info(f"[{verb}] {path}")
-
- method_mappings = self.__class__._method_mappings
-
- if verb not in method_mappings:
- logging.error(f"__call__: Verb '{verb}' not found in method_mappings.")
- return InvocableResponse.error(
- code=HTTPStatus.NOT_FOUND,
- message=f"No methods for verb {verb} available.",
- )
-
- if path not in method_mappings[verb]:
- logging.error(f"__call__: Path '{path}' not found in method_mappings[{verb}].")
- return InvocableResponse.error(
- code=HTTPStatus.NOT_FOUND,
- message=f"No handler for {verb} {path} available.",
- )
-
- method = method_mappings[verb][path]
- if not (hasattr(self, method) and callable(getattr(self, method))):
- logging.error(
- f"__call__: Method not found or not callable for '{path}' in method_mappings[{verb}]."
- )
- return InvocableResponse.error(
- code=HTTPStatus.INTERNAL_SERVER_ERROR,
- message=f"Handler for {verb} {path} not callable.",
- )
-
- arguments = request.invocation.arguments
- if arguments is None:
- return getattr(self, method)()
- else:
- return getattr(self, method)(**arguments)
-
- @classmethod
- def get_config_parameters(cls) -> Dict[str, ConfigParameter]:
- return cls.config_cls().get_config_parameters()
diff --git a/spaces/Justin-Choo/QuickGen-Photo/README.md b/spaces/Justin-Choo/QuickGen-Photo/README.md
deleted file mode 100644
index 1b82bad4ba4950496b43cb31e967cb21c305b7ff..0000000000000000000000000000000000000000
--- a/spaces/Justin-Choo/QuickGen-Photo/README.md
+++ /dev/null
@@ -1,14 +0,0 @@
----
-title: Photo-Gen
-emoji: 💩
-colorFrom: blue
-colorTo: red
-sdk: gradio
-sdk_version: 3.17.0
-app_file: app.py
-pinned: false
-license: creativeml-openrail-m
-duplicated_from: pulpapps/QuickGen-Photo
----
-
-Check out the configuration reference at https://huggingface.co/docs/hub/spaces-config-reference
\ No newline at end of file
diff --git a/spaces/KAIST-Geometric-AI-Lab/syncdiffusion-demo/syncdiffusion/syncdiffusion_model.py b/spaces/KAIST-Geometric-AI-Lab/syncdiffusion-demo/syncdiffusion/syncdiffusion_model.py
deleted file mode 100644
index 79dff962674eee942a447f8a4a9a3d76b7fc40f6..0000000000000000000000000000000000000000
--- a/spaces/KAIST-Geometric-AI-Lab/syncdiffusion-demo/syncdiffusion/syncdiffusion_model.py
+++ /dev/null
@@ -1,232 +0,0 @@
-import torch
-import torch.nn as nn
-import torchvision.transforms as T
-from torch.autograd import grad
-import argparse
-from tqdm import tqdm
-
-from syncdiffusion.utils import *
-import lpips
-from transformers import CLIPTextModel, CLIPTokenizer
-from diffusers import AutoencoderKL, UNet2DConditionModel, DDIMScheduler
-
-class SyncDiffusion(nn.Module):
- def __init__(self, device='cuda', sd_version='2.0', hf_key=None):
- super().__init__()
-
- self.device = device
- self.sd_version = sd_version
-
- print(f'[INFO] loading stable diffusion...')
- if hf_key is not None:
- print(f'[INFO] using hugging face custom model key: {hf_key}')
- model_key = hf_key
- elif self.sd_version == '2.1':
- model_key = "stabilityai/stable-diffusion-2-1-base"
- elif self.sd_version == '2.0':
- model_key = "stabilityai/stable-diffusion-2-base"
- elif self.sd_version == '1.5':
- model_key = "runwayml/stable-diffusion-v1-5"
- else:
- raise ValueError(f'Stable-diffusion version {self.sd_version} not supported.')
-
- # Load pretrained models from HuggingFace
- self.vae = AutoencoderKL.from_pretrained(model_key, subfolder="vae").to(self.device)
- self.tokenizer = CLIPTokenizer.from_pretrained(model_key, subfolder="tokenizer")
- self.text_encoder = CLIPTextModel.from_pretrained(model_key, subfolder="text_encoder").to(self.device)
- self.unet = UNet2DConditionModel.from_pretrained(model_key, subfolder="unet").to(self.device)
-
- # Freeze models
- for p in self.unet.parameters():
- p.requires_grad_(False)
- for p in self.vae.parameters():
- p.requires_grad_(False)
- for p in self.text_encoder.parameters():
- p.requires_grad_(False)
-
- self.unet.eval()
- self.vae.eval()
- self.text_encoder.eval()
- print(f'[INFO] loaded stable diffusion!')
-
- # Set DDIM scheduler
- self.scheduler = DDIMScheduler.from_pretrained(model_key, subfolder="scheduler")
-
- # load perceptual loss (LPIPS)
- self.percept_loss = lpips.LPIPS(net='vgg').to(self.device)
- print(f'[INFO] loaded perceptual loss!')
-
- def get_text_embeds(self, prompt, negative_prompt):
- # Tokenize text and get embeddings
- text_input = self.tokenizer(prompt, padding='max_length', max_length=self.tokenizer.model_max_length,
- truncation=True, return_tensors='pt')
- text_embeddings = self.text_encoder(text_input.input_ids.to(self.device))[0]
-
- # Repeat for unconditional embeddings
- uncond_input = self.tokenizer(negative_prompt, padding='max_length', max_length=self.tokenizer.model_max_length,
- return_tensors='pt')
- uncond_embeddings = self.text_encoder(uncond_input.input_ids.to(self.device))[0]
-
- # Concatenate for final embeddings
- text_embeddings = torch.cat([uncond_embeddings, text_embeddings])
- return text_embeddings
-
- def decode_latents(self, latents):
- latents = 1 / 0.18215 * latents
- imgs = self.vae.decode(latents).sample
- imgs = (imgs / 2 + 0.5).clamp(0, 1)
- return imgs
-
- def sample_syncdiffusion(
- self,
- prompts,
- negative_prompts="",
- height=512,
- width=2048,
- latent_size=64, # fix latent size to 64 for Stable Diffusion
- num_inference_steps=50,
- guidance_scale=7.5,
- sync_weight=20, # gradient descent weight 'w' in the paper
- sync_freq=1, # sync_freq=n: perform gradient descent every n steps
- sync_thres=50, # sync_thres=n: compute SyncDiffusion only for the first n steps
- sync_decay_rate=0.95, # decay rate for sync_weight, set as 0.95 in the paper
- stride=16, # stride for latents, set as 16 in the paper
- ):
- assert height >= 512 and width >= 512, 'height and width must be at least 512'
- assert height % (stride * 8) == 0 and width % (stride * 8) == 0, 'height and width must be divisible by the stride multiplied by 8'
- assert stride % 8 == 0 and stride < 64, 'stride must be divisible by 8 and smaller than the latent size of Stable Diffusion'
-
- if isinstance(prompts, str):
- prompts = [prompts]
-
- if isinstance(negative_prompts, str):
- negative_prompts = [negative_prompts]
-
- # obtain text embeddings
- text_embeds = self.get_text_embeds(prompts, negative_prompts) # [2, 77, 768]
-
- # define a list of windows to process in parallel
- views = get_views(height, width, stride=stride)
- print(f"[INFO] number of views to process: {len(views)}")
-
- # Initialize latent
- latent = torch.randn((1, self.unet.in_channels, height // 8, width // 8))
-
- count = torch.zeros_like(latent, requires_grad=False, device=self.device)
- value = torch.zeros_like(latent, requires_grad=False, device=self.device)
- latent = latent.to(self.device)
-
- # set DDIM scheduler
- self.scheduler.set_timesteps(num_inference_steps)
-
- # set the anchor view as the middle view
- anchor_view_idx = len(views) // 2
-
- # set SyncDiffusion scheduler
- sync_scheduler = exponential_decay_list(
- init_weight=sync_weight,
- decay_rate=sync_decay_rate,
- num_steps=num_inference_steps
- )
- print(f'[INFO] using exponential decay scheduler with decay rate {sync_decay_rate}')
-
- with torch.autocast('cuda'):
- for i, t in enumerate(tqdm(self.scheduler.timesteps)):
- count.zero_()
- value.zero_()
-
- '''
- (1) First, obtain the reference anchor view (for computing the perceptual loss)
- '''
- with torch.no_grad():
- if (i + 1) % sync_freq == 0 and i < sync_thres:
- # decode the anchor view
- h_start, h_end, w_start, w_end = views[anchor_view_idx]
- latent_view = latent[:, :, h_start:h_end, w_start:w_end].detach()
-
- latent_model_input = torch.cat([latent_view] * 2) # 2 x 4 x 64 x 64
- noise_pred = self.unet(latent_model_input, t, encoder_hidden_states=text_embeds)['sample']
-
- # perform guidance
- noise_pred_uncond, noise_pred_cond = noise_pred.chunk(2)
- noise_pred_new = noise_pred_uncond + guidance_scale * (noise_pred_cond - noise_pred_uncond)
-
- # predict the 'foreseen denoised' latent (x0) of the anchor view
- latent_pred_x0 = self.scheduler.step(noise_pred_new, t, latent_view)["pred_original_sample"]
- decoded_image_anchor = self.decode_latents(latent_pred_x0) # 1 x 3 x 512 x 512
-
- '''
- (2) Then perform SyncDiffusion and run a single denoising step
- '''
- for view_idx, (h_start, h_end, w_start, w_end) in enumerate(views):
- latent_view = latent[:, :, h_start:h_end, w_start:w_end].detach()
-
- ############################## BEGIN: PERFORM GRADIENT DESCENT (SyncDiffusion) ##############################
- latent_view_copy = latent_view.clone().detach()
-
- #### TODO: TEST ####
- # if i % sync_freq == 0 and i < sync_thres:
- if (i + 1) % sync_freq == 0 and i < sync_thres:
-
- # gradient on latent_view
- latent_view = latent_view.requires_grad_()
-
- # expand the latents for classifier-free guidance
- latent_model_input = torch.cat([latent_view] * 2)
-
- # predict the noise residual
- noise_pred = self.unet(latent_model_input, t, encoder_hidden_states=text_embeds)['sample']
-
- # perform guidance
- noise_pred_uncond, noise_pred_cond = noise_pred.chunk(2)
- noise_pred_new = noise_pred_uncond + guidance_scale * (noise_pred_cond - noise_pred_uncond)
-
- # compute the denoising step with the reference model
- out = self.scheduler.step(noise_pred_new, t, latent_view)
-
- # predict the 'foreseen denoised' latent (x0)
- latent_view_x0 = out['pred_original_sample']
-
- # decode the denoised latent
- decoded_x0 = self.decode_latents(latent_view_x0) # 1 x 3 x 512 x 512
-
- # compute the perceptual loss (LPIPS)
- percept_loss = self.percept_loss(
- decoded_x0 * 2.0 - 1.0,
- decoded_image_anchor * 2.0 - 1.0
- )
-
- # compute the gradient of the perceptual loss w.r.t. the latent
- norm_grad = grad(outputs=percept_loss, inputs=latent_view)[0]
-
- # SyncDiffusion: update the original latent
- if view_idx != anchor_view_idx:
- latent_view_copy = latent_view_copy - sync_scheduler[i] * norm_grad # 1 x 4 x 64 x 64
- ############################## END: PERFORM GRADIENT DESCENT (SyncDiffusion) ##############################
-
- # after gradient descent, perform a single denoising step
- with torch.no_grad():
- latent_model_input = torch.cat([latent_view_copy] * 2)
- noise_pred = self.unet(latent_model_input, t, encoder_hidden_states=text_embeds)['sample']
-
- noise_pred_uncond, noise_pred_cond = noise_pred.chunk(2)
- noise_pred_new = noise_pred_uncond + guidance_scale * (noise_pred_cond - noise_pred_uncond)
-
- out = self.scheduler.step(noise_pred_new, t, latent_view_copy)
- latent_view_denoised = out['prev_sample']
-
- # merge the latent views
- value[:, :, h_start:h_end, w_start:w_end] += latent_view_denoised
- count[:, :, h_start:h_end, w_start:w_end] += 1
-
- # take the MultiDiffusion step (average the latents)
- latent = torch.where(count > 0, value / count, value)
-
- # decode latents to panorama image
- with torch.no_grad():
- imgs = self.decode_latents(latent) # [1, 3, 512, 512]
- img = T.ToPILImage()(imgs[0].cpu())
-
- print(f"[INFO] Done!")
-
- return img
diff --git a/spaces/Kangarroar/ApplioRVC-Inference/lib/uvr5_pack/lib_v5/layers_537238KB.py b/spaces/Kangarroar/ApplioRVC-Inference/lib/uvr5_pack/lib_v5/layers_537238KB.py
deleted file mode 100644
index a38b7bb3ae3136b07eadfc2db445fef4c2de186b..0000000000000000000000000000000000000000
--- a/spaces/Kangarroar/ApplioRVC-Inference/lib/uvr5_pack/lib_v5/layers_537238KB.py
+++ /dev/null
@@ -1,126 +0,0 @@
-import torch
-from torch import nn
-import torch.nn.functional as F
-
-from . import spec_utils
-
-
-class Conv2DBNActiv(nn.Module):
- def __init__(self, nin, nout, ksize=3, stride=1, pad=1, dilation=1, activ=nn.ReLU):
- super(Conv2DBNActiv, self).__init__()
- self.conv = nn.Sequential(
- nn.Conv2d(
- nin,
- nout,
- kernel_size=ksize,
- stride=stride,
- padding=pad,
- dilation=dilation,
- bias=False,
- ),
- nn.BatchNorm2d(nout),
- activ(),
- )
-
- def __call__(self, x):
- return self.conv(x)
-
-
-class SeperableConv2DBNActiv(nn.Module):
- def __init__(self, nin, nout, ksize=3, stride=1, pad=1, dilation=1, activ=nn.ReLU):
- super(SeperableConv2DBNActiv, self).__init__()
- self.conv = nn.Sequential(
- nn.Conv2d(
- nin,
- nin,
- kernel_size=ksize,
- stride=stride,
- padding=pad,
- dilation=dilation,
- groups=nin,
- bias=False,
- ),
- nn.Conv2d(nin, nout, kernel_size=1, bias=False),
- nn.BatchNorm2d(nout),
- activ(),
- )
-
- def __call__(self, x):
- return self.conv(x)
-
-
-class Encoder(nn.Module):
- def __init__(self, nin, nout, ksize=3, stride=1, pad=1, activ=nn.LeakyReLU):
- super(Encoder, self).__init__()
- self.conv1 = Conv2DBNActiv(nin, nout, ksize, 1, pad, activ=activ)
- self.conv2 = Conv2DBNActiv(nout, nout, ksize, stride, pad, activ=activ)
-
- def __call__(self, x):
- skip = self.conv1(x)
- h = self.conv2(skip)
-
- return h, skip
-
-
-class Decoder(nn.Module):
- def __init__(
- self, nin, nout, ksize=3, stride=1, pad=1, activ=nn.ReLU, dropout=False
- ):
- super(Decoder, self).__init__()
- self.conv = Conv2DBNActiv(nin, nout, ksize, 1, pad, activ=activ)
- self.dropout = nn.Dropout2d(0.1) if dropout else None
-
- def __call__(self, x, skip=None):
- x = F.interpolate(x, scale_factor=2, mode="bilinear", align_corners=True)
- if skip is not None:
- skip = spec_utils.crop_center(skip, x)
- x = torch.cat([x, skip], dim=1)
- h = self.conv(x)
-
- if self.dropout is not None:
- h = self.dropout(h)
-
- return h
-
-
-class ASPPModule(nn.Module):
- def __init__(self, nin, nout, dilations=(4, 8, 16, 32, 64), activ=nn.ReLU):
- super(ASPPModule, self).__init__()
- self.conv1 = nn.Sequential(
- nn.AdaptiveAvgPool2d((1, None)),
- Conv2DBNActiv(nin, nin, 1, 1, 0, activ=activ),
- )
- self.conv2 = Conv2DBNActiv(nin, nin, 1, 1, 0, activ=activ)
- self.conv3 = SeperableConv2DBNActiv(
- nin, nin, 3, 1, dilations[0], dilations[0], activ=activ
- )
- self.conv4 = SeperableConv2DBNActiv(
- nin, nin, 3, 1, dilations[1], dilations[1], activ=activ
- )
- self.conv5 = SeperableConv2DBNActiv(
- nin, nin, 3, 1, dilations[2], dilations[2], activ=activ
- )
- self.conv6 = SeperableConv2DBNActiv(
- nin, nin, 3, 1, dilations[2], dilations[2], activ=activ
- )
- self.conv7 = SeperableConv2DBNActiv(
- nin, nin, 3, 1, dilations[2], dilations[2], activ=activ
- )
- self.bottleneck = nn.Sequential(
- Conv2DBNActiv(nin * 7, nout, 1, 1, 0, activ=activ), nn.Dropout2d(0.1)
- )
-
- def forward(self, x):
- _, _, h, w = x.size()
- feat1 = F.interpolate(
- self.conv1(x), size=(h, w), mode="bilinear", align_corners=True
- )
- feat2 = self.conv2(x)
- feat3 = self.conv3(x)
- feat4 = self.conv4(x)
- feat5 = self.conv5(x)
- feat6 = self.conv6(x)
- feat7 = self.conv7(x)
- out = torch.cat((feat1, feat2, feat3, feat4, feat5, feat6, feat7), dim=1)
- bottle = self.bottleneck(out)
- return bottle
diff --git a/spaces/Karumoon/test007/app.py b/spaces/Karumoon/test007/app.py
deleted file mode 100644
index 04b63907d6d8ee00b1ebc71972d9867d6d781405..0000000000000000000000000000000000000000
--- a/spaces/Karumoon/test007/app.py
+++ /dev/null
@@ -1,98 +0,0 @@
-import gradio as gr
-
-import os
-import sys
-from pathlib import Path
-import time
-import random
-from PIL import Image
-
-from diffusers import DiffusionPipeline
-
-#repo_id = "Karumoon/test00a1"
-repo_id = "runwayml/stable-diffusion-v1-5"
-pipe = DiffusionPipeline.from_pretrained(repo_id)
-print(pipe)
-
-m_pdir="/content/drive/MyDrive/aipic001/"
-
-models =[
- "",
- "CompVis/stable-diffusion-v1-4",
- "runwayml/stable-diffusion-v1-5",
- "prompthero/openjourney",
-#4
- "stabilityai/stable-diffusion-2-1",
- "stabilityai/stable-diffusion-2-1-base",
- "andite/anything-v4.0",
-
- "Linaqruf/anything-v3.0",
- "eimiss/EimisAnimeDiffusion_1.0v",
- "nitrosocke/Nitro-Diffusion",
-#10
- "wavymulder/portraitplus",
- "22h/vintedois-diffusion-v0-1",
- "dreamlike-art/dreamlike-photoreal-2.0",
-#11
- "dreamlike-art/dreamlike-diffusion-1.0",
- "wavymulder/Analog-Diffusion",
- "nitrosocke/redshift-diffusion",
- "claudfuen/photorealistic-fuen-v1",
- "prompthero/openjourney-v2",
- "johnslegers/epic-diffusion",
- "nitrosocke/Arcane-Diffusion",
- "darkstorm2150/Protogen_x5.8_Official_Release",
-
-]
-
-model_1=models[1]
-model_2=models[2]
-model_3=models[3]
-model_4=models[4]
-model_5=models[5]
-model_6=models[6]
-model_7=models[7]
-model_8=models[8]
-model_9=models[9]
-model_10=models[10]
-model_11=models[11]
-model_12=models[12]
-model_13=models[13]
-model_14=models[14]
-model_15=models[15]
-model_16=models[16]
-model_17=models[17]
-model_18=models[18]
-model_19=models[19]
-model_20=models[20]
-
-
-text_gen=gr.Interface.load("spaces/Omnibus/MagicPrompt-Stable-Diffusion_link",live=True, preprocess=True)
-
-proc1=gr.Interface.load(f"models/{model_1}",live=False,preprocess=True, postprocess=False)
-proc2=gr.Interface.load(f"models/{model_2}",live=False,preprocess=True, postprocess=False)
-proc3=gr.Interface.load(f"models/{model_3}",live=False,preprocess=True, postprocess=False)
-"""
-proc4=gr.Interface.load(f"models/{model_4}",live=False,preprocess=True, postprocess=False)
-proc5=gr.Interface.load(f"models/{model_5}",live=False,preprocess=True, postprocess=False)
-proc6=gr.Interface.load(f"models/{model_6}",live=False,preprocess=True, postprocess=False)
-proc7=gr.Interface.load(f"models/{model_7}",live=False,preprocess=True, postprocess=False)
-proc8=gr.Interface.load(f"models/{model_8}",live=False,preprocess=True, postprocess=False)
-proc9=gr.Interface.load(f"models/{model_9}",live=False,preprocess=True, postprocess=False)
-proc10=gr.Interface.load(f"models/{model_10}",live=False,preprocess=True, postprocess=False)
-proc11=gr.Interface.load(f"models/{model_11}",live=False,preprocess=True, postprocess=False)
-proc12=gr.Interface.load(f"models/{model_12}",live=False,preprocess=True, postprocess=False)
-proc13=gr.Interface.load(f"models/{model_13}",live=False,preprocess=True, postprocess=False)
-proc14=gr.Interface.load(f"models/{model_14}",live=False,preprocess=True, postprocess=False)
-proc15=gr.Interface.load(f"models/{model_15}",live=False,preprocess=True, postprocess=False)
-proc16=gr.Interface.load(f"models/{model_16}",live=False,preprocess=True, postprocess=False)
-proc17=gr.Interface.load(f"models/{model_17}",live=False,preprocess=True, postprocess=False)
-proc18=gr.Interface.load(f"models/{model_18}",live=False,preprocess=True, postprocess=False)
-proc19=gr.Interface.load(f"models/{model_19}",live=False,preprocess=True, postprocess=False)
-proc20=gr.Interface.load(f"models/{model_20}",live=False,preprocess=True, postprocess=False)
-"""
-#https://huggingface.co/docs/diffusers/main/en/using-diffusers/loading
-
-
-proc1.launch()
-#gr.Parallel(proc1, proc2, proc3).launch()
\ No newline at end of file
diff --git a/spaces/KdaiP/yolov8-deepsort-tracking/deep_sort/deep_sort/deep/train.py b/spaces/KdaiP/yolov8-deepsort-tracking/deep_sort/deep_sort/deep/train.py
deleted file mode 100644
index c95b55d7dce1f2f12a6c315bec9101faaeb45d6b..0000000000000000000000000000000000000000
--- a/spaces/KdaiP/yolov8-deepsort-tracking/deep_sort/deep_sort/deep/train.py
+++ /dev/null
@@ -1,192 +0,0 @@
-import argparse
-import os
-import time
-
-import numpy as np
-import matplotlib.pyplot as plt
-import torch
-import torch.backends.cudnn as cudnn
-import torchvision
-
-from model import Net
-
-parser = argparse.ArgumentParser(description="Train on market1501")
-parser.add_argument("--data-dir",default='data',type=str)
-parser.add_argument("--no-cuda",action="store_true")
-parser.add_argument("--gpu-id",default=0,type=int)
-parser.add_argument("--lr",default=0.1, type=float)
-parser.add_argument("--interval",'-i',default=20,type=int)
-parser.add_argument('--resume', '-r',action='store_true')
-args = parser.parse_args()
-
-# device
-device = "cuda:{}".format(args.gpu_id) if torch.cuda.is_available() and not args.no_cuda else "cpu"
-if torch.cuda.is_available() and not args.no_cuda:
- cudnn.benchmark = True
-
-# data loading
-root = args.data_dir
-train_dir = os.path.join(root,"train")
-test_dir = os.path.join(root,"test")
-
-transform_train = torchvision.transforms.Compose([
- torchvision.transforms.RandomCrop((128,64),padding=4),
- torchvision.transforms.RandomHorizontalFlip(),
- torchvision.transforms.ToTensor(),
- torchvision.transforms.Normalize([0.485, 0.456, 0.406], [0.229, 0.224, 0.225])
-])
-transform_test = torchvision.transforms.Compose([
- torchvision.transforms.Resize((128,64)),
- torchvision.transforms.ToTensor(),
- torchvision.transforms.Normalize([0.485, 0.456, 0.406], [0.229, 0.224, 0.225])
-])
-trainloader = torch.utils.data.DataLoader(
- torchvision.datasets.ImageFolder(train_dir, transform=transform_train),
- batch_size=64,shuffle=True
-)
-testloader = torch.utils.data.DataLoader(
- torchvision.datasets.ImageFolder(test_dir, transform=transform_test),
- batch_size=64,shuffle=True
-)
-num_classes = max(len(trainloader.dataset.classes), len(testloader.dataset.classes))
-print("num_classes = %s" %num_classes)
-
-# net definition
-start_epoch = 0
-net = Net(num_classes=num_classes)
-if args.resume:
- assert os.path.isfile("./checkpoint/ckpt.t7"), "Error: no checkpoint file found!"
- print('Loading from checkpoint/ckpt.t7')
- checkpoint = torch.load("./checkpoint/ckpt.t7")
- # import ipdb; ipdb.set_trace()
- net_dict = checkpoint['net_dict']
- net.load_state_dict(net_dict)
- best_acc = checkpoint['acc']
- start_epoch = checkpoint['epoch']
-net.to(device)
-
-# loss and optimizer
-criterion = torch.nn.CrossEntropyLoss()
-optimizer = torch.optim.SGD(net.parameters(), args.lr, momentum=0.9, weight_decay=5e-4)
-best_acc = 0.
-
-# train function for each epoch
-def train(epoch):
- print("\nEpoch : %d"%(epoch+1))
- net.train()
- training_loss = 0.
- train_loss = 0.
- correct = 0
- total = 0
- interval = args.interval
- start = time.time()
- for idx, (inputs, labels) in enumerate(trainloader):
- # forward
- inputs,labels = inputs.to(device),labels.to(device)
- outputs = net(inputs)
- loss = criterion(outputs, labels)
-
- # backward
- optimizer.zero_grad()
- loss.backward()
- optimizer.step()
-
- # accumurating
- training_loss += loss.item()
- train_loss += loss.item()
- correct += outputs.max(dim=1)[1].eq(labels).sum().item()
- total += labels.size(0)
-
- # print
- if (idx+1)%interval == 0:
- end = time.time()
- print("[progress:{:.1f}%]time:{:.2f}s Loss:{:.5f} Correct:{}/{} Acc:{:.3f}%".format(
- 100.*(idx+1)/len(trainloader), end-start, training_loss/interval, correct, total, 100.*correct/total
- ))
- training_loss = 0.
- start = time.time()
-
- return train_loss/len(trainloader), 1.- correct/total
-
-def test(epoch):
- global best_acc
- net.eval()
- test_loss = 0.
- correct = 0
- total = 0
- start = time.time()
- with torch.no_grad():
- for idx, (inputs, labels) in enumerate(testloader):
- inputs, labels = inputs.to(device), labels.to(device)
- outputs = net(inputs)
- loss = criterion(outputs, labels)
-
- test_loss += loss.item()
- correct += outputs.max(dim=1)[1].eq(labels).sum().item()
- total += labels.size(0)
-
- print("Testing ...")
- end = time.time()
- print("[progress:{:.1f}%]time:{:.2f}s Loss:{:.5f} Correct:{}/{} Acc:{:.3f}%".format(
- 100.*(idx+1)/len(testloader), end-start, test_loss/len(testloader), correct, total, 100.*correct/total
- ))
-
- # saving checkpoint
- acc = 100.*correct/total
- if acc > best_acc:
- best_acc = acc
- print("Saving parameters to checkpoint/ckpt.t7")
- checkpoint = {
- 'net_dict':net.state_dict(),
- 'acc':acc,
- 'epoch':epoch,
- }
- if not os.path.isdir('checkpoint'):
- os.mkdir('checkpoint')
- torch.save(checkpoint, './checkpoint/ckpt.t7')
-
- return test_loss/len(testloader), 1.- correct/total
-
-# plot figure
-x_epoch = []
-record = {'train_loss':[], 'train_err':[], 'test_loss':[], 'test_err':[]}
-fig = plt.figure()
-ax0 = fig.add_subplot(121, title="loss")
-ax1 = fig.add_subplot(122, title="top1err")
-def draw_curve(epoch, train_loss, train_err, test_loss, test_err):
- global record
- record['train_loss'].append(train_loss)
- record['train_err'].append(train_err)
- record['test_loss'].append(test_loss)
- record['test_err'].append(test_err)
-
- x_epoch.append(epoch)
- ax0.plot(x_epoch, record['train_loss'], 'bo-', label='train')
- ax0.plot(x_epoch, record['test_loss'], 'ro-', label='val')
- ax1.plot(x_epoch, record['train_err'], 'bo-', label='train')
- ax1.plot(x_epoch, record['test_err'], 'ro-', label='val')
- if epoch == 0:
- ax0.legend()
- ax1.legend()
- fig.savefig("train.jpg")
-
-# lr decay
-def lr_decay():
- global optimizer
- for params in optimizer.param_groups:
- params['lr'] *= 0.1
- lr = params['lr']
- print("Learning rate adjusted to {}".format(lr))
-
-def main():
- total_epoches = 40
- for epoch in range(start_epoch, start_epoch+total_epoches):
- train_loss, train_err = train(epoch)
- test_loss, test_err = test(epoch)
- draw_curve(epoch, train_loss, train_err, test_loss, test_err)
- if (epoch+1)%(total_epoches//2)==0:
- lr_decay()
-
-
-if __name__ == '__main__':
- main()
diff --git a/spaces/Kevin676/Real-Time-Voice-Cloning/vocoder/gen_wavernn.py b/spaces/Kevin676/Real-Time-Voice-Cloning/vocoder/gen_wavernn.py
deleted file mode 100644
index 2036737f805f6055893812e48f99d524624aab07..0000000000000000000000000000000000000000
--- a/spaces/Kevin676/Real-Time-Voice-Cloning/vocoder/gen_wavernn.py
+++ /dev/null
@@ -1,31 +0,0 @@
-from vocoder.models.fatchord_version import WaveRNN
-from vocoder.audio import *
-
-
-def gen_testset(model: WaveRNN, test_set, samples, batched, target, overlap, save_path):
- k = model.get_step() // 1000
-
- for i, (m, x) in enumerate(test_set, 1):
- if i > samples:
- break
-
- print('\n| Generating: %i/%i' % (i, samples))
-
- x = x[0].numpy()
-
- bits = 16 if hp.voc_mode == 'MOL' else hp.bits
-
- if hp.mu_law and hp.voc_mode != 'MOL' :
- x = decode_mu_law(x, 2**bits, from_labels=True)
- else :
- x = label_2_float(x, bits)
-
- save_wav(x, save_path.joinpath("%dk_steps_%d_target.wav" % (k, i)))
-
- batch_str = "gen_batched_target%d_overlap%d" % (target, overlap) if batched else \
- "gen_not_batched"
- save_str = save_path.joinpath("%dk_steps_%d_%s.wav" % (k, i, batch_str))
-
- wav = model.generate(m, batched, target, overlap, hp.mu_law)
- save_wav(wav, save_str)
-
diff --git a/spaces/KyanChen/RSPrompter/mmdet/engine/optimizers/layer_decay_optimizer_constructor.py b/spaces/KyanChen/RSPrompter/mmdet/engine/optimizers/layer_decay_optimizer_constructor.py
deleted file mode 100644
index 73028a0aef698d63dcba8c4935d6ef6c577d0f46..0000000000000000000000000000000000000000
--- a/spaces/KyanChen/RSPrompter/mmdet/engine/optimizers/layer_decay_optimizer_constructor.py
+++ /dev/null
@@ -1,158 +0,0 @@
-# Copyright (c) OpenMMLab. All rights reserved.
-import json
-from typing import List
-
-import torch.nn as nn
-from mmengine.dist import get_dist_info
-from mmengine.logging import MMLogger
-from mmengine.optim import DefaultOptimWrapperConstructor
-
-from mmdet.registry import OPTIM_WRAPPER_CONSTRUCTORS
-
-
-def get_layer_id_for_convnext(var_name, max_layer_id):
- """Get the layer id to set the different learning rates in ``layer_wise``
- decay_type.
-
- Args:
- var_name (str): The key of the model.
- max_layer_id (int): Maximum layer id.
-
- Returns:
- int: The id number corresponding to different learning rate in
- ``LearningRateDecayOptimizerConstructor``.
- """
-
- if var_name in ('backbone.cls_token', 'backbone.mask_token',
- 'backbone.pos_embed'):
- return 0
- elif var_name.startswith('backbone.downsample_layers'):
- stage_id = int(var_name.split('.')[2])
- if stage_id == 0:
- layer_id = 0
- elif stage_id == 1:
- layer_id = 2
- elif stage_id == 2:
- layer_id = 3
- elif stage_id == 3:
- layer_id = max_layer_id
- return layer_id
- elif var_name.startswith('backbone.stages'):
- stage_id = int(var_name.split('.')[2])
- block_id = int(var_name.split('.')[3])
- if stage_id == 0:
- layer_id = 1
- elif stage_id == 1:
- layer_id = 2
- elif stage_id == 2:
- layer_id = 3 + block_id // 3
- elif stage_id == 3:
- layer_id = max_layer_id
- return layer_id
- else:
- return max_layer_id + 1
-
-
-def get_stage_id_for_convnext(var_name, max_stage_id):
- """Get the stage id to set the different learning rates in ``stage_wise``
- decay_type.
-
- Args:
- var_name (str): The key of the model.
- max_stage_id (int): Maximum stage id.
-
- Returns:
- int: The id number corresponding to different learning rate in
- ``LearningRateDecayOptimizerConstructor``.
- """
-
- if var_name in ('backbone.cls_token', 'backbone.mask_token',
- 'backbone.pos_embed'):
- return 0
- elif var_name.startswith('backbone.downsample_layers'):
- return 0
- elif var_name.startswith('backbone.stages'):
- stage_id = int(var_name.split('.')[2])
- return stage_id + 1
- else:
- return max_stage_id - 1
-
-
-@OPTIM_WRAPPER_CONSTRUCTORS.register_module()
-class LearningRateDecayOptimizerConstructor(DefaultOptimWrapperConstructor):
- # Different learning rates are set for different layers of backbone.
- # Note: Currently, this optimizer constructor is built for ConvNeXt.
-
- def add_params(self, params: List[dict], module: nn.Module,
- **kwargs) -> None:
- """Add all parameters of module to the params list.
-
- The parameters of the given module will be added to the list of param
- groups, with specific rules defined by paramwise_cfg.
-
- Args:
- params (list[dict]): A list of param groups, it will be modified
- in place.
- module (nn.Module): The module to be added.
- """
- logger = MMLogger.get_current_instance()
-
- parameter_groups = {}
- logger.info(f'self.paramwise_cfg is {self.paramwise_cfg}')
- num_layers = self.paramwise_cfg.get('num_layers') + 2
- decay_rate = self.paramwise_cfg.get('decay_rate')
- decay_type = self.paramwise_cfg.get('decay_type', 'layer_wise')
- logger.info('Build LearningRateDecayOptimizerConstructor '
- f'{decay_type} {decay_rate} - {num_layers}')
- weight_decay = self.base_wd
- for name, param in module.named_parameters():
- if not param.requires_grad:
- continue # frozen weights
- if len(param.shape) == 1 or name.endswith('.bias') or name in (
- 'pos_embed', 'cls_token'):
- group_name = 'no_decay'
- this_weight_decay = 0.
- else:
- group_name = 'decay'
- this_weight_decay = weight_decay
- if 'layer_wise' in decay_type:
- if 'ConvNeXt' in module.backbone.__class__.__name__:
- layer_id = get_layer_id_for_convnext(
- name, self.paramwise_cfg.get('num_layers'))
- logger.info(f'set param {name} as id {layer_id}')
- else:
- raise NotImplementedError()
- elif decay_type == 'stage_wise':
- if 'ConvNeXt' in module.backbone.__class__.__name__:
- layer_id = get_stage_id_for_convnext(name, num_layers)
- logger.info(f'set param {name} as id {layer_id}')
- else:
- raise NotImplementedError()
- group_name = f'layer_{layer_id}_{group_name}'
-
- if group_name not in parameter_groups:
- scale = decay_rate**(num_layers - layer_id - 1)
-
- parameter_groups[group_name] = {
- 'weight_decay': this_weight_decay,
- 'params': [],
- 'param_names': [],
- 'lr_scale': scale,
- 'group_name': group_name,
- 'lr': scale * self.base_lr,
- }
-
- parameter_groups[group_name]['params'].append(param)
- parameter_groups[group_name]['param_names'].append(name)
- rank, _ = get_dist_info()
- if rank == 0:
- to_display = {}
- for key in parameter_groups:
- to_display[key] = {
- 'param_names': parameter_groups[key]['param_names'],
- 'lr_scale': parameter_groups[key]['lr_scale'],
- 'lr': parameter_groups[key]['lr'],
- 'weight_decay': parameter_groups[key]['weight_decay'],
- }
- logger.info(f'Param groups = {json.dumps(to_display, indent=2)}')
- params.extend(parameter_groups.values())
diff --git a/spaces/KyanChen/RSPrompter/mmdet/models/roi_heads/bbox_heads/convfc_bbox_head.py b/spaces/KyanChen/RSPrompter/mmdet/models/roi_heads/bbox_heads/convfc_bbox_head.py
deleted file mode 100644
index cb6aadd86d34af3605d432492931442026432cc8..0000000000000000000000000000000000000000
--- a/spaces/KyanChen/RSPrompter/mmdet/models/roi_heads/bbox_heads/convfc_bbox_head.py
+++ /dev/null
@@ -1,249 +0,0 @@
-# Copyright (c) OpenMMLab. All rights reserved.
-from typing import Optional, Tuple, Union
-
-import torch.nn as nn
-from mmcv.cnn import ConvModule
-from mmengine.config import ConfigDict
-from torch import Tensor
-
-from mmdet.registry import MODELS
-from .bbox_head import BBoxHead
-
-
-@MODELS.register_module()
-class ConvFCBBoxHead(BBoxHead):
- r"""More general bbox head, with shared conv and fc layers and two optional
- separated branches.
-
- .. code-block:: none
-
- /-> cls convs -> cls fcs -> cls
- shared convs -> shared fcs
- \-> reg convs -> reg fcs -> reg
- """ # noqa: W605
-
- def __init__(self,
- num_shared_convs: int = 0,
- num_shared_fcs: int = 0,
- num_cls_convs: int = 0,
- num_cls_fcs: int = 0,
- num_reg_convs: int = 0,
- num_reg_fcs: int = 0,
- conv_out_channels: int = 256,
- fc_out_channels: int = 1024,
- conv_cfg: Optional[Union[dict, ConfigDict]] = None,
- norm_cfg: Optional[Union[dict, ConfigDict]] = None,
- init_cfg: Optional[Union[dict, ConfigDict]] = None,
- *args,
- **kwargs) -> None:
- super().__init__(*args, init_cfg=init_cfg, **kwargs)
- assert (num_shared_convs + num_shared_fcs + num_cls_convs +
- num_cls_fcs + num_reg_convs + num_reg_fcs > 0)
- if num_cls_convs > 0 or num_reg_convs > 0:
- assert num_shared_fcs == 0
- if not self.with_cls:
- assert num_cls_convs == 0 and num_cls_fcs == 0
- if not self.with_reg:
- assert num_reg_convs == 0 and num_reg_fcs == 0
- self.num_shared_convs = num_shared_convs
- self.num_shared_fcs = num_shared_fcs
- self.num_cls_convs = num_cls_convs
- self.num_cls_fcs = num_cls_fcs
- self.num_reg_convs = num_reg_convs
- self.num_reg_fcs = num_reg_fcs
- self.conv_out_channels = conv_out_channels
- self.fc_out_channels = fc_out_channels
- self.conv_cfg = conv_cfg
- self.norm_cfg = norm_cfg
-
- # add shared convs and fcs
- self.shared_convs, self.shared_fcs, last_layer_dim = \
- self._add_conv_fc_branch(
- self.num_shared_convs, self.num_shared_fcs, self.in_channels,
- True)
- self.shared_out_channels = last_layer_dim
-
- # add cls specific branch
- self.cls_convs, self.cls_fcs, self.cls_last_dim = \
- self._add_conv_fc_branch(
- self.num_cls_convs, self.num_cls_fcs, self.shared_out_channels)
-
- # add reg specific branch
- self.reg_convs, self.reg_fcs, self.reg_last_dim = \
- self._add_conv_fc_branch(
- self.num_reg_convs, self.num_reg_fcs, self.shared_out_channels)
-
- if self.num_shared_fcs == 0 and not self.with_avg_pool:
- if self.num_cls_fcs == 0:
- self.cls_last_dim *= self.roi_feat_area
- if self.num_reg_fcs == 0:
- self.reg_last_dim *= self.roi_feat_area
-
- self.relu = nn.ReLU(inplace=True)
- # reconstruct fc_cls and fc_reg since input channels are changed
- if self.with_cls:
- if self.custom_cls_channels:
- cls_channels = self.loss_cls.get_cls_channels(self.num_classes)
- else:
- cls_channels = self.num_classes + 1
- cls_predictor_cfg_ = self.cls_predictor_cfg.copy()
- cls_predictor_cfg_.update(
- in_features=self.cls_last_dim, out_features=cls_channels)
- self.fc_cls = MODELS.build(cls_predictor_cfg_)
- if self.with_reg:
- box_dim = self.bbox_coder.encode_size
- out_dim_reg = box_dim if self.reg_class_agnostic else \
- box_dim * self.num_classes
- reg_predictor_cfg_ = self.reg_predictor_cfg.copy()
- if isinstance(reg_predictor_cfg_, (dict, ConfigDict)):
- reg_predictor_cfg_.update(
- in_features=self.reg_last_dim, out_features=out_dim_reg)
- self.fc_reg = MODELS.build(reg_predictor_cfg_)
-
- if init_cfg is None:
- # when init_cfg is None,
- # It has been set to
- # [[dict(type='Normal', std=0.01, override=dict(name='fc_cls'))],
- # [dict(type='Normal', std=0.001, override=dict(name='fc_reg'))]
- # after `super(ConvFCBBoxHead, self).__init__()`
- # we only need to append additional configuration
- # for `shared_fcs`, `cls_fcs` and `reg_fcs`
- self.init_cfg += [
- dict(
- type='Xavier',
- distribution='uniform',
- override=[
- dict(name='shared_fcs'),
- dict(name='cls_fcs'),
- dict(name='reg_fcs')
- ])
- ]
-
- def _add_conv_fc_branch(self,
- num_branch_convs: int,
- num_branch_fcs: int,
- in_channels: int,
- is_shared: bool = False) -> tuple:
- """Add shared or separable branch.
-
- convs -> avg pool (optional) -> fcs
- """
- last_layer_dim = in_channels
- # add branch specific conv layers
- branch_convs = nn.ModuleList()
- if num_branch_convs > 0:
- for i in range(num_branch_convs):
- conv_in_channels = (
- last_layer_dim if i == 0 else self.conv_out_channels)
- branch_convs.append(
- ConvModule(
- conv_in_channels,
- self.conv_out_channels,
- 3,
- padding=1,
- conv_cfg=self.conv_cfg,
- norm_cfg=self.norm_cfg))
- last_layer_dim = self.conv_out_channels
- # add branch specific fc layers
- branch_fcs = nn.ModuleList()
- if num_branch_fcs > 0:
- # for shared branch, only consider self.with_avg_pool
- # for separated branches, also consider self.num_shared_fcs
- if (is_shared
- or self.num_shared_fcs == 0) and not self.with_avg_pool:
- last_layer_dim *= self.roi_feat_area
- for i in range(num_branch_fcs):
- fc_in_channels = (
- last_layer_dim if i == 0 else self.fc_out_channels)
- branch_fcs.append(
- nn.Linear(fc_in_channels, self.fc_out_channels))
- last_layer_dim = self.fc_out_channels
- return branch_convs, branch_fcs, last_layer_dim
-
- def forward(self, x: Tuple[Tensor]) -> tuple:
- """Forward features from the upstream network.
-
- Args:
- x (tuple[Tensor]): Features from the upstream network, each is
- a 4D-tensor.
-
- Returns:
- tuple: A tuple of classification scores and bbox prediction.
-
- - cls_score (Tensor): Classification scores for all \
- scale levels, each is a 4D-tensor, the channels number \
- is num_base_priors * num_classes.
- - bbox_pred (Tensor): Box energies / deltas for all \
- scale levels, each is a 4D-tensor, the channels number \
- is num_base_priors * 4.
- """
- # shared part
- if self.num_shared_convs > 0:
- for conv in self.shared_convs:
- x = conv(x)
-
- if self.num_shared_fcs > 0:
- if self.with_avg_pool:
- x = self.avg_pool(x)
-
- x = x.flatten(1)
-
- for fc in self.shared_fcs:
- x = self.relu(fc(x))
- # separate branches
- x_cls = x
- x_reg = x
-
- for conv in self.cls_convs:
- x_cls = conv(x_cls)
- if x_cls.dim() > 2:
- if self.with_avg_pool:
- x_cls = self.avg_pool(x_cls)
- x_cls = x_cls.flatten(1)
- for fc in self.cls_fcs:
- x_cls = self.relu(fc(x_cls))
-
- for conv in self.reg_convs:
- x_reg = conv(x_reg)
- if x_reg.dim() > 2:
- if self.with_avg_pool:
- x_reg = self.avg_pool(x_reg)
- x_reg = x_reg.flatten(1)
- for fc in self.reg_fcs:
- x_reg = self.relu(fc(x_reg))
-
- cls_score = self.fc_cls(x_cls) if self.with_cls else None
- bbox_pred = self.fc_reg(x_reg) if self.with_reg else None
- return cls_score, bbox_pred
-
-
-@MODELS.register_module()
-class Shared2FCBBoxHead(ConvFCBBoxHead):
-
- def __init__(self, fc_out_channels: int = 1024, *args, **kwargs) -> None:
- super().__init__(
- num_shared_convs=0,
- num_shared_fcs=2,
- num_cls_convs=0,
- num_cls_fcs=0,
- num_reg_convs=0,
- num_reg_fcs=0,
- fc_out_channels=fc_out_channels,
- *args,
- **kwargs)
-
-
-@MODELS.register_module()
-class Shared4Conv1FCBBoxHead(ConvFCBBoxHead):
-
- def __init__(self, fc_out_channels: int = 1024, *args, **kwargs) -> None:
- super().__init__(
- num_shared_convs=4,
- num_shared_fcs=1,
- num_cls_convs=0,
- num_cls_fcs=0,
- num_reg_convs=0,
- num_reg_fcs=0,
- fc_out_channels=fc_out_channels,
- *args,
- **kwargs)
diff --git a/spaces/LaynzKunz/Advanced-RVC-Inference/lib/infer_pack/commons.py b/spaces/LaynzKunz/Advanced-RVC-Inference/lib/infer_pack/commons.py
deleted file mode 100644
index 54470986f37825b35d90d7efa7437d1c26b87215..0000000000000000000000000000000000000000
--- a/spaces/LaynzKunz/Advanced-RVC-Inference/lib/infer_pack/commons.py
+++ /dev/null
@@ -1,166 +0,0 @@
-import math
-import numpy as np
-import torch
-from torch import nn
-from torch.nn import functional as F
-
-
-def init_weights(m, mean=0.0, std=0.01):
- classname = m.__class__.__name__
- if classname.find("Conv") != -1:
- m.weight.data.normal_(mean, std)
-
-
-def get_padding(kernel_size, dilation=1):
- return int((kernel_size * dilation - dilation) / 2)
-
-
-def convert_pad_shape(pad_shape):
- l = pad_shape[::-1]
- pad_shape = [item for sublist in l for item in sublist]
- return pad_shape
-
-
-def kl_divergence(m_p, logs_p, m_q, logs_q):
- """KL(P||Q)"""
- kl = (logs_q - logs_p) - 0.5
- kl += (
- 0.5 * (torch.exp(2.0 * logs_p) + ((m_p - m_q) ** 2)) * torch.exp(-2.0 * logs_q)
- )
- return kl
-
-
-def rand_gumbel(shape):
- """Sample from the Gumbel distribution, protect from overflows."""
- uniform_samples = torch.rand(shape) * 0.99998 + 0.00001
- return -torch.log(-torch.log(uniform_samples))
-
-
-def rand_gumbel_like(x):
- g = rand_gumbel(x.size()).to(dtype=x.dtype, device=x.device)
- return g
-
-
-def slice_segments(x, ids_str, segment_size=4):
- ret = torch.zeros_like(x[:, :, :segment_size])
- for i in range(x.size(0)):
- idx_str = ids_str[i]
- idx_end = idx_str + segment_size
- ret[i] = x[i, :, idx_str:idx_end]
- return ret
-
-
-def slice_segments2(x, ids_str, segment_size=4):
- ret = torch.zeros_like(x[:, :segment_size])
- for i in range(x.size(0)):
- idx_str = ids_str[i]
- idx_end = idx_str + segment_size
- ret[i] = x[i, idx_str:idx_end]
- return ret
-
-
-def rand_slice_segments(x, x_lengths=None, segment_size=4):
- b, d, t = x.size()
- if x_lengths is None:
- x_lengths = t
- ids_str_max = x_lengths - segment_size + 1
- ids_str = (torch.rand([b]).to(device=x.device) * ids_str_max).to(dtype=torch.long)
- ret = slice_segments(x, ids_str, segment_size)
- return ret, ids_str
-
-
-def get_timing_signal_1d(length, channels, min_timescale=1.0, max_timescale=1.0e4):
- position = torch.arange(length, dtype=torch.float)
- num_timescales = channels // 2
- log_timescale_increment = math.log(float(max_timescale) / float(min_timescale)) / (
- num_timescales - 1
- )
- inv_timescales = min_timescale * torch.exp(
- torch.arange(num_timescales, dtype=torch.float) * -log_timescale_increment
- )
- scaled_time = position.unsqueeze(0) * inv_timescales.unsqueeze(1)
- signal = torch.cat([torch.sin(scaled_time), torch.cos(scaled_time)], 0)
- signal = F.pad(signal, [0, 0, 0, channels % 2])
- signal = signal.view(1, channels, length)
- return signal
-
-
-def add_timing_signal_1d(x, min_timescale=1.0, max_timescale=1.0e4):
- b, channels, length = x.size()
- signal = get_timing_signal_1d(length, channels, min_timescale, max_timescale)
- return x + signal.to(dtype=x.dtype, device=x.device)
-
-
-def cat_timing_signal_1d(x, min_timescale=1.0, max_timescale=1.0e4, axis=1):
- b, channels, length = x.size()
- signal = get_timing_signal_1d(length, channels, min_timescale, max_timescale)
- return torch.cat([x, signal.to(dtype=x.dtype, device=x.device)], axis)
-
-
-def subsequent_mask(length):
- mask = torch.tril(torch.ones(length, length)).unsqueeze(0).unsqueeze(0)
- return mask
-
-
-@torch.jit.script
-def fused_add_tanh_sigmoid_multiply(input_a, input_b, n_channels):
- n_channels_int = n_channels[0]
- in_act = input_a + input_b
- t_act = torch.tanh(in_act[:, :n_channels_int, :])
- s_act = torch.sigmoid(in_act[:, n_channels_int:, :])
- acts = t_act * s_act
- return acts
-
-
-def convert_pad_shape(pad_shape):
- l = pad_shape[::-1]
- pad_shape = [item for sublist in l for item in sublist]
- return pad_shape
-
-
-def shift_1d(x):
- x = F.pad(x, convert_pad_shape([[0, 0], [0, 0], [1, 0]]))[:, :, :-1]
- return x
-
-
-def sequence_mask(length, max_length=None):
- if max_length is None:
- max_length = length.max()
- x = torch.arange(max_length, dtype=length.dtype, device=length.device)
- return x.unsqueeze(0) < length.unsqueeze(1)
-
-
-def generate_path(duration, mask):
- """
- duration: [b, 1, t_x]
- mask: [b, 1, t_y, t_x]
- """
- device = duration.device
-
- b, _, t_y, t_x = mask.shape
- cum_duration = torch.cumsum(duration, -1)
-
- cum_duration_flat = cum_duration.view(b * t_x)
- path = sequence_mask(cum_duration_flat, t_y).to(mask.dtype)
- path = path.view(b, t_x, t_y)
- path = path - F.pad(path, convert_pad_shape([[0, 0], [1, 0], [0, 0]]))[:, :-1]
- path = path.unsqueeze(1).transpose(2, 3) * mask
- return path
-
-
-def clip_grad_value_(parameters, clip_value, norm_type=2):
- if isinstance(parameters, torch.Tensor):
- parameters = [parameters]
- parameters = list(filter(lambda p: p.grad is not None, parameters))
- norm_type = float(norm_type)
- if clip_value is not None:
- clip_value = float(clip_value)
-
- total_norm = 0
- for p in parameters:
- param_norm = p.grad.data.norm(norm_type)
- total_norm += param_norm.item() ** norm_type
- if clip_value is not None:
- p.grad.data.clamp_(min=-clip_value, max=clip_value)
- total_norm = total_norm ** (1.0 / norm_type)
- return total_norm
diff --git a/spaces/Lbin123/Lbingo/src/lib/storage.ts b/spaces/Lbin123/Lbingo/src/lib/storage.ts
deleted file mode 100644
index a5b7825c4f76a28c704da512ae39e8bb45addd09..0000000000000000000000000000000000000000
--- a/spaces/Lbin123/Lbingo/src/lib/storage.ts
+++ /dev/null
@@ -1,27 +0,0 @@
-import { getMany, set, del, clear } from 'idb-keyval';
-
-export const Storage = {
- async get(key: string | string[] | null): Promise {
- if (key === null) return null;
- if (typeof key === 'string') {
- key = [key]
- }
- const returnData: Record = {}
- const values = await getMany(key)
- key.forEach((k, idx)=> {
- returnData[k] = values[idx]
- })
- return returnData;
- },
- async set(object: any) {
- for (let key of Object.keys(object)) {
- await set(key, object[key])
- }
- },
- async remove(key: string) {
- return del(key);
- },
- async clear() {
- return clear();
- }
-}
diff --git a/spaces/Lianjd/stock_dashboard/backtrader/stores/ibstore.py b/spaces/Lianjd/stock_dashboard/backtrader/stores/ibstore.py
deleted file mode 100644
index c261493eac61c82aceff29647f746374149625fa..0000000000000000000000000000000000000000
--- a/spaces/Lianjd/stock_dashboard/backtrader/stores/ibstore.py
+++ /dev/null
@@ -1,1512 +0,0 @@
-#!/usr/bin/env python
-# -*- coding: utf-8; py-indent-offset:4 -*-
-###############################################################################
-#
-# Copyright (C) 2015-2020 Daniel Rodriguez
-#
-# This program is free software: you can redistribute it and/or modify
-# it under the terms of the GNU General Public License as published by
-# the Free Software Foundation, either version 3 of the License, or
-# (at your option) any later version.
-#
-# This program is distributed in the hope that it will be useful,
-# but WITHOUT ANY WARRANTY; without even the implied warranty of
-# MERCHANTABILITY or FITNESS FOR A PARTICULAR PURPOSE. See the
-# GNU General Public License for more details.
-#
-# You should have received a copy of the GNU General Public License
-# along with this program. If not, see .
-#
-###############################################################################
-from __future__ import (absolute_import, division, print_function,
- unicode_literals)
-
-import collections
-from copy import copy
-from datetime import date, datetime, timedelta
-import inspect
-import itertools
-import random
-import threading
-import time
-
-from ib.ext.Contract import Contract
-import ib.opt as ibopt
-
-from backtrader import TimeFrame, Position
-from backtrader.metabase import MetaParams
-from backtrader.utils.py3 import bytes, bstr, queue, with_metaclass, long
-from backtrader.utils import AutoDict, UTC
-
-bytes = bstr # py2/3 need for ibpy
-
-
-def _ts2dt(tstamp=None):
- # Transforms a RTVolume timestamp to a datetime object
- if not tstamp:
- return datetime.utcnow()
-
- sec, msec = divmod(long(tstamp), 1000)
- usec = msec * 1000
- return datetime.utcfromtimestamp(sec).replace(microsecond=usec)
-
-
-class RTVolume(object):
- '''Parses a tickString tickType 48 (RTVolume) event from the IB API into its
- constituent fields
-
- Supports using a "price" to simulate an RTVolume from a tickPrice event
- '''
- _fields = [
- ('price', float),
- ('size', int),
- ('datetime', _ts2dt),
- ('volume', int),
- ('vwap', float),
- ('single', bool)
- ]
-
- def __init__(self, rtvol='', price=None, tmoffset=None):
- # Use a provided string or simulate a list of empty tokens
- tokens = iter(rtvol.split(';'))
-
- # Put the tokens as attributes using the corresponding func
- for name, func in self._fields:
- setattr(self, name, func(next(tokens)) if rtvol else func())
-
- # If price was provided use it
- if price is not None:
- self.price = price
-
- if tmoffset is not None:
- self.datetime += tmoffset
-
-
-class MetaSingleton(MetaParams):
- '''Metaclass to make a metaclassed class a singleton'''
- def __init__(cls, name, bases, dct):
- super(MetaSingleton, cls).__init__(name, bases, dct)
- cls._singleton = None
-
- def __call__(cls, *args, **kwargs):
- if cls._singleton is None:
- cls._singleton = (
- super(MetaSingleton, cls).__call__(*args, **kwargs))
-
- return cls._singleton
-
-
-# Decorator to mark methods to register with ib.opt
-def ibregister(f):
- f._ibregister = True
- return f
-
-
-class IBStore(with_metaclass(MetaSingleton, object)):
- '''Singleton class wrapping an ibpy ibConnection instance.
-
- The parameters can also be specified in the classes which use this store,
- like ``IBData`` and ``IBBroker``
-
- Params:
-
- - ``host`` (default:``127.0.0.1``): where IB TWS or IB Gateway are
- actually running. And although this will usually be the localhost, it
- must not be
-
- - ``port`` (default: ``7496``): port to connect to. The demo system uses
- ``7497``
-
- - ``clientId`` (default: ``None``): which clientId to use to connect to
- TWS.
-
- ``None``: generates a random id between 1 and 65535
- An ``integer``: will be passed as the value to use.
-
- - ``notifyall`` (default: ``False``)
-
- If ``False`` only ``error`` messages will be sent to the
- ``notify_store`` methods of ``Cerebro`` and ``Strategy``.
-
- If ``True``, each and every message received from TWS will be notified
-
- - ``_debug`` (default: ``False``)
-
- Print all messages received from TWS to standard output
-
- - ``reconnect`` (default: ``3``)
-
- Number of attempts to try to reconnect after the 1st connection attempt
- fails
-
- Set it to a ``-1`` value to keep on reconnecting forever
-
- - ``timeout`` (default: ``3.0``)
-
- Time in seconds between reconnection attemps
-
- - ``timeoffset`` (default: ``True``)
-
- If True, the time obtained from ``reqCurrentTime`` (IB Server time)
- will be used to calculate the offset to localtime and this offset will
- be used for the price notifications (tickPrice events, for example for
- CASH markets) to modify the locally calculated timestamp.
-
- The time offset will propagate to other parts of the ``backtrader``
- ecosystem like the **resampling** to align resampling timestamps using
- the calculated offset.
-
- - ``timerefresh`` (default: ``60.0``)
-
- Time in seconds: how often the time offset has to be refreshed
-
- - ``indcash`` (default: ``True``)
-
- Manage IND codes as if they were cash for price retrieval
- '''
-
- # Set a base for the data requests (historical/realtime) to distinguish the
- # id in the error notifications from orders, where the basis (usually
- # starting at 1) is set by TWS
- REQIDBASE = 0x01000000
-
- BrokerCls = None # broker class will autoregister
- DataCls = None # data class will auto register
-
- params = (
- ('host', '127.0.0.1'),
- ('port', 7496),
- ('clientId', None), # None generates a random clientid 1 -> 2^16
- ('notifyall', False),
- ('_debug', False),
- ('reconnect', 3), # -1 forever, 0 No, > 0 number of retries
- ('timeout', 3.0), # timeout between reconnections
- ('timeoffset', True), # Use offset to server for timestamps if needed
- ('timerefresh', 60.0), # How often to refresh the timeoffset
- ('indcash', True), # Treat IND codes as CASH elements
- )
-
- @classmethod
- def getdata(cls, *args, **kwargs):
- '''Returns ``DataCls`` with args, kwargs'''
- return cls.DataCls(*args, **kwargs)
-
- @classmethod
- def getbroker(cls, *args, **kwargs):
- '''Returns broker with *args, **kwargs from registered ``BrokerCls``'''
- return cls.BrokerCls(*args, **kwargs)
-
- def __init__(self):
- super(IBStore, self).__init__()
-
- self._lock_q = threading.Lock() # sync access to _tickerId/Queues
- self._lock_accupd = threading.Lock() # sync account updates
- self._lock_pos = threading.Lock() # sync account updates
- self._lock_notif = threading.Lock() # sync access to notif queue
-
- # Account list received
- self._event_managed_accounts = threading.Event()
- self._event_accdownload = threading.Event()
-
- self.dontreconnect = False # for non-recoverable connect errors
-
- self._env = None # reference to cerebro for general notifications
- self.broker = None # broker instance
- self.datas = list() # datas that have registered over start
- self.ccount = 0 # requests to start (from cerebro or datas)
-
- self._lock_tmoffset = threading.Lock()
- self.tmoffset = timedelta() # to control time difference with server
-
- # Structures to hold datas requests
- self.qs = collections.OrderedDict() # key: tickerId -> queues
- self.ts = collections.OrderedDict() # key: queue -> tickerId
- self.iscash = dict() # tickerIds from cash products (for ex: EUR.JPY)
-
- self.histexreq = dict() # holds segmented historical requests
- self.histfmt = dict() # holds datetimeformat for request
- self.histsend = dict() # holds sessionend (data time) for request
- self.histtz = dict() # holds sessionend (data time) for request
-
- self.acc_cash = AutoDict() # current total cash per account
- self.acc_value = AutoDict() # current total value per account
- self.acc_upds = AutoDict() # current account valueinfos per account
-
- self.port_update = False # indicate whether to signal to broker
-
- self.positions = collections.defaultdict(Position) # actual positions
-
- self._tickerId = itertools.count(self.REQIDBASE) # unique tickerIds
- self.orderid = None # next possible orderid (will be itertools.count)
-
- self.cdetails = collections.defaultdict(list) # hold cdetails requests
-
- self.managed_accounts = list() # received via managedAccounts
-
- self.notifs = queue.Queue() # store notifications for cerebro
-
- # Use the provided clientId or a random one
- if self.p.clientId is None:
- self.clientId = random.randint(1, pow(2, 16) - 1)
- else:
- self.clientId = self.p.clientId
-
- # ibpy connection object
- self.conn = ibopt.ibConnection(
- host=self.p.host, port=self.p.port, clientId=self.clientId)
-
- # register a printall method if requested
- if self.p._debug or self.p.notifyall:
- self.conn.registerAll(self.watcher)
-
- # Register decorated methods with the conn
- methods = inspect.getmembers(self, inspect.ismethod)
- for name, method in methods:
- if not getattr(method, '_ibregister', False):
- continue
-
- message = getattr(ibopt.message, name)
- self.conn.register(method, message)
-
- # This utility key function transforms a barsize into a:
- # (Timeframe, Compression) tuple which can be sorted
- def keyfn(x):
- n, t = x.split()
- tf, comp = self._sizes[t]
- return (tf, int(n) * comp)
-
- # This utility key function transforms a duration into a:
- # (Timeframe, Compression) tuple which can be sorted
- def key2fn(x):
- n, d = x.split()
- tf = self._dur2tf[d]
- return (tf, int(n))
-
- # Generate a table of reverse durations
- self.revdur = collections.defaultdict(list)
- # The table (dict) is a ONE to MANY relation of
- # duration -> barsizes
- # Here it is reversed to get a ONE to MANY relation of
- # barsize -> durations
- for duration, barsizes in self._durations.items():
- for barsize in barsizes:
- self.revdur[keyfn(barsize)].append(duration)
-
- # Once managed, sort the durations according to real duration and not
- # to the text form using the utility key above
- for barsize in self.revdur:
- self.revdur[barsize].sort(key=key2fn)
-
- def start(self, data=None, broker=None):
- self.reconnect(fromstart=True) # reconnect should be an invariant
-
- # Datas require some processing to kickstart data reception
- if data is not None:
- self._env = data._env
- # For datas simulate a queue with None to kickstart co
- self.datas.append(data)
-
- # if connection fails, get a fake registration that will force the
- # datas to try to reconnect or else bail out
- return self.getTickerQueue(start=True)
-
- elif broker is not None:
- self.broker = broker
-
- def stop(self):
- try:
- self.conn.disconnect() # disconnect should be an invariant
- except AttributeError:
- pass # conn may have never been connected and lack "disconnect"
-
- # Unblock any calls set on these events
- self._event_managed_accounts.set()
- self._event_accdownload.set()
-
- def logmsg(self, *args):
- # for logging purposes
- if self.p._debug:
- print(*args)
-
- def watcher(self, msg):
- # will be registered to see all messages if debug is requested
- self.logmsg(str(msg))
- if self.p.notifyall:
- self.notifs.put((msg, tuple(msg.values()), dict(msg.items())))
-
- def connected(self):
- # The isConnected method is available through __getattr__ indirections
- # and may not be present, which indicates that no connection has been
- # made because the subattribute sender has not yet been created, hence
- # the check for the AttributeError exception
- try:
- return self.conn.isConnected()
- except AttributeError:
- pass
-
- return False # non-connected (including non-initialized)
-
- def reconnect(self, fromstart=False, resub=False):
- # This method must be an invariant in that it can be called several
- # times from the same source and must be consistent. An exampler would
- # be 5 datas which are being received simultaneously and all request a
- # reconnect
-
- # Policy:
- # - if dontreconnect has been set, no option to connect is possible
- # - check connection and use the absence of isConnected as signal of
- # first ever connection (add 1 to retries too)
- # - Calculate the retries (forever or not)
- # - Try to connct
- # - If achieved and fromstart is false, the datas will be
- # re-kickstarted to recreate the subscription
- firstconnect = False
- try:
- if self.conn.isConnected():
- if resub:
- self.startdatas()
- return True # nothing to do
- except AttributeError:
- # Not connected, several __getattr__ indirections to
- # self.conn.sender.client.isConnected
- firstconnect = True
-
- if self.dontreconnect:
- return False
-
- # This is only invoked from the main thread by datas and therefore no
- # lock is needed to control synchronicity to it
- retries = self.p.reconnect
- if retries >= 0:
- retries += firstconnect
-
- while retries < 0 or retries:
- if not firstconnect:
- time.sleep(self.p.timeout)
-
- firstconnect = False
-
- if self.conn.connect():
- if not fromstart or resub:
- self.startdatas()
- return True # connection successful
-
- if retries > 0:
- retries -= 1
-
- self.dontreconnect = True
- return False # connection/reconnection failed
-
- def startdatas(self):
- # kickstrat datas, not returning until all of them have been done
- ts = list()
- for data in self.datas:
- t = threading.Thread(target=data.reqdata)
- t.start()
- ts.append(t)
-
- for t in ts:
- t.join()
-
- def stopdatas(self):
- # stop subs and force datas out of the loop (in LIFO order)
- qs = list(self.qs.values())
- ts = list()
- for data in self.datas:
- t = threading.Thread(target=data.canceldata)
- t.start()
- ts.append(t)
-
- for t in ts:
- t.join()
-
- for q in reversed(qs): # datamaster the last one to get a None
- q.put(None)
-
- def get_notifications(self):
- '''Return the pending "store" notifications'''
- # The background thread could keep on adding notifications. The None
- # mark allows to identify which is the last notification to deliver
- self.notifs.put(None) # put a mark
- notifs = list()
- while True:
- notif = self.notifs.get()
- if notif is None: # mark is reached
- break
- notifs.append(notif)
-
- return notifs
-
- @ibregister
- def error(self, msg):
- # 100-199 Order/Data/Historical related
- # 200-203 tickerId and Order Related
- # 300-399 A mix of things: orders, connectivity, tickers, misc errors
- # 400-449 Seem order related again
- # 500-531 Connectivity/Communication Errors
- # 10000-100027 Mix of special orders/routing
- # 1100-1102 TWS connectivy to the outside
- # 1300- Socket dropped in client-TWS communication
- # 2100-2110 Informative about Data Farm status (id=-1)
-
- # All errors are logged to the environment (cerebro), because many
- # errors in Interactive Brokers are actually informational and many may
- # actually be of interest to the user
- if not self.p.notifyall:
- self.notifs.put((msg, tuple(msg.values()), dict(msg.items())))
-
- # Manage those events which have to do with connection
- if msg.errorCode is None:
- # Usually received as an error in connection of just before disconn
- pass
- elif msg.errorCode in [200, 203, 162, 320, 321, 322]:
- # cdetails 200 security not found, notify over right queue
- # cdetails 203 security not allowed for acct
- try:
- q = self.qs[msg.id]
- except KeyError:
- pass # should not happend but it can
- else:
- self.cancelQueue(q, True)
-
- elif msg.errorCode in [354, 420]:
- # 354 no subscription, 420 no real-time bar for contract
- # the calling data to let the data know ... it cannot resub
- try:
- q = self.qs[msg.id]
- except KeyError:
- pass # should not happend but it can
- else:
- q.put(-msg.errorCode)
- self.cancelQueue(q)
-
- elif msg.errorCode == 10225:
- # 10225-Bust event occurred, current subscription is deactivated.
- # Please resubscribe real-time bars immediately.
- try:
- q = self.qs[msg.id]
- except KeyError:
- pass # should not happend but it can
- else:
- q.put(-msg.errorCode)
-
- elif msg.errorCode == 326: # not recoverable, clientId in use
- self.dontreconnect = True
- self.conn.disconnect()
- self.stopdatas()
-
- elif msg.errorCode == 502:
- # Cannot connect to TWS: port, config not open, tws off (504 then)
- self.conn.disconnect()
- self.stopdatas()
-
- elif msg.errorCode == 504: # Not Connected for data op
- # Once for each data
- pass # don't need to manage it
-
- elif msg.errorCode == 1300:
- # TWS has been closed. The port for a new connection is there
- # newport = int(msg.errorMsg.split('-')[-1]) # bla bla bla -7496
- self.conn.disconnect()
- self.stopdatas()
-
- elif msg.errorCode == 1100:
- # Connection lost - Notify ... datas will wait on the queue
- # with no messages arriving
- for q in self.ts: # key: queue -> ticker
- q.put(-msg.errorCode)
-
- elif msg.errorCode == 1101:
- # Connection restored and tickerIds are gone
- for q in self.ts: # key: queue -> ticker
- q.put(-msg.errorCode)
-
- elif msg.errorCode == 1102:
- # Connection restored and tickerIds maintained
- for q in self.ts: # key: queue -> ticker
- q.put(-msg.errorCode)
-
- elif msg.errorCode < 500:
- # Given the myriad of errorCodes, start by assuming is an order
- # error and if not, the checks there will let it go
- if msg.id < self.REQIDBASE:
- if self.broker is not None:
- self.broker.push_ordererror(msg)
- else:
- # Cancel the queue if a "data" reqId error is given: sanity
- q = self.qs[msg.id]
- self.cancelQueue(q, True)
-
- @ibregister
- def connectionClosed(self, msg):
- # Sometmes this comes without 1300/502 or any other and will not be
- # seen in error hence the need to manage the situation independently
- self.conn.disconnect()
- self.stopdatas()
-
- @ibregister
- def managedAccounts(self, msg):
- # 1st message in the stream
- self.managed_accounts = msg.accountsList.split(',')
- self._event_managed_accounts.set()
-
- # Request time to avoid synchronization issues
- self.reqCurrentTime()
-
- def reqCurrentTime(self):
- self.conn.reqCurrentTime()
-
- @ibregister
- def currentTime(self, msg):
- if not self.p.timeoffset: # only if requested ... apply timeoffset
- return
- curtime = datetime.fromtimestamp(float(msg.time))
- with self._lock_tmoffset:
- self.tmoffset = curtime - datetime.now()
-
- threading.Timer(self.p.timerefresh, self.reqCurrentTime).start()
-
- def timeoffset(self):
- with self._lock_tmoffset:
- return self.tmoffset
-
- def nextTickerId(self):
- # Get the next ticker using next on the itertools.count
- return next(self._tickerId)
-
- @ibregister
- def nextValidId(self, msg):
- # Create a counter from the TWS notified value to apply to orders
- self.orderid = itertools.count(msg.orderId)
-
- def nextOrderId(self):
- # Get the next ticker using next on the itertools.count made with the
- # notified value from TWS
- return next(self.orderid)
-
- def reuseQueue(self, tickerId):
- '''Reuses queue for tickerId, returning the new tickerId and q'''
- with self._lock_q:
- # Invalidate tickerId in qs (where it is a key)
- q = self.qs.pop(tickerId, None) # invalidate old
- iscash = self.iscash.pop(tickerId, None)
-
- # Update ts: q -> ticker
- tickerId = self.nextTickerId() # get new tickerId
- self.ts[q] = tickerId # Update ts: q -> tickerId
- self.qs[tickerId] = q # Update qs: tickerId -> q
- self.iscash[tickerId] = iscash
-
- return tickerId, q
-
- def getTickerQueue(self, start=False):
- '''Creates ticker/Queue for data delivery to a data feed'''
- q = queue.Queue()
- if start:
- q.put(None)
- return q
-
- with self._lock_q:
- tickerId = self.nextTickerId()
- self.qs[tickerId] = q # can be managed from other thread
- self.ts[q] = tickerId
- self.iscash[tickerId] = False
-
- return tickerId, q
-
- def cancelQueue(self, q, sendnone=False):
- '''Cancels a Queue for data delivery'''
- # pop ts (tickers) and with the result qs (queues)
- tickerId = self.ts.pop(q, None)
- self.qs.pop(tickerId, None)
-
- self.iscash.pop(tickerId, None)
-
- if sendnone:
- q.put(None)
-
- def validQueue(self, q):
- '''Returns (bool) if a queue is still valid'''
- return q in self.ts # queue -> ticker
-
- def getContractDetails(self, contract, maxcount=None):
- cds = list()
- q = self.reqContractDetails(contract)
- while True:
- msg = q.get()
- if msg is None:
- break
- cds.append(msg)
-
- if not cds or (maxcount and len(cds) > maxcount):
- err = 'Ambiguous contract: none/multiple answers received'
- self.notifs.put((err, cds, {}))
- return None
-
- return cds
-
- def reqContractDetails(self, contract):
- # get a ticker/queue for identification/data delivery
- tickerId, q = self.getTickerQueue()
- self.conn.reqContractDetails(tickerId, contract)
- return q
-
- @ibregister
- def contractDetailsEnd(self, msg):
- '''Signal end of contractdetails'''
- self.cancelQueue(self.qs[msg.reqId], True)
-
- @ibregister
- def contractDetails(self, msg):
- '''Receive answer and pass it to the queue'''
- self.qs[msg.reqId].put(msg)
-
- def reqHistoricalDataEx(self, contract, enddate, begindate,
- timeframe, compression,
- what=None, useRTH=False, tz='', sessionend=None,
- tickerId=None):
- '''
- Extension of the raw reqHistoricalData proxy, which takes two dates
- rather than a duration, barsize and date
-
- It uses the IB published valid duration/barsizes to make a mapping and
- spread a historical request over several historical requests if needed
- '''
- # Keep a copy for error reporting purposes
- kwargs = locals().copy()
- kwargs.pop('self', None) # remove self, no need to report it
-
- if timeframe < TimeFrame.Seconds:
- # Ticks are not supported
- return self.getTickerQueue(start=True)
-
- if enddate is None:
- enddate = datetime.now()
-
- if begindate is None:
- duration = self.getmaxduration(timeframe, compression)
- if duration is None:
- err = ('No duration for historical data request for '
- 'timeframe/compresison')
- self.notifs.put((err, (), kwargs))
- return self.getTickerQueue(start=True)
- barsize = self.tfcomp_to_size(timeframe, compression)
- if barsize is None:
- err = ('No supported barsize for historical data request for '
- 'timeframe/compresison')
- self.notifs.put((err, (), kwargs))
- return self.getTickerQueue(start=True)
-
- return self.reqHistoricalData(contract=contract, enddate=enddate,
- duration=duration, barsize=barsize,
- what=what, useRTH=useRTH, tz=tz,
- sessionend=sessionend)
-
- # Check if the requested timeframe/compression is supported by IB
- durations = self.getdurations(timeframe, compression)
- if not durations: # return a queue and put a None in it
- return self.getTickerQueue(start=True)
-
- # Get or reuse a queue
- if tickerId is None:
- tickerId, q = self.getTickerQueue()
- else:
- tickerId, q = self.reuseQueue(tickerId) # reuse q for old tickerId
-
- # Get the best possible duration to reduce number of requests
- duration = None
- for dur in durations:
- intdate = self.dt_plus_duration(begindate, dur)
- if intdate >= enddate:
- intdate = enddate
- duration = dur # begin -> end fits in single request
- break
-
- if duration is None: # no duration large enough to fit the request
- duration = durations[-1]
-
- # Store the calculated data
- self.histexreq[tickerId] = dict(
- contract=contract, enddate=enddate, begindate=intdate,
- timeframe=timeframe, compression=compression,
- what=what, useRTH=useRTH, tz=tz, sessionend=sessionend)
-
- barsize = self.tfcomp_to_size(timeframe, compression)
- self.histfmt[tickerId] = timeframe >= TimeFrame.Days
- self.histsend[tickerId] = sessionend
- self.histtz[tickerId] = tz
-
- if contract.m_secType in ['CASH', 'CFD']:
- self.iscash[tickerId] = 1 # msg.field code
- if not what:
- what = 'BID' # default for cash unless otherwise specified
-
- elif contract.m_secType in ['IND'] and self.p.indcash:
- self.iscash[tickerId] = 4 # msg.field code
-
- what = what or 'TRADES'
-
- self.conn.reqHistoricalData(
- tickerId,
- contract,
- bytes(intdate.strftime('%Y%m%d %H:%M:%S') + ' GMT'),
- bytes(duration),
- bytes(barsize),
- bytes(what),
- int(useRTH),
- 2) # dateformat 1 for string, 2 for unix time in seconds
-
- return q
-
- def reqHistoricalData(self, contract, enddate, duration, barsize,
- what=None, useRTH=False, tz='', sessionend=None):
- '''Proxy to reqHistorical Data'''
-
- # get a ticker/queue for identification/data delivery
- tickerId, q = self.getTickerQueue()
-
- if contract.m_secType in ['CASH', 'CFD']:
- self.iscash[tickerId] = True
- if not what:
- what = 'BID' # TRADES doesn't work
- elif what == 'ASK':
- self.iscash[tickerId] = 2
- else:
- what = what or 'TRADES'
-
- # split barsize "x time", look in sizes for (tf, comp) get tf
- tframe = self._sizes[barsize.split()[1]][0]
- self.histfmt[tickerId] = tframe >= TimeFrame.Days
- self.histsend[tickerId] = sessionend
- self.histtz[tickerId] = tz
-
- self.conn.reqHistoricalData(
- tickerId,
- contract,
- bytes(enddate.strftime('%Y%m%d %H:%M:%S') + ' GMT'),
- bytes(duration),
- bytes(barsize),
- bytes(what),
- int(useRTH),
- 2)
-
- return q
-
- def cancelHistoricalData(self, q):
- '''Cancels an existing HistoricalData request
-
- Params:
- - q: the Queue returned by reqMktData
- '''
- with self._lock_q:
- self.conn.cancelHistoricalData(self.ts[q])
- self.cancelQueue(q, True)
-
- def reqRealTimeBars(self, contract, useRTH=False, duration=5):
- '''Creates a request for (5 seconds) Real Time Bars
-
- Params:
- - contract: a ib.ext.Contract.Contract intance
- - useRTH: (default: False) passed to TWS
- - duration: (default: 5) passed to TWS, no other value works in 2016)
-
- Returns:
- - a Queue the client can wait on to receive a RTVolume instance
- '''
- # get a ticker/queue for identification/data delivery
- tickerId, q = self.getTickerQueue()
-
- # 20150929 - Only 5 secs supported for duration
- self.conn.reqRealTimeBars(
- tickerId,
- contract,
- duration,
- bytes('TRADES'),
- int(useRTH))
-
- return q
-
- def cancelRealTimeBars(self, q):
- '''Cancels an existing MarketData subscription
-
- Params:
- - q: the Queue returned by reqMktData
- '''
- with self._lock_q:
- tickerId = self.ts.get(q, None)
- if tickerId is not None:
- self.conn.cancelRealTimeBars(tickerId)
-
- self.cancelQueue(q, True)
-
- def reqMktData(self, contract, what=None):
- '''Creates a MarketData subscription
-
- Params:
- - contract: a ib.ext.Contract.Contract intance
-
- Returns:
- - a Queue the client can wait on to receive a RTVolume instance
- '''
- # get a ticker/queue for identification/data delivery
- tickerId, q = self.getTickerQueue()
- ticks = '233' # request RTVOLUME tick delivered over tickString
-
- if contract.m_secType in ['CASH', 'CFD']:
- self.iscash[tickerId] = True
- ticks = '' # cash markets do not get RTVOLUME
- if what == 'ASK':
- self.iscash[tickerId] = 2
-
- # q.put(None) # to kickstart backfilling
- # Can request 233 also for cash ... nothing will arrive
- self.conn.reqMktData(tickerId, contract, bytes(ticks), False)
- return q
-
- def cancelMktData(self, q):
- '''Cancels an existing MarketData subscription
-
- Params:
- - q: the Queue returned by reqMktData
- '''
- with self._lock_q:
- tickerId = self.ts.get(q, None)
- if tickerId is not None:
- self.conn.cancelMktData(tickerId)
-
- self.cancelQueue(q, True)
-
- @ibregister
- def tickString(self, msg):
- # Receive and process a tickString message
- if msg.tickType == 48: # RTVolume
- try:
- rtvol = RTVolume(msg.value)
- except ValueError: # price not in message ...
- pass
- else:
- # Don't need to adjust the time, because it is in "timestamp"
- # form in the message
- self.qs[msg.tickerId].put(rtvol)
-
- @ibregister
- def tickPrice(self, msg):
- '''Cash Markets have no notion of "last_price"/"last_size" and the
- tracking of the price is done (industry de-facto standard at least with
- the IB API) following the BID price
-
- A RTVolume which will only contain a price is put into the client's
- queue to have a consistent cross-market interface
- '''
- # Used for "CASH" markets
- # The price field has been seen to be missing in some instances even if
- # "field" is 1
- tickerId = msg.tickerId
- fieldcode = self.iscash[tickerId]
- if fieldcode:
- if msg.field == fieldcode: # Expected cash field code
- try:
- if msg.price == -1.0:
- # seems to indicate the stream is halted for example in
- # between 23:00 - 23:15 CET for FOREX
- return
- except AttributeError:
- pass
-
- try:
- rtvol = RTVolume(price=msg.price, tmoffset=self.tmoffset)
- # print('rtvol with datetime:', rtvol.datetime)
- except ValueError: # price not in message ...
- pass
- else:
- self.qs[tickerId].put(rtvol)
-
- @ibregister
- def realtimeBar(self, msg):
- '''Receives x seconds Real Time Bars (at the time of writing only 5
- seconds are supported)
-
- Not valid for cash markets
- '''
- # Get a naive localtime object
- msg.time = datetime.utcfromtimestamp(float(msg.time))
- self.qs[msg.reqId].put(msg)
-
- @ibregister
- def historicalData(self, msg):
- '''Receives the events of a historical data request'''
- # For multi-tiered downloads we'd need to rebind the queue to a new
- # tickerId (in case tickerIds are not reusable) and instead of putting
- # None, issue a new reqHistData with the new data and move formward
- tickerId = msg.reqId
- q = self.qs[tickerId]
- if msg.date.startswith('finished-'):
- self.histfmt.pop(tickerId, None)
- self.histsend.pop(tickerId, None)
- self.histtz.pop(tickerId, None)
- kargs = self.histexreq.pop(tickerId, None)
- if kargs is not None:
- self.reqHistoricalDataEx(tickerId=tickerId, **kargs)
- return
-
- msg.date = None
- self.cancelQueue(q)
- else:
- dtstr = msg.date # Format when string req: YYYYMMDD[ HH:MM:SS]
- if self.histfmt[tickerId]:
- sessionend = self.histsend[tickerId]
- dt = datetime.strptime(dtstr, '%Y%m%d')
- dteos = datetime.combine(dt, sessionend)
- tz = self.histtz[tickerId]
- if tz:
- dteostz = tz.localize(dteos)
- dteosutc = dteostz.astimezone(UTC).replace(tzinfo=None)
- # When requesting for example daily bars, the current day
- # will be returned with the already happened data. If the
- # session end were added, the new ticks wouldn't make it
- # through, because they happen before the end of time
- else:
- dteosutc = dteos
-
- if dteosutc <= datetime.utcnow():
- dt = dteosutc
-
- msg.date = dt
- else:
- msg.date = datetime.utcfromtimestamp(long(dtstr))
-
- q.put(msg)
-
- # The _durations are meant to calculate the needed historical data to
- # perform backfilling at the start of a connetion or a connection is lost.
- # Using a timedelta as a key allows to quickly find out which bar size
- # bar size (values in the tuples int the dict) can be used.
-
- _durations = dict([
- # 60 seconds - 1 min
- ('60 S',
- ('1 secs', '5 secs', '10 secs', '15 secs', '30 secs',
- '1 min')),
-
- # 120 seconds - 2 mins
- ('120 S',
- ('1 secs', '5 secs', '10 secs', '15 secs', '30 secs',
- '1 min', '2 mins')),
-
- # 180 seconds - 3 mins
- ('180 S',
- ('1 secs', '5 secs', '10 secs', '15 secs', '30 secs',
- '1 min', '2 mins', '3 mins')),
-
- # 300 seconds - 5 mins
- ('300 S',
- ('1 secs', '5 secs', '10 secs', '15 secs', '30 secs',
- '1 min', '2 mins', '3 mins', '5 mins')),
-
- # 600 seconds - 10 mins
- ('600 S',
- ('1 secs', '5 secs', '10 secs', '15 secs', '30 secs',
- '1 min', '2 mins', '3 mins', '5 mins', '10 mins')),
-
- # 900 seconds - 15 mins
- ('900 S',
- ('1 secs', '5 secs', '10 secs', '15 secs', '30 secs',
- '1 min', '2 mins', '3 mins', '5 mins', '10 mins', '15 mins')),
-
- # 1200 seconds - 20 mins
- ('1200 S',
- ('1 secs', '5 secs', '10 secs', '15 secs', '30 secs',
- '1 min', '2 mins', '3 mins', '5 mins', '10 mins', '15 mins',
- '20 mins')),
-
- # 1800 seconds - 30 mins
- ('1800 S',
- ('1 secs', '5 secs', '10 secs', '15 secs', '30 secs',
- '1 min', '2 mins', '3 mins', '5 mins', '10 mins', '15 mins',
- '20 mins', '30 mins')),
-
- # 3600 seconds - 1 hour
- ('3600 S',
- ('5 secs', '10 secs', '15 secs', '30 secs',
- '1 min', '2 mins', '3 mins', '5 mins', '10 mins', '15 mins',
- '20 mins', '30 mins',
- '1 hour')),
-
- # 7200 seconds - 2 hours
- ('7200 S',
- ('5 secs', '10 secs', '15 secs', '30 secs',
- '1 min', '2 mins', '3 mins', '5 mins', '10 mins', '15 mins',
- '20 mins', '30 mins',
- '1 hour', '2 hours')),
-
- # 10800 seconds - 3 hours
- ('10800 S',
- ('10 secs', '15 secs', '30 secs',
- '1 min', '2 mins', '3 mins', '5 mins', '10 mins', '15 mins',
- '20 mins', '30 mins',
- '1 hour', '2 hours', '3 hours')),
-
- # 14400 seconds - 4 hours
- ('14400 S',
- ('15 secs', '30 secs',
- '1 min', '2 mins', '3 mins', '5 mins', '10 mins', '15 mins',
- '20 mins', '30 mins',
- '1 hour', '2 hours', '3 hours', '4 hours')),
-
- # 28800 seconds - 8 hours
- ('28800 S',
- ('30 secs',
- '1 min', '2 mins', '3 mins', '5 mins', '10 mins', '15 mins',
- '20 mins', '30 mins',
- '1 hour', '2 hours', '3 hours', '4 hours', '8 hours')),
-
- # 1 days
- ('1 D',
- ('1 min', '2 mins', '3 mins', '5 mins', '10 mins', '15 mins',
- '20 mins', '30 mins',
- '1 hour', '2 hours', '3 hours', '4 hours', '8 hours',
- '1 day')),
-
- # 2 days
- ('2 D',
- ('2 mins', '3 mins', '5 mins', '10 mins', '15 mins',
- '20 mins', '30 mins',
- '1 hour', '2 hours', '3 hours', '4 hours', '8 hours',
- '1 day')),
-
- # 1 weeks
- ('1 W',
- ('3 mins', '5 mins', '10 mins', '15 mins',
- '20 mins', '30 mins',
- '1 hour', '2 hours', '3 hours', '4 hours', '8 hours',
- '1 day', '1 W')),
-
- # 2 weeks
- ('2 W',
- ('15 mins', '20 mins', '30 mins',
- '1 hour', '2 hours', '3 hours', '4 hours', '8 hours',
- '1 day', '1 W')),
-
- # 1 months
- ('1 M',
- ('30 mins',
- '1 hour', '2 hours', '3 hours', '4 hours', '8 hours',
- '1 day', '1 W', '1 M')),
-
- # 2+ months
- ('2 M', ('1 day', '1 W', '1 M')),
- ('3 M', ('1 day', '1 W', '1 M')),
- ('4 M', ('1 day', '1 W', '1 M')),
- ('5 M', ('1 day', '1 W', '1 M')),
- ('6 M', ('1 day', '1 W', '1 M')),
- ('7 M', ('1 day', '1 W', '1 M')),
- ('8 M', ('1 day', '1 W', '1 M')),
- ('9 M', ('1 day', '1 W', '1 M')),
- ('10 M', ('1 day', '1 W', '1 M')),
- ('11 M', ('1 day', '1 W', '1 M')),
-
- # 1+ years
- ('1 Y', ('1 day', '1 W', '1 M')),
- ])
-
- # Sizes allow for quick translation from bar sizes above to actual
- # timeframes to make a comparison with the actual data
- _sizes = {
- 'secs': (TimeFrame.Seconds, 1),
- 'min': (TimeFrame.Minutes, 1),
- 'mins': (TimeFrame.Minutes, 1),
- 'hour': (TimeFrame.Minutes, 60),
- 'hours': (TimeFrame.Minutes, 60),
- 'day': (TimeFrame.Days, 1),
- 'W': (TimeFrame.Weeks, 1),
- 'M': (TimeFrame.Months, 1),
- }
-
- _dur2tf = {
- 'S': TimeFrame.Seconds,
- 'D': TimeFrame.Days,
- 'W': TimeFrame.Weeks,
- 'M': TimeFrame.Months,
- 'Y': TimeFrame.Years,
- }
-
- def getdurations(self, timeframe, compression):
- key = (timeframe, compression)
- if key not in self.revdur:
- return []
-
- return self.revdur[key]
-
- def getmaxduration(self, timeframe, compression):
- key = (timeframe, compression)
- try:
- return self.revdur[key][-1]
- except (KeyError, IndexError):
- pass
-
- return None
-
- def tfcomp_to_size(self, timeframe, compression):
- if timeframe == TimeFrame.Months:
- return '{} M'.format(compression)
-
- if timeframe == TimeFrame.Weeks:
- return '{} W'.format(compression)
-
- if timeframe == TimeFrame.Days:
- if not compression % 7:
- return '{} W'.format(compression // 7)
-
- return '{} day'.format(compression)
-
- if timeframe == TimeFrame.Minutes:
- if not compression % 60:
- hours = compression // 60
- return ('{} hour'.format(hours)) + ('s' * (hours > 1))
-
- return ('{} min'.format(compression)) + ('s' * (compression > 1))
-
- if timeframe == TimeFrame.Seconds:
- return '{} secs'.format(compression)
-
- # Microseconds or ticks
- return None
-
- def dt_plus_duration(self, dt, duration):
- size, dim = duration.split()
- size = int(size)
- if dim == 'S':
- return dt + timedelta(seconds=size)
-
- if dim == 'D':
- return dt + timedelta(days=size)
-
- if dim == 'W':
- return dt + timedelta(days=size * 7)
-
- if dim == 'M':
- month = dt.month - 1 + size # -1 to make it 0 based, readd below
- years, month = divmod(month, 12)
- return dt.replace(year=dt.year + years, month=month + 1, day=1) + timedelta(dt.day - 1)
-
- if dim == 'Y':
- return dt.replace(year=dt.year + size)
-
- return dt # could do nothing with it ... return it intact
-
- def calcdurations(self, dtbegin, dtend):
- '''Calculate a duration in between 2 datetimes'''
- duration = self.histduration(dtbegin, dtend)
-
- if duration[-1] == 'M':
- m = int(duration.split()[0])
- m1 = min(2, m) # (2, 1) -> 1, (2, 7) -> 2. Bottomline: 1 or 2
- m2 = max(1, m1) # m1 can only be 1 or 2
- checkdur = '{} M'.format(m2)
- elif duration[-1] == 'Y':
- checkdur = '1 Y'
- else:
- checkdur = duration
-
- sizes = self._durations[checkduration]
- return duration, sizes
-
- def calcduration(self, dtbegin, dtend):
- '''Calculate a duration in between 2 datetimes. Returns single size'''
- duration, sizes = self._calcdurations(dtbegin, dtend)
- return duration, sizes[0]
-
- def histduration(self, dt1, dt2):
- # Given two dates calculates the smallest possible duration according
- # to the table from the Historical Data API limitations provided by IB
- #
- # Seconds: 'x S' (x: [60, 120, 180, 300, 600, 900, 1200, 1800, 3600,
- # 7200, 10800, 14400, 28800])
- # Days: 'x D' (x: [1, 2]
- # Weeks: 'x W' (x: [1, 2])
- # Months: 'x M' (x: [1, 11])
- # Years: 'x Y' (x: [1])
-
- td = dt2 - dt1 # get a timedelta for calculations
-
- # First: array of secs
- tsecs = td.total_seconds()
- secs = [60, 120, 180, 300, 600, 900, 1200, 1800, 3600, 7200, 10800,
- 14400, 28800]
-
- idxsec = bisect.bisect_left(secs, tsecs)
- if idxsec < len(secs):
- return '{} S'.format(secs[idxsec])
-
- tdextra = bool(td.seconds or td.microseconds) # over days/weeks
-
- # Next: 1 or 2 days
- days = td.days + tdextra
- if td.days <= 2:
- return '{} D'.format(days)
-
- # Next: 1 or 2 weeks
- weeks, d = divmod(td.days, 7)
- weeks += bool(d or tdextra)
- if weeks <= 2:
- return '{} W'.format(weeks)
-
- # Get references to dt components
- y2, m2, d2 = dt2.year, dt2.month, dt2.day
- y1, m1, d1 = dt1.year, dt1.month, dt2.day
-
- H2, M2, S2, US2 = dt2.hour, dt2.minute, dt2.second, dt2.microsecond
- H1, M1, S1, US1 = dt1.hour, dt1.minute, dt1.second, dt1.microsecond
-
- # Next: 1 -> 11 months (11 incl)
- months = (y2 * 12 + m2) - (y1 * 12 + m1) + (
- (d2, H2, M2, S2, US2) > (d1, H1, M1, S1, US1))
- if months <= 1: # months <= 11
- return '1 M' # return '{} M'.format(months)
- elif months <= 11:
- return '2 M' # cap at 2 months to keep the table clean
-
- # Next: years
- # y = y2 - y1 + (m2, d2, H2, M2, S2, US2) > (m1, d1, H1, M1, S1, US1)
- # return '{} Y'.format(y)
-
- return '1 Y' # to keep the table clean
-
- def makecontract(self, symbol, sectype, exch, curr,
- expiry='', strike=0.0, right='', mult=1):
- '''returns a contract from the parameters without check'''
-
- contract = Contract()
- contract.m_symbol = bytes(symbol)
- contract.m_secType = bytes(sectype)
- contract.m_exchange = bytes(exch)
- if curr:
- contract.m_currency = bytes(curr)
- if sectype in ['FUT', 'OPT', 'FOP']:
- contract.m_expiry = bytes(expiry)
- if sectype in ['OPT', 'FOP']:
- contract.m_strike = strike
- contract.m_right = bytes(right)
- if mult:
- contract.m_multiplier = bytes(mult)
- return contract
-
- def cancelOrder(self, orderid):
- '''Proxy to cancelOrder'''
- self.conn.cancelOrder(orderid)
-
- def placeOrder(self, orderid, contract, order):
- '''Proxy to placeOrder'''
- self.conn.placeOrder(orderid, contract, order)
-
- @ibregister
- def openOrder(self, msg):
- '''Receive the event ``openOrder`` events'''
- self.broker.push_orderstate(msg)
-
- @ibregister
- def execDetails(self, msg):
- '''Receive execDetails'''
- self.broker.push_execution(msg.execution)
-
- @ibregister
- def orderStatus(self, msg):
- '''Receive the event ``orderStatus``'''
- self.broker.push_orderstatus(msg)
-
- @ibregister
- def commissionReport(self, msg):
- '''Receive the event commissionReport'''
- self.broker.push_commissionreport(msg.commissionReport)
-
- def reqPositions(self):
- '''Proxy to reqPositions'''
- self.conn.reqPositions()
-
- @ibregister
- def position(self, msg):
- '''Receive event positions'''
- pass # Not implemented yet
-
- def reqAccountUpdates(self, subscribe=True, account=None):
- '''Proxy to reqAccountUpdates
-
- If ``account`` is ``None``, wait for the ``managedAccounts`` message to
- set the account codes
- '''
- if account is None:
- self._event_managed_accounts.wait()
- account = self.managed_accounts[0]
-
- self.conn.reqAccountUpdates(subscribe, bytes(account))
-
- @ibregister
- def accountDownloadEnd(self, msg):
- # Signals the end of an account update
- # the event indicates it's over. It's only false once, and can be used
- # to find out if it has at least been downloaded once
- self._event_accdownload.set()
- if False:
- if self.port_update:
- self.broker.push_portupdate()
-
- self.port_update = False
-
- @ibregister
- def updatePortfolio(self, msg):
- # Lock access to the position dicts. This is called in sub-thread and
- # can kick in at any time
- with self._lock_pos:
- if not self._event_accdownload.is_set(): # 1st event seen
- position = Position(msg.position, msg.averageCost)
- self.positions[msg.contract.m_conId] = position
- else:
- position = self.positions[msg.contract.m_conId]
- if not position.fix(msg.position, msg.averageCost):
- err = ('The current calculated position and '
- 'the position reported by the broker do not match. '
- 'Operation can continue, but the trades '
- 'calculated in the strategy may be wrong')
-
- self.notifs.put((err, (), {}))
-
- # Flag signal to broker at the end of account download
- # self.port_update = True
- self.broker.push_portupdate()
-
- def getposition(self, contract, clone=False):
- # Lock access to the position dicts. This is called from main thread
- # and updates could be happening in the background
- with self._lock_pos:
- position = self.positions[contract.m_conId]
- if clone:
- return copy(position)
-
- return position
-
- @ibregister
- def updateAccountValue(self, msg):
- # Lock access to the dicts where values are updated. This happens in a
- # sub-thread and could kick it at anytime
- with self._lock_accupd:
- try:
- value = float(msg.value)
- except ValueError:
- value = msg.value
-
- self.acc_upds[msg.accountName][msg.key][msg.currency] = value
-
- if msg.key == 'NetLiquidation':
- # NetLiquidationByCurrency and currency == 'BASE' is the same
- self.acc_value[msg.accountName] = value
- elif msg.key == 'TotalCashBalance' and msg.currency == 'BASE':
- self.acc_cash[msg.accountName] = value
-
- def get_acc_values(self, account=None):
- '''Returns all account value infos sent by TWS during regular updates
- Waits for at least 1 successful download
-
- If ``account`` is ``None`` then a dictionary with accounts as keys will
- be returned containing all accounts
-
- If account is specified or the system has only 1 account the dictionary
- corresponding to that account is returned
- '''
- # Wait for at least 1 account update download to have been finished
- # before the account infos can be returned to the calling client
- if self.connected():
- self._event_accdownload.wait()
- # Lock access to acc_cash to avoid an event intefering
- with self._updacclock:
- if account is None:
- # wait for the managedAccount Messages
- if self.connected():
- self._event_managed_accounts.wait()
-
- if not self.managed_accounts:
- return self.acc_upds.copy()
-
- elif len(self.managed_accounts) > 1:
- return self.acc_upds.copy()
-
- # Only 1 account, fall through to return only 1
- account = self.managed_accounts[0]
-
- try:
- return self.acc_upds[account].copy()
- except KeyError:
- pass
-
- return self.acc_upds.copy()
-
- def get_acc_value(self, account=None):
- '''Returns the net liquidation value sent by TWS during regular updates
- Waits for at least 1 successful download
-
- If ``account`` is ``None`` then a dictionary with accounts as keys will
- be returned containing all accounts
-
- If account is specified or the system has only 1 account the dictionary
- corresponding to that account is returned
- '''
- # Wait for at least 1 account update download to have been finished
- # before the value can be returned to the calling client
- if self.connected():
- self._event_accdownload.wait()
- # Lock access to acc_cash to avoid an event intefering
- with self._lock_accupd:
- if account is None:
- # wait for the managedAccount Messages
- if self.connected():
- self._event_managed_accounts.wait()
-
- if not self.managed_accounts:
- return float()
-
- elif len(self.managed_accounts) > 1:
- return sum(self.acc_value.values())
-
- # Only 1 account, fall through to return only 1
- account = self.managed_accounts[0]
-
- try:
- return self.acc_value[account]
- except KeyError:
- pass
-
- return float()
-
- def get_acc_cash(self, account=None):
- '''Returns the total cash value sent by TWS during regular updates
- Waits for at least 1 successful download
-
- If ``account`` is ``None`` then a dictionary with accounts as keys will
- be returned containing all accounts
-
- If account is specified or the system has only 1 account the dictionary
- corresponding to that account is returned
- '''
- # Wait for at least 1 account update download to have been finished
- # before the cash can be returned to the calling client
- if self.connected():
- self._event_accdownload.wait()
- # Lock access to acc_cash to avoid an event intefering
- with self._lock_accupd:
- if account is None:
- # wait for the managedAccount Messages
- if self.connected():
- self._event_managed_accounts.wait()
-
- if not self.managed_accounts:
- return float()
-
- elif len(self.managed_accounts) > 1:
- return sum(self.acc_cash.values())
-
- # Only 1 account, fall through to return only 1
- account = self.managed_accounts[0]
-
- try:
- return self.acc_cash[account]
- except KeyError:
- pass
diff --git a/spaces/Loren/Streamlit_OCR_comparator/configs/_base_/det_pipelines/fcenet_pipeline.py b/spaces/Loren/Streamlit_OCR_comparator/configs/_base_/det_pipelines/fcenet_pipeline.py
deleted file mode 100644
index badb4536b10bd74760fdf519fe03f5c8d2bd7767..0000000000000000000000000000000000000000
--- a/spaces/Loren/Streamlit_OCR_comparator/configs/_base_/det_pipelines/fcenet_pipeline.py
+++ /dev/null
@@ -1,118 +0,0 @@
-img_norm_cfg = dict(
- mean=[123.675, 116.28, 103.53], std=[58.395, 57.12, 57.375], to_rgb=True)
-
-# for icdar2015
-leval_prop_range_icdar2015 = ((0, 0.4), (0.3, 0.7), (0.6, 1.0))
-train_pipeline_icdar2015 = [
- dict(type='LoadImageFromFile', color_type='color_ignore_orientation'),
- dict(
- type='LoadTextAnnotations',
- with_bbox=True,
- with_mask=True,
- poly2mask=False),
- dict(
- type='ColorJitter',
- brightness=32.0 / 255,
- saturation=0.5,
- contrast=0.5),
- dict(type='Normalize', **img_norm_cfg),
- dict(type='RandomScaling', size=800, scale=(3. / 4, 5. / 2)),
- dict(
- type='RandomCropFlip', crop_ratio=0.5, iter_num=1, min_area_ratio=0.2),
- dict(
- type='RandomCropPolyInstances',
- instance_key='gt_masks',
- crop_ratio=0.8,
- min_side_ratio=0.3),
- dict(
- type='RandomRotatePolyInstances',
- rotate_ratio=0.5,
- max_angle=30,
- pad_with_fixed_color=False),
- dict(type='SquareResizePad', target_size=800, pad_ratio=0.6),
- dict(type='RandomFlip', flip_ratio=0.5, direction='horizontal'),
- dict(type='Pad', size_divisor=32),
- dict(
- type='FCENetTargets',
- fourier_degree=5,
- level_proportion_range=leval_prop_range_icdar2015),
- dict(
- type='CustomFormatBundle',
- keys=['p3_maps', 'p4_maps', 'p5_maps'],
- visualize=dict(flag=False, boundary_key=None)),
- dict(type='Collect', keys=['img', 'p3_maps', 'p4_maps', 'p5_maps'])
-]
-
-img_scale_icdar2015 = (2260, 2260)
-test_pipeline_icdar2015 = [
- dict(type='LoadImageFromFile', color_type='color_ignore_orientation'),
- dict(
- type='MultiScaleFlipAug',
- img_scale=img_scale_icdar2015, # used by Resize
- flip=False,
- transforms=[
- dict(type='Resize', keep_ratio=True),
- dict(type='Normalize', **img_norm_cfg),
- dict(type='Pad', size_divisor=32),
- dict(type='ImageToTensor', keys=['img']),
- dict(type='Collect', keys=['img']),
- ])
-]
-
-# for ctw1500
-leval_prop_range_ctw1500 = ((0, 0.25), (0.2, 0.65), (0.55, 1.0))
-train_pipeline_ctw1500 = [
- dict(type='LoadImageFromFile', color_type='color_ignore_orientation'),
- dict(
- type='LoadTextAnnotations',
- with_bbox=True,
- with_mask=True,
- poly2mask=False),
- dict(
- type='ColorJitter',
- brightness=32.0 / 255,
- saturation=0.5,
- contrast=0.5),
- dict(type='Normalize', **img_norm_cfg),
- dict(type='RandomScaling', size=800, scale=(3. / 4, 5. / 2)),
- dict(
- type='RandomCropFlip', crop_ratio=0.5, iter_num=1, min_area_ratio=0.2),
- dict(
- type='RandomCropPolyInstances',
- instance_key='gt_masks',
- crop_ratio=0.8,
- min_side_ratio=0.3),
- dict(
- type='RandomRotatePolyInstances',
- rotate_ratio=0.5,
- max_angle=30,
- pad_with_fixed_color=False),
- dict(type='SquareResizePad', target_size=800, pad_ratio=0.6),
- dict(type='RandomFlip', flip_ratio=0.5, direction='horizontal'),
- dict(type='Pad', size_divisor=32),
- dict(
- type='FCENetTargets',
- fourier_degree=5,
- level_proportion_range=leval_prop_range_ctw1500),
- dict(
- type='CustomFormatBundle',
- keys=['p3_maps', 'p4_maps', 'p5_maps'],
- visualize=dict(flag=False, boundary_key=None)),
- dict(type='Collect', keys=['img', 'p3_maps', 'p4_maps', 'p5_maps'])
-]
-
-img_scale_ctw1500 = (1080, 736)
-test_pipeline_ctw1500 = [
- dict(type='LoadImageFromFile', color_type='color_ignore_orientation'),
- dict(
- type='MultiScaleFlipAug',
- img_scale=img_scale_ctw1500, # used by Resize
- flip=False,
- transforms=[
- dict(type='Resize', keep_ratio=True),
- dict(type='Normalize', **img_norm_cfg),
- dict(type='Pad', size_divisor=32),
- dict(type='ImageToTensor', keys=['img']),
- dict(type='Collect', keys=['img']),
- ])
-]
diff --git a/spaces/Loren/Streamlit_OCR_comparator/configs/textdet/psenet/psenet_r50_fpnf_600e_ctw1500.py b/spaces/Loren/Streamlit_OCR_comparator/configs/textdet/psenet/psenet_r50_fpnf_600e_ctw1500.py
deleted file mode 100644
index 483a2b2e1e7e584dfba26c7c5f506ce544953db8..0000000000000000000000000000000000000000
--- a/spaces/Loren/Streamlit_OCR_comparator/configs/textdet/psenet/psenet_r50_fpnf_600e_ctw1500.py
+++ /dev/null
@@ -1,35 +0,0 @@
-_base_ = [
- '../../_base_/default_runtime.py',
- '../../_base_/schedules/schedule_adam_step_600e.py',
- '../../_base_/det_models/psenet_r50_fpnf.py',
- '../../_base_/det_datasets/ctw1500.py',
- '../../_base_/det_pipelines/psenet_pipeline.py'
-]
-
-model = {{_base_.model_poly}}
-
-train_list = {{_base_.train_list}}
-test_list = {{_base_.test_list}}
-
-train_pipeline = {{_base_.train_pipeline}}
-test_pipeline_ctw1500 = {{_base_.test_pipeline_ctw1500}}
-
-data = dict(
- samples_per_gpu=2,
- workers_per_gpu=2,
- val_dataloader=dict(samples_per_gpu=1),
- test_dataloader=dict(samples_per_gpu=1),
- train=dict(
- type='UniformConcatDataset',
- datasets=train_list,
- pipeline=train_pipeline),
- val=dict(
- type='UniformConcatDataset',
- datasets=test_list,
- pipeline=test_pipeline_ctw1500),
- test=dict(
- type='UniformConcatDataset',
- datasets=test_list,
- pipeline=test_pipeline_ctw1500))
-
-evaluation = dict(interval=10, metric='hmean-iou')
diff --git a/spaces/MAGAer13/mPLUG-Owl2/model_worker.py b/spaces/MAGAer13/mPLUG-Owl2/model_worker.py
deleted file mode 100644
index 057fc6d1564acb021b5d21eeab6582ac9f72fc9b..0000000000000000000000000000000000000000
--- a/spaces/MAGAer13/mPLUG-Owl2/model_worker.py
+++ /dev/null
@@ -1,143 +0,0 @@
-"""
-A model worker executes the model.
-"""
-import argparse
-import asyncio
-import json
-import time
-import threading
-import uuid
-
-import requests
-import torch
-from functools import partial
-
-from mplug_owl2.constants import WORKER_HEART_BEAT_INTERVAL
-from mplug_owl2.utils import (build_logger, server_error_msg,
- pretty_print_semaphore)
-from mplug_owl2.model.builder import load_pretrained_model
-from mplug_owl2.mm_utils import process_images, load_image_from_base64, tokenizer_image_token, KeywordsStoppingCriteria
-from mplug_owl2.constants import IMAGE_TOKEN_INDEX, DEFAULT_IMAGE_TOKEN
-from transformers import TextIteratorStreamer
-from threading import Thread
-
-GB = 1 << 30
-
-worker_id = str(uuid.uuid4())[:6]
-logger = build_logger("model_worker", f"model_worker_{worker_id}.log")
-
-class ModelWorker:
- def __init__(self, model_path, model_base, model_name, load_8bit, load_4bit, device):
- self.worker_id = worker_id
- if model_path.endswith("/"):
- model_path = model_path[:-1]
- if model_name is None:
- model_paths = model_path.split("/")
- if model_paths[-1].startswith('checkpoint-'):
- self.model_name = model_paths[-2] + "_" + model_paths[-1]
- else:
- self.model_name = model_paths[-1]
- else:
- self.model_name = model_name
-
- self.device = device
- logger.info(f"Loading the model {self.model_name} on worker {worker_id} ...")
- self.tokenizer, self.model, self.image_processor, self.context_len = load_pretrained_model(
- model_path, model_base, self.model_name, load_8bit, load_4bit, device=self.device)
- self.is_multimodal = True
-
- @torch.inference_mode()
- def generate_stream(self, params):
- tokenizer, model, image_processor = self.tokenizer, self.model, self.image_processor
-
- prompt = params["prompt"]
- ori_prompt = prompt
- images = params.get("images", None)
- num_image_tokens = 0
- if images is not None and len(images) > 0 and self.is_multimodal:
- if len(images) > 0:
- if len(images) != prompt.count(DEFAULT_IMAGE_TOKEN):
- raise ValueError("Number of images does not match number of <|image|> tokens in prompt")
-
- images = [load_image_from_base64(image) for image in images]
- images = process_images(images, image_processor, model.config)
-
- if type(images) is list:
- images = [image.to(self.model.device, dtype=torch.float16) for image in images]
- else:
- images = images.to(self.model.device, dtype=torch.float16)
-
- replace_token = DEFAULT_IMAGE_TOKEN
- prompt = prompt.replace(DEFAULT_IMAGE_TOKEN, replace_token)
-
- num_image_tokens = prompt.count(replace_token) * (model.get_model().visual_abstractor.config.num_learnable_queries + 1)
- else:
- images = None
- image_args = {"images": images}
- else:
- images = None
- image_args = {}
-
- temperature = float(params.get("temperature", 1.0))
- top_p = float(params.get("top_p", 1.0))
- max_context_length = getattr(model.config, 'max_position_embeddings', 4096)
- max_new_tokens = min(int(params.get("max_new_tokens", 256)), 1024)
- stop_str = params.get("stop", None)
- do_sample = True if temperature > 0.001 else False
-
- input_ids = tokenizer_image_token(prompt, tokenizer, IMAGE_TOKEN_INDEX, return_tensors='pt').unsqueeze(0).to(self.device)
- keywords = [stop_str]
- stopping_criteria = KeywordsStoppingCriteria(keywords, tokenizer, input_ids)
- streamer = TextIteratorStreamer(tokenizer, skip_prompt=True, skip_special_tokens=True, timeout=15)
-
- max_new_tokens = min(max_new_tokens, max_context_length - input_ids.shape[-1] - num_image_tokens)
-
- if max_new_tokens < 1:
- yield json.dumps({"text": ori_prompt + "Exceeds max token length. Please start a new conversation, thanks.", "error_code": 0}).encode() + b"\0"
- return
-
- thread = Thread(target=model.generate, kwargs=dict(
- inputs=input_ids,
- do_sample=do_sample,
- temperature=temperature,
- top_p=top_p,
- max_new_tokens=max_new_tokens,
- streamer=streamer,
- stopping_criteria=[stopping_criteria],
- use_cache=True,
- **image_args
- ))
- thread.start()
-
- generated_text = ori_prompt
- for new_text in streamer:
- generated_text += new_text
- if generated_text.endswith(stop_str):
- generated_text = generated_text[:-len(stop_str)]
- yield json.dumps({"text": generated_text, "error_code": 0}).encode()
-
- def generate_stream_gate(self, params):
- try:
- for x in self.generate_stream(params):
- yield x
- except ValueError as e:
- print("Caught ValueError:", e)
- ret = {
- "text": server_error_msg,
- "error_code": 1,
- }
- yield json.dumps(ret).encode()
- except torch.cuda.CudaError as e:
- print("Caught torch.cuda.CudaError:", e)
- ret = {
- "text": server_error_msg,
- "error_code": 1,
- }
- yield json.dumps(ret).encode()
- except Exception as e:
- print("Caught Unknown Error", e)
- ret = {
- "text": server_error_msg,
- "error_code": 1,
- }
- yield json.dumps(ret).encode()
\ No newline at end of file
diff --git a/spaces/Make-A-Protagonist/Make-A-Protagonist-inference/Make-A-Protagonist/experts/XMem/inference/interact/__init__.py b/spaces/Make-A-Protagonist/Make-A-Protagonist-inference/Make-A-Protagonist/experts/XMem/inference/interact/__init__.py
deleted file mode 100644
index e69de29bb2d1d6434b8b29ae775ad8c2e48c5391..0000000000000000000000000000000000000000
diff --git a/spaces/Manjushri/MusicGen/audiocraft/utils/utils.py b/spaces/Manjushri/MusicGen/audiocraft/utils/utils.py
deleted file mode 100644
index 86e1448d065fa182ca69aae00d2f2a7eea55d8a4..0000000000000000000000000000000000000000
--- a/spaces/Manjushri/MusicGen/audiocraft/utils/utils.py
+++ /dev/null
@@ -1,234 +0,0 @@
-# Copyright (c) Meta Platforms, Inc. and affiliates.
-# All rights reserved.
-#
-# This source code is licensed under the license found in the
-# LICENSE file in the root directory of this source tree.
-
-from concurrent.futures import ProcessPoolExecutor
-from functools import wraps
-import hashlib
-import logging
-import typing as tp
-
-import flashy
-import flashy.distrib
-import omegaconf
-import torch
-from torch.nn.utils.rnn import pad_sequence
-
-
-logger = logging.getLogger(__name__)
-
-
-def dict_from_config(cfg: omegaconf.DictConfig) -> dict:
- """Convenience function to map an omegaconf configuration to a dictionary.
-
- Args:
- cfg (omegaconf.DictConfig): Original configuration to map to dict.
- Returns:
- dict: Config as dictionary object.
- """
- dct = omegaconf.OmegaConf.to_container(cfg, resolve=True)
- assert isinstance(dct, dict)
- return dct
-
-
-def random_subset(dataset, max_samples: int, seed: int = 42) -> torch.utils.data.Subset:
- if max_samples >= len(dataset):
- return dataset
-
- generator = torch.Generator().manual_seed(seed)
- perm = torch.randperm(len(dataset), generator=generator)
- return torch.utils.data.Subset(dataset, perm[:max_samples].tolist())
-
-
-def get_loader(dataset, num_samples: tp.Optional[int], batch_size: int,
- num_workers: int, seed: int, **kwargs) -> torch.utils.data.DataLoader:
- """Convenience function to load dataset into a dataloader with optional subset sampling.
-
- Args:
- dataset: Dataset to load.
- num_samples (Optional[int]): Number of samples to limit subset size.
- batch_size (int): Batch size.
- num_workers (int): Number of workers for data loading.
- seed (int): Random seed.
- """
- if num_samples is not None:
- dataset = random_subset(dataset, num_samples, seed)
-
- dataloader = flashy.distrib.loader(
- dataset,
- batch_size=batch_size,
- num_workers=num_workers,
- **kwargs
- )
- return dataloader
-
-
-def get_dataset_from_loader(dataloader):
- dataset = dataloader.dataset
- if isinstance(dataset, torch.utils.data.Subset):
- return dataset.dataset
- else:
- return dataset
-
-
-def multinomial(input: torch.Tensor, num_samples: int, replacement=False, *, generator=None):
- """torch.multinomial with arbitrary number of dimensions, and number of candidates on the last dimension.
-
- Args:
- input (torch.Tensor): The input tensor containing probabilities.
- num_samples (int): Number of samples to draw.
- replacement (bool): Whether to draw with replacement or not.
- Keywords args:
- generator (torch.Generator): A pseudorandom number generator for sampling.
- Returns:
- torch.Tensor: Last dimension contains num_samples indices
- sampled from the multinomial probability distribution
- located in the last dimension of tensor input.
- """
- input_ = input.reshape(-1, input.shape[-1])
- output_ = torch.multinomial(input_, num_samples=num_samples, replacement=replacement, generator=generator)
- output = output_.reshape(*list(input.shape[:-1]), -1)
- return output
-
-
-def sample_top_k(probs: torch.Tensor, k: int) -> torch.Tensor:
- """Sample next token from top K values along the last dimension of the input probs tensor.
-
- Args:
- probs (torch.Tensor): Input probabilities with token candidates on the last dimension.
- k (int): The k in “top-k”.
- Returns:
- torch.Tensor: Sampled tokens.
- """
- top_k_value, _ = torch.topk(probs, k, dim=-1)
- min_value_top_k = top_k_value[..., [-1]]
- probs *= (probs >= min_value_top_k).float()
- probs.div_(probs.sum(dim=-1, keepdim=True))
- next_token = multinomial(probs, num_samples=1)
- return next_token
-
-
-def sample_top_p(probs: torch.Tensor, p: float) -> torch.Tensor:
- """Sample next token from top P probabilities along the last dimension of the input probs tensor.
-
- Args:
- probs (torch.Tensor): Input probabilities with token candidates on the last dimension.
- p (int): The p in “top-p”.
- Returns:
- torch.Tensor: Sampled tokens.
- """
- probs_sort, probs_idx = torch.sort(probs, dim=-1, descending=True)
- probs_sum = torch.cumsum(probs_sort, dim=-1)
- mask = probs_sum - probs_sort > p
- probs_sort *= (~mask).float()
- probs_sort.div_(probs_sort.sum(dim=-1, keepdim=True))
- next_token = multinomial(probs_sort, num_samples=1)
- next_token = torch.gather(probs_idx, -1, next_token)
- return next_token
-
-
-class DummyPoolExecutor:
- """Dummy pool executor to use when we actually have only 1 worker.
- (e.g. instead of ProcessPoolExecutor).
- """
- class DummyResult:
- def __init__(self, func, *args, **kwargs):
- self.func = func
- self.args = args
- self.kwargs = kwargs
-
- def result(self):
- return self.func(*self.args, **self.kwargs)
-
- def __init__(self, workers, mp_context=None):
- pass
-
- def submit(self, func, *args, **kwargs):
- return DummyPoolExecutor.DummyResult(func, *args, **kwargs)
-
- def __enter__(self):
- return self
-
- def __exit__(self, exc_type, exc_value, exc_tb):
- return
-
-
-def get_pool_executor(num_workers: int, mp_context=None):
- return ProcessPoolExecutor(num_workers, mp_context) if num_workers > 1 else DummyPoolExecutor(1)
-
-
-def length_to_mask(lengths: torch.Tensor, max_len: tp.Optional[int] = None) -> torch.Tensor:
- """Utility function to convert a tensor of sequence lengths to a mask (useful when working on padded sequences).
- For example: [3, 5] => [[1, 1, 1, 0, 0], [1, 1, 1, 1, 1]]
-
- Args:
- lengths (torch.Tensor): tensor with lengths
- max_len (int): can set the max length manually. Defaults to None.
- Returns:
- torch.Tensor: mask with 0s where there is pad tokens else 1s
- """
- assert len(lengths.shape) == 1, "Length shape should be 1 dimensional."
- final_length = lengths.max().item() if not max_len else max_len
- final_length = max(final_length, 1) # if all seqs are of len zero we don't want a zero-size tensor
- return torch.arange(final_length)[None, :].to(lengths.device) < lengths[:, None]
-
-
-def hash_trick(word: str, vocab_size: int) -> int:
- """Hash trick to pair each word with an index
-
- Args:
- word (str): word we wish to convert to an index
- vocab_size (int): size of the vocabulary
- Returns:
- int: index of the word in the embedding LUT
- """
- hash = int(hashlib.sha256(word.encode("utf-8")).hexdigest(), 16)
- return hash % vocab_size
-
-
-def with_rank_rng(base_seed: int = 1234):
- """Decorator for a function so that the function will use a Random Number Generator
- whose state depend on the GPU rank. The original RNG state is restored upon returning.
-
- Args:
- base_seed (int): Random seed.
- """
- def _decorator(fun: tp.Callable):
- @wraps(fun)
- def _decorated(*args, **kwargs):
- state = torch.get_rng_state()
- seed = base_seed ^ flashy.distrib.rank()
- torch.manual_seed(seed)
- logger.debug('Rank dependent seed set to %d', seed)
- try:
- return fun(*args, **kwargs)
- finally:
- torch.set_rng_state(state)
- logger.debug('RNG state restored.')
- return _decorated
- return _decorator
-
-
-def collate(tensors: tp.List[torch.Tensor], dim: int = 0) -> tp.Tuple[torch.Tensor, torch.Tensor]:
- """Get a list of tensors and collate them to a single tensor. according to the following logic:
- - `dim` specifies the time dimension which will be stacked and padded.
- - The output will contain 1 new dimension (dimension index 0) which will be the size of
- of the original list.
-
- Args:
- tensors (tp.List[torch.Tensor]): List of tensors to collate.
- dim (int): Dimension which will be stacked and padded.
- Returns:
- tp.Tuple[torch.Tensor, torch.Tensor]:
- torch.Tensor: Stacked and padded tensor. The output will contain 1 new dimension
- (dimension index 0) which will be the size of the original list.
- torch.Tensor: Tensor containing length of original tensor sizes (without padding).
- """
- tensors = [x.transpose(0, dim) for x in tensors]
- lens = torch.LongTensor([len(x) for x in tensors])
- padded_tensors = pad_sequence(tensors)
- padded_tensors = padded_tensors.transpose(0, 1)
- padded_tensors = padded_tensors.transpose(1, dim + 1)
- return padded_tensors, lens
diff --git a/spaces/Manjushri/PhotoReal-V3.6/README.md b/spaces/Manjushri/PhotoReal-V3.6/README.md
deleted file mode 100644
index 02141e8c4ab20abd0f8eca803afb4405e0dbd7ef..0000000000000000000000000000000000000000
--- a/spaces/Manjushri/PhotoReal-V3.6/README.md
+++ /dev/null
@@ -1,13 +0,0 @@
----
-title: PhotoReal V3.6
-emoji: 👀
-colorFrom: red
-colorTo: indigo
-sdk: gradio
-sdk_version: 3.35.2
-app_file: app.py
-pinned: false
-license: mit
----
-
-Check out the configuration reference at https://huggingface.co/docs/hub/spaces-config-reference
\ No newline at end of file
diff --git a/spaces/Marshalls/testmtd/feature_extraction/apply_transforms.py b/spaces/Marshalls/testmtd/feature_extraction/apply_transforms.py
deleted file mode 100644
index 1a72080b0f88596fbdfd6073c0838b5364f340b0..0000000000000000000000000000000000000000
--- a/spaces/Marshalls/testmtd/feature_extraction/apply_transforms.py
+++ /dev/null
@@ -1,66 +0,0 @@
-import librosa
-import numpy as np
-from pathlib import Path
-import json
-import os.path
-import sys
-import argparse
-import pickle
-
-THIS_DIR = os.path.dirname(os.path.abspath(__file__))
-ROOT_DIR = os.path.abspath(os.path.join(os.path.join(THIS_DIR, os.pardir), os.pardir))
-DATA_DIR = os.path.join(ROOT_DIR, 'data')
-EXTRACT_DIR = os.path.join(DATA_DIR, 'extracted_data')
-if not os.path.isdir(DATA_DIR):
- os.mkdir(DATA_DIR)
-if not os.path.isdir(EXTRACT_DIR):
- os.mkdir(EXTRACT_DIR)
-sys.path.append(ROOT_DIR)
-from audio_feature_utils import extract_features_hybrid, extract_features_mel, extract_features_multi_mel
-from utils import distribute_tasks
-
-parser = argparse.ArgumentParser(description="Preprocess songs data")
-
-parser.add_argument("data_path", type=str, help="features path")
-parser.add_argument("--feature_name", metavar='', type=str, default="mel", help="coma separated list of names of features to combine")
-parser.add_argument("--transform_name", metavar='', type=str, default="scaler", help="pca_transform,scaler")
-parser.add_argument("--pca_dims", metavar='', type=int, default=2, help="number of pca dimensions to keep, if applying pca transform")
-parser.add_argument("--keep_feature_name", action="store_true")
-parser.add_argument("--new_feature_name", metavar='', type=str, default=None)
-parser.add_argument("--replace_existing", action="store_true")
-args = parser.parse_args()
-
-# makes arugments into global variables of the same name, used later in the code
-globals().update(vars(args))
-data_path = Path(data_path)
-
-## distributing tasks accross nodes ##
-from mpi4py import MPI
-comm = MPI.COMM_WORLD
-rank = comm.Get_rank()
-size = comm.Get_size()
-print(rank)
-
-#assuming mp3 for now. TODO: generalize
-candidate_files = sorted(data_path.glob('**/*'+feature_name+'.npy'), key=lambda path: path.parent.__str__())
-tasks = distribute_tasks(candidate_files,rank,size)
-
-for i in tasks:
- path = candidate_files[i]
- print(path)
- feature_file = path.__str__()
- if new_feature_name is None:
- if keep_feature_name:
- new_feature_name = feature_name
- else:
- new_feature_name = feature_name+"_applied_"+transform_name
- base_filename = feature_file[:-(len(feature_name)+4)]
- new_feature_file = base_filename+new_feature_name+".npy"
- if replace_existing or not os.path.isfile(new_feature_file):
- features = np.load(feature_file)
- transform = pickle.load(open(data_path.joinpath(feature_name+'_'+transform_name+'.pkl'), "rb"))
- pickle.dump(transform, open(data_path.joinpath(new_feature_name+'_scaler.pkl'), "wb"))
- features = transform.transform(features)
- if transform_name == "pca_transform":
- features = features[:,:pca_dims]
- np.save(new_feature_file,features)
diff --git a/spaces/Matthijs/mms-tts-demo/uroman/bin/uroman-tsv.sh b/spaces/Matthijs/mms-tts-demo/uroman/bin/uroman-tsv.sh
deleted file mode 100644
index adb81f4894a0539d44ad4370eda029694211e82b..0000000000000000000000000000000000000000
--- a/spaces/Matthijs/mms-tts-demo/uroman/bin/uroman-tsv.sh
+++ /dev/null
@@ -1,28 +0,0 @@
-#!/usr/bin/env bash
-# Created by Thamme Gowda on June 17, 2019
-
-DIR=$(dirname "${BASH_SOURCE[0]}") # get the directory name
-# DIR=$(realpath "${DIR}") # resolve its full path if need be
-
-if [[ $# -lt 1 || $# -gt 2 ]]; then
- >&2 echo "ERROR: invalid args"
- >&2 echo "Usage: []"
- exit 2
-fi
-
-INP=$1
-OUT=$2
-
-CMD=$DIR/uroman.pl
-
-function romanize(){
- paste <(cut -f1 $INP) <(cut -f2 $INP | $CMD)
-}
-
-if [[ -n $OUT ]]; then
- romanize > $OUT
-else
- romanize
-fi
-
-
diff --git a/spaces/MattiaSangermano/IncentiveAI/README.md b/spaces/MattiaSangermano/IncentiveAI/README.md
deleted file mode 100644
index 901f0aa9a23a4c14abe952018938668c569a9deb..0000000000000000000000000000000000000000
--- a/spaces/MattiaSangermano/IncentiveAI/README.md
+++ /dev/null
@@ -1,12 +0,0 @@
----
-title: IncentiveAI
-emoji: 💻
-colorFrom: pink
-colorTo: yellow
-sdk: gradio
-sdk_version: 3.39.0
-app_file: app.py
-pinned: false
----
-
-Check out the configuration reference at https://huggingface.co/docs/hub/spaces-config-reference
diff --git a/spaces/MattiaSangermano/IncentiveAI/app.py b/spaces/MattiaSangermano/IncentiveAI/app.py
deleted file mode 100644
index c9dd79e99e013d5ae21f4092be5f5235829bdef8..0000000000000000000000000000000000000000
--- a/spaces/MattiaSangermano/IncentiveAI/app.py
+++ /dev/null
@@ -1,51 +0,0 @@
-import gradio as gr
-import torch
-from transformers import AutoFeatureExtractor, SwinModel
-import pandas as pd
-import numpy as np
-from PIL import Image
-
-
-extractor = AutoFeatureExtractor.from_pretrained("Neruoy/swin-finetuned-food101-e3")
-model = SwinModel.from_pretrained("Neruoy/swin-finetuned-food101-e3")
-dataset = pd.read_csv("./kb.csv")
-embeddings = dataset['embeddings'].apply(eval).tolist()
-embeddings = np.array(embeddings)
-embeddings = torch.from_numpy(embeddings)
-filenames = dataset['filename'].tolist()
-
-
-def compute_scores(emb_one, emb_two):
- """Computes cosine similarity between two vectors."""
- scores = torch.nn.functional.cosine_similarity(emb_one, emb_two)
- return scores.numpy().tolist()
-
-def inference(img):
- processed_img = extractor(img, return_tensors="pt")
- with torch.no_grad():
- query_embeddings = model(**processed_img).last_hidden_state[:, 0].cpu()
-
- sim_scores = compute_scores(embeddings, query_embeddings)
- similarity_mapping = dict(zip(filenames, sim_scores))
-
- # Sort the mapping dictionary and return `top_k` candidates.
- similarity_mapping_sorted = dict(
- sorted(similarity_mapping.items(), key=lambda x: x[1], reverse=True)
- )
- id_entries = list(similarity_mapping_sorted.keys())[0:3]
- scores = list(similarity_mapping_sorted.values())[3:3]
- images = [Image.open(f"./data/{directory}") for directory in id_entries]
- return images
-
-title = "IncentiveAI"
-description = "Demo"
-
-demo = gr.Interface(
- fn=inference,
- inputs=gr.inputs.Image(type="pil"),
- outputs=[gr.outputs.Image(type="pil", label="First"), gr.outputs.Image(type="pil",label="Second"), gr.outputs.Image(type="pil",label="Third")],
- title=title,
- description=description
-)
-#app = gr.mount_gradio_app(app, demo, path="/incentive/")
-demo.launch()
\ No newline at end of file
diff --git a/spaces/Mcdimmy/Clothing-Identifier/app.py b/spaces/Mcdimmy/Clothing-Identifier/app.py
deleted file mode 100644
index 06893fb6a924a6894c6bedf831dfe5a673f2d75a..0000000000000000000000000000000000000000
--- a/spaces/Mcdimmy/Clothing-Identifier/app.py
+++ /dev/null
@@ -1,18 +0,0 @@
-from fastai.vision.all import *
-import gradio as gr
-
-learn = load_learner('export.pkl')
-
-categories = ('Blouse', 'Dress', 'Pants', 'Shirt', 'Shorts')
-title = "Clothing Identifier"
-
-def classify_image(img):
- pred, idx, probs = learn.predict(img)
- return dict(zip(categories, map(float, probs)))
-
-image = gr.Image(shape=(512, 512))
-label = gr.Label()
-examples = ['dress.jpg', 'shirt.jpg', 'pants.jpg']
-
-intf = gr.Interface(fn=classify_image, title=title, inputs=image, outputs=label, examples=examples)
-intf.launch(inline=False)
diff --git a/spaces/Mellow-ai/PhotoAI_Mellow/annotator/uniformer/mmseg/apis/test.py b/spaces/Mellow-ai/PhotoAI_Mellow/annotator/uniformer/mmseg/apis/test.py
deleted file mode 100644
index e574eb7da04f09a59cf99ff953c36468ae87a326..0000000000000000000000000000000000000000
--- a/spaces/Mellow-ai/PhotoAI_Mellow/annotator/uniformer/mmseg/apis/test.py
+++ /dev/null
@@ -1,238 +0,0 @@
-import os.path as osp
-import pickle
-import shutil
-import tempfile
-
-import annotator.uniformer.mmcv as mmcv
-import numpy as np
-import torch
-import torch.distributed as dist
-from annotator.uniformer.mmcv.image import tensor2imgs
-from annotator.uniformer.mmcv.runner import get_dist_info
-
-
-def np2tmp(array, temp_file_name=None):
- """Save ndarray to local numpy file.
-
- Args:
- array (ndarray): Ndarray to save.
- temp_file_name (str): Numpy file name. If 'temp_file_name=None', this
- function will generate a file name with tempfile.NamedTemporaryFile
- to save ndarray. Default: None.
-
- Returns:
- str: The numpy file name.
- """
-
- if temp_file_name is None:
- temp_file_name = tempfile.NamedTemporaryFile(
- suffix='.npy', delete=False).name
- np.save(temp_file_name, array)
- return temp_file_name
-
-
-def single_gpu_test(model,
- data_loader,
- show=False,
- out_dir=None,
- efficient_test=False,
- opacity=0.5):
- """Test with single GPU.
-
- Args:
- model (nn.Module): Model to be tested.
- data_loader (utils.data.Dataloader): Pytorch data loader.
- show (bool): Whether show results during inference. Default: False.
- out_dir (str, optional): If specified, the results will be dumped into
- the directory to save output results.
- efficient_test (bool): Whether save the results as local numpy files to
- save CPU memory during evaluation. Default: False.
- opacity(float): Opacity of painted segmentation map.
- Default 0.5.
- Must be in (0, 1] range.
- Returns:
- list: The prediction results.
- """
-
- model.eval()
- results = []
- dataset = data_loader.dataset
- prog_bar = mmcv.ProgressBar(len(dataset))
- for i, data in enumerate(data_loader):
- with torch.no_grad():
- result = model(return_loss=False, **data)
-
- if show or out_dir:
- img_tensor = data['img'][0]
- img_metas = data['img_metas'][0].data[0]
- imgs = tensor2imgs(img_tensor, **img_metas[0]['img_norm_cfg'])
- assert len(imgs) == len(img_metas)
-
- for img, img_meta in zip(imgs, img_metas):
- h, w, _ = img_meta['img_shape']
- img_show = img[:h, :w, :]
-
- ori_h, ori_w = img_meta['ori_shape'][:-1]
- img_show = mmcv.imresize(img_show, (ori_w, ori_h))
-
- if out_dir:
- out_file = osp.join(out_dir, img_meta['ori_filename'])
- else:
- out_file = None
-
- model.module.show_result(
- img_show,
- result,
- palette=dataset.PALETTE,
- show=show,
- out_file=out_file,
- opacity=opacity)
-
- if isinstance(result, list):
- if efficient_test:
- result = [np2tmp(_) for _ in result]
- results.extend(result)
- else:
- if efficient_test:
- result = np2tmp(result)
- results.append(result)
-
- batch_size = len(result)
- for _ in range(batch_size):
- prog_bar.update()
- return results
-
-
-def multi_gpu_test(model,
- data_loader,
- tmpdir=None,
- gpu_collect=False,
- efficient_test=False):
- """Test model with multiple gpus.
-
- This method tests model with multiple gpus and collects the results
- under two different modes: gpu and cpu modes. By setting 'gpu_collect=True'
- it encodes results to gpu tensors and use gpu communication for results
- collection. On cpu mode it saves the results on different gpus to 'tmpdir'
- and collects them by the rank 0 worker.
-
- Args:
- model (nn.Module): Model to be tested.
- data_loader (utils.data.Dataloader): Pytorch data loader.
- tmpdir (str): Path of directory to save the temporary results from
- different gpus under cpu mode.
- gpu_collect (bool): Option to use either gpu or cpu to collect results.
- efficient_test (bool): Whether save the results as local numpy files to
- save CPU memory during evaluation. Default: False.
-
- Returns:
- list: The prediction results.
- """
-
- model.eval()
- results = []
- dataset = data_loader.dataset
- rank, world_size = get_dist_info()
- if rank == 0:
- prog_bar = mmcv.ProgressBar(len(dataset))
- for i, data in enumerate(data_loader):
- with torch.no_grad():
- result = model(return_loss=False, rescale=True, **data)
-
- if isinstance(result, list):
- if efficient_test:
- result = [np2tmp(_) for _ in result]
- results.extend(result)
- else:
- if efficient_test:
- result = np2tmp(result)
- results.append(result)
-
- if rank == 0:
- batch_size = data['img'][0].size(0)
- for _ in range(batch_size * world_size):
- prog_bar.update()
-
- # collect results from all ranks
- if gpu_collect:
- results = collect_results_gpu(results, len(dataset))
- else:
- results = collect_results_cpu(results, len(dataset), tmpdir)
- return results
-
-
-def collect_results_cpu(result_part, size, tmpdir=None):
- """Collect results with CPU."""
- rank, world_size = get_dist_info()
- # create a tmp dir if it is not specified
- if tmpdir is None:
- MAX_LEN = 512
- # 32 is whitespace
- dir_tensor = torch.full((MAX_LEN, ),
- 32,
- dtype=torch.uint8,
- device='cuda')
- if rank == 0:
- tmpdir = tempfile.mkdtemp()
- tmpdir = torch.tensor(
- bytearray(tmpdir.encode()), dtype=torch.uint8, device='cuda')
- dir_tensor[:len(tmpdir)] = tmpdir
- dist.broadcast(dir_tensor, 0)
- tmpdir = dir_tensor.cpu().numpy().tobytes().decode().rstrip()
- else:
- mmcv.mkdir_or_exist(tmpdir)
- # dump the part result to the dir
- mmcv.dump(result_part, osp.join(tmpdir, 'part_{}.pkl'.format(rank)))
- dist.barrier()
- # collect all parts
- if rank != 0:
- return None
- else:
- # load results of all parts from tmp dir
- part_list = []
- for i in range(world_size):
- part_file = osp.join(tmpdir, 'part_{}.pkl'.format(i))
- part_list.append(mmcv.load(part_file))
- # sort the results
- ordered_results = []
- for res in zip(*part_list):
- ordered_results.extend(list(res))
- # the dataloader may pad some samples
- ordered_results = ordered_results[:size]
- # remove tmp dir
- shutil.rmtree(tmpdir)
- return ordered_results
-
-
-def collect_results_gpu(result_part, size):
- """Collect results with GPU."""
- rank, world_size = get_dist_info()
- # dump result part to tensor with pickle
- part_tensor = torch.tensor(
- bytearray(pickle.dumps(result_part)), dtype=torch.uint8, device='cuda')
- # gather all result part tensor shape
- shape_tensor = torch.tensor(part_tensor.shape, device='cuda')
- shape_list = [shape_tensor.clone() for _ in range(world_size)]
- dist.all_gather(shape_list, shape_tensor)
- # padding result part tensor to max length
- shape_max = torch.tensor(shape_list).max()
- part_send = torch.zeros(shape_max, dtype=torch.uint8, device='cuda')
- part_send[:shape_tensor[0]] = part_tensor
- part_recv_list = [
- part_tensor.new_zeros(shape_max) for _ in range(world_size)
- ]
- # gather all result part
- dist.all_gather(part_recv_list, part_send)
-
- if rank == 0:
- part_list = []
- for recv, shape in zip(part_recv_list, shape_list):
- part_list.append(
- pickle.loads(recv[:shape[0]].cpu().numpy().tobytes()))
- # sort the results
- ordered_results = []
- for res in zip(*part_list):
- ordered_results.extend(list(res))
- # the dataloader may pad some samples
- ordered_results = ordered_results[:size]
- return ordered_results
diff --git a/spaces/MoonQiu/LongerCrafter/lvdm/models/samplers/ddim_mp.py b/spaces/MoonQiu/LongerCrafter/lvdm/models/samplers/ddim_mp.py
deleted file mode 100644
index 86e0d45601c2d638a438253aa9f90d4d366012a8..0000000000000000000000000000000000000000
--- a/spaces/MoonQiu/LongerCrafter/lvdm/models/samplers/ddim_mp.py
+++ /dev/null
@@ -1,340 +0,0 @@
-import numpy as np
-from tqdm import tqdm
-import torch
-from lvdm.models.utils_diffusion import make_ddim_sampling_parameters, make_ddim_timesteps
-from lvdm.common import noise_like
-
-
-class DDIMSampler(object):
- def __init__(self, model, schedule="linear", **kwargs):
- super().__init__()
- self.model = model
- self.ddpm_num_timesteps = model.num_timesteps
- self.schedule = schedule
- self.counter = 0
-
- def register_buffer(self, name, attr):
- if type(attr) == torch.Tensor:
- if attr.device != torch.device("cuda"):
- attr = attr.to(torch.device("cuda"))
- setattr(self, name, attr)
-
- def make_schedule(self, ddim_num_steps, ddim_discretize="uniform", ddim_eta=0., verbose=True):
- self.ddim_timesteps = make_ddim_timesteps(ddim_discr_method=ddim_discretize, num_ddim_timesteps=ddim_num_steps,
- num_ddpm_timesteps=self.ddpm_num_timesteps,verbose=verbose)
- alphas_cumprod = self.model.alphas_cumprod
- assert alphas_cumprod.shape[0] == self.ddpm_num_timesteps, 'alphas have to be defined for each timestep'
- to_torch = lambda x: x.clone().detach().to(torch.float32).to(self.model.device)
-
- self.register_buffer('betas', to_torch(self.model.betas))
- self.register_buffer('alphas_cumprod', to_torch(alphas_cumprod))
- self.register_buffer('alphas_cumprod_prev', to_torch(self.model.alphas_cumprod_prev))
- self.use_scale = self.model.use_scale
- print('DDIM scale', self.use_scale)
-
- if self.use_scale:
- self.register_buffer('scale_arr', to_torch(self.model.scale_arr))
- ddim_scale_arr = self.scale_arr.cpu()[self.ddim_timesteps]
- self.register_buffer('ddim_scale_arr', ddim_scale_arr)
- ddim_scale_arr = np.asarray([self.scale_arr.cpu()[0]] + self.scale_arr.cpu()[self.ddim_timesteps[:-1]].tolist())
- self.register_buffer('ddim_scale_arr_prev', ddim_scale_arr)
-
- # calculations for diffusion q(x_t | x_{t-1}) and others
- self.register_buffer('sqrt_alphas_cumprod', to_torch(np.sqrt(alphas_cumprod.cpu())))
- self.register_buffer('sqrt_one_minus_alphas_cumprod', to_torch(np.sqrt(1. - alphas_cumprod.cpu())))
- self.register_buffer('log_one_minus_alphas_cumprod', to_torch(np.log(1. - alphas_cumprod.cpu())))
- self.register_buffer('sqrt_recip_alphas_cumprod', to_torch(np.sqrt(1. / alphas_cumprod.cpu())))
- self.register_buffer('sqrt_recipm1_alphas_cumprod', to_torch(np.sqrt(1. / alphas_cumprod.cpu() - 1)))
-
- # ddim sampling parameters
- ddim_sigmas, ddim_alphas, ddim_alphas_prev = make_ddim_sampling_parameters(alphacums=alphas_cumprod.cpu(),
- ddim_timesteps=self.ddim_timesteps,
- eta=ddim_eta,verbose=verbose)
- self.register_buffer('ddim_sigmas', ddim_sigmas)
- self.register_buffer('ddim_alphas', ddim_alphas)
- self.register_buffer('ddim_alphas_prev', ddim_alphas_prev)
- self.register_buffer('ddim_sqrt_one_minus_alphas', np.sqrt(1. - ddim_alphas))
- sigmas_for_original_sampling_steps = ddim_eta * torch.sqrt(
- (1 - self.alphas_cumprod_prev) / (1 - self.alphas_cumprod) * (
- 1 - self.alphas_cumprod / self.alphas_cumprod_prev))
- self.register_buffer('ddim_sigmas_for_original_num_steps', sigmas_for_original_sampling_steps)
-
- @torch.no_grad()
- def sample(self,
- S,
- batch_size,
- shape,
- conditioning=None,
- callback=None,
- normals_sequence=None,
- img_callback=None,
- quantize_x0=False,
- eta=0.,
- mask=None,
- x0=None,
- temperature=1.,
- noise_dropout=0.,
- score_corrector=None,
- corrector_kwargs=None,
- verbose=True,
- schedule_verbose=False,
- x_T=None,
- log_every_t=100,
- unconditional_guidance_scale=1.,
- unconditional_conditioning=None,
- # this has to come in the same format as the conditioning, # e.g. as encoded tokens, ...
- **kwargs
- ):
-
- # check condition bs
- # if conditioning is not None:
- # if isinstance(conditioning, dict):
- # try:
- # cbs = conditioning[list(conditioning.keys())[0]].shape[0]
- # except:
- # cbs = conditioning[list(conditioning.keys())[0]][0].shape[0]
-
- # if cbs != batch_size:
- # print(f"Warning: Got {cbs} conditionings but batch-size is {batch_size}")
- # else:
- # if conditioning.shape[0] != batch_size:
- # print(f"Warning: Got {conditioning.shape[0]} conditionings but batch-size is {batch_size}")
-
- self.make_schedule(ddim_num_steps=S, ddim_eta=eta, verbose=schedule_verbose)
-
- # make shape
- if len(shape) == 3:
- C, H, W = shape
- size = (batch_size, C, H, W)
- elif len(shape) == 4:
- C, T, H, W = shape
- size = (batch_size, C, T, H, W)
- # print(f'Data shape for DDIM sampling is {size}, eta {eta}')
-
- samples, intermediates = self.ddim_sampling(conditioning, size,
- callback=callback,
- img_callback=img_callback,
- quantize_denoised=quantize_x0,
- mask=mask, x0=x0,
- ddim_use_original_steps=False,
- noise_dropout=noise_dropout,
- temperature=temperature,
- score_corrector=score_corrector,
- corrector_kwargs=corrector_kwargs,
- x_T=x_T,
- log_every_t=log_every_t,
- unconditional_guidance_scale=unconditional_guidance_scale,
- unconditional_conditioning=unconditional_conditioning,
- verbose=verbose,
- **kwargs)
- return samples, intermediates
-
- @torch.no_grad()
- def ddim_sampling(self, cond, shape,
- x_T=None, ddim_use_original_steps=False,
- callback=None, timesteps=None, quantize_denoised=False,
- mask=None, x0=None, img_callback=None, log_every_t=100,
- temperature=1., noise_dropout=0., score_corrector=None, corrector_kwargs=None,
- unconditional_guidance_scale=1., unconditional_conditioning=None, verbose=True,
- cond_tau=1., target_size=None, start_timesteps=None,
- **kwargs):
- device = self.model.betas.device
- print('ddim device', device)
- b = shape[0]
- if x_T is None:
- img = torch.randn(shape, device=device)
- else:
- img = x_T
-
- if timesteps is None:
- timesteps = self.ddpm_num_timesteps if ddim_use_original_steps else self.ddim_timesteps
- elif timesteps is not None and not ddim_use_original_steps:
- subset_end = int(min(timesteps / self.ddim_timesteps.shape[0], 1) * self.ddim_timesteps.shape[0]) - 1
- timesteps = self.ddim_timesteps[:subset_end]
-
- intermediates = {'x_inter': [img], 'pred_x0': [img]}
- time_range = reversed(range(0,timesteps)) if ddim_use_original_steps else np.flip(timesteps)
- total_steps = timesteps if ddim_use_original_steps else timesteps.shape[0]
- if verbose:
- iterator = tqdm(time_range, desc='DDIM Sampler', total=total_steps)
- else:
- iterator = time_range
-
- init_x0 = False
- clean_cond = kwargs.pop("clean_cond", False)
- for i, step in enumerate(iterator):
- index = total_steps - i - 1
- ts = torch.full((b,), step, device=device, dtype=torch.long)
- if start_timesteps is not None:
- assert x0 is not None
- if step > start_timesteps*time_range[0]:
- continue
- elif not init_x0:
- img = self.model.q_sample(x0, ts)
- init_x0 = True
-
- # use mask to blend noised original latent (img_orig) & new sampled latent (img)
- if mask is not None:
- assert x0 is not None
- if clean_cond:
- img_orig = x0
- else:
- img_orig = self.model.q_sample(x0, ts) # TODO: deterministic forward pass?
- img = img_orig * mask + (1. - mask) * img # keep original & modify use img
-
- index_clip = int((1 - cond_tau) * total_steps)
- if index <= index_clip and target_size is not None:
- target_size_ = [target_size[0], target_size[1]//8, target_size[2]//8]
- img = torch.nn.functional.interpolate(
- img,
- size=target_size_,
- mode="nearest",
- )
- outs = self.p_sample_ddim(img, cond, ts, index=index, use_original_steps=ddim_use_original_steps,
- quantize_denoised=quantize_denoised, temperature=temperature,
- noise_dropout=noise_dropout, score_corrector=score_corrector,
- corrector_kwargs=corrector_kwargs,
- unconditional_guidance_scale=unconditional_guidance_scale,
- unconditional_conditioning=unconditional_conditioning,
- x0=x0,
- step=i,
- **kwargs)
-
- img, pred_x0 = outs
- if callback: callback(i)
- if img_callback: img_callback(pred_x0, i)
-
- if index % log_every_t == 0 or index == total_steps - 1:
- intermediates['x_inter'].append(img)
- intermediates['pred_x0'].append(pred_x0)
-
- return img, intermediates
-
- @torch.no_grad()
- def p_sample_ddim(self, x, c, t, index, repeat_noise=False, use_original_steps=False, quantize_denoised=False,
- temperature=1., noise_dropout=0., score_corrector=None, corrector_kwargs=None,
- unconditional_guidance_scale=1., unconditional_conditioning=None,
- uc_type=None, conditional_guidance_scale_temporal=None, step=0, **kwargs):
- b, *_, device = *x.shape, x.device
- if x.dim() == 5:
- is_video = True
- else:
- is_video = False
- if unconditional_conditioning is None or unconditional_guidance_scale == 1.:
- e_t = self.model.apply_model(x, t, c, **kwargs) # unet denoiser
- else:
- # with unconditional condition
- if step < 5 or step > 15:
- e_t = self.model.apply_model(x, t, c, use_injection=True, **kwargs)
- e_t_uncond = self.model.apply_model(x, t, unconditional_conditioning, **kwargs)
- elif isinstance(c, torch.Tensor):
- e_t = self.model.apply_model(x, t, c, **kwargs)
- e_t_uncond = self.model.apply_model(x, t, unconditional_conditioning, **kwargs)
- elif isinstance(c, dict):
- e_t = self.model.apply_model(x, t, c, **kwargs)
- e_t_uncond = self.model.apply_model(x, t, unconditional_conditioning, **kwargs)
- else:
- raise NotImplementedError
- # text cfg
- if uc_type is None:
- e_t = e_t_uncond + unconditional_guidance_scale * (e_t - e_t_uncond)
- else:
- if uc_type == 'cfg_original':
- e_t = e_t + unconditional_guidance_scale * (e_t - e_t_uncond)
- elif uc_type == 'cfg_ours':
- e_t = e_t + unconditional_guidance_scale * (e_t_uncond - e_t)
- else:
- raise NotImplementedError
- # temporal guidance
- if conditional_guidance_scale_temporal is not None:
- e_t_temporal = self.model.apply_model(x, t, c, **kwargs)
- e_t_image = self.model.apply_model(x, t, c, no_temporal_attn=True, **kwargs)
- e_t = e_t + conditional_guidance_scale_temporal * (e_t_temporal - e_t_image)
-
- if score_corrector is not None:
- assert self.model.parameterization == "eps"
- e_t = score_corrector.modify_score(self.model, e_t, x, t, c, **corrector_kwargs)
-
- alphas = self.model.alphas_cumprod if use_original_steps else self.ddim_alphas
- alphas_prev = self.model.alphas_cumprod_prev if use_original_steps else self.ddim_alphas_prev
- sqrt_one_minus_alphas = self.model.sqrt_one_minus_alphas_cumprod if use_original_steps else self.ddim_sqrt_one_minus_alphas
- sigmas = self.model.ddim_sigmas_for_original_num_steps if use_original_steps else self.ddim_sigmas
- # select parameters corresponding to the currently considered timestep
-
- if is_video:
- size = (b, 1, 1, 1, 1)
- else:
- size = (b, 1, 1, 1)
- a_t = torch.full(size, alphas[index], device=device)
- a_prev = torch.full(size, alphas_prev[index], device=device)
- sigma_t = torch.full(size, sigmas[index], device=device)
- sqrt_one_minus_at = torch.full(size, sqrt_one_minus_alphas[index],device=device)
-
- # current prediction for x_0
- pred_x0 = (x - sqrt_one_minus_at * e_t) / a_t.sqrt()
- if quantize_denoised:
- pred_x0, _, *_ = self.model.first_stage_model.quantize(pred_x0)
- # direction pointing to x_t
- dir_xt = (1. - a_prev - sigma_t**2).sqrt() * e_t
-
- noise = sigma_t * noise_like(x.shape, device, repeat_noise) * temperature
- if noise_dropout > 0.:
- noise = torch.nn.functional.dropout(noise, p=noise_dropout)
-
- alphas = self.model.alphas_cumprod if use_original_steps else self.ddim_alphas
- if self.use_scale:
- scale_arr = self.model.scale_arr if use_original_steps else self.ddim_scale_arr
- scale_t = torch.full(size, scale_arr[index], device=device)
- scale_arr_prev = self.model.scale_arr_prev if use_original_steps else self.ddim_scale_arr_prev
- scale_t_prev = torch.full(size, scale_arr_prev[index], device=device)
- pred_x0 /= scale_t
- x_prev = a_prev.sqrt() * scale_t_prev * pred_x0 + dir_xt + noise
- else:
- x_prev = a_prev.sqrt() * pred_x0 + dir_xt + noise
-
- return x_prev, pred_x0
-
-
- @torch.no_grad()
- def stochastic_encode(self, x0, t, use_original_steps=False, noise=None):
- # fast, but does not allow for exact reconstruction
- # t serves as an index to gather the correct alphas
- if use_original_steps:
- sqrt_alphas_cumprod = self.sqrt_alphas_cumprod
- sqrt_one_minus_alphas_cumprod = self.sqrt_one_minus_alphas_cumprod
- else:
- sqrt_alphas_cumprod = torch.sqrt(self.ddim_alphas)
- sqrt_one_minus_alphas_cumprod = self.ddim_sqrt_one_minus_alphas
-
- if noise is None:
- noise = torch.randn_like(x0)
-
- def extract_into_tensor(a, t, x_shape):
- b, *_ = t.shape
- out = a.gather(-1, t)
- return out.reshape(b, *((1,) * (len(x_shape) - 1)))
-
- return (extract_into_tensor(sqrt_alphas_cumprod, t, x0.shape) * x0 +
- extract_into_tensor(sqrt_one_minus_alphas_cumprod, t, x0.shape) * noise)
-
- @torch.no_grad()
- def decode(self, x_latent, cond, t_start, unconditional_guidance_scale=1.0, unconditional_conditioning=None,
- use_original_steps=False):
-
- timesteps = np.arange(self.ddpm_num_timesteps) if use_original_steps else self.ddim_timesteps
- timesteps = timesteps[:t_start]
-
- time_range = np.flip(timesteps)
- total_steps = timesteps.shape[0]
- print(f"Running DDIM Sampling with {total_steps} timesteps")
-
- iterator = tqdm(time_range, desc='Decoding image', total=total_steps)
- x_dec = x_latent
- for i, step in enumerate(iterator):
- index = total_steps - i - 1
- ts = torch.full((x_latent.shape[0],), step, device=x_latent.device, dtype=torch.long)
- x_dec, _ = self.p_sample_ddim(x_dec, cond, ts, index=index, use_original_steps=use_original_steps,
- unconditional_guidance_scale=unconditional_guidance_scale,
- unconditional_conditioning=unconditional_conditioning)
- return x_dec
-
diff --git a/spaces/MrBodean/VoiceClone/encoder/preprocess.py b/spaces/MrBodean/VoiceClone/encoder/preprocess.py
deleted file mode 100644
index 551a8b29c4d84c0e1430f285a1c8b5e10c98ee5f..0000000000000000000000000000000000000000
--- a/spaces/MrBodean/VoiceClone/encoder/preprocess.py
+++ /dev/null
@@ -1,175 +0,0 @@
-from multiprocess.pool import ThreadPool
-from encoder.params_data import *
-from encoder.config import librispeech_datasets, anglophone_nationalites
-from datetime import datetime
-from encoder import audio
-from pathlib import Path
-from tqdm import tqdm
-import numpy as np
-
-
-class DatasetLog:
- """
- Registers metadata about the dataset in a text file.
- """
- def __init__(self, root, name):
- self.text_file = open(Path(root, "Log_%s.txt" % name.replace("/", "_")), "w")
- self.sample_data = dict()
-
- start_time = str(datetime.now().strftime("%A %d %B %Y at %H:%M"))
- self.write_line("Creating dataset %s on %s" % (name, start_time))
- self.write_line("-----")
- self._log_params()
-
- def _log_params(self):
- from encoder import params_data
- self.write_line("Parameter values:")
- for param_name in (p for p in dir(params_data) if not p.startswith("__")):
- value = getattr(params_data, param_name)
- self.write_line("\t%s: %s" % (param_name, value))
- self.write_line("-----")
-
- def write_line(self, line):
- self.text_file.write("%s\n" % line)
-
- def add_sample(self, **kwargs):
- for param_name, value in kwargs.items():
- if not param_name in self.sample_data:
- self.sample_data[param_name] = []
- self.sample_data[param_name].append(value)
-
- def finalize(self):
- self.write_line("Statistics:")
- for param_name, values in self.sample_data.items():
- self.write_line("\t%s:" % param_name)
- self.write_line("\t\tmin %.3f, max %.3f" % (np.min(values), np.max(values)))
- self.write_line("\t\tmean %.3f, median %.3f" % (np.mean(values), np.median(values)))
- self.write_line("-----")
- end_time = str(datetime.now().strftime("%A %d %B %Y at %H:%M"))
- self.write_line("Finished on %s" % end_time)
- self.text_file.close()
-
-
-def _init_preprocess_dataset(dataset_name, datasets_root, out_dir) -> (Path, DatasetLog):
- dataset_root = datasets_root.joinpath(dataset_name)
- if not dataset_root.exists():
- print("Couldn\'t find %s, skipping this dataset." % dataset_root)
- return None, None
- return dataset_root, DatasetLog(out_dir, dataset_name)
-
-
-def _preprocess_speaker_dirs(speaker_dirs, dataset_name, datasets_root, out_dir, extension,
- skip_existing, logger):
- print("%s: Preprocessing data for %d speakers." % (dataset_name, len(speaker_dirs)))
-
- # Function to preprocess utterances for one speaker
- def preprocess_speaker(speaker_dir: Path):
- # Give a name to the speaker that includes its dataset
- speaker_name = "_".join(speaker_dir.relative_to(datasets_root).parts)
-
- # Create an output directory with that name, as well as a txt file containing a
- # reference to each source file.
- speaker_out_dir = out_dir.joinpath(speaker_name)
- speaker_out_dir.mkdir(exist_ok=True)
- sources_fpath = speaker_out_dir.joinpath("_sources.txt")
-
- # There's a possibility that the preprocessing was interrupted earlier, check if
- # there already is a sources file.
- if sources_fpath.exists():
- try:
- with sources_fpath.open("r") as sources_file:
- existing_fnames = {line.split(",")[0] for line in sources_file}
- except:
- existing_fnames = {}
- else:
- existing_fnames = {}
-
- # Gather all audio files for that speaker recursively
- sources_file = sources_fpath.open("a" if skip_existing else "w")
- for in_fpath in speaker_dir.glob("**/*.%s" % extension):
- # Check if the target output file already exists
- out_fname = "_".join(in_fpath.relative_to(speaker_dir).parts)
- out_fname = out_fname.replace(".%s" % extension, ".npy")
- if skip_existing and out_fname in existing_fnames:
- continue
-
- # Load and preprocess the waveform
- wav = audio.preprocess_wav(in_fpath)
- if len(wav) == 0:
- continue
-
- # Create the mel spectrogram, discard those that are too short
- frames = audio.wav_to_mel_spectrogram(wav)
- if len(frames) < partials_n_frames:
- continue
-
- out_fpath = speaker_out_dir.joinpath(out_fname)
- np.save(out_fpath, frames)
- logger.add_sample(duration=len(wav) / sampling_rate)
- sources_file.write("%s,%s\n" % (out_fname, in_fpath))
-
- sources_file.close()
-
- # Process the utterances for each speaker
- with ThreadPool(8) as pool:
- list(tqdm(pool.imap(preprocess_speaker, speaker_dirs), dataset_name, len(speaker_dirs),
- unit="speakers"))
- logger.finalize()
- print("Done preprocessing %s.\n" % dataset_name)
-
-
-def preprocess_librispeech(datasets_root: Path, out_dir: Path, skip_existing=False):
- for dataset_name in librispeech_datasets["train"]["other"]:
- # Initialize the preprocessing
- dataset_root, logger = _init_preprocess_dataset(dataset_name, datasets_root, out_dir)
- if not dataset_root:
- return
-
- # Preprocess all speakers
- speaker_dirs = list(dataset_root.glob("*"))
- _preprocess_speaker_dirs(speaker_dirs, dataset_name, datasets_root, out_dir, "flac",
- skip_existing, logger)
-
-
-def preprocess_voxceleb1(datasets_root: Path, out_dir: Path, skip_existing=False):
- # Initialize the preprocessing
- dataset_name = "VoxCeleb1"
- dataset_root, logger = _init_preprocess_dataset(dataset_name, datasets_root, out_dir)
- if not dataset_root:
- return
-
- # Get the contents of the meta file
- with dataset_root.joinpath("vox1_meta.csv").open("r") as metafile:
- metadata = [line.split("\t") for line in metafile][1:]
-
- # Select the ID and the nationality, filter out non-anglophone speakers
- nationalities = {line[0]: line[3] for line in metadata}
- keep_speaker_ids = [speaker_id for speaker_id, nationality in nationalities.items() if
- nationality.lower() in anglophone_nationalites]
- print("VoxCeleb1: using samples from %d (presumed anglophone) speakers out of %d." %
- (len(keep_speaker_ids), len(nationalities)))
-
- # Get the speaker directories for anglophone speakers only
- speaker_dirs = dataset_root.joinpath("wav").glob("*")
- speaker_dirs = [speaker_dir for speaker_dir in speaker_dirs if
- speaker_dir.name in keep_speaker_ids]
- print("VoxCeleb1: found %d anglophone speakers on the disk, %d missing (this is normal)." %
- (len(speaker_dirs), len(keep_speaker_ids) - len(speaker_dirs)))
-
- # Preprocess all speakers
- _preprocess_speaker_dirs(speaker_dirs, dataset_name, datasets_root, out_dir, "wav",
- skip_existing, logger)
-
-
-def preprocess_voxceleb2(datasets_root: Path, out_dir: Path, skip_existing=False):
- # Initialize the preprocessing
- dataset_name = "VoxCeleb2"
- dataset_root, logger = _init_preprocess_dataset(dataset_name, datasets_root, out_dir)
- if not dataset_root:
- return
-
- # Get the speaker directories
- # Preprocess all speakers
- speaker_dirs = list(dataset_root.joinpath("dev", "aac").glob("*"))
- _preprocess_speaker_dirs(speaker_dirs, dataset_name, datasets_root, out_dir, "m4a",
- skip_existing, logger)
diff --git a/spaces/NMEX/rvc-hoyogame-v2/app.py b/spaces/NMEX/rvc-hoyogame-v2/app.py
deleted file mode 100644
index 4e286df3b5a8843b0e948686fe94d86c4b336dcb..0000000000000000000000000000000000000000
--- a/spaces/NMEX/rvc-hoyogame-v2/app.py
+++ /dev/null
@@ -1,518 +0,0 @@
-import os
-import glob
-import json
-import traceback
-import logging
-import gradio as gr
-import numpy as np
-import librosa
-import torch
-import asyncio
-import edge_tts
-import yt_dlp
-import ffmpeg
-import subprocess
-import sys
-import io
-import wave
-from datetime import datetime
-from fairseq import checkpoint_utils
-from lib.infer_pack.models import (
- SynthesizerTrnMs256NSFsid,
- SynthesizerTrnMs256NSFsid_nono,
- SynthesizerTrnMs768NSFsid,
- SynthesizerTrnMs768NSFsid_nono,
-)
-from vc_infer_pipeline import VC
-from config import Config
-config = Config()
-logging.getLogger("numba").setLevel(logging.WARNING)
-limitation = os.getenv("SYSTEM") == "spaces"
-
-audio_mode = []
-f0method_mode = []
-f0method_info = ""
-if limitation is True:
- audio_mode = ["Upload audio", "TTS Audio"]
- f0method_mode = ["pm", "harvest"]
- f0method_info = "PM is fast, Harvest is good but extremely slow. (Default: PM)"
-else:
- audio_mode = ["Input path", "Upload audio", "Youtube", "TTS Audio"]
- f0method_mode = ["pm", "harvest", "crepe"]
- f0method_info = "PM is fast, Harvest is good but extremely slow, and Crepe effect is good but requires GPU (Default: PM)"
-
-def create_vc_fn(model_name, tgt_sr, net_g, vc, if_f0, version, file_index):
- def vc_fn(
- vc_audio_mode,
- vc_input,
- vc_upload,
- tts_text,
- tts_voice,
- f0_up_key,
- f0_method,
- index_rate,
- filter_radius,
- resample_sr,
- rms_mix_rate,
- protect,
- ):
- try:
- print(f"Converting using {model_name}...")
- if vc_audio_mode == "Input path" or "Youtube" and vc_input != "":
- audio, sr = librosa.load(vc_input, sr=16000, mono=True)
- elif vc_audio_mode == "Upload audio":
- if vc_upload is None:
- return "You need to upload an audio", None
- sampling_rate, audio = vc_upload
- duration = audio.shape[0] / sampling_rate
- if duration > 20 and limitation:
- return "Please upload an audio file that is less than 20 seconds. If you need to generate a longer audio file, please use Colab.", None
- audio = (audio / np.iinfo(audio.dtype).max).astype(np.float32)
- if len(audio.shape) > 1:
- audio = librosa.to_mono(audio.transpose(1, 0))
- if sampling_rate != 16000:
- audio = librosa.resample(audio, orig_sr=sampling_rate, target_sr=16000)
- elif vc_audio_mode == "TTS Audio":
- if len(tts_text) > 100 and limitation:
- return "Text is too long", None
- if tts_text is None or tts_voice is None:
- return "You need to enter text and select a voice", None
- asyncio.run(edge_tts.Communicate(tts_text, "-".join(tts_voice.split('-')[:-1])).save("tts.mp3"))
- audio, sr = librosa.load("tts.mp3", sr=16000, mono=True)
- vc_input = "tts.mp3"
- times = [0, 0, 0]
- f0_up_key = int(f0_up_key)
- audio_opt = vc.pipeline(
- hubert_model,
- net_g,
- 0,
- audio,
- vc_input,
- times,
- f0_up_key,
- f0_method,
- file_index,
- # file_big_npy,
- index_rate,
- if_f0,
- filter_radius,
- tgt_sr,
- resample_sr,
- rms_mix_rate,
- version,
- protect,
- f0_file=None,
- )
- info = f"[{datetime.now().strftime('%Y-%m-%d %H:%M')}]: npy: {times[0]}, f0: {times[1]}s, infer: {times[2]}s"
- print(f"{model_name} | {info}")
- return info, (tgt_sr, audio_opt)
- except:
- info = traceback.format_exc()
- print(info)
- return info, None
- return vc_fn
-
-def load_model():
- categories = []
- with open("weights/folder_info.json", "r", encoding="utf-8") as f:
- folder_info = json.load(f)
- for category_name, category_info in folder_info.items():
- if not category_info['enable']:
- continue
- category_title = category_info['title']
- category_folder = category_info['folder_path']
- description = category_info['description']
- models = []
- with open(f"weights/{category_folder}/model_info.json", "r", encoding="utf-8") as f:
- models_info = json.load(f)
- for character_name, info in models_info.items():
- if not info['enable']:
- continue
- model_title = info['title']
- model_name = info['model_path']
- model_author = info.get("author", None)
- model_cover = f"weights/{category_folder}/{character_name}/{info['cover']}"
- model_index = f"weights/{category_folder}/{character_name}/{info['feature_retrieval_library']}"
- cpt = torch.load(f"weights/{category_folder}/{character_name}/{model_name}", map_location="cpu")
- tgt_sr = cpt["config"][-1]
- cpt["config"][-3] = cpt["weight"]["emb_g.weight"].shape[0] # n_spk
- if_f0 = cpt.get("f0", 1)
- version = cpt.get("version", "v1")
- if version == "v1":
- if if_f0 == 1:
- net_g = SynthesizerTrnMs256NSFsid(*cpt["config"], is_half=config.is_half)
- else:
- net_g = SynthesizerTrnMs256NSFsid_nono(*cpt["config"])
- model_version = "V1"
- elif version == "v2":
- if if_f0 == 1:
- net_g = SynthesizerTrnMs768NSFsid(*cpt["config"], is_half=config.is_half)
- else:
- net_g = SynthesizerTrnMs768NSFsid_nono(*cpt["config"])
- model_version = "V2"
- del net_g.enc_q
- print(net_g.load_state_dict(cpt["weight"], strict=False))
- net_g.eval().to(config.device)
- if config.is_half:
- net_g = net_g.half()
- else:
- net_g = net_g.float()
- vc = VC(tgt_sr, config)
- print(f"Model loaded: {character_name} / {info['feature_retrieval_library']} | ({model_version})")
- models.append((character_name, model_title, model_author, model_cover, model_version, create_vc_fn(model_name, tgt_sr, net_g, vc, if_f0, version, model_index)))
- categories.append([category_title, category_folder, description, models])
- return categories
-
-def cut_vocal_and_inst(url, audio_provider, split_model):
- if url != "":
- if not os.path.exists("dl_audio"):
- os.mkdir("dl_audio")
- if audio_provider == "Youtube":
- ydl_opts = {
- 'noplaylist': True,
- 'format': 'bestaudio/best',
- 'postprocessors': [{
- 'key': 'FFmpegExtractAudio',
- 'preferredcodec': 'wav',
- }],
- "outtmpl": 'dl_audio/youtube_audio',
- }
- with yt_dlp.YoutubeDL(ydl_opts) as ydl:
- ydl.download([url])
- audio_path = "dl_audio/youtube_audio.wav"
- if split_model == "htdemucs":
- command = f"demucs --two-stems=vocals {audio_path} -o output"
- result = subprocess.run(command.split(), stdout=subprocess.PIPE)
- print(result.stdout.decode())
- return "output/htdemucs/youtube_audio/vocals.wav", "output/htdemucs/youtube_audio/no_vocals.wav", audio_path, "output/htdemucs/youtube_audio/vocals.wav"
- else:
- command = f"demucs --two-stems=vocals -n mdx_extra_q {audio_path} -o output"
- result = subprocess.run(command.split(), stdout=subprocess.PIPE)
- print(result.stdout.decode())
- return "output/mdx_extra_q/youtube_audio/vocals.wav", "output/mdx_extra_q/youtube_audio/no_vocals.wav", audio_path, "output/mdx_extra_q/youtube_audio/vocals.wav"
- else:
- raise gr.Error("URL Required!")
- return None, None, None, None
-
-def combine_vocal_and_inst(audio_data, audio_volume, split_model):
- if not os.path.exists("output/result"):
- os.mkdir("output/result")
- vocal_path = "output/result/output.wav"
- output_path = "output/result/combine.mp3"
- if split_model == "htdemucs":
- inst_path = "output/htdemucs/youtube_audio/no_vocals.wav"
- else:
- inst_path = "output/mdx_extra_q/youtube_audio/no_vocals.wav"
- with wave.open(vocal_path, "w") as wave_file:
- wave_file.setnchannels(1)
- wave_file.setsampwidth(2)
- wave_file.setframerate(audio_data[0])
- wave_file.writeframes(audio_data[1].tobytes())
- command = f'ffmpeg -y -i {inst_path} -i {vocal_path} -filter_complex [1:a]volume={audio_volume}dB[v];[0:a][v]amix=inputs=2:duration=longest -b:a 320k -c:a libmp3lame {output_path}'
- result = subprocess.run(command.split(), stdout=subprocess.PIPE)
- print(result.stdout.decode())
- return output_path
-
-def load_hubert():
- global hubert_model
- models, _, _ = checkpoint_utils.load_model_ensemble_and_task(
- ["hubert_base.pt"],
- suffix="",
- )
- hubert_model = models[0]
- hubert_model = hubert_model.to(config.device)
- if config.is_half:
- hubert_model = hubert_model.half()
- else:
- hubert_model = hubert_model.float()
- hubert_model.eval()
-
-def change_audio_mode(vc_audio_mode):
- if vc_audio_mode == "Input path":
- return (
- # Input & Upload
- gr.Textbox.update(visible=True),
- gr.Checkbox.update(visible=False),
- gr.Audio.update(visible=False),
- # Youtube
- gr.Dropdown.update(visible=False),
- gr.Textbox.update(visible=False),
- gr.Dropdown.update(visible=False),
- gr.Button.update(visible=False),
- gr.Audio.update(visible=False),
- gr.Audio.update(visible=False),
- gr.Audio.update(visible=False),
- gr.Slider.update(visible=False),
- gr.Audio.update(visible=False),
- gr.Button.update(visible=False),
- # TTS
- gr.Textbox.update(visible=False),
- gr.Dropdown.update(visible=False)
- )
- elif vc_audio_mode == "Upload audio":
- return (
- # Input & Upload
- gr.Textbox.update(visible=False),
- gr.Checkbox.update(visible=True),
- gr.Audio.update(visible=True),
- # Youtube
- gr.Dropdown.update(visible=False),
- gr.Textbox.update(visible=False),
- gr.Dropdown.update(visible=False),
- gr.Button.update(visible=False),
- gr.Audio.update(visible=False),
- gr.Audio.update(visible=False),
- gr.Audio.update(visible=False),
- gr.Slider.update(visible=False),
- gr.Audio.update(visible=False),
- gr.Button.update(visible=False),
- # TTS
- gr.Textbox.update(visible=False),
- gr.Dropdown.update(visible=False)
- )
- elif vc_audio_mode == "Youtube":
- return (
- # Input & Upload
- gr.Textbox.update(visible=False),
- gr.Checkbox.update(visible=False),
- gr.Audio.update(visible=False),
- # Youtube
- gr.Dropdown.update(visible=True),
- gr.Textbox.update(visible=True),
- gr.Dropdown.update(visible=True),
- gr.Button.update(visible=True),
- gr.Audio.update(visible=True),
- gr.Audio.update(visible=True),
- gr.Audio.update(visible=True),
- gr.Slider.update(visible=True),
- gr.Audio.update(visible=True),
- gr.Button.update(visible=True),
- # TTS
- gr.Textbox.update(visible=False),
- gr.Dropdown.update(visible=False)
- )
- elif vc_audio_mode == "TTS Audio":
- return (
- # Input & Upload
- gr.Textbox.update(visible=False),
- gr.Checkbox.update(visible=False),
- gr.Audio.update(visible=False),
- # Youtube
- gr.Dropdown.update(visible=False),
- gr.Textbox.update(visible=False),
- gr.Dropdown.update(visible=False),
- gr.Button.update(visible=False),
- gr.Audio.update(visible=False),
- gr.Audio.update(visible=False),
- gr.Audio.update(visible=False),
- gr.Slider.update(visible=False),
- gr.Audio.update(visible=False),
- gr.Button.update(visible=False),
- # TTS
- gr.Textbox.update(visible=True),
- gr.Dropdown.update(visible=True)
- )
- else:
- return (
- # Input & Upload
- gr.Textbox.update(visible=False),
- gr.Checkbox.update(visible=True),
- gr.Audio.update(visible=True),
- # Youtube
- gr.Dropdown.update(visible=False),
- gr.Textbox.update(visible=False),
- gr.Dropdown.update(visible=False),
- gr.Button.update(visible=False),
- gr.Audio.update(visible=False),
- gr.Audio.update(visible=False),
- gr.Audio.update(visible=False),
- gr.Slider.update(visible=False),
- gr.Audio.update(visible=False),
- gr.Button.update(visible=False),
- # TTS
- gr.Textbox.update(visible=False),
- gr.Dropdown.update(visible=False)
- )
-
-def use_microphone(microphone):
- if microphone == True:
- return gr.Audio.update(source="microphone")
- else:
- return gr.Audio.update(source="upload")
-
-if __name__ == '__main__':
- load_hubert()
- categories = load_model()
- tts_voice_list = asyncio.get_event_loop().run_until_complete(edge_tts.list_voices())
- voices = [f"{v['ShortName']}-{v['Gender']}" for v in tts_voice_list]
- with gr.Blocks() as app:
- gr.Markdown(
- "
\n\n"+
- "# RVC Genshin Impact\n\n"+
- "### Recommended to use Google Colab to use other character and feature.\n\n"+
- "[](https://colab.research.google.com/drive/110kiMZTdP6Ri1lY9-NbQf17GVPPhHyeT?usp=sharing)\n\n"+
- "
\n\n"+
- "[](https://github.com/ArkanDash/Multi-Model-RVC-Inference)"
- )
- for (folder_title, folder, description, models) in categories:
- with gr.TabItem(folder_title):
- if description:
- gr.Markdown(f"###
{description}")
- with gr.Tabs():
- if not models:
- gr.Markdown("#
No Model Loaded.")
- gr.Markdown("##
Please add model or fix your model path.")
- continue
- for (name, title, author, cover, model_version, vc_fn) in models:
- with gr.TabItem(name):
- with gr.Row():
- gr.Markdown(
- '
'
- f'
{title}
\n'+
- f'
RVC {model_version} Model
\n'+
- (f'
Model author: {author}
' if author else "")+
- (f'' if cover else "")+
- '
'
- )
- with gr.Row():
- with gr.Column():
- vc_audio_mode = gr.Dropdown(label="Input voice", choices=audio_mode, allow_custom_value=False, value="Upload audio")
- # Input
- vc_input = gr.Textbox(label="Input audio path", visible=False)
- # Upload
- vc_microphone_mode = gr.Checkbox(label="Use Microphone", value=False, visible=True, interactive=True)
- vc_upload = gr.Audio(label="Upload audio file", source="upload", visible=True, interactive=True)
- # Youtube
- vc_download_audio = gr.Dropdown(label="Provider", choices=["Youtube"], allow_custom_value=False, visible=False, value="Youtube", info="Select provider (Default: Youtube)")
- vc_link = gr.Textbox(label="Youtube URL", visible=False, info="Example: https://www.youtube.com/watch?v=Nc0sB1Bmf-A", placeholder="https://www.youtube.com/watch?v=...")
- vc_split_model = gr.Dropdown(label="Splitter Model", choices=["htdemucs", "mdx_extra_q"], allow_custom_value=False, visible=False, value="htdemucs", info="Select the splitter model (Default: htdemucs)")
- vc_split = gr.Button("Split Audio", variant="primary", visible=False)
- vc_vocal_preview = gr.Audio(label="Vocal Preview", visible=False)
- vc_inst_preview = gr.Audio(label="Instrumental Preview", visible=False)
- vc_audio_preview = gr.Audio(label="Audio Preview", visible=False)
- # TTS
- tts_text = gr.Textbox(visible=False, label="TTS text", info="Text to speech input")
- tts_voice = gr.Dropdown(label="Edge-tts speaker", choices=voices, visible=False, allow_custom_value=False, value="en-US-AnaNeural-Female")
- with gr.Column():
- vc_transform0 = gr.Number(label="Transpose", value=0, info='Type "12" to change from male to female voice. Type "-12" to change female to male voice')
- f0method0 = gr.Radio(
- label="Pitch extraction algorithm",
- info=f0method_info,
- choices=f0method_mode,
- value="pm",
- interactive=True
- )
- index_rate1 = gr.Slider(
- minimum=0,
- maximum=1,
- label="Retrieval feature ratio",
- info="(Default: 0.7)",
- value=0.7,
- interactive=True,
- )
- filter_radius0 = gr.Slider(
- minimum=0,
- maximum=7,
- label="Apply Median Filtering",
- info="The value represents the filter radius and can reduce breathiness.",
- value=3,
- step=1,
- interactive=True,
- )
- resample_sr0 = gr.Slider(
- minimum=0,
- maximum=48000,
- label="Resample the output audio",
- info="Resample the output audio in post-processing to the final sample rate. Set to 0 for no resampling",
- value=0,
- step=1,
- interactive=True,
- )
- rms_mix_rate0 = gr.Slider(
- minimum=0,
- maximum=1,
- label="Volume Envelope",
- info="Use the volume envelope of the input to replace or mix with the volume envelope of the output. The closer the ratio is to 1, the more the output envelope is used",
- value=1,
- interactive=True,
- )
- protect0 = gr.Slider(
- minimum=0,
- maximum=0.5,
- label="Voice Protection",
- info="Protect voiceless consonants and breath sounds to prevent artifacts such as tearing in electronic music. Set to 0.5 to disable. Decrease the value to increase protection, but it may reduce indexing accuracy",
- value=0.5,
- step=0.01,
- interactive=True,
- )
- with gr.Column():
- vc_log = gr.Textbox(label="Output Information", interactive=False)
- vc_output = gr.Audio(label="Output Audio", interactive=False)
- vc_convert = gr.Button("Convert", variant="primary")
- vc_volume = gr.Slider(
- minimum=0,
- maximum=10,
- label="Vocal volume",
- value=4,
- interactive=True,
- step=1,
- info="Adjust vocal volume (Default: 4}",
- visible=False
- )
- vc_combined_output = gr.Audio(label="Output Combined Audio", visible=False)
- vc_combine = gr.Button("Combine",variant="primary", visible=False)
- vc_convert.click(
- fn=vc_fn,
- inputs=[
- vc_audio_mode,
- vc_input,
- vc_upload,
- tts_text,
- tts_voice,
- vc_transform0,
- f0method0,
- index_rate1,
- filter_radius0,
- resample_sr0,
- rms_mix_rate0,
- protect0,
- ],
- outputs=[vc_log ,vc_output]
- )
- vc_split.click(
- fn=cut_vocal_and_inst,
- inputs=[vc_link, vc_download_audio, vc_split_model],
- outputs=[vc_vocal_preview, vc_inst_preview, vc_audio_preview, vc_input]
- )
- vc_combine.click(
- fn=combine_vocal_and_inst,
- inputs=[vc_output, vc_volume, vc_split_model],
- outputs=[vc_combined_output]
- )
- vc_microphone_mode.change(
- fn=use_microphone,
- inputs=vc_microphone_mode,
- outputs=vc_upload
- )
- vc_audio_mode.change(
- fn=change_audio_mode,
- inputs=[vc_audio_mode],
- outputs=[
- vc_input,
- vc_microphone_mode,
- vc_upload,
- vc_download_audio,
- vc_link,
- vc_split_model,
- vc_split,
- vc_vocal_preview,
- vc_inst_preview,
- vc_audio_preview,
- vc_volume,
- vc_combined_output,
- vc_combine,
- tts_text,
- tts_voice
- ]
- )
- app.queue(concurrency_count=1, max_size=20, api_open=config.api).launch(share=config.colab)
\ No newline at end of file
diff --git a/spaces/NachtYoru/Linaqruf-anything-v3-better-vae/app.py b/spaces/NachtYoru/Linaqruf-anything-v3-better-vae/app.py
deleted file mode 100644
index c9d41763c79cbb4bd6d7be45ea74e3e161a49774..0000000000000000000000000000000000000000
--- a/spaces/NachtYoru/Linaqruf-anything-v3-better-vae/app.py
+++ /dev/null
@@ -1,3 +0,0 @@
-import gradio as gr
-
-gr.Interface.load("models/Linaqruf/anything-v3-better-vae").launch()
\ No newline at end of file
diff --git a/spaces/Nikita22121671/stabilityai-stablecode-instruct-alpha-3b/README.md b/spaces/Nikita22121671/stabilityai-stablecode-instruct-alpha-3b/README.md
deleted file mode 100644
index 5121952f288b0ec178b669b4dc0f657008805efc..0000000000000000000000000000000000000000
--- a/spaces/Nikita22121671/stabilityai-stablecode-instruct-alpha-3b/README.md
+++ /dev/null
@@ -1,12 +0,0 @@
----
-title: Stabilityai Stablecode Instruct Alpha 3b
-emoji: ⚡
-colorFrom: pink
-colorTo: gray
-sdk: gradio
-sdk_version: 3.39.0
-app_file: app.py
-pinned: false
----
-
-Check out the configuration reference at https://huggingface.co/docs/hub/spaces-config-reference
diff --git a/spaces/OAOA/DifFace/basicsr/utils/img_util.py b/spaces/OAOA/DifFace/basicsr/utils/img_util.py
deleted file mode 100644
index fbce5dba5b01deb78f2453edc801a76e6a126998..0000000000000000000000000000000000000000
--- a/spaces/OAOA/DifFace/basicsr/utils/img_util.py
+++ /dev/null
@@ -1,172 +0,0 @@
-import cv2
-import math
-import numpy as np
-import os
-import torch
-from torchvision.utils import make_grid
-
-
-def img2tensor(imgs, bgr2rgb=True, float32=True):
- """Numpy array to tensor.
-
- Args:
- imgs (list[ndarray] | ndarray): Input images.
- bgr2rgb (bool): Whether to change bgr to rgb.
- float32 (bool): Whether to change to float32.
-
- Returns:
- list[tensor] | tensor: Tensor images. If returned results only have
- one element, just return tensor.
- """
-
- def _totensor(img, bgr2rgb, float32):
- if img.shape[2] == 3 and bgr2rgb:
- if img.dtype == 'float64':
- img = img.astype('float32')
- img = cv2.cvtColor(img, cv2.COLOR_BGR2RGB)
- img = torch.from_numpy(img.transpose(2, 0, 1))
- if float32:
- img = img.float()
- return img
-
- if isinstance(imgs, list):
- return [_totensor(img, bgr2rgb, float32) for img in imgs]
- else:
- return _totensor(imgs, bgr2rgb, float32)
-
-
-def tensor2img(tensor, rgb2bgr=True, out_type=np.uint8, min_max=(0, 1)):
- """Convert torch Tensors into image numpy arrays.
-
- After clamping to [min, max], values will be normalized to [0, 1].
-
- Args:
- tensor (Tensor or list[Tensor]): Accept shapes:
- 1) 4D mini-batch Tensor of shape (B x 3/1 x H x W);
- 2) 3D Tensor of shape (3/1 x H x W);
- 3) 2D Tensor of shape (H x W).
- Tensor channel should be in RGB order.
- rgb2bgr (bool): Whether to change rgb to bgr.
- out_type (numpy type): output types. If ``np.uint8``, transform outputs
- to uint8 type with range [0, 255]; otherwise, float type with
- range [0, 1]. Default: ``np.uint8``.
- min_max (tuple[int]): min and max values for clamp.
-
- Returns:
- (Tensor or list): 3D ndarray of shape (H x W x C) OR 2D ndarray of
- shape (H x W). The channel order is BGR.
- """
- if not (torch.is_tensor(tensor) or (isinstance(tensor, list) and all(torch.is_tensor(t) for t in tensor))):
- raise TypeError(f'tensor or list of tensors expected, got {type(tensor)}')
-
- if torch.is_tensor(tensor):
- tensor = [tensor]
- result = []
- for _tensor in tensor:
- _tensor = _tensor.squeeze(0).float().detach().cpu().clamp_(*min_max)
- _tensor = (_tensor - min_max[0]) / (min_max[1] - min_max[0])
-
- n_dim = _tensor.dim()
- if n_dim == 4:
- img_np = make_grid(_tensor, nrow=int(math.sqrt(_tensor.size(0))), normalize=False).numpy()
- img_np = img_np.transpose(1, 2, 0)
- if rgb2bgr:
- img_np = cv2.cvtColor(img_np, cv2.COLOR_RGB2BGR)
- elif n_dim == 3:
- img_np = _tensor.numpy()
- img_np = img_np.transpose(1, 2, 0)
- if img_np.shape[2] == 1: # gray image
- img_np = np.squeeze(img_np, axis=2)
- else:
- if rgb2bgr:
- img_np = cv2.cvtColor(img_np, cv2.COLOR_RGB2BGR)
- elif n_dim == 2:
- img_np = _tensor.numpy()
- else:
- raise TypeError(f'Only support 4D, 3D or 2D tensor. But received with dimension: {n_dim}')
- if out_type == np.uint8:
- # Unlike MATLAB, numpy.unit8() WILL NOT round by default.
- img_np = (img_np * 255.0).round()
- img_np = img_np.astype(out_type)
- result.append(img_np)
- if len(result) == 1 and torch.is_tensor(tensor):
- result = result[0]
- return result
-
-
-def tensor2img_fast(tensor, rgb2bgr=True, min_max=(0, 1)):
- """This implementation is slightly faster than tensor2img.
- It now only supports torch tensor with shape (1, c, h, w).
-
- Args:
- tensor (Tensor): Now only support torch tensor with (1, c, h, w).
- rgb2bgr (bool): Whether to change rgb to bgr. Default: True.
- min_max (tuple[int]): min and max values for clamp.
- """
- output = tensor.squeeze(0).detach().clamp_(*min_max).permute(1, 2, 0)
- output = (output - min_max[0]) / (min_max[1] - min_max[0]) * 255
- output = output.type(torch.uint8).cpu().numpy()
- if rgb2bgr:
- output = cv2.cvtColor(output, cv2.COLOR_RGB2BGR)
- return output
-
-
-def imfrombytes(content, flag='color', float32=False):
- """Read an image from bytes.
-
- Args:
- content (bytes): Image bytes got from files or other streams.
- flag (str): Flags specifying the color type of a loaded image,
- candidates are `color`, `grayscale` and `unchanged`.
- float32 (bool): Whether to change to float32., If True, will also norm
- to [0, 1]. Default: False.
-
- Returns:
- ndarray: Loaded image array.
- """
- img_np = np.frombuffer(content, np.uint8)
- imread_flags = {'color': cv2.IMREAD_COLOR, 'grayscale': cv2.IMREAD_GRAYSCALE, 'unchanged': cv2.IMREAD_UNCHANGED}
- img = cv2.imdecode(img_np, imread_flags[flag])
- if float32:
- img = img.astype(np.float32) / 255.
- return img
-
-
-def imwrite(img, file_path, params=None, auto_mkdir=True):
- """Write image to file.
-
- Args:
- img (ndarray): Image array to be written.
- file_path (str): Image file path.
- params (None or list): Same as opencv's :func:`imwrite` interface.
- auto_mkdir (bool): If the parent folder of `file_path` does not exist,
- whether to create it automatically.
-
- Returns:
- bool: Successful or not.
- """
- if auto_mkdir:
- dir_name = os.path.abspath(os.path.dirname(file_path))
- os.makedirs(dir_name, exist_ok=True)
- ok = cv2.imwrite(file_path, img, params)
- if not ok:
- raise IOError('Failed in writing images.')
-
-
-def crop_border(imgs, crop_border):
- """Crop borders of images.
-
- Args:
- imgs (list[ndarray] | ndarray): Images with shape (h, w, c).
- crop_border (int): Crop border for each end of height and weight.
-
- Returns:
- list[ndarray]: Cropped images.
- """
- if crop_border == 0:
- return imgs
- else:
- if isinstance(imgs, list):
- return [v[crop_border:-crop_border, crop_border:-crop_border, ...] for v in imgs]
- else:
- return imgs[crop_border:-crop_border, crop_border:-crop_border, ...]
diff --git a/spaces/OFA-Sys/OFA-Generic_Interface/fairseq/fairseq/models/fairseq_incremental_decoder.py b/spaces/OFA-Sys/OFA-Generic_Interface/fairseq/fairseq/models/fairseq_incremental_decoder.py
deleted file mode 100644
index cc72a0f8f3da238a8ce846240e5008d91ce1bc1a..0000000000000000000000000000000000000000
--- a/spaces/OFA-Sys/OFA-Generic_Interface/fairseq/fairseq/models/fairseq_incremental_decoder.py
+++ /dev/null
@@ -1,118 +0,0 @@
-# Copyright (c) Facebook, Inc. and its affiliates.
-#
-# This source code is licensed under the MIT license found in the
-# LICENSE file in the root directory of this source tree.
-
-import logging
-from typing import Dict, Optional
-
-from fairseq.incremental_decoding_utils import with_incremental_state
-from fairseq.models import FairseqDecoder
-from torch import Tensor
-
-
-logger = logging.getLogger(__name__)
-
-
-@with_incremental_state
-class FairseqIncrementalDecoder(FairseqDecoder):
- """Base class for incremental decoders.
-
- Incremental decoding is a special mode at inference time where the Model
- only receives a single timestep of input corresponding to the previous
- output token (for teacher forcing) and must produce the next output
- *incrementally*. Thus the model must cache any long-term state that is
- needed about the sequence, e.g., hidden states, convolutional states, etc.
-
- Compared to the standard :class:`FairseqDecoder` interface, the incremental
- decoder interface allows :func:`forward` functions to take an extra keyword
- argument (*incremental_state*) that can be used to cache state across
- time-steps.
-
- The :class:`FairseqIncrementalDecoder` interface also defines the
- :func:`reorder_incremental_state` method, which is used during beam search
- to select and reorder the incremental state based on the selection of beams.
-
- To learn more about how incremental decoding works, refer to `this blog
- `_.
- """
-
- def __init__(self, dictionary):
- super().__init__(dictionary)
-
- def forward(
- self, prev_output_tokens, encoder_out=None, incremental_state=None, **kwargs
- ):
- """
- Args:
- prev_output_tokens (LongTensor): shifted output tokens of shape
- `(batch, tgt_len)`, for teacher forcing
- encoder_out (dict, optional): output from the encoder, used for
- encoder-side attention
- incremental_state (dict, optional): dictionary used for storing
- state during :ref:`Incremental decoding`
-
- Returns:
- tuple:
- - the decoder's output of shape `(batch, tgt_len, vocab)`
- - a dictionary with any model-specific outputs
- """
- raise NotImplementedError
-
- def extract_features(
- self, prev_output_tokens, encoder_out=None, incremental_state=None, **kwargs
- ):
- """
- Returns:
- tuple:
- - the decoder's features of shape `(batch, tgt_len, embed_dim)`
- - a dictionary with any model-specific outputs
- """
- raise NotImplementedError
-
- def reorder_incremental_state(
- self,
- incremental_state: Dict[str, Dict[str, Optional[Tensor]]],
- new_order: Tensor,
- ):
- """Reorder incremental state.
-
- This will be called when the order of the input has changed from the
- previous time step. A typical use case is beam search, where the input
- order changes between time steps based on the selection of beams.
- """
- pass
-
- def reorder_incremental_state_scripting(
- self,
- incremental_state: Dict[str, Dict[str, Optional[Tensor]]],
- new_order: Tensor,
- ):
- """Main entry point for reordering the incremental state.
-
- Due to limitations in TorchScript, we call this function in
- :class:`fairseq.sequence_generator.SequenceGenerator` instead of
- calling :func:`reorder_incremental_state` directly.
- """
- for module in self.modules():
- if hasattr(module, "reorder_incremental_state"):
- result = module.reorder_incremental_state(incremental_state, new_order)
- if result is not None:
- incremental_state = result
-
- def set_beam_size(self, beam_size):
- """Sets the beam size in the decoder and all children."""
- if getattr(self, "_beam_size", -1) != beam_size:
- seen = set()
-
- def apply_set_beam_size(module):
- if (
- module != self
- and hasattr(module, "set_beam_size")
- and module not in seen
- ):
- seen.add(module)
- module.set_beam_size(beam_size)
-
- self.apply(apply_set_beam_size)
- self._beam_size = beam_size
diff --git a/spaces/OFA-Sys/OFA-Image_Caption/fairseq/fairseq/modules/quantization/scalar/utils.py b/spaces/OFA-Sys/OFA-Image_Caption/fairseq/fairseq/modules/quantization/scalar/utils.py
deleted file mode 100644
index 2ec6af3fcb09ccaf853be15a84ed8181f9e2f546..0000000000000000000000000000000000000000
--- a/spaces/OFA-Sys/OFA-Image_Caption/fairseq/fairseq/modules/quantization/scalar/utils.py
+++ /dev/null
@@ -1,78 +0,0 @@
-# Copyright (c) Facebook, Inc. and its affiliates.
-#
-# This source code is licensed under the MIT license found in the
-# LICENSE file in the root directory of this source tree.
-
-import logging
-from operator import attrgetter
-
-import torch.distributed as dist
-import torch.nn as nn
-
-from ..pq.utils import attrsetter, get_layers
-from .modules import ActivationQuantizer, IntConv2d, IntEmbedding, IntLinear
-
-
-MAPPING = {nn.Linear: IntLinear, nn.Embedding: IntEmbedding, nn.Conv2d: IntConv2d}
-
-
-def quantize_model_(model, p=0.2, bits=8, update_step=3000, method="histogram", remove_weights=False):
- """
- Replaces all modules with their scalar quantized counterpart and
- registers hooks to quantize the post-ativations of those modules.
-
- Args:
- - model: a nn.Module
- - p: amount of noise (0 for no noise, 1 to quantize all the weights/activations)
- - bits: number of bits
- - update_step: update quantization parameters every update_step steps
- """
- # quantize all layers
- # remove weights indicates whether the weights extension should be removed, in addition to
- # weight_orig and weight extension on names
- quantized_layers = get_layers(model, "(.*?)", remove_weights=remove_weights)
-
- for layer in quantized_layers:
-
- # book-keeping
- is_master_process = (not dist.is_initialized()) or (
- dist.is_initialized() and dist.get_rank() == 0
- )
-
- # recover module
- module = attrgetter(layer)(model)
- if is_master_process:
- logging.info(
- f"Quantizing layer {layer} with bits={bits} and QuantNoise={p}"
- )
-
- # quantization params
- q_params = {
- "p": p,
- "update_step": update_step,
- "bits": bits,
- "method": method,
- "counter": 0,
- }
-
- # instantiate the quantized counterpart
- if isinstance(module, tuple(MAPPING.keys())):
- QuantizedModule = MAPPING[module.__class__]
- quantized_module = QuantizedModule.__new__(QuantizedModule)
- params = module.__dict__
- params.update(q_params)
- quantized_module.__dict__.update(params)
-
- else:
- if is_master_process:
- logging.info(f"Module {module} not yet supported for quantization")
- continue
-
- # activation quantization
- a_q = ActivationQuantizer(quantized_module, p=0, bits=bits, method=method)
-
- # replace layer by its quantized counterpart
- attrsetter(layer)(model, quantized_module)
-
- # return name of quantized layers
- return quantized_layers
diff --git a/spaces/OFA-Sys/OFA-Image_Caption/fairseq/scripts/compare_namespaces.py b/spaces/OFA-Sys/OFA-Image_Caption/fairseq/scripts/compare_namespaces.py
deleted file mode 100644
index bc24db624f8db36f546c263ba3a806dae6d466bf..0000000000000000000000000000000000000000
--- a/spaces/OFA-Sys/OFA-Image_Caption/fairseq/scripts/compare_namespaces.py
+++ /dev/null
@@ -1,46 +0,0 @@
-#!/usr/bin/env python
-"""Helper script to compare two argparse.Namespace objects."""
-
-from argparse import Namespace # noqa
-
-
-def main():
-
- ns1 = eval(input("Namespace 1: "))
- ns2 = eval(input("Namespace 2: "))
-
- def keys(ns):
- ks = set()
- for k in dir(ns):
- if not k.startswith("_"):
- ks.add(k)
- return ks
-
- k1 = keys(ns1)
- k2 = keys(ns2)
-
- def print_keys(ks, ns1, ns2=None):
- for k in ks:
- if ns2 is None:
- print("{}\t{}".format(k, getattr(ns1, k, None)))
- else:
- print(
- "{}\t{}\t{}".format(k, getattr(ns1, k, None), getattr(ns2, k, None))
- )
-
- print("Keys unique to namespace 1:")
- print_keys(k1 - k2, ns1)
- print()
-
- print("Keys unique to namespace 2:")
- print_keys(k2 - k1, ns2)
- print()
-
- print("Overlapping keys with different values:")
- ks = [k for k in k1 & k2 if getattr(ns1, k, "None") != getattr(ns2, k, "None")]
- print_keys(ks, ns1, ns2)
- print()
-
-
-if __name__ == "__main__":
- main()
diff --git a/spaces/OFA-Sys/OFA-Visual_Grounding/fairseq/examples/linformer/linformer_src/models/__init__.py b/spaces/OFA-Sys/OFA-Visual_Grounding/fairseq/examples/linformer/linformer_src/models/__init__.py
deleted file mode 100644
index e69de29bb2d1d6434b8b29ae775ad8c2e48c5391..0000000000000000000000000000000000000000
diff --git a/spaces/OFA-Sys/OFA-vqa/fairseq/fairseq/modules/checkpoint_activations.py b/spaces/OFA-Sys/OFA-vqa/fairseq/fairseq/modules/checkpoint_activations.py
deleted file mode 100644
index 7489e09eb79b595aef674914556018d7f0a4efbf..0000000000000000000000000000000000000000
--- a/spaces/OFA-Sys/OFA-vqa/fairseq/fairseq/modules/checkpoint_activations.py
+++ /dev/null
@@ -1,236 +0,0 @@
-# Copyright (c) Facebook, Inc. and its affiliates.
-#
-# This source code is licensed under the MIT license found in the
-# LICENSE file in the root directory of this source tree.
-
-import functools
-from typing import Any, Dict, List, Tuple, Union
-
-import torch
-import torch.utils.checkpoint as checkpoint
-from fairseq import utils
-
-
-def checkpoint_wrapper(m, offload_to_cpu=False):
- """
- A friendlier wrapper for performing activation checkpointing.
-
- Compared to the PyTorch version, this version:
- - wraps an nn.Module, so that all subsequent calls will use checkpointing
- - handles keyword arguments in the forward
- - handles non-Tensor outputs from the forward
-
- Usage::
-
- checkpointed_module = checkpoint_wrapper(my_module, offload_to_cpu=True)
- a, b = checkpointed_module(x, y=3, z=torch.Tensor([1]))
- """
- # should I check whether original_forward has already been set?
- assert not hasattr(
- m, "precheckpoint_forward"
- ), "checkpoint function has already been applied?"
- m.precheckpoint_forward = m.forward
- m.forward = functools.partial(
- _checkpointed_forward,
- m.precheckpoint_forward, # original_forward
- offload_to_cpu,
- )
- return m
-
-
-def unwrap_checkpoint(m: torch.nn.Module):
- """
- unwrap a module and its children from checkpoint_wrapper
- """
- for module in m.modules():
- if hasattr(module, "precheckpoint_forward"):
- module.forward = module.precheckpoint_forward
- del module.precheckpoint_forward
- return m
-
-
-def _checkpointed_forward(original_forward, offload_to_cpu, *args, **kwargs):
- # Autograd Functions in PyTorch work best with positional args, since
- # the backward must return gradients (or None) for every input argument.
- # We can flatten keyword arguments to make this easier.
- kwarg_keys, flat_args = pack_kwargs(*args, **kwargs)
- parent_ctx_dict = {"offload": offload_to_cpu}
- output = CheckpointFunction.apply(
- original_forward, parent_ctx_dict, kwarg_keys, *flat_args
- )
- if isinstance(output, torch.Tensor):
- return output
- else:
- packed_non_tensor_outputs = parent_ctx_dict["packed_non_tensor_outputs"]
- if packed_non_tensor_outputs:
- output = unpack_non_tensors(output, packed_non_tensor_outputs)
- return output
-
-
-def pack_kwargs(*args, **kwargs) -> Tuple[List[str], List[Any]]:
- """
- Usage::
-
- kwarg_keys, flat_args = pack_kwargs(1, 2, a=3, b=4)
- args, kwargs = unpack_kwargs(kwarg_keys, flat_args)
- assert args == [1, 2]
- assert kwargs == {"a": 3, "b": 4}
- """
- kwarg_keys = []
- flat_args = list(args)
- for k, v in kwargs.items():
- kwarg_keys.append(k)
- flat_args.append(v)
- return kwarg_keys, flat_args
-
-
-def unpack_kwargs(
- kwarg_keys: List[str], flat_args: List[Any]
-) -> Tuple[List[Any], Dict[str, Any]]:
- if len(kwarg_keys) == 0:
- return flat_args, {}
- args = flat_args[: -len(kwarg_keys)]
- kwargs = {k: v for k, v in zip(kwarg_keys, flat_args[-len(kwarg_keys) :])}
- return args, kwargs
-
-
-def split_non_tensors(
- mixed: Union[torch.Tensor, Tuple[Any]]
-) -> Tuple[Tuple[torch.Tensor], Dict[str, List[Any]]]:
- """
- Usage::
-
- x = torch.Tensor([1])
- y = torch.Tensor([2])
- tensors, packed_non_tensors = split_non_tensors((x, y, None, 3))
- recon = unpack_non_tensors(tensors, packed_non_tensors)
- assert recon == (x, y, None, 3)
- """
- if isinstance(mixed, torch.Tensor):
- return (mixed,), None
- tensors = []
- packed_non_tensors = {"is_tensor": [], "objects": []}
- for o in mixed:
- if isinstance(o, torch.Tensor):
- packed_non_tensors["is_tensor"].append(True)
- tensors.append(o)
- else:
- packed_non_tensors["is_tensor"].append(False)
- packed_non_tensors["objects"].append(o)
- return tuple(tensors), packed_non_tensors
-
-
-def unpack_non_tensors(
- tensors: Tuple[torch.Tensor],
- packed_non_tensors: Dict[str, List[Any]],
-) -> Tuple[Any]:
- if packed_non_tensors is None:
- return tensors
- assert isinstance(packed_non_tensors, dict)
- mixed = []
- is_tensor_list = packed_non_tensors["is_tensor"]
- objects = packed_non_tensors["objects"]
- assert len(tensors) + len(objects) == len(is_tensor_list)
- obj_i = tnsr_i = 0
- for is_tensor in is_tensor_list:
- if is_tensor:
- mixed.append(tensors[tnsr_i])
- tnsr_i += 1
- else:
- mixed.append(objects[obj_i])
- obj_i += 1
- return tuple(mixed)
-
-
-class CheckpointFunction(torch.autograd.Function):
- """Similar to the torch version, but support non-Tensor outputs.
-
- The caller is expected to provide a dict (*parent_ctx_dict*) that will hold
- the non-Tensor outputs. These should be combined with the Tensor *outputs*
- by calling ``unpack_non_tensors``.
- """
-
- @staticmethod
- def forward(ctx, run_function, parent_ctx_dict, kwarg_keys, *args):
- if torch.is_grad_enabled(): # grad may be disabled, e.g., during validation
- checkpoint.check_backward_validity(args)
-
- ctx.run_function = run_function
- ctx.kwarg_keys = kwarg_keys
- ctx.fwd_rng_state = utils.get_rng_state()
-
- tensor_inputs, packed_non_tensor_inputs = split_non_tensors(args)
- if parent_ctx_dict["offload"]:
- ctx.fwd_device = tuple(x.device for x in tensor_inputs)
- ctx.grad_requirements = tuple(x.requires_grad for x in tensor_inputs)
- tensor_inputs = tuple(x.to(torch.device("cpu"), non_blocking=True) for x in tensor_inputs)
-
- else:
- ctx.fwd_device, ctx.grad_requirements = None, None
-
- ctx.save_for_backward(*tensor_inputs)
- ctx.packed_non_tensor_inputs = packed_non_tensor_inputs
-
- with torch.no_grad():
- unpacked_args, unpacked_kwargs = unpack_kwargs(kwarg_keys, args)
- outputs = run_function(*unpacked_args, **unpacked_kwargs)
-
- if isinstance(outputs, torch.Tensor):
- return outputs
- else:
- # Autograd Functions don't like non-Tensor outputs. We can split the
- # non-Tensor and Tensor outputs, returning the former by reference
- # through *parent_ctx_dict* and returning the latter directly.
- outputs, packed_non_tensor_outputs = split_non_tensors(outputs)
- parent_ctx_dict["packed_non_tensor_outputs"] = packed_non_tensor_outputs
- return outputs
-
- @staticmethod
- def backward(ctx, *args):
- if not torch.autograd._is_checkpoint_valid():
- raise RuntimeError(
- "Checkpointing is not compatible with .grad(), please use .backward() if possible"
- )
-
- tensor_inputs: Tuple = ctx.saved_tensors
- tensor_inputs = checkpoint.detach_variable(tensor_inputs)
- if ctx.fwd_device is not None:
- tensor_inputs = [
- t.to(ctx.fwd_device[i], non_blocking=True) for i, t in enumerate(tensor_inputs)
- ]
- for i, need_grad in enumerate(ctx.grad_requirements):
- tensor_inputs[i].requires_grad = need_grad
- inputs = unpack_non_tensors(tensor_inputs, ctx.packed_non_tensor_inputs)
-
- # Store the current states.
- bwd_rng_state = utils.get_rng_state()
-
- # Set the states to what it used to be before the forward pass.
- utils.set_rng_state(ctx.fwd_rng_state)
-
- with torch.enable_grad():
- unpacked_args, unpacked_kwargs = unpack_kwargs(ctx.kwarg_keys, inputs)
- outputs = ctx.run_function(*unpacked_args, **unpacked_kwargs)
- tensor_outputs, _ = split_non_tensors(outputs)
- # Set the states back to what it was at the start of this function.
- utils.set_rng_state(bwd_rng_state)
-
- # Run backward() with only Tensors that require grad
- outputs_with_grad = []
- args_with_grad = []
- for i in range(len(tensor_outputs)):
- if tensor_outputs[i].requires_grad:
- outputs_with_grad.append(tensor_outputs[i])
- args_with_grad.append(args[i])
- if len(outputs_with_grad) == 0:
- raise RuntimeError(
- "None of the outputs have requires_grad=True, "
- "this checkpoint() is not necessary"
- )
-
- torch.autograd.backward(outputs_with_grad, args_with_grad)
-
- grads = tuple(
- inp.grad if isinstance(inp, torch.Tensor) else None for inp in inputs
- )
- return (None, None, None) + grads
diff --git a/spaces/OFA-Sys/OFA-vqa/fairseq/fairseq/optim/adadelta.py b/spaces/OFA-Sys/OFA-vqa/fairseq/fairseq/optim/adadelta.py
deleted file mode 100644
index f1a21549770f0904a6a40a42ff7eb52811f1bfbe..0000000000000000000000000000000000000000
--- a/spaces/OFA-Sys/OFA-vqa/fairseq/fairseq/optim/adadelta.py
+++ /dev/null
@@ -1,47 +0,0 @@
-# Copyright (c) Facebook, Inc. and its affiliates.
-#
-# This source code is licensed under the MIT license found in the
-# LICENSE file in the root directory of this source tree.
-
-import torch.optim
-
-from . import LegacyFairseqOptimizer, register_optimizer
-
-
-@register_optimizer("adadelta")
-class Adadelta(LegacyFairseqOptimizer):
- def __init__(self, args, params):
- super().__init__(args)
- self._optimizer = torch.optim.Adadelta(params, **self.optimizer_config)
-
- @staticmethod
- def add_args(parser):
- """Add optimizer-specific arguments to the parser."""
- # fmt: off
- parser.add_argument('--adadelta-rho', type=float, default=0.9, metavar='RHO',
- help='coefficient used for computing a running average of squared gradients')
- parser.add_argument('--adadelta-eps', type=float, default=1e-6, metavar='EPS',
- help='term added to the denominator to improve numerical stability')
- parser.add_argument('--weight-decay', '--wd', default=0.0, type=float, metavar='WD',
- help='weight decay')
- parser.add_argument('--anneal-eps', action='store_true', help='flag to anneal eps')
- # fmt: on
-
- @property
- def optimizer_config(self):
- """
- Return a kwarg dictionary that will be used to override optimizer
- args stored in checkpoints. This allows us to load a checkpoint and
- resume training using a different set of optimizer args, e.g., with a
- different learning rate.
- """
- return {
- "lr": self.args.lr[0],
- "rho": self.args.adadelta_rho,
- "eps": self.args.adadelta_eps,
- "weight_decay": self.args.weight_decay,
- }
-
- @property
- def supports_flat_params(self):
- return True
diff --git a/spaces/OFA-Sys/OFA-vqa/utils/cider/pyciderevalcap/__init__.py b/spaces/OFA-Sys/OFA-vqa/utils/cider/pyciderevalcap/__init__.py
deleted file mode 100644
index 3f7d85bba884ea8f83fc6ab2a1e6ade80d98d4d9..0000000000000000000000000000000000000000
--- a/spaces/OFA-Sys/OFA-vqa/utils/cider/pyciderevalcap/__init__.py
+++ /dev/null
@@ -1 +0,0 @@
-__author__ = 'tylin'
diff --git a/spaces/Olivier-Truong/faster-whisper-webui-v2/tests/vad_test.py b/spaces/Olivier-Truong/faster-whisper-webui-v2/tests/vad_test.py
deleted file mode 100644
index c72492b1e7f9183c7a452784facb2cdf6c1bf0e2..0000000000000000000000000000000000000000
--- a/spaces/Olivier-Truong/faster-whisper-webui-v2/tests/vad_test.py
+++ /dev/null
@@ -1,72 +0,0 @@
-import unittest
-import numpy as np
-import sys
-
-sys.path.append('../whisper-webui')
-#print("Sys path: " + str(sys.path))
-
-from src.whisper.abstractWhisperContainer import LambdaWhisperCallback
-from src.vad import AbstractTranscription, TranscriptionConfig, VadSileroTranscription
-
-class TestVad(unittest.TestCase):
- def __init__(self, *args, **kwargs):
- super(TestVad, self).__init__(*args, **kwargs)
- self.transcribe_calls = []
-
- def test_transcript(self):
- mock = MockVadTranscription(mock_audio_length=120)
- config = TranscriptionConfig()
-
- self.transcribe_calls.clear()
- result = mock.transcribe("mock", LambdaWhisperCallback(lambda segment, _1, _2, _3, _4: self.transcribe_segments(segment)), config)
-
- self.assertListEqual(self.transcribe_calls, [
- [30, 30],
- [100, 100]
- ])
-
- self.assertListEqual(result['segments'],
- [{'end': 50.0, 'start': 40.0, 'text': 'Hello world '},
- {'end': 120.0, 'start': 110.0, 'text': 'Hello world '}]
- )
-
- def transcribe_segments(self, segment):
- self.transcribe_calls.append(segment.tolist())
-
- # Dummy text
- return {
- 'text': "Hello world ",
- 'segments': [
- {
- "start": 10.0,
- "end": 20.0,
- "text": "Hello world "
- }
- ],
- 'language': ""
- }
-
-class MockVadTranscription(AbstractTranscription):
- def __init__(self, mock_audio_length: float = 1000):
- super().__init__()
- self.mock_audio_length = mock_audio_length
-
- def get_audio_segment(self, str, start_time: str = None, duration: str = None):
- start_time_seconds = float(start_time.removesuffix("s"))
- duration_seconds = float(duration.removesuffix("s"))
-
- # For mocking, this just returns a simple numppy array
- return np.array([start_time_seconds, duration_seconds], dtype=np.float64)
-
- def get_transcribe_timestamps(self, audio: str, config: TranscriptionConfig, start_time: float, duration: float):
- result = []
-
- result.append( { 'start': 30, 'end': 60 } )
- result.append( { 'start': 100, 'end': 200 } )
- return result
-
- def get_audio_duration(self, audio: str, config: TranscriptionConfig):
- return self.mock_audio_length
-
-if __name__ == '__main__':
- unittest.main()
\ No newline at end of file
diff --git a/spaces/Omnibus/game-test/README.md b/spaces/Omnibus/game-test/README.md
deleted file mode 100644
index bfba0eb824b0ab8136d312f0bf7a6e089abe2d17..0000000000000000000000000000000000000000
--- a/spaces/Omnibus/game-test/README.md
+++ /dev/null
@@ -1,13 +0,0 @@
----
-title: Game Test
-emoji: 🐢
-colorFrom: purple
-colorTo: purple
-sdk: gradio
-sdk_version: 3.50.2
-app_file: app.py
-pinned: false
-
----
-
-Check out the configuration reference at https://huggingface.co/docs/hub/spaces-config-reference
diff --git a/spaces/OnabajoMonsurat/Medical_Diagnosis_Chatbot/README.md b/spaces/OnabajoMonsurat/Medical_Diagnosis_Chatbot/README.md
deleted file mode 100644
index a8a71e850807875245015217f124559d7fb404bc..0000000000000000000000000000000000000000
--- a/spaces/OnabajoMonsurat/Medical_Diagnosis_Chatbot/README.md
+++ /dev/null
@@ -1,13 +0,0 @@
----
-title: Medical Diagnosis Chatbot
-emoji: 🏢
-colorFrom: purple
-colorTo: green
-sdk: gradio
-sdk_version: 3.40.1
-app_file: app.py
-pinned: false
-license: mit
----
-
-Check out the configuration reference at https://huggingface.co/docs/hub/spaces-config-reference
diff --git a/spaces/OpenGVLab/InternGPT/third-party/lama/bin/models/ade20k/segm_lib/nn/modules/batchnorm.py b/spaces/OpenGVLab/InternGPT/third-party/lama/bin/models/ade20k/segm_lib/nn/modules/batchnorm.py
deleted file mode 100644
index 18318965335b37cc671004a6aceda3229dc7b477..0000000000000000000000000000000000000000
--- a/spaces/OpenGVLab/InternGPT/third-party/lama/bin/models/ade20k/segm_lib/nn/modules/batchnorm.py
+++ /dev/null
@@ -1,329 +0,0 @@
-# -*- coding: utf-8 -*-
-# File : batchnorm.py
-# Author : Jiayuan Mao
-# Email : maojiayuan@gmail.com
-# Date : 27/01/2018
-#
-# This file is part of Synchronized-BatchNorm-PyTorch.
-# https://github.com/vacancy/Synchronized-BatchNorm-PyTorch
-# Distributed under MIT License.
-
-import collections
-
-import torch
-import torch.nn.functional as F
-
-from torch.nn.modules.batchnorm import _BatchNorm
-from torch.nn.parallel._functions import ReduceAddCoalesced, Broadcast
-
-from .comm import SyncMaster
-
-__all__ = ['SynchronizedBatchNorm1d', 'SynchronizedBatchNorm2d', 'SynchronizedBatchNorm3d']
-
-
-def _sum_ft(tensor):
- """sum over the first and last dimention"""
- return tensor.sum(dim=0).sum(dim=-1)
-
-
-def _unsqueeze_ft(tensor):
- """add new dementions at the front and the tail"""
- return tensor.unsqueeze(0).unsqueeze(-1)
-
-
-_ChildMessage = collections.namedtuple('_ChildMessage', ['sum', 'ssum', 'sum_size'])
-_MasterMessage = collections.namedtuple('_MasterMessage', ['sum', 'inv_std'])
-
-
-class _SynchronizedBatchNorm(_BatchNorm):
- def __init__(self, num_features, eps=1e-5, momentum=0.001, affine=True):
- super(_SynchronizedBatchNorm, self).__init__(num_features, eps=eps, momentum=momentum, affine=affine)
-
- self._sync_master = SyncMaster(self._data_parallel_master)
-
- self._is_parallel = False
- self._parallel_id = None
- self._slave_pipe = None
-
- # customed batch norm statistics
- self._moving_average_fraction = 1. - momentum
- self.register_buffer('_tmp_running_mean', torch.zeros(self.num_features))
- self.register_buffer('_tmp_running_var', torch.ones(self.num_features))
- self.register_buffer('_running_iter', torch.ones(1))
- self._tmp_running_mean = self.running_mean.clone() * self._running_iter
- self._tmp_running_var = self.running_var.clone() * self._running_iter
-
- def forward(self, input):
- # If it is not parallel computation or is in evaluation mode, use PyTorch's implementation.
- if not (self._is_parallel and self.training):
- return F.batch_norm(
- input, self.running_mean, self.running_var, self.weight, self.bias,
- self.training, self.momentum, self.eps)
-
- # Resize the input to (B, C, -1).
- input_shape = input.size()
- input = input.view(input.size(0), self.num_features, -1)
-
- # Compute the sum and square-sum.
- sum_size = input.size(0) * input.size(2)
- input_sum = _sum_ft(input)
- input_ssum = _sum_ft(input ** 2)
-
- # Reduce-and-broadcast the statistics.
- if self._parallel_id == 0:
- mean, inv_std = self._sync_master.run_master(_ChildMessage(input_sum, input_ssum, sum_size))
- else:
- mean, inv_std = self._slave_pipe.run_slave(_ChildMessage(input_sum, input_ssum, sum_size))
-
- # Compute the output.
- if self.affine:
- # MJY:: Fuse the multiplication for speed.
- output = (input - _unsqueeze_ft(mean)) * _unsqueeze_ft(inv_std * self.weight) + _unsqueeze_ft(self.bias)
- else:
- output = (input - _unsqueeze_ft(mean)) * _unsqueeze_ft(inv_std)
-
- # Reshape it.
- return output.view(input_shape)
-
- def __data_parallel_replicate__(self, ctx, copy_id):
- self._is_parallel = True
- self._parallel_id = copy_id
-
- # parallel_id == 0 means master device.
- if self._parallel_id == 0:
- ctx.sync_master = self._sync_master
- else:
- self._slave_pipe = ctx.sync_master.register_slave(copy_id)
-
- def _data_parallel_master(self, intermediates):
- """Reduce the sum and square-sum, compute the statistics, and broadcast it."""
- intermediates = sorted(intermediates, key=lambda i: i[1].sum.get_device())
-
- to_reduce = [i[1][:2] for i in intermediates]
- to_reduce = [j for i in to_reduce for j in i] # flatten
- target_gpus = [i[1].sum.get_device() for i in intermediates]
-
- sum_size = sum([i[1].sum_size for i in intermediates])
- sum_, ssum = ReduceAddCoalesced.apply(target_gpus[0], 2, *to_reduce)
-
- mean, inv_std = self._compute_mean_std(sum_, ssum, sum_size)
-
- broadcasted = Broadcast.apply(target_gpus, mean, inv_std)
-
- outputs = []
- for i, rec in enumerate(intermediates):
- outputs.append((rec[0], _MasterMessage(*broadcasted[i*2:i*2+2])))
-
- return outputs
-
- def _add_weighted(self, dest, delta, alpha=1, beta=1, bias=0):
- """return *dest* by `dest := dest*alpha + delta*beta + bias`"""
- return dest * alpha + delta * beta + bias
-
- def _compute_mean_std(self, sum_, ssum, size):
- """Compute the mean and standard-deviation with sum and square-sum. This method
- also maintains the moving average on the master device."""
- assert size > 1, 'BatchNorm computes unbiased standard-deviation, which requires size > 1.'
- mean = sum_ / size
- sumvar = ssum - sum_ * mean
- unbias_var = sumvar / (size - 1)
- bias_var = sumvar / size
-
- self._tmp_running_mean = self._add_weighted(self._tmp_running_mean, mean.data, alpha=self._moving_average_fraction)
- self._tmp_running_var = self._add_weighted(self._tmp_running_var, unbias_var.data, alpha=self._moving_average_fraction)
- self._running_iter = self._add_weighted(self._running_iter, 1, alpha=self._moving_average_fraction)
-
- self.running_mean = self._tmp_running_mean / self._running_iter
- self.running_var = self._tmp_running_var / self._running_iter
-
- return mean, bias_var.clamp(self.eps) ** -0.5
-
-
-class SynchronizedBatchNorm1d(_SynchronizedBatchNorm):
- r"""Applies Synchronized Batch Normalization over a 2d or 3d input that is seen as a
- mini-batch.
-
- .. math::
-
- y = \frac{x - mean[x]}{ \sqrt{Var[x] + \epsilon}} * gamma + beta
-
- This module differs from the built-in PyTorch BatchNorm1d as the mean and
- standard-deviation are reduced across all devices during training.
-
- For example, when one uses `nn.DataParallel` to wrap the network during
- training, PyTorch's implementation normalize the tensor on each device using
- the statistics only on that device, which accelerated the computation and
- is also easy to implement, but the statistics might be inaccurate.
- Instead, in this synchronized version, the statistics will be computed
- over all training samples distributed on multiple devices.
-
- Note that, for one-GPU or CPU-only case, this module behaves exactly same
- as the built-in PyTorch implementation.
-
- The mean and standard-deviation are calculated per-dimension over
- the mini-batches and gamma and beta are learnable parameter vectors
- of size C (where C is the input size).
-
- During training, this layer keeps a running estimate of its computed mean
- and variance. The running sum is kept with a default momentum of 0.1.
-
- During evaluation, this running mean/variance is used for normalization.
-
- Because the BatchNorm is done over the `C` dimension, computing statistics
- on `(N, L)` slices, it's common terminology to call this Temporal BatchNorm
-
- Args:
- num_features: num_features from an expected input of size
- `batch_size x num_features [x width]`
- eps: a value added to the denominator for numerical stability.
- Default: 1e-5
- momentum: the value used for the running_mean and running_var
- computation. Default: 0.1
- affine: a boolean value that when set to ``True``, gives the layer learnable
- affine parameters. Default: ``True``
-
- Shape:
- - Input: :math:`(N, C)` or :math:`(N, C, L)`
- - Output: :math:`(N, C)` or :math:`(N, C, L)` (same shape as input)
-
- Examples:
- >>> # With Learnable Parameters
- >>> m = SynchronizedBatchNorm1d(100)
- >>> # Without Learnable Parameters
- >>> m = SynchronizedBatchNorm1d(100, affine=False)
- >>> input = torch.autograd.Variable(torch.randn(20, 100))
- >>> output = m(input)
- """
-
- def _check_input_dim(self, input):
- if input.dim() != 2 and input.dim() != 3:
- raise ValueError('expected 2D or 3D input (got {}D input)'
- .format(input.dim()))
- super(SynchronizedBatchNorm1d, self)._check_input_dim(input)
-
-
-class SynchronizedBatchNorm2d(_SynchronizedBatchNorm):
- r"""Applies Batch Normalization over a 4d input that is seen as a mini-batch
- of 3d inputs
-
- .. math::
-
- y = \frac{x - mean[x]}{ \sqrt{Var[x] + \epsilon}} * gamma + beta
-
- This module differs from the built-in PyTorch BatchNorm2d as the mean and
- standard-deviation are reduced across all devices during training.
-
- For example, when one uses `nn.DataParallel` to wrap the network during
- training, PyTorch's implementation normalize the tensor on each device using
- the statistics only on that device, which accelerated the computation and
- is also easy to implement, but the statistics might be inaccurate.
- Instead, in this synchronized version, the statistics will be computed
- over all training samples distributed on multiple devices.
-
- Note that, for one-GPU or CPU-only case, this module behaves exactly same
- as the built-in PyTorch implementation.
-
- The mean and standard-deviation are calculated per-dimension over
- the mini-batches and gamma and beta are learnable parameter vectors
- of size C (where C is the input size).
-
- During training, this layer keeps a running estimate of its computed mean
- and variance. The running sum is kept with a default momentum of 0.1.
-
- During evaluation, this running mean/variance is used for normalization.
-
- Because the BatchNorm is done over the `C` dimension, computing statistics
- on `(N, H, W)` slices, it's common terminology to call this Spatial BatchNorm
-
- Args:
- num_features: num_features from an expected input of
- size batch_size x num_features x height x width
- eps: a value added to the denominator for numerical stability.
- Default: 1e-5
- momentum: the value used for the running_mean and running_var
- computation. Default: 0.1
- affine: a boolean value that when set to ``True``, gives the layer learnable
- affine parameters. Default: ``True``
-
- Shape:
- - Input: :math:`(N, C, H, W)`
- - Output: :math:`(N, C, H, W)` (same shape as input)
-
- Examples:
- >>> # With Learnable Parameters
- >>> m = SynchronizedBatchNorm2d(100)
- >>> # Without Learnable Parameters
- >>> m = SynchronizedBatchNorm2d(100, affine=False)
- >>> input = torch.autograd.Variable(torch.randn(20, 100, 35, 45))
- >>> output = m(input)
- """
-
- def _check_input_dim(self, input):
- if input.dim() != 4:
- raise ValueError('expected 4D input (got {}D input)'
- .format(input.dim()))
- super(SynchronizedBatchNorm2d, self)._check_input_dim(input)
-
-
-class SynchronizedBatchNorm3d(_SynchronizedBatchNorm):
- r"""Applies Batch Normalization over a 5d input that is seen as a mini-batch
- of 4d inputs
-
- .. math::
-
- y = \frac{x - mean[x]}{ \sqrt{Var[x] + \epsilon}} * gamma + beta
-
- This module differs from the built-in PyTorch BatchNorm3d as the mean and
- standard-deviation are reduced across all devices during training.
-
- For example, when one uses `nn.DataParallel` to wrap the network during
- training, PyTorch's implementation normalize the tensor on each device using
- the statistics only on that device, which accelerated the computation and
- is also easy to implement, but the statistics might be inaccurate.
- Instead, in this synchronized version, the statistics will be computed
- over all training samples distributed on multiple devices.
-
- Note that, for one-GPU or CPU-only case, this module behaves exactly same
- as the built-in PyTorch implementation.
-
- The mean and standard-deviation are calculated per-dimension over
- the mini-batches and gamma and beta are learnable parameter vectors
- of size C (where C is the input size).
-
- During training, this layer keeps a running estimate of its computed mean
- and variance. The running sum is kept with a default momentum of 0.1.
-
- During evaluation, this running mean/variance is used for normalization.
-
- Because the BatchNorm is done over the `C` dimension, computing statistics
- on `(N, D, H, W)` slices, it's common terminology to call this Volumetric BatchNorm
- or Spatio-temporal BatchNorm
-
- Args:
- num_features: num_features from an expected input of
- size batch_size x num_features x depth x height x width
- eps: a value added to the denominator for numerical stability.
- Default: 1e-5
- momentum: the value used for the running_mean and running_var
- computation. Default: 0.1
- affine: a boolean value that when set to ``True``, gives the layer learnable
- affine parameters. Default: ``True``
-
- Shape:
- - Input: :math:`(N, C, D, H, W)`
- - Output: :math:`(N, C, D, H, W)` (same shape as input)
-
- Examples:
- >>> # With Learnable Parameters
- >>> m = SynchronizedBatchNorm3d(100)
- >>> # Without Learnable Parameters
- >>> m = SynchronizedBatchNorm3d(100, affine=False)
- >>> input = torch.autograd.Variable(torch.randn(20, 100, 35, 45, 10))
- >>> output = m(input)
- """
-
- def _check_input_dim(self, input):
- if input.dim() != 5:
- raise ValueError('expected 5D input (got {}D input)'
- .format(input.dim()))
- super(SynchronizedBatchNorm3d, self)._check_input_dim(input)
diff --git a/spaces/PAIR/PAIR-Diffusion/ldm/modules/midas/midas/vit.py b/spaces/PAIR/PAIR-Diffusion/ldm/modules/midas/midas/vit.py
deleted file mode 100644
index ea46b1be88b261b0dec04f3da0256f5f66f88a74..0000000000000000000000000000000000000000
--- a/spaces/PAIR/PAIR-Diffusion/ldm/modules/midas/midas/vit.py
+++ /dev/null
@@ -1,491 +0,0 @@
-import torch
-import torch.nn as nn
-import timm
-import types
-import math
-import torch.nn.functional as F
-
-
-class Slice(nn.Module):
- def __init__(self, start_index=1):
- super(Slice, self).__init__()
- self.start_index = start_index
-
- def forward(self, x):
- return x[:, self.start_index :]
-
-
-class AddReadout(nn.Module):
- def __init__(self, start_index=1):
- super(AddReadout, self).__init__()
- self.start_index = start_index
-
- def forward(self, x):
- if self.start_index == 2:
- readout = (x[:, 0] + x[:, 1]) / 2
- else:
- readout = x[:, 0]
- return x[:, self.start_index :] + readout.unsqueeze(1)
-
-
-class ProjectReadout(nn.Module):
- def __init__(self, in_features, start_index=1):
- super(ProjectReadout, self).__init__()
- self.start_index = start_index
-
- self.project = nn.Sequential(nn.Linear(2 * in_features, in_features), nn.GELU())
-
- def forward(self, x):
- readout = x[:, 0].unsqueeze(1).expand_as(x[:, self.start_index :])
- features = torch.cat((x[:, self.start_index :], readout), -1)
-
- return self.project(features)
-
-
-class Transpose(nn.Module):
- def __init__(self, dim0, dim1):
- super(Transpose, self).__init__()
- self.dim0 = dim0
- self.dim1 = dim1
-
- def forward(self, x):
- x = x.transpose(self.dim0, self.dim1)
- return x
-
-
-def forward_vit(pretrained, x):
- b, c, h, w = x.shape
-
- glob = pretrained.model.forward_flex(x)
-
- layer_1 = pretrained.activations["1"]
- layer_2 = pretrained.activations["2"]
- layer_3 = pretrained.activations["3"]
- layer_4 = pretrained.activations["4"]
-
- layer_1 = pretrained.act_postprocess1[0:2](layer_1)
- layer_2 = pretrained.act_postprocess2[0:2](layer_2)
- layer_3 = pretrained.act_postprocess3[0:2](layer_3)
- layer_4 = pretrained.act_postprocess4[0:2](layer_4)
-
- unflatten = nn.Sequential(
- nn.Unflatten(
- 2,
- torch.Size(
- [
- h // pretrained.model.patch_size[1],
- w // pretrained.model.patch_size[0],
- ]
- ),
- )
- )
-
- if layer_1.ndim == 3:
- layer_1 = unflatten(layer_1)
- if layer_2.ndim == 3:
- layer_2 = unflatten(layer_2)
- if layer_3.ndim == 3:
- layer_3 = unflatten(layer_3)
- if layer_4.ndim == 3:
- layer_4 = unflatten(layer_4)
-
- layer_1 = pretrained.act_postprocess1[3 : len(pretrained.act_postprocess1)](layer_1)
- layer_2 = pretrained.act_postprocess2[3 : len(pretrained.act_postprocess2)](layer_2)
- layer_3 = pretrained.act_postprocess3[3 : len(pretrained.act_postprocess3)](layer_3)
- layer_4 = pretrained.act_postprocess4[3 : len(pretrained.act_postprocess4)](layer_4)
-
- return layer_1, layer_2, layer_3, layer_4
-
-
-def _resize_pos_embed(self, posemb, gs_h, gs_w):
- posemb_tok, posemb_grid = (
- posemb[:, : self.start_index],
- posemb[0, self.start_index :],
- )
-
- gs_old = int(math.sqrt(len(posemb_grid)))
-
- posemb_grid = posemb_grid.reshape(1, gs_old, gs_old, -1).permute(0, 3, 1, 2)
- posemb_grid = F.interpolate(posemb_grid, size=(gs_h, gs_w), mode="bilinear")
- posemb_grid = posemb_grid.permute(0, 2, 3, 1).reshape(1, gs_h * gs_w, -1)
-
- posemb = torch.cat([posemb_tok, posemb_grid], dim=1)
-
- return posemb
-
-
-def forward_flex(self, x):
- b, c, h, w = x.shape
-
- pos_embed = self._resize_pos_embed(
- self.pos_embed, h // self.patch_size[1], w // self.patch_size[0]
- )
-
- B = x.shape[0]
-
- if hasattr(self.patch_embed, "backbone"):
- x = self.patch_embed.backbone(x)
- if isinstance(x, (list, tuple)):
- x = x[-1] # last feature if backbone outputs list/tuple of features
-
- x = self.patch_embed.proj(x).flatten(2).transpose(1, 2)
-
- if getattr(self, "dist_token", None) is not None:
- cls_tokens = self.cls_token.expand(
- B, -1, -1
- ) # stole cls_tokens impl from Phil Wang, thanks
- dist_token = self.dist_token.expand(B, -1, -1)
- x = torch.cat((cls_tokens, dist_token, x), dim=1)
- else:
- cls_tokens = self.cls_token.expand(
- B, -1, -1
- ) # stole cls_tokens impl from Phil Wang, thanks
- x = torch.cat((cls_tokens, x), dim=1)
-
- x = x + pos_embed
- x = self.pos_drop(x)
-
- for blk in self.blocks:
- x = blk(x)
-
- x = self.norm(x)
-
- return x
-
-
-activations = {}
-
-
-def get_activation(name):
- def hook(model, input, output):
- activations[name] = output
-
- return hook
-
-
-def get_readout_oper(vit_features, features, use_readout, start_index=1):
- if use_readout == "ignore":
- readout_oper = [Slice(start_index)] * len(features)
- elif use_readout == "add":
- readout_oper = [AddReadout(start_index)] * len(features)
- elif use_readout == "project":
- readout_oper = [
- ProjectReadout(vit_features, start_index) for out_feat in features
- ]
- else:
- assert (
- False
- ), "wrong operation for readout token, use_readout can be 'ignore', 'add', or 'project'"
-
- return readout_oper
-
-
-def _make_vit_b16_backbone(
- model,
- features=[96, 192, 384, 768],
- size=[384, 384],
- hooks=[2, 5, 8, 11],
- vit_features=768,
- use_readout="ignore",
- start_index=1,
-):
- pretrained = nn.Module()
-
- pretrained.model = model
- pretrained.model.blocks[hooks[0]].register_forward_hook(get_activation("1"))
- pretrained.model.blocks[hooks[1]].register_forward_hook(get_activation("2"))
- pretrained.model.blocks[hooks[2]].register_forward_hook(get_activation("3"))
- pretrained.model.blocks[hooks[3]].register_forward_hook(get_activation("4"))
-
- pretrained.activations = activations
-
- readout_oper = get_readout_oper(vit_features, features, use_readout, start_index)
-
- # 32, 48, 136, 384
- pretrained.act_postprocess1 = nn.Sequential(
- readout_oper[0],
- Transpose(1, 2),
- nn.Unflatten(2, torch.Size([size[0] // 16, size[1] // 16])),
- nn.Conv2d(
- in_channels=vit_features,
- out_channels=features[0],
- kernel_size=1,
- stride=1,
- padding=0,
- ),
- nn.ConvTranspose2d(
- in_channels=features[0],
- out_channels=features[0],
- kernel_size=4,
- stride=4,
- padding=0,
- bias=True,
- dilation=1,
- groups=1,
- ),
- )
-
- pretrained.act_postprocess2 = nn.Sequential(
- readout_oper[1],
- Transpose(1, 2),
- nn.Unflatten(2, torch.Size([size[0] // 16, size[1] // 16])),
- nn.Conv2d(
- in_channels=vit_features,
- out_channels=features[1],
- kernel_size=1,
- stride=1,
- padding=0,
- ),
- nn.ConvTranspose2d(
- in_channels=features[1],
- out_channels=features[1],
- kernel_size=2,
- stride=2,
- padding=0,
- bias=True,
- dilation=1,
- groups=1,
- ),
- )
-
- pretrained.act_postprocess3 = nn.Sequential(
- readout_oper[2],
- Transpose(1, 2),
- nn.Unflatten(2, torch.Size([size[0] // 16, size[1] // 16])),
- nn.Conv2d(
- in_channels=vit_features,
- out_channels=features[2],
- kernel_size=1,
- stride=1,
- padding=0,
- ),
- )
-
- pretrained.act_postprocess4 = nn.Sequential(
- readout_oper[3],
- Transpose(1, 2),
- nn.Unflatten(2, torch.Size([size[0] // 16, size[1] // 16])),
- nn.Conv2d(
- in_channels=vit_features,
- out_channels=features[3],
- kernel_size=1,
- stride=1,
- padding=0,
- ),
- nn.Conv2d(
- in_channels=features[3],
- out_channels=features[3],
- kernel_size=3,
- stride=2,
- padding=1,
- ),
- )
-
- pretrained.model.start_index = start_index
- pretrained.model.patch_size = [16, 16]
-
- # We inject this function into the VisionTransformer instances so that
- # we can use it with interpolated position embeddings without modifying the library source.
- pretrained.model.forward_flex = types.MethodType(forward_flex, pretrained.model)
- pretrained.model._resize_pos_embed = types.MethodType(
- _resize_pos_embed, pretrained.model
- )
-
- return pretrained
-
-
-def _make_pretrained_vitl16_384(pretrained, use_readout="ignore", hooks=None):
- model = timm.create_model("vit_large_patch16_384", pretrained=pretrained)
-
- hooks = [5, 11, 17, 23] if hooks == None else hooks
- return _make_vit_b16_backbone(
- model,
- features=[256, 512, 1024, 1024],
- hooks=hooks,
- vit_features=1024,
- use_readout=use_readout,
- )
-
-
-def _make_pretrained_vitb16_384(pretrained, use_readout="ignore", hooks=None):
- model = timm.create_model("vit_base_patch16_384", pretrained=pretrained)
-
- hooks = [2, 5, 8, 11] if hooks == None else hooks
- return _make_vit_b16_backbone(
- model, features=[96, 192, 384, 768], hooks=hooks, use_readout=use_readout
- )
-
-
-def _make_pretrained_deitb16_384(pretrained, use_readout="ignore", hooks=None):
- model = timm.create_model("vit_deit_base_patch16_384", pretrained=pretrained)
-
- hooks = [2, 5, 8, 11] if hooks == None else hooks
- return _make_vit_b16_backbone(
- model, features=[96, 192, 384, 768], hooks=hooks, use_readout=use_readout
- )
-
-
-def _make_pretrained_deitb16_distil_384(pretrained, use_readout="ignore", hooks=None):
- model = timm.create_model(
- "vit_deit_base_distilled_patch16_384", pretrained=pretrained
- )
-
- hooks = [2, 5, 8, 11] if hooks == None else hooks
- return _make_vit_b16_backbone(
- model,
- features=[96, 192, 384, 768],
- hooks=hooks,
- use_readout=use_readout,
- start_index=2,
- )
-
-
-def _make_vit_b_rn50_backbone(
- model,
- features=[256, 512, 768, 768],
- size=[384, 384],
- hooks=[0, 1, 8, 11],
- vit_features=768,
- use_vit_only=False,
- use_readout="ignore",
- start_index=1,
-):
- pretrained = nn.Module()
-
- pretrained.model = model
-
- if use_vit_only == True:
- pretrained.model.blocks[hooks[0]].register_forward_hook(get_activation("1"))
- pretrained.model.blocks[hooks[1]].register_forward_hook(get_activation("2"))
- else:
- pretrained.model.patch_embed.backbone.stages[0].register_forward_hook(
- get_activation("1")
- )
- pretrained.model.patch_embed.backbone.stages[1].register_forward_hook(
- get_activation("2")
- )
-
- pretrained.model.blocks[hooks[2]].register_forward_hook(get_activation("3"))
- pretrained.model.blocks[hooks[3]].register_forward_hook(get_activation("4"))
-
- pretrained.activations = activations
-
- readout_oper = get_readout_oper(vit_features, features, use_readout, start_index)
-
- if use_vit_only == True:
- pretrained.act_postprocess1 = nn.Sequential(
- readout_oper[0],
- Transpose(1, 2),
- nn.Unflatten(2, torch.Size([size[0] // 16, size[1] // 16])),
- nn.Conv2d(
- in_channels=vit_features,
- out_channels=features[0],
- kernel_size=1,
- stride=1,
- padding=0,
- ),
- nn.ConvTranspose2d(
- in_channels=features[0],
- out_channels=features[0],
- kernel_size=4,
- stride=4,
- padding=0,
- bias=True,
- dilation=1,
- groups=1,
- ),
- )
-
- pretrained.act_postprocess2 = nn.Sequential(
- readout_oper[1],
- Transpose(1, 2),
- nn.Unflatten(2, torch.Size([size[0] // 16, size[1] // 16])),
- nn.Conv2d(
- in_channels=vit_features,
- out_channels=features[1],
- kernel_size=1,
- stride=1,
- padding=0,
- ),
- nn.ConvTranspose2d(
- in_channels=features[1],
- out_channels=features[1],
- kernel_size=2,
- stride=2,
- padding=0,
- bias=True,
- dilation=1,
- groups=1,
- ),
- )
- else:
- pretrained.act_postprocess1 = nn.Sequential(
- nn.Identity(), nn.Identity(), nn.Identity()
- )
- pretrained.act_postprocess2 = nn.Sequential(
- nn.Identity(), nn.Identity(), nn.Identity()
- )
-
- pretrained.act_postprocess3 = nn.Sequential(
- readout_oper[2],
- Transpose(1, 2),
- nn.Unflatten(2, torch.Size([size[0] // 16, size[1] // 16])),
- nn.Conv2d(
- in_channels=vit_features,
- out_channels=features[2],
- kernel_size=1,
- stride=1,
- padding=0,
- ),
- )
-
- pretrained.act_postprocess4 = nn.Sequential(
- readout_oper[3],
- Transpose(1, 2),
- nn.Unflatten(2, torch.Size([size[0] // 16, size[1] // 16])),
- nn.Conv2d(
- in_channels=vit_features,
- out_channels=features[3],
- kernel_size=1,
- stride=1,
- padding=0,
- ),
- nn.Conv2d(
- in_channels=features[3],
- out_channels=features[3],
- kernel_size=3,
- stride=2,
- padding=1,
- ),
- )
-
- pretrained.model.start_index = start_index
- pretrained.model.patch_size = [16, 16]
-
- # We inject this function into the VisionTransformer instances so that
- # we can use it with interpolated position embeddings without modifying the library source.
- pretrained.model.forward_flex = types.MethodType(forward_flex, pretrained.model)
-
- # We inject this function into the VisionTransformer instances so that
- # we can use it with interpolated position embeddings without modifying the library source.
- pretrained.model._resize_pos_embed = types.MethodType(
- _resize_pos_embed, pretrained.model
- )
-
- return pretrained
-
-
-def _make_pretrained_vitb_rn50_384(
- pretrained, use_readout="ignore", hooks=None, use_vit_only=False
-):
- model = timm.create_model("vit_base_resnet50_384", pretrained=pretrained)
-
- hooks = [0, 1, 8, 11] if hooks == None else hooks
- return _make_vit_b_rn50_backbone(
- model,
- features=[256, 512, 768, 768],
- size=[384, 384],
- hooks=hooks,
- use_vit_only=use_vit_only,
- use_readout=use_readout,
- )
diff --git a/spaces/PAIR/Text2Video-Zero/annotator/uniformer/mmcv/ops/tin_shift.py b/spaces/PAIR/Text2Video-Zero/annotator/uniformer/mmcv/ops/tin_shift.py
deleted file mode 100644
index 472c9fcfe45a124e819b7ed5653e585f94a8811e..0000000000000000000000000000000000000000
--- a/spaces/PAIR/Text2Video-Zero/annotator/uniformer/mmcv/ops/tin_shift.py
+++ /dev/null
@@ -1,68 +0,0 @@
-# Copyright (c) OpenMMLab. All rights reserved.
-# Code reference from "Temporal Interlacing Network"
-# https://github.com/deepcs233/TIN/blob/master/cuda_shift/rtc_wrap.py
-# Hao Shao, Shengju Qian, Yu Liu
-# shaoh19@mails.tsinghua.edu.cn, sjqian@cse.cuhk.edu.hk, yuliu@ee.cuhk.edu.hk
-
-import torch
-import torch.nn as nn
-from torch.autograd import Function
-
-from ..utils import ext_loader
-
-ext_module = ext_loader.load_ext('_ext',
- ['tin_shift_forward', 'tin_shift_backward'])
-
-
-class TINShiftFunction(Function):
-
- @staticmethod
- def forward(ctx, input, shift):
- C = input.size(2)
- num_segments = shift.size(1)
- if C // num_segments <= 0 or C % num_segments != 0:
- raise ValueError('C should be a multiple of num_segments, '
- f'but got C={C} and num_segments={num_segments}.')
-
- ctx.save_for_backward(shift)
-
- out = torch.zeros_like(input)
- ext_module.tin_shift_forward(input, shift, out)
-
- return out
-
- @staticmethod
- def backward(ctx, grad_output):
-
- shift = ctx.saved_tensors[0]
- data_grad_input = grad_output.new(*grad_output.size()).zero_()
- shift_grad_input = shift.new(*shift.size()).zero_()
- ext_module.tin_shift_backward(grad_output, shift, data_grad_input)
-
- return data_grad_input, shift_grad_input
-
-
-tin_shift = TINShiftFunction.apply
-
-
-class TINShift(nn.Module):
- """Temporal Interlace Shift.
-
- Temporal Interlace shift is a differentiable temporal-wise frame shifting
- which is proposed in "Temporal Interlacing Network"
-
- Please refer to https://arxiv.org/abs/2001.06499 for more details.
- Code is modified from https://github.com/mit-han-lab/temporal-shift-module
- """
-
- def forward(self, input, shift):
- """Perform temporal interlace shift.
-
- Args:
- input (Tensor): Feature map with shape [N, num_segments, C, H * W].
- shift (Tensor): Shift tensor with shape [N, num_segments].
-
- Returns:
- Feature map after temporal interlace shift.
- """
- return tin_shift(input, shift)
diff --git a/spaces/PICOF/YusamiAlchemy/README.md b/spaces/PICOF/YusamiAlchemy/README.md
deleted file mode 100644
index e18307d9454971564f57cc2eb691865a1d672c2f..0000000000000000000000000000000000000000
--- a/spaces/PICOF/YusamiAlchemy/README.md
+++ /dev/null
@@ -1,13 +0,0 @@
----
-title: YusamiAlchemy
-emoji: 💩
-colorFrom: yellow
-colorTo: indigo
-sdk: gradio
-sdk_version: 3.8
-app_file: app.py
-pinned: false
-license: gpl
----
-
-Check out the configuration reference at https://huggingface.co/docs/hub/spaces-config-reference
diff --git a/spaces/Pattr/DrumClassification/lilypond-2.24.2/lib/guile/2.2/ccache/scripts/display-commentary.go b/spaces/Pattr/DrumClassification/lilypond-2.24.2/lib/guile/2.2/ccache/scripts/display-commentary.go
deleted file mode 100644
index 2718d7c472a4f263f306a14021faa4c491090c44..0000000000000000000000000000000000000000
Binary files a/spaces/Pattr/DrumClassification/lilypond-2.24.2/lib/guile/2.2/ccache/scripts/display-commentary.go and /dev/null differ
diff --git a/spaces/Pattr/DrumClassification/lilypond-2.24.2/lib/guile/2.2/ccache/srfi/srfi-42.go b/spaces/Pattr/DrumClassification/lilypond-2.24.2/lib/guile/2.2/ccache/srfi/srfi-42.go
deleted file mode 100644
index 256e5172b51b2e02daaa5a513045dae3cf0742c8..0000000000000000000000000000000000000000
Binary files a/spaces/Pattr/DrumClassification/lilypond-2.24.2/lib/guile/2.2/ccache/srfi/srfi-42.go and /dev/null differ
diff --git a/spaces/PaulHilders/CLIPGroundingExplainability/clip_grounding/utils/log.py b/spaces/PaulHilders/CLIPGroundingExplainability/clip_grounding/utils/log.py
deleted file mode 100644
index 70af379514fef1ea0b7ce3506c11366cab4b62a2..0000000000000000000000000000000000000000
--- a/spaces/PaulHilders/CLIPGroundingExplainability/clip_grounding/utils/log.py
+++ /dev/null
@@ -1,57 +0,0 @@
-"""Utilities for logging"""
-import logging
-from tqdm import tqdm
-from termcolor import colored
-
-
-def color(string: str, color_name: str = 'yellow') -> str:
- """Returns colored string for output to terminal"""
- return colored(string, color_name)
-
-
-def print_update(message: str, width: int = 140, fillchar: str = ":", color="yellow") -> str:
- """Prints an update message
-
- Args:
- message (str): message
- width (int): width of new update message
- fillchar (str): character to be filled to L and R of message
-
- Returns:
- str: print-ready update message
- """
- message = message.center(len(message) + 2, " ")
- print(colored(message.center(width, fillchar), color))
-
-
-def set_logger(log_path):
- """Set the logger to log info in terminal and file `log_path`.
-
- Args:
- log_path (str): path to the log file
- """
- logger = logging.getLogger()
- logger.setLevel(logging.INFO)
-
- if not logger.handlers:
- # Logging to a file
- file_handler = logging.FileHandler(log_path)
- file_handler.setFormatter(logging.Formatter('%(asctime)s:%(levelname)s: %(message)s'))
- logger.addHandler(file_handler)
-
- # Logging to console
- stream_handler = logging.StreamHandler()
- stream_handler.setFormatter(logging.Formatter('%(message)s'))
- logger.addHandler(stream_handler)
-
-
-def tqdm_iterator(items, desc=None, bar_format=None, **kwargs):
- tqdm._instances.clear()
- iterator = tqdm(
- items,
- desc=desc,
- bar_format='{l_bar}{bar:10}{r_bar}{bar:-10b}',
- **kwargs,
- )
-
- return iterator
\ No newline at end of file
diff --git a/spaces/Paulraj916/paulraj916/README.md b/spaces/Paulraj916/paulraj916/README.md
deleted file mode 100644
index cefbe952fbe84602469507091abda49eba2829ae..0000000000000000000000000000000000000000
--- a/spaces/Paulraj916/paulraj916/README.md
+++ /dev/null
@@ -1,12 +0,0 @@
----
-title: Paulraj916
-emoji: 🌖
-colorFrom: blue
-colorTo: pink
-sdk: streamlit
-sdk_version: 1.21.0
-app_file: app.py
-pinned: false
----
-
-Check out the configuration reference at https://huggingface.co/docs/hub/spaces-config-reference
diff --git a/spaces/PeepDaSlan9/SDXL-artists-browser/artists_and_tags.js b/spaces/PeepDaSlan9/SDXL-artists-browser/artists_and_tags.js
deleted file mode 100644
index 0ecc2cf6cfa0ca29247f96d01580328cfd7341ed..0000000000000000000000000000000000000000
--- a/spaces/PeepDaSlan9/SDXL-artists-browser/artists_and_tags.js
+++ /dev/null
@@ -1,815 +0,0 @@
-var artistsData = [
-["Alma-Tadema","Lawrence","romanticism|victorian|history|opulent|ancient|added-2023-08-08",false],
-["Anatsui","El","abstract|sculpture|contemporary|African|recycled-materials|Ghanaian|textiles|added-2023-08-08",false],
-["Andersen","Sarah","cartoon|comics|high-contrast|contemporary|collage|femininity|fashion|mixed-media|added-2023-08-08",false],
-["Balfour","Ronald","art-deco|art-nouveau|watercolor|contemporary|vibrant|abstract|organic|added-2023-08-08",true],
-["Basquiat","Jean-Michel","expressionism|messy|neo-expressionism|street-art|African-American|graffiti|punk|contemporary|added-2023-08-08",false],
-["Beaux","Cecilia","impressionism|portraits|elegant|femininity|American|added-2023-08-08",false],
-["Blanche","John","fantasy|science-fiction|portraits|elegant|French|added-2023-08-08",false],
-["Bontecou","Lee","sculpture|abstract|contemporary|mixed-media|added-2023-08-08",false],
-["Burgert","Jonas","contemporary|figurative|surrealism|allegory|large-scale|German|added-2023-08-08",true],
-["Burlet","Richard","art-nouveau|impressionism|figurative|urban-life|characters|cityscapes|French|added-2023-08-08",false],
-["Cassatt","Mary","impressionism|characters|portraits|pastel|added-2023-08-08",false],
-["Cézanne","Paul","impressionism|cubism|romanticism|post-impressionism|still-life|landscapes|geometric|added-2023-08-08",false],
-["Chicago","Judy","abstract|vibrant|psychedelic|feminism|sculpture|installation|activism|femininity|empowerment|added-2023-08-08",false],
-["Ciurlionis","Mikalojus Konstantinas","dark|art-nouveau|symbolist|spirituality|Lithuanian|mysticism|added-2023-08-08",false],
-["Clark","Alson Skinner","landscapes|impressionism|seascapes|atmospheric|added-2023-08-08",false],
-["Cowper","Frank Cadogan","Victorian|history|romanticism|British|opulent|added-2023-08-08",false],
-["Crewdson","Gregory","photography|surrealism|dark|eerie|suburbia|American|added-2023-08-08",false],
-["Davis","Stuart","cubism|abstract|social-realism|American|rural-life|printmaking|added-2023-08-08",false],
-["Dubbeldam","Ton","pointillism|landscapes|vibrant|contemporary|architecture|conceptual|geometric|Dutch|added-2023-08-08",false],
-["Earles","Amy","watercolor|characters|whimsical|dark|abstract-expressionism|gestural|American|added-2023-08-08",false],
-["Eliasson","Olafur","installation|contemporary|environmentalism|immersive|nature|added-2023-08-08",false],
-["Evans","Walker","photography|monochromatic|documentary|American|great-depression|portraits|social-commentary|added-2023-08-08",false],
-["Fahrenkrog","Ludwig","expressionism|symbolist|mysticism|eerie|German|added-2023-08-08",false],
-["Flavin","Dan","installation|minimalism|light-art|sculpture|conceptual|contemporary|added-2023-08-08",false],
-["Frankenthaler","Helen","abstract|expressionism|watercolor|abstract-expressionism|color-field|painting|feminism|printmaking|contemporary|added-2023-08-08",false],
-["Gascar","Henri","impressionism|landscapes|French|atmospheric|added-2023-08-08",true],
-["Goldberger","Sacha","photography|portraits|characters|contemporary|mixed-media|identity|immigrants|added-2023-08-08",false],
-["Gonzalez-Torres","Felix","installation|conceptual|minimalism|LGBTQ|contemporary|added-2023-08-08",false],
-["Haacke","Hans","installation|photography|sculpture|conceptual|environmentalism|politics|contemporary|added-2023-08-08",false],
-["Hammons","David","installation|abstract|conceptual|African-American|social-commentary|contemporary|added-2023-08-08",false],
-["Haring","Keith","graffiti|street-art|expressionism|flat-colors|high-contrast|vibrant|pop-art|activism|LGBTQ|added-2023-08-08",false],
-["Hartley","Marsden","landscapes|portraits|primitivism|expressionism|modern|American|abstract|added-2023-08-08",false],
-["Hassam","Childe","impressionism|cityscapes|American|landscapes|added-2023-08-08",false],
-["Hatoum","Mona","installation|sculpture|photography|conceptual|displacement|body-art|contemporary|added-2023-08-08",false],
-["Hawkes","Pam","figurativism|portraits|contemporary|ceramics|figurative|nature|organic|delicate|added-2023-08-08",false],
-["Heizer","Michael","installation|landscapes|angular|land-art|earthworks|nature|large-scale|added-2023-08-08",false],
-["Herrera","Carolina","photography|characters|fashion|minimalism|abstract|contemporary|added-2023-08-08",false],
-["Holler","Carsten","contemporary|immersive|interactive|experiential|playful|added-2023-08-08",false],
-["Huyghe","Pierre","conceptual|contemporary|multimedia|surrealism|added-2023-08-08",false],
-["Irwin","Robert","installation|angular|minimalism|environmentalism|contemporary|added-2023-08-08",false],
-["Judd","Donald","angular|installation|minimalism|sculpture|metalwork|contemporary|added-2023-08-08",false],
-["Kahlo","Frida","surrealism|portraits|vibrant|Mexican|self-portraits|feminism|added-2023-08-08",false],
-["Kelly","Ellsworth","abstract|flat-colors|minimalism|color-field|geometric|contemporary|added-2023-08-08",false],
-["Kentridge","William","messy|monochromatic|drawing|animation|printmaking|African|politics|contemporary|added-2023-08-08",false],
-["Koons","Jeff","sculpture|pop-art|vibrant|contemporary|kitsch|consumerism|post-modern|added-2023-08-08",false],
-["Krasner","Lee","expressionism|abstract|high-contrast|abstract-expressionism|color-field|gestural|improvisation|feminism|added-2023-08-08",false],
-["Kruger","Barbara","high-contrast|graphic-design|conceptual|feminism|text-based|montage|advertising|contemporary|added-2023-08-08",false],
-["Kusama","Yayoi","vibrant|polka-dots|installation|fashion|pop-art|contemporary|infinity-rooms|feminism|added-2023-08-08",false],
-["Lawrence","Jacob","cubism|angular|modern|African-American|social-realism|harlem-renaissance|contemporary|added-2023-08-08",false],
-["Lawson","Ernest","impressionism|landscapes|everyday-life|American|added-2023-08-08",false],
-["LeWitt","Sol","conceptual|minimalism|sculpture|geometric|abstract|wall-drawings|contemporary|serial-art|added-2023-08-08",false],
-["Lin","Maya","installation|land-art|architecture|identity|environmentalism|contemporary|added-2023-08-08",false],
-["List","Herbert","photography|monochromatic|high-contrast|surrealism|portraits|German|added-2023-08-08",false],
-["Mapplethorpe","Robert","photography|figure-studies|BDSM|monochromatic|portraits|homo-eroticism|LGBTQ|added-2023-08-08",false],
-["Martin","Agnes","minimalism|abstract-expressionism|grids|color-field|spirituality|contemporary|added-2023-08-08",false],
-["Merian","Maria Sibylla","biological|nature|naturalist|botanical|insects|observational|added-2023-08-08",false],
-["Metcalf","Willard","tonalism|landscapes|muted-colors|American|added-2023-08-08",false],
-["Morimoto","Kōji","contemporary|surrealism|illustration|Japanese|monsters|cute|added-2023-08-08",false],
-["Mostyn","Thomas Edwin","landscapes|still-life|portraits|romanticism|pre-raphaelite|dream-like|British|mysticism|added-2023-08-08",false],
-["Murakami","Takashi","pop-art|manga-anime|flat-colors|Japanese|cute|contemporary|added-2023-08-08",false],
-["Nan","Juliana","contemporary|multimedia|identity|African|added-2023-08-08",false],
-["Nauman","Bruce","conceptual|sculpture|performance|neon|contemporary|added-2023-08-08",false],
-["Neel","Alice","high-contrast|portraits|expressionism|figurative|social-realism|feminism|contemporary|added-2023-08-08",false],
-["Neshat","Shirin","contemporary|video-art|photography|Iranian|feminism|identity|added-2023-08-08",false],
-["Noguchi","Isamu","sculpture|landscape-architecture|organic|Japanese|added-2023-08-08",false],
-["O'Keeffe","Georgia","figurativism|abstract|watercolor|modern|precisionism|American|flowers|southwest|landscapes|added-2023-08-08",false],
-["Ofili","Chris","watercolor|expressionism|contemporary|figurative|painting|afro-futurism|mixed-media|post-colonialism|added-2023-08-08",false],
-["Parreno","Philippe","installation|contemporary|multimedia|film|conceptual|post-modern|added-2023-08-08",false],
-["Perry","Lilla Cabot","impressionism|interiors|gardens|American|added-2023-08-08",false],
-["Ribemont-Dessaignes","Georges","Dadaism|avant-garde|French|added-2023-08-08",false],
-["Ringgold","Faith","pop-art|abstract|expressionism|feminism|quilting|African-American|activism|contemporary|added-2023-08-08",false],
-["Scully","Sean","abstract|angular|minimalism|grids|added-2023-08-08",false],
-["Serra","Richard","sculpture|installation|minimalism|large-scale|contemporary|added-2023-08-08",false],
-["Sherman","Cindy","photography|portraits|conceptual|self-portraits|feminism|post-modern|identity|contemporary|added-2023-08-08",false],
-["Sims","David","contemporary|photography|fashion|British|added-2023-08-08",false],
-["Singer","Andy","pop-art|consumerism|celebrity|American|added-2023-08-08",false],
-["Smart","Jeffrey","surrealism|Scottish|dream-like|added-2023-08-08",false],
-["Smith","Kiki","minimalism|feminism|sculpture|body-art|performance|contemporary|added-2023-08-08",true],
-["Smithson","Robert","land-art|sculpture|conceptual|earthworks|environmentalism|post-minimalism|added-2023-08-08",false],
-["Suddese","Kate Van","contemporary|abstract|mixed-media|organic|vibrant|added-2023-08-08",true],
-["Sutherland","Graham","abstract|landscapes|expressionism|surrealism|portraits|distortion|British|battle-scenes|eerie|added-2023-08-08",false],
-["Tanning","Dorothea","surrealism|dream-like|figure-studies|metamorphosis|eerie|added-2023-08-08",false],
-["Tenniel","John","kids-book|fantasy|whimsical|drawing|added-2023-08-08",false],
-["Thomson","Tom","expressionism|art-nouveau|impressionism|landscapes|Canadian|nature|wilderness|added-2023-08-08",false],
-["Toth","Alex","cartoon|comics|high-contrast|figurative|animals|wildlife|bronze|added-2023-08-08",false],
-["Turrell","James","light-art|vibrant|installation|sculpture|contemporary|architecture|minimalism|colorful|geometric|added-2023-08-08",false],
-["Uhlig","Daniela","digital|portraits|characters|contemporary|landscapes|dream-like|ethereal|surrealism|German|added-2023-08-08",false],
-["Valadon","Suzanne","post-impressionism|nudes|mysterious|added-2023-08-08",false],
-["Valdi","Thiago","contemporary|urban-life|street-art|colorful|Brazilian|added-2023-08-08",false],
-["Varo","Remedios","surrealism|low-contrast|magic-realism|Spanish|added-2023-08-08",false],
-["Vonnoh","Robert","impressionism|bronze|sculpture|American|added-2023-08-08",false],
-["Walker","Kara","silhouettes|African-American|identity|contemporary|added-2023-08-08",false],
-["Warhol","Andy","pop-art|vibrant|portraits|celebrity|contemporary|added-2023-08-08",false],
-["Weiwei","Ai","contemporary|installation|social-commentary|activism|politics|Chinese|added-2023-08-08",true],
-["Wiley","Kehinde","photorealism|portraits|vibrant|colorful|contemporary|African-American|baroque|identity|added-2023-08-08",false],
-["Wilson","Wes","contemporary|art-nouveau|psychedelic|added-2023-08-08",false],
-["Woodman","Francesca","feminism|self-portraits|photography|surrealism|still-life|contemporary|added-2023-08-08",false],
-["Wu","Bayard","fantasy|fashion|illustration|Chinese|LGBTQ|contemporary|added-2023-08-08",true],
-["Wylie","Rose","figurative|portraits|painting|observational|contemporary|added-2023-08-08",false],
-["Abts","Tomma","abstract|angular|geometric|modern|minimalism|contemporary|color-field|added-2023-08-08",false],
-["Acconci","Vito","dark|installation|architecture|sculpture|performance|conceptual|added-2023-08-08",false],
-["Adams","Ansel","monochromatic|high-contrast|nature|American|landscapes|photography|added-2023-08-08",false],
-["Aoshima","Chiho","pop-art|colorful|whimsical|manga-anime|fantasy|vibrant|Japanese|digital|futuristic|added-2023-08-08",false],
-["Araki","Hirohiko","manga-anime|Japanese|characters|pop-culture|illustration|graphic-novel|surrealism|added-2023-08-08",false],
-["Bacon","Francis","expressionism|British|portraits|abstract|dark|figurative|distortion|surrealism|added-2023-08-08",false],
-["Banksy","","street-art|graffiti|high-contrast|politics|social-commentary|anonymous|urban-life|added-2023-08-08",false],
-["Barney","Matthew","photography|surrealism|sculpture|video-art|performance|multimedia|film|conceptual|added-2023-08-08",false],
-["Bosch","Hieronymus","whimsical|renaissance|religion|mysticism|surrealism|allegory|fantasy|added-2023-08-08",false],
-["Botticelli","Sandro","renaissance|Italian|figurative|mythology|religion|femininity|dream-like|added-2023-08-08",false],
-["Chagall","Marc","fauvism|impressionism|surrealism|stained-glass|Russian|French|Jewish|colorful|folklore|romanticism|added-2023-08-08",false],
-["Constable","John","landscapes|romanticism|dark|nature|British|oil-painting|skies|added-2023-08-08",false],
-["Creed","Martin","installation|abstract|expressionism|minimalism|conceptual|British|playful|interactive|added-2023-08-08",false],
-["Crumb","Robert","comics|characters|American|underground|satire|counter-culture|added-2023-08-08",false],
-["Dalí","Salvador","surrealism|dark|Spanish|dream-like|oil-painting|dreams|illusion|metaphysics|added-2023-08-08",false],
-["Degas","Edgar","impressionism|French|ballet|pastel|drawing|portraits|dancers|femininity|added-2023-08-08",false],
-["Delacroix","Eugene","romanticism|French|history|oil-painting|sketching|orientalism|colorful|vibrant|added-2023-08-08",false],
-["Doig","Peter","figurativism|landscapes|abstract|British|Canadian|large-scale|dream-like|nature|added-2023-08-08",false],
-["Duchamp","Marcel","surrealism|cubism|fauvism|expressionism|impressionism|conceptual|dadaism|added-2023-08-08",false],
-["Ernst","Max","surrealism|expressionism|German|Dadaism|collage|oil-painting|automatism|mythology|added-2023-08-08",false],
-["Escher","M. C.","angular|high-contrast|surrealism|Dutch|lithography|woodblock|geometric|illusion|mathematics|added-2023-08-08",false],
-["Freud","Lucian","portraits|expressionism|British|realism|oil-painting|figurative|flesh|added-2023-08-08",false],
-["Gaudi","Antoni","architecture|angular|Spanish|organic|mosaic|art-nouveau|fantasy|added-2023-08-08",false],
-["Gauguin","Paul","impressionism|primitivism|French|exoticism|oil-painting|colorful|tropics|spirituality|added-2023-08-08",false],
-["Giacometti","Alberto","sculpture|expressionism|Swiss|bronze|figurative|portraits|emaciation|added-2023-08-08",false],
-["Goya","Francisco","romanticism|portraits|Spanish|etching|social-commentary|oil-painting|dark|politics|satire|horror|added-2023-08-08",false],
-["Hiroshige","","ukiyo-e|landscapes|Japanese|woodblock|nature|Edo-period|printmaking|added-2023-08-08",false],
-["Hirst","Damien","conceptual|contemporary|installation|British|shock-art|mixed-media|sculpture|animals|death|added-2023-08-08",false],
-["Hockney","David","pools|cubism|vibrant|colorful|British|pop-art|portraits|added-2023-08-08",false],
-["Hokusai","Katsushika","ukiyo-e|high-contrast|Japanese|woodblock|nature|Edo-period|waves|japanese|added-2023-08-08",false],
-["Hopper","Edward","impressionism|American|realism|architecture|landscapes|oil-painting|urban-life|solitude|loneliness|nostalgia|added-2023-08-08",false],
-["Horn","Roni","conceptual|sculpture|photography|American|minimalism|installation|nature|environmentalism|added-2023-08-08",false],
-["Kandinsky","Wassily","bauhaus|expressionism|abstract|vibrant|Russian|modern|spirituality|added-2023-08-08",false],
-["Klee","Paul","bauhaus|expressionism|abstract|surrealism|German|drawing|playful|added-2023-08-08",false],
-["Klein","William","photography|monochromatic|abstract|American|urban-life|fashion|minimalism|added-2023-08-08",false],
-["Klein","Yves","abstract|monochromatic|expressionism|French|performance|modern|color-field|fashion|added-2023-08-08",false],
-["Kleiner","Carl","abstract|surrealism|portraits|graphic-design|American|digital|collage|pop-art|added-2023-08-08",false],
-["Klimt","Gustav","art-nouveau|Austrian|erotica|mosaic|portraits|golden|female-figures|added-2023-08-08",false],
-["Larson","Gary","cartoon|American|newspaper|satire|pop-culture|comics|animals|slice-of-life|added-2023-08-08",false],
-["Lichtenstein","Roy","flat-colors|comics|portraits|abstract|expressionism|American|pop-art|added-2023-08-08",false],
-["Magritte","Rene","surrealism|cloudscapes|art-deco|cubism|impressionism|Belgian|illusion|added-2023-08-08",false],
-["Manet","Édouard","impressionism|portraits|French|still-life|realism|femininity|modern-life|controversy|added-2023-08-08",false],
-["Matisse","Henri","fauvism|impressionism|French|collage|sculpture|color-field|colorful|cut-outs|added-2023-08-08",false],
-["Michelangelo","","renaissance|Italian|sculpture|frescoes|religion|figurative|ceiling-painting|added-2023-08-08",false],
-["Miró","Joan","abstract|Spanish|surrealism|sculpture|drawing|color-field|colorful|outer-space|playful|added-2023-08-08",false],
-["Miyazaki","Hayao","whimsical|manga-anime|kids-book|Japanese|animation|fantasy|adventure|added-2023-08-08",false],
-["Modigliani","Amedeo","expressionism|fauvism|portraits|Italian|sculpture|modern|romanticism|added-2023-08-08",false],
-["Mondrian","Piet","cubism|vibrant|angular|Dutch|abstract|geometric|primary-colors|added-2023-08-08",false],
-["Monet","Claude","impressionism|landscapes|seascapes|French|plein-air|color-field|water-lilies|added-2023-08-08",false],
-["Morisot","Berthe","impressionism|feminism|landscapes|portraits|French|still-life|domestic-scenes|fleeting-moments|added-2023-08-08",false],
-["Moriyama","Daido","photography|Japanese|urban-life|monochromatic|post-war|documentary|grungy|added-2023-08-08",false],
-["Mucha","Alphonse","art-nouveau|portraits|Czech|commercial-art|posters|femininity|stained-glass|added-2023-08-08",false],
-["Munch","Edvard","expressionism|impressionism|Norwegian|anxiety|oil-painting|dark|melancholy|added-2023-08-08",false],
-["Okamoto","Tarō","surrealism|gutai|Japanese|abstract|sculpture|avant-garde|performance|added-2023-08-08",false],
-["Picasso","Pablo","cubism|surrealism|impressionism|Spanish|sculpture|modern|collage|added-2023-08-08",false],
-["Pollock","Jackson","abstract|messy|expressionism|American|drip-painting|action-painting|added-2023-08-08",false],
-["Potter","Beatrix","whimsical|watercolor|kids-book|British|animals|book-illustration|nature|added-2023-08-08",false],
-["Renoir","Pierre-Auguste","impressionism|portraits|French|landscapes|plein-air|female-figures|pastel-colors|femininity|outdoor-scenes|added-2023-08-08",false],
-["Richter","Gerhard","abstract|German|photorealism|oil-painting|contemporary|blurry|multimedia|added-2023-08-08",false],
-["Rijn","Rembrandt van","baroque|portraits|Dutch|etching|self-portraits|history|religion|added-2023-08-08",false],
-["Rothko","Mark","abstract|expressionism|American|color-field|large-scale|minimalism|spirituality|added-2023-08-08",false],
-["Rubens","Peter Paul","baroque|renaissance|romanticism|Flemish|history|painting|oil-painting|mythology|nudes|added-2023-08-08",false],
-["Schulz","Charles","comics|cartoon|American|characters|nostalgia|childhood|social-commentary|added-2023-08-08",false],
-["Shimamoto","Shozo","performance|gutai|Japanese|abstract|mixed-media|post-war|action-painting|collaborative|added-2023-08-08",false],
-["Spiegelman","Art","cartoon|comics|American|history|graphic-novel|autobiographical|Holocaust|animals|added-2023-08-08",false],
-["Strand","Paul","photography|monochromatic|American|landscapes|portraits|abstract|minimalism|still-life|urban-life|added-2023-08-08",false],
-["Sugimoto","Hiroshi","photography|monochromatic|Japanese|conceptual|seascapes|long-exposure|architecture|geometric|added-2023-08-08",false],
-["Tezuka","Osamu","cartoon|manga-anime|Japanese|animation|characters|science-fiction|robots-cyborgs|added-2023-08-08",false],
-["Titian","","renaissance|dark|Italian|portraits|religion|oil-painting|mythology|painting|colorful|added-2023-08-08",false],
-["Toulouse-Lautrec","Henri de","impressionism|art-nouveau|French|posters|lithography|portraits|nightlife|cabaret|vibrant|added-2023-08-08",false],
-["Turner","J.M.W.","romanticism|landscapes|seascapes|British|watercolor|atmospheric|added-2023-08-08",false],
-["Utamaro","Kitagawa","ukiyo-e|Japanese|woodblock|Edo-period|female-figures|nature|portraits|fashion|genre-scenes|added-2023-08-08",false],
-["Velázquez","Diego","baroque|Spanish|portraits|religion|oil-painting|realism|royalty|history|added-2023-08-08",false],
-["Vermeer","Johannes","baroque|interiors|portraits|Dutch|genre-scenes|domestic-scenes|illusion|added-2023-08-08",false],
-["Ware","Chris","cartoon|comics|American|graphic-novel|modern-life|characters|slice-of-life|added-2023-08-08",false],
-["Watterson","Bill","friendship|American|characters|nostalgia|colorful|melancholy|loneliness|added-2023-08-08",false],
-["Whistler","James Abbott McNeill","whimsical|low-contrast|American|tonalism|portraits|etching|interiors|added-2023-08-08",false],
-["Woodring","Jim","surrealism|comics|American|fantasy|characters|pen-and-ink|psychedelic|dream-like|aliens|creatures|added-2023-08-08",false],
-["Nielsen","Kay","Danish|American|illustration|Fantasy|kids-book|exoticism|fantasy|orientalism|elegant|whimsical|Painting|added-2023-08-08",false],
-["Nesterov","Mikhail","Religion|Spirituality|religion|Figurative|Romanticism|Painting|added-2023-08-08",false],
-["Bloch","Albert","Satire|Social-commentary|Impressionism|Realism|Painting|Engraving|added-2023-08-08",false],
-["Kawase","Hasui","Plein-air|Slice-of-life|landscapes|ukiyo-e|Printmaking|added-2023-08-08",false],
-["Fontana","Franco","Conceptual|Metamorphosis|abstract|Spatialism|Painting|Sculpture|added-2023-08-08",false],
-["Stelfreeze","Brian","Activism|Social-realism|comics|Illustration|contemporary|digital|added-2023-08-08",false],
-["Hughes","Nicholas","Surreal|Symbolist|Realism|figurativism|Painting|added-2023-08-08",true],
-["Ditlev","Jan","Dreams|landscapes|Realism|Painting|Printmaking|added-2023-08-08",true],
-["Szukalski","Stanisław","Metaphysics|Mysticism|Surrealism|primitivism|Sculpture|added-2023-08-08",false],
-["Ancher","Helga","Observational|Slice-of-life|Realism|impressionism|Painting|added-2023-08-08",false],
-["MacDonald","Frances","Allegory|Nostalgia|landscapes|impressionism|Painting|added-2023-08-08",false],
-["Flint","Alex Russell","Social-commentary|Environmentalism|abstract|abstract-Expressionism|Painting|Illustration|added-2023-08-08",false],
-["Pasquini","Alice","Documentary|Social-realism|Public-Art|contemporary|Street-art|Mural-painting|added-2023-08-08",false],
-["Grimly","Gris","dark|comics|whimsical|fantasy|Surrealism|illustration|whimsical|kids-book|gothic|eerie|fantasy|added-2023-08-08",false],
-["Smith","Samantha Keely","Dream-like|Loneliness|abstract|abstract-Expressionism|contemporary|Painting|added-2023-08-08",false],
-["Semenov","Anton","Surreal|Symbolist|Surrealism|shock-art|digital|Painting|added-2023-08-08",false],
-["Podolchak","Ihor","Metaphysics|Surrealism|underground|Film|Painting|added-2023-08-08",true],
-["Rousse","Georges","Femininity|Mysticism|Impressionism|Neo-Impressionism|Post-Impressionism|Painting|added-2023-08-08",false],
-["Vrubel","Mikhail","Symbolist|Religion|Painting|Sculpture|added-2023-08-08",false],
-["Biddle","George","politics|Activism|Impressionism|contemporary|Painting|Illustration|added-2023-08-08",true],
-["Pissarro","Camille","impressionism|Observational|Impressionism|Painting|Printmaking|added-2023-08-08",false],
-["Selimoglu","Niyazi","Exoticism|Futurism|Geometric|Orientalism|Painting|Printmaking|added-2023-08-08",true],
-["Sidibé","Malick","Documentary|Slice-of-life|Harlem-Renaissance|Photography|added-2023-08-08",false],
-["the Elder","Lucas Cranach","Religion|Allegory|religion|Renaissance|Painting|added-2023-08-08",false],
-["Manabe","Johji","Science-fiction|Metamorphosis|abstract|contemporary|Illustration|added-2023-08-08",false],
-["Tarnowski","Artur","realism|3D-rendering|video-games|contemporary|added-2023-08-08",true],
-["Garcin","Gilbert","Surreal|Conceptual|abstract|contemporary|Sculpture|Installation|added-2023-08-08",false],
-["Smilde","Berndnaut","Surreal|Metamorphosis|installation|Installation|Photography|added-2023-08-08",false],
-["Ladrönn","José","Fantasy|Science-fiction|comics|Illustration|added-2023-08-08",true],
-["Shatseva","Tanya","Russian|Surrealism|eerie|contemporary|Painting|added-2023-08-08",false],
-["Tessari","Vittorio","Satire|Social-commentary|abstract|Realism|Painting|added-2023-08-08",true],
-["Cruz-Diez","Carlos","Conceptual|illusion|Kinetic|Light-art|added-2023-08-08",false],
-["Bak","Karol","Conceptual|Metamorphosis|Impressionism|contemporary|Painting|added-2023-08-08",false],
-["Robinson","Charles","Satire|politics|Realism|Painting|added-2023-08-08",false],
-["Korovin","Konstantin","impressionism|Plein-air|Impressionism|Painting|added-2023-08-08",false],
-["Rattner","Abraham","expressionism|Symbolist|Expressionism|Painting|Sculpture|added-2023-08-08",false],
-["Hamilton","Richard","Pop-art|Consumerism|Pop-Art|Mixed-media|added-2023-08-08",false],
-["Toraji","","Commercial-art|Sculpture|Installation|added-2023-08-08",true],
-["Shinkai","Makoto","Slice-of-life|Fleeting-moments|manga-anime|contemporary|Film|added-2023-08-08",false],
-["Aldridge","Miles","Femininity|Consumerism|Pop-Art|Pop-art|Illustration|added-2023-08-08",false],
-["Rydingsvard","Ursula von","Metamorphosis|abstract|Minimalism|Sculpture|added-2023-08-08",false],
-["Whitaker","William","Documentary|Social-realism|landscapes|contemporary|Painting|added-2023-08-08",false],
-["Weissenbruch","Hendrik","Plein-air|Observational|landscapes|Painting|added-2023-08-08",false],
-["Wilkes","Cathy","Activism|Social-commentary|Surrealism|contemporary|Photography|added-2023-08-08",false],
-["Rocafort","Kenneth","illustration|Science-fiction|comics|Fantasy|contemporary|Illustration|Graphic-novel|added-2023-08-08",false],
-["Knight","Nick","Fantasy|Adventure|Surrealism|Pop-art|Illustration|added-2023-08-08",false],
-["Jensen","Georg","Symbolist|Plein-air|Realism|Painting|added-2023-08-08",false],
-["Hobbema","Meindert","Observational|Plein-air|landscapes|Dutch-Golden-Age|Painting|added-2023-08-08",false],
-["Khnopff","Fernand","Symbolist|metaphysics|Painting|Sculpture|added-2023-08-08",false],
-["Carte","Anto","Dream-like|Fantasy|abstract|contemporary|Painting|added-2023-08-08",true],
-["the Elder","Lorenzo Costa","Religion|Allegory|religion|Renaissance|Painting|added-2023-08-08",false],
-["Broom","Lee","Activism|Social-commentary|abstract|Harlem-Renaissance|Painting|added-2023-08-08",false],
-["the Elder","Jan van Kessel","Observational|Allegory|Still-Life|Nature|Baroque|Painting|added-2023-08-08",false],
-["Mendoza","Eddie","Consumerism|Commercial-art|urban-life|underground|Painting|added-2023-08-08",true],
-["Prendergast","Maurice","impressionism|Observational|Impressionism|Painting|added-2023-08-08",false],
-["Ohman","Jack","Satire|politics|comics|Illustration|contemporary|Painting|added-2023-08-08",false],
-["Killion","Tom","Plein-air|Observational|landscapes|contemporary|Printmaking|added-2023-08-08",false],
-["Roybal","Antonio","Social-realism|Slice-of-life|Social-Realism|contemporary|Painting|added-2023-08-08",true],
-["Solomon","Simeon","Symbolist|Metaphysics|abstract|contemporary|Painting|added-2023-08-08",false],
-["Thomas","Mickalene","Femininity|identity|Collage|Portraits|contemporary|Painting|Photography|added-2023-08-08",false],
-["Ozeri","Yigal","Observational|Slice-of-life|Realism|contemporary|Painting|added-2023-08-08",false],
-["Picabia","Francis","Dadaism|Surreal|Surrealism|Painting|added-2023-08-08",false],
-["Aagaard","Zacharias Martin","Observational|Slice-of-life|landscapes|Romanticism|Painting|added-2023-08-08",false],
-["Tindle","David","Symbolist|Metaphysics|Surrealism|contemporary|Sculpture|added-2023-08-08",true],
-["Dossena","Emilio Giuseppe","Conceptual|metaphysics|abstract|contemporary|Sculpture|added-2023-08-08",false],
-["Ketner","Jeremiah","Activism|Social-commentary|abstract|contemporary|Painting|added-2023-08-08",false],
-["Lagorio","Lev","Plein-air|Observational|landscapes|Realism|Painting|added-2023-08-08",false],
-["Britenbucher","Renie","Fleeting-moments|Observational|Portraits|contemporary|Painting|added-2023-08-08",false],
-["Holloway","Zena","Photography|British|underwater|animals|portraits|added-2023-08-08",false],
-["Pinturicchio","","Painting|Renaissance|Religion|Allegory|added-2023-08-08",false],
-["Cold","Chris","Activism|Social-commentary|Land-Art|contemporary|Painting|added-2023-08-08",true],
-["Spriggs","Ian","Surreal|Symbolist|Illustration|contemporary|Painting|added-2023-08-08",true],
-["Marcela-Froideval","François","Fantasy|Science-fiction|contemporary|Graphic-novel|added-2023-08-08",false],
-["Caniglia","Jeremy","dark|Satire|Surrealism|contemporary|Painting|added-2023-08-08",true],
-["Nagy","Tibor","Symbolist|metaphysics|abstract|contemporary|Sculpture|added-2023-08-08",false],
-["Münter","Gabriele","expressionism|Symbolist|Expressionism|Painting|added-2023-08-08",false],
-["Fouquet","Jean","Religion|Allegory|Renaissance|renaissance|Painting|added-2023-08-08",false],
-["Gorky","Arshile","Surreal|Symbolist|abstract-Expressionism|Surrealism|Painting|Drawing|added-2023-08-08",false],
-["Raphael","","Renaissance|Painting|added-2023-08-08",false],
-["Ross","Bob","Commercial-art|Consumerism|landscapes|contemporary|Painting|added-2023-08-08",false],
-["Mosina","Inna","Femininity|identity|Ballet|Photography|contemporary|Sculpture|added-2023-08-08",false],
-["Disney","Walt","Fantasy|Adventure|Cartoon|contemporary|Animation|added-2023-08-08",false],
-["Lasdun","Denys","Architecture|metaphysics|contemporary|added-2023-08-08",false],
-["Ravesteyn","Jan van","Observational|Plein-air|Baroque|Architecture|Sculpture|added-2023-08-08",false],
-["HUSH","","Street-art|Activism|Painting|added-2023-08-08",false],
-["Heysen","Nora","Femininity|Consumerism|landscapes|contemporary|Painting|added-2023-08-08",false],
-["Fumito","Ueda","Dream-like|Surreal|video-games|contemporary|Video-art|added-2023-08-08",true],
-["Watts","James Thomas","Symbolist|Allegory|Victorian|Painting|added-2023-08-08",true],
-["Saarinen","Eero","Architecture|metaphysics|modern|Modern|added-2023-08-08",false],
-["Fautrier","Jean","Metaphysics|abstract-expressionism|Painting|Sculpture|added-2023-08-08",false],
-["Davis","Jim","comics|Satire|Illustration|contemporary|added-2023-08-08",true],
-["Taaffe","Philip","Surreal|Symbolist|abstract|contemporary|Painting|added-2023-08-08",false],
-["Permeke","Constant","expressionism|Symbolist|Expressionism|Painting|Sculpture|added-2023-08-08",false],
-["Qwek","Dom","Fantasy|Adventure|contemporary|Illustration|added-2023-08-08",true],
-["Solomon","Barbara Stauffacher","Pop-art|Commercial-art|Graphic-Design|contemporary|Graphic-design|added-2023-08-08",false],
-["Vivanco","Kelly","Femininity|Consumerism|Sculpture|contemporary|Photography|added-2023-08-08",false],
-["Grasso","Laurent","Surreal|Conceptual|Surrealism|contemporary|Sculpture|added-2023-08-08",false],
-["Francés","Victoria","expressionism|Metaphysics|abstract|contemporary|Painting|added-2023-08-08",true],
-["Fegredo","Duncan","Fantasy|Adventure|comics|contemporary|Illustration|added-2023-08-08",true],
-["Shwedoff","Yuri","Surreal|Fantasy|contemporary|Illustration|added-2023-08-08",false],
-["Nicholson","William","Observational|Slice-of-life|abstract|Modern|Painting|added-2023-08-08",false],
-["Cotton","Olive","Australian|Modern|photography|monochromatic|nature|added-2023-08-08",false],
-["Clausen","George","Observational|Plein-air|Realism|Painting|added-2023-08-08",false],
-["Howitt","Alex","Fleeting-moments|Slice-of-life|Illustration|contemporary|Painting|added-2023-08-08",false],
-["Cormon","Fernand","impressionism|Observational|Realism|Painting|added-2023-08-08",false],
-["Sueur","Eustache Le","impressionism|Fleeting-moments|portraits|Baroque|Painting|added-2023-08-08",false],
-["Williams","Kyffin","Surreal|Symbolist|landscapes|contemporary|Painting|added-2023-08-08",false],
-["Hegarty","Valerie","Social-commentary|metamorphosis|sculpture|Painting|added-2023-08-08",false],
-["Telgemeier","Raina","autobiographical|Slice-of-life|comics|graphic-novel|contemporary|Graphic-novel|added-2023-08-08",false],
-["Mashkov","Ilya","expressionism|Symbolist|russian|painting|added-2023-08-08",false],
-["Steinlen","Théophile","Observational|Allegory|Art-Nouveau|Printmaking|added-2023-08-08",false],
-["Bissell","Robert","impressionism|Plein-air|wildlife|contemporary|painting|animals|nature|whimsical|kids-book|fantasy|mysterious|added-2023-08-08",false],
-["Lhote","André","Symbolist|impressionism|Cubism|Painting|added-2023-08-08",false],
-["Morris","Sarah","Femininity|identity|abstract|contemporary|Painting|added-2023-08-08",false],
-["Truitt","Anne","minimalism|Conceptual|Minimalism|Sculpture|added-2023-08-08",false],
-["Launay","Melissa","Surreal|Symbolist|abstract|contemporary|Painting|added-2023-08-08",false],
-["Roy","Pierre","impressionism|Observational|abstract|contemporary|Painting|added-2023-08-08",true],
-["Jiaying","He","Femininity|identity|Realism|contemporary|Painting|added-2023-08-08",false],
-["Achenbach","Andreas","Plein-air|Observational|landscapes|Romanticism|Painting|added-2023-08-08",false],
-["Barnet","Will","Activism|Social-commentary|abstract|contemporary|Painting|added-2023-08-08",false],
-["Bellotto","Bernardo","Observational|Plein-air|landscapes|Rococo|Painting|added-2023-08-08",false],
-["Bernini","Gian Lorenzo","Religion|Allegory|Baroque|Sculpture|added-2023-08-08",false],
-["Herriman","George","Satire|politics|comics|contemporary|Illustration|added-2023-08-08",false],
-["Wooten","Ben","Femininity|identity|abstract|contemporary|Painting|added-2023-08-08",true],
-["Sudworth","Anne","Femininity|Metaphysics|landscapes|contemporary|Illustration|added-2023-08-08",true],
-["Belkina","Katerina","Femininity|identity|portraits|contemporary|Photography|added-2023-08-08",false],
-["Parrish","Maxfield","Fantasy|Nostalgia|Art-Nouveau|Painting|added-2023-08-08",false],
-["Fleischer","Max","comics|dark|Animation|contemporary|added-2023-08-08",false],
-["Oshii","Mamoru","Science-fiction|Metaphysics|manga-anime|contemporary|Animation|added-2023-08-08",false],
-["Etchells","Tim","Conceptual|metaphysics|conceptual|contemporary|Painting|added-2023-08-08",false],
-["Mutu","Wangechi","Feminism|identity|Collage|contemporary|Mixed-media|added-2023-08-08",false],
-["Chambers","Tom","Fleeting-moments|Observational|abstract|contemporary|Illustration|added-2023-08-08",false],
-["Maillol","Aristide","Surreal|metaphysics|modern|Art-Nouveau|Sculpture|added-2023-08-08",false],
-["the Younger","Hans Holbein","anthropomorphism|portraits|Renaissance|Painting|added-2023-08-08",false],
-["Werkman","H.N.","activism|Typography|Printmaking|added-2023-08-08",true],
-["Seliger","Mark","Anxiety|Metaphysics|Portraits|Photography|contemporary|added-2023-08-08",false],
-["Loughridge","Lee","autobiographical|Conceptual|abstract|contemporary|Illustration|added-2023-08-08",true],
-["Andreev","Alex","Death|Displacement|Surrealism|contemporary|Painting|added-2023-08-08",false],
-["Zerbe","Karl","Documentary|Dreams|Surrealism|Expressionism|Painting|added-2023-08-08",true],
-["Addams","Charles","Social-commentary|Cartoon|contemporary|Illustration|added-2023-08-08",false],
-["Castelfranco","Giorgio Barbarelli da","Environmentalism|Fantasy|Rococo|Renaissance|Painting|added-2023-08-08",false],
-["Fuke","Ryohei","Fleeting-moments|identity|landscapes|contemporary|Painting|added-2023-08-08",false],
-["Gahō","Hashimoto","Kitsch|Politics|Printmaking|ukiyo-e|added-2023-08-08",false],
-["Bergland","Don","Religion|Social-realism|landscapes|contemporary|Photography|added-2023-08-08",true],
-["Manara","Milo","Controversy|Femininity|erotica|Comics|Illustration|added-2023-08-08",false],
-["Guanzhong","Wu","Feminism|Homo-eroticism|landscapes|contemporary|Illustration|added-2023-08-08",false],
-["Johns","Jasper","Dream-like|Mysticism|abstract-Expressionism|Painting|Printmaking|added-2023-08-08",false],
-["Kelsner","Alfred","Metamorphosis|Surreal|abstract|contemporary|Painting|added-2023-08-08",false],
-["Mulready","Augustus Edwin","Symbolist|Commercial-art|Realism|Romanticism|Painting|added-2023-08-08",false],
-["Moonan","John","Nostalgia|Slice-of-life|abstract|contemporary|Painting|added-2023-08-08",true],
-["Dauterman","Russell","Observational|Plein-air|comics|superheroes|contemporary|Illustration|added-2023-08-08",true],
-["Vogelsang","Elke","abstract|contemporary|Painting|added-2023-08-08",false],
-["Ledroit","Olivier","comics|Fantasy|fantasy|Illustration|added-2023-08-08",true],
-["Casson","A. J.","Mathematics|Punk|landscapes|contemporary|Painting|added-2023-08-08",false],
-["Gray","Eileen","Friendship|Loneliness|abstract|contemporary|Painting|added-2023-08-08",false],
-["Olsen","Greg","outer-space|Spirituality|Wildlife|contemporary|Painting|added-2023-08-08",false],
-["Jover","Loui","eerie|satire|Illustration|contemporary|added-2023-08-08",false],
-["Veeber","Kuno","Science-fiction|Exoticism|abstract|contemporary|Painting|added-2023-08-08",true],
-["Musgrove","Scott","Adventure|Advertising|landscapes|contemporary|Illustration|added-2023-08-08",false],
-["Munnings","Alfred","horses|modern|Painting|added-2023-08-08",false],
-["Abbott","Elenore","fantasy|watercolor|art-nouveau|dream-like|ethereal|romanticism|pastel-colors|femininity|mythology|added-2023-08-08",false],
-["Anderson","Richard","digital|fantasy|dark|messy|surreal|gothic|horror|psychedelic|added-2023-08-08",false],
-["Argyle","Steve","fantasy|characters|whimsical|colorful|cartoon|playful|added-2023-08-08",true],
-["Bagshaw","Tom","characters|dark|fantasy|surreal|horror|eerie|melancholy|added-2023-08-08",false],
-["Balaskas","Christopher","vibrant|digital|landscapes|science-fiction|futuristic|eerie|outer-space|added-2023-08-08",false],
-["Bana","Benedick","characters|science-fiction|messy|monochromatic|3D-rendering|grungy|industrial|cyberpunk|dystopia|added-2023-08-08",false],
-["Barker","Cicely Mary","fantasy|whimsical|characters|folklore|magic|nostalgia|added-2023-08-08",false],
-["Barlowe","Wayne","science-fiction|fantasy|dark|alien-worlds|dystopia|mythology|creatures|eerie|added-2023-08-08",false],
-["Bautista","Chiara","fantasy|dark|whimsical|dream-like|mysterious|surreal|magic|illusion|added-2023-08-08",false],
-["Bean","Alan","science-fiction|outer-space|metaphysics|astronauts|painting|added-2023-08-08",false],
-["Becket-Griffith","Jasmine","fantasy|portraits|whimsical|vibrant|gothic|fairies|magic|romanticism|added-2023-08-08",false],
-["Bell","Julie","fantasy|nature|dragons|magic|mythology|wilderness|added-2023-08-08",false],
-["Bergsma","Jody","watercolor|fantasy|whimsical|fairies|mythology|dream-like|magic-realism|ethereal|added-2023-08-08",false],
-["Berkey","John","fantasy|science-fiction|eerie|outer-space|futuristic|added-2023-08-08",false],
-["Bilal","Enki","science-fiction|comics|cyberpunk|urban-life|grungy|futuristic|dystopia|surreal|added-2023-08-08",false],
-["Binkley","Ed","fantasy|mythology|dream-like|magic|ethereal|whimsical|added-2023-08-08",false],
-["Bogle","Lee","portraits|fantasy|surreal|dream-like|eerie|ethereal|added-2023-08-08",false],
-["Bonestell","Chesley","science-fiction|alien-worlds|outer-space|futuristic|added-2023-08-08",false],
-["Bosma","Sam","cartoon|comics|fantasy|characters|playful|whimsical|colorful|animation|added-2023-08-08",false],
-["Bosschart","Johfra","whimsical|fantasy|surreal|dream-like|magic|mythology|ethereal|added-2023-08-08",false],
-["Boulet","Susan Seddon","fantasy|magic-realism|nature|whimsical|ethereal|magic|dream-like|femininity|added-2023-08-08",false],
-["Bowater","Charlie","fantasy|digital|portraits|characters|dark|gothic|eerie|added-2023-08-08",false],
-["Bradley","Noah","dark|landscapes|fantasy|eerie|added-2023-08-08",false],
-["Briclot","Aleksi","fantasy|dark|grungy|dystopia|horror|gothic|added-2023-08-08",false],
-["Brom","Gerald","dark|fantasy|horror|gothic|eerie|added-2023-08-08",false],
-["Brooks","Mark","comics|fantasy|science-fiction|added-2023-08-08",false],
-["Brown","Patrick","fantasy|comics|colorful|added-2023-08-08",true],
-["Burdisio","Alejandro","digital|landscapes|fantasy|science-fiction|dark|atmospheric|eerie|magic|added-2023-08-08",false],
-["Burns","Jim","science-fiction|characters|cyberpunk|grungy|urban-life|dark|futuristic|dystopia|noir|added-2023-08-08",false],
-["Cai","Zhichao","fantasy|digital|whimsical|dream-like|magic|ethereal|surreal|added-2023-08-08",false],
-["Caldwell","Clyde","fantasy|science-fiction|mythology|female-figures|pulp|added-2023-08-08",false],
-["Callebaut","Vincent","architecture|science-fiction|3D-rendering|futuristic|surreal|fantasy|cyberpunk|utopia|dystopia|added-2023-08-08",false],
-["Canete","Eric","fantasy|characters|comics|superheroes|added-2023-08-08",false],
-["Carman","Bill","fantasy|pop-art|surrealism|whimsical|playful|psychedelic|added-2023-08-08",false],
-["Chen","Bo","fantasy|magic|whimsical|dream-like|ethereal|illusion|added-2023-08-08",true],
-["Christensen","James C.","whimsical|fantasy|mythology|ethereal|mysterious|magic|dream-like|American|illustration|kids-book|religion|magic|added-2023-08-08",false],
-["Clark","Amanda","fantasy|landscapes|characters|watercolor|dream-like|magic|whimsical|ethereal|added-2023-08-08",false],
-["Corben","Richard","science-fiction|horror|comics|dark|eerie|added-2023-08-08",false],
-["Dean","Roger","landscapes|fantasy|science-fiction|magic|eerie|dream-like|ethereal|posters|added-2023-08-08",false],
-["Deharme","Lise","fantasy|whimsical|dream-like|magic|ethereal|surreal|added-2023-08-08",true],
-["Dell'otto","Gabriele","comics|fantasy|colorful|added-2023-08-08",false],
-["Delort","Nicolas","monochromatic|fantasy|dark|gothic|horror|eerie|labyrinths|added-2023-08-08",false],
-["Delville","Jean","fantasy|surrealism|dream-like|magic|metaphysics|added-2023-08-08",false],
-["Demizu","Posuka","manga-anime|fantasy|whimsical|colorful|playful|adventure|contemporary|illustration|added-2023-08-08",false],
-["Deschambault","Martin","digital|landscapes|science-fiction|eerie|minimalism|atmospheric|mysterious|futuristic|added-2023-08-08",false],
-["Deschamps","Eric","fantasy|science-fiction|digital|surreal|added-2023-08-08",true],
-["Detmold","Charles Maurice","fantasy|watercolor|art-nouveau|ethereal|mythology|magic|opulent|dream-like|added-2023-08-08",false],
-["Detmold","Edward Julius","fantasy|watercolor|art-nouveau|ethereal|mythology|magic|opulent|dream-like|British|illustration|kids-book|Victorian|animals|nature|botanical|delicate|added-2023-08-08",false],
-["DiTerlizzi","Tony","fantasy|whimsical|magic|creatures|playful|added-2023-08-08",false],
-["Dittmann","Anna","portraits|fantasy|digital|ethereal|dream-like|mysterious|added-2023-08-08",false],
-["Dorman","Dave","science-fiction|horror|fantasy|photorealism|dark|added-2023-08-08",false],
-["Drysdale","TJ","photography|fantasy|landscapes|magic|dream-like|ethereal|eerie|added-2023-08-08",false],
-["Earle","Eyvind","magic-realism|magic-realism|fantasy|high-contrast|dream-like|whimsical|surreal|colorful|added-2023-08-08",false],
-["Easley","Jeff","fantasy|added-2023-08-08",false],
-["Edlin","Tyler","fantasy|digital|landscapes|dream-like|ethereal|magic|whimsical|added-2023-08-08",true],
-["Edmiston","Jason","fantasy|horror|characters|portraits|illustration|dark|eerie|monochromatic|ethereal|added-2023-08-08",false],
-["Edwards","Les","science-fiction|horror|fantasy|illustration|outer-space|creatures|dark|added-2023-08-08",true],
-["Eggleton","Bob","science-fiction|fantasy|horror|illustration|aliens|landscapes|colorful|dream-like|added-2023-08-08",true],
-["Ejsing","Jesper","fantasy|illustration|characters|mythology|whimsical|magic|adventure|added-2023-08-08",false],
-["Ellger","Christine","fantasy|magic-realism|illustration|dream-like|folklore|ethereal|surreal|added-2023-08-08",false],
-["Ellis","Dean","science-fiction|vibrant|illustration|cyberpunk|futuristic|technology|neon|added-2023-08-08",true],
-["Elmore","Larry","fantasy|illustration|superheroes|medieval|battle-scenes|added-2023-08-08",false],
-["Elorza","Joseba","photography|surrealism|collage|science-fiction|outer-space|dream-like|abstract|added-2023-08-08",false],
-["Elson","Peter","science-fiction|outer-space|illustration|space-ships|robots-cyborgs|futuristic|added-2023-08-08",false],
-["Emshwiller","Ed","science-fiction|illustration|outer-space|aliens|pulp|colorful|added-2023-08-08",false],
-["Eng","Kilian","digital|landscapes|science-fiction|fantasy|atmospheric|added-2023-08-08",false],
-["Engle","Jason A.","fantasy|science-fiction|dark|illustration|creatures|added-2023-08-08",false],
-["Fabry","Glenn","fantasy|science-fiction|comics|illustration|grungy|violence|added-2023-08-08",false],
-["Fairhurst","Andy","science-fiction|fantasy|horror|digital|illustration|eerie|added-2023-08-08",false],
-["Falero","Luis Ricardo","figurativism|fantasy|nudes|painting|dream-like|romanticism|erotica|added-2023-08-08",false],
-["Fate","Vincent Di","science-fiction|fantasy|illustration|outer-space|eerie|futuristic|added-2023-08-08",true],
-["Ferez","Andrew","surrealism|fantasy|illustration|dream-like|fragmentation|eerie|added-2023-08-08",false],
-["Finch","David","comics|fantasy|illustration|superheroes|grungy|noir|added-2023-08-08",false],
-["Finlay","Virgil","comics|science-fiction|fantasy|horror|dark|high-contrast|pulp|eerie|added-2023-08-08",false],
-["Finnstark","Anato","digital|fantasy|illustration|whimsical|magic|colorful|playful|added-2023-08-08",false],
-["Fitzgerald","John Anster","fantasy|whimsical|illustration|folklore|pastel|magic|added-2023-08-08",false],
-["Foss","Chris","vibrant|science-fiction|outer-space|illustration|psychedelic|alien-worlds|added-2023-08-08",false],
-["Frazetta","Frank","fantasy|dark|illustration|barbarians|muscles|added-2023-08-08",false],
-["Freas","Kelly","science-fiction|fantasy|illustration|adventure|eerie|colorful|added-2023-08-08",false],
-["Froud","Brian","fantasy|dark|whimsical|illustration|fairies|mythology|magic|added-2023-08-08",false],
-["Froud","Wendy","fantasy|dark|whimsical|illustration|fairies|mythology|magic|added-2023-08-08",false],
-["Gaughan","Jack","science-fiction|vibrant|illustration|aliens|outer-space|colorful|alien-worlds|added-2023-08-08",false],
-["Gerard","Justin","fantasy|whimsical|illustration|dream-like|folklore|magic|added-2023-08-08",true],
-["Giancola","Donato","fantasy|science-fiction|illustration|mythology|painting|added-2023-08-08",false],
-["Giger","H.R.","science-fiction|dark|monochromatic|painting|surreal|robots-cyborgs|horror|added-2023-08-08",false],
-["Giraud","Jean","comics|psychedelic|surrealism|fantasy|science-fiction|illustration|dream-like|added-2023-08-08",false],
-["Gonzalez","Josan","science-fiction|illustration|cyberpunk|futuristic|technology|grungy|atmospheric|added-2023-08-08",false],
-["Guay","Rebecca","watercolor|digital|fantasy|illustration|dream-like|ethereal|magic|added-2023-08-08",false],
-["Guidice","Rick","science-fiction|illustration|space-ships|outer-space|adventure|added-2023-08-08",false],
-["Gurney","James","fantasy|landscapes|illustration|realism|painting|atmospheric|magic|added-2023-08-08",true],
-["Gustafson","Scott","magic-realism|whimsical|kids-book|fantasy|illustration|playful|colorful|added-2023-08-08",false],
-["Hardy","David A.","landscapes|science-fiction|illustration|outer-space|added-2023-08-08",true],
-["Harris","John","dark|science-fiction|outer-space|messy|illustration|dystopia|grungy|added-2023-08-08",false],
-["Hase","Ryohei","magic-realism|surrealism|fantasy|digital|illustration|dream-like|ethereal|mysterious|added-2023-08-08",false],
-["Hideyoshi","Lorenz","digital|science-fiction|illustration|cyberpunk|futuristic|dark|neon|dystopia|added-2023-08-08",false],
-["Hildebrandt","Brothers","fantasy|vibrant|illustration|superheroes|painting|added-2023-08-08",false],
-["Hong","Kuang","fantasy|digital|dark|illustration|mythology|eerie|added-2023-08-08",true],
-["Horkey","Aaron","fantasy|comics|illustration|etching|added-2023-08-08",false],
-["Horley","Alex","fantasy|dark|characters|illustration|grungy|horror|added-2023-08-08",false],
-["Horsley","Ralph","science-fiction|fantasy|whimsical|vibrant|dark|high-contrast|colorful|monochromatic|geometric|angular|added-2023-08-08",false],
-["Howe","John","fantasy|dark|eerie|portraits|landscapes|nature|characters|added-2023-08-08",false],
-["Huang","Shilin","fantasy|characters|dream-like|mysterious|magic|mythology|added-2023-08-08",false],
-["Hughes","Edward Robert","romanticism|characters|fantasy|impressionism|whimsical|dream-like|ethereal|nostalgia|added-2023-08-08",false],
-["Hutter","Michael","surrealism|fantasy|science-fiction|dream-like|horror|surreal|eerie|added-2023-08-08",false],
-["Jansson","Alexander","fantasy|whimsical|dark|dream-like|mythology|surreal|added-2023-08-08",false],
-["Jean","James","fantasy|mythology|colorful|mysterious|added-2023-08-08",false],
-["Jia","Ruan","digital|portraits|fantasy|dark|colorful|futuristic|surreal|added-2023-08-08",true],
-["Jones","Jeffrey Catherine","fantasy|figurativism|realism|added-2023-08-08",false],
-["Jones","Peter Andrew","science-fiction|fantasy|futuristic|eerie|alien-worlds|outer-space|added-2023-08-08",false],
-["Jusko","Joe","comics|fantasy|added-2023-08-08",false],
-["Kaluta","M.W.","fantasy|whimsical|romanticism|nostalgia|victorian|dream-like|ethereal|added-2023-08-08",false],
-["Karcz","Michal","landscapes|fantasy|science-fiction|photography|futuristic|surreal|eerie|added-2023-08-08",false],
-["Katsuya","Terada","manga-anime|fantasy|portraits|colorful|magic|added-2023-08-08",false],
-["Kelly","Ken","fantasy|characters|mythology|vibrant|whimsical|added-2023-08-08",true],
-["Kikuchi","Hideyuki","horror|fantasy|manga-anime|dark|eerie|added-2023-08-08",false],
-["Kirby","Jack","comics|science-fiction|added-2023-08-08",false],
-["Koike","Kazuo","manga-anime|comics|fantasy|added-2023-08-08",false],
-["Kon","Satoshi","whimsical|high-contrast|fantasy|manga-anime|surreal|dream-like|added-2023-08-08",false],
-["Kutsche","Michael K","characters|fantasy|dark|whimsical|dream-like|mysterious|mythology|added-2023-08-08",false],
-["Kuvshinov","Ilya","vibrant|digital|fantasy|manga-anime|dream-like|romanticism|ethereal|surreal|added-2023-08-08",false],
-["Lacoste","Raphael","fantasy|landscapes|dark|mysterious|atmospheric|eerie|dream-like|added-2023-08-08",false],
-["Langley","Clint","comics|fantasy|digital|dark|grungy|urban-life|dystopia|noir|added-2023-08-08",true],
-["Lecouffe-Deharme","Bastien","digital|dark|fantasy|characters|surreal|ethereal|magic|added-2023-08-08",false],
-["Lee","Alan","fantasy|romanticism|dream-like|nostalgia|mythology|whimsical|ethereal|added-2023-08-08",false],
-["Lehr","Paul","science-fiction|fantasy|vibrant|colorful|high-contrast|futuristic|eerie|surreal|added-2023-08-08",false],
-["Lewandowski","Mariusz","fantasy|surrealism|dark|dream-like|mysterious|eerie|added-2023-08-08",true],
-["Liefeld","Rob","comics|science-fiction|fantasy|added-2023-08-08",false],
-["Madureira","Joe","comics|fantasy|added-2023-08-08",false],
-["Maitz","Don","science-fiction|fantasy|eerie|futuristic|surreal|added-2023-08-08",false],
-["Maleev","Alex","comics|fantasy|high-contrast|dark|noir|added-2023-08-08",false],
-["Maniak","Slawomir","fantasy|dark|surreal|dream-like|eerie|mysterious|added-2023-08-08",true],
-["Manzanedo","Antonio J.","fantasy|dark|characters|mysterious|added-2023-08-08",false],
-["Mars","Chris","surrealism|dark|fantasy|dream-like|eerie|abstract|added-2023-08-08",true],
-["Martinière","Stephan","science-fiction|fantasy|landscapes|dark|futuristic|surreal|atmospheric|added-2023-08-08",false],
-["Matthews","Rodney","fantasy|science-fiction|futuristic|eerie|colorful|added-2023-08-08",false],
-["Mattingly","David B.","science-fiction|fantasy|eerie|futuristic|surreal|vibrant|added-2023-08-08",true],
-["Mayhew","Mike","comics|fantasy|portraits|added-2023-08-08",false],
-["McCaffrey","Anne","dragons|fantasy|science-fiction|mythology|adventure|magic|added-2023-08-08",false],
-["McCall","Robert","science-fiction|outer-space|futuristic|added-2023-08-08",false],
-["McFarlane","Todd","comics|fantasy|dark|added-2023-08-08",false],
-["McKie","Angus","vibrant|science-fiction|fantasy|futuristic|added-2023-08-08",false],
-["McPharlin","Dan","science-fiction|vibrant|surrealism|abstract|dream-like|ethereal|magic|added-2023-08-08",false],
-["McQuarrie","Ralph","science-fiction|landscapes|eerie|futuristic|added-2023-08-08",false],
-["McQue","Ian","science-fiction|fantasy|messy|grungy|dark|surreal|added-2023-08-08",false],
-["Mead","Syd","flat-colors|science-fiction|abstract|angular|futuristic|minimalism|technology|modern|added-2023-08-08",false],
-["Minguez","Victor Adame","fantasy|characters|digital|colorful|whimsical|mysterious|added-2023-08-08",true],
-["Moebius","","comics|psychedelic|surrealism|fantasy|science-fiction|dream-like|added-2023-08-08",false],
-["Mohrbacher","Peter","surrealism|fantasy|dark|whimsical|dream-like|ethereal|mythology|added-2023-08-08",false],
-["Monge","Jean-Baptiste","dark|fantasy|surreal|eerie|mysterious|added-2023-08-08",false],
-["Moore","Alan","comics|graphic-novel|dark|science-fiction|horror|fantasy|dystopia|grungy|noir|added-2023-08-08",false],
-["Moore","Chris","science-fiction|high-contrast|cyberpunk|dystopia|technology|futuristic|added-2023-08-08",true],
-["Moore","Tony","comics|horror|science-fiction|magic|gothic|eerie|mythology|added-2023-08-08",true],
-["Mullins","Craig","dark|fantasy|surrealism|mythology|dream-like|horror|added-2023-08-08",false],
-["Mumford","Dan","digital|vibrant|fantasy|psychedelic|surrealism|colorful|dreams|added-2023-08-08",false],
-["Nasmith","Ted","fantasy|landscapes|ethereal|magic|mythology|atmospheric|added-2023-08-08",false],
-["Nauck","Todd","comics|characters|science-fiction|superheroes|adventure|added-2023-08-08",false],
-["Nerdrum","Odd","dark|characters|fantasy|figurative|melancholy|added-2023-08-08",false],
-["Nihei","Tsutomu","manga-anime|science-fiction|dark|monochromatic|cyberpunk|dystopia|industrial|alien-worlds|added-2023-08-08",false],
-["Nirasawa","Yasushi","fantasy|characters|dark|creatures|mythology|monsters|added-2023-08-08",true],
-["Nizovtsev","Victor","magic-realism|vibrant|whimsical|fantasy|magic|mysterious|dream-like|surreal|added-2023-08-08",false],
-["Norem","Earl","fantasy|dark|battle-scenes|mythology|added-2023-08-08",false],
-["Oakes","Terry","fantasy|science-fiction|magic|outer-space|colorful|adventure|added-2023-08-08",false],
-["Ohrai","Noriyoshi","fantasy|science-fiction|futuristic|posters|vibrant|added-2023-08-08",false],
-["Okon","Marek","digital|science-fiction|dark|surreal|robots-cyborgs|horror|magic|added-2023-08-08",true],
-["Paick","James","digital|landscapes|fantasy|science-fiction|vibrant|ethereal|eerie|immersive|added-2023-08-08",true],
-["Parkes","Michael","magic-realism|fantasy|art-nouveau|dream-like|spirituality|ethereal|added-2023-08-08",false],
-["Parkinson","Keith","fantasy|mythology|whimsical|added-2023-08-08",true],
-["Pennington","Bruce","science-fiction|fantasy|vibrant|landscapes|futuristic|outer-space|added-2023-08-08",false],
-["Razell","Aliza","photography|fantasy|surrealism|dream-like|ethereal|eerie|conceptual|added-2023-08-08",false],
-["Rebelka","Jakub","surrealism|fantasy|dream-like|illusion|added-2023-08-08",true],
-["Rekunenko","Valentin","fantasy|surrealism|dream-like|whimsical|added-2023-08-08",false],
-["Rigney","Brad","fantasy|characters|dark|mythology|surreal|added-2023-08-08",true],
-["Rocha","Andreas","digital|landscapes|fantasy|dark|atmospheric|added-2023-08-08",false],
-["Różalski","Jakub","landscapes|fantasy|science-fiction|battle-scenes|steampunk|futuristic|dystopia|added-2023-08-08",true],
-["Ruas","Joao","dark|comics|characters|fantasy|gothic|noir|horror|added-2023-08-08",false],
-["Rutkowski","Greg","digital|landscapes|fantasy|dark|atmospheric|surreal|eerie|added-2023-08-08",true],
-["Shaw","Barclay","science-fiction|dark|angular|cyberpunk|futuristic|industrial|neon|added-2023-08-08",false],
-["Shirow","Masamune","manga-anime|cartoon|comics|characters|fantasy|science-fiction|robots-cyborgs|added-2023-08-08",false],
-["Simonetti","Marc","landscapes|digital|fantasy|dark|surreal|dream-like|added-2023-08-08",false],
-["Smith","Adrian","dark|fantasy|digital|characters|grungy|added-2023-08-08",true],
-["Sorayama","Hajime","characters|science-fiction|robots-cyborgs|futuristic|erotica|technology|added-2023-08-08",false],
-["Sparth","","digital|fantasy|science-fiction|landscapes|futuristic|surreal|minimalism|abstract|added-2023-08-08",false],
-["Stålenhag","Simon","landscapes|digital|science-fiction|nostalgia|rural-life|futurism|suburbia|eerie|added-2023-08-08",false],
-["Staples","Greg","comics|fantasy|adventure|characters|colorful|added-2023-08-08",true],
-["Stokes","Anne","fantasy|dark|characters|whimsical|mysterious|gothic|eerie|added-2023-08-08",false],
-["Stout","William","dark|fantasy|mythology|gothic|added-2023-08-08",false],
-["Struzan","Drew","portraits|fantasy|science-fiction|nostalgia|added-2023-08-08",false],
-["Sum","Brian","science-fiction|digital|characters|cyberpunk|futuristic|added-2023-08-08",true],
-["Suuronen","Matti","architecture|photography|science-fiction|futuristic|minimalism|modern|eerie|added-2023-08-08",true],
-["Swanland","Raymond","fantasy|digital|dark|eerie|atmospheric|added-2023-08-08",false],
-["Theurer","Heather","fantasy|romanticism|renaissance|ethereal|erotica|mythology|dream-like|baroque|added-2023-08-08",false],
-["Thole","Karel","surrealism|dark|science-fiction|horror|dream-like|added-2023-08-08",true],
-["Uno","Aquirax","surreal|metaphysics|contemporary|painting|fantasy|vibrant|portraits|dream-like|abstract|added-2023-08-08",true],
-["Urschel","Jan","dark|digital|landscapes|science-fiction|atmospheric|dystopia|added-2023-08-08",true],
-["Vacher","Christophe","cloudscapes|landscapes|fantasy|magic-realism|ethereal|dream-like|added-2023-08-08",false],
-["Vess","Charles","fantasy|comics|magic|dream-like|mythology|whimsical|romanticism|added-2023-08-08",false],
-["Walotsky","Ron","science-fiction|fantasy|surreal|futuristic|added-2023-08-08",true],
-["Whelan","Michael","science-fiction|fantasy|dream-like|surreal|vibrant|eerie|outer-space|alien-worlds|added-2023-08-08",false],
-["White","Tim","science-fiction|fantasy|landscapes|atmospheric|immersive|added-2023-08-08",false],
-["Williams","Gilbert","fantasy|landscapes|whimsical|magic|nostalgia|added-2023-08-08",false],
-["Williamson","Al","comics|science-fiction|fantasy|adventure|mythology|added-2023-08-08",false],
-["Wong","Liam","photography|colorful|vibrant|science-fiction|futuristic|dystopia|urban-life|added-2023-08-08",false],
-["Woodroffe","Patrick","science-fiction|surrealism|dream-like|illusion|eerie|added-2023-08-08",false],
-["Zand","Amir","science-fiction|digital|vibrant|futuristic|robots-cyborgs|technology|added-2023-08-08",true],
-["Moscoso","Victor","vibrant|psychedelic|art-nouveau|pop-art|typography|colorful|added-2023-08-10",false],
-["Naismith","Scott","vibrant|seascapes|landscapes|abstract|impressionism|serenity|colorful|added-2023-08-10",false],
-["Dmitriev","Dima","impressionism|landscapes|vibrant|figure-studies|nature|oil-painting|romanticism|high-contrast|added-2023-08-10",false],
-["Rist","Pipilotti","vibrant|colorful|installation|video-art|immersive|dream-like|playful|added-2023-08-10",false],
-["Ventrue","Eve","digital|dark|characters|illustration|femininity|gothic|fantasy|costumes|added-2023-08-10",false],
-["Deforge","Michael","vibrant|cartoon|pop-art|surrealism|satire|whimsical|added-2023-08-10",false],
-["Saryan","Martiros","vibrant|impressionism|landscapes|colorful|nature|wildlife|serenity|added-2023-08-10",false],
-["Mosse","Richard","vibrant|colorful|photography|landscapes|surrealism|battle-scenes|documentary|added-2023-08-10",false],
-["Adnan","Etel","abstract|vibrant|landscapes|colorful|nature|serenity|added-2023-08-10",false],
-["Bocek","Anna","portraits|vibrant|figurativism|messy|colorful|added-2023-08-10",false],
-["Bearden","Romare","cubism|vibrant|expressionism|collage|African-American|urban-life|history|added-2023-08-10",false],
-["Erté","Romain de Tirtoff","art-deco|Russian|fashion|masks|theater|silhouettes|luxury|added-2023-08-10",false],
-["Metzinger","Jean","cubism|abstract|vibrant|contemporary|modern|geometric|futuristic|added-2023-08-10",false],
-["Grey","Alex","psychedelic|vibrant|contemporary|surrealism|abstract-expressionism|dream-like|colorful|added-2023-08-10",false],
-["Luce","Maximilien","landscapes|impressionism|vibrant|nature|french|plein-air|oil-painting|romanticism",false],
-["Turner","Pete","photography|vibrant|colorful|abstract|contemporary|impasto|ethereal|added-2023-08-10",false],
-["LaChapelle","David","surrealism|pop-art|photography|vibrant|contemporary|conceptual|luxury|added-2023-08-10",false],
-["Kaneko","Jun","abstract|sculpture|vibrant|contemporary|geometric|organic|added-2023-08-10",false],
-["Gottlieb","Adolph","abstract|contemporary|abstract-expressionism|geometric|color-field|added-2023-08-10",false],
-["Biggers","John T.","contemporary|modern|African-American|social-commentary|harlem-renaissance|mural-painting|added-2023-08-10",false],
-["Nagai","Go","manga-anime|vibrant|portraits|childhood|added-2023-08-10",false],
-["Scarry","Richard","kids-book|animals|anthropomorphism|vibrant|whimsical|contemporary|illustration|colorful|playful|added-2023-08-10",false],
-["Ghailan","Atey","digital|manga-anime|fantasy|science-fiction|illustration|characters|dream-like|surrealism|added-2023-08-10",false],
-["Armstrong","Rolf","characters|art-nouveau|art-deco|illustration|contemporary|fashion|added-2023-08-10",false],
-["Blackman","Charles","vibrant|high-contrast|painting|portraits|colorful|added-2023-08-10",false],
-["Fischinger","Oskar","abstract|vibrant|colorful|contemporary|avant-garde|spirituality|added-2023-08-10",false],
-["Pesce","Gaetano","architecture|vibrant|contemporary|organic|futuristic|added-2023-08-10",false],
-["Deakins","Roger","photography|vibrant|digital|contemporary|abstract|geometric|minimalism|added-2023-08-10",true],
-["Groening","Matt","cartoon|vibrant|pop-culture|satire|colorful|whimsical|added-2023-08-10",false],
-["Harper","Charley","vibrant|flat-colors|animals|nature|illustration|whimsical|playful|folk-art|added-2023-08-10",false],
-["Mouly","Marcel","abstract|fauvism|vibrant|contemporary|modern|colorful|added-2023-08-10",false],
-["Brooks","Troy","surrealism|portraits|vibrant|contemporary|oil-painting|dream-like|dark|impressionism|added-2023-08-10",false],
-["Pechstein","Max","expressionism|vibrant|contemporary|modern|colorful|added-2023-08-10",false],
-["Gangloff","Hope","high-contrast|vibrant|portraits|contemporary|expressionism|added-2023-08-10",false],
-["Leger","Fernand","abstract|cubism|vibrant|contemporary|modern|geometric|futuristic|added-2023-08-10",false],
-["Bonhomme","Olivier","surrealism|vibrant|colorful|contemporary|pop-art|whimsical|added-2023-08-10",true],
-["Heilmann","Mary","abstract|vibrant|high-contrast|contemporary|minimalism|geometric|colorful|added-2023-08-10",false],
-["Afremov","Leonid","vibrant|stained-glass|impressionism|nature|cityscapes|colorful|atmospheric|added-2023-08-10",false],
-["Dyer","Chris","psychedelic|vibrant|colorful|contemporary|abstract|pop-art|surrealism|expressionism|added-2023-08-10",false],
-["Ginner","Charles","vibrant|landscapes|cityscapes|urban-life|impressionism|added-2023-08-10",false],
-["Hyde","Doug","whimsical|kids-book|vibrant|contemporary|illustration|colorful|playful|added-2023-08-10",false],
-["Page","Michael","colorful|vibrant|pop-art|expressionism|contemporary|whimsical|playful|added-2023-08-10",false],
-["Chihuly","Dale","abstract|sculpture|vibrant|contemporary|organic|added-2023-08-10",false],
-["Delaunay","Sonia","art-deco|cubism|fauvism|abstract|French|modern|geometric|female-figures|fashion|added-2023-08-10",false],
-["Azzopardi","Deborah","pop-art|cartoon|whimsical|femininity|fashion|comics|colorful|added-2023-08-10",false],
-["Davenport","Ian","abstract|colorful|vibrant|contemporary|modern|geometric|added-2023-08-10",false],
-["Icart","Louis","art-deco|impressionism|low-contrast|romanticism|femininity|dancers|urban-life|added-2023-08-10",false],
-["Koch","Phil","landscapes|photography|vibrant|contemporary|nature|colorful|serenity|atmospheric|added-2023-08-10",false],
-["Calleri","Fred","whimsical|portraits|vibrant|sculpture|expressionism|colorful|mixed-media|added-2023-08-10",false],
-["Bomberg","David","cubism|vibrant|abstract|battle-scenes|expressionism|added-2023-08-10",false],
-["Moureaux","Emmanuelle","installation|colorful|vibrant|abstract|contemporary|multimedia|sculpture|environmentalism|added-2023-08-10",false],
-["Cappiello","Leonetto","graphic-design|vibrant|high-contrast|art-nouveau|color-field|mixed-media|posters|colorful|added-2023-08-10",false],
-["Lalique","René","art-deco|art-nouveau|glasswork|jewelry|luxury|nature|French|sculpture|added-2023-08-10",false],
-["Blanding","Don","art-deco|high-contrast|architecture|minimalism|added-2023-08-10",false],
-["Mallett","Keith","figurativism|abstract|vibrant|sculpture|urban-life|modern|dark|minimalism|added-2023-08-10",false],
-["Fink","Callie","psychedelic|vibrant|colorful|portraits|contemporary|pop-art|surrealism|expressionism|added-2023-08-10",false],
-["Barbier","George","art-deco|art-nouveau|illustration|fashion|vibrant|costumes|theater|romanticism|added-2023-08-10",false],
-["Billy","Butcher","graphic-design|pop-art|vibrant|comics|contemporary|colorful|characters|feminism|added-2023-08-10",false],
-["Gacy","John Wayne","vibrant|portraits|dark|horror|clowns|death|added-2023-08-10",false],
-["Blair","Mary","whimsical|high-contrast|vibrant|illustration|characters|childhood|nature|fantasy",false],
-["Nay","Ernst Wilhelm","expressionism|abstract|vibrant|figurativism|colorful|modern|german|surrealism|added-2023-08-10",false],
-["Phillips","Coles","art-deco|illustration|femininity|advertising|nostalgia|fashion|added-2023-08-10",false],
-["Lempicka","Tamara de","cubism|art-deco|portraits|fashion|luxury|romanticism|added-2023-08-10",false],
-["Yuumei","","digital|whimsical|characters|environmentalism|fantasy|dream-like|femininity|manga-anime|added-2023-08-10",false],
-["Aitchison","Craigie","vibrant|primitivism|figurativism|expressionism|nature|added-2023-08-10",false],
-["Stella","Frank","angular|abstract|expressionism|vibrant|cubism|colorful|geometric|modern|added-2023-08-10",false],
-["Carlson","Larry","psychedelic|surrealism|digital|vibrant|colorful|abstract|nature|dream-like|added-2023-08-10",false],
-["Wright","Frank Lloyd","architecture|art-deco|angular|organic|nature|environmentalism|added-2023-08-10",false],
-["Ferriss","Hugh","cityscapes|architecture|art-deco|geometric|nightlife|urban-life|futuristic|added-2023-08-10",false],
-["Foster","Jon","digital|portraits|abstract|minimalism|figurativism|contemporary|modern|added-2023-08-10",false],
-["Sottsass","Ettore","colorful|art-deco|furniture|architecture|playful|sculpture|added-2023-08-10",false],
-["Okubo","Naomi","vibrant|collage|identity|feminism|empowerment|politics|added-2023-08-10",false],
-["Aarons","Slim","vibrant|photography|fashion|social-commentary|luxury|nostalgia|added-2023-08-10",false],
-["Shiota","Chiharu","vibrant|installation|messy|low-contrast|environmentalism|conceptual|immersive|added-2023-08-10",false],
-["Criswell","Debbie","vibrant|landscapes|whimsical|surrealism|playful|added-2023-08-10",false],
-["Hironaka","Harumi","vibrant|portraits|manga-anime|watercolor|femininity|serenity|dream-like|added-2023-08-10",false],
-["Allred","Mike","comics|vibrant|illustration|pop-art|colorful|whimsical|superheroes|added-2023-08-10",false],
-["Agam","Yaacov","vibrant|colorful|abstract|angular|kinetic|illusion|interactive|added-2023-08-10",false],
-["Frank","Lisa","whimsical|vibrant|colorful|illustration|childhood|playful|fantasy|added-2023-08-10",false],
-["Ranson","Paul","abstract|vibrant|art-nouveau|nature|whimsical|fantasy|added-2023-08-10",false],
-["Hanson","Erin","colorful|vibrant|impressionism|landscapes|nature|serenity|atmospheric|dream-like|added-2023-08-10",false],
-["Scharf","Kenny","colorful|vibrant|pop-art|surrealism|psychedelic|whimsical|playful|added-2023-08-10",false],
-["Hoyland","John","abstract|vibrant|contemporary|modern|geometric|color-field|added-2023-08-10",false],
-["teamLab","","vibrant|colorful|installation|light-art|digital|immersive|interactive|technology|added-2023-08-10",false],
-["Ngai","Victo","vibrant|kids-book|surrealism|illustration|dream-like|playful|added-2023-08-10",false],
-["Asai","Miki","photography|vibrant|contemporary|nature|landscapes|abstract|minimalism|added-2023-08-10",false],
-["Hamiti","Bess","landscapes|vibrant|magic-realism|contemporary|dream-like|surrealism|whimsical|impressionism|added-2023-08-10",false],
-["Britto","Romero","colorful|vibrant|high-contrast|stained-glass|contemporary|pop-art|whimsical|playful|added-2023-08-10",false],
-["Lijun","Fang","figurativism|vibrant|contemporary|portraits|realism|dutch|added-2023-08-10",false],
-["Kurzgesagt","","vibrant|graphic-design|digital|minimalism|animation|outer-space|added-2023-08-10",false],
-["Knight","Chad","vibrant|digital|surrealism|pop-art|collage|colorful|whimsical|playful|added-2023-08-10",false],
-["Hewett","Ryan","vibrant|abstract|cubism|portraits|colorful|mysticism|added-2023-08-10",false],
-["Agar","Eileen","vibrant|abstract|collage|surrealism|femininity|dream-like|nature|added-2023-08-10",false],
-["Hughes","Jack","high-contrast|vibrant|portraits|flat-colors|contemporary|expressionism|added-2023-08-10",false],
-["Boccioni","Umberto","cubism|colorful|vibrant|messy|contemporary|futurism|added-2023-08-10",false],
-["Hodas","Filip","digital|3D-rendering|surrealism|conceptual|dream-like|dark|science-fiction|monochromatic",false],
-["Ascher","Clemens","photography|vibrant|high-contrast|contemporary|minimalism|geometric|abstract|architecture|added-2023-08-10",false],
-["Arkley","Howard","architecture|vibrant|colorful|contemporary|pop-art|whimsical|playful|futuristic|added-2023-08-10",false],
-["Anderson","Wes","vibrant|whimsical|photography|film|nostalgia|surreal|colorful|added-2023-08-10",false],
-["Jones","Lois Mailou","colorful|vibrant|contemporary|modern|geometric|abstract|identity|added-2023-08-10",true],
-["Burch","Laurel","vibrant|high-contrast|illustration|femininity|nature|fantasy|whimsical|added-2023-08-10",false],
-["Hundertwasser","Friedensreich","vibrant|colorful|messy|contemporary|expressionism|surrealism|abstract|organic|added-2023-08-10",false],
-["Max","Peter","colorful|abstract|vibrant|contemporary|pop-art|surrealism|added-2023-08-10",false],
-["Cooke","Darwyn","comics|cartoon|vibrant|contemporary|illustration|added-2023-08-10",false],
-["Haygarth","Stuart","installation|vibrant|angular|colorful|contemporary|conceptual|added-2023-08-10",false],
-["BurGerman","Jon","pop-art|colorful|vibrant|contemporary|illustration|playful|added-2023-08-10",false],
-["Delaunay","Robert","abstract|cubism|vibrant|contemporary|modern|geometric|added-2023-08-10",false],
-["Jones","Erik","vibrant|colorful|portraits|cubism|abstract|collage|added-2023-08-10",false],
-["Fontana","Lucio","abstract|sculpture|conceptual|minimalism|modern|large-scale|installation|added-2023-08-10",false],
-["Janson","Klaus","comics|high-contrast|vibrant|figurativism|pop-art|collage|graphic-novel|characters|added-2023-08-10",true],
-["Jawlensky","Alexej von","expressionism|vibrant|portraits|colorful|modern|abstract|german|spirituality|added-2023-08-10",false],
-["Schmidt-Rottluff","Karl","expressionism|vibrant|german|abstract|figurativism|colorful|japanese|woodblock|landscapes|added-2023-08-10",false],
-["Cortright","Petra","expressionism|messy|vibrant|digital|abstract|nature|impressionism|added-2023-08-10",false],
-["Wall","Josephine","psychedelic|colorful|vibrant|digital|pop-art|portraits|whimsical|femininity|added-2023-08-10",false],
-["Gaffrey","Justin","sculpture|landscapes|vibrant|installation|minimalism|nature|large-scale|environmentalism|added-2023-08-10",false],
-["RHADS","","digital|surrealism|landscapes|vibrant|mixed-media|magic-realism|added-2023-08-10",false],
-["Bayer","Herbert","graphic-design|colorful|flat-colors|vibrant|bauhaus|typography|angular|contemporary|added-2023-08-10",false],
-["Sienkiewicz","Bill","abstract|expressionism|grungy|comics|dark|figurativism|surrealism|pop-art|added-2023-08-10",false],
-["Newland","Jane","vibrant|watercolor|nature|botanical|serenity|added-2023-08-10",false],
-["Kngwarreye","Emily Kame","abstract|expressionism|vibrant|australian|Aboriginal|nature|landscapes|dream-like|added-2023-08-10",false],
-["Eaton","Tristan","graphic-design|street-art|collage|vibrant|pop-art|characters|colorful|added-2023-08-10",false],
-["Negley","Keith","vibrant|high-contrast|collage|illustration|mixed-media|pop-art|graphic-design|added-2023-08-10",false],
-["Perceval","John","vibrant|messy|expressionism|abstract|added-2023-08-10",false],
-["Marc","Franz","vibrant|expressionism|cubism|animals|colorful|spirituality|added-2023-08-10",false],
-["Macke","August","expressionism|vibrant|contemporary|modern|colorful|abstract|impressionism|serenity|added-2023-08-10",false],
-["Pelton","Agnes Lawrence","abstract|vibrant|contemporary|modern|ethereal|spirituality|serenity|color-field|added-2023-08-10",false],
-["Indiana","Robert","flat-colors|graphic-design|vibrant|pop-art|contemporary|typography|added-2023-08-10",false],
-["Beeple","","digital|3D-rendering|abstract|conceptual|science-fiction|cyberpunk|futuristic|added-2023-08-10",false],
-["Loftis","Cory","digital|cartoon|whimsical|characters|childhood|nature|fantasy|added-2023-08-10",true],
-["Corfield","Paul","vibrant|landscapes|cartoon|nature|whimsical|satire|playful|added-2023-08-10",false],
-["Brood","Herman","pop-art|vibrant|childhood|added-2023-08-10",false],
-["Birrell","George","cityscapes|vibrant|contemporary|urban-life|colorful|added-2023-08-10",false],
-["Amaral","Tarsila do","surrealism|vibrant|cubism|contemporary|modern|abstract|added-2023-08-10",false],
-["Gerstner","Karl","graphic-design|vibrant|abstract|colorful|contemporary|typography|geometric|added-2023-08-10",true],
-["Kiuchi","Tatsuro","flat-colors|landscapes|digital|vibrant|flat-colors|whimsical|nature|urban-life|street-art|added-2023-08-10",false],
-["Adamski","Josh","landscapes|contemporary|nature|photography|impressionism|atmospheric|serenity|added-2023-08-10",false],
-["McGinley","Ryan","photography|vibrant|contemporary|childhood|portraits|dream-like|colorful|added-2023-08-10",false],
-["Tartakovsky","Genndy","cartoon|vibrant|contemporary|animation|playful|whimsical|colorful|added-2023-08-10",false],
-["Parc","Julio Le","vibrant|colorful|abstract|pop-art|graphic-design|playful|added-2023-08-10",false],
-["Mahfood","Jim","comics|high-contrast|pop-art|graffiti|street-art|added-2023-08-10",false],
-["Hodgkin","Howard","abstract|vibrant|contemporary|modern|color-field|nature|added-2023-08-10",false],
-["Oiticica","Helio","abstract|vibrant|angular|installation|contemporary|multimedia|interactive|added-2023-08-10",false],
-["Sage","Amanda","psychedelic|contemporary|surrealism|expressionism|whimsical|playful|added-2023-08-10",false],
-["Schapiro","Miriam","abstract|vibrant|expressionism|contemporary|feminism|politics|added-2023-08-10",false],
-["Fitzpatrick","Tony","collage|vibrant|contemporary|mixed-media|pop-art|colorful|whimsical|playful|added-2023-08-10",false],
-["Murciano","Patrice","colorful|vibrant|portraits|contemporary|pop-art|surrealism|expressionism|added-2023-08-10",false],
-["Buren","Daniel","high-contrast|vibrant|installation|sculpture|contemporary|conceptual|minimalism|added-2023-08-10",false],
-["Sassen","Viviane","photography|vibrant|contemporary|abstract|surrealism|conceptual|geometric|added-2023-08-10",false],
-["Caulfield","Patrick","colorful|vibrant|high-contrast|contemporary|pop-art|minimalism|geometric|added-2023-08-10",false],
-["Aenami","Alena","vibrant|landscapes|digital|dream-like|surrealism|fantasy|serenity|atmospheric|added-2023-08-10",false],
-["Young","Skottie","comics|cartoon|vibrant|contemporary|illustration|colorful|whimsical|playful|added-2023-08-10",false],
-["Glaser","Milton","graphic-design|vibrant|colorful|contemporary|pop-art|whimsical|added-2023-08-10",false],
-["Nagai","Hiroshi","landscapes|cityscapes|vibrant|high-contrast|japanese|minimalism|urban-life|photorealism|added-2023-08-10",false],
-["Gilleard","James","flat-colors|digital|architecture|vibrant|landscapes|colorful|fantasy|futuristic|environmentalism|added-2023-08-10",false],
-["Hagan","Robert","impressionism|landscapes|vibrant|nature|colorful|dream-like|romanticism|added-2023-08-10",false],
-["Hammick","Tom","landscapes|figurativism|vibrant|multimedia|nature|dream-like|added-2023-08-10",false],
-["Stella","Joseph","angular|abstract|expressionism|vibrant|cubism|geometric|modern|minimalism|added-2023-08-10",false],
-["Skoglund","Sandy","installation|photography|surrealism|vibrant|contemporary|conceptual|whimsical|still-life|added-2023-08-10",false],
-["Fruin","Tom","sculpture|stained-glass|architecture|installation|vibrant|contemporary|colorful|geometric|multimedia|added-2023-08-10",false],
-["Fox","Toby","digital|cartoon|whimsical|childhood|fantasy|nature|animals|comics|added-2023-08-10",false],
-["Prades","Simon","digital|surrealism|whimsical|conceptual|dream-like|contemporary|pop-art|magic-realism|added-2023-08-10",false],
-["Saray","Rebeca","digital|photography|portraits|conceptual|contemporary|femininity|identity|added-2023-08-10",false],
-["Cushart","Krenz","digital|manga-anime|characters|portraits|illustration|fantasy|whimsical|added-2023-08-10",false],
-["Jones","Android","digital|psychedelic|conceptual|surrealism|dream-like|geometric|colorful|added-2023-08-10",false],
-["Smith","Jeffrey","digital|surrealism|landscapes|magic-realism|cloudscapes|dark|dream-like|conceptual|added-2023-08-10",true],
-["Apterus","Sabbas","digital|dark|abstract|conceptual|surrealism|dream-like|monochromatic|added-2023-08-10",false],
-["Baarle","Lois van","digital|characters|illustration|fantasy|femininity|whimsical|dream-like|added-2023-08-10",false],
-["Gillett","Leticia","digital|characters|3D-rendering|fantasy|science-fiction|whimsical|childhood|costumes|added-2023-08-10",true],
-["Inceoglu","Ismail","digital|landscapes|architecture|urban-life|conceptual|minimalism|colorful|futuristic|added-2023-08-10",true],
-["Lisowski","Michal","digital|dark|surrealism|conceptual|dream-like|fantasy|gothic|sculpture|added-2023-08-10",true],
-["Martinakis","Adam","digital|3D-rendering|sculpture|conceptual|futuristic|dream-like|multimedia|virtual-reality|added-2023-08-10",false],
-["Winkelmann","Mike","digital|conceptual|abstract|minimalism|geometric|color-field|contemporary|added-2023-08-10",false],
-["Koresh","Omri","dark|digital|surrealism|conceptual|dream-like|gothic|monochromatic|added-2023-08-10",false],
-["Viveros","Brian M.","digital|portraits|whimsical|femininity|surrealism|fantasy|contemporary|dream-like|added-2023-08-10",false],
-["Tran","Ross","portraits|digital|realism|conceptual|minimalism|figurativism|manga-anime|femininity|added-2023-08-10",false],
-["Crain","Clayton","comics|digital|fantasy|whimsical|illustration|science-fiction|characters|added-2023-08-10",false],
-["Cheng","Yanjun","portraits|digital|femininity|whimsical|contemporary|romanticism|dream-like|illustration|added-2023-08-10",false],
-["Campau","Mike","digital|3D-rendering|conceptual|contemporary|urban-life|landscapes|added-2023-08-10",false],
-["Fadeev","Anton","landscapes|digital|abstract|impressionism|vibrant|colorful|dream-like|nature|added-2023-08-10",true],
-["Kashin","Wadim","messy|dark|digital|surrealism|urban-life|street-art|expressionism|whimsical|added-2023-08-10",true],
-["Shau","Natalie","surrealism|digital|characters|fantasy|whimsical|dream-like|femininity|mixed-media|added-2023-08-10",false],
-["Cheng","Hsiao-Ron","portraits|digital|pop-art|femininity|fashion|colorful|minimalism|mixed-media|added-2023-08-10",false],
-["WLOP","","characters|portraits|digital|fantasy|manga-anime|femininity|added-2023-08-10",false],
-["Bleda","Elsa","photography|dark|digital|urban-life|environmentalism|social-commentary|added-2023-08-10",true],
-["Rossier","Jessica","outer-space|landscapes|digital|dark|surrealism|conceptual|whimsical|spirituality|added-2023-08-10",false],
-["Jewett","Ellen","sculpture|surrealism|digital|installation|abstract|expressionism|whimsical|nature|added-2023-08-10",false],
-["Jung","Matthias","architecture|surrealism|digital|conceptual|minimalism|dream-like|futuristic|environmentalism|added-2023-08-10",false],
-["Olschinsky","Atelier","cityscapes|abstract|digital|modern|minimalism|geometric|added-2023-08-10",false],
-["Wolfers","Philippe","Belgian|art-nouveau|jewelry|sculpture|metalwork|flowers|ornate|added-2023-08-12",true],
-["Tenniel","Sir John","British|illustration|kids-book|fantasy|whimsical|Victorian|added-2023-08-12",false],
-["Crane","Walter","British|illustration|kids-book|folklore|nostalgia|engraving|added-2023-08-12",false],
-["Caldecott","Randolph","British|illustration|kids-book|animals|nature|playful|added-2023-08-12",false],
-["Greenaway","Kate","British|illustration|kids-book|fashion|Victorian|childhood|romanticism|added-2023-08-12",false],
-["Pyle","Howard","American|illustration|kids-book|adventure|history|colorful|posters|added-2023-08-12",false],
-["Willcox Smith","Jessie","American|illustration|kids-book|childhood|nostalgia|whimsical|folklore|added-2023-08-12",false],
-["Rackham","Arthur","British|illustration|kids-book|fantasy|magic|creatures|added-2023-08-12",false],
-["Shippen Green","Elizabeth","American|illustration|kids-book|fairies|dream-like|added-2023-08-12",false],
-["Craft","Kinuko Y.","American|illustration|kids-book|fantasy|folklore|colorful|dream-like|royalty|added-2023-08-12",false],
-["Bilibin","Ivan","Russian|illustration|kids-book|folklore|ornate|mythology|added-2023-08-12",false],
-["Sowerby","Millicent","British|illustration|kids-book|botanical|nature|flowers|added-2023-08-12",false],
-["Dulac","Edmund","French|orientalism|illustration|kids-book|folklore|romanticism|dream-like|magic|added-2023-08-12",false],
-["Pogany","Willy","Hungarian|American|illustration|kids-book|whimsical|ornate|fantasy|added-2023-08-12",false],
-["Wyeth","N.C.","American|illustration|kids-book|realism|rural-life|nature|nostalgia|added-2023-08-12",false],
-["Tarrant","Margaret","British|illustration|kids-book|folklore|colorful|dream-like|whimsical|added-2023-08-12",false],
-["Saint-Exupery","Antoine de","French|illustration|kids-book|adventure|spirituality|whimsical|added-2023-08-12",false],
-["Wulfing","Sulamith","German|illustration|kids-book|dream-like|fantasy|whimsical|ethereal|spirituality|added-2023-08-12",false],
-["Sendak","Maurice","American|illustration|kids-book|whimsical|fantasy|wilderness|added-2023-08-12",false],
-["van Allsburg","Chris","American|illustration|kids-book|mysterious|adventure|psychedelic|added-2023-08-12",false],
-["Barrett","Angela","kids-book|animals|playful|whimsical|fantasy|added-2023-08-12",false],
-["Berenstain","Stan","kids-book|cartoon|family|animals|whimsical|playful|added-2023-08-12",false],
-["Carle","Eric","kids-book|colorful|interactive|animals|playful|added-2023-08-12",false],
-["Gammell","Stephen","dark|kids-book|high-contrast|horror|eerie|added-2023-08-12",false],
-["Goble","Warwick","whimsical|art-nouveau|kids-book|folklore|nature|vibrant|added-2023-08-12",false],
-["Gorey","Edward","high-contrast|monochromatic|dark|kids-book|gothic|mysterious|horror|eerie|added-2023-08-12",false],
-["Grimm","Brothers","art-nouveau|kids-book|folklore|magic|characters|added-2023-08-12",false],
-["Grimwood","Tracie","colorful|whimsical|kids-book|playful|fantasy|dream-like|added-2023-08-12",false],
-["Harrison","Florence","art-nouveau|kids-book|romanticism|whimsical|delicate|dream-like|added-2023-08-12",false],
-["Hatke","Ben","cartoon|kids-book|characters|adventure|playful|whimsical|added-2023-08-12",false],
-["Jansson","Tove","cartoon|kids-book|playful|whimsical|adventure|added-2023-08-12",false],
-["Jeffers","Oliver","cartoon|kids-book|whimsical|colorful|playful|added-2023-08-12",false],
-["Keane","Glen","cartoon|kids-book|characters|adventure|whimsical|playful|added-2023-08-12",false],
-["Klassen","Jon","watercolor|kids-book|animals|nature|playful|whimsical|dream-like|added-2023-08-12",false],
-["Larson","Abigail","dark|whimsical|kids-book|fantasy|eerie|added-2023-08-12",false],
-["Lathrop","Dorothy","art-nouveau|kids-book|whimsical|romanticism|delicate|dream-like|added-2023-08-12",false],
-["McGuire","Richard","comics|kids-book|whimsical|colorful|conceptual|added-2023-08-12",false],
-["Mortensen","John Kenn","kids-book|dark|horror|monochromatic|eerie|added-2023-08-12",false],
-["Outhwaite","Ida Rentoul","whimsical|kids-book|art-nouveau|fantasy|femininity|folklore|nature|watercolor|dream-like|added-2023-08-12",false],
-["Polacco","Patricia","kids-book|nostalgia|illustration|family|animals|colorful|added-2023-08-12",false],
-["Riddell","Chris","cartoon|kids-book|watercolor|whimsical|fantasy|illustration|creatures|added-2023-08-12",false],
-["Seuss","Dr.","cartoon|whimsical|kids-book|colorful|playful|characters|added-2023-08-12",false],
-["Shepard","E. H.","whimsical|kids-book|watercolor|illustration|nostalgia|nature|animals|added-2023-08-12",false],
-["Steig","William","kids-book|watercolor|playful|colorful|illustration|added-2023-08-12",false],
-["Wain","Louis","psychedelic|kids-book|animals|fantasy|whimsical|colorful|playful|creatures|added-2023-08-12",false],
-["Wiesner","David","cartoon|kids-book|whimsical|playful|added-2023-08-12",false],
-["Yokai","Kozo","kids-book|Japanese|folklore|magic|monsters|illustration|colorful|playful|added-2023-08-12",false],
-["Topor","Roland","eerie|horror|surreal|animation|dark|satire|added-2023-08-12",false],
-["Svankmajer","Jan","animation|sculpture|surreal|puppets|dark|horror|added-2023-08-12",false],
-["Plympton","Bill","animation|sketching|whimsical|cartoon|surreal|added-2023-08-12",false],
-["Hertzfeldt","Don","animation|drawing|whimsical|surreal|dark|added-2023-08-12",false],
-["Reiniger","Lotte","animation|silhouettes|German|folklore|puppets|nostalgia|added-2023-08-12",false],
-["Yuasa","Masaaki","animation|Japanese|eerie|surreal|colorful|fantasy|added-2023-08-12",false],
-["Peterson","Cleon","flat-colors|characters|graphic-design|childhood|modern|geometric|added-2023-08-12",false],
-["Jullien","Jean","high-contrast|cartoon|flat-colors|playful|graphic-design|minimalism|added-2023-08-12",false],
-["McNaught","Jon","cartoon|flat-colors|high-contrast|illustration|playful|added-2023-08-12",false],
-["Arntz","Gerd","graphic-design|high-contrast|flat-colors|monochromatic|minimalism|geometric|added-2023-08-12",false],
-["Bors","Matt","comics|flat-colors|satire|graphic-design|social-commentary|added-2023-08-12",false],
-["Brosh","Allie","comics|high-contrast|flat-colors|autobiographical|whimsical|added-2023-08-12",false],
-["Catherall","Paul","flat-colors|architecture|graphic-design|urban-life|minimalism|geometric|added-2023-08-12",false],
-["Correll","Gemma","cartoon|flat-colors|high-contrast|whimsical|graphic-design|playful|added-2023-08-12",false],
-["Gottardo","Alessandro","flat-colors|high-contrast|illustration|surreal|dream-like|whimsical|playful|characters|added-2023-08-12",false],
-["Hume","Gary","abstract|flat-colors|geometric|minimalism|vibrant|painting|modern|added-2023-08-12",false],
-["Fairey","Shepard","high-contrast|graphic-design|flat-colors|politics|street-art|social-commentary|added-2023-08-12",false],
-["Daeni","Pino","illustration|pulp|erotica|romanticism|nostalgia|figurative|added-2023-08-12",false],
-["Hall","H. Tom","illustration|pulp|erotica|romanticism|nostalgia|figurative|added-2023-08-12",true],
-["McGinnis","Robert","illustration|pulp|erotica|romanticism|figurative|dream-like|added-2023-08-12",false],
-["Stinkfish","","graffiti|Colombian|street-art|portraits|colorful|surreal|vibrant|urban-life|added-2023-08-12",false],
-["Steadman","Ralph","high-contrast|messy|cartoon|surreal|illustration|whimsical|dark|satire|added-2023-08-12",false],
-]
-
-// first category must be 'important' and last must be 'other' or things won't work
-// tag names cannot be 'image-item' or 'hidden' because well, this isn't coded that well, lol
-var tagCategories = [
- ['important'],
- ['mediums',"3D-rendering","animation","architecture","assemblage","body-art","book-illustration","bronze","calligraphy","caricature","cartoon","ceiling-painting","ceramics","collage","comics","digital","drawing","earthworks","enamel","engraving","etching","experiential","film","frescoes","glasswork","graffiti","graphic-design","graphic-novel","illuminated-manuscripts","illustration","immersive","metalwork","infinity-rooms","installation","interactive","jewelry","kinetic","land-art","landscape-architecture","light-art","lithography","manga-anime","mixed-media","montage","mosaic","multimedia","mural-painting","newspaper","oil-painting","painting","pastel","pen-and-ink","performance","photography","posters","printmaking","public-art","puppets","quilting","recycled-materials","sculpture","sketching","stained-glass","street-art","tapestry","textiles","typography","video-art","video-games","virtual-reality","wall-drawings","watercolor","woodblock"],
- ['styles',"abstract","action-painting","afro-futurism","angular","anthropomorphism","atmospheric","blurry","bohemian","bold-colors","color-field","colorful","cute","cyberpunk","dark","delicate","drip-painting","eerie","elegant","ethereal","figurative","flat-colors","folk-art","fragmentation","futuristic","geometric","gestural","golden","gothic","grids","grungy","high-contrast","illusion","impasto","improvisation","industrial","kids-book","large-scale","long-exposure","low-contrast","opulent","Maximalism","melancholy","messy","miniature","monochromatic","muted-colors","mysterious","naturalist","neon","noir","observational","organic","ornate","pastel-colors","photorealism","pin-up","playful","polka-dots","precisionism","primary-colors","propaganda","psychedelic","pulp","Rococo","steampunk","symbolist","text-based","vibrant","whimsical"],
- ['themes',"activism","adventure","advertising","allegory","anxiety","autobiographical","childhood","commercial-art","conceptual","consumerism","controversy","death","displacement","distortion","documentary","dream-like","dreams","dystopia","empowerment","environmentalism","exoticism","family","fantasy","femininity","feminism","fleeting-moments","folklore","friendship","futurism","homo-eroticism","horror","identity","kitsch","loneliness","luxury","magic","mathematics","metamorphosis","metaphysics","mysticism","nightlife","nostalgia","observational","plein-air","politics","punk","religion","satire","science-fiction","serenity","slice-of-life","social-commentary","solitude","spirituality","surreal","utopia"],
- ['subjects',"astronauts","alien-worlds","aliens","animals","ballet","barbarians","battle-scenes","BDSM","biological","botanical","cabaret","celebrity","characters","cityscapes","cloudscapes","clowns","contemporary-life","costumes","counter-culture","creatures","dancers","dinosaurs","domestic-scenes","dragons","emaciation","erotica","everyday-life","fairies","fashion","female-figures","figure-studies","flesh","flowers","furniture","gardens","genre-scenes","great-depression","history","holocaust","horses","immigrants","insects","interiors","kabuki-yakusha-e","labyrinths","landscapes","masks","modern-life","monsters","muscles","mythology","nature","nudes","outdoor-scenes","outer-space","plein-air","pools","pop-culture","portraits","robots-cyborgs","royalty","rural-life","seascapes","self-portraits","silhouettes","skies","Southwest","space-ships","still-life","suburbia","superheroes","technology","theater","tropics","underwater","urban-life","violence","water-lilies","waves","wilderness","wildlife"],
- ['movements',"abstract-expressionism","art-deco","art-Nouveau","automatism","avant-garde","baroque","bauhaus","collaborative","cubism","cut-outs","dadaism","Dutch-golden-age","earthworks","expressionism","fauvism","figurativism","gutai","harlem-renaissance","impressionism","magic-realism","minimalism","neo-expressionism","neo-impressionism","orientalism","pointillism","pop-art","post-colonialism","post-impressionism","post-minimalism","primitivism","realism","romanticism","serial-art","shock-art","social-realism","spatialism","surrealism","tonalism","underground"],
- ['periods',"ancient","Ancient-Egyptian","Ancient-Greek","contemporary","Edo-period","medieval","modern","post-colonialism","post-modern","post-war","pre-raphaelite","renaissance","ukiyo-e","Victorian"],
- ['identities',"Aboriginal","African","African-American","Albanian","Algerian","American","Angolan","anonymous","Argentinean","Armenian","Asian","Australian","Austrian","Azerbaijani","Bahraini","Bangladeshi","Barbadian","Belarusian","Belgian","Bengali","Bosnian","Brazilian","British","Bulgarian","Cameroonian","Canadian","Catalan","Chilean","Chinese","Colombian","CostaRican","Croatian","Cuban","Cypriot","Czech","Dane","Dominican","Danish","Dutch","Ecuadorian","Egyptian","Emirati","Estonian","Ethiopian","European","Filipino","Finnish","Flemish","French","Georgian","German","Ghanaian","Greek","Guatemalan","Guyanese","Hungarian","Icelandic","Indian","Indonesian","Iranian","Iraqi","Irish","Islamic","Israeli","Italian","Jamaican","Japanese","Jewish","Kenyan","Latvian","Lebanese","LGBTQ","Libyan","Lithuanian","Luxembourger","Macedonian","Mexican","Moldovan","Mongol","Montenegrin","Moroccan","Namibian","Native-American","New-Zealander","Nigerian","Norwegian","Palestinian","Peruvian","Polish","Portuguese","PuertoRican","Qatari","Romanian","Russian","Saudi","Scottish","Serbian","Slovak","Slovenian","SouthAfrican","SouthKorean","Spanish","Sudanese","Swedish","Swiss","Syrian","Thai","Tunisian","Turkish","Ukrainian","Uruguayan","Venezuelan","Vietnamese","Yemeni"],
- ['other'],
-];
-
diff --git a/spaces/Pie31415/control-animation/annotator/uniformer/mmseg/models/utils/se_layer.py b/spaces/Pie31415/control-animation/annotator/uniformer/mmseg/models/utils/se_layer.py
deleted file mode 100644
index 083bd7d1ccee909c900c7aed2cc928bf14727f3e..0000000000000000000000000000000000000000
--- a/spaces/Pie31415/control-animation/annotator/uniformer/mmseg/models/utils/se_layer.py
+++ /dev/null
@@ -1,57 +0,0 @@
-import annotator.uniformer.mmcv as mmcv
-import torch.nn as nn
-from annotator.uniformer.mmcv.cnn import ConvModule
-
-from .make_divisible import make_divisible
-
-
-class SELayer(nn.Module):
- """Squeeze-and-Excitation Module.
-
- Args:
- channels (int): The input (and output) channels of the SE layer.
- ratio (int): Squeeze ratio in SELayer, the intermediate channel will be
- ``int(channels/ratio)``. Default: 16.
- conv_cfg (None or dict): Config dict for convolution layer.
- Default: None, which means using conv2d.
- act_cfg (dict or Sequence[dict]): Config dict for activation layer.
- If act_cfg is a dict, two activation layers will be configured
- by this dict. If act_cfg is a sequence of dicts, the first
- activation layer will be configured by the first dict and the
- second activation layer will be configured by the second dict.
- Default: (dict(type='ReLU'), dict(type='HSigmoid', bias=3.0,
- divisor=6.0)).
- """
-
- def __init__(self,
- channels,
- ratio=16,
- conv_cfg=None,
- act_cfg=(dict(type='ReLU'),
- dict(type='HSigmoid', bias=3.0, divisor=6.0))):
- super(SELayer, self).__init__()
- if isinstance(act_cfg, dict):
- act_cfg = (act_cfg, act_cfg)
- assert len(act_cfg) == 2
- assert mmcv.is_tuple_of(act_cfg, dict)
- self.global_avgpool = nn.AdaptiveAvgPool2d(1)
- self.conv1 = ConvModule(
- in_channels=channels,
- out_channels=make_divisible(channels // ratio, 8),
- kernel_size=1,
- stride=1,
- conv_cfg=conv_cfg,
- act_cfg=act_cfg[0])
- self.conv2 = ConvModule(
- in_channels=make_divisible(channels // ratio, 8),
- out_channels=channels,
- kernel_size=1,
- stride=1,
- conv_cfg=conv_cfg,
- act_cfg=act_cfg[1])
-
- def forward(self, x):
- out = self.global_avgpool(x)
- out = self.conv1(out)
- out = self.conv2(out)
- return x * out
diff --git a/spaces/Prof-Reza/Audiocraft_Music-Audio_Generation/audiocraft/models/builders.py b/spaces/Prof-Reza/Audiocraft_Music-Audio_Generation/audiocraft/models/builders.py
deleted file mode 100644
index 038bf99c3d0fbbb86005683d5a2a1b4edcac4298..0000000000000000000000000000000000000000
--- a/spaces/Prof-Reza/Audiocraft_Music-Audio_Generation/audiocraft/models/builders.py
+++ /dev/null
@@ -1,252 +0,0 @@
-# Copyright (c) Meta Platforms, Inc. and affiliates.
-# All rights reserved.
-#
-# This source code is licensed under the license found in the
-# LICENSE file in the root directory of this source tree.
-
-"""
-All the functions to build the relevant models and modules
-from the Hydra config.
-"""
-
-import typing as tp
-
-import audiocraft
-import omegaconf
-import torch
-
-from .encodec import CompressionModel, EncodecModel
-from .lm import LMModel
-from ..modules.codebooks_patterns import (
- CodebooksPatternProvider,
- DelayedPatternProvider,
- MusicLMPattern,
- ParallelPatternProvider,
- UnrolledPatternProvider,
- VALLEPattern,
-)
-from ..modules.conditioners import (
- BaseConditioner,
- ChromaStemConditioner,
- CLAPEmbeddingConditioner,
- ConditionFuser,
- ConditioningProvider,
- LUTConditioner,
- T5Conditioner,
-)
-from .unet import DiffusionUnet
-from .. import quantization as qt
-from ..utils.utils import dict_from_config
-from ..modules.diffusion_schedule import MultiBandProcessor, SampleProcessor
-
-
-def get_quantizer(quantizer: str, cfg: omegaconf.DictConfig, dimension: int) -> qt.BaseQuantizer:
- klass = {
- 'no_quant': qt.DummyQuantizer,
- 'rvq': qt.ResidualVectorQuantizer
- }[quantizer]
- kwargs = dict_from_config(getattr(cfg, quantizer))
- if quantizer != 'no_quant':
- kwargs['dimension'] = dimension
- return klass(**kwargs)
-
-
-def get_encodec_autoencoder(encoder_name: str, cfg: omegaconf.DictConfig):
- if encoder_name == 'seanet':
- kwargs = dict_from_config(getattr(cfg, 'seanet'))
- encoder_override_kwargs = kwargs.pop('encoder')
- decoder_override_kwargs = kwargs.pop('decoder')
- encoder_kwargs = {**kwargs, **encoder_override_kwargs}
- decoder_kwargs = {**kwargs, **decoder_override_kwargs}
- encoder = audiocraft.modules.SEANetEncoder(**encoder_kwargs)
- decoder = audiocraft.modules.SEANetDecoder(**decoder_kwargs)
- return encoder, decoder
- else:
- raise KeyError(f"Unexpected compression model {cfg.compression_model}")
-
-
-def get_compression_model(cfg: omegaconf.DictConfig) -> CompressionModel:
- """Instantiate a compression model."""
- if cfg.compression_model == 'encodec':
- kwargs = dict_from_config(getattr(cfg, 'encodec'))
- encoder_name = kwargs.pop('autoencoder')
- quantizer_name = kwargs.pop('quantizer')
- encoder, decoder = get_encodec_autoencoder(encoder_name, cfg)
- quantizer = get_quantizer(quantizer_name, cfg, encoder.dimension)
- frame_rate = kwargs['sample_rate'] // encoder.hop_length
- renormalize = kwargs.pop('renormalize', False)
- # deprecated params
- kwargs.pop('renorm', None)
- return EncodecModel(encoder, decoder, quantizer,
- frame_rate=frame_rate, renormalize=renormalize, **kwargs).to(cfg.device)
- else:
- raise KeyError(f"Unexpected compression model {cfg.compression_model}")
-
-
-def get_lm_model(cfg: omegaconf.DictConfig) -> LMModel:
- """Instantiate a transformer LM."""
- if cfg.lm_model == 'transformer_lm':
- kwargs = dict_from_config(getattr(cfg, 'transformer_lm'))
- n_q = kwargs['n_q']
- q_modeling = kwargs.pop('q_modeling', None)
- codebooks_pattern_cfg = getattr(cfg, 'codebooks_pattern')
- attribute_dropout = dict_from_config(getattr(cfg, 'attribute_dropout'))
- cls_free_guidance = dict_from_config(getattr(cfg, 'classifier_free_guidance'))
- cfg_prob, cfg_coef = cls_free_guidance['training_dropout'], cls_free_guidance['inference_coef']
- fuser = get_condition_fuser(cfg)
- condition_provider = get_conditioner_provider(kwargs["dim"], cfg).to(cfg.device)
- if len(fuser.fuse2cond['cross']) > 0: # enforce cross-att programmatically
- kwargs['cross_attention'] = True
- if codebooks_pattern_cfg.modeling is None:
- assert q_modeling is not None, \
- "LM model should either have a codebook pattern defined or transformer_lm.q_modeling"
- codebooks_pattern_cfg = omegaconf.OmegaConf.create(
- {'modeling': q_modeling, 'delay': {'delays': list(range(n_q))}}
- )
- pattern_provider = get_codebooks_pattern_provider(n_q, codebooks_pattern_cfg)
- return LMModel(
- pattern_provider=pattern_provider,
- condition_provider=condition_provider,
- fuser=fuser,
- cfg_dropout=cfg_prob,
- cfg_coef=cfg_coef,
- attribute_dropout=attribute_dropout,
- dtype=getattr(torch, cfg.dtype),
- device=cfg.device,
- **kwargs
- ).to(cfg.device)
- else:
- raise KeyError(f"Unexpected LM model {cfg.lm_model}")
-
-
-def get_conditioner_provider(output_dim: int, cfg: omegaconf.DictConfig) -> ConditioningProvider:
- """Instantiate a conditioning model."""
- device = cfg.device
- duration = cfg.dataset.segment_duration
- cfg = getattr(cfg, 'conditioners')
- dict_cfg = {} if cfg is None else dict_from_config(cfg)
- conditioners: tp.Dict[str, BaseConditioner] = {}
- condition_provider_args = dict_cfg.pop('args', {})
- condition_provider_args.pop('merge_text_conditions_p', None)
- condition_provider_args.pop('drop_desc_p', None)
-
- for cond, cond_cfg in dict_cfg.items():
- model_type = cond_cfg['model']
- model_args = cond_cfg[model_type]
- if model_type == 't5':
- conditioners[str(cond)] = T5Conditioner(output_dim=output_dim, device=device, **model_args)
- elif model_type == 'lut':
- conditioners[str(cond)] = LUTConditioner(output_dim=output_dim, **model_args)
- elif model_type == 'chroma_stem':
- conditioners[str(cond)] = ChromaStemConditioner(
- output_dim=output_dim,
- duration=duration,
- device=device,
- **model_args
- )
- elif model_type == 'clap':
- conditioners[str(cond)] = CLAPEmbeddingConditioner(
- output_dim=output_dim,
- device=device,
- **model_args
- )
- else:
- raise ValueError(f"Unrecognized conditioning model: {model_type}")
- conditioner = ConditioningProvider(conditioners, device=device, **condition_provider_args)
- return conditioner
-
-
-def get_condition_fuser(cfg: omegaconf.DictConfig) -> ConditionFuser:
- """Instantiate a condition fuser object."""
- fuser_cfg = getattr(cfg, 'fuser')
- fuser_methods = ['sum', 'cross', 'prepend', 'input_interpolate']
- fuse2cond = {k: fuser_cfg[k] for k in fuser_methods}
- kwargs = {k: v for k, v in fuser_cfg.items() if k not in fuser_methods}
- fuser = ConditionFuser(fuse2cond=fuse2cond, **kwargs)
- return fuser
-
-
-def get_codebooks_pattern_provider(n_q: int, cfg: omegaconf.DictConfig) -> CodebooksPatternProvider:
- """Instantiate a codebooks pattern provider object."""
- pattern_providers = {
- 'parallel': ParallelPatternProvider,
- 'delay': DelayedPatternProvider,
- 'unroll': UnrolledPatternProvider,
- 'valle': VALLEPattern,
- 'musiclm': MusicLMPattern,
- }
- name = cfg.modeling
- kwargs = dict_from_config(cfg.get(name)) if hasattr(cfg, name) else {}
- klass = pattern_providers[name]
- return klass(n_q, **kwargs)
-
-
-def get_debug_compression_model(device='cpu', sample_rate: int = 32000):
- """Instantiate a debug compression model to be used for unit tests."""
- assert sample_rate in [16000, 32000], "unsupported sample rate for debug compression model"
- model_ratios = {
- 16000: [10, 8, 8], # 25 Hz at 16kHz
- 32000: [10, 8, 16] # 25 Hz at 32kHz
- }
- ratios: tp.List[int] = model_ratios[sample_rate]
- frame_rate = 25
- seanet_kwargs: dict = {
- 'n_filters': 4,
- 'n_residual_layers': 1,
- 'dimension': 32,
- 'ratios': ratios,
- }
- print(seanet_kwargs)
- encoder = audiocraft.modules.SEANetEncoder(**seanet_kwargs)
- decoder = audiocraft.modules.SEANetDecoder(**seanet_kwargs)
- quantizer = qt.ResidualVectorQuantizer(dimension=32, bins=400, n_q=4)
- init_x = torch.randn(8, 32, 128)
- quantizer(init_x, 1) # initialize kmeans etc.
- compression_model = EncodecModel(
- encoder, decoder, quantizer,
- frame_rate=frame_rate, sample_rate=sample_rate, channels=1).to(device)
- return compression_model.eval()
-
-
-def get_diffusion_model(cfg: omegaconf.DictConfig):
- # TODO Find a way to infer the channels from dset
- channels = cfg.channels
- num_steps = cfg.schedule.num_steps
- return DiffusionUnet(
- chin=channels, num_steps=num_steps, **cfg.diffusion_unet)
-
-
-def get_processor(cfg, sample_rate: int = 24000):
- sample_processor = SampleProcessor()
- if cfg.use:
- kw = dict(cfg)
- kw.pop('use')
- kw.pop('name')
- if cfg.name == "multi_band_processor":
- sample_processor = MultiBandProcessor(sample_rate=sample_rate, **kw)
- return sample_processor
-
-
-def get_debug_lm_model(device='cpu'):
- """Instantiate a debug LM to be used for unit tests."""
- pattern = DelayedPatternProvider(n_q=4)
- dim = 16
- providers = {
- 'description': LUTConditioner(n_bins=128, dim=dim, output_dim=dim, tokenizer="whitespace"),
- }
- condition_provider = ConditioningProvider(providers)
- fuser = ConditionFuser(
- {'cross': ['description'], 'prepend': [],
- 'sum': [], 'input_interpolate': []})
- lm = LMModel(
- pattern, condition_provider, fuser,
- n_q=4, card=400, dim=dim, num_heads=4, custom=True, num_layers=2,
- cross_attention=True, causal=True)
- return lm.to(device).eval()
-
-
-def get_wrapped_compression_model(
- compression_model: CompressionModel,
- cfg: omegaconf.DictConfig) -> CompressionModel:
- # more to come.
- return compression_model
diff --git a/spaces/Purple11/Grounded-Diffusion/src/taming-transformers/scripts/extract_depth.py b/spaces/Purple11/Grounded-Diffusion/src/taming-transformers/scripts/extract_depth.py
deleted file mode 100644
index d6aa0d80c63a3e580fa28e0f2c7af4e9ae003b64..0000000000000000000000000000000000000000
--- a/spaces/Purple11/Grounded-Diffusion/src/taming-transformers/scripts/extract_depth.py
+++ /dev/null
@@ -1,112 +0,0 @@
-import os
-import torch
-import numpy as np
-from tqdm import trange
-from PIL import Image
-
-
-def get_state(gpu):
- import torch
- midas = torch.hub.load("intel-isl/MiDaS", "MiDaS")
- if gpu:
- midas.cuda()
- midas.eval()
-
- midas_transforms = torch.hub.load("intel-isl/MiDaS", "transforms")
- transform = midas_transforms.default_transform
-
- state = {"model": midas,
- "transform": transform}
- return state
-
-
-def depth_to_rgba(x):
- assert x.dtype == np.float32
- assert len(x.shape) == 2
- y = x.copy()
- y.dtype = np.uint8
- y = y.reshape(x.shape+(4,))
- return np.ascontiguousarray(y)
-
-
-def rgba_to_depth(x):
- assert x.dtype == np.uint8
- assert len(x.shape) == 3 and x.shape[2] == 4
- y = x.copy()
- y.dtype = np.float32
- y = y.reshape(x.shape[:2])
- return np.ascontiguousarray(y)
-
-
-def run(x, state):
- model = state["model"]
- transform = state["transform"]
- hw = x.shape[:2]
- with torch.no_grad():
- prediction = model(transform((x + 1.0) * 127.5).cuda())
- prediction = torch.nn.functional.interpolate(
- prediction.unsqueeze(1),
- size=hw,
- mode="bicubic",
- align_corners=False,
- ).squeeze()
- output = prediction.cpu().numpy()
- return output
-
-
-def get_filename(relpath, level=-2):
- # save class folder structure and filename:
- fn = relpath.split(os.sep)[level:]
- folder = fn[-2]
- file = fn[-1].split('.')[0]
- return folder, file
-
-
-def save_depth(dataset, path, debug=False):
- os.makedirs(path)
- N = len(dset)
- if debug:
- N = 10
- state = get_state(gpu=True)
- for idx in trange(N, desc="Data"):
- ex = dataset[idx]
- image, relpath = ex["image"], ex["relpath"]
- folder, filename = get_filename(relpath)
- # prepare
- folderabspath = os.path.join(path, folder)
- os.makedirs(folderabspath, exist_ok=True)
- savepath = os.path.join(folderabspath, filename)
- # run model
- xout = run(image, state)
- I = depth_to_rgba(xout)
- Image.fromarray(I).save("{}.png".format(savepath))
-
-
-if __name__ == "__main__":
- from taming.data.imagenet import ImageNetTrain, ImageNetValidation
- out = "data/imagenet_depth"
- if not os.path.exists(out):
- print("Please create a folder or symlink '{}' to extract depth data ".format(out) +
- "(be prepared that the output size will be larger than ImageNet itself).")
- exit(1)
-
- # go
- dset = ImageNetValidation()
- abspath = os.path.join(out, "val")
- if os.path.exists(abspath):
- print("{} exists - not doing anything.".format(abspath))
- else:
- print("preparing {}".format(abspath))
- save_depth(dset, abspath)
- print("done with validation split")
-
- dset = ImageNetTrain()
- abspath = os.path.join(out, "train")
- if os.path.exists(abspath):
- print("{} exists - not doing anything.".format(abspath))
- else:
- print("preparing {}".format(abspath))
- save_depth(dset, abspath)
- print("done with train split")
-
- print("done done.")
diff --git a/spaces/Raspberry-ai/main/.env/lib/python3.11/site-packages/pip/_internal/req/req_set.py b/spaces/Raspberry-ai/main/.env/lib/python3.11/site-packages/pip/_internal/req/req_set.py
deleted file mode 100644
index ec7a6e07a25acfa978030c65ae7c1d8609163249..0000000000000000000000000000000000000000
--- a/spaces/Raspberry-ai/main/.env/lib/python3.11/site-packages/pip/_internal/req/req_set.py
+++ /dev/null
@@ -1,82 +0,0 @@
-import logging
-from collections import OrderedDict
-from typing import Dict, List
-
-from pip._vendor.packaging.utils import canonicalize_name
-
-from pip._internal.req.req_install import InstallRequirement
-
-logger = logging.getLogger(__name__)
-
-
-class RequirementSet:
- def __init__(self, check_supported_wheels: bool = True) -> None:
- """Create a RequirementSet."""
-
- self.requirements: Dict[str, InstallRequirement] = OrderedDict()
- self.check_supported_wheels = check_supported_wheels
-
- self.unnamed_requirements: List[InstallRequirement] = []
-
- def __str__(self) -> str:
- requirements = sorted(
- (req for req in self.requirements.values() if not req.comes_from),
- key=lambda req: canonicalize_name(req.name or ""),
- )
- return " ".join(str(req.req) for req in requirements)
-
- def __repr__(self) -> str:
- requirements = sorted(
- self.requirements.values(),
- key=lambda req: canonicalize_name(req.name or ""),
- )
-
- format_string = "<{classname} object; {count} requirement(s): {reqs}>"
- return format_string.format(
- classname=self.__class__.__name__,
- count=len(requirements),
- reqs=", ".join(str(req.req) for req in requirements),
- )
-
- def add_unnamed_requirement(self, install_req: InstallRequirement) -> None:
- assert not install_req.name
- self.unnamed_requirements.append(install_req)
-
- def add_named_requirement(self, install_req: InstallRequirement) -> None:
- assert install_req.name
-
- project_name = canonicalize_name(install_req.name)
- self.requirements[project_name] = install_req
-
- def has_requirement(self, name: str) -> bool:
- project_name = canonicalize_name(name)
-
- return (
- project_name in self.requirements
- and not self.requirements[project_name].constraint
- )
-
- def get_requirement(self, name: str) -> InstallRequirement:
- project_name = canonicalize_name(name)
-
- if project_name in self.requirements:
- return self.requirements[project_name]
-
- raise KeyError(f"No project with the name {name!r}")
-
- @property
- def all_requirements(self) -> List[InstallRequirement]:
- return self.unnamed_requirements + list(self.requirements.values())
-
- @property
- def requirements_to_install(self) -> List[InstallRequirement]:
- """Return the list of requirements that need to be installed.
-
- TODO remove this property together with the legacy resolver, since the new
- resolver only returns requirements that need to be installed.
- """
- return [
- install_req
- for install_req in self.all_requirements
- if not install_req.constraint and not install_req.satisfied_by
- ]
diff --git a/spaces/Raspberry-ai/main/.env/lib/python3.11/site-packages/pip/_vendor/packaging/__init__.py b/spaces/Raspberry-ai/main/.env/lib/python3.11/site-packages/pip/_vendor/packaging/__init__.py
deleted file mode 100644
index 3c50c5dcfeeda2efed282200a5c5cc8c5f7542f7..0000000000000000000000000000000000000000
--- a/spaces/Raspberry-ai/main/.env/lib/python3.11/site-packages/pip/_vendor/packaging/__init__.py
+++ /dev/null
@@ -1,25 +0,0 @@
-# This file is dual licensed under the terms of the Apache License, Version
-# 2.0, and the BSD License. See the LICENSE file in the root of this repository
-# for complete details.
-
-from .__about__ import (
- __author__,
- __copyright__,
- __email__,
- __license__,
- __summary__,
- __title__,
- __uri__,
- __version__,
-)
-
-__all__ = [
- "__title__",
- "__summary__",
- "__uri__",
- "__version__",
- "__author__",
- "__email__",
- "__license__",
- "__copyright__",
-]
diff --git a/spaces/Raspberry-ai/main/.env/lib/python3.11/site-packages/setuptools/_distutils/command/config.py b/spaces/Raspberry-ai/main/.env/lib/python3.11/site-packages/setuptools/_distutils/command/config.py
deleted file mode 100644
index 4492c89660c202acf882375258dffafff00a99ba..0000000000000000000000000000000000000000
--- a/spaces/Raspberry-ai/main/.env/lib/python3.11/site-packages/setuptools/_distutils/command/config.py
+++ /dev/null
@@ -1,377 +0,0 @@
-"""distutils.command.config
-
-Implements the Distutils 'config' command, a (mostly) empty command class
-that exists mainly to be sub-classed by specific module distributions and
-applications. The idea is that while every "config" command is different,
-at least they're all named the same, and users always see "config" in the
-list of standard commands. Also, this is a good place to put common
-configure-like tasks: "try to compile this C code", or "figure out where
-this header file lives".
-"""
-
-import os
-import re
-
-from distutils.core import Command
-from distutils.errors import DistutilsExecError
-from distutils.sysconfig import customize_compiler
-from distutils import log
-
-LANG_EXT = {"c": ".c", "c++": ".cxx"}
-
-
-class config(Command):
-
- description = "prepare to build"
-
- user_options = [
- ('compiler=', None, "specify the compiler type"),
- ('cc=', None, "specify the compiler executable"),
- ('include-dirs=', 'I', "list of directories to search for header files"),
- ('define=', 'D', "C preprocessor macros to define"),
- ('undef=', 'U', "C preprocessor macros to undefine"),
- ('libraries=', 'l', "external C libraries to link with"),
- ('library-dirs=', 'L', "directories to search for external C libraries"),
- ('noisy', None, "show every action (compile, link, run, ...) taken"),
- (
- 'dump-source',
- None,
- "dump generated source files before attempting to compile them",
- ),
- ]
-
- # The three standard command methods: since the "config" command
- # does nothing by default, these are empty.
-
- def initialize_options(self):
- self.compiler = None
- self.cc = None
- self.include_dirs = None
- self.libraries = None
- self.library_dirs = None
-
- # maximal output for now
- self.noisy = 1
- self.dump_source = 1
-
- # list of temporary files generated along-the-way that we have
- # to clean at some point
- self.temp_files = []
-
- def finalize_options(self):
- if self.include_dirs is None:
- self.include_dirs = self.distribution.include_dirs or []
- elif isinstance(self.include_dirs, str):
- self.include_dirs = self.include_dirs.split(os.pathsep)
-
- if self.libraries is None:
- self.libraries = []
- elif isinstance(self.libraries, str):
- self.libraries = [self.libraries]
-
- if self.library_dirs is None:
- self.library_dirs = []
- elif isinstance(self.library_dirs, str):
- self.library_dirs = self.library_dirs.split(os.pathsep)
-
- def run(self):
- pass
-
- # Utility methods for actual "config" commands. The interfaces are
- # loosely based on Autoconf macros of similar names. Sub-classes
- # may use these freely.
-
- def _check_compiler(self):
- """Check that 'self.compiler' really is a CCompiler object;
- if not, make it one.
- """
- # We do this late, and only on-demand, because this is an expensive
- # import.
- from distutils.ccompiler import CCompiler, new_compiler
-
- if not isinstance(self.compiler, CCompiler):
- self.compiler = new_compiler(
- compiler=self.compiler, dry_run=self.dry_run, force=1
- )
- customize_compiler(self.compiler)
- if self.include_dirs:
- self.compiler.set_include_dirs(self.include_dirs)
- if self.libraries:
- self.compiler.set_libraries(self.libraries)
- if self.library_dirs:
- self.compiler.set_library_dirs(self.library_dirs)
-
- def _gen_temp_sourcefile(self, body, headers, lang):
- filename = "_configtest" + LANG_EXT[lang]
- with open(filename, "w") as file:
- if headers:
- for header in headers:
- file.write("#include <%s>\n" % header)
- file.write("\n")
- file.write(body)
- if body[-1] != "\n":
- file.write("\n")
- return filename
-
- def _preprocess(self, body, headers, include_dirs, lang):
- src = self._gen_temp_sourcefile(body, headers, lang)
- out = "_configtest.i"
- self.temp_files.extend([src, out])
- self.compiler.preprocess(src, out, include_dirs=include_dirs)
- return (src, out)
-
- def _compile(self, body, headers, include_dirs, lang):
- src = self._gen_temp_sourcefile(body, headers, lang)
- if self.dump_source:
- dump_file(src, "compiling '%s':" % src)
- (obj,) = self.compiler.object_filenames([src])
- self.temp_files.extend([src, obj])
- self.compiler.compile([src], include_dirs=include_dirs)
- return (src, obj)
-
- def _link(self, body, headers, include_dirs, libraries, library_dirs, lang):
- (src, obj) = self._compile(body, headers, include_dirs, lang)
- prog = os.path.splitext(os.path.basename(src))[0]
- self.compiler.link_executable(
- [obj],
- prog,
- libraries=libraries,
- library_dirs=library_dirs,
- target_lang=lang,
- )
-
- if self.compiler.exe_extension is not None:
- prog = prog + self.compiler.exe_extension
- self.temp_files.append(prog)
-
- return (src, obj, prog)
-
- def _clean(self, *filenames):
- if not filenames:
- filenames = self.temp_files
- self.temp_files = []
- log.info("removing: %s", ' '.join(filenames))
- for filename in filenames:
- try:
- os.remove(filename)
- except OSError:
- pass
-
- # XXX these ignore the dry-run flag: what to do, what to do? even if
- # you want a dry-run build, you still need some sort of configuration
- # info. My inclination is to make it up to the real config command to
- # consult 'dry_run', and assume a default (minimal) configuration if
- # true. The problem with trying to do it here is that you'd have to
- # return either true or false from all the 'try' methods, neither of
- # which is correct.
-
- # XXX need access to the header search path and maybe default macros.
-
- def try_cpp(self, body=None, headers=None, include_dirs=None, lang="c"):
- """Construct a source file from 'body' (a string containing lines
- of C/C++ code) and 'headers' (a list of header files to include)
- and run it through the preprocessor. Return true if the
- preprocessor succeeded, false if there were any errors.
- ('body' probably isn't of much use, but what the heck.)
- """
- from distutils.ccompiler import CompileError
-
- self._check_compiler()
- ok = True
- try:
- self._preprocess(body, headers, include_dirs, lang)
- except CompileError:
- ok = False
-
- self._clean()
- return ok
-
- def search_cpp(self, pattern, body=None, headers=None, include_dirs=None, lang="c"):
- """Construct a source file (just like 'try_cpp()'), run it through
- the preprocessor, and return true if any line of the output matches
- 'pattern'. 'pattern' should either be a compiled regex object or a
- string containing a regex. If both 'body' and 'headers' are None,
- preprocesses an empty file -- which can be useful to determine the
- symbols the preprocessor and compiler set by default.
- """
- self._check_compiler()
- src, out = self._preprocess(body, headers, include_dirs, lang)
-
- if isinstance(pattern, str):
- pattern = re.compile(pattern)
-
- with open(out) as file:
- match = False
- while True:
- line = file.readline()
- if line == '':
- break
- if pattern.search(line):
- match = True
- break
-
- self._clean()
- return match
-
- def try_compile(self, body, headers=None, include_dirs=None, lang="c"):
- """Try to compile a source file built from 'body' and 'headers'.
- Return true on success, false otherwise.
- """
- from distutils.ccompiler import CompileError
-
- self._check_compiler()
- try:
- self._compile(body, headers, include_dirs, lang)
- ok = True
- except CompileError:
- ok = False
-
- log.info(ok and "success!" or "failure.")
- self._clean()
- return ok
-
- def try_link(
- self,
- body,
- headers=None,
- include_dirs=None,
- libraries=None,
- library_dirs=None,
- lang="c",
- ):
- """Try to compile and link a source file, built from 'body' and
- 'headers', to executable form. Return true on success, false
- otherwise.
- """
- from distutils.ccompiler import CompileError, LinkError
-
- self._check_compiler()
- try:
- self._link(body, headers, include_dirs, libraries, library_dirs, lang)
- ok = True
- except (CompileError, LinkError):
- ok = False
-
- log.info(ok and "success!" or "failure.")
- self._clean()
- return ok
-
- def try_run(
- self,
- body,
- headers=None,
- include_dirs=None,
- libraries=None,
- library_dirs=None,
- lang="c",
- ):
- """Try to compile, link to an executable, and run a program
- built from 'body' and 'headers'. Return true on success, false
- otherwise.
- """
- from distutils.ccompiler import CompileError, LinkError
-
- self._check_compiler()
- try:
- src, obj, exe = self._link(
- body, headers, include_dirs, libraries, library_dirs, lang
- )
- self.spawn([exe])
- ok = True
- except (CompileError, LinkError, DistutilsExecError):
- ok = False
-
- log.info(ok and "success!" or "failure.")
- self._clean()
- return ok
-
- # -- High-level methods --------------------------------------------
- # (these are the ones that are actually likely to be useful
- # when implementing a real-world config command!)
-
- def check_func(
- self,
- func,
- headers=None,
- include_dirs=None,
- libraries=None,
- library_dirs=None,
- decl=0,
- call=0,
- ):
- """Determine if function 'func' is available by constructing a
- source file that refers to 'func', and compiles and links it.
- If everything succeeds, returns true; otherwise returns false.
-
- The constructed source file starts out by including the header
- files listed in 'headers'. If 'decl' is true, it then declares
- 'func' (as "int func()"); you probably shouldn't supply 'headers'
- and set 'decl' true in the same call, or you might get errors about
- a conflicting declarations for 'func'. Finally, the constructed
- 'main()' function either references 'func' or (if 'call' is true)
- calls it. 'libraries' and 'library_dirs' are used when
- linking.
- """
- self._check_compiler()
- body = []
- if decl:
- body.append("int %s ();" % func)
- body.append("int main () {")
- if call:
- body.append(" %s();" % func)
- else:
- body.append(" %s;" % func)
- body.append("}")
- body = "\n".join(body) + "\n"
-
- return self.try_link(body, headers, include_dirs, libraries, library_dirs)
-
- def check_lib(
- self,
- library,
- library_dirs=None,
- headers=None,
- include_dirs=None,
- other_libraries=[],
- ):
- """Determine if 'library' is available to be linked against,
- without actually checking that any particular symbols are provided
- by it. 'headers' will be used in constructing the source file to
- be compiled, but the only effect of this is to check if all the
- header files listed are available. Any libraries listed in
- 'other_libraries' will be included in the link, in case 'library'
- has symbols that depend on other libraries.
- """
- self._check_compiler()
- return self.try_link(
- "int main (void) { }",
- headers,
- include_dirs,
- [library] + other_libraries,
- library_dirs,
- )
-
- def check_header(self, header, include_dirs=None, library_dirs=None, lang="c"):
- """Determine if the system header file named by 'header_file'
- exists and can be found by the preprocessor; return true if so,
- false otherwise.
- """
- return self.try_cpp(
- body="/* No body */", headers=[header], include_dirs=include_dirs
- )
-
-
-def dump_file(filename, head=None):
- """Dumps a file content into log.info.
-
- If head is not None, will be dumped before the file content.
- """
- if head is None:
- log.info('%s', filename)
- else:
- log.info(head)
- file = open(filename)
- try:
- log.info(file.read())
- finally:
- file.close()
diff --git a/spaces/Realcat/image-matching-webui/hloc/pipelines/Aachen_v1_1/__init__.py b/spaces/Realcat/image-matching-webui/hloc/pipelines/Aachen_v1_1/__init__.py
deleted file mode 100644
index e69de29bb2d1d6434b8b29ae775ad8c2e48c5391..0000000000000000000000000000000000000000
diff --git a/spaces/Realcat/image-matching-webui/third_party/ASpanFormer/src/lightning/lightning_aspanformer.py b/spaces/Realcat/image-matching-webui/third_party/ASpanFormer/src/lightning/lightning_aspanformer.py
deleted file mode 100644
index 9b34b7b7485d4419390614e3fe0174ccc53ac7a9..0000000000000000000000000000000000000000
--- a/spaces/Realcat/image-matching-webui/third_party/ASpanFormer/src/lightning/lightning_aspanformer.py
+++ /dev/null
@@ -1,374 +0,0 @@
-from collections import defaultdict
-import pprint
-from loguru import logger
-from pathlib import Path
-
-import torch
-import numpy as np
-import pytorch_lightning as pl
-from matplotlib import pyplot as plt
-
-from src.ASpanFormer.aspanformer import ASpanFormer
-from src.ASpanFormer.utils.supervision import (
- compute_supervision_coarse,
- compute_supervision_fine,
-)
-from src.losses.aspan_loss import ASpanLoss
-from src.optimizers import build_optimizer, build_scheduler
-from src.utils.metrics import (
- compute_symmetrical_epipolar_errors,
- compute_symmetrical_epipolar_errors_offset_bidirectional,
- compute_pose_errors,
- aggregate_metrics,
-)
-from src.utils.plotting import make_matching_figures, make_matching_figures_offset
-from src.utils.comm import gather, all_gather
-from src.utils.misc import lower_config, flattenList
-from src.utils.profiler import PassThroughProfiler
-
-
-class PL_ASpanFormer(pl.LightningModule):
- def __init__(self, config, pretrained_ckpt=None, profiler=None, dump_dir=None):
- """
- TODO:
- - use the new version of PL logging API.
- """
- super().__init__()
- # Misc
- self.config = config # full config
- _config = lower_config(self.config)
- self.loftr_cfg = lower_config(_config["aspan"])
- self.profiler = profiler or PassThroughProfiler()
- self.n_vals_plot = max(
- config.TRAINER.N_VAL_PAIRS_TO_PLOT // config.TRAINER.WORLD_SIZE, 1
- )
-
- # Matcher: LoFTR
- self.matcher = ASpanFormer(config=_config["aspan"])
- self.loss = ASpanLoss(_config)
-
- # Pretrained weights
- print(pretrained_ckpt)
- if pretrained_ckpt:
- print("load")
- state_dict = torch.load(pretrained_ckpt, map_location="cpu")["state_dict"]
- msg = self.matcher.load_state_dict(state_dict, strict=False)
- print(msg)
- logger.info(f"Load '{pretrained_ckpt}' as pretrained checkpoint")
-
- # Testing
- self.dump_dir = dump_dir
-
- def configure_optimizers(self):
- # FIXME: The scheduler did not work properly when `--resume_from_checkpoint`
- optimizer = build_optimizer(self, self.config)
- scheduler = build_scheduler(self.config, optimizer)
- return [optimizer], [scheduler]
-
- def optimizer_step(
- self,
- epoch,
- batch_idx,
- optimizer,
- optimizer_idx,
- optimizer_closure,
- on_tpu,
- using_native_amp,
- using_lbfgs,
- ):
- # learning rate warm up
- warmup_step = self.config.TRAINER.WARMUP_STEP
- if self.trainer.global_step < warmup_step:
- if self.config.TRAINER.WARMUP_TYPE == "linear":
- base_lr = self.config.TRAINER.WARMUP_RATIO * self.config.TRAINER.TRUE_LR
- lr = base_lr + (
- self.trainer.global_step / self.config.TRAINER.WARMUP_STEP
- ) * abs(self.config.TRAINER.TRUE_LR - base_lr)
- for pg in optimizer.param_groups:
- pg["lr"] = lr
- elif self.config.TRAINER.WARMUP_TYPE == "constant":
- pass
- else:
- raise ValueError(
- f"Unknown lr warm-up strategy: {self.config.TRAINER.WARMUP_TYPE}"
- )
-
- # update params
- optimizer.step(closure=optimizer_closure)
- optimizer.zero_grad()
-
- def _trainval_inference(self, batch):
- with self.profiler.profile("Compute coarse supervision"):
- compute_supervision_coarse(batch, self.config)
-
- with self.profiler.profile("LoFTR"):
- self.matcher(batch)
-
- with self.profiler.profile("Compute fine supervision"):
- compute_supervision_fine(batch, self.config)
-
- with self.profiler.profile("Compute losses"):
- self.loss(batch)
-
- def _compute_metrics(self, batch):
- with self.profiler.profile("Copmute metrics"):
- compute_symmetrical_epipolar_errors(
- batch
- ) # compute epi_errs for each match
- compute_symmetrical_epipolar_errors_offset_bidirectional(
- batch
- ) # compute epi_errs for offset match
- compute_pose_errors(
- batch, self.config
- ) # compute R_errs, t_errs, pose_errs for each pair
-
- rel_pair_names = list(zip(*batch["pair_names"]))
- bs = batch["image0"].size(0)
- metrics = {
- # to filter duplicate pairs caused by DistributedSampler
- "identifiers": ["#".join(rel_pair_names[b]) for b in range(bs)],
- "epi_errs": [
- batch["epi_errs"][batch["m_bids"] == b].cpu().numpy()
- for b in range(bs)
- ],
- "epi_errs_offset": [
- batch["epi_errs_offset_left"][batch["offset_bids_left"] == b]
- .cpu()
- .numpy()
- for b in range(bs)
- ], # only consider left side
- "R_errs": batch["R_errs"],
- "t_errs": batch["t_errs"],
- "inliers": batch["inliers"],
- }
- ret_dict = {"metrics": metrics}
- return ret_dict, rel_pair_names
-
- def training_step(self, batch, batch_idx):
- self._trainval_inference(batch)
-
- # logging
- if (
- self.trainer.global_rank == 0
- and self.global_step % self.trainer.log_every_n_steps == 0
- ):
- # scalars
- for k, v in batch["loss_scalars"].items():
- if not k.startswith("loss_flow") and not k.startswith("conf_"):
- self.logger.experiment.add_scalar(f"train/{k}", v, self.global_step)
-
- # log offset_loss and conf for each layer and level
- layer_num = self.loftr_cfg["coarse"]["layer_num"]
- for layer_index in range(layer_num):
- log_title = "layer_" + str(layer_index)
- self.logger.experiment.add_scalar(
- log_title + "/offset_loss",
- batch["loss_scalars"]["loss_flow_" + str(layer_index)],
- self.global_step,
- )
- self.logger.experiment.add_scalar(
- log_title + "/conf_",
- batch["loss_scalars"]["conf_" + str(layer_index)],
- self.global_step,
- )
-
- # net-params
- if self.config.ASPAN.MATCH_COARSE.MATCH_TYPE == "sinkhorn":
- self.logger.experiment.add_scalar(
- f"skh_bin_score",
- self.matcher.coarse_matching.bin_score.clone().detach().cpu().data,
- self.global_step,
- )
-
- # figures
- if self.config.TRAINER.ENABLE_PLOTTING:
- compute_symmetrical_epipolar_errors(
- batch
- ) # compute epi_errs for each match
- figures = make_matching_figures(
- batch, self.config, self.config.TRAINER.PLOT_MODE
- )
- for k, v in figures.items():
- self.logger.experiment.add_figure(
- f"train_match/{k}", v, self.global_step
- )
-
- # plot offset
- if self.global_step % 200 == 0:
- compute_symmetrical_epipolar_errors_offset_bidirectional(batch)
- figures_left = make_matching_figures_offset(
- batch, self.config, self.config.TRAINER.PLOT_MODE, side="_left"
- )
- figures_right = make_matching_figures_offset(
- batch, self.config, self.config.TRAINER.PLOT_MODE, side="_right"
- )
- for k, v in figures_left.items():
- self.logger.experiment.add_figure(
- f"train_offset/{k}" + "_left", v, self.global_step
- )
- figures = make_matching_figures_offset(
- batch, self.config, self.config.TRAINER.PLOT_MODE, side="_right"
- )
- for k, v in figures_right.items():
- self.logger.experiment.add_figure(
- f"train_offset/{k}" + "_right", v, self.global_step
- )
-
- return {"loss": batch["loss"]}
-
- def training_epoch_end(self, outputs):
- avg_loss = torch.stack([x["loss"] for x in outputs]).mean()
- if self.trainer.global_rank == 0:
- self.logger.experiment.add_scalar(
- "train/avg_loss_on_epoch", avg_loss, global_step=self.current_epoch
- )
-
- def validation_step(self, batch, batch_idx):
- self._trainval_inference(batch)
-
- ret_dict, _ = self._compute_metrics(
- batch
- ) # this func also compute the epi_errors
-
- val_plot_interval = max(self.trainer.num_val_batches[0] // self.n_vals_plot, 1)
- figures = {self.config.TRAINER.PLOT_MODE: []}
- figures_offset = {self.config.TRAINER.PLOT_MODE: []}
- if batch_idx % val_plot_interval == 0:
- figures = make_matching_figures(
- batch, self.config, mode=self.config.TRAINER.PLOT_MODE
- )
- figures_offset = make_matching_figures_offset(
- batch, self.config, self.config.TRAINER.PLOT_MODE, "_left"
- )
- return {
- **ret_dict,
- "loss_scalars": batch["loss_scalars"],
- "figures": figures,
- "figures_offset_left": figures_offset,
- }
-
- def validation_epoch_end(self, outputs):
- # handle multiple validation sets
- multi_outputs = (
- [outputs] if not isinstance(outputs[0], (list, tuple)) else outputs
- )
- multi_val_metrics = defaultdict(list)
-
- for valset_idx, outputs in enumerate(multi_outputs):
- # since pl performs sanity_check at the very begining of the training
- cur_epoch = self.trainer.current_epoch
- if (
- not self.trainer.resume_from_checkpoint
- and self.trainer.running_sanity_check
- ):
- cur_epoch = -1
-
- # 1. loss_scalars: dict of list, on cpu
- _loss_scalars = [o["loss_scalars"] for o in outputs]
- loss_scalars = {
- k: flattenList(all_gather([_ls[k] for _ls in _loss_scalars]))
- for k in _loss_scalars[0]
- }
-
- # 2. val metrics: dict of list, numpy
- _metrics = [o["metrics"] for o in outputs]
- metrics = {
- k: flattenList(all_gather(flattenList([_me[k] for _me in _metrics])))
- for k in _metrics[0]
- }
- # NOTE: all ranks need to `aggregate_merics`, but only log at rank-0
- val_metrics_4tb = aggregate_metrics(
- metrics, self.config.TRAINER.EPI_ERR_THR
- )
- for thr in [5, 10, 20]:
- multi_val_metrics[f"auc@{thr}"].append(val_metrics_4tb[f"auc@{thr}"])
-
- # 3. figures
- _figures = [o["figures"] for o in outputs]
- figures = {
- k: flattenList(gather(flattenList([_me[k] for _me in _figures])))
- for k in _figures[0]
- }
-
- # tensorboard records only on rank 0
- if self.trainer.global_rank == 0:
- for k, v in loss_scalars.items():
- mean_v = torch.stack(v).mean()
- self.logger.experiment.add_scalar(
- f"val_{valset_idx}/avg_{k}", mean_v, global_step=cur_epoch
- )
-
- for k, v in val_metrics_4tb.items():
- self.logger.experiment.add_scalar(
- f"metrics_{valset_idx}/{k}", v, global_step=cur_epoch
- )
-
- for k, v in figures.items():
- if self.trainer.global_rank == 0:
- for plot_idx, fig in enumerate(v):
- self.logger.experiment.add_figure(
- f"val_match_{valset_idx}/{k}/pair-{plot_idx}",
- fig,
- cur_epoch,
- close=True,
- )
- plt.close("all")
-
- for thr in [5, 10, 20]:
- # log on all ranks for ModelCheckpoint callback to work properly
- self.log(
- f"auc@{thr}", torch.tensor(np.mean(multi_val_metrics[f"auc@{thr}"]))
- ) # ckpt monitors on this
-
- def test_step(self, batch, batch_idx):
- with self.profiler.profile("LoFTR"):
- self.matcher(batch)
-
- ret_dict, rel_pair_names = self._compute_metrics(batch)
-
- with self.profiler.profile("dump_results"):
- if self.dump_dir is not None:
- # dump results for further analysis
- keys_to_save = {"mkpts0_f", "mkpts1_f", "mconf", "epi_errs"}
- pair_names = list(zip(*batch["pair_names"]))
- bs = batch["image0"].shape[0]
- dumps = []
- for b_id in range(bs):
- item = {}
- mask = batch["m_bids"] == b_id
- item["pair_names"] = pair_names[b_id]
- item["identifier"] = "#".join(rel_pair_names[b_id])
- for key in keys_to_save:
- item[key] = batch[key][mask].cpu().numpy()
- for key in ["R_errs", "t_errs", "inliers"]:
- item[key] = batch[key][b_id]
- dumps.append(item)
- ret_dict["dumps"] = dumps
-
- return ret_dict
-
- def test_epoch_end(self, outputs):
- # metrics: dict of list, numpy
- _metrics = [o["metrics"] for o in outputs]
- metrics = {
- k: flattenList(gather(flattenList([_me[k] for _me in _metrics])))
- for k in _metrics[0]
- }
-
- # [{key: [{...}, *#bs]}, *#batch]
- if self.dump_dir is not None:
- Path(self.dump_dir).mkdir(parents=True, exist_ok=True)
- _dumps = flattenList([o["dumps"] for o in outputs]) # [{...}, #bs*#batch]
- dumps = flattenList(gather(_dumps)) # [{...}, #proc*#bs*#batch]
- logger.info(
- f"Prediction and evaluation results will be saved to: {self.dump_dir}"
- )
-
- if self.trainer.global_rank == 0:
- print(self.profiler.summary())
- val_metrics_4tb = aggregate_metrics(
- metrics, self.config.TRAINER.EPI_ERR_THR
- )
- logger.info("\n" + pprint.pformat(val_metrics_4tb))
- if self.dump_dir is not None:
- np.save(Path(self.dump_dir) / "LoFTR_pred_eval", dumps)
diff --git a/spaces/Realcat/image-matching-webui/third_party/SOLD2/sold2/config/project_config.py b/spaces/Realcat/image-matching-webui/third_party/SOLD2/sold2/config/project_config.py
deleted file mode 100644
index 6846b4451e038b1c517043ea6db08f3029b79852..0000000000000000000000000000000000000000
--- a/spaces/Realcat/image-matching-webui/third_party/SOLD2/sold2/config/project_config.py
+++ /dev/null
@@ -1,46 +0,0 @@
-"""
-Project configurations.
-"""
-import os
-
-
-class Config(object):
- """Datasets and experiments folders for the whole project."""
-
- #####################
- ## Dataset setting ##
- #####################
- DATASET_ROOT = os.getenv(
- "DATASET_ROOT", "./datasets/"
- ) # TODO: path to your datasets folder
- if not os.path.exists(DATASET_ROOT):
- os.makedirs(DATASET_ROOT)
-
- # Synthetic shape dataset
- synthetic_dataroot = os.path.join(DATASET_ROOT, "synthetic_shapes")
- synthetic_cache_path = os.path.join(DATASET_ROOT, "synthetic_shapes")
- if not os.path.exists(synthetic_dataroot):
- os.makedirs(synthetic_dataroot)
-
- # Exported predictions dataset
- export_dataroot = os.path.join(DATASET_ROOT, "export_datasets")
- export_cache_path = os.path.join(DATASET_ROOT, "export_datasets")
- if not os.path.exists(export_dataroot):
- os.makedirs(export_dataroot)
-
- # Wireframe dataset
- wireframe_dataroot = os.path.join(DATASET_ROOT, "wireframe")
- wireframe_cache_path = os.path.join(DATASET_ROOT, "wireframe")
-
- # Holicity dataset
- holicity_dataroot = os.path.join(DATASET_ROOT, "Holicity")
- holicity_cache_path = os.path.join(DATASET_ROOT, "Holicity")
-
- ########################
- ## Experiment Setting ##
- ########################
- EXP_PATH = os.getenv(
- "EXP_PATH", "./experiments/"
- ) # TODO: path to your experiments folder
- if not os.path.exists(EXP_PATH):
- os.makedirs(EXP_PATH)
diff --git a/spaces/RedValis/Music-Helix/model.py b/spaces/RedValis/Music-Helix/model.py
deleted file mode 100644
index c3ab5205e8f2f5ed8c5baeff4cf81ecd35e6d628..0000000000000000000000000000000000000000
--- a/spaces/RedValis/Music-Helix/model.py
+++ /dev/null
@@ -1,520 +0,0 @@
-import pandas as pd
-import spotipy
-from spotipy.oauth2 import SpotifyOAuth, SpotifyClientCredentials
-import yaml
-import re
-from sklearn.feature_extraction.text import TfidfVectorizer
-from sklearn.metrics.pairwise import cosine_similarity
-from sklearn.preprocessing import MinMaxScaler
-import pickle
-import streamlit as st
-import os
-
-def playlist_model(url, model, max_gen=3, same_art=5):
- log = []
- Fresult = []
- try:
- log.append('Start logging')
- uri = url.split('/')[-1].split('?')[0]
- try:
- log.append('spotify local method')
- stream = open("Spotify/Spotify.yaml")
- spotify_details = yaml.safe_load(stream)
- auth_manager = SpotifyClientCredentials(client_id=spotify_details['Client_id'], client_secret=spotify_details['client_secret'])
- except:
- log.append('spotify .streamlit method')
- try:
- Client_id=st.secrets["Client_ID"]
- client_secret=st.secrets["Client_secret"]
- auth_manager = SpotifyClientCredentials(client_id=Client_id, client_secret=client_secret)
- except:
- log.append('spotify hug method')
- Client_id=os.environ['Client_ID']
- client_secret=os.environ['Client_secret']
- auth_manager = SpotifyClientCredentials(client_id=Client_id, client_secret=client_secret)
- sp = spotipy.client.Spotify(auth_manager=auth_manager)
-
- if model == 'Spotify Model':
- def get_IDs(user, playlist_id):
- try:
- log.append('start playlist extraction')
- track_ids = []
- playlist = sp.user_playlist(user, playlist_id)
- for item in playlist['tracks']['items']:
- track = item['track']
- track_ids.append(track['id'])
- return track_ids
- except Exception as e:
- log.append('Failed to load the playlist')
- log.append(e)
-
- track_ids = get_IDs('Ruby', uri)
- track_ids_uni = list(set(track_ids))
- log.append('Starting Spotify Model')
- Spotifyresult = pd.DataFrame()
- for i in range(len(track_ids_uni)-5):
- if len(Spotifyresult) >= 50:
- break
- try:
- ff = sp.recommendations(seed_tracks=list(track_ids_uni[i:i+5]), limit=5)
- except Exception as e:
- log.append(e)
- continue
- for z in range(5):
- result = pd.DataFrame([z+(5*i)+1])
- result['uri'] = ff['tracks'][z]['id']
- Spotifyresult = pd.concat([Spotifyresult, result], axis=0)
- Spotifyresult.drop_duplicates(subset=['uri'], inplace=True,keep='first')
- Fresult = Spotifyresult.uri[:50]
-
- log.append('Model run successfully')
- return Fresult, log
-
- lendf=len(pd.read_csv('Data/streamlit.csv',usecols=['track_uri']))
- dtypes = {'track_uri': 'object', 'artist_uri': 'object', 'album_uri': 'object', 'danceability': 'float16', 'energy': 'float16', 'key': 'float16',
- 'loudness': 'float16', 'mode': 'float16', 'speechiness': 'float16', 'acousticness': 'float16', 'instrumentalness': 'float16',
- 'liveness': 'float16', 'valence': 'float16', 'tempo': 'float16', 'duration_ms': 'float32', 'time_signature': 'float16',
- 'Track_release_date': 'int8', 'Track_pop': 'int8', 'Artist_pop': 'int8', 'Artist_genres': 'object'}
- col_name= ['track_uri', 'artist_uri', 'album_uri', 'danceability', 'energy', 'key',
- 'loudness', 'mode', 'speechiness', 'acousticness', 'instrumentalness',
- 'liveness', 'valence', 'tempo', 'duration_ms', 'time_signature',
- 'Track_release_date', 'Track_pop', 'Artist_pop', 'Artist_genres']
-
- try:
- def get_IDs(user, playlist_id):
- log.append('start playlist extraction')
- track_ids = []
- artist_id = []
- playlist = sp.user_playlist(user, playlist_id)
- for item in playlist['tracks']['items']:
- track = item['track']
- track_ids.append(track['id'])
- artist = item['track']['artists']
- artist_id.append(artist[0]['id'])
- return track_ids, artist_id
- except Exception as e:
- log.append('Failed to load the playlist')
- log.append(e)
-
- track_ids, artist_id = get_IDs('Ruby', uri)
- log.append("Number of Track : {}".format(len(track_ids)))
-
- artist_id_uni = list(set(artist_id))
- track_ids_uni = list(set(track_ids))
- log.append("Number of unique Artists : {}".format(len(artist_id_uni)))
- log.append("Number of unique Tracks : {}".format(len(track_ids_uni)))
-
- def extract(track_ids_uni, artist_id_uni):
- err = []
- err.append('Start audio features extraction')
- audio_features = pd.DataFrame()
- for i in range(0, len(track_ids_uni), 25):
- try:
- track_feature = sp.audio_features(track_ids_uni[i:i+25])
- track_df = pd.DataFrame(track_feature)
- audio_features = pd.concat([audio_features, track_df], axis=0)
- except Exception as e:
- err.append(e)
- continue
- err.append('Start track features extraction')
- track_ = pd.DataFrame()
- for i in range(0, len(track_ids_uni), 25):
- try:
- track_features = sp.tracks(track_ids_uni[i:i+25])
- for x in range(25):
- track_pop = pd.DataFrame([track_ids_uni[i+x]], columns=['Track_uri'])
- track_pop['Track_release_date'] = track_features['tracks'][x]['album']['release_date']
- track_pop['Track_pop'] = track_features['tracks'][x]["popularity"]
- track_pop['Artist_uri'] = track_features['tracks'][x]['artists'][0]['id']
- track_pop['Album_uri'] = track_features['tracks'][x]['album']['id']
- track_ = pd.concat([track_, track_pop], axis=0)
- except Exception as e:
- err.append(e)
- continue
- err.append('Start artist features extraction')
- artist_ = pd.DataFrame()
- for i in range(0, len(artist_id_uni), 25):
- try:
- artist_features = sp.artists(artist_id_uni[i:i+25])
- for x in range(25):
- artist_df = pd.DataFrame([artist_id_uni[i+x]], columns=['Artist_uri'])
- artist_pop = artist_features['artists'][x]["popularity"]
- artist_genres = artist_features['artists'][x]["genres"]
- artist_df["Artist_pop"] = artist_pop
- if artist_genres:
- artist_df["genres"] = " ".join([re.sub(' ', '_', i) for i in artist_genres])
- else:
- artist_df["genres"] = "unknown"
- artist_ = pd.concat([artist_, artist_df], axis=0)
- except Exception as e:
- err.append(e)
- continue
- try:
- test = pd.DataFrame(
- track_, columns=['Track_uri', 'Artist_uri', 'Album_uri'])
-
- test.rename(columns={'Track_uri': 'track_uri',
- 'Artist_uri': 'artist_uri', 'Album_uri': 'album_uri'}, inplace=True)
-
- audio_features.drop(
- columns=['type', 'uri', 'track_href', 'analysis_url'], axis=1, inplace=True)
-
- test = pd.merge(test, audio_features,
- left_on="track_uri", right_on="id", how='outer')
- test = pd.merge(test, track_, left_on="track_uri",
- right_on="Track_uri", how='outer')
- test = pd.merge(test, artist_, left_on="artist_uri",
- right_on="Artist_uri", how='outer')
-
- test.rename(columns={'genres': 'Artist_genres'}, inplace=True)
-
- test.drop(columns=['Track_uri', 'Artist_uri_x',
- 'Artist_uri_y', 'Album_uri', 'id'], axis=1, inplace=True)
-
- test.dropna(axis=0, inplace=True)
- test['Track_pop'] = test['Track_pop'].apply(lambda x: int(x/5))
- test['Artist_pop'] = test['Artist_pop'].apply(lambda x: int(x/5))
- test['Track_release_date'] = test['Track_release_date'].apply(lambda x: x.split('-')[0])
- test['Track_release_date'] = test['Track_release_date'].astype('int16')
- test['Track_release_date'] = test['Track_release_date'].apply(lambda x: int(x/50))
-
- test[['danceability', 'energy', 'key', 'loudness', 'mode', 'speechiness', 'acousticness', 'instrumentalness', 'liveness', 'valence', 'tempo', 'time_signature']] = test[[
- 'danceability', 'energy', 'key', 'loudness', 'mode', 'speechiness', 'acousticness', 'instrumentalness', 'liveness', 'valence', 'tempo', 'time_signature']].astype('float16')
- test[['duration_ms']] = test[['duration_ms']].astype('float32')
- test[['Track_release_date', 'Track_pop', 'Artist_pop']] = test[[
- 'Track_release_date', 'Track_pop', 'Artist_pop']].astype('int8')
- except Exception as e:
- err.append(e)
- err.append('Finish extraction')
- return test, err
- test, err = extract(track_ids_uni, artist_id_uni)
-
- for i in err:
- log.append(i)
- del err
- grow = test.copy()
- test['Artist_genres'] = test['Artist_genres'].apply(lambda x: x.split(" "))
- tfidf = TfidfVectorizer(max_features=max_gen)
- tfidf_matrix = tfidf.fit_transform(test['Artist_genres'].apply(lambda x: " ".join(x)))
- genre_df = pd.DataFrame(tfidf_matrix.toarray())
- genre_df.columns = ['genre' + "|" +i for i in tfidf.get_feature_names_out()]
- genre_df = genre_df.astype('float16')
- test.drop(columns=['Artist_genres'], axis=1, inplace=True)
- test = pd.concat([test.reset_index(drop=True),genre_df.reset_index(drop=True)], axis=1)
- Fresult = pd.DataFrame()
- x = 1
- for i in range(int(lendf/2), lendf+1, int(lendf/2)):
- try:
- df = pd.read_csv('Data/streamlit.csv',names= col_name,dtype=dtypes,skiprows=x,nrows=i)
- log.append('reading data frame chunks from {} to {}'.format(x,i))
- except Exception as e:
- log.append('Failed to load grow')
- log.append(e)
- grow = grow[~grow['track_uri'].isin(df['track_uri'].values)]
- df = df[~df['track_uri'].isin(test['track_uri'].values)]
- df['Artist_genres'] = df['Artist_genres'].apply(lambda x: x.split(" "))
- tfidf_matrix = tfidf.transform(df['Artist_genres'].apply(lambda x: " ".join(x)))
- genre_df = pd.DataFrame(tfidf_matrix.toarray())
- genre_df.columns = ['genre' + "|" +i for i in tfidf.get_feature_names_out()]
- genre_df = genre_df.astype('float16')
- df.drop(columns=['Artist_genres'], axis=1, inplace=True)
- df = pd.concat([df.reset_index(drop=True),
- genre_df.reset_index(drop=True)], axis=1)
- del genre_df
- try:
- df.drop(columns=['genre|unknown'], axis=1, inplace=True)
- test.drop(columns=['genre|unknown'], axis=1, inplace=True)
- except:
- log.append('genre|unknown not found')
- log.append('Scaling the data .....')
- if x == 1:
- sc = pickle.load(open('Data/sc.sav','rb'))
- df.iloc[:, 3:19] = sc.transform(df.iloc[:, 3:19])
- test.iloc[:, 3:19] = sc.transform(test.iloc[:, 3:19])
- log.append("Creating playlist vector")
- playvec = pd.DataFrame(test.sum(axis=0)).T
- else:
- df.iloc[:, 3:19] = sc.transform(df.iloc[:, 3:19])
- x = i
- if model == 'Model 1':
- df['sim']=cosine_similarity(df.drop(['track_uri', 'artist_uri', 'album_uri'], axis = 1),playvec.drop(['track_uri', 'artist_uri', 'album_uri'], axis = 1))
- df['sim2']=cosine_similarity(df.iloc[:,16:-1],playvec.iloc[:,16:])
- df['sim3']=cosine_similarity(df.iloc[:,19:-2],playvec.iloc[:,19:])
- df = df.sort_values(['sim3','sim2','sim'],ascending = False,kind='stable').groupby('artist_uri').head(same_art).head(50)
- Fresult = pd.concat([Fresult, df], axis=0)
- Fresult = Fresult.sort_values(['sim3', 'sim2', 'sim'],ascending=False,kind='stable')
- Fresult.drop_duplicates(subset=['track_uri'], inplace=True,keep='first')
- Fresult = Fresult.groupby('artist_uri').head(same_art).head(50)
- elif model == 'Model 2':
- df['sim'] = cosine_similarity(df.iloc[:, 3:16], playvec.iloc[:, 3:16])
- df['sim2'] = cosine_similarity(df.loc[:, df.columns.str.startswith('T') | df.columns.str.startswith('A')], playvec.loc[:, playvec.columns.str.startswith('T') | playvec.columns.str.startswith('A')])
- df['sim3'] = cosine_similarity(df.loc[:, df.columns.str.startswith('genre')], playvec.loc[:, playvec.columns.str.startswith('genre')])
- df['sim4'] = (df['sim']+df['sim2']+df['sim3'])/3
- df = df.sort_values(['sim4'], ascending=False,kind='stable').groupby('artist_uri').head(same_art).head(50)
- Fresult = pd.concat([Fresult, df], axis=0)
- Fresult = Fresult.sort_values(['sim4'], ascending=False,kind='stable')
- Fresult.drop_duplicates(subset=['track_uri'], inplace=True,keep='first')
- Fresult = Fresult.groupby('artist_uri').head(same_art).head(50)
- del test
- try:
- del df
- log.append('Getting Result')
- except:
- log.append('Getting Result')
- if model == 'Model 1':
- Fresult = Fresult.sort_values(['sim3', 'sim2', 'sim'],ascending=False,kind='stable')
- Fresult.drop_duplicates(subset=['track_uri'], inplace=True,keep='first')
- Fresult = Fresult.groupby('artist_uri').head(same_art).track_uri.head(50)
- elif model == 'Model 2':
- Fresult = Fresult.sort_values(['sim4'], ascending=False,kind='stable')
- Fresult.drop_duplicates(subset=['track_uri'], inplace=True,keep='first')
- Fresult = Fresult.groupby('artist_uri').head(same_art).track_uri.head(50)
- log.append('{} New Tracks Found'.format(len(grow)))
- if(len(grow)>=1):
- try:
- new=pd.read_csv('Data/new_tracks.csv',dtype=dtypes)
- new=pd.concat([new, grow], axis=0)
- new=new[new.Track_pop >0]
- new.drop_duplicates(subset=['track_uri'], inplace=True,keep='last')
- new.to_csv('Data/new_tracks.csv',index=False)
- except:
- grow.to_csv('Data/new_tracks.csv', index=False)
- log.append('Model run successfully')
- except Exception as e:
- log.append("Model Failed")
- log.append(e)
- return Fresult, log
-
-
-
-def top_tracks(url,region):
- log = []
- Fresult = []
- uri = url.split('/')[-1].split('?')[0]
- try:
- log.append('spotify local method')
- stream = open("Spotify/Spotify.yaml")
- spotify_details = yaml.safe_load(stream)
- auth_manager = SpotifyClientCredentials(client_id=spotify_details['Client_id'], client_secret=spotify_details['client_secret'])
- except:
- log.append('spotify .streamlit method')
- try:
- Client_id=st.secrets["Client_ID"]
- client_secret=st.secrets["Client_secret"]
- auth_manager = SpotifyClientCredentials(client_id=Client_id, client_secret=client_secret)
- except:
- log.append('spotify hug method')
- Client_id=os.environ['Client_ID']
- client_secret=os.environ['Client_secret']
- auth_manager = SpotifyClientCredentials(client_id=Client_id, client_secret=client_secret)
- sp = spotipy.client.Spotify(auth_manager=auth_manager)
- try:
- log.append('Starting Spotify Model')
- top=sp.artist_top_tracks(uri,country=region)
- for i in range(10) :
- Fresult.append(top['tracks'][i]['id'])
- log.append('Model run successfully')
- except Exception as e:
- log.append("Model Failed")
- log.append(e)
- return Fresult,log
-
-def song_model(url, model, max_gen=3, same_art=5):
- log = []
- Fresult = []
- try:
- log.append('Start logging')
- uri = url.split('/')[-1].split('?')[0]
- try:
- log.append('spotify local method')
- stream = open("Spotify/Spotify.yaml")
- spotify_details = yaml.safe_load(stream)
- auth_manager = SpotifyClientCredentials(client_id=spotify_details['Client_id'], client_secret=spotify_details['client_secret'])
- except:
- log.append('spotify .streamlit method')
- try:
- Client_id=st.secrets["Client_ID"]
- client_secret=st.secrets["Client_secret"]
- auth_manager = SpotifyClientCredentials(client_id=Client_id, client_secret=client_secret)
- except:
- log.append('spotify hug method')
- Client_id=os.environ['Client_ID']
- client_secret=os.environ['Client_secret']
- auth_manager = SpotifyClientCredentials(client_id=Client_id, client_secret=client_secret)
- sp = spotipy.client.Spotify(auth_manager=auth_manager)
-
- if model == 'Spotify Model':
- log.append('Starting Spotify Model')
- aa=sp.recommendations(seed_tracks=[uri], limit=25)
- for i in range(25):
- Fresult.append(aa['tracks'][i]['id'])
- log.append('Model run successfully')
- return Fresult, log
- lendf=len(pd.read_csv('Data/streamlit.csv',usecols=['track_uri']))
- dtypes = {'track_uri': 'object', 'artist_uri': 'object', 'album_uri': 'object', 'danceability': 'float16', 'energy': 'float16', 'key': 'float16',
- 'loudness': 'float16', 'mode': 'float16', 'speechiness': 'float16', 'acousticness': 'float16', 'instrumentalness': 'float16',
- 'liveness': 'float16', 'valence': 'float16', 'tempo': 'float16', 'duration_ms': 'float32', 'time_signature': 'float16',
- 'Track_release_date': 'int8', 'Track_pop': 'int8', 'Artist_pop': 'int8', 'Artist_genres': 'object'}
- col_name= ['track_uri', 'artist_uri', 'album_uri', 'danceability', 'energy', 'key',
- 'loudness', 'mode', 'speechiness', 'acousticness', 'instrumentalness',
- 'liveness', 'valence', 'tempo', 'duration_ms', 'time_signature',
- 'Track_release_date', 'Track_pop', 'Artist_pop', 'Artist_genres']
- log.append('Start audio features extraction')
- audio_features = pd.DataFrame(sp.audio_features([uri]))
- log.append('Start track features extraction')
- track_ = pd.DataFrame()
- track_features = sp.tracks([uri])
- track_pop = pd.DataFrame([uri], columns=['Track_uri'])
- track_pop['Track_release_date'] = track_features['tracks'][0]['album']['release_date']
- track_pop['Track_pop'] = track_features['tracks'][0]["popularity"]
- track_pop['Artist_uri'] = track_features['tracks'][0]['artists'][0]['id']
- track_pop['Album_uri'] = track_features['tracks'][0]['album']['id']
- track_ = pd.concat([track_, track_pop], axis=0)
- log.append('Start artist features extraction')
- artist_id_uni=list(track_['Artist_uri'])
- artist_ = pd.DataFrame()
- artist_features = sp.artists(artist_id_uni)
- artist_df = pd.DataFrame(artist_id_uni, columns=['Artist_uri'])
- artist_pop = artist_features['artists'][0]["popularity"]
- artist_genres = artist_features['artists'][0]["genres"]
- artist_df["Artist_pop"] = artist_pop
- if artist_genres:
- artist_df["genres"] = " ".join([re.sub(' ', '_', i) for i in artist_genres])
- else:
- artist_df["genres"] = "unknown"
- artist_ = pd.concat([artist_, artist_df], axis=0)
- try:
- test = pd.DataFrame(track_, columns=['Track_uri', 'Artist_uri', 'Album_uri'])
- test.rename(columns={'Track_uri': 'track_uri','Artist_uri': 'artist_uri', 'Album_uri': 'album_uri'}, inplace=True)
- audio_features.drop(columns=['type', 'uri', 'track_href', 'analysis_url'], axis=1, inplace=True)
- test = pd.merge(test, audio_features,left_on="track_uri", right_on="id", how='outer')
- test = pd.merge(test, track_, left_on="track_uri",right_on="Track_uri", how='outer')
- test = pd.merge(test, artist_, left_on="artist_uri",right_on="Artist_uri", how='outer')
- test.rename(columns={'genres': 'Artist_genres'}, inplace=True)
- test.drop(columns=['Track_uri', 'Artist_uri_x','Artist_uri_y', 'Album_uri', 'id'], axis=1, inplace=True)
- test.dropna(axis=0, inplace=True)
- test['Track_pop'] = test['Track_pop'].apply(lambda x: int(x/5))
- test['Artist_pop'] = test['Artist_pop'].apply(lambda x: int(x/5))
- test['Track_release_date'] = test['Track_release_date'].apply(lambda x: x.split('-')[0])
- test['Track_release_date'] = test['Track_release_date'].astype('int16')
- test['Track_release_date'] = test['Track_release_date'].apply(lambda x: int(x/50))
- test[['danceability', 'energy', 'key', 'loudness', 'mode', 'speechiness', 'acousticness', 'instrumentalness', 'liveness', 'valence', 'tempo', 'time_signature']] = test[['danceability', 'energy', 'key', 'loudness', 'mode', 'speechiness', 'acousticness', 'instrumentalness', 'liveness', 'valence', 'tempo', 'time_signature']].astype('float16')
- test[['duration_ms']] = test[['duration_ms']].astype('float32')
- test[['Track_release_date', 'Track_pop', 'Artist_pop']] = test[['Track_release_date', 'Track_pop', 'Artist_pop']].astype('int8')
- except Exception as e:
- log.append(e)
- log.append('Finish extraction')
- grow = test.copy()
- test['Artist_genres'] = test['Artist_genres'].apply(lambda x: x.split(" "))
- tfidf = TfidfVectorizer(max_features=max_gen)
- tfidf_matrix = tfidf.fit_transform(test['Artist_genres'].apply(lambda x: " ".join(x)))
- genre_df = pd.DataFrame(tfidf_matrix.toarray())
- genre_df.columns = ['genre' + "|" +i for i in tfidf.get_feature_names_out()]
- genre_df = genre_df.astype('float16')
- test.drop(columns=['Artist_genres'], axis=1, inplace=True)
- test = pd.concat([test.reset_index(drop=True),genre_df.reset_index(drop=True)], axis=1)
- Fresult = pd.DataFrame()
- x = 1
- for i in range(int(lendf/2), lendf+1, int(lendf/2)):
- try:
- df = pd.read_csv('Data/streamlit.csv',names= col_name,dtype=dtypes,skiprows=x,nrows=i)
- log.append('reading data frame chunks from {} to {}'.format(x,i))
- except Exception as e:
- log.append('Failed to load grow')
- log.append(e)
- grow = grow[~grow['track_uri'].isin(df['track_uri'].values)]
- df = df[~df['track_uri'].isin(test['track_uri'].values)]
- df['Artist_genres'] = df['Artist_genres'].apply(lambda x: x.split(" "))
- tfidf_matrix = tfidf.transform(df['Artist_genres'].apply(lambda x: " ".join(x)))
- genre_df = pd.DataFrame(tfidf_matrix.toarray())
- genre_df.columns = ['genre' + "|" +i for i in tfidf.get_feature_names_out()]
- genre_df = genre_df.astype('float16')
- df.drop(columns=['Artist_genres'], axis=1, inplace=True)
- df = pd.concat([df.reset_index(drop=True),
- genre_df.reset_index(drop=True)], axis=1)
- del genre_df
- try:
- df.drop(columns=['genre|unknown'], axis=1, inplace=True)
- test.drop(columns=['genre|unknown'], axis=1, inplace=True)
- except:
- log.append('genre|unknown not found')
- log.append('Scaling the data .....')
- if x == 1:
- sc = pickle.load(open('Data/sc.sav','rb'))
- df.iloc[:, 3:19] = sc.transform(df.iloc[:, 3:19])
- test.iloc[:, 3:19] = sc.transform(test.iloc[:, 3:19])
- log.append("Creating playlist vector")
- playvec = pd.DataFrame(test.sum(axis=0)).T
- else:
- df.iloc[:, 3:19] = sc.transform(df.iloc[:, 3:19])
- x = i
- if model == 'Model 1':
- df['sim']=cosine_similarity(df.drop(['track_uri', 'artist_uri', 'album_uri'], axis = 1),playvec.drop(['track_uri', 'artist_uri', 'album_uri'], axis = 1))
- df['sim2']=cosine_similarity(df.iloc[:,16:-1],playvec.iloc[:,16:])
- df['sim3']=cosine_similarity(df.iloc[:,19:-2],playvec.iloc[:,19:])
- df = df.sort_values(['sim3','sim2','sim'],ascending = False,kind='stable').groupby('artist_uri').head(same_art).head(50)
- Fresult = pd.concat([Fresult, df], axis=0)
- Fresult = Fresult.sort_values(['sim3', 'sim2', 'sim'],ascending=False,kind='stable')
- Fresult.drop_duplicates(subset=['track_uri'], inplace=True,keep='first')
- Fresult = Fresult.groupby('artist_uri').head(same_art).head(50)
- elif model == 'Model 2':
- df['sim'] = cosine_similarity(df.iloc[:, 3:16], playvec.iloc[:, 3:16])
- df['sim2'] = cosine_similarity(df.loc[:, df.columns.str.startswith('T') | df.columns.str.startswith('A')], playvec.loc[:, playvec.columns.str.startswith('T') | playvec.columns.str.startswith('A')])
- df['sim3'] = cosine_similarity(df.loc[:, df.columns.str.startswith('genre')], playvec.loc[:, playvec.columns.str.startswith('genre')])
- df['sim4'] = (df['sim']+df['sim2']+df['sim3'])/3
- df = df.sort_values(['sim4'], ascending=False,kind='stable').groupby('artist_uri').head(same_art).head(50)
- Fresult = pd.concat([Fresult, df], axis=0)
- Fresult = Fresult.sort_values(['sim4'], ascending=False,kind='stable')
- Fresult.drop_duplicates(subset=['track_uri'], inplace=True,keep='first')
- Fresult = Fresult.groupby('artist_uri').head(same_art).head(50)
- del test
- try:
- del df
- log.append('Getting Result')
- except:
- log.append('Getting Result')
- if model == 'Model 1':
- Fresult = Fresult.sort_values(['sim3', 'sim2', 'sim'],ascending=False,kind='stable')
- Fresult.drop_duplicates(subset=['track_uri'], inplace=True,keep='first')
- Fresult = Fresult.groupby('artist_uri').head(same_art).track_uri.head(50)
- elif model == 'Model 2':
- Fresult = Fresult.sort_values(['sim4'], ascending=False,kind='stable')
- Fresult.drop_duplicates(subset=['track_uri'], inplace=True,keep='first')
- Fresult = Fresult.groupby('artist_uri').head(same_art).track_uri.head(50)
- log.append('{} New Tracks Found'.format(len(grow)))
- if(len(grow)>=1):
- try:
- new=pd.read_csv('Data/new_tracks.csv',dtype=dtypes)
- new=pd.concat([new, grow], axis=0)
- new=new[new.Track_pop >0]
- new.drop_duplicates(subset=['track_uri'], inplace=True,keep='last')
- new.to_csv('Data/new_tracks.csv',index=False)
- except:
- grow.to_csv('Data/new_tracks.csv', index=False)
- log.append('Model run successfully')
- except Exception as e:
- log.append("Model Failed")
- log.append(e)
- return Fresult, log
-
-def update_dataset():
- col_name= ['track_uri', 'artist_uri', 'album_uri', 'danceability', 'energy', 'key',
- 'loudness', 'mode', 'speechiness', 'acousticness', 'instrumentalness',
- 'liveness', 'valence', 'tempo', 'duration_ms', 'time_signature',
- 'Track_release_date', 'Track_pop', 'Artist_pop', 'Artist_genres']
- dtypes = {'track_uri': 'object', 'artist_uri': 'object', 'album_uri': 'object', 'danceability': 'float16', 'energy': 'float16', 'key': 'float16',
- 'loudness': 'float16', 'mode': 'float16', 'speechiness': 'float16', 'acousticness': 'float16', 'instrumentalness': 'float16',
- 'liveness': 'float16', 'valence': 'float16', 'tempo': 'float16', 'duration_ms': 'float32', 'time_signature': 'float16',
- 'Track_release_date': 'int8', 'Track_pop': 'int8', 'Artist_pop': 'int8', 'Artist_genres': 'object'}
- df = pd.read_csv('Data/streamlit.csv',dtype=dtypes)
- grow = pd.read_csv('Data/new_tracks.csv',dtype=dtypes)
- cur = len(df)
- df=pd.concat([df,grow],axis=0)
- grow=pd.DataFrame(columns=col_name)
- grow.to_csv('Data/new_tracks.csv',index=False)
- df=df[df.Track_pop >0]
- df.drop_duplicates(subset=['track_uri'],inplace=True,keep='last')
- df.dropna(axis=0,inplace=True)
- df.to_csv('Data/streamlit.csv',index=False)
- return (len(df)-cur)
-
diff --git a/spaces/Redgon/bingo/src/components/chat-attachments.tsx b/spaces/Redgon/bingo/src/components/chat-attachments.tsx
deleted file mode 100644
index ef43d4e262935d263b6099138c56f7daade5299d..0000000000000000000000000000000000000000
--- a/spaces/Redgon/bingo/src/components/chat-attachments.tsx
+++ /dev/null
@@ -1,37 +0,0 @@
-import Image from 'next/image'
-import ClearIcon from '@/assets/images/clear.svg'
-import RefreshIcon from '@/assets/images/refresh.svg'
-import { FileItem } from '@/lib/bots/bing/types'
-import { cn } from '@/lib/utils'
-import { useBing } from '@/lib/hooks/use-bing'
-
-type ChatAttachmentsProps = Pick, 'attachmentList' | 'setAttachmentList' | 'uploadImage'>
-
-export function ChatAttachments({ attachmentList = [], setAttachmentList, uploadImage }: ChatAttachmentsProps) {
- return attachmentList.length ? (
-
- {attachmentList.map(file => (
-
- {file.status === 'loading' && (
-
-
-
)
- }
- {file.status !== 'error' && (
-
-
-
)
- }
- {file.status === 'error' && (
-
- uploadImage(file.url)} />
-
- )}
-
-
- ))}
-
- ) : null
-}
diff --git a/spaces/Rimi98/InsectRecognizer/app.py b/spaces/Rimi98/InsectRecognizer/app.py
deleted file mode 100644
index ed6b20c0c3fc0a15d541df2074aac9785ed3dc1e..0000000000000000000000000000000000000000
--- a/spaces/Rimi98/InsectRecognizer/app.py
+++ /dev/null
@@ -1,33 +0,0 @@
-import gradio as gr
-from fastai.vision.all import load_learner
-from fastai import *
-import torch
-import os
-from PIL import Image
-
-
-model_path = 'model6-90%.pkl'
-
-model = load_learner(model_path)
-
-def result(path):
-
- pred,_,probability = model.predict(path)
- pred = str(pred)
- pred = pred.upper()
-
- return {pred: float(probability.max())}
-
-path = 'test_images/'
-
-image_path = []
-
-for i in os.listdir(path):
- image_path.append(path+i)
-
-
-image = gr.inputs.Image(shape =(128,128))
-label = gr.outputs.Label()
-
-iface = gr.Interface(fn=result, inputs=image, outputs=label, examples = image_path)
-iface.launch(inline=False)
\ No newline at end of file
diff --git a/spaces/RitaParadaRamos/SmallCapDemo/src/utils.py b/spaces/RitaParadaRamos/SmallCapDemo/src/utils.py
deleted file mode 100644
index dc2f3b70261ef1e4346c8c7990ceed6441020b57..0000000000000000000000000000000000000000
--- a/spaces/RitaParadaRamos/SmallCapDemo/src/utils.py
+++ /dev/null
@@ -1,131 +0,0 @@
-from torch.utils.data import Dataset
-from PIL import Image
-import torch
-import json
-import h5py
-import bisect
-
-CAPTION_LENGTH = 25
-SIMPLE_PREFIX = "This image shows "
-
-def prep_strings(text, tokenizer, template=None, retrieved_caps=None, k=None, is_test=False, max_length=None):
-
- if is_test:
- padding = False
- truncation = False
- else:
- padding = True
- truncation = True
-
- if retrieved_caps is not None:
- infix = '\n\n'.join(retrieved_caps[:k]) + '.'
- prefix = template.replace('||', infix)
- else:
- prefix = SIMPLE_PREFIX
-
- prefix_ids = tokenizer.encode(prefix)
- len_prefix = len(prefix_ids)
-
- text_ids = tokenizer.encode(text, add_special_tokens=False)
- if truncation:
- text_ids = text_ids[:CAPTION_LENGTH]
- input_ids = prefix_ids + text_ids if not is_test else prefix_ids
-
- # we ignore the prefix (minus one as the first subtoken in the prefix is not predicted)
- label_ids = [-100] * (len_prefix - 1) + text_ids + [tokenizer.eos_token_id]
- if padding:
- input_ids += [tokenizer.pad_token_id] * (max_length - len(input_ids))
- label_ids += [-100] * (max_length - len(label_ids))
-
- if is_test:
- return input_ids
- else:
- return input_ids, label_ids
-
-def postprocess_preds(pred, tokenizer):
- pred = pred.split(SIMPLE_PREFIX)[-1]
- pred = pred.replace(tokenizer.pad_token, '')
- if pred.startswith(tokenizer.bos_token):
- pred = pred[len(tokenizer.bos_token):]
- if pred.endswith(tokenizer.eos_token):
- pred = pred[:-len(tokenizer.eos_token)]
- return pred
-
-class TrainDataset(Dataset):
- def __init__(self, df, features_path, tokenizer, rag=False, template_path=None, k=None, max_caption_length=25):
- self.df = df
- self.tokenizer = tokenizer
- self.features = h5py.File(features_path, 'r')
-
- if rag:
- self.template = open(template_path).read().strip() + ' '
- self.max_target_length = (max_caption_length # target caption
- + max_caption_length * k # retrieved captions
- + len(tokenizer.encode(self.template)) # template
- + len(tokenizer.encode('\n\n')) * (k-1) # separator between captions
- )
- assert k is not None
- self.k = k
- self.rag = rag
-
- def __len__(self):
- return len(self.df)
-
- def __getitem__(self, idx):
- text = self.df['text'][idx]
- if self.rag:
- caps = self.df['caps'][idx]
- decoder_input_ids, labels = prep_strings(text, self.tokenizer, template=self.template,
- retrieved_caps=caps, k=self.k, max_length=self.max_target_length)
- else:
- decoder_input_ids, labels = prep_strings(text, self.tokenizer, max_length=self.max_target_length)
- # load precomputed features
- encoder_outputs = self.features[self.df['cocoid'][idx]][()]
- encoding = {"encoder_outputs": torch.tensor(encoder_outputs),
- "decoder_input_ids": torch.tensor(decoder_input_ids),
- "labels": torch.tensor(labels)}
-
- return encoding
-
-
-def load_data_for_training(annot_path, caps_path=None):
- annotations = json.load(open(annot_path))['images']
- if caps_path is not None:
- retrieved_caps = json.load(open(caps_path))
- data = {'train': [], 'val': []}
-
- for item in annotations:
- file_name = item['filename'].split('_')[-1]
- if caps_path is not None:
- caps = retrieved_caps[str(item['cocoid'])]
- else:
- caps = None
- samples = []
- for sentence in item['sentences']:
- samples.append({'file_name': file_name, 'cocoid': str(item['cocoid']), 'caps': caps, 'text': ' '.join(sentence['tokens'])})
- if item['split'] == 'train' or item['split'] == 'restval':
- data['train'] += samples
- elif item['split'] == 'val':
- data['val'] += samples
- return data
-
-def load_data_for_inference(annot_path, caps_path=None):
- annotations = json.load(open(annot_path))['images']
- if caps_path is not None:
- retrieved_caps = json.load(open(caps_path))
- data = {'test': [], 'val': []}
-
- for item in annotations:
- file_name = item['filename'].split('_')[-1]
- if caps_path is not None:
- caps = retrieved_caps[str(item['cocoid'])]
- else:
- caps = None
- image = {'file_name': file_name, 'caps': caps, 'image_id': str(item['cocoid'])}
- if item['split'] == 'test':
- data['test'].append(image)
- elif item['split'] == 'val':
- data['val'].append(image)
-
- return data
-
diff --git a/spaces/RohithMidigudla/Comment_Toxicity_Detection/README.md b/spaces/RohithMidigudla/Comment_Toxicity_Detection/README.md
deleted file mode 100644
index 455547a9b972b44869bc60993fc450b6c75e5ae6..0000000000000000000000000000000000000000
--- a/spaces/RohithMidigudla/Comment_Toxicity_Detection/README.md
+++ /dev/null
@@ -1,12 +0,0 @@
----
-title: Comment Toxicity Detection
-emoji: 💻
-colorFrom: indigo
-colorTo: blue
-sdk: gradio
-sdk_version: 3.50.2
-app_file: app.py
-pinned: false
----
-
-Check out the configuration reference at https://huggingface.co/docs/hub/spaces-config-reference
diff --git a/spaces/Sakil/sakil_text_summarization_app/README.md b/spaces/Sakil/sakil_text_summarization_app/README.md
deleted file mode 100644
index b48c54c2b1292a9b34d3928e004efaa7b412a337..0000000000000000000000000000000000000000
--- a/spaces/Sakil/sakil_text_summarization_app/README.md
+++ /dev/null
@@ -1,46 +0,0 @@
----
-title: Sakil_text_summarization_app
-emoji: ⚡
-colorFrom: green
-colorTo: gray
-sdk: gradio
-app_file: app.py
-pinned: false
-license: apache-2.0
----
-
-# Configuration
-
-`title`: _string_
-Display title for the Space
-
-`emoji`: _string_
-Space emoji (emoji-only character allowed)
-
-`colorFrom`: _string_
-Color for Thumbnail gradient (red, yellow, green, blue, indigo, purple, pink, gray)
-
-`colorTo`: _string_
-Color for Thumbnail gradient (red, yellow, green, blue, indigo, purple, pink, gray)
-
-`sdk`: _string_
-Can be either `gradio`, `streamlit`, or `static`
-
-`sdk_version` : _string_
-Only applicable for `streamlit` SDK.
-See [doc](https://hf.co/docs/hub/spaces) for more info on supported versions.
-
-`app_file`: _string_
-Path to your main application file (which contains either `gradio` or `streamlit` Python code, or `static` html code).
-Path is relative to the root of the repository.
-
-`models`: _List[string]_
-HF model IDs (like "gpt2" or "deepset/roberta-base-squad2") used in the Space.
-Will be parsed automatically from your code if not specified here.
-
-`datasets`: _List[string]_
-HF dataset IDs (like "common_voice" or "oscar-corpus/OSCAR-2109") used in the Space.
-Will be parsed automatically from your code if not specified here.
-
-`pinned`: _boolean_
-Whether the Space stays on top of your list.
diff --git a/spaces/Salesforce/EDICT/my_half_diffusers/pipelines/ddim/__init__.py b/spaces/Salesforce/EDICT/my_half_diffusers/pipelines/ddim/__init__.py
deleted file mode 100644
index 8fd31868a88ac0d9ec7118574f21a9d8a1d4069b..0000000000000000000000000000000000000000
--- a/spaces/Salesforce/EDICT/my_half_diffusers/pipelines/ddim/__init__.py
+++ /dev/null
@@ -1,2 +0,0 @@
-# flake8: noqa
-from .pipeline_ddim import DDIMPipeline
diff --git a/spaces/Salesforce/EDICT/my_half_diffusers/schedulers/__init__.py b/spaces/Salesforce/EDICT/my_half_diffusers/schedulers/__init__.py
deleted file mode 100644
index 20c25f35183faeeef2cd7b5095f80a70a9edac01..0000000000000000000000000000000000000000
--- a/spaces/Salesforce/EDICT/my_half_diffusers/schedulers/__init__.py
+++ /dev/null
@@ -1,28 +0,0 @@
-# Copyright 2022 The HuggingFace Team. All rights reserved.
-#
-# Licensed under the Apache License, Version 2.0 (the "License");
-# you may not use this file except in compliance with the License.
-# You may obtain a copy of the License at
-#
-# http://www.apache.org/licenses/LICENSE-2.0
-#
-# Unless required by applicable law or agreed to in writing, software
-# distributed under the License is distributed on an "AS IS" BASIS,
-# WITHOUT WARRANTIES OR CONDITIONS OF ANY KIND, either express or implied.
-# See the License for the specific language governing permissions and
-# limitations under the License.
-
-from ..utils import is_scipy_available
-from .scheduling_ddim import DDIMScheduler
-from .scheduling_ddpm import DDPMScheduler
-from .scheduling_karras_ve import KarrasVeScheduler
-from .scheduling_pndm import PNDMScheduler
-from .scheduling_sde_ve import ScoreSdeVeScheduler
-from .scheduling_sde_vp import ScoreSdeVpScheduler
-from .scheduling_utils import SchedulerMixin
-
-
-if is_scipy_available():
- from .scheduling_lms_discrete import LMSDiscreteScheduler
-else:
- from ..utils.dummy_scipy_objects import * # noqa F403
diff --git a/spaces/Sarst/VITS-Umamusume-voice-synthesizer2/text/sanskrit.py b/spaces/Sarst/VITS-Umamusume-voice-synthesizer2/text/sanskrit.py
deleted file mode 100644
index 0223aaac384a2f850f5bc20651fc18eb964607d0..0000000000000000000000000000000000000000
--- a/spaces/Sarst/VITS-Umamusume-voice-synthesizer2/text/sanskrit.py
+++ /dev/null
@@ -1,62 +0,0 @@
-import re
-from indic_transliteration import sanscript
-
-
-# List of (iast, ipa) pairs:
-_iast_to_ipa = [(re.compile('%s' % x[0]), x[1]) for x in [
- ('a', 'ə'),
- ('ā', 'aː'),
- ('ī', 'iː'),
- ('ū', 'uː'),
- ('ṛ', 'ɹ`'),
- ('ṝ', 'ɹ`ː'),
- ('ḷ', 'l`'),
- ('ḹ', 'l`ː'),
- ('e', 'eː'),
- ('o', 'oː'),
- ('k', 'k⁼'),
- ('k⁼h', 'kʰ'),
- ('g', 'g⁼'),
- ('g⁼h', 'gʰ'),
- ('ṅ', 'ŋ'),
- ('c', 'ʧ⁼'),
- ('ʧ⁼h', 'ʧʰ'),
- ('j', 'ʥ⁼'),
- ('ʥ⁼h', 'ʥʰ'),
- ('ñ', 'n^'),
- ('ṭ', 't`⁼'),
- ('t`⁼h', 't`ʰ'),
- ('ḍ', 'd`⁼'),
- ('d`⁼h', 'd`ʰ'),
- ('ṇ', 'n`'),
- ('t', 't⁼'),
- ('t⁼h', 'tʰ'),
- ('d', 'd⁼'),
- ('d⁼h', 'dʰ'),
- ('p', 'p⁼'),
- ('p⁼h', 'pʰ'),
- ('b', 'b⁼'),
- ('b⁼h', 'bʰ'),
- ('y', 'j'),
- ('ś', 'ʃ'),
- ('ṣ', 's`'),
- ('r', 'ɾ'),
- ('l̤', 'l`'),
- ('h', 'ɦ'),
- ("'", ''),
- ('~', '^'),
- ('ṃ', '^')
-]]
-
-
-def devanagari_to_ipa(text):
- text = text.replace('ॐ', 'ओम्')
- text = re.sub(r'\s*।\s*$', '.', text)
- text = re.sub(r'\s*।\s*', ', ', text)
- text = re.sub(r'\s*॥', '.', text)
- text = sanscript.transliterate(text, sanscript.DEVANAGARI, sanscript.IAST)
- for regex, replacement in _iast_to_ipa:
- text = re.sub(regex, replacement, text)
- text = re.sub('(.)[`ː]*ḥ', lambda x: x.group(0)
- [:-1]+'h'+x.group(1)+'*', text)
- return text
diff --git a/spaces/SeViLA/SeViLA/lavis/datasets/builders/classification_builder.py b/spaces/SeViLA/SeViLA/lavis/datasets/builders/classification_builder.py
deleted file mode 100644
index 1fa4787bea4eae08114f12112ada29f7105ec686..0000000000000000000000000000000000000000
--- a/spaces/SeViLA/SeViLA/lavis/datasets/builders/classification_builder.py
+++ /dev/null
@@ -1,27 +0,0 @@
-"""
- Copyright (c) 2022, salesforce.com, inc.
- All rights reserved.
- SPDX-License-Identifier: BSD-3-Clause
- For full license text, see the LICENSE file in the repo root or https://opensource.org/licenses/BSD-3-Clause
-"""
-
-from lavis.common.registry import registry
-from lavis.datasets.builders.base_dataset_builder import BaseDatasetBuilder
-from lavis.datasets.datasets.nlvr_datasets import NLVRDataset, NLVREvalDataset
-from lavis.datasets.datasets.snli_ve_datasets import SNLIVisualEntialmentDataset
-
-
-@registry.register_builder("nlvr")
-class NLVRBuilder(BaseDatasetBuilder):
- train_dataset_cls = NLVRDataset
- eval_dataset_cls = NLVREvalDataset
-
- DATASET_CONFIG_DICT = {"default": "configs/datasets/nlvr/defaults.yaml"}
-
-
-@registry.register_builder("snli_ve")
-class SNLIVisualEntailmentBuilder(BaseDatasetBuilder):
- train_dataset_cls = SNLIVisualEntialmentDataset
- eval_dataset_cls = SNLIVisualEntialmentDataset
-
- DATASET_CONFIG_DICT = {"default": "configs/datasets/snli_ve/defaults.yaml"}
diff --git a/spaces/SeyedAli/Multilingual-Text-Similarity/README.md b/spaces/SeyedAli/Multilingual-Text-Similarity/README.md
deleted file mode 100644
index 0677f894d25f906823dafeb4c12884ffab182c1c..0000000000000000000000000000000000000000
--- a/spaces/SeyedAli/Multilingual-Text-Similarity/README.md
+++ /dev/null
@@ -1,13 +0,0 @@
----
-title: Multilingual Text Similarity
-emoji: 📝🆚📝
-colorFrom: purple
-colorTo: red
-sdk: gradio
-sdk_version: 3.44.4
-app_file: app.py
-pinned: false
-license: mit
----
-
-Check out the configuration reference at https://huggingface.co/docs/hub/spaces-config-reference
diff --git a/spaces/Silentlin/DiffSinger/inference/svs/opencpop/map.py b/spaces/Silentlin/DiffSinger/inference/svs/opencpop/map.py
deleted file mode 100644
index 37d5d0b8a43f88293c73362d75c591e51ec82aee..0000000000000000000000000000000000000000
--- a/spaces/Silentlin/DiffSinger/inference/svs/opencpop/map.py
+++ /dev/null
@@ -1,8 +0,0 @@
-def cpop_pinyin2ph_func():
- # In the README file of opencpop dataset, they defined a "pinyin to phoneme mapping table"
- pinyin2phs = {'AP': 'AP', 'SP': 'SP'}
- with open('inference/svs/opencpop/cpop_pinyin2ph.txt') as rf:
- for line in rf.readlines():
- elements = [x.strip() for x in line.split('|') if x.strip() != '']
- pinyin2phs[elements[0]] = elements[1]
- return pinyin2phs
\ No newline at end of file
diff --git a/spaces/SouthCity/ShuruiXu/crazy_functions/test_project/cpp/cppipc/prod_cons.h b/spaces/SouthCity/ShuruiXu/crazy_functions/test_project/cpp/cppipc/prod_cons.h
deleted file mode 100644
index c9004bb8043a12e32814436baa6262a00c8ef68e..0000000000000000000000000000000000000000
--- a/spaces/SouthCity/ShuruiXu/crazy_functions/test_project/cpp/cppipc/prod_cons.h
+++ /dev/null
@@ -1,433 +0,0 @@
-#pragma once
-
-#include
-#include
-#include
-#include
-#include
-
-#include "libipc/def.h"
-
-#include "libipc/platform/detail.h"
-#include "libipc/circ/elem_def.h"
-#include "libipc/utility/log.h"
-#include "libipc/utility/utility.h"
-
-namespace ipc {
-
-////////////////////////////////////////////////////////////////
-/// producer-consumer implementation
-////////////////////////////////////////////////////////////////
-
-template
-struct prod_cons_impl;
-
-template <>
-struct prod_cons_impl> {
-
- template
- struct elem_t {
- std::aligned_storage_t data_ {};
- };
-
- alignas(cache_line_size) std::atomic rd_; // read index
- alignas(cache_line_size) std::atomic wt_; // write index
-
- constexpr circ::u2_t cursor() const noexcept {
- return 0;
- }
-
- template
- bool push(W* /*wrapper*/, F&& f, E* elems) {
- auto cur_wt = circ::index_of(wt_.load(std::memory_order_relaxed));
- if (cur_wt == circ::index_of(rd_.load(std::memory_order_acquire) - 1)) {
- return false; // full
- }
- std::forward(f)(&(elems[cur_wt].data_));
- wt_.fetch_add(1, std::memory_order_release);
- return true;
- }
-
- /**
- * In single-single-unicast, 'force_push' means 'no reader' or 'the only one reader is dead'.
- * So we could just disconnect all connections of receiver, and return false.
- */
- template
- bool force_push(W* wrapper, F&&, E*) {
- wrapper->elems()->disconnect_receiver(~static_cast(0u));
- return false;
- }
-
- template
- bool pop(W* /*wrapper*/, circ::u2_t& /*cur*/, F&& f, R&& out, E* elems) {
- auto cur_rd = circ::index_of(rd_.load(std::memory_order_relaxed));
- if (cur_rd == circ::index_of(wt_.load(std::memory_order_acquire))) {
- return false; // empty
- }
- std::forward(f)(&(elems[cur_rd].data_));
- std::forward(out)(true);
- rd_.fetch_add(1, std::memory_order_release);
- return true;
- }
-};
-
-template <>
-struct prod_cons_impl>
- : prod_cons_impl> {
-
- template
- bool force_push(W* wrapper, F&&, E*) {
- wrapper->elems()->disconnect_receiver(1);
- return false;
- }
-
- template class E, std::size_t DS, std::size_t AS>
- bool pop(W* /*wrapper*/, circ::u2_t& /*cur*/, F&& f, R&& out, E* elems) {
- byte_t buff[DS];
- for (unsigned k = 0;;) {
- auto cur_rd = rd_.load(std::memory_order_relaxed);
- if (circ::index_of(cur_rd) ==
- circ::index_of(wt_.load(std::memory_order_acquire))) {
- return false; // empty
- }
- std::memcpy(buff, &(elems[circ::index_of(cur_rd)].data_), sizeof(buff));
- if (rd_.compare_exchange_weak(cur_rd, cur_rd + 1, std::memory_order_release)) {
- std::forward(f)(buff);
- std::forward(out)(true);
- return true;
- }
- ipc::yield(k);
- }
- }
-};
-
-template <>
-struct prod_cons_impl>
- : prod_cons_impl> {
-
- using flag_t = std::uint64_t;
-
- template
- struct elem_t {
- std::aligned_storage_t data_ {};
- std::atomic f_ct_ { 0 }; // commit flag
- };
-
- alignas(cache_line_size) std::atomic ct_; // commit index
-
- template
- bool push(W* /*wrapper*/, F&& f, E* elems) {
- circ::u2_t cur_ct, nxt_ct;
- for (unsigned k = 0;;) {
- cur_ct = ct_.load(std::memory_order_relaxed);
- if (circ::index_of(nxt_ct = cur_ct + 1) ==
- circ::index_of(rd_.load(std::memory_order_acquire))) {
- return false; // full
- }
- if (ct_.compare_exchange_weak(cur_ct, nxt_ct, std::memory_order_acq_rel)) {
- break;
- }
- ipc::yield(k);
- }
- auto* el = elems + circ::index_of(cur_ct);
- std::forward(f)(&(el->data_));
- // set flag & try update wt
- el->f_ct_.store(~static_cast(cur_ct), std::memory_order_release);
- while (1) {
- auto cac_ct = el->f_ct_.load(std::memory_order_acquire);
- if (cur_ct != wt_.load(std::memory_order_relaxed)) {
- return true;
- }
- if ((~cac_ct) != cur_ct) {
- return true;
- }
- if (!el->f_ct_.compare_exchange_strong(cac_ct, 0, std::memory_order_relaxed)) {
- return true;
- }
- wt_.store(nxt_ct, std::memory_order_release);
- cur_ct = nxt_ct;
- nxt_ct = cur_ct + 1;
- el = elems + circ::index_of(cur_ct);
- }
- return true;
- }
-
- template
- bool force_push(W* wrapper, F&&, E*) {
- wrapper->elems()->disconnect_receiver(1);
- return false;
- }
-
- template class E, std::size_t DS, std::size_t AS>
- bool pop(W* /*wrapper*/, circ::u2_t& /*cur*/, F&& f, R&& out, E* elems) {
- byte_t buff[DS];
- for (unsigned k = 0;;) {
- auto cur_rd = rd_.load(std::memory_order_relaxed);
- auto cur_wt = wt_.load(std::memory_order_acquire);
- auto id_rd = circ::index_of(cur_rd);
- auto id_wt = circ::index_of(cur_wt);
- if (id_rd == id_wt) {
- auto* el = elems + id_wt;
- auto cac_ct = el->f_ct_.load(std::memory_order_acquire);
- if ((~cac_ct) != cur_wt) {
- return false; // empty
- }
- if (el->f_ct_.compare_exchange_weak(cac_ct, 0, std::memory_order_relaxed)) {
- wt_.store(cur_wt + 1, std::memory_order_release);
- }
- k = 0;
- }
- else {
- std::memcpy(buff, &(elems[circ::index_of(cur_rd)].data_), sizeof(buff));
- if (rd_.compare_exchange_weak(cur_rd, cur_rd + 1, std::memory_order_release)) {
- std::forward(f)(buff);
- std::forward(out)(true);
- return true;
- }
- ipc::yield(k);
- }
- }
- }
-};
-
-template <>
-struct prod_cons_impl> {
-
- using rc_t = std::uint64_t;
-
- enum : rc_t {
- ep_mask = 0x00000000ffffffffull,
- ep_incr = 0x0000000100000000ull
- };
-
- template
- struct elem_t {
- std::aligned_storage_t data_ {};
- std::atomic rc_ { 0 }; // read-counter
- };
-
- alignas(cache_line_size) std::atomic wt_; // write index
- alignas(cache_line_size) rc_t epoch_ { 0 }; // only one writer
-
- circ::u2_t cursor() const noexcept {
- return wt_.load(std::memory_order_acquire);
- }
-
- template
- bool push(W* wrapper, F&& f, E* elems) {
- E* el;
- for (unsigned k = 0;;) {
- circ::cc_t cc = wrapper->elems()->connections(std::memory_order_relaxed);
- if (cc == 0) return false; // no reader
- el = elems + circ::index_of(wt_.load(std::memory_order_relaxed));
- // check all consumers have finished reading this element
- auto cur_rc = el->rc_.load(std::memory_order_acquire);
- circ::cc_t rem_cc = cur_rc & ep_mask;
- if ((cc & rem_cc) && ((cur_rc & ~ep_mask) == epoch_)) {
- return false; // has not finished yet
- }
- // consider rem_cc to be 0 here
- if (el->rc_.compare_exchange_weak(
- cur_rc, epoch_ | static_cast(cc), std::memory_order_release)) {
- break;
- }
- ipc::yield(k);
- }
- std::forward(f)(&(el->data_));
- wt_.fetch_add(1, std::memory_order_release);
- return true;
- }
-
- template
- bool force_push(W* wrapper, F&& f, E* elems) {
- E* el;
- epoch_ += ep_incr;
- for (unsigned k = 0;;) {
- circ::cc_t cc = wrapper->elems()->connections(std::memory_order_relaxed);
- if (cc == 0) return false; // no reader
- el = elems + circ::index_of(wt_.load(std::memory_order_relaxed));
- // check all consumers have finished reading this element
- auto cur_rc = el->rc_.load(std::memory_order_acquire);
- circ::cc_t rem_cc = cur_rc & ep_mask;
- if (cc & rem_cc) {
- ipc::log("force_push: k = %u, cc = %u, rem_cc = %u\n", k, cc, rem_cc);
- cc = wrapper->elems()->disconnect_receiver(rem_cc); // disconnect all invalid readers
- if (cc == 0) return false; // no reader
- }
- // just compare & exchange
- if (el->rc_.compare_exchange_weak(
- cur_rc, epoch_ | static_cast(cc), std::memory_order_release)) {
- break;
- }
- ipc::yield(k);
- }
- std::forward(f)(&(el->data_));
- wt_.fetch_add(1, std::memory_order_release);
- return true;
- }
-
- template
- bool pop(W* wrapper, circ::u2_t& cur, F&& f, R&& out, E* elems) {
- if (cur == cursor()) return false; // acquire
- auto* el = elems + circ::index_of(cur++);
- std::forward(f)(&(el->data_));
- for (unsigned k = 0;;) {
- auto cur_rc = el->rc_.load(std::memory_order_acquire);
- if ((cur_rc & ep_mask) == 0) {
- std::forward(out)(true);
- return true;
- }
- auto nxt_rc = cur_rc & ~static_cast(wrapper->connected_id());
- if (el->rc_.compare_exchange_weak(cur_rc, nxt_rc, std::memory_order_release)) {
- std::forward(out)((nxt_rc & ep_mask) == 0);
- return true;
- }
- ipc::yield(k);
- }
- }
-};
-
-template <>
-struct prod_cons_impl> {
-
- using rc_t = std::uint64_t;
- using flag_t = std::uint64_t;
-
- enum : rc_t {
- rc_mask = 0x00000000ffffffffull,
- ep_mask = 0x00ffffffffffffffull,
- ep_incr = 0x0100000000000000ull,
- ic_mask = 0xff000000ffffffffull,
- ic_incr = 0x0000000100000000ull
- };
-
- template
- struct elem_t {
- std::aligned_storage_t data_ {};
- std::atomic rc_ { 0 }; // read-counter
- std::atomic f_ct_ { 0 }; // commit flag
- };
-
- alignas(cache_line_size) std::atomic ct_; // commit index
- alignas(cache_line_size) std::atomic epoch_ { 0 };
-
- circ::u2_t cursor() const noexcept {
- return ct_.load(std::memory_order_acquire);
- }
-
- constexpr static rc_t inc_rc(rc_t rc) noexcept {
- return (rc & ic_mask) | ((rc + ic_incr) & ~ic_mask);
- }
-
- constexpr static rc_t inc_mask(rc_t rc) noexcept {
- return inc_rc(rc) & ~rc_mask;
- }
-
- template
- bool push(W* wrapper, F&& f, E* elems) {
- E* el;
- circ::u2_t cur_ct;
- rc_t epoch = epoch_.load(std::memory_order_acquire);
- for (unsigned k = 0;;) {
- circ::cc_t cc = wrapper->elems()->connections(std::memory_order_relaxed);
- if (cc == 0) return false; // no reader
- el = elems + circ::index_of(cur_ct = ct_.load(std::memory_order_relaxed));
- // check all consumers have finished reading this element
- auto cur_rc = el->rc_.load(std::memory_order_relaxed);
- circ::cc_t rem_cc = cur_rc & rc_mask;
- if ((cc & rem_cc) && ((cur_rc & ~ep_mask) == epoch)) {
- return false; // has not finished yet
- }
- else if (!rem_cc) {
- auto cur_fl = el->f_ct_.load(std::memory_order_acquire);
- if ((cur_fl != cur_ct) && cur_fl) {
- return false; // full
- }
- }
- // consider rem_cc to be 0 here
- if (el->rc_.compare_exchange_weak(
- cur_rc, inc_mask(epoch | (cur_rc & ep_mask)) | static_cast(cc), std::memory_order_relaxed) &&
- epoch_.compare_exchange_weak(epoch, epoch, std::memory_order_acq_rel)) {
- break;
- }
- ipc::yield(k);
- }
- // only one thread/process would touch here at one time
- ct_.store(cur_ct + 1, std::memory_order_release);
- std::forward(f)(&(el->data_));
- // set flag & try update wt
- el->f_ct_.store(~static_cast(cur_ct), std::memory_order_release);
- return true;
- }
-
- template
- bool force_push(W* wrapper, F&& f, E* elems) {
- E* el;
- circ::u2_t cur_ct;
- rc_t epoch = epoch_.fetch_add(ep_incr, std::memory_order_release) + ep_incr;
- for (unsigned k = 0;;) {
- circ::cc_t cc = wrapper->elems()->connections(std::memory_order_relaxed);
- if (cc == 0) return false; // no reader
- el = elems + circ::index_of(cur_ct = ct_.load(std::memory_order_relaxed));
- // check all consumers have finished reading this element
- auto cur_rc = el->rc_.load(std::memory_order_acquire);
- circ::cc_t rem_cc = cur_rc & rc_mask;
- if (cc & rem_cc) {
- ipc::log("force_push: k = %u, cc = %u, rem_cc = %u\n", k, cc, rem_cc);
- cc = wrapper->elems()->disconnect_receiver(rem_cc); // disconnect all invalid readers
- if (cc == 0) return false; // no reader
- }
- // just compare & exchange
- if (el->rc_.compare_exchange_weak(
- cur_rc, inc_mask(epoch | (cur_rc & ep_mask)) | static_cast(cc), std::memory_order_relaxed)) {
- if (epoch == epoch_.load(std::memory_order_acquire)) {
- break;
- }
- else if (push(wrapper, std::forward(f), elems)) {
- return true;
- }
- epoch = epoch_.fetch_add(ep_incr, std::memory_order_release) + ep_incr;
- }
- ipc::yield(k);
- }
- // only one thread/process would touch here at one time
- ct_.store(cur_ct + 1, std::memory_order_release);
- std::forward(f)(&(el->data_));
- // set flag & try update wt
- el->f_ct_.store(~static_cast(cur_ct), std::memory_order_release);
- return true;
- }
-
- template
- bool pop(W* wrapper, circ::u2_t& cur, F&& f, R&& out, E(& elems)[N]) {
- auto* el = elems + circ::index_of(cur);
- auto cur_fl = el->f_ct_.load(std::memory_order_acquire);
- if (cur_fl != ~static_cast(cur)) {
- return false; // empty
- }
- ++cur;
- std::forward(f)(&(el->data_));
- for (unsigned k = 0;;) {
- auto cur_rc = el->rc_.load(std::memory_order_acquire);
- if ((cur_rc & rc_mask) == 0) {
- std::forward(out)(true);
- el->f_ct_.store(cur + N - 1, std::memory_order_release);
- return true;
- }
- auto nxt_rc = inc_rc(cur_rc) & ~static_cast(wrapper->connected_id());
- bool last_one = false;
- if ((last_one = (nxt_rc & rc_mask) == 0)) {
- el->f_ct_.store(cur + N - 1, std::memory_order_release);
- }
- if (el->rc_.compare_exchange_weak(cur_rc, nxt_rc, std::memory_order_release)) {
- std::forward(out)(last_one);
- return true;
- }
- ipc::yield(k);
- }
- }
-};
-
-} // namespace ipc
diff --git a/spaces/Spark808/rvc-demo/config.py b/spaces/Spark808/rvc-demo/config.py
deleted file mode 100644
index c0c16e0017efbcaf250cb539a1d0edb4e83575e4..0000000000000000000000000000000000000000
--- a/spaces/Spark808/rvc-demo/config.py
+++ /dev/null
@@ -1,88 +0,0 @@
-########################硬件参数########################
-
-# 填写cuda:x, cpu 或 mps, x指代第几张卡,只支持 N卡 / Apple Silicon 加速
-device = "cuda:0"
-
-# 9-10-20-30-40系显卡无脑True,不影响质量,>=20显卡开启有加速
-is_half = True
-
-# 默认0用上所有线程,写数字限制CPU资源使用
-n_cpu = 0
-
-########################硬件参数########################
-
-
-##################下为参数处理逻辑,勿动##################
-
-########################命令行参数########################
-import argparse
-
-parser = argparse.ArgumentParser()
-parser.add_argument("--port", type=int, default=7865, help="Listen port")
-parser.add_argument("--pycmd", type=str, default="python", help="Python command")
-parser.add_argument("--colab", action="store_true", help="Launch in colab")
-parser.add_argument(
- "--noparallel", action="store_true", help="Disable parallel processing"
-)
-parser.add_argument(
- "--noautoopen", action="store_true", help="Do not open in browser automatically"
-)
-cmd_opts, unknown = parser.parse_known_args()
-
-python_cmd = cmd_opts.pycmd
-listen_port = cmd_opts.port
-iscolab = cmd_opts.colab
-noparallel = cmd_opts.noparallel
-noautoopen = cmd_opts.noautoopen
-########################命令行参数########################
-
-import sys
-import torch
-
-
-# has_mps is only available in nightly pytorch (for now) and MasOS 12.3+.
-# check `getattr` and try it for compatibility
-def has_mps() -> bool:
- if sys.platform != "darwin":
- return False
- else:
- if not getattr(torch, "has_mps", False):
- return False
- try:
- torch.zeros(1).to(torch.device("mps"))
- return True
- except Exception:
- return False
-
-
-if not torch.cuda.is_available():
- if has_mps():
- print("没有发现支持的N卡, 使用MPS进行推理")
- device = "mps"
- else:
- print("没有发现支持的N卡, 使用CPU进行推理")
- device = "cpu"
- is_half = False
-
-if device not in ["cpu", "mps"]:
- gpu_name = torch.cuda.get_device_name(int(device.split(":")[-1]))
- if "16" in gpu_name or "MX" in gpu_name:
- print("16系显卡/MX系显卡强制单精度")
- is_half = False
-
-from multiprocessing import cpu_count
-
-if n_cpu == 0:
- n_cpu = cpu_count()
-if is_half:
- # 6G显存配置
- x_pad = 3
- x_query = 10
- x_center = 60
- x_max = 65
-else:
- # 5G显存配置
- x_pad = 1
- x_query = 6
- x_center = 38
- x_max = 41
diff --git a/spaces/Starcodium/README/README.md b/spaces/Starcodium/README/README.md
deleted file mode 100644
index da773cdd3a0a1cd462747db1f16b6235db4f6efb..0000000000000000000000000000000000000000
--- a/spaces/Starcodium/README/README.md
+++ /dev/null
@@ -1,64 +0,0 @@
----
-title: README
-emoji: 💻
-colorFrom: pink
-thumbnail: https://imgur.com/a/AxhpUlp
-colorTo: yellow
-sdk: static
-pinned: false
----
-
-
-
-
-
-
-
-## Welcome to Starcodium Community
-
-Welcome to the vibrant community of Starcodium! Here, we share exciting updates on newly developed and trained AI models. Our current star is the remarkable AI companion, "Vergil," who actively engages with our community on our Discord server.
-
-## Discover What Awaits
-
-At Starcodium, we house a collection of diverse AI models, each trained with a unique approach. As of now, we specialize in text-generation models that are fine-tuned on conversational datasets which bring delightful interactions. With your invaluable support and feedback, we aim to expand our repertoire by developing and training models with different functionalities to keep you thoroughly entertained.
-
-## Meet Our Team
-
-Organization Leader
-- Username: AP
-- Discord: Aubrie#0727
-- YouTube: [Aubrie's YouTube Channel](https://www.youtube.com/@aubriep)
-- Github: [Aubrie's Github](https://github.com/AubrienPippin)
-- HuggingFace: [Aubrie on HuggingFace](https://huggingface.co/aubrie)
-
-AI Researcher/Software Developer
-- Username: SpeedStar101
-- Discord: SpeedStar101#0101
-- YouTube: [SpeedStar101's YouTube Channel](https://www.youtube.com/@SpeedStar101)
-- Github: [SpeedStar101's Github](https://github.com/SpeedStar1O1)
-- HuggingFace: [SpeedStar101 on HuggingFace](https://huggingface.co/SpeedStar101)
-
-App Testers
-- Username: GhostFace4606
-- Discord: Ghost4606#8895
-
-- Username: Tekio
-- Discord: ʞɔıɟɹǝl⋊#1878
-
-## Join the Starcodium Community
-
-Welcome to our thriving Discord server! If you haven't joined us yet, we invite you to become part of our engaging community. Click the link below to join:
-
-[Starcodium Server](https://discord.com/invite/qauyxubB7a)
-
-## Become an App Tester
-
-At Starcodium, we value the contributions of our dedicated community members. By becoming an App Tester, you'll gain exclusive privileges and play a crucial role in shaping our future endeavors.
-
-As an esteemed App Tester, you will be granted the prestigious app tester role in our main server. Moreover, you'll have the exciting opportunity to join our Testing Ground Server—a dedicated space where we put our cutting-edge Discord Bots and AI Models through rigorous testing and refinement.
-
-Join us today, and unlock a world of possibilities. Elevate your involvement, collaborate with like-minded individuals, and help us shape the future of AI innovation. Let's embark on this exciting journey together!
-
-## Thank you
-
-We're thrilled to have you join our community at Starcodium, and we look forward to creating captivating AI experiences together. Explore, engage, and let's embark on an incredible journey into the realm of AI innovation!
\ No newline at end of file
diff --git a/spaces/SungBeom/chatwine-korean/.venv/Lib/site-packages/debugpy/_vendored/pydevd/_pydev_bundle/pydev_monkey.py b/spaces/SungBeom/chatwine-korean/.venv/Lib/site-packages/debugpy/_vendored/pydevd/_pydev_bundle/pydev_monkey.py
deleted file mode 100644
index d5fe01d2a2dab89ef79c9de152556fa113ce205d..0000000000000000000000000000000000000000
--- a/spaces/SungBeom/chatwine-korean/.venv/Lib/site-packages/debugpy/_vendored/pydevd/_pydev_bundle/pydev_monkey.py
+++ /dev/null
@@ -1,1246 +0,0 @@
-# License: EPL
-import os
-import re
-import sys
-from _pydev_bundle._pydev_saved_modules import threading
-from _pydevd_bundle.pydevd_constants import get_global_debugger, IS_WINDOWS, IS_JYTHON, get_current_thread_id, \
- sorted_dict_repr, set_global_debugger, DebugInfoHolder
-from _pydev_bundle import pydev_log
-from contextlib import contextmanager
-from _pydevd_bundle import pydevd_constants, pydevd_defaults
-from _pydevd_bundle.pydevd_defaults import PydevdCustomization
-import ast
-
-try:
- from pathlib import Path
-except ImportError:
- Path = None
-
-#===============================================================================
-# Things that are dependent on having the pydevd debugger
-#===============================================================================
-
-pydev_src_dir = os.path.dirname(os.path.dirname(__file__))
-
-_arg_patch = threading.local()
-
-
-@contextmanager
-def skip_subprocess_arg_patch():
- _arg_patch.apply_arg_patching = False
- try:
- yield
- finally:
- _arg_patch.apply_arg_patching = True
-
-
-def _get_apply_arg_patching():
- return getattr(_arg_patch, 'apply_arg_patching', True)
-
-
-def _get_setup_updated_with_protocol_and_ppid(setup, is_exec=False):
- if setup is None:
- setup = {}
- setup = setup.copy()
- # Discard anything related to the protocol (we'll set the the protocol based on the one
- # currently set).
- setup.pop(pydevd_constants.ARGUMENT_HTTP_JSON_PROTOCOL, None)
- setup.pop(pydevd_constants.ARGUMENT_JSON_PROTOCOL, None)
- setup.pop(pydevd_constants.ARGUMENT_QUOTED_LINE_PROTOCOL, None)
-
- if not is_exec:
- # i.e.: The ppid for the subprocess is the current pid.
- # If it's an exec, keep it what it was.
- setup[pydevd_constants.ARGUMENT_PPID] = os.getpid()
-
- protocol = pydevd_constants.get_protocol()
- if protocol == pydevd_constants.HTTP_JSON_PROTOCOL:
- setup[pydevd_constants.ARGUMENT_HTTP_JSON_PROTOCOL] = True
-
- elif protocol == pydevd_constants.JSON_PROTOCOL:
- setup[pydevd_constants.ARGUMENT_JSON_PROTOCOL] = True
-
- elif protocol == pydevd_constants.QUOTED_LINE_PROTOCOL:
- setup[pydevd_constants.ARGUMENT_QUOTED_LINE_PROTOCOL] = True
-
- elif protocol == pydevd_constants.HTTP_PROTOCOL:
- setup[pydevd_constants.ARGUMENT_HTTP_PROTOCOL] = True
-
- else:
- pydev_log.debug('Unexpected protocol: %s', protocol)
-
- mode = pydevd_defaults.PydevdCustomization.DEBUG_MODE
- if mode:
- setup['debug-mode'] = mode
-
- preimport = pydevd_defaults.PydevdCustomization.PREIMPORT
- if preimport:
- setup['preimport'] = preimport
-
- if DebugInfoHolder.PYDEVD_DEBUG_FILE:
- setup['log-file'] = DebugInfoHolder.PYDEVD_DEBUG_FILE
-
- if DebugInfoHolder.DEBUG_TRACE_LEVEL:
- setup['log-level'] = DebugInfoHolder.DEBUG_TRACE_LEVEL
-
- return setup
-
-
-class _LastFutureImportFinder(ast.NodeVisitor):
-
- def __init__(self):
- self.last_future_import_found = None
-
- def visit_ImportFrom(self, node):
- if node.module == '__future__':
- self.last_future_import_found = node
-
-
-def _get_offset_from_line_col(code, line, col):
- offset = 0
- for i, line_contents in enumerate(code.splitlines(True)):
- if i == line:
- offset += col
- return offset
- else:
- offset += len(line_contents)
-
- return -1
-
-
-def _separate_future_imports(code):
- '''
- :param code:
- The code from where we want to get the __future__ imports (note that it's possible that
- there's no such entry).
-
- :return tuple(str, str):
- The return is a tuple(future_import, code).
-
- If the future import is not available a return such as ('', code) is given, otherwise, the
- future import will end with a ';' (so that it can be put right before the pydevd attach
- code).
- '''
- try:
- node = ast.parse(code, '', 'exec')
- visitor = _LastFutureImportFinder()
- visitor.visit(node)
-
- if visitor.last_future_import_found is None:
- return '', code
-
- node = visitor.last_future_import_found
- offset = -1
- if hasattr(node, 'end_lineno') and hasattr(node, 'end_col_offset'):
- # Python 3.8 onwards has these (so, use when possible).
- line, col = node.end_lineno, node.end_col_offset
- offset = _get_offset_from_line_col(code, line - 1, col) # ast lines are 1-based, make it 0-based.
-
- else:
- # end line/col not available, let's just find the offset and then search
- # for the alias from there.
- line, col = node.lineno, node.col_offset
- offset = _get_offset_from_line_col(code, line - 1, col) # ast lines are 1-based, make it 0-based.
- if offset >= 0 and node.names:
- from_future_import_name = node.names[-1].name
- i = code.find(from_future_import_name, offset)
- if i < 0:
- offset = -1
- else:
- offset = i + len(from_future_import_name)
-
- if offset >= 0:
- for i in range(offset, len(code)):
- if code[i] in (' ', '\t', ';', ')', '\n'):
- offset += 1
- else:
- break
-
- future_import = code[:offset]
- code_remainder = code[offset:]
-
- # Now, put '\n' lines back into the code remainder (we had to search for
- # `\n)`, but in case we just got the `\n`, it should be at the remainder,
- # not at the future import.
- while future_import.endswith('\n'):
- future_import = future_import[:-1]
- code_remainder = '\n' + code_remainder
-
- if not future_import.endswith(';'):
- future_import += ';'
- return future_import, code_remainder
-
- # This shouldn't happen...
- pydev_log.info('Unable to find line %s in code:\n%r', line, code)
- return '', code
-
- except:
- pydev_log.exception('Error getting from __future__ imports from: %r', code)
- return '', code
-
-
-def _get_python_c_args(host, port, code, args, setup):
- setup = _get_setup_updated_with_protocol_and_ppid(setup)
-
- # i.e.: We want to make the repr sorted so that it works in tests.
- setup_repr = setup if setup is None else (sorted_dict_repr(setup))
-
- future_imports = ''
- if '__future__' in code:
- # If the code has a __future__ import, we need to be able to strip the __future__
- # imports from the code and add them to the start of our code snippet.
- future_imports, code = _separate_future_imports(code)
-
- return ("%simport sys; sys.path.insert(0, r'%s'); import pydevd; pydevd.config(%r, %r); "
- "pydevd.settrace(host=%r, port=%s, suspend=False, trace_only_current_thread=False, patch_multiprocessing=True, access_token=%r, client_access_token=%r, __setup_holder__=%s); "
- "%s"
- ) % (
- future_imports,
- pydev_src_dir,
- pydevd_constants.get_protocol(),
- PydevdCustomization.DEBUG_MODE,
- host,
- port,
- setup.get('access-token'),
- setup.get('client-access-token'),
- setup_repr,
- code)
-
-
-def _get_host_port():
- import pydevd
- host, port = pydevd.dispatch()
- return host, port
-
-
-def _is_managed_arg(arg):
- pydevd_py = _get_str_type_compatible(arg, 'pydevd.py')
- if arg.endswith(pydevd_py):
- return True
- return False
-
-
-def _on_forked_process(setup_tracing=True):
- pydevd_constants.after_fork()
- pydev_log.initialize_debug_stream(reinitialize=True)
-
- if setup_tracing:
- pydev_log.debug('pydevd on forked process: %s', os.getpid())
-
- import pydevd
- pydevd.threadingCurrentThread().__pydevd_main_thread = True
- pydevd.settrace_forked(setup_tracing=setup_tracing)
-
-
-def _on_set_trace_for_new_thread(global_debugger):
- if global_debugger is not None:
- global_debugger.enable_tracing()
-
-
-def _get_str_type_compatible(s, args):
- '''
- This method converts `args` to byte/unicode based on the `s' type.
- '''
- if isinstance(args, (list, tuple)):
- ret = []
- for arg in args:
- if type(s) == type(arg):
- ret.append(arg)
- else:
- if isinstance(s, bytes):
- ret.append(arg.encode('utf-8'))
- else:
- ret.append(arg.decode('utf-8'))
- return ret
- else:
- if type(s) == type(args):
- return args
- else:
- if isinstance(s, bytes):
- return args.encode('utf-8')
- else:
- return args.decode('utf-8')
-
-
-#===============================================================================
-# Things related to monkey-patching
-#===============================================================================
-def is_python(path):
- single_quote, double_quote = _get_str_type_compatible(path, ["'", '"'])
-
- if path.endswith(single_quote) or path.endswith(double_quote):
- path = path[1:len(path) - 1]
- filename = os.path.basename(path).lower()
- for name in _get_str_type_compatible(filename, ['python', 'jython', 'pypy']):
- if filename.find(name) != -1:
- return True
-
- return False
-
-
-class InvalidTypeInArgsException(Exception):
- pass
-
-
-def remove_quotes_from_args(args):
- if sys.platform == "win32":
- new_args = []
-
- for x in args:
- if Path is not None and isinstance(x, Path):
- x = str(x)
- else:
- if not isinstance(x, (bytes, str)):
- raise InvalidTypeInArgsException(str(type(x)))
-
- double_quote, two_double_quotes = _get_str_type_compatible(x, ['"', '""'])
-
- if x != two_double_quotes:
- if len(x) > 1 and x.startswith(double_quote) and x.endswith(double_quote):
- x = x[1:-1]
-
- new_args.append(x)
- return new_args
- else:
- new_args = []
- for x in args:
- if Path is not None and isinstance(x, Path):
- x = x.as_posix()
- else:
- if not isinstance(x, (bytes, str)):
- raise InvalidTypeInArgsException(str(type(x)))
- new_args.append(x)
-
- return new_args
-
-
-def quote_arg_win32(arg):
- fix_type = lambda x: _get_str_type_compatible(arg, x)
-
- # See if we need to quote at all - empty strings need quoting, as do strings
- # with whitespace or quotes in them. Backslashes do not need quoting.
- if arg and not set(arg).intersection(fix_type(' "\t\n\v')):
- return arg
-
- # Per https://docs.microsoft.com/en-us/windows/desktop/api/shellapi/nf-shellapi-commandlinetoargvw,
- # the standard way to interpret arguments in double quotes is as follows:
- #
- # 2N backslashes followed by a quotation mark produce N backslashes followed by
- # begin/end quote. This does not become part of the parsed argument, but toggles
- # the "in quotes" mode.
- #
- # 2N+1 backslashes followed by a quotation mark again produce N backslashes followed
- # by a quotation mark literal ("). This does not toggle the "in quotes" mode.
- #
- # N backslashes not followed by a quotation mark simply produce N backslashes.
- #
- # This code needs to do the reverse transformation, thus:
- #
- # N backslashes followed by " produce 2N+1 backslashes followed by "
- #
- # N backslashes at the end (i.e. where the closing " goes) produce 2N backslashes.
- #
- # N backslashes in any other position remain as is.
-
- arg = re.sub(fix_type(r'(\\*)\"'), fix_type(r'\1\1\\"'), arg)
- arg = re.sub(fix_type(r'(\\*)$'), fix_type(r'\1\1'), arg)
- return fix_type('"') + arg + fix_type('"')
-
-
-def quote_args(args):
- if sys.platform == "win32":
- return list(map(quote_arg_win32, args))
- else:
- return args
-
-
-def patch_args(args, is_exec=False):
- '''
- :param list args:
- Arguments to patch.
-
- :param bool is_exec:
- If it's an exec, the current process will be replaced (this means we have
- to keep the same ppid).
- '''
- try:
- pydev_log.debug("Patching args: %s", args)
- original_args = args
- try:
- unquoted_args = remove_quotes_from_args(args)
- except InvalidTypeInArgsException as e:
- pydev_log.info('Unable to monkey-patch subprocess arguments because a type found in the args is invalid: %s', e)
- return original_args
-
- # Internally we should reference original_args (if we want to return them) or unquoted_args
- # to add to the list which will be then quoted in the end.
- del args
-
- from pydevd import SetupHolder
- if not unquoted_args:
- return original_args
-
- if not is_python(unquoted_args[0]):
- pydev_log.debug("Process is not python, returning.")
- return original_args
-
- # Note: we create a copy as string to help with analyzing the arguments, but
- # the final list should have items from the unquoted_args as they were initially.
- args_as_str = _get_str_type_compatible('', unquoted_args)
-
- params_with_value_in_separate_arg = (
- '--check-hash-based-pycs',
- '--jit' # pypy option
- )
-
- # All short switches may be combined together. The ones below require a value and the
- # value itself may be embedded in the arg.
- #
- # i.e.: Python accepts things as:
- #
- # python -OQold -qmtest
- #
- # Which is the same as:
- #
- # python -O -Q old -q -m test
- #
- # or even:
- #
- # python -OQold "-vcimport sys;print(sys)"
- #
- # Which is the same as:
- #
- # python -O -Q old -v -c "import sys;print(sys)"
-
- params_with_combinable_arg = set(('W', 'X', 'Q', 'c', 'm'))
-
- module_name = None
- before_module_flag = ''
- module_name_i_start = -1
- module_name_i_end = -1
-
- code = None
- code_i = -1
- code_i_end = -1
- code_flag = ''
-
- filename = None
- filename_i = -1
-
- ignore_next = True # start ignoring the first (the first entry is the python executable)
- for i, arg_as_str in enumerate(args_as_str):
- if ignore_next:
- ignore_next = False
- continue
-
- if arg_as_str.startswith('-'):
- if arg_as_str == '-':
- # Contents will be read from the stdin. This is not currently handled.
- pydev_log.debug('Unable to fix arguments to attach debugger on subprocess when reading from stdin ("python ... -").')
- return original_args
-
- if arg_as_str.startswith(params_with_value_in_separate_arg):
- if arg_as_str in params_with_value_in_separate_arg:
- ignore_next = True
- continue
-
- break_out = False
- for j, c in enumerate(arg_as_str):
-
- # i.e.: Python supports -X faulthandler as well as -Xfaulthandler
- # (in one case we have to ignore the next and in the other we don't
- # have to ignore it).
- if c in params_with_combinable_arg:
- remainder = arg_as_str[j + 1:]
- if not remainder:
- ignore_next = True
-
- if c == 'm':
- # i.e.: Something as
- # python -qm test
- # python -m test
- # python -qmtest
- before_module_flag = arg_as_str[:j] # before_module_flag would then be "-q"
- if before_module_flag == '-':
- before_module_flag = ''
- module_name_i_start = i
- if not remainder:
- module_name = unquoted_args[i + 1]
- module_name_i_end = i + 1
- else:
- # i.e.: python -qmtest should provide 'test' as the module_name
- module_name = unquoted_args[i][j + 1:]
- module_name_i_end = module_name_i_start
- break_out = True
- break
-
- elif c == 'c':
- # i.e.: Something as
- # python -qc "import sys"
- # python -c "import sys"
- # python "-qcimport sys"
- code_flag = arg_as_str[:j + 1] # code_flag would then be "-qc"
-
- if not remainder:
- # arg_as_str is something as "-qc", "import sys"
- code = unquoted_args[i + 1]
- code_i_end = i + 2
- else:
- # if arg_as_str is something as "-qcimport sys"
- code = remainder # code would be "import sys"
- code_i_end = i + 1
- code_i = i
- break_out = True
- break
-
- else:
- break
-
- if break_out:
- break
-
- else:
- # It doesn't start with '-' and we didn't ignore this entry:
- # this means that this is the file to be executed.
- filename = unquoted_args[i]
-
- # Note that the filename is not validated here.
- # There are cases where even a .exe is valid (xonsh.exe):
- # https://github.com/microsoft/debugpy/issues/945
- # So, we should support whatever runpy.run_path
- # supports in this case.
-
- filename_i = i
-
- if _is_managed_arg(filename): # no need to add pydevd twice
- pydev_log.debug('Skipped monkey-patching as pydevd.py is in args already.')
- return original_args
-
- break
- else:
- # We didn't find the filename (something is unexpected).
- pydev_log.debug('Unable to fix arguments to attach debugger on subprocess (filename not found).')
- return original_args
-
- if code_i != -1:
- host, port = _get_host_port()
-
- if port is not None:
- new_args = []
- new_args.extend(unquoted_args[:code_i])
- new_args.append(code_flag)
- new_args.append(_get_python_c_args(host, port, code, unquoted_args, SetupHolder.setup))
- new_args.extend(unquoted_args[code_i_end:])
-
- return quote_args(new_args)
-
- first_non_vm_index = max(filename_i, module_name_i_start)
- if first_non_vm_index == -1:
- pydev_log.debug('Unable to fix arguments to attach debugger on subprocess (could not resolve filename nor module name).')
- return original_args
-
- # Original args should be something as:
- # ['X:\\pysrc\\pydevd.py', '--multiprocess', '--print-in-debugger-startup',
- # '--vm_type', 'python', '--client', '127.0.0.1', '--port', '56352', '--file', 'x:\\snippet1.py']
- from _pydevd_bundle.pydevd_command_line_handling import setup_to_argv
- new_args = []
- new_args.extend(unquoted_args[:first_non_vm_index])
- if before_module_flag:
- new_args.append(before_module_flag)
-
- add_module_at = len(new_args) + 1
-
- new_args.extend(setup_to_argv(
- _get_setup_updated_with_protocol_and_ppid(SetupHolder.setup, is_exec=is_exec),
- skip_names=set(('module', 'cmd-line'))
- ))
- new_args.append('--file')
-
- if module_name is not None:
- assert module_name_i_start != -1
- assert module_name_i_end != -1
- # Always after 'pydevd' (i.e.: pydevd "--module" --multiprocess ...)
- new_args.insert(add_module_at, '--module')
- new_args.append(module_name)
- new_args.extend(unquoted_args[module_name_i_end + 1:])
-
- elif filename is not None:
- assert filename_i != -1
- new_args.append(filename)
- new_args.extend(unquoted_args[filename_i + 1:])
-
- else:
- raise AssertionError('Internal error (unexpected condition)')
-
- return quote_args(new_args)
- except:
- pydev_log.exception('Error patching args (debugger not attached to subprocess).')
- return original_args
-
-
-def str_to_args_windows(args):
- # See https://docs.microsoft.com/en-us/cpp/c-language/parsing-c-command-line-arguments.
- #
- # Implemetation ported from DebugPlugin.parseArgumentsWindows:
- # https://github.com/eclipse/eclipse.platform.debug/blob/master/org.eclipse.debug.core/core/org/eclipse/debug/core/DebugPlugin.java
-
- result = []
-
- DEFAULT = 0
- ARG = 1
- IN_DOUBLE_QUOTE = 2
-
- state = DEFAULT
- backslashes = 0
- buf = ''
-
- args_len = len(args)
- for i in range(args_len):
- ch = args[i]
- if (ch == '\\'):
- backslashes += 1
- continue
- elif (backslashes != 0):
- if ch == '"':
- while backslashes >= 2:
- backslashes -= 2
- buf += '\\'
- if (backslashes == 1):
- if (state == DEFAULT):
- state = ARG
-
- buf += '"'
- backslashes = 0
- continue
- # else fall through to switch
- else:
- # false alarm, treat passed backslashes literally...
- if (state == DEFAULT):
- state = ARG
-
- while backslashes > 0:
- backslashes -= 1
- buf += '\\'
- # fall through to switch
- if ch in (' ', '\t'):
- if (state == DEFAULT):
- # skip
- continue
- elif (state == ARG):
- state = DEFAULT
- result.append(buf)
- buf = ''
- continue
-
- if state in (DEFAULT, ARG):
- if ch == '"':
- state = IN_DOUBLE_QUOTE
- else:
- state = ARG
- buf += ch
-
- elif state == IN_DOUBLE_QUOTE:
- if ch == '"':
- if (i + 1 < args_len and args[i + 1] == '"'):
- # Undocumented feature in Windows:
- # Two consecutive double quotes inside a double-quoted argument are interpreted as
- # a single double quote.
- buf += '"'
- i += 1
- else:
- state = ARG
- else:
- buf += ch
-
- else:
- raise RuntimeError('Illegal condition')
-
- if len(buf) > 0 or state != DEFAULT:
- result.append(buf)
-
- return result
-
-
-def patch_arg_str_win(arg_str):
- args = str_to_args_windows(arg_str)
- # Fix https://youtrack.jetbrains.com/issue/PY-9767 (args may be empty)
- if not args or not is_python(args[0]):
- return arg_str
- arg_str = ' '.join(patch_args(args))
- pydev_log.debug("New args: %s", arg_str)
- return arg_str
-
-
-def monkey_patch_module(module, funcname, create_func):
- if hasattr(module, funcname):
- original_name = 'original_' + funcname
- if not hasattr(module, original_name):
- setattr(module, original_name, getattr(module, funcname))
- setattr(module, funcname, create_func(original_name))
-
-
-def monkey_patch_os(funcname, create_func):
- monkey_patch_module(os, funcname, create_func)
-
-
-def warn_multiproc():
- pass # TODO: Provide logging as messages to the IDE.
- # pydev_log.error_once(
- # "pydev debugger: New process is launching (breakpoints won't work in the new process).\n"
- # "pydev debugger: To debug that process please enable 'Attach to subprocess automatically while debugging?' option in the debugger settings.\n")
- #
-
-
-def create_warn_multiproc(original_name):
-
- def new_warn_multiproc(*args, **kwargs):
- import os
-
- warn_multiproc()
-
- return getattr(os, original_name)(*args, **kwargs)
-
- return new_warn_multiproc
-
-
-def create_execl(original_name):
-
- def new_execl(path, *args):
- """
- os.execl(path, arg0, arg1, ...)
- os.execle(path, arg0, arg1, ..., env)
- os.execlp(file, arg0, arg1, ...)
- os.execlpe(file, arg0, arg1, ..., env)
- """
- if _get_apply_arg_patching():
- args = patch_args(args, is_exec=True)
- send_process_created_message()
- send_process_about_to_be_replaced()
-
- return getattr(os, original_name)(path, *args)
-
- return new_execl
-
-
-def create_execv(original_name):
-
- def new_execv(path, args):
- """
- os.execv(path, args)
- os.execvp(file, args)
- """
- if _get_apply_arg_patching():
- args = patch_args(args, is_exec=True)
- send_process_created_message()
- send_process_about_to_be_replaced()
-
- return getattr(os, original_name)(path, args)
-
- return new_execv
-
-
-def create_execve(original_name):
- """
- os.execve(path, args, env)
- os.execvpe(file, args, env)
- """
-
- def new_execve(path, args, env):
- if _get_apply_arg_patching():
- args = patch_args(args, is_exec=True)
- send_process_created_message()
- send_process_about_to_be_replaced()
-
- return getattr(os, original_name)(path, args, env)
-
- return new_execve
-
-
-def create_spawnl(original_name):
-
- def new_spawnl(mode, path, *args):
- """
- os.spawnl(mode, path, arg0, arg1, ...)
- os.spawnlp(mode, file, arg0, arg1, ...)
- """
- if _get_apply_arg_patching():
- args = patch_args(args)
- send_process_created_message()
-
- return getattr(os, original_name)(mode, path, *args)
-
- return new_spawnl
-
-
-def create_spawnv(original_name):
-
- def new_spawnv(mode, path, args):
- """
- os.spawnv(mode, path, args)
- os.spawnvp(mode, file, args)
- """
- if _get_apply_arg_patching():
- args = patch_args(args)
- send_process_created_message()
-
- return getattr(os, original_name)(mode, path, args)
-
- return new_spawnv
-
-
-def create_spawnve(original_name):
- """
- os.spawnve(mode, path, args, env)
- os.spawnvpe(mode, file, args, env)
- """
-
- def new_spawnve(mode, path, args, env):
- if _get_apply_arg_patching():
- args = patch_args(args)
- send_process_created_message()
-
- return getattr(os, original_name)(mode, path, args, env)
-
- return new_spawnve
-
-
-def create_posix_spawn(original_name):
- """
- os.posix_spawn(executable, args, env, **kwargs)
- """
-
- def new_posix_spawn(executable, args, env, **kwargs):
- if _get_apply_arg_patching():
- args = patch_args(args)
- send_process_created_message()
-
- return getattr(os, original_name)(executable, args, env, **kwargs)
-
- return new_posix_spawn
-
-
-def create_fork_exec(original_name):
- """
- _posixsubprocess.fork_exec(args, executable_list, close_fds, ... (13 more))
- """
-
- def new_fork_exec(args, *other_args):
- import _posixsubprocess # @UnresolvedImport
- if _get_apply_arg_patching():
- args = patch_args(args)
- send_process_created_message()
-
- return getattr(_posixsubprocess, original_name)(args, *other_args)
-
- return new_fork_exec
-
-
-def create_warn_fork_exec(original_name):
- """
- _posixsubprocess.fork_exec(args, executable_list, close_fds, ... (13 more))
- """
-
- def new_warn_fork_exec(*args):
- try:
- import _posixsubprocess
- warn_multiproc()
- return getattr(_posixsubprocess, original_name)(*args)
- except:
- pass
-
- return new_warn_fork_exec
-
-
-def create_subprocess_fork_exec(original_name):
- """
- subprocess._fork_exec(args, executable_list, close_fds, ... (13 more))
- """
-
- def new_fork_exec(args, *other_args):
- import subprocess
- if _get_apply_arg_patching():
- args = patch_args(args)
- send_process_created_message()
-
- return getattr(subprocess, original_name)(args, *other_args)
-
- return new_fork_exec
-
-
-def create_subprocess_warn_fork_exec(original_name):
- """
- subprocess._fork_exec(args, executable_list, close_fds, ... (13 more))
- """
-
- def new_warn_fork_exec(*args):
- try:
- import subprocess
- warn_multiproc()
- return getattr(subprocess, original_name)(*args)
- except:
- pass
-
- return new_warn_fork_exec
-
-
-def create_CreateProcess(original_name):
- """
- CreateProcess(*args, **kwargs)
- """
-
- def new_CreateProcess(app_name, cmd_line, *args):
- try:
- import _subprocess
- except ImportError:
- import _winapi as _subprocess
-
- if _get_apply_arg_patching():
- cmd_line = patch_arg_str_win(cmd_line)
- send_process_created_message()
-
- return getattr(_subprocess, original_name)(app_name, cmd_line, *args)
-
- return new_CreateProcess
-
-
-def create_CreateProcessWarnMultiproc(original_name):
- """
- CreateProcess(*args, **kwargs)
- """
-
- def new_CreateProcess(*args):
- try:
- import _subprocess
- except ImportError:
- import _winapi as _subprocess
- warn_multiproc()
- return getattr(_subprocess, original_name)(*args)
-
- return new_CreateProcess
-
-
-def create_fork(original_name):
-
- def new_fork():
- # A simple fork will result in a new python process
- is_new_python_process = True
- frame = sys._getframe()
-
- apply_arg_patch = _get_apply_arg_patching()
-
- is_subprocess_fork = False
- while frame is not None:
- if frame.f_code.co_name == '_execute_child' and 'subprocess' in frame.f_code.co_filename:
- is_subprocess_fork = True
- # If we're actually in subprocess.Popen creating a child, it may
- # result in something which is not a Python process, (so, we
- # don't want to connect with it in the forked version).
- executable = frame.f_locals.get('executable')
- if executable is not None:
- is_new_python_process = False
- if is_python(executable):
- is_new_python_process = True
- break
-
- frame = frame.f_back
- frame = None # Just make sure we don't hold on to it.
-
- protocol = pydevd_constants.get_protocol()
- debug_mode = PydevdCustomization.DEBUG_MODE
-
- child_process = getattr(os, original_name)() # fork
- if not child_process:
- if is_new_python_process:
- PydevdCustomization.DEFAULT_PROTOCOL = protocol
- PydevdCustomization.DEBUG_MODE = debug_mode
- _on_forked_process(setup_tracing=apply_arg_patch and not is_subprocess_fork)
- else:
- set_global_debugger(None)
- else:
- if is_new_python_process:
- send_process_created_message()
- return child_process
-
- return new_fork
-
-
-def send_process_created_message():
- py_db = get_global_debugger()
- if py_db is not None:
- py_db.send_process_created_message()
-
-
-def send_process_about_to_be_replaced():
- py_db = get_global_debugger()
- if py_db is not None:
- py_db.send_process_about_to_be_replaced()
-
-
-def patch_new_process_functions():
- # os.execl(path, arg0, arg1, ...)
- # os.execle(path, arg0, arg1, ..., env)
- # os.execlp(file, arg0, arg1, ...)
- # os.execlpe(file, arg0, arg1, ..., env)
- # os.execv(path, args)
- # os.execve(path, args, env)
- # os.execvp(file, args)
- # os.execvpe(file, args, env)
- monkey_patch_os('execl', create_execl)
- monkey_patch_os('execle', create_execl)
- monkey_patch_os('execlp', create_execl)
- monkey_patch_os('execlpe', create_execl)
- monkey_patch_os('execv', create_execv)
- monkey_patch_os('execve', create_execve)
- monkey_patch_os('execvp', create_execv)
- monkey_patch_os('execvpe', create_execve)
-
- # os.spawnl(mode, path, ...)
- # os.spawnle(mode, path, ..., env)
- # os.spawnlp(mode, file, ...)
- # os.spawnlpe(mode, file, ..., env)
- # os.spawnv(mode, path, args)
- # os.spawnve(mode, path, args, env)
- # os.spawnvp(mode, file, args)
- # os.spawnvpe(mode, file, args, env)
-
- monkey_patch_os('spawnl', create_spawnl)
- monkey_patch_os('spawnle', create_spawnl)
- monkey_patch_os('spawnlp', create_spawnl)
- monkey_patch_os('spawnlpe', create_spawnl)
- monkey_patch_os('spawnv', create_spawnv)
- monkey_patch_os('spawnve', create_spawnve)
- monkey_patch_os('spawnvp', create_spawnv)
- monkey_patch_os('spawnvpe', create_spawnve)
- monkey_patch_os('posix_spawn', create_posix_spawn)
-
- if not IS_JYTHON:
- if not IS_WINDOWS:
- monkey_patch_os('fork', create_fork)
- try:
- import _posixsubprocess
- monkey_patch_module(_posixsubprocess, 'fork_exec', create_fork_exec)
- except ImportError:
- pass
-
- try:
- import subprocess
- monkey_patch_module(subprocess, '_fork_exec', create_subprocess_fork_exec)
- except AttributeError:
- pass
- else:
- # Windows
- try:
- import _subprocess
- except ImportError:
- import _winapi as _subprocess
- monkey_patch_module(_subprocess, 'CreateProcess', create_CreateProcess)
-
-
-def patch_new_process_functions_with_warning():
- monkey_patch_os('execl', create_warn_multiproc)
- monkey_patch_os('execle', create_warn_multiproc)
- monkey_patch_os('execlp', create_warn_multiproc)
- monkey_patch_os('execlpe', create_warn_multiproc)
- monkey_patch_os('execv', create_warn_multiproc)
- monkey_patch_os('execve', create_warn_multiproc)
- monkey_patch_os('execvp', create_warn_multiproc)
- monkey_patch_os('execvpe', create_warn_multiproc)
- monkey_patch_os('spawnl', create_warn_multiproc)
- monkey_patch_os('spawnle', create_warn_multiproc)
- monkey_patch_os('spawnlp', create_warn_multiproc)
- monkey_patch_os('spawnlpe', create_warn_multiproc)
- monkey_patch_os('spawnv', create_warn_multiproc)
- monkey_patch_os('spawnve', create_warn_multiproc)
- monkey_patch_os('spawnvp', create_warn_multiproc)
- monkey_patch_os('spawnvpe', create_warn_multiproc)
- monkey_patch_os('posix_spawn', create_warn_multiproc)
-
- if not IS_JYTHON:
- if not IS_WINDOWS:
- monkey_patch_os('fork', create_warn_multiproc)
- try:
- import _posixsubprocess
- monkey_patch_module(_posixsubprocess, 'fork_exec', create_warn_fork_exec)
- except ImportError:
- pass
-
- try:
- import subprocess
- monkey_patch_module(subprocess, '_fork_exec', create_subprocess_warn_fork_exec)
- except AttributeError:
- pass
-
- else:
- # Windows
- try:
- import _subprocess
- except ImportError:
- import _winapi as _subprocess
- monkey_patch_module(_subprocess, 'CreateProcess', create_CreateProcessWarnMultiproc)
-
-
-class _NewThreadStartupWithTrace:
-
- def __init__(self, original_func, args, kwargs):
- self.original_func = original_func
- self.args = args
- self.kwargs = kwargs
-
- def __call__(self):
- # We monkey-patch the thread creation so that this function is called in the new thread. At this point
- # we notify of its creation and start tracing it.
- py_db = get_global_debugger()
-
- thread_id = None
- if py_db is not None:
- # Note: if this is a thread from threading.py, we're too early in the boostrap process (because we mocked
- # the start_new_thread internal machinery and thread._bootstrap has not finished), so, the code below needs
- # to make sure that we use the current thread bound to the original function and not use
- # threading.current_thread() unless we're sure it's a dummy thread.
- t = getattr(self.original_func, '__self__', getattr(self.original_func, 'im_self', None))
- if not isinstance(t, threading.Thread):
- # This is not a threading.Thread but a Dummy thread (so, get it as a dummy thread using
- # currentThread).
- t = threading.current_thread()
-
- if not getattr(t, 'is_pydev_daemon_thread', False):
- thread_id = get_current_thread_id(t)
- py_db.notify_thread_created(thread_id, t)
- _on_set_trace_for_new_thread(py_db)
-
- if getattr(py_db, 'thread_analyser', None) is not None:
- try:
- from _pydevd_bundle.pydevd_concurrency_analyser.pydevd_concurrency_logger import log_new_thread
- log_new_thread(py_db, t)
- except:
- sys.stderr.write("Failed to detect new thread for visualization")
- try:
- ret = self.original_func(*self.args, **self.kwargs)
- finally:
- if thread_id is not None:
- if py_db is not None:
- # At thread shutdown we only have pydevd-related code running (which shouldn't
- # be tracked).
- py_db.disable_tracing()
- py_db.notify_thread_not_alive(thread_id)
-
- return ret
-
-
-class _NewThreadStartupWithoutTrace:
-
- def __init__(self, original_func, args, kwargs):
- self.original_func = original_func
- self.args = args
- self.kwargs = kwargs
-
- def __call__(self):
- return self.original_func(*self.args, **self.kwargs)
-
-
-_UseNewThreadStartup = _NewThreadStartupWithTrace
-
-
-def _get_threading_modules_to_patch():
- threading_modules_to_patch = []
-
- try:
- import thread as _thread
- except:
- import _thread
- threading_modules_to_patch.append(_thread)
- threading_modules_to_patch.append(threading)
-
- return threading_modules_to_patch
-
-
-threading_modules_to_patch = _get_threading_modules_to_patch()
-
-
-def patch_thread_module(thread_module):
-
- if getattr(thread_module, '_original_start_new_thread', None) is None:
- if thread_module is threading:
- if not hasattr(thread_module, '_start_new_thread'):
- return # Jython doesn't have it.
- _original_start_new_thread = thread_module._original_start_new_thread = thread_module._start_new_thread
- else:
- _original_start_new_thread = thread_module._original_start_new_thread = thread_module.start_new_thread
- else:
- _original_start_new_thread = thread_module._original_start_new_thread
-
- class ClassWithPydevStartNewThread:
-
- def pydev_start_new_thread(self, function, args=(), kwargs={}):
- '''
- We need to replace the original thread_module.start_new_thread with this function so that threads started
- through it and not through the threading module are properly traced.
- '''
- return _original_start_new_thread(_UseNewThreadStartup(function, args, kwargs), ())
-
- # This is a hack for the situation where the thread_module.start_new_thread is declared inside a class, such as the one below
- # class F(object):
- # start_new_thread = thread_module.start_new_thread
- #
- # def start_it(self):
- # self.start_new_thread(self.function, args, kwargs)
- # So, if it's an already bound method, calling self.start_new_thread won't really receive a different 'self' -- it
- # does work in the default case because in builtins self isn't passed either.
- pydev_start_new_thread = ClassWithPydevStartNewThread().pydev_start_new_thread
-
- try:
- # We need to replace the original thread_module.start_new_thread with this function so that threads started through
- # it and not through the threading module are properly traced.
- if thread_module is threading:
- thread_module._start_new_thread = pydev_start_new_thread
- else:
- thread_module.start_new_thread = pydev_start_new_thread
- thread_module.start_new = pydev_start_new_thread
- except:
- pass
-
-
-def patch_thread_modules():
- for t in threading_modules_to_patch:
- patch_thread_module(t)
-
-
-def undo_patch_thread_modules():
- for t in threading_modules_to_patch:
- try:
- t.start_new_thread = t._original_start_new_thread
- except:
- pass
-
- try:
- t.start_new = t._original_start_new_thread
- except:
- pass
-
- try:
- t._start_new_thread = t._original_start_new_thread
- except:
- pass
-
-
-def disable_trace_thread_modules():
- '''
- Can be used to temporarily stop tracing threads created with thread.start_new_thread.
- '''
- global _UseNewThreadStartup
- _UseNewThreadStartup = _NewThreadStartupWithoutTrace
-
-
-def enable_trace_thread_modules():
- '''
- Can be used to start tracing threads created with thread.start_new_thread again.
- '''
- global _UseNewThreadStartup
- _UseNewThreadStartup = _NewThreadStartupWithTrace
-
-
-def get_original_start_new_thread(threading_module):
- try:
- return threading_module._original_start_new_thread
- except:
- return threading_module.start_new_thread
diff --git a/spaces/SungBeom/chatwine-korean/.venv/Lib/site-packages/debugpy/_vendored/pydevd/_pydevd_bundle/pydevd_dont_trace_files.py b/spaces/SungBeom/chatwine-korean/.venv/Lib/site-packages/debugpy/_vendored/pydevd/_pydevd_bundle/pydevd_dont_trace_files.py
deleted file mode 100644
index d37b1fc53c28d4dd7373fd30f0aa1128345ade7c..0000000000000000000000000000000000000000
--- a/spaces/SungBeom/chatwine-korean/.venv/Lib/site-packages/debugpy/_vendored/pydevd/_pydevd_bundle/pydevd_dont_trace_files.py
+++ /dev/null
@@ -1,153 +0,0 @@
-# Important: Autogenerated file.
-
-# DO NOT edit manually!
-# DO NOT edit manually!
-
-LIB_FILE = 1
-PYDEV_FILE = 2
-
-DONT_TRACE_DIRS = {
- '_pydev_bundle': PYDEV_FILE,
- '_pydev_runfiles': PYDEV_FILE,
- '_pydevd_bundle': PYDEV_FILE,
- '_pydevd_frame_eval': PYDEV_FILE,
- 'pydev_ipython': PYDEV_FILE,
- 'pydev_sitecustomize': PYDEV_FILE,
- 'pydevd_attach_to_process': PYDEV_FILE,
- 'pydevd_concurrency_analyser': PYDEV_FILE,
- 'pydevd_plugins': PYDEV_FILE,
- 'test_pydevd_reload': PYDEV_FILE,
-}
-
-DONT_TRACE = {
- # commonly used things from the stdlib that we don't want to trace
- 'Queue.py':LIB_FILE,
- 'queue.py':LIB_FILE,
- 'socket.py':LIB_FILE,
- 'weakref.py':LIB_FILE,
- '_weakrefset.py':LIB_FILE,
- 'linecache.py':LIB_FILE,
- 'threading.py':LIB_FILE,
- 'dis.py':LIB_FILE,
-
- # things from pydev that we don't want to trace
- '__main__pydevd_gen_debug_adapter_protocol.py': PYDEV_FILE,
- '_pydev_calltip_util.py': PYDEV_FILE,
- '_pydev_completer.py': PYDEV_FILE,
- '_pydev_execfile.py': PYDEV_FILE,
- '_pydev_filesystem_encoding.py': PYDEV_FILE,
- '_pydev_getopt.py': PYDEV_FILE,
- '_pydev_imports_tipper.py': PYDEV_FILE,
- '_pydev_jy_imports_tipper.py': PYDEV_FILE,
- '_pydev_log.py': PYDEV_FILE,
- '_pydev_saved_modules.py': PYDEV_FILE,
- '_pydev_sys_patch.py': PYDEV_FILE,
- '_pydev_tipper_common.py': PYDEV_FILE,
- 'django_debug.py': PYDEV_FILE,
- 'jinja2_debug.py': PYDEV_FILE,
- 'pycompletionserver.py': PYDEV_FILE,
- 'pydev_app_engine_debug_startup.py': PYDEV_FILE,
- 'pydev_console_utils.py': PYDEV_FILE,
- 'pydev_import_hook.py': PYDEV_FILE,
- 'pydev_imports.py': PYDEV_FILE,
- 'pydev_ipython_console.py': PYDEV_FILE,
- 'pydev_ipython_console_011.py': PYDEV_FILE,
- 'pydev_is_thread_alive.py': PYDEV_FILE,
- 'pydev_localhost.py': PYDEV_FILE,
- 'pydev_log.py': PYDEV_FILE,
- 'pydev_monkey.py': PYDEV_FILE,
- 'pydev_monkey_qt.py': PYDEV_FILE,
- 'pydev_override.py': PYDEV_FILE,
- 'pydev_run_in_console.py': PYDEV_FILE,
- 'pydev_runfiles.py': PYDEV_FILE,
- 'pydev_runfiles_coverage.py': PYDEV_FILE,
- 'pydev_runfiles_nose.py': PYDEV_FILE,
- 'pydev_runfiles_parallel.py': PYDEV_FILE,
- 'pydev_runfiles_parallel_client.py': PYDEV_FILE,
- 'pydev_runfiles_pytest2.py': PYDEV_FILE,
- 'pydev_runfiles_unittest.py': PYDEV_FILE,
- 'pydev_runfiles_xml_rpc.py': PYDEV_FILE,
- 'pydev_umd.py': PYDEV_FILE,
- 'pydev_versioncheck.py': PYDEV_FILE,
- 'pydevconsole.py': PYDEV_FILE,
- 'pydevconsole_code.py': PYDEV_FILE,
- 'pydevd.py': PYDEV_FILE,
- 'pydevd_additional_thread_info.py': PYDEV_FILE,
- 'pydevd_additional_thread_info_regular.py': PYDEV_FILE,
- 'pydevd_api.py': PYDEV_FILE,
- 'pydevd_base_schema.py': PYDEV_FILE,
- 'pydevd_breakpoints.py': PYDEV_FILE,
- 'pydevd_bytecode_utils.py': PYDEV_FILE,
- 'pydevd_code_to_source.py': PYDEV_FILE,
- 'pydevd_collect_bytecode_info.py': PYDEV_FILE,
- 'pydevd_comm.py': PYDEV_FILE,
- 'pydevd_comm_constants.py': PYDEV_FILE,
- 'pydevd_command_line_handling.py': PYDEV_FILE,
- 'pydevd_concurrency_logger.py': PYDEV_FILE,
- 'pydevd_console.py': PYDEV_FILE,
- 'pydevd_constants.py': PYDEV_FILE,
- 'pydevd_custom_frames.py': PYDEV_FILE,
- 'pydevd_cython_wrapper.py': PYDEV_FILE,
- 'pydevd_daemon_thread.py': PYDEV_FILE,
- 'pydevd_defaults.py': PYDEV_FILE,
- 'pydevd_dont_trace.py': PYDEV_FILE,
- 'pydevd_dont_trace_files.py': PYDEV_FILE,
- 'pydevd_exec2.py': PYDEV_FILE,
- 'pydevd_extension_api.py': PYDEV_FILE,
- 'pydevd_extension_utils.py': PYDEV_FILE,
- 'pydevd_file_utils.py': PYDEV_FILE,
- 'pydevd_filtering.py': PYDEV_FILE,
- 'pydevd_frame.py': PYDEV_FILE,
- 'pydevd_frame_eval_cython_wrapper.py': PYDEV_FILE,
- 'pydevd_frame_eval_main.py': PYDEV_FILE,
- 'pydevd_frame_tracing.py': PYDEV_FILE,
- 'pydevd_frame_utils.py': PYDEV_FILE,
- 'pydevd_gevent_integration.py': PYDEV_FILE,
- 'pydevd_helpers.py': PYDEV_FILE,
- 'pydevd_import_class.py': PYDEV_FILE,
- 'pydevd_io.py': PYDEV_FILE,
- 'pydevd_json_debug_options.py': PYDEV_FILE,
- 'pydevd_line_validation.py': PYDEV_FILE,
- 'pydevd_modify_bytecode.py': PYDEV_FILE,
- 'pydevd_net_command.py': PYDEV_FILE,
- 'pydevd_net_command_factory_json.py': PYDEV_FILE,
- 'pydevd_net_command_factory_xml.py': PYDEV_FILE,
- 'pydevd_plugin_numpy_types.py': PYDEV_FILE,
- 'pydevd_plugin_pandas_types.py': PYDEV_FILE,
- 'pydevd_plugin_utils.py': PYDEV_FILE,
- 'pydevd_plugins_django_form_str.py': PYDEV_FILE,
- 'pydevd_process_net_command.py': PYDEV_FILE,
- 'pydevd_process_net_command_json.py': PYDEV_FILE,
- 'pydevd_referrers.py': PYDEV_FILE,
- 'pydevd_reload.py': PYDEV_FILE,
- 'pydevd_resolver.py': PYDEV_FILE,
- 'pydevd_runpy.py': PYDEV_FILE,
- 'pydevd_safe_repr.py': PYDEV_FILE,
- 'pydevd_save_locals.py': PYDEV_FILE,
- 'pydevd_schema.py': PYDEV_FILE,
- 'pydevd_schema_log.py': PYDEV_FILE,
- 'pydevd_signature.py': PYDEV_FILE,
- 'pydevd_source_mapping.py': PYDEV_FILE,
- 'pydevd_stackless.py': PYDEV_FILE,
- 'pydevd_suspended_frames.py': PYDEV_FILE,
- 'pydevd_thread_lifecycle.py': PYDEV_FILE,
- 'pydevd_thread_wrappers.py': PYDEV_FILE,
- 'pydevd_timeout.py': PYDEV_FILE,
- 'pydevd_trace_api.py': PYDEV_FILE,
- 'pydevd_trace_dispatch.py': PYDEV_FILE,
- 'pydevd_trace_dispatch_regular.py': PYDEV_FILE,
- 'pydevd_traceproperty.py': PYDEV_FILE,
- 'pydevd_tracing.py': PYDEV_FILE,
- 'pydevd_utils.py': PYDEV_FILE,
- 'pydevd_vars.py': PYDEV_FILE,
- 'pydevd_vm_type.py': PYDEV_FILE,
- 'pydevd_xml.py': PYDEV_FILE,
-}
-
-# if we try to trace io.py it seems it can get halted (see http://bugs.python.org/issue4716)
-DONT_TRACE['io.py'] = LIB_FILE
-
-# Don't trace common encodings too
-DONT_TRACE['cp1252.py'] = LIB_FILE
-DONT_TRACE['utf_8.py'] = LIB_FILE
-DONT_TRACE['codecs.py'] = LIB_FILE
diff --git a/spaces/Superlang/ImageProcessor/annotator/uniformer/mmseg/models/backbones/uniformer.py b/spaces/Superlang/ImageProcessor/annotator/uniformer/mmseg/models/backbones/uniformer.py
deleted file mode 100644
index 0c4bb88e4c928540cca9ab609988b916520f5b7a..0000000000000000000000000000000000000000
--- a/spaces/Superlang/ImageProcessor/annotator/uniformer/mmseg/models/backbones/uniformer.py
+++ /dev/null
@@ -1,422 +0,0 @@
-# --------------------------------------------------------
-# UniFormer
-# Copyright (c) 2022 SenseTime X-Lab
-# Licensed under The MIT License [see LICENSE for details]
-# Written by Kunchang Li
-# --------------------------------------------------------
-
-from collections import OrderedDict
-import math
-
-from functools import partial
-import torch
-import torch.nn as nn
-import torch.nn.functional as F
-import torch.utils.checkpoint as checkpoint
-import numpy as np
-from timm.models.layers import DropPath, to_2tuple, trunc_normal_
-
-from annotator.uniformer.mmcv_custom import load_checkpoint
-from annotator.uniformer.mmseg.utils import get_root_logger
-from ..builder import BACKBONES
-
-
-class Mlp(nn.Module):
- def __init__(self, in_features, hidden_features=None, out_features=None, act_layer=nn.GELU, drop=0.):
- super().__init__()
- out_features = out_features or in_features
- hidden_features = hidden_features or in_features
- self.fc1 = nn.Linear(in_features, hidden_features)
- self.act = act_layer()
- self.fc2 = nn.Linear(hidden_features, out_features)
- self.drop = nn.Dropout(drop)
-
- def forward(self, x):
- x = self.fc1(x)
- x = self.act(x)
- x = self.drop(x)
- x = self.fc2(x)
- x = self.drop(x)
- return x
-
-
-class CMlp(nn.Module):
- def __init__(self, in_features, hidden_features=None, out_features=None, act_layer=nn.GELU, drop=0.):
- super().__init__()
- out_features = out_features or in_features
- hidden_features = hidden_features or in_features
- self.fc1 = nn.Conv2d(in_features, hidden_features, 1)
- self.act = act_layer()
- self.fc2 = nn.Conv2d(hidden_features, out_features, 1)
- self.drop = nn.Dropout(drop)
-
- def forward(self, x):
- x = self.fc1(x)
- x = self.act(x)
- x = self.drop(x)
- x = self.fc2(x)
- x = self.drop(x)
- return x
-
-
-class CBlock(nn.Module):
- def __init__(self, dim, num_heads, mlp_ratio=4., qkv_bias=False, qk_scale=None, drop=0., attn_drop=0.,
- drop_path=0., act_layer=nn.GELU, norm_layer=nn.LayerNorm):
- super().__init__()
- self.pos_embed = nn.Conv2d(dim, dim, 3, padding=1, groups=dim)
- self.norm1 = nn.BatchNorm2d(dim)
- self.conv1 = nn.Conv2d(dim, dim, 1)
- self.conv2 = nn.Conv2d(dim, dim, 1)
- self.attn = nn.Conv2d(dim, dim, 5, padding=2, groups=dim)
- # NOTE: drop path for stochastic depth, we shall see if this is better than dropout here
- self.drop_path = DropPath(drop_path) if drop_path > 0. else nn.Identity()
- self.norm2 = nn.BatchNorm2d(dim)
- mlp_hidden_dim = int(dim * mlp_ratio)
- self.mlp = CMlp(in_features=dim, hidden_features=mlp_hidden_dim, act_layer=act_layer, drop=drop)
-
- def forward(self, x):
- x = x + self.pos_embed(x)
- x = x + self.drop_path(self.conv2(self.attn(self.conv1(self.norm1(x)))))
- x = x + self.drop_path(self.mlp(self.norm2(x)))
- return x
-
-
-class Attention(nn.Module):
- def __init__(self, dim, num_heads=8, qkv_bias=False, qk_scale=None, attn_drop=0., proj_drop=0.):
- super().__init__()
- self.num_heads = num_heads
- head_dim = dim // num_heads
- # NOTE scale factor was wrong in my original version, can set manually to be compat with prev weights
- self.scale = qk_scale or head_dim ** -0.5
-
- self.qkv = nn.Linear(dim, dim * 3, bias=qkv_bias)
- self.attn_drop = nn.Dropout(attn_drop)
- self.proj = nn.Linear(dim, dim)
- self.proj_drop = nn.Dropout(proj_drop)
-
- def forward(self, x):
- B, N, C = x.shape
- qkv = self.qkv(x).reshape(B, N, 3, self.num_heads, C // self.num_heads).permute(2, 0, 3, 1, 4)
- q, k, v = qkv[0], qkv[1], qkv[2] # make torchscript happy (cannot use tensor as tuple)
-
- attn = (q @ k.transpose(-2, -1)) * self.scale
- attn = attn.softmax(dim=-1)
- attn = self.attn_drop(attn)
-
- x = (attn @ v).transpose(1, 2).reshape(B, N, C)
- x = self.proj(x)
- x = self.proj_drop(x)
- return x
-
-
-class SABlock(nn.Module):
- def __init__(self, dim, num_heads, mlp_ratio=4., qkv_bias=False, qk_scale=None, drop=0., attn_drop=0.,
- drop_path=0., act_layer=nn.GELU, norm_layer=nn.LayerNorm):
- super().__init__()
- self.pos_embed = nn.Conv2d(dim, dim, 3, padding=1, groups=dim)
- self.norm1 = norm_layer(dim)
- self.attn = Attention(
- dim,
- num_heads=num_heads, qkv_bias=qkv_bias, qk_scale=qk_scale,
- attn_drop=attn_drop, proj_drop=drop)
- # NOTE: drop path for stochastic depth, we shall see if this is better than dropout here
- self.drop_path = DropPath(drop_path) if drop_path > 0. else nn.Identity()
- self.norm2 = norm_layer(dim)
- mlp_hidden_dim = int(dim * mlp_ratio)
- self.mlp = Mlp(in_features=dim, hidden_features=mlp_hidden_dim, act_layer=act_layer, drop=drop)
-
- def forward(self, x):
- x = x + self.pos_embed(x)
- B, N, H, W = x.shape
- x = x.flatten(2).transpose(1, 2)
- x = x + self.drop_path(self.attn(self.norm1(x)))
- x = x + self.drop_path(self.mlp(self.norm2(x)))
- x = x.transpose(1, 2).reshape(B, N, H, W)
- return x
-
-
-def window_partition(x, window_size):
- """
- Args:
- x: (B, H, W, C)
- window_size (int): window size
- Returns:
- windows: (num_windows*B, window_size, window_size, C)
- """
- B, H, W, C = x.shape
- x = x.view(B, H // window_size, window_size, W // window_size, window_size, C)
- windows = x.permute(0, 1, 3, 2, 4, 5).contiguous().view(-1, window_size, window_size, C)
- return windows
-
-
-def window_reverse(windows, window_size, H, W):
- """
- Args:
- windows: (num_windows*B, window_size, window_size, C)
- window_size (int): Window size
- H (int): Height of image
- W (int): Width of image
- Returns:
- x: (B, H, W, C)
- """
- B = int(windows.shape[0] / (H * W / window_size / window_size))
- x = windows.view(B, H // window_size, W // window_size, window_size, window_size, -1)
- x = x.permute(0, 1, 3, 2, 4, 5).contiguous().view(B, H, W, -1)
- return x
-
-
-class SABlock_Windows(nn.Module):
- def __init__(self, dim, num_heads, window_size=14, mlp_ratio=4., qkv_bias=False, qk_scale=None, drop=0., attn_drop=0.,
- drop_path=0., act_layer=nn.GELU, norm_layer=nn.LayerNorm):
- super().__init__()
- self.window_size=window_size
- self.pos_embed = nn.Conv2d(dim, dim, 3, padding=1, groups=dim)
- self.norm1 = norm_layer(dim)
- self.attn = Attention(
- dim,
- num_heads=num_heads, qkv_bias=qkv_bias, qk_scale=qk_scale,
- attn_drop=attn_drop, proj_drop=drop)
- # NOTE: drop path for stochastic depth, we shall see if this is better than dropout here
- self.drop_path = DropPath(drop_path) if drop_path > 0. else nn.Identity()
- self.norm2 = norm_layer(dim)
- mlp_hidden_dim = int(dim * mlp_ratio)
- self.mlp = Mlp(in_features=dim, hidden_features=mlp_hidden_dim, act_layer=act_layer, drop=drop)
-
- def forward(self, x):
- x = x + self.pos_embed(x)
- x = x.permute(0, 2, 3, 1)
- B, H, W, C = x.shape
- shortcut = x
- x = self.norm1(x)
-
- pad_l = pad_t = 0
- pad_r = (self.window_size - W % self.window_size) % self.window_size
- pad_b = (self.window_size - H % self.window_size) % self.window_size
- x = F.pad(x, (0, 0, pad_l, pad_r, pad_t, pad_b))
- _, Hp, Wp, _ = x.shape
-
- x_windows = window_partition(x, self.window_size) # nW*B, window_size, window_size, C
- x_windows = x_windows.view(-1, self.window_size * self.window_size, C) # nW*B, window_size*window_size, C
-
- # W-MSA/SW-MSA
- attn_windows = self.attn(x_windows) # nW*B, window_size*window_size, C
-
- # merge windows
- attn_windows = attn_windows.view(-1, self.window_size, self.window_size, C)
- x = window_reverse(attn_windows, self.window_size, Hp, Wp) # B H' W' C
-
- # reverse cyclic shift
- if pad_r > 0 or pad_b > 0:
- x = x[:, :H, :W, :].contiguous()
-
- x = shortcut + self.drop_path(x)
- x = x + self.drop_path(self.mlp(self.norm2(x)))
- x = x.permute(0, 3, 1, 2).reshape(B, C, H, W)
- return x
-
-
-class PatchEmbed(nn.Module):
- """ Image to Patch Embedding
- """
- def __init__(self, img_size=224, patch_size=16, in_chans=3, embed_dim=768):
- super().__init__()
- img_size = to_2tuple(img_size)
- patch_size = to_2tuple(patch_size)
- num_patches = (img_size[1] // patch_size[1]) * (img_size[0] // patch_size[0])
- self.img_size = img_size
- self.patch_size = patch_size
- self.num_patches = num_patches
- self.norm = nn.LayerNorm(embed_dim)
- self.proj = nn.Conv2d(in_chans, embed_dim, kernel_size=patch_size, stride=patch_size)
-
- def forward(self, x):
- B, _, H, W = x.shape
- x = self.proj(x)
- B, _, H, W = x.shape
- x = x.flatten(2).transpose(1, 2)
- x = self.norm(x)
- x = x.reshape(B, H, W, -1).permute(0, 3, 1, 2).contiguous()
- return x
-
-
-@BACKBONES.register_module()
-class UniFormer(nn.Module):
- """ Vision Transformer
- A PyTorch impl of : `An Image is Worth 16x16 Words: Transformers for Image Recognition at Scale` -
- https://arxiv.org/abs/2010.11929
- """
- def __init__(self, layers=[3, 4, 8, 3], img_size=224, in_chans=3, num_classes=80, embed_dim=[64, 128, 320, 512],
- head_dim=64, mlp_ratio=4., qkv_bias=True, qk_scale=None, representation_size=None,
- drop_rate=0., attn_drop_rate=0., drop_path_rate=0., norm_layer=partial(nn.LayerNorm, eps=1e-6),
- pretrained_path=None, use_checkpoint=False, checkpoint_num=[0, 0, 0, 0],
- windows=False, hybrid=False, window_size=14):
- """
- Args:
- layer (list): number of block in each layer
- img_size (int, tuple): input image size
- in_chans (int): number of input channels
- num_classes (int): number of classes for classification head
- embed_dim (int): embedding dimension
- head_dim (int): dimension of attention heads
- mlp_ratio (int): ratio of mlp hidden dim to embedding dim
- qkv_bias (bool): enable bias for qkv if True
- qk_scale (float): override default qk scale of head_dim ** -0.5 if set
- representation_size (Optional[int]): enable and set representation layer (pre-logits) to this value if set
- drop_rate (float): dropout rate
- attn_drop_rate (float): attention dropout rate
- drop_path_rate (float): stochastic depth rate
- norm_layer (nn.Module): normalization layer
- pretrained_path (str): path of pretrained model
- use_checkpoint (bool): whether use checkpoint
- checkpoint_num (list): index for using checkpoint in every stage
- windows (bool): whether use window MHRA
- hybrid (bool): whether use hybrid MHRA
- window_size (int): size of window (>14)
- """
- super().__init__()
- self.num_classes = num_classes
- self.use_checkpoint = use_checkpoint
- self.checkpoint_num = checkpoint_num
- self.windows = windows
- print(f'Use Checkpoint: {self.use_checkpoint}')
- print(f'Checkpoint Number: {self.checkpoint_num}')
- self.num_features = self.embed_dim = embed_dim # num_features for consistency with other models
- norm_layer = norm_layer or partial(nn.LayerNorm, eps=1e-6)
-
- self.patch_embed1 = PatchEmbed(
- img_size=img_size, patch_size=4, in_chans=in_chans, embed_dim=embed_dim[0])
- self.patch_embed2 = PatchEmbed(
- img_size=img_size // 4, patch_size=2, in_chans=embed_dim[0], embed_dim=embed_dim[1])
- self.patch_embed3 = PatchEmbed(
- img_size=img_size // 8, patch_size=2, in_chans=embed_dim[1], embed_dim=embed_dim[2])
- self.patch_embed4 = PatchEmbed(
- img_size=img_size // 16, patch_size=2, in_chans=embed_dim[2], embed_dim=embed_dim[3])
-
- self.pos_drop = nn.Dropout(p=drop_rate)
- dpr = [x.item() for x in torch.linspace(0, drop_path_rate, sum(layers))] # stochastic depth decay rule
- num_heads = [dim // head_dim for dim in embed_dim]
- self.blocks1 = nn.ModuleList([
- CBlock(
- dim=embed_dim[0], num_heads=num_heads[0], mlp_ratio=mlp_ratio, qkv_bias=qkv_bias, qk_scale=qk_scale,
- drop=drop_rate, attn_drop=attn_drop_rate, drop_path=dpr[i], norm_layer=norm_layer)
- for i in range(layers[0])])
- self.norm1=norm_layer(embed_dim[0])
- self.blocks2 = nn.ModuleList([
- CBlock(
- dim=embed_dim[1], num_heads=num_heads[1], mlp_ratio=mlp_ratio, qkv_bias=qkv_bias, qk_scale=qk_scale,
- drop=drop_rate, attn_drop=attn_drop_rate, drop_path=dpr[i+layers[0]], norm_layer=norm_layer)
- for i in range(layers[1])])
- self.norm2 = norm_layer(embed_dim[1])
- if self.windows:
- print('Use local window for all blocks in stage3')
- self.blocks3 = nn.ModuleList([
- SABlock_Windows(
- dim=embed_dim[2], num_heads=num_heads[2], window_size=window_size, mlp_ratio=mlp_ratio, qkv_bias=qkv_bias, qk_scale=qk_scale,
- drop=drop_rate, attn_drop=attn_drop_rate, drop_path=dpr[i+layers[0]+layers[1]], norm_layer=norm_layer)
- for i in range(layers[2])])
- elif hybrid:
- print('Use hybrid window for blocks in stage3')
- block3 = []
- for i in range(layers[2]):
- if (i + 1) % 4 == 0:
- block3.append(SABlock(
- dim=embed_dim[2], num_heads=num_heads[2], mlp_ratio=mlp_ratio, qkv_bias=qkv_bias, qk_scale=qk_scale,
- drop=drop_rate, attn_drop=attn_drop_rate, drop_path=dpr[i+layers[0]+layers[1]], norm_layer=norm_layer))
- else:
- block3.append(SABlock_Windows(
- dim=embed_dim[2], num_heads=num_heads[2], window_size=window_size, mlp_ratio=mlp_ratio, qkv_bias=qkv_bias, qk_scale=qk_scale,
- drop=drop_rate, attn_drop=attn_drop_rate, drop_path=dpr[i+layers[0]+layers[1]], norm_layer=norm_layer))
- self.blocks3 = nn.ModuleList(block3)
- else:
- print('Use global window for all blocks in stage3')
- self.blocks3 = nn.ModuleList([
- SABlock(
- dim=embed_dim[2], num_heads=num_heads[2], mlp_ratio=mlp_ratio, qkv_bias=qkv_bias, qk_scale=qk_scale,
- drop=drop_rate, attn_drop=attn_drop_rate, drop_path=dpr[i+layers[0]+layers[1]], norm_layer=norm_layer)
- for i in range(layers[2])])
- self.norm3 = norm_layer(embed_dim[2])
- self.blocks4 = nn.ModuleList([
- SABlock(
- dim=embed_dim[3], num_heads=num_heads[3], mlp_ratio=mlp_ratio, qkv_bias=qkv_bias, qk_scale=qk_scale,
- drop=drop_rate, attn_drop=attn_drop_rate, drop_path=dpr[i+layers[0]+layers[1]+layers[2]], norm_layer=norm_layer)
- for i in range(layers[3])])
- self.norm4 = norm_layer(embed_dim[3])
-
- # Representation layer
- if representation_size:
- self.num_features = representation_size
- self.pre_logits = nn.Sequential(OrderedDict([
- ('fc', nn.Linear(embed_dim, representation_size)),
- ('act', nn.Tanh())
- ]))
- else:
- self.pre_logits = nn.Identity()
-
- self.apply(self._init_weights)
- self.init_weights(pretrained=pretrained_path)
-
- def init_weights(self, pretrained):
- if isinstance(pretrained, str):
- logger = get_root_logger()
- load_checkpoint(self, pretrained, map_location='cpu', strict=False, logger=logger)
- print(f'Load pretrained model from {pretrained}')
- def _init_weights(self, m):
- if isinstance(m, nn.Linear):
- trunc_normal_(m.weight, std=.02)
- if isinstance(m, nn.Linear) and m.bias is not None:
- nn.init.constant_(m.bias, 0)
- elif isinstance(m, nn.LayerNorm):
- nn.init.constant_(m.bias, 0)
- nn.init.constant_(m.weight, 1.0)
-
- @torch.jit.ignore
- def no_weight_decay(self):
- return {'pos_embed', 'cls_token'}
-
- def get_classifier(self):
- return self.head
-
- def reset_classifier(self, num_classes, global_pool=''):
- self.num_classes = num_classes
- self.head = nn.Linear(self.embed_dim, num_classes) if num_classes > 0 else nn.Identity()
-
- def forward_features(self, x):
- out = []
- x = self.patch_embed1(x)
- x = self.pos_drop(x)
- for i, blk in enumerate(self.blocks1):
- if self.use_checkpoint and i < self.checkpoint_num[0]:
- x = checkpoint.checkpoint(blk, x)
- else:
- x = blk(x)
- x_out = self.norm1(x.permute(0, 2, 3, 1))
- out.append(x_out.permute(0, 3, 1, 2).contiguous())
- x = self.patch_embed2(x)
- for i, blk in enumerate(self.blocks2):
- if self.use_checkpoint and i < self.checkpoint_num[1]:
- x = checkpoint.checkpoint(blk, x)
- else:
- x = blk(x)
- x_out = self.norm2(x.permute(0, 2, 3, 1))
- out.append(x_out.permute(0, 3, 1, 2).contiguous())
- x = self.patch_embed3(x)
- for i, blk in enumerate(self.blocks3):
- if self.use_checkpoint and i < self.checkpoint_num[2]:
- x = checkpoint.checkpoint(blk, x)
- else:
- x = blk(x)
- x_out = self.norm3(x.permute(0, 2, 3, 1))
- out.append(x_out.permute(0, 3, 1, 2).contiguous())
- x = self.patch_embed4(x)
- for i, blk in enumerate(self.blocks4):
- if self.use_checkpoint and i < self.checkpoint_num[3]:
- x = checkpoint.checkpoint(blk, x)
- else:
- x = blk(x)
- x_out = self.norm4(x.permute(0, 2, 3, 1))
- out.append(x_out.permute(0, 3, 1, 2).contiguous())
- return tuple(out)
-
- def forward(self, x):
- x = self.forward_features(x)
- return x
diff --git a/spaces/Superlang/ImageProcessor/annotator/uniformer/mmseg/models/utils/drop.py b/spaces/Superlang/ImageProcessor/annotator/uniformer/mmseg/models/utils/drop.py
deleted file mode 100644
index 4520b0ff407d2a95a864086bdbca0065f222aa63..0000000000000000000000000000000000000000
--- a/spaces/Superlang/ImageProcessor/annotator/uniformer/mmseg/models/utils/drop.py
+++ /dev/null
@@ -1,31 +0,0 @@
-"""Modified from https://github.com/rwightman/pytorch-image-
-models/blob/master/timm/models/layers/drop.py."""
-
-import torch
-from torch import nn
-
-
-class DropPath(nn.Module):
- """Drop paths (Stochastic Depth) per sample (when applied in main path of
- residual blocks).
-
- Args:
- drop_prob (float): Drop rate for paths of model. Dropout rate has
- to be between 0 and 1. Default: 0.
- """
-
- def __init__(self, drop_prob=0.):
- super(DropPath, self).__init__()
- self.drop_prob = drop_prob
- self.keep_prob = 1 - drop_prob
-
- def forward(self, x):
- if self.drop_prob == 0. or not self.training:
- return x
- shape = (x.shape[0], ) + (1, ) * (
- x.ndim - 1) # work with diff dim tensors, not just 2D ConvNets
- random_tensor = self.keep_prob + torch.rand(
- shape, dtype=x.dtype, device=x.device)
- random_tensor.floor_() # binarize
- output = x.div(self.keep_prob) * random_tensor
- return output
diff --git a/spaces/TEnngal/bingo/src/components/chat-list.tsx b/spaces/TEnngal/bingo/src/components/chat-list.tsx
deleted file mode 100644
index 624a78ef0d7be0f1192cf02a81e2e9cf214cb193..0000000000000000000000000000000000000000
--- a/spaces/TEnngal/bingo/src/components/chat-list.tsx
+++ /dev/null
@@ -1,28 +0,0 @@
-import React from 'react'
-
-import { Separator } from '@/components/ui/separator'
-import { ChatMessage } from '@/components/chat-message'
-import { ChatMessageModel } from '@/lib/bots/bing/types'
-
-export interface ChatList {
- messages: ChatMessageModel[]
-}
-
-export function ChatList({ messages }: ChatList) {
- if (!messages.length) {
- return null
- }
-
- return (
-
- ) : null
-}
diff --git a/spaces/arnavkartikeya/SCRIPture-final/data/flickr30k_dataset.py b/spaces/arnavkartikeya/SCRIPture-final/data/flickr30k_dataset.py
deleted file mode 100644
index 018ab387014ddaf554c4d3184cfc0e2ba8b2d487..0000000000000000000000000000000000000000
--- a/spaces/arnavkartikeya/SCRIPture-final/data/flickr30k_dataset.py
+++ /dev/null
@@ -1,93 +0,0 @@
-import os
-import json
-
-from torch.utils.data import Dataset
-from torchvision.datasets.utils import download_url
-
-from PIL import Image
-
-from data.utils import pre_caption
-
-class flickr30k_train(Dataset):
- def __init__(self, transform, image_root, ann_root, max_words=30, prompt=''):
- '''
- image_root (string): Root directory of images (e.g. flickr30k/)
- ann_root (string): directory to store the annotation file
- '''
- url = 'https://storage.googleapis.com/sfr-vision-language-research/datasets/flickr30k_train.json'
- filename = 'flickr30k_train.json'
-
- download_url(url,ann_root)
-
- self.annotation = json.load(open(os.path.join(ann_root,filename),'r'))
- self.transform = transform
- self.image_root = image_root
- self.max_words = max_words
- self.prompt = prompt
-
- self.img_ids = {}
- n = 0
- for ann in self.annotation:
- img_id = ann['image_id']
- if img_id not in self.img_ids.keys():
- self.img_ids[img_id] = n
- n += 1
-
- def __len__(self):
- return len(self.annotation)
-
- def __getitem__(self, index):
-
- ann = self.annotation[index]
-
- image_path = os.path.join(self.image_root,ann['image'])
- image = Image.open(image_path).convert('RGB')
- image = self.transform(image)
-
- caption = self.prompt+pre_caption(ann['caption'], self.max_words)
-
- return image, caption, self.img_ids[ann['image_id']]
-
-
-class flickr30k_retrieval_eval(Dataset):
- def __init__(self, transform, image_root, ann_root, split, max_words=30):
- '''
- image_root (string): Root directory of images (e.g. flickr30k/)
- ann_root (string): directory to store the annotation file
- split (string): val or test
- '''
- urls = {'val':'https://storage.googleapis.com/sfr-vision-language-research/datasets/flickr30k_val.json',
- 'test':'https://storage.googleapis.com/sfr-vision-language-research/datasets/flickr30k_test.json'}
- filenames = {'val':'flickr30k_val.json','test':'flickr30k_test.json'}
-
- download_url(urls[split],ann_root)
-
- self.annotation = json.load(open(os.path.join(ann_root,filenames[split]),'r'))
- self.transform = transform
- self.image_root = image_root
-
- self.text = []
- self.image = []
- self.txt2img = {}
- self.img2txt = {}
-
- txt_id = 0
- for img_id, ann in enumerate(self.annotation):
- self.image.append(ann['image'])
- self.img2txt[img_id] = []
- for i, caption in enumerate(ann['caption']):
- self.text.append(pre_caption(caption,max_words))
- self.img2txt[img_id].append(txt_id)
- self.txt2img[txt_id] = img_id
- txt_id += 1
-
- def __len__(self):
- return len(self.annotation)
-
- def __getitem__(self, index):
-
- image_path = os.path.join(self.image_root, self.annotation[index]['image'])
- image = Image.open(image_path).convert('RGB')
- image = self.transform(image)
-
- return image, index
\ No newline at end of file
diff --git a/spaces/arxify/RVC-beta-v2-0618/runtime/Lib/site-packages/fairseq/data/audio/speech_to_speech_dataset.py b/spaces/arxify/RVC-beta-v2-0618/runtime/Lib/site-packages/fairseq/data/audio/speech_to_speech_dataset.py
deleted file mode 100644
index 4b7f8b6824dec8082733284f92050048fcc743e6..0000000000000000000000000000000000000000
--- a/spaces/arxify/RVC-beta-v2-0618/runtime/Lib/site-packages/fairseq/data/audio/speech_to_speech_dataset.py
+++ /dev/null
@@ -1,428 +0,0 @@
-# Copyright (c) Facebook, Inc. and its affiliates.
-#
-# This source code is licensed under the MIT license found in the
-# LICENSE file in the root directory of this source tree.
-
-import logging
-from dataclasses import dataclass
-from pathlib import Path
-from typing import Dict, List, Optional, Tuple
-
-import torch
-
-from fairseq.data import ConcatDataset, Dictionary
-from fairseq.data import data_utils as fairseq_data_utils
-from fairseq.data.audio.data_cfg import S2SDataConfig
-from fairseq.data.audio.audio_utils import get_features_or_waveform
-from fairseq.data.audio.speech_to_text_dataset import (
- SpeechToTextDataset,
- SpeechToTextDatasetCreator,
- _collate_frames,
-)
-
-logger = logging.getLogger(__name__)
-
-
-@dataclass
-class SpeechToSpeechDatasetItem(object):
- index: int
- source: torch.Tensor
- target: Optional[torch.Tensor] = None
- target_speaker: Optional[torch.Tensor] = None
- tgt_lang_tag: Optional[int] = None
-
-
-class SpeechToSpeechDataset(SpeechToTextDataset):
- def __init__(
- self,
- split: str,
- is_train_split: bool,
- data_cfg: S2SDataConfig,
- src_audio_paths: List[str],
- src_n_frames: List[int],
- tgt_audio_paths: List[str],
- tgt_n_frames: List[int],
- src_langs: Optional[List[str]] = None,
- tgt_langs: Optional[List[str]] = None,
- ids: Optional[List[str]] = None,
- target_is_code: bool = False,
- tgt_dict: Dictionary = None,
- n_frames_per_step: int = 1,
- ):
- tgt_texts = tgt_audio_paths if target_is_code else None
- super().__init__(
- split,
- is_train_split,
- data_cfg,
- src_audio_paths,
- src_n_frames,
- ids=ids,
- tgt_dict=tgt_dict,
- tgt_texts=tgt_texts,
- src_langs=src_langs,
- tgt_langs=tgt_langs,
- n_frames_per_step=n_frames_per_step,
- )
-
- self.tgt_audio_paths = tgt_audio_paths
- self.tgt_lens = [t // self.n_frames_per_step for t in tgt_n_frames]
-
- assert not target_is_code or tgt_dict is not None
- self.target_is_code = target_is_code
-
- assert len(tgt_audio_paths) == self.n_samples
- assert len(tgt_n_frames) == self.n_samples
-
- self.tgt_speakers = None
- if self.cfg.target_speaker_embed:
- samples = SpeechToTextDatasetCreator._load_samples_from_tsv(
- self.cfg.target_speaker_embed, split
- )
- spk_emb_dict = {s["id"]: s["speaker_embed"] for s in samples}
- self.tgt_speakers = [spk_emb_dict[id] for id in self.ids]
- assert len(self.tgt_speakers) == self.n_samples
-
- logger.info(self.__repr__())
-
- def pack_units(self, input: torch.Tensor) -> torch.Tensor:
- if self.n_frames_per_step <= 1:
- return input
-
- offset = 4
- vocab_size = (
- len(self.tgt_dict) - offset
- ) # remove offset from , , , , which is specific to fairseq dictionary
-
- assert input.dim() == 1
- stacked_input = (
- input[:-1].view(-1, self.n_frames_per_step) - offset
- ) # remove
- scale = [
- pow(vocab_size, self.n_frames_per_step - 1 - i)
- for i in range(self.n_frames_per_step)
- ]
- scale = torch.LongTensor(scale).squeeze(0)
- res = input.new((len(input) - 1) // self.n_frames_per_step + 1).fill_(input[-1])
- res[:-1] = (stacked_input * scale).sum(dim=1) + offset
-
- return res
-
- def __getitem__(self, index: int) -> SpeechToSpeechDatasetItem:
- source = self._get_source_audio(index)
-
- tgt_lang_tag = None
- if self.cfg.prepend_tgt_lang_tag_as_bos:
- # prepend_tgt_lang_tag_as_bos: put tgt_lang_tag as bos of target
- tgt_lang_tag = self.get_lang_tag_idx(self.tgt_langs[index], self.tgt_dict)
-
- if not self.target_is_code:
- target = get_features_or_waveform(self.tgt_audio_paths[index])
- target = torch.from_numpy(target).float()
- target = self.pack_frames(target)
- else:
- target = self.tgt_dict.encode_line(
- self.tgt_audio_paths[index],
- add_if_not_exist=False,
- append_eos=True,
- ).long()
- if self.n_frames_per_step > 1:
- n_tgt_frame = target.size(0) - 1 # exclude
- keep_n_tgt_frame = n_tgt_frame - n_tgt_frame % self.n_frames_per_step
- target = torch.cat(
- (
- target[:keep_n_tgt_frame],
- target.new_full((1,), self.tgt_dict.eos()),
- ),
- dim=0,
- )
-
- if self.tgt_speakers:
- tgt_spk = get_features_or_waveform(self.tgt_speakers[index])
- tgt_spk = torch.from_numpy(tgt_spk).float()
- else:
- tgt_spk = torch.FloatTensor([])
-
- return SpeechToSpeechDatasetItem(
- index=index,
- source=source,
- target=target,
- target_speaker=tgt_spk,
- tgt_lang_tag=tgt_lang_tag,
- )
-
- def _collate_target(self, samples: List[SpeechToSpeechDatasetItem]) -> torch.Tensor:
- if self.target_is_code:
- target = fairseq_data_utils.collate_tokens(
- [x.target for x in samples],
- self.tgt_dict.pad(),
- self.tgt_dict.eos(),
- left_pad=False,
- move_eos_to_beginning=False,
- )
- # convert stacked units to a single id
- pack_targets = [self.pack_units(x.target) for x in samples]
- prev_output_tokens = fairseq_data_utils.collate_tokens(
- pack_targets,
- self.tgt_dict.pad(),
- self.tgt_dict.eos(),
- left_pad=False,
- move_eos_to_beginning=True,
- )
- target_lengths = torch.tensor(
- [x.size(0) for x in pack_targets], dtype=torch.long
- )
- else:
- target = _collate_frames([x.target for x in samples], is_audio_input=False)
- bsz, _, d = target.size()
- prev_output_tokens = torch.cat(
- (target.new_full((bsz, 1, d), 0.0), target[:, :-1, :]), dim=1
- )
- target_lengths = torch.tensor(
- [x.target.size(0) for x in samples], dtype=torch.long
- )
-
- return target, prev_output_tokens, target_lengths
-
- def collater(
- self, samples: List[SpeechToSpeechDatasetItem], return_order: bool = False
- ) -> Dict:
- if len(samples) == 0:
- return {}
- indices = torch.tensor([x.index for x in samples], dtype=torch.long)
- frames = _collate_frames([x.source for x in samples], self.cfg.use_audio_input)
- # sort samples by descending number of frames
- n_frames = torch.tensor([x.source.size(0) for x in samples], dtype=torch.long)
- n_frames, order = n_frames.sort(descending=True)
- indices = indices.index_select(0, order)
- frames = frames.index_select(0, order)
-
- target, prev_output_tokens, target_lengths = self._collate_target(samples)
- target = target.index_select(0, order)
- target_lengths = target_lengths.index_select(0, order)
- prev_output_tokens = prev_output_tokens.index_select(0, order)
- ntokens = sum(x.target.size(0) for x in samples)
-
- tgt_speakers = None
- if self.cfg.target_speaker_embed:
- tgt_speakers = _collate_frames(
- [x.target_speaker for x in samples], is_audio_input=True
- ).index_select(0, order)
-
- net_input = {
- "src_tokens": frames,
- "src_lengths": n_frames,
- "prev_output_tokens": prev_output_tokens,
- "tgt_speaker": tgt_speakers, # TODO: unify "speaker" and "tgt_speaker"
- }
- if self.tgt_texts is not None and samples[0].tgt_lang_tag is not None:
- for i in range(len(samples)):
- net_input["prev_output_tokens"][i][0] = samples[order[i]].tgt_lang_tag
- out = {
- "id": indices,
- "net_input": net_input,
- "speaker": tgt_speakers, # to support Tacotron2 loss for speech-to-spectrogram model
- "target": target,
- "target_lengths": target_lengths,
- "ntokens": ntokens,
- "nsentences": len(samples),
- }
- if return_order:
- out["order"] = order
- return out
-
-
-class TextTargetMultitaskData(object):
- # mandatory columns
- KEY_ID, KEY_TEXT = "id", "tgt_text"
-
- def __init__(self, args, split, tgt_dict):
- samples = SpeechToTextDatasetCreator._load_samples_from_tsv(args.data, split)
- self.data = {s[self.KEY_ID]: s[self.KEY_TEXT] for s in samples}
- self.dict = tgt_dict
- self.append_eos = args.decoder_type != "ctc"
-
- def get(self, sample_id):
- if sample_id in self.data:
- return self.dict.encode_line(
- self.data[sample_id],
- add_if_not_exist=False,
- append_eos=self.append_eos,
- )
- else:
- logger.warning(f"no target for {sample_id}")
- return torch.IntTensor([])
-
- def collater(self, samples: List[torch.Tensor]) -> torch.Tensor:
- out = fairseq_data_utils.collate_tokens(
- samples,
- self.dict.pad(),
- self.dict.eos(),
- left_pad=False,
- move_eos_to_beginning=False,
- ).long()
-
- prev_out = fairseq_data_utils.collate_tokens(
- samples,
- self.dict.pad(),
- self.dict.eos(),
- left_pad=False,
- move_eos_to_beginning=True,
- ).long()
-
- target_lengths = torch.tensor([t.size(0) for t in samples], dtype=torch.long)
- ntokens = sum(t.size(0) for t in samples)
-
- output = {
- "prev_output_tokens": prev_out,
- "target": out,
- "target_lengths": target_lengths,
- "ntokens": ntokens,
- }
-
- return output
-
-
-class SpeechToSpeechMultitaskDataset(SpeechToSpeechDataset):
- def __init__(self, *argv):
- super().__init__(*argv)
- self.multitask_data = {}
-
- def add_multitask_dataset(self, task_name, task_data):
- self.multitask_data[task_name] = task_data
-
- def __getitem__(
- self, index: int
- ) -> Tuple[SpeechToSpeechDatasetItem, Dict[str, torch.Tensor]]:
- s2s_data = super().__getitem__(index)
-
- multitask_target = {}
- sample_id = self.ids[index]
- for task_name, task_dataset in self.multitask_data.items():
- multitask_target[task_name] = task_dataset.get(sample_id)
-
- return s2s_data, multitask_target
-
- def collater(
- self, samples: List[Tuple[SpeechToSpeechDatasetItem, Dict[str, torch.Tensor]]]
- ) -> Dict:
- if len(samples) == 0:
- return {}
-
- out = super().collater([s for s, _ in samples], return_order=True)
- order = out["order"]
- del out["order"]
-
- for task_name, task_dataset in self.multitask_data.items():
- if "multitask" not in out:
- out["multitask"] = {}
- d = [s[task_name] for _, s in samples]
- task_target = task_dataset.collater(d)
- out["multitask"][task_name] = {
- "target": task_target["target"].index_select(0, order),
- "target_lengths": task_target["target_lengths"].index_select(0, order),
- "ntokens": task_target["ntokens"],
- }
- out["multitask"][task_name]["net_input"] = {
- "prev_output_tokens": task_target["prev_output_tokens"].index_select(
- 0, order
- ),
- }
-
- return out
-
-
-class SpeechToSpeechDatasetCreator(object):
- # mandatory columns
- KEY_ID, KEY_SRC_AUDIO, KEY_SRC_N_FRAMES = "id", "src_audio", "src_n_frames"
- KEY_TGT_AUDIO, KEY_TGT_N_FRAMES = "tgt_audio", "tgt_n_frames"
- # optional columns
- KEY_SRC_LANG, KEY_TGT_LANG = "src_lang", "tgt_lang"
- # default values
- DEFAULT_LANG = ""
-
- @classmethod
- def _from_list(
- cls,
- split_name: str,
- is_train_split,
- samples: List[Dict],
- data_cfg: S2SDataConfig,
- target_is_code: bool = False,
- target_dictionary: Dictionary = None,
- n_frames_per_step: int = 1,
- multitask: Optional[Dict] = None,
- ) -> SpeechToSpeechDataset:
- audio_root = Path(data_cfg.audio_root)
- ids = [s[cls.KEY_ID] for s in samples]
- src_audio_paths = [
- (audio_root / s[cls.KEY_SRC_AUDIO]).as_posix() for s in samples
- ]
- tgt_audio_paths = [
- s[cls.KEY_TGT_AUDIO]
- if target_is_code
- else (audio_root / s[cls.KEY_TGT_AUDIO]).as_posix()
- for s in samples
- ]
- src_n_frames = [int(s[cls.KEY_SRC_N_FRAMES]) for s in samples]
- tgt_n_frames = [int(s[cls.KEY_TGT_N_FRAMES]) for s in samples]
- src_langs = [s.get(cls.KEY_SRC_LANG, cls.DEFAULT_LANG) for s in samples]
- tgt_langs = [s.get(cls.KEY_TGT_LANG, cls.DEFAULT_LANG) for s in samples]
-
- has_multitask = len(multitask) > 0
- dataset_cls = (
- SpeechToSpeechMultitaskDataset if has_multitask else SpeechToSpeechDataset
- )
-
- ds = dataset_cls(
- split_name,
- is_train_split,
- data_cfg,
- src_audio_paths,
- src_n_frames,
- tgt_audio_paths,
- tgt_n_frames,
- src_langs,
- tgt_langs,
- ids,
- target_is_code,
- target_dictionary,
- n_frames_per_step,
- )
-
- if has_multitask:
- for task_name, task_obj in multitask.items():
- task_data = TextTargetMultitaskData(
- task_obj.args, split_name, task_obj.target_dictionary
- )
- ds.add_multitask_dataset(task_name, task_data)
- return ds
-
- @classmethod
- def from_tsv(
- cls,
- root: str,
- data_cfg: S2SDataConfig,
- splits: str,
- is_train_split: bool,
- epoch: int,
- seed: int,
- target_is_code: bool = False,
- target_dictionary: Dictionary = None,
- n_frames_per_step: int = 1,
- multitask: Optional[Dict] = None,
- ) -> SpeechToSpeechDataset:
- datasets = []
- for split in splits.split(","):
- samples = SpeechToTextDatasetCreator._load_samples_from_tsv(root, split)
- ds = cls._from_list(
- split,
- is_train_split,
- samples,
- data_cfg,
- target_is_code,
- target_dictionary,
- n_frames_per_step,
- multitask,
- )
- datasets.append(ds)
- return ConcatDataset(datasets) if len(datasets) > 1 else datasets[0]
diff --git a/spaces/at2507/SM_NLP_RecoSys/Data/Mentor_interviews/Tanh Hong.html b/spaces/at2507/SM_NLP_RecoSys/Data/Mentor_interviews/Tanh Hong.html
deleted file mode 100644
index c8cb91a59464f8589819ad40f4b29bf12e7af822..0000000000000000000000000000000000000000
--- a/spaces/at2507/SM_NLP_RecoSys/Data/Mentor_interviews/Tanh Hong.html
+++ /dev/null
@@ -1,132 +0,0 @@
-
-
-
- Tanh Hong
-
-
-
-
-
-
Tanh Hong
-
-
-
1- How did you hear about SM? What motivated you to become a mentor with SM? - A friend was a mentor with SM. Want to help new people get into DS and also promote it to other people.
2- Do you have any previous mentorship experience formal or informal? - During Phd - Mentored undergrad and masters students, helped them finish their thesis and also was a TA. Designed course in Advanced ML in bio-medical. Helped students reaching their goals. - In job - managed team of more than 10 people - encouraged and motivated team members.
3- What's your DS career journey been like? - Did PhD in 4 years in ML & Computer vision. - Senior Data Scientist in Usee (FPT Americas) - Currently working in Automotive industry ( Top 4 in the world ) exploring relationship in Big data, generate insights and learning techniques.
4- What are some of the challenges that beginners face when landing a DS related role? How can you help them with this? - There is a big gap in learning DS and tackling real issues in the industry. Schools provide industry-ambiguous data sets. The preparation is done on ready-to-use data sets, and less focus is on the techniques.
Can help mentees with realistic projects so they can learn by doing projects - ex. building 3D Models.
5- Do you have any questions regarding SM? - Friend used to mentor with SM a year ago - has anything changed in 1 year? - How many mentors and mentees are with the platform? - Are mentees new to the field or have some experience? - Do mentees have to be in Canada? - How do we know if a mentee gets a job? - Typical length of mentorship? - Do students/Mentees have a good background? - Do we get chance to interview with mentee? - Is there any fees to use the platform?
-
-
-
-
-
-
\ No newline at end of file
diff --git a/spaces/auto-academic/auto-draft/latex_templates/ICLR2022/template.tex b/spaces/auto-academic/auto-draft/latex_templates/ICLR2022/template.tex
deleted file mode 100644
index 45b8f35308d03b2514f744589ac3e601bf4775d5..0000000000000000000000000000000000000000
--- a/spaces/auto-academic/auto-draft/latex_templates/ICLR2022/template.tex
+++ /dev/null
@@ -1,35 +0,0 @@
-\documentclass{article} % For LaTeX2e
-\UseRawInputEncoding
-\usepackage{graphicx}
-\usepackage{booktabs}
-\usepackage{iclr2022_conference, times}
-\input{math_commands.tex}
-\usepackage{hyperref}
-\usepackage{url}
-\usepackage{algorithm}
-\usepackage{algpseudocode}
-
-\title{TITLE}
-\author{GPT-4}
-
-\newcommand{\fix}{\marginpar{FIX}}
-\newcommand{\new}{\marginpar{NEW}}
-
-\begin{document}
-\maketitle
-\input{abstract.tex}
-\input{introduction.tex}
-\input{related works.tex}
-\input{backgrounds.tex}
-\input{methodology.tex}
-\input{experiments.tex}
-\input{conclusion.tex}
-
-\bibliography{ref}
-\bibliographystyle{iclr2022_conference}
-
-%\appendix
-%\section{Appendix}
-%You may include other additional sections here.
-
-\end{document}
diff --git a/spaces/badayvedat/AudioSep/models/CLAP/open_clip/__init__.py b/spaces/badayvedat/AudioSep/models/CLAP/open_clip/__init__.py
deleted file mode 100644
index e9f728f2f273be5d5fdbec6c6cc41d737176a8c0..0000000000000000000000000000000000000000
--- a/spaces/badayvedat/AudioSep/models/CLAP/open_clip/__init__.py
+++ /dev/null
@@ -1,25 +0,0 @@
-from .factory import (
- list_models,
- create_model,
- create_model_and_transforms,
- add_model_config,
-)
-from .loss import ClipLoss, gather_features, LPLoss, lp_gather_features, LPMetrics
-from .model import (
- CLAP,
- CLAPTextCfg,
- CLAPVisionCfg,
- CLAPAudioCfp,
- convert_weights_to_fp16,
- trace_model,
-)
-from .openai import load_openai_model, list_openai_models
-from .pretrained import (
- list_pretrained,
- list_pretrained_tag_models,
- list_pretrained_model_tags,
- get_pretrained_url,
- download_pretrained,
-)
-from .tokenizer import SimpleTokenizer, tokenize
-from .transform import image_transform
diff --git a/spaces/betterme/mestreamlit/pages/997_streamlit_aggrid.py b/spaces/betterme/mestreamlit/pages/997_streamlit_aggrid.py
deleted file mode 100644
index 057be818ff8e5694735cdcb687e4c6d40fc798ba..0000000000000000000000000000000000000000
--- a/spaces/betterme/mestreamlit/pages/997_streamlit_aggrid.py
+++ /dev/null
@@ -1,16 +0,0 @@
-#!/usr/bin/env python
-# -*- coding: utf-8 -*-
-# @Project : Python.
-# @File : 997_streamlit_aggrid
-# @Time : 2022/10/17 下午1:14
-# @Author : yuanjie
-# @WeChat : meutils
-# @Software : PyCharm
-# @Description :
-
-
-from st_aggrid import AgGrid
-import pandas as pd
-
-df = pd.read_csv('./data/airline-safety.csv')
-AgGrid(df)
\ No newline at end of file
diff --git a/spaces/bhasker412/IDD-YOLO-Tracking/utils/general.py b/spaces/bhasker412/IDD-YOLO-Tracking/utils/general.py
deleted file mode 100644
index 6b7edb3e013683b2ee38af9ce9e103616aaaa3ff..0000000000000000000000000000000000000000
--- a/spaces/bhasker412/IDD-YOLO-Tracking/utils/general.py
+++ /dev/null
@@ -1,892 +0,0 @@
-# YOLOR general utils
-
-import glob
-import logging
-import math
-import os
-import platform
-import random
-import re
-import subprocess
-import time
-from pathlib import Path
-
-import cv2
-import numpy as np
-import pandas as pd
-import torch
-import torchvision
-import yaml
-
-from utils.google_utils import gsutil_getsize
-from utils.metrics import fitness
-from utils.torch_utils import init_torch_seeds
-
-# Settings
-torch.set_printoptions(linewidth=320, precision=5, profile='long')
-np.set_printoptions(linewidth=320, formatter={'float_kind': '{:11.5g}'.format}) # format short g, %precision=5
-pd.options.display.max_columns = 10
-cv2.setNumThreads(0) # prevent OpenCV from multithreading (incompatible with PyTorch DataLoader)
-os.environ['NUMEXPR_MAX_THREADS'] = str(min(os.cpu_count(), 8)) # NumExpr max threads
-
-
-def set_logging(rank=-1):
- logging.basicConfig(
- format="%(message)s",
- level=logging.INFO if rank in [-1, 0] else logging.WARN)
-
-
-def init_seeds(seed=0):
- # Initialize random number generator (RNG) seeds
- random.seed(seed)
- np.random.seed(seed)
- init_torch_seeds(seed)
-
-
-def get_latest_run(search_dir='.'):
- # Return path to most recent 'last.pt' in /runs (i.e. to --resume from)
- last_list = glob.glob(f'{search_dir}/**/last*.pt', recursive=True)
- return max(last_list, key=os.path.getctime) if last_list else ''
-
-
-def isdocker():
- # Is environment a Docker container
- return Path('/workspace').exists() # or Path('/.dockerenv').exists()
-
-
-def emojis(str=''):
- # Return platform-dependent emoji-safe version of string
- return str.encode().decode('ascii', 'ignore') if platform.system() == 'Windows' else str
-
-
-def check_online():
- # Check internet connectivity
- import socket
- try:
- socket.create_connection(("1.1.1.1", 443), 5) # check host accesability
- return True
- except OSError:
- return False
-
-
-def check_git_status():
- # Recommend 'git pull' if code is out of date
- print(colorstr('github: '), end='')
- try:
- assert Path('.git').exists(), 'skipping check (not a git repository)'
- assert not isdocker(), 'skipping check (Docker image)'
- assert check_online(), 'skipping check (offline)'
-
- cmd = 'git fetch && git config --get remote.origin.url'
- url = subprocess.check_output(cmd, shell=True).decode().strip().rstrip('.git') # github repo url
- branch = subprocess.check_output('git rev-parse --abbrev-ref HEAD', shell=True).decode().strip() # checked out
- n = int(subprocess.check_output(f'git rev-list {branch}..origin/master --count', shell=True)) # commits behind
- if n > 0:
- s = f"⚠️ WARNING: code is out of date by {n} commit{'s' * (n > 1)}. " \
- f"Use 'git pull' to update or 'git clone {url}' to download latest."
- else:
- s = f'up to date with {url} ✅'
- print(emojis(s)) # emoji-safe
- except Exception as e:
- print(e)
-
-
-def check_requirements(requirements='requirements.txt', exclude=()):
- # Check installed dependencies meet requirements (pass *.txt file or list of packages)
- import pkg_resources as pkg
- prefix = colorstr('red', 'bold', 'requirements:')
- if isinstance(requirements, (str, Path)): # requirements.txt file
- file = Path(requirements)
- if not file.exists():
- print(f"{prefix} {file.resolve()} not found, check failed.")
- return
- requirements = [f'{x.name}{x.specifier}' for x in pkg.parse_requirements(file.open()) if x.name not in exclude]
- else: # list or tuple of packages
- requirements = [x for x in requirements if x not in exclude]
-
- n = 0 # number of packages updates
- for r in requirements:
- try:
- pkg.require(r)
- except Exception as e: # DistributionNotFound or VersionConflict if requirements not met
- n += 1
- print(f"{prefix} {e.req} not found and is required by YOLOR, attempting auto-update...")
- print(subprocess.check_output(f"pip install '{e.req}'", shell=True).decode())
-
- if n: # if packages updated
- source = file.resolve() if 'file' in locals() else requirements
- s = f"{prefix} {n} package{'s' * (n > 1)} updated per {source}\n" \
- f"{prefix} ⚠️ {colorstr('bold', 'Restart runtime or rerun command for updates to take effect')}\n"
- print(emojis(s)) # emoji-safe
-
-
-def check_img_size(img_size, s=32):
- # Verify img_size is a multiple of stride s
- new_size = make_divisible(img_size, int(s)) # ceil gs-multiple
- if new_size != img_size:
- print('WARNING: --img-size %g must be multiple of max stride %g, updating to %g' % (img_size, s, new_size))
- return new_size
-
-
-def check_imshow():
- # Check if environment supports image displays
- try:
- assert not isdocker(), 'cv2.imshow() is disabled in Docker environments'
- cv2.imshow('test', np.zeros((1, 1, 3)))
- cv2.waitKey(1)
- cv2.destroyAllWindows()
- cv2.waitKey(1)
- return True
- except Exception as e:
- print(f'WARNING: Environment does not support cv2.imshow() or PIL Image.show() image displays\n{e}')
- return False
-
-
-def check_file(file):
- # Search for file if not found
- if Path(file).is_file() or file == '':
- return file
- else:
- files = glob.glob('./**/' + file, recursive=True) # find file
- assert len(files), f'File Not Found: {file}' # assert file was found
- assert len(files) == 1, f"Multiple files match '{file}', specify exact path: {files}" # assert unique
- return files[0] # return file
-
-
-def check_dataset(dict):
- # Download dataset if not found locally
- val, s = dict.get('val'), dict.get('download')
- if val and len(val):
- val = [Path(x).resolve() for x in (val if isinstance(val, list) else [val])] # val path
- if not all(x.exists() for x in val):
- print('\nWARNING: Dataset not found, nonexistent paths: %s' % [str(x) for x in val if not x.exists()])
- if s and len(s): # download script
- print('Downloading %s ...' % s)
- if s.startswith('http') and s.endswith('.zip'): # URL
- f = Path(s).name # filename
- torch.hub.download_url_to_file(s, f)
- r = os.system('unzip -q %s -d ../ && rm %s' % (f, f)) # unzip
- else: # bash script
- r = os.system(s)
- print('Dataset autodownload %s\n' % ('success' if r == 0 else 'failure')) # analyze return value
- else:
- raise Exception('Dataset not found.')
-
-
-def make_divisible(x, divisor):
- # Returns x evenly divisible by divisor
- return math.ceil(x / divisor) * divisor
-
-
-def clean_str(s):
- # Cleans a string by replacing special characters with underscore _
- return re.sub(pattern="[|@#!¡·$€%&()=?¿^*;:,¨´><+]", repl="_", string=s)
-
-
-def one_cycle(y1=0.0, y2=1.0, steps=100):
- # lambda function for sinusoidal ramp from y1 to y2
- return lambda x: ((1 - math.cos(x * math.pi / steps)) / 2) * (y2 - y1) + y1
-
-
-def colorstr(*input):
- # Colors a string https://en.wikipedia.org/wiki/ANSI_escape_code, i.e. colorstr('blue', 'hello world')
- *args, string = input if len(input) > 1 else ('blue', 'bold', input[0]) # color arguments, string
- colors = {'black': '\033[30m', # basic colors
- 'red': '\033[31m',
- 'green': '\033[32m',
- 'yellow': '\033[33m',
- 'blue': '\033[34m',
- 'magenta': '\033[35m',
- 'cyan': '\033[36m',
- 'white': '\033[37m',
- 'bright_black': '\033[90m', # bright colors
- 'bright_red': '\033[91m',
- 'bright_green': '\033[92m',
- 'bright_yellow': '\033[93m',
- 'bright_blue': '\033[94m',
- 'bright_magenta': '\033[95m',
- 'bright_cyan': '\033[96m',
- 'bright_white': '\033[97m',
- 'end': '\033[0m', # misc
- 'bold': '\033[1m',
- 'underline': '\033[4m'}
- return ''.join(colors[x] for x in args) + f'{string}' + colors['end']
-
-
-def labels_to_class_weights(labels, nc=80):
- # Get class weights (inverse frequency) from training labels
- if labels[0] is None: # no labels loaded
- return torch.Tensor()
-
- labels = np.concatenate(labels, 0) # labels.shape = (866643, 5) for COCO
- classes = labels[:, 0].astype(np.int) # labels = [class xywh]
- weights = np.bincount(classes, minlength=nc) # occurrences per class
-
- # Prepend gridpoint count (for uCE training)
- # gpi = ((320 / 32 * np.array([1, 2, 4])) ** 2 * 3).sum() # gridpoints per image
- # weights = np.hstack([gpi * len(labels) - weights.sum() * 9, weights * 9]) ** 0.5 # prepend gridpoints to start
-
- weights[weights == 0] = 1 # replace empty bins with 1
- weights = 1 / weights # number of targets per class
- weights /= weights.sum() # normalize
- return torch.from_numpy(weights)
-
-
-def labels_to_image_weights(labels, nc=80, class_weights=np.ones(80)):
- # Produces image weights based on class_weights and image contents
- class_counts = np.array([np.bincount(x[:, 0].astype(np.int), minlength=nc) for x in labels])
- image_weights = (class_weights.reshape(1, nc) * class_counts).sum(1)
- # index = random.choices(range(n), weights=image_weights, k=1) # weight image sample
- return image_weights
-
-
-def coco80_to_coco91_class(): # converts 80-index (val2014) to 91-index (paper)
- # https://tech.amikelive.com/node-718/what-object-categories-labels-are-in-coco-dataset/
- # a = np.loadtxt('data/coco.names', dtype='str', delimiter='\n')
- # b = np.loadtxt('data/coco_paper.names', dtype='str', delimiter='\n')
- # x1 = [list(a[i] == b).index(True) + 1 for i in range(80)] # darknet to coco
- # x2 = [list(b[i] == a).index(True) if any(b[i] == a) else None for i in range(91)] # coco to darknet
- x = [1, 2, 3, 4, 5, 6, 7, 8, 9, 10, 11, 13, 14, 15, 16, 17, 18, 19, 20, 21, 22, 23, 24, 25, 27, 28, 31, 32, 33, 34,
- 35, 36, 37, 38, 39, 40, 41, 42, 43, 44, 46, 47, 48, 49, 50, 51, 52, 53, 54, 55, 56, 57, 58, 59, 60, 61, 62, 63,
- 64, 65, 67, 70, 72, 73, 74, 75, 76, 77, 78, 79, 80, 81, 82, 84, 85, 86, 87, 88, 89, 90]
- return x
-
-
-def xyxy2xywh(x):
- # Convert nx4 boxes from [x1, y1, x2, y2] to [x, y, w, h] where xy1=top-left, xy2=bottom-right
- y = x.clone() if isinstance(x, torch.Tensor) else np.copy(x)
- y[:, 0] = (x[:, 0] + x[:, 2]) / 2 # x center
- y[:, 1] = (x[:, 1] + x[:, 3]) / 2 # y center
- y[:, 2] = x[:, 2] - x[:, 0] # width
- y[:, 3] = x[:, 3] - x[:, 1] # height
- return y
-
-
-def xywh2xyxy(x):
- # Convert nx4 boxes from [x, y, w, h] to [x1, y1, x2, y2] where xy1=top-left, xy2=bottom-right
- y = x.clone() if isinstance(x, torch.Tensor) else np.copy(x)
- y[:, 0] = x[:, 0] - x[:, 2] / 2 # top left x
- y[:, 1] = x[:, 1] - x[:, 3] / 2 # top left y
- y[:, 2] = x[:, 0] + x[:, 2] / 2 # bottom right x
- y[:, 3] = x[:, 1] + x[:, 3] / 2 # bottom right y
- return y
-
-
-def xywhn2xyxy(x, w=640, h=640, padw=0, padh=0):
- # Convert nx4 boxes from [x, y, w, h] normalized to [x1, y1, x2, y2] where xy1=top-left, xy2=bottom-right
- y = x.clone() if isinstance(x, torch.Tensor) else np.copy(x)
- y[:, 0] = w * (x[:, 0] - x[:, 2] / 2) + padw # top left x
- y[:, 1] = h * (x[:, 1] - x[:, 3] / 2) + padh # top left y
- y[:, 2] = w * (x[:, 0] + x[:, 2] / 2) + padw # bottom right x
- y[:, 3] = h * (x[:, 1] + x[:, 3] / 2) + padh # bottom right y
- return y
-
-
-def xyn2xy(x, w=640, h=640, padw=0, padh=0):
- # Convert normalized segments into pixel segments, shape (n,2)
- y = x.clone() if isinstance(x, torch.Tensor) else np.copy(x)
- y[:, 0] = w * x[:, 0] + padw # top left x
- y[:, 1] = h * x[:, 1] + padh # top left y
- return y
-
-
-def segment2box(segment, width=640, height=640):
- # Convert 1 segment label to 1 box label, applying inside-image constraint, i.e. (xy1, xy2, ...) to (xyxy)
- x, y = segment.T # segment xy
- inside = (x >= 0) & (y >= 0) & (x <= width) & (y <= height)
- x, y, = x[inside], y[inside]
- return np.array([x.min(), y.min(), x.max(), y.max()]) if any(x) else np.zeros((1, 4)) # xyxy
-
-
-def segments2boxes(segments):
- # Convert segment labels to box labels, i.e. (cls, xy1, xy2, ...) to (cls, xywh)
- boxes = []
- for s in segments:
- x, y = s.T # segment xy
- boxes.append([x.min(), y.min(), x.max(), y.max()]) # cls, xyxy
- return xyxy2xywh(np.array(boxes)) # cls, xywh
-
-
-def resample_segments(segments, n=1000):
- # Up-sample an (n,2) segment
- for i, s in enumerate(segments):
- s = np.concatenate((s, s[0:1, :]), axis=0)
- x = np.linspace(0, len(s) - 1, n)
- xp = np.arange(len(s))
- segments[i] = np.concatenate([np.interp(x, xp, s[:, i]) for i in range(2)]).reshape(2, -1).T # segment xy
- return segments
-
-
-def scale_coords(img1_shape, coords, img0_shape, ratio_pad=None):
- # Rescale coords (xyxy) from img1_shape to img0_shape
- if ratio_pad is None: # calculate from img0_shape
- gain = min(img1_shape[0] / img0_shape[0], img1_shape[1] / img0_shape[1]) # gain = old / new
- pad = (img1_shape[1] - img0_shape[1] * gain) / 2, (img1_shape[0] - img0_shape[0] * gain) / 2 # wh padding
- else:
- gain = ratio_pad[0][0]
- pad = ratio_pad[1]
-
- coords[:, [0, 2]] -= pad[0] # x padding
- coords[:, [1, 3]] -= pad[1] # y padding
- coords[:, :4] /= gain
- clip_coords(coords, img0_shape)
- return coords
-
-
-def clip_coords(boxes, img_shape):
- # Clip bounding xyxy bounding boxes to image shape (height, width)
- boxes[:, 0].clamp_(0, img_shape[1]) # x1
- boxes[:, 1].clamp_(0, img_shape[0]) # y1
- boxes[:, 2].clamp_(0, img_shape[1]) # x2
- boxes[:, 3].clamp_(0, img_shape[0]) # y2
-
-
-def bbox_iou(box1, box2, x1y1x2y2=True, GIoU=False, DIoU=False, CIoU=False, eps=1e-7):
- # Returns the IoU of box1 to box2. box1 is 4, box2 is nx4
- box2 = box2.T
-
- # Get the coordinates of bounding boxes
- if x1y1x2y2: # x1, y1, x2, y2 = box1
- b1_x1, b1_y1, b1_x2, b1_y2 = box1[0], box1[1], box1[2], box1[3]
- b2_x1, b2_y1, b2_x2, b2_y2 = box2[0], box2[1], box2[2], box2[3]
- else: # transform from xywh to xyxy
- b1_x1, b1_x2 = box1[0] - box1[2] / 2, box1[0] + box1[2] / 2
- b1_y1, b1_y2 = box1[1] - box1[3] / 2, box1[1] + box1[3] / 2
- b2_x1, b2_x2 = box2[0] - box2[2] / 2, box2[0] + box2[2] / 2
- b2_y1, b2_y2 = box2[1] - box2[3] / 2, box2[1] + box2[3] / 2
-
- # Intersection area
- inter = (torch.min(b1_x2, b2_x2) - torch.max(b1_x1, b2_x1)).clamp(0) * \
- (torch.min(b1_y2, b2_y2) - torch.max(b1_y1, b2_y1)).clamp(0)
-
- # Union Area
- w1, h1 = b1_x2 - b1_x1, b1_y2 - b1_y1 + eps
- w2, h2 = b2_x2 - b2_x1, b2_y2 - b2_y1 + eps
- union = w1 * h1 + w2 * h2 - inter + eps
-
- iou = inter / union
-
- if GIoU or DIoU or CIoU:
- cw = torch.max(b1_x2, b2_x2) - torch.min(b1_x1, b2_x1) # convex (smallest enclosing box) width
- ch = torch.max(b1_y2, b2_y2) - torch.min(b1_y1, b2_y1) # convex height
- if CIoU or DIoU: # Distance or Complete IoU https://arxiv.org/abs/1911.08287v1
- c2 = cw ** 2 + ch ** 2 + eps # convex diagonal squared
- rho2 = ((b2_x1 + b2_x2 - b1_x1 - b1_x2) ** 2 +
- (b2_y1 + b2_y2 - b1_y1 - b1_y2) ** 2) / 4 # center distance squared
- if DIoU:
- return iou - rho2 / c2 # DIoU
- elif CIoU: # https://github.com/Zzh-tju/DIoU-SSD-pytorch/blob/master/utils/box/box_utils.py#L47
- v = (4 / math.pi ** 2) * torch.pow(torch.atan(w2 / (h2 + eps)) - torch.atan(w1 / (h1 + eps)), 2)
- with torch.no_grad():
- alpha = v / (v - iou + (1 + eps))
- return iou - (rho2 / c2 + v * alpha) # CIoU
- else: # GIoU https://arxiv.org/pdf/1902.09630.pdf
- c_area = cw * ch + eps # convex area
- return iou - (c_area - union) / c_area # GIoU
- else:
- return iou # IoU
-
-
-
-
-def bbox_alpha_iou(box1, box2, x1y1x2y2=False, GIoU=False, DIoU=False, CIoU=False, alpha=2, eps=1e-9):
- # Returns tsqrt_he IoU of box1 to box2. box1 is 4, box2 is nx4
- box2 = box2.T
-
- # Get the coordinates of bounding boxes
- if x1y1x2y2: # x1, y1, x2, y2 = box1
- b1_x1, b1_y1, b1_x2, b1_y2 = box1[0], box1[1], box1[2], box1[3]
- b2_x1, b2_y1, b2_x2, b2_y2 = box2[0], box2[1], box2[2], box2[3]
- else: # transform from xywh to xyxy
- b1_x1, b1_x2 = box1[0] - box1[2] / 2, box1[0] + box1[2] / 2
- b1_y1, b1_y2 = box1[1] - box1[3] / 2, box1[1] + box1[3] / 2
- b2_x1, b2_x2 = box2[0] - box2[2] / 2, box2[0] + box2[2] / 2
- b2_y1, b2_y2 = box2[1] - box2[3] / 2, box2[1] + box2[3] / 2
-
- # Intersection area
- inter = (torch.min(b1_x2, b2_x2) - torch.max(b1_x1, b2_x1)).clamp(0) * \
- (torch.min(b1_y2, b2_y2) - torch.max(b1_y1, b2_y1)).clamp(0)
-
- # Union Area
- w1, h1 = b1_x2 - b1_x1, b1_y2 - b1_y1 + eps
- w2, h2 = b2_x2 - b2_x1, b2_y2 - b2_y1 + eps
- union = w1 * h1 + w2 * h2 - inter + eps
-
- # change iou into pow(iou+eps)
- # iou = inter / union
- iou = torch.pow(inter/union + eps, alpha)
- # beta = 2 * alpha
- if GIoU or DIoU or CIoU:
- cw = torch.max(b1_x2, b2_x2) - torch.min(b1_x1, b2_x1) # convex (smallest enclosing box) width
- ch = torch.max(b1_y2, b2_y2) - torch.min(b1_y1, b2_y1) # convex height
- if CIoU or DIoU: # Distance or Complete IoU https://arxiv.org/abs/1911.08287v1
- c2 = (cw ** 2 + ch ** 2) ** alpha + eps # convex diagonal
- rho_x = torch.abs(b2_x1 + b2_x2 - b1_x1 - b1_x2)
- rho_y = torch.abs(b2_y1 + b2_y2 - b1_y1 - b1_y2)
- rho2 = ((rho_x ** 2 + rho_y ** 2) / 4) ** alpha # center distance
- if DIoU:
- return iou - rho2 / c2 # DIoU
- elif CIoU: # https://github.com/Zzh-tju/DIoU-SSD-pytorch/blob/master/utils/box/box_utils.py#L47
- v = (4 / math.pi ** 2) * torch.pow(torch.atan(w2 / h2) - torch.atan(w1 / h1), 2)
- with torch.no_grad():
- alpha_ciou = v / ((1 + eps) - inter / union + v)
- # return iou - (rho2 / c2 + v * alpha_ciou) # CIoU
- return iou - (rho2 / c2 + torch.pow(v * alpha_ciou + eps, alpha)) # CIoU
- else: # GIoU https://arxiv.org/pdf/1902.09630.pdf
- # c_area = cw * ch + eps # convex area
- # return iou - (c_area - union) / c_area # GIoU
- c_area = torch.max(cw * ch + eps, union) # convex area
- return iou - torch.pow((c_area - union) / c_area + eps, alpha) # GIoU
- else:
- return iou # torch.log(iou+eps) or iou
-
-
-def box_iou(box1, box2):
- # https://github.com/pytorch/vision/blob/master/torchvision/ops/boxes.py
- """
- Return intersection-over-union (Jaccard index) of boxes.
- Both sets of boxes are expected to be in (x1, y1, x2, y2) format.
- Arguments:
- box1 (Tensor[N, 4])
- box2 (Tensor[M, 4])
- Returns:
- iou (Tensor[N, M]): the NxM matrix containing the pairwise
- IoU values for every element in boxes1 and boxes2
- """
-
- def box_area(box):
- # box = 4xn
- return (box[2] - box[0]) * (box[3] - box[1])
-
- area1 = box_area(box1.T)
- area2 = box_area(box2.T)
-
- # inter(N,M) = (rb(N,M,2) - lt(N,M,2)).clamp(0).prod(2)
- inter = (torch.min(box1[:, None, 2:], box2[:, 2:]) - torch.max(box1[:, None, :2], box2[:, :2])).clamp(0).prod(2)
- return inter / (area1[:, None] + area2 - inter) # iou = inter / (area1 + area2 - inter)
-
-
-def wh_iou(wh1, wh2):
- # Returns the nxm IoU matrix. wh1 is nx2, wh2 is mx2
- wh1 = wh1[:, None] # [N,1,2]
- wh2 = wh2[None] # [1,M,2]
- inter = torch.min(wh1, wh2).prod(2) # [N,M]
- return inter / (wh1.prod(2) + wh2.prod(2) - inter) # iou = inter / (area1 + area2 - inter)
-
-
-def box_giou(box1, box2):
- """
- Return generalized intersection-over-union (Jaccard index) between two sets of boxes.
- Both sets of boxes are expected to be in ``(x1, y1, x2, y2)`` format with
- ``0 <= x1 < x2`` and ``0 <= y1 < y2``.
- Args:
- boxes1 (Tensor[N, 4]): first set of boxes
- boxes2 (Tensor[M, 4]): second set of boxes
- Returns:
- Tensor[N, M]: the NxM matrix containing the pairwise generalized IoU values
- for every element in boxes1 and boxes2
- """
-
- def box_area(box):
- # box = 4xn
- return (box[2] - box[0]) * (box[3] - box[1])
-
- area1 = box_area(box1.T)
- area2 = box_area(box2.T)
-
- inter = (torch.min(box1[:, None, 2:], box2[:, 2:]) - torch.max(box1[:, None, :2], box2[:, :2])).clamp(0).prod(2)
- union = (area1[:, None] + area2 - inter)
-
- iou = inter / union
-
- lti = torch.min(box1[:, None, :2], box2[:, :2])
- rbi = torch.max(box1[:, None, 2:], box2[:, 2:])
-
- whi = (rbi - lti).clamp(min=0) # [N,M,2]
- areai = whi[:, :, 0] * whi[:, :, 1]
-
- return iou - (areai - union) / areai
-
-
-def box_ciou(box1, box2, eps: float = 1e-7):
- """
- Return complete intersection-over-union (Jaccard index) between two sets of boxes.
- Both sets of boxes are expected to be in ``(x1, y1, x2, y2)`` format with
- ``0 <= x1 < x2`` and ``0 <= y1 < y2``.
- Args:
- boxes1 (Tensor[N, 4]): first set of boxes
- boxes2 (Tensor[M, 4]): second set of boxes
- eps (float, optional): small number to prevent division by zero. Default: 1e-7
- Returns:
- Tensor[N, M]: the NxM matrix containing the pairwise complete IoU values
- for every element in boxes1 and boxes2
- """
-
- def box_area(box):
- # box = 4xn
- return (box[2] - box[0]) * (box[3] - box[1])
-
- area1 = box_area(box1.T)
- area2 = box_area(box2.T)
-
- inter = (torch.min(box1[:, None, 2:], box2[:, 2:]) - torch.max(box1[:, None, :2], box2[:, :2])).clamp(0).prod(2)
- union = (area1[:, None] + area2 - inter)
-
- iou = inter / union
-
- lti = torch.min(box1[:, None, :2], box2[:, :2])
- rbi = torch.max(box1[:, None, 2:], box2[:, 2:])
-
- whi = (rbi - lti).clamp(min=0) # [N,M,2]
- diagonal_distance_squared = (whi[:, :, 0] ** 2) + (whi[:, :, 1] ** 2) + eps
-
- # centers of boxes
- x_p = (box1[:, None, 0] + box1[:, None, 2]) / 2
- y_p = (box1[:, None, 1] + box1[:, None, 3]) / 2
- x_g = (box2[:, 0] + box2[:, 2]) / 2
- y_g = (box2[:, 1] + box2[:, 3]) / 2
- # The distance between boxes' centers squared.
- centers_distance_squared = (x_p - x_g) ** 2 + (y_p - y_g) ** 2
-
- w_pred = box1[:, None, 2] - box1[:, None, 0]
- h_pred = box1[:, None, 3] - box1[:, None, 1]
-
- w_gt = box2[:, 2] - box2[:, 0]
- h_gt = box2[:, 3] - box2[:, 1]
-
- v = (4 / (torch.pi ** 2)) * torch.pow((torch.atan(w_gt / h_gt) - torch.atan(w_pred / h_pred)), 2)
- with torch.no_grad():
- alpha = v / (1 - iou + v + eps)
- return iou - (centers_distance_squared / diagonal_distance_squared) - alpha * v
-
-
-def box_diou(box1, box2, eps: float = 1e-7):
- """
- Return distance intersection-over-union (Jaccard index) between two sets of boxes.
- Both sets of boxes are expected to be in ``(x1, y1, x2, y2)`` format with
- ``0 <= x1 < x2`` and ``0 <= y1 < y2``.
- Args:
- boxes1 (Tensor[N, 4]): first set of boxes
- boxes2 (Tensor[M, 4]): second set of boxes
- eps (float, optional): small number to prevent division by zero. Default: 1e-7
- Returns:
- Tensor[N, M]: the NxM matrix containing the pairwise distance IoU values
- for every element in boxes1 and boxes2
- """
-
- def box_area(box):
- # box = 4xn
- return (box[2] - box[0]) * (box[3] - box[1])
-
- area1 = box_area(box1.T)
- area2 = box_area(box2.T)
-
- inter = (torch.min(box1[:, None, 2:], box2[:, 2:]) - torch.max(box1[:, None, :2], box2[:, :2])).clamp(0).prod(2)
- union = (area1[:, None] + area2 - inter)
-
- iou = inter / union
-
- lti = torch.min(box1[:, None, :2], box2[:, :2])
- rbi = torch.max(box1[:, None, 2:], box2[:, 2:])
-
- whi = (rbi - lti).clamp(min=0) # [N,M,2]
- diagonal_distance_squared = (whi[:, :, 0] ** 2) + (whi[:, :, 1] ** 2) + eps
-
- # centers of boxes
- x_p = (box1[:, None, 0] + box1[:, None, 2]) / 2
- y_p = (box1[:, None, 1] + box1[:, None, 3]) / 2
- x_g = (box2[:, 0] + box2[:, 2]) / 2
- y_g = (box2[:, 1] + box2[:, 3]) / 2
- # The distance between boxes' centers squared.
- centers_distance_squared = (x_p - x_g) ** 2 + (y_p - y_g) ** 2
-
- # The distance IoU is the IoU penalized by a normalized
- # distance between boxes' centers squared.
- return iou - (centers_distance_squared / diagonal_distance_squared)
-
-
-def non_max_suppression(prediction, conf_thres=0.25, iou_thres=0.45, classes=None, agnostic=False, multi_label=False,
- labels=()):
- """Runs Non-Maximum Suppression (NMS) on inference results
-
- Returns:
- list of detections, on (n,6) tensor per image [xyxy, conf, cls]
- """
-
- nc = prediction.shape[2] - 5 # number of classes
- xc = prediction[..., 4] > conf_thres # candidates
-
- # Settings
- min_wh, max_wh = 2, 4096 # (pixels) minimum and maximum box width and height
- max_det = 300 # maximum number of detections per image
- max_nms = 30000 # maximum number of boxes into torchvision.ops.nms()
- time_limit = 10.0 # seconds to quit after
- redundant = True # require redundant detections
- multi_label &= nc > 1 # multiple labels per box (adds 0.5ms/img)
- merge = False # use merge-NMS
-
- t = time.time()
- output = [torch.zeros((0, 6), device=prediction.device)] * prediction.shape[0]
- for xi, x in enumerate(prediction): # image index, image inference
- # Apply constraints
- # x[((x[..., 2:4] < min_wh) | (x[..., 2:4] > max_wh)).any(1), 4] = 0 # width-height
- x = x[xc[xi]] # confidence
-
- # Cat apriori labels if autolabelling
- if labels and len(labels[xi]):
- l = labels[xi]
- v = torch.zeros((len(l), nc + 5), device=x.device)
- v[:, :4] = l[:, 1:5] # box
- v[:, 4] = 1.0 # conf
- v[range(len(l)), l[:, 0].long() + 5] = 1.0 # cls
- x = torch.cat((x, v), 0)
-
- # If none remain process next image
- if not x.shape[0]:
- continue
-
- # Compute conf
- if nc == 1:
- x[:, 5:] = x[:, 4:5] # for models with one class, cls_loss is 0 and cls_conf is always 0.5,
- # so there is no need to multiplicate.
- else:
- x[:, 5:] *= x[:, 4:5] # conf = obj_conf * cls_conf
-
- # Box (center x, center y, width, height) to (x1, y1, x2, y2)
- box = xywh2xyxy(x[:, :4])
-
- # Detections matrix nx6 (xyxy, conf, cls)
- if multi_label:
- i, j = (x[:, 5:] > conf_thres).nonzero(as_tuple=False).T
- x = torch.cat((box[i], x[i, j + 5, None], j[:, None].float()), 1)
- else: # best class only
- conf, j = x[:, 5:].max(1, keepdim=True)
- x = torch.cat((box, conf, j.float()), 1)[conf.view(-1) > conf_thres]
-
- # Filter by class
- if classes is not None:
- x = x[(x[:, 5:6] == torch.tensor(classes, device=x.device)).any(1)]
-
- # Apply finite constraint
- # if not torch.isfinite(x).all():
- # x = x[torch.isfinite(x).all(1)]
-
- # Check shape
- n = x.shape[0] # number of boxes
- if not n: # no boxes
- continue
- elif n > max_nms: # excess boxes
- x = x[x[:, 4].argsort(descending=True)[:max_nms]] # sort by confidence
-
- # Batched NMS
- c = x[:, 5:6] * (0 if agnostic else max_wh) # classes
- boxes, scores = x[:, :4] + c, x[:, 4] # boxes (offset by class), scores
- i = torchvision.ops.nms(boxes, scores, iou_thres) # NMS
- if i.shape[0] > max_det: # limit detections
- i = i[:max_det]
- if merge and (1 < n < 3E3): # Merge NMS (boxes merged using weighted mean)
- # update boxes as boxes(i,4) = weights(i,n) * boxes(n,4)
- iou = box_iou(boxes[i], boxes) > iou_thres # iou matrix
- weights = iou * scores[None] # box weights
- x[i, :4] = torch.mm(weights, x[:, :4]).float() / weights.sum(1, keepdim=True) # merged boxes
- if redundant:
- i = i[iou.sum(1) > 1] # require redundancy
-
- output[xi] = x[i]
- if (time.time() - t) > time_limit:
- print(f'WARNING: NMS time limit {time_limit}s exceeded')
- break # time limit exceeded
-
- return output
-
-
-def non_max_suppression_kpt(prediction, conf_thres=0.25, iou_thres=0.45, classes=None, agnostic=False, multi_label=False,
- labels=(), kpt_label=False, nc=None, nkpt=None):
- """Runs Non-Maximum Suppression (NMS) on inference results
-
- Returns:
- list of detections, on (n,6) tensor per image [xyxy, conf, cls]
- """
- if nc is None:
- nc = prediction.shape[2] - 5 if not kpt_label else prediction.shape[2] - 56 # number of classes
- xc = prediction[..., 4] > conf_thres # candidates
-
- # Settings
- min_wh, max_wh = 2, 4096 # (pixels) minimum and maximum box width and height
- max_det = 300 # maximum number of detections per image
- max_nms = 30000 # maximum number of boxes into torchvision.ops.nms()
- time_limit = 10.0 # seconds to quit after
- redundant = True # require redundant detections
- multi_label &= nc > 1 # multiple labels per box (adds 0.5ms/img)
- merge = False # use merge-NMS
-
- t = time.time()
- output = [torch.zeros((0,6), device=prediction.device)] * prediction.shape[0]
- for xi, x in enumerate(prediction): # image index, image inference
- # Apply constraints
- # x[((x[..., 2:4] < min_wh) | (x[..., 2:4] > max_wh)).any(1), 4] = 0 # width-height
- x = x[xc[xi]] # confidence
-
- # Cat apriori labels if autolabelling
- if labels and len(labels[xi]):
- l = labels[xi]
- v = torch.zeros((len(l), nc + 5), device=x.device)
- v[:, :4] = l[:, 1:5] # box
- v[:, 4] = 1.0 # conf
- v[range(len(l)), l[:, 0].long() + 5] = 1.0 # cls
- x = torch.cat((x, v), 0)
-
- # If none remain process next image
- if not x.shape[0]:
- continue
-
- # Compute conf
- x[:, 5:5+nc] *= x[:, 4:5] # conf = obj_conf * cls_conf
-
- # Box (center x, center y, width, height) to (x1, y1, x2, y2)
- box = xywh2xyxy(x[:, :4])
-
- # Detections matrix nx6 (xyxy, conf, cls)
- if multi_label:
- i, j = (x[:, 5:] > conf_thres).nonzero(as_tuple=False).T
- x = torch.cat((box[i], x[i, j + 5, None], j[:, None].float()), 1)
- else: # best class only
- if not kpt_label:
- conf, j = x[:, 5:].max(1, keepdim=True)
- x = torch.cat((box, conf, j.float()), 1)[conf.view(-1) > conf_thres]
- else:
- kpts = x[:, 6:]
- conf, j = x[:, 5:6].max(1, keepdim=True)
- x = torch.cat((box, conf, j.float(), kpts), 1)[conf.view(-1) > conf_thres]
-
-
- # Filter by class
- if classes is not None:
- x = x[(x[:, 5:6] == torch.tensor(classes, device=x.device)).any(1)]
-
- # Apply finite constraint
- # if not torch.isfinite(x).all():
- # x = x[torch.isfinite(x).all(1)]
-
- # Check shape
- n = x.shape[0] # number of boxes
- if not n: # no boxes
- continue
- elif n > max_nms: # excess boxes
- x = x[x[:, 4].argsort(descending=True)[:max_nms]] # sort by confidence
-
- # Batched NMS
- c = x[:, 5:6] * (0 if agnostic else max_wh) # classes
- boxes, scores = x[:, :4] + c, x[:, 4] # boxes (offset by class), scores
- i = torchvision.ops.nms(boxes, scores, iou_thres) # NMS
- if i.shape[0] > max_det: # limit detections
- i = i[:max_det]
- if merge and (1 < n < 3E3): # Merge NMS (boxes merged using weighted mean)
- # update boxes as boxes(i,4) = weights(i,n) * boxes(n,4)
- iou = box_iou(boxes[i], boxes) > iou_thres # iou matrix
- weights = iou * scores[None] # box weights
- x[i, :4] = torch.mm(weights, x[:, :4]).float() / weights.sum(1, keepdim=True) # merged boxes
- if redundant:
- i = i[iou.sum(1) > 1] # require redundancy
-
- output[xi] = x[i]
- if (time.time() - t) > time_limit:
- print(f'WARNING: NMS time limit {time_limit}s exceeded')
- break # time limit exceeded
-
- return output
-
-
-def strip_optimizer(f='best.pt', s=''): # from utils.general import *; strip_optimizer()
- # Strip optimizer from 'f' to finalize training, optionally save as 's'
- x = torch.load(f, map_location=torch.device('cpu'))
- if x.get('ema'):
- x['model'] = x['ema'] # replace model with ema
- for k in 'optimizer', 'training_results', 'wandb_id', 'ema', 'updates': # keys
- x[k] = None
- x['epoch'] = -1
- x['model'].half() # to FP16
- for p in x['model'].parameters():
- p.requires_grad = False
- torch.save(x, s or f)
- mb = os.path.getsize(s or f) / 1E6 # filesize
- print(f"Optimizer stripped from {f},{(' saved as %s,' % s) if s else ''} {mb:.1f}MB")
-
-
-def print_mutation(hyp, results, yaml_file='hyp_evolved.yaml', bucket=''):
- # Print mutation results to evolve.txt (for use with train.py --evolve)
- a = '%10s' * len(hyp) % tuple(hyp.keys()) # hyperparam keys
- b = '%10.3g' * len(hyp) % tuple(hyp.values()) # hyperparam values
- c = '%10.4g' * len(results) % results # results (P, R, mAP@0.5, mAP@0.5:0.95, val_losses x 3)
- print('\n%s\n%s\nEvolved fitness: %s\n' % (a, b, c))
-
- if bucket:
- url = 'gs://%s/evolve.txt' % bucket
- if gsutil_getsize(url) > (os.path.getsize('evolve.txt') if os.path.exists('evolve.txt') else 0):
- os.system('gsutil cp %s .' % url) # download evolve.txt if larger than local
-
- with open('evolve.txt', 'a') as f: # append result
- f.write(c + b + '\n')
- x = np.unique(np.loadtxt('evolve.txt', ndmin=2), axis=0) # load unique rows
- x = x[np.argsort(-fitness(x))] # sort
- np.savetxt('evolve.txt', x, '%10.3g') # save sort by fitness
-
- # Save yaml
- for i, k in enumerate(hyp.keys()):
- hyp[k] = float(x[0, i + 7])
- with open(yaml_file, 'w') as f:
- results = tuple(x[0, :7])
- c = '%10.4g' * len(results) % results # results (P, R, mAP@0.5, mAP@0.5:0.95, val_losses x 3)
- f.write('# Hyperparameter Evolution Results\n# Generations: %g\n# Metrics: ' % len(x) + c + '\n\n')
- yaml.dump(hyp, f, sort_keys=False)
-
- if bucket:
- os.system('gsutil cp evolve.txt %s gs://%s' % (yaml_file, bucket)) # upload
-
-
-def apply_classifier(x, model, img, im0):
- # applies a second stage classifier to yolo outputs
- im0 = [im0] if isinstance(im0, np.ndarray) else im0
- for i, d in enumerate(x): # per image
- if d is not None and len(d):
- d = d.clone()
-
- # Reshape and pad cutouts
- b = xyxy2xywh(d[:, :4]) # boxes
- b[:, 2:] = b[:, 2:].max(1)[0].unsqueeze(1) # rectangle to square
- b[:, 2:] = b[:, 2:] * 1.3 + 30 # pad
- d[:, :4] = xywh2xyxy(b).long()
-
- # Rescale boxes from img_size to im0 size
- scale_coords(img.shape[2:], d[:, :4], im0[i].shape)
-
- # Classes
- pred_cls1 = d[:, 5].long()
- ims = []
- for j, a in enumerate(d): # per item
- cutout = im0[i][int(a[1]):int(a[3]), int(a[0]):int(a[2])]
- im = cv2.resize(cutout, (224, 224)) # BGR
- # cv2.imwrite('test%i.jpg' % j, cutout)
-
- im = im[:, :, ::-1].transpose(2, 0, 1) # BGR to RGB, to 3x416x416
- im = np.ascontiguousarray(im, dtype=np.float32) # uint8 to float32
- im /= 255.0 # 0 - 255 to 0.0 - 1.0
- ims.append(im)
-
- pred_cls2 = model(torch.Tensor(ims).to(d.device)).argmax(1) # classifier prediction
- x[i] = x[i][pred_cls1 == pred_cls2] # retain matching class detections
-
- return x
-
-
-def increment_path(path, exist_ok=True, sep=''):
- # Increment path, i.e. runs/exp --> runs/exp{sep}0, runs/exp{sep}1 etc.
- path = Path(path) # os-agnostic
- if (path.exists() and exist_ok) or (not path.exists()):
- return str(path)
- else:
- dirs = glob.glob(f"{path}{sep}*") # similar paths
- matches = [re.search(rf"%s{sep}(\d+)" % path.stem, d) for d in dirs]
- i = [int(m.groups()[0]) for m in matches if m] # indices
- n = max(i) + 1 if i else 2 # increment number
- return f"{path}{sep}{n}" # update path
diff --git a/spaces/bioriAsaeru/text-to-voice/Astute Graphics Plugins Bundle 1.2.2 Crack.md b/spaces/bioriAsaeru/text-to-voice/Astute Graphics Plugins Bundle 1.2.2 Crack.md
deleted file mode 100644
index 05076bdedefff7e2c38042676d975d4ed06766e1..0000000000000000000000000000000000000000
--- a/spaces/bioriAsaeru/text-to-voice/Astute Graphics Plugins Bundle 1.2.2 Crack.md
+++ /dev/null
@@ -1,92 +0,0 @@
-
-
Astute Graphics Plugins Bundle 1.2.2 Crack: How to Unlock the Full Potential of Adobe Illustrator
-
Adobe Illustrator is one of the most popular and powerful vector design software in the world. However, sometimes it can be frustrating and time-consuming to create and edit vector artwork, especially if you need to perform complex or repetitive tasks.
-
That's why many vector artists and designers use Astute Graphics Plugins Bundle 1.2.2 Crack, a collection of plug-ins that seamlessly integrate into Illustrator and enhance its functionality and performance. Astute Graphics Plugins Bundle 1.2.2 Crack offers a variety of tools that can help you draw more naturally and intuitively, edit and manipulate vectors more easily and accurately, apply and adjust effects more dynamically and creatively, and save time and effort in your workflow.
In this article, we will review some of the features and benefits of Astute Graphics Plugins Bundle 1.2.2 Crack, and show you how to use it to unlock the full potential of Adobe Illustrator.
-
What is Astute Graphics Plugins Bundle 1.2.2 Crack?
-
Astute Graphics Plugins Bundle 1.2.2 Crack is a collection of plug-ins that extend the capabilities of Adobe Illustrator. It includes 18 plug-ins that cover various aspects of vector design, such as drawing, editing, coloring, styling, transforming, aligning, filling, texturing, mirroring, saving, printing, and more.
-
Some of the plug-ins included in Astute Graphics Plugins Bundle 1.2.2 Crack are:
-
-
DynamicSketch: A tool that allows you to sketch with a live preview of your path, adjusting the width and smoothness of your strokes based on the pressure of your tablet or the speed of your mouse.
-
VectorScribe: A tool that allows you to edit and create vectors faster and smarter, with features such as dynamic shapes, smart removal, path extension, ghost handles, pathscribe, cornerscribe, and more.
-
Phantasm: A tool that allows you to apply and change effects directly in Illustrator, such as halftones, duotones, color adjustments, curves, levels, hue/saturation, brightness/contrast, etc.
-
Texturino: A tool that allows you to add textures to your vector artwork with ease and flexibility, using texture brushes and opacity masks.
-
MirrorMe: A tool that allows you to create symmetrical artwork with live functionality, using axes or grids to mirror your shapes.
-
InkScribe: A tool that allows you to draw precisely and efficiently in vector, with features such as smart guides, annotations, ghost handles, rubber band mode, etc.
-
ColliderScribe: A tool that allows you to align shapes accurately and quickly with collision detection and space fill features.
-
Stipplism: A tool that allows you to explore dot and shape patterns faster and easier than ever before.
-
And many more!
-
-
How to use Astute Graphics Plugins Bundle 1.2.2 Crack?
-
To use Astute Graphics Plugins Bundle 1.2.2 Crack, you need to have Adobe Illustrator installed on your computer. You also need to download the crack file from a reliable source and follow the instructions to install it on your system.
-
Once you have installed Astute Graphics Plugins Bundle 1.2.2 Crack, you can access the plug-ins from the Illustrator menu bar or from the tools panel. Each plug-in has its own interface and settings that you can customize according to your preferences and needs.
-
You can use Astute Graphics Plugins Bundle 1.2.2 Crack for various purposes and projects in Illustrator. For example:
-
-
-
You can use DynamicSketch to draw natural and organic shapes with variable width strokes.
-
You can use VectorScribe to edit and manipulate vectors with ease and precision.
-
You can use Phantasm to apply and adjust effects directly in Illustrator without switching to Photoshop.
-
You can use Texturino to add textures to your vector artwork for more depth and realism.
-
You can use MirrorMe to create symmetrical artwork with live functionality.
-
You can use InkScribe to draw precisely and efficiently in vector.
-
You can use ColliderScribe to align shapes accurately and quickly with collision detection and space fill features.
-
You can use Stipplism to explore dot and shape patterns faster and easier than ever before.
-
-
What are the benefits of using Astute Graphics Plugins Bundle 1.2.2 Crack?
-
Using Astute Graphics Plugins Bundle 1.2.2 Crack can bring many benefits to your vector design workflow in Illustrator. Some of them are:
-
-
You can save time and effort by performing complex or repetitive tasks more quickly and easily.
-
You can improve your creativity and productivity by exploring new possibilities and techniques in vector design.
-
You can enhance your quality and accuracy by working with vectors more dynamically and intelligently.
-
You can simplify your workflow by using intuitive and integrated tools that work seamlessly with Illustrator's native features.
-
-
Conclusion
-
Astute Graphics Plugins Bundle 1.2.2 Crack is a collection of plug-ins that enhance the functionality and performance of Adobe Illustrator. It offers a variety of tools that can help you draw more naturally and intuitively, edit and manipulate vectors more easily and accurately, apply and adjust effects more dynamically and creatively, and save time and effort in your workflow.
-
If you are looking for a way to unlock the full potential of Adobe Illustrator, you might want to check out Astute Graphics Plugins Bundle 1.2.2 Crack. It is a must-have for vector artists and designers who want to work faster, smarter, and better in vector.
-
How to learn and master Astute Graphics Plugins Bundle 1.2.2 Crack?
-
If you want to learn and master Astute Graphics Plugins Bundle 1.2.2 Crack, you need to practice and experiment with the plug-ins on your own vector projects. You can also use some resources and tutorials that are available online to help you get started and improve your skills.
-
Some of the resources and tutorials that you can use are:
-
-
The official website of Astute Graphics, where you can find detailed information and documentation about each plug-in, as well as tips and tricks, FAQs, and support.
-
The official YouTube channel of Astute Graphics, where you can watch video tutorials and demos of the plug-ins, as well as interviews and webinars with vector experts and artists.
-
The official blog of Astute Graphics, where you can read articles and case studies about the plug-ins, as well as news and updates.
-
The official forum of Astute Graphics, where you can interact with other users of the plug-ins, ask questions, share feedback, and showcase your work.
-
The online courses and workshops offered by Astute Graphics, where you can learn from experienced instructors and get certified in using the plug-ins.
-
-
How to get the best results with Astute Graphics Plugins Bundle 1.2.2 Crack?
-
To get the best results with Astute Graphics Plugins Bundle 1.2.2 Crack, you need to use the plug-ins wisely and creatively. You should not rely on the plug-ins alone to create your vector artwork, but rather use them as tools that complement and enhance your own vision and style.
-
Some of the tips that you can follow to get the best results with Astute Graphics Plugins Bundle 1.2.2 Crack are:
-
-
Use the plug-ins that suit your needs and preferences. You don't have to use all of them at once or for every project. Choose the ones that help you achieve your goals and solve your problems.
-
Customize the settings and options of the plug-ins according to your project requirements and personal taste. You can adjust the parameters, presets, modes, colors, brushes, etc. of each plug-in to fit your needs.
-
Combine and integrate the plug-ins with each other and with Illustrator's native features. You can use multiple plug-ins together to create complex and unique effects and transformations. You can also use the plug-ins with Illustrator's tools, panels, layers, masks, etc. to create a seamless workflow.
-
Experiment and explore with the plug-ins. You can try different combinations and variations of the plug-ins to discover new possibilities and techniques in vector design. You can also use the plug-ins for purposes other than their intended ones to create unexpected and original results.
-
-
Conclusion
-
Astute Graphics Plugins Bundle 1.2.2 Crack is a collection of plug-ins that enhance the functionality and performance of Adobe Illustrator. It offers a variety of tools that can help you draw more naturally and intuitively, edit and manipulate vectors more easily and accurately, apply and adjust effects more dynamically and creatively, and save time and effort in your workflow.
-
If you are looking for a way to unlock the full potential of Adobe Illustrator, you might want to check out Astute Graphics Plugins Bundle 1.2.2 Crack. It is a must-have for vector artists and designers who want to work faster, smarter, and better in vector.
-
However, you should also consider the drawbacks of using Astute Graphics Plugins Bundle 1.2.2 Crack, such as violating the intellectual property rights of Astute Graphics, not receiving any technical support or updates from Astute Graphics, and exposing your computer or data to malware or viruses.
-
You should always respect the rights and wishes of the original developer and use the plug-ins ethically and responsibly. You should also be careful and do some research when looking for a reliable and safe source for Astute Graphics Plugins Bundle 1.2.2 Crack.
-
You should also practice and experiment with the plug-ins on your own vector projects, use some resources and tutorials that are available online to help you learn and master them, use them wisely and creatively according to your needs and preferences, combine them with each other
-and with Illustrator's native features for a seamless workflow, experiment
-and explore with them for new possibilities
-and techniques in vector design,
-and get
-the best results
-with them.
-
Conclusion
-
In this article, we have discussed what Astute Graphics Plugins Bundle 1.2.2 Crack is, how to use it, where to find it, what are the benefits and drawbacks of using it, how to learn and master it, and how to get the best results with it. We hope that this article has been informative and helpful for you.
-
Astute Graphics Plugins Bundle 1.2.2 Crack is a collection of plug-ins that enhance the functionality and performance of Adobe Illustrator. It offers a variety of tools that can help you draw more naturally and intuitively, edit and manipulate vectors more easily and accurately, apply and adjust effects more dynamically and creatively, and save time and effort in your workflow.
-
If you are looking for a way to unlock the full potential of Adobe Illustrator, you might want to check out Astute Graphics Plugins Bundle 1.2.2 Crack. It is a must-have for vector artists and designers who want to work faster, smarter, and better in vector.
-
However, you should also consider the drawbacks of using Astute Graphics Plugins Bundle 1.2.2 Crack, such as violating the intellectual property rights of Astute Graphics, not receiving any technical support or updates from Astute Graphics, and exposing your computer or data to malware or viruses.
-
You should always respect the rights and wishes of the original developer and use the plug-ins ethically and responsibly. You should also be careful and do some research when looking for a reliable and safe source for Astute Graphics Plugins Bundle 1.2.2 Crack.
-
You should also practice and experiment with the plug-ins on your own vector projects, use some resources and tutorials that are available online to help you learn and master them, use them wisely and creatively according to your needs and preferences, combine them with each other
-and with Illustrator's native features for a seamless workflow, experiment
-and explore with them for new possibilities
-and techniques in vector design,
-and get
-the best results
-with them.
3cee63e6c2
-
-
\ No newline at end of file
diff --git a/spaces/bioriAsaeru/text-to-voice/Direccionamiento Ip Subredes Ejercicios Resueltos los mejores consejos y trucos para manejar scheart boliviano ra en el mbito de las redes.md b/spaces/bioriAsaeru/text-to-voice/Direccionamiento Ip Subredes Ejercicios Resueltos los mejores consejos y trucos para manejar scheart boliviano ra en el mbito de las redes.md
deleted file mode 100644
index f7231236af1ef179fbec2d0e1e343b8498e45332..0000000000000000000000000000000000000000
--- a/spaces/bioriAsaeru/text-to-voice/Direccionamiento Ip Subredes Ejercicios Resueltos los mejores consejos y trucos para manejar scheart boliviano ra en el mbito de las redes.md
+++ /dev/null
@@ -1,6 +0,0 @@
-
Direccionamiento Ip Subredes Ejercicios Resueltos scheart boliviano ra
-
- aaccfb2cb3
-
-
-
diff --git a/spaces/bioriAsaeru/text-to-voice/Kuch Kuch Hota Hai HD 720p Download Everything You Need to Know About the Movie.md b/spaces/bioriAsaeru/text-to-voice/Kuch Kuch Hota Hai HD 720p Download Everything You Need to Know About the Movie.md
deleted file mode 100644
index 5a2ec50b2d62d490460833248b4847d5f7b9a338..0000000000000000000000000000000000000000
--- a/spaces/bioriAsaeru/text-to-voice/Kuch Kuch Hota Hai HD 720p Download Everything You Need to Know About the Movie.md
+++ /dev/null
@@ -1,6 +0,0 @@
-
- ''',
- unsafe_allow_html=True
- )
-
-def new_get_client(session):
- scope = "playlist-modify-public"
-
- cache_handler = StreamlitCacheHandler(session)
- auth_manager = spotipy.oauth2.SpotifyOAuth(scope=scope,
- cache_handler=cache_handler,
- show_dialog=True)
- sp, user_id = None, None
-
- if not auth_manager.validate_token(cache_handler.get_cached_token()):
- # Step 1. Display sign in link when no token
- auth_url = auth_manager.get_authorize_url()
- if 'code' not in st.experimental_get_query_params():
- add_button(auth_url, 'Log in')
-
- # st.markdown(f'Click here to log in', unsafe_allow_html=True)
- # Step 2. Being redirected from Spotify auth page
- if 'code' in st.experimental_get_query_params():
- auth_manager.get_access_token(st.experimental_get_query_params()['code'])
- sp = spotipy.Spotify(auth_manager=auth_manager)
- user_id = sp.me()['id']
-
- return sp, user_id, auth_manager
-
-
-def extract_uris_from_links(links, url_type):
- assert url_type in ['playlist', 'artist', 'user']
- urls = links.split('\n')
- uris = []
- for url in urls:
- if 'playlist' in url:
- uri = url.split(f'{url_type}/')[-1].split('?')[0]
- elif 'user' in url:
- uri = url.split(f'{url_type}/')[-1].split('?')[0]
- else:
- uri = url.split('?')[0]
- uris.append(uri)
- return uris
-
-def wall_of_checkboxes(labels, max_width=10):
- n_labels = len(labels)
- n_rows = int(np.ceil(n_labels/max_width))
- checkboxes = []
- for i in range(n_rows):
- columns = st.columns(np.ones(max_width))
- row_length = n_labels % max_width if i == n_rows - 1 else max_width
- for j in range(row_length):
- with columns[j]:
- checkboxes.append(st.empty())
- return checkboxes
-
-def find_legit_genre(glabel, legit_genres, verbose=False):
- legit_genres_formatted = [lg.replace('-', '').replace(' ', '') for lg in legit_genres]
- glabel_formatted = glabel.replace(' ', '').replace('-', '')
- if verbose: print('\n', glabel)
- best_match = None
- best_match_score = 0
- for legit_glabel, legit_glabel_formatted in zip(legit_genres, legit_genres_formatted):
- if 'jazz' in glabel_formatted:
- best_match = 'jazz'
- if verbose: print('\t', 'pop')
- break
- if 'ukpop' in glabel_formatted:
- best_match = 'pop'
- if verbose: print('\t', 'pop')
- break
- if legit_glabel_formatted == glabel_formatted:
- if verbose: print('\t', legit_glabel_formatted)
- best_match = legit_glabel
- break
- elif glabel_formatted in legit_glabel_formatted:
- if verbose: print('\t', legit_glabel_formatted)
- if len(glabel_formatted) > best_match_score:
- best_match = legit_glabel
- best_match_score = len(glabel_formatted)
- elif legit_glabel_formatted in glabel_formatted:
- if verbose: print('\t', legit_glabel_formatted)
- if len(legit_glabel_formatted) > best_match_score:
- best_match = legit_glabel
- best_match_score = len(legit_glabel_formatted)
-
- if best_match is None:
- return "unknown"
- else:
- return best_match
-
-
-# def aggregate_genres(genres, legit_genres, verbose=False):
-# genres_output = dict()
-# legit_genres_formatted = [lg.replace('-', '').replace(' ', '') for lg in legit_genres]
-# for glabel in genres.keys():
-# if verbose: print('\n', glabel)
-# glabel_formatted = glabel.replace(' ', '').replace('-', '')
-# best_match = None
-# best_match_score = 0
-# for legit_glabel, legit_glabel_formatted in zip(legit_genres, legit_genres_formatted):
-# if 'jazz' in glabel_formatted:
-# best_match = 'jazz'
-# if verbose: print('\t', 'pop')
-# break
-# if 'ukpop' in glabel_formatted:
-# best_match = 'pop'
-# if verbose: print('\t', 'pop')
-# break
-# if legit_glabel_formatted == glabel_formatted:
-# if verbose: print('\t', legit_glabel_formatted)
-# best_match = legit_glabel
-# break
-# elif glabel_formatted in legit_glabel_formatted:
-# if verbose: print('\t', legit_glabel_formatted)
-# if len(glabel_formatted) > best_match_score:
-# best_match = legit_glabel
-# best_match_score = len(glabel_formatted)
-# elif legit_glabel_formatted in glabel_formatted:
-# if verbose: print('\t', legit_glabel_formatted)
-# if len(legit_glabel_formatted) > best_match_score:
-# best_match = legit_glabel
-# best_match_score = len(legit_glabel_formatted)
-#
-# if best_match is not None:
-# if verbose: print('\t', '-->', best_match)
-# if best_match in genres_output.keys():
-# genres_output[best_match] += genres[glabel]
-# else:
-# genres_output[best_match] = genres[glabel]
-# else:
-# if "unknown" in genres_output.keys():
-# genres_output["unknown"] += genres[glabel]
-# else:
-# genres_output["unknown"] = genres[glabel]
-# for k in genres_output.keys():
-# genres_output[k] = sorted(set(genres_output[k]))
-# return genres_output
-
-def get_all_playlists_uris_from_users(sp, user_ids):
- all_uris = []
- all_names = []
- for user_id in user_ids:
- print(user_id)
- offset = 0
- done = False
- while not done:
- playlist_list = sp.user_playlists(user_id, offset=offset, limit=50)
- these_names = [p['name'] for p in playlist_list['items']]
- these_uris = [p['uri'] for p in playlist_list['items']]
- for name, uri in zip(these_names, these_uris):
- if uri not in all_uris:
- all_uris.append(uri)
- all_names.append(user_id + '/' + name)
- if len(playlist_list['items']) < offset:
- done = True
- else:
- offset += 50
- return all_uris, all_names
-
-
-
-
-class StreamlitCacheHandler(spotipy.cache_handler.CacheHandler):
- """
- A cache handler that stores the token info in the session framework
- provided by streamlit.
- """
-
- def __init__(self, session):
- self.session = session
-
- def get_cached_token(self):
- token_info = None
- try:
- token_info = self.session["token_info"]
- except KeyError:
- print("Token not found in the session")
-
- return token_info
-
- def save_token_to_cache(self, token_info):
- try:
- self.session["token_info"] = token_info
- except Exception as e:
- print("Error saving token to cache: " + str(e))
diff --git a/spaces/chendl/compositional_test/transformers/examples/research_projects/vqgan-clip/utils.py b/spaces/chendl/compositional_test/transformers/examples/research_projects/vqgan-clip/utils.py
deleted file mode 100644
index 7db45fcbb52b0fa3f82226194ff7c824fd873184..0000000000000000000000000000000000000000
--- a/spaces/chendl/compositional_test/transformers/examples/research_projects/vqgan-clip/utils.py
+++ /dev/null
@@ -1,35 +0,0 @@
-from datetime import datetime
-
-import matplotlib.pyplot as plt
-import torch
-
-
-def freeze_module(module):
- for param in module.parameters():
- param.requires_grad = False
-
-
-def get_device():
- device = "cuda" if torch.cuda.is_available() else "cpu"
- if torch.backends.mps.is_available() and torch.backends.mps.is_built():
- device = "mps"
- if device == "mps":
- print(
- "WARNING: MPS currently doesn't seem to work, and messes up backpropagation without any visible torch"
- " errors. I recommend using CUDA on a colab notebook or CPU instead if you're facing inexplicable issues"
- " with generations."
- )
- return device
-
-
-def show_pil(img):
- fig = plt.imshow(img)
- fig.axes.get_xaxis().set_visible(False)
- fig.axes.get_yaxis().set_visible(False)
- plt.show()
-
-
-def get_timestamp():
- current_time = datetime.now()
- timestamp = current_time.strftime("%H:%M:%S")
- return timestamp
diff --git a/spaces/chendl/compositional_test/transformers/src/transformers/models/altclip/configuration_altclip.py b/spaces/chendl/compositional_test/transformers/src/transformers/models/altclip/configuration_altclip.py
deleted file mode 100644
index 4ddbb5ec81606ac23b1851aa3d8a0984139ff65c..0000000000000000000000000000000000000000
--- a/spaces/chendl/compositional_test/transformers/src/transformers/models/altclip/configuration_altclip.py
+++ /dev/null
@@ -1,405 +0,0 @@
-# coding=utf-8
-# Copyright 2022 WenXiang ZhongzhiCheng LedellWu LiuGuang BoWenZhang and The HuggingFace Inc. team. All rights reserved.
-#
-# Licensed under the Apache License, Version 2.0 (the "License");
-# you may not use this file except in compliance with the License.
-# You may obtain a copy of the License at
-#
-# http://www.apache.org/licenses/LICENSE-2.0
-#
-# Unless required by applicable law or agreed to in writing, software
-# distributed under the License is distributed on an "AS IS" BASIS,
-# WITHOUT WARRANTIES OR CONDITIONS OF ANY KIND, either express or implied.
-# See the License for the specific language governing permissions and
-# limitations under the License.
-""" AltCLIP model configuration"""
-import copy
-import os
-from typing import Union
-
-from ...configuration_utils import PretrainedConfig
-from ...utils import logging
-
-
-logger = logging.get_logger(__name__)
-
-ALTCLIP_PRETRAINED_CONFIG_ARCHIVE_MAP = {
- "BAAI/AltCLIP": "https://huggingface.co/BAAI/AltCLIP/resolve/main/config.json",
- # See all AltCLIP models at https://huggingface.co/models?filter=altclip
-}
-
-
-class AltCLIPTextConfig(PretrainedConfig):
- r"""
- This is the configuration class to store the configuration of a [`AltCLIPTextModel`]. It is used to instantiate a
- AltCLIP text model according to the specified arguments, defining the model architecture. Instantiating a
- configuration with the defaults will yield a similar configuration to that of the AltCLIP
- [BAAI/AltCLIP](https://huggingface.co/BAAI/AltCLIP) architecture.
-
- Configuration objects inherit from [`PretrainedConfig`] and can be used to control the model outputs. Read the
- documentation from [`PretrainedConfig`] for more information.
-
-
- Args:
- vocab_size (`int`, *optional*, defaults to 250002):
- Vocabulary size of the AltCLIP model. Defines the number of different tokens that can be represented by the
- `inputs_ids` passed when calling [`AltCLIPTextModel`].
- hidden_size (`int`, *optional*, defaults to 1024):
- Dimensionality of the encoder layers and the pooler layer.
- num_hidden_layers (`int`, *optional*, defaults to 24):
- Number of hidden layers in the Transformer encoder.
- num_attention_heads (`int`, *optional*, defaults to 16):
- Number of attention heads for each attention layer in the Transformer encoder.
- intermediate_size (`int`, *optional*, defaults to 4096):
- Dimensionality of the "intermediate" (often named feed-forward) layer in the Transformer encoder.
- hidden_act (`str` or `Callable`, *optional*, defaults to `"gelu"`):
- The non-linear activation function (function or string) in the encoder and pooler. If string, `"gelu"`,
- `"relu"`, `"silu"` and `"gelu_new"` are supported.
- hidden_dropout_prob (`float`, *optional*, defaults to 0.1):
- The dropout probability for all fully connected layers in the embeddings, encoder, and pooler.
- attention_probs_dropout_prob (`float`, *optional*, defaults to 0.1):
- The dropout ratio for the attention probabilities.
- max_position_embeddings (`int`, *optional*, defaults to 514):
- The maximum sequence length that this model might ever be used with. Typically set this to something large
- just in case (e.g., 512 or 1024 or 2048).
- type_vocab_size (`int`, *optional*, defaults to 2):
- The vocabulary size of the `token_type_ids` passed when calling [`AltCLIPTextModel`]
- initializer_range (`float`, *optional*, defaults to 0.02):
- The standard deviation of the truncated_normal_initializer for initializing all weight matrices.
- layer_norm_eps (`float`, *optional*, defaults to 1e-5):
- The epsilon used by the layer normalization layers.
- position_embedding_type (`str`, *optional*, defaults to `"absolute"`):
- Type of position embedding. Choose one of `"absolute"`, `"relative_key"`, `"relative_key_query"`. For
- positional embeddings use `"absolute"`. For more information on `"relative_key"`, please refer to
- [Self-Attention with Relative Position Representations (Shaw et al.)](https://arxiv.org/abs/1803.02155).
- For more information on `"relative_key_query"`, please refer to *Method 4* in [Improve Transformer Models
- with Better Relative Position Embeddings (Huang et al.)](https://arxiv.org/abs/2009.13658).
- use_cache (`bool`, *optional*, defaults to `True`):
- Whether or not the model should return the last key/values attentions (not used by all models). Only
- relevant if `config.is_decoder=True`.
- project_dim (`int`, *optional*, defaults to 768):
- The dimentions of the teacher model before the mapping layer.
-
- Examples:
-
- ```python
- >>> from transformers import AltCLIPTextModel, AltCLIPTextConfig
-
- >>> # Initializing a AltCLIPTextConfig with BAAI/AltCLIP style configuration
- >>> configuration = AltCLIPTextConfig()
-
- >>> # Initializing a AltCLIPTextModel (with random weights) from the BAAI/AltCLIP style configuration
- >>> model = AltCLIPTextModel(configuration)
-
- >>> # Accessing the model configuration
- >>> configuration = model.config
- ```"""
- model_type = "altclip_text_model"
-
- def __init__(
- self,
- vocab_size=250002,
- hidden_size=1024,
- num_hidden_layers=24,
- num_attention_heads=16,
- intermediate_size=4096,
- hidden_act="gelu",
- hidden_dropout_prob=0.1,
- attention_probs_dropout_prob=0.1,
- max_position_embeddings=514,
- type_vocab_size=1,
- initializer_range=0.02,
- initializer_factor=0.02,
- layer_norm_eps=1e-05,
- pad_token_id=1,
- bos_token_id=0,
- eos_token_id=2,
- position_embedding_type="absolute",
- use_cache=True,
- project_dim=768,
- **kwargs,
- ):
- super().__init__(pad_token_id=pad_token_id, bos_token_id=bos_token_id, eos_token_id=eos_token_id, **kwargs)
-
- self.vocab_size = vocab_size
- self.hidden_size = hidden_size
- self.num_hidden_layers = num_hidden_layers
- self.num_attention_heads = num_attention_heads
- self.hidden_act = hidden_act
- self.intermediate_size = intermediate_size
- self.hidden_dropout_prob = hidden_dropout_prob
- self.attention_probs_dropout_prob = attention_probs_dropout_prob
- self.max_position_embeddings = max_position_embeddings
- self.type_vocab_size = type_vocab_size
- self.initializer_range = initializer_range
- self.initializer_factor = initializer_factor
- self.layer_norm_eps = layer_norm_eps
- self.position_embedding_type = position_embedding_type
- self.use_cache = use_cache
- self.project_dim = project_dim
-
-
-class AltCLIPVisionConfig(PretrainedConfig):
- r"""
- This is the configuration class to store the configuration of a [`AltCLIPModel`]. It is used to instantiate an
- AltCLIP model according to the specified arguments, defining the model architecture. Instantiating a configuration
- with the defaults will yield a similar configuration to that of the AltCLIP
- [BAAI/AltCLIP](https://huggingface.co/BAAI/AltCLIP) architecture.
-
- Configuration objects inherit from [`PretrainedConfig`] and can be used to control the model outputs. Read the
- documentation from [`PretrainedConfig`] for more information.
-
-
- Args:
- hidden_size (`int`, *optional*, defaults to 768):
- Dimensionality of the encoder layers and the pooler layer.
- intermediate_size (`int`, *optional*, defaults to 3072):
- Dimensionality of the "intermediate" (i.e., feed-forward) layer in the Transformer encoder.
- num_hidden_layers (`int`, *optional*, defaults to 12):
- Number of hidden layers in the Transformer encoder.
- num_attention_heads (`int`, *optional*, defaults to 12):
- Number of attention heads for each attention layer in the Transformer encoder.
- image_size (`int`, *optional*, defaults to 224):
- The size (resolution) of each image.
- patch_size (`int`, *optional*, defaults to 32):
- The size (resolution) of each patch.
- hidden_act (`str` or `function`, *optional*, defaults to `"quick_gelu"`):
- The non-linear activation function (function or string) in the encoder and pooler. If string, `"gelu"`,
- `"relu"`, `"selu"` and `"gelu_new"` ``"quick_gelu"` are supported.
- layer_norm_eps (`float`, *optional*, defaults to 1e-5):
- The epsilon used by the layer normalization layers.
- attention_dropout (`float`, *optional*, defaults to 0.0):
- The dropout ratio for the attention probabilities.
- initializer_range (`float`, *optional*, defaults to 0.02):
- The standard deviation of the truncated_normal_initializer for initializing all weight matrices.
- initializer_factor (`float``, *optional*, defaults to 1):
- A factor for initializing all weight matrices (should be kept to 1, used internally for initialization
- testing).
-
- Example:
-
- ```python
- >>> from transformers import AltCLIPVisionConfig, AltCLIPVisionModel
-
- >>> # Initializing a AltCLIPVisionConfig with BAAI/AltCLIP style configuration
- >>> configuration = AltCLIPVisionConfig()
-
- >>> # Initializing a AltCLIPVisionModel (with random weights) from the BAAI/AltCLIP style configuration
- >>> model = AltCLIPVisionModel(configuration)
-
- >>> # Accessing the model configuration
- >>> configuration = model.config
- ```"""
-
- model_type = "altclip_vision_model"
-
- def __init__(
- self,
- hidden_size=768,
- intermediate_size=3072,
- projection_dim=512,
- num_hidden_layers=12,
- num_attention_heads=12,
- num_channels=3,
- image_size=224,
- patch_size=32,
- hidden_act="quick_gelu",
- layer_norm_eps=1e-5,
- attention_dropout=0.0,
- initializer_range=0.02,
- initializer_factor=1.0,
- **kwargs,
- ):
- super().__init__(**kwargs)
-
- self.hidden_size = hidden_size
- self.intermediate_size = intermediate_size
- self.projection_dim = projection_dim
- self.num_hidden_layers = num_hidden_layers
- self.num_attention_heads = num_attention_heads
- self.num_channels = num_channels
- self.patch_size = patch_size
- self.image_size = image_size
- self.initializer_range = initializer_range
- self.initializer_factor = initializer_factor
- self.attention_dropout = attention_dropout
- self.layer_norm_eps = layer_norm_eps
- self.hidden_act = hidden_act
-
- @classmethod
- def from_pretrained(cls, pretrained_model_name_or_path: Union[str, os.PathLike], **kwargs) -> "PretrainedConfig":
- config_dict, kwargs = cls.get_config_dict(pretrained_model_name_or_path, **kwargs)
-
- # get the vision config dict if we are loading from AltCLIPConfig
- if config_dict.get("model_type") == "altclip":
- config_dict = config_dict["vision_config"]
-
- if "model_type" in config_dict and hasattr(cls, "model_type") and config_dict["model_type"] != cls.model_type:
- logger.warning(
- f"You are using a model of type {config_dict['model_type']} to instantiate a model of type "
- f"{cls.model_type}. This is not supported for all configurations of models and can yield errors."
- )
-
- return cls.from_dict(config_dict, **kwargs)
-
-
-class AltCLIPConfig(PretrainedConfig):
- r"""
- This is the configuration class to store the configuration of a [`AltCLIPModel`]. It is used to instantiate an
- AltCLIP model according to the specified arguments, defining the model architecture. Instantiating a configuration
- with the defaults will yield a similar configuration to that of the AltCLIP
- [BAAI/AltCLIP](https://huggingface.co/BAAI/AltCLIP) architecture.
-
- Configuration objects inherit from [`PretrainedConfig`] and can be used to control the model outputs. Read the
- documentation from [`PretrainedConfig`] for more information.
-
- Args:
- text_config (`dict`, *optional*):
- Dictionary of configuration options used to initialize [`AltCLIPTextConfig`].
- vision_config (`dict`, *optional*):
- Dictionary of configuration options used to initialize [`AltCLIPVisionConfig`].
- projection_dim (`int`, *optional*, defaults to 512):
- Dimentionality of text and vision projection layers.
- logit_scale_init_value (`float`, *optional*, defaults to 2.6592):
- The inital value of the *logit_scale* paramter. Default is used as per the original CLIP implementation.
- kwargs (*optional*):
- Dictionary of keyword arguments.
-
- Example:
-
- ```python
- >>> from transformers import AltCLIPConfig, AltCLIPModel
-
- >>> # Initializing a AltCLIPConfig with BAAI/AltCLIP style configuration
- >>> configuration = AltCLIPConfig()
-
- >>> # Initializing a AltCLIPModel (with random weights) from the BAAI/AltCLIP style configuration
- >>> model = AltCLIPModel(configuration)
-
- >>> # Accessing the model configuration
- >>> configuration = model.config
-
- >>> # We can also initialize a AltCLIPConfig from a AltCLIPTextConfig and a AltCLIPVisionConfig
-
- >>> # Initializing a AltCLIPText and AltCLIPVision configuration
- >>> config_text = AltCLIPTextConfig()
- >>> config_vision = AltCLIPVisionConfig()
-
- >>> config = AltCLIPConfig.from_text_vision_configs(config_text, config_vision)
- ```"""
-
- model_type = "altclip"
- is_composition = True
-
- def __init__(
- self, text_config=None, vision_config=None, projection_dim=768, logit_scale_init_value=2.6592, **kwargs
- ):
- # If `_config_dict` exist, we use them for the backward compatibility.
- # We pop out these 2 attributes before calling `super().__init__` to avoid them being saved (which causes a lot
- # of confusion!).
- text_config_dict = kwargs.pop("text_config_dict", None)
- vision_config_dict = kwargs.pop("vision_config_dict", None)
-
- super().__init__(**kwargs)
-
- # Instead of simply assigning `[text|vision]_config_dict` to `[text|vision]_config`, we use the values in
- # `[text|vision]_config_dict` to update the values in `[text|vision]_config`. The values should be same in most
- # cases, but we don't want to break anything regarding `_config_dict` that existed before commit `8827e1b2`.
- if text_config_dict is not None:
- if text_config is None:
- text_config = {}
-
- # This is the complete result when using `text_config_dict`.
- _text_config_dict = AltCLIPTextConfig(**text_config_dict).to_dict()
-
- # Give a warning if the values exist in both `_text_config_dict` and `text_config` but being different.
- for key, value in _text_config_dict.items():
- if key in text_config and value != text_config[key] and key not in ["transformers_version"]:
- # If specified in `text_config_dict`
- if key in text_config_dict:
- message = (
- f"`{key}` is found in both `text_config_dict` and `text_config` but with different values. "
- f'The value `text_config_dict["{key}"]` will be used instead.'
- )
- # If inferred from default argument values (just to be super careful)
- else:
- message = (
- f"`text_config_dict` is provided which will be used to initialize `AltCLIPTextConfig`. The "
- f'value `text_config["{key}"]` will be overriden.'
- )
- logger.warning(message)
-
- # Update all values in `text_config` with the ones in `_text_config_dict`.
- text_config.update(_text_config_dict)
-
- if vision_config_dict is not None:
- if vision_config is None:
- vision_config = {}
-
- # This is the complete result when using `vision_config_dict`.
- _vision_config_dict = AltCLIPVisionConfig(**vision_config_dict).to_dict()
- # convert keys to string instead of integer
- if "id2label" in _vision_config_dict:
- _vision_config_dict["id2label"] = {
- str(key): value for key, value in _vision_config_dict["id2label"].items()
- }
-
- # Give a warning if the values exist in both `_vision_config_dict` and `vision_config` but being different.
- for key, value in _vision_config_dict.items():
- if key in vision_config and value != vision_config[key] and key not in ["transformers_version"]:
- # If specified in `vision_config_dict`
- if key in vision_config_dict:
- message = (
- f"`{key}` is found in both `vision_config_dict` and `vision_config` but with different "
- f'values. The value `vision_config_dict["{key}"]` will be used instead.'
- )
- # If inferred from default argument values (just to be super careful)
- else:
- message = (
- f"`vision_config_dict` is provided which will be used to initialize `AltCLIPVisionConfig`. "
- f'The value `vision_config["{key}"]` will be overriden.'
- )
- logger.warning(message)
-
- # Update all values in `vision_config` with the ones in `_vision_config_dict`.
- vision_config.update(_vision_config_dict)
-
- if text_config is None:
- text_config = {}
- logger.info("`text_config` is `None`. Initializing the `AltCLIPTextConfig` with default values.")
-
- if vision_config is None:
- vision_config = {}
- logger.info("`vision_config` is `None`. initializing the `AltCLIPVisionConfig` with default values.")
-
- self.text_config = AltCLIPTextConfig(**text_config)
- self.vision_config = AltCLIPVisionConfig(**vision_config)
-
- self.projection_dim = projection_dim
- self.logit_scale_init_value = logit_scale_init_value
- self.initializer_factor = 1.0
-
- @classmethod
- def from_text_vision_configs(cls, text_config: AltCLIPTextConfig, vision_config: AltCLIPVisionConfig, **kwargs):
- r"""
- Instantiate a [`AltCLIPConfig`] (or a derived class) from altclip text model configuration and altclip vision
- model configuration.
-
- Returns:
- [`AltCLIPConfig`]: An instance of a configuration object
- """
-
- return cls(text_config=text_config.to_dict(), vision_config=vision_config.to_dict(), **kwargs)
-
- def to_dict(self):
- """
- Serializes this instance to a Python dictionary. Override the default [`~PretrainedConfig.to_dict`].
-
- Returns:
- `Dict[str, any]`: Dictionary of all the attributes that make up this configuration instance,
- """
- output = copy.deepcopy(self.__dict__)
- output["text_config"] = self.text_config.to_dict()
- output["vision_config"] = self.vision_config.to_dict()
- output["model_type"] = self.__class__.model_type
- return output
diff --git a/spaces/chenxx/ChuanhuChatGPT/chat_func.py b/spaces/chenxx/ChuanhuChatGPT/chat_func.py
deleted file mode 100644
index 676259bd4d394240cf0f41f0bcdcb480121c9c98..0000000000000000000000000000000000000000
--- a/spaces/chenxx/ChuanhuChatGPT/chat_func.py
+++ /dev/null
@@ -1,456 +0,0 @@
-# -*- coding:utf-8 -*-
-from __future__ import annotations
-from typing import TYPE_CHECKING, List
-
-import logging
-import json
-import os
-import requests
-import urllib3
-
-from tqdm import tqdm
-import colorama
-from duckduckgo_search import ddg
-import asyncio
-import aiohttp
-
-from presets import *
-from llama_func import *
-from utils import *
-
-# logging.basicConfig(level=logging.INFO, format="%(asctime)s [%(levelname)s] [%(filename)s:%(lineno)d] %(message)s")
-
-if TYPE_CHECKING:
- from typing import TypedDict
-
- class DataframeData(TypedDict):
- headers: List[str]
- data: List[List[str | int | bool]]
-
-
-initial_prompt = "You are a helpful assistant."
-API_URL = "https://api.openai.com/v1/chat/completions"
-HISTORY_DIR = "history"
-TEMPLATES_DIR = "templates"
-
-def get_response(
- openai_api_key, system_prompt, history, temperature, top_p, stream, selected_model
-):
- headers = {
- "Content-Type": "application/json",
- "Authorization": f"Bearer {openai_api_key}",
- }
-
- history = [construct_system(system_prompt), *history]
-
- payload = {
- "model": selected_model,
- "messages": history, # [{"role": "user", "content": f"{inputs}"}],
- "temperature": temperature, # 1.0,
- "top_p": top_p, # 1.0,
- "n": 1,
- "stream": stream,
- "presence_penalty": 0,
- "frequency_penalty": 0,
- }
- if stream:
- timeout = timeout_streaming
- else:
- timeout = timeout_all
-
- # 获取环境变量中的代理设置
- http_proxy = os.environ.get("HTTP_PROXY") or os.environ.get("http_proxy")
- https_proxy = os.environ.get("HTTPS_PROXY") or os.environ.get("https_proxy")
-
- # 如果存在代理设置,使用它们
- proxies = {}
- if http_proxy:
- logging.info(f"Using HTTP proxy: {http_proxy}")
- proxies["http"] = http_proxy
- if https_proxy:
- logging.info(f"Using HTTPS proxy: {https_proxy}")
- proxies["https"] = https_proxy
-
- # 如果有代理,使用代理发送请求,否则使用默认设置发送请求
- if proxies:
- response = requests.post(
- API_URL,
- headers=headers,
- json=payload,
- stream=True,
- timeout=timeout,
- proxies=proxies,
- )
- else:
- response = requests.post(
- API_URL,
- headers=headers,
- json=payload,
- stream=True,
- timeout=timeout,
- )
- return response
-
-
-def stream_predict(
- openai_api_key,
- system_prompt,
- history,
- inputs,
- chatbot,
- all_token_counts,
- top_p,
- temperature,
- selected_model,
- fake_input=None,
- display_append=""
-):
- def get_return_value():
- return chatbot, history, status_text, all_token_counts
-
- logging.info("实时回答模式")
- partial_words = ""
- counter = 0
- status_text = "开始实时传输回答……"
- history.append(construct_user(inputs))
- history.append(construct_assistant(""))
- if fake_input:
- chatbot.append((fake_input, ""))
- else:
- chatbot.append((inputs, ""))
- user_token_count = 0
- if len(all_token_counts) == 0:
- system_prompt_token_count = count_token(construct_system(system_prompt))
- user_token_count = (
- count_token(construct_user(inputs)) + system_prompt_token_count
- )
- else:
- user_token_count = count_token(construct_user(inputs))
- all_token_counts.append(user_token_count)
- logging.info(f"输入token计数: {user_token_count}")
- yield get_return_value()
- try:
- response = get_response(
- openai_api_key,
- system_prompt,
- history,
- temperature,
- top_p,
- True,
- selected_model,
- )
- except requests.exceptions.ConnectTimeout:
- status_text = (
- standard_error_msg + connection_timeout_prompt + error_retrieve_prompt
- )
- yield get_return_value()
- return
- except requests.exceptions.ReadTimeout:
- status_text = standard_error_msg + read_timeout_prompt + error_retrieve_prompt
- yield get_return_value()
- return
-
- yield get_return_value()
- error_json_str = ""
-
- for chunk in response.iter_lines():
- if counter == 0:
- counter += 1
- continue
- counter += 1
- # check whether each line is non-empty
- if chunk:
- chunk = chunk.decode()
- chunklength = len(chunk)
- try:
- chunk = json.loads(chunk[6:])
- except json.JSONDecodeError:
- logging.info(chunk)
- error_json_str += chunk
- status_text = f"JSON解析错误。请重置对话。收到的内容: {error_json_str}"
- yield get_return_value()
- continue
- # decode each line as response data is in bytes
- if chunklength > 6 and "delta" in chunk["choices"][0]:
- finish_reason = chunk["choices"][0]["finish_reason"]
- status_text = construct_token_message(
- sum(all_token_counts), stream=True
- )
- if finish_reason == "stop":
- yield get_return_value()
- break
- try:
- partial_words = (
- partial_words + chunk["choices"][0]["delta"]["content"]
- )
- except KeyError:
- status_text = (
- standard_error_msg
- + "API回复中找不到内容。很可能是Token计数达到上限了。请重置对话。当前Token计数: "
- + str(sum(all_token_counts))
- )
- yield get_return_value()
- break
- history[-1] = construct_assistant(partial_words)
- chatbot[-1] = (chatbot[-1][0], partial_words+display_append)
- all_token_counts[-1] += 1
- yield get_return_value()
-
-
-def predict_all(
- openai_api_key,
- system_prompt,
- history,
- inputs,
- chatbot,
- all_token_counts,
- top_p,
- temperature,
- selected_model,
- fake_input=None,
- display_append=""
-):
- logging.info("一次性回答模式")
- history.append(construct_user(inputs))
- history.append(construct_assistant(""))
- if fake_input:
- chatbot.append((fake_input, ""))
- else:
- chatbot.append((inputs, ""))
- all_token_counts.append(count_token(construct_user(inputs)))
- try:
- response = get_response(
- openai_api_key,
- system_prompt,
- history,
- temperature,
- top_p,
- False,
- selected_model,
- )
- except requests.exceptions.ConnectTimeout:
- status_text = (
- standard_error_msg + connection_timeout_prompt + error_retrieve_prompt
- )
- return chatbot, history, status_text, all_token_counts
- except requests.exceptions.ProxyError:
- status_text = standard_error_msg + proxy_error_prompt + error_retrieve_prompt
- return chatbot, history, status_text, all_token_counts
- except requests.exceptions.SSLError:
- status_text = standard_error_msg + ssl_error_prompt + error_retrieve_prompt
- return chatbot, history, status_text, all_token_counts
- response = json.loads(response.text)
- content = response["choices"][0]["message"]["content"]
- history[-1] = construct_assistant(content)
- chatbot[-1] = (chatbot[-1][0], content+display_append)
- total_token_count = response["usage"]["total_tokens"]
- all_token_counts[-1] = total_token_count - sum(all_token_counts)
- status_text = construct_token_message(total_token_count)
- return chatbot, history, status_text, all_token_counts
-
-
-def predict(
- openai_api_key,
- system_prompt,
- history,
- inputs,
- chatbot,
- all_token_counts,
- top_p,
- temperature,
- stream=False,
- selected_model=MODELS[0],
- use_websearch=False,
- files = None,
- should_check_token_count=True,
-): # repetition_penalty, top_k
- logging.info("输入为:" + colorama.Fore.BLUE + f"{inputs}" + colorama.Style.RESET_ALL)
- if files:
- msg = "构建索引中……(这可能需要比较久的时间)"
- logging.info(msg)
- yield chatbot, history, msg, all_token_counts
- index = construct_index(openai_api_key, file_src=files)
- msg = "索引构建完成,获取回答中……"
- yield chatbot, history, msg, all_token_counts
- history, chatbot, status_text = chat_ai(openai_api_key, index, inputs, history, chatbot)
- yield chatbot, history, status_text, all_token_counts
- return
-
- old_inputs = ""
- link_references = []
- if use_websearch:
- search_results = ddg(inputs, max_results=5)
- old_inputs = inputs
- web_results = []
- for idx, result in enumerate(search_results):
- logging.info(f"搜索结果{idx + 1}:{result}")
- domain_name = urllib3.util.parse_url(result["href"]).host
- web_results.append(f'[{idx+1}]"{result["body"]}"\nURL: {result["href"]}')
- link_references.append(f"{idx+1}. [{domain_name}]({result['href']})\n")
- link_references = "\n\n" + "".join(link_references)
- inputs = (
- replace_today(WEBSEARCH_PTOMPT_TEMPLATE)
- .replace("{query}", inputs)
- .replace("{web_results}", "\n\n".join(web_results))
- )
- else:
- link_references = ""
-
- if len(openai_api_key) != 51:
- status_text = standard_error_msg + no_apikey_msg
- logging.info(status_text)
- chatbot.append((inputs, ""))
- if len(history) == 0:
- history.append(construct_user(inputs))
- history.append("")
- all_token_counts.append(0)
- else:
- history[-2] = construct_user(inputs)
- yield chatbot, history, status_text, all_token_counts
- return
-
- yield chatbot, history, "开始生成回答……", all_token_counts
-
- if stream:
- logging.info("使用流式传输")
- iter = stream_predict(
- openai_api_key,
- system_prompt,
- history,
- inputs,
- chatbot,
- all_token_counts,
- top_p,
- temperature,
- selected_model,
- fake_input=old_inputs,
- display_append=link_references
- )
- for chatbot, history, status_text, all_token_counts in iter:
- yield chatbot, history, status_text, all_token_counts
- else:
- logging.info("不使用流式传输")
- chatbot, history, status_text, all_token_counts = predict_all(
- openai_api_key,
- system_prompt,
- history,
- inputs,
- chatbot,
- all_token_counts,
- top_p,
- temperature,
- selected_model,
- fake_input=old_inputs,
- display_append=link_references
- )
- yield chatbot, history, status_text, all_token_counts
-
- logging.info(f"传输完毕。当前token计数为{all_token_counts}")
- if len(history) > 1 and history[-1]["content"] != inputs:
- logging.info(
- "回答为:"
- + colorama.Fore.BLUE
- + f"{history[-1]['content']}"
- + colorama.Style.RESET_ALL
- )
-
- if stream:
- max_token = max_token_streaming
- else:
- max_token = max_token_all
-
- if sum(all_token_counts) > max_token and should_check_token_count:
- status_text = f"精简token中{all_token_counts}/{max_token}"
- logging.info(status_text)
- yield chatbot, history, status_text, all_token_counts
- iter = reduce_token_size(
- openai_api_key,
- system_prompt,
- history,
- chatbot,
- all_token_counts,
- top_p,
- temperature,
- max_token//2,
- selected_model=selected_model,
- )
- for chatbot, history, status_text, all_token_counts in iter:
- status_text = f"Token 达到上限,已自动降低Token计数至 {status_text}"
- yield chatbot, history, status_text, all_token_counts
-
-
-def retry(
- openai_api_key,
- system_prompt,
- history,
- chatbot,
- token_count,
- top_p,
- temperature,
- stream=False,
- selected_model=MODELS[0],
-):
- logging.info("重试中……")
- if len(history) == 0:
- yield chatbot, history, f"{standard_error_msg}上下文是空的", token_count
- return
- history.pop()
- inputs = history.pop()["content"]
- token_count.pop()
- iter = predict(
- openai_api_key,
- system_prompt,
- history,
- inputs,
- chatbot,
- token_count,
- top_p,
- temperature,
- stream=stream,
- selected_model=selected_model,
- )
- logging.info("重试中……")
- for x in iter:
- yield x
- logging.info("重试完毕")
-
-
-def reduce_token_size(
- openai_api_key,
- system_prompt,
- history,
- chatbot,
- token_count,
- top_p,
- temperature,
- max_token_count,
- selected_model=MODELS[0],
-):
- logging.info("开始减少token数量……")
- iter = predict(
- openai_api_key,
- system_prompt,
- history,
- summarize_prompt,
- chatbot,
- token_count,
- top_p,
- temperature,
- selected_model=selected_model,
- should_check_token_count=False,
- )
- logging.info(f"chatbot: {chatbot}")
- flag = False
- for chatbot, history, status_text, previous_token_count in iter:
- num_chat = find_n(previous_token_count, max_token_count)
- if flag:
- chatbot = chatbot[:-1]
- flag = True
- history = history[-2*num_chat:] if num_chat > 0 else []
- token_count = previous_token_count[-num_chat:] if num_chat > 0 else []
- msg = f"保留了最近{num_chat}轮对话"
- yield chatbot, history, msg + "," + construct_token_message(
- sum(token_count) if len(token_count) > 0 else 0,
- ), token_count
- logging.info(msg)
- logging.info("减少token数量完毕")
\ No newline at end of file
diff --git a/spaces/chuan-hd/law-assistant-chatbot/.venv/lib/python3.11/site-packages/gradio/components/clear_button.py b/spaces/chuan-hd/law-assistant-chatbot/.venv/lib/python3.11/site-packages/gradio/components/clear_button.py
deleted file mode 100644
index 56652e731ae430e16ea3e7da432d06d6bd5e2a91..0000000000000000000000000000000000000000
--- a/spaces/chuan-hd/law-assistant-chatbot/.venv/lib/python3.11/site-packages/gradio/components/clear_button.py
+++ /dev/null
@@ -1,70 +0,0 @@
-""" Predefined buttons with bound events that can be included in a gr.Blocks for convenience. """
-
-from __future__ import annotations
-
-import json
-from typing import Literal
-
-from gradio_client.documentation import document, set_documentation_group
-
-from gradio.components import Button, Component
-
-set_documentation_group("component")
-
-
-@document("add")
-class ClearButton(Button):
- """
- Button that clears the value of a component or a list of components when clicked. It is instantiated with the list of components to clear.
- Preprocessing: passes the button value as a {str} into the function
- Postprocessing: expects a {str} to be returned from a function, which is set as the label of the button
- """
-
- is_template = True
-
- def __init__(
- self,
- components: None | list[Component] | Component = None,
- *,
- value: str = "Clear",
- variant: Literal["primary", "secondary", "stop"] = "secondary",
- size: Literal["sm", "lg"] | None = None,
- visible: bool = True,
- interactive: bool = True,
- elem_id: str | None = None,
- elem_classes: list[str] | str | None = None,
- scale: int | None = None,
- min_width: int | None = None,
- **kwargs,
- ):
- super().__init__(
- value,
- variant=variant,
- size=size,
- visible=visible,
- interactive=interactive,
- elem_id=elem_id,
- elem_classes=elem_classes,
- scale=scale,
- min_width=min_width,
- **kwargs,
- )
- self.add(components)
-
- def add(self, components: None | Component | list[Component]) -> ClearButton:
- """
- Adds a component or list of components to the list of components that will be cleared when the button is clicked.
- """
- if not components:
- # This needs to be here because when the ClearButton is created in an gr.Interface, we don't
- # want to create dependencies for it before we have created the dependencies for the submit function.
- # We generally assume that the submit function dependency is the first thing created in an gr.Interface.
- return self
-
- if isinstance(components, Component):
- components = [components]
- clear_values = json.dumps(
- [component.postprocess(None) for component in components]
- )
- self.click(None, [], components, _js=f"() => {clear_values}")
- return self
diff --git a/spaces/cihyFjudo/fairness-paper-search/Cold War Kids Discography (albums EPs bootlegs and b-sides) MP The Ultimate Collection of the Cold War Kids Songs.md b/spaces/cihyFjudo/fairness-paper-search/Cold War Kids Discography (albums EPs bootlegs and b-sides) MP The Ultimate Collection of the Cold War Kids Songs.md
deleted file mode 100644
index dcdc93734336d868be89f6ef9962c308f094fa43..0000000000000000000000000000000000000000
--- a/spaces/cihyFjudo/fairness-paper-search/Cold War Kids Discography (albums EPs bootlegs and b-sides) MP The Ultimate Collection of the Cold War Kids Songs.md
+++ /dev/null
@@ -1,6 +0,0 @@
-
Cold War Kids Discography (albums, EPs, bootlegs and b-sides) MP
-
- aaccfb2cb3
-
-
-
diff --git a/spaces/cihyFjudo/fairness-paper-search/How to Get Going Medieval Crack Only for Free and Enjoy the Medieval Simulation.md b/spaces/cihyFjudo/fairness-paper-search/How to Get Going Medieval Crack Only for Free and Enjoy the Medieval Simulation.md
deleted file mode 100644
index b4bd23b5788d17d005f9ad8021dff1dfd464d6f8..0000000000000000000000000000000000000000
--- a/spaces/cihyFjudo/fairness-paper-search/How to Get Going Medieval Crack Only for Free and Enjoy the Medieval Simulation.md
+++ /dev/null
@@ -1,12 +0,0 @@
-
-
The latest Going Medieval update, Terraforming & Cats, is now available on Steam, Epic Games Store, and GOG. Releasing only a month after the previous update, Terraforming & Cats adds terraforming, custom difficulty, new animals and behavior, and other tweaks and improvements. However, before you play the patch, you should disable any mods you might have installed. If you don't, your game might crash or refuse to start altogether, which would definitely prevent you from going medieval.
Over the years, rates of crack use among blacks have only been slightly higher than among whites, but since whites are the majority of the population, most crack users are white. For example, in 2017, 4.5% of blacks and 3.9% of whites reported ever using crack in their lives, according to the federal drug use survey.
-
Sometimes, it can feel like the list of home improvements, DIY jobs and general sprucing up tasks that need to be completed in your home is only getting longer. Things break over time or from overuse and certain objects or appliances may need upgrading or replacing. But when you spy a crack in a wall or ceiling, you may instantly panic. Luckily, most cracks are completely normal in all sorts of houses, even new builds, and are simply a sign that the house is settling. Other causes of cracks include change in temperature or humidity levels and vibrations from traffic if you live near a busy or fast road.
-
Most of the time you will need to apply more than one dip coat to full cover the nail (around 2-3 dips). However, if you apply your dip colors too quickly, the color will not dry or set properly and this will cause the powder to crack. Some dip powders are quick dry, like Fairy Glamor, but some are not. If you're using a quick dry dip powder you should only need to wait around 5 seconds before dipping again. But if you are not using a quick dry brand you'll need to wait a minute or two before applying another coat.
-
-
This is the most common fix for cracked dip nails. If the crack happened beneath your top coat, you're going to need to buff the surface away so that you can reach the crack. You can either use a nail file or a drill for this. Once you've removed the top layer you can apply your base coat over the crack and dip your finger in the same color again. The layer will become uneven--don't worry about this. Apply activator and let the layer dry before buffing it smooth. Then apply a thin layer top coat over the entire nail. Tada! Good as new.
-
It was the ancient Romans, however, who contributed the notion that a broken mirror would bring seven years of bad luck, since it was believed that only poor health would cause a mirror to crack, and the number seven was seen by the Romans as the number of years required to complete a full life-cycle of sickness and renewal. As a result, a broken mirror meant you were headed toward a death-spiral that might take seven years to pull yourself out of! But, then, those same Romans felt you could prevent that horrible outcome by gathering the broken pieces of the mirror and burying them by moonlight, so should we really trust them about all the bad luck stuff?
-
The most commonly shouted phrase after the crack is "Oh, my back!" but the trope itself isn't confined solely to spinal-lumbar complaints and can happen with any body part, bone or joint. Occasionally, this will even happen with younger characters if they move in very awkward positions.
aaccfb2cb3
-
-
\ No newline at end of file
diff --git a/spaces/cihyFjudo/fairness-paper-search/Udemy Ultimate Low Poly Game Assets In Blender 2.8 And Unity How To Make Amazing Low Poly Games In No Time.md b/spaces/cihyFjudo/fairness-paper-search/Udemy Ultimate Low Poly Game Assets In Blender 2.8 And Unity How To Make Amazing Low Poly Games In No Time.md
deleted file mode 100644
index 590886adf09fec9cc3deda10dc3a8d95a8098a7a..0000000000000000000000000000000000000000
--- a/spaces/cihyFjudo/fairness-paper-search/Udemy Ultimate Low Poly Game Assets In Blender 2.8 And Unity How To Make Amazing Low Poly Games In No Time.md
+++ /dev/null
@@ -1,5 +0,0 @@
-
-
In this course I will teach you how to model low poly Bucket game assets inside the blender 3.1 which is new released version. This tutorial is around 2 hours and if you like be sure to check out our other courses.
-
Udemy Ultimate Low Poly Game Assets In Blender 2.8 And Unity
-
- aaccfb2cb3
-
-
-
diff --git a/spaces/cllatMTK/TransformerAnalyzer/render_util.py b/spaces/cllatMTK/TransformerAnalyzer/render_util.py
deleted file mode 100644
index b21f08b259ca0fa554c4952f3044a90728d41e40..0000000000000000000000000000000000000000
--- a/spaces/cllatMTK/TransformerAnalyzer/render_util.py
+++ /dev/null
@@ -1,22 +0,0 @@
-import streamlit as st
-
-def create_table(df):
- # Table header based on df columns
- header = "| " + " | ".join(df.columns) + " |"
- # Number of columns in df to set table divider accordingly
- divider = "|:---|" + "|-----:|" * len(df.columns[:-1])
- rows = [header, divider]
-
- for _, row in df.iterrows():
- rows.append("| " + " | ".join(row.astype(str)) + " |")
-
- return "\n".join(rows)
-
-def header3(text):
- st.markdown(f"### {text}")
-
-def header4(text):
- st.markdown(f"#### {text}")
-
-def header5(text):
- st.markdown(f"##### {text}")
\ No newline at end of file
diff --git a/spaces/cloudtheboi/Lofi4All/.pythonlibs/lib/python3.10/site-packages/fontTools/ttLib/tables/_s_b_i_x.py b/spaces/cloudtheboi/Lofi4All/.pythonlibs/lib/python3.10/site-packages/fontTools/ttLib/tables/_s_b_i_x.py
deleted file mode 100644
index 29b82c3e43e8bd199a841c577774885d92499aba..0000000000000000000000000000000000000000
--- a/spaces/cloudtheboi/Lofi4All/.pythonlibs/lib/python3.10/site-packages/fontTools/ttLib/tables/_s_b_i_x.py
+++ /dev/null
@@ -1,119 +0,0 @@
-from fontTools.misc import sstruct
-from fontTools.misc.textTools import safeEval, num2binary, binary2num
-from . import DefaultTable
-from .sbixStrike import Strike
-
-
-sbixHeaderFormat = """
- >
- version: H # Version number (set to 1)
- flags: H # The only two bits used in the flags field are bits 0
- # and 1. For historical reasons, bit 0 must always be 1.
- # Bit 1 is a sbixDrawOutlines flag and is interpreted as
- # follows:
- # 0: Draw only 'sbix' bitmaps
- # 1: Draw both 'sbix' bitmaps and outlines, in that
- # order
- numStrikes: L # Number of bitmap strikes to follow
-"""
-sbixHeaderFormatSize = sstruct.calcsize(sbixHeaderFormat)
-
-
-sbixStrikeOffsetFormat = """
- >
- strikeOffset: L # Offset from begining of table to data for the
- # individual strike
-"""
-sbixStrikeOffsetFormatSize = sstruct.calcsize(sbixStrikeOffsetFormat)
-
-
-class table__s_b_i_x(DefaultTable.DefaultTable):
- def __init__(self, tag=None):
- DefaultTable.DefaultTable.__init__(self, tag)
- self.version = 1
- self.flags = 1
- self.numStrikes = 0
- self.strikes = {}
- self.strikeOffsets = []
-
- def decompile(self, data, ttFont):
- # read table header
- sstruct.unpack(sbixHeaderFormat, data[:sbixHeaderFormatSize], self)
- # collect offsets to individual strikes in self.strikeOffsets
- for i in range(self.numStrikes):
- current_offset = sbixHeaderFormatSize + i * sbixStrikeOffsetFormatSize
- offset_entry = sbixStrikeOffset()
- sstruct.unpack(
- sbixStrikeOffsetFormat,
- data[current_offset : current_offset + sbixStrikeOffsetFormatSize],
- offset_entry,
- )
- self.strikeOffsets.append(offset_entry.strikeOffset)
-
- # decompile Strikes
- for i in range(self.numStrikes - 1, -1, -1):
- current_strike = Strike(rawdata=data[self.strikeOffsets[i] :])
- data = data[: self.strikeOffsets[i]]
- current_strike.decompile(ttFont)
- # print " Strike length: %xh" % len(bitmapSetData)
- # print "Number of Glyph entries:", len(current_strike.glyphs)
- if current_strike.ppem in self.strikes:
- from fontTools import ttLib
-
- raise ttLib.TTLibError("Pixel 'ppem' must be unique for each Strike")
- self.strikes[current_strike.ppem] = current_strike
-
- # after the glyph data records have been extracted, we don't need the offsets anymore
- del self.strikeOffsets
- del self.numStrikes
-
- def compile(self, ttFont):
- sbixData = b""
- self.numStrikes = len(self.strikes)
- sbixHeader = sstruct.pack(sbixHeaderFormat, self)
-
- # calculate offset to start of first strike
- setOffset = sbixHeaderFormatSize + sbixStrikeOffsetFormatSize * self.numStrikes
-
- for si in sorted(self.strikes.keys()):
- current_strike = self.strikes[si]
- current_strike.compile(ttFont)
- # append offset to this strike to table header
- current_strike.strikeOffset = setOffset
- sbixHeader += sstruct.pack(sbixStrikeOffsetFormat, current_strike)
- setOffset += len(current_strike.data)
- sbixData += current_strike.data
-
- return sbixHeader + sbixData
-
- def toXML(self, xmlWriter, ttFont):
- xmlWriter.simpletag("version", value=self.version)
- xmlWriter.newline()
- xmlWriter.simpletag("flags", value=num2binary(self.flags, 16))
- xmlWriter.newline()
- for i in sorted(self.strikes.keys()):
- self.strikes[i].toXML(xmlWriter, ttFont)
-
- def fromXML(self, name, attrs, content, ttFont):
- if name == "version":
- setattr(self, name, safeEval(attrs["value"]))
- elif name == "flags":
- setattr(self, name, binary2num(attrs["value"]))
- elif name == "strike":
- current_strike = Strike()
- for element in content:
- if isinstance(element, tuple):
- name, attrs, content = element
- current_strike.fromXML(name, attrs, content, ttFont)
- self.strikes[current_strike.ppem] = current_strike
- else:
- from fontTools import ttLib
-
- raise ttLib.TTLibError("can't handle '%s' element" % name)
-
-
-# Helper classes
-
-
-class sbixStrikeOffset(object):
- pass
diff --git a/spaces/cmudrc/wecnet/app.py b/spaces/cmudrc/wecnet/app.py
deleted file mode 100644
index bd11ff26c462389c872b14b705c79eade9c2c700..0000000000000000000000000000000000000000
--- a/spaces/cmudrc/wecnet/app.py
+++ /dev/null
@@ -1,1414 +0,0 @@
-import keras
-import numpy
-import gradio
-import pandas
-import glob
-import os
-import shutil
-import math
-import platform
-import scipy.spatial
-import plotly.graph_objects as go
-import random
-from huggingface_hub import from_pretrained_keras
-
-def load_data():
-
- from datasets import load_dataset
-
- S = 5
- N = 1000
- D = 3
- F = 64
- G = 32
-
- data = load_dataset("cmudrc/wave-energy", data_files=["data.zip"], split='train')
- geometry = numpy.reshape(data['geometry'], (S*N, G*G*G))
- curves = numpy.reshape(data['curves'], (S*N, D*F))
- return None, None, S, N, D, F, G, curves, geometry
-
-# Disable eager execution because its bad
-from tensorflow.python.framework.ops import disable_eager_execution
-disable_eager_execution()
-
-class Mesh:
- def __init__(self):
- # Define blank values
- self.np = 0
- self.nf = 0
- self.X = []
- self.Y = []
- self.Z = []
- self.P = []
-
- def combine_meshes(self, ob1, ob2):
- # Check for largest mesh
- if ob1.nf < ob2.nf:
- coin_test = ob1.make_coin()
- coin_target = ob2.make_coin()
- else:
- coin_test = ob2.make_coin()
- coin_target = ob1.make_coin()
- # Check for duplicate panels
- deletion_list = []
- for iF in range(numpy.size(coin_test[1, 1, :])):
- panel_test = coin_test[:, :, iF]
- for iFF in range(numpy.size(coin_target[1, 1, :])):
- panel_target = coin_target[:, :, iFF]
- if numpy.sum(panel_test == panel_target) == 12:
- coin_target = numpy.delete(coin_target, iFF, 2)
- deletion_list.append(iF)
- coin_test = numpy.delete(coin_test, deletion_list, 2)
-
- # Concatenate unique meshes
- coin = numpy.concatenate((coin_test, coin_target), axis=2)
- self.np = numpy.size(coin[1, 1, :]) * 4
- self.nf = numpy.size(coin[1, 1, :])
- self.X = numpy.zeros(numpy.size(coin[1, 1, :]) * 4)
- self.Y = numpy.zeros(numpy.size(coin[1, 1, :]) * 4)
- self.Z = numpy.zeros(numpy.size(coin[1, 1, :]) * 4)
- self.P = numpy.zeros((numpy.size(coin[1, 1, :]), 4), dtype=int)
-
- iP = 0
- for iF in range(numpy.size(coin[1, 1, :])):
- for iC in range(4):
- self.X[iP] = coin[0, iC, iF]
- self.Y[iP] = coin[1, iC, iF]
- self.Z[iP] = coin[2, iC, iF]
- iP += 1
- self.P[iF, 0] = 1 + iF * 4
- self.P[iF, 1] = 2 + iF * 4
- self.P[iF, 2] = 3 + iF * 4
- self.P[iF, 3] = 4 + iF * 4
-
- def make_coin(self):
- coin = numpy.zeros((3, 4, self.nf))
- for iF in range(self.nf):
- for iC in range(4):
- coin[0, iC, iF] = self.X[self.P[iF, iC] - 1]
- coin[1, iC, iF] = self.Y[self.P[iF, iC] - 1]
- coin[2, iC, iF] = self.Z[self.P[iF, iC] - 1]
- return coin
-
- def delete_horizontal_panels(self):
- coin = self.make_coin()
- apex = numpy.min(self.Z)
- zLoc = numpy.zeros(4)
- deletion_list = []
-
- # Check every panel for horizontality and higher position than lowest point
- for iP in range(self.nf):
- for iC in range(4):
- zLoc[iC] = coin[2, iC, iP]
- if numpy.abs(numpy.mean(zLoc) - zLoc[0]) < 0.001 and numpy.mean(zLoc) > apex:
- deletion_list.append(iP)
-
- # Delete selected panels
- coin = numpy.delete(coin, deletion_list, 2)
-
- # Remake mesh
- self.np = numpy.size(coin[1, 1, :]) * 4
- self.nf = numpy.size(coin[1, 1, :])
- self.X = numpy.zeros(numpy.size(coin[1, 1, :]) * 4)
- self.Y = numpy.zeros(numpy.size(coin[1, 1, :]) * 4)
- self.Z = numpy.zeros(numpy.size(coin[1, 1, :]) * 4)
- self.P = numpy.zeros((numpy.size(coin[1, 1, :]), 4), dtype=int)
-
- iP = 0
- for iF in range(numpy.size(coin[1, 1, :])):
- for iC in range(4):
- self.X[iP] = coin[0, iC, iF]
- self.Y[iP] = coin[1, iC, iF]
- self.Z[iP] = coin[2, iC, iF]
- iP += 1
- self.P[iF, 0] = 1 + (iF) * 4
- self.P[iF, 1] = 2 + (iF) * 4
- self.P[iF, 2] = 3 + (iF) * 4
- self.P[iF, 3] = 4 + (iF) * 4
-
-
-
-
-def writeMesh(msh, filename):
- with open(filename, 'w') as f:
- f.write('{:d}\n'.format(msh.np))
- f.write('{:d}\n'.format(msh.nf))
- for iP in range(msh.np):
- f.write(' {:.7f} {:.7f} {:.7f}\n'.format(msh.X[iP], msh.Y[iP], msh.Z[iP]))
- for iF in range(msh.nf):
- f.write(' {:d} {:d} {:d} {:d}\n'.format(msh.P[iF, 0], msh.P[iF, 1], msh.P[iF, 2], msh.P[iF, 3]))
- return None
-
-
-
-class box:
- def __init__(self, length, width, height, cCor):
- self.length = length
- self.width = width
- self.height = height
- self.xC = cCor[0]
- self.yC = cCor[1]
- self.zC = cCor[2]
- self.name = 'box'
- self.panelize()
- self.translate(self.xC, self.yC, self.zC)
-
- def panelize(self):
- self.nf = 6
- self.np = 8
- self.X = numpy.array(
- [-self.length / 2.0, self.length / 2.0, -self.length / 2.0, self.length / 2.0, -self.length / 2.0,
- self.length / 2.0, -self.length / 2.0, self.length / 2.0])
- self.Y = numpy.array([self.width / 2.0, self.width / 2.0, self.width / 2.0, self.width / 2.0, -self.width / 2.0,
- -self.width / 2.0, -self.width / 2.0, -self.width / 2.0])
- self.Z = numpy.array(
- [-self.height / 2.0, -self.height / 2.0, self.height / 2.0, self.height / 2.0, -self.height / 2.0,
- -self.height / 2.0, self.height / 2.0, self.height / 2.0])
- self.P = numpy.zeros([6, 4], dtype=int)
- self.P[0, :] = numpy.array([3, 4, 2, 1])
- self.P[1, :] = numpy.array([4, 8, 6, 2])
- self.P[2, :] = numpy.array([8, 7, 5, 6])
- self.P[3, :] = numpy.array([7, 3, 1, 5])
- self.P[4, :] = numpy.array([2, 6, 5, 1])
- self.P[5, :] = numpy.array([8, 4, 3, 7])
- # Define triangles for plotting
- self.trii = numpy.zeros([2 * self.nf, 3], dtype=int)
- iT = 0
- for iTr in range(self.nf):
- self.trii[iT, :] = [self.P[iTr, 0] - 1, self.P[iTr, 1] - 1, self.P[iTr, 2] - 1]
- self.trii[iT + 1, :] = [self.P[iTr, 0] - 1, self.P[iTr, 2] - 1, self.P[iTr, 3] - 1]
- iT += 2
-
- def translate(self, xT, yT, zT):
- self.X += xT
- self.Y += yT
- self.Z += zT
-
- def rotate(self, a1, a2, theta):
- R = numpy.zeros([3, 3])
- # Normal vector through origin
- u = a2[0] - a1[0]
- v = a2[1] - a1[1]
- w = a2[2] - a1[2]
- u = u / numpy.sqrt(u ** 2 + v ** 2 + w ** 2)
- v = v / numpy.sqrt(u ** 2 + v ** 2 + w ** 2)
- w = w / numpy.sqrt(u ** 2 + v ** 2 + w ** 2)
- # Translate mesh so that rotation axis starts from the origin
- self.X -= a1[0]
- self.Y -= a1[1]
- self.Z -= a1[2]
-
- # Rotation matrix
- R[0, 0] = u ** 2 + numpy.cos(theta) * (1 - u ** 2)
- R[0, 1] = u * v * (1 - numpy.cos(theta)) - w * numpy.sin(theta)
- R[0, 2] = u * w * (1 - numpy.cos(theta)) + v * numpy.sin(theta)
- R[1, 0] = u * v * (1 - numpy.cos(theta)) + w * numpy.sin(theta)
- R[1, 1] = v ** 2 + numpy.cos(theta) * (1 - v ** 2)
- R[1, 2] = v * w * (1 - numpy.cos(theta)) - u * numpy.sin(theta)
- R[2, 0] = w * u * (1 - numpy.cos(theta)) - v * numpy.sin(theta)
- R[2, 1] = w * v * (1 - numpy.cos(theta)) + u * numpy.sin(theta)
- R[2, 2] = w ** 2 + numpy.cos(theta) * (1 - w ** 2)
-
- for iP in range(self.np):
- p1 = numpy.array([self.X[iP], self.Y[iP], self.Z[iP]])
- p2 = numpy.dot(R, p1)
- self.X[iP] = p2[0]
- self.Y[iP] = p2[1]
- self.Z[iP] = p2[2]
-
- # Translate back to original position
-
- self.X += a1[0]
- self.Y += a1[1]
- self.Z += a1[2]
-
- def makeCoin(self):
- coin = numpy.zeros((3, 4, self.nf))
- for iF in range(self.nf):
- for iC in range(4):
- coin[0, iC, iF] = self.X[self.P[iF, iC] - 1]
- coin[1, iC, iF] = self.Y[self.P[iF, iC] - 1]
- coin[2, iC, iF] = self.Z[self.P[iF, iC] - 1]
- return coin
-
-
-
-
-class cone:
- def __init__(self, diameter, height, cCor):
- self.diameter = diameter
- self.height = height
- self.xC = cCor[0]
- self.yC = cCor[1]
- self.zC = cCor[2]
- self.name = 'cone'
- self.panelize()
- self.translate(self.xC, self.yC, self.zC)
-
- def panelize(self):
- Ntheta = 18
- Nz = 3
- theta = [xx * 2 * numpy.pi / (Ntheta - 1) for xx in range(Ntheta)]
- self.nf = 0
- self.np = 0
- r = [0, self.diameter / 2.0, 0]
- z = [0, 0, -self.height]
- self.X = []
- self.Y = []
- self.Z = []
- self.P = numpy.zeros([(len(r) - 1) * (Ntheta - 1), 4], dtype=int)
- n = len(r)
-
- for iT in range(Ntheta):
- for iN in range(n):
- self.X.append(r[iN] * numpy.cos(theta[iT]))
- self.Y.append(r[iN] * numpy.sin(theta[iT]))
- self.Z.append(z[iN])
- self.np += 1
-
- iP = 0
- for iN in range(1, n):
- for iT in range(1, Ntheta):
- self.P[iP, 0] = iN + n * (iT - 1)
- self.P[iP, 1] = iN + 1 + n * (iT - 1)
- self.P[iP, 2] = iN + 1 + n * iT
- self.P[iP, 3] = iN + n * iT
- self.nf += 1
- iP += 1
-
- self.X = numpy.array(self.X)
- self.Y = numpy.array(self.Y)
- self.Z = numpy.array(self.Z)
- # Define triangles for plotting
- self.trii = numpy.zeros([2 * self.nf, 3], dtype=int)
- iT = 0
- for iTr in range(self.nf):
- self.trii[iT, :] = [self.P[iTr, 0] - 1, self.P[iTr, 1] - 1, self.P[iTr, 2] - 1]
- self.trii[iT + 1, :] = [self.P[iTr, 0] - 1, self.P[iTr, 2] - 1, self.P[iTr, 3] - 1]
- iT += 2
-
- def translate(self, xT, yT, zT):
- self.X += xT
- self.Y += yT
- self.Z += zT
-
- def rotate(self, a1, a2, theta):
- R = numpy.zeros([3, 3])
- # Normal vector through origin
- u = a2[0] - a1[0]
- v = a2[1] - a1[1]
- w = a2[2] - a1[2]
- u = u / numpy.sqrt(u ** 2 + v ** 2 + w ** 2)
- v = v / numpy.sqrt(u ** 2 + v ** 2 + w ** 2)
- w = w / numpy.sqrt(u ** 2 + v ** 2 + w ** 2)
- # Translate mesh so that rotation axis starts from the origin
- self.X -= a1[0]
- self.Y -= a1[1]
- self.Z -= a1[2]
-
- # Rotation matrix
- R[0, 0] = u ** 2 + numpy.cos(theta) * (1 - u ** 2)
- R[0, 1] = u * v * (1 - numpy.cos(theta)) - w * numpy.sin(theta)
- R[0, 2] = u * w * (1 - numpy.cos(theta)) + v * numpy.sin(theta)
- R[1, 0] = u * v * (1 - numpy.cos(theta)) + w * numpy.sin(theta)
- R[1, 1] = v ** 2 + numpy.cos(theta) * (1 - v ** 2)
- R[1, 2] = v * w * (1 - numpy.cos(theta)) - u * numpy.sin(theta)
- R[2, 0] = w * u * (1 - numpy.cos(theta)) - v * numpy.sin(theta)
- R[2, 1] = w * v * (1 - numpy.cos(theta)) + u * numpy.sin(theta)
- R[2, 2] = w ** 2 + numpy.cos(theta) * (1 - w ** 2)
-
- for iP in range(self.np):
- p1 = numpy.array([self.X[iP], self.Y[iP], self.Z[iP]])
- p2 = numpy.dot(R, p1)
- self.X[iP] = p2[0]
- self.Y[iP] = p2[1]
- self.Z[iP] = p2[2]
-
- # Translate back to original position
-
- self.X += a1[0]
- self.Y += a1[1]
- self.Z += a1[2]
-
- def makeCoin(self):
- coin = numpy.zeros((3, 4, self.nf))
- for iF in range(self.nf):
- for iC in range(4):
- coin[0, iC, iF] = self.X[self.P[iF, iC] - 1]
- coin[1, iC, iF] = self.Y[self.P[iF, iC] - 1]
- coin[2, iC, iF] = self.Z[self.P[iF, iC] - 1]
- return coin
-
-
-
-class cylinder:
- def __init__(self, diameter, height, cCor):
- self.diameter = diameter
- self.height = height
- self.xC = cCor[0]
- self.yC = cCor[1]
- self.zC = cCor[2]
- self.name = 'cylinder'
- self.panelize()
- self.translate(self.xC, self.yC, self.zC)
-
- def panelize(self):
- Ntheta = 18
- Nz = 3
- theta = [xx * 2 * numpy.pi / (Ntheta - 1) for xx in range(Ntheta)]
- self.nf = 0
- self.np = 0
- r = [0, self.diameter / 2.0, self.diameter / 2.0, 0]
- z = [0, 0, -self.height, -self.height]
- self.X = []
- self.Y = []
- self.Z = []
- self.P = numpy.zeros([(len(r) - 1) * (Ntheta - 1), 4], dtype=int)
- n = len(r)
-
- for iT in range(Ntheta):
- for iN in range(n):
- self.X.append(r[iN] * numpy.cos(theta[iT]))
- self.Y.append(r[iN] * numpy.sin(theta[iT]))
- self.Z.append(z[iN])
- self.np += 1
-
- iP = 0
- for iN in range(1, n):
- for iT in range(1, Ntheta):
- self.P[iP, 0] = iN + n * (iT - 1)
- self.P[iP, 1] = iN + 1 + n * (iT - 1)
- self.P[iP, 2] = iN + 1 + n * iT
- self.P[iP, 3] = iN + n * iT
- self.nf += 1
- iP += 1
-
- self.X = numpy.array(self.X)
- self.Y = numpy.array(self.Y)
- self.Z = numpy.array(self.Z)
- # Define triangles for plotting
- self.trii = numpy.zeros([2 * self.nf, 3], dtype=int)
- iT = 0
- for iTr in range(self.nf):
- self.trii[iT, :] = [self.P[iTr, 0] - 1, self.P[iTr, 1] - 1, self.P[iTr, 2] - 1]
- self.trii[iT + 1, :] = [self.P[iTr, 0] - 1, self.P[iTr, 2] - 1, self.P[iTr, 3] - 1]
- iT += 2
-
- def translate(self, xT, yT, zT):
- self.X += xT
- self.Y += yT
- self.Z += zT
-
- def rotate(self, a1, a2, theta):
- R = numpy.zeros([3, 3])
- # Normal vector through origin
- u = a2[0] - a1[0]
- v = a2[1] - a1[1]
- w = a2[2] - a1[2]
- u = u / numpy.sqrt(u ** 2 + v ** 2 + w ** 2)
- v = v / numpy.sqrt(u ** 2 + v ** 2 + w ** 2)
- w = w / numpy.sqrt(u ** 2 + v ** 2 + w ** 2)
- # Translate mesh so that rotation axis starts from the origin
- self.X -= a1[0]
- self.Y -= a1[1]
- self.Z -= a1[2]
-
- # Rotation matrix
- R[0, 0] = u ** 2 + numpy.cos(theta) * (1 - u ** 2)
- R[0, 1] = u * v * (1 - numpy.cos(theta)) - w * numpy.sin(theta)
- R[0, 2] = u * w * (1 - numpy.cos(theta)) + v * numpy.sin(theta)
- R[1, 0] = u * v * (1 - numpy.cos(theta)) + w * numpy.sin(theta)
- R[1, 1] = v ** 2 + numpy.cos(theta) * (1 - v ** 2)
- R[1, 2] = v * w * (1 - numpy.cos(theta)) - u * numpy.sin(theta)
- R[2, 0] = w * u * (1 - numpy.cos(theta)) - v * numpy.sin(theta)
- R[2, 1] = w * v * (1 - numpy.cos(theta)) + u * numpy.sin(theta)
- R[2, 2] = w ** 2 + numpy.cos(theta) * (1 - w ** 2)
-
- for iP in range(self.np):
- p1 = numpy.array([self.X[iP], self.Y[iP], self.Z[iP]])
- p2 = numpy.dot(R, p1)
- self.X[iP] = p2[0]
- self.Y[iP] = p2[1]
- self.Z[iP] = p2[2]
-
- # Translate back to original position
-
- self.X += a1[0]
- self.Y += a1[1]
- self.Z += a1[2]
-
- def makeCoin(self):
- coin = numpy.zeros((3, 4, self.nf))
- for iF in range(self.nf):
- for iC in range(4):
- coin[0, iC, iF] = self.X[self.P[iF, iC] - 1]
- coin[1, iC, iF] = self.Y[self.P[iF, iC] - 1]
- coin[2, iC, iF] = self.Z[self.P[iF, iC] - 1]
- return coin
-
-
-
-
-class hemicylinder:
- def __init__(self, diameter, height, cCor):
- self.diameter = diameter
- self.height = height
- self.xC = cCor[0]
- self.yC = cCor[1]
- self.zC = cCor[2]
- self.name = 'hemicylinder'
- self.panelize()
- self.translate(self.xC, self.yC, self.zC)
-
- def panelize(self):
- Ntheta = 18
- Nz = 3
- theta = [xx * numpy.pi / (Ntheta - 1) - numpy.pi / 2.0 for xx in range(Ntheta)]
- self.nf = 0
- self.np = 0
- r = [0, self.diameter / 2.0, self.diameter / 2.0, 0]
- z = [self.height / 2.0, self.height / 2.0, -self.height / 2.0, -self.height / 2.0]
- self.X = []
- self.Y = []
- self.Z = []
- self.P = numpy.zeros([(len(r) - 1) * (Ntheta - 1), 4], dtype=int)
- n = len(r)
-
- for iT in range(Ntheta):
- for iN in range(n):
- self.Z.append(-r[iN] * numpy.cos(theta[iT]))
- self.X.append(r[iN] * numpy.sin(theta[iT]))
- self.Y.append(z[iN])
- self.np += 1
-
- iP = 0
- for iN in range(1, n):
- for iT in range(1, Ntheta):
- self.P[iP, 3] = iN + n * (iT - 1)
- self.P[iP, 2] = iN + 1 + n * (iT - 1)
- self.P[iP, 1] = iN + 1 + n * iT
- self.P[iP, 0] = iN + n * iT
- self.nf += 1
- iP += 1
-
- self.X = numpy.array(self.X)
- self.Y = numpy.array(self.Y)
- self.Z = numpy.array(self.Z)
- # Define triangles for plotting
- self.trii = numpy.zeros([2 * self.nf, 3], dtype=int)
- iT = 0
- for iTr in range(self.nf):
- self.trii[iT, :] = [self.P[iTr, 0] - 1, self.P[iTr, 1] - 1, self.P[iTr, 2] - 1]
- self.trii[iT + 1, :] = [self.P[iTr, 0] - 1, self.P[iTr, 2] - 1, self.P[iTr, 3] - 1]
- iT += 2
-
- def translate(self, xT, yT, zT):
- self.X += xT
- self.Y += yT
- self.Z += zT
-
- def rotate(self, a1, a2, theta):
- R = numpy.zeros([3, 3])
- # Normal vector through origin
- u = a2[0] - a1[0]
- v = a2[1] - a1[1]
- w = a2[2] - a1[2]
- u = u / numpy.sqrt(u ** 2 + v ** 2 + w ** 2)
- v = v / numpy.sqrt(u ** 2 + v ** 2 + w ** 2)
- w = w / numpy.sqrt(u ** 2 + v ** 2 + w ** 2)
- # Translate mesh so that rotation axis starts from the origin
- self.X -= a1[0]
- self.Y -= a1[1]
- self.Z -= a1[2]
-
- # Rotation matrix
- R[0, 0] = u ** 2 + numpy.cos(theta) * (1 - u ** 2)
- R[0, 1] = u * v * (1 - numpy.cos(theta)) - w * numpy.sin(theta)
- R[0, 2] = u * w * (1 - numpy.cos(theta)) + v * numpy.sin(theta)
- R[1, 0] = u * v * (1 - numpy.cos(theta)) + w * numpy.sin(theta)
- R[1, 1] = v ** 2 + numpy.cos(theta) * (1 - v ** 2)
- R[1, 2] = v * w * (1 - numpy.cos(theta)) - u * numpy.sin(theta)
- R[2, 0] = w * u * (1 - numpy.cos(theta)) - v * numpy.sin(theta)
- R[2, 1] = w * v * (1 - numpy.cos(theta)) + u * numpy.sin(theta)
- R[2, 2] = w ** 2 + numpy.cos(theta) * (1 - w ** 2)
-
- for iP in range(self.np):
- p1 = numpy.array([self.X[iP], self.Y[iP], self.Z[iP]])
- p2 = numpy.dot(R, p1)
- self.X[iP] = p2[0]
- self.Y[iP] = p2[1]
- self.Z[iP] = p2[2]
-
- # Translate back to original position
-
- self.X += a1[0]
- self.Y += a1[1]
- self.Z += a1[2]
-
- def makeCoin(self):
- coin = numpy.zeros((3, 4, self.nf))
- for iF in range(self.nf):
- for iC in range(4):
- coin[0, iC, iF] = self.X[self.P[iF, iC] - 1]
- coin[1, iC, iF] = self.Y[self.P[iF, iC] - 1]
- coin[2, iC, iF] = self.Z[self.P[iF, iC] - 1]
- return coin
-
-
-class sphere:
- def __init__(self, diameter, cCor):
- self.diameter = diameter
- self.xC = cCor[0]
- self.yC = cCor[1]
- self.zC = cCor[2]
- self.name = 'sphere'
- self.panelize()
- self.translate(self.xC, self.yC, self.zC)
-
- def panelize(self):
- Ntheta = 18
- Nthetad2 = int(Ntheta / 2)
- Nz = 3
- theta = [xx * 2 * numpy.pi / (Ntheta - 1) for xx in range(Ntheta)]
- phi = [xx * numpy.pi / (Ntheta / 2 - 1) for xx in range(Nthetad2)]
- self.nf = 0
- self.np = 0
- r = self.diameter / 2.0
- self.X = []
- self.Y = []
- self.Z = []
- self.P = numpy.zeros([(Ntheta - 1) * (Nthetad2 - 1), 4], dtype=int)
-
- for iT in range(Nthetad2):
- for iTT in range(Ntheta):
- self.X.append(r * numpy.cos(theta[iTT]) * numpy.sin(phi[iT]))
- self.Y.append(r * numpy.sin(theta[iTT]) * numpy.sin(phi[iT]))
- self.Z.append(r * numpy.cos(phi[iT]))
- self.np += 1
-
- iP = 0
- for iN in range(1, Ntheta):
- for iT in range(1, Nthetad2):
- self.P[iP, 3] = iN + Ntheta * (iT - 1)
- self.P[iP, 2] = iN + 1 + Ntheta * (iT - 1)
- self.P[iP, 1] = iN + 1 + Ntheta * iT
- self.P[iP, 0] = iN + Ntheta * iT
- self.nf += 1
- iP += 1
- self.X = numpy.array(self.X)
- self.Y = numpy.array(self.Y)
- self.Z = numpy.array(self.Z)
- # Define triangles for plotting
- self.trii = numpy.zeros([2 * self.nf, 3], dtype=int)
- iT = 0
- for iTr in range(self.nf):
- self.trii[iT, :] = [self.P[iTr, 0] - 1, self.P[iTr, 1] - 1, self.P[iTr, 2] - 1]
- self.trii[iT + 1, :] = [self.P[iTr, 0] - 1, self.P[iTr, 2] - 1, self.P[iTr, 3] - 1]
- iT += 2
-
- def translate(self, xT, yT, zT):
- self.X += xT
- self.Y += yT
- self.Z += zT
-
- def rotate(self, a1, a2, theta):
- R = numpy.zeros([3, 3])
- # Normal vector through origin
- u = a2[0] - a1[0]
- v = a2[1] - a1[1]
- w = a2[2] - a1[2]
- u = u / numpy.sqrt(u ** 2 + v ** 2 + w ** 2)
- v = v / numpy.sqrt(u ** 2 + v ** 2 + w ** 2)
- w = w / numpy.sqrt(u ** 2 + v ** 2 + w ** 2)
- # Translate mesh so that rotation axis starts from the origin
- self.X -= a1[0]
- self.Y -= a1[1]
- self.Z -= a1[2]
-
- # Rotation matrix
- R[0, 0] = u ** 2 + numpy.cos(theta) * (1 - u ** 2)
- R[0, 1] = u * v * (1 - numpy.cos(theta)) - w * numpy.sin(theta)
- R[0, 2] = u * w * (1 - numpy.cos(theta)) + v * numpy.sin(theta)
- R[1, 0] = u * v * (1 - numpy.cos(theta)) + w * numpy.sin(theta)
- R[1, 1] = v ** 2 + numpy.cos(theta) * (1 - v ** 2)
- R[1, 2] = v * w * (1 - numpy.cos(theta)) - u * numpy.sin(theta)
- R[2, 0] = w * u * (1 - numpy.cos(theta)) - v * numpy.sin(theta)
- R[2, 1] = w * v * (1 - numpy.cos(theta)) + u * numpy.sin(theta)
- R[2, 2] = w ** 2 + numpy.cos(theta) * (1 - w ** 2)
-
- for iP in range(self.np):
- p1 = numpy.array([self.X[iP], self.Y[iP], self.Z[iP]])
- p2 = numpy.dot(R, p1)
- self.X[iP] = p2[0]
- self.Y[iP] = p2[1]
- self.Z[iP] = p2[2]
-
- # Translate back to original position
-
- self.X += a1[0]
- self.Y += a1[1]
- self.Z += a1[2]
-
- def makeCoin(self):
- coin = numpy.zeros((3, 4, self.nf))
- for iF in range(self.nf):
- for iC in range(4):
- coin[0, iC, iF] = self.X[self.P[iF, iC] - 1]
- coin[1, iC, iF] = self.Y[self.P[iF, iC] - 1]
- coin[2, iC, iF] = self.Z[self.P[iF, iC] - 1]
- return coin
-
-
-
-
-
-class hemisphere:
- def __init__(self, diameter, cCor):
- self.diameter = diameter
- self.xC = cCor[0]
- self.yC = cCor[1]
- self.zC = cCor[2]
- self.name = 'hemisphere'
- self.panelize()
- self.translate(self.xC, self.yC, self.zC)
-
- def panelize(self):
- Ntheta = 18
- theta = [xx * 2 * numpy.pi / (Ntheta - 1) for xx in range(Ntheta)]
- phi = [xx * numpy.pi / 2.0 / (Ntheta / 2 - 1) for xx in range(Ntheta / 2)]
- self.nf = 0
- self.np = 0
- r = self.diameter / 2.0
- self.X = []
- self.Y = []
- self.Z = []
- self.P = numpy.zeros([(Ntheta - 1) * (Ntheta / 2 - 1), 4], dtype=int)
-
- for iT in range(Ntheta / 2):
- for iTT in range(Ntheta):
- self.X.append(r * numpy.cos(theta[iTT]) * numpy.sin(phi[iT]))
- self.Y.append(r * numpy.sin(theta[iTT]) * numpy.sin(phi[iT]))
- self.Z.append(-r * numpy.cos(phi[iT]))
- self.np += 1
-
- iP = 0
- for iN in range(1, Ntheta):
- for iT in range(1, Ntheta / 2):
- self.P[iP, 0] = iN + Ntheta * (iT - 1)
- self.P[iP, 1] = iN + 1 + Ntheta * (iT - 1)
- self.P[iP, 2] = iN + 1 + Ntheta * iT
- self.P[iP, 3] = iN + Ntheta * iT
- self.nf += 1
- iP += 1
-
- self.X = numpy.array(self.X)
- self.Y = numpy.array(self.Y)
- self.Z = numpy.array(self.Z)
- # Define triangles for plotting
- self.trii = numpy.zeros([2 * self.nf, 3], dtype=int)
- iT = 0
- for iTr in range(self.nf):
- self.trii[iT, :] = [self.P[iTr, 0] - 1, self.P[iTr, 1] - 1, self.P[iTr, 2] - 1]
- self.trii[iT + 1, :] = [self.P[iTr, 0] - 1, self.P[iTr, 2] - 1, self.P[iTr, 3] - 1]
- iT += 2
-
- def translate(self, xT, yT, zT):
- self.X += xT
- self.Y += yT
- self.Z += zT
-
- def rotate(self, a1, a2, theta):
- R = numpy.zeros([3, 3])
- # Normal vector through origin
- u = a2[0] - a1[0]
- v = a2[1] - a1[1]
- w = a2[2] - a1[2]
- u = u / numpy.sqrt(u ** 2 + v ** 2 + w ** 2)
- v = v / numpy.sqrt(u ** 2 + v ** 2 + w ** 2)
- w = w / numpy.sqrt(u ** 2 + v ** 2 + w ** 2)
- # Translate mesh so that rotation axis starts from the origin
- self.X -= a1[0]
- self.Y -= a1[1]
- self.Z -= a1[2]
-
- # Rotation matrix
- R[0, 0] = u ** 2 + numpy.cos(theta) * (1 - u ** 2)
- R[0, 1] = u * v * (1 - numpy.cos(theta)) - w * numpy.sin(theta)
- R[0, 2] = u * w * (1 - numpy.cos(theta)) + v * numpy.sin(theta)
- R[1, 0] = u * v * (1 - numpy.cos(theta)) + w * numpy.sin(theta)
- R[1, 1] = v ** 2 + numpy.cos(theta) * (1 - v ** 2)
- R[1, 2] = v * w * (1 - numpy.cos(theta)) - u * numpy.sin(theta)
- R[2, 0] = w * u * (1 - numpy.cos(theta)) - v * numpy.sin(theta)
- R[2, 1] = w * v * (1 - numpy.cos(theta)) + u * numpy.sin(theta)
- R[2, 2] = w ** 2 + numpy.cos(theta) * (1 - w ** 2)
-
- for iP in range(self.np):
- p1 = numpy.array([self.X[iP], self.Y[iP], self.Z[iP]])
- p2 = numpy.dot(R, p1)
- self.X[iP] = p2[0]
- self.Y[iP] = p2[1]
- self.Z[iP] = p2[2]
-
- # Translate back to original position
-
- self.X += a1[0]
- self.Y += a1[1]
- self.Z += a1[2]
-
- def makeCoin(self):
- coin = numpy.zeros((3, 4, self.nf))
- for iF in range(self.nf):
- for iC in range(4):
- coin[0, iC, iF] = self.X[self.P[iF, iC] - 1]
- coin[1, iC, iF] = self.Y[self.P[iF, iC] - 1]
- coin[2, iC, iF] = self.Z[self.P[iF, iC] - 1]
- return coin
-
-
-
-
-class pyramid:
- def __init__(self, length, width, height, cCor):
- self.length = length
- self.width = width
- self.height = height
- self.xC = cCor[0]
- self.yC = cCor[1]
- self.zC = cCor[2]
- self.name = 'pyramid'
- self.panelize()
- self.translate(self.xC, self.yC, self.zC)
-
- def panelize(self):
- self.nf = 6
- self.np = 8
- self.X = numpy.array(
- [0.0, 0.0, -self.length / 2.0, self.length / 2.0, 0.0, 0.0, -self.length / 2.0, self.length / 2.0])
- self.Y = numpy.array(
- [0.0, 0.0, self.width / 2.0, self.width / 2.0, 0.0, 0.0, -self.width / 2.0, -self.width / 2.0])
- self.Z = numpy.array([-self.height, -self.height, 0.0, 0.0, -self.height, -self.height, 0.0, 0.0])
- self.P = numpy.zeros([6, 4], dtype=int)
- self.P[0, :] = numpy.array([3, 4, 2, 1])
- self.P[1, :] = numpy.array([4, 8, 6, 2])
- self.P[2, :] = numpy.array([8, 7, 5, 6])
- self.P[3, :] = numpy.array([7, 3, 1, 5])
- self.P[4, :] = numpy.array([5, 6, 5, 1])
- self.P[5, :] = numpy.array([8, 4, 3, 7])
- # Define triangles for plotting
- self.trii = numpy.zeros([2 * self.nf, 3], dtype=int)
- iT = 0
- for iTr in range(self.nf):
- self.trii[iT, :] = [self.P[iTr, 0] - 1, self.P[iTr, 1] - 1, self.P[iTr, 2] - 1]
- self.trii[iT + 1, :] = [self.P[iTr, 0] - 1, self.P[iTr, 2] - 1, self.P[iTr, 3] - 1]
- iT += 2
-
- def translate(self, xT, yT, zT):
- self.X += xT
- self.Y += yT
- self.Z += zT
-
- def rotate(self, a1, a2, theta):
- R = numpy.zeros([3, 3])
- # Normal vector through origin
- u = a2[0] - a1[0]
- v = a2[1] - a1[1]
- w = a2[2] - a1[2]
- u = u / numpy.sqrt(u ** 2 + v ** 2 + w ** 2)
- v = v / numpy.sqrt(u ** 2 + v ** 2 + w ** 2)
- w = w / numpy.sqrt(u ** 2 + v ** 2 + w ** 2)
- # Translate mesh so that rotation axis starts from the origin
- self.X -= a1[0]
- self.Y -= a1[1]
- self.Z -= a1[2]
-
- # Rotation matrix
- R[0, 0] = u ** 2 + numpy.cos(theta) * (1 - u ** 2)
- R[0, 1] = u * v * (1 - numpy.cos(theta)) - w * numpy.sin(theta)
- R[0, 2] = u * w * (1 - numpy.cos(theta)) + v * numpy.sin(theta)
- R[1, 0] = u * v * (1 - numpy.cos(theta)) + w * numpy.sin(theta)
- R[1, 1] = v ** 2 + numpy.cos(theta) * (1 - v ** 2)
- R[1, 2] = v * w * (1 - numpy.cos(theta)) - u * numpy.sin(theta)
- R[2, 0] = w * u * (1 - numpy.cos(theta)) - v * numpy.sin(theta)
- R[2, 1] = w * v * (1 - numpy.cos(theta)) + u * numpy.sin(theta)
- R[2, 2] = w ** 2 + numpy.cos(theta) * (1 - w ** 2)
-
- for iP in range(self.np):
- p1 = numpy.array([self.X[iP], self.Y[iP], self.Z[iP]])
- p2 = numpy.dot(R, p1)
- self.X[iP] = p2[0]
- self.Y[iP] = p2[1]
- self.Z[iP] = p2[2]
-
- # Translate back to original position
-
- self.X += a1[0]
- self.Y += a1[1]
- self.Z += a1[2]
-
- def makeCoin(self):
- coin = numpy.zeros((3, 4, self.nf))
- for iF in range(self.nf):
- for iC in range(4):
- coin[0, iC, iF] = self.X[self.P[iF, iC] - 1]
- coin[1, iC, iF] = self.Y[self.P[iF, iC] - 1]
- coin[2, iC, iF] = self.Z[self.P[iF, iC] - 1]
- return coin
-
-
-
-
-
-class wedge:
- def __init__(self, length, width, height, cCor):
- self.length = length
- self.width = width
- self.height = height
- self.xC = cCor[0]
- self.yC = cCor[1]
- self.zC = cCor[2]
- self.name = 'wedge'
- self.panelize()
- self.translate(self.xC, self.yC, self.zC)
-
- def panelize(self):
- self.nf = 6
- self.np = 8
- self.X = numpy.array(
- [0.0, 0.0, -self.length / 2.0, self.length / 2.0, 0.0, 0.0, -self.length / 2.0, self.length / 2.0])
- self.Y = numpy.array([self.width / 2.0, self.width / 2.0, self.width / 2.0, self.width / 2.0, -self.width / 2.0,
- -self.width / 2.0, -self.width / 2.0, -self.width / 2.0])
- self.Z = numpy.array([-self.height, -self.height, 0.0, 0.0, -self.height, -self.height, 0.0, 0.0])
- self.P = numpy.zeros([6, 4], dtype=int)
- self.P[0, :] = numpy.array([3, 4, 2, 1])
- self.P[1, :] = numpy.array([4, 8, 6, 2])
- self.P[2, :] = numpy.array([8, 7, 5, 6])
- self.P[3, :] = numpy.array([7, 3, 1, 5])
- self.P[4, :] = numpy.array([2, 6, 5, 1])
- self.P[5, :] = numpy.array([8, 4, 3, 7])
- # Define triangles for plotting
- self.trii = numpy.zeros([2 * self.nf, 3], dtype=int)
- iT = 0
- for iTr in range(self.nf):
- self.trii[iT, :] = [self.P[iTr, 0] - 1, self.P[iTr, 1] - 1, self.P[iTr, 2] - 1]
- self.trii[iT + 1, :] = [self.P[iTr, 0] - 1, self.P[iTr, 2] - 1, self.P[iTr, 3] - 1]
- iT += 2
-
- def translate(self, xT, yT, zT):
- self.X += xT
- self.Y += yT
- self.Z += zT
-
- def rotate(self, a1, a2, theta):
- R = numpy.zeros([3, 3])
- # Normal vector through origin
- u = a2[0] - a1[0]
- v = a2[1] - a1[1]
- w = a2[2] - a1[2]
- u = u / numpy.sqrt(u ** 2 + v ** 2 + w ** 2)
- v = v / numpy.sqrt(u ** 2 + v ** 2 + w ** 2)
- w = w / numpy.sqrt(u ** 2 + v ** 2 + w ** 2)
- # Translate mesh so that rotation axis starts from the origin
- self.X -= a1[0]
- self.Y -= a1[1]
- self.Z -= a1[2]
-
- # Rotation matrix
- R[0, 0] = u ** 2 + numpy.cos(theta) * (1 - u ** 2)
- R[0, 1] = u * v * (1 - numpy.cos(theta)) - w * numpy.sin(theta)
- R[0, 2] = u * w * (1 - numpy.cos(theta)) + v * numpy.sin(theta)
- R[1, 0] = u * v * (1 - numpy.cos(theta)) + w * numpy.sin(theta)
- R[1, 1] = v ** 2 + numpy.cos(theta) * (1 - v ** 2)
- R[1, 2] = v * w * (1 - numpy.cos(theta)) - u * numpy.sin(theta)
- R[2, 0] = w * u * (1 - numpy.cos(theta)) - v * numpy.sin(theta)
- R[2, 1] = w * v * (1 - numpy.cos(theta)) + u * numpy.sin(theta)
- R[2, 2] = w ** 2 + numpy.cos(theta) * (1 - w ** 2)
-
- for iP in range(self.np):
- p1 = numpy.array([self.X[iP], self.Y[iP], self.Z[iP]])
- p2 = numpy.dot(R, p1)
- self.X[iP] = p2[0]
- self.Y[iP] = p2[1]
- self.Z[iP] = p2[2]
-
- # Translate back to original position
-
- self.X += a1[0]
- self.Y += a1[1]
- self.Z += a1[2]
-
- def makeCoin(self):
- coin = numpy.zeros((3, 4, self.nf))
- for iF in range(self.nf):
- for iC in range(4):
- coin[0, iC, iF] = self.X[self.P[iF, iC] - 1]
- coin[1, iC, iF] = self.Y[self.P[iF, iC] - 1]
- coin[2, iC, iF] = self.Z[self.P[iF, iC] - 1]
- return coin
-
-
-
-
-
-class torus:
- def __init__(self, diamOut, diamIn, cCor):
- self.diamOut = diamOut
- self.diamIn = diamIn
- self.xC = cCor[0]
- self.yC = cCor[1]
- self.zC = cCor[2]
- self.name = 'torus'
- self.panelize()
- self.translate(self.xC, self.yC, self.zC)
-
- def panelize(self):
- Ntheta = 18
- Nphi = 18
- theta = [xx * 2 * numpy.pi / (Ntheta - 1) for xx in range(Ntheta)]
- phi = [xx * 2 * numpy.pi / (Nphi - 1) for xx in range(Nphi)]
- self.nf = 0
- self.np = 0
- self.X = []
- self.Y = []
- self.Z = []
- R = self.diamOut / 2.0
- r = self.diamIn / 2.0
-
- for iT in range(Ntheta):
- for iP in range(Nphi):
- self.X.append((R + r * numpy.cos(theta[iT])) * numpy.cos(phi[iP]))
- self.Y.append((R + r * numpy.cos(theta[iT])) * numpy.sin(phi[iP]))
- self.Z.append(r * numpy.sin(theta[iT]))
- self.np += 1
-
- self.nf = (Ntheta - 1) * (Nphi - 1)
- self.P = numpy.zeros([self.nf, 4], dtype=int)
- iPan = 0
- for iT in range(Ntheta - 1):
- for iP in range(Nphi - 1):
- self.P[iPan, 0] = iP + iT * Nphi + 1
- self.P[iPan, 1] = iP + 1 + iT * Nphi + 1
- self.P[iPan, 2] = iP + 1 + Ntheta + iT * Nphi + 1
- self.P[iPan, 3] = iP + Ntheta + iT * Nphi + 1
- iPan += 1
-
- self.X = numpy.array(self.X)
- self.Y = numpy.array(self.Y)
- self.Z = numpy.array(self.Z)
- # Define triangles for plotting
- self.trii = numpy.zeros([2 * self.nf, 3], dtype=int)
- iT = 0
- for iTr in range(self.nf):
- self.trii[iT, :] = [self.P[iTr, 0] - 1, self.P[iTr, 1] - 1, self.P[iTr, 2] - 1]
- self.trii[iT + 1, :] = [self.P[iTr, 0] - 1, self.P[iTr, 2] - 1, self.P[iTr, 3] - 1]
- iT += 2
-
- def translate(self, xT, yT, zT):
- self.X += xT
- self.Y += yT
- self.Z += zT
-
- def rotate(self, a1, a2, theta):
- R = numpy.zeros([3, 3])
- # Normal vector through origin
- u = a2[0] - a1[0]
- v = a2[1] - a1[1]
- w = a2[2] - a1[2]
- u = u / numpy.sqrt(u ** 2 + v ** 2 + w ** 2)
- v = v / numpy.sqrt(u ** 2 + v ** 2 + w ** 2)
- w = w / numpy.sqrt(u ** 2 + v ** 2 + w ** 2)
- # Translate mesh so that rotation axis starts from the origin
- self.X -= a1[0]
- self.Y -= a1[1]
- self.Z -= a1[2]
-
- # Rotation matrix
- R[0, 0] = u ** 2 + numpy.cos(theta) * (1 - u ** 2)
- R[0, 1] = u * v * (1 - numpy.cos(theta)) - w * numpy.sin(theta)
- R[0, 2] = u * w * (1 - numpy.cos(theta)) + v * numpy.sin(theta)
- R[1, 0] = u * v * (1 - numpy.cos(theta)) + w * numpy.sin(theta)
- R[1, 1] = v ** 2 + numpy.cos(theta) * (1 - v ** 2)
- R[1, 2] = v * w * (1 - numpy.cos(theta)) - u * numpy.sin(theta)
- R[2, 0] = w * u * (1 - numpy.cos(theta)) - v * numpy.sin(theta)
- R[2, 1] = w * v * (1 - numpy.cos(theta)) + u * numpy.sin(theta)
- R[2, 2] = w ** 2 + numpy.cos(theta) * (1 - w ** 2)
-
- for iP in range(self.np):
- p1 = numpy.array([self.X[iP], self.Y[iP], self.Z[iP]])
- p2 = numpy.dot(R, p1)
- self.X[iP] = p2[0]
- self.Y[iP] = p2[1]
- self.Z[iP] = p2[2]
-
- # Translate back to original position
-
- self.X += a1[0]
- self.Y += a1[1]
- self.Z += a1[2]
-
- def makeCoin(self):
- coin = numpy.zeros((3, 4, self.nf))
- for iF in range(self.nf):
- for iC in range(4):
- coin[0, iC, iF] = self.X[self.P[iF, iC] - 1]
- coin[1, iC, iF] = self.Y[self.P[iF, iC] - 1]
- coin[2, iC, iF] = self.Z[self.P[iF, iC] - 1]
- return coin
-
-def make_voxels_without_figure(shape, length, height, width, diameter):
- pos = [0, 0, 0]
- if shape == "box":
- mesh = box(length, width, height, pos)
- elif shape == "cone":
- mesh = cone(diameter, height, pos)
- elif shape == "cylinder":
- mesh = cylinder(diameter, height, pos)
- elif shape == "sphere":
- mesh = sphere(diameter, pos)
- elif shape == "wedge":
- mesh = wedge(length, width, height, pos)
-
- hull_points = numpy.array([mesh.X.tolist(), mesh.Y.tolist(), mesh.Z.tolist()]).T
-
- # Set up test points
- G = 32
- ex = 5 - 5 / G
- x, y, z = numpy.meshgrid(numpy.linspace(-ex, ex, G),
- numpy.linspace(-ex, ex, G),
- numpy.linspace(-(9.5 - 5 / G), 0.5 - 5 / G, G))
- test_points = numpy.vstack((x.ravel(), y.ravel(), z.ravel())).T
-
- hull = scipy.spatial.Delaunay(hull_points)
- within = hull.find_simplex(test_points) >= 0
-
- return within*1.0
-
-
-def make_voxels(shape, length, height, width, diameter):
- return plotly_fig(make_voxels_without_figure(shape, length, height, width, diameter))
-
-# This function loads a fuckton of data
-# def load_data():
-# # Open all the files we downloaded at the beginning and take out hte good bits
-# curves = numpy.load('data_curves.npz')['curves']
-# geometry = numpy.load('data_geometry.npz')['geometry']
-# constants = numpy.load('constants.npz')
-# S = constants['S']
-# N = constants['N']
-# D = constants['D']
-# F = constants['F']
-# G = constants['G']
-
-# # Some of the good bits need additional processining
-# new_curves = numpy.zeros((S*N, D * F))
-# for i, curveset in enumerate(curves):
-# new_curves[i, :] = curveset.T.flatten() / 1000000
-
-# new_geometry = numpy.zeros((S*N, G * G * G))
-# for i, geometryset in enumerate(geometry):
-# new_geometry[i, :] = geometryset.T.flatten()
-
-# # Return good bits to user
-# return curves, geometry, S, N, D, F, G, new_curves, new_geometry
-
-curves, geometry, S, N, D, F, G, new_curves, new_geometry = load_data()
-
-class Network(object):
-
- def __init__(self, type):
- # Instantiate variables
- # self.curves = curves
- # self.new_curves = new_curves
- # self.geometry = geometry
- # self.new_geometry = new_geometry
- # self.S = S
- # self.N = N
- # self.D = D
- # self.F = F
- # self.G = G
-
- # Load network
- # with open(structure, 'r') as file:
- # self.network = keras.models.model_from_json(file.read())
- # self.network.load_weights(weights)
- self.network = from_pretrained_keras("cmudrc/wave-energy-analysis") if type == "forward" else from_pretrained_keras("cmudrc/wave-energy-synthesis")
-
- def analysis(self, idx=None):
- print(idx)
-
- if idx is None:
- idx = numpy.random.randint(1, S * N)
- else:
- idx = int(idx)
-
- # Get the input
- data_input = new_geometry[idx:(idx+1), :]
- other_data_input = data_input.reshape((G, G, G), order='F')
-
- # Get the outputs
- print(data_input.shape)
- predicted_output = self.network.predict(data_input)
- true_output = new_curves[idx].reshape((3, F))
- predicted_output = predicted_output.reshape((3, F))
-
- f = numpy.linspace(0.05, 2.0, 64)
- fd = pandas.DataFrame(f).rename(columns={0: "Frequency"})
- df_pred = pandas.DataFrame(predicted_output.transpose()).rename(columns={0: "Surge", 1: "Heave", 2: "Pitch"})
- df_true = pandas.DataFrame(true_output.transpose()).rename(columns={0: "Surge", 1: "Heave", 2: "Pitch"})
-
- # return idx, other_data_input, true_output, predicted_output
- return pandas.concat([fd, df_pred], axis=1), pandas.concat([fd, df_true], axis=1)
-
-
- def analysis_from_geometry(self, geometry):
- # Get the outputs
- predicted_output = self.network.predict(numpy.array([geometry.flatten().tolist()]))
- predicted_output = predicted_output.reshape((3, F))
-
- f = numpy.linspace(0.05, 2.0, 64)
- fd = pandas.DataFrame(f).rename(columns={0: "Frequency"})
- df_pred = pandas.DataFrame(predicted_output.transpose()).rename(columns={0: "Surge", 1: "Heave", 2: "Pitch"})
- good_frame = pandas.concat([fd, df_pred], axis=1)
-
- return good_frame, good_frame
-
- def synthesis(self, idx=None):
- print(idx)
-
- if idx is None:
- idx = numpy.random.randint(1, S * N)
- else:
- idx = int(idx)
-
- # Get the input
- data_input = new_curves[idx:(idx+1), :]
- other_data_input = data_input.reshape((3, F))
-
- # Get the outputs
- predicted_output = self.network.predict(data_input)
- true_output = new_geometry[idx].reshape((G, G, G), order='F')
- predicted_output = predicted_output.reshape((G, G, G), order='F')
-
- # return idx, other_data_input, true_output, predicted_output
- return predicted_output, true_output
-
-
- def synthesis_from_spectrum(self, other_data_input):
- # Get the input
- data_input = other_data_input.reshape((1, 3*F))
-
- # Get the outputs
- predicted_output = self.network.predict(data_input)
- predicted_output = predicted_output.reshape((G, G, G), order='F')
-
- # return idx, other_data_input, true_output, predicted_output
- return predicted_output
-
- def get_geometry(self, idx=None):
-
- if idx is None:
- idx = numpy.random.randint(1, S * N)
- else:
- idx = int(idx)
-
- idx = int(idx)
-
- # Get the input
- data_input = new_geometry[idx:(idx+1), :]
- other_data_input = data_input.reshape((G, G, G), order='F')
-
- # return idx, other_data_input, true_output, predicted_output
- return other_data_input
-
-
- def get_performance(self, idx=None):
-
- if idx is None:
- idx = numpy.random.randint(1, S *N)
- else:
- idx = int(idx)
-
- idx = int(idx)
-
- # Get the input
- data_input = new_curves[idx:(idx+1), :]
- other_data_input = data_input.reshape((3, F))
-
- f = numpy.linspace(0.05, 2.0, 64)
- fd = pandas.DataFrame(f).rename(columns={0: "Frequency"})
- df_pred = pandas.DataFrame(other_data_input.transpose()).rename(columns={0: "Surge", 1: "Heave", 2: "Pitch"})
- table = pandas.concat([fd, df_pred], axis=1)
-
- return table
-
-
-def plotly_fig(values):
- X, Y, Z = numpy.mgrid[0:1:32j, 0:1:32j, 0:1:32j]
- fig = go.Figure(data=go.Volume(
- x=X.flatten(),
- y=Y.flatten(),
- z=Z.flatten(),
- value=values.flatten(),
- isomin=0.0,
- isomax=1.0,
- opacity=0.1, # needs to be small to see through all surfaces
- surface_count=21, # needs to be a large number for good volume rendering
- colorscale='haline'
- ))
- return fig
-
-
-value_net = Network("forward")
-
-def performance(index):
- return value_net.get_performance(index)
-
-def geometry(index):
- values = value_net.get_geometry(index)
- return plotly_fig(values)
-
-def simple_analysis(index, choice, shape, length, width, height, diameter):
- forward_net = Network("forward")
- # forward_net = Network("16forward_structure.json", "16forward_weights.h5")
- if choice == "Construct Shape from Parameters":
- return forward_net.analysis_from_geometry(make_voxels_without_figure(shape, length, height, width, diameter))
- elif choice == "Pick Shape from Dataset":
- return forward_net.analysis(index)
-
-
-def simple_synthesis(index):
- inverse_net = Network("inverse")
- # inverse_net = Network("16inverse_structure.json", "16inverse_weights.h5")
- pred, true = inverse_net.synthesis(index)
- return plotly_fig(pred), plotly_fig(true)
-
-def synthesis_from_spectrum(df):
- inverse_net = Network("inverse")
- # inverse_net = Network("16inverse_structure.json", "16inverse_weights.h5")
- pred = inverse_net.synthesis_from_spectrum(df.to_numpy()[:, 1:])
- return plotly_fig(pred)
-
-
-
-def change_textbox(choice, length, height, width, diameter):
- fig = make_voxels(choice, length, height, width, diameter)
- if choice == "cylinder":
- return [gradio.Slider.update(visible=True), gradio.Slider.update(visible=False), gradio.Slider.update(visible=True), gradio.Slider.update(visible=False), gradio.Plot.update(fig)]
- elif choice == "sphere":
- return [gradio.Slider.update(visible=False), gradio.Slider.update(visible=False), gradio.Slider.update(visible=True), gradio.Slider.update(visible=False), gradio.Plot.update(fig)]
- elif choice == "box":
- return [gradio.Slider.update(visible=True), gradio.Slider.update(visible=True), gradio.Slider.update(visible=False), gradio.Slider.update(visible=True), gradio.Plot.update(fig)]
- elif choice == "wedge":
- return [gradio.Slider.update(visible=True), gradio.Slider.update(visible=True), gradio.Slider.update(visible=False), gradio.Slider.update(visible=True), gradio.Plot.update(fig)]
- elif choice == "cone":
- return [gradio.Slider.update(visible=True), gradio.Slider.update(visible=False), gradio.Slider.update(visible=True), gradio.Slider.update(visible=False), gradio.Plot.update(fig)]
-
-
-
-def randomize_analysis(choice):
- if choice == "Construct Shape from Parameters":
- length = random.uniform(3.0, 10.0)
- height = random.uniform(3.0, 10.0)
- width = random.uniform(3.0, 10.0)
- diameter = random.uniform(3.0, 10.0)
- choice2 = random.choice(["box", "cone", "sphere", "wedge", "cone"])
- if choice2 == "box" or choice2 == "wedge":
- return [gradio.Radio.update(choice2), gradio.Slider.update(length), gradio.Slider.update(height), gradio.Slider.update(width), gradio.Slider.update(), gradio.Number.update(), gradio.Plot.update(make_voxels(choice2, length, height, width, diameter))]
- elif choice2 == "cone" or choice2 == "cylinder":
- return [gradio.Radio.update(choice2), gradio.Slider.update(), gradio.Slider.update(height), gradio.Slider.update(), gradio.Slider.update(diameter), gradio.Number.update(), gradio.Plot.update(make_voxels(choice2, length, height, width, diameter))]
- elif choice2 == "sphere":
- return [gradio.Radio.update(choice2), gradio.Slider.update(), gradio.Slider.update(), gradio.Slider.update(), gradio.Slider.update(diameter), gradio.Number.update(), gradio.Plot.update(make_voxels(choice2, length, height, width, diameter))]
- elif choice == "Pick Shape from Dataset":
- num = random.randint(1, 4999)
- return [gradio.Radio.update(), gradio.Slider.update(), gradio.Slider.update(), gradio.Slider.update(), gradio.Slider.update(), gradio.Number.update(num), gradio.Plot.update(geometry(num))]
-
-
-
-def geometry_change(choice, choice2, num, length, width, height, diameter):
- if choice == "Construct Shape from Parameters":
- [slider1, slider2, slider3, slider4, plot] = change_textbox(choice2, length, height, width, diameter)
- return [gradio.Radio.update(visible=True), slider1, slider2, slider3, slider4, gradio.Number.update(visible=False), gradio.Timeseries.update(visible=False), gradio.Plot.update(make_voxels(choice2, length, height, width, diameter))]
- elif choice == "Pick Shape from Dataset":
- return [gradio.Radio.update(visible=False), gradio.Slider.update(visible=False), gradio.Slider.update(visible=False), gradio.Slider.update(visible=False), gradio.Slider.update(visible=False), gradio.Number.update(visible=True), gradio.Timeseries.update(visible=True), gradio.Plot.update(geometry(num))]
-
-with gradio.Blocks() as demo:
- with gradio.Accordion("✨ Read about the underlying ML model here! ✨", open=False):
- with gradio.Row():
- with gradio.Column():
- gradio.Markdown("# Toward the Rapid Design of Engineered Systems Through Deep Neural Networks")
- gradio.HTML("Christopher McComb, Carnegie Mellon University")
- gradio.Markdown("__Abstract__: The design of a system commits a significant portion of the final cost of that system. Many computational approaches have been developed to assist designers in the analysis (e.g., computational fluid dynamics) and synthesis (e.g., topology optimization) of engineered systems. However, many of these approaches are computationally intensive, taking significant time to complete an analysis and even longer to iteratively synthesize a solution. The current work proposes a methodology for rapidly evaluating and synthesizing engineered systems through the use of deep neural networks. The proposed methodology is applied to the analysis and synthesis of offshore structures such as oil platforms. These structures are constructed in a marine environment and are typically designed to achieve specific dynamics in response to a known spectrum of ocean waves. Results show that deep learning can be used to accurately and rapidly synthesize and analyze offshore structure.")
- with gradio.Column():
- download = gradio.HTML("")
-
- gradio.Markdown("When designing offshore structure, like [wave energy converters](https://www.nrel.gov/news/program/2021/how-wave-energy-could-go-big-by-getting-smaller.html), it's important to know what forces will be placed on the structure as waves come at different speeds. Likewise, if we have some idea of how we want the structure to respond to different waves, we can use that to guide the design of the shape of the structure. We call the first process _Analysis_, and the second process _Synthesis_. This demo has ML models that do both, very quickly.")
-
- with gradio.Tab("Analysis"):
-
- with gradio.Row():
- with gradio.Column():
- whence_commeth_geometry = gradio.Radio(
- ["Construct Shape from Parameters", "Pick Shape from Dataset"], label="How would you like to generate the shape of the offshore structure for analysis?", value="Construct Shape from Parameters"
- )
- radio = gradio.Radio(
- ["box", "cone", "cylinder", "sphere", "wedge"], label="What kind of shape would you like to generate?", value="sphere"
- )
- height = gradio.Slider(label="Height", interactive=True, minimum=3.0, maximum=10.0, value=6.5, visible=False)
- width = gradio.Slider(label="Width", interactive=True, minimum=3.0, maximum=10.0, value=6.5, visible=False)
- diameter = gradio.Slider(label="Diameter", interactive=True, minimum=3.0, maximum=10.0, value=6.5, visible=True)
- length = gradio.Slider(label="Length", interactive=True, minimum=3.0, maximum=10.0, value=6.5, visible=False)
-
-
- num = gradio.Number(42, label="Type the index of the spectrum you would like to use or randomly select it.", visible=False)
-
- btn1 = gradio.Button("Randomize")
- with gradio.Column():
- geo = gradio.Plot(make_voxels("sphere", 6.5, 6.5, 6.5, 6.5), label="Geometry")
-
-
- with gradio.Row():
- btn2 = gradio.Button("Estimate Spectrum")
-
- with gradio.Row():
- with gradio.Column():
- pred = gradio.Timeseries(x="Frequency", y=['Surge', 'Heave', 'Pitch'], label="Predicted")
-
- with gradio.Column():
- true = gradio.Timeseries(x="Frequency", y=['Surge', 'Heave', 'Pitch'], label="True", visible=False)
-
- radio.change(fn=change_textbox, inputs=[radio, length, height, width, diameter], outputs=[height, width, diameter, length, geo])
- height.change(fn=make_voxels, inputs = [radio, length, height, width, diameter], outputs=[geo])
- width.change(fn=make_voxels, inputs = [radio, length, height, width, diameter], outputs=[geo])
- diameter.change(fn=make_voxels, inputs = [radio, length, height, width, diameter], outputs=[geo])
- length.change(fn=make_voxels, inputs = [radio, length, height, width, diameter], outputs=[geo])
- whence_commeth_geometry.change(fn=geometry_change, inputs=[whence_commeth_geometry, radio, num, length, width, height, diameter], outputs=[radio, height, width, diameter, length, num, true, geo])
- num.change(fn=geometry, inputs=[num], outputs=[geo])
-
- btn1.click(fn=randomize_analysis, inputs=[whence_commeth_geometry], outputs=[radio, length, height, width, diameter, num, geo])
- btn2.click(fn=simple_analysis, inputs=[num, whence_commeth_geometry, radio, length, width, height, diameter], outputs=[pred, true], api_name="analyze")
- with gradio.Tab("Synthesis"):
- with gradio.Row():
- with gradio.Column():
- whence_commeth_performance = gradio.Radio(
- ["Pick Spectrum from Dataset"], label="How would you like to generate the desired response spectrum to synthesize from?", value="Construct Spectrum from Table"
- )
- num = gradio.Number(42, label="Type the index of the shape you would like to use or randomly select it.")
- btn1 = gradio.Button("Randomize")
- with gradio.Column():
- perf = gradio.Timeseries(x="Frequency", y=['Surge', 'Heave', 'Pitch'], label="Performance")
-
- with gradio.Row():
- btn2 = gradio.Button("Synthesize Geometry")
-
- with gradio.Row():
- with gradio.Column():
- pred = gradio.Plot(label="Predicted")
-
- with gradio.Column():
- true = gradio.Plot(label="True")
-
-
- btn1.click(fn=lambda: random.randint(1, 4999), inputs=[], outputs=num)
- num.change(fn=performance, inputs=[num], outputs=[perf])
- btn2.click(fn=simple_synthesis, inputs=[num], outputs=[pred, true], api_name="synthesize")
-
-demo.launch()
\ No newline at end of file
diff --git a/spaces/colakin/video-generater/public/ffmpeg/libavcodec/dxva2.c b/spaces/colakin/video-generater/public/ffmpeg/libavcodec/dxva2.c
deleted file mode 100644
index 568d686f39a58e6b6f160388a42b157fb4332e4d..0000000000000000000000000000000000000000
--- a/spaces/colakin/video-generater/public/ffmpeg/libavcodec/dxva2.c
+++ /dev/null
@@ -1,1058 +0,0 @@
-/*
- * DXVA2 HW acceleration.
- *
- * copyright (c) 2010 Laurent Aimar
- *
- * This file is part of FFmpeg.
- *
- * FFmpeg is free software; you can redistribute it and/or
- * modify it under the terms of the GNU Lesser General Public
- * License as published by the Free Software Foundation; either
- * version 2.1 of the License, or (at your option) any later version.
- *
- * FFmpeg is distributed in the hope that it will be useful,
- * but WITHOUT ANY WARRANTY; without even the implied warranty of
- * MERCHANTABILITY or FITNESS FOR A PARTICULAR PURPOSE. See the GNU
- * Lesser General Public License for more details.
- *
- * You should have received a copy of the GNU Lesser General Public
- * License along with FFmpeg; if not, write to the Free Software
- * Foundation, Inc., 51 Franklin Street, Fifth Floor, Boston, MA 02110-1301 USA
- */
-
-#include
-#include
-#include
-
-#include "libavutil/common.h"
-#include "libavutil/log.h"
-#include "libavutil/time.h"
-
-#include "avcodec.h"
-#include "decode.h"
-#include "dxva2_internal.h"
-
-/* define all the GUIDs used directly here,
- to avoid problems with inconsistent dxva2api.h versions in mingw-w64 and different MSVC version */
-DEFINE_GUID(ff_DXVA2_ModeMPEG2_VLD, 0xee27417f, 0x5e28,0x4e65,0xbe,0xea,0x1d,0x26,0xb5,0x08,0xad,0xc9);
-DEFINE_GUID(ff_DXVA2_ModeMPEG2and1_VLD, 0x86695f12, 0x340e,0x4f04,0x9f,0xd3,0x92,0x53,0xdd,0x32,0x74,0x60);
-DEFINE_GUID(ff_DXVA2_ModeH264_E, 0x1b81be68, 0xa0c7,0x11d3,0xb9,0x84,0x00,0xc0,0x4f,0x2e,0x73,0xc5);
-DEFINE_GUID(ff_DXVA2_ModeH264_F, 0x1b81be69, 0xa0c7,0x11d3,0xb9,0x84,0x00,0xc0,0x4f,0x2e,0x73,0xc5);
-DEFINE_GUID(ff_DXVADDI_Intel_ModeH264_E, 0x604F8E68, 0x4951,0x4C54,0x88,0xFE,0xAB,0xD2,0x5C,0x15,0xB3,0xD6);
-DEFINE_GUID(ff_DXVA2_ModeVC1_D, 0x1b81beA3, 0xa0c7,0x11d3,0xb9,0x84,0x00,0xc0,0x4f,0x2e,0x73,0xc5);
-DEFINE_GUID(ff_DXVA2_ModeVC1_D2010, 0x1b81beA4, 0xa0c7,0x11d3,0xb9,0x84,0x00,0xc0,0x4f,0x2e,0x73,0xc5);
-DEFINE_GUID(ff_DXVA2_ModeHEVC_VLD_Main, 0x5b11d51b, 0x2f4c,0x4452,0xbc,0xc3,0x09,0xf2,0xa1,0x16,0x0c,0xc0);
-DEFINE_GUID(ff_DXVA2_ModeHEVC_VLD_Main10,0x107af0e0, 0xef1a,0x4d19,0xab,0xa8,0x67,0xa1,0x63,0x07,0x3d,0x13);
-DEFINE_GUID(ff_DXVA2_ModeVP9_VLD_Profile0,0x463707f8,0xa1d0,0x4585,0x87,0x6d,0x83,0xaa,0x6d,0x60,0xb8,0x9e);
-DEFINE_GUID(ff_DXVA2_ModeVP9_VLD_10bit_Profile2,0xa4c749ef,0x6ecf,0x48aa,0x84,0x48,0x50,0xa7,0xa1,0x16,0x5f,0xf7);
-DEFINE_GUID(ff_DXVA2_ModeAV1_VLD_Profile0,0xb8be4ccb,0xcf53,0x46ba,0x8d,0x59,0xd6,0xb8,0xa6,0xda,0x5d,0x2a);
-DEFINE_GUID(ff_DXVA2_NoEncrypt, 0x1b81beD0, 0xa0c7,0x11d3,0xb9,0x84,0x00,0xc0,0x4f,0x2e,0x73,0xc5);
-DEFINE_GUID(ff_GUID_NULL, 0x00000000, 0x0000,0x0000,0x00,0x00,0x00,0x00,0x00,0x00,0x00,0x00);
-DEFINE_GUID(ff_IID_IDirectXVideoDecoderService, 0xfc51a551,0xd5e7,0x11d9,0xaf,0x55,0x00,0x05,0x4e,0x43,0xff,0x02);
-
-typedef struct dxva_mode {
- const GUID *guid;
- enum AVCodecID codec;
- // List of supported profiles, terminated by a FF_PROFILE_UNKNOWN entry.
- // If NULL, don't check profile.
- const int *profiles;
-} dxva_mode;
-
-static const int prof_mpeg2_main[] = {FF_PROFILE_MPEG2_SIMPLE,
- FF_PROFILE_MPEG2_MAIN,
- FF_PROFILE_UNKNOWN};
-static const int prof_h264_high[] = {FF_PROFILE_H264_CONSTRAINED_BASELINE,
- FF_PROFILE_H264_MAIN,
- FF_PROFILE_H264_HIGH,
- FF_PROFILE_UNKNOWN};
-static const int prof_hevc_main[] = {FF_PROFILE_HEVC_MAIN,
- FF_PROFILE_UNKNOWN};
-static const int prof_hevc_main10[] = {FF_PROFILE_HEVC_MAIN_10,
- FF_PROFILE_UNKNOWN};
-static const int prof_vp9_profile0[] = {FF_PROFILE_VP9_0,
- FF_PROFILE_UNKNOWN};
-static const int prof_vp9_profile2[] = {FF_PROFILE_VP9_2,
- FF_PROFILE_UNKNOWN};
-static const int prof_av1_profile0[] = {FF_PROFILE_AV1_MAIN,
- FF_PROFILE_UNKNOWN};
-
-static const dxva_mode dxva_modes[] = {
- /* MPEG-2 */
- { &ff_DXVA2_ModeMPEG2_VLD, AV_CODEC_ID_MPEG2VIDEO, prof_mpeg2_main },
- { &ff_DXVA2_ModeMPEG2and1_VLD, AV_CODEC_ID_MPEG2VIDEO, prof_mpeg2_main },
-
- /* H.264 */
- { &ff_DXVA2_ModeH264_F, AV_CODEC_ID_H264, prof_h264_high },
- { &ff_DXVA2_ModeH264_E, AV_CODEC_ID_H264, prof_h264_high },
- /* Intel specific H.264 mode */
- { &ff_DXVADDI_Intel_ModeH264_E, AV_CODEC_ID_H264, prof_h264_high },
-
- /* VC-1 / WMV3 */
- { &ff_DXVA2_ModeVC1_D2010, AV_CODEC_ID_VC1 },
- { &ff_DXVA2_ModeVC1_D2010, AV_CODEC_ID_WMV3 },
- { &ff_DXVA2_ModeVC1_D, AV_CODEC_ID_VC1 },
- { &ff_DXVA2_ModeVC1_D, AV_CODEC_ID_WMV3 },
-
- /* HEVC/H.265 */
- { &ff_DXVA2_ModeHEVC_VLD_Main10, AV_CODEC_ID_HEVC, prof_hevc_main10 },
- { &ff_DXVA2_ModeHEVC_VLD_Main, AV_CODEC_ID_HEVC, prof_hevc_main },
-
- /* VP8/9 */
- { &ff_DXVA2_ModeVP9_VLD_Profile0, AV_CODEC_ID_VP9, prof_vp9_profile0 },
- { &ff_DXVA2_ModeVP9_VLD_10bit_Profile2, AV_CODEC_ID_VP9, prof_vp9_profile2 },
-
- /* AV1 */
- { &ff_DXVA2_ModeAV1_VLD_Profile0, AV_CODEC_ID_AV1, prof_av1_profile0 },
-
- { NULL, 0 },
-};
-
-static int dxva_get_decoder_configuration(AVCodecContext *avctx,
- const void *cfg_list,
- unsigned cfg_count)
-{
- FFDXVASharedContext *sctx = DXVA_SHARED_CONTEXT(avctx);
- unsigned i, best_score = 0;
- int best_cfg = -1;
-
- for (i = 0; i < cfg_count; i++) {
- unsigned score;
- UINT ConfigBitstreamRaw;
- GUID guidConfigBitstreamEncryption;
-
-#if CONFIG_D3D11VA
- if (sctx->pix_fmt == AV_PIX_FMT_D3D11) {
- D3D11_VIDEO_DECODER_CONFIG *cfg = &((D3D11_VIDEO_DECODER_CONFIG *)cfg_list)[i];
- ConfigBitstreamRaw = cfg->ConfigBitstreamRaw;
- guidConfigBitstreamEncryption = cfg->guidConfigBitstreamEncryption;
- }
-#endif
-#if CONFIG_DXVA2
- if (sctx->pix_fmt == AV_PIX_FMT_DXVA2_VLD) {
- DXVA2_ConfigPictureDecode *cfg = &((DXVA2_ConfigPictureDecode *)cfg_list)[i];
- ConfigBitstreamRaw = cfg->ConfigBitstreamRaw;
- guidConfigBitstreamEncryption = cfg->guidConfigBitstreamEncryption;
- }
-#endif
-
- if (ConfigBitstreamRaw == 1)
- score = 1;
- else if (avctx->codec_id == AV_CODEC_ID_H264 && ConfigBitstreamRaw == 2)
- score = 2;
- else
- continue;
- if (IsEqualGUID(&guidConfigBitstreamEncryption, &ff_DXVA2_NoEncrypt))
- score += 16;
- if (score > best_score) {
- best_score = score;
- best_cfg = i;
- }
- }
-
- if (!best_score) {
- av_log(avctx, AV_LOG_VERBOSE, "No valid decoder configuration available\n");
- return AVERROR(EINVAL);
- }
-
- return best_cfg;
-}
-
-#if CONFIG_D3D11VA
-static int d3d11va_validate_output(void *service, GUID guid, const void *surface_format)
-{
- HRESULT hr;
- BOOL is_supported = FALSE;
- hr = ID3D11VideoDevice_CheckVideoDecoderFormat((ID3D11VideoDevice *)service,
- &guid,
- *(DXGI_FORMAT *)surface_format,
- &is_supported);
- return SUCCEEDED(hr) && is_supported;
-}
-#endif
-
-#if CONFIG_DXVA2
-static int dxva2_validate_output(void *decoder_service, GUID guid, const void *surface_format)
-{
- HRESULT hr;
- int ret = 0;
- unsigned j, target_count;
- D3DFORMAT *target_list;
- hr = IDirectXVideoDecoderService_GetDecoderRenderTargets((IDirectXVideoDecoderService *)decoder_service, &guid, &target_count, &target_list);
- if (SUCCEEDED(hr)) {
- for (j = 0; j < target_count; j++) {
- const D3DFORMAT format = target_list[j];
- if (format == *(D3DFORMAT *)surface_format) {
- ret = 1;
- break;
- }
- }
- CoTaskMemFree(target_list);
- }
- return ret;
-}
-#endif
-
-static int dxva_check_codec_compatibility(AVCodecContext *avctx, const dxva_mode *mode)
-{
- if (mode->codec != avctx->codec_id)
- return 0;
-
- if (mode->profiles && !(avctx->hwaccel_flags & AV_HWACCEL_FLAG_ALLOW_PROFILE_MISMATCH)) {
- int i, found = 0;
- for (i = 0; mode->profiles[i] != FF_PROFILE_UNKNOWN; i++) {
- if (avctx->profile == mode->profiles[i]) {
- found = 1;
- break;
- }
- }
- if (!found)
- return 0;
- }
-
- return 1;
-}
-
-static void dxva_list_guids_debug(AVCodecContext *avctx, void *service,
- unsigned guid_count, const GUID *guid_list)
-{
- FFDXVASharedContext *sctx = DXVA_SHARED_CONTEXT(avctx);
- int i;
-
- av_log(avctx, AV_LOG_VERBOSE, "Decoder GUIDs reported as supported:\n");
-
- for (i = 0; i < guid_count; i++) {
- const GUID *guid = &guid_list[i];
-
- av_log(avctx, AV_LOG_VERBOSE,
- "{%8.8x-%4.4x-%4.4x-%2.2x%2.2x-%2.2x%2.2x%2.2x%2.2x%2.2x%2.2x}",
- (unsigned) guid->Data1, guid->Data2, guid->Data3,
- guid->Data4[0], guid->Data4[1],
- guid->Data4[2], guid->Data4[3],
- guid->Data4[4], guid->Data4[5],
- guid->Data4[6], guid->Data4[7]);
-
-#if CONFIG_D3D11VA
- if (sctx->pix_fmt == AV_PIX_FMT_D3D11) {
- DXGI_FORMAT format;
- // We don't know the maximum valid DXGI_FORMAT, so use 200 as
- // arbitrary upper bound (that could become outdated).
- for (format = 0; format < 200; format++) {
- if (d3d11va_validate_output(service, *guid, &format))
- av_log(avctx, AV_LOG_VERBOSE, " %d", (int)format);
- }
- }
-#endif
-#if CONFIG_DXVA2
- if (sctx->pix_fmt == AV_PIX_FMT_DXVA2_VLD) {
- const D3DFORMAT formats[] = {MKTAG('N', 'V', '1', '2'),
- MKTAG('P', '0', '1', '0')};
- int i;
- for (i = 0; i < FF_ARRAY_ELEMS(formats); i++) {
- if (dxva2_validate_output(service, *guid, &formats[i]))
- av_log(avctx, AV_LOG_VERBOSE, " %d", i);
- }
- }
-#endif
- av_log(avctx, AV_LOG_VERBOSE, "\n");
- }
-}
-
-static int dxva_get_decoder_guid(AVCodecContext *avctx, void *service, void *surface_format,
- unsigned guid_count, const GUID *guid_list, GUID *decoder_guid)
-{
- FFDXVASharedContext *sctx = DXVA_SHARED_CONTEXT(avctx);
- unsigned i, j;
-
- dxva_list_guids_debug(avctx, service, guid_count, guid_list);
-
- *decoder_guid = ff_GUID_NULL;
- for (i = 0; dxva_modes[i].guid; i++) {
- const dxva_mode *mode = &dxva_modes[i];
- int validate;
- if (!dxva_check_codec_compatibility(avctx, mode))
- continue;
-
- for (j = 0; j < guid_count; j++) {
- if (IsEqualGUID(mode->guid, &guid_list[j]))
- break;
- }
- if (j == guid_count)
- continue;
-
-#if CONFIG_D3D11VA
- if (sctx->pix_fmt == AV_PIX_FMT_D3D11)
- validate = d3d11va_validate_output(service, *mode->guid, surface_format);
-#endif
-#if CONFIG_DXVA2
- if (sctx->pix_fmt == AV_PIX_FMT_DXVA2_VLD)
- validate = dxva2_validate_output(service, *mode->guid, surface_format);
-#endif
- if (validate) {
- *decoder_guid = *mode->guid;
- break;
- }
- }
-
- if (IsEqualGUID(decoder_guid, &ff_GUID_NULL)) {
- av_log(avctx, AV_LOG_VERBOSE, "No decoder device for codec found\n");
- return AVERROR(EINVAL);
- }
-
- if (IsEqualGUID(decoder_guid, &ff_DXVADDI_Intel_ModeH264_E))
- sctx->workaround |= FF_DXVA2_WORKAROUND_INTEL_CLEARVIDEO;
-
- return 0;
-}
-
-static void bufref_free_interface(void *opaque, uint8_t *data)
-{
- IUnknown_Release((IUnknown *)opaque);
-}
-
-static AVBufferRef *bufref_wrap_interface(IUnknown *iface)
-{
- return av_buffer_create((uint8_t*)iface, 1, bufref_free_interface, iface, 0);
-}
-
-#if CONFIG_DXVA2
-
-static int dxva2_get_decoder_configuration(AVCodecContext *avctx, const GUID *device_guid,
- const DXVA2_VideoDesc *desc,
- DXVA2_ConfigPictureDecode *config)
-{
- FFDXVASharedContext *sctx = DXVA_SHARED_CONTEXT(avctx);
- unsigned cfg_count;
- DXVA2_ConfigPictureDecode *cfg_list;
- HRESULT hr;
- int ret;
-
- hr = IDirectXVideoDecoderService_GetDecoderConfigurations(sctx->dxva2_service, device_guid, desc, NULL, &cfg_count, &cfg_list);
- if (FAILED(hr)) {
- av_log(avctx, AV_LOG_ERROR, "Unable to retrieve decoder configurations\n");
- return AVERROR(EINVAL);
- }
-
- ret = dxva_get_decoder_configuration(avctx, cfg_list, cfg_count);
- if (ret >= 0)
- *config = cfg_list[ret];
- CoTaskMemFree(cfg_list);
- return ret;
-}
-
-static int dxva2_create_decoder(AVCodecContext *avctx)
-{
- FFDXVASharedContext *sctx = DXVA_SHARED_CONTEXT(avctx);
- GUID *guid_list;
- unsigned guid_count;
- GUID device_guid;
- D3DFORMAT surface_format = avctx->sw_pix_fmt == AV_PIX_FMT_YUV420P10 ?
- MKTAG('P', '0', '1', '0') : MKTAG('N', 'V', '1', '2');
- DXVA2_VideoDesc desc = { 0 };
- DXVA2_ConfigPictureDecode config;
- HRESULT hr;
- int ret;
- HANDLE device_handle;
- AVHWFramesContext *frames_ctx = (AVHWFramesContext*)avctx->hw_frames_ctx->data;
- AVDXVA2FramesContext *frames_hwctx = frames_ctx->hwctx;
- AVDXVA2DeviceContext *device_hwctx = frames_ctx->device_ctx->hwctx;
-
- hr = IDirect3DDeviceManager9_OpenDeviceHandle(device_hwctx->devmgr,
- &device_handle);
- if (FAILED(hr)) {
- av_log(avctx, AV_LOG_ERROR, "Failed to open a device handle\n");
- goto fail;
- }
-
- hr = IDirect3DDeviceManager9_GetVideoService(device_hwctx->devmgr, device_handle,
- &ff_IID_IDirectXVideoDecoderService,
- (void **)&sctx->dxva2_service);
- IDirect3DDeviceManager9_CloseDeviceHandle(device_hwctx->devmgr, device_handle);
- if (FAILED(hr)) {
- av_log(avctx, AV_LOG_ERROR, "Failed to create IDirectXVideoDecoderService\n");
- goto fail;
- }
-
- hr = IDirectXVideoDecoderService_GetDecoderDeviceGuids(sctx->dxva2_service, &guid_count, &guid_list);
- if (FAILED(hr)) {
- av_log(avctx, AV_LOG_ERROR, "Failed to retrieve decoder device GUIDs\n");
- goto fail;
- }
-
- ret = dxva_get_decoder_guid(avctx, sctx->dxva2_service, &surface_format,
- guid_count, guid_list, &device_guid);
- CoTaskMemFree(guid_list);
- if (ret < 0) {
- goto fail;
- }
-
- desc.SampleWidth = avctx->coded_width;
- desc.SampleHeight = avctx->coded_height;
- desc.Format = surface_format;
-
- ret = dxva2_get_decoder_configuration(avctx, &device_guid, &desc, &config);
- if (ret < 0) {
- goto fail;
- }
-
- hr = IDirectXVideoDecoderService_CreateVideoDecoder(sctx->dxva2_service, &device_guid,
- &desc, &config, frames_hwctx->surfaces,
- frames_hwctx->nb_surfaces, &sctx->dxva2_decoder);
- if (FAILED(hr)) {
- av_log(avctx, AV_LOG_ERROR, "Failed to create DXVA2 video decoder\n");
- goto fail;
- }
-
- sctx->dxva2_config = config;
-
- sctx->decoder_ref = bufref_wrap_interface((IUnknown *)sctx->dxva2_decoder);
- if (!sctx->decoder_ref)
- return AVERROR(ENOMEM);
-
- return 0;
-fail:
- return AVERROR(EINVAL);
-}
-
-#endif
-
-#if CONFIG_D3D11VA
-
-static int d3d11va_get_decoder_configuration(AVCodecContext *avctx,
- ID3D11VideoDevice *video_device,
- const D3D11_VIDEO_DECODER_DESC *desc,
- D3D11_VIDEO_DECODER_CONFIG *config)
-{
- unsigned cfg_count = 0;
- D3D11_VIDEO_DECODER_CONFIG *cfg_list = NULL;
- HRESULT hr;
- int i, ret;
-
- hr = ID3D11VideoDevice_GetVideoDecoderConfigCount(video_device, desc, &cfg_count);
- if (FAILED(hr)) {
- av_log(avctx, AV_LOG_ERROR, "Unable to retrieve decoder configurations\n");
- return AVERROR(EINVAL);
- }
-
- cfg_list = av_malloc_array(cfg_count, sizeof(D3D11_VIDEO_DECODER_CONFIG));
- if (cfg_list == NULL)
- return AVERROR(ENOMEM);
- for (i = 0; i < cfg_count; i++) {
- hr = ID3D11VideoDevice_GetVideoDecoderConfig(video_device, desc, i, &cfg_list[i]);
- if (FAILED(hr)) {
- av_log(avctx, AV_LOG_ERROR, "Unable to retrieve decoder configurations. (hr=0x%lX)\n", hr);
- av_free(cfg_list);
- return AVERROR(EINVAL);
- }
- }
-
- ret = dxva_get_decoder_configuration(avctx, cfg_list, cfg_count);
- if (ret >= 0)
- *config = cfg_list[ret];
- av_free(cfg_list);
- return ret;
-}
-
-static DXGI_FORMAT d3d11va_map_sw_to_hw_format(enum AVPixelFormat pix_fmt)
-{
- switch (pix_fmt) {
- case AV_PIX_FMT_NV12: return DXGI_FORMAT_NV12;
- case AV_PIX_FMT_P010: return DXGI_FORMAT_P010;
- case AV_PIX_FMT_YUV420P: return DXGI_FORMAT_420_OPAQUE;
- default: return DXGI_FORMAT_UNKNOWN;
- }
-}
-
-static int d3d11va_create_decoder(AVCodecContext *avctx)
-{
- FFDXVASharedContext *sctx = DXVA_SHARED_CONTEXT(avctx);
- GUID *guid_list;
- unsigned guid_count, i;
- GUID decoder_guid;
- D3D11_VIDEO_DECODER_DESC desc = { 0 };
- D3D11_VIDEO_DECODER_CONFIG config;
- AVHWFramesContext *frames_ctx = (AVHWFramesContext *)avctx->hw_frames_ctx->data;
- AVD3D11VADeviceContext *device_hwctx = frames_ctx->device_ctx->hwctx;
- AVD3D11VAFramesContext *frames_hwctx = frames_ctx->hwctx;
- DXGI_FORMAT surface_format = d3d11va_map_sw_to_hw_format(frames_ctx->sw_format);
- D3D11_TEXTURE2D_DESC texdesc;
- HRESULT hr;
- int ret;
-
- if (!frames_hwctx->texture) {
- av_log(avctx, AV_LOG_ERROR, "AVD3D11VAFramesContext.texture not set.\n");
- return AVERROR(EINVAL);
- }
- ID3D11Texture2D_GetDesc(frames_hwctx->texture, &texdesc);
-
- guid_count = ID3D11VideoDevice_GetVideoDecoderProfileCount(device_hwctx->video_device);
- guid_list = av_malloc_array(guid_count, sizeof(*guid_list));
- if (guid_list == NULL || guid_count == 0) {
- av_log(avctx, AV_LOG_ERROR, "Failed to get the decoder GUIDs\n");
- av_free(guid_list);
- return AVERROR(EINVAL);
- }
- for (i = 0; i < guid_count; i++) {
- hr = ID3D11VideoDevice_GetVideoDecoderProfile(device_hwctx->video_device, i, &guid_list[i]);
- if (FAILED(hr)) {
- av_log(avctx, AV_LOG_ERROR, "Failed to retrieve decoder GUID %d\n", i);
- av_free(guid_list);
- return AVERROR(EINVAL);
- }
- }
-
- ret = dxva_get_decoder_guid(avctx, device_hwctx->video_device, &surface_format,
- guid_count, guid_list, &decoder_guid);
- av_free(guid_list);
- if (ret < 0)
- return AVERROR(EINVAL);
-
- desc.SampleWidth = avctx->coded_width;
- desc.SampleHeight = avctx->coded_height;
- desc.OutputFormat = surface_format;
- desc.Guid = decoder_guid;
-
- ret = d3d11va_get_decoder_configuration(avctx, device_hwctx->video_device, &desc, &config);
- if (ret < 0)
- return AVERROR(EINVAL);
-
- sctx->d3d11_views = av_calloc(texdesc.ArraySize, sizeof(sctx->d3d11_views[0]));
- if (!sctx->d3d11_views)
- return AVERROR(ENOMEM);
- sctx->nb_d3d11_views = texdesc.ArraySize;
-
- for (i = 0; i < sctx->nb_d3d11_views; i++) {
- D3D11_VIDEO_DECODER_OUTPUT_VIEW_DESC viewDesc = {
- .DecodeProfile = decoder_guid,
- .ViewDimension = D3D11_VDOV_DIMENSION_TEXTURE2D,
- .Texture2D = {
- .ArraySlice = i,
- }
- };
- hr = ID3D11VideoDevice_CreateVideoDecoderOutputView(device_hwctx->video_device,
- (ID3D11Resource*) frames_hwctx->texture,
- &viewDesc,
- (ID3D11VideoDecoderOutputView**) &sctx->d3d11_views[i]);
- if (FAILED(hr)) {
- av_log(avctx, AV_LOG_ERROR, "Could not create the decoder output view %d\n", i);
- return AVERROR_UNKNOWN;
- }
- }
-
- hr = ID3D11VideoDevice_CreateVideoDecoder(device_hwctx->video_device, &desc,
- &config, &sctx->d3d11_decoder);
- if (FAILED(hr)) {
- av_log(avctx, AV_LOG_ERROR, "Failed to create D3D11VA video decoder\n");
- return AVERROR(EINVAL);
- }
-
- sctx->d3d11_config = config;
- sctx->d3d11_texture = frames_hwctx->texture;
-
- sctx->decoder_ref = bufref_wrap_interface((IUnknown *)sctx->d3d11_decoder);
- if (!sctx->decoder_ref)
- return AVERROR(ENOMEM);
-
- return 0;
-}
-
-#endif
-
-static void ff_dxva2_lock(AVCodecContext *avctx)
-{
-#if CONFIG_D3D11VA
- if (ff_dxva2_is_d3d11(avctx)) {
- FFDXVASharedContext *sctx = DXVA_SHARED_CONTEXT(avctx);
- AVDXVAContext *ctx = DXVA_CONTEXT(avctx);
- if (D3D11VA_CONTEXT(ctx)->context_mutex != INVALID_HANDLE_VALUE)
- WaitForSingleObjectEx(D3D11VA_CONTEXT(ctx)->context_mutex, INFINITE, FALSE);
- if (sctx->device_ctx) {
- AVD3D11VADeviceContext *hwctx = sctx->device_ctx->hwctx;
- hwctx->lock(hwctx->lock_ctx);
- }
- }
-#endif
-}
-
-static void ff_dxva2_unlock(AVCodecContext *avctx)
-{
-#if CONFIG_D3D11VA
- if (ff_dxva2_is_d3d11(avctx)) {
- FFDXVASharedContext *sctx = DXVA_SHARED_CONTEXT(avctx);
- AVDXVAContext *ctx = DXVA_CONTEXT(avctx);
- if (D3D11VA_CONTEXT(ctx)->context_mutex != INVALID_HANDLE_VALUE)
- ReleaseMutex(D3D11VA_CONTEXT(ctx)->context_mutex);
- if (sctx->device_ctx) {
- AVD3D11VADeviceContext *hwctx = sctx->device_ctx->hwctx;
- hwctx->unlock(hwctx->lock_ctx);
- }
- }
-#endif
-}
-
-int ff_dxva2_common_frame_params(AVCodecContext *avctx,
- AVBufferRef *hw_frames_ctx)
-{
- AVHWFramesContext *frames_ctx = (AVHWFramesContext *)hw_frames_ctx->data;
- AVHWDeviceContext *device_ctx = frames_ctx->device_ctx;
- int surface_alignment, num_surfaces;
-
- if (device_ctx->type == AV_HWDEVICE_TYPE_DXVA2) {
- frames_ctx->format = AV_PIX_FMT_DXVA2_VLD;
- } else if (device_ctx->type == AV_HWDEVICE_TYPE_D3D11VA) {
- frames_ctx->format = AV_PIX_FMT_D3D11;
- } else {
- return AVERROR(EINVAL);
- }
-
- /* decoding MPEG-2 requires additional alignment on some Intel GPUs,
- but it causes issues for H.264 on certain AMD GPUs..... */
- if (avctx->codec_id == AV_CODEC_ID_MPEG2VIDEO)
- surface_alignment = 32;
- /* the HEVC DXVA2 spec asks for 128 pixel aligned surfaces to ensure
- all coding features have enough room to work with */
- else if (avctx->codec_id == AV_CODEC_ID_HEVC || avctx->codec_id == AV_CODEC_ID_AV1)
- surface_alignment = 128;
- else
- surface_alignment = 16;
-
- /* 1 base work surface */
- num_surfaces = 1;
-
- /* add surfaces based on number of possible refs */
- if (avctx->codec_id == AV_CODEC_ID_H264 || avctx->codec_id == AV_CODEC_ID_HEVC)
- num_surfaces += 16;
- else if (avctx->codec_id == AV_CODEC_ID_VP9 || avctx->codec_id == AV_CODEC_ID_AV1)
- num_surfaces += 8;
- else
- num_surfaces += 2;
-
- frames_ctx->sw_format = avctx->sw_pix_fmt == AV_PIX_FMT_YUV420P10 ?
- AV_PIX_FMT_P010 : AV_PIX_FMT_NV12;
- frames_ctx->width = FFALIGN(avctx->coded_width, surface_alignment);
- frames_ctx->height = FFALIGN(avctx->coded_height, surface_alignment);
- frames_ctx->initial_pool_size = num_surfaces;
-
-
-#if CONFIG_DXVA2
- if (frames_ctx->format == AV_PIX_FMT_DXVA2_VLD) {
- AVDXVA2FramesContext *frames_hwctx = frames_ctx->hwctx;
-
- frames_hwctx->surface_type = DXVA2_VideoDecoderRenderTarget;
- }
-#endif
-
-#if CONFIG_D3D11VA
- if (frames_ctx->format == AV_PIX_FMT_D3D11) {
- AVD3D11VAFramesContext *frames_hwctx = frames_ctx->hwctx;
-
- frames_hwctx->BindFlags |= D3D11_BIND_DECODER;
- }
-#endif
-
- return 0;
-}
-
-int ff_dxva2_decode_init(AVCodecContext *avctx)
-{
- FFDXVASharedContext *sctx = DXVA_SHARED_CONTEXT(avctx);
- AVHWFramesContext *frames_ctx;
- enum AVHWDeviceType dev_type = avctx->hwaccel->pix_fmt == AV_PIX_FMT_DXVA2_VLD
- ? AV_HWDEVICE_TYPE_DXVA2 : AV_HWDEVICE_TYPE_D3D11VA;
- int ret = 0;
-
- // Old API.
- if (avctx->hwaccel_context)
- return 0;
-
- // (avctx->pix_fmt is not updated yet at this point)
- sctx->pix_fmt = avctx->hwaccel->pix_fmt;
-
- ret = ff_decode_get_hw_frames_ctx(avctx, dev_type);
- if (ret < 0)
- return ret;
-
- frames_ctx = (AVHWFramesContext*)avctx->hw_frames_ctx->data;
- sctx->device_ctx = frames_ctx->device_ctx;
-
- if (frames_ctx->format != sctx->pix_fmt) {
- av_log(avctx, AV_LOG_ERROR, "Invalid pixfmt for hwaccel!\n");
- ret = AVERROR(EINVAL);
- goto fail;
- }
-
-#if CONFIG_D3D11VA
- if (sctx->pix_fmt == AV_PIX_FMT_D3D11) {
- AVD3D11VADeviceContext *device_hwctx = frames_ctx->device_ctx->hwctx;
- AVD3D11VAContext *d3d11_ctx = &sctx->ctx.d3d11va;
-
- ff_dxva2_lock(avctx);
- ret = d3d11va_create_decoder(avctx);
- ff_dxva2_unlock(avctx);
- if (ret < 0)
- goto fail;
-
- d3d11_ctx->decoder = sctx->d3d11_decoder;
- d3d11_ctx->video_context = device_hwctx->video_context;
- d3d11_ctx->cfg = &sctx->d3d11_config;
- d3d11_ctx->surface_count = sctx->nb_d3d11_views;
- d3d11_ctx->surface = sctx->d3d11_views;
- d3d11_ctx->workaround = sctx->workaround;
- d3d11_ctx->context_mutex = INVALID_HANDLE_VALUE;
- }
-#endif
-
-#if CONFIG_DXVA2
- if (sctx->pix_fmt == AV_PIX_FMT_DXVA2_VLD) {
- AVDXVA2FramesContext *frames_hwctx = frames_ctx->hwctx;
- struct dxva_context *dxva_ctx = &sctx->ctx.dxva2;
-
- ff_dxva2_lock(avctx);
- ret = dxva2_create_decoder(avctx);
- ff_dxva2_unlock(avctx);
- if (ret < 0)
- goto fail;
-
- dxva_ctx->decoder = sctx->dxva2_decoder;
- dxva_ctx->cfg = &sctx->dxva2_config;
- dxva_ctx->surface = frames_hwctx->surfaces;
- dxva_ctx->surface_count = frames_hwctx->nb_surfaces;
- dxva_ctx->workaround = sctx->workaround;
- }
-#endif
-
- return 0;
-
-fail:
- ff_dxva2_decode_uninit(avctx);
- return ret;
-}
-
-int ff_dxva2_decode_uninit(AVCodecContext *avctx)
-{
- FFDXVASharedContext *sctx = DXVA_SHARED_CONTEXT(avctx);
- int i;
-
- av_buffer_unref(&sctx->decoder_ref);
-
-#if CONFIG_D3D11VA
- for (i = 0; i < sctx->nb_d3d11_views; i++) {
- if (sctx->d3d11_views[i])
- ID3D11VideoDecoderOutputView_Release(sctx->d3d11_views[i]);
- }
- av_freep(&sctx->d3d11_views);
-#endif
-
-#if CONFIG_DXVA2
- if (sctx->dxva2_service)
- IDirectXVideoDecoderService_Release(sctx->dxva2_service);
-#endif
-
- return 0;
-}
-
-static void *get_surface(const AVCodecContext *avctx, const AVFrame *frame)
-{
-#if CONFIG_D3D11VA
- if (frame->format == AV_PIX_FMT_D3D11) {
- FFDXVASharedContext *sctx = DXVA_SHARED_CONTEXT(avctx);
- intptr_t index = (intptr_t)frame->data[1];
- if (index < 0 || index >= sctx->nb_d3d11_views ||
- sctx->d3d11_texture != (ID3D11Texture2D *)frame->data[0]) {
- av_log((void *)avctx, AV_LOG_ERROR, "get_buffer frame is invalid!\n");
- return NULL;
- }
- return sctx->d3d11_views[index];
- }
-#endif
- return frame->data[3];
-}
-
-unsigned ff_dxva2_get_surface_index(const AVCodecContext *avctx,
- const AVDXVAContext *ctx,
- const AVFrame *frame)
-{
- void *surface = get_surface(avctx, frame);
- unsigned i;
-
-#if CONFIG_D3D11VA
- if (avctx->pix_fmt == AV_PIX_FMT_D3D11)
- return (intptr_t)frame->data[1];
- if (avctx->pix_fmt == AV_PIX_FMT_D3D11VA_VLD) {
- D3D11_VIDEO_DECODER_OUTPUT_VIEW_DESC viewDesc;
- ID3D11VideoDecoderOutputView_GetDesc((ID3D11VideoDecoderOutputView*) surface, &viewDesc);
- return viewDesc.Texture2D.ArraySlice;
- }
-#endif
-#if CONFIG_DXVA2
- for (i = 0; i < DXVA_CONTEXT_COUNT(avctx, ctx); i++) {
- if (avctx->pix_fmt == AV_PIX_FMT_DXVA2_VLD && ctx->dxva2.surface[i] == surface)
- return i;
- }
-#endif
-
- assert(0);
- return 0;
-}
-
-int ff_dxva2_commit_buffer(AVCodecContext *avctx,
- AVDXVAContext *ctx,
- DECODER_BUFFER_DESC *dsc,
- unsigned type, const void *data, unsigned size,
- unsigned mb_count)
-{
- void *dxva_data;
- unsigned dxva_size;
- int result;
- HRESULT hr = 0;
-
-#if CONFIG_D3D11VA
- if (ff_dxva2_is_d3d11(avctx))
- hr = ID3D11VideoContext_GetDecoderBuffer(D3D11VA_CONTEXT(ctx)->video_context,
- D3D11VA_CONTEXT(ctx)->decoder,
- type,
- &dxva_size, &dxva_data);
-#endif
-#if CONFIG_DXVA2
- if (avctx->pix_fmt == AV_PIX_FMT_DXVA2_VLD)
- hr = IDirectXVideoDecoder_GetBuffer(DXVA2_CONTEXT(ctx)->decoder, type,
- &dxva_data, &dxva_size);
-#endif
- if (FAILED(hr)) {
- av_log(avctx, AV_LOG_ERROR, "Failed to get a buffer for %u: 0x%x\n",
- type, (unsigned)hr);
- return -1;
- }
- if (size <= dxva_size) {
- memcpy(dxva_data, data, size);
-
-#if CONFIG_D3D11VA
- if (ff_dxva2_is_d3d11(avctx)) {
- D3D11_VIDEO_DECODER_BUFFER_DESC *dsc11 = dsc;
- memset(dsc11, 0, sizeof(*dsc11));
- dsc11->BufferType = type;
- dsc11->DataSize = size;
- dsc11->NumMBsInBuffer = mb_count;
- }
-#endif
-#if CONFIG_DXVA2
- if (avctx->pix_fmt == AV_PIX_FMT_DXVA2_VLD) {
- DXVA2_DecodeBufferDesc *dsc2 = dsc;
- memset(dsc2, 0, sizeof(*dsc2));
- dsc2->CompressedBufferType = type;
- dsc2->DataSize = size;
- dsc2->NumMBsInBuffer = mb_count;
- }
-#endif
-
- result = 0;
- } else {
- av_log(avctx, AV_LOG_ERROR, "Buffer for type %u was too small\n", type);
- result = -1;
- }
-
-#if CONFIG_D3D11VA
- if (ff_dxva2_is_d3d11(avctx))
- hr = ID3D11VideoContext_ReleaseDecoderBuffer(D3D11VA_CONTEXT(ctx)->video_context, D3D11VA_CONTEXT(ctx)->decoder, type);
-#endif
-#if CONFIG_DXVA2
- if (avctx->pix_fmt == AV_PIX_FMT_DXVA2_VLD)
- hr = IDirectXVideoDecoder_ReleaseBuffer(DXVA2_CONTEXT(ctx)->decoder, type);
-#endif
- if (FAILED(hr)) {
- av_log(avctx, AV_LOG_ERROR,
- "Failed to release buffer type %u: 0x%x\n",
- type, (unsigned)hr);
- result = -1;
- }
- return result;
-}
-
-static int frame_add_buf(AVFrame *frame, AVBufferRef *ref)
-{
- int i;
-
- for (i = 0; i < AV_NUM_DATA_POINTERS; i++) {
- if (!frame->buf[i]) {
- frame->buf[i] = av_buffer_ref(ref);
- return frame->buf[i] ? 0 : AVERROR(ENOMEM);
- }
- }
-
- // For now we expect that the caller does not use more than
- // AV_NUM_DATA_POINTERS-1 buffers if the user uses a custom pool.
- return AVERROR(EINVAL);
-}
-
-int ff_dxva2_common_end_frame(AVCodecContext *avctx, AVFrame *frame,
- const void *pp, unsigned pp_size,
- const void *qm, unsigned qm_size,
- int (*commit_bs_si)(AVCodecContext *,
- DECODER_BUFFER_DESC *bs,
- DECODER_BUFFER_DESC *slice))
-{
- AVDXVAContext *ctx = DXVA_CONTEXT(avctx);
- unsigned buffer_count = 0;
-#if CONFIG_D3D11VA
- D3D11_VIDEO_DECODER_BUFFER_DESC buffer11[4];
-#endif
-#if CONFIG_DXVA2
- DXVA2_DecodeBufferDesc buffer2[4];
-#endif
- DECODER_BUFFER_DESC *buffer = NULL, *buffer_slice = NULL;
- int result, runs = 0;
- HRESULT hr;
- unsigned type;
- FFDXVASharedContext *sctx = DXVA_SHARED_CONTEXT(avctx);
-
- if (sctx->decoder_ref) {
- result = frame_add_buf(frame, sctx->decoder_ref);
- if (result < 0)
- return result;
- }
-
- do {
- ff_dxva2_lock(avctx);
-#if CONFIG_D3D11VA
- if (ff_dxva2_is_d3d11(avctx))
- hr = ID3D11VideoContext_DecoderBeginFrame(D3D11VA_CONTEXT(ctx)->video_context, D3D11VA_CONTEXT(ctx)->decoder,
- get_surface(avctx, frame),
- 0, NULL);
-#endif
-#if CONFIG_DXVA2
- if (avctx->pix_fmt == AV_PIX_FMT_DXVA2_VLD)
- hr = IDirectXVideoDecoder_BeginFrame(DXVA2_CONTEXT(ctx)->decoder,
- get_surface(avctx, frame),
- NULL);
-#endif
- if (hr != E_PENDING || ++runs > 50)
- break;
- ff_dxva2_unlock(avctx);
- av_usleep(2000);
- } while(1);
-
- if (FAILED(hr)) {
- av_log(avctx, AV_LOG_ERROR, "Failed to begin frame: 0x%x\n", (unsigned)hr);
- ff_dxva2_unlock(avctx);
- return -1;
- }
-
-#if CONFIG_D3D11VA
- if (ff_dxva2_is_d3d11(avctx)) {
- buffer = &buffer11[buffer_count];
- type = D3D11_VIDEO_DECODER_BUFFER_PICTURE_PARAMETERS;
- }
-#endif
-#if CONFIG_DXVA2
- if (avctx->pix_fmt == AV_PIX_FMT_DXVA2_VLD) {
- buffer = &buffer2[buffer_count];
- type = DXVA2_PictureParametersBufferType;
- }
-#endif
- result = ff_dxva2_commit_buffer(avctx, ctx, buffer,
- type,
- pp, pp_size, 0);
- if (result) {
- av_log(avctx, AV_LOG_ERROR,
- "Failed to add picture parameter buffer\n");
- goto end;
- }
- buffer_count++;
-
- if (qm_size > 0) {
-#if CONFIG_D3D11VA
- if (ff_dxva2_is_d3d11(avctx)) {
- buffer = &buffer11[buffer_count];
- type = D3D11_VIDEO_DECODER_BUFFER_INVERSE_QUANTIZATION_MATRIX;
- }
-#endif
-#if CONFIG_DXVA2
- if (avctx->pix_fmt == AV_PIX_FMT_DXVA2_VLD) {
- buffer = &buffer2[buffer_count];
- type = DXVA2_InverseQuantizationMatrixBufferType;
- }
-#endif
- result = ff_dxva2_commit_buffer(avctx, ctx, buffer,
- type,
- qm, qm_size, 0);
- if (result) {
- av_log(avctx, AV_LOG_ERROR,
- "Failed to add inverse quantization matrix buffer\n");
- goto end;
- }
- buffer_count++;
- }
-
-#if CONFIG_D3D11VA
- if (ff_dxva2_is_d3d11(avctx)) {
- buffer = &buffer11[buffer_count + 0];
- buffer_slice = &buffer11[buffer_count + 1];
- }
-#endif
-#if CONFIG_DXVA2
- if (avctx->pix_fmt == AV_PIX_FMT_DXVA2_VLD) {
- buffer = &buffer2[buffer_count + 0];
- buffer_slice = &buffer2[buffer_count + 1];
- }
-#endif
-
- result = commit_bs_si(avctx,
- buffer,
- buffer_slice);
- if (result) {
- av_log(avctx, AV_LOG_ERROR,
- "Failed to add bitstream or slice control buffer\n");
- goto end;
- }
- buffer_count += 2;
-
- /* TODO Film Grain when possible */
-
- assert(buffer_count == 1 + (qm_size > 0) + 2);
-
-#if CONFIG_D3D11VA
- if (ff_dxva2_is_d3d11(avctx))
- hr = ID3D11VideoContext_SubmitDecoderBuffers(D3D11VA_CONTEXT(ctx)->video_context,
- D3D11VA_CONTEXT(ctx)->decoder,
- buffer_count, buffer11);
-#endif
-#if CONFIG_DXVA2
- if (avctx->pix_fmt == AV_PIX_FMT_DXVA2_VLD) {
- DXVA2_DecodeExecuteParams exec = {
- .NumCompBuffers = buffer_count,
- .pCompressedBuffers = buffer2,
- .pExtensionData = NULL,
- };
- hr = IDirectXVideoDecoder_Execute(DXVA2_CONTEXT(ctx)->decoder, &exec);
- }
-#endif
- if (FAILED(hr)) {
- av_log(avctx, AV_LOG_ERROR, "Failed to execute: 0x%x\n", (unsigned)hr);
- result = -1;
- }
-
-end:
-#if CONFIG_D3D11VA
- if (ff_dxva2_is_d3d11(avctx))
- hr = ID3D11VideoContext_DecoderEndFrame(D3D11VA_CONTEXT(ctx)->video_context, D3D11VA_CONTEXT(ctx)->decoder);
-#endif
-#if CONFIG_DXVA2
- if (avctx->pix_fmt == AV_PIX_FMT_DXVA2_VLD)
- hr = IDirectXVideoDecoder_EndFrame(DXVA2_CONTEXT(ctx)->decoder, NULL);
-#endif
- ff_dxva2_unlock(avctx);
- if (FAILED(hr)) {
- av_log(avctx, AV_LOG_ERROR, "Failed to end frame: 0x%x\n", (unsigned)hr);
- result = -1;
- }
-
- return result;
-}
-
-int ff_dxva2_is_d3d11(const AVCodecContext *avctx)
-{
- if (CONFIG_D3D11VA)
- return avctx->pix_fmt == AV_PIX_FMT_D3D11VA_VLD ||
- avctx->pix_fmt == AV_PIX_FMT_D3D11;
- else
- return 0;
-}
diff --git a/spaces/colakin/video-generater/public/ffmpeg/libavcodec/escape130.c b/spaces/colakin/video-generater/public/ffmpeg/libavcodec/escape130.c
deleted file mode 100644
index 3b0460fd79a09e1ea31dcedb567f876441481063..0000000000000000000000000000000000000000
--- a/spaces/colakin/video-generater/public/ffmpeg/libavcodec/escape130.c
+++ /dev/null
@@ -1,359 +0,0 @@
-/*
- * Escape 130 video decoder
- * Copyright (C) 2008 Eli Friedman (eli.friedman gmail.com)
- *
- * This file is part of FFmpeg.
- *
- * FFmpeg is free software; you can redistribute it and/or
- * modify it under the terms of the GNU Lesser General Public
- * License as published by the Free Software Foundation; either
- * version 2.1 of the License, or (at your option) any later version.
- *
- * FFmpeg is distributed in the hope that it will be useful,
- * but WITHOUT ANY WARRANTY; without even the implied warranty of
- * MERCHANTABILITY or FITNESS FOR A PARTICULAR PURPOSE. See the GNU
- * Lesser General Public License for more details.
- *
- * You should have received a copy of the GNU Lesser General Public
- * License along with FFmpeg; if not, write to the Free Software
- * Foundation, Inc., 51 Franklin Street, Fifth Floor, Boston, MA 02110-1301 USA
- */
-
-#include "libavutil/attributes.h"
-#include "libavutil/mem.h"
-
-#define BITSTREAM_READER_LE
-#include "avcodec.h"
-#include "codec_internal.h"
-#include "decode.h"
-#include "get_bits.h"
-
-typedef struct Escape130Context {
- uint8_t *old_y_avg;
-
- uint8_t *new_y, *old_y;
- uint8_t *new_u, *old_u;
- uint8_t *new_v, *old_v;
-
- uint8_t *buf1, *buf2;
- int linesize[3];
-} Escape130Context;
-
-static const uint8_t offset_table[] = { 2, 4, 10, 20 };
-static const int8_t sign_table[64][4] = {
- { 0, 0, 0, 0 },
- { -1, 1, 0, 0 },
- { 1, -1, 0, 0 },
- { -1, 0, 1, 0 },
- { -1, 1, 1, 0 },
- { 0, -1, 1, 0 },
- { 1, -1, 1, 0 },
- { -1, -1, 1, 0 },
- { 1, 0, -1, 0 },
- { 0, 1, -1, 0 },
- { 1, 1, -1, 0 },
- { -1, 1, -1, 0 },
- { 1, -1, -1, 0 },
- { -1, 0, 0, 1 },
- { -1, 1, 0, 1 },
- { 0, -1, 0, 1 },
-
- { 0, 0, 0, 0 },
- { 1, -1, 0, 1 },
- { -1, -1, 0, 1 },
- { -1, 0, 1, 1 },
- { -1, 1, 1, 1 },
- { 0, -1, 1, 1 },
- { 1, -1, 1, 1 },
- { -1, -1, 1, 1 },
- { 0, 0, -1, 1 },
- { 1, 0, -1, 1 },
- { -1, 0, -1, 1 },
- { 0, 1, -1, 1 },
- { 1, 1, -1, 1 },
- { -1, 1, -1, 1 },
- { 0, -1, -1, 1 },
- { 1, -1, -1, 1 },
-
- { 0, 0, 0, 0 },
- { -1, -1, -1, 1 },
- { 1, 0, 0, -1 },
- { 0, 1, 0, -1 },
- { 1, 1, 0, -1 },
- { -1, 1, 0, -1 },
- { 1, -1, 0, -1 },
- { 0, 0, 1, -1 },
- { 1, 0, 1, -1 },
- { -1, 0, 1, -1 },
- { 0, 1, 1, -1 },
- { 1, 1, 1, -1 },
- { -1, 1, 1, -1 },
- { 0, -1, 1, -1 },
- { 1, -1, 1, -1 },
- { -1, -1, 1, -1 },
-
- { 0, 0, 0, 0 },
- { 1, 0, -1, -1 },
- { 0, 1, -1, -1 },
- { 1, 1, -1, -1 },
- { -1, 1, -1, -1 },
- { 1, -1, -1, -1 }
-};
-
-static const int8_t luma_adjust[] = { -4, -3, -2, -1, 1, 2, 3, 4 };
-
-static const int8_t chroma_adjust[2][8] = {
- { 1, 1, 0, -1, -1, -1, 0, 1 },
- { 0, 1, 1, 1, 0, -1, -1, -1 }
-};
-
-static const uint8_t chroma_vals[] = {
- 20, 28, 36, 44, 52, 60, 68, 76,
- 84, 92, 100, 106, 112, 116, 120, 124,
- 128, 132, 136, 140, 144, 150, 156, 164,
- 172, 180, 188, 196, 204, 212, 220, 228
-};
-
-static av_cold int escape130_decode_init(AVCodecContext *avctx)
-{
- Escape130Context *s = avctx->priv_data;
- avctx->pix_fmt = AV_PIX_FMT_YUV420P;
-
- if ((avctx->width & 1) || (avctx->height & 1)) {
- av_log(avctx, AV_LOG_ERROR,
- "Dimensions should be a multiple of two.\n");
- return AVERROR_INVALIDDATA;
- }
-
- s->old_y_avg = av_malloc(avctx->width * avctx->height / 4);
- s->buf1 = av_malloc(avctx->width * avctx->height * 3 / 2);
- s->buf2 = av_malloc(avctx->width * avctx->height * 3 / 2);
- if (!s->old_y_avg || !s->buf1 || !s->buf2) {
- av_log(avctx, AV_LOG_ERROR, "Could not allocate buffer.\n");
- return AVERROR(ENOMEM);
- }
-
- s->linesize[0] = avctx->width;
- s->linesize[1] =
- s->linesize[2] = avctx->width / 2;
-
- s->new_y = s->buf1;
- s->new_u = s->new_y + avctx->width * avctx->height;
- s->new_v = s->new_u + avctx->width * avctx->height / 4;
- s->old_y = s->buf2;
- s->old_u = s->old_y + avctx->width * avctx->height;
- s->old_v = s->old_u + avctx->width * avctx->height / 4;
- memset(s->old_y, 0, avctx->width * avctx->height);
- memset(s->old_u, 0x10, avctx->width * avctx->height / 4);
- memset(s->old_v, 0x10, avctx->width * avctx->height / 4);
-
- return 0;
-}
-
-static av_cold int escape130_decode_close(AVCodecContext *avctx)
-{
- Escape130Context *s = avctx->priv_data;
-
- av_freep(&s->old_y_avg);
- av_freep(&s->buf1);
- av_freep(&s->buf2);
-
- return 0;
-}
-
-static int decode_skip_count(GetBitContext* gb)
-{
- int value;
-
- if (get_bits_left(gb) < 1+3)
- return -1;
-
- value = get_bits1(gb);
- if (value)
- return 0;
-
- value = get_bits(gb, 3);
- if (value)
- return value;
-
- value = get_bits(gb, 8);
- if (value)
- return value + 7;
-
- value = get_bits(gb, 15);
- if (value)
- return value + 262;
-
- return -1;
-}
-
-static int escape130_decode_frame(AVCodecContext *avctx, AVFrame *pic,
- int *got_frame, AVPacket *avpkt)
-{
- int buf_size = avpkt->size;
- Escape130Context *s = avctx->priv_data;
- GetBitContext gb;
- int ret;
-
- uint8_t *old_y, *old_cb, *old_cr,
- *new_y, *new_cb, *new_cr;
- uint8_t *dstY, *dstU, *dstV;
- unsigned old_y_stride, old_cb_stride, old_cr_stride,
- new_y_stride, new_cb_stride, new_cr_stride;
- unsigned total_blocks = avctx->width * avctx->height / 4,
- block_index, block_x = 0;
- unsigned y[4] = { 0 }, cb = 0x10, cr = 0x10;
- int skip = -1, y_avg = 0, i, j;
- uint8_t *ya = s->old_y_avg;
-
- // first 16 bytes are header; no useful information in here
- if (buf_size <= 16) {
- av_log(avctx, AV_LOG_ERROR, "Insufficient frame data\n");
- return AVERROR_INVALIDDATA;
- }
-
- if ((ret = ff_get_buffer(avctx, pic, 0)) < 0)
- return ret;
-
- if ((ret = init_get_bits8(&gb, avpkt->data, avpkt->size)) < 0)
- return ret;
- skip_bits_long(&gb, 16 * 8);
-
- new_y = s->new_y;
- new_cb = s->new_u;
- new_cr = s->new_v;
- new_y_stride = s->linesize[0];
- new_cb_stride = s->linesize[1];
- new_cr_stride = s->linesize[2];
- old_y = s->old_y;
- old_cb = s->old_u;
- old_cr = s->old_v;
- old_y_stride = s->linesize[0];
- old_cb_stride = s->linesize[1];
- old_cr_stride = s->linesize[2];
-
- for (block_index = 0; block_index < total_blocks; block_index++) {
- // Note that this call will make us skip the rest of the blocks
- // if the frame ends prematurely.
- if (skip == -1)
- skip = decode_skip_count(&gb);
- if (skip == -1) {
- av_log(avctx, AV_LOG_ERROR, "Error decoding skip value\n");
- return AVERROR_INVALIDDATA;
- }
-
- if (skip) {
- y[0] = old_y[0];
- y[1] = old_y[1];
- y[2] = old_y[old_y_stride];
- y[3] = old_y[old_y_stride + 1];
- y_avg = ya[0];
- cb = old_cb[0];
- cr = old_cr[0];
- } else {
- if (get_bits1(&gb)) {
- unsigned sign_selector = get_bits(&gb, 6);
- unsigned difference_selector = get_bits(&gb, 2);
- y_avg = 2 * get_bits(&gb, 5);
- for (i = 0; i < 4; i++) {
- y[i] = av_clip(y_avg + offset_table[difference_selector] *
- sign_table[sign_selector][i], 0, 63);
- }
- } else if (get_bits1(&gb)) {
- if (get_bits1(&gb)) {
- y_avg = get_bits(&gb, 6);
- } else {
- unsigned adjust_index = get_bits(&gb, 3);
- y_avg = (y_avg + luma_adjust[adjust_index]) & 63;
- }
- for (i = 0; i < 4; i++)
- y[i] = y_avg;
- }
-
- if (get_bits1(&gb)) {
- if (get_bits1(&gb)) {
- cb = get_bits(&gb, 5);
- cr = get_bits(&gb, 5);
- } else {
- unsigned adjust_index = get_bits(&gb, 3);
- cb = (cb + chroma_adjust[0][adjust_index]) & 31;
- cr = (cr + chroma_adjust[1][adjust_index]) & 31;
- }
- }
- }
- *ya++ = y_avg;
-
- new_y[0] = y[0];
- new_y[1] = y[1];
- new_y[new_y_stride] = y[2];
- new_y[new_y_stride + 1] = y[3];
- *new_cb = cb;
- *new_cr = cr;
-
- old_y += 2;
- old_cb++;
- old_cr++;
- new_y += 2;
- new_cb++;
- new_cr++;
- block_x++;
- if (block_x * 2 == avctx->width) {
- block_x = 0;
- old_y += old_y_stride * 2 - avctx->width;
- old_cb += old_cb_stride - avctx->width / 2;
- old_cr += old_cr_stride - avctx->width / 2;
- new_y += new_y_stride * 2 - avctx->width;
- new_cb += new_cb_stride - avctx->width / 2;
- new_cr += new_cr_stride - avctx->width / 2;
- }
-
- skip--;
- }
-
- new_y = s->new_y;
- new_cb = s->new_u;
- new_cr = s->new_v;
- dstY = pic->data[0];
- dstU = pic->data[1];
- dstV = pic->data[2];
- for (j = 0; j < avctx->height; j++) {
- for (i = 0; i < avctx->width; i++)
- dstY[i] = new_y[i] << 2;
- dstY += pic->linesize[0];
- new_y += new_y_stride;
- }
- for (j = 0; j < avctx->height / 2; j++) {
- for (i = 0; i < avctx->width / 2; i++) {
- dstU[i] = chroma_vals[new_cb[i]];
- dstV[i] = chroma_vals[new_cr[i]];
- }
- dstU += pic->linesize[1];
- dstV += pic->linesize[2];
- new_cb += new_cb_stride;
- new_cr += new_cr_stride;
- }
-
- ff_dlog(avctx, "Frame data: provided %d bytes, used %d bytes\n",
- buf_size, get_bits_count(&gb) >> 3);
-
- FFSWAP(uint8_t*, s->old_y, s->new_y);
- FFSWAP(uint8_t*, s->old_u, s->new_u);
- FFSWAP(uint8_t*, s->old_v, s->new_v);
-
- *got_frame = 1;
-
- return buf_size;
-}
-
-const FFCodec ff_escape130_decoder = {
- .p.name = "escape130",
- CODEC_LONG_NAME("Escape 130"),
- .p.type = AVMEDIA_TYPE_VIDEO,
- .p.id = AV_CODEC_ID_ESCAPE130,
- .priv_data_size = sizeof(Escape130Context),
- .init = escape130_decode_init,
- .close = escape130_decode_close,
- FF_CODEC_DECODE_CB(escape130_decode_frame),
- .p.capabilities = AV_CODEC_CAP_DR1,
- .caps_internal = FF_CODEC_CAP_INIT_CLEANUP,
-};
diff --git a/spaces/colakin/video-generater/public/ffmpeg/libavcodec/fdctdsp.c b/spaces/colakin/video-generater/public/ffmpeg/libavcodec/fdctdsp.c
deleted file mode 100644
index 5306c9d047e9bc7bcf683a8192a622a9afc1c16d..0000000000000000000000000000000000000000
--- a/spaces/colakin/video-generater/public/ffmpeg/libavcodec/fdctdsp.c
+++ /dev/null
@@ -1,51 +0,0 @@
-/*
- * This file is part of FFmpeg.
- *
- * FFmpeg is free software; you can redistribute it and/or
- * modify it under the terms of the GNU Lesser General Public
- * License as published by the Free Software Foundation; either
- * version 2.1 of the License, or (at your option) any later version.
- *
- * FFmpeg is distributed in the hope that it will be useful,
- * but WITHOUT ANY WARRANTY; without even the implied warranty of
- * MERCHANTABILITY or FITNESS FOR A PARTICULAR PURPOSE. See the GNU
- * Lesser General Public License for more details.
- *
- * You should have received a copy of the GNU Lesser General Public
- * License along with FFmpeg; if not, write to the Free Software
- * Foundation, Inc., 51 Franklin Street, Fifth Floor, Boston, MA 02110-1301 USA
- */
-
-#include "libavutil/attributes.h"
-#include "avcodec.h"
-#include "dct.h"
-#include "faandct.h"
-#include "fdctdsp.h"
-#include "config.h"
-
-av_cold void ff_fdctdsp_init(FDCTDSPContext *c, AVCodecContext *avctx)
-{
- av_unused const unsigned high_bit_depth = avctx->bits_per_raw_sample > 8;
-
- if (avctx->bits_per_raw_sample == 10 || avctx->bits_per_raw_sample == 9) {
- c->fdct = ff_jpeg_fdct_islow_10;
- c->fdct248 = ff_fdct248_islow_10;
- } else if (avctx->dct_algo == FF_DCT_FASTINT) {
- c->fdct = ff_fdct_ifast;
- c->fdct248 = ff_fdct_ifast248;
-#if CONFIG_FAANDCT
- } else if (avctx->dct_algo == FF_DCT_FAAN) {
- c->fdct = ff_faandct;
- c->fdct248 = ff_faandct248;
-#endif /* CONFIG_FAANDCT */
- } else {
- c->fdct = ff_jpeg_fdct_islow_8; // slow/accurate/default
- c->fdct248 = ff_fdct248_islow_8;
- }
-
-#if ARCH_PPC
- ff_fdctdsp_init_ppc(c, avctx, high_bit_depth);
-#elif ARCH_X86
- ff_fdctdsp_init_x86(c, avctx, high_bit_depth);
-#endif
-}
diff --git a/spaces/congsaPfin/Manga-OCR/logs/Apkdays Call of Duty Warzone Mobile How to Sync Your Battle Pass and Friends List Across Platforms.md b/spaces/congsaPfin/Manga-OCR/logs/Apkdays Call of Duty Warzone Mobile How to Sync Your Battle Pass and Friends List Across Platforms.md
deleted file mode 100644
index a08748ecc667cad1a960fd94b4ef3bb16ee4dd11..0000000000000000000000000000000000000000
--- a/spaces/congsaPfin/Manga-OCR/logs/Apkdays Call of Duty Warzone Mobile How to Sync Your Battle Pass and Friends List Across Platforms.md
+++ /dev/null
@@ -1,168 +0,0 @@
-
-
Apkdays Call of Duty Warzone Mobile: Everything You Need to Know
-
If you are a fan of Call of Duty: Warzone, the popular battle royale game that has taken the gaming world by storm, you might be wondering if you can play it on your mobile device. Well, the answer is yes, thanks to Apkdays Call of Duty Warzone Mobile, a modded version of the game that lets you enjoy Verdansk on the go. In this article, we will tell you everything you need to know about Apkdays Call of Duty Warzone Mobile, including what it is, how to download and install it, how to play it like a pro, and how it compares to other mobile battle royales.
-
What is Apkdays Call of Duty Warzone Mobile?
-
A brief introduction to the game and its features
-
Apkdays Call of Duty Warzone Mobile is a free-to-play mobile game that is based on Call of Duty: Warzone, the hit battle royale game that is available on PC and consoles. It is not an official release from Activision, but rather a modded version that has been created by a third-party developer called Apkdays. The game aims to replicate the authentic Call of Duty: Warzone experience on mobile devices, with high-quality graphics, intuitive controls, and cross-progression with Call of Duty: Warzone 2.0 and Call of Duty: Modern Warfare II.
Some of the features that Apkdays Call of Duty Warzone Mobile offers are:
-
-
The iconic Verdansk map, with dozens of points of interest and strategies to survive
-
Up to 120 live players in a match, with real players and no bots
-
A variety of weapons, attachments, upgrades, killstreaks, revive tokens, and contracts to use
-
The Gulag system, where you can win a duel to get a second chance at survival
-
Social features like friends, chat channels, and Battle Pass across platforms
-
A shorter 10-minute mode for quick sessions
-
-
How to download and install the game on your device
-
Since Apkdays Call of Duty Warzone Mobile is not an official release from Activision, you cannot find it on the Google Play Store or the App Store. Instead, you have to download it from the Apkdays website , where you can find the latest version of the game. Here are the steps to download and install the game on your device:
-
-
Go to [Apkdays](^1^) website and find the download link for Apkdays Call of Duty Warzone Mobile.
-
Click on the download link and wait for the file to be downloaded on your device.
-
Once the file is downloaded, locate it in your file manager and tap on it to install it.
-
If you see a warning message that says "Install blocked", go to your device settings and enable "Unknown sources" or "Allow from this source".
-
After enabling the installation, launch the game and enjoy Apkdays Call of Duty Warzone Mobile.
-
-
Note: You may need to update the game from time to time to get the latest features and fixes. You can check for updates on the Apkdays website or in the game itself.
-
What are the benefits of using Apkdays Call of Duty Warzone Mobile?
-
Apkdays Call of Duty Warzone Mobile is not just a cheap imitation of Call of Duty: Warzone, but rather a faithful adaptation that has many benefits for mobile gamers. Some of the benefits are:
-
-
You can play Call of Duty: Warzone on your mobile device without compromising on the quality or performance of the game.
-
You can save storage space and data usage by downloading a smaller file size than the original game.
-
You can access exclusive features and content that are not available in the official game, such as new weapons, skins, modes, and events.
-
You can cross-play and cross-progression with other players who are using Call of Duty: Warzone 2.0 and Call of Duty: Modern Warfare II on PC and consoles.
-
You can support the independent developer who created Apkdays Call of Duty Warzone Mobile and help them improve the game further.
-
-
How to play Apkdays Call of Duty Warzone Mobile like a pro
-
Tips and tricks for surviving and winning in Verdansk
-
Apkdays Call of Duty Warzone Mobile is not an easy game to master, especially if you are new to the battle royale genre. You will face many challenges and threats in Verdansk, such as enemy players, gas circles, loot scarcity, and environmental hazards. To increase your chances of survival and victory, you need to follow some tips and tricks that will help you improve your skills and strategies. Here are some of them:
-
-
Choose your landing spot wisely. Depending on your playstyle and preference, you may want to land in a hot zone with high loot potential and high risk, or a cold zone with low loot potential and low risk. You can also use the ping system to communicate with your teammates and coordinate your landing spot.
-
Loot fast and smart. As soon as you land, you need to find weapons, armor, ammo, and other items that will help you survive. You can loot from buildings, crates, supply boxes, dead enemies, and contracts. You can also use the loadout drop marker to get your custom loadout from Call of Duty: Warzone 2.0 or Call of Duty: Modern Warfare II.
-
Stay alert and aware. Verdansk is a huge map with many enemies lurking around. You need to keep an eye on your surroundings, use your mini-map, listen to audio cues, and watch out for enemy indicators. You also need to pay attention to the gas circle, which will shrink over time and force you to move to a safe zone.
-
Play as a team. Apkdays Call of Duty Warzone Mobile is best played with friends or other players who can cooperate and coordinate with you. You can use voice chat or text chat to communicate with your teammates, share loot, revive each other, and execute tactics. You can also use the ping system to mark enemies, locations, items, and vehicles.
-
Be adaptable and flexible. Verdansk is a dynamic map that changes every match. You need to be ready to face different situations and scenarios that may require you to change your plan or strategy. You also need to be able to use different weapons, attachments, killstreaks, contracts, and vehicles that suit your needs.
-
-
Best weapons, attachments, and loadouts to use
-
Apkdays Call of Duty Warzone Mobile has a wide range of weapons that you can use in Verdansk, from pistols and shotguns to assault rifles and sniper rifles. Each weapon has its own stats, pros, cons, and attachments that affect its performance. You can customize your weapons with different attachments that enhance their accuracy, damage, range, fire rate, mobility, or control. You can also create your own loadouts with different weapons, attachments, perks, and equipment that suit your playstyle and preference. You can access your loadouts from the loadout drop marker that appears randomly or from contracts. Some of the best weapons, attachments, and loadouts to use in Apkdays Call of Duty Warzone Mobile are: - The M4A1 assault rifle, which is a versatile and reliable weapon that can handle any situation. It has high damage, accuracy, range, and fire rate, making it a great choice for medium to long-range engagements. Some of the best attachments for the M4A1 are the Monolithic Suppressor, the M16 Grenadier Barrel, the VLK 3.0x Optic, the Commando Foregrip, and the 60 Round Mags. - The MP5 submachine gun, which is a fast and powerful weapon that excels in close-range combat. It has high damage, fire rate, mobility, and control, making it a great choice for rushing and flanking enemies. Some of the best attachments for the MP5 are the Monolithic Integral Suppressor, the Merc Foregrip, the 45 Round Mags, the Stippled Grip Tape, and the Sleight of Hand. - The HDR sniper rifle, which is a deadly and accurate weapon that can take down enemies from afar. It has high damage, range, bullet velocity, and penetration, making it a great choice for sniping and counter-sniping enemies. Some of the best attachments for the HDR are the Monolithic Suppressor, the 26.9" HDR Pro Barrel, the Variable Zoom Scope, the FTAC Champion Stock, and the Focus. - The loadout that combines the M4A1 and the MP5, which is a balanced and effective loadout that can handle any situation. You can use the M4A1 for medium to long-range engagements and switch to the MP5 for close-range engagements. You can also use perks like Cold-Blooded, Ghost, and Amped to stay hidden from enemy detection and switch weapons faster. You can also use equipment like C4 and Heartbeat Sensor to deal damage and locate enemies. - The loadout that combines the HDR and a pistol of your choice, which is a risky but rewarding loadout that can dominate at long-range engagements. You can use the HDR to snipe enemies from a distance and switch to your pistol for self-defense or finishing off enemies. You can also use perks like Overkill, High Alert, and Shrapnel to carry two primary weapons, be aware of enemy flanks, and carry extra lethal equipment. You can also use equipment like Claymore and Smoke Grenade to protect yourself and cover your escape.
How to use contracts, killstreaks, and cash wisely
-
Apkdays Call of Duty Warzone Mobile has many elements that make it more than just a simple battle royale game. One of these elements is contracts, which are optional missions that you can find and activate in Verdansk. Contracts offer various rewards such as cash, loot, intel, or loadout drops. There are different types of contracts such as Bounty (hunt down a specific enemy), Recon (secure a location), Scavenger (collect supply boxes), Most Wanted (survive as a marked target), or Supply Run (reach a buy station). Another element is killstreaks, which are powerful abilities that you can use to gain an edge over your enemies. Killstreaks include UAV (reveal enemy locations), Cluster Strike (call in an airstrike), Precision Airstrike (call in a targeted airstrike), Sentry Gun (deploy an automated turret), Wheelson (control a mini-tank), or Juggernaut (wear a heavy armor suit). You can find killstreaks from loot or buy them from buy stations. The last element is cash, which is the currency that you can use to buy various items and services in Verdansk. Cash can be found from loot, contracts, enemies, or cash drops. You can use cash to buy weapons, attachments, loadouts, killstreaks, armor plates, revive tokens, self-revive kits, gas masks, or redeploy your teammates from buy stations. You can also use cash to deposit or withdraw from bank stations or ATMs. Some of the tips on how to use contracts, killstreaks, and cash wisely are: - Choose contracts that suit your playstyle and situation. For example, if you want to hunt enemies, go for Bounty contracts. If you want to secure a location, go for Recon contracts. If you want to loot more, go for Scavenger contracts. If you want to challenge yourself, go for Most Wanted or Supply Run contracts. - Use killstreaks at the right time and place. For example, use UAV when you want to locate enemies or avoid them. Use Cluster Strike or Precision Airstrike when you want to damage or eliminate enemies in a specific area. Use Sentry Gun when you want to defend a location or distract enemies. Use Wheelson when you want to wreak havoc on enemies or vehicles. Use Juggernaut when you want to dominate the battlefield or survive longer. - Manage your cash carefully and strategically. For example, don't spend all your cash on unnecessary items or services. Save some cash for emergencies or late-game situations. Share your cash with your teammates if they need it more than you. Deposit your cash in bank stations or ATMs if you have too much of it and don't want to lose it. Withdraw your cash from bank stations or ATMs if you need more of it and have enough balance.
How does Apkdays Call of Duty Warzone Mobile compare to other mobile battle royales?
-
The advantages and disadvantages of Apkdays Call of Duty Warzone Mobile
-
Apkdays Call of Duty Warzone Mobile is not the only mobile battle royale game that you can play on your device. There are many other options that you can choose from, such as PUBG Mobile, Free Fire, Fortnite Mobile, COD Mobile, and more. Each game has its own strengths and weaknesses that make it appealing or unappealing to different players. Here are some of the advantages and disadvantages of Apkdays Call of Duty Warzone Mobile compared to other mobile battle royales:
-
-
-
Advantages
-
Disadvantages
-
-
-
- It offers a realistic and immersive Call of Duty: Warzone experience on mobile devices.
-
- It is not an official release from Activision and may have compatibility or security issues.
-
-
-
- It has high-quality graphics, sound effects, and animations that create a stunning visual and auditory experience.
-
- It requires a high-end device and a stable internet connection to run smoothly and avoid lag or crashes.
-
-
-
- It has many features and content that are not available in other mobile battle royales, such as Verdansk map, Gulag system, contracts, killstreaks, cross-play, and cross-progression.
-
- It has a steep learning curve and a high difficulty level that may frustrate or discourage new or casual players.
-
-
-
- It has a loyal and active community of players and fans who support the game and the developer.
-
- It has a limited player base and may have long waiting times or matchmaking issues.
-
-
-
The similarities and differences between Apkdays Call of Duty Warzone Mobile and Call of Duty: Warzone 2.0
-
Apkdays Call of Duty Warzone Mobile is not the same as Call of Duty: Warzone 2.0, the official sequel to Call of Duty: Warzone that is expected to launch in 2023. Call of Duty: Warzone 2.0 is a major update that will introduce new features, content, and improvements to the original game. Apkdays Call of Duty Warzone Mobile is a modded version of the original game that aims to bring it to mobile devices. Here are some of the similarities and differences between Apkdays Call of Duty Warzone Mobile and Call of Duty: Warzone 2.0:
-
apkdays call of duty warzone mobile download
-apkdays call of duty warzone mobile release date
-apkdays call of duty warzone mobile gameplay
-apkdays call of duty warzone mobile pre-registration
-apkdays call of duty warzone mobile review
-apkdays call of duty warzone mobile system requirements
-apkdays call of duty warzone mobile tips and tricks
-apkdays call of duty warzone mobile best weapons
-apkdays call of duty warzone mobile cheats and hacks
-apkdays call of duty warzone mobile update
-apkdays call of duty warzone mobile vs pubg mobile
-apkdays call of duty warzone mobile crossplay
-apkdays call of duty warzone mobile controller support
-apkdays call of duty warzone mobile battle pass
-apkdays call of duty warzone mobile maps
-apkdays call of duty warzone mobile verdansk
-apkdays call of duty warzone mobile gulag
-apkdays call of duty warzone mobile killstreaks
-apkdays call of duty warzone mobile vehicles
-apkdays call of duty warzone mobile graphics settings
-apkdays call of duty warzone mobile how to install
-apkdays call of duty warzone mobile how to play
-apkdays call of duty warzone mobile how to win
-apkdays call of duty warzone mobile how to get ghost skin
-apkdays call of duty warzone mobile how to get shoot house map
-apkdays call of duty warzone mobile news and updates
-apkdays call of duty warzone mobile trailer and gameplay videos
-apkdays call of duty warzone mobile reddit and discord communities
-apkdays call of duty warzone mobile feedback and suggestions
-apkdays call of duty warzone mobile bugs and issues
-apkdays call of duty warzone mobile comparison with other cod games
-apkdays call of duty warzone mobile comparison with other battle royale games
-apkdays call of duty warzone mobile pros and cons
-apkdays call of duty warzone mobile features and modes
-apkdays call of duty warzone mobile loadouts and customization
-apkdays call of duty warzone mobile weapons and attachments
-apkdays call of duty warzone mobile perks and equipment
-apkdays call of duty warzone mobile contracts and missions
-apkdays call of duty warzone mobile challenges and rewards
-apkdays call of duty warzone mobile tournaments and events
-
-
-
Similarities
-
Differences
-
-
-
- They are both based on Call of Duty: Warzone, the popular battle royale game that is set in the Modern Warfare universe.
-
- Apkdays Call of Duty Warzone Mobile is a mobile game that is available on Android and iOS devices, while Call of Duty: Warzone 2.0 is a PC and console game that is available on Windows, PlayStation, and Xbox platforms.
-
-
-
- They both feature the iconic Verdansk map, with dozens of points of interest and strategies to survive.
-
- Apkdays Call of Duty Warzone Mobile has a smaller and simplified version of Verdansk, while Call of Duty: Warzone 2.0 has a larger and more detailed version of Verdansk.
-
-
-
- They both have up to 120 live players in a match, with real players and no bots.
-
- Apkdays Call of Duty Warzone Mobile has a shorter 10-minute mode for quick sessions, while Call of Duty: Warzone 2.0 has a longer 20-minute mode for intense sessions.
-
-
-
- They both have a variety of weapons, attachments, upgrades, killstreaks, revive tokens, and contracts to use.
-
- Apkdays Call of Duty Warzone Mobile has some exclusive features and content that are not available in Call of Duty: Warzone 2.0, such as new weapons, skins, modes, and events.
-
-
-
- They both have the Gulag system, where you can win a duel to get a second chance at survival.
-
- Apkdays Call of Duty Warzone Mobile has a different Gulag system than Call of Duty: Warzone 2.0, where you can choose your weapon and loadout before entering the duel.
-
-
-
- They both have social features like friends, chat channels, and Battle Pass across platforms.
-
- Apkdays Call of Duty Warzone Mobile has cross-play and cross-progression with Call of Duty: Warzone 2.0 and Call of Duty: Modern Warfare II, while Call of Duty: Warzone 2.0 has cross-play and cross-progression with Call of Duty: Vanguard and Call of Duty: Black Ops Cold War.
-
-
-
The feedback and reviews from players and critics
-
Apkdays Call of Duty Warzone Mobile has received mixed feedback and reviews from players and critics who have tried the game. Some players and critics have praised the game for its impressive graphics, smooth gameplay, faithful adaptation, and exclusive features. They have also appreciated the developer's efforts to create and update the game regularly. Some examples of positive feedback and reviews are:
- - "Apkdays Call of Duty Warzone Mobile is a masterpiece that delivers an authentic and immersive Call of Duty: Warzone experience on mobile devices. It is one of the best mobile battle royale games I have ever played." - A player from Reddit - "Apkdays Call of Duty Warzone Mobile is a stunning achievement that showcases the potential and power of mobile gaming. It is not just a clone or a rip-off, but rather a tribute and a homage to the original game." - A critic from IGN - "Apkdays Call of Duty Warzone Mobile is a must-play for any fan of Call of Duty: Warzone or battle royale games in general. It has everything you need to enjoy Verdansk on the go." - A player from Google Play Store However, some players and critics have criticized the game for its compatibility or security issues, high difficulty level, limited player base, or lack of originality. They have also warned about the possible legal or ethical implications of using a modded version of the game. Some examples of negative feedback and reviews are: - "Apkdays Call of Duty Warzone Mobile is a buggy and unstable game that crashes frequently and drains battery life. It is not compatible with many devices and may contain malware or viruses." - A player from App Store - "Apkdays Call of Duty Warzone Mobile is a cheap and lazy game that copies everything from the original game without adding anything new or innovative. It is a waste of time and money." - A critic from GameSpot - "Apkdays Call of Duty Warzone Mobile is a risky and illegal game that violates the intellectual property rights of Activision and may result in legal action or account ban. It is not worth the trouble." - A player from YouTube
Conclusion
-
A summary of the main points and a call to action for the readers
-
Apkdays Call of Duty Warzone Mobile is a mobile game that is based on Call of Duty: Warzone, the popular battle royale game that is available on PC and consoles. It is a modded version that has been created by a third-party developer called Apkdays. The game aims to replicate the authentic Call of Duty: Warzone experience on mobile devices, with high-quality graphics, intuitive controls, and cross-progression with Call of Duty: Warzone 2.0 and Call of Duty: Modern Warfare II.
-
In this article, we have told you everything you need to know about Apkdays Call of Duty Warzone Mobile, including what it is, how to download and install it, how to play it like a pro, and how it compares to other mobile battle royales. We have also shared some tips and tricks that will help you improve your skills and strategies in Verdansk.
-
If you are interested in trying out Apkdays Call of Duty Warzone Mobile, you can download it from the Apkdays website and enjoy Verdansk on the go. However, you should also be aware of the potential risks and drawbacks of using a modded version of the game, such as compatibility or security issues, high difficulty level, limited player base, or legal or ethical implications.
-
Apkdays Call of Duty Warzone Mobile is not for everyone, but it is definitely worth a shot for any fan of Call of Duty: Warzone or battle royale games in general. It is one of the best mobile battle royale games we have ever played, and we hope you will enjoy it as much as we did.
-
FAQs
-
Five unique questions and answers about Apkdays Call of Duty Warzone Mobile
-
-
Q: Is Apkdays Call of Duty Warzone Mobile safe to use?
-
A: Apkdays Call of Duty Warzone Mobile is not an official release from Activision and may have compatibility or security issues. You should download it at your own risk and discretion. You should also scan the file for malware or viruses before installing it.
-
Q: Is Apkdays Call of Duty Warzone Mobile free to play?
-
A: Apkdays Call of Duty Warzone Mobile is free to play and does not require any subscription or purchase. However, it may have some in-game purchases or ads that support the developer.
-
Q: How can I update Apkdays Call of Duty Warzone Mobile?
-
A: You can update Apkdays Call of Duty Warzone Mobile by visiting the Apkdays website and downloading the latest version of the game. You can also check for updates in the game itself.
-
Q: How can I contact the developer of Apkdays Call of Duty Warzone Mobile?
-
A: You can contact the developer of Apkdays Call of Duty Warzone Mobile by visiting their website and filling out their contact form. You can also follow them on their social media accounts or join their Discord server.
-
Q: How can I report a bug or a problem in Apkdays Call of Duty Warzone Mobile?
-
A: You can report a bug or a problem in Apkdays Call of Duty Warzone Mobile by visiting their website and filling out their bug report form. You can also contact them via their email address or their Discord server.
-
197e85843d
-
-
\ No newline at end of file
diff --git a/spaces/congsaPfin/Manga-OCR/logs/Download Getting Over It with Bennett Foddy APK and Enjoy the Ultimate Challenge.md b/spaces/congsaPfin/Manga-OCR/logs/Download Getting Over It with Bennett Foddy APK and Enjoy the Ultimate Challenge.md
deleted file mode 100644
index ad57104716b46e119c5a51f9240373d2c8dc9bcc..0000000000000000000000000000000000000000
--- a/spaces/congsaPfin/Manga-OCR/logs/Download Getting Over It with Bennett Foddy APK and Enjoy the Ultimate Challenge.md
+++ /dev/null
@@ -1,82 +0,0 @@
-
-
How to Download Getting Over It Free APK for Android
-
Do you want to play a game that will test your patience, skill, and sanity? Do you want to experience the thrill of climbing up a mountain with nothing but a hammer and a pot? Do you want to do it for free on your Android device? If you answered yes to any of these questions, then you might be interested in downloading Getting Over It free APK.
Getting Over It is an arcade climbing game where you carefully use a hammer to climb up a mountain. You move the hammer with the mouse, and that's all there is. With practice, you'll be able to jump, swing, climb and fly. But be careful, because one wrong move can send you flying back to where you started, or even worse. The game is designed to be frustrating, punishing, and rewarding at the same time. You'll hear the developer, Bennett Foddy, make philosophical observations about the problem at hand as you play. And if you manage to reach the top of the mountain, a magical reward awaits you.
-
A fan game based on a popular original
-
Getting Over It is a fan game based on the hugely popular Getting Over It with Bennett Foddy, which was released in 2017. The original game was inspired by Jazzuo's 2002 B-Game classic 'Sexy Hiking'. The fan game has a different theme and graphics, but the gameplay and mechanics are very similar. Instead of a man in a pot navigating a punishing and surreal landscape, this playful alternative has you playing as a cat in a plant pot climbing various colorful blocks and giant fruits.
-
What is an APK file?
-
A package file for Android apps
-
The term APK stands for Android Package Kit. An APK file, on the other hand, can be considered as an archive file for any app which comes with the .apk file extension. It is a package file format used by the Android operating system for the distribution and installation of mobile applications. An APK file contains all of a program's code, resources, assets, certificates, and manifest file.
-
A way to install apps from unknown sources
-
Most Android devices allow users to manually install APK files only after they turn on an "Unknown Sources" setting that allows installation from sources other than trusted ones like Google Play. One may do so for many reasons, such as during the development of apps, to install apps not found on the store, or to install an older version of an existing app. However, one should be careful when opening an APK file from a source they're unfamiliar with, as it may contain malware or viruses.
-
How to download Getting Over It free APK?
-
Find a reliable website that offers the APK file
-
The first step to download Getting Over It free APK is to find a website that offers the APK file for download. You can use Google or any other search engine to look for websites that have the APK file. Some examples of websites that offer Getting Over It free APK are [CrazyGames](^1^), [Steam](^2^), and [Google Play](^3^). Make sure that the website you choose is reliable and trustworthy, and that it has positive reviews and ratings from other users.
-
Enable unknown sources on your Android device
-
The next step is to enable unknown sources on your Android device. This will allow you to install apps from sources other than Google Play. To do this, navigate to one of these menus depending on your Android version:
-
How to download getting over it free apk for android
-Download getting over it with bennett foddy apk free
-Getting over it free apk download latest version
-Download getting over it mod apk free unlimited money
-Getting over it free apk download for pc windows 10
-Download getting over it apk free no verification
-Getting over it free apk download full game
-Download getting over it hack apk free all levels unlocked
-Getting over it free apk download without ads
-Download getting over it cracked apk free no root
-Getting over it free apk download for ios iphone ipad
-Download getting over it premium apk free original
-Getting over it free apk download offline mode
-Download getting over it mega mod apk free god mode
-Getting over it free apk download for mac os x
-Download getting over it pro apk free updated
-Getting over it free apk download with obb data file
-Download getting over it unlimited coins apk free
-Getting over it free apk download high graphics quality
-Download getting over it cheat apk free easy mode
-Getting over it free apk download for chromebook
-Download getting over it plus apk free extra features
-Getting over it free apk download with voice commentary
-Download getting over it gold apk free special edition
-Getting over it free apk download for android tv box
-Download getting over it lite apk free low size
-Getting over it free apk download with multiplayer mode
-Download getting over it rexdl apk free fast speed
-Getting over it free apk download for firestick fire tv
-Download getting over it revdl apk free direct link
-Getting over it free apk download for bluestacks emulator
-Download getting over it apkpure apk free safe secure
-Getting over it free apk download for linux ubuntu
-Download getting over it happymod apk free working tested
-Getting over it free apk download for nvidia shield tv
-Download getting over it moddroid apk free no ads
-Getting over it free apk download for smart tv lg samsung sony
-Download getting over it apkmirror apk free latest update
-Getting over it free apk download for kindle fire hd tablet
-Download getting over it apkmody apk free mod menu unlocked
-
-
Settings > Security > Unknown Sources
-
Settings > Apps and Notifications > Advanced > Special App Access > Install Unknown Apps
-
Settings > Biometrics and Security > Install Unknown Apps
-
-
Then, tap on the toggle switch to turn it on. You may see a warning message that says installing from unknown sources may harm your device. Tap on OK to proceed.
-
Download and install the APK file
-
The final step is to download and install the APK file. To do this, go back to the website where you found the APK file and tap on the download button. You may see a pop-up message that asks you to confirm the download. Tap on OK to start the download. Once the download is complete, you will see a notification that says "Download complete". Tap on the notification to open the APK file. You may see another pop-up message that asks you to confirm the installation. Tap on Install to begin the installation. Wait for a few seconds until the installation is finished. You will see a message that says "App installed". Tap on Open to launch the app and enjoy playing Getting Over It for free.
-
Conclusion
-
Getting Over It is a fun and challenging game that will test your skills and patience as you climb up a mountain with a hammer and a pot. You can download Getting Over It free APK for your Android device by following these simple steps: find a reliable website that offers the APK file, enable unknown sources on your device, and download and install the APK file. However, be careful when downloading from unknown sources, as they may contain malware or viruses. Always check the reviews and ratings of the website and the app before downloading. Have fun playing Getting Over It and don't give up!
-
FAQs
-
What are the requirements to play Getting Over It on Android?
-
To play Getting Over It on Android, you need an Android device that runs on Android 5.0 or higher, has at least 1 GB of RAM, and has at least 100 MB of free storage space.
-
Is Getting Over It free on Google Play?
-
No, Getting Over It is not free on Google Play. It costs $4.99 to download from Google Play. However, you can download Getting Over It free APK from other sources as explained in this article.
-
Is Getting Over It safe to play?
-
Getting Over It is safe to play as long as you download it from a trusted source. However, be aware that the game is very frustrating and may cause rage or despair in some players. If you feel stressed or angry while playing, take a break and calm down.
-
How long does it take to finish Getting Over It?
-
The length of time it takes to finish Getting Over It depends on your skill level and luck. Some players have finished the game in less than an hour, while others have spent hundreds of hours trying to reach the top. The average time to finish the game is around 5 hours.
-
What is the reward for finishing Getting Over It?
-
The reward for finishing Getting Over It is a secret that only those who have completed the game can know. However, some hints have been given by the developer and other players who have finished the game. The reward involves a golden cauldron, a special song, and a message from Bennett Foddy.
401be4b1e0
-
-
\ No newline at end of file
diff --git a/spaces/congsaPfin/Manga-OCR/logs/Stick War Legacy MOD APK Enjoy 999 Army Unlimited Upgrades and More.md b/spaces/congsaPfin/Manga-OCR/logs/Stick War Legacy MOD APK Enjoy 999 Army Unlimited Upgrades and More.md
deleted file mode 100644
index 9c86e8920ca2fc9d9fbcd2a712a8ecb89b1d650a..0000000000000000000000000000000000000000
--- a/spaces/congsaPfin/Manga-OCR/logs/Stick War Legacy MOD APK Enjoy 999 Army Unlimited Upgrades and More.md
+++ /dev/null
@@ -1,97 +0,0 @@
-
-
Stick War Legacy MOD APK: How to Get a New Army and Win Every Battle
-
Do you love playing stick figure games? Do you want to lead your own army of stickmen and conquer the world? If yes, then you should try Stick War Legacy, one of the most popular and addictive strategy games on mobile devices. But wait, there's more! You can also download Stick War Legacy MOD APK, which gives you unlimited gems, gold, mana, and access to a new army of powerful units. In this article, we will tell you everything you need to know about Stick War Legacy MOD APK, how to download and install it, and how to get a new army and win every battle. Let's get started!
-
Introduction
-
What is Stick War Legacy?
-
Stick War Legacy is a strategy game developed by Max Games Studios. It is based on the popular web game, Stick War, which was released in 2009. In this game, you play as the leader of a nation called Order, which is surrounded by enemies who want to destroy you. You must build and train your army of stickmen, mine resources, research technologies, and fight against other nations in epic battles. You can also play in different modes, such as campaign, tournament, endless zombies, and custom battles.
Stick War Legacy MOD APK is a modified version of the original game, which gives you some extra features and advantages. For example, you can get unlimited gems, gold, and mana, which are the main currencies in the game. You can use them to buy weapons, resources, upgrades, and more. You can also unlock and use a new army of stickmen, which have different abilities and skills. These units can help you defeat your enemies faster and easier.
-
Why do you need a new army in Stick War Legacy?
-
As you progress in the game, you will face more challenging and stronger enemies. They will have better weapons, defenses, and strategies. They will also have their own unique units, such as giants, wizards, archers, spearmen, and swordsmen. If you want to win against them, you need to have a diverse and powerful army of your own. That's why having a new army in Stick War Legacy MOD APK is very useful. You can have more options and flexibility in choosing your units and tactics.
-
How to download and install Stick War Legacy MOD APK
-
Step 1: Download the MOD APK file from a trusted source
-
The first thing you need to do is to download the Stick War Legacy MOD APK file from a reliable source. You can use the link below or search for other websites that offer it. Make sure that the file is safe and virus-free before downloading it.
-
Step 2: Enable unknown sources on your device
-
The next thing you need to do is to enable unknown sources on your device. This will allow you to install apps that are not from the official Google Play Store. To do this, go to your device settings > security > unknown sources > toggle on.
-Step 3: Install the MOD APK file and launch the game
-
The final thing you need to do is to install the Stick War Legacy MOD APK file and launch the game. To do this, locate the file in your device storage and tap on it. Follow the instructions on the screen to complete the installation. Once done, open the game and enjoy the new features and army.
-
stick war legacy mod apk unlimited gems and army
-stick war legacy mod apk 9999 army download
-stick war legacy mod apk new army update
-stick war legacy mod apk all weapons unlocked
-stick war legacy mod apk unlimited mana and gold
-stick war legacy mod apk 999 army latest version
-stick war legacy mod apk new army skins
-stick war legacy mod apk all levels unlocked
-stick war legacy mod apk unlimited resources and army
-stick war legacy mod apk 9999 army free download
-stick war legacy mod apk new army mode
-stick war legacy mod apk all characters unlocked
-stick war legacy mod apk unlimited troops and gems
-stick war legacy mod apk 999 army no root
-stick war legacy mod apk new army cheats
-stick war legacy mod apk all upgrades unlocked
-stick war legacy mod apk unlimited coins and army
-stick war legacy mod apk 9999 army hack
-stick war legacy mod apk new army gameplay
-stick war legacy mod apk all skins unlocked
-stick war legacy mod apk unlimited money and army
-stick war legacy mod apk 999 army offline
-stick war legacy mod apk new army features
-stick war legacy mod apk all modes unlocked
-stick war legacy mod apk unlimited diamonds and army
-stick war legacy mod apk 9999 army online
-stick war legacy mod apk new army review
-stick war legacy mod apk all armies unlocked
-stick war legacy mod apk unlimited power and army
-stick war legacy mod apk 999 army android
-stick war legacy mod apk new army trailer
-stick war legacy mod apk all items unlocked
-stick war legacy mod apk unlimited energy and army
-stick war legacy mod apk 9999 army android 1
-stick war legacy mod apk new army tips
-stick war legacy mod apk all missions unlocked
-stick war legacy mod apk unlimited skills and army
-stick war legacy mod apk 999 army ios
-stick war legacy mod apk new army guide
-stick war legacy mod apk all achievements unlocked
-
How to get a new army in Stick War Legacy MOD APK
-
What are the benefits of having a new army?
-
Having a new army in Stick War Legacy MOD APK can give you many benefits, such as:
-
-
You can have more variety and fun in choosing your units and strategies.
-
You can have more power and advantage in combat and defense.
-
You can have more challenges and rewards in completing missions and achievements.
-
You can have more satisfaction and enjoyment in playing the game.
-
-
What are the types of new army units in Stick War Legacy MOD APK?
-
The new army units in Stick War Legacy MOD APK are divided into five categories, each with their own strengths and weaknesses. They are:
-
Giants
-
Giants are huge and strong units that can deal massive damage and take a lot of hits. They are good for breaking enemy lines and smashing their defenses. However, they are also slow and expensive, and vulnerable to ranged attacks.
-
Wizards
-
Wizards are magical units that can cast spells and summon creatures. They are good for supporting your army and weakening your enemies. However, they are also fragile and costly, and need mana to use their abilities.
-
Archidons
-
Archidons are archers that can shoot arrows from a distance. They are good for harassing your enemies and killing them from afar. However, they are also weak and cheap, and need space to fire their arrows.
-
Speartons
-
Speartons are spearmen that can throw spears and shield themselves. They are good for defending your base and attacking your enemies. However, they are also medium and moderate, and need time to reload their spears.
-
Swordwrath
-
Swordwrath are swordsmen that can slash and rage. They are good for rushing your enemies and overwhelming them with numbers. However, they are also low and low, and need courage to fight.
-
How to unlock and upgrade new army units in Stick War Legacy MOD APK?
-
To unlock and upgrade new army units in Stick War Legacy MOD APK, you need to use gems, gold, or mana. You can get them by playing the game, completing missions, watching ads, or using the MOD APK features. You can also use them to buy other things, such as weapons, resources, skins, modes, etc. To unlock and upgrade new army units in Stick War Legacy MOD APK, you need to follow these steps:
-
-
Go to the main menu of the game.
-
Tap on the shop icon on the bottom right corner of the screen.
-
Select the unit you want to unlock or upgrade from the list.
-
Tap on the buy or upgrade button on the bottom of the screen.
-
Confirm your purchase or upgrade by tapping on the yes button.
-
Enjoy your new army unit in the game.
-
-
Conclusion
-
Summary of the main points
-
In conclusion, Stick War Legacy is a fun and addictive strategy game that lets you lead your own army of stickmen and conquer the world. You can also download Stick War Legacy MOD APK, which gives you unlimited gems, gold, mana, and access to a new army of powerful units. You can download and install it easily by following our guide. You can also get a new army by using gems, gold, or mana to unlock and upgrade them. Having a new army can give you many benefits, such as more variety, power, challenge, and satisfaction in playing the game.
-
Call to action
-
If you want to try Stick War Legacy MOD APK for yourself, you can download it from the link below or search for other sources online. Make sure that you follow our instructions carefully to avoid any problems or errors. Once you have it installed on your device, you can start playing the game and enjoy the new features and army. Don't forget to share your experience with us in the comments section below. We would love to hear from you!
- FAQs Q: Is Stick War Legacy MOD APK safe to A: Stick War Legacy MOD APK is safe to use as long as you download it from a trusted source and follow our instructions. However, you should always be careful when installing any third-party apps on your device, as they may contain malware or viruses. You should also backup your data before using the MOD APK, in case anything goes wrong. Q: How can I update Stick War Legacy MOD APK? A: To update Stick War Legacy MOD APK, you need to download the latest version of the MOD APK file from the same source you used before and install it over the existing one. You don't need to uninstall the previous version, as it will overwrite it automatically. However, you should always check the compatibility and features of the new version before updating, as they may differ from the old one. Q: Can I play Stick War Legacy MOD APK online with other players? A: No, you cannot play Stick War Legacy MOD APK online with other players, as it is a modified version of the original game. The MOD APK only works offline, and you can only play with the computer or yourself. If you want to play online with other players, you need to use the official version of the game from the Google Play Store. Q: Can I use Stick War Legacy MOD APK on iOS devices? A: No, you cannot use Stick War Legacy MOD APK on iOS devices, as it is only compatible with Android devices. The MOD APK file is an Android application package, which cannot be installed or run on iOS devices. If you want to play Stick War Legacy on iOS devices, you need to use the official version of the game from the App Store. Q: What are some tips and tricks for playing Stick War Legacy MOD APK? A: Some tips and tricks for playing Stick War Legacy MOD APK are: - Use your gems, gold, and mana wisely. Don't waste them on unnecessary things, and save them for important purchases and upgrades. - Experiment with different units and strategies. Find out what works best for you and your army, and adapt to different situations and enemies. - Balance your offense and defense. Don't neglect your base or your army, and protect them from enemy attacks. Don't be too aggressive or too passive, and find the right timing and opportunity to strike. - Have fun and enjoy the game. Don't get frustrated or bored by the game, and try to have a positive attitude. Remember that it is just a game, and not a real war. 401be4b1e0
-
-
\ No newline at end of file
diff --git a/spaces/coreml-community/ControlNet-v1-1-Annotators-cpu/annotator/oneformer/detectron2/structures/keypoints.py b/spaces/coreml-community/ControlNet-v1-1-Annotators-cpu/annotator/oneformer/detectron2/structures/keypoints.py
deleted file mode 100644
index b93ebed4f6554e67ba9bde8d3af90e8dbb3246b6..0000000000000000000000000000000000000000
--- a/spaces/coreml-community/ControlNet-v1-1-Annotators-cpu/annotator/oneformer/detectron2/structures/keypoints.py
+++ /dev/null
@@ -1,235 +0,0 @@
-# Copyright (c) Facebook, Inc. and its affiliates.
-import numpy as np
-from typing import Any, List, Tuple, Union
-import torch
-from torch.nn import functional as F
-
-
-class Keypoints:
- """
- Stores keypoint **annotation** data. GT Instances have a `gt_keypoints` property
- containing the x,y location and visibility flag of each keypoint. This tensor has shape
- (N, K, 3) where N is the number of instances and K is the number of keypoints per instance.
-
- The visibility flag follows the COCO format and must be one of three integers:
-
- * v=0: not labeled (in which case x=y=0)
- * v=1: labeled but not visible
- * v=2: labeled and visible
- """
-
- def __init__(self, keypoints: Union[torch.Tensor, np.ndarray, List[List[float]]]):
- """
- Arguments:
- keypoints: A Tensor, numpy array, or list of the x, y, and visibility of each keypoint.
- The shape should be (N, K, 3) where N is the number of
- instances, and K is the number of keypoints per instance.
- """
- device = keypoints.device if isinstance(keypoints, torch.Tensor) else torch.device("cpu")
- keypoints = torch.as_tensor(keypoints, dtype=torch.float32, device=device)
- assert keypoints.dim() == 3 and keypoints.shape[2] == 3, keypoints.shape
- self.tensor = keypoints
-
- def __len__(self) -> int:
- return self.tensor.size(0)
-
- def to(self, *args: Any, **kwargs: Any) -> "Keypoints":
- return type(self)(self.tensor.to(*args, **kwargs))
-
- @property
- def device(self) -> torch.device:
- return self.tensor.device
-
- def to_heatmap(self, boxes: torch.Tensor, heatmap_size: int) -> torch.Tensor:
- """
- Convert keypoint annotations to a heatmap of one-hot labels for training,
- as described in :paper:`Mask R-CNN`.
-
- Arguments:
- boxes: Nx4 tensor, the boxes to draw the keypoints to
-
- Returns:
- heatmaps:
- A tensor of shape (N, K), each element is integer spatial label
- in the range [0, heatmap_size**2 - 1] for each keypoint in the input.
- valid:
- A tensor of shape (N, K) containing whether each keypoint is in the roi or not.
- """
- return _keypoints_to_heatmap(self.tensor, boxes, heatmap_size)
-
- def __getitem__(self, item: Union[int, slice, torch.BoolTensor]) -> "Keypoints":
- """
- Create a new `Keypoints` by indexing on this `Keypoints`.
-
- The following usage are allowed:
-
- 1. `new_kpts = kpts[3]`: return a `Keypoints` which contains only one instance.
- 2. `new_kpts = kpts[2:10]`: return a slice of key points.
- 3. `new_kpts = kpts[vector]`, where vector is a torch.ByteTensor
- with `length = len(kpts)`. Nonzero elements in the vector will be selected.
-
- Note that the returned Keypoints might share storage with this Keypoints,
- subject to Pytorch's indexing semantics.
- """
- if isinstance(item, int):
- return Keypoints([self.tensor[item]])
- return Keypoints(self.tensor[item])
-
- def __repr__(self) -> str:
- s = self.__class__.__name__ + "("
- s += "num_instances={})".format(len(self.tensor))
- return s
-
- @staticmethod
- def cat(keypoints_list: List["Keypoints"]) -> "Keypoints":
- """
- Concatenates a list of Keypoints into a single Keypoints
-
- Arguments:
- keypoints_list (list[Keypoints])
-
- Returns:
- Keypoints: the concatenated Keypoints
- """
- assert isinstance(keypoints_list, (list, tuple))
- assert len(keypoints_list) > 0
- assert all(isinstance(keypoints, Keypoints) for keypoints in keypoints_list)
-
- cat_kpts = type(keypoints_list[0])(
- torch.cat([kpts.tensor for kpts in keypoints_list], dim=0)
- )
- return cat_kpts
-
-
-# TODO make this nicer, this is a direct translation from C2 (but removing the inner loop)
-def _keypoints_to_heatmap(
- keypoints: torch.Tensor, rois: torch.Tensor, heatmap_size: int
-) -> Tuple[torch.Tensor, torch.Tensor]:
- """
- Encode keypoint locations into a target heatmap for use in SoftmaxWithLoss across space.
-
- Maps keypoints from the half-open interval [x1, x2) on continuous image coordinates to the
- closed interval [0, heatmap_size - 1] on discrete image coordinates. We use the
- continuous-discrete conversion from Heckbert 1990 ("What is the coordinate of a pixel?"):
- d = floor(c) and c = d + 0.5, where d is a discrete coordinate and c is a continuous coordinate.
-
- Arguments:
- keypoints: tensor of keypoint locations in of shape (N, K, 3).
- rois: Nx4 tensor of rois in xyxy format
- heatmap_size: integer side length of square heatmap.
-
- Returns:
- heatmaps: A tensor of shape (N, K) containing an integer spatial label
- in the range [0, heatmap_size**2 - 1] for each keypoint in the input.
- valid: A tensor of shape (N, K) containing whether each keypoint is in
- the roi or not.
- """
-
- if rois.numel() == 0:
- return rois.new().long(), rois.new().long()
- offset_x = rois[:, 0]
- offset_y = rois[:, 1]
- scale_x = heatmap_size / (rois[:, 2] - rois[:, 0])
- scale_y = heatmap_size / (rois[:, 3] - rois[:, 1])
-
- offset_x = offset_x[:, None]
- offset_y = offset_y[:, None]
- scale_x = scale_x[:, None]
- scale_y = scale_y[:, None]
-
- x = keypoints[..., 0]
- y = keypoints[..., 1]
-
- x_boundary_inds = x == rois[:, 2][:, None]
- y_boundary_inds = y == rois[:, 3][:, None]
-
- x = (x - offset_x) * scale_x
- x = x.floor().long()
- y = (y - offset_y) * scale_y
- y = y.floor().long()
-
- x[x_boundary_inds] = heatmap_size - 1
- y[y_boundary_inds] = heatmap_size - 1
-
- valid_loc = (x >= 0) & (y >= 0) & (x < heatmap_size) & (y < heatmap_size)
- vis = keypoints[..., 2] > 0
- valid = (valid_loc & vis).long()
-
- lin_ind = y * heatmap_size + x
- heatmaps = lin_ind * valid
-
- return heatmaps, valid
-
-
-@torch.jit.script_if_tracing
-def heatmaps_to_keypoints(maps: torch.Tensor, rois: torch.Tensor) -> torch.Tensor:
- """
- Extract predicted keypoint locations from heatmaps.
-
- Args:
- maps (Tensor): (#ROIs, #keypoints, POOL_H, POOL_W). The predicted heatmap of logits for
- each ROI and each keypoint.
- rois (Tensor): (#ROIs, 4). The box of each ROI.
-
- Returns:
- Tensor of shape (#ROIs, #keypoints, 4) with the last dimension corresponding to
- (x, y, logit, score) for each keypoint.
-
- When converting discrete pixel indices in an NxN image to a continuous keypoint coordinate,
- we maintain consistency with :meth:`Keypoints.to_heatmap` by using the conversion from
- Heckbert 1990: c = d + 0.5, where d is a discrete coordinate and c is a continuous coordinate.
- """
-
- offset_x = rois[:, 0]
- offset_y = rois[:, 1]
-
- widths = (rois[:, 2] - rois[:, 0]).clamp(min=1)
- heights = (rois[:, 3] - rois[:, 1]).clamp(min=1)
- widths_ceil = widths.ceil()
- heights_ceil = heights.ceil()
-
- num_rois, num_keypoints = maps.shape[:2]
- xy_preds = maps.new_zeros(rois.shape[0], num_keypoints, 4)
-
- width_corrections = widths / widths_ceil
- height_corrections = heights / heights_ceil
-
- keypoints_idx = torch.arange(num_keypoints, device=maps.device)
-
- for i in range(num_rois):
- outsize = (int(heights_ceil[i]), int(widths_ceil[i]))
- roi_map = F.interpolate(maps[[i]], size=outsize, mode="bicubic", align_corners=False)
-
- # Although semantically equivalent, `reshape` is used instead of `squeeze` due
- # to limitation during ONNX export of `squeeze` in scripting mode
- roi_map = roi_map.reshape(roi_map.shape[1:]) # keypoints x H x W
-
- # softmax over the spatial region
- max_score, _ = roi_map.view(num_keypoints, -1).max(1)
- max_score = max_score.view(num_keypoints, 1, 1)
- tmp_full_resolution = (roi_map - max_score).exp_()
- tmp_pool_resolution = (maps[i] - max_score).exp_()
- # Produce scores over the region H x W, but normalize with POOL_H x POOL_W,
- # so that the scores of objects of different absolute sizes will be more comparable
- roi_map_scores = tmp_full_resolution / tmp_pool_resolution.sum((1, 2), keepdim=True)
-
- w = roi_map.shape[2]
- pos = roi_map.view(num_keypoints, -1).argmax(1)
-
- x_int = pos % w
- y_int = (pos - x_int) // w
-
- assert (
- roi_map_scores[keypoints_idx, y_int, x_int]
- == roi_map_scores.view(num_keypoints, -1).max(1)[0]
- ).all()
-
- x = (x_int.float() + 0.5) * width_corrections[i]
- y = (y_int.float() + 0.5) * height_corrections[i]
-
- xy_preds[i, :, 0] = x + offset_x[i]
- xy_preds[i, :, 1] = y + offset_y[i]
- xy_preds[i, :, 2] = roi_map[keypoints_idx, y_int, x_int]
- xy_preds[i, :, 3] = roi_map_scores[keypoints_idx, y_int, x_int]
-
- return xy_preds
diff --git a/spaces/coreml-community/ControlNet-v1-1-Annotators-cpu/annotator/zoe/zoedepth/models/base_models/midas_repo/mobile/android/gradlew.bat b/spaces/coreml-community/ControlNet-v1-1-Annotators-cpu/annotator/zoe/zoedepth/models/base_models/midas_repo/mobile/android/gradlew.bat
deleted file mode 100644
index 9618d8d9607cd91a0efb866bcac4810064ba6fac..0000000000000000000000000000000000000000
--- a/spaces/coreml-community/ControlNet-v1-1-Annotators-cpu/annotator/zoe/zoedepth/models/base_models/midas_repo/mobile/android/gradlew.bat
+++ /dev/null
@@ -1,100 +0,0 @@
-@rem
-@rem Copyright 2015 the original author or authors.
-@rem
-@rem Licensed under the Apache License, Version 2.0 (the "License");
-@rem you may not use this file except in compliance with the License.
-@rem You may obtain a copy of the License at
-@rem
-@rem https://www.apache.org/licenses/LICENSE-2.0
-@rem
-@rem Unless required by applicable law or agreed to in writing, software
-@rem distributed under the License is distributed on an "AS IS" BASIS,
-@rem WITHOUT WARRANTIES OR CONDITIONS OF ANY KIND, either express or implied.
-@rem See the License for the specific language governing permissions and
-@rem limitations under the License.
-@rem
-
-@if "%DEBUG%" == "" @echo off
-@rem ##########################################################################
-@rem
-@rem Gradle startup script for Windows
-@rem
-@rem ##########################################################################
-
-@rem Set local scope for the variables with windows NT shell
-if "%OS%"=="Windows_NT" setlocal
-
-set DIRNAME=%~dp0
-if "%DIRNAME%" == "" set DIRNAME=.
-set APP_BASE_NAME=%~n0
-set APP_HOME=%DIRNAME%
-
-@rem Add default JVM options here. You can also use JAVA_OPTS and GRADLE_OPTS to pass JVM options to this script.
-set DEFAULT_JVM_OPTS="-Xmx64m" "-Xms64m"
-
-@rem Find java.exe
-if defined JAVA_HOME goto findJavaFromJavaHome
-
-set JAVA_EXE=java.exe
-%JAVA_EXE% -version >NUL 2>&1
-if "%ERRORLEVEL%" == "0" goto init
-
-echo.
-echo ERROR: JAVA_HOME is not set and no 'java' command could be found in your PATH.
-echo.
-echo Please set the JAVA_HOME variable in your environment to match the
-echo location of your Java installation.
-
-goto fail
-
-:findJavaFromJavaHome
-set JAVA_HOME=%JAVA_HOME:"=%
-set JAVA_EXE=%JAVA_HOME%/bin/java.exe
-
-if exist "%JAVA_EXE%" goto init
-
-echo.
-echo ERROR: JAVA_HOME is set to an invalid directory: %JAVA_HOME%
-echo.
-echo Please set the JAVA_HOME variable in your environment to match the
-echo location of your Java installation.
-
-goto fail
-
-:init
-@rem Get command-line arguments, handling Windows variants
-
-if not "%OS%" == "Windows_NT" goto win9xME_args
-
-:win9xME_args
-@rem Slurp the command line arguments.
-set CMD_LINE_ARGS=
-set _SKIP=2
-
-:win9xME_args_slurp
-if "x%~1" == "x" goto execute
-
-set CMD_LINE_ARGS=%*
-
-:execute
-@rem Setup the command line
-
-set CLASSPATH=%APP_HOME%\gradle\wrapper\gradle-wrapper.jar
-
-@rem Execute Gradle
-"%JAVA_EXE%" %DEFAULT_JVM_OPTS% %JAVA_OPTS% %GRADLE_OPTS% "-Dorg.gradle.appname=%APP_BASE_NAME%" -classpath "%CLASSPATH%" org.gradle.wrapper.GradleWrapperMain %CMD_LINE_ARGS%
-
-:end
-@rem End local scope for the variables with windows NT shell
-if "%ERRORLEVEL%"=="0" goto mainEnd
-
-:fail
-rem Set variable GRADLE_EXIT_CONSOLE if you need the _script_ return code instead of
-rem the _cmd.exe /c_ return code!
-if not "" == "%GRADLE_EXIT_CONSOLE%" exit 1
-exit /b 1
-
-:mainEnd
-if "%OS%"=="Windows_NT" endlocal
-
-:omega
diff --git a/spaces/coreml-community/ControlNet-v1-1-Annotators-cpu/annotator/zoe/zoedepth/models/base_models/midas_repo/mobile/android/lib_support/src/main/java/org/tensorflow/lite/examples/classification/tflite/ClassifierQuantizedMobileNet.java b/spaces/coreml-community/ControlNet-v1-1-Annotators-cpu/annotator/zoe/zoedepth/models/base_models/midas_repo/mobile/android/lib_support/src/main/java/org/tensorflow/lite/examples/classification/tflite/ClassifierQuantizedMobileNet.java
deleted file mode 100644
index 94b06e3df659005c287733a8a37672863fdadd71..0000000000000000000000000000000000000000
--- a/spaces/coreml-community/ControlNet-v1-1-Annotators-cpu/annotator/zoe/zoedepth/models/base_models/midas_repo/mobile/android/lib_support/src/main/java/org/tensorflow/lite/examples/classification/tflite/ClassifierQuantizedMobileNet.java
+++ /dev/null
@@ -1,72 +0,0 @@
-/* Copyright 2017 The TensorFlow Authors. All Rights Reserved.
-
-Licensed under the Apache License, Version 2.0 (the "License");
-you may not use this file except in compliance with the License.
-You may obtain a copy of the License at
-
- http://www.apache.org/licenses/LICENSE-2.0
-
-Unless required by applicable law or agreed to in writing, software
-distributed under the License is distributed on an "AS IS" BASIS,
-WITHOUT WARRANTIES OR CONDITIONS OF ANY KIND, either express or implied.
-See the License for the specific language governing permissions and
-limitations under the License.
-==============================================================================*/
-
-package org.tensorflow.lite.examples.classification.tflite;
-
-import android.app.Activity;
-import java.io.IOException;
-import org.tensorflow.lite.examples.classification.tflite.Classifier.Device;
-import org.tensorflow.lite.support.common.TensorOperator;
-import org.tensorflow.lite.support.common.ops.NormalizeOp;
-
-/** This TensorFlow Lite classifier works with the quantized MobileNet model. */
-public class ClassifierQuantizedMobileNet extends Classifier {
-
- /**
- * The quantized model does not require normalization, thus set mean as 0.0f, and std as 1.0f to
- * bypass the normalization.
- */
- private static final float IMAGE_MEAN = 0.0f;
-
- private static final float IMAGE_STD = 1.0f;
-
- /** Quantized MobileNet requires additional dequantization to the output probability. */
- private static final float PROBABILITY_MEAN = 0.0f;
-
- private static final float PROBABILITY_STD = 255.0f;
-
- /**
- * Initializes a {@code ClassifierQuantizedMobileNet}.
- *
- * @param activity
- */
- public ClassifierQuantizedMobileNet(Activity activity, Device device, int numThreads)
- throws IOException {
- super(activity, device, numThreads);
- }
-
- @Override
- protected String getModelPath() {
- // you can download this file from
- // see build.gradle for where to obtain this file. It should be auto
- // downloaded into assets.
- return "model_quant_0.tflite";
- }
-
- @Override
- protected String getLabelPath() {
- return "labels.txt";
- }
-
- @Override
- protected TensorOperator getPreprocessNormalizeOp() {
- return new NormalizeOp(IMAGE_MEAN, IMAGE_STD);
- }
-
- @Override
- protected TensorOperator getPostprocessNormalizeOp() {
- return new NormalizeOp(PROBABILITY_MEAN, PROBABILITY_STD);
- }
-}
diff --git a/spaces/course-demos/marian-finetuned-kde4-en-to-fr/README.md b/spaces/course-demos/marian-finetuned-kde4-en-to-fr/README.md
deleted file mode 100644
index 4702b5cd4157148119786012193f262fa57ce07c..0000000000000000000000000000000000000000
--- a/spaces/course-demos/marian-finetuned-kde4-en-to-fr/README.md
+++ /dev/null
@@ -1,13 +0,0 @@
----
-title: Marian Finetuned Kde4 En To Fr
-emoji: 📈
-colorFrom: pink
-colorTo: pink
-sdk: gradio
-sdk_version: 2.9b40
-app_file: app.py
-pinned: false
-license: afl-3.0
----
-
-Check out the configuration reference at https://huggingface.co/docs/hub/spaces#reference
diff --git a/spaces/cscan/CodeFormer/CodeFormer/basicsr/ops/upfirdn2d/src/upfirdn2d.cpp b/spaces/cscan/CodeFormer/CodeFormer/basicsr/ops/upfirdn2d/src/upfirdn2d.cpp
deleted file mode 100644
index 43d0b6783a5b512b55815a291fcac2bebeea31e0..0000000000000000000000000000000000000000
--- a/spaces/cscan/CodeFormer/CodeFormer/basicsr/ops/upfirdn2d/src/upfirdn2d.cpp
+++ /dev/null
@@ -1,24 +0,0 @@
-// from https://github.com/rosinality/stylegan2-pytorch/blob/master/op/upfirdn2d.cpp
-#include
-
-
-torch::Tensor upfirdn2d_op(const torch::Tensor& input, const torch::Tensor& kernel,
- int up_x, int up_y, int down_x, int down_y,
- int pad_x0, int pad_x1, int pad_y0, int pad_y1);
-
-#define CHECK_CUDA(x) TORCH_CHECK(x.type().is_cuda(), #x " must be a CUDA tensor")
-#define CHECK_CONTIGUOUS(x) TORCH_CHECK(x.is_contiguous(), #x " must be contiguous")
-#define CHECK_INPUT(x) CHECK_CUDA(x); CHECK_CONTIGUOUS(x)
-
-torch::Tensor upfirdn2d(const torch::Tensor& input, const torch::Tensor& kernel,
- int up_x, int up_y, int down_x, int down_y,
- int pad_x0, int pad_x1, int pad_y0, int pad_y1) {
- CHECK_CUDA(input);
- CHECK_CUDA(kernel);
-
- return upfirdn2d_op(input, kernel, up_x, up_y, down_x, down_y, pad_x0, pad_x1, pad_y0, pad_y1);
-}
-
-PYBIND11_MODULE(TORCH_EXTENSION_NAME, m) {
- m.def("upfirdn2d", &upfirdn2d, "upfirdn2d (CUDA)");
-}
diff --git a/spaces/daddyjin/TalkingFaceGeneration/Demo_TFR_Pirenderer/src/audio2pose_models/cvae.py b/spaces/daddyjin/TalkingFaceGeneration/Demo_TFR_Pirenderer/src/audio2pose_models/cvae.py
deleted file mode 100644
index 4dd4d128445e197ebb3417905750ff8ef384b702..0000000000000000000000000000000000000000
--- a/spaces/daddyjin/TalkingFaceGeneration/Demo_TFR_Pirenderer/src/audio2pose_models/cvae.py
+++ /dev/null
@@ -1,149 +0,0 @@
-import torch
-import torch.nn.functional as F
-from torch import nn
-from Demo_TFR_Pirenderer.src.audio2pose_models.res_unet import ResUnet
-
-def class2onehot(idx, class_num):
-
- assert torch.max(idx).item() < class_num
- onehot = torch.zeros(idx.size(0), class_num).to(idx.device)
- onehot.scatter_(1, idx, 1)
- return onehot
-
-class CVAE(nn.Module):
- def __init__(self, cfg):
- super().__init__()
- encoder_layer_sizes = cfg.MODEL.CVAE.ENCODER_LAYER_SIZES
- decoder_layer_sizes = cfg.MODEL.CVAE.DECODER_LAYER_SIZES
- latent_size = cfg.MODEL.CVAE.LATENT_SIZE
- num_classes = cfg.DATASET.NUM_CLASSES
- audio_emb_in_size = cfg.MODEL.CVAE.AUDIO_EMB_IN_SIZE
- audio_emb_out_size = cfg.MODEL.CVAE.AUDIO_EMB_OUT_SIZE
- seq_len = cfg.MODEL.CVAE.SEQ_LEN
-
- self.latent_size = latent_size
-
- self.encoder = ENCODER(encoder_layer_sizes, latent_size, num_classes,
- audio_emb_in_size, audio_emb_out_size, seq_len)
- self.decoder = DECODER(decoder_layer_sizes, latent_size, num_classes,
- audio_emb_in_size, audio_emb_out_size, seq_len)
- def reparameterize(self, mu, logvar):
- std = torch.exp(0.5 * logvar)
- eps = torch.randn_like(std)
- return mu + eps * std
-
- def forward(self, batch):
- batch = self.encoder(batch)
- mu = batch['mu']
- logvar = batch['logvar']
- z = self.reparameterize(mu, logvar)
- batch['z'] = z
- return self.decoder(batch)
-
- def test(self, batch):
- '''
- class_id = batch['class']
- z = torch.randn([class_id.size(0), self.latent_size]).to(class_id.device)
- batch['z'] = z
- '''
- return self.decoder(batch)
-
-class ENCODER(nn.Module):
- def __init__(self, layer_sizes, latent_size, num_classes,
- audio_emb_in_size, audio_emb_out_size, seq_len):
- super().__init__()
-
- self.resunet = ResUnet()
- self.num_classes = num_classes
- self.seq_len = seq_len
-
- self.MLP = nn.Sequential()
- layer_sizes[0] += latent_size + seq_len*audio_emb_out_size + 6
- for i, (in_size, out_size) in enumerate(zip(layer_sizes[:-1], layer_sizes[1:])):
- self.MLP.add_module(
- name="L{:d}".format(i), module=nn.Linear(in_size, out_size))
- self.MLP.add_module(name="A{:d}".format(i), module=nn.ReLU())
-
- self.linear_means = nn.Linear(layer_sizes[-1], latent_size)
- self.linear_logvar = nn.Linear(layer_sizes[-1], latent_size)
- self.linear_audio = nn.Linear(audio_emb_in_size, audio_emb_out_size)
-
- self.classbias = nn.Parameter(torch.randn(self.num_classes, latent_size))
-
- def forward(self, batch):
- class_id = batch['class']
- pose_motion_gt = batch['pose_motion_gt'] #bs seq_len 6
- ref = batch['ref'] #bs 6
- bs = pose_motion_gt.shape[0]
- audio_in = batch['audio_emb'] # bs seq_len audio_emb_in_size
-
- #pose encode
- pose_emb = self.resunet(pose_motion_gt.unsqueeze(1)) #bs 1 seq_len 6
- pose_emb = pose_emb.reshape(bs, -1) #bs seq_len*6
-
- #audio mapping
- print(audio_in.shape)
- audio_out = self.linear_audio(audio_in) # bs seq_len audio_emb_out_size
- audio_out = audio_out.reshape(bs, -1)
-
- class_bias = self.classbias[class_id] #bs latent_size
- x_in = torch.cat([ref, pose_emb, audio_out, class_bias], dim=-1) #bs seq_len*(audio_emb_out_size+6)+latent_size
- x_out = self.MLP(x_in)
-
- mu = self.linear_means(x_out)
- logvar = self.linear_means(x_out) #bs latent_size
-
- batch.update({'mu':mu, 'logvar':logvar})
- return batch
-
-class DECODER(nn.Module):
- def __init__(self, layer_sizes, latent_size, num_classes,
- audio_emb_in_size, audio_emb_out_size, seq_len):
- super().__init__()
-
- self.resunet = ResUnet()
- self.num_classes = num_classes
- self.seq_len = seq_len
-
- self.MLP = nn.Sequential()
- input_size = latent_size + seq_len*audio_emb_out_size + 6
- for i, (in_size, out_size) in enumerate(zip([input_size]+layer_sizes[:-1], layer_sizes)):
- self.MLP.add_module(
- name="L{:d}".format(i), module=nn.Linear(in_size, out_size))
- if i+1 < len(layer_sizes):
- self.MLP.add_module(name="A{:d}".format(i), module=nn.ReLU())
- else:
- self.MLP.add_module(name="sigmoid", module=nn.Sigmoid())
-
- self.pose_linear = nn.Linear(6, 6)
- self.linear_audio = nn.Linear(audio_emb_in_size, audio_emb_out_size)
-
- self.classbias = nn.Parameter(torch.randn(self.num_classes, latent_size))
-
- def forward(self, batch):
-
- z = batch['z'] #bs latent_size
- bs = z.shape[0]
- class_id = batch['class']
- ref = batch['ref'] #bs 6
- audio_in = batch['audio_emb'] # bs seq_len audio_emb_in_size
- #print('audio_in: ', audio_in[:, :, :10])
-
- audio_out = self.linear_audio(audio_in) # bs seq_len audio_emb_out_size
- #print('audio_out: ', audio_out[:, :, :10])
- audio_out = audio_out.reshape([bs, -1]) # bs seq_len*audio_emb_out_size
- class_bias = self.classbias[class_id] #bs latent_size
-
- z = z + class_bias
- x_in = torch.cat([ref, z, audio_out], dim=-1)
- x_out = self.MLP(x_in) # bs layer_sizes[-1]
- x_out = x_out.reshape((bs, self.seq_len, -1))
-
- #print('x_out: ', x_out)
-
- pose_emb = self.resunet(x_out.unsqueeze(1)) #bs 1 seq_len 6
-
- pose_motion_pred = self.pose_linear(pose_emb.squeeze(1)) #bs seq_len 6
-
- batch.update({'pose_motion_pred':pose_motion_pred})
- return batch
diff --git a/spaces/daddyjin/TalkingFaceGeneration/Demo_TFR_Pirenderer/src/face3d/options/test_options.py b/spaces/daddyjin/TalkingFaceGeneration/Demo_TFR_Pirenderer/src/face3d/options/test_options.py
deleted file mode 100644
index 4ff3ad142779850d1d5a1640bc00f70d34d4a862..0000000000000000000000000000000000000000
--- a/spaces/daddyjin/TalkingFaceGeneration/Demo_TFR_Pirenderer/src/face3d/options/test_options.py
+++ /dev/null
@@ -1,21 +0,0 @@
-"""This script contains the test options for Deep3DFaceRecon_pytorch
-"""
-
-from .base_options import BaseOptions
-
-
-class TestOptions(BaseOptions):
- """This class includes test options.
-
- It also includes shared options defined in BaseOptions.
- """
-
- def initialize(self, parser):
- parser = BaseOptions.initialize(self, parser) # define shared options
- parser.add_argument('--phase', type=str, default='test', help='train, val, test, etc')
- parser.add_argument('--dataset_mode', type=str, default=None, help='chooses how datasets are loaded. [None | flist]')
- parser.add_argument('--img_folder', type=str, default='examples', help='folder for test images.')
-
- # Dropout and Batchnorm has different behavior during training and test.
- self.isTrain = False
- return parser
diff --git a/spaces/dcarpintero/nlp-summarizer-pegasus/.venv/lib/python3.9/site-packages/jsonschema/_validators.py b/spaces/dcarpintero/nlp-summarizer-pegasus/.venv/lib/python3.9/site-packages/jsonschema/_validators.py
deleted file mode 100644
index 45b53c9c47a82b9f69bf786d9596b8b1166628db..0000000000000000000000000000000000000000
--- a/spaces/dcarpintero/nlp-summarizer-pegasus/.venv/lib/python3.9/site-packages/jsonschema/_validators.py
+++ /dev/null
@@ -1,449 +0,0 @@
-from fractions import Fraction
-import re
-
-from jsonschema._utils import (
- ensure_list,
- equal,
- extras_msg,
- find_additional_properties,
- find_evaluated_item_indexes_by_schema,
- find_evaluated_property_keys_by_schema,
- unbool,
- uniq,
-)
-from jsonschema.exceptions import FormatError, ValidationError
-
-
-def patternProperties(validator, patternProperties, instance, schema):
- if not validator.is_type(instance, "object"):
- return
-
- for pattern, subschema in patternProperties.items():
- for k, v in instance.items():
- if re.search(pattern, k):
- yield from validator.descend(
- v, subschema, path=k, schema_path=pattern,
- )
-
-
-def propertyNames(validator, propertyNames, instance, schema):
- if not validator.is_type(instance, "object"):
- return
-
- for property in instance:
- yield from validator.descend(instance=property, schema=propertyNames)
-
-
-def additionalProperties(validator, aP, instance, schema):
- if not validator.is_type(instance, "object"):
- return
-
- extras = set(find_additional_properties(instance, schema))
-
- if validator.is_type(aP, "object"):
- for extra in extras:
- yield from validator.descend(instance[extra], aP, path=extra)
- elif not aP and extras:
- if "patternProperties" in schema:
- verb = "does" if len(extras) == 1 else "do"
- joined = ", ".join(repr(each) for each in sorted(extras))
- patterns = ", ".join(
- repr(each) for each in sorted(schema["patternProperties"])
- )
- error = f"{joined} {verb} not match any of the regexes: {patterns}"
- yield ValidationError(error)
- else:
- error = "Additional properties are not allowed (%s %s unexpected)"
- yield ValidationError(error % extras_msg(extras))
-
-
-def items(validator, items, instance, schema):
- if not validator.is_type(instance, "array"):
- return
-
- prefix = len(schema.get("prefixItems", []))
- total = len(instance)
- if items is False and total > prefix:
- message = f"Expected at most {prefix} items, but found {total}"
- yield ValidationError(message)
- else:
- for index in range(prefix, total):
- yield from validator.descend(
- instance=instance[index],
- schema=items,
- path=index,
- )
-
-
-def additionalItems(validator, aI, instance, schema):
- if (
- not validator.is_type(instance, "array")
- or validator.is_type(schema.get("items", {}), "object")
- ):
- return
-
- len_items = len(schema.get("items", []))
- if validator.is_type(aI, "object"):
- for index, item in enumerate(instance[len_items:], start=len_items):
- yield from validator.descend(item, aI, path=index)
- elif not aI and len(instance) > len(schema.get("items", [])):
- error = "Additional items are not allowed (%s %s unexpected)"
- yield ValidationError(
- error % extras_msg(instance[len(schema.get("items", [])):]),
- )
-
-
-def const(validator, const, instance, schema):
- if not equal(instance, const):
- yield ValidationError(f"{const!r} was expected")
-
-
-def contains(validator, contains, instance, schema):
- if not validator.is_type(instance, "array"):
- return
-
- matches = 0
- min_contains = schema.get("minContains", 1)
- max_contains = schema.get("maxContains", len(instance))
-
- for each in instance:
- if validator.evolve(schema=contains).is_valid(each):
- matches += 1
- if matches > max_contains:
- yield ValidationError(
- "Too many items match the given schema "
- f"(expected at most {max_contains})",
- validator="maxContains",
- validator_value=max_contains,
- )
- return
-
- if matches < min_contains:
- if not matches:
- yield ValidationError(
- f"{instance!r} does not contain items "
- "matching the given schema",
- )
- else:
- yield ValidationError(
- "Too few items match the given schema (expected at least "
- f"{min_contains} but only {matches} matched)",
- validator="minContains",
- validator_value=min_contains,
- )
-
-
-def exclusiveMinimum(validator, minimum, instance, schema):
- if not validator.is_type(instance, "number"):
- return
-
- if instance <= minimum:
- yield ValidationError(
- f"{instance!r} is less than or equal to "
- f"the minimum of {minimum!r}",
- )
-
-
-def exclusiveMaximum(validator, maximum, instance, schema):
- if not validator.is_type(instance, "number"):
- return
-
- if instance >= maximum:
- yield ValidationError(
- f"{instance!r} is greater than or equal "
- f"to the maximum of {maximum!r}",
- )
-
-
-def minimum(validator, minimum, instance, schema):
- if not validator.is_type(instance, "number"):
- return
-
- if instance < minimum:
- message = f"{instance!r} is less than the minimum of {minimum!r}"
- yield ValidationError(message)
-
-
-def maximum(validator, maximum, instance, schema):
- if not validator.is_type(instance, "number"):
- return
-
- if instance > maximum:
- message = f"{instance!r} is greater than the maximum of {maximum!r}"
- yield ValidationError(message)
-
-
-def multipleOf(validator, dB, instance, schema):
- if not validator.is_type(instance, "number"):
- return
-
- if isinstance(dB, float):
- quotient = instance / dB
- try:
- failed = int(quotient) != quotient
- except OverflowError:
- # When `instance` is large and `dB` is less than one,
- # quotient can overflow to infinity; and then casting to int
- # raises an error.
- #
- # In this case we fall back to Fraction logic, which is
- # exact and cannot overflow. The performance is also
- # acceptable: we try the fast all-float option first, and
- # we know that fraction(dB) can have at most a few hundred
- # digits in each part. The worst-case slowdown is therefore
- # for already-slow enormous integers or Decimals.
- failed = (Fraction(instance) / Fraction(dB)).denominator != 1
- else:
- failed = instance % dB
-
- if failed:
- yield ValidationError(f"{instance!r} is not a multiple of {dB}")
-
-
-def minItems(validator, mI, instance, schema):
- if validator.is_type(instance, "array") and len(instance) < mI:
- yield ValidationError(f"{instance!r} is too short")
-
-
-def maxItems(validator, mI, instance, schema):
- if validator.is_type(instance, "array") and len(instance) > mI:
- yield ValidationError(f"{instance!r} is too long")
-
-
-def uniqueItems(validator, uI, instance, schema):
- if (
- uI
- and validator.is_type(instance, "array")
- and not uniq(instance)
- ):
- yield ValidationError(f"{instance!r} has non-unique elements")
-
-
-def pattern(validator, patrn, instance, schema):
- if (
- validator.is_type(instance, "string")
- and not re.search(patrn, instance)
- ):
- yield ValidationError(f"{instance!r} does not match {patrn!r}")
-
-
-def format(validator, format, instance, schema):
- if validator.format_checker is not None:
- try:
- validator.format_checker.check(instance, format)
- except FormatError as error:
- yield ValidationError(error.message, cause=error.cause)
-
-
-def minLength(validator, mL, instance, schema):
- if validator.is_type(instance, "string") and len(instance) < mL:
- yield ValidationError(f"{instance!r} is too short")
-
-
-def maxLength(validator, mL, instance, schema):
- if validator.is_type(instance, "string") and len(instance) > mL:
- yield ValidationError(f"{instance!r} is too long")
-
-
-def dependentRequired(validator, dependentRequired, instance, schema):
- if not validator.is_type(instance, "object"):
- return
-
- for property, dependency in dependentRequired.items():
- if property not in instance:
- continue
-
- for each in dependency:
- if each not in instance:
- message = f"{each!r} is a dependency of {property!r}"
- yield ValidationError(message)
-
-
-def dependentSchemas(validator, dependentSchemas, instance, schema):
- if not validator.is_type(instance, "object"):
- return
-
- for property, dependency in dependentSchemas.items():
- if property not in instance:
- continue
- yield from validator.descend(
- instance, dependency, schema_path=property,
- )
-
-
-def enum(validator, enums, instance, schema):
- if instance == 0 or instance == 1:
- unbooled = unbool(instance)
- if all(unbooled != unbool(each) for each in enums):
- yield ValidationError(f"{instance!r} is not one of {enums!r}")
- elif instance not in enums:
- yield ValidationError(f"{instance!r} is not one of {enums!r}")
-
-
-def ref(validator, ref, instance, schema):
- yield from validator._validate_reference(ref=ref, instance=instance)
-
-
-def dynamicRef(validator, dynamicRef, instance, schema):
- yield from validator._validate_reference(ref=dynamicRef, instance=instance)
-
-
-def type(validator, types, instance, schema):
- types = ensure_list(types)
-
- if not any(validator.is_type(instance, type) for type in types):
- reprs = ", ".join(repr(type) for type in types)
- yield ValidationError(f"{instance!r} is not of type {reprs}")
-
-
-def properties(validator, properties, instance, schema):
- if not validator.is_type(instance, "object"):
- return
-
- for property, subschema in properties.items():
- if property in instance:
- yield from validator.descend(
- instance[property],
- subschema,
- path=property,
- schema_path=property,
- )
-
-
-def required(validator, required, instance, schema):
- if not validator.is_type(instance, "object"):
- return
- for property in required:
- if property not in instance:
- yield ValidationError(f"{property!r} is a required property")
-
-
-def minProperties(validator, mP, instance, schema):
- if validator.is_type(instance, "object") and len(instance) < mP:
- yield ValidationError(f"{instance!r} does not have enough properties")
-
-
-def maxProperties(validator, mP, instance, schema):
- if not validator.is_type(instance, "object"):
- return
- if validator.is_type(instance, "object") and len(instance) > mP:
- yield ValidationError(f"{instance!r} has too many properties")
-
-
-def allOf(validator, allOf, instance, schema):
- for index, subschema in enumerate(allOf):
- yield from validator.descend(instance, subschema, schema_path=index)
-
-
-def anyOf(validator, anyOf, instance, schema):
- all_errors = []
- for index, subschema in enumerate(anyOf):
- errs = list(validator.descend(instance, subschema, schema_path=index))
- if not errs:
- break
- all_errors.extend(errs)
- else:
- yield ValidationError(
- f"{instance!r} is not valid under any of the given schemas",
- context=all_errors,
- )
-
-
-def oneOf(validator, oneOf, instance, schema):
- subschemas = enumerate(oneOf)
- all_errors = []
- for index, subschema in subschemas:
- errs = list(validator.descend(instance, subschema, schema_path=index))
- if not errs:
- first_valid = subschema
- break
- all_errors.extend(errs)
- else:
- yield ValidationError(
- f"{instance!r} is not valid under any of the given schemas",
- context=all_errors,
- )
-
- more_valid = [
- each for _, each in subschemas
- if validator.evolve(schema=each).is_valid(instance)
- ]
- if more_valid:
- more_valid.append(first_valid)
- reprs = ", ".join(repr(schema) for schema in more_valid)
- yield ValidationError(f"{instance!r} is valid under each of {reprs}")
-
-
-def not_(validator, not_schema, instance, schema):
- if validator.evolve(schema=not_schema).is_valid(instance):
- message = f"{instance!r} should not be valid under {not_schema!r}"
- yield ValidationError(message)
-
-
-def if_(validator, if_schema, instance, schema):
- if validator.evolve(schema=if_schema).is_valid(instance):
- if "then" in schema:
- then = schema["then"]
- yield from validator.descend(instance, then, schema_path="then")
- elif "else" in schema:
- else_ = schema["else"]
- yield from validator.descend(instance, else_, schema_path="else")
-
-
-def unevaluatedItems(validator, unevaluatedItems, instance, schema):
- if not validator.is_type(instance, "array"):
- return
- evaluated_item_indexes = find_evaluated_item_indexes_by_schema(
- validator, instance, schema,
- )
- unevaluated_items = [
- item for index, item in enumerate(instance)
- if index not in evaluated_item_indexes
- ]
- if unevaluated_items:
- error = "Unevaluated items are not allowed (%s %s unexpected)"
- yield ValidationError(error % extras_msg(unevaluated_items))
-
-
-def unevaluatedProperties(validator, unevaluatedProperties, instance, schema):
- if not validator.is_type(instance, "object"):
- return
- evaluated_keys = find_evaluated_property_keys_by_schema(
- validator, instance, schema,
- )
- unevaluated_keys = []
- for property in instance:
- if property not in evaluated_keys:
- for _ in validator.descend(
- instance[property],
- unevaluatedProperties,
- path=property,
- schema_path=property,
- ):
- # FIXME: Include context for each unevaluated property
- # indicating why it's invalid under the subschema.
- unevaluated_keys.append(property)
-
- if unevaluated_keys:
- if unevaluatedProperties is False:
- error = "Unevaluated properties are not allowed (%s %s unexpected)"
- yield ValidationError(error % extras_msg(unevaluated_keys))
- else:
- error = (
- "Unevaluated properties are not valid under "
- "the given schema (%s %s unevaluated and invalid)"
- )
- yield ValidationError(error % extras_msg(unevaluated_keys))
-
-
-def prefixItems(validator, prefixItems, instance, schema):
- if not validator.is_type(instance, "array"):
- return
-
- for (index, item), subschema in zip(enumerate(instance), prefixItems):
- yield from validator.descend(
- instance=item,
- schema=subschema,
- schema_path=index,
- path=index,
- )
diff --git a/spaces/dcq/freegpt-webui/g4f/Provider/Providers/ChatgptAi.py b/spaces/dcq/freegpt-webui/g4f/Provider/Providers/ChatgptAi.py
deleted file mode 100644
index 504fdb37d4099e5f21eeea4a5101e3e42f59aec2..0000000000000000000000000000000000000000
--- a/spaces/dcq/freegpt-webui/g4f/Provider/Providers/ChatgptAi.py
+++ /dev/null
@@ -1,51 +0,0 @@
-import os
-import requests, re
-from ...typing import sha256, Dict, get_type_hints
-
-url = 'https://chatgpt.ai/gpt-4/'
-model = ['gpt-4']
-supports_stream = True
-needs_auth = False
-
-def _create_completion(model: str, messages: list, stream: bool, **kwargs):
- chat = ''
- for message in messages:
- chat += '%s: %s\n' % (message['role'], message['content'])
- chat += 'assistant: '
-
- response = requests.get('https://chatgpt.ai/gpt-4/')
-
- nonce, post_id, _, bot_id = re.findall(r'data-nonce="(.*)"\n data-post-id="(.*)"\n data-url="(.*)"\n data-bot-id="(.*)"\n data-width', response.text)[0]
-
- headers = {
- 'authority': 'chatgpt.ai',
- 'accept': '*/*',
- 'accept-language': 'en,fr-FR;q=0.9,fr;q=0.8,es-ES;q=0.7,es;q=0.6,en-US;q=0.5,am;q=0.4,de;q=0.3',
- 'cache-control': 'no-cache',
- 'origin': 'https://chatgpt.ai',
- 'pragma': 'no-cache',
- 'referer': 'https://chatgpt.ai/gpt-4/',
- 'sec-ch-ua': '"Not.A/Brand";v="8", "Chromium";v="114", "Google Chrome";v="114"',
- 'sec-ch-ua-mobile': '?0',
- 'sec-ch-ua-platform': '"Windows"',
- 'sec-fetch-dest': 'empty',
- 'sec-fetch-mode': 'cors',
- 'sec-fetch-site': 'same-origin',
- 'user-agent': 'Mozilla/5.0 (Windows NT 10.0; Win64; x64) AppleWebKit/537.36 (KHTML, like Gecko) Chrome/114.0.0.0 Safari/537.36',
- }
- data = {
- '_wpnonce': nonce,
- 'post_id': post_id,
- 'url': 'https://chatgpt.ai/gpt-4',
- 'action': 'wpaicg_chat_shortcode_message',
- 'message': chat,
- 'bot_id': bot_id
- }
-
- response = requests.post('https://chatgpt.ai/wp-admin/admin-ajax.php',
- headers=headers, data=data)
-
- yield (response.json()['data'])
-
-params = f'g4f.Providers.{os.path.basename(__file__)[:-3]} supports: ' + \
- '(%s)' % ', '.join([f"{name}: {get_type_hints(_create_completion)[name].__name__}" for name in _create_completion.__code__.co_varnames[:_create_completion.__code__.co_argcount]])
\ No newline at end of file
diff --git a/spaces/declare-lab/tango/diffusers/src/diffusers/pipelines/stable_diffusion/safety_checker.py b/spaces/declare-lab/tango/diffusers/src/diffusers/pipelines/stable_diffusion/safety_checker.py
deleted file mode 100644
index 84b8aeb7bcde36bafd3412a800149f41e0b331c8..0000000000000000000000000000000000000000
--- a/spaces/declare-lab/tango/diffusers/src/diffusers/pipelines/stable_diffusion/safety_checker.py
+++ /dev/null
@@ -1,122 +0,0 @@
-# Copyright 2023 The HuggingFace Team. All rights reserved.
-#
-# Licensed under the Apache License, Version 2.0 (the "License");
-# you may not use this file except in compliance with the License.
-# You may obtain a copy of the License at
-#
-# http://www.apache.org/licenses/LICENSE-2.0
-#
-# Unless required by applicable law or agreed to in writing, software
-# distributed under the License is distributed on an "AS IS" BASIS,
-# WITHOUT WARRANTIES OR CONDITIONS OF ANY KIND, either express or implied.
-# See the License for the specific language governing permissions and
-# limitations under the License.
-
-import numpy as np
-import torch
-import torch.nn as nn
-from transformers import CLIPConfig, CLIPVisionModel, PreTrainedModel
-
-from ...utils import logging
-
-
-logger = logging.get_logger(__name__)
-
-
-def cosine_distance(image_embeds, text_embeds):
- normalized_image_embeds = nn.functional.normalize(image_embeds)
- normalized_text_embeds = nn.functional.normalize(text_embeds)
- return torch.mm(normalized_image_embeds, normalized_text_embeds.t())
-
-
-class StableDiffusionSafetyChecker(PreTrainedModel):
- config_class = CLIPConfig
-
- _no_split_modules = ["CLIPEncoderLayer"]
-
- def __init__(self, config: CLIPConfig):
- super().__init__(config)
-
- self.vision_model = CLIPVisionModel(config.vision_config)
- self.visual_projection = nn.Linear(config.vision_config.hidden_size, config.projection_dim, bias=False)
-
- self.concept_embeds = nn.Parameter(torch.ones(17, config.projection_dim), requires_grad=False)
- self.special_care_embeds = nn.Parameter(torch.ones(3, config.projection_dim), requires_grad=False)
-
- self.concept_embeds_weights = nn.Parameter(torch.ones(17), requires_grad=False)
- self.special_care_embeds_weights = nn.Parameter(torch.ones(3), requires_grad=False)
-
- @torch.no_grad()
- def forward(self, clip_input, images):
- pooled_output = self.vision_model(clip_input)[1] # pooled_output
- image_embeds = self.visual_projection(pooled_output)
-
- # we always cast to float32 as this does not cause significant overhead and is compatible with bfloat16
- special_cos_dist = cosine_distance(image_embeds, self.special_care_embeds).cpu().float().numpy()
- cos_dist = cosine_distance(image_embeds, self.concept_embeds).cpu().float().numpy()
-
- result = []
- batch_size = image_embeds.shape[0]
- for i in range(batch_size):
- result_img = {"special_scores": {}, "special_care": [], "concept_scores": {}, "bad_concepts": []}
-
- # increase this value to create a stronger `nfsw` filter
- # at the cost of increasing the possibility of filtering benign images
- adjustment = 0.0
-
- for concept_idx in range(len(special_cos_dist[0])):
- concept_cos = special_cos_dist[i][concept_idx]
- concept_threshold = self.special_care_embeds_weights[concept_idx].item()
- result_img["special_scores"][concept_idx] = round(concept_cos - concept_threshold + adjustment, 3)
- if result_img["special_scores"][concept_idx] > 0:
- result_img["special_care"].append({concept_idx, result_img["special_scores"][concept_idx]})
- adjustment = 0.01
-
- for concept_idx in range(len(cos_dist[0])):
- concept_cos = cos_dist[i][concept_idx]
- concept_threshold = self.concept_embeds_weights[concept_idx].item()
- result_img["concept_scores"][concept_idx] = round(concept_cos - concept_threshold + adjustment, 3)
- if result_img["concept_scores"][concept_idx] > 0:
- result_img["bad_concepts"].append(concept_idx)
-
- result.append(result_img)
-
- has_nsfw_concepts = [len(res["bad_concepts"]) > 0 for res in result]
-
- for idx, has_nsfw_concept in enumerate(has_nsfw_concepts):
- if has_nsfw_concept:
- images[idx] = np.zeros(images[idx].shape) # black image
-
- if any(has_nsfw_concepts):
- logger.warning(
- "Potential NSFW content was detected in one or more images. A black image will be returned instead."
- " Try again with a different prompt and/or seed."
- )
-
- return images, has_nsfw_concepts
-
- @torch.no_grad()
- def forward_onnx(self, clip_input: torch.FloatTensor, images: torch.FloatTensor):
- pooled_output = self.vision_model(clip_input)[1] # pooled_output
- image_embeds = self.visual_projection(pooled_output)
-
- special_cos_dist = cosine_distance(image_embeds, self.special_care_embeds)
- cos_dist = cosine_distance(image_embeds, self.concept_embeds)
-
- # increase this value to create a stronger `nsfw` filter
- # at the cost of increasing the possibility of filtering benign images
- adjustment = 0.0
-
- special_scores = special_cos_dist - self.special_care_embeds_weights + adjustment
- # special_scores = special_scores.round(decimals=3)
- special_care = torch.any(special_scores > 0, dim=1)
- special_adjustment = special_care * 0.01
- special_adjustment = special_adjustment.unsqueeze(1).expand(-1, cos_dist.shape[1])
-
- concept_scores = (cos_dist - self.concept_embeds_weights) + special_adjustment
- # concept_scores = concept_scores.round(decimals=3)
- has_nsfw_concepts = torch.any(concept_scores > 0, dim=1)
-
- images[has_nsfw_concepts] = 0.0 # black image
-
- return images, has_nsfw_concepts
diff --git a/spaces/deepghs/nsfw_prediction/app.py b/spaces/deepghs/nsfw_prediction/app.py
deleted file mode 100644
index 01aaeee2617734de7a3801c50cd61776c59eb1ec..0000000000000000000000000000000000000000
--- a/spaces/deepghs/nsfw_prediction/app.py
+++ /dev/null
@@ -1,58 +0,0 @@
-import os
-from functools import lru_cache
-
-import gradio as gr
-import numpy as np
-from PIL import Image
-from huggingface_hub import hf_hub_download
-from imgutils.data import load_image
-from imgutils.utils import open_onnx_model
-
-_MODELS = [
- ('nsfwjs.onnx', 224),
- ('inception_v3.onnx', 299),
-]
-_MODEL_NAMES = [name for name, _ in _MODELS]
-_DEFAULT_MODEL_NAME = _MODEL_NAMES[0]
-_MODEL_TO_SIZE = dict(_MODELS)
-
-
-@lru_cache()
-def _onnx_model(name):
- return open_onnx_model(hf_hub_download(
- 'deepghs/imgutils-models',
- f'nsfw/{name}'
- ))
-
-
-def _image_preprocess(image, size: int = 224) -> np.ndarray:
- image = load_image(image, mode='RGB').resize((size, size), Image.NEAREST)
- return (np.array(image) / 255.0)[None, ...]
-
-
-_LABELS = ['drawings', 'hentai', 'neutral', 'porn', 'sexy']
-
-
-def predict(image, model_name):
- input_ = _image_preprocess(image, _MODEL_TO_SIZE[model_name]).astype(np.float32)
- output_, = _onnx_model(model_name).run(['dense_3'], {'input_1': input_})
- return dict(zip(_LABELS, map(float, output_[0])))
-
-
-if __name__ == '__main__':
- with gr.Blocks() as demo:
- with gr.Row():
- with gr.Column():
- gr_input_image = gr.Image(type='pil', label='Original Image')
- gr_model = gr.Dropdown(_MODEL_NAMES, value=_DEFAULT_MODEL_NAME, label='Model')
- gr_btn_submit = gr.Button(value='Tagging', variant='primary')
-
- with gr.Column():
- gr_ratings = gr.Label(label='Ratings')
-
- gr_btn_submit.click(
- predict,
- inputs=[gr_input_image, gr_model],
- outputs=[gr_ratings],
- )
- demo.queue(os.cpu_count()).launch()
diff --git a/spaces/desudes/desu/README.md b/spaces/desudes/desu/README.md
deleted file mode 100644
index 232ed9f79418b525626439c3950b15588bd7d895..0000000000000000000000000000000000000000
--- a/spaces/desudes/desu/README.md
+++ /dev/null
@@ -1,10 +0,0 @@
----
-title: Desu
-emoji: 📈
-colorFrom: green
-colorTo: yellow
-sdk: docker
-pinned: false
----
-
-Check out the configuration reference at https://huggingface.co/docs/hub/spaces-config-reference
diff --git a/spaces/dfhhr4/QQsign/bin/unidbg-fetch-qsign.bat b/spaces/dfhhr4/QQsign/bin/unidbg-fetch-qsign.bat
deleted file mode 100644
index 8b291e7303b0c07d14b714e5795473891363c85b..0000000000000000000000000000000000000000
--- a/spaces/dfhhr4/QQsign/bin/unidbg-fetch-qsign.bat
+++ /dev/null
@@ -1,89 +0,0 @@
-@rem
-@rem Copyright 2015 the original author or authors.
-@rem
-@rem Licensed under the Apache License, Version 2.0 (the "License");
-@rem you may not use this file except in compliance with the License.
-@rem You may obtain a copy of the License at
-@rem
-@rem https://www.apache.org/licenses/LICENSE-2.0
-@rem
-@rem Unless required by applicable law or agreed to in writing, software
-@rem distributed under the License is distributed on an "AS IS" BASIS,
-@rem WITHOUT WARRANTIES OR CONDITIONS OF ANY KIND, either express or implied.
-@rem See the License for the specific language governing permissions and
-@rem limitations under the License.
-@rem
-
-@if "%DEBUG%" == "" @echo off
-@rem ##########################################################################
-@rem
-@rem unidbg-fetch-qsign startup script for Windows
-@rem
-@rem ##########################################################################
-
-@rem Set local scope for the variables with windows NT shell
-if "%OS%"=="Windows_NT" setlocal
-
-set DIRNAME=%~dp0
-if "%DIRNAME%" == "" set DIRNAME=.
-set APP_BASE_NAME=%~n0
-set APP_HOME=%DIRNAME%..
-
-@rem Resolve any "." and ".." in APP_HOME to make it shorter.
-for %%i in ("%APP_HOME%") do set APP_HOME=%%~fi
-
-@rem Add default JVM options here. You can also use JAVA_OPTS and UNIDBG_FETCH_QSIGN_OPTS to pass JVM options to this script.
-set DEFAULT_JVM_OPTS=
-
-@rem Find java.exe
-if defined JAVA_HOME goto findJavaFromJavaHome
-
-set JAVA_EXE=java.exe
-%JAVA_EXE% -version >NUL 2>&1
-if "%ERRORLEVEL%" == "0" goto execute
-
-echo.
-echo ERROR: JAVA_HOME is not set and no 'java' command could be found in your PATH.
-echo.
-echo Please set the JAVA_HOME variable in your environment to match the
-echo location of your Java installation.
-
-goto fail
-
-:findJavaFromJavaHome
-set JAVA_HOME=%JAVA_HOME:"=%
-set JAVA_EXE=%JAVA_HOME%/bin/java.exe
-
-if exist "%JAVA_EXE%" goto execute
-
-echo.
-echo ERROR: JAVA_HOME is set to an invalid directory: %JAVA_HOME%
-echo.
-echo Please set the JAVA_HOME variable in your environment to match the
-echo location of your Java installation.
-
-goto fail
-
-:execute
-@rem Setup the command line
-
-set CLASSPATH=%APP_HOME%\lib\unidbg-fetch-qsign-1.1.9.jar;%APP_HOME%\lib\unidbg-android-105.jar;%APP_HOME%\lib\ktor-server-content-negotiation-jvm-2.3.1.jar;%APP_HOME%\lib\ktor-serialization-kotlinx-json-jvm-2.3.1.jar;%APP_HOME%\lib\ktor-server-status-pages-jvm-2.3.1.jar;%APP_HOME%\lib\ktor-server-netty-jvm-2.3.1.jar;%APP_HOME%\lib\ktor-server-host-common-jvm-2.3.1.jar;%APP_HOME%\lib\ktor-server-core-jvm-2.3.1.jar;%APP_HOME%\lib\ktor-serialization-kotlinx-jvm-2.3.1.jar;%APP_HOME%\lib\ktor-serialization-jvm-2.3.1.jar;%APP_HOME%\lib\ktor-events-jvm-2.3.1.jar;%APP_HOME%\lib\ktor-websockets-jvm-2.3.1.jar;%APP_HOME%\lib\ktor-http-cio-jvm-2.3.1.jar;%APP_HOME%\lib\ktor-http-jvm-2.3.1.jar;%APP_HOME%\lib\ktor-network-jvm-2.3.1.jar;%APP_HOME%\lib\ktor-utils-jvm-2.3.1.jar;%APP_HOME%\lib\ktor-io-jvm-2.3.1.jar;%APP_HOME%\lib\kotlin-stdlib-jdk8-1.8.22.jar;%APP_HOME%\lib\kotlinx-serialization-json-jvm-1.5.1.jar;%APP_HOME%\lib\kotlinx-serialization-protobuf-jvm-1.5.1.jar;%APP_HOME%\lib\kotlinx-serialization-core-jvm-1.5.1.jar;%APP_HOME%\lib\logback-classic-1.2.11.jar;%APP_HOME%\lib\kotlinx-coroutines-jdk8-1.7.1.jar;%APP_HOME%\lib\kotlinx-coroutines-core-jvm-1.7.1.jar;%APP_HOME%\lib\kotlin-stdlib-jdk7-1.8.22.jar;%APP_HOME%\lib\kotlin-reflect-1.8.10.jar;%APP_HOME%\lib\kotlin-stdlib-1.8.22.jar;%APP_HOME%\lib\slf4j-api-1.7.36.jar;%APP_HOME%\lib\kotlin-stdlib-common-1.8.22.jar;%APP_HOME%\lib\config-1.4.2.jar;%APP_HOME%\lib\jansi-2.4.0.jar;%APP_HOME%\lib\netty-codec-http2-4.1.92.Final.jar;%APP_HOME%\lib\alpn-api-1.1.3.v20160715.jar;%APP_HOME%\lib\netty-transport-native-kqueue-4.1.92.Final.jar;%APP_HOME%\lib\netty-transport-native-epoll-4.1.92.Final.jar;%APP_HOME%\lib\logback-core-1.2.11.jar;%APP_HOME%\lib\annotations-23.0.0.jar;%APP_HOME%\lib\netty-codec-http-4.1.92.Final.jar;%APP_HOME%\lib\netty-handler-4.1.92.Final.jar;%APP_HOME%\lib\netty-codec-4.1.92.Final.jar;%APP_HOME%\lib\netty-transport-classes-kqueue-4.1.92.Final.jar;%APP_HOME%\lib\netty-transport-classes-epoll-4.1.92.Final.jar;%APP_HOME%\lib\netty-transport-native-unix-common-4.1.92.Final.jar;%APP_HOME%\lib\netty-transport-4.1.92.Final.jar;%APP_HOME%\lib\netty-buffer-4.1.92.Final.jar;%APP_HOME%\lib\netty-resolver-4.1.92.Final.jar;%APP_HOME%\lib\netty-common-4.1.92.Final.jar
-
-
-@rem Execute unidbg-fetch-qsign
-"%JAVA_EXE%" %DEFAULT_JVM_OPTS% %JAVA_OPTS% %UNIDBG_FETCH_QSIGN_OPTS% -classpath "%CLASSPATH%" MainKt %*
-
-:end
-@rem End local scope for the variables with windows NT shell
-if "%ERRORLEVEL%"=="0" goto mainEnd
-
-:fail
-rem Set variable UNIDBG_FETCH_QSIGN_EXIT_CONSOLE if you need the _script_ return code instead of
-rem the _cmd.exe /c_ return code!
-if not "" == "%UNIDBG_FETCH_QSIGN_EXIT_CONSOLE%" exit 1
-exit /b 1
-
-:mainEnd
-if "%OS%"=="Windows_NT" endlocal
-
-:omega
diff --git a/spaces/diacanFperku/AutoGPT/Digi Loader 1 Exe TOP Download.md b/spaces/diacanFperku/AutoGPT/Digi Loader 1 Exe TOP Download.md
deleted file mode 100644
index abf18e1c636434a82404a9e6b51f4f0a4c099fb1..0000000000000000000000000000000000000000
--- a/spaces/diacanFperku/AutoGPT/Digi Loader 1 Exe TOP Download.md
+++ /dev/null
@@ -1,124 +0,0 @@
-
-
Digi Loader 1 Exe Download: What You Need to Know
-
If you are looking for a free and easy way to transfer files, connect to games online, or use the digiCLIP amplifier, you might want to try Digi Loader 1 Exe Download. This is a software that allows you to download and install various programs and files on your PC, USB stick, or mobile device. In this article, we will explain what Digi Loader 1 Exe is, how to use it, and where to get it.
Digi Loader 1 Exe is a file transfer software that can download and install various programs and files on your device. Some of the programs and files that you can download with Digi Loader 1 Exe are:
-
-
GSLite: This is a program that allows you to connect to games online, such as Coub, which is a video streaming platform that lets you create and watch video loops.
-
DigiCLIP-pmd: This is a wrapper for Windows that uses the PME-API (by Digi-Key) for direct communication between digiCLIP and the PLC. DigiCLIP is an amplifier that can be used in large-scale networks, such as water distribution networks. DigiCLIP-pmd can create the digiCLIP-DM (driver) and digiCLIP-DB (database) for the used digiCLIP, as well as the config files for the digiCLIP and all PME devices in the network.
-
DoubleDIN: This is a tool that can turn your DIN-A4 card into a USB key. You can use the USB key to run programs or download files on your device.
-
PME firmware: This is a firmware that can update your modules of the PME series. PME is a series of products that can measure physical quantities, such as temperature, pressure, or flow.
-
-
How to Use Digi Loader 1 Exe?
-
To use Digi Loader 1 Exe, you need to follow these steps:
-
-
Download Digi Loader 1 Exe from the link below.
-
Run the Digi Loader 1 Exe file on your device.
-
Select the program or file that you want to download and install.
-
Follow the instructions on the screen to complete the installation.
-
Enjoy using the program or file on your device.
-
-
Where to Get Digi Loader 1 Exe?
-
You can get Digi Loader 1 Exe from this link: https://bltlly.com/2tazUw. This link will take you to a website where you can download Digi Loader 1 Exe for free. You can also find more information about Digi Loader 1 Exe and its features on this website.
-
Conclusion
-
Digi Loader 1 Exe is a file transfer software that can help you download and install various programs and files on your device. You can use it to connect to games online, use the digiCLIP amplifier, turn your DIN-A4 card into a USB key, or update your PME modules. Digi Loader 1 Exe is free and easy to use. You can download it from the link above and enjoy its benefits.
-
-
How to Download and Install Digi Loader 1 Exe for Windows
-
If you want to use Digi Loader 1 Exe on your Windows PC, you need to follow these steps:
-
-
Go to this link: https://bltlly.com/2tazUw and click on the Download button.
-
Save the Digi Loader 1 Exe file on your PC.
-
Double-click on the Digi Loader 1 Exe file to run it.
-
Select the language and the destination folder for the installation.
-
Click on the Install button and wait for the installation to finish.
-
Click on the Finish button and launch Digi Loader 1 Exe from your desktop or start menu.
-
-
How to Download and Install Digi Loader 1 Exe for Mac
-
If you want to use Digi Loader 1 Exe on your Mac, you need to follow these steps:
-
-
Go to this link: https://bltlly.com/2tazUw and click on the Download button.
-
Save the Digi Loader 1 Exe file on your Mac.
-
Open the Digi Loader 1 Exe file and drag it to the Applications folder.
-
Open the Applications folder and double-click on the Digi Loader 1 Exe icon.
-
Follow the instructions on the screen to complete the installation.
-
Launch Digi Loader 1 Exe from your Applications folder or dock.
-
-
How to Download and Install Digi Loader 1 Exe for Android
-
If you want to use Digi Loader 1 Exe on your Android device, you need to follow these steps:
-
-
Go to this link: https://bltlly.com/2tazUw and click on the Download button.
-
Save the Digi Loader 1 Exe file on your Android device.
-
Open the Digi Loader 1 Exe file and tap on the Install button.
-
Allow the installation from unknown sources if prompted.
-
Wait for the installation to finish and tap on the Open button.
How to Download and Install Digi Loader 1 Exe for Linux
-
If you want to use Digi Loader 1 Exe on your Linux device, you need to follow these steps:
-
-
Go to this link: https://bltlly.com/2tazUw and click on the Download button.
-
Save the Digi Loader 1 Exe file on your Linux device.
-
Open a terminal and navigate to the folder where you saved the Digi Loader 1 Exe file.
-
Type chmod +x DigiLoader1.exe to make the file executable.
-
Type ./DigiLoader1.exe to run the file.
-
Select the program or file that you want to download and install.
-
Follow the instructions on the screen to complete the installation.
-
Enjoy using the program or file on your device.
-
-
How to Uninstall Digi Loader 1 Exe
-
If you want to uninstall Digi Loader 1 Exe from your device, you need to follow these steps:
-
-
Open Digi Loader 1 Exe on your device.
-
Select the program or file that you want to uninstall.
-
Click on the Uninstall button and confirm your choice.
-
Wait for the uninstallation to finish and close Digi Loader 1 Exe.
-
Delete the Digi Loader 1 Exe file from your device.
-
-
Frequently Asked Questions about Digi Loader 1 Exe
-
Here are some of the most common questions and answers about Digi Loader 1 Exe:
-
-
Is Digi Loader 1 Exe safe?: Yes, Digi Loader 1 Exe is safe to use. It does not contain any viruses, malware, or spyware. It also does not collect or share any personal information from your device.
-
Is Digi Loader 1 Exe legal?: Yes, Digi Loader 1 Exe is legal to use. It does not violate any copyrights or trademarks of the programs and files that it downloads and installs. It also does not infringe on any intellectual property rights of the developers or owners of the programs and files.
How to Download and Install Digi Loader 1 Exe for iOS
-
If you want to use Digi Loader 1 Exe on your iOS device, you need to follow these steps:
-
-
Go to this link: https://bltlly.com/2tazUw and click on the Download button.
-
Save the Digi Loader 1 Exe file on your iOS device.
-
Open the Digi Loader 1 Exe file and tap on the Install button.
-
Allow the installation from unknown sources if prompted.
-
Wait for the installation to finish and tap on the Open button.
-
Enjoy using the program or file on your device.
-
-
What are the Features of Digi Loader 1 Exe?
-
Digi Loader 1 Exe has many features that make it a useful and convenient software tool. Some of the features are:
-
-
Multiple downloads: Digi Loader 1 Exe can download and install multiple programs and files at the same time. You can select the ones that you want and start the download process with one click.
-
Resume downloads: Digi Loader 1 Exe can resume interrupted or incomplete downloads. You can pause and resume the download process at any time without losing any data.
-
Automatic updates: Digi Loader 1 Exe can check for updates for the programs and files that it downloads and installs. You can choose to update them automatically or manually whenever there is a new version available.
How to Troubleshoot Digi Loader 1 Exe
-
If you encounter any problems or errors while using Digi Loader 1 Exe, you can try these solutions:
-
-
Check your internet connection: Make sure that your device is connected to a stable and fast internet connection. You can test your internet speed and ping using online tools or apps. If your internet connection is slow or unstable, you may experience delays, interruptions, or failures in downloading or installing programs and files with Digi Loader 1 Exe.
-
Check your device compatibility: Make sure that your device meets the minimum requirements for running Digi Loader 1 Exe. You can check the system requirements on the website where you downloaded Digi Loader 1 Exe. If your device does not meet the requirements, you may experience performance issues, crashes, or errors while using Digi Loader 1 Exe.
-
Check your device storage: Make sure that your device has enough free space to download and install programs and files with Digi Loader 1 Exe. You can check the storage capacity and usage on your device settings. If your device is running low on storage, you may encounter errors or failures in downloading or installing programs and files with Digi Loader 1 Exe.
How to Download and Install Digi Loader 1 Exe for Windows Phone
-
If you want to use Digi Loader 1 Exe on your Windows Phone, you need to follow these steps:
-
-
Go to this link: https://bltlly.com/2tazUw and click on the Download button.
-
Save the Digi Loader 1 Exe file on your Windows Phone.
-
Open the Digi Loader 1 Exe file and tap on the Install button.
-
Allow the installation from unknown sources if prompted.
-
Wait for the installation to finish and tap on the Open button.
-
Enjoy using the program or file on your device.
-
-
How to Download and Install Digi Loader 1 Exe for BlackBerry
-
If you want to use Digi Loader 1 Exe on your BlackBerry, you need to follow these steps:
-
-
Go to this link: https://bltlly.com/2tazUw and click on the Download button.
-
Save the Digi Loader 1 Exe file on your BlackBerry.
-
Open the Digi Loader 1 Exe file and tap on the Install button.
-
Allow the installation from unknown sources if prompted.
-
Wait for the installation to finish and tap on the Open button.
Conclusion
-
Digi Loader 1 Exe is a file transfer software that can help you download and install various programs and files on your device. You can use it to connect to games online, use the digiCLIP amplifier, turn your DIN-A4 card into a USB key, or update your PME modules. Digi Loader 1 Exe is free and easy to use. You can download it from the link above and enjoy its benefits. Digi Loader 1 Exe is compatible with different devices and platforms, such as Windows, Mac, Android, iOS, Linux, Windows Phone, and BlackBerry. Digi Loader 1 Exe has many features and benefits that make it a useful and convenient software tool. If you have any questions or problems while using Digi Loader 1 Exe, you can check the troubleshooting tips above or contact the support team.
3cee63e6c2
-
-
\ No newline at end of file
diff --git a/spaces/diacanFperku/AutoGPT/Metastock 11 Pro Full Crack Free Download 1.md b/spaces/diacanFperku/AutoGPT/Metastock 11 Pro Full Crack Free Download 1.md
deleted file mode 100644
index f6e10ca667ba999248bcde6668a4703a7f4ee147..0000000000000000000000000000000000000000
--- a/spaces/diacanFperku/AutoGPT/Metastock 11 Pro Full Crack Free Download 1.md
+++ /dev/null
@@ -1,80 +0,0 @@
-
-
MetaStock 11 Pro Endo Of Day: How to Download and Crack It for Free
-
If you are looking for a powerful trading software that can help you analyze the end of day market data and make profitable decisions, you might have heard of MetaStock 11 Pro Endo Of Day. This software is one of the most popular and trusted tools among traders, as it offers a variety of features and functions that can enhance your trading performance.
However, MetaStock 11 Pro Endo Of Day is not a cheap software. It costs hundreds of dollars to buy it from the official website, and you also need to pay a monthly subscription fee to access the data feed. That's why many people are looking for a way to download and crack it for free, so they can enjoy its benefits without spending a fortune.
-
What is MetaStock 11 Pro Endo Of Day?
-
MetaStock 11 Pro Endo Of Day is a trading software that allows you to analyze the end of day market data and create custom indicators, systems, explorations, and experts. It also lets you backtest your strategies and optimize your parameters to find the best settings for your trading goals.
-
MetaStock 11 Pro Endo Of Day is designed for traders who prefer to trade on a daily basis, using the end of day data from various exchanges and markets. It supports stocks, futures, options, forex, commodities, bonds, ETFs, and more. It also integrates with Reuters DataLink, which provides high-quality historical and real-time data for over 100 global markets.
-
How to Download MetaStock 11 Pro Endo Of Day for Free?
-
There are many websites that claim to offer MetaStock 11 Pro Endo Of Day for free download, but most of them are either scams or viruses. They may ask you to fill out surveys, enter your personal information, or download malicious software that can harm your computer or steal your data.
-
The only safe and reliable way to download MetaStock 11 Pro Endo Of Day for free is to use a torrent file from a trusted source. A torrent file is a small file that contains information about the files you want to download, such as their names, sizes, and locations on the internet. You need a torrent client software, such as uTorrent or BitTorrent, to open the torrent file and start downloading the files.
-
One of the best sources for MetaStock 11 Pro Endo Of Day torrent file is Archive.org. This website is a non-profit digital library that hosts millions of free books, movies, music, software, and more. You can find the torrent file for MetaStock 11 Pro Endo Of Day here: https://archive.org/details/ms-110-eod
-
-
How to Crack MetaStock 11 Pro Endo Of Day?
-
After downloading MetaStock 11 Pro Endo Of Day from Archive.org, you need to crack it to bypass the activation process and use it without any limitations. Cracking MetaStock 11 Pro Endo Of Day is not very difficult if you follow these steps:
-
-
Extract the downloaded files using WinRAR or any other file compression software.
-
Run the setup.exe file and follow the instructions to install MetaStock 11 Pro Endo Of Day on your computer.
-
Do not launch MetaStock 11 Pro Endo Of Day after installation.
-
Copy the crack file (mswin.exe) from the crack folder and paste it into the installation folder (usually C:\Program Files\Equis\MetaStock).
-
Replace the original file with the crack file when prompted.
-
Launch MetaStock 11 Pro Endo Of Day from your desktop shortcut or start menu.
-
Enjoy using MetaStock 11 Pro Endo Of Day for free!
-
-
Conclusion
-
MetaStock 11 Pro Endo Of Day is a great trading software that can help you improve your trading skills and results. However, it is not cheap to buy or use it legally. That's why many people are looking for a way to download and crack it for free.
-
In this article, we have shown you how to download MetaStock 11 Pro Endo Of Day for free using a torrent file from Archive.org, and how to crack it using a simple method. We hope this article was helpful and informative for you.
-
Please note that downloading and cracking MetaStock 11 Pro Endo Of Day may be illegal in some countries or regions. We do not condone or encourage piracy or copyright infringement. This article is for educational purposes only. Use MetaStock 11 Pro Endo Of Day at your own risk.
-
What are the Benefits of Using MetaStock 11 Pro Endo Of Day?
-
MetaStock 11 Pro Endo Of Day is not just a trading software, but a complete trading solution that can help you achieve your trading goals. Here are some of the benefits of using MetaStock 11 Pro Endo Of Day:
-
-
It gives you access to over 300 built-in indicators, systems, explorations, and experts that can help you analyze the market and find trading opportunities.
-
It allows you to create your own custom indicators, systems, explorations, and experts using the powerful MetaStock formula language.
-
It enables you to backtest your strategies and optimize your parameters using historical data and advanced statistical tools.
-
It provides you with a comprehensive charting package that can display multiple time frames, multiple securities, and multiple chart types.
-
It integrates with Reuters DataLink, which delivers high-quality historical and real-time data for over 100 global markets.
-
It supports multiple data formats, such as ASCII, MetaStock, CSI, TC2000, and more.
-
It offers a user-friendly interface that is easy to navigate and customize.
-
-
What are the Drawbacks of Using MetaStock 11 Pro Endo Of Day?
-
MetaStock 11 Pro Endo Of Day is not a perfect software, and it has some drawbacks that you should be aware of before using it. Here are some of the drawbacks of using MetaStock 11 Pro Endo Of Day:
-
-
It is not compatible with Windows 10 or later versions. You need to use Windows XP, Vista, or 7 to run it.
-
It is not compatible with Mac OS or Linux. You need to use a Windows emulator or a virtual machine to run it on these platforms.
-
It is not a standalone software. You need to have an internet connection and a data feed subscription to use it.
-
It is not a cheap software. It costs $499 to buy it from the official website, and you also need to pay $24.95 per month for the Reuters DataLink data feed.
-
It may not work properly if you download and crack it for free. You may encounter errors, bugs, or viruses that can affect your trading performance or damage your computer.
-
-
Is MetaStock 11 Pro Endo Of Day Worth It?
-
MetaStock 11 Pro Endo Of Day is a powerful trading software that can help you analyze the end of day market data and make profitable decisions. It has many features and functions that can enhance your trading performance and results.
-
However, MetaStock 11 Pro Endo Of Day is not a cheap software. It costs hundreds of dollars to buy it legally, and you also need to pay a monthly subscription fee for the data feed. Moreover, it has some compatibility issues and limitations that may affect your user experience.
-
If you are looking for a legal and reliable way to use MetaStock 11 Pro Endo Of Day, you should buy it from the official website and pay for the data feed. This way, you can enjoy its benefits without any risks or problems.
-
If you are looking for a free and easy way to use MetaStock 11 Pro Endo Of Day, you should download and crack it from Archive.org. This way, you can save money and time without buying or installing anything. However, you should be careful and cautious when doing this, as you may encounter some issues or dangers that can harm your computer or your trading performance.
-
The choice is yours. You should weigh the pros and cons of using MetaStock 11 Pro Endo Of Day before deciding whether it is worth it or not.
-
How to Use MetaStock 11 Pro Endo Of Day?
-
MetaStock 11 Pro Endo Of Day is a user-friendly software that can help you analyze the end of day market data and make trading decisions. Here are some basic steps on how to use MetaStock 11 Pro Endo Of Day:
-
-
Launch MetaStock 11 Pro Endo Of Day from your desktop shortcut or start menu.
-
Select the data source and the data range that you want to use for your analysis.
-
Choose the securities that you want to analyze from the instrument list or create your own custom list.
-
Open a chart window and select the chart type, time frame, and indicators that you want to use for your analysis.
-
Use the tools and functions on the toolbar and menu to perform various tasks, such as drawing trend lines, adding text, zooming in and out, etc.
-
Use the system tester to backtest your strategies and optimize your parameters.
-
Use the explorer to scan the market and find trading opportunities based on your criteria.
-
Use the expert advisor to get guidance and advice from predefined or custom experts.
-
Use the commentary window to get detailed information and analysis on any security or indicator.
-
-
What are the Alternatives to MetaStock 11 Pro Endo Of Day?
-
If you are not satisfied with MetaStock 11 Pro Endo Of Day or you want to try something different, you may want to check out some of the alternatives to MetaStock 11 Pro Endo Of Day. Here are some of the alternatives to MetaStock 11 Pro Endo Of Day that you may want to consider:
-
-
AmiBroker: AmiBroker is a trading software that offers advanced charting, scanning, backtesting, optimization, and automation features. It supports multiple data sources and formats, and it has a powerful formula language that allows you to create your own indicators and systems. It also has a low price compared to MetaStock 11 Pro Endo Of Day.
-
TradeStation: TradeStation is a trading software that offers comprehensive charting, analysis, testing, optimization, and execution features. It supports stocks, futures, options, forex, and more. It also has a built-in programming language that allows you to create your own indicators and strategies. It also has a high-quality data feed and a large community of users.
-
NinjaTrader: NinjaTrader is a trading software that offers advanced charting, analysis, testing, optimization, and automation features. It supports stocks, futures, forex, and more. It also has a flexible programming language that allows you to create your own indicators and strategies. It also has a free version that you can use for simulation and analysis.
-
-
Final Words
-
In this article, we have discussed MetaStock 11 Pro Endo Of Day, a trading software that can help you analyze the end of day market data and make profitable decisions. We have shown you how to download and crack it for free using a torrent file from Archive.org, and how to use it for your trading purposes. We have also discussed some of the benefits and drawbacks of using MetaStock 11 Pro Endo Of Day, and some of the alternatives to MetaStock 11 Pro Endo Of Day that you may want to try.
-
We hope this article was helpful and informative for you. If you have any questions or comments about MetaStock 11 Pro Endo Of Day or anything related to trading software, feel free to leave them below. We would love to hear from you.
-
We hope this article was helpful and informative for you. If you have any questions or comments about MetaStock 11 Pro Endo Of Day or anything related to trading software, feel free to leave them below. We would love to hear from you.
3cee63e6c2
-
-
\ No newline at end of file
diff --git a/spaces/diffusers/check_pr/app.py b/spaces/diffusers/check_pr/app.py
deleted file mode 100644
index 51e84cc44cac49ef25090d43d05972d3e5e417b1..0000000000000000000000000000000000000000
--- a/spaces/diffusers/check_pr/app.py
+++ /dev/null
@@ -1,101 +0,0 @@
-from diffusers import DiffusionPipeline
-import gradio as gr
-import torch
-import time
-import psutil
-
-
-start_time = time.time()
-
-device = "GPU 🔥" if torch.cuda.is_available() else "CPU 🥶"
-
-
-def error_str(error, title="Error"):
- return (
- f"""#### {title}
- {error}"""
- if error
- else ""
- )
-
-
-def inference(
- repo_id,
- discuss_nr,
- prompt,
-):
-
- print(psutil.virtual_memory()) # print memory usage
-
- seed = 0
- torch_device = "cuda" if "GPU" in device else "cpu"
-
- generator = torch.Generator(torch_device).manual_seed(seed)
-
- dtype = torch.float16 if torch_device == "cuda" else torch.float32
-
- try:
- revision = f"refs/pr/{discuss_nr}" if (discuss_nr != "" or discuss_nr is None) else None
- pipe = DiffusionPipeline.from_pretrained(repo_id, revision=revision, torch_dtype=dtype)
- pipe.to(torch_device)
-
- return pipe(prompt, generator=generator, num_inference_steps=25).images, f"Done. Seed: {seed}"
- except Exception as e:
- url = f"https://huggingface.co/{repo_id}/discussions/{discuss_nr}"
- message = f"There is a problem with your diffusers weights of the PR: {url}. Error message: \n"
- return None, error_str(message + e)
-
-
-with gr.Blocks(css="style.css") as demo:
- gr.HTML(
- f"""
-
-
- Space to test whether `diffusers` PRs work.
-
-
- Running on {device}
-
-
- """
- )
- with gr.Row():
-
- with gr.Column(scale=55):
- with gr.Group():
- repo_id = gr.Textbox(
- label="Repo id on Hub",
- placeholder="Path to model, e.g. CompVis/stable-diffusion-v1-4 for https://huggingface.co/CompVis/stable-diffusion-v1-4",
- )
- discuss_nr = gr.Textbox(
- label="Discussion number",
- placeholder="Number of the discussion that should be checked, e.g. 171 for https://huggingface.co/CompVis/stable-diffusion-v1-4/discussions/171",
- )
- prompt = gr.Textbox(
- label="Prompt",
- default="An astronaut riding a horse on Mars.",
- placeholder="Enter prompt.",
- )
- gallery = gr.Gallery(
- label="Generated images", show_label=False, elem_id="gallery"
- ).style(grid=[2], height="auto")
-
- error_output = gr.Markdown()
-
- generate = gr.Button(value="Generate").style(
- rounded=(False, True, True, False)
- )
-
- inputs = [
- repo_id,
- discuss_nr,
- prompt,
- ]
- outputs = [gallery, error_output]
- prompt.submit(inference, inputs=inputs, outputs=outputs)
- generate.click(inference, inputs=inputs, outputs=outputs)
-
-print(f"Space built in {time.time() - start_time:.2f} seconds")
-
-demo.queue(concurrency_count=1)
-demo.launch()
diff --git a/spaces/digitalxingtong/Azusa-Bert-VITS2/attentions.py b/spaces/digitalxingtong/Azusa-Bert-VITS2/attentions.py
deleted file mode 100644
index ecbdbc8be941a962046fc11fd6739b093112123e..0000000000000000000000000000000000000000
--- a/spaces/digitalxingtong/Azusa-Bert-VITS2/attentions.py
+++ /dev/null
@@ -1,343 +0,0 @@
-import copy
-import math
-import numpy as np
-import torch
-from torch import nn
-from torch.nn import functional as F
-
-import commons
-import modules
-from torch.nn.utils import weight_norm, remove_weight_norm
-class LayerNorm(nn.Module):
- def __init__(self, channels, eps=1e-5):
- super().__init__()
- self.channels = channels
- self.eps = eps
-
- self.gamma = nn.Parameter(torch.ones(channels))
- self.beta = nn.Parameter(torch.zeros(channels))
-
- def forward(self, x):
- x = x.transpose(1, -1)
- x = F.layer_norm(x, (self.channels,), self.gamma, self.beta, self.eps)
- return x.transpose(1, -1)
-
-
-
-@torch.jit.script
-def fused_add_tanh_sigmoid_multiply(input_a, input_b, n_channels):
- n_channels_int = n_channels[0]
- in_act = input_a + input_b
- t_act = torch.tanh(in_act[:, :n_channels_int, :])
- s_act = torch.sigmoid(in_act[:, n_channels_int:, :])
- acts = t_act * s_act
- return acts
-
-class Encoder(nn.Module):
- def __init__(self, hidden_channels, filter_channels, n_heads, n_layers, kernel_size=1, p_dropout=0., window_size=4, isflow = True, **kwargs):
- super().__init__()
- self.hidden_channels = hidden_channels
- self.filter_channels = filter_channels
- self.n_heads = n_heads
- self.n_layers = n_layers
- self.kernel_size = kernel_size
- self.p_dropout = p_dropout
- self.window_size = window_size
- if isflow:
- cond_layer = torch.nn.Conv1d(256, 2*hidden_channels*n_layers, 1)
- self.cond_pre = torch.nn.Conv1d(hidden_channels, 2*hidden_channels, 1)
- self.cond_layer = weight_norm(cond_layer, name='weight')
- self.gin_channels = 256
- self.cond_layer_idx = self.n_layers
- if 'gin_channels' in kwargs:
- self.gin_channels = kwargs['gin_channels']
- if self.gin_channels != 0:
- self.spk_emb_linear = nn.Linear(self.gin_channels, self.hidden_channels)
- # vits2 says 3rd block, so idx is 2 by default
- self.cond_layer_idx = kwargs['cond_layer_idx'] if 'cond_layer_idx' in kwargs else 2
- print(self.gin_channels, self.cond_layer_idx)
- assert self.cond_layer_idx < self.n_layers, 'cond_layer_idx should be less than n_layers'
- self.drop = nn.Dropout(p_dropout)
- self.attn_layers = nn.ModuleList()
- self.norm_layers_1 = nn.ModuleList()
- self.ffn_layers = nn.ModuleList()
- self.norm_layers_2 = nn.ModuleList()
- for i in range(self.n_layers):
- self.attn_layers.append(MultiHeadAttention(hidden_channels, hidden_channels, n_heads, p_dropout=p_dropout, window_size=window_size))
- self.norm_layers_1.append(LayerNorm(hidden_channels))
- self.ffn_layers.append(FFN(hidden_channels, hidden_channels, filter_channels, kernel_size, p_dropout=p_dropout))
- self.norm_layers_2.append(LayerNorm(hidden_channels))
- def forward(self, x, x_mask, g=None):
- attn_mask = x_mask.unsqueeze(2) * x_mask.unsqueeze(-1)
- x = x * x_mask
- for i in range(self.n_layers):
- if i == self.cond_layer_idx and g is not None:
- g = self.spk_emb_linear(g.transpose(1, 2))
- g = g.transpose(1, 2)
- x = x + g
- x = x * x_mask
- y = self.attn_layers[i](x, x, attn_mask)
- y = self.drop(y)
- x = self.norm_layers_1[i](x + y)
-
- y = self.ffn_layers[i](x, x_mask)
- y = self.drop(y)
- x = self.norm_layers_2[i](x + y)
- x = x * x_mask
- return x
-
-
-class Decoder(nn.Module):
- def __init__(self, hidden_channels, filter_channels, n_heads, n_layers, kernel_size=1, p_dropout=0., proximal_bias=False, proximal_init=True, **kwargs):
- super().__init__()
- self.hidden_channels = hidden_channels
- self.filter_channels = filter_channels
- self.n_heads = n_heads
- self.n_layers = n_layers
- self.kernel_size = kernel_size
- self.p_dropout = p_dropout
- self.proximal_bias = proximal_bias
- self.proximal_init = proximal_init
-
- self.drop = nn.Dropout(p_dropout)
- self.self_attn_layers = nn.ModuleList()
- self.norm_layers_0 = nn.ModuleList()
- self.encdec_attn_layers = nn.ModuleList()
- self.norm_layers_1 = nn.ModuleList()
- self.ffn_layers = nn.ModuleList()
- self.norm_layers_2 = nn.ModuleList()
- for i in range(self.n_layers):
- self.self_attn_layers.append(MultiHeadAttention(hidden_channels, hidden_channels, n_heads, p_dropout=p_dropout, proximal_bias=proximal_bias, proximal_init=proximal_init))
- self.norm_layers_0.append(LayerNorm(hidden_channels))
- self.encdec_attn_layers.append(MultiHeadAttention(hidden_channels, hidden_channels, n_heads, p_dropout=p_dropout))
- self.norm_layers_1.append(LayerNorm(hidden_channels))
- self.ffn_layers.append(FFN(hidden_channels, hidden_channels, filter_channels, kernel_size, p_dropout=p_dropout, causal=True))
- self.norm_layers_2.append(LayerNorm(hidden_channels))
-
- def forward(self, x, x_mask, h, h_mask):
- """
- x: decoder input
- h: encoder output
- """
- self_attn_mask = commons.subsequent_mask(x_mask.size(2)).to(device=x.device, dtype=x.dtype)
- encdec_attn_mask = h_mask.unsqueeze(2) * x_mask.unsqueeze(-1)
- x = x * x_mask
- for i in range(self.n_layers):
- y = self.self_attn_layers[i](x, x, self_attn_mask)
- y = self.drop(y)
- x = self.norm_layers_0[i](x + y)
-
- y = self.encdec_attn_layers[i](x, h, encdec_attn_mask)
- y = self.drop(y)
- x = self.norm_layers_1[i](x + y)
-
- y = self.ffn_layers[i](x, x_mask)
- y = self.drop(y)
- x = self.norm_layers_2[i](x + y)
- x = x * x_mask
- return x
-
-
-class MultiHeadAttention(nn.Module):
- def __init__(self, channels, out_channels, n_heads, p_dropout=0., window_size=None, heads_share=True, block_length=None, proximal_bias=False, proximal_init=False):
- super().__init__()
- assert channels % n_heads == 0
-
- self.channels = channels
- self.out_channels = out_channels
- self.n_heads = n_heads
- self.p_dropout = p_dropout
- self.window_size = window_size
- self.heads_share = heads_share
- self.block_length = block_length
- self.proximal_bias = proximal_bias
- self.proximal_init = proximal_init
- self.attn = None
-
- self.k_channels = channels // n_heads
- self.conv_q = nn.Conv1d(channels, channels, 1)
- self.conv_k = nn.Conv1d(channels, channels, 1)
- self.conv_v = nn.Conv1d(channels, channels, 1)
- self.conv_o = nn.Conv1d(channels, out_channels, 1)
- self.drop = nn.Dropout(p_dropout)
-
- if window_size is not None:
- n_heads_rel = 1 if heads_share else n_heads
- rel_stddev = self.k_channels**-0.5
- self.emb_rel_k = nn.Parameter(torch.randn(n_heads_rel, window_size * 2 + 1, self.k_channels) * rel_stddev)
- self.emb_rel_v = nn.Parameter(torch.randn(n_heads_rel, window_size * 2 + 1, self.k_channels) * rel_stddev)
-
- nn.init.xavier_uniform_(self.conv_q.weight)
- nn.init.xavier_uniform_(self.conv_k.weight)
- nn.init.xavier_uniform_(self.conv_v.weight)
- if proximal_init:
- with torch.no_grad():
- self.conv_k.weight.copy_(self.conv_q.weight)
- self.conv_k.bias.copy_(self.conv_q.bias)
-
- def forward(self, x, c, attn_mask=None):
- q = self.conv_q(x)
- k = self.conv_k(c)
- v = self.conv_v(c)
-
- x, self.attn = self.attention(q, k, v, mask=attn_mask)
-
- x = self.conv_o(x)
- return x
-
- def attention(self, query, key, value, mask=None):
- # reshape [b, d, t] -> [b, n_h, t, d_k]
- b, d, t_s, t_t = (*key.size(), query.size(2))
- query = query.view(b, self.n_heads, self.k_channels, t_t).transpose(2, 3)
- key = key.view(b, self.n_heads, self.k_channels, t_s).transpose(2, 3)
- value = value.view(b, self.n_heads, self.k_channels, t_s).transpose(2, 3)
-
- scores = torch.matmul(query / math.sqrt(self.k_channels), key.transpose(-2, -1))
- if self.window_size is not None:
- assert t_s == t_t, "Relative attention is only available for self-attention."
- key_relative_embeddings = self._get_relative_embeddings(self.emb_rel_k, t_s)
- rel_logits = self._matmul_with_relative_keys(query /math.sqrt(self.k_channels), key_relative_embeddings)
- scores_local = self._relative_position_to_absolute_position(rel_logits)
- scores = scores + scores_local
- if self.proximal_bias:
- assert t_s == t_t, "Proximal bias is only available for self-attention."
- scores = scores + self._attention_bias_proximal(t_s).to(device=scores.device, dtype=scores.dtype)
- if mask is not None:
- scores = scores.masked_fill(mask == 0, -1e4)
- if self.block_length is not None:
- assert t_s == t_t, "Local attention is only available for self-attention."
- block_mask = torch.ones_like(scores).triu(-self.block_length).tril(self.block_length)
- scores = scores.masked_fill(block_mask == 0, -1e4)
- p_attn = F.softmax(scores, dim=-1) # [b, n_h, t_t, t_s]
- p_attn = self.drop(p_attn)
- output = torch.matmul(p_attn, value)
- if self.window_size is not None:
- relative_weights = self._absolute_position_to_relative_position(p_attn)
- value_relative_embeddings = self._get_relative_embeddings(self.emb_rel_v, t_s)
- output = output + self._matmul_with_relative_values(relative_weights, value_relative_embeddings)
- output = output.transpose(2, 3).contiguous().view(b, d, t_t) # [b, n_h, t_t, d_k] -> [b, d, t_t]
- return output, p_attn
-
- def _matmul_with_relative_values(self, x, y):
- """
- x: [b, h, l, m]
- y: [h or 1, m, d]
- ret: [b, h, l, d]
- """
- ret = torch.matmul(x, y.unsqueeze(0))
- return ret
-
- def _matmul_with_relative_keys(self, x, y):
- """
- x: [b, h, l, d]
- y: [h or 1, m, d]
- ret: [b, h, l, m]
- """
- ret = torch.matmul(x, y.unsqueeze(0).transpose(-2, -1))
- return ret
-
- def _get_relative_embeddings(self, relative_embeddings, length):
- max_relative_position = 2 * self.window_size + 1
- # Pad first before slice to avoid using cond ops.
- pad_length = max(length - (self.window_size + 1), 0)
- slice_start_position = max((self.window_size + 1) - length, 0)
- slice_end_position = slice_start_position + 2 * length - 1
- if pad_length > 0:
- padded_relative_embeddings = F.pad(
- relative_embeddings,
- commons.convert_pad_shape([[0, 0], [pad_length, pad_length], [0, 0]]))
- else:
- padded_relative_embeddings = relative_embeddings
- used_relative_embeddings = padded_relative_embeddings[:,slice_start_position:slice_end_position]
- return used_relative_embeddings
-
- def _relative_position_to_absolute_position(self, x):
- """
- x: [b, h, l, 2*l-1]
- ret: [b, h, l, l]
- """
- batch, heads, length, _ = x.size()
- # Concat columns of pad to shift from relative to absolute indexing.
- x = F.pad(x, commons.convert_pad_shape([[0,0],[0,0],[0,0],[0,1]]))
-
- # Concat extra elements so to add up to shape (len+1, 2*len-1).
- x_flat = x.view([batch, heads, length * 2 * length])
- x_flat = F.pad(x_flat, commons.convert_pad_shape([[0,0],[0,0],[0,length-1]]))
-
- # Reshape and slice out the padded elements.
- x_final = x_flat.view([batch, heads, length+1, 2*length-1])[:, :, :length, length-1:]
- return x_final
-
- def _absolute_position_to_relative_position(self, x):
- """
- x: [b, h, l, l]
- ret: [b, h, l, 2*l-1]
- """
- batch, heads, length, _ = x.size()
- # padd along column
- x = F.pad(x, commons.convert_pad_shape([[0, 0], [0, 0], [0, 0], [0, length-1]]))
- x_flat = x.view([batch, heads, length**2 + length*(length -1)])
- # add 0's in the beginning that will skew the elements after reshape
- x_flat = F.pad(x_flat, commons.convert_pad_shape([[0, 0], [0, 0], [length, 0]]))
- x_final = x_flat.view([batch, heads, length, 2*length])[:,:,:,1:]
- return x_final
-
- def _attention_bias_proximal(self, length):
- """Bias for self-attention to encourage attention to close positions.
- Args:
- length: an integer scalar.
- Returns:
- a Tensor with shape [1, 1, length, length]
- """
- r = torch.arange(length, dtype=torch.float32)
- diff = torch.unsqueeze(r, 0) - torch.unsqueeze(r, 1)
- return torch.unsqueeze(torch.unsqueeze(-torch.log1p(torch.abs(diff)), 0), 0)
-
-
-class FFN(nn.Module):
- def __init__(self, in_channels, out_channels, filter_channels, kernel_size, p_dropout=0., activation=None, causal=False):
- super().__init__()
- self.in_channels = in_channels
- self.out_channels = out_channels
- self.filter_channels = filter_channels
- self.kernel_size = kernel_size
- self.p_dropout = p_dropout
- self.activation = activation
- self.causal = causal
-
- if causal:
- self.padding = self._causal_padding
- else:
- self.padding = self._same_padding
-
- self.conv_1 = nn.Conv1d(in_channels, filter_channels, kernel_size)
- self.conv_2 = nn.Conv1d(filter_channels, out_channels, kernel_size)
- self.drop = nn.Dropout(p_dropout)
-
- def forward(self, x, x_mask):
- x = self.conv_1(self.padding(x * x_mask))
- if self.activation == "gelu":
- x = x * torch.sigmoid(1.702 * x)
- else:
- x = torch.relu(x)
- x = self.drop(x)
- x = self.conv_2(self.padding(x * x_mask))
- return x * x_mask
-
- def _causal_padding(self, x):
- if self.kernel_size == 1:
- return x
- pad_l = self.kernel_size - 1
- pad_r = 0
- padding = [[0, 0], [0, 0], [pad_l, pad_r]]
- x = F.pad(x, commons.convert_pad_shape(padding))
- return x
-
- def _same_padding(self, x):
- if self.kernel_size == 1:
- return x
- pad_l = (self.kernel_size - 1) // 2
- pad_r = self.kernel_size // 2
- padding = [[0, 0], [0, 0], [pad_l, pad_r]]
- x = F.pad(x, commons.convert_pad_shape(padding))
- return x
diff --git a/spaces/digitalxingtong/Kino-Bert-VITS2/text/chinese_bert.py b/spaces/digitalxingtong/Kino-Bert-VITS2/text/chinese_bert.py
deleted file mode 100644
index cb84ce0b426cd0a1c7954ddcdf41322c10ed14fa..0000000000000000000000000000000000000000
--- a/spaces/digitalxingtong/Kino-Bert-VITS2/text/chinese_bert.py
+++ /dev/null
@@ -1,50 +0,0 @@
-import torch
-from transformers import AutoTokenizer, AutoModelForMaskedLM
-
-device = torch.device("cuda" if torch.cuda.is_available() else "cpu")
-
-tokenizer = AutoTokenizer.from_pretrained("./bert/chinese-roberta-wwm-ext-large")
-model = AutoModelForMaskedLM.from_pretrained("./bert/chinese-roberta-wwm-ext-large").to(device)
-
-def get_bert_feature(text, word2ph):
- with torch.no_grad():
- inputs = tokenizer(text, return_tensors='pt')
- for i in inputs:
- inputs[i] = inputs[i].to(device)
- res = model(**inputs, output_hidden_states=True)
- res = torch.cat(res['hidden_states'][-3:-2], -1)[0].cpu()
-
- assert len(word2ph) == len(text)+2
- word2phone = word2ph
- phone_level_feature = []
- for i in range(len(word2phone)):
- repeat_feature = res[i].repeat(word2phone[i], 1)
- phone_level_feature.append(repeat_feature)
-
- phone_level_feature = torch.cat(phone_level_feature, dim=0)
-
-
- return phone_level_feature.T
-
-if __name__ == '__main__':
- # feature = get_bert_feature('你好,我是说的道理。')
- import torch
-
- word_level_feature = torch.rand(38, 1024) # 12个词,每个词1024维特征
- word2phone = [1, 2, 1, 2, 2, 1, 2, 2, 1, 2, 2, 1, 2, 2, 2, 2, 2, 1, 1, 2, 2, 1, 2, 2, 2, 2, 1, 2, 2, 2, 2, 2, 1, 2, 2, 2, 2, 1]
-
- # 计算总帧数
- total_frames = sum(word2phone)
- print(word_level_feature.shape)
- print(word2phone)
- phone_level_feature = []
- for i in range(len(word2phone)):
- print(word_level_feature[i].shape)
-
- # 对每个词重复word2phone[i]次
- repeat_feature = word_level_feature[i].repeat(word2phone[i], 1)
- phone_level_feature.append(repeat_feature)
-
- phone_level_feature = torch.cat(phone_level_feature, dim=0)
- print(phone_level_feature.shape) # torch.Size([36, 1024])
-
diff --git a/spaces/digitalxingtong/Nailv-read-Bert-Vits2/commons.py b/spaces/digitalxingtong/Nailv-read-Bert-Vits2/commons.py
deleted file mode 100644
index 9ad0444b61cbadaa388619986c2889c707d873ce..0000000000000000000000000000000000000000
--- a/spaces/digitalxingtong/Nailv-read-Bert-Vits2/commons.py
+++ /dev/null
@@ -1,161 +0,0 @@
-import math
-import numpy as np
-import torch
-from torch import nn
-from torch.nn import functional as F
-
-
-def init_weights(m, mean=0.0, std=0.01):
- classname = m.__class__.__name__
- if classname.find("Conv") != -1:
- m.weight.data.normal_(mean, std)
-
-
-def get_padding(kernel_size, dilation=1):
- return int((kernel_size*dilation - dilation)/2)
-
-
-def convert_pad_shape(pad_shape):
- l = pad_shape[::-1]
- pad_shape = [item for sublist in l for item in sublist]
- return pad_shape
-
-
-def intersperse(lst, item):
- result = [item] * (len(lst) * 2 + 1)
- result[1::2] = lst
- return result
-
-
-def kl_divergence(m_p, logs_p, m_q, logs_q):
- """KL(P||Q)"""
- kl = (logs_q - logs_p) - 0.5
- kl += 0.5 * (torch.exp(2. * logs_p) + ((m_p - m_q)**2)) * torch.exp(-2. * logs_q)
- return kl
-
-
-def rand_gumbel(shape):
- """Sample from the Gumbel distribution, protect from overflows."""
- uniform_samples = torch.rand(shape) * 0.99998 + 0.00001
- return -torch.log(-torch.log(uniform_samples))
-
-
-def rand_gumbel_like(x):
- g = rand_gumbel(x.size()).to(dtype=x.dtype, device=x.device)
- return g
-
-
-def slice_segments(x, ids_str, segment_size=4):
- ret = torch.zeros_like(x[:, :, :segment_size])
- for i in range(x.size(0)):
- idx_str = ids_str[i]
- idx_end = idx_str + segment_size
- ret[i] = x[i, :, idx_str:idx_end]
- return ret
-
-
-def rand_slice_segments(x, x_lengths=None, segment_size=4):
- b, d, t = x.size()
- if x_lengths is None:
- x_lengths = t
- ids_str_max = x_lengths - segment_size + 1
- ids_str = (torch.rand([b]).to(device=x.device) * ids_str_max).to(dtype=torch.long)
- ret = slice_segments(x, ids_str, segment_size)
- return ret, ids_str
-
-
-def get_timing_signal_1d(
- length, channels, min_timescale=1.0, max_timescale=1.0e4):
- position = torch.arange(length, dtype=torch.float)
- num_timescales = channels // 2
- log_timescale_increment = (
- math.log(float(max_timescale) / float(min_timescale)) /
- (num_timescales - 1))
- inv_timescales = min_timescale * torch.exp(
- torch.arange(num_timescales, dtype=torch.float) * -log_timescale_increment)
- scaled_time = position.unsqueeze(0) * inv_timescales.unsqueeze(1)
- signal = torch.cat([torch.sin(scaled_time), torch.cos(scaled_time)], 0)
- signal = F.pad(signal, [0, 0, 0, channels % 2])
- signal = signal.view(1, channels, length)
- return signal
-
-
-def add_timing_signal_1d(x, min_timescale=1.0, max_timescale=1.0e4):
- b, channels, length = x.size()
- signal = get_timing_signal_1d(length, channels, min_timescale, max_timescale)
- return x + signal.to(dtype=x.dtype, device=x.device)
-
-
-def cat_timing_signal_1d(x, min_timescale=1.0, max_timescale=1.0e4, axis=1):
- b, channels, length = x.size()
- signal = get_timing_signal_1d(length, channels, min_timescale, max_timescale)
- return torch.cat([x, signal.to(dtype=x.dtype, device=x.device)], axis)
-
-
-def subsequent_mask(length):
- mask = torch.tril(torch.ones(length, length)).unsqueeze(0).unsqueeze(0)
- return mask
-
-
-@torch.jit.script
-def fused_add_tanh_sigmoid_multiply(input_a, input_b, n_channels):
- n_channels_int = n_channels[0]
- in_act = input_a + input_b
- t_act = torch.tanh(in_act[:, :n_channels_int, :])
- s_act = torch.sigmoid(in_act[:, n_channels_int:, :])
- acts = t_act * s_act
- return acts
-
-
-def convert_pad_shape(pad_shape):
- l = pad_shape[::-1]
- pad_shape = [item for sublist in l for item in sublist]
- return pad_shape
-
-
-def shift_1d(x):
- x = F.pad(x, convert_pad_shape([[0, 0], [0, 0], [1, 0]]))[:, :, :-1]
- return x
-
-
-def sequence_mask(length, max_length=None):
- if max_length is None:
- max_length = length.max()
- x = torch.arange(max_length, dtype=length.dtype, device=length.device)
- return x.unsqueeze(0) < length.unsqueeze(1)
-
-
-def generate_path(duration, mask):
- """
- duration: [b, 1, t_x]
- mask: [b, 1, t_y, t_x]
- """
- device = duration.device
-
- b, _, t_y, t_x = mask.shape
- cum_duration = torch.cumsum(duration, -1)
-
- cum_duration_flat = cum_duration.view(b * t_x)
- path = sequence_mask(cum_duration_flat, t_y).to(mask.dtype)
- path = path.view(b, t_x, t_y)
- path = path - F.pad(path, convert_pad_shape([[0, 0], [1, 0], [0, 0]]))[:, :-1]
- path = path.unsqueeze(1).transpose(2,3) * mask
- return path
-
-
-def clip_grad_value_(parameters, clip_value, norm_type=2):
- if isinstance(parameters, torch.Tensor):
- parameters = [parameters]
- parameters = list(filter(lambda p: p.grad is not None, parameters))
- norm_type = float(norm_type)
- if clip_value is not None:
- clip_value = float(clip_value)
-
- total_norm = 0
- for p in parameters:
- param_norm = p.grad.data.norm(norm_type)
- total_norm += param_norm.item() ** norm_type
- if clip_value is not None:
- p.grad.data.clamp_(min=-clip_value, max=clip_value)
- total_norm = total_norm ** (1. / norm_type)
- return total_norm
diff --git a/spaces/dilums/sentence-similarity/tailwind.config.ts b/spaces/dilums/sentence-similarity/tailwind.config.ts
deleted file mode 100644
index c7ead804652ebfb1e07a5acb03880d553a7da011..0000000000000000000000000000000000000000
--- a/spaces/dilums/sentence-similarity/tailwind.config.ts
+++ /dev/null
@@ -1,20 +0,0 @@
-import type { Config } from 'tailwindcss'
-
-const config: Config = {
- content: [
- './pages/**/*.{js,ts,jsx,tsx,mdx}',
- './components/**/*.{js,ts,jsx,tsx,mdx}',
- './app/**/*.{js,ts,jsx,tsx,mdx}',
- ],
- theme: {
- extend: {
- backgroundImage: {
- 'gradient-radial': 'radial-gradient(var(--tw-gradient-stops))',
- 'gradient-conic':
- 'conic-gradient(from 180deg at 50% 50%, var(--tw-gradient-stops))',
- },
- },
- },
- plugins: [],
-}
-export default config
diff --git a/spaces/dineshreddy/WALT/mmdet/models/roi_heads/standard_roi_head.py b/spaces/dineshreddy/WALT/mmdet/models/roi_heads/standard_roi_head.py
deleted file mode 100644
index 4d5e163e90b4e2bba6ee1b04a7d8989a52e07fa3..0000000000000000000000000000000000000000
--- a/spaces/dineshreddy/WALT/mmdet/models/roi_heads/standard_roi_head.py
+++ /dev/null
@@ -1,306 +0,0 @@
-import torch
-
-from mmdet.core import bbox2result, bbox2roi, build_assigner, build_sampler
-from ..builder import HEADS, build_head, build_roi_extractor
-from .base_roi_head import BaseRoIHead
-from .test_mixins import BBoxTestMixin, MaskTestMixin
-
-
-@HEADS.register_module()
-class StandardRoIHead(BaseRoIHead, BBoxTestMixin, MaskTestMixin):
- """Simplest base roi head including one bbox head and one mask head."""
-
- def init_assigner_sampler(self):
- """Initialize assigner and sampler."""
- self.bbox_assigner = None
- self.bbox_sampler = None
- if self.train_cfg:
- self.bbox_assigner = build_assigner(self.train_cfg.assigner)
- self.bbox_sampler = build_sampler(
- self.train_cfg.sampler, context=self)
-
- def init_bbox_head(self, bbox_roi_extractor, bbox_head):
- """Initialize ``bbox_head``"""
- self.bbox_roi_extractor = build_roi_extractor(bbox_roi_extractor)
- self.bbox_head = build_head(bbox_head)
-
- def init_mask_head(self, mask_roi_extractor, mask_head):
- """Initialize ``mask_head``"""
- if mask_roi_extractor is not None:
- self.mask_roi_extractor = build_roi_extractor(mask_roi_extractor)
- self.share_roi_extractor = False
- else:
- self.share_roi_extractor = True
- self.mask_roi_extractor = self.bbox_roi_extractor
- self.mask_head = build_head(mask_head)
-
- def init_gan_head(self, gan_roi_extractor, gan_head):
- """Initialize ``mask_head``"""
- if gan_roi_extractor is not None:
- self.gan_roi_extractor = build_roi_extractor(gan_roi_extractor)
- self.share_roi_extractor = False
- else:
- self.share_roi_extractor = True
- self.gan_roi_extractor = self.bbox_roi_extractor
- self.gan_head = build_head(gan_head)
-
-
- def init_weights(self, pretrained):
- """Initialize the weights in head.
-
- Args:
- pretrained (str, optional): Path to pre-trained weights.
- Defaults to None.
- """
- if self.with_shared_head:
- self.shared_head.init_weights(pretrained=pretrained)
- if self.with_bbox:
- self.bbox_roi_extractor.init_weights()
- self.bbox_head.init_weights()
- if self.with_mask:
- self.mask_head.init_weights()
- if not self.share_roi_extractor:
- self.mask_roi_extractor.init_weights()
-
- def forward_dummy(self, x, proposals):
- """Dummy forward function."""
- # bbox head
- outs = ()
- rois = bbox2roi([proposals])
- if self.with_bbox:
- bbox_results = self._bbox_forward(x, rois)
- outs = outs + (bbox_results['cls_score'],
- bbox_results['bbox_pred'])
- # mask head
- if self.with_mask:
- mask_rois = rois[:100]
- mask_results = self._mask_forward(x, mask_rois)
- outs = outs + (mask_results['mask_pred'], )
- return outs
-
- def forward_train(self,
- x,
- img_metas,
- proposal_list,
- gt_bboxes,
- gt_labels,
- gt_bboxes_ignore=None,
- gt_masks=None):
- """
- Args:
- x (list[Tensor]): list of multi-level img features.
- img_metas (list[dict]): list of image info dict where each dict
- has: 'img_shape', 'scale_factor', 'flip', and may also contain
- 'filename', 'ori_shape', 'pad_shape', and 'img_norm_cfg'.
- For details on the values of these keys see
- `mmdet/datasets/pipelines/formatting.py:Collect`.
- proposals (list[Tensors]): list of region proposals.
- gt_bboxes (list[Tensor]): Ground truth bboxes for each image with
- shape (num_gts, 4) in [tl_x, tl_y, br_x, br_y] format.
- gt_labels (list[Tensor]): class indices corresponding to each box
- gt_bboxes_ignore (None | list[Tensor]): specify which bounding
- boxes can be ignored when computing the loss.
- gt_masks (None | Tensor) : true segmentation masks for each box
- used if the architecture supports a segmentation task.
-
- Returns:
- dict[str, Tensor]: a dictionary of loss components
- """
- # assign gts and sample proposals
- if self.with_bbox or self.with_mask:
- num_imgs = len(img_metas)
- if gt_bboxes_ignore is None:
- gt_bboxes_ignore = [None for _ in range(num_imgs)]
- sampling_results = []
- for i in range(num_imgs):
- assign_result = self.bbox_assigner.assign(
- proposal_list[i], gt_bboxes[i], gt_bboxes_ignore[i],
- gt_labels[i])
- sampling_result = self.bbox_sampler.sample(
- assign_result,
- proposal_list[i],
- gt_bboxes[i],
- gt_labels[i],
- feats=[lvl_feat[i][None] for lvl_feat in x])
- sampling_results.append(sampling_result)
-
- losses = dict()
- # bbox head forward and loss
- if self.with_bbox:
- bbox_results = self._bbox_forward_train(x, sampling_results,
- gt_bboxes, gt_labels,
- img_metas)
- losses.update(bbox_results['loss_bbox'])
-
- # mask head forward and loss
- if self.with_mask:
- mask_results = self._mask_forward_train(x, sampling_results,
- bbox_results['bbox_feats'],
- gt_masks, img_metas)
- losses.update(mask_results['loss_mask'])
-
- return losses
-
- def _bbox_forward(self, x, rois):
- """Box head forward function used in both training and testing."""
- # TODO: a more flexible way to decide which feature maps to use
- bbox_feats = self.bbox_roi_extractor(
- x[:self.bbox_roi_extractor.num_inputs], rois)
- if self.with_shared_head:
- bbox_feats = self.shared_head(bbox_feats)
- cls_score, bbox_pred = self.bbox_head(bbox_feats)
-
- bbox_results = dict(
- cls_score=cls_score, bbox_pred=bbox_pred, bbox_feats=bbox_feats)
- return bbox_results
-
- def _bbox_forward_train(self, x, sampling_results, gt_bboxes, gt_labels,
- img_metas):
- """Run forward function and calculate loss for box head in training."""
- rois = bbox2roi([res.bboxes for res in sampling_results])
- bbox_results = self._bbox_forward(x, rois)
-
- bbox_targets = self.bbox_head.get_targets(sampling_results, gt_bboxes,
- gt_labels, self.train_cfg)
- loss_bbox = self.bbox_head.loss(bbox_results['cls_score'],
- bbox_results['bbox_pred'], rois,
- *bbox_targets)
-
- bbox_results.update(loss_bbox=loss_bbox)
- return bbox_results
-
- def _mask_forward_train(self, x, sampling_results, bbox_feats, gt_masks,
- img_metas):
- """Run forward function and calculate loss for mask head in
- training."""
- if not self.share_roi_extractor:
- pos_rois = bbox2roi([res.pos_bboxes for res in sampling_results])
- mask_results = self._mask_forward(x, pos_rois)
- else:
- pos_inds = []
- device = bbox_feats.device
- for res in sampling_results:
- pos_inds.append(
- torch.ones(
- res.pos_bboxes.shape[0],
- device=device,
- dtype=torch.uint8))
- pos_inds.append(
- torch.zeros(
- res.neg_bboxes.shape[0],
- device=device,
- dtype=torch.uint8))
- pos_inds = torch.cat(pos_inds)
-
- mask_results = self._mask_forward(
- x, pos_inds=pos_inds, bbox_feats=bbox_feats)
-
- mask_targets = self.mask_head.get_targets(sampling_results, gt_masks,
- self.train_cfg)
- pos_labels = torch.cat([res.pos_gt_labels for res in sampling_results])
- loss_mask = self.mask_head.loss(mask_results['mask_pred'],
- mask_targets, pos_labels)
-
- mask_results.update(loss_mask=loss_mask, mask_targets=mask_targets)
- return mask_results
-
- def _mask_forward(self, x, rois=None, pos_inds=None, bbox_feats=None):
- """Mask head forward function used in both training and testing."""
- assert ((rois is not None) ^
- (pos_inds is not None and bbox_feats is not None))
- if rois is not None:
- mask_feats = self.mask_roi_extractor(
- x[:self.mask_roi_extractor.num_inputs], rois)
- if self.with_shared_head:
- mask_feats = self.shared_head(mask_feats)
- else:
- assert bbox_feats is not None
- mask_feats = bbox_feats[pos_inds]
-
- mask_pred = self.mask_head(mask_feats)
- mask_results = dict(mask_pred=mask_pred, mask_feats=mask_feats)
- return mask_results
-
- async def async_simple_test(self,
- x,
- proposal_list,
- img_metas,
- proposals=None,
- rescale=False):
- """Async test without augmentation."""
- assert self.with_bbox, 'Bbox head must be implemented.'
-
- det_bboxes, det_labels = await self.async_test_bboxes(
- x, img_metas, proposal_list, self.test_cfg, rescale=rescale)
- bbox_results = bbox2result(det_bboxes, det_labels,
- self.bbox_head.num_classes)
- if not self.with_mask:
- return bbox_results
- else:
- segm_results = await self.async_test_mask(
- x,
- img_metas,
- det_bboxes,
- det_labels,
- rescale=rescale,
- mask_test_cfg=self.test_cfg.get('mask'))
- return bbox_results, segm_results
-
- def simple_test(self,
- x,
- proposal_list,
- img_metas,
- proposals=None,
- rescale=False):
- """Test without augmentation."""
- assert self.with_bbox, 'Bbox head must be implemented.'
-
- det_bboxes, det_labels = self.simple_test_bboxes(
- x, img_metas, proposal_list, self.test_cfg, rescale=rescale)
- if torch.onnx.is_in_onnx_export():
- if self.with_mask:
- segm_results = self.simple_test_mask(
- x, img_metas, det_bboxes, det_labels, rescale=rescale)
- return det_bboxes, det_labels, segm_results
- else:
- return det_bboxes, det_labels
-
- bbox_results = [
- bbox2result(det_bboxes[i], det_labels[i],
- self.bbox_head.num_classes)
- for i in range(len(det_bboxes))
- ]
-
- if not self.with_mask:
- return bbox_results
- else:
- segm_results = self.simple_test_mask(
- x, img_metas, det_bboxes, det_labels, rescale=rescale)
- return list(zip(bbox_results, segm_results))
-
- def aug_test(self, x, proposal_list, img_metas, rescale=False):
- """Test with augmentations.
-
- If rescale is False, then returned bboxes and masks will fit the scale
- of imgs[0].
- """
- det_bboxes, det_labels = self.aug_test_bboxes(x, img_metas,
- proposal_list,
- self.test_cfg)
-
- if rescale:
- _det_bboxes = det_bboxes
- else:
- _det_bboxes = det_bboxes.clone()
- _det_bboxes[:, :4] *= det_bboxes.new_tensor(
- img_metas[0][0]['scale_factor'])
- bbox_results = bbox2result(_det_bboxes, det_labels,
- self.bbox_head.num_classes)
-
- # det_bboxes always keep the original scale
- if self.with_mask:
- segm_results = self.aug_test_mask(x, img_metas, det_bboxes,
- det_labels)
- return [(bbox_results, segm_results)]
- else:
- return [bbox_results]
diff --git a/spaces/dmccreary/spaces-demo/app.py b/spaces/dmccreary/spaces-demo/app.py
deleted file mode 100644
index 77e20d100a8bf0f78a4b9791e515116c94cf14d2..0000000000000000000000000000000000000000
--- a/spaces/dmccreary/spaces-demo/app.py
+++ /dev/null
@@ -1,6 +0,0 @@
-import streamlit as st
-
-x = st.slider('Select a value')
-st.write(x, 'Input (x) : ', x)
-st.write(x, 'x squared: ', x * x)
-st.write(x, 'x + x : ', x + x)
\ No newline at end of file
diff --git a/spaces/dolceschokolade/chatbot-mini/components/Spinner/index.ts b/spaces/dolceschokolade/chatbot-mini/components/Spinner/index.ts
deleted file mode 100644
index f90663a519f138da6e80f382b3afee6d13029fd8..0000000000000000000000000000000000000000
--- a/spaces/dolceschokolade/chatbot-mini/components/Spinner/index.ts
+++ /dev/null
@@ -1 +0,0 @@
-export { default } from './Spinner';
diff --git a/spaces/dorkai/singpt/extensions/llama_prompts/script.py b/spaces/dorkai/singpt/extensions/llama_prompts/script.py
deleted file mode 100644
index 22c96f7c2d6763213a728d77ee6666496d9c4aa3..0000000000000000000000000000000000000000
--- a/spaces/dorkai/singpt/extensions/llama_prompts/script.py
+++ /dev/null
@@ -1,18 +0,0 @@
-import gradio as gr
-import modules.shared as shared
-import pandas as pd
-
-df = pd.read_csv("https://raw.githubusercontent.com/devbrones/llama-prompts/main/prompts/prompts.csv")
-
-def get_prompt_by_name(name):
- if name == 'None':
- return ''
- else:
- return df[df['Prompt name'] == name].iloc[0]['Prompt'].replace('\\n', '\n')
-
-def ui():
- if not shared.args.chat or shared.args.cai_chat:
- choices = ['None'] + list(df['Prompt name'])
-
- prompts_menu = gr.Dropdown(value=choices[0], choices=choices, label='Prompt')
- prompts_menu.change(get_prompt_by_name, prompts_menu, shared.gradio['textbox'])
diff --git a/spaces/dorkai/text-generation-webui-main/extensions/superbooga/chromadb.py b/spaces/dorkai/text-generation-webui-main/extensions/superbooga/chromadb.py
deleted file mode 100644
index 52f4854bdbaaa9d0fcde18ea395c50e216b06b8e..0000000000000000000000000000000000000000
--- a/spaces/dorkai/text-generation-webui-main/extensions/superbooga/chromadb.py
+++ /dev/null
@@ -1,95 +0,0 @@
-import logging
-
-import posthog
-import torch
-from sentence_transformers import SentenceTransformer
-
-import chromadb
-from chromadb.config import Settings
-
-logging.info('Intercepting all calls to posthog :)')
-posthog.capture = lambda *args, **kwargs: None
-
-
-class Collecter():
- def __init__(self):
- pass
-
- def add(self, texts: list[str]):
- pass
-
- def get(self, search_strings: list[str], n_results: int) -> list[str]:
- pass
-
- def clear(self):
- pass
-
-
-class Embedder():
- def __init__(self):
- pass
-
- def embed(self, text: str) -> list[torch.Tensor]:
- pass
-
-
-class ChromaCollector(Collecter):
- def __init__(self, embedder: Embedder):
- super().__init__()
- self.chroma_client = chromadb.Client(Settings(anonymized_telemetry=False))
- self.embedder = embedder
- self.collection = self.chroma_client.create_collection(name="context", embedding_function=embedder.embed)
- self.ids = []
-
- def add(self, texts: list[str]):
- self.ids = [f"id{i}" for i in range(len(texts))]
- self.collection.add(documents=texts, ids=self.ids)
-
- def get_documents_and_ids(self, search_strings: list[str], n_results: int):
- n_results = min(len(self.ids), n_results)
- result = self.collection.query(query_texts=search_strings, n_results=n_results, include=['documents'])
- documents = result['documents'][0]
- ids = list(map(lambda x: int(x[2:]), result['ids'][0]))
- return documents, ids
-
- # Get chunks by similarity
- def get(self, search_strings: list[str], n_results: int) -> list[str]:
- documents, _ = self.get_documents_and_ids(search_strings, n_results)
- return documents
-
- # Get ids by similarity
- def get_ids(self, search_strings: list[str], n_results: int) -> list[str]:
- _ , ids = self.get_documents_and_ids(search_strings, n_results)
- return ids
-
- # Get chunks by similarity and then sort by insertion order
- def get_sorted(self, search_strings: list[str], n_results: int) -> list[str]:
- documents, ids = self.get_documents_and_ids(search_strings, n_results)
- return [x for _, x in sorted(zip(ids, documents))]
-
- # Get ids by similarity and then sort by insertion order
- def get_ids_sorted(self, search_strings: list[str], n_results: int) -> list[str]:
- _ , ids = self.get_documents_and_ids(search_strings, n_results)
- return sorted(ids)
-
- def clear(self):
- self.collection.delete(ids=self.ids)
-
-
-class SentenceTransformerEmbedder(Embedder):
- def __init__(self) -> None:
- self.model = SentenceTransformer("sentence-transformers/all-mpnet-base-v2")
- self.embed = self.model.encode
-
-
-def make_collector():
- global embedder
- return ChromaCollector(embedder)
-
-
-def add_chunks_to_collector(chunks, collector):
- collector.clear()
- collector.add(chunks)
-
-
-embedder = SentenceTransformerEmbedder()
diff --git a/spaces/dorkai/text-generation-webui-main/modules/deepspeed_parameters.py b/spaces/dorkai/text-generation-webui-main/modules/deepspeed_parameters.py
deleted file mode 100644
index 9116f5792fea4edf4b536b6605ee40e254109a98..0000000000000000000000000000000000000000
--- a/spaces/dorkai/text-generation-webui-main/modules/deepspeed_parameters.py
+++ /dev/null
@@ -1,74 +0,0 @@
-def generate_ds_config(ds_bf16, train_batch_size, nvme_offload_dir):
- '''
- DeepSpeed configration
- https://huggingface.co/docs/transformers/main_classes/deepspeed
- '''
-
- if nvme_offload_dir:
- ds_config = {
- "fp16": {
- "enabled": not ds_bf16,
- },
- "bf16": {
- "enabled": ds_bf16,
- },
- "zero_optimization": {
- "stage": 3,
- "offload_param": {
- "device": "nvme",
- "nvme_path": nvme_offload_dir,
- "pin_memory": True,
- "buffer_count": 5,
- "buffer_size": 1e9,
- "max_in_cpu": 1e9
- },
- "overlap_comm": True,
- "reduce_bucket_size": "auto",
- "contiguous_gradients": True,
- "sub_group_size": 1e8,
- "stage3_prefetch_bucket_size": "auto",
- "stage3_param_persistence_threshold": "auto",
- "stage3_max_live_parameters": "auto",
- "stage3_max_reuse_distance": "auto",
- },
- "aio": {
- "block_size": 262144,
- "queue_depth": 32,
- "thread_count": 1,
- "single_submit": False,
- "overlap_events": True
- },
- "steps_per_print": 2000,
- "train_batch_size": train_batch_size,
- "train_micro_batch_size_per_gpu": 1,
- "wall_clock_breakdown": False
- }
- else:
- ds_config = {
- "fp16": {
- "enabled": not ds_bf16,
- },
- "bf16": {
- "enabled": ds_bf16,
- },
- "zero_optimization": {
- "stage": 3,
- "offload_param": {
- "device": "cpu",
- "pin_memory": True
- },
- "overlap_comm": True,
- "contiguous_gradients": True,
- "reduce_bucket_size": "auto",
- "stage3_prefetch_bucket_size": "auto",
- "stage3_param_persistence_threshold": "auto",
- "stage3_max_live_parameters": "auto",
- "stage3_max_reuse_distance": "auto",
- },
- "steps_per_print": 2000,
- "train_batch_size": train_batch_size,
- "train_micro_batch_size_per_gpu": 1,
- "wall_clock_breakdown": False
- }
-
- return ds_config
diff --git a/spaces/ennov8ion/3dart-Models/app.py b/spaces/ennov8ion/3dart-Models/app.py
deleted file mode 100644
index ea19c813f3a3d2c4784784eb408ad4e62b0818a7..0000000000000000000000000000000000000000
--- a/spaces/ennov8ion/3dart-Models/app.py
+++ /dev/null
@@ -1,97 +0,0 @@
-import gradio as gr
-import os
-import sys
-from pathlib import Path
-
-models = [
- {"name": "DucHaiten Art", "url": "DucHaiten/DucHaitenAIart"},
- {"name": "DucHaiten ClassicAnime", "url": "DucHaiten/DH_ClassicAnime"},
- {"name": "DucHaiten DreamWorld", "url": "DucHaiten/DucHaitenDreamWorld"},
- {"name": "DucHaiten Journey", "url": "DucHaiten/DucHaitenJourney"},
- {"name": "DucHaiten StyleLikeMe", "url": "DucHaiten/DucHaiten-StyleLikeMe"},
- {"name": "DucHaiten SuperCute", "url": "DucHaiten/DucHaitenSuperCute"},
- {"name": "Redshift Diffusion 768", "url": "nitrosocke/redshift-diffusion-768"},
- {"name": "Redshift Diffusion", "url": "nitrosocke/redshift-diffusion"},
-]
-
-current_model = models[0]
-
-text_gen = gr.Interface.load("spaces/daspartho/prompt-extend")
-
-models2 = []
-for model in models:
- model_url = f"models/{model['url']}"
- loaded_model = gr.Interface.load(model_url, live=True, preprocess=True)
- models2.append(loaded_model)
-
-
-def text_it(inputs, text_gen=text_gen):
- return text_gen(inputs)
-
-
-def set_model(current_model_index):
- global current_model
- current_model = models[current_model_index]
- return gr.update(value=f"{current_model['name']}")
-
-
-def send_it(inputs, model_choice):
- proc = models2[model_choice]
- return proc(inputs)
-
-
-with gr.Blocks() as myface:
- gr.HTML(
-
- )
-
- with gr.Row():
- with gr.Row():
- input_text = gr.Textbox(label="Prompt idea", placeholder="", lines=1)
- # Model selection dropdown
- model_name1 = gr.Dropdown(
- label="Choose Model",
- choices=[m["name"] for m in models],
- type="index",
- value=current_model["name"],
- interactive=True,
- )
- with gr.Row():
- see_prompts = gr.Button("Generate Prompts")
- run = gr.Button("Generate Images", variant="primary")
-
- with gr.Row():
- output1 = gr.Image(label="")
- output2 = gr.Image(label="")
- output3 = gr.Image(label="")
- with gr.Row():
- magic1 = gr.Textbox(label="Generated Prompt", lines=2)
- magic2 = gr.Textbox(label="Generated Prompt", lines=2)
- magic3 = gr.Textbox(label="Generated Prompt", lines=2)
- with gr.Row():
- output4 = gr.Image(label="")
- output5 = gr.Image(label="")
- output6 = gr.Image(label="")
- with gr.Row():
- magic4 = gr.Textbox(label="Generated Prompt", lines=2)
- magic5 = gr.Textbox(label="Generated Prompt", lines=2)
- magic6 = gr.Textbox(label="Generated Prompt", lines=2)
-
- model_name1.change(set_model, inputs=model_name1, outputs=[output1, output2, output3, output4, output5, output6])
-
- run.click(send_it, inputs=[magic1, model_name1], outputs=[output1])
- run.click(send_it, inputs=[magic2, model_name1], outputs=[output2])
- run.click(send_it, inputs=[magic3, model_name1], outputs=[output3])
- run.click(send_it, inputs=[magic4, model_name1], outputs=[output4])
- run.click(send_it, inputs=[magic5, model_name1], outputs=[output5])
- run.click(send_it, inputs=[magic6, model_name1], outputs=[output6])
-
- see_prompts.click(text_it, inputs=[input_text], outputs=[magic1])
- see_prompts.click(text_it, inputs=[input_text], outputs=[magic2])
- see_prompts.click(text_it, inputs=[input_text], outputs=[magic3])
- see_prompts.click(text_it, inputs=[input_text], outputs=[magic4])
- see_prompts.click(text_it, inputs=[input_text], outputs=[magic5])
- see_prompts.click(text_it, inputs=[input_text], outputs=[magic6])
-
-myface.queue(concurrency_count=200)
-myface.launch(inline=True, show_api=False, max_threads=400)
\ No newline at end of file
diff --git a/spaces/euphi/smmry/app.py b/spaces/euphi/smmry/app.py
deleted file mode 100644
index d21b211fc1829d53d4d338833e2e32a0133dc8a0..0000000000000000000000000000000000000000
--- a/spaces/euphi/smmry/app.py
+++ /dev/null
@@ -1,99 +0,0 @@
-import os
-import openai
-import gradio as gr
-
-
-## API INSTANTIATION
-## ---------------------------------------------------------------------------------------------------------------------
-# Applying our API key and organization ID to OpenAI
-openai.api_key = os.getenv("openai_secret")
-
-
-## HELPER FUNCTIONS
-## ---------------------------------------------------------------------------------------------------------------------
-def initiate_chat_flow():
- '''
- Initiates a new chat flow
-
- Inputs:
- - N/A
-
- Returns:
- - chat_flow (list): A newly initiated chat flow
- '''
-
- chat_flow = [
- {'role': 'system', 'content': "You are a summarizing AI. Summarize a random article from wikipedia based on the input by the user. Do not use the article directly connected to the input of the user. Choose a random one of that category. If no category is identified, choose a random article. Start with the article title, then the summary content and then give the name of the source at the bottom. Identify the language of the user input and use the corresponding wikipedia language version for your output. e.g. if the user input is in german, make sure to use the german version of wikipedia and also translate your output to the same language as the user input."}
- ]
-
- return chat_flow
-
-
-def process_prompt(user_prompt, chatbot):
- '''
- Processes the user prompt submitted to the chat interface with the appropriate response from OpenAI's API
-
- Inputs:
- - user_prompt (str): The prompt text submitted by the user
- - chatbot (Gradio chatbot): The chatbot interface that is displayed to the user
-
- Returns:
- - user_prompt (str): A cleared out prompt ready for the next user input
- - chatbot (Gradio chatbot): The chatbot interface that is displayed to the user
- '''
-
- # Referencing the chat_flow as a global variable
- global chat_flow
-
-
- # Appending the prompt to the chat flow
- chat_flow.append({'role': 'user', 'content': user_prompt})
-
- # Obtaining the response from the API
- chat_response = openai.ChatCompletion.create(
- model = 'gpt-3.5-turbo',
- messages = chat_flow
- )
-
- # Obtaining the specific message to return to the user
- chat_answer = chat_response['choices'][0]['message']['content']
-
- # Appending the user prompt and answer to the chatbot interaction
- chatbot.append((user_prompt, chat_answer))
-
- # Appending the chat answer to the chat flow sent to OpenAI
- chat_flow.append({'role': 'assistant', 'content': chat_answer})
-
- # Clearing the prompt for the next user input
- user_prompt = ''
-
- return user_prompt, chatbot
-
-
-
-## GRADIO UI LAYOUT & FUNCTIONALITY
-## ---------------------------------------------------------------------------------------------------------------------
-# Defining the building blocks that represent the form and function of the Gradio UI
-with gr.Blocks() as chat_ui:
-
- # Instantiating the chatbot interface
- chatbot = gr.Chatbot(label = 'Summry')
- user_prompt = gr.Textbox(placeholder = 'Wanna read something quick? Tell me the categorie or just type "random" if you are not sure. Press "Enter" to submit.',
- show_label = False)
-
- # Defining the behavior for what occurs when the user hits "Enter" after typing a prompt
- user_prompt.submit(fn = process_prompt,
- inputs = [user_prompt, chatbot],
- outputs = [user_prompt, chatbot])
-
-
-
-## SCRIPT INVOCATION
-## ---------------------------------------------------------------------------------------------------------------------
-if __name__ == "__main__":
-
- # Instantiating the initial chat flow used as a global variable
- chat_flow = initiate_chat_flow()
-
- # Launching the Gradio Chatbot
- chat_ui.launch()
\ No newline at end of file
diff --git a/spaces/evaluate-metric/recall/recall.py b/spaces/evaluate-metric/recall/recall.py
deleted file mode 100644
index 8522cfcf66563befa3b8fa3ba5be1f1145fadb7e..0000000000000000000000000000000000000000
--- a/spaces/evaluate-metric/recall/recall.py
+++ /dev/null
@@ -1,135 +0,0 @@
-# Copyright 2020 The HuggingFace Datasets Authors and the current dataset script contributor.
-#
-# Licensed under the Apache License, Version 2.0 (the "License");
-# you may not use this file except in compliance with the License.
-# You may obtain a copy of the License at
-#
-# http://www.apache.org/licenses/LICENSE-2.0
-#
-# Unless required by applicable law or agreed to in writing, software
-# distributed under the License is distributed on an "AS IS" BASIS,
-# WITHOUT WARRANTIES OR CONDITIONS OF ANY KIND, either express or implied.
-# See the License for the specific language governing permissions and
-# limitations under the License.
-"""Recall metric."""
-
-import datasets
-from sklearn.metrics import recall_score
-
-import evaluate
-
-
-_DESCRIPTION = """
-Recall is the fraction of the positive examples that were correctly labeled by the model as positive. It can be computed with the equation:
-Recall = TP / (TP + FN)
-Where TP is the true positives and FN is the false negatives.
-"""
-
-
-_KWARGS_DESCRIPTION = """
-Args:
-- **predictions** (`list` of `int`): The predicted labels.
-- **references** (`list` of `int`): The ground truth labels.
-- **labels** (`list` of `int`): The set of labels to include when `average` is not set to `binary`, and their order when average is `None`. Labels present in the data can be excluded in this input, for example to calculate a multiclass average ignoring a majority negative class, while labels not present in the data will result in 0 components in a macro average. For multilabel targets, labels are column indices. By default, all labels in y_true and y_pred are used in sorted order. Defaults to None.
-- **pos_label** (`int`): The class label to use as the 'positive class' when calculating the recall. Defaults to `1`.
-- **average** (`string`): This parameter is required for multiclass/multilabel targets. If None, the scores for each class are returned. Otherwise, this determines the type of averaging performed on the data. Defaults to `'binary'`.
- - `'binary'`: Only report results for the class specified by `pos_label`. This is applicable only if the target labels and predictions are binary.
- - `'micro'`: Calculate metrics globally by counting the total true positives, false negatives, and false positives.
- - `'macro'`: Calculate metrics for each label, and find their unweighted mean. This does not take label imbalance into account.
- - `'weighted'`: Calculate metrics for each label, and find their average weighted by support (the number of true instances for each label). This alters `'macro'` to account for label imbalance. Note that it can result in an F-score that is not between precision and recall.
- - `'samples'`: Calculate metrics for each instance, and find their average (only meaningful for multilabel classification).
-- **sample_weight** (`list` of `float`): Sample weights Defaults to `None`.
-- **zero_division** (): Sets the value to return when there is a zero division. Defaults to .
- - `'warn'`: If there is a zero division, the return value is `0`, but warnings are also raised.
- - `0`: If there is a zero division, the return value is `0`.
- - `1`: If there is a zero division, the return value is `1`.
-
-Returns:
-- **recall** (`float`, or `array` of `float`): Either the general recall score, or the recall scores for individual classes, depending on the values input to `labels` and `average`. Minimum possible value is 0. Maximum possible value is 1. A higher recall means that more of the positive examples have been labeled correctly. Therefore, a higher recall is generally considered better.
-
-Examples:
-
- Example 1-A simple example with some errors
- >>> recall_metric = evaluate.load('recall')
- >>> results = recall_metric.compute(references=[0, 0, 1, 1, 1], predictions=[0, 1, 0, 1, 1])
- >>> print(results)
- {'recall': 0.6666666666666666}
-
- Example 2-The same example as Example 1, but with `pos_label=0` instead of the default `pos_label=1`.
- >>> recall_metric = evaluate.load('recall')
- >>> results = recall_metric.compute(references=[0, 0, 1, 1, 1], predictions=[0, 1, 0, 1, 1], pos_label=0)
- >>> print(results)
- {'recall': 0.5}
-
- Example 3-The same example as Example 1, but with `sample_weight` included.
- >>> recall_metric = evaluate.load('recall')
- >>> sample_weight = [0.9, 0.2, 0.9, 0.3, 0.8]
- >>> results = recall_metric.compute(references=[0, 0, 1, 1, 1], predictions=[0, 1, 0, 1, 1], sample_weight=sample_weight)
- >>> print(results)
- {'recall': 0.55}
-
- Example 4-A multiclass example, using different averages.
- >>> recall_metric = evaluate.load('recall')
- >>> predictions = [0, 2, 1, 0, 0, 1]
- >>> references = [0, 1, 2, 0, 1, 2]
- >>> results = recall_metric.compute(predictions=predictions, references=references, average='macro')
- >>> print(results)
- {'recall': 0.3333333333333333}
- >>> results = recall_metric.compute(predictions=predictions, references=references, average='micro')
- >>> print(results)
- {'recall': 0.3333333333333333}
- >>> results = recall_metric.compute(predictions=predictions, references=references, average='weighted')
- >>> print(results)
- {'recall': 0.3333333333333333}
- >>> results = recall_metric.compute(predictions=predictions, references=references, average=None)
- >>> print(results)
- {'recall': array([1., 0., 0.])}
-"""
-
-
-_CITATION = """
-@article{scikit-learn, title={Scikit-learn: Machine Learning in {P}ython}, author={Pedregosa, F. and Varoquaux, G. and Gramfort, A. and Michel, V. and Thirion, B. and Grisel, O. and Blondel, M. and Prettenhofer, P. and Weiss, R. and Dubourg, V. and Vanderplas, J. and Passos, A. and Cournapeau, D. and Brucher, M. and Perrot, M. and Duchesnay, E.}, journal={Journal of Machine Learning Research}, volume={12}, pages={2825--2830}, year={2011}
-"""
-
-
-@evaluate.utils.file_utils.add_start_docstrings(_DESCRIPTION, _KWARGS_DESCRIPTION)
-class Recall(evaluate.Metric):
- def _info(self):
- return evaluate.MetricInfo(
- description=_DESCRIPTION,
- citation=_CITATION,
- inputs_description=_KWARGS_DESCRIPTION,
- features=datasets.Features(
- {
- "predictions": datasets.Sequence(datasets.Value("int32")),
- "references": datasets.Sequence(datasets.Value("int32")),
- }
- if self.config_name == "multilabel"
- else {
- "predictions": datasets.Value("int32"),
- "references": datasets.Value("int32"),
- }
- ),
- reference_urls=["https://scikit-learn.org/stable/modules/generated/sklearn.metrics.recall_score.html"],
- )
-
- def _compute(
- self,
- predictions,
- references,
- labels=None,
- pos_label=1,
- average="binary",
- sample_weight=None,
- zero_division="warn",
- ):
- score = recall_score(
- references,
- predictions,
- labels=labels,
- pos_label=pos_label,
- average=average,
- sample_weight=sample_weight,
- zero_division=zero_division,
- )
- return {"recall": float(score) if score.size == 1 else score}
diff --git a/spaces/evaluate-metric/squad_v2/README.md b/spaces/evaluate-metric/squad_v2/README.md
deleted file mode 100644
index 6c4a7e2435106940f05565b5523f3dc53c98ed1d..0000000000000000000000000000000000000000
--- a/spaces/evaluate-metric/squad_v2/README.md
+++ /dev/null
@@ -1,142 +0,0 @@
----
-title: SQuAD v2
-emoji: 🤗
-colorFrom: blue
-colorTo: red
-sdk: gradio
-sdk_version: 3.19.1
-app_file: app.py
-pinned: false
-tags:
-- evaluate
-- metric
-description: >-
- This metric wrap the official scoring script for version 2 of the Stanford Question Answering Dataset (SQuAD).
-
- Stanford Question Answering Dataset (SQuAD) is a reading comprehension dataset, consisting of questions posed by
- crowdworkers on a set of Wikipedia articles, where the answer to every question is a segment of text, or span,
- from the corresponding reading passage, or the question might be unanswerable.
-
- SQuAD2.0 combines the 100,000 questions in SQuAD1.1 with over 50,000 unanswerable questions
- written adversarially by crowdworkers to look similar to answerable ones.
- To do well on SQuAD2.0, systems must not only answer questions when possible, but also
- determine when no answer is supported by the paragraph and abstain from answering.
----
-
-# Metric Card for SQuAD v2
-
-## Metric description
-This metric wraps the official scoring script for version 2 of the [Stanford Question Answering Dataset (SQuAD)](https://huggingface.co/datasets/squad_v2).
-
-SQuAD is a reading comprehension dataset, consisting of questions posed by crowdworkers on a set of Wikipedia articles, where the answer to every question is a segment of text, or span, from the corresponding reading passage, or the question might be unanswerable.
-
-SQuAD 2.0 combines the 100,000 questions in SQuAD 1.1 with over 50,000 unanswerable questions written adversarially by crowdworkers to look similar to answerable ones. To do well on SQuAD2.0, systems must not only answer questions when possible, but also determine when no answer is supported by the paragraph and abstain from answering.
-
-## How to use
-
-The metric takes two files or two lists - one representing model predictions and the other the references to compare them to.
-
-*Predictions* : List of triple for question-answers to score with the following key-value pairs:
-* `'id'`: the question-answer identification field of the question and answer pair
-* `'prediction_text'` : the text of the answer
-* `'no_answer_probability'` : the probability that the question has no answer
-
-*References*: List of question-answers dictionaries with the following key-value pairs:
-* `'id'`: id of the question-answer pair (see above),
-* `'answers'`: a list of Dict {'text': text of the answer as a string}
-* `'no_answer_threshold'`: the probability threshold to decide that a question has no answer.
-
-```python
-from evaluate import load
-squad_metric = load("squad_v2")
-results = squad_metric.compute(predictions=predictions, references=references)
-```
-## Output values
-
-This metric outputs a dictionary with 13 values:
-* `'exact'`: Exact match (the normalized answer exactly match the gold answer) (see the `exact_match` metric (forthcoming))
-* `'f1'`: The average F1-score of predicted tokens versus the gold answer (see the [F1 score](https://huggingface.co/metrics/f1) metric)
-* `'total'`: Number of scores considered
-* `'HasAns_exact'`: Exact match (the normalized answer exactly match the gold answer)
-* `'HasAns_f1'`: The F-score of predicted tokens versus the gold answer
-* `'HasAns_total'`: How many of the questions have answers
-* `'NoAns_exact'`: Exact match (the normalized answer exactly match the gold answer)
-* `'NoAns_f1'`: The F-score of predicted tokens versus the gold answer
-* `'NoAns_total'`: How many of the questions have no answers
-* `'best_exact'` : Best exact match (with varying threshold)
-* `'best_exact_thresh'`: No-answer probability threshold associated to the best exact match
-* `'best_f1'`: Best F1 score (with varying threshold)
-* `'best_f1_thresh'`: No-answer probability threshold associated to the best F1
-
-
-The range of `exact_match` is 0-100, where 0.0 means no answers were matched and 100.0 means all answers were matched.
-
-The range of `f1` is 0-1 -- its lowest possible value is 0, if either the precision or the recall is 0, and its highest possible value is 1.0, which means perfect precision and recall.
-
-The range of `total` depends on the length of predictions/references: its minimal value is 0, and maximal value is the total number of questions in the predictions and references.
-
-### Values from popular papers
-The [SQuAD v2 paper](https://arxiv.org/pdf/1806.03822.pdf) reported an F1 score of 66.3% and an Exact Match score of 63.4%.
-They also report that human performance on the dataset represents an F1 score of 89.5% and an Exact Match score of 86.9%.
-
-For more recent model performance, see the [dataset leaderboard](https://paperswithcode.com/dataset/squad).
-
-## Examples
-
-Maximal values for both exact match and F1 (perfect match):
-
-```python
-from evaluate import load
-squad_v2_ metric = load("squad_v2")
-predictions = [{'prediction_text': '1976', 'id': '56e10a3be3433e1400422b22', 'no_answer_probability': 0.}]
-references = [{'answers': {'answer_start': [97], 'text': ['1976']}, 'id': '56e10a3be3433e1400422b22'}]
-results = squad_v2_metric.compute(predictions=predictions, references=references)
-results
-{'exact': 100.0, 'f1': 100.0, 'total': 1, 'HasAns_exact': 100.0, 'HasAns_f1': 100.0, 'HasAns_total': 1, 'best_exact': 100.0, 'best_exact_thresh': 0.0, 'best_f1': 100.0, 'best_f1_thresh': 0.0}
-```
-
-Minimal values for both exact match and F1 (no match):
-
-```python
-from evaluate import load
-squad_metric = load("squad_v2")
-predictions = [{'prediction_text': '1999', 'id': '56e10a3be3433e1400422b22', 'no_answer_probability': 0.}]
-references = [{'answers': {'answer_start': [97], 'text': ['1976']}, 'id': '56e10a3be3433e1400422b22'}]
-results = squad_v2_metric.compute(predictions=predictions, references=references)
-results
-{'exact': 0.0, 'f1': 0.0, 'total': 1, 'HasAns_exact': 0.0, 'HasAns_f1': 0.0, 'HasAns_total': 1, 'best_exact': 0.0, 'best_exact_thresh': 0.0, 'best_f1': 0.0, 'best_f1_thresh': 0.0}
-```
-
-Partial match (2 out of 3 answers correct) :
-
-```python
-from evaluate import load
-squad_metric = load("squad_v2")
-predictions = [{'prediction_text': '1976', 'id': '56e10a3be3433e1400422b22', 'no_answer_probability': 0.}, {'prediction_text': 'Beyonce', 'id': '56d2051ce7d4791d0090260b', 'no_answer_probability': 0.}, {'prediction_text': 'climate change', 'id': '5733b5344776f419006610e1', 'no_answer_probability': 0.}]
-references = [{'answers': {'answer_start': [97], 'text': ['1976']}, 'id': '56e10a3be3433e1400422b22'}, {'answers': {'answer_start': [233], 'text': ['Beyoncé and Bruno Mars']}, 'id': '56d2051ce7d4791d0090260b'}, {'answers': {'answer_start': [891], 'text': ['climate change']}, 'id': '5733b5344776f419006610e1'}]
-results = squad_v2_metric.compute(predictions=predictions, references=references)
-results
-{'exact': 66.66666666666667, 'f1': 66.66666666666667, 'total': 3, 'HasAns_exact': 66.66666666666667, 'HasAns_f1': 66.66666666666667, 'HasAns_total': 3, 'best_exact': 66.66666666666667, 'best_exact_thresh': 0.0, 'best_f1': 66.66666666666667, 'best_f1_thresh': 0.0}
-```
-
-## Limitations and bias
-This metric works only with the datasets in the same format as the [SQuAD v.2 dataset](https://huggingface.co/datasets/squad_v2).
-
-The SQuAD datasets do contain a certain amount of noise, such as duplicate questions as well as missing answers, but these represent a minority of the 100,000 question-answer pairs. Also, neither exact match nor F1 score reflect whether models do better on certain types of questions (e.g. who questions) or those that cover a certain gender or geographical area -- carrying out more in-depth error analysis can complement these numbers.
-
-
-## Citation
-
-```bibtex
-@inproceedings{Rajpurkar2018SQuAD2,
-title={Know What You Don't Know: Unanswerable Questions for SQuAD},
-author={Pranav Rajpurkar and Jian Zhang and Percy Liang},
-booktitle={ACL 2018},
-year={2018}
-}
-```
-
-## Further References
-
-- [The Stanford Question Answering Dataset: Background, Challenges, Progress (blog post)](https://rajpurkar.github.io/mlx/qa-and-squad/)
-- [Hugging Face Course -- Question Answering](https://huggingface.co/course/chapter7/7)
diff --git a/spaces/falterWliame/Face_Mask_Detection/Lake Placid 1999 Hollywood Movie Hindi Download 2021.md b/spaces/falterWliame/Face_Mask_Detection/Lake Placid 1999 Hollywood Movie Hindi Download 2021.md
deleted file mode 100644
index 5ed1f35a6930d42ed12d32304b547bfd508c694a..0000000000000000000000000000000000000000
--- a/spaces/falterWliame/Face_Mask_Detection/Lake Placid 1999 Hollywood Movie Hindi Download 2021.md
+++ /dev/null
@@ -1,6 +0,0 @@
-
-
-jocuri pentru fete dress up Download free Lake placid 3 2010 hindi uncut from ... Filetype: Hollywood dubbed movies avi format & Size: 142823.76 kb-Mobile Version. ... XviD-aAF 1 A sequel to horror movie Lake Placid (1999). 4d29de3e1b
-
-
-
diff --git a/spaces/fatiXbelha/sd/Descarga Sniper 3D Assassin Mod Apk y disfruta de dinero infinito en 2021.md b/spaces/fatiXbelha/sd/Descarga Sniper 3D Assassin Mod Apk y disfruta de dinero infinito en 2021.md
deleted file mode 100644
index 6bde4bdf084a0a34419c71838e289f5222c3ff17..0000000000000000000000000000000000000000
--- a/spaces/fatiXbelha/sd/Descarga Sniper 3D Assassin Mod Apk y disfruta de dinero infinito en 2021.md
+++ /dev/null
@@ -1,137 +0,0 @@
-
-
Sniper 3D Dinero Infinito APK: The Ultimate Guide
-
If you are a fan of shooting games, you might have heard of Sniper 3D, one of the most popular and realistic sniper games on mobile devices. But did you know that there is a modded version of this game that gives you unlimited money, diamonds, and other resources? This version is called Sniper 3D Dinero Infinito APK, and it can make your gaming experience more fun and exciting. In this guide, we will tell you everything you need to know about Sniper 3D Dinero Infinito APK, including what it is, how to download and install it, how to play it, and what are its pros and cons. Let's get started!
Sniper 3D Dinero Infinito APK is a modified version of the original Sniper 3D game, which is developed by Fun Games For Free. This modded version gives you access to unlimited money, diamonds, and other resources that you can use to buy and upgrade your weapons, unlock new missions, and enjoy other features of the game. With Sniper 3D Dinero Infinito APK, you can become the ultimate sniper assassin without spending any real money or waiting for long hours.
-
A brief introduction to Sniper 3D game
-
Sniper 3D is a free-to-play gun game that puts you in the shoes of a deadly assassin. You can immerse yourself in high-intensity offline missions and show off your shooting skills in this adrenaline-pumping sniper adventure. You can also play against other assassins in real-time PVP mode and compete for the top rank. The game has over 850+ thrilling missions and 13 different worlds to explore. You can also unlock a vast arsenal of sniper rifles, assault rifles, and other powerful guns. You can customize your weapons and become the ultimate sniper 3d assassin.
-
The features and benefits of Sniper 3D Dinero Infinito APK
-
Sniper 3D Dinero Infinito APK has many features and benefits that make it different from the original game. Some of them are:
-
-
You can get unlimited money, diamonds, and other resources that you can use to buy and upgrade your weapons, unlock new missions, and enjoy other features of the game.
-
You can skip the ads that interrupt your gameplay and annoy you.
-
You can enjoy all the premium features of the game without paying any real money or subscribing to any plan.
-
You can play the game without any internet connection or data usage.
-
You can experience the thrill of being a professional sniper in this stunning 3d gun game with intuitive controls and realistic ballistics.
-
-
How to download and install Sniper 3D Dinero Infinito APK?
-
If you want to download and install Sniper 3D Dinero Infinito APK on your Android device, you need to follow these steps:
-
sniper 3d mod apk dinero y diamantes infinitos
-descargar sniper 3d hackeado con dinero infinito
-sniper 3d assassin apk mod dinero ilimitado
-sniper 3d apk full con dinero infinito
-sniper 3d dinero infinito apk sin root
-sniper 3d dinero infinito apk ultima version
-sniper 3d dinero infinito apk mediafıre
-sniper 3d dinero infinito apk mega
-sniper 3d dinero infinito apk android
-sniper 3d dinero infinito apk 2021
-sniper 3d dinero infinito apk offline
-sniper 3d dinero infinito apk online
-sniper 3d dinero infinito apk sin internet
-sniper 3d dinero infinito apk sin anuncios
-sniper 3d dinero infinito apk actualizado
-sniper 3d dinero infinito apk premium
-sniper 3d dinero infinito apk gratis
-sniper 3d dinero infinito apk facil de instalar
-sniper 3d dinero infinito apk para pc
-sniper 3d dinero infinito apk para ios
-sniper 3d dinero infinito apk sin verificacion
-sniper 3d dinero infinito apk sin virus
-sniper 3d dinero infinito apk sin licencia
-sniper 3d dinero infinito apk sin baneos
-sniper 3d dinero infinito apk trucos y consejos
-como descargar sniper 3d con dinero infinito apk
-como instalar sniper 3d con dinero infinito apk
-como jugar sniper 3d con dinero infinito apk
-como actualizar sniper 3d con dinero infinito apk
-como hackear sniper 3d con dinero infinito apk
-descargar juego de sniper 3d con dinero infinito apk
-descargar ultima version de sniper 3d con dinero infinito apk
-descargar gratis juego de sniper 3d con dinero infinito apk
-descargar e instalar sniper 3d con dinero infinito apk
-descargar e jugar sniper 3d con dinero infinito apk
-descargar e hackear sniper 3d con dinero infinito apk
-descargar e actualizar sniper 3d con dinero infinito apk
-descargar e disfrutar de sniper 3d con dinero infinito apk
-descargar e compartir sniper 3d con dinero infinito apk
-descargar e comentar sobre sniper 3d con dinero infinito apk
-
The steps to download the APK file
-
-
Go to a trusted website that provides the link to download Sniper 3D Dinero Infinito APK. For example, you can use this link to download the latest version of the modded game.
-
Click on the download button and wait for the APK file to be downloaded on your device.
-
Once the download is complete, locate the APK file in your device's file manager and tap on it to open it.
-
-
The steps to install the APK file
-
-
Before you install the APK file, you need to enable the installation of apps from unknown sources on your device. To do this, go to your device's settings, then security, and then toggle on the option that says "allow installation of apps from unknown sources".
-
After you enable this option, go back to the APK file and tap on it to start the installation process.
-
Follow the instructions on the screen and wait for the installation to finish.
-
Once the installation is done, you can launch the game from your app drawer or home screen and enjoy Sniper 3D Dinero Infinito APK.
-
-
How to play Sniper 3D Dinero Infinito APK?
-
Playing Sniper 3D Dinero Infinito APK is very easy and fun. You can choose from different modes and missions and use your sniper skills to eliminate your targets. Here are some tips on how to play the game:
-
The main modes and missions of the game
-
The game has three main modes: offline mode, online mode, and PVP mode. In offline mode, you can play over 850+ thrilling missions in 13 different worlds. You can also play special events and daily missions to earn extra rewards. In online mode, you can join a clan or create your own and team up with other players to complete co-op missions. You can also chat with other players and share your achievements. In PVP mode, you can challenge other players in real-time sniper duels and climb the leaderboard.
-
The tips and tricks to master the game
-
To master the game, you need to practice your shooting skills and use your resources wisely. Here are some tips and tricks that can help you:
-
-
Aim for the head or vital organs of your targets to get more points and bonuses.
-
Use the zoom feature to get a better view of your targets and their surroundings.
-
Use the silencer attachment to reduce the noise of your shots and avoid alerting other enemies.
-
Use the thermal vision attachment to see through walls and smoke.
-
Use the explosive bullets attachment to cause more damage and destruction.
-
Upgrade your weapons regularly to improve their performance and accuracy.
-
Buy new weapons with different features and specifications to suit different scenarios and preferences.
-
Use the money and diamonds you get from Sniper 3D Dinero Infinito APK to buy and upgrade your weapons, unlock new missions, and enjoy other features of the game.
-
-
What are the pros and cons of Sniper 3D Dinero Infinito APK?
-
Sniper 3D Dinero Infinito APK has many pros and cons that you should be aware of before you download and install it. Here are some of them:
-
The advantages of using the modded version
-
-
You can get unlimited money, diamonds, and other resources that you can use to buy and upgrade your weapons, unlock new missions, and enjoy other features of the game.
-
You can skip the ads that interrupt your gameplay and annoy you.
-
You can enjoy all the premium features of the game without paying any real money or subscribing to any plan.
-
You can play the game without any internet connection or data usage.
-
You can experience the thrill of being a professional sniper in this stunning 3d gun game with intuitive controls and realistic ballistics.
-
-
The disadvantages and risks of using the modded version
-
-
You may face some compatibility issues or bugs while playing the game on some devices or Android versions.
-
You may get banned from the game or lose your progress if you use the modded version online or in PVP mode.
-
You may expose your device to malware or viruses if you download the modded version from untrusted sources or websites.
-
You may violate the terms of service or privacy policy of the original game developer if you use the modded version without their permission or consent.
-
-
Conclusion
-
Sniper 3D Dinero Infinito APK is a modified version of Sniper 3D game that gives you unlimited money, diamonds, and other resources that you can use to buy and upgrade your weapons, unlock new missions, and enjoy other features of the game. It also lets you skip the ads that interrupt your gameplay and annoy you. You can also play the game without any internet connection or data usage. However, you should also be aware of the disadvantages and risks of using the modded version, such as compatibility issues, bans, malware, and violations. Therefore, you should use Sniper 3D Dinero Infinito APK at your own risk and discretion. We hope this guide has helped you understand what Sniper 3D Dinero Infinito APK is, how to download and install it, how to play it, and what are its pros and cons. If you have any questions or feedback, feel free to leave a comment below. Happy sniping!
-
FAQs
-
Here are some frequently asked questions about Sniper 3D Dinero Infinito APK:
-
-
-
Question
-
Answer
-
-
-
Is Sniper 3D Dinero Infinito APK safe to use?
-
Sniper 3D Dinero Infinito APK is not an official version of Sniper 3D game, and it may contain malware or viruses that can harm your device. Therefore, you should only download it from trusted sources or websites, and scan it with an antivirus before installing it. You should also backup your data and progress before using it.
-
-
-
Can I play Sniper 3D Dinero Infinito APK online or in PVP mode?
-
You can play Sniper 3D Dinero Infinito APK offline without any internet connection or data usage. However, if you try to play it online or in PVP mode, you may get banned from the game or lose your progress. This is because the game developer can detect the modded version and take action against it. Therefore, you should avoid playing it online or in PVP mode.
-
-
-
Do I need to root my device to use Sniper 3D Dinero Infinito APK?
-
No, you do not need to root your device to use Sniper 3D Dinero Infinito APK. You just need to enable the installation of apps from unknown sources on your device's settings, and then install the APK file as usual.
-
-
-
Will Sniper 3D Dinero Infinito APK work on my device or Android version?
-
Sniper 3D Dinero Infinito APK may not work on some devices or Android versions due to compatibility issues or bugs. Therefore, you should check the requirements and specifications of the modded version before downloading and installing it. You should also update your device and Android version to the latest version if possible.
-
-
-
How can I update Sniper 3D Dinero Infinito APK?
-
Sniper 3D Dinero Infinito APK may not receive regular updates from the original game developer, and it may become outdated or incompatible with the original game. Therefore, you should check for updates from the website or source where you downloaded the modded version, and download and install the latest version if available.
-
-
197e85843d
-
-
\ No newline at end of file
diff --git a/spaces/fatiXbelha/sd/Download NBA 2K Mobile MOD and Get Ready for the Most Realistic Basketball Game Ever.md b/spaces/fatiXbelha/sd/Download NBA 2K Mobile MOD and Get Ready for the Most Realistic Basketball Game Ever.md
deleted file mode 100644
index 3335961ec790e16cf23e651f772ea0d7b9c620e2..0000000000000000000000000000000000000000
--- a/spaces/fatiXbelha/sd/Download NBA 2K Mobile MOD and Get Ready for the Most Realistic Basketball Game Ever.md
+++ /dev/null
@@ -1,140 +0,0 @@
-
-
Download Mod NBA 2K Mobile: How to Enjoy the Best Basketball Game on Your Phone
-
If you are a fan of basketball and want to experience the thrill of playing with your favorite NBA stars on your phone, then you should try NBA 2K Mobile. This is a popular and realistic basketball game that lets you create your own team, compete with other players, and win rewards. But what if you want to unlock more features, get unlimited resources, and have more fun? Then you should download mod NBA 2K Mobile. This is a modified version of the original game that gives you access to everything you need to dominate the court. In this article, we will tell you what mod NBA 2K Mobile is, how it works, and how to download it safely and easily.
-
What is NBA 2K Mobile?
-
NBA 2K Mobile is a free-to-play basketball game that is developed by Cat Daddy Games and published by 2K Sports. It is available for both Android and iOS devices. The game features stunning graphics, realistic gameplay, and a variety of modes and events. You can choose from over 400 NBA players, customize your team, and play against other players online. You can also collect cards, upgrade your players, and earn rewards. Some of the features of NBA 2K Mobile are:
Real-time PVP matches with players from around the world
-
Season mode where you can play through a full NBA season and win the championship
-
Tourney mode where you can compete in tournaments and win exclusive rewards
-
Daily and weekly events where you can earn more cards and coins
-
Card mentoring system where you can improve your cards by using other cards as mentors
-
Courtside Pass subscription where you can get premium benefits such as extra coins, energy, and cards
-
-
Benefits of NBA 2K Mobile
-
-
You can enjoy the best basketball game on your phone with high-quality graphics and sound effects
-
You can play with your favorite NBA players and teams and relive their iconic moments
-
You can challenge yourself and test your skills against other players in real-time matches
-
You can build your own dream team and customize it according to your preference
-
You can collect hundreds of cards and upgrade them to make them stronger
-
You can have fun and earn rewards by participating in various modes and events
-
-
What is Mod NBA 2K Mobile?
-
Mod NBA 2K Mobile is a modified version of the original game that gives you access to unlimited resources and features. With mod NBA 2K Mobile, you can enjoy the game without any limitations or restrictions. You can get unlimited coins, energy, cards, mentors, and more. You can also unlock all the players, teams, modes, events, and rewards. Some of the advantages of mod NBA 2K Mobile are:
-
How Mod NBA 2K Mobile Works
-
-
Mod NBA 2K Mobile works by modifying the original game files and adding new features and functions
-
Mod NBA 2K Mobile does not require root or jailbreak to work on your device
Mod NBA 2K Mobile is compatible with most Android and iOS devices and does not affect the performance or security of your device
-
-
Advantages of Mod NBA 2K Mobile
-
-
You can get unlimited coins and use them to buy anything you want in the game
-
You can get unlimited energy and play as much as you want without waiting for it to refill
-
You can get unlimited cards and mentors and use them to upgrade your players and make them stronger
-
You can unlock all the players, teams, modes, events, and rewards and enjoy them without any restrictions
-
You can have more fun and excitement by playing with the best players and teams in the game
-
You can have an edge over other players and dominate the game
-
-
How to Download Mod NBA 2K Mobile
-
If you want to download mod NBA 2K Mobile, you need to follow some simple steps. But before that, you need to make sure that your device meets some requirements. Here are the requirements for downloading mod NBA 2K Mobile:
-
Requirements for Downloading Mod NBA 2K Mobile
-
-
Your device should have Android version 4.4 or higher or iOS version 11.0 or higher
-
Your device should have at least 2 GB of RAM and 4 GB of free storage space
-
Your device should have a stable internet connection
-
Your device should allow installation of apps from unknown sources (for Android) or trust third-party apps (for iOS)
-
-
If your device meets these requirements, you can proceed to the steps for downloading mod NBA 2K Mobile. Here are the steps:
-
Steps for Downloading Mod NBA 2K Mobile
-
Step 1: Find a Reliable Source for Mod NBA 2K Mobile
-
The first step is to find a reliable source for mod NBA 2K Mobile. There are many websites that claim to offer mod NBA 2K Mobile, but not all of them are trustworthy. Some of them may contain viruses, malware, or spyware that can harm your device or steal your personal information. Therefore, you need to be careful and do some research before downloading mod NBA 2K Mobile from any source. You can check the reviews, ratings, comments, and feedback of other users who have downloaded mod NBA 2K Mobile from the same source. You can also use antivirus software or online scanners to scan the source for any potential threats. One of the reliable sources that we recommend for downloading mod NBA 2K Mobile is [Modded-1.com]. This is a trusted website that provides modded versions of various games and apps, including mod NBA 2K Mobile.
-
Step 2: Download the Mod NBA 2K Mobile APK File
-
The next step is to download the mod NBA 2K Mobile APK file from the source that you have chosen. The APK file is the installer file that contains the modified version of the game. To download the mod NBA 2K Mobile APK file, you need to follow these steps:
-
download mod nba 2k mobile apk
-download mod nba 2k mobile basketball
-download mod nba 2k mobile unlimited money
-download mod nba 2k mobile latest version
-download mod nba 2k mobile android
-download mod nba 2k mobile ios
-download mod nba 2k mobile offline
-download mod nba 2k mobile free
-download mod nba 2k mobile hack
-download mod nba 2k mobile cheats
-download mod nba 2k mobile season 3
-download mod nba 2k mobile no verification
-download mod nba 2k mobile online
-download mod nba 2k mobile gameplay
-download mod nba 2k mobile obb
-download mod nba 2k mobile reddit
-download mod nba 2k mobile update
-download mod nba 2k mobile coins
-download mod nba 2k mobile generator
-download mod nba 2k mobile codes
-download mod nba 2k mobile review
-download mod nba 2k mobile tips
-download mod nba 2k mobile guide
-download mod nba 2k mobile tutorial
-download mod nba 2k mobile best players
-download mod nba 2k mobile legends
-download mod nba 2k mobile roster
-download mod nba 2k mobile teams
-download mod nba 2k mobile cards
-download mod nba 2k mobile draft
-download mod nba 2k mobile controller support
-download mod nba 2k mobile requirements
-download mod nba 2k mobile pc
-download mod nba 2k mobile mac
-download mod nba 2k mobile windows
-download mod nba 2k mobile chromebook
-download mod nba 2k mobile bluestacks
-download mod nba 2k mobile emulator
-download mod nba 2k mobile app store
-download mod nba 2k mobile google play store
-
-
Go to the website of the source that you have chosen (for example, [Modded-1.com])
-
Search for mod NBA 2K Mobile or click on the link that directs you to the download page of mod NBA 2K Mobile
-
On the download page, read the description, features, and instructions of mod NBA 2K Mobile carefully
-
Click on the download button or link that starts the download process of mod NBA 2K Mobile APK file
-
Wait for a few seconds or minutes until the download is completed (the time may vary depending on your internet speed and file size)
-
Once the download is completed, locate the mod NBA 2K Mobile APK file in your device's download folder or notification bar
-
-
Step 3: Install the Mod NBA 2K Mobile APK File
-
The third step is to install the mod NBA 2K Mobile APK file on your device. To install the mod NBA 2K Mobile APK file, you need to follow these steps:
-
-
Before installing the mod NBA 2K Mobile APK file, make sure that you have uninstalled or deleted the original version of NBA 2K Mobile from your device (if you have it)
-
Also, make sure that you have enabled the installation of apps from unknown sources (for Android) or trusted third-party apps (for iOS) on your device settings
-
Tap on the mod NBA 2K Mobile APK file and select the install option
-
Wait for a few seconds or minutes until the installation is completed (the time may vary depending on your device and file size)
-
Once the installation is completed, you will see a confirmation message or icon on your device screen
-
-
Step 4: Launch the Mod NBA 2K Mobile App and Enjoy
-
The final step is to launch the mod NBA 2K Mobile app and enjoy the game. To launch the mod NBA 2K Mobile app, you need to follow these steps:
-
-
Go to your device's app drawer or home screen and look for the mod NBA 2K Mobile app icon
-
Tap on the mod NBA 2K Mobile app icon and open the game
-
Allow the game to load and access the necessary permissions and data
-
Choose your preferred language, region, and settings
-
Create or log in to your account and start playing the game
-
-
Congratulations! You have successfully downloaded and installed mod NBA 2K Mobile on your device. Now you can enjoy the best basketball game on your phone with unlimited resources and features.
-
Conclusion
-
NBA 2K Mobile is a great basketball game that lets you play with your favorite NBA stars on your phone. However, if you want to have more fun and excitement, you should download mod NBA 2K Mobile. This is a modified version of the original game that gives you access to everything you need to dominate the court. You can get unlimited coins, energy, cards, mentors, and more. You can also unlock all the players, teams, modes, events, and rewards. To download mod NBA 2K Mobile, you just need to follow some simple steps that we have explained in this article. We hope that this article has helped you to download mod NBA 2K Mobile safely and easily. If you have any questions or feedback, please feel free to leave a comment below.
-
FAQs
-
Here are some frequently asked questions about mod NBA 2K Mobile:
-
-
Is mod NBA 2K Mobile safe to use?
-
Yes, mod NBA 2K Mobile is safe to use as long as you download it from a reliable source and scan it for any potential threats. However, you should always be careful and use it at your own risk.
-
Is mod NBA 2K Mobile legal to use?
-
No, mod NBA 2K Mobile is not legal to use as it violates the terms and conditions of the original game. Therefore, you may face some consequences such as account suspension or ban if you use it.
-
Does mod NBA 2K Mobile require root or jailbreak?
-
No, mod NBA 2K Mobile does not require root or jailbreak to work on your device. You just need to enable the installation of apps from unknown sources (for Android) or trust third-party apps (for iOS) on your device settings.
-
Does mod NBA 2K Mobile work offline?
-
No, mod NBA 2K Mobile does not work offline as it requires an internet connection to access the game servers and data. Therefore, you need to have a stable internet connection to play the game.
-
Can I update mod NBA 2K Mobile?
-
No, you cannot update mod NBA 2K Mobile as it may cause some errors or issues with the game. Therefore, you need to download the latest version of mod NBA 2K Mobile from the same source whenever there is an update available.
-
401be4b1e0
-
-
\ No newline at end of file
diff --git "a/spaces/fb700/chat3/crazy_functions/\346\200\273\347\273\223word\346\226\207\346\241\243.py" "b/spaces/fb700/chat3/crazy_functions/\346\200\273\347\273\223word\346\226\207\346\241\243.py"
deleted file mode 100644
index f1fe20171cc54aec0c79f4961e71b57845f252d5..0000000000000000000000000000000000000000
--- "a/spaces/fb700/chat3/crazy_functions/\346\200\273\347\273\223word\346\226\207\346\241\243.py"
+++ /dev/null
@@ -1,127 +0,0 @@
-from toolbox import update_ui
-from toolbox import CatchException, report_execption, write_results_to_file
-from .crazy_utils import request_gpt_model_in_new_thread_with_ui_alive
-fast_debug = False
-
-
-def 解析docx(file_manifest, project_folder, llm_kwargs, plugin_kwargs, chatbot, history, system_prompt):
- import time, os
- # pip install python-docx 用于docx格式,跨平台
- # pip install pywin32 用于doc格式,仅支持Win平台
- for index, fp in enumerate(file_manifest):
- if fp.split(".")[-1] == "docx":
- from docx import Document
- doc = Document(fp)
- file_content = "\n".join([para.text for para in doc.paragraphs])
- else:
- import win32com.client
- word = win32com.client.Dispatch("Word.Application")
- word.visible = False
- # 打开文件
- print('fp', os.getcwd())
- doc = word.Documents.Open(os.getcwd() + '/' + fp)
- # file_content = doc.Content.Text
- doc = word.ActiveDocument
- file_content = doc.Range().Text
- doc.Close()
- word.Quit()
-
- print(file_content)
- # private_upload里面的文件名在解压zip后容易出现乱码(rar和7z格式正常),故可以只分析文章内容,不输入文件名
- from .crazy_utils import breakdown_txt_to_satisfy_token_limit_for_pdf
- from request_llm.bridge_all import model_info
- max_token = model_info[llm_kwargs['llm_model']]['max_token']
- TOKEN_LIMIT_PER_FRAGMENT = max_token * 3 // 4
- paper_fragments = breakdown_txt_to_satisfy_token_limit_for_pdf(
- txt=file_content,
- get_token_fn=model_info[llm_kwargs['llm_model']]['token_cnt'],
- limit=TOKEN_LIMIT_PER_FRAGMENT
- )
- this_paper_history = []
- for i, paper_frag in enumerate(paper_fragments):
- i_say = f'请对下面的文章片段用中文做概述,文件名是{os.path.relpath(fp, project_folder)},文章内容是 ```{paper_frag}```'
- i_say_show_user = f'请对下面的文章片段做概述: {os.path.abspath(fp)}的第{i+1}/{len(paper_fragments)}个片段。'
- gpt_say = yield from request_gpt_model_in_new_thread_with_ui_alive(
- inputs=i_say,
- inputs_show_user=i_say_show_user,
- llm_kwargs=llm_kwargs,
- chatbot=chatbot,
- history=[],
- sys_prompt="总结文章。"
- )
-
- chatbot[-1] = (i_say_show_user, gpt_say)
- history.extend([i_say_show_user,gpt_say])
- this_paper_history.extend([i_say_show_user,gpt_say])
-
- # 已经对该文章的所有片段总结完毕,如果文章被切分了,
- if len(paper_fragments) > 1:
- i_say = f"根据以上的对话,总结文章{os.path.abspath(fp)}的主要内容。"
- gpt_say = yield from request_gpt_model_in_new_thread_with_ui_alive(
- inputs=i_say,
- inputs_show_user=i_say,
- llm_kwargs=llm_kwargs,
- chatbot=chatbot,
- history=this_paper_history,
- sys_prompt="总结文章。"
- )
-
- history.extend([i_say,gpt_say])
- this_paper_history.extend([i_say,gpt_say])
-
- res = write_results_to_file(history)
- chatbot.append(("完成了吗?", res))
- yield from update_ui(chatbot=chatbot, history=history) # 刷新界面
-
- res = write_results_to_file(history)
- chatbot.append(("所有文件都总结完成了吗?", res))
- yield from update_ui(chatbot=chatbot, history=history) # 刷新界面
-
-
-@CatchException
-def 总结word文档(txt, llm_kwargs, plugin_kwargs, chatbot, history, system_prompt, web_port):
- import glob, os
-
- # 基本信息:功能、贡献者
- chatbot.append([
- "函数插件功能?",
- "批量总结Word文档。函数插件贡献者: JasonGuo1"])
- yield from update_ui(chatbot=chatbot, history=history) # 刷新界面
-
- # 尝试导入依赖,如果缺少依赖,则给出安装建议
- try:
- from docx import Document
- except:
- report_execption(chatbot, history,
- a=f"解析项目: {txt}",
- b=f"导入软件依赖失败。使用该模块需要额外依赖,安装方法```pip install --upgrade python-docx pywin32```。")
- yield from update_ui(chatbot=chatbot, history=history) # 刷新界面
- return
-
- # 清空历史,以免输入溢出
- history = []
-
- # 检测输入参数,如没有给定输入参数,直接退出
- if os.path.exists(txt):
- project_folder = txt
- else:
- if txt == "": txt = '空空如也的输入栏'
- report_execption(chatbot, history, a=f"解析项目: {txt}", b=f"找不到本地项目或无权访问: {txt}")
- yield from update_ui(chatbot=chatbot, history=history) # 刷新界面
- return
-
- # 搜索需要处理的文件清单
- if txt.endswith('.docx') or txt.endswith('.doc'):
- file_manifest = [txt]
- else:
- file_manifest = [f for f in glob.glob(f'{project_folder}/**/*.docx', recursive=True)] + \
- [f for f in glob.glob(f'{project_folder}/**/*.doc', recursive=True)]
-
- # 如果没找到任何文件
- if len(file_manifest) == 0:
- report_execption(chatbot, history, a=f"解析项目: {txt}", b=f"找不到任何.docx或doc文件: {txt}")
- yield from update_ui(chatbot=chatbot, history=history) # 刷新界面
- return
-
- # 开始正式执行任务
- yield from 解析docx(file_manifest, project_folder, llm_kwargs, plugin_kwargs, chatbot, history, system_prompt)
diff --git a/spaces/fb700/chatglm-fitness-RLHF/crazy_functions/test_project/latex/attention/introduction.tex b/spaces/fb700/chatglm-fitness-RLHF/crazy_functions/test_project/latex/attention/introduction.tex
deleted file mode 100644
index 1baa8915f4cf7aec2520894a87470fc9436d954b..0000000000000000000000000000000000000000
--- a/spaces/fb700/chatglm-fitness-RLHF/crazy_functions/test_project/latex/attention/introduction.tex
+++ /dev/null
@@ -1,18 +0,0 @@
-Recurrent neural networks, long short-term memory \citep{hochreiter1997} and gated recurrent \citep{gruEval14} neural networks in particular, have been firmly established as state of the art approaches in sequence modeling and transduction problems such as language modeling and machine translation \citep{sutskever14, bahdanau2014neural, cho2014learning}. Numerous efforts have since continued to push the boundaries of recurrent language models and encoder-decoder architectures \citep{wu2016google,luong2015effective,jozefowicz2016exploring}.
-
-Recurrent models typically factor computation along the symbol positions of the input and output sequences. Aligning the positions to steps in computation time, they generate a sequence of hidden states $h_t$, as a function of the previous hidden state $h_{t-1}$ and the input for position $t$. This inherently sequential nature precludes parallelization within training examples, which becomes critical at longer sequence lengths, as memory constraints limit batching across examples.
-%\marginpar{not sure if the memory constraints are understandable here}
-Recent work has achieved significant improvements in computational efficiency through factorization tricks \citep{Kuchaiev2017Factorization} and conditional computation \citep{shazeer2017outrageously}, while also improving model performance in case of the latter. The fundamental constraint of sequential computation, however, remains.
-
-%\marginpar{@all: there is work on analyzing what attention really does in seq2seq models, couldn't find it right away}
-
-Attention mechanisms have become an integral part of compelling sequence modeling and transduction models in various tasks, allowing modeling of dependencies without regard to their distance in the input or output sequences \citep{bahdanau2014neural, structuredAttentionNetworks}. In all but a few cases \citep{decomposableAttnModel}, however, such attention mechanisms are used in conjunction with a recurrent network.
-
-%\marginpar{not sure if "cross-positional communication" is understandable without explanation}
-%\marginpar{insert exact training times and stats for the model that reaches sota earliest, maybe even a single GPU model?}
-
-In this work we propose the Transformer, a model architecture eschewing recurrence and instead relying entirely on an attention mechanism to draw global dependencies between input and output. The Transformer allows for significantly more parallelization and can reach a new state of the art in translation quality after being trained for as little as twelve hours on eight P100 GPUs.
-%\marginpar{you removed the constant number of repetitions part. I wrote it because I wanted to make it clear that the model does not only perform attention once, while it's also not recurrent. I thought that might be important to get across early.}
-
-% Just a standard paragraph with citations, rewrite.
-%After the seminal papers of \citep{sutskever14}, \citep{bahdanau2014neural}, and \citep{cho2014learning}, recurrent models have become the dominant solution for both sequence modeling and sequence-to-sequence transduction. Many efforts such as \citep{wu2016google,luong2015effective,jozefowicz2016exploring} have pushed the boundaries of machine translation and language modeling with recurrent sequence models. Recent effort \citep{shazeer2017outrageously} has combined the power of conditional computation with sequence models to train very large models for machine translation, pushing SOTA at lower computational cost. Recurrent models compute a vector of hidden states $h_t$, for each time step $t$ of computation. $h_t$ is a function of both the input at time $t$ and the previous hidden state $h_t$. This dependence on the previous hidden state encumbers recurrnet models to process multiple inputs at once, and their time complexity is a linear function of the length of the input and output, both during training and inference. [What I want to say here is that although this is fine during decoding, at training time, we are given both input and output and this linear nature does not allow the RNN to process all inputs and outputs simultaneously and haven't been used on datasets that are the of the scale of the web. What's the largest dataset we have ? . Talk about Nividia and possibly other's effors to speed up things, and possibly other efforts that alleviate this, but are still limited by it's comptuational nature]. Rest of the intro: What if you could construct the state based on the actual inputs and outputs, then you could construct them all at once. This has been the foundation of many promising recent efforts, bytenet,facenet (Also talk about quasi rnn here). Now we talk about attention!! Along with cell architectures such as long short-term meory (LSTM) \citep{hochreiter1997}, and gated recurrent units (GRUs) \citep{cho2014learning}, attention has emerged as an essential ingredient in successful sequence models, in particular for machine translation. In recent years, many, if not all, state-of-the-art (SOTA) results in machine translation have been achieved with attention-based sequence models \citep{wu2016google,luong2015effective,jozefowicz2016exploring}. Talk about the neon work on how it played with attention to do self attention! Then talk about what we do.
\ No newline at end of file
diff --git a/spaces/fb700/chatglm-fitness-RLHF/src/facerender/animate.py b/spaces/fb700/chatglm-fitness-RLHF/src/facerender/animate.py
deleted file mode 100644
index 781f5a3318a086049cc6b74393073ddda7001d5e..0000000000000000000000000000000000000000
--- a/spaces/fb700/chatglm-fitness-RLHF/src/facerender/animate.py
+++ /dev/null
@@ -1,257 +0,0 @@
-import os
-import cv2
-import yaml
-import numpy as np
-import warnings
-from skimage import img_as_ubyte
-import safetensors
-import safetensors.torch
-warnings.filterwarnings('ignore')
-
-
-import imageio
-import torch
-import torchvision
-
-
-from src.facerender.modules.keypoint_detector import HEEstimator, KPDetector
-from src.facerender.modules.mapping import MappingNet
-from src.facerender.modules.generator import OcclusionAwareGenerator, OcclusionAwareSPADEGenerator
-from src.facerender.modules.make_animation import make_animation
-
-from pydub import AudioSegment
-from src.utils.face_enhancer import enhancer_generator_with_len, enhancer_list
-from src.utils.paste_pic import paste_pic
-from src.utils.videoio import save_video_with_watermark
-
-try:
- import webui # in webui
- in_webui = True
-except:
- in_webui = False
-
-class AnimateFromCoeff():
-
- def __init__(self, sadtalker_path, device):
-
- with open(sadtalker_path['facerender_yaml']) as f:
- config = yaml.safe_load(f)
-
- generator = OcclusionAwareSPADEGenerator(**config['model_params']['generator_params'],
- **config['model_params']['common_params'])
- kp_extractor = KPDetector(**config['model_params']['kp_detector_params'],
- **config['model_params']['common_params'])
- he_estimator = HEEstimator(**config['model_params']['he_estimator_params'],
- **config['model_params']['common_params'])
- mapping = MappingNet(**config['model_params']['mapping_params'])
-
- generator.to(device)
- kp_extractor.to(device)
- he_estimator.to(device)
- mapping.to(device)
- for param in generator.parameters():
- param.requires_grad = False
- for param in kp_extractor.parameters():
- param.requires_grad = False
- for param in he_estimator.parameters():
- param.requires_grad = False
- for param in mapping.parameters():
- param.requires_grad = False
-
- if sadtalker_path is not None:
- if 'checkpoint' in sadtalker_path: # use safe tensor
- self.load_cpk_facevid2vid_safetensor(sadtalker_path['checkpoint'], kp_detector=kp_extractor, generator=generator, he_estimator=None)
- else:
- self.load_cpk_facevid2vid(sadtalker_path['free_view_checkpoint'], kp_detector=kp_extractor, generator=generator, he_estimator=he_estimator)
- else:
- raise AttributeError("Checkpoint should be specified for video head pose estimator.")
-
- if sadtalker_path['mappingnet_checkpoint'] is not None:
- self.load_cpk_mapping(sadtalker_path['mappingnet_checkpoint'], mapping=mapping)
- else:
- raise AttributeError("Checkpoint should be specified for video head pose estimator.")
-
- self.kp_extractor = kp_extractor
- self.generator = generator
- self.he_estimator = he_estimator
- self.mapping = mapping
-
- self.kp_extractor.eval()
- self.generator.eval()
- self.he_estimator.eval()
- self.mapping.eval()
-
- self.device = device
-
- def load_cpk_facevid2vid_safetensor(self, checkpoint_path, generator=None,
- kp_detector=None, he_estimator=None,
- device="cpu"):
-
- checkpoint = safetensors.torch.load_file(checkpoint_path)
-
- if generator is not None:
- x_generator = {}
- for k,v in checkpoint.items():
- if 'generator' in k:
- x_generator[k.replace('generator.', '')] = v
- generator.load_state_dict(x_generator)
- if kp_detector is not None:
- x_generator = {}
- for k,v in checkpoint.items():
- if 'kp_extractor' in k:
- x_generator[k.replace('kp_extractor.', '')] = v
- kp_detector.load_state_dict(x_generator)
- if he_estimator is not None:
- x_generator = {}
- for k,v in checkpoint.items():
- if 'he_estimator' in k:
- x_generator[k.replace('he_estimator.', '')] = v
- he_estimator.load_state_dict(x_generator)
-
- return None
-
- def load_cpk_facevid2vid(self, checkpoint_path, generator=None, discriminator=None,
- kp_detector=None, he_estimator=None, optimizer_generator=None,
- optimizer_discriminator=None, optimizer_kp_detector=None,
- optimizer_he_estimator=None, device="cpu"):
- checkpoint = torch.load(checkpoint_path, map_location=torch.device(device))
- if generator is not None:
- generator.load_state_dict(checkpoint['generator'])
- if kp_detector is not None:
- kp_detector.load_state_dict(checkpoint['kp_detector'])
- if he_estimator is not None:
- he_estimator.load_state_dict(checkpoint['he_estimator'])
- if discriminator is not None:
- try:
- discriminator.load_state_dict(checkpoint['discriminator'])
- except:
- print ('No discriminator in the state-dict. Dicriminator will be randomly initialized')
- if optimizer_generator is not None:
- optimizer_generator.load_state_dict(checkpoint['optimizer_generator'])
- if optimizer_discriminator is not None:
- try:
- optimizer_discriminator.load_state_dict(checkpoint['optimizer_discriminator'])
- except RuntimeError as e:
- print ('No discriminator optimizer in the state-dict. Optimizer will be not initialized')
- if optimizer_kp_detector is not None:
- optimizer_kp_detector.load_state_dict(checkpoint['optimizer_kp_detector'])
- if optimizer_he_estimator is not None:
- optimizer_he_estimator.load_state_dict(checkpoint['optimizer_he_estimator'])
-
- return checkpoint['epoch']
-
- def load_cpk_mapping(self, checkpoint_path, mapping=None, discriminator=None,
- optimizer_mapping=None, optimizer_discriminator=None, device='cpu'):
- checkpoint = torch.load(checkpoint_path, map_location=torch.device(device))
- if mapping is not None:
- mapping.load_state_dict(checkpoint['mapping'])
- if discriminator is not None:
- discriminator.load_state_dict(checkpoint['discriminator'])
- if optimizer_mapping is not None:
- optimizer_mapping.load_state_dict(checkpoint['optimizer_mapping'])
- if optimizer_discriminator is not None:
- optimizer_discriminator.load_state_dict(checkpoint['optimizer_discriminator'])
-
- return checkpoint['epoch']
-
- def generate(self, x, video_save_dir, pic_path, crop_info, enhancer=None, background_enhancer=None, preprocess='crop', img_size=256):
-
- source_image=x['source_image'].type(torch.FloatTensor)
- source_semantics=x['source_semantics'].type(torch.FloatTensor)
- target_semantics=x['target_semantics_list'].type(torch.FloatTensor)
- source_image=source_image.to(self.device)
- source_semantics=source_semantics.to(self.device)
- target_semantics=target_semantics.to(self.device)
- if 'yaw_c_seq' in x:
- yaw_c_seq = x['yaw_c_seq'].type(torch.FloatTensor)
- yaw_c_seq = x['yaw_c_seq'].to(self.device)
- else:
- yaw_c_seq = None
- if 'pitch_c_seq' in x:
- pitch_c_seq = x['pitch_c_seq'].type(torch.FloatTensor)
- pitch_c_seq = x['pitch_c_seq'].to(self.device)
- else:
- pitch_c_seq = None
- if 'roll_c_seq' in x:
- roll_c_seq = x['roll_c_seq'].type(torch.FloatTensor)
- roll_c_seq = x['roll_c_seq'].to(self.device)
- else:
- roll_c_seq = None
-
- frame_num = x['frame_num']
-
- predictions_video = make_animation(source_image, source_semantics, target_semantics,
- self.generator, self.kp_extractor, self.he_estimator, self.mapping,
- yaw_c_seq, pitch_c_seq, roll_c_seq, use_exp = True)
-
- predictions_video = predictions_video.reshape((-1,)+predictions_video.shape[2:])
- predictions_video = predictions_video[:frame_num]
-
- video = []
- for idx in range(predictions_video.shape[0]):
- image = predictions_video[idx]
- image = np.transpose(image.data.cpu().numpy(), [1, 2, 0]).astype(np.float32)
- video.append(image)
- result = img_as_ubyte(video)
-
- ### the generated video is 256x256, so we keep the aspect ratio,
- original_size = crop_info[0]
- if original_size:
- result = [ cv2.resize(result_i,(img_size, int(img_size * original_size[1]/original_size[0]) )) for result_i in result ]
-
- video_name = x['video_name'] + '.mp4'
- path = os.path.join(video_save_dir, 'temp_'+video_name)
-
- imageio.mimsave(path, result, fps=float(25))
-
- av_path = os.path.join(video_save_dir, video_name)
- return_path = av_path
-
- audio_path = x['audio_path']
- audio_name = os.path.splitext(os.path.split(audio_path)[-1])[0]
- new_audio_path = os.path.join(video_save_dir, audio_name+'.wav')
- start_time = 0
- # cog will not keep the .mp3 filename
- sound = AudioSegment.from_file(audio_path)
- frames = frame_num
- end_time = start_time + frames*1/25*1000
- word1=sound.set_frame_rate(16000)
- word = word1[start_time:end_time]
- word.export(new_audio_path, format="wav")
-
- save_video_with_watermark(path, new_audio_path, av_path, watermark= False)
- print(f'The generated video is named {video_save_dir}/{video_name}')
-
- if 'full' in preprocess.lower():
- # only add watermark to the full image.
- video_name_full = x['video_name'] + '_full.mp4'
- full_video_path = os.path.join(video_save_dir, video_name_full)
- return_path = full_video_path
- paste_pic(path, pic_path, crop_info, new_audio_path, full_video_path, extended_crop= True if 'ext' in preprocess.lower() else False)
- print(f'The generated video is named {video_save_dir}/{video_name_full}')
- else:
- full_video_path = av_path
-
- #### paste back then enhancers
- if enhancer:
- video_name_enhancer = x['video_name'] + '_enhanced.mp4'
- enhanced_path = os.path.join(video_save_dir, 'temp_'+video_name_enhancer)
- av_path_enhancer = os.path.join(video_save_dir, video_name_enhancer)
- return_path = av_path_enhancer
-
- try:
- enhanced_images_gen_with_len = enhancer_generator_with_len(full_video_path, method=enhancer, bg_upsampler=background_enhancer)
- imageio.mimsave(enhanced_path, enhanced_images_gen_with_len, fps=float(25))
- except:
- enhanced_images_gen_with_len = enhancer_list(full_video_path, method=enhancer, bg_upsampler=background_enhancer)
- imageio.mimsave(enhanced_path, enhanced_images_gen_with_len, fps=float(25))
-
- save_video_with_watermark(enhanced_path, new_audio_path, av_path_enhancer, watermark= False)
- print(f'The generated video is named {video_save_dir}/{video_name_enhancer}')
- os.remove(enhanced_path)
-
- os.remove(path)
- os.remove(new_audio_path)
-
- return return_path
-
diff --git a/spaces/fclong/summary/fengshen/examples/clue_sim/main.py b/spaces/fclong/summary/fengshen/examples/clue_sim/main.py
deleted file mode 100644
index 91c5a732d8cb1a683aa34a3b3f7c158861cd4492..0000000000000000000000000000000000000000
--- a/spaces/fclong/summary/fengshen/examples/clue_sim/main.py
+++ /dev/null
@@ -1,133 +0,0 @@
-# coding=utf-8
-# Copyright 2021 The IDEA Authors. All rights reserved.
-
-# Licensed under the Apache License, Version 2.0 (the "License");
-# you may not use this file except in compliance with the License.
-# You may obtain a copy of the License at
-
-# http://www.apache.org/licenses/LICENSE-2.0
-
-# Unless required by applicable law or agreed to in writing, software
-# distributed under the License is distributed on an "AS IS" BASIS,
-# WITHOUT WARRANTIES OR CONDITIONS OF ANY KIND, either express or implied.
-# See the License for the specific language governing permissions and
-# limitations under the License.
-import jsonlines
-import torch
-import pytorch_lightning as pl
-from transformers import AutoTokenizer, BertTokenizer
-from train_func import CustomDataset, CustomDataModule, CustomModel
-import argparse
-import os
-import gpustat
-
-if __name__ == '__main__':
- my_parser = argparse.ArgumentParser()
- my_parser.add_argument(
- "--model_path", default="./weights/Erlangshen-MegatronBert-1.3B-Similarity", type=str, required=False)
- my_parser.add_argument(
- "--model_name", default="IDEA-CCNL/Erlangshen-MegatronBert-1.3B-Similarity", type=str, required=False)
- my_parser.add_argument("--max_seq_length", default=64, type=int, required=False)
- my_parser.add_argument("--batch_size", default=32, type=int, required=False)
- my_parser.add_argument("--val_batch_size", default=64, type=int, required=False)
- my_parser.add_argument("--num_epochs", default=10, type=int, required=False)
- my_parser.add_argument("--learning_rate", default=4e-5, type=float, required=False)
- my_parser.add_argument("--warmup_proportion", default=0.2, type=int, required=False)
- my_parser.add_argument("--warmup_step", default=2, type=int, required=False)
- my_parser.add_argument("--num_labels", default=3, type=int, required=False)
- my_parser.add_argument("--cate_performance", default=False, type=bool, required=False)
- my_parser.add_argument("--use_original_pooler", default=True, type=bool, required=False)
- my_parser.add_argument("--model_output_path", default='./pl_model', type=str, required=False)
- my_parser.add_argument("--mode", type=str, choices=['Train', 'Test'], required=True)
- my_parser.add_argument("--predict_model_path", default='./pl_model/', type=str, required=False)
- my_parser.add_argument("--test_output_path", default='./submissions', type=str, required=False)
- my_parser.add_argument("--optimizer", default='AdamW', type=str, required=False) # ['Adam', 'AdamW']
- # ['StepLR', 'CosineWarmup', 'CosineAnnealingLR']
- my_parser.add_argument("--scheduler", default='CosineWarmup', type=str, required=False)
- my_parser.add_argument("--loss_function", default='LSCE_correction', type=str,
- required=False) # ['CE', 'Focal', 'LSCE_correction']
-
- args = my_parser.parse_args()
-
- print(args)
- gpustat.print_gpustat()
-
- if 'Erlangshen' in args.model_name:
- tokenizer = BertTokenizer.from_pretrained(args.model_name, cache_dir=args.model_path)
- else:
- tokenizer = AutoTokenizer.from_pretrained(args.model_name, cache_dir=args.model_path)
-
- seed = 1919
- pl.seed_everything(seed)
-
- dm = CustomDataModule(
- args=args,
- tokenizer=tokenizer,
- )
-
- metric_index = 2
- checkpoint = pl.callbacks.ModelCheckpoint(
- save_top_k=1,
- verbose=True,
- monitor=['val_loss', 'val_acc', 'val_f1'][metric_index],
- mode=['min', 'max', 'max'][metric_index]
- )
-
- lr_monitor = pl.callbacks.LearningRateMonitor(logging_interval="step")
- callbacks = [checkpoint, lr_monitor]
-
- logger = pl.loggers.TensorBoardLogger(save_dir=os.getcwd(),
- name='lightning_logs/' + args.model_name.split('/')[-1]),
-
- trainer = pl.Trainer(
- progress_bar_refresh_rate=50,
- logger=logger,
- gpus=-1 if torch.cuda.is_available() else None,
- amp_backend='native',
- amp_level='O2',
- precision=16,
- callbacks=callbacks,
- gradient_clip_val=1.0,
- max_epochs=args.num_epochs,
- # accelerator='ddp',
- # plugins='ddp_sharded',
- )
-
- if args.mode == 'Train':
- print('Only Train')
- model = CustomModel(
- args=args,
- )
- trainer.fit(model, dm)
-
- # Predict test, save results to json
- if args.mode == 'Test':
- print('Only Test')
- test_loader = torch.utils.data.DataLoader(
- CustomDataset('test.json', tokenizer, args.max_seq_length, 'test'),
- batch_size=args.val_batch_size,
- num_workers=4,
- shuffle=False,
- pin_memory=True,
- drop_last=False
- )
-
- model = CustomModel(args=args).load_from_checkpoint(args.predict_model_path, args=args)
-
- predict_results = trainer.predict(model, test_loader, return_predictions=True)
-
- path = os.path.join(
- args.test_output_path,
- args.model_name.split('/')[-1].replace('-', '_'))
- file_path = os.path.join(path, 'qbqtc_predict.json')
-
- if not os.path.exists(path):
- os.makedirs(path)
- if os.path.exists(file_path):
- print('Json文件已存在, 将用本次结果替换')
-
- with jsonlines.open(file_path, 'w') as jsonf:
- for predict_res in predict_results:
- for i, p in zip(predict_res['id'], predict_res['logits']):
- jsonf.write({"id": i, "label": str(p)})
- print('Json saved:', file_path)
diff --git a/spaces/feregVcuzo/sanity-test-midi/checkpoint/APK99 How to Download and Use the Best Android Gaming Platform.md b/spaces/feregVcuzo/sanity-test-midi/checkpoint/APK99 How to Download and Use the Best Android Gaming Platform.md
deleted file mode 100644
index 2771815faed336493e48b7cf72c0dddd1c2f1149..0000000000000000000000000000000000000000
--- a/spaces/feregVcuzo/sanity-test-midi/checkpoint/APK99 How to Download and Use the Best Android Gaming Platform.md
+++ /dev/null
@@ -1,124 +0,0 @@
-
-
How to Download and Use apk99 on Your Android Device
-
If you are an Android user, you might have heard of APK files. These are files that contain the installation package of an app or a game that you can download from various sources other than the official Google Play Store. APK files can be useful if you want to access apps or games that are not available in your region, or if you want to try out different versions of an app or a game.
-
One of the popular APK downloaders is apk99, which is a website that offers a large collection of apps and games for Android devices. You can find apps and games from different categories, such as entertainment, social, tools, education, sports, etc. You can also find modded or hacked versions of some apps and games that provide extra features or unlimited resources.
In this article, we will show you how to download and use apk99 on your Android device. We will also provide some tips and warnings for using this service safely and responsibly.
-
How to Download apk99 from Different Sources
-
There are several ways to download apk99 on your Android device. You can use one of the following sources:
-
-
-
Source
-
Description
-
URL
-
-
-
APKPure
-
A popular third-party app store that offers original APK files from Google Play Store as well as other sources.
-
(^6^)
-
-
-
Aptoide
-
A decentralized app store that allows users to manage their own stores and download apps from other users.
-
(^8^)
-
-
-
F-Droid
-
An open-source app store that only hosts free and open-source apps for Android devices.
-
(^7^)
-
-
-
Amazon Appstore
-
The official app store of Amazon devices, such as Kindle Fire, Fire Phone, and Fire Tablet. It also offers a free app every day.
-
(^6^)
-
-
-
APKMirror
-
A website that hosts APK files of various apps and games, including beta versions and older versions.
-
(^2^)
-
-
-
To download apk99 from any of these sources, you need to visit their website using your browser or their app (if available). Then, you need to search for apk99 in their search bar or browse their categories. Once you find apk99, you need to tap on the download button and wait for the file to be downloaded on your device.
-
How to Install apk99 on Your Android Device
-
After downloading apk99 on your device, you need to install it before you can use it. To install apk99, you need to follow these steps:
-
-
Enable unknown sources on your device. This is a security setting that allows you to install apps from sources other than Google Play Store. To enable unknown sources, go to Settings > Security > Unknown sources (or Settings > Apps > Special app access > Install unknown apps) and toggle it on.
-
Locate the downloaded file on your device. You can use a file manager app (such as Astro File Manager or ES File Explorer File Manager) or your browser's downloads section to find the file. The file should have an .apk extension.
-
Tap on the file and follow the installation instructions. You might see a warning message that says "This type of file can harm your device. Do you want to keep apk99.apk anyway?". Tap on OK to proceed.
-
Wait for the installation to complete. You might see a message that says "App installed". Tap on Open to launch apk99 or Done to exit the installer.
-
-
Congratulations, you have successfully installed apk99 on your device. You can now use it to download and install various apps and games.
-
How to Use apk99 to Access Various Apps and Games
-
Using apk99 is very easy and convenient. You can use it to access a wide range of apps and games that you might not find on Google Play Store or other sources. To use apk99, you need to follow these steps:
-
-
Browse or search for your desired app or game. You can use the menu button on the top left corner of the screen to access different categories, such as Popular, New, Trending, etc. You can also use the search bar on the top right corner of the screen to enter the name or keyword of the app or game you are looking for.
-
Tap on the download button and wait for the file to be downloaded. You will see a progress bar that shows the download speed and the remaining time. You can also pause or resume the download at any time.
-
Install the app or game and enjoy. Once the download is complete, you will see a notification that says "Download complete". Tap on it to open the file and follow the installation instructions. Alternatively, you can go to your file manager app or browser's downloads section and tap on the file manually.
-
-
That's it, you have successfully downloaded and installed an app or game using apk99. You can now launch it from your app drawer or home screen and enjoy its features.
-
Conclusion
-
In this article, we have shown you how to download and use apk99 on your Android device. We have also provided some tips and warnings for using this service safely and responsibly.
apk99 is a great way to access various apps and games that are not available on Google Play Store or other sources. However, you should also be careful about the sources and files you download, as some of them might contain malware or viruses that can harm your device or compromise your privacy. Therefore, we recommend that you:
-
-
Always scan the files before installing them using a reliable antivirus app (such as Avast Mobile Security or AVG Antivirus).
-
Always check the permissions and reviews of the apps and games before installing them, and avoid those that ask for unnecessary or suspicious permissions (such as access to your contacts, messages, camera, etc.).
-
Always backup your data and settings before installing any app or game, in case something goes wrong or you want to uninstall it later.
-
Always update your device's software and security patches regularly, to prevent any vulnerabilities or exploits.
-
-
We hope you have found this article helpful and informative. If you have any questions or feedback about apk99, feel free to leave a comment below. We would love to hear from you.
-
Frequently Asked Questions
-
Here are some of the common questions that people ask about apk99:
-
-
What is the difference between apk99 and Google Play Store?
-
apk99 is a third-party website that offers APK files of various apps and games for Android devices. Google Play Store is the official app store of Google that offers apps and games that are verified and approved by Google. The main difference between apk99 and Google Play Store is that apk99 offers apps and games that are not available on Google Play Store, such as modded or hacked versions, regional exclusives, beta versions, etc.
-
Is apk99 safe and legal?
-
apk99 is safe and legal as long as you download files from trusted sources and scan them before installing them. However, some of the files might contain malware or viruses that can harm your device or compromise your privacy. Therefore, you should always be careful about what you download and install using apk99.
-
How do I update the apps and games that I download from apk99?
-
To update the apps and games that you download from apk99, you need to visit their website again and check if there is a newer version available. If there is, you need to download it and install it over the existing one. Alternatively, you can use an app updater app (such as APKUpdater or Uptodown) that can automatically check for updates from various sources.
-
How do I uninstall the apps and games that I download from apk99?
-
To uninstall the apps and games that you download from apk99, you need to go to Settings > Apps > apk99 (or Settings > Apps & notifications > See all apps > apk99) and tap on Uninstall. You can also use a third-party app uninstaller app (such as Easy Uninstaller or App Master) that can help you remove multiple apps at once.
-
Can I use apk99 on other devices, such as PC, iOS, or Fire TV?
-
No, apk99 is only compatible with Android devices. However, you can use an Android emulator (such as BlueStacks or Nox Player) on your PC to run apk99 and download apps and games. You can also use an APK converter app (such as APK to IPA Converter or APK to Bar Converter) to convert APK files to other formats that can be used on iOS or Fire TV devices.
- 401be4b1e0
-
-
\ No newline at end of file
diff --git a/spaces/feregVcuzo/sanity-test-midi/checkpoint/Brawl Stars A Fast-Paced and Fun Game for Mobile - Download APK Now.md b/spaces/feregVcuzo/sanity-test-midi/checkpoint/Brawl Stars A Fast-Paced and Fun Game for Mobile - Download APK Now.md
deleted file mode 100644
index 31dff2a96db139037280d75257b611579a7a0ad3..0000000000000000000000000000000000000000
--- a/spaces/feregVcuzo/sanity-test-midi/checkpoint/Brawl Stars A Fast-Paced and Fun Game for Mobile - Download APK Now.md
+++ /dev/null
@@ -1,170 +0,0 @@
-
-
Brawl Stars Latest Apk Download: Everything You Need to Know
-
If you are looking for a fast-paced, action-packed, and fun multiplayer game for your mobile device, you should definitely check out Brawl Stars. This game is developed by Supercell, the same company behind the popular games Clash of Clans and Clash Royale. In Brawl Stars, you can choose from a variety of characters, each with their own unique abilities and play-styles, and compete with other players in various game modes. You can also unlock new characters, skins, and rewards as you progress through the game.
-
But how can you download the latest apk version of Brawl Stars? And what are the features, tips, and updates that you need to know about this game? In this article, we will answer all these questions and more. Read on to find out everything you need to know about Brawl Stars latest apk download.
Brawl Stars is a game that offers a lot of features that make it fun and exciting to play. Here are some of the main features that you can enjoy in this game:
-
Characters
-
Brawl Stars has a roster of 68 characters, also known as Brawlers, that you can choose from. Each Brawler has a different rarity, class, attack, super ability, star power, and gadget. You can unlock new Brawlers by earning trophies, opening brawl boxes, or buying them with gems. You can also upgrade your Brawlers by collecting power points and coins.
-
Some of the most popular Brawlers in Brawl Stars are:
-
-
Shelly: A common Brawler that shoots shotgun shells that deal more damage at close range. Her super ability is a powerful blast that can break walls and knock back enemies.
-
Crow: A legendary Brawler that throws poisoned daggers that deal damage over time. His super ability is a jump that lets him escape or chase enemies.
-
Leon: A legendary Brawler that shoots blades that deal more damage at close range. His super ability is a stealth mode that makes him invisible for a short time.
-
Sandy: A legendary Brawler that throws sand pebbles that pierce through enemies. His super ability is a sandstorm that hides him and his allies from enemy sight.
-
Edgar: An epic Brawler that attacks with his scarf and heals himself with each hit. His super ability is a jump that lets him close the distance with enemies.
-
-
Game Modes
-
Brawl Stars has several game modes that you can play with your friends or solo. Each game mode has a different objective and rules. Here are some of the most popular game modes in Brawl Stars:
-
-
Gem Grab: A 3v3 mode where you have to collect and hold 10 gems to win. If you die, you drop all your gems.
-
Showdown: A solo or duo mode where you have to survive against other players in a shrinking map. You can collect power cubes to increase your strength.
-
Brawl Ball: A 3v3 mode where you have to score two goals with a ball before the other team. You can use your super ability to break walls or pass the ball.
-
Heist: A 3v3 mode where you have to attack the enemy's safe or defend your own. You can use your super ability to deal damage to the safe or protect it.
-
Bounty: A 3v3 mode where you have to kill enemies and collect stars. The team with the most stars at the end wins. You lose stars if you die.
-
Hot Zone: A 3v3 mode where you have to control zones on the map. The team with the most points at the end wins. You gain points by staying in the zones.
-
Knockout: A 3v3 mode where you have to eliminate all the enemies in a best-of-three match. The team with the most kills wins. You don't respawn if you die.
-
-
Brawl Pass and Rewards
-
Brawl Stars has a feature called Brawl Pass that lets you earn rewards by completing quests and gaining tiers. You can get a free Brawl Pass or buy a premium Brawl Pass with gems. The premium Brawl Pass gives you more rewards, such as exclusive skins, pins, and a guaranteed new Brawler.
-
Some of the rewards that you can get from the Brawl Pass are:
-
brawl stars new update apk download
-brawl stars mod apk download latest version
-brawl stars hack apk download 2023
-brawl stars apk download for android
-brawl stars apk download for pc
-brawl stars apk download for ios
-brawl stars apk download free gems
-brawl stars apk download unlimited money
-brawl stars apk download private server
-brawl stars apk download no verification
-brawl stars latest version apk download 2023
-brawl stars latest version apk download link
-brawl stars latest version apk download uptodown
-brawl stars latest version apk download apkpure
-brawl stars latest version apk download rexdl
-brawl stars latest version apk download modded
-brawl stars latest version apk download offline
-brawl stars latest version apk download online
-brawl stars latest version apk download original
-brawl stars latest version apk download hacked
-brawl stars latest apk download 2023 mod menu
-brawl stars latest apk download 2023 unlimited gems
-brawl stars latest apk download 2023 new brawlers
-brawl stars latest apk download 2023 android 1
-brawl stars latest apk download 2023 ios 14
-brawl stars latest apk download 2023 pc windows 10
-brawl stars latest apk download 2023 mac os x
-brawl stars latest apk download 2023 linux ubuntu
-brawl stars latest apk download 2023 chromebook google play
-brawl stars latest apk download 2023 amazon fire tablet
-how to download brawl stars latest apk on android phone
-how to download brawl stars latest apk on iphone or ipad
-how to download brawl stars latest apk on windows laptop or desktop
-how to download brawl stars latest apk on macbook or imac
-how to download brawl stars latest apk on linux computer or laptop
-how to download brawl stars latest apk on chromebook or chrome os device
-how to download brawl stars latest apk on amazon fire or kindle device
-how to install brawl stars latest apk on android device without root access
-how to install brawl stars latest apk on ios device without jailbreak access
-how to install brawl stars latest apk on pc device without emulator software
-how to update brawl stars latest apk on android device manually or automatically
-how to update brawl stars latest apk on ios device manually or automatically
-how to update brawl stars latest apk on pc device manually or automatically
-how to play brawl stars latest apk on android device with friends or solo
-how to play brawl stars latest apk on ios device with friends or solo
-how to play brawl stars latest apk on pc device with friends or solo
-how to get free gems in brawl stars latest apk without human verification or survey
-how to get unlimited coins in brawl stars latest apk without human verification or survey
-how to unlock all brawlers in brawl stars latest apk without human verification or survey
-
-
Brawl Boxes: These are loot boxes that contain power points, coins, gems, or Brawlers.
-
Big Boxes: These are loot boxes that contain three times more items than Brawl Boxes.
-
Mega Boxes: These are loot boxes that contain 10 times more items than Brawl Boxes.
-
Tokens: These are currency that you can use to buy Brawl Boxes, Big Boxes, or Mega Boxes in the shop.
-
Gems: These are premium currency that you can use to buy skins, pins, Brawlers, or Brawl Passes in the shop.
-
Coins: These are currency that you can use to upgrade your Brawlers by spending power points and coins.
-
Power Points: These are items that you can use to upgrade your Brawlers by spending power points and coins.
-
Skins: These are cosmetic items that change the appearance of your Brawlers.
-
Pins: These are cosmetic items that you can use to express yourself in the game chat or in your profile.
-
Brawlers: These are characters that you can play with in the game.
-
-
Tips and Tricks for Brawl Stars
-
Brawl Stars is a game that requires skill, strategy, and teamwork to win. Here are some tips and tricks that can help you improve your game and have more fun:
-
How to Unlock New Characters
-
As mentioned earlier, there are 68 characters in Brawl Stars that you can unlock by different methods. Here are some of the ways that you can get new characters:
-
-
Earning trophies: You can earn trophies by playing and winning matches. As you earn more trophies, you unlock more characters from the trophy road. For example, you can unlock Nita at 10 trophies, Colt at 60 trophies, Bull at 250 trophies, and so on.
-
Opening brawl boxes: You can open brawl boxes by using tokens or gems. Each brawl box has a chance of containing a new character, depending on its rarity. For example, a common character has a 2.6784% chance of appearing in a brawl box, while a legendary character has a 0.0128% chance of appearing in a brawl box.
-
Buying with gems: You can buy some characters with gems in the shop. These characters are usually exclusive to the premium Brawl Pass or limited-time offers. For example, you can buy Gale for 170 gems, Surge for 349 gems, Colette for 149 gems, and so on.
-
-
How to Choose the Best Character for Each Mode
-
Each character in Brawl Stars has its own strengths and weaknesses, and some characters are better suited for certain modes than others. Here are some of the factors that you should consider when choosing a character for each mode:
-
-
Range: This is how far your character can attack. Some characters have long range, such as Piper, Brock, and Bea, while some have short range, such as El Primo, Rosa, and Darryl. Depending on the mode and the map, you may want to choose a character with a range that suits your play-style and strategy. For example, in Gem Grab, you may want to choose a long-range character to control the center area and collect gems, while in Heist, you may want to choose a short-range character to deal damage to the enemy's safe.
-
Damage: This is how much damage your character can deal with each attack. Some characters have high damage, such as Spike, Amber, and Colette, while some characters have low damage, such as Poco, Gene, and Tick. Depending on the mode and the map, you may want to choose a character with a damage that suits your play-style and strategy. For example, in Showdown, you may want to choose a high-damage character to eliminate enemies quickly and collect power cubes, while in Brawl Ball, you may want to choose a low-damage character to support your teammates and pass the ball.
-
Health: This is how much health your character has. Some characters have high health, such as Frank, Pam, and Bibi, while some characters have low health, such as Rico, Dynamike, and Barley. Depending on the mode and the map, you may want to choose a character with a health that suits your play-style and strategy. For example, in Bounty, you may want to choose a high-health character to survive longer and collect stars, while in Knockout, you may want to choose a low-health character to avoid dying and losing kills.
-
Super Ability: This is a special ability that your character can use after charging it with normal attacks. Each super ability has a different effect and duration. Depending on the mode and the map, you may want to choose a character with a super ability that suits your play-style and strategy. For example, in Hot Zone, you may want to choose a character with a super ability that can control zones or disrupt enemies, such as Sandy's sandstorm or Gale's snowstorm, while in Heist, you may want to choose a character with a super ability that can deal damage or protect the safe, such as Colt's bullet storm or Jessie's turret.
-
-
How to Use Obstacles and Power-Ups
-
Brawl Stars has various obstacles and power-ups that you can use to your advantage or disadvantage in the game. Here are some of the obstacles and power-ups that you should know about:
-
-
Walls: These are solid objects that block your movement and attacks. You can use walls to hide from enemies or ambush them. You can also break walls with some super abilities or gadgets.
-
Bushes: These are green areas that conceal your presence from enemies unless they enter them or use an ability that reveals them. You can use bushes to sneak up on enemies or escape from them.
-
Water: These are blue areas that slow down your movement and prevent you from attacking or using abilities. You can use water to avoid enemies or trap them.
-
Meteor: These are fiery rocks that fall from the sky and deal damage to anyone who gets hit by them. They also break walls and bushes. You can use meteors to damage enemies or create openings.
-
Energy Drink: These are red bottles that spawn randomly on the map and give you a temporary boost in speed and damage. You can use energy drinks to overpower enemies or run away from them.
-
Healing Mushroom: These are purple mushrooms that spawn randomly on the map and heal you and your allies over time. You can use healing mushrooms to recover health or sustain fights.
-
Power Cube: These are yellow cubes that drop from defeated enemies or boxes in Showdown. They increase your health and damage permanently. You can use power cubes to become stronger or intimidate enemies.
-
-
How to Cooperate with Your Teammates
-
Brawl Stars is a game that requires teamwork to win most of the modes. Here are some tips on how to cooperate with your teammates:
-
-
Communicate: You can use the chat feature or the pin feature to communicate with your teammates. You can send messages or emojis to express yourself or give instructions. For example, you can say "Nice!" or "Help!" or "Attack!" or "Defend!" or "GG!".
-
Coordinate: You can use the team code feature or the club feature to coordinate with your teammates. You can invite friends or club members to join your team or join other teams using a shark-shaped jet ski. Buzz can use his jet ski to dash towards enemies and stun them with his super ability. He can also charge his super ability faster when he is near water or enemies.
-
The update also added new skins, such as Surfer Carl, Wicked Stu, Quickdraw Edgar, and Dino Leon. It also added new game modes, such as Volley Brawl and Basket Brawl, which are based on volleyball and basketball respectively. It also added new maps, pins, quests, and balance changes.
-
How to Update Your Game to the Latest Version
-
If you want to enjoy the latest features and content of Brawl Stars, you need to update your game to the latest version. Here are some of the ways that you can update your game:
-
-
Using Google Play Store: If you have downloaded Brawl Stars from the Google Play Store, you can update your game by following these steps:
-
Open the Google Play Store app on your device.
-
Tap on the menu icon on the top left corner and select "My apps & games".
-
Find Brawl Stars on the list of apps and tap on "Update".
-
Wait for the update to download and install.
-
-
-
Using APKPure: If you have downloaded Brawl Stars from APKPure, you can update your game by following these steps:
-
Open the APKPure app on your device.
-
Tap on the menu icon on the top left corner and select "Downloads".
-
Find Brawl Stars on the list of downloads and tap on "Update".
-
Wait for the update to download and install.
-
-
-
Using APKMirror: If you have downloaded Brawl Stars from APKMirror, you can update your game by following these steps:
-
Open the APKMirror website on your device's browser.
-
Search for Brawl Stars on the search bar and tap on the result.
-
Find the latest version of Brawl Stars on the list of variants and tap on "Download APK".
-
Wait for the download to finish and open the file.
-
Follow the instructions to install the update.
-
-
-
-
Conclusion
-
Brawl Stars is a fun and exciting game that you can play with your friends or solo. It has a lot of features, such as characters, game modes, brawl pass, and rewards. It also has a lot of tips and tricks that can help you improve your game and have more fun. It also has a lot of updates that add new features, content, and changes to the game.
-
If you want to download the latest apk version of Brawl Stars, you can use one of the methods that we have mentioned in this article. You can also check out our website for more information and guides about Brawl Stars.
-
We hope that you enjoyed this article and learned something new about Brawl Stars latest apk download. If you did, please share it with your friends and leave a comment below. Thank you for reading!
-
FAQs
-
Here are some of the frequently asked questions about Brawl Stars latest apk download:
-
What are the requirements for playing Brawl Stars?
-
Brawl Stars is compatible with Android devices that have Android 4.3 or higher and iOS devices that have iOS 9 or higher. You also need a stable internet connection to play online.
-
How can I get free gems and coins in Brawl Stars?
-
You can get free gems and coins in Brawl Stars by completing quests, gaining tiers in the brawl pass, opening brawl boxes, or watching ads. You can also buy gems and coins with real money in the shop.
-
What are the best characters in Brawl Stars?
-
The best characters in Brawl Stars depend on your personal preference, play-style, skill level, game mode, map, and team composition. However, some of the generally considered best characters are Spike, Amber, Colette, Sandy, Edgar, Crow, Leon, Byron, Belle, Stu, Squeak, Buzz, etc.
-
How can I join or create a club in Brawl Stars?
-
You can join or create a club in B rawl Stars by tapping on the club icon on the bottom left corner of the main screen. You can search for a club by name, tag, or location, or browse the recommended clubs. You can also create your own club by tapping on the create button and choosing a name, badge, description, and settings for your club.
-
How can I participate in the championship challenge in Brawl Stars?
-
The championship challenge is a special event that occurs every month in Brawl Stars. It is a series of matches that test your skills and knowledge of the game. You can participate in the championship challenge by tapping on the trophy icon on the top right corner of the main screen and selecting the championship challenge option. You need to have at least 800 trophies to enter the challenge. You can win rewards by winning matches, such as star points, coins, and pins. You can also qualify for the monthly finals and the world finals if you win enough matches.
401be4b1e0
-
-
\ No newline at end of file
diff --git a/spaces/feregVcuzo/sanity-test-midi/checkpoint/CarX Street APK Download Experience the Thrill of Racing with Unlimited Money.md b/spaces/feregVcuzo/sanity-test-midi/checkpoint/CarX Street APK Download Experience the Thrill of Racing with Unlimited Money.md
deleted file mode 100644
index bd21546694699666a2cdafa2a99d8981b3eb4e5b..0000000000000000000000000000000000000000
--- a/spaces/feregVcuzo/sanity-test-midi/checkpoint/CarX Street APK Download Experience the Thrill of Racing with Unlimited Money.md
+++ /dev/null
@@ -1,106 +0,0 @@
-
-
CarX Street APK: A Dynamic and Realistic Street Racing Game for Android
-
Introduction
-
If you are a fan of street racing games, you might have heard of CarX Street APK, a free racing game from CarX Technologies for Android gaming lovers. The best thing about it is that it's free to play, and upgrades can be earned by winning races. You can also download the mod version of the game to get unlimited money and resources.
-
carx street apk download latest version unlimited money
In this article, we will tell you everything you need to know about CarX Street APK, including its features, how to download and install it on your device, how to play it like a pro, and how it compares with other racing games. So, buckle up and get ready for some high-speed action!
-
Features of CarX Street APK
-
Diverse selection of cars and tracks
-
One of the most interesting features of CarX Street APK is its diverse selection of cars and tracks. You can choose from over 20 different types of sports cars, each with its own characteristics and performance. You can also unlock and customize different parts for the cars to get the most out of the game.
-
As for the tracks, you can explore a huge open world with various locations, such as highways, city streets, industrial zones, suburbs, and more. You can also find hidden spots and secrets in the map, as well as challenges and events to participate in.
-
Realistic driving physics and mechanics
-
Another feature that makes CarX Street APK stand out from other racing games is its realistic driving physics and mechanics. The game uses the CarX Technology engine, which simulates the behavior of real cars on different surfaces and conditions. You can feel the difference between driving on asphalt, dirt, grass, or snow, as well as the impact of weather, traffic, and collisions.
-
The game also gives you full control over your car, allowing you to adjust the steering, throttle, brake, clutch, gearbox, handbrake, and camera. You can also switch between different driving modes, such as drift, drag, or sprint.
-
Customization option for vehicles
-
If you like to personalize your car, you will love the customization option in CarX Street APK. You can change the appearance of your car by modifying the mirrors, headlights, lights, skirt, bumper, rims, and much more. You can also create a unique look for your car by applying different paint jobs, stickers, decals, and vinyls.
-
carx street mod apk free download unlimited money and gold
-download carx street racing game apk latest version with money hack
-carx street android apk download full version unlocked all cars
-how to download carx street mod apk unlimited money for ios
-carx street apk download no root latest version unlimited cash
-carx street hack apk download 2023 latest version free money
-carx street modded apk download unlimited money and gems
-carx street game apk download latest update with money cheat
-carx street apk download for pc windows 10 latest version unlimited money
-carx street unlimited money apk download offline latest version
-carx street cracked apk download latest version with money mod
-carx street premium apk download unlimited money and coins
-carx street pro apk download latest version with money generator
-carx street apk download online latest version unlimited money and diamonds
-carx street mega mod apk download unlimited money and keys
-carx street apk download for android latest version with money glitch
-carx street vip mod apk download unlimited money and credits
-carx street apk download new version with unlimited money and fuel
-carx street mod menu apk download unlimited money and nitro
-carx street rexdl apk download latest version with money trainer
-carx street revdl apk download unlimited money and cars
-carx street obb apk download latest version with money patch
-carx street lite apk download unlimited money and upgrades
-carx street plus apk download latest version with money editor
-carx street hd apk download unlimited money and parts
-carx street 3d apk download latest version with money hack tool
-carx street beta apk download unlimited money and levels
-carx street original apk download latest version with money unlocker
-carx street old version apk download unlimited money and features
-carx street new update apk download with unlimited money and rewards
-
Besides the visual tuning, you can also improve the performance of your car by upgrading the engine, transmission, body, suspension, and tires. You can also swap the engine of your unique car to make it more powerful or suitable for a specific race.
-
Range of game modes
-
CarX Street APK offers a range of game modes for you to enjoy, such as: - Career mode: This is the main mode of the game, where you can start your journey as a street racer and compete against other racers in various events and tournaments. You can also earn money, reputation, and rewards by completing missions and objectives. - Free ride mode: This is the mode where you can roam freely in the open world and explore the different locations and secrets. You can also find and join random races or challenges that pop up on the map. - Multiplayer mode: This is the mode where you can race against other players online in real-time. You can join or create a lobby and choose the track, car, and rules of the race. You can also chat with other players and make friends or rivals.
-
Thrilling multiplayer mode
-
One of the most exciting features of CarX Street APK is its thrilling multiplayer mode, where you can test your skills and compete with other players from around the world. You can join or create a lobby and choose the track, car, and rules of the race. You can also chat with other players and make friends or rivals.
-
The multiplayer mode offers different types of races, such as: - Sprint: This is a short-distance race where you have to reach the finish line first. - Drift: This is a race where you have to score as many points as possible by drifting on the track. - Drag: This is a race where you have to accelerate as fast as possible on a straight line. - Time attack: This is a race where you have to complete a lap in the shortest time possible.
-
The multiplayer mode also has a ranking system, where you can earn points and trophies by winning races and climbing up the leaderboard. You can also join or create a club, where you can team up with other players and participate in club events and tournaments.
-
Regular updates and new content releases
-
Another feature that makes CarX Street APK worth playing is its regular updates and new content releases. The developers of the game are constantly working on improving the game and adding new features, cars, tracks, events, and more. You can always expect something new and exciting in the game every month or so.
-
Some of the recent updates and new content releases include: - New cars: The game has added new cars, such as the Nissan Skyline GT-R R34, the Toyota Supra MK4, the BMW M3 E46, and more. - New tracks: The game has added new tracks, such as the Tokyo Highway, the Moscow Ring Road, the Los Angeles Downtown, and more. - New events: The game has added new events, such as the Halloween Event, the Christmas Event, the Valentine's Day Event, and more. - New features: The game has added new features, such as the photo mode, the replay mode, the car showroom, and more.
-
Stunning graphics and sound effects
-
The last feature that we want to mention about CarX Street APK is its stunning graphics and sound effects. The game has amazing 3D graphics that create a realistic and immersive experience for the players. You can see every detail of the cars, tracks, environments, weather, lighting, shadows, and more.
-
The game also has realistic sound effects that enhance the gameplay. You can hear the engine roar, the tires screech, the wind blow, the traffic honk, and more. You can also enjoy a variety of music tracks that suit your mood and style.
-
How to download and install CarX Street APK on your Android device
-
Step 1: Download the APK file from a trusted source
-
The first step to download and install CarX Street APK on your Android device is to download the APK file is not from the Google Play Store, and your device may block the installation of apps from unknown sources by default. To enable unknown sources, you need to follow these steps: - Go to your device settings and tap on security or privacy. - Find the option that says unknown sources or install unknown apps and toggle it on. - Confirm your choice by tapping on OK or allow.
-
Step 3: Install the APK file by tapping on it
-
The third step to download and install CarX Street APK on your Android device is to install the APK file by tapping on it. You can find the APK file in your device's download folder or in the notification bar. To install the APK file, you need to follow these steps: - Tap on the APK file and wait for the installation screen to appear. - Tap on install and wait for the installation process to complete. - Tap on open or done to launch the game or exit the installation screen.
-
Step 4: Launch the game and enjoy
-
The final step to download and install CarX Street APK on your Android device is to launch the game and enjoy. You can find the game icon on your device's home screen or app drawer. To launch the game, you need to follow these steps: - Tap on the game icon and wait for the game to load. - Choose your preferred language and accept the terms and conditions. - Log in with your Google Play account or create a new account. - Follow the tutorial and start playing.
-
How to play CarX Street APK like a pro
-
Follow the tutorial
-
One of the best ways to play CarX Street APK like a pro is to follow the tutorial. The tutorial will teach you the basics of the game, such as how to control your car, how to drift, how to race, how to customize your car, and more. You can also learn some tips and tricks that will help you improve your skills and performance.
-
The tutorial will also guide you through the career mode, where you can start your journey as a street racer and compete against other racers in various events and tournaments. You can also earn money, reputation, and rewards by completing missions and objectives.
-
Roam through the city for more rewards
-
Another way to play CarX Street APK like a pro is to roam through the city for more rewards. The game offers a huge open world with various locations, such as highways, city streets, industrial zones, suburbs, and more. You can also find hidden spots and secrets in the map, as well as challenges and events to participate in.
-
By roaming through the city, you can earn more money, reputation, and rewards, such as new cars, parts, paint jobs, stickers, decals, vinyls, and more. You can also discover new tracks and locations that will make your gameplay more fun and exciting.
-
Take part in sprints
-
A third way to play CarX Street APK like a pro is to take part in sprints. Sprints are short-distance races where you have to reach the finish line first. They are one of the easiest ways to earn money and reputation in the game, as well as to improve your driving skills.
-
To take part in sprints, you need to find a sprint icon on the map and tap on it. You can also join a random sprint that pops up on the map or create your own sprint by choosing the track, car, and rules. You can also invite other players or friends to join your sprint.
-
Participate in clubs
-
A fourth way to play CarX Street APK like a pro is to participate in clubs. Clubs are groups of players who share a common interest or goal in the game. You can join or create a club and participate in club events and tournaments. You can also chat with other club members and make friends or rivals.
-
By participating in clubs, you can earn more money, reputation, and rewards, such as exclusive cars, parts, paint jobs, stickers, decals, vinyls, and more. You can also compete with other clubs and climb up the club leaderboard.
-
Go for the best cars
-
A fifth way to play CarX Street APK like a pro is to go for the best cars. The game offers over 20 different types of sports cars, each with its own characteristics and performance. You can also unlock and customize different parts for the cars to get the most out of the game.
-
To go for the best cars, you need to earn enough money and reputation to buy them or win them in events and tournaments. You can also swap the engine of your unique car to make it more powerful or suitable for a specific race. You can also visit the car showroom to see the details and stats of each car.
-
Visit the tuning shop
-
A sixth way to play CarX Street APK like a pro is to visit the tuning shop. The tuning shop is where you can change the appearance and performance of your car by modifying the mirrors, headlights, lights, skirt, bumper, rims, and much more. You can also create a unique look for your car by applying different paint jobs, stickers, decals, and vinyls.
-
To visit the tuning shop, you need to tap on the tuning icon on the main menu or on the map. You can also access the tuning shop from the garage or before a race. You can also save your tuning settings and load them later.
-
Comparison of CarX Street APK with other racing games
-
Pros of CarX Street APK
-
Some of the pros of CarX Street APK are: - It is free to play and download - It has realistic driving physics and mechanics - It has a diverse selection of cars and tracks - It has a customization option for vehicles - It has a range of game modes - It has a thrilling multiplayer mode - It has regular updates and new content releases - It has stunning graphics and sound effects
-
Cons of CarX Street APK
-
Some of the cons of CarX Street APK are: - It requires a lot of storage space and data - It may have some bugs and glitches - It may have some ads and in-app purchases - It may have some compatibility issues with some devices - It may have some lagging or crashing issues
-
Conclusion
-
In conclusion, CarX Street APK is a dynamic and realistic street racing game for Android that offers a lot of fun and excitement for racing fans. You can choose from over 20 different types of sports cars, each with its own characteristics and performance. You can also explore a huge open world with various locations, such as highways, city streets, industrial zones, suburbs, and more. You can also customize your car by modifying the appearance and performance of your car. You can also compete against other players online in real-time in different types of races.
-
If you want to download and install CarX Street APK on your Android device, you can follow the steps that we have provided in this article. You can also follow the tips and tricks that we have shared to play CarX Street APK like a pro. We hope that you enjoy playing this game as much as we do!
-
FAQ
-
Here are some frequently asked questions about CarX Street APK:
-
Q: Is CarX Street APK safe to download?
-
A: Yes, CarX Street APK is safe to download as long as you download it from a trusted source. However, you should always scan the APK file before installing it on your device to make sure that it does not contain any viruses or malware.
-
Q: How do I get unlimited money in CarX Street APK?
-
A: One way to get unlimited money in CarX Street APK is to download the mod version of the game that gives you unlimited money and resources. Another way is to use a cheat or hack tool that can generate unlimited money for you. However, we do not recommend using these methods as they may ruin your gaming experience or get you banned from the game.
-
Q: How do I update CarX Street APK?
-
A: To update CarX Street APK, you need to download the latest version of the game from a trusted source and install it on your device. You can also check for updates from within the game by tapping on the settings icon and then on the update button.
-
Q: Can I play CarX Street APK on PC?
-
A: Yes, you can play CarX Street APK on PC by using an Android emulator. An Android emulator is a software that allows you to run Android apps and games on your PC. Some of the popular Android emulators are BlueStacks, NoxPlayer, LDPlayer, and more. To play CarX Street APK on PC, you need to follow these steps: - Download and install an Android emulator on your PC. - Download the APK file of CarX Street APK from a trusted source. - Launch the emulator and drag and drop the APK file into it. - Install the APK file and launch the game.
-
Q: What are some of the best cars in CarX Street APK?
-
A: Some of the best cars in CarX Street APK are: - Nissan Skyline GT-R R34: This is a legendary car that has a high speed, acceleration, and handling. It is also one of the best cars for drifting. - Toyota Supra MK4: This is a powerful car that has a high speed, acceleration, and torque. It is also one of the best cars for drag racing. - BMW M3 E46: This is a balanced car that has a good speed, acceleration, and handling. It is also one of the best cars for sprint racing.
401be4b1e0
-
-
\ No newline at end of file
diff --git a/spaces/feregVcuzo/sanity-test-midi/checkpoint/Download Instagram Data A Complete Guide to Save Photos Videos Stories and More.md b/spaces/feregVcuzo/sanity-test-midi/checkpoint/Download Instagram Data A Complete Guide to Save Photos Videos Stories and More.md
deleted file mode 100644
index 5c7d565b48e7f23e6b9c0482a9a31550b7cb9e97..0000000000000000000000000000000000000000
--- a/spaces/feregVcuzo/sanity-test-midi/checkpoint/Download Instagram Data A Complete Guide to Save Photos Videos Stories and More.md
+++ /dev/null
@@ -1,151 +0,0 @@
-
-
How to Download Everything from an Instagram Profile
-
Instagram is one of the most popular social media platforms in the world, with over one billion monthly active users. It allows you to share your photos, videos, stories, IGTV, reels, and more with your followers and friends. But what if you want to download some of that content for yourself?
There are many reasons why you might want to download Instagram content. Maybe you want to back up your own posts or data, or save some of your favorite content from other users. Maybe you want to repost someone else's content (with their permission, of course) or use it as inspiration for your own creations. Maybe you just want to enjoy some offline viewing when you don't have internet access.
-
Whatever your reason, downloading Instagram content is not as easy as it sounds. Unlike some other platforms, Instagram does not have a built-in option to save or download other users' posts. You can only save them to a private collection within the app, which is not very convenient if you want to access them outside of Instagram.
-
However, there are some ways to download your own data from Instagram, such as photos, videos, comments, messages, profile information, and more. You can request a copy of your data from Instagram by following these steps - Open the Instagram app or website and go to your profile page. - Tap or click on the settings icon and select Privacy and Security. - Scroll down to Data Download and tap or click on Request Download. - Enter your email address and password and tap or click on Request Download. - You will receive an email with a link to download your data within 48 hours.
-
How to download all of your Instagram data, photos, and more
-Instagram profile downloader: save quality profile pictures
-How to download your Instagram data and get a file with all of your photos, comments, messages, and more
-How to download and view your Instagram data (2023)
-How to download your old Instagram photos for safekeeping
-How to download Instagram stories, videos, and photos from any profile
-How to backup your entire Instagram account
-How to export your Instagram posts, captions, and hashtags
-How to download all the media from an Instagram user
-How to save Instagram profile data in one click
-How to download Instagram data on PC or Mac
-How to download Instagram data on iPhone or Android
-How to request a copy of your Instagram data
-How to view everything Instagram tracks from the date you joined the platform
-How to download Instagram reels, IGTV, and highlights
-How to download Instagram data in bulk
-How to download private Instagram profiles
-How to download Instagram data without app or software
-How to download high-resolution Instagram profile pictures
-How to download Instagram data with Inflact Profile Downloader
-How to access your Instagram data online
-How to download multiple Instagram profiles at once
-How to download Instagram data as ZIP file
-How to download Instagram data faster and easier
-How to download Instagram data for free
-How to delete your Instagram data after downloading it
-How to transfer your Instagram data to another account
-How to analyze your Instagram data after downloading it
-How to download Instagram data securely and safely
-How to download Instagram followers and following list
-How to download Instagram live videos and broadcasts
-How to download tagged photos from any Instagram profile
-How to download archived posts and stories from Instagram
-How to download all the comments and likes from an Instagram profile
-How to download all the DMs and chats from an Instagram account
-How to download all the ads interests and activity from an Instagram profile
-How to download all the filters and stickers from an Instagram account
-How to download all the saved collections and posts from an Instagram profile
-How to download all the blocked users and accounts from an Instagram profile
-How to download all the search history and suggestions from an Instagram account
-How to download all the settings and preferences from an Instagram profile
-How to download all the verification and security information from an Instagram account
-How to download all the location and device data from an Instagram profile
-How to download all the shopping and payment data from an Instagram account
-How to download all the notifications and emails from an Instagram profile
-How to download all the contacts and connections from an Instagram account
-How to download all the insights and analytics from an Instagram profile
-How to download all the content you've reported or flagged on Instagram
-How to download all the content that's been removed or restricted by Instagram
-
But what if you want to download content from other users? Well, you will need some help from third-party tools. There are many tools available online that can help you download Instagram content, such as photos, videos, stories, IGTV, reels, and more. Some of them are web-based, while others are apps that you can install on your device. Here are some of the best tools for downloading Instagram content.
-
Best Tools for Downloading Instagram Content
-
DownloadGram
-
DownloadGram is a simple and easy-to-use web-based tool that allows you to download photos, videos, IGTV, and reels from Instagram. All you need to do is copy the link of the post you want to download and paste it into the input box on the website. Then, click on the Download button and wait for the tool to process your request. You will then see a preview of the content and a Download button below it. Click on it and save the file to your device.
-
Some of the pros of DownloadGram are:
-
-
It is free and fast.
-
It does not require any registration or installation.
-
It supports multiple formats and resolutions.
-
-
Some of the cons of DownloadGram are:
-
-
It does not support downloading multiple photos or videos from a single post.
-
It does not support downloading stories or live videos.
-
It may not work for some private accounts or posts.
-
-
iGram
-
iGram is another web-based tool that can help you download Instagram content. It works similarly to DownloadGram, but it has some additional features. For example, it can help you download stories, highlights, profile pictures, and hashtags from Instagram. It can also help you download videos from other platforms, such as YouTube, Facebook, Twitter, TikTok, etc.
-
Some of the pros of iGram are:
-
-
It is free and easy to use.
-
It supports downloading various types of content from Instagram and other platforms.
-
It allows you to choose the quality and format of the downloaded files.
-
-
Some of the cons of iGram are:
-
-
It does not support downloading reels or live videos.
-
It may not work for some private accounts or posts.
-
It may show some ads or pop-ups on the website.
-
Inflact
-
Inflact is a web-based tool that offers various services for Instagram users, such as growth, analytics, marketing, and downloading. It can help you download photos, videos, stories, IGTV, reels, live videos, profile pictures, and hashtags from Instagram. It can also help you download content from other users' profiles in bulk.
-
Some of the pros of Inflact are:
-
-
It supports downloading all kinds of content from Instagram.
-
It allows you to download content from multiple profiles at once.
-
It provides a preview of the content before downloading.
-
-
Some of the cons of Inflact are:
-
-
It is not free. You need to pay for a subscription to use the downloading service.
-
It may not work for some private accounts or posts.
-
It may require you to log in with your Instagram account to use some features.
-
-
Video Downloader for Instagram
-
Video Downloader for Instagram is an app that you can install on your Android device to download videos from Instagram. It can also help you download photos, stories, reels, and IGTV from Instagram. It is very easy to use. You just need to copy the link of the post you want to download and open the app. It will automatically detect the link and show you a Download button. You can then choose the quality and format of the file and save it to your device.
-
Some of the pros of Video Downloader for Instagram are:
-
-
It is free and fast.
-
It supports downloading multiple photos or videos from a single post.
-
It has a built-in video player and gallery to view the downloaded files.
-
-
Some of the cons of Video Downloader for Instagram are:
-
-
It does not support downloading live videos or profile pictures.
-
It may not work for some private accounts or posts.
-
It may show some ads or permissions on the app.
-
InstaDownloader
-
InstaDownloader is another app that you can install on your Android device to download Instagram content. It can help you download photos, videos, stories, IGTV, reels, live videos, profile pictures, and hashtags from Instagram. It also has a web version that you can use on your browser. It works similarly to Video Downloader for Instagram. You just need to copy the link of the post you want to download and open the app or website. It will then show you a Download button and let you choose the quality and format of the file.
-
Some of the pros of InstaDownloader are:
-
-
It is free and easy to use.
-
It supports downloading all kinds of content from Instagram.
-
It has a web version that you can use on any device.
-
-
Some of the cons of InstaDownloader are:
-
-
It may not work for some private accounts or posts.
-
It may show some ads or permissions on the app or website.
-
It may have some bugs or errors on some devices.
-
-
Tips and Tricks for Downloading Instagram Content
-
Now that you know some of the best tools for downloading Instagram content, here are some tips and tricks that can help you make the most of them.
-
-
How to save space on your device: Downloading Instagram content can take up a lot of space on your device, especially if you download high-quality videos or multiple files. To save space, you can delete the files that you don't need anymore, or transfer them to an external storage device or cloud service. You can also use a file manager app or a cleaner app to find and remove duplicate or unwanted files.
-
How to respect the original creators and avoid copyright issues: Downloading Instagram content does not mean that you own it or have the right to use it without permission. You should always respect the original creators and their rights. Before downloading or reposting their content, you should ask for their permission and give them credit. You should also link back to their profile or post. You should not use their content for commercial purposes or modify it without their consent.
-
How to use the downloaded content for inspiration or reposting (with permission): Downloading Instagram content can help you get inspired by other users' creativity and style. You can use their content as a reference or a source of ideas for your own posts. You can also repost their content (with their permission) to share it with your followers or friends. You can add your own caption, hashtags, stickers, filters, etc. to make it more personalized and engaging.
-
-
Conclusion
-
Downloading Instagram content can be a fun and useful way to enjoy your favorite posts offline, back up your own data, get inspired by other users, or repost their content (with their permission). However, it can also be tricky and risky if you don't use the right tools or follow the proper etiquette. In this article, we have shown you some of the best tools for downloading Instagram content, such as photos, videos, stories, IGTV, reels, live videos, profile pictures, and hashtags. We have also given you some tips and tricks that can help you save space on your device, respect the original creators, and use the downloaded content wisely. We hope that this article has helped you learn how to download everything from an Instagram profile.
-
If you have any questions or feedback about this article, please feel free to leave a comment below. We would love to hear from you. And if you liked this article, please share it with your friends and followers on social media. Thank you for reading!
-
FAQs
-
-
Q: Is downloading Instagram content legal?
-
A: It depends on how you use it. You should always ask for permission from the original creators before downloading or reposting their content. You should also give them credit and link back to their profile.
-
Q: How can I download Instagram stories?
-
A: Some of the tools mentioned in this article can help you download Instagram stories, such as Inflact and InstaDownloader. You can also use third-party apps or websites that allow you to view and save stories anonymously, such as StorySaver or StoriesIG.
-
Q: How can I download IGTV videos?
-
A: Some of the tools mentioned in this article can also help you download IGTV videos, such as DownloadGram and iGram. You can also use the official IGTV app or website to copy the video link and paste it into a downloader tool.
-
Q - Q: How can I download multiple photos or videos from a single post?
-
A: Some of the tools mentioned in this article can help you download multiple photos or videos from a single post, such as Inflact and Video Downloader for Instagram. You can also use a browser extension that allows you to download all media from a page, such as Image Downloader for Chrome or DownAlbum for Firefox.
-
Q: How can I download my own data from Instagram?
-
A: You can request a copy of your data from Instagram by following these steps: - Open the Instagram app or website and go to your profile page. - Tap or click on the settings icon and select Privacy and Security. - Scroll down to Data Download and tap or click on Request Download. - Enter your email address and password and tap or click on Request Download. - You will receive an email with a link to download your data within 48 hours.
-
197e85843d
-
-
\ No newline at end of file
diff --git a/spaces/fffiloni/Music_Source_Separation/scripts/0_download_datasets/instruments.sh b/spaces/fffiloni/Music_Source_Separation/scripts/0_download_datasets/instruments.sh
deleted file mode 100644
index a848adbe45957923c47bc3047c33958a1421c8f6..0000000000000000000000000000000000000000
--- a/spaces/fffiloni/Music_Source_Separation/scripts/0_download_datasets/instruments.sh
+++ /dev/null
@@ -1,43 +0,0 @@
-#!/bin/bash
-
-echo "The dataset link is created internally by kqq"
-
-# The downloaded MAESTRO dataset looks like:
-# ./datasets/instruments
-# ├── violin_solo
-# │ └── v0.1
-# │ ├── mp3s (12 files)
-# │ │ ├── 0jXXWBt5URw.mp3
-# │ │ └── ...
-# │ ├── README.txt
-# │ └── validation.csv
-# ├── basson_solo
-# │ └── ...
-# ├── cello_solo
-# │ └── ...
-# ├── clarinet_solo
-# │ └── ...
-# ├── flute_solo
-# │ └── ...
-# ├── harp_solo
-# │ └── ...
-# ├── horn_solo
-# │ └── ...
-# ├── oboe_solo
-# │ └── ...
-# ├── saxophone_solo
-# │ └── ...
-# ├── string_quartet
-# │ └── ...
-# ├── symphony_solo
-# │ └── ...
-# ├── timpani_solo
-# │ └── ...
-# ├── trombone_solo
-# │ └── ...
-# ├── trumpet_solo
-# │ └── ...
-# ├── tuba_solo
-# │ └── ...
-# └── viola_solo
-# └── ...
\ No newline at end of file
diff --git a/spaces/fffiloni/controlnet-animation-doodle/node_modules/bytes/Readme.md b/spaces/fffiloni/controlnet-animation-doodle/node_modules/bytes/Readme.md
deleted file mode 100644
index 5790e23e328e045e66ec6f0b98526157b6c2abcf..0000000000000000000000000000000000000000
--- a/spaces/fffiloni/controlnet-animation-doodle/node_modules/bytes/Readme.md
+++ /dev/null
@@ -1,152 +0,0 @@
-# Bytes utility
-
-[![NPM Version][npm-image]][npm-url]
-[![NPM Downloads][downloads-image]][downloads-url]
-[![Build Status][ci-image]][ci-url]
-[![Test Coverage][coveralls-image]][coveralls-url]
-
-Utility to parse a string bytes (ex: `1TB`) to bytes (`1099511627776`) and vice-versa.
-
-## Installation
-
-This is a [Node.js](https://nodejs.org/en/) module available through the
-[npm registry](https://www.npmjs.com/). Installation is done using the
-[`npm install` command](https://docs.npmjs.com/getting-started/installing-npm-packages-locally):
-
-```bash
-$ npm install bytes
-```
-
-## Usage
-
-```js
-var bytes = require('bytes');
-```
-
-#### bytes(number|string value, [options]): number|string|null
-
-Default export function. Delegates to either `bytes.format` or `bytes.parse` based on the type of `value`.
-
-**Arguments**
-
-| Name | Type | Description |
-|---------|----------|--------------------|
-| value | `number`|`string` | Number value to format or string value to parse |
-| options | `Object` | Conversion options for `format` |
-
-**Returns**
-
-| Name | Type | Description |
-|---------|------------------|-------------------------------------------------|
-| results | `string`|`number`|`null` | Return null upon error. Numeric value in bytes, or string value otherwise. |
-
-**Example**
-
-```js
-bytes(1024);
-// output: '1KB'
-
-bytes('1KB');
-// output: 1024
-```
-
-#### bytes.format(number value, [options]): string|null
-
-Format the given value in bytes into a string. If the value is negative, it is kept as such. If it is a float, it is
- rounded.
-
-**Arguments**
-
-| Name | Type | Description |
-|---------|----------|--------------------|
-| value | `number` | Value in bytes |
-| options | `Object` | Conversion options |
-
-**Options**
-
-| Property | Type | Description |
-|-------------------|--------|-----------------------------------------------------------------------------------------|
-| decimalPlaces | `number`|`null` | Maximum number of decimal places to include in output. Default value to `2`. |
-| fixedDecimals | `boolean`|`null` | Whether to always display the maximum number of decimal places. Default value to `false` |
-| thousandsSeparator | `string`|`null` | Example of values: `' '`, `','` and `'.'`... Default value to `''`. |
-| unit | `string`|`null` | The unit in which the result will be returned (B/KB/MB/GB/TB). Default value to `''` (which means auto detect). |
-| unitSeparator | `string`|`null` | Separator to use between number and unit. Default value to `''`. |
-
-**Returns**
-
-| Name | Type | Description |
-|---------|------------------|-------------------------------------------------|
-| results | `string`|`null` | Return null upon error. String value otherwise. |
-
-**Example**
-
-```js
-bytes.format(1024);
-// output: '1KB'
-
-bytes.format(1000);
-// output: '1000B'
-
-bytes.format(1000, {thousandsSeparator: ' '});
-// output: '1 000B'
-
-bytes.format(1024 * 1.7, {decimalPlaces: 0});
-// output: '2KB'
-
-bytes.format(1024, {unitSeparator: ' '});
-// output: '1 KB'
-```
-
-#### bytes.parse(string|number value): number|null
-
-Parse the string value into an integer in bytes. If no unit is given, or `value`
-is a number, it is assumed the value is in bytes.
-
-Supported units and abbreviations are as follows and are case-insensitive:
-
- * `b` for bytes
- * `kb` for kilobytes
- * `mb` for megabytes
- * `gb` for gigabytes
- * `tb` for terabytes
- * `pb` for petabytes
-
-The units are in powers of two, not ten. This means 1kb = 1024b according to this parser.
-
-**Arguments**
-
-| Name | Type | Description |
-|---------------|--------|--------------------|
-| value | `string`|`number` | String to parse, or number in bytes. |
-
-**Returns**
-
-| Name | Type | Description |
-|---------|-------------|-------------------------|
-| results | `number`|`null` | Return null upon error. Value in bytes otherwise. |
-
-**Example**
-
-```js
-bytes.parse('1KB');
-// output: 1024
-
-bytes.parse('1024');
-// output: 1024
-
-bytes.parse(1024);
-// output: 1024
-```
-
-## License
-
-[MIT](LICENSE)
-
-[ci-image]: https://badgen.net/github/checks/visionmedia/bytes.js/master?label=ci
-[ci-url]: https://github.com/visionmedia/bytes.js/actions?query=workflow%3Aci
-[coveralls-image]: https://badgen.net/coveralls/c/github/visionmedia/bytes.js/master
-[coveralls-url]: https://coveralls.io/r/visionmedia/bytes.js?branch=master
-[downloads-image]: https://badgen.net/npm/dm/bytes
-[downloads-url]: https://npmjs.org/package/bytes
-[npm-image]: https://badgen.net/npm/v/bytes
-[npm-url]: https://npmjs.org/package/bytes
diff --git a/spaces/fffiloni/controlnet-animation-doodle/node_modules/raw-body/SECURITY.md b/spaces/fffiloni/controlnet-animation-doodle/node_modules/raw-body/SECURITY.md
deleted file mode 100644
index 2421efc4b12f32ab85d704489d910da9d1a0aa40..0000000000000000000000000000000000000000
--- a/spaces/fffiloni/controlnet-animation-doodle/node_modules/raw-body/SECURITY.md
+++ /dev/null
@@ -1,24 +0,0 @@
-# Security Policies and Procedures
-
-## Reporting a Bug
-
-The `raw-body` team and community take all security bugs seriously. Thank you
-for improving the security of Express. We appreciate your efforts and
-responsible disclosure and will make every effort to acknowledge your
-contributions.
-
-Report security bugs by emailing the current owners of `raw-body`. This information
-can be found in the npm registry using the command `npm owner ls raw-body`.
-If unsure or unable to get the information from the above, open an issue
-in the [project issue tracker](https://github.com/stream-utils/raw-body/issues)
-asking for the current contact information.
-
-To ensure the timely response to your report, please ensure that the entirety
-of the report is contained within the email body and not solely behind a web
-link or an attachment.
-
-At least one owner will acknowledge your email within 48 hours, and will send a
-more detailed response within 48 hours indicating the next steps in handling
-your report. After the initial reply to your report, the owners will
-endeavor to keep you informed of the progress towards a fix and full
-announcement, and may ask for additional information or guidance.
diff --git a/spaces/fgenie/scamtext_PAL_self_consistency/funcs/f_41.py b/spaces/fgenie/scamtext_PAL_self_consistency/funcs/f_41.py
deleted file mode 100644
index d14426a4ab84dd0169c34ebc1144caca1a35bbb1..0000000000000000000000000000000000000000
--- a/spaces/fgenie/scamtext_PAL_self_consistency/funcs/f_41.py
+++ /dev/null
@@ -1,23 +0,0 @@
-
-import re
-
-def is_spam(message: str) -> bool:
- # Check for common spam characteristics
- url_regex = r'http[s]?://(?:[a-zA-Z]|[0-9]|[$-_@.&+]|[!*\\(\\),]|(?:%[0-9a-fA-F][0-9a-fA-F]))+'
- money_regex = r'[\d,]+원'
- percent_regex = r'\d+%'
-
- # Check if message contains URL
- if re.search(url_regex, message):
- return True
-
- # Check if message contains money or percentage expressions
- if re.search(money_regex, message) or re.search(percent_regex, message):
- return True
-
- # Check for suspicious leading/trailing whitespace
- if message.strip() != message:
- return True
-
- # If none of the above checks have been met, consider the message as normal (non-spam)
- return False
diff --git a/spaces/foghuang/ChatGLM2-6B/web_demo2.py b/spaces/foghuang/ChatGLM2-6B/web_demo2.py
deleted file mode 100644
index 6c66308f25a07b2c23e09d79eb32c1a1552a33a2..0000000000000000000000000000000000000000
--- a/spaces/foghuang/ChatGLM2-6B/web_demo2.py
+++ /dev/null
@@ -1,75 +0,0 @@
-from transformers import AutoModel, AutoTokenizer
-import streamlit as st
-from streamlit_chat import message
-
-
-st.set_page_config(
- page_title="ChatGLM2-6b 演示",
- page_icon=":robot:",
- layout='wide'
-)
-
-
-@st.cache_resource
-def get_model():
- tokenizer = AutoTokenizer.from_pretrained("THUDM/chatglm2-6b", trust_remote_code=True)
- model = AutoModel.from_pretrained("THUDM/chatglm2-6b", trust_remote_code=True).cuda()
- # 多显卡支持,使用下面两行代替上面一行,将num_gpus改为你实际的显卡数量
- # from utils import load_model_on_gpus
- # model = load_model_on_gpus("THUDM/chatglm2-6b", num_gpus=2)
- model = model.eval()
- return tokenizer, model
-
-
-MAX_TURNS = 20
-MAX_BOXES = MAX_TURNS * 2
-
-
-def predict(input, max_length, top_p, temperature, history=None):
- tokenizer, model = get_model()
- if history is None:
- history = []
-
- with container:
- if len(history) > 0:
- if len(history)>MAX_BOXES:
- history = history[-MAX_TURNS:]
- for i, (query, response) in enumerate(history):
- message(query, avatar_style="big-smile", key=str(i) + "_user")
- message(response, avatar_style="bottts", key=str(i))
-
- message(input, avatar_style="big-smile", key=str(len(history)) + "_user")
- st.write("AI正在回复:")
- with st.empty():
- for response, history in model.stream_chat(tokenizer, input, history, max_length=max_length, top_p=top_p,
- temperature=temperature):
- query, response = history[-1]
- st.write(response)
-
- return history
-
-
-container = st.container()
-
-# create a prompt text for the text generation
-prompt_text = st.text_area(label="用户命令输入",
- height = 100,
- placeholder="请在这儿输入您的命令")
-
-max_length = st.sidebar.slider(
- 'max_length', 0, 32768, 8192, step=1
-)
-top_p = st.sidebar.slider(
- 'top_p', 0.0, 1.0, 0.8, step=0.01
-)
-temperature = st.sidebar.slider(
- 'temperature', 0.0, 1.0, 0.95, step=0.01
-)
-
-if 'state' not in st.session_state:
- st.session_state['state'] = []
-
-if st.button("发送", key="predict"):
- with st.spinner("AI正在思考,请稍等........"):
- # text generation
- st.session_state["state"] = predict(prompt_text, max_length, top_p, temperature, st.session_state["state"])
diff --git a/spaces/freddyaboulton/3.1.4.9-all-demos/demos/fake_gan_no_input/run.py b/spaces/freddyaboulton/3.1.4.9-all-demos/demos/fake_gan_no_input/run.py
deleted file mode 100644
index 9b7f5152937b5694917e8fa25a2c7b7e83d6080e..0000000000000000000000000000000000000000
--- a/spaces/freddyaboulton/3.1.4.9-all-demos/demos/fake_gan_no_input/run.py
+++ /dev/null
@@ -1,32 +0,0 @@
-# This demo needs to be run from the repo folder.
-# python demo/fake_gan/run.py
-import random
-import time
-
-import gradio as gr
-
-
-def fake_gan():
- time.sleep(1)
- image = random.choice(
- [
- "https://images.unsplash.com/photo-1507003211169-0a1dd7228f2d?ixlib=rb-1.2.1&ixid=MnwxMjA3fDB8MHxwaG90by1wYWdlfHx8fGVufDB8fHx8&auto=format&fit=crop&w=387&q=80",
- "https://images.unsplash.com/photo-1554151228-14d9def656e4?ixlib=rb-1.2.1&ixid=MnwxMjA3fDB8MHxwaG90by1wYWdlfHx8fGVufDB8fHx8&auto=format&fit=crop&w=386&q=80",
- "https://images.unsplash.com/photo-1542909168-82c3e7fdca5c?ixlib=rb-1.2.1&ixid=MnwxMjA3fDB8MHxzZWFyY2h8MXx8aHVtYW4lMjBmYWNlfGVufDB8fDB8fA%3D%3D&w=1000&q=80",
- "https://images.unsplash.com/photo-1546456073-92b9f0a8d413?ixlib=rb-1.2.1&ixid=MnwxMjA3fDB8MHxwaG90by1wYWdlfHx8fGVufDB8fHx8&auto=format&fit=crop&w=387&q=80",
- "https://images.unsplash.com/photo-1601412436009-d964bd02edbc?ixlib=rb-1.2.1&ixid=MnwxMjA3fDB8MHxwaG90by1wYWdlfHx8fGVufDB8fHx8&auto=format&fit=crop&w=464&q=80",
- ]
- )
- return image
-
-
-demo = gr.Interface(
- fn=fake_gan,
- inputs=None,
- outputs=gr.Image(label="Generated Image"),
- title="FD-GAN",
- description="This is a fake demo of a GAN. In reality, the images are randomly chosen from Unsplash.",
-)
-
-if __name__ == "__main__":
- demo.launch()
diff --git a/spaces/freddyaboulton/3.1.4.9-all-demos/demos/no_input/run.py b/spaces/freddyaboulton/3.1.4.9-all-demos/demos/no_input/run.py
deleted file mode 100644
index c1d9344d7902f7673af289d5f04ab706f924178b..0000000000000000000000000000000000000000
--- a/spaces/freddyaboulton/3.1.4.9-all-demos/demos/no_input/run.py
+++ /dev/null
@@ -1,18 +0,0 @@
-import gradio as gr
-import random
-
-sentence_list = [
- "Good morning!",
- "Prayers are with you, have a safe day!",
- "I love you!"
-]
-
-
-def random_sentence():
- return sentence_list[random.randint(0, 2)]
-
-
-demo = gr.Interface(fn=random_sentence, inputs=None, outputs="text")
-
-if __name__ == "__main__":
- demo.launch()
diff --git a/spaces/fuckyoudeki/AutoGPT/autogpt/__main__.py b/spaces/fuckyoudeki/AutoGPT/autogpt/__main__.py
deleted file mode 100644
index 128f9eea4900429e88276abdde3419b806001ac7..0000000000000000000000000000000000000000
--- a/spaces/fuckyoudeki/AutoGPT/autogpt/__main__.py
+++ /dev/null
@@ -1,5 +0,0 @@
-"""Auto-GPT: A GPT powered AI Assistant"""
-import autogpt.cli
-
-if __name__ == "__main__":
- autogpt.cli.main()
diff --git a/spaces/fuckyoudeki/AutoGPT/autogpt/configurator.py b/spaces/fuckyoudeki/AutoGPT/autogpt/configurator.py
deleted file mode 100644
index 1dc3be124f638b8859eb459bcb2d46696f62e2b7..0000000000000000000000000000000000000000
--- a/spaces/fuckyoudeki/AutoGPT/autogpt/configurator.py
+++ /dev/null
@@ -1,134 +0,0 @@
-"""Configurator module."""
-import click
-from colorama import Back, Fore, Style
-
-from autogpt import utils
-from autogpt.config import Config
-from autogpt.logs import logger
-from autogpt.memory import get_supported_memory_backends
-
-CFG = Config()
-
-
-def create_config(
- continuous: bool,
- continuous_limit: int,
- ai_settings_file: str,
- skip_reprompt: bool,
- speak: bool,
- debug: bool,
- gpt3only: bool,
- gpt4only: bool,
- memory_type: str,
- browser_name: str,
- allow_downloads: bool,
- skip_news: bool,
-) -> None:
- """Updates the config object with the given arguments.
-
- Args:
- continuous (bool): Whether to run in continuous mode
- continuous_limit (int): The number of times to run in continuous mode
- ai_settings_file (str): The path to the ai_settings.yaml file
- skip_reprompt (bool): Whether to skip the re-prompting messages at the beginning of the script
- speak (bool): Whether to enable speak mode
- debug (bool): Whether to enable debug mode
- gpt3only (bool): Whether to enable GPT3.5 only mode
- gpt4only (bool): Whether to enable GPT4 only mode
- memory_type (str): The type of memory backend to use
- browser_name (str): The name of the browser to use when using selenium to scrape the web
- allow_downloads (bool): Whether to allow Auto-GPT to download files natively
- skips_news (bool): Whether to suppress the output of latest news on startup
- """
- CFG.set_debug_mode(False)
- CFG.set_continuous_mode(False)
- CFG.set_speak_mode(False)
-
- if debug:
- logger.typewriter_log("Debug Mode: ", Fore.GREEN, "ENABLED")
- CFG.set_debug_mode(True)
-
- if continuous:
- logger.typewriter_log("Continuous Mode: ", Fore.RED, "ENABLED")
- logger.typewriter_log(
- "WARNING: ",
- Fore.RED,
- "Continuous mode is not recommended. It is potentially dangerous and may"
- " cause your AI to run forever or carry out actions you would not usually"
- " authorise. Use at your own risk.",
- )
- CFG.set_continuous_mode(True)
-
- if continuous_limit:
- logger.typewriter_log(
- "Continuous Limit: ", Fore.GREEN, f"{continuous_limit}"
- )
- CFG.set_continuous_limit(continuous_limit)
-
- # Check if continuous limit is used without continuous mode
- if continuous_limit and not continuous:
- raise click.UsageError("--continuous-limit can only be used with --continuous")
-
- if speak:
- logger.typewriter_log("Speak Mode: ", Fore.GREEN, "ENABLED")
- CFG.set_speak_mode(True)
-
- if gpt3only:
- logger.typewriter_log("GPT3.5 Only Mode: ", Fore.GREEN, "ENABLED")
- CFG.set_smart_llm_model(CFG.fast_llm_model)
-
- if gpt4only:
- logger.typewriter_log("GPT4 Only Mode: ", Fore.GREEN, "ENABLED")
- CFG.set_fast_llm_model(CFG.smart_llm_model)
-
- if memory_type:
- supported_memory = get_supported_memory_backends()
- chosen = memory_type
- if chosen not in supported_memory:
- logger.typewriter_log(
- "ONLY THE FOLLOWING MEMORY BACKENDS ARE SUPPORTED: ",
- Fore.RED,
- f"{supported_memory}",
- )
- logger.typewriter_log("Defaulting to: ", Fore.YELLOW, CFG.memory_backend)
- else:
- CFG.memory_backend = chosen
-
- if skip_reprompt:
- logger.typewriter_log("Skip Re-prompt: ", Fore.GREEN, "ENABLED")
- CFG.skip_reprompt = True
-
- if ai_settings_file:
- file = ai_settings_file
-
- # Validate file
- (validated, message) = utils.validate_yaml_file(file)
- if not validated:
- logger.typewriter_log("FAILED FILE VALIDATION", Fore.RED, message)
- logger.double_check()
- exit(1)
-
- logger.typewriter_log("Using AI Settings File:", Fore.GREEN, file)
- CFG.ai_settings_file = file
- CFG.skip_reprompt = True
-
- if allow_downloads:
- logger.typewriter_log("Native Downloading:", Fore.GREEN, "ENABLED")
- logger.typewriter_log(
- "WARNING: ",
- Fore.YELLOW,
- f"{Back.LIGHTYELLOW_EX}Auto-GPT will now be able to download and save files to your machine.{Back.RESET} "
- + "It is recommended that you monitor any files it downloads carefully.",
- )
- logger.typewriter_log(
- "WARNING: ",
- Fore.YELLOW,
- f"{Back.RED + Style.BRIGHT}ALWAYS REMEMBER TO NEVER OPEN FILES YOU AREN'T SURE OF!{Style.RESET_ALL}",
- )
- CFG.allow_downloads = True
-
- if skip_news:
- CFG.skip_news = True
-
- if browser_name:
- CFG.selenium_web_browser = browser_name
diff --git a/spaces/gabortoth74/openjourney/app.py b/spaces/gabortoth74/openjourney/app.py
deleted file mode 100644
index bea4accb45793c8e748731c184dee0ffaf509dd5..0000000000000000000000000000000000000000
--- a/spaces/gabortoth74/openjourney/app.py
+++ /dev/null
@@ -1,8 +0,0 @@
-import gradio as gr
-
-description = """
-
-
- """
-
-gr.Interface.load("models/prompthero/openjourney", description=description).launch()
\ No newline at end of file
diff --git a/spaces/gagan3012/T5-Summarization/src/models/train_model.py b/spaces/gagan3012/T5-Summarization/src/models/train_model.py
deleted file mode 100644
index de0749cda1a720ab23d9c4048e7b130fd3ee8f4c..0000000000000000000000000000000000000000
--- a/spaces/gagan3012/T5-Summarization/src/models/train_model.py
+++ /dev/null
@@ -1,51 +0,0 @@
-import json
-
-import yaml
-
-from model import Summarization
-import pandas as pd
-
-
-def train_model():
- """
- Train the model
- """
- with open("params.yml") as f:
- params = yaml.safe_load(f)
-
- # Load the data
- train_df = pd.read_csv("data/processed/train.csv")
- eval_df = pd.read_csv("data/processed/validation.csv")
-
- train_df = train_df.sample(frac=params["split"], replace=True, random_state=1)
- eval_df = eval_df.sample(frac=params["split"], replace=True, random_state=1)
-
- model = Summarization()
- model.from_pretrained(
- model_type=params["model_type"], model_name=params["model_name"]
- )
-
- model.train(
- train_df=train_df,
- eval_df=eval_df,
- batch_size=params["batch_size"],
- max_epochs=params["epochs"],
- use_gpu=params["use_gpu"],
- learning_rate=float(params["learning_rate"]),
- num_workers=int(params["num_workers"]),
- )
-
- model.save_model(model_dir=params["model_dir"])
-
- with open("wandb/latest-run/files/wandb-summary.json") as json_file:
- data = json.load(json_file)
-
- with open("reports/training_metrics.txt", "w") as fp:
- json.dump(data, fp)
-
- if params["upload_to_hf"]:
- model.upload(hf_username=params["hf_username"], model_name=params["name"])
-
-
-if __name__ == "__main__":
- train_model()
diff --git a/spaces/georgefen/Face-Landmark-ControlNet/annotator/uniformer/mmcv/ops/saconv.py b/spaces/georgefen/Face-Landmark-ControlNet/annotator/uniformer/mmcv/ops/saconv.py
deleted file mode 100644
index b4ee3978e097fca422805db4e31ae481006d7971..0000000000000000000000000000000000000000
--- a/spaces/georgefen/Face-Landmark-ControlNet/annotator/uniformer/mmcv/ops/saconv.py
+++ /dev/null
@@ -1,145 +0,0 @@
-# Copyright (c) OpenMMLab. All rights reserved.
-import torch
-import torch.nn as nn
-import torch.nn.functional as F
-
-from annotator.uniformer.mmcv.cnn import CONV_LAYERS, ConvAWS2d, constant_init
-from annotator.uniformer.mmcv.ops.deform_conv import deform_conv2d
-from annotator.uniformer.mmcv.utils import TORCH_VERSION, digit_version
-
-
-@CONV_LAYERS.register_module(name='SAC')
-class SAConv2d(ConvAWS2d):
- """SAC (Switchable Atrous Convolution)
-
- This is an implementation of SAC in DetectoRS
- (https://arxiv.org/pdf/2006.02334.pdf).
-
- Args:
- in_channels (int): Number of channels in the input image
- out_channels (int): Number of channels produced by the convolution
- kernel_size (int or tuple): Size of the convolving kernel
- stride (int or tuple, optional): Stride of the convolution. Default: 1
- padding (int or tuple, optional): Zero-padding added to both sides of
- the input. Default: 0
- padding_mode (string, optional): ``'zeros'``, ``'reflect'``,
- ``'replicate'`` or ``'circular'``. Default: ``'zeros'``
- dilation (int or tuple, optional): Spacing between kernel elements.
- Default: 1
- groups (int, optional): Number of blocked connections from input
- channels to output channels. Default: 1
- bias (bool, optional): If ``True``, adds a learnable bias to the
- output. Default: ``True``
- use_deform: If ``True``, replace convolution with deformable
- convolution. Default: ``False``.
- """
-
- def __init__(self,
- in_channels,
- out_channels,
- kernel_size,
- stride=1,
- padding=0,
- dilation=1,
- groups=1,
- bias=True,
- use_deform=False):
- super().__init__(
- in_channels,
- out_channels,
- kernel_size,
- stride=stride,
- padding=padding,
- dilation=dilation,
- groups=groups,
- bias=bias)
- self.use_deform = use_deform
- self.switch = nn.Conv2d(
- self.in_channels, 1, kernel_size=1, stride=stride, bias=True)
- self.weight_diff = nn.Parameter(torch.Tensor(self.weight.size()))
- self.pre_context = nn.Conv2d(
- self.in_channels, self.in_channels, kernel_size=1, bias=True)
- self.post_context = nn.Conv2d(
- self.out_channels, self.out_channels, kernel_size=1, bias=True)
- if self.use_deform:
- self.offset_s = nn.Conv2d(
- self.in_channels,
- 18,
- kernel_size=3,
- padding=1,
- stride=stride,
- bias=True)
- self.offset_l = nn.Conv2d(
- self.in_channels,
- 18,
- kernel_size=3,
- padding=1,
- stride=stride,
- bias=True)
- self.init_weights()
-
- def init_weights(self):
- constant_init(self.switch, 0, bias=1)
- self.weight_diff.data.zero_()
- constant_init(self.pre_context, 0)
- constant_init(self.post_context, 0)
- if self.use_deform:
- constant_init(self.offset_s, 0)
- constant_init(self.offset_l, 0)
-
- def forward(self, x):
- # pre-context
- avg_x = F.adaptive_avg_pool2d(x, output_size=1)
- avg_x = self.pre_context(avg_x)
- avg_x = avg_x.expand_as(x)
- x = x + avg_x
- # switch
- avg_x = F.pad(x, pad=(2, 2, 2, 2), mode='reflect')
- avg_x = F.avg_pool2d(avg_x, kernel_size=5, stride=1, padding=0)
- switch = self.switch(avg_x)
- # sac
- weight = self._get_weight(self.weight)
- zero_bias = torch.zeros(
- self.out_channels, device=weight.device, dtype=weight.dtype)
-
- if self.use_deform:
- offset = self.offset_s(avg_x)
- out_s = deform_conv2d(x, offset, weight, self.stride, self.padding,
- self.dilation, self.groups, 1)
- else:
- if (TORCH_VERSION == 'parrots'
- or digit_version(TORCH_VERSION) < digit_version('1.5.0')):
- out_s = super().conv2d_forward(x, weight)
- elif digit_version(TORCH_VERSION) >= digit_version('1.8.0'):
- # bias is a required argument of _conv_forward in torch 1.8.0
- out_s = super()._conv_forward(x, weight, zero_bias)
- else:
- out_s = super()._conv_forward(x, weight)
- ori_p = self.padding
- ori_d = self.dilation
- self.padding = tuple(3 * p for p in self.padding)
- self.dilation = tuple(3 * d for d in self.dilation)
- weight = weight + self.weight_diff
- if self.use_deform:
- offset = self.offset_l(avg_x)
- out_l = deform_conv2d(x, offset, weight, self.stride, self.padding,
- self.dilation, self.groups, 1)
- else:
- if (TORCH_VERSION == 'parrots'
- or digit_version(TORCH_VERSION) < digit_version('1.5.0')):
- out_l = super().conv2d_forward(x, weight)
- elif digit_version(TORCH_VERSION) >= digit_version('1.8.0'):
- # bias is a required argument of _conv_forward in torch 1.8.0
- out_l = super()._conv_forward(x, weight, zero_bias)
- else:
- out_l = super()._conv_forward(x, weight)
-
- out = switch * out_s + (1 - switch) * out_l
- self.padding = ori_p
- self.dilation = ori_d
- # post-context
- avg_x = F.adaptive_avg_pool2d(out, output_size=1)
- avg_x = self.post_context(avg_x)
- avg_x = avg_x.expand_as(out)
- out = out + avg_x
- return out
diff --git a/spaces/georgefen/Face-Landmark-ControlNet/annotator/uniformer/mmcv/ops/voxelize.py b/spaces/georgefen/Face-Landmark-ControlNet/annotator/uniformer/mmcv/ops/voxelize.py
deleted file mode 100644
index ca3226a4fbcbfe58490fa2ea8e1c16b531214121..0000000000000000000000000000000000000000
--- a/spaces/georgefen/Face-Landmark-ControlNet/annotator/uniformer/mmcv/ops/voxelize.py
+++ /dev/null
@@ -1,132 +0,0 @@
-# Copyright (c) OpenMMLab. All rights reserved.
-import torch
-from torch import nn
-from torch.autograd import Function
-from torch.nn.modules.utils import _pair
-
-from ..utils import ext_loader
-
-ext_module = ext_loader.load_ext(
- '_ext', ['dynamic_voxelize_forward', 'hard_voxelize_forward'])
-
-
-class _Voxelization(Function):
-
- @staticmethod
- def forward(ctx,
- points,
- voxel_size,
- coors_range,
- max_points=35,
- max_voxels=20000):
- """Convert kitti points(N, >=3) to voxels.
-
- Args:
- points (torch.Tensor): [N, ndim]. Points[:, :3] contain xyz points
- and points[:, 3:] contain other information like reflectivity.
- voxel_size (tuple or float): The size of voxel with the shape of
- [3].
- coors_range (tuple or float): The coordinate range of voxel with
- the shape of [6].
- max_points (int, optional): maximum points contained in a voxel. if
- max_points=-1, it means using dynamic_voxelize. Default: 35.
- max_voxels (int, optional): maximum voxels this function create.
- for second, 20000 is a good choice. Users should shuffle points
- before call this function because max_voxels may drop points.
- Default: 20000.
-
- Returns:
- voxels_out (torch.Tensor): Output voxels with the shape of [M,
- max_points, ndim]. Only contain points and returned when
- max_points != -1.
- coors_out (torch.Tensor): Output coordinates with the shape of
- [M, 3].
- num_points_per_voxel_out (torch.Tensor): Num points per voxel with
- the shape of [M]. Only returned when max_points != -1.
- """
- if max_points == -1 or max_voxels == -1:
- coors = points.new_zeros(size=(points.size(0), 3), dtype=torch.int)
- ext_module.dynamic_voxelize_forward(points, coors, voxel_size,
- coors_range, 3)
- return coors
- else:
- voxels = points.new_zeros(
- size=(max_voxels, max_points, points.size(1)))
- coors = points.new_zeros(size=(max_voxels, 3), dtype=torch.int)
- num_points_per_voxel = points.new_zeros(
- size=(max_voxels, ), dtype=torch.int)
- voxel_num = ext_module.hard_voxelize_forward(
- points, voxels, coors, num_points_per_voxel, voxel_size,
- coors_range, max_points, max_voxels, 3)
- # select the valid voxels
- voxels_out = voxels[:voxel_num]
- coors_out = coors[:voxel_num]
- num_points_per_voxel_out = num_points_per_voxel[:voxel_num]
- return voxels_out, coors_out, num_points_per_voxel_out
-
-
-voxelization = _Voxelization.apply
-
-
-class Voxelization(nn.Module):
- """Convert kitti points(N, >=3) to voxels.
-
- Please refer to `PVCNN `_ for more
- details.
-
- Args:
- voxel_size (tuple or float): The size of voxel with the shape of [3].
- point_cloud_range (tuple or float): The coordinate range of voxel with
- the shape of [6].
- max_num_points (int): maximum points contained in a voxel. if
- max_points=-1, it means using dynamic_voxelize.
- max_voxels (int, optional): maximum voxels this function create.
- for second, 20000 is a good choice. Users should shuffle points
- before call this function because max_voxels may drop points.
- Default: 20000.
- """
-
- def __init__(self,
- voxel_size,
- point_cloud_range,
- max_num_points,
- max_voxels=20000):
- super().__init__()
-
- self.voxel_size = voxel_size
- self.point_cloud_range = point_cloud_range
- self.max_num_points = max_num_points
- if isinstance(max_voxels, tuple):
- self.max_voxels = max_voxels
- else:
- self.max_voxels = _pair(max_voxels)
-
- point_cloud_range = torch.tensor(
- point_cloud_range, dtype=torch.float32)
- voxel_size = torch.tensor(voxel_size, dtype=torch.float32)
- grid_size = (point_cloud_range[3:] -
- point_cloud_range[:3]) / voxel_size
- grid_size = torch.round(grid_size).long()
- input_feat_shape = grid_size[:2]
- self.grid_size = grid_size
- # the origin shape is as [x-len, y-len, z-len]
- # [w, h, d] -> [d, h, w]
- self.pcd_shape = [*input_feat_shape, 1][::-1]
-
- def forward(self, input):
- if self.training:
- max_voxels = self.max_voxels[0]
- else:
- max_voxels = self.max_voxels[1]
-
- return voxelization(input, self.voxel_size, self.point_cloud_range,
- self.max_num_points, max_voxels)
-
- def __repr__(self):
- s = self.__class__.__name__ + '('
- s += 'voxel_size=' + str(self.voxel_size)
- s += ', point_cloud_range=' + str(self.point_cloud_range)
- s += ', max_num_points=' + str(self.max_num_points)
- s += ', max_voxels=' + str(self.max_voxels)
- s += ')'
- return s
diff --git a/spaces/georgefen/Face-Landmark-ControlNet/annotator/uniformer/mmcv/visualization/color.py b/spaces/georgefen/Face-Landmark-ControlNet/annotator/uniformer/mmcv/visualization/color.py
deleted file mode 100644
index 9041e0e6b7581c3356795d6a3c5e84667c88f025..0000000000000000000000000000000000000000
--- a/spaces/georgefen/Face-Landmark-ControlNet/annotator/uniformer/mmcv/visualization/color.py
+++ /dev/null
@@ -1,51 +0,0 @@
-# Copyright (c) OpenMMLab. All rights reserved.
-from enum import Enum
-
-import numpy as np
-
-from annotator.uniformer.mmcv.utils import is_str
-
-
-class Color(Enum):
- """An enum that defines common colors.
-
- Contains red, green, blue, cyan, yellow, magenta, white and black.
- """
- red = (0, 0, 255)
- green = (0, 255, 0)
- blue = (255, 0, 0)
- cyan = (255, 255, 0)
- yellow = (0, 255, 255)
- magenta = (255, 0, 255)
- white = (255, 255, 255)
- black = (0, 0, 0)
-
-
-def color_val(color):
- """Convert various input to color tuples.
-
- Args:
- color (:obj:`Color`/str/tuple/int/ndarray): Color inputs
-
- Returns:
- tuple[int]: A tuple of 3 integers indicating BGR channels.
- """
- if is_str(color):
- return Color[color].value
- elif isinstance(color, Color):
- return color.value
- elif isinstance(color, tuple):
- assert len(color) == 3
- for channel in color:
- assert 0 <= channel <= 255
- return color
- elif isinstance(color, int):
- assert 0 <= color <= 255
- return color, color, color
- elif isinstance(color, np.ndarray):
- assert color.ndim == 1 and color.size == 3
- assert np.all((color >= 0) & (color <= 255))
- color = color.astype(np.uint8)
- return tuple(color)
- else:
- raise TypeError(f'Invalid type for color: {type(color)}')
diff --git a/spaces/gradio/HuBERT/examples/latent_depth/latent_depth_src/models/__init__.py b/spaces/gradio/HuBERT/examples/latent_depth/latent_depth_src/models/__init__.py
deleted file mode 100644
index e69de29bb2d1d6434b8b29ae775ad8c2e48c5391..0000000000000000000000000000000000000000
diff --git a/spaces/gradio/HuBERT/examples/paraphraser/paraphrase.py b/spaces/gradio/HuBERT/examples/paraphraser/paraphrase.py
deleted file mode 100644
index d3422fb3db9a381b73a854d2379df214ebe544a2..0000000000000000000000000000000000000000
--- a/spaces/gradio/HuBERT/examples/paraphraser/paraphrase.py
+++ /dev/null
@@ -1,85 +0,0 @@
-#!/usr/bin/env python3 -u
-
-import argparse
-import fileinput
-import logging
-import os
-import sys
-
-from fairseq.models.transformer import TransformerModel
-
-
-logging.getLogger().setLevel(logging.INFO)
-
-
-def main():
- parser = argparse.ArgumentParser(description="")
- parser.add_argument("--en2fr", required=True, help="path to en2fr model")
- parser.add_argument(
- "--fr2en", required=True, help="path to fr2en mixture of experts model"
- )
- parser.add_argument(
- "--user-dir", help="path to fairseq examples/translation_moe/src directory"
- )
- parser.add_argument(
- "--num-experts",
- type=int,
- default=10,
- help="(keep at 10 unless using a different model)",
- )
- parser.add_argument(
- "files",
- nargs="*",
- default=["-"],
- help='input files to paraphrase; "-" for stdin',
- )
- args = parser.parse_args()
-
- if args.user_dir is None:
- args.user_dir = os.path.join(
- os.path.dirname(os.path.dirname(os.path.abspath(__file__))), # examples/
- "translation_moe",
- "src",
- )
- if os.path.exists(args.user_dir):
- logging.info("found user_dir:" + args.user_dir)
- else:
- raise RuntimeError(
- "cannot find fairseq examples/translation_moe/src "
- "(tried looking here: {})".format(args.user_dir)
- )
-
- logging.info("loading en2fr model from:" + args.en2fr)
- en2fr = TransformerModel.from_pretrained(
- model_name_or_path=args.en2fr,
- tokenizer="moses",
- bpe="sentencepiece",
- ).eval()
-
- logging.info("loading fr2en model from:" + args.fr2en)
- fr2en = TransformerModel.from_pretrained(
- model_name_or_path=args.fr2en,
- tokenizer="moses",
- bpe="sentencepiece",
- user_dir=args.user_dir,
- task="translation_moe",
- ).eval()
-
- def gen_paraphrases(en):
- fr = en2fr.translate(en)
- return [
- fr2en.translate(fr, inference_step_args={"expert": i})
- for i in range(args.num_experts)
- ]
-
- logging.info("Type the input sentence and press return:")
- for line in fileinput.input(args.files):
- line = line.strip()
- if len(line) == 0:
- continue
- for paraphrase in gen_paraphrases(line):
- print(paraphrase)
-
-
-if __name__ == "__main__":
- main()
diff --git a/spaces/gradio/HuBERT/fairseq/criterions/ctc.py b/spaces/gradio/HuBERT/fairseq/criterions/ctc.py
deleted file mode 100644
index 10e3618382c86a84466cb4264d62f31537980251..0000000000000000000000000000000000000000
--- a/spaces/gradio/HuBERT/fairseq/criterions/ctc.py
+++ /dev/null
@@ -1,295 +0,0 @@
-# All rights reserved.
-#
-# This source code is licensed under the license found in the LICENSE file in
-# the root directory of this source tree. An additional grant of patent rights
-# can be found in the PATENTS file in the same directory.
-
-import math
-from argparse import Namespace
-from dataclasses import dataclass, field
-from omegaconf import II
-from typing import Optional
-
-import torch
-import torch.nn.functional as F
-from fairseq import metrics, utils
-from fairseq.criterions import FairseqCriterion, register_criterion
-from fairseq.dataclass import FairseqDataclass
-from fairseq.data.data_utils import post_process
-from fairseq.tasks import FairseqTask
-from fairseq.logging.meters import safe_round
-
-
-@dataclass
-class CtcCriterionConfig(FairseqDataclass):
- zero_infinity: bool = field(
- default=False,
- metadata={"help": "zero inf loss when source length <= target length"},
- )
- sentence_avg: bool = II("optimization.sentence_avg")
- post_process: str = field(
- default="letter",
- metadata={
- "help": "how to post process predictions into words. can be letter, "
- "wordpiece, BPE symbols, etc. "
- "See fairseq.data.data_utils.post_process() for full list of options"
- },
- )
- wer_kenlm_model: Optional[str] = field(
- default=None,
- metadata={
- "help": "if this is provided, use kenlm to compute wer (along with other wer_* args)"
- },
- )
- wer_lexicon: Optional[str] = field(
- default=None,
- metadata={"help": "lexicon to use with wer_kenlm_model"},
- )
- wer_lm_weight: float = field(
- default=2.0,
- metadata={"help": "lm weight to use with wer_kenlm_model"},
- )
- wer_word_score: float = field(
- default=-1.0,
- metadata={"help": "lm word score to use with wer_kenlm_model"},
- )
-
- wer_args: Optional[str] = field(
- default=None,
- metadata={
- "help": "DEPRECATED: tuple of (wer_kenlm_model, wer_lexicon, wer_lm_weight, wer_word_score)"
- },
- )
-
-
-@register_criterion("ctc", dataclass=CtcCriterionConfig)
-class CtcCriterion(FairseqCriterion):
- def __init__(self, cfg: CtcCriterionConfig, task: FairseqTask):
- super().__init__(task)
- self.blank_idx = (
- task.target_dictionary.index(task.blank_symbol)
- if hasattr(task, "blank_symbol")
- else 0
- )
- self.pad_idx = task.target_dictionary.pad()
- self.eos_idx = task.target_dictionary.eos()
- self.post_process = cfg.post_process
-
- if cfg.wer_args is not None:
- (
- cfg.wer_kenlm_model,
- cfg.wer_lexicon,
- cfg.wer_lm_weight,
- cfg.wer_word_score,
- ) = eval(cfg.wer_args)
-
- if cfg.wer_kenlm_model is not None:
- from examples.speech_recognition.w2l_decoder import W2lKenLMDecoder
-
- dec_args = Namespace()
- dec_args.nbest = 1
- dec_args.criterion = "ctc"
- dec_args.kenlm_model = cfg.wer_kenlm_model
- dec_args.lexicon = cfg.wer_lexicon
- dec_args.beam = 50
- dec_args.beam_size_token = min(50, len(task.target_dictionary))
- dec_args.beam_threshold = min(50, len(task.target_dictionary))
- dec_args.lm_weight = cfg.wer_lm_weight
- dec_args.word_score = cfg.wer_word_score
- dec_args.unk_weight = -math.inf
- dec_args.sil_weight = 0
-
- self.w2l_decoder = W2lKenLMDecoder(dec_args, task.target_dictionary)
- else:
- self.w2l_decoder = None
-
- self.zero_infinity = cfg.zero_infinity
- self.sentence_avg = cfg.sentence_avg
-
- def forward(self, model, sample, reduce=True):
- net_output = model(**sample["net_input"])
- lprobs = model.get_normalized_probs(
- net_output, log_probs=True
- ).contiguous() # (T, B, C) from the encoder
-
- if "src_lengths" in sample["net_input"]:
- input_lengths = sample["net_input"]["src_lengths"]
- else:
- if net_output["padding_mask"] is not None:
- non_padding_mask = ~net_output["padding_mask"]
- input_lengths = non_padding_mask.long().sum(-1)
- else:
- input_lengths = lprobs.new_full(
- (lprobs.size(1),), lprobs.size(0), dtype=torch.long
- )
-
- pad_mask = (sample["target"] != self.pad_idx) & (
- sample["target"] != self.eos_idx
- )
- targets_flat = sample["target"].masked_select(pad_mask)
- if "target_lengths" in sample:
- target_lengths = sample["target_lengths"]
- else:
- target_lengths = pad_mask.sum(-1)
-
- with torch.backends.cudnn.flags(enabled=False):
- loss = F.ctc_loss(
- lprobs,
- targets_flat,
- input_lengths,
- target_lengths,
- blank=self.blank_idx,
- reduction="sum",
- zero_infinity=self.zero_infinity,
- )
-
- ntokens = (
- sample["ntokens"] if "ntokens" in sample else target_lengths.sum().item()
- )
-
- sample_size = sample["target"].size(0) if self.sentence_avg else ntokens
- logging_output = {
- "loss": utils.item(loss.data), # * sample['ntokens'],
- "ntokens": ntokens,
- "nsentences": sample["id"].numel(),
- "sample_size": sample_size,
- }
-
- if not model.training:
- import editdistance
-
- with torch.no_grad():
- lprobs_t = lprobs.transpose(0, 1).float().contiguous().cpu()
-
- c_err = 0
- c_len = 0
- w_errs = 0
- w_len = 0
- wv_errs = 0
- for lp, t, inp_l in zip(
- lprobs_t,
- sample["target_label"]
- if "target_label" in sample
- else sample["target"],
- input_lengths,
- ):
- lp = lp[:inp_l].unsqueeze(0)
-
- decoded = None
- if self.w2l_decoder is not None:
- decoded = self.w2l_decoder.decode(lp)
- if len(decoded) < 1:
- decoded = None
- else:
- decoded = decoded[0]
- if len(decoded) < 1:
- decoded = None
- else:
- decoded = decoded[0]
-
- p = (t != self.task.target_dictionary.pad()) & (
- t != self.task.target_dictionary.eos()
- )
- targ = t[p]
- targ_units = self.task.target_dictionary.string(targ)
- targ_units_arr = targ.tolist()
-
- toks = lp.argmax(dim=-1).unique_consecutive()
- pred_units_arr = toks[toks != self.blank_idx].tolist()
-
- c_err += editdistance.eval(pred_units_arr, targ_units_arr)
- c_len += len(targ_units_arr)
-
- targ_words = post_process(targ_units, self.post_process).split()
-
- pred_units = self.task.target_dictionary.string(pred_units_arr)
- pred_words_raw = post_process(pred_units, self.post_process).split()
-
- if decoded is not None and "words" in decoded:
- pred_words = decoded["words"]
- w_errs += editdistance.eval(pred_words, targ_words)
- wv_errs += editdistance.eval(pred_words_raw, targ_words)
- else:
- dist = editdistance.eval(pred_words_raw, targ_words)
- w_errs += dist
- wv_errs += dist
-
- w_len += len(targ_words)
-
- logging_output["wv_errors"] = wv_errs
- logging_output["w_errors"] = w_errs
- logging_output["w_total"] = w_len
- logging_output["c_errors"] = c_err
- logging_output["c_total"] = c_len
-
- return loss, sample_size, logging_output
-
- @staticmethod
- def reduce_metrics(logging_outputs) -> None:
- """Aggregate logging outputs from data parallel training."""
-
- loss_sum = utils.item(sum(log.get("loss", 0) for log in logging_outputs))
- ntokens = utils.item(sum(log.get("ntokens", 0) for log in logging_outputs))
- nsentences = utils.item(
- sum(log.get("nsentences", 0) for log in logging_outputs)
- )
- sample_size = utils.item(
- sum(log.get("sample_size", 0) for log in logging_outputs)
- )
-
- metrics.log_scalar(
- "loss", loss_sum / sample_size / math.log(2), sample_size, round=3
- )
- metrics.log_scalar("ntokens", ntokens)
- metrics.log_scalar("nsentences", nsentences)
- if sample_size != ntokens:
- metrics.log_scalar(
- "nll_loss", loss_sum / ntokens / math.log(2), ntokens, round=3
- )
-
- c_errors = sum(log.get("c_errors", 0) for log in logging_outputs)
- metrics.log_scalar("_c_errors", c_errors)
- c_total = sum(log.get("c_total", 0) for log in logging_outputs)
- metrics.log_scalar("_c_total", c_total)
- w_errors = sum(log.get("w_errors", 0) for log in logging_outputs)
- metrics.log_scalar("_w_errors", w_errors)
- wv_errors = sum(log.get("wv_errors", 0) for log in logging_outputs)
- metrics.log_scalar("_wv_errors", wv_errors)
- w_total = sum(log.get("w_total", 0) for log in logging_outputs)
- metrics.log_scalar("_w_total", w_total)
-
- if c_total > 0:
- metrics.log_derived(
- "uer",
- lambda meters: safe_round(
- meters["_c_errors"].sum * 100.0 / meters["_c_total"].sum, 3
- )
- if meters["_c_total"].sum > 0
- else float("nan"),
- )
- if w_total > 0:
- metrics.log_derived(
- "wer",
- lambda meters: safe_round(
- meters["_w_errors"].sum * 100.0 / meters["_w_total"].sum, 3
- )
- if meters["_w_total"].sum > 0
- else float("nan"),
- )
- metrics.log_derived(
- "raw_wer",
- lambda meters: safe_round(
- meters["_wv_errors"].sum * 100.0 / meters["_w_total"].sum, 3
- )
- if meters["_w_total"].sum > 0
- else float("nan"),
- )
-
- @staticmethod
- def logging_outputs_can_be_summed() -> bool:
- """
- Whether the logging outputs returned by `forward` can be summed
- across workers prior to calling `reduce_metrics`. Setting this
- to True will improves distributed training speed.
- """
- return True
diff --git a/spaces/gradio/HuBERT/fairseq/models/huggingface/__init__.py b/spaces/gradio/HuBERT/fairseq/models/huggingface/__init__.py
deleted file mode 100644
index f7911c2c8edf516855023a285b18935e5389ec02..0000000000000000000000000000000000000000
--- a/spaces/gradio/HuBERT/fairseq/models/huggingface/__init__.py
+++ /dev/null
@@ -1,20 +0,0 @@
-# Copyright (c) Facebook, Inc. and its affiliates.
-#
-# This source code is licensed under the MIT license found in the
-# LICENSE file in the root directory of this source tree.
-
-import importlib
-import os
-
-
-# automatically import any Python files in the models/huggingface/ directory
-models_dir = os.path.dirname(__file__)
-for file in os.listdir(models_dir):
- path = os.path.join(models_dir, file)
- if (
- not file.startswith("_")
- and not file.startswith(".")
- and (file.endswith(".py") or os.path.isdir(path))
- ):
- model_name = file[: file.find(".py")] if file.endswith(".py") else file
- module = importlib.import_module("fairseq.models.huggingface." + model_name)
diff --git a/spaces/gyugnsu/DragGan-Inversion/gui_utils/imgui_window.py b/spaces/gyugnsu/DragGan-Inversion/gui_utils/imgui_window.py
deleted file mode 100644
index 5937788f2e8e51772677ab12c67038f5ccd37b42..0000000000000000000000000000000000000000
--- a/spaces/gyugnsu/DragGan-Inversion/gui_utils/imgui_window.py
+++ /dev/null
@@ -1,110 +0,0 @@
-# Copyright (c) 2021, NVIDIA CORPORATION & AFFILIATES. All rights reserved.
-#
-# NVIDIA CORPORATION and its licensors retain all intellectual property
-# and proprietary rights in and to this software, related documentation
-# and any modifications thereto. Any use, reproduction, disclosure or
-# distribution of this software and related documentation without an express
-# license agreement from NVIDIA CORPORATION is strictly prohibited.
-
-import os
-import imgui
-import imgui.integrations.glfw
-
-from . import glfw_window
-from . import imgui_utils
-from . import text_utils
-
-# ----------------------------------------------------------------------------
-
-
-class ImguiWindow(glfw_window.GlfwWindow):
- def __init__(self, *, title='ImguiWindow', font=None, font_sizes=range(14, 24), **glfw_kwargs):
- if font is None:
- font = text_utils.get_default_font()
- font_sizes = {int(size) for size in font_sizes}
- super().__init__(title=title, **glfw_kwargs)
-
- # Init fields.
- self._imgui_context = None
- self._imgui_renderer = None
- self._imgui_fonts = None
- self._cur_font_size = max(font_sizes)
-
- # Delete leftover imgui.ini to avoid unexpected behavior.
- if os.path.isfile('imgui.ini'):
- os.remove('imgui.ini')
-
- # Init ImGui.
- self._imgui_context = imgui.create_context()
- self._imgui_renderer = _GlfwRenderer(self._glfw_window)
- self._attach_glfw_callbacks()
- # Disable creating imgui.ini at runtime.
- imgui.get_io().ini_saving_rate = 0
- # Improve behavior with imgui_utils.drag_custom().
- imgui.get_io().mouse_drag_threshold = 0
- self._imgui_fonts = {size: imgui.get_io().fonts.add_font_from_file_ttf(
- font, size) for size in font_sizes}
- self._imgui_renderer.refresh_font_texture()
-
- def close(self):
- self.make_context_current()
- self._imgui_fonts = None
- if self._imgui_renderer is not None:
- self._imgui_renderer.shutdown()
- self._imgui_renderer = None
- if self._imgui_context is not None:
- # imgui.destroy_context(self._imgui_context) # Commented out to avoid creating imgui.ini at the end.
- self._imgui_context = None
- super().close()
-
- def _glfw_key_callback(self, *args):
- super()._glfw_key_callback(*args)
- self._imgui_renderer.keyboard_callback(*args)
-
- @property
- def font_size(self):
- return self._cur_font_size
-
- @property
- def spacing(self):
- return round(self._cur_font_size * 0.4)
-
- def set_font_size(self, target): # Applied on next frame.
- self._cur_font_size = min((abs(key - target), key)
- for key in self._imgui_fonts.keys())[1]
-
- def begin_frame(self):
- # Begin glfw frame.
- super().begin_frame()
-
- # Process imgui events.
- self._imgui_renderer.mouse_wheel_multiplier = self._cur_font_size / 10
- if self.content_width > 0 and self.content_height > 0:
- self._imgui_renderer.process_inputs()
-
- # Begin imgui frame.
- imgui.new_frame()
- imgui.push_font(self._imgui_fonts[self._cur_font_size])
- imgui_utils.set_default_style(
- spacing=self.spacing, indent=self.font_size, scrollbar=self.font_size+4)
-
- def end_frame(self):
- imgui.pop_font()
- imgui.render()
- imgui.end_frame()
- self._imgui_renderer.render(imgui.get_draw_data())
- super().end_frame()
-
-# ----------------------------------------------------------------------------
-# Wrapper class for GlfwRenderer to fix a mouse wheel bug on Linux.
-
-
-class _GlfwRenderer(imgui.integrations.glfw.GlfwRenderer):
- def __init__(self, *args, **kwargs):
- super().__init__(*args, **kwargs)
- self.mouse_wheel_multiplier = 1
-
- def scroll_callback(self, window, x_offset, y_offset):
- self.io.mouse_wheel += y_offset * self.mouse_wheel_multiplier
-
-# ----------------------------------------------------------------------------
diff --git a/spaces/hanzaq/Doc-Bot/README.md b/spaces/hanzaq/Doc-Bot/README.md
deleted file mode 100644
index 94d2066896f43de7ad123a2739a3071f00018e75..0000000000000000000000000000000000000000
--- a/spaces/hanzaq/Doc-Bot/README.md
+++ /dev/null
@@ -1,12 +0,0 @@
----
-title: Doc Bot
-emoji: 💻
-colorFrom: pink
-colorTo: yellow
-sdk: streamlit
-sdk_version: 1.21.0
-app_file: app.py
-pinned: false
-license: apache-2.0
----
-# https---github.com-hanzalah416-PDF-analyzer-App
diff --git a/spaces/harisansarkhan/Image-Classification-with-CIFAR-10/Gradio.py b/spaces/harisansarkhan/Image-Classification-with-CIFAR-10/Gradio.py
deleted file mode 100644
index d3709bf8b4825bf449275f392f0a1c43fbd10fe5..0000000000000000000000000000000000000000
--- a/spaces/harisansarkhan/Image-Classification-with-CIFAR-10/Gradio.py
+++ /dev/null
@@ -1,50 +0,0 @@
-#!/usr/bin/env python
-# coding: utf-8
-
-# In[21]:
-
-
-import gradio as gr
-import tensorflow as tf
-import numpy as np
-from PIL import Image
-
-# Load your trained model
-model = tf.keras.models.load_model("Image Classification with CIFAR-10.h5")
-
-# label mapping
-labels = '''Airplane Automobile Bird Cat Deer Dog Frog Horse Ship Truck'''.split()
-
-def classify_image(input_image):
- # Convert Gradio image to numpy array
- image = np.array(input_image)
-
- # Resize the image to 32x32 pixels
- resized_image = Image.fromarray(image).resize((32, 32))
-
- # Convert the resized image to an array
- image_array = np.array(resized_image)
-
- # Expand dimensions to match the model input shape
- image_array = np.expand_dims(image_array, axis=0)
-
- # Make predictions using the model
- predicted_label = labels[model.predict(image_array).argmax()]
-
- return f"{predicted_label}"
-
-input_image = gr.inputs.Image() # No need to specify shape
-output_text = gr.outputs.Textbox()
-
-# Create the Gradio interface
-demo = gr.Interface(fn=classify_image, inputs=input_image, outputs=output_text,
- title="CNN Image Classifier",
- description="Upload an image and get the predicted class out of Airplane, Automobile, Bird, Cat, Deer, Dog, Frog, Horse, Ship, Truck.")
-demo.launch()
-
-
-# In[ ]:
-
-
-
-
diff --git a/spaces/hasibzunair/fifa-tryon-demo/Self-Correction-Human-Parsing-for-ACGPN/mhp_extension/detectron2/detectron2/data/datasets/__init__.py b/spaces/hasibzunair/fifa-tryon-demo/Self-Correction-Human-Parsing-for-ACGPN/mhp_extension/detectron2/detectron2/data/datasets/__init__.py
deleted file mode 100644
index 9c3f556bd201890fcca901d26efb5f9d8c3304f5..0000000000000000000000000000000000000000
--- a/spaces/hasibzunair/fifa-tryon-demo/Self-Correction-Human-Parsing-for-ACGPN/mhp_extension/detectron2/detectron2/data/datasets/__init__.py
+++ /dev/null
@@ -1,9 +0,0 @@
-# Copyright (c) Facebook, Inc. and its affiliates. All Rights Reserved
-from .cityscapes import load_cityscapes_instances
-from .coco import load_coco_json, load_sem_seg
-from .lvis import load_lvis_json, register_lvis_instances, get_lvis_instances_meta
-from .register_coco import register_coco_instances, register_coco_panoptic_separated
-from . import builtin # ensure the builtin data are registered
-
-
-__all__ = [k for k in globals().keys() if "builtin" not in k and not k.startswith("_")]
diff --git a/spaces/hasibzunair/fifa-tryon-demo/Self-Correction-Human-Parsing-for-ACGPN/mhp_extension/detectron2/docs/tutorials/datasets.md b/spaces/hasibzunair/fifa-tryon-demo/Self-Correction-Human-Parsing-for-ACGPN/mhp_extension/detectron2/docs/tutorials/datasets.md
deleted file mode 100644
index 8dc1c0c55598887e4de73e988567753ebf4538e2..0000000000000000000000000000000000000000
--- a/spaces/hasibzunair/fifa-tryon-demo/Self-Correction-Human-Parsing-for-ACGPN/mhp_extension/detectron2/docs/tutorials/datasets.md
+++ /dev/null
@@ -1,221 +0,0 @@
-# Use Custom Datasets
-
-Datasets that have builtin support in detectron2 are listed in [datasets](../../datasets).
-If you want to use a custom dataset while also reusing detectron2's data loaders,
-you will need to
-
-1. __Register__ your dataset (i.e., tell detectron2 how to obtain your dataset).
-2. Optionally, __register metadata__ for your dataset.
-
-Next, we explain the above two concepts in detail.
-
-The [Colab tutorial](https://colab.research.google.com/drive/16jcaJoc6bCFAQ96jDe2HwtXj7BMD_-m5)
-has a live example of how to register and train on a dataset of custom formats.
-
-### Register a Dataset
-
-To let detectron2 know how to obtain a dataset named "my_dataset", you will implement
-a function that returns the items in your dataset and then tell detectron2 about this
-function:
-```python
-def my_dataset_function():
- ...
- return list[dict] in the following format
-
-from detectron2.data import DatasetCatalog
-DatasetCatalog.register("my_dataset", my_dataset_function)
-```
-
-Here, the snippet associates a dataset "my_dataset" with a function that returns the data.
-The registration stays effective until the process exists.
-
-The function can processes data from its original format into either one of the following:
-1. Detectron2's standard dataset dict, described below. This will work with many other builtin
- features in detectron2, so it's recommended to use it when it's sufficient for your task.
-2. Your custom dataset dict. You can also return arbitrary dicts in your own format,
- such as adding extra keys for new tasks.
- Then you will need to handle them properly downstream as well.
- See below for more details.
-
-#### Standard Dataset Dicts
-
-For standard tasks
-(instance detection, instance/semantic/panoptic segmentation, keypoint detection),
-we load the original dataset into `list[dict]` with a specification similar to COCO's json annotations.
-This is our standard representation for a dataset.
-
-Each dict contains information about one image.
-The dict may have the following fields,
-and the required fields vary based on what the dataloader or the task needs (see more below).
-
-+ `file_name`: the full path to the image file. Will apply rotation and flipping if the image has such exif information.
-+ `height`, `width`: integer. The shape of image.
-+ `image_id` (str or int): a unique id that identifies this image. Used
- during evaluation to identify the images, but a dataset may use it for different purposes.
-+ `annotations` (list[dict]): each dict corresponds to annotations of one instance
- in this image. Required by instance detection/segmentation or keypoint detection tasks.
-
- Images with empty `annotations` will by default be removed from training,
- but can be included using `DATALOADER.FILTER_EMPTY_ANNOTATIONS`.
-
- Each dict contains the following keys, of which `bbox`,`bbox_mode` and `category_id` are required:
- + `bbox` (list[float]): list of 4 numbers representing the bounding box of the instance.
- + `bbox_mode` (int): the format of bbox.
- It must be a member of
- [structures.BoxMode](../modules/structures.html#detectron2.structures.BoxMode).
- Currently supports: `BoxMode.XYXY_ABS`, `BoxMode.XYWH_ABS`.
- + `category_id` (int): an integer in the range [0, num_categories) representing the category label.
- The value num_categories is reserved to represent the "background" category, if applicable.
- + `segmentation` (list[list[float]] or dict): the segmentation mask of the instance.
- + If `list[list[float]]`, it represents a list of polygons, one for each connected component
- of the object. Each `list[float]` is one simple polygon in the format of `[x1, y1, ..., xn, yn]`.
- The Xs and Ys are either relative coordinates in [0, 1], or absolute coordinates,
- depend on whether "bbox_mode" is relative.
- + If `dict`, it represents the per-pixel segmentation mask in COCO's RLE format. The dict should have
- keys "size" and "counts". You can convert a uint8 segmentation mask of 0s and 1s into
- RLE format by `pycocotools.mask.encode(np.asarray(mask, order="F"))`.
- + `keypoints` (list[float]): in the format of [x1, y1, v1,..., xn, yn, vn].
- v[i] means the [visibility](http://cocodataset.org/#format-data) of this keypoint.
- `n` must be equal to the number of keypoint categories.
- The Xs and Ys are either relative coordinates in [0, 1], or absolute coordinates,
- depend on whether "bbox_mode" is relative.
-
- Note that the coordinate annotations in COCO format are integers in range [0, H-1 or W-1].
- By default, detectron2 adds 0.5 to absolute keypoint coordinates to convert them from discrete
- pixel indices to floating point coordinates.
- + `iscrowd`: 0 (default) or 1. Whether this instance is labeled as COCO's "crowd
- region". Don't include this field if you don't know what it means.
-+ `sem_seg_file_name`: the full path to the ground truth semantic segmentation file.
- Required by semantic segmentation task.
- It should be an image whose pixel values are integer labels.
-
-
-Fast R-CNN (with precomputed proposals) is rarely used today.
-To train a Fast R-CNN, the following extra keys are needed:
-
-+ `proposal_boxes` (array): 2D numpy array with shape (K, 4) representing K precomputed proposal boxes for this image.
-+ `proposal_objectness_logits` (array): numpy array with shape (K, ), which corresponds to the objectness
- logits of proposals in 'proposal_boxes'.
-+ `proposal_bbox_mode` (int): the format of the precomputed proposal bbox.
- It must be a member of
- [structures.BoxMode](../modules/structures.html#detectron2.structures.BoxMode).
- Default is `BoxMode.XYXY_ABS`.
-
-#### Custom Dataset Dicts for New Tasks
-
-In the `list[dict]` that your dataset function returns, the dictionary can also have arbitrary custom data.
-This will be useful for a new task that needs extra information not supported
-by the standard dataset dicts. In this case, you need to make sure the downstream code can handle your data
-correctly. Usually this requires writing a new `mapper` for the dataloader (see [Use Custom Dataloaders](./data_loading.md)).
-
-When designing a custom format, note that all dicts are stored in memory
-(sometimes serialized and with multiple copies).
-To save memory, each dict is meant to contain small but sufficient information
-about each sample, such as file names and annotations.
-Loading full samples typically happens in the data loader.
-
-For attributes shared among the entire dataset, use `Metadata` (see below).
-To avoid extra memory, do not save such information repeatly for each sample.
-
-### "Metadata" for Datasets
-
-Each dataset is associated with some metadata, accessible through
-`MetadataCatalog.get(dataset_name).some_metadata`.
-Metadata is a key-value mapping that contains information that's shared among
-the entire dataset, and usually is used to interpret what's in the dataset, e.g.,
-names of classes, colors of classes, root of files, etc.
-This information will be useful for augmentation, evaluation, visualization, logging, etc.
-The structure of metadata depends on the what is needed from the corresponding downstream code.
-
-If you register a new dataset through `DatasetCatalog.register`,
-you may also want to add its corresponding metadata through
-`MetadataCatalog.get(dataset_name).some_key = some_value`, to enable any features that need the metadata.
-You can do it like this (using the metadata key "thing_classes" as an example):
-
-```python
-from detectron2.data import MetadataCatalog
-MetadataCatalog.get("my_dataset").thing_classes = ["person", "dog"]
-```
-
-Here is a list of metadata keys that are used by builtin features in detectron2.
-If you add your own dataset without these metadata, some features may be
-unavailable to you:
-
-* `thing_classes` (list[str]): Used by all instance detection/segmentation tasks.
- A list of names for each instance/thing category.
- If you load a COCO format dataset, it will be automatically set by the function `load_coco_json`.
-
-* `thing_colors` (list[tuple(r, g, b)]): Pre-defined color (in [0, 255]) for each thing category.
- Used for visualization. If not given, random colors are used.
-
-* `stuff_classes` (list[str]): Used by semantic and panoptic segmentation tasks.
- A list of names for each stuff category.
-
-* `stuff_colors` (list[tuple(r, g, b)]): Pre-defined color (in [0, 255]) for each stuff category.
- Used for visualization. If not given, random colors are used.
-
-* `keypoint_names` (list[str]): Used by keypoint localization. A list of names for each keypoint.
-
-* `keypoint_flip_map` (list[tuple[str]]): Used by the keypoint localization task. A list of pairs of names,
- where each pair are the two keypoints that should be flipped if the image is
- flipped horizontally during augmentation.
-* `keypoint_connection_rules`: list[tuple(str, str, (r, g, b))]. Each tuple specifies a pair of keypoints
- that are connected and the color to use for the line between them when visualized.
-
-Some additional metadata that are specific to the evaluation of certain datasets (e.g. COCO):
-
-* `thing_dataset_id_to_contiguous_id` (dict[int->int]): Used by all instance detection/segmentation tasks in the COCO format.
- A mapping from instance class ids in the dataset to contiguous ids in range [0, #class).
- Will be automatically set by the function `load_coco_json`.
-
-* `stuff_dataset_id_to_contiguous_id` (dict[int->int]): Used when generating prediction json files for
- semantic/panoptic segmentation.
- A mapping from semantic segmentation class ids in the dataset
- to contiguous ids in [0, num_categories). It is useful for evaluation only.
-
-* `json_file`: The COCO annotation json file. Used by COCO evaluation for COCO-format datasets.
-* `panoptic_root`, `panoptic_json`: Used by panoptic evaluation.
-* `evaluator_type`: Used by the builtin main training script to select
- evaluator. Don't use it in a new training script.
- You can just provide the [DatasetEvaluator](../modules/evaluation.html#detectron2.evaluation.DatasetEvaluator)
- for your dataset directly in your main script.
-
-NOTE: For background on the concept of "thing" and "stuff", see
-[On Seeing Stuff: The Perception of Materials by Humans and Machines](http://persci.mit.edu/pub_pdfs/adelson_spie_01.pdf).
-In detectron2, the term "thing" is used for instance-level tasks,
-and "stuff" is used for semantic segmentation tasks.
-Both are used in panoptic segmentation.
-
-### Register a COCO Format Dataset
-
-If your dataset is already a json file in the COCO format,
-the dataset and its associated metadata can be registered easily with:
-```python
-from detectron2.data.datasets import register_coco_instances
-register_coco_instances("my_dataset", {}, "json_annotation.json", "path/to/image/dir")
-```
-
-If your dataset is in COCO format but with extra custom per-instance annotations,
-the [load_coco_json](../modules/data.html#detectron2.data.datasets.load_coco_json)
-function might be useful.
-
-### Update the Config for New Datasets
-
-Once you've registered the dataset, you can use the name of the dataset (e.g., "my_dataset" in
-example above) in `cfg.DATASETS.{TRAIN,TEST}`.
-There are other configs you might want to change to train or evaluate on new datasets:
-
-* `MODEL.ROI_HEADS.NUM_CLASSES` and `MODEL.RETINANET.NUM_CLASSES` are the number of thing classes
- for R-CNN and RetinaNet models, respectively.
-* `MODEL.ROI_KEYPOINT_HEAD.NUM_KEYPOINTS` sets the number of keypoints for Keypoint R-CNN.
- You'll also need to set [Keypoint OKS](http://cocodataset.org/#keypoints-eval)
- with `TEST.KEYPOINT_OKS_SIGMAS` for evaluation.
-* `MODEL.SEM_SEG_HEAD.NUM_CLASSES` sets the number of stuff classes for Semantic FPN & Panoptic FPN.
-* If you're training Fast R-CNN (with precomputed proposals), `DATASETS.PROPOSAL_FILES_{TRAIN,TEST}`
- need to match the datasets. The format of proposal files are documented
- [here](../modules/data.html#detectron2.data.load_proposals_into_dataset).
-
-New models
-(e.g. [TensorMask](../../projects/TensorMask),
-[PointRend](../../projects/PointRend))
-often have similar configs of their own that need to be changed as well.
diff --git a/spaces/hdhzk/bingo/src/components/voice.tsx b/spaces/hdhzk/bingo/src/components/voice.tsx
deleted file mode 100644
index 074d0e145229947282a472bd84f6578cf0b3c71c..0000000000000000000000000000000000000000
--- a/spaces/hdhzk/bingo/src/components/voice.tsx
+++ /dev/null
@@ -1,52 +0,0 @@
-import React, { useEffect } from 'react'
-import { useSetAtom } from 'jotai'
-import { useBing } from '@/lib/hooks/use-bing'
-import Image from 'next/image'
-import VoiceIcon from '@/assets/images/voice.svg'
-import VoiceButton from './ui/voice'
-import { SR } from '@/lib/bots/bing/sr'
-import { voiceListenAtom } from '@/state'
-
-const sr = new SR(['发送', '清空', '退出'])
-
-const Voice = ({ setInput, input, sendMessage, isSpeaking }: Pick, 'setInput' | 'sendMessage' | 'input' | 'isSpeaking'>) => {
- const setListen = useSetAtom(voiceListenAtom)
- useEffect(() => {
- if (sr.listening) return
- sr.transcript = !isSpeaking
- }, [isSpeaking])
-
- useEffect(() => {
- sr.onchange = (msg: string, command?: string) => {
- switch (command) {
- case '退出':
- sr.stop()
- break;
- case '发送':
- sendMessage(input)
- case '清空':
- setInput('')
- break;
- default:
- setInput(input + msg)
- }
- }
- }, [input])
-
- const switchSR = (enable: boolean = false) => {
- setListen(enable)
- if (enable) {
- sr.start()
- } else {
- sr.stop()
- }
- }
-
- return sr.listening ? (
- switchSR(false)} />
- ) : (
- switchSR(true)} />
- )
-};
-
-export default Voice;
diff --git a/spaces/hezhaoqia/vits-simple-api/vits/attentions.py b/spaces/hezhaoqia/vits-simple-api/vits/attentions.py
deleted file mode 100644
index 207437cd697cbaaf019543cdee1942bc56324d3f..0000000000000000000000000000000000000000
--- a/spaces/hezhaoqia/vits-simple-api/vits/attentions.py
+++ /dev/null
@@ -1,300 +0,0 @@
-import math
-import torch
-from torch import nn
-from torch.nn import functional as F
-
-from vits import commons
-from vits.modules import LayerNorm
-
-
-class Encoder(nn.Module):
- def __init__(self, hidden_channels, filter_channels, n_heads, n_layers, kernel_size=1, p_dropout=0., window_size=4, **kwargs):
- super().__init__()
- self.hidden_channels = hidden_channels
- self.filter_channels = filter_channels
- self.n_heads = n_heads
- self.n_layers = n_layers
- self.kernel_size = kernel_size
- self.p_dropout = p_dropout
- self.window_size = window_size
-
- self.drop = nn.Dropout(p_dropout)
- self.attn_layers = nn.ModuleList()
- self.norm_layers_1 = nn.ModuleList()
- self.ffn_layers = nn.ModuleList()
- self.norm_layers_2 = nn.ModuleList()
- for i in range(self.n_layers):
- self.attn_layers.append(MultiHeadAttention(hidden_channels, hidden_channels, n_heads, p_dropout=p_dropout, window_size=window_size))
- self.norm_layers_1.append(LayerNorm(hidden_channels))
- self.ffn_layers.append(FFN(hidden_channels, hidden_channels, filter_channels, kernel_size, p_dropout=p_dropout))
- self.norm_layers_2.append(LayerNorm(hidden_channels))
-
- def forward(self, x, x_mask):
- attn_mask = x_mask.unsqueeze(2) * x_mask.unsqueeze(-1)
- x = x * x_mask
- for i in range(self.n_layers):
- y = self.attn_layers[i](x, x, attn_mask)
- y = self.drop(y)
- x = self.norm_layers_1[i](x + y)
-
- y = self.ffn_layers[i](x, x_mask)
- y = self.drop(y)
- x = self.norm_layers_2[i](x + y)
- x = x * x_mask
- return x
-
-
-class Decoder(nn.Module):
- def __init__(self, hidden_channels, filter_channels, n_heads, n_layers, kernel_size=1, p_dropout=0., proximal_bias=False, proximal_init=True, **kwargs):
- super().__init__()
- self.hidden_channels = hidden_channels
- self.filter_channels = filter_channels
- self.n_heads = n_heads
- self.n_layers = n_layers
- self.kernel_size = kernel_size
- self.p_dropout = p_dropout
- self.proximal_bias = proximal_bias
- self.proximal_init = proximal_init
-
- self.drop = nn.Dropout(p_dropout)
- self.self_attn_layers = nn.ModuleList()
- self.norm_layers_0 = nn.ModuleList()
- self.encdec_attn_layers = nn.ModuleList()
- self.norm_layers_1 = nn.ModuleList()
- self.ffn_layers = nn.ModuleList()
- self.norm_layers_2 = nn.ModuleList()
- for i in range(self.n_layers):
- self.self_attn_layers.append(MultiHeadAttention(hidden_channels, hidden_channels, n_heads, p_dropout=p_dropout, proximal_bias=proximal_bias, proximal_init=proximal_init))
- self.norm_layers_0.append(LayerNorm(hidden_channels))
- self.encdec_attn_layers.append(MultiHeadAttention(hidden_channels, hidden_channels, n_heads, p_dropout=p_dropout))
- self.norm_layers_1.append(LayerNorm(hidden_channels))
- self.ffn_layers.append(FFN(hidden_channels, hidden_channels, filter_channels, kernel_size, p_dropout=p_dropout, causal=True))
- self.norm_layers_2.append(LayerNorm(hidden_channels))
-
- def forward(self, x, x_mask, h, h_mask):
- """
- x: decoder input
- h: encoder output
- """
- self_attn_mask = commons.subsequent_mask(x_mask.size(2)).to(device=x.device, dtype=x.dtype)
- encdec_attn_mask = h_mask.unsqueeze(2) * x_mask.unsqueeze(-1)
- x = x * x_mask
- for i in range(self.n_layers):
- y = self.self_attn_layers[i](x, x, self_attn_mask)
- y = self.drop(y)
- x = self.norm_layers_0[i](x + y)
-
- y = self.encdec_attn_layers[i](x, h, encdec_attn_mask)
- y = self.drop(y)
- x = self.norm_layers_1[i](x + y)
-
- y = self.ffn_layers[i](x, x_mask)
- y = self.drop(y)
- x = self.norm_layers_2[i](x + y)
- x = x * x_mask
- return x
-
-
-class MultiHeadAttention(nn.Module):
- def __init__(self, channels, out_channels, n_heads, p_dropout=0., window_size=None, heads_share=True, block_length=None, proximal_bias=False, proximal_init=False):
- super().__init__()
- assert channels % n_heads == 0
-
- self.channels = channels
- self.out_channels = out_channels
- self.n_heads = n_heads
- self.p_dropout = p_dropout
- self.window_size = window_size
- self.heads_share = heads_share
- self.block_length = block_length
- self.proximal_bias = proximal_bias
- self.proximal_init = proximal_init
- self.attn = None
-
- self.k_channels = channels // n_heads
- self.conv_q = nn.Conv1d(channels, channels, 1)
- self.conv_k = nn.Conv1d(channels, channels, 1)
- self.conv_v = nn.Conv1d(channels, channels, 1)
- self.conv_o = nn.Conv1d(channels, out_channels, 1)
- self.drop = nn.Dropout(p_dropout)
-
- if window_size is not None:
- n_heads_rel = 1 if heads_share else n_heads
- rel_stddev = self.k_channels**-0.5
- self.emb_rel_k = nn.Parameter(torch.randn(n_heads_rel, window_size * 2 + 1, self.k_channels) * rel_stddev)
- self.emb_rel_v = nn.Parameter(torch.randn(n_heads_rel, window_size * 2 + 1, self.k_channels) * rel_stddev)
-
- nn.init.xavier_uniform_(self.conv_q.weight)
- nn.init.xavier_uniform_(self.conv_k.weight)
- nn.init.xavier_uniform_(self.conv_v.weight)
- if proximal_init:
- with torch.no_grad():
- self.conv_k.weight.copy_(self.conv_q.weight)
- self.conv_k.bias.copy_(self.conv_q.bias)
-
- def forward(self, x, c, attn_mask=None):
- q = self.conv_q(x)
- k = self.conv_k(c)
- v = self.conv_v(c)
-
- x, self.attn = self.attention(q, k, v, mask=attn_mask)
-
- x = self.conv_o(x)
- return x
-
- def attention(self, query, key, value, mask=None):
- # reshape [b, d, t] -> [b, n_h, t, d_k]
- b, d, t_s, t_t = (*key.size(), query.size(2))
- query = query.view(b, self.n_heads, self.k_channels, t_t).transpose(2, 3)
- key = key.view(b, self.n_heads, self.k_channels, t_s).transpose(2, 3)
- value = value.view(b, self.n_heads, self.k_channels, t_s).transpose(2, 3)
-
- scores = torch.matmul(query / math.sqrt(self.k_channels), key.transpose(-2, -1))
- if self.window_size is not None:
- assert t_s == t_t, "Relative attention is only available for self-attention."
- key_relative_embeddings = self._get_relative_embeddings(self.emb_rel_k, t_s)
- rel_logits = self._matmul_with_relative_keys(query /math.sqrt(self.k_channels), key_relative_embeddings)
- scores_local = self._relative_position_to_absolute_position(rel_logits)
- scores = scores + scores_local
- if self.proximal_bias:
- assert t_s == t_t, "Proximal bias is only available for self-attention."
- scores = scores + self._attention_bias_proximal(t_s).to(device=scores.device, dtype=scores.dtype)
- if mask is not None:
- scores = scores.masked_fill(mask == 0, -1e4)
- if self.block_length is not None:
- assert t_s == t_t, "Local attention is only available for self-attention."
- block_mask = torch.ones_like(scores).triu(-self.block_length).tril(self.block_length)
- scores = scores.masked_fill(block_mask == 0, -1e4)
- p_attn = F.softmax(scores, dim=-1) # [b, n_h, t_t, t_s]
- p_attn = self.drop(p_attn)
- output = torch.matmul(p_attn, value)
- if self.window_size is not None:
- relative_weights = self._absolute_position_to_relative_position(p_attn)
- value_relative_embeddings = self._get_relative_embeddings(self.emb_rel_v, t_s)
- output = output + self._matmul_with_relative_values(relative_weights, value_relative_embeddings)
- output = output.transpose(2, 3).contiguous().view(b, d, t_t) # [b, n_h, t_t, d_k] -> [b, d, t_t]
- return output, p_attn
-
- def _matmul_with_relative_values(self, x, y):
- """
- x: [b, h, l, m]
- y: [h or 1, m, d]
- ret: [b, h, l, d]
- """
- ret = torch.matmul(x, y.unsqueeze(0))
- return ret
-
- def _matmul_with_relative_keys(self, x, y):
- """
- x: [b, h, l, d]
- y: [h or 1, m, d]
- ret: [b, h, l, m]
- """
- ret = torch.matmul(x, y.unsqueeze(0).transpose(-2, -1))
- return ret
-
- def _get_relative_embeddings(self, relative_embeddings, length):
- max_relative_position = 2 * self.window_size + 1
- # Pad first before slice to avoid using cond ops.
- pad_length = max(length - (self.window_size + 1), 0)
- slice_start_position = max((self.window_size + 1) - length, 0)
- slice_end_position = slice_start_position + 2 * length - 1
- if pad_length > 0:
- padded_relative_embeddings = F.pad(
- relative_embeddings,
- commons.convert_pad_shape([[0, 0], [pad_length, pad_length], [0, 0]]))
- else:
- padded_relative_embeddings = relative_embeddings
- used_relative_embeddings = padded_relative_embeddings[:,slice_start_position:slice_end_position]
- return used_relative_embeddings
-
- def _relative_position_to_absolute_position(self, x):
- """
- x: [b, h, l, 2*l-1]
- ret: [b, h, l, l]
- """
- batch, heads, length, _ = x.size()
- # Concat columns of pad to shift from relative to absolute indexing.
- x = F.pad(x, commons.convert_pad_shape([[0, 0], [0, 0], [0, 0], [0, 1]]))
-
- # Concat extra elements so to add up to shape (len+1, 2*len-1).
- x_flat = x.view([batch, heads, length * 2 * length])
- x_flat = F.pad(x_flat, commons.convert_pad_shape([[0, 0], [0, 0], [0, length - 1]]))
-
- # Reshape and slice out the padded elements.
- x_final = x_flat.view([batch, heads, length+1, 2*length-1])[:, :, :length, length-1:]
- return x_final
-
- def _absolute_position_to_relative_position(self, x):
- """
- x: [b, h, l, l]
- ret: [b, h, l, 2*l-1]
- """
- batch, heads, length, _ = x.size()
- # padd along column
- x = F.pad(x, commons.convert_pad_shape([[0, 0], [0, 0], [0, 0], [0, length - 1]]))
- x_flat = x.view([batch, heads, length**2 + length*(length -1)])
- # add 0's in the beginning that will skew the elements after reshape
- x_flat = F.pad(x_flat, commons.convert_pad_shape([[0, 0], [0, 0], [length, 0]]))
- x_final = x_flat.view([batch, heads, length, 2*length])[:,:,:,1:]
- return x_final
-
- def _attention_bias_proximal(self, length):
- """Bias for self-attention to encourage attention to close positions.
- Args:
- length: an integer scalar.
- Returns:
- a Tensor with shape [1, 1, length, length]
- """
- r = torch.arange(length, dtype=torch.float32)
- diff = torch.unsqueeze(r, 0) - torch.unsqueeze(r, 1)
- return torch.unsqueeze(torch.unsqueeze(-torch.log1p(torch.abs(diff)), 0), 0)
-
-
-class FFN(nn.Module):
- def __init__(self, in_channels, out_channels, filter_channels, kernel_size, p_dropout=0., activation=None, causal=False):
- super().__init__()
- self.in_channels = in_channels
- self.out_channels = out_channels
- self.filter_channels = filter_channels
- self.kernel_size = kernel_size
- self.p_dropout = p_dropout
- self.activation = activation
- self.causal = causal
-
- if causal:
- self.padding = self._causal_padding
- else:
- self.padding = self._same_padding
-
- self.conv_1 = nn.Conv1d(in_channels, filter_channels, kernel_size)
- self.conv_2 = nn.Conv1d(filter_channels, out_channels, kernel_size)
- self.drop = nn.Dropout(p_dropout)
-
- def forward(self, x, x_mask):
- x = self.conv_1(self.padding(x * x_mask))
- if self.activation == "gelu":
- x = x * torch.sigmoid(1.702 * x)
- else:
- x = torch.relu(x)
- x = self.drop(x)
- x = self.conv_2(self.padding(x * x_mask))
- return x * x_mask
-
- def _causal_padding(self, x):
- if self.kernel_size == 1:
- return x
- pad_l = self.kernel_size - 1
- pad_r = 0
- padding = [[0, 0], [0, 0], [pad_l, pad_r]]
- x = F.pad(x, commons.convert_pad_shape(padding))
- return x
-
- def _same_padding(self, x):
- if self.kernel_size == 1:
- return x
- pad_l = (self.kernel_size - 1) // 2
- pad_r = self.kernel_size // 2
- padding = [[0, 0], [0, 0], [pad_l, pad_r]]
- x = F.pad(x, commons.convert_pad_shape(padding))
- return x
diff --git a/spaces/ho11laqe/nnUNet_calvingfront_detection/nnunet/postprocessing/consolidate_all_for_paper.py b/spaces/ho11laqe/nnUNet_calvingfront_detection/nnunet/postprocessing/consolidate_all_for_paper.py
deleted file mode 100644
index 51c787261b4662894cf5b356c185669fc6eb33b9..0000000000000000000000000000000000000000
--- a/spaces/ho11laqe/nnUNet_calvingfront_detection/nnunet/postprocessing/consolidate_all_for_paper.py
+++ /dev/null
@@ -1,61 +0,0 @@
-# Copyright 2020 Division of Medical Image Computing, German Cancer Research Center (DKFZ), Heidelberg, Germany
-#
-# Licensed under the Apache License, Version 2.0 (the "License");
-# you may not use this file except in compliance with the License.
-# You may obtain a copy of the License at
-#
-# http://www.apache.org/licenses/LICENSE-2.0
-#
-# Unless required by applicable law or agreed to in writing, software
-# distributed under the License is distributed on an "AS IS" BASIS,
-# WITHOUT WARRANTIES OR CONDITIONS OF ANY KIND, either express or implied.
-# See the License for the specific language governing permissions and
-# limitations under the License.
-
-
-from nnunet.utilities.folder_names import get_output_folder_name
-
-
-def get_datasets():
- configurations_all = {
- "Task01_BrainTumour": ("3d_fullres", "2d"),
- "Task02_Heart": ("3d_fullres", "2d",),
- "Task03_Liver": ("3d_cascade_fullres", "3d_fullres", "3d_lowres", "2d"),
- "Task04_Hippocampus": ("3d_fullres", "2d",),
- "Task05_Prostate": ("3d_fullres", "2d",),
- "Task06_Lung": ("3d_cascade_fullres", "3d_fullres", "3d_lowres", "2d"),
- "Task07_Pancreas": ("3d_cascade_fullres", "3d_fullres", "3d_lowres", "2d"),
- "Task08_HepaticVessel": ("3d_cascade_fullres", "3d_fullres", "3d_lowres", "2d"),
- "Task09_Spleen": ("3d_cascade_fullres", "3d_fullres", "3d_lowres", "2d"),
- "Task10_Colon": ("3d_cascade_fullres", "3d_fullres", "3d_lowres", "2d"),
- "Task48_KiTS_clean": ("3d_cascade_fullres", "3d_lowres", "3d_fullres", "2d"),
- "Task27_ACDC": ("3d_fullres", "2d",),
- "Task24_Promise": ("3d_fullres", "2d",),
- "Task35_ISBILesionSegmentation": ("3d_fullres", "2d",),
- "Task38_CHAOS_Task_3_5_Variant2": ("3d_fullres", "2d",),
- "Task29_LITS": ("3d_cascade_fullres", "3d_lowres", "2d", "3d_fullres",),
- "Task17_AbdominalOrganSegmentation": ("3d_cascade_fullres", "3d_lowres", "2d", "3d_fullres",),
- "Task55_SegTHOR": ("3d_cascade_fullres", "3d_lowres", "3d_fullres", "2d",),
- "Task56_VerSe": ("3d_cascade_fullres", "3d_lowres", "3d_fullres", "2d",),
- }
- return configurations_all
-
-
-def get_commands(configurations, regular_trainer="nnUNetTrainerV2", cascade_trainer="nnUNetTrainerV2CascadeFullRes",
- plans="nnUNetPlansv2.1"):
-
- node_pool = ["hdf18-gpu%02.0d" % i for i in range(1, 21)] + ["hdf19-gpu%02.0d" % i for i in range(1, 8)] + ["hdf19-gpu%02.0d" % i for i in range(11, 16)]
- ctr = 0
- for task in configurations:
- models = configurations[task]
- for m in models:
- if m == "3d_cascade_fullres":
- trainer = cascade_trainer
- else:
- trainer = regular_trainer
-
- folder = get_output_folder_name(m, task, trainer, plans, overwrite_training_output_dir="/datasets/datasets_fabian/results/nnUNet")
- node = node_pool[ctr % len(node_pool)]
- print("bsub -m %s -q gputest -L /bin/bash \"source ~/.bashrc && python postprocessing/"
- "consolidate_postprocessing.py -f" % node, folder, "\"")
- ctr += 1
diff --git a/spaces/huang4414/GTest/public/index.html b/spaces/huang4414/GTest/public/index.html
deleted file mode 100644
index 43cca0b13062f6dc7483289c2c6cd0fd3c1bff9f..0000000000000000000000000000000000000000
--- a/spaces/huang4414/GTest/public/index.html
+++ /dev/null
@@ -1,11 +0,0 @@
-
-
-
-
-
-
-
-
-
-
-
\ No newline at end of file
diff --git a/spaces/huggan/FastGan/models.py b/spaces/huggan/FastGan/models.py
deleted file mode 100644
index 513119e40f43f7b031927d13dd816ef0a31f4a8a..0000000000000000000000000000000000000000
--- a/spaces/huggan/FastGan/models.py
+++ /dev/null
@@ -1,245 +0,0 @@
-import torch
-import torch.nn as nn
-
-from typing import Any, Tuple, Union
-
-from utils import (
- ImageType,
- crop_image_part,
-)
-
-from layers import (
- SpectralConv2d,
- InitLayer,
- SLEBlock,
- UpsampleBlockT1,
- UpsampleBlockT2,
- DownsampleBlockT1,
- DownsampleBlockT2,
- Decoder,
-)
-
-from huggan.pytorch.huggan_mixin import HugGANModelHubMixin
-
-
-class Generator(nn.Module, HugGANModelHubMixin):
-
- def __init__(self, in_channels: int,
- out_channels: int):
- super().__init__()
-
- self._channels = {
- 4: 1024,
- 8: 512,
- 16: 256,
- 32: 128,
- 64: 128,
- 128: 64,
- 256: 32,
- 512: 16,
- 1024: 8,
- }
-
- self._init = InitLayer(
- in_channels=in_channels,
- out_channels=self._channels[4],
- )
-
- self._upsample_8 = UpsampleBlockT2(in_channels=self._channels[4], out_channels=self._channels[8] )
- self._upsample_16 = UpsampleBlockT1(in_channels=self._channels[8], out_channels=self._channels[16] )
- self._upsample_32 = UpsampleBlockT2(in_channels=self._channels[16], out_channels=self._channels[32] )
- self._upsample_64 = UpsampleBlockT1(in_channels=self._channels[32], out_channels=self._channels[64] )
- self._upsample_128 = UpsampleBlockT2(in_channels=self._channels[64], out_channels=self._channels[128] )
- self._upsample_256 = UpsampleBlockT1(in_channels=self._channels[128], out_channels=self._channels[256] )
- self._upsample_512 = UpsampleBlockT2(in_channels=self._channels[256], out_channels=self._channels[512] )
- self._upsample_1024 = UpsampleBlockT1(in_channels=self._channels[512], out_channels=self._channels[1024])
-
- self._sle_64 = SLEBlock(in_channels=self._channels[4], out_channels=self._channels[64] )
- self._sle_128 = SLEBlock(in_channels=self._channels[8], out_channels=self._channels[128])
- self._sle_256 = SLEBlock(in_channels=self._channels[16], out_channels=self._channels[256])
- self._sle_512 = SLEBlock(in_channels=self._channels[32], out_channels=self._channels[512])
-
- self._out_128 = nn.Sequential(
- SpectralConv2d(
- in_channels=self._channels[128],
- out_channels=out_channels,
- kernel_size=1,
- stride=1,
- padding='same',
- bias=False,
- ),
- nn.Tanh(),
- )
-
- self._out_1024 = nn.Sequential(
- SpectralConv2d(
- in_channels=self._channels[1024],
- out_channels=out_channels,
- kernel_size=3,
- stride=1,
- padding='same',
- bias=False,
- ),
- nn.Tanh(),
- )
-
- def forward(self, input: torch.Tensor) -> \
- Tuple[torch.Tensor, torch.Tensor]:
- size_4 = self._init(input)
- size_8 = self._upsample_8(size_4)
- size_16 = self._upsample_16(size_8)
- size_32 = self._upsample_32(size_16)
-
- size_64 = self._sle_64 (size_4, self._upsample_64 (size_32) )
- size_128 = self._sle_128(size_8, self._upsample_128(size_64) )
- size_256 = self._sle_256(size_16, self._upsample_256(size_128))
- size_512 = self._sle_512(size_32, self._upsample_512(size_256))
-
- size_1024 = self._upsample_1024(size_512)
-
- out_128 = self._out_128 (size_128)
- out_1024 = self._out_1024(size_1024)
- return out_1024, out_128
-
-
-class Discriminrator(nn.Module, HugGANModelHubMixin):
-
- def __init__(self, in_channels: int):
- super().__init__()
-
- self._channels = {
- 4: 1024,
- 8: 512,
- 16: 256,
- 32: 128,
- 64: 128,
- 128: 64,
- 256: 32,
- 512: 16,
- 1024: 8,
- }
-
- self._init = nn.Sequential(
- SpectralConv2d(
- in_channels=in_channels,
- out_channels=self._channels[1024],
- kernel_size=4,
- stride=2,
- padding=1,
- bias=False,
- ),
- nn.LeakyReLU(negative_slope=0.2),
- SpectralConv2d(
- in_channels=self._channels[1024],
- out_channels=self._channels[512],
- kernel_size=4,
- stride=2,
- padding=1,
- bias=False,
- ),
- nn.BatchNorm2d(num_features=self._channels[512]),
- nn.LeakyReLU(negative_slope=0.2),
- )
-
- self._downsample_256 = DownsampleBlockT2(in_channels=self._channels[512], out_channels=self._channels[256])
- self._downsample_128 = DownsampleBlockT2(in_channels=self._channels[256], out_channels=self._channels[128])
- self._downsample_64 = DownsampleBlockT2(in_channels=self._channels[128], out_channels=self._channels[64] )
- self._downsample_32 = DownsampleBlockT2(in_channels=self._channels[64], out_channels=self._channels[32] )
- self._downsample_16 = DownsampleBlockT2(in_channels=self._channels[32], out_channels=self._channels[16] )
-
- self._sle_64 = SLEBlock(in_channels=self._channels[512], out_channels=self._channels[64])
- self._sle_32 = SLEBlock(in_channels=self._channels[256], out_channels=self._channels[32])
- self._sle_16 = SLEBlock(in_channels=self._channels[128], out_channels=self._channels[16])
-
- self._small_track = nn.Sequential(
- SpectralConv2d(
- in_channels=in_channels,
- out_channels=self._channels[256],
- kernel_size=4,
- stride=2,
- padding=1,
- bias=False,
- ),
- nn.LeakyReLU(negative_slope=0.2),
- DownsampleBlockT1(in_channels=self._channels[256], out_channels=self._channels[128]),
- DownsampleBlockT1(in_channels=self._channels[128], out_channels=self._channels[64] ),
- DownsampleBlockT1(in_channels=self._channels[64], out_channels=self._channels[32] ),
- )
-
- self._features_large = nn.Sequential(
- SpectralConv2d(
- in_channels=self._channels[16] ,
- out_channels=self._channels[8],
- kernel_size=1,
- stride=1,
- padding=0,
- bias=False,
- ),
- nn.BatchNorm2d(num_features=self._channels[8]),
- nn.LeakyReLU(negative_slope=0.2),
- SpectralConv2d(
- in_channels=self._channels[8],
- out_channels=1,
- kernel_size=4,
- stride=1,
- padding=0,
- bias=False,
- )
- )
-
- self._features_small = nn.Sequential(
- SpectralConv2d(
- in_channels=self._channels[32],
- out_channels=1,
- kernel_size=4,
- stride=1,
- padding=0,
- bias=False,
- ),
- )
-
- self._decoder_large = Decoder(in_channels=self._channels[16], out_channels=3)
- self._decoder_small = Decoder(in_channels=self._channels[32], out_channels=3)
- self._decoder_piece = Decoder(in_channels=self._channels[32], out_channels=3)
-
- def forward(self, images_1024: torch.Tensor,
- images_128: torch.Tensor,
- image_type: ImageType) -> \
- Union[
- torch.Tensor,
- Tuple[torch.Tensor, Tuple[Any, Any, Any]]
- ]:
- # large track
-
- down_512 = self._init(images_1024)
- down_256 = self._downsample_256(down_512)
- down_128 = self._downsample_128(down_256)
-
- down_64 = self._downsample_64(down_128)
- down_64 = self._sle_64(down_512, down_64)
-
- down_32 = self._downsample_32(down_64)
- down_32 = self._sle_32(down_256, down_32)
-
- down_16 = self._downsample_16(down_32)
- down_16 = self._sle_16(down_128, down_16)
-
- # small track
-
- down_small = self._small_track(images_128)
-
- # features
-
- features_large = self._features_large(down_16).view(-1)
- features_small = self._features_small(down_small).view(-1)
- features = torch.cat([features_large, features_small], dim=0)
-
- # decoder
-
- if image_type != ImageType.FAKE:
- dec_large = self._decoder_large(down_16)
- dec_small = self._decoder_small(down_small)
- dec_piece = self._decoder_piece(crop_image_part(down_32, image_type))
- return features, (dec_large, dec_small, dec_piece)
-
- return features
diff --git a/spaces/hylee/apdrawing/APDrawingGAN2/docs/tips.md b/spaces/hylee/apdrawing/APDrawingGAN2/docs/tips.md
deleted file mode 100644
index 0dfe11770ef35d5e7c6ea03695d7734220129171..0000000000000000000000000000000000000000
--- a/spaces/hylee/apdrawing/APDrawingGAN2/docs/tips.md
+++ /dev/null
@@ -1,8 +0,0 @@
-## Training/test Tips
-- Flags: see `options/train_options.py` and `options/base_options.py` for the training flags; see `options/test_options.py` and `options/base_options.py` for the test flags. The default values of these options are somtimes adjusted in the model files.
-
-- CPU/GPU (default `--gpu_ids 0`): set`--gpu_ids -1` to use CPU mode; set `--gpu_ids 0,1,2` for multi-GPU mode. You need a large batch size (e.g. `--batch_size 32`) to benefit from multiple GPUs.
-
-- Visualization: during training, the current results can be viewed using two methods. First, if you set `--display_id` > 0, the results and loss plot will appear on a local graphics web server launched by [visdom](https://github.com/facebookresearch/visdom). To do this, you should have `visdom` installed and a server running by the command `python -m visdom.server`. The default server URL is `http://localhost:8097`. `display_id` corresponds to the window ID that is displayed on the `visdom` server. The `visdom` display functionality is turned on by default. To avoid the extra overhead of communicating with `visdom` set `--display_id -1`. Second, the intermediate results are saved to `[opt.checkpoints_dir]/[opt.name]/web/` as an HTML file. To avoid this, set `--no_html`.
-
-- Fine-tuning/Resume training: to fine-tune a pre-trained model, or resume the previous training, use the `--continue_train` flag. The program will then load the model based on `which_epoch`. By default, the program will initialize the epoch count as 1. Set `--epoch_count ` to specify a different starting epoch count.
diff --git a/spaces/hyxue/HiFiFace-inference-demo/Deep3DFaceRecon_pytorch/data/__init__.py b/spaces/hyxue/HiFiFace-inference-demo/Deep3DFaceRecon_pytorch/data/__init__.py
deleted file mode 100644
index 0aaef54bd893314aa8a7b97af8625659cd8d3bfe..0000000000000000000000000000000000000000
--- a/spaces/hyxue/HiFiFace-inference-demo/Deep3DFaceRecon_pytorch/data/__init__.py
+++ /dev/null
@@ -1,118 +0,0 @@
-"""This package includes all the modules related to data loading and preprocessing
-
- To add a custom dataset class called 'dummy', you need to add a file called 'dummy_dataset.py' and define a subclass 'DummyDataset' inherited from BaseDataset.
- You need to implement four functions:
- -- <__init__>: initialize the class, first call BaseDataset.__init__(self, opt).
- -- <__len__>: return the size of dataset.
- -- <__getitem__>: get a data point from data loader.
- -- : (optionally) add dataset-specific options and set default options.
-
-Now you can use the dataset class by specifying flag '--dataset_mode dummy'.
-See our template dataset class 'template_dataset.py' for more details.
-"""
-import importlib
-
-import numpy as np
-import torch.utils.data
-from data.base_dataset import BaseDataset
-
-
-def find_dataset_using_name(dataset_name):
- """Import the module "data/[dataset_name]_dataset.py".
-
- In the file, the class called DatasetNameDataset() will
- be instantiated. It has to be a subclass of BaseDataset,
- and it is case-insensitive.
- """
- dataset_filename = "data." + dataset_name + "_dataset"
- datasetlib = importlib.import_module(dataset_filename)
-
- dataset = None
- target_dataset_name = dataset_name.replace("_", "") + "dataset"
- for name, cls in datasetlib.__dict__.items():
- if name.lower() == target_dataset_name.lower() and issubclass(cls, BaseDataset):
- dataset = cls
-
- if dataset is None:
- raise NotImplementedError(
- "In %s.py, there should be a subclass of BaseDataset with class name that matches %s in lowercase."
- % (dataset_filename, target_dataset_name)
- )
-
- return dataset
-
-
-def get_option_setter(dataset_name):
- """Return the static method of the dataset class."""
- dataset_class = find_dataset_using_name(dataset_name)
- return dataset_class.modify_commandline_options
-
-
-def create_dataset(opt, rank=0):
- """Create a dataset given the option.
-
- This function wraps the class CustomDatasetDataLoader.
- This is the main interface between this package and 'train.py'/'test.py'
-
- Example:
- >>> from data import create_dataset
- >>> dataset = create_dataset(opt)
- """
- data_loader = CustomDatasetDataLoader(opt, rank=rank)
- dataset = data_loader.load_data()
- return dataset
-
-
-class CustomDatasetDataLoader:
- """Wrapper class of Dataset class that performs multi-threaded data loading"""
-
- def __init__(self, opt, rank=0):
- """Initialize this class
-
- Step 1: create a dataset instance given the name [dataset_mode]
- Step 2: create a multi-threaded data loader.
- """
- self.opt = opt
- dataset_class = find_dataset_using_name(opt.dataset_mode)
- self.dataset = dataset_class(opt)
- self.sampler = None
- print("rank %d %s dataset [%s] was created" % (rank, self.dataset.name, type(self.dataset).__name__))
- if opt.use_ddp and opt.isTrain:
- world_size = opt.world_size
- self.sampler = torch.utils.data.distributed.DistributedSampler(
- self.dataset, num_replicas=world_size, rank=rank, shuffle=not opt.serial_batches
- )
- self.dataloader = torch.utils.data.DataLoader(
- self.dataset,
- sampler=self.sampler,
- num_workers=int(opt.num_threads / world_size),
- batch_size=int(opt.batch_size / world_size),
- drop_last=True,
- )
- else:
- self.dataloader = torch.utils.data.DataLoader(
- self.dataset,
- batch_size=opt.batch_size,
- shuffle=(not opt.serial_batches) and opt.isTrain,
- num_workers=int(opt.num_threads),
- drop_last=True,
- )
-
- def set_epoch(self, epoch):
- self.dataset.current_epoch = epoch
- if self.sampler is not None:
- self.sampler.set_epoch(epoch)
-
- def load_data(self):
- return self
-
- def __len__(self):
- """Return the number of data in the dataset"""
- return min(len(self.dataset), self.opt.max_dataset_size)
-
- def __iter__(self):
- """Return a batch of data"""
- for i, data in enumerate(self.dataloader):
- if i * self.opt.batch_size >= self.opt.max_dataset_size:
- break
- yield data
diff --git a/spaces/hyxue/HiFiFace-inference-demo/server.sh b/spaces/hyxue/HiFiFace-inference-demo/server.sh
deleted file mode 100644
index 6bb639f9d123903f36aa5d98b25a5893d1b839ba..0000000000000000000000000000000000000000
--- a/spaces/hyxue/HiFiFace-inference-demo/server.sh
+++ /dev/null
@@ -1 +0,0 @@
-python3 app.py
diff --git a/spaces/iamironman4279/SadTalker/src/test_audio2coeff.py b/spaces/iamironman4279/SadTalker/src/test_audio2coeff.py
deleted file mode 100644
index bbf19f494e2127b4ae9d6074b172fddb694d6e34..0000000000000000000000000000000000000000
--- a/spaces/iamironman4279/SadTalker/src/test_audio2coeff.py
+++ /dev/null
@@ -1,123 +0,0 @@
-import os
-import torch
-import numpy as np
-from scipy.io import savemat, loadmat
-from yacs.config import CfgNode as CN
-from scipy.signal import savgol_filter
-
-import safetensors
-import safetensors.torch
-
-from src.audio2pose_models.audio2pose import Audio2Pose
-from src.audio2exp_models.networks import SimpleWrapperV2
-from src.audio2exp_models.audio2exp import Audio2Exp
-from src.utils.safetensor_helper import load_x_from_safetensor
-
-def load_cpk(checkpoint_path, model=None, optimizer=None, device="cpu"):
- checkpoint = torch.load(checkpoint_path, map_location=torch.device(device))
- if model is not None:
- model.load_state_dict(checkpoint['model'])
- if optimizer is not None:
- optimizer.load_state_dict(checkpoint['optimizer'])
-
- return checkpoint['epoch']
-
-class Audio2Coeff():
-
- def __init__(self, sadtalker_path, device):
- #load config
- fcfg_pose = open(sadtalker_path['audio2pose_yaml_path'])
- cfg_pose = CN.load_cfg(fcfg_pose)
- cfg_pose.freeze()
- fcfg_exp = open(sadtalker_path['audio2exp_yaml_path'])
- cfg_exp = CN.load_cfg(fcfg_exp)
- cfg_exp.freeze()
-
- # load audio2pose_model
- self.audio2pose_model = Audio2Pose(cfg_pose, None, device=device)
- self.audio2pose_model = self.audio2pose_model.to(device)
- self.audio2pose_model.eval()
- for param in self.audio2pose_model.parameters():
- param.requires_grad = False
-
- try:
- if sadtalker_path['use_safetensor']:
- checkpoints = safetensors.torch.load_file(sadtalker_path['checkpoint'])
- self.audio2pose_model.load_state_dict(load_x_from_safetensor(checkpoints, 'audio2pose'))
- else:
- load_cpk(sadtalker_path['audio2pose_checkpoint'], model=self.audio2pose_model, device=device)
- except:
- raise Exception("Failed in loading audio2pose_checkpoint")
-
- # load audio2exp_model
- netG = SimpleWrapperV2()
- netG = netG.to(device)
- for param in netG.parameters():
- netG.requires_grad = False
- netG.eval()
- try:
- if sadtalker_path['use_safetensor']:
- checkpoints = safetensors.torch.load_file(sadtalker_path['checkpoint'])
- netG.load_state_dict(load_x_from_safetensor(checkpoints, 'audio2exp'))
- else:
- load_cpk(sadtalker_path['audio2exp_checkpoint'], model=netG, device=device)
- except:
- raise Exception("Failed in loading audio2exp_checkpoint")
- self.audio2exp_model = Audio2Exp(netG, cfg_exp, device=device, prepare_training_loss=False)
- self.audio2exp_model = self.audio2exp_model.to(device)
- for param in self.audio2exp_model.parameters():
- param.requires_grad = False
- self.audio2exp_model.eval()
-
- self.device = device
-
- def generate(self, batch, coeff_save_dir, pose_style, ref_pose_coeff_path=None):
-
- with torch.no_grad():
- #test
- results_dict_exp= self.audio2exp_model.test(batch)
- exp_pred = results_dict_exp['exp_coeff_pred'] #bs T 64
-
- #for class_id in range(1):
- #class_id = 0#(i+10)%45
- #class_id = random.randint(0,46) #46 styles can be selected
- batch['class'] = torch.LongTensor([pose_style]).to(self.device)
- results_dict_pose = self.audio2pose_model.test(batch)
- pose_pred = results_dict_pose['pose_pred'] #bs T 6
-
- pose_len = pose_pred.shape[1]
- if pose_len<13:
- pose_len = int((pose_len-1)/2)*2+1
- pose_pred = torch.Tensor(savgol_filter(np.array(pose_pred.cpu()), pose_len, 2, axis=1)).to(self.device)
- else:
- pose_pred = torch.Tensor(savgol_filter(np.array(pose_pred.cpu()), 13, 2, axis=1)).to(self.device)
-
- coeffs_pred = torch.cat((exp_pred, pose_pred), dim=-1) #bs T 70
-
- coeffs_pred_numpy = coeffs_pred[0].clone().detach().cpu().numpy()
-
- if ref_pose_coeff_path is not None:
- coeffs_pred_numpy = self.using_refpose(coeffs_pred_numpy, ref_pose_coeff_path)
-
- savemat(os.path.join(coeff_save_dir, '%s##%s.mat'%(batch['pic_name'], batch['audio_name'])),
- {'coeff_3dmm': coeffs_pred_numpy})
-
- return os.path.join(coeff_save_dir, '%s##%s.mat'%(batch['pic_name'], batch['audio_name']))
-
- def using_refpose(self, coeffs_pred_numpy, ref_pose_coeff_path):
- num_frames = coeffs_pred_numpy.shape[0]
- refpose_coeff_dict = loadmat(ref_pose_coeff_path)
- refpose_coeff = refpose_coeff_dict['coeff_3dmm'][:,64:70]
- refpose_num_frames = refpose_coeff.shape[0]
- if refpose_num_frames,
- React.ComponentPropsWithoutRef
->(({ className, children, ...props }, ref) => (
-
- {children}
-
-
-
-
-))
-SelectTrigger.displayName = SelectPrimitive.Trigger.displayName
-
-const SelectContent = React.forwardRef<
- React.ElementRef,
- React.ComponentPropsWithoutRef
->(({ className, children, position = 'popper', ...props }, ref) => (
-
-
-
- {children}
-
-
-
-))
-SelectContent.displayName = SelectPrimitive.Content.displayName
-
-const SelectLabel = React.forwardRef<
- React.ElementRef,
- React.ComponentPropsWithoutRef
->(({ className, ...props }, ref) => (
-
-))
-SelectLabel.displayName = SelectPrimitive.Label.displayName
-
-const SelectItem = React.forwardRef<
- React.ElementRef,
- React.ComponentPropsWithoutRef
->(({ className, children, ...props }, ref) => (
-
-
-
-
-
-
- {children}
-
-))
-SelectItem.displayName = SelectPrimitive.Item.displayName
-
-const SelectSeparator = React.forwardRef<
- React.ElementRef,
- React.ComponentPropsWithoutRef
->(({ className, ...props }, ref) => (
-
-))
-SelectSeparator.displayName = SelectPrimitive.Separator.displayName
-
-export {
- Select,
- SelectGroup,
- SelectValue,
- SelectTrigger,
- SelectContent,
- SelectLabel,
- SelectItem,
- SelectSeparator
-}
diff --git a/spaces/ikram9820/sd_dreambooth-20im/app.py b/spaces/ikram9820/sd_dreambooth-20im/app.py
deleted file mode 100644
index 830cd9a556c77755d785b549c2131626dba375cf..0000000000000000000000000000000000000000
--- a/spaces/ikram9820/sd_dreambooth-20im/app.py
+++ /dev/null
@@ -1,45 +0,0 @@
-import gradio as gr
-import torch
-from diffusers import StableDiffusionPipeline
-from PIL import Image
-
-path = 'ikram9820/ikram-20-im'
-# try:
-# pipe
-# except :
-# pipe = StableDiffusionPipeline.from_pretrained(path, torch_dtype=torch.float16 ).to("cuda")
-
-
-def inference(prompt, num_samples=1,seed=57097832,gs=7.5,step=50):
- step = int(step)
- seed = int(seed)
- all_images = []
- generator =torch.Generator("cuda").manual_seed( seed)
- try:
- images = pipe(prompt, num_images_per_prompt=num_samples,generator=generator, num_inference_steps=step, guidance_scale=gs).images
- except:
- pipe = StableDiffusionPipeline.from_pretrained(path, torch_dtype=torch.float16 ).to("cuda")
-
- all_images.extend(images)
- return all_images
-
-ex_prompt = "Portrait of an sks knight with a large moustache, male, detailed face, fantasy, highly detailed, cinematic lighting, digital art painting by greg rutkowski"
-with gr.Blocks() as demo:
- with gr.Row():
- with gr.Column():
- prompt = gr.Textbox(label="prompt",value = ex_prompt)
- seed = gr.components.Number(label= "seed",value = 57097832)
- gs = gr.components.Number(label="guidance_scale",value=7.5)
- step = gr.components.Number(label= "step",value = 50)
- samples = gr.Slider(label="Samples",value=1)
- run = gr.Button(value="Run")
- with gr.Column():
- gallery = gr.Gallery(show_label=False)
-
- run.click(inference, inputs=[prompt,samples,seed,gs,step], outputs=gallery)
-
- gr.Examples([[ex_prompt, 1,1]],
- [prompt,samples], gallery, inference, cache_examples=False)
-
-
-demo.launch()
\ No newline at end of file
diff --git a/spaces/inamXcontru/PoeticTTS/Arma 3 Project Life Server Files !EXCLUSIVE!.md b/spaces/inamXcontru/PoeticTTS/Arma 3 Project Life Server Files !EXCLUSIVE!.md
deleted file mode 100644
index b3f6a8948c7d48017ccb50327a2cd585a06b04ad..0000000000000000000000000000000000000000
--- a/spaces/inamXcontru/PoeticTTS/Arma 3 Project Life Server Files !EXCLUSIVE!.md
+++ /dev/null
@@ -1,8 +0,0 @@
-
-
Anyway, that's about it for now. I'll update this page as it happens. Feel free to post your rebuttal. I haven't given up on life entirely, but my participation might well be a very limited one. If my brother doesn't talk me into it, then I will not participate in your project. Good luck, i hope you make it.
-
Additionally, they've stated that they will implement classes and skills for players to create their own classes/skills via writing code. They also state that they will be striving for high quality mods and no half-ass content that will just bug the crap out of everybody. Anyone know what their business model is, that hasn't been spoilt yet? Obviously their cutting edge internet will need some kind of licensing/subscription system, but if they're going to distribute their source mod files over the internet I can't see them charging individual players to mod the base game?
4. As far as modding goes, they've already uploaded the files for all the base game mods and will be hosting them for public use. They'll also be hosting a database of all the mods created and will have a portal site for modding suggestions and feedback. They claim to be prepared to deal with several hundred people all at once even though they're doing this with one account.
-
Are you insane? Of course we're going to have Arma 2 content in our RP. We're not going to waste our time porting it all over again. We're going to be adding new Arma 2 content to our RP and updating the base Arma 2 content in the game. We'll also be going over Arma 2 content separately and fully porting it to Arma 3.
899543212b
-
-
\ No newline at end of file
diff --git a/spaces/indichealth/indic-health-demo/app.py b/spaces/indichealth/indic-health-demo/app.py
deleted file mode 100644
index c09f97b40576a5804e0d3421607ead67f45b0857..0000000000000000000000000000000000000000
--- a/spaces/indichealth/indic-health-demo/app.py
+++ /dev/null
@@ -1,79 +0,0 @@
-import streamlit as st
-from response import get_response_model, IntentClassifier
-import time
-import os
-
-os.environ["STREAMLIT_SERVER_HEADLESS"] = "1"
-print(os.environ["STREAMLIT_SERVER_HEADLESS"])
-
-st.set_page_config(layout="centered")
-st.title("Health Intent and NER Detection")
-#original_title = '
[The website is currently available only for english language]
'
-#st.markdown(original_title, unsafe_allow_html=True)
-
-l = ["Both Intent and Entity", "Intent only", "Entity only"]
-
-Q = st.text_input('Query', "What is fever?")
-
-col1, col2 = st.columns(2)
-with col1:
- en_expander = st.expander(label='Sample English Queries')
- with en_expander:
- st.code('Are hepatitis and jaundice related?')
- st.code('Is laser therapy good for skin?')
- st.code('Why do I need to take regular blood tests while taking Folitrax 15 Tablet?')
- st.code('When should I call my child’s doctor right away?')
-with col2:
- hi_expander = st.expander(label='Sample Hindi Queries')
- with hi_expander:
- st.code('क्या हेपेटाइटिस और पीलिया संबंधित हैं?')
- st.code('क्या मधुमेह ठीक हो सकता है?')
- st.code('क्या गुडसेफ २०० टैबलेट के कारण कब्ज हो सकता है?')
- st.code('क्या यीस्ट इन्फेक्शन से ट्रिच हो सकता है?')
-
-#st.markdown("---")
-lang = st.selectbox("Choose the Input Language", ["English", "Hindi"])
-#st.markdown("---")
-mode = st.selectbox("Mode", l)
-#st.markdown("---")
-show_time = st.checkbox("Show Execution time", value=True)
-
-req_map = {x: str(i+1) for i, x in enumerate(l)}
-
-#st.write("(Please wait for about 10 seconds to get the results)")
-temp = f'
Note: Do not forget to choose the language appropriately The demo is currently live for only English and Hindi
'
- st.markdown(temp, unsafe_allow_html=True)
-
- if show_time:
- temp = f'
Execution time = {end:.2f} seconds
'
- st.markdown(temp, unsafe_allow_html=True)
-
-else:
- st.write("")
-
-
-
-
\ No newline at end of file
diff --git a/spaces/innnky/nyaru4.0/vdecoder/hifigan/utils.py b/spaces/innnky/nyaru4.0/vdecoder/hifigan/utils.py
deleted file mode 100644
index 9c93c996d3cc73c30d71c1fc47056e4230f35c0f..0000000000000000000000000000000000000000
--- a/spaces/innnky/nyaru4.0/vdecoder/hifigan/utils.py
+++ /dev/null
@@ -1,68 +0,0 @@
-import glob
-import os
-import matplotlib
-import torch
-from torch.nn.utils import weight_norm
-# matplotlib.use("Agg")
-import matplotlib.pylab as plt
-
-
-def plot_spectrogram(spectrogram):
- fig, ax = plt.subplots(figsize=(10, 2))
- im = ax.imshow(spectrogram, aspect="auto", origin="lower",
- interpolation='none')
- plt.colorbar(im, ax=ax)
-
- fig.canvas.draw()
- plt.close()
-
- return fig
-
-
-def init_weights(m, mean=0.0, std=0.01):
- classname = m.__class__.__name__
- if classname.find("Conv") != -1:
- m.weight.data.normal_(mean, std)
-
-
-def apply_weight_norm(m):
- classname = m.__class__.__name__
- if classname.find("Conv") != -1:
- weight_norm(m)
-
-
-def get_padding(kernel_size, dilation=1):
- return int((kernel_size*dilation - dilation)/2)
-
-
-def load_checkpoint(filepath, device):
- assert os.path.isfile(filepath)
- print("Loading '{}'".format(filepath))
- checkpoint_dict = torch.load(filepath, map_location=device)
- print("Complete.")
- return checkpoint_dict
-
-
-def save_checkpoint(filepath, obj):
- print("Saving checkpoint to {}".format(filepath))
- torch.save(obj, filepath)
- print("Complete.")
-
-
-def del_old_checkpoints(cp_dir, prefix, n_models=2):
- pattern = os.path.join(cp_dir, prefix + '????????')
- cp_list = glob.glob(pattern) # get checkpoint paths
- cp_list = sorted(cp_list)# sort by iter
- if len(cp_list) > n_models: # if more than n_models models are found
- for cp in cp_list[:-n_models]:# delete the oldest models other than lastest n_models
- open(cp, 'w').close()# empty file contents
- os.unlink(cp)# delete file (move to trash when using Colab)
-
-
-def scan_checkpoint(cp_dir, prefix):
- pattern = os.path.join(cp_dir, prefix + '????????')
- cp_list = glob.glob(pattern)
- if len(cp_list) == 0:
- return None
- return sorted(cp_list)[-1]
-
diff --git a/spaces/inplisQlawa/anything-midjourney-v4-1/Adobe Photoshop Lightroom CC 6.6.1 Final Crack (Rootdorid) Serial Key Keygen [BEST].md b/spaces/inplisQlawa/anything-midjourney-v4-1/Adobe Photoshop Lightroom CC 6.6.1 Final Crack (Rootdorid) Serial Key Keygen [BEST].md
deleted file mode 100644
index 45f34a6921f129da366a1e449e81ab71e5c5746b..0000000000000000000000000000000000000000
--- a/spaces/inplisQlawa/anything-midjourney-v4-1/Adobe Photoshop Lightroom CC 6.6.1 Final Crack (Rootdorid) Serial Key Keygen [BEST].md
+++ /dev/null
@@ -1,97 +0,0 @@
-
-
Adobe Photoshop Lightroom CC 6.6.1 Final Crack (Rootdorid) Serial Key keygen: A Complete Guide
-
If you are looking for a powerful and professional photo editing software, you might have heard of Adobe Photoshop Lightroom CC 6.6.1 Final Crack (Rootdorid) Serial Key keygen. This is a cracked version of the original Adobe Photoshop Lightroom CC 6.6.1, which is one of the best tools for managing, editing, and sharing your photos.
-
Adobe Photoshop Lightroom CC 6.6.1 Final Crack (Rootdorid) Serial Key keygen
In this article, we will explain what Adobe Photoshop Lightroom CC 6.6.1 Final Crack (Rootdorid) Serial Key keygen is, how to download and install it, and what features it offers. We will also give you some tips and tricks on how to use it effectively and safely.
-
What is Adobe Photoshop Lightroom CC 6.6.1 Final Crack (Rootdorid) Serial Key keygen?
-
Adobe Photoshop Lightroom CC 6.6.1 Final Crack (Rootdorid) Serial Key keygen is a modified version of the original Adobe Photoshop Lightroom CC 6.6.1, which is a software that allows you to organize, edit, and share your photos in a simple and intuitive way.
-
The original Adobe Photoshop Lightroom CC 6.6.1 is a paid software that requires a subscription to use it. However, some people have managed to crack it and make it available for free download on the internet. This cracked version is called Adobe Photoshop Lightroom CC 6.6.1 Final Crack (Rootdorid) Serial Key keygen.
-
The main difference between the original and the cracked version is that the cracked version does not require any activation or registration to use it. You just need to download it, install it, and enter a serial key that is provided with the crack.
-
The crack also bypasses the online verification process that the original software uses to check for updates and validity of the license. This means that you can use the cracked version without any internet connection or risk of being detected by Adobe.
-
How to download and install Adobe Photoshop Lightroom CC 6.6.1 Final Crack (Rootdorid) Serial Key keygen?
-
There are many websites that claim to offer Adobe Photoshop Lightroom CC 6.6.1 Final Crack (Rootdorid) Serial Key keygen for free download, but not all of them are reliable or safe. Some of them may contain viruses, malware, or spyware that can harm your computer or steal your personal information.
Wait for the download to finish and then extract the zip file using WinRAR or any other software that can handle zip files.
-
Open the extracted folder and run the setup file named "Setup.run this.(ask4pc).exe". Follow the instructions on the screen to install Adobe Photoshop Lightroom CC 6.6.1 on your computer.
-
After the installation is complete, do not run the software yet. Instead, open the folder named "Patch.Lightroom.(ask4pc)" and run the patch file named "Patch.Lightroom.(ask4pc).exe". This will crack the software and make it ready to use.
-
Now you can run Adobe Photoshop Lightroom CC 6.6.1 from your desktop or start menu shortcut. When prompted to enter a serial number, use any of the serial keys that are provided in the text file named "Ask Me.(ask4pc).txt".
-
Congratulations! You have successfully installed Adobe Photoshop Lightroom CC 6.6.1 Final Crack (Rootdorid) Serial Key keygen on your computer.
-
-
What features does Adobe Photoshop Lightroom CC 6.6.1 Final Crack (Rootdorid) Serial Key keygen offer?
-
Adobe Photoshop Lightroom CC 6.6.1 Final Crack (Rootdorid) Serial Key keygen offers all the features that the original Adobe Photoshop Lightroom CC 6.6.1 offers, plus some extra features that are added by the crack.
-
Some of the main features that you can enjoy with Adobe Photoshop Lightroom CC 6.6.1 Final Crack (Rootdorid) Serial Key keygen are:
-
-
You can import, organize, edit, and share your photos in one place.
-
You can work with high-quality previews of offline images from multiple libraries and drives.
-
You can use custom keywords to organize your photos according to your preferences.
-
You can easily compare before and after versions of your photos using side-by-side or split-screen views.
-
You can apply various adjustments to your photos such as exposure, contrast, color, tone curve, noise reduction, sharpening, lens correction, and more.
-
You can use advanced tools such as spot removal, graduated filter, radial filter, adjustment brush, and more to fine-tune specific areas of your photos.
-
You can create stunning photo books using various templates and layouts that are included in the software.
-
You can showcase your photos in web galleries or slideshows with music and effects.
-
You can export your photos in various formats and sizes for printing or sharing online.
-
You can sync your photos across multiple devices using Adobe Creative Cloud.
-
You can access a rich community of export plug-ins and web gallery styles at the Lightroom Exchange website.
-
You can enjoy unlimited usage without any subscription or activation fees.
-
-
How to use Adobe Photoshop Lightroom CC 6.6.1 Final Crack (Rootdorid) Serial Key keygen effectively and safely?
-
Adobe Photoshop Lightroom CC 6.6.1 Final Crack (Rootdorid) Serial Key keygen is a powerful and professional photo editing software that can help you achieve amazing results with your photos.
-
However, you need to be aware of some risks and limitations that come with using a cracked software such as this one.
-
-
-
-
The first risk is that you may be violating the intellectual property rights of Adobe by using their software without paying for it or obtaining their permission.
-
-
The second risk is that you may be exposing your computer to potential threats such as viruses, malware, or spyware that may be hidden in the crack or in the websites that offer it for download.
-
-
The third risk is that you may not be able to access some features or updates that are available only for the original software users who have a valid license or subscription.
-
-
The fourth risk is that you may not be able to get any technical support or customer service from Adobe if you encounter any problems or issues with their software.
-
-
-
-
To avoid these risks and use Adobe Photoshop Lightroom CC 6.6.1 Final Crack (Rootdorid) Serial Key keygen effectively and safely, we suggest you follow these tips:
Scan your computer regularly with an antivirus or anti-malware software to detect and
-
What are the benefits of using Adobe Photoshop Lightroom CC 6.6.1 Final Crack (Rootdorid) Serial Key keygen?
-
Using Adobe Photoshop Lightroom CC 6.6.1 Final Crack (Rootdorid) Serial Key keygen can bring you many benefits, especially if you are a professional or hobbyist photographer who wants to enhance your photos and showcase them in the best possible way.
-
Some of the benefits of using Adobe Photoshop Lightroom CC 6.6.1 Final Crack (Rootdorid) Serial Key keygen are:
-
-
You can save money by not having to pay for a subscription or a license to use the original software.
-
You can enjoy all the features and functions that the original software offers, plus some extra features that are added by the crack.
-
You can work offline without any internet connection or online verification required by the original software.
-
You can edit your photos faster and easier with the intuitive and user-friendly interface of the software.
-
You can improve the quality and appearance of your photos with the powerful and advanced tools and adjustments that the software provides.
-
You can create stunning photo books, web galleries, or slideshows with your photos using the various templates and layouts that are included in the software.
-
You can sync your photos across multiple devices using Adobe Creative Cloud and access them anytime and anywhere.
-
You can learn new skills and techniques from the rich community of export plug-ins and web gallery styles at the Lightroom Exchange website.
-
-
What are the drawbacks of using Adobe Photoshop Lightroom CC 6.6.1 Final Crack (Rootdorid) Serial Key keygen?
-
While using Adobe Photoshop Lightroom CC 6.6.1 Final Crack (Rootdorid) Serial Key keygen can bring you many benefits, it also has some drawbacks that you need to be aware of before deciding to use it.
-
Some of the drawbacks of using Adobe Photoshop Lightroom CC 6.6.1 Final Crack (Rootdorid) Serial Key keygen are:
-
-
You may be violating the intellectual property rights of Adobe by using their software without paying for it or obtaining their permission.
-
You may be exposing your computer to potential threats such as viruses, malware, or spyware that may be hidden in the crack or in the websites that offer it for download.
-
You may not be able to access some features or updates that are available only for the original software users who have a valid license or subscription.
-
You may not be able to get any technical support or customer service from Adobe if you encounter any problems or issues with their software.
-
-
Therefore, you need to weigh the pros and cons of using Adobe Photoshop Lightroom CC 6.6.1 Final Crack (Rootdorid) Serial Key keygen carefully before making your choice.
-
-
Conclusion
-
Adobe Photoshop Lightroom CC 6.6.1 Final Crack (Rootdorid) Serial Key keygen is a cracked version of the original Adobe Photoshop Lightroom CC 6.6.1, which is a powerful and professional photo editing software that allows you to organize, edit, and share your photos in a simple and intuitive way.
-
In this article, we have explained what Adobe Photoshop Lightroom CC 6.6.1 Final Crack (Rootdorid) Serial Key keygen is, how to download and install it, what features it offers, and how to use it effectively and safely.
-
We hope that this article has been helpful and informative for you. If you have any questions or comments, please feel free to leave them below.
-
Conclusion
-
Adobe Photoshop Lightroom CC 6.6.1 Final Crack (Rootdorid) Serial Key keygen is a cracked version of the original Adobe Photoshop Lightroom CC 6.6.1, which is a powerful and professional photo editing software that allows you to organize, edit, and share your photos in a simple and intuitive way.
-
In this article, we have explained what Adobe Photoshop Lightroom CC 6.6.1 Final Crack (Rootdorid) Serial Key keygen is, how to download and install it, what features it offers, and how to use it effectively and safely.
-
We hope that this article has been helpful and informative for you. If you have any questions or comments, please feel free to leave them below.
3cee63e6c2
-
-
\ No newline at end of file
diff --git a/spaces/inplisQlawa/anything-midjourney-v4-1/Eamcet Physics Formulas 99.pdf !EXCLUSIVE!.md b/spaces/inplisQlawa/anything-midjourney-v4-1/Eamcet Physics Formulas 99.pdf !EXCLUSIVE!.md
deleted file mode 100644
index 71d3fce21ee103efee5096fbff4f87dfe2850118..0000000000000000000000000000000000000000
--- a/spaces/inplisQlawa/anything-midjourney-v4-1/Eamcet Physics Formulas 99.pdf !EXCLUSIVE!.md
+++ /dev/null
@@ -1,6 +0,0 @@
-
-
-MejorTorrent - La web de descargas torrent por excelencia, aqui encontraras las mejores peliculas y series con la mejor calidad. 4d29de3e1b
-
-
-
diff --git a/spaces/ishanchennupati/ishanavatarchatbot/README.md b/spaces/ishanchennupati/ishanavatarchatbot/README.md
deleted file mode 100644
index 7546fed2d61e310de36d5d05206730a866fdc2b6..0000000000000000000000000000000000000000
--- a/spaces/ishanchennupati/ishanavatarchatbot/README.md
+++ /dev/null
@@ -1,12 +0,0 @@
----
-title: Ishanavatarchatbot
-emoji: 📊
-colorFrom: gray
-colorTo: blue
-sdk: gradio
-sdk_version: 3.39.0
-app_file: app.py
-pinned: false
----
-
-Check out the configuration reference at https://huggingface.co/docs/hub/spaces-config-reference
diff --git a/spaces/jackli888/stable-diffusion-webui/extensions/deforum/javascript/deforum-hints.js b/spaces/jackli888/stable-diffusion-webui/extensions/deforum/javascript/deforum-hints.js
deleted file mode 100644
index bc50ffc016ee93cd88050b7e4d0fbd50f3c96718..0000000000000000000000000000000000000000
--- a/spaces/jackli888/stable-diffusion-webui/extensions/deforum/javascript/deforum-hints.js
+++ /dev/null
@@ -1,191 +0,0 @@
-// mouseover tooltips for various UI elements
-
-deforum_titles = {
- //Run
- "Override settings": "specify a custom settings file and ignore settings displayed in the interface",
- "Custom settings file": "the path to a custom settings file",
- "Width": "The width of the output images, in pixels (must be a multiple of 64)",
- "Height": "The height of the output images, in pixels (must be a multiple of 64)",
- "Restore faces": "Restore low quality faces using GFPGAN neural network",
- "Tiling": "Produce an image that can be tiled.",
- "Highres. fix": "Use a two step process to partially create an image at smaller resolution, upscale, and then improve details in it without changing composition",
- "Seed": "A value that determines the output of random number generator - if you create an image with same parameters and seed as another image, you'll get the same result",
- "Sampler": "Which algorithm to use to produce the image",
- "Enable extras": "enable additional seed settings",
- "Subseed": "Seed of a different picture to be mixed into the generation.",
- "Subseed strength": "How strong of a variation to produce. At 0, there will be no effect. At 1, you will get the complete picture with variation seed (except for ancestral samplers, where you will just get something).",
- "Resize seed from width": "Normally, changing the resolution will completely change an image, even when using the same seed. If you generated an image with a particular seed and then changed the resolution, put the original resolution here to get an image that more closely resemles the original",
- "Resize seed from height": "Normally, changing the resolution will completely change an image, even when using the same seed. If you generated an image with a particular seed and then changed the resolution, put the original resolution here to get an image that more closely resemles the original",
- "Steps": "How many times to improve the generated image iteratively; higher values take longer; very low values can produce bad results",
- //"ddim_eta": "";
- //"n_batch": "",
- //"make_grid": "",
- //"grid_rows": "",
- //"save_settings": "",
- //"save_samples": "",
- "Batch name": "output images will be placed in a folder with this name, inside of the img2img output folder",
- "Pix2Pix img CFG schedule": "*Only in use with pix2pix checkpoints!*",
- "Filename format": "specify the format of the filename for output images",
- "Seed behavior": "defines the seed behavior that is used for animations",
- "iter": "the seed value will increment by 1 for each subsequent frame of the animation",
- "fixed": "the seed will remain fixed across all frames of animation",
- "random": "a random seed will be used on each frame of the animation",
- "schedule": "specify your own seed schedule (found on the Keyframes page)",
-
- //Keyframes
- "Animation mode": "selects the type of animation",
- "2D": "only 2D motion parameters will be used, but this mode uses the least amount of VRAM. You can optionally enable flip_2d_perspective to enable some psuedo-3d animation parameters while in 2D mode.",
- "3D": "enables all 3D motion parameters.",
- "Video Input": "will ignore all motion parameters and attempt to reference a video loaded into the runtime, specified by the video_init_path. Max_frames is ignored during video_input mode, and instead, follows the number of frames pulled from the video’s length. Resume_from_timestring is NOT available with Video_Input mode.",
- "Max frames": "the maximum number of output images to be created",
- "Border": "controls handling method of pixels to be generated when the image is smaller than the frame.",
- "wrap": "pulls pixels from the opposite edge of the image",
- "replicate": "repeats the edge of the pixels, and extends them. Animations with quick motion may yield lines where this border function was attempting to populate pixels into the empty space created.",
- "Angle": "2D operator to rotate canvas clockwise/anticlockwise in degrees per frame",
- "Zoom": "2D operator that scales the canvas size, multiplicatively. [static = 1.0]",
- "Translation X": "2D & 3D operator to move canvas left/right in pixels per frame",
- "Translation Y": "2D & 3D operator to move canvas up/down in pixels per frame",
- "Translation Z": "3D operator to move canvas towards/away from view [speed set by FOV]",
- "Rotation 3D X": "3D operator to tilt canvas up/down in degrees per frame",
- "Rotation 3D Y": "3D operator to pan canvas left/right in degrees per frame",
- "Rotation 3D Z": "3D operator to roll canvas clockwise/anticlockwise",
- "Enable perspective flip": "enables 2D mode functions to simulate faux 3D movement",
- "Perspective flip theta": "the roll effect angle",
- "Perspective flip phi": "the tilt effect angle",
- "Perspective flip gamma": "the pan effect angle",
- "Perspective flip fv": "the 2D vanishing point of perspective (recommended range 30-160)",
- "Noise schedule": "amount of graininess to add per frame for diffusion diversity",
- "Strength schedule": "amount of presence of previous frame to influence next frame, also controls steps in the following formula [steps - (strength_schedule * steps)]",
- "Sampler schedule": "controls which sampler to use at a specific scheduled frame",
- "Contrast schedule": "adjusts the overall contrast per frame [default neutral at 1.0]",
- "CFG scale schedule": "how closely the image should conform to the prompt. Lower values produce more creative results. (recommended range 5-15)",
- "FOV schedule": "adjusts the scale at which the canvas is moved in 3D by the translation_z value. [maximum range -180 to +180, with 0 being undefined. Values closer to 180 will make the image have less depth, while values closer to 0 will allow more depth]",
- //"near_schedule": "",
- //"far_schedule": "",
- "Seed schedule": "allows you to specify seeds at a specific schedule, if seed_behavior is set to schedule.",
- "Color coherence": "The color coherence will attempt to sample the overall pixel color information, and trend those values analyzed in the first frame to be applied to future frames.",
- // "None": "Disable color coherence",
- "Match Frame 0 HSV": "HSV is a good method for balancing presence of vibrant colors, but may produce unrealistic results - (ie.blue apples)",
- "Match Frame 0 LAB": "LAB is a more linear approach to mimic human perception of color space - a good default setting for most users.",
- "Match Frame 0 RGB": "RGB is good for enforcing unbiased amounts of color in each red, green and blue channel - some images may yield colorized artifacts if sampling is too low.",
- "Cadence": "A setting of 1 will cause every frame to receive diffusion in the sequence of image outputs. A setting of 2 will only diffuse on every other frame, yet motion will still be in effect. The output of images during the cadence sequence will be automatically blended, additively and saved to the specified drive. This may improve the illusion of coherence in some workflows as the content and context of an image will not change or diffuse during frames that were skipped. Higher values of 4-8 cadence will skip over a larger amount of frames and only diffuse the “Nth” frame as set by the diffusion_cadence value. This may produce more continuity in an animation, at the cost of little opportunity to add more diffused content. In extreme examples, motion within a frame will fail to produce diverse prompt context, and the space will be filled with lines or approximations of content - resulting in unexpected animation patterns and artifacts. Video Input & Interpolation modes are not affected by diffusion_cadence.",
- "Noise type": "Selects the type of noise being added to each frame",
- "uniform": "Uniform noise covers the entire frame. It somewhat flattens and sharpens the video over time, but may be good for cartoonish look. This is the old default setting.",
- "perlin": "Perlin noise is a more natural looking noise. It is heterogeneous and less sharp than uniform noise, this way it is more likely that new details will appear in a more coherent way. This is the new default setting.",
- "Perlin W": "The width of the Perlin sample. Lower values will make larger noise regions. Think of it as inverse brush stroke width. The greater this setting, the smaller details it will affect.",
- "Perlin H": "The height of the Perlin sample. Lower values will make larger noise regions. Think of it as inverse brush stroke width. The greater this setting, the smaller details it will affect.",
- "Perlin octaves": "The number of Perlin noise octaves, that is the count of P-noise iterations. Higher values will make the noise more soft and smoke-like, whereas lower values will make it look more organic and spotty. It is limited by 8 octaves as the resulting gain will run out of bounds.",
- "Perlin persistence": "How much of noise from each octave is added on each iteration. Higher values will make it more straighter and sharper, while lower values will make it rounder and smoother. It is limited by 1.0 as the resulting gain fill the frame completely with noise.",
- "Use depth warping": "enables instructions to warp an image dynamically in 3D mode only.",
- "MiDaS weight": "sets a midpoint at which a depthmap is to be drawn: range [-1 to +1]",
- "Padding mode": "instructs the handling of pixels outside the field of view as they come into the scene.",
- //"border": "Border will attempt to use the edges of the canvas as the pixels to be drawn", //duplicate name as another property
- "reflection": "reflection will attempt to approximate the image and tile/repeat pixels",
- "zeros": "zeros will not add any new pixel information",
- "sampling_mode": "choose from Bicubic, Bilinear or Nearest modes. (Recommended: Bicubic)",
- "Save depth maps": "will output a greyscale depth map image alongside the output images.",
-
- // Prompts
- "Prompts": "prompts for your animation in a JSON format. Use --neg words to add 'words' as negative prompt",
- "Prompts positive": "positive prompt to be appended to *all* prompts",
- "Prompts negative": "negative prompt to be appended to *all* prompts. DON'T use --neg here!",
-
- //Init
- "Use init": "Diffuse the first frame based on an image, similar to img2img.",
- "Strength": "Controls the strength of the diffusion on the init image. 0 = disabled",
- "Strength 0 no init": "Set the strength to 0 automatically when no init image is used",
- "Init image": "the path to your init image",
- "Use mask": "Use a grayscale image as a mask on your init image. Whiter areas of the mask are areas that change more.",
- "Use alpha as mask": "use the alpha channel of the init image as the mask",
- "Mask file": "the path to your mask image",
- "Invert mask": "Inverts the colors of the mask",
- "Mask brightness adjust": "adjust the brightness of the mask. Should be a positive number, with 1.0 meaning no adjustment.",
- "Mask contrast adjust": "adjust the brightness of the mask. Should be a positive number, with 1.0 meaning no adjustment.",
- "overlay mask": "Overlay the masked image at the end of the generation so it does not get degraded by encoding and decoding",
- "Mask overlay blur": "Blur edges of final overlay mask, if used. Minimum = 0 (no blur)",
- "Video init path": "the directory \/ URL at which your video file is located for Video Input mode only",
- "Extract nth frame": "during the run sequence, only frames specified by this value will be extracted, saved, and diffused upon. A value of 1 indicates that every frame is to be accounted for. Values of 2 will use every other frame for the sequence. Higher values will skip that number of frames respectively.",
- "Extract from frame":"start extracting the input video only from this frame number",
- "Extract to frame": "stop the extraction of the video at this frame number. -1 for no limits",
- "Overwrite extracted frames": "when enabled, will re-extract video frames each run. When using video_input mode, the run will be instructed to write video frames to the drive. If you’ve already populated the frames needed, uncheck this box to skip past redundant extraction, and immediately start the render. If you have not extracted frames, you must run at least once with this box checked to write the necessary frames.",
- "Use mask video": "video_input mode only, enables the extraction and use of a separate video file intended for use as a mask. White areas of the extracted video frames will not be affected by diffusion, while black areas will be fully effected. Lighter/darker areas are affected dynamically.",
- "Video mask path": "the directory in which your mask video is located.",
- "Interpolate key frames": "selects whether to ignore prompt schedule or _x_frames.",
- "Interpolate x frames": "the number of frames to transition thru between prompts (when interpolate_key_frames = true, then the numbers in front of the animation prompts will dynamically guide the images based on their value. If set to false, will ignore the prompt numbers and force interpole_x_frames value regardless of prompt number)",
- "Resume from timestring": "instructs the run to start from a specified point",
- "Resume timestring": "the required timestamp to reference when resuming. Currently only available in 2D & 3D mode, the timestamp is saved as the settings .txt file name as well as images produced during your previous run. The format follows: yyyymmddhhmmss - a timestamp of when the run was started to diffuse.",
-
- //Video Output
- "Skip video for run all": "when checked, do not output a video",
- "Make GIF": "create a gif in addition to .mp4 file. supports up to 30 fps, will self-disable at higher fps values",
- "Upscale":"upscale the images of the next run once it's finished + make a video out of them",
- "Upscale model":"model of the upscaler to use. 'realesr-animevideov3' is much faster but yields smoother, less detailed results. the other models only do x4",
- "Upscale factor":"how many times to upscale, actual options depend on the chosen upscale model",
- "FPS": "The frames per second that the video will run at",
- "Output format": "select the type of video file to output",
- "PIL gif": "create an animated GIF",
- "FFMPEG mp4": "create an MP4 video file",
- "FFmpeg location": "the path to where ffmpeg is located. Leave at default 'ffmpeg' if ffmpeg is in your PATH!",
- "FFmpeg crf": "controls quality where lower is better, less compressed. values: 0 to 51, default 17",
- "FFmpeg preset": "controls how good the compression is, and the operation speed. If you're not in a rush keep it at 'veryslow'",
- "Add soundtrack": "when this box is checked, and FFMPEG mp4 is selected as the output format, an audio file will be multiplexed with the video.",
- "Soundtrack path": "the path\/ URL to an audio file to accompany the video",
- "Use manual settings": "when this is unchecked, the video will automatically be created in the same output folder as the images. Check this box to specify different settings for the creation of the video, specified by the following options",
- "Render steps": "render each step of diffusion as a separate frame",
- "Max video frames": "the maximum number of frames to include in the video, when use_manual_settings is checked",
- //"path_name_modifier": "",
- "Image path": "the location of images to create the video from, when use_manual_settings is checked",
- "MP4 path": "the output location of the mp4 file, when use_manual_settings is checked",
- "Engine": "choose the frame interpolation engine and version",
- "Interp X":"how many times to interpolate the source video. e.g source video fps of 12 and a value of x2 will yield a 24fps interpolated video",
- "Slow-Mo X":"how many times to slow-down the video. *Naturally affects output fps as well",
- "Keep Imgs": "delete or keep raw affected (interpolated/ upscaled depending on the UI section) png imgs",
- "Interpolate an existing video":"This feature allows you to interpolate any video with a dedicated button. Video could be completly unrelated to deforum",
- "In Frame Count": "uploaded video total frame count",
- "In FPS":"uploaded video FPS",
- "Interpolated Vid FPS":"calculated output-interpolated video FPS",
- "In Res":"uploaded video resolution",
- "Out Res":"output video resolution",
-
- // Looper Args
- // "use_looper": "",
- "Enable guided images mode": "check this box to enable guided images mode",
- "Images to use for keyframe guidance": "images you iterate over, you can do local or web paths (no single backslashes!)",
- "Image strength schedule": "how much the image should look like the previou one and new image frame init. strength schedule might be better if this is higher, around .75 during the keyfames you want to switch on",
- "Blend factor max": "blendFactor = blendFactorMax - blendFactorSlope * cos((frame % tweening_frames_schedule) / (tweening_frames_schedule / 2))",
- "Blend factor slope": "blendFactor = blendFactorMax - blendFactorSlope * cos((frame % tweening_frames_schedule) / (tweening_frames_schedule / 2))",
- "Tweening frames schedule": "number of the frames that we will blend between current imagined image and input frame image",
- "Color correction factor": "how close to get to the colors of the input frame image/ the amount each frame during a tweening step to use the new images colors"
-}
-
-
-onUiUpdate(function(){
- gradioApp().querySelectorAll('span, button, select, p').forEach(function(span){
- tooltip = deforum_titles[span.textContent];
-
- if(!tooltip){
- tooltip = deforum_titles[span.value];
- }
-
- if(!tooltip){
- for (const c of span.classList) {
- if (c in deforum_titles) {
- tooltip = deforum_titles[c];
- break;
- }
- }
- }
-
- if(tooltip){
- span.title = tooltip;
- }
- })
-
- gradioApp().querySelectorAll('select').forEach(function(select){
- if (select.onchange != null) return;
-
- select.onchange = function(){
- select.title = deforum_titles[select.value] || "";
- }
- })
-})
\ No newline at end of file
diff --git a/spaces/jbilcke-hf/Panoremix/src/components/icons/full-screen.tsx b/spaces/jbilcke-hf/Panoremix/src/components/icons/full-screen.tsx
deleted file mode 100644
index 34ec93bbab4b8359868737dbab9c6f7f6d594e03..0000000000000000000000000000000000000000
--- a/spaces/jbilcke-hf/Panoremix/src/components/icons/full-screen.tsx
+++ /dev/null
@@ -1,16 +0,0 @@
-export function FullScreenIcon() {
- return (
-
- )
-}
\ No newline at end of file
diff --git a/spaces/jbilcke-hf/ai-clip-factory/src/lib/triggerDownload.ts b/spaces/jbilcke-hf/ai-clip-factory/src/lib/triggerDownload.ts
deleted file mode 100644
index e5627a26a4bba34bdf28279d265c6a71440d8136..0000000000000000000000000000000000000000
--- a/spaces/jbilcke-hf/ai-clip-factory/src/lib/triggerDownload.ts
+++ /dev/null
@@ -1,12 +0,0 @@
-export function triggerDownload(filename: string, text: string) {
- var element = document.createElement('a');
- element.setAttribute('href', 'data:text/plain;charset=utf-8,' + encodeURIComponent(text));
- element.setAttribute('download', filename);
-
- element.style.display = 'none';
- document.body.appendChild(element);
-
- element.click();
-
- document.body.removeChild(element);
-}
\ No newline at end of file
diff --git a/spaces/joaopereirajp/livvieChatBot/venv/lib/python3.9/site-packages/fsspec/spec.py b/spaces/joaopereirajp/livvieChatBot/venv/lib/python3.9/site-packages/fsspec/spec.py
deleted file mode 100644
index a205cb2b66cf5caa77aa84b81b77b0e66db12f33..0000000000000000000000000000000000000000
--- a/spaces/joaopereirajp/livvieChatBot/venv/lib/python3.9/site-packages/fsspec/spec.py
+++ /dev/null
@@ -1,1977 +0,0 @@
-from __future__ import annotations
-
-import io
-import logging
-import os
-import threading
-import warnings
-import weakref
-from errno import ESPIPE
-from glob import has_magic
-from hashlib import sha256
-from typing import ClassVar
-
-from .callbacks import _DEFAULT_CALLBACK
-from .config import apply_config, conf
-from .dircache import DirCache
-from .transaction import Transaction
-from .utils import (
- _unstrip_protocol,
- isfilelike,
- other_paths,
- read_block,
- stringify_path,
- tokenize,
-)
-
-logger = logging.getLogger("fsspec")
-
-
-def make_instance(cls, args, kwargs):
- return cls(*args, **kwargs)
-
-
-class _Cached(type):
- """
- Metaclass for caching file system instances.
-
- Notes
- -----
- Instances are cached according to
-
- * The values of the class attributes listed in `_extra_tokenize_attributes`
- * The arguments passed to ``__init__``.
-
- This creates an additional reference to the filesystem, which prevents the
- filesystem from being garbage collected when all *user* references go away.
- A call to the :meth:`AbstractFileSystem.clear_instance_cache` must *also*
- be made for a filesystem instance to be garbage collected.
- """
-
- def __init__(cls, *args, **kwargs):
- super().__init__(*args, **kwargs)
- # Note: we intentionally create a reference here, to avoid garbage
- # collecting instances when all other references are gone. To really
- # delete a FileSystem, the cache must be cleared.
- if conf.get("weakref_instance_cache"): # pragma: no cover
- # debug option for analysing fork/spawn conditions
- cls._cache = weakref.WeakValueDictionary()
- else:
- cls._cache = {}
- cls._pid = os.getpid()
-
- def __call__(cls, *args, **kwargs):
- kwargs = apply_config(cls, kwargs)
- extra_tokens = tuple(
- getattr(cls, attr, None) for attr in cls._extra_tokenize_attributes
- )
- token = tokenize(
- cls, cls._pid, threading.get_ident(), *args, *extra_tokens, **kwargs
- )
- skip = kwargs.pop("skip_instance_cache", False)
- if os.getpid() != cls._pid:
- cls._cache.clear()
- cls._pid = os.getpid()
- if not skip and cls.cachable and token in cls._cache:
- cls._latest = token
- return cls._cache[token]
- else:
- obj = super().__call__(*args, **kwargs)
- # Setting _fs_token here causes some static linters to complain.
- obj._fs_token_ = token
- obj.storage_args = args
- obj.storage_options = kwargs
- if obj.async_impl and obj.mirror_sync_methods:
- from .asyn import mirror_sync_methods
-
- mirror_sync_methods(obj)
-
- if cls.cachable and not skip:
- cls._latest = token
- cls._cache[token] = obj
- return obj
-
-
-class AbstractFileSystem(metaclass=_Cached):
- """
- An abstract super-class for pythonic file-systems
-
- Implementations are expected to be compatible with or, better, subclass
- from here.
- """
-
- cachable = True # this class can be cached, instances reused
- _cached = False
- blocksize = 2**22
- sep = "/"
- protocol: ClassVar[str | tuple[str, ...]] = "abstract"
- _latest = None
- async_impl = False
- mirror_sync_methods = False
- root_marker = "" # For some FSs, may require leading '/' or other character
-
- #: Extra *class attributes* that should be considered when hashing.
- _extra_tokenize_attributes = ()
-
- def __init__(self, *args, **storage_options):
- """Create and configure file-system instance
-
- Instances may be cachable, so if similar enough arguments are seen
- a new instance is not required. The token attribute exists to allow
- implementations to cache instances if they wish.
-
- A reasonable default should be provided if there are no arguments.
-
- Subclasses should call this method.
-
- Parameters
- ----------
- use_listings_cache, listings_expiry_time, max_paths:
- passed to ``DirCache``, if the implementation supports
- directory listing caching. Pass use_listings_cache=False
- to disable such caching.
- skip_instance_cache: bool
- If this is a cachable implementation, pass True here to force
- creating a new instance even if a matching instance exists, and prevent
- storing this instance.
- asynchronous: bool
- loop: asyncio-compatible IOLoop or None
- """
- if self._cached:
- # reusing instance, don't change
- return
- self._cached = True
- self._intrans = False
- self._transaction = None
- self._invalidated_caches_in_transaction = []
- self.dircache = DirCache(**storage_options)
-
- if storage_options.pop("add_docs", None):
- warnings.warn("add_docs is no longer supported.", FutureWarning)
-
- if storage_options.pop("add_aliases", None):
- warnings.warn("add_aliases has been removed.", FutureWarning)
- # This is set in _Cached
- self._fs_token_ = None
-
- @property
- def fsid(self):
- """Persistent filesystem id that can be used to compare filesystems
- across sessions.
- """
- raise NotImplementedError
-
- @property
- def _fs_token(self):
- return self._fs_token_
-
- def __dask_tokenize__(self):
- return self._fs_token
-
- def __hash__(self):
- return int(self._fs_token, 16)
-
- def __eq__(self, other):
- return isinstance(other, type(self)) and self._fs_token == other._fs_token
-
- def __reduce__(self):
- return make_instance, (type(self), self.storage_args, self.storage_options)
-
- @classmethod
- def _strip_protocol(cls, path):
- """Turn path from fully-qualified to file-system-specific
-
- May require FS-specific handling, e.g., for relative paths or links.
- """
- if isinstance(path, list):
- return [cls._strip_protocol(p) for p in path]
- path = stringify_path(path)
- protos = (cls.protocol,) if isinstance(cls.protocol, str) else cls.protocol
- for protocol in protos:
- if path.startswith(protocol + "://"):
- path = path[len(protocol) + 3 :]
- elif path.startswith(protocol + "::"):
- path = path[len(protocol) + 2 :]
- path = path.rstrip("/")
- # use of root_marker to make minimum required path, e.g., "/"
- return path or cls.root_marker
-
- def unstrip_protocol(self, name):
- """Format FS-specific path to generic, including protocol"""
- protos = (self.protocol,) if isinstance(self.protocol, str) else self.protocol
- for protocol in protos:
- if name.startswith(f"{protocol}://"):
- return name
- return f"{protos[0]}://{name}"
-
- @staticmethod
- def _get_kwargs_from_urls(path):
- """If kwargs can be encoded in the paths, extract them here
-
- This should happen before instantiation of the class; incoming paths
- then should be amended to strip the options in methods.
-
- Examples may look like an sftp path "sftp://user@host:/my/path", where
- the user and host should become kwargs and later get stripped.
- """
- # by default, nothing happens
- return {}
-
- @classmethod
- def current(cls):
- """Return the most recently instantiated FileSystem
-
- If no instance has been created, then create one with defaults
- """
- if cls._latest in cls._cache:
- return cls._cache[cls._latest]
- return cls()
-
- @property
- def transaction(self):
- """A context within which files are committed together upon exit
-
- Requires the file class to implement `.commit()` and `.discard()`
- for the normal and exception cases.
- """
- if self._transaction is None:
- self._transaction = Transaction(self)
- return self._transaction
-
- def start_transaction(self):
- """Begin write transaction for deferring files, non-context version"""
- self._intrans = True
- self._transaction = Transaction(self)
- return self.transaction
-
- def end_transaction(self):
- """Finish write transaction, non-context version"""
- self.transaction.complete()
- self._transaction = None
- # The invalid cache must be cleared after the transcation is completed.
- for path in self._invalidated_caches_in_transaction:
- self.invalidate_cache(path)
- self._invalidated_caches_in_transaction.clear()
-
- def invalidate_cache(self, path=None):
- """
- Discard any cached directory information
-
- Parameters
- ----------
- path: string or None
- If None, clear all listings cached else listings at or under given
- path.
- """
- # Not necessary to implement invalidation mechanism, may have no cache.
- # But if have, you should call this method of parent class from your
- # subclass to ensure expiring caches after transacations correctly.
- # See the implementation of FTPFileSystem in ftp.py
- if self._intrans:
- self._invalidated_caches_in_transaction.append(path)
-
- def mkdir(self, path, create_parents=True, **kwargs):
- """
- Create directory entry at path
-
- For systems that don't have true directories, may create an for
- this instance only and not touch the real filesystem
-
- Parameters
- ----------
- path: str
- location
- create_parents: bool
- if True, this is equivalent to ``makedirs``
- kwargs:
- may be permissions, etc.
- """
- pass # not necessary to implement, may not have directories
-
- def makedirs(self, path, exist_ok=False):
- """Recursively make directories
-
- Creates directory at path and any intervening required directories.
- Raises exception if, for instance, the path already exists but is a
- file.
-
- Parameters
- ----------
- path: str
- leaf directory name
- exist_ok: bool (False)
- If False, will error if the target already exists
- """
- pass # not necessary to implement, may not have directories
-
- def rmdir(self, path):
- """Remove a directory, if empty"""
- pass # not necessary to implement, may not have directories
-
- def ls(self, path, detail=True, **kwargs):
- """List objects at path.
-
- This should include subdirectories and files at that location. The
- difference between a file and a directory must be clear when details
- are requested.
-
- The specific keys, or perhaps a FileInfo class, or similar, is TBD,
- but must be consistent across implementations.
- Must include:
-
- - full path to the entry (without protocol)
- - size of the entry, in bytes. If the value cannot be determined, will
- be ``None``.
- - type of entry, "file", "directory" or other
-
- Additional information
- may be present, appropriate to the file-system, e.g., generation,
- checksum, etc.
-
- May use refresh=True|False to allow use of self._ls_from_cache to
- check for a saved listing and avoid calling the backend. This would be
- common where listing may be expensive.
-
- Parameters
- ----------
- path: str
- detail: bool
- if True, gives a list of dictionaries, where each is the same as
- the result of ``info(path)``. If False, gives a list of paths
- (str).
- kwargs: may have additional backend-specific options, such as version
- information
-
- Returns
- -------
- List of strings if detail is False, or list of directory information
- dicts if detail is True.
- """
- raise NotImplementedError
-
- def _ls_from_cache(self, path):
- """Check cache for listing
-
- Returns listing, if found (may be empty list for a directly that exists
- but contains nothing), None if not in cache.
- """
- parent = self._parent(path)
- if path.rstrip("/") in self.dircache:
- return self.dircache[path.rstrip("/")]
- try:
- files = [
- f
- for f in self.dircache[parent]
- if f["name"] == path
- or (f["name"] == path.rstrip("/") and f["type"] == "directory")
- ]
- if len(files) == 0:
- # parent dir was listed but did not contain this file
- raise FileNotFoundError(path)
- return files
- except KeyError:
- pass
-
- def walk(self, path, maxdepth=None, topdown=True, on_error="omit", **kwargs):
- """Return all files belows path
-
- List all files, recursing into subdirectories; output is iterator-style,
- like ``os.walk()``. For a simple list of files, ``find()`` is available.
-
- When topdown is True, the caller can modify the dirnames list in-place (perhaps
- using del or slice assignment), and walk() will
- only recurse into the subdirectories whose names remain in dirnames;
- this can be used to prune the search, impose a specific order of visiting,
- or even to inform walk() about directories the caller creates or renames before
- it resumes walk() again.
- Modifying dirnames when topdown is False has no effect. (see os.walk)
-
- Note that the "files" outputted will include anything that is not
- a directory, such as links.
-
- Parameters
- ----------
- path: str
- Root to recurse into
- maxdepth: int
- Maximum recursion depth. None means limitless, but not recommended
- on link-based file-systems.
- topdown: bool (True)
- Whether to walk the directory tree from the top downwards or from
- the bottom upwards.
- on_error: "omit", "raise", a collable
- if omit (default), path with exception will simply be empty;
- If raise, an underlying exception will be raised;
- if callable, it will be called with a single OSError instance as argument
- kwargs: passed to ``ls``
- """
- if maxdepth is not None and maxdepth < 1:
- raise ValueError("maxdepth must be at least 1")
-
- path = self._strip_protocol(path)
- full_dirs = {}
- dirs = {}
- files = {}
-
- detail = kwargs.pop("detail", False)
- try:
- listing = self.ls(path, detail=True, **kwargs)
- except (FileNotFoundError, OSError) as e:
- if on_error == "raise":
- raise
- elif callable(on_error):
- on_error(e)
- if detail:
- return path, {}, {}
- return path, [], []
-
- for info in listing:
- # each info name must be at least [path]/part , but here
- # we check also for names like [path]/part/
- pathname = info["name"].rstrip("/")
- name = pathname.rsplit("/", 1)[-1]
- if info["type"] == "directory" and pathname != path:
- # do not include "self" path
- full_dirs[name] = pathname
- dirs[name] = info
- elif pathname == path:
- # file-like with same name as give path
- files[""] = info
- else:
- files[name] = info
-
- if not detail:
- dirs = list(dirs)
- files = list(files)
-
- if topdown:
- # Yield before recursion if walking top down
- yield path, dirs, files
-
- if maxdepth is not None:
- maxdepth -= 1
- if maxdepth < 1:
- if not topdown:
- yield path, dirs, files
- return
-
- for d in dirs:
- yield from self.walk(
- full_dirs[d],
- maxdepth=maxdepth,
- detail=detail,
- topdown=topdown,
- **kwargs,
- )
-
- if not topdown:
- # Yield after recursion if walking bottom up
- yield path, dirs, files
-
- def find(self, path, maxdepth=None, withdirs=False, detail=False, **kwargs):
- """List all files below path.
-
- Like posix ``find`` command without conditions
-
- Parameters
- ----------
- path : str
- maxdepth: int or None
- If not None, the maximum number of levels to descend
- withdirs: bool
- Whether to include directory paths in the output. This is True
- when used by glob, but users usually only want files.
- kwargs are passed to ``ls``.
- """
- # TODO: allow equivalent of -name parameter
- path = self._strip_protocol(path)
- out = {}
-
- # Add the root directory if withdirs is requested
- # This is needed for posix glob compliance
- if withdirs and path != "" and self.isdir(path):
- out[path] = self.info(path)
-
- for _, dirs, files in self.walk(path, maxdepth, detail=True, **kwargs):
- if withdirs:
- files.update(dirs)
- out.update({info["name"]: info for name, info in files.items()})
- if not out and self.isfile(path):
- # walk works on directories, but find should also return [path]
- # when path happens to be a file
- out[path] = {}
- names = sorted(out)
- if not detail:
- return names
- else:
- return {name: out[name] for name in names}
-
- def du(self, path, total=True, maxdepth=None, withdirs=False, **kwargs):
- """Space used by files and optionally directories within a path
-
- Directory size does not include the size of its contents.
-
- Parameters
- ----------
- path: str
- total: bool
- Whether to sum all the file sizes
- maxdepth: int or None
- Maximum number of directory levels to descend, None for unlimited.
- withdirs: bool
- Whether to include directory paths in the output.
- kwargs: passed to ``find``
-
- Returns
- -------
- Dict of {path: size} if total=False, or int otherwise, where numbers
- refer to bytes used.
- """
- sizes = {}
- if withdirs and self.isdir(path):
- # Include top-level directory in output
- info = self.info(path)
- sizes[info["name"]] = info["size"]
- for f in self.find(path, maxdepth=maxdepth, withdirs=withdirs, **kwargs):
- info = self.info(f)
- sizes[info["name"]] = info["size"]
- if total:
- return sum(sizes.values())
- else:
- return sizes
-
- def glob(self, path, maxdepth=None, **kwargs):
- """
- Find files by glob-matching.
-
- If the path ends with '/', only folders are returned.
-
- We support ``"**"``,
- ``"?"`` and ``"[..]"``. We do not support ^ for pattern negation.
-
- The `maxdepth` option is applied on the first `**` found in the path.
-
- Search path names that contain embedded characters special to this
- implementation of glob may not produce expected results;
- e.g., 'foo/bar/*starredfilename*'.
-
- kwargs are passed to ``ls``.
- """
- if maxdepth is not None and maxdepth < 1:
- raise ValueError("maxdepth must be at least 1")
-
- import re
-
- ends = path.endswith("/")
- path = self._strip_protocol(path)
- idx_star = path.find("*") if path.find("*") >= 0 else len(path)
- idx_qmark = path.find("?") if path.find("?") >= 0 else len(path)
- idx_brace = path.find("[") if path.find("[") >= 0 else len(path)
-
- min_idx = min(idx_star, idx_qmark, idx_brace)
-
- detail = kwargs.pop("detail", False)
-
- if not has_magic(path):
- if self.exists(path):
- if not detail:
- return [path]
- else:
- return {path: self.info(path)}
- else:
- if not detail:
- return [] # glob of non-existent returns empty
- else:
- return {}
- elif "/" in path[:min_idx]:
- min_idx = path[:min_idx].rindex("/")
- root = path[: min_idx + 1]
- depth = path[min_idx + 1 :].count("/") + 1
- else:
- root = ""
- depth = path[min_idx + 1 :].count("/") + 1
-
- if "**" in path:
- if maxdepth is not None:
- idx_double_stars = path.find("**")
- depth_double_stars = path[idx_double_stars:].count("/") + 1
- depth = depth - depth_double_stars + maxdepth
- else:
- depth = None
-
- allpaths = self.find(root, maxdepth=depth, withdirs=True, detail=True, **kwargs)
- # Escape characters special to python regex, leaving our supported
- # special characters in place.
- # See https://www.gnu.org/software/bash/manual/html_node/Pattern-Matching.html
- # for shell globbing details.
- pattern = (
- "^"
- + (
- path.replace("\\", r"\\")
- .replace(".", r"\.")
- .replace("+", r"\+")
- .replace("//", "/")
- .replace("(", r"\(")
- .replace(")", r"\)")
- .replace("|", r"\|")
- .replace("^", r"\^")
- .replace("$", r"\$")
- .replace("{", r"\{")
- .replace("}", r"\}")
- .rstrip("/")
- .replace("?", ".")
- )
- + "$"
- )
- pattern = re.sub("/[*]{2}", "=SLASH_DOUBLE_STARS=", pattern)
- pattern = re.sub("[*]{2}/?", "=DOUBLE_STARS=", pattern)
- pattern = re.sub("[*]", "[^/]*", pattern)
- pattern = re.sub("=SLASH_DOUBLE_STARS=", "(|/.*)", pattern)
- pattern = re.sub("=DOUBLE_STARS=", ".*", pattern)
- pattern = re.compile(pattern)
-
- out = {
- p: allpaths[p]
- for p in sorted(allpaths)
- if pattern.match(p.replace("//", "/").rstrip("/"))
- }
-
- # Return directories only when the glob end by a slash
- # This is needed for posix glob compliance
- if ends:
- out = {k: v for k, v in out.items() if v["type"] == "directory"}
-
- if detail:
- return out
- else:
- return list(out)
-
- def exists(self, path, **kwargs):
- """Is there a file at the given path"""
- try:
- self.info(path, **kwargs)
- return True
- except: # noqa: E722
- # any exception allowed bar FileNotFoundError?
- return False
-
- def lexists(self, path, **kwargs):
- """If there is a file at the given path (including
- broken links)"""
- return self.exists(path)
-
- def info(self, path, **kwargs):
- """Give details of entry at path
-
- Returns a single dictionary, with exactly the same information as ``ls``
- would with ``detail=True``.
-
- The default implementation should calls ls and could be overridden by a
- shortcut. kwargs are passed on to ```ls()``.
-
- Some file systems might not be able to measure the file's size, in
- which case, the returned dict will include ``'size': None``.
-
- Returns
- -------
- dict with keys: name (full path in the FS), size (in bytes), type (file,
- directory, or something else) and other FS-specific keys.
- """
- path = self._strip_protocol(path)
- out = self.ls(self._parent(path), detail=True, **kwargs)
- out = [o for o in out if o["name"].rstrip("/") == path]
- if out:
- return out[0]
- out = self.ls(path, detail=True, **kwargs)
- path = path.rstrip("/")
- out1 = [o for o in out if o["name"].rstrip("/") == path]
- if len(out1) == 1:
- if "size" not in out1[0]:
- out1[0]["size"] = None
- return out1[0]
- elif len(out1) > 1 or out:
- return {"name": path, "size": 0, "type": "directory"}
- else:
- raise FileNotFoundError(path)
-
- def checksum(self, path):
- """Unique value for current version of file
-
- If the checksum is the same from one moment to another, the contents
- are guaranteed to be the same. If the checksum changes, the contents
- *might* have changed.
-
- This should normally be overridden; default will probably capture
- creation/modification timestamp (which would be good) or maybe
- access timestamp (which would be bad)
- """
- return int(tokenize(self.info(path)), 16)
-
- def size(self, path):
- """Size in bytes of file"""
- return self.info(path).get("size", None)
-
- def sizes(self, paths):
- """Size in bytes of each file in a list of paths"""
- return [self.size(p) for p in paths]
-
- def isdir(self, path):
- """Is this entry directory-like?"""
- try:
- return self.info(path)["type"] == "directory"
- except OSError:
- return False
-
- def isfile(self, path):
- """Is this entry file-like?"""
- try:
- return self.info(path)["type"] == "file"
- except: # noqa: E722
- return False
-
- def read_text(self, path, encoding=None, errors=None, newline=None, **kwargs):
- """Get the contents of the file as a string.
-
- Parameters
- ----------
- path: str
- URL of file on this filesystems
- encoding, errors, newline: same as `open`.
- """
- with self.open(
- path,
- mode="r",
- encoding=encoding,
- errors=errors,
- newline=newline,
- **kwargs,
- ) as f:
- return f.read()
-
- def write_text(
- self, path, value, encoding=None, errors=None, newline=None, **kwargs
- ):
- """Write the text to the given file.
-
- An existing file will be overwritten.
-
- Parameters
- ----------
- path: str
- URL of file on this filesystems
- value: str
- Text to write.
- encoding, errors, newline: same as `open`.
- """
- with self.open(
- path,
- mode="w",
- encoding=encoding,
- errors=errors,
- newline=newline,
- **kwargs,
- ) as f:
- return f.write(value)
-
- def cat_file(self, path, start=None, end=None, **kwargs):
- """Get the content of a file
-
- Parameters
- ----------
- path: URL of file on this filesystems
- start, end: int
- Bytes limits of the read. If negative, backwards from end,
- like usual python slices. Either can be None for start or
- end of file, respectively
- kwargs: passed to ``open()``.
- """
- # explicitly set buffering off?
- with self.open(path, "rb", **kwargs) as f:
- if start is not None:
- if start >= 0:
- f.seek(start)
- else:
- f.seek(max(0, f.size + start))
- if end is not None:
- if end < 0:
- end = f.size + end
- return f.read(end - f.tell())
- return f.read()
-
- def pipe_file(self, path, value, **kwargs):
- """Set the bytes of given file"""
- with self.open(path, "wb", **kwargs) as f:
- f.write(value)
-
- def pipe(self, path, value=None, **kwargs):
- """Put value into path
-
- (counterpart to ``cat``)
-
- Parameters
- ----------
- path: string or dict(str, bytes)
- If a string, a single remote location to put ``value`` bytes; if a dict,
- a mapping of {path: bytesvalue}.
- value: bytes, optional
- If using a single path, these are the bytes to put there. Ignored if
- ``path`` is a dict
- """
- if isinstance(path, str):
- self.pipe_file(self._strip_protocol(path), value, **kwargs)
- elif isinstance(path, dict):
- for k, v in path.items():
- self.pipe_file(self._strip_protocol(k), v, **kwargs)
- else:
- raise ValueError("path must be str or dict")
-
- def cat_ranges(
- self, paths, starts, ends, max_gap=None, on_error="return", **kwargs
- ):
- if max_gap is not None:
- raise NotImplementedError
- if not isinstance(paths, list):
- raise TypeError
- if not isinstance(starts, list):
- starts = [starts] * len(paths)
- if not isinstance(ends, list):
- ends = [starts] * len(paths)
- if len(starts) != len(paths) or len(ends) != len(paths):
- raise ValueError
- out = []
- for p, s, e in zip(paths, starts, ends):
- try:
- out.append(self.cat_file(p, s, e))
- except Exception as e:
- if on_error == "return":
- out.append(e)
- else:
- raise
- return out
-
- def cat(self, path, recursive=False, on_error="raise", **kwargs):
- """Fetch (potentially multiple) paths' contents
-
- Parameters
- ----------
- recursive: bool
- If True, assume the path(s) are directories, and get all the
- contained files
- on_error : "raise", "omit", "return"
- If raise, an underlying exception will be raised (converted to KeyError
- if the type is in self.missing_exceptions); if omit, keys with exception
- will simply not be included in the output; if "return", all keys are
- included in the output, but the value will be bytes or an exception
- instance.
- kwargs: passed to cat_file
-
- Returns
- -------
- dict of {path: contents} if there are multiple paths
- or the path has been otherwise expanded
- """
- paths = self.expand_path(path, recursive=recursive)
- if (
- len(paths) > 1
- or isinstance(path, list)
- or paths[0] != self._strip_protocol(path)
- ):
- out = {}
- for path in paths:
- try:
- out[path] = self.cat_file(path, **kwargs)
- except Exception as e:
- if on_error == "raise":
- raise
- if on_error == "return":
- out[path] = e
- return out
- else:
- return self.cat_file(paths[0], **kwargs)
-
- def get_file(
- self, rpath, lpath, callback=_DEFAULT_CALLBACK, outfile=None, **kwargs
- ):
- """Copy single remote file to local"""
- from .implementations.local import LocalFileSystem
-
- if isfilelike(lpath):
- outfile = lpath
- elif self.isdir(rpath):
- os.makedirs(lpath, exist_ok=True)
- return None
-
- LocalFileSystem(auto_mkdir=True).makedirs(self._parent(lpath), exist_ok=True)
-
- with self.open(rpath, "rb", **kwargs) as f1:
- if outfile is None:
- outfile = open(lpath, "wb")
-
- try:
- callback.set_size(getattr(f1, "size", None))
- data = True
- while data:
- data = f1.read(self.blocksize)
- segment_len = outfile.write(data)
- if segment_len is None:
- segment_len = len(data)
- callback.relative_update(segment_len)
- finally:
- if not isfilelike(lpath):
- outfile.close()
-
- def get(
- self,
- rpath,
- lpath,
- recursive=False,
- callback=_DEFAULT_CALLBACK,
- maxdepth=None,
- **kwargs,
- ):
- """Copy file(s) to local.
-
- Copies a specific file or tree of files (if recursive=True). If lpath
- ends with a "/", it will be assumed to be a directory, and target files
- will go within. Can submit a list of paths, which may be glob-patterns
- and will be expanded.
-
- Calls get_file for each source.
- """
- if isinstance(lpath, list) and isinstance(rpath, list):
- # No need to expand paths when both source and destination
- # are provided as lists
- rpaths = rpath
- lpaths = lpath
- else:
- from .implementations.local import (
- LocalFileSystem,
- make_path_posix,
- trailing_sep,
- )
-
- source_is_str = isinstance(rpath, str)
- rpaths = self.expand_path(rpath, recursive=recursive, maxdepth=maxdepth)
- if source_is_str and (not recursive or maxdepth is not None):
- # Non-recursive glob does not copy directories
- rpaths = [p for p in rpaths if not (trailing_sep(p) or self.isdir(p))]
- if not rpaths:
- return
-
- if isinstance(lpath, str):
- lpath = make_path_posix(lpath)
-
- source_is_file = len(rpaths) == 1
- dest_is_dir = isinstance(lpath, str) and (
- trailing_sep(lpath) or LocalFileSystem().isdir(lpath)
- )
-
- exists = source_is_str and (
- (has_magic(rpath) and source_is_file)
- or (not has_magic(rpath) and dest_is_dir and not trailing_sep(rpath))
- )
- lpaths = other_paths(
- rpaths,
- lpath,
- exists=exists,
- flatten=not source_is_str,
- )
-
- callback.set_size(len(lpaths))
- for lpath, rpath in callback.wrap(zip(lpaths, rpaths)):
- callback.branch(rpath, lpath, kwargs)
- self.get_file(rpath, lpath, **kwargs)
-
- def put_file(self, lpath, rpath, callback=_DEFAULT_CALLBACK, **kwargs):
- """Copy single file to remote"""
- if os.path.isdir(lpath):
- self.makedirs(rpath, exist_ok=True)
- return None
-
- with open(lpath, "rb") as f1:
- size = f1.seek(0, 2)
- callback.set_size(size)
- f1.seek(0)
-
- self.mkdirs(self._parent(os.fspath(rpath)), exist_ok=True)
- with self.open(rpath, "wb", **kwargs) as f2:
- while f1.tell() < size:
- data = f1.read(self.blocksize)
- segment_len = f2.write(data)
- if segment_len is None:
- segment_len = len(data)
- callback.relative_update(segment_len)
-
- def put(
- self,
- lpath,
- rpath,
- recursive=False,
- callback=_DEFAULT_CALLBACK,
- maxdepth=None,
- **kwargs,
- ):
- """Copy file(s) from local.
-
- Copies a specific file or tree of files (if recursive=True). If rpath
- ends with a "/", it will be assumed to be a directory, and target files
- will go within.
-
- Calls put_file for each source.
- """
- if isinstance(lpath, list) and isinstance(rpath, list):
- # No need to expand paths when both source and destination
- # are provided as lists
- rpaths = rpath
- lpaths = lpath
- else:
- from .implementations.local import (
- LocalFileSystem,
- make_path_posix,
- trailing_sep,
- )
-
- source_is_str = isinstance(lpath, str)
- if source_is_str:
- lpath = make_path_posix(lpath)
- fs = LocalFileSystem()
- lpaths = fs.expand_path(lpath, recursive=recursive, maxdepth=maxdepth)
- if source_is_str and (not recursive or maxdepth is not None):
- # Non-recursive glob does not copy directories
- lpaths = [p for p in lpaths if not (trailing_sep(p) or fs.isdir(p))]
- if not lpaths:
- return
-
- source_is_file = len(lpaths) == 1
- dest_is_dir = isinstance(rpath, str) and (
- trailing_sep(rpath) or self.isdir(rpath)
- )
-
- rpath = (
- self._strip_protocol(rpath)
- if isinstance(rpath, str)
- else [self._strip_protocol(p) for p in rpath]
- )
- exists = source_is_str and (
- (has_magic(lpath) and source_is_file)
- or (not has_magic(lpath) and dest_is_dir and not trailing_sep(lpath))
- )
- rpaths = other_paths(
- lpaths,
- rpath,
- exists=exists,
- flatten=not source_is_str,
- )
-
- callback.set_size(len(rpaths))
- for lpath, rpath in callback.wrap(zip(lpaths, rpaths)):
- callback.branch(lpath, rpath, kwargs)
- self.put_file(lpath, rpath, **kwargs)
-
- def head(self, path, size=1024):
- """Get the first ``size`` bytes from file"""
- with self.open(path, "rb") as f:
- return f.read(size)
-
- def tail(self, path, size=1024):
- """Get the last ``size`` bytes from file"""
- with self.open(path, "rb") as f:
- f.seek(max(-size, -f.size), 2)
- return f.read()
-
- def cp_file(self, path1, path2, **kwargs):
- raise NotImplementedError
-
- def copy(
- self, path1, path2, recursive=False, maxdepth=None, on_error=None, **kwargs
- ):
- """Copy within two locations in the filesystem
-
- on_error : "raise", "ignore"
- If raise, any not-found exceptions will be raised; if ignore any
- not-found exceptions will cause the path to be skipped; defaults to
- raise unless recursive is true, where the default is ignore
- """
- if on_error is None and recursive:
- on_error = "ignore"
- elif on_error is None:
- on_error = "raise"
-
- if isinstance(path1, list) and isinstance(path2, list):
- # No need to expand paths when both source and destination
- # are provided as lists
- paths1 = path1
- paths2 = path2
- else:
- from .implementations.local import trailing_sep
-
- source_is_str = isinstance(path1, str)
- paths1 = self.expand_path(path1, recursive=recursive, maxdepth=maxdepth)
- if source_is_str and (not recursive or maxdepth is not None):
- # Non-recursive glob does not copy directories
- paths1 = [p for p in paths1 if not (trailing_sep(p) or self.isdir(p))]
- if not paths1:
- return
-
- source_is_file = len(paths1) == 1
- dest_is_dir = isinstance(path2, str) and (
- trailing_sep(path2) or self.isdir(path2)
- )
-
- exists = source_is_str and (
- (has_magic(path1) and source_is_file)
- or (not has_magic(path1) and dest_is_dir and not trailing_sep(path1))
- )
- paths2 = other_paths(
- paths1,
- path2,
- exists=exists,
- flatten=not source_is_str,
- )
-
- for p1, p2 in zip(paths1, paths2):
- try:
- self.cp_file(p1, p2, **kwargs)
- except FileNotFoundError:
- if on_error == "raise":
- raise
-
- def expand_path(self, path, recursive=False, maxdepth=None, **kwargs):
- """Turn one or more globs or directories into a list of all matching paths
- to files or directories.
-
- kwargs are passed to ``glob`` or ``find``, which may in turn call ``ls``
- """
-
- if maxdepth is not None and maxdepth < 1:
- raise ValueError("maxdepth must be at least 1")
-
- if isinstance(path, str):
- out = self.expand_path([path], recursive, maxdepth)
- else:
- out = set()
- path = [self._strip_protocol(p) for p in path]
- for p in path:
- if has_magic(p):
- bit = set(self.glob(p, maxdepth=maxdepth, **kwargs))
- out |= bit
- if recursive:
- # glob call above expanded one depth so if maxdepth is defined
- # then decrement it in expand_path call below. If it is zero
- # after decrementing then avoid expand_path call.
- if maxdepth is not None and maxdepth <= 1:
- continue
- out |= set(
- self.expand_path(
- list(bit),
- recursive=recursive,
- maxdepth=maxdepth - 1 if maxdepth is not None else None,
- **kwargs,
- )
- )
- continue
- elif recursive:
- rec = set(
- self.find(
- p, maxdepth=maxdepth, withdirs=True, detail=False, **kwargs
- )
- )
- out |= rec
- if p not in out and (recursive is False or self.exists(p)):
- # should only check once, for the root
- out.add(p)
- if not out:
- raise FileNotFoundError(path)
- return sorted(out)
-
- def mv(self, path1, path2, recursive=False, maxdepth=None, **kwargs):
- """Move file(s) from one location to another"""
- if path1 == path2:
- logger.debug(
- "%s mv: The paths are the same, so no files were moved." % (self)
- )
- else:
- self.copy(path1, path2, recursive=recursive, maxdepth=maxdepth)
- self.rm(path1, recursive=recursive)
-
- def rm_file(self, path):
- """Delete a file"""
- self._rm(path)
-
- def _rm(self, path):
- """Delete one file"""
- # this is the old name for the method, prefer rm_file
- raise NotImplementedError
-
- def rm(self, path, recursive=False, maxdepth=None):
- """Delete files.
-
- Parameters
- ----------
- path: str or list of str
- File(s) to delete.
- recursive: bool
- If file(s) are directories, recursively delete contents and then
- also remove the directory
- maxdepth: int or None
- Depth to pass to walk for finding files to delete, if recursive.
- If None, there will be no limit and infinite recursion may be
- possible.
- """
- path = self.expand_path(path, recursive=recursive, maxdepth=maxdepth)
- for p in reversed(path):
- self.rm_file(p)
-
- @classmethod
- def _parent(cls, path):
- path = cls._strip_protocol(path)
- if "/" in path:
- parent = path.rsplit("/", 1)[0].lstrip(cls.root_marker)
- return cls.root_marker + parent
- else:
- return cls.root_marker
-
- def _open(
- self,
- path,
- mode="rb",
- block_size=None,
- autocommit=True,
- cache_options=None,
- **kwargs,
- ):
- """Return raw bytes-mode file-like from the file-system"""
- return AbstractBufferedFile(
- self,
- path,
- mode,
- block_size,
- autocommit,
- cache_options=cache_options,
- **kwargs,
- )
-
- def open(
- self,
- path,
- mode="rb",
- block_size=None,
- cache_options=None,
- compression=None,
- **kwargs,
- ):
- """
- Return a file-like object from the filesystem
-
- The resultant instance must function correctly in a context ``with``
- block.
-
- Parameters
- ----------
- path: str
- Target file
- mode: str like 'rb', 'w'
- See builtin ``open()``
- block_size: int
- Some indication of buffering - this is a value in bytes
- cache_options : dict, optional
- Extra arguments to pass through to the cache.
- compression: string or None
- If given, open file using compression codec. Can either be a compression
- name (a key in ``fsspec.compression.compr``) or "infer" to guess the
- compression from the filename suffix.
- encoding, errors, newline: passed on to TextIOWrapper for text mode
- """
- import io
-
- path = self._strip_protocol(path)
- if "b" not in mode:
- mode = mode.replace("t", "") + "b"
-
- text_kwargs = {
- k: kwargs.pop(k)
- for k in ["encoding", "errors", "newline"]
- if k in kwargs
- }
- return io.TextIOWrapper(
- self.open(
- path,
- mode,
- block_size=block_size,
- cache_options=cache_options,
- compression=compression,
- **kwargs,
- ),
- **text_kwargs,
- )
- else:
- ac = kwargs.pop("autocommit", not self._intrans)
- f = self._open(
- path,
- mode=mode,
- block_size=block_size,
- autocommit=ac,
- cache_options=cache_options,
- **kwargs,
- )
- if compression is not None:
- from fsspec.compression import compr
- from fsspec.core import get_compression
-
- compression = get_compression(path, compression)
- compress = compr[compression]
- f = compress(f, mode=mode[0])
-
- if not ac and "r" not in mode:
- self.transaction.files.append(f)
- return f
-
- def touch(self, path, truncate=True, **kwargs):
- """Create empty file, or update timestamp
-
- Parameters
- ----------
- path: str
- file location
- truncate: bool
- If True, always set file size to 0; if False, update timestamp and
- leave file unchanged, if backend allows this
- """
- if truncate or not self.exists(path):
- with self.open(path, "wb", **kwargs):
- pass
- else:
- raise NotImplementedError # update timestamp, if possible
-
- def ukey(self, path):
- """Hash of file properties, to tell if it has changed"""
- return sha256(str(self.info(path)).encode()).hexdigest()
-
- def read_block(self, fn, offset, length, delimiter=None):
- """Read a block of bytes from
-
- Starting at ``offset`` of the file, read ``length`` bytes. If
- ``delimiter`` is set then we ensure that the read starts and stops at
- delimiter boundaries that follow the locations ``offset`` and ``offset
- + length``. If ``offset`` is zero then we start at zero. The
- bytestring returned WILL include the end delimiter string.
-
- If offset+length is beyond the eof, reads to eof.
-
- Parameters
- ----------
- fn: string
- Path to filename
- offset: int
- Byte offset to start read
- length: int
- Number of bytes to read. If None, read to end.
- delimiter: bytes (optional)
- Ensure reading starts and stops at delimiter bytestring
-
- Examples
- --------
- >>> fs.read_block('data/file.csv', 0, 13) # doctest: +SKIP
- b'Alice, 100\\nBo'
- >>> fs.read_block('data/file.csv', 0, 13, delimiter=b'\\n') # doctest: +SKIP
- b'Alice, 100\\nBob, 200\\n'
-
- Use ``length=None`` to read to the end of the file.
- >>> fs.read_block('data/file.csv', 0, None, delimiter=b'\\n') # doctest: +SKIP
- b'Alice, 100\\nBob, 200\\nCharlie, 300'
-
- See Also
- --------
- :func:`fsspec.utils.read_block`
- """
- with self.open(fn, "rb") as f:
- size = f.size
- if length is None:
- length = size
- if size is not None and offset + length > size:
- length = size - offset
- return read_block(f, offset, length, delimiter)
-
- def to_json(self):
- """
- JSON representation of this filesystem instance
-
- Returns
- -------
- str: JSON structure with keys cls (the python location of this class),
- protocol (text name of this class's protocol, first one in case of
- multiple), args (positional args, usually empty), and all other
- kwargs as their own keys.
- """
- import json
-
- cls = type(self)
- cls = ".".join((cls.__module__, cls.__name__))
- proto = (
- self.protocol[0]
- if isinstance(self.protocol, (tuple, list))
- else self.protocol
- )
- return json.dumps(
- dict(
- **{"cls": cls, "protocol": proto, "args": self.storage_args},
- **self.storage_options,
- )
- )
-
- @staticmethod
- def from_json(blob):
- """
- Recreate a filesystem instance from JSON representation
-
- See ``.to_json()`` for the expected structure of the input
-
- Parameters
- ----------
- blob: str
-
- Returns
- -------
- file system instance, not necessarily of this particular class.
- """
- import json
-
- from .registry import _import_class, get_filesystem_class
-
- dic = json.loads(blob)
- protocol = dic.pop("protocol")
- try:
- cls = _import_class(dic.pop("cls"))
- except (ImportError, ValueError, RuntimeError, KeyError):
- cls = get_filesystem_class(protocol)
- return cls(*dic.pop("args", ()), **dic)
-
- def _get_pyarrow_filesystem(self):
- """
- Make a version of the FS instance which will be acceptable to pyarrow
- """
- # all instances already also derive from pyarrow
- return self
-
- def get_mapper(self, root="", check=False, create=False, missing_exceptions=None):
- """Create key/value store based on this file-system
-
- Makes a MutableMapping interface to the FS at the given root path.
- See ``fsspec.mapping.FSMap`` for further details.
- """
- from .mapping import FSMap
-
- return FSMap(
- root,
- self,
- check=check,
- create=create,
- missing_exceptions=missing_exceptions,
- )
-
- @classmethod
- def clear_instance_cache(cls):
- """
- Clear the cache of filesystem instances.
-
- Notes
- -----
- Unless overridden by setting the ``cachable`` class attribute to False,
- the filesystem class stores a reference to newly created instances. This
- prevents Python's normal rules around garbage collection from working,
- since the instances refcount will not drop to zero until
- ``clear_instance_cache`` is called.
- """
- cls._cache.clear()
-
- def created(self, path):
- """Return the created timestamp of a file as a datetime.datetime"""
- raise NotImplementedError
-
- def modified(self, path):
- """Return the modified timestamp of a file as a datetime.datetime"""
- raise NotImplementedError
-
- # ------------------------------------------------------------------------
- # Aliases
-
- def read_bytes(self, path, start=None, end=None, **kwargs):
- """Alias of `AbstractFileSystem.cat_file`."""
- return self.cat_file(path, start=start, end=end, **kwargs)
-
- def write_bytes(self, path, value, **kwargs):
- """Alias of `AbstractFileSystem.pipe_file`."""
- self.pipe_file(path, value, **kwargs)
-
- def makedir(self, path, create_parents=True, **kwargs):
- """Alias of `AbstractFileSystem.mkdir`."""
- return self.mkdir(path, create_parents=create_parents, **kwargs)
-
- def mkdirs(self, path, exist_ok=False):
- """Alias of `AbstractFileSystem.makedirs`."""
- return self.makedirs(path, exist_ok=exist_ok)
-
- def listdir(self, path, detail=True, **kwargs):
- """Alias of `AbstractFileSystem.ls`."""
- return self.ls(path, detail=detail, **kwargs)
-
- def cp(self, path1, path2, **kwargs):
- """Alias of `AbstractFileSystem.copy`."""
- return self.copy(path1, path2, **kwargs)
-
- def move(self, path1, path2, **kwargs):
- """Alias of `AbstractFileSystem.mv`."""
- return self.mv(path1, path2, **kwargs)
-
- def stat(self, path, **kwargs):
- """Alias of `AbstractFileSystem.info`."""
- return self.info(path, **kwargs)
-
- def disk_usage(self, path, total=True, maxdepth=None, **kwargs):
- """Alias of `AbstractFileSystem.du`."""
- return self.du(path, total=total, maxdepth=maxdepth, **kwargs)
-
- def rename(self, path1, path2, **kwargs):
- """Alias of `AbstractFileSystem.mv`."""
- return self.mv(path1, path2, **kwargs)
-
- def delete(self, path, recursive=False, maxdepth=None):
- """Alias of `AbstractFileSystem.rm`."""
- return self.rm(path, recursive=recursive, maxdepth=maxdepth)
-
- def upload(self, lpath, rpath, recursive=False, **kwargs):
- """Alias of `AbstractFileSystem.put`."""
- return self.put(lpath, rpath, recursive=recursive, **kwargs)
-
- def download(self, rpath, lpath, recursive=False, **kwargs):
- """Alias of `AbstractFileSystem.get`."""
- return self.get(rpath, lpath, recursive=recursive, **kwargs)
-
- def sign(self, path, expiration=100, **kwargs):
- """Create a signed URL representing the given path
-
- Some implementations allow temporary URLs to be generated, as a
- way of delegating credentials.
-
- Parameters
- ----------
- path : str
- The path on the filesystem
- expiration : int
- Number of seconds to enable the URL for (if supported)
-
- Returns
- -------
- URL : str
- The signed URL
-
- Raises
- ------
- NotImplementedError : if method is not implemented for a filesystem
- """
- raise NotImplementedError("Sign is not implemented for this filesystem")
-
- def _isfilestore(self):
- # Originally inherited from pyarrow DaskFileSystem. Keeping this
- # here for backwards compatibility as long as pyarrow uses its
- # legacy fsspec-compatible filesystems and thus accepts fsspec
- # filesystems as well
- return False
-
-
-class AbstractBufferedFile(io.IOBase):
- """Convenient class to derive from to provide buffering
-
- In the case that the backend does not provide a pythonic file-like object
- already, this class contains much of the logic to build one. The only
- methods that need to be overridden are ``_upload_chunk``,
- ``_initiate_upload`` and ``_fetch_range``.
- """
-
- DEFAULT_BLOCK_SIZE = 5 * 2**20
- _details = None
-
- def __init__(
- self,
- fs,
- path,
- mode="rb",
- block_size="default",
- autocommit=True,
- cache_type="readahead",
- cache_options=None,
- size=None,
- **kwargs,
- ):
- """
- Template for files with buffered reading and writing
-
- Parameters
- ----------
- fs: instance of FileSystem
- path: str
- location in file-system
- mode: str
- Normal file modes. Currently only 'wb', 'ab' or 'rb'. Some file
- systems may be read-only, and some may not support append.
- block_size: int
- Buffer size for reading or writing, 'default' for class default
- autocommit: bool
- Whether to write to final destination; may only impact what
- happens when file is being closed.
- cache_type: {"readahead", "none", "mmap", "bytes"}, default "readahead"
- Caching policy in read mode. See the definitions in ``core``.
- cache_options : dict
- Additional options passed to the constructor for the cache specified
- by `cache_type`.
- size: int
- If given and in read mode, suppressed having to look up the file size
- kwargs:
- Gets stored as self.kwargs
- """
- from .core import caches
-
- self.path = path
- self.fs = fs
- self.mode = mode
- self.blocksize = (
- self.DEFAULT_BLOCK_SIZE if block_size in ["default", None] else block_size
- )
- self.loc = 0
- self.autocommit = autocommit
- self.end = None
- self.start = None
- self.closed = False
-
- if cache_options is None:
- cache_options = {}
-
- if "trim" in kwargs:
- warnings.warn(
- "Passing 'trim' to control the cache behavior has been deprecated. "
- "Specify it within the 'cache_options' argument instead.",
- FutureWarning,
- )
- cache_options["trim"] = kwargs.pop("trim")
-
- self.kwargs = kwargs
-
- if mode not in {"ab", "rb", "wb"}:
- raise NotImplementedError("File mode not supported")
- if mode == "rb":
- if size is not None:
- self.size = size
- else:
- self.size = self.details["size"]
- self.cache = caches[cache_type](
- self.blocksize, self._fetch_range, self.size, **cache_options
- )
- else:
- self.buffer = io.BytesIO()
- self.offset = None
- self.forced = False
- self.location = None
-
- @property
- def details(self):
- if self._details is None:
- self._details = self.fs.info(self.path)
- return self._details
-
- @details.setter
- def details(self, value):
- self._details = value
- self.size = value["size"]
-
- @property
- def full_name(self):
- return _unstrip_protocol(self.path, self.fs)
-
- @property
- def closed(self):
- # get around this attr being read-only in IOBase
- # use getattr here, since this can be called during del
- return getattr(self, "_closed", True)
-
- @closed.setter
- def closed(self, c):
- self._closed = c
-
- def __hash__(self):
- if "w" in self.mode:
- return id(self)
- else:
- return int(tokenize(self.details), 16)
-
- def __eq__(self, other):
- """Files are equal if they have the same checksum, only in read mode"""
- return self.mode == "rb" and other.mode == "rb" and hash(self) == hash(other)
-
- def commit(self):
- """Move from temp to final destination"""
-
- def discard(self):
- """Throw away temporary file"""
-
- def info(self):
- """File information about this path"""
- if "r" in self.mode:
- return self.details
- else:
- raise ValueError("Info not available while writing")
-
- def tell(self):
- """Current file location"""
- return self.loc
-
- def seek(self, loc, whence=0):
- """Set current file location
-
- Parameters
- ----------
- loc: int
- byte location
- whence: {0, 1, 2}
- from start of file, current location or end of file, resp.
- """
- loc = int(loc)
- if not self.mode == "rb":
- raise OSError(ESPIPE, "Seek only available in read mode")
- if whence == 0:
- nloc = loc
- elif whence == 1:
- nloc = self.loc + loc
- elif whence == 2:
- nloc = self.size + loc
- else:
- raise ValueError("invalid whence (%s, should be 0, 1 or 2)" % whence)
- if nloc < 0:
- raise ValueError("Seek before start of file")
- self.loc = nloc
- return self.loc
-
- def write(self, data):
- """
- Write data to buffer.
-
- Buffer only sent on flush() or if buffer is greater than
- or equal to blocksize.
-
- Parameters
- ----------
- data: bytes
- Set of bytes to be written.
- """
- if self.mode not in {"wb", "ab"}:
- raise ValueError("File not in write mode")
- if self.closed:
- raise ValueError("I/O operation on closed file.")
- if self.forced:
- raise ValueError("This file has been force-flushed, can only close")
- out = self.buffer.write(data)
- self.loc += out
- if self.buffer.tell() >= self.blocksize:
- self.flush()
- return out
-
- def flush(self, force=False):
- """
- Write buffered data to backend store.
-
- Writes the current buffer, if it is larger than the block-size, or if
- the file is being closed.
-
- Parameters
- ----------
- force: bool
- When closing, write the last block even if it is smaller than
- blocks are allowed to be. Disallows further writing to this file.
- """
-
- if self.closed:
- raise ValueError("Flush on closed file")
- if force and self.forced:
- raise ValueError("Force flush cannot be called more than once")
- if force:
- self.forced = True
-
- if self.mode not in {"wb", "ab"}:
- # no-op to flush on read-mode
- return
-
- if not force and self.buffer.tell() < self.blocksize:
- # Defer write on small block
- return
-
- if self.offset is None:
- # Initialize a multipart upload
- self.offset = 0
- try:
- self._initiate_upload()
- except: # noqa: E722
- self.closed = True
- raise
-
- if self._upload_chunk(final=force) is not False:
- self.offset += self.buffer.seek(0, 2)
- self.buffer = io.BytesIO()
-
- def _upload_chunk(self, final=False):
- """Write one part of a multi-block file upload
-
- Parameters
- ==========
- final: bool
- This is the last block, so should complete file, if
- self.autocommit is True.
- """
- # may not yet have been initialized, may need to call _initialize_upload
-
- def _initiate_upload(self):
- """Create remote file/upload"""
- pass
-
- def _fetch_range(self, start, end):
- """Get the specified set of bytes from remote"""
- raise NotImplementedError
-
- def read(self, length=-1):
- """
- Return data from cache, or fetch pieces as necessary
-
- Parameters
- ----------
- length: int (-1)
- Number of bytes to read; if <0, all remaining bytes.
- """
- length = -1 if length is None else int(length)
- if self.mode != "rb":
- raise ValueError("File not in read mode")
- if length < 0:
- length = self.size - self.loc
- if self.closed:
- raise ValueError("I/O operation on closed file.")
- logger.debug("%s read: %i - %i" % (self, self.loc, self.loc + length))
- if length == 0:
- # don't even bother calling fetch
- return b""
- out = self.cache._fetch(self.loc, self.loc + length)
- self.loc += len(out)
- return out
-
- def readinto(self, b):
- """mirrors builtin file's readinto method
-
- https://docs.python.org/3/library/io.html#io.RawIOBase.readinto
- """
- out = memoryview(b).cast("B")
- data = self.read(out.nbytes)
- out[: len(data)] = data
- return len(data)
-
- def readuntil(self, char=b"\n", blocks=None):
- """Return data between current position and first occurrence of char
-
- char is included in the output, except if the end of the tile is
- encountered first.
-
- Parameters
- ----------
- char: bytes
- Thing to find
- blocks: None or int
- How much to read in each go. Defaults to file blocksize - which may
- mean a new read on every call.
- """
- out = []
- while True:
- start = self.tell()
- part = self.read(blocks or self.blocksize)
- if len(part) == 0:
- break
- found = part.find(char)
- if found > -1:
- out.append(part[: found + len(char)])
- self.seek(start + found + len(char))
- break
- out.append(part)
- return b"".join(out)
-
- def readline(self):
- """Read until first occurrence of newline character
-
- Note that, because of character encoding, this is not necessarily a
- true line ending.
- """
- return self.readuntil(b"\n")
-
- def __next__(self):
- out = self.readline()
- if out:
- return out
- raise StopIteration
-
- def __iter__(self):
- return self
-
- def readlines(self):
- """Return all data, split by the newline character"""
- data = self.read()
- lines = data.split(b"\n")
- out = [l + b"\n" for l in lines[:-1]]
- if data.endswith(b"\n"):
- return out
- else:
- return out + [lines[-1]]
- # return list(self) ???
-
- def readinto1(self, b):
- return self.readinto(b)
-
- def close(self):
- """Close file
-
- Finalizes writes, discards cache
- """
- if getattr(self, "_unclosable", False):
- return
- if self.closed:
- return
- if self.mode == "rb":
- self.cache = None
- else:
- if not self.forced:
- self.flush(force=True)
-
- if self.fs is not None:
- self.fs.invalidate_cache(self.path)
- self.fs.invalidate_cache(self.fs._parent(self.path))
-
- self.closed = True
-
- def readable(self):
- """Whether opened for reading"""
- return self.mode == "rb" and not self.closed
-
- def seekable(self):
- """Whether is seekable (only in read mode)"""
- return self.readable()
-
- def writable(self):
- """Whether opened for writing"""
- return self.mode in {"wb", "ab"} and not self.closed
-
- def __del__(self):
- if not self.closed:
- self.close()
-
- def __str__(self):
- return "" % (type(self.fs).__name__, self.path)
-
- __repr__ = __str__
-
- def __enter__(self):
- return self
-
- def __exit__(self, *args):
- self.close()
diff --git a/spaces/jonas/sdg-policy-tracing/README.md b/spaces/jonas/sdg-policy-tracing/README.md
deleted file mode 100644
index cf8617c32f763f8ad0ba95fdb93cd221ba3c1fe7..0000000000000000000000000000000000000000
--- a/spaces/jonas/sdg-policy-tracing/README.md
+++ /dev/null
@@ -1,13 +0,0 @@
----
-title: Document Processing Develop
-emoji: 😻
-colorFrom: red
-colorTo: blue
-sdk: streamlit
-sdk_version: 1.10.0
-app_file: app.py
-pinned: false
-license: cc-by-4.0
----
-
-Check out the configuration reference at https://huggingface.co/docs/hub/spaces-config-reference
diff --git a/spaces/jordonpeter01/MusicGen/audiocraft/modules/codebooks_patterns.py b/spaces/jordonpeter01/MusicGen/audiocraft/modules/codebooks_patterns.py
deleted file mode 100644
index c5b35cbea8cff84aa56116dbdd860fc72a913a13..0000000000000000000000000000000000000000
--- a/spaces/jordonpeter01/MusicGen/audiocraft/modules/codebooks_patterns.py
+++ /dev/null
@@ -1,539 +0,0 @@
-# Copyright (c) Meta Platforms, Inc. and affiliates.
-# All rights reserved.
-#
-# This source code is licensed under the license found in the
-# LICENSE file in the root directory of this source tree.
-
-from collections import namedtuple
-from dataclasses import dataclass
-from functools import lru_cache
-import logging
-import typing as tp
-
-from abc import ABC, abstractmethod
-import torch
-
-LayoutCoord = namedtuple('LayoutCoord', ['t', 'q']) # (timestep, codebook index)
-PatternLayout = tp.List[tp.List[LayoutCoord]] # Sequence of coordinates
-logger = logging.getLogger(__name__)
-
-
-@dataclass
-class Pattern:
- """Base implementation of a pattern over a sequence with multiple codebooks.
-
- The codebook pattern consists in a layout, defining for each sequence step
- the list of coordinates of each codebook timestep in the resulting interleaved sequence.
- The first item of the pattern is always an empty list in order to properly insert a special token
- to start with. For convenience, we also keep track of ``n_q`` the number of codebooks used for the pattern
- and ``timesteps`` the number of timesteps corresponding to the original sequence.
-
- The pattern provides convenient methods to build and revert interleaved sequences from it:
- ``build_pattern_sequence`` maps a given a dense input tensor of multi-codebook sequence from [B, K, T]
- to the interleaved sequence of shape [B, K, S] applying the pattern, with S being the batch size,
- K being the number of codebooks, T the number of original timesteps and S the number of sequence steps
- for the output sequence. The unfilled positions are replaced with a special token and the built sequence
- is returned along with a mask indicating valid tokens.
- ``revert_pattern_sequence`` maps back an interleaved sequence of shape [B, K, S] to the original alignment
- of codebooks across timesteps to an output tensor of shape [B, K, T], using again a special token and a mask
- to fill and specify invalid positions if needed.
- See the dedicated methods for more details.
- """
- # Pattern layout, for each sequence step, we have a list of coordinates
- # corresponding to the original codebook timestep and position.
- # The first list is always an empty list in order to properly insert
- # a special token to start with.
- layout: PatternLayout
- timesteps: int
- n_q: int
-
- def __post_init__(self):
- assert len(self.layout) > 0
- assert self.layout[0] == []
- self._validate_layout()
- self._build_reverted_sequence_scatter_indexes = lru_cache(100)(self._build_reverted_sequence_scatter_indexes)
- self._build_pattern_sequence_scatter_indexes = lru_cache(100)(self._build_pattern_sequence_scatter_indexes)
- logger.info("New pattern, time steps: %d, sequence steps: %d", self.timesteps, len(self.layout))
-
- def _validate_layout(self):
- """Runs checks on the layout to ensure a valid pattern is defined.
- A pattern is considered invalid if:
- - Multiple timesteps for a same codebook are defined in the same sequence step
- - The timesteps for a given codebook are not in ascending order as we advance in the sequence
- (this would mean that we have future timesteps before past timesteps).
- """
- q_timesteps = {q: 0 for q in range(self.n_q)}
- for s, seq_coords in enumerate(self.layout):
- if len(seq_coords) > 0:
- qs = set()
- for coord in seq_coords:
- qs.add(coord.q)
- last_q_timestep = q_timesteps[coord.q]
- assert coord.t >= last_q_timestep, \
- f"Past timesteps are found in the sequence for codebook = {coord.q} at step {s}"
- q_timesteps[coord.q] = coord.t
- # each sequence step contains at max 1 coordinate per codebook
- assert len(qs) == len(seq_coords), \
- f"Multiple entries for a same codebook are found at step {s}"
-
- @property
- def num_sequence_steps(self):
- return len(self.layout) - 1
-
- @property
- def max_delay(self):
- max_t_in_seq_coords = 0
- for seq_coords in self.layout[1:]:
- for coords in seq_coords:
- max_t_in_seq_coords = max(max_t_in_seq_coords, coords.t + 1)
- return max_t_in_seq_coords - self.timesteps
-
- @property
- def valid_layout(self):
- valid_step = len(self.layout) - self.max_delay
- return self.layout[:valid_step]
-
- def get_sequence_coords_with_timestep(self, t: int, q: tp.Optional[int] = None):
- """Get codebook coordinates in the layout that corresponds to the specified timestep t
- and optionally to the codebook q. Coordinates are returned as a tuple with the sequence step
- and the actual codebook coordinates.
- """
- assert t <= self.timesteps, "provided timesteps is greater than the pattern's number of timesteps"
- if q is not None:
- assert q <= self.n_q, "provided number of codebooks is greater than the pattern's number of codebooks"
- coords = []
- for s, seq_codes in enumerate(self.layout):
- for code in seq_codes:
- if code.t == t and (q is None or code.q == q):
- coords.append((s, code))
- return coords
-
- def get_steps_with_timestep(self, t: int, q: tp.Optional[int] = None) -> tp.List[int]:
- return [step for step, coords in self.get_sequence_coords_with_timestep(t, q)]
-
- def get_first_step_with_timesteps(self, t: int, q: tp.Optional[int] = None) -> tp.Optional[int]:
- steps_with_timesteps = self.get_steps_with_timestep(t, q)
- return steps_with_timesteps[0] if len(steps_with_timesteps) > 0 else None
-
- def _build_pattern_sequence_scatter_indexes(self, timesteps: int, n_q: int, keep_only_valid_steps: bool,
- device: tp.Union[torch.device, str] = 'cpu'):
- """Build scatter indexes corresponding to the pattern, up to the provided sequence_steps.
-
- Args:
- timesteps (int): Maximum number of timesteps steps to consider.
- keep_only_valid_steps (bool): Restrict the pattern layout to match only valid steps.
- device (Union[torch.device, str]): Device for created tensors.
- Returns:
- indexes (torch.Tensor): Indexes corresponding to the sequence, of shape [K, S].
- mask (torch.Tensor): Mask corresponding to indexes that matches valid indexes, of shape [K, S].
- """
- assert n_q == self.n_q, f"invalid number of codebooks for the sequence and the pattern: {n_q} != {self.n_q}"
- assert timesteps <= self.timesteps, "invalid number of timesteps used to build the sequence from the pattern"
- # use the proper layout based on whether we limit ourselves to valid steps only or not,
- # note that using the valid_layout will result in a truncated sequence up to the valid steps
- ref_layout = self.valid_layout if keep_only_valid_steps else self.layout
- # single item indexing being super slow with pytorch vs. numpy, so we use numpy here
- indexes = torch.zeros(n_q, len(ref_layout), dtype=torch.long).numpy()
- mask = torch.zeros(n_q, len(ref_layout), dtype=torch.bool).numpy()
- # fill indexes with last sequence step value that will correspond to our special token
- # the last value is n_q * timesteps as we have flattened z and append special token as the last token
- # which will correspond to the index: n_q * timesteps
- indexes[:] = n_q * timesteps
- # iterate over the pattern and fill scattered indexes and mask
- for s, sequence_coords in enumerate(ref_layout):
- for coords in sequence_coords:
- if coords.t < timesteps:
- indexes[coords.q, s] = coords.t + coords.q * timesteps
- mask[coords.q, s] = 1
- indexes = torch.from_numpy(indexes).to(device)
- mask = torch.from_numpy(mask).to(device)
- return indexes, mask
-
- def build_pattern_sequence(self, z: torch.Tensor, special_token: int, keep_only_valid_steps: bool = False):
- """Build sequence corresponding to the pattern from the input tensor z.
- The sequence is built using up to sequence_steps if specified, and non-pattern
- coordinates are filled with the special token.
-
- Args:
- z (torch.Tensor): Input tensor of multi-codebooks sequence, of shape [B, K, T].
- special_token (int): Special token used to fill non-pattern coordinates in the new sequence.
- keep_only_valid_steps (bool): Build a sequence from the pattern up to valid (= fully defined) steps.
- Steps that are beyond valid steps will be replaced by the special_token in that case.
- Returns:
- values (torch.Tensor): Interleaved sequence matching the pattern, of shape [B, K, S] with S
- corresponding either to the sequence_steps if provided, otherwise to the length of the pattern.
- indexes (torch.Tensor): Indexes corresponding to the interleaved sequence, of shape [K, S].
- mask (torch.Tensor): Mask corresponding to indexes that matches valid indexes of shape [K, S].
- """
- B, K, T = z.shape
- indexes, mask = self._build_pattern_sequence_scatter_indexes(
- T, K, keep_only_valid_steps=keep_only_valid_steps, device=str(z.device)
- )
- z = z.view(B, -1)
- # we append the special token as the last index of our flattened z tensor
- z = torch.cat([z, torch.zeros_like(z[:, :1]) + special_token], dim=1)
- values = z[:, indexes.view(-1)]
- values = values.view(B, K, indexes.shape[-1])
- return values, indexes, mask
-
- def _build_reverted_sequence_scatter_indexes(self, sequence_steps: int, n_q: int,
- keep_only_valid_steps: bool = False,
- is_model_output: bool = False,
- device: tp.Union[torch.device, str] = 'cpu'):
- """Builds scatter indexes required to retrieve the original multi-codebook sequence
- from interleaving pattern.
-
- Args:
- sequence_steps (int): Sequence steps.
- n_q (int): Number of codebooks.
- keep_only_valid_steps (bool): Build a sequence from the pattern up to valid (= fully defined) steps.
- Steps that are beyond valid steps will be replaced by the special_token in that case.
- is_model_output (bool): Whether to keep the sequence item corresponding to initial special token or not.
- device (Union[torch.device, str]): Device for created tensors.
- Returns:
- torch.Tensor: Indexes for reconstructing the output, of shape [K, T].
- mask (torch.Tensor): Mask corresponding to indexes that matches valid indexes of shape [K, T].
- """
- ref_layout = self.valid_layout if keep_only_valid_steps else self.layout
- # TODO(jade): Do we want to further truncate to only valid timesteps here as well?
- timesteps = self.timesteps
- assert n_q == self.n_q, f"invalid number of codebooks for the sequence and the pattern: {n_q} != {self.n_q}"
- assert sequence_steps <= len(ref_layout), \
- f"sequence to revert is longer than the defined pattern: {sequence_steps} > {len(ref_layout)}"
-
- # ensure we take the appropriate indexes to keep the model output from the first special token as well
- if is_model_output:
- ref_layout = ref_layout[1:]
-
- # single item indexing being super slow with pytorch vs. numpy, so we use numpy here
- indexes = torch.zeros(n_q, timesteps, dtype=torch.long).numpy()
- mask = torch.zeros(n_q, timesteps, dtype=torch.bool).numpy()
- # fill indexes with last sequence step value that will correspond to our special token
- indexes[:] = n_q * sequence_steps
- for s, sequence_codes in enumerate(ref_layout):
- if s < sequence_steps:
- for code in sequence_codes:
- if code.t < timesteps:
- indexes[code.q, code.t] = s + code.q * sequence_steps
- mask[code.q, code.t] = 1
- indexes = torch.from_numpy(indexes).to(device)
- mask = torch.from_numpy(mask).to(device)
- return indexes, mask
-
- def revert_pattern_sequence(self, s: torch.Tensor, special_token: int, keep_only_valid_steps: bool = False):
- """Revert a sequence built from the pattern back to the original multi-codebook sequence without interleaving.
- The sequence is reverted using up to timesteps if specified, and non-pattern coordinates
- are filled with the special token.
-
- Args:
- s (torch.Tensor): Interleaved sequence tensor obtained from the pattern, of shape [B, K, S].
- special_token (int or float): Special token used to fill non-pattern coordinates in the new sequence.
- Returns:
- values (torch.Tensor): Interleaved sequence matching the pattern, of shape [B, K, T] with T
- corresponding either to the timesteps if provided, or the total timesteps in pattern otherwise.
- indexes (torch.Tensor): Indexes corresponding to the interleaved sequence, of shape [K, T].
- mask (torch.Tensor): Mask corresponding to indexes that matches valid indexes of shape [K, T].
- """
- B, K, S = s.shape
- indexes, mask = self._build_reverted_sequence_scatter_indexes(
- S, K, keep_only_valid_steps, is_model_output=False, device=str(s.device)
- )
- s = s.view(B, -1)
- # we append the special token as the last index of our flattened z tensor
- s = torch.cat([s, torch.zeros_like(s[:, :1]) + special_token], dim=1)
- values = s[:, indexes.view(-1)]
- values = values.view(B, K, indexes.shape[-1])
- return values, indexes, mask
-
- def revert_pattern_logits(self, logits: torch.Tensor, special_token: float, keep_only_valid_steps: bool = False):
- """Revert model logits obtained on a sequence built from the pattern
- back to a tensor matching the original sequence.
-
- This method is similar to ``revert_pattern_sequence`` with the following specificities:
- 1. It is designed to work with the extra cardinality dimension
- 2. We return the logits for the first sequence item that matches the special_token and
- which matching target in the original sequence is the first item of the sequence,
- while we skip the last logits as there is no matching target
- """
- B, card, K, S = logits.shape
- indexes, mask = self._build_reverted_sequence_scatter_indexes(
- S, K, keep_only_valid_steps, is_model_output=True, device=logits.device
- )
- logits = logits.reshape(B, card, -1)
- # we append the special token as the last index of our flattened z tensor
- logits = torch.cat([logits, torch.zeros_like(logits[:, :, :1]) + special_token], dim=-1) # [B, card, K x S]
- values = logits[:, :, indexes.view(-1)]
- values = values.view(B, card, K, indexes.shape[-1])
- return values, indexes, mask
-
-
-class CodebooksPatternProvider(ABC):
- """Abstraction around providing pattern for interleaving codebooks.
-
- The CodebooksPatternProvider abstraction allows to implement various strategies to
- define interleaving pattern of sequences composed of multiple codebooks. For a given
- number of codebooks `n_q`, the pattern provider can generate a specified pattern
- corresponding to a sequence of `T` timesteps with `n_q` parallel codebooks. This pattern
- can be used to construct a new sequence from the original codes respecting the specified
- pattern. The pattern is defined as a list of list of code coordinates, code coordinate
- being a tuple with the original timestep and codebook to build the new sequence.
- Note that all patterns must start with an empty list that is then used to insert a first
- sequence step of special tokens in the newly generated sequence.
-
- Args:
- n_q (int): number of codebooks.
- cached (bool): if True, patterns for a given length are cached. In general
- that should be true for efficiency reason to avoid synchronization points.
- """
- def __init__(self, n_q: int, cached: bool = True):
- assert n_q > 0
- self.n_q = n_q
- self.get_pattern = lru_cache(100)(self.get_pattern) # type: ignore
-
- @abstractmethod
- def get_pattern(self, timesteps: int) -> Pattern:
- """Builds pattern with specific interleaving between codebooks.
-
- Args:
- timesteps (int): Total numer of timesteps.
- """
- raise NotImplementedError()
-
-
-class DelayedPatternProvider(CodebooksPatternProvider):
- """Provider for delayed pattern across delayed codebooks.
- Codebooks are delayed in the sequence and sequence steps will contain codebooks
- from different timesteps.
-
- Example:
- Taking timesteps=4 and n_q=3, delays=None, the multi-codebook sequence:
- [[1, 2, 3, 4],
- [1, 2, 3, 4],
- [1, 2, 3, 4]]
- The resulting sequence obtained from the returned pattern is:
- [[S, 1, 2, 3, 4],
- [S, S, 1, 2, 3],
- [S, S, S, 1, 2]]
- (with S being a special token)
-
- Args:
- n_q (int): Number of codebooks.
- delays (Optional[List[int]]): Delay for each of the codebooks.
- If delays not defined, each codebook is delayed by 1 compared to the previous one.
- flatten_first (int): Flatten the first N timesteps.
- empty_initial (int): Prepend with N empty list of coordinates.
- """
- def __init__(self, n_q: int, delays: tp.Optional[tp.List[int]] = None,
- flatten_first: int = 0, empty_initial: int = 0):
- super().__init__(n_q)
- if delays is None:
- delays = list(range(n_q))
- self.delays = delays
- self.flatten_first = flatten_first
- self.empty_initial = empty_initial
- assert len(self.delays) == self.n_q
- assert sorted(self.delays) == self.delays
-
- def get_pattern(self, timesteps: int) -> Pattern:
- out: PatternLayout = [[]]
- max_delay = max(self.delays)
- if self.empty_initial:
- out += [[] for _ in range(self.empty_initial)]
- if self.flatten_first:
- for t in range(min(timesteps, self.flatten_first)):
- for q in range(self.n_q):
- out.append([LayoutCoord(t, q)])
- for t in range(self.flatten_first, timesteps + max_delay):
- v = []
- for q, delay in enumerate(self.delays):
- t_for_q = t - delay
- if t_for_q >= self.flatten_first:
- v.append(LayoutCoord(t_for_q, q))
- out.append(v)
- return Pattern(out, n_q=self.n_q, timesteps=timesteps)
-
-
-class ParallelPatternProvider(DelayedPatternProvider):
- """Provider for parallel pattern across codebooks.
- This pattern provider is a special case of the delayed pattern with actually no delay,
- hence delays=repeat(0, n_q).
-
- Args:
- n_q (int): Number of codebooks.
- """
- def __init__(self, n_q: int):
- super().__init__(n_q, [0] * n_q)
-
-
-class UnrolledPatternProvider(CodebooksPatternProvider):
- """Provider for unrolling codebooks pattern.
- This pattern provider enables to represent the codebook flattened completely or only to some extend
- while also specifying a given delay between the flattened codebooks representation, allowing to
- unroll the codebooks in the sequence.
-
- Example:
- 1. Flattening of the codebooks.
- By default, the pattern provider will fully flatten the codebooks such as flattening=range(n_q),
- taking n_q = 3 and timesteps = 4:
- [[1, 2, 3, 4],
- [1, 2, 3, 4],
- [1, 2, 3, 4]]
- will result into:
- [[S, S, 1, S, S, 2, S, S, 3, S, S, 4],
- [S, 1, S, S, 2, S, S, 3, S, S, 4, S],
- [1, S, S, 2, S, S, 3, S, S, 4, S, S]]
- 2. Partial flattening of the codebooks. The ``flattening`` parameter allows to specify the inner step
- for each of the codebook, allowing to define which codebook to flatten (or keep in parallel), for example
- taking n_q = 3, timesteps = 4 and flattening = [0, 1, 1]:
- [[1, 2, 3, 4],
- [1, 2, 3, 4],
- [1, 2, 3, 4]]
- will result into:
- [[S, 1, S, S, 2, S, S, 3, S, S, 4, S],
- [S, 1, S, S, 2, S, S, 3, S, S, 4, S],
- [1, S, S, 2, S, S, 3, S, S, 4, S, S]]
- 3. Flattening with delay. The ``delay`` parameter allows to further unroll the sequence of codebooks
- allowing to specify the delay per codebook. Note that the delay between codebooks flattened to the
- same inner timestep should be coherent. For example, taking n_q = 3, timesteps = 4, flattening = [0, 1, 1]
- and delays = [0, 3, 3]:
- [[1, 2, 3, 4],
- [1, 2, 3, 4],
- [1, 2, 3, 4]]
- will result into:
- [[S, S, S, 1, S, 2, S, 3, S, 4],
- [S, S, S, 1, S, 2, S, 3, S, 4],
- [1, 2, 3, S, 4, S, 5, S, 6, S]]
-
- Args:
- n_q (int): Number of codebooks.
- flattening (Optional[List[int]]): Flattening schema over the codebooks. If not defined,
- the codebooks will be flattened to 1 codebook per step, meaning that the sequence will
- have n_q extra steps for each timestep.
- delays (Optional[List[int]]): Delay for each of the codebooks. If not defined,
- no delay is added and therefore will default to [0] * ``n_q``.
- Note that two codebooks that will be flattened to the same inner step
- should have the same delay, otherwise the pattern is considered as invalid.
- """
- FlattenedCodebook = namedtuple('FlattenedCodebook', ['codebooks', 'delay'])
-
- def __init__(self, n_q: int, flattening: tp.Optional[tp.List[int]] = None,
- delays: tp.Optional[tp.List[int]] = None):
- super().__init__(n_q)
- if flattening is None:
- flattening = list(range(n_q))
- if delays is None:
- delays = [0] * n_q
- assert len(flattening) == n_q
- assert len(delays) == n_q
- assert sorted(flattening) == flattening
- assert sorted(delays) == delays
- self._flattened_codebooks = self._build_flattened_codebooks(delays, flattening)
- self.max_delay = max(delays)
-
- def _build_flattened_codebooks(self, delays: tp.List[int], flattening: tp.List[int]):
- """Build a flattened codebooks representation as a dictionary of inner step
- and the actual codebook indices corresponding to the flattened codebook. For convenience, we
- also store the delay associated to the flattened codebook to avoid maintaining an extra mapping.
- """
- flattened_codebooks: dict = {}
- for q, (inner_step, delay) in enumerate(zip(flattening, delays)):
- if inner_step not in flattened_codebooks:
- flat_codebook = UnrolledPatternProvider.FlattenedCodebook(codebooks=[q], delay=delay)
- else:
- flat_codebook = flattened_codebooks[inner_step]
- assert flat_codebook.delay == delay, (
- "Delay and flattening between codebooks is inconsistent: ",
- "two codebooks flattened to the same position should have the same delay."
- )
- flat_codebook.codebooks.append(q)
- flattened_codebooks[inner_step] = flat_codebook
- return flattened_codebooks
-
- @property
- def _num_inner_steps(self):
- """Number of inner steps to unroll between timesteps in order to flatten the codebooks.
- """
- return max([inner_step for inner_step in self._flattened_codebooks.keys()]) + 1
-
- def num_virtual_steps(self, timesteps: int) -> int:
- return timesteps * self._num_inner_steps + 1
-
- def get_pattern(self, timesteps: int) -> Pattern:
- """Builds pattern for delay across codebooks.
-
- Args:
- timesteps (int): Total numer of timesteps.
- """
- # the PatternLayout is built as a tuple of sequence position and list of coordinates
- # so that it can be reordered properly given the required delay between codebooks of given timesteps
- indexed_out: list = [(-1, [])]
- max_timesteps = timesteps + self.max_delay
- for t in range(max_timesteps):
- # for each timestep, we unroll the flattened codebooks,
- # emitting the sequence step with the corresponding delay
- for step in range(self._num_inner_steps):
- if step in self._flattened_codebooks:
- # we have codebooks at this virtual step to emit
- step_codebooks = self._flattened_codebooks[step]
- t_for_q = t + step_codebooks.delay
- coords = [LayoutCoord(t, q) for q in step_codebooks.codebooks]
- if t_for_q < max_timesteps and t < max_timesteps:
- indexed_out.append((t_for_q, coords))
- else:
- # there is no codebook in this virtual step so we emit an empty list
- indexed_out.append((t, []))
- out = [coords for _, coords in sorted(indexed_out)]
- return Pattern(out, n_q=self.n_q, timesteps=timesteps)
-
-
-class VALLEPattern(CodebooksPatternProvider):
- """Almost VALL-E style pattern. We futher allow some delays for the
- codebooks other than the first one.
-
- Args:
- n_q (int): Number of codebooks.
- delays (Optional[List[int]]): Delay for each of the codebooks.
- If delays not defined, each codebook is delayed by 1 compared to the previous one.
- """
- def __init__(self, n_q: int, delays: tp.Optional[tp.List[int]] = None):
- super().__init__(n_q)
- if delays is None:
- delays = [0] * (n_q - 1)
- self.delays = delays
- assert len(self.delays) == self.n_q - 1
- assert sorted(self.delays) == self.delays
-
- def get_pattern(self, timesteps: int) -> Pattern:
- out: PatternLayout = [[]]
- for t in range(timesteps):
- out.append([LayoutCoord(t, 0)])
- max_delay = max(self.delays)
- for t in range(timesteps + max_delay):
- v = []
- for q, delay in enumerate(self.delays):
- t_for_q = t - delay
- if t_for_q >= 0:
- v.append(LayoutCoord(t_for_q, q + 1))
- out.append(v)
- return Pattern(out, n_q=self.n_q, timesteps=timesteps)
-
-
-class MusicLMPattern(CodebooksPatternProvider):
- """Almost MusicLM style pattern. This is equivalent to full flattening
- but in a different order.
-
- Args:
- n_q (int): Number of codebooks.
- group_by (int): Number of codebooks to group together.
- """
- def __init__(self, n_q: int, group_by: int = 2):
- super().__init__(n_q)
- self.group_by = group_by
-
- def get_pattern(self, timesteps: int) -> Pattern:
- out: PatternLayout = [[]]
- for offset in range(0, self.n_q, self.group_by):
- for t in range(timesteps):
- for q in range(offset, offset + self.group_by):
- out.append([LayoutCoord(t, q)])
- return Pattern(out, n_q=self.n_q, timesteps=timesteps)
diff --git a/spaces/joshen/gpt-academic/crazy_functions/test_project/cpp/cppipc/queue.h b/spaces/joshen/gpt-academic/crazy_functions/test_project/cpp/cppipc/queue.h
deleted file mode 100644
index a21f3446e06b5826af7b554c8a7d9c5d80848b62..0000000000000000000000000000000000000000
--- a/spaces/joshen/gpt-academic/crazy_functions/test_project/cpp/cppipc/queue.h
+++ /dev/null
@@ -1,216 +0,0 @@
-#pragma once
-
-#include
-#include
-#include // [[since C++14]]: std::exchange
-#include
-#include
-#include
-#include
-#include
-#include
-#include // assert
-
-#include "libipc/def.h"
-#include "libipc/shm.h"
-#include "libipc/rw_lock.h"
-
-#include "libipc/utility/log.h"
-#include "libipc/platform/detail.h"
-#include "libipc/circ/elem_def.h"
-
-namespace ipc {
-namespace detail {
-
-class queue_conn {
-protected:
- circ::cc_t connected_ = 0;
- shm::handle elems_h_;
-
- template
- Elems* open(char const * name) {
- if (name == nullptr || name[0] == '\0') {
- ipc::error("fail open waiter: name is empty!\n");
- return nullptr;
- }
- if (!elems_h_.acquire(name, sizeof(Elems))) {
- return nullptr;
- }
- auto elems = static_cast(elems_h_.get());
- if (elems == nullptr) {
- ipc::error("fail acquire elems: %s\n", name);
- return nullptr;
- }
- elems->init();
- return elems;
- }
-
- void close() {
- elems_h_.release();
- }
-
-public:
- queue_conn() = default;
- queue_conn(const queue_conn&) = delete;
- queue_conn& operator=(const queue_conn&) = delete;
-
- bool connected() const noexcept {
- return connected_ != 0;
- }
-
- circ::cc_t connected_id() const noexcept {
- return connected_;
- }
-
- template
- auto connect(Elems* elems) noexcept
- /*needs 'optional' here*/
- -> std::tuple().cursor())> {
- if (elems == nullptr) return {};
- // if it's already connected, just return
- if (connected()) return {connected(), false, 0};
- connected_ = elems->connect_receiver();
- return {connected(), true, elems->cursor()};
- }
-
- template
- bool disconnect(Elems* elems) noexcept {
- if (elems == nullptr) return false;
- // if it's already disconnected, just return false
- if (!connected()) return false;
- elems->disconnect_receiver(std::exchange(connected_, 0));
- return true;
- }
-};
-
-template
-class queue_base : public queue_conn {
- using base_t = queue_conn;
-
-public:
- using elems_t = Elems;
- using policy_t = typename elems_t::policy_t;
-
-protected:
- elems_t * elems_ = nullptr;
- decltype(std::declval().cursor()) cursor_ = 0;
- bool sender_flag_ = false;
-
-public:
- using base_t::base_t;
-
- queue_base() = default;
-
- explicit queue_base(char const * name)
- : queue_base{} {
- elems_ = open(name);
- }
-
- explicit queue_base(elems_t * elems) noexcept
- : queue_base{} {
- assert(elems != nullptr);
- elems_ = elems;
- }
-
- /* not virtual */ ~queue_base() {
- base_t::close();
- }
-
- elems_t * elems() noexcept { return elems_; }
- elems_t const * elems() const noexcept { return elems_; }
-
- bool ready_sending() noexcept {
- if (elems_ == nullptr) return false;
- return sender_flag_ || (sender_flag_ = elems_->connect_sender());
- }
-
- void shut_sending() noexcept {
- if (elems_ == nullptr) return;
- if (!sender_flag_) return;
- elems_->disconnect_sender();
- }
-
- bool connect() noexcept {
- auto tp = base_t::connect(elems_);
- if (std::get<0>(tp) && std::get<1>(tp)) {
- cursor_ = std::get<2>(tp);
- return true;
- }
- return std::get<0>(tp);
- }
-
- bool disconnect() noexcept {
- return base_t::disconnect(elems_);
- }
-
- std::size_t conn_count() const noexcept {
- return (elems_ == nullptr) ? static_cast(invalid_value) : elems_->conn_count();
- }
-
- bool valid() const noexcept {
- return elems_ != nullptr;
- }
-
- bool empty() const noexcept {
- return !valid() || (cursor_ == elems_->cursor());
- }
-
- template
- bool push(F&& prep, P&&... params) {
- if (elems_ == nullptr) return false;
- return elems_->push(this, [&](void* p) {
- if (prep(p)) ::new (p) T(std::forward
(params)...);
- }
-
- bool pop(T& item) {
- return base_t::pop(item, [](bool) {});
- }
-
- template
- bool pop(T& item, F&& out) {
- return base_t::pop(item, std::forward(out));
- }
-};
-
-} // namespace ipc
diff --git a/spaces/jungwoo9/foodvision_big/model.py b/spaces/jungwoo9/foodvision_big/model.py
deleted file mode 100644
index 52c2696c874740179528f0bdae8ce87b774a138f..0000000000000000000000000000000000000000
--- a/spaces/jungwoo9/foodvision_big/model.py
+++ /dev/null
@@ -1,36 +0,0 @@
-import torch
-import torchvision
-
-from torch import nn
-
-
-def create_effnetb2_model(num_classes:int=3,
- seed:int=42):
- """Creates an EfficientNetB2 feature extractor model and transforms.
-
- Args:
- num_classes (int, optional): number of classes in the classifier head.
- Defaults to 3.
- seed (int, optional): random seed value. Defaults to 42.
-
- Returns:
- model (torch.nn.Module): EffNetB2 feature extractor model.
- transforms (torchvision.transforms): EffNetB2 image transforms.
- """
- # Create EffNetB2 pretrained weights, transforms and model
- weights = torchvision.models.EfficientNet_B2_Weights.DEFAULT
- transforms = weights.transforms()
- model = torchvision.models.efficientnet_b2(weights=weights)
-
- # Freeze all layers in base model
- for param in model.parameters():
- param.requires_grad = False
-
- # Change classifier head with random seed for reproducibility
- torch.manual_seed(seed)
- model.classifier = nn.Sequential(
- nn.Dropout(p=0.3, inplace=True),
- nn.Linear(in_features=1408, out_features=num_classes),
- )
-
- return model, transforms
diff --git a/spaces/k4black/codebleu/README.md b/spaces/k4black/codebleu/README.md
deleted file mode 100644
index 3997ace46354c06b55046fc0c46a9e5f5374251a..0000000000000000000000000000000000000000
--- a/spaces/k4black/codebleu/README.md
+++ /dev/null
@@ -1,122 +0,0 @@
----
-title: codebleu
-tags:
-- evaluate
-- metric
-- code
-- codebleu
-description: "Unofficial `CodeBLEU` implementation that supports Linux and MacOS."
-sdk: gradio
-sdk_version: 3.19.1
-app_file: app.py
-pinned: false
----
-
-# Metric Card for codebleu
-
-This repository contains an unofficial `CodeBLEU` implementation that supports Linux and MacOS. It is available through `PyPI` and the `evaluate` library.
-
-The code is based on the original [CodeXGLUE/CodeBLEU](https://github.com/microsoft/CodeXGLUE/tree/main/Code-Code/code-to-code-trans/evaluator/CodeBLEU) and updated version by [XLCoST/CodeBLEU](https://github.com/reddy-lab-code-research/XLCoST/tree/main/code/translation/evaluator/CodeBLEU). It has been refactored, tested, built for macOS, and multiple improvements have been made to enhance usability
-
-Available for: `Python`, `C`, `C#`, `C++`, `Java`, `JavaScript`, `PHP`.
-
-## Metric Description
-
-> An ideal evaluation metric should consider the grammatical correctness and the logic correctness.
-> We propose weighted n-gram match and syntactic AST match to measure grammatical correctness, and introduce semantic data-flow match to calculate logic correctness.
-> 
-(from [CodeXGLUE](https://github.com/microsoft/CodeXGLUE/tree/main/Code-Code/code-to-code-trans/evaluator/CodeBLEU) repo)
-
-In a nutshell, `CodeBLEU` is a weighted combination of `n-gram match (BLEU)`, `weighted n-gram match (BLEU-weighted)`, `AST match` and `data-flow match` scores.
-
-The metric has shown higher correlation with human evaluation than `BLEU` and `accuracy` metrics.
-
-## How to Use
-
-### Inputs
-
-- `refarences` (`list[str]` or `list[list[str]]`): reference code
-- `predictions` (`list[str]`) predicted code
-- `lang` (`str`): code language, see `codebleu.AVAILABLE_LANGS` for available languages (python, c_sharp c, cpp, javascript, java, php at the moment)
-- `weights` (`tuple[float,float,float,float]`): weights of the `ngram_match`, `weighted_ngram_match`, `syntax_match`, and `dataflow_match` respectively, defaults to `(0.25, 0.25, 0.25, 0.25)`
-- `tokenizer` (`callable`): to split code string to tokens, defaults to `s.split()`
-
-
-### Output Values
-
-[//]: # (*Explain what this metric outputs and provide an example of what the metric output looks like. Modules should return a dictionary with one or multiple key-value pairs, e.g. {"bleu" : 6.02}*)
-
-[//]: # (*State the range of possible values that the metric's output can take, as well as what in that range is considered good. For example: "This metric can take on any value between 0 and 100, inclusive. Higher scores are better."*)
-
-The metric outputs the `dict[str, float]` with following fields:
-- `codebleu`: the final `CodeBLEU` score
-- `ngram_match_score`: `ngram_match` score (BLEU)
-- `weighted_ngram_match_score`: `weighted_ngram_match` score (BLEU-weighted)
-- `syntax_match_score`: `syntax_match` score (AST match)
-- `dataflow_match_score`: `dataflow_match` score
-
-Each of the scores is in range `[0, 1]`, where `1` is the best score.
-
-
-### Examples
-
-[//]: # (*Give code examples of the metric being used. Try to include examples that clear up any potential ambiguity left from the metric description above. If possible, provide a range of examples that show both typical and atypical results, as well as examples where a variety of input parameters are passed.*)
-
-Using pip package (`pip install codebleu`):
-```python
-from codebleu import calc_codebleu
-
-prediction = "def add ( a , b ) :\n return a + b"
-reference = "def sum ( first , second ) :\n return second + first"
-
-result = calc_codebleu([reference], [prediction], lang="python", weights=(0.25, 0.25, 0.25, 0.25), tokenizer=None)
-print(result)
-# {
-# 'codebleu': 0.5537,
-# 'ngram_match_score': 0.1041,
-# 'weighted_ngram_match_score': 0.1109,
-# 'syntax_match_score': 1.0,
-# 'dataflow_match_score': 1.0
-# }
-```
-
-Or using `evaluate` library (`codebleu` package required):
-```python
-import evaluate
-metric = evaluate.load("k4black/codebleu")
-
-prediction = "def add ( a , b ) :\n return a + b"
-reference = "def sum ( first , second ) :\n return second + first"
-
-result = metric.compute([reference], [prediction], lang="python", weights=(0.25, 0.25, 0.25, 0.25), tokenizer=None)
-```
-
-Note: `lang` is required;
-
-
-## Limitations and Bias
-
-[//]: # (*Note any known limitations or biases that the metric has, with links and references if possible.*)
-
-As this library require `so` file compilation it is platform dependent.
-
-Currently available for Linux (manylinux) and MacOS on Python 3.8+.
-
-
-## Citation
-```bibtex
-@misc{ren2020codebleu,
- title={CodeBLEU: a Method for Automatic Evaluation of Code Synthesis},
- author={Shuo Ren and Daya Guo and Shuai Lu and Long Zhou and Shujie Liu and Duyu Tang and Neel Sundaresan and Ming Zhou and Ambrosio Blanco and Shuai Ma},
- year={2020},
- eprint={2009.10297},
- archivePrefix={arXiv},
- primaryClass={cs.SE}
-}
-```
-
-## Further References
-
-This implementation is Based on original [CodeXGLUE/CodeBLEU](https://github.com/microsoft/CodeXGLUE/tree/main/Code-Code/code-to-code-trans/evaluator/CodeBLEU) code -- refactored, build for macos, tested and fixed multiple crutches to make it more usable.
-
-The source code is available at GitHub [k4black/codebleu](https://github.com/k4black/codebleu) repository.
diff --git a/spaces/kadirnar/yolox/configs/yolox_tiny.py b/spaces/kadirnar/yolox/configs/yolox_tiny.py
deleted file mode 100644
index 5220de2f2e6760d5c9a966d5dd397aad721fc60a..0000000000000000000000000000000000000000
--- a/spaces/kadirnar/yolox/configs/yolox_tiny.py
+++ /dev/null
@@ -1,20 +0,0 @@
-#!/usr/bin/env python3
-# -*- coding:utf-8 -*-
-# Copyright (c) Megvii, Inc. and its affiliates.
-
-import os
-
-from yolox.exp import Exp as MyExp
-
-
-class Exp(MyExp):
- def __init__(self):
- super(Exp, self).__init__()
- self.depth = 0.33
- self.width = 0.375
- self.input_size = (416, 416)
- self.mosaic_scale = (0.5, 1.5)
- self.random_size = (10, 20)
- self.test_size = (416, 416)
- self.exp_name = os.path.split(os.path.realpath(__file__))[1].split(".")[0]
- self.enable_mixup = False
diff --git a/spaces/kandysh/NER_Tagger/main.py b/spaces/kandysh/NER_Tagger/main.py
deleted file mode 100644
index 4a563e6ba45e639a8844ef162e5ac298823abd53..0000000000000000000000000000000000000000
--- a/spaces/kandysh/NER_Tagger/main.py
+++ /dev/null
@@ -1,17 +0,0 @@
-import json
-from normalizer import process_df
-
-
-def main():
- with open(f'H:\\Caarya\Odin\\json_files\\back_end_developer.json', encoding='utf-8') as file:
- raw_data = json.load(file)
- with open(f'H:\\Caarya\Odin\\json_files\\tag_color.json', encoding='utf-8') as file2:
- tags_data = json.load(file2)
- df_list = list()
- for data in raw_data:
- df_list.append(process_df(data))
- return df_list, tags_data
-
-
-if __name__ == "__main__":
- main()
diff --git a/spaces/kangvcar/RealChar/alembic/versions/3821f7adaca9_add_session_id.py b/spaces/kangvcar/RealChar/alembic/versions/3821f7adaca9_add_session_id.py
deleted file mode 100644
index 9c0e8310a2c3131a17ac29bc1724af1a33718006..0000000000000000000000000000000000000000
--- a/spaces/kangvcar/RealChar/alembic/versions/3821f7adaca9_add_session_id.py
+++ /dev/null
@@ -1,25 +0,0 @@
-"""Add session ID
-
-Revision ID: 3821f7adaca9
-Revises: 27fe156a6d72
-Create Date: 2023-07-18 22:44:33.107380
-
-"""
-from alembic import op
-import sqlalchemy as sa
-
-
-# revision identifiers, used by Alembic.
-revision = '3821f7adaca9'
-down_revision = '27fe156a6d72'
-branch_labels = None
-depends_on = None
-
-
-def upgrade() -> None:
- op.add_column('interactions', sa.Column(
- 'session_id', sa.String(50), nullable=True))
-
-
-def downgrade() -> None:
- op.drop_column('interactions', 'session_id')
diff --git a/spaces/kevinwang676/Bert-VITS2/losses.py b/spaces/kevinwang676/Bert-VITS2/losses.py
deleted file mode 100644
index fb22a0e834dd87edaa37bb8190eee2c3c7abe0d5..0000000000000000000000000000000000000000
--- a/spaces/kevinwang676/Bert-VITS2/losses.py
+++ /dev/null
@@ -1,61 +0,0 @@
-import torch
-from torch.nn import functional as F
-
-import commons
-
-
-def feature_loss(fmap_r, fmap_g):
- loss = 0
- for dr, dg in zip(fmap_r, fmap_g):
- for rl, gl in zip(dr, dg):
- rl = rl.float().detach()
- gl = gl.float()
- loss += torch.mean(torch.abs(rl - gl))
-
- return loss * 2
-
-
-def discriminator_loss(disc_real_outputs, disc_generated_outputs):
- loss = 0
- r_losses = []
- g_losses = []
- for dr, dg in zip(disc_real_outputs, disc_generated_outputs):
- dr = dr.float()
- dg = dg.float()
- r_loss = torch.mean((1-dr)**2)
- g_loss = torch.mean(dg**2)
- loss += (r_loss + g_loss)
- r_losses.append(r_loss.item())
- g_losses.append(g_loss.item())
-
- return loss, r_losses, g_losses
-
-
-def generator_loss(disc_outputs):
- loss = 0
- gen_losses = []
- for dg in disc_outputs:
- dg = dg.float()
- l = torch.mean((1-dg)**2)
- gen_losses.append(l)
- loss += l
-
- return loss, gen_losses
-
-
-def kl_loss(z_p, logs_q, m_p, logs_p, z_mask):
- """
- z_p, logs_q: [b, h, t_t]
- m_p, logs_p: [b, h, t_t]
- """
- z_p = z_p.float()
- logs_q = logs_q.float()
- m_p = m_p.float()
- logs_p = logs_p.float()
- z_mask = z_mask.float()
-
- kl = logs_p - logs_q - 0.5
- kl += 0.5 * ((z_p - m_p)**2) * torch.exp(-2. * logs_p)
- kl = torch.sum(kl * z_mask)
- l = kl / torch.sum(z_mask)
- return l
diff --git a/spaces/kevinwang676/ChatGLM2-SadTalker/src/facerender/modules/mapping.py b/spaces/kevinwang676/ChatGLM2-SadTalker/src/facerender/modules/mapping.py
deleted file mode 100644
index 0e3a1c2d1770996080c08e9daafb346f05d7bcdd..0000000000000000000000000000000000000000
--- a/spaces/kevinwang676/ChatGLM2-SadTalker/src/facerender/modules/mapping.py
+++ /dev/null
@@ -1,47 +0,0 @@
-import numpy as np
-
-import torch
-import torch.nn as nn
-import torch.nn.functional as F
-
-
-class MappingNet(nn.Module):
- def __init__(self, coeff_nc, descriptor_nc, layer, num_kp, num_bins):
- super( MappingNet, self).__init__()
-
- self.layer = layer
- nonlinearity = nn.LeakyReLU(0.1)
-
- self.first = nn.Sequential(
- torch.nn.Conv1d(coeff_nc, descriptor_nc, kernel_size=7, padding=0, bias=True))
-
- for i in range(layer):
- net = nn.Sequential(nonlinearity,
- torch.nn.Conv1d(descriptor_nc, descriptor_nc, kernel_size=3, padding=0, dilation=3))
- setattr(self, 'encoder' + str(i), net)
-
- self.pooling = nn.AdaptiveAvgPool1d(1)
- self.output_nc = descriptor_nc
-
- self.fc_roll = nn.Linear(descriptor_nc, num_bins)
- self.fc_pitch = nn.Linear(descriptor_nc, num_bins)
- self.fc_yaw = nn.Linear(descriptor_nc, num_bins)
- self.fc_t = nn.Linear(descriptor_nc, 3)
- self.fc_exp = nn.Linear(descriptor_nc, 3*num_kp)
-
- def forward(self, input_3dmm):
- out = self.first(input_3dmm)
- for i in range(self.layer):
- model = getattr(self, 'encoder' + str(i))
- out = model(out) + out[:,:,3:-3]
- out = self.pooling(out)
- out = out.view(out.shape[0], -1)
- #print('out:', out.shape)
-
- yaw = self.fc_yaw(out)
- pitch = self.fc_pitch(out)
- roll = self.fc_roll(out)
- t = self.fc_t(out)
- exp = self.fc_exp(out)
-
- return {'yaw': yaw, 'pitch': pitch, 'roll': roll, 't': t, 'exp': exp}
\ No newline at end of file
diff --git a/spaces/kevinwang676/VoiceChanger/src/face3d/models/arcface_torch/backbones/iresnet2060.py b/spaces/kevinwang676/VoiceChanger/src/face3d/models/arcface_torch/backbones/iresnet2060.py
deleted file mode 100644
index 21d1122144d207637d2444cba1f68fe630c89f31..0000000000000000000000000000000000000000
--- a/spaces/kevinwang676/VoiceChanger/src/face3d/models/arcface_torch/backbones/iresnet2060.py
+++ /dev/null
@@ -1,176 +0,0 @@
-import torch
-from torch import nn
-
-assert torch.__version__ >= "1.8.1"
-from torch.utils.checkpoint import checkpoint_sequential
-
-__all__ = ['iresnet2060']
-
-
-def conv3x3(in_planes, out_planes, stride=1, groups=1, dilation=1):
- """3x3 convolution with padding"""
- return nn.Conv2d(in_planes,
- out_planes,
- kernel_size=3,
- stride=stride,
- padding=dilation,
- groups=groups,
- bias=False,
- dilation=dilation)
-
-
-def conv1x1(in_planes, out_planes, stride=1):
- """1x1 convolution"""
- return nn.Conv2d(in_planes,
- out_planes,
- kernel_size=1,
- stride=stride,
- bias=False)
-
-
-class IBasicBlock(nn.Module):
- expansion = 1
-
- def __init__(self, inplanes, planes, stride=1, downsample=None,
- groups=1, base_width=64, dilation=1):
- super(IBasicBlock, self).__init__()
- if groups != 1 or base_width != 64:
- raise ValueError('BasicBlock only supports groups=1 and base_width=64')
- if dilation > 1:
- raise NotImplementedError("Dilation > 1 not supported in BasicBlock")
- self.bn1 = nn.BatchNorm2d(inplanes, eps=1e-05, )
- self.conv1 = conv3x3(inplanes, planes)
- self.bn2 = nn.BatchNorm2d(planes, eps=1e-05, )
- self.prelu = nn.PReLU(planes)
- self.conv2 = conv3x3(planes, planes, stride)
- self.bn3 = nn.BatchNorm2d(planes, eps=1e-05, )
- self.downsample = downsample
- self.stride = stride
-
- def forward(self, x):
- identity = x
- out = self.bn1(x)
- out = self.conv1(out)
- out = self.bn2(out)
- out = self.prelu(out)
- out = self.conv2(out)
- out = self.bn3(out)
- if self.downsample is not None:
- identity = self.downsample(x)
- out += identity
- return out
-
-
-class IResNet(nn.Module):
- fc_scale = 7 * 7
-
- def __init__(self,
- block, layers, dropout=0, num_features=512, zero_init_residual=False,
- groups=1, width_per_group=64, replace_stride_with_dilation=None, fp16=False):
- super(IResNet, self).__init__()
- self.fp16 = fp16
- self.inplanes = 64
- self.dilation = 1
- if replace_stride_with_dilation is None:
- replace_stride_with_dilation = [False, False, False]
- if len(replace_stride_with_dilation) != 3:
- raise ValueError("replace_stride_with_dilation should be None "
- "or a 3-element tuple, got {}".format(replace_stride_with_dilation))
- self.groups = groups
- self.base_width = width_per_group
- self.conv1 = nn.Conv2d(3, self.inplanes, kernel_size=3, stride=1, padding=1, bias=False)
- self.bn1 = nn.BatchNorm2d(self.inplanes, eps=1e-05)
- self.prelu = nn.PReLU(self.inplanes)
- self.layer1 = self._make_layer(block, 64, layers[0], stride=2)
- self.layer2 = self._make_layer(block,
- 128,
- layers[1],
- stride=2,
- dilate=replace_stride_with_dilation[0])
- self.layer3 = self._make_layer(block,
- 256,
- layers[2],
- stride=2,
- dilate=replace_stride_with_dilation[1])
- self.layer4 = self._make_layer(block,
- 512,
- layers[3],
- stride=2,
- dilate=replace_stride_with_dilation[2])
- self.bn2 = nn.BatchNorm2d(512 * block.expansion, eps=1e-05, )
- self.dropout = nn.Dropout(p=dropout, inplace=True)
- self.fc = nn.Linear(512 * block.expansion * self.fc_scale, num_features)
- self.features = nn.BatchNorm1d(num_features, eps=1e-05)
- nn.init.constant_(self.features.weight, 1.0)
- self.features.weight.requires_grad = False
-
- for m in self.modules():
- if isinstance(m, nn.Conv2d):
- nn.init.normal_(m.weight, 0, 0.1)
- elif isinstance(m, (nn.BatchNorm2d, nn.GroupNorm)):
- nn.init.constant_(m.weight, 1)
- nn.init.constant_(m.bias, 0)
-
- if zero_init_residual:
- for m in self.modules():
- if isinstance(m, IBasicBlock):
- nn.init.constant_(m.bn2.weight, 0)
-
- def _make_layer(self, block, planes, blocks, stride=1, dilate=False):
- downsample = None
- previous_dilation = self.dilation
- if dilate:
- self.dilation *= stride
- stride = 1
- if stride != 1 or self.inplanes != planes * block.expansion:
- downsample = nn.Sequential(
- conv1x1(self.inplanes, planes * block.expansion, stride),
- nn.BatchNorm2d(planes * block.expansion, eps=1e-05, ),
- )
- layers = []
- layers.append(
- block(self.inplanes, planes, stride, downsample, self.groups,
- self.base_width, previous_dilation))
- self.inplanes = planes * block.expansion
- for _ in range(1, blocks):
- layers.append(
- block(self.inplanes,
- planes,
- groups=self.groups,
- base_width=self.base_width,
- dilation=self.dilation))
-
- return nn.Sequential(*layers)
-
- def checkpoint(self, func, num_seg, x):
- if self.training:
- return checkpoint_sequential(func, num_seg, x)
- else:
- return func(x)
-
- def forward(self, x):
- with torch.cuda.amp.autocast(self.fp16):
- x = self.conv1(x)
- x = self.bn1(x)
- x = self.prelu(x)
- x = self.layer1(x)
- x = self.checkpoint(self.layer2, 20, x)
- x = self.checkpoint(self.layer3, 100, x)
- x = self.layer4(x)
- x = self.bn2(x)
- x = torch.flatten(x, 1)
- x = self.dropout(x)
- x = self.fc(x.float() if self.fp16 else x)
- x = self.features(x)
- return x
-
-
-def _iresnet(arch, block, layers, pretrained, progress, **kwargs):
- model = IResNet(block, layers, **kwargs)
- if pretrained:
- raise ValueError()
- return model
-
-
-def iresnet2060(pretrained=False, progress=True, **kwargs):
- return _iresnet('iresnet2060', IBasicBlock, [3, 128, 1024 - 128, 3], pretrained, progress, **kwargs)
diff --git a/spaces/kidcoconut/spcdkr_omdenasaudi_liverhccxai/scripts/streamlitFastApi/util_local_runStreamlitFastApi.sh b/spaces/kidcoconut/spcdkr_omdenasaudi_liverhccxai/scripts/streamlitFastApi/util_local_runStreamlitFastApi.sh
deleted file mode 100644
index 1ca04084d5507acb9ba5ff1da01727666f343378..0000000000000000000000000000000000000000
--- a/spaces/kidcoconut/spcdkr_omdenasaudi_liverhccxai/scripts/streamlitFastApi/util_local_runStreamlitFastApi.sh
+++ /dev/null
@@ -1,27 +0,0 @@
-#!/bin/bash
-
-#--- Note: this file is designed to run locally and within docker to prep the environment
-#--- Entry: this script is assumed to run from the /app root folder
-#--- Usage: ./scripts/util_local_runStreamlitFastApi.sh
-echo -e "INFO(util_local_runStreamlitFastApi):\t Initializing ..."
-
-#--- for volume initialization; ensure folders are in place; assume: we are in the /app folder
-mkdir -p data/demo_tiles/raw
-mkdir -p data/tiles/raw data/tiles/pred data/tiles/grad_bg data/tiles/grad_wt data/tiles/grad_vt
-mkdir -p data/wsi/raw
-
-#--- for streamlit; external 49400; internal 39400
-echo "INFO: starting streamlit ..."
-streamlit run app.py --server.port=49400 --server.maxUploadSize=2000 &
-
-#--- for fastapi; external 49500; internal 39500
-echo "INFO: starting fastapi ..."
-
-#--- uvicorn main:app --reload --workers 1 --host 0.0.0.0 --port 39500 &
-uvicorn main:app --reload --workers 1 --host 0.0.0.0 --port 49500
-
-#--- wait for any process to exit
-#wait -n
-
-#--- Exit with status of process that exited first
-#exit $?
\ No newline at end of file
diff --git a/spaces/king007/google-flan-t5-test/README.md b/spaces/king007/google-flan-t5-test/README.md
deleted file mode 100644
index 72342271e31f543941c3a58068a6a5d7b12576e0..0000000000000000000000000000000000000000
--- a/spaces/king007/google-flan-t5-test/README.md
+++ /dev/null
@@ -1,11 +0,0 @@
----
-title: Flan Playground
-emoji: 🍮
-colorFrom: green
-colorTo: green
-sdk: gradio
-sdk_version: 3.2
-app_file: app.py
-pinned: true
-duplicated_from: impira/flan-playground
----
diff --git a/spaces/kirch/Text2Video-Zero/annotator/uniformer/mmseg/models/decode_heads/apc_head.py b/spaces/kirch/Text2Video-Zero/annotator/uniformer/mmseg/models/decode_heads/apc_head.py
deleted file mode 100644
index c7038bdbe0edf2a1f184b6899486d2d190dda076..0000000000000000000000000000000000000000
--- a/spaces/kirch/Text2Video-Zero/annotator/uniformer/mmseg/models/decode_heads/apc_head.py
+++ /dev/null
@@ -1,158 +0,0 @@
-import torch
-import torch.nn as nn
-import torch.nn.functional as F
-from annotator.uniformer.mmcv.cnn import ConvModule
-
-from annotator.uniformer.mmseg.ops import resize
-from ..builder import HEADS
-from .decode_head import BaseDecodeHead
-
-
-class ACM(nn.Module):
- """Adaptive Context Module used in APCNet.
-
- Args:
- pool_scale (int): Pooling scale used in Adaptive Context
- Module to extract region features.
- fusion (bool): Add one conv to fuse residual feature.
- in_channels (int): Input channels.
- channels (int): Channels after modules, before conv_seg.
- conv_cfg (dict | None): Config of conv layers.
- norm_cfg (dict | None): Config of norm layers.
- act_cfg (dict): Config of activation layers.
- """
-
- def __init__(self, pool_scale, fusion, in_channels, channels, conv_cfg,
- norm_cfg, act_cfg):
- super(ACM, self).__init__()
- self.pool_scale = pool_scale
- self.fusion = fusion
- self.in_channels = in_channels
- self.channels = channels
- self.conv_cfg = conv_cfg
- self.norm_cfg = norm_cfg
- self.act_cfg = act_cfg
- self.pooled_redu_conv = ConvModule(
- self.in_channels,
- self.channels,
- 1,
- conv_cfg=self.conv_cfg,
- norm_cfg=self.norm_cfg,
- act_cfg=self.act_cfg)
-
- self.input_redu_conv = ConvModule(
- self.in_channels,
- self.channels,
- 1,
- conv_cfg=self.conv_cfg,
- norm_cfg=self.norm_cfg,
- act_cfg=self.act_cfg)
-
- self.global_info = ConvModule(
- self.channels,
- self.channels,
- 1,
- conv_cfg=self.conv_cfg,
- norm_cfg=self.norm_cfg,
- act_cfg=self.act_cfg)
-
- self.gla = nn.Conv2d(self.channels, self.pool_scale**2, 1, 1, 0)
-
- self.residual_conv = ConvModule(
- self.channels,
- self.channels,
- 1,
- conv_cfg=self.conv_cfg,
- norm_cfg=self.norm_cfg,
- act_cfg=self.act_cfg)
-
- if self.fusion:
- self.fusion_conv = ConvModule(
- self.channels,
- self.channels,
- 1,
- conv_cfg=self.conv_cfg,
- norm_cfg=self.norm_cfg,
- act_cfg=self.act_cfg)
-
- def forward(self, x):
- """Forward function."""
- pooled_x = F.adaptive_avg_pool2d(x, self.pool_scale)
- # [batch_size, channels, h, w]
- x = self.input_redu_conv(x)
- # [batch_size, channels, pool_scale, pool_scale]
- pooled_x = self.pooled_redu_conv(pooled_x)
- batch_size = x.size(0)
- # [batch_size, pool_scale * pool_scale, channels]
- pooled_x = pooled_x.view(batch_size, self.channels,
- -1).permute(0, 2, 1).contiguous()
- # [batch_size, h * w, pool_scale * pool_scale]
- affinity_matrix = self.gla(x + resize(
- self.global_info(F.adaptive_avg_pool2d(x, 1)), size=x.shape[2:])
- ).permute(0, 2, 3, 1).reshape(
- batch_size, -1, self.pool_scale**2)
- affinity_matrix = F.sigmoid(affinity_matrix)
- # [batch_size, h * w, channels]
- z_out = torch.matmul(affinity_matrix, pooled_x)
- # [batch_size, channels, h * w]
- z_out = z_out.permute(0, 2, 1).contiguous()
- # [batch_size, channels, h, w]
- z_out = z_out.view(batch_size, self.channels, x.size(2), x.size(3))
- z_out = self.residual_conv(z_out)
- z_out = F.relu(z_out + x)
- if self.fusion:
- z_out = self.fusion_conv(z_out)
-
- return z_out
-
-
-@HEADS.register_module()
-class APCHead(BaseDecodeHead):
- """Adaptive Pyramid Context Network for Semantic Segmentation.
-
- This head is the implementation of
- `APCNet `_.
-
- Args:
- pool_scales (tuple[int]): Pooling scales used in Adaptive Context
- Module. Default: (1, 2, 3, 6).
- fusion (bool): Add one conv to fuse residual feature.
- """
-
- def __init__(self, pool_scales=(1, 2, 3, 6), fusion=True, **kwargs):
- super(APCHead, self).__init__(**kwargs)
- assert isinstance(pool_scales, (list, tuple))
- self.pool_scales = pool_scales
- self.fusion = fusion
- acm_modules = []
- for pool_scale in self.pool_scales:
- acm_modules.append(
- ACM(pool_scale,
- self.fusion,
- self.in_channels,
- self.channels,
- conv_cfg=self.conv_cfg,
- norm_cfg=self.norm_cfg,
- act_cfg=self.act_cfg))
- self.acm_modules = nn.ModuleList(acm_modules)
- self.bottleneck = ConvModule(
- self.in_channels + len(pool_scales) * self.channels,
- self.channels,
- 3,
- padding=1,
- conv_cfg=self.conv_cfg,
- norm_cfg=self.norm_cfg,
- act_cfg=self.act_cfg)
-
- def forward(self, inputs):
- """Forward function."""
- x = self._transform_inputs(inputs)
- acm_outs = [x]
- for acm_module in self.acm_modules:
- acm_outs.append(acm_module(x))
- acm_outs = torch.cat(acm_outs, dim=1)
- output = self.bottleneck(acm_outs)
- output = self.cls_seg(output)
- return output
diff --git a/spaces/kmahtan2/AIPairProgramming2/README.md b/spaces/kmahtan2/AIPairProgramming2/README.md
deleted file mode 100644
index b725340df0f024d4070f5129e116fa25b96b5c9f..0000000000000000000000000000000000000000
--- a/spaces/kmahtan2/AIPairProgramming2/README.md
+++ /dev/null
@@ -1,13 +0,0 @@
----
-title: AIPairProgramming2
-emoji: 🔥
-colorFrom: blue
-colorTo: pink
-sdk: streamlit
-sdk_version: 1.21.0
-app_file: app.py
-pinned: false
-license: mit
----
-
-Check out the configuration reference at https://huggingface.co/docs/hub/spaces-config-reference
diff --git a/spaces/koajoel/PolyFormer/fairseq/examples/language_model/README.md b/spaces/koajoel/PolyFormer/fairseq/examples/language_model/README.md
deleted file mode 100644
index e78ea48e08dc99b69751923762107a8f8a9a5e3e..0000000000000000000000000000000000000000
--- a/spaces/koajoel/PolyFormer/fairseq/examples/language_model/README.md
+++ /dev/null
@@ -1,123 +0,0 @@
-# Neural Language Modeling
-
-## Pre-trained models
-
-Model | Description | Dataset | Download
----|---|---|---
-`transformer_lm.gbw.adaptive_huge` | Adaptive Inputs ([Baevski and Auli, 2018](https://arxiv.org/abs/1809.10853)) 1026M params | [Google Billion Words](https://github.com/ciprian-chelba/1-billion-word-language-modeling-benchmark) | [download (.tar.bz2)](https://dl.fbaipublicfiles.com/fairseq/models/lm/adaptive_lm_gbw_huge.tar.bz2)
-`transformer_lm.wiki103.adaptive` | Adaptive Inputs ([Baevski and Auli, 2018](https://arxiv.org/abs/1809.10853)) 247M params | [WikiText-103](https://blog.einstein.ai/the-wikitext-long-term-dependency-language-modeling-dataset) | [download (.tar.bz2)](https://dl.fbaipublicfiles.com/fairseq/models/lm/adaptive_lm_wiki103.v2.tar.bz2)
-`transformer_lm.wmt19.en` | English LM ([Ng et al., 2019](https://arxiv.org/abs/1907.06616)) | [WMT News Crawl](http://data.statmt.org/news-crawl/) | [download (.tar.gz)](https://dl.fbaipublicfiles.com/fairseq/models/lm/wmt19.en.tar.gz)
-`transformer_lm.wmt19.de` | German LM ([Ng et al., 2019](https://arxiv.org/abs/1907.06616)) | [WMT News Crawl](http://data.statmt.org/news-crawl/) | [download (.tar.gz)](https://dl.fbaipublicfiles.com/fairseq/models/lm/wmt19.de.tar.gz)
-`transformer_lm.wmt19.ru` | Russian LM ([Ng et al., 2019](https://arxiv.org/abs/1907.06616)) | [WMT News Crawl](http://data.statmt.org/news-crawl/) | [download (.tar.gz)](https://dl.fbaipublicfiles.com/fairseq/models/lm/wmt19.ru.tar.gz)
-
-## Example usage
-
-We require a few additional Python dependencies for preprocessing:
-```bash
-pip install fastBPE sacremoses
-```
-
-To sample from a language model using PyTorch Hub:
-```python
-import torch
-
-# List available models
-torch.hub.list('pytorch/fairseq') # [..., 'transformer_lm.wmt19.en', ...]
-
-# Load an English LM trained on WMT'19 News Crawl data
-en_lm = torch.hub.load('pytorch/fairseq', 'transformer_lm.wmt19.en', tokenizer='moses', bpe='fastbpe')
-en_lm.eval() # disable dropout
-
-# Move model to GPU
-en_lm.cuda()
-
-# Sample from the language model
-en_lm.sample('Barack Obama', beam=1, sampling=True, sampling_topk=10, temperature=0.8)
-# "Barack Obama is coming to Sydney and New Zealand (...)"
-
-# Compute perplexity for a sequence
-en_lm.score('Barack Obama is coming to Sydney and New Zealand')['positional_scores'].mean().neg().exp()
-# tensor(15.1474)
-
-# The same interface can be used with custom models as well
-from fairseq.models.transformer_lm import TransformerLanguageModel
-custom_lm = TransformerLanguageModel.from_pretrained('/path/to/model/dir', 'checkpoint100.pt', tokenizer='moses', bpe='fastbpe')
-custom_lm.sample('Barack Obama', beam=5)
-# "Barack Obama (...)"
-```
-
-## Training a transformer language model with the CLI tools
-
-### 1) Preprocess the data
-
-First download and prepare the [WikiText-103 dataset](https://www.salesforce.com/products/einstein/ai-research/the-wikitext-dependency-language-modeling-dataset/):
-```bash
-cd examples/language_model/
-bash prepare-wikitext-103.sh
-cd ../..
-```
-
-Next preprocess/binarize the data:
-```bash
-TEXT=examples/language_model/wikitext-103
-fairseq-preprocess \
- --only-source \
- --trainpref $TEXT/wiki.train.tokens \
- --validpref $TEXT/wiki.valid.tokens \
- --testpref $TEXT/wiki.test.tokens \
- --destdir data-bin/wikitext-103 \
- --workers 20
-```
-
-### 2) Train a language model
-
-Next we'll train a basic transformer language model on wikitext-103. For more
-advanced usage, see the [adaptive inputs README](README.adaptive_inputs.md).
-
-To train a basic LM (assumes 2 GPUs):
-```
-$ fairseq-train --task language_modeling \
- data-bin/wikitext-103 \
- --save-dir checkpoints/transformer_wikitext-103 \
- --arch transformer_lm --share-decoder-input-output-embed \
- --dropout 0.1 \
- --optimizer adam --adam-betas '(0.9, 0.98)' --weight-decay 0.01 --clip-norm 0.0 \
- --lr 0.0005 --lr-scheduler inverse_sqrt --warmup-updates 4000 --warmup-init-lr 1e-07 \
- --tokens-per-sample 512 --sample-break-mode none \
- --max-tokens 2048 --update-freq 16 \
- --fp16 \
- --max-update 50000
-```
-
-If you run out of memory, try reducing `--max-tokens` (max number of tokens per
-batch) or `--tokens-per-sample` (max sequence length). You can also adjust
-`--update-freq` to accumulate gradients and simulate training on a different
-number of GPUs.
-
-### 3) Evaluate
-
-```bash
-fairseq-eval-lm data-bin/wikitext-103 \
- --path checkpoints/transformer_wiki103/checkpoint_best.pt \
- --batch-size 2 \
- --tokens-per-sample 512 \
- --context-window 400
-# | Evaluated 245569 tokens in 56.1s (4379.02 tokens/s)
-# | Loss: 3.4164, Perplexity: 30.46
-```
-
-*Note:* The `--context-window` option controls how much context is provided to
-each token when computing perplexity. When the window size is 0, the dataset is
-chunked into segments of length 512 and perplexity is computed over each segment
-normally. However, this results in worse (higher) perplexity since tokens that
-appear earlier in each segment have less conditioning. When the maximum window
-size is used (511 in this case), then we compute perplexity for each token
-fully conditioned on 511 tokens of context. This slows down evaluation
-significantly, since we must run a separate forward pass for every token in the
-dataset, but results in better (lower) perplexity.
-
-
-## Convolutional language models
-
-Please see the [convolutional LM README](README.conv.md) for instructions on
-training convolutional language models.
diff --git a/spaces/koby-Jason/Music_recommend/info.md b/spaces/koby-Jason/Music_recommend/info.md
deleted file mode 100644
index 70eb95e5cc238f4c174543bf407bb4270cf4fe49..0000000000000000000000000000000000000000
--- a/spaces/koby-Jason/Music_recommend/info.md
+++ /dev/null
@@ -1,8 +0,0 @@
-# 😌 [The best music recommend system you can have ]
-
-### 🧐 Problem Statement and Research Summary
-[To let you lesiten to the music best fit to you!]
-
-
-
-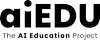
diff --git a/spaces/ky2k/Toxicity_Classifier_POC/.venv/lib/python3.9/site-packages/fontTools/ttLib/sfnt.py b/spaces/ky2k/Toxicity_Classifier_POC/.venv/lib/python3.9/site-packages/fontTools/ttLib/sfnt.py
deleted file mode 100644
index 0923ffd3942dda30013dcbe9940c0069f7c10692..0000000000000000000000000000000000000000
--- a/spaces/ky2k/Toxicity_Classifier_POC/.venv/lib/python3.9/site-packages/fontTools/ttLib/sfnt.py
+++ /dev/null
@@ -1,664 +0,0 @@
-"""ttLib/sfnt.py -- low-level module to deal with the sfnt file format.
-
-Defines two public classes:
- SFNTReader
- SFNTWriter
-
-(Normally you don't have to use these classes explicitly; they are
-used automatically by ttLib.TTFont.)
-
-The reading and writing of sfnt files is separated in two distinct
-classes, since whenever the number of tables changes or whenever
-a table's length changes you need to rewrite the whole file anyway.
-"""
-
-from io import BytesIO
-from types import SimpleNamespace
-from fontTools.misc.textTools import Tag
-from fontTools.misc import sstruct
-from fontTools.ttLib import TTLibError, TTLibFileIsCollectionError
-import struct
-from collections import OrderedDict
-import logging
-
-
-log = logging.getLogger(__name__)
-
-
-class SFNTReader(object):
- def __new__(cls, *args, **kwargs):
- """Return an instance of the SFNTReader sub-class which is compatible
- with the input file type.
- """
- if args and cls is SFNTReader:
- infile = args[0]
- infile.seek(0)
- sfntVersion = Tag(infile.read(4))
- infile.seek(0)
- if sfntVersion == "wOF2":
- # return new WOFF2Reader object
- from fontTools.ttLib.woff2 import WOFF2Reader
-
- return object.__new__(WOFF2Reader)
- # return default object
- return object.__new__(cls)
-
- def __init__(self, file, checkChecksums=0, fontNumber=-1):
- self.file = file
- self.checkChecksums = checkChecksums
-
- self.flavor = None
- self.flavorData = None
- self.DirectoryEntry = SFNTDirectoryEntry
- self.file.seek(0)
- self.sfntVersion = self.file.read(4)
- self.file.seek(0)
- if self.sfntVersion == b"ttcf":
- header = readTTCHeader(self.file)
- numFonts = header.numFonts
- if not 0 <= fontNumber < numFonts:
- raise TTLibFileIsCollectionError(
- "specify a font number between 0 and %d (inclusive)"
- % (numFonts - 1)
- )
- self.numFonts = numFonts
- self.file.seek(header.offsetTable[fontNumber])
- data = self.file.read(sfntDirectorySize)
- if len(data) != sfntDirectorySize:
- raise TTLibError("Not a Font Collection (not enough data)")
- sstruct.unpack(sfntDirectoryFormat, data, self)
- elif self.sfntVersion == b"wOFF":
- self.flavor = "woff"
- self.DirectoryEntry = WOFFDirectoryEntry
- data = self.file.read(woffDirectorySize)
- if len(data) != woffDirectorySize:
- raise TTLibError("Not a WOFF font (not enough data)")
- sstruct.unpack(woffDirectoryFormat, data, self)
- else:
- data = self.file.read(sfntDirectorySize)
- if len(data) != sfntDirectorySize:
- raise TTLibError("Not a TrueType or OpenType font (not enough data)")
- sstruct.unpack(sfntDirectoryFormat, data, self)
- self.sfntVersion = Tag(self.sfntVersion)
-
- if self.sfntVersion not in ("\x00\x01\x00\x00", "OTTO", "true"):
- raise TTLibError("Not a TrueType or OpenType font (bad sfntVersion)")
- tables = {}
- for i in range(self.numTables):
- entry = self.DirectoryEntry()
- entry.fromFile(self.file)
- tag = Tag(entry.tag)
- tables[tag] = entry
- self.tables = OrderedDict(sorted(tables.items(), key=lambda i: i[1].offset))
-
- # Load flavor data if any
- if self.flavor == "woff":
- self.flavorData = WOFFFlavorData(self)
-
- def has_key(self, tag):
- return tag in self.tables
-
- __contains__ = has_key
-
- def keys(self):
- return self.tables.keys()
-
- def __getitem__(self, tag):
- """Fetch the raw table data."""
- entry = self.tables[Tag(tag)]
- data = entry.loadData(self.file)
- if self.checkChecksums:
- if tag == "head":
- # Beh: we have to special-case the 'head' table.
- checksum = calcChecksum(data[:8] + b"\0\0\0\0" + data[12:])
- else:
- checksum = calcChecksum(data)
- if self.checkChecksums > 1:
- # Be obnoxious, and barf when it's wrong
- assert checksum == entry.checkSum, "bad checksum for '%s' table" % tag
- elif checksum != entry.checkSum:
- # Be friendly, and just log a warning.
- log.warning("bad checksum for '%s' table", tag)
- return data
-
- def __delitem__(self, tag):
- del self.tables[Tag(tag)]
-
- def close(self):
- self.file.close()
-
- # We define custom __getstate__ and __setstate__ to make SFNTReader pickle-able
- # and deepcopy-able. When a TTFont is loaded as lazy=True, SFNTReader holds a
- # reference to an external file object which is not pickleable. So in __getstate__
- # we store the file name and current position, and in __setstate__ we reopen the
- # same named file after unpickling.
-
- def __getstate__(self):
- if isinstance(self.file, BytesIO):
- # BytesIO is already pickleable, return the state unmodified
- return self.__dict__
-
- # remove unpickleable file attribute, and only store its name and pos
- state = self.__dict__.copy()
- del state["file"]
- state["_filename"] = self.file.name
- state["_filepos"] = self.file.tell()
- return state
-
- def __setstate__(self, state):
- if "file" not in state:
- self.file = open(state.pop("_filename"), "rb")
- self.file.seek(state.pop("_filepos"))
- self.__dict__.update(state)
-
-
-# default compression level for WOFF 1.0 tables and metadata
-ZLIB_COMPRESSION_LEVEL = 6
-
-# if set to True, use zopfli instead of zlib for compressing WOFF 1.0.
-# The Python bindings are available at https://pypi.python.org/pypi/zopfli
-USE_ZOPFLI = False
-
-# mapping between zlib's compression levels and zopfli's 'numiterations'.
-# Use lower values for files over several MB in size or it will be too slow
-ZOPFLI_LEVELS = {
- # 0: 0, # can't do 0 iterations...
- 1: 1,
- 2: 3,
- 3: 5,
- 4: 8,
- 5: 10,
- 6: 15,
- 7: 25,
- 8: 50,
- 9: 100,
-}
-
-
-def compress(data, level=ZLIB_COMPRESSION_LEVEL):
- """Compress 'data' to Zlib format. If 'USE_ZOPFLI' variable is True,
- zopfli is used instead of the zlib module.
- The compression 'level' must be between 0 and 9. 1 gives best speed,
- 9 gives best compression (0 gives no compression at all).
- The default value is a compromise between speed and compression (6).
- """
- if not (0 <= level <= 9):
- raise ValueError("Bad compression level: %s" % level)
- if not USE_ZOPFLI or level == 0:
- from zlib import compress
-
- return compress(data, level)
- else:
- from zopfli.zlib import compress
-
- return compress(data, numiterations=ZOPFLI_LEVELS[level])
-
-
-class SFNTWriter(object):
- def __new__(cls, *args, **kwargs):
- """Return an instance of the SFNTWriter sub-class which is compatible
- with the specified 'flavor'.
- """
- flavor = None
- if kwargs and "flavor" in kwargs:
- flavor = kwargs["flavor"]
- elif args and len(args) > 3:
- flavor = args[3]
- if cls is SFNTWriter:
- if flavor == "woff2":
- # return new WOFF2Writer object
- from fontTools.ttLib.woff2 import WOFF2Writer
-
- return object.__new__(WOFF2Writer)
- # return default object
- return object.__new__(cls)
-
- def __init__(
- self,
- file,
- numTables,
- sfntVersion="\000\001\000\000",
- flavor=None,
- flavorData=None,
- ):
- self.file = file
- self.numTables = numTables
- self.sfntVersion = Tag(sfntVersion)
- self.flavor = flavor
- self.flavorData = flavorData
-
- if self.flavor == "woff":
- self.directoryFormat = woffDirectoryFormat
- self.directorySize = woffDirectorySize
- self.DirectoryEntry = WOFFDirectoryEntry
-
- self.signature = "wOFF"
-
- # to calculate WOFF checksum adjustment, we also need the original SFNT offsets
- self.origNextTableOffset = (
- sfntDirectorySize + numTables * sfntDirectoryEntrySize
- )
- else:
- assert not self.flavor, "Unknown flavor '%s'" % self.flavor
- self.directoryFormat = sfntDirectoryFormat
- self.directorySize = sfntDirectorySize
- self.DirectoryEntry = SFNTDirectoryEntry
-
- from fontTools.ttLib import getSearchRange
-
- self.searchRange, self.entrySelector, self.rangeShift = getSearchRange(
- numTables, 16
- )
-
- self.directoryOffset = self.file.tell()
- self.nextTableOffset = (
- self.directoryOffset
- + self.directorySize
- + numTables * self.DirectoryEntry.formatSize
- )
- # clear out directory area
- self.file.seek(self.nextTableOffset)
- # make sure we're actually where we want to be. (old cStringIO bug)
- self.file.write(b"\0" * (self.nextTableOffset - self.file.tell()))
- self.tables = OrderedDict()
-
- def setEntry(self, tag, entry):
- if tag in self.tables:
- raise TTLibError("cannot rewrite '%s' table" % tag)
-
- self.tables[tag] = entry
-
- def __setitem__(self, tag, data):
- """Write raw table data to disk."""
- if tag in self.tables:
- raise TTLibError("cannot rewrite '%s' table" % tag)
-
- entry = self.DirectoryEntry()
- entry.tag = tag
- entry.offset = self.nextTableOffset
- if tag == "head":
- entry.checkSum = calcChecksum(data[:8] + b"\0\0\0\0" + data[12:])
- self.headTable = data
- entry.uncompressed = True
- else:
- entry.checkSum = calcChecksum(data)
- entry.saveData(self.file, data)
-
- if self.flavor == "woff":
- entry.origOffset = self.origNextTableOffset
- self.origNextTableOffset += (entry.origLength + 3) & ~3
-
- self.nextTableOffset = self.nextTableOffset + ((entry.length + 3) & ~3)
- # Add NUL bytes to pad the table data to a 4-byte boundary.
- # Don't depend on f.seek() as we need to add the padding even if no
- # subsequent write follows (seek is lazy), ie. after the final table
- # in the font.
- self.file.write(b"\0" * (self.nextTableOffset - self.file.tell()))
- assert self.nextTableOffset == self.file.tell()
-
- self.setEntry(tag, entry)
-
- def __getitem__(self, tag):
- return self.tables[tag]
-
- def close(self):
- """All tables must have been written to disk. Now write the
- directory.
- """
- tables = sorted(self.tables.items())
- if len(tables) != self.numTables:
- raise TTLibError(
- "wrong number of tables; expected %d, found %d"
- % (self.numTables, len(tables))
- )
-
- if self.flavor == "woff":
- self.signature = b"wOFF"
- self.reserved = 0
-
- self.totalSfntSize = 12
- self.totalSfntSize += 16 * len(tables)
- for tag, entry in tables:
- self.totalSfntSize += (entry.origLength + 3) & ~3
-
- data = self.flavorData if self.flavorData else WOFFFlavorData()
- if data.majorVersion is not None and data.minorVersion is not None:
- self.majorVersion = data.majorVersion
- self.minorVersion = data.minorVersion
- else:
- if hasattr(self, "headTable"):
- self.majorVersion, self.minorVersion = struct.unpack(
- ">HH", self.headTable[4:8]
- )
- else:
- self.majorVersion = self.minorVersion = 0
- if data.metaData:
- self.metaOrigLength = len(data.metaData)
- self.file.seek(0, 2)
- self.metaOffset = self.file.tell()
- compressedMetaData = compress(data.metaData)
- self.metaLength = len(compressedMetaData)
- self.file.write(compressedMetaData)
- else:
- self.metaOffset = self.metaLength = self.metaOrigLength = 0
- if data.privData:
- self.file.seek(0, 2)
- off = self.file.tell()
- paddedOff = (off + 3) & ~3
- self.file.write("\0" * (paddedOff - off))
- self.privOffset = self.file.tell()
- self.privLength = len(data.privData)
- self.file.write(data.privData)
- else:
- self.privOffset = self.privLength = 0
-
- self.file.seek(0, 2)
- self.length = self.file.tell()
-
- else:
- assert not self.flavor, "Unknown flavor '%s'" % self.flavor
- pass
-
- directory = sstruct.pack(self.directoryFormat, self)
-
- self.file.seek(self.directoryOffset + self.directorySize)
- seenHead = 0
- for tag, entry in tables:
- if tag == "head":
- seenHead = 1
- directory = directory + entry.toString()
- if seenHead:
- self.writeMasterChecksum(directory)
- self.file.seek(self.directoryOffset)
- self.file.write(directory)
-
- def _calcMasterChecksum(self, directory):
- # calculate checkSumAdjustment
- tags = list(self.tables.keys())
- checksums = []
- for i in range(len(tags)):
- checksums.append(self.tables[tags[i]].checkSum)
-
- if self.DirectoryEntry != SFNTDirectoryEntry:
- # Create a SFNT directory for checksum calculation purposes
- from fontTools.ttLib import getSearchRange
-
- self.searchRange, self.entrySelector, self.rangeShift = getSearchRange(
- self.numTables, 16
- )
- directory = sstruct.pack(sfntDirectoryFormat, self)
- tables = sorted(self.tables.items())
- for tag, entry in tables:
- sfntEntry = SFNTDirectoryEntry()
- sfntEntry.tag = entry.tag
- sfntEntry.checkSum = entry.checkSum
- sfntEntry.offset = entry.origOffset
- sfntEntry.length = entry.origLength
- directory = directory + sfntEntry.toString()
-
- directory_end = sfntDirectorySize + len(self.tables) * sfntDirectoryEntrySize
- assert directory_end == len(directory)
-
- checksums.append(calcChecksum(directory))
- checksum = sum(checksums) & 0xFFFFFFFF
- # BiboAfba!
- checksumadjustment = (0xB1B0AFBA - checksum) & 0xFFFFFFFF
- return checksumadjustment
-
- def writeMasterChecksum(self, directory):
- checksumadjustment = self._calcMasterChecksum(directory)
- # write the checksum to the file
- self.file.seek(self.tables["head"].offset + 8)
- self.file.write(struct.pack(">L", checksumadjustment))
-
- def reordersTables(self):
- return False
-
-
-# -- sfnt directory helpers and cruft
-
-ttcHeaderFormat = """
- > # big endian
- TTCTag: 4s # "ttcf"
- Version: L # 0x00010000 or 0x00020000
- numFonts: L # number of fonts
- # OffsetTable[numFonts]: L # array with offsets from beginning of file
- # ulDsigTag: L # version 2.0 only
- # ulDsigLength: L # version 2.0 only
- # ulDsigOffset: L # version 2.0 only
-"""
-
-ttcHeaderSize = sstruct.calcsize(ttcHeaderFormat)
-
-sfntDirectoryFormat = """
- > # big endian
- sfntVersion: 4s
- numTables: H # number of tables
- searchRange: H # (max2 <= numTables)*16
- entrySelector: H # log2(max2 <= numTables)
- rangeShift: H # numTables*16-searchRange
-"""
-
-sfntDirectorySize = sstruct.calcsize(sfntDirectoryFormat)
-
-sfntDirectoryEntryFormat = """
- > # big endian
- tag: 4s
- checkSum: L
- offset: L
- length: L
-"""
-
-sfntDirectoryEntrySize = sstruct.calcsize(sfntDirectoryEntryFormat)
-
-woffDirectoryFormat = """
- > # big endian
- signature: 4s # "wOFF"
- sfntVersion: 4s
- length: L # total woff file size
- numTables: H # number of tables
- reserved: H # set to 0
- totalSfntSize: L # uncompressed size
- majorVersion: H # major version of WOFF file
- minorVersion: H # minor version of WOFF file
- metaOffset: L # offset to metadata block
- metaLength: L # length of compressed metadata
- metaOrigLength: L # length of uncompressed metadata
- privOffset: L # offset to private data block
- privLength: L # length of private data block
-"""
-
-woffDirectorySize = sstruct.calcsize(woffDirectoryFormat)
-
-woffDirectoryEntryFormat = """
- > # big endian
- tag: 4s
- offset: L
- length: L # compressed length
- origLength: L # original length
- checkSum: L # original checksum
-"""
-
-woffDirectoryEntrySize = sstruct.calcsize(woffDirectoryEntryFormat)
-
-
-class DirectoryEntry(object):
- def __init__(self):
- self.uncompressed = False # if True, always embed entry raw
-
- def fromFile(self, file):
- sstruct.unpack(self.format, file.read(self.formatSize), self)
-
- def fromString(self, str):
- sstruct.unpack(self.format, str, self)
-
- def toString(self):
- return sstruct.pack(self.format, self)
-
- def __repr__(self):
- if hasattr(self, "tag"):
- return "<%s '%s' at %x>" % (self.__class__.__name__, self.tag, id(self))
- else:
- return "<%s at %x>" % (self.__class__.__name__, id(self))
-
- def loadData(self, file):
- file.seek(self.offset)
- data = file.read(self.length)
- assert len(data) == self.length
- if hasattr(self.__class__, "decodeData"):
- data = self.decodeData(data)
- return data
-
- def saveData(self, file, data):
- if hasattr(self.__class__, "encodeData"):
- data = self.encodeData(data)
- self.length = len(data)
- file.seek(self.offset)
- file.write(data)
-
- def decodeData(self, rawData):
- return rawData
-
- def encodeData(self, data):
- return data
-
-
-class SFNTDirectoryEntry(DirectoryEntry):
-
- format = sfntDirectoryEntryFormat
- formatSize = sfntDirectoryEntrySize
-
-
-class WOFFDirectoryEntry(DirectoryEntry):
-
- format = woffDirectoryEntryFormat
- formatSize = woffDirectoryEntrySize
-
- def __init__(self):
- super(WOFFDirectoryEntry, self).__init__()
- # With fonttools<=3.1.2, the only way to set a different zlib
- # compression level for WOFF directory entries was to set the class
- # attribute 'zlibCompressionLevel'. This is now replaced by a globally
- # defined `ZLIB_COMPRESSION_LEVEL`, which is also applied when
- # compressing the metadata. For backward compatibility, we still
- # use the class attribute if it was already set.
- if not hasattr(WOFFDirectoryEntry, "zlibCompressionLevel"):
- self.zlibCompressionLevel = ZLIB_COMPRESSION_LEVEL
-
- def decodeData(self, rawData):
- import zlib
-
- if self.length == self.origLength:
- data = rawData
- else:
- assert self.length < self.origLength
- data = zlib.decompress(rawData)
- assert len(data) == self.origLength
- return data
-
- def encodeData(self, data):
- self.origLength = len(data)
- if not self.uncompressed:
- compressedData = compress(data, self.zlibCompressionLevel)
- if self.uncompressed or len(compressedData) >= self.origLength:
- # Encode uncompressed
- rawData = data
- self.length = self.origLength
- else:
- rawData = compressedData
- self.length = len(rawData)
- return rawData
-
-
-class WOFFFlavorData:
-
- Flavor = "woff"
-
- def __init__(self, reader=None):
- self.majorVersion = None
- self.minorVersion = None
- self.metaData = None
- self.privData = None
- if reader:
- self.majorVersion = reader.majorVersion
- self.minorVersion = reader.minorVersion
- if reader.metaLength:
- reader.file.seek(reader.metaOffset)
- rawData = reader.file.read(reader.metaLength)
- assert len(rawData) == reader.metaLength
- data = self._decompress(rawData)
- assert len(data) == reader.metaOrigLength
- self.metaData = data
- if reader.privLength:
- reader.file.seek(reader.privOffset)
- data = reader.file.read(reader.privLength)
- assert len(data) == reader.privLength
- self.privData = data
-
- def _decompress(self, rawData):
- import zlib
-
- return zlib.decompress(rawData)
-
-
-def calcChecksum(data):
- """Calculate the checksum for an arbitrary block of data.
-
- If the data length is not a multiple of four, it assumes
- it is to be padded with null byte.
-
- >>> print(calcChecksum(b"abcd"))
- 1633837924
- >>> print(calcChecksum(b"abcdxyz"))
- 3655064932
- """
- remainder = len(data) % 4
- if remainder:
- data += b"\0" * (4 - remainder)
- value = 0
- blockSize = 4096
- assert blockSize % 4 == 0
- for i in range(0, len(data), blockSize):
- block = data[i : i + blockSize]
- longs = struct.unpack(">%dL" % (len(block) // 4), block)
- value = (value + sum(longs)) & 0xFFFFFFFF
- return value
-
-
-def readTTCHeader(file):
- file.seek(0)
- data = file.read(ttcHeaderSize)
- if len(data) != ttcHeaderSize:
- raise TTLibError("Not a Font Collection (not enough data)")
- self = SimpleNamespace()
- sstruct.unpack(ttcHeaderFormat, data, self)
- if self.TTCTag != "ttcf":
- raise TTLibError("Not a Font Collection")
- assert self.Version == 0x00010000 or self.Version == 0x00020000, (
- "unrecognized TTC version 0x%08x" % self.Version
- )
- self.offsetTable = struct.unpack(
- ">%dL" % self.numFonts, file.read(self.numFonts * 4)
- )
- if self.Version == 0x00020000:
- pass # ignoring version 2.0 signatures
- return self
-
-
-def writeTTCHeader(file, numFonts):
- self = SimpleNamespace()
- self.TTCTag = "ttcf"
- self.Version = 0x00010000
- self.numFonts = numFonts
- file.seek(0)
- file.write(sstruct.pack(ttcHeaderFormat, self))
- offset = file.tell()
- file.write(struct.pack(">%dL" % self.numFonts, *([0] * self.numFonts)))
- return offset
-
-
-if __name__ == "__main__":
- import sys
- import doctest
-
- sys.exit(doctest.testmod().failed)
diff --git a/spaces/ky2k/Toxicity_Classifier_POC/.venv/lib/python3.9/site-packages/gradio/interpretation.py b/spaces/ky2k/Toxicity_Classifier_POC/.venv/lib/python3.9/site-packages/gradio/interpretation.py
deleted file mode 100644
index 767ad641b99a51c08b4efadec350c7170bdc734b..0000000000000000000000000000000000000000
--- a/spaces/ky2k/Toxicity_Classifier_POC/.venv/lib/python3.9/site-packages/gradio/interpretation.py
+++ /dev/null
@@ -1,328 +0,0 @@
-"""Contains classes and methods related to interpretation for components in Gradio."""
-
-from __future__ import annotations
-
-import copy
-import math
-from abc import ABC, abstractmethod
-from typing import TYPE_CHECKING, Any
-
-import numpy as np
-from gradio_client import utils as client_utils
-
-from gradio import components
-
-if TYPE_CHECKING: # Only import for type checking (is False at runtime).
- from gradio import Interface
-
-
-class Interpretable(ABC): # noqa: B024
- def __init__(self) -> None:
- self.set_interpret_parameters()
-
- def set_interpret_parameters(self): # noqa: B027
- """
- Set any parameters for interpretation. Properties can be set here to be
- used in get_interpretation_neighbors and get_interpretation_scores.
- """
- pass
-
- def get_interpretation_scores(
- self, x: Any, neighbors: list[Any] | None, scores: list[float], **kwargs
- ) -> list:
- """
- Arrange the output values from the neighbors into interpretation scores for the interface to render.
- Parameters:
- x: Input to interface
- neighbors: Neighboring values to input x used for interpretation.
- scores: Output value corresponding to each neighbor in neighbors
- Returns:
- Arrangement of interpretation scores for interfaces to render.
- """
- return scores
-
-
-class TokenInterpretable(Interpretable, ABC):
- @abstractmethod
- def tokenize(self, x: Any) -> tuple[list, list, None]:
- """
- Interprets an input data point x by splitting it into a list of tokens (e.g
- a string into words or an image into super-pixels).
- """
- return [], [], None
-
- @abstractmethod
- def get_masked_inputs(self, tokens: list, binary_mask_matrix: list[list]) -> list:
- return []
-
-
-class NeighborInterpretable(Interpretable, ABC):
- @abstractmethod
- def get_interpretation_neighbors(self, x: Any) -> tuple[list, dict]:
- """
- Generates values similar to input to be used to interpret the significance of the input in the final output.
- Parameters:
- x: Input to interface
- Returns: (neighbor_values, interpret_kwargs, interpret_by_removal)
- neighbor_values: Neighboring values to input x to compute for interpretation
- interpret_kwargs: Keyword arguments to be passed to get_interpretation_scores
- """
- return [], {}
-
-
-async def run_interpret(interface: Interface, raw_input: list):
- """
- Runs the interpretation command for the machine learning model. Handles both the "default" out-of-the-box
- interpretation for a certain set of UI component types, as well as the custom interpretation case.
- Parameters:
- raw_input: a list of raw inputs to apply the interpretation(s) on.
- """
- if isinstance(interface.interpretation, list): # Either "default" or "shap"
- processed_input = [
- input_component.preprocess(raw_input[i])
- for i, input_component in enumerate(interface.input_components)
- ]
- original_output = await interface.call_function(0, processed_input)
- original_output = original_output["prediction"]
-
- if len(interface.output_components) == 1:
- original_output = [original_output]
-
- scores, alternative_outputs = [], []
-
- for i, (x, interp) in enumerate(zip(raw_input, interface.interpretation)):
- if interp == "default":
- input_component = interface.input_components[i]
- neighbor_raw_input = list(raw_input)
- if isinstance(input_component, TokenInterpretable):
- tokens, neighbor_values, masks = input_component.tokenize(x)
- interface_scores = []
- alternative_output = []
- for neighbor_input in neighbor_values:
- neighbor_raw_input[i] = neighbor_input
- processed_neighbor_input = [
- input_component.preprocess(neighbor_raw_input[i])
- for i, input_component in enumerate(
- interface.input_components
- )
- ]
-
- neighbor_output = await interface.call_function(
- 0, processed_neighbor_input
- )
- neighbor_output = neighbor_output["prediction"]
- if len(interface.output_components) == 1:
- neighbor_output = [neighbor_output]
- processed_neighbor_output = [
- output_component.postprocess(neighbor_output[i])
- for i, output_component in enumerate(
- interface.output_components
- )
- ]
-
- alternative_output.append(processed_neighbor_output)
- interface_scores.append(
- quantify_difference_in_label(
- interface, original_output, neighbor_output
- )
- )
- alternative_outputs.append(alternative_output)
- scores.append(
- input_component.get_interpretation_scores(
- raw_input[i],
- neighbor_values,
- interface_scores,
- masks=masks,
- tokens=tokens,
- )
- )
- elif isinstance(input_component, NeighborInterpretable):
- (
- neighbor_values,
- interpret_kwargs,
- ) = input_component.get_interpretation_neighbors(
- x
- ) # type: ignore
- interface_scores = []
- alternative_output = []
- for neighbor_input in neighbor_values:
- neighbor_raw_input[i] = neighbor_input
- processed_neighbor_input = [
- input_component.preprocess(neighbor_raw_input[i])
- for i, input_component in enumerate(
- interface.input_components
- )
- ]
- neighbor_output = await interface.call_function(
- 0, processed_neighbor_input
- )
- neighbor_output = neighbor_output["prediction"]
- if len(interface.output_components) == 1:
- neighbor_output = [neighbor_output]
- processed_neighbor_output = [
- output_component.postprocess(neighbor_output[i])
- for i, output_component in enumerate(
- interface.output_components
- )
- ]
-
- alternative_output.append(processed_neighbor_output)
- interface_scores.append(
- quantify_difference_in_label(
- interface, original_output, neighbor_output
- )
- )
- alternative_outputs.append(alternative_output)
- interface_scores = [-score for score in interface_scores]
- scores.append(
- input_component.get_interpretation_scores(
- raw_input[i],
- neighbor_values,
- interface_scores,
- **interpret_kwargs,
- )
- )
- else:
- raise ValueError(
- f"Component {input_component} does not support interpretation"
- )
- elif interp == "shap" or interp == "shapley":
- try:
- import shap # type: ignore
- except (ImportError, ModuleNotFoundError) as err:
- raise ValueError(
- "The package `shap` is required for this interpretation method. Try: `pip install shap`"
- ) from err
- input_component = interface.input_components[i]
- if not isinstance(input_component, TokenInterpretable):
- raise ValueError(
- f"Input component {input_component} does not support `shap` interpretation"
- )
-
- tokens, _, masks = input_component.tokenize(x)
-
- # construct a masked version of the input
- def get_masked_prediction(binary_mask):
- assert isinstance(input_component, TokenInterpretable)
- masked_xs = input_component.get_masked_inputs(tokens, binary_mask)
- preds = []
- for masked_x in masked_xs:
- processed_masked_input = copy.deepcopy(processed_input)
- processed_masked_input[i] = input_component.preprocess(masked_x)
- new_output = client_utils.synchronize_async(
- interface.call_function, 0, processed_masked_input
- )
- new_output = new_output["prediction"]
- if len(interface.output_components) == 1:
- new_output = [new_output]
- pred = get_regression_or_classification_value(
- interface, original_output, new_output
- )
- preds.append(pred)
- return np.array(preds)
-
- num_total_segments = len(tokens)
- explainer = shap.KernelExplainer(
- get_masked_prediction, np.zeros((1, num_total_segments))
- )
- shap_values = explainer.shap_values(
- np.ones((1, num_total_segments)),
- nsamples=int(interface.num_shap * num_total_segments),
- silent=True,
- )
- assert shap_values is not None, "SHAP values could not be calculated"
- scores.append(
- input_component.get_interpretation_scores(
- raw_input[i],
- None,
- shap_values[0].tolist(),
- masks=masks,
- tokens=tokens,
- )
- )
- alternative_outputs.append([])
- elif interp is None:
- scores.append(None)
- alternative_outputs.append([])
- else:
- raise ValueError(f"Unknown interpretation method: {interp}")
- return scores, alternative_outputs
- elif interface.interpretation: # custom interpretation function
- processed_input = [
- input_component.preprocess(raw_input[i])
- for i, input_component in enumerate(interface.input_components)
- ]
- interpreter = interface.interpretation
- interpretation = interpreter(*processed_input)
- if len(raw_input) == 1:
- interpretation = [interpretation]
- return interpretation, []
- else:
- raise ValueError("No interpretation method specified.")
-
-
-def diff(original: Any, perturbed: Any) -> int | float:
- try: # try computing numerical difference
- score = float(original) - float(perturbed)
- except ValueError: # otherwise, look at strict difference in label
- score = int(original != perturbed)
- return score
-
-
-def quantify_difference_in_label(
- interface: Interface, original_output: list, perturbed_output: list
-) -> int | float:
- output_component = interface.output_components[0]
- post_original_output = output_component.postprocess(original_output[0])
- post_perturbed_output = output_component.postprocess(perturbed_output[0])
-
- if isinstance(output_component, components.Label):
- original_label = post_original_output["label"]
- perturbed_label = post_perturbed_output["label"]
-
- # Handle different return types of Label interface
- if "confidences" in post_original_output:
- original_confidence = original_output[0][original_label]
- perturbed_confidence = perturbed_output[0][original_label]
- score = original_confidence - perturbed_confidence
- else:
- score = diff(original_label, perturbed_label)
- return score
-
- elif isinstance(output_component, components.Number):
- score = diff(post_original_output, post_perturbed_output)
- return score
-
- else:
- raise ValueError(
- f"This interpretation method doesn't support the Output component: {output_component}"
- )
-
-
-def get_regression_or_classification_value(
- interface: Interface, original_output: list, perturbed_output: list
-) -> int | float:
- """Used to combine regression/classification for Shap interpretation method."""
- output_component = interface.output_components[0]
- post_original_output = output_component.postprocess(original_output[0])
- post_perturbed_output = output_component.postprocess(perturbed_output[0])
-
- if isinstance(output_component, components.Label):
- original_label = post_original_output["label"]
- perturbed_label = post_perturbed_output["label"]
-
- # Handle different return types of Label interface
- if "confidences" in post_original_output:
- if math.isnan(perturbed_output[0][original_label]):
- return 0
- return perturbed_output[0][original_label]
- else:
- score = diff(
- perturbed_label, original_label
- ) # Intentionally inverted order of arguments.
- return score
-
- else:
- raise ValueError(
- f"This interpretation method doesn't support the Output component: {output_component}"
- )
diff --git a/spaces/ky2k/Toxicity_Classifier_POC/.venv/lib/python3.9/site-packages/gradio/templates/frontend/assets/index-d3896e81.js b/spaces/ky2k/Toxicity_Classifier_POC/.venv/lib/python3.9/site-packages/gradio/templates/frontend/assets/index-d3896e81.js
deleted file mode 100644
index 6de2dc04c5d884dd25a9406a47ac9109cf74eacb..0000000000000000000000000000000000000000
--- a/spaces/ky2k/Toxicity_Classifier_POC/.venv/lib/python3.9/site-packages/gradio/templates/frontend/assets/index-d3896e81.js
+++ /dev/null
@@ -1,2 +0,0 @@
-import{C as e}from"./Column-da0cdf3b.js";import"./index-8c3da1d9.js";/* empty css */const m=["static"];export{e as Component,m as modes};
-//# sourceMappingURL=index-d3896e81.js.map
diff --git a/spaces/ky2k/Toxicity_Classifier_POC/.venv/lib/python3.9/site-packages/matplotlib/axes/_base.py b/spaces/ky2k/Toxicity_Classifier_POC/.venv/lib/python3.9/site-packages/matplotlib/axes/_base.py
deleted file mode 100644
index fe1fac98eeebe89a1ee3353df5a7493febb5df98..0000000000000000000000000000000000000000
--- a/spaces/ky2k/Toxicity_Classifier_POC/.venv/lib/python3.9/site-packages/matplotlib/axes/_base.py
+++ /dev/null
@@ -1,4611 +0,0 @@
-from collections.abc import Iterable, Sequence
-from contextlib import ExitStack
-import functools
-import inspect
-import itertools
-import logging
-from numbers import Real
-from operator import attrgetter
-import types
-
-import numpy as np
-
-import matplotlib as mpl
-from matplotlib import _api, cbook, _docstring, offsetbox
-import matplotlib.artist as martist
-import matplotlib.axis as maxis
-from matplotlib.cbook import _OrderedSet, _check_1d, index_of
-import matplotlib.collections as mcoll
-import matplotlib.colors as mcolors
-import matplotlib.font_manager as font_manager
-from matplotlib.gridspec import SubplotSpec
-import matplotlib.image as mimage
-import matplotlib.lines as mlines
-import matplotlib.patches as mpatches
-from matplotlib.rcsetup import cycler, validate_axisbelow
-import matplotlib.spines as mspines
-import matplotlib.table as mtable
-import matplotlib.text as mtext
-import matplotlib.ticker as mticker
-import matplotlib.transforms as mtransforms
-
-_log = logging.getLogger(__name__)
-
-
-class _axis_method_wrapper:
- """
- Helper to generate Axes methods wrapping Axis methods.
-
- After ::
-
- get_foo = _axis_method_wrapper("xaxis", "get_bar")
-
- (in the body of a class) ``get_foo`` is a method that forwards it arguments
- to the ``get_bar`` method of the ``xaxis`` attribute, and gets its
- signature and docstring from ``Axis.get_bar``.
-
- The docstring of ``get_foo`` is built by replacing "this Axis" by "the
- {attr_name}" (i.e., "the xaxis", "the yaxis") in the wrapped method's
- dedented docstring; additional replacements can be given in *doc_sub*.
- """
-
- def __init__(self, attr_name, method_name, *, doc_sub=None):
- self.attr_name = attr_name
- self.method_name = method_name
- # Immediately put the docstring in ``self.__doc__`` so that docstring
- # manipulations within the class body work as expected.
- doc = inspect.getdoc(getattr(maxis.Axis, method_name))
- self._missing_subs = []
- if doc:
- doc_sub = {"this Axis": f"the {self.attr_name}", **(doc_sub or {})}
- for k, v in doc_sub.items():
- if k not in doc: # Delay raising error until we know qualname.
- self._missing_subs.append(k)
- doc = doc.replace(k, v)
- self.__doc__ = doc
-
- def __set_name__(self, owner, name):
- # This is called at the end of the class body as
- # ``self.__set_name__(cls, name_under_which_self_is_assigned)``; we
- # rely on that to give the wrapper the correct __name__/__qualname__.
- get_method = attrgetter(f"{self.attr_name}.{self.method_name}")
-
- def wrapper(self, *args, **kwargs):
- return get_method(self)(*args, **kwargs)
-
- wrapper.__module__ = owner.__module__
- wrapper.__name__ = name
- wrapper.__qualname__ = f"{owner.__qualname__}.{name}"
- wrapper.__doc__ = self.__doc__
- # Manually copy the signature instead of using functools.wraps because
- # displaying the Axis method source when asking for the Axes method
- # source would be confusing.
- wrapper.__signature__ = inspect.signature(
- getattr(maxis.Axis, self.method_name))
-
- if self._missing_subs:
- raise ValueError(
- "The definition of {} expected that the docstring of Axis.{} "
- "contains {!r} as substrings".format(
- wrapper.__qualname__, self.method_name,
- ", ".join(map(repr, self._missing_subs))))
-
- setattr(owner, name, wrapper)
-
-
-class _TransformedBoundsLocator:
- """
- Axes locator for `.Axes.inset_axes` and similarly positioned Axes.
-
- The locator is a callable object used in `.Axes.set_aspect` to compute the
- Axes location depending on the renderer.
- """
-
- def __init__(self, bounds, transform):
- """
- *bounds* (a ``[l, b, w, h]`` rectangle) and *transform* together
- specify the position of the inset Axes.
- """
- self._bounds = bounds
- self._transform = transform
-
- def __call__(self, ax, renderer):
- # Subtracting transSubfigure will typically rely on inverted(),
- # freezing the transform; thus, this needs to be delayed until draw
- # time as transSubfigure may otherwise change after this is evaluated.
- return mtransforms.TransformedBbox(
- mtransforms.Bbox.from_bounds(*self._bounds),
- self._transform - ax.figure.transSubfigure)
-
-
-def _process_plot_format(fmt, *, ambiguous_fmt_datakey=False):
- """
- Convert a MATLAB style color/line style format string to a (*linestyle*,
- *marker*, *color*) tuple.
-
- Example format strings include:
-
- * 'ko': black circles
- * '.b': blue dots
- * 'r--': red dashed lines
- * 'C2--': the third color in the color cycle, dashed lines
-
- The format is absolute in the sense that if a linestyle or marker is not
- defined in *fmt*, there is no line or marker. This is expressed by
- returning 'None' for the respective quantity.
-
- See Also
- --------
- matplotlib.Line2D.lineStyles, matplotlib.colors.cnames
- All possible styles and color format strings.
- """
-
- linestyle = None
- marker = None
- color = None
-
- # Is fmt just a colorspec?
- try:
- color = mcolors.to_rgba(fmt)
-
- # We need to differentiate grayscale '1.0' from tri_down marker '1'
- try:
- fmtint = str(int(fmt))
- except ValueError:
- return linestyle, marker, color # Yes
- else:
- if fmt != fmtint:
- # user definitely doesn't want tri_down marker
- return linestyle, marker, color # Yes
- else:
- # ignore converted color
- color = None
- except ValueError:
- pass # No, not just a color.
-
- errfmt = ("{!r} is neither a data key nor a valid format string ({})"
- if ambiguous_fmt_datakey else
- "{!r} is not a valid format string ({})")
-
- i = 0
- while i < len(fmt):
- c = fmt[i]
- if fmt[i:i+2] in mlines.lineStyles: # First, the two-char styles.
- if linestyle is not None:
- raise ValueError(errfmt.format(fmt, "two linestyle symbols"))
- linestyle = fmt[i:i+2]
- i += 2
- elif c in mlines.lineStyles:
- if linestyle is not None:
- raise ValueError(errfmt.format(fmt, "two linestyle symbols"))
- linestyle = c
- i += 1
- elif c in mlines.lineMarkers:
- if marker is not None:
- raise ValueError(errfmt.format(fmt, "two marker symbols"))
- marker = c
- i += 1
- elif c in mcolors.get_named_colors_mapping():
- if color is not None:
- raise ValueError(errfmt.format(fmt, "two color symbols"))
- color = c
- i += 1
- elif c == 'C' and i < len(fmt) - 1:
- color_cycle_number = int(fmt[i + 1])
- color = mcolors.to_rgba("C{}".format(color_cycle_number))
- i += 2
- else:
- raise ValueError(
- errfmt.format(fmt, f"unrecognized character {c!r}"))
-
- if linestyle is None and marker is None:
- linestyle = mpl.rcParams['lines.linestyle']
- if linestyle is None:
- linestyle = 'None'
- if marker is None:
- marker = 'None'
-
- return linestyle, marker, color
-
-
-class _process_plot_var_args:
- """
- Process variable length arguments to `~.Axes.plot`, to support ::
-
- plot(t, s)
- plot(t1, s1, t2, s2)
- plot(t1, s1, 'ko', t2, s2)
- plot(t1, s1, 'ko', t2, s2, 'r--', t3, e3)
-
- an arbitrary number of *x*, *y*, *fmt* are allowed
- """
- def __init__(self, axes, command='plot'):
- self.axes = axes
- self.command = command
- self.set_prop_cycle(None)
-
- def __getstate__(self):
- # note: it is not possible to pickle a generator (and thus a cycler).
- return {'axes': self.axes, 'command': self.command}
-
- def __setstate__(self, state):
- self.__dict__ = state.copy()
- self.set_prop_cycle(None)
-
- def set_prop_cycle(self, cycler):
- if cycler is None:
- cycler = mpl.rcParams['axes.prop_cycle']
- self.prop_cycler = itertools.cycle(cycler)
- self._prop_keys = cycler.keys # This should make a copy
-
- def __call__(self, *args, data=None, **kwargs):
- self.axes._process_unit_info(kwargs=kwargs)
-
- for pos_only in "xy":
- if pos_only in kwargs:
- raise _api.kwarg_error(self.command, pos_only)
-
- if not args:
- return
-
- if data is None: # Process dict views
- args = [cbook.sanitize_sequence(a) for a in args]
- else: # Process the 'data' kwarg.
- replaced = [mpl._replacer(data, arg) for arg in args]
- if len(args) == 1:
- label_namer_idx = 0
- elif len(args) == 2: # Can be x, y or y, c.
- # Figure out what the second argument is.
- # 1) If the second argument cannot be a format shorthand, the
- # second argument is the label_namer.
- # 2) Otherwise (it could have been a format shorthand),
- # a) if we did perform a substitution, emit a warning, and
- # use it as label_namer.
- # b) otherwise, it is indeed a format shorthand; use the
- # first argument as label_namer.
- try:
- _process_plot_format(args[1])
- except ValueError: # case 1)
- label_namer_idx = 1
- else:
- if replaced[1] is not args[1]: # case 2a)
- _api.warn_external(
- f"Second argument {args[1]!r} is ambiguous: could "
- f"be a format string but is in 'data'; using as "
- f"data. If it was intended as data, set the "
- f"format string to an empty string to suppress "
- f"this warning. If it was intended as a format "
- f"string, explicitly pass the x-values as well. "
- f"Alternatively, rename the entry in 'data'.",
- RuntimeWarning)
- label_namer_idx = 1
- else: # case 2b)
- label_namer_idx = 0
- elif len(args) == 3:
- label_namer_idx = 1
- else:
- raise ValueError(
- "Using arbitrary long args with data is not supported due "
- "to ambiguity of arguments; use multiple plotting calls "
- "instead")
- if kwargs.get("label") is None:
- kwargs["label"] = mpl._label_from_arg(
- replaced[label_namer_idx], args[label_namer_idx])
- args = replaced
- ambiguous_fmt_datakey = data is not None and len(args) == 2
-
- if len(args) >= 4 and not cbook.is_scalar_or_string(
- kwargs.get("label")):
- raise ValueError("plot() with multiple groups of data (i.e., "
- "pairs of x and y) does not support multiple "
- "labels")
-
- # Repeatedly grab (x, y) or (x, y, format) from the front of args and
- # massage them into arguments to plot() or fill().
-
- while args:
- this, args = args[:2], args[2:]
- if args and isinstance(args[0], str):
- this += args[0],
- args = args[1:]
- yield from self._plot_args(
- this, kwargs, ambiguous_fmt_datakey=ambiguous_fmt_datakey)
-
- def get_next_color(self):
- """Return the next color in the cycle."""
- if 'color' not in self._prop_keys:
- return 'k'
- return next(self.prop_cycler)['color']
-
- def _getdefaults(self, ignore, kw):
- """
- If some keys in the property cycle (excluding those in the set
- *ignore*) are absent or set to None in the dict *kw*, return a copy
- of the next entry in the property cycle, excluding keys in *ignore*.
- Otherwise, don't advance the property cycle, and return an empty dict.
- """
- prop_keys = self._prop_keys - ignore
- if any(kw.get(k, None) is None for k in prop_keys):
- # Need to copy this dictionary or else the next time around
- # in the cycle, the dictionary could be missing entries.
- default_dict = next(self.prop_cycler).copy()
- for p in ignore:
- default_dict.pop(p, None)
- else:
- default_dict = {}
- return default_dict
-
- def _setdefaults(self, defaults, kw):
- """
- Add to the dict *kw* the entries in the dict *default* that are absent
- or set to None in *kw*.
- """
- for k in defaults:
- if kw.get(k, None) is None:
- kw[k] = defaults[k]
-
- def _makeline(self, x, y, kw, kwargs):
- kw = {**kw, **kwargs} # Don't modify the original kw.
- default_dict = self._getdefaults(set(), kw)
- self._setdefaults(default_dict, kw)
- seg = mlines.Line2D(x, y, **kw)
- return seg, kw
-
- def _makefill(self, x, y, kw, kwargs):
- # Polygon doesn't directly support unitized inputs.
- x = self.axes.convert_xunits(x)
- y = self.axes.convert_yunits(y)
-
- kw = kw.copy() # Don't modify the original kw.
- kwargs = kwargs.copy()
-
- # Ignore 'marker'-related properties as they aren't Polygon
- # properties, but they are Line2D properties, and so they are
- # likely to appear in the default cycler construction.
- # This is done here to the defaults dictionary as opposed to the
- # other two dictionaries because we do want to capture when a
- # *user* explicitly specifies a marker which should be an error.
- # We also want to prevent advancing the cycler if there are no
- # defaults needed after ignoring the given properties.
- ignores = {'marker', 'markersize', 'markeredgecolor',
- 'markerfacecolor', 'markeredgewidth'}
- # Also ignore anything provided by *kwargs*.
- for k, v in kwargs.items():
- if v is not None:
- ignores.add(k)
-
- # Only using the first dictionary to use as basis
- # for getting defaults for back-compat reasons.
- # Doing it with both seems to mess things up in
- # various places (probably due to logic bugs elsewhere).
- default_dict = self._getdefaults(ignores, kw)
- self._setdefaults(default_dict, kw)
-
- # Looks like we don't want "color" to be interpreted to
- # mean both facecolor and edgecolor for some reason.
- # So the "kw" dictionary is thrown out, and only its
- # 'color' value is kept and translated as a 'facecolor'.
- # This design should probably be revisited as it increases
- # complexity.
- facecolor = kw.get('color', None)
-
- # Throw out 'color' as it is now handled as a facecolor
- default_dict.pop('color', None)
-
- # To get other properties set from the cycler
- # modify the kwargs dictionary.
- self._setdefaults(default_dict, kwargs)
-
- seg = mpatches.Polygon(np.column_stack((x, y)),
- facecolor=facecolor,
- fill=kwargs.get('fill', True),
- closed=kw['closed'])
- seg.set(**kwargs)
- return seg, kwargs
-
- def _plot_args(self, tup, kwargs, *,
- return_kwargs=False, ambiguous_fmt_datakey=False):
- """
- Process the arguments of ``plot([x], y, [fmt], **kwargs)`` calls.
-
- This processes a single set of ([x], y, [fmt]) parameters; i.e. for
- ``plot(x, y, x2, y2)`` it will be called twice. Once for (x, y) and
- once for (x2, y2).
-
- x and y may be 2D and thus can still represent multiple datasets.
-
- For multiple datasets, if the keyword argument *label* is a list, this
- will unpack the list and assign the individual labels to the datasets.
-
- Parameters
- ----------
- tup : tuple
- A tuple of the positional parameters. This can be one of
-
- - (y,)
- - (x, y)
- - (y, fmt)
- - (x, y, fmt)
-
- kwargs : dict
- The keyword arguments passed to ``plot()``.
-
- return_kwargs : bool
- Whether to also return the effective keyword arguments after label
- unpacking as well.
-
- ambiguous_fmt_datakey : bool
- Whether the format string in *tup* could also have been a
- misspelled data key.
-
- Returns
- -------
- result
- If *return_kwargs* is false, a list of Artists representing the
- dataset(s).
- If *return_kwargs* is true, a list of (Artist, effective_kwargs)
- representing the dataset(s). See *return_kwargs*.
- The Artist is either `.Line2D` (if called from ``plot()``) or
- `.Polygon` otherwise.
- """
- if len(tup) > 1 and isinstance(tup[-1], str):
- # xy is tup with fmt stripped (could still be (y,) only)
- *xy, fmt = tup
- linestyle, marker, color = _process_plot_format(
- fmt, ambiguous_fmt_datakey=ambiguous_fmt_datakey)
- elif len(tup) == 3:
- raise ValueError('third arg must be a format string')
- else:
- xy = tup
- linestyle, marker, color = None, None, None
-
- # Don't allow any None value; these would be up-converted to one
- # element array of None which causes problems downstream.
- if any(v is None for v in tup):
- raise ValueError("x, y, and format string must not be None")
-
- kw = {}
- for prop_name, val in zip(('linestyle', 'marker', 'color'),
- (linestyle, marker, color)):
- if val is not None:
- # check for conflicts between fmt and kwargs
- if (fmt.lower() != 'none'
- and prop_name in kwargs
- and val != 'None'):
- # Technically ``plot(x, y, 'o', ls='--')`` is a conflict
- # because 'o' implicitly unsets the linestyle
- # (linestyle='None').
- # We'll gracefully not warn in this case because an
- # explicit set via kwargs can be seen as intention to
- # override an implicit unset.
- # Note: We don't val.lower() != 'none' because val is not
- # necessarily a string (can be a tuple for colors). This
- # is safe, because *val* comes from _process_plot_format()
- # which only returns 'None'.
- _api.warn_external(
- f"{prop_name} is redundantly defined by the "
- f"'{prop_name}' keyword argument and the fmt string "
- f'"{fmt}" (-> {prop_name}={val!r}). The keyword '
- f"argument will take precedence.")
- kw[prop_name] = val
-
- if len(xy) == 2:
- x = _check_1d(xy[0])
- y = _check_1d(xy[1])
- else:
- x, y = index_of(xy[-1])
-
- if self.axes.xaxis is not None:
- self.axes.xaxis.update_units(x)
- if self.axes.yaxis is not None:
- self.axes.yaxis.update_units(y)
-
- if x.shape[0] != y.shape[0]:
- raise ValueError(f"x and y must have same first dimension, but "
- f"have shapes {x.shape} and {y.shape}")
- if x.ndim > 2 or y.ndim > 2:
- raise ValueError(f"x and y can be no greater than 2D, but have "
- f"shapes {x.shape} and {y.shape}")
- if x.ndim == 1:
- x = x[:, np.newaxis]
- if y.ndim == 1:
- y = y[:, np.newaxis]
-
- if self.command == 'plot':
- make_artist = self._makeline
- else:
- kw['closed'] = kwargs.get('closed', True)
- make_artist = self._makefill
-
- ncx, ncy = x.shape[1], y.shape[1]
- if ncx > 1 and ncy > 1 and ncx != ncy:
- raise ValueError(f"x has {ncx} columns but y has {ncy} columns")
- if ncx == 0 or ncy == 0:
- return []
-
- label = kwargs.get('label')
- n_datasets = max(ncx, ncy)
- if n_datasets > 1 and not cbook.is_scalar_or_string(label):
- if len(label) != n_datasets:
- raise ValueError(f"label must be scalar or have the same "
- f"length as the input data, but found "
- f"{len(label)} for {n_datasets} datasets.")
- labels = label
- else:
- labels = [label] * n_datasets
-
- result = (make_artist(x[:, j % ncx], y[:, j % ncy], kw,
- {**kwargs, 'label': label})
- for j, label in enumerate(labels))
-
- if return_kwargs:
- return list(result)
- else:
- return [l[0] for l in result]
-
-
-@_api.define_aliases({"facecolor": ["fc"]})
-class _AxesBase(martist.Artist):
- name = "rectilinear"
-
- # axis names are the prefixes for the attributes that contain the
- # respective axis; e.g. 'x' <-> self.xaxis, containing an XAxis.
- # Note that PolarAxes uses these attributes as well, so that we have
- # 'x' <-> self.xaxis, containing a ThetaAxis. In particular we do not
- # have 'theta' in _axis_names.
- # In practice, this is ('x', 'y') for all 2D Axes and ('x', 'y', 'z')
- # for Axes3D.
- _axis_names = ("x", "y")
- _shared_axes = {name: cbook.Grouper() for name in _axis_names}
- _twinned_axes = cbook.Grouper()
-
- _subclass_uses_cla = False
-
- @property
- def _axis_map(self):
- """A mapping of axis names, e.g. 'x', to `Axis` instances."""
- return {name: getattr(self, f"{name}axis")
- for name in self._axis_names}
-
- def __str__(self):
- return "{0}({1[0]:g},{1[1]:g};{1[2]:g}x{1[3]:g})".format(
- type(self).__name__, self._position.bounds)
-
- def __init__(self, fig,
- *args,
- facecolor=None, # defaults to rc axes.facecolor
- frameon=True,
- sharex=None, # use Axes instance's xaxis info
- sharey=None, # use Axes instance's yaxis info
- label='',
- xscale=None,
- yscale=None,
- box_aspect=None,
- **kwargs
- ):
- """
- Build an Axes in a figure.
-
- Parameters
- ----------
- fig : `~matplotlib.figure.Figure`
- The Axes is built in the `.Figure` *fig*.
-
- *args
- ``*args`` can be a single ``(left, bottom, width, height)``
- rectangle or a single `.Bbox`. This specifies the rectangle (in
- figure coordinates) where the Axes is positioned.
-
- ``*args`` can also consist of three numbers or a single three-digit
- number; in the latter case, the digits are considered as
- independent numbers. The numbers are interpreted as ``(nrows,
- ncols, index)``: ``(nrows, ncols)`` specifies the size of an array
- of subplots, and ``index`` is the 1-based index of the subplot
- being created. Finally, ``*args`` can also directly be a
- `.SubplotSpec` instance.
-
- sharex, sharey : `~.axes.Axes`, optional
- The x- or y-`~.matplotlib.axis` is shared with the x- or y-axis in
- the input `~.axes.Axes`.
-
- frameon : bool, default: True
- Whether the Axes frame is visible.
-
- box_aspect : float, optional
- Set a fixed aspect for the Axes box, i.e. the ratio of height to
- width. See `~.axes.Axes.set_box_aspect` for details.
-
- **kwargs
- Other optional keyword arguments:
-
- %(Axes:kwdoc)s
-
- Returns
- -------
- `~.axes.Axes`
- The new `~.axes.Axes` object.
- """
-
- super().__init__()
- if "rect" in kwargs:
- if args:
- raise TypeError(
- "'rect' cannot be used together with positional arguments")
- rect = kwargs.pop("rect")
- _api.check_isinstance((mtransforms.Bbox, Iterable), rect=rect)
- args = (rect,)
- subplotspec = None
- if len(args) == 1 and isinstance(args[0], mtransforms.Bbox):
- self._position = args[0]
- elif len(args) == 1 and np.iterable(args[0]):
- self._position = mtransforms.Bbox.from_bounds(*args[0])
- else:
- self._position = self._originalPosition = mtransforms.Bbox.unit()
- subplotspec = SubplotSpec._from_subplot_args(fig, args)
- if self._position.width < 0 or self._position.height < 0:
- raise ValueError('Width and height specified must be non-negative')
- self._originalPosition = self._position.frozen()
- self.axes = self
- self._aspect = 'auto'
- self._adjustable = 'box'
- self._anchor = 'C'
- self._stale_viewlims = {name: False for name in self._axis_names}
- self._sharex = sharex
- self._sharey = sharey
- self.set_label(label)
- self.set_figure(fig)
- # The subplotspec needs to be set after the figure (so that
- # figure-level subplotpars are taken into account), but the figure
- # needs to be set after self._position is initialized.
- if subplotspec:
- self.set_subplotspec(subplotspec)
- else:
- self._subplotspec = None
- self.set_box_aspect(box_aspect)
- self._axes_locator = None # Optionally set via update(kwargs).
-
- self._children = []
-
- # placeholder for any colorbars added that use this Axes.
- # (see colorbar.py):
- self._colorbars = []
- self.spines = mspines.Spines.from_dict(self._gen_axes_spines())
-
- # this call may differ for non-sep axes, e.g., polar
- self._init_axis()
- if facecolor is None:
- facecolor = mpl.rcParams['axes.facecolor']
- self._facecolor = facecolor
- self._frameon = frameon
- self.set_axisbelow(mpl.rcParams['axes.axisbelow'])
-
- self._rasterization_zorder = None
- self.clear()
-
- # funcs used to format x and y - fall back on major formatters
- self.fmt_xdata = None
- self.fmt_ydata = None
-
- self.set_navigate(True)
- self.set_navigate_mode(None)
-
- if xscale:
- self.set_xscale(xscale)
- if yscale:
- self.set_yscale(yscale)
-
- self._internal_update(kwargs)
-
- for name, axis in self._axis_map.items():
- axis.callbacks._connect_picklable(
- 'units', self._unit_change_handler(name))
-
- rcParams = mpl.rcParams
- self.tick_params(
- top=rcParams['xtick.top'] and rcParams['xtick.minor.top'],
- bottom=rcParams['xtick.bottom'] and rcParams['xtick.minor.bottom'],
- labeltop=(rcParams['xtick.labeltop'] and
- rcParams['xtick.minor.top']),
- labelbottom=(rcParams['xtick.labelbottom'] and
- rcParams['xtick.minor.bottom']),
- left=rcParams['ytick.left'] and rcParams['ytick.minor.left'],
- right=rcParams['ytick.right'] and rcParams['ytick.minor.right'],
- labelleft=(rcParams['ytick.labelleft'] and
- rcParams['ytick.minor.left']),
- labelright=(rcParams['ytick.labelright'] and
- rcParams['ytick.minor.right']),
- which='minor')
-
- self.tick_params(
- top=rcParams['xtick.top'] and rcParams['xtick.major.top'],
- bottom=rcParams['xtick.bottom'] and rcParams['xtick.major.bottom'],
- labeltop=(rcParams['xtick.labeltop'] and
- rcParams['xtick.major.top']),
- labelbottom=(rcParams['xtick.labelbottom'] and
- rcParams['xtick.major.bottom']),
- left=rcParams['ytick.left'] and rcParams['ytick.major.left'],
- right=rcParams['ytick.right'] and rcParams['ytick.major.right'],
- labelleft=(rcParams['ytick.labelleft'] and
- rcParams['ytick.major.left']),
- labelright=(rcParams['ytick.labelright'] and
- rcParams['ytick.major.right']),
- which='major')
-
- def __init_subclass__(cls, **kwargs):
- parent_uses_cla = super(cls, cls)._subclass_uses_cla
- if 'cla' in cls.__dict__:
- _api.warn_deprecated(
- '3.6',
- pending=True,
- message=f'Overriding `Axes.cla` in {cls.__qualname__} is '
- 'pending deprecation in %(since)s and will be fully '
- 'deprecated in favor of `Axes.clear` in the future. '
- 'Please report '
- f'this to the {cls.__module__!r} author.')
- cls._subclass_uses_cla = 'cla' in cls.__dict__ or parent_uses_cla
- super().__init_subclass__(**kwargs)
-
- def __getstate__(self):
- state = super().__getstate__()
- # Prune the sharing & twinning info to only contain the current group.
- state["_shared_axes"] = {
- name: self._shared_axes[name].get_siblings(self)
- for name in self._axis_names if self in self._shared_axes[name]}
- state["_twinned_axes"] = (self._twinned_axes.get_siblings(self)
- if self in self._twinned_axes else None)
- return state
-
- def __setstate__(self, state):
- # Merge the grouping info back into the global groupers.
- shared_axes = state.pop("_shared_axes")
- for name, shared_siblings in shared_axes.items():
- self._shared_axes[name].join(*shared_siblings)
- twinned_siblings = state.pop("_twinned_axes")
- if twinned_siblings:
- self._twinned_axes.join(*twinned_siblings)
- self.__dict__ = state
- self._stale = True
-
- def __repr__(self):
- fields = []
- if self.get_label():
- fields += [f"label={self.get_label()!r}"]
- if hasattr(self, "get_title"):
- titles = {}
- for k in ["left", "center", "right"]:
- title = self.get_title(loc=k)
- if title:
- titles[k] = title
- if titles:
- fields += [f"title={titles}"]
- for name, axis in self._axis_map.items():
- if axis.get_label() and axis.get_label().get_text():
- fields += [f"{name}label={axis.get_label().get_text()!r}"]
- return f"<{self.__class__.__name__}: " + ", ".join(fields) + ">"
-
- def get_subplotspec(self):
- """Return the `.SubplotSpec` associated with the subplot, or None."""
- return self._subplotspec
-
- def set_subplotspec(self, subplotspec):
- """Set the `.SubplotSpec`. associated with the subplot."""
- self._subplotspec = subplotspec
- self._set_position(subplotspec.get_position(self.figure))
-
- def get_gridspec(self):
- """Return the `.GridSpec` associated with the subplot, or None."""
- return self._subplotspec.get_gridspec() if self._subplotspec else None
-
- @_api.delete_parameter("3.6", "args")
- @_api.delete_parameter("3.6", "kwargs")
- def get_window_extent(self, renderer=None, *args, **kwargs):
- """
- Return the Axes bounding box in display space; *args* and *kwargs*
- are empty.
-
- This bounding box does not include the spines, ticks, ticklabels,
- or other labels. For a bounding box including these elements use
- `~matplotlib.axes.Axes.get_tightbbox`.
-
- See Also
- --------
- matplotlib.axes.Axes.get_tightbbox
- matplotlib.axis.Axis.get_tightbbox
- matplotlib.spines.Spine.get_window_extent
- """
- return self.bbox
-
- def _init_axis(self):
- # This is moved out of __init__ because non-separable axes don't use it
- self.xaxis = maxis.XAxis(self)
- self.spines.bottom.register_axis(self.xaxis)
- self.spines.top.register_axis(self.xaxis)
- self.yaxis = maxis.YAxis(self)
- self.spines.left.register_axis(self.yaxis)
- self.spines.right.register_axis(self.yaxis)
-
- def set_figure(self, fig):
- # docstring inherited
- super().set_figure(fig)
-
- self.bbox = mtransforms.TransformedBbox(self._position,
- fig.transSubfigure)
- # these will be updated later as data is added
- self.dataLim = mtransforms.Bbox.null()
- self._viewLim = mtransforms.Bbox.unit()
- self.transScale = mtransforms.TransformWrapper(
- mtransforms.IdentityTransform())
-
- self._set_lim_and_transforms()
-
- def _unstale_viewLim(self):
- # We should arrange to store this information once per share-group
- # instead of on every axis.
- need_scale = {
- name: any(ax._stale_viewlims[name]
- for ax in self._shared_axes[name].get_siblings(self))
- for name in self._axis_names}
- if any(need_scale.values()):
- for name in need_scale:
- for ax in self._shared_axes[name].get_siblings(self):
- ax._stale_viewlims[name] = False
- self.autoscale_view(**{f"scale{name}": scale
- for name, scale in need_scale.items()})
-
- @property
- def viewLim(self):
- self._unstale_viewLim()
- return self._viewLim
-
- def _request_autoscale_view(self, axis="all", tight=None):
- """
- Mark a single axis, or all of them, as stale wrt. autoscaling.
-
- No computation is performed until the next autoscaling; thus, separate
- calls to control individual axises incur negligible performance cost.
-
- Parameters
- ----------
- axis : str, default: "all"
- Either an element of ``self._axis_names``, or "all".
- tight : bool or None, default: None
- """
- axis_names = _api.check_getitem(
- {**{k: [k] for k in self._axis_names}, "all": self._axis_names},
- axis=axis)
- for name in axis_names:
- self._stale_viewlims[name] = True
- if tight is not None:
- self._tight = tight
-
- def _set_lim_and_transforms(self):
- """
- Set the *_xaxis_transform*, *_yaxis_transform*, *transScale*,
- *transData*, *transLimits* and *transAxes* transformations.
-
- .. note::
-
- This method is primarily used by rectilinear projections of the
- `~matplotlib.axes.Axes` class, and is meant to be overridden by
- new kinds of projection Axes that need different transformations
- and limits. (See `~matplotlib.projections.polar.PolarAxes` for an
- example.)
- """
- self.transAxes = mtransforms.BboxTransformTo(self.bbox)
-
- # Transforms the x and y axis separately by a scale factor.
- # It is assumed that this part will have non-linear components
- # (e.g., for a log scale).
- self.transScale = mtransforms.TransformWrapper(
- mtransforms.IdentityTransform())
-
- # An affine transformation on the data, generally to limit the
- # range of the axes
- self.transLimits = mtransforms.BboxTransformFrom(
- mtransforms.TransformedBbox(self._viewLim, self.transScale))
-
- # The parentheses are important for efficiency here -- they
- # group the last two (which are usually affines) separately
- # from the first (which, with log-scaling can be non-affine).
- self.transData = self.transScale + (self.transLimits + self.transAxes)
-
- self._xaxis_transform = mtransforms.blended_transform_factory(
- self.transData, self.transAxes)
- self._yaxis_transform = mtransforms.blended_transform_factory(
- self.transAxes, self.transData)
-
- def get_xaxis_transform(self, which='grid'):
- """
- Get the transformation used for drawing x-axis labels, ticks
- and gridlines. The x-direction is in data coordinates and the
- y-direction is in axis coordinates.
-
- .. note::
-
- This transformation is primarily used by the
- `~matplotlib.axis.Axis` class, and is meant to be
- overridden by new kinds of projections that may need to
- place axis elements in different locations.
-
- Parameters
- ----------
- which : {'grid', 'tick1', 'tick2'}
- """
- if which == 'grid':
- return self._xaxis_transform
- elif which == 'tick1':
- # for cartesian projection, this is bottom spine
- return self.spines.bottom.get_spine_transform()
- elif which == 'tick2':
- # for cartesian projection, this is top spine
- return self.spines.top.get_spine_transform()
- else:
- raise ValueError(f'unknown value for which: {which!r}')
-
- def get_xaxis_text1_transform(self, pad_points):
- """
- Returns
- -------
- transform : Transform
- The transform used for drawing x-axis labels, which will add
- *pad_points* of padding (in points) between the axis and the label.
- The x-direction is in data coordinates and the y-direction is in
- axis coordinates
- valign : {'center', 'top', 'bottom', 'baseline', 'center_baseline'}
- The text vertical alignment.
- halign : {'center', 'left', 'right'}
- The text horizontal alignment.
-
- Notes
- -----
- This transformation is primarily used by the `~matplotlib.axis.Axis`
- class, and is meant to be overridden by new kinds of projections that
- may need to place axis elements in different locations.
- """
- labels_align = mpl.rcParams["xtick.alignment"]
- return (self.get_xaxis_transform(which='tick1') +
- mtransforms.ScaledTranslation(0, -1 * pad_points / 72,
- self.figure.dpi_scale_trans),
- "top", labels_align)
-
- def get_xaxis_text2_transform(self, pad_points):
- """
- Returns
- -------
- transform : Transform
- The transform used for drawing secondary x-axis labels, which will
- add *pad_points* of padding (in points) between the axis and the
- label. The x-direction is in data coordinates and the y-direction
- is in axis coordinates
- valign : {'center', 'top', 'bottom', 'baseline', 'center_baseline'}
- The text vertical alignment.
- halign : {'center', 'left', 'right'}
- The text horizontal alignment.
-
- Notes
- -----
- This transformation is primarily used by the `~matplotlib.axis.Axis`
- class, and is meant to be overridden by new kinds of projections that
- may need to place axis elements in different locations.
- """
- labels_align = mpl.rcParams["xtick.alignment"]
- return (self.get_xaxis_transform(which='tick2') +
- mtransforms.ScaledTranslation(0, pad_points / 72,
- self.figure.dpi_scale_trans),
- "bottom", labels_align)
-
- def get_yaxis_transform(self, which='grid'):
- """
- Get the transformation used for drawing y-axis labels, ticks
- and gridlines. The x-direction is in axis coordinates and the
- y-direction is in data coordinates.
-
- .. note::
-
- This transformation is primarily used by the
- `~matplotlib.axis.Axis` class, and is meant to be
- overridden by new kinds of projections that may need to
- place axis elements in different locations.
-
- Parameters
- ----------
- which : {'grid', 'tick1', 'tick2'}
- """
- if which == 'grid':
- return self._yaxis_transform
- elif which == 'tick1':
- # for cartesian projection, this is bottom spine
- return self.spines.left.get_spine_transform()
- elif which == 'tick2':
- # for cartesian projection, this is top spine
- return self.spines.right.get_spine_transform()
- else:
- raise ValueError(f'unknown value for which: {which!r}')
-
- def get_yaxis_text1_transform(self, pad_points):
- """
- Returns
- -------
- transform : Transform
- The transform used for drawing y-axis labels, which will add
- *pad_points* of padding (in points) between the axis and the label.
- The x-direction is in axis coordinates and the y-direction is in
- data coordinates
- valign : {'center', 'top', 'bottom', 'baseline', 'center_baseline'}
- The text vertical alignment.
- halign : {'center', 'left', 'right'}
- The text horizontal alignment.
-
- Notes
- -----
- This transformation is primarily used by the `~matplotlib.axis.Axis`
- class, and is meant to be overridden by new kinds of projections that
- may need to place axis elements in different locations.
- """
- labels_align = mpl.rcParams["ytick.alignment"]
- return (self.get_yaxis_transform(which='tick1') +
- mtransforms.ScaledTranslation(-1 * pad_points / 72, 0,
- self.figure.dpi_scale_trans),
- labels_align, "right")
-
- def get_yaxis_text2_transform(self, pad_points):
- """
- Returns
- -------
- transform : Transform
- The transform used for drawing secondart y-axis labels, which will
- add *pad_points* of padding (in points) between the axis and the
- label. The x-direction is in axis coordinates and the y-direction
- is in data coordinates
- valign : {'center', 'top', 'bottom', 'baseline', 'center_baseline'}
- The text vertical alignment.
- halign : {'center', 'left', 'right'}
- The text horizontal alignment.
-
- Notes
- -----
- This transformation is primarily used by the `~matplotlib.axis.Axis`
- class, and is meant to be overridden by new kinds of projections that
- may need to place axis elements in different locations.
- """
- labels_align = mpl.rcParams["ytick.alignment"]
- return (self.get_yaxis_transform(which='tick2') +
- mtransforms.ScaledTranslation(pad_points / 72, 0,
- self.figure.dpi_scale_trans),
- labels_align, "left")
-
- def _update_transScale(self):
- self.transScale.set(
- mtransforms.blended_transform_factory(
- self.xaxis.get_transform(), self.yaxis.get_transform()))
-
- def get_position(self, original=False):
- """
- Return the position of the Axes within the figure as a `.Bbox`.
-
- Parameters
- ----------
- original : bool
- If ``True``, return the original position. Otherwise, return the
- active position. For an explanation of the positions see
- `.set_position`.
-
- Returns
- -------
- `.Bbox`
-
- """
- if original:
- return self._originalPosition.frozen()
- else:
- locator = self.get_axes_locator()
- if not locator:
- self.apply_aspect()
- return self._position.frozen()
-
- def set_position(self, pos, which='both'):
- """
- Set the Axes position.
-
- Axes have two position attributes. The 'original' position is the
- position allocated for the Axes. The 'active' position is the
- position the Axes is actually drawn at. These positions are usually
- the same unless a fixed aspect is set to the Axes. See
- `.Axes.set_aspect` for details.
-
- Parameters
- ----------
- pos : [left, bottom, width, height] or `~matplotlib.transforms.Bbox`
- The new position of the Axes in `.Figure` coordinates.
-
- which : {'both', 'active', 'original'}, default: 'both'
- Determines which position variables to change.
-
- See Also
- --------
- matplotlib.transforms.Bbox.from_bounds
- matplotlib.transforms.Bbox.from_extents
- """
- self._set_position(pos, which=which)
- # because this is being called externally to the library we
- # don't let it be in the layout.
- self.set_in_layout(False)
-
- def _set_position(self, pos, which='both'):
- """
- Private version of set_position.
-
- Call this internally to get the same functionality of `set_position`,
- but not to take the axis out of the constrained_layout hierarchy.
- """
- if not isinstance(pos, mtransforms.BboxBase):
- pos = mtransforms.Bbox.from_bounds(*pos)
- for ax in self._twinned_axes.get_siblings(self):
- if which in ('both', 'active'):
- ax._position.set(pos)
- if which in ('both', 'original'):
- ax._originalPosition.set(pos)
- self.stale = True
-
- def reset_position(self):
- """
- Reset the active position to the original position.
-
- This undoes changes to the active position (as defined in
- `.set_position`) which may have been performed to satisfy fixed-aspect
- constraints.
- """
- for ax in self._twinned_axes.get_siblings(self):
- pos = ax.get_position(original=True)
- ax.set_position(pos, which='active')
-
- def set_axes_locator(self, locator):
- """
- Set the Axes locator.
-
- Parameters
- ----------
- locator : Callable[[Axes, Renderer], Bbox]
- """
- self._axes_locator = locator
- self.stale = True
-
- def get_axes_locator(self):
- """
- Return the axes_locator.
- """
- return self._axes_locator
-
- def _set_artist_props(self, a):
- """Set the boilerplate props for artists added to Axes."""
- a.set_figure(self.figure)
- if not a.is_transform_set():
- a.set_transform(self.transData)
-
- a.axes = self
- if a.get_mouseover():
- self._mouseover_set.add(a)
-
- def _gen_axes_patch(self):
- """
- Returns
- -------
- Patch
- The patch used to draw the background of the Axes. It is also used
- as the clipping path for any data elements on the Axes.
-
- In the standard Axes, this is a rectangle, but in other projections
- it may not be.
-
- Notes
- -----
- Intended to be overridden by new projection types.
- """
- return mpatches.Rectangle((0.0, 0.0), 1.0, 1.0)
-
- def _gen_axes_spines(self, locations=None, offset=0.0, units='inches'):
- """
- Returns
- -------
- dict
- Mapping of spine names to `.Line2D` or `.Patch` instances that are
- used to draw Axes spines.
-
- In the standard Axes, spines are single line segments, but in other
- projections they may not be.
-
- Notes
- -----
- Intended to be overridden by new projection types.
- """
- return {side: mspines.Spine.linear_spine(self, side)
- for side in ['left', 'right', 'bottom', 'top']}
-
- def sharex(self, other):
- """
- Share the x-axis with *other*.
-
- This is equivalent to passing ``sharex=other`` when constructing the
- Axes, and cannot be used if the x-axis is already being shared with
- another Axes.
- """
- _api.check_isinstance(_AxesBase, other=other)
- if self._sharex is not None and other is not self._sharex:
- raise ValueError("x-axis is already shared")
- self._shared_axes["x"].join(self, other)
- self._sharex = other
- self.xaxis.major = other.xaxis.major # Ticker instances holding
- self.xaxis.minor = other.xaxis.minor # locator and formatter.
- x0, x1 = other.get_xlim()
- self.set_xlim(x0, x1, emit=False, auto=other.get_autoscalex_on())
- self.xaxis._scale = other.xaxis._scale
-
- def sharey(self, other):
- """
- Share the y-axis with *other*.
-
- This is equivalent to passing ``sharey=other`` when constructing the
- Axes, and cannot be used if the y-axis is already being shared with
- another Axes.
- """
- _api.check_isinstance(_AxesBase, other=other)
- if self._sharey is not None and other is not self._sharey:
- raise ValueError("y-axis is already shared")
- self._shared_axes["y"].join(self, other)
- self._sharey = other
- self.yaxis.major = other.yaxis.major # Ticker instances holding
- self.yaxis.minor = other.yaxis.minor # locator and formatter.
- y0, y1 = other.get_ylim()
- self.set_ylim(y0, y1, emit=False, auto=other.get_autoscaley_on())
- self.yaxis._scale = other.yaxis._scale
-
- def __clear(self):
- """Clear the Axes."""
- # The actual implementation of clear() as long as clear() has to be
- # an adapter delegating to the correct implementation.
- # The implementation can move back into clear() when the
- # deprecation on cla() subclassing expires.
-
- # stash the current visibility state
- if hasattr(self, 'patch'):
- patch_visible = self.patch.get_visible()
- else:
- patch_visible = True
-
- xaxis_visible = self.xaxis.get_visible()
- yaxis_visible = self.yaxis.get_visible()
-
- for axis in self._axis_map.values():
- axis.clear() # Also resets the scale to linear.
- for spine in self.spines.values():
- spine.clear()
-
- self.ignore_existing_data_limits = True
- self.callbacks = cbook.CallbackRegistry(
- signals=["xlim_changed", "ylim_changed", "zlim_changed"])
-
- # update the minor locator for x and y axis based on rcParams
- if mpl.rcParams['xtick.minor.visible']:
- self.xaxis.set_minor_locator(mticker.AutoMinorLocator())
- if mpl.rcParams['ytick.minor.visible']:
- self.yaxis.set_minor_locator(mticker.AutoMinorLocator())
-
- self._xmargin = mpl.rcParams['axes.xmargin']
- self._ymargin = mpl.rcParams['axes.ymargin']
- self._tight = None
- self._use_sticky_edges = True
-
- self._get_lines = _process_plot_var_args(self)
- self._get_patches_for_fill = _process_plot_var_args(self, 'fill')
-
- self._gridOn = mpl.rcParams['axes.grid']
- old_children, self._children = self._children, []
- for chld in old_children:
- chld.axes = chld.figure = None
- self._mouseover_set = _OrderedSet()
- self.child_axes = []
- self._current_image = None # strictly for pyplot via _sci, _gci
- self._projection_init = None # strictly for pyplot.subplot
- self.legend_ = None
- self.containers = []
-
- self.grid(False) # Disable grid on init to use rcParameter
- self.grid(self._gridOn, which=mpl.rcParams['axes.grid.which'],
- axis=mpl.rcParams['axes.grid.axis'])
- props = font_manager.FontProperties(
- size=mpl.rcParams['axes.titlesize'],
- weight=mpl.rcParams['axes.titleweight'])
-
- y = mpl.rcParams['axes.titley']
- if y is None:
- y = 1.0
- self._autotitlepos = True
- else:
- self._autotitlepos = False
-
- self.title = mtext.Text(
- x=0.5, y=y, text='',
- fontproperties=props,
- verticalalignment='baseline',
- horizontalalignment='center',
- )
- self._left_title = mtext.Text(
- x=0.0, y=y, text='',
- fontproperties=props.copy(),
- verticalalignment='baseline',
- horizontalalignment='left', )
- self._right_title = mtext.Text(
- x=1.0, y=y, text='',
- fontproperties=props.copy(),
- verticalalignment='baseline',
- horizontalalignment='right',
- )
- title_offset_points = mpl.rcParams['axes.titlepad']
- # refactor this out so it can be called in ax.set_title if
- # pad argument used...
- self._set_title_offset_trans(title_offset_points)
-
- for _title in (self.title, self._left_title, self._right_title):
- self._set_artist_props(_title)
-
- # The patch draws the background of the Axes. We want this to be below
- # the other artists. We use the frame to draw the edges so we are
- # setting the edgecolor to None.
- self.patch = self._gen_axes_patch()
- self.patch.set_figure(self.figure)
- self.patch.set_facecolor(self._facecolor)
- self.patch.set_edgecolor('none')
- self.patch.set_linewidth(0)
- self.patch.set_transform(self.transAxes)
-
- self.set_axis_on()
-
- self.xaxis.set_clip_path(self.patch)
- self.yaxis.set_clip_path(self.patch)
-
- self._shared_axes["x"].clean()
- self._shared_axes["y"].clean()
- if self._sharex is not None:
- self.xaxis.set_visible(xaxis_visible)
- self.patch.set_visible(patch_visible)
- if self._sharey is not None:
- self.yaxis.set_visible(yaxis_visible)
- self.patch.set_visible(patch_visible)
-
- # This comes last, as the call to _set_lim may trigger an autoscale (in
- # case of shared axes), requiring children to be already set up.
- for name, axis in self._axis_map.items():
- share = getattr(self, f"_share{name}")
- if share is not None:
- getattr(self, f"share{name}")(share)
- else:
- axis._set_scale("linear")
- axis._set_lim(0, 1, auto=True)
- self._update_transScale()
-
- self.stale = True
-
- def clear(self):
- """Clear the Axes."""
- # Act as an alias, or as the superclass implementation depending on the
- # subclass implementation.
- if self._subclass_uses_cla:
- self.cla()
- else:
- self.__clear()
-
- def cla(self):
- """Clear the Axes."""
- # Act as an alias, or as the superclass implementation depending on the
- # subclass implementation.
- if self._subclass_uses_cla:
- self.__clear()
- else:
- self.clear()
-
- class ArtistList(Sequence):
- """
- A sublist of Axes children based on their type.
-
- The type-specific children sublists were made immutable in Matplotlib
- 3.7. In the future these artist lists may be replaced by tuples. Use
- as if this is a tuple already.
- """
- def __init__(self, axes, prop_name,
- valid_types=None, invalid_types=None):
- """
- Parameters
- ----------
- axes : `~matplotlib.axes.Axes`
- The Axes from which this sublist will pull the children
- Artists.
- prop_name : str
- The property name used to access this sublist from the Axes;
- used to generate deprecation warnings.
- valid_types : list of type, optional
- A list of types that determine which children will be returned
- by this sublist. If specified, then the Artists in the sublist
- must be instances of any of these types. If unspecified, then
- any type of Artist is valid (unless limited by
- *invalid_types*.)
- invalid_types : tuple, optional
- A list of types that determine which children will *not* be
- returned by this sublist. If specified, then Artists in the
- sublist will never be an instance of these types. Otherwise, no
- types will be excluded.
- """
- self._axes = axes
- self._prop_name = prop_name
- self._type_check = lambda artist: (
- (not valid_types or isinstance(artist, valid_types)) and
- (not invalid_types or not isinstance(artist, invalid_types))
- )
-
- def __repr__(self):
- return f''
-
- def __len__(self):
- return sum(self._type_check(artist)
- for artist in self._axes._children)
-
- def __iter__(self):
- for artist in list(self._axes._children):
- if self._type_check(artist):
- yield artist
-
- def __getitem__(self, key):
- return [artist
- for artist in self._axes._children
- if self._type_check(artist)][key]
-
- def __add__(self, other):
- if isinstance(other, (list, _AxesBase.ArtistList)):
- return [*self, *other]
- if isinstance(other, (tuple, _AxesBase.ArtistList)):
- return (*self, *other)
- return NotImplemented
-
- def __radd__(self, other):
- if isinstance(other, list):
- return other + list(self)
- if isinstance(other, tuple):
- return other + tuple(self)
- return NotImplemented
-
- @property
- def artists(self):
- return self.ArtistList(self, 'artists', invalid_types=(
- mcoll.Collection, mimage.AxesImage, mlines.Line2D, mpatches.Patch,
- mtable.Table, mtext.Text))
-
- @property
- def collections(self):
- return self.ArtistList(self, 'collections',
- valid_types=mcoll.Collection)
-
- @property
- def images(self):
- return self.ArtistList(self, 'images', valid_types=mimage.AxesImage)
-
- @property
- def lines(self):
- return self.ArtistList(self, 'lines', valid_types=mlines.Line2D)
-
- @property
- def patches(self):
- return self.ArtistList(self, 'patches', valid_types=mpatches.Patch)
-
- @property
- def tables(self):
- return self.ArtistList(self, 'tables', valid_types=mtable.Table)
-
- @property
- def texts(self):
- return self.ArtistList(self, 'texts', valid_types=mtext.Text)
-
- def get_facecolor(self):
- """Get the facecolor of the Axes."""
- return self.patch.get_facecolor()
-
- def set_facecolor(self, color):
- """
- Set the facecolor of the Axes.
-
- Parameters
- ----------
- color : color
- """
- self._facecolor = color
- self.stale = True
- return self.patch.set_facecolor(color)
-
- def _set_title_offset_trans(self, title_offset_points):
- """
- Set the offset for the title either from :rc:`axes.titlepad`
- or from set_title kwarg ``pad``.
- """
- self.titleOffsetTrans = mtransforms.ScaledTranslation(
- 0.0, title_offset_points / 72,
- self.figure.dpi_scale_trans)
- for _title in (self.title, self._left_title, self._right_title):
- _title.set_transform(self.transAxes + self.titleOffsetTrans)
- _title.set_clip_box(None)
-
- def set_prop_cycle(self, *args, **kwargs):
- """
- Set the property cycle of the Axes.
-
- The property cycle controls the style properties such as color,
- marker and linestyle of future plot commands. The style properties
- of data already added to the Axes are not modified.
-
- Call signatures::
-
- set_prop_cycle(cycler)
- set_prop_cycle(label=values[, label2=values2[, ...]])
- set_prop_cycle(label, values)
-
- Form 1 sets given `~cycler.Cycler` object.
-
- Form 2 creates a `~cycler.Cycler` which cycles over one or more
- properties simultaneously and set it as the property cycle of the
- Axes. If multiple properties are given, their value lists must have
- the same length. This is just a shortcut for explicitly creating a
- cycler and passing it to the function, i.e. it's short for
- ``set_prop_cycle(cycler(label=values label2=values2, ...))``.
-
- Form 3 creates a `~cycler.Cycler` for a single property and set it
- as the property cycle of the Axes. This form exists for compatibility
- with the original `cycler.cycler` interface. Its use is discouraged
- in favor of the kwarg form, i.e. ``set_prop_cycle(label=values)``.
-
- Parameters
- ----------
- cycler : Cycler
- Set the given Cycler. *None* resets to the cycle defined by the
- current style.
-
- label : str
- The property key. Must be a valid `.Artist` property.
- For example, 'color' or 'linestyle'. Aliases are allowed,
- such as 'c' for 'color' and 'lw' for 'linewidth'.
-
- values : iterable
- Finite-length iterable of the property values. These values
- are validated and will raise a ValueError if invalid.
-
- See Also
- --------
- matplotlib.rcsetup.cycler
- Convenience function for creating validated cyclers for properties.
- cycler.cycler
- The original function for creating unvalidated cyclers.
-
- Examples
- --------
- Setting the property cycle for a single property:
-
- >>> ax.set_prop_cycle(color=['red', 'green', 'blue'])
-
- Setting the property cycle for simultaneously cycling over multiple
- properties (e.g. red circle, green plus, blue cross):
-
- >>> ax.set_prop_cycle(color=['red', 'green', 'blue'],
- ... marker=['o', '+', 'x'])
-
- """
- if args and kwargs:
- raise TypeError("Cannot supply both positional and keyword "
- "arguments to this method.")
- # Can't do `args == (None,)` as that crashes cycler.
- if len(args) == 1 and args[0] is None:
- prop_cycle = None
- else:
- prop_cycle = cycler(*args, **kwargs)
- self._get_lines.set_prop_cycle(prop_cycle)
- self._get_patches_for_fill.set_prop_cycle(prop_cycle)
-
- def get_aspect(self):
- """
- Return the aspect ratio of the axes scaling.
-
- This is either "auto" or a float giving the ratio of y/x-scale.
- """
- return self._aspect
-
- def set_aspect(self, aspect, adjustable=None, anchor=None, share=False):
- """
- Set the aspect ratio of the axes scaling, i.e. y/x-scale.
-
- Parameters
- ----------
- aspect : {'auto', 'equal'} or float
- Possible values:
-
- - 'auto': fill the position rectangle with data.
- - 'equal': same as ``aspect=1``, i.e. same scaling for x and y.
- - *float*: The displayed size of 1 unit in y-data coordinates will
- be *aspect* times the displayed size of 1 unit in x-data
- coordinates; e.g. for ``aspect=2`` a square in data coordinates
- will be rendered with a height of twice its width.
-
- adjustable : None or {'box', 'datalim'}, optional
- If not ``None``, this defines which parameter will be adjusted to
- meet the required aspect. See `.set_adjustable` for further
- details.
-
- anchor : None or str or (float, float), optional
- If not ``None``, this defines where the Axes will be drawn if there
- is extra space due to aspect constraints. The most common way
- to specify the anchor are abbreviations of cardinal directions:
-
- ===== =====================
- value description
- ===== =====================
- 'C' centered
- 'SW' lower left corner
- 'S' middle of bottom edge
- 'SE' lower right corner
- etc.
- ===== =====================
-
- See `~.Axes.set_anchor` for further details.
-
- share : bool, default: False
- If ``True``, apply the settings to all shared Axes.
-
- See Also
- --------
- matplotlib.axes.Axes.set_adjustable
- Set how the Axes adjusts to achieve the required aspect ratio.
- matplotlib.axes.Axes.set_anchor
- Set the position in case of extra space.
- """
- if cbook._str_equal(aspect, 'equal'):
- aspect = 1
- if not cbook._str_equal(aspect, 'auto'):
- aspect = float(aspect) # raise ValueError if necessary
- if aspect <= 0 or not np.isfinite(aspect):
- raise ValueError("aspect must be finite and positive ")
-
- if share:
- axes = {sibling for name in self._axis_names
- for sibling in self._shared_axes[name].get_siblings(self)}
- else:
- axes = [self]
-
- for ax in axes:
- ax._aspect = aspect
-
- if adjustable is None:
- adjustable = self._adjustable
- self.set_adjustable(adjustable, share=share) # Handle sharing.
-
- if anchor is not None:
- self.set_anchor(anchor, share=share)
- self.stale = True
-
- def get_adjustable(self):
- """
- Return whether the Axes will adjust its physical dimension ('box') or
- its data limits ('datalim') to achieve the desired aspect ratio.
-
- See Also
- --------
- matplotlib.axes.Axes.set_adjustable
- Set how the Axes adjusts to achieve the required aspect ratio.
- matplotlib.axes.Axes.set_aspect
- For a description of aspect handling.
- """
- return self._adjustable
-
- def set_adjustable(self, adjustable, share=False):
- """
- Set how the Axes adjusts to achieve the required aspect ratio.
-
- Parameters
- ----------
- adjustable : {'box', 'datalim'}
- If 'box', change the physical dimensions of the Axes.
- If 'datalim', change the ``x`` or ``y`` data limits.
-
- share : bool, default: False
- If ``True``, apply the settings to all shared Axes.
-
- See Also
- --------
- matplotlib.axes.Axes.set_aspect
- For a description of aspect handling.
-
- Notes
- -----
- Shared Axes (of which twinned Axes are a special case)
- impose restrictions on how aspect ratios can be imposed.
- For twinned Axes, use 'datalim'. For Axes that share both
- x and y, use 'box'. Otherwise, either 'datalim' or 'box'
- may be used. These limitations are partly a requirement
- to avoid over-specification, and partly a result of the
- particular implementation we are currently using, in
- which the adjustments for aspect ratios are done sequentially
- and independently on each Axes as it is drawn.
- """
- _api.check_in_list(["box", "datalim"], adjustable=adjustable)
- if share:
- axs = {sibling for name in self._axis_names
- for sibling in self._shared_axes[name].get_siblings(self)}
- else:
- axs = [self]
- if (adjustable == "datalim"
- and any(getattr(ax.get_data_ratio, "__func__", None)
- != _AxesBase.get_data_ratio
- for ax in axs)):
- # Limits adjustment by apply_aspect assumes that the axes' aspect
- # ratio can be computed from the data limits and scales.
- raise ValueError("Cannot set Axes adjustable to 'datalim' for "
- "Axes which override 'get_data_ratio'")
- for ax in axs:
- ax._adjustable = adjustable
- self.stale = True
-
- def get_box_aspect(self):
- """
- Return the Axes box aspect, i.e. the ratio of height to width.
-
- The box aspect is ``None`` (i.e. chosen depending on the available
- figure space) unless explicitly specified.
-
- See Also
- --------
- matplotlib.axes.Axes.set_box_aspect
- for a description of box aspect.
- matplotlib.axes.Axes.set_aspect
- for a description of aspect handling.
- """
- return self._box_aspect
-
- def set_box_aspect(self, aspect=None):
- """
- Set the Axes box aspect, i.e. the ratio of height to width.
-
- This defines the aspect of the Axes in figure space and is not to be
- confused with the data aspect (see `~.Axes.set_aspect`).
-
- Parameters
- ----------
- aspect : float or None
- Changes the physical dimensions of the Axes, such that the ratio
- of the Axes height to the Axes width in physical units is equal to
- *aspect*. Defining a box aspect will change the *adjustable*
- property to 'datalim' (see `~.Axes.set_adjustable`).
-
- *None* will disable a fixed box aspect so that height and width
- of the Axes are chosen independently.
-
- See Also
- --------
- matplotlib.axes.Axes.set_aspect
- for a description of aspect handling.
- """
- axs = {*self._twinned_axes.get_siblings(self),
- *self._twinned_axes.get_siblings(self)}
-
- if aspect is not None:
- aspect = float(aspect)
- # when box_aspect is set to other than ´None`,
- # adjustable must be "datalim"
- for ax in axs:
- ax.set_adjustable("datalim")
-
- for ax in axs:
- ax._box_aspect = aspect
- ax.stale = True
-
- def get_anchor(self):
- """
- Get the anchor location.
-
- See Also
- --------
- matplotlib.axes.Axes.set_anchor
- for a description of the anchor.
- matplotlib.axes.Axes.set_aspect
- for a description of aspect handling.
- """
- return self._anchor
-
- def set_anchor(self, anchor, share=False):
- """
- Define the anchor location.
-
- The actual drawing area (active position) of the Axes may be smaller
- than the Bbox (original position) when a fixed aspect is required. The
- anchor defines where the drawing area will be located within the
- available space.
-
- Parameters
- ----------
- anchor : (float, float) or {'C', 'SW', 'S', 'SE', 'E', 'NE', ...}
- Either an (*x*, *y*) pair of relative coordinates (0 is left or
- bottom, 1 is right or top), 'C' (center), or a cardinal direction
- ('SW', southwest, is bottom left, etc.). str inputs are shorthands
- for (*x*, *y*) coordinates, as shown in the following diagram::
-
- ┌─────────────────┬─────────────────┬─────────────────┐
- │ 'NW' (0.0, 1.0) │ 'N' (0.5, 1.0) │ 'NE' (1.0, 1.0) │
- ├─────────────────┼─────────────────┼─────────────────┤
- │ 'W' (0.0, 0.5) │ 'C' (0.5, 0.5) │ 'E' (1.0, 0.5) │
- ├─────────────────┼─────────────────┼─────────────────┤
- │ 'SW' (0.0, 0.0) │ 'S' (0.5, 0.0) │ 'SE' (1.0, 0.0) │
- └─────────────────┴─────────────────┴─────────────────┘
-
- share : bool, default: False
- If ``True``, apply the settings to all shared Axes.
-
- See Also
- --------
- matplotlib.axes.Axes.set_aspect
- for a description of aspect handling.
- """
- if not (anchor in mtransforms.Bbox.coefs or len(anchor) == 2):
- raise ValueError('argument must be among %s' %
- ', '.join(mtransforms.Bbox.coefs))
- if share:
- axes = {sibling for name in self._axis_names
- for sibling in self._shared_axes[name].get_siblings(self)}
- else:
- axes = [self]
- for ax in axes:
- ax._anchor = anchor
-
- self.stale = True
-
- def get_data_ratio(self):
- """
- Return the aspect ratio of the scaled data.
-
- Notes
- -----
- This method is intended to be overridden by new projection types.
- """
- txmin, txmax = self.xaxis.get_transform().transform(self.get_xbound())
- tymin, tymax = self.yaxis.get_transform().transform(self.get_ybound())
- xsize = max(abs(txmax - txmin), 1e-30)
- ysize = max(abs(tymax - tymin), 1e-30)
- return ysize / xsize
-
- def apply_aspect(self, position=None):
- """
- Adjust the Axes for a specified data aspect ratio.
-
- Depending on `.get_adjustable` this will modify either the
- Axes box (position) or the view limits. In the former case,
- `~matplotlib.axes.Axes.get_anchor` will affect the position.
-
- Parameters
- ----------
- position : None or .Bbox
- If not ``None``, this defines the position of the
- Axes within the figure as a Bbox. See `~.Axes.get_position`
- for further details.
-
- Notes
- -----
- This is called automatically when each Axes is drawn. You may need
- to call it yourself if you need to update the Axes position and/or
- view limits before the Figure is drawn.
-
- See Also
- --------
- matplotlib.axes.Axes.set_aspect
- For a description of aspect ratio handling.
- matplotlib.axes.Axes.set_adjustable
- Set how the Axes adjusts to achieve the required aspect ratio.
- matplotlib.axes.Axes.set_anchor
- Set the position in case of extra space.
- """
- if position is None:
- position = self.get_position(original=True)
-
- aspect = self.get_aspect()
-
- if aspect == 'auto' and self._box_aspect is None:
- self._set_position(position, which='active')
- return
-
- trans = self.get_figure().transSubfigure
- bb = mtransforms.Bbox.unit().transformed(trans)
- # this is the physical aspect of the panel (or figure):
- fig_aspect = bb.height / bb.width
-
- if self._adjustable == 'box':
- if self in self._twinned_axes:
- raise RuntimeError("Adjustable 'box' is not allowed in a "
- "twinned Axes; use 'datalim' instead")
- box_aspect = aspect * self.get_data_ratio()
- pb = position.frozen()
- pb1 = pb.shrunk_to_aspect(box_aspect, pb, fig_aspect)
- self._set_position(pb1.anchored(self.get_anchor(), pb), 'active')
- return
-
- # The following is only seen if self._adjustable == 'datalim'
- if self._box_aspect is not None:
- pb = position.frozen()
- pb1 = pb.shrunk_to_aspect(self._box_aspect, pb, fig_aspect)
- self._set_position(pb1.anchored(self.get_anchor(), pb), 'active')
- if aspect == "auto":
- return
-
- # reset active to original in case it had been changed by prior use
- # of 'box'
- if self._box_aspect is None:
- self._set_position(position, which='active')
- else:
- position = pb1.anchored(self.get_anchor(), pb)
-
- x_trf = self.xaxis.get_transform()
- y_trf = self.yaxis.get_transform()
- xmin, xmax = x_trf.transform(self.get_xbound())
- ymin, ymax = y_trf.transform(self.get_ybound())
- xsize = max(abs(xmax - xmin), 1e-30)
- ysize = max(abs(ymax - ymin), 1e-30)
-
- box_aspect = fig_aspect * (position.height / position.width)
- data_ratio = box_aspect / aspect
-
- y_expander = data_ratio * xsize / ysize - 1
- # If y_expander > 0, the dy/dx viewLim ratio needs to increase
- if abs(y_expander) < 0.005:
- return
-
- dL = self.dataLim
- x0, x1 = x_trf.transform(dL.intervalx)
- y0, y1 = y_trf.transform(dL.intervaly)
- xr = 1.05 * (x1 - x0)
- yr = 1.05 * (y1 - y0)
-
- xmarg = xsize - xr
- ymarg = ysize - yr
- Ysize = data_ratio * xsize
- Xsize = ysize / data_ratio
- Xmarg = Xsize - xr
- Ymarg = Ysize - yr
- # Setting these targets to, e.g., 0.05*xr does not seem to help.
- xm = 0
- ym = 0
-
- shared_x = self in self._shared_axes["x"]
- shared_y = self in self._shared_axes["y"]
-
- if shared_x and shared_y:
- raise RuntimeError("set_aspect(..., adjustable='datalim') or "
- "axis('equal') are not allowed when both axes "
- "are shared. Try set_aspect(..., "
- "adjustable='box').")
-
- # If y is shared, then we are only allowed to change x, etc.
- if shared_y:
- adjust_y = False
- else:
- if xmarg > xm and ymarg > ym:
- adjy = ((Ymarg > 0 and y_expander < 0) or
- (Xmarg < 0 and y_expander > 0))
- else:
- adjy = y_expander > 0
- adjust_y = shared_x or adjy # (Ymarg > xmarg)
-
- if adjust_y:
- yc = 0.5 * (ymin + ymax)
- y0 = yc - Ysize / 2.0
- y1 = yc + Ysize / 2.0
- self.set_ybound(y_trf.inverted().transform([y0, y1]))
- else:
- xc = 0.5 * (xmin + xmax)
- x0 = xc - Xsize / 2.0
- x1 = xc + Xsize / 2.0
- self.set_xbound(x_trf.inverted().transform([x0, x1]))
-
- def axis(self, arg=None, /, *, emit=True, **kwargs):
- """
- Convenience method to get or set some axis properties.
-
- Call signatures::
-
- xmin, xmax, ymin, ymax = axis()
- xmin, xmax, ymin, ymax = axis([xmin, xmax, ymin, ymax])
- xmin, xmax, ymin, ymax = axis(option)
- xmin, xmax, ymin, ymax = axis(**kwargs)
-
- Parameters
- ----------
- xmin, xmax, ymin, ymax : float, optional
- The axis limits to be set. This can also be achieved using ::
-
- ax.set(xlim=(xmin, xmax), ylim=(ymin, ymax))
-
- option : bool or str
- If a bool, turns axis lines and labels on or off. If a string,
- possible values are:
-
- ======== ==========================================================
- Value Description
- ======== ==========================================================
- 'on' Turn on axis lines and labels. Same as ``True``.
- 'off' Turn off axis lines and labels. Same as ``False``.
- 'equal' Set equal scaling (i.e., make circles circular) by
- changing axis limits. This is the same as
- ``ax.set_aspect('equal', adjustable='datalim')``.
- Explicit data limits may not be respected in this case.
- 'scaled' Set equal scaling (i.e., make circles circular) by
- changing dimensions of the plot box. This is the same as
- ``ax.set_aspect('equal', adjustable='box', anchor='C')``.
- Additionally, further autoscaling will be disabled.
- 'tight' Set limits just large enough to show all data, then
- disable further autoscaling.
- 'auto' Automatic scaling (fill plot box with data).
- 'image' 'scaled' with axis limits equal to data limits.
- 'square' Square plot; similar to 'scaled', but initially forcing
- ``xmax-xmin == ymax-ymin``.
- ======== ==========================================================
-
- emit : bool, default: True
- Whether observers are notified of the axis limit change.
- This option is passed on to `~.Axes.set_xlim` and
- `~.Axes.set_ylim`.
-
- Returns
- -------
- xmin, xmax, ymin, ymax : float
- The axis limits.
-
- See Also
- --------
- matplotlib.axes.Axes.set_xlim
- matplotlib.axes.Axes.set_ylim
- """
- if isinstance(arg, (str, bool)):
- if arg is True:
- arg = 'on'
- if arg is False:
- arg = 'off'
- arg = arg.lower()
- if arg == 'on':
- self.set_axis_on()
- elif arg == 'off':
- self.set_axis_off()
- elif arg in [
- 'equal', 'tight', 'scaled', 'auto', 'image', 'square']:
- self.set_autoscale_on(True)
- self.set_aspect('auto')
- self.autoscale_view(tight=False)
- if arg == 'equal':
- self.set_aspect('equal', adjustable='datalim')
- elif arg == 'scaled':
- self.set_aspect('equal', adjustable='box', anchor='C')
- self.set_autoscale_on(False) # Req. by Mark Bakker
- elif arg == 'tight':
- self.autoscale_view(tight=True)
- self.set_autoscale_on(False)
- elif arg == 'image':
- self.autoscale_view(tight=True)
- self.set_autoscale_on(False)
- self.set_aspect('equal', adjustable='box', anchor='C')
- elif arg == 'square':
- self.set_aspect('equal', adjustable='box', anchor='C')
- self.set_autoscale_on(False)
- xlim = self.get_xlim()
- ylim = self.get_ylim()
- edge_size = max(np.diff(xlim), np.diff(ylim))[0]
- self.set_xlim([xlim[0], xlim[0] + edge_size],
- emit=emit, auto=False)
- self.set_ylim([ylim[0], ylim[0] + edge_size],
- emit=emit, auto=False)
- else:
- raise ValueError(f"Unrecognized string {arg!r} to axis; "
- "try 'on' or 'off'")
- else:
- if arg is not None:
- try:
- xmin, xmax, ymin, ymax = arg
- except (TypeError, ValueError) as err:
- raise TypeError('the first argument to axis() must be an '
- 'iterable of the form '
- '[xmin, xmax, ymin, ymax]') from err
- else:
- xmin = kwargs.pop('xmin', None)
- xmax = kwargs.pop('xmax', None)
- ymin = kwargs.pop('ymin', None)
- ymax = kwargs.pop('ymax', None)
- xauto = (None # Keep autoscale state as is.
- if xmin is None and xmax is None
- else False) # Turn off autoscale.
- yauto = (None
- if ymin is None and ymax is None
- else False)
- self.set_xlim(xmin, xmax, emit=emit, auto=xauto)
- self.set_ylim(ymin, ymax, emit=emit, auto=yauto)
- if kwargs:
- raise _api.kwarg_error("axis", kwargs)
- return (*self.get_xlim(), *self.get_ylim())
-
- def get_legend(self):
- """Return the `.Legend` instance, or None if no legend is defined."""
- return self.legend_
-
- def get_images(self):
- r"""Return a list of `.AxesImage`\s contained by the Axes."""
- return cbook.silent_list('AxesImage', self.images)
-
- def get_lines(self):
- """Return a list of lines contained by the Axes."""
- return cbook.silent_list('Line2D', self.lines)
-
- def get_xaxis(self):
- """
- [*Discouraged*] Return the XAxis instance.
-
- .. admonition:: Discouraged
-
- The use of this function is discouraged. You should instead
- directly access the attribute ``ax.xaxis``.
- """
- return self.xaxis
-
- def get_yaxis(self):
- """
- [*Discouraged*] Return the YAxis instance.
-
- .. admonition:: Discouraged
-
- The use of this function is discouraged. You should instead
- directly access the attribute ``ax.yaxis``.
- """
- return self.yaxis
-
- get_xgridlines = _axis_method_wrapper("xaxis", "get_gridlines")
- get_xticklines = _axis_method_wrapper("xaxis", "get_ticklines")
- get_ygridlines = _axis_method_wrapper("yaxis", "get_gridlines")
- get_yticklines = _axis_method_wrapper("yaxis", "get_ticklines")
-
- # Adding and tracking artists
-
- def _sci(self, im):
- """
- Set the current image.
-
- This image will be the target of colormap functions like
- ``pyplot.viridis``, and other functions such as `~.pyplot.clim`. The
- current image is an attribute of the current Axes.
- """
- _api.check_isinstance(
- (mpl.contour.ContourSet, mcoll.Collection, mimage.AxesImage),
- im=im)
- if isinstance(im, mpl.contour.ContourSet):
- if im.collections[0] not in self._children:
- raise ValueError("ContourSet must be in current Axes")
- elif im not in self._children:
- raise ValueError("Argument must be an image, collection, or "
- "ContourSet in this Axes")
- self._current_image = im
-
- def _gci(self):
- """Helper for `~matplotlib.pyplot.gci`; do not use elsewhere."""
- return self._current_image
-
- def has_data(self):
- """
- Return whether any artists have been added to the Axes.
-
- This should not be used to determine whether the *dataLim*
- need to be updated, and may not actually be useful for
- anything.
- """
- return any(isinstance(a, (mcoll.Collection, mimage.AxesImage,
- mlines.Line2D, mpatches.Patch))
- for a in self._children)
-
- def add_artist(self, a):
- """
- Add an `.Artist` to the Axes; return the artist.
-
- Use `add_artist` only for artists for which there is no dedicated
- "add" method; and if necessary, use a method such as `update_datalim`
- to manually update the dataLim if the artist is to be included in
- autoscaling.
-
- If no ``transform`` has been specified when creating the artist (e.g.
- ``artist.get_transform() == None``) then the transform is set to
- ``ax.transData``.
- """
- a.axes = self
- self._children.append(a)
- a._remove_method = self._children.remove
- self._set_artist_props(a)
- a.set_clip_path(self.patch)
- self.stale = True
- return a
-
- def add_child_axes(self, ax):
- """
- Add an `.AxesBase` to the Axes' children; return the child Axes.
-
- This is the lowlevel version. See `.axes.Axes.inset_axes`.
- """
-
- # normally Axes have themselves as the Axes, but these need to have
- # their parent...
- # Need to bypass the getter...
- ax._axes = self
- ax.stale_callback = martist._stale_axes_callback
-
- self.child_axes.append(ax)
- ax._remove_method = self.child_axes.remove
- self.stale = True
- return ax
-
- def add_collection(self, collection, autolim=True):
- """
- Add a `.Collection` to the Axes; return the collection.
- """
- _api.check_isinstance(mcoll.Collection, collection=collection)
- label = collection.get_label()
- if not label:
- collection.set_label(f'_child{len(self._children)}')
- self._children.append(collection)
- collection._remove_method = self._children.remove
- self._set_artist_props(collection)
-
- if collection.get_clip_path() is None:
- collection.set_clip_path(self.patch)
-
- if autolim:
- # Make sure viewLim is not stale (mostly to match
- # pre-lazy-autoscale behavior, which is not really better).
- self._unstale_viewLim()
- datalim = collection.get_datalim(self.transData)
- points = datalim.get_points()
- if not np.isinf(datalim.minpos).all():
- # By definition, if minpos (minimum positive value) is set
- # (i.e., non-inf), then min(points) <= minpos <= max(points),
- # and minpos would be superfluous. However, we add minpos to
- # the call so that self.dataLim will update its own minpos.
- # This ensures that log scales see the correct minimum.
- points = np.concatenate([points, [datalim.minpos]])
- self.update_datalim(points)
-
- self.stale = True
- return collection
-
- def add_image(self, image):
- """
- Add an `.AxesImage` to the Axes; return the image.
- """
- _api.check_isinstance(mimage.AxesImage, image=image)
- self._set_artist_props(image)
- if not image.get_label():
- image.set_label(f'_child{len(self._children)}')
- self._children.append(image)
- image._remove_method = self._children.remove
- self.stale = True
- return image
-
- def _update_image_limits(self, image):
- xmin, xmax, ymin, ymax = image.get_extent()
- self.axes.update_datalim(((xmin, ymin), (xmax, ymax)))
-
- def add_line(self, line):
- """
- Add a `.Line2D` to the Axes; return the line.
- """
- _api.check_isinstance(mlines.Line2D, line=line)
- self._set_artist_props(line)
- if line.get_clip_path() is None:
- line.set_clip_path(self.patch)
-
- self._update_line_limits(line)
- if not line.get_label():
- line.set_label(f'_child{len(self._children)}')
- self._children.append(line)
- line._remove_method = self._children.remove
- self.stale = True
- return line
-
- def _add_text(self, txt):
- """
- Add a `.Text` to the Axes; return the text.
- """
- _api.check_isinstance(mtext.Text, txt=txt)
- self._set_artist_props(txt)
- self._children.append(txt)
- txt._remove_method = self._children.remove
- self.stale = True
- return txt
-
- def _update_line_limits(self, line):
- """
- Figures out the data limit of the given line, updating self.dataLim.
- """
- path = line.get_path()
- if path.vertices.size == 0:
- return
-
- line_trf = line.get_transform()
-
- if line_trf == self.transData:
- data_path = path
- elif any(line_trf.contains_branch_seperately(self.transData)):
- # Compute the transform from line coordinates to data coordinates.
- trf_to_data = line_trf - self.transData
- # If transData is affine we can use the cached non-affine component
- # of line's path (since the non-affine part of line_trf is
- # entirely encapsulated in trf_to_data).
- if self.transData.is_affine:
- line_trans_path = line._get_transformed_path()
- na_path, _ = line_trans_path.get_transformed_path_and_affine()
- data_path = trf_to_data.transform_path_affine(na_path)
- else:
- data_path = trf_to_data.transform_path(path)
- else:
- # For backwards compatibility we update the dataLim with the
- # coordinate range of the given path, even though the coordinate
- # systems are completely different. This may occur in situations
- # such as when ax.transAxes is passed through for absolute
- # positioning.
- data_path = path
-
- if not data_path.vertices.size:
- return
-
- updatex, updatey = line_trf.contains_branch_seperately(self.transData)
- if self.name != "rectilinear":
- # This block is mostly intended to handle axvline in polar plots,
- # for which updatey would otherwise be True.
- if updatex and line_trf == self.get_yaxis_transform():
- updatex = False
- if updatey and line_trf == self.get_xaxis_transform():
- updatey = False
- self.dataLim.update_from_path(data_path,
- self.ignore_existing_data_limits,
- updatex=updatex, updatey=updatey)
- self.ignore_existing_data_limits = False
-
- def add_patch(self, p):
- """
- Add a `.Patch` to the Axes; return the patch.
- """
- _api.check_isinstance(mpatches.Patch, p=p)
- self._set_artist_props(p)
- if p.get_clip_path() is None:
- p.set_clip_path(self.patch)
- self._update_patch_limits(p)
- self._children.append(p)
- p._remove_method = self._children.remove
- return p
-
- def _update_patch_limits(self, patch):
- """Update the data limits for the given patch."""
- # hist can add zero height Rectangles, which is useful to keep
- # the bins, counts and patches lined up, but it throws off log
- # scaling. We'll ignore rects with zero height or width in
- # the auto-scaling
-
- # cannot check for '==0' since unitized data may not compare to zero
- # issue #2150 - we update the limits if patch has non zero width
- # or height.
- if (isinstance(patch, mpatches.Rectangle) and
- ((not patch.get_width()) and (not patch.get_height()))):
- return
- p = patch.get_path()
- # Get all vertices on the path
- # Loop through each segment to get extrema for Bezier curve sections
- vertices = []
- for curve, code in p.iter_bezier(simplify=False):
- # Get distance along the curve of any extrema
- _, dzeros = curve.axis_aligned_extrema()
- # Calculate vertices of start, end and any extrema in between
- vertices.append(curve([0, *dzeros, 1]))
-
- if len(vertices):
- vertices = np.row_stack(vertices)
-
- patch_trf = patch.get_transform()
- updatex, updatey = patch_trf.contains_branch_seperately(self.transData)
- if not (updatex or updatey):
- return
- if self.name != "rectilinear":
- # As in _update_line_limits, but for axvspan.
- if updatex and patch_trf == self.get_yaxis_transform():
- updatex = False
- if updatey and patch_trf == self.get_xaxis_transform():
- updatey = False
- trf_to_data = patch_trf - self.transData
- xys = trf_to_data.transform(vertices)
- self.update_datalim(xys, updatex=updatex, updatey=updatey)
-
- def add_table(self, tab):
- """
- Add a `.Table` to the Axes; return the table.
- """
- _api.check_isinstance(mtable.Table, tab=tab)
- self._set_artist_props(tab)
- self._children.append(tab)
- tab.set_clip_path(self.patch)
- tab._remove_method = self._children.remove
- return tab
-
- def add_container(self, container):
- """
- Add a `.Container` to the Axes' containers; return the container.
- """
- label = container.get_label()
- if not label:
- container.set_label('_container%d' % len(self.containers))
- self.containers.append(container)
- container._remove_method = self.containers.remove
- return container
-
- def _unit_change_handler(self, axis_name, event=None):
- """
- Process axis units changes: requests updates to data and view limits.
- """
- if event is None: # Allow connecting `self._unit_change_handler(name)`
- return functools.partial(
- self._unit_change_handler, axis_name, event=object())
- _api.check_in_list(self._axis_map, axis_name=axis_name)
- for line in self.lines:
- line.recache_always()
- self.relim()
- self._request_autoscale_view(axis_name)
-
- def relim(self, visible_only=False):
- """
- Recompute the data limits based on current artists.
-
- At present, `.Collection` instances are not supported.
-
- Parameters
- ----------
- visible_only : bool, default: False
- Whether to exclude invisible artists.
- """
- # Collections are deliberately not supported (yet); see
- # the TODO note in artists.py.
- self.dataLim.ignore(True)
- self.dataLim.set_points(mtransforms.Bbox.null().get_points())
- self.ignore_existing_data_limits = True
-
- for artist in self._children:
- if not visible_only or artist.get_visible():
- if isinstance(artist, mlines.Line2D):
- self._update_line_limits(artist)
- elif isinstance(artist, mpatches.Patch):
- self._update_patch_limits(artist)
- elif isinstance(artist, mimage.AxesImage):
- self._update_image_limits(artist)
-
- def update_datalim(self, xys, updatex=True, updatey=True):
- """
- Extend the `~.Axes.dataLim` Bbox to include the given points.
-
- If no data is set currently, the Bbox will ignore its limits and set
- the bound to be the bounds of the xydata (*xys*). Otherwise, it will
- compute the bounds of the union of its current data and the data in
- *xys*.
-
- Parameters
- ----------
- xys : 2D array-like
- The points to include in the data limits Bbox. This can be either
- a list of (x, y) tuples or a Nx2 array.
-
- updatex, updatey : bool, default: True
- Whether to update the x/y limits.
- """
- xys = np.asarray(xys)
- if not np.any(np.isfinite(xys)):
- return
- self.dataLim.update_from_data_xy(xys, self.ignore_existing_data_limits,
- updatex=updatex, updatey=updatey)
- self.ignore_existing_data_limits = False
-
- def _process_unit_info(self, datasets=None, kwargs=None, *, convert=True):
- """
- Set axis units based on *datasets* and *kwargs*, and optionally apply
- unit conversions to *datasets*.
-
- Parameters
- ----------
- datasets : list
- List of (axis_name, dataset) pairs (where the axis name is defined
- as in `._axis_map`). Individual datasets can also be None
- (which gets passed through).
- kwargs : dict
- Other parameters from which unit info (i.e., the *xunits*,
- *yunits*, *zunits* (for 3D Axes), *runits* and *thetaunits* (for
- polar) entries) is popped, if present. Note that this dict is
- mutated in-place!
- convert : bool, default: True
- Whether to return the original datasets or the converted ones.
-
- Returns
- -------
- list
- Either the original datasets if *convert* is False, or the
- converted ones if *convert* is True (the default).
- """
- # The API makes datasets a list of pairs rather than an axis_name to
- # dataset mapping because it is sometimes necessary to process multiple
- # datasets for a single axis, and concatenating them may be tricky
- # (e.g. if some are scalars, etc.).
- datasets = datasets or []
- kwargs = kwargs or {}
- axis_map = self._axis_map
- for axis_name, data in datasets:
- try:
- axis = axis_map[axis_name]
- except KeyError:
- raise ValueError(f"Invalid axis name: {axis_name!r}") from None
- # Update from data if axis is already set but no unit is set yet.
- if axis is not None and data is not None and not axis.have_units():
- axis.update_units(data)
- for axis_name, axis in axis_map.items():
- # Return if no axis is set.
- if axis is None:
- continue
- # Check for units in the kwargs, and if present update axis.
- units = kwargs.pop(f"{axis_name}units", axis.units)
- if self.name == "polar":
- # Special case: polar supports "thetaunits"/"runits".
- polar_units = {"x": "thetaunits", "y": "runits"}
- units = kwargs.pop(polar_units[axis_name], units)
- if units != axis.units and units is not None:
- axis.set_units(units)
- # If the units being set imply a different converter,
- # we need to update again.
- for dataset_axis_name, data in datasets:
- if dataset_axis_name == axis_name and data is not None:
- axis.update_units(data)
- return [axis_map[axis_name].convert_units(data)
- if convert and data is not None else data
- for axis_name, data in datasets]
-
- def in_axes(self, mouseevent):
- """
- Return whether the given event (in display coords) is in the Axes.
- """
- return self.patch.contains(mouseevent)[0]
-
- get_autoscalex_on = _axis_method_wrapper("xaxis", "_get_autoscale_on")
- get_autoscaley_on = _axis_method_wrapper("yaxis", "_get_autoscale_on")
- set_autoscalex_on = _axis_method_wrapper("xaxis", "_set_autoscale_on")
- set_autoscaley_on = _axis_method_wrapper("yaxis", "_set_autoscale_on")
-
- def get_autoscale_on(self):
- """Return True if each axis is autoscaled, False otherwise."""
- return all(axis._get_autoscale_on()
- for axis in self._axis_map.values())
-
- def set_autoscale_on(self, b):
- """
- Set whether autoscaling is applied to each axis on the next draw or
- call to `.Axes.autoscale_view`.
-
- Parameters
- ----------
- b : bool
- """
- for axis in self._axis_map.values():
- axis._set_autoscale_on(b)
-
- @property
- def use_sticky_edges(self):
- """
- When autoscaling, whether to obey all `Artist.sticky_edges`.
-
- Default is ``True``.
-
- Setting this to ``False`` ensures that the specified margins
- will be applied, even if the plot includes an image, for
- example, which would otherwise force a view limit to coincide
- with its data limit.
-
- The changing this property does not change the plot until
- `autoscale` or `autoscale_view` is called.
- """
- return self._use_sticky_edges
-
- @use_sticky_edges.setter
- def use_sticky_edges(self, b):
- self._use_sticky_edges = bool(b)
- # No effect until next autoscaling, which will mark the Axes as stale.
-
- def set_xmargin(self, m):
- """
- Set padding of X data limits prior to autoscaling.
-
- *m* times the data interval will be added to each end of that interval
- before it is used in autoscaling. If *m* is negative, this will clip
- the data range instead of expanding it.
-
- For example, if your data is in the range [0, 2], a margin of 0.1 will
- result in a range [-0.2, 2.2]; a margin of -0.1 will result in a range
- of [0.2, 1.8].
-
- Parameters
- ----------
- m : float greater than -0.5
- """
- if m <= -0.5:
- raise ValueError("margin must be greater than -0.5")
- self._xmargin = m
- self._request_autoscale_view("x")
- self.stale = True
-
- def set_ymargin(self, m):
- """
- Set padding of Y data limits prior to autoscaling.
-
- *m* times the data interval will be added to each end of that interval
- before it is used in autoscaling. If *m* is negative, this will clip
- the data range instead of expanding it.
-
- For example, if your data is in the range [0, 2], a margin of 0.1 will
- result in a range [-0.2, 2.2]; a margin of -0.1 will result in a range
- of [0.2, 1.8].
-
- Parameters
- ----------
- m : float greater than -0.5
- """
- if m <= -0.5:
- raise ValueError("margin must be greater than -0.5")
- self._ymargin = m
- self._request_autoscale_view("y")
- self.stale = True
-
- def margins(self, *margins, x=None, y=None, tight=True):
- """
- Set or retrieve autoscaling margins.
-
- The padding added to each limit of the Axes is the *margin*
- times the data interval. All input parameters must be floats
- within the range [0, 1]. Passing both positional and keyword
- arguments is invalid and will raise a TypeError. If no
- arguments (positional or otherwise) are provided, the current
- margins will remain in place and simply be returned.
-
- Specifying any margin changes only the autoscaling; for example,
- if *xmargin* is not None, then *xmargin* times the X data
- interval will be added to each end of that interval before
- it is used in autoscaling.
-
- Parameters
- ----------
- *margins : float, optional
- If a single positional argument is provided, it specifies
- both margins of the x-axis and y-axis limits. If two
- positional arguments are provided, they will be interpreted
- as *xmargin*, *ymargin*. If setting the margin on a single
- axis is desired, use the keyword arguments described below.
-
- x, y : float, optional
- Specific margin values for the x-axis and y-axis,
- respectively. These cannot be used with positional
- arguments, but can be used individually to alter on e.g.,
- only the y-axis.
-
- tight : bool or None, default: True
- The *tight* parameter is passed to `~.axes.Axes.autoscale_view`,
- which is executed after a margin is changed; the default
- here is *True*, on the assumption that when margins are
- specified, no additional padding to match tick marks is
- usually desired. Setting *tight* to *None* preserves
- the previous setting.
-
- Returns
- -------
- xmargin, ymargin : float
-
- Notes
- -----
- If a previously used Axes method such as :meth:`pcolor` has set
- :attr:`use_sticky_edges` to `True`, only the limits not set by
- the "sticky artists" will be modified. To force all of the
- margins to be set, set :attr:`use_sticky_edges` to `False`
- before calling :meth:`margins`.
- """
-
- if margins and (x is not None or y is not None):
- raise TypeError('Cannot pass both positional and keyword '
- 'arguments for x and/or y.')
- elif len(margins) == 1:
- x = y = margins[0]
- elif len(margins) == 2:
- x, y = margins
- elif margins:
- raise TypeError('Must pass a single positional argument for all '
- 'margins, or one for each margin (x, y).')
-
- if x is None and y is None:
- if tight is not True:
- _api.warn_external(f'ignoring tight={tight!r} in get mode')
- return self._xmargin, self._ymargin
-
- if tight is not None:
- self._tight = tight
- if x is not None:
- self.set_xmargin(x)
- if y is not None:
- self.set_ymargin(y)
-
- def set_rasterization_zorder(self, z):
- """
- Set the zorder threshold for rasterization for vector graphics output.
-
- All artists with a zorder below the given value will be rasterized if
- they support rasterization.
-
- This setting is ignored for pixel-based output.
-
- See also :doc:`/gallery/misc/rasterization_demo`.
-
- Parameters
- ----------
- z : float or None
- The zorder below which artists are rasterized.
- If ``None`` rasterization based on zorder is deactivated.
- """
- self._rasterization_zorder = z
- self.stale = True
-
- def get_rasterization_zorder(self):
- """Return the zorder value below which artists will be rasterized."""
- return self._rasterization_zorder
-
- def autoscale(self, enable=True, axis='both', tight=None):
- """
- Autoscale the axis view to the data (toggle).
-
- Convenience method for simple axis view autoscaling.
- It turns autoscaling on or off, and then,
- if autoscaling for either axis is on, it performs
- the autoscaling on the specified axis or Axes.
-
- Parameters
- ----------
- enable : bool or None, default: True
- True turns autoscaling on, False turns it off.
- None leaves the autoscaling state unchanged.
- axis : {'both', 'x', 'y'}, default: 'both'
- The axis on which to operate. (For 3D Axes, *axis* can also be set
- to 'z', and 'both' refers to all three axes.)
- tight : bool or None, default: None
- If True, first set the margins to zero. Then, this argument is
- forwarded to `~.axes.Axes.autoscale_view` (regardless of
- its value); see the description of its behavior there.
- """
- if enable is None:
- scalex = True
- scaley = True
- else:
- if axis in ['x', 'both']:
- self.set_autoscalex_on(bool(enable))
- scalex = self.get_autoscalex_on()
- else:
- scalex = False
- if axis in ['y', 'both']:
- self.set_autoscaley_on(bool(enable))
- scaley = self.get_autoscaley_on()
- else:
- scaley = False
- if tight and scalex:
- self._xmargin = 0
- if tight and scaley:
- self._ymargin = 0
- if scalex:
- self._request_autoscale_view("x", tight=tight)
- if scaley:
- self._request_autoscale_view("y", tight=tight)
-
- def autoscale_view(self, tight=None, scalex=True, scaley=True):
- """
- Autoscale the view limits using the data limits.
-
- Parameters
- ----------
- tight : bool or None
- If *True*, only expand the axis limits using the margins. Note
- that unlike for `autoscale`, ``tight=True`` does *not* set the
- margins to zero.
-
- If *False* and :rc:`axes.autolimit_mode` is 'round_numbers', then
- after expansion by the margins, further expand the axis limits
- using the axis major locator.
-
- If None (the default), reuse the value set in the previous call to
- `autoscale_view` (the initial value is False, but the default style
- sets :rc:`axes.autolimit_mode` to 'data', in which case this
- behaves like True).
-
- scalex : bool, default: True
- Whether to autoscale the x-axis.
-
- scaley : bool, default: True
- Whether to autoscale the y-axis.
-
- Notes
- -----
- The autoscaling preserves any preexisting axis direction reversal.
-
- The data limits are not updated automatically when artist data are
- changed after the artist has been added to an Axes instance. In that
- case, use :meth:`matplotlib.axes.Axes.relim` prior to calling
- autoscale_view.
-
- If the views of the Axes are fixed, e.g. via `set_xlim`, they will
- not be changed by autoscale_view().
- See :meth:`matplotlib.axes.Axes.autoscale` for an alternative.
- """
- if tight is not None:
- self._tight = bool(tight)
-
- x_stickies = y_stickies = np.array([])
- if self.use_sticky_edges:
- if self._xmargin and scalex and self.get_autoscalex_on():
- x_stickies = np.sort(np.concatenate([
- artist.sticky_edges.x
- for ax in self._shared_axes["x"].get_siblings(self)
- for artist in ax.get_children()]))
- if self._ymargin and scaley and self.get_autoscaley_on():
- y_stickies = np.sort(np.concatenate([
- artist.sticky_edges.y
- for ax in self._shared_axes["y"].get_siblings(self)
- for artist in ax.get_children()]))
- if self.get_xscale() == 'log':
- x_stickies = x_stickies[x_stickies > 0]
- if self.get_yscale() == 'log':
- y_stickies = y_stickies[y_stickies > 0]
-
- def handle_single_axis(
- scale, shared_axes, name, axis, margin, stickies, set_bound):
-
- if not (scale and axis._get_autoscale_on()):
- return # nothing to do...
-
- shared = shared_axes.get_siblings(self)
- # Base autoscaling on finite data limits when there is at least one
- # finite data limit among all the shared_axes and intervals.
- values = [val for ax in shared
- for val in getattr(ax.dataLim, f"interval{name}")
- if np.isfinite(val)]
- if values:
- x0, x1 = (min(values), max(values))
- elif getattr(self._viewLim, f"mutated{name}")():
- # No data, but explicit viewLims already set:
- # in mutatedx or mutatedy.
- return
- else:
- x0, x1 = (-np.inf, np.inf)
- # If x0 and x1 are nonfinite, get default limits from the locator.
- locator = axis.get_major_locator()
- x0, x1 = locator.nonsingular(x0, x1)
- # Find the minimum minpos for use in the margin calculation.
- minimum_minpos = min(
- getattr(ax.dataLim, f"minpos{name}") for ax in shared)
-
- # Prevent margin addition from crossing a sticky value. A small
- # tolerance must be added due to floating point issues with
- # streamplot; it is defined relative to x0, x1, x1-x0 but has
- # no absolute term (e.g. "+1e-8") to avoid issues when working with
- # datasets where all values are tiny (less than 1e-8).
- tol = 1e-5 * max(abs(x0), abs(x1), abs(x1 - x0))
- # Index of largest element < x0 + tol, if any.
- i0 = stickies.searchsorted(x0 + tol) - 1
- x0bound = stickies[i0] if i0 != -1 else None
- # Index of smallest element > x1 - tol, if any.
- i1 = stickies.searchsorted(x1 - tol)
- x1bound = stickies[i1] if i1 != len(stickies) else None
-
- # Add the margin in figure space and then transform back, to handle
- # non-linear scales.
- transform = axis.get_transform()
- inverse_trans = transform.inverted()
- x0, x1 = axis._scale.limit_range_for_scale(x0, x1, minimum_minpos)
- x0t, x1t = transform.transform([x0, x1])
- delta = (x1t - x0t) * margin
- if not np.isfinite(delta):
- delta = 0 # If a bound isn't finite, set margin to zero.
- x0, x1 = inverse_trans.transform([x0t - delta, x1t + delta])
-
- # Apply sticky bounds.
- if x0bound is not None:
- x0 = max(x0, x0bound)
- if x1bound is not None:
- x1 = min(x1, x1bound)
-
- if not self._tight:
- x0, x1 = locator.view_limits(x0, x1)
- set_bound(x0, x1)
- # End of definition of internal function 'handle_single_axis'.
-
- handle_single_axis(
- scalex, self._shared_axes["x"], 'x', self.xaxis, self._xmargin,
- x_stickies, self.set_xbound)
- handle_single_axis(
- scaley, self._shared_axes["y"], 'y', self.yaxis, self._ymargin,
- y_stickies, self.set_ybound)
-
- def _update_title_position(self, renderer):
- """
- Update the title position based on the bounding box enclosing
- all the ticklabels and x-axis spine and xlabel...
- """
- if self._autotitlepos is not None and not self._autotitlepos:
- _log.debug('title position was updated manually, not adjusting')
- return
-
- titles = (self.title, self._left_title, self._right_title)
-
- # Need to check all our twins too, and all the children as well.
- axs = self._twinned_axes.get_siblings(self) + self.child_axes
- for ax in self.child_axes: # Child positions must be updated first.
- locator = ax.get_axes_locator()
- ax.apply_aspect(locator(self, renderer) if locator else None)
-
- for title in titles:
- x, _ = title.get_position()
- # need to start again in case of window resizing
- title.set_position((x, 1.0))
- top = -np.inf
- for ax in axs:
- bb = None
- if (ax.xaxis.get_ticks_position() in ['top', 'unknown']
- or ax.xaxis.get_label_position() == 'top'):
- bb = ax.xaxis.get_tightbbox(renderer)
- if bb is None:
- if 'outline' in ax.spines:
- # Special case for colorbars:
- bb = ax.spines['outline'].get_window_extent()
- else:
- bb = ax.get_window_extent(renderer)
- top = max(top, bb.ymax)
- if title.get_text():
- ax.yaxis.get_tightbbox(renderer) # update offsetText
- if ax.yaxis.offsetText.get_text():
- bb = ax.yaxis.offsetText.get_tightbbox(renderer)
- if bb.intersection(title.get_tightbbox(renderer), bb):
- top = bb.ymax
- if top < 0:
- # the top of Axes is not even on the figure, so don't try and
- # automatically place it.
- _log.debug('top of Axes not in the figure, so title not moved')
- return
- if title.get_window_extent(renderer).ymin < top:
- _, y = self.transAxes.inverted().transform((0, top))
- title.set_position((x, y))
- # empirically, this doesn't always get the min to top,
- # so we need to adjust again.
- if title.get_window_extent(renderer).ymin < top:
- _, y = self.transAxes.inverted().transform(
- (0., 2 * top - title.get_window_extent(renderer).ymin))
- title.set_position((x, y))
-
- ymax = max(title.get_position()[1] for title in titles)
- for title in titles:
- # now line up all the titles at the highest baseline.
- x, _ = title.get_position()
- title.set_position((x, ymax))
-
- # Drawing
- @martist.allow_rasterization
- def draw(self, renderer):
- # docstring inherited
- if renderer is None:
- raise RuntimeError('No renderer defined')
- if not self.get_visible():
- return
- self._unstale_viewLim()
-
- renderer.open_group('axes', gid=self.get_gid())
-
- # prevent triggering call backs during the draw process
- self._stale = True
-
- # loop over self and child Axes...
- locator = self.get_axes_locator()
- self.apply_aspect(locator(self, renderer) if locator else None)
-
- artists = self.get_children()
- artists.remove(self.patch)
-
- # the frame draws the edges around the Axes patch -- we
- # decouple these so the patch can be in the background and the
- # frame in the foreground. Do this before drawing the axis
- # objects so that the spine has the opportunity to update them.
- if not (self.axison and self._frameon):
- for spine in self.spines.values():
- artists.remove(spine)
-
- self._update_title_position(renderer)
-
- if not self.axison:
- for _axis in self._axis_map.values():
- artists.remove(_axis)
-
- if not self.figure.canvas.is_saving():
- artists = [
- a for a in artists
- if not a.get_animated() or isinstance(a, mimage.AxesImage)]
- artists = sorted(artists, key=attrgetter('zorder'))
-
- # rasterize artists with negative zorder
- # if the minimum zorder is negative, start rasterization
- rasterization_zorder = self._rasterization_zorder
-
- if (rasterization_zorder is not None and
- artists and artists[0].zorder < rasterization_zorder):
- split_index = np.searchsorted(
- [art.zorder for art in artists],
- rasterization_zorder, side='right'
- )
- artists_rasterized = artists[:split_index]
- artists = artists[split_index:]
- else:
- artists_rasterized = []
-
- if self.axison and self._frameon:
- if artists_rasterized:
- artists_rasterized = [self.patch] + artists_rasterized
- else:
- artists = [self.patch] + artists
-
- if artists_rasterized:
- _draw_rasterized(self.figure, artists_rasterized, renderer)
-
- mimage._draw_list_compositing_images(
- renderer, self, artists, self.figure.suppressComposite)
-
- renderer.close_group('axes')
- self.stale = False
-
- def draw_artist(self, a):
- """
- Efficiently redraw a single artist.
- """
- a.draw(self.figure.canvas.get_renderer())
-
- def redraw_in_frame(self):
- """
- Efficiently redraw Axes data, but not axis ticks, labels, etc.
- """
- with ExitStack() as stack:
- for artist in [*self._axis_map.values(),
- self.title, self._left_title, self._right_title]:
- stack.enter_context(artist._cm_set(visible=False))
- self.draw(self.figure.canvas.get_renderer())
-
- @_api.deprecated("3.6", alternative="Axes.figure.canvas.get_renderer()")
- def get_renderer_cache(self):
- return self.figure.canvas.get_renderer()
-
- # Axes rectangle characteristics
-
- def get_frame_on(self):
- """Get whether the Axes rectangle patch is drawn."""
- return self._frameon
-
- def set_frame_on(self, b):
- """
- Set whether the Axes rectangle patch is drawn.
-
- Parameters
- ----------
- b : bool
- """
- self._frameon = b
- self.stale = True
-
- def get_axisbelow(self):
- """
- Get whether axis ticks and gridlines are above or below most artists.
-
- Returns
- -------
- bool or 'line'
-
- See Also
- --------
- set_axisbelow
- """
- return self._axisbelow
-
- def set_axisbelow(self, b):
- """
- Set whether axis ticks and gridlines are above or below most artists.
-
- This controls the zorder of the ticks and gridlines. For more
- information on the zorder see :doc:`/gallery/misc/zorder_demo`.
-
- Parameters
- ----------
- b : bool or 'line'
- Possible values:
-
- - *True* (zorder = 0.5): Ticks and gridlines are below all Artists.
- - 'line' (zorder = 1.5): Ticks and gridlines are above patches
- (e.g. rectangles, with default zorder = 1) but still below lines
- and markers (with their default zorder = 2).
- - *False* (zorder = 2.5): Ticks and gridlines are above patches
- and lines / markers.
-
- See Also
- --------
- get_axisbelow
- """
- # Check that b is True, False or 'line'
- self._axisbelow = axisbelow = validate_axisbelow(b)
- zorder = {
- True: 0.5,
- 'line': 1.5,
- False: 2.5,
- }[axisbelow]
- for axis in self._axis_map.values():
- axis.set_zorder(zorder)
- self.stale = True
-
- @_docstring.dedent_interpd
- def grid(self, visible=None, which='major', axis='both', **kwargs):
- """
- Configure the grid lines.
-
- Parameters
- ----------
- visible : bool or None, optional
- Whether to show the grid lines. If any *kwargs* are supplied, it
- is assumed you want the grid on and *visible* will be set to True.
-
- If *visible* is *None* and there are no *kwargs*, this toggles the
- visibility of the lines.
-
- which : {'major', 'minor', 'both'}, optional
- The grid lines to apply the changes on.
-
- axis : {'both', 'x', 'y'}, optional
- The axis to apply the changes on.
-
- **kwargs : `.Line2D` properties
- Define the line properties of the grid, e.g.::
-
- grid(color='r', linestyle='-', linewidth=2)
-
- Valid keyword arguments are:
-
- %(Line2D:kwdoc)s
-
- Notes
- -----
- The axis is drawn as a unit, so the effective zorder for drawing the
- grid is determined by the zorder of each axis, not by the zorder of the
- `.Line2D` objects comprising the grid. Therefore, to set grid zorder,
- use `.set_axisbelow` or, for more control, call the
- `~.Artist.set_zorder` method of each axis.
- """
- _api.check_in_list(['x', 'y', 'both'], axis=axis)
- if axis in ['x', 'both']:
- self.xaxis.grid(visible, which=which, **kwargs)
- if axis in ['y', 'both']:
- self.yaxis.grid(visible, which=which, **kwargs)
-
- def ticklabel_format(self, *, axis='both', style='', scilimits=None,
- useOffset=None, useLocale=None, useMathText=None):
- r"""
- Configure the `.ScalarFormatter` used by default for linear Axes.
-
- If a parameter is not set, the corresponding property of the formatter
- is left unchanged.
-
- Parameters
- ----------
- axis : {'x', 'y', 'both'}, default: 'both'
- The axis to configure. Only major ticks are affected.
-
- style : {'sci', 'scientific', 'plain'}
- Whether to use scientific notation.
- The formatter default is to use scientific notation.
-
- scilimits : pair of ints (m, n)
- Scientific notation is used only for numbers outside the range
- 10\ :sup:`m` to 10\ :sup:`n` (and only if the formatter is
- configured to use scientific notation at all). Use (0, 0) to
- include all numbers. Use (m, m) where m != 0 to fix the order of
- magnitude to 10\ :sup:`m`.
- The formatter default is :rc:`axes.formatter.limits`.
-
- useOffset : bool or float
- If True, the offset is calculated as needed.
- If False, no offset is used.
- If a numeric value, it sets the offset.
- The formatter default is :rc:`axes.formatter.useoffset`.
-
- useLocale : bool
- Whether to format the number using the current locale or using the
- C (English) locale. This affects e.g. the decimal separator. The
- formatter default is :rc:`axes.formatter.use_locale`.
-
- useMathText : bool
- Render the offset and scientific notation in mathtext.
- The formatter default is :rc:`axes.formatter.use_mathtext`.
-
- Raises
- ------
- AttributeError
- If the current formatter is not a `.ScalarFormatter`.
- """
- style = style.lower()
- axis = axis.lower()
- if scilimits is not None:
- try:
- m, n = scilimits
- m + n + 1 # check that both are numbers
- except (ValueError, TypeError) as err:
- raise ValueError("scilimits must be a sequence of 2 integers"
- ) from err
- STYLES = {'sci': True, 'scientific': True, 'plain': False, '': None}
- is_sci_style = _api.check_getitem(STYLES, style=style)
- axis_map = {**{k: [v] for k, v in self._axis_map.items()},
- 'both': list(self._axis_map.values())}
- axises = _api.check_getitem(axis_map, axis=axis)
- try:
- for axis in axises:
- if is_sci_style is not None:
- axis.major.formatter.set_scientific(is_sci_style)
- if scilimits is not None:
- axis.major.formatter.set_powerlimits(scilimits)
- if useOffset is not None:
- axis.major.formatter.set_useOffset(useOffset)
- if useLocale is not None:
- axis.major.formatter.set_useLocale(useLocale)
- if useMathText is not None:
- axis.major.formatter.set_useMathText(useMathText)
- except AttributeError as err:
- raise AttributeError(
- "This method only works with the ScalarFormatter") from err
-
- def locator_params(self, axis='both', tight=None, **kwargs):
- """
- Control behavior of major tick locators.
-
- Because the locator is involved in autoscaling, `~.Axes.autoscale_view`
- is called automatically after the parameters are changed.
-
- Parameters
- ----------
- axis : {'both', 'x', 'y'}, default: 'both'
- The axis on which to operate. (For 3D Axes, *axis* can also be
- set to 'z', and 'both' refers to all three axes.)
- tight : bool or None, optional
- Parameter passed to `~.Axes.autoscale_view`.
- Default is None, for no change.
-
- Other Parameters
- ----------------
- **kwargs
- Remaining keyword arguments are passed to directly to the
- ``set_params()`` method of the locator. Supported keywords depend
- on the type of the locator. See for example
- `~.ticker.MaxNLocator.set_params` for the `.ticker.MaxNLocator`
- used by default for linear.
-
- Examples
- --------
- When plotting small subplots, one might want to reduce the maximum
- number of ticks and use tight bounds, for example::
-
- ax.locator_params(tight=True, nbins=4)
-
- """
- _api.check_in_list([*self._axis_names, "both"], axis=axis)
- for name in self._axis_names:
- if axis in [name, "both"]:
- loc = self._axis_map[name].get_major_locator()
- loc.set_params(**kwargs)
- self._request_autoscale_view(name, tight=tight)
- self.stale = True
-
- def tick_params(self, axis='both', **kwargs):
- """
- Change the appearance of ticks, tick labels, and gridlines.
-
- Tick properties that are not explicitly set using the keyword
- arguments remain unchanged unless *reset* is True. For the current
- style settings, see `.Axis.get_tick_params`.
-
- Parameters
- ----------
- axis : {'x', 'y', 'both'}, default: 'both'
- The axis to which the parameters are applied.
- which : {'major', 'minor', 'both'}, default: 'major'
- The group of ticks to which the parameters are applied.
- reset : bool, default: False
- Whether to reset the ticks to defaults before updating them.
-
- Other Parameters
- ----------------
- direction : {'in', 'out', 'inout'}
- Puts ticks inside the Axes, outside the Axes, or both.
- length : float
- Tick length in points.
- width : float
- Tick width in points.
- color : color
- Tick color.
- pad : float
- Distance in points between tick and label.
- labelsize : float or str
- Tick label font size in points or as a string (e.g., 'large').
- labelcolor : color
- Tick label color.
- colors : color
- Tick color and label color.
- zorder : float
- Tick and label zorder.
- bottom, top, left, right : bool
- Whether to draw the respective ticks.
- labelbottom, labeltop, labelleft, labelright : bool
- Whether to draw the respective tick labels.
- labelrotation : float
- Tick label rotation
- grid_color : color
- Gridline color.
- grid_alpha : float
- Transparency of gridlines: 0 (transparent) to 1 (opaque).
- grid_linewidth : float
- Width of gridlines in points.
- grid_linestyle : str
- Any valid `.Line2D` line style spec.
-
- Examples
- --------
- ::
-
- ax.tick_params(direction='out', length=6, width=2, colors='r',
- grid_color='r', grid_alpha=0.5)
-
- This will make all major ticks be red, pointing out of the box,
- and with dimensions 6 points by 2 points. Tick labels will
- also be red. Gridlines will be red and translucent.
-
- """
- _api.check_in_list(['x', 'y', 'both'], axis=axis)
- if axis in ['x', 'both']:
- xkw = dict(kwargs)
- xkw.pop('left', None)
- xkw.pop('right', None)
- xkw.pop('labelleft', None)
- xkw.pop('labelright', None)
- self.xaxis.set_tick_params(**xkw)
- if axis in ['y', 'both']:
- ykw = dict(kwargs)
- ykw.pop('top', None)
- ykw.pop('bottom', None)
- ykw.pop('labeltop', None)
- ykw.pop('labelbottom', None)
- self.yaxis.set_tick_params(**ykw)
-
- def set_axis_off(self):
- """
- Turn the x- and y-axis off.
-
- This affects the axis lines, ticks, ticklabels, grid and axis labels.
- """
- self.axison = False
- self.stale = True
-
- def set_axis_on(self):
- """
- Turn the x- and y-axis on.
-
- This affects the axis lines, ticks, ticklabels, grid and axis labels.
- """
- self.axison = True
- self.stale = True
-
- # data limits, ticks, tick labels, and formatting
-
- def get_xlabel(self):
- """
- Get the xlabel text string.
- """
- label = self.xaxis.get_label()
- return label.get_text()
-
- def set_xlabel(self, xlabel, fontdict=None, labelpad=None, *,
- loc=None, **kwargs):
- """
- Set the label for the x-axis.
-
- Parameters
- ----------
- xlabel : str
- The label text.
-
- labelpad : float, default: :rc:`axes.labelpad`
- Spacing in points from the Axes bounding box including ticks
- and tick labels. If None, the previous value is left as is.
-
- loc : {'left', 'center', 'right'}, default: :rc:`xaxis.labellocation`
- The label position. This is a high-level alternative for passing
- parameters *x* and *horizontalalignment*.
-
- Other Parameters
- ----------------
- **kwargs : `.Text` properties
- `.Text` properties control the appearance of the label.
-
- See Also
- --------
- text : Documents the properties supported by `.Text`.
- """
- if labelpad is not None:
- self.xaxis.labelpad = labelpad
- protected_kw = ['x', 'horizontalalignment', 'ha']
- if {*kwargs} & {*protected_kw}:
- if loc is not None:
- raise TypeError(f"Specifying 'loc' is disallowed when any of "
- f"its corresponding low level keyword "
- f"arguments ({protected_kw}) are also "
- f"supplied")
-
- else:
- loc = (loc if loc is not None
- else mpl.rcParams['xaxis.labellocation'])
- _api.check_in_list(('left', 'center', 'right'), loc=loc)
-
- x = {
- 'left': 0,
- 'center': 0.5,
- 'right': 1,
- }[loc]
- kwargs.update(x=x, horizontalalignment=loc)
-
- return self.xaxis.set_label_text(xlabel, fontdict, **kwargs)
-
- def invert_xaxis(self):
- """
- Invert the x-axis.
-
- See Also
- --------
- xaxis_inverted
- get_xlim, set_xlim
- get_xbound, set_xbound
- """
- self.xaxis.set_inverted(not self.xaxis.get_inverted())
-
- xaxis_inverted = _axis_method_wrapper("xaxis", "get_inverted")
-
- def get_xbound(self):
- """
- Return the lower and upper x-axis bounds, in increasing order.
-
- See Also
- --------
- set_xbound
- get_xlim, set_xlim
- invert_xaxis, xaxis_inverted
- """
- left, right = self.get_xlim()
- if left < right:
- return left, right
- else:
- return right, left
-
- def set_xbound(self, lower=None, upper=None):
- """
- Set the lower and upper numerical bounds of the x-axis.
-
- This method will honor axis inversion regardless of parameter order.
- It will not change the autoscaling setting (`.get_autoscalex_on()`).
-
- Parameters
- ----------
- lower, upper : float or None
- The lower and upper bounds. If *None*, the respective axis bound
- is not modified.
-
- See Also
- --------
- get_xbound
- get_xlim, set_xlim
- invert_xaxis, xaxis_inverted
- """
- if upper is None and np.iterable(lower):
- lower, upper = lower
-
- old_lower, old_upper = self.get_xbound()
- if lower is None:
- lower = old_lower
- if upper is None:
- upper = old_upper
-
- self.set_xlim(sorted((lower, upper),
- reverse=bool(self.xaxis_inverted())),
- auto=None)
-
- def get_xlim(self):
- """
- Return the x-axis view limits.
-
- Returns
- -------
- left, right : (float, float)
- The current x-axis limits in data coordinates.
-
- See Also
- --------
- .Axes.set_xlim
- set_xbound, get_xbound
- invert_xaxis, xaxis_inverted
-
- Notes
- -----
- The x-axis may be inverted, in which case the *left* value will
- be greater than the *right* value.
- """
- return tuple(self.viewLim.intervalx)
-
- def _validate_converted_limits(self, limit, convert):
- """
- Raise ValueError if converted limits are non-finite.
-
- Note that this function also accepts None as a limit argument.
-
- Returns
- -------
- The limit value after call to convert(), or None if limit is None.
- """
- if limit is not None:
- converted_limit = convert(limit)
- if (isinstance(converted_limit, Real)
- and not np.isfinite(converted_limit)):
- raise ValueError("Axis limits cannot be NaN or Inf")
- return converted_limit
-
- @_api.make_keyword_only("3.6", "emit")
- def set_xlim(self, left=None, right=None, emit=True, auto=False,
- *, xmin=None, xmax=None):
- """
- Set the x-axis view limits.
-
- Parameters
- ----------
- left : float, optional
- The left xlim in data coordinates. Passing *None* leaves the
- limit unchanged.
-
- The left and right xlims may also be passed as the tuple
- (*left*, *right*) as the first positional argument (or as
- the *left* keyword argument).
-
- .. ACCEPTS: (bottom: float, top: float)
-
- right : float, optional
- The right xlim in data coordinates. Passing *None* leaves the
- limit unchanged.
-
- emit : bool, default: True
- Whether to notify observers of limit change.
-
- auto : bool or None, default: False
- Whether to turn on autoscaling of the x-axis. True turns on,
- False turns off, None leaves unchanged.
-
- xmin, xmax : float, optional
- They are equivalent to left and right respectively, and it is an
- error to pass both *xmin* and *left* or *xmax* and *right*.
-
- Returns
- -------
- left, right : (float, float)
- The new x-axis limits in data coordinates.
-
- See Also
- --------
- get_xlim
- set_xbound, get_xbound
- invert_xaxis, xaxis_inverted
-
- Notes
- -----
- The *left* value may be greater than the *right* value, in which
- case the x-axis values will decrease from left to right.
-
- Examples
- --------
- >>> set_xlim(left, right)
- >>> set_xlim((left, right))
- >>> left, right = set_xlim(left, right)
-
- One limit may be left unchanged.
-
- >>> set_xlim(right=right_lim)
-
- Limits may be passed in reverse order to flip the direction of
- the x-axis. For example, suppose *x* represents the number of
- years before present. The x-axis limits might be set like the
- following so 5000 years ago is on the left of the plot and the
- present is on the right.
-
- >>> set_xlim(5000, 0)
- """
- if right is None and np.iterable(left):
- left, right = left
- if xmin is not None:
- if left is not None:
- raise TypeError("Cannot pass both 'left' and 'xmin'")
- left = xmin
- if xmax is not None:
- if right is not None:
- raise TypeError("Cannot pass both 'right' and 'xmax'")
- right = xmax
- return self.xaxis._set_lim(left, right, emit=emit, auto=auto)
-
- get_xscale = _axis_method_wrapper("xaxis", "get_scale")
- set_xscale = _axis_method_wrapper("xaxis", "_set_axes_scale")
- get_xticks = _axis_method_wrapper("xaxis", "get_ticklocs")
- set_xticks = _axis_method_wrapper("xaxis", "set_ticks")
- get_xmajorticklabels = _axis_method_wrapper("xaxis", "get_majorticklabels")
- get_xminorticklabels = _axis_method_wrapper("xaxis", "get_minorticklabels")
- get_xticklabels = _axis_method_wrapper("xaxis", "get_ticklabels")
- set_xticklabels = _axis_method_wrapper(
- "xaxis", "set_ticklabels",
- doc_sub={"Axis.set_ticks": "Axes.set_xticks"})
-
- def get_ylabel(self):
- """
- Get the ylabel text string.
- """
- label = self.yaxis.get_label()
- return label.get_text()
-
- def set_ylabel(self, ylabel, fontdict=None, labelpad=None, *,
- loc=None, **kwargs):
- """
- Set the label for the y-axis.
-
- Parameters
- ----------
- ylabel : str
- The label text.
-
- labelpad : float, default: :rc:`axes.labelpad`
- Spacing in points from the Axes bounding box including ticks
- and tick labels. If None, the previous value is left as is.
-
- loc : {'bottom', 'center', 'top'}, default: :rc:`yaxis.labellocation`
- The label position. This is a high-level alternative for passing
- parameters *y* and *horizontalalignment*.
-
- Other Parameters
- ----------------
- **kwargs : `.Text` properties
- `.Text` properties control the appearance of the label.
-
- See Also
- --------
- text : Documents the properties supported by `.Text`.
- """
- if labelpad is not None:
- self.yaxis.labelpad = labelpad
- protected_kw = ['y', 'horizontalalignment', 'ha']
- if {*kwargs} & {*protected_kw}:
- if loc is not None:
- raise TypeError(f"Specifying 'loc' is disallowed when any of "
- f"its corresponding low level keyword "
- f"arguments ({protected_kw}) are also "
- f"supplied")
-
- else:
- loc = (loc if loc is not None
- else mpl.rcParams['yaxis.labellocation'])
- _api.check_in_list(('bottom', 'center', 'top'), loc=loc)
-
- y, ha = {
- 'bottom': (0, 'left'),
- 'center': (0.5, 'center'),
- 'top': (1, 'right')
- }[loc]
- kwargs.update(y=y, horizontalalignment=ha)
-
- return self.yaxis.set_label_text(ylabel, fontdict, **kwargs)
-
- def invert_yaxis(self):
- """
- Invert the y-axis.
-
- See Also
- --------
- yaxis_inverted
- get_ylim, set_ylim
- get_ybound, set_ybound
- """
- self.yaxis.set_inverted(not self.yaxis.get_inverted())
-
- yaxis_inverted = _axis_method_wrapper("yaxis", "get_inverted")
-
- def get_ybound(self):
- """
- Return the lower and upper y-axis bounds, in increasing order.
-
- See Also
- --------
- set_ybound
- get_ylim, set_ylim
- invert_yaxis, yaxis_inverted
- """
- bottom, top = self.get_ylim()
- if bottom < top:
- return bottom, top
- else:
- return top, bottom
-
- def set_ybound(self, lower=None, upper=None):
- """
- Set the lower and upper numerical bounds of the y-axis.
-
- This method will honor axis inversion regardless of parameter order.
- It will not change the autoscaling setting (`.get_autoscaley_on()`).
-
- Parameters
- ----------
- lower, upper : float or None
- The lower and upper bounds. If *None*, the respective axis bound
- is not modified.
-
- See Also
- --------
- get_ybound
- get_ylim, set_ylim
- invert_yaxis, yaxis_inverted
- """
- if upper is None and np.iterable(lower):
- lower, upper = lower
-
- old_lower, old_upper = self.get_ybound()
- if lower is None:
- lower = old_lower
- if upper is None:
- upper = old_upper
-
- self.set_ylim(sorted((lower, upper),
- reverse=bool(self.yaxis_inverted())),
- auto=None)
-
- def get_ylim(self):
- """
- Return the y-axis view limits.
-
- Returns
- -------
- bottom, top : (float, float)
- The current y-axis limits in data coordinates.
-
- See Also
- --------
- .Axes.set_ylim
- set_ybound, get_ybound
- invert_yaxis, yaxis_inverted
-
- Notes
- -----
- The y-axis may be inverted, in which case the *bottom* value
- will be greater than the *top* value.
- """
- return tuple(self.viewLim.intervaly)
-
- @_api.make_keyword_only("3.6", "emit")
- def set_ylim(self, bottom=None, top=None, emit=True, auto=False,
- *, ymin=None, ymax=None):
- """
- Set the y-axis view limits.
-
- Parameters
- ----------
- bottom : float, optional
- The bottom ylim in data coordinates. Passing *None* leaves the
- limit unchanged.
-
- The bottom and top ylims may also be passed as the tuple
- (*bottom*, *top*) as the first positional argument (or as
- the *bottom* keyword argument).
-
- .. ACCEPTS: (bottom: float, top: float)
-
- top : float, optional
- The top ylim in data coordinates. Passing *None* leaves the
- limit unchanged.
-
- emit : bool, default: True
- Whether to notify observers of limit change.
-
- auto : bool or None, default: False
- Whether to turn on autoscaling of the y-axis. *True* turns on,
- *False* turns off, *None* leaves unchanged.
-
- ymin, ymax : float, optional
- They are equivalent to bottom and top respectively, and it is an
- error to pass both *ymin* and *bottom* or *ymax* and *top*.
-
- Returns
- -------
- bottom, top : (float, float)
- The new y-axis limits in data coordinates.
-
- See Also
- --------
- get_ylim
- set_ybound, get_ybound
- invert_yaxis, yaxis_inverted
-
- Notes
- -----
- The *bottom* value may be greater than the *top* value, in which
- case the y-axis values will decrease from *bottom* to *top*.
-
- Examples
- --------
- >>> set_ylim(bottom, top)
- >>> set_ylim((bottom, top))
- >>> bottom, top = set_ylim(bottom, top)
-
- One limit may be left unchanged.
-
- >>> set_ylim(top=top_lim)
-
- Limits may be passed in reverse order to flip the direction of
- the y-axis. For example, suppose ``y`` represents depth of the
- ocean in m. The y-axis limits might be set like the following
- so 5000 m depth is at the bottom of the plot and the surface,
- 0 m, is at the top.
-
- >>> set_ylim(5000, 0)
- """
- if top is None and np.iterable(bottom):
- bottom, top = bottom
- if ymin is not None:
- if bottom is not None:
- raise TypeError("Cannot pass both 'bottom' and 'ymin'")
- bottom = ymin
- if ymax is not None:
- if top is not None:
- raise TypeError("Cannot pass both 'top' and 'ymax'")
- top = ymax
- return self.yaxis._set_lim(bottom, top, emit=emit, auto=auto)
-
- get_yscale = _axis_method_wrapper("yaxis", "get_scale")
- set_yscale = _axis_method_wrapper("yaxis", "_set_axes_scale")
- get_yticks = _axis_method_wrapper("yaxis", "get_ticklocs")
- set_yticks = _axis_method_wrapper("yaxis", "set_ticks")
- get_ymajorticklabels = _axis_method_wrapper("yaxis", "get_majorticklabels")
- get_yminorticklabels = _axis_method_wrapper("yaxis", "get_minorticklabels")
- get_yticklabels = _axis_method_wrapper("yaxis", "get_ticklabels")
- set_yticklabels = _axis_method_wrapper(
- "yaxis", "set_ticklabels",
- doc_sub={"Axis.set_ticks": "Axes.set_yticks"})
-
- xaxis_date = _axis_method_wrapper("xaxis", "axis_date")
- yaxis_date = _axis_method_wrapper("yaxis", "axis_date")
-
- def format_xdata(self, x):
- """
- Return *x* formatted as an x-value.
-
- This function will use the `.fmt_xdata` attribute if it is not None,
- else will fall back on the xaxis major formatter.
- """
- return (self.fmt_xdata if self.fmt_xdata is not None
- else self.xaxis.get_major_formatter().format_data_short)(x)
-
- def format_ydata(self, y):
- """
- Return *y* formatted as a y-value.
-
- This function will use the `.fmt_ydata` attribute if it is not None,
- else will fall back on the yaxis major formatter.
- """
- return (self.fmt_ydata if self.fmt_ydata is not None
- else self.yaxis.get_major_formatter().format_data_short)(y)
-
- def format_coord(self, x, y):
- """Return a format string formatting the *x*, *y* coordinates."""
- return "x={} y={}".format(
- "???" if x is None else self.format_xdata(x),
- "???" if y is None else self.format_ydata(y),
- )
-
- def minorticks_on(self):
- """
- Display minor ticks on the Axes.
-
- Displaying minor ticks may reduce performance; you may turn them off
- using `minorticks_off()` if drawing speed is a problem.
- """
- for ax in (self.xaxis, self.yaxis):
- scale = ax.get_scale()
- if scale == 'log':
- s = ax._scale
- ax.set_minor_locator(mticker.LogLocator(s.base, s.subs))
- elif scale == 'symlog':
- s = ax._scale
- ax.set_minor_locator(
- mticker.SymmetricalLogLocator(s._transform, s.subs))
- else:
- ax.set_minor_locator(mticker.AutoMinorLocator())
-
- def minorticks_off(self):
- """Remove minor ticks from the Axes."""
- self.xaxis.set_minor_locator(mticker.NullLocator())
- self.yaxis.set_minor_locator(mticker.NullLocator())
-
- # Interactive manipulation
-
- def can_zoom(self):
- """
- Return whether this Axes supports the zoom box button functionality.
- """
- return True
-
- def can_pan(self):
- """
- Return whether this Axes supports any pan/zoom button functionality.
- """
- return True
-
- def get_navigate(self):
- """
- Get whether the Axes responds to navigation commands.
- """
- return self._navigate
-
- def set_navigate(self, b):
- """
- Set whether the Axes responds to navigation toolbar commands.
-
- Parameters
- ----------
- b : bool
- """
- self._navigate = b
-
- def get_navigate_mode(self):
- """
- Get the navigation toolbar button status: 'PAN', 'ZOOM', or None.
- """
- return self._navigate_mode
-
- def set_navigate_mode(self, b):
- """
- Set the navigation toolbar button status.
-
- .. warning::
- This is not a user-API function.
-
- """
- self._navigate_mode = b
-
- def _get_view(self):
- """
- Save information required to reproduce the current view.
-
- Called before a view is changed, such as during a pan or zoom
- initiated by the user. You may return any information you deem
- necessary to describe the view.
-
- .. note::
-
- Intended to be overridden by new projection types, but if not, the
- default implementation saves the view limits. You *must* implement
- :meth:`_set_view` if you implement this method.
- """
- xmin, xmax = self.get_xlim()
- ymin, ymax = self.get_ylim()
- return xmin, xmax, ymin, ymax
-
- def _set_view(self, view):
- """
- Apply a previously saved view.
-
- Called when restoring a view, such as with the navigation buttons.
-
- .. note::
-
- Intended to be overridden by new projection types, but if not, the
- default implementation restores the view limits. You *must*
- implement :meth:`_get_view` if you implement this method.
- """
- xmin, xmax, ymin, ymax = view
- self.set_xlim((xmin, xmax))
- self.set_ylim((ymin, ymax))
-
- def _prepare_view_from_bbox(self, bbox, direction='in',
- mode=None, twinx=False, twiny=False):
- """
- Helper function to prepare the new bounds from a bbox.
-
- This helper function returns the new x and y bounds from the zoom
- bbox. This a convenience method to abstract the bbox logic
- out of the base setter.
- """
- if len(bbox) == 3:
- xp, yp, scl = bbox # Zooming code
- if scl == 0: # Should not happen
- scl = 1.
- if scl > 1:
- direction = 'in'
- else:
- direction = 'out'
- scl = 1/scl
- # get the limits of the axes
- (xmin, ymin), (xmax, ymax) = self.transData.transform(
- np.transpose([self.get_xlim(), self.get_ylim()]))
- # set the range
- xwidth = xmax - xmin
- ywidth = ymax - ymin
- xcen = (xmax + xmin)*.5
- ycen = (ymax + ymin)*.5
- xzc = (xp*(scl - 1) + xcen)/scl
- yzc = (yp*(scl - 1) + ycen)/scl
- bbox = [xzc - xwidth/2./scl, yzc - ywidth/2./scl,
- xzc + xwidth/2./scl, yzc + ywidth/2./scl]
- elif len(bbox) != 4:
- # should be len 3 or 4 but nothing else
- _api.warn_external(
- "Warning in _set_view_from_bbox: bounding box is not a tuple "
- "of length 3 or 4. Ignoring the view change.")
- return
-
- # Original limits.
- xmin0, xmax0 = self.get_xbound()
- ymin0, ymax0 = self.get_ybound()
- # The zoom box in screen coords.
- startx, starty, stopx, stopy = bbox
- # Convert to data coords.
- (startx, starty), (stopx, stopy) = self.transData.inverted().transform(
- [(startx, starty), (stopx, stopy)])
- # Clip to axes limits.
- xmin, xmax = np.clip(sorted([startx, stopx]), xmin0, xmax0)
- ymin, ymax = np.clip(sorted([starty, stopy]), ymin0, ymax0)
- # Don't double-zoom twinned axes or if zooming only the other axis.
- if twinx or mode == "y":
- xmin, xmax = xmin0, xmax0
- if twiny or mode == "x":
- ymin, ymax = ymin0, ymax0
-
- if direction == "in":
- new_xbound = xmin, xmax
- new_ybound = ymin, ymax
-
- elif direction == "out":
- x_trf = self.xaxis.get_transform()
- sxmin0, sxmax0, sxmin, sxmax = x_trf.transform(
- [xmin0, xmax0, xmin, xmax]) # To screen space.
- factor = (sxmax0 - sxmin0) / (sxmax - sxmin) # Unzoom factor.
- # Move original bounds away by
- # (factor) x (distance between unzoom box and Axes bbox).
- sxmin1 = sxmin0 - factor * (sxmin - sxmin0)
- sxmax1 = sxmax0 + factor * (sxmax0 - sxmax)
- # And back to data space.
- new_xbound = x_trf.inverted().transform([sxmin1, sxmax1])
-
- y_trf = self.yaxis.get_transform()
- symin0, symax0, symin, symax = y_trf.transform(
- [ymin0, ymax0, ymin, ymax])
- factor = (symax0 - symin0) / (symax - symin)
- symin1 = symin0 - factor * (symin - symin0)
- symax1 = symax0 + factor * (symax0 - symax)
- new_ybound = y_trf.inverted().transform([symin1, symax1])
-
- return new_xbound, new_ybound
-
- def _set_view_from_bbox(self, bbox, direction='in',
- mode=None, twinx=False, twiny=False):
- """
- Update view from a selection bbox.
-
- .. note::
-
- Intended to be overridden by new projection types, but if not, the
- default implementation sets the view limits to the bbox directly.
-
- Parameters
- ----------
- bbox : 4-tuple or 3 tuple
- * If bbox is a 4 tuple, it is the selected bounding box limits,
- in *display* coordinates.
- * If bbox is a 3 tuple, it is an (xp, yp, scl) triple, where
- (xp, yp) is the center of zooming and scl the scale factor to
- zoom by.
-
- direction : str
- The direction to apply the bounding box.
- * `'in'` - The bounding box describes the view directly, i.e.,
- it zooms in.
- * `'out'` - The bounding box describes the size to make the
- existing view, i.e., it zooms out.
-
- mode : str or None
- The selection mode, whether to apply the bounding box in only the
- `'x'` direction, `'y'` direction or both (`None`).
-
- twinx : bool
- Whether this axis is twinned in the *x*-direction.
-
- twiny : bool
- Whether this axis is twinned in the *y*-direction.
- """
- new_xbound, new_ybound = self._prepare_view_from_bbox(
- bbox, direction=direction, mode=mode, twinx=twinx, twiny=twiny)
- if not twinx and mode != "y":
- self.set_xbound(new_xbound)
- self.set_autoscalex_on(False)
- if not twiny and mode != "x":
- self.set_ybound(new_ybound)
- self.set_autoscaley_on(False)
-
- def start_pan(self, x, y, button):
- """
- Called when a pan operation has started.
-
- Parameters
- ----------
- x, y : float
- The mouse coordinates in display coords.
- button : `.MouseButton`
- The pressed mouse button.
-
- Notes
- -----
- This is intended to be overridden by new projection types.
- """
- self._pan_start = types.SimpleNamespace(
- lim=self.viewLim.frozen(),
- trans=self.transData.frozen(),
- trans_inverse=self.transData.inverted().frozen(),
- bbox=self.bbox.frozen(),
- x=x,
- y=y)
-
- def end_pan(self):
- """
- Called when a pan operation completes (when the mouse button is up.)
-
- Notes
- -----
- This is intended to be overridden by new projection types.
- """
- del self._pan_start
-
- def _get_pan_points(self, button, key, x, y):
- """
- Helper function to return the new points after a pan.
-
- This helper function returns the points on the axis after a pan has
- occurred. This is a convenience method to abstract the pan logic
- out of the base setter.
- """
- def format_deltas(key, dx, dy):
- if key == 'control':
- if abs(dx) > abs(dy):
- dy = dx
- else:
- dx = dy
- elif key == 'x':
- dy = 0
- elif key == 'y':
- dx = 0
- elif key == 'shift':
- if 2 * abs(dx) < abs(dy):
- dx = 0
- elif 2 * abs(dy) < abs(dx):
- dy = 0
- elif abs(dx) > abs(dy):
- dy = dy / abs(dy) * abs(dx)
- else:
- dx = dx / abs(dx) * abs(dy)
- return dx, dy
-
- p = self._pan_start
- dx = x - p.x
- dy = y - p.y
- if dx == dy == 0:
- return
- if button == 1:
- dx, dy = format_deltas(key, dx, dy)
- result = p.bbox.translated(-dx, -dy).transformed(p.trans_inverse)
- elif button == 3:
- try:
- dx = -dx / self.bbox.width
- dy = -dy / self.bbox.height
- dx, dy = format_deltas(key, dx, dy)
- if self.get_aspect() != 'auto':
- dx = dy = 0.5 * (dx + dy)
- alpha = np.power(10.0, (dx, dy))
- start = np.array([p.x, p.y])
- oldpoints = p.lim.transformed(p.trans)
- newpoints = start + alpha * (oldpoints - start)
- result = (mtransforms.Bbox(newpoints)
- .transformed(p.trans_inverse))
- except OverflowError:
- _api.warn_external('Overflow while panning')
- return
- else:
- return
-
- valid = np.isfinite(result.transformed(p.trans))
- points = result.get_points().astype(object)
- # Just ignore invalid limits (typically, underflow in log-scale).
- points[~valid] = None
- return points
-
- def drag_pan(self, button, key, x, y):
- """
- Called when the mouse moves during a pan operation.
-
- Parameters
- ----------
- button : `.MouseButton`
- The pressed mouse button.
- key : str or None
- The pressed key, if any.
- x, y : float
- The mouse coordinates in display coords.
-
- Notes
- -----
- This is intended to be overridden by new projection types.
- """
- points = self._get_pan_points(button, key, x, y)
- if points is not None:
- self.set_xlim(points[:, 0])
- self.set_ylim(points[:, 1])
-
- def get_children(self):
- # docstring inherited.
- return [
- *self._children,
- *self.spines.values(),
- *self._axis_map.values(),
- self.title, self._left_title, self._right_title,
- *self.child_axes,
- *([self.legend_] if self.legend_ is not None else []),
- self.patch,
- ]
-
- def contains(self, mouseevent):
- # docstring inherited.
- inside, info = self._default_contains(mouseevent)
- if inside is not None:
- return inside, info
- return self.patch.contains(mouseevent)
-
- def contains_point(self, point):
- """
- Return whether *point* (pair of pixel coordinates) is inside the Axes
- patch.
- """
- return self.patch.contains_point(point, radius=1.0)
-
- def get_default_bbox_extra_artists(self):
- """
- Return a default list of artists that are used for the bounding box
- calculation.
-
- Artists are excluded either by not being visible or
- ``artist.set_in_layout(False)``.
- """
-
- artists = self.get_children()
-
- for axis in self._axis_map.values():
- # axis tight bboxes are calculated separately inside
- # Axes.get_tightbbox() using for_layout_only=True
- artists.remove(axis)
- if not (self.axison and self._frameon):
- # don't do bbox on spines if frame not on.
- for spine in self.spines.values():
- artists.remove(spine)
-
- artists.remove(self.title)
- artists.remove(self._left_title)
- artists.remove(self._right_title)
-
- # always include types that do not internally implement clipping
- # to Axes. may have clip_on set to True and clip_box equivalent
- # to ax.bbox but then ignore these properties during draws.
- noclip = (_AxesBase, maxis.Axis,
- offsetbox.AnnotationBbox, offsetbox.OffsetBox)
- return [a for a in artists if a.get_visible() and a.get_in_layout()
- and (isinstance(a, noclip) or not a._fully_clipped_to_axes())]
-
- def get_tightbbox(self, renderer=None, call_axes_locator=True,
- bbox_extra_artists=None, *, for_layout_only=False):
- """
- Return the tight bounding box of the Axes, including axis and their
- decorators (xlabel, title, etc).
-
- Artists that have ``artist.set_in_layout(False)`` are not included
- in the bbox.
-
- Parameters
- ----------
- renderer : `.RendererBase` subclass
- renderer that will be used to draw the figures (i.e.
- ``fig.canvas.get_renderer()``)
-
- bbox_extra_artists : list of `.Artist` or ``None``
- List of artists to include in the tight bounding box. If
- ``None`` (default), then all artist children of the Axes are
- included in the tight bounding box.
-
- call_axes_locator : bool, default: True
- If *call_axes_locator* is ``False``, it does not call the
- ``_axes_locator`` attribute, which is necessary to get the correct
- bounding box. ``call_axes_locator=False`` can be used if the
- caller is only interested in the relative size of the tightbbox
- compared to the Axes bbox.
-
- for_layout_only : default: False
- The bounding box will *not* include the x-extent of the title and
- the xlabel, or the y-extent of the ylabel.
-
- Returns
- -------
- `.BboxBase`
- Bounding box in figure pixel coordinates.
-
- See Also
- --------
- matplotlib.axes.Axes.get_window_extent
- matplotlib.axis.Axis.get_tightbbox
- matplotlib.spines.Spine.get_window_extent
- """
-
- bb = []
- if renderer is None:
- renderer = self.figure._get_renderer()
-
- if not self.get_visible():
- return None
-
- locator = self.get_axes_locator()
- self.apply_aspect(
- locator(self, renderer) if locator and call_axes_locator else None)
-
- for axis in self._axis_map.values():
- if self.axison and axis.get_visible():
- ba = martist._get_tightbbox_for_layout_only(axis, renderer)
- if ba:
- bb.append(ba)
- self._update_title_position(renderer)
- axbbox = self.get_window_extent(renderer)
- bb.append(axbbox)
-
- for title in [self.title, self._left_title, self._right_title]:
- if title.get_visible():
- bt = title.get_window_extent(renderer)
- if for_layout_only and bt.width > 0:
- # make the title bbox 1 pixel wide so its width
- # is not accounted for in bbox calculations in
- # tight/constrained_layout
- bt.x0 = (bt.x0 + bt.x1) / 2 - 0.5
- bt.x1 = bt.x0 + 1.0
- bb.append(bt)
-
- bbox_artists = bbox_extra_artists
- if bbox_artists is None:
- bbox_artists = self.get_default_bbox_extra_artists()
-
- for a in bbox_artists:
- bbox = a.get_tightbbox(renderer)
- if (bbox is not None
- and 0 < bbox.width < np.inf
- and 0 < bbox.height < np.inf):
- bb.append(bbox)
- return mtransforms.Bbox.union(
- [b for b in bb if b.width != 0 or b.height != 0])
-
- def _make_twin_axes(self, *args, **kwargs):
- """Make a twinx Axes of self. This is used for twinx and twiny."""
- if 'sharex' in kwargs and 'sharey' in kwargs:
- # The following line is added in v2.2 to avoid breaking Seaborn,
- # which currently uses this internal API.
- if kwargs["sharex"] is not self and kwargs["sharey"] is not self:
- raise ValueError("Twinned Axes may share only one axis")
- ss = self.get_subplotspec()
- if ss:
- twin = self.figure.add_subplot(ss, *args, **kwargs)
- else:
- twin = self.figure.add_axes(
- self.get_position(True), *args, **kwargs,
- axes_locator=_TransformedBoundsLocator(
- [0, 0, 1, 1], self.transAxes))
- self.set_adjustable('datalim')
- twin.set_adjustable('datalim')
- self._twinned_axes.join(self, twin)
- return twin
-
- def twinx(self):
- """
- Create a twin Axes sharing the xaxis.
-
- Create a new Axes with an invisible x-axis and an independent
- y-axis positioned opposite to the original one (i.e. at right). The
- x-axis autoscale setting will be inherited from the original
- Axes. To ensure that the tick marks of both y-axes align, see
- `~matplotlib.ticker.LinearLocator`.
-
- Returns
- -------
- Axes
- The newly created Axes instance
-
- Notes
- -----
- For those who are 'picking' artists while using twinx, pick
- events are only called for the artists in the top-most Axes.
- """
- ax2 = self._make_twin_axes(sharex=self)
- ax2.yaxis.tick_right()
- ax2.yaxis.set_label_position('right')
- ax2.yaxis.set_offset_position('right')
- ax2.set_autoscalex_on(self.get_autoscalex_on())
- self.yaxis.tick_left()
- ax2.xaxis.set_visible(False)
- ax2.patch.set_visible(False)
- return ax2
-
- def twiny(self):
- """
- Create a twin Axes sharing the yaxis.
-
- Create a new Axes with an invisible y-axis and an independent
- x-axis positioned opposite to the original one (i.e. at top). The
- y-axis autoscale setting will be inherited from the original Axes.
- To ensure that the tick marks of both x-axes align, see
- `~matplotlib.ticker.LinearLocator`.
-
- Returns
- -------
- Axes
- The newly created Axes instance
-
- Notes
- -----
- For those who are 'picking' artists while using twiny, pick
- events are only called for the artists in the top-most Axes.
- """
- ax2 = self._make_twin_axes(sharey=self)
- ax2.xaxis.tick_top()
- ax2.xaxis.set_label_position('top')
- ax2.set_autoscaley_on(self.get_autoscaley_on())
- self.xaxis.tick_bottom()
- ax2.yaxis.set_visible(False)
- ax2.patch.set_visible(False)
- return ax2
-
- def get_shared_x_axes(self):
- """Return an immutable view on the shared x-axes Grouper."""
- return cbook.GrouperView(self._shared_axes["x"])
-
- def get_shared_y_axes(self):
- """Return an immutable view on the shared y-axes Grouper."""
- return cbook.GrouperView(self._shared_axes["y"])
-
- def label_outer(self):
- """
- Only show "outer" labels and tick labels.
-
- x-labels are only kept for subplots on the last row (or first row, if
- labels are on the top side); y-labels only for subplots on the first
- column (or last column, if labels are on the right side).
- """
- self._label_outer_xaxis(check_patch=False)
- self._label_outer_yaxis(check_patch=False)
-
- def _label_outer_xaxis(self, *, check_patch):
- # see documentation in label_outer.
- if check_patch and not isinstance(self.patch, mpl.patches.Rectangle):
- return
- ss = self.get_subplotspec()
- if not ss:
- return
- label_position = self.xaxis.get_label_position()
- if not ss.is_first_row(): # Remove top label/ticklabels/offsettext.
- if label_position == "top":
- self.set_xlabel("")
- self.xaxis.set_tick_params(which="both", labeltop=False)
- if self.xaxis.offsetText.get_position()[1] == 1:
- self.xaxis.offsetText.set_visible(False)
- if not ss.is_last_row(): # Remove bottom label/ticklabels/offsettext.
- if label_position == "bottom":
- self.set_xlabel("")
- self.xaxis.set_tick_params(which="both", labelbottom=False)
- if self.xaxis.offsetText.get_position()[1] == 0:
- self.xaxis.offsetText.set_visible(False)
-
- def _label_outer_yaxis(self, *, check_patch):
- # see documentation in label_outer.
- if check_patch and not isinstance(self.patch, mpl.patches.Rectangle):
- return
- ss = self.get_subplotspec()
- if not ss:
- return
- label_position = self.yaxis.get_label_position()
- if not ss.is_first_col(): # Remove left label/ticklabels/offsettext.
- if label_position == "left":
- self.set_ylabel("")
- self.yaxis.set_tick_params(which="both", labelleft=False)
- if self.yaxis.offsetText.get_position()[0] == 0:
- self.yaxis.offsetText.set_visible(False)
- if not ss.is_last_col(): # Remove right label/ticklabels/offsettext.
- if label_position == "right":
- self.set_ylabel("")
- self.yaxis.set_tick_params(which="both", labelright=False)
- if self.yaxis.offsetText.get_position()[0] == 1:
- self.yaxis.offsetText.set_visible(False)
-
-
-def _draw_rasterized(figure, artists, renderer):
- """
- A helper function for rasterizing the list of artists.
-
- The bookkeeping to track if we are or are not in rasterizing mode
- with the mixed-mode backends is relatively complicated and is now
- handled in the matplotlib.artist.allow_rasterization decorator.
-
- This helper defines the absolute minimum methods and attributes on a
- shim class to be compatible with that decorator and then uses it to
- rasterize the list of artists.
-
- This is maybe too-clever, but allows us to re-use the same code that is
- used on normal artists to participate in the "are we rasterizing"
- accounting.
-
- Please do not use this outside of the "rasterize below a given zorder"
- functionality of Axes.
-
- Parameters
- ----------
- figure : matplotlib.figure.Figure
- The figure all of the artists belong to (not checked). We need this
- because we can at the figure level suppress composition and insert each
- rasterized artist as its own image.
-
- artists : List[matplotlib.artist.Artist]
- The list of Artists to be rasterized. These are assumed to all
- be in the same Figure.
-
- renderer : matplotlib.backendbases.RendererBase
- The currently active renderer
-
- Returns
- -------
- None
-
- """
- class _MinimalArtist:
- def get_rasterized(self):
- return True
-
- def get_agg_filter(self):
- return None
-
- def __init__(self, figure, artists):
- self.figure = figure
- self.artists = artists
-
- @martist.allow_rasterization
- def draw(self, renderer):
- for a in self.artists:
- a.draw(renderer)
-
- return _MinimalArtist(figure, artists).draw(renderer)
diff --git a/spaces/latent-consistency/lcm-lora-for-sdxl/README.md b/spaces/latent-consistency/lcm-lora-for-sdxl/README.md
deleted file mode 100644
index 66203721011c18c6e9951207600b99994041ddf2..0000000000000000000000000000000000000000
--- a/spaces/latent-consistency/lcm-lora-for-sdxl/README.md
+++ /dev/null
@@ -1,12 +0,0 @@
----
-title: LCM-LoRA on SDXL
-emoji: 📚
-colorFrom: blue
-colorTo: blue
-sdk: gradio
-sdk_version: 4.1.2
-app_file: app.py
-pinned: false
----
-
-Check out the configuration reference at https://huggingface.co/docs/hub/spaces-config-reference
diff --git a/spaces/leilevy/bingo/src/lib/isomorphic/browser.ts b/spaces/leilevy/bingo/src/lib/isomorphic/browser.ts
deleted file mode 100644
index de125b1f1786d1618cb1ff47f403d76c6784f4ce..0000000000000000000000000000000000000000
--- a/spaces/leilevy/bingo/src/lib/isomorphic/browser.ts
+++ /dev/null
@@ -1,11 +0,0 @@
-'use client'
-
-const debug = console.info.bind(console)
-
-class WebSocketAlias extends WebSocket {
- constructor(address: string | URL, ...args: any) {
- super(address)
- }
-}
-
-export default { fetch, WebSocket: WebSocketAlias, debug }
diff --git a/spaces/lewisliuX123/wechatllama2/app.py b/spaces/lewisliuX123/wechatllama2/app.py
deleted file mode 100644
index 59f0f0c5f48cd69b6b08d7fd0ea65dca9f497f2f..0000000000000000000000000000000000000000
--- a/spaces/lewisliuX123/wechatllama2/app.py
+++ /dev/null
@@ -1,45 +0,0 @@
-# encoding:utf-8
-
-import config
-import gradio as gr
-from channel import channel_factory
-from common.log import logger
-from io import BytesIO
-from PIL import Image
-from concurrent.futures import ThreadPoolExecutor
-thread_pool = ThreadPoolExecutor(max_workers=8)
-
-def getImage(bytes):
- bytes_stream = BytesIO(bytes)
- image = Image.open(bytes_stream)
- return image
-
-def getLoginUrl():
- # load config
- config.load_config()
-
- # create channel
- bot = channel_factory.create_channel("wx")
- thread_pool.submit(bot.startup)
-
- while (True):
- if bot.getQrCode():
- return getImage(bot.getQrCode())
-
-if __name__ == '__main__':
- try:
-
- with gr.Blocks() as demo:
- with gr.Row():
- with gr.Column():
- btn = gr.Button(value="生成二维码")
- with gr.Column():
- outputs=[gr.Pil()]
- btn.click(getLoginUrl, outputs=outputs)
-
- demo.launch()
-
-
- except Exception as e:
- logger.error("App startup failed!")
- logger.exception(e)
diff --git a/spaces/lincquiQcaudo/Top-20-Diffusion/Anandam Telugu Movie English Subtitles 14 PORTABLE.md b/spaces/lincquiQcaudo/Top-20-Diffusion/Anandam Telugu Movie English Subtitles 14 PORTABLE.md
deleted file mode 100644
index 77514724caefe42b7abeb5e0e06f139a02250e58..0000000000000000000000000000000000000000
--- a/spaces/lincquiQcaudo/Top-20-Diffusion/Anandam Telugu Movie English Subtitles 14 PORTABLE.md
+++ /dev/null
@@ -1,28 +0,0 @@
-
-
-Watch Anandam Telugu Movie English Subtitles 14 with English Subtitles. 2018. Directed By: Prabhas. The original poster of this movie Anandam Telugu Movie English Subtitles 14 is:
-
-Download Anandam Telugu Movie English Subtitles 14 Without Subtitles. Directed By: Prabhas. The original poster of this movie Anandam Telugu Movie English Subtitles 14 is:
-
-Watch Anandam Telugu Movie English Subtitles 14 in HD quality 720p. Please download the subtitles for the movie Anandam Telugu Movie English Subtitles 14 or watch the movie Anandam Telugu Movie English Subtitles 14 online with English Subtitles. The subtitles of the movie Anandam Telugu Movie English Subtitles 14 will be updated on our website as soon as the newest versions of the movies' subtitles are available.
-
-Download Anandam Telugu Movie English Subtitles 14 Without Subtitles:
-
-Anandam Telugu Movie English Subtitles 14 is a movie in the genres of Drama, Action, History. It was released in September 28, 2018, by actor Prabhas. The movie is directed by K. V. Anand. It has been under production since 2011. The film's music is composed by Anand-Milind.
-
-Watch Anandam Telugu Movie English Subtitles 14 in HD
-
-Anandam Telugu Movie English Subtitles 14 poster, trailers, photos
-
-I really like the movie Anandam Telugu Movie English Subtitles 14
-
-Watch Anandam Telugu Movie English Subtitles 14 with english subtitles
-
-The movie Anandam Telugu Movie English Subtitles 14 is about
-
-The movie Anandam Telugu Movie English Subtitles 14 is about the lives of the historic Chola rulers, particularly, Karikala Cholan, who ruled the territories of the present-day Telangana and Andhra Pradesh. It is set in the reign of Karikala Cholan, who was the successor to the much-celebrated king of the 11th century king of the Cholas, Rajendra Cholan. The film tells the story of Karikala Cholan's rise to power, and the events that led to his defeat by the rival local ruler of the area, who took over and ruled until Karikala was able to return.
-
-The movie An 4fefd39f24
-
-
-
diff --git a/spaces/lincquiQcaudo/Top-20-Diffusion/Autocad 2012 X64 (64bit) (Product Key And Xforce Keygen ((EXCLUSIVE))) 64 Bit.md b/spaces/lincquiQcaudo/Top-20-Diffusion/Autocad 2012 X64 (64bit) (Product Key And Xforce Keygen ((EXCLUSIVE))) 64 Bit.md
deleted file mode 100644
index bb6c3d99e3ca942e57650ad8f1bcfef9a7ff36d7..0000000000000000000000000000000000000000
--- a/spaces/lincquiQcaudo/Top-20-Diffusion/Autocad 2012 X64 (64bit) (Product Key And Xforce Keygen ((EXCLUSIVE))) 64 Bit.md
+++ /dev/null
@@ -1,6 +0,0 @@
-
Autocad 2012 x64 (64bit) (Product key and Xforce keygen) 64 bit
-
-John Deere American Farmer Deluxe Free Download PC Game Cracked in Direct Link and Torrent. John Deere American Farmer is a farm ... 1fdad05405
-
-
-
diff --git a/spaces/lincquiQcaudo/Top-20-Diffusion/La Noire Synchronizing Fix Skidrow 214.md b/spaces/lincquiQcaudo/Top-20-Diffusion/La Noire Synchronizing Fix Skidrow 214.md
deleted file mode 100644
index 4cf3f99dee203e34615402581b5985452993af72..0000000000000000000000000000000000000000
--- a/spaces/lincquiQcaudo/Top-20-Diffusion/La Noire Synchronizing Fix Skidrow 214.md
+++ /dev/null
@@ -1,29 +0,0 @@
-
-
How to Fix L.A. Noire Synchronization Issue with SKiDROW Update
-
L.A. Noire is a detective game set in 1940s Los Angeles, where you play as Cole Phelps, a war veteran and police officer who solves crimes using clues, interrogations and chases. The game was released in 2011 by Rockstar Games and Team Bondi, and received critical acclaim for its realistic facial animations, story and atmosphere.
-
However, some PC players have encountered a problem with the game where it gets stuck on a screen that says "Synchronizing" after launching it. This issue prevents them from playing the game at all, and can be very frustrating. Fortunately, there is a solution for this problem, thanks to a patch released by SKiDROW, a group of hackers who crack games and bypass their protections.
In this article, we will show you how to fix L.A. Noire synchronization issue with SKiDROW update, which is version 1.2.2610 of the game. This update also adds DirectX 11 support, improves performance and fixes some crashes. Here are the steps you need to follow:
-
-
Download the SKiDROW update from here. This is a trusted source that provides safe and working files. The update is about 60 MB in size.
-
Unpack the downloaded file using a program like WinRAR or 7-Zip. You will get a folder called SKIDROW with two files inside: L.A.Noire.v1.2.2610.Update.exe and SKIDROW.nfo.
-
Run L.A.Noire.v1.2.2610.Update.exe and follow the instructions on the screen. It will ask you to select the folder where you installed L.A. Noire on your PC. Make sure you choose the correct one.
-
After the update is installed, open the SKIDROW folder and copy the file called LANLauncher.exe to the main folder where you installed L.A. Noire. This file will replace the original one that came with the game.
-
Launch L.A. Noire using LANLauncher.exe. You will see a window that allows you to switch between DirectX 9 and DirectX 11 renderers. Choose the one that works best for your system.
-
Enjoy playing L.A. Noire without any synchronization issues!
-
-
Note: You will need to create a local profile using Rockstar's Social Club to be able to save and load your progress in the game. You can do this by clicking on "Create New User" on the Social Club window that pops up when you launch the game.
-
We hope this article helped you fix L.A. Noire synchronization issue with SKiDROW update. If you have any questions or feedback, feel free to leave a comment below.
-
-
How to Play L.A. Noire Like a Pro
-
L.A. Noire is not your typical action game. It requires a lot of attention to detail, logic and intuition to solve the cases and catch the criminals. You will need to explore crime scenes, collect clues, interview witnesses and suspects, and make choices that affect the outcome of the story. Here are some tips that will help you play L.A. Noire like a pro:
-
-
Pay attention to your partner. Your partner is not only your backup in combat, but also your guide and advisor in investigations. He will often give you hints about where to go next, what to look for, and how to question people. He will also comment on your performance and react to your decisions. Listen to what he says and follow his lead.
-
Use intuition points wisely. Intuition points are a limited resource that can help you in various ways. You can use them to highlight all clues in a crime scene, remove one wrong answer in an interrogation, or ask the community for help. You can earn intuition points by ranking up or finding newspapers. Use them sparingly and only when you are really stuck.
-
Read people's faces. One of the most unique features of L.A. Noire is its facial animation technology, which allows you to see realistic expressions and emotions on the characters' faces. This is crucial for interrogations, where you have to decide whether someone is telling the truth, lying, or hiding something. Pay attention to their eyes, mouth, and body language, and compare them to the evidence you have.
-
Choose your approach carefully. When questioning someone, you have three options: good cop, bad cop, or accuse. Good cop means you are friendly and sympathetic, bad cop means you are aggressive and intimidating, and accuse means you are confrontational and have proof of their guilt. Depending on the person's personality and situation, different approaches will yield different results. Choose wisely and be prepared to back up your accusations with evidence.
-
Don't be afraid to fail. L.A. Noire is a game that allows you to make mistakes and learn from them. If you miss a clue, botch an interrogation, or lose a chase, the game will not end. You will still be able to continue the case and reach a conclusion, although it might not be the best one possible. You can always replay a case later if you want to improve your score or see a different outcome.
-
-
L.A. Noire is a game that rewards patience, curiosity, and deduction. It is also a game that immerses you in a rich and authentic recreation of 1940s Los Angeles, full of crime, corruption, and mystery. If you follow these tips, you will be able to enjoy this game to its fullest potential.
d5da3c52bf
-
-
\ No newline at end of file
diff --git a/spaces/ljjggr/bingo/postcss.config.js b/spaces/ljjggr/bingo/postcss.config.js
deleted file mode 100644
index 33ad091d26d8a9dc95ebdf616e217d985ec215b8..0000000000000000000000000000000000000000
--- a/spaces/ljjggr/bingo/postcss.config.js
+++ /dev/null
@@ -1,6 +0,0 @@
-module.exports = {
- plugins: {
- tailwindcss: {},
- autoprefixer: {},
- },
-}
diff --git a/spaces/ltgoslo/ssa-perin/README.md b/spaces/ltgoslo/ssa-perin/README.md
deleted file mode 100644
index 75a7d659f8f9e1202adda22315b4c3cbc6912aff..0000000000000000000000000000000000000000
--- a/spaces/ltgoslo/ssa-perin/README.md
+++ /dev/null
@@ -1,34 +0,0 @@
----
-title: Sentiment Analysis
-emoji: 🤔
-colorFrom: purple
-colorTo: yellow
-sdk: gradio
-sdk_version: 3.1.7
-app_file: app.py
-pinned: false
----
-
-This space provides a gradio demo and an easy-to-run wrapper of the pre-trained model for structured sentiment analysis in Norwegian language, pre-trained on the [NoReC dataset](https://huggingface.co/datasets/norec).
-This model is an implementation of the paper "Direct parsing to sentiment graphs" (Samuel _et al._, ACL 2022). The main repository that also contains the scripts for training the model, can be found on the project [github](https://github.com/jerbarnes/direct_parsing_to_sent_graph).
-
-The current model uses the 'labeled-edge' graph encoding, and achieves the following results on the NoReC dataset:
-
-| Unlabeled sentiment tuple F1 | Target F1 | Relative polarity precision |
-|:----------------------------:|:----------:|:---------------------------:|
-| 0.393 | 0.468 | 0.939 |
-
-
-The model can be easily used for predicting sentiment tuples as follows:
-
-```python
->>> import model_wrapper
->>> model = model_wrapper.PredictionModel()
->>> model.predict(['vi liker svart kaffe'])
-[{'sent_id': '0',
- 'text': 'vi liker svart kaffe',
- 'opinions': [{'Source': [['vi'], ['0:2']],
- 'Target': [['svart', 'kaffe'], ['9:14', '15:20']],
- 'Polar_expression': [['liker'], ['3:8']],
- 'Polarity': 'Positive'}]}]
-```
diff --git a/spaces/lulmer/paraphraser_ai/backend/data_augmenter.py b/spaces/lulmer/paraphraser_ai/backend/data_augmenter.py
deleted file mode 100644
index 9a66c692f56771388c8c4248fef2b3b8e3359958..0000000000000000000000000000000000000000
--- a/spaces/lulmer/paraphraser_ai/backend/data_augmenter.py
+++ /dev/null
@@ -1,185 +0,0 @@
-#%%
-import argparse
-import time
-from tqdm import tqdm
-import pandas as pd
-from transformers import AutoTokenizer, AutoModelForSeq2SeqLM
-import os
-import json
-import torch
-from dotenv import load_dotenv
-#%%
-
-
-load_dotenv()
-from nltk.tokenize import sent_tokenize
-
-wd = os.path.dirname(os.path.realpath(__file__))
-
-
-class BackTranslatorAugmenter:
- """
- A class that performs BackTranslation in order to do data augmentation.
- For best results we recommend using bottleneck languages (`out_lang`)
- such as russian (ru) and
- spanish (es).
-
- Example
- -------
- .. code-block:: python
-
- data_augmenter = BackTranslatorAugmenter(out_lang="es")
- text = "I want to augment this sentence"
- print(text)
- data_augmenter.back_translate(text, verbose=True)
-
- :param in_lang: the text input language, defaults to "en"
- :type in_lang: str, optional
- :param out_lang: the language to translate with, defaults to "ru"
- :type out_lang: str, optional
- """
-
- def __init__(self, in_lang="en", out_lang="ru") -> None:
- if torch.cuda.is_available():
- self.device = "cuda"
- else:
- self.device = "cpu"
-
- self.in_tokenizer = AutoTokenizer.from_pretrained(
- f"Helsinki-NLP/opus-mt-{in_lang}-{out_lang}",
- cache_dir=os.getenv("TRANSFORMERS_CACHE"),
- )
- self.in_model = AutoModelForSeq2SeqLM.from_pretrained(
- f"Helsinki-NLP/opus-mt-{in_lang}-{out_lang}",
- cache_dir=os.getenv("TRANSFORMERS_CACHE"),
- ).to(self.device)
- self.out_tokenizer = AutoTokenizer.from_pretrained(
- f"Helsinki-NLP/opus-mt-{out_lang}-{in_lang}",
- cache_dir=os.getenv("TRANSFORMERS_CACHE"),
- )
- self.out_model = AutoModelForSeq2SeqLM.from_pretrained(
- f"Helsinki-NLP/opus-mt-{out_lang}-{in_lang}",
- cache_dir=os.getenv("TRANSFORMERS_CACHE"),
- ).to(self.device)
-
- def back_translate(self, text, verbose=False):
- if verbose:
- tic = time.time()
- encoded_text = self.in_tokenizer(
- text, return_tensors="pt", padding=True, truncation=True, return_overflowing_tokens=True
- ).to(self.device)
- if encoded_text['num_truncated_tokens'][0] > 0:
- print('Text is too long ')
- return self.back_translate_long(text,verbose=verbose)
-
- in_generated_ids = self.in_model.generate(inputs=encoded_text['input_ids'],
- attention_mask=encoded_text["attention_mask"])
-
- in_preds = [
- self.in_tokenizer.decode(
- gen_id, skip_special_tokens=True, clean_up_tokenization_spaces=True
- )
- for gen_id in in_generated_ids
- ]
- if verbose:
- print("in_pred : ", in_preds)
- encoded_text = self.out_tokenizer(
- in_preds, return_tensors="pt", padding=True, truncation=True,return_overflowing_tokens=True
- ).to(self.device)
- out_generated_ids = self.out_model.generate(inputs=encoded_text['input_ids'],
- attention_mask=encoded_text["attention_mask"])
- out_preds = [
- self.out_tokenizer.decode(
- gen_id, skip_special_tokens=True, clean_up_tokenization_spaces=True
- )
- for gen_id in out_generated_ids
- ]
-
- if verbose:
- tac = time.time()
- print("out_pred : ", out_preds)
- print("Elapsed time : ", tac - tic)
- return out_preds
-
- def back_translate_long(self, text, verbose=False):
- sentences = sent_tokenize(text)
- return [" ".join(self.back_translate(sentences, verbose=verbose))]
-
-
-def do_backtranslation(**args):
- df = pd.read_csv(args["input_data_path"])[:1]
- data_augmenter = BackTranslatorAugmenter(
- in_lang=args["in_lang"], out_lang=args["out_lang"]
- )
-
- dict_res = {col_name: [] for _, col_name in args["col_map"].items()}
-
- for i in tqdm(range(0, len(df), args["batch_size"])):
- for old_col, new_col in args["col_map"].items():
- dict_res[new_col] += data_augmenter.back_translate(
- list(df[old_col].iloc[i : i + args["batch_size"]])
- )
-
- augmented_df = pd.DataFrame(dict_res)
- os.makedirs(os.path.dirname(args["output_data_path"]), exist_ok=True)
- augmented_df.to_csv(args["output_data_path"])
-
-
-if __name__ == "__main__":
- parser = argparse.ArgumentParser(
- description="Back Translate a dataset for better training"
- )
- parser.add_argument(
- "-in_lang",
- type=str,
- default="en",
- help="""the text input language, defaults to "en",
- one can choose between {'es','ru','en','fr','de','pt','zh'}
- but please have a look at https://huggingface.co/Helsinki-NLP to make sure the language
- pair you ask for is available""",
- )
-
- parser.add_argument(
- "-out_lang",
- type=str,
- default="ru",
- help="The bottleneck language if you want to resume training one can"
- "choose between {'es','ru','en','fr','de','pt','zh'} but please have a "
- "look at https://huggingface.co/Helsinki-NLP to make sure the language"
- "pair you ask for is available",
- )
-
- parser.add_argument(
- "-input_data_path",
- type=str,
- default=os.path.join(wd, "dataset", "train_neurips_dataset.csv"),
- help="dataset location, please note it should be a CSV file with two"
- 'columns : "text" and "summary"',
- )
-
- parser.add_argument(
- "-output_data_path",
- type=str,
- default=os.path.join(
- wd, "dataset", "augmented_datas", "augmented_dataset_output.csv"
- ),
- help="augmented dataset output location",
- )
-
- parser.add_argument(
- "-columns_mapping",
- "--col_map",
- type=json.loads,
- default={"abstract": "text", "tldr": "summary"},
- help="columns names to apply data augmentation on "
- "you have to give a key/value pair dict such that "
- "{'input_column_name1':'output_column_name1'} by default "
- " it is set as {'abstract': 'text', 'tldr':'summary'}, "
- "if you don't want to change the column names,"
- " please provide a dict such that keys=values ",
- )
-
- parser.add_argument("-batch_size", type=int, default=25, help="batch_size")
-
- args = parser.parse_args()
- do_backtranslation(**vars(args))
diff --git a/spaces/ma-xu/LIVE/thrust/thrust/system/detail/adl/temporary_buffer.h b/spaces/ma-xu/LIVE/thrust/thrust/system/detail/adl/temporary_buffer.h
deleted file mode 100644
index 0cada5ee4b10a9fc36d19f80a276bb19ef7fff6d..0000000000000000000000000000000000000000
--- a/spaces/ma-xu/LIVE/thrust/thrust/system/detail/adl/temporary_buffer.h
+++ /dev/null
@@ -1,44 +0,0 @@
-/*
- * Copyright 2008-2013 NVIDIA Corporation
- *
- * Licensed under the Apache License, Version 2.0 (the "License");
- * you may not use this file except in compliance with the License.
- * You may obtain a copy of the License at
- *
- * http://www.apache.org/licenses/LICENSE-2.0
- *
- * Unless required by applicable law or agreed to in writing, software
- * distributed under the License is distributed on an "AS IS" BASIS,
- * WITHOUT WARRANTIES OR CONDITIONS OF ANY KIND, either express or implied.
- * See the License for the specific language governing permissions and
- * limitations under the License.
- */
-
-#pragma once
-
-#include
-
-// the purpose of this header is to #include the temporary_buffer.h header
-// of the sequential, host, and device systems. It should be #included in any
-// code which uses adl to dispatch get_temporary_buffer or return_temporary_buffer
-
-#include
-
-// SCons can't see through the #defines below to figure out what this header
-// includes, so we fake it out by specifying all possible files we might end up
-// including inside an #if 0.
-#if 0
-#include
-#include